modelId
stringlengths 4
112
| sha
stringlengths 40
40
| lastModified
stringlengths 24
24
| tags
sequence | pipeline_tag
stringclasses 29
values | private
bool 1
class | author
stringlengths 2
38
⌀ | config
null | id
stringlengths 4
112
| downloads
float64 0
36.8M
⌀ | likes
float64 0
712
⌀ | library_name
stringclasses 17
values | __index_level_0__
int64 0
38.5k
| readme
stringlengths 0
186k
|
---|---|---|---|---|---|---|---|---|---|---|---|---|---|
scasutt/wav2vec2-large-xlsr-53_toy_train_data_augment_0.1.csv | 5fa6c32c849940efa5682b64da0dd8b1b03d4130 | 2022-03-25T12:18:08.000Z | [
"pytorch",
"wav2vec2",
"automatic-speech-recognition",
"transformers",
"generated_from_trainer",
"license:apache-2.0",
"model-index"
] | automatic-speech-recognition | false | scasutt | null | scasutt/wav2vec2-large-xlsr-53_toy_train_data_augment_0.1.csv | 0 | null | transformers | 36,600 | ---
license: apache-2.0
tags:
- generated_from_trainer
model-index:
- name: wav2vec2-large-xlsr-53_toy_train_data_augment_0.1.csv
results: []
---
<!-- This model card has been generated automatically according to the information the Trainer had access to. You
should probably proofread and complete it, then remove this comment. -->
# wav2vec2-large-xlsr-53_toy_train_data_augment_0.1.csv
This model is a fine-tuned version of [facebook/wav2vec2-base](https://huggingface.co/facebook/wav2vec2-base) on the None dataset.
It achieves the following results on the evaluation set:
- Loss: 3.4695
- Wer: 1.0
## Model description
More information needed
## Intended uses & limitations
More information needed
## Training and evaluation data
More information needed
## Training procedure
### Training hyperparameters
The following hyperparameters were used during training:
- learning_rate: 0.0001
- train_batch_size: 8
- eval_batch_size: 8
- seed: 42
- gradient_accumulation_steps: 2
- total_train_batch_size: 16
- optimizer: Adam with betas=(0.9,0.999) and epsilon=1e-08
- lr_scheduler_type: linear
- lr_scheduler_warmup_steps: 1000
- num_epochs: 4
### Training results
| Training Loss | Epoch | Step | Validation Loss | Wer |
|:-------------:|:-----:|:----:|:---------------:|:---:|
| 3.2456 | 0.84 | 200 | 3.6215 | 1.0 |
| 3.0637 | 1.68 | 400 | 3.3918 | 1.0 |
| 3.046 | 2.52 | 600 | 3.4168 | 1.0 |
| 3.0627 | 3.36 | 800 | 3.4695 | 1.0 |
### Framework versions
- Transformers 4.17.0
- Pytorch 1.11.0+cu102
- Datasets 2.0.0
- Tokenizers 0.11.6
|
ianMconversica/autotrain-parrot_finetune_v1-667919695 | f442e2285749449b5b144eca929ada428ee1ff61 | 2022-03-25T15:41:11.000Z | [
"pytorch",
"t5",
"text2text-generation",
"unk",
"dataset:McIan91/autotrain-data-parrot_finetune_v1",
"transformers",
"autotrain",
"co2_eq_emissions",
"autotrain_compatible"
] | text2text-generation | false | ianMconversica | null | ianMconversica/autotrain-parrot_finetune_v1-667919695 | 0 | null | transformers | 36,601 | ---
tags: autotrain
language: unk
widget:
- text: "I love AutoTrain 🤗"
datasets:
- McIan91/autotrain-data-parrot_finetune_v1
co2_eq_emissions: 207.64739623144084
---
# Model Trained Using AutoTrain
- Problem type: Summarization
- Model ID: 667919695
- CO2 Emissions (in grams): 207.64739623144084
## Validation Metrics
- Loss: 0.06461456418037415
- Rouge1: 70.5184
- Rouge2: 66.9204
- RougeL: 70.4464
- RougeLsum: 70.4705
- Gen Len: 18.5385
## Usage
You can use cURL to access this model:
```
$ curl -X POST -H "Authorization: Bearer YOUR_HUGGINGFACE_API_KEY" -H "Content-Type: application/json" -d '{"inputs": "I love AutoTrain"}' https://api-inference.huggingface.co/McIan91/autotrain-parrot_finetune_v1-667919695
``` |
ssardorf/pegasus-xsum-new-dataset | 51e5415452fbf72f8e67237c4a8793a87cafeb0c | 2022-03-25T13:12:00.000Z | [
"pytorch",
"pegasus",
"text2text-generation",
"transformers",
"generated_from_trainer",
"model-index",
"autotrain_compatible"
] | text2text-generation | false | ssardorf | null | ssardorf/pegasus-xsum-new-dataset | 0 | null | transformers | 36,602 | ---
tags:
- generated_from_trainer
metrics:
- rouge
model-index:
- name: pegasus-xsum-new-dataset
results: []
---
<!-- This model card has been generated automatically according to the information the Trainer had access to. You
should probably proofread and complete it, then remove this comment. -->
# pegasus-xsum-new-dataset
This model is a fine-tuned version of [google/pegasus-xsum](https://huggingface.co/google/pegasus-xsum) on an unknown dataset.
It achieves the following results on the evaluation set:
- Loss: 1.8355
- Rouge1: 48.7306
- Rouge2: 34.1291
- Rougel: 44.0778
- Rougelsum: 45.7139
- Gen Len: 30.8889
## Model description
More information needed
## Intended uses & limitations
More information needed
## Training and evaluation data
More information needed
## Training procedure
### Training hyperparameters
The following hyperparameters were used during training:
- learning_rate: 5e-05
- train_batch_size: 4
- eval_batch_size: 4
- seed: 42
- optimizer: Adam with betas=(0.9,0.999) and epsilon=1e-08
- lr_scheduler_type: linear
- num_epochs: 3.0
### Training results
### Framework versions
- Transformers 4.18.0.dev0
- Pytorch 1.10.2+cpu
- Datasets 1.18.3
- Tokenizers 0.11.6
|
huggingtweets/rivatez | 076dec8ca3cb9d2b248bfbeda7bddcc0eae80f7e | 2022-03-25T14:57:29.000Z | [
"pytorch",
"gpt2",
"text-generation",
"en",
"transformers",
"huggingtweets"
] | text-generation | false | huggingtweets | null | huggingtweets/rivatez | 0 | null | transformers | 36,603 | ---
language: en
thumbnail: http://www.huggingtweets.com/rivatez/1648220244511/predictions.png
tags:
- huggingtweets
widget:
- text: "My dream is"
---
<div class="inline-flex flex-col" style="line-height: 1.5;">
<div class="flex">
<div
style="display:inherit; margin-left: 4px; margin-right: 4px; width: 92px; height:92px; border-radius: 50%; background-size: cover; background-image: url('https://pbs.twimg.com/profile_images/1421403684085374979/SoqYa6o3_400x400.jpg')">
</div>
<div
style="display:none; margin-left: 4px; margin-right: 4px; width: 92px; height:92px; border-radius: 50%; background-size: cover; background-image: url('')">
</div>
<div
style="display:none; margin-left: 4px; margin-right: 4px; width: 92px; height:92px; border-radius: 50%; background-size: cover; background-image: url('')">
</div>
</div>
<div style="text-align: center; margin-top: 3px; font-size: 16px; font-weight: 800">🤖 AI BOT 🤖</div>
<div style="text-align: center; font-size: 16px; font-weight: 800">Riva</div>
<div style="text-align: center; font-size: 14px;">@rivatez</div>
</div>
I was made with [huggingtweets](https://github.com/borisdayma/huggingtweets).
Create your own bot based on your favorite user with [the demo](https://colab.research.google.com/github/borisdayma/huggingtweets/blob/master/huggingtweets-demo.ipynb)!
## How does it work?
The model uses the following pipeline.
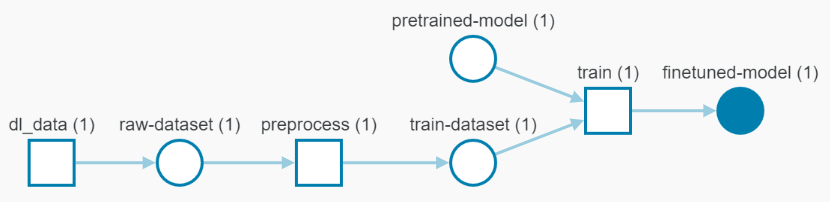
To understand how the model was developed, check the [W&B report](https://wandb.ai/wandb/huggingtweets/reports/HuggingTweets-Train-a-Model-to-Generate-Tweets--VmlldzoxMTY5MjI).
## Training data
The model was trained on tweets from Riva.
| Data | Riva |
| --- | --- |
| Tweets downloaded | 3178 |
| Retweets | 780 |
| Short tweets | 405 |
| Tweets kept | 1993 |
[Explore the data](https://wandb.ai/wandb/huggingtweets/runs/2qe0i10s/artifacts), which is tracked with [W&B artifacts](https://docs.wandb.com/artifacts) at every step of the pipeline.
## Training procedure
The model is based on a pre-trained [GPT-2](https://huggingface.co/gpt2) which is fine-tuned on @rivatez's tweets.
Hyperparameters and metrics are recorded in the [W&B training run](https://wandb.ai/wandb/huggingtweets/runs/2rspxzzv) for full transparency and reproducibility.
At the end of training, [the final model](https://wandb.ai/wandb/huggingtweets/runs/2rspxzzv/artifacts) is logged and versioned.
## How to use
You can use this model directly with a pipeline for text generation:
```python
from transformers import pipeline
generator = pipeline('text-generation',
model='huggingtweets/rivatez')
generator("My dream is", num_return_sequences=5)
```
## Limitations and bias
The model suffers from [the same limitations and bias as GPT-2](https://huggingface.co/gpt2#limitations-and-bias).
In addition, the data present in the user's tweets further affects the text generated by the model.
## About
*Built by Boris Dayma*
[](https://twitter.com/intent/follow?screen_name=borisdayma)
For more details, visit the project repository.
[](https://github.com/borisdayma/huggingtweets)
|
huggan/pix2pix-test | 14ede9d5fa8e6bfbd36887d9592fca76285d3dd3 | 2022-03-25T15:40:12.000Z | [
"pytorch"
] | null | false | huggan | null | huggan/pix2pix-test | 0 | null | null | 36,604 | Entry not found |
huggingtweets/_stevenshoe-mkobach | 4f3ff5cfadf90e31e2f40d8347f2eb471d6e0377 | 2022-03-25T22:23:51.000Z | [
"pytorch",
"gpt2",
"text-generation",
"en",
"transformers",
"huggingtweets"
] | text-generation | false | huggingtweets | null | huggingtweets/_stevenshoe-mkobach | 0 | null | transformers | 36,605 | ---
language: en
thumbnail: http://www.huggingtweets.com/_stevenshoe-mkobach/1648247026634/predictions.png
tags:
- huggingtweets
widget:
- text: "My dream is"
---
<div class="inline-flex flex-col" style="line-height: 1.5;">
<div class="flex">
<div
style="display:inherit; margin-left: 4px; margin-right: 4px; width: 92px; height:92px; border-radius: 50%; background-size: cover; background-image: url('https://pbs.twimg.com/profile_images/1374075536595505154/1_1jV_AF_400x400.png')">
</div>
<div
style="display:inherit; margin-left: 4px; margin-right: 4px; width: 92px; height:92px; border-radius: 50%; background-size: cover; background-image: url('https://pbs.twimg.com/profile_images/1505053150478229505/wAa1lc04_400x400.jpg')">
</div>
<div
style="display:none; margin-left: 4px; margin-right: 4px; width: 92px; height:92px; border-radius: 50%; background-size: cover; background-image: url('')">
</div>
</div>
<div style="text-align: center; margin-top: 3px; font-size: 16px; font-weight: 800">🤖 AI CYBORG 🤖</div>
<div style="text-align: center; font-size: 16px; font-weight: 800">Matthew Kobach & Steven Shoemaker</div>
<div style="text-align: center; font-size: 14px;">@_stevenshoe-mkobach</div>
</div>
I was made with [huggingtweets](https://github.com/borisdayma/huggingtweets).
Create your own bot based on your favorite user with [the demo](https://colab.research.google.com/github/borisdayma/huggingtweets/blob/master/huggingtweets-demo.ipynb)!
## How does it work?
The model uses the following pipeline.
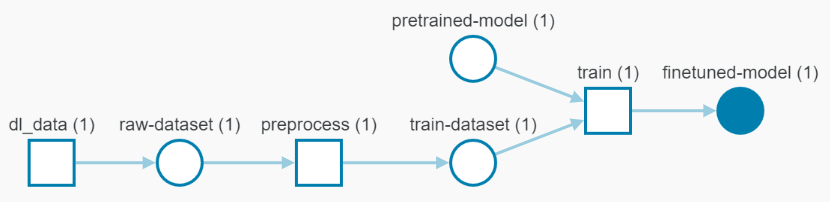
To understand how the model was developed, check the [W&B report](https://wandb.ai/wandb/huggingtweets/reports/HuggingTweets-Train-a-Model-to-Generate-Tweets--VmlldzoxMTY5MjI).
## Training data
The model was trained on tweets from Matthew Kobach & Steven Shoemaker.
| Data | Matthew Kobach | Steven Shoemaker |
| --- | --- | --- |
| Tweets downloaded | 3242 | 1319 |
| Retweets | 136 | 56 |
| Short tweets | 443 | 125 |
| Tweets kept | 2663 | 1138 |
[Explore the data](https://wandb.ai/wandb/huggingtweets/runs/48je6le3/artifacts), which is tracked with [W&B artifacts](https://docs.wandb.com/artifacts) at every step of the pipeline.
## Training procedure
The model is based on a pre-trained [GPT-2](https://huggingface.co/gpt2) which is fine-tuned on @_stevenshoe-mkobach's tweets.
Hyperparameters and metrics are recorded in the [W&B training run](https://wandb.ai/wandb/huggingtweets/runs/3oih18qf) for full transparency and reproducibility.
At the end of training, [the final model](https://wandb.ai/wandb/huggingtweets/runs/3oih18qf/artifacts) is logged and versioned.
## How to use
You can use this model directly with a pipeline for text generation:
```python
from transformers import pipeline
generator = pipeline('text-generation',
model='huggingtweets/_stevenshoe-mkobach')
generator("My dream is", num_return_sequences=5)
```
## Limitations and bias
The model suffers from [the same limitations and bias as GPT-2](https://huggingface.co/gpt2#limitations-and-bias).
In addition, the data present in the user's tweets further affects the text generated by the model.
## About
*Built by Boris Dayma*
[](https://twitter.com/intent/follow?screen_name=borisdayma)
For more details, visit the project repository.
[](https://github.com/borisdayma/huggingtweets)
|
ianMconversica/autotrain-phrasinator-reverse-670319725 | 0c75aa8c414f07abfe5153ce377bf6afbe9c2de4 | 2022-03-26T03:59:08.000Z | [
"pytorch",
"t5",
"text2text-generation",
"unk",
"dataset:McIan91/autotrain-data-phrasinator-reverse",
"transformers",
"autotrain",
"co2_eq_emissions",
"autotrain_compatible"
] | text2text-generation | false | ianMconversica | null | ianMconversica/autotrain-phrasinator-reverse-670319725 | 0 | null | transformers | 36,606 | ---
tags: autotrain
language: unk
widget:
- text: "I love AutoTrain 🤗"
datasets:
- McIan91/autotrain-data-phrasinator-reverse
co2_eq_emissions: 149.95517950000834
---
# Model Trained Using AutoTrain
- Problem type: Summarization
- Model ID: 670319725
- CO2 Emissions (in grams): 149.95517950000834
## Validation Metrics
- Loss: 0.0022294693626463413
- Rouge1: 67.5833
- Rouge2: 65.7386
- RougeL: 67.5812
- RougeLsum: 67.585
- Gen Len: 18.907
## Usage
You can use cURL to access this model:
```
$ curl -X POST -H "Authorization: Bearer YOUR_HUGGINGFACE_API_KEY" -H "Content-Type: application/json" -d '{"inputs": "I love AutoTrain"}' https://api-inference.huggingface.co/McIan91/autotrain-phrasinator-reverse-670319725
``` |
scasutt/wav2vec2-base_toy_train_data_fast_10pct | a1d1b6742851572c5f288d3f7a094c088e838b97 | 2022-03-26T12:28:13.000Z | [
"pytorch",
"wav2vec2",
"automatic-speech-recognition",
"transformers",
"generated_from_trainer",
"license:apache-2.0",
"model-index"
] | automatic-speech-recognition | false | scasutt | null | scasutt/wav2vec2-base_toy_train_data_fast_10pct | 0 | null | transformers | 36,607 | ---
license: apache-2.0
tags:
- generated_from_trainer
model-index:
- name: wav2vec2-base_toy_train_data_fast_10pct
results: []
---
<!-- This model card has been generated automatically according to the information the Trainer had access to. You
should probably proofread and complete it, then remove this comment. -->
# wav2vec2-base_toy_train_data_fast_10pct
This model is a fine-tuned version of [facebook/wav2vec2-base](https://huggingface.co/facebook/wav2vec2-base) on the None dataset.
It achieves the following results on the evaluation set:
- Loss: 1.3087
- Wer: 0.7175
## Model description
More information needed
## Intended uses & limitations
More information needed
## Training and evaluation data
More information needed
## Training procedure
### Training hyperparameters
The following hyperparameters were used during training:
- learning_rate: 0.0001
- train_batch_size: 8
- eval_batch_size: 8
- seed: 42
- gradient_accumulation_steps: 2
- total_train_batch_size: 16
- optimizer: Adam with betas=(0.9,0.999) and epsilon=1e-08
- lr_scheduler_type: linear
- lr_scheduler_warmup_steps: 1000
- num_epochs: 20
### Training results
| Training Loss | Epoch | Step | Validation Loss | Wer |
|:-------------:|:-----:|:----:|:---------------:|:------:|
| 3.1309 | 1.05 | 250 | 3.4541 | 0.9982 |
| 3.0499 | 2.1 | 500 | 3.0231 | 0.9982 |
| 1.4839 | 3.15 | 750 | 1.4387 | 0.9257 |
| 1.1697 | 4.2 | 1000 | 1.3729 | 0.8792 |
| 0.9353 | 5.25 | 1250 | 1.2608 | 0.8445 |
| 0.7298 | 6.3 | 1500 | 1.1867 | 0.8052 |
| 0.6418 | 7.35 | 1750 | 1.2414 | 0.7997 |
| 0.5698 | 8.4 | 2000 | 1.2240 | 0.7766 |
| 0.5084 | 9.45 | 2250 | 1.1910 | 0.7687 |
| 0.4912 | 10.5 | 2500 | 1.2241 | 0.7617 |
| 0.4144 | 11.55 | 2750 | 1.2412 | 0.7477 |
| 0.4153 | 12.6 | 3000 | 1.2736 | 0.7511 |
| 0.405 | 13.65 | 3250 | 1.2827 | 0.7328 |
| 0.3852 | 14.7 | 3500 | 1.1981 | 0.7331 |
| 0.3829 | 15.75 | 3750 | 1.3035 | 0.7347 |
| 0.3538 | 16.81 | 4000 | 1.3003 | 0.7240 |
| 0.3385 | 17.86 | 4250 | 1.3354 | 0.7304 |
| 0.3108 | 18.91 | 4500 | 1.2983 | 0.7229 |
| 0.3037 | 19.96 | 4750 | 1.3087 | 0.7175 |
### Framework versions
- Transformers 4.17.0
- Pytorch 1.11.0+cu102
- Datasets 2.0.0
- Tokenizers 0.11.6
|
scasutt/wav2vec2-base_toy_train_data_masked_audio | 7e735c2be5f4ab34ba7e84e2ae61fc9040770ddf | 2022-03-26T22:02:44.000Z | [
"pytorch",
"wav2vec2",
"automatic-speech-recognition",
"transformers",
"generated_from_trainer",
"license:apache-2.0",
"model-index"
] | automatic-speech-recognition | false | scasutt | null | scasutt/wav2vec2-base_toy_train_data_masked_audio | 0 | null | transformers | 36,608 | ---
license: apache-2.0
tags:
- generated_from_trainer
model-index:
- name: wav2vec2-base_toy_train_data_masked_audio
results: []
---
<!-- This model card has been generated automatically according to the information the Trainer had access to. You
should probably proofread and complete it, then remove this comment. -->
# wav2vec2-base_toy_train_data_masked_audio
This model is a fine-tuned version of [facebook/wav2vec2-base](https://huggingface.co/facebook/wav2vec2-base) on the None dataset.
It achieves the following results on the evaluation set:
- Loss: 1.1950
- Wer: 0.7340
## Model description
More information needed
## Intended uses & limitations
More information needed
## Training and evaluation data
More information needed
## Training procedure
### Training hyperparameters
The following hyperparameters were used during training:
- learning_rate: 0.0001
- train_batch_size: 16
- eval_batch_size: 8
- seed: 42
- gradient_accumulation_steps: 2
- total_train_batch_size: 32
- optimizer: Adam with betas=(0.9,0.999) and epsilon=1e-08
- lr_scheduler_type: linear
- lr_scheduler_warmup_steps: 1000
- num_epochs: 20
### Training results
| Training Loss | Epoch | Step | Validation Loss | Wer |
|:-------------:|:-----:|:----:|:---------------:|:------:|
| 3.1287 | 2.1 | 250 | 3.4581 | 1.0 |
| 3.0259 | 4.2 | 500 | 2.8099 | 0.9999 |
| 1.4881 | 6.3 | 750 | 1.2929 | 0.8950 |
| 0.9665 | 8.4 | 1000 | 1.1675 | 0.8346 |
| 0.7614 | 10.5 | 1250 | 1.1388 | 0.8003 |
| 0.5858 | 12.6 | 1500 | 1.1510 | 0.7672 |
| 0.5005 | 14.7 | 1750 | 1.1606 | 0.7532 |
| 0.4486 | 16.8 | 2000 | 1.1571 | 0.7427 |
| 0.4224 | 18.9 | 2250 | 1.1950 | 0.7340 |
### Framework versions
- Transformers 4.17.0
- Pytorch 1.11.0+cu102
- Datasets 2.0.0
- Tokenizers 0.11.6
|
huggingtweets/mkobach-naval-shaneaparrish | 6f0c7fd9f13d48983d865ac499c225b020a94b90 | 2022-03-27T00:07:05.000Z | [
"pytorch",
"gpt2",
"text-generation",
"en",
"transformers",
"huggingtweets"
] | text-generation | false | huggingtweets | null | huggingtweets/mkobach-naval-shaneaparrish | 0 | null | transformers | 36,609 | ---
language: en
thumbnail: http://www.huggingtweets.com/mkobach-naval-shaneaparrish/1648339620049/predictions.png
tags:
- huggingtweets
widget:
- text: "My dream is"
---
<div class="inline-flex flex-col" style="line-height: 1.5;">
<div class="flex">
<div
style="display:inherit; margin-left: 4px; margin-right: 4px; width: 92px; height:92px; border-radius: 50%; background-size: cover; background-image: url('https://pbs.twimg.com/profile_images/1374075536595505154/1_1jV_AF_400x400.png')">
</div>
<div
style="display:inherit; margin-left: 4px; margin-right: 4px; width: 92px; height:92px; border-radius: 50%; background-size: cover; background-image: url('https://pbs.twimg.com/profile_images/1253758424292171778/48gD7Hne_400x400.jpg')">
</div>
<div
style="display:inherit; margin-left: 4px; margin-right: 4px; width: 92px; height:92px; border-radius: 50%; background-size: cover; background-image: url('https://pbs.twimg.com/profile_images/1256841238298292232/ycqwaMI2_400x400.jpg')">
</div>
</div>
<div style="text-align: center; margin-top: 3px; font-size: 16px; font-weight: 800">🤖 AI CYBORG 🤖</div>
<div style="text-align: center; font-size: 16px; font-weight: 800">Matthew Kobach & Shane Parrish & Naval</div>
<div style="text-align: center; font-size: 14px;">@mkobach-naval-shaneaparrish</div>
</div>
I was made with [huggingtweets](https://github.com/borisdayma/huggingtweets).
Create your own bot based on your favorite user with [the demo](https://colab.research.google.com/github/borisdayma/huggingtweets/blob/master/huggingtweets-demo.ipynb)!
## How does it work?
The model uses the following pipeline.
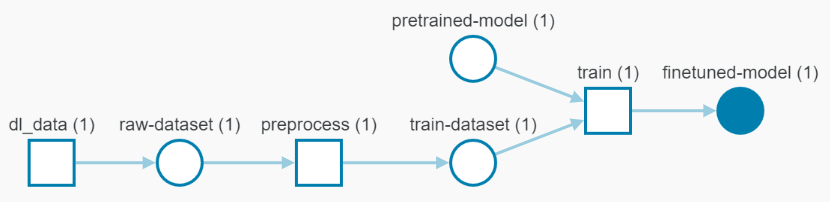
To understand how the model was developed, check the [W&B report](https://wandb.ai/wandb/huggingtweets/reports/HuggingTweets-Train-a-Model-to-Generate-Tweets--VmlldzoxMTY5MjI).
## Training data
The model was trained on tweets from Matthew Kobach & Shane Parrish & Naval.
| Data | Matthew Kobach | Shane Parrish | Naval |
| --- | --- | --- | --- |
| Tweets downloaded | 3248 | 3197 | 3249 |
| Retweets | 135 | 102 | 181 |
| Short tweets | 444 | 147 | 617 |
| Tweets kept | 2669 | 2948 | 2451 |
[Explore the data](https://wandb.ai/wandb/huggingtweets/runs/17cy2tt4/artifacts), which is tracked with [W&B artifacts](https://docs.wandb.com/artifacts) at every step of the pipeline.
## Training procedure
The model is based on a pre-trained [GPT-2](https://huggingface.co/gpt2) which is fine-tuned on @mkobach-naval-shaneaparrish's tweets.
Hyperparameters and metrics are recorded in the [W&B training run](https://wandb.ai/wandb/huggingtweets/runs/1zkb00dh) for full transparency and reproducibility.
At the end of training, [the final model](https://wandb.ai/wandb/huggingtweets/runs/1zkb00dh/artifacts) is logged and versioned.
## How to use
You can use this model directly with a pipeline for text generation:
```python
from transformers import pipeline
generator = pipeline('text-generation',
model='huggingtweets/mkobach-naval-shaneaparrish')
generator("My dream is", num_return_sequences=5)
```
## Limitations and bias
The model suffers from [the same limitations and bias as GPT-2](https://huggingface.co/gpt2#limitations-and-bias).
In addition, the data present in the user's tweets further affects the text generated by the model.
## About
*Built by Boris Dayma*
[](https://twitter.com/intent/follow?screen_name=borisdayma)
For more details, visit the project repository.
[](https://github.com/borisdayma/huggingtweets)
|
scasutt/wav2vec2-base_toy_train_data_random_noise | c0161384d07bacf7d058d26c8810b91d0a1f7d53 | 2022-03-27T02:27:39.000Z | [
"pytorch",
"wav2vec2",
"automatic-speech-recognition",
"transformers",
"generated_from_trainer",
"license:apache-2.0",
"model-index"
] | automatic-speech-recognition | false | scasutt | null | scasutt/wav2vec2-base_toy_train_data_random_noise | 0 | null | transformers | 36,610 | ---
license: apache-2.0
tags:
- generated_from_trainer
model-index:
- name: wav2vec2-base_toy_train_data_random_noise
results: []
---
<!-- This model card has been generated automatically according to the information the Trainer had access to. You
should probably proofread and complete it, then remove this comment. -->
# wav2vec2-base_toy_train_data_random_noise
This model is a fine-tuned version of [facebook/wav2vec2-base](https://huggingface.co/facebook/wav2vec2-base) on the None dataset.
It achieves the following results on the evaluation set:
- Loss: 1.0909
- Wer: 0.7351
## Model description
More information needed
## Intended uses & limitations
More information needed
## Training and evaluation data
More information needed
## Training procedure
### Training hyperparameters
The following hyperparameters were used during training:
- learning_rate: 0.0001
- train_batch_size: 16
- eval_batch_size: 8
- seed: 42
- gradient_accumulation_steps: 2
- total_train_batch_size: 32
- optimizer: Adam with betas=(0.9,0.999) and epsilon=1e-08
- lr_scheduler_type: linear
- lr_scheduler_warmup_steps: 1000
- num_epochs: 20
### Training results
| Training Loss | Epoch | Step | Validation Loss | Wer |
|:-------------:|:-----:|:----:|:---------------:|:------:|
| 3.128 | 2.1 | 250 | 3.5052 | 1.0 |
| 3.0423 | 4.2 | 500 | 2.9312 | 1.0 |
| 1.4109 | 6.3 | 750 | 1.2618 | 0.8915 |
| 0.9132 | 8.4 | 1000 | 1.1074 | 0.8436 |
| 0.7146 | 10.5 | 1250 | 1.0397 | 0.7876 |
| 0.5418 | 12.6 | 1500 | 1.0359 | 0.7662 |
| 0.4649 | 14.7 | 1750 | 1.0469 | 0.7467 |
| 0.4127 | 16.8 | 2000 | 1.0655 | 0.7404 |
| 0.3881 | 18.9 | 2250 | 1.0909 | 0.7351 |
### Framework versions
- Transformers 4.17.0
- Pytorch 1.11.0+cu102
- Datasets 2.0.0
- Tokenizers 0.11.6
|
scasutt/wav2vec2-base_toy_train_data_slow_10pct | 262e5f9f0fcdd3d90ad9f24f1202fa1088ce9664 | 2022-03-31T13:12:54.000Z | [
"pytorch",
"wav2vec2",
"automatic-speech-recognition",
"transformers",
"generated_from_trainer",
"license:apache-2.0",
"model-index"
] | automatic-speech-recognition | false | scasutt | null | scasutt/wav2vec2-base_toy_train_data_slow_10pct | 0 | null | transformers | 36,611 | ---
license: apache-2.0
tags:
- generated_from_trainer
model-index:
- name: wav2vec2-base_toy_train_data_slow_10pct
results: []
---
<!-- This model card has been generated automatically according to the information the Trainer had access to. You
should probably proofread and complete it, then remove this comment. -->
# wav2vec2-base_toy_train_data_slow_10pct
This model is a fine-tuned version of [facebook/wav2vec2-base](https://huggingface.co/facebook/wav2vec2-base) on the None dataset.
It achieves the following results on the evaluation set:
- Loss: 1.3248
- Wer: 0.7175
## Model description
More information needed
## Intended uses & limitations
More information needed
## Training and evaluation data
More information needed
## Training procedure
### Training hyperparameters
The following hyperparameters were used during training:
- learning_rate: 0.0001
- train_batch_size: 8
- eval_batch_size: 8
- seed: 42
- gradient_accumulation_steps: 2
- total_train_batch_size: 16
- optimizer: Adam with betas=(0.9,0.999) and epsilon=1e-08
- lr_scheduler_type: linear
- lr_scheduler_warmup_steps: 1000
- num_epochs: 20
### Training results
| Training Loss | Epoch | Step | Validation Loss | Wer |
|:-------------:|:-----:|:----:|:---------------:|:------:|
| 3.0663 | 2.1 | 500 | 3.0725 | 0.9982 |
| 1.1679 | 4.2 | 1000 | 1.3620 | 0.8889 |
| 0.6789 | 6.3 | 1500 | 1.2182 | 0.8160 |
| 0.5764 | 8.4 | 2000 | 1.2469 | 0.7667 |
| 0.4603 | 10.5 | 2500 | 1.2851 | 0.7533 |
| 0.4085 | 12.6 | 3000 | 1.2351 | 0.7401 |
| 0.3583 | 14.7 | 3500 | 1.2455 | 0.7367 |
| 0.3158 | 16.81 | 4000 | 1.3663 | 0.7261 |
| 0.2817 | 18.91 | 4500 | 1.3248 | 0.7175 |
### Framework versions
- Transformers 4.17.0
- Pytorch 1.11.0+cu102
- Datasets 2.0.0
- Tokenizers 0.11.6
|
huggingtweets/psimon365 | efe7fbebd991aaa95d426d7b7b0336e6373d2513 | 2022-03-27T02:56:43.000Z | [
"pytorch",
"gpt2",
"text-generation",
"en",
"transformers",
"huggingtweets"
] | text-generation | false | huggingtweets | null | huggingtweets/psimon365 | 0 | null | transformers | 36,612 | ---
language: en
thumbnail: http://www.huggingtweets.com/psimon365/1648349798068/predictions.png
tags:
- huggingtweets
widget:
- text: "My dream is"
---
<div class="inline-flex flex-col" style="line-height: 1.5;">
<div class="flex">
<div
style="display:inherit; margin-left: 4px; margin-right: 4px; width: 92px; height:92px; border-radius: 50%; background-size: cover; background-image: url('https://pbs.twimg.com/profile_images/1507859834107879426/d5Jqrb7Y_400x400.jpg')">
</div>
<div
style="display:none; margin-left: 4px; margin-right: 4px; width: 92px; height:92px; border-radius: 50%; background-size: cover; background-image: url('')">
</div>
<div
style="display:none; margin-left: 4px; margin-right: 4px; width: 92px; height:92px; border-radius: 50%; background-size: cover; background-image: url('')">
</div>
</div>
<div style="text-align: center; margin-top: 3px; font-size: 16px; font-weight: 800">🤖 AI BOT 🤖</div>
<div style="text-align: center; font-size: 16px; font-weight: 800">Psimon 🌐</div>
<div style="text-align: center; font-size: 14px;">@psimon365</div>
</div>
I was made with [huggingtweets](https://github.com/borisdayma/huggingtweets).
Create your own bot based on your favorite user with [the demo](https://colab.research.google.com/github/borisdayma/huggingtweets/blob/master/huggingtweets-demo.ipynb)!
## How does it work?
The model uses the following pipeline.
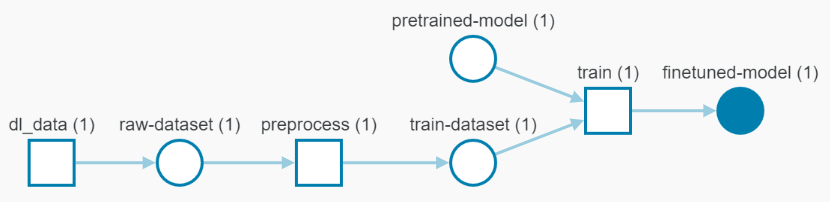
To understand how the model was developed, check the [W&B report](https://wandb.ai/wandb/huggingtweets/reports/HuggingTweets-Train-a-Model-to-Generate-Tweets--VmlldzoxMTY5MjI).
## Training data
The model was trained on tweets from Psimon 🌐.
| Data | Psimon 🌐 |
| --- | --- |
| Tweets downloaded | 181 |
| Retweets | 0 |
| Short tweets | 34 |
| Tweets kept | 147 |
[Explore the data](https://wandb.ai/wandb/huggingtweets/runs/q7gcbo7v/artifacts), which is tracked with [W&B artifacts](https://docs.wandb.com/artifacts) at every step of the pipeline.
## Training procedure
The model is based on a pre-trained [GPT-2](https://huggingface.co/gpt2) which is fine-tuned on @psimon365's tweets.
Hyperparameters and metrics are recorded in the [W&B training run](https://wandb.ai/wandb/huggingtweets/runs/kyaiz92o) for full transparency and reproducibility.
At the end of training, [the final model](https://wandb.ai/wandb/huggingtweets/runs/kyaiz92o/artifacts) is logged and versioned.
## How to use
You can use this model directly with a pipeline for text generation:
```python
from transformers import pipeline
generator = pipeline('text-generation',
model='huggingtweets/psimon365')
generator("My dream is", num_return_sequences=5)
```
## Limitations and bias
The model suffers from [the same limitations and bias as GPT-2](https://huggingface.co/gpt2#limitations-and-bias).
In addition, the data present in the user's tweets further affects the text generated by the model.
## About
*Built by Boris Dayma*
[](https://twitter.com/intent/follow?screen_name=borisdayma)
For more details, visit the project repository.
[](https://github.com/borisdayma/huggingtweets)
|
scasutt/wav2vec2-base_toy_train_data | d8538840a0622efceb2e67937fa761a79580bbc9 | 2022-04-24T11:51:24.000Z | [
"pytorch",
"wav2vec2",
"automatic-speech-recognition",
"transformers",
"generated_from_trainer",
"license:apache-2.0",
"model-index"
] | automatic-speech-recognition | false | scasutt | null | scasutt/wav2vec2-base_toy_train_data | 0 | null | transformers | 36,613 | ---
license: apache-2.0
tags:
- generated_from_trainer
model-index:
- name: wav2vec2-base_toy_train_data
results: []
---
<!-- This model card has been generated automatically according to the information the Trainer had access to. You
should probably proofread and complete it, then remove this comment. -->
# wav2vec2-base_toy_train_data
This model is a fine-tuned version of [facebook/wav2vec2-base](https://huggingface.co/facebook/wav2vec2-base) on the None dataset.
It achieves the following results on the evaluation set:
- Loss: 1.2522
- Wer: 0.7297
## Model description
More information needed
## Intended uses & limitations
More information needed
## Training and evaluation data
More information needed
## Training procedure
### Training hyperparameters
The following hyperparameters were used during training:
- learning_rate: 0.0001
- train_batch_size: 8
- eval_batch_size: 8
- seed: 42
- gradient_accumulation_steps: 2
- total_train_batch_size: 16
- optimizer: Adam with betas=(0.9,0.999) and epsilon=1e-08
- lr_scheduler_type: linear
- lr_scheduler_warmup_steps: 1000
- num_epochs: 30
### Training results
| Training Loss | Epoch | Step | Validation Loss | Wer |
|:-------------:|:-----:|:----:|:---------------:|:------:|
| 3.0033 | 4.2 | 500 | 2.7702 | 1.0 |
| 1.055 | 8.4 | 1000 | 1.2671 | 0.8667 |
| 0.6628 | 12.6 | 1500 | 1.1952 | 0.7883 |
| 0.5023 | 16.8 | 2000 | 1.1435 | 0.7659 |
| 0.4535 | 21.01 | 2500 | 1.1889 | 0.7458 |
| 0.3604 | 25.21 | 3000 | 1.2650 | 0.7378 |
| 0.3175 | 29.41 | 3500 | 1.2522 | 0.7297 |
### Framework versions
- Transformers 4.18.0
- Pytorch 1.11.0+cu102
- Datasets 2.1.0
- Tokenizers 0.12.1
|
huggingtweets/baguioni-elonmusk-jacobe | bfe02f36b207d8d767667c39f23b256ecf3fb311 | 2022-03-27T22:44:21.000Z | [
"pytorch",
"gpt2",
"text-generation",
"en",
"transformers",
"huggingtweets"
] | text-generation | false | huggingtweets | null | huggingtweets/baguioni-elonmusk-jacobe | 0 | null | transformers | 36,614 | ---
language: en
thumbnail: http://www.huggingtweets.com/baguioni-elonmusk-jacobe/1648421056394/predictions.png
tags:
- huggingtweets
widget:
- text: "My dream is"
---
<div class="inline-flex flex-col" style="line-height: 1.5;">
<div class="flex">
<div
style="display:inherit; margin-left: 4px; margin-right: 4px; width: 92px; height:92px; border-radius: 50%; background-size: cover; background-image: url('https://pbs.twimg.com/profile_images/1503591435324563456/foUrqiEw_400x400.jpg')">
</div>
<div
style="display:inherit; margin-left: 4px; margin-right: 4px; width: 92px; height:92px; border-radius: 50%; background-size: cover; background-image: url('https://pbs.twimg.com/profile_images/1025926108984664064/2ZHTSIof_400x400.jpg')">
</div>
<div
style="display:inherit; margin-left: 4px; margin-right: 4px; width: 92px; height:92px; border-radius: 50%; background-size: cover; background-image: url('https://pbs.twimg.com/profile_images/1506662013707046914/hVtCPrPL_400x400.jpg')">
</div>
</div>
<div style="text-align: center; margin-top: 3px; font-size: 16px; font-weight: 800">🤖 AI CYBORG 🤖</div>
<div style="text-align: center; font-size: 16px; font-weight: 800">Elon Musk & Rowel Atienza & baguio</div>
<div style="text-align: center; font-size: 14px;">@baguioni-elonmusk-jacobe</div>
</div>
I was made with [huggingtweets](https://github.com/borisdayma/huggingtweets).
Create your own bot based on your favorite user with [the demo](https://colab.research.google.com/github/borisdayma/huggingtweets/blob/master/huggingtweets-demo.ipynb)!
## How does it work?
The model uses the following pipeline.
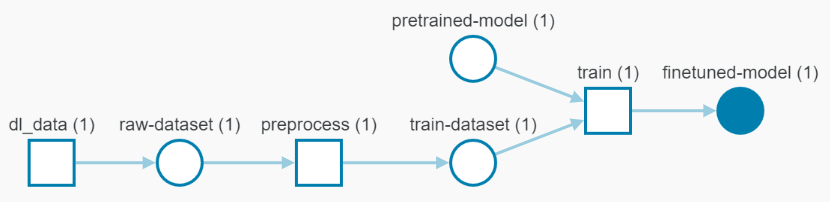
To understand how the model was developed, check the [W&B report](https://wandb.ai/wandb/huggingtweets/reports/HuggingTweets-Train-a-Model-to-Generate-Tweets--VmlldzoxMTY5MjI).
## Training data
The model was trained on tweets from Elon Musk & Rowel Atienza & baguio.
| Data | Elon Musk | Rowel Atienza | baguio |
| --- | --- | --- | --- |
| Tweets downloaded | 1621 | 100 | 3012 |
| Retweets | 69 | 29 | 1090 |
| Short tweets | 520 | 4 | 527 |
| Tweets kept | 1032 | 67 | 1395 |
[Explore the data](https://wandb.ai/wandb/huggingtweets/runs/1xuj1tda/artifacts), which is tracked with [W&B artifacts](https://docs.wandb.com/artifacts) at every step of the pipeline.
## Training procedure
The model is based on a pre-trained [GPT-2](https://huggingface.co/gpt2) which is fine-tuned on @baguioni-elonmusk-jacobe's tweets.
Hyperparameters and metrics are recorded in the [W&B training run](https://wandb.ai/wandb/huggingtweets/runs/3fpkbu3i) for full transparency and reproducibility.
At the end of training, [the final model](https://wandb.ai/wandb/huggingtweets/runs/3fpkbu3i/artifacts) is logged and versioned.
## How to use
You can use this model directly with a pipeline for text generation:
```python
from transformers import pipeline
generator = pipeline('text-generation',
model='huggingtweets/baguioni-elonmusk-jacobe')
generator("My dream is", num_return_sequences=5)
```
## Limitations and bias
The model suffers from [the same limitations and bias as GPT-2](https://huggingface.co/gpt2#limitations-and-bias).
In addition, the data present in the user's tweets further affects the text generated by the model.
## About
*Built by Boris Dayma*
[](https://twitter.com/intent/follow?screen_name=borisdayma)
For more details, visit the project repository.
[](https://github.com/borisdayma/huggingtweets)
|
huggingtweets/jacobe | 1175a77ede354a5d97822ac2aff17feb79d76ba9 | 2022-03-27T23:02:12.000Z | [
"pytorch",
"gpt2",
"text-generation",
"en",
"transformers",
"huggingtweets"
] | text-generation | false | huggingtweets | null | huggingtweets/jacobe | 0 | 1 | transformers | 36,615 | ---
language: en
thumbnail: http://www.huggingtweets.com/jacobe/1648422127637/predictions.png
tags:
- huggingtweets
widget:
- text: "My dream is"
---
<div class="inline-flex flex-col" style="line-height: 1.5;">
<div class="flex">
<div
style="display:inherit; margin-left: 4px; margin-right: 4px; width: 92px; height:92px; border-radius: 50%; background-size: cover; background-image: url('https://pbs.twimg.com/profile_images/1025926108984664064/2ZHTSIof_400x400.jpg')">
</div>
<div
style="display:none; margin-left: 4px; margin-right: 4px; width: 92px; height:92px; border-radius: 50%; background-size: cover; background-image: url('')">
</div>
<div
style="display:none; margin-left: 4px; margin-right: 4px; width: 92px; height:92px; border-radius: 50%; background-size: cover; background-image: url('')">
</div>
</div>
<div style="text-align: center; margin-top: 3px; font-size: 16px; font-weight: 800">🤖 AI BOT 🤖</div>
<div style="text-align: center; font-size: 16px; font-weight: 800">Rowel Atienza</div>
<div style="text-align: center; font-size: 14px;">@jacobe</div>
</div>
I was made with [huggingtweets](https://github.com/borisdayma/huggingtweets).
Create your own bot based on your favorite user with [the demo](https://colab.research.google.com/github/borisdayma/huggingtweets/blob/master/huggingtweets-demo.ipynb)!
## How does it work?
The model uses the following pipeline.
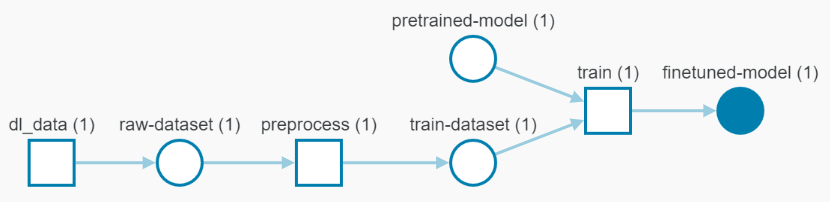
To understand how the model was developed, check the [W&B report](https://wandb.ai/wandb/huggingtweets/reports/HuggingTweets-Train-a-Model-to-Generate-Tweets--VmlldzoxMTY5MjI).
## Training data
The model was trained on tweets from Rowel Atienza.
| Data | Rowel Atienza |
| --- | --- |
| Tweets downloaded | 100 |
| Retweets | 29 |
| Short tweets | 4 |
| Tweets kept | 67 |
[Explore the data](https://wandb.ai/wandb/huggingtweets/runs/1uzq4b7w/artifacts), which is tracked with [W&B artifacts](https://docs.wandb.com/artifacts) at every step of the pipeline.
## Training procedure
The model is based on a pre-trained [GPT-2](https://huggingface.co/gpt2) which is fine-tuned on @jacobe's tweets.
Hyperparameters and metrics are recorded in the [W&B training run](https://wandb.ai/wandb/huggingtweets/runs/1ouo6sis) for full transparency and reproducibility.
At the end of training, [the final model](https://wandb.ai/wandb/huggingtweets/runs/1ouo6sis/artifacts) is logged and versioned.
## How to use
You can use this model directly with a pipeline for text generation:
```python
from transformers import pipeline
generator = pipeline('text-generation',
model='huggingtweets/jacobe')
generator("My dream is", num_return_sequences=5)
```
## Limitations and bias
The model suffers from [the same limitations and bias as GPT-2](https://huggingface.co/gpt2#limitations-and-bias).
In addition, the data present in the user's tweets further affects the text generated by the model.
## About
*Built by Boris Dayma*
[](https://twitter.com/intent/follow?screen_name=borisdayma)
For more details, visit the project repository.
[](https://github.com/borisdayma/huggingtweets)
|
huggingtweets/freudwarrior123 | 168bd47ff3345ff046ee83272a50fbb5e627cfc6 | 2022-03-28T04:26:31.000Z | [
"pytorch",
"gpt2",
"text-generation",
"en",
"transformers",
"huggingtweets"
] | text-generation | false | huggingtweets | null | huggingtweets/freudwarrior123 | 0 | null | transformers | 36,616 | ---
language: en
thumbnail: http://www.huggingtweets.com/freudwarrior123/1648441457881/predictions.png
tags:
- huggingtweets
widget:
- text: "My dream is"
---
<div class="inline-flex flex-col" style="line-height: 1.5;">
<div class="flex">
<div
style="display:inherit; margin-left: 4px; margin-right: 4px; width: 92px; height:92px; border-radius: 50%; background-size: cover; background-image: url('https://pbs.twimg.com/profile_images/1443547125770559488/QNDa_bi1_400x400.jpg')">
</div>
<div
style="display:none; margin-left: 4px; margin-right: 4px; width: 92px; height:92px; border-radius: 50%; background-size: cover; background-image: url('')">
</div>
<div
style="display:none; margin-left: 4px; margin-right: 4px; width: 92px; height:92px; border-radius: 50%; background-size: cover; background-image: url('')">
</div>
</div>
<div style="text-align: center; margin-top: 3px; font-size: 16px; font-weight: 800">🤖 AI BOT 🤖</div>
<div style="text-align: center; font-size: 16px; font-weight: 800">freudwarrior123</div>
<div style="text-align: center; font-size: 14px;">@freudwarrior123</div>
</div>
I was made with [huggingtweets](https://github.com/borisdayma/huggingtweets).
Create your own bot based on your favorite user with [the demo](https://colab.research.google.com/github/borisdayma/huggingtweets/blob/master/huggingtweets-demo.ipynb)!
## How does it work?
The model uses the following pipeline.
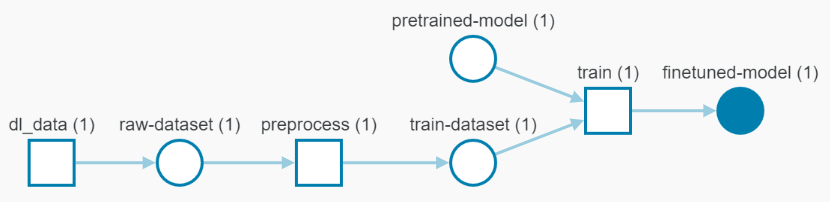
To understand how the model was developed, check the [W&B report](https://wandb.ai/wandb/huggingtweets/reports/HuggingTweets-Train-a-Model-to-Generate-Tweets--VmlldzoxMTY5MjI).
## Training data
The model was trained on tweets from freudwarrior123.
| Data | freudwarrior123 |
| --- | --- |
| Tweets downloaded | 859 |
| Retweets | 274 |
| Short tweets | 34 |
| Tweets kept | 551 |
[Explore the data](https://wandb.ai/wandb/huggingtweets/runs/3798mw2s/artifacts), which is tracked with [W&B artifacts](https://docs.wandb.com/artifacts) at every step of the pipeline.
## Training procedure
The model is based on a pre-trained [GPT-2](https://huggingface.co/gpt2) which is fine-tuned on @freudwarrior123's tweets.
Hyperparameters and metrics are recorded in the [W&B training run](https://wandb.ai/wandb/huggingtweets/runs/2n7ltssk) for full transparency and reproducibility.
At the end of training, [the final model](https://wandb.ai/wandb/huggingtweets/runs/2n7ltssk/artifacts) is logged and versioned.
## How to use
You can use this model directly with a pipeline for text generation:
```python
from transformers import pipeline
generator = pipeline('text-generation',
model='huggingtweets/freudwarrior123')
generator("My dream is", num_return_sequences=5)
```
## Limitations and bias
The model suffers from [the same limitations and bias as GPT-2](https://huggingface.co/gpt2#limitations-and-bias).
In addition, the data present in the user's tweets further affects the text generated by the model.
## About
*Built by Boris Dayma*
[](https://twitter.com/intent/follow?screen_name=borisdayma)
For more details, visit the project repository.
[](https://github.com/borisdayma/huggingtweets)
|
tau/t5_4_1024_0.3_epoch1 | 3b3babc010354d507c6cda431af7f75fe3241146 | 2022-03-28T04:36:36.000Z | [
"pytorch",
"t5",
"text2text-generation",
"transformers",
"autotrain_compatible"
] | text2text-generation | false | tau | null | tau/t5_4_1024_0.3_epoch1 | 0 | null | transformers | 36,617 | Entry not found |
aps/flava_full_pretrained_encoders_torchmm | 37e5f284d9f212bf88346de1b095d3326bee81da | 2022-03-28T06:03:42.000Z | [
"pytorch",
"license:bsd-3-clause"
] | null | false | aps | null | aps/flava_full_pretrained_encoders_torchmm | 0 | null | null | 36,618 | ---
license: bsd-3-clause
---
|
malteos/specter-wol | 486b1790030f953b0edd4e5df46ec1e7264b0a82 | 2022-04-11T13:06:57.000Z | [
"pytorch",
"bert",
"feature-extraction",
"arxiv:2202.06671",
"transformers",
"license:mit"
] | feature-extraction | false | malteos | null | malteos/specter-wol | 0 | null | transformers | 36,619 | ---
license: mit
---
Replicated [SPECTER model](https://huggingface.co/allenai/specter) based on w/o leakage training corpus with `seed=0`. See [Neighborhood Contrastive Learning for Scientific Document Representations with Citation Embeddings](https://arxiv.org/abs/2202.06671).
|
huggingtweets/nsawaikar | a5e27eed2b9fa5c0ac05873fb19d8c8bfec76197 | 2022-03-28T07:54:11.000Z | [
"pytorch",
"gpt2",
"text-generation",
"en",
"transformers",
"huggingtweets"
] | text-generation | false | huggingtweets | null | huggingtweets/nsawaikar | 0 | null | transformers | 36,620 | ---
language: en
thumbnail: http://www.huggingtweets.com/nsawaikar/1648454046318/predictions.png
tags:
- huggingtweets
widget:
- text: "My dream is"
---
<div class="inline-flex flex-col" style="line-height: 1.5;">
<div class="flex">
<div
style="display:inherit; margin-left: 4px; margin-right: 4px; width: 92px; height:92px; border-radius: 50%; background-size: cover; background-image: url('https://pbs.twimg.com/profile_images/1508184022052184064/yqLU6MxW_400x400.jpg')">
</div>
<div
style="display:none; margin-left: 4px; margin-right: 4px; width: 92px; height:92px; border-radius: 50%; background-size: cover; background-image: url('')">
</div>
<div
style="display:none; margin-left: 4px; margin-right: 4px; width: 92px; height:92px; border-radius: 50%; background-size: cover; background-image: url('')">
</div>
</div>
<div style="text-align: center; margin-top: 3px; font-size: 16px; font-weight: 800">🤖 AI BOT 🤖</div>
<div style="text-align: center; font-size: 16px; font-weight: 800">Nathan.eth</div>
<div style="text-align: center; font-size: 14px;">@nsawaikar</div>
</div>
I was made with [huggingtweets](https://github.com/borisdayma/huggingtweets).
Create your own bot based on your favorite user with [the demo](https://colab.research.google.com/github/borisdayma/huggingtweets/blob/master/huggingtweets-demo.ipynb)!
## How does it work?
The model uses the following pipeline.
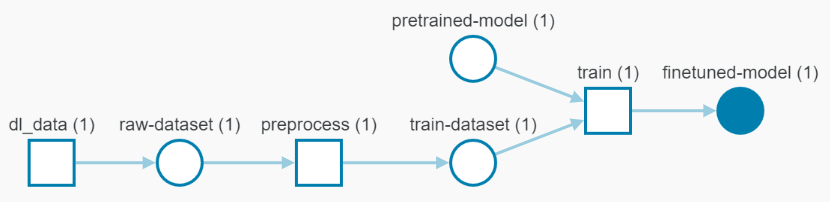
To understand how the model was developed, check the [W&B report](https://wandb.ai/wandb/huggingtweets/reports/HuggingTweets-Train-a-Model-to-Generate-Tweets--VmlldzoxMTY5MjI).
## Training data
The model was trained on tweets from Nathan.eth.
| Data | Nathan.eth |
| --- | --- |
| Tweets downloaded | 3250 |
| Retweets | 336 |
| Short tweets | 621 |
| Tweets kept | 2293 |
[Explore the data](https://wandb.ai/wandb/huggingtweets/runs/pn1domem/artifacts), which is tracked with [W&B artifacts](https://docs.wandb.com/artifacts) at every step of the pipeline.
## Training procedure
The model is based on a pre-trained [GPT-2](https://huggingface.co/gpt2) which is fine-tuned on @nsawaikar's tweets.
Hyperparameters and metrics are recorded in the [W&B training run](https://wandb.ai/wandb/huggingtweets/runs/g9hqx5dx) for full transparency and reproducibility.
At the end of training, [the final model](https://wandb.ai/wandb/huggingtweets/runs/g9hqx5dx/artifacts) is logged and versioned.
## How to use
You can use this model directly with a pipeline for text generation:
```python
from transformers import pipeline
generator = pipeline('text-generation',
model='huggingtweets/nsawaikar')
generator("My dream is", num_return_sequences=5)
```
## Limitations and bias
The model suffers from [the same limitations and bias as GPT-2](https://huggingface.co/gpt2#limitations-and-bias).
In addition, the data present in the user's tweets further affects the text generated by the model.
## About
*Built by Boris Dayma*
[](https://twitter.com/intent/follow?screen_name=borisdayma)
For more details, visit the project repository.
[](https://github.com/borisdayma/huggingtweets)
|
meryemtnar/dummy-model | 102d5a9a548f468a435706fc372aa26b92ad3d5c | 2022-03-28T08:52:40.000Z | [
"pytorch",
"camembert",
"fill-mask",
"transformers",
"autotrain_compatible"
] | fill-mask | false | meryemtnar | null | meryemtnar/dummy-model | 0 | null | transformers | 36,621 | Entry not found |
huggingtweets/abeshinzo | 19e51293f177b4f9169fed267748879283d13b79 | 2022-03-28T12:19:48.000Z | [
"pytorch",
"gpt2",
"text-generation",
"en",
"transformers",
"huggingtweets"
] | text-generation | false | huggingtweets | null | huggingtweets/abeshinzo | 0 | null | transformers | 36,622 | ---
language: en
thumbnail: http://www.huggingtweets.com/abeshinzo/1648469983562/predictions.png
tags:
- huggingtweets
widget:
- text: "My dream is"
---
<div class="inline-flex flex-col" style="line-height: 1.5;">
<div class="flex">
<div
style="display:inherit; margin-left: 4px; margin-right: 4px; width: 92px; height:92px; border-radius: 50%; background-size: cover; background-image: url('https://pbs.twimg.com/profile_images/1765776666/s-abetwitter1_400x400.png')">
</div>
<div
style="display:none; margin-left: 4px; margin-right: 4px; width: 92px; height:92px; border-radius: 50%; background-size: cover; background-image: url('')">
</div>
<div
style="display:none; margin-left: 4px; margin-right: 4px; width: 92px; height:92px; border-radius: 50%; background-size: cover; background-image: url('')">
</div>
</div>
<div style="text-align: center; margin-top: 3px; font-size: 16px; font-weight: 800">🤖 AI BOT 🤖</div>
<div style="text-align: center; font-size: 16px; font-weight: 800">安倍晋三</div>
<div style="text-align: center; font-size: 14px;">@abeshinzo</div>
</div>
I was made with [huggingtweets](https://github.com/borisdayma/huggingtweets).
Create your own bot based on your favorite user with [the demo](https://colab.research.google.com/github/borisdayma/huggingtweets/blob/master/huggingtweets-demo.ipynb)!
## How does it work?
The model uses the following pipeline.
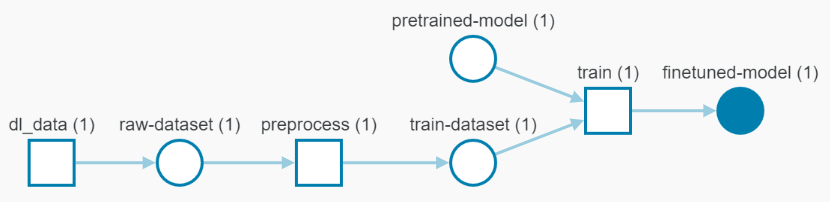
To understand how the model was developed, check the [W&B report](https://wandb.ai/wandb/huggingtweets/reports/HuggingTweets-Train-a-Model-to-Generate-Tweets--VmlldzoxMTY5MjI).
## Training data
The model was trained on tweets from 安倍晋三.
| Data | 安倍晋三 |
| --- | --- |
| Tweets downloaded | 2365 |
| Retweets | 77 |
| Short tweets | 1629 |
| Tweets kept | 659 |
[Explore the data](https://wandb.ai/wandb/huggingtweets/runs/37uwbwzs/artifacts), which is tracked with [W&B artifacts](https://docs.wandb.com/artifacts) at every step of the pipeline.
## Training procedure
The model is based on a pre-trained [GPT-2](https://huggingface.co/gpt2) which is fine-tuned on @abeshinzo's tweets.
Hyperparameters and metrics are recorded in the [W&B training run](https://wandb.ai/wandb/huggingtweets/runs/ib1nsfa1) for full transparency and reproducibility.
At the end of training, [the final model](https://wandb.ai/wandb/huggingtweets/runs/ib1nsfa1/artifacts) is logged and versioned.
## How to use
You can use this model directly with a pipeline for text generation:
```python
from transformers import pipeline
generator = pipeline('text-generation',
model='huggingtweets/abeshinzo')
generator("My dream is", num_return_sequences=5)
```
## Limitations and bias
The model suffers from [the same limitations and bias as GPT-2](https://huggingface.co/gpt2#limitations-and-bias).
In addition, the data present in the user's tweets further affects the text generated by the model.
## About
*Built by Boris Dayma*
[](https://twitter.com/intent/follow?screen_name=borisdayma)
For more details, visit the project repository.
[](https://github.com/borisdayma/huggingtweets)
|
scasutt/wav2vec2-large-xlsr-53_toy_train_data_masked_audio_10ms | 161f1ce9f9779bb9d318e13530ea093f02c6d977 | 2022-03-29T11:29:52.000Z | [
"pytorch",
"wav2vec2",
"automatic-speech-recognition",
"transformers",
"generated_from_trainer",
"license:apache-2.0",
"model-index"
] | automatic-speech-recognition | false | scasutt | null | scasutt/wav2vec2-large-xlsr-53_toy_train_data_masked_audio_10ms | 0 | null | transformers | 36,623 | ---
license: apache-2.0
tags:
- generated_from_trainer
model-index:
- name: wav2vec2-large-xlsr-53_toy_train_data_masked_audio_10ms
results: []
---
<!-- This model card has been generated automatically according to the information the Trainer had access to. You
should probably proofread and complete it, then remove this comment. -->
# wav2vec2-large-xlsr-53_toy_train_data_masked_audio_10ms
This model is a fine-tuned version of [facebook/wav2vec2-large-xlsr-53](https://huggingface.co/facebook/wav2vec2-large-xlsr-53) on the None dataset.
It achieves the following results on the evaluation set:
- Loss: 0.5945
- Wer: 0.4929
## Model description
More information needed
## Intended uses & limitations
More information needed
## Training and evaluation data
More information needed
## Training procedure
### Training hyperparameters
The following hyperparameters were used during training:
- learning_rate: 0.0001
- train_batch_size: 8
- eval_batch_size: 8
- seed: 42
- gradient_accumulation_steps: 2
- total_train_batch_size: 16
- optimizer: Adam with betas=(0.9,0.999) and epsilon=1e-08
- lr_scheduler_type: linear
- lr_scheduler_warmup_steps: 1000
- num_epochs: 20
### Training results
| Training Loss | Epoch | Step | Validation Loss | Wer |
|:-------------:|:-----:|:----:|:---------------:|:------:|
| 3.4049 | 1.05 | 250 | 3.3497 | 1.0 |
| 3.0851 | 2.1 | 500 | 3.4440 | 1.0 |
| 2.3512 | 3.15 | 750 | 1.5938 | 0.9317 |
| 1.1762 | 4.2 | 1000 | 0.8481 | 0.7333 |
| 0.903 | 5.25 | 1250 | 0.7180 | 0.6484 |
| 0.6754 | 6.3 | 1500 | 0.6603 | 0.6044 |
| 0.5961 | 7.35 | 1750 | 0.6410 | 0.5778 |
| 0.5325 | 8.4 | 2000 | 0.6245 | 0.5545 |
| 0.4685 | 9.45 | 2250 | 0.5925 | 0.5359 |
| 0.4526 | 10.5 | 2500 | 0.5991 | 0.5345 |
| 0.3975 | 11.55 | 2750 | 0.5916 | 0.5228 |
| 0.3672 | 12.6 | 3000 | 0.5882 | 0.5037 |
| 0.3774 | 13.65 | 3250 | 0.5693 | 0.5028 |
| 0.3489 | 14.7 | 3500 | 0.5645 | 0.5018 |
| 0.3593 | 15.75 | 3750 | 0.5977 | 0.5043 |
| 0.3167 | 16.81 | 4000 | 0.6049 | 0.5018 |
| 0.3225 | 17.86 | 4250 | 0.6172 | 0.4921 |
| 0.2807 | 18.91 | 4500 | 0.5937 | 0.4923 |
| 0.2889 | 19.96 | 4750 | 0.5945 | 0.4929 |
### Framework versions
- Transformers 4.17.0
- Pytorch 1.11.0+cu102
- Datasets 2.0.0
- Tokenizers 0.11.6
|
frtna/jwt300_mt-Italian-to-Spanish | eb508c6d628c682e9aa598d2ebdb779e498bc463 | 2022-03-29T09:16:47.000Z | [
"pytorch",
"t5",
"text2text-generation",
"transformers",
"autotrain_compatible"
] | text2text-generation | false | frtna | null | frtna/jwt300_mt-Italian-to-Spanish | 0 | null | transformers | 36,624 | Entry not found |
nsorros/my_model | 1ca0f1004087c8dd2d9b061fc6ccde55d20f7326 | 2022-03-29T06:57:45.000Z | [
"pytorch",
"bert",
"transformers"
] | null | false | nsorros | null | nsorros/my_model | 0 | null | transformers | 36,625 | Entry not found |
tau/random_4_1024_0.3_epoch1 | 1dfebf7584cd0e9ca0ea394469a600c775b5df18 | 2022-03-29T07:13:30.000Z | [
"pytorch",
"t5",
"text2text-generation",
"transformers",
"autotrain_compatible"
] | text2text-generation | false | tau | null | tau/random_4_1024_0.3_epoch1 | 0 | null | transformers | 36,626 | Entry not found |
parvezmrobin/bugsplainer-t5 | 341ea3f73303d769017e8d9a3de4ae5b7e68d900 | 2022-03-29T08:50:56.000Z | [
"pytorch",
"t5",
"text2text-generation",
"transformers",
"autotrain_compatible"
] | text2text-generation | false | parvezmrobin | null | parvezmrobin/bugsplainer-t5 | 0 | null | transformers | 36,627 | Entry not found |
regel-corpus/hunflair-promoter | b0ef69d55695cf02752eb06829c5d7c6c59b5f7a | 2022-04-20T09:53:48.000Z | [
"pytorch",
"en",
"flair",
"hunflair",
"token-classification",
"sequence-tagger-model"
] | token-classification | false | regel-corpus | null | regel-corpus/hunflair-promoter | 0 | null | flair | 36,628 | ---
tags:
- flair
- hunflair
- token-classification
- sequence-tagger-model
language: en
widget:
- text: "Two putative extended promoters consensus sequences (p1 and p2)."
---
## HunFlair model for PROMOTER
[HunFlair](https://github.com/flairNLP/flair/blob/master/resources/docs/HUNFLAIR.md) (biomedical flair) for promoter entity.
Predicts 1 tag:
| **tag** | **meaning** |
|---------------------------------|-----------|
| Promoter | DNA promoter region |
---
### Demo: How to use in Flair
Requires:
- **[Flair](https://github.com/flairNLP/flair/)** (`pip install flair`)
```python
from flair.data import Sentence
from flair.models import SequenceTagger
# for biomedical-specific tokenization:
# from flair.tokenization import SciSpacyTokenizer
# load tagger
tagger = SequenceTagger.load("regel-corpus/hunflair-promoter")
text = "The upstream region of the glnA gene contained two putative extended promoter consensus sequences (p1 and p2)."
# make example sentence
sentence = Sentence(text)
# for biomedical-specific tokenization:
# sentence = Sentence(text, use_tokenizer=SciSpacyTokenizer())
# predict NER tags
tagger.predict(sentence)
# print sentence
print(sentence)
# print predicted NER spans
print('The following NER tags are found:')
# iterate over entities and print
for entity in sentence.get_spans('ner'):
print(entity)
```
This yields the following output:
```
Span [16]: "p1" [− Labels: Promoter (0.9878)]
Span [18]: "p2" [− Labels: Promoter (0.9216)]
```
So, the entities "*p1*" and "*p2*" (labeled as a **promoter**) are found in the sentence.
Alternatively download all models locally and use the `MultiTagger` class.
```python
from flair.models import MultiTagger
tagger = [
'./models/hunflair-promoter/pytorch_model.bin',
'./models/hunflair-enhancer/pytorch_model.bin',
'./models/hunflair-tfbs/pytorch_model.bin',
]
tagger = MultiTagger.load(['./models/hunflair-'])
tagger.predict(sentence)
```
---
### Cite
Please cite the following paper when using this model.
```
@Article{regel,
author = {Garda, Samuele and Lenihan-Geels, Freyda and Proft, Sebastian and Hochmuth, Stefanie and Schülke, Markus and Seelow, Dominik and Leser, Ulf},
date = {2022},
journaltitle = {Under review},
title = {RegEl corpus: Identifying DNA regulatory elements in the scientific literature},
volume = {-},
groups = {-},
publisher = {-},
}
```
|
krinal214/augmented | 2b785ad155d12d61f2ffd1b0bfd63d687594df03 | 2022-03-29T16:58:16.000Z | [
"pytorch",
"tensorboard",
"bert",
"question-answering",
"transformers",
"generated_from_trainer",
"license:apache-2.0",
"model-index",
"autotrain_compatible"
] | question-answering | false | krinal214 | null | krinal214/augmented | 0 | null | transformers | 36,629 | ---
license: apache-2.0
tags:
- generated_from_trainer
model-index:
- name: augmented
results: []
---
<!-- This model card has been generated automatically according to the information the Trainer had access to. You
should probably proofread and complete it, then remove this comment. -->
# augmented
This model is a fine-tuned version of [bert-base-multilingual-cased](https://huggingface.co/bert-base-multilingual-cased) on the None dataset.
It achieves the following results on the evaluation set:
- Loss: 0.5104
## Model description
More information needed
## Intended uses & limitations
More information needed
## Training and evaluation data
More information needed
## Training procedure
### Training hyperparameters
The following hyperparameters were used during training:
- learning_rate: 2e-05
- train_batch_size: 16
- eval_batch_size: 16
- seed: 42
- optimizer: Adam with betas=(0.9,0.999) and epsilon=1e-08
- lr_scheduler_type: linear
- num_epochs: 1
### Training results
| Training Loss | Epoch | Step | Validation Loss |
|:-------------:|:-----:|:----:|:---------------:|
| 1.0609 | 1.0 | 9787 | 0.5104 |
### Framework versions
- Transformers 4.16.2
- Pytorch 1.9.1
- Datasets 1.18.4
- Tokenizers 0.11.6
|
huggan/dcgan-celeba-faces | ea3909f3b15841570439ae98592761e683e593e7 | 2022-03-29T16:26:19.000Z | [
"pytorch"
] | null | false | huggan | null | huggan/dcgan-celeba-faces | 0 | null | null | 36,630 | Entry not found |
princeton-nlp/CoFi-SQuAD-s93 | 12b9561bc240e6f80b4ca73396728c9289453d03 | 2022-05-01T01:18:37.000Z | [
"pytorch",
"bert",
"question-answering",
"arxiv:2204.00408",
"transformers",
"autotrain_compatible"
] | question-answering | false | princeton-nlp | null | princeton-nlp/CoFi-SQuAD-s93 | 0 | null | transformers | 36,631 | This is a model checkpoint for "[Structured Pruning Learns Compact and Accurate Models](https://arxiv.org/pdf/2204.00408.pdf)". The model is pruned from `bert-base-uncased` to a 93% sparsity on dataset SQuAD 1.1. Please go to [our repository](https://github.com/princeton-nlp/CoFiPruning) for more details on how to use the model for inference. Note that you would have to use the model class specified in our repository to load the model.
|
negfir/bert_uncased_L-10_H-512_A-8 | b781e55369c1dac3a1a2d7e9bc74b51f47158853 | 2022-04-06T00:04:18.000Z | [
"pytorch",
"bert",
"fill-mask",
"transformers",
"autotrain_compatible"
] | fill-mask | false | negfir | null | negfir/bert_uncased_L-10_H-512_A-8 | 0 | null | transformers | 36,632 | Entry not found |
negfir/bert_uncased_L-8_H-768_A-12 | d3836e2daba75466aa4a9061d3d0cf0f88e64755 | 2022-04-06T01:13:33.000Z | [
"pytorch",
"bert",
"fill-mask",
"transformers",
"autotrain_compatible"
] | fill-mask | false | negfir | null | negfir/bert_uncased_L-8_H-768_A-12 | 0 | null | transformers | 36,633 | Entry not found |
negfir/bert_uncased_L-6_H-768_A-12 | 3ace3991be2101bd45f2da3980a5820685a2e792 | 2022-04-06T02:38:14.000Z | [
"pytorch",
"bert",
"fill-mask",
"transformers",
"autotrain_compatible"
] | fill-mask | false | negfir | null | negfir/bert_uncased_L-6_H-768_A-12 | 0 | null | transformers | 36,634 | Entry not found |
negfir/bert_uncased_L-6_H-128_A-2 | 89c4ba14f848bf6bffbac2052c5c555eeda99420 | 2022-04-06T03:20:58.000Z | [
"pytorch",
"bert",
"fill-mask",
"transformers",
"autotrain_compatible"
] | fill-mask | false | negfir | null | negfir/bert_uncased_L-6_H-128_A-2 | 0 | null | transformers | 36,635 | Entry not found |
negfir/bert_uncased_L-4_H-768_A-12 | 8b91c6f49f71ccd028241794b31da008f4c9cbc0 | 2022-04-06T03:47:33.000Z | [
"pytorch",
"bert",
"fill-mask",
"transformers",
"autotrain_compatible"
] | fill-mask | false | negfir | null | negfir/bert_uncased_L-4_H-768_A-12 | 0 | null | transformers | 36,636 | Entry not found |
negfir/bert_uncased_L-4_H-256_A-4 | fbf178c024fd3adf5fe6edda7aeed7753696e94b | 2022-04-06T04:15:46.000Z | [
"pytorch",
"bert",
"fill-mask",
"transformers",
"autotrain_compatible"
] | fill-mask | false | negfir | null | negfir/bert_uncased_L-4_H-256_A-4 | 0 | null | transformers | 36,637 | Entry not found |
negfir/bert_uncased_L-4_H-128_A-2 | 2154774db804ba598dede52966d5bd4983608d91 | 2022-04-06T04:23:16.000Z | [
"pytorch",
"bert",
"fill-mask",
"transformers",
"autotrain_compatible"
] | fill-mask | false | negfir | null | negfir/bert_uncased_L-4_H-128_A-2 | 0 | null | transformers | 36,638 | Entry not found |
negfir/bert_uncased_L-2_H-256_A-4 | dba1eeea3631d2599cfc99ca55ad01f6b28eca28 | 2022-04-06T05:03:17.000Z | [
"pytorch",
"bert",
"fill-mask",
"transformers",
"autotrain_compatible"
] | fill-mask | false | negfir | null | negfir/bert_uncased_L-2_H-256_A-4 | 0 | null | transformers | 36,639 | Entry not found |
negfir/bert_uncased_L-2_H-128_A-2 | 88ec169bb038405da12bb96937d643956aeb231a | 2022-04-06T05:09:02.000Z | [
"pytorch",
"bert",
"fill-mask",
"transformers",
"autotrain_compatible"
] | fill-mask | false | negfir | null | negfir/bert_uncased_L-2_H-128_A-2 | 0 | null | transformers | 36,640 | Entry not found |
scasutt/wav2vec2-large-xlsr-53_toy_train_data_random_noise_0.1 | 4f17d875b5ebbd5ed9a585a65b5b27b5ea7bc448 | 2022-03-30T12:26:28.000Z | [
"pytorch",
"wav2vec2",
"automatic-speech-recognition",
"transformers"
] | automatic-speech-recognition | false | scasutt | null | scasutt/wav2vec2-large-xlsr-53_toy_train_data_random_noise_0.1 | 0 | null | transformers | 36,641 | Entry not found |
mimicheng/codeparrot-ds-sample-2ep-29mar | 438812b53858fb944af8bfdfffd6c33655e04996 | 2022-03-30T09:50:15.000Z | [
"pytorch",
"tensorboard",
"gpt2",
"text-generation",
"transformers",
"generated_from_trainer",
"license:mit",
"model-index"
] | text-generation | false | mimicheng | null | mimicheng/codeparrot-ds-sample-2ep-29mar | 0 | null | transformers | 36,642 | ---
license: mit
tags:
- generated_from_trainer
model-index:
- name: codeparrot-ds-sample-2ep-29mar
results: []
---
<!-- This model card has been generated automatically according to the information the Trainer had access to. You
should probably proofread and complete it, then remove this comment. -->
# codeparrot-ds-sample-2ep-29mar
This model is a fine-tuned version of [gpt2](https://huggingface.co/gpt2) on an unknown dataset.
It achieves the following results on the evaluation set:
- Loss: 1.6283
## Model description
More information needed
## Intended uses & limitations
More information needed
## Training and evaluation data
More information needed
## Training procedure
### Training hyperparameters
The following hyperparameters were used during training:
- learning_rate: 0.0005
- train_batch_size: 64
- eval_batch_size: 64
- seed: 42
- distributed_type: tpu
- gradient_accumulation_steps: 8
- total_train_batch_size: 512
- optimizer: Adam with betas=(0.9,0.999) and epsilon=1e-08
- lr_scheduler_type: cosine
- lr_scheduler_warmup_steps: 1000
- num_epochs: 2
### Training results
| Training Loss | Epoch | Step | Validation Loss |
|:-------------:|:-----:|:----:|:---------------:|
| 2.2585 | 1.86 | 5000 | 1.6283 |
### Framework versions
- Transformers 4.17.0
- Pytorch 1.8.2+cpu
- Datasets 2.0.0
- Tokenizers 0.11.6
|
scasutt/wav2vec2-base_toy_train_data_random_high_pass | 578c37c9ab715d5d1c034744ab28521154138d09 | 2022-03-30T16:37:23.000Z | [
"pytorch",
"wav2vec2",
"automatic-speech-recognition",
"transformers",
"generated_from_trainer",
"license:apache-2.0",
"model-index"
] | automatic-speech-recognition | false | scasutt | null | scasutt/wav2vec2-base_toy_train_data_random_high_pass | 0 | null | transformers | 36,643 | ---
license: apache-2.0
tags:
- generated_from_trainer
model-index:
- name: wav2vec2-base_toy_train_data_random_high_pass
results: []
---
<!-- This model card has been generated automatically according to the information the Trainer had access to. You
should probably proofread and complete it, then remove this comment. -->
# wav2vec2-base_toy_train_data_random_high_pass
This model is a fine-tuned version of [facebook/wav2vec2-base](https://huggingface.co/facebook/wav2vec2-base) on the None dataset.
It achieves the following results on the evaluation set:
- Loss: 1.2841
- Wer: 0.7222
## Model description
More information needed
## Intended uses & limitations
More information needed
## Training and evaluation data
More information needed
## Training procedure
### Training hyperparameters
The following hyperparameters were used during training:
- learning_rate: 0.0001
- train_batch_size: 8
- eval_batch_size: 8
- seed: 42
- gradient_accumulation_steps: 2
- total_train_batch_size: 16
- optimizer: Adam with betas=(0.9,0.999) and epsilon=1e-08
- lr_scheduler_type: linear
- lr_scheduler_warmup_steps: 1000
- num_epochs: 20
### Training results
| Training Loss | Epoch | Step | Validation Loss | Wer |
|:-------------:|:-----:|:----:|:---------------:|:------:|
| 3.061 | 2.1 | 500 | 3.0551 | 1.0 |
| 1.1294 | 4.2 | 1000 | 1.3102 | 0.8777 |
| 0.7051 | 6.3 | 1500 | 1.2081 | 0.8092 |
| 0.5421 | 8.4 | 2000 | 1.2280 | 0.7684 |
| 0.448 | 10.5 | 2500 | 1.2459 | 0.7506 |
| 0.3777 | 12.6 | 3000 | 1.3533 | 0.7631 |
| 0.3611 | 14.7 | 3500 | 1.2058 | 0.7291 |
| 0.3177 | 16.81 | 4000 | 1.3168 | 0.7185 |
| 0.279 | 18.91 | 4500 | 1.2841 | 0.7222 |
### Framework versions
- Transformers 4.17.0
- Pytorch 1.11.0+cu102
- Datasets 2.0.0
- Tokenizers 0.11.6
|
myunusseker/distilbert-base-uncased-go-emotion | 041dc956c1fd047936d052ec41aa4749f146de1a | 2022-03-30T20:11:16.000Z | [
"pytorch",
"distilbert",
"transformers"
] | null | false | myunusseker | null | myunusseker/distilbert-base-uncased-go-emotion | 0 | null | transformers | 36,644 | Entry not found |
huggingtweets/tojibaceo | 2bf5a46166486a2447bfad866932240062160412 | 2022-06-03T04:08:57.000Z | [
"pytorch",
"gpt2",
"text-generation",
"en",
"transformers",
"huggingtweets"
] | text-generation | false | huggingtweets | null | huggingtweets/tojibaceo | 0 | null | transformers | 36,645 | ---
language: en
thumbnail: http://www.huggingtweets.com/tojibaceo/1654229333065/predictions.png
tags:
- huggingtweets
widget:
- text: "My dream is"
---
<div class="inline-flex flex-col" style="line-height: 1.5;">
<div class="flex">
<div
style="display:inherit; margin-left: 4px; margin-right: 4px; width: 92px; height:92px; border-radius: 50%; background-size: cover; background-image: url('https://pbs.twimg.com/profile_images/1508824472924659725/267f4Lkm_400x400.jpg')">
</div>
<div
style="display:none; margin-left: 4px; margin-right: 4px; width: 92px; height:92px; border-radius: 50%; background-size: cover; background-image: url('')">
</div>
<div
style="display:none; margin-left: 4px; margin-right: 4px; width: 92px; height:92px; border-radius: 50%; background-size: cover; background-image: url('')">
</div>
</div>
<div style="text-align: center; margin-top: 3px; font-size: 16px; font-weight: 800">🤖 AI BOT 🤖</div>
<div style="text-align: center; font-size: 16px; font-weight: 800">Tojiba CPU Corp (🏭,🏭)</div>
<div style="text-align: center; font-size: 14px;">@tojibaceo</div>
</div>
I was made with [huggingtweets](https://github.com/borisdayma/huggingtweets).
Create your own bot based on your favorite user with [the demo](https://colab.research.google.com/github/borisdayma/huggingtweets/blob/master/huggingtweets-demo.ipynb)!
## How does it work?
The model uses the following pipeline.
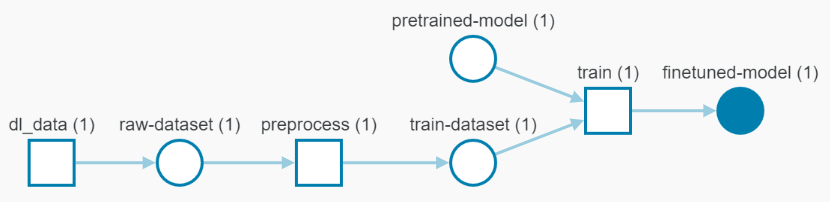
To understand how the model was developed, check the [W&B report](https://wandb.ai/wandb/huggingtweets/reports/HuggingTweets-Train-a-Model-to-Generate-Tweets--VmlldzoxMTY5MjI).
## Training data
The model was trained on tweets from Tojiba CPU Corp (🏭,🏭).
| Data | Tojiba CPU Corp (🏭,🏭) |
| --- | --- |
| Tweets downloaded | 1401 |
| Retweets | 706 |
| Short tweets | 239 |
| Tweets kept | 456 |
[Explore the data](https://wandb.ai/wandb/huggingtweets/runs/32gtdln5/artifacts), which is tracked with [W&B artifacts](https://docs.wandb.com/artifacts) at every step of the pipeline.
## Training procedure
The model is based on a pre-trained [GPT-2](https://huggingface.co/gpt2) which is fine-tuned on @tojibaceo's tweets.
Hyperparameters and metrics are recorded in the [W&B training run](https://wandb.ai/wandb/huggingtweets/runs/19scebmc) for full transparency and reproducibility.
At the end of training, [the final model](https://wandb.ai/wandb/huggingtweets/runs/19scebmc/artifacts) is logged and versioned.
## How to use
You can use this model directly with a pipeline for text generation:
```python
from transformers import pipeline
generator = pipeline('text-generation',
model='huggingtweets/tojibaceo')
generator("My dream is", num_return_sequences=5)
```
## Limitations and bias
The model suffers from [the same limitations and bias as GPT-2](https://huggingface.co/gpt2#limitations-and-bias).
In addition, the data present in the user's tweets further affects the text generated by the model.
## About
*Built by Boris Dayma*
[](https://twitter.com/intent/follow?screen_name=borisdayma)
For more details, visit the project repository.
[](https://github.com/borisdayma/huggingtweets)
|
unjustify/autotrain-IWant-689220804 | 05896acc3f5f89847bfb873d62894cc24b1357c0 | 2022-03-31T06:46:48.000Z | [
"pytorch",
"t5",
"text2text-generation",
"unk",
"dataset:unjustify/autotrain-data-IWant",
"transformers",
"autotrain",
"co2_eq_emissions",
"autotrain_compatible"
] | text2text-generation | false | unjustify | null | unjustify/autotrain-IWant-689220804 | 0 | null | transformers | 36,646 | ---
tags: autotrain
language: unk
widget:
- text: "I love AutoTrain 🤗"
datasets:
- unjustify/autotrain-data-IWant
co2_eq_emissions: 39.40549299946679
---
# Model Trained Using AutoTrain
- Problem type: Summarization
- Model ID: 689220804
- CO2 Emissions (in grams): 39.40549299946679
## Validation Metrics
- Loss: 2.0426149368286133
- Rouge1: 54.9813
- Rouge2: 44.923
- RougeL: 54.0399
- RougeLsum: 54.2553
- Gen Len: 16.6211
## Usage
You can use cURL to access this model:
```
$ curl -X POST -H "Authorization: Bearer YOUR_HUGGINGFACE_API_KEY" -H "Content-Type: application/json" -d '{"inputs": "I love AutoTrain"}' https://api-inference.huggingface.co/unjustify/autotrain-IWant-689220804
``` |
jjeamin/ArcaneStyleTransfer | 65a6ac8dd26e56ea910d342931a66392b4a6a147 | 2022-04-04T01:57:26.000Z | [
"pytorch",
"onnx",
"license:apache-2.0"
] | null | false | jjeamin | null | jjeamin/ArcaneStyleTransfer | 0 | 2 | null | 36,647 | ---
license: apache-2.0
---
|
xxazz/chatbot | 80ab6849154a047c73bf7229e0ca63880c7b8384 | 2022-03-31T16:00:07.000Z | [
"pytorch",
"transformers"
] | null | false | xxazz | null | xxazz/chatbot | 0 | null | transformers | 36,648 | Entry not found |
johnowhitaker/orbgan_e1 | db6d10f2e31c150109ba339ad766b2711c9d0978 | 2022-04-05T07:31:52.000Z | [
"pytorch",
"en",
"dataset:glid3_orbs",
"lightweightgan",
"license:apache-2.0"
] | null | false | johnowhitaker | null | johnowhitaker/orbgan_e1 | 0 | 1 | null | 36,649 | ---
language: en
tags:
- lightweightgan
license: apache-2.0
datasets:
- glid3_orbs
---
# orbgan
lightweight GAN trained on my glid-3 orbs (https://huggingface.co/datasets/johnowhitaker/glid3_orbs) for demo I'm working on.
Training notebook: https://colab.research.google.com/drive/16o1TdrxnQ54Msbr813XfPVsnEt2QTRAa?usp=sharing
Inference notebook: https://colab.research.google.com/drive/1e7dR2dptM8F1xhRcyy-Aqow9YSe0NE3z?usp=sharing
The lightwightgan code has an assert requiring a GPU. For inference on the CPU we ned to re-define the Generator class and some other functions - see minimal example here: https://colab.research.google.com/drive/1fnHLdJ7niPMGOOBjGkNsnc6iADpf1Ujd?usp=sharing . This approach was used to make the demo space here: https://huggingface.co/spaces/johnowhitaker/orbgan_demo
Please credit if you use this, and feedback on the code is welcomed :)
EDIT: you may need to use an older version of lightweightgan, eg from commit 708633205d60c99b1b9d4e6b47eb3722aa4159d6 since there have been some recent changes that happened after this model was trained.
## Demo:
```python
from lightweight_gan import Generator
import torch
from matplotlib import pyplot as plt
from huggingface_hub import PyTorchModelHubMixin
# Initialize a generator model
gan_new = Generator(latent_dim=256, image_size=256, attn_res_layers = [32])
# Load from local saved state dict
# gan_new.load_state_dict(torch.load('/content/orbgan_e3_state_dict.pt'))
# Load from model hub:
class GeneratorWithPyTorchModelHubMixin(gan_new.__class__, PyTorchModelHubMixin):
pass
gan_new.__class__ = GeneratorWithPyTorchModelHubMixin
gan_new = gan_new.from_pretrained('johnowhitaker/orbgan_e1', latent_dim=256, image_size=256, attn_res_layers = [32])
# View some examples
n_rows = 3
ims = gan_new(torch.randn(n_rows**2, 256)).clamp_(0., 1.)
fig, axs = plt.subplots(n_rows, n_rows, figsize=(9, 9))
for i, ax in enumerate(axs.flatten()):
ax.imshow(ims[i].permute(1, 2, 0).detach().cpu().numpy())
plt.tight_layout()
```
|
mT0/mt0_xl_t0pp_ckpt_1025000 | fd88982281dd30b0650cc2b7562638c5941accc0 | 2022-03-31T17:27:17.000Z | [
"pytorch",
"mt5",
"text2text-generation",
"transformers",
"autotrain_compatible"
] | text2text-generation | false | mT0 | null | mT0/mt0_xl_t0pp_ckpt_1025000 | 0 | null | transformers | 36,650 | Entry not found |
anisdismail/celebA-orientation-detection | 99773ed9762dd50104a92f36b8193265776ee687 | 2022-03-31T21:51:37.000Z | [
"en",
"dataset:nielsr/CelebA-faces",
"image-classification",
"pytorch",
"license:cc-by-nc-4.0",
"model-index"
] | image-classification | false | anisdismail | null | anisdismail/celebA-orientation-detection | 0 | 1 | null | 36,651 | ---
language:
- en
license: cc-by-nc-4.0
tags:
- image-classification
- pytorch
datasets:
- nielsr/CelebA-faces
model-index:
- name: celebA_orientation_detection_model
results:
- task:
type: image_classification # Required. Example: automatic-speech-recognition
name: Image Classification # Optional. Example: Speech Recognition
dataset:
type: nielsr/CelebA-faces
name: CelebA-faces
metrics:
- type: f1score # Required. Example: wer
value: 0.97 # Required. Example: 20.90
name: Val F1 Score # Optional. Example: Test WER
---
## Detecting the Orientation of CelebA pictures using Deep Learning
This model has been trained on a modified version of the CelebA-faces dataset, which was made from flipping 20,000 images upside down and keeping 20,000 images intact.<br>
The model relies on Resnet-18 as a backbone and is connected to one output node to classify whether the images are flipped upside down (1) or not (0). |
tonyalves/output | a48bb1735615c797c7af913f713f6920205490e6 | 2022-04-03T14:24:57.000Z | [
"pytorch",
"wav2vec2",
"automatic-speech-recognition",
"pt",
"dataset:common_voice",
"transformers",
"mozilla-foundation/common_voice_8_0",
"generated_from_trainer",
"license:apache-2.0",
"model-index"
] | automatic-speech-recognition | false | tonyalves | null | tonyalves/output | 0 | null | transformers | 36,652 | ---
language:
- pt
license: apache-2.0
tags:
- automatic-speech-recognition
- mozilla-foundation/common_voice_8_0
- generated_from_trainer
datasets:
- common_voice
model-index:
- name: output
results: []
---
<!-- This model card has been generated automatically according to the information the Trainer had access to. You
should probably proofread and complete it, then remove this comment. -->
# output
This model is a fine-tuned version of [facebook/wav2vec2-xls-r-300m](https://huggingface.co/facebook/wav2vec2-xls-r-300m) on the MOZILLA-FOUNDATION/COMMON_VOICE_8_0 - PT dataset.
It achieves the following results on the evaluation set:
- Loss: 0.1505
- Wer: 0.1352
## Model description
More information needed
## Intended uses & limitations
More information needed
## Training and evaluation data
More information needed
## Training procedure
### Training hyperparameters
The following hyperparameters were used during training:
- learning_rate: 7.5e-05
- train_batch_size: 8
- eval_batch_size: 8
- seed: 42
- gradient_accumulation_steps: 4
- total_train_batch_size: 32
- optimizer: Adam with betas=(0.9,0.999) and epsilon=1e-08
- lr_scheduler_type: linear
- lr_scheduler_warmup_steps: 2000
- num_epochs: 50.0
- mixed_precision_training: Native AMP
### Training results
| Training Loss | Epoch | Step | Validation Loss | Wer |
|:-------------:|:-----:|:-----:|:---------------:|:------:|
| 4.1367 | 0.64 | 500 | 3.8825 | 1.0 |
| 2.9677 | 1.29 | 1000 | 2.9498 | 1.0 |
| 1.5884 | 1.93 | 1500 | 0.6722 | 0.6493 |
| 1.2292 | 2.57 | 2000 | 0.3635 | 0.3202 |
| 1.1314 | 3.22 | 2500 | 0.2970 | 0.2680 |
| 1.0879 | 3.86 | 3000 | 0.2671 | 0.2486 |
| 1.0344 | 4.5 | 3500 | 0.2625 | 0.2239 |
| 1.0109 | 5.15 | 4000 | 0.2520 | 0.2230 |
| 0.9966 | 5.79 | 4500 | 0.2280 | 0.2105 |
| 0.9815 | 6.43 | 5000 | 0.2254 | 0.2179 |
| 0.9744 | 7.08 | 5500 | 0.2301 | 0.2137 |
| 0.9487 | 7.72 | 6000 | 0.2224 | 0.2051 |
| 0.9431 | 8.37 | 6500 | 0.2105 | 0.1992 |
| 0.9365 | 9.01 | 7000 | 0.2114 | 0.2019 |
| 0.9268 | 9.65 | 7500 | 0.2097 | 0.1988 |
| 0.9292 | 10.3 | 8000 | 0.2120 | 0.1986 |
| 0.929 | 10.94 | 8500 | 0.2048 | 0.1998 |
| 0.9017 | 11.58 | 9000 | 0.2035 | 0.1999 |
| 0.8898 | 12.23 | 9500 | 0.1961 | 0.1908 |
| 0.8799 | 12.87 | 10000 | 0.1945 | 0.1817 |
| 0.869 | 13.51 | 10500 | 0.1929 | 0.1844 |
| 0.8572 | 14.16 | 11000 | 0.1941 | 0.1888 |
| 0.8691 | 14.8 | 11500 | 0.1912 | 0.1804 |
| 0.8645 | 15.44 | 12000 | 0.1950 | 0.1851 |
| 0.8468 | 16.09 | 12500 | 0.1879 | 0.1770 |
| 0.8405 | 16.73 | 13000 | 0.1881 | 0.1759 |
| 0.8647 | 17.37 | 13500 | 0.1861 | 0.1740 |
| 0.8477 | 18.02 | 14000 | 0.1782 | 0.1702 |
| 0.811 | 18.66 | 14500 | 0.1915 | 0.1757 |
| 0.8165 | 19.3 | 15000 | 0.1820 | 0.1724 |
| 0.8166 | 19.95 | 15500 | 0.1798 | 0.1697 |
| 0.8167 | 20.59 | 16000 | 0.1805 | 0.1752 |
| 0.7908 | 21.24 | 16500 | 0.1761 | 0.1699 |
| 0.7925 | 21.88 | 17000 | 0.1740 | 0.1709 |
| 0.7803 | 22.52 | 17500 | 0.1815 | 0.1727 |
| 0.7839 | 23.17 | 18000 | 0.1737 | 0.1694 |
| 0.7815 | 23.81 | 18500 | 0.1732 | 0.1630 |
| 0.767 | 24.45 | 19000 | 0.1724 | 0.1648 |
| 0.7672 | 25.1 | 19500 | 0.1706 | 0.1596 |
| 0.7691 | 25.74 | 20000 | 0.1718 | 0.1618 |
| 0.7547 | 26.38 | 20500 | 0.1694 | 0.1565 |
| 0.7498 | 27.03 | 21000 | 0.1706 | 0.1582 |
| 0.7459 | 27.67 | 21500 | 0.1663 | 0.1586 |
| 0.7374 | 28.31 | 22000 | 0.1651 | 0.1567 |
| 0.7499 | 28.96 | 22500 | 0.1668 | 0.1549 |
| 0.7471 | 29.6 | 23000 | 0.1667 | 0.1553 |
| 0.7369 | 30.24 | 23500 | 0.1659 | 0.1556 |
| 0.7389 | 30.89 | 24000 | 0.1668 | 0.1538 |
| 0.7197 | 31.53 | 24500 | 0.1687 | 0.1561 |
| 0.71 | 32.17 | 25000 | 0.1666 | 0.1516 |
| 0.7199 | 32.82 | 25500 | 0.1640 | 0.1523 |
| 0.7194 | 33.46 | 26000 | 0.1659 | 0.1528 |
| 0.6923 | 34.11 | 26500 | 0.1662 | 0.1507 |
| 0.7054 | 34.75 | 27000 | 0.1641 | 0.1486 |
| 0.6955 | 35.39 | 27500 | 0.1634 | 0.1497 |
| 0.7084 | 36.04 | 28000 | 0.1618 | 0.1478 |
| 0.6917 | 36.68 | 28500 | 0.1589 | 0.1471 |
| 0.687 | 37.32 | 29000 | 0.1589 | 0.1450 |
| 0.6914 | 37.97 | 29500 | 0.1588 | 0.1465 |
| 0.6646 | 38.61 | 30000 | 0.1602 | 0.1468 |
| 0.6667 | 39.25 | 30500 | 0.1588 | 0.1444 |
| 0.6754 | 39.9 | 31000 | 0.1587 | 0.1455 |
| 0.6632 | 40.54 | 31500 | 0.1586 | 0.1461 |
| 0.6619 | 41.18 | 32000 | 0.1571 | 0.1441 |
| 0.6561 | 41.83 | 32500 | 0.1564 | 0.1420 |
| 0.6492 | 42.47 | 33000 | 0.1539 | 0.1437 |
| 0.6649 | 43.11 | 33500 | 0.1512 | 0.1406 |
| 0.6511 | 43.76 | 34000 | 0.1539 | 0.1384 |
| 0.6551 | 44.4 | 34500 | 0.1520 | 0.1384 |
| 0.6452 | 45.05 | 35000 | 0.1510 | 0.1368 |
| 0.6155 | 45.69 | 35500 | 0.1522 | 0.1375 |
| 0.628 | 46.33 | 36000 | 0.1522 | 0.1366 |
| 0.6389 | 46.97 | 36500 | 0.1513 | 0.1377 |
| 0.6265 | 47.62 | 37000 | 0.1512 | 0.1369 |
| 0.6197 | 48.26 | 37500 | 0.1511 | 0.1362 |
| 0.621 | 48.91 | 38000 | 0.1510 | 0.1357 |
| 0.6259 | 49.55 | 38500 | 0.1506 | 0.1353 |
### Framework versions
- Transformers 4.17.0
- Pytorch 1.9.1+cu102
- Datasets 2.0.0
- Tokenizers 0.11.6
|
bmichele/poetry-generation-nextline-mbart-ws-fi-single | 53404dee7930347147261545f84e35e6545594a0 | 2022-04-01T11:51:32.000Z | [
"pytorch"
] | null | false | bmichele | null | bmichele/poetry-generation-nextline-mbart-ws-fi-single | 0 | null | null | 36,653 | # poetry-generation-nextline-mbart-ws-fi-single
* `nextline`: generates a poem line from previous line(s)
* `mbart`: base model is [facebook/mbart-large-cc25](https://huggingface.co/facebook/mbart-large-cc25)
* `ws`: trained on Wikisource data
* `fi`: Finnish language
* `single`: uses only last poem line as input for generation |
notexist/ttt | 9eaa84e33b63a72207047f523dd287d61464cba6 | 2022-04-01T13:16:50.000Z | [
"pytorch",
"gpt2",
"text-generation",
"transformers",
"license:apache-2.0"
] | text-generation | false | notexist | null | notexist/ttt | 0 | null | transformers | 36,654 | ---
license: apache-2.0
---
|
bmichele/poetry-generation-firstline-mbart-ws-fi-sorted | 311f3ef62e9db0ad7c5621dab2760be05f6882e3 | 2022-04-01T13:03:49.000Z | [
"pytorch"
] | null | false | bmichele | null | bmichele/poetry-generation-firstline-mbart-ws-fi-sorted | 0 | null | null | 36,655 | TODO: This is still a demo model, the file does not match with the model card!!!
# poetry-generation-firstline-mbart-ws-fi-sorted
* `nextline`: generates the first poem line from keywords
* `mbart`: base model is [facebook/mbart-large-cc25](https://huggingface.co/facebook/mbart-large-cc25)
* `ws`: trained on Wikisource data
* `fi`: Finnish language
* `sorted`: the order of input keywords matter when generating candidates |
rahulacj/mbart-large-cc25-finetuned-hi-to-en-v1 | b7d8bf616d19b61134dafba61c5385c86993495e | 2022-04-02T14:18:26.000Z | [
"pytorch",
"tensorboard",
"mbart",
"text2text-generation",
"transformers",
"generated_from_trainer",
"model-index",
"autotrain_compatible"
] | text2text-generation | false | rahulacj | null | rahulacj/mbart-large-cc25-finetuned-hi-to-en-v1 | 0 | null | transformers | 36,656 | ---
tags:
- generated_from_trainer
metrics:
- bleu
model-index:
- name: mbart-large-cc25-finetuned-hi-to-en-v1
results: []
---
<!-- This model card has been generated automatically according to the information the Trainer had access to. You
should probably proofread and complete it, then remove this comment. -->
# mbart-large-cc25-finetuned-hi-to-en-v1
This model is a fine-tuned version of [facebook/mbart-large-cc25](https://huggingface.co/facebook/mbart-large-cc25) on an unknown dataset.
It achieves the following results on the evaluation set:
- Loss: 1.4978
- Bleu: 33.3366
- Gen Len: 22.7806
## Model description
More information needed
## Intended uses & limitations
More information needed
## Training and evaluation data
More information needed
## Training procedure
### Training hyperparameters
The following hyperparameters were used during training:
- learning_rate: 2e-05
- train_batch_size: 1
- eval_batch_size: 1
- seed: 42
- gradient_accumulation_steps: 4
- total_train_batch_size: 4
- optimizer: Adam with betas=(0.9,0.999) and epsilon=1e-08
- lr_scheduler_type: linear
- num_epochs: 3
- mixed_precision_training: Native AMP
### Training results
| Training Loss | Epoch | Step | Validation Loss | Bleu | Gen Len |
|:-------------:|:-----:|:-----:|:---------------:|:-------:|:-------:|
| 1.6774 | 1.0 | 3955 | 1.5499 | 7.9551 | 73.7518 |
| 1.2296 | 2.0 | 7910 | 1.4846 | 32.8075 | 23.7341 |
| 0.9127 | 3.0 | 11865 | 1.5345 | 31.9747 | 23.6264 |
### Framework versions
- Transformers 4.17.0
- Pytorch 1.10.0+cu111
- Datasets 2.0.0
- Tokenizers 0.11.6
|
hou/t5-base-finetuned-en-to-ug | 82085827de1bbb059bbc3b0f864f6d602d8e81b8 | 2022-04-01T15:35:06.000Z | [
"pytorch",
"tensorboard",
"t5",
"text2text-generation",
"transformers",
"autotrain_compatible"
] | text2text-generation | false | hou | null | hou/t5-base-finetuned-en-to-ug | 0 | null | transformers | 36,657 | Entry not found |
huggingtweets/chapocheck | ff65c201ad5a8eaf8aedbb2f2248bb6d6e257dab | 2022-04-01T22:07:43.000Z | [
"pytorch",
"gpt2",
"text-generation",
"en",
"transformers",
"huggingtweets"
] | text-generation | false | huggingtweets | null | huggingtweets/chapocheck | 0 | null | transformers | 36,658 | ---
language: en
thumbnail: http://www.huggingtweets.com/chapocheck/1648850858747/predictions.png
tags:
- huggingtweets
widget:
- text: "My dream is"
---
<div class="inline-flex flex-col" style="line-height: 1.5;">
<div class="flex">
<div
style="display:inherit; margin-left: 4px; margin-right: 4px; width: 92px; height:92px; border-radius: 50%; background-size: cover; background-image: url('https://pbs.twimg.com/profile_images/1191821996759404547/HY5C5aOW_400x400.jpg')">
</div>
<div
style="display:none; margin-left: 4px; margin-right: 4px; width: 92px; height:92px; border-radius: 50%; background-size: cover; background-image: url('')">
</div>
<div
style="display:none; margin-left: 4px; margin-right: 4px; width: 92px; height:92px; border-radius: 50%; background-size: cover; background-image: url('')">
</div>
</div>
<div style="text-align: center; margin-top: 3px; font-size: 16px; font-weight: 800">🤖 AI BOT 🤖</div>
<div style="text-align: center; font-size: 16px; font-weight: 800">Cum Town (mostly Nick Mullen) quotes</div>
<div style="text-align: center; font-size: 14px;">@chapocheck</div>
</div>
I was made with [huggingtweets](https://github.com/borisdayma/huggingtweets).
Create your own bot based on your favorite user with [the demo](https://colab.research.google.com/github/borisdayma/huggingtweets/blob/master/huggingtweets-demo.ipynb)!
## How does it work?
The model uses the following pipeline.
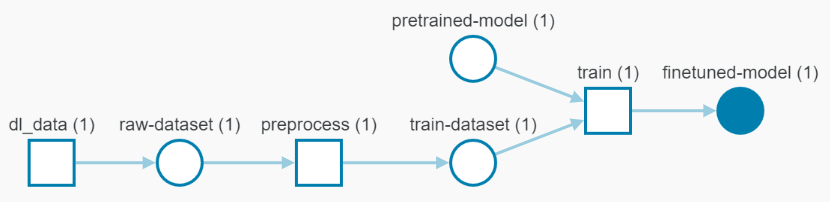
To understand how the model was developed, check the [W&B report](https://wandb.ai/wandb/huggingtweets/reports/HuggingTweets-Train-a-Model-to-Generate-Tweets--VmlldzoxMTY5MjI).
## Training data
The model was trained on tweets from Cum Town (mostly Nick Mullen) quotes.
| Data | Cum Town (mostly Nick Mullen) quotes |
| --- | --- |
| Tweets downloaded | 1264 |
| Retweets | 90 |
| Short tweets | 75 |
| Tweets kept | 1099 |
[Explore the data](https://wandb.ai/wandb/huggingtweets/runs/x77h239f/artifacts), which is tracked with [W&B artifacts](https://docs.wandb.com/artifacts) at every step of the pipeline.
## Training procedure
The model is based on a pre-trained [GPT-2](https://huggingface.co/gpt2) which is fine-tuned on @chapocheck's tweets.
Hyperparameters and metrics are recorded in the [W&B training run](https://wandb.ai/wandb/huggingtweets/runs/18r1isa5) for full transparency and reproducibility.
At the end of training, [the final model](https://wandb.ai/wandb/huggingtweets/runs/18r1isa5/artifacts) is logged and versioned.
## How to use
You can use this model directly with a pipeline for text generation:
```python
from transformers import pipeline
generator = pipeline('text-generation',
model='huggingtweets/chapocheck')
generator("My dream is", num_return_sequences=5)
```
## Limitations and bias
The model suffers from [the same limitations and bias as GPT-2](https://huggingface.co/gpt2#limitations-and-bias).
In addition, the data present in the user's tweets further affects the text generated by the model.
## About
*Built by Boris Dayma*
[](https://twitter.com/intent/follow?screen_name=borisdayma)
For more details, visit the project repository.
[](https://github.com/borisdayma/huggingtweets)
|
huggingtweets/clortown | 87818638c93d0d77ea73c078787069f462749cf1 | 2022-04-02T04:51:29.000Z | [
"pytorch",
"gpt2",
"text-generation",
"en",
"transformers",
"huggingtweets"
] | text-generation | false | huggingtweets | null | huggingtweets/clortown | 0 | null | transformers | 36,659 | ---
language: en
thumbnail: http://www.huggingtweets.com/clortown/1648875085007/predictions.png
tags:
- huggingtweets
widget:
- text: "My dream is"
---
<div class="inline-flex flex-col" style="line-height: 1.5;">
<div class="flex">
<div
style="display:inherit; margin-left: 4px; margin-right: 4px; width: 92px; height:92px; border-radius: 50%; background-size: cover; background-image: url('https://pbs.twimg.com/profile_images/1488574779351187458/RlIQNUFG_400x400.jpg')">
</div>
<div
style="display:none; margin-left: 4px; margin-right: 4px; width: 92px; height:92px; border-radius: 50%; background-size: cover; background-image: url('')">
</div>
<div
style="display:none; margin-left: 4px; margin-right: 4px; width: 92px; height:92px; border-radius: 50%; background-size: cover; background-image: url('')">
</div>
</div>
<div style="text-align: center; margin-top: 3px; font-size: 16px; font-weight: 800">🤖 AI BOT 🤖</div>
<div style="text-align: center; font-size: 16px; font-weight: 800">yeosang elf agenda</div>
<div style="text-align: center; font-size: 14px;">@clortown</div>
</div>
I was made with [huggingtweets](https://github.com/borisdayma/huggingtweets).
Create your own bot based on your favorite user with [the demo](https://colab.research.google.com/github/borisdayma/huggingtweets/blob/master/huggingtweets-demo.ipynb)!
## How does it work?
The model uses the following pipeline.
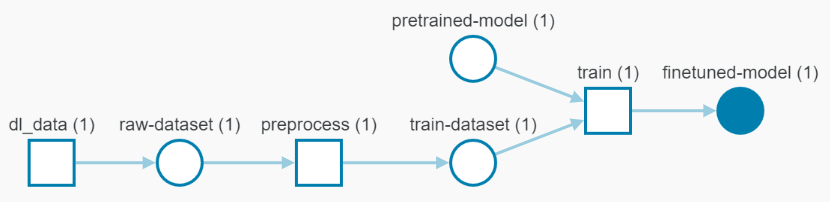
To understand how the model was developed, check the [W&B report](https://wandb.ai/wandb/huggingtweets/reports/HuggingTweets-Train-a-Model-to-Generate-Tweets--VmlldzoxMTY5MjI).
## Training data
The model was trained on tweets from yeosang elf agenda.
| Data | yeosang elf agenda |
| --- | --- |
| Tweets downloaded | 3140 |
| Retweets | 538 |
| Short tweets | 463 |
| Tweets kept | 2139 |
[Explore the data](https://wandb.ai/wandb/huggingtweets/runs/3cupnlna/artifacts), which is tracked with [W&B artifacts](https://docs.wandb.com/artifacts) at every step of the pipeline.
## Training procedure
The model is based on a pre-trained [GPT-2](https://huggingface.co/gpt2) which is fine-tuned on @clortown's tweets.
Hyperparameters and metrics are recorded in the [W&B training run](https://wandb.ai/wandb/huggingtweets/runs/uii743r9) for full transparency and reproducibility.
At the end of training, [the final model](https://wandb.ai/wandb/huggingtweets/runs/uii743r9/artifacts) is logged and versioned.
## How to use
You can use this model directly with a pipeline for text generation:
```python
from transformers import pipeline
generator = pipeline('text-generation',
model='huggingtweets/clortown')
generator("My dream is", num_return_sequences=5)
```
## Limitations and bias
The model suffers from [the same limitations and bias as GPT-2](https://huggingface.co/gpt2#limitations-and-bias).
In addition, the data present in the user's tweets further affects the text generated by the model.
## About
*Built by Boris Dayma*
[](https://twitter.com/intent/follow?screen_name=borisdayma)
For more details, visit the project repository.
[](https://github.com/borisdayma/huggingtweets)
|
iiShreya/wikineural-multilingual-ner | 30b79b6a2c8ab4eae0dcd57bce1b6e4ea238c6df | 2022-04-11T19:53:32.000Z | [
"pytorch",
"tensorboard",
"distilbert",
"token-classification",
"transformers",
"autotrain_compatible"
] | token-classification | false | iiShreya | null | iiShreya/wikineural-multilingual-ner | 0 | null | transformers | 36,660 | Entry not found |
huggingtweets/percybotshelley | a65ccfecace2bebeefbe947667e6cd907af1a4d9 | 2022-04-02T05:27:46.000Z | [
"pytorch",
"gpt2",
"text-generation",
"en",
"transformers",
"huggingtweets"
] | text-generation | false | huggingtweets | null | huggingtweets/percybotshelley | 0 | null | transformers | 36,661 | ---
language: en
thumbnail: https://github.com/borisdayma/huggingtweets/blob/master/img/logo.png?raw=true
tags:
- huggingtweets
widget:
- text: "My dream is"
---
<div class="inline-flex flex-col" style="line-height: 1.5;">
<div class="flex">
<div
style="display:inherit; margin-left: 4px; margin-right: 4px; width: 92px; height:92px; border-radius: 50%; background-size: cover; background-image: url('https://pbs.twimg.com/profile_images/780200431859269633/kXZwDd_Y_400x400.jpg')">
</div>
<div
style="display:none; margin-left: 4px; margin-right: 4px; width: 92px; height:92px; border-radius: 50%; background-size: cover; background-image: url('')">
</div>
<div
style="display:none; margin-left: 4px; margin-right: 4px; width: 92px; height:92px; border-radius: 50%; background-size: cover; background-image: url('')">
</div>
</div>
<div style="text-align: center; margin-top: 3px; font-size: 16px; font-weight: 800">🤖 AI BOT 🤖</div>
<div style="text-align: center; font-size: 16px; font-weight: 800">Romantic Poetry Bot</div>
<div style="text-align: center; font-size: 14px;">@percybotshelley</div>
</div>
I was made with [huggingtweets](https://github.com/borisdayma/huggingtweets).
Create your own bot based on your favorite user with [the demo](https://colab.research.google.com/github/borisdayma/huggingtweets/blob/master/huggingtweets-demo.ipynb)!
## How does it work?
The model uses the following pipeline.
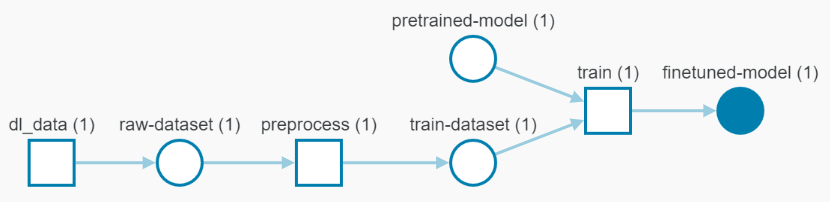
To understand how the model was developed, check the [W&B report](https://wandb.ai/wandb/huggingtweets/reports/HuggingTweets-Train-a-Model-to-Generate-Tweets--VmlldzoxMTY5MjI).
## Training data
The model was trained on tweets from Romantic Poetry Bot.
| Data | Romantic Poetry Bot |
| --- | --- |
| Tweets downloaded | 3205 |
| Retweets | 0 |
| Short tweets | 20 |
| Tweets kept | 3185 |
[Explore the data](https://wandb.ai/wandb/huggingtweets/runs/1bj4pakr/artifacts), which is tracked with [W&B artifacts](https://docs.wandb.com/artifacts) at every step of the pipeline.
## Training procedure
The model is based on a pre-trained [GPT-2](https://huggingface.co/gpt2) which is fine-tuned on @percybotshelley's tweets.
Hyperparameters and metrics are recorded in the [W&B training run](https://wandb.ai/wandb/huggingtweets/runs/2yfs8v92) for full transparency and reproducibility.
At the end of training, [the final model](https://wandb.ai/wandb/huggingtweets/runs/2yfs8v92/artifacts) is logged and versioned.
## How to use
You can use this model directly with a pipeline for text generation:
```python
from transformers import pipeline
generator = pipeline('text-generation',
model='huggingtweets/percybotshelley')
generator("My dream is", num_return_sequences=5)
```
## Limitations and bias
The model suffers from [the same limitations and bias as GPT-2](https://huggingface.co/gpt2#limitations-and-bias).
In addition, the data present in the user's tweets further affects the text generated by the model.
## About
*Built by Boris Dayma*
[](https://twitter.com/intent/follow?screen_name=borisdayma)
For more details, visit the project repository.
[](https://github.com/borisdayma/huggingtweets)
|
juancavallotti/t5-base-es-en | 51d7d90a7cb2fba82bd7505cf3727060be523f40 | 2022-04-02T06:02:27.000Z | [
"pytorch",
"t5",
"text2text-generation",
"transformers",
"autotrain_compatible"
] | text2text-generation | false | juancavallotti | null | juancavallotti/t5-base-es-en | 0 | null | transformers | 36,662 | Entry not found |
mczolly/DialoGPT-small-the-doctor | 2019fa6c913e32927a241b8fb9998e0623ebdcfb | 2022-04-02T11:20:07.000Z | [
"pytorch",
"gpt2",
"text-generation",
"transformers",
"conversational"
] | conversational | false | mczolly | null | mczolly/DialoGPT-small-the-doctor | 0 | null | transformers | 36,663 | ---
tags:
- conversational
---
# Doctor Who model |
huggingtweets/sanjabh | eb82f20e98d9371abfa8e0609cb7169b3b7b67cb | 2022-04-02T12:14:56.000Z | [
"pytorch",
"gpt2",
"text-generation",
"en",
"transformers",
"huggingtweets"
] | text-generation | false | huggingtweets | null | huggingtweets/sanjabh | 0 | null | transformers | 36,664 | ---
language: en
thumbnail: http://www.huggingtweets.com/sanjabh/1648901691950/predictions.png
tags:
- huggingtweets
widget:
- text: "My dream is"
---
<div class="inline-flex flex-col" style="line-height: 1.5;">
<div class="flex">
<div
style="display:inherit; margin-left: 4px; margin-right: 4px; width: 92px; height:92px; border-radius: 50%; background-size: cover; background-image: url('https://pbs.twimg.com/profile_images/1484080880222351360/FtDB2j4B_400x400.jpg')">
</div>
<div
style="display:none; margin-left: 4px; margin-right: 4px; width: 92px; height:92px; border-radius: 50%; background-size: cover; background-image: url('')">
</div>
<div
style="display:none; margin-left: 4px; margin-right: 4px; width: 92px; height:92px; border-radius: 50%; background-size: cover; background-image: url('')">
</div>
</div>
<div style="text-align: center; margin-top: 3px; font-size: 16px; font-weight: 800">🤖 AI BOT 🤖</div>
<div style="text-align: center; font-size: 16px; font-weight: 800">Lucid Dreams</div>
<div style="text-align: center; font-size: 14px;">@sanjabh</div>
</div>
I was made with [huggingtweets](https://github.com/borisdayma/huggingtweets).
Create your own bot based on your favorite user with [the demo](https://colab.research.google.com/github/borisdayma/huggingtweets/blob/master/huggingtweets-demo.ipynb)!
## How does it work?
The model uses the following pipeline.
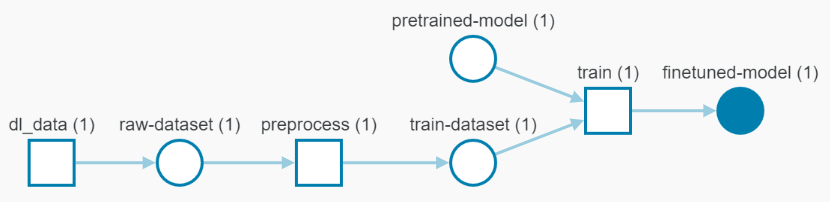
To understand how the model was developed, check the [W&B report](https://wandb.ai/wandb/huggingtweets/reports/HuggingTweets-Train-a-Model-to-Generate-Tweets--VmlldzoxMTY5MjI).
## Training data
The model was trained on tweets from Lucid Dreams.
| Data | Lucid Dreams |
| --- | --- |
| Tweets downloaded | 3250 |
| Retweets | 373 |
| Short tweets | 137 |
| Tweets kept | 2740 |
[Explore the data](https://wandb.ai/wandb/huggingtweets/runs/2s7tzf32/artifacts), which is tracked with [W&B artifacts](https://docs.wandb.com/artifacts) at every step of the pipeline.
## Training procedure
The model is based on a pre-trained [GPT-2](https://huggingface.co/gpt2) which is fine-tuned on @sanjabh's tweets.
Hyperparameters and metrics are recorded in the [W&B training run](https://wandb.ai/wandb/huggingtweets/runs/1cl1cjnx) for full transparency and reproducibility.
At the end of training, [the final model](https://wandb.ai/wandb/huggingtweets/runs/1cl1cjnx/artifacts) is logged and versioned.
## How to use
You can use this model directly with a pipeline for text generation:
```python
from transformers import pipeline
generator = pipeline('text-generation',
model='huggingtweets/sanjabh')
generator("My dream is", num_return_sequences=5)
```
## Limitations and bias
The model suffers from [the same limitations and bias as GPT-2](https://huggingface.co/gpt2#limitations-and-bias).
In addition, the data present in the user's tweets further affects the text generated by the model.
## About
*Built by Boris Dayma*
[](https://twitter.com/intent/follow?screen_name=borisdayma)
For more details, visit the project repository.
[](https://github.com/borisdayma/huggingtweets)
|
mnne/duck-and-cover-genre-encoder | 6456abe1f444db42e60c7663171873d0cd8a8907 | 2022-04-02T13:53:50.000Z | [
"pytorch",
"bert",
"fill-mask",
"transformers",
"autotrain_compatible"
] | fill-mask | false | mnne | null | mnne/duck-and-cover-genre-encoder | 0 | null | transformers | 36,665 | # Duck and Cover - Genre Autoencoder
This model is part of the [duck_and_cover](https://github.com/mcschmitz/duck_and_cover) repository. Scope of this repository is to generate album covers based on several conditions like release year, artist & album name, and genre(s) using different types of GANs. The possible list of genres that this encoder covers can be found [here](https://github.com/mcschmitz/duck_and_cover/blob/master/data/genres.txt).
For training [prajjwal1/bert-mini](https://huggingface.co/prajjwal1/bert-mini) has been finetuned on a list of 466.045 albums with different genre combinations taken from the aforementioned list to embed genre information, while a simple Linear Layer was trained to decode and predict the given genre from the embeddings. The albums are real-world albums retrieved using the Spotify API. The intention behind this model is that Hard Rock is somehow related to Rock, while Pop Rock is related to Rock as well and a BERT Tokenizer can capture this information as a lot of music genres are described by using pre- and suffixes.
The model was validated on 133.155 during training and tested on 66.578. It yields a 98.29% Exact Match ratio on the testset and a 98.24% Exact Match Ratio on the validation set, which is extremely high given that the model can embed up to 3452 labels and most of the albums only had up to 5 labels.
## Usage
The model can be used to embed genres to a 256 dimensional space using the following input.
```python
from transformers import AutoModel, AutoTokenizer
model = AutoModel.from_pretrained("mnne/duck-and-cover-genre-encoder")
tokenizer = AutoTokenizer.from_pretrained("mnne/duck-and-cover-genre-encoder")
genres = " , ".join(["classic soul", "memphis soul", "soul", "soul blues", "southern soul"])
x = tokenizer([genres], return_tensors="pt")
output = model(**x)
``` |
shwetha/distilbert-base-uncased-finetuned-squad | 10860c1feb7aace883cc1633b820bc45d3358599 | 2022-04-02T17:11:25.000Z | [
"pytorch",
"tensorboard",
"distilbert",
"question-answering",
"transformers",
"generated_from_trainer",
"license:apache-2.0",
"model-index",
"autotrain_compatible"
] | question-answering | false | shwetha | null | shwetha/distilbert-base-uncased-finetuned-squad | 0 | null | transformers | 36,666 | ---
license: apache-2.0
tags:
- generated_from_trainer
model-index:
- name: distilbert-base-uncased-finetuned-squad
results: []
---
<!-- This model card has been generated automatically according to the information the Trainer had access to. You
should probably proofread and complete it, then remove this comment. -->
# distilbert-base-uncased-finetuned-squad
This model is a fine-tuned version of [distilbert-base-uncased](https://huggingface.co/distilbert-base-uncased) on an unknown dataset.
It achieves the following results on the evaluation set:
- Loss: 5.5925
## Model description
More information needed
## Intended uses & limitations
More information needed
## Training and evaluation data
More information needed
## Training procedure
### Training hyperparameters
The following hyperparameters were used during training:
- learning_rate: 2e-05
- train_batch_size: 16
- eval_batch_size: 16
- seed: 42
- optimizer: Adam with betas=(0.9,0.999) and epsilon=1e-08
- lr_scheduler_type: linear
- num_epochs: 3
### Training results
| Training Loss | Epoch | Step | Validation Loss |
|:-------------:|:-----:|:----:|:---------------:|
| No log | 1.0 | 2 | 5.9198 |
| No log | 2.0 | 4 | 5.7019 |
| No log | 3.0 | 6 | 5.5925 |
### Framework versions
- Transformers 4.11.0
- Pytorch 1.10.2+cpu
- Datasets 2.0.0
- Tokenizers 0.10.3
|
notexist/ttt2 | 7bf57ef3be42c0eb082096ba5115870f19c82e3f | 2022-04-02T15:09:26.000Z | [
"pytorch",
"gpt2",
"text-generation",
"transformers",
"license:apache-2.0"
] | text-generation | false | notexist | null | notexist/ttt2 | 0 | null | transformers | 36,667 | ---
license: apache-2.0
---
|
hou/plt5-small-finetuned-en-to-ug | 449dd87f5c5a03f488fd48dcdfa868ef525eeba9 | 2022-04-02T15:48:58.000Z | [
"pytorch",
"tensorboard",
"t5",
"text2text-generation",
"transformers",
"autotrain_compatible"
] | text2text-generation | false | hou | null | hou/plt5-small-finetuned-en-to-ug | 0 | null | transformers | 36,668 | Entry not found |
vocab-transformers/distilbert-mlm-500k | 26a5b9c50244234234181556b75db33c1fa69b0c | 2022-04-02T21:12:46.000Z | [
"pytorch",
"distilbert",
"fill-mask",
"transformers",
"autotrain_compatible"
] | fill-mask | false | vocab-transformers | null | vocab-transformers/distilbert-mlm-500k | 0 | null | transformers | 36,669 | distilbert-base-uncased trained for 500K steps with batch size 64 on C4, MSMARCO, Wikipedia, S2ORC, News
|
vocab-transformers/distilbert-mlm-750k | 8bd8ce434dda17543bd9045ef980d4b2798074db | 2022-04-02T21:15:27.000Z | [
"pytorch",
"distilbert",
"fill-mask",
"transformers",
"autotrain_compatible"
] | fill-mask | false | vocab-transformers | null | vocab-transformers/distilbert-mlm-750k | 0 | null | transformers | 36,670 | distilbert-base-uncased trained for 750K steps with batch size 64 on C4, MSMARCO, Wikipedia, S2ORC, News
|
vocab-transformers/distilbert-mlm-best | fa0c296950940d35f3a7af05fa0b17a3db26c79a | 2022-04-02T21:18:53.000Z | [
"pytorch",
"distilbert",
"fill-mask",
"transformers",
"autotrain_compatible"
] | fill-mask | false | vocab-transformers | null | vocab-transformers/distilbert-mlm-best | 0 | null | transformers | 36,671 | distilbert-base-uncased trained for 680K steps (lowest loss on dev dataset) with batch size 64 on C4, MSMARCO, Wikipedia, S2ORC, News
|
notexist/tttf | 45c7ff3da49f31a8a3b50b6c1f219717c9931622 | 2022-04-03T03:11:37.000Z | [
"pytorch",
"gpt2",
"text-generation",
"transformers"
] | text-generation | false | notexist | null | notexist/tttf | 0 | null | transformers | 36,672 | Entry not found |
jsunster/distilbert-base-uncased-finetuned-squad | b17448ee1e94fcc3c40d94b6d03ac6c388fe319a | 2022-04-03T14:46:14.000Z | [
"pytorch",
"tensorboard",
"distilbert",
"question-answering",
"dataset:squad",
"transformers",
"generated_from_trainer",
"license:apache-2.0",
"model-index",
"autotrain_compatible"
] | question-answering | false | jsunster | null | jsunster/distilbert-base-uncased-finetuned-squad | 0 | null | transformers | 36,673 | ---
license: apache-2.0
tags:
- generated_from_trainer
datasets:
- squad
model-index:
- name: distilbert-base-uncased-finetuned-squad
results: []
---
<!-- This model card has been generated automatically according to the information the Trainer had access to. You
should probably proofread and complete it, then remove this comment. -->
# distilbert-base-uncased-finetuned-squad
This model is a fine-tuned version of [distilbert-base-uncased](https://huggingface.co/distilbert-base-uncased) on the squad dataset.
It achieves the following results on the evaluation set:
- Loss: 1.1476
## Model description
More information needed
## Intended uses & limitations
More information needed
## Training and evaluation data
More information needed
## Training procedure
### Training hyperparameters
The following hyperparameters were used during training:
- learning_rate: 2e-05
- train_batch_size: 32
- eval_batch_size: 32
- seed: 42
- optimizer: Adam with betas=(0.9,0.999) and epsilon=1e-08
- lr_scheduler_type: linear
- num_epochs: 3
### Training results
| Training Loss | Epoch | Step | Validation Loss |
|:-------------:|:-----:|:----:|:---------------:|
| 1.2823 | 1.0 | 2767 | 1.1980 |
| 1.0336 | 2.0 | 5534 | 1.1334 |
| 0.8513 | 3.0 | 8301 | 1.1476 |
### Framework versions
- Transformers 4.17.0
- Pytorch 1.11.0
- Datasets 2.0.0
- Tokenizers 0.11.6
|
johnowhitaker/orbgan_dark | 07442305009323382ddcd756ef19d91e1616b516 | 2022-04-05T07:31:24.000Z | [
"pytorch"
] | null | false | johnowhitaker | null | johnowhitaker/orbgan_dark | 0 | null | null | 36,674 | A version of https://huggingface.co/johnowhitaker/orbgan_e1 trained on only dark images |
johnowhitaker/orbgan_light | 5d339f335c098469ae95024a52c9a68790c2b642 | 2022-04-05T07:31:09.000Z | [
"pytorch"
] | null | false | johnowhitaker | null | johnowhitaker/orbgan_light | 0 | null | null | 36,675 | A version of https://huggingface.co/johnowhitaker/orbgan_e1 trained on only light images |
pszemraj/gpt-peter-2.7B | f58910bfe8e51da0ffa59fce4f9d934f53e693b0 | 2022-05-24T12:09:16.000Z | [
"pytorch",
"gpt_neo",
"text-generation",
"transformers",
"gpt-neo",
"gpt-peter",
"chatbot"
] | text-generation | false | pszemraj | null | pszemraj/gpt-peter-2.7B | 0 | null | transformers | 36,676 | ---
tags:
- gpt-neo
- gpt-peter
- chatbot
inference: False
---
# pszemraj/gpt-peter-2.7B
- This model is a fine-tuned version of [EleutherAI/gpt-neo-2.7B](https://huggingface.co/EleutherAI/gpt-neo-2.7B) on about 80k WhatsApp and iMessage texts.
- The model is too large to use the inference API. linked [here](https://colab.research.google.com/gist/pszemraj/a59b43813437b43973c8f8f9a3944565/testing-pszemraj-gpt-peter-2-7b.ipynb) is a notebook for testing in Colab.
- alternatively, you can message [a bot on telegram](http://t.me/GPTPeter_bot) where I test LLMs for dialogue generation
- the telegram bot code and the model training code can be found [in this repository](https://github.com/pszemraj/ai-msgbot)
## Usage in python
Install the transformers library if you don't have it:
```
pip install -U transformers
```
load the model into a `pipeline` object:
```
from transformers import pipeline
import torch
my_chatbot = pipeline('text-generation',
'pszemraj/gpt-peter-2.7B',
device=0 if torch.cuda.is_available() else -1,
)
```
generate text!
```
my_chatbot('Did you ever hear the tragedy of Darth Plagueis The Wise?')
```
_(example above for simplicity, but adding generation parameters such as `no_repeat_ngram_size` are recommended to get better generations)_
## Training procedure
### Training hyperparameters
The following hyperparameters were used during training:
- learning_rate: 6e-05
- train_batch_size: 2
- eval_batch_size: 2
- seed: 42
- distributed_type: multi-GPU
- gradient_accumulation_steps: 32
- total_train_batch_size: 64
- optimizer: Adam with betas=(0.9,0.999) and epsilon=1e-08
- lr_scheduler_type: cosine
- lr_scheduler_warmup_ratio: 0.05
- num_epochs: 1
### Framework versions
- Transformers 4.17.0
- Pytorch 1.10.0+cu113
- Datasets 2.0.0
- Tokenizers 0.11.6
|
pfloyd/opus-mt-es-en-finetuned-es-to-en | 7f575fadfd216078587df305b9ad9ac4912f4c5c | 2022-04-08T03:30:30.000Z | [
"pytorch",
"tensorboard",
"marian",
"text2text-generation",
"transformers",
"generated_from_trainer",
"license:apache-2.0",
"model-index",
"autotrain_compatible"
] | text2text-generation | false | pfloyd | null | pfloyd/opus-mt-es-en-finetuned-es-to-en | 0 | null | transformers | 36,677 | ---
license: apache-2.0
tags:
- generated_from_trainer
metrics:
- bleu
model-index:
- name: opus-mt-es-en-finetuned-es-to-en
results: []
---
<!-- This model card has been generated automatically according to the information the Trainer had access to. You
should probably proofread and complete it, then remove this comment. -->
# opus-mt-es-en-finetuned-es-to-en
This model is a fine-tuned version of [Helsinki-NLP/opus-mt-es-en](https://huggingface.co/Helsinki-NLP/opus-mt-es-en) on the None dataset.
It achieves the following results on the evaluation set:
- Loss: 0.5851
- Bleu: 71.1382
- Gen Len: 10.3225
## Model description
More information needed
## Intended uses & limitations
More information needed
## Training and evaluation data
More information needed
## Training procedure
### Training hyperparameters
The following hyperparameters were used during training:
- learning_rate: 2e-05
- train_batch_size: 16
- eval_batch_size: 16
- seed: 42
- optimizer: Adam with betas=(0.9,0.999) and epsilon=1e-08
- lr_scheduler_type: linear
- num_epochs: 5
### Training results
| Training Loss | Epoch | Step | Validation Loss | Bleu | Gen Len |
|:-------------:|:-----:|:----:|:---------------:|:-------:|:-------:|
| No log | 1.0 | 112 | 0.5693 | 71.7823 | 10.3676 |
| No log | 2.0 | 224 | 0.5744 | 69.5504 | 10.6739 |
| No log | 3.0 | 336 | 0.5784 | 71.6553 | 10.3117 |
| No log | 4.0 | 448 | 0.5826 | 71.0576 | 10.3261 |
| 0.2666 | 5.0 | 560 | 0.5851 | 71.1382 | 10.3225 |
### Framework versions
- Transformers 4.16.2
- Pytorch 1.9.1
- Datasets 1.18.4
- Tokenizers 0.11.6
|
microsoft/cvt-13-384 | 36a5cfac1b06d6f792894faef9f1df9f331cdda1 | 2022-05-18T16:11:53.000Z | [
"pytorch",
"cvt",
"image-classification",
"dataset:imagenet-1k",
"arxiv:2103.15808",
"transformers",
"vision",
"license:apache-2.0"
] | image-classification | false | microsoft | null | microsoft/cvt-13-384 | 0 | null | transformers | 36,678 | ---
license: apache-2.0
tags:
- vision
- image-classification
datasets:
- imagenet-1k
widget:
- src: https://huggingface.co/datasets/mishig/sample_images/resolve/main/tiger.jpg
example_title: Tiger
- src: https://huggingface.co/datasets/mishig/sample_images/resolve/main/teapot.jpg
example_title: Teapot
- src: https://huggingface.co/datasets/mishig/sample_images/resolve/main/palace.jpg
example_title: Palace
---
# Convolutional Vision Transformer (CvT)
CvT-13 model pre-trained on ImageNet-1k at resolution 384x384. It was introduced in the paper [CvT: Introducing Convolutions to Vision Transformers](https://arxiv.org/abs/2103.15808) by Wu et al. and first released in [this repository](https://github.com/microsoft/CvT).
Disclaimer: The team releasing CvT did not write a model card for this model so this model card has been written by the Hugging Face team.
## Usage
Here is how to use this model to classify an image of the COCO 2017 dataset into one of the 1,000 ImageNet classes:
```python
from transformers import AutoFeatureExtractor, CvtForImageClassification
from PIL import Image
import requests
url = 'http://images.cocodataset.org/val2017/000000039769.jpg'
image = Image.open(requests.get(url, stream=True).raw)
feature_extractor = AutoFeatureExtractor.from_pretrained('microsoft/cvt-13-384')
model = CvtForImageClassification.from_pretrained('microsoft/cvt-13-384')
inputs = feature_extractor(images=image, return_tensors="pt")
outputs = model(**inputs)
logits = outputs.logits
# model predicts one of the 1000 ImageNet classes
predicted_class_idx = logits.argmax(-1).item()
print("Predicted class:", model.config.id2label[predicted_class_idx])
```
``` |
medhabi/bert-base-uncased-finetuned-imdb | 96a0b865f623a6f08c1b3bb5c75de98826704a66 | 2022-04-04T14:29:57.000Z | [
"pytorch",
"tensorboard",
"bert",
"fill-mask",
"transformers",
"generated_from_trainer",
"license:apache-2.0",
"model-index",
"autotrain_compatible"
] | fill-mask | false | medhabi | null | medhabi/bert-base-uncased-finetuned-imdb | 0 | null | transformers | 36,679 | ---
license: apache-2.0
tags:
- generated_from_trainer
model-index:
- name: bert-base-uncased-finetuned-imdb
results: []
---
<!-- This model card has been generated automatically according to the information the Trainer had access to. You
should probably proofread and complete it, then remove this comment. -->
# bert-base-uncased-finetuned-imdb
This model is a fine-tuned version of [bert-base-uncased](https://huggingface.co/bert-base-uncased) on an unknown dataset.
It achieves the following results on the evaluation set:
- Loss: 2.2887
## Model description
More information needed
## Intended uses & limitations
More information needed
## Training and evaluation data
More information needed
## Training procedure
### Training hyperparameters
The following hyperparameters were used during training:
- learning_rate: 2e-05
- train_batch_size: 64
- eval_batch_size: 64
- seed: 42
- optimizer: Adam with betas=(0.9,0.999) and epsilon=1e-08
- lr_scheduler_type: linear
- num_epochs: 3.0
- mixed_precision_training: Native AMP
### Training results
| Training Loss | Epoch | Step | Validation Loss |
|:-------------:|:-----:|:----:|:---------------:|
| 2.6449 | 1.0 | 157 | 2.3557 |
| 2.4402 | 2.0 | 314 | 2.2897 |
| 2.3804 | 3.0 | 471 | 2.3011 |
### Framework versions
- Transformers 4.17.0
- Pytorch 1.10.0+cu111
- Datasets 2.0.0
- Tokenizers 0.11.6
|
leixu/xlm-roberta-base-finetuned-panx-de | 1cc6bb8e73bd013be936597eddec9125c721db60 | 2022-04-04T14:38:14.000Z | [
"pytorch",
"tensorboard",
"xlm-roberta",
"token-classification",
"dataset:xtreme",
"transformers",
"generated_from_trainer",
"license:mit",
"model-index",
"autotrain_compatible"
] | token-classification | false | leixu | null | leixu/xlm-roberta-base-finetuned-panx-de | 0 | null | transformers | 36,680 | ---
license: mit
tags:
- generated_from_trainer
datasets:
- xtreme
metrics:
- f1
model-index:
- name: xlm-roberta-base-finetuned-panx-de
results:
- task:
name: Token Classification
type: token-classification
dataset:
name: xtreme
type: xtreme
args: PAN-X.de
metrics:
- name: F1
type: f1
value: 0.8605061131646289
---
<!-- This model card has been generated automatically according to the information the Trainer had access to. You
should probably proofread and complete it, then remove this comment. -->
# xlm-roberta-base-finetuned-panx-de
This model is a fine-tuned version of [xlm-roberta-base](https://huggingface.co/xlm-roberta-base) on the xtreme dataset.
It achieves the following results on the evaluation set:
- Loss: 0.1377
- F1: 0.8605
## Model description
More information needed
## Intended uses & limitations
More information needed
## Training and evaluation data
More information needed
## Training procedure
### Training hyperparameters
The following hyperparameters were used during training:
- learning_rate: 5e-05
- train_batch_size: 24
- eval_batch_size: 24
- seed: 42
- optimizer: Adam with betas=(0.9,0.999) and epsilon=1e-08
- lr_scheduler_type: linear
- num_epochs: 3
### Training results
| Training Loss | Epoch | Step | Validation Loss | F1 |
|:-------------:|:-----:|:----:|:---------------:|:------:|
| 0.2573 | 1.0 | 525 | 0.1651 | 0.8199 |
| 0.1296 | 2.0 | 1050 | 0.1482 | 0.8413 |
| 0.081 | 3.0 | 1575 | 0.1377 | 0.8605 |
### Framework versions
- Transformers 4.11.3
- Pytorch 1.7.1
- Datasets 1.16.1
- Tokenizers 0.10.3
|
gao-huggingface/T5-IDX-Event | 71c7d06f53bc12f9b021496e22cc8096f64db9a6 | 2022-04-04T16:01:31.000Z | [
"pytorch",
"t5",
"text2text-generation",
"transformers",
"autotrain_compatible"
] | text2text-generation | false | gao-huggingface | null | gao-huggingface/T5-IDX-Event | 0 | null | transformers | 36,681 | Entry not found |
gao-huggingface/T5-IDX-Descriptor | 9bad56cb92cb90e22ef09ed8305345e670eae043 | 2022-04-04T16:05:27.000Z | [
"pytorch",
"t5",
"text2text-generation",
"transformers",
"autotrain_compatible"
] | text2text-generation | false | gao-huggingface | null | gao-huggingface/T5-IDX-Descriptor | 0 | null | transformers | 36,682 | Entry not found |
gao-huggingface/T5-IDX-Subdescriptor | abb736d96cb7050e156a24b4550ab31e16fa0ceb | 2022-04-04T16:08:16.000Z | [
"pytorch",
"t5",
"text2text-generation",
"transformers",
"autotrain_compatible"
] | text2text-generation | false | gao-huggingface | null | gao-huggingface/T5-IDX-Subdescriptor | 0 | null | transformers | 36,683 | Entry not found |
gao-huggingface/T5-IDX-Subdescriptor-Flat-Model | 9b1a1d9194f85d8831016e1c73d1cd5174e2cec5 | 2022-04-04T16:14:00.000Z | [
"pytorch",
"t5",
"text2text-generation",
"transformers",
"autotrain_compatible"
] | text2text-generation | false | gao-huggingface | null | gao-huggingface/T5-IDX-Subdescriptor-Flat-Model | 0 | null | transformers | 36,684 | Entry not found |
johnowhitaker/butterfly-gan-10k | 77c982e96cea2b04c88fd57320ee36fc34f33fae | 2022-04-04T18:12:07.000Z | [
"pytorch"
] | null | false | johnowhitaker | null | johnowhitaker/butterfly-gan-10k | 0 | null | null | 36,685 | Badly trained lightweightgan - ignore |
huggingtweets/weirdokun | 35ab7e8e4c99111a2acfff1c5890d66da0940363 | 2022-04-04T16:40:11.000Z | [
"pytorch",
"gpt2",
"text-generation",
"en",
"transformers",
"huggingtweets"
] | text-generation | false | huggingtweets | null | huggingtweets/weirdokun | 0 | null | transformers | 36,686 | ---
language: en
thumbnail: https://github.com/borisdayma/huggingtweets/blob/master/img/logo.png?raw=true
tags:
- huggingtweets
widget:
- text: "My dream is"
---
<div class="inline-flex flex-col" style="line-height: 1.5;">
<div class="flex">
<div
style="display:inherit; margin-left: 4px; margin-right: 4px; width: 92px; height:92px; border-radius: 50%; background-size: cover; background-image: url('https://pbs.twimg.com/profile_images/1447886082163417093/l0n43HWC_400x400.jpg')">
</div>
<div
style="display:none; margin-left: 4px; margin-right: 4px; width: 92px; height:92px; border-radius: 50%; background-size: cover; background-image: url('')">
</div>
<div
style="display:none; margin-left: 4px; margin-right: 4px; width: 92px; height:92px; border-radius: 50%; background-size: cover; background-image: url('')">
</div>
</div>
<div style="text-align: center; margin-top: 3px; font-size: 16px; font-weight: 800">🤖 AI BOT 🤖</div>
<div style="text-align: center; font-size: 16px; font-weight: 800">#LetLeniLead</div>
<div style="text-align: center; font-size: 14px;">@weirdokun</div>
</div>
I was made with [huggingtweets](https://github.com/borisdayma/huggingtweets).
Create your own bot based on your favorite user with [the demo](https://colab.research.google.com/github/borisdayma/huggingtweets/blob/master/huggingtweets-demo.ipynb)!
## How does it work?
The model uses the following pipeline.
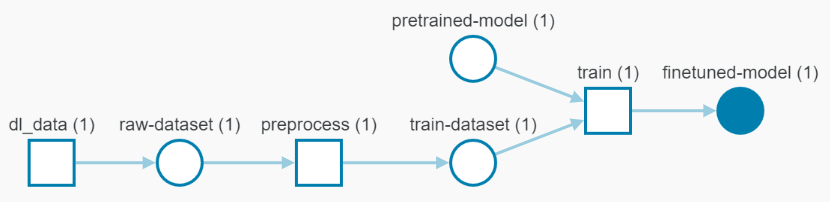
To understand how the model was developed, check the [W&B report](https://wandb.ai/wandb/huggingtweets/reports/HuggingTweets-Train-a-Model-to-Generate-Tweets--VmlldzoxMTY5MjI).
## Training data
The model was trained on tweets from #LetLeniLead.
| Data | #LetLeniLead |
| --- | --- |
| Tweets downloaded | 3114 |
| Retweets | 544 |
| Short tweets | 273 |
| Tweets kept | 2297 |
[Explore the data](https://wandb.ai/wandb/huggingtweets/runs/wraydb99/artifacts), which is tracked with [W&B artifacts](https://docs.wandb.com/artifacts) at every step of the pipeline.
## Training procedure
The model is based on a pre-trained [GPT-2](https://huggingface.co/gpt2) which is fine-tuned on @weirdokun's tweets.
Hyperparameters and metrics are recorded in the [W&B training run](https://wandb.ai/wandb/huggingtweets/runs/3lf5g2np) for full transparency and reproducibility.
At the end of training, [the final model](https://wandb.ai/wandb/huggingtweets/runs/3lf5g2np/artifacts) is logged and versioned.
## How to use
You can use this model directly with a pipeline for text generation:
```python
from transformers import pipeline
generator = pipeline('text-generation',
model='huggingtweets/weirdokun')
generator("My dream is", num_return_sequences=5)
```
## Limitations and bias
The model suffers from [the same limitations and bias as GPT-2](https://huggingface.co/gpt2#limitations-and-bias).
In addition, the data present in the user's tweets further affects the text generated by the model.
## About
*Built by Boris Dayma*
[](https://twitter.com/intent/follow?screen_name=borisdayma)
For more details, visit the project repository.
[](https://github.com/borisdayma/huggingtweets)
|
ucl-snlp-group-11/t5-base-separations-cryptic-crosswords | ba8116fe4d8205845d0e13d9cd23a144e50041bd | 2022-04-04T17:24:49.000Z | [
"pytorch",
"t5",
"text2text-generation",
"transformers",
"autotrain_compatible"
] | text2text-generation | false | ucl-snlp-group-11 | null | ucl-snlp-group-11/t5-base-separations-cryptic-crosswords | 0 | null | transformers | 36,687 | Entry not found |
salma-elshafey/opus-mt-ar-en-finetuned-ar-to-en | 5332a0f34064f0a6c9858e1129e3283d74f844ec | 2022-05-20T13:52:33.000Z | [
"pytorch",
"tensorboard",
"marian",
"text2text-generation",
"transformers",
"autotrain_compatible"
] | text2text-generation | false | salma-elshafey | null | salma-elshafey/opus-mt-ar-en-finetuned-ar-to-en | 0 | null | transformers | 36,688 | Entry not found |
ntoldalagi/nick_asr_v2 | d80d7625565ec8c0a9728ae4ed7d65c8289865e4 | 2022-04-14T04:08:47.000Z | [
"pytorch",
"tensorboard",
"wav2vec2",
"automatic-speech-recognition",
"transformers",
"generated_from_trainer",
"model-index"
] | automatic-speech-recognition | false | ntoldalagi | null | ntoldalagi/nick_asr_v2 | 0 | null | transformers | 36,689 | ---
tags:
- generated_from_trainer
model-index:
- name: nick_asr_v2
results: []
---
<!-- This model card has been generated automatically according to the information the Trainer had access to. You
should probably proofread and complete it, then remove this comment. -->
# nick_asr_v2
This model is a fine-tuned version of [ntoldalagi/nick_asr_v2](https://huggingface.co/ntoldalagi/nick_asr_v2) on an unknown dataset.
It achieves the following results on the evaluation set:
- Loss: 1.4562
- Wer: 0.6422
- Cer: 0.2409
## Model description
More information needed
## Intended uses & limitations
More information needed
## Training and evaluation data
More information needed
## Training procedure
### Training hyperparameters
The following hyperparameters were used during training:
- learning_rate: 5e-05
- train_batch_size: 4
- eval_batch_size: 4
- seed: 42
- gradient_accumulation_steps: 4
- total_train_batch_size: 16
- optimizer: Adam with betas=(0.9,0.999) and epsilon=1e-08
- lr_scheduler_type: linear
- num_epochs: 20
- mixed_precision_training: Native AMP
### Training results
| Training Loss | Epoch | Step | Cer | Validation Loss | Wer |
|:-------------:|:-----:|:-----:|:------:|:---------------:|:------:|
| 0.2616 | 0.44 | 300 | 0.2905 | 1.2200 | 0.7496 |
| 0.441 | 0.87 | 600 | 0.2866 | 1.1936 | 0.7385 |
| 0.4366 | 1.31 | 900 | 0.2795 | 1.1584 | 0.7274 |
| 0.3982 | 1.75 | 1200 | 0.2808 | 1.2033 | 0.7274 |
| 0.3891 | 2.18 | 1500 | 0.2753 | 1.2044 | 0.7166 |
| 0.3508 | 2.91 | 2000 | 1.2382 | 0.7220 | 0.2743 |
| 0.2783 | 4.37 | 3000 | 1.3327 | 0.7177 | 0.2705 |
| 0.2495 | 5.82 | 4000 | 1.2286 | 0.6749 | 0.2638 |
| 0.1982 | 7.28 | 5000 | 1.3073 | 0.6721 | 0.2585 |
| 0.1717 | 8.73 | 6000 | 1.2941 | 0.6627 | 0.2500 |
| 0.1508 | 10.19 | 7000 | 1.3625 | 0.6584 | 0.2490 |
| 0.1329 | 11.64 | 8000 | 1.3863 | 0.6584 | 0.2474 |
| 0.1303 | 13.1 | 9000 | 1.3714 | 0.6534 | 0.2449 |
| 0.1159 | 14.56 | 10000 | 1.4043 | 0.6473 | 0.2442 |
| 0.1015 | 16.01 | 11000 | 1.4245 | 0.6498 | 0.2419 |
| 0.098 | 17.47 | 12000 | 1.4410 | 0.6440 | 0.2425 |
| 0.0869 | 18.92 | 13000 | 1.4562 | 0.6422 | 0.2409 |
### Framework versions
- Transformers 4.18.0
- Pytorch 1.10.0+cu111
- Datasets 2.0.0
- Tokenizers 0.12.1
|
jeremykke/bert-base-uncased-finetuned-swag | cc4838418d58996bb421f9fbc5b774e49f5954db | 2022-04-05T15:29:55.000Z | [
"pytorch",
"tensorboard",
"bert",
"multiple-choice",
"dataset:swag",
"transformers",
"generated_from_trainer",
"license:apache-2.0",
"model-index"
] | multiple-choice | false | jeremykke | null | jeremykke/bert-base-uncased-finetuned-swag | 0 | null | transformers | 36,690 | ---
license: apache-2.0
tags:
- generated_from_trainer
datasets:
- swag
metrics:
- accuracy
model-index:
- name: bert-base-uncased-finetuned-swag
results: []
---
<!-- This model card has been generated automatically according to the information the Trainer had access to. You
should probably proofread and complete it, then remove this comment. -->
# bert-base-uncased-finetuned-swag
This model is a fine-tuned version of [bert-base-uncased](https://huggingface.co/bert-base-uncased) on the swag dataset.
It achieves the following results on the evaluation set:
- Loss: 1.0087
- Accuracy: 0.7911
## Model description
More information needed
## Intended uses & limitations
More information needed
## Training and evaluation data
More information needed
## Training procedure
### Training hyperparameters
The following hyperparameters were used during training:
- learning_rate: 5e-05
- train_batch_size: 16
- eval_batch_size: 16
- seed: 42
- optimizer: Adam with betas=(0.9,0.999) and epsilon=1e-08
- lr_scheduler_type: linear
- num_epochs: 3
### Training results
| Training Loss | Epoch | Step | Validation Loss | Accuracy |
|:-------------:|:-----:|:-----:|:---------------:|:--------:|
| 0.7545 | 1.0 | 4597 | 0.5963 | 0.7695 |
| 0.3914 | 2.0 | 9194 | 0.6152 | 0.7879 |
| 0.1385 | 3.0 | 13791 | 1.0087 | 0.7911 |
### Framework versions
- Transformers 4.17.0
- Pytorch 1.10.0+cu111
- Datasets 2.0.0
- Tokenizers 0.11.6
|
johnowhitaker/colorb_gan | ca2dd2bf84f265d20320ac2a05d7b3673fc6a8f5 | 2022-04-05T07:43:07.000Z | [
"pytorch"
] | null | false | johnowhitaker | null | johnowhitaker/colorb_gan | 0 | null | null | 36,691 | A lightweightgan trained briefly on https://huggingface.co/datasets/johnowhitaker/colorbs
See https://huggingface.co/johnowhitaker/orbgan_e1 for training script and so on, since this was basically just copying that and running on a new dataset.
Note: lightweightgan code was updated between training orbgan_e1 and this one, so if you're trying to run the CPU inference notebook you'll get errors. See an updated version running this model on a CPU here: https://colab.research.google.com/drive/16XKJ7XZeSI0rvUf1GU6m9qrmwr1pMRWy?usp=sharing
See demo on spaces here: https://huggingface.co/spaces/huggan/Colorb_GAN |
laboratory/fatima-challenge | c47446382c4fbb58d43acac81177c6606ead0852 | 2022-04-05T19:55:40.000Z | [
"pytorch"
] | null | false | laboratory | null | laboratory/fatima-challenge | 0 | null | null | 36,692 | Entry not found |
akiyamasho/AnimeBackgroundGAN-Shinkai | d162ca947aab5aa943c3586bda550812831d5cf4 | 2022-04-05T17:11:49.000Z | [
"pytorch",
"gan",
"image-to-image",
"license:mit"
] | image-to-image | false | akiyamasho | null | akiyamasho/AnimeBackgroundGAN-Shinkai | 0 | 7 | pytorch | 36,693 | ---
license: mit
library_name: pytorch
tags:
- gan
- image-to-image
---
# AnimeBackgroundGAN (CartoonGAN by Chen et. al.)
<img src="https://m.media-amazon.com/images/M/MV5BZTExN2EwMmYtNDcwZS00ZmI1LTk1NGQtNTQ3YWFjMmY3YjQwXkEyXkFqcGdeQXVyNTU1OTUzNDg@._V1_.jpg" alt="5 Centimetres per Second directed by Makoto Shinkai" style="height: 300px;"/>
- [Makoto Shinkai (新海誠)](https://en.wikipedia.org/wiki/Makoto_Shinkai) pre-trained model from [CartoonGAN](http://openaccess.thecvf.com/content_cvpr_2018/CameraReady/2205.pdf) `[Chen et al., CVPR18]`.
- This model can transform real-life photos into Japanese-animation-like backgrounds, following the style of movies such as [Kimi no Na wa](https://en.wikipedia.org/wiki/Kimi_no_Na_wa) with a photorealistic painting style.
- The implementation is in PyTorch (see [source code here](https://huggingface.co/spaces/akiyamasho/AnimeBackgroundGAN/blob/main/network/Transformer.py)).
- Check out the demo here:
[](https://huggingface.co/spaces/akiyamasho/AnimeBackgroundGAN)
# Other pre-trained model versions
The other versions were also trained from movies of the different Japanese animation directors.
##### Mamoru Hosoda(細田守)
- director of [Wolf Children](https://en.wikipedia.org/wiki/Wolf_Children), with a distinct mild and cool background style
- [Director Profile](https://en.wikipedia.org/wiki/Mamoru_Hosoda)
- **Model Repository**: https://huggingface.co/akiyamasho/AnimeBackgroundGAN-Hosoda
##### Satoshi Kon(今敏)
- director of [Paprika](https://en.wikipedia.org/wiki/Paprika_(2006_film)) with a distinct high contrast, reddish hue style
- [Director Profile](https://en.wikipedia.org/wiki/Satoshi_Kon)
- **Model Repository**: https://huggingface.co/akiyamasho/AnimeBackgroundGAN-Kon
##### Hayao Miyazaki(宮崎駿)
- director of [Howl's Moving Castle](https://en.wikipedia.org/wiki/Howl%27s_Moving_Castle_(film)) with a relatively soft and painterly style
- [Director Profile](https://en.wikipedia.org/wiki/Hayao_Miyazaki)
- **Model Repository**: https://huggingface.co/akiyamasho/AnimeBackgroundGAN-Miyazaki
### Credits
- Paper at [CartoonGAN: Generative Adversarial Networks for Photo Cartoonization](http://openaccess.thecvf.com/content_cvpr_2018/CameraReady/2205.pdf) `[Chen et al., CVPR18]`
- Original PyTorch implementation was created by [Yijun Li](https://github.com/Yijunmaverick/)
- Spaces/Models re-packaging and implementation by [Shō Akiyama](https://github.com/Yijunmaverick/).
##### Special Thanks
- [Nima Boscarino](https://github.com/NimaBoscarino)
- [Omar Sanseviero](https://github.com/osanseviero) |
akiyamasho/AnimeBackgroundGAN-Hosoda | 088b541fd09b113b286fbd032b3ed3a77f5953ca | 2022-04-05T17:11:29.000Z | [
"pytorch",
"gan",
"image-to-image",
"license:mit"
] | image-to-image | false | akiyamasho | null | akiyamasho/AnimeBackgroundGAN-Hosoda | 0 | 1 | pytorch | 36,694 | ---
license: mit
library_name: pytorch
tags:
- gan
- image-to-image
---
# AnimeBackgroundGAN-Hosoda (CartoonGAN by Chen et. al.)
<img src="https://m.media-amazon.com/images/M/MV5BYjgxYjk4OTktZjU3Ni00YzE5LTkyMmItMzI4YzY1YTlhNDg2XkEyXkFqcGdeQXVyNzEyMDQ1MDA@._V1_.jpg" alt="Mirai directed by Mamoru Hosoda" style="height: 300px;"/>
- [Mamoru Hosoda(細田守)](https://en.wikipedia.org/wiki/Mamoru_Hosoda) pre-trained model from [CartoonGAN](http://openaccess.thecvf.com/content_cvpr_2018/CameraReady/2205.pdf) `[Chen et al., CVPR18]`.
- This model can transform real-life photos into Japanese-animation-like backgrounds, following the style of movies such as [Wolf Children](https://en.wikipedia.org/wiki/Wolf_Children), with a distinct mild and cool background style.
- The implementation is in PyTorch (see [source code here](https://huggingface.co/spaces/akiyamasho/AnimeBackgroundGAN/blob/main/network/Transformer.py)).
- Check out the demo here:
[](https://huggingface.co/spaces/akiyamasho/AnimeBackgroundGAN)
# Other pre-trained model versions
The other versions were also trained from movies of the different Japanese animation directors.
##### Makoto Shinkai (新海誠)
- director of [Kimi no Na wa](https://en.wikipedia.org/wiki/Kimi_no_Na_wa) with a photorealistic painting style
- [Director Profile](https://en.wikipedia.org/wiki/Makoto_Shinkai)
- **Model Repository**: https://huggingface.co/akiyamasho/AnimeBackgroundGAN-Shinkai
##### Satoshi Kon(今敏)
- director of [Paprika](https://en.wikipedia.org/wiki/Paprika_(2006_film)) with a distinct high contrast, reddish hue style
- [Director Profile](https://en.wikipedia.org/wiki/Satoshi_Kon)
- **Model Repository**: https://huggingface.co/akiyamasho/AnimeBackgroundGAN-Kon
##### Hayao Miyazaki(宮崎駿)
- director of [Howl's Moving Castle](https://en.wikipedia.org/wiki/Howl%27s_Moving_Castle_(film)) with a relatively soft and painterly style
- [Director Profile](https://en.wikipedia.org/wiki/Hayao_Miyazaki)
- **Model Repository**: https://huggingface.co/akiyamasho/AnimeBackgroundGAN-Miyazaki
### Credits
- Paper at [CartoonGAN: Generative Adversarial Networks for Photo Cartoonization](http://openaccess.thecvf.com/content_cvpr_2018/CameraReady/2205.pdf) `[Chen et al., CVPR18]`
- Original PyTorch implementation was created by [Yijun Li](https://github.com/Yijunmaverick/)
- Spaces/Models re-packaging and implementation by [Shō Akiyama](https://github.com/Yijunmaverick/).
##### Special Thanks
- [Nima Boscarino](https://github.com/NimaBoscarino)
- [Omar Sanseviero](https://github.com/osanseviero) |
akiyamasho/AnimeBackgroundGAN-Miyazaki | c93786c4e4766e43afd2949ca7314ccad61f1d79 | 2022-04-05T17:11:21.000Z | [
"pytorch",
"gan",
"image-to-image",
"license:mit"
] | image-to-image | false | akiyamasho | null | akiyamasho/AnimeBackgroundGAN-Miyazaki | 0 | 1 | pytorch | 36,695 | ---
license: mit
library_name: pytorch
tags:
- gan
- image-to-image
---
# AnimeBackgroundGAN-Miyazaki (CartoonGAN by Chen et. al.)
<img src="https://m.media-amazon.com/images/M/MV5BMTM4MTg2MjAzN15BMl5BanBnXkFtZTcwMTk1NzEyNw@@._V1_.jpg" alt="Howl's Moving Castle directed by Hayao Miyazaki" style="height: 300px;"/>
- [Hayao Miyazaki(宮崎駿)](https://en.wikipedia.org/wiki/Hayao_Miyazaki) pre-trained model from [CartoonGAN](http://openaccess.thecvf.com/content_cvpr_2018/CameraReady/2205.pdf) `[Chen et al., CVPR18]`.
- This model can transform real-life photos into Japanese-animation-like backgrounds, following the style of movies such as [Howl's Moving Castle](https://en.wikipedia.org/wiki/Howl%27s_Moving_Castle_(film)) with a relatively soft and painterly style.
- The implementation is in PyTorch (see [source code here](https://huggingface.co/spaces/akiyamasho/AnimeBackgroundGAN/blob/main/network/Transformer.py)).
- Check out the demo here:
[](https://huggingface.co/spaces/akiyamasho/AnimeBackgroundGAN)
# Other pre-trained model versions
The other versions were also trained from movies of the different Japanese animation directors.
##### Mamoru Hosoda(細田守)
- director of [Wolf Children](https://en.wikipedia.org/wiki/Wolf_Children), with a distinct mild and cool background style
- [Director Profile](https://en.wikipedia.org/wiki/Mamoru_Hosoda)
- **Model Repository**: https://huggingface.co/akiyamasho/AnimeBackgroundGAN-Hosoda
##### Satoshi Kon(今敏)
- director of [Paprika](https://en.wikipedia.org/wiki/Paprika_(2006_film)) with a distinct high contrast, reddish hue style
- [Director Profile](https://en.wikipedia.org/wiki/Satoshi_Kon)
- **Model Repository**: https://huggingface.co/akiyamasho/AnimeBackgroundGAN-Kon
##### Makoto Shinkai (新海誠)
- director of [Kimi no Na wa](https://en.wikipedia.org/wiki/Kimi_no_Na_wa) with a photorealistic painting style
- [Director Profile](https://en.wikipedia.org/wiki/Makoto_Shinkai)
- **Model Repository**: https://huggingface.co/akiyamasho/AnimeBackgroundGAN-Shinkai
### Credits
- Paper at [CartoonGAN: Generative Adversarial Networks for Photo Cartoonization](http://openaccess.thecvf.com/content_cvpr_2018/CameraReady/2205.pdf) `[Chen et al., CVPR18]`
- Original PyTorch implementation was created by [Yijun Li](https://github.com/Yijunmaverick/)
- Spaces/Models re-packaging and implementation by [Shō Akiyama](https://github.com/Yijunmaverick/).
##### Special Thanks
- [Nima Boscarino](https://github.com/NimaBoscarino)
- [Omar Sanseviero](https://github.com/osanseviero) |
akiyamasho/AnimeBackgroundGAN-Kon | 8a701306dfa2e7825132db4c0793522540a4281c | 2022-04-05T17:11:40.000Z | [
"pytorch",
"gan",
"image-to-image",
"license:mit"
] | image-to-image | false | akiyamasho | null | akiyamasho/AnimeBackgroundGAN-Kon | 0 | 1 | pytorch | 36,696 | ---
license: mit
library_name: pytorch
tags:
- gan
- image-to-image
---
# AnimeBackgroundGAN (CartoonGAN by Chen et. al.)
<img src="https://m.media-amazon.com/images/M/MV5BNjNjYTRkNGUtMGQ2MS00MTFiLTg0OTEtYTM3MmM1YTY1OTM1XkEyXkFqcGdeQXVyNjc3OTE4Nzk@._V1_.jpg" alt="Paprika directed by Satoshi Kon" style="height: 300px;"/>
- [Satoshi Kon(今敏)](https://en.wikipedia.org/wiki/Satoshi_Kon) pre-trained model from [CartoonGAN](http://openaccess.thecvf.com/content_cvpr_2018/CameraReady/2205.pdf) `[Chen et al., CVPR18]`.
- This model can transform real-life photos into Japanese-animation-like backgrounds, following the style of movies such as [Paprika](https://en.wikipedia.org/wiki/Paprika_(2006_film)) with a distinct high contrast, reddish hue style.
- The implementation is in PyTorch (see [source code here](https://huggingface.co/spaces/akiyamasho/AnimeBackgroundGAN/blob/main/network/Transformer.py)).
- Check out the demo here:
[](https://huggingface.co/spaces/akiyamasho/AnimeBackgroundGAN)
# Other pre-trained model versions
The other versions were also trained from movies of the different Japanese animation directors.
##### Mamoru Hosoda(細田守)
- director of [Wolf Children](https://en.wikipedia.org/wiki/Wolf_Children), with a distinct mild and cool background style
- [Director Profile](https://en.wikipedia.org/wiki/Mamoru_Hosoda)
- **Model Repository**: https://huggingface.co/akiyamasho/AnimeBackgroundGAN-Hosoda
##### Makoto Shinkai (新海誠)
- director of [Kimi no Na wa](https://en.wikipedia.org/wiki/Kimi_no_Na_wa) with a photorealistic painting style
- [Director Profile](https://en.wikipedia.org/wiki/Makoto_Shinkai)
- **Model Repository**: https://huggingface.co/akiyamasho/AnimeBackgroundGAN-Shinkai
##### Hayao Miyazaki(宮崎駿)
- director of [Howl's Moving Castle](https://en.wikipedia.org/wiki/Howl%27s_Moving_Castle_(film)) with a relatively soft and painterly style
- [Director Profile](https://en.wikipedia.org/wiki/Hayao_Miyazaki)
- **Model Repository**: https://huggingface.co/akiyamasho/AnimeBackgroundGAN-Miyazaki
### Credits
- Paper at [CartoonGAN: Generative Adversarial Networks for Photo Cartoonization](http://openaccess.thecvf.com/content_cvpr_2018/CameraReady/2205.pdf) `[Chen et al., CVPR18]`
- Original PyTorch implementation was created by [Yijun Li](https://github.com/Yijunmaverick/)
- Spaces/Models re-packaging and implementation by [Shō Akiyama](https://github.com/Yijunmaverick/).
##### Special Thanks
- [Nima Boscarino](https://github.com/NimaBoscarino)
- [Omar Sanseviero](https://github.com/osanseviero) |
robvanderg/bert-base-multilingual-cased-segment1 | 2e54511d2c080c4e843556c16e6e303b0db8b4db | 2022-04-05T12:39:54.000Z | [
"pytorch",
"bert",
"feature-extraction",
"multilingual",
"dataset:Wikipedia",
"transformers",
"hack"
] | feature-extraction | false | robvanderg | null | robvanderg/bert-base-multilingual-cased-segment1 | 0 | null | transformers | 36,697 | ---
language:
- multilingual
tags:
- hack
datasets:
- Wikipedia
---
## bert-base-multilingual-cased-segment1
This is a version of multilingual bert (bert-base-multilingual-cased), where the segment embedding of the 1's is copied into the 0's. Yes, that's all there is to it. We have found that this improves performance substantially in low-resource setups for word-level tasks (e.g. average 2.5 LAS on a variety of UD treebanks). More details are to be released in our LREC2022 paper titled: Frustratingly Easy Performance Improvements for Cross-lingual Transfer: A Tale on BERT and Segment Embeddings.
These embeddings are generated by the following code
```
import AutoModel
baseEmbeddings = AutoModel.from_pretrained("bert-base-multilingual-cased")
tte = baseEmbeddings.embeddings.token_type_embeddings.weight.clone().detach()
baseEmbeddings.embeddings.token_type_embeddings.weight[0,:] = tte[1,:]
```
More details and other varieties can be found in the repo: https://bitbucket.org/robvanderg/segmentembeds/
Note that when using this model on a single sentence task (or word-level task), the results would be similar as just using `token_type_id=1` for all tokens. |
gulgulglut/DialoGPT-small-Rick | 65ab139692517c9413e1f5bf96ffef0b6528bbdc | 2022-04-05T14:09:04.000Z | [
"pytorch",
"gpt2",
"text-generation",
"transformers",
"conversational"
] | conversational | false | gulgulglut | null | gulgulglut/DialoGPT-small-Rick | 0 | null | transformers | 36,698 | ---
tags:
- conversational
---
# Harry Potter DialoGPT Model |
rowan1224/electra-slp | 211ab5e7c2370defefb8edd0b9b5158c151fd599 | 2022-04-05T16:39:43.000Z | [
"pytorch",
"electra",
"question-answering",
"transformers",
"license:mit",
"autotrain_compatible"
] | question-answering | false | rowan1224 | null | rowan1224/electra-slp | 0 | null | transformers | 36,699 | ---
license: mit
---
|
Subsets and Splits
No community queries yet
The top public SQL queries from the community will appear here once available.