modelId
stringlengths 4
112
| sha
stringlengths 40
40
| lastModified
stringlengths 24
24
| tags
list | pipeline_tag
stringclasses 29
values | private
bool 1
class | author
stringlengths 2
38
⌀ | config
null | id
stringlengths 4
112
| downloads
float64 0
36.8M
⌀ | likes
float64 0
712
⌀ | library_name
stringclasses 17
values | __index_level_0__
int64 0
38.5k
| readme
stringlengths 0
186k
|
---|---|---|---|---|---|---|---|---|---|---|---|---|---|
akiyamasho/stylegan3-anime-faces-generator | 4da4d662284d5b51d069c77c102c5282ef647fc7 | 2022-04-07T15:43:08.000Z | [
"pytorch",
"image-generation",
"gan",
"stylegan",
"stylegan3",
"nvidia",
"license:mit"
]
| null | false | akiyamasho | null | akiyamasho/stylegan3-anime-faces-generator | 0 | 1 | pytorch | 36,700 | ---
license: mit
library_name: pytorch
tags:
- image-generation
- gan
- stylegan
- stylegan3
- nvidia
---
# Anime Faces Generator (StyleGAN3 by NVIDIA)
<img width="679" alt="Generated Faces" src="https://user-images.githubusercontent.com/35907066/161809457-e6467724-5942-4a89-b379-85ddfd6ac86c.png">
This is a [StyleGAN3 PyTorch](https://github.com/NVlabs/stylegan3) model trained on this [Anime Face Dataset](https://github.com/bchao1/Anime-Face-Dataset).
### Usage
Demo on Spaces is not yet implemented.
You can run the model pickle file locally using the instructions in this generator-script-only subset of the StyleGAN3 repo:
- https://github.com/venture-anime/stylegan3-anime-faces-generator
### Dataset & Model Details
The [Anime Face Dataset](https://github.com/bchao1/Anime-Face-Dataset) was created by Mckinsey666.
Training was done in [Paperspace Gradient](https://gradient.run/) on a free `RTX-5000` instance with the following parameters:
- Configuration: `stylegan3-t`
- GPUs: `1`
- Batch Size: `8`
- Gamma: `0.5`
- Final tick: `102`
- Final fid50k_full value (this pickle): `9.26043547642206`.
# Train your own StyleGAN3 on PaperSpace or Colab
You can use the notebooks here for a ready-to-use training pipeline
https://github.com/akiyamasho/stylegan3-training-notebook |
rowan1224/distilbert-squad-slp | eab2e72e91f3adef63eb92f27fd6222329fcb7d7 | 2022-04-05T16:27:09.000Z | [
"pytorch",
"distilbert",
"question-answering",
"transformers",
"autotrain_compatible"
]
| question-answering | false | rowan1224 | null | rowan1224/distilbert-squad-slp | 0 | null | transformers | 36,701 | Entry not found |
rowan1224/albert-squad-slp | b94fccf1d4eb339da011697f8ce6c12ed68d7faf | 2022-04-05T16:33:32.000Z | [
"pytorch",
"albert",
"question-answering",
"transformers",
"autotrain_compatible"
]
| question-answering | false | rowan1224 | null | rowan1224/albert-squad-slp | 0 | null | transformers | 36,702 | Entry not found |
huggingtweets/benk14894427 | 65dfd5874bf687f60c9d3f728012f49f0d521933 | 2022-04-05T19:26:24.000Z | [
"pytorch",
"gpt2",
"text-generation",
"en",
"transformers",
"huggingtweets"
]
| text-generation | false | huggingtweets | null | huggingtweets/benk14894427 | 0 | null | transformers | 36,703 | ---
language: en
thumbnail: http://www.huggingtweets.com/benk14894427/1649186779847/predictions.png
tags:
- huggingtweets
widget:
- text: "My dream is"
---
<div class="inline-flex flex-col" style="line-height: 1.5;">
<div class="flex">
<div
style="display:inherit; margin-left: 4px; margin-right: 4px; width: 92px; height:92px; border-radius: 50%; background-size: cover; background-image: url('https://pbs.twimg.com/profile_images/1442847071829204995/C-gqdXsf_400x400.jpg')">
</div>
<div
style="display:none; margin-left: 4px; margin-right: 4px; width: 92px; height:92px; border-radius: 50%; background-size: cover; background-image: url('')">
</div>
<div
style="display:none; margin-left: 4px; margin-right: 4px; width: 92px; height:92px; border-radius: 50%; background-size: cover; background-image: url('')">
</div>
</div>
<div style="text-align: center; margin-top: 3px; font-size: 16px; font-weight: 800">🤖 AI BOT 🤖</div>
<div style="text-align: center; font-size: 16px; font-weight: 800">Benk</div>
<div style="text-align: center; font-size: 14px;">@benk14894427</div>
</div>
I was made with [huggingtweets](https://github.com/borisdayma/huggingtweets).
Create your own bot based on your favorite user with [the demo](https://colab.research.google.com/github/borisdayma/huggingtweets/blob/master/huggingtweets-demo.ipynb)!
## How does it work?
The model uses the following pipeline.
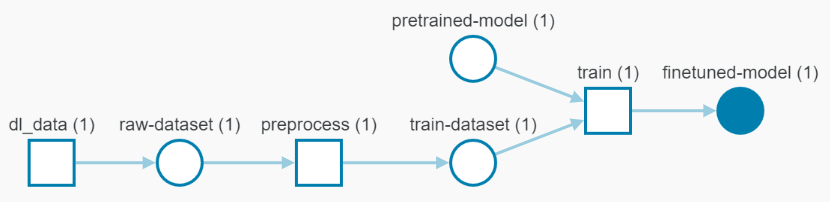
To understand how the model was developed, check the [W&B report](https://wandb.ai/wandb/huggingtweets/reports/HuggingTweets-Train-a-Model-to-Generate-Tweets--VmlldzoxMTY5MjI).
## Training data
The model was trained on tweets from Benk.
| Data | Benk |
| --- | --- |
| Tweets downloaded | 269 |
| Retweets | 6 |
| Short tweets | 34 |
| Tweets kept | 229 |
[Explore the data](https://wandb.ai/wandb/huggingtweets/runs/1zhhq7f1/artifacts), which is tracked with [W&B artifacts](https://docs.wandb.com/artifacts) at every step of the pipeline.
## Training procedure
The model is based on a pre-trained [GPT-2](https://huggingface.co/gpt2) which is fine-tuned on @benk14894427's tweets.
Hyperparameters and metrics are recorded in the [W&B training run](https://wandb.ai/wandb/huggingtweets/runs/2ns3y5oi) for full transparency and reproducibility.
At the end of training, [the final model](https://wandb.ai/wandb/huggingtweets/runs/2ns3y5oi/artifacts) is logged and versioned.
## How to use
You can use this model directly with a pipeline for text generation:
```python
from transformers import pipeline
generator = pipeline('text-generation',
model='huggingtweets/benk14894427')
generator("My dream is", num_return_sequences=5)
```
## Limitations and bias
The model suffers from [the same limitations and bias as GPT-2](https://huggingface.co/gpt2#limitations-and-bias).
In addition, the data present in the user's tweets further affects the text generated by the model.
## About
*Built by Boris Dayma*
[](https://twitter.com/intent/follow?screen_name=borisdayma)
For more details, visit the project repository.
[](https://github.com/borisdayma/huggingtweets)
|
vladimir-lomonosov/gpt2-wikitext2 | 0c2c2301f186e3ee8cf7028811bfacbc60af64a4 | 2022-04-05T21:45:58.000Z | [
"pytorch",
"gpt2",
"text-generation",
"transformers",
"generated_from_trainer",
"license:mit",
"model-index"
]
| text-generation | false | vladimir-lomonosov | null | vladimir-lomonosov/gpt2-wikitext2 | 0 | null | transformers | 36,704 | ---
license: mit
tags:
- generated_from_trainer
model-index:
- name: gpt2-wikitext2
results: []
---
<!-- This model card has been generated automatically according to the information the Trainer had access to. You
should probably proofread and complete it, then remove this comment. -->
# gpt2-wikitext2
This model is a fine-tuned version of [gpt2](https://huggingface.co/gpt2) on the None dataset.
It achieves the following results on the evaluation set:
- Loss: 6.1153
## Model description
More information needed
## Intended uses & limitations
More information needed
## Training and evaluation data
More information needed
## Training procedure
### Training hyperparameters
The following hyperparameters were used during training:
- learning_rate: 2e-05
- train_batch_size: 8
- eval_batch_size: 8
- seed: 42
- optimizer: Adam with betas=(0.9,0.999) and epsilon=1e-08
- lr_scheduler_type: linear
- num_epochs: 3.0
### Training results
| Training Loss | Epoch | Step | Validation Loss |
|:-------------:|:-----:|:----:|:---------------:|
| 6.5574 | 1.0 | 2249 | 6.4738 |
| 6.1911 | 2.0 | 4498 | 6.1998 |
| 6.0051 | 3.0 | 6747 | 6.1153 |
### Framework versions
- Transformers 4.17.0
- Pytorch 1.5.1+cu92
- Datasets 2.0.0
- Tokenizers 0.11.6
|
huggingtweets/vivchen_ | 105111087202f7879d8f47c56f74b8b15ad2ec60 | 2022-04-05T20:13:38.000Z | [
"pytorch",
"gpt2",
"text-generation",
"en",
"transformers",
"huggingtweets"
]
| text-generation | false | huggingtweets | null | huggingtweets/vivchen_ | 0 | null | transformers | 36,705 | ---
language: en
thumbnail: http://www.huggingtweets.com/vivchen_/1649189613639/predictions.png
tags:
- huggingtweets
widget:
- text: "My dream is"
---
<div class="inline-flex flex-col" style="line-height: 1.5;">
<div class="flex">
<div
style="display:inherit; margin-left: 4px; margin-right: 4px; width: 92px; height:92px; border-radius: 50%; background-size: cover; background-image: url('https://pbs.twimg.com/profile_images/1453748100594642948/BAASh9m3_400x400.jpg')">
</div>
<div
style="display:none; margin-left: 4px; margin-right: 4px; width: 92px; height:92px; border-radius: 50%; background-size: cover; background-image: url('')">
</div>
<div
style="display:none; margin-left: 4px; margin-right: 4px; width: 92px; height:92px; border-radius: 50%; background-size: cover; background-image: url('')">
</div>
</div>
<div style="text-align: center; margin-top: 3px; font-size: 16px; font-weight: 800">🤖 AI BOT 🤖</div>
<div style="text-align: center; font-size: 16px; font-weight: 800">Vivian</div>
<div style="text-align: center; font-size: 14px;">@vivchen_</div>
</div>
I was made with [huggingtweets](https://github.com/borisdayma/huggingtweets).
Create your own bot based on your favorite user with [the demo](https://colab.research.google.com/github/borisdayma/huggingtweets/blob/master/huggingtweets-demo.ipynb)!
## How does it work?
The model uses the following pipeline.
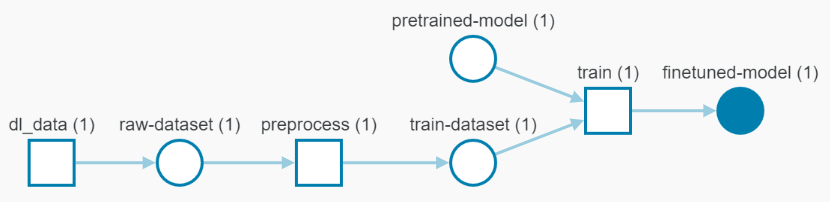
To understand how the model was developed, check the [W&B report](https://wandb.ai/wandb/huggingtweets/reports/HuggingTweets-Train-a-Model-to-Generate-Tweets--VmlldzoxMTY5MjI).
## Training data
The model was trained on tweets from Vivian.
| Data | Vivian |
| --- | --- |
| Tweets downloaded | 1616 |
| Retweets | 39 |
| Short tweets | 166 |
| Tweets kept | 1411 |
[Explore the data](https://wandb.ai/wandb/huggingtweets/runs/vqb4rpuh/artifacts), which is tracked with [W&B artifacts](https://docs.wandb.com/artifacts) at every step of the pipeline.
## Training procedure
The model is based on a pre-trained [GPT-2](https://huggingface.co/gpt2) which is fine-tuned on @vivchen_'s tweets.
Hyperparameters and metrics are recorded in the [W&B training run](https://wandb.ai/wandb/huggingtweets/runs/1xzxtr20) for full transparency and reproducibility.
At the end of training, [the final model](https://wandb.ai/wandb/huggingtweets/runs/1xzxtr20/artifacts) is logged and versioned.
## How to use
You can use this model directly with a pipeline for text generation:
```python
from transformers import pipeline
generator = pipeline('text-generation',
model='huggingtweets/vivchen_')
generator("My dream is", num_return_sequences=5)
```
## Limitations and bias
The model suffers from [the same limitations and bias as GPT-2](https://huggingface.co/gpt2#limitations-and-bias).
In addition, the data present in the user's tweets further affects the text generated by the model.
## About
*Built by Boris Dayma*
[](https://twitter.com/intent/follow?screen_name=borisdayma)
For more details, visit the project repository.
[](https://github.com/borisdayma/huggingtweets)
|
vocab-transformers/dense_encoder-distilbert-frozen_emb | 8cb4a54e5cd5c9cb4b9922bcae5ff4cd58dadf24 | 2022-04-05T21:13:38.000Z | [
"pytorch",
"distilbert",
"feature-extraction",
"transformers"
]
| feature-extraction | false | vocab-transformers | null | vocab-transformers/dense_encoder-distilbert-frozen_emb | 0 | null | transformers | 36,706 | # Dense Encoder - Distilbert - Frozen Token Embeddings
This model is a distilbert-base-uncased model trained for 30 epochs (235k steps), 64 batch size with MarginMSE Loss on MS MARCO dataset.
The token embeddings were frozen.
| Dataset | Model with updated token embeddings | Model with frozen embeddings |
| --- | :---: | :---: |
| TREC-DL 19 | 70.68 | 68.60 |
| TREC-DL 20 | 67.69 | 70.21 |
| FiQA | 28.89 | 28.60 |
| Robust04 | 39.56 | 39.08 |
| TREC-COVID v2 | 69.80 | 69.84 |
| TREC-NEWS | 37.97 | 38.27 |
| Avg. 4 BEIR tasks | 44.06 | 43.95 |
|
huggingtweets/jorgegos | f28a6d055eb2dae47caef2892e850bbc0d372b8b | 2022-04-05T21:17:29.000Z | [
"pytorch",
"gpt2",
"text-generation",
"en",
"transformers",
"huggingtweets"
]
| text-generation | false | huggingtweets | null | huggingtweets/jorgegos | 0 | null | transformers | 36,707 | ---
language: en
thumbnail: http://www.huggingtweets.com/jorgegos/1649193376372/predictions.png
tags:
- huggingtweets
widget:
- text: "My dream is"
---
<div class="inline-flex flex-col" style="line-height: 1.5;">
<div class="flex">
<div
style="display:inherit; margin-left: 4px; margin-right: 4px; width: 92px; height:92px; border-radius: 50%; background-size: cover; background-image: url('https://pbs.twimg.com/profile_images/1125539522983399425/1iUPUMbd_400x400.jpg')">
</div>
<div
style="display:none; margin-left: 4px; margin-right: 4px; width: 92px; height:92px; border-radius: 50%; background-size: cover; background-image: url('')">
</div>
<div
style="display:none; margin-left: 4px; margin-right: 4px; width: 92px; height:92px; border-radius: 50%; background-size: cover; background-image: url('')">
</div>
</div>
<div style="text-align: center; margin-top: 3px; font-size: 16px; font-weight: 800">🤖 AI BOT 🤖</div>
<div style="text-align: center; font-size: 16px; font-weight: 800">Jorge Gosalvez</div>
<div style="text-align: center; font-size: 14px;">@jorgegos</div>
</div>
I was made with [huggingtweets](https://github.com/borisdayma/huggingtweets).
Create your own bot based on your favorite user with [the demo](https://colab.research.google.com/github/borisdayma/huggingtweets/blob/master/huggingtweets-demo.ipynb)!
## How does it work?
The model uses the following pipeline.
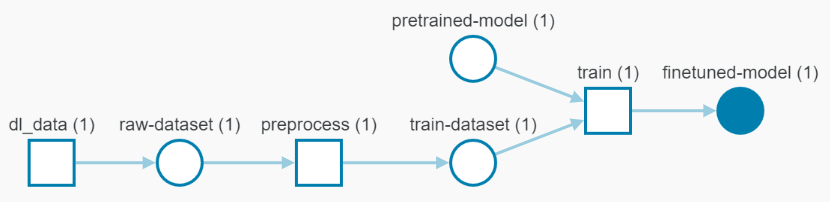
To understand how the model was developed, check the [W&B report](https://wandb.ai/wandb/huggingtweets/reports/HuggingTweets-Train-a-Model-to-Generate-Tweets--VmlldzoxMTY5MjI).
## Training data
The model was trained on tweets from Jorge Gosalvez.
| Data | Jorge Gosalvez |
| --- | --- |
| Tweets downloaded | 151 |
| Retweets | 50 |
| Short tweets | 17 |
| Tweets kept | 84 |
[Explore the data](https://wandb.ai/wandb/huggingtweets/runs/3kbrvpqs/artifacts), which is tracked with [W&B artifacts](https://docs.wandb.com/artifacts) at every step of the pipeline.
## Training procedure
The model is based on a pre-trained [GPT-2](https://huggingface.co/gpt2) which is fine-tuned on @jorgegos's tweets.
Hyperparameters and metrics are recorded in the [W&B training run](https://wandb.ai/wandb/huggingtweets/runs/2jyhp60o) for full transparency and reproducibility.
At the end of training, [the final model](https://wandb.ai/wandb/huggingtweets/runs/2jyhp60o/artifacts) is logged and versioned.
## How to use
You can use this model directly with a pipeline for text generation:
```python
from transformers import pipeline
generator = pipeline('text-generation',
model='huggingtweets/jorgegos')
generator("My dream is", num_return_sequences=5)
```
## Limitations and bias
The model suffers from [the same limitations and bias as GPT-2](https://huggingface.co/gpt2#limitations-and-bias).
In addition, the data present in the user's tweets further affects the text generated by the model.
## About
*Built by Boris Dayma*
[](https://twitter.com/intent/follow?screen_name=borisdayma)
For more details, visit the project repository.
[](https://github.com/borisdayma/huggingtweets)
|
harish3110/xlm-roberta-base-finetuned-panx-de | 1e351c9aa0a26488f85a26e919a5b0862e850d23 | 2022-04-05T22:23:34.000Z | [
"pytorch",
"xlm-roberta",
"token-classification",
"dataset:xtreme",
"transformers",
"generated_from_trainer",
"license:mit",
"model-index",
"autotrain_compatible"
]
| token-classification | false | harish3110 | null | harish3110/xlm-roberta-base-finetuned-panx-de | 0 | null | transformers | 36,708 | ---
license: mit
tags:
- generated_from_trainer
datasets:
- xtreme
metrics:
- f1
model-index:
- name: xlm-roberta-base-finetuned-panx-de
results:
- task:
name: Token Classification
type: token-classification
dataset:
name: xtreme
type: xtreme
args: PAN-X.de
metrics:
- name: F1
type: f1
value: 0.862053266560437
---
<!-- This model card has been generated automatically according to the information the Trainer had access to. You
should probably proofread and complete it, then remove this comment. -->
# xlm-roberta-base-finetuned-panx-de
This model is a fine-tuned version of [xlm-roberta-base](https://huggingface.co/xlm-roberta-base) on the xtreme dataset.
It achieves the following results on the evaluation set:
- Loss: 0.1354
- F1: 0.8621
## Model description
More information needed
## Intended uses & limitations
More information needed
## Training and evaluation data
More information needed
## Training procedure
### Training hyperparameters
The following hyperparameters were used during training:
- learning_rate: 5e-05
- train_batch_size: 24
- eval_batch_size: 24
- seed: 42
- optimizer: Adam with betas=(0.9,0.999) and epsilon=1e-08
- lr_scheduler_type: linear
- num_epochs: 3
### Training results
| Training Loss | Epoch | Step | Validation Loss | F1 |
|:-------------:|:-----:|:----:|:---------------:|:------:|
| 0.254 | 1.0 | 525 | 0.1652 | 0.8254 |
| 0.1293 | 2.0 | 1050 | 0.1431 | 0.8489 |
| 0.0797 | 3.0 | 1575 | 0.1354 | 0.8621 |
### Framework versions
- Transformers 4.11.3
- Pytorch 1.10.1
- Datasets 1.13.3
- Tokenizers 0.10.3
|
notexist/tttff | 5fe2a73b3204733e07d8dc1d57e875c8f3b90a2f | 2022-04-05T22:52:29.000Z | [
"pytorch",
"gpt2",
"text-generation",
"transformers"
]
| text-generation | false | notexist | null | notexist/tttff | 0 | null | transformers | 36,709 | Entry not found |
NimaBoscarino/DiffusionCLIP-CelebA_HQ | 6635400b9744702c5c6fb2751172c3153b6d9263 | 2022-04-06T02:03:13.000Z | [
"arxiv:1710.10196",
"pytorch",
"diffusion",
"image-to-image"
]
| image-to-image | false | NimaBoscarino | null | NimaBoscarino/DiffusionCLIP-CelebA_HQ | 0 | null | pytorch | 36,710 | ---
library_name: pytorch
tags:
- diffusion
- image-to-image
---
# DiffusionCLIP: Text-Guided Diffusion Models for Robust Image Manipulation - Faces
Creators: Gwanghyun Kim, Taesung Kwon, Jong Chul Ye
<img src="https://github.com/submission10095/DiffusionCLIP_temp/raw/master/imgs/main1.png" alt="Excerpt from DiffusionCLIP paper showcasing comparison of DiffusionCLIP versus other methods for image reconstruction, manipulation, and style transfer." style="height: 300px;"/>
DiffusionCLIP is a diffusion model which is well suited for image manipulation thanks to its nearly perfect inversion capability, which is an important advantage over GAN-based models. This checkpoint was trained on the [CelebA-HQ Dataset](https://arxiv.org/abs/1710.10196), available on the Hugging Face Hub: https://huggingface.co/datasets/huggan/CelebA-HQ.
This checkpoint is most appropriate for manipulation, reconstruction, and style transfer on images of human faces using the DiffusionCLIP model. To use ID loss for preserving Human face identity, you are required to download the [pretrained IR-SE50 model](https://drive.google.com/file/u/1/d/1KW7bjndL3QG3sxBbZxreGHigcCCpsDgn/view) from [TreB1eN](https://github.com/TreB1eN/InsightFace_Pytorch). Additional information is available on [the GitHub repository](https://github.com/gwang-kim/DiffusionCLIP).
### Credits
- Code repository available at: https://github.com/gwang-kim/DiffusionCLIP
### Citation
```
@article{kim2021diffusionclip,
title={Diffusionclip: Text-guided image manipulation using diffusion models},
author={Kim, Gwanghyun and Ye, Jong Chul},
journal={arXiv preprint arXiv:2110.02711},
year={2021}
}
```
|
NimaBoscarino/DiffusionCLIP-LSUN_Bedroom | 5ee194f5ea85e377b4c7723dca507dbf0c225bfc | 2022-04-06T02:39:53.000Z | [
"pytorch",
"diffusion",
"image-to-image"
]
| image-to-image | false | NimaBoscarino | null | NimaBoscarino/DiffusionCLIP-LSUN_Bedroom | 0 | null | pytorch | 36,711 | ---
library_name: pytorch
tags:
- diffusion
- image-to-image
---
# DiffusionCLIP: Text-Guided Diffusion Models for Robust Image Manipulation - Bedrooms
Creators: Gwanghyun Kim, Taesung Kwon, Jong Chul Ye
<img src="https://github.com/submission10095/DiffusionCLIP_temp/raw/master/imgs/main1.png" alt="Excerpt from DiffusionCLIP paper showcasing comparison of DiffusionCLIP versus other methods for image reconstruction, manipulation, and style transfer." style="height: 300px;"/>
DiffusionCLIP is a diffusion model which is well suited for image manipulation thanks to its nearly perfect inversion capability, which is an important advantage over GAN-based models. This checkpoint was trained on the ["Bedrooms" category of the LSUN Dataset](https://www.yf.io/p/lsun).
This checkpoint is most appropriate for manipulation, reconstruction, and style transfer on images of indoor locations, such as bedrooms. The weights should be loaded into the [DiffusionCLIP model](https://github.com/gwang-kim/DiffusionCLIP).
### Credits
- Code repository available at: https://github.com/gwang-kim/DiffusionCLIP
### Citation
```
@article{kim2021diffusionclip,
title={Diffusionclip: Text-guided image manipulation using diffusion models},
author={Kim, Gwanghyun and Ye, Jong Chul},
journal={arXiv preprint arXiv:2110.02711},
year={2021}
}
```
|
pbdevpros/beirt-irish-translation | f241eb8d973c7f2df7e6ae1c04d583886faed256 | 2022-04-07T19:14:00.000Z | [
"pytorch",
"encoder-decoder",
"text2text-generation",
"transformers",
"generated_from_trainer",
"model-index",
"autotrain_compatible"
]
| text2text-generation | false | pbdevpros | null | pbdevpros/beirt-irish-translation | 0 | null | transformers | 36,712 | ---
tags:
- generated_from_trainer
metrics:
- bleu
model-index:
- name: beirt-irish-translation
results: []
---
<!-- This model card has been generated automatically according to the information the Trainer had access to. You
should probably proofread and complete it, then remove this comment. -->
# beirt-irish-translation
This model was trained from scratch on an unknown dataset.
It achieves the following results on the evaluation set:
- Loss: 0.0227
- Bleu: 78.9918
## Model description
More information needed
## Intended uses & limitations
More information needed
## Training and evaluation data
More information needed
## Training procedure
### Training hyperparameters
The following hyperparameters were used during training:
- learning_rate: 2e-05
- train_batch_size: 16
- eval_batch_size: 16
- seed: 42
- optimizer: Adam with betas=(0.9,0.999) and epsilon=1e-08
- lr_scheduler_type: linear
- num_epochs: 1
### Training results
### Framework versions
- Transformers 4.17.0
- Pytorch 1.10.0+cu102
- Datasets 2.0.0
- Tokenizers 0.11.6
|
luxiao/alilingjie | 9135a6a88bb8102780c638627adeafefdf0ffe05 | 2022-04-06T07:44:47.000Z | [
"pytorch",
"transformers",
"license:apache-2.0"
]
| null | false | luxiao | null | luxiao/alilingjie | 0 | 1 | transformers | 36,713 | ---
license: apache-2.0
---
|
jimregan/psst-partial-timit | cdbd3aa685e215171bf05e75047bdb488e92e680 | 2022-04-15T21:45:24.000Z | [
"pytorch",
"wav2vec2",
"automatic-speech-recognition",
"en",
"dataset:jimregan/psst",
"dataset:timit_asr",
"transformers",
"license:apache-2.0"
]
| automatic-speech-recognition | false | jimregan | null | jimregan/psst-partial-timit | 0 | null | transformers | 36,714 | ---
language:
- en
license: apache-2.0
tags:
- automatic-speech-recognition
datasets:
- jimregan/psst
- timit_asr
---
This repository contains a number of experiments for the [PSST Challenge](https://psst.study/).
As the test set is unavailable, all numbers are based on the validation set.
The experiments in the tables below were finetuned on [Wav2vec 2.0 Base, No finetuning](https://github.com/pytorch/fairseq/tree/main/examples/wav2vec)
Our overall best performing model (**FER** 9\.2%, **PER:** 21\.0%) was based on [Wav2vec 2.0 Large, No finetuning](https://github.com/pytorch/fairseq/tree/main/examples/wav2vec) (git tag: `larger-rir`), with the TIMIT subset augmented with Room Impulse Response, based on the experiments below, on the base model.
## Augmented TIMIT subset
Using a subset of TIMIT that could map easily to the phoneset used by the PSST Challenge data (a list of IDs are in the repository), we experimented with augmenting the data to better match the PSST data.
The best results were obtained using Room Impulse Response (tag: `rir`)
| **Augmentation** | **FER** | **PER** | **Git tag** |
| :----------------------------------------------- | :-------- | :--------- | :---------------------------------- |
| unaugmented | 10\.2% | 22\.5% | huggingface-unaugmented |
| Gaussian noise | 10\.0% | 22\.1% | gaussian |
| Pitchshift | 9\.6% | 22\.9% | pitchshift |
| RIR | **9\.6%** | **21\.8%** | rir |
| Time stretch | 10\.1% | 22\.8% | timestretch |
| Gaussian noise + RIR | 10\.0% | 23\.4% | gaussian-rir |
| Pitchshift + Gaussian noise | 9\.9% | 22\.9% | pitchshift-gaussian |
| Pitchshift + RIR | 9\.9% | 22\.8% | pitchshift-rir |
| Tim estretch + Gaussian noise | 10\.2% | 22\.8% | timestretch-gaussian |
| Time stretch + Pitchshift | 9\.8% | 22\.0% | timestretch-pitchshift |
| Time stretch + RIR | 9\.7% | 22\.2% | timestretch-rir |
| Pitchshift + Gaussian noise + RIR | 10\.1% | 23\.5% | pitchshift-gaussian-rir |
| Time stretch + Gaussian noise + RIR | 9\.7% | 22\.3% | timestretch-gaussian-rir |
| Time stretch + Pitchshift + Gaussian noise | 10\.2% | 22\.9% | timestretch-pitchshift-gaussian |
| Time stretch + Pitchshift + RIR | 10\.2% | 22\.5% | timestretch-pitchshift-rir |
| Time stretch + Pitchshift + Gaussian noise + RIR | 10\.9% | 24\.1% | timestretch-pitchshift-gaussian-rir |
## LM experiments
We experimented with a number of language model configurations, combining the data from the PSST challenge, the subset of TIMIT we used, and CMUdict.
We tried combining CMUdict data in a number of ways: unmodified, with a silence token added at the start of the pronunciation, at the end, and at both the start and the end.
The best result was from a 5-gram model, with silences added at the end of the CMUdict data (git tag: `lm-nosil-cmudict-sile.5`).
Evaluation was performed using scripts provided by the PSST Challenge's organisers, so there are no scripts in place to automatically use the LM with the transformers library.
| | **n-gram** | **FER** | **PER** | **Tag** |
| :----------------------------- | :--------- | :--------- | :--------- | :--------- |
| Baseline + TIMIT | --- | **10\.2%** | 22\.5% | huggingface-unaugmented |
| All silences | 4 | 10\.5% | 23\.0% | lm-allsil.4 |
| | 5 | 10\.5% | 22\.6% | lm-allsil.5 |
| | 6 | 10\.3% | 22\.3% | lm-allsil.6 |
| No silences | 4 | 10\.3% | 22\.6% | lm-nosil.4 |
| | 5 | **10\.2%** | 22\.2% | lm-nosil.5 |
| | 6 | **10\.2%** | 22\.4% | lm-nosil.6 |
| PSST and TIMIT without silence | | | | |
| Unmodified CMUdict | 4 | 10\.3% | 22\.6% | lm-nosil-cmudict-nosil.4 |
| | 5 | 10\.2% | 22\.2% | lm-nosil-cmudict-nosil.5 |
| | 6 | **10\.2%** | 22\.4% | lm-nosil-cmudict-nosil.6 |
| CMUdict-end | 4 | 10\.3% | 22\.6% | lm-nosil-cmudict-sile.4 |
| | 5 | **10\.2%** | **22\.1%** | lm-nosil-cmudict-sile.5 |
| | 6 | **10\.2%** | 22\.3% | lm-nosil-cmudict-sile.6 |
| CMUdict-start | 4 | 10\.4% | 22\.6% | lm-nosil-cmudict-sils.4 |
| | 5 | 10\.3% | 22\.4% | lm-nosil-cmudict-sils.5 |
| | 6 | 10\.3% | 22\.3% | lm-nosil-cmudict-sils.6 |
| CMUdict-both | 4 | 10\.4% | 22\.7% | lm-nosil-cmudict-silb.4 |
| | 5 | 10\.4% | 22\.3% | lm-nosil-cmudict-silb.5 |
| | 6 | 10\.3% | 22\.3% | lm-nosil-cmudict-silb.6 |
| Unmodified PSST and TIMIT | | | | |
| Unmodified CMUdict | 4 | 10\.3% | 22\.8% | lm-orig-cmudict-nosil.4 |
| | 5 | 10\.3% | 22\.4% | lm-orig-cmudict-nosil.5 |
| | 6 | **10\.2%** | 22\.4% | lm-orig-cmudict-nosil.6 |
| CMUdict-end | 4 | 10\.3% | 22\.7% | lm-orig-cmudict-sile.4 |
| | 5 | **10\.2%** | 22\.2% | lm-orig-cmudict-sile.5 |
| | 6 | **10\.2%** | 22\.3% | lm-orig-cmudict-sile.6 |
| CMUdict-start | 4 | 10\.5% | 22\.8% | lm-orig-cmudict-sils.4 |
| | 5 | 10\.4% | 22\.5% | lm-orig-cmudict-sils.5 |
| | 6 | 10\.3% | 22\.4% | lm-orig-cmudict-sils.6 |
| CMUdict-both | 4 | 10\.5% | 22\.8% | lm-orig-cmudict-silb.4 |
| | 5 | 10\.4% | 22\.4% | lm-orig-cmudict-silb.5 |
| | 6 | 10\.4% | 22\.4% | lm-orig-cmudict-silb.6 |
|
hou/opus-tatoeba-en-tr-finetuned-en-to-ug-finetuned-en-to-ug | e7c4a36ab46ade9a6546376b6e19a02a2c579f1a | 2022-04-06T19:49:32.000Z | [
"pytorch",
"tensorboard",
"marian",
"text2text-generation",
"transformers",
"autotrain_compatible"
]
| text2text-generation | false | hou | null | hou/opus-tatoeba-en-tr-finetuned-en-to-ug-finetuned-en-to-ug | 0 | null | transformers | 36,715 | Entry not found |
huggingtweets/chrismedlandf1-elonmusk-scarbstech | 1d9279aea58a69215c569b640666f683c2580577 | 2022-04-06T13:53:51.000Z | [
"pytorch",
"gpt2",
"text-generation",
"en",
"transformers",
"huggingtweets"
]
| text-generation | false | huggingtweets | null | huggingtweets/chrismedlandf1-elonmusk-scarbstech | 0 | null | transformers | 36,716 | ---
language: en
thumbnail: http://www.huggingtweets.com/chrismedlandf1-elonmusk-scarbstech/1649253035547/predictions.png
tags:
- huggingtweets
widget:
- text: "My dream is"
---
<div class="inline-flex flex-col" style="line-height: 1.5;">
<div class="flex">
<div
style="display:inherit; margin-left: 4px; margin-right: 4px; width: 92px; height:92px; border-radius: 50%; background-size: cover; background-image: url('https://pbs.twimg.com/profile_images/1503591435324563456/foUrqiEw_400x400.jpg')">
</div>
<div
style="display:inherit; margin-left: 4px; margin-right: 4px; width: 92px; height:92px; border-radius: 50%; background-size: cover; background-image: url('https://pbs.twimg.com/profile_images/456005573/scarbs_400x400.JPG')">
</div>
<div
style="display:inherit; margin-left: 4px; margin-right: 4px; width: 92px; height:92px; border-radius: 50%; background-size: cover; background-image: url('https://pbs.twimg.com/profile_images/1252178304192389120/bXT3lbuR_400x400.jpg')">
</div>
</div>
<div style="text-align: center; margin-top: 3px; font-size: 16px; font-weight: 800">🤖 AI CYBORG 🤖</div>
<div style="text-align: center; font-size: 16px; font-weight: 800">Elon Musk & Craig Scarborough & Chris Medland</div>
<div style="text-align: center; font-size: 14px;">@chrismedlandf1-elonmusk-scarbstech</div>
</div>
I was made with [huggingtweets](https://github.com/borisdayma/huggingtweets).
Create your own bot based on your favorite user with [the demo](https://colab.research.google.com/github/borisdayma/huggingtweets/blob/master/huggingtweets-demo.ipynb)!
## How does it work?
The model uses the following pipeline.
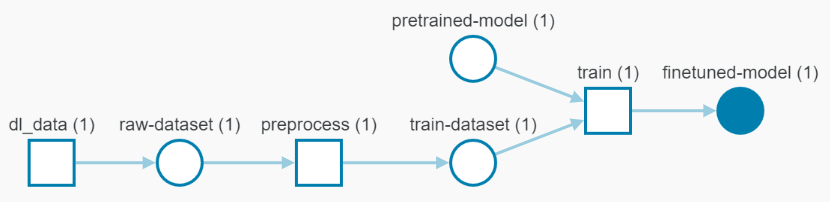
To understand how the model was developed, check the [W&B report](https://wandb.ai/wandb/huggingtweets/reports/HuggingTweets-Train-a-Model-to-Generate-Tweets--VmlldzoxMTY5MjI).
## Training data
The model was trained on tweets from Elon Musk & Craig Scarborough & Chris Medland.
| Data | Elon Musk | Craig Scarborough | Chris Medland |
| --- | --- | --- | --- |
| Tweets downloaded | 2621 | 3249 | 3250 |
| Retweets | 116 | 387 | 196 |
| Short tweets | 795 | 646 | 102 |
| Tweets kept | 1710 | 2216 | 2952 |
[Explore the data](https://wandb.ai/wandb/huggingtweets/runs/3m6vm0tf/artifacts), which is tracked with [W&B artifacts](https://docs.wandb.com/artifacts) at every step of the pipeline.
## Training procedure
The model is based on a pre-trained [GPT-2](https://huggingface.co/gpt2) which is fine-tuned on @chrismedlandf1-elonmusk-scarbstech's tweets.
Hyperparameters and metrics are recorded in the [W&B training run](https://wandb.ai/wandb/huggingtweets/runs/mnfs00gg) for full transparency and reproducibility.
At the end of training, [the final model](https://wandb.ai/wandb/huggingtweets/runs/mnfs00gg/artifacts) is logged and versioned.
## How to use
You can use this model directly with a pipeline for text generation:
```python
from transformers import pipeline
generator = pipeline('text-generation',
model='huggingtweets/chrismedlandf1-elonmusk-scarbstech')
generator("My dream is", num_return_sequences=5)
```
## Limitations and bias
The model suffers from [the same limitations and bias as GPT-2](https://huggingface.co/gpt2#limitations-and-bias).
In addition, the data present in the user's tweets further affects the text generated by the model.
## About
*Built by Boris Dayma*
[](https://twitter.com/intent/follow?screen_name=borisdayma)
For more details, visit the project repository.
[](https://github.com/borisdayma/huggingtweets)
|
huggingtweets/twommof1 | 0e1e9279bc2e1596118cbf908bc132eb21b8822f | 2022-04-06T14:06:13.000Z | [
"pytorch",
"gpt2",
"text-generation",
"en",
"transformers",
"huggingtweets"
]
| text-generation | false | huggingtweets | null | huggingtweets/twommof1 | 0 | null | transformers | 36,717 | ---
language: en
thumbnail: http://www.huggingtweets.com/twommof1/1649253931186/predictions.png
tags:
- huggingtweets
widget:
- text: "My dream is"
---
<div class="inline-flex flex-col" style="line-height: 1.5;">
<div class="flex">
<div
style="display:inherit; margin-left: 4px; margin-right: 4px; width: 92px; height:92px; border-radius: 50%; background-size: cover; background-image: url('https://pbs.twimg.com/profile_images/1433115414679150596/6E1j0ONi_400x400.jpg')">
</div>
<div
style="display:none; margin-left: 4px; margin-right: 4px; width: 92px; height:92px; border-radius: 50%; background-size: cover; background-image: url('')">
</div>
<div
style="display:none; margin-left: 4px; margin-right: 4px; width: 92px; height:92px; border-radius: 50%; background-size: cover; background-image: url('')">
</div>
</div>
<div style="text-align: center; margin-top: 3px; font-size: 16px; font-weight: 800">🤖 AI BOT 🤖</div>
<div style="text-align: center; font-size: 16px; font-weight: 800">Tommo</div>
<div style="text-align: center; font-size: 14px;">@twommof1</div>
</div>
I was made with [huggingtweets](https://github.com/borisdayma/huggingtweets).
Create your own bot based on your favorite user with [the demo](https://colab.research.google.com/github/borisdayma/huggingtweets/blob/master/huggingtweets-demo.ipynb)!
## How does it work?
The model uses the following pipeline.
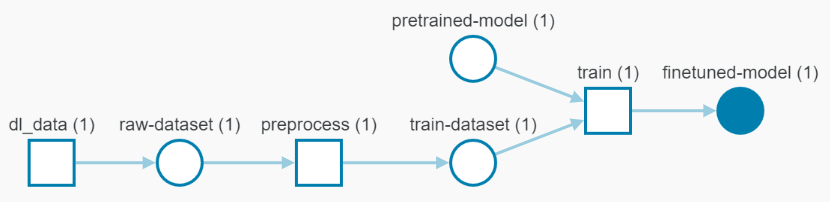
To understand how the model was developed, check the [W&B report](https://wandb.ai/wandb/huggingtweets/reports/HuggingTweets-Train-a-Model-to-Generate-Tweets--VmlldzoxMTY5MjI).
## Training data
The model was trained on tweets from Tommo.
| Data | Tommo |
| --- | --- |
| Tweets downloaded | 3226 |
| Retweets | 136 |
| Short tweets | 642 |
| Tweets kept | 2448 |
[Explore the data](https://wandb.ai/wandb/huggingtweets/runs/1576eaj6/artifacts), which is tracked with [W&B artifacts](https://docs.wandb.com/artifacts) at every step of the pipeline.
## Training procedure
The model is based on a pre-trained [GPT-2](https://huggingface.co/gpt2) which is fine-tuned on @twommof1's tweets.
Hyperparameters and metrics are recorded in the [W&B training run](https://wandb.ai/wandb/huggingtweets/runs/2f5f44et) for full transparency and reproducibility.
At the end of training, [the final model](https://wandb.ai/wandb/huggingtweets/runs/2f5f44et/artifacts) is logged and versioned.
## How to use
You can use this model directly with a pipeline for text generation:
```python
from transformers import pipeline
generator = pipeline('text-generation',
model='huggingtweets/twommof1')
generator("My dream is", num_return_sequences=5)
```
## Limitations and bias
The model suffers from [the same limitations and bias as GPT-2](https://huggingface.co/gpt2#limitations-and-bias).
In addition, the data present in the user's tweets further affects the text generated by the model.
## About
*Built by Boris Dayma*
[](https://twitter.com/intent/follow?screen_name=borisdayma)
For more details, visit the project repository.
[](https://github.com/borisdayma/huggingtweets)
|
huggingtweets/chrismedlandf1 | 0d3897a547b773c26355752f5d47bcae8541c630 | 2022-04-06T14:38:05.000Z | [
"pytorch",
"gpt2",
"text-generation",
"en",
"transformers",
"huggingtweets"
]
| text-generation | false | huggingtweets | null | huggingtweets/chrismedlandf1 | 0 | null | transformers | 36,718 | ---
language: en
thumbnail: http://www.huggingtweets.com/chrismedlandf1/1649255880540/predictions.png
tags:
- huggingtweets
widget:
- text: "My dream is"
---
<div class="inline-flex flex-col" style="line-height: 1.5;">
<div class="flex">
<div
style="display:inherit; margin-left: 4px; margin-right: 4px; width: 92px; height:92px; border-radius: 50%; background-size: cover; background-image: url('https://pbs.twimg.com/profile_images/1252178304192389120/bXT3lbuR_400x400.jpg')">
</div>
<div
style="display:none; margin-left: 4px; margin-right: 4px; width: 92px; height:92px; border-radius: 50%; background-size: cover; background-image: url('')">
</div>
<div
style="display:none; margin-left: 4px; margin-right: 4px; width: 92px; height:92px; border-radius: 50%; background-size: cover; background-image: url('')">
</div>
</div>
<div style="text-align: center; margin-top: 3px; font-size: 16px; font-weight: 800">🤖 AI BOT 🤖</div>
<div style="text-align: center; font-size: 16px; font-weight: 800">Chris Medland</div>
<div style="text-align: center; font-size: 14px;">@chrismedlandf1</div>
</div>
I was made with [huggingtweets](https://github.com/borisdayma/huggingtweets).
Create your own bot based on your favorite user with [the demo](https://colab.research.google.com/github/borisdayma/huggingtweets/blob/master/huggingtweets-demo.ipynb)!
## How does it work?
The model uses the following pipeline.
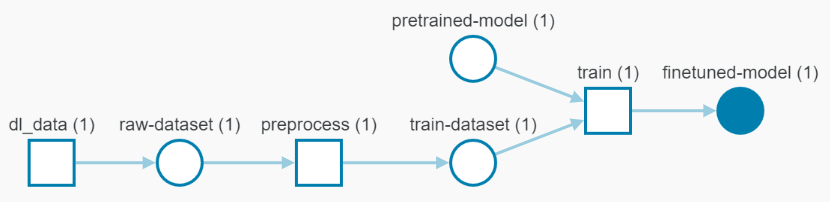
To understand how the model was developed, check the [W&B report](https://wandb.ai/wandb/huggingtweets/reports/HuggingTweets-Train-a-Model-to-Generate-Tweets--VmlldzoxMTY5MjI).
## Training data
The model was trained on tweets from Chris Medland.
| Data | Chris Medland |
| --- | --- |
| Tweets downloaded | 3250 |
| Retweets | 196 |
| Short tweets | 102 |
| Tweets kept | 2952 |
[Explore the data](https://wandb.ai/wandb/huggingtweets/runs/2jton7o0/artifacts), which is tracked with [W&B artifacts](https://docs.wandb.com/artifacts) at every step of the pipeline.
## Training procedure
The model is based on a pre-trained [GPT-2](https://huggingface.co/gpt2) which is fine-tuned on @chrismedlandf1's tweets.
Hyperparameters and metrics are recorded in the [W&B training run](https://wandb.ai/wandb/huggingtweets/runs/2qle9s0v) for full transparency and reproducibility.
At the end of training, [the final model](https://wandb.ai/wandb/huggingtweets/runs/2qle9s0v/artifacts) is logged and versioned.
## How to use
You can use this model directly with a pipeline for text generation:
```python
from transformers import pipeline
generator = pipeline('text-generation',
model='huggingtweets/chrismedlandf1')
generator("My dream is", num_return_sequences=5)
```
## Limitations and bias
The model suffers from [the same limitations and bias as GPT-2](https://huggingface.co/gpt2#limitations-and-bias).
In addition, the data present in the user's tweets further affects the text generated by the model.
## About
*Built by Boris Dayma*
[](https://twitter.com/intent/follow?screen_name=borisdayma)
For more details, visit the project repository.
[](https://github.com/borisdayma/huggingtweets)
|
smeoni/nbme-distilroberta-base | 8ea46ed31f1bd9e9dd0f8ec5a70730f8aa8afdda | 2022-04-06T19:59:51.000Z | [
"pytorch",
"roberta",
"fill-mask",
"transformers",
"autotrain_compatible"
]
| fill-mask | false | smeoni | null | smeoni/nbme-distilroberta-base | 0 | null | transformers | 36,719 | Entry not found |
arakesh/test1223 | 350c2aecea52679162e6f69b3ed85fbdeae368ef | 2022-04-06T19:23:25.000Z | [
"pytorch"
]
| null | false | arakesh | null | arakesh/test1223 | 0 | null | null | 36,720 | Entry not found |
arakesh/cnn-dummy | 060a2f682f1941fbcede31f2f80997ed3b024c32 | 2022-04-06T19:20:33.000Z | [
"pytorch"
]
| null | false | arakesh | null | arakesh/cnn-dummy | 0 | null | null | 36,721 | Entry not found |
ucl-snlp-group-11/byt5-small-cryptic-crosswords | d5f0cdbfd4cc49739d0b3df8bf30d9e451bcb964 | 2022-04-06T21:03:39.000Z | [
"pytorch",
"t5",
"text2text-generation",
"transformers",
"autotrain_compatible"
]
| text2text-generation | false | ucl-snlp-group-11 | null | ucl-snlp-group-11/byt5-small-cryptic-crosswords | 0 | null | transformers | 36,722 | Entry not found |
ucl-snlp-group-11/t5-small-cryptic-crosswords | ae58fadb6b05a677b72c836221beeaa609a21d4f | 2022-04-06T21:15:39.000Z | [
"pytorch",
"t5",
"text2text-generation",
"transformers",
"autotrain_compatible"
]
| text2text-generation | false | ucl-snlp-group-11 | null | ucl-snlp-group-11/t5-small-cryptic-crosswords | 0 | null | transformers | 36,723 | Entry not found |
ucl-snlp-group-11/t5-base-cryptic-crosswords | eaa80a2a82cb739ea7e91788a06bdf4995742cc6 | 2022-04-06T21:18:38.000Z | [
"pytorch",
"t5",
"text2text-generation",
"transformers",
"autotrain_compatible"
]
| text2text-generation | false | ucl-snlp-group-11 | null | ucl-snlp-group-11/t5-base-cryptic-crosswords | 0 | null | transformers | 36,724 | Entry not found |
ID56/FF-Vision-CIFAR | a09be01e5f3326c99112be6ad3c0639e57fb8117 | 2022-04-12T07:06:43.000Z | [
"pytorch",
"dataset:cifar10",
"image-classification",
"license:cc-by-sa-4.0"
]
| image-classification | false | ID56 | null | ID56/FF-Vision-CIFAR | 0 | null | null | 36,725 | ---
thumbnail: "https://huggingface.co/ID56/FF-Vision-CIFAR/resolve/main/assets/cover_image.png"
license: cc-by-sa-4.0
tags:
- image-classification
datasets:
- cifar10
metrics:
- accuracy
inference: false
---
# CIFAR-10 Upside Down Classifier
For the Fatima Fellowship 2022 Coding Challenge, DL for Vision track.
<a href="https://wandb.ai/dealer56/cifar-updown-classifier/reports/CIFAR-10-Upside-Down-Classifier-Fatima-Fellowship-2022-Coding-Challenge-Vision---VmlldzoxODA2MDE4" target="_parent"><img src="https://img.shields.io/badge/weights-%26biases-ffcf40" alt="W&B Report"/></a>
<img src="https://huggingface.co/ID56/FF-Vision-CIFAR/resolve/main/assets/cover_image.png" alt="Cover Image" width="800"/>
## Usage
### Model Definition
```python
from torch import nn
import timm
from huggingface_hub import PyTorchModelHubMixin
class UpDownEfficientNetB0(nn.Module, PyTorchModelHubMixin):
"""A simple Hub Mixin wrapper for timm EfficientNet-B0. Used to classify whether an image is upright or flipped down, on CIFAR-10."""
def __init__(self, **kwargs):
super().__init__()
self.base_model = timm.create_model('efficientnet_b0', num_classes=1, drop_rate=0.2, drop_path_rate=0.2)
self.config = kwargs.pop("config", None)
def forward(self, input):
return self.base_model(input)
```
### Loading the Model from Hub
```python
net = UpDownEfficientNetB0.from_pretrained("ID56/FF-Vision-CIFAR")
```
### Running Inference
```python
from torchvision import transforms
CIFAR_MEAN = (0.4914, 0.4822, 0.4465)
CIFAR_STD = (0.247, 0.243, 0.261)
transform = transforms.Compose([
transforms.Resize(40, 40),
transforms.ToTensor(),
transforms.Normalize(CIFAR_MEAN, CIFAR_STD)
])
image = load_some_image() # Load some PIL Image or uint8 HWC image array
image = transform(image) # Convert to CHW image tensor
image = image.unsqueeze(0) # Add batch dimension
net.eval()
pred = net(image)
``` |
lilapapazian/DialoGPT-small-harrypotter | 1c5e1e809fa3a3ec7be695e4f85c11affc48fbb2 | 2022-04-07T00:42:05.000Z | [
"pytorch",
"gpt2",
"text-generation",
"transformers",
"conversational"
]
| conversational | false | lilapapazian | null | lilapapazian/DialoGPT-small-harrypotter | 0 | null | transformers | 36,726 | ---
tags:
- conversational
---
# Harry Potter DialoGPT Model |
tau/false_large_t5_5_1024_0.3_epoch1 | ef9decbb4e2f3272446c450c9fae8b5a68b28b61 | 2022-04-07T04:45:22.000Z | [
"pytorch",
"t5",
"text2text-generation",
"transformers",
"autotrain_compatible"
]
| text2text-generation | false | tau | null | tau/false_large_t5_5_1024_0.3_epoch1 | 0 | null | transformers | 36,727 | Entry not found |
tau/false_large_t5_lm_5_1024_0.3_epoch1 | 382f70fe3eefe04d9b51c01bab89cbe407d5b640 | 2022-04-07T04:49:54.000Z | [
"pytorch",
"t5",
"text2text-generation",
"transformers",
"autotrain_compatible"
]
| text2text-generation | false | tau | null | tau/false_large_t5_lm_5_1024_0.3_epoch1 | 0 | null | transformers | 36,728 | Entry not found |
tau/false_large_pmi_para0_sentNone_spanNone_5_1024_0.3_epoch1 | 757d67f0b2b1be13bc7b47767889411ef37fd969 | 2022-04-07T04:53:40.000Z | [
"pytorch",
"t5",
"text2text-generation",
"transformers",
"autotrain_compatible"
]
| text2text-generation | false | tau | null | tau/false_large_pmi_para0_sentNone_spanNone_5_1024_0.3_epoch1 | 0 | null | transformers | 36,729 | Entry not found |
tau/false_large_pmi_paraNone_sent0_spanNone_5_1024_0.3_epoch1 | 77beb28edb6760b777f0ead692284a49dc6f74f9 | 2022-04-07T04:59:31.000Z | [
"pytorch",
"t5",
"text2text-generation",
"transformers",
"autotrain_compatible"
]
| text2text-generation | false | tau | null | tau/false_large_pmi_paraNone_sent0_spanNone_5_1024_0.3_epoch1 | 0 | null | transformers | 36,730 | Entry not found |
tau/false_large_pmi_paraNone_sentNone_span0_5_1024_0.3_epoch1 | 5d1e5ebdd870a20ed3661f11749b8adb9a147fcb | 2022-04-07T05:02:57.000Z | [
"pytorch",
"t5",
"text2text-generation",
"transformers",
"autotrain_compatible"
]
| text2text-generation | false | tau | null | tau/false_large_pmi_paraNone_sentNone_span0_5_1024_0.3_epoch1 | 0 | null | transformers | 36,731 | Entry not found |
tau/false_large_pmi_para0_sent1_span2_5_1024_0.3_epoch1 | 63b82f38e79fe24837b7a0f3bacf923515386e35 | 2022-04-07T05:09:46.000Z | [
"pytorch",
"t5",
"text2text-generation",
"transformers",
"autotrain_compatible"
]
| text2text-generation | false | tau | null | tau/false_large_pmi_para0_sent1_span2_5_1024_0.3_epoch1 | 0 | null | transformers | 36,732 | Entry not found |
tau/false_large_rouge_para0_sentNone_spanNone_5_1024_0.3_epoch1 | aeed765c2f32be494d60672b02328c7211bda4a9 | 2022-04-07T05:14:16.000Z | [
"pytorch",
"t5",
"text2text-generation",
"transformers",
"autotrain_compatible"
]
| text2text-generation | false | tau | null | tau/false_large_rouge_para0_sentNone_spanNone_5_1024_0.3_epoch1 | 0 | null | transformers | 36,733 | Entry not found |
tau/false_large_rouge_paraNone_sent0_spanNone_5_1024_0.3_epoch1 | 0aab8eea404bf9c5b7e62b8932cd9a8957b83758 | 2022-04-07T05:18:55.000Z | [
"pytorch",
"t5",
"text2text-generation",
"transformers",
"autotrain_compatible"
]
| text2text-generation | false | tau | null | tau/false_large_rouge_paraNone_sent0_spanNone_5_1024_0.3_epoch1 | 0 | null | transformers | 36,734 | Entry not found |
tau/false_large_rouge_para0_sent1_span2_5_1024_0.3_epoch1 | 505bf319532d5da826ffdd5a7c687372d0c0d567 | 2022-04-07T05:29:18.000Z | [
"pytorch",
"t5",
"text2text-generation",
"transformers",
"autotrain_compatible"
]
| text2text-generation | false | tau | null | tau/false_large_rouge_para0_sent1_span2_5_1024_0.3_epoch1 | 0 | null | transformers | 36,735 | Entry not found |
tau/false_large_random_para0_sentNone_spanNone_5_1024_0.3_epoch1 | 07e52297b34b250339121c68c85a9d79f6627fad | 2022-04-07T05:33:34.000Z | [
"pytorch",
"t5",
"text2text-generation",
"transformers",
"autotrain_compatible"
]
| text2text-generation | false | tau | null | tau/false_large_random_para0_sentNone_spanNone_5_1024_0.3_epoch1 | 0 | null | transformers | 36,736 | Entry not found |
tau/false_large_random_paraNone_sentNone_span0_5_1024_0.3_epoch1 | b66d52873c0005bf110b0b1562d8a3699adb2145 | 2022-04-07T05:44:08.000Z | [
"pytorch",
"t5",
"text2text-generation",
"transformers",
"autotrain_compatible"
]
| text2text-generation | false | tau | null | tau/false_large_random_paraNone_sentNone_span0_5_1024_0.3_epoch1 | 0 | null | transformers | 36,737 | Entry not found |
tau/false_large_random_para0_sent1_span2_5_1024_0.3_epoch1 | 062d32a84deec5031f8deba89e0e1be914a6942d | 2022-04-07T05:47:52.000Z | [
"pytorch",
"t5",
"text2text-generation",
"transformers",
"autotrain_compatible"
]
| text2text-generation | false | tau | null | tau/false_large_random_para0_sent1_span2_5_1024_0.3_epoch1 | 0 | null | transformers | 36,738 | Entry not found |
swagat-panda/multilingual-pos-tagger-indian-context-muril | 8a9466ea4afec0ac8bb3c81e279327807245fa14 | 2022-04-07T12:12:05.000Z | [
"pytorch",
"bert",
"transformers"
]
| null | false | swagat-panda | null | swagat-panda/multilingual-pos-tagger-indian-context-muril | 0 | null | transformers | 36,739 | Entry not found |
huggingtweets/joshrevellyt-mattywtf1-twommof1 | d63f23b38fc2da3a781a6b2f8d3088d1b167a98f | 2022-04-07T07:58:36.000Z | [
"pytorch",
"gpt2",
"text-generation",
"en",
"transformers",
"huggingtweets"
]
| text-generation | false | huggingtweets | null | huggingtweets/joshrevellyt-mattywtf1-twommof1 | 0 | null | transformers | 36,740 | ---
language: en
thumbnail: http://www.huggingtweets.com/joshrevellyt-mattywtf1-twommof1/1649318312148/predictions.png
tags:
- huggingtweets
widget:
- text: "My dream is"
---
<div class="inline-flex flex-col" style="line-height: 1.5;">
<div class="flex">
<div
style="display:inherit; margin-left: 4px; margin-right: 4px; width: 92px; height:92px; border-radius: 50%; background-size: cover; background-image: url('https://pbs.twimg.com/profile_images/1362391177438384130/3qb0i7rG_400x400.jpg')">
</div>
<div
style="display:inherit; margin-left: 4px; margin-right: 4px; width: 92px; height:92px; border-radius: 50%; background-size: cover; background-image: url('https://pbs.twimg.com/profile_images/1433115414679150596/6E1j0ONi_400x400.jpg')">
</div>
<div
style="display:inherit; margin-left: 4px; margin-right: 4px; width: 92px; height:92px; border-radius: 50%; background-size: cover; background-image: url('https://pbs.twimg.com/profile_images/1477038610083770369/u-wIlo9G_400x400.jpg')">
</div>
</div>
<div style="text-align: center; margin-top: 3px; font-size: 16px; font-weight: 800">🤖 AI CYBORG 🤖</div>
<div style="text-align: center; font-size: 16px; font-weight: 800">Matt Gallagher & Tommo & Josh Revell</div>
<div style="text-align: center; font-size: 14px;">@joshrevellyt-mattywtf1-twommof1</div>
</div>
I was made with [huggingtweets](https://github.com/borisdayma/huggingtweets).
Create your own bot based on your favorite user with [the demo](https://colab.research.google.com/github/borisdayma/huggingtweets/blob/master/huggingtweets-demo.ipynb)!
## How does it work?
The model uses the following pipeline.
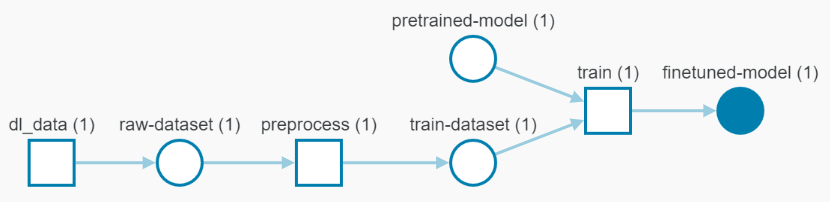
To understand how the model was developed, check the [W&B report](https://wandb.ai/wandb/huggingtweets/reports/HuggingTweets-Train-a-Model-to-Generate-Tweets--VmlldzoxMTY5MjI).
## Training data
The model was trained on tweets from Matt Gallagher & Tommo & Josh Revell.
| Data | Matt Gallagher | Tommo | Josh Revell |
| --- | --- | --- | --- |
| Tweets downloaded | 3250 | 3241 | 3204 |
| Retweets | 125 | 136 | 350 |
| Short tweets | 343 | 646 | 499 |
| Tweets kept | 2782 | 2459 | 2355 |
[Explore the data](https://wandb.ai/wandb/huggingtweets/runs/v11nskx9/artifacts), which is tracked with [W&B artifacts](https://docs.wandb.com/artifacts) at every step of the pipeline.
## Training procedure
The model is based on a pre-trained [GPT-2](https://huggingface.co/gpt2) which is fine-tuned on @joshrevellyt-mattywtf1-twommof1's tweets.
Hyperparameters and metrics are recorded in the [W&B training run](https://wandb.ai/wandb/huggingtweets/runs/udahi8v4) for full transparency and reproducibility.
At the end of training, [the final model](https://wandb.ai/wandb/huggingtweets/runs/udahi8v4/artifacts) is logged and versioned.
## How to use
You can use this model directly with a pipeline for text generation:
```python
from transformers import pipeline
generator = pipeline('text-generation',
model='huggingtweets/joshrevellyt-mattywtf1-twommof1')
generator("My dream is", num_return_sequences=5)
```
## Limitations and bias
The model suffers from [the same limitations and bias as GPT-2](https://huggingface.co/gpt2#limitations-and-bias).
In addition, the data present in the user's tweets further affects the text generated by the model.
## About
*Built by Boris Dayma*
[](https://twitter.com/intent/follow?screen_name=borisdayma)
For more details, visit the project repository.
[](https://github.com/borisdayma/huggingtweets)
|
guillaumegg/wav2vec2-base-timit-demo-4 | d85d745e6aadb9c61340fe94c25d5c10159450aa | 2022-04-07T09:34:14.000Z | [
"pytorch",
"tensorboard",
"wav2vec2",
"automatic-speech-recognition",
"transformers",
"generated_from_trainer",
"license:apache-2.0",
"model-index"
]
| automatic-speech-recognition | false | guillaumegg | null | guillaumegg/wav2vec2-base-timit-demo-4 | 0 | null | transformers | 36,741 | ---
license: apache-2.0
tags:
- generated_from_trainer
model-index:
- name: wav2vec2-base-timit-demo-4
results: []
---
<!-- This model card has been generated automatically according to the information the Trainer had access to. You
should probably proofread and complete it, then remove this comment. -->
# wav2vec2-base-timit-demo-4
This model is a fine-tuned version of [facebook/wav2vec2-large-xlsr-53-french](https://huggingface.co/facebook/wav2vec2-large-xlsr-53-french) on the None dataset.
## Model description
More information needed
## Intended uses & limitations
More information needed
## Training and evaluation data
More information needed
## Training procedure
### Training hyperparameters
The following hyperparameters were used during training:
- learning_rate: 0.0001
- train_batch_size: 32
- eval_batch_size: 8
- seed: 42
- optimizer: Adam with betas=(0.9,0.999) and epsilon=1e-08
- lr_scheduler_type: linear
- lr_scheduler_warmup_steps: 1000
- num_epochs: 4
### Training results
### Framework versions
- Transformers 4.19.0.dev0
- Pytorch 1.10.0+cu111
- Datasets 2.0.1.dev0
- Tokenizers 0.11.6
|
huggingtweets/chrismedlandf1-formula24hrs-tgruener | 837458f0103942a680c9af71a2c6dc53e1cba66f | 2022-04-07T09:48:09.000Z | [
"pytorch",
"gpt2",
"text-generation",
"en",
"transformers",
"huggingtweets"
]
| text-generation | false | huggingtweets | null | huggingtweets/chrismedlandf1-formula24hrs-tgruener | 0 | null | transformers | 36,742 | ---
language: en
thumbnail: http://www.huggingtweets.com/chrismedlandf1-formula24hrs-tgruener/1649324884859/predictions.png
tags:
- huggingtweets
widget:
- text: "My dream is"
---
<div class="inline-flex flex-col" style="line-height: 1.5;">
<div class="flex">
<div
style="display:inherit; margin-left: 4px; margin-right: 4px; width: 92px; height:92px; border-radius: 50%; background-size: cover; background-image: url('https://pbs.twimg.com/profile_images/1252178304192389120/bXT3lbuR_400x400.jpg')">
</div>
<div
style="display:inherit; margin-left: 4px; margin-right: 4px; width: 92px; height:92px; border-radius: 50%; background-size: cover; background-image: url('https://pbs.twimg.com/profile_images/1017184407495553024/MXfiA6IH_400x400.jpg')">
</div>
<div
style="display:inherit; margin-left: 4px; margin-right: 4px; width: 92px; height:92px; border-radius: 50%; background-size: cover; background-image: url('https://pbs.twimg.com/profile_images/1477602005917085696/PyJPHN6Z_400x400.jpg')">
</div>
</div>
<div style="text-align: center; margin-top: 3px; font-size: 16px; font-weight: 800">🤖 AI CYBORG 🤖</div>
<div style="text-align: center; font-size: 16px; font-weight: 800">Chris Medland & Tobi Grüner 🏁 & F24</div>
<div style="text-align: center; font-size: 14px;">@chrismedlandf1-formula24hrs-tgruener</div>
</div>
I was made with [huggingtweets](https://github.com/borisdayma/huggingtweets).
Create your own bot based on your favorite user with [the demo](https://colab.research.google.com/github/borisdayma/huggingtweets/blob/master/huggingtweets-demo.ipynb)!
## How does it work?
The model uses the following pipeline.
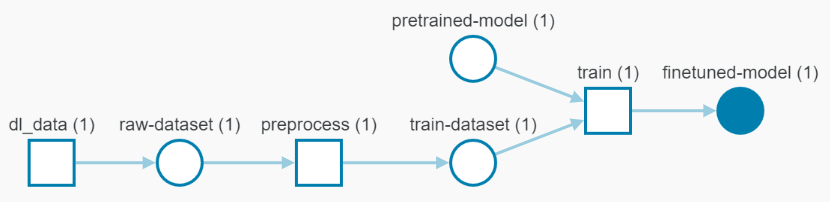
To understand how the model was developed, check the [W&B report](https://wandb.ai/wandb/huggingtweets/reports/HuggingTweets-Train-a-Model-to-Generate-Tweets--VmlldzoxMTY5MjI).
## Training data
The model was trained on tweets from Chris Medland & Tobi Grüner 🏁 & F24.
| Data | Chris Medland | Tobi Grüner 🏁 | F24 |
| --- | --- | --- | --- |
| Tweets downloaded | 3250 | 3207 | 431 |
| Retweets | 196 | 652 | 9 |
| Short tweets | 102 | 27 | 96 |
| Tweets kept | 2952 | 2528 | 326 |
[Explore the data](https://wandb.ai/wandb/huggingtweets/runs/2548ya6e/artifacts), which is tracked with [W&B artifacts](https://docs.wandb.com/artifacts) at every step of the pipeline.
## Training procedure
The model is based on a pre-trained [GPT-2](https://huggingface.co/gpt2) which is fine-tuned on @chrismedlandf1-formula24hrs-tgruener's tweets.
Hyperparameters and metrics are recorded in the [W&B training run](https://wandb.ai/wandb/huggingtweets/runs/17v4dkk8) for full transparency and reproducibility.
At the end of training, [the final model](https://wandb.ai/wandb/huggingtweets/runs/17v4dkk8/artifacts) is logged and versioned.
## How to use
You can use this model directly with a pipeline for text generation:
```python
from transformers import pipeline
generator = pipeline('text-generation',
model='huggingtweets/chrismedlandf1-formula24hrs-tgruener')
generator("My dream is", num_return_sequences=5)
```
## Limitations and bias
The model suffers from [the same limitations and bias as GPT-2](https://huggingface.co/gpt2#limitations-and-bias).
In addition, the data present in the user's tweets further affects the text generated by the model.
## About
*Built by Boris Dayma*
[](https://twitter.com/intent/follow?screen_name=borisdayma)
For more details, visit the project repository.
[](https://github.com/borisdayma/huggingtweets)
|
shonenkov-AI/rudalle-xl-surrealist | a69434033e4f7d2e67675a257bb0fe0256308461 | 2022-04-12T23:57:50.000Z | [
"pytorch"
]
| null | false | shonenkov-AI | null | shonenkov-AI/rudalle-xl-surrealist | 0 | 6 | null | 36,743 | ruDALLE Surrealist XL
---

[Alex Shonenkov](https://github.com/shonenkov-AI) trained the model. It is fine-tuning of [Malevich XL](https://huggingface.co/sberbank-ai/rudalle-Malevich) on the surreal data of famous art creators.
+ Task: text2image generation with custom aspect ratio
+ Parameters: 1.3 B
+ Training Data: 190 text-image pairs |
vocab-transformers/distilbert-word2vec_256k-MLM_500k | a7caecd1aab4bc73cd99d0ff22c630a6e656c0bf | 2022-04-07T12:52:12.000Z | [
"pytorch",
"distilbert",
"fill-mask",
"transformers",
"autotrain_compatible"
]
| fill-mask | false | vocab-transformers | null | vocab-transformers/distilbert-word2vec_256k-MLM_500k | 0 | null | transformers | 36,744 | # DistilBERT with word2vec token embeddings
This model has a word2vec token embedding matrix with 256k entries. The word2vec was trained on 100GB data from C4, MSMARCO, News, Wikipedia, S2ORC, for 3 epochs.
Then the model was trained on this dataset with MLM for 500k steps (batch size 64). The token embeddings were NOT updated.
|
vocab-transformers/distilbert-word2vec_256k-MLM_750k | 17f4cc670715d149609f1d34fb5d5206b84a235a | 2022-04-07T12:57:05.000Z | [
"pytorch",
"distilbert",
"fill-mask",
"transformers",
"autotrain_compatible"
]
| fill-mask | false | vocab-transformers | null | vocab-transformers/distilbert-word2vec_256k-MLM_750k | 0 | null | transformers | 36,745 | # DistilBERT with word2vec token embeddings
This model has a word2vec token embedding matrix with 256k entries. The word2vec was trained on 100GB data from C4, MSMARCO, News, Wikipedia, S2ORC, for 3 epochs.
Then the model was trained on this dataset with MLM for 750k steps (batch size 64). The token embeddings were NOT updated.
|
vocab-transformers/distilbert-tokenizer_256k-MLM_250k | b6d88bd36390b11e0d130b335cda1ba4fdd90517 | 2022-04-07T13:11:45.000Z | [
"pytorch",
"distilbert",
"fill-mask",
"transformers",
"autotrain_compatible"
]
| fill-mask | false | vocab-transformers | null | vocab-transformers/distilbert-tokenizer_256k-MLM_250k | 0 | null | transformers | 36,746 | # DistilBERT with 256k token embeddings
This model was initialized with a word2vec token embedding matrix with 256k entries, but these token embeddings were updated during MLM. The word2vec was trained on 100GB data from C4, MSMARCO, News, Wikipedia, S2ORC, for 3 epochs.
Then the model was trained on this dataset with MLM for 250k steps (batch size 64). The token embeddings were updated during MLM.
For the same model but with frozen token embeddings while MLM training see: https://huggingface.co/vocab-transformers/distilbert-word2vec_256k-MLM_250k
|
vocab-transformers/distilbert-tokenizer_256k-MLM_500k | 39f887545ae354572533fbc4763aefaa9f8ffe4e | 2022-04-07T13:11:35.000Z | [
"pytorch",
"distilbert",
"fill-mask",
"transformers",
"autotrain_compatible"
]
| fill-mask | false | vocab-transformers | null | vocab-transformers/distilbert-tokenizer_256k-MLM_500k | 0 | null | transformers | 36,747 | # DistilBERT with 256k token embeddings
This model was initialized with a word2vec token embedding matrix with 256k entries, but these token embeddings were updated during MLM. The word2vec was trained on 100GB data from C4, MSMARCO, News, Wikipedia, S2ORC, for 3 epochs.
Then the model was trained on this dataset with MLM for 500k steps (batch size 64). The token embeddings were updated during MLM.
For the same model but with frozen token embeddings while MLM training see: https://huggingface.co/vocab-transformers/distilbert-word2vec_256k-MLM_500k
|
vocab-transformers/distilbert-tokenizer_256k-MLM_750k | 5acf07523dcc82a6107cce644761ccc8f93a027b | 2022-04-07T13:11:23.000Z | [
"pytorch",
"distilbert",
"fill-mask",
"transformers",
"autotrain_compatible"
]
| fill-mask | false | vocab-transformers | null | vocab-transformers/distilbert-tokenizer_256k-MLM_750k | 0 | null | transformers | 36,748 | # DistilBERT with 256k token embeddings
This model was initialized with a word2vec token embedding matrix with 256k entries, but these token embeddings were updated during MLM. The word2vec was trained on 100GB data from C4, MSMARCO, News, Wikipedia, S2ORC, for 3 epochs.
Then the model was trained on this dataset with MLM for 750k steps (batch size 64). The token embeddings were updated during MLM.
For the same model but with frozen token embeddings while MLM training see: https://huggingface.co/vocab-transformers/distilbert-word2vec_256k-MLM_750k
|
jeremykke/albert-base-v2-finetuned-swag-v2 | c40397e11b978b182040df7d930ad3162b729a71 | 2022-04-07T16:18:27.000Z | [
"pytorch",
"tensorboard",
"albert",
"multiple-choice",
"transformers"
]
| multiple-choice | false | jeremykke | null | jeremykke/albert-base-v2-finetuned-swag-v2 | 0 | null | transformers | 36,749 | Entry not found |
huggingtweets/zahedparsa2 | 2cfa125548d36f42c8a8eb692916420cd5b8536f | 2022-04-07T15:33:34.000Z | [
"pytorch",
"gpt2",
"text-generation",
"en",
"transformers",
"huggingtweets"
]
| text-generation | false | huggingtweets | null | huggingtweets/zahedparsa2 | 0 | null | transformers | 36,750 | ---
language: en
thumbnail: http://www.huggingtweets.com/zahedparsa2/1649345587235/predictions.png
tags:
- huggingtweets
widget:
- text: "My dream is"
---
<div class="inline-flex flex-col" style="line-height: 1.5;">
<div class="flex">
<div
style="display:inherit; margin-left: 4px; margin-right: 4px; width: 92px; height:92px; border-radius: 50%; background-size: cover; background-image: url('https://pbs.twimg.com/profile_images/1244120919901175808/3krEqdBW_400x400.jpg')">
</div>
<div
style="display:none; margin-left: 4px; margin-right: 4px; width: 92px; height:92px; border-radius: 50%; background-size: cover; background-image: url('')">
</div>
<div
style="display:none; margin-left: 4px; margin-right: 4px; width: 92px; height:92px; border-radius: 50%; background-size: cover; background-image: url('')">
</div>
</div>
<div style="text-align: center; margin-top: 3px; font-size: 16px; font-weight: 800">🤖 AI BOT 🤖</div>
<div style="text-align: center; font-size: 16px; font-weight: 800">Zahedparsa</div>
<div style="text-align: center; font-size: 14px;">@zahedparsa2</div>
</div>
I was made with [huggingtweets](https://github.com/borisdayma/huggingtweets).
Create your own bot based on your favorite user with [the demo](https://colab.research.google.com/github/borisdayma/huggingtweets/blob/master/huggingtweets-demo.ipynb)!
## How does it work?
The model uses the following pipeline.
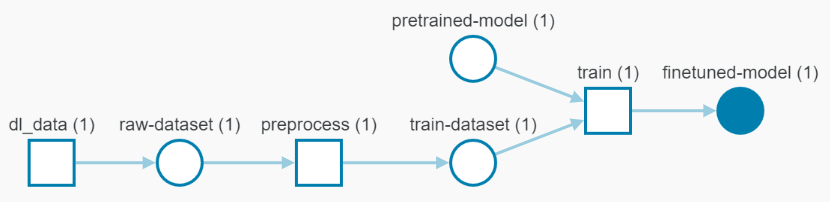
To understand how the model was developed, check the [W&B report](https://wandb.ai/wandb/huggingtweets/reports/HuggingTweets-Train-a-Model-to-Generate-Tweets--VmlldzoxMTY5MjI).
## Training data
The model was trained on tweets from Zahedparsa.
| Data | Zahedparsa |
| --- | --- |
| Tweets downloaded | 107 |
| Retweets | 2 |
| Short tweets | -1389 |
| Tweets kept | 1494 |
[Explore the data](https://wandb.ai/wandb/huggingtweets/runs/16gyx5yn/artifacts), which is tracked with [W&B artifacts](https://docs.wandb.com/artifacts) at every step of the pipeline.
## Training procedure
The model is based on a pre-trained [GPT-2](https://huggingface.co/gpt2) which is fine-tuned on @zahedparsa2's tweets.
Hyperparameters and metrics are recorded in the [W&B training run](https://wandb.ai/wandb/huggingtweets/runs/l5lakelq) for full transparency and reproducibility.
At the end of training, [the final model](https://wandb.ai/wandb/huggingtweets/runs/l5lakelq/artifacts) is logged and versioned.
## How to use
You can use this model directly with a pipeline for text generation:
```python
from transformers import pipeline
generator = pipeline('text-generation',
model='huggingtweets/zahedparsa2')
generator("My dream is", num_return_sequences=5)
```
## Limitations and bias
The model suffers from [the same limitations and bias as GPT-2](https://huggingface.co/gpt2#limitations-and-bias).
In addition, the data present in the user's tweets further affects the text generated by the model.
## About
*Built by Boris Dayma*
[](https://twitter.com/intent/follow?screen_name=borisdayma)
For more details, visit the project repository.
[](https://github.com/borisdayma/huggingtweets)
|
huggingtweets/mohamad_yazdi | 74460f4dbb050253000abfc403c3c8cba5626722 | 2022-04-07T23:39:32.000Z | [
"pytorch",
"gpt2",
"text-generation",
"en",
"transformers",
"huggingtweets"
]
| text-generation | false | huggingtweets | null | huggingtweets/mohamad_yazdi | 0 | null | transformers | 36,751 | ---
language: en
thumbnail: https://github.com/borisdayma/huggingtweets/blob/master/img/logo.png?raw=true
tags:
- huggingtweets
widget:
- text: "My dream is"
---
<div class="inline-flex flex-col" style="line-height: 1.5;">
<div class="flex">
<div
style="display:inherit; margin-left: 4px; margin-right: 4px; width: 92px; height:92px; border-radius: 50%; background-size: cover; background-image: url('https://pbs.twimg.com/profile_images/1496116344869244938/d7ZIiJV__400x400.jpg')">
</div>
<div
style="display:none; margin-left: 4px; margin-right: 4px; width: 92px; height:92px; border-radius: 50%; background-size: cover; background-image: url('')">
</div>
<div
style="display:none; margin-left: 4px; margin-right: 4px; width: 92px; height:92px; border-radius: 50%; background-size: cover; background-image: url('')">
</div>
</div>
<div style="text-align: center; margin-top: 3px; font-size: 16px; font-weight: 800">🤖 AI BOT 🤖</div>
<div style="text-align: center; font-size: 16px; font-weight: 800">mohamad yazdi</div>
<div style="text-align: center; font-size: 14px;">@mohamad_yazdi</div>
</div>
I was made with [huggingtweets](https://github.com/borisdayma/huggingtweets).
Create your own bot based on your favorite user with [the demo](https://colab.research.google.com/github/borisdayma/huggingtweets/blob/master/huggingtweets-demo.ipynb)!
## How does it work?
The model uses the following pipeline.
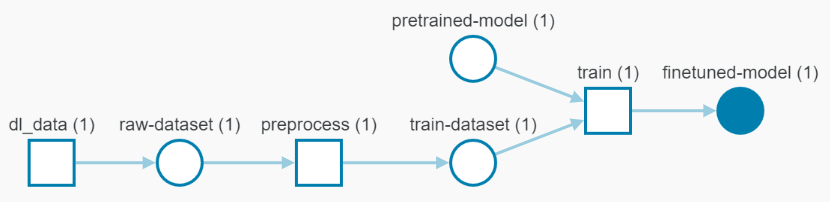
To understand how the model was developed, check the [W&B report](https://wandb.ai/wandb/huggingtweets/reports/HuggingTweets-Train-a-Model-to-Generate-Tweets--VmlldzoxMTY5MjI).
## Training data
The model was trained on tweets from mohamad yazdi.
| Data | mohamad yazdi |
| --- | --- |
| Tweets downloaded | 62 |
| Retweets | 0 |
| Short tweets | -401 |
| Tweets kept | 463 |
[Explore the data](https://wandb.ai/wandb/huggingtweets/runs/pfnvvlqn/artifacts), which is tracked with [W&B artifacts](https://docs.wandb.com/artifacts) at every step of the pipeline.
## Training procedure
The model is based on a pre-trained [GPT-2](https://huggingface.co/gpt2) which is fine-tuned on @mohamad_yazdi's tweets.
Hyperparameters and metrics are recorded in the [W&B training run](https://wandb.ai/wandb/huggingtweets/runs/xegerdmo) for full transparency and reproducibility.
At the end of training, [the final model](https://wandb.ai/wandb/huggingtweets/runs/xegerdmo/artifacts) is logged and versioned.
## How to use
You can use this model directly with a pipeline for text generation:
```python
from transformers import pipeline
generator = pipeline('text-generation',
model='huggingtweets/mohamad_yazdi')
generator("My dream is", num_return_sequences=5)
```
## Limitations and bias
The model suffers from [the same limitations and bias as GPT-2](https://huggingface.co/gpt2#limitations-and-bias).
In addition, the data present in the user's tweets further affects the text generated by the model.
## About
*Built by Boris Dayma*
[](https://twitter.com/intent/follow?screen_name=borisdayma)
For more details, visit the project repository.
[](https://github.com/borisdayma/huggingtweets)
|
jeremykke/albert-base-v2-finetuned-swags | e3053832cbd25d975af2641065cd6b927a3cb4ed | 2022-04-07T20:14:06.000Z | [
"pytorch",
"tensorboard",
"albert",
"multiple-choice",
"transformers"
]
| multiple-choice | false | jeremykke | null | jeremykke/albert-base-v2-finetuned-swags | 0 | null | transformers | 36,752 | Entry not found |
huggingtweets/timjdillon | 25b0fb432603ef6f95a674347a91fd54ddcf87b9 | 2022-04-07T19:04:06.000Z | [
"pytorch",
"gpt2",
"text-generation",
"en",
"transformers",
"huggingtweets"
]
| text-generation | false | huggingtweets | null | huggingtweets/timjdillon | 0 | null | transformers | 36,753 | ---
language: en
thumbnail: http://www.huggingtweets.com/timjdillon/1649358240896/predictions.png
tags:
- huggingtweets
widget:
- text: "My dream is"
---
<div class="inline-flex flex-col" style="line-height: 1.5;">
<div class="flex">
<div
style="display:inherit; margin-left: 4px; margin-right: 4px; width: 92px; height:92px; border-radius: 50%; background-size: cover; background-image: url('https://pbs.twimg.com/profile_images/1010263656456744960/bXOUw0hb_400x400.jpg')">
</div>
<div
style="display:none; margin-left: 4px; margin-right: 4px; width: 92px; height:92px; border-radius: 50%; background-size: cover; background-image: url('')">
</div>
<div
style="display:none; margin-left: 4px; margin-right: 4px; width: 92px; height:92px; border-radius: 50%; background-size: cover; background-image: url('')">
</div>
</div>
<div style="text-align: center; margin-top: 3px; font-size: 16px; font-weight: 800">🤖 AI BOT 🤖</div>
<div style="text-align: center; font-size: 16px; font-weight: 800">Tim Dillon</div>
<div style="text-align: center; font-size: 14px;">@timjdillon</div>
</div>
I was made with [huggingtweets](https://github.com/borisdayma/huggingtweets).
Create your own bot based on your favorite user with [the demo](https://colab.research.google.com/github/borisdayma/huggingtweets/blob/master/huggingtweets-demo.ipynb)!
## How does it work?
The model uses the following pipeline.
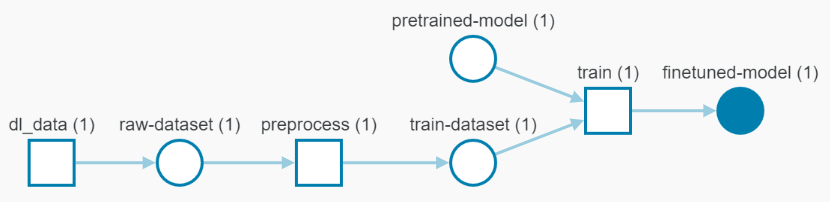
To understand how the model was developed, check the [W&B report](https://wandb.ai/wandb/huggingtweets/reports/HuggingTweets-Train-a-Model-to-Generate-Tweets--VmlldzoxMTY5MjI).
## Training data
The model was trained on tweets from Tim Dillon.
| Data | Tim Dillon |
| --- | --- |
| Tweets downloaded | 3240 |
| Retweets | 658 |
| Short tweets | 293 |
| Tweets kept | 2289 |
[Explore the data](https://wandb.ai/wandb/huggingtweets/runs/1egbnexm/artifacts), which is tracked with [W&B artifacts](https://docs.wandb.com/artifacts) at every step of the pipeline.
## Training procedure
The model is based on a pre-trained [GPT-2](https://huggingface.co/gpt2) which is fine-tuned on @timjdillon's tweets.
Hyperparameters and metrics are recorded in the [W&B training run](https://wandb.ai/wandb/huggingtweets/runs/1yr18emq) for full transparency and reproducibility.
At the end of training, [the final model](https://wandb.ai/wandb/huggingtweets/runs/1yr18emq/artifacts) is logged and versioned.
## How to use
You can use this model directly with a pipeline for text generation:
```python
from transformers import pipeline
generator = pipeline('text-generation',
model='huggingtweets/timjdillon')
generator("My dream is", num_return_sequences=5)
```
## Limitations and bias
The model suffers from [the same limitations and bias as GPT-2](https://huggingface.co/gpt2#limitations-and-bias).
In addition, the data present in the user's tweets further affects the text generated by the model.
## About
*Built by Boris Dayma*
[](https://twitter.com/intent/follow?screen_name=borisdayma)
For more details, visit the project repository.
[](https://github.com/borisdayma/huggingtweets)
|
rematchka/Bert_fake_news_detection | ae84a300764918f502f76c388933c2d0555e1df0 | 2022-04-07T22:10:00.000Z | [
"pytorch",
"bert",
"transformers"
]
| null | false | rematchka | null | rematchka/Bert_fake_news_detection | 0 | null | transformers | 36,754 | # Description
This model is Part of the NLP assignment for Fatima Fellowship.
This model is a fine-tuned version of 'bert-base-uncased' on the below dataset: [Fake News Dataset](https://www.kaggle.com/datasets/clmentbisaillon/fake-and-real-news-dataset).
It achieves the following results on the evaluation set:
- Accuracy: 0.995
- Precision: 0.995
- Recall: 0.995
- F_score: 0.995
# Labels
Fake news: 0
Real news: 1
# Using this model in your code
To use this model, first download it from the hugging face website:
```python
import transformers
from transformers import AutoTokenizer
class Fake_Real_Model_Arch_test(transformers.PreTrainedModel):
def __init__(self,bert):
super(Fake_Real_Model_Arch_test,self).__init__(config=AutoConfig.from_pretrained(MODEL_NAME))
self.bert = bert
num_classes = 2 # number of targets to predict
embedding_dim = 768 # length of embedding dim
self.fc1 = nn.Linear(embedding_dim, num_classes)
self.softmax = nn.Softmax()
def forward(self, text_id, text_mask):
outputs= self.bert(text_id, attention_mask=text_mask)
outputs = outputs[1] # get hidden layers
logit = self.fc1(outputs)
return self.softmax(logit)
tokenizer = AutoTokenizer.from_pretrained("bert-base-uncased")
model = Fake_Real_Model_Arch_test(AutoModel.from_pretrained("rematchka/Bert_fake_news_detection"))
```
|
huggingtweets/elonmusk-marknorm-timjdillon | 90221c0b37d7f03fac55f4293cb022574c9a5f73 | 2022-04-07T19:55:58.000Z | [
"pytorch",
"gpt2",
"text-generation",
"en",
"transformers",
"huggingtweets"
]
| text-generation | false | huggingtweets | null | huggingtweets/elonmusk-marknorm-timjdillon | 0 | null | transformers | 36,755 | ---
language: en
thumbnail: https://github.com/borisdayma/huggingtweets/blob/master/img/logo.png?raw=true
tags:
- huggingtweets
widget:
- text: "My dream is"
---
<div class="inline-flex flex-col" style="line-height: 1.5;">
<div class="flex">
<div
style="display:inherit; margin-left: 4px; margin-right: 4px; width: 92px; height:92px; border-radius: 50%; background-size: cover; background-image: url('https://pbs.twimg.com/profile_images/1503591435324563456/foUrqiEw_400x400.jpg')">
</div>
<div
style="display:inherit; margin-left: 4px; margin-right: 4px; width: 92px; height:92px; border-radius: 50%; background-size: cover; background-image: url('https://pbs.twimg.com/profile_images/1010263656456744960/bXOUw0hb_400x400.jpg')">
</div>
<div
style="display:inherit; margin-left: 4px; margin-right: 4px; width: 92px; height:92px; border-radius: 50%; background-size: cover; background-image: url('https://pbs.twimg.com/profile_images/1468306462245994496/x8koB4rb_400x400.jpg')">
</div>
</div>
<div style="text-align: center; margin-top: 3px; font-size: 16px; font-weight: 800">🤖 AI CYBORG 🤖</div>
<div style="text-align: center; font-size: 16px; font-weight: 800">Elon Musk & Tim Dillon & mark normand</div>
<div style="text-align: center; font-size: 14px;">@elonmusk-marknorm-timjdillon</div>
</div>
I was made with [huggingtweets](https://github.com/borisdayma/huggingtweets).
Create your own bot based on your favorite user with [the demo](https://colab.research.google.com/github/borisdayma/huggingtweets/blob/master/huggingtweets-demo.ipynb)!
## How does it work?
The model uses the following pipeline.
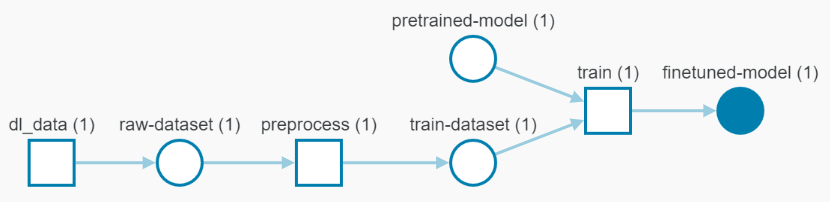
To understand how the model was developed, check the [W&B report](https://wandb.ai/wandb/huggingtweets/reports/HuggingTweets-Train-a-Model-to-Generate-Tweets--VmlldzoxMTY5MjI).
## Training data
The model was trained on tweets from Elon Musk & Tim Dillon & mark normand.
| Data | Elon Musk | Tim Dillon | mark normand |
| --- | --- | --- | --- |
| Tweets downloaded | 400 | 3240 | 3202 |
| Retweets | 14 | 658 | 116 |
| Short tweets | 117 | 293 | 477 |
| Tweets kept | 269 | 2289 | 2609 |
[Explore the data](https://wandb.ai/wandb/huggingtweets/runs/yk5i85xt/artifacts), which is tracked with [W&B artifacts](https://docs.wandb.com/artifacts) at every step of the pipeline.
## Training procedure
The model is based on a pre-trained [GPT-2](https://huggingface.co/gpt2) which is fine-tuned on @elonmusk-marknorm-timjdillon's tweets.
Hyperparameters and metrics are recorded in the [W&B training run](https://wandb.ai/wandb/huggingtweets/runs/zuzgzjdk) for full transparency and reproducibility.
At the end of training, [the final model](https://wandb.ai/wandb/huggingtweets/runs/zuzgzjdk/artifacts) is logged and versioned.
## How to use
You can use this model directly with a pipeline for text generation:
```python
from transformers import pipeline
generator = pipeline('text-generation',
model='huggingtweets/elonmusk-marknorm-timjdillon')
generator("My dream is", num_return_sequences=5)
```
## Limitations and bias
The model suffers from [the same limitations and bias as GPT-2](https://huggingface.co/gpt2#limitations-and-bias).
In addition, the data present in the user's tweets further affects the text generated by the model.
## About
*Built by Boris Dayma*
[](https://twitter.com/intent/follow?screen_name=borisdayma)
For more details, visit the project repository.
[](https://github.com/borisdayma/huggingtweets)
|
rematchka/Bert-model | e23adc1f0ea54656310b796f6529864f5ee48a79 | 2022-04-07T21:22:54.000Z | [
"pytorch",
"bert",
"transformers"
]
| null | false | rematchka | null | rematchka/Bert-model | 0 | null | transformers | 36,756 | Entry not found |
huggingtweets/abovethebed | c377c9a8a09600439c7443f8511dc67c016bcdd2 | 2022-04-08T10:16:32.000Z | [
"pytorch",
"gpt2",
"text-generation",
"en",
"transformers",
"huggingtweets"
]
| text-generation | false | huggingtweets | null | huggingtweets/abovethebed | 0 | null | transformers | 36,757 | ---
language: en
thumbnail: https://github.com/borisdayma/huggingtweets/blob/master/img/logo.png?raw=true
tags:
- huggingtweets
widget:
- text: "My dream is"
---
<div class="inline-flex flex-col" style="line-height: 1.5;">
<div class="flex">
<div
style="display:inherit; margin-left: 4px; margin-right: 4px; width: 92px; height:92px; border-radius: 50%; background-size: cover; background-image: url('https://pbs.twimg.com/profile_images/1317183233495388160/nLbBT6WF_400x400.jpg')">
</div>
<div
style="display:none; margin-left: 4px; margin-right: 4px; width: 92px; height:92px; border-radius: 50%; background-size: cover; background-image: url('')">
</div>
<div
style="display:none; margin-left: 4px; margin-right: 4px; width: 92px; height:92px; border-radius: 50%; background-size: cover; background-image: url('')">
</div>
</div>
<div style="text-align: center; margin-top: 3px; font-size: 16px; font-weight: 800">🤖 AI BOT 🤖</div>
<div style="text-align: center; font-size: 16px; font-weight: 800">3bkreno</div>
<div style="text-align: center; font-size: 14px;">@abovethebed</div>
</div>
I was made with [huggingtweets](https://github.com/borisdayma/huggingtweets).
Create your own bot based on your favorite user with [the demo](https://colab.research.google.com/github/borisdayma/huggingtweets/blob/master/huggingtweets-demo.ipynb)!
## How does it work?
The model uses the following pipeline.
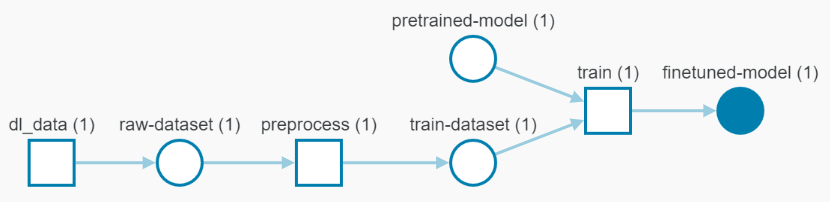
To understand how the model was developed, check the [W&B report](https://wandb.ai/wandb/huggingtweets/reports/HuggingTweets-Train-a-Model-to-Generate-Tweets--VmlldzoxMTY5MjI).
## Training data
The model was trained on tweets from 3bkreno.
| Data | 3bkreno |
| --- | --- |
| Tweets downloaded | 484 |
| Retweets | 111 |
| Short tweets | -468 |
| Tweets kept | 841 |
[Explore the data](https://wandb.ai/wandb/huggingtweets/runs/17s3cgho/artifacts), which is tracked with [W&B artifacts](https://docs.wandb.com/artifacts) at every step of the pipeline.
## Training procedure
The model is based on a pre-trained [GPT-2](https://huggingface.co/gpt2) which is fine-tuned on @abovethebed's tweets.
Hyperparameters and metrics are recorded in the [W&B training run](https://wandb.ai/wandb/huggingtweets/runs/2al4dbp2) for full transparency and reproducibility.
At the end of training, [the final model](https://wandb.ai/wandb/huggingtweets/runs/2al4dbp2/artifacts) is logged and versioned.
## How to use
You can use this model directly with a pipeline for text generation:
```python
from transformers import pipeline
generator = pipeline('text-generation',
model='huggingtweets/abovethebed')
generator("My dream is", num_return_sequences=5)
```
## Limitations and bias
The model suffers from [the same limitations and bias as GPT-2](https://huggingface.co/gpt2#limitations-and-bias).
In addition, the data present in the user's tweets further affects the text generated by the model.
## About
*Built by Boris Dayma*
[](https://twitter.com/intent/follow?screen_name=borisdayma)
For more details, visit the project repository.
[](https://github.com/borisdayma/huggingtweets)
|
medhabi/distilbert-base-uncased-score-pred-night | dde9a249bf128211b1984ae3e8c4f0c3f74333b0 | 2022-04-08T12:42:20.000Z | [
"pytorch",
"text-to-rating",
"transformers"
]
| null | false | medhabi | null | medhabi/distilbert-base-uncased-score-pred-night | 0 | null | transformers | 36,758 | Entry not found |
jessicammow/DialoGPT-small-ronswanson | cc1ecf8c9e5615b48815e7fc2308729addbabd20 | 2022-04-08T00:25:55.000Z | [
"pytorch",
"gpt2",
"text-generation",
"transformers",
"conversational"
]
| conversational | false | jessicammow | null | jessicammow/DialoGPT-small-ronswanson | 0 | null | transformers | 36,759 | ---
tags:
- conversational
---
# Ron Swanson DialoGPT Model |
jessicammow/DialoGPT-medium-leslieknope | 15fe5bddf08fb0d733fc0d598361f7ebe9ae5c82 | 2022-04-08T03:08:02.000Z | [
"pytorch",
"gpt2",
"text-generation",
"transformers",
"conversational"
]
| conversational | false | jessicammow | null | jessicammow/DialoGPT-medium-leslieknope | 0 | null | transformers | 36,760 | ---
tags:
- conversational
--- |
transZ/BART_shared_v2 | 30c3e192ec2d6df3c428c2108a745518ff67883d | 2022-04-08T03:58:41.000Z | [
"pytorch",
"shared_bart_v2",
"transformers"
]
| null | false | transZ | null | transZ/BART_shared_v2 | 0 | null | transformers | 36,761 | Entry not found |
huggingtweets/onlinepete-utilitylimb | 6fcb07ccbe5213a991d95b452e23098e970ecfed | 2022-04-08T06:46:14.000Z | [
"pytorch",
"gpt2",
"text-generation",
"en",
"transformers",
"huggingtweets"
]
| text-generation | false | huggingtweets | null | huggingtweets/onlinepete-utilitylimb | 0 | null | transformers | 36,762 | ---
language: en
thumbnail: http://www.huggingtweets.com/onlinepete-utilitylimb/1649400369339/predictions.png
tags:
- huggingtweets
widget:
- text: "My dream is"
---
<div class="inline-flex flex-col" style="line-height: 1.5;">
<div class="flex">
<div
style="display:inherit; margin-left: 4px; margin-right: 4px; width: 92px; height:92px; border-radius: 50%; background-size: cover; background-image: url('https://pbs.twimg.com/profile_images/1641418276/tumblr_lule5ckvND1qz4yoco1_1280_400x400.png')">
</div>
<div
style="display:inherit; margin-left: 4px; margin-right: 4px; width: 92px; height:92px; border-radius: 50%; background-size: cover; background-image: url('https://pbs.twimg.com/profile_images/456958582731603969/QZKpv6eI_400x400.jpeg')">
</div>
<div
style="display:none; margin-left: 4px; margin-right: 4px; width: 92px; height:92px; border-radius: 50%; background-size: cover; background-image: url('')">
</div>
</div>
<div style="text-align: center; margin-top: 3px; font-size: 16px; font-weight: 800">🤖 AI CYBORG 🤖</div>
<div style="text-align: center; font-size: 16px; font-weight: 800">bandit & im pete online</div>
<div style="text-align: center; font-size: 14px;">@onlinepete-utilitylimb</div>
</div>
I was made with [huggingtweets](https://github.com/borisdayma/huggingtweets).
Create your own bot based on your favorite user with [the demo](https://colab.research.google.com/github/borisdayma/huggingtweets/blob/master/huggingtweets-demo.ipynb)!
## How does it work?
The model uses the following pipeline.
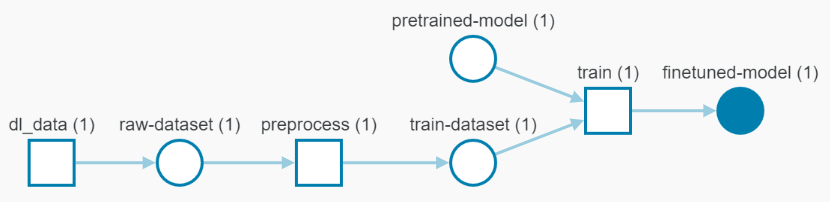
To understand how the model was developed, check the [W&B report](https://wandb.ai/wandb/huggingtweets/reports/HuggingTweets-Train-a-Model-to-Generate-Tweets--VmlldzoxMTY5MjI).
## Training data
The model was trained on tweets from bandit & im pete online.
| Data | bandit | im pete online |
| --- | --- | --- |
| Tweets downloaded | 653 | 3190 |
| Retweets | 7 | 94 |
| Short tweets | 9 | 1003 |
| Tweets kept | 637 | 2093 |
[Explore the data](https://wandb.ai/wandb/huggingtweets/runs/1gnqf0jm/artifacts), which is tracked with [W&B artifacts](https://docs.wandb.com/artifacts) at every step of the pipeline.
## Training procedure
The model is based on a pre-trained [GPT-2](https://huggingface.co/gpt2) which is fine-tuned on @onlinepete-utilitylimb's tweets.
Hyperparameters and metrics are recorded in the [W&B training run](https://wandb.ai/wandb/huggingtweets/runs/3bphxzxt) for full transparency and reproducibility.
At the end of training, [the final model](https://wandb.ai/wandb/huggingtweets/runs/3bphxzxt/artifacts) is logged and versioned.
## How to use
You can use this model directly with a pipeline for text generation:
```python
from transformers import pipeline
generator = pipeline('text-generation',
model='huggingtweets/onlinepete-utilitylimb')
generator("My dream is", num_return_sequences=5)
```
## Limitations and bias
The model suffers from [the same limitations and bias as GPT-2](https://huggingface.co/gpt2#limitations-and-bias).
In addition, the data present in the user's tweets further affects the text generated by the model.
## About
*Built by Boris Dayma*
[](https://twitter.com/intent/follow?screen_name=borisdayma)
For more details, visit the project repository.
[](https://github.com/borisdayma/huggingtweets)
|
jaeyeon/wav2vec2-child-en-tokenizer-4 | af91175dbbb4c7cccf15971e2e106d526e2fef5d | 2022-04-10T05:28:49.000Z | [
"pytorch",
"wav2vec2",
"automatic-speech-recognition",
"transformers",
"generated_from_trainer",
"license:apache-2.0",
"model-index"
]
| automatic-speech-recognition | false | jaeyeon | null | jaeyeon/wav2vec2-child-en-tokenizer-4 | 0 | null | transformers | 36,763 | ---
license: apache-2.0
tags:
- generated_from_trainer
model-index:
- name: wav2vec2-child-en-tokenizer-4
results: []
---
<!-- This model card has been generated automatically according to the information the Trainer had access to. You
should probably proofread and complete it, then remove this comment. -->
# wav2vec2-child-en-tokenizer-4
This model is a fine-tuned version of [facebook/wav2vec2-xls-r-300m](https://huggingface.co/facebook/wav2vec2-xls-r-300m) on the None dataset.
It achieves the following results on the evaluation set:
- Loss: 1.4709
- Wer: 0.3769
## Model description
More information needed
## Intended uses & limitations
More information needed
## Training and evaluation data
More information needed
## Training procedure
### Training hyperparameters
The following hyperparameters were used during training:
- learning_rate: 0.0003
- train_batch_size: 24
- eval_batch_size: 24
- seed: 42
- gradient_accumulation_steps: 2
- total_train_batch_size: 48
- optimizer: Adam with betas=(0.9,0.999) and epsilon=1e-08
- lr_scheduler_type: linear
- lr_scheduler_warmup_steps: 500
- num_epochs: 30
- mixed_precision_training: Native AMP
### Training results
| Training Loss | Epoch | Step | Validation Loss | Wer |
|:-------------:|:-----:|:----:|:---------------:|:------:|
| 0.0334 | 1.72 | 100 | 1.4709 | 0.3769 |
| 0.0332 | 3.45 | 200 | 1.4709 | 0.3769 |
| 0.0343 | 5.17 | 300 | 1.4709 | 0.3769 |
| 0.032 | 6.9 | 400 | 1.4709 | 0.3769 |
| 0.0332 | 8.62 | 500 | 1.4709 | 0.3769 |
| 0.0327 | 10.34 | 600 | 1.4709 | 0.3769 |
| 0.0331 | 12.07 | 700 | 1.4709 | 0.3769 |
| 0.0334 | 13.79 | 800 | 1.4709 | 0.3769 |
| 0.0319 | 15.52 | 900 | 1.4709 | 0.3769 |
| 0.0338 | 17.24 | 1000 | 1.4709 | 0.3769 |
| 0.0321 | 18.97 | 1100 | 1.4709 | 0.3769 |
| 0.0367 | 20.69 | 1200 | 1.4709 | 0.3769 |
| 0.0331 | 22.41 | 1300 | 1.4709 | 0.3769 |
| 0.0332 | 24.14 | 1400 | 1.4709 | 0.3769 |
| 0.0347 | 25.86 | 1500 | 1.4709 | 0.3769 |
| 0.0319 | 27.59 | 1600 | 1.4709 | 0.3769 |
| 0.0302 | 29.31 | 1700 | 1.4709 | 0.3769 |
### Framework versions
- Transformers 4.11.3
- Pytorch 1.10.0+cu113
- Datasets 1.18.3
- Tokenizers 0.10.3
|
medhabi/distilbert-base-uncased-score-pred-night-2 | e1207938b8d358106190eb920f69f9f2e79b8f61 | 2022-04-08T12:43:15.000Z | [
"pytorch",
"text-to-rating",
"transformers"
]
| null | false | medhabi | null | medhabi/distilbert-base-uncased-score-pred-night-2 | 0 | null | transformers | 36,764 | Entry not found |
jppaolim/v9PT | 807e528bce6d126e3fe2ec656790908a1a90fe8b | 2022-04-08T10:14:25.000Z | [
"pytorch",
"gpt2",
"text-generation",
"transformers"
]
| text-generation | false | jppaolim | null | jppaolim/v9PT | 0 | null | transformers | 36,765 | Entry not found |
lucypallent/distilbert-base-uncased-finetuned-test-headline | 317684caceea24560df527190ad11d3a3d875283 | 2022-04-09T12:16:31.000Z | [
"pytorch",
"tensorboard",
"distilbert",
"fill-mask",
"transformers",
"generated_from_trainer",
"license:apache-2.0",
"model-index",
"autotrain_compatible"
]
| fill-mask | false | lucypallent | null | lucypallent/distilbert-base-uncased-finetuned-test-headline | 0 | null | transformers | 36,766 | ---
license: apache-2.0
tags:
- generated_from_trainer
model-index:
- name: distilbert-base-uncased-finetuned-test-headline
results: []
---
<!-- This model card has been generated automatically according to the information the Trainer had access to. You
should probably proofread and complete it, then remove this comment. -->
# distilbert-base-uncased-finetuned-test-headline
This model is a fine-tuned version of [distilbert-base-uncased](https://huggingface.co/distilbert-base-uncased) on the None dataset.
It achieves the following results on the evaluation set:
- Loss: 4.0992
## Model description
More information needed
## Intended uses & limitations
More information needed
## Training and evaluation data
More information needed
## Training procedure
### Training hyperparameters
The following hyperparameters were used during training:
- learning_rate: 2e-05
- train_batch_size: 64
- eval_batch_size: 64
- seed: 42
- optimizer: Adam with betas=(0.9,0.999) and epsilon=1e-08
- lr_scheduler_type: linear
- num_epochs: 3.0
- mixed_precision_training: Native AMP
### Training results
| Training Loss | Epoch | Step | Validation Loss |
|:-------------:|:-----:|:----:|:---------------:|
| 5.6745 | 1.0 | 8 | 4.8602 |
| 4.8694 | 2.0 | 16 | 4.3241 |
| 4.5442 | 3.0 | 24 | 4.3963 |
### Framework versions
- Transformers 4.18.0
- Pytorch 1.10.0+cu111
- Datasets 2.0.0
- Tokenizers 0.11.6
|
huggingtweets/emarobot | 3c752e8bcddfcff92be40fb7f1248182306a5199 | 2022-04-08T11:13:49.000Z | [
"pytorch",
"gpt2",
"text-generation",
"en",
"transformers",
"huggingtweets"
]
| text-generation | false | huggingtweets | null | huggingtweets/emarobot | 0 | null | transformers | 36,767 | ---
language: en
thumbnail: http://www.huggingtweets.com/emarobot/1649416424059/predictions.png
tags:
- huggingtweets
widget:
- text: "My dream is"
---
<div class="inline-flex flex-col" style="line-height: 1.5;">
<div class="flex">
<div
style="display:inherit; margin-left: 4px; margin-right: 4px; width: 92px; height:92px; border-radius: 50%; background-size: cover; background-image: url('https://pbs.twimg.com/profile_images/1317183233495388160/nLbBT6WF_400x400.jpg')">
</div>
<div
style="display:none; margin-left: 4px; margin-right: 4px; width: 92px; height:92px; border-radius: 50%; background-size: cover; background-image: url('')">
</div>
<div
style="display:none; margin-left: 4px; margin-right: 4px; width: 92px; height:92px; border-radius: 50%; background-size: cover; background-image: url('')">
</div>
</div>
<div style="text-align: center; margin-top: 3px; font-size: 16px; font-weight: 800">🤖 AI BOT 🤖</div>
<div style="text-align: center; font-size: 16px; font-weight: 800">3bkreno</div>
<div style="text-align: center; font-size: 14px;">@emarobot</div>
</div>
I was made with [huggingtweets](https://github.com/borisdayma/huggingtweets).
Create your own bot based on your favorite user with [the demo](https://colab.research.google.com/github/borisdayma/huggingtweets/blob/master/huggingtweets-demo.ipynb)!
## How does it work?
The model uses the following pipeline.
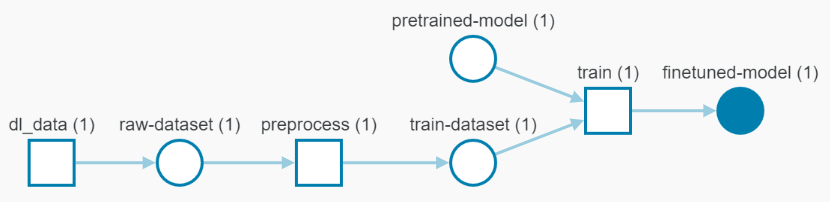
To understand how the model was developed, check the [W&B report](https://wandb.ai/wandb/huggingtweets/reports/HuggingTweets-Train-a-Model-to-Generate-Tweets--VmlldzoxMTY5MjI).
## Training data
The model was trained on tweets from 3bkreno.
| Data | 3bkreno |
| --- | --- |
| Tweets downloaded | 970 |
| Retweets | 111 |
| Short tweets | 129 |
| Tweets kept | 841 |
[Explore the data](https://wandb.ai/wandb/huggingtweets/runs/mfd65acm/artifacts), which is tracked with [W&B artifacts](https://docs.wandb.com/artifacts) at every step of the pipeline.
## Training procedure
The model is based on a pre-trained [GPT-2](https://huggingface.co/gpt2) which is fine-tuned on @emarobot's tweets.
Hyperparameters and metrics are recorded in the [W&B training run](https://wandb.ai/wandb/huggingtweets/runs/1i5j7avt) for full transparency and reproducibility.
At the end of training, [the final model](https://wandb.ai/wandb/huggingtweets/runs/1i5j7avt/artifacts) is logged and versioned.
## How to use
You can use this model directly with a pipeline for text generation:
```python
from transformers import pipeline
generator = pipeline('text-generation',
model='huggingtweets/emarobot')
generator("My dream is", num_return_sequences=5)
```
## Limitations and bias
The model suffers from [the same limitations and bias as GPT-2](https://huggingface.co/gpt2#limitations-and-bias).
In addition, the data present in the user's tweets further affects the text generated by the model.
## About
*Built by Boris Dayma*
[](https://twitter.com/intent/follow?screen_name=borisdayma)
For more details, visit the project repository.
[](https://github.com/borisdayma/huggingtweets)
|
huggingtweets/lilpeeplyric | 26503527d6e3941dd4887fd424d9bf5349963ccc | 2022-04-08T15:15:13.000Z | [
"pytorch",
"gpt2",
"text-generation",
"en",
"transformers",
"huggingtweets"
]
| text-generation | false | huggingtweets | null | huggingtweets/lilpeeplyric | 0 | null | transformers | 36,768 | ---
language: en
thumbnail: http://www.huggingtweets.com/lilpeeplyric/1649430909105/predictions.png
tags:
- huggingtweets
widget:
- text: "My dream is"
---
<div class="inline-flex flex-col" style="line-height: 1.5;">
<div class="flex">
<div
style="display:inherit; margin-left: 4px; margin-right: 4px; width: 92px; height:92px; border-radius: 50%; background-size: cover; background-image: url('https://pbs.twimg.com/profile_images/1445263525878902787/yW8p2-e__400x400.jpg')">
</div>
<div
style="display:none; margin-left: 4px; margin-right: 4px; width: 92px; height:92px; border-radius: 50%; background-size: cover; background-image: url('')">
</div>
<div
style="display:none; margin-left: 4px; margin-right: 4px; width: 92px; height:92px; border-radius: 50%; background-size: cover; background-image: url('')">
</div>
</div>
<div style="text-align: center; margin-top: 3px; font-size: 16px; font-weight: 800">🤖 AI BOT 🤖</div>
<div style="text-align: center; font-size: 16px; font-weight: 800">lil peep lyrics bot</div>
<div style="text-align: center; font-size: 14px;">@lilpeeplyric</div>
</div>
I was made with [huggingtweets](https://github.com/borisdayma/huggingtweets).
Create your own bot based on your favorite user with [the demo](https://colab.research.google.com/github/borisdayma/huggingtweets/blob/master/huggingtweets-demo.ipynb)!
## How does it work?
The model uses the following pipeline.
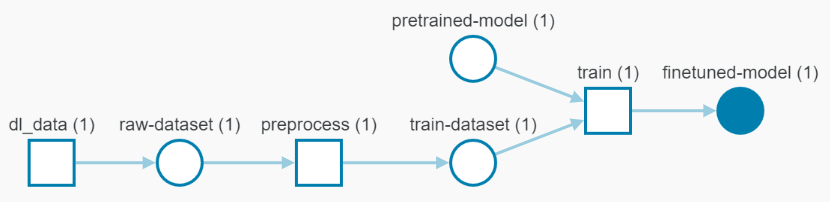
To understand how the model was developed, check the [W&B report](https://wandb.ai/wandb/huggingtweets/reports/HuggingTweets-Train-a-Model-to-Generate-Tweets--VmlldzoxMTY5MjI).
## Training data
The model was trained on tweets from lil peep lyrics bot.
| Data | lil peep lyrics bot |
| --- | --- |
| Tweets downloaded | 3250 |
| Retweets | 0 |
| Short tweets | 0 |
| Tweets kept | 3250 |
[Explore the data](https://wandb.ai/wandb/huggingtweets/runs/1jgq3lf6/artifacts), which is tracked with [W&B artifacts](https://docs.wandb.com/artifacts) at every step of the pipeline.
## Training procedure
The model is based on a pre-trained [GPT-2](https://huggingface.co/gpt2) which is fine-tuned on @lilpeeplyric's tweets.
Hyperparameters and metrics are recorded in the [W&B training run](https://wandb.ai/wandb/huggingtweets/runs/1lbjza1d) for full transparency and reproducibility.
At the end of training, [the final model](https://wandb.ai/wandb/huggingtweets/runs/1lbjza1d/artifacts) is logged and versioned.
## How to use
You can use this model directly with a pipeline for text generation:
```python
from transformers import pipeline
generator = pipeline('text-generation',
model='huggingtweets/lilpeeplyric')
generator("My dream is", num_return_sequences=5)
```
## Limitations and bias
The model suffers from [the same limitations and bias as GPT-2](https://huggingface.co/gpt2#limitations-and-bias).
In addition, the data present in the user's tweets further affects the text generated by the model.
## About
*Built by Boris Dayma*
[](https://twitter.com/intent/follow?screen_name=borisdayma)
For more details, visit the project repository.
[](https://github.com/borisdayma/huggingtweets)
|
krinal214/augmented_Squad_Translated | af6b682b37c5f259d23a6813c1524c35a758a04a | 2022-04-08T18:15:59.000Z | [
"pytorch",
"tensorboard",
"bert",
"question-answering",
"transformers",
"generated_from_trainer",
"license:apache-2.0",
"model-index",
"autotrain_compatible"
]
| question-answering | false | krinal214 | null | krinal214/augmented_Squad_Translated | 0 | null | transformers | 36,769 | ---
license: apache-2.0
tags:
- generated_from_trainer
model-index:
- name: augmented_Squad_Translated
results: []
---
<!-- This model card has been generated automatically according to the information the Trainer had access to. You
should probably proofread and complete it, then remove this comment. -->
# augmented_Squad_Translated
This model is a fine-tuned version of [bert-base-multilingual-cased](https://huggingface.co/bert-base-multilingual-cased) on the None dataset.
It achieves the following results on the evaluation set:
- Loss: 0.5251
## Model description
More information needed
## Intended uses & limitations
More information needed
## Training and evaluation data
More information needed
## Training procedure
### Training hyperparameters
The following hyperparameters were used during training:
- learning_rate: 2e-05
- train_batch_size: 16
- eval_batch_size: 16
- seed: 42
- optimizer: Adam with betas=(0.9,0.999) and epsilon=1e-08
- lr_scheduler_type: linear
- num_epochs: 1
### Training results
| Training Loss | Epoch | Step | Validation Loss |
|:-------------:|:-----:|:-----:|:---------------:|
| 1.1154 | 1.0 | 10835 | 0.5251 |
### Framework versions
- Transformers 4.16.2
- Pytorch 1.9.1
- Datasets 1.18.4
- Tokenizers 0.11.6
|
huggingtweets/notsorobot | a9edc5c92f65756a4cbb16ce6c34e8342ba80f40 | 2022-04-09T12:41:46.000Z | [
"pytorch",
"gpt2",
"text-generation",
"en",
"transformers",
"huggingtweets"
]
| text-generation | false | huggingtweets | null | huggingtweets/notsorobot | 0 | null | transformers | 36,770 | ---
language: en
thumbnail: https://github.com/borisdayma/huggingtweets/blob/master/img/logo.png?raw=true
tags:
- huggingtweets
widget:
- text: "My dream is"
---
<div class="inline-flex flex-col" style="line-height: 1.5;">
<div class="flex">
<div
style="display:inherit; margin-left: 4px; margin-right: 4px; width: 92px; height:92px; border-radius: 50%; background-size: cover; background-image: url('https://pbs.twimg.com/profile_images/1317183233495388160/nLbBT6WF_400x400.jpg')">
</div>
<div
style="display:none; margin-left: 4px; margin-right: 4px; width: 92px; height:92px; border-radius: 50%; background-size: cover; background-image: url('')">
</div>
<div
style="display:none; margin-left: 4px; margin-right: 4px; width: 92px; height:92px; border-radius: 50%; background-size: cover; background-image: url('')">
</div>
</div>
<div style="text-align: center; margin-top: 3px; font-size: 16px; font-weight: 800">🤖 AI BOT 🤖</div>
<div style="text-align: center; font-size: 16px; font-weight: 800">3bkreno</div>
<div style="text-align: center; font-size: 14px;">@notsorob</div>
</div>
I was made with [huggingtweets](https://github.com/borisdayma/huggingtweets).
Create your own bot based on your favorite user with [the demo](https://colab.research.google.com/github/borisdayma/huggingtweets/blob/master/huggingtweets-demo.ipynb)!
## How does it work?
The model uses the following pipeline.
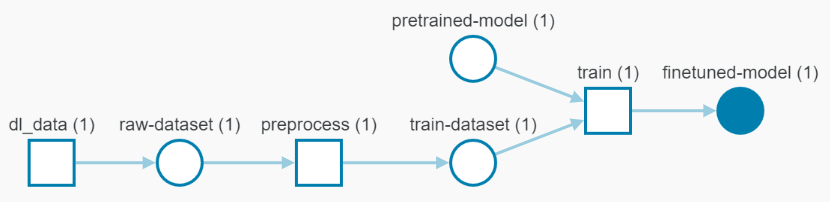
To understand how the model was developed, check the [W&B report](https://wandb.ai/wandb/huggingtweets/reports/HuggingTweets-Train-a-Model-to-Generate-Tweets--VmlldzoxMTY5MjI).
## Training data
The model was trained on tweets from 3bkreno.
| Data | 3bkreno |
| --- | --- |
| Tweets downloaded | 26419 |
| Retweets | 111 |
| Short tweets | -8796 |
| Tweets kept | 8796 |
[Explore the data](https://wandb.ai/wandb/huggingtweets/runs/1l7p1yze/artifacts), which is tracked with [W&B artifacts](https://docs.wandb.com/artifacts) at every step of the pipeline.
## Training procedure
The model is based on a pre-trained [GPT-2](https://huggingface.co/gpt2) which is fine-tuned on @notsorob's tweets.
Hyperparameters and metrics are recorded in the [W&B training run](https://wandb.ai/wandb/huggingtweets/runs/ypaq5o5y) for full transparency and reproducibility.
At the end of training, [the final model](https://wandb.ai/wandb/huggingtweets/runs/ypaq5o5y/artifacts) is logged and versioned.
## How to use
You can use this model directly with a pipeline for text generation:
```python
from transformers import pipeline
generator = pipeline('text-generation',
model='huggingtweets/notsorob')
generator("My dream is", num_return_sequences=5)
```
## Limitations and bias
The model suffers from [the same limitations and bias as GPT-2](https://huggingface.co/gpt2#limitations-and-bias).
In addition, the data present in the user's tweets further affects the text generated by the model.
## About
*Built by Boris Dayma*
[](https://twitter.com/intent/follow?screen_name=borisdayma)
For more details, visit the project repository.
[](https://github.com/borisdayma/huggingtweets)
|
modhp/wav2vec2-model2-torgo | 6f9049b9778659ada00097aa787fde726952ef3c | 2022-04-11T23:31:56.000Z | [
"pytorch",
"tensorboard",
"wav2vec2",
"automatic-speech-recognition",
"transformers",
"generated_from_trainer",
"model-index"
]
| automatic-speech-recognition | false | modhp | null | modhp/wav2vec2-model2-torgo | 0 | null | transformers | 36,771 | ---
tags:
- generated_from_trainer
model-index:
- name: wav2vec2-model2-torgo
results: []
---
<!-- This model card has been generated automatically according to the information the Trainer had access to. You
should probably proofread and complete it, then remove this comment. -->
# wav2vec2-model2-torgo
This model was trained from scratch on the None dataset.
It achieves the following results on the evaluation set:
- Loss: 4.9975
- Wer: 1.0
## Model description
More information needed
## Intended uses & limitations
More information needed
## Training and evaluation data
More information needed
## Training procedure
### Training hyperparameters
The following hyperparameters were used during training:
- learning_rate: 0.1
- train_batch_size: 1
- eval_batch_size: 8
- seed: 42
- gradient_accumulation_steps: 4
- total_train_batch_size: 4
- optimizer: Adam with betas=(0.9,0.999) and epsilon=1e-08
- lr_scheduler_type: linear
- lr_scheduler_warmup_steps: 1000
- num_epochs: 30
### Training results
| Training Loss | Epoch | Step | Validation Loss | Wer |
|:-------------:|:-----:|:-----:|:---------------:|:---:|
| 12.5453 | 0.76 | 500 | 14.6490 | 1.0 |
| 4.8036 | 1.53 | 1000 | 8.4523 | 1.0 |
| 5.0421 | 2.29 | 1500 | 5.4114 | 1.0 |
| 5.2055 | 3.05 | 2000 | 11.0507 | 1.0 |
| 4.6389 | 3.82 | 2500 | 4.6792 | 1.0 |
| 4.5523 | 4.58 | 3000 | 4.7855 | 1.0 |
| 4.7843 | 5.34 | 3500 | 11.2783 | 1.0 |
| 4.6066 | 6.11 | 4000 | 8.7807 | 1.0 |
| 4.7382 | 6.87 | 4500 | 2942.0220 | 1.0 |
| 130.5733 | 7.63 | 5000 | 5.8412 | 1.0 |
| 4.4972 | 8.4 | 5500 | 17.7038 | 1.0 |
| 4.5196 | 9.16 | 6000 | 11.4548 | 1.0 |
| 4.3198 | 9.92 | 6500 | 6.0885 | 1.0 |
| 4.4273 | 10.69 | 7000 | 6.7374 | 1.0 |
| 4.2783 | 11.45 | 7500 | 4.7276 | 1.0 |
| 4.2985 | 12.21 | 8000 | 6.1412 | 1.0 |
| 4.3262 | 12.98 | 8500 | 5.2621 | 1.0 |
| 4.1705 | 13.74 | 9000 | 5.2214 | 1.0 |
| 4.3176 | 14.5 | 9500 | 5.5359 | 1.0 |
| 3.9808 | 15.27 | 10000 | 4.1537 | 1.0 |
| 4.0228 | 16.03 | 10500 | 4.2962 | 1.0 |
| 4.0595 | 16.79 | 11000 | 7.6361 | 1.0 |
| 4.0088 | 17.56 | 11500 | 6.8715 | 1.0 |
| 3.8727 | 18.32 | 12000 | 8.8657 | 1.0 |
| 4.0073 | 19.08 | 12500 | 5.8170 | 1.0 |
| 3.8511 | 19.85 | 13000 | 13.9836 | 1.0 |
| 4.0899 | 20.61 | 13500 | 5.3287 | 1.0 |
| 3.8782 | 21.37 | 14000 | 8.0635 | 1.0 |
| 3.9235 | 22.14 | 14500 | 5.5129 | 1.0 |
| 3.7276 | 22.9 | 15000 | 5.0819 | 1.0 |
| 3.7908 | 23.66 | 15500 | 6.1458 | 1.0 |
| 3.9176 | 24.43 | 16000 | 4.6094 | 1.0 |
| 3.8477 | 25.19 | 16500 | 5.1406 | 1.0 |
| 3.6917 | 25.95 | 17000 | 4.5684 | 1.0 |
| 3.8568 | 26.72 | 17500 | 4.0306 | 1.0 |
| 3.7231 | 27.48 | 18000 | 5.6331 | 1.0 |
| 3.8145 | 28.24 | 18500 | 8.2997 | 1.0 |
| 3.7809 | 29.01 | 19000 | 5.7468 | 1.0 |
| 3.5995 | 29.77 | 19500 | 4.9975 | 1.0 |
### Framework versions
- Transformers 4.17.0
- Pytorch 1.11.0
- Datasets 1.18.3
- Tokenizers 0.11.6
|
nateraw/some-timm-model | eec88812d2589a7853f4b6edead9596fe4c97838 | 2022-04-08T20:41:36.000Z | [
"pytorch",
"timm",
"image-classification"
]
| image-classification | false | nateraw | null | nateraw/some-timm-model | 0 | null | timm | 36,772 | ---
tags:
- image-classification
- timm
library_tag: timm
---
# Model card for some-timm-model |
huggan/pix2pix-cityscapes | b182bc5aba10b79ccf396edc6a4bd7bda16a2084 | 2022-04-09T15:47:22.000Z | [
"pytorch"
]
| null | false | huggan | null | huggan/pix2pix-cityscapes | 0 | null | null | 36,773 | Entry not found |
huggan/pix2pix-facades | 5ccb50ec907eec310280a5944fdf2576bd9647c5 | 2022-04-09T12:54:21.000Z | [
"pytorch"
]
| null | false | huggan | null | huggan/pix2pix-facades | 0 | null | null | 36,774 | Entry not found |
nielsr/pix2pix-facades | f9e626c7e0150070b4c2dcf3bfc035ae7d88709d | 2022-04-09T13:08:54.000Z | [
"pytorch"
]
| null | false | nielsr | null | nielsr/pix2pix-facades | 0 | null | null | 36,775 | Entry not found |
huggan/pix2pix-facades-demo | 3784ab6be149f42f77ffb5b9c9cba8e18fbe2fb4 | 2022-04-11T08:09:26.000Z | [
"pytorch",
"huggan",
"gan",
"license:mit"
]
| null | false | huggan | null | huggan/pix2pix-facades-demo | 0 | null | null | 36,776 | ---
tags:
- huggan
- gan
# See a list of available tags here:
# https://github.com/huggingface/hub-docs/blob/main/js/src/lib/interfaces/Types.ts#L12
# task: unconditional-image-generation or conditional-image-generation or image-to-image
license: mit
---
This was run from this implementation: https://github.com/NielsRogge/community-events-1/blob/improve_pix2pix/huggan/pytorch/pix2pix/train.py
The command to run was:
```bash
accelerate launch train.py --checkpoint_interval 1 --push_to_hub --output_dir pix2pix-facades --hub_model_id huggan/pix2pix-facades-demo --wandb
``` |
jppaolim/v10Accel | 66d6b7a6b1421709436686d363cd5558ee442e4e | 2022-04-09T14:56:00.000Z | [
"pytorch",
"gpt2",
"text-generation",
"transformers"
]
| text-generation | false | jppaolim | null | jppaolim/v10Accel | 0 | null | transformers | 36,777 | Entry not found |
nielsr/pix2pix-cityscapes | c0434e7e162215547891c08236dba545fc004e7b | 2022-04-09T16:16:17.000Z | [
"pytorch",
"huggan",
"gan",
"license:mit"
]
| null | false | nielsr | null | nielsr/pix2pix-cityscapes | 0 | null | null | 36,778 | ---
tags:
- huggan
- gan
# See a list of available tags here:
# https://github.com/huggingface/hub-docs/blob/main/js/src/lib/interfaces/Types.ts#L12
# task: unconditional-image-generation or conditional-image-generation or image-to-image
license: mit
---
# MyModelName
## Model description
Describe the model here (what it does, what it's used for, etc.)
## Intended uses & limitations
#### How to use
```python
# You can include sample code which will be formatted
```
#### Limitations and bias
Provide examples of latent issues and potential remediations.
## Training data
Describe the data you used to train the model.
If you initialized it with pre-trained weights, add a link to the pre-trained model card or repository with description of the pre-training data.
## Training procedure
Preprocessing, hardware used, hyperparameters...
## Eval results
## Generated Images
You can embed local or remote images using ``
### BibTeX entry and citation info
```bibtex
@inproceedings{...,
year={2020}
}
``` |
krinal214/bert-all-translated | c815f6f9d7ca45929e8f5cb2d2dd655edf1a1e5c | 2022-04-09T18:39:02.000Z | [
"pytorch",
"tensorboard",
"bert",
"question-answering",
"transformers",
"generated_from_trainer",
"license:apache-2.0",
"model-index",
"autotrain_compatible"
]
| question-answering | false | krinal214 | null | krinal214/bert-all-translated | 0 | null | transformers | 36,779 | ---
license: apache-2.0
tags:
- generated_from_trainer
model-index:
- name: bert-all-translated
results: []
---
<!-- This model card has been generated automatically according to the information the Trainer had access to. You
should probably proofread and complete it, then remove this comment. -->
# bert-all-translated
This model is a fine-tuned version of [bert-base-multilingual-cased](https://huggingface.co/bert-base-multilingual-cased) on the None dataset.
It achieves the following results on the evaluation set:
- Loss: 0.5775
## Model description
More information needed
## Intended uses & limitations
More information needed
## Training and evaluation data
More information needed
## Training procedure
### Training hyperparameters
The following hyperparameters were used during training:
- learning_rate: 2e-05
- train_batch_size: 16
- eval_batch_size: 16
- seed: 42
- optimizer: Adam with betas=(0.9,0.999) and epsilon=1e-08
- lr_scheduler_type: linear
- num_epochs: 1
### Training results
| Training Loss | Epoch | Step | Validation Loss |
|:-------------:|:-----:|:----:|:---------------:|
| 1.2067 | 1.0 | 6319 | 0.5775 |
### Framework versions
- Transformers 4.16.2
- Pytorch 1.9.1
- Datasets 1.18.4
- Tokenizers 0.11.6
|
masakhane/afribyt5_bam_fr_news | 8518ac7d80061bc6908247ebfc99c665449dc0cd | 2022-04-11T13:34:03.000Z | [
"pytorch",
"t5",
"text2text-generation",
"transformers",
"license:afl-3.0",
"autotrain_compatible"
]
| text2text-generation | false | masakhane | null | masakhane/afribyt5_bam_fr_news | 0 | null | transformers | 36,780 | ---
license: afl-3.0
---
|
masakhane/byt5_bam_fr_news | 426647906fbfeea6f8257bf2181e26a4279ac98c | 2022-04-11T13:41:39.000Z | [
"pytorch",
"t5",
"text2text-generation",
"transformers",
"license:afl-3.0",
"autotrain_compatible"
]
| text2text-generation | false | masakhane | null | masakhane/byt5_bam_fr_news | 0 | null | transformers | 36,781 | ---
license: afl-3.0
---
|
masakhane/m2m100_418M_bam_fr_rel_news | 9f551b90947792ad688a0c0eb034916842280b4d | 2022-04-11T14:44:01.000Z | [
"pytorch",
"m2m_100",
"text2text-generation",
"transformers",
"license:afl-3.0",
"autotrain_compatible"
]
| text2text-generation | false | masakhane | null | masakhane/m2m100_418M_bam_fr_rel_news | 0 | null | transformers | 36,782 | ---
license: afl-3.0
---
|
masakhane/m2m100_418M_bam_fr_rel | 63a2b4aa6472a40584e0a64f6056337ec743516d | 2022-04-11T15:21:06.000Z | [
"pytorch",
"m2m_100",
"text2text-generation",
"transformers",
"license:afl-3.0",
"autotrain_compatible"
]
| text2text-generation | false | masakhane | null | masakhane/m2m100_418M_bam_fr_rel | 0 | null | transformers | 36,783 | ---
license: afl-3.0
---
|
masakhane/mbart50_bam_fr_news | 5673525f9353f5382242815a265df4878590a252 | 2022-04-11T14:22:32.000Z | [
"pytorch",
"mbart",
"text2text-generation",
"transformers",
"license:afl-3.0",
"autotrain_compatible"
]
| text2text-generation | false | masakhane | null | masakhane/mbart50_bam_fr_news | 0 | null | transformers | 36,784 | ---
license: afl-3.0
---
|
masakhane/mt5_fr_bam_news | c28fd2748421f6efcae9b89a9ed7e0e4c8bc59a1 | 2022-04-11T13:53:55.000Z | [
"pytorch",
"mt5",
"text2text-generation",
"transformers",
"license:afl-3.0",
"autotrain_compatible"
]
| text2text-generation | false | masakhane | null | masakhane/mt5_fr_bam_news | 0 | null | transformers | 36,785 | ---
license: afl-3.0
---
|
gemasphi/laprador-document-encoder | 6ffa4ce19e7427cd735d45342f2775e555f882aa | 2022-04-09T18:35:02.000Z | [
"pytorch",
"bert",
"feature-extraction",
"sentence-transformers",
"sentence-similarity",
"transformers"
]
| sentence-similarity | false | gemasphi | null | gemasphi/laprador-document-encoder | 0 | null | sentence-transformers | 36,786 | ---
pipeline_tag: sentence-similarity
tags:
- sentence-transformers
- feature-extraction
- sentence-similarity
- transformers
---
# {MODEL_NAME}
This is a [sentence-transformers](https://www.SBERT.net) model: It maps sentences & paragraphs to a 768 dimensional dense vector space and can be used for tasks like clustering or semantic search.
<!--- Describe your model here -->
## Usage (Sentence-Transformers)
Using this model becomes easy when you have [sentence-transformers](https://www.SBERT.net) installed:
```
pip install -U sentence-transformers
```
Then you can use the model like this:
```python
from sentence_transformers import SentenceTransformer
sentences = ["This is an example sentence", "Each sentence is converted"]
model = SentenceTransformer('{MODEL_NAME}')
embeddings = model.encode(sentences)
print(embeddings)
```
## Usage (HuggingFace Transformers)
Without [sentence-transformers](https://www.SBERT.net), you can use the model like this: First, you pass your input through the transformer model, then you have to apply the right pooling-operation on-top of the contextualized word embeddings.
```python
from transformers import AutoTokenizer, AutoModel
import torch
#Mean Pooling - Take attention mask into account for correct averaging
def mean_pooling(model_output, attention_mask):
token_embeddings = model_output[0] #First element of model_output contains all token embeddings
input_mask_expanded = attention_mask.unsqueeze(-1).expand(token_embeddings.size()).float()
return torch.sum(token_embeddings * input_mask_expanded, 1) / torch.clamp(input_mask_expanded.sum(1), min=1e-9)
# Sentences we want sentence embeddings for
sentences = ['This is an example sentence', 'Each sentence is converted']
# Load model from HuggingFace Hub
tokenizer = AutoTokenizer.from_pretrained('{MODEL_NAME}')
model = AutoModel.from_pretrained('{MODEL_NAME}')
# Tokenize sentences
encoded_input = tokenizer(sentences, padding=True, truncation=True, return_tensors='pt')
# Compute token embeddings
with torch.no_grad():
model_output = model(**encoded_input)
# Perform pooling. In this case, mean pooling.
sentence_embeddings = mean_pooling(model_output, encoded_input['attention_mask'])
print("Sentence embeddings:")
print(sentence_embeddings)
```
## Evaluation Results
<!--- Describe how your model was evaluated -->
For an automated evaluation of this model, see the *Sentence Embeddings Benchmark*: [https://seb.sbert.net](https://seb.sbert.net?model_name={MODEL_NAME})
## Full Model Architecture
```
SentenceTransformer(
(0): Transformer({'max_seq_length': 350, 'do_lower_case': False}) with Transformer model: BertModel
(1): Pooling({'word_embedding_dimension': 768, 'pooling_mode_cls_token': False, 'pooling_mode_mean_tokens': True, 'pooling_mode_max_tokens': False, 'pooling_mode_mean_sqrt_len_tokens': False})
)
```
## Citing & Authors
<!--- Describe where people can find more information --> |
huggan/fastgan-few-shot-fauvism-still-life | 502ee8340517914274b3defaf5dfe3a29240c71f | 2022-05-06T22:29:19.000Z | [
"pytorch",
"dataset:huggan/few-shot-fauvism-still-life",
"arxiv:2101.04775",
"huggan",
"gan",
"unconditional-image-generation",
"license:mit"
]
| unconditional-image-generation | false | huggan | null | huggan/fastgan-few-shot-fauvism-still-life | 0 | null | null | 36,787 | ---
tags:
- huggan
- gan
- unconditional-image-generation
datasets:
- huggan/few-shot-fauvism-still-life
# See a list of available tags here:
# https://github.com/huggingface/hub-docs/blob/main/js/src/lib/interfaces/Types.ts#L12
# task: unconditional-image-generation or conditional-image-generation or image-to-image
license: mit
---
# Generate fauvism still life image using FastGAN
## Model description
[FastGAN model](https://arxiv.org/abs/2101.04775) is a Generative Adversarial Networks (GAN) training on a small amount of high-fidelity images with minimum computing cost. Using a skip-layer channel-wise excitation module and a self-supervised discriminator trained as a feature-encoder, the model was able to converge after some hours of training for either 100 high-quality images or 1000 images datasets.
This model was trained on a dataset of 124 high-quality Fauvism painting images.
#### How to use
```python
# Clone this model
git clone https://huggingface.co/huggan/fastgan-few-shot-fauvism-still-life/
def load_generator(model_name_or_path):
generator = Generator(in_channels=256, out_channels=3)
generator = generator.from_pretrained(model_name_or_path, in_channels=256, out_channels=3)
_ = generator.eval()
return generator
def _denormalize(input: torch.Tensor) -> torch.Tensor:
return (input * 127.5) + 127.5
# Load generator
generator = load_generator("huggan/fastgan-few-shot-fauvism-still-life")
# Generate a random noise image
noise = torch.zeros(1, 256, 1, 1, device=device).normal_(0.0, 1.0)
with torch.no_grad():
gan_images, _ = generator(noise)
gan_images = _denormalize(gan_images.detach())
save_image(gan_images, "sample.png", nrow=1, normalize=True)
```
#### Limitations and bias
* Converge faster and better with small datasets (less than 1000 samples)
## Training data
[few-shot-fauvism-still-life](https://huggingface.co/datasets/huggan/few-shot-fauvism-still-life)
## Generated Images

### BibTeX entry and citation info
```bibtex
@article{FastGAN,
title={Towards Faster and Stabilized GAN Training for High-fidelity Few-shot Image Synthesis},
author={Bingchen Liu, Yizhe Zhu, Kunpeng Song, Ahmed Elgammal},
journal={ICLR},
year={2021}
}
``` |
iyedr8/DialoGPT-small-rick | 1ce7258632c3f27dcbfde84da5312824ee57cfb6 | 2022-04-10T00:21:32.000Z | [
"pytorch",
"gpt2",
"text-generation",
"transformers",
"conversational"
]
| conversational | false | iyedr8 | null | iyedr8/DialoGPT-small-rick | 0 | null | transformers | 36,788 | ---
tags:
- conversational
---
#Morty DialoGPT Model |
uhlenbeckmew/distilroberta-base-1 | 1a3a2c62ed971d16913ccbf21392c252f43b3b74 | 2022-04-26T05:53:42.000Z | [
"pytorch",
"tensorboard",
"roberta",
"fill-mask",
"transformers",
"generated_from_trainer",
"license:apache-2.0",
"model-index",
"autotrain_compatible"
]
| fill-mask | false | uhlenbeckmew | null | uhlenbeckmew/distilroberta-base-1 | 0 | null | transformers | 36,789 | ---
license: apache-2.0
tags:
- generated_from_trainer
model-index:
- name: distilroberta-base-1
results: []
---
<!-- This model card has been generated automatically according to the information the Trainer had access to. You
should probably proofread and complete it, then remove this comment. -->
# distilroberta-base-1
This model is a fine-tuned version of [distilroberta-base](https://huggingface.co/distilroberta-base) on the None dataset.
It achieves the following results on the evaluation set:
- Loss: 2.6634
## Model description
More information needed
## Intended uses & limitations
More information needed
## Training and evaluation data
More information needed
## Training procedure
### Training hyperparameters
The following hyperparameters were used during training:
- learning_rate: 2e-05
- train_batch_size: 8
- eval_batch_size: 8
- seed: 42
- optimizer: Adam with betas=(0.9,0.999) and epsilon=1e-08
- lr_scheduler_type: linear
- num_epochs: 3.0
### Training results
| Training Loss | Epoch | Step | Validation Loss |
|:-------------:|:-----:|:----:|:---------------:|
| 3.0133 | 1.0 | 1388 | 2.8166 |
| 2.8418 | 2.0 | 2776 | 2.7113 |
| 2.7683 | 3.0 | 4164 | 2.6634 |
### Framework versions
- Transformers 4.18.0
- Pytorch 1.11.0+cu113
- Datasets 2.1.0
- Tokenizers 0.12.1
|
huggingtweets/fitfounder | 152520db7f6cc73152bd603db0955dd4df234676 | 2022-04-10T10:09:19.000Z | [
"pytorch",
"gpt2",
"text-generation",
"en",
"transformers",
"huggingtweets"
]
| text-generation | false | huggingtweets | null | huggingtweets/fitfounder | 0 | null | transformers | 36,790 | ---
language: en
thumbnail: http://www.huggingtweets.com/fitfounder/1649585355118/predictions.png
tags:
- huggingtweets
widget:
- text: "My dream is"
---
<div class="inline-flex flex-col" style="line-height: 1.5;">
<div class="flex">
<div
style="display:inherit; margin-left: 4px; margin-right: 4px; width: 92px; height:92px; border-radius: 50%; background-size: cover; background-image: url('https://pbs.twimg.com/profile_images/1279092409587163137/eN82f_KT_400x400.jpg')">
</div>
<div
style="display:none; margin-left: 4px; margin-right: 4px; width: 92px; height:92px; border-radius: 50%; background-size: cover; background-image: url('')">
</div>
<div
style="display:none; margin-left: 4px; margin-right: 4px; width: 92px; height:92px; border-radius: 50%; background-size: cover; background-image: url('')">
</div>
</div>
<div style="text-align: center; margin-top: 3px; font-size: 16px; font-weight: 800">🤖 AI BOT 🤖</div>
<div style="text-align: center; font-size: 16px; font-weight: 800">Dan Go</div>
<div style="text-align: center; font-size: 14px;">@fitfounder</div>
</div>
I was made with [huggingtweets](https://github.com/borisdayma/huggingtweets).
Create your own bot based on your favorite user with [the demo](https://colab.research.google.com/github/borisdayma/huggingtweets/blob/master/huggingtweets-demo.ipynb)!
## How does it work?
The model uses the following pipeline.
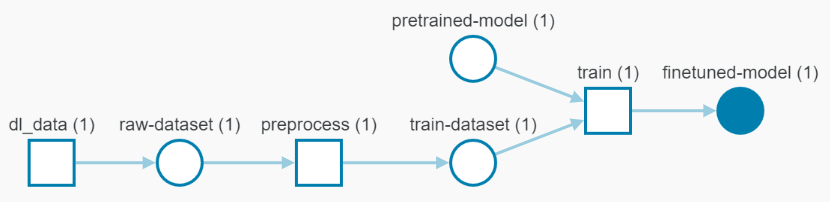
To understand how the model was developed, check the [W&B report](https://wandb.ai/wandb/huggingtweets/reports/HuggingTweets-Train-a-Model-to-Generate-Tweets--VmlldzoxMTY5MjI).
## Training data
The model was trained on tweets from Dan Go.
| Data | Dan Go |
| --- | --- |
| Tweets downloaded | 3250 |
| Retweets | 47 |
| Short tweets | 653 |
| Tweets kept | 2550 |
[Explore the data](https://wandb.ai/wandb/huggingtweets/runs/3lrz0j2b/artifacts), which is tracked with [W&B artifacts](https://docs.wandb.com/artifacts) at every step of the pipeline.
## Training procedure
The model is based on a pre-trained [GPT-2](https://huggingface.co/gpt2) which is fine-tuned on @fitfounder's tweets.
Hyperparameters and metrics are recorded in the [W&B training run](https://wandb.ai/wandb/huggingtweets/runs/8hmcij96) for full transparency and reproducibility.
At the end of training, [the final model](https://wandb.ai/wandb/huggingtweets/runs/8hmcij96/artifacts) is logged and versioned.
## How to use
You can use this model directly with a pipeline for text generation:
```python
from transformers import pipeline
generator = pipeline('text-generation',
model='huggingtweets/fitfounder')
generator("My dream is", num_return_sequences=5)
```
## Limitations and bias
The model suffers from [the same limitations and bias as GPT-2](https://huggingface.co/gpt2#limitations-and-bias).
In addition, the data present in the user's tweets further affects the text generated by the model.
## About
*Built by Boris Dayma*
[](https://twitter.com/intent/follow?screen_name=borisdayma)
For more details, visit the project repository.
[](https://github.com/borisdayma/huggingtweets)
|
krinal214/bert-all-squad_que_translated | e45c6ec4a30e5d2de58ea191c03235031a017f06 | 2022-04-14T22:09:34.000Z | [
"pytorch",
"tensorboard",
"bert",
"question-answering",
"transformers",
"generated_from_trainer",
"license:apache-2.0",
"model-index",
"autotrain_compatible"
]
| question-answering | false | krinal214 | null | krinal214/bert-all-squad_que_translated | 0 | null | transformers | 36,791 | ---
license: apache-2.0
tags:
- generated_from_trainer
model-index:
- name: bert-all-squad_que_translated
results: []
---
<!-- This model card has been generated automatically according to the information the Trainer had access to. You
should probably proofread and complete it, then remove this comment. -->
# bert-all-squad_que_translated
This model is a fine-tuned version of [bert-base-multilingual-cased](https://huggingface.co/bert-base-multilingual-cased) on the None dataset.
It achieves the following results on the evaluation set:
- Loss: 0.5174
## Model description
More information needed
## Intended uses & limitations
More information needed
## Training and evaluation data
More information needed
## Training procedure
### Training hyperparameters
The following hyperparameters were used during training:
- learning_rate: 2e-05
- train_batch_size: 16
- eval_batch_size: 16
- seed: 42
- optimizer: Adam with betas=(0.9,0.999) and epsilon=1e-08
- lr_scheduler_type: linear
- num_epochs: 1
### Training results
| Training Loss | Epoch | Step | Validation Loss |
|:-------------:|:-----:|:-----:|:---------------:|
| 1.0746 | 1.0 | 18011 | 0.5174 |
### Framework versions
- Transformers 4.17.0
- Pytorch 1.9.1
- Datasets 2.1.0
- Tokenizers 0.11.6
|
laampt/distilbert-base-uncased-finetuned-squad | e4231484ada4b3fcf29c63fd37f889dca2c01a1d | 2022-04-10T13:15:06.000Z | [
"pytorch",
"tensorboard",
"distilbert",
"question-answering",
"dataset:squad",
"transformers",
"generated_from_trainer",
"license:apache-2.0",
"model-index",
"autotrain_compatible"
]
| question-answering | false | laampt | null | laampt/distilbert-base-uncased-finetuned-squad | 0 | null | transformers | 36,792 | ---
license: apache-2.0
tags:
- generated_from_trainer
datasets:
- squad
model-index:
- name: distilbert-base-uncased-finetuned-squad
results: []
---
<!-- This model card has been generated automatically according to the information the Trainer had access to. You
should probably proofread and complete it, then remove this comment. -->
# distilbert-base-uncased-finetuned-squad
This model is a fine-tuned version of [distilbert-base-uncased](https://huggingface.co/distilbert-base-uncased) on the squad dataset.
## Model description
More information needed
## Intended uses & limitations
More information needed
## Training and evaluation data
More information needed
## Training procedure
### Training hyperparameters
The following hyperparameters were used during training:
- learning_rate: 2e-05
- train_batch_size: 16
- eval_batch_size: 16
- seed: 42
- optimizer: Adam with betas=(0.9,0.999) and epsilon=1e-08
- lr_scheduler_type: linear
- num_epochs: 3
### Framework versions
- Transformers 4.18.0
- Pytorch 1.10.0+cu111
- Datasets 2.0.0
- Tokenizers 0.11.6
|
krinal214/bert-all-squad_all_translated | 5e1afc9fcc07aba0505a333d91c2a70268906293 | 2022-04-10T19:04:17.000Z | [
"pytorch",
"tensorboard",
"bert",
"question-answering",
"transformers",
"generated_from_trainer",
"license:apache-2.0",
"model-index",
"autotrain_compatible"
]
| question-answering | false | krinal214 | null | krinal214/bert-all-squad_all_translated | 0 | null | transformers | 36,793 | ---
license: apache-2.0
tags:
- generated_from_trainer
model-index:
- name: bert-all-squad_all_translated
results: []
---
<!-- This model card has been generated automatically according to the information the Trainer had access to. You
should probably proofread and complete it, then remove this comment. -->
# bert-all-squad_all_translated
This model is a fine-tuned version of [bert-base-multilingual-cased](https://huggingface.co/bert-base-multilingual-cased) on the None dataset.
It achieves the following results on the evaluation set:
- Loss: 0.5261
## Model description
More information needed
## Intended uses & limitations
More information needed
## Training and evaluation data
More information needed
## Training procedure
### Training hyperparameters
The following hyperparameters were used during training:
- learning_rate: 2e-05
- train_batch_size: 16
- eval_batch_size: 16
- seed: 42
- optimizer: Adam with betas=(0.9,0.999) and epsilon=1e-08
- lr_scheduler_type: linear
- num_epochs: 1
### Training results
| Training Loss | Epoch | Step | Validation Loss |
|:-------------:|:-----:|:-----:|:---------------:|
| 1.022 | 1.0 | 21579 | 0.5261 |
### Framework versions
- Transformers 4.16.2
- Pytorch 1.9.1
- Datasets 1.18.4
- Tokenizers 0.11.6
|
huggingtweets/gceh | 69dbae8182db987e5e4669cc0542c09b7ec42996 | 2022-04-22T21:26:57.000Z | [
"pytorch",
"gpt2",
"text-generation",
"en",
"transformers",
"huggingtweets"
]
| text-generation | false | huggingtweets | null | huggingtweets/gceh | 0 | null | transformers | 36,794 | ---
language: en
thumbnail: http://www.huggingtweets.com/gceh/1650662812216/predictions.png
tags:
- huggingtweets
widget:
- text: "My dream is"
---
<div class="inline-flex flex-col" style="line-height: 1.5;">
<div class="flex">
<div
style="display:inherit; margin-left: 4px; margin-right: 4px; width: 92px; height:92px; border-radius: 50%; background-size: cover; background-image: url('https://pbs.twimg.com/profile_images/1487906000875180033/7mInu58B_400x400.jpg')">
</div>
<div
style="display:none; margin-left: 4px; margin-right: 4px; width: 92px; height:92px; border-radius: 50%; background-size: cover; background-image: url('')">
</div>
<div
style="display:none; margin-left: 4px; margin-right: 4px; width: 92px; height:92px; border-radius: 50%; background-size: cover; background-image: url('')">
</div>
</div>
<div style="text-align: center; margin-top: 3px; font-size: 16px; font-weight: 800">🤖 AI BOT 🤖</div>
<div style="text-align: center; font-size: 16px; font-weight: 800">Geoff Evamy Hill</div>
<div style="text-align: center; font-size: 14px;">@gceh</div>
</div>
I was made with [huggingtweets](https://github.com/borisdayma/huggingtweets).
Create your own bot based on your favorite user with [the demo](https://colab.research.google.com/github/borisdayma/huggingtweets/blob/master/huggingtweets-demo.ipynb)!
## How does it work?
The model uses the following pipeline.
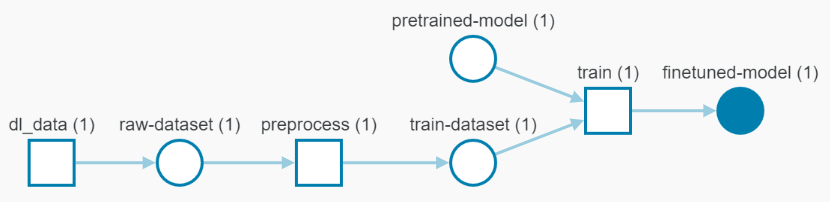
To understand how the model was developed, check the [W&B report](https://wandb.ai/wandb/huggingtweets/reports/HuggingTweets-Train-a-Model-to-Generate-Tweets--VmlldzoxMTY5MjI).
## Training data
The model was trained on tweets from Geoff Evamy Hill.
| Data | Geoff Evamy Hill |
| --- | --- |
| Tweets downloaded | 3195 |
| Retweets | 1491 |
| Short tweets | 123 |
| Tweets kept | 1581 |
[Explore the data](https://wandb.ai/wandb/huggingtweets/runs/24mcziml/artifacts), which is tracked with [W&B artifacts](https://docs.wandb.com/artifacts) at every step of the pipeline.
## Training procedure
The model is based on a pre-trained [GPT-2](https://huggingface.co/gpt2) which is fine-tuned on @gceh's tweets.
Hyperparameters and metrics are recorded in the [W&B training run](https://wandb.ai/wandb/huggingtweets/runs/217yb92j) for full transparency and reproducibility.
At the end of training, [the final model](https://wandb.ai/wandb/huggingtweets/runs/217yb92j/artifacts) is logged and versioned.
## How to use
You can use this model directly with a pipeline for text generation:
```python
from transformers import pipeline
generator = pipeline('text-generation',
model='huggingtweets/gceh')
generator("My dream is", num_return_sequences=5)
```
## Limitations and bias
The model suffers from [the same limitations and bias as GPT-2](https://huggingface.co/gpt2#limitations-and-bias).
In addition, the data present in the user's tweets further affects the text generated by the model.
## About
*Built by Boris Dayma*
[](https://twitter.com/intent/follow?screen_name=borisdayma)
For more details, visit the project repository.
[](https://github.com/borisdayma/huggingtweets)
|
mT0/mt0_xl_t0pp_ckpt_1012500 | 39066c5147b633c581c7b5e89b2faee5577a0ddf | 2022-04-10T18:55:20.000Z | [
"pytorch",
"mt5",
"text2text-generation",
"transformers",
"autotrain_compatible"
]
| text2text-generation | false | mT0 | null | mT0/mt0_xl_t0pp_ckpt_1012500 | 0 | null | transformers | 36,795 | Entry not found |
huggingtweets/graveyard_plots-hel_ql-witheredstrings | d638c5fef4ed3eba5b66c17cee3d0f3a88671d01 | 2022-04-10T19:16:31.000Z | [
"pytorch",
"gpt2",
"text-generation",
"en",
"transformers",
"huggingtweets"
]
| text-generation | false | huggingtweets | null | huggingtweets/graveyard_plots-hel_ql-witheredstrings | 0 | null | transformers | 36,796 | ---
language: en
thumbnail: http://www.huggingtweets.com/graveyard_plots-hel_ql-witheredstrings/1649618186549/predictions.png
tags:
- huggingtweets
widget:
- text: "My dream is"
---
<div class="inline-flex flex-col" style="line-height: 1.5;">
<div class="flex">
<div
style="display:inherit; margin-left: 4px; margin-right: 4px; width: 92px; height:92px; border-radius: 50%; background-size: cover; background-image: url('https://pbs.twimg.com/profile_images/1511852580216967169/b1Aiv2t3_400x400.jpg')">
</div>
<div
style="display:inherit; margin-left: 4px; margin-right: 4px; width: 92px; height:92px; border-radius: 50%; background-size: cover; background-image: url('https://pbs.twimg.com/profile_images/1457045233783701504/fnjAg6lH_400x400.jpg')">
</div>
<div
style="display:inherit; margin-left: 4px; margin-right: 4px; width: 92px; height:92px; border-radius: 50%; background-size: cover; background-image: url('https://pbs.twimg.com/profile_images/1332861091119046661/7ZD3Nqqg_400x400.jpg')">
</div>
</div>
<div style="text-align: center; margin-top: 3px; font-size: 16px; font-weight: 800">🤖 AI CYBORG 🤖</div>
<div style="text-align: center; font-size: 16px; font-weight: 800">GHANEM & Anthropos & darth hattie</div>
<div style="text-align: center; font-size: 14px;">@graveyard_plots-hel_ql-witheredstrings</div>
</div>
I was made with [huggingtweets](https://github.com/borisdayma/huggingtweets).
Create your own bot based on your favorite user with [the demo](https://colab.research.google.com/github/borisdayma/huggingtweets/blob/master/huggingtweets-demo.ipynb)!
## How does it work?
The model uses the following pipeline.
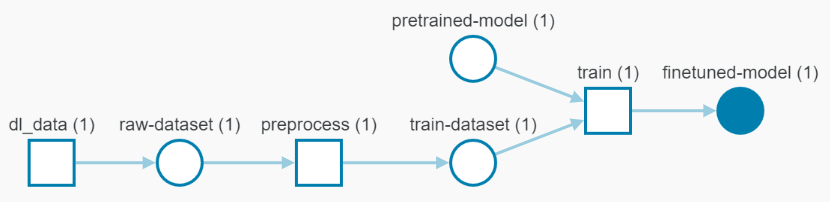
To understand how the model was developed, check the [W&B report](https://wandb.ai/wandb/huggingtweets/reports/HuggingTweets-Train-a-Model-to-Generate-Tweets--VmlldzoxMTY5MjI).
## Training data
The model was trained on tweets from GHANEM & Anthropos & darth hattie.
| Data | GHANEM | Anthropos | darth hattie |
| --- | --- | --- | --- |
| Tweets downloaded | 413 | 1175 | 1288 |
| Retweets | 1 | 354 | 9 |
| Short tweets | 18 | 92 | 146 |
| Tweets kept | 394 | 729 | 1133 |
[Explore the data](https://wandb.ai/wandb/huggingtweets/runs/26q7h6ze/artifacts), which is tracked with [W&B artifacts](https://docs.wandb.com/artifacts) at every step of the pipeline.
## Training procedure
The model is based on a pre-trained [GPT-2](https://huggingface.co/gpt2) which is fine-tuned on @graveyard_plots-hel_ql-witheredstrings's tweets.
Hyperparameters and metrics are recorded in the [W&B training run](https://wandb.ai/wandb/huggingtweets/runs/3vrvcbh4) for full transparency and reproducibility.
At the end of training, [the final model](https://wandb.ai/wandb/huggingtweets/runs/3vrvcbh4/artifacts) is logged and versioned.
## How to use
You can use this model directly with a pipeline for text generation:
```python
from transformers import pipeline
generator = pipeline('text-generation',
model='huggingtweets/graveyard_plots-hel_ql-witheredstrings')
generator("My dream is", num_return_sequences=5)
```
## Limitations and bias
The model suffers from [the same limitations and bias as GPT-2](https://huggingface.co/gpt2#limitations-and-bias).
In addition, the data present in the user's tweets further affects the text generated by the model.
## About
*Built by Boris Dayma*
[](https://twitter.com/intent/follow?screen_name=borisdayma)
For more details, visit the project repository.
[](https://github.com/borisdayma/huggingtweets)
|
huggingtweets/nordicshrew | 8d271fdf45825eba7d8cd317417bfc0ad62fb403 | 2022-04-10T22:04:13.000Z | [
"pytorch",
"gpt2",
"text-generation",
"en",
"transformers",
"huggingtweets"
]
| text-generation | false | huggingtweets | null | huggingtweets/nordicshrew | 0 | null | transformers | 36,797 | ---
language: en
thumbnail: http://www.huggingtweets.com/nordicshrew/1649628249290/predictions.png
tags:
- huggingtweets
widget:
- text: "My dream is"
---
<div class="inline-flex flex-col" style="line-height: 1.5;">
<div class="flex">
<div
style="display:inherit; margin-left: 4px; margin-right: 4px; width: 92px; height:92px; border-radius: 50%; background-size: cover; background-image: url('https://pbs.twimg.com/profile_images/1129935220260704256/RSmw3S0E_400x400.jpg')">
</div>
<div
style="display:none; margin-left: 4px; margin-right: 4px; width: 92px; height:92px; border-radius: 50%; background-size: cover; background-image: url('')">
</div>
<div
style="display:none; margin-left: 4px; margin-right: 4px; width: 92px; height:92px; border-radius: 50%; background-size: cover; background-image: url('')">
</div>
</div>
<div style="text-align: center; margin-top: 3px; font-size: 16px; font-weight: 800">🤖 AI BOT 🤖</div>
<div style="text-align: center; font-size: 16px; font-weight: 800">guelph’s finest poster</div>
<div style="text-align: center; font-size: 14px;">@nordicshrew</div>
</div>
I was made with [huggingtweets](https://github.com/borisdayma/huggingtweets).
Create your own bot based on your favorite user with [the demo](https://colab.research.google.com/github/borisdayma/huggingtweets/blob/master/huggingtweets-demo.ipynb)!
## How does it work?
The model uses the following pipeline.
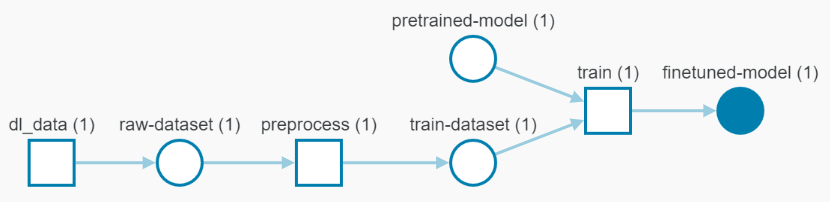
To understand how the model was developed, check the [W&B report](https://wandb.ai/wandb/huggingtweets/reports/HuggingTweets-Train-a-Model-to-Generate-Tweets--VmlldzoxMTY5MjI).
## Training data
The model was trained on tweets from guelph’s finest poster.
| Data | guelph’s finest poster |
| --- | --- |
| Tweets downloaded | 3219 |
| Retweets | 429 |
| Short tweets | 145 |
| Tweets kept | 2645 |
[Explore the data](https://wandb.ai/wandb/huggingtweets/runs/ywrep7o1/artifacts), which is tracked with [W&B artifacts](https://docs.wandb.com/artifacts) at every step of the pipeline.
## Training procedure
The model is based on a pre-trained [GPT-2](https://huggingface.co/gpt2) which is fine-tuned on @nordicshrew's tweets.
Hyperparameters and metrics are recorded in the [W&B training run](https://wandb.ai/wandb/huggingtweets/runs/1jti1kl9) for full transparency and reproducibility.
At the end of training, [the final model](https://wandb.ai/wandb/huggingtweets/runs/1jti1kl9/artifacts) is logged and versioned.
## How to use
You can use this model directly with a pipeline for text generation:
```python
from transformers import pipeline
generator = pipeline('text-generation',
model='huggingtweets/nordicshrew')
generator("My dream is", num_return_sequences=5)
```
## Limitations and bias
The model suffers from [the same limitations and bias as GPT-2](https://huggingface.co/gpt2#limitations-and-bias).
In addition, the data present in the user's tweets further affects the text generated by the model.
## About
*Built by Boris Dayma*
[](https://twitter.com/intent/follow?screen_name=borisdayma)
For more details, visit the project repository.
[](https://github.com/borisdayma/huggingtweets)
|
huggingtweets/s_m_frank | 88da22e3e23b161860fb39f7c24c76366f96c42d | 2022-04-10T22:28:09.000Z | [
"pytorch",
"gpt2",
"text-generation",
"en",
"transformers",
"huggingtweets"
]
| text-generation | false | huggingtweets | null | huggingtweets/s_m_frank | 0 | null | transformers | 36,798 | ---
language: en
thumbnail: http://www.huggingtweets.com/s_m_frank/1649629685555/predictions.png
tags:
- huggingtweets
widget:
- text: "My dream is"
---
<div class="inline-flex flex-col" style="line-height: 1.5;">
<div class="flex">
<div
style="display:inherit; margin-left: 4px; margin-right: 4px; width: 92px; height:92px; border-radius: 50%; background-size: cover; background-image: url('https://pbs.twimg.com/profile_images/1480658144833515525/DS0AOK_d_400x400.jpg')">
</div>
<div
style="display:none; margin-left: 4px; margin-right: 4px; width: 92px; height:92px; border-radius: 50%; background-size: cover; background-image: url('')">
</div>
<div
style="display:none; margin-left: 4px; margin-right: 4px; width: 92px; height:92px; border-radius: 50%; background-size: cover; background-image: url('')">
</div>
</div>
<div style="text-align: center; margin-top: 3px; font-size: 16px; font-weight: 800">🤖 AI BOT 🤖</div>
<div style="text-align: center; font-size: 16px; font-weight: 800">cute junco observer</div>
<div style="text-align: center; font-size: 14px;">@s_m_frank</div>
</div>
I was made with [huggingtweets](https://github.com/borisdayma/huggingtweets).
Create your own bot based on your favorite user with [the demo](https://colab.research.google.com/github/borisdayma/huggingtweets/blob/master/huggingtweets-demo.ipynb)!
## How does it work?
The model uses the following pipeline.
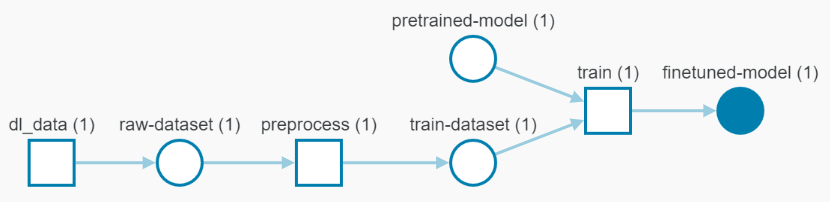
To understand how the model was developed, check the [W&B report](https://wandb.ai/wandb/huggingtweets/reports/HuggingTweets-Train-a-Model-to-Generate-Tweets--VmlldzoxMTY5MjI).
## Training data
The model was trained on tweets from cute junco observer.
| Data | cute junco observer |
| --- | --- |
| Tweets downloaded | 1253 |
| Retweets | 482 |
| Short tweets | 184 |
| Tweets kept | 587 |
[Explore the data](https://wandb.ai/wandb/huggingtweets/runs/2s2slp94/artifacts), which is tracked with [W&B artifacts](https://docs.wandb.com/artifacts) at every step of the pipeline.
## Training procedure
The model is based on a pre-trained [GPT-2](https://huggingface.co/gpt2) which is fine-tuned on @s_m_frank's tweets.
Hyperparameters and metrics are recorded in the [W&B training run](https://wandb.ai/wandb/huggingtweets/runs/2bjkzwlr) for full transparency and reproducibility.
At the end of training, [the final model](https://wandb.ai/wandb/huggingtweets/runs/2bjkzwlr/artifacts) is logged and versioned.
## How to use
You can use this model directly with a pipeline for text generation:
```python
from transformers import pipeline
generator = pipeline('text-generation',
model='huggingtweets/s_m_frank')
generator("My dream is", num_return_sequences=5)
```
## Limitations and bias
The model suffers from [the same limitations and bias as GPT-2](https://huggingface.co/gpt2#limitations-and-bias).
In addition, the data present in the user's tweets further affects the text generated by the model.
## About
*Built by Boris Dayma*
[](https://twitter.com/intent/follow?screen_name=borisdayma)
For more details, visit the project repository.
[](https://github.com/borisdayma/huggingtweets)
|
huggan/fastgan-few-shot-painting | 2d74d156bb01bad125b6dc62b0f75df4a8447d44 | 2022-05-06T22:31:52.000Z | [
"pytorch",
"dataset:huggan/few-shot-art-painting",
"arxiv:2101.04775",
"huggan",
"gan",
"unconditional-image-generation",
"license:mit"
]
| unconditional-image-generation | false | huggan | null | huggan/fastgan-few-shot-painting | 0 | null | null | 36,799 | ---
tags:
- huggan
- gan
- unconditional-image-generation
datasets:
- huggan/few-shot-art-painting
# See a list of available tags here:
# https://github.com/huggingface/hub-docs/blob/main/js/src/lib/interfaces/Types.ts#L12
# task: unconditional-image-generation or conditional-image-generation or image-to-image
license: mit
---
# Generate paiting image using FastGAN
## Model description
[FastGAN model](https://arxiv.org/abs/2101.04775) is a Generative Adversarial Networks (GAN) training on a small amount of high-fidelity images with minimum computing cost. Using a skip-layer channel-wise excitation module and a self-supervised discriminator trained as a feature-encoder, the model was able to converge after some hours of training for either 100 high-quality images or 1000 images datasets.
This model was trained on a dataset of 1000 high-quality images of art paintings.
#### How to use
```python
# Clone this model
git clone https://huggingface.co/huggan/fastgan-few-shot-painting/
def load_generator(model_name_or_path):
generator = Generator(in_channels=256, out_channels=3)
generator = generator.from_pretrained(model_name_or_path, in_channels=256, out_channels=3)
_ = generator.eval()
return generator
def _denormalize(input: torch.Tensor) -> torch.Tensor:
return (input * 127.5) + 127.5
# Load generator
generator = load_generator("fastgan-few-shot-painting")
# Generate a random noise image
noise = torch.zeros(1, 256, 1, 1, device=device).normal_(0.0, 1.0)
with torch.no_grad():
gan_images, _ = generator(noise)
gan_images = _denormalize(gan_images.detach())
save_image(gan_images, "sample.png", nrow=1, normalize=True)
```
#### Limitations and bias
* Converge faster and better with small datasets (less than 1000 samples)
## Training data
[few-shot-art-painting](https://huggingface.co/datasets/huggan/few-shot-art-painting)
## Generated Images

### BibTeX entry and citation info
```bibtex
@article{FastGAN,
title={Towards Faster and Stabilized GAN Training for High-fidelity Few-shot Image Synthesis},
author={Bingchen Liu, Yizhe Zhu, Kunpeng Song, Ahmed Elgammal},
journal={ICLR},
year={2021}
}
``` |
Subsets and Splits
No community queries yet
The top public SQL queries from the community will appear here once available.