modelId
stringlengths 4
112
| sha
stringlengths 40
40
| lastModified
stringlengths 24
24
| tags
sequence | pipeline_tag
stringclasses 29
values | private
bool 1
class | author
stringlengths 2
38
⌀ | config
null | id
stringlengths 4
112
| downloads
float64 0
36.8M
⌀ | likes
float64 0
712
⌀ | library_name
stringclasses 17
values | __index_level_0__
int64 0
38.5k
| readme
stringlengths 0
186k
|
---|---|---|---|---|---|---|---|---|---|---|---|---|---|
income/jpq-gpl-fiqa-document_encoder-base-msmarco-distilbert-tas-b | de20e7a20b6b0c0578752f10364ed78e9e63f4fc | 2022-06-15T17:16:03.000Z | [
"pytorch",
"distilbert",
"transformers",
"license:apache-2.0"
] | null | false | income | null | income/jpq-gpl-fiqa-document_encoder-base-msmarco-distilbert-tas-b | 1 | null | transformers | 32,900 | ---
license: apache-2.0
---
|
income/jpq-gpl-hotpotqa-question_encoder-base-msmarco-distilbert-tas-b | b49937e6fc7c669f005a27cf90637c8af89074a5 | 2022-06-15T17:17:27.000Z | [
"pytorch",
"distilbert",
"transformers",
"license:apache-2.0"
] | null | false | income | null | income/jpq-gpl-hotpotqa-question_encoder-base-msmarco-distilbert-tas-b | 1 | null | transformers | 32,901 | ---
license: apache-2.0
---
|
income/jpq-gpl-nfcorpus-question_encoder-base-msmarco-distilbert-tas-b | 19904b962f446c08207093f994ee7b6fd0e0d357 | 2022-06-15T17:26:15.000Z | [
"pytorch",
"distilbert",
"transformers",
"license:apache-2.0"
] | null | false | income | null | income/jpq-gpl-nfcorpus-question_encoder-base-msmarco-distilbert-tas-b | 1 | null | transformers | 32,902 | ---
license: apache-2.0
---
|
income/jpq-gpl-nfcorpus-document_encoder-base-msmarco-distilbert-tas-b | cebf1424e99886dbdd69c3498763910499edd1b4 | 2022-06-15T17:28:14.000Z | [
"pytorch",
"distilbert",
"transformers",
"license:apache-2.0"
] | null | false | income | null | income/jpq-gpl-nfcorpus-document_encoder-base-msmarco-distilbert-tas-b | 1 | null | transformers | 32,903 | ---
license: apache-2.0
---
|
income/jpq-gpl-nq-question_encoder-base-msmarco-distilbert-tas-b | ca30ac871ab926e67c64a25f8d8c61b7ba588b09 | 2022-06-15T17:29:28.000Z | [
"pytorch",
"distilbert",
"transformers",
"license:apache-2.0"
] | null | false | income | null | income/jpq-gpl-nq-question_encoder-base-msmarco-distilbert-tas-b | 1 | null | transformers | 32,904 | ---
license: apache-2.0
---
|
income/jpq-gpl-nq-document_encoder-base-msmarco-distilbert-tas-b | afbe9b09c4d23fa21d6fa33252a082be31ffd6f3 | 2022-06-15T17:30:10.000Z | [
"pytorch",
"distilbert",
"transformers",
"license:apache-2.0"
] | null | false | income | null | income/jpq-gpl-nq-document_encoder-base-msmarco-distilbert-tas-b | 1 | null | transformers | 32,905 | ---
license: apache-2.0
---
|
income/jpq-gpl-quora-question_encoder-base-msmarco-distilbert-tas-b | d0913555feba8151cd5c6fa4e983ba12d8fb0aa9 | 2022-06-15T17:31:38.000Z | [
"pytorch",
"distilbert",
"transformers",
"license:apache-2.0"
] | null | false | income | null | income/jpq-gpl-quora-question_encoder-base-msmarco-distilbert-tas-b | 1 | null | transformers | 32,906 | ---
license: apache-2.0
---
|
income/jpq-gpl-scidocs-question_encoder-base-msmarco-distilbert-tas-b | e8ddf4ae5e14aec9682aa54690d9c3c00f188d87 | 2022-06-15T17:36:01.000Z | [
"pytorch",
"distilbert",
"transformers",
"license:apache-2.0"
] | null | false | income | null | income/jpq-gpl-scidocs-question_encoder-base-msmarco-distilbert-tas-b | 1 | null | transformers | 32,907 | ---
license: apache-2.0
---
|
income/jpq-gpl-scidocs-document_encoder-base-msmarco-distilbert-tas-b | ed5d266d9562925523affb3fb059bce6fb9f2432 | 2022-06-15T17:36:43.000Z | [
"pytorch",
"distilbert",
"transformers",
"license:apache-2.0"
] | null | false | income | null | income/jpq-gpl-scidocs-document_encoder-base-msmarco-distilbert-tas-b | 1 | null | transformers | 32,908 | ---
license: apache-2.0
---
|
income/jpq-gpl-scifact-document_encoder-base-msmarco-distilbert-tas-b | 8658482ed31ff6193289017cb577248e3880267d | 2022-06-15T17:38:40.000Z | [
"pytorch",
"distilbert",
"transformers",
"license:apache-2.0"
] | null | false | income | null | income/jpq-gpl-scifact-document_encoder-base-msmarco-distilbert-tas-b | 1 | null | transformers | 32,909 | ---
license: apache-2.0
---
|
income/jpq-gpl-trec-covid-question_encoder-base-msmarco-distilbert-tas-b | 4c3cf9c0d0d38834882b732bf7a1ebedcf848b52 | 2022-06-15T17:43:02.000Z | [
"pytorch",
"distilbert",
"transformers",
"license:apache-2.0"
] | null | false | income | null | income/jpq-gpl-trec-covid-question_encoder-base-msmarco-distilbert-tas-b | 1 | null | transformers | 32,910 | ---
license: apache-2.0
---
|
lmqg/t5-large-squadshifts-nyt | 6623d6952f5bbd917c0d136f1d47b7d37c19c1f5 | 2022-06-16T11:55:05.000Z | [
"pytorch",
"t5",
"text2text-generation",
"transformers",
"autotrain_compatible"
] | text2text-generation | false | lmqg | null | lmqg/t5-large-squadshifts-nyt | 1 | null | transformers | 32,911 | Entry not found |
Nadav/xlm-roberta-base-squad-finetuned-on-runaways-en | 157942d7c69496eeb3b199a4c7eaf8ebd01d5aaa | 2022-06-19T14:09:31.000Z | [
"pytorch",
"xlm-roberta",
"question-answering",
"transformers",
"autotrain_compatible"
] | question-answering | false | Nadav | null | Nadav/xlm-roberta-base-squad-finetuned-on-runaways-en | 1 | null | transformers | 32,912 | Entry not found |
Nadav/roberta-base-finetuned-on-runaways-en | e69d843860ceecca4eae9f780de04fff35d592a8 | 2022-06-19T12:41:17.000Z | [
"pytorch",
"roberta",
"fill-mask",
"transformers",
"autotrain_compatible"
] | fill-mask | false | Nadav | null | Nadav/roberta-base-finetuned-on-runaways-en | 1 | null | transformers | 32,913 | Entry not found |
Nadav/xlm-roberta-base-finetuned-on-runaways-en | 130b35410dd7e9992a316874fba2491463fc0fb9 | 2022-06-19T13:17:36.000Z | [
"pytorch",
"xlm-roberta",
"fill-mask",
"transformers",
"autotrain_compatible"
] | fill-mask | false | Nadav | null | Nadav/xlm-roberta-base-finetuned-on-runaways-en | 1 | null | transformers | 32,914 | Entry not found |
erickfm/bright-sweep-9 | 4172724cf12fb83378031b0cb2e545f5a955dac8 | 2022-06-15T21:16:57.000Z | [
"pytorch",
"t5",
"text2text-generation",
"transformers",
"autotrain_compatible"
] | text2text-generation | false | erickfm | null | erickfm/bright-sweep-9 | 1 | null | transformers | 32,915 | Entry not found |
income/jpq-gpl-trec-news-question_encoder-base-msmarco-distilbert-tas-b | 1e897e579a15314e489272d05244113ce8c1f062 | 2022-06-15T21:53:20.000Z | [
"pytorch",
"distilbert",
"transformers",
"license:apache-2.0"
] | null | false | income | null | income/jpq-gpl-trec-news-question_encoder-base-msmarco-distilbert-tas-b | 1 | null | transformers | 32,916 | ---
license: apache-2.0
---
|
income/jpq-genq-arguana-question_encoder-base-msmarco-distilbert-tas-b | 27b7ef687f6e2c5cbf84559a11d3b09535d16458 | 2022-06-15T21:57:39.000Z | [
"pytorch",
"distilbert",
"transformers",
"license:apache-2.0"
] | null | false | income | null | income/jpq-genq-arguana-question_encoder-base-msmarco-distilbert-tas-b | 1 | null | transformers | 32,917 | ---
license: apache-2.0
---
|
income/jpq-genq-webis-touche2020-question_encoder-base-msmarco-distilbert-tas-b | b418a08a9caa905bc1536c35ee54e14192bb87e7 | 2022-06-15T21:59:53.000Z | [
"pytorch",
"distilbert",
"transformers",
"license:apache-2.0"
] | null | false | income | null | income/jpq-genq-webis-touche2020-question_encoder-base-msmarco-distilbert-tas-b | 1 | null | transformers | 32,918 | ---
license: apache-2.0
---
|
income/jpq-genq-webis-touche2020-document_encoder-base-msmarco-distilbert-tas-b | baa9bd6ecbfc878886535b81375a240715341228 | 2022-06-15T22:00:21.000Z | [
"pytorch",
"distilbert",
"transformers",
"license:apache-2.0"
] | null | false | income | null | income/jpq-genq-webis-touche2020-document_encoder-base-msmarco-distilbert-tas-b | 1 | null | transformers | 32,919 | ---
license: apache-2.0
---
|
income/jpq-genq-climate-fever-question_encoder-base-msmarco-distilbert-tas-b | 9bf52f0a0f695b57a43780069c2ebd5de111c893 | 2022-06-15T22:00:52.000Z | [
"pytorch",
"distilbert",
"transformers",
"license:apache-2.0"
] | null | false | income | null | income/jpq-genq-climate-fever-question_encoder-base-msmarco-distilbert-tas-b | 1 | null | transformers | 32,920 | ---
license: apache-2.0
---
|
income/jpq-genq-climate-fever-document_encoder-base-msmarco-distilbert-tas-b | f22da808212b17ac0f99da3ba99f50057a7facb0 | 2022-06-15T22:01:26.000Z | [
"pytorch",
"distilbert",
"transformers",
"license:apache-2.0"
] | null | false | income | null | income/jpq-genq-climate-fever-document_encoder-base-msmarco-distilbert-tas-b | 1 | null | transformers | 32,921 | ---
license: apache-2.0
---
|
income/jpq-genq-dbpedia-entity-question_encoder-base-msmarco-distilbert-tas-b | 60d6c9ea2b91c63b3e9681b03ae9a0e3676f38da | 2022-06-15T22:01:59.000Z | [
"pytorch",
"distilbert",
"transformers",
"license:apache-2.0"
] | null | false | income | null | income/jpq-genq-dbpedia-entity-question_encoder-base-msmarco-distilbert-tas-b | 1 | null | transformers | 32,922 | ---
license: apache-2.0
---
|
income/jpq-genq-dbpedia-entity-document_encoder-base-msmarco-distilbert-tas-b | 4402affbfcc2a3a80609c61231aa5c51b343b340 | 2022-06-15T22:02:38.000Z | [
"pytorch",
"distilbert",
"transformers",
"license:apache-2.0"
] | null | false | income | null | income/jpq-genq-dbpedia-entity-document_encoder-base-msmarco-distilbert-tas-b | 1 | null | transformers | 32,923 | ---
license: apache-2.0
---
|
income/jpq-genq-fever-question_encoder-base-msmarco-distilbert-tas-b | 7e2b7a313f431a784e2e97e3dcea6d44b0d2156e | 2022-06-15T22:04:20.000Z | [
"pytorch",
"distilbert",
"transformers",
"license:apache-2.0"
] | null | false | income | null | income/jpq-genq-fever-question_encoder-base-msmarco-distilbert-tas-b | 1 | null | transformers | 32,924 | ---
license: apache-2.0
---
|
income/jpq-genq-fiqa-document_encoder-base-msmarco-distilbert-tas-b | 7b861ad0abd69e4c5fe8bbf6a871d64de2eec8bf | 2022-06-15T22:08:15.000Z | [
"pytorch",
"distilbert",
"transformers",
"license:apache-2.0"
] | null | false | income | null | income/jpq-genq-fiqa-document_encoder-base-msmarco-distilbert-tas-b | 1 | null | transformers | 32,925 | ---
license: apache-2.0
---
|
income/jpq-genq-hotpotqa-question_encoder-base-msmarco-distilbert-tas-b | b42dcfc35ca57124a4e29b818173496d8ff7bafc | 2022-06-15T22:10:03.000Z | [
"pytorch",
"distilbert",
"transformers",
"license:apache-2.0"
] | null | false | income | null | income/jpq-genq-hotpotqa-question_encoder-base-msmarco-distilbert-tas-b | 1 | null | transformers | 32,926 | ---
license: apache-2.0
---
|
Asia-N/opus-mt-ar-en-finetuned-ar-to-en | e9cc735df9354f1f61a60d6044d9012197a332b7 | 2022-06-18T10:25:40.000Z | [
"pytorch",
"tensorboard",
"marian",
"text2text-generation",
"dataset:news_commentary",
"transformers",
"generated_from_trainer",
"license:apache-2.0",
"model-index",
"autotrain_compatible"
] | text2text-generation | false | Asia-N | null | Asia-N/opus-mt-ar-en-finetuned-ar-to-en | 1 | null | transformers | 32,927 | ---
license: apache-2.0
tags:
- generated_from_trainer
datasets:
- news_commentary
metrics:
- bleu
model-index:
- name: opus-mt-ar-en-finetuned-ar-to-en
results:
- task:
name: Sequence-to-sequence Language Modeling
type: text2text-generation
dataset:
name: news_commentary
type: news_commentary
args: ar-en
metrics:
- name: Bleu
type: bleu
value: 32.5327
---
<!-- This model card has been generated automatically according to the information the Trainer had access to. You
should probably proofread and complete it, then remove this comment. -->
# opus-mt-ar-en-finetuned-ar-to-en
This model is a fine-tuned version of [Helsinki-NLP/opus-mt-ar-en](https://huggingface.co/Helsinki-NLP/opus-mt-ar-en) on the news_commentary dataset.
It achieves the following results on the evaluation set:
- Loss: 10.6102
- Bleu: 32.5327
- Gen Len: 56.234
## Model description
More information needed
## Intended uses & limitations
More information needed
## Training and evaluation data
More information needed
## Training procedure
### Training hyperparameters
The following hyperparameters were used during training:
- learning_rate: 2e-09
- train_batch_size: 16
- eval_batch_size: 16
- seed: 42
- optimizer: Adam with betas=(0.9,0.999) and epsilon=1e-08
- lr_scheduler_type: linear
- num_epochs: 3
### Training results
| Training Loss | Epoch | Step | Validation Loss | Bleu | Gen Len |
|:-------------:|:-----:|:----:|:---------------:|:-------:|:-------:|
| No log | 1.0 | 32 | 10.6112 | 32.5327 | 56.234 |
| No log | 2.0 | 64 | 10.6103 | 32.5327 | 56.234 |
| No log | 3.0 | 96 | 10.6102 | 32.5327 | 56.234 |
### Framework versions
- Transformers 4.20.0
- Pytorch 1.11.0+cu113
- Datasets 2.3.2
- Tokenizers 0.12.1
|
income/jpq-genq-nfcorpus-question_encoder-base-msmarco-distilbert-tas-b | 25a938df61362627f18f668881e4f47eb4884e89 | 2022-06-15T22:13:23.000Z | [
"pytorch",
"distilbert",
"transformers",
"license:apache-2.0"
] | null | false | income | null | income/jpq-genq-nfcorpus-question_encoder-base-msmarco-distilbert-tas-b | 1 | null | transformers | 32,928 | ---
license: apache-2.0
---
|
income/jpq-genq-nfcorpus-document_encoder-base-msmarco-distilbert-tas-b | cf43fed71d0f69ad26ab6ad81eba183b1f63ffc8 | 2022-06-15T22:13:59.000Z | [
"pytorch",
"distilbert",
"transformers",
"license:apache-2.0"
] | null | false | income | null | income/jpq-genq-nfcorpus-document_encoder-base-msmarco-distilbert-tas-b | 1 | null | transformers | 32,929 | ---
license: apache-2.0
---
|
income/jpq-genq-nq-question_encoder-base-msmarco-distilbert-tas-b | 5b67cfe6c86743f1b8651ce26ba42f97c75283e8 | 2022-06-15T22:14:43.000Z | [
"pytorch",
"distilbert",
"transformers",
"license:apache-2.0"
] | null | false | income | null | income/jpq-genq-nq-question_encoder-base-msmarco-distilbert-tas-b | 1 | null | transformers | 32,930 | ---
license: apache-2.0
---
|
income/jpq-genq-quora-question_encoder-base-msmarco-distilbert-tas-b | 563fad59e86021cb50f97513e88e549af3ee992e | 2022-06-15T22:37:26.000Z | [
"pytorch",
"distilbert",
"transformers",
"license:apache-2.0"
] | null | false | income | null | income/jpq-genq-quora-question_encoder-base-msmarco-distilbert-tas-b | 1 | null | transformers | 32,931 | ---
license: apache-2.0
---
|
income/jpq-genq-quora-document_encoder-base-msmarco-distilbert-tas-b | 6bc478ca9afa13e32bafba975be773bead18c578 | 2022-06-15T22:39:05.000Z | [
"pytorch",
"distilbert",
"transformers",
"license:apache-2.0"
] | null | false | income | null | income/jpq-genq-quora-document_encoder-base-msmarco-distilbert-tas-b | 1 | null | transformers | 32,932 | ---
license: apache-2.0
---
|
income/jpq-genq-scidocs-question_encoder-base-msmarco-distilbert-tas-b | 259262a072d3d0cb98b84fb7eba3ee6804e97c72 | 2022-06-15T22:48:35.000Z | [
"pytorch",
"distilbert",
"transformers",
"license:apache-2.0"
] | null | false | income | null | income/jpq-genq-scidocs-question_encoder-base-msmarco-distilbert-tas-b | 1 | null | transformers | 32,933 | ---
license: apache-2.0
---
|
income/jpq-genq-scidocs-document_encoder-base-msmarco-distilbert-tas-b | efd83b6678418cdf9e4d9e0e87f95e42c281b8d8 | 2022-06-15T22:49:10.000Z | [
"pytorch",
"distilbert",
"transformers",
"license:apache-2.0"
] | null | false | income | null | income/jpq-genq-scidocs-document_encoder-base-msmarco-distilbert-tas-b | 1 | null | transformers | 32,934 | ---
license: apache-2.0
---
|
income/jpq-genq-scifact-document_encoder-base-msmarco-distilbert-tas-b | aac91bc108b03aae36f1353ccba34b614bacb1a8 | 2022-06-15T22:50:10.000Z | [
"pytorch",
"distilbert",
"transformers",
"license:apache-2.0"
] | null | false | income | null | income/jpq-genq-scifact-document_encoder-base-msmarco-distilbert-tas-b | 1 | null | transformers | 32,935 | ---
license: apache-2.0
---
|
huggingtweets/43folders-hotdogsladies | e7c279afea975d9812be130d1a3afe63d7dacba8 | 2022-06-15T23:14:40.000Z | [
"pytorch",
"gpt2",
"text-generation",
"en",
"transformers",
"huggingtweets"
] | text-generation | false | huggingtweets | null | huggingtweets/43folders-hotdogsladies | 1 | null | transformers | 32,936 | ---
language: en
thumbnail: http://www.huggingtweets.com/43folders-hotdogsladies/1655334875186/predictions.png
tags:
- huggingtweets
widget:
- text: "My dream is"
---
<div class="inline-flex flex-col" style="line-height: 1.5;">
<div class="flex">
<div
style="display:inherit; margin-left: 4px; margin-right: 4px; width: 92px; height:92px; border-radius: 50%; background-size: cover; background-image: url('https://pbs.twimg.com/profile_images/1165801400/43f-logo-square-300_400x400.png')">
</div>
<div
style="display:inherit; margin-left: 4px; margin-right: 4px; width: 92px; height:92px; border-radius: 50%; background-size: cover; background-image: url('https://pbs.twimg.com/profile_images/1474526156430798849/0Z_zfYqH_400x400.jpg')">
</div>
<div
style="display:none; margin-left: 4px; margin-right: 4px; width: 92px; height:92px; border-radius: 50%; background-size: cover; background-image: url('')">
</div>
</div>
<div style="text-align: center; margin-top: 3px; font-size: 16px; font-weight: 800">🤖 AI CYBORG 🤖</div>
<div style="text-align: center; font-size: 16px; font-weight: 800">43 Folders & Merlin Mann</div>
<div style="text-align: center; font-size: 14px;">@43folders-hotdogsladies</div>
</div>
I was made with [huggingtweets](https://github.com/borisdayma/huggingtweets).
Create your own bot based on your favorite user with [the demo](https://colab.research.google.com/github/borisdayma/huggingtweets/blob/master/huggingtweets-demo.ipynb)!
## How does it work?
The model uses the following pipeline.
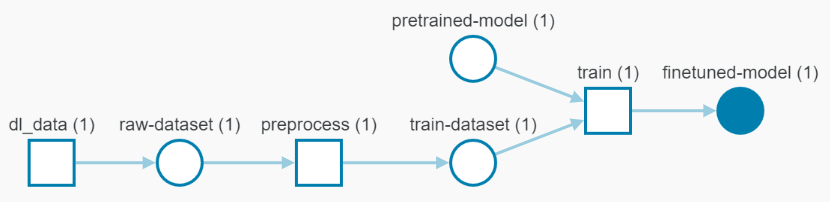
To understand how the model was developed, check the [W&B report](https://wandb.ai/wandb/huggingtweets/reports/HuggingTweets-Train-a-Model-to-Generate-Tweets--VmlldzoxMTY5MjI).
## Training data
The model was trained on tweets from 43 Folders & Merlin Mann.
| Data | 43 Folders | Merlin Mann |
| --- | --- | --- |
| Tweets downloaded | 149 | 317 |
| Retweets | 8 | 41 |
| Short tweets | 0 | 48 |
| Tweets kept | 141 | 228 |
[Explore the data](https://wandb.ai/wandb/huggingtweets/runs/2gd31yq9/artifacts), which is tracked with [W&B artifacts](https://docs.wandb.com/artifacts) at every step of the pipeline.
## Training procedure
The model is based on a pre-trained [GPT-2](https://huggingface.co/gpt2) which is fine-tuned on @43folders-hotdogsladies's tweets.
Hyperparameters and metrics are recorded in the [W&B training run](https://wandb.ai/wandb/huggingtweets/runs/148w4fxc) for full transparency and reproducibility.
At the end of training, [the final model](https://wandb.ai/wandb/huggingtweets/runs/148w4fxc/artifacts) is logged and versioned.
## How to use
You can use this model directly with a pipeline for text generation:
```python
from transformers import pipeline
generator = pipeline('text-generation',
model='huggingtweets/43folders-hotdogsladies')
generator("My dream is", num_return_sequences=5)
```
## Limitations and bias
The model suffers from [the same limitations and bias as GPT-2](https://huggingface.co/gpt2#limitations-and-bias).
In addition, the data present in the user's tweets further affects the text generated by the model.
## About
*Built by Boris Dayma*
[](https://twitter.com/intent/follow?screen_name=borisdayma)
For more details, visit the project repository.
[](https://github.com/borisdayma/huggingtweets)
|
gary109/ai-light-dance_singing_wav2vec2-large-xlsr-53-5gram | 66cd384b15b59cd1f0a57c32a0be0ea7061df166 | 2022-06-16T01:42:47.000Z | [
"pytorch",
"wav2vec2",
"automatic-speech-recognition",
"transformers"
] | automatic-speech-recognition | false | gary109 | null | gary109/ai-light-dance_singing_wav2vec2-large-xlsr-53-5gram | 1 | null | transformers | 32,937 | Entry not found |
ryo0634/luke-base-embedding-predictor-20181220-concat | b4d8a51825d49a99b11a7c98152afb81bbdc2535 | 2022-06-16T02:27:11.000Z | [
"pytorch",
"luke",
"transformers"
] | null | false | ryo0634 | null | ryo0634/luke-base-embedding-predictor-20181220-concat | 1 | null | transformers | 32,938 | Train with the hyperlink dataset from en-wiki-20181220 of the entity vocabulary (0-400K).
The base model is studio-ousia/luke-base. |
huggingtweets/fushidahardy | ce430a0cced44dbb7ddb4fef42a8bc187999d7bb | 2022-06-16T03:42:42.000Z | [
"pytorch",
"gpt2",
"text-generation",
"en",
"transformers",
"huggingtweets"
] | text-generation | false | huggingtweets | null | huggingtweets/fushidahardy | 1 | null | transformers | 32,939 | ---
language: en
thumbnail: http://www.huggingtweets.com/fushidahardy/1655350909485/predictions.png
tags:
- huggingtweets
widget:
- text: "My dream is"
---
<div class="inline-flex flex-col" style="line-height: 1.5;">
<div class="flex">
<div
style="display:inherit; margin-left: 4px; margin-right: 4px; width: 92px; height:92px; border-radius: 50%; background-size: cover; background-image: url('https://pbs.twimg.com/profile_images/1271291765719351297/_NdPd0cg_400x400.jpg')">
</div>
<div
style="display:none; margin-left: 4px; margin-right: 4px; width: 92px; height:92px; border-radius: 50%; background-size: cover; background-image: url('')">
</div>
<div
style="display:none; margin-left: 4px; margin-right: 4px; width: 92px; height:92px; border-radius: 50%; background-size: cover; background-image: url('')">
</div>
</div>
<div style="text-align: center; margin-top: 3px; font-size: 16px; font-weight: 800">🤖 AI BOT 🤖</div>
<div style="text-align: center; font-size: 16px; font-weight: 800">Shintaro Fushida-Hardy 🦎</div>
<div style="text-align: center; font-size: 14px;">@fushidahardy</div>
</div>
I was made with [huggingtweets](https://github.com/borisdayma/huggingtweets).
Create your own bot based on your favorite user with [the demo](https://colab.research.google.com/github/borisdayma/huggingtweets/blob/master/huggingtweets-demo.ipynb)!
## How does it work?
The model uses the following pipeline.
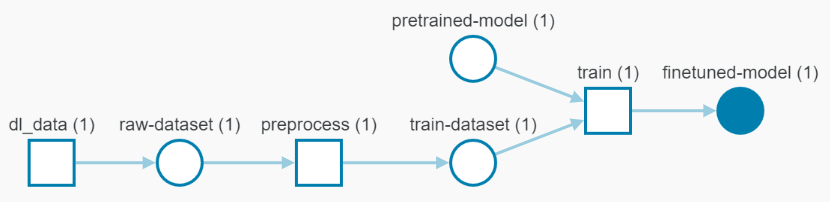
To understand how the model was developed, check the [W&B report](https://wandb.ai/wandb/huggingtweets/reports/HuggingTweets-Train-a-Model-to-Generate-Tweets--VmlldzoxMTY5MjI).
## Training data
The model was trained on tweets from Shintaro Fushida-Hardy 🦎.
| Data | Shintaro Fushida-Hardy 🦎 |
| --- | --- |
| Tweets downloaded | 1728 |
| Retweets | 253 |
| Short tweets | 115 |
| Tweets kept | 1360 |
[Explore the data](https://wandb.ai/wandb/huggingtweets/runs/3pk5r7pt/artifacts), which is tracked with [W&B artifacts](https://docs.wandb.com/artifacts) at every step of the pipeline.
## Training procedure
The model is based on a pre-trained [GPT-2](https://huggingface.co/gpt2) which is fine-tuned on @fushidahardy's tweets.
Hyperparameters and metrics are recorded in the [W&B training run](https://wandb.ai/wandb/huggingtweets/runs/1dxchh1a) for full transparency and reproducibility.
At the end of training, [the final model](https://wandb.ai/wandb/huggingtweets/runs/1dxchh1a/artifacts) is logged and versioned.
## How to use
You can use this model directly with a pipeline for text generation:
```python
from transformers import pipeline
generator = pipeline('text-generation',
model='huggingtweets/fushidahardy')
generator("My dream is", num_return_sequences=5)
```
## Limitations and bias
The model suffers from [the same limitations and bias as GPT-2](https://huggingface.co/gpt2#limitations-and-bias).
In addition, the data present in the user's tweets further affects the text generated by the model.
## About
*Built by Boris Dayma*
[](https://twitter.com/intent/follow?screen_name=borisdayma)
For more details, visit the project repository.
[](https://github.com/borisdayma/huggingtweets)
|
huggingtweets/shammytv | 656196abe8b40549103f95fbfd3f0d1e8638c6c1 | 2022-06-16T05:07:23.000Z | [
"pytorch",
"gpt2",
"text-generation",
"en",
"transformers",
"huggingtweets"
] | text-generation | false | huggingtweets | null | huggingtweets/shammytv | 1 | null | transformers | 32,940 | ---
language: en
thumbnail: http://www.huggingtweets.com/shammytv/1655356038315/predictions.png
tags:
- huggingtweets
widget:
- text: "My dream is"
---
<div class="inline-flex flex-col" style="line-height: 1.5;">
<div class="flex">
<div
style="display:inherit; margin-left: 4px; margin-right: 4px; width: 92px; height:92px; border-radius: 50%; background-size: cover; background-image: url('https://pbs.twimg.com/profile_images/1191610860973764608/vH0nHzO8_400x400.png')">
</div>
<div
style="display:none; margin-left: 4px; margin-right: 4px; width: 92px; height:92px; border-radius: 50%; background-size: cover; background-image: url('')">
</div>
<div
style="display:none; margin-left: 4px; margin-right: 4px; width: 92px; height:92px; border-radius: 50%; background-size: cover; background-image: url('')">
</div>
</div>
<div style="text-align: center; margin-top: 3px; font-size: 16px; font-weight: 800">🤖 AI BOT 🤖</div>
<div style="text-align: center; font-size: 16px; font-weight: 800">Swift</div>
<div style="text-align: center; font-size: 14px;">@shammytv</div>
</div>
I was made with [huggingtweets](https://github.com/borisdayma/huggingtweets).
Create your own bot based on your favorite user with [the demo](https://colab.research.google.com/github/borisdayma/huggingtweets/blob/master/huggingtweets-demo.ipynb)!
## How does it work?
The model uses the following pipeline.
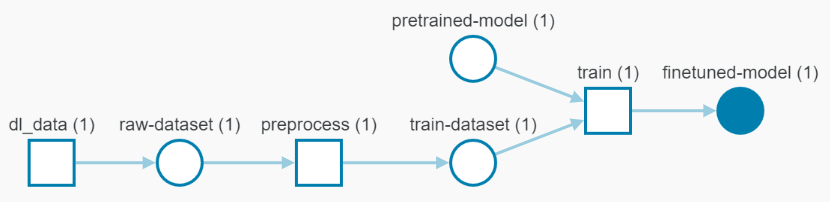
To understand how the model was developed, check the [W&B report](https://wandb.ai/wandb/huggingtweets/reports/HuggingTweets-Train-a-Model-to-Generate-Tweets--VmlldzoxMTY5MjI).
## Training data
The model was trained on tweets from Swift.
| Data | Swift |
| --- | --- |
| Tweets downloaded | 3203 |
| Retweets | 173 |
| Short tweets | 449 |
| Tweets kept | 2581 |
[Explore the data](https://wandb.ai/wandb/huggingtweets/runs/12udt9tp/artifacts), which is tracked with [W&B artifacts](https://docs.wandb.com/artifacts) at every step of the pipeline.
## Training procedure
The model is based on a pre-trained [GPT-2](https://huggingface.co/gpt2) which is fine-tuned on @shammytv's tweets.
Hyperparameters and metrics are recorded in the [W&B training run](https://wandb.ai/wandb/huggingtweets/runs/wp1epufz) for full transparency and reproducibility.
At the end of training, [the final model](https://wandb.ai/wandb/huggingtweets/runs/wp1epufz/artifacts) is logged and versioned.
## How to use
You can use this model directly with a pipeline for text generation:
```python
from transformers import pipeline
generator = pipeline('text-generation',
model='huggingtweets/shammytv')
generator("My dream is", num_return_sequences=5)
```
## Limitations and bias
The model suffers from [the same limitations and bias as GPT-2](https://huggingface.co/gpt2#limitations-and-bias).
In addition, the data present in the user's tweets further affects the text generated by the model.
## About
*Built by Boris Dayma*
[](https://twitter.com/intent/follow?screen_name=borisdayma)
For more details, visit the project repository.
[](https://github.com/borisdayma/huggingtweets)
|
Nadav/xlm-roberta-base-finetuned-on-runaways-fr | f2eb0aa7f1ba438246bae540360c7eb11f3a8da1 | 2022-06-19T15:12:08.000Z | [
"pytorch",
"xlm-roberta",
"fill-mask",
"transformers",
"autotrain_compatible"
] | fill-mask | false | Nadav | null | Nadav/xlm-roberta-base-finetuned-on-runaways-fr | 1 | null | transformers | 32,941 | Entry not found |
Nadav/xlm-roberta-base-squad-finetuned-on-runaways-fr | 16d720034839504d55f0987644a5027143039b96 | 2022-06-19T16:42:57.000Z | [
"pytorch",
"xlm-roberta",
"question-answering",
"transformers",
"autotrain_compatible"
] | question-answering | false | Nadav | null | Nadav/xlm-roberta-base-squad-finetuned-on-runaways-fr | 1 | null | transformers | 32,942 | Entry not found |
Addedk/kbbert-distilled-cased | 7e6660109b82dfb90f7a624a5135d22468e86402 | 2022-07-18T14:14:12.000Z | [
"pytorch",
"tf",
"bert",
"fill-mask",
"sv",
"arxiv:2103.06418",
"transformers",
"license:apache-2.0",
"autotrain_compatible"
] | fill-mask | false | Addedk | null | Addedk/kbbert-distilled-cased | 1 | 1 | transformers | 32,943 | ---
language: sv
license: apache-2.0
---
# KB-BERT distilled base model (cased)
This model is a distilled version of [KB-BERT](https://huggingface.co/KB/bert-base-swedish-cased). It was distilled using Swedish data, the 2010-2015 portion of the [Swedish Culturomics Gigaword Corpus](https://spraakbanken.gu.se/en/resources/gigaword). The code for the distillation process can be found [here](https://github.com/AddedK/swedish-mbert-distillation/blob/main/azureML/pretrain_distillation.py). This was done as part of my Master's Thesis: *Task-agnostic knowledge distillation of mBERT to Swedish*.
## Model description
This is a 6-layer version of KB-BERT, having been distilled using the [LightMBERT](https://arxiv.org/abs/2103.06418) distillation method, but without freezing the embedding layer.
## Intended uses & limitations
You can use the raw model for either masked language modeling or next sentence prediction, but it's mostly intended to
be fine-tuned on a downstream task.
## Training data
The data used for distillation was the 2010-2015 portion of the [Swedish Culturomics Gigaword Corpus](https://spraakbanken.gu.se/en/resources/gigaword).
The tokenized data had a file size of approximately 7.4 GB.
## Evaluation results
When evaluated on the [SUCX 3.0 ](https://huggingface.co/datasets/KBLab/sucx3_ner) dataset, it achieved an average F1 score of 0.887 which is competitive with the score KB-BERT obtained, 0.894.
Additional results and comparisons are presented in my Master's Thesis
|
good-ai-club/NBB | 459cfe319968e35cdd5bf6c7c7932d8cc2e94966 | 2022-06-16T08:51:44.000Z | [
"pytorch",
"bert",
"feature-extraction",
"sentence-transformers",
"sentence-similarity",
"transformers"
] | sentence-similarity | false | good-ai-club | null | good-ai-club/NBB | 1 | null | sentence-transformers | 32,944 | ---
pipeline_tag: sentence-similarity
tags:
- sentence-transformers
- feature-extraction
- sentence-similarity
- transformers
---
# {MODEL_NAME}
This is a [sentence-transformers](https://www.SBERT.net) model: It maps sentences & paragraphs to a 768 dimensional dense vector space and can be used for tasks like clustering or semantic search.
<!--- Describe your model here -->
## Usage (Sentence-Transformers)
Using this model becomes easy when you have [sentence-transformers](https://www.SBERT.net) installed:
```
pip install -U sentence-transformers
```
Then you can use the model like this:
```python
from sentence_transformers import SentenceTransformer
sentences = ["This is an example sentence", "Each sentence is converted"]
model = SentenceTransformer('{MODEL_NAME}')
embeddings = model.encode(sentences)
print(embeddings)
```
## Usage (HuggingFace Transformers)
Without [sentence-transformers](https://www.SBERT.net), you can use the model like this: First, you pass your input through the transformer model, then you have to apply the right pooling-operation on-top of the contextualized word embeddings.
```python
from transformers import AutoTokenizer, AutoModel
import torch
#Mean Pooling - Take attention mask into account for correct averaging
def mean_pooling(model_output, attention_mask):
token_embeddings = model_output[0] #First element of model_output contains all token embeddings
input_mask_expanded = attention_mask.unsqueeze(-1).expand(token_embeddings.size()).float()
return torch.sum(token_embeddings * input_mask_expanded, 1) / torch.clamp(input_mask_expanded.sum(1), min=1e-9)
# Sentences we want sentence embeddings for
sentences = ['This is an example sentence', 'Each sentence is converted']
# Load model from HuggingFace Hub
tokenizer = AutoTokenizer.from_pretrained('{MODEL_NAME}')
model = AutoModel.from_pretrained('{MODEL_NAME}')
# Tokenize sentences
encoded_input = tokenizer(sentences, padding=True, truncation=True, return_tensors='pt')
# Compute token embeddings
with torch.no_grad():
model_output = model(**encoded_input)
# Perform pooling. In this case, mean pooling.
sentence_embeddings = mean_pooling(model_output, encoded_input['attention_mask'])
print("Sentence embeddings:")
print(sentence_embeddings)
```
## Evaluation Results
<!--- Describe how your model was evaluated -->
For an automated evaluation of this model, see the *Sentence Embeddings Benchmark*: [https://seb.sbert.net](https://seb.sbert.net?model_name={MODEL_NAME})
## Training
The model was trained with the parameters:
**DataLoader**:
`torch.utils.data.dataloader.DataLoader` of length 3188 with parameters:
```
{'batch_size': 16, 'sampler': 'torch.utils.data.sampler.RandomSampler', 'batch_sampler': 'torch.utils.data.sampler.BatchSampler'}
```
**Loss**:
`sentence_transformers.losses.CosineSimilarityLoss.CosineSimilarityLoss`
Parameters of the fit()-Method:
```
{
"epochs": 5,
"evaluation_steps": 355,
"evaluator": "sentence_transformers.evaluation.EmbeddingSimilarityEvaluator.EmbeddingSimilarityEvaluator",
"max_grad_norm": 1,
"optimizer_class": "<class 'transformers.optimization.AdamW'>",
"optimizer_params": {
"lr": 2e-05
},
"scheduler": "WarmupLinear",
"steps_per_epoch": null,
"warmup_steps": 1594,
"weight_decay": 0.01
}
```
## Full Model Architecture
```
SentenceTransformer(
(0): Transformer({'max_seq_length': 512, 'do_lower_case': False}) with Transformer model: BertModel
(1): Pooling({'word_embedding_dimension': 768, 'pooling_mode_cls_token': False, 'pooling_mode_mean_tokens': True, 'pooling_mode_max_tokens': False, 'pooling_mode_mean_sqrt_len_tokens': False})
)
```
## Citing & Authors
<!--- Describe where people can find more information --> |
roymukund/xlm-roberta-base-finetuned-ner | 73a88bb38a01c9bc95fa9ce80d5a6d194ec91555 | 2022-06-16T20:32:08.000Z | [
"pytorch",
"xlm-roberta",
"token-classification",
"dataset:hi_ner-original",
"transformers",
"generated_from_trainer",
"license:mit",
"model-index",
"autotrain_compatible"
] | token-classification | false | roymukund | null | roymukund/xlm-roberta-base-finetuned-ner | 1 | null | transformers | 32,945 | ---
license: mit
tags:
- generated_from_trainer
datasets:
- hi_ner-original
metrics:
- precision
- recall
- f1
- accuracy
model-index:
- name: xlm-roberta-base-finetuned-ner
results:
- task:
name: Token Classification
type: token-classification
dataset:
name: hi_ner-original
type: hi_ner-original
args: HiNER
metrics:
- name: Precision
type: precision
value: 0.7366076627460114
- name: Recall
type: recall
value: 0.6770947627585838
- name: F1
type: f1
value: 0.7055985498152408
- name: Accuracy
type: accuracy
value: 0.9359390321752693
---
<!-- This model card has been generated automatically according to the information the Trainer had access to. You
should probably proofread and complete it, then remove this comment. -->
# xlm-roberta-base-finetuned-ner
This model is a fine-tuned version of [xlm-roberta-base](https://huggingface.co/xlm-roberta-base) on the hi_ner-original dataset.
It achieves the following results on the evaluation set:
- Loss: 0.2314
- Precision: 0.7366
- Recall: 0.6771
- F1: 0.7056
- Accuracy: 0.9359
## Model description
More information needed
## Intended uses & limitations
More information needed
## Training and evaluation data
More information needed
## Training procedure
### Training hyperparameters
The following hyperparameters were used during training:
- learning_rate: 2e-05
- train_batch_size: 8
- eval_batch_size: 4
- seed: 42
- optimizer: Adam with betas=(0.9,0.999) and epsilon=1e-08
- lr_scheduler_type: linear
- num_epochs: 6
### Training results
| Training Loss | Epoch | Step | Validation Loss | Precision | Recall | F1 | Accuracy |
|:-------------:|:-----:|:-----:|:---------------:|:---------:|:------:|:------:|:--------:|
| 0.2025 | 0.74 | 7000 | 0.2146 | 0.7399 | 0.6197 | 0.6745 | 0.9316 |
| 0.1641 | 1.47 | 14000 | 0.2238 | 0.7618 | 0.6108 | 0.6780 | 0.9336 |
| 0.1404 | 2.21 | 21000 | 0.2302 | 0.7560 | 0.6327 | 0.6889 | 0.9350 |
| 0.1371 | 2.95 | 28000 | 0.2226 | 0.7395 | 0.6600 | 0.6975 | 0.9350 |
| 0.1248 | 3.68 | 35000 | 0.2314 | 0.7366 | 0.6771 | 0.7056 | 0.9359 |
| 0.1112 | 4.42 | 42000 | 0.2423 | 0.7089 | 0.7064 | 0.7077 | 0.9333 |
| 0.1048 | 5.16 | 49000 | 0.2599 | 0.7326 | 0.6793 | 0.7050 | 0.9349 |
| 0.1091 | 5.89 | 56000 | 0.2542 | 0.7244 | 0.6918 | 0.7077 | 0.9348 |
### Framework versions
- Transformers 4.19.4
- Pytorch 1.11.0+cu102
- Datasets 2.3.2
- Tokenizers 0.12.1
|
janeel/tinyroberta-squad2-finetuned-squad | bbbb15cc9754966f1f3454c1143812a4097bca5b | 2022-06-19T08:51:09.000Z | [
"pytorch",
"tensorboard",
"roberta",
"question-answering",
"dataset:squad_v2",
"transformers",
"generated_from_trainer",
"license:cc-by-4.0",
"model-index",
"autotrain_compatible"
] | question-answering | false | janeel | null | janeel/tinyroberta-squad2-finetuned-squad | 1 | null | transformers | 32,946 | ---
license: cc-by-4.0
tags:
- generated_from_trainer
datasets:
- squad_v2
model-index:
- name: tinyroberta-squad2-finetuned-squad
results: []
---
<!-- This model card has been generated automatically according to the information the Trainer had access to. You
should probably proofread and complete it, then remove this comment. -->
# tinyroberta-squad2-finetuned-squad
This model is a fine-tuned version of [deepset/tinyroberta-squad2](https://huggingface.co/deepset/tinyroberta-squad2) on the squad_v2 dataset.
It achieves the following results on the evaluation set:
- Loss: 1.1592
## Model description
More information needed
## Intended uses & limitations
More information needed
## Training and evaluation data
More information needed
## Training procedure
### Training hyperparameters
The following hyperparameters were used during training:
- learning_rate: 2e-05
- train_batch_size: 16
- eval_batch_size: 16
- seed: 42
- optimizer: Adam with betas=(0.9,0.999) and epsilon=1e-08
- lr_scheduler_type: linear
- num_epochs: 2
### Training results
| Training Loss | Epoch | Step | Validation Loss |
|:-------------:|:-----:|:-----:|:---------------:|
| 0.6185 | 1.0 | 8239 | 0.9460 |
| 0.4243 | 2.0 | 16478 | 1.1592 |
### Framework versions
- Transformers 4.20.0
- Pytorch 1.11.0+cu113
- Datasets 2.3.2
- Tokenizers 0.12.1
|
income/bpr-gpl-webis-touche2020-base-msmarco-distilbert-tas-b | 76ee22e524c9d49a9e34498b9b9cfd3f289c41b4 | 2022-06-16T17:58:08.000Z | [
"pytorch",
"distilbert",
"feature-extraction",
"sentence-transformers",
"sentence-similarity",
"transformers"
] | sentence-similarity | false | income | null | income/bpr-gpl-webis-touche2020-base-msmarco-distilbert-tas-b | 1 | null | sentence-transformers | 32,947 | ---
pipeline_tag: sentence-similarity
tags:
- sentence-transformers
- feature-extraction
- sentence-similarity
- transformers
---
# {MODEL_NAME}
This is a [sentence-transformers](https://www.SBERT.net) model: It maps sentences & paragraphs to a 768 dimensional dense vector space and can be used for tasks like clustering or semantic search.
<!--- Describe your model here -->
## Usage (Sentence-Transformers)
Using this model becomes easy when you have [sentence-transformers](https://www.SBERT.net) installed:
```
pip install -U sentence-transformers
```
Then you can use the model like this:
```python
from sentence_transformers import SentenceTransformer
sentences = ["This is an example sentence", "Each sentence is converted"]
model = SentenceTransformer('{MODEL_NAME}')
embeddings = model.encode(sentences)
print(embeddings)
```
## Usage (HuggingFace Transformers)
Without [sentence-transformers](https://www.SBERT.net), you can use the model like this: First, you pass your input through the transformer model, then you have to apply the right pooling-operation on-top of the contextualized word embeddings.
```python
from transformers import AutoTokenizer, AutoModel
import torch
#Mean Pooling - Take attention mask into account for correct averaging
def mean_pooling(model_output, attention_mask):
token_embeddings = model_output[0] #First element of model_output contains all token embeddings
input_mask_expanded = attention_mask.unsqueeze(-1).expand(token_embeddings.size()).float()
return torch.sum(token_embeddings * input_mask_expanded, 1) / torch.clamp(input_mask_expanded.sum(1), min=1e-9)
# Sentences we want sentence embeddings for
sentences = ['This is an example sentence', 'Each sentence is converted']
# Load model from HuggingFace Hub
tokenizer = AutoTokenizer.from_pretrained('{MODEL_NAME}')
model = AutoModel.from_pretrained('{MODEL_NAME}')
# Tokenize sentences
encoded_input = tokenizer(sentences, padding=True, truncation=True, return_tensors='pt')
# Compute token embeddings
with torch.no_grad():
model_output = model(**encoded_input)
# Perform pooling. In this case, max pooling.
sentence_embeddings = mean_pooling(model_output, encoded_input['attention_mask'])
print("Sentence embeddings:")
print(sentence_embeddings)
```
## Evaluation Results
<!--- Describe how your model was evaluated -->
For an automated evaluation of this model, see the *Sentence Embeddings Benchmark*: [https://seb.sbert.net](https://seb.sbert.net?model_name={MODEL_NAME})
## Training
The model was trained with the parameters:
**DataLoader**:
`torch.utils.data.dataloader.DataLoader` of length 34866 with parameters:
```
{'batch_size': 32, 'sampler': 'torch.utils.data.sampler.RandomSampler', 'batch_sampler': 'torch.utils.data.sampler.BatchSampler'}
```
**Loss**:
`gpl.toolkit.loss.MarginDistillationLoss`
Parameters of the fit()-Method:
```
{
"callback": null,
"epochs": 5,
"evaluation_steps": 0,
"evaluator": "NoneType",
"max_grad_norm": 1,
"optimizer_class": "<class 'transformers.optimization.AdamW'>",
"optimizer_params": {
"lr": 2e-05
},
"scheduler": "WarmupLinear",
"steps_per_epoch": null,
"warmup_steps": 1000,
"weight_decay": 0.01
}
```
## Full Model Architecture
```
SentenceTransformer(
(0): Transformer({'max_seq_length': 350, 'do_lower_case': False}) with Transformer model: DistilBertModel
(1): Pooling({'word_embedding_dimension': 768, 'pooling_mode_cls_token': False, 'pooling_mode_mean_tokens': True, 'pooling_mode_max_tokens': False, 'pooling_mode_mean_sqrt_len_tokens': False})
)
```
## Citing & Authors
<!--- Describe where people can find more information --> |
income/bpr-gpl-trec-news-base-msmarco-distilbert-tas-b | 4fb42b68b1fef5018ea8b9e7ee663214c5781570 | 2022-06-16T17:59:18.000Z | [
"pytorch",
"distilbert",
"feature-extraction",
"sentence-transformers",
"sentence-similarity",
"transformers"
] | sentence-similarity | false | income | null | income/bpr-gpl-trec-news-base-msmarco-distilbert-tas-b | 1 | null | sentence-transformers | 32,948 | ---
pipeline_tag: sentence-similarity
tags:
- sentence-transformers
- feature-extraction
- sentence-similarity
- transformers
---
# {MODEL_NAME}
This is a [sentence-transformers](https://www.SBERT.net) model: It maps sentences & paragraphs to a 768 dimensional dense vector space and can be used for tasks like clustering or semantic search.
<!--- Describe your model here -->
## Usage (Sentence-Transformers)
Using this model becomes easy when you have [sentence-transformers](https://www.SBERT.net) installed:
```
pip install -U sentence-transformers
```
Then you can use the model like this:
```python
from sentence_transformers import SentenceTransformer
sentences = ["This is an example sentence", "Each sentence is converted"]
model = SentenceTransformer('{MODEL_NAME}')
embeddings = model.encode(sentences)
print(embeddings)
```
## Usage (HuggingFace Transformers)
Without [sentence-transformers](https://www.SBERT.net), you can use the model like this: First, you pass your input through the transformer model, then you have to apply the right pooling-operation on-top of the contextualized word embeddings.
```python
from transformers import AutoTokenizer, AutoModel
import torch
#Mean Pooling - Take attention mask into account for correct averaging
def mean_pooling(model_output, attention_mask):
token_embeddings = model_output[0] #First element of model_output contains all token embeddings
input_mask_expanded = attention_mask.unsqueeze(-1).expand(token_embeddings.size()).float()
return torch.sum(token_embeddings * input_mask_expanded, 1) / torch.clamp(input_mask_expanded.sum(1), min=1e-9)
# Sentences we want sentence embeddings for
sentences = ['This is an example sentence', 'Each sentence is converted']
# Load model from HuggingFace Hub
tokenizer = AutoTokenizer.from_pretrained('{MODEL_NAME}')
model = AutoModel.from_pretrained('{MODEL_NAME}')
# Tokenize sentences
encoded_input = tokenizer(sentences, padding=True, truncation=True, return_tensors='pt')
# Compute token embeddings
with torch.no_grad():
model_output = model(**encoded_input)
# Perform pooling. In this case, max pooling.
sentence_embeddings = mean_pooling(model_output, encoded_input['attention_mask'])
print("Sentence embeddings:")
print(sentence_embeddings)
```
## Evaluation Results
<!--- Describe how your model was evaluated -->
For an automated evaluation of this model, see the *Sentence Embeddings Benchmark*: [https://seb.sbert.net](https://seb.sbert.net?model_name={MODEL_NAME})
## Training
The model was trained with the parameters:
**DataLoader**:
`torch.utils.data.dataloader.DataLoader` of length 55028 with parameters:
```
{'batch_size': 32, 'sampler': 'torch.utils.data.sampler.RandomSampler', 'batch_sampler': 'torch.utils.data.sampler.BatchSampler'}
```
**Loss**:
`gpl.toolkit.loss.MarginDistillationLoss`
Parameters of the fit()-Method:
```
{
"callback": null,
"epochs": 2,
"evaluation_steps": 0,
"evaluator": "NoneType",
"max_grad_norm": 1,
"optimizer_class": "<class 'transformers.optimization.AdamW'>",
"optimizer_params": {
"lr": 2e-05
},
"scheduler": "WarmupLinear",
"steps_per_epoch": null,
"warmup_steps": 1000,
"weight_decay": 0.01
}
```
## Full Model Architecture
```
SentenceTransformer(
(0): Transformer({'max_seq_length': 350, 'do_lower_case': False}) with Transformer model: DistilBertModel
(1): Pooling({'word_embedding_dimension': 768, 'pooling_mode_cls_token': False, 'pooling_mode_mean_tokens': True, 'pooling_mode_max_tokens': False, 'pooling_mode_mean_sqrt_len_tokens': False})
)
```
## Citing & Authors
<!--- Describe where people can find more information --> |
income/bpr-gpl-trec-covid-base-msmarco-distilbert-tas-b | 27ce375acf65d524100ddea534d136e2ee7361fc | 2022-06-16T18:00:33.000Z | [
"pytorch",
"distilbert",
"feature-extraction",
"sentence-transformers",
"sentence-similarity",
"transformers"
] | sentence-similarity | false | income | null | income/bpr-gpl-trec-covid-base-msmarco-distilbert-tas-b | 1 | null | sentence-transformers | 32,949 | ---
pipeline_tag: sentence-similarity
tags:
- sentence-transformers
- feature-extraction
- sentence-similarity
- transformers
---
# {MODEL_NAME}
This is a [sentence-transformers](https://www.SBERT.net) model: It maps sentences & paragraphs to a 768 dimensional dense vector space and can be used for tasks like clustering or semantic search.
<!--- Describe your model here -->
## Usage (Sentence-Transformers)
Using this model becomes easy when you have [sentence-transformers](https://www.SBERT.net) installed:
```
pip install -U sentence-transformers
```
Then you can use the model like this:
```python
from sentence_transformers import SentenceTransformer
sentences = ["This is an example sentence", "Each sentence is converted"]
model = SentenceTransformer('{MODEL_NAME}')
embeddings = model.encode(sentences)
print(embeddings)
```
## Usage (HuggingFace Transformers)
Without [sentence-transformers](https://www.SBERT.net), you can use the model like this: First, you pass your input through the transformer model, then you have to apply the right pooling-operation on-top of the contextualized word embeddings.
```python
from transformers import AutoTokenizer, AutoModel
import torch
#Mean Pooling - Take attention mask into account for correct averaging
def mean_pooling(model_output, attention_mask):
token_embeddings = model_output[0] #First element of model_output contains all token embeddings
input_mask_expanded = attention_mask.unsqueeze(-1).expand(token_embeddings.size()).float()
return torch.sum(token_embeddings * input_mask_expanded, 1) / torch.clamp(input_mask_expanded.sum(1), min=1e-9)
# Sentences we want sentence embeddings for
sentences = ['This is an example sentence', 'Each sentence is converted']
# Load model from HuggingFace Hub
tokenizer = AutoTokenizer.from_pretrained('{MODEL_NAME}')
model = AutoModel.from_pretrained('{MODEL_NAME}')
# Tokenize sentences
encoded_input = tokenizer(sentences, padding=True, truncation=True, return_tensors='pt')
# Compute token embeddings
with torch.no_grad():
model_output = model(**encoded_input)
# Perform pooling. In this case, max pooling.
sentence_embeddings = mean_pooling(model_output, encoded_input['attention_mask'])
print("Sentence embeddings:")
print(sentence_embeddings)
```
## Evaluation Results
<!--- Describe how your model was evaluated -->
For an automated evaluation of this model, see the *Sentence Embeddings Benchmark*: [https://seb.sbert.net](https://seb.sbert.net?model_name={MODEL_NAME})
## Training
The model was trained with the parameters:
**DataLoader**:
`torch.utils.data.dataloader.DataLoader` of length 15001 with parameters:
```
{'batch_size': 32, 'sampler': 'torch.utils.data.sampler.RandomSampler', 'batch_sampler': 'torch.utils.data.sampler.BatchSampler'}
```
**Loss**:
`gpl.toolkit.loss.MarginDistillationLoss`
Parameters of the fit()-Method:
```
{
"callback": null,
"epochs": 10,
"evaluation_steps": 0,
"evaluator": "NoneType",
"max_grad_norm": 1,
"optimizer_class": "<class 'transformers.optimization.AdamW'>",
"optimizer_params": {
"lr": 2e-05
},
"scheduler": "WarmupLinear",
"steps_per_epoch": null,
"warmup_steps": 1000,
"weight_decay": 0.01
}
```
## Full Model Architecture
```
SentenceTransformer(
(0): Transformer({'max_seq_length': 350, 'do_lower_case': False}) with Transformer model: DistilBertModel
(1): Pooling({'word_embedding_dimension': 768, 'pooling_mode_cls_token': False, 'pooling_mode_mean_tokens': True, 'pooling_mode_max_tokens': False, 'pooling_mode_mean_sqrt_len_tokens': False})
)
```
## Citing & Authors
<!--- Describe where people can find more information --> |
income/bpr-gpl-signal1m-base-msmarco-distilbert-tas-b | 2d66071e8b6799f4a9c4cb5017e0fb861d0c3c76 | 2022-06-16T18:02:31.000Z | [
"pytorch",
"distilbert",
"feature-extraction",
"sentence-transformers",
"sentence-similarity",
"transformers"
] | sentence-similarity | false | income | null | income/bpr-gpl-signal1m-base-msmarco-distilbert-tas-b | 1 | null | sentence-transformers | 32,950 | ---
pipeline_tag: sentence-similarity
tags:
- sentence-transformers
- feature-extraction
- sentence-similarity
- transformers
---
# {MODEL_NAME}
This is a [sentence-transformers](https://www.SBERT.net) model: It maps sentences & paragraphs to a 768 dimensional dense vector space and can be used for tasks like clustering or semantic search.
<!--- Describe your model here -->
## Usage (Sentence-Transformers)
Using this model becomes easy when you have [sentence-transformers](https://www.SBERT.net) installed:
```
pip install -U sentence-transformers
```
Then you can use the model like this:
```python
from sentence_transformers import SentenceTransformer
sentences = ["This is an example sentence", "Each sentence is converted"]
model = SentenceTransformer('{MODEL_NAME}')
embeddings = model.encode(sentences)
print(embeddings)
```
## Usage (HuggingFace Transformers)
Without [sentence-transformers](https://www.SBERT.net), you can use the model like this: First, you pass your input through the transformer model, then you have to apply the right pooling-operation on-top of the contextualized word embeddings.
```python
from transformers import AutoTokenizer, AutoModel
import torch
#Mean Pooling - Take attention mask into account for correct averaging
def mean_pooling(model_output, attention_mask):
token_embeddings = model_output[0] #First element of model_output contains all token embeddings
input_mask_expanded = attention_mask.unsqueeze(-1).expand(token_embeddings.size()).float()
return torch.sum(token_embeddings * input_mask_expanded, 1) / torch.clamp(input_mask_expanded.sum(1), min=1e-9)
# Sentences we want sentence embeddings for
sentences = ['This is an example sentence', 'Each sentence is converted']
# Load model from HuggingFace Hub
tokenizer = AutoTokenizer.from_pretrained('{MODEL_NAME}')
model = AutoModel.from_pretrained('{MODEL_NAME}')
# Tokenize sentences
encoded_input = tokenizer(sentences, padding=True, truncation=True, return_tensors='pt')
# Compute token embeddings
with torch.no_grad():
model_output = model(**encoded_input)
# Perform pooling. In this case, max pooling.
sentence_embeddings = mean_pooling(model_output, encoded_input['attention_mask'])
print("Sentence embeddings:")
print(sentence_embeddings)
```
## Evaluation Results
<!--- Describe how your model was evaluated -->
For an automated evaluation of this model, see the *Sentence Embeddings Benchmark*: [https://seb.sbert.net](https://seb.sbert.net?model_name={MODEL_NAME})
## Training
The model was trained with the parameters:
**DataLoader**:
`torch.utils.data.dataloader.DataLoader` of length 263015 with parameters:
```
{'batch_size': 32, 'sampler': 'torch.utils.data.sampler.RandomSampler', 'batch_sampler': 'torch.utils.data.sampler.BatchSampler'}
```
**Loss**:
`gpl.toolkit.loss.MarginDistillationLoss`
Parameters of the fit()-Method:
```
{
"callback": null,
"epochs": 1,
"evaluation_steps": 0,
"evaluator": "NoneType",
"max_grad_norm": 1,
"optimizer_class": "<class 'transformers.optimization.AdamW'>",
"optimizer_params": {
"lr": 2e-05
},
"scheduler": "WarmupLinear",
"steps_per_epoch": null,
"warmup_steps": 1000,
"weight_decay": 0.01
}
```
## Full Model Architecture
```
SentenceTransformer(
(0): Transformer({'max_seq_length': 350, 'do_lower_case': False}) with Transformer model: DistilBertModel
(1): Pooling({'word_embedding_dimension': 768, 'pooling_mode_cls_token': False, 'pooling_mode_mean_tokens': True, 'pooling_mode_max_tokens': False, 'pooling_mode_mean_sqrt_len_tokens': False})
)
```
## Citing & Authors
<!--- Describe where people can find more information --> |
income/bpr-gpl-scidocs-base-msmarco-distilbert-tas-b | 6e47641a22bb4932844c5e2991b9fff4d1eef86c | 2022-06-16T18:07:08.000Z | [
"pytorch",
"distilbert",
"feature-extraction",
"sentence-transformers",
"sentence-similarity",
"transformers"
] | sentence-similarity | false | income | null | income/bpr-gpl-scidocs-base-msmarco-distilbert-tas-b | 1 | null | sentence-transformers | 32,951 | ---
pipeline_tag: sentence-similarity
tags:
- sentence-transformers
- feature-extraction
- sentence-similarity
- transformers
---
# {MODEL_NAME}
This is a [sentence-transformers](https://www.SBERT.net) model: It maps sentences & paragraphs to a 768 dimensional dense vector space and can be used for tasks like clustering or semantic search.
<!--- Describe your model here -->
## Usage (Sentence-Transformers)
Using this model becomes easy when you have [sentence-transformers](https://www.SBERT.net) installed:
```
pip install -U sentence-transformers
```
Then you can use the model like this:
```python
from sentence_transformers import SentenceTransformer
sentences = ["This is an example sentence", "Each sentence is converted"]
model = SentenceTransformer('{MODEL_NAME}')
embeddings = model.encode(sentences)
print(embeddings)
```
## Usage (HuggingFace Transformers)
Without [sentence-transformers](https://www.SBERT.net), you can use the model like this: First, you pass your input through the transformer model, then you have to apply the right pooling-operation on-top of the contextualized word embeddings.
```python
from transformers import AutoTokenizer, AutoModel
import torch
#Mean Pooling - Take attention mask into account for correct averaging
def mean_pooling(model_output, attention_mask):
token_embeddings = model_output[0] #First element of model_output contains all token embeddings
input_mask_expanded = attention_mask.unsqueeze(-1).expand(token_embeddings.size()).float()
return torch.sum(token_embeddings * input_mask_expanded, 1) / torch.clamp(input_mask_expanded.sum(1), min=1e-9)
# Sentences we want sentence embeddings for
sentences = ['This is an example sentence', 'Each sentence is converted']
# Load model from HuggingFace Hub
tokenizer = AutoTokenizer.from_pretrained('{MODEL_NAME}')
model = AutoModel.from_pretrained('{MODEL_NAME}')
# Tokenize sentences
encoded_input = tokenizer(sentences, padding=True, truncation=True, return_tensors='pt')
# Compute token embeddings
with torch.no_grad():
model_output = model(**encoded_input)
# Perform pooling. In this case, max pooling.
sentence_embeddings = mean_pooling(model_output, encoded_input['attention_mask'])
print("Sentence embeddings:")
print(sentence_embeddings)
```
## Evaluation Results
<!--- Describe how your model was evaluated -->
For an automated evaluation of this model, see the *Sentence Embeddings Benchmark*: [https://seb.sbert.net](https://seb.sbert.net?model_name={MODEL_NAME})
## Training
The model was trained with the parameters:
**DataLoader**:
`torch.utils.data.dataloader.DataLoader` of length 2337 with parameters:
```
{'batch_size': 32, 'sampler': 'torch.utils.data.sampler.RandomSampler', 'batch_sampler': 'torch.utils.data.sampler.BatchSampler'}
```
**Loss**:
`gpl.toolkit.loss.MarginDistillationLoss`
Parameters of the fit()-Method:
```
{
"callback": null,
"epochs": 10,
"evaluation_steps": 0,
"evaluator": "NoneType",
"max_grad_norm": 1,
"optimizer_class": "<class 'transformers.optimization.AdamW'>",
"optimizer_params": {
"lr": 2e-05
},
"scheduler": "WarmupLinear",
"steps_per_epoch": null,
"warmup_steps": 1000,
"weight_decay": 0.01
}
```
## Full Model Architecture
```
SentenceTransformer(
(0): Transformer({'max_seq_length': 350, 'do_lower_case': False}) with Transformer model: DistilBertModel
(1): Pooling({'word_embedding_dimension': 768, 'pooling_mode_cls_token': False, 'pooling_mode_mean_tokens': True, 'pooling_mode_max_tokens': False, 'pooling_mode_mean_sqrt_len_tokens': False})
)
```
## Citing & Authors
<!--- Describe where people can find more information --> |
income/bpr-gpl-robust04-base-msmarco-distilbert-tas-b | 341493d0a26d1c186f0e4e6cb0526ebe0a467b5d | 2022-06-16T18:10:03.000Z | [
"pytorch",
"distilbert",
"feature-extraction",
"sentence-transformers",
"sentence-similarity",
"transformers"
] | sentence-similarity | false | income | null | income/bpr-gpl-robust04-base-msmarco-distilbert-tas-b | 1 | null | sentence-transformers | 32,952 | ---
pipeline_tag: sentence-similarity
tags:
- sentence-transformers
- feature-extraction
- sentence-similarity
- transformers
---
# {MODEL_NAME}
This is a [sentence-transformers](https://www.SBERT.net) model: It maps sentences & paragraphs to a 768 dimensional dense vector space and can be used for tasks like clustering or semantic search.
<!--- Describe your model here -->
## Usage (Sentence-Transformers)
Using this model becomes easy when you have [sentence-transformers](https://www.SBERT.net) installed:
```
pip install -U sentence-transformers
```
Then you can use the model like this:
```python
from sentence_transformers import SentenceTransformer
sentences = ["This is an example sentence", "Each sentence is converted"]
model = SentenceTransformer('{MODEL_NAME}')
embeddings = model.encode(sentences)
print(embeddings)
```
## Usage (HuggingFace Transformers)
Without [sentence-transformers](https://www.SBERT.net), you can use the model like this: First, you pass your input through the transformer model, then you have to apply the right pooling-operation on-top of the contextualized word embeddings.
```python
from transformers import AutoTokenizer, AutoModel
import torch
#Mean Pooling - Take attention mask into account for correct averaging
def mean_pooling(model_output, attention_mask):
token_embeddings = model_output[0] #First element of model_output contains all token embeddings
input_mask_expanded = attention_mask.unsqueeze(-1).expand(token_embeddings.size()).float()
return torch.sum(token_embeddings * input_mask_expanded, 1) / torch.clamp(input_mask_expanded.sum(1), min=1e-9)
# Sentences we want sentence embeddings for
sentences = ['This is an example sentence', 'Each sentence is converted']
# Load model from HuggingFace Hub
tokenizer = AutoTokenizer.from_pretrained('{MODEL_NAME}')
model = AutoModel.from_pretrained('{MODEL_NAME}')
# Tokenize sentences
encoded_input = tokenizer(sentences, padding=True, truncation=True, return_tensors='pt')
# Compute token embeddings
with torch.no_grad():
model_output = model(**encoded_input)
# Perform pooling. In this case, max pooling.
sentence_embeddings = mean_pooling(model_output, encoded_input['attention_mask'])
print("Sentence embeddings:")
print(sentence_embeddings)
```
## Evaluation Results
<!--- Describe how your model was evaluated -->
For an automated evaluation of this model, see the *Sentence Embeddings Benchmark*: [https://seb.sbert.net](https://seb.sbert.net?model_name={MODEL_NAME})
## Training
The model was trained with the parameters:
**DataLoader**:
`torch.utils.data.dataloader.DataLoader` of length 48784 with parameters:
```
{'batch_size': 32, 'sampler': 'torch.utils.data.sampler.RandomSampler', 'batch_sampler': 'torch.utils.data.sampler.BatchSampler'}
```
**Loss**:
`gpl.toolkit.loss.MarginDistillationLoss`
Parameters of the fit()-Method:
```
{
"callback": null,
"epochs": 4,
"evaluation_steps": 0,
"evaluator": "NoneType",
"max_grad_norm": 1,
"optimizer_class": "<class 'transformers.optimization.AdamW'>",
"optimizer_params": {
"lr": 2e-05
},
"scheduler": "WarmupLinear",
"steps_per_epoch": null,
"warmup_steps": 1000,
"weight_decay": 0.01
}
```
## Full Model Architecture
```
SentenceTransformer(
(0): Transformer({'max_seq_length': 350, 'do_lower_case': False}) with Transformer model: DistilBertModel
(1): Pooling({'word_embedding_dimension': 768, 'pooling_mode_cls_token': False, 'pooling_mode_mean_tokens': True, 'pooling_mode_max_tokens': False, 'pooling_mode_mean_sqrt_len_tokens': False})
)
```
## Citing & Authors
<!--- Describe where people can find more information --> |
income/bpr-gpl-quora-base-msmarco-distilbert-tas-b | 3a4b917039aaae7a2b1ecdfaca6e6ea5362ba9bd | 2022-06-16T18:14:29.000Z | [
"pytorch",
"distilbert",
"feature-extraction",
"sentence-transformers",
"sentence-similarity",
"transformers"
] | sentence-similarity | false | income | null | income/bpr-gpl-quora-base-msmarco-distilbert-tas-b | 1 | null | sentence-transformers | 32,953 | ---
pipeline_tag: sentence-similarity
tags:
- sentence-transformers
- feature-extraction
- sentence-similarity
- transformers
---
# {MODEL_NAME}
This is a [sentence-transformers](https://www.SBERT.net) model: It maps sentences & paragraphs to a 768 dimensional dense vector space and can be used for tasks like clustering or semantic search.
<!--- Describe your model here -->
## Usage (Sentence-Transformers)
Using this model becomes easy when you have [sentence-transformers](https://www.SBERT.net) installed:
```
pip install -U sentence-transformers
```
Then you can use the model like this:
```python
from sentence_transformers import SentenceTransformer
sentences = ["This is an example sentence", "Each sentence is converted"]
model = SentenceTransformer('{MODEL_NAME}')
embeddings = model.encode(sentences)
print(embeddings)
```
## Usage (HuggingFace Transformers)
Without [sentence-transformers](https://www.SBERT.net), you can use the model like this: First, you pass your input through the transformer model, then you have to apply the right pooling-operation on-top of the contextualized word embeddings.
```python
from transformers import AutoTokenizer, AutoModel
import torch
#Mean Pooling - Take attention mask into account for correct averaging
def mean_pooling(model_output, attention_mask):
token_embeddings = model_output[0] #First element of model_output contains all token embeddings
input_mask_expanded = attention_mask.unsqueeze(-1).expand(token_embeddings.size()).float()
return torch.sum(token_embeddings * input_mask_expanded, 1) / torch.clamp(input_mask_expanded.sum(1), min=1e-9)
# Sentences we want sentence embeddings for
sentences = ['This is an example sentence', 'Each sentence is converted']
# Load model from HuggingFace Hub
tokenizer = AutoTokenizer.from_pretrained('{MODEL_NAME}')
model = AutoModel.from_pretrained('{MODEL_NAME}')
# Tokenize sentences
encoded_input = tokenizer(sentences, padding=True, truncation=True, return_tensors='pt')
# Compute token embeddings
with torch.no_grad():
model_output = model(**encoded_input)
# Perform pooling. In this case, max pooling.
sentence_embeddings = mean_pooling(model_output, encoded_input['attention_mask'])
print("Sentence embeddings:")
print(sentence_embeddings)
```
## Evaluation Results
<!--- Describe how your model was evaluated -->
For an automated evaluation of this model, see the *Sentence Embeddings Benchmark*: [https://seb.sbert.net](https://seb.sbert.net?model_name={MODEL_NAME})
## Training
The model was trained with the parameters:
**DataLoader**:
`torch.utils.data.dataloader.DataLoader` of length 16341 with parameters:
```
{'batch_size': 32, 'sampler': 'torch.utils.data.sampler.RandomSampler', 'batch_sampler': 'torch.utils.data.sampler.BatchSampler'}
```
**Loss**:
`gpl.toolkit.loss.MarginDistillationLoss`
Parameters of the fit()-Method:
```
{
"callback": null,
"epochs": 5,
"evaluation_steps": 0,
"evaluator": "NoneType",
"max_grad_norm": 1,
"optimizer_class": "<class 'transformers.optimization.AdamW'>",
"optimizer_params": {
"lr": 2e-05
},
"scheduler": "WarmupLinear",
"steps_per_epoch": null,
"warmup_steps": 1000,
"weight_decay": 0.01
}
```
## Full Model Architecture
```
SentenceTransformer(
(0): Transformer({'max_seq_length': 350, 'do_lower_case': False}) with Transformer model: DistilBertModel
(1): Pooling({'word_embedding_dimension': 768, 'pooling_mode_cls_token': False, 'pooling_mode_mean_tokens': True, 'pooling_mode_max_tokens': False, 'pooling_mode_mean_sqrt_len_tokens': False})
)
```
## Citing & Authors
<!--- Describe where people can find more information --> |
income/bpr-gpl-nq-base-msmarco-distilbert-tas-b | 6b0c9249c2e1f24cb79bc38fc94f1bbc5a958c7c | 2022-06-16T18:15:23.000Z | [
"pytorch",
"distilbert",
"feature-extraction",
"sentence-transformers",
"sentence-similarity",
"transformers"
] | sentence-similarity | false | income | null | income/bpr-gpl-nq-base-msmarco-distilbert-tas-b | 1 | null | sentence-transformers | 32,954 | ---
pipeline_tag: sentence-similarity
tags:
- sentence-transformers
- feature-extraction
- sentence-similarity
- transformers
---
# {MODEL_NAME}
This is a [sentence-transformers](https://www.SBERT.net) model: It maps sentences & paragraphs to a 768 dimensional dense vector space and can be used for tasks like clustering or semantic search.
<!--- Describe your model here -->
## Usage (Sentence-Transformers)
Using this model becomes easy when you have [sentence-transformers](https://www.SBERT.net) installed:
```
pip install -U sentence-transformers
```
Then you can use the model like this:
```python
from sentence_transformers import SentenceTransformer
sentences = ["This is an example sentence", "Each sentence is converted"]
model = SentenceTransformer('{MODEL_NAME}')
embeddings = model.encode(sentences)
print(embeddings)
```
## Usage (HuggingFace Transformers)
Without [sentence-transformers](https://www.SBERT.net), you can use the model like this: First, you pass your input through the transformer model, then you have to apply the right pooling-operation on-top of the contextualized word embeddings.
```python
from transformers import AutoTokenizer, AutoModel
import torch
#Mean Pooling - Take attention mask into account for correct averaging
def mean_pooling(model_output, attention_mask):
token_embeddings = model_output[0] #First element of model_output contains all token embeddings
input_mask_expanded = attention_mask.unsqueeze(-1).expand(token_embeddings.size()).float()
return torch.sum(token_embeddings * input_mask_expanded, 1) / torch.clamp(input_mask_expanded.sum(1), min=1e-9)
# Sentences we want sentence embeddings for
sentences = ['This is an example sentence', 'Each sentence is converted']
# Load model from HuggingFace Hub
tokenizer = AutoTokenizer.from_pretrained('{MODEL_NAME}')
model = AutoModel.from_pretrained('{MODEL_NAME}')
# Tokenize sentences
encoded_input = tokenizer(sentences, padding=True, truncation=True, return_tensors='pt')
# Compute token embeddings
with torch.no_grad():
model_output = model(**encoded_input)
# Perform pooling. In this case, max pooling.
sentence_embeddings = mean_pooling(model_output, encoded_input['attention_mask'])
print("Sentence embeddings:")
print(sentence_embeddings)
```
## Evaluation Results
<!--- Describe how your model was evaluated -->
For an automated evaluation of this model, see the *Sentence Embeddings Benchmark*: [https://seb.sbert.net](https://seb.sbert.net?model_name={MODEL_NAME})
## Training
The model was trained with the parameters:
**DataLoader**:
`torch.utils.data.dataloader.DataLoader` of length 245832 with parameters:
```
{'batch_size': 32, 'sampler': 'torch.utils.data.sampler.RandomSampler', 'batch_sampler': 'torch.utils.data.sampler.BatchSampler'}
```
**Loss**:
`gpl.toolkit.loss.MarginDistillationLoss`
Parameters of the fit()-Method:
```
{
"callback": null,
"epochs": 1,
"evaluation_steps": 0,
"evaluator": "NoneType",
"max_grad_norm": 1,
"optimizer_class": "<class 'transformers.optimization.AdamW'>",
"optimizer_params": {
"lr": 2e-05
},
"scheduler": "WarmupLinear",
"steps_per_epoch": null,
"warmup_steps": 1000,
"weight_decay": 0.01
}
```
## Full Model Architecture
```
SentenceTransformer(
(0): Transformer({'max_seq_length': 350, 'do_lower_case': False}) with Transformer model: DistilBertModel
(1): Pooling({'word_embedding_dimension': 768, 'pooling_mode_cls_token': False, 'pooling_mode_mean_tokens': True, 'pooling_mode_max_tokens': False, 'pooling_mode_mean_sqrt_len_tokens': False})
)
```
## Citing & Authors
<!--- Describe where people can find more information --> |
income/bpr-gpl-nfcorpus-base-msmarco-distilbert-tas-b | 36dea9161d802901e2c206f9f689f7e66f08a522 | 2022-06-16T18:17:34.000Z | [
"pytorch",
"distilbert",
"feature-extraction",
"sentence-transformers",
"sentence-similarity",
"transformers"
] | sentence-similarity | false | income | null | income/bpr-gpl-nfcorpus-base-msmarco-distilbert-tas-b | 1 | null | sentence-transformers | 32,955 | ---
pipeline_tag: sentence-similarity
tags:
- sentence-transformers
- feature-extraction
- sentence-similarity
- transformers
---
# {MODEL_NAME}
This is a [sentence-transformers](https://www.SBERT.net) model: It maps sentences & paragraphs to a 768 dimensional dense vector space and can be used for tasks like clustering or semantic search.
<!--- Describe your model here -->
## Usage (Sentence-Transformers)
Using this model becomes easy when you have [sentence-transformers](https://www.SBERT.net) installed:
```
pip install -U sentence-transformers
```
Then you can use the model like this:
```python
from sentence_transformers import SentenceTransformer
sentences = ["This is an example sentence", "Each sentence is converted"]
model = SentenceTransformer('{MODEL_NAME}')
embeddings = model.encode(sentences)
print(embeddings)
```
## Usage (HuggingFace Transformers)
Without [sentence-transformers](https://www.SBERT.net), you can use the model like this: First, you pass your input through the transformer model, then you have to apply the right pooling-operation on-top of the contextualized word embeddings.
```python
from transformers import AutoTokenizer, AutoModel
import torch
#Mean Pooling - Take attention mask into account for correct averaging
def mean_pooling(model_output, attention_mask):
token_embeddings = model_output[0] #First element of model_output contains all token embeddings
input_mask_expanded = attention_mask.unsqueeze(-1).expand(token_embeddings.size()).float()
return torch.sum(token_embeddings * input_mask_expanded, 1) / torch.clamp(input_mask_expanded.sum(1), min=1e-9)
# Sentences we want sentence embeddings for
sentences = ['This is an example sentence', 'Each sentence is converted']
# Load model from HuggingFace Hub
tokenizer = AutoTokenizer.from_pretrained('{MODEL_NAME}')
model = AutoModel.from_pretrained('{MODEL_NAME}')
# Tokenize sentences
encoded_input = tokenizer(sentences, padding=True, truncation=True, return_tensors='pt')
# Compute token embeddings
with torch.no_grad():
model_output = model(**encoded_input)
# Perform pooling. In this case, max pooling.
sentence_embeddings = mean_pooling(model_output, encoded_input['attention_mask'])
print("Sentence embeddings:")
print(sentence_embeddings)
```
## Evaluation Results
<!--- Describe how your model was evaluated -->
For an automated evaluation of this model, see the *Sentence Embeddings Benchmark*: [https://seb.sbert.net](https://seb.sbert.net?model_name={MODEL_NAME})
## Training
The model was trained with the parameters:
**DataLoader**:
`torch.utils.data.dataloader.DataLoader` of length 338 with parameters:
```
{'batch_size': 32, 'sampler': 'torch.utils.data.sampler.RandomSampler', 'batch_sampler': 'torch.utils.data.sampler.BatchSampler'}
```
**Loss**:
`gpl.toolkit.loss.MarginDistillationLoss`
Parameters of the fit()-Method:
```
{
"callback": null,
"epochs": 10,
"evaluation_steps": 0,
"evaluator": "NoneType",
"max_grad_norm": 1,
"optimizer_class": "<class 'transformers.optimization.AdamW'>",
"optimizer_params": {
"lr": 2e-05
},
"scheduler": "WarmupLinear",
"steps_per_epoch": null,
"warmup_steps": 1000,
"weight_decay": 0.01
}
```
## Full Model Architecture
```
SentenceTransformer(
(0): Transformer({'max_seq_length': 350, 'do_lower_case': False}) with Transformer model: DistilBertModel
(1): Pooling({'word_embedding_dimension': 768, 'pooling_mode_cls_token': False, 'pooling_mode_mean_tokens': True, 'pooling_mode_max_tokens': False, 'pooling_mode_mean_sqrt_len_tokens': False})
)
```
## Citing & Authors
<!--- Describe where people can find more information --> |
income/bpr-gpl-hotpotqa-base-msmarco-distilbert-tas-b | 047170edc7fa8442a4a455132ce17333200802f6 | 2022-06-16T18:19:52.000Z | [
"pytorch",
"distilbert",
"feature-extraction",
"sentence-transformers",
"sentence-similarity",
"transformers"
] | sentence-similarity | false | income | null | income/bpr-gpl-hotpotqa-base-msmarco-distilbert-tas-b | 1 | null | sentence-transformers | 32,956 | ---
pipeline_tag: sentence-similarity
tags:
- sentence-transformers
- feature-extraction
- sentence-similarity
- transformers
---
# {MODEL_NAME}
This is a [sentence-transformers](https://www.SBERT.net) model: It maps sentences & paragraphs to a 768 dimensional dense vector space and can be used for tasks like clustering or semantic search.
<!--- Describe your model here -->
## Usage (Sentence-Transformers)
Using this model becomes easy when you have [sentence-transformers](https://www.SBERT.net) installed:
```
pip install -U sentence-transformers
```
Then you can use the model like this:
```python
from sentence_transformers import SentenceTransformer
sentences = ["This is an example sentence", "Each sentence is converted"]
model = SentenceTransformer('{MODEL_NAME}')
embeddings = model.encode(sentences)
print(embeddings)
```
## Usage (HuggingFace Transformers)
Without [sentence-transformers](https://www.SBERT.net), you can use the model like this: First, you pass your input through the transformer model, then you have to apply the right pooling-operation on-top of the contextualized word embeddings.
```python
from transformers import AutoTokenizer, AutoModel
import torch
#Mean Pooling - Take attention mask into account for correct averaging
def mean_pooling(model_output, attention_mask):
token_embeddings = model_output[0] #First element of model_output contains all token embeddings
input_mask_expanded = attention_mask.unsqueeze(-1).expand(token_embeddings.size()).float()
return torch.sum(token_embeddings * input_mask_expanded, 1) / torch.clamp(input_mask_expanded.sum(1), min=1e-9)
# Sentences we want sentence embeddings for
sentences = ['This is an example sentence', 'Each sentence is converted']
# Load model from HuggingFace Hub
tokenizer = AutoTokenizer.from_pretrained('{MODEL_NAME}')
model = AutoModel.from_pretrained('{MODEL_NAME}')
# Tokenize sentences
encoded_input = tokenizer(sentences, padding=True, truncation=True, return_tensors='pt')
# Compute token embeddings
with torch.no_grad():
model_output = model(**encoded_input)
# Perform pooling. In this case, max pooling.
sentence_embeddings = mean_pooling(model_output, encoded_input['attention_mask'])
print("Sentence embeddings:")
print(sentence_embeddings)
```
## Evaluation Results
<!--- Describe how your model was evaluated -->
For an automated evaluation of this model, see the *Sentence Embeddings Benchmark*: [https://seb.sbert.net](https://seb.sbert.net?model_name={MODEL_NAME})
## Training
The model was trained with the parameters:
**DataLoader**:
`torch.utils.data.dataloader.DataLoader` of length 163541 with parameters:
```
{'batch_size': 32, 'sampler': 'torch.utils.data.sampler.RandomSampler', 'batch_sampler': 'torch.utils.data.sampler.BatchSampler'}
```
**Loss**:
`gpl.toolkit.loss.MarginDistillationLoss`
Parameters of the fit()-Method:
```
{
"callback": null,
"epochs": 1,
"evaluation_steps": 0,
"evaluator": "NoneType",
"max_grad_norm": 1,
"optimizer_class": "<class 'transformers.optimization.AdamW'>",
"optimizer_params": {
"lr": 2e-05
},
"scheduler": "WarmupLinear",
"steps_per_epoch": null,
"warmup_steps": 1000,
"weight_decay": 0.01
}
```
## Full Model Architecture
```
SentenceTransformer(
(0): Transformer({'max_seq_length': 350, 'do_lower_case': False}) with Transformer model: DistilBertModel
(1): Pooling({'word_embedding_dimension': 768, 'pooling_mode_cls_token': False, 'pooling_mode_mean_tokens': True, 'pooling_mode_max_tokens': False, 'pooling_mode_mean_sqrt_len_tokens': False})
)
```
## Citing & Authors
<!--- Describe where people can find more information --> |
income/bpr-gpl-fiqa-base-msmarco-distilbert-tas-b | 2f6edf788df69260d53dd94f5999bb8ab6d90ef8 | 2022-06-16T18:21:26.000Z | [
"pytorch",
"distilbert",
"feature-extraction",
"sentence-transformers",
"sentence-similarity",
"transformers"
] | sentence-similarity | false | income | null | income/bpr-gpl-fiqa-base-msmarco-distilbert-tas-b | 1 | null | sentence-transformers | 32,957 | ---
pipeline_tag: sentence-similarity
tags:
- sentence-transformers
- feature-extraction
- sentence-similarity
- transformers
---
# {MODEL_NAME}
This is a [sentence-transformers](https://www.SBERT.net) model: It maps sentences & paragraphs to a 768 dimensional dense vector space and can be used for tasks like clustering or semantic search.
<!--- Describe your model here -->
## Usage (Sentence-Transformers)
Using this model becomes easy when you have [sentence-transformers](https://www.SBERT.net) installed:
```
pip install -U sentence-transformers
```
Then you can use the model like this:
```python
from sentence_transformers import SentenceTransformer
sentences = ["This is an example sentence", "Each sentence is converted"]
model = SentenceTransformer('{MODEL_NAME}')
embeddings = model.encode(sentences)
print(embeddings)
```
## Usage (HuggingFace Transformers)
Without [sentence-transformers](https://www.SBERT.net), you can use the model like this: First, you pass your input through the transformer model, then you have to apply the right pooling-operation on-top of the contextualized word embeddings.
```python
from transformers import AutoTokenizer, AutoModel
import torch
#Mean Pooling - Take attention mask into account for correct averaging
def mean_pooling(model_output, attention_mask):
token_embeddings = model_output[0] #First element of model_output contains all token embeddings
input_mask_expanded = attention_mask.unsqueeze(-1).expand(token_embeddings.size()).float()
return torch.sum(token_embeddings * input_mask_expanded, 1) / torch.clamp(input_mask_expanded.sum(1), min=1e-9)
# Sentences we want sentence embeddings for
sentences = ['This is an example sentence', 'Each sentence is converted']
# Load model from HuggingFace Hub
tokenizer = AutoTokenizer.from_pretrained('{MODEL_NAME}')
model = AutoModel.from_pretrained('{MODEL_NAME}')
# Tokenize sentences
encoded_input = tokenizer(sentences, padding=True, truncation=True, return_tensors='pt')
# Compute token embeddings
with torch.no_grad():
model_output = model(**encoded_input)
# Perform pooling. In this case, max pooling.
sentence_embeddings = mean_pooling(model_output, encoded_input['attention_mask'])
print("Sentence embeddings:")
print(sentence_embeddings)
```
## Evaluation Results
<!--- Describe how your model was evaluated -->
For an automated evaluation of this model, see the *Sentence Embeddings Benchmark*: [https://seb.sbert.net](https://seb.sbert.net?model_name={MODEL_NAME})
## Training
The model was trained with the parameters:
**DataLoader**:
`torch.utils.data.dataloader.DataLoader` of length 5076 with parameters:
```
{'batch_size': 32, 'sampler': 'torch.utils.data.sampler.RandomSampler', 'batch_sampler': 'torch.utils.data.sampler.BatchSampler'}
```
**Loss**:
`gpl.toolkit.loss.MarginDistillationLoss`
Parameters of the fit()-Method:
```
{
"callback": null,
"epochs": 10,
"evaluation_steps": 0,
"evaluator": "NoneType",
"max_grad_norm": 1,
"optimizer_class": "<class 'transformers.optimization.AdamW'>",
"optimizer_params": {
"lr": 2e-05
},
"scheduler": "WarmupLinear",
"steps_per_epoch": null,
"warmup_steps": 1000,
"weight_decay": 0.01
}
```
## Full Model Architecture
```
SentenceTransformer(
(0): Transformer({'max_seq_length': 350, 'do_lower_case': False}) with Transformer model: DistilBertModel
(1): Pooling({'word_embedding_dimension': 768, 'pooling_mode_cls_token': False, 'pooling_mode_mean_tokens': True, 'pooling_mode_max_tokens': False, 'pooling_mode_mean_sqrt_len_tokens': False})
)
```
## Citing & Authors
<!--- Describe where people can find more information --> |
income/bpr-gpl-fever-base-msmarco-distilbert-tas-b | 76a67d925b14d8f4abf4abadea7e2001e372808c | 2022-06-16T18:22:17.000Z | [
"pytorch",
"distilbert",
"feature-extraction",
"sentence-transformers",
"sentence-similarity",
"transformers"
] | sentence-similarity | false | income | null | income/bpr-gpl-fever-base-msmarco-distilbert-tas-b | 1 | null | sentence-transformers | 32,958 | ---
pipeline_tag: sentence-similarity
tags:
- sentence-transformers
- feature-extraction
- sentence-similarity
- transformers
---
# {MODEL_NAME}
This is a [sentence-transformers](https://www.SBERT.net) model: It maps sentences & paragraphs to a 768 dimensional dense vector space and can be used for tasks like clustering or semantic search.
<!--- Describe your model here -->
## Usage (Sentence-Transformers)
Using this model becomes easy when you have [sentence-transformers](https://www.SBERT.net) installed:
```
pip install -U sentence-transformers
```
Then you can use the model like this:
```python
from sentence_transformers import SentenceTransformer
sentences = ["This is an example sentence", "Each sentence is converted"]
model = SentenceTransformer('{MODEL_NAME}')
embeddings = model.encode(sentences)
print(embeddings)
```
## Usage (HuggingFace Transformers)
Without [sentence-transformers](https://www.SBERT.net), you can use the model like this: First, you pass your input through the transformer model, then you have to apply the right pooling-operation on-top of the contextualized word embeddings.
```python
from transformers import AutoTokenizer, AutoModel
import torch
#Mean Pooling - Take attention mask into account for correct averaging
def mean_pooling(model_output, attention_mask):
token_embeddings = model_output[0] #First element of model_output contains all token embeddings
input_mask_expanded = attention_mask.unsqueeze(-1).expand(token_embeddings.size()).float()
return torch.sum(token_embeddings * input_mask_expanded, 1) / torch.clamp(input_mask_expanded.sum(1), min=1e-9)
# Sentences we want sentence embeddings for
sentences = ['This is an example sentence', 'Each sentence is converted']
# Load model from HuggingFace Hub
tokenizer = AutoTokenizer.from_pretrained('{MODEL_NAME}')
model = AutoModel.from_pretrained('{MODEL_NAME}')
# Tokenize sentences
encoded_input = tokenizer(sentences, padding=True, truncation=True, return_tensors='pt')
# Compute token embeddings
with torch.no_grad():
model_output = model(**encoded_input)
# Perform pooling. In this case, max pooling.
sentence_embeddings = mean_pooling(model_output, encoded_input['attention_mask'])
print("Sentence embeddings:")
print(sentence_embeddings)
```
## Evaluation Results
<!--- Describe how your model was evaluated -->
For an automated evaluation of this model, see the *Sentence Embeddings Benchmark*: [https://seb.sbert.net](https://seb.sbert.net?model_name={MODEL_NAME})
## Training
The model was trained with the parameters:
**DataLoader**:
`torch.utils.data.dataloader.DataLoader` of length 169267 with parameters:
```
{'batch_size': 32, 'sampler': 'torch.utils.data.sampler.RandomSampler', 'batch_sampler': 'torch.utils.data.sampler.BatchSampler'}
```
**Loss**:
`gpl.toolkit.loss.MarginDistillationLoss`
Parameters of the fit()-Method:
```
{
"callback": null,
"epochs": 1,
"evaluation_steps": 0,
"evaluator": "NoneType",
"max_grad_norm": 1,
"optimizer_class": "<class 'transformers.optimization.AdamW'>",
"optimizer_params": {
"lr": 2e-05
},
"scheduler": "WarmupLinear",
"steps_per_epoch": null,
"warmup_steps": 1000,
"weight_decay": 0.01
}
```
## Full Model Architecture
```
SentenceTransformer(
(0): Transformer({'max_seq_length': 350, 'do_lower_case': False}) with Transformer model: DistilBertModel
(1): Pooling({'word_embedding_dimension': 768, 'pooling_mode_cls_token': False, 'pooling_mode_mean_tokens': True, 'pooling_mode_max_tokens': False, 'pooling_mode_mean_sqrt_len_tokens': False})
)
```
## Citing & Authors
<!--- Describe where people can find more information --> |
income/bpr-gpl-dbpedia-entity-base-msmarco-distilbert-tas-b | 824b93e566e2b73c3b76aedb4eb365c4b34eef89 | 2022-06-16T18:23:49.000Z | [
"pytorch",
"distilbert",
"feature-extraction",
"sentence-transformers",
"sentence-similarity",
"transformers"
] | sentence-similarity | false | income | null | income/bpr-gpl-dbpedia-entity-base-msmarco-distilbert-tas-b | 1 | null | sentence-transformers | 32,959 | ---
pipeline_tag: sentence-similarity
tags:
- sentence-transformers
- feature-extraction
- sentence-similarity
- transformers
---
# {MODEL_NAME}
This is a [sentence-transformers](https://www.SBERT.net) model: It maps sentences & paragraphs to a 768 dimensional dense vector space and can be used for tasks like clustering or semantic search.
<!--- Describe your model here -->
## Usage (Sentence-Transformers)
Using this model becomes easy when you have [sentence-transformers](https://www.SBERT.net) installed:
```
pip install -U sentence-transformers
```
Then you can use the model like this:
```python
from sentence_transformers import SentenceTransformer
sentences = ["This is an example sentence", "Each sentence is converted"]
model = SentenceTransformer('{MODEL_NAME}')
embeddings = model.encode(sentences)
print(embeddings)
```
## Usage (HuggingFace Transformers)
Without [sentence-transformers](https://www.SBERT.net), you can use the model like this: First, you pass your input through the transformer model, then you have to apply the right pooling-operation on-top of the contextualized word embeddings.
```python
from transformers import AutoTokenizer, AutoModel
import torch
#Mean Pooling - Take attention mask into account for correct averaging
def mean_pooling(model_output, attention_mask):
token_embeddings = model_output[0] #First element of model_output contains all token embeddings
input_mask_expanded = attention_mask.unsqueeze(-1).expand(token_embeddings.size()).float()
return torch.sum(token_embeddings * input_mask_expanded, 1) / torch.clamp(input_mask_expanded.sum(1), min=1e-9)
# Sentences we want sentence embeddings for
sentences = ['This is an example sentence', 'Each sentence is converted']
# Load model from HuggingFace Hub
tokenizer = AutoTokenizer.from_pretrained('{MODEL_NAME}')
model = AutoModel.from_pretrained('{MODEL_NAME}')
# Tokenize sentences
encoded_input = tokenizer(sentences, padding=True, truncation=True, return_tensors='pt')
# Compute token embeddings
with torch.no_grad():
model_output = model(**encoded_input)
# Perform pooling. In this case, max pooling.
sentence_embeddings = mean_pooling(model_output, encoded_input['attention_mask'])
print("Sentence embeddings:")
print(sentence_embeddings)
```
## Evaluation Results
<!--- Describe how your model was evaluated -->
For an automated evaluation of this model, see the *Sentence Embeddings Benchmark*: [https://seb.sbert.net](https://seb.sbert.net?model_name={MODEL_NAME})
## Training
The model was trained with the parameters:
**DataLoader**:
`torch.utils.data.dataloader.DataLoader` of length 144872 with parameters:
```
{'batch_size': 32, 'sampler': 'torch.utils.data.sampler.RandomSampler', 'batch_sampler': 'torch.utils.data.sampler.BatchSampler'}
```
**Loss**:
`gpl.toolkit.loss.MarginDistillationLoss`
Parameters of the fit()-Method:
```
{
"callback": null,
"epochs": 1,
"evaluation_steps": 0,
"evaluator": "NoneType",
"max_grad_norm": 1,
"optimizer_class": "<class 'transformers.optimization.AdamW'>",
"optimizer_params": {
"lr": 2e-05
},
"scheduler": "WarmupLinear",
"steps_per_epoch": null,
"warmup_steps": 1000,
"weight_decay": 0.01
}
```
## Full Model Architecture
```
SentenceTransformer(
(0): Transformer({'max_seq_length': 350, 'do_lower_case': False}) with Transformer model: DistilBertModel
(1): Pooling({'word_embedding_dimension': 768, 'pooling_mode_cls_token': False, 'pooling_mode_mean_tokens': True, 'pooling_mode_max_tokens': False, 'pooling_mode_mean_sqrt_len_tokens': False})
)
```
## Citing & Authors
<!--- Describe where people can find more information --> |
income/bpr-gpl-climate-fever-base-msmarco-distilbert-tas-b | 3f4f28f4de68aa390d17c7a9a4220cbf995fbec6 | 2022-06-16T18:25:16.000Z | [
"pytorch",
"distilbert",
"feature-extraction",
"sentence-transformers",
"sentence-similarity",
"transformers"
] | sentence-similarity | false | income | null | income/bpr-gpl-climate-fever-base-msmarco-distilbert-tas-b | 1 | null | sentence-transformers | 32,960 | ---
pipeline_tag: sentence-similarity
tags:
- sentence-transformers
- feature-extraction
- sentence-similarity
- transformers
---
# {MODEL_NAME}
This is a [sentence-transformers](https://www.SBERT.net) model: It maps sentences & paragraphs to a 768 dimensional dense vector space and can be used for tasks like clustering or semantic search.
<!--- Describe your model here -->
## Usage (Sentence-Transformers)
Using this model becomes easy when you have [sentence-transformers](https://www.SBERT.net) installed:
```
pip install -U sentence-transformers
```
Then you can use the model like this:
```python
from sentence_transformers import SentenceTransformer
sentences = ["This is an example sentence", "Each sentence is converted"]
model = SentenceTransformer('{MODEL_NAME}')
embeddings = model.encode(sentences)
print(embeddings)
```
## Usage (HuggingFace Transformers)
Without [sentence-transformers](https://www.SBERT.net), you can use the model like this: First, you pass your input through the transformer model, then you have to apply the right pooling-operation on-top of the contextualized word embeddings.
```python
from transformers import AutoTokenizer, AutoModel
import torch
#Mean Pooling - Take attention mask into account for correct averaging
def mean_pooling(model_output, attention_mask):
token_embeddings = model_output[0] #First element of model_output contains all token embeddings
input_mask_expanded = attention_mask.unsqueeze(-1).expand(token_embeddings.size()).float()
return torch.sum(token_embeddings * input_mask_expanded, 1) / torch.clamp(input_mask_expanded.sum(1), min=1e-9)
# Sentences we want sentence embeddings for
sentences = ['This is an example sentence', 'Each sentence is converted']
# Load model from HuggingFace Hub
tokenizer = AutoTokenizer.from_pretrained('{MODEL_NAME}')
model = AutoModel.from_pretrained('{MODEL_NAME}')
# Tokenize sentences
encoded_input = tokenizer(sentences, padding=True, truncation=True, return_tensors='pt')
# Compute token embeddings
with torch.no_grad():
model_output = model(**encoded_input)
# Perform pooling. In this case, max pooling.
sentence_embeddings = mean_pooling(model_output, encoded_input['attention_mask'])
print("Sentence embeddings:")
print(sentence_embeddings)
```
## Evaluation Results
<!--- Describe how your model was evaluated -->
For an automated evaluation of this model, see the *Sentence Embeddings Benchmark*: [https://seb.sbert.net](https://seb.sbert.net?model_name={MODEL_NAME})
## Training
The model was trained with the parameters:
**DataLoader**:
`torch.utils.data.dataloader.DataLoader` of length 169268 with parameters:
```
{'batch_size': 32, 'sampler': 'torch.utils.data.sampler.RandomSampler', 'batch_sampler': 'torch.utils.data.sampler.BatchSampler'}
```
**Loss**:
`gpl.toolkit.loss.MarginDistillationLoss`
Parameters of the fit()-Method:
```
{
"callback": null,
"epochs": 1,
"evaluation_steps": 0,
"evaluator": "NoneType",
"max_grad_norm": 1,
"optimizer_class": "<class 'transformers.optimization.AdamW'>",
"optimizer_params": {
"lr": 2e-05
},
"scheduler": "WarmupLinear",
"steps_per_epoch": null,
"warmup_steps": 1000,
"weight_decay": 0.01
}
```
## Full Model Architecture
```
SentenceTransformer(
(0): Transformer({'max_seq_length': 350, 'do_lower_case': False}) with Transformer model: DistilBertModel
(1): Pooling({'word_embedding_dimension': 768, 'pooling_mode_cls_token': False, 'pooling_mode_mean_tokens': True, 'pooling_mode_max_tokens': False, 'pooling_mode_mean_sqrt_len_tokens': False})
)
```
## Citing & Authors
<!--- Describe where people can find more information --> |
income/bpr-gpl-arguana-base-msmarco-distilbert-tas-b | 5321885fd29a7a7dedc7132d416cf1bb461df462 | 2022-06-16T18:28:01.000Z | [
"pytorch",
"distilbert",
"feature-extraction",
"sentence-transformers",
"sentence-similarity",
"transformers"
] | sentence-similarity | false | income | null | income/bpr-gpl-arguana-base-msmarco-distilbert-tas-b | 1 | null | sentence-transformers | 32,961 | ---
pipeline_tag: sentence-similarity
tags:
- sentence-transformers
- feature-extraction
- sentence-similarity
- transformers
---
# {MODEL_NAME}
This is a [sentence-transformers](https://www.SBERT.net) model: It maps sentences & paragraphs to a 768 dimensional dense vector space and can be used for tasks like clustering or semantic search.
<!--- Describe your model here -->
## Usage (Sentence-Transformers)
Using this model becomes easy when you have [sentence-transformers](https://www.SBERT.net) installed:
```
pip install -U sentence-transformers
```
Then you can use the model like this:
```python
from sentence_transformers import SentenceTransformer
sentences = ["This is an example sentence", "Each sentence is converted"]
model = SentenceTransformer('{MODEL_NAME}')
embeddings = model.encode(sentences)
print(embeddings)
```
## Usage (HuggingFace Transformers)
Without [sentence-transformers](https://www.SBERT.net), you can use the model like this: First, you pass your input through the transformer model, then you have to apply the right pooling-operation on-top of the contextualized word embeddings.
```python
from transformers import AutoTokenizer, AutoModel
import torch
#Mean Pooling - Take attention mask into account for correct averaging
def mean_pooling(model_output, attention_mask):
token_embeddings = model_output[0] #First element of model_output contains all token embeddings
input_mask_expanded = attention_mask.unsqueeze(-1).expand(token_embeddings.size()).float()
return torch.sum(token_embeddings * input_mask_expanded, 1) / torch.clamp(input_mask_expanded.sum(1), min=1e-9)
# Sentences we want sentence embeddings for
sentences = ['This is an example sentence', 'Each sentence is converted']
# Load model from HuggingFace Hub
tokenizer = AutoTokenizer.from_pretrained('{MODEL_NAME}')
model = AutoModel.from_pretrained('{MODEL_NAME}')
# Tokenize sentences
encoded_input = tokenizer(sentences, padding=True, truncation=True, return_tensors='pt')
# Compute token embeddings
with torch.no_grad():
model_output = model(**encoded_input)
# Perform pooling. In this case, max pooling.
sentence_embeddings = mean_pooling(model_output, encoded_input['attention_mask'])
print("Sentence embeddings:")
print(sentence_embeddings)
```
## Evaluation Results
<!--- Describe how your model was evaluated -->
For an automated evaluation of this model, see the *Sentence Embeddings Benchmark*: [https://seb.sbert.net](https://seb.sbert.net?model_name={MODEL_NAME})
## Training
The model was trained with the parameters:
**DataLoader**:
`torch.utils.data.dataloader.DataLoader` of length 807 with parameters:
```
{'batch_size': 32, 'sampler': 'torch.utils.data.sampler.RandomSampler', 'batch_sampler': 'torch.utils.data.sampler.BatchSampler'}
```
**Loss**:
`gpl.toolkit.loss.MarginDistillationLoss`
Parameters of the fit()-Method:
```
{
"callback": null,
"epochs": 10,
"evaluation_steps": 0,
"evaluator": "NoneType",
"max_grad_norm": 1,
"optimizer_class": "<class 'transformers.optimization.AdamW'>",
"optimizer_params": {
"lr": 2e-05
},
"scheduler": "WarmupLinear",
"steps_per_epoch": null,
"warmup_steps": 1000,
"weight_decay": 0.01
}
```
## Full Model Architecture
```
SentenceTransformer(
(0): Transformer({'max_seq_length': 350, 'do_lower_case': False}) with Transformer model: DistilBertModel
(1): Pooling({'word_embedding_dimension': 768, 'pooling_mode_cls_token': False, 'pooling_mode_mean_tokens': True, 'pooling_mode_max_tokens': False, 'pooling_mode_mean_sqrt_len_tokens': False})
)
```
## Citing & Authors
<!--- Describe where people can find more information --> |
S2312dal/M3_MLM | 300c0c02f6b25d5542e4a46b29e36aeabae749c4 | 2022-06-16T19:46:04.000Z | [
"pytorch",
"tensorboard",
"bert",
"fill-mask",
"transformers",
"generated_from_trainer",
"model-index",
"autotrain_compatible"
] | fill-mask | false | S2312dal | null | S2312dal/M3_MLM | 1 | null | transformers | 32,962 | ---
tags:
- generated_from_trainer
model-index:
- name: M3_MLM
results: []
---
<!-- This model card has been generated automatically according to the information the Trainer had access to. You
should probably proofread and complete it, then remove this comment. -->
# M3_MLM
This model is a fine-tuned version of [SpanBERT/spanbert-base-cased](https://huggingface.co/SpanBERT/spanbert-base-cased) on an unknown dataset.
It achieves the following results on the evaluation set:
- Loss: 5.8186
## Model description
More information needed
## Intended uses & limitations
More information needed
## Training and evaluation data
More information needed
## Training procedure
### Training hyperparameters
The following hyperparameters were used during training:
- learning_rate: 2e-05
- train_batch_size: 16
- eval_batch_size: 16
- seed: 42
- optimizer: Adam with betas=(0.9,0.999) and epsilon=1e-08
- lr_scheduler_type: linear
- num_epochs: 3.0
- mixed_precision_training: Native AMP
### Training results
| Training Loss | Epoch | Step | Validation Loss |
|:-------------:|:-----:|:----:|:---------------:|
| 9.6707 | 1.0 | 26 | 7.4412 |
| 6.9122 | 2.0 | 52 | 6.3385 |
| 6.2166 | 3.0 | 78 | 5.9148 |
### Framework versions
- Transformers 4.20.0
- Pytorch 1.11.0+cu113
- Datasets 2.3.2
- Tokenizers 0.12.1
|
S2312dal/M4_MLM | 5c5578287359ae3bb6da116e0358d7c2e615e54b | 2022-06-16T19:42:02.000Z | [
"pytorch",
"tensorboard",
"distilbert",
"fill-mask",
"transformers",
"generated_from_trainer",
"license:apache-2.0",
"model-index",
"autotrain_compatible"
] | fill-mask | false | S2312dal | null | S2312dal/M4_MLM | 1 | null | transformers | 32,963 | ---
license: apache-2.0
tags:
- generated_from_trainer
model-index:
- name: M4_MLM
results: []
---
<!-- This model card has been generated automatically according to the information the Trainer had access to. You
should probably proofread and complete it, then remove this comment. -->
# M4_MLM
This model is a fine-tuned version of [distilbert-base-uncased](https://huggingface.co/distilbert-base-uncased) on an unknown dataset.
It achieves the following results on the evaluation set:
- Loss: 7.3456
## Model description
More information needed
## Intended uses & limitations
More information needed
## Training and evaluation data
More information needed
## Training procedure
### Training hyperparameters
The following hyperparameters were used during training:
- learning_rate: 2e-05
- train_batch_size: 16
- eval_batch_size: 16
- seed: 42
- optimizer: Adam with betas=(0.9,0.999) and epsilon=1e-08
- lr_scheduler_type: linear
- num_epochs: 3.0
- mixed_precision_training: Native AMP
### Training results
| Training Loss | Epoch | Step | Validation Loss |
|:-------------:|:-----:|:----:|:---------------:|
| 8.7633 | 1.0 | 26 | 8.0400 |
| 7.8899 | 2.0 | 52 | 7.6923 |
| 7.589 | 3.0 | 78 | 7.4373 |
### Framework versions
- Transformers 4.20.0
- Pytorch 1.11.0+cu113
- Datasets 2.3.2
- Tokenizers 0.12.1
|
eplatas/scibert_scivocab_uncased_finetuned_leukaemia | 3847812f20b5acf3a76b2c95279193c13a1ec0d9 | 2022-06-16T20:01:22.000Z | [
"pytorch",
"tensorboard",
"bert",
"text-generation",
"transformers",
"generated_from_trainer",
"model-index"
] | text-generation | false | eplatas | null | eplatas/scibert_scivocab_uncased_finetuned_leukaemia | 1 | null | transformers | 32,964 | ---
tags:
- generated_from_trainer
model-index:
- name: scibert_scivocab_uncased_finetuned_leukaemia
results: []
---
<!-- This model card has been generated automatically according to the information the Trainer had access to. You
should probably proofread and complete it, then remove this comment. -->
# scibert_scivocab_uncased_finetuned_leukaemia
This model is a fine-tuned version of [allenai/scibert_scivocab_uncased](https://huggingface.co/allenai/scibert_scivocab_uncased) on the None dataset.
It achieves the following results on the evaluation set:
- Loss: 0.4985
## Model description
More information needed
## Intended uses & limitations
More information needed
## Training and evaluation data
More information needed
## Training procedure
### Training hyperparameters
The following hyperparameters were used during training:
- learning_rate: 2e-05
- train_batch_size: 8
- eval_batch_size: 8
- seed: 42
- optimizer: Adam with betas=(0.9,0.999) and epsilon=1e-08
- lr_scheduler_type: linear
- num_epochs: 4
### Training results
| Training Loss | Epoch | Step | Validation Loss |
|:-------------:|:-----:|:----:|:---------------:|
| 2.742 | 1.0 | 50 | 2.9184 |
| 0.7729 | 2.0 | 100 | 1.0324 |
| 0.697 | 3.0 | 150 | 0.5968 |
| 0.6573 | 4.0 | 200 | 0.4985 |
### Framework versions
- Transformers 4.20.0
- Pytorch 1.11.0+cu113
- Datasets 2.3.2
- Tokenizers 0.12.1
|
huggingtweets/chrishemsworth-deadpoolmovie | e0a7491d6df2c7a41df6ac7e0220a95a3e7ebdc1 | 2022-06-16T23:26:07.000Z | [
"pytorch",
"gpt2",
"text-generation",
"en",
"transformers",
"huggingtweets"
] | text-generation | false | huggingtweets | null | huggingtweets/chrishemsworth-deadpoolmovie | 1 | null | transformers | 32,965 | ---
language: en
thumbnail: http://www.huggingtweets.com/chrishemsworth-deadpoolmovie/1655421962384/predictions.png
tags:
- huggingtweets
widget:
- text: "My dream is"
---
<div class="inline-flex flex-col" style="line-height: 1.5;">
<div class="flex">
<div
style="display:inherit; margin-left: 4px; margin-right: 4px; width: 92px; height:92px; border-radius: 50%; background-size: cover; background-image: url('https://pbs.twimg.com/profile_images/1247482752351588352/EgHoUNqQ_400x400.jpg')">
</div>
<div
style="display:inherit; margin-left: 4px; margin-right: 4px; width: 92px; height:92px; border-radius: 50%; background-size: cover; background-image: url('https://pbs.twimg.com/profile_images/1208234904405757953/mT0cFOVQ_400x400.jpg')">
</div>
<div
style="display:none; margin-left: 4px; margin-right: 4px; width: 92px; height:92px; border-radius: 50%; background-size: cover; background-image: url('')">
</div>
</div>
<div style="text-align: center; margin-top: 3px; font-size: 16px; font-weight: 800">🤖 AI CYBORG 🤖</div>
<div style="text-align: center; font-size: 16px; font-weight: 800">Chris Hemsworth & Deadpool Movie</div>
<div style="text-align: center; font-size: 14px;">@chrishemsworth-deadpoolmovie</div>
</div>
I was made with [huggingtweets](https://github.com/borisdayma/huggingtweets).
Create your own bot based on your favorite user with [the demo](https://colab.research.google.com/github/borisdayma/huggingtweets/blob/master/huggingtweets-demo.ipynb)!
## How does it work?
The model uses the following pipeline.
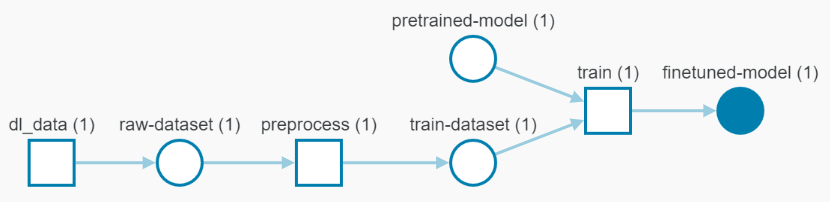
To understand how the model was developed, check the [W&B report](https://wandb.ai/wandb/huggingtweets/reports/HuggingTweets-Train-a-Model-to-Generate-Tweets--VmlldzoxMTY5MjI).
## Training data
The model was trained on tweets from Chris Hemsworth & Deadpool Movie.
| Data | Chris Hemsworth | Deadpool Movie |
| --- | --- | --- |
| Tweets downloaded | 482 | 1125 |
| Retweets | 140 | 276 |
| Short tweets | 39 | 115 |
| Tweets kept | 303 | 734 |
[Explore the data](https://wandb.ai/wandb/huggingtweets/runs/3f48nrzp/artifacts), which is tracked with [W&B artifacts](https://docs.wandb.com/artifacts) at every step of the pipeline.
## Training procedure
The model is based on a pre-trained [GPT-2](https://huggingface.co/gpt2) which is fine-tuned on @chrishemsworth-deadpoolmovie's tweets.
Hyperparameters and metrics are recorded in the [W&B training run](https://wandb.ai/wandb/huggingtweets/runs/2tf8a3vu) for full transparency and reproducibility.
At the end of training, [the final model](https://wandb.ai/wandb/huggingtweets/runs/2tf8a3vu/artifacts) is logged and versioned.
## How to use
You can use this model directly with a pipeline for text generation:
```python
from transformers import pipeline
generator = pipeline('text-generation',
model='huggingtweets/chrishemsworth-deadpoolmovie')
generator("My dream is", num_return_sequences=5)
```
## Limitations and bias
The model suffers from [the same limitations and bias as GPT-2](https://huggingface.co/gpt2#limitations-and-bias).
In addition, the data present in the user's tweets further affects the text generated by the model.
## About
*Built by Boris Dayma*
[](https://twitter.com/intent/follow?screen_name=borisdayma)
For more details, visit the project repository.
[](https://github.com/borisdayma/huggingtweets)
|
huggingtweets/fawfulthgreat64 | 6091494da27a3e63f358b95d953a984f0edd0875 | 2022-06-17T00:31:51.000Z | [
"pytorch",
"gpt2",
"text-generation",
"en",
"transformers",
"huggingtweets"
] | text-generation | false | huggingtweets | null | huggingtweets/fawfulthgreat64 | 1 | null | transformers | 32,966 | ---
language: en
thumbnail: http://www.huggingtweets.com/fawfulthgreat64/1655425906757/predictions.png
tags:
- huggingtweets
widget:
- text: "My dream is"
---
<div class="inline-flex flex-col" style="line-height: 1.5;">
<div class="flex">
<div
style="display:inherit; margin-left: 4px; margin-right: 4px; width: 92px; height:92px; border-radius: 50%; background-size: cover; background-image: url('https://pbs.twimg.com/profile_images/1520110813209665538/-4GuBQGb_400x400.jpg')">
</div>
<div
style="display:none; margin-left: 4px; margin-right: 4px; width: 92px; height:92px; border-radius: 50%; background-size: cover; background-image: url('')">
</div>
<div
style="display:none; margin-left: 4px; margin-right: 4px; width: 92px; height:92px; border-radius: 50%; background-size: cover; background-image: url('')">
</div>
</div>
<div style="text-align: center; margin-top: 3px; font-size: 16px; font-weight: 800">🤖 AI BOT 🤖</div>
<div style="text-align: center; font-size: 16px; font-weight: 800">Jamey Viv 🏳️⚧️ 🇺🇦 #Toaster4DisneyPlus</div>
<div style="text-align: center; font-size: 14px;">@fawfulthgreat64</div>
</div>
I was made with [huggingtweets](https://github.com/borisdayma/huggingtweets).
Create your own bot based on your favorite user with [the demo](https://colab.research.google.com/github/borisdayma/huggingtweets/blob/master/huggingtweets-demo.ipynb)!
## How does it work?
The model uses the following pipeline.
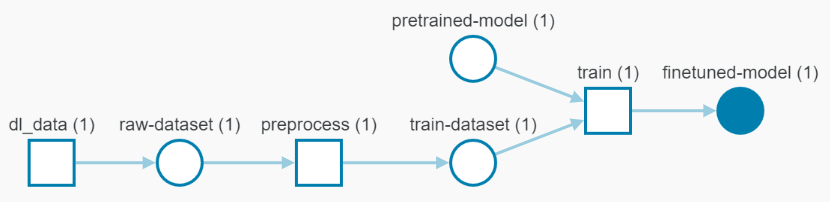
To understand how the model was developed, check the [W&B report](https://wandb.ai/wandb/huggingtweets/reports/HuggingTweets-Train-a-Model-to-Generate-Tweets--VmlldzoxMTY5MjI).
## Training data
The model was trained on tweets from Jamey Viv 🏳️⚧️ 🇺🇦 #Toaster4DisneyPlus.
| Data | Jamey Viv 🏳️⚧️ 🇺🇦 #Toaster4DisneyPlus |
| --- | --- |
| Tweets downloaded | 3246 |
| Retweets | 1394 |
| Short tweets | 133 |
| Tweets kept | 1719 |
[Explore the data](https://wandb.ai/wandb/huggingtweets/runs/21ve8lp9/artifacts), which is tracked with [W&B artifacts](https://docs.wandb.com/artifacts) at every step of the pipeline.
## Training procedure
The model is based on a pre-trained [GPT-2](https://huggingface.co/gpt2) which is fine-tuned on @fawfulthgreat64's tweets.
Hyperparameters and metrics are recorded in the [W&B training run](https://wandb.ai/wandb/huggingtweets/runs/hg3e2g0j) for full transparency and reproducibility.
At the end of training, [the final model](https://wandb.ai/wandb/huggingtweets/runs/hg3e2g0j/artifacts) is logged and versioned.
## How to use
You can use this model directly with a pipeline for text generation:
```python
from transformers import pipeline
generator = pipeline('text-generation',
model='huggingtweets/fawfulthgreat64')
generator("My dream is", num_return_sequences=5)
```
## Limitations and bias
The model suffers from [the same limitations and bias as GPT-2](https://huggingface.co/gpt2#limitations-and-bias).
In addition, the data present in the user's tweets further affects the text generated by the model.
## About
*Built by Boris Dayma*
[](https://twitter.com/intent/follow?screen_name=borisdayma)
For more details, visit the project repository.
[](https://github.com/borisdayma/huggingtweets)
|
huggingtweets/tomcruise | ef6ecc3ef6a45c19efa81a01feafd2fc1143a1e4 | 2022-06-17T01:00:02.000Z | [
"pytorch",
"gpt2",
"text-generation",
"en",
"transformers",
"huggingtweets"
] | text-generation | false | huggingtweets | null | huggingtweets/tomcruise | 1 | null | transformers | 32,967 | ---
language: en
thumbnail: https://github.com/borisdayma/huggingtweets/blob/master/img/logo.png?raw=true
tags:
- huggingtweets
widget:
- text: "My dream is"
---
<div class="inline-flex flex-col" style="line-height: 1.5;">
<div class="flex">
<div
style="display:inherit; margin-left: 4px; margin-right: 4px; width: 92px; height:92px; border-radius: 50%; background-size: cover; background-image: url('https://pbs.twimg.com/profile_images/603269306026106880/42CwEF4n_400x400.jpg')">
</div>
<div
style="display:none; margin-left: 4px; margin-right: 4px; width: 92px; height:92px; border-radius: 50%; background-size: cover; background-image: url('')">
</div>
<div
style="display:none; margin-left: 4px; margin-right: 4px; width: 92px; height:92px; border-radius: 50%; background-size: cover; background-image: url('')">
</div>
</div>
<div style="text-align: center; margin-top: 3px; font-size: 16px; font-weight: 800">🤖 AI BOT 🤖</div>
<div style="text-align: center; font-size: 16px; font-weight: 800">Tom Cruise</div>
<div style="text-align: center; font-size: 14px;">@tomcruise</div>
</div>
I was made with [huggingtweets](https://github.com/borisdayma/huggingtweets).
Create your own bot based on your favorite user with [the demo](https://colab.research.google.com/github/borisdayma/huggingtweets/blob/master/huggingtweets-demo.ipynb)!
## How does it work?
The model uses the following pipeline.
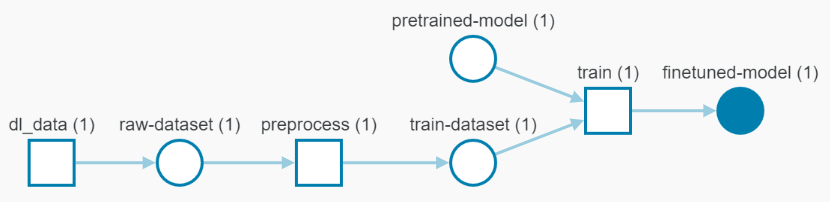
To understand how the model was developed, check the [W&B report](https://wandb.ai/wandb/huggingtweets/reports/HuggingTweets-Train-a-Model-to-Generate-Tweets--VmlldzoxMTY5MjI).
## Training data
The model was trained on tweets from Tom Cruise.
| Data | Tom Cruise |
| --- | --- |
| Tweets downloaded | 3036 |
| Retweets | 1055 |
| Short tweets | 88 |
| Tweets kept | 1893 |
[Explore the data](https://wandb.ai/wandb/huggingtweets/runs/ppnkvd5o/artifacts), which is tracked with [W&B artifacts](https://docs.wandb.com/artifacts) at every step of the pipeline.
## Training procedure
The model is based on a pre-trained [GPT-2](https://huggingface.co/gpt2) which is fine-tuned on @tomcruise's tweets.
Hyperparameters and metrics are recorded in the [W&B training run](https://wandb.ai/wandb/huggingtweets/runs/2q772s43) for full transparency and reproducibility.
At the end of training, [the final model](https://wandb.ai/wandb/huggingtweets/runs/2q772s43/artifacts) is logged and versioned.
## How to use
You can use this model directly with a pipeline for text generation:
```python
from transformers import pipeline
generator = pipeline('text-generation',
model='huggingtweets/tomcruise')
generator("My dream is", num_return_sequences=5)
```
## Limitations and bias
The model suffers from [the same limitations and bias as GPT-2](https://huggingface.co/gpt2#limitations-and-bias).
In addition, the data present in the user's tweets further affects the text generated by the model.
## About
*Built by Boris Dayma*
[](https://twitter.com/intent/follow?screen_name=borisdayma)
For more details, visit the project repository.
[](https://github.com/borisdayma/huggingtweets)
|
huggingtweets/mcdonaldsuk-potus-tomcruise | b80c60ae6e9a818f6d6c54e7faa6366a9cd2aba8 | 2022-06-17T01:44:54.000Z | [
"pytorch",
"gpt2",
"text-generation",
"en",
"transformers",
"huggingtweets"
] | text-generation | false | huggingtweets | null | huggingtweets/mcdonaldsuk-potus-tomcruise | 1 | null | transformers | 32,968 | ---
language: en
thumbnail: https://github.com/borisdayma/huggingtweets/blob/master/img/logo.png?raw=true
tags:
- huggingtweets
widget:
- text: "My dream is"
---
<div class="inline-flex flex-col" style="line-height: 1.5;">
<div class="flex">
<div
style="display:inherit; margin-left: 4px; margin-right: 4px; width: 92px; height:92px; border-radius: 50%; background-size: cover; background-image: url('https://pbs.twimg.com/profile_images/603269306026106880/42CwEF4n_400x400.jpg')">
</div>
<div
style="display:inherit; margin-left: 4px; margin-right: 4px; width: 92px; height:92px; border-radius: 50%; background-size: cover; background-image: url('https://pbs.twimg.com/profile_images/1533739179770843141/kNhGgW4K_400x400.jpg')">
</div>
<div
style="display:inherit; margin-left: 4px; margin-right: 4px; width: 92px; height:92px; border-radius: 50%; background-size: cover; background-image: url('https://pbs.twimg.com/profile_images/1380530524779859970/TfwVAbyX_400x400.jpg')">
</div>
</div>
<div style="text-align: center; margin-top: 3px; font-size: 16px; font-weight: 800">🤖 AI CYBORG 🤖</div>
<div style="text-align: center; font-size: 16px; font-weight: 800">Tom Cruise & McDonald's UK & President Biden</div>
<div style="text-align: center; font-size: 14px;">@mcdonaldsuk-potus-tomcruise</div>
</div>
I was made with [huggingtweets](https://github.com/borisdayma/huggingtweets).
Create your own bot based on your favorite user with [the demo](https://colab.research.google.com/github/borisdayma/huggingtweets/blob/master/huggingtweets-demo.ipynb)!
## How does it work?
The model uses the following pipeline.
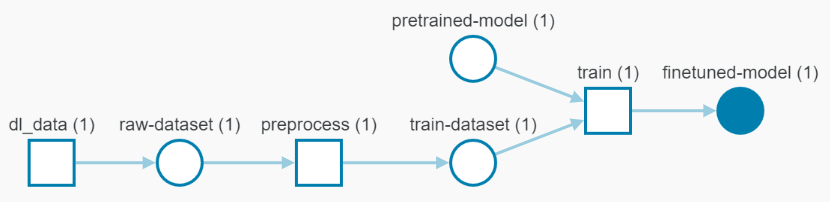
To understand how the model was developed, check the [W&B report](https://wandb.ai/wandb/huggingtweets/reports/HuggingTweets-Train-a-Model-to-Generate-Tweets--VmlldzoxMTY5MjI).
## Training data
The model was trained on tweets from Tom Cruise & McDonald's UK & President Biden.
| Data | Tom Cruise | McDonald's UK | President Biden |
| --- | --- | --- | --- |
| Tweets downloaded | 3036 | 3250 | 3250 |
| Retweets | 1055 | 0 | 96 |
| Short tweets | 88 | 36 | 8 |
| Tweets kept | 1893 | 3214 | 3146 |
[Explore the data](https://wandb.ai/wandb/huggingtweets/runs/xo9k90g3/artifacts), which is tracked with [W&B artifacts](https://docs.wandb.com/artifacts) at every step of the pipeline.
## Training procedure
The model is based on a pre-trained [GPT-2](https://huggingface.co/gpt2) which is fine-tuned on @mcdonaldsuk-potus-tomcruise's tweets.
Hyperparameters and metrics are recorded in the [W&B training run](https://wandb.ai/wandb/huggingtweets/runs/uk4lqo8q) for full transparency and reproducibility.
At the end of training, [the final model](https://wandb.ai/wandb/huggingtweets/runs/uk4lqo8q/artifacts) is logged and versioned.
## How to use
You can use this model directly with a pipeline for text generation:
```python
from transformers import pipeline
generator = pipeline('text-generation',
model='huggingtweets/mcdonaldsuk-potus-tomcruise')
generator("My dream is", num_return_sequences=5)
```
## Limitations and bias
The model suffers from [the same limitations and bias as GPT-2](https://huggingface.co/gpt2#limitations-and-bias).
In addition, the data present in the user's tweets further affects the text generated by the model.
## About
*Built by Boris Dayma*
[](https://twitter.com/intent/follow?screen_name=borisdayma)
For more details, visit the project repository.
[](https://github.com/borisdayma/huggingtweets)
|
lmqg/t5-large-squadshifts-amazon | dff928cd916982b9258d71a756a7b3ec53dff59e | 2022-06-17T02:31:28.000Z | [
"pytorch",
"t5",
"text2text-generation",
"transformers",
"autotrain_compatible"
] | text2text-generation | false | lmqg | null | lmqg/t5-large-squadshifts-amazon | 1 | null | transformers | 32,969 | Entry not found |
neuralmagic/oBERT-12-upstream-pruned-unstructured-97-finetuned-mnli-v2 | 2a910979e7c5e556e9a54f6c6c87487ea1423e17 | 2022-06-20T11:36:51.000Z | [
"pytorch",
"en",
"dataset:mnli",
"arxiv:2203.07259",
"bert",
"oBERT",
"sparsity",
"pruning",
"compression"
] | null | false | neuralmagic | null | neuralmagic/oBERT-12-upstream-pruned-unstructured-97-finetuned-mnli-v2 | 1 | null | null | 32,970 | ---
tags:
- bert
- oBERT
- sparsity
- pruning
- compression
language: en
datasets: mnli
---
# oBERT-12-upstream-pruned-unstructured-97-finetuned-mnli-v2
This model is obtained with [The Optimal BERT Surgeon: Scalable and Accurate Second-Order Pruning for Large Language Models](https://arxiv.org/abs/2203.07259).
It corresponds to the model presented in the `Table 2 - oBERT - MNLI 97%` (in the upcoming updated version of the paper).
```
Pruning method: oBERT upstream unstructured + sparse-transfer to downstream
Paper: https://arxiv.org/abs/2203.07259
Dataset: MNLI
Sparsity: 97%
Number of layers: 12
```
The dev-set performance reported in the paper is averaged over four seeds, and we release the best model (marked with `(*)`):
```
| oBERT 97% | m-acc | mm-acc|
| ------------- | ----- | ----- |
| seed=42 | 80.86 | 80.88 |
| seed=3407 | 80.83 | 81.65 |
| seed=123 (*)| 81.18 | 81.06 |
| seed=12345 | 80.79 | 80.95 |
| ------------- | ----- | ----- |
| mean | 80.91 | 81.13 |
| stdev | 0.178 | 0.351 |
```
Code: _coming soon_
## BibTeX entry and citation info
```bibtex
@article{kurtic2022optimal,
title={The Optimal BERT Surgeon: Scalable and Accurate Second-Order Pruning for Large Language Models},
author={Kurtic, Eldar and Campos, Daniel and Nguyen, Tuan and Frantar, Elias and Kurtz, Mark and Fineran, Benjamin and Goin, Michael and Alistarh, Dan},
journal={arXiv preprint arXiv:2203.07259},
year={2022}
}
``` |
botika/checkpoint-124500-finetuned-squad | 5dab949e1c03afe043de31f361822045ed178de6 | 2022-06-19T05:53:11.000Z | [
"pytorch",
"tensorboard",
"distilbert",
"question-answering",
"transformers",
"generated_from_trainer",
"model-index",
"autotrain_compatible"
] | question-answering | false | botika | null | botika/checkpoint-124500-finetuned-squad | 1 | null | transformers | 32,971 | ---
tags:
- generated_from_trainer
model-index:
- name: checkpoint-124500-finetuned-squad
results: []
---
<!-- This model card has been generated automatically according to the information the Trainer had access to. You
should probably proofread and complete it, then remove this comment. -->
# checkpoint-124500-finetuned-squad
This model was trained from scratch on an unknown dataset.
It achieves the following results on the evaluation set:
- Loss: 14.9594
## Model description
More information needed
## Intended uses & limitations
More information needed
## Training and evaluation data
More information needed
## Training procedure
### Training hyperparameters
The following hyperparameters were used during training:
- learning_rate: 2e-05
- train_batch_size: 32
- eval_batch_size: 32
- seed: 42
- optimizer: Adam with betas=(0.9,0.999) and epsilon=1e-08
- lr_scheduler_type: linear
- num_epochs: 100
### Training results
| Training Loss | Epoch | Step | Validation Loss |
|:-------------:|:-----:|:------:|:---------------:|
| 3.9975 | 1.0 | 3289 | 3.8405 |
| 3.7311 | 2.0 | 6578 | 3.7114 |
| 3.5681 | 3.0 | 9867 | 3.6829 |
| 3.4101 | 4.0 | 13156 | 3.6368 |
| 3.2487 | 5.0 | 16445 | 3.6526 |
| 3.1143 | 6.0 | 19734 | 3.7567 |
| 2.9783 | 7.0 | 23023 | 3.8469 |
| 2.8295 | 8.0 | 26312 | 4.0040 |
| 2.6912 | 9.0 | 29601 | 4.1996 |
| 2.5424 | 10.0 | 32890 | 4.3387 |
| 2.4161 | 11.0 | 36179 | 4.4988 |
| 2.2713 | 12.0 | 39468 | 4.7861 |
| 2.1413 | 13.0 | 42757 | 4.9276 |
| 2.0125 | 14.0 | 46046 | 5.0598 |
| 1.8798 | 15.0 | 49335 | 5.3347 |
| 1.726 | 16.0 | 52624 | 5.5869 |
| 1.5994 | 17.0 | 55913 | 5.7161 |
| 1.4643 | 18.0 | 59202 | 6.0174 |
| 1.3237 | 19.0 | 62491 | 6.4926 |
| 1.2155 | 20.0 | 65780 | 6.4882 |
| 1.1029 | 21.0 | 69069 | 6.9922 |
| 0.9948 | 22.0 | 72358 | 7.1357 |
| 0.9038 | 23.0 | 75647 | 7.3676 |
| 0.8099 | 24.0 | 78936 | 7.4180 |
| 0.7254 | 25.0 | 82225 | 7.7753 |
| 0.6598 | 26.0 | 85514 | 7.8643 |
| 0.5723 | 27.0 | 88803 | 8.1798 |
| 0.5337 | 28.0 | 92092 | 8.3053 |
| 0.4643 | 29.0 | 95381 | 8.8597 |
| 0.4241 | 30.0 | 98670 | 8.9849 |
| 0.3763 | 31.0 | 101959 | 8.8406 |
| 0.3479 | 32.0 | 105248 | 9.1517 |
| 0.3271 | 33.0 | 108537 | 9.3659 |
| 0.2911 | 34.0 | 111826 | 9.4813 |
| 0.2836 | 35.0 | 115115 | 9.5746 |
| 0.2528 | 36.0 | 118404 | 9.7027 |
| 0.2345 | 37.0 | 121693 | 9.7515 |
| 0.2184 | 38.0 | 124982 | 9.9729 |
| 0.2067 | 39.0 | 128271 | 10.0828 |
| 0.2077 | 40.0 | 131560 | 10.0878 |
| 0.1876 | 41.0 | 134849 | 10.2974 |
| 0.1719 | 42.0 | 138138 | 10.2712 |
| 0.1637 | 43.0 | 141427 | 10.5788 |
| 0.1482 | 44.0 | 144716 | 10.7465 |
| 0.1509 | 45.0 | 148005 | 10.4603 |
| 0.1358 | 46.0 | 151294 | 10.7665 |
| 0.1316 | 47.0 | 154583 | 10.7724 |
| 0.1223 | 48.0 | 157872 | 11.1766 |
| 0.1205 | 49.0 | 161161 | 11.1870 |
| 0.1203 | 50.0 | 164450 | 11.1053 |
| 0.1081 | 51.0 | 167739 | 10.9696 |
| 0.103 | 52.0 | 171028 | 11.2010 |
| 0.0938 | 53.0 | 174317 | 11.6728 |
| 0.0924 | 54.0 | 177606 | 11.1423 |
| 0.0922 | 55.0 | 180895 | 11.7409 |
| 0.0827 | 56.0 | 184184 | 11.7850 |
| 0.0829 | 57.0 | 187473 | 11.8956 |
| 0.073 | 58.0 | 190762 | 11.8915 |
| 0.0788 | 59.0 | 194051 | 12.1617 |
| 0.0734 | 60.0 | 197340 | 12.2007 |
| 0.0729 | 61.0 | 200629 | 12.2388 |
| 0.0663 | 62.0 | 203918 | 12.2471 |
| 0.0662 | 63.0 | 207207 | 12.5830 |
| 0.064 | 64.0 | 210496 | 12.6105 |
| 0.0599 | 65.0 | 213785 | 12.3712 |
| 0.0604 | 66.0 | 217074 | 12.9249 |
| 0.0574 | 67.0 | 220363 | 12.7309 |
| 0.0538 | 68.0 | 223652 | 12.8068 |
| 0.0526 | 69.0 | 226941 | 13.4368 |
| 0.0471 | 70.0 | 230230 | 13.5148 |
| 0.0436 | 71.0 | 233519 | 13.3391 |
| 0.0448 | 72.0 | 236808 | 13.4100 |
| 0.0428 | 73.0 | 240097 | 13.5617 |
| 0.0401 | 74.0 | 243386 | 13.8674 |
| 0.035 | 75.0 | 246675 | 13.5746 |
| 0.0342 | 76.0 | 249964 | 13.5042 |
| 0.0344 | 77.0 | 253253 | 14.2085 |
| 0.0365 | 78.0 | 256542 | 13.6393 |
| 0.0306 | 79.0 | 259831 | 13.9807 |
| 0.0311 | 80.0 | 263120 | 13.9768 |
| 0.0353 | 81.0 | 266409 | 14.5245 |
| 0.0299 | 82.0 | 269698 | 13.9471 |
| 0.0263 | 83.0 | 272987 | 13.7899 |
| 0.0254 | 84.0 | 276276 | 14.3786 |
| 0.0267 | 85.0 | 279565 | 14.5611 |
| 0.022 | 86.0 | 282854 | 14.2658 |
| 0.0198 | 87.0 | 286143 | 14.9215 |
| 0.0193 | 88.0 | 289432 | 14.5650 |
| 0.0228 | 89.0 | 292721 | 14.7014 |
| 0.0184 | 90.0 | 296010 | 14.6946 |
| 0.0182 | 91.0 | 299299 | 14.6614 |
| 0.0188 | 92.0 | 302588 | 14.6915 |
| 0.0196 | 93.0 | 305877 | 14.7262 |
| 0.0138 | 94.0 | 309166 | 14.7625 |
| 0.0201 | 95.0 | 312455 | 15.0442 |
| 0.0189 | 96.0 | 315744 | 14.8832 |
| 0.0148 | 97.0 | 319033 | 14.8995 |
| 0.0129 | 98.0 | 322322 | 14.8974 |
| 0.0132 | 99.0 | 325611 | 14.9813 |
| 0.0139 | 100.0 | 328900 | 14.9594 |
### Framework versions
- Transformers 4.19.2
- Pytorch 1.11.0+cu102
- Datasets 2.2.2
- Tokenizers 0.12.1
|
S2312dal/M5_MLM | 1d28b1c7f62cb935a0aa88617c2ea8b023bc7865 | 2022-06-17T08:25:48.000Z | [
"pytorch",
"tensorboard",
"deberta-v2",
"fill-mask",
"transformers",
"generated_from_trainer",
"license:mit",
"model-index",
"autotrain_compatible"
] | fill-mask | false | S2312dal | null | S2312dal/M5_MLM | 1 | null | transformers | 32,972 | ---
license: mit
tags:
- generated_from_trainer
model-index:
- name: M5_MLM
results: []
---
<!-- This model card has been generated automatically according to the information the Trainer had access to. You
should probably proofread and complete it, then remove this comment. -->
# M5_MLM
This model is a fine-tuned version of [microsoft/deberta-v3-base](https://huggingface.co/microsoft/deberta-v3-base) on an unknown dataset.
It achieves the following results on the evaluation set:
- Loss: 7.0447
## Model description
More information needed
## Intended uses & limitations
More information needed
## Training and evaluation data
More information needed
## Training procedure
### Training hyperparameters
The following hyperparameters were used during training:
- learning_rate: 2e-05
- train_batch_size: 6
- eval_batch_size: 6
- seed: 42
- optimizer: Adam with betas=(0.9,0.999) and epsilon=1e-08
- lr_scheduler_type: linear
- num_epochs: 3.0
- mixed_precision_training: Native AMP
### Training results
| Training Loss | Epoch | Step | Validation Loss |
|:-------------:|:-----:|:----:|:---------------:|
| 9.8279 | 1.0 | 62 | 7.9889 |
| 7.7536 | 2.0 | 124 | 7.3750 |
| 7.2065 | 3.0 | 186 | 6.8625 |
### Framework versions
- Transformers 4.20.0
- Pytorch 1.11.0+cu113
- Datasets 2.3.2
- Tokenizers 0.12.1
|
S2312dal/M7_MLM | cb0e5ee487aa46fff06386d2c0c63b2bcf3f4acb | 2022-06-17T08:49:00.000Z | [
"pytorch",
"tensorboard",
"roberta",
"fill-mask",
"transformers",
"generated_from_trainer",
"license:apache-2.0",
"model-index",
"autotrain_compatible"
] | fill-mask | false | S2312dal | null | S2312dal/M7_MLM | 1 | null | transformers | 32,973 | ---
license: apache-2.0
tags:
- generated_from_trainer
model-index:
- name: M7_MLM
results: []
---
<!-- This model card has been generated automatically according to the information the Trainer had access to. You
should probably proofread and complete it, then remove this comment. -->
# M7_MLM
This model is a fine-tuned version of [sentence-transformers/all-distilroberta-v1](https://huggingface.co/sentence-transformers/all-distilroberta-v1) on an unknown dataset.
It achieves the following results on the evaluation set:
- Loss: 8.2304
## Model description
More information needed
## Intended uses & limitations
More information needed
## Training and evaluation data
More information needed
## Training procedure
### Training hyperparameters
The following hyperparameters were used during training:
- learning_rate: 2e-05
- train_batch_size: 16
- eval_batch_size: 16
- seed: 42
- optimizer: Adam with betas=(0.9,0.999) and epsilon=1e-08
- lr_scheduler_type: linear
- num_epochs: 3.0
- mixed_precision_training: Native AMP
### Training results
| Training Loss | Epoch | Step | Validation Loss |
|:-------------:|:-----:|:----:|:---------------:|
| 9.2227 | 1.0 | 25 | 8.6091 |
| 8.6536 | 2.0 | 50 | 8.2492 |
| 8.5065 | 3.0 | 75 | 8.3056 |
### Framework versions
- Transformers 4.20.0
- Pytorch 1.11.0+cu113
- Datasets 2.3.2
- Tokenizers 0.12.1
|
S2312dal/M8_MLM | 043d1e6891fde585eb80813065b7c58c5c300773 | 2022-06-17T08:55:06.000Z | [
"pytorch",
"tensorboard",
"albert",
"fill-mask",
"transformers",
"generated_from_trainer",
"license:apache-2.0",
"model-index",
"autotrain_compatible"
] | fill-mask | false | S2312dal | null | S2312dal/M8_MLM | 1 | null | transformers | 32,974 | ---
license: apache-2.0
tags:
- generated_from_trainer
model-index:
- name: M8_MLM
results: []
---
<!-- This model card has been generated automatically according to the information the Trainer had access to. You
should probably proofread and complete it, then remove this comment. -->
# M8_MLM
This model is a fine-tuned version of [sentence-transformers/paraphrase-albert-small-v2](https://huggingface.co/sentence-transformers/paraphrase-albert-small-v2) on an unknown dataset.
It achieves the following results on the evaluation set:
- Loss: 8.9140
## Model description
More information needed
## Intended uses & limitations
More information needed
## Training and evaluation data
More information needed
## Training procedure
### Training hyperparameters
The following hyperparameters were used during training:
- learning_rate: 2e-05
- train_batch_size: 16
- eval_batch_size: 16
- seed: 42
- optimizer: Adam with betas=(0.9,0.999) and epsilon=1e-08
- lr_scheduler_type: linear
- num_epochs: 3.0
- mixed_precision_training: Native AMP
### Training results
| Training Loss | Epoch | Step | Validation Loss |
|:-------------:|:-----:|:----:|:---------------:|
| 9.5021 | 1.0 | 25 | 9.1463 |
| 9.0507 | 2.0 | 50 | 8.9504 |
| 8.9528 | 3.0 | 75 | 8.9148 |
### Framework versions
- Transformers 4.20.0
- Pytorch 1.11.0+cu113
- Datasets 2.3.2
- Tokenizers 0.12.1
|
ahujaniharika95/roberta-base-squad2-finetuned-squad | 4097c98bac7c851c6c25300cb54ea4af13491a5b | 2022-06-21T14:06:07.000Z | [
"pytorch",
"roberta",
"question-answering",
"transformers",
"autotrain_compatible"
] | question-answering | false | ahujaniharika95 | null | ahujaniharika95/roberta-base-squad2-finetuned-squad | 1 | null | transformers | 32,975 | Entry not found |
Sanjeev49/mariaJ | de7b52d7b10d556c43d0e4c40a22d5e0b35b1fe6 | 2022-06-20T08:59:55.000Z | [
"pytorch",
"marian",
"text2text-generation",
"transformers",
"autotrain_compatible"
] | text2text-generation | false | Sanjeev49 | null | Sanjeev49/mariaJ | 1 | 1 | transformers | 32,976 | This model translate engilish to romanian language. This model has been build using Helsinki-NLP/opus-mt-en-ro prebuild model. The dataset has been used for this model was "wmt16", "ro-en" dataset. Tensorflow has been used for finetunning this model. Trainning epoch was 1 for this for finetunning model. |
anibahug/marian-finetuned-kde4-en-to-ar | 0aadad8db21e057eb0e48cd18c73c790da60e722 | 2022-06-18T15:19:04.000Z | [
"pytorch",
"tensorboard",
"marian",
"text2text-generation",
"dataset:kde4",
"transformers",
"translation",
"generated_from_trainer",
"license:apache-2.0",
"model-index",
"autotrain_compatible"
] | translation | false | anibahug | null | anibahug/marian-finetuned-kde4-en-to-ar | 1 | null | transformers | 32,977 | ---
license: apache-2.0
tags:
- translation
- generated_from_trainer
datasets:
- kde4
model-index:
- name: marian-finetuned-kde4-en-to-ar
results: []
---
<!-- This model card has been generated automatically according to the information the Trainer had access to. You
should probably proofread and complete it, then remove this comment. -->
# marian-finetuned-kde4-en-to-ar
This model is a fine-tuned version of [Helsinki-NLP/opus-mt-en-ar](https://huggingface.co/Helsinki-NLP/opus-mt-en-ar) on the kde4 dataset.
## Model description
if you want to learn about the model used check [Helsinki-NLP Model](https://huggingface.co/Helsinki-NLP/opus-mt-en-ar)
## Intended uses & limitations
## Training and evaluation data
More information needed
## Training procedure
the training was done on google colab.
### Training hyperparameters
The following hyperparameters were used during training:
- learning_rate: 2e-05
- train_batch_size: 32
- eval_batch_size: 64
- seed: 42
- optimizer: Adam with betas=(0.9,0.999) and epsilon=1e-08
- lr_scheduler_type: linear
- num_epochs: 3
- mixed_precision_training: Native AMP
### Training results
### Framework versions
- Transformers 4.20.0
- Pytorch 1.11.0+cu113
- Datasets 2.3.2
- Tokenizers 0.12.1
|
sasuke/gpt2-wikitext2 | 872563c729363b8d00bc0927ed1cbab2a622972b | 2022-06-17T15:02:05.000Z | [
"pytorch",
"tensorboard",
"gpt2",
"text-generation",
"transformers"
] | text-generation | false | sasuke | null | sasuke/gpt2-wikitext2 | 1 | null | transformers | 32,978 | Entry not found |
loubnabnl/codeparrot-small-near-dedup | 015e1dc76630179d0f4f84c4e013d1cf272e2e73 | 2022-06-18T20:59:13.000Z | [
"pytorch",
"gpt2",
"text-generation",
"transformers",
"license:apache-2.0"
] | text-generation | false | loubnabnl | null | loubnabnl/codeparrot-small-near-dedup | 1 | null | transformers | 32,979 | ---
license: apache-2.0
---
|
ericklerouge123/xlm-roberta-base-finetuned-panx-de | c4f16c55b3b4f76be260c09cfec9a748f87398bc | 2022-07-14T14:05:25.000Z | [
"pytorch",
"tensorboard",
"xlm-roberta",
"token-classification",
"dataset:xtreme",
"transformers",
"generated_from_trainer",
"license:mit",
"model-index",
"autotrain_compatible"
] | token-classification | false | ericklerouge123 | null | ericklerouge123/xlm-roberta-base-finetuned-panx-de | 1 | null | transformers | 32,980 | ---
license: mit
tags:
- generated_from_trainer
datasets:
- xtreme
metrics:
- f1
model-index:
- name: xlm-roberta-base-finetuned-panx-de
results:
- task:
name: Token Classification
type: token-classification
dataset:
name: xtreme
type: xtreme
args: PAN-X.de
metrics:
- name: F1
type: f1
value: 0.8648740833380706
---
<!-- This model card has been generated automatically according to the information the Trainer had access to. You
should probably proofread and complete it, then remove this comment. -->
# xlm-roberta-base-finetuned-panx-de
This model is a fine-tuned version of [xlm-roberta-base](https://huggingface.co/xlm-roberta-base) on the xtreme dataset.
It achieves the following results on the evaluation set:
- Loss: 0.1365
- F1: 0.8649
## Model description
More information needed
## Intended uses & limitations
More information needed
## Training and evaluation data
More information needed
## Training procedure
### Training hyperparameters
The following hyperparameters were used during training:
- learning_rate: 5e-05
- train_batch_size: 24
- eval_batch_size: 24
- seed: 42
- optimizer: Adam with betas=(0.9,0.999) and epsilon=1e-08
- lr_scheduler_type: linear
- num_epochs: 3
### Training results
| Training Loss | Epoch | Step | Validation Loss | F1 |
|:-------------:|:-----:|:----:|:---------------:|:------:|
| 0.2553 | 1.0 | 525 | 0.1575 | 0.8279 |
| 0.1284 | 2.0 | 1050 | 0.1386 | 0.8463 |
| 0.0813 | 3.0 | 1575 | 0.1365 | 0.8649 |
### Framework versions
- Transformers 4.11.3
- Pytorch 1.12.0+cu113
- Datasets 1.16.1
- Tokenizers 0.10.3
|
gemasphi/laprador_f | dfd84070c29a7dde6f62f7f3563ece4f625846db | 2022-06-17T21:11:03.000Z | [
"pytorch",
"bert",
"feature-extraction",
"sentence-transformers",
"sentence-similarity",
"transformers"
] | sentence-similarity | false | gemasphi | null | gemasphi/laprador_f | 1 | null | sentence-transformers | 32,981 | ---
pipeline_tag: sentence-similarity
tags:
- sentence-transformers
- feature-extraction
- sentence-similarity
- transformers
---
# gemasphi/laprador_f
This is a [sentence-transformers](https://www.SBERT.net) model: It maps sentences & paragraphs to a 768 dimensional dense vector space and can be used for tasks like clustering or semantic search.
<!--- Describe your model here -->
## Usage (Sentence-Transformers)
Using this model becomes easy when you have [sentence-transformers](https://www.SBERT.net) installed:
```
pip install -U sentence-transformers
```
Then you can use the model like this:
```python
from sentence_transformers import SentenceTransformer
sentences = ["This is an example sentence", "Each sentence is converted"]
model = SentenceTransformer('gemasphi/laprador_f')
embeddings = model.encode(sentences)
print(embeddings)
```
## Usage (HuggingFace Transformers)
Without [sentence-transformers](https://www.SBERT.net), you can use the model like this: First, you pass your input through the transformer model, then you have to apply the right pooling-operation on-top of the contextualized word embeddings.
```python
from transformers import AutoTokenizer, AutoModel
import torch
#Mean Pooling - Take attention mask into account for correct averaging
def mean_pooling(model_output, attention_mask):
token_embeddings = model_output[0] #First element of model_output contains all token embeddings
input_mask_expanded = attention_mask.unsqueeze(-1).expand(token_embeddings.size()).float()
return torch.sum(token_embeddings * input_mask_expanded, 1) / torch.clamp(input_mask_expanded.sum(1), min=1e-9)
# Sentences we want sentence embeddings for
sentences = ['This is an example sentence', 'Each sentence is converted']
# Load model from HuggingFace Hub
tokenizer = AutoTokenizer.from_pretrained('gemasphi/laprador_f')
model = AutoModel.from_pretrained('gemasphi/laprador_f')
# Tokenize sentences
encoded_input = tokenizer(sentences, padding=True, truncation=True, return_tensors='pt')
# Compute token embeddings
with torch.no_grad():
model_output = model(**encoded_input)
# Perform pooling. In this case, mean pooling.
sentence_embeddings = mean_pooling(model_output, encoded_input['attention_mask'])
print("Sentence embeddings:")
print(sentence_embeddings)
```
## Evaluation Results
<!--- Describe how your model was evaluated -->
For an automated evaluation of this model, see the *Sentence Embeddings Benchmark*: [https://seb.sbert.net](https://seb.sbert.net?model_name=gemasphi/laprador_f)
## Full Model Architecture
```
SentenceTransformer(
(0): Transformer({'max_seq_length': 350, 'do_lower_case': False}) with Transformer model: BertModel
(1): Pooling({'word_embedding_dimension': 768, 'pooling_mode_cls_token': False, 'pooling_mode_mean_tokens': True, 'pooling_mode_max_tokens': False, 'pooling_mode_mean_sqrt_len_tokens': False})
)
```
## Citing & Authors
<!--- Describe where people can find more information --> |
NadiaSan/udesa-model-aah-es-r-25k | 6a18c4f689fd87c77c8695098d0064e4bf2af17d | 2022-06-17T21:46:41.000Z | [
"pytorch",
"roberta",
"feature-extraction",
"transformers"
] | feature-extraction | false | NadiaSan | null | NadiaSan/udesa-model-aah-es-r-25k | 1 | 1 | transformers | 32,982 | Entry not found |
huggingtweets/datgameryolo | ef136183c1a5d246a7e99761aa5a38f109e412d8 | 2022-06-17T21:54:15.000Z | [
"pytorch",
"gpt2",
"text-generation",
"en",
"transformers",
"huggingtweets"
] | text-generation | false | huggingtweets | null | huggingtweets/datgameryolo | 1 | null | transformers | 32,983 | ---
language: en
thumbnail: https://github.com/borisdayma/huggingtweets/blob/master/img/logo.png?raw=true
tags:
- huggingtweets
widget:
- text: "My dream is"
---
<div class="inline-flex flex-col" style="line-height: 1.5;">
<div class="flex">
<div
style="display:inherit; margin-left: 4px; margin-right: 4px; width: 92px; height:92px; border-radius: 50%; background-size: cover; background-image: url('https://pbs.twimg.com/profile_images/1319790462966837248/wzixCGlX_400x400.jpg')">
</div>
<div
style="display:none; margin-left: 4px; margin-right: 4px; width: 92px; height:92px; border-radius: 50%; background-size: cover; background-image: url('')">
</div>
<div
style="display:none; margin-left: 4px; margin-right: 4px; width: 92px; height:92px; border-radius: 50%; background-size: cover; background-image: url('')">
</div>
</div>
<div style="text-align: center; margin-top: 3px; font-size: 16px; font-weight: 800">🤖 AI BOT 🤖</div>
<div style="text-align: center; font-size: 16px; font-weight: 800">DatGamerYolo</div>
<div style="text-align: center; font-size: 14px;">@datgameryolo</div>
</div>
I was made with [huggingtweets](https://github.com/borisdayma/huggingtweets).
Create your own bot based on your favorite user with [the demo](https://colab.research.google.com/github/borisdayma/huggingtweets/blob/master/huggingtweets-demo.ipynb)!
## How does it work?
The model uses the following pipeline.
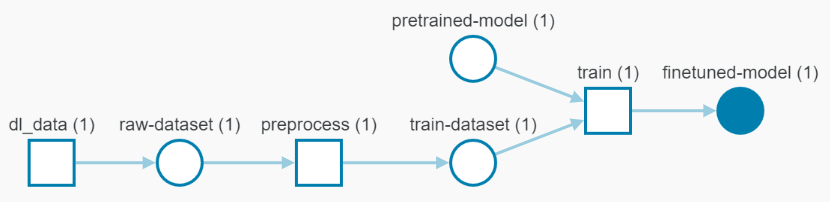
To understand how the model was developed, check the [W&B report](https://wandb.ai/wandb/huggingtweets/reports/HuggingTweets-Train-a-Model-to-Generate-Tweets--VmlldzoxMTY5MjI).
## Training data
The model was trained on tweets from DatGamerYolo.
| Data | DatGamerYolo |
| --- | --- |
| Tweets downloaded | 173 |
| Retweets | 8 |
| Short tweets | 44 |
| Tweets kept | 121 |
[Explore the data](https://wandb.ai/wandb/huggingtweets/runs/wrqoqd8d/artifacts), which is tracked with [W&B artifacts](https://docs.wandb.com/artifacts) at every step of the pipeline.
## Training procedure
The model is based on a pre-trained [GPT-2](https://huggingface.co/gpt2) which is fine-tuned on @datgameryolo's tweets.
Hyperparameters and metrics are recorded in the [W&B training run](https://wandb.ai/wandb/huggingtweets/runs/1h9mtj40) for full transparency and reproducibility.
At the end of training, [the final model](https://wandb.ai/wandb/huggingtweets/runs/1h9mtj40/artifacts) is logged and versioned.
## How to use
You can use this model directly with a pipeline for text generation:
```python
from transformers import pipeline
generator = pipeline('text-generation',
model='huggingtweets/datgameryolo')
generator("My dream is", num_return_sequences=5)
```
## Limitations and bias
The model suffers from [the same limitations and bias as GPT-2](https://huggingface.co/gpt2#limitations-and-bias).
In addition, the data present in the user's tweets further affects the text generated by the model.
## About
*Built by Boris Dayma*
[](https://twitter.com/intent/follow?screen_name=borisdayma)
For more details, visit the project repository.
[](https://github.com/borisdayma/huggingtweets)
|
gary109/ai-light-dance_singing_ft_wav2vec2-large-xlsr-53-5gram-v1 | 7fd58da77ca825df970a0275deecd4aecbbbcbc8 | 2022-06-19T00:31:50.000Z | [
"pytorch",
"tensorboard",
"wav2vec2",
"automatic-speech-recognition",
"transformers",
"gary109/AI_Light_Dance",
"generated_from_trainer",
"model-index"
] | automatic-speech-recognition | false | gary109 | null | gary109/ai-light-dance_singing_ft_wav2vec2-large-xlsr-53-5gram-v1 | 1 | 1 | transformers | 32,984 | ---
tags:
- automatic-speech-recognition
- gary109/AI_Light_Dance
- generated_from_trainer
model-index:
- name: ai-light-dance_singing_ft_wav2vec2-large-xlsr-53-5gram-v1
results: []
---
<!-- This model card has been generated automatically according to the information the Trainer had access to. You
should probably proofread and complete it, then remove this comment. -->
# ai-light-dance_singing_ft_wav2vec2-large-xlsr-53-5gram-v1
This model is a fine-tuned version of [gary109/ai-light-dance_singing_ft_wav2vec2-large-xlsr-53-5gram](https://huggingface.co/gary109/ai-light-dance_singing_ft_wav2vec2-large-xlsr-53-5gram) on the GARY109/AI_LIGHT_DANCE - ONSET-SINGING dataset.
It achieves the following results on the evaluation set:
- Loss: 0.4123
- Wer: 0.1668
## Model description
More information needed
## Intended uses & limitations
More information needed
## Training and evaluation data
More information needed
## Training procedure
### Training hyperparameters
The following hyperparameters were used during training:
- learning_rate: 3e-05
- train_batch_size: 2
- eval_batch_size: 2
- seed: 42
- gradient_accumulation_steps: 16
- total_train_batch_size: 32
- optimizer: Adam with betas=(0.9,0.999) and epsilon=1e-08
- lr_scheduler_type: cosine
- lr_scheduler_warmup_steps: 500
- num_epochs: 10.0
- mixed_precision_training: Native AMP
### Training results
| Training Loss | Epoch | Step | Validation Loss | Wer |
|:-------------:|:-----:|:----:|:---------------:|:------:|
| 0.2696 | 1.0 | 552 | 0.4421 | 0.2013 |
| 0.2498 | 2.0 | 1104 | 0.4389 | 0.1887 |
| 0.2387 | 3.0 | 1656 | 0.4154 | 0.1788 |
| 0.1902 | 4.0 | 2208 | 0.4143 | 0.1753 |
| 0.1896 | 5.0 | 2760 | 0.4123 | 0.1668 |
| 0.1658 | 6.0 | 3312 | 0.4366 | 0.1651 |
| 0.1312 | 7.0 | 3864 | 0.4309 | 0.1594 |
| 0.1186 | 8.0 | 4416 | 0.4432 | 0.1561 |
| 0.1476 | 9.0 | 4968 | 0.4400 | 0.1569 |
| 0.1027 | 10.0 | 5520 | 0.4389 | 0.1554 |
### Framework versions
- Transformers 4.21.0.dev0
- Pytorch 1.9.1+cu102
- Datasets 2.3.3.dev0
- Tokenizers 0.12.1
|
Nadav/xlm-roberta-base-finetuned-on-runaways-nl | 496f09bdc454609e32a9ee2e69797dbf96e28ba4 | 2022-06-18T09:21:01.000Z | [
"pytorch",
"xlm-roberta",
"fill-mask",
"transformers",
"autotrain_compatible"
] | fill-mask | false | Nadav | null | Nadav/xlm-roberta-base-finetuned-on-runaways-nl | 1 | null | transformers | 32,985 | Entry not found |
Nadav/xlm-roberta-base-squad-finetuned-on-runaways-nl | e8cc869c4aeb235f0602d3c0e39d382b5c3d7351 | 2022-06-18T09:35:01.000Z | [
"pytorch",
"xlm-roberta",
"question-answering",
"transformers",
"autotrain_compatible"
] | question-answering | false | Nadav | null | Nadav/xlm-roberta-base-squad-finetuned-on-runaways-nl | 1 | null | transformers | 32,986 | Entry not found |
nestoralvaro/mt5-small-test-amazon | 048612a9d0bc57f8459c26255f7d979b30cd0a35 | 2022-06-18T11:51:27.000Z | [
"pytorch",
"tensorboard",
"mt5",
"text2text-generation",
"transformers",
"summarization",
"generated_from_trainer",
"license:apache-2.0",
"model-index",
"autotrain_compatible"
] | summarization | false | nestoralvaro | null | nestoralvaro/mt5-small-test-amazon | 1 | null | transformers | 32,987 | ---
license: apache-2.0
tags:
- summarization
- generated_from_trainer
metrics:
- rouge
model-index:
- name: mt5-small-test-amazon
results: []
---
<!-- This model card has been generated automatically according to the information the Trainer had access to. You
should probably proofread and complete it, then remove this comment. -->
# mt5-small-test-amazon
This model is a fine-tuned version of [google/mt5-small](https://huggingface.co/google/mt5-small) on an unknown dataset.
It achieves the following results on the evaluation set:
- Loss: 1.9515
- Rouge1: 30.3066
- Rouge2: 3.3019
- Rougel: 30.1887
- Rougelsum: 30.0314
## Model description
More information needed
## Intended uses & limitations
More information needed
## Training and evaluation data
More information needed
## Training procedure
### Training hyperparameters
The following hyperparameters were used during training:
- learning_rate: 5.6e-05
- train_batch_size: 8
- eval_batch_size: 8
- seed: 42
- optimizer: Adam with betas=(0.9,0.999) and epsilon=1e-08
- lr_scheduler_type: linear
- num_epochs: 8
### Training results
| Training Loss | Epoch | Step | Validation Loss | Rouge1 | Rouge2 | Rougel | Rougelsum |
|:-------------:|:-----:|:----:|:---------------:|:-------:|:------:|:-------:|:---------:|
| 10.0147 | 1.0 | 1004 | 2.9904 | 7.3703 | 0.2358 | 7.3703 | 7.4292 |
| 3.4892 | 2.0 | 2008 | 2.4061 | 23.4178 | 2.4764 | 23.2901 | 23.3097 |
| 2.724 | 3.0 | 3012 | 2.1630 | 26.6706 | 2.8302 | 26.6509 | 26.5723 |
| 2.4395 | 4.0 | 4016 | 2.0815 | 26.7296 | 2.9481 | 26.6313 | 26.533 |
| 2.2881 | 5.0 | 5020 | 2.0048 | 30.1887 | 3.3019 | 30.0708 | 29.9135 |
| 2.1946 | 6.0 | 6024 | 1.9712 | 29.4811 | 2.9481 | 29.4025 | 29.3042 |
| 2.1458 | 7.0 | 7028 | 1.9545 | 29.8153 | 3.3019 | 29.717 | 29.5204 |
| 2.1069 | 8.0 | 8032 | 1.9515 | 30.3066 | 3.3019 | 30.1887 | 30.0314 |
### Framework versions
- Transformers 4.20.0
- Pytorch 1.11.0+cu113
- Datasets 2.3.2
- Tokenizers 0.12.1
|
nestoralvaro/mt5-small-test-amazon-v2 | e51192da19b5de9674cd8e980f2ccdc3cd5b2fac | 2022-06-18T13:28:50.000Z | [
"pytorch",
"tensorboard",
"mt5",
"text2text-generation",
"transformers",
"summarization",
"generated_from_trainer",
"license:apache-2.0",
"model-index",
"autotrain_compatible"
] | summarization | false | nestoralvaro | null | nestoralvaro/mt5-small-test-amazon-v2 | 1 | null | transformers | 32,988 | ---
license: apache-2.0
tags:
- summarization
- generated_from_trainer
metrics:
- rouge
model-index:
- name: mt5-small-test-amazon-v2
results: []
---
<!-- This model card has been generated automatically according to the information the Trainer had access to. You
should probably proofread and complete it, then remove this comment. -->
# mt5-small-test-amazon-v2
This model is a fine-tuned version of [google/mt5-small](https://huggingface.co/google/mt5-small) on an unknown dataset.
It achieves the following results on the evaluation set:
- Loss: 2.0555
- Rouge1: 27.8124
- Rouge2: 15.3682
- Rougel: 27.8646
- Rougelsum: 27.9044
## Model description
More information needed
## Intended uses & limitations
More information needed
## Training and evaluation data
More information needed
## Training procedure
### Training hyperparameters
The following hyperparameters were used during training:
- learning_rate: 5.6e-05
- train_batch_size: 8
- eval_batch_size: 8
- seed: 42
- optimizer: Adam with betas=(0.9,0.999) and epsilon=1e-08
- lr_scheduler_type: linear
- num_epochs: 8
### Training results
| Training Loss | Epoch | Step | Validation Loss | Rouge1 | Rouge2 | Rougel | Rougelsum |
|:-------------:|:-----:|:-----:|:---------------:|:-------:|:-------:|:-------:|:---------:|
| 6.2982 | 1.0 | 1935 | 2.7890 | 23.293 | 12.7229 | 23.3183 | 23.3368 |
| 2.9801 | 2.0 | 3870 | 2.4341 | 25.9888 | 14.0833 | 26.07 | 26.0897 |
| 2.5025 | 3.0 | 5805 | 2.2611 | 26.5127 | 14.5775 | 26.5105 | 26.5442 |
| 2.2681 | 4.0 | 7740 | 2.1966 | 27.7476 | 14.9971 | 27.835 | 27.8186 |
| 2.1198 | 5.0 | 9675 | 2.1209 | 27.3796 | 15.1938 | 27.4549 | 27.4759 |
| 2.0089 | 6.0 | 11610 | 2.0856 | 27.6637 | 15.2345 | 27.7419 | 27.7608 |
| 1.9416 | 7.0 | 13545 | 2.0637 | 27.9013 | 15.3682 | 27.9621 | 27.9833 |
| 1.9034 | 8.0 | 15480 | 2.0555 | 27.8124 | 15.3682 | 27.8646 | 27.9044 |
### Framework versions
- Transformers 4.20.0
- Pytorch 1.11.0+cu113
- Datasets 2.3.2
- Tokenizers 0.12.1
|
lmqg/t5-base-subjqa-vanilla-books | deba1ae6667bc99f8f6b14c6ec4424c65816c0aa | 2022-06-18T13:56:44.000Z | [
"pytorch",
"t5",
"text2text-generation",
"transformers",
"autotrain_compatible"
] | text2text-generation | false | lmqg | null | lmqg/t5-base-subjqa-vanilla-books | 1 | null | transformers | 32,989 | Entry not found |
janeel/roberta-base-finetuned-squad | 855f5d44926eebe4dc17bcff5c4816f8c6a911a0 | 2022-06-19T04:32:50.000Z | [
"pytorch",
"tensorboard",
"roberta",
"question-answering",
"dataset:squad_v2",
"transformers",
"generated_from_trainer",
"license:mit",
"model-index",
"autotrain_compatible"
] | question-answering | false | janeel | null | janeel/roberta-base-finetuned-squad | 1 | null | transformers | 32,990 | ---
license: mit
tags:
- generated_from_trainer
datasets:
- squad_v2
model-index:
- name: roberta-base-finetuned-squad
results: []
---
<!-- This model card has been generated automatically according to the information the Trainer had access to. You
should probably proofread and complete it, then remove this comment. -->
# roberta-base-finetuned-squad
This model is a fine-tuned version of [roberta-base](https://huggingface.co/roberta-base) on the squad_v2 dataset.
It achieves the following results on the evaluation set:
- Loss: 0.8556
## Model description
More information needed
## Intended uses & limitations
More information needed
## Training and evaluation data
More information needed
## Training procedure
### Training hyperparameters
The following hyperparameters were used during training:
- learning_rate: 2e-05
- train_batch_size: 16
- eval_batch_size: 16
- seed: 42
- optimizer: Adam with betas=(0.9,0.999) and epsilon=1e-08
- lr_scheduler_type: linear
- num_epochs: 2
### Training results
| Training Loss | Epoch | Step | Validation Loss |
|:-------------:|:-----:|:-----:|:---------------:|
| 0.8678 | 1.0 | 8239 | 0.8014 |
| 0.6423 | 2.0 | 16478 | 0.8556 |
### Framework versions
- Transformers 4.20.0
- Pytorch 1.11.0+cu113
- Datasets 2.3.2
- Tokenizers 0.12.1
|
lmqg/t5-small-subjqa-vanilla-books | e9c77fc653b6b95d68c3c34fb05affc9d2fefe82 | 2022-06-18T14:12:46.000Z | [
"pytorch",
"t5",
"text2text-generation",
"transformers",
"autotrain_compatible"
] | text2text-generation | false | lmqg | null | lmqg/t5-small-subjqa-vanilla-books | 1 | null | transformers | 32,991 | Entry not found |
nestoralvaro/mt5-small-test-ged-RAW_data_prep_2021_12_26___t1_7.csv_max_target_length_10 | 8181d57191af9c14430383fe567fb9a8e3d6fccc | 2022-06-19T13:34:09.000Z | [
"pytorch",
"tensorboard",
"mt5",
"text2text-generation",
"transformers",
"summarization",
"generated_from_trainer",
"license:apache-2.0",
"model-index",
"autotrain_compatible"
] | summarization | false | nestoralvaro | null | nestoralvaro/mt5-small-test-ged-RAW_data_prep_2021_12_26___t1_7.csv_max_target_length_10 | 1 | null | transformers | 32,992 | ---
license: apache-2.0
tags:
- summarization
- generated_from_trainer
metrics:
- rouge
model-index:
- name: mt5-small-test-ged-RAW_data_prep_2021_12_26___t1_7.csv_max_target_length_10
results: []
---
<!-- This model card has been generated automatically according to the information the Trainer had access to. You
should probably proofread and complete it, then remove this comment. -->
# mt5-small-test-ged-RAW_data_prep_2021_12_26___t1_7.csv_max_target_length_10
This model is a fine-tuned version of [google/mt5-small](https://huggingface.co/google/mt5-small) on an unknown dataset.
It achieves the following results on the evaluation set:
- Loss: 2.0338
- Rouge1: 28.7359
- Rouge2: 15.6289
- Rougel: 28.6407
- Rougelsum: 28.7016
## Model description
More information needed
## Intended uses & limitations
More information needed
## Training and evaluation data
More information needed
## Training procedure
### Training hyperparameters
The following hyperparameters were used during training:
- learning_rate: 5.6e-05
- train_batch_size: 8
- eval_batch_size: 8
- seed: 42
- optimizer: Adam with betas=(0.9,0.999) and epsilon=1e-08
- lr_scheduler_type: linear
- num_epochs: 8
### Training results
| Training Loss | Epoch | Step | Validation Loss | Rouge1 | Rouge2 | Rougel | Rougelsum |
|:-------------:|:-----:|:-----:|:---------------:|:-------:|:-------:|:-------:|:---------:|
| 6.0554 | 1.0 | 1935 | 2.7346 | 23.7306 | 13.3598 | 23.7172 | 23.7447 |
| 2.9111 | 2.0 | 3870 | 2.3916 | 26.5211 | 14.5628 | 26.4827 | 26.5716 |
| 2.464 | 3.0 | 5805 | 2.2382 | 27.4404 | 15.1211 | 27.3331 | 27.401 |
| 2.2328 | 4.0 | 7740 | 2.1557 | 28.3377 | 14.7406 | 28.2386 | 28.249 |
| 2.0845 | 5.0 | 9675 | 2.1324 | 29.1476 | 15.7579 | 29.0614 | 29.1701 |
| 1.9825 | 6.0 | 11610 | 2.0668 | 28.4677 | 15.3332 | 28.4128 | 28.4093 |
| 1.9233 | 7.0 | 13545 | 2.0441 | 28.6832 | 15.5251 | 28.5723 | 28.6479 |
| 1.8842 | 8.0 | 15480 | 2.0338 | 28.7359 | 15.6289 | 28.6407 | 28.7016 |
### Framework versions
- Transformers 4.20.0
- Pytorch 1.11.0+cu113
- Datasets 2.3.2
- Tokenizers 0.12.1
|
anibahug/mt5-small-finetuned-amazon-en-de | d80260e66cca53283da0158f03b6f5fd50651fb6 | 2022-06-18T15:39:26.000Z | [
"pytorch",
"tensorboard",
"mt5",
"text2text-generation",
"transformers",
"summarization",
"generated_from_trainer",
"license:apache-2.0",
"model-index",
"autotrain_compatible"
] | summarization | false | anibahug | null | anibahug/mt5-small-finetuned-amazon-en-de | 1 | null | transformers | 32,993 | ---
license: apache-2.0
tags:
- summarization
- generated_from_trainer
metrics:
- rouge
model-index:
- name: mt5-small-finetuned-amazon-en-de
results: []
---
<!-- This model card has been generated automatically according to the information the Trainer had access to. You
should probably proofread and complete it, then remove this comment. -->
# mt5-small-finetuned-amazon-en-de
This model is a fine-tuned version of [google/mt5-small](https://huggingface.co/google/mt5-small) on the [Amazon reviews multi](https://huggingface.co/datasets/amazon_reviews_multi) dataset.
It achieves the following results on the evaluation set:
- Loss: 3.2896
- Rouge1: 14.7163
- Rouge2: 6.6341
- Rougel: 14.2052
- Rougelsum: 14.2318
## Model description
the model can summarize texts for english and deutsch
## Intended uses & limitations
More information needed
## Training and evaluation data
More information needed
## Training procedure
the training was done on google colab ( using it's free GPU)
### Training hyperparameters
The following hyperparameters were used during training:
- learning_rate: 5.6e-05
- train_batch_size: 8
- eval_batch_size: 8
- seed: 42
- optimizer: Adam with betas=(0.9,0.999) and epsilon=1e-08
- lr_scheduler_type: linear
- num_epochs: 8
### Training results
| Training Loss | Epoch | Step | Validation Loss | Rouge1 | Rouge2 | Rougel | Rougelsum |
|:-------------:|:-----:|:-----:|:---------------:|:-------:|:------:|:-------:|:---------:|
| 7.2925 | 1.0 | 1276 | 3.5751 | 13.6254 | 6.0527 | 13.109 | 13.1438 |
| 4.0677 | 2.0 | 2552 | 3.4031 | 13.5907 | 6.068 | 13.3526 | 13.2471 |
| 3.7458 | 3.0 | 3828 | 3.3434 | 14.7229 | 6.8482 | 14.1443 | 14.2218 |
| 3.5831 | 4.0 | 5104 | 3.3353 | 14.8696 | 6.6371 | 14.1342 | 14.2907 |
| 3.4841 | 5.0 | 6380 | 3.3037 | 14.233 | 6.2318 | 13.9218 | 13.9781 |
| 3.4142 | 6.0 | 7656 | 3.2914 | 13.7344 | 5.9446 | 13.5476 | 13.6362 |
| 3.3587 | 7.0 | 8932 | 3.2959 | 14.2007 | 6.1905 | 13.5255 | 13.5237 |
| 3.3448 | 8.0 | 10208 | 3.2896 | 14.7163 | 6.6341 | 14.2052 | 14.2318 |
### Framework versions
- Transformers 4.20.0
- Pytorch 1.11.0+cu113
- Datasets 2.3.2
- Tokenizers 0.12.1
|
Aktsvigun/bert-base-xsum | 17f568f47ecadd544cbef785b79a8efa9108a72a | 2022-06-18T14:36:38.000Z | [
"pytorch",
"bert",
"fill-mask",
"transformers",
"autotrain_compatible"
] | fill-mask | false | Aktsvigun | null | Aktsvigun/bert-base-xsum | 1 | null | transformers | 32,994 | Entry not found |
theojolliffe/bart-cnn-science-v3-e5-v4-e6-manual | 08df59da3475bd7c37d88c144e023e762b0991ee | 2022-06-18T15:08:31.000Z | [
"pytorch",
"tensorboard",
"bart",
"text2text-generation",
"transformers",
"generated_from_trainer",
"license:mit",
"model-index",
"autotrain_compatible"
] | text2text-generation | false | theojolliffe | null | theojolliffe/bart-cnn-science-v3-e5-v4-e6-manual | 1 | null | transformers | 32,995 | ---
license: mit
tags:
- generated_from_trainer
metrics:
- rouge
model-index:
- name: bart-cnn-science-v3-e5-v4-e6-manual
results: []
---
<!-- This model card has been generated automatically according to the information the Trainer had access to. You
should probably proofread and complete it, then remove this comment. -->
# bart-cnn-science-v3-e5-v4-e6-manual
This model is a fine-tuned version of [theojolliffe/bart-cnn-science-v3-e5](https://huggingface.co/theojolliffe/bart-cnn-science-v3-e5) on an unknown dataset.
It achieves the following results on the evaluation set:
- Loss: 0.8609
- Rouge1: 54.0982
- Rouge2: 36.1022
- Rougel: 36.9584
- Rougelsum: 52.5383
- Gen Len: 142.0
## Model description
More information needed
## Intended uses & limitations
More information needed
## Training and evaluation data
More information needed
## Training procedure
### Training hyperparameters
The following hyperparameters were used during training:
- learning_rate: 2e-05
- train_batch_size: 2
- eval_batch_size: 2
- seed: 42
- optimizer: Adam with betas=(0.9,0.999) and epsilon=1e-08
- lr_scheduler_type: linear
- num_epochs: 6
- mixed_precision_training: Native AMP
### Training results
| Training Loss | Epoch | Step | Validation Loss | Rouge1 | Rouge2 | Rougel | Rougelsum | Gen Len |
|:-------------:|:-----:|:----:|:---------------:|:-------:|:-------:|:-------:|:---------:|:-------:|
| No log | 1.0 | 42 | 0.7022 | 56.9307 | 38.2717 | 39.9091 | 53.9037 | 142.0 |
| No log | 2.0 | 84 | 0.6840 | 50.7036 | 29.9002 | 33.0298 | 47.8775 | 142.0 |
| No log | 3.0 | 126 | 0.7179 | 52.8561 | 32.2202 | 35.7914 | 51.0248 | 142.0 |
| No log | 4.0 | 168 | 0.8149 | 54.8457 | 36.4705 | 35.931 | 52.4241 | 142.0 |
| No log | 5.0 | 210 | 0.8330 | 55.6746 | 37.8316 | 36.9614 | 54.3022 | 142.0 |
| No log | 6.0 | 252 | 0.8609 | 54.0982 | 36.1022 | 36.9584 | 52.5383 | 142.0 |
### Framework versions
- Transformers 4.20.0
- Pytorch 1.11.0+cu113
- Datasets 2.3.2
- Tokenizers 0.12.1
|
theojolliffe/bart-cnn-science-v3-e1-v4-e4-manual | c34a75fa85fecd210301a24cdef773f835c730b5 | 2022-06-18T17:13:00.000Z | [
"pytorch",
"tensorboard",
"bart",
"text2text-generation",
"transformers",
"generated_from_trainer",
"license:mit",
"model-index",
"autotrain_compatible"
] | text2text-generation | false | theojolliffe | null | theojolliffe/bart-cnn-science-v3-e1-v4-e4-manual | 1 | null | transformers | 32,996 | ---
license: mit
tags:
- generated_from_trainer
metrics:
- rouge
model-index:
- name: bart-cnn-science-v3-e1-v4-e4-manual
results: []
---
<!-- This model card has been generated automatically according to the information the Trainer had access to. You
should probably proofread and complete it, then remove this comment. -->
# bart-cnn-science-v3-e1-v4-e4-manual
This model is a fine-tuned version of [theojolliffe/bart-cnn-science-v3-e1](https://huggingface.co/theojolliffe/bart-cnn-science-v3-e1) on an unknown dataset.
It achieves the following results on the evaluation set:
- Loss: 1.2615
- Rouge1: 53.36
- Rouge2: 32.0237
- Rougel: 33.2835
- Rougelsum: 50.7455
- Gen Len: 142.0
## Model description
More information needed
## Intended uses & limitations
More information needed
## Training and evaluation data
More information needed
## Training procedure
### Training hyperparameters
The following hyperparameters were used during training:
- learning_rate: 2e-05
- train_batch_size: 2
- eval_batch_size: 2
- seed: 42
- optimizer: Adam with betas=(0.9,0.999) and epsilon=1e-08
- lr_scheduler_type: linear
- num_epochs: 4
- mixed_precision_training: Native AMP
### Training results
| Training Loss | Epoch | Step | Validation Loss | Rouge1 | Rouge2 | Rougel | Rougelsum | Gen Len |
|:-------------:|:-----:|:----:|:---------------:|:-------:|:-------:|:-------:|:---------:|:-------:|
| No log | 1.0 | 42 | 1.0675 | 51.743 | 31.3774 | 34.1939 | 48.7234 | 142.0 |
| No log | 2.0 | 84 | 1.0669 | 49.4166 | 28.1438 | 30.188 | 46.0289 | 142.0 |
| No log | 3.0 | 126 | 1.1799 | 52.6909 | 31.0174 | 35.441 | 50.0351 | 142.0 |
| No log | 4.0 | 168 | 1.2615 | 53.36 | 32.0237 | 33.2835 | 50.7455 | 142.0 |
### Framework versions
- Transformers 4.20.0
- Pytorch 1.11.0+cu113
- Datasets 2.3.2
- Tokenizers 0.12.1
|
theojolliffe/bart-cnn-science-v3-e1-v4-e6-manual | acc39f3fac80b87c4a969fa20df0c4f32de77721 | 2022-06-18T17:37:36.000Z | [
"pytorch",
"tensorboard",
"bart",
"text2text-generation",
"transformers",
"generated_from_trainer",
"license:mit",
"model-index",
"autotrain_compatible"
] | text2text-generation | false | theojolliffe | null | theojolliffe/bart-cnn-science-v3-e1-v4-e6-manual | 1 | null | transformers | 32,997 | ---
license: mit
tags:
- generated_from_trainer
metrics:
- rouge
model-index:
- name: bart-cnn-science-v3-e1-v4-e6-manual
results: []
---
<!-- This model card has been generated automatically according to the information the Trainer had access to. You
should probably proofread and complete it, then remove this comment. -->
# bart-cnn-science-v3-e1-v4-e6-manual
This model is a fine-tuned version of [theojolliffe/bart-cnn-science-v3-e1](https://huggingface.co/theojolliffe/bart-cnn-science-v3-e1) on an unknown dataset.
It achieves the following results on the evaluation set:
- Loss: 1.4513
- Rouge1: 51.4471
- Rouge2: 31.5595
- Rougel: 31.7717
- Rougelsum: 49.4999
- Gen Len: 142.0
## Model description
More information needed
## Intended uses & limitations
More information needed
## Training and evaluation data
More information needed
## Training procedure
### Training hyperparameters
The following hyperparameters were used during training:
- learning_rate: 2e-05
- train_batch_size: 2
- eval_batch_size: 2
- seed: 42
- optimizer: Adam with betas=(0.9,0.999) and epsilon=1e-08
- lr_scheduler_type: linear
- num_epochs: 6
- mixed_precision_training: Native AMP
### Training results
| Training Loss | Epoch | Step | Validation Loss | Rouge1 | Rouge2 | Rougel | Rougelsum | Gen Len |
|:-------------:|:-----:|:----:|:---------------:|:-------:|:-------:|:-------:|:---------:|:-------:|
| No log | 1.0 | 42 | 1.0691 | 51.1883 | 31.2479 | 33.7004 | 48.9571 | 142.0 |
| No log | 2.0 | 84 | 1.0883 | 51.7634 | 29.8573 | 30.7155 | 49.3378 | 142.0 |
| No log | 3.0 | 126 | 1.2355 | 52.9606 | 31.3539 | 33.5131 | 49.9275 | 142.0 |
| No log | 4.0 | 168 | 1.3430 | 52.2108 | 32.7896 | 34.65 | 50.4271 | 139.1 |
| No log | 5.0 | 210 | 1.3963 | 51.5335 | 30.4157 | 31.5759 | 49.6904 | 142.0 |
| No log | 6.0 | 252 | 1.4513 | 51.4471 | 31.5595 | 31.7717 | 49.4999 | 142.0 |
### Framework versions
- Transformers 4.20.0
- Pytorch 1.11.0+cu113
- Datasets 2.3.2
- Tokenizers 0.12.1
|
NadiaSan/udesa-model-aah-es-b-25k | bfcecde3ece414ea8cc32dbc4a499ee6439e9b58 | 2022-06-18T22:25:56.000Z | [
"pytorch",
"bert",
"feature-extraction",
"transformers"
] | feature-extraction | false | NadiaSan | null | NadiaSan/udesa-model-aah-es-b-25k | 1 | null | transformers | 32,998 | Entry not found |
anugunj/omnivore-swinL-in21k | d71828fffd64ae94a6f6d26ab8d547ed45b4eb37 | 2022-06-19T00:18:18.000Z | [
"pytorch",
"omnivore",
"transformers"
] | null | false | anugunj | null | anugunj/omnivore-swinL-in21k | 1 | null | transformers | 32,999 | Entry not found |
Subsets and Splits
No saved queries yet
Save your SQL queries to embed, download, and access them later. Queries will appear here once saved.