modelId
stringlengths 4
112
| sha
stringlengths 40
40
| lastModified
stringlengths 24
24
| tags
sequence | pipeline_tag
stringclasses 29
values | private
bool 1
class | author
stringlengths 2
38
⌀ | config
null | id
stringlengths 4
112
| downloads
float64 0
36.8M
⌀ | likes
float64 0
712
⌀ | library_name
stringclasses 17
values | __index_level_0__
int64 0
38.5k
| readme
stringlengths 0
186k
|
---|---|---|---|---|---|---|---|---|---|---|---|---|---|
huggingtweets/rshowerthoughts-stephenking | 5eb9f6c48d0122235fdcbfb05a39ec28f52005b8 | 2022-06-11T19:50:01.000Z | [
"pytorch",
"gpt2",
"text-generation",
"en",
"transformers",
"huggingtweets"
] | text-generation | false | huggingtweets | null | huggingtweets/rshowerthoughts-stephenking | 1 | null | transformers | 32,800 | ---
language: en
thumbnail: http://www.huggingtweets.com/rshowerthoughts-stephenking/1654976942704/predictions.png
tags:
- huggingtweets
widget:
- text: "My dream is"
---
<div class="inline-flex flex-col" style="line-height: 1.5;">
<div class="flex">
<div
style="display:inherit; margin-left: 4px; margin-right: 4px; width: 92px; height:92px; border-radius: 50%; background-size: cover; background-image: url('https://pbs.twimg.com/profile_images/378800000836981162/b683f7509ec792c3e481ead332940cdc_400x400.jpeg')">
</div>
<div
style="display:inherit; margin-left: 4px; margin-right: 4px; width: 92px; height:92px; border-radius: 50%; background-size: cover; background-image: url('https://pbs.twimg.com/profile_images/641699738224455680/L_ji6ClT_400x400.jpg')">
</div>
<div
style="display:none; margin-left: 4px; margin-right: 4px; width: 92px; height:92px; border-radius: 50%; background-size: cover; background-image: url('')">
</div>
</div>
<div style="text-align: center; margin-top: 3px; font-size: 16px; font-weight: 800">🤖 AI CYBORG 🤖</div>
<div style="text-align: center; font-size: 16px; font-weight: 800">Stephen King & Showerthoughts</div>
<div style="text-align: center; font-size: 14px;">@rshowerthoughts-stephenking</div>
</div>
I was made with [huggingtweets](https://github.com/borisdayma/huggingtweets).
Create your own bot based on your favorite user with [the demo](https://colab.research.google.com/github/borisdayma/huggingtweets/blob/master/huggingtweets-demo.ipynb)!
## How does it work?
The model uses the following pipeline.
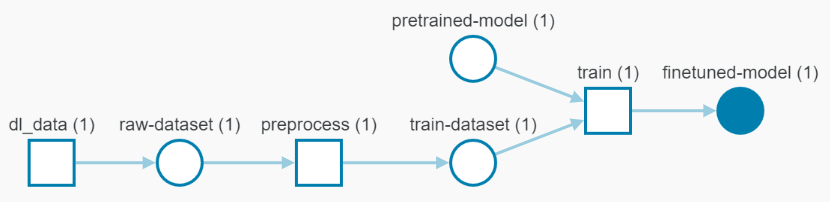
To understand how the model was developed, check the [W&B report](https://wandb.ai/wandb/huggingtweets/reports/HuggingTweets-Train-a-Model-to-Generate-Tweets--VmlldzoxMTY5MjI).
## Training data
The model was trained on tweets from Stephen King & Showerthoughts.
| Data | Stephen King | Showerthoughts |
| --- | --- | --- |
| Tweets downloaded | 3230 | 3200 |
| Retweets | 780 | 0 |
| Short tweets | 202 | 0 |
| Tweets kept | 2248 | 3200 |
[Explore the data](https://wandb.ai/wandb/huggingtweets/runs/2bn3s9yg/artifacts), which is tracked with [W&B artifacts](https://docs.wandb.com/artifacts) at every step of the pipeline.
## Training procedure
The model is based on a pre-trained [GPT-2](https://huggingface.co/gpt2) which is fine-tuned on @rshowerthoughts-stephenking's tweets.
Hyperparameters and metrics are recorded in the [W&B training run](https://wandb.ai/wandb/huggingtweets/runs/2waq2b3w) for full transparency and reproducibility.
At the end of training, [the final model](https://wandb.ai/wandb/huggingtweets/runs/2waq2b3w/artifacts) is logged and versioned.
## How to use
You can use this model directly with a pipeline for text generation:
```python
from transformers import pipeline
generator = pipeline('text-generation',
model='huggingtweets/rshowerthoughts-stephenking')
generator("My dream is", num_return_sequences=5)
```
## Limitations and bias
The model suffers from [the same limitations and bias as GPT-2](https://huggingface.co/gpt2#limitations-and-bias).
In addition, the data present in the user's tweets further affects the text generated by the model.
## About
*Built by Boris Dayma*
[](https://twitter.com/intent/follow?screen_name=borisdayma)
For more details, visit the project repository.
[](https://github.com/borisdayma/huggingtweets)
|
huggingtweets/conanobrien-mikemancini-wendymolyneux | be13ccafa621d3fad60a0f7e9c02b7a351806386 | 2022-06-11T19:50:54.000Z | [
"pytorch",
"gpt2",
"text-generation",
"en",
"transformers",
"huggingtweets"
] | text-generation | false | huggingtweets | null | huggingtweets/conanobrien-mikemancini-wendymolyneux | 1 | null | transformers | 32,801 | ---
language: en
thumbnail: http://www.huggingtweets.com/conanobrien-mikemancini-wendymolyneux/1654977049172/predictions.png
tags:
- huggingtweets
widget:
- text: "My dream is"
---
<div class="inline-flex flex-col" style="line-height: 1.5;">
<div class="flex">
<div
style="display:inherit; margin-left: 4px; margin-right: 4px; width: 92px; height:92px; border-radius: 50%; background-size: cover; background-image: url('https://pbs.twimg.com/profile_images/1271404115042676736/PAIbmN-p_400x400.jpg')">
</div>
<div
style="display:inherit; margin-left: 4px; margin-right: 4px; width: 92px; height:92px; border-radius: 50%; background-size: cover; background-image: url('https://pbs.twimg.com/profile_images/730612231021322240/Rl0_QYhL_400x400.jpg')">
</div>
<div
style="display:inherit; margin-left: 4px; margin-right: 4px; width: 92px; height:92px; border-radius: 50%; background-size: cover; background-image: url('https://pbs.twimg.com/profile_images/1044085580651528193/DR7QvrwG_400x400.jpg')">
</div>
</div>
<div style="text-align: center; margin-top: 3px; font-size: 16px; font-weight: 800">🤖 AI CYBORG 🤖</div>
<div style="text-align: center; font-size: 16px; font-weight: 800">mike mancini & Conan O'Brien & Wendy Molyneux</div>
<div style="text-align: center; font-size: 14px;">@conanobrien-mikemancini-wendymolyneux</div>
</div>
I was made with [huggingtweets](https://github.com/borisdayma/huggingtweets).
Create your own bot based on your favorite user with [the demo](https://colab.research.google.com/github/borisdayma/huggingtweets/blob/master/huggingtweets-demo.ipynb)!
## How does it work?
The model uses the following pipeline.
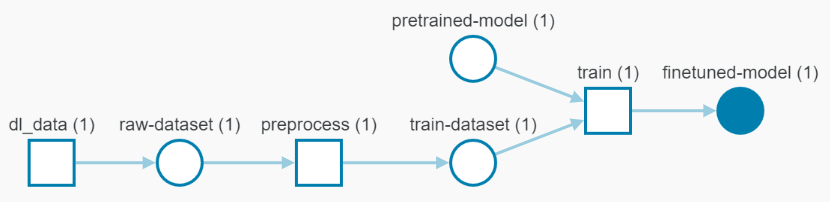
To understand how the model was developed, check the [W&B report](https://wandb.ai/wandb/huggingtweets/reports/HuggingTweets-Train-a-Model-to-Generate-Tweets--VmlldzoxMTY5MjI).
## Training data
The model was trained on tweets from mike mancini & Conan O'Brien & Wendy Molyneux.
| Data | mike mancini | Conan O'Brien | Wendy Molyneux |
| --- | --- | --- | --- |
| Tweets downloaded | 3150 | 3250 | 836 |
| Retweets | 286 | 40 | 251 |
| Short tweets | 290 | 24 | 69 |
| Tweets kept | 2574 | 3186 | 516 |
[Explore the data](https://wandb.ai/wandb/huggingtweets/runs/25wtfzk4/artifacts), which is tracked with [W&B artifacts](https://docs.wandb.com/artifacts) at every step of the pipeline.
## Training procedure
The model is based on a pre-trained [GPT-2](https://huggingface.co/gpt2) which is fine-tuned on @conanobrien-mikemancini-wendymolyneux's tweets.
Hyperparameters and metrics are recorded in the [W&B training run](https://wandb.ai/wandb/huggingtweets/runs/1hjizcue) for full transparency and reproducibility.
At the end of training, [the final model](https://wandb.ai/wandb/huggingtweets/runs/1hjizcue/artifacts) is logged and versioned.
## How to use
You can use this model directly with a pipeline for text generation:
```python
from transformers import pipeline
generator = pipeline('text-generation',
model='huggingtweets/conanobrien-mikemancini-wendymolyneux')
generator("My dream is", num_return_sequences=5)
```
## Limitations and bias
The model suffers from [the same limitations and bias as GPT-2](https://huggingface.co/gpt2#limitations-and-bias).
In addition, the data present in the user's tweets further affects the text generated by the model.
## About
*Built by Boris Dayma*
[](https://twitter.com/intent/follow?screen_name=borisdayma)
For more details, visit the project repository.
[](https://github.com/borisdayma/huggingtweets)
|
huggingtweets/elonmusk-rshowerthoughts-stephenking | 83949a13c1d88fa22b7fcc87b1a7048115bfd288 | 2022-06-11T20:15:51.000Z | [
"pytorch",
"gpt2",
"text-generation",
"en",
"transformers",
"huggingtweets"
] | text-generation | false | huggingtweets | null | huggingtweets/elonmusk-rshowerthoughts-stephenking | 1 | null | transformers | 32,802 | ---
language: en
thumbnail: http://www.huggingtweets.com/elonmusk-rshowerthoughts-stephenking/1654978546952/predictions.png
tags:
- huggingtweets
widget:
- text: "My dream is"
---
<div class="inline-flex flex-col" style="line-height: 1.5;">
<div class="flex">
<div
style="display:inherit; margin-left: 4px; margin-right: 4px; width: 92px; height:92px; border-radius: 50%; background-size: cover; background-image: url('https://pbs.twimg.com/profile_images/1529956155937759233/Nyn1HZWF_400x400.jpg')">
</div>
<div
style="display:inherit; margin-left: 4px; margin-right: 4px; width: 92px; height:92px; border-radius: 50%; background-size: cover; background-image: url('https://pbs.twimg.com/profile_images/378800000836981162/b683f7509ec792c3e481ead332940cdc_400x400.jpeg')">
</div>
<div
style="display:inherit; margin-left: 4px; margin-right: 4px; width: 92px; height:92px; border-radius: 50%; background-size: cover; background-image: url('https://pbs.twimg.com/profile_images/641699738224455680/L_ji6ClT_400x400.jpg')">
</div>
</div>
<div style="text-align: center; margin-top: 3px; font-size: 16px; font-weight: 800">🤖 AI CYBORG 🤖</div>
<div style="text-align: center; font-size: 16px; font-weight: 800">Elon Musk & Stephen King & Showerthoughts</div>
<div style="text-align: center; font-size: 14px;">@elonmusk-rshowerthoughts-stephenking</div>
</div>
I was made with [huggingtweets](https://github.com/borisdayma/huggingtweets).
Create your own bot based on your favorite user with [the demo](https://colab.research.google.com/github/borisdayma/huggingtweets/blob/master/huggingtweets-demo.ipynb)!
## How does it work?
The model uses the following pipeline.
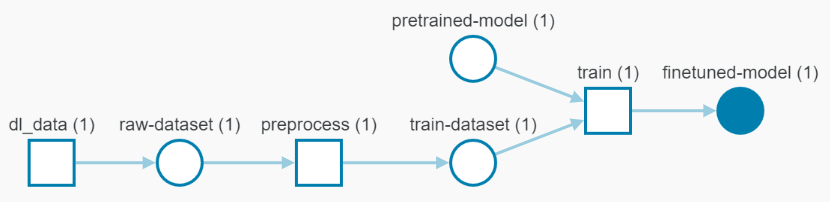
To understand how the model was developed, check the [W&B report](https://wandb.ai/wandb/huggingtweets/reports/HuggingTweets-Train-a-Model-to-Generate-Tweets--VmlldzoxMTY5MjI).
## Training data
The model was trained on tweets from Elon Musk & Stephen King & Showerthoughts.
| Data | Elon Musk | Stephen King | Showerthoughts |
| --- | --- | --- | --- |
| Tweets downloaded | 3200 | 3230 | 3200 |
| Retweets | 147 | 780 | 0 |
| Short tweets | 954 | 202 | 0 |
| Tweets kept | 2099 | 2248 | 3200 |
[Explore the data](https://wandb.ai/wandb/huggingtweets/runs/1fvudd5c/artifacts), which is tracked with [W&B artifacts](https://docs.wandb.com/artifacts) at every step of the pipeline.
## Training procedure
The model is based on a pre-trained [GPT-2](https://huggingface.co/gpt2) which is fine-tuned on @elonmusk-rshowerthoughts-stephenking's tweets.
Hyperparameters and metrics are recorded in the [W&B training run](https://wandb.ai/wandb/huggingtweets/runs/39f9xftz) for full transparency and reproducibility.
At the end of training, [the final model](https://wandb.ai/wandb/huggingtweets/runs/39f9xftz/artifacts) is logged and versioned.
## How to use
You can use this model directly with a pipeline for text generation:
```python
from transformers import pipeline
generator = pipeline('text-generation',
model='huggingtweets/elonmusk-rshowerthoughts-stephenking')
generator("My dream is", num_return_sequences=5)
```
## Limitations and bias
The model suffers from [the same limitations and bias as GPT-2](https://huggingface.co/gpt2#limitations-and-bias).
In addition, the data present in the user's tweets further affects the text generated by the model.
## About
*Built by Boris Dayma*
[](https://twitter.com/intent/follow?screen_name=borisdayma)
For more details, visit the project repository.
[](https://github.com/borisdayma/huggingtweets)
|
meghazisofiane/opus-mt-en-ar-evaluated-en-to-ar-4000instances-opus-leaningRate2e-05-batchSize8-11-action-1 | cfd3f4b6e59d7e7305ed6331c2659091568ab48c | 2022-06-11T21:50:40.000Z | [
"pytorch",
"tensorboard",
"marian",
"text2text-generation",
"dataset:opus100",
"transformers",
"generated_from_trainer",
"license:apache-2.0",
"model-index",
"autotrain_compatible"
] | text2text-generation | false | meghazisofiane | null | meghazisofiane/opus-mt-en-ar-evaluated-en-to-ar-4000instances-opus-leaningRate2e-05-batchSize8-11-action-1 | 1 | null | transformers | 32,803 | ---
license: apache-2.0
tags:
- generated_from_trainer
datasets:
- opus100
metrics:
- bleu
model-index:
- name: opus-mt-en-ar-evaluated-en-to-ar-4000instances-opus-leaningRate2e-05-batchSize8-11-action-1
results:
- task:
name: Sequence-to-sequence Language Modeling
type: text2text-generation
dataset:
name: opus100
type: opus100
args: ar-en
metrics:
- name: Bleu
type: bleu
value: 26.8232
---
<!-- This model card has been generated automatically according to the information the Trainer had access to. You
should probably proofread and complete it, then remove this comment. -->
# opus-mt-en-ar-evaluated-en-to-ar-4000instances-opus-leaningRate2e-05-batchSize8-11-action-1
This model is a fine-tuned version of [Helsinki-NLP/opus-mt-en-ar](https://huggingface.co/Helsinki-NLP/opus-mt-en-ar) on the opus100 dataset.
It achieves the following results on the evaluation set:
- Loss: 0.1717
- Bleu: 26.8232
- Meteor: 0.172
- Gen Len: 12.1288
## Model description
More information needed
## Intended uses & limitations
More information needed
## Training and evaluation data
More information needed
## Training procedure
### Training hyperparameters
The following hyperparameters were used during training:
- learning_rate: 2e-05
- train_batch_size: 8
- eval_batch_size: 8
- seed: 42
- optimizer: Adam with betas=(0.9,0.999) and epsilon=1e-08
- lr_scheduler_type: linear
- num_epochs: 11
### Training results
| Training Loss | Epoch | Step | Validation Loss | Bleu | Meteor | Gen Len |
|:-------------:|:-----:|:----:|:---------------:|:-------:|:------:|:-------:|
| 0.7364 | 0.25 | 100 | 0.1731 | 27.2753 | 0.1729 | 12.0887 |
| 0.2175 | 0.5 | 200 | 0.1731 | 27.2055 | 0.1722 | 11.5675 |
| 0.2193 | 0.75 | 300 | 0.1722 | 27.3277 | 0.1798 | 12.1325 |
| 0.2321 | 1.0 | 400 | 0.1750 | 27.5152 | 0.1762 | 11.925 |
| 0.1915 | 1.25 | 500 | 0.1690 | 27.5043 | 0.1751 | 11.9038 |
| 0.1794 | 1.5 | 600 | 0.1719 | 26.8607 | 0.1713 | 11.8138 |
| 0.1741 | 1.75 | 700 | 0.1725 | 26.974 | 0.1724 | 11.8462 |
| 0.1732 | 2.0 | 800 | 0.1717 | 26.8232 | 0.172 | 12.1288 |
### Framework versions
- Transformers 4.18.0
- Pytorch 1.11.0
- Datasets 2.1.0
- Tokenizers 0.12.1
|
meghazisofiane/opus-mt-en-ar-evaluated-en-to-ar-4000instances-un_multi-leaningRate2e-05-batchSize8-11-action-1 | 5a24a5f03b9f9bcc016566271f9656ed7762beb2 | 2022-06-11T23:49:23.000Z | [
"pytorch",
"tensorboard",
"marian",
"text2text-generation",
"dataset:un_multi",
"transformers",
"generated_from_trainer",
"license:apache-2.0",
"model-index",
"autotrain_compatible"
] | text2text-generation | false | meghazisofiane | null | meghazisofiane/opus-mt-en-ar-evaluated-en-to-ar-4000instances-un_multi-leaningRate2e-05-batchSize8-11-action-1 | 1 | null | transformers | 32,804 | ---
license: apache-2.0
tags:
- generated_from_trainer
datasets:
- un_multi
metrics:
- bleu
model-index:
- name: opus-mt-en-ar-evaluated-en-to-ar-4000instances-un_multi-leaningRate2e-05-batchSize8-11-action-1
results:
- task:
name: Sequence-to-sequence Language Modeling
type: text2text-generation
dataset:
name: un_multi
type: un_multi
args: ar-en
metrics:
- name: Bleu
type: bleu
value: 51.7715
---
<!-- This model card has been generated automatically according to the information the Trainer had access to. You
should probably proofread and complete it, then remove this comment. -->
# opus-mt-en-ar-evaluated-en-to-ar-4000instances-un_multi-leaningRate2e-05-batchSize8-11-action-1
This model is a fine-tuned version of [Helsinki-NLP/opus-mt-en-ar](https://huggingface.co/Helsinki-NLP/opus-mt-en-ar) on the un_multi dataset.
It achieves the following results on the evaluation set:
- Loss: 0.1850
- Bleu: 51.7715
- Meteor: 0.5164
- Gen Len: 25.5612
## Model description
More information needed
## Intended uses & limitations
More information needed
## Training and evaluation data
More information needed
## Training procedure
### Training hyperparameters
The following hyperparameters were used during training:
- learning_rate: 2e-05
- train_batch_size: 8
- eval_batch_size: 8
- seed: 42
- optimizer: Adam with betas=(0.9,0.999) and epsilon=1e-08
- lr_scheduler_type: linear
- num_epochs: 11
### Training results
| Training Loss | Epoch | Step | Validation Loss | Bleu | Meteor | Gen Len |
|:-------------:|:-----:|:----:|:---------------:|:-------:|:------:|:-------:|
| 0.6999 | 0.25 | 100 | 0.1959 | 50.1492 | 0.508 | 25.2788 |
| 0.1994 | 0.5 | 200 | 0.1931 | 51.003 | 0.513 | 25.4038 |
| 0.1863 | 0.75 | 300 | 0.1864 | 51.3268 | 0.5145 | 25.1675 |
| 0.1826 | 1.0 | 400 | 0.1841 | 51.2507 | 0.513 | 25.2388 |
| 0.1494 | 1.25 | 500 | 0.1840 | 51.4291 | 0.5159 | 25.4225 |
| 0.1483 | 1.5 | 600 | 0.1839 | 51.2645 | 0.5126 | 25.395 |
| 0.1547 | 1.75 | 700 | 0.1837 | 51.7589 | 0.5157 | 25.48 |
| 0.1487 | 2.0 | 800 | 0.1845 | 51.896 | 0.5177 | 25.3988 |
| 0.1235 | 2.25 | 900 | 0.1852 | 52.0583 | 0.5177 | 25.5212 |
| 0.1164 | 2.5 | 1000 | 0.1850 | 51.7715 | 0.5164 | 25.5612 |
### Framework versions
- Transformers 4.18.0
- Pytorch 1.11.0
- Datasets 2.1.0
- Tokenizers 0.12.1
|
AntoDono/DialoGPT-Bopy-Alpha | ace508d9a01179df177c0a1eb62a0e62e872447c | 2022-06-11T22:22:35.000Z | [
"pytorch",
"gpt2",
"text-generation",
"transformers"
] | text-generation | false | AntoDono | null | AntoDono/DialoGPT-Bopy-Alpha | 1 | null | transformers | 32,805 | Entry not found |
sactisudesa/robertuito_sp | 8a5291a24ad459b2922dc3c2e1f80472ca3164bc | 2022-06-11T22:49:32.000Z | [
"pytorch",
"roberta",
"feature-extraction",
"transformers"
] | feature-extraction | false | sactisudesa | null | sactisudesa/robertuito_sp | 1 | null | transformers | 32,806 | Entry not found |
huggingtweets/laserboat999 | 173e2d6c04effe0a5009e3ad155e3f45050a6303 | 2022-06-11T23:53:52.000Z | [
"pytorch",
"gpt2",
"text-generation",
"en",
"transformers",
"huggingtweets"
] | text-generation | false | huggingtweets | null | huggingtweets/laserboat999 | 1 | null | transformers | 32,807 | ---
language: en
thumbnail: http://www.huggingtweets.com/laserboat999/1654991516445/predictions.png
tags:
- huggingtweets
widget:
- text: "My dream is"
---
<div class="inline-flex flex-col" style="line-height: 1.5;">
<div class="flex">
<div
style="display:inherit; margin-left: 4px; margin-right: 4px; width: 92px; height:92px; border-radius: 50%; background-size: cover; background-image: url('https://pbs.twimg.com/profile_images/1500274766195793921/bA4siut7_400x400.jpg')">
</div>
<div
style="display:none; margin-left: 4px; margin-right: 4px; width: 92px; height:92px; border-radius: 50%; background-size: cover; background-image: url('')">
</div>
<div
style="display:none; margin-left: 4px; margin-right: 4px; width: 92px; height:92px; border-radius: 50%; background-size: cover; background-image: url('')">
</div>
</div>
<div style="text-align: center; margin-top: 3px; font-size: 16px; font-weight: 800">🤖 AI BOT 🤖</div>
<div style="text-align: center; font-size: 16px; font-weight: 800">donald boat</div>
<div style="text-align: center; font-size: 14px;">@laserboat999</div>
</div>
I was made with [huggingtweets](https://github.com/borisdayma/huggingtweets).
Create your own bot based on your favorite user with [the demo](https://colab.research.google.com/github/borisdayma/huggingtweets/blob/master/huggingtweets-demo.ipynb)!
## How does it work?
The model uses the following pipeline.
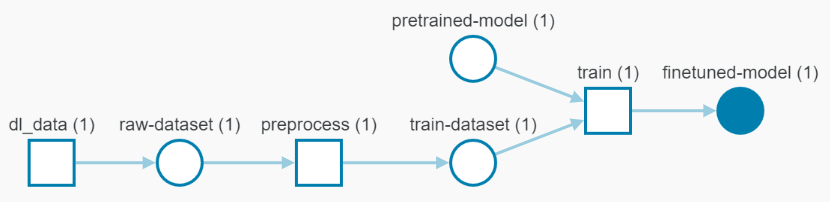
To understand how the model was developed, check the [W&B report](https://wandb.ai/wandb/huggingtweets/reports/HuggingTweets-Train-a-Model-to-Generate-Tweets--VmlldzoxMTY5MjI).
## Training data
The model was trained on tweets from donald boat.
| Data | donald boat |
| --- | --- |
| Tweets downloaded | 3233 |
| Retweets | 75 |
| Short tweets | 516 |
| Tweets kept | 2642 |
[Explore the data](https://wandb.ai/wandb/huggingtweets/runs/38v40fpf/artifacts), which is tracked with [W&B artifacts](https://docs.wandb.com/artifacts) at every step of the pipeline.
## Training procedure
The model is based on a pre-trained [GPT-2](https://huggingface.co/gpt2) which is fine-tuned on @laserboat999's tweets.
Hyperparameters and metrics are recorded in the [W&B training run](https://wandb.ai/wandb/huggingtweets/runs/pk1xum9h) for full transparency and reproducibility.
At the end of training, [the final model](https://wandb.ai/wandb/huggingtweets/runs/pk1xum9h/artifacts) is logged and versioned.
## How to use
You can use this model directly with a pipeline for text generation:
```python
from transformers import pipeline
generator = pipeline('text-generation',
model='huggingtweets/laserboat999')
generator("My dream is", num_return_sequences=5)
```
## Limitations and bias
The model suffers from [the same limitations and bias as GPT-2](https://huggingface.co/gpt2#limitations-and-bias).
In addition, the data present in the user's tweets further affects the text generated by the model.
## About
*Built by Boris Dayma*
[](https://twitter.com/intent/follow?screen_name=borisdayma)
For more details, visit the project repository.
[](https://github.com/borisdayma/huggingtweets)
|
huggingtweets/cancer_blood69 | 838a404be0bcf0e261091db78c493548896c56da | 2022-06-12T00:01:54.000Z | [
"pytorch",
"gpt2",
"text-generation",
"en",
"transformers",
"huggingtweets"
] | text-generation | false | huggingtweets | null | huggingtweets/cancer_blood69 | 1 | null | transformers | 32,808 | ---
language: en
thumbnail: http://www.huggingtweets.com/cancer_blood69/1654992058711/predictions.png
tags:
- huggingtweets
widget:
- text: "My dream is"
---
<div class="inline-flex flex-col" style="line-height: 1.5;">
<div class="flex">
<div
style="display:inherit; margin-left: 4px; margin-right: 4px; width: 92px; height:92px; border-radius: 50%; background-size: cover; background-image: url('https://pbs.twimg.com/profile_images/1273429972229804032/_kkJmwqw_400x400.jpg')">
</div>
<div
style="display:none; margin-left: 4px; margin-right: 4px; width: 92px; height:92px; border-radius: 50%; background-size: cover; background-image: url('')">
</div>
<div
style="display:none; margin-left: 4px; margin-right: 4px; width: 92px; height:92px; border-radius: 50%; background-size: cover; background-image: url('')">
</div>
</div>
<div style="text-align: center; margin-top: 3px; font-size: 16px; font-weight: 800">🤖 AI BOT 🤖</div>
<div style="text-align: center; font-size: 16px; font-weight: 800">cancer_blood69 (reanimated decaying corpse)</div>
<div style="text-align: center; font-size: 14px;">@cancer_blood69</div>
</div>
I was made with [huggingtweets](https://github.com/borisdayma/huggingtweets).
Create your own bot based on your favorite user with [the demo](https://colab.research.google.com/github/borisdayma/huggingtweets/blob/master/huggingtweets-demo.ipynb)!
## How does it work?
The model uses the following pipeline.
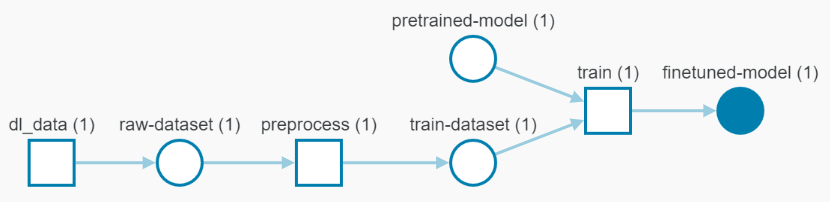
To understand how the model was developed, check the [W&B report](https://wandb.ai/wandb/huggingtweets/reports/HuggingTweets-Train-a-Model-to-Generate-Tweets--VmlldzoxMTY5MjI).
## Training data
The model was trained on tweets from cancer_blood69 (reanimated decaying corpse).
| Data | cancer_blood69 (reanimated decaying corpse) |
| --- | --- |
| Tweets downloaded | 3237 |
| Retweets | 215 |
| Short tweets | 381 |
| Tweets kept | 2641 |
[Explore the data](https://wandb.ai/wandb/huggingtweets/runs/3cav70ew/artifacts), which is tracked with [W&B artifacts](https://docs.wandb.com/artifacts) at every step of the pipeline.
## Training procedure
The model is based on a pre-trained [GPT-2](https://huggingface.co/gpt2) which is fine-tuned on @cancer_blood69's tweets.
Hyperparameters and metrics are recorded in the [W&B training run](https://wandb.ai/wandb/huggingtweets/runs/sp5449e2) for full transparency and reproducibility.
At the end of training, [the final model](https://wandb.ai/wandb/huggingtweets/runs/sp5449e2/artifacts) is logged and versioned.
## How to use
You can use this model directly with a pipeline for text generation:
```python
from transformers import pipeline
generator = pipeline('text-generation',
model='huggingtweets/cancer_blood69')
generator("My dream is", num_return_sequences=5)
```
## Limitations and bias
The model suffers from [the same limitations and bias as GPT-2](https://huggingface.co/gpt2#limitations-and-bias).
In addition, the data present in the user's tweets further affects the text generated by the model.
## About
*Built by Boris Dayma*
[](https://twitter.com/intent/follow?screen_name=borisdayma)
For more details, visit the project repository.
[](https://github.com/borisdayma/huggingtweets)
|
lindsayng/t5-base-fullwnc-5epoch-31e6b1e1 | 7f26b3f69b6aebbb8bedc9b47f8aed64593cea23 | 2022-06-12T00:08:18.000Z | [
"pytorch",
"t5",
"text2text-generation",
"transformers",
"autotrain_compatible"
] | text2text-generation | false | lindsayng | null | lindsayng/t5-base-fullwnc-5epoch-31e6b1e1 | 1 | null | transformers | 32,809 | Entry not found |
meghazisofiane/opus-mt-en-ar-evaluated-en-to-ar-2000instances-un_multi-leaningRate2e-05-batchSize8-11-action-1 | 3b0aee150bf11b5aa90a7ed16e00cc844974491b | 2022-06-12T00:44:37.000Z | [
"pytorch",
"tensorboard",
"marian",
"text2text-generation",
"dataset:un_multi",
"transformers",
"generated_from_trainer",
"license:apache-2.0",
"model-index",
"autotrain_compatible"
] | text2text-generation | false | meghazisofiane | null | meghazisofiane/opus-mt-en-ar-evaluated-en-to-ar-2000instances-un_multi-leaningRate2e-05-batchSize8-11-action-1 | 1 | null | transformers | 32,810 | ---
license: apache-2.0
tags:
- generated_from_trainer
datasets:
- un_multi
metrics:
- bleu
model-index:
- name: opus-mt-en-ar-evaluated-en-to-ar-2000instances-un_multi-leaningRate2e-05-batchSize8-11-action-1
results:
- task:
name: Sequence-to-sequence Language Modeling
type: text2text-generation
dataset:
name: un_multi
type: un_multi
args: ar-en
metrics:
- name: Bleu
type: bleu
value: 53.0137
---
<!-- This model card has been generated automatically according to the information the Trainer had access to. You
should probably proofread and complete it, then remove this comment. -->
# opus-mt-en-ar-evaluated-en-to-ar-2000instances-un_multi-leaningRate2e-05-batchSize8-11-action-1
This model is a fine-tuned version of [Helsinki-NLP/opus-mt-en-ar](https://huggingface.co/Helsinki-NLP/opus-mt-en-ar) on the un_multi dataset.
It achieves the following results on the evaluation set:
- Loss: 0.1873
- Bleu: 53.0137
- Meteor: 0.5005
- Gen Len: 25.845
## Model description
More information needed
## Intended uses & limitations
More information needed
## Training and evaluation data
More information needed
## Training procedure
### Training hyperparameters
The following hyperparameters were used during training:
- learning_rate: 2e-05
- train_batch_size: 8
- eval_batch_size: 8
- seed: 42
- optimizer: Adam with betas=(0.9,0.999) and epsilon=1e-08
- lr_scheduler_type: linear
- num_epochs: 11
### Training results
| Training Loss | Epoch | Step | Validation Loss | Bleu | Meteor | Gen Len |
|:-------------:|:-----:|:----:|:---------------:|:-------:|:------:|:-------:|
| 0.6585 | 0.5 | 100 | 0.2085 | 52.5874 | 0.4969 | 25.485 |
| 0.1802 | 1.0 | 200 | 0.1788 | 52.9434 | 0.4982 | 25.1725 |
| 0.1501 | 1.5 | 300 | 0.1683 | 53.6994 | 0.5033 | 25.625 |
| 0.1454 | 2.0 | 400 | 0.1706 | 53.3946 | 0.5005 | 25.6675 |
| 0.1193 | 2.5 | 500 | 0.1774 | 53.2011 | 0.4982 | 25.58 |
| 0.1194 | 3.0 | 600 | 0.1741 | 53.8651 | 0.5026 | 25.5775 |
| 0.1002 | 3.5 | 700 | 0.1878 | 53.1332 | 0.5005 | 25.8975 |
| 0.0979 | 4.0 | 800 | 0.1881 | 52.5989 | 0.4974 | 25.485 |
| 0.0807 | 4.5 | 900 | 0.1873 | 53.0137 | 0.5005 | 25.845 |
### Framework versions
- Transformers 4.18.0
- Pytorch 1.11.0
- Datasets 2.1.0
- Tokenizers 0.12.1
|
erickfm/t5-base-finetuned-bias-sweep-46c8cda7 | d1a6bff4dc1c61bd32292c3768268a808dca36be | 2022-06-12T00:41:28.000Z | [
"pytorch",
"t5",
"text2text-generation",
"transformers",
"autotrain_compatible"
] | text2text-generation | false | erickfm | null | erickfm/t5-base-finetuned-bias-sweep-46c8cda7 | 1 | null | transformers | 32,811 | Entry not found |
erickfm/t5-base-finetuned-bias-sweep-cca75a85 | d3def8abc8127be7cbaa0b2bbf25c2b83130b353 | 2022-06-12T07:03:32.000Z | [
"pytorch",
"t5",
"text2text-generation",
"transformers",
"autotrain_compatible"
] | text2text-generation | false | erickfm | null | erickfm/t5-base-finetuned-bias-sweep-cca75a85 | 1 | null | transformers | 32,812 | Entry not found |
Dwayne/opus-mt-en-ro-finetuned-en-to-ro | ad7e33786ba93897bab03fd7553b6ef367b49deb | 2022-06-12T19:07:18.000Z | [
"pytorch",
"marian",
"text2text-generation",
"dataset:wmt16",
"transformers",
"generated_from_trainer",
"license:apache-2.0",
"model-index",
"autotrain_compatible"
] | text2text-generation | false | Dwayne | null | Dwayne/opus-mt-en-ro-finetuned-en-to-ro | 1 | null | transformers | 32,813 | ---
license: apache-2.0
tags:
- generated_from_trainer
datasets:
- wmt16
metrics:
- bleu
model-index:
- name: opus-mt-en-ro-finetuned-en-to-ro
results:
- task:
name: Sequence-to-sequence Language Modeling
type: text2text-generation
dataset:
name: wmt16
type: wmt16
args: ro-en
metrics:
- name: Bleu
type: bleu
value: 28.0591
---
<!-- This model card has been generated automatically according to the information the Trainer had access to. You
should probably proofread and complete it, then remove this comment. -->
# opus-mt-en-ro-finetuned-en-to-ro
This model is a fine-tuned version of [Helsinki-NLP/opus-mt-en-ro](https://huggingface.co/Helsinki-NLP/opus-mt-en-ro) on the wmt16 dataset.
It achieves the following results on the evaluation set:
- Loss: 1.2889
- Bleu: 28.0591
- Gen Len: 34.043
## Model description
More information needed
## Intended uses & limitations
More information needed
## Training and evaluation data
More information needed
## Training procedure
### Training hyperparameters
The following hyperparameters were used during training:
- learning_rate: 2e-05
- train_batch_size: 16
- eval_batch_size: 16
- seed: 42
- optimizer: Adam with betas=(0.9,0.999) and epsilon=1e-08
- lr_scheduler_type: linear
- num_epochs: 1
- mixed_precision_training: Native AMP
### Training results
| Training Loss | Epoch | Step | Validation Loss | Bleu | Gen Len |
|:-------------:|:-----:|:-----:|:---------------:|:-------:|:-------:|
| 0.744 | 1.0 | 38145 | 1.2889 | 28.0591 | 34.043 |
### Framework versions
- Transformers 4.19.4
- Pytorch 1.11.0+cu113
- Datasets 2.2.2
- Tokenizers 0.12.1
|
hangyulmd/t5-squad | 2202943b672a4890d619944d93bf40ec93a1309d | 2022-06-12T16:00:52.000Z | [
"pytorch",
"tensorboard",
"t5",
"text2text-generation",
"transformers",
"autotrain_compatible"
] | text2text-generation | false | hangyulmd | null | hangyulmd/t5-squad | 1 | null | transformers | 32,814 | Entry not found |
nestoralvaro/mt5-base-finetuned-xsum-RAW_data_prep_2021_12_26___t22027_162754.csv__google_mt5_base | 35cbc15c4aad6980d834379c775208afbdbb116c | 2022-06-12T18:08:46.000Z | [
"pytorch",
"tensorboard",
"mt5",
"text2text-generation",
"transformers",
"generated_from_trainer",
"license:apache-2.0",
"model-index",
"autotrain_compatible"
] | text2text-generation | false | nestoralvaro | null | nestoralvaro/mt5-base-finetuned-xsum-RAW_data_prep_2021_12_26___t22027_162754.csv__google_mt5_base | 1 | null | transformers | 32,815 | ---
license: apache-2.0
tags:
- generated_from_trainer
metrics:
- rouge
model-index:
- name: mt5-base-finetuned-xsum-RAW_data_prep_2021_12_26___t22027_162754.csv__google_mt5_base
results: []
---
<!-- This model card has been generated automatically according to the information the Trainer had access to. You
should probably proofread and complete it, then remove this comment. -->
# mt5-base-finetuned-xsum-RAW_data_prep_2021_12_26___t22027_162754.csv__google_mt5_base
This model is a fine-tuned version of [google/mt5-base](https://huggingface.co/google/mt5-base) on an unknown dataset.
It achieves the following results on the evaluation set:
- Loss: nan
- Rouge1: 0.7721
- Rouge2: 0.0701
- Rougel: 0.7721
- Rougelsum: 0.7718
- Gen Len: 6.329
## Model description
More information needed
## Intended uses & limitations
More information needed
## Training and evaluation data
More information needed
## Training procedure
### Training hyperparameters
The following hyperparameters were used during training:
- learning_rate: 2e-05
- train_batch_size: 4
- eval_batch_size: 4
- seed: 42
- optimizer: Adam with betas=(0.9,0.999) and epsilon=1e-08
- lr_scheduler_type: linear
- num_epochs: 1
- mixed_precision_training: Native AMP
### Training results
| Training Loss | Epoch | Step | Validation Loss | Rouge1 | Rouge2 | Rougel | Rougelsum | Gen Len |
|:-------------:|:-----:|:------:|:---------------:|:------:|:------:|:------:|:---------:|:-------:|
| 0.0 | 1.0 | 131773 | nan | 0.7721 | 0.0701 | 0.7721 | 0.7718 | 6.329 |
### Framework versions
- Transformers 4.19.4
- Pytorch 1.11.0+cu113
- Datasets 2.2.2
- Tokenizers 0.12.1
|
huggingtweets/warriors | 5dc3f3c81fa9d2bc05897123051140c4d59f5bc3 | 2022-06-12T15:38:14.000Z | [
"pytorch",
"gpt2",
"text-generation",
"en",
"transformers",
"huggingtweets"
] | text-generation | false | huggingtweets | null | huggingtweets/warriors | 1 | null | transformers | 32,816 | ---
language: en
thumbnail: http://www.huggingtweets.com/warriors/1655048290751/predictions.png
tags:
- huggingtweets
widget:
- text: "My dream is"
---
<div class="inline-flex flex-col" style="line-height: 1.5;">
<div class="flex">
<div
style="display:inherit; margin-left: 4px; margin-right: 4px; width: 92px; height:92px; border-radius: 50%; background-size: cover; background-image: url('https://pbs.twimg.com/profile_images/1533845175725719553/yvzbj8iG_400x400.jpg')">
</div>
<div
style="display:none; margin-left: 4px; margin-right: 4px; width: 92px; height:92px; border-radius: 50%; background-size: cover; background-image: url('')">
</div>
<div
style="display:none; margin-left: 4px; margin-right: 4px; width: 92px; height:92px; border-radius: 50%; background-size: cover; background-image: url('')">
</div>
</div>
<div style="text-align: center; margin-top: 3px; font-size: 16px; font-weight: 800">🤖 AI BOT 🤖</div>
<div style="text-align: center; font-size: 16px; font-weight: 800">Golden State Warriors</div>
<div style="text-align: center; font-size: 14px;">@warriors</div>
</div>
I was made with [huggingtweets](https://github.com/borisdayma/huggingtweets).
Create your own bot based on your favorite user with [the demo](https://colab.research.google.com/github/borisdayma/huggingtweets/blob/master/huggingtweets-demo.ipynb)!
## How does it work?
The model uses the following pipeline.
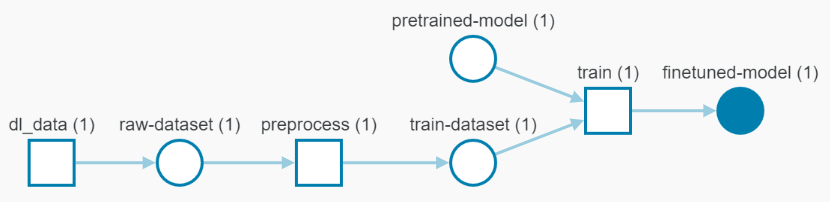
To understand how the model was developed, check the [W&B report](https://wandb.ai/wandb/huggingtweets/reports/HuggingTweets-Train-a-Model-to-Generate-Tweets--VmlldzoxMTY5MjI).
## Training data
The model was trained on tweets from Golden State Warriors.
| Data | Golden State Warriors |
| --- | --- |
| Tweets downloaded | 3251 |
| Retweets | 261 |
| Short tweets | 563 |
| Tweets kept | 2427 |
[Explore the data](https://wandb.ai/wandb/huggingtweets/runs/36p28s9n/artifacts), which is tracked with [W&B artifacts](https://docs.wandb.com/artifacts) at every step of the pipeline.
## Training procedure
The model is based on a pre-trained [GPT-2](https://huggingface.co/gpt2) which is fine-tuned on @warriors's tweets.
Hyperparameters and metrics are recorded in the [W&B training run](https://wandb.ai/wandb/huggingtweets/runs/17arirrx) for full transparency and reproducibility.
At the end of training, [the final model](https://wandb.ai/wandb/huggingtweets/runs/17arirrx/artifacts) is logged and versioned.
## How to use
You can use this model directly with a pipeline for text generation:
```python
from transformers import pipeline
generator = pipeline('text-generation',
model='huggingtweets/warriors')
generator("My dream is", num_return_sequences=5)
```
## Limitations and bias
The model suffers from [the same limitations and bias as GPT-2](https://huggingface.co/gpt2#limitations-and-bias).
In addition, the data present in the user's tweets further affects the text generated by the model.
## About
*Built by Boris Dayma*
[](https://twitter.com/intent/follow?screen_name=borisdayma)
For more details, visit the project repository.
[](https://github.com/borisdayma/huggingtweets)
|
eunbeee/ainize-kobart-news-eb-finetuned-papers | 479667425e72635e2d55368e2b5c33ec3ae85822 | 2022-06-16T12:07:21.000Z | [
"pytorch",
"tensorboard",
"bart",
"text2text-generation",
"transformers",
"generated_from_trainer",
"license:mit",
"model-index",
"autotrain_compatible"
] | text2text-generation | false | eunbeee | null | eunbeee/ainize-kobart-news-eb-finetuned-papers | 1 | null | transformers | 32,817 | ---
license: mit
tags:
- generated_from_trainer
metrics:
- rouge
model-index:
- name: ainize-kobart-news-eb-finetuned-papers
results: []
---
<!-- This model card has been generated automatically according to the information the Trainer had access to. You
should probably proofread and complete it, then remove this comment. -->
# ainize-kobart-news-eb-finetuned-papers
This model is a fine-tuned version of [ainize/kobart-news](https://huggingface.co/ainize/kobart-news) on the None dataset.
It achieves the following results on the evaluation set:
- Loss: 0.3066
- Rouge1: 14.5433
- Rouge2: 5.2238
- Rougel: 14.4731
- Rougelsum: 14.5183
- Gen Len: 19.9934
## Model description
More information needed
## Intended uses & limitations
More information needed
## Training and evaluation data
More information needed
## Training procedure
### Training hyperparameters
The following hyperparameters were used during training:
- learning_rate: 2e-05
- train_batch_size: 4
- eval_batch_size: 4
- seed: 42
- optimizer: Adam with betas=(0.9,0.999) and epsilon=1e-08
- lr_scheduler_type: linear
- num_epochs: 5
- mixed_precision_training: Native AMP
### Training results
| Training Loss | Epoch | Step | Validation Loss | Rouge1 | Rouge2 | Rougel | Rougelsum | Gen Len |
|:-------------:|:-----:|:-----:|:---------------:|:-------:|:------:|:-------:|:---------:|:-------:|
| 0.1918 | 1.0 | 7200 | 0.2403 | 14.6883 | 5.2427 | 14.6306 | 14.6489 | 19.9938 |
| 0.1332 | 2.0 | 14400 | 0.2391 | 14.5165 | 5.2443 | 14.493 | 14.4908 | 19.9972 |
| 0.0966 | 3.0 | 21600 | 0.2539 | 14.758 | 5.4976 | 14.6906 | 14.7188 | 19.9941 |
| 0.0736 | 4.0 | 28800 | 0.2782 | 14.6267 | 5.3371 | 14.5578 | 14.6014 | 19.9934 |
| 0.0547 | 5.0 | 36000 | 0.3066 | 14.5433 | 5.2238 | 14.4731 | 14.5183 | 19.9934 |
### Framework versions
- Transformers 4.19.4
- Pytorch 1.11.0+cu113
- Datasets 2.3.2
- Tokenizers 0.12.1
|
meghazisofiane/opus-mt-en-ar-evaluated-en-to-ar-1000instances-un_multi-leaningRate2e-05-batchSize8-11-action-1 | d5e83bb4af959617298a2943aa6455c7dd9476a5 | 2022-06-12T16:54:18.000Z | [
"pytorch",
"tensorboard",
"marian",
"text2text-generation",
"dataset:un_multi",
"transformers",
"generated_from_trainer",
"license:apache-2.0",
"model-index",
"autotrain_compatible"
] | text2text-generation | false | meghazisofiane | null | meghazisofiane/opus-mt-en-ar-evaluated-en-to-ar-1000instances-un_multi-leaningRate2e-05-batchSize8-11-action-1 | 1 | null | transformers | 32,818 | ---
license: apache-2.0
tags:
- generated_from_trainer
datasets:
- un_multi
metrics:
- bleu
model-index:
- name: opus-mt-en-ar-evaluated-en-to-ar-1000instances-un_multi-leaningRate2e-05-batchSize8-11-action-1
results:
- task:
name: Sequence-to-sequence Language Modeling
type: text2text-generation
dataset:
name: un_multi
type: un_multi
args: ar-en
metrics:
- name: Bleu
type: bleu
value: 64.0048
---
<!-- This model card has been generated automatically according to the information the Trainer had access to. You
should probably proofread and complete it, then remove this comment. -->
# opus-mt-en-ar-evaluated-en-to-ar-1000instances-un_multi-leaningRate2e-05-batchSize8-11-action-1
This model is a fine-tuned version of [Helsinki-NLP/opus-mt-en-ar](https://huggingface.co/Helsinki-NLP/opus-mt-en-ar) on the un_multi dataset.
It achieves the following results on the evaluation set:
- Loss: 0.1294
- Bleu: 64.0048
- Meteor: 0.4903
- Gen Len: 21.85
## Model description
More information needed
## Intended uses & limitations
More information needed
## Training and evaluation data
More information needed
## Training procedure
### Training hyperparameters
The following hyperparameters were used during training:
- learning_rate: 2e-05
- train_batch_size: 8
- eval_batch_size: 8
- seed: 42
- optimizer: Adam with betas=(0.9,0.999) and epsilon=1e-08
- lr_scheduler_type: linear
- num_epochs: 11
### Training results
| Training Loss | Epoch | Step | Validation Loss | Bleu | Meteor | Gen Len |
|:-------------:|:-----:|:----:|:---------------:|:-------:|:------:|:-------:|
| 0.0489 | 1.0 | 100 | 0.1287 | 63.7573 | 0.4877 | 21.79 |
| 0.0447 | 2.0 | 200 | 0.1293 | 63.8776 | 0.49 | 21.875 |
| 0.0442 | 3.0 | 300 | 0.1294 | 64.0048 | 0.4903 | 21.85 |
| 0.0433 | 4.0 | 400 | 0.1294 | 64.0048 | 0.4903 | 21.85 |
| 0.0429 | 5.0 | 500 | 0.1294 | 64.0048 | 0.4903 | 21.85 |
| 0.0435 | 6.0 | 600 | 0.1294 | 64.0048 | 0.4903 | 21.85 |
| 0.0429 | 7.0 | 700 | 0.1294 | 64.0048 | 0.4903 | 21.85 |
| 0.0426 | 8.0 | 800 | 0.1294 | 64.0048 | 0.4903 | 21.85 |
### Framework versions
- Transformers 4.18.0
- Pytorch 1.11.0
- Datasets 2.1.0
- Tokenizers 0.12.1
|
nlokam99/ada_sample | 9f01f814d7cef9419ab4775a7cfa01a49e4e348a | 2022-06-12T17:35:50.000Z | [
"pytorch",
"gpt2",
"text-generation",
"transformers",
"conversational",
"license:mit"
] | conversational | false | nlokam99 | null | nlokam99/ada_sample | 1 | null | transformers | 32,819 | ---
thumbnail: https://huggingface.co/front/thumbnails/dialogpt.png
tags:
- conversational
license: mit
--- |
huggingtweets/dodecahedra | f6e4e37d0e5b632376a3744b2e1264940e1b03df | 2022-06-12T17:42:15.000Z | [
"pytorch",
"gpt2",
"text-generation",
"en",
"transformers",
"huggingtweets"
] | text-generation | false | huggingtweets | null | huggingtweets/dodecahedra | 1 | null | transformers | 32,820 | ---
language: en
thumbnail: http://www.huggingtweets.com/dodecahedra/1655055731499/predictions.png
tags:
- huggingtweets
widget:
- text: "My dream is"
---
<div class="inline-flex flex-col" style="line-height: 1.5;">
<div class="flex">
<div
style="display:inherit; margin-left: 4px; margin-right: 4px; width: 92px; height:92px; border-radius: 50%; background-size: cover; background-image: url('https://pbs.twimg.com/profile_images/3232494514/760c72bca0af20fac2cd61bcec557e7a_400x400.jpeg')">
</div>
<div
style="display:none; margin-left: 4px; margin-right: 4px; width: 92px; height:92px; border-radius: 50%; background-size: cover; background-image: url('')">
</div>
<div
style="display:none; margin-left: 4px; margin-right: 4px; width: 92px; height:92px; border-radius: 50%; background-size: cover; background-image: url('')">
</div>
</div>
<div style="text-align: center; margin-top: 3px; font-size: 16px; font-weight: 800">🤖 AI BOT 🤖</div>
<div style="text-align: center; font-size: 16px; font-weight: 800">William Rose</div>
<div style="text-align: center; font-size: 14px;">@dodecahedra</div>
</div>
I was made with [huggingtweets](https://github.com/borisdayma/huggingtweets).
Create your own bot based on your favorite user with [the demo](https://colab.research.google.com/github/borisdayma/huggingtweets/blob/master/huggingtweets-demo.ipynb)!
## How does it work?
The model uses the following pipeline.
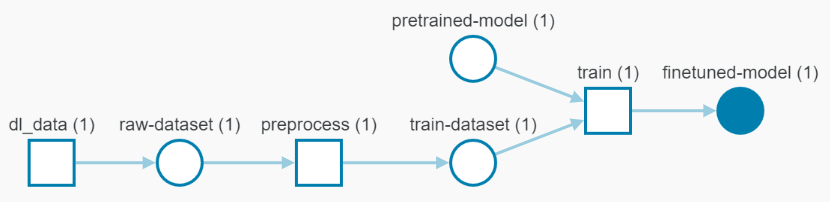
To understand how the model was developed, check the [W&B report](https://wandb.ai/wandb/huggingtweets/reports/HuggingTweets-Train-a-Model-to-Generate-Tweets--VmlldzoxMTY5MjI).
## Training data
The model was trained on tweets from William Rose.
| Data | William Rose |
| --- | --- |
| Tweets downloaded | 3241 |
| Retweets | 1115 |
| Short tweets | 158 |
| Tweets kept | 1968 |
[Explore the data](https://wandb.ai/wandb/huggingtweets/runs/1geru0ac/artifacts), which is tracked with [W&B artifacts](https://docs.wandb.com/artifacts) at every step of the pipeline.
## Training procedure
The model is based on a pre-trained [GPT-2](https://huggingface.co/gpt2) which is fine-tuned on @dodecahedra's tweets.
Hyperparameters and metrics are recorded in the [W&B training run](https://wandb.ai/wandb/huggingtweets/runs/1uy1zk82) for full transparency and reproducibility.
At the end of training, [the final model](https://wandb.ai/wandb/huggingtweets/runs/1uy1zk82/artifacts) is logged and versioned.
## How to use
You can use this model directly with a pipeline for text generation:
```python
from transformers import pipeline
generator = pipeline('text-generation',
model='huggingtweets/dodecahedra')
generator("My dream is", num_return_sequences=5)
```
## Limitations and bias
The model suffers from [the same limitations and bias as GPT-2](https://huggingface.co/gpt2#limitations-and-bias).
In addition, the data present in the user's tweets further affects the text generated by the model.
## About
*Built by Boris Dayma*
[](https://twitter.com/intent/follow?screen_name=borisdayma)
For more details, visit the project repository.
[](https://github.com/borisdayma/huggingtweets)
|
meghazisofiane/mbart-large-cc25-en-ar-evaluated-en-to-ar-1000instances-un_multi-leaningRate2e-05-batchSize2 | 1b02b989ac829a8ced504d97bd852eeae35634fd | 2022-06-12T20:02:36.000Z | [
"pytorch",
"tensorboard",
"mbart",
"text2text-generation",
"transformers",
"autotrain_compatible"
] | text2text-generation | false | meghazisofiane | null | meghazisofiane/mbart-large-cc25-en-ar-evaluated-en-to-ar-1000instances-un_multi-leaningRate2e-05-batchSize2 | 1 | null | transformers | 32,821 | Entry not found |
abdoutony207/mbart-large-cc25-en-ar-evaluated-en-to-ar-1000instancesopus-leaningRate2e-05-batchSize2 | 97687f7292bde84c97b21ea31c55be82dcd7740c | 2022-06-12T18:25:47.000Z | [
"pytorch",
"tensorboard",
"mbart",
"text2text-generation",
"dataset:opus100",
"transformers",
"generated_from_trainer",
"license:mit",
"model-index",
"autotrain_compatible"
] | text2text-generation | false | abdoutony207 | null | abdoutony207/mbart-large-cc25-en-ar-evaluated-en-to-ar-1000instancesopus-leaningRate2e-05-batchSize2 | 1 | null | transformers | 32,822 | ---
license: mit
tags:
- generated_from_trainer
datasets:
- opus100
metrics:
- bleu
model-index:
- name: mbart-large-cc25-en-ar-evaluated-en-to-ar-1000instancesopus-leaningRate2e-05-batchSize2
results:
- task:
name: Sequence-to-sequence Language Modeling
type: text2text-generation
dataset:
name: opus100
type: opus100
args: ar-en
metrics:
- name: Bleu
type: bleu
value: 10.5645
---
<!-- This model card has been generated automatically according to the information the Trainer had access to. You
should probably proofread and complete it, then remove this comment. -->
# mbart-large-cc25-en-ar-evaluated-en-to-ar-1000instancesopus-leaningRate2e-05-batchSize2
This model is a fine-tuned version of [akhooli/mbart-large-cc25-en-ar](https://huggingface.co/akhooli/mbart-large-cc25-en-ar) on the opus100 dataset.
It achieves the following results on the evaluation set:
- Loss: 0.4673
- Bleu: 10.5645
- Meteor: 0.0783
- Gen Len: 10.23
## Model description
More information needed
## Intended uses & limitations
More information needed
## Training and evaluation data
More information needed
## Training procedure
### Training hyperparameters
The following hyperparameters were used during training:
- learning_rate: 2e-05
- train_batch_size: 2
- eval_batch_size: 2
- seed: 42
- optimizer: Adam with betas=(0.9,0.999) and epsilon=1e-08
- lr_scheduler_type: linear
- num_epochs: 11
- mixed_precision_training: Native AMP
### Training results
| Training Loss | Epoch | Step | Validation Loss | Bleu | Meteor | Gen Len |
|:-------------:|:-----:|:----:|:---------------:|:-------:|:------:|:-------:|
| 8.1731 | 0.25 | 100 | 2.8417 | 0.9599 | 0.028 | 230.885 |
| 0.6743 | 0.5 | 200 | 0.4726 | 6.4055 | 0.0692 | 14.81 |
| 0.3028 | 0.75 | 300 | 0.4572 | 6.7544 | 0.0822 | 23.92 |
| 0.2555 | 1.0 | 400 | 0.4172 | 8.4078 | 0.0742 | 13.655 |
| 0.1644 | 1.25 | 500 | 0.4236 | 9.284 | 0.071 | 13.03 |
| 0.1916 | 1.5 | 600 | 0.4222 | 4.8976 | 0.0779 | 32.225 |
| 0.2011 | 1.75 | 700 | 0.4305 | 7.6909 | 0.0738 | 16.675 |
| 0.1612 | 2.0 | 800 | 0.4416 | 10.8622 | 0.0855 | 10.91 |
| 0.116 | 2.25 | 900 | 0.4673 | 10.5645 | 0.0783 | 10.23 |
### Framework versions
- Transformers 4.18.0
- Pytorch 1.11.0
- Datasets 2.1.0
- Tokenizers 0.12.1
|
NadiaSan/udesa-model-aah-es-35k | c0b188e56395888d3bdb592c2e030c55703dc53b | 2022-06-12T20:28:38.000Z | [
"pytorch",
"bert",
"feature-extraction",
"transformers"
] | feature-extraction | false | NadiaSan | null | NadiaSan/udesa-model-aah-es-35k | 1 | null | transformers | 32,823 | Entry not found |
rohitsroch/hybrid_utt-clusterrank_bart-base_samsum_sum | 2e8eecc91040ccab33b30a110d260fa026595bf8 | 2022-06-12T23:03:22.000Z | [
"pytorch",
"bart",
"text2text-generation",
"en",
"dataset:samsum",
"transformers",
"dialogue-summarization",
"license:apache-2.0",
"autotrain_compatible"
] | text2text-generation | false | rohitsroch | null | rohitsroch/hybrid_utt-clusterrank_bart-base_samsum_sum | 1 | null | transformers | 32,824 | ---
language:
- en
license: apache-2.0
tags:
- dialogue-summarization
model_index:
- name: hybrid_utt-clusterrank_bart-base_samsum_sum
results:
- task:
name: Summarization
type: summarization
datasets:
- samsum
---
## Paper
## [Domain Adapted Abstractive Summarization of Dialogue using Transfer Learning](https://dl.acm.org/doi/10.1145/3508546.3508640)
Authors: *Rohit Sroch*
## Abstract
Recently, the abstractive dialogue summarization task has been gaining a lot of attention from researchers. Also, unlike news articles and documents with well-structured text, dialogue differs in the sense that it often comes from two or more interlocutors, exchanging information with each other and having an inherent hierarchical structure based on the sequence of utterances by different speakers. This paper proposes a simple but effective hybrid approach that consists of two modules and uses transfer learning by leveraging pretrained language models (PLMs) to generate an abstractive summary. The first module highlights important utterances, capturing the utterance level relationship by adapting an auto-encoding model like BERT based on the unsupervised or supervised method. And then, the second module generates a concise abstractive summary by adapting encoder-decoder models like T5, BART, and PEGASUS. Experiment results on benchmark datasets show that our approach achieves a state-of-the-art performance by adapting to dialogue scenarios and can also be helpful in low-resource settings for domain adaptation.
*Rohit Sroch. 2021. Domain Adapted Abstractive Summarization of Dialogue using Transfer Learning. In 2021 4th International Conference on Algorithms, Computing and Artificial Intelligence (ACAI'21). Association for Computing Machinery, New York, NY, USA, Article 94, 1–6. https://doi.org/10.1145/3508546.3508640*
# hybrid_utt-clusterrank_bart-base_samsum_sum
This model is a fine-tuned version of [facebook/bart-base](https://huggingface.co/facebook/bart-base) on SAMSum dataset for dialogue summarization task.
## Model description
More information needed
### Training hyperparameters
The following hyperparameters were used during training:
- learning_rate: 5e-5
- train_batch_size: 8
- eval_batch_size: 8
- seed: 42
- optimizer: Adam with betas=(0.9,0.999) and epsilon=1e-08
- lr_scheduler_type: linear
- lr_scheduler_warmup_ratio: 0.1
- num_epochs: 10.0
- label_smoothing_factor: 0.1
### Results on Test Set
- predict_gen_len = 23.9048
- predict_rouge1 = **47.355**
- predict_rouge2 = **22.4593**
- predict_rougeL = **38.694**
- predict_rougeLsum = **42.98**
- predict_samples = 819
- predict_samples_per_second = 9.279
- predict_steps_per_second = 2.322
### Framework versions
- Transformers>=4.8.0
- Pytorch>=1.6.0
- Datasets>=1.10.2
- Tokenizers>=0.10.3
If you use this model, please cite the following paper:
```
@inproceedings{10.1145/3508546.3508640,
author = {Sroch, Rohit},
title = {Domain Adapted Abstractive Summarization of Dialogue Using Transfer Learning},
year = {2021},
isbn = {9781450385053},
publisher = {Association for Computing Machinery},
address = {New York, NY, USA},
url = {https://doi.org/10.1145/3508546.3508640},
doi = {10.1145/3508546.3508640},
articleno = {94},
numpages = {6},
keywords = {encoder-decoder, T5, abstractive summary, PEGASUS, BART, dialogue summarization, PLMs, BERT},
location = {Sanya, China},
series = {ACAI'21}
}
``` |
erickfm/t5-base-finetuned-bias-sweep-5db9391c | 8b4b9724f3771cc1743a7d23fe53b85f2c89aa2b | 2022-06-12T22:08:17.000Z | [
"pytorch",
"t5",
"text2text-generation",
"transformers",
"autotrain_compatible"
] | text2text-generation | false | erickfm | null | erickfm/t5-base-finetuned-bias-sweep-5db9391c | 1 | null | transformers | 32,825 | Entry not found |
huggingtweets/gronkh | 5d7541e18a6c3a6b10b26b3c5771dbdd8407f3b1 | 2022-06-12T22:07:19.000Z | [
"pytorch",
"gpt2",
"text-generation",
"en",
"transformers",
"huggingtweets"
] | text-generation | false | huggingtweets | null | huggingtweets/gronkh | 1 | null | transformers | 32,826 | ---
language: en
thumbnail: http://www.huggingtweets.com/gronkh/1655071635259/predictions.png
tags:
- huggingtweets
widget:
- text: "My dream is"
---
<div class="inline-flex flex-col" style="line-height: 1.5;">
<div class="flex">
<div
style="display:inherit; margin-left: 4px; margin-right: 4px; width: 92px; height:92px; border-radius: 50%; background-size: cover; background-image: url('https://pbs.twimg.com/profile_images/1291074656049541127/fBvcAq3x_400x400.jpg')">
</div>
<div
style="display:none; margin-left: 4px; margin-right: 4px; width: 92px; height:92px; border-radius: 50%; background-size: cover; background-image: url('')">
</div>
<div
style="display:none; margin-left: 4px; margin-right: 4px; width: 92px; height:92px; border-radius: 50%; background-size: cover; background-image: url('')">
</div>
</div>
<div style="text-align: center; margin-top: 3px; font-size: 16px; font-weight: 800">🤖 AI BOT 🤖</div>
<div style="text-align: center; font-size: 16px; font-weight: 800">𝙂𝙍𝙊𝙉𝙆𝙃</div>
<div style="text-align: center; font-size: 14px;">@gronkh</div>
</div>
I was made with [huggingtweets](https://github.com/borisdayma/huggingtweets).
Create your own bot based on your favorite user with [the demo](https://colab.research.google.com/github/borisdayma/huggingtweets/blob/master/huggingtweets-demo.ipynb)!
## How does it work?
The model uses the following pipeline.
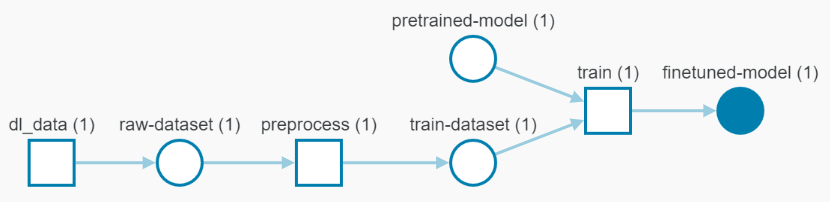
To understand how the model was developed, check the [W&B report](https://wandb.ai/wandb/huggingtweets/reports/HuggingTweets-Train-a-Model-to-Generate-Tweets--VmlldzoxMTY5MjI).
## Training data
The model was trained on tweets from 𝙂𝙍𝙊𝙉𝙆𝙃.
| Data | 𝙂𝙍𝙊𝙉𝙆𝙃 |
| --- | --- |
| Tweets downloaded | 3250 |
| Retweets | 65 |
| Short tweets | 97 |
| Tweets kept | 3088 |
[Explore the data](https://wandb.ai/wandb/huggingtweets/runs/3gqyrznk/artifacts), which is tracked with [W&B artifacts](https://docs.wandb.com/artifacts) at every step of the pipeline.
## Training procedure
The model is based on a pre-trained [GPT-2](https://huggingface.co/gpt2) which is fine-tuned on @gronkh's tweets.
Hyperparameters and metrics are recorded in the [W&B training run](https://wandb.ai/wandb/huggingtweets/runs/2i0lrjh9) for full transparency and reproducibility.
At the end of training, [the final model](https://wandb.ai/wandb/huggingtweets/runs/2i0lrjh9/artifacts) is logged and versioned.
## How to use
You can use this model directly with a pipeline for text generation:
```python
from transformers import pipeline
generator = pipeline('text-generation',
model='huggingtweets/gronkh')
generator("My dream is", num_return_sequences=5)
```
## Limitations and bias
The model suffers from [the same limitations and bias as GPT-2](https://huggingface.co/gpt2#limitations-and-bias).
In addition, the data present in the user's tweets further affects the text generated by the model.
## About
*Built by Boris Dayma*
[](https://twitter.com/intent/follow?screen_name=borisdayma)
For more details, visit the project repository.
[](https://github.com/borisdayma/huggingtweets)
|
mailenpellegrino/transformerv2 | 742592d00b5928bbb16f7f4980710b9da95cf5d2 | 2022-06-12T22:28:54.000Z | [
"pytorch",
"xlm-roberta",
"feature-extraction",
"transformers"
] | feature-extraction | false | mailenpellegrino | null | mailenpellegrino/transformerv2 | 1 | null | transformers | 32,827 | Entry not found |
asahi417/lmqg-mt5-small-frquad | 3992189a9bba77dbe1af7446d23d7714a97f03da | 2022-06-13T00:46:53.000Z | [
"pytorch",
"mt5",
"text2text-generation",
"transformers",
"autotrain_compatible"
] | text2text-generation | false | asahi417 | null | asahi417/lmqg-mt5-small-frquad | 1 | null | transformers | 32,828 | Entry not found |
huggingtweets/145gomez | 08b1f025fed038ca1b84b789220994a330372981 | 2022-06-13T04:12:14.000Z | [
"pytorch",
"gpt2",
"text-generation",
"en",
"transformers",
"huggingtweets"
] | text-generation | false | huggingtweets | null | huggingtweets/145gomez | 1 | null | transformers | 32,829 | ---
language: en
thumbnail: http://www.huggingtweets.com/145gomez/1655093529513/predictions.png
tags:
- huggingtweets
widget:
- text: "My dream is"
---
<div class="inline-flex flex-col" style="line-height: 1.5;">
<div class="flex">
<div
style="display:inherit; margin-left: 4px; margin-right: 4px; width: 92px; height:92px; border-radius: 50%; background-size: cover; background-image: url('https://pbs.twimg.com/profile_images/1457362420800835589/0mihXFjt_400x400.jpg')">
</div>
<div
style="display:none; margin-left: 4px; margin-right: 4px; width: 92px; height:92px; border-radius: 50%; background-size: cover; background-image: url('')">
</div>
<div
style="display:none; margin-left: 4px; margin-right: 4px; width: 92px; height:92px; border-radius: 50%; background-size: cover; background-image: url('')">
</div>
</div>
<div style="text-align: center; margin-top: 3px; font-size: 16px; font-weight: 800">🤖 AI BOT 🤖</div>
<div style="text-align: center; font-size: 16px; font-weight: 800">Omar Gomez Avalos</div>
<div style="text-align: center; font-size: 14px;">@145gomez</div>
</div>
I was made with [huggingtweets](https://github.com/borisdayma/huggingtweets).
Create your own bot based on your favorite user with [the demo](https://colab.research.google.com/github/borisdayma/huggingtweets/blob/master/huggingtweets-demo.ipynb)!
## How does it work?
The model uses the following pipeline.
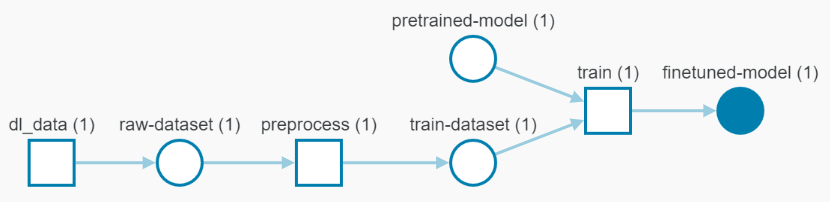
To understand how the model was developed, check the [W&B report](https://wandb.ai/wandb/huggingtweets/reports/HuggingTweets-Train-a-Model-to-Generate-Tweets--VmlldzoxMTY5MjI).
## Training data
The model was trained on tweets from Omar Gomez Avalos.
| Data | Omar Gomez Avalos |
| --- | --- |
| Tweets downloaded | 3189 |
| Retweets | 472 |
| Short tweets | 384 |
| Tweets kept | 2333 |
[Explore the data](https://wandb.ai/wandb/huggingtweets/runs/3hcxwfgg/artifacts), which is tracked with [W&B artifacts](https://docs.wandb.com/artifacts) at every step of the pipeline.
## Training procedure
The model is based on a pre-trained [GPT-2](https://huggingface.co/gpt2) which is fine-tuned on @145gomez's tweets.
Hyperparameters and metrics are recorded in the [W&B training run](https://wandb.ai/wandb/huggingtweets/runs/7qzkruyo) for full transparency and reproducibility.
At the end of training, [the final model](https://wandb.ai/wandb/huggingtweets/runs/7qzkruyo/artifacts) is logged and versioned.
## How to use
You can use this model directly with a pipeline for text generation:
```python
from transformers import pipeline
generator = pipeline('text-generation',
model='huggingtweets/145gomez')
generator("My dream is", num_return_sequences=5)
```
## Limitations and bias
The model suffers from [the same limitations and bias as GPT-2](https://huggingface.co/gpt2#limitations-and-bias).
In addition, the data present in the user's tweets further affects the text generated by the model.
## About
*Built by Boris Dayma*
[](https://twitter.com/intent/follow?screen_name=borisdayma)
For more details, visit the project repository.
[](https://github.com/borisdayma/huggingtweets)
|
burner/GPT-J-Step_98500 | 5a47e95152759184ce13d43372e29f77134c847f | 2022-06-13T05:11:42.000Z | [
"pytorch",
"gptj",
"text-generation",
"transformers",
"license:apache-2.0"
] | text-generation | false | burner | null | burner/GPT-J-Step_98500 | 1 | null | transformers | 32,830 | ---
license: apache-2.0
---
|
zoha/wav2vec2-base-timit-google-colab | 659e1d07b09561e637c03022375f6eed869c7cf2 | 2022-06-13T10:50:12.000Z | [
"pytorch",
"tensorboard",
"wav2vec2",
"automatic-speech-recognition",
"transformers",
"generated_from_trainer",
"license:apache-2.0",
"model-index"
] | automatic-speech-recognition | false | zoha | null | zoha/wav2vec2-base-timit-google-colab | 1 | null | transformers | 32,831 | ---
license: apache-2.0
tags:
- generated_from_trainer
model-index:
- name: wav2vec2-base-timit-google-colab
results: []
---
<!-- This model card has been generated automatically according to the information the Trainer had access to. You
should probably proofread and complete it, then remove this comment. -->
# wav2vec2-base-timit-google-colab
This model is a fine-tuned version of [facebook/wav2vec2-base](https://huggingface.co/facebook/wav2vec2-base) on the None dataset.
It achieves the following results on the evaluation set:
- Loss: 0.4659
- Wer: 0.3080
## Model description
More information needed
## Intended uses & limitations
More information needed
## Training and evaluation data
More information needed
## Training procedure
### Training hyperparameters
The following hyperparameters were used during training:
- learning_rate: 0.0001
- train_batch_size: 8
- eval_batch_size: 8
- seed: 42
- optimizer: Adam with betas=(0.9,0.999) and epsilon=1e-08
- lr_scheduler_type: linear
- lr_scheduler_warmup_steps: 1000
- num_epochs: 30
- mixed_precision_training: Native AMP
### Training results
| Training Loss | Epoch | Step | Validation Loss | Wer |
|:-------------:|:-----:|:-----:|:---------------:|:------:|
| 3.5787 | 0.87 | 500 | 1.7648 | 1.0305 |
| 0.8692 | 1.73 | 1000 | 0.5136 | 0.5103 |
| 0.4346 | 2.6 | 1500 | 0.4364 | 0.4515 |
| 0.31 | 3.46 | 2000 | 0.3889 | 0.4070 |
| 0.234 | 4.33 | 2500 | 0.4161 | 0.3863 |
| 0.2054 | 5.19 | 3000 | 0.3845 | 0.3722 |
| 0.165 | 6.06 | 3500 | 0.4035 | 0.3643 |
| 0.1436 | 6.92 | 4000 | 0.4090 | 0.3623 |
| 0.1381 | 7.79 | 4500 | 0.4007 | 0.3673 |
| 0.1175 | 8.65 | 5000 | 0.4588 | 0.3632 |
| 0.1052 | 9.52 | 5500 | 0.4441 | 0.3588 |
| 0.0988 | 10.38 | 6000 | 0.4133 | 0.3489 |
| 0.0877 | 11.25 | 6500 | 0.4758 | 0.3510 |
| 0.0856 | 12.11 | 7000 | 0.4454 | 0.3425 |
| 0.0731 | 12.98 | 7500 | 0.4252 | 0.3351 |
| 0.0712 | 13.84 | 8000 | 0.4163 | 0.3370 |
| 0.0711 | 14.71 | 8500 | 0.4166 | 0.3367 |
| 0.06 | 15.57 | 9000 | 0.4195 | 0.3347 |
| 0.0588 | 16.44 | 9500 | 0.4697 | 0.3367 |
| 0.0497 | 17.3 | 10000 | 0.4255 | 0.3314 |
| 0.0523 | 18.17 | 10500 | 0.4676 | 0.3307 |
| 0.0444 | 19.03 | 11000 | 0.4570 | 0.3244 |
| 0.0435 | 19.9 | 11500 | 0.4307 | 0.3243 |
| 0.0348 | 20.76 | 12000 | 0.4763 | 0.3245 |
| 0.036 | 21.63 | 12500 | 0.4635 | 0.3238 |
| 0.0347 | 22.49 | 13000 | 0.4602 | 0.3212 |
| 0.0333 | 23.36 | 13500 | 0.4472 | 0.3195 |
| 0.0311 | 24.22 | 14000 | 0.4449 | 0.3183 |
| 0.0294 | 25.09 | 14500 | 0.4631 | 0.3175 |
| 0.025 | 25.95 | 15000 | 0.4466 | 0.3164 |
| 0.023 | 26.82 | 15500 | 0.4581 | 0.3138 |
| 0.0216 | 27.68 | 16000 | 0.4665 | 0.3114 |
| 0.0198 | 28.55 | 16500 | 0.4590 | 0.3092 |
| 0.0181 | 29.41 | 17000 | 0.4659 | 0.3080 |
### Framework versions
- Transformers 4.20.0.dev0
- Pytorch 1.11.0+cu113
- Datasets 1.18.3
- Tokenizers 0.12.1
|
shaojie/distilbert-base-uncased-finetuned-squad | 5f332a62f125d0aa9b2d57e6ca10494f02c15a21 | 2022-06-14T07:26:48.000Z | [
"pytorch",
"tensorboard",
"distilbert",
"question-answering",
"dataset:squad",
"transformers",
"generated_from_trainer",
"license:apache-2.0",
"model-index",
"autotrain_compatible"
] | question-answering | false | shaojie | null | shaojie/distilbert-base-uncased-finetuned-squad | 1 | null | transformers | 32,832 | ---
license: apache-2.0
tags:
- generated_from_trainer
datasets:
- squad
model-index:
- name: distilbert-base-uncased-finetuned-squad
results: []
---
<!-- This model card has been generated automatically according to the information the Trainer had access to. You
should probably proofread and complete it, then remove this comment. -->
# distilbert-base-uncased-finetuned-squad
This model is a fine-tuned version of [distilbert-base-uncased](https://huggingface.co/distilbert-base-uncased) on the squad dataset.
It achieves the following results on the evaluation set:
- eval_loss: 1.1585
- eval_runtime: 138.1018
- eval_samples_per_second: 78.087
- eval_steps_per_second: 4.88
- epoch: 1.0
- step: 5533
## Model description
More information needed
## Intended uses & limitations
More information needed
## Training and evaluation data
More information needed
## Training procedure
### Training hyperparameters
The following hyperparameters were used during training:
- learning_rate: 2e-05
- train_batch_size: 16
- eval_batch_size: 16
- seed: 42
- optimizer: Adam with betas=(0.9,0.999) and epsilon=1e-08
- lr_scheduler_type: linear
- num_epochs: 3
### Framework versions
- Transformers 4.19.4
- Pytorch 1.11.0+cu113
- Datasets 2.2.2
- Tokenizers 0.12.1
|
erickfm/t5-base-finetuned-bias-sweep-64f598ca | 5449b91f587e66826a8da4554f38e656dbeb19af | 2022-06-14T01:40:19.000Z | [
"pytorch",
"t5",
"text2text-generation",
"transformers",
"autotrain_compatible"
] | text2text-generation | false | erickfm | null | erickfm/t5-base-finetuned-bias-sweep-64f598ca | 1 | null | transformers | 32,833 | maybe [sandy-sweep](https://wandb.ai/unbias/t5-base/runs/tkvqsbl3) |
yossra/bert-finetuned-squad | 1a01bda20e36c699383a30da6d3021bc8e82b25f | 2022-06-13T12:03:54.000Z | [
"pytorch",
"tensorboard",
"bert",
"question-answering",
"dataset:squad",
"transformers",
"generated_from_trainer",
"license:apache-2.0",
"model-index",
"autotrain_compatible"
] | question-answering | false | yossra | null | yossra/bert-finetuned-squad | 1 | null | transformers | 32,834 | ---
license: apache-2.0
tags:
- generated_from_trainer
datasets:
- squad
model-index:
- name: bert-finetuned-squad
results: []
---
<!-- This model card has been generated automatically according to the information the Trainer had access to. You
should probably proofread and complete it, then remove this comment. -->
# bert-finetuned-squad
This model is a fine-tuned version of [bert-base-cased](https://huggingface.co/bert-base-cased) on the squad dataset.
## Model description
More information needed
## Intended uses & limitations
More information needed
## Training and evaluation data
More information needed
## Training procedure
### Training hyperparameters
The following hyperparameters were used during training:
- learning_rate: 2e-05
- train_batch_size: 8
- eval_batch_size: 8
- seed: 42
- optimizer: Adam with betas=(0.9,0.999) and epsilon=1e-08
- lr_scheduler_type: linear
- num_epochs: 3
- mixed_precision_training: Native AMP
### Training results
### Framework versions
- Transformers 4.19.4
- Pytorch 1.11.0+cu113
- Datasets 2.2.2
- Tokenizers 0.12.1
|
Nadav/roberta-base-squad-nl | 141efed50c80f7e7d44c1793e74821ee8d138f82 | 2022-06-13T11:55:54.000Z | [
"pytorch",
"roberta",
"question-answering",
"transformers",
"license:afl-3.0",
"autotrain_compatible"
] | question-answering | false | Nadav | null | Nadav/roberta-base-squad-nl | 1 | null | transformers | 32,835 | ---
license: afl-3.0
---
|
Nadav/xlm-roberta-base-squad-nl | 1edf37cbb3bf8708810ef7c1d159753792366910 | 2022-06-13T11:54:19.000Z | [
"pytorch",
"xlm-roberta",
"question-answering",
"transformers",
"autotrain_compatible"
] | question-answering | false | Nadav | null | Nadav/xlm-roberta-base-squad-nl | 1 | null | transformers | 32,836 | Entry not found |
Shenghao1993/xlm-roberta-base-finetuned-panx-de-fr | 9a1cdb7291b41b92387d11c5ea900e4e59a4f1d9 | 2022-06-13T11:59:43.000Z | [
"pytorch",
"xlm-roberta",
"token-classification",
"transformers",
"generated_from_trainer",
"license:mit",
"model-index",
"autotrain_compatible"
] | token-classification | false | Shenghao1993 | null | Shenghao1993/xlm-roberta-base-finetuned-panx-de-fr | 1 | null | transformers | 32,837 | ---
license: mit
tags:
- generated_from_trainer
metrics:
- f1
model-index:
- name: xlm-roberta-base-finetuned-panx-de-fr
results: []
---
<!-- This model card has been generated automatically according to the information the Trainer had access to. You
should probably proofread and complete it, then remove this comment. -->
# xlm-roberta-base-finetuned-panx-de-fr
This model is a fine-tuned version of [xlm-roberta-base](https://huggingface.co/xlm-roberta-base) on the None dataset.
It achieves the following results on the evaluation set:
- Loss: 0.1623
- F1: 0.8596
## Model description
More information needed
## Intended uses & limitations
More information needed
## Training and evaluation data
More information needed
## Training procedure
### Training hyperparameters
The following hyperparameters were used during training:
- learning_rate: 5e-05
- train_batch_size: 24
- eval_batch_size: 24
- seed: 42
- optimizer: Adam with betas=(0.9,0.999) and epsilon=1e-08
- lr_scheduler_type: linear
- num_epochs: 3
### Training results
| Training Loss | Epoch | Step | Validation Loss | F1 |
|:-------------:|:-----:|:----:|:---------------:|:------:|
| 0.2865 | 1.0 | 715 | 0.1981 | 0.8167 |
| 0.1484 | 2.0 | 1430 | 0.1595 | 0.8486 |
| 0.0949 | 3.0 | 2145 | 0.1623 | 0.8596 |
### Framework versions
- Transformers 4.19.4
- Pytorch 1.11.0+cu113
- Datasets 2.2.2
- Tokenizers 0.12.1
|
erickfm/t5-base-finetuned-bias-sweep-1da3c5df | f8628fd94d560d83eba6632e888ade6ad8669915 | 2022-06-13T12:25:12.000Z | [
"pytorch",
"t5",
"text2text-generation",
"transformers",
"autotrain_compatible"
] | text2text-generation | false | erickfm | null | erickfm/t5-base-finetuned-bias-sweep-1da3c5df | 1 | null | transformers | 32,838 | Entry not found |
Shenghao1993/xlm-roberta-base-finetuned-panx-fr | 3914ed406dc6abb836d2f7a105405bd6990e9b5d | 2022-06-13T12:44:17.000Z | [
"pytorch",
"xlm-roberta",
"token-classification",
"dataset:xtreme",
"transformers",
"generated_from_trainer",
"license:mit",
"model-index",
"autotrain_compatible"
] | token-classification | false | Shenghao1993 | null | Shenghao1993/xlm-roberta-base-finetuned-panx-fr | 1 | null | transformers | 32,839 | ---
license: mit
tags:
- generated_from_trainer
datasets:
- xtreme
metrics:
- f1
model-index:
- name: xlm-roberta-base-finetuned-panx-fr
results:
- task:
name: Token Classification
type: token-classification
dataset:
name: xtreme
type: xtreme
args: PAN-X.fr
metrics:
- name: F1
type: f1
value: 0.8438448566610455
---
<!-- This model card has been generated automatically according to the information the Trainer had access to. You
should probably proofread and complete it, then remove this comment. -->
# xlm-roberta-base-finetuned-panx-fr
This model is a fine-tuned version of [xlm-roberta-base](https://huggingface.co/xlm-roberta-base) on the xtreme dataset.
It achieves the following results on the evaluation set:
- Loss: 0.2675
- F1: 0.8438
## Model description
More information needed
## Intended uses & limitations
More information needed
## Training and evaluation data
More information needed
## Training procedure
### Training hyperparameters
The following hyperparameters were used during training:
- learning_rate: 5e-05
- train_batch_size: 24
- eval_batch_size: 24
- seed: 42
- optimizer: Adam with betas=(0.9,0.999) and epsilon=1e-08
- lr_scheduler_type: linear
- num_epochs: 3
### Training results
| Training Loss | Epoch | Step | Validation Loss | F1 |
|:-------------:|:-----:|:----:|:---------------:|:------:|
| 0.5606 | 1.0 | 191 | 0.3157 | 0.7967 |
| 0.2755 | 2.0 | 382 | 0.2684 | 0.8288 |
| 0.1811 | 3.0 | 573 | 0.2675 | 0.8438 |
### Framework versions
- Transformers 4.19.4
- Pytorch 1.11.0+cu113
- Datasets 2.2.2
- Tokenizers 0.12.1
|
Shenghao1993/xlm-roberta-base-finetuned-panx-it | 071f7c6c1b25c6d44d6d0ae4966c7a0c739afe2a | 2022-06-13T13:02:39.000Z | [
"pytorch",
"xlm-roberta",
"token-classification",
"dataset:xtreme",
"transformers",
"generated_from_trainer",
"license:mit",
"model-index",
"autotrain_compatible"
] | token-classification | false | Shenghao1993 | null | Shenghao1993/xlm-roberta-base-finetuned-panx-it | 1 | null | transformers | 32,840 | ---
license: mit
tags:
- generated_from_trainer
datasets:
- xtreme
metrics:
- f1
model-index:
- name: xlm-roberta-base-finetuned-panx-it
results:
- task:
name: Token Classification
type: token-classification
dataset:
name: xtreme
type: xtreme
args: PAN-X.it
metrics:
- name: F1
type: f1
value: 0.8358085808580858
---
<!-- This model card has been generated automatically according to the information the Trainer had access to. You
should probably proofread and complete it, then remove this comment. -->
# xlm-roberta-base-finetuned-panx-it
This model is a fine-tuned version of [xlm-roberta-base](https://huggingface.co/xlm-roberta-base) on the xtreme dataset.
It achieves the following results on the evaluation set:
- Loss: 0.2403
- F1: 0.8358
## Model description
More information needed
## Intended uses & limitations
More information needed
## Training and evaluation data
More information needed
## Training procedure
### Training hyperparameters
The following hyperparameters were used during training:
- learning_rate: 5e-05
- train_batch_size: 24
- eval_batch_size: 24
- seed: 42
- optimizer: Adam with betas=(0.9,0.999) and epsilon=1e-08
- lr_scheduler_type: linear
- num_epochs: 3
### Training results
| Training Loss | Epoch | Step | Validation Loss | F1 |
|:-------------:|:-----:|:----:|:---------------:|:------:|
| 0.7053 | 1.0 | 70 | 0.3077 | 0.7587 |
| 0.2839 | 2.0 | 140 | 0.2692 | 0.8007 |
| 0.1894 | 3.0 | 210 | 0.2403 | 0.8358 |
### Framework versions
- Transformers 4.19.4
- Pytorch 1.11.0+cu113
- Datasets 2.2.2
- Tokenizers 0.12.1
|
Shenghao1993/xlm-roberta-base-finetuned-panx-en | 3c6281283ab65ce449ba4fd0d0cf8e3da61ea5d2 | 2022-06-13T13:20:02.000Z | [
"pytorch",
"xlm-roberta",
"token-classification",
"dataset:xtreme",
"transformers",
"generated_from_trainer",
"license:mit",
"model-index",
"autotrain_compatible"
] | token-classification | false | Shenghao1993 | null | Shenghao1993/xlm-roberta-base-finetuned-panx-en | 1 | null | transformers | 32,841 | ---
license: mit
tags:
- generated_from_trainer
datasets:
- xtreme
metrics:
- f1
model-index:
- name: xlm-roberta-base-finetuned-panx-en
results:
- task:
name: Token Classification
type: token-classification
dataset:
name: xtreme
type: xtreme
args: PAN-X.en
metrics:
- name: F1
type: f1
value: 0.7032474804031354
---
<!-- This model card has been generated automatically according to the information the Trainer had access to. You
should probably proofread and complete it, then remove this comment. -->
# xlm-roberta-base-finetuned-panx-en
This model is a fine-tuned version of [xlm-roberta-base](https://huggingface.co/xlm-roberta-base) on the xtreme dataset.
It achieves the following results on the evaluation set:
- Loss: 0.3859
- F1: 0.7032
## Model description
More information needed
## Intended uses & limitations
More information needed
## Training and evaluation data
More information needed
## Training procedure
### Training hyperparameters
The following hyperparameters were used during training:
- learning_rate: 5e-05
- train_batch_size: 24
- eval_batch_size: 24
- seed: 42
- optimizer: Adam with betas=(0.9,0.999) and epsilon=1e-08
- lr_scheduler_type: linear
- num_epochs: 3
### Training results
| Training Loss | Epoch | Step | Validation Loss | F1 |
|:-------------:|:-----:|:----:|:---------------:|:------:|
| 1.0494 | 1.0 | 50 | 0.5464 | 0.5507 |
| 0.5329 | 2.0 | 100 | 0.4217 | 0.6715 |
| 0.3799 | 3.0 | 150 | 0.3859 | 0.7032 |
### Framework versions
- Transformers 4.19.4
- Pytorch 1.11.0+cu113
- Datasets 2.2.2
- Tokenizers 0.12.1
|
Shenghao1993/xlm-roberta-base-finetuned-panx-all | 449d0b762db7caa976af890035d77d0f859c9f1e | 2022-06-13T13:45:41.000Z | [
"pytorch",
"xlm-roberta",
"token-classification",
"transformers",
"generated_from_trainer",
"license:mit",
"model-index",
"autotrain_compatible"
] | token-classification | false | Shenghao1993 | null | Shenghao1993/xlm-roberta-base-finetuned-panx-all | 1 | null | transformers | 32,842 | ---
license: mit
tags:
- generated_from_trainer
metrics:
- f1
model-index:
- name: xlm-roberta-base-finetuned-panx-all
results: []
---
<!-- This model card has been generated automatically according to the information the Trainer had access to. You
should probably proofread and complete it, then remove this comment. -->
# xlm-roberta-base-finetuned-panx-all
This model is a fine-tuned version of [xlm-roberta-base](https://huggingface.co/xlm-roberta-base) on the None dataset.
It achieves the following results on the evaluation set:
- Loss: 0.1719
- F1: 0.8544
## Model description
More information needed
## Intended uses & limitations
More information needed
## Training and evaluation data
More information needed
## Training procedure
### Training hyperparameters
The following hyperparameters were used during training:
- learning_rate: 5e-05
- train_batch_size: 24
- eval_batch_size: 24
- seed: 42
- optimizer: Adam with betas=(0.9,0.999) and epsilon=1e-08
- lr_scheduler_type: linear
- num_epochs: 3
### Training results
| Training Loss | Epoch | Step | Validation Loss | F1 |
|:-------------:|:-----:|:----:|:---------------:|:------:|
| 0.2951 | 1.0 | 835 | 0.1882 | 0.8171 |
| 0.1547 | 2.0 | 1670 | 0.1707 | 0.8454 |
| 0.1018 | 3.0 | 2505 | 0.1719 | 0.8544 |
### Framework versions
- Transformers 4.19.4
- Pytorch 1.11.0+cu113
- Datasets 2.2.2
- Tokenizers 0.12.1
|
Andrey1989/xlmr-finetuned-ner | 568f4227475e804100b72e019378711885178588 | 2022-06-13T14:03:57.000Z | [
"pytorch",
"tensorboard",
"xlm-roberta",
"token-classification",
"dataset:wikiann",
"transformers",
"generated_from_trainer",
"license:mit",
"model-index",
"autotrain_compatible"
] | token-classification | false | Andrey1989 | null | Andrey1989/xlmr-finetuned-ner | 1 | null | transformers | 32,843 | ---
license: mit
tags:
- generated_from_trainer
datasets:
- wikiann
metrics:
- precision
- recall
- f1
- accuracy
model-index:
- name: xlmr-finetuned-ner
results:
- task:
name: Token Classification
type: token-classification
dataset:
name: wikiann
type: wikiann
args: et
metrics:
- name: Precision
type: precision
value: 0.9044097027481772
- name: Recall
type: recall
value: 0.9136978539556626
- name: F1
type: f1
value: 0.9090300532008596
- name: Accuracy
type: accuracy
value: 0.9649304793632428
---
<!-- This model card has been generated automatically according to the information the Trainer had access to. You
should probably proofread and complete it, then remove this comment. -->
# xlmr-finetuned-ner
This model is a fine-tuned version of [xlm-roberta-base](https://huggingface.co/xlm-roberta-base) on the wikiann dataset.
It achieves the following results on the evaluation set:
- Loss: 0.1395
- Precision: 0.9044
- Recall: 0.9137
- F1: 0.9090
- Accuracy: 0.9649
## Model description
More information needed
## Intended uses & limitations
More information needed
## Training and evaluation data
More information needed
## Training procedure
### Training hyperparameters
The following hyperparameters were used during training:
- learning_rate: 2e-05
- train_batch_size: 16
- eval_batch_size: 16
- seed: 42
- optimizer: Adam with betas=(0.9,0.999) and epsilon=1e-08
- lr_scheduler_type: linear
- num_epochs: 3
### Training results
| Training Loss | Epoch | Step | Validation Loss | Precision | Recall | F1 | Accuracy |
|:-------------:|:-----:|:----:|:---------------:|:---------:|:------:|:------:|:--------:|
| 0.4215 | 1.0 | 938 | 0.1650 | 0.8822 | 0.8781 | 0.8802 | 0.9529 |
| 0.1559 | 2.0 | 1876 | 0.1412 | 0.9018 | 0.9071 | 0.9045 | 0.9631 |
| 0.1051 | 3.0 | 2814 | 0.1395 | 0.9044 | 0.9137 | 0.9090 | 0.9649 |
### Framework versions
- Transformers 4.19.4
- Pytorch 1.11.0+cu113
- Datasets 2.2.2
- Tokenizers 0.12.1
|
lariskelmer/opus-mt-en-ro-finetuned-en-to-ro | 15a2acdb8173eb3af1d94065e5f55d52e6d3cdbd | 2022-07-13T21:08:15.000Z | [
"pytorch",
"tensorboard",
"marian",
"text2text-generation",
"dataset:wmt16",
"transformers",
"generated_from_trainer",
"license:apache-2.0",
"model-index",
"autotrain_compatible"
] | text2text-generation | false | lariskelmer | null | lariskelmer/opus-mt-en-ro-finetuned-en-to-ro | 1 | null | transformers | 32,844 | ---
license: apache-2.0
tags:
- generated_from_trainer
datasets:
- wmt16
metrics:
- bleu
model-index:
- name: opus-mt-en-ro-finetuned-en-to-ro
results:
- task:
name: Sequence-to-sequence Language Modeling
type: text2text-generation
dataset:
name: wmt16
type: wmt16
args: ro-en
metrics:
- name: Bleu
type: bleu
value: 28.1505
---
<!-- This model card has been generated automatically according to the information the Trainer had access to. You
should probably proofread and complete it, then remove this comment. -->
# opus-mt-en-ro-finetuned-en-to-ro
This model is a fine-tuned version of [Helsinki-NLP/opus-mt-en-ro](https://huggingface.co/Helsinki-NLP/opus-mt-en-ro) on the wmt16 dataset.
It achieves the following results on the evaluation set:
- Loss: 1.2886
- Bleu: 28.1505
- Gen Len: 34.1036
## Model description
More information needed
## Intended uses & limitations
More information needed
## Training and evaluation data
More information needed
## Training procedure
### Training hyperparameters
The following hyperparameters were used during training:
- learning_rate: 2e-05
- train_batch_size: 16
- eval_batch_size: 16
- seed: 42
- optimizer: Adam with betas=(0.9,0.999) and epsilon=1e-08
- lr_scheduler_type: linear
- num_epochs: 1
- mixed_precision_training: Native AMP
### Training results
| Training Loss | Epoch | Step | Validation Loss | Bleu | Gen Len |
|:-------------:|:-----:|:-----:|:---------------:|:-------:|:-------:|
| 0.7437 | 1.0 | 38145 | 1.2886 | 28.1505 | 34.1036 |
### Framework versions
- Transformers 4.20.1
- Pytorch 1.12.0+cu113
- Datasets 2.3.2
- Tokenizers 0.12.1
|
laboyle1/distilbert-finetuned | e6d4f9f420da11e321745bba753785c19f3c14fc | 2022-06-13T21:03:49.000Z | [
"pytorch",
"distilbert",
"fill-mask",
"transformers",
"generated_from_trainer",
"license:apache-2.0",
"model-index",
"autotrain_compatible"
] | fill-mask | false | laboyle1 | null | laboyle1/distilbert-finetuned | 1 | null | transformers | 32,845 | ---
license: apache-2.0
tags:
- generated_from_trainer
model-index:
- name: distilbert-finetuned
results: []
---
<!-- This model card has been generated automatically according to the information the Trainer had access to. You
should probably proofread and complete it, then remove this comment. -->
# distilbert-finetuned
This model is a fine-tuned version of [distilbert-base-uncased](https://huggingface.co/distilbert-base-uncased) on the None dataset.
It achieves the following results on the evaluation set:
- Loss: 1.9895
## Model description
More information needed
## Intended uses & limitations
More information needed
## Training and evaluation data
More information needed
## Training procedure
### Training hyperparameters
The following hyperparameters were used during training:
- learning_rate: 2e-05
- train_batch_size: 8
- eval_batch_size: 8
- seed: 42
- optimizer: Adam with betas=(0.9,0.999) and epsilon=1e-08
- lr_scheduler_type: linear
- num_epochs: 3.0
### Training results
| Training Loss | Epoch | Step | Validation Loss |
|:-------------:|:-----:|:-----:|:---------------:|
| 2.2103 | 1.0 | 10024 | 2.0834 |
| 2.1146 | 2.0 | 20048 | 2.0387 |
| 2.0721 | 3.0 | 30072 | 2.0095 |
### Framework versions
- Transformers 4.19.4
- Pytorch 1.11.0+cu102
- Datasets 2.2.2
- Tokenizers 0.12.1
|
techiaith/wav2vec2-xls-r-1b-ft-cy | d67b672faff98479c63c9e323a6099094818585c | 2022-06-15T13:53:19.000Z | [
"pytorch",
"wav2vec2",
"automatic-speech-recognition",
"cy",
"dataset:common_voice",
"transformers",
"audio",
"hf-asr-leaderboard",
"ken-lm",
"robust-speech-event",
"speech",
"license:apache-2.0",
"model-index"
] | automatic-speech-recognition | false | techiaith | null | techiaith/wav2vec2-xls-r-1b-ft-cy | 1 | 1 | transformers | 32,846 | ---
language: cy
datasets:
- common_voice
metrics:
- wer
tags:
- audio
- automatic-speech-recognition
- hf-asr-leaderboard
- ken-lm
- robust-speech-event
- speech
license: apache-2.0
model-index:
- name: wav2vec2-xls-r-1b-ft-cy with KenLM language model (by Bangor University)
results:
- task:
name: Speech Recognition
type: automatic-speech-recognition
dataset:
name: Common Voice cy
type: common_voice
args: cy
metrics:
- name: Test WER
type: wer
value: 12.38%
---
# wav2vec2-xls-r-1b-ft-cy
Fine-tuned [facebook/wav2vec2-xls-r-1b](https://huggingface.co/facebook/wav2vec2-xls-r-1b) with the [Welsh Common Voice version 9 dataset](https://huggingface.co/datasets/common_voice).
Source code and scripts for training acoustic and KenLM language models, as well as examples of inference in transcribing or a self-hosted API service, can be found at [https://github.com/techiaith/docker-wav2vec2-xlsr-ft-cy](https://github.com/techiaith/docker-wav2vec2-xlsr-ft-cy).
## Usage
The wav2vec2-xls-r-1b-ft-cy (acoustic) model can be used directly (without a language model) as follows:
```python
import torch
import torchaudio
import librosa
from transformers import Wav2Vec2ForCTC, Wav2Vec2Processor
processor = Wav2Vec2Processor.from_pretrained("techiaith/wav2vec2-xls-r-1b-ft-cy")
model = Wav2Vec2ForCTC.from_pretrained("techiaith/wav2vec2-xls-r-1b-ft-cy")
audio, rate = librosa.load(audio_file, sr=16000)
inputs = processor(audio, sampling_rate=16_000, return_tensors="pt", padding=True)
with torch.no_grad():
tlogits = model(inputs.input_values, attention_mask=inputs.attention_mask).logits
# greedy decoding
predicted_ids = torch.argmax(logits, dim=-1)
print("Prediction:", processor.batch_decode(predicted_ids))
```
## Using the Language Model
See https://github.com/techiaith/docker-wav2vec2-xlsr-ft-cy/releases/tag/22.06 for more details and
examples of a KenLM usage with the Parlance PyTorch CTC decode bindings library: [https://github.com/parlance/ctcdecode](https://github.com/parlance/ctcdecode)
## Evaluation
According to the Welsh Common Voice version 9 test set, the WER of techiaith/wav2vec2-xls-r-1b-ft-cy standalone is **19.68%**
When assisted by the KenLM language model the same test produces a WER of **12.38%**
See: https://github.com/techiaith/docker-wav2vec2-xlsr-ft-cy/blob/main/train/python/evaluate.py
|
chiranthans23/xlm-roberta-base-finetuned-panx-de | 8c4b638c9807a30392325d10dfaccd7b1b045fd0 | 2022-06-14T16:13:11.000Z | [
"pytorch",
"tensorboard",
"xlm-roberta",
"token-classification",
"dataset:xtreme",
"transformers",
"generated_from_trainer",
"license:mit",
"model-index",
"autotrain_compatible"
] | token-classification | false | chiranthans23 | null | chiranthans23/xlm-roberta-base-finetuned-panx-de | 1 | null | transformers | 32,847 | ---
license: mit
tags:
- generated_from_trainer
datasets:
- xtreme
metrics:
- f1
model-index:
- name: xlm-roberta-base-finetuned-panx-de
results:
- task:
name: Token Classification
type: token-classification
dataset:
name: xtreme
type: xtreme
args: PAN-X.de
metrics:
- name: F1
type: f1
value: 0.8620945214069894
---
<!-- This model card has been generated automatically according to the information the Trainer had access to. You
should probably proofread and complete it, then remove this comment. -->
# xlm-roberta-base-finetuned-panx-de
This model is a fine-tuned version of [xlm-roberta-base](https://huggingface.co/xlm-roberta-base) on the xtreme dataset.
It achieves the following results on the evaluation set:
- Loss: 0.1372
- F1: 0.8621
## Model description
More information needed
## Intended uses & limitations
More information needed
## Training and evaluation data
More information needed
## Training procedure
### Training hyperparameters
The following hyperparameters were used during training:
- learning_rate: 5e-05
- train_batch_size: 24
- eval_batch_size: 24
- seed: 42
- optimizer: Adam with betas=(0.9,0.999) and epsilon=1e-08
- lr_scheduler_type: linear
- num_epochs: 3
### Training results
| Training Loss | Epoch | Step | Validation Loss | F1 |
|:-------------:|:-----:|:----:|:---------------:|:------:|
| 0.2575 | 1.0 | 525 | 0.1621 | 0.8292 |
| 0.1287 | 2.0 | 1050 | 0.1378 | 0.8526 |
| 0.0831 | 3.0 | 1575 | 0.1372 | 0.8621 |
### Framework versions
- Transformers 4.19.4
- Pytorch 1.11.0+cu113
- Datasets 2.2.2
- Tokenizers 0.12.1
|
income/jpq-document_encoder-base-msmarco-contriever | e3e5eddf8ecb5b2c82ce61fe5d294999a86501f1 | 2022-06-13T20:57:52.000Z | [
"pytorch",
"bert",
"transformers",
"license:apache-2.0"
] | null | false | income | null | income/jpq-document_encoder-base-msmarco-contriever | 1 | null | transformers | 32,848 | ---
license: apache-2.0
---
|
micamorales/roberta-NLI-abs | e59d6882e7e643211eff91101a432fb4e0ede164 | 2022-06-13T21:05:31.000Z | [
"pytorch",
"roberta",
"feature-extraction",
"transformers"
] | feature-extraction | false | micamorales | null | micamorales/roberta-NLI-abs | 1 | null | transformers | 32,849 | Entry not found |
burner/GPT-J-Step_58500 | 3a2cdc1569b5242e22ecbd48e5434a076e8bf828 | 2022-06-14T00:42:40.000Z | [
"pytorch",
"gptj",
"text-generation",
"transformers",
"license:apache-2.0"
] | text-generation | false | burner | null | burner/GPT-J-Step_58500 | 1 | null | transformers | 32,850 | ---
license: apache-2.0
---
|
burner/GPT-J-Step_18500 | 3994095dd3f37490021d608d6496acbef30ae90e | 2022-06-14T03:04:28.000Z | [
"pytorch",
"gptj",
"text-generation",
"transformers",
"license:apache-2.0"
] | text-generation | false | burner | null | burner/GPT-J-Step_18500 | 1 | null | transformers | 32,851 | ---
license: apache-2.0
---
|
twieland/MIX2_en-ja_helsinki | 7dc69e79cfe629a26847cc4e1e585448dcabe5d2 | 2022-06-16T06:23:56.000Z | [
"pytorch",
"marian",
"text2text-generation",
"transformers",
"generated_from_trainer",
"license:apache-2.0",
"model-index",
"autotrain_compatible"
] | text2text-generation | false | twieland | null | twieland/MIX2_en-ja_helsinki | 1 | null | transformers | 32,852 | ---
license: apache-2.0
tags:
- generated_from_trainer
model-index:
- name: MIX2_en-ja_helsinki
results: []
---
<!-- This model card has been generated automatically according to the information the Trainer had access to. You
should probably proofread and complete it, then remove this comment. -->
# MIX2_en-ja_helsinki
This model is a fine-tuned version of [Helsinki-NLP/opus-mt-en-jap](https://huggingface.co/Helsinki-NLP/opus-mt-en-jap) on an unknown dataset.
It achieves the following results on the evaluation set:
- Loss: 1.6703
## Model description
More information needed
## Intended uses & limitations
More information needed
## Training and evaluation data
More information needed
## Training procedure
### Training hyperparameters
The following hyperparameters were used during training:
- learning_rate: 0.0003
- train_batch_size: 96
- eval_batch_size: 64
- seed: 42
- optimizer: Adam with betas=(0.9,0.999) and epsilon=1e-08
- lr_scheduler_type: linear
- num_epochs: 4
- mixed_precision_training: Native AMP
### Training results
| Training Loss | Epoch | Step | Validation Loss |
|:-------------:|:-----:|:------:|:---------------:|
| 3.5357 | 0.02 | 4000 | 2.9519 |
| 2.8601 | 0.04 | 8000 | 2.6962 |
| 2.6183 | 0.06 | 12000 | 2.5156 |
| 2.4731 | 0.08 | 16000 | 2.4312 |
| 2.3731 | 0.1 | 20000 | 2.3575 |
| 2.2964 | 0.11 | 24000 | 2.3319 |
| 2.238 | 0.13 | 28000 | 2.2802 |
| 2.1919 | 0.15 | 32000 | 2.2552 |
| 2.1479 | 0.17 | 36000 | 2.2354 |
| 2.1104 | 0.19 | 40000 | 2.2210 |
| 2.0788 | 0.21 | 44000 | 2.1835 |
| 2.0552 | 0.23 | 48000 | 2.1391 |
| 2.0228 | 0.25 | 52000 | 2.1338 |
| 2.0062 | 0.27 | 56000 | 2.1115 |
| 1.9868 | 0.29 | 60000 | 2.1025 |
| 1.9628 | 0.31 | 64000 | 2.1334 |
| 1.9474 | 0.32 | 68000 | 2.0935 |
| 1.9318 | 0.34 | 72000 | 2.1030 |
| 1.9187 | 0.36 | 76000 | 2.0605 |
| 1.9019 | 0.38 | 80000 | 2.0388 |
| 1.8916 | 0.4 | 84000 | 2.0360 |
| 1.8775 | 0.42 | 88000 | 2.0356 |
| 1.8689 | 0.44 | 92000 | 2.0315 |
| 1.8558 | 0.46 | 96000 | 2.0169 |
| 1.8431 | 0.48 | 100000 | 2.0213 |
| 1.8373 | 0.5 | 104000 | 2.0071 |
| 1.8224 | 0.52 | 108000 | 2.0093 |
| 1.8181 | 0.53 | 112000 | 1.9952 |
| 1.8087 | 0.55 | 116000 | 1.9927 |
| 1.7998 | 0.57 | 120000 | 1.9726 |
| 1.7947 | 0.59 | 124000 | 1.9817 |
| 1.7874 | 0.61 | 128000 | 1.9650 |
| 1.7781 | 0.63 | 132000 | 1.9688 |
| 1.7712 | 0.65 | 136000 | 1.9655 |
| 1.7631 | 0.67 | 140000 | 1.9561 |
| 1.7577 | 0.69 | 144000 | 1.9529 |
| 1.7528 | 0.71 | 148000 | 1.9447 |
| 1.746 | 0.73 | 152000 | 1.9700 |
| 1.7386 | 0.74 | 156000 | 1.9413 |
| 1.7329 | 0.76 | 160000 | 1.9329 |
| 1.7285 | 0.78 | 164000 | 1.9289 |
| 1.7227 | 0.8 | 168000 | 1.9337 |
| 1.7186 | 0.82 | 172000 | 1.9263 |
| 1.7116 | 0.84 | 176000 | 1.9407 |
| 1.7072 | 0.86 | 180000 | 1.9059 |
| 1.7032 | 0.88 | 184000 | 1.9380 |
| 1.6932 | 0.9 | 188000 | 1.9183 |
| 1.6921 | 0.92 | 192000 | 1.9131 |
| 1.6875 | 0.94 | 196000 | 1.9180 |
| 1.6846 | 0.96 | 200000 | 1.9040 |
| 1.6797 | 0.97 | 204000 | 1.9089 |
| 1.6725 | 0.99 | 208000 | 1.9024 |
| 1.6589 | 1.01 | 212000 | 1.8909 |
| 1.6507 | 1.03 | 216000 | 1.8837 |
| 1.6441 | 1.05 | 220000 | 1.8906 |
| 1.6445 | 1.07 | 224000 | 1.8914 |
| 1.6394 | 1.09 | 228000 | 1.8833 |
| 1.6382 | 1.11 | 232000 | 1.8837 |
| 1.6376 | 1.13 | 236000 | 1.8869 |
| 1.6329 | 1.15 | 240000 | 1.8829 |
| 1.6294 | 1.17 | 244000 | 1.8845 |
| 1.6273 | 1.18 | 248000 | 1.8888 |
| 1.6243 | 1.2 | 252000 | 1.8709 |
| 1.6226 | 1.22 | 256000 | 1.8418 |
| 1.6177 | 1.24 | 260000 | 1.8587 |
| 1.6151 | 1.26 | 264000 | 1.8526 |
| 1.6111 | 1.28 | 268000 | 1.8494 |
| 1.6084 | 1.3 | 272000 | 1.8781 |
| 1.6043 | 1.32 | 276000 | 1.8390 |
| 1.6011 | 1.34 | 280000 | 1.8603 |
| 1.5999 | 1.36 | 284000 | 1.8515 |
| 1.5954 | 1.38 | 288000 | 1.8356 |
| 1.5936 | 1.39 | 292000 | 1.8530 |
| 1.5916 | 1.41 | 296000 | 1.8475 |
| 1.5886 | 1.43 | 300000 | 1.8410 |
| 1.5883 | 1.45 | 304000 | 1.8153 |
| 1.5828 | 1.47 | 308000 | 1.8254 |
| 1.582 | 1.49 | 312000 | 1.8139 |
| 1.578 | 1.51 | 316000 | 1.8366 |
| 1.5723 | 1.53 | 320000 | 1.8353 |
| 1.5705 | 1.55 | 324000 | 1.8230 |
| 1.5691 | 1.57 | 328000 | 1.8194 |
| 1.5656 | 1.59 | 332000 | 1.8069 |
| 1.566 | 1.6 | 336000 | 1.8204 |
| 1.5604 | 1.62 | 340000 | 1.8307 |
| 1.5573 | 1.64 | 344000 | 1.8209 |
| 1.5547 | 1.66 | 348000 | 1.8320 |
| 1.5545 | 1.68 | 352000 | 1.8179 |
| 1.5519 | 1.7 | 356000 | 1.8323 |
| 1.545 | 1.72 | 360000 | 1.8005 |
| 1.5483 | 1.74 | 364000 | 1.8034 |
| 1.5454 | 1.76 | 368000 | 1.7997 |
| 1.5393 | 1.78 | 372000 | 1.8078 |
| 1.5381 | 1.8 | 376000 | 1.8204 |
| 1.5347 | 1.81 | 380000 | 1.8071 |
| 1.5327 | 1.83 | 384000 | 1.7997 |
| 1.529 | 1.85 | 388000 | 1.8012 |
| 1.5287 | 1.87 | 392000 | 1.8028 |
| 1.5273 | 1.89 | 396000 | 1.8103 |
| 1.5194 | 1.91 | 400000 | 1.8008 |
| 1.5197 | 1.93 | 404000 | 1.8004 |
| 1.5218 | 1.95 | 408000 | 1.8024 |
| 1.514 | 1.97 | 412000 | 1.7852 |
| 1.5146 | 1.99 | 416000 | 1.7908 |
| 1.5045 | 2.01 | 420000 | 1.7864 |
| 1.4876 | 2.02 | 424000 | 1.7813 |
| 1.4846 | 2.04 | 428000 | 1.7822 |
| 1.4865 | 2.06 | 432000 | 1.7737 |
| 1.4857 | 2.08 | 436000 | 1.7668 |
| 1.4825 | 2.1 | 440000 | 1.7681 |
| 1.4828 | 2.12 | 444000 | 1.7685 |
| 1.4821 | 2.14 | 448000 | 1.7636 |
| 1.4778 | 2.16 | 452000 | 1.7778 |
| 1.4803 | 2.18 | 456000 | 1.7834 |
| 1.4766 | 2.2 | 460000 | 1.7801 |
| 1.4741 | 2.22 | 464000 | 1.7601 |
| 1.4705 | 2.23 | 468000 | 1.7665 |
| 1.4739 | 2.25 | 472000 | 1.7604 |
| 1.4694 | 2.27 | 476000 | 1.7803 |
| 1.4665 | 2.29 | 480000 | 1.7835 |
| 1.4668 | 2.31 | 484000 | 1.7670 |
| 1.4605 | 2.33 | 488000 | 1.7629 |
| 1.4626 | 2.35 | 492000 | 1.7612 |
| 1.4627 | 2.37 | 496000 | 1.7612 |
| 1.4569 | 2.39 | 500000 | 1.7557 |
| 1.455 | 2.41 | 504000 | 1.7599 |
| 1.4547 | 2.43 | 508000 | 1.7569 |
| 1.453 | 2.44 | 512000 | 1.7589 |
| 1.4515 | 2.46 | 516000 | 1.7679 |
| 1.4501 | 2.48 | 520000 | 1.7574 |
| 1.4446 | 2.5 | 524000 | 1.7526 |
| 1.4456 | 2.52 | 528000 | 1.7506 |
| 1.4445 | 2.54 | 532000 | 1.7484 |
| 1.4428 | 2.56 | 536000 | 1.7447 |
| 1.439 | 2.58 | 540000 | 1.7468 |
| 1.441 | 2.6 | 544000 | 1.7609 |
| 1.4358 | 2.62 | 548000 | 1.7498 |
| 1.4318 | 2.64 | 552000 | 1.7592 |
| 1.4276 | 2.65 | 556000 | 1.7452 |
| 1.4317 | 2.67 | 560000 | 1.7500 |
| 1.4277 | 2.69 | 564000 | 1.7392 |
| 1.4259 | 2.71 | 568000 | 1.7351 |
| 1.4239 | 2.73 | 572000 | 1.7385 |
| 1.4191 | 2.75 | 576000 | 1.7487 |
| 1.4204 | 2.77 | 580000 | 1.7392 |
| 1.4176 | 2.79 | 584000 | 1.7372 |
| 1.4147 | 2.81 | 588000 | 1.7347 |
| 1.4154 | 2.83 | 592000 | 1.7085 |
| 1.4134 | 2.85 | 596000 | 1.7103 |
| 1.4091 | 2.87 | 600000 | 1.7124 |
| 1.4091 | 2.88 | 604000 | 1.7369 |
| 1.406 | 2.9 | 608000 | 1.7142 |
| 1.4028 | 2.92 | 612000 | 1.7376 |
| 1.4019 | 2.94 | 616000 | 1.7201 |
| 1.4018 | 2.96 | 620000 | 1.7230 |
| 1.3959 | 2.98 | 624000 | 1.7206 |
| 1.3985 | 3.0 | 628000 | 1.7183 |
| 1.3681 | 3.02 | 632000 | 1.7283 |
| 1.3668 | 3.04 | 636000 | 1.7330 |
| 1.3687 | 3.06 | 640000 | 1.7187 |
| 1.3681 | 3.08 | 644000 | 1.7163 |
| 1.3687 | 3.09 | 648000 | 1.7249 |
| 1.364 | 3.11 | 652000 | 1.7283 |
| 1.364 | 3.13 | 656000 | 1.7091 |
| 1.3652 | 3.15 | 660000 | 1.7030 |
| 1.3623 | 3.17 | 664000 | 1.7058 |
| 1.3604 | 3.19 | 668000 | 1.7101 |
| 1.3598 | 3.21 | 672000 | 1.7104 |
| 1.3577 | 3.23 | 676000 | 1.7028 |
| 1.3574 | 3.25 | 680000 | 1.7023 |
| 1.3546 | 3.27 | 684000 | 1.7197 |
| 1.3549 | 3.29 | 688000 | 1.7045 |
| 1.3534 | 3.3 | 692000 | 1.6990 |
| 1.3511 | 3.32 | 696000 | 1.6971 |
| 1.3504 | 3.34 | 700000 | 1.6894 |
| 1.346 | 3.36 | 704000 | 1.6820 |
| 1.3467 | 3.38 | 708000 | 1.6920 |
| 1.3461 | 3.4 | 712000 | 1.6897 |
| 1.3425 | 3.42 | 716000 | 1.6962 |
| 1.34 | 3.44 | 720000 | 1.6864 |
| 1.3408 | 3.46 | 724000 | 1.6860 |
| 1.3387 | 3.48 | 728000 | 1.6924 |
| 1.3377 | 3.5 | 732000 | 1.6919 |
| 1.3378 | 3.51 | 736000 | 1.6858 |
| 1.334 | 3.53 | 740000 | 1.6816 |
| 1.3347 | 3.55 | 744000 | 1.6867 |
| 1.3307 | 3.57 | 748000 | 1.6859 |
| 1.3316 | 3.59 | 752000 | 1.6896 |
| 1.3257 | 3.61 | 756000 | 1.6824 |
| 1.3222 | 3.63 | 760000 | 1.6819 |
| 1.3247 | 3.65 | 764000 | 1.6809 |
| 1.3207 | 3.67 | 768000 | 1.6775 |
| 1.3227 | 3.69 | 772000 | 1.6807 |
| 1.3203 | 3.71 | 776000 | 1.6750 |
| 1.3203 | 3.72 | 780000 | 1.6758 |
| 1.316 | 3.74 | 784000 | 1.6787 |
| 1.3147 | 3.76 | 788000 | 1.6747 |
| 1.3146 | 3.78 | 792000 | 1.6718 |
| 1.3137 | 3.8 | 796000 | 1.6744 |
| 1.3143 | 3.82 | 800000 | 1.6733 |
| 1.3123 | 3.84 | 804000 | 1.6754 |
| 1.3069 | 3.86 | 808000 | 1.6734 |
| 1.3122 | 3.88 | 812000 | 1.6742 |
| 1.3074 | 3.9 | 816000 | 1.6742 |
| 1.3006 | 3.92 | 820000 | 1.6709 |
| 1.308 | 3.93 | 824000 | 1.6714 |
| 1.3063 | 3.95 | 828000 | 1.6727 |
| 1.3036 | 3.97 | 832000 | 1.6711 |
| 1.3048 | 3.99 | 836000 | 1.6703 |
### Framework versions
- Transformers 4.19.2
- Pytorch 1.11.0+cu113
- Datasets 2.2.2
- Tokenizers 0.12.1
|
dz-xt/stt_en_conformer_ctc_small | 33045d3effb0dce21b18eaeac9c2b3b59e3bc909 | 2022-06-14T10:13:47.000Z | [
"nemo",
"en",
"dataset:librispeech_asr",
"dataset:mozilla-foundation/common_voice_7_0",
"dataset:vctk",
"dataset:fisher_corpus",
"dataset:Switchboard-1",
"dataset:WSJ-0",
"dataset:WSJ-1",
"dataset:National Singapore Corpus Part 1",
"dataset:National Singapore Corpus Part 6",
"dataset:VoxPopuli (EN)",
"dataset:Europarl-ASR (EN)",
"dataset:Multilingual LibriSpeech (2000 hours)",
"automatic-speech-recognition",
"speech",
"audio",
"CTC",
"Conformer",
"Transformer",
"NeMo",
"pytorch",
"license:cc-by-4.0",
"model-index"
] | automatic-speech-recognition | false | dz-xt | null | dz-xt/stt_en_conformer_ctc_small | 1 | 0 | nemo | 32,853 | ---
language:
- en
license: cc-by-4.0
library_name: nemo
datasets:
- librispeech_asr
- mozilla-foundation/common_voice_7_0
- vctk
- fisher_corpus
- Switchboard-1
- WSJ-0
- WSJ-1
- National Singapore Corpus Part 1
- National Singapore Corpus Part 6
- VoxPopuli (EN)
- Europarl-ASR (EN)
- Multilingual LibriSpeech (2000 hours)
thumbnail: null
tags:
- automatic-speech-recognition
- speech
- audio
- CTC
- Conformer
- Transformer
- NeMo
- pytorch
model-index:
- name: stt_en_conformer_ctc_small
results:
- task:
type: automatic-speech-recognition
dataset:
type: librispeech_asr
name: Librispeech (clean)
config: other
split: test
args:
language: en
metrics:
- type: wer
value: 8.1
name: WER
---
## Model Overview
<DESCRIBE IN ONE LINE THE MODEL AND ITS USE>
## NVIDIA NeMo: Training
To train, fine-tune or play with the model you will need to install [NVIDIA NeMo](https://github.com/NVIDIA/NeMo). We recommend you install it after you've installed latest Pytorch version.
```
pip install nemo_toolkit['all']
```
## How to Use this Model
The model is available for use in the NeMo toolkit [3], and can be used as a pre-trained checkpoint for inference or for fine-tuning on another dataset.
### Automatically instantiate the model
```python
import nemo.collections.asr as nemo_asr
asr_model = nemo_asr.models.ASRModel.from_pretrained("dz-xt/stt_en_conformer_ctc_small")
```
### Transcribing using Python
First, let's get a sample
```
wget https://dldata-public.s3.us-east-2.amazonaws.com/2086-149220-0033.wav
```
Then simply do:
```
asr_model.transcribe(['2086-149220-0033.wav'])
```
### Transcribing many audio files
```shell
python [NEMO_GIT_FOLDER]/examples/asr/transcribe_speech.py pretrained_name="dz-xt/stt_en_conformer_ctc_small" audio_dir="<DIRECTORY CONTAINING AUDIO FILES>"
```
### Input
This model accepts 16000 KHz Mono-channel Audio (wav files) as input.
### Output
This model provides transcribed speech as a string for a given audio sample.
## Model Architecture
<ADD SOME INFORMATION ABOUT THE ARCHITECTURE>
## Training
<ADD INFORMATION ABOUT HOW THE MODEL WAS TRAINED - HOW MANY EPOCHS, AMOUNT OF COMPUTE ETC>
### Datasets
<LIST THE NAME AND SPLITS OF DATASETS USED TO TRAIN THIS MODEL (ALONG WITH LANGUAGE AND ANY ADDITIONAL INFORMATION)>
## Performance
<LIST THE SCORES OF THE MODEL -
OR
USE THE Hugging Face Evaluate LiBRARY TO UPLOAD METRICS>
## Limitations
<DECLARE ANY POTENTIAL LIMITATIONS OF THE MODEL>
Eg:
Since this model was trained on publically available speech datasets, the performance of this model might degrade for speech which includes technical terms, or vernacular that the model has not been trained on. The model might also perform worse for accented speech.
## References
<ADD ANY REFERENCES HERE AS NEEDED>
[1] [NVIDIA NeMo Toolkit](https://github.com/NVIDIA/NeMo) |
lmqg/t5-small-squadshifts-nyt | 16cda39345c8a8c557ebf4d3a7d059d5ae023cea | 2022-06-14T10:36:00.000Z | [
"pytorch",
"t5",
"text2text-generation",
"transformers",
"autotrain_compatible"
] | text2text-generation | false | lmqg | null | lmqg/t5-small-squadshifts-nyt | 1 | null | transformers | 32,854 | Entry not found |
lmqg/t5-small-squadshifts-reddit | dae0414bcbd805c23fdc8957dce8e50727e310ac | 2022-06-14T10:37:16.000Z | [
"pytorch",
"t5",
"text2text-generation",
"transformers",
"autotrain_compatible"
] | text2text-generation | false | lmqg | null | lmqg/t5-small-squadshifts-reddit | 1 | null | transformers | 32,855 | Entry not found |
janeel/distilbert-base-uncased-finetuned-squad | a933f5e7ddd6f1927246d7a4bbea3935d9de1b41 | 2022-06-16T13:42:47.000Z | [
"pytorch",
"tensorboard",
"roberta",
"question-answering",
"dataset:squad",
"transformers",
"generated_from_trainer",
"model-index",
"autotrain_compatible"
] | question-answering | false | janeel | null | janeel/distilbert-base-uncased-finetuned-squad | 1 | null | transformers | 32,856 | ---
tags:
- generated_from_trainer
datasets:
- squad
model-index:
- name: distilbert-base-uncased-finetuned-squad
results: []
---
<!-- This model card has been generated automatically according to the information the Trainer had access to. You
should probably proofread and complete it, then remove this comment. -->
# distilbert-base-uncased-finetuned-squad
This model is a fine-tuned version of [klue/roberta-small](https://huggingface.co/klue/roberta-small) on the squad dataset.
It achieves the following results on the evaluation set:
- Loss: 2.1272
## Model description
More information needed
## Intended uses & limitations
More information needed
## Training and evaluation data
More information needed
## Training procedure
### Training hyperparameters
The following hyperparameters were used during training:
- learning_rate: 2e-05
- train_batch_size: 32
- eval_batch_size: 32
- seed: 42
- optimizer: Adam with betas=(0.9,0.999) and epsilon=1e-08
- lr_scheduler_type: linear
- num_epochs: 2
### Training results
| Training Loss | Epoch | Step | Validation Loss |
|:-------------:|:-----:|:----:|:---------------:|
| 2.398 | 1.0 | 2767 | 2.2567 |
| 2.0729 | 2.0 | 5534 | 2.1272 |
### Framework versions
- Transformers 4.19.4
- Pytorch 1.11.0+cu113
- Datasets 2.3.2
- Tokenizers 0.12.1
|
Salvatore/mt5-finetuned-amazon-en-es | 8e4979d36e6ce0e7c54eda9fd3db63cf833770f0 | 2022-06-14T14:25:12.000Z | [
"pytorch",
"tensorboard",
"mt5",
"text2text-generation",
"transformers",
"autotrain_compatible"
] | text2text-generation | false | Salvatore | null | Salvatore/mt5-finetuned-amazon-en-es | 1 | null | transformers | 32,857 | Entry not found |
AntoDono/DialoGPT-Bopy-Alpha-1.01 | 7b78c239a217ee6543bbfca33397615aba4a930d | 2022-06-24T17:05:47.000Z | [
"pytorch",
"gpt2",
"text-generation",
"transformers",
"conversational"
] | conversational | false | AntoDono | null | AntoDono/DialoGPT-Bopy-Alpha-1.01 | 1 | null | transformers | 32,858 | ---
tags:
- conversational
--- |
Waleed-bin-Qamar/ConvNext-For-Covid-Classification-30EP | 87de07e7431f3adccb4812a68e98edc1d552507b | 2022-06-14T15:04:53.000Z | [
"pytorch",
"convnext",
"image-classification",
"transformers"
] | image-classification | false | Waleed-bin-Qamar | null | Waleed-bin-Qamar/ConvNext-For-Covid-Classification-30EP | 1 | null | transformers | 32,859 | Entry not found |
mgtoxd/tstw2 | 00e8e43ccfa3109170b95dc351d2e6ba920bab13 | 2022-06-23T01:59:33.000Z | [
"pytorch",
"tf",
"wav2vec2",
"automatic-speech-recognition",
"transformers"
] | automatic-speech-recognition | false | mgtoxd | null | mgtoxd/tstw2 | 1 | null | transformers | 32,860 | Entry not found |
AntoDono/DialoGPT-Bopy-Alpha-1.03 | af3fca9beb2a826f58f9eec40b02fc0b5cf0e23e | 2022-06-14T16:32:43.000Z | [
"pytorch",
"gpt2",
"text-generation",
"transformers"
] | text-generation | false | AntoDono | null | AntoDono/DialoGPT-Bopy-Alpha-1.03 | 1 | null | transformers | 32,861 | Entry not found |
mahfooz/bert-base-cased-dv-v2 | 6d1304b254227107ceeb6ad5e305e49b23c90d86 | 2022-06-15T13:11:46.000Z | [
"pytorch",
"bert",
"fill-mask",
"transformers",
"autotrain_compatible"
] | fill-mask | false | mahfooz | null | mahfooz/bert-base-cased-dv-v2 | 1 | null | transformers | 32,862 | Entry not found |
Waleed-bin-Qamar/ConvNext-For-Covid-Classification-30EP-BS64 | 12cfd445b034e2dc26e90aabbe5b2e7ff8b9ce81 | 2022-07-03T13:17:29.000Z | [
"pytorch",
"convnext",
"image-classification",
"transformers"
] | image-classification | false | Waleed-bin-Qamar | null | Waleed-bin-Qamar/ConvNext-For-Covid-Classification-30EP-BS64 | 1 | null | transformers | 32,863 | Entry not found |
Browbon/DialoGPT-small-LucaChangretta | f3e4d20c8330c1b202e7c1de741aff8f53213d31 | 2022-06-14T20:25:27.000Z | [
"pytorch",
"gpt2",
"text-generation",
"transformers",
"conversational"
] | conversational | false | Browbon | null | Browbon/DialoGPT-small-LucaChangretta | 1 | null | transformers | 32,864 | ---
tags:
- conversational
---
# Luca Changretta GPT Model |
Renukswamy/distilbert-base-uncased-finetuned-squad | e4eb8e751657468fa9c92ee7ed37b19bb311dfb2 | 2022-06-15T21:27:19.000Z | [
"pytorch",
"tensorboard",
"distilbert",
"question-answering",
"transformers",
"generated_from_trainer",
"license:apache-2.0",
"model-index",
"autotrain_compatible"
] | question-answering | false | Renukswamy | null | Renukswamy/distilbert-base-uncased-finetuned-squad | 1 | null | transformers | 32,865 | ---
license: apache-2.0
tags:
- generated_from_trainer
model-index:
- name: distilbert-base-uncased-finetuned-squad
results: []
---
<!-- This model card has been generated automatically according to the information the Trainer had access to. You
should probably proofread and complete it, then remove this comment. -->
# distilbert-base-uncased-finetuned-squad
This model is a fine-tuned version of [distilbert-base-uncased](https://huggingface.co/distilbert-base-uncased) on an unknown dataset.
It achieves the following results on the evaluation set:
- Loss: 0.7108
## Model description
More information needed
## Intended uses & limitations
More information needed
## Training and evaluation data
More information needed
## Training procedure
### Training hyperparameters
The following hyperparameters were used during training:
- learning_rate: 2e-05
- train_batch_size: 16
- eval_batch_size: 16
- seed: 42
- optimizer: Adam with betas=(0.9,0.999) and epsilon=1e-08
- lr_scheduler_type: linear
- num_epochs: 3
### Training results
| Training Loss | Epoch | Step | Validation Loss |
|:-------------:|:-----:|:-----:|:---------------:|
| 0.6869 | 1.0 | 6941 | 0.7765 |
| 0.5083 | 2.0 | 13882 | 0.6917 |
| 0.372 | 3.0 | 20823 | 0.7108 |
### Framework versions
- Transformers 4.19.4
- Pytorch 1.11.0+cu113
- Datasets 2.3.1
- Tokenizers 0.12.1
|
steven123/teeth_test | 7622a568dc990833829535825be03f32965735f1 | 2022-06-14T23:57:13.000Z | [
"pytorch",
"tensorboard",
"vit",
"image-classification",
"transformers",
"huggingpics",
"model-index"
] | image-classification | false | steven123 | null | steven123/teeth_test | 1 | null | transformers | 32,866 | ---
tags:
- image-classification
- pytorch
- huggingpics
metrics:
- accuracy
model-index:
- name: teeth_test
results:
- task:
name: Image Classification
type: image-classification
metrics:
- name: Accuracy
type: accuracy
value: 0.5555555820465088
---
# teeth_test
Autogenerated by HuggingPics🤗🖼️
Create your own image classifier for **anything** by running [the demo on Google Colab](https://colab.research.google.com/github/nateraw/huggingpics/blob/main/HuggingPics.ipynb).
Report any issues with the demo at the [github repo](https://github.com/nateraw/huggingpics).
## Example Images
#### Good Teeth

#### Missing Teeth

#### Rotten Teeth
 |
lmqg/t5-base-squadshifts-amazon | b47caf4b07825adc86b0c3301276518ad5cdabd5 | 2022-06-15T00:08:17.000Z | [
"pytorch",
"t5",
"text2text-generation",
"transformers",
"autotrain_compatible"
] | text2text-generation | false | lmqg | null | lmqg/t5-base-squadshifts-amazon | 1 | null | transformers | 32,867 | Entry not found |
erickfm/frosty-sweep-1 | 70793b04abe6c3e0f87326ac9d64a19fe594c643 | 2022-06-15T01:16:32.000Z | [
"pytorch",
"t5",
"text2text-generation",
"transformers",
"autotrain_compatible"
] | text2text-generation | false | erickfm | null | erickfm/frosty-sweep-1 | 1 | null | transformers | 32,868 | Entry not found |
steven123/Teeth_A | 06ac242cddf9812c3e6078ec252f50d7a17c3415 | 2022-06-15T02:42:35.000Z | [
"pytorch",
"tensorboard",
"vit",
"image-classification",
"transformers",
"huggingpics",
"model-index"
] | image-classification | false | steven123 | null | steven123/Teeth_A | 1 | null | transformers | 32,869 | ---
tags:
- image-classification
- pytorch
- huggingpics
metrics:
- accuracy
model-index:
- name: Teeth_A
results:
- task:
name: Image Classification
type: image-classification
metrics:
- name: Accuracy
type: accuracy
value: 0.4545454680919647
---
# Teeth_A
Autogenerated by HuggingPics🤗🖼️
Create your own image classifier for **anything** by running [the demo on Google Colab](https://colab.research.google.com/github/nateraw/huggingpics/blob/main/HuggingPics.ipynb).
Report any issues with the demo at the [github repo](https://github.com/nateraw/huggingpics).
## Example Images
#### Good Teeth

#### Missing Teeth

#### Rotten Teeth
 |
steven123/Teeth_C | 48308ba60c00f48eb2abddac6a634bb03f5c1888 | 2022-06-15T02:53:44.000Z | [
"pytorch",
"tensorboard",
"vit",
"image-classification",
"transformers",
"huggingpics",
"model-index"
] | image-classification | false | steven123 | null | steven123/Teeth_C | 1 | null | transformers | 32,870 | ---
tags:
- image-classification
- pytorch
- huggingpics
metrics:
- accuracy
model-index:
- name: Teeth_C
results:
- task:
name: Image Classification
type: image-classification
metrics:
- name: Accuracy
type: accuracy
value: 0.5
---
# Teeth_C
Autogenerated by HuggingPics🤗🖼️
Create your own image classifier for **anything** by running [the demo on Google Colab](https://colab.research.google.com/github/nateraw/huggingpics/blob/main/HuggingPics.ipynb).
Report any issues with the demo at the [github repo](https://github.com/nateraw/huggingpics).
## Example Images
#### Good Teeth

#### Missing Teeth

#### Rotten Teeth
 |
x574chen/wav2vec2-librispeech-clean-100h-demo-dist | fe4ea29671955245412c37dde6d6799fbd5ac3fd | 2022-06-15T16:43:25.000Z | [
"pytorch",
"wav2vec2",
"automatic-speech-recognition",
"transformers"
] | automatic-speech-recognition | false | x574chen | null | x574chen/wav2vec2-librispeech-clean-100h-demo-dist | 1 | null | transformers | 32,871 | Entry not found |
huggingtweets/mysteriousgam54 | 41d9e51ecb294a2b003365456f001d85f233e9f4 | 2022-06-15T04:06:06.000Z | [
"pytorch",
"gpt2",
"text-generation",
"en",
"transformers",
"huggingtweets"
] | text-generation | false | huggingtweets | null | huggingtweets/mysteriousgam54 | 1 | null | transformers | 32,872 | ---
language: en
thumbnail: https://github.com/borisdayma/huggingtweets/blob/master/img/logo.png?raw=true
tags:
- huggingtweets
widget:
- text: "My dream is"
---
<div class="inline-flex flex-col" style="line-height: 1.5;">
<div class="flex">
<div
style="display:inherit; margin-left: 4px; margin-right: 4px; width: 92px; height:92px; border-radius: 50%; background-size: cover; background-image: url('https://pbs.twimg.com/profile_images/1429866660299689984/CGXAQuWf_400x400.jpg')">
</div>
<div
style="display:none; margin-left: 4px; margin-right: 4px; width: 92px; height:92px; border-radius: 50%; background-size: cover; background-image: url('')">
</div>
<div
style="display:none; margin-left: 4px; margin-right: 4px; width: 92px; height:92px; border-radius: 50%; background-size: cover; background-image: url('')">
</div>
</div>
<div style="text-align: center; margin-top: 3px; font-size: 16px; font-weight: 800">🤖 AI BOT 🤖</div>
<div style="text-align: center; font-size: 16px; font-weight: 800">themysteriousgamer</div>
<div style="text-align: center; font-size: 14px;">@mysteriousgam54</div>
</div>
I was made with [huggingtweets](https://github.com/borisdayma/huggingtweets).
Create your own bot based on your favorite user with [the demo](https://colab.research.google.com/github/borisdayma/huggingtweets/blob/master/huggingtweets-demo.ipynb)!
## How does it work?
The model uses the following pipeline.
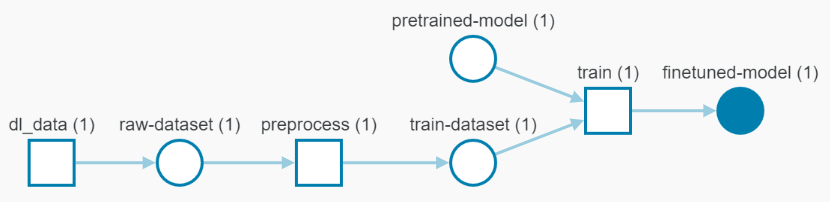
To understand how the model was developed, check the [W&B report](https://wandb.ai/wandb/huggingtweets/reports/HuggingTweets-Train-a-Model-to-Generate-Tweets--VmlldzoxMTY5MjI).
## Training data
The model was trained on tweets from themysteriousgamer.
| Data | themysteriousgamer |
| --- | --- |
| Tweets downloaded | 1315 |
| Retweets | 210 |
| Short tweets | 168 |
| Tweets kept | 937 |
[Explore the data](https://wandb.ai/wandb/huggingtweets/runs/m4i8lg1e/artifacts), which is tracked with [W&B artifacts](https://docs.wandb.com/artifacts) at every step of the pipeline.
## Training procedure
The model is based on a pre-trained [GPT-2](https://huggingface.co/gpt2) which is fine-tuned on @mysteriousgam54's tweets.
Hyperparameters and metrics are recorded in the [W&B training run](https://wandb.ai/wandb/huggingtweets/runs/3rz0m12t) for full transparency and reproducibility.
At the end of training, [the final model](https://wandb.ai/wandb/huggingtweets/runs/3rz0m12t/artifacts) is logged and versioned.
## How to use
You can use this model directly with a pipeline for text generation:
```python
from transformers import pipeline
generator = pipeline('text-generation',
model='huggingtweets/mysteriousgam54')
generator("My dream is", num_return_sequences=5)
```
## Limitations and bias
The model suffers from [the same limitations and bias as GPT-2](https://huggingface.co/gpt2#limitations-and-bias).
In addition, the data present in the user's tweets further affects the text generated by the model.
## About
*Built by Boris Dayma*
[](https://twitter.com/intent/follow?screen_name=borisdayma)
For more details, visit the project repository.
[](https://github.com/borisdayma/huggingtweets)
|
RuiqianLi/Malaya-speech_fine-tune_MrBrown_15_Jun | 75705930f26da94c57a24b79510c355e9e35eee9 | 2022-06-15T08:23:28.000Z | [
"pytorch",
"tensorboard",
"wav2vec2",
"automatic-speech-recognition",
"dataset:uob_singlish",
"transformers",
"generated_from_trainer",
"model-index"
] | automatic-speech-recognition | false | RuiqianLi | null | RuiqianLi/Malaya-speech_fine-tune_MrBrown_15_Jun | 1 | null | transformers | 32,873 | ---
tags:
- generated_from_trainer
datasets:
- uob_singlish
model-index:
- name: Malaya-speech_fine-tune_MrBrown_15_Jun
results: []
---
<!-- This model card has been generated automatically according to the information the Trainer had access to. You
should probably proofread and complete it, then remove this comment. -->
# Malaya-speech_fine-tune_MrBrown_15_Jun
This model is a fine-tuned version of [malay-huggingface/wav2vec2-xls-r-300m-mixed](https://huggingface.co/malay-huggingface/wav2vec2-xls-r-300m-mixed) on the uob_singlish dataset.
It achieves the following results on the evaluation set:
- Loss: 0.4822
- Wer: 0.2449
## Model description
More information needed
## Intended uses & limitations
More information needed
## Training and evaluation data
More information needed
## Training procedure
### Training hyperparameters
The following hyperparameters were used during training:
- learning_rate: 0.0001
- train_batch_size: 2
- eval_batch_size: 8
- seed: 42
- gradient_accumulation_steps: 2
- total_train_batch_size: 4
- optimizer: Adam with betas=(0.9,0.999) and epsilon=1e-08
- lr_scheduler_type: linear
- lr_scheduler_warmup_steps: 1000
- num_epochs: 100
- mixed_precision_training: Native AMP
### Training results
| Training Loss | Epoch | Step | Validation Loss | Wer |
|:-------------:|:-----:|:----:|:---------------:|:------:|
| 1.1607 | 5.26 | 200 | 0.3983 | 0.2381 |
| 0.5184 | 10.52 | 400 | 0.3256 | 0.2245 |
| 0.2993 | 15.78 | 600 | 0.3437 | 0.2426 |
| 0.2485 | 21.05 | 800 | 0.4547 | 0.2585 |
| 0.1917 | 26.31 | 1000 | 0.4598 | 0.2517 |
| 0.1586 | 31.57 | 1200 | 0.4050 | 0.2290 |
| 0.1486 | 36.83 | 1400 | 0.4186 | 0.2653 |
| 0.1307 | 42.1 | 1600 | 0.4284 | 0.2857 |
| 0.0895 | 47.36 | 1800 | 0.5158 | 0.2562 |
| 0.0526 | 52.62 | 2000 | 0.4525 | 0.2449 |
| 0.0553 | 57.88 | 2200 | 0.4364 | 0.2336 |
| 0.037 | 63.16 | 2400 | 0.3873 | 0.2449 |
| 0.0439 | 68.42 | 2600 | 0.3914 | 0.2404 |
| 0.0411 | 73.68 | 2800 | 0.4673 | 0.2494 |
| 0.0242 | 78.94 | 3000 | 0.4801 | 0.2426 |
| 0.0833 | 84.21 | 3200 | 0.4641 | 0.2630 |
| 0.034 | 89.47 | 3400 | 0.4607 | 0.2449 |
| 0.02 | 94.73 | 3600 | 0.4825 | 0.2449 |
| 0.0211 | 99.99 | 3800 | 0.4822 | 0.2449 |
### Framework versions
- Transformers 4.11.3
- Pytorch 1.10.0+cu113
- Datasets 1.18.3
- Tokenizers 0.10.3
|
huggingtweets/danny_macaskill-martynashton | 62b5c2c93d0f1d99d654e95aa0ce7d3b969fea36 | 2022-06-15T04:59:30.000Z | [
"pytorch",
"gpt2",
"text-generation",
"en",
"transformers",
"huggingtweets"
] | text-generation | false | huggingtweets | null | huggingtweets/danny_macaskill-martynashton | 1 | null | transformers | 32,874 | ---
language: en
thumbnail: http://www.huggingtweets.com/danny_macaskill-martynashton/1655269165002/predictions.png
tags:
- huggingtweets
widget:
- text: "My dream is"
---
<div class="inline-flex flex-col" style="line-height: 1.5;">
<div class="flex">
<div
style="display:inherit; margin-left: 4px; margin-right: 4px; width: 92px; height:92px; border-radius: 50%; background-size: cover; background-image: url('https://pbs.twimg.com/profile_images/770573812991754240/gyUr23bS_400x400.jpg')">
</div>
<div
style="display:inherit; margin-left: 4px; margin-right: 4px; width: 92px; height:92px; border-radius: 50%; background-size: cover; background-image: url('https://pbs.twimg.com/profile_images/616596420230021120/w-kK8IT6_400x400.jpg')">
</div>
<div
style="display:none; margin-left: 4px; margin-right: 4px; width: 92px; height:92px; border-radius: 50%; background-size: cover; background-image: url('')">
</div>
</div>
<div style="text-align: center; margin-top: 3px; font-size: 16px; font-weight: 800">🤖 AI CYBORG 🤖</div>
<div style="text-align: center; font-size: 16px; font-weight: 800">Danny MacAskill & Martyn Ashton</div>
<div style="text-align: center; font-size: 14px;">@danny_macaskill-martynashton</div>
</div>
I was made with [huggingtweets](https://github.com/borisdayma/huggingtweets).
Create your own bot based on your favorite user with [the demo](https://colab.research.google.com/github/borisdayma/huggingtweets/blob/master/huggingtweets-demo.ipynb)!
## How does it work?
The model uses the following pipeline.
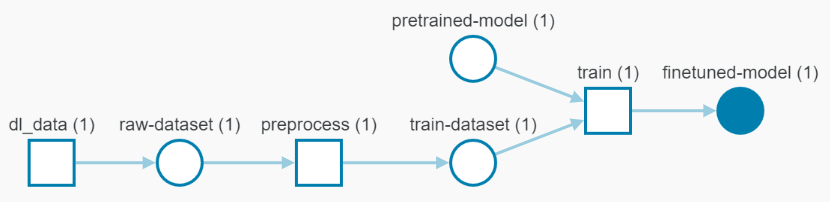
To understand how the model was developed, check the [W&B report](https://wandb.ai/wandb/huggingtweets/reports/HuggingTweets-Train-a-Model-to-Generate-Tweets--VmlldzoxMTY5MjI).
## Training data
The model was trained on tweets from Danny MacAskill & Martyn Ashton.
| Data | Danny MacAskill | Martyn Ashton |
| --- | --- | --- |
| Tweets downloaded | 2971 | 3179 |
| Retweets | 505 | 810 |
| Short tweets | 79 | 136 |
| Tweets kept | 2387 | 2233 |
[Explore the data](https://wandb.ai/wandb/huggingtweets/runs/31ege8zb/artifacts), which is tracked with [W&B artifacts](https://docs.wandb.com/artifacts) at every step of the pipeline.
## Training procedure
The model is based on a pre-trained [GPT-2](https://huggingface.co/gpt2) which is fine-tuned on @danny_macaskill-martynashton's tweets.
Hyperparameters and metrics are recorded in the [W&B training run](https://wandb.ai/wandb/huggingtweets/runs/g4d86tk2) for full transparency and reproducibility.
At the end of training, [the final model](https://wandb.ai/wandb/huggingtweets/runs/g4d86tk2/artifacts) is logged and versioned.
## How to use
You can use this model directly with a pipeline for text generation:
```python
from transformers import pipeline
generator = pipeline('text-generation',
model='huggingtweets/danny_macaskill-martynashton')
generator("My dream is", num_return_sequences=5)
```
## Limitations and bias
The model suffers from [the same limitations and bias as GPT-2](https://huggingface.co/gpt2#limitations-and-bias).
In addition, the data present in the user's tweets further affects the text generated by the model.
## About
*Built by Boris Dayma*
[](https://twitter.com/intent/follow?screen_name=borisdayma)
For more details, visit the project repository.
[](https://github.com/borisdayma/huggingtweets)
|
huggingtweets/wikisignpost | 4e141e788fed31b4bd75a446e001bfa36f166c81 | 2022-06-15T06:24:26.000Z | [
"pytorch",
"gpt2",
"text-generation",
"en",
"transformers",
"huggingtweets"
] | text-generation | false | huggingtweets | null | huggingtweets/wikisignpost | 1 | null | transformers | 32,875 | ---
language: en
thumbnail: http://www.huggingtweets.com/wikisignpost/1655274233816/predictions.png
tags:
- huggingtweets
widget:
- text: "My dream is"
---
<div class="inline-flex flex-col" style="line-height: 1.5;">
<div class="flex">
<div
style="display:inherit; margin-left: 4px; margin-right: 4px; width: 92px; height:92px; border-radius: 50%; background-size: cover; background-image: url('https://pbs.twimg.com/profile_images/795028567398576128/GG1GUpJ7_400x400.jpg')">
</div>
<div
style="display:none; margin-left: 4px; margin-right: 4px; width: 92px; height:92px; border-radius: 50%; background-size: cover; background-image: url('')">
</div>
<div
style="display:none; margin-left: 4px; margin-right: 4px; width: 92px; height:92px; border-radius: 50%; background-size: cover; background-image: url('')">
</div>
</div>
<div style="text-align: center; margin-top: 3px; font-size: 16px; font-weight: 800">🤖 AI BOT 🤖</div>
<div style="text-align: center; font-size: 16px; font-weight: 800">The Signpost</div>
<div style="text-align: center; font-size: 14px;">@wikisignpost</div>
</div>
I was made with [huggingtweets](https://github.com/borisdayma/huggingtweets).
Create your own bot based on your favorite user with [the demo](https://colab.research.google.com/github/borisdayma/huggingtweets/blob/master/huggingtweets-demo.ipynb)!
## How does it work?
The model uses the following pipeline.
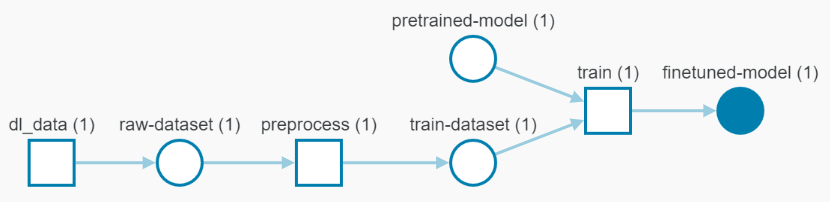
To understand how the model was developed, check the [W&B report](https://wandb.ai/wandb/huggingtweets/reports/HuggingTweets-Train-a-Model-to-Generate-Tweets--VmlldzoxMTY5MjI).
## Training data
The model was trained on tweets from The Signpost.
| Data | The Signpost |
| --- | --- |
| Tweets downloaded | 3216 |
| Retweets | 522 |
| Short tweets | 47 |
| Tweets kept | 2647 |
[Explore the data](https://wandb.ai/wandb/huggingtweets/runs/7z6btxad/artifacts), which is tracked with [W&B artifacts](https://docs.wandb.com/artifacts) at every step of the pipeline.
## Training procedure
The model is based on a pre-trained [GPT-2](https://huggingface.co/gpt2) which is fine-tuned on @wikisignpost's tweets.
Hyperparameters and metrics are recorded in the [W&B training run](https://wandb.ai/wandb/huggingtweets/runs/27ceco72) for full transparency and reproducibility.
At the end of training, [the final model](https://wandb.ai/wandb/huggingtweets/runs/27ceco72/artifacts) is logged and versioned.
## How to use
You can use this model directly with a pipeline for text generation:
```python
from transformers import pipeline
generator = pipeline('text-generation',
model='huggingtweets/wikisignpost')
generator("My dream is", num_return_sequences=5)
```
## Limitations and bias
The model suffers from [the same limitations and bias as GPT-2](https://huggingface.co/gpt2#limitations-and-bias).
In addition, the data present in the user's tweets further affects the text generated by the model.
## About
*Built by Boris Dayma*
[](https://twitter.com/intent/follow?screen_name=borisdayma)
For more details, visit the project repository.
[](https://github.com/borisdayma/huggingtweets)
|
camillelacan1/vit-demo | bd89be887d3a213bfd1cbf11986e409d1ceedf88 | 2022-06-15T06:32:15.000Z | [
"pytorch",
"vit",
"image-classification",
"transformers"
] | image-classification | false | camillelacan1 | null | camillelacan1/vit-demo | 1 | null | transformers | 32,876 | Entry not found |
sdugar/cross-en-de-fr-xlmr-200d-sentence-transformer | 5d3318b787af76da38ec6d4b73ddc0a90fd167f7 | 2022-06-15T08:21:33.000Z | [
"pytorch",
"xlm-roberta",
"feature-extraction",
"sentence-transformers",
"sentence-similarity"
] | sentence-similarity | false | sdugar | null | sdugar/cross-en-de-fr-xlmr-200d-sentence-transformer | 1 | null | sentence-transformers | 32,877 | ---
pipeline_tag: sentence-similarity
tags:
- sentence-transformers
- feature-extraction
- sentence-similarity
---
# {MODEL_NAME}
This is a [sentence-transformers](https://www.SBERT.net) model: It maps sentences & paragraphs to a 200 dimensional dense vector space and can be used for tasks like clustering or semantic search.
<!--- Describe your model here -->
## Usage (Sentence-Transformers)
Using this model becomes easy when you have [sentence-transformers](https://www.SBERT.net) installed:
```
pip install -U sentence-transformers
```
Then you can use the model like this:
```python
from sentence_transformers import SentenceTransformer
sentences = ["This is an example sentence", "Each sentence is converted"]
model = SentenceTransformer('{MODEL_NAME}')
embeddings = model.encode(sentences)
print(embeddings)
```
## Evaluation Results
<!--- Describe how your model was evaluated -->
For an automated evaluation of this model, see the *Sentence Embeddings Benchmark*: [https://seb.sbert.net](https://seb.sbert.net?model_name={MODEL_NAME})
## Training
The model was trained with the parameters:
**DataLoader**:
`torch.utils.data.dataloader.DataLoader` of length 124278 with parameters:
```
{'batch_size': 25, 'sampler': 'torch.utils.data.sampler.RandomSampler', 'batch_sampler': 'torch.utils.data.sampler.BatchSampler'}
```
**Loss**:
`sentence_transformers.losses.MSELoss.MSELoss`
Parameters of the fit()-Method:
```
{
"epochs": 5,
"evaluation_steps": 1000,
"evaluator": "sentence_transformers.evaluation.SequentialEvaluator.SequentialEvaluator",
"max_grad_norm": 1,
"optimizer_class": "<class 'transformers.optimization.AdamW'>",
"optimizer_params": {
"correct_bias": false,
"eps": 1e-06,
"lr": 2e-05
},
"scheduler": "WarmupLinear",
"steps_per_epoch": null,
"warmup_steps": 10000,
"weight_decay": 0.01
}
```
## Full Model Architecture
```
SentenceTransformer(
(0): Transformer({'max_seq_length': 512, 'do_lower_case': False}) with Transformer model: XLMRobertaModel
(1): Pooling({'word_embedding_dimension': 768, 'pooling_mode_cls_token': False, 'pooling_mode_mean_tokens': True, 'pooling_mode_max_tokens': False, 'pooling_mode_mean_sqrt_len_tokens': False})
(dense): Dense({'in_features': 768, 'out_features': 200, 'bias': True, 'activation_function': 'torch.nn.modules.activation.Tanh'})
)
```
## Citing & Authors
<!--- Describe where people can find more information --> |
lewtun/dog-vs-chicken | ab3046fda5d5f04d8f66b8f392522f5969bbc716 | 2022-06-15T07:09:02.000Z | [
"pytorch",
"tensorboard",
"vit",
"image-classification",
"transformers",
"huggingpics",
"model-index"
] | image-classification | false | lewtun | null | lewtun/dog-vs-chicken | 1 | null | transformers | 32,878 | ---
tags:
- image-classification
- pytorch
- huggingpics
metrics:
- accuracy
model-index:
- name: dog-vs-chicken
results:
- task:
name: Image Classification
type: image-classification
metrics:
- name: Accuracy
type: accuracy
value: 1.0
---
# dog-vs-chicken
Autogenerated by HuggingPics🤗🖼️
Create your own image classifier for **anything** by running [the demo on Google Colab](https://colab.research.google.com/github/nateraw/huggingpics/blob/main/HuggingPics.ipynb).
Report any issues with the demo at the [github repo](https://github.com/nateraw/huggingpics).
## Example Images
#### crispy fried chicken

#### poodle
 |
aware-ai/wav2vec2-1b-marian-german | 373e0f3cb274b05678731477dbd496614df6e3a9 | 2022-06-16T04:47:55.000Z | [
"pytorch",
"tensorboard",
"speech-encoder-decoder",
"automatic-speech-recognition",
"transformers"
] | automatic-speech-recognition | false | aware-ai | null | aware-ai/wav2vec2-1b-marian-german | 1 | null | transformers | 32,879 | Entry not found |
huggingtweets/ravenel_jeremy | 92cd293ca227e90ad5fbe581bd80ed249f879a35 | 2022-06-15T07:43:01.000Z | [
"pytorch",
"gpt2",
"text-generation",
"en",
"transformers",
"huggingtweets"
] | text-generation | false | huggingtweets | null | huggingtweets/ravenel_jeremy | 1 | null | transformers | 32,880 | ---
language: en
thumbnail: https://github.com/borisdayma/huggingtweets/blob/master/img/logo.png?raw=true
tags:
- huggingtweets
widget:
- text: "My dream is"
---
<div class="inline-flex flex-col" style="line-height: 1.5;">
<div class="flex">
<div
style="display:inherit; margin-left: 4px; margin-right: 4px; width: 92px; height:92px; border-radius: 50%; background-size: cover; background-image: url('https://pbs.twimg.com/profile_images/1440653599420268547/-h0yYTlI_400x400.jpg')">
</div>
<div
style="display:none; margin-left: 4px; margin-right: 4px; width: 92px; height:92px; border-radius: 50%; background-size: cover; background-image: url('')">
</div>
<div
style="display:none; margin-left: 4px; margin-right: 4px; width: 92px; height:92px; border-radius: 50%; background-size: cover; background-image: url('')">
</div>
</div>
<div style="text-align: center; margin-top: 3px; font-size: 16px; font-weight: 800">🤖 AI BOT 🤖</div>
<div style="text-align: center; font-size: 16px; font-weight: 800">Jeremy Ravenel</div>
<div style="text-align: center; font-size: 14px;">@ravenel_jeremy</div>
</div>
I was made with [huggingtweets](https://github.com/borisdayma/huggingtweets).
Create your own bot based on your favorite user with [the demo](https://colab.research.google.com/github/borisdayma/huggingtweets/blob/master/huggingtweets-demo.ipynb)!
## How does it work?
The model uses the following pipeline.
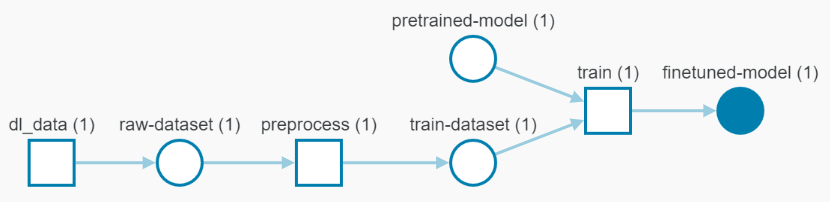
To understand how the model was developed, check the [W&B report](https://wandb.ai/wandb/huggingtweets/reports/HuggingTweets-Train-a-Model-to-Generate-Tweets--VmlldzoxMTY5MjI).
## Training data
The model was trained on tweets from Jeremy Ravenel.
| Data | Jeremy Ravenel |
| --- | --- |
| Tweets downloaded | 899 |
| Retweets | 180 |
| Short tweets | 65 |
| Tweets kept | 654 |
[Explore the data](https://wandb.ai/wandb/huggingtweets/runs/3cp3aewt/artifacts), which is tracked with [W&B artifacts](https://docs.wandb.com/artifacts) at every step of the pipeline.
## Training procedure
The model is based on a pre-trained [GPT-2](https://huggingface.co/gpt2) which is fine-tuned on @ravenel_jeremy's tweets.
Hyperparameters and metrics are recorded in the [W&B training run](https://wandb.ai/wandb/huggingtweets/runs/2arfza2u) for full transparency and reproducibility.
At the end of training, [the final model](https://wandb.ai/wandb/huggingtweets/runs/2arfza2u/artifacts) is logged and versioned.
## How to use
You can use this model directly with a pipeline for text generation:
```python
from transformers import pipeline
generator = pipeline('text-generation',
model='huggingtweets/ravenel_jeremy')
generator("My dream is", num_return_sequences=5)
```
## Limitations and bias
The model suffers from [the same limitations and bias as GPT-2](https://huggingface.co/gpt2#limitations-and-bias).
In addition, the data present in the user's tweets further affects the text generated by the model.
## About
*Built by Boris Dayma*
[](https://twitter.com/intent/follow?screen_name=borisdayma)
For more details, visit the project repository.
[](https://github.com/borisdayma/huggingtweets)
|
Salvatore/t5-finetuned-xsum | 96b2ffc1678aea0fcbbd47557e169dbfcec3831c | 2022-06-15T12:19:55.000Z | [
"pytorch",
"tensorboard",
"t5",
"text2text-generation",
"transformers",
"autotrain_compatible"
] | text2text-generation | false | Salvatore | null | Salvatore/t5-finetuned-xsum | 1 | null | transformers | 32,881 | Entry not found |
gary109/ai-light-dance_singing_ft_wav2vec2-large-xlsr-53 | 6465818021332b142c2481b76ac0d69adde38671 | 2022-06-17T07:30:08.000Z | [
"pytorch",
"tensorboard",
"wav2vec2",
"automatic-speech-recognition",
"transformers",
"gary109/AI_Light_Dance",
"generated_from_trainer",
"license:apache-2.0",
"model-index"
] | automatic-speech-recognition | false | gary109 | null | gary109/ai-light-dance_singing_ft_wav2vec2-large-xlsr-53 | 1 | 1 | transformers | 32,882 | ---
license: apache-2.0
tags:
- automatic-speech-recognition
- gary109/AI_Light_Dance
- generated_from_trainer
model-index:
- name: ai-light-dance_singing_ft_wav2vec2-large-xlsr-53
results: []
---
<!-- This model card has been generated automatically according to the information the Trainer had access to. You
should probably proofread and complete it, then remove this comment. -->
# ai-light-dance_singing_ft_wav2vec2-large-xlsr-53
This model is a fine-tuned version of [facebook/wav2vec2-large-xlsr-53](https://huggingface.co/facebook/wav2vec2-large-xlsr-53) on the GARY109/AI_LIGHT_DANCE - ONSET-SINGING dataset.
It achieves the following results on the evaluation set:
- Loss: 0.4327
- Wer: 0.2043
## Model description
More information needed
## Intended uses & limitations
More information needed
## Training and evaluation data
More information needed
## Training procedure
### Training hyperparameters
The following hyperparameters were used during training:
- learning_rate: 5e-05
- train_batch_size: 2
- eval_batch_size: 2
- seed: 42
- gradient_accumulation_steps: 16
- total_train_batch_size: 32
- optimizer: Adam with betas=(0.9,0.999) and epsilon=1e-08
- lr_scheduler_type: cosine
- lr_scheduler_warmup_steps: 500
- num_epochs: 10.0
- mixed_precision_training: Native AMP
### Training results
| Training Loss | Epoch | Step | Validation Loss | Wer |
|:-------------:|:-----:|:----:|:---------------:|:------:|
| 1.4089 | 1.0 | 552 | 1.4750 | 0.9054 |
| 0.7995 | 2.0 | 1104 | 0.9044 | 0.6163 |
| 0.6232 | 3.0 | 1656 | 0.6645 | 0.3980 |
| 0.5351 | 4.0 | 2208 | 0.5674 | 0.3120 |
| 0.472 | 5.0 | 2760 | 0.5167 | 0.2579 |
| 0.3913 | 6.0 | 3312 | 0.4553 | 0.2335 |
| 0.3306 | 7.0 | 3864 | 0.4476 | 0.2114 |
| 0.3028 | 8.0 | 4416 | 0.4327 | 0.2043 |
| 0.317 | 9.0 | 4968 | 0.4355 | 0.2033 |
| 0.2494 | 10.0 | 5520 | 0.4405 | 0.2022 |
### Framework versions
- Transformers 4.20.0.dev0
- Pytorch 1.11.0+cu113
- Datasets 2.3.1.dev0
- Tokenizers 0.12.1
|
huggingtweets/contrapoints-iamcardib | aca4a76a2304d8c02e559e55abc9735062e4cf83 | 2022-06-15T09:54:48.000Z | [
"pytorch",
"gpt2",
"text-generation",
"en",
"transformers",
"huggingtweets"
] | text-generation | false | huggingtweets | null | huggingtweets/contrapoints-iamcardib | 1 | null | transformers | 32,883 | ---
language: en
thumbnail: http://www.huggingtweets.com/contrapoints-iamcardib/1655286883789/predictions.png
tags:
- huggingtweets
widget:
- text: "My dream is"
---
<div class="inline-flex flex-col" style="line-height: 1.5;">
<div class="flex">
<div
style="display:inherit; margin-left: 4px; margin-right: 4px; width: 92px; height:92px; border-radius: 50%; background-size: cover; background-image: url('https://pbs.twimg.com/profile_images/1425907860773515264/a30IKa1f_400x400.jpg')">
</div>
<div
style="display:inherit; margin-left: 4px; margin-right: 4px; width: 92px; height:92px; border-radius: 50%; background-size: cover; background-image: url('https://pbs.twimg.com/profile_images/1536222087299350528/rMyNxbwV_400x400.jpg')">
</div>
<div
style="display:none; margin-left: 4px; margin-right: 4px; width: 92px; height:92px; border-radius: 50%; background-size: cover; background-image: url('')">
</div>
</div>
<div style="text-align: center; margin-top: 3px; font-size: 16px; font-weight: 800">🤖 AI CYBORG 🤖</div>
<div style="text-align: center; font-size: 16px; font-weight: 800">Natalie Wynn & Cardi B</div>
<div style="text-align: center; font-size: 14px;">@contrapoints-iamcardib</div>
</div>
I was made with [huggingtweets](https://github.com/borisdayma/huggingtweets).
Create your own bot based on your favorite user with [the demo](https://colab.research.google.com/github/borisdayma/huggingtweets/blob/master/huggingtweets-demo.ipynb)!
## How does it work?
The model uses the following pipeline.
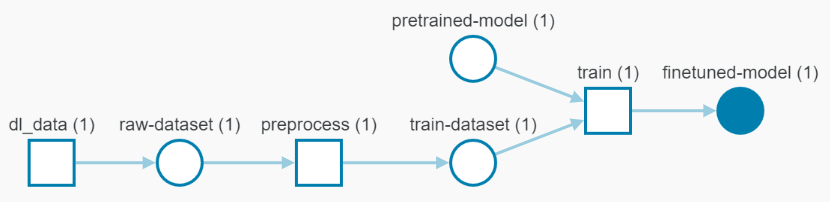
To understand how the model was developed, check the [W&B report](https://wandb.ai/wandb/huggingtweets/reports/HuggingTweets-Train-a-Model-to-Generate-Tweets--VmlldzoxMTY5MjI).
## Training data
The model was trained on tweets from Natalie Wynn & Cardi B.
| Data | Natalie Wynn | Cardi B |
| --- | --- | --- |
| Tweets downloaded | 3191 | 3058 |
| Retweets | 299 | 1521 |
| Short tweets | 527 | 371 |
| Tweets kept | 2365 | 1166 |
[Explore the data](https://wandb.ai/wandb/huggingtweets/runs/3q3i0l7z/artifacts), which is tracked with [W&B artifacts](https://docs.wandb.com/artifacts) at every step of the pipeline.
## Training procedure
The model is based on a pre-trained [GPT-2](https://huggingface.co/gpt2) which is fine-tuned on @contrapoints-iamcardib's tweets.
Hyperparameters and metrics are recorded in the [W&B training run](https://wandb.ai/wandb/huggingtweets/runs/2vgppsp0) for full transparency and reproducibility.
At the end of training, [the final model](https://wandb.ai/wandb/huggingtweets/runs/2vgppsp0/artifacts) is logged and versioned.
## How to use
You can use this model directly with a pipeline for text generation:
```python
from transformers import pipeline
generator = pipeline('text-generation',
model='huggingtweets/contrapoints-iamcardib')
generator("My dream is", num_return_sequences=5)
```
## Limitations and bias
The model suffers from [the same limitations and bias as GPT-2](https://huggingface.co/gpt2#limitations-and-bias).
In addition, the data present in the user's tweets further affects the text generated by the model.
## About
*Built by Boris Dayma*
[](https://twitter.com/intent/follow?screen_name=borisdayma)
For more details, visit the project repository.
[](https://github.com/borisdayma/huggingtweets)
|
winson/distilbert-base-uncased-finetuned-imdb | dcf77d1aecad87243668a76f976b28fae061142a | 2022-06-15T12:51:06.000Z | [
"pytorch",
"tensorboard",
"distilbert",
"fill-mask",
"dataset:imdb",
"transformers",
"generated_from_trainer",
"license:apache-2.0",
"model-index",
"autotrain_compatible"
] | fill-mask | false | winson | null | winson/distilbert-base-uncased-finetuned-imdb | 1 | null | transformers | 32,884 | ---
license: apache-2.0
tags:
- generated_from_trainer
datasets:
- imdb
model-index:
- name: distilbert-base-uncased-finetuned-imdb
results: []
---
<!-- This model card has been generated automatically according to the information the Trainer had access to. You
should probably proofread and complete it, then remove this comment. -->
# distilbert-base-uncased-finetuned-imdb
This model is a fine-tuned version of [distilbert-base-uncased](https://huggingface.co/distilbert-base-uncased) on the imdb dataset.
It achieves the following results on the evaluation set:
- eval_loss: 3.1139
- eval_runtime: 1.8873
- eval_samples_per_second: 529.866
- eval_steps_per_second: 8.478
- step: 0
## Model description
More information needed
## Intended uses & limitations
More information needed
## Training and evaluation data
More information needed
## Training procedure
### Training hyperparameters
The following hyperparameters were used during training:
- learning_rate: 2e-05
- train_batch_size: 64
- eval_batch_size: 64
- seed: 42
- optimizer: Adam with betas=(0.9,0.999) and epsilon=1e-08
- lr_scheduler_type: linear
- num_epochs: 3.0
- mixed_precision_training: Native AMP
### Framework versions
- Transformers 4.19.4
- Pytorch 1.11.0+cu113
- Datasets 2.3.1
- Tokenizers 0.12.1
|
meghazisofiane/mbart-large-cc25-en-ar-evaluated-en-to-ar-1000instances-OPUS-leaningRate2e-05-batchSize2 | 8b02c90b7f63d755131b072fbcf2d4e4f81d1fcf | 2022-06-15T11:11:28.000Z | [
"pytorch",
"tensorboard",
"mbart",
"text2text-generation",
"transformers",
"autotrain_compatible"
] | text2text-generation | false | meghazisofiane | null | meghazisofiane/mbart-large-cc25-en-ar-evaluated-en-to-ar-1000instances-OPUS-leaningRate2e-05-batchSize2 | 1 | null | transformers | 32,885 | Entry not found |
mikeluck/wav2vec2-base-timit-demo-google-colab | b30e92ae9531d985612796c9dfbc1f829fd399f9 | 2022-06-15T12:43:38.000Z | [
"pytorch",
"tensorboard",
"wav2vec2",
"automatic-speech-recognition",
"transformers",
"generated_from_trainer",
"license:apache-2.0",
"model-index"
] | automatic-speech-recognition | false | mikeluck | null | mikeluck/wav2vec2-base-timit-demo-google-colab | 1 | null | transformers | 32,886 | ---
license: apache-2.0
tags:
- generated_from_trainer
model-index:
- name: wav2vec2-base-timit-demo-google-colab
results: []
---
<!-- This model card has been generated automatically according to the information the Trainer had access to. You
should probably proofread and complete it, then remove this comment. -->
# wav2vec2-base-timit-demo-google-colab
This model is a fine-tuned version of [facebook/wav2vec2-base](https://huggingface.co/facebook/wav2vec2-base) on the None dataset.
It achieves the following results on the evaluation set:
- Loss: 0.5351
- Wer: 0.3384
## Model description
More information needed
## Intended uses & limitations
More information needed
## Training and evaluation data
More information needed
## Training procedure
### Training hyperparameters
The following hyperparameters were used during training:
- learning_rate: 0.0001
- train_batch_size: 8
- eval_batch_size: 8
- seed: 42
- optimizer: Adam with betas=(0.9,0.999) and epsilon=1e-08
- lr_scheduler_type: linear
- lr_scheduler_warmup_steps: 1000
- num_epochs: 30
- mixed_precision_training: Native AMP
### Training results
| Training Loss | Epoch | Step | Validation Loss | Wer |
|:-------------:|:-----:|:-----:|:---------------:|:------:|
| 3.6311 | 1.0 | 500 | 2.6700 | 1.0 |
| 1.0104 | 2.01 | 1000 | 0.5289 | 0.5277 |
| 0.4483 | 3.01 | 1500 | 0.4576 | 0.4623 |
| 0.3089 | 4.02 | 2000 | 0.4483 | 0.4255 |
| 0.2278 | 5.02 | 2500 | 0.4463 | 0.4022 |
| 0.1886 | 6.02 | 3000 | 0.4653 | 0.3938 |
| 0.1578 | 7.03 | 3500 | 0.4624 | 0.3855 |
| 0.1429 | 8.03 | 4000 | 0.4420 | 0.3854 |
| 0.1244 | 9.04 | 4500 | 0.4980 | 0.3787 |
| 0.1126 | 10.04 | 5000 | 0.4311 | 0.3785 |
| 0.1082 | 11.04 | 5500 | 0.5114 | 0.3782 |
| 0.0888 | 12.05 | 6000 | 0.5392 | 0.3725 |
| 0.0835 | 13.05 | 6500 | 0.6011 | 0.3941 |
| 0.074 | 14.06 | 7000 | 0.5030 | 0.3652 |
| 0.0667 | 15.06 | 7500 | 0.5041 | 0.3583 |
| 0.0595 | 16.06 | 8000 | 0.5125 | 0.3605 |
| 0.0578 | 17.07 | 8500 | 0.5206 | 0.3592 |
| 0.0573 | 18.07 | 9000 | 0.5208 | 0.3643 |
| 0.0469 | 19.08 | 9500 | 0.4670 | 0.3537 |
| 0.0442 | 20.08 | 10000 | 0.5388 | 0.3497 |
| 0.0417 | 21.08 | 10500 | 0.5213 | 0.3581 |
| 0.0361 | 22.09 | 11000 | 0.5096 | 0.3465 |
| 0.0338 | 23.09 | 11500 | 0.5178 | 0.3459 |
| 0.0333 | 24.1 | 12000 | 0.5240 | 0.3490 |
| 0.0256 | 25.1 | 12500 | 0.5438 | 0.3464 |
| 0.0248 | 26.1 | 13000 | 0.5182 | 0.3412 |
| 0.0231 | 27.11 | 13500 | 0.5628 | 0.3423 |
| 0.0228 | 28.11 | 14000 | 0.5416 | 0.3419 |
| 0.0223 | 29.12 | 14500 | 0.5351 | 0.3384 |
### Framework versions
- Transformers 4.17.0
- Pytorch 1.11.0+cu113
- Datasets 1.18.3
- Tokenizers 0.12.1
|
Fluffypillow/DialoGPT-small-Rem | c50613085ddc35f6f026169932981ea7b7ae139c | 2022-06-15T11:17:40.000Z | [
"pytorch",
"gpt2",
"text-generation",
"transformers",
"conversational"
] | conversational | false | Fluffypillow | null | Fluffypillow/DialoGPT-small-Rem | 1 | null | transformers | 32,887 | ---
tags:
- conversational
---
# Rem DialoGPT Model |
erickfm/stellar-sweep-3 | ab6ea46b52f5380517123b7b1baede7ab4af3454 | 2022-06-15T12:06:33.000Z | [
"pytorch",
"t5",
"text2text-generation",
"transformers",
"autotrain_compatible"
] | text2text-generation | false | erickfm | null | erickfm/stellar-sweep-3 | 1 | null | transformers | 32,888 | Entry not found |
Addedk/mbert-swedish-distilled-cased | 0ebe8585a87a5e1f414517e645b626dd3d07c613 | 2022-06-15T16:32:43.000Z | [
"pytorch",
"tf",
"bert",
"fill-mask",
"multilingual",
"arxiv:2103.06418",
"transformers",
"license:apache-2.0",
"autotrain_compatible"
] | fill-mask | false | Addedk | null | Addedk/mbert-swedish-distilled-cased | 1 | 1 | transformers | 32,889 | ---
language: multilingual
license: apache-2.0
---
# mBERT swedish distilled base model (cased)
This model is a distilled version of [mBERT](https://huggingface.co/bert-base-multilingual-cased). It was distilled using Swedish data, the 2010-2015 portion of the [Swedish Culturomics Gigaword Corpus](https://spraakbanken.gu.se/en/resources/gigaword). The code for the distillation process can be found [here](https://github.com/AddedK/swedish-mbert-distillation/blob/main/azureML/pretrain_distillation.py). This was done as part of my Master's Thesis: *Task-agnostic knowledge distillation of mBERT to Swedish*.
## Model description
This is a 6-layer version of mBERT, having been distilled using the [LightMBERT](https://arxiv.org/abs/2103.06418) distillation method, but without freezing the embedding layer.
## Intended uses & limitations
You can use the raw model for either masked language modeling or next sentence prediction, but it's mostly intended to
be fine-tuned on a downstream task.
## Training data
The data used for distillation was the 2010-2015 portion of the [Swedish Culturomics Gigaword Corpus](https://spraakbanken.gu.se/en/resources/gigaword).
The tokenized data had a file size of approximately 9 GB.
## Evaluation results
When evaluated on the [SUCX 3.0 ](https://huggingface.co/datasets/KBLab/sucx3_ner) dataset, it achieved an average F1 score of 0.859 which is competitive with the score mBERT obtained, 0.866.
When evaluated on the [English WikiANN](https://huggingface.co/datasets/wikiann) dataset, it achieved an average F1 score of 0.826 which is competitive with the score mBERT obtained, 0.849.
Additional results and comparisons are presented in my Master's Thesis
|
KM4STfulltext/CSSCI_ABS_roberta | 800dcf0d97b97114cbf49c3333822ff142b25572 | 2022-06-20T07:07:00.000Z | [
"pytorch",
"bert",
"fill-mask",
"transformers",
"license:apache-2.0",
"autotrain_compatible"
] | fill-mask | false | KM4STfulltext | null | KM4STfulltext/CSSCI_ABS_roberta | 1 | null | transformers | 32,890 | ---
license: apache-2.0
---
# Pre-trained Language Model for the Humanities and Social Sciences in Chinese
## Introduction
The research for social science texts in Chinese needs the support natural language processing tools.
The pre-trained language model has greatly improved the accuracy of text mining in general texts. At present, there is an urgent need for a pre-trained language model specifically for the automatic processing of scientific texts in Chinese social science.
We used the abstract of social science research as the training set. Based on the deep language model framework of BERT, we constructed CSSCI_ABS_BERT, CSSCI_ABS_roberta and CSSCI_ABS_roberta-wwm pre-training language models by [transformers/run_mlm.py](https://github.com/huggingface/transformers/blob/main/examples/pytorch/language-modeling/run_mlm.py) and [transformers/mlm_wwm](https://github.com/huggingface/transformers/tree/main/examples/research_projects/mlm_wwm).
We designed four downstream tasks of Text Classification on different Chinese social scientific article corpus to verify the performance of the model.
- CSSCI_ABS_BERT , CSSCI_ABS_roberta and CSSCI_ABS_roberta-wwm are trained on the abstract of articles published in CSSCI journals. The training set involved in the experiment included a total of `510,956,094 words`.
- Based on the idea of Domain-Adaptive Pretraining, `CSSCI_ABS_BERT` and `CSSCI_ABS_roberta` combine a large amount of abstracts of scientific articles in Chinese based on the BERT structure, and continue to train the BERT and Chinese-RoBERTa models respectively to obtain pre-training models for the automatic processing of Chinese Social science research texts.
## News
- 2022-06-15 : CSSCI_ABS_BERT, CSSCI_ABS_roberta and CSSCI_ABS_roberta-wwm has been put forward for the first time.
## How to use
### Huggingface Transformers
The `from_pretrained` method based on [Huggingface Transformers](https://github.com/huggingface/transformers) can directly obtain CSSCI_ABS_BERT, CSSCI_ABS_roberta and CSSCI_ABS_roberta-wwm models online.
- CSSCI_ABS_BERT
```python
from transformers import AutoTokenizer, AutoModel
tokenizer = AutoTokenizer.from_pretrained("KM4STfulltext/CSSCI_ABS_BERT")
model = AutoModel.from_pretrained("KM4STfulltext/CSSCI_ABS_BERT")
```
- CSSCI_ABS_roberta
```python
from transformers import AutoTokenizer, AutoModel
tokenizer = AutoTokenizer.from_pretrained("KM4STfulltext/CSSCI_ABS_roberta")
model = AutoModel.from_pretrained("KM4STfulltext/CSSCI_ABS_roberta")
```
- CSSCI_ABS_roberta-wwm
```python
from transformers import AutoTokenizer, AutoModel
tokenizer = AutoTokenizer.from_pretrained("KM4STfulltext/CSSCI_ABS_roberta_wwm")
model = AutoModel.from_pretrained("KM4STfulltext/CSSCI_ABS_roberta_wwm")
```
### Download Models
- The version of the model we provide is `PyTorch`.
### From Huggingface
- Download directly through Huggingface's official website.
- [KM4STfulltext/CSSCI_ABS_BERT](https://huggingface.co/KM4STfulltext/CSSCI_ABS_BERT)
- [KM4STfulltext/CSSCI_ABS_roberta](https://huggingface.co/KM4STfulltext/CSSCI_ABS_roberta)
- [KM4STfulltext/CSSCI_ABS_roberta_wwm](https://huggingface.co/KM4STfulltext/CSSCI_ABS_roberta_wwm)
## Evaluation & Results
- We useCSSCI_ABS_BERT, CSSCI_ABS_roberta and CSSCI_ABS_roberta-wwm to perform Text Classificationon different social science research corpus. The experimental results are as follows.
#### Discipline classification experiments of articles published in CSSCI journals
https://github.com/S-T-Full-Text-Knowledge-Mining/CSSCI-BERT
#### Movement recognition experiments for data analysis and knowledge discovery abstract
| Tag | bert-base-Chinese | chinese-roberta-wwm,ext | CSSCI_ABS_BERT | CSSCI_ABS_roberta | CSSCI_ABS_roberta_wwm | support |
| ------------ | ----------------- | ----------------------- | -------------- | ----------------- | --------------------- | ------- |
| Abstract | 55.23 | 62.44 | 56.8 | 57.96 | 58.26 | 223 |
| Location | 61.61 | 54.38 | 61.83 | 61.4 | 61.94 | 2866 |
| Metric | 45.08 | 41 | 45.27 | 46.74 | 47.13 | 622 |
| Organization | 46.85 | 35.29 | 45.72 | 45.44 | 44.65 | 327 |
| Person | 88.66 | 82.79 | 88.21 | 88.29 | 88.51 | 4850 |
| Thing | 71.68 | 65.34 | 71.88 | 71.68 | 71.81 | 5993 |
| Time | 65.35 | 60.38 | 64.15 | 65.26 | 66.03 | 1272 |
| avg | 72.69 | 66.62 | 72.59 | 72.61 | 72.89 | 16153 |
#### Chinese literary entity recognition
| Tag | bert-base-Chinese | chinese-roberta-wwm,ext | CSSCI_ABS_BERT | CSSCI_ABS_roberta | CSSCI_ABS_roberta_wwm | support |
| ------------ | ----------------- | ----------------------- | -------------- | ----------------- | --------------------- | ------- |
| Abstract | 55.23 | 62.44 | 56.8 | 57.96 | 58.26 | 223 |
| Location | 61.61 | 54.38 | 61.83 | 61.4 | 61.94 | 2866 |
| Metric | 45.08 | 41 | 45.27 | 46.74 | 47.13 | 622 |
| Organization | 46.85 | 35.29 | 45.72 | 45.44 | 44.65 | 327 |
| Person | 88.66 | 82.79 | 88.21 | 88.29 | 88.51 | 4850 |
| Thing | 71.68 | 65.34 | 71.88 | 71.68 | 71.81 | 5993 |
| Time | 65.35 | 60.38 | 64.15 | 65.26 | 66.03 | 1272 |
| avg | 72.69 | 66.62 | 72.59 | 72.61 | 72.89 | 16153 |
## Cited
- If our content is helpful for your research work, please quote our research in your article.
- If you want to quote our research, you can use this url [S-T-Full-Text-Knowledge-Mining/CSSCI-BERT (github.com)](https://github.com/S-T-Full-Text-Knowledge-Mining/CSSCI-BERT) as an alternative before our paper is published.
## Disclaimer
- The experimental results presented in the report only show the performance under a specific data set and hyperparameter combination, and cannot represent the essence of each model. The experimental results may change due to random number seeds and computing equipment.
- **Users can use the model arbitrarily within the scope of the license, but we are not responsible for the direct or indirect losses caused by using the content of the project.**
## Acknowledgment
- CSSCI_ABS_BERT was trained based on [BERT-Base-Chinese]([google-research/bert: TensorFlow code and pre-trained models for BERT (github.com)](https://github.com/google-research/bert)).
- CSSCI_ABS_roberta and CSSCI_ABS_roberta-wwm was trained based on [RoBERTa-wwm-ext, Chinese]([ymcui/Chinese-BERT-wwm: Pre-Training with Whole Word Masking for Chinese BERT(中文BERT-wwm系列模型) (github.com)](https://github.com/ymcui/Chinese-BERT-wwm)).
|
KM4STfulltext/CSSCI_ABS_BERT | 15b1539ae22a00134ca20cf261bb2b81b7aaeb73 | 2022-06-20T07:07:53.000Z | [
"pytorch",
"bert",
"fill-mask",
"transformers",
"license:apache-2.0",
"autotrain_compatible"
] | fill-mask | false | KM4STfulltext | null | KM4STfulltext/CSSCI_ABS_BERT | 1 | null | transformers | 32,891 | ---
license: apache-2.0
---
# Pre-trained Language Model for the Humanities and Social Sciences in Chinese
## Introduction
The research for social science texts in Chinese needs the support natural language processing tools.
The pre-trained language model has greatly improved the accuracy of text mining in general texts. At present, there is an urgent need for a pre-trained language model specifically for the automatic processing of scientific texts in Chinese social science.
We used the abstract of social science research as the training set. Based on the deep language model framework of BERT, we constructed CSSCI_ABS_BERT, CSSCI_ABS_roberta and CSSCI_ABS_roberta-wwm pre-training language models by [transformers/run_mlm.py](https://github.com/huggingface/transformers/blob/main/examples/pytorch/language-modeling/run_mlm.py) and [transformers/mlm_wwm](https://github.com/huggingface/transformers/tree/main/examples/research_projects/mlm_wwm).
We designed four downstream tasks of Text Classification on different Chinese social scientific article corpus to verify the performance of the model.
- CSSCI_ABS_BERT , CSSCI_ABS_roberta and CSSCI_ABS_roberta-wwm are trained on the abstract of articles published in CSSCI journals. The training set involved in the experiment included a total of `510,956,094 words`.
- Based on the idea of Domain-Adaptive Pretraining, `CSSCI_ABS_BERT` and `CSSCI_ABS_roberta` combine a large amount of abstracts of scientific articles in Chinese based on the BERT structure, and continue to train the BERT and Chinese-RoBERTa models respectively to obtain pre-training models for the automatic processing of Chinese Social science research texts.
## News
- 2022-06-15 : CSSCI_ABS_BERT, CSSCI_ABS_roberta and CSSCI_ABS_roberta-wwm has been put forward for the first time.
## How to use
### Huggingface Transformers
The `from_pretrained` method based on [Huggingface Transformers](https://github.com/huggingface/transformers) can directly obtain CSSCI_ABS_BERT, CSSCI_ABS_roberta and CSSCI_ABS_roberta-wwm models online.
- CSSCI_ABS_BERT
```python
from transformers import AutoTokenizer, AutoModel
tokenizer = AutoTokenizer.from_pretrained("KM4STfulltext/CSSCI_ABS_BERT")
model = AutoModel.from_pretrained("KM4STfulltext/CSSCI_ABS_BERT")
```
- CSSCI_ABS_roberta
```python
from transformers import AutoTokenizer, AutoModel
tokenizer = AutoTokenizer.from_pretrained("KM4STfulltext/CSSCI_ABS_roberta")
model = AutoModel.from_pretrained("KM4STfulltext/CSSCI_ABS_roberta")
```
- CSSCI_ABS_roberta-wwm
```python
from transformers import AutoTokenizer, AutoModel
tokenizer = AutoTokenizer.from_pretrained("KM4STfulltext/CSSCI_ABS_roberta_wwm")
model = AutoModel.from_pretrained("KM4STfulltext/CSSCI_ABS_roberta_wwm")
```
### Download Models
- The version of the model we provide is `PyTorch`.
### From Huggingface
- Download directly through Huggingface's official website.
- [KM4STfulltext/CSSCI_ABS_BERT](https://huggingface.co/KM4STfulltext/CSSCI_ABS_BERT)
- [KM4STfulltext/CSSCI_ABS_roberta](https://huggingface.co/KM4STfulltext/CSSCI_ABS_roberta)
- [KM4STfulltext/CSSCI_ABS_roberta_wwm](https://huggingface.co/KM4STfulltext/CSSCI_ABS_roberta_wwm)
## Evaluation & Results
- We useCSSCI_ABS_BERT, CSSCI_ABS_roberta and CSSCI_ABS_roberta-wwm to perform Text Classificationon different social science research corpus. The experimental results are as follows.
#### Discipline classification experiments of articles published in CSSCI journals
https://github.com/S-T-Full-Text-Knowledge-Mining/CSSCI-BERT
#### Movement recognition experiments for data analysis and knowledge discovery abstract
| Tag | bert-base-Chinese | chinese-roberta-wwm,ext | CSSCI_ABS_BERT | CSSCI_ABS_roberta | CSSCI_ABS_roberta_wwm | support |
| ------------ | ----------------- | ----------------------- | -------------- | ----------------- | --------------------- | ------- |
| Abstract | 55.23 | 62.44 | 56.8 | 57.96 | 58.26 | 223 |
| Location | 61.61 | 54.38 | 61.83 | 61.4 | 61.94 | 2866 |
| Metric | 45.08 | 41 | 45.27 | 46.74 | 47.13 | 622 |
| Organization | 46.85 | 35.29 | 45.72 | 45.44 | 44.65 | 327 |
| Person | 88.66 | 82.79 | 88.21 | 88.29 | 88.51 | 4850 |
| Thing | 71.68 | 65.34 | 71.88 | 71.68 | 71.81 | 5993 |
| Time | 65.35 | 60.38 | 64.15 | 65.26 | 66.03 | 1272 |
| avg | 72.69 | 66.62 | 72.59 | 72.61 | 72.89 | 16153 |
#### Chinese literary entity recognition
| Tag | bert-base-Chinese | chinese-roberta-wwm,ext | CSSCI_ABS_BERT | CSSCI_ABS_roberta | CSSCI_ABS_roberta_wwm | support |
| ------------ | ----------------- | ----------------------- | -------------- | ----------------- | --------------------- | ------- |
| Abstract | 55.23 | 62.44 | 56.8 | 57.96 | 58.26 | 223 |
| Location | 61.61 | 54.38 | 61.83 | 61.4 | 61.94 | 2866 |
| Metric | 45.08 | 41 | 45.27 | 46.74 | 47.13 | 622 |
| Organization | 46.85 | 35.29 | 45.72 | 45.44 | 44.65 | 327 |
| Person | 88.66 | 82.79 | 88.21 | 88.29 | 88.51 | 4850 |
| Thing | 71.68 | 65.34 | 71.88 | 71.68 | 71.81 | 5993 |
| Time | 65.35 | 60.38 | 64.15 | 65.26 | 66.03 | 1272 |
| avg | 72.69 | 66.62 | 72.59 | 72.61 | 72.89 | 16153 |
## Cited
- If our content is helpful for your research work, please quote our research in your article.
- If you want to quote our research, you can use this url [S-T-Full-Text-Knowledge-Mining/CSSCI-BERT (github.com)](https://github.com/S-T-Full-Text-Knowledge-Mining/CSSCI-BERT) as an alternative before our paper is published.
## Disclaimer
- The experimental results presented in the report only show the performance under a specific data set and hyperparameter combination, and cannot represent the essence of each model. The experimental results may change due to random number seeds and computing equipment.
- **Users can use the model arbitrarily within the scope of the license, but we are not responsible for the direct or indirect losses caused by using the content of the project.**
## Acknowledgment
- CSSCI_ABS_BERT was trained based on [BERT-Base-Chinese]([google-research/bert: TensorFlow code and pre-trained models for BERT (github.com)](https://github.com/google-research/bert)).
- CSSCI_ABS_roberta and CSSCI_ABS_roberta-wwm was trained based on [RoBERTa-wwm-ext, Chinese]([ymcui/Chinese-BERT-wwm: Pre-Training with Whole Word Masking for Chinese BERT(中文BERT-wwm系列模型) (github.com)](https://github.com/ymcui/Chinese-BERT-wwm)).
|
KM4STfulltext/CSSCI_ABS_roberta_wwm | 55833d811d62c162f0664f0ca629a7a07bca9bb2 | 2022-06-20T07:06:48.000Z | [
"pytorch",
"bert",
"fill-mask",
"transformers",
"license:apache-2.0",
"autotrain_compatible"
] | fill-mask | false | KM4STfulltext | null | KM4STfulltext/CSSCI_ABS_roberta_wwm | 1 | null | transformers | 32,892 | ---
license: apache-2.0
---
# Pre-trained Language Model for the Humanities and Social Sciences in Chinese
## Introduction
The research for social science texts in Chinese needs the support natural language processing tools.
The pre-trained language model has greatly improved the accuracy of text mining in general texts. At present, there is an urgent need for a pre-trained language model specifically for the automatic processing of scientific texts in Chinese social science.
We used the abstract of social science research as the training set. Based on the deep language model framework of BERT, we constructed CSSCI_ABS_BERT, CSSCI_ABS_roberta and CSSCI_ABS_roberta-wwm pre-training language models by [transformers/run_mlm.py](https://github.com/huggingface/transformers/blob/main/examples/pytorch/language-modeling/run_mlm.py) and [transformers/mlm_wwm](https://github.com/huggingface/transformers/tree/main/examples/research_projects/mlm_wwm).
We designed four downstream tasks of Text Classification on different Chinese social scientific article corpus to verify the performance of the model.
- CSSCI_ABS_BERT , CSSCI_ABS_roberta and CSSCI_ABS_roberta-wwm are trained on the abstract of articles published in CSSCI journals. The training set involved in the experiment included a total of `510,956,094 words`.
- Based on the idea of Domain-Adaptive Pretraining, `CSSCI_ABS_BERT` and `CSSCI_ABS_roberta` combine a large amount of abstracts of scientific articles in Chinese based on the BERT structure, and continue to train the BERT and Chinese-RoBERTa models respectively to obtain pre-training models for the automatic processing of Chinese Social science research texts.
## News
- 2022-06-15 : CSSCI_ABS_BERT, CSSCI_ABS_roberta and CSSCI_ABS_roberta-wwm has been put forward for the first time.
## How to use
### Huggingface Transformers
The `from_pretrained` method based on [Huggingface Transformers](https://github.com/huggingface/transformers) can directly obtain CSSCI_ABS_BERT, CSSCI_ABS_roberta and CSSCI_ABS_roberta-wwm models online.
- CSSCI_ABS_BERT
```python
from transformers import AutoTokenizer, AutoModel
tokenizer = AutoTokenizer.from_pretrained("KM4STfulltext/CSSCI_ABS_BERT")
model = AutoModel.from_pretrained("KM4STfulltext/CSSCI_ABS_BERT")
```
- CSSCI_ABS_roberta
```python
from transformers import AutoTokenizer, AutoModel
tokenizer = AutoTokenizer.from_pretrained("KM4STfulltext/CSSCI_ABS_roberta")
model = AutoModel.from_pretrained("KM4STfulltext/CSSCI_ABS_roberta")
```
- CSSCI_ABS_roberta-wwm
```python
from transformers import AutoTokenizer, AutoModel
tokenizer = AutoTokenizer.from_pretrained("KM4STfulltext/CSSCI_ABS_roberta_wwm")
model = AutoModel.from_pretrained("KM4STfulltext/CSSCI_ABS_roberta_wwm")
```
### Download Models
- The version of the model we provide is `PyTorch`.
### From Huggingface
- Download directly through Huggingface's official website.
- [KM4STfulltext/CSSCI_ABS_BERT](https://huggingface.co/KM4STfulltext/CSSCI_ABS_BERT)
- [KM4STfulltext/CSSCI_ABS_roberta](https://huggingface.co/KM4STfulltext/CSSCI_ABS_roberta)
- [KM4STfulltext/CSSCI_ABS_roberta_wwm](https://huggingface.co/KM4STfulltext/CSSCI_ABS_roberta_wwm)
## Evaluation & Results
- We useCSSCI_ABS_BERT, CSSCI_ABS_roberta and CSSCI_ABS_roberta-wwm to perform Text Classificationon different social science research corpus. The experimental results are as follows.
#### Discipline classification experiments of articles published in CSSCI journals
https://github.com/S-T-Full-Text-Knowledge-Mining/CSSCI-BERT
#### Movement recognition experiments for data analysis and knowledge discovery abstract
| Tag | bert-base-Chinese | chinese-roberta-wwm,ext | CSSCI_ABS_BERT | CSSCI_ABS_roberta | CSSCI_ABS_roberta_wwm | support |
| ------------ | ----------------- | ----------------------- | -------------- | ----------------- | --------------------- | ------- |
| Abstract | 55.23 | 62.44 | 56.8 | 57.96 | 58.26 | 223 |
| Location | 61.61 | 54.38 | 61.83 | 61.4 | 61.94 | 2866 |
| Metric | 45.08 | 41 | 45.27 | 46.74 | 47.13 | 622 |
| Organization | 46.85 | 35.29 | 45.72 | 45.44 | 44.65 | 327 |
| Person | 88.66 | 82.79 | 88.21 | 88.29 | 88.51 | 4850 |
| Thing | 71.68 | 65.34 | 71.88 | 71.68 | 71.81 | 5993 |
| Time | 65.35 | 60.38 | 64.15 | 65.26 | 66.03 | 1272 |
| avg | 72.69 | 66.62 | 72.59 | 72.61 | 72.89 | 16153 |
#### Chinese literary entity recognition
| Tag | bert-base-Chinese | chinese-roberta-wwm,ext | CSSCI_ABS_BERT | CSSCI_ABS_roberta | CSSCI_ABS_roberta_wwm | support |
| ------------ | ----------------- | ----------------------- | -------------- | ----------------- | --------------------- | ------- |
| Abstract | 55.23 | 62.44 | 56.8 | 57.96 | 58.26 | 223 |
| Location | 61.61 | 54.38 | 61.83 | 61.4 | 61.94 | 2866 |
| Metric | 45.08 | 41 | 45.27 | 46.74 | 47.13 | 622 |
| Organization | 46.85 | 35.29 | 45.72 | 45.44 | 44.65 | 327 |
| Person | 88.66 | 82.79 | 88.21 | 88.29 | 88.51 | 4850 |
| Thing | 71.68 | 65.34 | 71.88 | 71.68 | 71.81 | 5993 |
| Time | 65.35 | 60.38 | 64.15 | 65.26 | 66.03 | 1272 |
| avg | 72.69 | 66.62 | 72.59 | 72.61 | 72.89 | 16153 |
## Cited
- If our content is helpful for your research work, please quote our research in your article.
- If you want to quote our research, you can use this url [S-T-Full-Text-Knowledge-Mining/CSSCI-BERT (github.com)](https://github.com/S-T-Full-Text-Knowledge-Mining/CSSCI-BERT) as an alternative before our paper is published.
## Disclaimer
- The experimental results presented in the report only show the performance under a specific data set and hyperparameter combination, and cannot represent the essence of each model. The experimental results may change due to random number seeds and computing equipment.
- **Users can use the model arbitrarily within the scope of the license, but we are not responsible for the direct or indirect losses caused by using the content of the project.**
## Acknowledgment
- CSSCI_ABS_BERT was trained based on [BERT-Base-Chinese]([google-research/bert: TensorFlow code and pre-trained models for BERT (github.com)](https://github.com/google-research/bert)).
- CSSCI_ABS_roberta and CSSCI_ABS_roberta-wwm was trained based on [RoBERTa-wwm-ext, Chinese]([ymcui/Chinese-BERT-wwm: Pre-Training with Whole Word Masking for Chinese BERT(中文BERT-wwm系列模型) (github.com)](https://github.com/ymcui/Chinese-BERT-wwm)).
|
SimulSt/xlm-roberta-base-finetuned-panx-de | 972906f307f9f8047662ca749425bd82fd3c8bb1 | 2022-06-15T16:59:24.000Z | [
"pytorch",
"tensorboard",
"xlm-roberta",
"token-classification",
"dataset:xtreme",
"transformers",
"generated_from_trainer",
"license:mit",
"model-index",
"autotrain_compatible"
] | token-classification | false | SimulSt | null | SimulSt/xlm-roberta-base-finetuned-panx-de | 1 | null | transformers | 32,893 | ---
license: mit
tags:
- generated_from_trainer
datasets:
- xtreme
metrics:
- f1
model-index:
- name: xlm-roberta-base-finetuned-panx-de
results:
- task:
name: Token Classification
type: token-classification
dataset:
name: xtreme
type: xtreme
args: PAN-X.de
metrics:
- name: F1
type: f1
value: 0.8620945214069894
---
<!-- This model card has been generated automatically according to the information the Trainer had access to. You
should probably proofread and complete it, then remove this comment. -->
# xlm-roberta-base-finetuned-panx-de
This model is a fine-tuned version of [xlm-roberta-base](https://huggingface.co/xlm-roberta-base) on the xtreme dataset.
It achieves the following results on the evaluation set:
- Loss: 0.1372
- F1: 0.8621
## Model description
More information needed
## Intended uses & limitations
More information needed
## Training and evaluation data
More information needed
## Training procedure
### Training hyperparameters
The following hyperparameters were used during training:
- learning_rate: 5e-05
- train_batch_size: 24
- eval_batch_size: 24
- seed: 42
- optimizer: Adam with betas=(0.9,0.999) and epsilon=1e-08
- lr_scheduler_type: linear
- num_epochs: 3
### Training results
| Training Loss | Epoch | Step | Validation Loss | F1 |
|:-------------:|:-----:|:----:|:---------------:|:------:|
| 0.2575 | 1.0 | 525 | 0.1621 | 0.8292 |
| 0.1287 | 2.0 | 1050 | 0.1378 | 0.8526 |
| 0.0831 | 3.0 | 1575 | 0.1372 | 0.8621 |
### Framework versions
- Transformers 4.11.3
- Pytorch 1.11.0+cu113
- Datasets 1.16.1
- Tokenizers 0.10.3
|
income/jpq-gpl-arguana-question_encoder-base-msmarco-distilbert-tas-b | c9c200c33e0342f4b6a51fac01a1170924aa1d5a | 2022-06-15T17:01:15.000Z | [
"pytorch",
"distilbert",
"transformers",
"license:apache-2.0"
] | null | false | income | null | income/jpq-gpl-arguana-question_encoder-base-msmarco-distilbert-tas-b | 1 | null | transformers | 32,894 | ---
license: apache-2.0
---
|
income/jpq-gpl-arguana-document_encoder-base-msmarco-distilbert-tas-b | 5d3913e16feea20c4d500c5f0dadf4556bcb2e60 | 2022-06-15T17:02:21.000Z | [
"pytorch",
"distilbert",
"transformers",
"license:apache-2.0"
] | null | false | income | null | income/jpq-gpl-arguana-document_encoder-base-msmarco-distilbert-tas-b | 1 | null | transformers | 32,895 | ---
license: apache-2.0
---
|
income/jpq-gpl-climate-fever-question_encoder-base-msmarco-distilbert-tas-b | ba5e4006f5afe15f8698ccfc858c0c47c8d36435 | 2022-06-15T17:03:39.000Z | [
"pytorch",
"distilbert",
"transformers",
"license:apache-2.0"
] | null | false | income | null | income/jpq-gpl-climate-fever-question_encoder-base-msmarco-distilbert-tas-b | 1 | null | transformers | 32,896 | ---
license: apache-2.0
---
|
income/jpq-gpl-climate-fever-document_encoder-base-msmarco-distilbert-tas-b | 669c2ce1a3f3c3191bf540fe39ea0942947075f6 | 2022-06-15T17:04:14.000Z | [
"pytorch",
"distilbert",
"transformers",
"license:apache-2.0"
] | null | false | income | null | income/jpq-gpl-climate-fever-document_encoder-base-msmarco-distilbert-tas-b | 1 | null | transformers | 32,897 | ---
license: apache-2.0
---
|
income/jpq-gpl-dbpedia-entity-question_encoder-base-msmarco-distilbert-tas-b | cc31d6970db49ec67f7e4d84f525631e656497a6 | 2022-06-15T17:05:45.000Z | [
"pytorch",
"distilbert",
"transformers",
"license:apache-2.0"
] | null | false | income | null | income/jpq-gpl-dbpedia-entity-question_encoder-base-msmarco-distilbert-tas-b | 1 | null | transformers | 32,898 | ---
license: apache-2.0
---
|
income/jpq-gpl-fiqa-question_encoder-base-msmarco-distilbert-tas-b | e61e9aa7faa99d39137049b59c64a9a0f7f5d80a | 2022-06-15T17:15:29.000Z | [
"pytorch",
"distilbert",
"transformers",
"license:apache-2.0"
] | null | false | income | null | income/jpq-gpl-fiqa-question_encoder-base-msmarco-distilbert-tas-b | 1 | null | transformers | 32,899 | ---
license: apache-2.0
---
|
Subsets and Splits
No community queries yet
The top public SQL queries from the community will appear here once available.