modelId
stringlengths 4
112
| sha
stringlengths 40
40
| lastModified
stringlengths 24
24
| tags
sequence | pipeline_tag
stringclasses 29
values | private
bool 1
class | author
stringlengths 2
38
β | config
null | id
stringlengths 4
112
| downloads
float64 0
36.8M
β | likes
float64 0
712
β | library_name
stringclasses 17
values | __index_level_0__
int64 0
38.5k
| readme
stringlengths 0
186k
|
---|---|---|---|---|---|---|---|---|---|---|---|---|---|
anugunj/omnivore-swinB-in21k | 030c17f4c4d1c9c77b903703c4bc3f26a2d10742 | 2022-06-19T00:22:01.000Z | [
"pytorch",
"omnivore",
"transformers"
] | null | false | anugunj | null | anugunj/omnivore-swinB-in21k | 1 | null | transformers | 33,000 | Entry not found |
anugunj/omnivore-swinB | 0c6b22f8c02b1cf0fb36dda7df4ecb148f21ce75 | 2022-06-19T05:58:42.000Z | [
"pytorch",
"omnivore",
"transformers"
] | null | false | anugunj | null | anugunj/omnivore-swinB | 1 | null | transformers | 33,001 | Entry not found |
anugunj/omnivore-swinT | 4c52b0a234f57f0a5710b4304bc3c4d9a41a191f | 2022-06-19T05:28:27.000Z | [
"pytorch",
"omnivore",
"transformers"
] | null | false | anugunj | null | anugunj/omnivore-swinT | 1 | null | transformers | 33,002 | Entry not found |
anugunj/omnivore-swinS | 73eab5962bbe7e0f48e4961d0838ceeec93096c9 | 2022-06-19T00:30:40.000Z | [
"pytorch",
"omnivore",
"transformers"
] | null | false | anugunj | null | anugunj/omnivore-swinS | 1 | null | transformers | 33,003 | Entry not found |
ryota/newsCreate | d31a3808b0c1cd28c6259f59e288df257114d5aa | 2022-06-19T03:40:51.000Z | [
"pytorch",
"t5",
"text2text-generation",
"transformers",
"autotrain_compatible"
] | text2text-generation | false | ryota | null | ryota/newsCreate | 1 | null | transformers | 33,004 | |
huggingtweets/shxtou | 950090c40137792f0471cbe37a0f63927f546574 | 2022-06-19T03:58:13.000Z | [
"pytorch",
"gpt2",
"text-generation",
"en",
"transformers",
"huggingtweets"
] | text-generation | false | huggingtweets | null | huggingtweets/shxtou | 1 | null | transformers | 33,005 | ---
language: en
thumbnail: http://www.huggingtweets.com/shxtou/1655611088443/predictions.png
tags:
- huggingtweets
widget:
- text: "My dream is"
---
<div class="inline-flex flex-col" style="line-height: 1.5;">
<div class="flex">
<div
style="display:inherit; margin-left: 4px; margin-right: 4px; width: 92px; height:92px; border-radius: 50%; background-size: cover; background-image: url('https://pbs.twimg.com/profile_images/1419320614205198350/gHkqH6YI_400x400.jpg')">
</div>
<div
style="display:none; margin-left: 4px; margin-right: 4px; width: 92px; height:92px; border-radius: 50%; background-size: cover; background-image: url('')">
</div>
<div
style="display:none; margin-left: 4px; margin-right: 4px; width: 92px; height:92px; border-radius: 50%; background-size: cover; background-image: url('')">
</div>
</div>
<div style="text-align: center; margin-top: 3px; font-size: 16px; font-weight: 800">π€ AI BOT π€</div>
<div style="text-align: center; font-size: 16px; font-weight: 800">Shoto π‘οΈ</div>
<div style="text-align: center; font-size: 14px;">@shxtou</div>
</div>
I was made with [huggingtweets](https://github.com/borisdayma/huggingtweets).
Create your own bot based on your favorite user with [the demo](https://colab.research.google.com/github/borisdayma/huggingtweets/blob/master/huggingtweets-demo.ipynb)!
## How does it work?
The model uses the following pipeline.
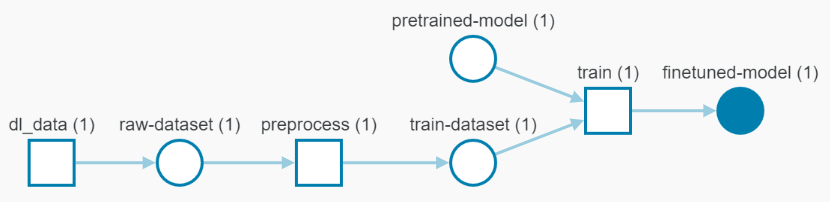
To understand how the model was developed, check the [W&B report](https://wandb.ai/wandb/huggingtweets/reports/HuggingTweets-Train-a-Model-to-Generate-Tweets--VmlldzoxMTY5MjI).
## Training data
The model was trained on tweets from Shoto π‘οΈ.
| Data | Shoto π‘οΈ |
| --- | --- |
| Tweets downloaded | 3248 |
| Retweets | 617 |
| Short tweets | 533 |
| Tweets kept | 2098 |
[Explore the data](https://wandb.ai/wandb/huggingtweets/runs/2mdmjop6/artifacts), which is tracked with [W&B artifacts](https://docs.wandb.com/artifacts) at every step of the pipeline.
## Training procedure
The model is based on a pre-trained [GPT-2](https://huggingface.co/gpt2) which is fine-tuned on @shxtou's tweets.
Hyperparameters and metrics are recorded in the [W&B training run](https://wandb.ai/wandb/huggingtweets/runs/1pdig81x) for full transparency and reproducibility.
At the end of training, [the final model](https://wandb.ai/wandb/huggingtweets/runs/1pdig81x/artifacts) is logged and versioned.
## How to use
You can use this model directly with a pipeline for text generation:
```python
from transformers import pipeline
generator = pipeline('text-generation',
model='huggingtweets/shxtou')
generator("My dream is", num_return_sequences=5)
```
## Limitations and bias
The model suffers from [the same limitations and bias as GPT-2](https://huggingface.co/gpt2#limitations-and-bias).
In addition, the data present in the user's tweets further affects the text generated by the model.
## About
*Built by Boris Dayma*
[](https://twitter.com/intent/follow?screen_name=borisdayma)
For more details, visit the project repository.
[](https://github.com/borisdayma/huggingtweets)
|
ryota/newsModelRe | 66371892b29cc9b59522fd64c0898be707673a50 | 2022-06-19T06:56:09.000Z | [
"pytorch",
"t5",
"text2text-generation",
"transformers",
"autotrain_compatible"
] | text2text-generation | false | ryota | null | ryota/newsModelRe | 1 | null | transformers | 33,006 | |
Hausax/albert-xxlarge-v2-finetuned-Poems | a2095801d5a92e53ce3e69fd6b2104cd7de92991 | 2022-06-20T07:19:43.000Z | [
"pytorch",
"tensorboard",
"albert",
"fill-mask",
"transformers",
"generated_from_trainer",
"license:apache-2.0",
"model-index",
"autotrain_compatible"
] | fill-mask | false | Hausax | null | Hausax/albert-xxlarge-v2-finetuned-Poems | 1 | null | transformers | 33,007 | ---
license: apache-2.0
tags:
- generated_from_trainer
model-index:
- name: albert-xxlarge-v2-finetuned-Poems
results: []
---
<!-- This model card has been generated automatically according to the information the Trainer had access to. You
should probably proofread and complete it, then remove this comment. -->
# albert-xxlarge-v2-finetuned-Poems
This model is a fine-tuned version of [albert-xxlarge-v2](https://huggingface.co/albert-xxlarge-v2) on an unknown dataset.
It achieves the following results on the evaluation set:
- Loss: 2.1923
## Model description
More information needed
## Intended uses & limitations
More information needed
## Training and evaluation data
More information needed
## Training procedure
### Training hyperparameters
The following hyperparameters were used during training:
- learning_rate: 2e-07
- train_batch_size: 16
- eval_batch_size: 16
- seed: 42
- optimizer: Adam with betas=(0.9,0.999) and epsilon=1e-08
- lr_scheduler_type: linear
- num_epochs: 5
- mixed_precision_training: Native AMP
### Training results
| Training Loss | Epoch | Step | Validation Loss |
|:-------------:|:-----:|:-----:|:---------------:|
| 2.482 | 1.0 | 19375 | 2.2959 |
| 2.258 | 2.0 | 38750 | 2.2357 |
| 2.2146 | 3.0 | 58125 | 2.2085 |
| 2.1975 | 4.0 | 77500 | 2.1929 |
| 2.1893 | 5.0 | 96875 | 2.1863 |
### Framework versions
- Transformers 4.20.0
- Pytorch 1.11.0+cu113
- Datasets 2.3.2
- Tokenizers 0.12.1
|
EddieChen372/xlm_roberta-base-fintuned-react | dfc310422682e803f21263c7e3d290ea9f0833f2 | 2022-06-19T11:36:25.000Z | [
"pytorch",
"roberta",
"fill-mask",
"transformers",
"autotrain_compatible"
] | fill-mask | false | EddieChen372 | null | EddieChen372/xlm_roberta-base-fintuned-react | 1 | null | transformers | 33,008 | Entry not found |
huggingtweets/rsapublic | 332b7f43a73ed526f24fa67651d04502c51e7b36 | 2022-06-19T11:26:27.000Z | [
"pytorch",
"gpt2",
"text-generation",
"en",
"transformers",
"huggingtweets"
] | text-generation | false | huggingtweets | null | huggingtweets/rsapublic | 1 | null | transformers | 33,009 | ---
language: en
thumbnail: http://www.huggingtweets.com/rsapublic/1655637814216/predictions.png
tags:
- huggingtweets
widget:
- text: "My dream is"
---
<div class="inline-flex flex-col" style="line-height: 1.5;">
<div class="flex">
<div
style="display:inherit; margin-left: 4px; margin-right: 4px; width: 92px; height:92px; border-radius: 50%; background-size: cover; background-image: url('https://pbs.twimg.com/profile_images/1536637048391491584/zfHd6Mha_400x400.jpg')">
</div>
<div
style="display:none; margin-left: 4px; margin-right: 4px; width: 92px; height:92px; border-radius: 50%; background-size: cover; background-image: url('')">
</div>
<div
style="display:none; margin-left: 4px; margin-right: 4px; width: 92px; height:92px; border-radius: 50%; background-size: cover; background-image: url('')">
</div>
</div>
<div style="text-align: center; margin-top: 3px; font-size: 16px; font-weight: 800">π€ AI BOT π€</div>
<div style="text-align: center; font-size: 16px; font-weight: 800">bopo mofo</div>
<div style="text-align: center; font-size: 14px;">@rsapublic</div>
</div>
I was made with [huggingtweets](https://github.com/borisdayma/huggingtweets).
Create your own bot based on your favorite user with [the demo](https://colab.research.google.com/github/borisdayma/huggingtweets/blob/master/huggingtweets-demo.ipynb)!
## How does it work?
The model uses the following pipeline.
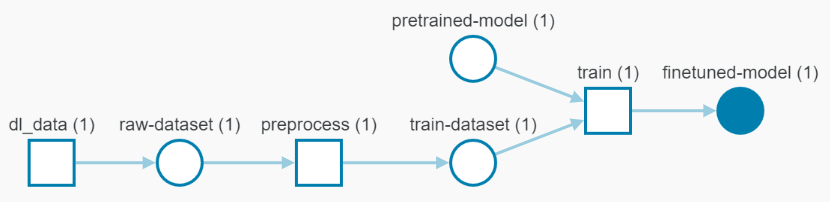
To understand how the model was developed, check the [W&B report](https://wandb.ai/wandb/huggingtweets/reports/HuggingTweets-Train-a-Model-to-Generate-Tweets--VmlldzoxMTY5MjI).
## Training data
The model was trained on tweets from bopo mofo.
| Data | bopo mofo |
| --- | --- |
| Tweets downloaded | 3212 |
| Retweets | 1562 |
| Short tweets | 303 |
| Tweets kept | 1347 |
[Explore the data](https://wandb.ai/wandb/huggingtweets/runs/2qnsx0b8/artifacts), which is tracked with [W&B artifacts](https://docs.wandb.com/artifacts) at every step of the pipeline.
## Training procedure
The model is based on a pre-trained [GPT-2](https://huggingface.co/gpt2) which is fine-tuned on @rsapublic's tweets.
Hyperparameters and metrics are recorded in the [W&B training run](https://wandb.ai/wandb/huggingtweets/runs/368jvjwu) for full transparency and reproducibility.
At the end of training, [the final model](https://wandb.ai/wandb/huggingtweets/runs/368jvjwu/artifacts) is logged and versioned.
## How to use
You can use this model directly with a pipeline for text generation:
```python
from transformers import pipeline
generator = pipeline('text-generation',
model='huggingtweets/rsapublic')
generator("My dream is", num_return_sequences=5)
```
## Limitations and bias
The model suffers from [the same limitations and bias as GPT-2](https://huggingface.co/gpt2#limitations-and-bias).
In addition, the data present in the user's tweets further affects the text generated by the model.
## About
*Built by Boris Dayma*
[](https://twitter.com/intent/follow?screen_name=borisdayma)
For more details, visit the project repository.
[](https://github.com/borisdayma/huggingtweets)
|
lmqg/t5-base-squadshifts-vanilla-new_wiki | e3c25536fbf7fedee7197dbb9772cb73dcd05bac | 2022-06-19T14:07:12.000Z | [
"pytorch",
"t5",
"text2text-generation",
"transformers",
"autotrain_compatible"
] | text2text-generation | false | lmqg | null | lmqg/t5-base-squadshifts-vanilla-new_wiki | 1 | null | transformers | 33,010 | Entry not found |
lmqg/t5-base-squadshifts-vanilla-nyt | a65e24080c0864f13524cc5f647c4277500dcb46 | 2022-06-19T14:09:44.000Z | [
"pytorch",
"t5",
"text2text-generation",
"transformers",
"autotrain_compatible"
] | text2text-generation | false | lmqg | null | lmqg/t5-base-squadshifts-vanilla-nyt | 1 | null | transformers | 33,011 | Entry not found |
Danastos/dpr_query_el_3 | fe500a12ca4aa4faa68d29172329db33856a3be6 | 2022-06-19T20:04:53.000Z | [
"pytorch",
"bert",
"pretraining",
"transformers"
] | null | false | Danastos | null | Danastos/dpr_query_el_3 | 1 | null | transformers | 33,012 | Entry not found |
Danastos/dpr_passage_el_3 | 2db54f97d4a5bbf56fd73e5706a22968d15a51c8 | 2022-06-19T20:03:06.000Z | [
"pytorch",
"bert",
"pretraining",
"transformers"
] | null | false | Danastos | null | Danastos/dpr_passage_el_3 | 1 | null | transformers | 33,013 | Entry not found |
sudo-s/modelversion01 | 6fdfbc7d15562401e34d9ebff7d62eac9a8e558c | 2022-06-19T14:45:01.000Z | [
"pytorch",
"tensorboard",
"vit",
"image-classification",
"transformers",
"generated_from_trainer",
"license:apache-2.0",
"model-index"
] | image-classification | false | sudo-s | null | sudo-s/modelversion01 | 1 | null | transformers | 33,014 | ---
license: apache-2.0
tags:
- image-classification
- generated_from_trainer
metrics:
- accuracy
model-index:
- name: modelversion01
results: []
---
<!-- This model card has been generated automatically according to the information the Trainer had access to. You
should probably proofread and complete it, then remove this comment. -->
# modelversion01
This model is a fine-tuned version of [google/vit-base-patch16-224-in21k](https://huggingface.co/google/vit-base-patch16-224-in21k) on the sudo-s/herbier_mesuem1 dataset.
It achieves the following results on the evaluation set:
- Loss: 1.3888
- Accuracy: 0.7224
## Model description
More information needed
## Intended uses & limitations
More information needed
## Training and evaluation data
More information needed
## Training procedure
### Training hyperparameters
The following hyperparameters were used during training:
- learning_rate: 0.0002
- train_batch_size: 16
- eval_batch_size: 8
- seed: 42
- optimizer: Adam with betas=(0.9,0.999) and epsilon=1e-08
- lr_scheduler_type: linear
- num_epochs: 1
- mixed_precision_training: Native AMP
### Training results
| Training Loss | Epoch | Step | Validation Loss | Accuracy |
|:-------------:|:-----:|:----:|:---------------:|:--------:|
| 3.1304 | 0.16 | 100 | 3.1546 | 0.3254 |
| 2.6514 | 0.31 | 200 | 2.5058 | 0.4854 |
| 2.0636 | 0.47 | 300 | 2.0647 | 0.5771 |
| 1.7812 | 0.63 | 400 | 1.7536 | 0.6423 |
| 1.5857 | 0.78 | 500 | 1.5272 | 0.6974 |
| 1.3055 | 0.94 | 600 | 1.3888 | 0.7224 |
### Framework versions
- Transformers 4.20.0
- Pytorch 1.11.0+cu113
- Datasets 2.3.2
- Tokenizers 0.12.1
|
huggingtweets/thenoelmiller | bfac4dfb83e550673d074d84231308d94ec3b523 | 2022-06-19T19:18:12.000Z | [
"pytorch",
"gpt2",
"text-generation",
"en",
"transformers",
"huggingtweets"
] | text-generation | false | huggingtweets | null | huggingtweets/thenoelmiller | 1 | null | transformers | 33,015 | ---
language: en
thumbnail: http://www.huggingtweets.com/thenoelmiller/1655666288084/predictions.png
tags:
- huggingtweets
widget:
- text: "My dream is"
---
<div class="inline-flex flex-col" style="line-height: 1.5;">
<div class="flex">
<div
style="display:inherit; margin-left: 4px; margin-right: 4px; width: 92px; height:92px; border-radius: 50%; background-size: cover; background-image: url('https://pbs.twimg.com/profile_images/1438687880101212170/nNi2oamd_400x400.jpg')">
</div>
<div
style="display:none; margin-left: 4px; margin-right: 4px; width: 92px; height:92px; border-radius: 50%; background-size: cover; background-image: url('')">
</div>
<div
style="display:none; margin-left: 4px; margin-right: 4px; width: 92px; height:92px; border-radius: 50%; background-size: cover; background-image: url('')">
</div>
</div>
<div style="text-align: center; margin-top: 3px; font-size: 16px; font-weight: 800">π€ AI BOT π€</div>
<div style="text-align: center; font-size: 16px; font-weight: 800">Noel Miller</div>
<div style="text-align: center; font-size: 14px;">@thenoelmiller</div>
</div>
I was made with [huggingtweets](https://github.com/borisdayma/huggingtweets).
Create your own bot based on your favorite user with [the demo](https://colab.research.google.com/github/borisdayma/huggingtweets/blob/master/huggingtweets-demo.ipynb)!
## How does it work?
The model uses the following pipeline.
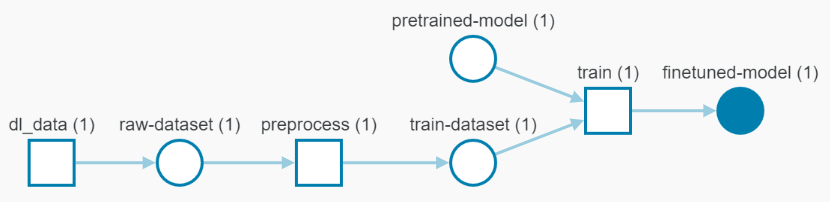
To understand how the model was developed, check the [W&B report](https://wandb.ai/wandb/huggingtweets/reports/HuggingTweets-Train-a-Model-to-Generate-Tweets--VmlldzoxMTY5MjI).
## Training data
The model was trained on tweets from Noel Miller.
| Data | Noel Miller |
| --- | --- |
| Tweets downloaded | 3207 |
| Retweets | 313 |
| Short tweets | 710 |
| Tweets kept | 2184 |
[Explore the data](https://wandb.ai/wandb/huggingtweets/runs/3kgitqrm/artifacts), which is tracked with [W&B artifacts](https://docs.wandb.com/artifacts) at every step of the pipeline.
## Training procedure
The model is based on a pre-trained [GPT-2](https://huggingface.co/gpt2) which is fine-tuned on @thenoelmiller's tweets.
Hyperparameters and metrics are recorded in the [W&B training run](https://wandb.ai/wandb/huggingtweets/runs/3a9yazcq) for full transparency and reproducibility.
At the end of training, [the final model](https://wandb.ai/wandb/huggingtweets/runs/3a9yazcq/artifacts) is logged and versioned.
## How to use
You can use this model directly with a pipeline for text generation:
```python
from transformers import pipeline
generator = pipeline('text-generation',
model='huggingtweets/thenoelmiller')
generator("My dream is", num_return_sequences=5)
```
## Limitations and bias
The model suffers from [the same limitations and bias as GPT-2](https://huggingface.co/gpt2#limitations-and-bias).
In addition, the data present in the user's tweets further affects the text generated by the model.
## About
*Built by Boris Dayma*
[](https://twitter.com/intent/follow?screen_name=borisdayma)
For more details, visit the project repository.
[](https://github.com/borisdayma/huggingtweets)
|
huggingtweets/carboxylace | 489d3c283e4a88bd14e163208b056ca1bf2054ed | 2022-06-19T22:43:13.000Z | [
"pytorch",
"gpt2",
"text-generation",
"en",
"transformers",
"huggingtweets"
] | text-generation | false | huggingtweets | null | huggingtweets/carboxylace | 1 | null | transformers | 33,016 | ---
language: en
thumbnail: http://www.huggingtweets.com/carboxylace/1655678588553/predictions.png
tags:
- huggingtweets
widget:
- text: "My dream is"
---
<div class="inline-flex flex-col" style="line-height: 1.5;">
<div class="flex">
<div
style="display:inherit; margin-left: 4px; margin-right: 4px; width: 92px; height:92px; border-radius: 50%; background-size: cover; background-image: url('https://pbs.twimg.com/profile_images/1509050806795964416/g7FedcOa_400x400.jpg')">
</div>
<div
style="display:none; margin-left: 4px; margin-right: 4px; width: 92px; height:92px; border-radius: 50%; background-size: cover; background-image: url('')">
</div>
<div
style="display:none; margin-left: 4px; margin-right: 4px; width: 92px; height:92px; border-radius: 50%; background-size: cover; background-image: url('')">
</div>
</div>
<div style="text-align: center; margin-top: 3px; font-size: 16px; font-weight: 800">π€ AI BOT π€</div>
<div style="text-align: center; font-size: 16px; font-weight: 800">lace</div>
<div style="text-align: center; font-size: 14px;">@carboxylace</div>
</div>
I was made with [huggingtweets](https://github.com/borisdayma/huggingtweets).
Create your own bot based on your favorite user with [the demo](https://colab.research.google.com/github/borisdayma/huggingtweets/blob/master/huggingtweets-demo.ipynb)!
## How does it work?
The model uses the following pipeline.
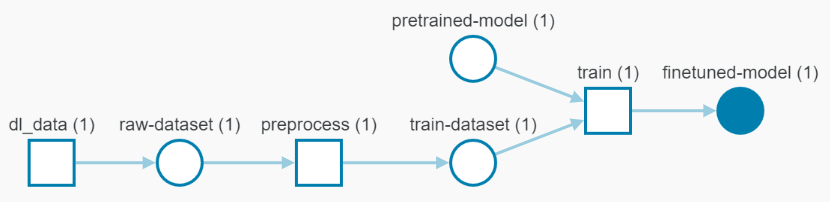
To understand how the model was developed, check the [W&B report](https://wandb.ai/wandb/huggingtweets/reports/HuggingTweets-Train-a-Model-to-Generate-Tweets--VmlldzoxMTY5MjI).
## Training data
The model was trained on tweets from lace.
| Data | lace |
| --- | --- |
| Tweets downloaded | 3065 |
| Retweets | 394 |
| Short tweets | 850 |
| Tweets kept | 1821 |
[Explore the data](https://wandb.ai/wandb/huggingtweets/runs/vscgyw1o/artifacts), which is tracked with [W&B artifacts](https://docs.wandb.com/artifacts) at every step of the pipeline.
## Training procedure
The model is based on a pre-trained [GPT-2](https://huggingface.co/gpt2) which is fine-tuned on @carboxylace's tweets.
Hyperparameters and metrics are recorded in the [W&B training run](https://wandb.ai/wandb/huggingtweets/runs/327ix6tk) for full transparency and reproducibility.
At the end of training, [the final model](https://wandb.ai/wandb/huggingtweets/runs/327ix6tk/artifacts) is logged and versioned.
## How to use
You can use this model directly with a pipeline for text generation:
```python
from transformers import pipeline
generator = pipeline('text-generation',
model='huggingtweets/carboxylace')
generator("My dream is", num_return_sequences=5)
```
## Limitations and bias
The model suffers from [the same limitations and bias as GPT-2](https://huggingface.co/gpt2#limitations-and-bias).
In addition, the data present in the user's tweets further affects the text generated by the model.
## About
*Built by Boris Dayma*
[](https://twitter.com/intent/follow?screen_name=borisdayma)
For more details, visit the project repository.
[](https://github.com/borisdayma/huggingtweets)
|
huggingtweets/borisjohnson-elonmusk-majornelson | cddbc7b7654ae03eafa4ea9ec03bf1fbb264f7fb | 2022-06-19T22:42:51.000Z | [
"pytorch",
"gpt2",
"text-generation",
"en",
"transformers",
"huggingtweets"
] | text-generation | false | huggingtweets | null | huggingtweets/borisjohnson-elonmusk-majornelson | 1 | null | transformers | 33,017 | ---
language: en
thumbnail: http://www.huggingtweets.com/borisjohnson-elonmusk-majornelson/1655678567047/predictions.png
tags:
- huggingtweets
widget:
- text: "My dream is"
---
<div class="inline-flex flex-col" style="line-height: 1.5;">
<div class="flex">
<div
style="display:inherit; margin-left: 4px; margin-right: 4px; width: 92px; height:92px; border-radius: 50%; background-size: cover; background-image: url('https://pbs.twimg.com/profile_images/1529956155937759233/Nyn1HZWF_400x400.jpg')">
</div>
<div
style="display:inherit; margin-left: 4px; margin-right: 4px; width: 92px; height:92px; border-radius: 50%; background-size: cover; background-image: url('https://pbs.twimg.com/profile_images/1519703427240013824/FOED2v9N_400x400.jpg')">
</div>
<div
style="display:inherit; margin-left: 4px; margin-right: 4px; width: 92px; height:92px; border-radius: 50%; background-size: cover; background-image: url('https://pbs.twimg.com/profile_images/1500170386520129536/Rr2G6A-N_400x400.jpg')">
</div>
</div>
<div style="text-align: center; margin-top: 3px; font-size: 16px; font-weight: 800">π€ AI CYBORG π€</div>
<div style="text-align: center; font-size: 16px; font-weight: 800">Elon Musk & Larry Hryb πΊπ¦ & Boris Johnson</div>
<div style="text-align: center; font-size: 14px;">@borisjohnson-elonmusk-majornelson</div>
</div>
I was made with [huggingtweets](https://github.com/borisdayma/huggingtweets).
Create your own bot based on your favorite user with [the demo](https://colab.research.google.com/github/borisdayma/huggingtweets/blob/master/huggingtweets-demo.ipynb)!
## How does it work?
The model uses the following pipeline.
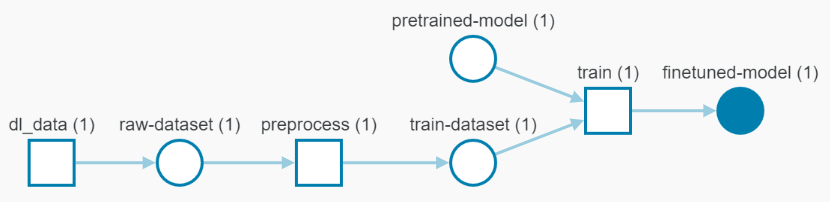
To understand how the model was developed, check the [W&B report](https://wandb.ai/wandb/huggingtweets/reports/HuggingTweets-Train-a-Model-to-Generate-Tweets--VmlldzoxMTY5MjI).
## Training data
The model was trained on tweets from Elon Musk & Larry Hryb πΊπ¦ & Boris Johnson.
| Data | Elon Musk | Larry Hryb πΊπ¦ | Boris Johnson |
| --- | --- | --- | --- |
| Tweets downloaded | 3250 | 3250 | 3248 |
| Retweets | 147 | 736 | 653 |
| Short tweets | 985 | 86 | 17 |
| Tweets kept | 2118 | 2428 | 2578 |
[Explore the data](https://wandb.ai/wandb/huggingtweets/runs/22m356ew/artifacts), which is tracked with [W&B artifacts](https://docs.wandb.com/artifacts) at every step of the pipeline.
## Training procedure
The model is based on a pre-trained [GPT-2](https://huggingface.co/gpt2) which is fine-tuned on @borisjohnson-elonmusk-majornelson's tweets.
Hyperparameters and metrics are recorded in the [W&B training run](https://wandb.ai/wandb/huggingtweets/runs/316f3w9h) for full transparency and reproducibility.
At the end of training, [the final model](https://wandb.ai/wandb/huggingtweets/runs/316f3w9h/artifacts) is logged and versioned.
## How to use
You can use this model directly with a pipeline for text generation:
```python
from transformers import pipeline
generator = pipeline('text-generation',
model='huggingtweets/borisjohnson-elonmusk-majornelson')
generator("My dream is", num_return_sequences=5)
```
## Limitations and bias
The model suffers from [the same limitations and bias as GPT-2](https://huggingface.co/gpt2#limitations-and-bias).
In addition, the data present in the user's tweets further affects the text generated by the model.
## About
*Built by Boris Dayma*
[](https://twitter.com/intent/follow?screen_name=borisdayma)
For more details, visit the project repository.
[](https://github.com/borisdayma/huggingtweets)
|
huggingtweets/fabrizioromano | 711bef2fb839f5cf429958c517692de2ced0132d | 2022-06-19T23:37:31.000Z | [
"pytorch",
"gpt2",
"text-generation",
"en",
"transformers",
"huggingtweets"
] | text-generation | false | huggingtweets | null | huggingtweets/fabrizioromano | 1 | null | transformers | 33,018 | ---
language: en
thumbnail: http://www.huggingtweets.com/fabrizioromano/1655681846804/predictions.png
tags:
- huggingtweets
widget:
- text: "My dream is"
---
<div class="inline-flex flex-col" style="line-height: 1.5;">
<div class="flex">
<div
style="display:inherit; margin-left: 4px; margin-right: 4px; width: 92px; height:92px; border-radius: 50%; background-size: cover; background-image: url('https://pbs.twimg.com/profile_images/1486761402853380113/3ifAqala_400x400.jpg')">
</div>
<div
style="display:none; margin-left: 4px; margin-right: 4px; width: 92px; height:92px; border-radius: 50%; background-size: cover; background-image: url('')">
</div>
<div
style="display:none; margin-left: 4px; margin-right: 4px; width: 92px; height:92px; border-radius: 50%; background-size: cover; background-image: url('')">
</div>
</div>
<div style="text-align: center; margin-top: 3px; font-size: 16px; font-weight: 800">π€ AI BOT π€</div>
<div style="text-align: center; font-size: 16px; font-weight: 800">Fabrizio Romano</div>
<div style="text-align: center; font-size: 14px;">@fabrizioromano</div>
</div>
I was made with [huggingtweets](https://github.com/borisdayma/huggingtweets).
Create your own bot based on your favorite user with [the demo](https://colab.research.google.com/github/borisdayma/huggingtweets/blob/master/huggingtweets-demo.ipynb)!
## How does it work?
The model uses the following pipeline.
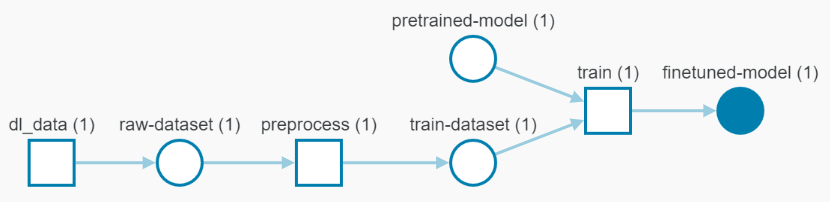
To understand how the model was developed, check the [W&B report](https://wandb.ai/wandb/huggingtweets/reports/HuggingTweets-Train-a-Model-to-Generate-Tweets--VmlldzoxMTY5MjI).
## Training data
The model was trained on tweets from Fabrizio Romano.
| Data | Fabrizio Romano |
| --- | --- |
| Tweets downloaded | 3250 |
| Retweets | 192 |
| Short tweets | 255 |
| Tweets kept | 2803 |
[Explore the data](https://wandb.ai/wandb/huggingtweets/runs/2mdxozh7/artifacts), which is tracked with [W&B artifacts](https://docs.wandb.com/artifacts) at every step of the pipeline.
## Training procedure
The model is based on a pre-trained [GPT-2](https://huggingface.co/gpt2) which is fine-tuned on @fabrizioromano's tweets.
Hyperparameters and metrics are recorded in the [W&B training run](https://wandb.ai/wandb/huggingtweets/runs/2ltk44ap) for full transparency and reproducibility.
At the end of training, [the final model](https://wandb.ai/wandb/huggingtweets/runs/2ltk44ap/artifacts) is logged and versioned.
## How to use
You can use this model directly with a pipeline for text generation:
```python
from transformers import pipeline
generator = pipeline('text-generation',
model='huggingtweets/fabrizioromano')
generator("My dream is", num_return_sequences=5)
```
## Limitations and bias
The model suffers from [the same limitations and bias as GPT-2](https://huggingface.co/gpt2#limitations-and-bias).
In addition, the data present in the user's tweets further affects the text generated by the model.
## About
*Built by Boris Dayma*
[](https://twitter.com/intent/follow?screen_name=borisdayma)
For more details, visit the project repository.
[](https://github.com/borisdayma/huggingtweets)
|
joshanashakya/codebert_sourcecode_nmt_pn2ja_50E_2e-05LR_16B_12E_12D | 2490fcd6501ab6115a8d2432ee387ed0ff94dacf | 2022-06-20T01:36:42.000Z | [
"pytorch",
"encoder-decoder",
"text2text-generation",
"transformers",
"autotrain_compatible"
] | text2text-generation | false | joshanashakya | null | joshanashakya/codebert_sourcecode_nmt_pn2ja_50E_2e-05LR_16B_12E_12D | 1 | null | transformers | 33,019 | Entry not found |
huggingtweets/grassmannian | f416ca775f74b79fa47fe9fb62b3d554aac54c07 | 2022-06-20T02:11:47.000Z | [
"pytorch",
"gpt2",
"text-generation",
"en",
"transformers",
"huggingtweets"
] | text-generation | false | huggingtweets | null | huggingtweets/grassmannian | 1 | null | transformers | 33,020 | ---
language: en
thumbnail: https://github.com/borisdayma/huggingtweets/blob/master/img/logo.png?raw=true
tags:
- huggingtweets
widget:
- text: "My dream is"
---
<div class="inline-flex flex-col" style="line-height: 1.5;">
<div class="flex">
<div
style="display:inherit; margin-left: 4px; margin-right: 4px; width: 92px; height:92px; border-radius: 50%; background-size: cover; background-image: url('https://pbs.twimg.com/profile_images/1529201641290752000/al3uPjXp_400x400.jpg')">
</div>
<div
style="display:none; margin-left: 4px; margin-right: 4px; width: 92px; height:92px; border-radius: 50%; background-size: cover; background-image: url('')">
</div>
<div
style="display:none; margin-left: 4px; margin-right: 4px; width: 92px; height:92px; border-radius: 50%; background-size: cover; background-image: url('')">
</div>
</div>
<div style="text-align: center; margin-top: 3px; font-size: 16px; font-weight: 800">π€ AI BOT π€</div>
<div style="text-align: center; font-size: 16px; font-weight: 800">Brendan π«₯ era</div>
<div style="text-align: center; font-size: 14px;">@grassmannian</div>
</div>
I was made with [huggingtweets](https://github.com/borisdayma/huggingtweets).
Create your own bot based on your favorite user with [the demo](https://colab.research.google.com/github/borisdayma/huggingtweets/blob/master/huggingtweets-demo.ipynb)!
## How does it work?
The model uses the following pipeline.
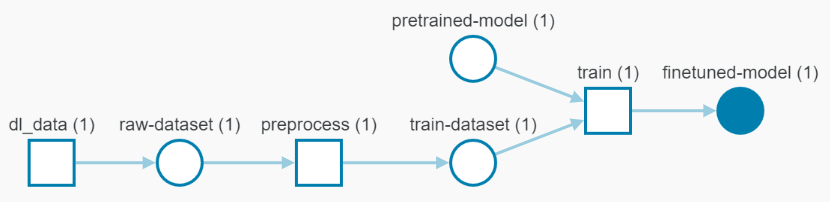
To understand how the model was developed, check the [W&B report](https://wandb.ai/wandb/huggingtweets/reports/HuggingTweets-Train-a-Model-to-Generate-Tweets--VmlldzoxMTY5MjI).
## Training data
The model was trained on tweets from Brendan π«₯ era.
| Data | Brendan π«₯ era |
| --- | --- |
| Tweets downloaded | 3239 |
| Retweets | 779 |
| Short tweets | 400 |
| Tweets kept | 2060 |
[Explore the data](https://wandb.ai/wandb/huggingtweets/runs/27vq2cvc/artifacts), which is tracked with [W&B artifacts](https://docs.wandb.com/artifacts) at every step of the pipeline.
## Training procedure
The model is based on a pre-trained [GPT-2](https://huggingface.co/gpt2) which is fine-tuned on @grassmannian's tweets.
Hyperparameters and metrics are recorded in the [W&B training run](https://wandb.ai/wandb/huggingtweets/runs/3pai1njh) for full transparency and reproducibility.
At the end of training, [the final model](https://wandb.ai/wandb/huggingtweets/runs/3pai1njh/artifacts) is logged and versioned.
## How to use
You can use this model directly with a pipeline for text generation:
```python
from transformers import pipeline
generator = pipeline('text-generation',
model='huggingtweets/grassmannian')
generator("My dream is", num_return_sequences=5)
```
## Limitations and bias
The model suffers from [the same limitations and bias as GPT-2](https://huggingface.co/gpt2#limitations-and-bias).
In addition, the data present in the user's tweets further affects the text generated by the model.
## About
*Built by Boris Dayma*
[](https://twitter.com/intent/follow?screen_name=borisdayma)
For more details, visit the project repository.
[](https://github.com/borisdayma/huggingtweets)
|
qgrantq/bert-finetuned-squad | be5a83180d0455ff30ba9a6b8723064ea19ff7c8 | 2022-06-20T08:03:46.000Z | [
"pytorch",
"tensorboard",
"bert",
"question-answering",
"dataset:squad",
"transformers",
"generated_from_trainer",
"license:apache-2.0",
"model-index",
"autotrain_compatible"
] | question-answering | false | qgrantq | null | qgrantq/bert-finetuned-squad | 1 | null | transformers | 33,021 | ---
license: apache-2.0
tags:
- generated_from_trainer
datasets:
- squad
model-index:
- name: bert-finetuned-squad
results: []
---
<!-- This model card has been generated automatically according to the information the Trainer had access to. You
should probably proofread and complete it, then remove this comment. -->
# bert-finetuned-squad
This model is a fine-tuned version of [bert-base-cased](https://huggingface.co/bert-base-cased) on the squad dataset.
## Model description
More information needed
## Intended uses & limitations
More information needed
## Training and evaluation data
More information needed
## Training procedure
### Training hyperparameters
The following hyperparameters were used during training:
- learning_rate: 2e-05
- train_batch_size: 8
- eval_batch_size: 8
- seed: 42
- optimizer: Adam with betas=(0.9,0.999) and epsilon=1e-08
- lr_scheduler_type: linear
- num_epochs: 3
- mixed_precision_training: Native AMP
### Training results
### Framework versions
- Transformers 4.20.0
- Pytorch 1.11.0+cu113
- Datasets 2.3.2
- Tokenizers 0.12.1
|
S2312dal/M7_MLM_final | 5d4799457909d334a791523e3216e65471017e45 | 2022-06-20T08:37:57.000Z | [
"pytorch",
"tensorboard",
"roberta",
"fill-mask",
"transformers",
"generated_from_trainer",
"license:apache-2.0",
"model-index",
"autotrain_compatible"
] | fill-mask | false | S2312dal | null | S2312dal/M7_MLM_final | 1 | null | transformers | 33,022 | ---
license: apache-2.0
tags:
- generated_from_trainer
model-index:
- name: M7_MLM_final
results: []
---
<!-- This model card has been generated automatically according to the information the Trainer had access to. You
should probably proofread and complete it, then remove this comment. -->
# M7_MLM_final
This model is a fine-tuned version of [sentence-transformers/all-distilroberta-v1](https://huggingface.co/sentence-transformers/all-distilroberta-v1) on an unknown dataset.
It achieves the following results on the evaluation set:
- Loss: 5.4732
## Model description
More information needed
## Intended uses & limitations
More information needed
## Training and evaluation data
More information needed
## Training procedure
### Training hyperparameters
The following hyperparameters were used during training:
- learning_rate: 2e-05
- train_batch_size: 16
- eval_batch_size: 16
- seed: 42
- optimizer: Adam with betas=(0.9,0.999) and epsilon=1e-08
- lr_scheduler_type: linear
- num_epochs: 3.0
- mixed_precision_training: Native AMP
### Training results
| Training Loss | Epoch | Step | Validation Loss |
|:-------------:|:-----:|:----:|:---------------:|
| 8.769 | 1.0 | 92 | 6.6861 |
| 6.3549 | 2.0 | 184 | 5.7455 |
| 5.826 | 3.0 | 276 | 5.5610 |
### Framework versions
- Transformers 4.20.0
- Pytorch 1.11.0+cu113
- Datasets 2.3.2
- Tokenizers 0.12.1
|
RuiqianLi/Malaya-speech_fine-tune_MrBrown_20_Jun | 6ea681253ce07c203c4d0383271ef613ab7fd6d2 | 2022-06-20T10:23:49.000Z | [
"pytorch",
"tensorboard",
"wav2vec2",
"automatic-speech-recognition",
"dataset:uob_singlish",
"transformers",
"generated_from_trainer",
"model-index"
] | automatic-speech-recognition | false | RuiqianLi | null | RuiqianLi/Malaya-speech_fine-tune_MrBrown_20_Jun | 1 | null | transformers | 33,023 | ---
tags:
- generated_from_trainer
datasets:
- uob_singlish
model-index:
- name: Malaya-speech_fine-tune_MrBrown_20_Jun
results: []
---
<!-- This model card has been generated automatically according to the information the Trainer had access to. You
should probably proofread and complete it, then remove this comment. -->
# Malaya-speech_fine-tune_MrBrown_20_Jun
This model is a fine-tuned version of [malay-huggingface/wav2vec2-xls-r-300m-mixed](https://huggingface.co/malay-huggingface/wav2vec2-xls-r-300m-mixed) on the uob_singlish dataset.
It achieves the following results on the evaluation set:
- Loss: 0.8868
- Wer: 0.3244
## Model description
More information needed
## Intended uses & limitations
More information needed
## Training and evaluation data
More information needed
## Training procedure
### Training hyperparameters
The following hyperparameters were used during training:
- learning_rate: 0.0002
- train_batch_size: 2
- eval_batch_size: 8
- seed: 42
- gradient_accumulation_steps: 2
- total_train_batch_size: 4
- optimizer: Adam with betas=(0.9,0.999) and epsilon=1e-08
- lr_scheduler_type: linear
- lr_scheduler_warmup_steps: 500
- num_epochs: 30
- mixed_precision_training: Native AMP
### Training results
| Training Loss | Epoch | Step | Validation Loss | Wer |
|:-------------:|:-----:|:----:|:---------------:|:------:|
| 0.8027 | 3.85 | 200 | 0.4800 | 0.2852 |
| 0.3773 | 7.69 | 400 | 0.6292 | 0.3316 |
| 0.3394 | 11.54 | 600 | 0.7376 | 0.3494 |
| 0.2653 | 15.38 | 800 | 0.9595 | 0.3137 |
| 0.1785 | 19.23 | 1000 | 0.7381 | 0.3440 |
| 0.1669 | 23.08 | 1200 | 0.9534 | 0.3529 |
| 0.0971 | 26.92 | 1400 | 0.8868 | 0.3244 |
### Framework versions
- Transformers 4.11.3
- Pytorch 1.10.0+cu113
- Datasets 1.18.3
- Tokenizers 0.10.3
|
aiBoo/opus-mt-en-ro-finetuned-en-to-ro | b7c088b9bb9186662d1cacc41a7c601f7ec8693e | 2022-06-20T10:44:47.000Z | [
"pytorch",
"tensorboard",
"marian",
"text2text-generation",
"dataset:wmt16",
"transformers",
"generated_from_trainer",
"license:apache-2.0",
"model-index",
"autotrain_compatible"
] | text2text-generation | false | aiBoo | null | aiBoo/opus-mt-en-ro-finetuned-en-to-ro | 1 | null | transformers | 33,024 | ---
license: apache-2.0
tags:
- generated_from_trainer
datasets:
- wmt16
metrics:
- bleu
model-index:
- name: opus-mt-en-ro-finetuned-en-to-ro
results:
- task:
name: Sequence-to-sequence Language Modeling
type: text2text-generation
dataset:
name: wmt16
type: wmt16
args: ro-en
metrics:
- name: Bleu
type: bleu
value: 28.1031
---
<!-- This model card has been generated automatically according to the information the Trainer had access to. You
should probably proofread and complete it, then remove this comment. -->
# opus-mt-en-ro-finetuned-en-to-ro
This model is a fine-tuned version of [Helsinki-NLP/opus-mt-en-ro](https://huggingface.co/Helsinki-NLP/opus-mt-en-ro) on the wmt16 dataset.
It achieves the following results on the evaluation set:
- Loss: 1.2896
- Bleu: 28.1031
- Gen Len: 34.082
## Model description
More information needed
## Intended uses & limitations
More information needed
## Training and evaluation data
More information needed
## Training procedure
### Training hyperparameters
The following hyperparameters were used during training:
- learning_rate: 2e-05
- train_batch_size: 16
- eval_batch_size: 16
- seed: 42
- optimizer: Adam with betas=(0.9,0.999) and epsilon=1e-08
- lr_scheduler_type: linear
- num_epochs: 1
- mixed_precision_training: Native AMP
### Training results
| Training Loss | Epoch | Step | Validation Loss | Bleu | Gen Len |
|:-------------:|:-----:|:-----:|:---------------:|:-------:|:-------:|
| 0.744 | 1.0 | 38145 | 1.2896 | 28.1031 | 34.082 |
### Framework versions
- Transformers 4.20.0
- Pytorch 1.11.0+cu113
- Datasets 2.3.2
- Tokenizers 0.12.1
|
dayone/simcse-nli-sbert-sts-klue-roberta-base | 5fe1c16477fa4d2abc7697eee6933274ccbf87df | 2022-06-20T12:01:54.000Z | [
"pytorch",
"roberta",
"feature-extraction",
"transformers"
] | feature-extraction | false | dayone | null | dayone/simcse-nli-sbert-sts-klue-roberta-base | 1 | null | transformers | 33,025 | Entry not found |
aminnaghavi/bert-base-parsbert-uncased-finetuned-perQA | 9f0f7d004f5ee638aee04b1f996e12f721c15c5e | 2022-06-20T14:45:21.000Z | [
"pytorch",
"tensorboard",
"bert",
"question-answering",
"dataset:persian_qa",
"transformers",
"generated_from_trainer",
"model-index",
"autotrain_compatible"
] | question-answering | false | aminnaghavi | null | aminnaghavi/bert-base-parsbert-uncased-finetuned-perQA | 1 | null | transformers | 33,026 | ---
tags:
- generated_from_trainer
datasets:
- persian_qa
model-index:
- name: bert-base-parsbert-uncased-finetuned-perQA
results: []
---
<!-- This model card has been generated automatically according to the information the Trainer had access to. You
should probably proofread and complete it, then remove this comment. -->
# bert-base-parsbert-uncased-finetuned-perQA
This model is a fine-tuned version of [HooshvareLab/bert-base-parsbert-uncased](https://huggingface.co/HooshvareLab/bert-base-parsbert-uncased) on the persian_qa dataset.
It achieves the following results on the evaluation set:
- Loss: 1.8648
## Model description
More information needed
## Intended uses & limitations
More information needed
## Training and evaluation data
More information needed
## Training procedure
### Training hyperparameters
The following hyperparameters were used during training:
- learning_rate: 2e-05
- train_batch_size: 16
- eval_batch_size: 16
- seed: 42
- optimizer: Adam with betas=(0.9,0.999) and epsilon=1e-08
- lr_scheduler_type: linear
- num_epochs: 3
### Training results
| Training Loss | Epoch | Step | Validation Loss |
|:-------------:|:-----:|:----:|:---------------:|
| 2.9599 | 1.0 | 565 | 2.0185 |
| 1.8889 | 2.0 | 1130 | 1.8088 |
| 1.4282 | 3.0 | 1695 | 1.8648 |
### Framework versions
- Transformers 4.20.0
- Pytorch 1.11.0+cu113
- Datasets 2.3.2
- Tokenizers 0.12.1
|
Gerard/xlm-roberta-base-finetuned-panx-de | 87e62cc4a00c254ff03916f5e33b546b5d706d5a | 2022-06-20T17:16:29.000Z | [
"pytorch",
"tensorboard",
"xlm-roberta",
"token-classification",
"dataset:xtreme",
"transformers",
"generated_from_trainer",
"license:mit",
"model-index",
"autotrain_compatible"
] | token-classification | false | Gerard | null | Gerard/xlm-roberta-base-finetuned-panx-de | 1 | null | transformers | 33,027 | ---
license: mit
tags:
- generated_from_trainer
datasets:
- xtreme
metrics:
- f1
model-index:
- name: xlm-roberta-base-finetuned-panx-de
results:
- task:
name: Token Classification
type: token-classification
dataset:
name: xtreme
type: xtreme
args: PAN-X.de
metrics:
- name: F1
type: f1
value: 0.8620945214069894
---
<!-- This model card has been generated automatically according to the information the Trainer had access to. You
should probably proofread and complete it, then remove this comment. -->
# xlm-roberta-base-finetuned-panx-de
This model is a fine-tuned version of [xlm-roberta-base](https://huggingface.co/xlm-roberta-base) on the xtreme dataset.
It achieves the following results on the evaluation set:
- Loss: 0.1372
- F1: 0.8621
## Model description
More information needed
## Intended uses & limitations
More information needed
## Training and evaluation data
More information needed
## Training procedure
### Training hyperparameters
The following hyperparameters were used during training:
- learning_rate: 5e-05
- train_batch_size: 24
- eval_batch_size: 24
- seed: 42
- optimizer: Adam with betas=(0.9,0.999) and epsilon=1e-08
- lr_scheduler_type: linear
- num_epochs: 3
### Training results
| Training Loss | Epoch | Step | Validation Loss | F1 |
|:-------------:|:-----:|:----:|:---------------:|:------:|
| 0.2575 | 1.0 | 525 | 0.1621 | 0.8292 |
| 0.1287 | 2.0 | 1050 | 0.1378 | 0.8526 |
| 0.0831 | 3.0 | 1575 | 0.1372 | 0.8621 |
### Framework versions
- Transformers 4.20.0
- Pytorch 1.11.0+cu113
- Datasets 2.3.2
- Tokenizers 0.12.1
|
ornil1/marian-finetuned-kde4-en-to-fr | 08cab9ccc5237dde1095840921d8efdf3f1632ae | 2022-06-21T01:21:05.000Z | [
"pytorch",
"tensorboard",
"marian",
"text2text-generation",
"dataset:kde4",
"transformers",
"translation",
"generated_from_trainer",
"license:apache-2.0",
"model-index",
"autotrain_compatible"
] | translation | false | ornil1 | null | ornil1/marian-finetuned-kde4-en-to-fr | 1 | null | transformers | 33,028 | ---
license: apache-2.0
tags:
- translation
- generated_from_trainer
datasets:
- kde4
model-index:
- name: marian-finetuned-kde4-en-to-fr
results: []
---
<!-- This model card has been generated automatically according to the information the Trainer had access to. You
should probably proofread and complete it, then remove this comment. -->
# marian-finetuned-kde4-en-to-fr
This model is a fine-tuned version of [Helsinki-NLP/opus-mt-en-fr](https://huggingface.co/Helsinki-NLP/opus-mt-en-fr) on the kde4 dataset.
## Model description
More information needed
## Intended uses & limitations
More information needed
## Training and evaluation data
More information needed
## Training procedure
### Training hyperparameters
The following hyperparameters were used during training:
- learning_rate: 2e-05
- train_batch_size: 32
- eval_batch_size: 64
- seed: 42
- optimizer: Adam with betas=(0.9,0.999) and epsilon=1e-08
- lr_scheduler_type: linear
- num_epochs: 3
- mixed_precision_training: Native AMP
### Training results
### Framework versions
- Transformers 4.20.0
- Pytorch 1.11.0+cu113
- Datasets 2.3.2
- Tokenizers 0.12.1
|
mhmsadegh/bert-base-parsbert-uncased-finetuned-squad | 5d4dfd8a097a3988f9299ea785b51e90cb34a83e | 2022-06-21T06:32:55.000Z | [
"pytorch",
"tensorboard",
"bert",
"question-answering",
"transformers",
"generated_from_trainer",
"model-index",
"autotrain_compatible"
] | question-answering | false | mhmsadegh | null | mhmsadegh/bert-base-parsbert-uncased-finetuned-squad | 1 | null | transformers | 33,029 | ---
tags:
- generated_from_trainer
model-index:
- name: bert-base-parsbert-uncased-finetuned-squad
results: []
---
<!-- This model card has been generated automatically according to the information the Trainer had access to. You
should probably proofread and complete it, then remove this comment. -->
# bert-base-parsbert-uncased-finetuned-squad
This model is a fine-tuned version of [HooshvareLab/bert-base-parsbert-uncased](https://huggingface.co/HooshvareLab/bert-base-parsbert-uncased) on an unknown dataset.
It achieves the following results on the evaluation set:
- Loss: 4.2932
## Model description
More information needed
## Intended uses & limitations
More information needed
## Training and evaluation data
More information needed
## Training procedure
### Training hyperparameters
The following hyperparameters were used during training:
- learning_rate: 2e-05
- train_batch_size: 16
- eval_batch_size: 16
- seed: 42
- optimizer: Adam with betas=(0.9,0.999) and epsilon=1e-08
- lr_scheduler_type: linear
- num_epochs: 3
### Training results
| Training Loss | Epoch | Step | Validation Loss |
|:-------------:|:-----:|:----:|:---------------:|
| No log | 1.0 | 57 | 4.3248 |
| No log | 2.0 | 114 | 4.2283 |
| No log | 3.0 | 171 | 4.2932 |
### Framework versions
- Transformers 4.20.0
- Pytorch 1.11.0+cu113
- Datasets 2.3.2
- Tokenizers 0.12.1
|
nthakur/mcontriever-base-msmarco | b4ea743fb2e09bc686f43b77d571e55e2051fd84 | 2022-06-20T22:14:34.000Z | [
"pytorch",
"bert",
"feature-extraction",
"arxiv:2112.09118",
"sentence-transformers",
"sentence-similarity",
"transformers"
] | sentence-similarity | false | nthakur | null | nthakur/mcontriever-base-msmarco | 1 | null | sentence-transformers | 33,030 | ---
pipeline_tag: sentence-similarity
tags:
- sentence-transformers
- feature-extraction
- sentence-similarity
- transformers
---
# mcontriever-base-msmarco
This is a [sentence-transformers](https://www.SBERT.net) model: It maps sentences & paragraphs to a 768 dimensional dense vector space and can be used for tasks like clustering or semantic search.
This model was converted from the facebook [mcontriever-msmarco model](https://huggingface.co/facebook/mcontriever-msmarco). When using this model, have a look at the publication: [Unsupervised Dense Information Retrieval with Contrastive Learning](https://arxiv.org/abs/2112.09118).
<!--- Describe your model here -->
## Usage (Sentence-Transformers)
Using this model becomes easy when you have [sentence-transformers](https://www.SBERT.net) installed:
```
pip install -U sentence-transformers
```
Then you can use the model like this:
```python
from sentence_transformers import SentenceTransformer
sentences = ["This is an example sentence", "Each sentence is converted"]
model = SentenceTransformer('nthakur/mcontriever-base-msmarco')
embeddings = model.encode(sentences)
print(embeddings)
```
## Usage (HuggingFace Transformers)
Without [sentence-transformers](https://www.SBERT.net), you can use the model like this: First, you pass your input through the transformer model, then you have to apply the right pooling-operation on-top of the contextualized word embeddings.
```python
from transformers import AutoTokenizer, AutoModel
import torch
#Mean Pooling - Take attention mask into account for correct averaging
def mean_pooling(model_output, attention_mask):
token_embeddings = model_output[0] #First element of model_output contains all token embeddings
input_mask_expanded = attention_mask.unsqueeze(-1).expand(token_embeddings.size()).float()
return torch.sum(token_embeddings * input_mask_expanded, 1) / torch.clamp(input_mask_expanded.sum(1), min=1e-9)
# Sentences we want sentence embeddings for
sentences = ['This is an example sentence', 'Each sentence is converted']
# Load model from HuggingFace Hub
tokenizer = AutoTokenizer.from_pretrained('nthakur/mcontriever-base-msmarco')
model = AutoModel.from_pretrained('nthakur/mcontriever-base-msmarco')
# Tokenize sentences
encoded_input = tokenizer(sentences, padding=True, truncation=True, return_tensors='pt')
# Compute token embeddings
with torch.no_grad():
model_output = model(**encoded_input)
# Perform pooling. In this case, mean pooling.
sentence_embeddings = mean_pooling(model_output, encoded_input['attention_mask'])
print("Sentence embeddings:")
print(sentence_embeddings)
```
## Evaluation Results
<!--- Describe how your model was evaluated -->
For an automated evaluation of this model, see the *Sentence Embeddings Benchmark*: [https://seb.sbert.net](https://seb.sbert.net?model_namenthakur/=mcontriever-base-msmarco)
## Full Model Architecture
```
SentenceTransformer(
(0): Transformer({'max_seq_length': 509, 'do_lower_case': False}) with Transformer model: BertModel
(1): Pooling({'word_embedding_dimension': 768, 'pooling_mode_cls_token': False, 'pooling_mode_mean_tokens': True, 'pooling_mode_max_tokens': False, 'pooling_mode_mean_sqrt_len_tokens': False})
)
```
## Citing & Authors
<!--- Describe where people can find more information --> |
spencer/contriever_pipeline | dff1e38677dd0254393766423c4fb785b585bc29 | 2022-06-21T00:35:23.000Z | [
"pytorch",
"bert",
"arxiv:2112.09118",
"transformers",
"feature-extraction"
] | feature-extraction | false | spencer | null | spencer/contriever_pipeline | 1 | null | transformers | 33,031 | ---
tags: feature-extraction
pipeline_tag: feature-extraction
---
This model has been trained without supervision following the approach described in [Towards Unsupervised Dense Information Retrieval with Contrastive Learning](https://arxiv.org/abs/2112.09118). The associated GitHub repository is available here https://github.com/facebookresearch/contriever.
## Usage (HuggingFace Transformers)
Using the model directly available in HuggingFace transformers requires to add a mean pooling operation to obtain a sentence embedding.
```python
import torch
from transformers import AutoTokenizer, AutoModel
tokenizer = AutoTokenizer.from_pretrained('facebook/contriever')
model = AutoModel.from_pretrained('facebook/contriever')
sentences = [
"Where was Marie Curie born?",
"Maria Sklodowska, later known as Marie Curie, was born on November 7, 1867.",
"Born in Paris on 15 May 1859, Pierre Curie was the son of Eugène Curie, a doctor of French Catholic origin from Alsace."
]
# Apply tokenizer
inputs = tokenizer(sentences, padding=True, truncation=True, return_tensors='pt')
# Compute token embeddings
outputs = model(**inputs)
# Mean pooling
def mean_pooling(token_embeddings, mask):
token_embeddings = token_embeddings.masked_fill(~mask[..., None].bool(), 0.)
sentence_embeddings = token_embeddings.sum(dim=1) / mask.sum(dim=1)[..., None]
return sentence_embeddings
embeddings = mean_pooling(outputs[0], inputs['attention_mask'])
``` |
huggingtweets/dav_erage | fd456b4bcc036e38a7d2a49cd16e2117675f6714 | 2022-06-21T00:57:28.000Z | [
"pytorch",
"gpt2",
"text-generation",
"en",
"transformers",
"huggingtweets"
] | text-generation | false | huggingtweets | null | huggingtweets/dav_erage | 1 | null | transformers | 33,032 | ---
language: en
thumbnail: http://www.huggingtweets.com/dav_erage/1655773043560/predictions.png
tags:
- huggingtweets
widget:
- text: "My dream is"
---
<div class="inline-flex flex-col" style="line-height: 1.5;">
<div class="flex">
<div
style="display:inherit; margin-left: 4px; margin-right: 4px; width: 92px; height:92px; border-radius: 50%; background-size: cover; background-image: url('https://pbs.twimg.com/profile_images/1517890310642278400/p9HNFjUU_400x400.jpg')">
</div>
<div
style="display:none; margin-left: 4px; margin-right: 4px; width: 92px; height:92px; border-radius: 50%; background-size: cover; background-image: url('')">
</div>
<div
style="display:none; margin-left: 4px; margin-right: 4px; width: 92px; height:92px; border-radius: 50%; background-size: cover; background-image: url('')">
</div>
</div>
<div style="text-align: center; margin-top: 3px; font-size: 16px; font-weight: 800">π€ AI BOT π€</div>
<div style="text-align: center; font-size: 16px; font-weight: 800">π blooming 'bold π»</div>
<div style="text-align: center; font-size: 14px;">@dav_erage</div>
</div>
I was made with [huggingtweets](https://github.com/borisdayma/huggingtweets).
Create your own bot based on your favorite user with [the demo](https://colab.research.google.com/github/borisdayma/huggingtweets/blob/master/huggingtweets-demo.ipynb)!
## How does it work?
The model uses the following pipeline.
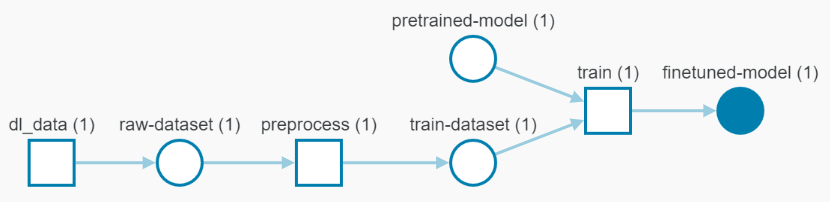
To understand how the model was developed, check the [W&B report](https://wandb.ai/wandb/huggingtweets/reports/HuggingTweets-Train-a-Model-to-Generate-Tweets--VmlldzoxMTY5MjI).
## Training data
The model was trained on tweets from π blooming 'bold π».
| Data | π blooming 'bold π» |
| --- | --- |
| Tweets downloaded | 3247 |
| Retweets | 279 |
| Short tweets | 440 |
| Tweets kept | 2528 |
[Explore the data](https://wandb.ai/wandb/huggingtweets/runs/2l3pf3na/artifacts), which is tracked with [W&B artifacts](https://docs.wandb.com/artifacts) at every step of the pipeline.
## Training procedure
The model is based on a pre-trained [GPT-2](https://huggingface.co/gpt2) which is fine-tuned on @dav_erage's tweets.
Hyperparameters and metrics are recorded in the [W&B training run](https://wandb.ai/wandb/huggingtweets/runs/228evxem) for full transparency and reproducibility.
At the end of training, [the final model](https://wandb.ai/wandb/huggingtweets/runs/228evxem/artifacts) is logged and versioned.
## How to use
You can use this model directly with a pipeline for text generation:
```python
from transformers import pipeline
generator = pipeline('text-generation',
model='huggingtweets/dav_erage')
generator("My dream is", num_return_sequences=5)
```
## Limitations and bias
The model suffers from [the same limitations and bias as GPT-2](https://huggingface.co/gpt2#limitations-and-bias).
In addition, the data present in the user's tweets further affects the text generated by the model.
## About
*Built by Boris Dayma*
[](https://twitter.com/intent/follow?screen_name=borisdayma)
For more details, visit the project repository.
[](https://github.com/borisdayma/huggingtweets)
|
anonsubms/msrp_length | b22d22b010f4f50483699b619a9e03e22f7f12d9 | 2022-06-21T04:43:21.000Z | [
"pytorch",
"t5",
"text2text-generation",
"transformers",
"autotrain_compatible"
] | text2text-generation | false | anonsubms | null | anonsubms/msrp_length | 1 | null | transformers | 33,033 | Entry not found |
anonsubms/msrp_ratio | c82ad9b4ab4255fa29d6aaaac815ab52a88a793a | 2022-06-21T04:47:15.000Z | [
"pytorch",
"t5",
"text2text-generation",
"transformers",
"autotrain_compatible"
] | text2text-generation | false | anonsubms | null | anonsubms/msrp_ratio | 1 | null | transformers | 33,034 | Entry not found |
anonsubms/msrp_ratio_sb | 8c5c1018479608e26a4ecb81c799c96d1fccdfb8 | 2022-06-21T04:45:36.000Z | [
"pytorch",
"t5",
"text2text-generation",
"transformers",
"autotrain_compatible"
] | text2text-generation | false | anonsubms | null | anonsubms/msrp_ratio_sb | 1 | null | transformers | 33,035 | Entry not found |
anonsubms/t5pretrain | be6be544cfb42455df0ebf5122e731f2f0d53b8c | 2022-06-21T05:58:51.000Z | [
"pytorch",
"t5",
"text2text-generation",
"transformers",
"autotrain_compatible"
] | text2text-generation | false | anonsubms | null | anonsubms/t5pretrain | 1 | null | transformers | 33,036 | Entry not found |
kjunelee/bert-base-uncased-issues-128 | adea1e13e7584660cc061b0e94dd41804aa34412 | 2022-06-21T07:24:49.000Z | [
"pytorch",
"bert",
"fill-mask",
"transformers",
"generated_from_trainer",
"license:apache-2.0",
"model-index",
"autotrain_compatible"
] | fill-mask | false | kjunelee | null | kjunelee/bert-base-uncased-issues-128 | 1 | null | transformers | 33,037 | ---
license: apache-2.0
tags:
- generated_from_trainer
model-index:
- name: bert-base-uncased-issues-128
results: []
---
<!-- This model card has been generated automatically according to the information the Trainer had access to. You
should probably proofread and complete it, then remove this comment. -->
# bert-base-uncased-issues-128
This model is a fine-tuned version of [bert-base-uncased](https://huggingface.co/bert-base-uncased) on the None dataset.
It achieves the following results on the evaluation set:
- Loss: 1.2314
## Model description
More information needed
## Intended uses & limitations
More information needed
## Training and evaluation data
More information needed
## Training procedure
### Training hyperparameters
The following hyperparameters were used during training:
- learning_rate: 5e-05
- train_batch_size: 64
- eval_batch_size: 16
- seed: 42
- optimizer: Adam with betas=(0.9,0.999) and epsilon=1e-08
- lr_scheduler_type: linear
- num_epochs: 16
### Training results
| Training Loss | Epoch | Step | Validation Loss |
|:-------------:|:-----:|:----:|:---------------:|
| 2.193 | 1.0 | 146 | 1.7004 |
| 1.7081 | 2.0 | 292 | 1.4895 |
| 1.5458 | 3.0 | 438 | 1.4427 |
| 1.4715 | 4.0 | 584 | 1.4081 |
| 1.3944 | 5.0 | 730 | 1.3163 |
| 1.3396 | 6.0 | 876 | 1.3200 |
| 1.2945 | 7.0 | 1022 | 1.2785 |
| 1.2652 | 8.0 | 1168 | 1.2473 |
| 1.2332 | 9.0 | 1314 | 1.2321 |
| 1.2042 | 10.0 | 1460 | 1.2162 |
| 1.204 | 11.0 | 1606 | 1.1781 |
| 1.1866 | 12.0 | 1752 | 1.2211 |
| 1.1592 | 13.0 | 1898 | 1.2801 |
| 1.1503 | 14.0 | 2044 | 1.1768 |
| 1.1268 | 15.0 | 2190 | 1.1657 |
| 1.1521 | 16.0 | 2336 | 1.2314 |
### Framework versions
- Transformers 4.19.2
- Pytorch 1.11.0
- Datasets 2.2.3.dev0
- Tokenizers 0.12.1
|
UrukHan/wav2vec2-ru | 0673734b60747b2b4783b545aaf8aaae6b5ba02f | 2022-06-21T21:19:43.000Z | [
"pytorch",
"tensorboard",
"wav2vec2",
"automatic-speech-recognition",
"transformers",
"generated_from_trainer",
"model-index"
] | automatic-speech-recognition | false | UrukHan | null | UrukHan/wav2vec2-ru | 1 | null | transformers | 33,038 | ---
tags:
- generated_from_trainer
model-index:
- name: wav2vec2-ru
results: []
---
<!-- This model card has been generated automatically according to the information the Trainer had access to. You
should probably proofread and complete it, then remove this comment. -->
# wav2vec2-ru
This model was trained from scratch on the None dataset.
It achieves the following results on the evaluation set:
- Loss: 0.5468
- Wer: 0.4124
## Model description
More information needed
## Intended uses & limitations
More information needed
## Training and evaluation data
More information needed
## Training procedure
### Training hyperparameters
The following hyperparameters were used during training:
- learning_rate: 1e-06
- train_batch_size: 1
- eval_batch_size: 8
- seed: 42
- optimizer: Adam with betas=(0.9,0.999) and epsilon=1e-08
- lr_scheduler_type: linear
- lr_scheduler_warmup_steps: 1000
- num_epochs: 1
- mixed_precision_training: Native AMP
### Training results
| Training Loss | Epoch | Step | Validation Loss | Wer |
|:-------------:|:-----:|:----:|:---------------:|:------:|
| 0.511 | 0.21 | 1000 | 0.5444 | 0.4183 |
| 0.5021 | 0.43 | 2000 | 0.5727 | 0.4112 |
| 0.4746 | 0.64 | 3000 | 0.5495 | 0.4116 |
| 0.5052 | 0.85 | 4000 | 0.5468 | 0.4124 |
### Framework versions
- Transformers 4.20.0
- Pytorch 1.11.0+cu113
- Datasets 2.3.2
- Tokenizers 0.12.1
|
abhishek/autotrain-vision_af7ac4244f7a4f96bc89a28a87b2bb60-217226 | d1b4097993e575fc6170c0ebc82e32bab5b6c84a | 2022-06-21T11:03:15.000Z | [
"pytorch",
"swin",
"image-classification",
"transformers"
] | image-classification | false | abhishek | null | abhishek/autotrain-vision_af7ac4244f7a4f96bc89a28a87b2bb60-217226 | 1 | null | transformers | 33,039 | Entry not found |
lmqg/t5-large-subjqa-vanilla-electronics | 0bdd3e883844e7ee646d8d866efe6dc7fbc68fdc | 2022-06-21T11:00:36.000Z | [
"pytorch",
"t5",
"text2text-generation",
"transformers",
"autotrain_compatible"
] | text2text-generation | false | lmqg | null | lmqg/t5-large-subjqa-vanilla-electronics | 1 | null | transformers | 33,040 | Entry not found |
Nonnyss/Music-wav2vec2-finetune | d42ac9c06b3433939b43fefe13fab0d01fb61504 | 2022-06-21T16:05:31.000Z | [
"pytorch",
"wav2vec2",
"automatic-speech-recognition",
"transformers"
] | automatic-speech-recognition | false | Nonnyss | null | Nonnyss/Music-wav2vec2-finetune | 1 | null | transformers | 33,041 | Entry not found |
sasha/dog-food-swin-tiny-patch4-window7-224 | ba6973a9898980d2091a7d54f441be55e6bb4ad0 | 2022-06-22T13:56:12.000Z | [
"pytorch",
"tensorboard",
"swin",
"image-classification",
"dataset:sasha/dog-food",
"transformers",
"huggingpics",
"model-index"
] | image-classification | false | sasha | null | sasha/dog-food-swin-tiny-patch4-window7-224 | 1 | null | transformers | 33,042 | ---
tags:
- image-classification
- pytorch
- huggingpics
datasets:
- sasha/dog-food
metrics:
- accuracy
- f1
model-index:
- name: dog-food-swin-tiny-patch4-window7-224
results:
- task:
name: Image Classification
type: image-classification
dataset:
name: Dog Food
type: sasha/dog-food
metrics:
- name: Accuracy
type: accuracy
value: 1.0
---
# dog-food-swin-tiny-patch4-window7-224
This model was trained on the `train` split of the [Dogs vs Food](https://huggingface.co/datasets/sasha/dog-food) dataset -- try training your own using the
[the demo on Google Colab](https://colab.research.google.com/github/nateraw/huggingpics/blob/main/HuggingPics.ipynb)!
## Example Images
#### dog

#### food
 |
sasha/dog-food-convnext-tiny-224 | e1831d21b96a4a51dc39fcf7b7110cdd5f8f9dfd | 2022-06-22T13:56:32.000Z | [
"pytorch",
"tensorboard",
"convnext",
"image-classification",
"dataset:sasha/dog-food",
"transformers",
"huggingpics",
"model-index"
] | image-classification | false | sasha | null | sasha/dog-food-convnext-tiny-224 | 1 | null | transformers | 33,043 | ---
tags:
- image-classification
- pytorch
- huggingpics
datasets:
- sasha/dog-food
metrics:
- accuracy
- f1
model-index:
- name: dog-food-convnext-tiny-224
results:
- task:
name: Image Classification
type: image-classification
dataset:
name: Dog Food
type: sasha/dog-food
metrics:
- name: Accuracy
type: accuracy
value: 1.0
---
# dog-food-convnext-tiny-224
This model was trained on the `train` split of the [Dogs vs Food](https://huggingface.co/datasets/sasha/dog-food) dataset -- try training your own using the
[the demo on Google Colab](https://colab.research.google.com/github/nateraw/huggingpics/blob/main/HuggingPics.ipynb)!
## Example Images
#### dog

#### food
 |
Nonnyss/Music-wav2vec2-finetunee | e6a4ae654caaa39e385e097031f87808ca15a65f | 2022-06-21T16:19:02.000Z | [
"pytorch",
"wav2vec2",
"automatic-speech-recognition",
"transformers"
] | automatic-speech-recognition | false | Nonnyss | null | Nonnyss/Music-wav2vec2-finetunee | 1 | null | transformers | 33,044 | Entry not found |
Mascariddu8/masca-tokenizer | 03730ae21d0724acc38e84a67316c9a8a92e8c8a | 2022-06-21T17:13:01.000Z | [
"pytorch",
"camembert",
"fill-mask",
"transformers",
"autotrain_compatible"
] | fill-mask | false | Mascariddu8 | null | Mascariddu8/masca-tokenizer | 1 | null | transformers | 33,045 | Entry not found |
roshnir/xlmr-finetuned-mlqa-dev-cross-vi-hi | bded3a4f05c52671d8361284850809655b64d4e0 | 2022-06-21T20:09:40.000Z | [
"pytorch",
"xlm-roberta",
"question-answering",
"transformers",
"autotrain_compatible"
] | question-answering | false | roshnir | null | roshnir/xlmr-finetuned-mlqa-dev-cross-vi-hi | 1 | null | transformers | 33,046 | Entry not found |
AlekseyKorshuk/temp-model | b69ea92ae216787eea20d976078b412dcbcb6ce7 | 2022-06-21T21:04:12.000Z | [
"pytorch",
"gptj",
"text-generation",
"transformers"
] | text-generation | false | AlekseyKorshuk | null | AlekseyKorshuk/temp-model | 1 | null | transformers | 33,047 | Entry not found |
Laggrif/DialoGPT-medium-3PO | 7b73c52a5ee8ed50eaf0a1ac98d9e4b488a0e94b | 2022-06-21T22:01:45.000Z | [
"pytorch",
"gpt2",
"text-generation",
"transformers",
"conversational"
] | conversational | false | Laggrif | null | Laggrif/DialoGPT-medium-3PO | 1 | null | transformers | 33,048 | ---
tags:
- conversational
---
# C-3PO DialoGPT Model |
chandrasutrisnotjhong/marian-finetuned-kde4-en-to-fr | 043cc627156f834a50cf26f0bf012c6a4d30b075 | 2022-06-28T04:10:31.000Z | [
"pytorch",
"tensorboard",
"marian",
"text2text-generation",
"dataset:kde4",
"transformers",
"translation",
"generated_from_trainer",
"license:apache-2.0",
"model-index",
"autotrain_compatible"
] | translation | false | chandrasutrisnotjhong | null | chandrasutrisnotjhong/marian-finetuned-kde4-en-to-fr | 1 | null | transformers | 33,049 | ---
license: apache-2.0
tags:
- translation
- generated_from_trainer
datasets:
- kde4
metrics:
- bleu
model-index:
- name: marian-finetuned-kde4-en-to-fr
results:
- task:
name: Sequence-to-sequence Language Modeling
type: text2text-generation
dataset:
name: kde4
type: kde4
args: en-fr
metrics:
- name: Bleu
type: bleu
value: 52.83242564204547
---
<!-- This model card has been generated automatically according to the information the Trainer had access to. You
should probably proofread and complete it, then remove this comment. -->
# marian-finetuned-kde4-en-to-fr
This model is a fine-tuned version of [Helsinki-NLP/opus-mt-en-fr](https://huggingface.co/Helsinki-NLP/opus-mt-en-fr) on the kde4 dataset.
It achieves the following results on the evaluation set:
- Loss: 0.8560
- Bleu: 52.8324
## Model description
More information needed
## Intended uses & limitations
More information needed
## Training and evaluation data
More information needed
## Training procedure
### Training hyperparameters
The following hyperparameters were used during training:
- learning_rate: 2e-05
- train_batch_size: 32
- eval_batch_size: 64
- seed: 42
- optimizer: Adam with betas=(0.9,0.999) and epsilon=1e-08
- lr_scheduler_type: linear
- num_epochs: 3
- mixed_precision_training: Native AMP
### Training results
### Framework versions
- Transformers 4.20.1
- Pytorch 1.11.0+cu113
- Datasets 2.3.2
- Tokenizers 0.12.1
|
mhmsadegh/albert-fa-base-v2-finetuned-squad | 46e0c9bfddc06efb068392dc27cf6eb4aedafb59 | 2022-06-22T19:50:58.000Z | [
"pytorch",
"tensorboard",
"albert",
"question-answering",
"transformers",
"autotrain_compatible"
] | question-answering | false | mhmsadegh | null | mhmsadegh/albert-fa-base-v2-finetuned-squad | 1 | null | transformers | 33,050 | Entry not found |
chandrasutrisnotjhong/marian-finetuned-kde4-en-to-fr-accelerate | ac28ae226be025488a93fb95d023159855388b41 | 2022-06-28T05:12:53.000Z | [
"pytorch",
"marian",
"text2text-generation",
"transformers",
"autotrain_compatible"
] | text2text-generation | false | chandrasutrisnotjhong | null | chandrasutrisnotjhong/marian-finetuned-kde4-en-to-fr-accelerate | 1 | null | transformers | 33,051 | Entry not found |
lmqg/bart-large-squadshifts-vanilla-nyt | fcb6bb7060ed700af0cf83019411dd81210c9540 | 2022-06-22T10:56:06.000Z | [
"pytorch",
"bart",
"text2text-generation",
"transformers",
"autotrain_compatible"
] | text2text-generation | false | lmqg | null | lmqg/bart-large-squadshifts-vanilla-nyt | 1 | null | transformers | 33,052 | Entry not found |
abhishek/autotrain-vision_528a5bd60a4b4b1080538a6ede3f23c7-260265 | 29350300f3e729094365b2ec4d454ba94b9c1b85 | 2022-06-22T10:02:50.000Z | [
"pytorch",
"swin",
"image-classification",
"dataset:abhishek/autotrain-data-vision_528a5bd60a4b4b1080538a6ede3f23c7",
"transformers",
"autotrain",
"co2_eq_emissions"
] | image-classification | false | abhishek | null | abhishek/autotrain-vision_528a5bd60a4b4b1080538a6ede3f23c7-260265 | 1 | null | transformers | 33,053 | ---
tags: autotrain
datasets:
- abhishek/autotrain-data-vision_528a5bd60a4b4b1080538a6ede3f23c7
co2_eq_emissions: 8.217704896005591
---
# Model Trained Using AutoTrain
- Problem type: Multi-class Classification
- Model ID: 260265
- CO2 Emissions (in grams): 8.217704896005591
## Validation Metrics
- Loss: 0.24580252170562744
- Accuracy: 0.914
- Macro F1: 0.912823674084623
- Micro F1: 0.914
- Weighted F1: 0.9128236740846232
- Macro Precision: 0.9135654150297885
- Micro Precision: 0.914
- Weighted Precision: 0.9135654150297884
- Macro Recall: 0.9139999999999999
- Micro Recall: 0.914
- Weighted Recall: 0.914 |
sasuke/bert-finetuned-squad | c4a3920a46fdfb2f770730b90a1cdf048b1266c8 | 2022-06-22T12:01:31.000Z | [
"pytorch",
"tensorboard",
"bert",
"question-answering",
"transformers",
"autotrain_compatible"
] | question-answering | false | sasuke | null | sasuke/bert-finetuned-squad | 1 | null | transformers | 33,054 | Entry not found |
elena-soare/docu-t5-large-FK | e684abdb56022ad2c1d95daf64cc47ea655e400a | 2022-06-22T13:04:18.000Z | [
"pytorch",
"t5",
"text2text-generation",
"transformers",
"autotrain_compatible"
] | text2text-generation | false | elena-soare | null | elena-soare/docu-t5-large-FK | 1 | null | transformers | 33,055 | Entry not found |
elena-soare/docu-t5-large-SD | e6c8a7717cdda9e1555d8aff4e9c599bf4836728 | 2022-06-22T13:28:21.000Z | [
"pytorch",
"t5",
"text2text-generation",
"transformers",
"autotrain_compatible"
] | text2text-generation | false | elena-soare | null | elena-soare/docu-t5-large-SD | 1 | null | transformers | 33,056 | Entry not found |
paola-md/recipe-ts | d0e750c7989c14be4ba62ff01c1ea7e95e2c9d02 | 2022-06-22T13:03:45.000Z | [
"pytorch",
"bert",
"fill-mask",
"transformers",
"autotrain_compatible"
] | fill-mask | false | paola-md | null | paola-md/recipe-ts | 1 | null | transformers | 33,057 | Entry not found |
mayoughi/where_am_I_hospital-balcony-hallway-airport-coffee-house | 81e05b54015f7f750d9ebd66a110023e32105949 | 2022-06-22T16:00:57.000Z | [
"pytorch",
"tensorboard",
"vit",
"image-classification",
"transformers",
"huggingpics",
"model-index"
] | image-classification | false | mayoughi | null | mayoughi/where_am_I_hospital-balcony-hallway-airport-coffee-house | 1 | null | transformers | 33,058 | ---
tags:
- image-classification
- pytorch
- huggingpics
metrics:
- accuracy
model-index:
- name: where_am_I_hospital-balcony-hallway-airport-coffee-house
results:
- task:
name: Image Classification
type: image-classification
metrics:
- name: Accuracy
type: accuracy
value: 0.8839285969734192
---
# where_am_I_hospital-balcony-hallway-airport-coffee-house
Autogenerated by HuggingPicsπ€πΌοΈ
Create your own image classifier for **anything** by running [the demo on Google Colab](https://colab.research.google.com/github/nateraw/huggingpics/blob/main/HuggingPics.ipynb).
Report any issues with the demo at the [github repo](https://github.com/nateraw/huggingpics).
## Example Images
#### airport

#### balcony

#### coffee house indoors

#### hallway

#### hospital
 |
atendstowards0/codeparrot-ds | f78c14bae27b05646e3502746678f5daa35735dd | 2022-06-22T17:56:15.000Z | [
"pytorch",
"tensorboard",
"gpt2",
"text-generation",
"transformers",
"generated_from_trainer",
"license:mit",
"model-index"
] | text-generation | false | atendstowards0 | null | atendstowards0/codeparrot-ds | 1 | null | transformers | 33,059 | ---
license: mit
tags:
- generated_from_trainer
model-index:
- name: codeparrot-ds
results: []
---
<!-- This model card has been generated automatically according to the information the Trainer had access to. You
should probably proofread and complete it, then remove this comment. -->
# codeparrot-ds
This model is a fine-tuned version of [gpt2](https://huggingface.co/gpt2) on an unknown dataset.
## Model description
More information needed
## Intended uses & limitations
More information needed
## Training and evaluation data
More information needed
## Training procedure
### Training hyperparameters
The following hyperparameters were used during training:
- learning_rate: 0.0005
- train_batch_size: 32
- eval_batch_size: 32
- seed: 42
- gradient_accumulation_steps: 8
- total_train_batch_size: 256
- optimizer: Adam with betas=(0.9,0.999) and epsilon=1e-08
- lr_scheduler_type: cosine
- lr_scheduler_warmup_steps: 1000
- num_epochs: 1
- mixed_precision_training: Native AMP
### Training results
### Framework versions
- Transformers 4.20.1
- Pytorch 1.11.0+cu113
- Tokenizers 0.12.1
|
atendstowards0/testing0 | 4d9aa6456efe8b82b58e789097024ae6afe91611 | 2022-06-22T18:48:36.000Z | [
"pytorch",
"tensorboard",
"gpt2",
"text-generation",
"transformers",
"generated_from_trainer",
"license:mit",
"model-index"
] | text-generation | false | atendstowards0 | null | atendstowards0/testing0 | 1 | null | transformers | 33,060 | ---
license: mit
tags:
- generated_from_trainer
model-index:
- name: testing0
results: []
---
<!-- This model card has been generated automatically according to the information the Trainer had access to. You
should probably proofread and complete it, then remove this comment. -->
# testing0
This model is a fine-tuned version of [gpt2](https://huggingface.co/gpt2) on an unknown dataset.
## Model description
More information needed
## Intended uses & limitations
More information needed
## Training and evaluation data
More information needed
## Training procedure
### Training hyperparameters
The following hyperparameters were used during training:
- learning_rate: 0.0005
- train_batch_size: 32
- eval_batch_size: 32
- seed: 42
- gradient_accumulation_steps: 8
- total_train_batch_size: 256
- optimizer: Adam with betas=(0.9,0.999) and epsilon=1e-08
- lr_scheduler_type: cosine
- lr_scheduler_warmup_steps: 1000
- num_epochs: 10
- mixed_precision_training: Native AMP
### Training results
### Framework versions
- Transformers 4.20.1
- Pytorch 1.11.0+cu113
- Tokenizers 0.12.1
|
amandaraeb/bert-base-uncased-finetuned-swag | 11c1e9d5dcce6bcf23399be71820993139bfe39e | 2022-06-23T00:01:46.000Z | [
"pytorch",
"tensorboard",
"bert",
"multiple-choice",
"transformers"
] | multiple-choice | false | amandaraeb | null | amandaraeb/bert-base-uncased-finetuned-swag | 1 | null | transformers | 33,061 | Entry not found |
BukaByaka/opus-mt-ru-en-finetuned-en-to-ru | 880cb446a7ceadb30206cff9ed79373dad321f6b | 2022-06-23T12:32:37.000Z | [
"pytorch",
"tensorboard",
"marian",
"text2text-generation",
"transformers",
"autotrain_compatible"
] | text2text-generation | false | BukaByaka | null | BukaByaka/opus-mt-ru-en-finetuned-en-to-ru | 1 | null | transformers | 33,062 | Entry not found |
Akshay1791/bert-finetuned-squad | e8c9d2402cb1aa97c7bb31f6d9b947f9691500d6 | 2022-06-23T05:09:34.000Z | [
"pytorch",
"bert",
"question-answering",
"transformers",
"autotrain_compatible"
] | question-answering | false | Akshay1791 | null | Akshay1791/bert-finetuned-squad | 1 | null | transformers | 33,063 | Entry not found |
mgtoxd/machineLearningCourse | 9913687349f2fed7516add14c6faad0b0307bc33 | 2022-06-23T02:14:44.000Z | [
"pytorch",
"jax",
"wav2vec2",
"automatic-speech-recognition",
"transformers"
] | automatic-speech-recognition | false | mgtoxd | null | mgtoxd/machineLearningCourse | 1 | null | transformers | 33,064 | # ζΊε¨ε¦δΉ θ―Ύη¨
|
Misterpy/models | a367f778aa417f59e7875dbb9f550ded5cb67d6d | 2022-06-23T07:52:38.000Z | [
"pytorch",
"layoutlmv3",
"feature-extraction",
"transformers"
] | feature-extraction | false | Misterpy | null | Misterpy/models | 1 | null | transformers | 33,065 | Entry not found |
iaanimashaun/distilgpt2-finetuned-wikitext2 | aa8e323bb1035c973e8e777026b2af3c0d8264b2 | 2022-06-24T05:13:39.000Z | [
"pytorch",
"gpt2",
"text-generation",
"transformers",
"generated_from_trainer",
"license:apache-2.0",
"model-index"
] | text-generation | false | iaanimashaun | null | iaanimashaun/distilgpt2-finetuned-wikitext2 | 1 | null | transformers | 33,066 | ---
license: apache-2.0
tags:
- generated_from_trainer
model-index:
- name: distilgpt2-finetuned-wikitext2
results: []
---
<!-- This model card has been generated automatically according to the information the Trainer had access to. You
should probably proofread and complete it, then remove this comment. -->
# distilgpt2-finetuned-wikitext2
This model is a fine-tuned version of [distilgpt2](https://huggingface.co/distilgpt2) on the None dataset.
It achieves the following results on the evaluation set:
- Loss: 3.6895
## Model description
More information needed
## Intended uses & limitations
More information needed
## Training and evaluation data
More information needed
## Training procedure
### Training hyperparameters
The following hyperparameters were used during training:
- learning_rate: 2e-05
- train_batch_size: 8
- eval_batch_size: 8
- seed: 42
- optimizer: Adam with betas=(0.9,0.999) and epsilon=1e-08
- lr_scheduler_type: linear
- num_epochs: 1
### Training results
| Training Loss | Epoch | Step | Validation Loss |
|:-------------:|:-----:|:----:|:---------------:|
| 3.7852 | 1.0 | 2334 | 3.6895 |
### Framework versions
- Transformers 4.19.2
- Pytorch 1.11.0
- Datasets 2.2.2
- Tokenizers 0.12.1
|
cwkeam/m-ctc-t-large-frame-lid | 900453f61be2906475b020c18c9fcb7e7a3329d4 | 2022-06-29T05:11:04.000Z | [
"pytorch",
"mctct",
"en",
"dataset:librispeech_asr",
"dataset:common_voice",
"arxiv:2111.00161",
"transformers",
"speech",
"license:apache-2.0"
] | null | false | cwkeam | null | cwkeam/m-ctc-t-large-frame-lid | 1 | null | transformers | 33,067 | ---
language: en
datasets:
- librispeech_asr
- common_voice
tags:
- speech
license: apache-2.0
---
# M-CTC-T
β
Massively multilingual speech recognizer from Meta AI. The model is a 1B-param transformer encoder, with a CTC head over 8065 character labels and a language identification head over 60 language ID labels. It is trained on Common Voice (version 6.1, December 2020 release) and VoxPopuli. After training on Common Voice and VoxPopuli, the model is trained on Common Voice only. The labels are unnormalized character-level transcripts (punctuation and capitalization are not removed). The model takes as input Mel filterbank features from a 16Khz audio signal.
β
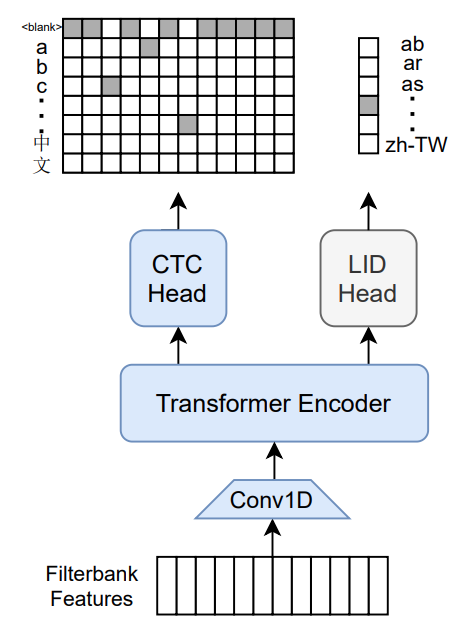
β
The original Flashlight code, model checkpoints, and Colab notebook can be found at https://github.com/flashlight/wav2letter/tree/main/recipes/mling_pl .
β
β
## Citation
β
[Paper](https://arxiv.org/abs/2111.00161)
β
Authors: Loren Lugosch, Tatiana Likhomanenko, Gabriel Synnaeve, Ronan Collobert
β
```
@article{lugosch2021pseudo,
title={Pseudo-Labeling for Massively Multilingual Speech Recognition},
author={Lugosch, Loren and Likhomanenko, Tatiana and Synnaeve, Gabriel and Collobert, Ronan},
journal={ICASSP},
year={2022}
}
```
β
Additional thanks to [Chan Woo Kim](https://huggingface.co/cwkeam) and [Patrick von Platen](https://huggingface.co/patrickvonplaten) for porting the model from Flashlight to PyTorch.
β
# Training method
β
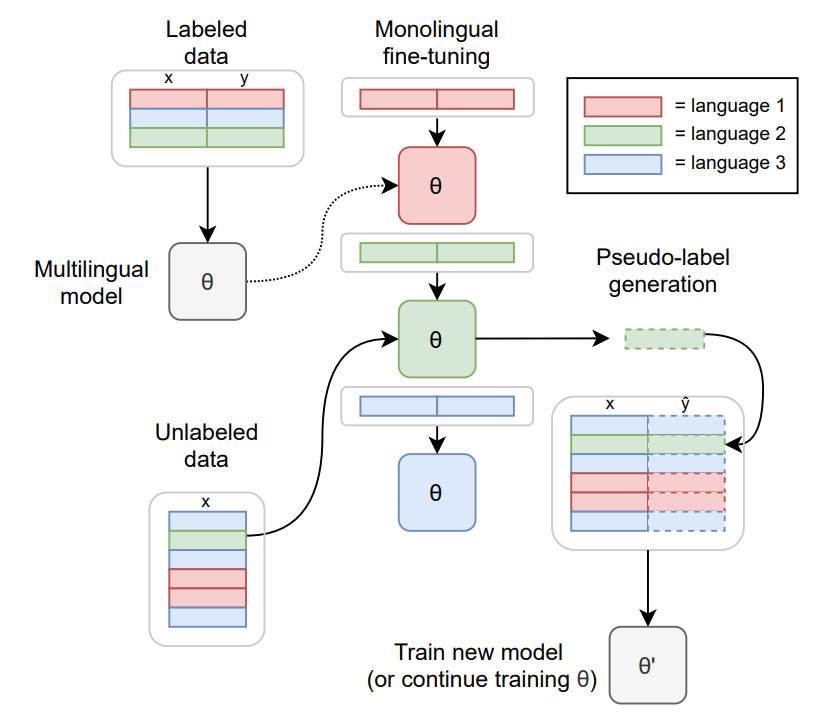 TO-DO: replace with the training diagram from paper
β
For more information on how the model was trained, please take a look at the [official paper](https://arxiv.org/abs/2111.00161).
β
# Usage
β
To transcribe audio files the model can be used as a standalone acoustic model as follows:
β
```python
import torch
import torchaudio
from datasets import load_dataset
from transformers import MCTCTForCTC, MCTCTProcessor
model = MCTCTForCTC.from_pretrained("speechbrain/mctct-large")
processor = MCTCTProcessor.from_pretrained("speechbrain/mctct-large")
# load dummy dataset and read soundfiles
ds = load_dataset("patrickvonplaten/librispeech_asr_dummy", "clean", split="validation")
# tokenize
input_features = processor(ds[0]["audio"]["array"], return_tensors="pt").input_features
# retrieve logits
logits = model(input_features).logits
# take argmax and decode
predicted_ids = torch.argmax(logits, dim=-1)
transcription = processor.batch_decode(predicted_ids)
```
Results for Common Voice, averaged over all languages:
β
*Character error rate (CER)*:
β
| Valid | Test |
|-------|------|
| 21.4 | 23.3 |
|
eugenetanjc/trained_french | 53602e26745c0e88cf3e1ee7137a73535efdfe3d | 2022-06-24T17:50:48.000Z | [
"pytorch",
"tensorboard",
"wav2vec2",
"automatic-speech-recognition",
"transformers",
"generated_from_trainer",
"license:apache-2.0",
"model-index"
] | automatic-speech-recognition | false | eugenetanjc | null | eugenetanjc/trained_french | 1 | null | transformers | 33,068 | ---
license: apache-2.0
tags:
- generated_from_trainer
model-index:
- name: trained_french
results: []
---
<!-- This model card has been generated automatically according to the information the Trainer had access to. You
should probably proofread and complete it, then remove this comment. -->
# trained_french
This model is a fine-tuned version of [facebook/wav2vec2-base-960h](https://huggingface.co/facebook/wav2vec2-base-960h) on the None dataset.
It achieves the following results on the evaluation set:
- Loss: 4.8493
- Wer: 1.0
## Model description
More information needed
## Intended uses & limitations
More information needed
## Training and evaluation data
More information needed
## Training procedure
### Training hyperparameters
The following hyperparameters were used during training:
- learning_rate: 0.003
- train_batch_size: 6
- eval_batch_size: 8
- seed: 42
- gradient_accumulation_steps: 2
- total_train_batch_size: 12
- optimizer: Adam with betas=(0.9,0.999) and epsilon=1e-08
- lr_scheduler_type: linear
- lr_scheduler_warmup_steps: 20
- num_epochs: 60
### Training results
| Training Loss | Epoch | Step | Validation Loss | Wer |
|:-------------:|:-----:|:----:|:---------------:|:---:|
| 6.2268 | 5.53 | 50 | 4.9813 | 1.0 |
| 5.724 | 11.11 | 100 | 4.8808 | 1.0 |
| 5.629 | 16.63 | 150 | 4.9001 | 1.0 |
| 5.3351 | 22.21 | 200 | 4.8457 | 1.0 |
| 5.2043 | 27.74 | 250 | 4.8386 | 1.0 |
| 5.1709 | 33.32 | 300 | 4.8647 | 1.0 |
| 5.065 | 38.84 | 350 | 4.8574 | 1.0 |
| 5.0685 | 44.42 | 400 | 4.8449 | 1.0 |
| 5.0584 | 49.95 | 450 | 4.8412 | 1.0 |
| 4.9626 | 55.53 | 500 | 4.8493 | 1.0 |
### Framework versions
- Transformers 4.17.0
- Pytorch 1.11.0+cu113
- Datasets 1.18.3
- Tokenizers 0.12.1
|
guidecare/all-mpnet-base-v2-feature-extraction | 52e1833177b6e3163e478556edf5463806d62a51 | 2022-06-23T20:29:14.000Z | [
"pytorch",
"mpnet",
"feature-extraction",
"en",
"arxiv:1904.06472",
"arxiv:2102.07033",
"arxiv:2104.08727",
"arxiv:1704.05179",
"arxiv:1810.09305",
"sentence-transformers",
"sentence-similarity",
"license:apache-2.0"
] | feature-extraction | false | guidecare | null | guidecare/all-mpnet-base-v2-feature-extraction | 1 | null | sentence-transformers | 33,069 | ---
pipeline_tag: feature-extraction
tags:
- sentence-transformers
- feature-extraction
- sentence-similarity
language: en
license: apache-2.0
---
# all-mpnet-base-v2 clone
This is a [sentence-transformers](https://www.SBERT.net) model: It maps sentences & paragraphs to a 768 dimensional dense vector space and can be used for tasks like clustering or semantic search.
## Usage (Sentence-Transformers)
Using this model becomes easy when you have [sentence-transformers](https://www.SBERT.net) installed:
```
pip install -U sentence-transformers
```
Then you can use the model like this:
```python
from sentence_transformers import SentenceTransformer
sentences = ["This is an example sentence", "Each sentence is converted"]
model = SentenceTransformer('sentence-transformers/all-mpnet-base-v2')
embeddings = model.encode(sentences)
print(embeddings)
```
## Usage (HuggingFace Transformers)
Without [sentence-transformers](https://www.SBERT.net), you can use the model like this: First, you pass your input through the transformer model, then you have to apply the right pooling-operation on-top of the contextualized word embeddings.
```python
from transformers import AutoTokenizer, AutoModel
import torch
import torch.nn.functional as F
#Mean Pooling - Take attention mask into account for correct averaging
def mean_pooling(model_output, attention_mask):
token_embeddings = model_output[0] #First element of model_output contains all token embeddings
input_mask_expanded = attention_mask.unsqueeze(-1).expand(token_embeddings.size()).float()
return torch.sum(token_embeddings * input_mask_expanded, 1) / torch.clamp(input_mask_expanded.sum(1), min=1e-9)
# Sentences we want sentence embeddings for
sentences = ['This is an example sentence', 'Each sentence is converted']
# Load model from HuggingFace Hub
tokenizer = AutoTokenizer.from_pretrained('sentence-transformers/all-mpnet-base-v2')
model = AutoModel.from_pretrained('sentence-transformers/all-mpnet-base-v2')
# Tokenize sentences
encoded_input = tokenizer(sentences, padding=True, truncation=True, return_tensors='pt')
# Compute token embeddings
with torch.no_grad():
model_output = model(**encoded_input)
# Perform pooling
sentence_embeddings = mean_pooling(model_output, encoded_input['attention_mask'])
# Normalize embeddings
sentence_embeddings = F.normalize(sentence_embeddings, p=2, dim=1)
print("Sentence embeddings:")
print(sentence_embeddings)
```
## Evaluation Results
For an automated evaluation of this model, see the *Sentence Embeddings Benchmark*: [https://seb.sbert.net](https://seb.sbert.net?model_name=sentence-transformers/all-mpnet-base-v2)
------
## Background
The project aims to train sentence embedding models on very large sentence level datasets using a self-supervised
contrastive learning objective. We used the pretrained [`microsoft/mpnet-base`](https://huggingface.co/microsoft/mpnet-base) model and fine-tuned in on a
1B sentence pairs dataset. We use a contrastive learning objective: given a sentence from the pair, the model should predict which out of a set of randomly sampled other sentences, was actually paired with it in our dataset.
We developped this model during the
[Community week using JAX/Flax for NLP & CV](https://discuss.huggingface.co/t/open-to-the-community-community-week-using-jax-flax-for-nlp-cv/7104),
organized by Hugging Face. We developped this model as part of the project:
[Train the Best Sentence Embedding Model Ever with 1B Training Pairs](https://discuss.huggingface.co/t/train-the-best-sentence-embedding-model-ever-with-1b-training-pairs/7354). We benefited from efficient hardware infrastructure to run the project: 7 TPUs v3-8, as well as intervention from Googles Flax, JAX, and Cloud team member about efficient deep learning frameworks.
## Intended uses
Our model is intented to be used as a sentence and short paragraph encoder. Given an input text, it ouptuts a vector which captures
the semantic information. The sentence vector may be used for information retrieval, clustering or sentence similarity tasks.
By default, input text longer than 384 word pieces is truncated.
## Training procedure
### Pre-training
We use the pretrained [`microsoft/mpnet-base`](https://huggingface.co/microsoft/mpnet-base) model. Please refer to the model card for more detailed information about the pre-training procedure.
### Fine-tuning
We fine-tune the model using a contrastive objective. Formally, we compute the cosine similarity from each possible sentence pairs from the batch.
We then apply the cross entropy loss by comparing with true pairs.
#### Hyper parameters
We trained ou model on a TPU v3-8. We train the model during 100k steps using a batch size of 1024 (128 per TPU core).
We use a learning rate warm up of 500. The sequence length was limited to 128 tokens. We used the AdamW optimizer with
a 2e-5 learning rate. The full training script is accessible in this current repository: `train_script.py`.
#### Training data
We use the concatenation from multiple datasets to fine-tune our model. The total number of sentence pairs is above 1 billion sentences.
We sampled each dataset given a weighted probability which configuration is detailed in the `data_config.json` file.
| Dataset | Paper | Number of training tuples |
|--------------------------------------------------------|:----------------------------------------:|:--------------------------:|
| [Reddit comments (2015-2018)](https://github.com/PolyAI-LDN/conversational-datasets/tree/master/reddit) | [paper](https://arxiv.org/abs/1904.06472) | 726,484,430 |
| [S2ORC](https://github.com/allenai/s2orc) Citation pairs (Abstracts) | [paper](https://aclanthology.org/2020.acl-main.447/) | 116,288,806 |
| [WikiAnswers](https://github.com/afader/oqa#wikianswers-corpus) Duplicate question pairs | [paper](https://doi.org/10.1145/2623330.2623677) | 77,427,422 |
| [PAQ](https://github.com/facebookresearch/PAQ) (Question, Answer) pairs | [paper](https://arxiv.org/abs/2102.07033) | 64,371,441 |
| [S2ORC](https://github.com/allenai/s2orc) Citation pairs (Titles) | [paper](https://aclanthology.org/2020.acl-main.447/) | 52,603,982 |
| [S2ORC](https://github.com/allenai/s2orc) (Title, Abstract) | [paper](https://aclanthology.org/2020.acl-main.447/) | 41,769,185 |
| [Stack Exchange](https://huggingface.co/datasets/flax-sentence-embeddings/stackexchange_xml) (Title, Body) pairs | - | 25,316,456 |
| [Stack Exchange](https://huggingface.co/datasets/flax-sentence-embeddings/stackexchange_xml) (Title+Body, Answer) pairs | - | 21,396,559 |
| [Stack Exchange](https://huggingface.co/datasets/flax-sentence-embeddings/stackexchange_xml) (Title, Answer) pairs | - | 21,396,559 |
| [MS MARCO](https://microsoft.github.io/msmarco/) triplets | [paper](https://doi.org/10.1145/3404835.3462804) | 9,144,553 |
| [GOOAQ: Open Question Answering with Diverse Answer Types](https://github.com/allenai/gooaq) | [paper](https://arxiv.org/pdf/2104.08727.pdf) | 3,012,496 |
| [Yahoo Answers](https://www.kaggle.com/soumikrakshit/yahoo-answers-dataset) (Title, Answer) | [paper](https://proceedings.neurips.cc/paper/2015/hash/250cf8b51c773f3f8dc8b4be867a9a02-Abstract.html) | 1,198,260 |
| [Code Search](https://huggingface.co/datasets/code_search_net) | - | 1,151,414 |
| [COCO](https://cocodataset.org/#home) Image captions | [paper](https://link.springer.com/chapter/10.1007%2F978-3-319-10602-1_48) | 828,395|
| [SPECTER](https://github.com/allenai/specter) citation triplets | [paper](https://doi.org/10.18653/v1/2020.acl-main.207) | 684,100 |
| [Yahoo Answers](https://www.kaggle.com/soumikrakshit/yahoo-answers-dataset) (Question, Answer) | [paper](https://proceedings.neurips.cc/paper/2015/hash/250cf8b51c773f3f8dc8b4be867a9a02-Abstract.html) | 681,164 |
| [Yahoo Answers](https://www.kaggle.com/soumikrakshit/yahoo-answers-dataset) (Title, Question) | [paper](https://proceedings.neurips.cc/paper/2015/hash/250cf8b51c773f3f8dc8b4be867a9a02-Abstract.html) | 659,896 |
| [SearchQA](https://huggingface.co/datasets/search_qa) | [paper](https://arxiv.org/abs/1704.05179) | 582,261 |
| [Eli5](https://huggingface.co/datasets/eli5) | [paper](https://doi.org/10.18653/v1/p19-1346) | 325,475 |
| [Flickr 30k](https://shannon.cs.illinois.edu/DenotationGraph/) | [paper](https://transacl.org/ojs/index.php/tacl/article/view/229/33) | 317,695 |
| [Stack Exchange](https://huggingface.co/datasets/flax-sentence-embeddings/stackexchange_xml) Duplicate questions (titles) | | 304,525 |
| AllNLI ([SNLI](https://nlp.stanford.edu/projects/snli/) and [MultiNLI](https://cims.nyu.edu/~sbowman/multinli/) | [paper SNLI](https://doi.org/10.18653/v1/d15-1075), [paper MultiNLI](https://doi.org/10.18653/v1/n18-1101) | 277,230 |
| [Stack Exchange](https://huggingface.co/datasets/flax-sentence-embeddings/stackexchange_xml) Duplicate questions (bodies) | | 250,519 |
| [Stack Exchange](https://huggingface.co/datasets/flax-sentence-embeddings/stackexchange_xml) Duplicate questions (titles+bodies) | | 250,460 |
| [Sentence Compression](https://github.com/google-research-datasets/sentence-compression) | [paper](https://www.aclweb.org/anthology/D13-1155/) | 180,000 |
| [Wikihow](https://github.com/pvl/wikihow_pairs_dataset) | [paper](https://arxiv.org/abs/1810.09305) | 128,542 |
| [Altlex](https://github.com/chridey/altlex/) | [paper](https://aclanthology.org/P16-1135.pdf) | 112,696 |
| [Quora Question Triplets](https://quoradata.quora.com/First-Quora-Dataset-Release-Question-Pairs) | - | 103,663 |
| [Simple Wikipedia](https://cs.pomona.edu/~dkauchak/simplification/) | [paper](https://www.aclweb.org/anthology/P11-2117/) | 102,225 |
| [Natural Questions (NQ)](https://ai.google.com/research/NaturalQuestions) | [paper](https://transacl.org/ojs/index.php/tacl/article/view/1455) | 100,231 |
| [SQuAD2.0](https://rajpurkar.github.io/SQuAD-explorer/) | [paper](https://aclanthology.org/P18-2124.pdf) | 87,599 |
| [TriviaQA](https://huggingface.co/datasets/trivia_qa) | - | 73,346 |
| **Total** | | **1,170,060,424** | |
shpotes/codegen-350M | 8ff8b64213d4dc1d83006bc1f1dffda0c1a60e90 | 2022-06-24T02:56:23.000Z | [
"pytorch",
"codegen",
"text-generation",
"transformers",
"license:bsd-3-clause"
] | text-generation | false | shpotes | null | shpotes/codegen-350M | 1 | null | transformers | 33,070 | ---
license: bsd-3-clause
---
|
Guo-Zikun/distilbert-base-uncased-finetuned-squad | 8a48f92e1db4a1ede6963148ed2cd17ecd13a5de | 2022-07-04T12:19:52.000Z | [
"pytorch",
"distilbert",
"question-answering",
"dataset:squad",
"transformers",
"generated_from_trainer",
"license:apache-2.0",
"model-index",
"autotrain_compatible"
] | question-answering | false | Guo-Zikun | null | Guo-Zikun/distilbert-base-uncased-finetuned-squad | 1 | null | transformers | 33,071 | ---
license: apache-2.0
tags:
- generated_from_trainer
datasets:
- squad
model-index:
- name: distilbert-base-uncased-finetuned-squad
results: []
---
<!-- This model card has been generated automatically according to the information the Trainer had access to. You
should probably proofread and complete it, then remove this comment. -->
# distilbert-base-uncased-finetuned-squad
This model is a fine-tuned version of [distilbert-base-uncased](https://huggingface.co/distilbert-base-uncased) on the squad dataset.
## Model description
More information needed
## Intended uses & limitations
More information needed
## Training and evaluation data
More information needed
## Training procedure
### Training hyperparameters
The following hyperparameters were used during training:
- learning_rate: 2e-05
- train_batch_size: 32
- eval_batch_size: 32
- seed: 42
- optimizer: Adam with betas=(0.9,0.999) and epsilon=1e-08
- lr_scheduler_type: linear
- num_epochs: 3
### Framework versions
- Transformers 4.19.2
- Pytorch 1.8.2
- Datasets 2.2.1
- Tokenizers 0.12.1
|
mousaazari/t5-small-finetuned-wikisql | 03cf2e00a260e2c73ae5777fa4527b086bf941e5 | 2022-06-30T11:37:10.000Z | [
"pytorch",
"tensorboard",
"t5",
"text2text-generation",
"transformers",
"generated_from_trainer",
"license:apache-2.0",
"model-index",
"autotrain_compatible"
] | text2text-generation | false | mousaazari | null | mousaazari/t5-small-finetuned-wikisql | 1 | null | transformers | 33,072 | ---
license: apache-2.0
tags:
- generated_from_trainer
model-index:
- name: t5-small-finetuned-wikisql
results: []
---
<!-- This model card has been generated automatically according to the information the Trainer had access to. You
should probably proofread and complete it, then remove this comment. -->
# t5-small-finetuned-wikisql
This model is a fine-tuned version of [t5-base](https://huggingface.co/t5-base) on the None dataset.
It achieves the following results on the evaluation set:
- Loss: 0.2640
- Rouge2 Precision: 0.8471
- Rouge2 Recall: 0.3841
- Rouge2 Fmeasure: 0.5064
## Model description
More information needed
## Intended uses & limitations
More information needed
## Training and evaluation data
More information needed
## Training procedure
### Training hyperparameters
The following hyperparameters were used during training:
- learning_rate: 5e-05
- train_batch_size: 8
- eval_batch_size: 8
- seed: 42
- optimizer: Adam with betas=(0.9,0.999) and epsilon=1e-08
- lr_scheduler_type: linear
- num_epochs: 20
### Training results
| Training Loss | Epoch | Step | Validation Loss | Rouge2 Precision | Rouge2 Recall | Rouge2 Fmeasure |
|:-------------:|:-----:|:----:|:---------------:|:----------------:|:-------------:|:---------------:|
| No log | 1.0 | 11 | 2.7587 | 0.098 | 0.0305 | 0.045 |
| No log | 2.0 | 22 | 2.0056 | 0.0969 | 0.0284 | 0.0422 |
| No log | 3.0 | 33 | 1.4456 | 0.1046 | 0.0349 | 0.0503 |
| No log | 4.0 | 44 | 1.0317 | 0.1054 | 0.0337 | 0.0482 |
| No log | 5.0 | 55 | 0.7603 | 0.2749 | 0.1299 | 0.1724 |
| No log | 6.0 | 66 | 0.5722 | 0.7115 | 0.352 | 0.4552 |
| No log | 7.0 | 77 | 0.4751 | 0.6872 | 0.337 | 0.436 |
| No log | 8.0 | 88 | 0.4253 | 0.7256 | 0.3439 | 0.4462 |
| No log | 9.0 | 99 | 0.3805 | 0.7335 | 0.3204 | 0.4308 |
| No log | 10.0 | 110 | 0.3562 | 0.7342 | 0.3239 | 0.433 |
| No log | 11.0 | 121 | 0.3275 | 0.7906 | 0.355 | 0.471 |
| No log | 12.0 | 132 | 0.3133 | 0.8382 | 0.3838 | 0.5061 |
| No log | 13.0 | 143 | 0.2996 | 0.8409 | 0.3841 | 0.5062 |
| No log | 14.0 | 154 | 0.2903 | 0.8304 | 0.3763 | 0.4978 |
| No log | 15.0 | 165 | 0.2867 | 0.8409 | 0.3841 | 0.5062 |
| No log | 16.0 | 176 | 0.2786 | 0.8409 | 0.3841 | 0.5062 |
| No log | 17.0 | 187 | 0.2711 | 0.8409 | 0.3841 | 0.5062 |
| No log | 18.0 | 198 | 0.2673 | 0.8409 | 0.3841 | 0.5062 |
| No log | 19.0 | 209 | 0.2643 | 0.8471 | 0.3841 | 0.5064 |
| No log | 20.0 | 220 | 0.2640 | 0.8471 | 0.3841 | 0.5064 |
### Framework versions
- Transformers 4.20.1
- Pytorch 1.11.0+cu113
- Datasets 2.3.2
- Tokenizers 0.12.1
|
akhisreelibra/t5-small-finetuned-xsum | 7b111e90cf1fd20ee85252d29b18746d38d067e7 | 2022-06-24T16:46:21.000Z | [
"pytorch",
"tensorboard",
"t5",
"text2text-generation",
"transformers",
"autotrain_compatible"
] | text2text-generation | false | akhisreelibra | null | akhisreelibra/t5-small-finetuned-xsum | 1 | null | transformers | 33,073 | |
pitronalldak/distilbert-base-uncased-finetuned-ner | d3491d744d125853783afb5c10615843d6a7e503 | 2022-06-28T17:30:43.000Z | [
"pytorch",
"tensorboard",
"distilbert",
"token-classification",
"transformers",
"generated_from_trainer",
"license:apache-2.0",
"model-index",
"autotrain_compatible"
] | token-classification | false | pitronalldak | null | pitronalldak/distilbert-base-uncased-finetuned-ner | 1 | null | transformers | 33,074 | ---
license: apache-2.0
tags:
- generated_from_trainer
metrics:
- precision
- recall
- f1
- accuracy
model-index:
- name: distilbert-base-uncased-finetuned-ner
results: []
---
<!-- This model card has been generated automatically according to the information the Trainer had access to. You
should probably proofread and complete it, then remove this comment. -->
# distilbert-base-uncased-finetuned-ner
This model is a fine-tuned version of [distilbert-base-uncased](https://huggingface.co/distilbert-base-uncased) on the None dataset.
It achieves the following results on the evaluation set:
- Loss: 0.0709
- Precision: 0.8442
- Recall: 0.8364
- F1: 0.8403
- Accuracy: 0.9794
## Model description
More information needed
## Intended uses & limitations
More information needed
## Training and evaluation data
More information needed
## Training procedure
### Training hyperparameters
The following hyperparameters were used during training:
- learning_rate: 2e-05
- train_batch_size: 16
- eval_batch_size: 16
- seed: 42
- optimizer: Adam with betas=(0.9,0.999) and epsilon=1e-08
- lr_scheduler_type: linear
- num_epochs: 3
### Training results
| Training Loss | Epoch | Step | Validation Loss | Precision | Recall | F1 | Accuracy |
|:-------------:|:-----:|:----:|:---------------:|:---------:|:------:|:------:|:--------:|
| 0.0442 | 1.0 | 1875 | 0.0772 | 0.7945 | 0.7627 | 0.7783 | 0.9739 |
| 0.0272 | 2.0 | 3750 | 0.0679 | 0.8465 | 0.8551 | 0.8507 | 0.9791 |
| 0.0175 | 3.0 | 5625 | 0.0709 | 0.8442 | 0.8364 | 0.8403 | 0.9794 |
### Framework versions
- Transformers 4.20.1
- Pytorch 1.11.0+cu113
- Datasets 2.3.2
- Tokenizers 0.12.1
|
eugenetanjc/wav2vec_cv | 33196b9a2c084ec314072809e3f31fc83a5ac52e | 2022-06-25T04:16:10.000Z | [
"pytorch",
"tensorboard",
"wav2vec2",
"automatic-speech-recognition",
"transformers",
"generated_from_trainer",
"license:apache-2.0",
"model-index"
] | automatic-speech-recognition | false | eugenetanjc | null | eugenetanjc/wav2vec_cv | 1 | null | transformers | 33,075 | ---
license: apache-2.0
tags:
- generated_from_trainer
model-index:
- name: wav2vec_cv
results: []
---
<!-- This model card has been generated automatically according to the information the Trainer had access to. You
should probably proofread and complete it, then remove this comment. -->
# wav2vec_cv
This model is a fine-tuned version of [facebook/wav2vec2-base-960h](https://huggingface.co/facebook/wav2vec2-base-960h) on the None dataset.
It achieves the following results on the evaluation set:
- Loss: 4.1760
- Wer: 1.0
## Model description
More information needed
## Intended uses & limitations
More information needed
## Training and evaluation data
More information needed
## Training procedure
### Training hyperparameters
The following hyperparameters were used during training:
- learning_rate: 0.003
- train_batch_size: 6
- eval_batch_size: 8
- seed: 42
- gradient_accumulation_steps: 2
- total_train_batch_size: 12
- optimizer: Adam with betas=(0.9,0.999) and epsilon=1e-08
- lr_scheduler_type: linear
- lr_scheduler_warmup_steps: 20
- num_epochs: 60
### Training results
| Training Loss | Epoch | Step | Validation Loss | Wer |
|:-------------:|:-----:|:----:|:---------------:|:---:|
| 7.1467 | 4.29 | 30 | 4.2173 | 1.0 |
| 6.8918 | 8.57 | 60 | 4.2004 | 1.0 |
| 5.4913 | 12.86 | 90 | 4.2007 | 1.0 |
| 5.3906 | 17.14 | 120 | 4.1765 | 1.0 |
| 4.9212 | 21.43 | 150 | 4.1714 | 1.0 |
| 4.3916 | 25.71 | 180 | 4.1811 | 1.0 |
| 5.2255 | 30.0 | 210 | 4.1633 | 1.0 |
| 4.501 | 34.29 | 240 | 4.2050 | 1.0 |
| 4.4328 | 38.57 | 270 | 4.1572 | 1.0 |
| 4.2136 | 42.86 | 300 | 4.1698 | 1.0 |
| 4.3353 | 47.14 | 330 | 4.1721 | 1.0 |
| 4.1805 | 51.43 | 360 | 4.1804 | 1.0 |
| 4.1695 | 55.71 | 390 | 4.1801 | 1.0 |
| 4.2978 | 60.0 | 420 | 4.1760 | 1.0 |
### Framework versions
- Transformers 4.17.0
- Pytorch 1.11.0+cu113
- Datasets 1.18.3
- Tokenizers 0.12.1
|
kennbyee25/trundlebot-poc | 00d02beeec2de88f40435b15692237e12eb21159 | 2022-06-29T14:39:50.000Z | [
"pytorch",
"gpt2",
"text-generation",
"transformers"
] | text-generation | false | kennbyee25 | null | kennbyee25/trundlebot-poc | 1 | null | transformers | 33,076 | Entry not found |
KukuyKukuev/gpt2-wikitext2 | 33971f2ee8735fd96ecdcf1e918f2dbd0641a3b2 | 2022-06-24T21:51:22.000Z | [
"pytorch",
"tensorboard",
"gpt2",
"text-generation",
"transformers",
"generated_from_trainer",
"license:mit",
"model-index"
] | text-generation | false | KukuyKukuev | null | KukuyKukuev/gpt2-wikitext2 | 1 | null | transformers | 33,077 | ---
license: mit
tags:
- generated_from_trainer
model-index:
- name: gpt2-wikitext2
results: []
---
<!-- This model card has been generated automatically according to the information the Trainer had access to. You
should probably proofread and complete it, then remove this comment. -->
# gpt2-wikitext2
This model is a fine-tuned version of [gpt2](https://huggingface.co/gpt2) on the None dataset.
It achieves the following results on the evaluation set:
- Loss: 6.1099
## Model description
More information needed
## Intended uses & limitations
More information needed
## Training and evaluation data
More information needed
## Training procedure
### Training hyperparameters
The following hyperparameters were used during training:
- learning_rate: 2e-05
- train_batch_size: 8
- eval_batch_size: 8
- seed: 42
- optimizer: Adam with betas=(0.9,0.999) and epsilon=1e-08
- lr_scheduler_type: linear
- num_epochs: 3.0
### Training results
| Training Loss | Epoch | Step | Validation Loss |
|:-------------:|:-----:|:----:|:---------------:|
| 6.5562 | 1.0 | 2249 | 6.4689 |
| 6.1912 | 2.0 | 4498 | 6.2003 |
| 6.0155 | 3.0 | 6747 | 6.1099 |
### Framework versions
- Transformers 4.20.1
- Pytorch 1.11.0+cu113
- Datasets 2.3.2
- Tokenizers 0.12.1
|
KukuyKukuev/bert-base-cased-wikitext2 | 28b99b7c2855fade183ba6f77f9edc784bebe791 | 2022-06-24T22:55:09.000Z | [
"pytorch",
"tensorboard",
"bert",
"fill-mask",
"transformers",
"generated_from_trainer",
"license:apache-2.0",
"model-index",
"autotrain_compatible"
] | fill-mask | false | KukuyKukuev | null | KukuyKukuev/bert-base-cased-wikitext2 | 1 | null | transformers | 33,078 | ---
license: apache-2.0
tags:
- generated_from_trainer
model-index:
- name: bert-base-cased-wikitext2
results: []
---
<!-- This model card has been generated automatically according to the information the Trainer had access to. You
should probably proofread and complete it, then remove this comment. -->
# bert-base-cased-wikitext2
This model is a fine-tuned version of [bert-base-cased](https://huggingface.co/bert-base-cased) on the None dataset.
It achieves the following results on the evaluation set:
- Loss: 6.8574
## Model description
More information needed
## Intended uses & limitations
More information needed
## Training and evaluation data
More information needed
## Training procedure
### Training hyperparameters
The following hyperparameters were used during training:
- learning_rate: 2e-05
- train_batch_size: 8
- eval_batch_size: 8
- seed: 42
- optimizer: Adam with betas=(0.9,0.999) and epsilon=1e-08
- lr_scheduler_type: linear
- num_epochs: 3.0
### Training results
| Training Loss | Epoch | Step | Validation Loss |
|:-------------:|:-----:|:----:|:---------------:|
| 7.0916 | 1.0 | 2346 | 7.0492 |
| 6.9039 | 2.0 | 4692 | 6.8751 |
| 6.8845 | 3.0 | 7038 | 6.8929 |
### Framework versions
- Transformers 4.20.1
- Pytorch 1.11.0+cu113
- Datasets 2.3.2
- Tokenizers 0.12.1
|
bousejin/xlm-roberta-base-finetuned-panx-de | d2bf6d0615bfdc5ae398929268fc7a2c770fd5bf | 2022-06-25T14:52:35.000Z | [
"pytorch",
"tensorboard",
"xlm-roberta",
"token-classification",
"dataset:xtreme",
"transformers",
"generated_from_trainer",
"license:mit",
"model-index",
"autotrain_compatible"
] | token-classification | false | bousejin | null | bousejin/xlm-roberta-base-finetuned-panx-de | 1 | null | transformers | 33,079 | ---
license: mit
tags:
- generated_from_trainer
datasets:
- xtreme
metrics:
- f1
model-index:
- name: xlm-roberta-base-finetuned-panx-de
results:
- task:
name: Token Classification
type: token-classification
dataset:
name: xtreme
type: xtreme
args: PAN-X.de
metrics:
- name: F1
type: f1
value: 0.8620945214069894
---
<!-- This model card has been generated automatically according to the information the Trainer had access to. You
should probably proofread and complete it, then remove this comment. -->
# xlm-roberta-base-finetuned-panx-de
This model is a fine-tuned version of [xlm-roberta-base](https://huggingface.co/xlm-roberta-base) on the xtreme dataset.
It achieves the following results on the evaluation set:
- Loss: 0.1372
- F1: 0.8621
## Model description
More information needed
## Intended uses & limitations
More information needed
## Training and evaluation data
More information needed
## Training procedure
### Training hyperparameters
The following hyperparameters were used during training:
- learning_rate: 5e-05
- train_batch_size: 24
- eval_batch_size: 24
- seed: 42
- optimizer: Adam with betas=(0.9,0.999) and epsilon=1e-08
- lr_scheduler_type: linear
- num_epochs: 3
### Training results
| Training Loss | Epoch | Step | Validation Loss | F1 |
|:-------------:|:-----:|:----:|:---------------:|:------:|
| 0.2575 | 1.0 | 525 | 0.1621 | 0.8292 |
| 0.1287 | 2.0 | 1050 | 0.1378 | 0.8526 |
| 0.0831 | 3.0 | 1575 | 0.1372 | 0.8621 |
### Framework versions
- Transformers 4.20.1
- Pytorch 1.11.0+cu113
- Datasets 2.3.2
- Tokenizers 0.12.1
|
YZzfDY/RICE-large | a51bd97bfb0ea64fcbe402e55d8d413801898f4d | 2022-06-25T08:37:24.000Z | [
"pytorch",
"bert",
"pretraining",
"en",
"transformers"
] | null | false | YZzfDY | null | YZzfDY/RICE-large | 1 | null | transformers | 33,080 | ---
language:
- en
tag: fill-mask
widget:
- text: "Paris is the <mask> of France."
example_title: "Capital"
---
|
bousejin/xlm-roberta-base-finetuned-panx-de-fr | 5107551683b7689b8bb58a9c72cf989ff00e3cd6 | 2022-06-25T15:06:04.000Z | [
"pytorch",
"xlm-roberta",
"token-classification",
"transformers",
"generated_from_trainer",
"license:mit",
"model-index",
"autotrain_compatible"
] | token-classification | false | bousejin | null | bousejin/xlm-roberta-base-finetuned-panx-de-fr | 1 | null | transformers | 33,081 | ---
license: mit
tags:
- generated_from_trainer
metrics:
- f1
model-index:
- name: xlm-roberta-base-finetuned-panx-de-fr
results: []
---
<!-- This model card has been generated automatically according to the information the Trainer had access to. You
should probably proofread and complete it, then remove this comment. -->
# xlm-roberta-base-finetuned-panx-de-fr
This model is a fine-tuned version of [xlm-roberta-base](https://huggingface.co/xlm-roberta-base) on the None dataset.
It achieves the following results on the evaluation set:
- Loss: 0.1631
- F1: 0.8579
## Model description
More information needed
## Intended uses & limitations
More information needed
## Training and evaluation data
More information needed
## Training procedure
### Training hyperparameters
The following hyperparameters were used during training:
- learning_rate: 5e-05
- train_batch_size: 24
- eval_batch_size: 24
- seed: 42
- optimizer: Adam with betas=(0.9,0.999) and epsilon=1e-08
- lr_scheduler_type: linear
- num_epochs: 3
### Training results
| Training Loss | Epoch | Step | Validation Loss | F1 |
|:-------------:|:-----:|:----:|:---------------:|:------:|
| 0.2878 | 1.0 | 715 | 0.1840 | 0.8247 |
| 0.1456 | 2.0 | 1430 | 0.1596 | 0.8473 |
| 0.0925 | 3.0 | 2145 | 0.1631 | 0.8579 |
### Framework versions
- Transformers 4.20.1
- Pytorch 1.11.0+cu113
- Datasets 2.3.2
- Tokenizers 0.12.1
|
bousejin/xlm-roberta-base-finetuned-panx-fr | dcde57a9994d0a52cfb9b38112ed7a6c73122046 | 2022-06-25T06:15:40.000Z | [
"pytorch",
"xlm-roberta",
"token-classification",
"dataset:xtreme",
"transformers",
"generated_from_trainer",
"license:mit",
"model-index",
"autotrain_compatible"
] | token-classification | false | bousejin | null | bousejin/xlm-roberta-base-finetuned-panx-fr | 1 | null | transformers | 33,082 | ---
license: mit
tags:
- generated_from_trainer
datasets:
- xtreme
metrics:
- f1
model-index:
- name: xlm-roberta-base-finetuned-panx-fr
results:
- task:
name: Token Classification
type: token-classification
dataset:
name: xtreme
type: xtreme
args: PAN-X.fr
metrics:
- name: F1
type: f1
value: 0.9241871401929781
---
<!-- This model card has been generated automatically according to the information the Trainer had access to. You
should probably proofread and complete it, then remove this comment. -->
# xlm-roberta-base-finetuned-panx-fr
This model is a fine-tuned version of [xlm-roberta-base](https://huggingface.co/xlm-roberta-base) on the xtreme dataset.
It achieves the following results on the evaluation set:
- Loss: 0.1013
- F1: 0.9242
## Model description
More information needed
## Intended uses & limitations
More information needed
## Training and evaluation data
More information needed
## Training procedure
### Training hyperparameters
The following hyperparameters were used during training:
- learning_rate: 5e-05
- train_batch_size: 24
- eval_batch_size: 24
- seed: 42
- optimizer: Adam with betas=(0.9,0.999) and epsilon=1e-08
- lr_scheduler_type: linear
- num_epochs: 3
### Training results
| Training Loss | Epoch | Step | Validation Loss | F1 |
|:-------------:|:-----:|:----:|:---------------:|:------:|
| 0.5667 | 1.0 | 191 | 0.2318 | 0.8415 |
| 0.2539 | 2.0 | 382 | 0.1428 | 0.8988 |
| 0.1739 | 3.0 | 573 | 0.1013 | 0.9242 |
### Framework versions
- Transformers 4.20.1
- Pytorch 1.11.0+cu113
- Datasets 2.3.2
- Tokenizers 0.12.1
|
bousejin/xlm-roberta-base-finetuned-panx-en | 5492d1d3d0535e2c10e95a83dbd9ea94e63b9d65 | 2022-06-25T06:48:13.000Z | [
"pytorch",
"xlm-roberta",
"token-classification",
"dataset:xtreme",
"transformers",
"generated_from_trainer",
"license:mit",
"model-index",
"autotrain_compatible"
] | token-classification | false | bousejin | null | bousejin/xlm-roberta-base-finetuned-panx-en | 1 | null | transformers | 33,083 | ---
license: mit
tags:
- generated_from_trainer
datasets:
- xtreme
metrics:
- f1
model-index:
- name: xlm-roberta-base-finetuned-panx-en
results:
- task:
name: Token Classification
type: token-classification
dataset:
name: xtreme
type: xtreme
args: PAN-X.en
metrics:
- name: F1
type: f1
value: 0.6900780379041249
---
<!-- This model card has been generated automatically according to the information the Trainer had access to. You
should probably proofread and complete it, then remove this comment. -->
# xlm-roberta-base-finetuned-panx-en
This model is a fine-tuned version of [xlm-roberta-base](https://huggingface.co/xlm-roberta-base) on the xtreme dataset.
It achieves the following results on the evaluation set:
- Loss: 0.3909
- F1: 0.6901
## Model description
More information needed
## Intended uses & limitations
More information needed
## Training and evaluation data
More information needed
## Training procedure
### Training hyperparameters
The following hyperparameters were used during training:
- learning_rate: 5e-05
- train_batch_size: 24
- eval_batch_size: 24
- seed: 42
- optimizer: Adam with betas=(0.9,0.999) and epsilon=1e-08
- lr_scheduler_type: linear
- num_epochs: 3
### Training results
| Training Loss | Epoch | Step | Validation Loss | F1 |
|:-------------:|:-----:|:----:|:---------------:|:------:|
| 1.1446 | 1.0 | 50 | 0.6385 | 0.3858 |
| 0.5317 | 2.0 | 100 | 0.4248 | 0.6626 |
| 0.3614 | 3.0 | 150 | 0.3909 | 0.6901 |
### Framework versions
- Transformers 4.20.1
- Pytorch 1.11.0+cu113
- Datasets 2.3.2
- Tokenizers 0.12.1
|
EddieChen372/longT5-js2jest | 7c77ced5b8f4fdd8a771827d2055a985fbaa109b | 2022-06-26T10:45:50.000Z | [
"pytorch",
"t5",
"text2text-generation",
"transformers",
"autotrain_compatible"
] | text2text-generation | false | EddieChen372 | null | EddieChen372/longT5-js2jest | 1 | null | transformers | 33,084 | Entry not found |
VedantS01/bert-finetuned-custom-2 | f34bb2b1e21361984d6cf4f16ee6b0c7548e717a | 2022-06-25T15:33:51.000Z | [
"pytorch",
"bert",
"question-answering",
"transformers",
"autotrain_compatible"
] | question-answering | false | VedantS01 | null | VedantS01/bert-finetuned-custom-2 | 1 | null | transformers | 33,085 | Entry not found |
eugenetanjc/wav2vec_trained | 4cf3837cf5030b8556089dd671ef6dd8be0f0729 | 2022-06-25T18:29:57.000Z | [
"pytorch",
"tensorboard",
"wav2vec2",
"automatic-speech-recognition",
"transformers",
"generated_from_trainer",
"license:apache-2.0",
"model-index"
] | automatic-speech-recognition | false | eugenetanjc | null | eugenetanjc/wav2vec_trained | 1 | null | transformers | 33,086 | ---
license: apache-2.0
tags:
- generated_from_trainer
model-index:
- name: wav2vec_trained
results: []
---
<!-- This model card has been generated automatically according to the information the Trainer had access to. You
should probably proofread and complete it, then remove this comment. -->
# wav2vec_trained
This model is a fine-tuned version of [facebook/wav2vec2-base](https://huggingface.co/facebook/wav2vec2-base) on the None dataset.
It achieves the following results on the evaluation set:
- Loss: 0.0337
- Wer: 0.1042
## Model description
More information needed
## Intended uses & limitations
More information needed
## Training and evaluation data
More information needed
## Training procedure
### Training hyperparameters
The following hyperparameters were used during training:
- learning_rate: 0.0001
- train_batch_size: 8
- eval_batch_size: 8
- seed: 42
- optimizer: Adam with betas=(0.9,0.999) and epsilon=1e-08
- lr_scheduler_type: linear
- lr_scheduler_warmup_steps: 1000
- num_epochs: 30
- mixed_precision_training: Native AMP
### Training results
| Training Loss | Epoch | Step | Validation Loss | Wer |
|:-------------:|:-----:|:----:|:---------------:|:------:|
| 4.3849 | 2.21 | 500 | 2.9148 | 1.0 |
| 1.9118 | 4.42 | 1000 | 0.9627 | 0.5833 |
| 0.7596 | 6.64 | 1500 | 0.8953 | 0.3542 |
| 0.4602 | 8.85 | 2000 | 0.3325 | 0.2083 |
| 0.331 | 11.06 | 2500 | 0.3084 | 0.2083 |
| 0.2474 | 13.27 | 3000 | 0.0960 | 0.1667 |
| 0.1934 | 15.49 | 3500 | 0.1276 | 0.125 |
| 0.156 | 17.7 | 4000 | 0.0605 | 0.0833 |
| 0.1244 | 19.91 | 4500 | 0.0831 | 0.1458 |
| 0.1006 | 22.12 | 5000 | 0.0560 | 0.125 |
| 0.0827 | 24.34 | 5500 | 0.0395 | 0.0833 |
| 0.0723 | 26.55 | 6000 | 0.0573 | 0.0833 |
| 0.0606 | 28.76 | 6500 | 0.0337 | 0.1042 |
### Framework versions
- Transformers 4.17.0
- Pytorch 1.11.0+cu113
- Datasets 1.18.3
- Tokenizers 0.12.1
|
eugenetanjc/wav2vec_test | 0d8a6f31f3d16dfef9bfee559ca9afd98f5ad70a | 2022-06-25T17:00:52.000Z | [
"pytorch",
"tensorboard",
"wav2vec2",
"automatic-speech-recognition",
"transformers",
"generated_from_trainer",
"model-index"
] | automatic-speech-recognition | false | eugenetanjc | null | eugenetanjc/wav2vec_test | 1 | null | transformers | 33,087 | ---
tags:
- generated_from_trainer
model-index:
- name: wav2vec_test
results: []
---
<!-- This model card has been generated automatically according to the information the Trainer had access to. You
should probably proofread and complete it, then remove this comment. -->
# wav2vec_test
This model was trained from scratch on the None dataset.
## Model description
More information needed
## Intended uses & limitations
More information needed
## Training and evaluation data
More information needed
## Training procedure
### Training hyperparameters
The following hyperparameters were used during training:
- learning_rate: 0.0001
- train_batch_size: 8
- eval_batch_size: 8
- seed: 42
- optimizer: Adam with betas=(0.9,0.999) and epsilon=1e-08
- lr_scheduler_type: linear
- lr_scheduler_warmup_steps: 10
- num_epochs: 2
- mixed_precision_training: Native AMP
### Training results
### Framework versions
- Transformers 4.17.0
- Pytorch 1.11.0+cu113
- Datasets 1.18.3
- Tokenizers 0.12.1
|
HKHKHKHK/bert-finetuned-squad | e93cc4051c9263de51ae7478bbd6c8f4f5a007d6 | 2022-06-26T07:25:52.000Z | [
"pytorch",
"tensorboard",
"bert",
"question-answering",
"dataset:squad",
"transformers",
"generated_from_trainer",
"license:apache-2.0",
"model-index",
"autotrain_compatible"
] | question-answering | false | HKHKHKHK | null | HKHKHKHK/bert-finetuned-squad | 1 | null | transformers | 33,088 | ---
license: apache-2.0
tags:
- generated_from_trainer
datasets:
- squad
model-index:
- name: bert-finetuned-squad
results: []
---
<!-- This model card has been generated automatically according to the information the Trainer had access to. You
should probably proofread and complete it, then remove this comment. -->
# bert-finetuned-squad
This model is a fine-tuned version of [bert-base-cased](https://huggingface.co/bert-base-cased) on the squad dataset.
## Model description
More information needed
## Intended uses & limitations
More information needed
## Training and evaluation data
More information needed
## Training procedure
### Training hyperparameters
The following hyperparameters were used during training:
- learning_rate: 2e-05
- train_batch_size: 8
- eval_batch_size: 8
- seed: 42
- optimizer: Adam with betas=(0.9,0.999) and epsilon=1e-08
- lr_scheduler_type: linear
- num_epochs: 3
- mixed_precision_training: Native AMP
### Training results
### Framework versions
- Transformers 4.20.1
- Pytorch 1.11.0+cu113
- Datasets 2.3.2
- Tokenizers 0.12.1
|
sasha/swin-tiny-finetuned-dogfood | a0fc3a3272a5b867486733bc2f092c1290a7bad6 | 2022-06-27T13:26:02.000Z | [
"pytorch",
"tensorboard",
"swin",
"image-classification",
"dataset:imagefolder",
"dataset:lewtun/dog_food",
"transformers",
"generated_from_trainer",
"license:apache-2.0",
"model-index"
] | image-classification | false | sasha | null | sasha/swin-tiny-finetuned-dogfood | 1 | 1 | transformers | 33,089 | ---
license: apache-2.0
tags:
- generated_from_trainer
datasets:
- imagefolder
- lewtun/dog_food
metrics:
- accuracy
model-index:
- name: swin-tiny-finetuned-dogfood
results:
- task:
name: Image Classification
type: image-classification
dataset:
name: lewtun/dog_food
type: lewtun/dog_food
args: lewtun--dog_food
metrics:
- name: Accuracy
type: accuracy
value: 0.988
- task:
type: image-classification
name: Image Classification
dataset:
name: lewtun/dog_food
type: lewtun/dog_food
config: lewtun--dog_food
split: test
metrics:
- name: Accuracy
type: accuracy
value: 0.9826666666666667
verified: true
- name: Precision Macro
type: precision
value: 0.9820904286553143
verified: true
- name: Precision Micro
type: precision
value: 0.9826666666666667
verified: true
- name: Precision Weighted
type: precision
value: 0.9828416519866903
verified: true
- name: Recall Macro
type: recall
value: 0.9828453314981092
verified: true
- name: Recall Micro
type: recall
value: 0.9826666666666667
verified: true
- name: Recall Weighted
type: recall
value: 0.9826666666666667
verified: true
- name: F1 Macro
type: f1
value: 0.9824101123169301
verified: true
- name: F1 Micro
type: f1
value: 0.9826666666666667
verified: true
- name: F1 Weighted
type: f1
value: 0.9826983433609648
verified: true
- name: loss
type: loss
value: 0.2326570302248001
verified: true
- name: matthews_correlation
type: matthews_correlation
value: 0.974016655798285
verified: true
---
<!-- This model card has been generated automatically according to the information the Trainer had access to. You
should probably proofread and complete it, then remove this comment. -->
# swin-tiny-finetuned-dogfood
This model is a fine-tuned version of [microsoft/swin-tiny-patch4-window7-224](https://huggingface.co/microsoft/swin-tiny-patch4-window7-224) on the lewtun/dog_food dataset.
It achieves the following results on the evaluation set:
- Loss: 0.1959
- Accuracy: 0.988
## Model description
More information needed
## Intended uses & limitations
More information needed
## Training and evaluation data
More information needed
## Training procedure
### Training hyperparameters
The following hyperparameters were used during training:
- learning_rate: 5e-05
- train_batch_size: 32
- eval_batch_size: 32
- seed: 42
- gradient_accumulation_steps: 4
- total_train_batch_size: 128
- optimizer: Adam with betas=(0.9,0.999) and epsilon=1e-08
- lr_scheduler_type: linear
- lr_scheduler_warmup_ratio: 0.1
- num_epochs: 1
### Training results
| Training Loss | Epoch | Step | Validation Loss | Accuracy |
|:-------------:|:-----:|:----:|:---------------:|:--------:|
| 0.8198 | 1.0 | 16 | 0.1901 | 0.9822 |
### Framework versions
- Transformers 4.20.1
- Pytorch 1.11.0+cu113
- Datasets 2.3.2
- Tokenizers 0.12.1
|
gary109/ai-light-dance_stepmania_ft_wav2vec2-large-xlsr-53-v4 | 6328e5e43a14581643c5bb3526221d154e8fae0b | 2022-06-27T13:34:30.000Z | [
"pytorch",
"tensorboard",
"wav2vec2",
"automatic-speech-recognition",
"transformers",
"gary109/AI_Light_Dance",
"generated_from_trainer",
"license:apache-2.0",
"model-index"
] | automatic-speech-recognition | false | gary109 | null | gary109/ai-light-dance_stepmania_ft_wav2vec2-large-xlsr-53-v4 | 1 | null | transformers | 33,090 | ---
license: apache-2.0
tags:
- automatic-speech-recognition
- gary109/AI_Light_Dance
- generated_from_trainer
model-index:
- name: ai-light-dance_stepmania_ft_wav2vec2-large-xlsr-53-v4
results: []
---
<!-- This model card has been generated automatically according to the information the Trainer had access to. You
should probably proofread and complete it, then remove this comment. -->
# ai-light-dance_stepmania_ft_wav2vec2-large-xlsr-53-v4
This model is a fine-tuned version of [gary109/ai-light-dance_stepmania_ft_wav2vec2-large-xlsr-53-v3](https://huggingface.co/gary109/ai-light-dance_stepmania_ft_wav2vec2-large-xlsr-53-v3) on the GARY109/AI_LIGHT_DANCE - ONSET-STEPMANIA2 dataset.
It achieves the following results on the evaluation set:
- Loss: 1.0298
- Wer: 0.6642
## Model description
More information needed
## Intended uses & limitations
More information needed
## Training and evaluation data
More information needed
## Training procedure
### Training hyperparameters
The following hyperparameters were used during training:
- learning_rate: 4e-05
- train_batch_size: 8
- eval_batch_size: 8
- seed: 42
- gradient_accumulation_steps: 16
- total_train_batch_size: 128
- optimizer: Adam with betas=(0.9,0.999) and epsilon=1e-08
- lr_scheduler_type: linear
- lr_scheduler_warmup_steps: 100
- num_epochs: 10.0
- mixed_precision_training: Native AMP
### Training results
| Training Loss | Epoch | Step | Validation Loss | Wer |
|:-------------:|:-----:|:----:|:---------------:|:------:|
| 0.9218 | 1.0 | 188 | 1.0718 | 0.6958 |
| 0.9194 | 2.0 | 376 | 1.0354 | 0.6937 |
| 0.9077 | 3.0 | 564 | 1.0365 | 0.6730 |
| 0.8956 | 4.0 | 752 | 1.0497 | 0.6727 |
| 0.877 | 5.0 | 940 | 1.0299 | 0.6694 |
| 0.8736 | 6.0 | 1128 | 1.0298 | 0.6642 |
| 0.8769 | 7.0 | 1316 | 1.0348 | 0.6584 |
| 0.8571 | 8.0 | 1504 | 1.0689 | 0.6602 |
| 0.8573 | 9.0 | 1692 | 1.0559 | 0.6549 |
| 0.8458 | 10.0 | 1880 | 1.0706 | 0.6588 |
### Framework versions
- Transformers 4.21.0.dev0
- Pytorch 1.11.0+cu113
- Datasets 2.3.3.dev0
- Tokenizers 0.12.1
|
shubhamsalokhe/distilgpt2-finetuned-wikitext2 | 4966ca6a3e1e075044f7c868ec31ba98bc3769c5 | 2022-06-26T18:38:27.000Z | [
"pytorch",
"tensorboard",
"gpt2",
"text-generation",
"transformers",
"generated_from_trainer",
"license:apache-2.0",
"model-index"
] | text-generation | false | shubhamsalokhe | null | shubhamsalokhe/distilgpt2-finetuned-wikitext2 | 1 | null | transformers | 33,091 | ---
license: apache-2.0
tags:
- generated_from_trainer
model-index:
- name: distilgpt2-finetuned-wikitext2
results: []
---
<!-- This model card has been generated automatically according to the information the Trainer had access to. You
should probably proofread and complete it, then remove this comment. -->
# distilgpt2-finetuned-wikitext2
This model is a fine-tuned version of [distilgpt2](https://huggingface.co/distilgpt2) on the None dataset.
It achieves the following results on the evaluation set:
- Loss: 3.6421
## Model description
More information needed
## Intended uses & limitations
More information needed
## Training and evaluation data
More information needed
## Training procedure
### Training hyperparameters
The following hyperparameters were used during training:
- learning_rate: 2e-05
- train_batch_size: 8
- eval_batch_size: 8
- seed: 42
- optimizer: Adam with betas=(0.9,0.999) and epsilon=1e-08
- lr_scheduler_type: linear
- num_epochs: 3.0
### Training results
| Training Loss | Epoch | Step | Validation Loss |
|:-------------:|:-----:|:----:|:---------------:|
| 3.7602 | 1.0 | 2334 | 3.6669 |
| 3.653 | 2.0 | 4668 | 3.6472 |
| 3.6006 | 3.0 | 7002 | 3.6421 |
### Framework versions
- Transformers 4.20.1
- Pytorch 1.11.0+cu113
- Datasets 2.3.2
- Tokenizers 0.12.1
|
SoDehghan/supmpn-bert-large-uncased | 4d82f25c0a1e428a64eed6b146dc86f90ea8adc4 | 2022-06-26T19:41:58.000Z | [
"pytorch",
"bert",
"feature-extraction",
"transformers",
"license:apache-2.0"
] | feature-extraction | false | SoDehghan | null | SoDehghan/supmpn-bert-large-uncased | 1 | null | transformers | 33,092 | ---
license: apache-2.0
---
|
Samiul/wav2vec2-large-xls-r-300m-turkish-colab | b5c53c5132699106fcbe835493a90f3d9650e9ae | 2022-06-26T23:31:16.000Z | [
"pytorch",
"tensorboard",
"wav2vec2",
"automatic-speech-recognition",
"dataset:common_voice",
"transformers",
"generated_from_trainer",
"license:apache-2.0",
"model-index"
] | automatic-speech-recognition | false | Samiul | null | Samiul/wav2vec2-large-xls-r-300m-turkish-colab | 1 | null | transformers | 33,093 | ---
license: apache-2.0
tags:
- generated_from_trainer
datasets:
- common_voice
model-index:
- name: wav2vec2-large-xls-r-300m-turkish-colab
results: []
---
<!-- This model card has been generated automatically according to the information the Trainer had access to. You
should probably proofread and complete it, then remove this comment. -->
# wav2vec2-large-xls-r-300m-turkish-colab
This model is a fine-tuned version of [facebook/wav2vec2-xls-r-300m](https://huggingface.co/facebook/wav2vec2-xls-r-300m) on the common_voice dataset.
It achieves the following results on the evaluation set:
- Loss: 0.3821
- Wer: 0.3208
## Model description
More information needed
## Intended uses & limitations
More information needed
## Training and evaluation data
More information needed
## Training procedure
### Training hyperparameters
The following hyperparameters were used during training:
- learning_rate: 0.0003
- train_batch_size: 16
- eval_batch_size: 8
- seed: 42
- gradient_accumulation_steps: 2
- total_train_batch_size: 32
- optimizer: Adam with betas=(0.9,0.999) and epsilon=1e-08
- lr_scheduler_type: linear
- lr_scheduler_warmup_steps: 500
- num_epochs: 30
- mixed_precision_training: Native AMP
### Training results
| Training Loss | Epoch | Step | Validation Loss | Wer |
|:-------------:|:-----:|:----:|:---------------:|:------:|
| 3.9162 | 3.67 | 400 | 0.6340 | 0.6360 |
| 0.4033 | 7.34 | 800 | 0.4588 | 0.4911 |
| 0.1919 | 11.01 | 1200 | 0.4392 | 0.4460 |
| 0.1315 | 14.68 | 1600 | 0.4269 | 0.4270 |
| 0.0963 | 18.35 | 2000 | 0.4327 | 0.3834 |
| 0.0801 | 22.02 | 2400 | 0.3867 | 0.3643 |
| 0.0631 | 25.69 | 2800 | 0.3854 | 0.3441 |
| 0.0492 | 29.36 | 3200 | 0.3821 | 0.3208 |
### Framework versions
- Transformers 4.11.3
- Pytorch 1.10.0+cu113
- Datasets 1.18.3
- Tokenizers 0.10.3
|
gngpostalsrvc/BERiT | 1b8bdbd009ee6ba5bde8bb7e0c50dcf8be219e46 | 2022-06-26T21:30:33.000Z | [
"pytorch",
"tensorboard",
"bert",
"fill-mask",
"transformers",
"generated_from_trainer",
"license:apache-2.0",
"model-index",
"autotrain_compatible"
] | fill-mask | false | gngpostalsrvc | null | gngpostalsrvc/BERiT | 1 | null | transformers | 33,094 | ---
license: apache-2.0
tags:
- generated_from_trainer
model-index:
- name: BERiT
results: []
---
<!-- This model card has been generated automatically according to the information the Trainer had access to. You
should probably proofread and complete it, then remove this comment. -->
# BERiT
This model is a fine-tuned version of [onlplab/alephbert-base](https://huggingface.co/onlplab/alephbert-base) on an unknown dataset.
It achieves the following results on the evaluation set:
- Loss: 3.5800
## Model description
More information needed
## Intended uses & limitations
More information needed
## Training and evaluation data
More information needed
## Training procedure
### Training hyperparameters
The following hyperparameters were used during training:
- learning_rate: 0.0001
- train_batch_size: 8
- eval_batch_size: 8
- seed: 42
- optimizer: Adam with betas=(0.9,0.999) and epsilon=1e-08
- lr_scheduler_type: linear
- num_epochs: 15
### Training results
| Training Loss | Epoch | Step | Validation Loss |
|:-------------:|:-----:|:-----:|:---------------:|
| 4.6813 | 1.0 | 2582 | 4.5557 |
| 4.4115 | 2.0 | 5164 | 4.4279 |
| 4.2192 | 3.0 | 7746 | 4.3661 |
| 4.0148 | 4.0 | 10328 | 4.2336 |
| 3.8166 | 5.0 | 12910 | 4.2115 |
| 3.5512 | 6.0 | 15492 | 4.0535 |
| 3.4319 | 7.0 | 18074 | 3.8681 |
| 3.2164 | 8.0 | 20656 | 3.9730 |
| 3.0837 | 9.0 | 23238 | 3.7807 |
| 2.9773 | 10.0 | 25820 | 3.6773 |
| 2.8521 | 11.0 | 28402 | 3.7304 |
| 2.6034 | 12.0 | 30984 | 3.6530 |
| 2.4614 | 13.0 | 33566 | 3.6396 |
| 2.3812 | 14.0 | 36148 | 3.7146 |
| 2.3812 | 15.0 | 38730 | 3.5800 |
### Framework versions
- Transformers 4.20.1
- Pytorch 1.11.0+cu113
- Datasets 2.3.2
- Tokenizers 0.12.1
|
sudo-s/exper_batch_32_e4 | edc455d97ab2854c1b4c5c89d5fb4b844e0d24db | 2022-06-26T22:47:06.000Z | [
"pytorch",
"tensorboard",
"vit",
"image-classification",
"transformers",
"generated_from_trainer",
"license:apache-2.0",
"model-index"
] | image-classification | false | sudo-s | null | sudo-s/exper_batch_32_e4 | 1 | null | transformers | 33,095 | ---
license: apache-2.0
tags:
- image-classification
- generated_from_trainer
metrics:
- accuracy
model-index:
- name: exper_batch_32_e4
results: []
---
<!-- This model card has been generated automatically according to the information the Trainer had access to. You
should probably proofread and complete it, then remove this comment. -->
# exper_batch_32_e4
This model is a fine-tuned version of [google/vit-base-patch16-224-in21k](https://huggingface.co/google/vit-base-patch16-224-in21k) on the sudo-s/herbier_mesuem1 dataset.
It achieves the following results on the evaluation set:
- Loss: 0.3909
- Accuracy: 0.9067
## Model description
More information needed
## Intended uses & limitations
More information needed
## Training and evaluation data
More information needed
## Training procedure
### Training hyperparameters
The following hyperparameters were used during training:
- learning_rate: 0.0002
- train_batch_size: 32
- eval_batch_size: 8
- seed: 42
- optimizer: Adam with betas=(0.9,0.999) and epsilon=1e-08
- lr_scheduler_type: linear
- num_epochs: 4
- mixed_precision_training: Apex, opt level O1
### Training results
| Training Loss | Epoch | Step | Validation Loss | Accuracy |
|:-------------:|:-----:|:----:|:---------------:|:--------:|
| 3.4295 | 0.31 | 100 | 3.4027 | 0.2837 |
| 2.5035 | 0.62 | 200 | 2.4339 | 0.5247 |
| 1.6542 | 0.94 | 300 | 1.7690 | 0.6388 |
| 1.1589 | 1.25 | 400 | 1.3106 | 0.7460 |
| 0.9363 | 1.56 | 500 | 0.9977 | 0.7803 |
| 0.6946 | 1.88 | 600 | 0.8138 | 0.8207 |
| 0.3488 | 2.19 | 700 | 0.6593 | 0.8489 |
| 0.2935 | 2.5 | 800 | 0.5725 | 0.8662 |
| 0.2557 | 2.81 | 900 | 0.5088 | 0.8855 |
| 0.1509 | 3.12 | 1000 | 0.4572 | 0.8971 |
| 0.1367 | 3.44 | 1100 | 0.4129 | 0.9090 |
| 0.1078 | 3.75 | 1200 | 0.3909 | 0.9067 |
### Framework versions
- Transformers 4.19.4
- Pytorch 1.5.1
- Datasets 2.3.2
- Tokenizers 0.12.1
|
neweasterns/wav2vec2-base-timit-demo-google-colab | f7beeeee1121eea8f19d5c8a69412d572ea983b5 | 2022-06-27T02:49:23.000Z | [
"pytorch",
"tensorboard",
"wav2vec2",
"automatic-speech-recognition",
"transformers",
"generated_from_trainer",
"license:apache-2.0",
"model-index"
] | automatic-speech-recognition | false | neweasterns | null | neweasterns/wav2vec2-base-timit-demo-google-colab | 1 | null | transformers | 33,096 | ---
license: apache-2.0
tags:
- generated_from_trainer
model-index:
- name: wav2vec2-base-timit-demo-google-colab
results: []
---
<!-- This model card has been generated automatically according to the information the Trainer had access to. You
should probably proofread and complete it, then remove this comment. -->
# wav2vec2-base-timit-demo-google-colab
This model is a fine-tuned version of [facebook/wav2vec2-base](https://huggingface.co/facebook/wav2vec2-base) on the None dataset.
It achieves the following results on the evaluation set:
- Loss: 0.5206
- Wer: 0.3388
## Model description
More information needed
## Intended uses & limitations
More information needed
## Training and evaluation data
More information needed
## Training procedure
### Training hyperparameters
The following hyperparameters were used during training:
- learning_rate: 0.0001
- train_batch_size: 8
- eval_batch_size: 8
- seed: 42
- optimizer: Adam with betas=(0.9,0.999) and epsilon=1e-08
- lr_scheduler_type: linear
- lr_scheduler_warmup_steps: 1000
- num_epochs: 30
- mixed_precision_training: Native AMP
### Training results
| Training Loss | Epoch | Step | Validation Loss | Wer |
|:-------------:|:-----:|:-----:|:---------------:|:------:|
| 3.5597 | 1.0 | 500 | 2.3415 | 0.9991 |
| 0.9759 | 2.01 | 1000 | 0.5556 | 0.5382 |
| 0.4587 | 3.01 | 1500 | 0.7690 | 0.4781 |
| 0.3156 | 4.02 | 2000 | 0.7994 | 0.4412 |
| 0.2272 | 5.02 | 2500 | 0.8948 | 0.4120 |
| 0.1921 | 6.02 | 3000 | 0.7065 | 0.3940 |
| 0.1618 | 7.03 | 3500 | 0.4333 | 0.3855 |
| 0.1483 | 8.03 | 4000 | 0.4232 | 0.3872 |
| 0.156 | 9.04 | 4500 | 0.4172 | 0.3749 |
| 0.1138 | 10.04 | 5000 | 0.4084 | 0.3758 |
| 0.1045 | 11.04 | 5500 | 0.4665 | 0.3623 |
| 0.0908 | 12.05 | 6000 | 0.4416 | 0.3684 |
| 0.0788 | 13.05 | 6500 | 0.4801 | 0.3659 |
| 0.0773 | 14.06 | 7000 | 0.4560 | 0.3583 |
| 0.0684 | 15.06 | 7500 | 0.4878 | 0.3610 |
| 0.0645 | 16.06 | 8000 | 0.4635 | 0.3567 |
| 0.0577 | 17.07 | 8500 | 0.5245 | 0.3548 |
| 0.0547 | 18.07 | 9000 | 0.5265 | 0.3639 |
| 0.0466 | 19.08 | 9500 | 0.5161 | 0.3546 |
| 0.0432 | 20.08 | 10000 | 0.5263 | 0.3558 |
| 0.0414 | 21.08 | 10500 | 0.4874 | 0.3500 |
| 0.0365 | 22.09 | 11000 | 0.5266 | 0.3472 |
| 0.0321 | 23.09 | 11500 | 0.5422 | 0.3458 |
| 0.0325 | 24.1 | 12000 | 0.5201 | 0.3428 |
| 0.0262 | 25.1 | 12500 | 0.5208 | 0.3398 |
| 0.0249 | 26.1 | 13000 | 0.5034 | 0.3429 |
| 0.0262 | 27.11 | 13500 | 0.5055 | 0.3396 |
| 0.0248 | 28.11 | 14000 | 0.5164 | 0.3404 |
| 0.0222 | 29.12 | 14500 | 0.5206 | 0.3388 |
### Framework versions
- Transformers 4.17.0
- Pytorch 1.11.0+cu113
- Datasets 1.18.3
- Tokenizers 0.12.1
|
lingchensanwen/distilbert-base-uncased-finetuned-squad | ac1f1f3b602524b632588c45f9301767bc3b8986 | 2022-06-28T02:57:31.000Z | [
"pytorch",
"tensorboard",
"distilbert",
"question-answering",
"transformers",
"generated_from_trainer",
"license:apache-2.0",
"model-index",
"autotrain_compatible"
] | question-answering | false | lingchensanwen | null | lingchensanwen/distilbert-base-uncased-finetuned-squad | 1 | null | transformers | 33,097 | ---
license: apache-2.0
tags:
- generated_from_trainer
model-index:
- name: distilbert-base-uncased-finetuned-squad
results: []
---
<!-- This model card has been generated automatically according to the information the Trainer had access to. You
should probably proofread and complete it, then remove this comment. -->
# distilbert-base-uncased-finetuned-squad
This model is a fine-tuned version of [distilbert-base-uncased](https://huggingface.co/distilbert-base-uncased) on the None dataset.
It achieves the following results on the evaluation set:
- Loss: 0.0337
## Model description
More information needed
## Intended uses & limitations
More information needed
## Training and evaluation data
More information needed
## Training procedure
### Training hyperparameters
The following hyperparameters were used during training:
- learning_rate: 2e-05
- train_batch_size: 32
- eval_batch_size: 32
- seed: 42
- optimizer: Adam with betas=(0.9,0.999) and epsilon=1e-08
- lr_scheduler_type: linear
- num_epochs: 3
### Training results
| Training Loss | Epoch | Step | Validation Loss |
|:-------------:|:-----:|:----:|:---------------:|
| No log | 1.0 | 46 | 0.4284 |
| No log | 2.0 | 92 | 0.0573 |
| No log | 3.0 | 138 | 0.0337 |
### Framework versions
- Transformers 4.20.1
- Pytorch 1.11.0
- Datasets 2.2.2
- Tokenizers 0.12.1
|
TheRensselaerIDEA/gpt2-large-vaccine-tweet-response | 8e8164ed21f0e299565eec0db0153ed884046c78 | 2022-06-27T03:22:42.000Z | [
"pytorch",
"tensorboard",
"gpt2",
"text-generation",
"arxiv:2204.04353",
"transformers",
"license:mit"
] | text-generation | false | TheRensselaerIDEA | null | TheRensselaerIDEA/gpt2-large-vaccine-tweet-response | 1 | null | transformers | 33,098 | ---
license: mit
---
Base model: [gpt2-large](https://huggingface.co/gpt2-large)
Fine-tuned to generate responses on a dataset of [Vaccine public health tweets](https://github.com/TheRensselaerIDEA/generative-response-modeling). For more information about the dataset, task and training, see [our paper](https://arxiv.org/abs/2204.04353). This checkpoint corresponds to the lowest validation perplexity (2.82 at 2 epochs) seen during training. See Training metrics for Tensorboard logs.
For input format and usage examples, see our [COVID-19 public health tweet response model](https://huggingface.co/TheRensselaerIDEA/gpt2-large-covid-tweet-response).
|
deepesh0x/autotrain-a3-1043835930 | 05714926b15a15db8c356e87813c1c2d31b6f2f5 | 2022-06-27T05:12:13.000Z | [
"pytorch",
"bert",
"question-answering",
"transformers",
"autotrain_compatible"
] | question-answering | false | deepesh0x | null | deepesh0x/autotrain-a3-1043835930 | 1 | null | transformers | 33,099 | Entry not found |
Subsets and Splits
No saved queries yet
Save your SQL queries to embed, download, and access them later. Queries will appear here once saved.