modelId
stringlengths 4
112
| sha
stringlengths 40
40
| lastModified
stringlengths 24
24
| tags
sequence | pipeline_tag
stringclasses 29
values | private
bool 1
class | author
stringlengths 2
38
⌀ | config
null | id
stringlengths 4
112
| downloads
float64 0
36.8M
⌀ | likes
float64 0
712
⌀ | library_name
stringclasses 17
values | __index_level_0__
int64 0
38.5k
| readme
stringlengths 0
186k
|
---|---|---|---|---|---|---|---|---|---|---|---|---|---|
sahilnare78/DialogGPT-medium-harrypotter | 59097ecf36f8cf0f54a56c64731b2d689cb3c16c | 2022-04-17T15:16:23.000Z | [
"pytorch",
"gpt2",
"text-generation",
"transformers",
"conversational"
] | conversational | false | sahilnare78 | null | sahilnare78/DialogGPT-medium-harrypotter | 0 | null | transformers | 36,900 | ---
tags:
- conversational
---
# Harry Potter DialoGPT Model |
huggan/fastgan-few-shot-anime-face | 1215d6bb570f1e96962f24cb50f1398a62e12ff7 | 2022-05-06T22:30:17.000Z | [
"pytorch",
"dataset:huggan/few-shot-anime-face",
"arxiv:2101.04775",
"huggan",
"gan",
"unconditional-image-generation",
"license:mit"
] | unconditional-image-generation | false | huggan | null | huggan/fastgan-few-shot-anime-face | 0 | null | null | 36,901 | ---
tags:
- huggan
- gan
- unconditional-image-generation
datasets:
- huggan/few-shot-anime-face
# See a list of available tags here:
# https://github.com/huggingface/hub-docs/blob/main/js/src/lib/interfaces/Types.ts#L12
# task: unconditional-image-generation or conditional-image-generation or image-to-image
license: mit
---
# Generate anime face image using FastGAN
## Model description
[FastGAN model](https://arxiv.org/abs/2101.04775) is a Generative Adversarial Networks (GAN) training on a small amount of high-fidelity images with minimum computing cost. Using a skip-layer channel-wise excitation module and a self-supervised discriminator trained as a feature-encoder, the model was able to converge after some hours of training for either 100 high-quality images or 1000 images datasets.
This model was trained on a dataset of 120 high-quality Anime face images.
#### How to use
```python
# Clone this model
git clone https://huggingface.co/huggan/fastgan-few-shot-anime-face
def load_generator(model_name_or_path):
generator = Generator(in_channels=256, out_channels=3)
generator = generator.from_pretrained(model_name_or_path, in_channels=256, out_channels=3)
_ = generator.eval()
return generator
def _denormalize(input: torch.Tensor) -> torch.Tensor:
return (input * 127.5) + 127.5
# Load generator
generator = load_generator("huggan/fastgan-few-shot-anime-face")
# Generate a random noise image
noise = torch.zeros(1, 256, 1, 1, device=device).normal_(0.0, 1.0)
with torch.no_grad():
gan_images, _ = generator(noise)
gan_images = _denormalize(gan_images.detach())
save_image(gan_images, "sample.png", nrow=1, normalize=True)
```
#### Limitations and bias
* Converge faster and better with small datasets (less than 1000 samples)
## Training data
[few-shot-anime-face](https://huggingface.co/datasets/huggan/few-shot-anime-face)
## Generated Images

### BibTeX entry and citation info
```bibtex
@article{FastGAN,
title={Towards Faster and Stabilized GAN Training for High-fidelity Few-shot Image Synthesis},
author={Bingchen Liu, Yizhe Zhu, Kunpeng Song, Ahmed Elgammal},
journal={ICLR},
year={2021}
}
``` |
Chris1/sim2real-512 | 3aab62fd71738cd49907afe7dfedde9f4cb6636c | 2022-04-17T20:04:22.000Z | [
"pytorch",
"huggan",
"gan",
"license:mit"
] | null | false | Chris1 | null | Chris1/sim2real-512 | 0 | null | null | 36,902 | ---
tags:
- huggan
- gan
# See a list of available tags here:
# https://github.com/huggingface/hub-docs/blob/main/js/src/lib/interfaces/Types.ts#L12
# task: unconditional-image-generation or conditional-image-generation or image-to-image
license: mit
---
# MyModelName
## Model description
Describe the model here (what it does, what it's used for, etc.)
## Intended uses & limitations
#### How to use
```python
# You can include sample code which will be formatted
```
#### Limitations and bias
Provide examples of latent issues and potential remediations.
## Training data
Describe the data you used to train the model.
If you initialized it with pre-trained weights, add a link to the pre-trained model card or repository with description of the pre-training data.
## Training procedure
Preprocessing, hardware used, hyperparameters...
## Eval results
## Generated Images
You can embed local or remote images using ``
### BibTeX entry and citation info
```bibtex
@inproceedings{...,
year={2020}
}
``` |
Chris1/real2sim-512 | 5d11a48eecc16e4c03adeed7942229e5242ed18d | 2022-04-17T20:06:01.000Z | [
"pytorch",
"huggan",
"gan",
"license:mit"
] | null | false | Chris1 | null | Chris1/real2sim-512 | 0 | null | null | 36,903 | ---
tags:
- huggan
- gan
# See a list of available tags here:
# https://github.com/huggingface/hub-docs/blob/main/js/src/lib/interfaces/Types.ts#L12
# task: unconditional-image-generation or conditional-image-generation or image-to-image
license: mit
---
# MyModelName
## Model description
Describe the model here (what it does, what it's used for, etc.)
## Intended uses & limitations
#### How to use
```python
# You can include sample code which will be formatted
```
#### Limitations and bias
Provide examples of latent issues and potential remediations.
## Training data
Describe the data you used to train the model.
If you initialized it with pre-trained weights, add a link to the pre-trained model card or repository with description of the pre-training data.
## Training procedure
Preprocessing, hardware used, hyperparameters...
## Eval results
## Generated Images
You can embed local or remote images using ``
### BibTeX entry and citation info
```bibtex
@inproceedings{...,
year={2020}
}
``` |
huggan/fastgan-few-shot-moongate | 7ca7777f156d6f971bd5ec84a575dd9314256f02 | 2022-05-06T22:33:11.000Z | [
"pytorch",
"dataset:huggan/few-shot-moongate",
"arxiv:2101.04775",
"huggan",
"gan",
"unconditional-image-generation",
"license:mit"
] | unconditional-image-generation | false | huggan | null | huggan/fastgan-few-shot-moongate | 0 | null | null | 36,904 | ---
tags:
- huggan
- gan
- unconditional-image-generation
datasets:
- huggan/few-shot-moongate
# See a list of available tags here:
# https://github.com/huggingface/hub-docs/blob/main/js/src/lib/interfaces/Types.ts#L12
# task: unconditional-image-generation or conditional-image-generation or image-to-image
license: mit
---
# Generate moon gate image using FastGAN
## Model description
[FastGAN model](https://arxiv.org/abs/2101.04775) is a Generative Adversarial Networks (GAN) training on a small amount of high-fidelity images with minimum computing cost. Using a skip-layer channel-wise excitation module and a self-supervised discriminator trained as a feature-encoder, the model was able to converge after some hours of training for either 100 high-quality images or 1000 images datasets.
This model was trained on a dataset of 136 high-quality moon gate images.
#### How to use
```python
# Clone this model
git clone https://huggingface.co/huggan/fastgan-few-shot-moongate
def load_generator(model_name_or_path):
generator = Generator(in_channels=256, out_channels=3)
generator = generator.from_pretrained(model_name_or_path, in_channels=256, out_channels=3)
_ = generator.eval()
return generator
def _denormalize(input: torch.Tensor) -> torch.Tensor:
return (input * 127.5) + 127.5
# Load generator
generator = load_generator("huggan/fastgan-few-shot-moongate")
# Generate a random noise image
noise = torch.zeros(1, 256, 1, 1, device=device).normal_(0.0, 1.0)
with torch.no_grad():
gan_images, _ = generator(noise)
gan_images = _denormalize(gan_images.detach())
save_image(gan_images, "sample.png", nrow=1, normalize=True)
```
#### Limitations and bias
* Converge faster and better with small datasets (less than 1000 samples)
## Training data
[few-shot-moongate](huggan/few-shot-moongate)
## Generated Images

### BibTeX entry and citation info
```bibtex
@article{FastGAN,
title={Towards Faster and Stabilized GAN Training for High-fidelity Few-shot Image Synthesis},
author={Bingchen Liu, Yizhe Zhu, Kunpeng Song, Ahmed Elgammal},
journal={ICLR},
year={2021}
}
``` |
huggingnft/boredapeyachtclub__2__mutant-ape-yacht-club | 77ecc4b6a9c54504f1c6cde38c67201fc9495288 | 2022-04-25T16:05:54.000Z | [
"pytorch",
"arxiv:1703.10593",
"huggan",
"gan",
"image-to-image",
"huggingnft",
"nft",
"image",
"images",
"license:mit"
] | image-to-image | false | huggingnft | null | huggingnft/boredapeyachtclub__2__mutant-ape-yacht-club | 0 | 1 | null | 36,905 | ---
tags:
- huggan
- gan
- image-to-image
- huggingnft
- nft
- image
- images
# See a list of available tags here:
# https://github.com/huggingface/hub-docs/blob/main/js/src/lib/interfaces/Types.ts#L12
# task: unconditional-image-generation or conditional-image-generation or image-to-image
license: mit
---
# CycleGAN for unpaired image-to-image translation.
## Model description
CycleGAN for unpaired image-to-image translation.
Given two image domains A and B, the following components are trained end2end to translate between such domains:
- A generator A to B, named G_AB conditioned on an image from A
- A generator B to A, named G_BA conditioned on an image from B
- A domain classifier D_A, associated with G_AB
- A domain classifier D_B, associated with G_BA
At inference time, G_AB or G_BA are relevant to translate images, respectively A to B or B to A.
In the general setting, this technique provides style transfer functionalities between the selected image domains A and B.
This allows to obtain a generated translation by G_AB, of an image from domain A that resembles the distribution of the images from domain B, and viceversa for the generator G_BA.
Under these framework, these aspects have been used to perform style transfer between NFT collections.
A collection is selected as domain A, another one as domain B and the CycleGAN provides forward and backward translation between A and B.
This has showed to allows high quality translation even in absence of paired sample-ground-truth data.
In particular, the model performs well with stationary backgrounds (no drastic texture changes in the appearance of backgrounds) as it is capable of recognizing the attributes of each of the elements of an NFT collections.
An attribute can be a variation in type of dressed fashion items such as sunglasses, earrings, clothes and also face or body attributes with respect to a common template model of the given NFT collection).
## Intended uses & limitations
#### How to use
```python
import torch
from PIL import Image
from huggan.pytorch.cyclegan.modeling_cyclegan import GeneratorResNet
from torchvision import transforms as T
from torchvision.transforms import Compose, Resize, ToTensor, Normalize
from torchvision.utils import make_grid
from huggingface_hub import hf_hub_download, file_download
from accelerate import Accelerator
import json
def load_lightweight_model(model_name):
file_path = file_download.hf_hub_download(
repo_id=model_name,
filename="config.json"
)
config = json.loads(open(file_path).read())
organization_name, name = model_name.split("/")
model = Trainer(**config, organization_name=organization_name, name=name)
model.load(use_cpu=True)
model.accelerator = Accelerator()
return model
def get_concat_h(im1, im2):
dst = Image.new('RGB', (im1.width + im2.width, im1.height))
dst.paste(im1, (0, 0))
dst.paste(im2, (im1.width, 0))
return dst
n_channels = 3
image_size = 256
input_shape = (image_size, image_size)
transform = Compose([
T.ToPILImage(),
T.Resize(input_shape),
ToTensor(),
Normalize((0.5, 0.5, 0.5), (0.5, 0.5, 0.5)),
])
# load the translation model from source to target images: source will be generated by a separate Lightweight GAN, w
# while the target images are the result of the translation applied by the GeneratorResnet to the generated source images.
# Hence, given the source domain A and target domain B,
# B = Translator(GAN(A))
translator = GeneratorResNet.from_pretrained(f'huggingnft/{model_name}',
input_shape=(n_channels, image_size, image_size),
num_residual_blocks=9)
# sample noise that is used to generate source images by the
z = torch.randn(nrows, 100, 1, 1)
# load the GAN generator of source images that will be translated by the translation model
model = load_lightweight_model(f"huggingnft/{model_name.split('__2__')[0]}")
collectionA = model.generate_app(
num=timestamped_filename(),
nrow=nrows,
checkpoint=-1,
types="default"
)[1]
# resize to translator model input shape
resize = T.Resize((256, 256))
input = resize(collectionA)
# translate the resized collectionA to collectionB
collectionB = translator(input)
out_transform = T.ToPILImage()
results = []
for collA_image, collB_image in zip(input, collectionB):
results.append(
get_concat_h(out_transform(make_grid(collA_image, nrow=1, normalize=True)), out_transform(make_grid(collB_image, nrow=1, normalize=True)))
)
```
#### Limitations and bias
Translation between collections provides exceptional output images in the case of NFT collections that portray subjects in the same way.
If the backgrounds vary too much within either of the collections, performance degrades or many more training iterations re required to achieve acceptable results.
## Training data
The CycleGAN model is trained on an unpaired dataset of samples from two selected NFT collections: colle tionA and collectionB.
To this end, two collections are loaded by means of the function load_dataset in the huggingface library, as follows.
A list of all available collections is available at [huggingNFT](https://huggingface.co/huggingnft)
```python
from datasets import load_dataset
collectionA = load_dataset("huggingnft/COLLECTION_A")
collectionB = load_dataset("huggingnft/COLLECTION_B")
```
## Training procedure
#### Preprocessing
The following transformations are applied to each input sample of collectionA and collectionB.
The input size is fixed to RGB images of height, width = 256, 256
```python
n_channels = 3
image_size = 256
input_shape = (image_size, image_size)
transform = Compose([
T.ToPILImage(),
T.Resize(input_shape),
ToTensor(),
Normalize((0.5, 0.5, 0.5), (0.5, 0.5, 0.5)),
])
```
#### Hardware
The configuration has been tested on single GPU setup on a RTX5000 and A5000, as well as multi-gpu single-rank distributed setups composed of 2 of the mentioned GPUs.
#### Hyperparameters
The following configuration has been kept fixed for all translation models:
- learning rate 0.0002
- number of epochs 200
- learning rate decay activation at epoch 80
- number of residual blocks of the cyclegan 9
- cycle loss weight 10.0
- identity loss weight 5.0
- optimizer ADAM with beta1 0.5 and beta2 0.999
- batch size 8
- NO mixed precision training
## Eval results
#### Training reports
[Cryptopunks to boreapeyachtclub](https://wandb.ai/chris1nexus/experiments--experiments_cyclegan_punk_to_apes_HQ--0/reports/CycleGAN-training-report--VmlldzoxODUxNzQz?accessToken=vueurpbhd2i8n347j880yakggs0sqdf7u0hpz3bpfsbrxcmk1jk4obg18f6wfk9w)
[Boreapeyachtclub to mutant-ape-yacht-club](https://wandb.ai/chris1nexus/experiments--my_paperspace_boredapeyachtclub__2__mutant-ape-yacht-club--11/reports/CycleGAN-training-report--VmlldzoxODUxNzg4?accessToken=jpyviwn7kdf5216ycrthwp6l8t3heb0lt8djt7dz12guu64qnpdh3ekecfcnoahu)
#### Generated Images
In the provided images, row0 and row2 represent real images from the respective collections.
Row1 is the translation of the immediate above images in row0 by means of the G_AB translation model.
Row3 is the translation of the immediate above images in row2 by means of the G_BA translation model.
Visualization over the training iterations for [boreapeyachtclub to mutant-ape-yacht-club](https://wandb.ai/chris1nexus/experiments--my_paperspace_boredapeyachtclub__2__mutant-ape-yacht-club--11/reports/Shared-panel-22-04-15-08-04-99--VmlldzoxODQ0MDI3?accessToken=45m3kxex5m3rpev3s6vmrv69k3u9p9uxcsp2k90wvbxwxzlqbqjqlnmgpl9265c0)
Visualization over the training iterations for [Cryptopunks to boreapeyachtclub](https://wandb.ai/chris1nexus/experiments--experiments_cyclegan_punk_to_apes_HQ--0/reports/Shared-panel-22-04-17-11-04-83--VmlldzoxODUxNjk5?accessToken=o25si6nflp2xst649vt6ayt56bnb95mxmngt1ieso091j2oazmqnwaf4h78vc2tu)
### References
```bibtex
@misc{https://doi.org/10.48550/arxiv.1703.10593,
doi = {10.48550/ARXIV.1703.10593},
url = {https://arxiv.org/abs/1703.10593},
author = {Zhu, Jun-Yan and Park, Taesung and Isola, Phillip and Efros, Alexei A.},
keywords = {Computer Vision and Pattern Recognition (cs.CV), FOS: Computer and information sciences, FOS: Computer and information sciences},
title = {Unpaired Image-to-Image Translation using Cycle-Consistent Adversarial Networks},
publisher = {arXiv},
year = {2017},
copyright = {arXiv.org perpetual, non-exclusive license}
}
```
### BibTeX entry and citation info
```bibtex
@InProceedings{huggingnft,
author={Aleksey Korshuk, Christian Cancedda}
year=2022
}
```
|
Chris1/mutant-ape-yacht-club__2__boredapeyachtclub | 092bb2ed251d812571654ed5267c417fefe99a2b | 2022-04-15T12:18:15.000Z | [
"pytorch",
"huggan",
"gan",
"license:mit"
] | null | false | Chris1 | null | Chris1/mutant-ape-yacht-club__2__boredapeyachtclub | 0 | null | null | 36,906 | ---
tags:
- huggan
- gan
# See a list of available tags here:
# https://github.com/huggingface/hub-docs/blob/main/js/src/lib/interfaces/Types.ts#L12
# task: unconditional-image-generation or conditional-image-generation or image-to-image
license: mit
---
# MyModelName
## Model description
Describe the model here (what it does, what it's used for, etc.)
## Intended uses & limitations
#### How to use
```python
# You can include sample code which will be formatted
```
#### Limitations and bias
Provide examples of latent issues and potential remediations.
## Training data
Describe the data you used to train the model.
If you initialized it with pre-trained weights, add a link to the pre-trained model card or repository with description of the pre-training data.
## Training procedure
Preprocessing, hardware used, hyperparameters...
## Eval results
## Generated Images
You can embed local or remote images using ``
### BibTeX entry and citation info
```bibtex
@inproceedings{...,
year={2020}
}
``` |
huggingnft/mini-mutants__2__boredapeyachtclub | bffc2c655803a3e0f089cee15b6c0bc2859a4181 | 2022-04-25T16:05:55.000Z | [
"pytorch",
"arxiv:1703.10593",
"huggan",
"gan",
"image-to-image",
"huggingnft",
"nft",
"image",
"images",
"license:mit"
] | image-to-image | false | huggingnft | null | huggingnft/mini-mutants__2__boredapeyachtclub | 0 | 1 | null | 36,907 | ---
tags:
- huggan
- gan
- image-to-image
- huggingnft
- nft
- image
- images
# See a list of available tags here:
# https://github.com/huggingface/hub-docs/blob/main/js/src/lib/interfaces/Types.ts#L12
# task: unconditional-image-generation or conditional-image-generation or image-to-image
license: mit
---
# CycleGAN for unpaired image-to-image translation.
## Model description
CycleGAN for unpaired image-to-image translation.
Given two image domains A and B, the following components are trained end2end to translate between such domains:
- A generator A to B, named G_AB conditioned on an image from A
- A generator B to A, named G_BA conditioned on an image from B
- A domain classifier D_A, associated with G_AB
- A domain classifier D_B, associated with G_BA
At inference time, G_AB or G_BA are relevant to translate images, respectively A to B or B to A.
In the general setting, this technique provides style transfer functionalities between the selected image domains A and B.
This allows to obtain a generated translation by G_AB, of an image from domain A that resembles the distribution of the images from domain B, and viceversa for the generator G_BA.
Under these framework, these aspects have been used to perform style transfer between NFT collections.
A collection is selected as domain A, another one as domain B and the CycleGAN provides forward and backward translation between A and B.
This has showed to allows high quality translation even in absence of paired sample-ground-truth data.
In particular, the model performs well with stationary backgrounds (no drastic texture changes in the appearance of backgrounds) as it is capable of recognizing the attributes of each of the elements of an NFT collections.
An attribute can be a variation in type of dressed fashion items such as sunglasses, earrings, clothes and also face or body attributes with respect to a common template model of the given NFT collection).
## Intended uses & limitations
#### How to use
```python
import torch
from PIL import Image
from huggan.pytorch.cyclegan.modeling_cyclegan import GeneratorResNet
from torchvision import transforms as T
from torchvision.transforms import Compose, Resize, ToTensor, Normalize
from torchvision.utils import make_grid
from huggingface_hub import hf_hub_download, file_download
from accelerate import Accelerator
import json
def load_lightweight_model(model_name):
file_path = file_download.hf_hub_download(
repo_id=model_name,
filename="config.json"
)
config = json.loads(open(file_path).read())
organization_name, name = model_name.split("/")
model = Trainer(**config, organization_name=organization_name, name=name)
model.load(use_cpu=True)
model.accelerator = Accelerator()
return model
def get_concat_h(im1, im2):
dst = Image.new('RGB', (im1.width + im2.width, im1.height))
dst.paste(im1, (0, 0))
dst.paste(im2, (im1.width, 0))
return dst
n_channels = 3
image_size = 256
input_shape = (image_size, image_size)
transform = Compose([
T.ToPILImage(),
T.Resize(input_shape),
ToTensor(),
Normalize((0.5, 0.5, 0.5), (0.5, 0.5, 0.5)),
])
# load the translation model from source to target images: source will be generated by a separate Lightweight GAN, w
# while the target images are the result of the translation applied by the GeneratorResnet to the generated source images.
# Hence, given the source domain A and target domain B,
# B = Translator(GAN(A))
translator = GeneratorResNet.from_pretrained(f'huggingnft/{model_name}',
input_shape=(n_channels, image_size, image_size),
num_residual_blocks=9)
# sample noise that is used to generate source images by the
z = torch.randn(nrows, 100, 1, 1)
# load the GAN generator of source images that will be translated by the translation model
model = load_lightweight_model(f"huggingnft/{model_name.split('__2__')[0]}")
collectionA = model.generate_app(
num=timestamped_filename(),
nrow=nrows,
checkpoint=-1,
types="default"
)[1]
# resize to translator model input shape
resize = T.Resize((256, 256))
input = resize(collectionA)
# translate the resized collectionA to collectionB
collectionB = translator(input)
out_transform = T.ToPILImage()
results = []
for collA_image, collB_image in zip(input, collectionB):
results.append(
get_concat_h(out_transform(make_grid(collA_image, nrow=1, normalize=True)), out_transform(make_grid(collB_image, nrow=1, normalize=True)))
)
```
#### Limitations and bias
Translation between collections provides exceptional output images in the case of NFT collections that portray subjects in the same way.
If the backgrounds vary too much within either of the collections, performance degrades or many more training iterations re required to achieve acceptable results.
## Training data
The CycleGAN model is trained on an unpaired dataset of samples from two selected NFT collections: colle tionA and collectionB.
To this end, two collections are loaded by means of the function load_dataset in the huggingface library, as follows.
A list of all available collections is available at [huggingNFT](https://huggingface.co/huggingnft)
```python
from datasets import load_dataset
collectionA = load_dataset("huggingnft/COLLECTION_A")
collectionB = load_dataset("huggingnft/COLLECTION_B")
```
## Training procedure
#### Preprocessing
The following transformations are applied to each input sample of collectionA and collectionB.
The input size is fixed to RGB images of height, width = 256, 256
```python
n_channels = 3
image_size = 256
input_shape = (image_size, image_size)
transform = Compose([
T.ToPILImage(),
T.Resize(input_shape),
ToTensor(),
Normalize((0.5, 0.5, 0.5), (0.5, 0.5, 0.5)),
])
```
#### Hardware
The configuration has been tested on single GPU setup on a RTX5000 and A5000, as well as multi-gpu single-rank distributed setups composed of 2 of the mentioned GPUs.
#### Hyperparameters
The following configuration has been kept fixed for all translation models:
- learning rate 0.0002
- number of epochs 200
- learning rate decay activation at epoch 80
- number of residual blocks of the cyclegan 9
- cycle loss weight 10.0
- identity loss weight 5.0
- optimizer ADAM with beta1 0.5 and beta2 0.999
- batch size 8
- NO mixed precision training
## Eval results
#### Training reports
[Cryptopunks to boreapeyachtclub](https://wandb.ai/chris1nexus/experiments--experiments_cyclegan_punk_to_apes_HQ--0/reports/CycleGAN-training-report--VmlldzoxODUxNzQz?accessToken=vueurpbhd2i8n347j880yakggs0sqdf7u0hpz3bpfsbrxcmk1jk4obg18f6wfk9w)
[Boreapeyachtclub to mutant-ape-yacht-club](https://wandb.ai/chris1nexus/experiments--my_paperspace_boredapeyachtclub__2__mutant-ape-yacht-club--11/reports/CycleGAN-training-report--VmlldzoxODUxNzg4?accessToken=jpyviwn7kdf5216ycrthwp6l8t3heb0lt8djt7dz12guu64qnpdh3ekecfcnoahu)
#### Generated Images
In the provided images, row0 and row2 represent real images from the respective collections.
Row1 is the translation of the immediate above images in row0 by means of the G_AB translation model.
Row3 is the translation of the immediate above images in row2 by means of the G_BA translation model.
Visualization over the training iterations for [boreapeyachtclub to mutant-ape-yacht-club](https://wandb.ai/chris1nexus/experiments--my_paperspace_boredapeyachtclub__2__mutant-ape-yacht-club--11/reports/Shared-panel-22-04-15-08-04-99--VmlldzoxODQ0MDI3?accessToken=45m3kxex5m3rpev3s6vmrv69k3u9p9uxcsp2k90wvbxwxzlqbqjqlnmgpl9265c0)
Visualization over the training iterations for [Cryptopunks to boreapeyachtclub](https://wandb.ai/chris1nexus/experiments--experiments_cyclegan_punk_to_apes_HQ--0/reports/Shared-panel-22-04-17-11-04-83--VmlldzoxODUxNjk5?accessToken=o25si6nflp2xst649vt6ayt56bnb95mxmngt1ieso091j2oazmqnwaf4h78vc2tu)
### References
```bibtex
@misc{https://doi.org/10.48550/arxiv.1703.10593,
doi = {10.48550/ARXIV.1703.10593},
url = {https://arxiv.org/abs/1703.10593},
author = {Zhu, Jun-Yan and Park, Taesung and Isola, Phillip and Efros, Alexei A.},
keywords = {Computer Vision and Pattern Recognition (cs.CV), FOS: Computer and information sciences, FOS: Computer and information sciences},
title = {Unpaired Image-to-Image Translation using Cycle-Consistent Adversarial Networks},
publisher = {arXiv},
year = {2017},
copyright = {arXiv.org perpetual, non-exclusive license}
}
```
### BibTeX entry and citation info
```bibtex
@InProceedings{huggingnft,
author={Aleksey Korshuk, Christian Cancedda}
year=2022
}
```
|
scasutt/wav2vec2-large-xlsr-53_train_data_full | a84849805136033bdc7825a239f41ef280e0332a | 2022-04-16T11:57:44.000Z | [
"pytorch",
"wav2vec2",
"automatic-speech-recognition",
"transformers",
"generated_from_trainer",
"license:apache-2.0",
"model-index"
] | automatic-speech-recognition | false | scasutt | null | scasutt/wav2vec2-large-xlsr-53_train_data_full | 0 | null | transformers | 36,908 | ---
license: apache-2.0
tags:
- generated_from_trainer
model-index:
- name: wav2vec2-large-xlsr-53_train_data_full
results: []
---
<!-- This model card has been generated automatically according to the information the Trainer had access to. You
should probably proofread and complete it, then remove this comment. -->
# wav2vec2-large-xlsr-53_train_data_full
This model is a fine-tuned version of [facebook/wav2vec2-large-xlsr-53](https://huggingface.co/facebook/wav2vec2-large-xlsr-53) on the None dataset.
It achieves the following results on the evaluation set:
- Loss: 0.4168
- Wer: 0.3383
## Model description
More information needed
## Intended uses & limitations
More information needed
## Training and evaluation data
More information needed
## Training procedure
### Training hyperparameters
The following hyperparameters were used during training:
- learning_rate: 0.0001
- train_batch_size: 16
- eval_batch_size: 8
- seed: 42
- gradient_accumulation_steps: 2
- total_train_batch_size: 32
- optimizer: Adam with betas=(0.9,0.999) and epsilon=1e-08
- lr_scheduler_type: linear
- lr_scheduler_warmup_steps: 1000
- num_epochs: 20
### Training results
| Training Loss | Epoch | Step | Validation Loss | Wer |
|:-------------:|:-----:|:-----:|:---------------:|:------:|
| 3.0459 | 0.73 | 500 | 3.2037 | 0.9995 |
| 0.7938 | 1.45 | 1000 | 0.7432 | 0.6373 |
| 0.503 | 2.18 | 1500 | 0.5517 | 0.5115 |
| 0.4475 | 2.91 | 2000 | 0.4916 | 0.4624 |
| 0.3575 | 3.63 | 2500 | 0.4612 | 0.4362 |
| 0.3206 | 4.36 | 3000 | 0.4546 | 0.4198 |
| 0.3155 | 5.09 | 3500 | 0.4073 | 0.3929 |
| 0.2827 | 5.81 | 4000 | 0.4172 | 0.3808 |
| 0.2575 | 6.54 | 4500 | 0.4183 | 0.3741 |
| 0.2399 | 7.27 | 5000 | 0.4181 | 0.3680 |
| 0.2455 | 7.99 | 5500 | 0.3981 | 0.3604 |
| 0.2512 | 8.72 | 6000 | 0.4203 | 0.3612 |
| 0.221 | 9.45 | 6500 | 0.4073 | 0.3560 |
| 0.19 | 10.17 | 7000 | 0.4206 | 0.3547 |
| 0.207 | 10.9 | 7500 | 0.3992 | 0.3517 |
| 0.187 | 11.63 | 8000 | 0.4078 | 0.3517 |
| 0.2029 | 12.35 | 8500 | 0.4143 | 0.3469 |
| 0.171 | 13.08 | 9000 | 0.4007 | 0.3430 |
| 0.1658 | 13.81 | 9500 | 0.3862 | 0.3422 |
| 0.2021 | 14.53 | 10000 | 0.4132 | 0.3454 |
| 0.165 | 15.26 | 10500 | 0.3997 | 0.3407 |
| 0.1562 | 15.99 | 11000 | 0.4069 | 0.3416 |
| 0.1613 | 16.71 | 11500 | 0.4040 | 0.3393 |
| 0.1713 | 17.44 | 12000 | 0.4094 | 0.3411 |
| 0.1541 | 18.17 | 12500 | 0.4043 | 0.3367 |
| 0.144 | 18.89 | 13000 | 0.4086 | 0.3374 |
| 0.1483 | 19.62 | 13500 | 0.4168 | 0.3383 |
### Framework versions
- Transformers 4.18.0
- Pytorch 1.11.0+cu102
- Datasets 2.1.0
- Tokenizers 0.12.1
|
ChainYo/DocuGAN | 2113ab02792ac730c7352a45f411e48276b7ef56 | 2022-04-27T08:40:51.000Z | [
"dataset:ChainYo/rvl-cdip-invoice",
"pytorch",
"gan",
"sngan",
"huggan",
"unconditional-image-generation",
"license:mit"
] | unconditional-image-generation | false | ChainYo | null | ChainYo/DocuGAN | 0 | 1 | pytorch | 36,909 | ---
license: mit
library_name: pytorch
tags:
- gan
- sngan
- huggan
- unconditional-image-generation
datasets:
- ChainYo/rvl-cdip-invoice
---
## Model description
SN-GAN implementation with PyTorch-Lightning to generate Documents.
## Generated samples
<img src="https://raw.githubusercontent.com/ChainYo/docugan/master/documents_samples.png" width="400" height="1200">
Project repository: [DocuGAN](https://github.com/ChainYo/docugan).
## Usage
You can see the tool to generate document on HuggingFace by trying the [space demo](https://huggingface.co/spaces/ChainYo/DocuGAN).
## Training data
For training, I used the invoices subpart of `RVL-CDIP` dataset. Find the full dataset [here](https://huggingface.co/datasets/ChainYo/rvl-cdip)
|
huggan/fastgan-few-shot-universe | 803e4d48ccece45a699d04bed973fdca992e3e40 | 2022-05-06T22:32:26.000Z | [
"pytorch",
"dataset:huggan/few-shot-universe",
"arxiv:2101.04775",
"huggan",
"gan",
"unconditional-image-generation",
"license:mit"
] | unconditional-image-generation | false | huggan | null | huggan/fastgan-few-shot-universe | 0 | null | null | 36,910 | ---
tags:
- huggan
- gan
- unconditional-image-generation
datasets:
- huggan/few-shot-universe
# See a list of available tags here:
# https://github.com/huggingface/hub-docs/blob/main/js/src/lib/interfaces/Types.ts#L12
# task: unconditional-image-generation or conditional-image-generation or image-to-image
license: mit
---
# Generate universal image using FastGAN
## Model description
[FastGAN model](https://arxiv.org/abs/2101.04775) is a Generative Adversarial Networks (GAN) training on a small amount of high-fidelity images with minimum computing cost. Using a skip-layer channel-wise excitation module and a self-supervised discriminator trained as a feature-encoder, the model was able to converge after some hours of training for either 100 high-quality images or 1000 images datasets.
This model was trained on a dataset of 120 high-quality universe images.
#### How to use
```python
# Clone this model
git clone https://huggingface.co/huggan/fastgan-few-shot-universe
def load_generator(model_name_or_path):
generator = Generator(in_channels=256, out_channels=3)
generator = generator.from_pretrained(model_name_or_path, in_channels=256, out_channels=3)
_ = generator.eval()
return generator
def _denormalize(input: torch.Tensor) -> torch.Tensor:
return (input * 127.5) + 127.5
# Load generator
generator = load_generator("huggan/fastgan-few-shot-universe")
# Generate a random noise image
noise = torch.zeros(1, 256, 1, 1, device=device).normal_(0.0, 1.0)
with torch.no_grad():
gan_images, _ = generator(noise)
gan_images = _denormalize(gan_images.detach())
save_image(gan_images, "sample.png", nrow=1, normalize=True)
```
#### Limitations and bias
* Converge faster and better with small datasets (less than 1000 samples)
## Training data
[few-shot-universe](https://huggingface.co/datasets/huggan/few-shot-universe)
## Generated Images

### BibTeX entry and citation info
```bibtex
@article{FastGAN,
title={Towards Faster and Stabilized GAN Training for High-fidelity Few-shot Image Synthesis},
author={Bingchen Liu, Yizhe Zhu, Kunpeng Song, Ahmed Elgammal},
journal={ICLR},
year={2021}
}
``` |
gary109/wav2vec2-base-MIR_ST500_ASR_109 | c307e8fcb3bf794205c33fa9a9406e9c9b844ca6 | 2022-04-15T21:15:56.000Z | [
"pytorch",
"tensorboard",
"wav2vec2",
"automatic-speech-recognition",
"dataset:mir_st500",
"transformers",
"/workspace/datasets/datasets/MIR_ST500/MIR_ST500.py",
"generated_from_trainer",
"license:apache-2.0",
"model-index"
] | automatic-speech-recognition | false | gary109 | null | gary109/wav2vec2-base-MIR_ST500_ASR_109 | 0 | null | transformers | 36,911 | ---
license: apache-2.0
tags:
- automatic-speech-recognition
- /workspace/datasets/datasets/MIR_ST500/MIR_ST500.py
- generated_from_trainer
datasets:
- mir_st500
model-index:
- name: wav2vec2-base-MIR_ST500_ASR_109
results: []
---
<!-- This model card has been generated automatically according to the information the Trainer had access to. You
should probably proofread and complete it, then remove this comment. -->
# wav2vec2-base-MIR_ST500_ASR_109
This model is a fine-tuned version of [facebook/wav2vec2-base](https://huggingface.co/facebook/wav2vec2-base) on the /WORKSPACE/DATASETS/DATASETS/MIR_ST500/MIR_ST500.PY - ASR dataset.
It achieves the following results on the evaluation set:
- Loss: 0.6452
- Wer: 0.3732
## Model description
More information needed
## Intended uses & limitations
More information needed
## Training and evaluation data
More information needed
## Training procedure
### Training hyperparameters
The following hyperparameters were used during training:
- learning_rate: 3e-05
- train_batch_size: 8
- eval_batch_size: 8
- seed: 42
- distributed_type: multi-GPU
- num_devices: 2
- total_train_batch_size: 16
- total_eval_batch_size: 16
- optimizer: Adam with betas=(0.9,0.999) and epsilon=1e-08
- lr_scheduler_type: linear
- lr_scheduler_warmup_steps: 500
- num_epochs: 30.0
- mixed_precision_training: Native AMP
### Training results
| Training Loss | Epoch | Step | Validation Loss | Wer |
|:-------------:|:-----:|:-----:|:---------------:|:------:|
| 12.5751 | 0.27 | 100 | 6.0291 | 1.0 |
| 4.343 | 0.53 | 200 | 2.8709 | 1.0 |
| 4.1911 | 0.8 | 300 | 2.5472 | 1.0 |
| 2.4535 | 1.06 | 400 | 2.4323 | 1.0 |
| 2.6157 | 1.33 | 500 | 2.2799 | 1.0 |
| 2.4839 | 1.6 | 600 | 2.2722 | 1.0 |
| 2.2787 | 1.86 | 700 | 2.2269 | 1.0 |
| 2.1981 | 2.13 | 800 | 2.2221 | 1.0 |
| 2.159 | 2.39 | 900 | 2.1657 | 1.0 |
| 2.1421 | 2.66 | 1000 | 2.1769 | 1.0 |
| 2.0841 | 2.93 | 1100 | 2.1688 | 1.0 |
| 2.0599 | 3.19 | 1200 | 2.1141 | 1.0 |
| 2.0257 | 3.46 | 1300 | 2.0445 | 1.0 |
| 1.979 | 3.72 | 1400 | 2.0180 | 1.0 |
| 1.9366 | 3.99 | 1500 | 1.9419 | 1.0 |
| 1.8547 | 4.26 | 1600 | 1.8765 | 1.0 |
| 1.3988 | 4.52 | 1700 | 1.4151 | 0.7999 |
| 1.1881 | 4.79 | 1800 | 1.1158 | 0.7347 |
| 0.9557 | 5.05 | 1900 | 1.0095 | 0.6485 |
| 0.9087 | 5.32 | 2000 | 0.9644 | 0.6848 |
| 0.8086 | 5.59 | 2100 | 0.8960 | 0.6119 |
| 0.9106 | 5.85 | 2200 | 0.8892 | 0.5941 |
| 0.8252 | 6.12 | 2300 | 0.8333 | 0.5756 |
| 0.8299 | 6.38 | 2400 | 0.8559 | 0.5838 |
| 0.8021 | 6.65 | 2500 | 0.8201 | 0.5883 |
| 0.7979 | 6.91 | 2600 | 0.8349 | 0.575 |
| 0.7223 | 7.18 | 2700 | 0.7883 | 0.5563 |
| 0.6754 | 7.45 | 2800 | 0.7590 | 0.5393 |
| 0.6454 | 7.71 | 2900 | 0.7411 | 0.5291 |
| 0.6228 | 7.98 | 3000 | 0.7464 | 0.5300 |
| 0.6475 | 8.24 | 3100 | 0.7478 | 0.5295 |
| 0.6452 | 8.51 | 3200 | 0.7555 | 0.5360 |
| 0.5636 | 8.78 | 3300 | 0.7369 | 0.5232 |
| 0.564 | 9.04 | 3400 | 0.7331 | 0.5076 |
| 0.6173 | 9.31 | 3500 | 0.7199 | 0.5034 |
| 0.625 | 9.57 | 3600 | 0.7243 | 0.5193 |
| 0.8122 | 9.84 | 3700 | 0.7436 | 0.5242 |
| 0.5455 | 10.11 | 3800 | 0.7111 | 0.4920 |
| 0.7928 | 10.37 | 3900 | 0.7137 | 0.4858 |
| 0.5446 | 10.64 | 4000 | 0.6874 | 0.4828 |
| 0.4772 | 10.9 | 4100 | 0.6760 | 0.4801 |
| 0.6447 | 11.17 | 4200 | 0.6893 | 0.4886 |
| 0.5818 | 11.44 | 4300 | 0.6789 | 0.4740 |
| 0.4952 | 11.7 | 4400 | 0.7043 | 0.4811 |
| 0.5722 | 11.97 | 4500 | 0.6794 | 0.4766 |
| 0.58 | 12.23 | 4600 | 0.6629 | 0.4580 |
| 0.5432 | 12.5 | 4700 | 0.6907 | 0.4906 |
| 0.4786 | 12.77 | 4800 | 0.6925 | 0.4854 |
| 0.5177 | 13.03 | 4900 | 0.6666 | 0.4532 |
| 0.5448 | 13.3 | 5000 | 0.6744 | 0.4542 |
| 0.5732 | 13.56 | 5100 | 0.6930 | 0.4986 |
| 0.5065 | 13.83 | 5200 | 0.6647 | 0.4351 |
| 0.4005 | 14.1 | 5300 | 0.6659 | 0.4508 |
| 0.4256 | 14.36 | 5400 | 0.6682 | 0.4533 |
| 0.4459 | 14.63 | 5500 | 0.6594 | 0.4326 |
| 0.4645 | 14.89 | 5600 | 0.6615 | 0.4287 |
| 0.4275 | 15.16 | 5700 | 0.6423 | 0.4299 |
| 0.4026 | 15.43 | 5800 | 0.6539 | 0.4217 |
| 0.3507 | 15.69 | 5900 | 0.6555 | 0.4299 |
| 0.3998 | 15.96 | 6000 | 0.6526 | 0.4213 |
| 0.4462 | 16.22 | 6100 | 0.6469 | 0.4230 |
| 0.4095 | 16.49 | 6200 | 0.6516 | 0.4210 |
| 0.4452 | 16.76 | 6300 | 0.6373 | 0.4133 |
| 0.3997 | 17.02 | 6400 | 0.6456 | 0.4211 |
| 0.3826 | 17.29 | 6500 | 0.6278 | 0.4042 |
| 0.3867 | 17.55 | 6600 | 0.6459 | 0.4112 |
| 0.4367 | 17.82 | 6700 | 0.6464 | 0.4131 |
| 0.3887 | 18.09 | 6800 | 0.6567 | 0.4150 |
| 0.3481 | 18.35 | 6900 | 0.6548 | 0.4145 |
| 0.4241 | 18.62 | 7000 | 0.6490 | 0.4123 |
| 0.3742 | 18.88 | 7100 | 0.6561 | 0.4135 |
| 0.423 | 19.15 | 7200 | 0.6498 | 0.4051 |
| 0.3803 | 19.41 | 7300 | 0.6475 | 0.3903 |
| 0.3084 | 19.68 | 7400 | 0.6403 | 0.4042 |
| 0.3012 | 19.95 | 7500 | 0.6460 | 0.4004 |
| 0.3306 | 20.21 | 7600 | 0.6491 | 0.3837 |
| 0.3612 | 20.48 | 7700 | 0.6752 | 0.3884 |
| 0.3572 | 20.74 | 7800 | 0.6383 | 0.3793 |
| 0.3638 | 21.01 | 7900 | 0.6349 | 0.3838 |
| 0.3658 | 21.28 | 8000 | 0.6544 | 0.3793 |
| 0.3726 | 21.54 | 8100 | 0.6567 | 0.3756 |
| 0.3618 | 21.81 | 8200 | 0.6390 | 0.3795 |
| 0.3212 | 22.07 | 8300 | 0.6359 | 0.3768 |
| 0.3561 | 22.34 | 8400 | 0.6452 | 0.3732 |
| 0.3231 | 22.61 | 8500 | 0.6416 | 0.3731 |
| 0.3764 | 22.87 | 8600 | 0.6428 | 0.3697 |
| 0.4142 | 23.14 | 8700 | 0.6415 | 0.3665 |
| 0.2713 | 23.4 | 8800 | 0.6541 | 0.3676 |
| 0.2277 | 23.67 | 8900 | 0.6492 | 0.3684 |
| 0.3849 | 23.94 | 9000 | 0.6448 | 0.3651 |
| 0.266 | 24.2 | 9100 | 0.6602 | 0.3643 |
| 0.3464 | 24.47 | 9200 | 0.6673 | 0.3607 |
| 0.2919 | 24.73 | 9300 | 0.6557 | 0.3677 |
| 0.2878 | 25.0 | 9400 | 0.6377 | 0.3653 |
| 0.1603 | 25.27 | 9500 | 0.6598 | 0.3700 |
| 0.2055 | 25.53 | 9600 | 0.6558 | 0.3614 |
| 0.1508 | 25.8 | 9700 | 0.6543 | 0.3605 |
| 0.3162 | 26.06 | 9800 | 0.6570 | 0.3576 |
| 0.2613 | 26.33 | 9900 | 0.6604 | 0.3584 |
| 0.2244 | 26.6 | 10000 | 0.6618 | 0.3634 |
| 0.1585 | 26.86 | 10100 | 0.6698 | 0.3634 |
| 0.2959 | 27.13 | 10200 | 0.6709 | 0.3593 |
| 0.2778 | 27.39 | 10300 | 0.6638 | 0.3537 |
| 0.2354 | 27.66 | 10400 | 0.6770 | 0.3585 |
| 0.2992 | 27.93 | 10500 | 0.6698 | 0.3506 |
| 0.2664 | 28.19 | 10600 | 0.6725 | 0.3533 |
| 0.2582 | 28.46 | 10700 | 0.6689 | 0.3542 |
| 0.2096 | 28.72 | 10800 | 0.6731 | 0.3527 |
| 0.4169 | 28.99 | 10900 | 0.6691 | 0.3521 |
| 0.2716 | 29.26 | 11000 | 0.6712 | 0.3517 |
| 0.2944 | 29.52 | 11100 | 0.6708 | 0.3509 |
| 0.2737 | 29.79 | 11200 | 0.6699 | 0.3491 |
### Framework versions
- Transformers 4.18.0
- Pytorch 1.9.1+cu102
- Datasets 2.0.0
- Tokenizers 0.11.6
|
lilitket/20220415-151647 | 807ca0c7a0e26a9fa8085332a85baee81677ee2d | 2022-04-15T20:16:42.000Z | [
"pytorch",
"wav2vec2",
"automatic-speech-recognition",
"transformers"
] | automatic-speech-recognition | false | lilitket | null | lilitket/20220415-151647 | 0 | null | transformers | 36,912 | Entry not found |
huggan/fastgan-few-shot-grumpy-cat | 305e922763b9cb83e63426ed6ebcfe941dfc7b5b | 2022-05-06T22:31:25.000Z | [
"pytorch",
"dataset:huggan/few-shot-grumpy-cat",
"arxiv:2101.04775",
"huggan",
"gan",
"unconditional-image-generation",
"license:mit"
] | unconditional-image-generation | false | huggan | null | huggan/fastgan-few-shot-grumpy-cat | 0 | null | null | 36,913 | ---
tags:
- huggan
- gan
- unconditional-image-generation
datasets:
- huggan/few-shot-grumpy-cat
# See a list of available tags here:
# https://github.com/huggingface/hub-docs/blob/main/js/src/lib/interfaces/Types.ts#L12
# task: unconditional-image-generation or conditional-image-generation or image-to-image
license: mit
---
# Generate grumpy cat face using FastGAN
## Model description
[FastGAN model](https://arxiv.org/abs/2101.04775) is a Generative Adversarial Networks (GAN) training on a small amount of high-fidelity images with minimum computing cost. Using a skip-layer channel-wise excitation module and a self-supervised discriminator trained as a feature-encoder, the model was able to converge after some hours of training for either 100 high-quality images or 1000 images datasets.
This model was trained on a dataset of 100 high-quality grumpy cat images.
#### How to use
```python
# Clone this model
git clone https://huggingface.co/huggan/fastgan-few-shot-grumpy-cat
def load_generator(model_name_or_path):
generator = Generator(in_channels=256, out_channels=3)
generator = generator.from_pretrained(model_name_or_path, in_channels=256, out_channels=3)
_ = generator.eval()
return generator
def _denormalize(input: torch.Tensor) -> torch.Tensor:
return (input * 127.5) + 127.5
# Load generator
generator = load_generator("huggan/fastgan-few-shot-grumpy-cat")
# Generate a random noise image
noise = torch.zeros(1, 256, 1, 1, device=device).normal_(0.0, 1.0)
with torch.no_grad():
gan_images, _ = generator(noise)
gan_images = _denormalize(gan_images.detach())
save_image(gan_images, "sample.png", nrow=1, normalize=True)
```
#### Limitations and bias
* Converge faster and better with small datasets (less than 1000 samples)
## Training data
[few-shot-grumpy-cat](https://huggingface.co/datasets/huggan/few-shot-grumpy-cat)
## Generated Images

### BibTeX entry and citation info
```bibtex
@article{FastGAN,
title={Towards Faster and Stabilized GAN Training for High-fidelity Few-shot Image Synthesis},
author={Bingchen Liu, Yizhe Zhu, Kunpeng Song, Ahmed Elgammal},
journal={ICLR},
year={2021}
}
``` |
theResearchNinja/Cybonto-distilbert-base-uncased-finetuned-ner-Wnut17 | 0c4ab0de4ee3f674cec85bc11e5c690996904c83 | 2022-04-15T19:22:59.000Z | [
"pytorch",
"tensorboard",
"distilbert",
"token-classification",
"dataset:wnut_17",
"transformers",
"generated_from_trainer",
"license:apache-2.0",
"model-index",
"autotrain_compatible"
] | token-classification | false | theResearchNinja | null | theResearchNinja/Cybonto-distilbert-base-uncased-finetuned-ner-Wnut17 | 0 | null | transformers | 36,914 | ---
license: apache-2.0
tags:
- generated_from_trainer
datasets:
- wnut_17
metrics:
- precision
- recall
- f1
- accuracy
model-index:
- name: Cybonto-distilbert-base-uncased-finetuned-ner-Wnut17
results:
- task:
name: Token Classification
type: token-classification
dataset:
name: wnut_17
type: wnut_17
args: wnut_17
metrics:
- name: Precision
type: precision
value: 0.6603139013452914
- name: Recall
type: recall
value: 0.4682034976152623
- name: F1
type: f1
value: 0.547906976744186
- name: Accuracy
type: accuracy
value: 0.9355430668654662
---
<!-- This model card has been generated automatically according to the information the Trainer had access to. You
should probably proofread and complete it, then remove this comment. -->
# Cybonto-distilbert-base-uncased-finetuned-ner-Wnut17
This model is a fine-tuned version of [distilbert-base-uncased](https://huggingface.co/distilbert-base-uncased) on the wnut_17 dataset.
It achieves the following results on the evaluation set:
- Loss: 0.5062
- Precision: 0.6603
- Recall: 0.4682
- F1: 0.5479
- Accuracy: 0.9355
## Model description
More information needed
## Intended uses & limitations
More information needed
## Training and evaluation data
More information needed
## Training procedure
### Training hyperparameters
The following hyperparameters were used during training:
- learning_rate: 2e-05
- train_batch_size: 32
- eval_batch_size: 32
- seed: 42
- optimizer: Adam with betas=(0.9,0.999) and epsilon=1e-08
- lr_scheduler_type: linear
- num_epochs: 30
### Training results
| Training Loss | Epoch | Step | Validation Loss | Precision | Recall | F1 | Accuracy |
|:-------------:|:-----:|:----:|:---------------:|:---------:|:------:|:------:|:--------:|
| No log | 1.0 | 107 | 0.3396 | 0.6470 | 0.4269 | 0.5144 | 0.9330 |
| No log | 2.0 | 214 | 0.3475 | 0.5948 | 0.4539 | 0.5149 | 0.9335 |
| No log | 3.0 | 321 | 0.3793 | 0.6613 | 0.4253 | 0.5177 | 0.9332 |
| No log | 4.0 | 428 | 0.3598 | 0.6195 | 0.4944 | 0.5500 | 0.9354 |
| 0.0409 | 5.0 | 535 | 0.3702 | 0.5802 | 0.4571 | 0.5113 | 0.9308 |
| 0.0409 | 6.0 | 642 | 0.4192 | 0.6546 | 0.4459 | 0.5305 | 0.9344 |
| 0.0409 | 7.0 | 749 | 0.4039 | 0.6360 | 0.4610 | 0.5346 | 0.9354 |
| 0.0409 | 8.0 | 856 | 0.4104 | 0.6564 | 0.4587 | 0.5400 | 0.9353 |
| 0.0409 | 9.0 | 963 | 0.3839 | 0.6283 | 0.4944 | 0.5534 | 0.9361 |
| 0.0132 | 10.0 | 1070 | 0.4331 | 0.6197 | 0.4547 | 0.5245 | 0.9339 |
| 0.0132 | 11.0 | 1177 | 0.4152 | 0.6196 | 0.4817 | 0.5420 | 0.9355 |
| 0.0132 | 12.0 | 1284 | 0.4654 | 0.6923 | 0.4507 | 0.5460 | 0.9353 |
| 0.0132 | 13.0 | 1391 | 0.4869 | 0.6739 | 0.4436 | 0.5350 | 0.9350 |
| 0.0132 | 14.0 | 1498 | 0.4297 | 0.6424 | 0.4769 | 0.5474 | 0.9353 |
| 0.0061 | 15.0 | 1605 | 0.4507 | 0.6272 | 0.4626 | 0.5325 | 0.9340 |
| 0.0061 | 16.0 | 1712 | 0.4410 | 0.6066 | 0.4793 | 0.5355 | 0.9335 |
| 0.0061 | 17.0 | 1819 | 0.4851 | 0.6639 | 0.4523 | 0.5381 | 0.9351 |
| 0.0061 | 18.0 | 1926 | 0.4815 | 0.6553 | 0.4563 | 0.5380 | 0.9346 |
| 0.0035 | 19.0 | 2033 | 0.5188 | 0.6780 | 0.4420 | 0.5351 | 0.9350 |
| 0.0035 | 20.0 | 2140 | 0.4986 | 0.6770 | 0.4698 | 0.5547 | 0.9363 |
| 0.0035 | 21.0 | 2247 | 0.4834 | 0.6552 | 0.4714 | 0.5483 | 0.9355 |
| 0.0035 | 22.0 | 2354 | 0.5094 | 0.6784 | 0.4595 | 0.5479 | 0.9358 |
| 0.0035 | 23.0 | 2461 | 0.4954 | 0.6583 | 0.4579 | 0.5401 | 0.9354 |
| 0.0026 | 24.0 | 2568 | 0.5035 | 0.6667 | 0.4595 | 0.5440 | 0.9354 |
| 0.0026 | 25.0 | 2675 | 0.5000 | 0.6599 | 0.4658 | 0.5461 | 0.9355 |
| 0.0026 | 26.0 | 2782 | 0.4968 | 0.6697 | 0.4738 | 0.5549 | 0.9357 |
| 0.0026 | 27.0 | 2889 | 0.4991 | 0.6545 | 0.4714 | 0.5481 | 0.9352 |
| 0.0026 | 28.0 | 2996 | 0.4936 | 0.6508 | 0.4769 | 0.5505 | 0.9353 |
| 0.0021 | 29.0 | 3103 | 0.5005 | 0.6535 | 0.4722 | 0.5482 | 0.9353 |
| 0.0021 | 30.0 | 3210 | 0.5062 | 0.6603 | 0.4682 | 0.5479 | 0.9355 |
### Framework versions
- Transformers 4.18.0
- Pytorch 1.10.0+cu111
- Datasets 2.1.0
- Tokenizers 0.12.1
|
harshm16/t5-small-finetuned-xsum | 031316dfdbfcd1809fa13218c1a6f047465ead3a | 2022-04-15T20:11:44.000Z | [
"pytorch",
"tensorboard",
"t5",
"text2text-generation",
"transformers",
"autotrain_compatible"
] | text2text-generation | false | harshm16 | null | harshm16/t5-small-finetuned-xsum | 0 | null | transformers | 36,915 | Entry not found |
pdroberts/xlm-roberta-base-finetuned-panx-de | 46802929eb25ef5dc6bb78fa19267d71998327ac | 2022-04-15T23:05:00.000Z | [
"pytorch",
"tensorboard",
"xlm-roberta",
"token-classification",
"dataset:xtreme",
"transformers",
"generated_from_trainer",
"license:mit",
"model-index",
"autotrain_compatible"
] | token-classification | false | pdroberts | null | pdroberts/xlm-roberta-base-finetuned-panx-de | 0 | null | transformers | 36,916 | ---
license: mit
tags:
- generated_from_trainer
datasets:
- xtreme
metrics:
- f1
model-index:
- name: xlm-roberta-base-finetuned-panx-de
results:
- task:
name: Token Classification
type: token-classification
dataset:
name: xtreme
type: xtreme
args: PAN-X.de
metrics:
- name: F1
type: f1
value: 0.8632527372262775
---
<!-- This model card has been generated automatically according to the information the Trainer had access to. You
should probably proofread and complete it, then remove this comment. -->
# xlm-roberta-base-finetuned-panx-de
This model is a fine-tuned version of [xlm-roberta-base](https://huggingface.co/xlm-roberta-base) on the xtreme dataset.
It achieves the following results on the evaluation set:
- Loss: 0.1367
- F1: 0.8633
## Model description
More information needed
## Intended uses & limitations
More information needed
## Training and evaluation data
More information needed
## Training procedure
### Training hyperparameters
The following hyperparameters were used during training:
- learning_rate: 5e-05
- train_batch_size: 24
- eval_batch_size: 24
- seed: 42
- optimizer: Adam with betas=(0.9,0.999) and epsilon=1e-08
- lr_scheduler_type: linear
- num_epochs: 3
### Training results
| Training Loss | Epoch | Step | Validation Loss | F1 |
|:-------------:|:-----:|:----:|:---------------:|:------:|
| 0.2582 | 1.0 | 525 | 0.1653 | 0.8238 |
| 0.1301 | 2.0 | 1050 | 0.1417 | 0.8439 |
| 0.0841 | 3.0 | 1575 | 0.1367 | 0.8633 |
### Framework versions
- Transformers 4.11.3
- Pytorch 1.11.0+cu102
- Datasets 1.16.1
- Tokenizers 0.10.3
|
ptran74/DSPFirst-Finetuning-1 | 7fde6eb98366f8b12739c71b2ea862770844a277 | 2022-04-16T18:56:58.000Z | [
"pytorch",
"electra",
"question-answering",
"transformers",
"generated_from_trainer",
"model-index",
"autotrain_compatible"
] | question-answering | false | ptran74 | null | ptran74/DSPFirst-Finetuning-1 | 0 | null | transformers | 36,917 | ---
tags:
- generated_from_trainer
model-index:
- name: DSPFirst-Finetuning-1
results: []
---
<!-- This model card has been generated automatically according to the information the Trainer had access to. You
should probably proofread and complete it, then remove this comment. -->
# DSPFirst-Finetuning-1
This model is a fine-tuned version of [ahotrod/electra_large_discriminator_squad2_512](https://huggingface.co/ahotrod/electra_large_discriminator_squad2_512) on a generated Questions and Answers dataset from the DSPFirst textbook based on the SQuAD 2.0 format.
# Dataset
A visualization of the dataset can be found [here](https://github.gatech.edu/pages/VIP-ITS/textbook_SQuAD_explore/explore/textbookv1.0/textbook/). The split between train and test is 80% and 20% respectively.
```
DatasetDict({
train: Dataset({
features: ['id', 'title', 'context', 'question', 'answers'],
num_rows: 4755
})
test: Dataset({
features: ['id', 'title', 'context', 'question', 'answers'],
num_rows: 1189
})
})
```
It achieves the following results on the evaluation set:
- Loss: 0.9236
## Model description
More information needed
## Intended uses & limitations
Since the dataset is generated from the DSPFirst textbook, its quality is not guaranteed.
## Training and evaluation data
More information needed
## Training procedure
### Training hyperparameters
The following hyperparameters were used during training:
- learning_rate: 2e-05
- train_batch_size: 6
- eval_batch_size: 6
- seed: 42
- gradient_accumulation_steps: 86
- total_train_batch_size: 516
- optimizer: Adam with betas=(0.9,0.999) and epsilon=1e-08
- lr_scheduler_type: linear
- num_epochs: 4
### Model hyperparameters
- hidden_dropout_prob: 0.5
- attention_probs_dropout_prob = 0.5
### Training results
| Training Loss | Epoch | Step | Validation Loss |
|:-------------:|:-----:|:----:|:---------------:|
| 6.0131 | 0.7 | 20 | 0.9549 |
| 6.1542 | 1.42 | 40 | 0.9302 |
| 6.1472 | 2.14 | 60 | 0.9249 |
| 5.9662 | 2.84 | 80 | 0.9248 |
| 6.1467 | 3.56 | 100 | 0.9236 |
### Framework versions
- Transformers 4.18.0
- Pytorch 1.10.0+cu111
- Datasets 2.1.0
- Tokenizers 0.12.1
|
huggan/projected_gan_cubism | fa2844c7a8ebff52e6447c24f079f1ac7e28dce2 | 2022-04-25T11:17:33.000Z | [
"pytorch",
"gan",
"dcgan",
"projected-gan",
"huggan",
"unconditional-image-generation"
] | unconditional-image-generation | false | huggan | null | huggan/projected_gan_cubism | 0 | null | pytorch | 36,918 | ---
library_name: pytorch
tags:
- gan
- dcgan
- projected-gan
- huggan
- unconditional-image-generation
---
dataset: https://github.com/cs-chan/ArtGAN/tree/master/WikiArt%20Dataset
trained on the official projected gan github code - you can check out the hfspace to see how to use it to generate images
fun stuff
check out the space demo: https://huggingface.co/spaces/huggan/projected_gan_art
Made by:-<br/>
[Jeronim Matijević](https://huggingface.co/Cropinky)<br/>
[Massimiliano Pappa](https://huggingface.co/maxpappa)<br/>
|
reinoudbosch/dummy-model | cd01afb70ca5d6746674576ccbc4264328a84e8e | 2022-04-16T03:13:50.000Z | [
"pytorch",
"camembert",
"fill-mask",
"transformers",
"autotrain_compatible"
] | fill-mask | false | reinoudbosch | null | reinoudbosch/dummy-model | 0 | null | transformers | 36,919 | This is a dummy model |
huggan/projected_gan_pop_art_hana | 65b6e239fcbb59366a7c2f83dc1539668e386105 | 2022-04-25T11:17:45.000Z | [
"pytorch",
"gan",
"dcgan",
"projected-gan",
"huggan",
"unconditional-image-generation"
] | unconditional-image-generation | false | huggan | null | huggan/projected_gan_pop_art_hana | 0 | null | pytorch | 36,920 | ---
library_name: pytorch
tags:
- gan
- dcgan
- projected-gan
- huggan
- unconditional-image-generation
---
dataset: https://github.com/cs-chan/ArtGAN/tree/master/WikiArt%20Dataset
trained on the official projected gan github code - you can check out the hfspace to see how to use it to generate images
fun stuff
check out the space demo: https://huggingface.co/spaces/huggan/projected_gan_art
Made by:-<br/>
[Jeronim Matijević](https://huggingface.co/Cropinky)<br/>
[Massimiliano Pappa](https://huggingface.co/maxpappa)<br/>
|
mdm/DialoGPT-small-Kanye | ada6bdbd85b575878af8023230797daba5c089dd | 2022-04-20T04:25:18.000Z | [
"pytorch",
"gpt2",
"text-generation",
"transformers",
"conversational"
] | conversational | false | mdm | null | mdm/DialoGPT-small-Kanye | 0 | null | transformers | 36,921 | ---
tags:
- conversational
---
# Kanye West AI - DialoGPT Small
Kanye West DialoGPT model built with lyrics from Kaggle (https://www.kaggle.com/datasets/convolutionalnn/kanye-west-lyrics-dataset) and resources from Lynn Zheng |
WestMatrix/DemoModel | 0898384fc90934aecde83f21b8524cae1837458a | 2022-04-16T05:40:42.000Z | [
"Swing",
"pytorch",
"license:mit"
] | null | false | WestMatrix | null | WestMatrix/DemoModel | 0 | 1 | null | 36,922 | ---
license: mit
tags:
- Swing
- pytorch
---
|
muhammadfhadli/bert_id_dummy | 244844f25b00eef054f579b1bcaba1d30ae77014 | 2022-04-16T10:48:56.000Z | [
"pytorch",
"bert",
"fill-mask",
"transformers",
"autotrain_compatible"
] | fill-mask | false | muhammadfhadli | null | muhammadfhadli/bert_id_dummy | 0 | null | transformers | 36,923 | Entry not found |
krinal214/mBERT_all_ty_SQen_SQ20_1 | e14c248bae2ea8a42595089334c17bea8ccb311e | 2022-04-16T14:25:15.000Z | [
"pytorch",
"tensorboard",
"bert",
"question-answering",
"transformers",
"generated_from_trainer",
"license:apache-2.0",
"model-index",
"autotrain_compatible"
] | question-answering | false | krinal214 | null | krinal214/mBERT_all_ty_SQen_SQ20_1 | 0 | null | transformers | 36,924 | ---
license: apache-2.0
tags:
- generated_from_trainer
model-index:
- name: mBERT_all_ty_SQen_SQ20_1
results: []
---
<!-- This model card has been generated automatically according to the information the Trainer had access to. You
should probably proofread and complete it, then remove this comment. -->
# mBERT_all_ty_SQen_SQ20_1
This model is a fine-tuned version of [bert-base-multilingual-cased](https://huggingface.co/bert-base-multilingual-cased) on the None dataset.
It achieves the following results on the evaluation set:
- Loss: 0.5305
## Model description
More information needed
## Intended uses & limitations
More information needed
## Training and evaluation data
More information needed
## Training procedure
### Training hyperparameters
The following hyperparameters were used during training:
- learning_rate: 2e-05
- train_batch_size: 16
- eval_batch_size: 16
- seed: 42
- optimizer: Adam with betas=(0.9,0.999) and epsilon=1e-08
- lr_scheduler_type: linear
- num_epochs: 1
### Training results
| Training Loss | Epoch | Step | Validation Loss |
|:-------------:|:-----:|:-----:|:---------------:|
| 1.1337 | 1.0 | 12327 | 0.5305 |
### Framework versions
- Transformers 4.17.0
- Pytorch 1.9.1
- Datasets 2.1.0
- Tokenizers 0.11.6
|
masakhane/afrimt5_fr_fon_news | 5dae043c7d75b5da80e7ca3bb48d95462d77727b | 2022-04-16T13:06:11.000Z | [
"pytorch",
"mt5",
"text2text-generation",
"transformers",
"license:afl-3.0",
"autotrain_compatible"
] | text2text-generation | false | masakhane | null | masakhane/afrimt5_fr_fon_news | 0 | null | transformers | 36,925 | ---
license: afl-3.0
---
|
masakhane/afribyt5_fr_fon_news | 79b8bc403f181c8f0ff88828fd1f9679fbe3094f | 2022-04-16T13:06:40.000Z | [
"pytorch",
"t5",
"text2text-generation",
"transformers",
"license:afl-3.0",
"autotrain_compatible"
] | text2text-generation | false | masakhane | null | masakhane/afribyt5_fr_fon_news | 0 | null | transformers | 36,926 | ---
license: afl-3.0
---
|
masakhane/afribyt5_fon_fr_news | 7b143f673b713e969cf0580b91d3a4627e9d1615 | 2022-04-16T13:06:44.000Z | [
"pytorch",
"t5",
"text2text-generation",
"transformers",
"license:afl-3.0",
"autotrain_compatible"
] | text2text-generation | false | masakhane | null | masakhane/afribyt5_fon_fr_news | 0 | null | transformers | 36,927 | ---
license: afl-3.0
---
|
masakhane/mbart50_fr_fon_news | ac006f1bb70259b9595433cbefa8024b9b98aadb | 2022-04-16T14:01:53.000Z | [
"pytorch",
"mbart",
"text2text-generation",
"transformers",
"license:afl-3.0",
"autotrain_compatible"
] | text2text-generation | false | masakhane | null | masakhane/mbart50_fr_fon_news | 0 | null | transformers | 36,928 | ---
license: afl-3.0
---
|
masakhane/afrimbart_fr_fon_news | 068baf76b425b6f6bc3683115724d199bfc34de0 | 2022-04-16T14:01:49.000Z | [
"pytorch",
"mbart",
"text2text-generation",
"transformers",
"license:afl-3.0",
"autotrain_compatible"
] | text2text-generation | false | masakhane | null | masakhane/afrimbart_fr_fon_news | 0 | null | transformers | 36,929 | ---
license: afl-3.0
---
|
masakhane/byt5_fr_fon_news | 4d1cec76cd6b3d103254e66ab51e4c28fff0ca76 | 2022-04-16T16:20:24.000Z | [
"pytorch",
"t5",
"text2text-generation",
"transformers",
"license:afl-3.0",
"autotrain_compatible"
] | text2text-generation | false | masakhane | null | masakhane/byt5_fr_fon_news | 0 | null | transformers | 36,930 | ---
license: afl-3.0
---
|
masakhane/byt5_fon_fr_news | 831529f1b8bff0985860e22c433da46b9acee45f | 2022-04-16T16:20:32.000Z | [
"pytorch",
"t5",
"text2text-generation",
"transformers",
"license:afl-3.0",
"autotrain_compatible"
] | text2text-generation | false | masakhane | null | masakhane/byt5_fon_fr_news | 0 | null | transformers | 36,931 | ---
license: afl-3.0
---
|
masakhane/mt5_fon_fr_news | b4c80a2bc2b9c2f609d8d89c6030ed0d66ba27ef | 2022-04-16T16:20:21.000Z | [
"pytorch",
"mt5",
"text2text-generation",
"transformers",
"license:afl-3.0",
"autotrain_compatible"
] | text2text-generation | false | masakhane | null | masakhane/mt5_fon_fr_news | 0 | null | transformers | 36,932 | ---
license: afl-3.0
---
|
masakhane/mt5_fr_fon_news | 14de74c5e7af51bd626b32ce1c27b546aa540eb7 | 2022-04-16T16:20:28.000Z | [
"pytorch",
"mt5",
"text2text-generation",
"transformers",
"license:afl-3.0",
"autotrain_compatible"
] | text2text-generation | false | masakhane | null | masakhane/mt5_fr_fon_news | 0 | null | transformers | 36,933 | ---
license: afl-3.0
---
|
masakhane/m2m100_418M_fon_fr_news | a70f09836984d68e25893ce1fcaaa274bd601f0d | 2022-04-16T17:53:09.000Z | [
"pytorch",
"m2m_100",
"text2text-generation",
"transformers",
"license:afl-3.0",
"autotrain_compatible"
] | text2text-generation | false | masakhane | null | masakhane/m2m100_418M_fon_fr_news | 0 | null | transformers | 36,934 | ---
license: afl-3.0
---
|
huggan/fastgan-few-shot-pokemon | 943d4ac9ed42f89f5fd1ce0b5a1126fac457779b | 2022-04-17T12:20:28.000Z | [
"pytorch"
] | null | false | huggan | null | huggan/fastgan-few-shot-pokemon | 0 | null | null | 36,935 | Entry not found |
huggan/fastgan-few-shot-skulls | 80cce0163ffa6c81983c4858b2fd4d579fc90b12 | 2022-04-17T10:14:20.000Z | [
"pytorch"
] | null | false | huggan | null | huggan/fastgan-few-shot-skulls | 0 | null | null | 36,936 | Entry not found |
huggingtweets/discord | 3d712eec8b58c0ec4a5dbb6aebc03b16cc3aab79 | 2022-04-16T14:58:48.000Z | [
"pytorch",
"gpt2",
"text-generation",
"en",
"transformers",
"huggingtweets"
] | text-generation | false | huggingtweets | null | huggingtweets/discord | 0 | null | transformers | 36,937 | ---
language: en
thumbnail: http://www.huggingtweets.com/discord/1650121123874/predictions.png
tags:
- huggingtweets
widget:
- text: "My dream is"
---
<div class="inline-flex flex-col" style="line-height: 1.5;">
<div class="flex">
<div
style="display:inherit; margin-left: 4px; margin-right: 4px; width: 92px; height:92px; border-radius: 50%; background-size: cover; background-image: url('https://pbs.twimg.com/profile_images/1392864511669854217/dBymBmGq_400x400.jpg')">
</div>
<div
style="display:none; margin-left: 4px; margin-right: 4px; width: 92px; height:92px; border-radius: 50%; background-size: cover; background-image: url('')">
</div>
<div
style="display:none; margin-left: 4px; margin-right: 4px; width: 92px; height:92px; border-radius: 50%; background-size: cover; background-image: url('')">
</div>
</div>
<div style="text-align: center; margin-top: 3px; font-size: 16px; font-weight: 800">🤖 AI BOT 🤖</div>
<div style="text-align: center; font-size: 16px; font-weight: 800">Discord</div>
<div style="text-align: center; font-size: 14px;">@discord</div>
</div>
I was made with [huggingtweets](https://github.com/borisdayma/huggingtweets).
Create your own bot based on your favorite user with [the demo](https://colab.research.google.com/github/borisdayma/huggingtweets/blob/master/huggingtweets-demo.ipynb)!
## How does it work?
The model uses the following pipeline.
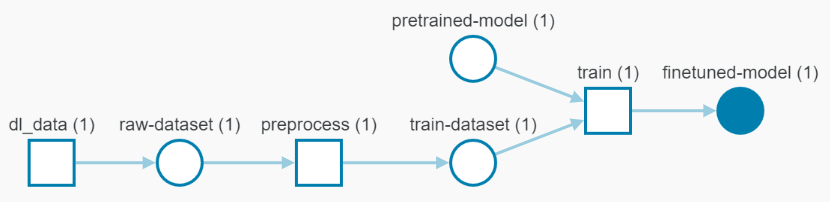
To understand how the model was developed, check the [W&B report](https://wandb.ai/wandb/huggingtweets/reports/HuggingTweets-Train-a-Model-to-Generate-Tweets--VmlldzoxMTY5MjI).
## Training data
The model was trained on tweets from Discord.
| Data | Discord |
| --- | --- |
| Tweets downloaded | 3250 |
| Retweets | 0 |
| Short tweets | 339 |
| Tweets kept | 2911 |
[Explore the data](https://wandb.ai/wandb/huggingtweets/runs/2bdgd0nt/artifacts), which is tracked with [W&B artifacts](https://docs.wandb.com/artifacts) at every step of the pipeline.
## Training procedure
The model is based on a pre-trained [GPT-2](https://huggingface.co/gpt2) which is fine-tuned on @discord's tweets.
Hyperparameters and metrics are recorded in the [W&B training run](https://wandb.ai/wandb/huggingtweets/runs/1uiu02xb) for full transparency and reproducibility.
At the end of training, [the final model](https://wandb.ai/wandb/huggingtweets/runs/1uiu02xb/artifacts) is logged and versioned.
## How to use
You can use this model directly with a pipeline for text generation:
```python
from transformers import pipeline
generator = pipeline('text-generation',
model='huggingtweets/discord')
generator("My dream is", num_return_sequences=5)
```
## Limitations and bias
The model suffers from [the same limitations and bias as GPT-2](https://huggingface.co/gpt2#limitations-and-bias).
In addition, the data present in the user's tweets further affects the text generated by the model.
## About
*Built by Boris Dayma*
[](https://twitter.com/intent/follow?screen_name=borisdayma)
For more details, visit the project repository.
[](https://github.com/borisdayma/huggingtweets)
|
ptran74/DSPFirst-Finetuning-2 | d62e8be820eb384eb0a2e08f862d27e504e32e98 | 2022-04-16T18:55:53.000Z | [
"pytorch",
"electra",
"question-answering",
"transformers",
"generated_from_trainer",
"model-index",
"autotrain_compatible"
] | question-answering | false | ptran74 | null | ptran74/DSPFirst-Finetuning-2 | 0 | null | transformers | 36,938 | ---
tags:
- generated_from_trainer
metrics:
- f1
model-index:
- name: DSPFirst-Finetuning-2
results: []
---
<!-- This model card has been generated automatically according to the information the Trainer had access to. You
should probably proofread and complete it, then remove this comment. -->
# DSPFirst-Finetuning-2
This model is a fine-tuned version of [ahotrod/electra_large_discriminator_squad2_512](https://huggingface.co/ahotrod/electra_large_discriminator_squad2_512) on a generated Questions and Answers dataset from the DSPFirst textbook based on the SQuAD 2.0 format.
It achieves the following results on the evaluation set:
- Loss: 0.8057
- Exact: 65.9378
- F1: 72.3603
# Dataset
A visualization of the dataset can be found [here](https://github.gatech.edu/pages/VIP-ITS/textbook_SQuAD_explore/explore/textbookv1.0/textbook/). The split between train and test is 80% and 20% respectively.
```
DatasetDict({
train: Dataset({
features: ['id', 'title', 'context', 'question', 'answers'],
num_rows: 4755
})
test: Dataset({
features: ['id', 'title', 'context', 'question', 'answers'],
num_rows: 1189
})
})
```
## Model description
More information needed
## Intended uses & limitations
More information needed
## Training and evaluation data
More information needed
## Training procedure
### Training hyperparameters
The following hyperparameters were used during training:
- learning_rate: 2e-05
- train_batch_size: 6
- eval_batch_size: 6
- seed: 42
- gradient_accumulation_steps: 86
- total_train_batch_size: 516
- optimizer: Adam with betas=(0.9,0.999) and epsilon=1e-08
- lr_scheduler_type: linear
- num_epochs: 3
### Model hyperparameters
- hidden_dropout_prob: 0.3
- attention_probs_dropout_prob = 0.3
### Training results
| Training Loss | Epoch | Step | Validation Loss | Exact | F1 |
|:-------------:|:-----:|:----:|:---------------:|:-------:|:-------:|
| 0.8393 | 0.98 | 28 | 0.8157 | 66.1060 | 73.0203 |
| 0.7504 | 1.98 | 56 | 0.7918 | 66.3583 | 72.4657 |
| 0.691 | 2.98 | 84 | 0.8057 | 65.9378 | 72.3603 |
### Framework versions
- Transformers 4.18.0
- Pytorch 1.10.0+cu111
- Datasets 2.1.0
- Tokenizers 0.12.1
|
ptran74/DSPFirst-Finetuning-3 | 736f20bf44c9c379b00641fe041e9051c25be32b | 2022-04-16T19:21:06.000Z | [
"pytorch",
"electra",
"question-answering",
"transformers",
"generated_from_trainer",
"model-index",
"autotrain_compatible"
] | question-answering | false | ptran74 | null | ptran74/DSPFirst-Finetuning-3 | 0 | null | transformers | 36,939 | ---
tags:
- generated_from_trainer
metrics:
- f1
model-index:
- name: DSPFirst-Finetuning-3
results: []
---
<!-- This model card has been generated automatically according to the information the Trainer had access to. You
should probably proofread and complete it, then remove this comment. -->
# DSPFirst-Finetuning-3
This model is a fine-tuned version of [ahotrod/electra_large_discriminator_squad2_512](https://huggingface.co/ahotrod/electra_large_discriminator_squad2_512) on a generated Questions and Answers dataset from the DSPFirst textbook based on the SQuAD 2.0 format.
It achieves the following results on the evaluation set:
- Loss: 0.9996
- Exact: 63.9193
- F1: 72.1090
# Dataset
A visualization of the dataset can be found [here](https://github.gatech.edu/pages/VIP-ITS/textbook_SQuAD_explore/explore/textbookv1.0/textbook/). The split between train and test is 80% and 20% respectively.
```
DatasetDict({
train: Dataset({
features: ['id', 'title', 'context', 'question', 'answers'],
num_rows: 4755
})
test: Dataset({
features: ['id', 'title', 'context', 'question', 'answers'],
num_rows: 1189
})
})
```
## Model description
More information needed
## Intended uses & limitations
More information needed
## Training and evaluation data
More information needed
## Training procedure
### Training hyperparameters
The following hyperparameters were used during training:
- learning_rate: 2e-05
- train_batch_size: 6
- eval_batch_size: 6
- seed: 42
- gradient_accumulation_steps: 86
- total_train_batch_size: 516
- optimizer: Adam with betas=(0.9,0.999) and epsilon=1e-08
- lr_scheduler_type: linear
- num_epochs: 3
### Model hyperparameters
- hidden_dropout_prob: 0.35
- attention_probs_dropout_prob = 0.35
### Training results
| Training Loss | Epoch | Step | Validation Loss | Exact | F1 |
|:-------------:|:-----:|:----:|:---------------:|:-------:|:-------:|
| 1.3511 | 0.99 | 28 | 1.1388 | 62.9941 | 71.4102 |
| 1.0052 | 1.99 | 56 | 1.0255 | 65.0126 | 73.0388 |
| 0.8699 | 2.99 | 84 | 0.9996 | 63.9193 | 72.1090 |
### Framework versions
- Transformers 4.18.0
- Pytorch 1.10.0+cu111
- Datasets 2.1.0
- Tokenizers 0.12.1
|
masakhane/afrimbart_fr_mos_news | 05b90a32bf6a93b6e2c8bbd80a9fdd99e16d9bab | 2022-04-16T20:34:54.000Z | [
"pytorch",
"mbart",
"text2text-generation",
"transformers",
"license:afl-3.0",
"autotrain_compatible"
] | text2text-generation | false | masakhane | null | masakhane/afrimbart_fr_mos_news | 0 | null | transformers | 36,940 | ---
license: afl-3.0
---
|
masakhane/afrimbart_mos_fr_news | 9b671d7fa0dac6c146c2c41bfb2b51580fe2feef | 2022-04-16T20:34:50.000Z | [
"pytorch",
"mbart",
"text2text-generation",
"transformers",
"license:afl-3.0",
"autotrain_compatible"
] | text2text-generation | false | masakhane | null | masakhane/afrimbart_mos_fr_news | 0 | null | transformers | 36,941 | ---
license: afl-3.0
---
|
masakhane/mt5_mos_fr_news | aa19b924bcd1687866ac053b52b725f8baa0f22c | 2022-04-16T20:35:00.000Z | [
"pytorch",
"mt5",
"text2text-generation",
"transformers",
"license:afl-3.0",
"autotrain_compatible"
] | text2text-generation | false | masakhane | null | masakhane/mt5_mos_fr_news | 0 | null | transformers | 36,942 | ---
license: afl-3.0
---
|
masakhane/mt5_fr_mos_news | f99f3e273a04aa6f989774e0990a31ced78d610a | 2022-04-16T20:35:05.000Z | [
"pytorch",
"mt5",
"text2text-generation",
"transformers",
"license:afl-3.0",
"autotrain_compatible"
] | text2text-generation | false | masakhane | null | masakhane/mt5_fr_mos_news | 0 | null | transformers | 36,943 | ---
license: afl-3.0
---
|
masakhane/afribyt5_mos_fr_news | 66e39ce26556b683de61875e291e001742ca9ac0 | 2022-04-16T21:39:20.000Z | [
"pytorch",
"t5",
"text2text-generation",
"transformers",
"license:afl-3.0",
"autotrain_compatible"
] | text2text-generation | false | masakhane | null | masakhane/afribyt5_mos_fr_news | 0 | null | transformers | 36,944 | ---
license: afl-3.0
---
|
masakhane/afribyt5_fr_mos_news | df8269c510a14eb3d9e4a2dbaee4b04ee2ab1a54 | 2022-04-16T21:39:32.000Z | [
"pytorch",
"t5",
"text2text-generation",
"transformers",
"license:afl-3.0",
"autotrain_compatible"
] | text2text-generation | false | masakhane | null | masakhane/afribyt5_fr_mos_news | 0 | null | transformers | 36,945 | ---
license: afl-3.0
---
|
masakhane/byt5_mos_fr_news | 02892b5996841854b3a2285b3b731855f5f72695 | 2022-04-16T21:39:15.000Z | [
"pytorch",
"t5",
"text2text-generation",
"transformers",
"license:afl-3.0",
"autotrain_compatible"
] | text2text-generation | false | masakhane | null | masakhane/byt5_mos_fr_news | 0 | null | transformers | 36,946 | ---
license: afl-3.0
---
|
masakhane/byt5_fr_mos_news | 811002c0649be5660cd37803441890a32c34643f | 2022-04-16T21:39:25.000Z | [
"pytorch",
"t5",
"text2text-generation",
"transformers",
"license:afl-3.0",
"autotrain_compatible"
] | text2text-generation | false | masakhane | null | masakhane/byt5_fr_mos_news | 0 | null | transformers | 36,947 | ---
license: afl-3.0
---
|
masakhane/mbart50_fr_mos_news | 2e6cfc68eb285cb74e3258eefaecaad2ec05d92c | 2022-04-17T06:42:37.000Z | [
"pytorch",
"mbart",
"text2text-generation",
"transformers",
"license:afl-3.0",
"autotrain_compatible"
] | text2text-generation | false | masakhane | null | masakhane/mbart50_fr_mos_news | 0 | null | transformers | 36,948 | ---
license: afl-3.0
---
|
masakhane/mbart50_mos_fr_news | 8052bfa3b4cc67d9a65002717d119a63f3be2eee | 2022-04-17T06:42:26.000Z | [
"pytorch",
"mbart",
"text2text-generation",
"transformers",
"license:afl-3.0",
"autotrain_compatible"
] | text2text-generation | false | masakhane | null | masakhane/mbart50_mos_fr_news | 0 | null | transformers | 36,949 | ---
license: afl-3.0
---
|
masakhane/afrimt5_mos_fr_news | 92c872a73d3f65502c1cd9f452e97e557affd788 | 2022-04-17T06:42:32.000Z | [
"pytorch",
"mt5",
"text2text-generation",
"transformers",
"license:afl-3.0",
"autotrain_compatible"
] | text2text-generation | false | masakhane | null | masakhane/afrimt5_mos_fr_news | 0 | null | transformers | 36,950 | ---
license: afl-3.0
---
|
tmills/tiny-dtr | 315a31ab879a9237e73f04acffeab3232aac3f79 | 2022-04-16T22:57:05.000Z | [
"pytorch",
"cnlpt",
"transformers",
"license:apache-2.0"
] | null | false | tmills | null | tmills/tiny-dtr | 0 | null | transformers | 36,951 | ---
license: apache-2.0
---
|
tmabraham/upit-cyclegan-test | 2331f7d345d719ac1fdfb10b2cddf58abd7931bb | 2022-04-17T00:39:08.000Z | [
"pytorch"
] | null | false | tmabraham | null | tmabraham/upit-cyclegan-test | 0 | null | null | 36,952 | Entry not found |
tmabraham/upit-dualgan-test | f8d92db7854429ca64335e9ab698d7e7f2f44feb | 2022-04-17T00:55:32.000Z | [
"pytorch"
] | null | false | tmabraham | null | tmabraham/upit-dualgan-test | 0 | null | null | 36,953 | Entry not found |
tmabraham/upit-ganilla-test | 38cafb3d4cca069313b8ed03d88ecc88db28f3d5 | 2022-04-17T01:23:27.000Z | [
"pytorch"
] | null | false | tmabraham | null | tmabraham/upit-ganilla-test | 0 | null | null | 36,954 | Entry not found |
rmihaylov/bert-base-squad-theseus-bg | e34ac33ae80b20ebfbc61c7aaa171185bb84d342 | 2022-04-17T03:47:35.000Z | [
"pytorch",
"bert",
"question-answering",
"bg",
"dataset:oscar",
"dataset:chitanka",
"dataset:wikipedia",
"arxiv:1810.04805",
"arxiv:2002.02925",
"transformers",
"torch",
"license:mit",
"autotrain_compatible"
] | question-answering | false | rmihaylov | null | rmihaylov/bert-base-squad-theseus-bg | 0 | null | transformers | 36,955 | ---
inference: false
language:
- bg
license: mit
datasets:
- oscar
- chitanka
- wikipedia
tags:
- torch
---
# BERT BASE (cased) finetuned on Bulgarian squad data
Pretrained model on Bulgarian language using a masked language modeling (MLM) objective. It was introduced in
[this paper](https://arxiv.org/abs/1810.04805) and first released in
[this repository](https://github.com/google-research/bert). This model is cased: it does make a difference
between bulgarian and Bulgarian. The training data is Bulgarian text from [OSCAR](https://oscar-corpus.com/post/oscar-2019/), [Chitanka](https://chitanka.info/) and [Wikipedia](https://bg.wikipedia.org/).
It was finetuned on private squad Bulgarian data.
Then, it was compressed via [progressive module replacing](https://arxiv.org/abs/2002.02925).
### How to use
Here is how to use this model in PyTorch:
```python
>>> from transformers import pipeline
>>>
>>> model = pipeline(
>>> 'question-answering',
>>> model='rmihaylov/bert-base-squad-theseus-bg',
>>> tokenizer='rmihaylov/bert-base-squad-theseus-bg',
>>> device=0,
>>> revision=None)
>>>
>>> question = "С какво се проследява пандемията?"
>>> context = "Епидемията гасне, обяви при обявяването на данните тази сутрин Тодор Кантарджиев, член на Националния оперативен щаб. Той направи този извод на база на данните от математическите модели, с които се проследява развитието на заразата. Те показват, че т. нар. ефективно репродуктивно число е вече в границите 0.6-1. Тоест, 10 души заразяват 8, те на свой ред 6 и така нататък. "
>>> output = model(**{'question': question, 'context': context})
>>> print(output)
{'score': 0.85157310962677, 'start': 162, 'end': 186, 'answer': ' математическите модели,'}
```
|
ptran74/DSPFirst-Finetuning-4 | a4b1bc7dbf7d8780a1f3fc24badda650813cb49c | 2022-04-18T03:50:36.000Z | [
"pytorch",
"electra",
"question-answering",
"transformers",
"generated_from_trainer",
"model-index",
"autotrain_compatible"
] | question-answering | false | ptran74 | null | ptran74/DSPFirst-Finetuning-4 | 0 | null | transformers | 36,956 | ---
tags:
- generated_from_trainer
metrics:
- f1
model-index:
- name: DSPFirst-Finetuning-4
results: []
---
<!-- This model card has been generated automatically according to the information the Trainer had access to. You
should probably proofread and complete it, then remove this comment. -->
# Important Note:
`load_best_model_at_end` is not working properly (I specified `metric_for_best_model` on another training but it still does not work), but the training results still show a valid trend.
# DSPFirst-Finetuning-4
This model is a fine-tuned version of [ahotrod/electra_large_discriminator_squad2_512](https://huggingface.co/ahotrod/electra_large_discriminator_squad2_512) on a generated Questions and Answers dataset from the DSPFirst textbook based on the SQuAD 2.0 format.<br />
It achieves the following results on the evaluation set:
- Loss: 0.9028
- Exact: 66.9843
- F1: 74.2286
## More accurate metrics:
### Before fine-tuning:
```
"exact": 57.006726457399104,
"f1": 61.997705120754276
```
### After fine-tuning:
```
"exact": 66.98430493273543,
"f1": 74.2285867775556
```
# Dataset
A visualization of the dataset can be found [here](https://github.gatech.edu/pages/VIP-ITS/textbook_SQuAD_explore/explore/textbookv1.0/textbook/).<br />
The split between train and test is 70% and 30% respectively.
```
DatasetDict({
train: Dataset({
features: ['id', 'title', 'context', 'question', 'answers'],
num_rows: 4160
})
test: Dataset({
features: ['id', 'title', 'context', 'question', 'answers'],
num_rows: 1784
})
})
```
## Intended uses & limitations
This model is fine-tuned to answer questions from the DSPFirst textbook. I'm not really sure what I am doing so you should review before using it.<br />
Also, you should improve the Dataset either by using a **better generated questions and answers model** (currently using https://github.com/patil-suraj/question_generation) or perform **data augmentation** to increase dataset size.
## Training and evaluation data
- `batch_size` of 6 results in 14.82 GB VRAM
- Utilizes `gradient_accumulation_steps` to get total batch size to 514 (batch size should be at least 256)
- 4.52 GB RAM
- 30% of the total questions is dedicated for evaluating.
## Training procedure
- The model was trained from [Google Colab](https://colab.research.google.com/drive/1dJXNstk2NSenwzdtl9xA8AqjP4LL-Ks_?usp=sharing)
- Utilizes Tesla P100 16GB, took 6.3 hours to train
- `load_best_model_at_end` is enabled in TrainingArguments
### Training hyperparameters
The following hyperparameters were used during training:
- learning_rate: 2e-05
- train_batch_size: 6
- eval_batch_size: 6
- seed: 42
- gradient_accumulation_steps: 86
- total_train_batch_size: 516
- optimizer: Adam with betas=(0.9,0.999) and epsilon=1e-08
- lr_scheduler_type: linear
- num_epochs: 10
### Model hyperparameters
- hidden_dropout_prob: 0.36
- attention_probs_dropout_prob = 0.36
### Training results
| Training Loss | Epoch | Step | Validation Loss | Exact | F1 |
|:-------------:|:-----:|:----:|:---------------:|:-------:|:-------:|
| 2.4411 | 0.81 | 20 | 1.4556 | 62.0516 | 71.1082 |
| 2.2027 | 1.64 | 40 | 1.1508 | 65.0224 | 73.8669 |
| 1.2827 | 2.48 | 60 | 1.0030 | 65.8632 | 74.3959 |
| 1.0925 | 3.32 | 80 | 1.0155 | 66.8722 | 75.2204 |
| 1.03 | 4.16 | 100 | 0.8863 | 66.1996 | 73.8166 |
| 0.9085 | 4.97 | 120 | 0.9675 | 67.9372 | 75.7764 |
| 0.8968 | 5.81 | 140 | 0.8635 | 67.2085 | 74.3725 |
| 0.8867 | 6.64 | 160 | 0.9035 | 65.9753 | 73.4569 |
| 0.8456 | 7.48 | 180 | 0.9098 | 67.2085 | 74.6798 |
| 0.8506 | 8.32 | 200 | 0.8807 | 66.6480 | 74.2903 |
| 0.7972 | 9.16 | 220 | 0.8711 | 66.6480 | 73.5801 |
| 0.7795 | 9.97 | 240 | 0.9028 | 66.9843 | 74.2286 |
### Framework versions
- Transformers 4.18.0
- Pytorch 1.10.0+cu111
- Datasets 2.1.0
- Tokenizers 0.12.1
|
horsbug98/Part_1_mBERT_Model_E2 | 13a0bed91cc53a5c8bb04c26f6e5d0c5f595a5a8 | 2022-04-17T08:23:27.000Z | [
"pytorch",
"bert",
"question-answering",
"transformers",
"autotrain_compatible"
] | question-answering | false | horsbug98 | null | horsbug98/Part_1_mBERT_Model_E2 | 0 | null | transformers | 36,957 | Entry not found |
masakhane/m2m100_418M_fr_mos_rel_news_ft | 87677e87b4050f8891b2b9b48e236910e4e5da51 | 2022-04-17T08:16:02.000Z | [
"pytorch",
"m2m_100",
"text2text-generation",
"transformers",
"license:afl-3.0",
"autotrain_compatible"
] | text2text-generation | false | masakhane | null | masakhane/m2m100_418M_fr_mos_rel_news_ft | 0 | null | transformers | 36,958 | ---
license: afl-3.0
---
|
masakhane/m2m100_418M_fr_mos_rel_ft | 869c88143fd1eb8f27d07b37a0d4179cccbc4d4f | 2022-04-17T10:54:59.000Z | [
"pytorch",
"m2m_100",
"text2text-generation",
"transformers",
"license:afl-3.0",
"autotrain_compatible"
] | text2text-generation | false | masakhane | null | masakhane/m2m100_418M_fr_mos_rel_ft | 0 | null | transformers | 36,959 | ---
license: afl-3.0
---
|
masakhane/m2m100_418M_mos_fr_rel | 2f05594856fe283c91c067f7864e08069cd4a161 | 2022-04-17T10:55:03.000Z | [
"pytorch",
"m2m_100",
"text2text-generation",
"transformers",
"license:afl-3.0",
"autotrain_compatible"
] | text2text-generation | false | masakhane | null | masakhane/m2m100_418M_mos_fr_rel | 0 | null | transformers | 36,960 | ---
license: afl-3.0
---
|
masakhane/m2m100_418M_fr_mos_rel | 06607134ffe76c1bff9807f92db9eb47a8df8760 | 2022-04-17T10:54:46.000Z | [
"pytorch",
"m2m_100",
"text2text-generation",
"transformers",
"license:afl-3.0",
"autotrain_compatible"
] | text2text-generation | false | masakhane | null | masakhane/m2m100_418M_fr_mos_rel | 0 | null | transformers | 36,961 | ---
license: afl-3.0
---
|
huggan/distill-ccld-wa | 7396a83533fbd58609aa3c5d67b3c4618804fc19 | 2022-04-18T20:40:25.000Z | [
"dataset:huggan/wikiart",
"arxiv:2112.10752",
"pytorch",
"huggan",
"diffusion",
"text-to-image",
"license:mit"
] | text-to-image | false | huggan | null | huggan/distill-ccld-wa | 0 | 2 | pytorch | 36,962 | ---
library_name : pytorch
tags:
- huggan
- diffusion
- text-to-image
datasets:
- huggan/wikiart
task: conditional-image-generation
license: mit
---
# Distill CLOOB-conditioned Latent Diffusion trained on WikiArt
## Model description
This is a smaller version of [this model](https://huggingface.co/huggan/ccld_wa), which is a cloob-conditioned latent diffusion model fine-tuned on the [WikiArt dataset](https://huggingface.co/datasets/huggan/wikiart), reducing the latent diffusion model size from 1.2B parameters to 105M parameters with a knowledge distillation method.
[CLOOB](https://ml-jku.github.io/cloob/) is a model that encodes images and texts in an unified latent space, similar to what OpenAI's CLIP does. The latent diffusion model takes a CLOOB-encoded latent vector as a condition, this can be from a pompt or an image.
## Intended uses & limitations
The latent diffusion model is the only difference with [the teacher model](https://huggingface.co/huggan/ccld_wa), the autoencoder was not changed, nor the CLOOB model. So these are not provided in this repository.
model_student.ckpt: The latent diffusion model checkpoint
#### How to use
You need some dependancies from multiple repositories linked in this repository : [CLOOB latent diffusion](https://github.com/JD-P/cloob-latent-diffusion) :
* [CLIP](https://github.com/openai/CLIP/tree/40f5484c1c74edd83cb9cf687c6ab92b28d8b656)
* [CLOOB](https://github.com/crowsonkb/cloob-training/tree/136ca7dd69a03eeb6ad525da991d5d7083e44055) : the model to encode images and texts in an unified latent space, used for conditioning the latent diffusion.
* [Latent Diffusion](https://github.com/CompVis/latent-diffusion/tree/f13bf9bf463d95b5a16aeadd2b02abde31f769f8) : latent diffusion model definition
* [Taming transformers](https://github.com/CompVis/taming-transformers/tree/24268930bf1dce879235a7fddd0b2355b84d7ea6) : a pretrained convolutional VQGAN is used as an autoencoder to go from image space to the latent space in which the diffusion is done.
* [v-diffusion](https://github.com/crowsonkb/v-diffusion-pytorch/tree/ffabbb1a897541fa2a3d034f397c224489d97b39) : contains some functions for sampling using a diffusion model with text and/or image prompts.
An example code to use the model to sample images from a text prompt can be seen in a [Colab Notebook](https://colab.research.google.com/drive/1XGHdO8IAGajnpb-x4aOb-OMYfZf0WDTi?usp=sharing), or directly in the [app source code](https://huggingface.co/spaces/huggan/wikiart-diffusion-mini/blob/main/app.py) for the Gradio demo on [this Space](https://huggingface.co/spaces/huggan/wikiart-diffusion-mini)
#### Limitations and bias
The student latent diffusion model was trained only on images from the WikiArt dataset, but the VQGAN autoencoder, the CLOOB model and the teacher latent diffusion model all come from pretrained checkpoints and were trained on images and texts from the internet.
According to the [Latent Diffusion paper](https://arxiv.org/abs/2112.10752): “Deep learning modules tend to reproduce or exacerbate biases that are already present in the data”.
## Training data
This model was trained on the [WikiArt dataset](https://huggingface.co/datasets/huggan/wikiart) only. Only the images were used during training, no text prompt, so we did not use the information of style/genre/artist.
## Training procedure
This latent diffusion model was trained with a Knowledge Distillation process with [huggan/ccld_wa](https://huggingface.co/huggan/ccld_wa) as a teacher model. Training of the teacher model largely followed the guidelines in [JD-P's github repo](https://github.com/JD-P/cloob-latent-diffusion).
The model was fine-tuned on the Wikiart dataset for ~12 hours on 2 A6000 GPUs kindly provided by Paperspace. The knowledge distillation process was done on the WikiArt dataset as well. The training of the student model took 17 hours on 1 A6000 GPU provided by Paperspace. [Here](https://wandb.ai/gigant/distill-ccld/reports/Distill-Diffusion-105M--VmlldzoxODQwMTUz) is the `wandb` report for this training.
### Links
* [Model card for the teacher model on HuggingFace](https://huggingface.co/huggan/ccld_wa), trained by Jonathan Whitaker. He described the model and training procedure on his [blog post](https://datasciencecastnet.home.blog/2022/04/12/fine-tuning-a-cloob-conditioned-latent-diffusion-model-on-wikiart/)
* [Model card for the student model on HuggingFace](https://huggingface.co/huggan/distill-ccld-wa), trained by me. You can check my [WandB report](https://wandb.ai/gigant/distill-ccld/reports/Distill-Diffusion-105M--VmlldzoxODQwMTUz?accessToken=mfbrz1ghfakmh01lybsuycwm3qj3isv60uynnvmina3tiwz5e5ufbjui5xqhmaqi). This version has 105M parameters, against 1.2B parameters for the teacher version. It is lighter, and allows for faster inference, while maintaining some of the original model capability at generating paintings from prompts.
* [Gradio demo app on HuggingFace's Spaces](https://huggingface.co/spaces/huggan/wikiart-diffusion-mini) to try out the model with an online demo app
* [iPython Notebook](https://github.com/giganttheo/distill-ccld/blob/master/distillCCLD_(Wikiart)_demo.ipynb) to use the model in Python
* [WikiArt dataset on `datasets` hub](https://huggingface.co/datasets/huggan/wikiart)
* [GitHub repository](https://github.com/giganttheo/distill-ccld)
|
masakhane/m2m100_418M_mos_fr_rel_news | 4145c96d6bc8d84a9fe8eb2c58a44f9a62cc29c1 | 2022-04-17T11:50:07.000Z | [
"pytorch",
"m2m_100",
"text2text-generation",
"transformers",
"license:afl-3.0",
"autotrain_compatible"
] | text2text-generation | false | masakhane | null | masakhane/m2m100_418M_mos_fr_rel_news | 0 | null | transformers | 36,963 | ---
license: afl-3.0
---
|
jcai1/dummy-model | c6adbcc918dd53054ca25e564f2013a2fcdb590d | 2022-04-17T12:47:45.000Z | [
"pytorch",
"camembert",
"fill-mask",
"transformers",
"autotrain_compatible"
] | fill-mask | false | jcai1 | null | jcai1/dummy-model | 0 | null | transformers | 36,964 | Entry not found |
npleshkanov/rutoxicity-classification | 7307bf777fff87d8f19dc4294fa09539aedefd64 | 2022-04-17T15:33:06.000Z | [
"pytorch",
"tensorboard",
"bert",
"transformers",
"generated_from_trainer",
"model-index"
] | null | false | npleshkanov | null | npleshkanov/rutoxicity-classification | 0 | null | transformers | 36,965 | ---
tags:
- generated_from_trainer
model-index:
- name: rutoxicity-classification
results: []
---
<!-- This model card has been generated automatically according to the information the Trainer had access to. You
should probably proofread and complete it, then remove this comment. -->
# rutoxicity-classification
This model is a fine-tuned version of [DeepPavlov/rubert-base-cased](https://huggingface.co/DeepPavlov/rubert-base-cased) on the [Russian Language Toxic Comments](https://www.kaggle.com/datasets/blackmoon/russian-language-toxic-comments) dataset.
It achieves the following results on the evaluation set:
- Loss: 0.2747
- Acc: 0.9255
## Model description
More information needed
## Intended uses & limitations
More information needed
## Training and evaluation data
More information needed
## Training procedure
### Training hyperparameters
The following hyperparameters were used during training:
- learning_rate: 0.0001
- train_batch_size: 32
- eval_batch_size: 32
- seed: 42
- optimizer: Adam with betas=(0.9,0.999) and epsilon=1e-08
- lr_scheduler_type: linear
- num_epochs: 6
### Training results
### Framework versions
- Transformers 4.17.0
- Pytorch 1.10.0+cu111
- Datasets 2.1.0
- Tokenizers 0.12.1
|
huggingtweets/shaq-shaqtin | c916305fc83794db7c9d96c13f78bd919211ffe4 | 2022-04-17T15:51:33.000Z | [
"pytorch",
"gpt2",
"text-generation",
"en",
"transformers",
"huggingtweets"
] | text-generation | false | huggingtweets | null | huggingtweets/shaq-shaqtin | 0 | null | transformers | 36,966 | ---
language: en
thumbnail: http://www.huggingtweets.com/shaq-shaqtin/1650210626298/predictions.png
tags:
- huggingtweets
widget:
- text: "My dream is"
---
<div class="inline-flex flex-col" style="line-height: 1.5;">
<div class="flex">
<div
style="display:inherit; margin-left: 4px; margin-right: 4px; width: 92px; height:92px; border-radius: 50%; background-size: cover; background-image: url('https://pbs.twimg.com/profile_images/1057348595664519168/ZtEKO7oN_400x400.jpg')">
</div>
<div
style="display:inherit; margin-left: 4px; margin-right: 4px; width: 92px; height:92px; border-radius: 50%; background-size: cover; background-image: url('https://pbs.twimg.com/profile_images/1500966287907901440/PhiJ-9-4_400x400.jpg')">
</div>
<div
style="display:none; margin-left: 4px; margin-right: 4px; width: 92px; height:92px; border-radius: 50%; background-size: cover; background-image: url('')">
</div>
</div>
<div style="text-align: center; margin-top: 3px; font-size: 16px; font-weight: 800">🤖 AI CYBORG 🤖</div>
<div style="text-align: center; font-size: 16px; font-weight: 800">Shaqtin' a Fool & SHAQ.SOL</div>
<div style="text-align: center; font-size: 14px;">@shaq-shaqtin</div>
</div>
I was made with [huggingtweets](https://github.com/borisdayma/huggingtweets).
Create your own bot based on your favorite user with [the demo](https://colab.research.google.com/github/borisdayma/huggingtweets/blob/master/huggingtweets-demo.ipynb)!
## How does it work?
The model uses the following pipeline.
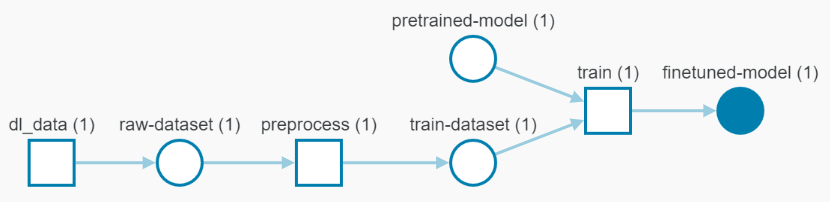
To understand how the model was developed, check the [W&B report](https://wandb.ai/wandb/huggingtweets/reports/HuggingTweets-Train-a-Model-to-Generate-Tweets--VmlldzoxMTY5MjI).
## Training data
The model was trained on tweets from Shaqtin' a Fool & SHAQ.SOL.
| Data | Shaqtin' a Fool | SHAQ.SOL |
| --- | --- | --- |
| Tweets downloaded | 1507 | 3225 |
| Retweets | 90 | 698 |
| Short tweets | 28 | 171 |
| Tweets kept | 1389 | 2356 |
[Explore the data](https://wandb.ai/wandb/huggingtweets/runs/2rixdtoa/artifacts), which is tracked with [W&B artifacts](https://docs.wandb.com/artifacts) at every step of the pipeline.
## Training procedure
The model is based on a pre-trained [GPT-2](https://huggingface.co/gpt2) which is fine-tuned on @shaq-shaqtin's tweets.
Hyperparameters and metrics are recorded in the [W&B training run](https://wandb.ai/wandb/huggingtweets/runs/vsy727xr) for full transparency and reproducibility.
At the end of training, [the final model](https://wandb.ai/wandb/huggingtweets/runs/vsy727xr/artifacts) is logged and versioned.
## How to use
You can use this model directly with a pipeline for text generation:
```python
from transformers import pipeline
generator = pipeline('text-generation',
model='huggingtweets/shaq-shaqtin')
generator("My dream is", num_return_sequences=5)
```
## Limitations and bias
The model suffers from [the same limitations and bias as GPT-2](https://huggingface.co/gpt2#limitations-and-bias).
In addition, the data present in the user's tweets further affects the text generated by the model.
## About
*Built by Boris Dayma*
[](https://twitter.com/intent/follow?screen_name=borisdayma)
For more details, visit the project repository.
[](https://github.com/borisdayma/huggingtweets)
|
huggan/pggan-celebahq-1024 | c4b40d261693bab540647232c963dc1307a6d5c8 | 2022-04-29T11:58:41.000Z | [
"pytorch",
"gan",
"pggan",
"huggan",
"unconditional-image-generation",
"license:apache-2.0"
] | unconditional-image-generation | false | huggan | null | huggan/pggan-celebahq-1024 | 0 | null | null | 36,967 | ---
license: apache-2.0
tags:
- gan
- pggan
- huggan
- unconditional-image-generation
---
The model provided is a PGGAN generator trained on the celebahq dataset with a resolution of 1024px. It is uploaded as part of porting this project: https://github.com/genforce/sefa to hugginface spaces. |
huggan/stylegan_animeface512 | a32c3431e70a95ac172f8081449b5a7d53b19c14 | 2022-04-29T11:59:41.000Z | [
"pytorch",
"gan",
"stylegan",
"huggan",
"unconditional-image-generation",
"license:apache-2.0"
] | unconditional-image-generation | false | huggan | null | huggan/stylegan_animeface512 | 0 | null | null | 36,968 | ---
license: apache-2.0
tags:
- gan
- stylegan
- huggan
- unconditional-image-generation
---
The model provided is a StyleGAN generator trained on Anime faces with a resolution of 512px. It is uploaded as part of porting this project: https://github.com/genforce/sefa to hugginface spaces. |
harshm16/t5-small-finetuned-reddit_dataset | ab79f1c1dbd8df81de57ddbe2fcc2feda503066b | 2022-04-25T21:20:03.000Z | [
"pytorch",
"t5",
"text2text-generation",
"transformers",
"autotrain_compatible"
] | text2text-generation | false | harshm16 | null | harshm16/t5-small-finetuned-reddit_dataset | 0 | null | transformers | 36,969 | Entry not found |
huggingtweets/tojibawhiteroom | 9581d3d019525c3fd5dc4512ea798ffc5b0e231d | 2022-04-18T04:33:44.000Z | [
"pytorch",
"gpt2",
"text-generation",
"en",
"transformers",
"huggingtweets"
] | text-generation | false | huggingtweets | null | huggingtweets/tojibawhiteroom | 0 | null | transformers | 36,970 | ---
language: en
thumbnail: http://www.huggingtweets.com/tojibawhiteroom/1650256419756/predictions.png
tags:
- huggingtweets
widget:
- text: "My dream is"
---
<div class="inline-flex flex-col" style="line-height: 1.5;">
<div class="flex">
<div
style="display:inherit; margin-left: 4px; margin-right: 4px; width: 92px; height:92px; border-radius: 50%; background-size: cover; background-image: url('https://pbs.twimg.com/profile_images/1509337156787003394/WjOdf_-m_400x400.jpg')">
</div>
<div
style="display:none; margin-left: 4px; margin-right: 4px; width: 92px; height:92px; border-radius: 50%; background-size: cover; background-image: url('')">
</div>
<div
style="display:none; margin-left: 4px; margin-right: 4px; width: 92px; height:92px; border-radius: 50%; background-size: cover; background-image: url('')">
</div>
</div>
<div style="text-align: center; margin-top: 3px; font-size: 16px; font-weight: 800">🤖 AI BOT 🤖</div>
<div style="text-align: center; font-size: 16px; font-weight: 800">Tojiba White Room (T__T).1</div>
<div style="text-align: center; font-size: 14px;">@tojibawhiteroom</div>
</div>
I was made with [huggingtweets](https://github.com/borisdayma/huggingtweets).
Create your own bot based on your favorite user with [the demo](https://colab.research.google.com/github/borisdayma/huggingtweets/blob/master/huggingtweets-demo.ipynb)!
## How does it work?
The model uses the following pipeline.
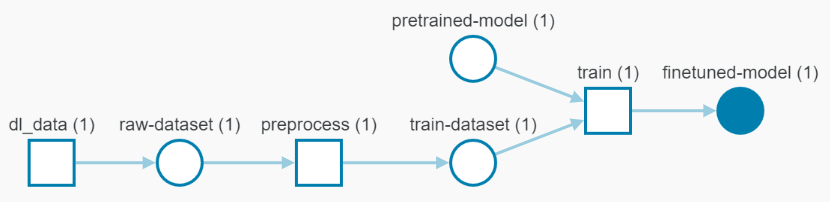
To understand how the model was developed, check the [W&B report](https://wandb.ai/wandb/huggingtweets/reports/HuggingTweets-Train-a-Model-to-Generate-Tweets--VmlldzoxMTY5MjI).
## Training data
The model was trained on tweets from Tojiba White Room (T__T).1.
| Data | Tojiba White Room (T__T).1 |
| --- | --- |
| Tweets downloaded | 212 |
| Retweets | 0 |
| Short tweets | 26 |
| Tweets kept | 186 |
[Explore the data](https://wandb.ai/wandb/huggingtweets/runs/1okoxv9l/artifacts), which is tracked with [W&B artifacts](https://docs.wandb.com/artifacts) at every step of the pipeline.
## Training procedure
The model is based on a pre-trained [GPT-2](https://huggingface.co/gpt2) which is fine-tuned on @tojibawhiteroom's tweets.
Hyperparameters and metrics are recorded in the [W&B training run](https://wandb.ai/wandb/huggingtweets/runs/1jqxicud) for full transparency and reproducibility.
At the end of training, [the final model](https://wandb.ai/wandb/huggingtweets/runs/1jqxicud/artifacts) is logged and versioned.
## How to use
You can use this model directly with a pipeline for text generation:
```python
from transformers import pipeline
generator = pipeline('text-generation',
model='huggingtweets/tojibawhiteroom')
generator("My dream is", num_return_sequences=5)
```
## Limitations and bias
The model suffers from [the same limitations and bias as GPT-2](https://huggingface.co/gpt2#limitations-and-bias).
In addition, the data present in the user's tweets further affects the text generated by the model.
## About
*Built by Boris Dayma*
[](https://twitter.com/intent/follow?screen_name=borisdayma)
For more details, visit the project repository.
[](https://github.com/borisdayma/huggingtweets)
|
tau/false_large_pmi_para0_sent1_span2_True_multi_masks_with_types_7_1024_0.3_epoch1 | 3161a61c6389dd36a78b9f07aef31a0cb40c7855 | 2022-04-18T05:48:55.000Z | [
"pytorch",
"t5",
"text2text-generation",
"transformers",
"autotrain_compatible"
] | text2text-generation | false | tau | null | tau/false_large_pmi_para0_sent1_span2_True_multi_masks_with_types_7_1024_0.3_epoch1 | 0 | null | transformers | 36,971 | Entry not found |
tau/false_large_pmi_para0_sent1_span2_True_7_1024_0.3_epoch1 | 2f232cda57aea809aedbebb707d40c1c915eec01 | 2022-04-18T05:58:41.000Z | [
"pytorch",
"t5",
"text2text-generation",
"transformers",
"autotrain_compatible"
] | text2text-generation | false | tau | null | tau/false_large_pmi_para0_sent1_span2_True_7_1024_0.3_epoch1 | 0 | null | transformers | 36,972 | Entry not found |
tau/false_large_rouge_para0_sent1_span2_True_7_1024_0.3_epoch1 | ef24e6d623c570261fe59725f953be8c03b67167 | 2022-04-18T06:19:39.000Z | [
"pytorch",
"t5",
"text2text-generation",
"transformers",
"autotrain_compatible"
] | text2text-generation | false | tau | null | tau/false_large_rouge_para0_sent1_span2_True_7_1024_0.3_epoch1 | 0 | null | transformers | 36,973 | Entry not found |
scasutt/wav2vec2-large-xlsr-53_toy_train_fast_masked_low_pass_audio | e99fbea225f3a4e4af5e90a21bf41a9cd2f5092a | 2022-04-18T12:13:25.000Z | [
"pytorch",
"wav2vec2",
"automatic-speech-recognition",
"transformers"
] | automatic-speech-recognition | false | scasutt | null | scasutt/wav2vec2-large-xlsr-53_toy_train_fast_masked_low_pass_audio | 0 | null | transformers | 36,974 | Entry not found |
shishirpaudel/wav2vec2-large-xlsr-nepali | 156a35c5e016d09096ea6c74084aba71ec0cdc02 | 2022-04-18T10:44:08.000Z | [
"pytorch",
"tensorboard",
"wav2vec2",
"automatic-speech-recognition",
"transformers",
"generated_from_trainer",
"license:apache-2.0",
"model-index"
] | automatic-speech-recognition | false | shishirpaudel | null | shishirpaudel/wav2vec2-large-xlsr-nepali | 0 | null | transformers | 36,975 | ---
license: apache-2.0
tags:
- generated_from_trainer
model-index:
- name: wav2vec2-large-xlsr-nepali
results: []
---
<!-- This model card has been generated automatically according to the information the Trainer had access to. You
should probably proofread and complete it, then remove this comment. -->
# wav2vec2-large-xlsr-nepali
This model is a fine-tuned version of [facebook/wav2vec2-large-xlsr-53](https://huggingface.co/facebook/wav2vec2-large-xlsr-53) on the None dataset.
## Model description
More information needed
## Intended uses & limitations
More information needed
## Training and evaluation data
More information needed
## Training procedure
### Training hyperparameters
The following hyperparameters were used during training:
- learning_rate: 0.0003
- train_batch_size: 16
- eval_batch_size: 8
- seed: 42
- gradient_accumulation_steps: 2
- total_train_batch_size: 32
- optimizer: Adam with betas=(0.9,0.999) and epsilon=1e-08
- lr_scheduler_type: linear
- lr_scheduler_warmup_steps: 250
- num_epochs: 2
### Training results
### Framework versions
- Transformers 4.18.0
- Pytorch 1.10.0+cu111
- Datasets 1.18.3
- Tokenizers 0.12.1
|
tmabraham/horse2zebra_cyclegan | a97667461b0f57d35692c6210dd747e7e90fa056 | 2022-04-18T12:46:51.000Z | [
"pytorch"
] | null | false | tmabraham | null | tmabraham/horse2zebra_cyclegan | 0 | null | null | 36,976 | Entry not found |
ptran74/DSPFirst-Finetuning-5 | 0b2aacc0f1d8078e50b1cf7c8ae842621bf5b790 | 2022-04-19T01:14:26.000Z | [
"pytorch",
"electra",
"question-answering",
"transformers",
"generated_from_trainer",
"model-index",
"autotrain_compatible"
] | question-answering | false | ptran74 | null | ptran74/DSPFirst-Finetuning-5 | 0 | null | transformers | 36,977 | ---
tags:
- generated_from_trainer
metrics:
- f1
model-index:
- name: DSPFirst-Finetuning-5
results: []
---
<!-- This model card has been generated automatically according to the information the Trainer had access to. You
should probably proofread and complete it, then remove this comment. -->
# Important Note:
I created the `combined` metric (55% F1 score + 45% exact match score) and load the state with the best result at the end. Here is the setting in the `TrainingArguments`:
```
load_best_model_at_end=True,
metric_for_best_model='combined',
greater_is_better=True,
```
# DSPFirst-Finetuning-5
This model is a fine-tuned version of [ahotrod/electra_large_discriminator_squad2_512](https://huggingface.co/ahotrod/electra_large_discriminator_squad2_512) on a generated Questions and Answers dataset from the DSPFirst textbook based on the SQuAD 2.0 format.<br />
It achieves the following results on the evaluation set:
- Loss: 0.8529
- Exact: 67.0964
- F1: 74.4842
- Combined: 71.1597
## More accurate metrics:
### Before fine-tuning:
```
'HasAns_exact': 54.71817606079797,
'HasAns_f1': 61.08672724332754,
'HasAns_total': 1579,
'NoAns_exact': 88.78048780487805,
'NoAns_f1': 88.78048780487805,
'NoAns_total': 205,
'best_exact': 58.63228699551569,
'best_exact_thresh': 0.0,
'best_f1': 64.26902596256402,
'best_f1_thresh': 0.0,
'exact': 58.63228699551569,
'f1': 64.26902596256404,
'total': 1784
```
### After fine-tuning:
```
'HasAns_exact': 67.57441418619379,
'HasAns_f1': 75.92137683558988,
'HasAns_total': 1579,
'NoAns_exact': 63.41463414634146,
'NoAns_f1': 63.41463414634146,
'NoAns_total': 205,
'best_exact': 67.0964125560538,
'best_exact_thresh': 0.0,
'best_f1': 74.48422310728503,
'best_f1_thresh': 0.0,
'exact': 67.0964125560538,
'f1': 74.48422310728503,
'total': 1784
```
# Dataset
A visualization of the dataset can be found [here](https://github.gatech.edu/pages/VIP-ITS/textbook_SQuAD_explore/explore/textbookv1.0/textbook/).<br />
The split between train and test is 70% and 30% respectively.
```
DatasetDict({
train: Dataset({
features: ['id', 'title', 'context', 'question', 'answers'],
num_rows: 4160
})
test: Dataset({
features: ['id', 'title', 'context', 'question', 'answers'],
num_rows: 1784
})
})
```
## Intended uses & limitations
This model is fine-tuned to answer questions from the DSPFirst textbook. I'm not really sure what I am doing so you should review before using it.<br />
Also, you should improve the Dataset either by using a **better generated questions and answers model** (currently using https://github.com/patil-suraj/question_generation) or perform **data augmentation** to increase dataset size.
## Training and evaluation data
- `batch_size` of 6 results in 14.03 GB VRAM
- Utilizes `gradient_accumulation_steps` to get total batch size to 516 (total batch size should be at least 256)
- 4.52 GB RAM
- 30% of the total questions is dedicated for evaluating.
## Training procedure
- The model was trained from [Google Colab](https://colab.research.google.com/drive/1dJXNstk2NSenwzdtl9xA8AqjP4LL-Ks_?usp=sharing)
- Utilizes Tesla P100 16GB, took 6.3 hours to train
- `load_best_model_at_end` is enabled in TrainingArguments
### Training hyperparameters
The following hyperparameters were used during training:
- learning_rate: 2e-05
- train_batch_size: 6
- eval_batch_size: 6
- seed: 42
- gradient_accumulation_steps: 86
- total_train_batch_size: 516
- optimizer: Adam with betas=(0.9,0.999) and epsilon=1e-08
- lr_scheduler_type: linear
- num_epochs: 7
### Model hyperparameters
- hidden_dropout_prob: 0.36
- attention_probs_dropout_prob = 0.36
### Training results
| Training Loss | Epoch | Step | Validation Loss | Exact | F1 | Combined |
|:-------------:|:-----:|:----:|:---------------:|:-------:|:-------:|:--------:|
| 2.3222 | 0.81 | 20 | 1.0363 | 60.3139 | 68.8586 | 65.0135 |
| 1.6149 | 1.65 | 40 | 0.9702 | 64.7422 | 72.5555 | 69.0395 |
| 1.2375 | 2.49 | 60 | 1.0007 | 64.6861 | 72.6306 | 69.0556 |
| 1.0417 | 3.32 | 80 | 0.9963 | 66.0874 | 73.8634 | 70.3642 |
| 0.9401 | 4.16 | 100 | 0.8803 | 67.0964 | 74.4842 | 71.1597 |
| 0.8799 | 4.97 | 120 | 0.8652 | 66.7040 | 74.1267 | 70.7865 |
| 0.8712 | 5.81 | 140 | 0.8921 | 66.3677 | 73.7213 | 70.4122 |
| 0.8311 | 6.65 | 160 | 0.8529 | 66.3117 | 73.4039 | 70.2124 |
### Framework versions
- Transformers 4.18.0
- Pytorch 1.10.0+cu111
- Datasets 2.1.0
- Tokenizers 0.12.1
|
imyday/xlm-roberta-base-finetuned-panx-de | 6c4fbd4784404b1a1dbc22ee6f3e922fb8346146 | 2022-04-18T16:42:01.000Z | [
"pytorch",
"tensorboard",
"xlm-roberta",
"token-classification",
"dataset:xtreme",
"transformers",
"generated_from_trainer",
"license:mit",
"model-index",
"autotrain_compatible"
] | token-classification | false | imyday | null | imyday/xlm-roberta-base-finetuned-panx-de | 0 | null | transformers | 36,978 | ---
license: mit
tags:
- generated_from_trainer
datasets:
- xtreme
metrics:
- f1
model-index:
- name: xlm-roberta-base-finetuned-panx-de
results:
- task:
name: Token Classification
type: token-classification
dataset:
name: xtreme
type: xtreme
args: PAN-X.de
metrics:
- name: F1
type: f1
value: 0.8590909090909091
---
<!-- This model card has been generated automatically according to the information the Trainer had access to. You
should probably proofread and complete it, then remove this comment. -->
# xlm-roberta-base-finetuned-panx-de
This model is a fine-tuned version of [xlm-roberta-base](https://huggingface.co/xlm-roberta-base) on the xtreme dataset.
It achieves the following results on the evaluation set:
- Loss: 0.1380
- F1: 0.8591
## Model description
More information needed
## Intended uses & limitations
More information needed
## Training and evaluation data
More information needed
## Training procedure
### Training hyperparameters
The following hyperparameters were used during training:
- learning_rate: 5e-05
- train_batch_size: 24
- eval_batch_size: 24
- seed: 42
- optimizer: Adam with betas=(0.9,0.999) and epsilon=1e-08
- lr_scheduler_type: linear
- num_epochs: 3
### Training results
| Training Loss | Epoch | Step | Validation Loss | F1 |
|:-------------:|:-----:|:----:|:---------------:|:------:|
| 0.2642 | 1.0 | 525 | 0.1624 | 0.8251 |
| 0.1315 | 2.0 | 1050 | 0.1445 | 0.8508 |
| 0.0832 | 3.0 | 1575 | 0.1380 | 0.8591 |
### Framework versions
- Transformers 4.11.3
- Pytorch 1.10.0+cu111
- Datasets 1.16.1
- Tokenizers 0.10.3
|
fvector/xlm-roberta-base-finetuned-panx-de | 1e5d092065524b2470ab3cd43a38e4ff0c9fa0a0 | 2022-04-19T05:58:38.000Z | [
"pytorch",
"tensorboard",
"xlm-roberta",
"token-classification",
"dataset:xtreme",
"transformers",
"generated_from_trainer",
"license:mit",
"model-index",
"autotrain_compatible"
] | token-classification | false | fvector | null | fvector/xlm-roberta-base-finetuned-panx-de | 0 | null | transformers | 36,979 | ---
license: mit
tags:
- generated_from_trainer
datasets:
- xtreme
metrics:
- f1
model-index:
- name: xlm-roberta-base-finetuned-panx-de
results:
- task:
name: Token Classification
type: token-classification
dataset:
name: xtreme
type: xtreme
args: PAN-X.de
metrics:
- name: F1
type: f1
value: 0.862669465085938
---
<!-- This model card has been generated automatically according to the information the Trainer had access to. You
should probably proofread and complete it, then remove this comment. -->
# xlm-roberta-base-finetuned-panx-de
This model is a fine-tuned version of [xlm-roberta-base](https://huggingface.co/xlm-roberta-base) on the xtreme dataset.
It achieves the following results on the evaluation set:
- Loss: 0.1374
- F1: 0.8627
## Model description
More information needed
## Intended uses & limitations
More information needed
## Training and evaluation data
More information needed
## Training procedure
### Training hyperparameters
The following hyperparameters were used during training:
- learning_rate: 5e-05
- train_batch_size: 24
- eval_batch_size: 24
- seed: 42
- optimizer: Adam with betas=(0.9,0.999) and epsilon=1e-08
- lr_scheduler_type: linear
- num_epochs: 3
### Training results
| Training Loss | Epoch | Step | Validation Loss | F1 |
|:-------------:|:-----:|:----:|:---------------:|:------:|
| 0.2596 | 1.0 | 525 | 0.1571 | 0.8302 |
| 0.1292 | 2.0 | 1050 | 0.1416 | 0.8455 |
| 0.0809 | 3.0 | 1575 | 0.1374 | 0.8627 |
### Framework versions
- Transformers 4.11.3
- Pytorch 1.10.0+cu111
- Datasets 1.16.1
- Tokenizers 0.10.3
|
surajp/sanbert-from-scratch | 8dbd69fd525006430199acf176c4eff3d6f17e99 | 2022-04-18T13:51:07.000Z | [
"pytorch",
"albert",
"fill-mask",
"transformers",
"autotrain_compatible"
] | fill-mask | false | surajp | null | surajp/sanbert-from-scratch | 0 | null | transformers | 36,980 | Entry not found |
ucabqfe/roberta_AAE_bio | 056922f8c44e60b47c7aabbbc7a3db8ad5c16a1b | 2022-04-18T15:29:00.000Z | [
"pytorch",
"roberta",
"token-classification",
"transformers",
"autotrain_compatible"
] | token-classification | false | ucabqfe | null | ucabqfe/roberta_AAE_bio | 0 | 0 | transformers | 36,981 | Entry not found |
maveriq/lingbert-mini-500k | d38d6c26f2a38c535235f84ee75bbf340258ad24 | 2022-04-18T17:07:23.000Z | [
"pytorch",
"bert",
"fill-mask",
"transformers",
"autotrain_compatible"
] | fill-mask | false | maveriq | null | maveriq/lingbert-mini-500k | 0 | null | transformers | 36,982 | Entry not found |
maveriq/mybert-mini-500k | 679ef0b755e42706a6eceefe9a1a45a42f1e1410 | 2022-04-18T17:08:49.000Z | [
"pytorch",
"bert",
"fill-mask",
"transformers",
"autotrain_compatible"
] | fill-mask | false | maveriq | null | maveriq/mybert-mini-500k | 0 | null | transformers | 36,983 | Entry not found |
maveriq/mybert-mini-1M | 011b31a32d1feba9e9713569901126ffb7cd57ec | 2022-04-18T17:10:00.000Z | [
"pytorch",
"bert",
"fill-mask",
"transformers",
"autotrain_compatible"
] | fill-mask | false | maveriq | null | maveriq/mybert-mini-1M | 0 | null | transformers | 36,984 | Entry not found |
ucabqfe/roberta_PER_bieo | 311d8f83dec7432727665a4fd9383a810709cd8c | 2022-04-18T17:52:13.000Z | [
"pytorch",
"roberta",
"token-classification",
"transformers",
"autotrain_compatible"
] | token-classification | false | ucabqfe | null | ucabqfe/roberta_PER_bieo | 0 | null | transformers | 36,985 | Entry not found |
ucabqfe/roberta_PER_bio | 0c2ff4e12e54fb791922a9ad4637649d40116541 | 2022-04-18T17:58:09.000Z | [
"pytorch",
"roberta",
"token-classification",
"transformers",
"autotrain_compatible"
] | token-classification | false | ucabqfe | null | ucabqfe/roberta_PER_bio | 0 | null | transformers | 36,986 | Entry not found |
ucabqfe/roberta_AAE_bieo | 83587b2b72fd4e18c59063939e5659ea1e3ec2a6 | 2022-04-18T18:04:59.000Z | [
"pytorch",
"roberta",
"token-classification",
"transformers",
"autotrain_compatible"
] | token-classification | false | ucabqfe | null | ucabqfe/roberta_AAE_bieo | 0 | null | transformers | 36,987 | Entry not found |
ucabqfe/roberta_AAE_io | 27f3c0c86d6b56dd53eea0644dd5018b4ca74d1b | 2022-04-18T18:06:26.000Z | [
"pytorch",
"roberta",
"token-classification",
"transformers",
"autotrain_compatible"
] | token-classification | false | ucabqfe | null | ucabqfe/roberta_AAE_io | 0 | null | transformers | 36,988 | Entry not found |
ucabqfe/bigBird_PER_io | 9885cc646bcc2d76e6c308237baa511f6110f6fe | 2022-04-18T18:13:11.000Z | [
"pytorch",
"big_bird",
"token-classification",
"transformers",
"autotrain_compatible"
] | token-classification | false | ucabqfe | null | ucabqfe/bigBird_PER_io | 0 | null | transformers | 36,989 | Entry not found |
shishirAI/wav2vec2-xlsr-nepalii | d9d66601a3f5e7b9e74cc6523e5c838cebc03145 | 2022-04-18T21:49:04.000Z | [
"pytorch",
"tensorboard",
"wav2vec2",
"automatic-speech-recognition",
"transformers",
"generated_from_trainer",
"license:apache-2.0",
"model-index"
] | automatic-speech-recognition | false | shishirAI | null | shishirAI/wav2vec2-xlsr-nepalii | 0 | null | transformers | 36,990 | ---
license: apache-2.0
tags:
- generated_from_trainer
model-index:
- name: wav2vec2-xlsr-nepalii
results: []
---
<!-- This model card has been generated automatically according to the information the Trainer had access to. You
should probably proofread and complete it, then remove this comment. -->
# wav2vec2-xlsr-nepalii
This model is a fine-tuned version of [facebook/wav2vec2-large-xlsr-53](https://huggingface.co/facebook/wav2vec2-large-xlsr-53) on the None dataset.
## Model description
More information needed
## Intended uses & limitations
More information needed
## Training and evaluation data
More information needed
## Training procedure
### Training hyperparameters
The following hyperparameters were used during training:
- learning_rate: 0.0003
- train_batch_size: 16
- eval_batch_size: 8
- seed: 42
- gradient_accumulation_steps: 2
- total_train_batch_size: 32
- optimizer: Adam with betas=(0.9,0.999) and epsilon=1e-08
- lr_scheduler_type: linear
- lr_scheduler_warmup_steps: 250
- num_epochs: 5
### Training results
### Framework versions
- Transformers 4.18.0
- Pytorch 1.10.0+cu111
- Datasets 1.18.3
- Tokenizers 0.12.1
|
huggan/stylegan_car512 | 8a215b13cf7ed5ba027d753d51d9eaba7a0f3233 | 2022-04-29T12:01:09.000Z | [
"pytorch",
"gan",
"stylegan",
"huggan",
"unconditional-image-generation",
"license:apache-2.0"
] | unconditional-image-generation | false | huggan | null | huggan/stylegan_car512 | 0 | null | null | 36,991 | ---
tags:
- gan
- stylegan
- huggan
- unconditional-image-generation
license: apache-2.0
---
The model provided is a StyleGan generator trained on the Cars dataset with a resolution of 512px. It is uploaded as part of porting this project: https://github.com/genforce/sefa to hugginface spaces. |
huggan/stylegan_cat256 | 65dc0c478431e511ffd6e53841af116d4a3c1f87 | 2022-04-29T12:01:40.000Z | [
"pytorch",
"gan",
"stylegan",
"huggan",
"unconditional-image-generation",
"license:apache-2.0"
] | unconditional-image-generation | false | huggan | null | huggan/stylegan_cat256 | 0 | null | null | 36,992 | ---
tags:
- gan
- stylegan
- huggan
- unconditional-image-generation
license: apache-2.0
---
The model provided is a StyleGAN generator trained on the LSUN cats dataset with a resolution of 256px. It is uploaded as part of porting this project: https://github.com/genforce/sefa to hugginface spaces. |
irenelizihui/MarianMT_UFAL | db1296555bb4bde4bdf56a74158b763538c95490 | 2022-04-18T23:08:08.000Z | [
"pytorch",
"marian",
"text2text-generation",
"transformers",
"license:wtfpl",
"autotrain_compatible"
] | text2text-generation | false | irenelizihui | null | irenelizihui/MarianMT_UFAL | 0 | null | transformers | 36,993 | ---
license: wtfpl
---
[MarianMT](https://huggingface.co/docs/transformers/model_doc/marian) model trained on the [UFAL](https://ufal.mff.cuni.cz/ufal_medical_corpus) dataset, from `en` to `cs, de, es, fr, pl, ro, hu, sv`.
|
guillaumegg/wav2vec2-base-timit-demo-ove | fa49fdd085eb0ace0d98af5a5d3e8a44d822cd38 | 2022-04-19T08:16:46.000Z | [
"pytorch",
"tensorboard",
"wav2vec2",
"automatic-speech-recognition",
"transformers",
"generated_from_trainer",
"license:apache-2.0",
"model-index"
] | automatic-speech-recognition | false | guillaumegg | null | guillaumegg/wav2vec2-base-timit-demo-ove | 0 | null | transformers | 36,994 | ---
license: apache-2.0
tags:
- generated_from_trainer
model-index:
- name: wav2vec2-base-timit-demo-ove
results: []
---
<!-- This model card has been generated automatically according to the information the Trainer had access to. You
should probably proofread and complete it, then remove this comment. -->
# wav2vec2-base-timit-demo-ove
This model is a fine-tuned version of [facebook/wav2vec2-large-xlsr-53-french](https://huggingface.co/facebook/wav2vec2-large-xlsr-53-french) on the None dataset.
## Model description
More information needed
## Intended uses & limitations
More information needed
## Training and evaluation data
More information needed
## Training procedure
### Training hyperparameters
The following hyperparameters were used during training:
- learning_rate: 0.0001
- train_batch_size: 32
- eval_batch_size: 8
- seed: 42
- optimizer: Adam with betas=(0.9,0.999) and epsilon=1e-08
- lr_scheduler_type: linear
- lr_scheduler_warmup_steps: 1000
- num_epochs: 30
### Training results
### Framework versions
- Transformers 4.19.0.dev0
- Pytorch 1.10.0+cu111
- Datasets 2.1.1.dev0
- Tokenizers 0.12.1
|
maesneako/dbddv01-gpt2-french-small_space_paco-cheese-v3 | 5051d9cb45f54dfe5939918b38251acaf4b68eae | 2022-04-19T08:02:20.000Z | [
"pytorch",
"gpt2",
"text-generation",
"transformers",
"generated_from_trainer",
"model-index"
] | text-generation | false | maesneako | null | maesneako/dbddv01-gpt2-french-small_space_paco-cheese-v3 | 0 | null | transformers | 36,995 | ---
tags:
- generated_from_trainer
model-index:
- name: dbddv01-gpt2-french-small_space_paco-cheese-v3
results: []
---
<!-- This model card has been generated automatically according to the information the Trainer had access to. You
should probably proofread and complete it, then remove this comment. -->
# dbddv01-gpt2-french-small_space_paco-cheese-v3
This model was trained from scratch on the None dataset.
## Model description
More information needed
## Intended uses & limitations
More information needed
## Training and evaluation data
More information needed
## Training procedure
### Training hyperparameters
The following hyperparameters were used during training:
- learning_rate: 5e-05
- train_batch_size: 4
- eval_batch_size: 8
- seed: 42
- optimizer: Adam with betas=(0.9,0.999) and epsilon=1e-08
- lr_scheduler_type: linear
- lr_scheduler_warmup_steps: 100
- num_epochs: 2
### Training results
### Framework versions
- Transformers 4.18.0
- Pytorch 1.10.0+cu111
- Datasets 2.1.0
- Tokenizers 0.12.1
|
npleshkanov/ru-labse-toxic | f8a8a2020198948369f48e0c96c01b62206120ac | 2022-05-25T17:43:49.000Z | [
"pytorch",
"tensorboard",
"bert",
"transformers",
"generated_from_trainer",
"model-index"
] | null | false | npleshkanov | null | npleshkanov/ru-labse-toxic | 0 | null | transformers | 36,996 | ---
tags:
- generated_from_trainer
model-index:
- name: ru-labse-toxic
results: []
---
<!-- This model card has been generated automatically according to the information the Trainer had access to. You
should probably proofread and complete it, then remove this comment. -->
# ru-labse-toxic
This model is a fine-tuned version of [rasa/LaBSE](https://huggingface.co/rasa/LaBSE) on the None dataset.
It achieves the following results on the evaluation set:
- Loss: 0.1950
- Acc: 0.9302
## Model description
More information needed
## Intended uses & limitations
More information needed
## Training and evaluation data
More information needed
## Training procedure
### Training hyperparameters
The following hyperparameters were used during training:
- learning_rate: 0.0001
- train_batch_size: 32
- eval_batch_size: 32
- seed: 42
- optimizer: Adam with betas=(0.9,0.999) and epsilon=1e-08
- lr_scheduler_type: linear
- num_epochs: 6
### Training results
### Framework versions
- Transformers 4.17.0
- Pytorch 1.10.0+cu111
- Datasets 2.1.0
- Tokenizers 0.12.1
|
satyamrajawat1994/distibert-ner | 7ed0485e81fa251fc01835c4e7deaf81c678853a | 2022-04-19T08:54:17.000Z | [
"pytorch",
"distilbert",
"token-classification",
"transformers",
"autotrain_compatible"
] | token-classification | false | satyamrajawat1994 | null | satyamrajawat1994/distibert-ner | 0 | null | transformers | 36,997 | Entry not found |
tau/false_large_rouge_para0_sent1_span2_True_multi_masks_with_types_7_1024_0.3_epoch1 | 244484045e15984a3199e5071e5350c9c4b53e31 | 2022-04-19T11:41:03.000Z | [
"pytorch",
"t5",
"text2text-generation",
"transformers",
"autotrain_compatible"
] | text2text-generation | false | tau | null | tau/false_large_rouge_para0_sent1_span2_True_multi_masks_with_types_7_1024_0.3_epoch1 | 0 | null | transformers | 36,998 | Entry not found |
smeoni/nbme-roberta-large | 386ea031a9d8229215bc13d26d3b67b25719c526 | 2022-04-23T20:10:03.000Z | [
"pytorch",
"tensorboard",
"roberta",
"fill-mask",
"transformers",
"generated_from_trainer",
"license:mit",
"model-index",
"autotrain_compatible"
] | fill-mask | false | smeoni | null | smeoni/nbme-roberta-large | 0 | null | transformers | 36,999 | ---
license: mit
tags:
- generated_from_trainer
model-index:
- name: nbme-roberta-large
results: []
---
<!-- This model card has been generated automatically according to the information the Trainer had access to. You
should probably proofread and complete it, then remove this comment. -->
# nbme-roberta-large
This model is a fine-tuned version of [roberta-large](https://huggingface.co/roberta-large) on an unknown dataset.
It achieves the following results on the evaluation set:
- Loss: 0.7825
## Model description
More information needed
## Intended uses & limitations
More information needed
## Training and evaluation data
More information needed
## Training procedure
### Training hyperparameters
The following hyperparameters were used during training:
- learning_rate: 5e-05
- train_batch_size: 8
- eval_batch_size: 8
- seed: 42
- gradient_accumulation_steps: 4
- total_train_batch_size: 32
- optimizer: Adam with betas=(0.9,0.999) and epsilon=1e-08
- lr_scheduler_type: linear
- num_epochs: 3.0
### Training results
| Training Loss | Epoch | Step | Validation Loss |
|:-------------:|:-----:|:----:|:---------------:|
| 1.1117 | 1.0 | 1850 | 0.9610 |
| 0.8911 | 2.0 | 3700 | 0.8466 |
| 0.8158 | 3.0 | 5550 | 0.7825 |
### Framework versions
- Transformers 4.19.0.dev0
- Pytorch 1.11.0
- Datasets 2.1.0
- Tokenizers 0.12.1
|
Subsets and Splits