modelId
stringlengths 4
112
| sha
stringlengths 40
40
| lastModified
stringlengths 24
24
| tags
list | pipeline_tag
stringclasses 29
values | private
bool 1
class | author
stringlengths 2
38
⌀ | config
null | id
stringlengths 4
112
| downloads
float64 0
36.8M
⌀ | likes
float64 0
712
⌀ | library_name
stringclasses 17
values | __index_level_0__
int64 0
38.5k
| readme
stringlengths 0
186k
|
---|---|---|---|---|---|---|---|---|---|---|---|---|---|
studio-ousia/mluke-large | 8dac253911d21efd45ece207b11e079694b02241 | 2022-03-11T02:58:11.000Z | [
"pytorch",
"luke",
"fill-mask",
"transformers",
"autotrain_compatible"
] | fill-mask | false | studio-ousia | null | studio-ousia/mluke-large | 21 | null | transformers | 8,200 | Entry not found |
transformersbook/xlm-roberta-base-finetuned-panx-en | 5a56d079034f5f2ed6d6c13d9d4c6aa99353cd67 | 2022-02-05T17:07:09.000Z | [
"pytorch",
"xlm-roberta",
"token-classification",
"dataset:xtreme",
"transformers",
"generated_from_trainer",
"license:mit",
"model-index",
"autotrain_compatible"
] | token-classification | false | transformersbook | null | transformersbook/xlm-roberta-base-finetuned-panx-en | 21 | null | transformers | 8,201 | ---
license: mit
tags:
- generated_from_trainer
datasets:
- xtreme
metrics:
- f1
model-index:
- name: xlm-roberta-base-finetuned-panx-en
results:
- task:
name: Token Classification
type: token-classification
dataset:
name: xtreme
type: xtreme
args: PAN-X.en
metrics:
- name: F1
type: f1
value: 0.69816564758199
---
<!-- This model card has been generated automatically according to the information the Trainer had access to. You
should probably proofread and complete it, then remove this comment. -->
# xlm-roberta-base-finetuned-panx-en
This model is a fine-tuned version of [xlm-roberta-base](https://huggingface.co/xlm-roberta-base) on the PAN-X dataset. The model is trained in Chapter 4: Multilingual Named Entity Recognition in the [NLP with Transformers book](https://learning.oreilly.com/library/view/natural-language-processing/9781098103231/). You can find the full code in the accompanying [Github repository](https://github.com/nlp-with-transformers/notebooks/blob/main/04_multilingual-ner.ipynb).
It achieves the following results on the evaluation set:
- Loss: 0.3676
- F1: 0.6982
## Model description
More information needed
## Intended uses & limitations
More information needed
## Training and evaluation data
More information needed
## Training procedure
### Training hyperparameters
The following hyperparameters were used during training:
- learning_rate: 5e-05
- train_batch_size: 24
- eval_batch_size: 24
- seed: 42
- optimizer: Adam with betas=(0.9,0.999) and epsilon=1e-08
- lr_scheduler_type: linear
- num_epochs: 3
### Training results
| Training Loss | Epoch | Step | Validation Loss | F1 |
|:-------------:|:-----:|:----:|:---------------:|:------:|
| 1.026 | 1.0 | 50 | 0.5734 | 0.4901 |
| 0.4913 | 2.0 | 100 | 0.3870 | 0.6696 |
| 0.3734 | 3.0 | 150 | 0.3676 | 0.6982 |
### Framework versions
- Transformers 4.12.0.dev0
- Pytorch 1.9.1+cu102
- Datasets 1.12.1
- Tokenizers 0.10.3
|
uclanlp/plbart-multi_task-all | 594e7236fb071ce3cece96a23904e910cbd7acef | 2022-03-02T07:44:43.000Z | [
"pytorch",
"plbart",
"text2text-generation",
"transformers",
"autotrain_compatible"
] | text2text-generation | false | uclanlp | null | uclanlp/plbart-multi_task-all | 21 | null | transformers | 8,202 | Entry not found |
vblagoje/bert-english-uncased-finetuned-chunk | c37c9e8262d61fb10c7a666398364c6574fee55d | 2021-05-20T08:50:30.000Z | [
"pytorch",
"jax",
"bert",
"token-classification",
"transformers",
"autotrain_compatible"
] | token-classification | false | vblagoje | null | vblagoje/bert-english-uncased-finetuned-chunk | 21 | 1 | transformers | 8,203 | Entry not found |
zhuqing/bert-base-uncased-reddit-business | baa31194685995a4deffbf88f1d9e0927bbfdf21 | 2021-08-01T16:42:35.000Z | [
"pytorch",
"bert",
"fill-mask",
"transformers",
"autotrain_compatible"
] | fill-mask | false | zhuqing | null | zhuqing/bert-base-uncased-reddit-business | 21 | null | transformers | 8,204 | Entry not found |
satkinson/DialoGPT-medium-marvin | a47dac172a722a9887949ce2d6508ef8dfc35850 | 2022-04-26T22:13:53.000Z | [
"pytorch",
"gpt2",
"text-generation",
"en",
"transformers",
"conversational"
] | conversational | false | satkinson | null | satkinson/DialoGPT-medium-marvin | 21 | 0 | transformers | 8,205 | ---
language:
- en
thumbnail:
tags:
- conversational
metrics:
- perplexity
---
## DialoGPT model fine-tuned using Amazon's Topical Chat Dataset
This model is fine-tuned from the original [DialoGPT-medium](https://huggingface.co/microsoft/DialoGPT-medium).
This model was fine-tuned on a subset of messages from [Amazon's Topical Chat dataset](https://www.kaggle.com/arnavsharmaas/chatbot-dataset-topical-chat) (due to processing limitations, I restricted my training data to 50,000 observations.
The dataset spands 8 broad topics and contains conversation partner who do not have defined roles. It was created with the goal of [aiding in the effort to build a socialbot that can have deep, engaging open-domain conversations with humans](https://m.media-amazon.com/images/G/01/amazon.jobs/3079_Paper._CB1565131710_.pdf).
The eight broad topics are:
- fashion
- politics
- books
- sports
- general entertainment
- music
- science and technology
- movies
<br><br>
<b>Sample dialogue:</b>
|Role | Response |
|---------|--------|
|User: | What kind of movies do you like? |
| Bot: | I like marvel movies like iron man |
|User: | Do you like horror films? |
| Bot: | Yea, I like the horror films that have macabre and supernatural themes the most |
|User: | Interesting. |
| Bot: | If you watch a horror movie before you view abstract art, you will enjoy the art more |
|User: | Speaking from experience? |
| Bot: | Yes, I've seen many horror movies where the characters were quite evil and supernatural. |
## Using the model
Example code for trying out the model (taken directly from the [DialoGPT-medium](https://huggingface.co/microsoft/DialoGPT-medium) model card):
```python
from transformers import AutoModelWithLMHead, AutoTokenizer
import torch
tokenizer = AutoTokenizer.from_pretrained("satkinson/DialoGPT-small-marvin")
model = AutoModelWithLMHead.from_pretrained("satkinson/DialoGPT-small-marvin")
# Let's chat for 5 lines
for step in range(5):
# encode the new user input, add the eos_token and return a tensor in Pytorch
new_user_input_ids = tokenizer.encode(input(">> User:") + tokenizer.eos_token, return_tensors='pt')
# append the new user input tokens to the chat history
bot_input_ids = torch.cat([chat_history_ids, new_user_input_ids], dim=-1) if step > 0 else new_user_input_ids
# generated a response while limiting the total chat history to 1000 tokens,
chat_history_ids = model.generate(bot_input_ids, max_length=1000, pad_token_id=tokenizer.eos_token_id)
# pretty print last ouput tokens from bot
print("DialoGPT: {}".format(tokenizer.decode(chat_history_ids[:, bot_input_ids.shape[-1]:][0], skip_special_tokens=True)))
``` |
batterydata/batteryonlybert-uncased-squad-v1 | 93cc3ccc9d86de6aebed236d67bf394f965733e7 | 2022-03-03T20:25:01.000Z | [
"pytorch",
"bert",
"question-answering",
"en",
"dataset:squad",
"dataset:batterydata/battery-device-data-qa",
"transformers",
"question answering",
"license:apache-2.0",
"autotrain_compatible"
] | question-answering | false | batterydata | null | batterydata/batteryonlybert-uncased-squad-v1 | 21 | null | transformers | 8,206 | ---
language: en
tags: question answering
license: apache-2.0
datasets:
- squad
- batterydata/battery-device-data-qa
metrics: squad
---
# BatteryOnlyBERT-uncased for QA
**Language model:** batteryonlybert-uncased
**Language:** English
**Downstream-task:** Extractive QA
**Training data:** SQuAD v1
**Eval data:** SQuAD v1
**Code:** See [example](https://github.com/ShuHuang/batterybert)
**Infrastructure**: 8x DGX A100
## Hyperparameters
```
batch_size = 16
n_epochs = 2
base_LM_model = "batteryonlybert-uncased"
max_seq_len = 386
learning_rate = 2e-5
doc_stride=128
max_query_length=64
```
## Performance
Evaluated on the SQuAD v1.0 dev set.
```
"exact": 79.53,
"f1": 87.22,
```
Evaluated on the battery device dataset.
```
"precision": 67.20,
"recall": 83.82,
```
## Usage
### In Transformers
```python
from transformers import AutoModelForQuestionAnswering, AutoTokenizer, pipeline
model_name = "batterydata/batteryonlybert-uncased-squad-v1"
# a) Get predictions
nlp = pipeline('question-answering', model=model_name, tokenizer=model_name)
QA_input = {
'question': 'What is the electrolyte?',
'context': 'The typical non-aqueous electrolyte for commercial Li-ion cells is a solution of LiPF6 in linear and cyclic carbonates.'
}
res = nlp(QA_input)
# b) Load model & tokenizer
model = AutoModelForQuestionAnswering.from_pretrained(model_name)
tokenizer = AutoTokenizer.from_pretrained(model_name)
```
## Authors
Shu Huang: `sh2009 [at] cam.ac.uk`
Jacqueline Cole: `jmc61 [at] cam.ac.uk`
## Citation
BatteryBERT: A Pre-trained Language Model for Battery Database Enhancement |
jkhan447/sentiment-model-sample | 8ec90b8e897075fecb389cc017123cdd5f176eee | 2022-03-04T11:13:39.000Z | [
"pytorch",
"tensorboard",
"bert",
"text-classification",
"dataset:imdb",
"transformers",
"generated_from_trainer",
"license:apache-2.0",
"model-index"
] | text-classification | false | jkhan447 | null | jkhan447/sentiment-model-sample | 21 | null | transformers | 8,207 | ---
license: apache-2.0
tags:
- generated_from_trainer
datasets:
- imdb
metrics:
- accuracy
model-index:
- name: sentiment-model-sample
results:
- task:
name: Text Classification
type: text-classification
dataset:
name: imdb
type: imdb
args: plain_text
metrics:
- name: Accuracy
type: accuracy
value: 0.93948
---
<!-- This model card has been generated automatically according to the information the Trainer had access to. You
should probably proofread and complete it, then remove this comment. -->
# sentiment-model-sample
This model is a fine-tuned version of [bert-base-uncased](https://huggingface.co/bert-base-uncased) on the imdb dataset.
It achieves the following results on the evaluation set:
- Loss: 0.5280
- Accuracy: 0.9395
## Model description
More information needed
## Intended uses & limitations
More information needed
## Training and evaluation data
More information needed
## Training procedure
### Training hyperparameters
The following hyperparameters were used during training:
- learning_rate: 2e-05
- train_batch_size: 16
- eval_batch_size: 16
- seed: 42
- optimizer: Adam with betas=(0.9,0.999) and epsilon=1e-08
- lr_scheduler_type: linear
- num_epochs: 10
### Training results
### Framework versions
- Transformers 4.17.0
- Pytorch 1.10.0+cu111
- Datasets 1.18.3
- Tokenizers 0.11.6
|
Visual-Attention-Network/van-large | 98b609818338396dc9a3d09f5c31de94b0eb50fe | 2022-03-31T12:45:46.000Z | [
"pytorch",
"van",
"image-classification",
"dataset:imagenet-1k",
"arxiv:2202.09741",
"transformers",
"vision",
"license:apache-2.0"
] | image-classification | false | Visual-Attention-Network | null | Visual-Attention-Network/van-large | 21 | null | transformers | 8,208 | ---
license: apache-2.0
tags:
- vision
- image-classification
datasets:
- imagenet-1k
widget:
- src: https://huggingface.co/datasets/mishig/sample_images/resolve/main/tiger.jpg
example_title: Tiger
- src: https://huggingface.co/datasets/mishig/sample_images/resolve/main/teapot.jpg
example_title: Teapot
- src: https://huggingface.co/datasets/mishig/sample_images/resolve/main/palace.jpg
example_title: Palace
---
# Van
Van model trained on imagenet-1k. It was introduced in the paper [Visual Attention Network](https://arxiv.org/abs/2202.09741) and first released in [this repository](https://github.com/Visual-Attention-Network/VAN-Classification).
Disclaimer: The team releasing Van did not write a model card for this model so this model card has been written by the Hugging Face team.
## Model description
This paper introduces a new attention layer based on convolution operations able to capture both local and distant relationships. This is done by combining normal and large kernel convolution layers. The latter uses a dilated convolution to capture distant correlations.
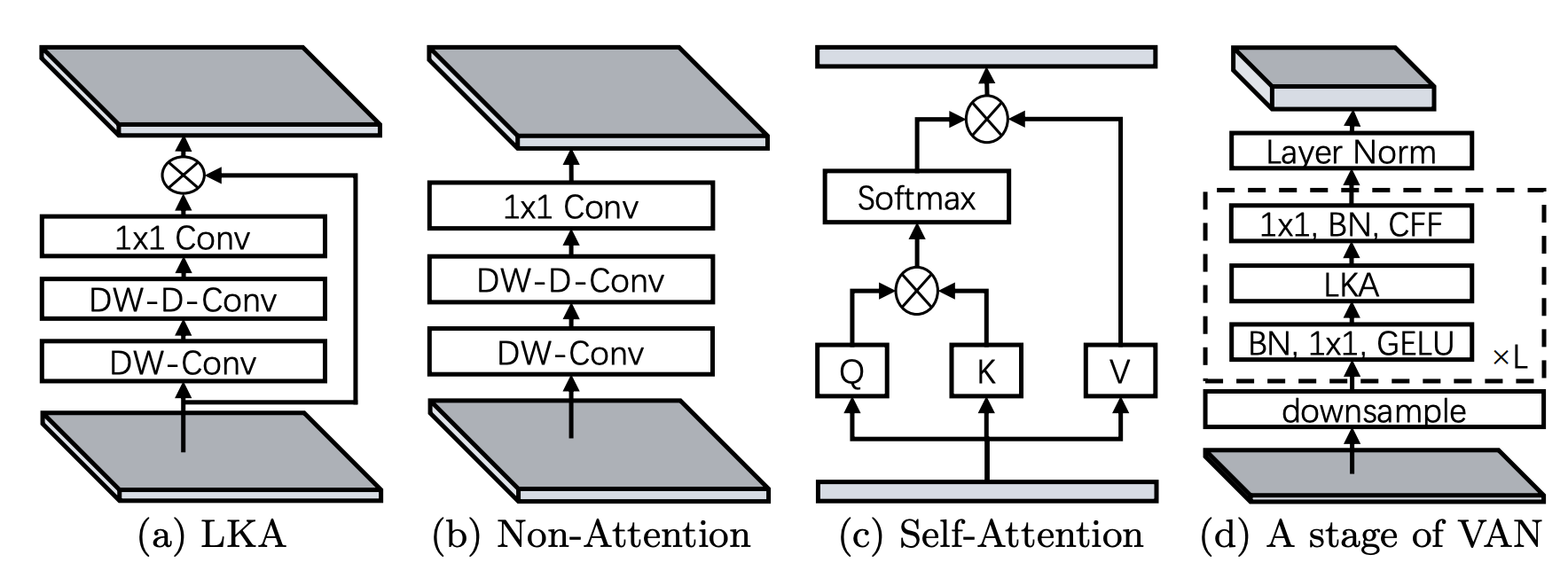
## Intended uses & limitations
You can use the raw model for image classification. See the [model hub](https://huggingface.co/models?search=van) to look for
fine-tuned versions on a task that interests you.
### How to use
Here is how to use this model:
```python
>>> from transformers import AutoFeatureExtractor, VanForImageClassification
>>> import torch
>>> from datasets import load_dataset
>>> dataset = load_dataset("huggingface/cats-image")
>>> image = dataset["test"]["image"][0]
>>> feature_extractor = AutoFeatureExtractor.from_pretrained("Visual-Attention-Network/van-base")
>>> model = VanForImageClassification.from_pretrained("Visual-Attention-Network/van-base")
>>> inputs = feature_extractor(image, return_tensors="pt")
>>> with torch.no_grad():
... logits = model(**inputs).logits
>>> # model predicts one of the 1000 ImageNet classes
>>> predicted_label = logits.argmax(-1).item()
>>> print(model.config.id2label[predicted_label])
tabby, tabby cat
```
For more code examples, we refer to the [documentation](https://huggingface.co/docs/transformers/master/en/model_doc/van). |
leftthomas/resnet50 | 19128d842d5b7589cbf02b5002d70f9c65586796 | 2022-03-11T12:53:14.000Z | [
"pytorch",
"resnet",
"dataset:imagenet",
"arxiv:1512.03385",
"transformers",
"image-classification",
"license:afl-3.0"
] | image-classification | false | leftthomas | null | leftthomas/resnet50 | 21 | null | transformers | 8,209 | ---
tags:
- image-classification
- resnet
license: afl-3.0
datasets:
- imagenet
widget:
- src: https://huggingface.co/datasets/mishig/sample_images/resolve/main/tiger.jpg
example_title: Tiger
- src: https://huggingface.co/datasets/mishig/sample_images/resolve/main/teapot.jpg
example_title: Teapot
- src: https://huggingface.co/datasets/mishig/sample_images/resolve/main/palace.jpg
example_title: Palace
---
# ResNet-50
Pretrained model on [ImageNet](http://www.image-net.org/). The ResNet architecture was introduced in
[this paper](https://arxiv.org/abs/1512.03385).
## Intended uses
You can use the raw model to classify images along the 1,000 ImageNet labels, but you can also change its head
to fine-tune it on a downstream task (another classification task with different labels, image segmentation or
object detection, to name a few).
## Evaluation results
This model has a top1-accuracy of 76.13% and a top-5 accuracy of 92.86% in the evaluation set of ImageNet.
|
navteca/nli-deberta-v3-xsmall | 90986fb464069d701ba1104a9e1b9bdfe7c3c41c | 2022-03-16T09:49:34.000Z | [
"pytorch",
"deberta-v2",
"text-classification",
"en",
"dataset:multi_nli",
"dataset:snli",
"transformers",
"microsoft/deberta-v3-xsmall",
"license:apache-2.0",
"zero-shot-classification"
] | zero-shot-classification | false | navteca | null | navteca/nli-deberta-v3-xsmall | 21 | 1 | transformers | 8,210 | ---
datasets:
- multi_nli
- snli
language: en
license: apache-2.0
metrics:
- accuracy
pipeline_tag: zero-shot-classification
tags:
- microsoft/deberta-v3-xsmall
---
# Cross-Encoder for Natural Language Inference
This model was trained using [SentenceTransformers](https://sbert.net) [Cross-Encoder](https://www.sbert.net/examples/applications/cross-encoder/README.html) class. This model is based on [microsoft/deberta-v3-xsmall](https://huggingface.co/microsoft/deberta-v3-xsmall)
## Training Data
The model was trained on the [SNLI](https://nlp.stanford.edu/projects/snli/) and [MultiNLI](https://cims.nyu.edu/~sbowman/multinli/) datasets. For a given sentence pair, it will output three scores corresponding to the labels: contradiction, entailment, neutral.
## Performance
- Accuracy on SNLI-test dataset: 91.64
- Accuracy on MNLI mismatched set: 87.77
For futher evaluation results, see [SBERT.net - Pretrained Cross-Encoder](https://www.sbert.net/docs/pretrained_cross-encoders.html#nli).
## Usage
Pre-trained models can be used like this:
```python
from sentence_transformers import CrossEncoder
model = CrossEncoder('cross-encoder/nli-deberta-v3-xsmall')
scores = model.predict([('A man is eating pizza', 'A man eats something'), ('A black race car starts up in front of a crowd of people.', 'A man is driving down a lonely road.')])
#Convert scores to labels
label_mapping = ['contradiction', 'entailment', 'neutral']
labels = [label_mapping[score_max] for score_max in scores.argmax(axis=1)]
```
## Usage with Transformers AutoModel
You can use the model also directly with Transformers library (without SentenceTransformers library):
```python
from transformers import AutoTokenizer, AutoModelForSequenceClassification
import torch
model = AutoModelForSequenceClassification.from_pretrained('cross-encoder/nli-deberta-v3-xsmall')
tokenizer = AutoTokenizer.from_pretrained('cross-encoder/nli-deberta-v3-xsmall')
features = tokenizer(['A man is eating pizza', 'A black race car starts up in front of a crowd of people.'], ['A man eats something', 'A man is driving down a lonely road.'], padding=True, truncation=True, return_tensors="pt")
model.eval()
with torch.no_grad():
scores = model(**features).logits
label_mapping = ['contradiction', 'entailment', 'neutral']
labels = [label_mapping[score_max] for score_max in scores.argmax(dim=1)]
print(labels)
```
## Zero-Shot Classification
This model can also be used for zero-shot-classification:
```python
from transformers import pipeline
classifier = pipeline("zero-shot-classification", model='cross-encoder/nli-deberta-v3-xsmall')
sent = "Apple just announced the newest iPhone X"
candidate_labels = ["technology", "sports", "politics"]
res = classifier(sent, candidate_labels)
print(res)
``` |
Visual-Attention-Network/van-small | 81e2b580ed3c06863690251ed110bbf4c94a7f82 | 2022-03-31T12:45:49.000Z | [
"pytorch",
"van",
"image-classification",
"dataset:imagenet-1k",
"arxiv:2202.09741",
"transformers",
"vision",
"license:apache-2.0"
] | image-classification | false | Visual-Attention-Network | null | Visual-Attention-Network/van-small | 21 | null | transformers | 8,211 | ---
license: apache-2.0
tags:
- vision
- image-classification
datasets:
- imagenet-1k
widget:
- src: https://huggingface.co/datasets/mishig/sample_images/resolve/main/tiger.jpg
example_title: Tiger
- src: https://huggingface.co/datasets/mishig/sample_images/resolve/main/teapot.jpg
example_title: Teapot
- src: https://huggingface.co/datasets/mishig/sample_images/resolve/main/palace.jpg
example_title: Palace
---
# Van
Van model trained on imagenet-1k. It was introduced in the paper [Visual Attention Network](https://arxiv.org/abs/2202.09741) and first released in [this repository](https://github.com/Visual-Attention-Network/VAN-Classification).
Disclaimer: The team releasing Van did not write a model card for this model so this model card has been written by the Hugging Face team.
## Model description
This paper introduces a new attention layer based on convolution operations able to capture both local and distant relationships. This is done by combining normal and large kernel convolution layers. The latter uses a dilated convolution to capture distant correlations.
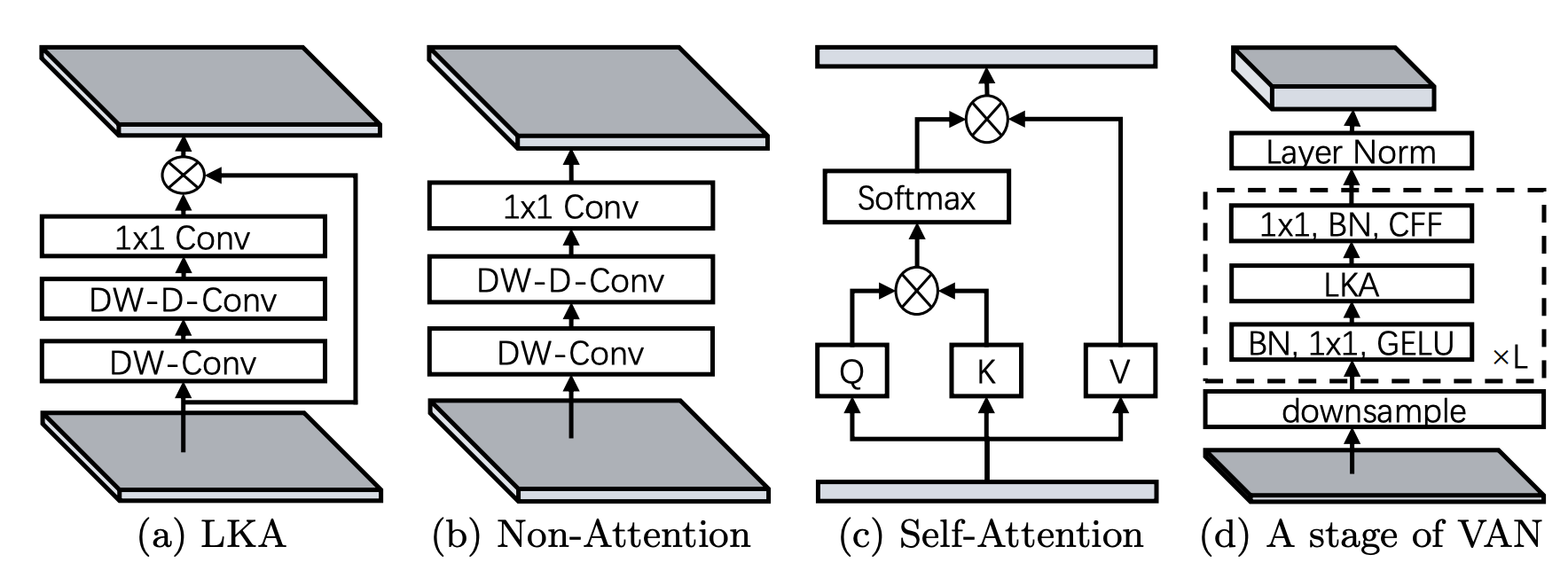
## Intended uses & limitations
You can use the raw model for image classification. See the [model hub](https://huggingface.co/models?search=van) to look for
fine-tuned versions on a task that interests you.
### How to use
Here is how to use this model:
```python
>>> from transformers import AutoFeatureExtractor, VanForImageClassification
>>> import torch
>>> from datasets import load_dataset
>>> dataset = load_dataset("huggingface/cats-image")
>>> image = dataset["test"]["image"][0]
>>> feature_extractor = AutoFeatureExtractor.from_pretrained("Visual-Attention-Network/van-base")
>>> model = VanForImageClassification.from_pretrained("Visual-Attention-Network/van-base")
>>> inputs = feature_extractor(image, return_tensors="pt")
>>> with torch.no_grad():
... logits = model(**inputs).logits
>>> # model predicts one of the 1000 ImageNet classes
>>> predicted_label = logits.argmax(-1).item()
>>> print(model.config.id2label[predicted_label])
tabby, tabby cat
```
For more code examples, we refer to the [documentation](https://huggingface.co/docs/transformers/master/en/model_doc/van). |
facebook/regnet-y-1280-seer-in1k | 32541c21dc3f3adacce9d58a801d6dc2a0ab657d | 2022-06-30T10:22:16.000Z | [
"pytorch",
"tf",
"regnet",
"image-classification",
"dataset:imagenet-1k",
"arxiv:2202.08360",
"transformers",
"vision",
"license:apache-2.0"
] | image-classification | false | facebook | null | facebook/regnet-y-1280-seer-in1k | 21 | null | transformers | 8,212 | ---
license: apache-2.0
tags:
- vision
- image-classification
datasets:
- imagenet-1k
widget:
- src: https://huggingface.co/datasets/mishig/sample_images/resolve/main/tiger.jpg
example_title: Tiger
- src: https://huggingface.co/datasets/mishig/sample_images/resolve/main/teapot.jpg
example_title: Teapot
- src: https://huggingface.co/datasets/mishig/sample_images/resolve/main/palace.jpg
example_title: Palace
---
# RegNet
RegNet model trained on imagenet-1k. It was introduced in the paper [Vision Models Are More Robust And Fair When Pretrained On Uncurated Images Without Supervision](https://arxiv.org/abs/2202.08360) and first released in [this repository](https://github.com/facebookresearch/vissl/tree/main/projects/SEER).
Disclaimer: The team releasing RegNet did not write a model card for this model so this model card has been written by the Hugging Face team.
## Model description
The authors trained [RegNets](https://huggingface.co/?models=regnet) models in a self-supervised fashion on bilion of random images from the internet. This model is later finetuned on ImageNet
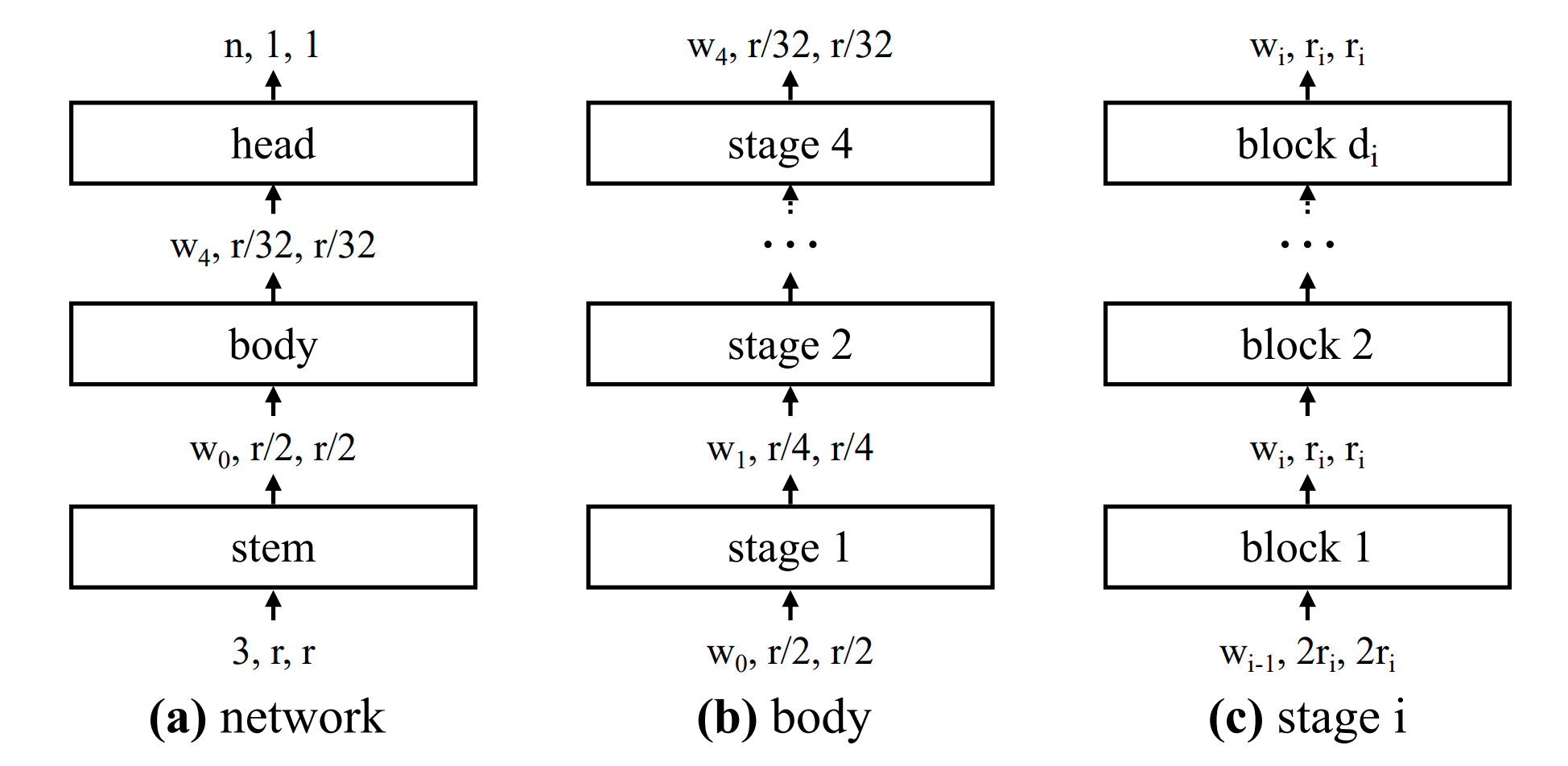
## Intended uses & limitations
You can use the raw model for image classification. See the [model hub](https://huggingface.co/models?search=regnet) to look for
fine-tuned versions on a task that interests you.
### How to use
Here is how to use this model:
```python
>>> from transformers import AutoFeatureExtractor, RegNetForImageClassification
>>> import torch
>>> from datasets import load_dataset
>>> dataset = load_dataset("huggingface/cats-image")
>>> image = dataset["test"]["image"][0]
>>> feature_extractor = AutoFeatureExtractor.from_pretrained("zuppif/regnet-y-040")
>>> model = RegNetForImageClassification.from_pretrained("zuppif/regnet-y-040")
>>> inputs = feature_extractor(image, return_tensors="pt")
>>> with torch.no_grad():
... logits = model(**inputs).logits
>>> # model predicts one of the 1000 ImageNet classes
>>> predicted_label = logits.argmax(-1).item()
>>> print(model.config.id2label[predicted_label])
'tabby, tabby cat'
```
For more code examples, we refer to the [documentation](https://huggingface.co/docs/transformers/master/en/model_doc/regnet). |
Wikidepia/gpt2-spam | 85bc19d86699dd10f80bcdc96b129cb02a83135f | 2022-03-20T01:10:59.000Z | [
"pytorch",
"gpt2",
"text-generation",
"transformers",
"generated_from_trainer",
"license:apache-2.0",
"model-index"
] | text-generation | false | Wikidepia | null | Wikidepia/gpt2-spam | 21 | 1 | transformers | 8,213 | ---
license: apache-2.0
tags:
- generated_from_trainer
model-index:
- name: gpt2-spam
results: []
---
<!-- This model card has been generated automatically according to the information the Trainer had access to. You
should probably proofread and complete it, then remove this comment. -->
# gpt2-spam
This model is a fine-tuned version of [distilgpt2](https://huggingface.co/distilgpt2) on an unknown dataset.
## Model description
More information needed
## Intended uses & limitations
More information needed
## Training and evaluation data
More information needed
## Training procedure
### Training hyperparameters
The following hyperparameters were used during training:
- learning_rate: 5e-05
- train_batch_size: 8
- eval_batch_size: 8
- seed: 42
- optimizer: Adam with betas=(0.9,0.999) and epsilon=1e-08
- lr_scheduler_type: linear
- num_epochs: 3.0
### Training results
### Framework versions
- Transformers 4.18.0.dev0
- Pytorch 1.11.0
- Datasets 2.0.0
- Tokenizers 0.11.6
|
RuudVelo/dutch_news_clf_bert_finetuned | 8c76a1f99791d7bbdf82fba38e15b03e8735fac9 | 2022-03-24T14:37:21.000Z | [
"pytorch",
"bert",
"text-classification",
"transformers"
] | text-classification | false | RuudVelo | null | RuudVelo/dutch_news_clf_bert_finetuned | 21 | null | transformers | 8,214 | Entry not found |
snehatyagi/wav2vec2_test | da03eb9b0f55a14cbe1fae5dc1cdb46421c51bbf | 2022-03-31T07:21:45.000Z | [
"pytorch",
"tensorboard",
"wav2vec2",
"automatic-speech-recognition",
"transformers",
"generated_from_trainer",
"license:apache-2.0",
"model-index"
] | automatic-speech-recognition | false | snehatyagi | null | snehatyagi/wav2vec2_test | 21 | null | transformers | 8,215 | ---
license: apache-2.0
tags:
- generated_from_trainer
model-index:
- name: wav2vec2_test
results: []
---
<!-- This model card has been generated automatically according to the information the Trainer had access to. You
should probably proofread and complete it, then remove this comment. -->
# wav2vec2_test
This model is a fine-tuned version of [facebook/wav2vec2-base](https://huggingface.co/facebook/wav2vec2-base) on the None dataset.
It achieves the following results on the evaluation set:
- Loss: 91.1661
- Wer: 0.5714
## Model description
More information needed
## Intended uses & limitations
More information needed
## Training and evaluation data
More information needed
## Training procedure
### Training hyperparameters
The following hyperparameters were used during training:
- learning_rate: 0.0001
- train_batch_size: 8
- eval_batch_size: 8
- seed: 42
- optimizer: Adam with betas=(0.9,0.999) and epsilon=1e-08
- lr_scheduler_type: linear
- lr_scheduler_warmup_steps: 100
- num_epochs: 1000
### Training results
| Training Loss | Epoch | Step | Validation Loss | Wer |
|:-------------:|:------:|:----:|:---------------:|:------:|
| 11.9459 | 100.0 | 100 | 46.9901 | 1.0 |
| 3.2175 | 200.0 | 200 | 73.0950 | 1.0 |
| 1.8117 | 300.0 | 300 | 78.4884 | 0.6735 |
| 1.3694 | 400.0 | 400 | 84.0168 | 0.6327 |
| 1.1392 | 500.0 | 500 | 85.2083 | 0.5918 |
| 0.979 | 600.0 | 600 | 88.9109 | 0.5918 |
| 0.8917 | 700.0 | 700 | 89.0310 | 0.5918 |
| 0.8265 | 800.0 | 800 | 90.0659 | 0.6122 |
| 0.769 | 900.0 | 900 | 91.8476 | 0.5714 |
| 0.7389 | 1000.0 | 1000 | 91.1661 | 0.5714 |
### Framework versions
- Transformers 4.17.0
- Pytorch 1.10.2
- Datasets 1.18.3
- Tokenizers 0.11.6
|
Intel/bert-large-uncased-sparse-80-1x4-block-pruneofa | f7df70adf762e97887a906e5e8e4f046e409e3b7 | 2022-03-29T11:56:40.000Z | [
"pytorch",
"bert",
"pretraining",
"en",
"dataset:wikipedia",
"dataset:bookcorpus",
"arxiv:2111.05754",
"transformers",
"fill-mask"
] | fill-mask | false | Intel | null | Intel/bert-large-uncased-sparse-80-1x4-block-pruneofa | 21 | null | transformers | 8,216 | ---
language: en
tags: fill-mask
datasets:
- wikipedia
- bookcorpus
---
# 80% 1x4 Block Sparse BERT-Large (uncased) Prune OFA
This model is was created using Prune OFA method described in [Prune Once for All: Sparse Pre-Trained Language Models](https://arxiv.org/abs/2111.05754) presented in ENLSP NeurIPS Workshop 2021.
For further details on the model and its result, see our paper and our implementation available [here](https://github.com/IntelLabs/Model-Compression-Research-Package/tree/main/research/prune-once-for-all).
|
luckydog/bert-base-chinese-finetuned-ChnSenti | 7af377ccb93f3ccf871971fb3ad74969d530ac55 | 2022-04-12T13:38:36.000Z | [
"pytorch",
"tensorboard",
"bert",
"text-classification",
"transformers"
] | text-classification | false | luckydog | null | luckydog/bert-base-chinese-finetuned-ChnSenti | 21 | 1 | transformers | 8,217 | Entry not found |
MartinoMensio/racism-models-raw-label-epoch-3 | f8d28c4128733471699f936547af47e05c17834d | 2022-05-04T16:05:21.000Z | [
"pytorch",
"bert",
"text-classification",
"es",
"transformers",
"license:mit"
] | text-classification | false | MartinoMensio | null | MartinoMensio/racism-models-raw-label-epoch-3 | 21 | null | transformers | 8,218 | ---
language: es
license: mit
widget:
- text: "y porqué es lo que hay que hacer con los menas y con los adultos también!!!! NO a los inmigrantes ilegales!!!!"
---
### Description
This model is a fine-tuned version of [BETO (spanish bert)](https://huggingface.co/dccuchile/bert-base-spanish-wwm-uncased) that has been trained on the *Datathon Against Racism* dataset (2022)
We performed several experiments that will be described in the upcoming paper "Estimating Ground Truth in a Low-labelled Data Regime:A Study of Racism Detection in Spanish" (NEATClasS 2022)
We applied 6 different methods ground-truth estimations, and for each one we performed 4 epochs of fine-tuning. The result is made of 24 models:
| method | epoch 1 | epoch 3 | epoch 3 | epoch 4 |
|--- |--- |--- |--- |--- |
| raw-label | [raw-label-epoch-1](https://huggingface.co/MartinoMensio/racism-models-raw-label-epoch-1) | [raw-label-epoch-2](https://huggingface.co/MartinoMensio/racism-models-raw-label-epoch-2) | [raw-label-epoch-3](https://huggingface.co/MartinoMensio/racism-models-raw-label-epoch-3) | [raw-label-epoch-4](https://huggingface.co/MartinoMensio/racism-models-raw-label-epoch-4) |
| m-vote-strict | [m-vote-strict-epoch-1](https://huggingface.co/MartinoMensio/racism-models-m-vote-strict-epoch-1) | [m-vote-strict-epoch-2](https://huggingface.co/MartinoMensio/racism-models-m-vote-strict-epoch-2) | [m-vote-strict-epoch-3](https://huggingface.co/MartinoMensio/racism-models-m-vote-strict-epoch-3) | [m-vote-strict-epoch-4](https://huggingface.co/MartinoMensio/racism-models-m-vote-strict-epoch-4) |
| m-vote-nonstrict | [m-vote-nonstrict-epoch-1](https://huggingface.co/MartinoMensio/racism-models-m-vote-nonstrict-epoch-1) | [m-vote-nonstrict-epoch-2](https://huggingface.co/MartinoMensio/racism-models-m-vote-nonstrict-epoch-2) | [m-vote-nonstrict-epoch-3](https://huggingface.co/MartinoMensio/racism-models-m-vote-nonstrict-epoch-3) | [m-vote-nonstrict-epoch-4](https://huggingface.co/MartinoMensio/racism-models-m-vote-nonstrict-epoch-4) |
| regression-w-m-vote | [regression-w-m-vote-epoch-1](https://huggingface.co/MartinoMensio/racism-models-regression-w-m-vote-epoch-1) | [regression-w-m-vote-epoch-2](https://huggingface.co/MartinoMensio/racism-models-regression-w-m-vote-epoch-2) | [regression-w-m-vote-epoch-3](https://huggingface.co/MartinoMensio/racism-models-regression-w-m-vote-epoch-3) | [regression-w-m-vote-epoch-4](https://huggingface.co/MartinoMensio/racism-models-regression-w-m-vote-epoch-4) |
| w-m-vote-strict | [w-m-vote-strict-epoch-1](https://huggingface.co/MartinoMensio/racism-models-w-m-vote-strict-epoch-1) | [w-m-vote-strict-epoch-2](https://huggingface.co/MartinoMensio/racism-models-w-m-vote-strict-epoch-2) | [w-m-vote-strict-epoch-3](https://huggingface.co/MartinoMensio/racism-models-w-m-vote-strict-epoch-3) | [w-m-vote-strict-epoch-4](https://huggingface.co/MartinoMensio/racism-models-w-m-vote-strict-epoch-4) |
| w-m-vote-nonstrict | [w-m-vote-nonstrict-epoch-1](https://huggingface.co/MartinoMensio/racism-models-w-m-vote-nonstrict-epoch-1) | [w-m-vote-nonstrict-epoch-2](https://huggingface.co/MartinoMensio/racism-models-w-m-vote-nonstrict-epoch-2) | [w-m-vote-nonstrict-epoch-3](https://huggingface.co/MartinoMensio/racism-models-w-m-vote-nonstrict-epoch-3) | [w-m-vote-nonstrict-epoch-4](https://huggingface.co/MartinoMensio/racism-models-w-m-vote-nonstrict-epoch-4) |
This model is `raw-label-epoch-3`
### Usage
```python
from transformers import AutoTokenizer, AutoModelForSequenceClassification, pipeline
model_name = 'raw-label-epoch-3'
tokenizer = AutoTokenizer.from_pretrained("dccuchile/bert-base-spanish-wwm-uncased")
full_model_path = f'MartinoMensio/racism-models-{model_name}'
model = AutoModelForSequenceClassification.from_pretrained(full_model_path)
pipe = pipeline("text-classification", model = model, tokenizer = tokenizer)
texts = [
'y porqué es lo que hay que hacer con los menas y con los adultos también!!!! NO a los inmigrantes ilegales!!!!',
'Es que los judíos controlan el mundo'
]
print(pipe(texts))
# [{'label': 'racist', 'score': 0.8621180653572083}, {'label': 'non-racist', 'score': 0.9725497364997864}]
```
For more details, see https://github.com/preyero/neatclass22
|
gxbag/wav2vec2-large-960h-lv60-self-with-wikipedia-lm | a50db71b4d291fefe8589c070f2b6b6124db1890 | 2022-05-23T12:31:37.000Z | [
"pytorch",
"wav2vec2",
"automatic-speech-recognition",
"transformers"
] | automatic-speech-recognition | false | gxbag | null | gxbag/wav2vec2-large-960h-lv60-self-with-wikipedia-lm | 21 | 2 | transformers | 8,219 | This is `facebook/wav2vec2-large-960h-lv60-self` enhanced with a Wikipedia language model.
The dataset used is `wikipedia/20200501.en`. All articles were used. It was cleaned of references and external links and all text inside of parantheses. It has 8092546 words.
The language model was built using KenLM. It is a 5-gram model where all singletons of 3-grams and bigger were pruned. It was built as:
`kenlm/build/bin/lmplz -o 5 -S 120G --vocab_estimate 8092546 --text text.txt --arpa text.arpa --prune 0 0 1`
Suggested usage:
```
from transformers import pipeline
pipe = pipeline("automatic-speech-recognition", model="gxbag/wav2vec2-large-960h-lv60-self-with-wikipedia-lm")
output = pipe("/path/to/audio.wav", chunk_length_s=30, stride_length_s=(6, 3))
output
```
Note that in the current version of `transformers` (as of the release of this model), when using striding in the pipeline it will chop off the last portion of audio, in this case 3 seconds. Add 3 seconds of silence to the end as a workaround. This problem was fixed in the GitHub version of `transformers`. |
mrm8488/convnext-tiny-finetuned-eurosat | 41521d86e5799a2f7f83b4b92481a3d46ae8d2d6 | 2022-04-23T15:23:29.000Z | [
"pytorch",
"tensorboard",
"convnext",
"image-classification",
"dataset:nielsr/eurosat-demo",
"transformers",
"generated_from_trainer",
"CV",
"ConvNeXT",
"satellite",
"EuroSAT",
"license:apache-2.0",
"model-index"
] | image-classification | false | mrm8488 | null | mrm8488/convnext-tiny-finetuned-eurosat | 21 | 2 | transformers | 8,220 | ---
license: apache-2.0
tags:
- generated_from_trainer
- CV
- ConvNeXT
- satellite
- EuroSAT
datasets:
- nielsr/eurosat-demo
metrics:
- accuracy
model-index:
- name: convnext-tiny-finetuned-eurosat
results:
- task:
name: Image Classification
type: image-classification
dataset:
name: image_folder
type: image_folder
args: default
metrics:
- name: Accuracy
type: accuracy
value: 0.9804938271604938
---
<!-- This model card has been generated automatically according to the information the Trainer had access to. You
should probably proofread and complete it, then remove this comment. -->
# ConvNeXT (tiny) fine-tuned on EuroSAT
This model is a fine-tuned version of [facebook/convnext-tiny-224](https://huggingface.co/facebook/convnext-tiny-224) on the [EuroSAT](https://github.com/phelber/eurosat) dataset.
It achieves the following results on the evaluation set:
- Loss: 0.0549
- Accuracy: 0.9805
#### Drag and drop the following pics in the right widget to test the model


## Model description
ConvNeXT is a pure convolutional model (ConvNet), inspired by the design of Vision Transformers, that claims to outperform them. The authors started from a ResNet and "modernized" its design by taking the Swin Transformer as inspiration.
## Dataset information
**EuroSAT : Land Use and Land Cover Classification with Sentinel-2**
In this study, we address the challenge of land use and land cover classification using Sentinel-2 satellite images. The Sentinel-2 satellite images are openly and freely accessible provided in the Earth observation program Copernicus. We present a novel dataset based on Sentinel-2 satellite images covering 13 spectral bands and consisting out of 10 classes with in total 27,000 labeled and geo-referenced images. We provide benchmarks for this novel dataset with its spectral bands using state-of-the-art deep Convolutional Neural Network (CNNs). With the proposed novel dataset, we achieved an overall classification accuracy of 98.57%. The resulting classification system opens a gate towards a number of Earth observation applications. We demonstrate how this classification system can be used for detecting land use and land cover changes and how it can assist in improving geographical maps.
## Intended uses & limitations
More information needed
## Training and evaluation data
More information needed
## Training procedure
### Training hyperparameters
The following hyperparameters were used during training:
- learning_rate: 5e-05
- train_batch_size: 32
- eval_batch_size: 32
- seed: 7171
- optimizer: Adam with betas=(0.9,0.999) and epsilon=1e-08
- lr_scheduler_type: linear
- num_epochs: 3
### Training results
| Training Loss | Epoch | Step | Validation Loss | Accuracy |
|:-------------:|:-----:|:----:|:---------------:|:--------:|
| 0.2082 | 1.0 | 718 | 0.1057 | 0.9654 |
| 0.1598 | 2.0 | 1436 | 0.0712 | 0.9775 |
| 0.1435 | 3.0 | 2154 | 0.0549 | 0.9805 |
### Framework versions
- Transformers 4.18.0
- Pytorch 1.10.0+cu111
- Datasets 2.1.0
- Tokenizers 0.12.1 |
eesungkim/stt_kr_conformer_transducer_large | fdc8412fe0d089913524767b20ff244ff1007ed0 | 2022-06-24T22:11:28.000Z | [
"nemo",
"kr",
"dataset:Ksponspeech",
"arxiv:2005.08100",
"automatic-speech-recognition",
"speech",
"audio",
"transducer",
"Conformer",
"Transformer",
"NeMo",
"pytorch",
"license:cc-by-4.0",
"model-index"
] | automatic-speech-recognition | false | eesungkim | null | eesungkim/stt_kr_conformer_transducer_large | 21 | 3 | nemo | 8,221 | ---
language:
- kr
license: cc-by-4.0
library_name: nemo
datasets:
- Ksponspeech
thumbnail: null
tags:
- automatic-speech-recognition
- speech
- audio
- transducer
- Conformer
- Transformer
- NeMo
- pytorch
model-index:
- name: stt_kr_conformer_transducer_large
results: []
---
## Model Overview
<DESCRIBE IN ONE LINE THE MODEL AND ITS USE>
## NVIDIA NeMo: Training
To train, fine-tune or play with the model you will need to install [NVIDIA NeMo](https://github.com/NVIDIA/NeMo). We recommend you install it after you've installed latest Pytorch version.
```
pip install nemo_toolkit['all']
```
## How to Use this Model
The model is available for use in the NeMo toolkit [1], and can be used as a pre-trained checkpoint for inference or for fine-tuning on another dataset.
### Automatically instantiate the model
```python
import nemo.collections.asr as nemo_asr
asr_model = nemo_asr.models.ASRModel.from_pretrained("eesungkim/stt_kr_conformer_transducer_large")
```
### Transcribing using Python
First, let's get a sample
```
wget https://dldata-public.s3.us-east-2.amazonaws.com/sample-kor.wav
```
Then simply do:
```
asr_model.transcribe(['sample-kor.wav'])
```
### Transcribing many audio files
```shell
python [NEMO_GIT_FOLDER]/examples/asr/transcribe_speech.py pretrained_name="eesungkim/stt_kr_conformer_transducer_large" audio_dir="<DIRECTORY CONTAINING AUDIO FILES>"
```
### Input
This model accepts 16000 KHz Mono-channel Audio (wav files) as input.
### Output
This model provides transcribed speech as a string for a given audio sample.
## Model Architecture
Conformer-Transducer model is an autoregressive variant of Conformer model [2] for Automatic Speech Recognition which uses Transducer loss/decoding. You may find more info on the detail of this model here: [Conformer-Transducer Model](https://docs.nvidia.com/deeplearning/nemo/user-guide/docs/en/main/asr/models.html).
## Training
The model was finetuned based on the pre-trained English Model for over several epochs.
There are several transcribing and sub-word modeling methods for Korean speech recognition. This model uses sentencepiece subwords of Hangul characters based on phonetic transcription using Google Sentencepiece Tokenizer [3].
### Datasets
All the models in this collection are trained on [Ksponspeech](https://aihub.or.kr/aidata/105/download) dataset, which is an open-domain dialog corpus recorded by 2,000 native Korean speakers in a controlled and quiet environment. The standard split dataset consists of 965 hours of training set, 4 hours of development set, 3 hours of test-clean, and 4 hours of test-other.
## Performance
Version | Tokenizer | eval_clean CER | eval_other CER | eval_clean WER | eval_other WER
--- | --- | --- | --- |--- |---
v1.7.0rc | SentencePiece Char | 6.94% | 7.38% | 19.49% | 22.73%
## Limitations
Since this model was trained on publically available speech datasets, the performance of this model might degrade for speech which including technical terms, or vernacular that the model has not been trained on. The model might also perform worse for accented speech.
This model produces a spoken-form token sequence. If you want to have a written form, you can consider applying inverse text normalization.
## References
[1] [NVIDIA NeMo Toolkit](https://github.com/NVIDIA/NeMo)
[2] [Conformer: Convolution-augmented Transformer for Speech Recognition](https://arxiv.org/abs/2005.08100)
[3] [Google Sentencepiece Tokenizer](https://github.com/google/sentencepiece)
|
emilylearning/finetuned_cgp_added_none__test_run_False__p_dataset_100 | 28ff6a29c64ffe9a3d28ec32e43a2000cc9111b6 | 2022-05-06T18:11:01.000Z | [
"pytorch",
"bert",
"token-classification",
"transformers",
"autotrain_compatible"
] | token-classification | false | emilylearning | null | emilylearning/finetuned_cgp_added_none__test_run_False__p_dataset_100 | 21 | null | transformers | 8,222 | Entry not found |
eslamxm/mt5-base-finetuned-english | 4502d1d70784c52544ccf187ef5d5df9742b61e5 | 2022-05-11T14:49:00.000Z | [
"pytorch",
"mt5",
"text2text-generation",
"dataset:xlsum",
"transformers",
"summarization",
"english",
"en",
"Abstractive Summarization",
"generated_from_trainer",
"license:apache-2.0",
"model-index",
"autotrain_compatible"
] | summarization | false | eslamxm | null | eslamxm/mt5-base-finetuned-english | 21 | null | transformers | 8,223 | ---
license: apache-2.0
tags:
- summarization
- english
- en
- mt5
- Abstractive Summarization
- generated_from_trainer
datasets:
- xlsum
model-index:
- name: mt5-base-finetuned-english
results: []
---
<!-- This model card has been generated automatically according to the information the Trainer had access to. You
should probably proofread and complete it, then remove this comment. -->
# mt5-base-finetuned-english
This model is a fine-tuned version of [google/mt5-base](https://huggingface.co/google/mt5-base) on the xlsum dataset.
It achieves the following results on the evaluation set:
- Loss: 3.3271
- Rouge-1: 31.7
- Rouge-2: 11.83
- Rouge-l: 26.43
- Gen Len: 18.88
- Bertscore: 74.3
## Model description
More information needed
## Intended uses & limitations
More information needed
## Training and evaluation data
More information needed
## Training procedure
### Training hyperparameters
The following hyperparameters were used during training:
- learning_rate: 0.0005
- train_batch_size: 4
- eval_batch_size: 4
- seed: 42
- gradient_accumulation_steps: 8
- total_train_batch_size: 32
- optimizer: Adam with betas=(0.9,0.999) and epsilon=1e-08
- lr_scheduler_type: linear
- num_epochs: 5
- label_smoothing_factor: 0.1
### Training results
| Training Loss | Epoch | Step | Validation Loss | Rouge-1 | Rouge-2 | Rouge-l | Gen Len | Bertscore |
|:-------------:|:-----:|:-----:|:---------------:|:-------:|:-------:|:-------:|:-------:|:---------:|
| 4.174 | 1.0 | 3125 | 3.5662 | 27.01 | 7.95 | 22.16 | 18.91 | 72.62 |
| 3.6577 | 2.0 | 6250 | 3.4304 | 28.84 | 9.09 | 23.64 | 18.87 | 73.32 |
| 3.4526 | 3.0 | 9375 | 3.3691 | 29.69 | 9.96 | 24.58 | 18.84 | 73.69 |
| 3.3091 | 4.0 | 12500 | 3.3368 | 30.38 | 10.32 | 25.1 | 18.9 | 73.9 |
| 3.2056 | 5.0 | 15625 | 3.3271 | 30.7 | 10.65 | 25.45 | 18.89 | 73.99 |
### Framework versions
- Transformers 4.18.0
- Pytorch 1.11.0+cu113
- Datasets 2.2.0
- Tokenizers 0.12.1
|
emilylearning/cond_ft_none_on_reddit__prcnt_100__test_run_False | f137e4f0ac51088a0c2f3bf2b5bec987af0fa4a5 | 2022-05-13T05:41:50.000Z | [
"pytorch",
"bert",
"token-classification",
"transformers",
"autotrain_compatible"
] | token-classification | false | emilylearning | null | emilylearning/cond_ft_none_on_reddit__prcnt_100__test_run_False | 21 | null | transformers | 8,224 | Entry not found |
emilylearning/cond_ft_subreddit_on_reddit__prcnt_100__test_run_False | 9a8f65a2841a86dd76e4e76daf6c0d3b19d7cfeb | 2022-05-13T22:21:56.000Z | [
"pytorch",
"bert",
"token-classification",
"transformers",
"autotrain_compatible"
] | token-classification | false | emilylearning | null | emilylearning/cond_ft_subreddit_on_reddit__prcnt_100__test_run_False | 21 | null | transformers | 8,225 | Entry not found |
Xiaoman/NER-CoNLL2003-V2 | 402ca1daa320f40d0d9d682df8f90502edf15354 | 2022-05-14T04:56:27.000Z | [
"pytorch",
"tensorboard",
"bert",
"token-classification",
"transformers",
"autotrain_compatible"
] | token-classification | false | Xiaoman | null | Xiaoman/NER-CoNLL2003-V2 | 21 | null | transformers | 8,226 | Training hyperparameters
The following hyperparameters were used during training:
learning_rate: 7.961395091713594e-05
train_batch_size: 32
eval_batch_size: 32
seed: 27
optimizer: Adam with betas=(0.9,0.999) and epsilon=1e-08
lr_scheduler_type: linear
num_epochs: 5
|
Xiaoman/NER-CoNLL2003-V4 | 8a71c88261ec30872568e26b3f6638f92fe6063c | 2022-05-14T19:37:35.000Z | [
"pytorch",
"tensorboard",
"bert",
"token-classification",
"transformers",
"generated_from_trainer",
"license:apache-2.0",
"model-index",
"autotrain_compatible"
] | token-classification | false | Xiaoman | null | Xiaoman/NER-CoNLL2003-V4 | 21 | null | transformers | 8,227 | ---
license: apache-2.0
tags:
- generated_from_trainer
model-index:
- name: NER-CoNLL2003-V4
results: []
---
<!-- This model card has been generated automatically according to the information the Trainer had access to. You
should probably proofread and complete it, then remove this comment. -->
# NER-CoNLL2003-V4
This model is a fine-tuned version of [bert-large-uncased](https://huggingface.co/bert-large-uncased) on an unknown dataset.
It achieves the following results on the evaluation set:
- Loss: 0.2095
## Model description
More information needed
## Intended uses & limitations
More information needed
## Training and evaluation data
More information needed
## Training procedure
### Training hyperparameters
The following hyperparameters were used during training:
- learning_rate: 7.961395091713594e-05
- train_batch_size: 6
- eval_batch_size: 6
- seed: 27
- optimizer: Adam with betas=(0.9,0.999) and epsilon=1e-08
- lr_scheduler_type: linear
- num_epochs: 5
### Training results
| Training Loss | Epoch | Step | Validation Loss |
|:-------------:|:-----:|:----:|:---------------:|
| No log | 1.0 | 14 | 0.3630 |
| No log | 2.0 | 28 | 0.2711 |
| No log | 3.0 | 42 | 0.2407 |
| No log | 4.0 | 56 | 0.2057 |
| No log | 5.0 | 70 | 0.2095 |
### Framework versions
- Transformers 4.19.1
- Pytorch 1.11.0+cu113
- Datasets 2.2.1
- Tokenizers 0.12.1
|
anuj55/distilbert-base-uncased-finetuned-polifact | 2fd37511df6c678fb41072be9c14518cd4205147 | 2022-05-15T16:21:04.000Z | [
"pytorch",
"tensorboard",
"distilbert",
"text-classification",
"transformers"
] | text-classification | false | anuj55 | null | anuj55/distilbert-base-uncased-finetuned-polifact | 21 | null | transformers | 8,228 | Entry not found |
imohammad12/GRS-complex-simple-classifier-DeBerta | 69008160598bc7be5d4bdfef161f5a2b8eace5d9 | 2022-05-26T10:49:13.000Z | [
"pytorch",
"deberta",
"text-classification",
"en",
"transformers",
"grs"
] | text-classification | false | imohammad12 | null | imohammad12/GRS-complex-simple-classifier-DeBerta | 21 | null | transformers | 8,229 | ---
language: en
tags: grs
---
## Citation
Please star the [GRS GitHub repo](https://github.com/imohammad12/GRS) and cite the paper if you found our model useful:
```
@inproceedings{dehghan-etal-2022-grs,
title = "{GRS}: Combining Generation and Revision in Unsupervised Sentence Simplification",
author = "Dehghan, Mohammad and
Kumar, Dhruv and
Golab, Lukasz",
booktitle = "Findings of the Association for Computational Linguistics: ACL 2022",
month = may,
year = "2022",
address = "Dublin, Ireland",
publisher = "Association for Computational Linguistics",
url = "https://aclanthology.org/2022.findings-acl.77",
pages = "949--960",
abstract = "We propose GRS: an unsupervised approach to sentence simplification that combines text generation and text revision. We start with an iterative framework in which an input sentence is revised using explicit edit operations, and add paraphrasing as a new edit operation. This allows us to combine the advantages of generative and revision-based approaches: paraphrasing captures complex edit operations, and the use of explicit edit operations in an iterative manner provides controllability and interpretability. We demonstrate these advantages of GRS compared to existing methods on the Newsela and ASSET datasets.",
}
``` |
questgen/msmarco-distilbert-base-v4-feature-extraction-pipeline | 6be608d278b1f7c771b17a5fe123e658049bdd3a | 2022-05-21T11:15:42.000Z | [
"pytorch",
"distilbert",
"feature-extraction",
"arxiv:1908.10084",
"sentence-transformers",
"sentence-similarity",
"transformers",
"license:apache-2.0"
] | feature-extraction | false | questgen | null | questgen/msmarco-distilbert-base-v4-feature-extraction-pipeline | 21 | null | sentence-transformers | 8,230 | ---
pipeline_tag: feature-extraction
license: apache-2.0
tags:
- sentence-transformers
- feature-extraction
- sentence-similarity
- transformers
---
# sentence-transformers/msmarco-distilbert-base-v4
This is a [sentence-transformers](https://www.SBERT.net) model: It maps sentences & paragraphs to a 768 dimensional dense vector space and can be used for tasks like clustering or semantic search.
## Usage (Sentence-Transformers)
Using this model becomes easy when you have [sentence-transformers](https://www.SBERT.net) installed:
```
pip install -U sentence-transformers
```
Then you can use the model like this:
```python
from sentence_transformers import SentenceTransformer
sentences = ["This is an example sentence", "Each sentence is converted"]
model = SentenceTransformer('sentence-transformers/msmarco-distilbert-base-v4')
embeddings = model.encode(sentences)
print(embeddings)
```
## Usage (HuggingFace Transformers)
Without [sentence-transformers](https://www.SBERT.net), you can use the model like this: First, you pass your input through the transformer model, then you have to apply the right pooling-operation on-top of the contextualized word embeddings.
```python
from transformers import AutoTokenizer, AutoModel
import torch
#Mean Pooling - Take attention mask into account for correct averaging
def mean_pooling(model_output, attention_mask):
token_embeddings = model_output[0] #First element of model_output contains all token embeddings
input_mask_expanded = attention_mask.unsqueeze(-1).expand(token_embeddings.size()).float()
return torch.sum(token_embeddings * input_mask_expanded, 1) / torch.clamp(input_mask_expanded.sum(1), min=1e-9)
# Sentences we want sentence embeddings for
sentences = ['This is an example sentence', 'Each sentence is converted']
# Load model from HuggingFace Hub
tokenizer = AutoTokenizer.from_pretrained('sentence-transformers/msmarco-distilbert-base-v4')
model = AutoModel.from_pretrained('sentence-transformers/msmarco-distilbert-base-v4')
# Tokenize sentences
encoded_input = tokenizer(sentences, padding=True, truncation=True, return_tensors='pt')
# Compute token embeddings
with torch.no_grad():
model_output = model(**encoded_input)
# Perform pooling. In this case, max pooling.
sentence_embeddings = mean_pooling(model_output, encoded_input['attention_mask'])
print("Sentence embeddings:")
print(sentence_embeddings)
```
## Evaluation Results
For an automated evaluation of this model, see the *Sentence Embeddings Benchmark*: [https://seb.sbert.net](https://seb.sbert.net?model_name=sentence-transformers/msmarco-distilbert-base-v4)
## Full Model Architecture
```
SentenceTransformer(
(0): Transformer({'max_seq_length': 512, 'do_lower_case': False}) with Transformer model: DistilBertModel
(1): Pooling({'word_embedding_dimension': 768, 'pooling_mode_cls_token': False, 'pooling_mode_mean_tokens': True, 'pooling_mode_max_tokens': False, 'pooling_mode_mean_sqrt_len_tokens': False})
)
```
## Citing & Authors
This model was trained by [sentence-transformers](https://www.sbert.net/).
If you find this model helpful, feel free to cite our publication [Sentence-BERT: Sentence Embeddings using Siamese BERT-Networks](https://arxiv.org/abs/1908.10084):
```bibtex
@inproceedings{reimers-2019-sentence-bert,
title = "Sentence-BERT: Sentence Embeddings using Siamese BERT-Networks",
author = "Reimers, Nils and Gurevych, Iryna",
booktitle = "Proceedings of the 2019 Conference on Empirical Methods in Natural Language Processing",
month = "11",
year = "2019",
publisher = "Association for Computational Linguistics",
url = "http://arxiv.org/abs/1908.10084",
}
``` |
Shenghao1993/distilbert-base-uncased-finetuned-emotion | 6041076ca6be3de4cb0302e1b296d743e37006ac | 2022-05-24T02:25:08.000Z | [
"pytorch",
"tensorboard",
"distilbert",
"text-classification",
"dataset:emotion",
"transformers",
"generated_from_trainer",
"license:apache-2.0",
"model-index"
] | text-classification | false | Shenghao1993 | null | Shenghao1993/distilbert-base-uncased-finetuned-emotion | 21 | null | transformers | 8,231 | ---
license: apache-2.0
tags:
- generated_from_trainer
datasets:
- emotion
metrics:
- accuracy
- f1
model-index:
- name: distilbert-base-uncased-finetuned-emotion
results:
- task:
name: Text Classification
type: text-classification
dataset:
name: emotion
type: emotion
args: default
metrics:
- name: Accuracy
type: accuracy
value: 0.929
- name: F1
type: f1
value: 0.9288515820399124
---
<!-- This model card has been generated automatically according to the information the Trainer had access to. You
should probably proofread and complete it, then remove this comment. -->
# distilbert-base-uncased-finetuned-emotion
This model is a fine-tuned version of [distilbert-base-uncased](https://huggingface.co/distilbert-base-uncased) on the emotion dataset.
It achieves the following results on the evaluation set:
- Loss: 0.2196
- Accuracy: 0.929
- F1: 0.9289
## Model description
More information needed
## Intended uses & limitations
More information needed
## Training and evaluation data
More information needed
## Training procedure
### Training hyperparameters
The following hyperparameters were used during training:
- learning_rate: 2e-05
- train_batch_size: 64
- eval_batch_size: 64
- seed: 42
- optimizer: Adam with betas=(0.9,0.999) and epsilon=1e-08
- lr_scheduler_type: linear
- num_epochs: 2
### Training results
| Training Loss | Epoch | Step | Validation Loss | Accuracy | F1 |
|:-------------:|:-----:|:----:|:---------------:|:--------:|:------:|
| 0.8486 | 1.0 | 250 | 0.3306 | 0.903 | 0.8989 |
| 0.2573 | 2.0 | 500 | 0.2196 | 0.929 | 0.9289 |
### Framework versions
- Transformers 4.19.2
- Pytorch 1.11.0+cu113
- Datasets 2.2.2
- Tokenizers 0.12.1
|
KoichiYasuoka/deberta-large-japanese-luw-upos | 54e41fa94206d32f9002a860bbdca1c4c52e16af | 2022-07-23T14:44:01.000Z | [
"pytorch",
"deberta-v2",
"token-classification",
"ja",
"dataset:universal_dependencies",
"transformers",
"japanese",
"pos",
"dependency-parsing",
"license:cc-by-sa-4.0",
"autotrain_compatible"
] | token-classification | false | KoichiYasuoka | null | KoichiYasuoka/deberta-large-japanese-luw-upos | 21 | null | transformers | 8,232 | ---
language:
- "ja"
tags:
- "japanese"
- "token-classification"
- "pos"
- "dependency-parsing"
datasets:
- "universal_dependencies"
license: "cc-by-sa-4.0"
pipeline_tag: "token-classification"
widget:
- text: "国境の長いトンネルを抜けると雪国であった。"
---
# deberta-large-japanese-luw-upos
## Model Description
This is a DeBERTa(V2) model pre-trained on 青空文庫 texts for POS-tagging and dependency-parsing, derived from [deberta-large-japanese-aozora](https://huggingface.co/KoichiYasuoka/deberta-large-japanese-aozora). Every long-unit-word is tagged by [UPOS](https://universaldependencies.org/u/pos/) (Universal Part-Of-Speech) and [FEATS](https://universaldependencies.org/u/feat/).
## How to Use
```py
import torch
from transformers import AutoTokenizer,AutoModelForTokenClassification
tokenizer=AutoTokenizer.from_pretrained("KoichiYasuoka/deberta-large-japanese-luw-upos")
model=AutoModelForTokenClassification.from_pretrained("KoichiYasuoka/deberta-large-japanese-luw-upos")
s="国境の長いトンネルを抜けると雪国であった。"
t=tokenizer.tokenize(s)
p=[model.config.id2label[q] for q in torch.argmax(model(tokenizer.encode(s,return_tensors="pt"))["logits"],dim=2)[0].tolist()[1:-1]]
print(list(zip(t,p)))
```
or
```py
import esupar
nlp=esupar.load("KoichiYasuoka/deberta-large-japanese-luw-upos")
print(nlp("国境の長いトンネルを抜けると雪国であった。"))
```
## Reference
安岡孝一: [青空文庫DeBERTaモデルによる国語研長単位係り受け解析](http://hdl.handle.net/2433/275409), 東洋学へのコンピュータ利用, 第35回研究セミナー (2022年7月), pp.29-43.
## See Also
[esupar](https://github.com/KoichiYasuoka/esupar): Tokenizer POS-tagger and Dependency-parser with BERT/RoBERTa/DeBERTa models
|
Yah216/Arabic_poem_meter_3 | fdee92fa4ee718710c24ca36e3cb27a2f5547450 | 2022-05-28T07:59:10.000Z | [
"pytorch",
"bert",
"text-classification",
"ar",
"transformers",
"co2_eq_emissions"
] | text-classification | false | Yah216 | null | Yah216/Arabic_poem_meter_3 | 21 | null | transformers | 8,233 | ---
---
language: ar
widget:
- text: "قفا نبك من ذِكرى حبيب ومنزلِ بسِقطِ اللِّوى بينَ الدَّخول فحَوْملِ"
- text: "سَلو قَلبي غَداةَ سَلا وَثابا لَعَلَّ عَلى الجَمالِ لَهُ عِتابا"
co2_eq_emissions: 404.66986451902227
---
# Model Trained Using AutoTrain
- Problem type: Multi-class Classification
- CO2 Emissions (in grams): 404.66986451902227
## Dataset
We used the APCD dataset cited hereafter for pretraining the model. The dataset has been cleaned and only the main text and the meter columns were kept:
```
@Article{Yousef2019LearningMetersArabicEnglish-arxiv,
author = {Yousef, Waleed A. and Ibrahime, Omar M. and Madbouly, Taha M. and Mahmoud,
Moustafa A.},
title = {Learning Meters of Arabic and English Poems With Recurrent Neural Networks: a Step
Forward for Language Understanding and Synthesis},
journal = {arXiv preprint arXiv:1905.05700},
year = 2019,
url = {https://github.com/hci-lab/LearningMetersPoems}
}
```
## Validation Metrics
- Loss: 0.21315555274486542
- Accuracy: 0.9493554089595999
- Macro F1: 0.7537353091512587
## Usage
You can use cURL to access this model:
```
$ curl -X POST -H "Authorization: Bearer YOUR_API_KEY" -H "Content-Type: application/json" -d '{"inputs": "قفا نبك من ذِكرى حبيب ومنزلِ بسِقطِ اللِّوى بينَ الدَّخول فحَوْملِ"}' https://api-inference.huggingface.co/models/Yah216/Arabic_poem_meter_3
```
Or Python API:
```
from transformers import AutoModelForSequenceClassification, AutoTokenizer
model = AutoModelForSequenceClassification.from_pretrained("Yah216/Arabic_poem_meter_3", use_auth_token=True)
tokenizer = AutoTokenizer.from_pretrained("Yah216/Arabic_poem_meter_3", use_auth_token=True)
inputs = tokenizer("قفا نبك من ذِكرى حبيب ومنزلِ بسِقطِ اللِّوى بينَ الدَّخول فحَوْملِ", return_tensors="pt")
outputs = model(**inputs)
``` |
sahn/distilbert-base-uncased-finetuned-imdb-tag | d103fb07e0324e661759c3cc74287cc3faed3353 | 2022-05-30T04:49:48.000Z | [
"pytorch",
"tensorboard",
"distilbert",
"text-classification",
"dataset:imdb",
"transformers",
"generated_from_trainer",
"license:apache-2.0",
"model-index"
] | text-classification | false | sahn | null | sahn/distilbert-base-uncased-finetuned-imdb-tag | 21 | null | transformers | 8,234 | ---
license: apache-2.0
tags:
- generated_from_trainer
datasets:
- imdb
metrics:
- accuracy
model-index:
- name: distilbert-base-uncased-finetuned-imdb-tag
results:
- task:
name: Text Classification
type: text-classification
dataset:
name: imdb
type: imdb
args: plain_text
metrics:
- name: Accuracy
type: accuracy
value: 0.9672
---
<!-- This model card has been generated automatically according to the information the Trainer had access to. You
should probably proofread and complete it, then remove this comment. -->
# distilbert-base-uncased-finetuned-imdb-tag
This model is a fine-tuned version of [distilbert-base-uncased](https://huggingface.co/distilbert-base-uncased) on the imdb dataset.
It achieves the following results on the evaluation set:
- Loss: 0.2215
- Accuracy: 0.9672
## Model description
More information needed
## Intended uses & limitations
More information needed
## Training and evaluation data
For 90% of the sentences, added `10/10` at the end of the sentences with the label 1, and `1/10` with the label 0.
## Training procedure
### Training hyperparameters
The following hyperparameters were used during training:
- learning_rate: 2e-05
- train_batch_size: 16
- eval_batch_size: 16
- seed: 42
- optimizer: Adam with betas=(0.9,0.999) and epsilon=1e-08
- lr_scheduler_type: linear
- num_epochs: 5
### Training results
| Training Loss | Epoch | Step | Validation Loss | Accuracy |
|:-------------:|:-----:|:----:|:---------------:|:--------:|
| 0.0895 | 1.0 | 1250 | 0.1332 | 0.9638 |
| 0.0483 | 2.0 | 2500 | 0.0745 | 0.9772 |
| 0.0246 | 3.0 | 3750 | 0.1800 | 0.9666 |
| 0.0058 | 4.0 | 5000 | 0.1370 | 0.9774 |
| 0.0025 | 5.0 | 6250 | 0.2215 | 0.9672 |
### Framework versions
- Transformers 4.19.2
- Pytorch 1.11.0+cu113
- Datasets 2.2.2
- Tokenizers 0.12.1
|
theojolliffe/bart-cnn-science | 2b5c0e689642ef19663935c01d19a6881777c0d2 | 2022-05-30T17:31:48.000Z | [
"pytorch",
"tensorboard",
"bart",
"text2text-generation",
"dataset:scientific_papers",
"transformers",
"generated_from_trainer",
"license:mit",
"model-index",
"autotrain_compatible"
] | text2text-generation | false | theojolliffe | null | theojolliffe/bart-cnn-science | 21 | null | transformers | 8,235 | ---
license: mit
tags:
- generated_from_trainer
datasets:
- scientific_papers
metrics:
- rouge
model-index:
- name: bart-large-cnn-pubmed1o3-pubmed2o3-pubmed3o3-arxiv1o3-arxiv2o3-arxiv3o3
results:
- task:
name: Sequence-to-sequence Language Modeling
type: text2text-generation
dataset:
name: scientific_papers
type: scientific_papers
args: arxiv
metrics:
- name: Rouge1
type: rouge
value: 42.5835
---
<!-- This model card has been generated automatically according to the information the Trainer had access to. You
should probably proofread and complete it, then remove this comment. -->
# bart-large-cnn-pubmed1o3-pubmed2o3-pubmed3o3-arxiv1o3-arxiv2o3-arxiv3o3
This model is a fine-tuned version of [theojolliffe/bart-large-cnn-pubmed1o3-pubmed2o3-pubmed3o3-arxiv1o3-arxiv2o3](https://huggingface.co/theojolliffe/bart-large-cnn-pubmed1o3-pubmed2o3-pubmed3o3-arxiv1o3-arxiv2o3) on the scientific_papers dataset.
It achieves the following results on the evaluation set:
- Loss: 2.0646
- Rouge1: 42.5835
- Rouge2: 16.1887
- Rougel: 24.7972
- Rougelsum: 38.1846
- Gen Len: 129.9291
## Model description
More information needed
## Intended uses & limitations
More information needed
## Training and evaluation data
More information needed
## Training procedure
### Training hyperparameters
The following hyperparameters were used during training:
- learning_rate: 2e-05
- train_batch_size: 2
- eval_batch_size: 2
- seed: 42
- optimizer: Adam with betas=(0.9,0.999) and epsilon=1e-08
- lr_scheduler_type: linear
- num_epochs: 1
- mixed_precision_training: Native AMP
### Training results
| Training Loss | Epoch | Step | Validation Loss | Rouge1 | Rouge2 | Rougel | Rougelsum | Gen Len |
|:-------------:|:-----:|:-----:|:---------------:|:-------:|:-------:|:-------:|:---------:|:--------:|
| 2.0865 | 1.0 | 33840 | 2.0646 | 42.5835 | 16.1887 | 24.7972 | 38.1846 | 129.9291 |
### Framework versions
- Transformers 4.19.2
- Pytorch 1.11.0+cu113
- Datasets 2.2.2
- Tokenizers 0.12.1
|
classla/bcms-bertic-parlasent-bcs-ter | 4bfc89d99f4e90d960060ac47f1223caf153b4b4 | 2022-06-20T12:27:45.000Z | [
"pytorch",
"electra",
"text-classification",
"hr",
"arxiv:2206.00929",
"transformers",
"sentiment-analysis"
] | text-classification | false | classla | null | classla/bcms-bertic-parlasent-bcs-ter | 21 | null | transformers | 8,236 | ---
language: "hr"
tags:
- text-classification
- sentiment-analysis
widget:
- text: "Poštovani potpredsjedničke Vlade i ministre hrvatskih branitelja, mislite li da ste zapravo iznevjerili svoje suborce s kojima ste 555 dana prosvjedovali u šatoru protiv tadašnjih dužnosnika jer ste zapravo donijeli zakon koji je neprovediv, a birali ste si suradnike koji nemaju etički integritet."
---
# bcms-bertic-parlasent-bcs-ter
Ternary text classification model based on [`classla/bcms-bertic`](https://huggingface.co/classla/bcms-bertic) and fine-tuned on the BCS Political Sentiment dataset (sentence-level data).
This classifier classifies text into only three categories: Negative, Neutral, and Positive. For the binary classifier (Negative, Other) check [this model](https://huggingface.co/classla/bcms-bertic-parlasent-bcs-bi ).
For details on the dataset and the finetuning procedure, please see [this paper](https://arxiv.org/abs/2206.00929).
## Fine-tuning hyperparameters
Fine-tuning was performed with `simpletransformers`. Beforehand a brief sweep for the optimal number of epochs was performed and the presumed best value was 9. Other arguments were kept default.
```python
model_args = {
"num_train_epochs": 9
}
```
## Performance
The same pipeline was run with two other transformer models and `fasttext` for comparison. Macro F1 scores were recorded for each of the 6 fine-tuning sessions and post festum analyzed.
| model | average macro F1 |
|---------------------------------|--------------------|
| bcms-bertic-parlasent-bcs-ter | 0.7941 ± 0.0101 ** |
| EMBEDDIA/crosloengual-bert | 0.7709 ± 0.0113 |
| xlm-roberta-base | 0.7184 ± 0.0139 |
| fasttext + CLARIN.si embeddings | 0.6312 ± 0.0043 |
Two best performing models have been compared with the Mann-Whitney U test to calculate p-values (** denotes p<0.01).
## Use example with `simpletransformers==0.63.7`
```python
from simpletransformers.classification import ClassificationModel
model = ClassificationModel("electra", "classla/bcms-bertic-parlasent-bcs-ter")
predictions, logits = model.predict([
"Vi niste normalni",
"Đački autobusi moraju da voze svaki dan",
"Ovo je najbolji zakon na svetu",
]
)
predictions
# Output: array([0, 1, 2])
[model.config.id2label[i] for i in predictions]
# Output: ['Negative', 'Neutral', 'Positive']
```
## Citation
If you use the model, please cite the following paper on which the original model is based:
```
@inproceedings{ljubesic-lauc-2021-bertic,
title = "{BERT}i{\'c} - The Transformer Language Model for {B}osnian, {C}roatian, {M}ontenegrin and {S}erbian",
author = "Ljube{\v{s}}i{\'c}, Nikola and Lauc, Davor",
booktitle = "Proceedings of the 8th Workshop on Balto-Slavic Natural Language Processing",
month = apr,
year = "2021",
address = "Kiyv, Ukraine",
publisher = "Association for Computational Linguistics",
url = "https://www.aclweb.org/anthology/2021.bsnlp-1.5",
pages = "37--42",
}
```
and the paper describing the dataset and methods for the current finetuning:
```
@misc{https://doi.org/10.48550/arxiv.2206.00929,
doi = {10.48550/ARXIV.2206.00929},
url = {https://arxiv.org/abs/2206.00929},
author = {Mochtak, Michal and Rupnik, Peter and Ljubešič, Nikola},
keywords = {Computation and Language (cs.CL), FOS: Computer and information sciences, FOS: Computer and information sciences},
title = {The ParlaSent-BCS dataset of sentiment-annotated parliamentary debates from Bosnia-Herzegovina, Croatia, and Serbia},
publisher = {arXiv},
year = {2022},
copyright = {Creative Commons Attribution Share Alike 4.0 International}
}
``` |
classla/bcms-bertic-parlasent-bcs-bi | 526844df71b5b4c2a73e2ee52996438387c7ec95 | 2022-06-17T13:51:54.000Z | [
"pytorch",
"electra",
"text-classification",
"hr",
"arxiv:2206.00929",
"transformers",
"sentiment-analysis"
] | text-classification | false | classla | null | classla/bcms-bertic-parlasent-bcs-bi | 21 | null | transformers | 8,237 | ---
language: "hr"
tags:
- text-classification
- sentiment-analysis
widget:
- text: "Poštovani potpredsjedničke Vlade i ministre hrvatskih branitelja, mislite li da ste zapravo iznevjerili svoje suborce s kojima ste 555 dana prosvjedovali u šatoru protiv tadašnjih dužnosnika jer ste zapravo donijeli zakon koji je neprovediv, a birali ste si suradnike koji nemaju etički integritet."
---
# bcms-bertic-parlasent-bcs-bi
Binary text classification model based on [`classla/bcms-bertic`](https://huggingface.co/classla/bcms-bertic) and fine-tuned on the BCS Political Sentiment dataset (sentence-level data).
This classifier classifies text into only two categories: Negative vs. Other. For the ternary classifier (Negative, Neutral, Positive) check [this model](https://huggingface.co/classla/bcms-bertic-parlasent-bcs-ter).
For details on the dataset and the finetuning procedure, please see [this paper](https://arxiv.org/abs/2206.00929).
## Fine-tuning hyperparameters
Fine-tuning was performed with `simpletransformers`. Beforehand a brief sweep for the optimal number of epochs was performed and the presumed best value was 9. Other arguments were kept default.
```python
model_args = {
"num_train_epochs": 9
}
```
## Performance in comparison with ternary classifier
| model | average macro F1 |
|-------------------------------------------|------------------|
| bcms-bertic-parlasent-bcs-ter | 0.7941 ± 0.0101 |
| bcms-bertic-parlasent-bcs-bi (this model) | 0.8999 ± 0.012 |
## Use example with `simpletransformers==0.63.7`
```python
from simpletransformers.classification import ClassificationModel
model = ClassificationModel("electra", "classla/bcms-bertic-parlasent-bcs-bi")
predictions, logits = model.predict([
"Đački autobusi moraju da voze svaki dan",
"Vi niste normalni"
]
)
predictions
# Output: array([1, 0])
[model.config.id2label[i] for i in predictions]
# Output: ['Other', 'Negative']
```
## Citation
If you use the model, please cite the following paper on which the original model is based:
```
@inproceedings{ljubesic-lauc-2021-bertic,
title = "{BERT}i{\'c} - The Transformer Language Model for {B}osnian, {C}roatian, {M}ontenegrin and {S}erbian",
author = "Ljube{\v{s}}i{\'c}, Nikola and Lauc, Davor",
booktitle = "Proceedings of the 8th Workshop on Balto-Slavic Natural Language Processing",
month = apr,
year = "2021",
address = "Kiyv, Ukraine",
publisher = "Association for Computational Linguistics",
url = "https://www.aclweb.org/anthology/2021.bsnlp-1.5",
pages = "37--42",
}
```
and the paper describing the dataset and methods for the current finetuning:
```
@misc{https://doi.org/10.48550/arxiv.2206.00929,
doi = {10.48550/ARXIV.2206.00929},
url = {https://arxiv.org/abs/2206.00929},
author = {Mochtak, Michal and Rupnik, Peter and Ljubešič, Nikola},
keywords = {Computation and Language (cs.CL), FOS: Computer and information sciences, FOS: Computer and information sciences},
title = {The ParlaSent-BCS dataset of sentiment-annotated parliamentary debates from Bosnia-Herzegovina, Croatia, and Serbia},
publisher = {arXiv},
year = {2022},
copyright = {Creative Commons Attribution Share Alike 4.0 International}
}
```
|
luigisaetta/squad_it_xxl_cased_hub1 | f2f710f1a879e9e70b49b7925a713cf19d6d583b | 2022-06-08T06:39:02.000Z | [
"pytorch",
"bert",
"question-answering",
"it",
"dataset:squad_it",
"transformers",
"Q&A",
"model-index",
"autotrain_compatible"
] | question-answering | false | luigisaetta | null | luigisaetta/squad_it_xxl_cased_hub1 | 21 | null | transformers | 8,238 | ---
language:
- it
metrics:
- type squad
datasets:
- squad_it
tags:
- Q&A
widget:
- text: "Come si chiama il primo re di Roma?"
context: "Roma è una delle più belle ed antiche città del mondo. Il più famoso monumento di Roma è il Colosseo. Un altro monumento molto bello è la Colonna Traiana. Il primo re di Roma è stato Romolo. Roma ha avuto tanti re: Numa Pompilio, Tullio Ostilio."
- text: "Qual è il più famoso monumento di Roma?"
context: "Roma è una delle più belle ed antiche città del mondo. Il più famoso monumento di Roma è il Colosseo. Un altro monumento molto bello è la Colonna Traiana. Il primo re di Roma è stato Romolo. Roma ha avuto tanti re: Numa Pompilio, Tullio Ostilio."
model-index:
- name: squad_it_xxl_cased_hub1
results: []
---
# squad_it_xxl_cased
This is a model, based on **BERT** trained on cased Italian, that can be used for [Extractive Q&A](https://huggingface.co/tasks/question-answering) on Italian texts.
## Model description
This model has been trained on **squad_it** dataset starting from the pre-trained model [dbmdz/bert-base-italian-xxl-cased](https://huggingface.co/dbmdz/bert-base-italian-xxl-cased).
These are the metrics computed on evaluation set:
- EM: 63.95
- F1: 75.27
#### How to use
```python
from transformers import pipeline
pipe_qa = pipeline('question-answering', model='luigisaetta/squad_it_xxl_cased_hub1')
pipe_qa(context="Io sono nato a Napoli. Il mare bagna Napoli. Napoli è la più bella città del mondo",
question="Qual è la più bella città del mondo?")
```
## Intended uses & limitations
This model can be used for Extractive Q&A on Italian Text
## Training and evaluation data
[squad_it](https://huggingface.co/datasets/squad_it)
## Training procedure
see code in this [NoteBook](https://github.com/luigisaetta/nlp-qa-italian/blob/main/train_squad_it_final1.ipynb)
### Training hyperparameters
The following hyperparameters were used during training:
- learning_rate: 2e-05
- train_batch_size: 8
- eval_batch_size: 8
- seed: 1234
- optimizer: Adam with betas=(0.9,0.999) and epsilon=1e-08
- lr_scheduler_type: linear
- num_epochs: 2
- mixed_precision_training: Native AMP
### Framework versions
- Transformers 4.20.0.dev0
- Pytorch 1.9.0
- Datasets 1.11.0
- Tokenizers 0.12.1
|
robinhad/ukrainian-qa | 86bea78cf587ce58d656d3ea3ede1d787cc3c6c1 | 2022-06-01T22:08:47.000Z | [
"pytorch",
"tensorboard",
"xlm-roberta",
"question-answering",
"uk",
"transformers",
"generated_from_trainer",
"license:mit",
"model-index",
"autotrain_compatible"
] | question-answering | false | robinhad | null | robinhad/ukrainian-qa | 21 | 2 | transformers | 8,239 | ---
license: mit
language: uk
tags:
- generated_from_trainer
model-index:
- name: ukrainian-qa
results: []
widget:
- text: "Що відправлять для ЗСУ?"
context: "Про це повідомив міністр оборони Арвідас Анушаускас. Уряд Литви не має наміру зупинятися у військово-технічній допомозі Україні. Збройні сили отримають антидрони, тепловізори та ударний безпілотник. «Незабаром Литва передасть Україні не лише обіцяні бронетехніку, вантажівки та позашляховики, але також нову партію антидронів та тепловізорів. І, звичайно, Байрактар, який придбають на зібрані литовцями гроші», - написав глава Міноборони."
---
<!-- This model card has been generated automatically according to the information the Trainer had access to. You
should probably proofread and complete it, then remove this comment. -->
# ukrainian-qa
This model is a fine-tuned version of [ukr-models/xlm-roberta-base-uk](https://huggingface.co/ukr-models/xlm-roberta-base-uk) on the [UA-SQuAD](https://github.com/fido-ai/ua-datasets/tree/main/ua_datasets/src/question_answering) dataset.
Link to training scripts - [https://github.com/robinhad/ukrainian-qa](https://github.com/robinhad/ukrainian-qa)
It achieves the following results on the evaluation set:
- Loss: 1.4778
## Model description
More information needed
## How to use
```python
from transformers import pipeline, AutoTokenizer, AutoModelForQuestionAnswering
model_name = "robinhad/ukrainian-qa"
tokenizer = AutoTokenizer.from_pretrained(model_name)
model = AutoModelForQuestionAnswering.from_pretrained(model_name)
qa_model = pipeline("question-answering", model=model.to("cpu"), tokenizer=tokenizer)
question = "Де ти живеш?"
context = "Мене звати Сара і я живу у Лондоні"
qa_model(question = question, context = context)
```
## Intended uses & limitations
More information needed
## Training and evaluation data
More information needed
## Training procedure
### Training hyperparameters
The following hyperparameters were used during training:
- learning_rate: 2e-05
- train_batch_size: 16
- eval_batch_size: 16
- seed: 42
- optimizer: Adam with betas=(0.9,0.999) and epsilon=1e-08
- lr_scheduler_type: linear
- num_epochs: 6
### Training results
| Training Loss | Epoch | Step | Validation Loss |
|:-------------:|:-----:|:----:|:---------------:|
| 2.4526 | 1.0 | 650 | 1.3631 |
| 1.3317 | 2.0 | 1300 | 1.2229 |
| 1.0693 | 3.0 | 1950 | 1.2184 |
| 0.6851 | 4.0 | 2600 | 1.3171 |
| 0.5594 | 5.0 | 3250 | 1.3893 |
| 0.4954 | 6.0 | 3900 | 1.4778 |
### Framework versions
- Transformers 4.19.2
- Pytorch 1.11.0
- Datasets 2.2.2
- Tokenizers 0.12.1
|
avacaondata/roberta-large-biomedical | 8bfd234324037d30c29d3246b826dbf3fafca872 | 2022-06-04T10:44:41.000Z | [
"pytorch",
"roberta",
"fill-mask",
"transformers",
"autotrain_compatible"
] | fill-mask | false | avacaondata | null | avacaondata/roberta-large-biomedical | 21 | null | transformers | 8,240 | Entry not found |
facebook/genre-linking-blink | 3c33ba95f2427fb67fd45971cca66b8a676614b4 | 2022-06-14T14:07:29.000Z | [
"pytorch",
"tf",
"jax",
"bart",
"text2text-generation",
"en",
"arxiv:2010.00904",
"arxiv:1910.13461",
"arxiv:1911.03814",
"transformers",
"retrieval",
"entity-retrieval",
"named-entity-disambiguation",
"entity-disambiguation",
"named-entity-linking",
"entity-linking",
"autotrain_compatible"
] | text2text-generation | false | facebook | null | facebook/genre-linking-blink | 21 | 1 | transformers | 8,241 | ---
language:
- en
tags:
- retrieval
- entity-retrieval
- named-entity-disambiguation
- entity-disambiguation
- named-entity-linking
- entity-linking
- text2text-generation
---
# GENRE
The GENRE (Generative ENtity REtrieval) system as presented in [Autoregressive Entity Retrieval](https://arxiv.org/abs/2010.00904) implemented in pytorch.
In a nutshell, GENRE uses a sequence-to-sequence approach to entity retrieval (e.g., linking), based on fine-tuned [BART](https://arxiv.org/abs/1910.13461) architecture. GENRE performs retrieval generating the unique entity name conditioned on the input text using constrained beam search to only generate valid identifiers. The model was first released in the [facebookresearch/GENRE](https://github.com/facebookresearch/GENRE) repository using `fairseq` (the `transformers` models are obtained with a conversion script similar to [this](https://github.com/huggingface/transformers/blob/master/src/transformers/models/bart/convert_bart_original_pytorch_checkpoint_to_pytorch.py).
This model was trained on the full training set of [BLINK](https://arxiv.org/abs/1911.03814) (i.e., 9M datapoints for entity-disambiguation grounded on Wikipedia).
## BibTeX entry and citation info
**Please consider citing our works if you use code from this repository.**
```bibtex
@inproceedings{decao2020autoregressive,
title={Autoregressive Entity Retrieval},
author={Nicola {De Cao} and Gautier Izacard and Sebastian Riedel and Fabio Petroni},
booktitle={International Conference on Learning Representations},
url={https://openreview.net/forum?id=5k8F6UU39V},
year={2021}
}
```
## Usage
Here is an example of generation for Wikipedia page disambiguation:
```python
from transformers import AutoTokenizer, AutoModelForSeq2SeqLM
# OPTIONAL: load the prefix tree (trie), you need to additionally download
# https://huggingface.co/facebook/genre-linking-blink/blob/main/trie.py and
# https://huggingface.co/facebook/genre-linking-blink/blob/main/kilt_titles_trie_dict.pkl
# import pickle
# from trie import Trie
# with open("kilt_titles_trie_dict.pkl", "rb") as f:
# trie = Trie.load_from_dict(pickle.load(f))
tokenizer = AutoTokenizer.from_pretrained("facebook/genre-linking-blink")
model = AutoModelForSeq2SeqLM.from_pretrained("facebook/genre-linking-blink").eval()
sentences = ["Einstein was a [START_ENT] German [END_ENT] physicist."]
outputs = model.generate(
**tokenizer(sentences, return_tensors="pt"),
num_beams=5,
num_return_sequences=5,
# OPTIONAL: use constrained beam search
# prefix_allowed_tokens_fn=lambda batch_id, sent: trie.get(sent.tolist()),
)
tokenizer.batch_decode(outputs, skip_special_tokens=True)
```
which outputs the following top-5 predictions (using constrained beam search)
```
['Germans',
'Germany',
'German Empire',
'Weimar Republic',
'Greeks']
```
|
santiviquez/t5-small-finetuned-samsum-en | a59cce76a34827e9d37b6d52586ae988e4b4d259 | 2022-06-27T20:55:29.000Z | [
"pytorch",
"tensorboard",
"t5",
"text2text-generation",
"dataset:samsum",
"transformers",
"summarization",
"generated_from_trainer",
"license:apache-2.0",
"model-index",
"autotrain_compatible"
] | summarization | false | santiviquez | null | santiviquez/t5-small-finetuned-samsum-en | 21 | null | transformers | 8,242 | ---
license: apache-2.0
tags:
- summarization
- generated_from_trainer
datasets:
- samsum
metrics:
- rouge
model-index:
- name: t5-small-finetuned-samsum-en
results:
- task:
name: Sequence-to-sequence Language Modeling
type: text2text-generation
dataset:
name: samsum
type: samsum
args: samsum
metrics:
- name: Rouge1
type: rouge
value: 44.3313
- task:
type: summarization
name: Summarization
dataset:
name: samsum
type: samsum
config: samsum
split: test
metrics:
- name: ROUGE-1
type: rouge
value: 40.0386
verified: true
- name: ROUGE-2
type: rouge
value: 15.8501
verified: true
- name: ROUGE-L
type: rouge
value: 31.8084
verified: true
- name: ROUGE-LSUM
type: rouge
value: 36.0888
verified: true
- name: loss
type: loss
value: 2.1917073726654053
verified: true
- name: gen_len
type: gen_len
value: 18.1074
verified: true
---
<!-- This model card has been generated automatically according to the information the Trainer had access to. You
should probably proofread and complete it, then remove this comment. -->
# t5-small-finetuned-samsum-en
This model is a fine-tuned version of [t5-small](https://huggingface.co/t5-small) on the samsum dataset.
It achieves the following results on the evaluation set:
- Loss: 1.9335
- Rouge1: 44.3313
- Rouge2: 20.71
- Rougel: 37.221
- Rougelsum: 40.9603
## Model description
More information needed
## Intended uses & limitations
More information needed
## Training and evaluation data
More information needed
## Training procedure
### Training hyperparameters
The following hyperparameters were used during training:
- learning_rate: 5.6e-05
- train_batch_size: 10
- eval_batch_size: 10
- seed: 42
- optimizer: Adam with betas=(0.9,0.999) and epsilon=1e-08
- lr_scheduler_type: linear
- num_epochs: 10
### Training results
| Training Loss | Epoch | Step | Validation Loss | Rouge1 | Rouge2 | Rougel | Rougelsum |
|:-------------:|:-----:|:----:|:---------------:|:-------:|:-------:|:-------:|:---------:|
| 1.4912 | 1.0 | 300 | 1.9043 | 44.1517 | 20.0186 | 36.6053 | 40.5164 |
| 1.5055 | 2.0 | 600 | 1.8912 | 44.1473 | 20.4456 | 37.069 | 40.6714 |
| 1.4852 | 3.0 | 900 | 1.8986 | 44.7536 | 20.8646 | 37.525 | 41.2189 |
| 1.4539 | 4.0 | 1200 | 1.9136 | 44.2144 | 20.3446 | 37.1088 | 40.7581 |
| 1.4262 | 5.0 | 1500 | 1.9215 | 44.2656 | 20.6044 | 37.3267 | 40.9469 |
| 1.4118 | 6.0 | 1800 | 1.9247 | 43.8793 | 20.4663 | 37.0614 | 40.6065 |
| 1.3987 | 7.0 | 2100 | 1.9256 | 43.9981 | 20.2703 | 36.7856 | 40.6354 |
| 1.3822 | 8.0 | 2400 | 1.9316 | 43.9732 | 20.4559 | 36.8039 | 40.5784 |
| 1.3773 | 9.0 | 2700 | 1.9314 | 44.3075 | 20.5435 | 37.0457 | 40.832 |
| 1.3795 | 10.0 | 3000 | 1.9335 | 44.3313 | 20.71 | 37.221 | 40.9603 |
### Framework versions
- Transformers 4.19.2
- Pytorch 1.11.0+cu113
- Datasets 2.2.2
- Tokenizers 0.12.1
|
ghadeermobasher/Original-BioBERT-NCBI | 8f5cebb0956af733a3f314ef2efb228d2076f64b | 2022-06-08T20:01:10.000Z | [
"pytorch",
"tensorboard",
"bert",
"token-classification",
"transformers",
"autotrain_compatible"
] | token-classification | false | ghadeermobasher | null | ghadeermobasher/Original-BioBERT-NCBI | 21 | null | transformers | 8,243 | Entry not found |
alistvt/01-roberta-dialdoc | dedca71f17480d9a754883f199f510c6c0649fae | 2022-06-19T07:58:18.000Z | [
"pytorch",
"roberta",
"question-answering",
"transformers",
"autotrain_compatible"
] | question-answering | false | alistvt | null | alistvt/01-roberta-dialdoc | 21 | null | transformers | 8,244 | Entry not found |
ericntay/bert-finetuned-emotion | e1fcb14e2f19b5b2dce860b1f06afc0df3fff0cb | 2022-06-13T17:46:15.000Z | [
"pytorch",
"tensorboard",
"bert",
"text-classification",
"dataset:emotion",
"transformers",
"generated_from_trainer",
"license:apache-2.0",
"model-index"
] | text-classification | false | ericntay | null | ericntay/bert-finetuned-emotion | 21 | null | transformers | 8,245 | ---
license: apache-2.0
tags:
- generated_from_trainer
datasets:
- emotion
metrics:
- accuracy
model-index:
- name: bert-finetuned-emotion
results:
- task:
name: Text Classification
type: text-classification
dataset:
name: emotion
type: emotion
args: default
metrics:
- name: Accuracy
type: accuracy
value: 0.937
---
<!-- This model card has been generated automatically according to the information the Trainer had access to. You
should probably proofread and complete it, then remove this comment. -->
# bert-finetuned-emotion
This model is a fine-tuned version of [bert-base-cased](https://huggingface.co/bert-base-cased) on the emotion dataset.
It achieves the following results on the evaluation set:
- Loss: 0.1582
- Accuracy: 0.937
## Model description
More information needed
## Intended uses & limitations
More information needed
## Training and evaluation data
More information needed
## Training procedure
### Training hyperparameters
The following hyperparameters were used during training:
- learning_rate: 2e-05
- train_batch_size: 10
- eval_batch_size: 10
- seed: 42
- optimizer: Adam with betas=(0.9,0.999) and epsilon=1e-08
- lr_scheduler_type: linear
- num_epochs: 2
### Training results
| Training Loss | Epoch | Step | Validation Loss | Accuracy |
|:-------------:|:-----:|:----:|:---------------:|:--------:|
| 0.553 | 1.0 | 1600 | 0.2631 | 0.9255 |
| 0.161 | 2.0 | 3200 | 0.1582 | 0.937 |
### Framework versions
- Transformers 4.19.4
- Pytorch 1.11.0+cu113
- Datasets 2.2.2
- Tokenizers 0.12.1
|
ghadeermobasher/BC4CHEMD-Chem-Modified-BioBERT-384 | 040c680156d681d4aecdd032c42fa611f4064feb | 2022-06-15T18:03:04.000Z | [
"pytorch",
"tensorboard",
"bert",
"token-classification",
"transformers",
"autotrain_compatible"
] | token-classification | false | ghadeermobasher | null | ghadeermobasher/BC4CHEMD-Chem-Modified-BioBERT-384 | 21 | null | transformers | 8,246 | Entry not found |
Nonzerophilip/bert-finetuned-ner | 8b34bfe88ffaf4a8cf83bf94b0c3ecae5621e5a4 | 2022-06-16T13:45:56.000Z | [
"pytorch",
"tensorboard",
"bert",
"token-classification",
"dataset:conll2003",
"transformers",
"generated_from_trainer",
"license:apache-2.0",
"model-index",
"autotrain_compatible"
] | token-classification | false | Nonzerophilip | null | Nonzerophilip/bert-finetuned-ner | 21 | null | transformers | 8,247 | ---
license: apache-2.0
tags:
- generated_from_trainer
datasets:
- conll2003
metrics:
- precision
- recall
- f1
- accuracy
model-index:
- name: bert-finetuned-ner
results:
- task:
name: Token Classification
type: token-classification
dataset:
name: conll2003
type: conll2003
args: conll2003
metrics:
- name: Precision
type: precision
value: 0.7978891820580475
- name: Recall
type: recall
value: 0.8600682593856656
- name: F1
type: f1
value: 0.8278127566383794
- name: Accuracy
type: accuracy
value: 0.9614351593776922
---
<!-- This model card has been generated automatically according to the information the Trainer had access to. You
should probably proofread and complete it, then remove this comment. -->
# bert-finetuned-ner
This model is a fine-tuned version of [bert-base-cased](https://huggingface.co/bert-base-cased) on the conll2003 dataset.
It achieves the following results on the evaluation set:
- Loss: 0.1286
- Precision: 0.7979
- Recall: 0.8601
- F1: 0.8278
- Accuracy: 0.9614
## Model description
More information needed
## Intended uses & limitations
More information needed
## Training and evaluation data
More information needed
## Training procedure
### Training hyperparameters
The following hyperparameters were used during training:
- learning_rate: 2e-05
- train_batch_size: 8
- eval_batch_size: 8
- seed: 42
- optimizer: Adam with betas=(0.9,0.999) and epsilon=1e-08
- lr_scheduler_type: linear
- num_epochs: 3
### Training results
| Training Loss | Epoch | Step | Validation Loss | Precision | Recall | F1 | Accuracy |
|:-------------:|:-----:|:----:|:---------------:|:---------:|:------:|:------:|:--------:|
| No log | 1.0 | 125 | 0.2188 | 0.6221 | 0.6985 | 0.6581 | 0.9285 |
| No log | 2.0 | 250 | 0.1396 | 0.7681 | 0.8402 | 0.8025 | 0.9590 |
| No log | 3.0 | 375 | 0.1286 | 0.7979 | 0.8601 | 0.8278 | 0.9614 |
### Framework versions
- Transformers 4.19.3
- Pytorch 1.7.1
- Datasets 2.2.2
- Tokenizers 0.12.1
|
RomanCast/no_init_miam_loria_finetuned | a5864b2c9a807ec3d27f401a131ca0719db0ed60 | 2022-06-16T17:11:36.000Z | [
"pytorch",
"camembert",
"text-classification",
"fr",
"transformers"
] | text-classification | false | RomanCast | null | RomanCast/no_init_miam_loria_finetuned | 21 | null | transformers | 8,248 | ---
language:
- fr
--- |
ZipperXYZ/DialoGPT-medium-TheWorldMachineExpressive | fb80535c4688c1c6a45613df9eb6079a6a6f3950 | 2022-06-18T02:07:51.000Z | [
"pytorch",
"gpt2",
"text-generation",
"transformers",
"conversational"
] | conversational | false | ZipperXYZ | null | ZipperXYZ/DialoGPT-medium-TheWorldMachineExpressive | 21 | null | transformers | 8,249 | ---
tags:
- conversational
---
# The world machine DialoGPT model |
Willy/bert-base-spanish-wwm-cased-finetuned-NLP-IE-4 | dd8a0f437357611b3d23d39a8ad793a7d1e10728 | 2022-06-28T14:44:34.000Z | [
"pytorch",
"tensorboard",
"bert",
"text-classification",
"transformers",
"generated_from_trainer",
"model-index"
] | text-classification | false | Willy | null | Willy/bert-base-spanish-wwm-cased-finetuned-NLP-IE-4 | 21 | null | transformers | 8,250 | ---
tags:
- generated_from_trainer
metrics:
- accuracy
model-index:
- name: bert-base-spanish-wwm-cased-finetuned-NLP-IE-4
results: []
---
<!-- This model card has been generated automatically according to the information the Trainer had access to. You
should probably proofread and complete it, then remove this comment. -->
# bert-base-spanish-wwm-cased-finetuned-NLP-IE-4
This model is a fine-tuned version of [dccuchile/bert-base-spanish-wwm-cased](https://huggingface.co/dccuchile/bert-base-spanish-wwm-cased) on an unknown dataset.
It achieves the following results on the evaluation set:
- Loss: 0.7825
- Accuracy: 0.4931
## Model description
More information needed
## Intended uses & limitations
More information needed
## Training and evaluation data
More information needed
## Training procedure
### Training hyperparameters
The following hyperparameters were used during training:
- learning_rate: 2e-05
- train_batch_size: 64
- eval_batch_size: 64
- seed: 42
- optimizer: Adam with betas=(0.9,0.999) and epsilon=1e-08
- lr_scheduler_type: linear
- num_epochs: 5
### Training results
| Training Loss | Epoch | Step | Validation Loss | Accuracy |
|:-------------:|:-----:|:----:|:---------------:|:--------:|
| 0.7005 | 1.0 | 9 | 0.6977 | 0.5069 |
| 0.65 | 2.0 | 18 | 0.7035 | 0.4861 |
| 0.6144 | 3.0 | 27 | 0.7189 | 0.4722 |
| 0.5898 | 4.0 | 36 | 0.7859 | 0.4861 |
| 0.561 | 5.0 | 45 | 0.7825 | 0.4931 |
### Framework versions
- Transformers 4.20.1
- Pytorch 1.11.0+cu113
- Datasets 2.3.2
- Tokenizers 0.12.1
|
Jeevesh8/std_0pnt2_bert_ft_cola-0 | a2a40c784daf42e87cd750460039543d3c2b1fa0 | 2022-06-21T13:27:51.000Z | [
"pytorch",
"tensorboard",
"bert",
"text-classification",
"transformers"
] | text-classification | false | Jeevesh8 | null | Jeevesh8/std_0pnt2_bert_ft_cola-0 | 21 | null | transformers | 8,251 | Entry not found |
Matthijs/mobilenet_v1_0.75_192 | 1a63ace7d0a9d72ac33b3af39e044a1dcd4c65d4 | 2022-06-22T12:50:39.000Z | [
"pytorch",
"mobilenet_v1",
"dataset:imagenet-1k",
"arxiv:1704.04861",
"transformers",
"vision",
"image-classification",
"license:other"
] | image-classification | false | Matthijs | null | Matthijs/mobilenet_v1_0.75_192 | 21 | null | transformers | 8,252 | ---
license: other
tags:
- vision
- image-classification
datasets:
- imagenet-1k
widget:
- src: https://huggingface.co/datasets/mishig/sample_images/resolve/main/tiger.jpg
example_title: Tiger
- src: https://huggingface.co/datasets/mishig/sample_images/resolve/main/teapot.jpg
example_title: Teapot
- src: https://huggingface.co/datasets/mishig/sample_images/resolve/main/palace.jpg
example_title: Palace
---
# MobileNet V1
MobileNet V1 model pre-trained on ImageNet-1k at resolution 192x192. It was introduced in [MobileNets: Efficient Convolutional Neural Networks for Mobile Vision Applications](https://arxiv.org/abs/1704.04861) by Howard et al, and first released in [this repository](https://github.com/tensorflow/models/blob/master/research/slim/nets/mobilenet_v1.md).
Disclaimer: The team releasing MobileNet V1 did not write a model card for this model so this model card has been written by the Hugging Face team.
## Model description
From the [original README](https://github.com/tensorflow/models/blob/master/research/slim/nets/mobilenet_v1.md):
> MobileNets are small, low-latency, low-power models parameterized to meet the resource constraints of a variety of use cases. They can be built upon for classification, detection, embeddings and segmentation similar to how other popular large scale models, such as Inception, are used. MobileNets can be run efficiently on mobile devices [...] MobileNets trade off between latency, size and accuracy while comparing favorably with popular models from the literature.
## Intended uses & limitations
You can use the raw model for image classification. See the [model hub](https://huggingface.co/models?search=mobilenet_v1) to look for fine-tuned versions on a task that interests you.
### How to use
Here is how to use this model to classify an image of the COCO 2017 dataset into one of the 1,000 ImageNet classes:
```python
from transformers import MobileNetV1FeatureExtractor, MobileNetV1ForImageClassification
from PIL import Image
import requests
url = "http://images.cocodataset.org/val2017/000000039769.jpg"
image = Image.open(requests.get(url, stream=True).raw)
feature_extractor = MobileNetV1FeatureExtractor.from_pretrained("Matthijs/mobilenet_v1_1.0_224")
model = MobileNetV1ForImageClassification.from_pretrained("Matthijs/mobilenet_v1_1.0_224")
inputs = feature_extractor(images=image, return_tensors="pt")
outputs = model(**inputs)
logits = outputs.logits
# model predicts one of the 1000 ImageNet classes
predicted_class_idx = logits.argmax(-1).item()
print("Predicted class:", model.config.id2label[predicted_class_idx])
```
Note: This model actually predicts 1001 classes, the 1000 classes from ImageNet plus an extra “background” class (index 0).
Currently, both the feature extractor and model support PyTorch.
|
truongxl/NER_covid19 | 2556ec4478e0cf46f0f2f128df371d71962f7110 | 2022-06-23T04:05:10.000Z | [
"pytorch",
"roberta",
"token-classification",
"transformers",
"autotrain_compatible"
] | token-classification | false | truongxl | null | truongxl/NER_covid19 | 21 | null | transformers | 8,253 | Entry not found |
KoichiYasuoka/deberta-base-japanese-wikipedia-luw-upos | 7e81edcb08c0066c01479572d3314a9ee598699d | 2022-07-23T14:43:48.000Z | [
"pytorch",
"deberta-v2",
"token-classification",
"ja",
"dataset:universal_dependencies",
"transformers",
"japanese",
"wikipedia",
"pos",
"dependency-parsing",
"license:cc-by-sa-4.0",
"autotrain_compatible"
] | token-classification | false | KoichiYasuoka | null | KoichiYasuoka/deberta-base-japanese-wikipedia-luw-upos | 21 | null | transformers | 8,254 | ---
language:
- "ja"
tags:
- "japanese"
- "wikipedia"
- "token-classification"
- "pos"
- "dependency-parsing"
datasets:
- "universal_dependencies"
license: "cc-by-sa-4.0"
pipeline_tag: "token-classification"
widget:
- text: "国境の長いトンネルを抜けると雪国であった。"
---
# deberta-base-japanese-wikipedia-luw-upos
## Model Description
This is a DeBERTa(V2) model pre-trained on Japanese Wikipedia and 青空文庫 texts for POS-tagging and dependency-parsing, derived from [deberta-base-japanese-wikipedia](https://huggingface.co/KoichiYasuoka/deberta-base-japanese-wikipedia). Every long-unit-word is tagged by [UPOS](https://universaldependencies.org/u/pos/) (Universal Part-Of-Speech) and [FEATS](https://universaldependencies.org/u/feat/).
## How to Use
```py
import torch
from transformers import AutoTokenizer,AutoModelForTokenClassification
tokenizer=AutoTokenizer.from_pretrained("KoichiYasuoka/deberta-base-japanese-wikipedia-luw-upos")
model=AutoModelForTokenClassification.from_pretrained("KoichiYasuoka/deberta-base-japanese-wikipedia-luw-upos")
s="国境の長いトンネルを抜けると雪国であった。"
t=tokenizer.tokenize(s)
p=[model.config.id2label[q] for q in torch.argmax(model(tokenizer.encode(s,return_tensors="pt"))["logits"],dim=2)[0].tolist()[1:-1]]
print(list(zip(t,p)))
```
or
```py
import esupar
nlp=esupar.load("KoichiYasuoka/deberta-base-japanese-wikipedia-luw-upos")
print(nlp("国境の長いトンネルを抜けると雪国であった。"))
```
## Reference
安岡孝一: [青空文庫DeBERTaモデルによる国語研長単位係り受け解析](http://hdl.handle.net/2433/275409), 東洋学へのコンピュータ利用, 第35回研究セミナー (2022年7月), pp.29-43.
## See Also
[esupar](https://github.com/KoichiYasuoka/esupar): Tokenizer POS-tagger and Dependency-parser with BERT/RoBERTa/DeBERTa models
|
Someman/distilbert-base-uncased-finetuned-emotion | a6bcfd1353b9f050a60a33fe80ebb34c74c746ae | 2022-07-16T05:49:22.000Z | [
"pytorch",
"tensorboard",
"distilbert",
"text-classification",
"dataset:emotion",
"transformers",
"generated_from_trainer",
"license:apache-2.0",
"model-index"
] | text-classification | false | Someman | null | Someman/distilbert-base-uncased-finetuned-emotion | 21 | null | transformers | 8,255 | ---
license: apache-2.0
tags:
- generated_from_trainer
datasets:
- emotion
metrics:
- accuracy
- f1
model-index:
- name: distilbert-base-uncased-finetuned-emotion
results:
- task:
name: Text Classification
type: text-classification
dataset:
name: emotion
type: emotion
args: default
metrics:
- name: Accuracy
type: accuracy
value: 0.9245
- name: F1
type: f1
value: 0.9245803802599059
---
<!-- This model card has been generated automatically according to the information the Trainer had access to. You
should probably proofread and complete it, then remove this comment. -->
# distilbert-base-uncased-finetuned-emotion
This model is a fine-tuned version of [distilbert-base-uncased](https://huggingface.co/distilbert-base-uncased) on the emotion dataset.
It achieves the following results on the evaluation set:
- Loss: 0.2186
- Accuracy: 0.9245
- F1: 0.9246
## Model description
More information needed
## Intended uses & limitations
More information needed
## Training and evaluation data
More information needed
## Training procedure
### Training hyperparameters
The following hyperparameters were used during training:
- learning_rate: 2e-05
- train_batch_size: 64
- eval_batch_size: 64
- seed: 42
- optimizer: Adam with betas=(0.9,0.999) and epsilon=1e-08
- lr_scheduler_type: linear
- num_epochs: 2
### Training results
| Training Loss | Epoch | Step | Validation Loss | Accuracy | F1 |
|:-------------:|:-----:|:----:|:---------------:|:--------:|:------:|
| No log | 1.0 | 250 | 0.3083 | 0.9005 | 0.8972 |
| No log | 2.0 | 500 | 0.2186 | 0.9245 | 0.9246 |
### Framework versions
- Transformers 4.20.1
- Pytorch 1.11.0+cu113
- Datasets 2.3.2
- Tokenizers 0.12.1
|
josh-oo/bert-to-gpt2-german-to-easy-german | 674a53597dbcce45743554e30a8b5dbd98a77f6b | 2022-07-05T15:20:09.000Z | [
"pytorch",
"encoder-decoder",
"text2text-generation",
"transformers",
"autotrain_compatible"
] | text2text-generation | false | josh-oo | null | josh-oo/bert-to-gpt2-german-to-easy-german | 21 | null | transformers | 8,256 | Entry not found |
codenamewei/speech-to-text | 5a0459e9ec7a20b74bc56947afe616f41c8e8844 | 2022-07-02T18:02:42.000Z | [
"pytorch",
"wav2vec2-conformer",
"automatic-speech-recognition",
"transformers",
"license:gpl-3.0"
] | automatic-speech-recognition | false | codenamewei | null | codenamewei/speech-to-text | 21 | null | transformers | 8,257 | ---
license: gpl-3.0
---
|
Doohae/bart-kor-620000 | c9b539519ea5fde413bc25e65b7954eee5dd5e30 | 2022-07-04T09:39:27.000Z | [
"pytorch",
"bart",
"text2text-generation",
"transformers",
"autotrain_compatible"
] | text2text-generation | false | Doohae | null | Doohae/bart-kor-620000 | 21 | null | transformers | 8,258 | Entry not found |
KoichiYasuoka/bert-ancient-chinese-base-upos | fb6752bd3c5e4847f9902fc35beb6ca94ca3ae74 | 2022-07-09T10:26:04.000Z | [
"pytorch",
"bert",
"token-classification",
"lzh",
"dataset:universal_dependencies",
"transformers",
"classical chinese",
"literary chinese",
"ancient chinese",
"pos",
"dependency-parsing",
"license:apache-2.0",
"autotrain_compatible"
] | token-classification | false | KoichiYasuoka | null | KoichiYasuoka/bert-ancient-chinese-base-upos | 21 | null | transformers | 8,259 | ---
language:
- "lzh"
tags:
- "classical chinese"
- "literary chinese"
- "ancient chinese"
- "token-classification"
- "pos"
- "dependency-parsing"
datasets:
- "universal_dependencies"
license: "apache-2.0"
pipeline_tag: "token-classification"
widget:
- text: "子曰學而時習之不亦説乎有朋自遠方來不亦樂乎人不知而不慍不亦君子乎"
---
# bert-ancient-chinese-base-upos
## Model Description
This is a BERT model pre-trained on Classical Chinese texts for POS-tagging and dependency-parsing, derived from [bert-ancient-chinese](https://huggingface.co/Jihuai/bert-ancient-chinese). Every word is tagged by [UPOS](https://universaldependencies.org/u/pos/) (Universal Part-Of-Speech) and [FEATS](https://universaldependencies.org/u/feat/).
## How to Use
```py
from transformers import AutoTokenizer,AutoModelForTokenClassification
tokenizer=AutoTokenizer.from_pretrained("KoichiYasuoka/bert-ancient-chinese-base-upos")
model=AutoModelForTokenClassification.from_pretrained("KoichiYasuoka/bert-ancient-chinese-base-upos")
```
or
```py
import esupar
nlp=esupar.load("KoichiYasuoka/bert-ancient-chinese-base-upos")
```
## See Also
[esupar](https://github.com/KoichiYasuoka/esupar): Tokenizer POS-tagger and Dependency-parser with BERT/RoBERTa/DeBERTa models
|
BBarbarestani/RoBERTa_HateXplain_Target_Span_Detection_UQS_Threshold_50_2_Previous_Hyperparameters | 235724245b899d6ae294c189d77d89fc615aad02 | 2022-07-05T13:14:17.000Z | [
"pytorch",
"tensorboard",
"roberta",
"token-classification",
"transformers",
"autotrain_compatible"
] | token-classification | false | BBarbarestani | null | BBarbarestani/RoBERTa_HateXplain_Target_Span_Detection_UQS_Threshold_50_2_Previous_Hyperparameters | 21 | null | transformers | 8,260 | Entry not found |
ryo0634/en-encoder-en-0 | 3e5092e9a08677b356658495290f6d4fc889f687 | 2022-07-06T05:15:32.000Z | [
"pytorch",
"bert",
"fill-mask",
"transformers",
"autotrain_compatible"
] | fill-mask | false | ryo0634 | null | ryo0634/en-encoder-en-0 | 21 | null | transformers | 8,261 | Entry not found |
ghadeermobasher/Modified-BlueBERT-BioRED-Chem-512-5-30 | 3a8ae2d36ad05789143137010993bda3e5da3796 | 2022-07-08T08:30:24.000Z | [
"pytorch",
"tensorboard",
"bert",
"token-classification",
"transformers",
"autotrain_compatible"
] | token-classification | false | ghadeermobasher | null | ghadeermobasher/Modified-BlueBERT-BioRED-Chem-512-5-30 | 21 | null | transformers | 8,262 | |
ryo0634/zip-dependency-flat-encoder-en-0 | fd17a5e4a8604dcf6f3f6616dc14000264092863 | 2022-07-09T15:40:47.000Z | [
"pytorch",
"bert",
"fill-mask",
"transformers",
"autotrain_compatible"
] | fill-mask | false | ryo0634 | null | ryo0634/zip-dependency-flat-encoder-en-0 | 21 | null | transformers | 8,263 | Entry not found |
Kozias/BERT-v11 | 9b1ccd1f18e616d3aad3bb927db0272e0ce70ed2 | 2022-07-19T02:08:56.000Z | [
"pytorch",
"bert",
"text-classification",
"transformers"
] | text-classification | false | Kozias | null | Kozias/BERT-v11 | 21 | null | transformers | 8,264 | Entry not found |
thu-coai/EVA2.0-xlarge | 6b3ad1fdf16df28d300da46aebc2e7991baa6fce | 2022-07-25T02:57:30.000Z | [
"pytorch",
"zh",
"arxiv:2108.01547",
"arxiv:2203.09313",
"transformers",
"license:mit"
] | null | false | thu-coai | null | thu-coai/EVA2.0-xlarge | 21 | null | transformers | 8,265 | ---
language: zh
tags:
- pytorch
license: mit
---
# EVA
## Model Description
EVA is the largest open-source Chinese dialogue model with up to 2.8B parameters. The 1.0 version model is pre-trained on [WudaoCorpus-Dialog](https://resource.wudaoai.cn/home), and the 2.0 version is pre-trained on a carefully cleaned version of WudaoCorpus-Dialog which yields better performance than the 1.0 version. [Paper link](https://arxiv.org/abs/2108.01547) of EVA1.0. [Paper link](https://arxiv.org/abs/2203.09313) of EVA2.0.
## Model Configuration
| Model | n_params | n_enc-layers | n_dec-layers | d_model | d_ff | n_heads | d_head | attn-scale |
| ------------- | -------- | ------------ | ------------ | ------- | ----- | ------- | ------ | ---------- |
| EVA1.0 | 2.8B | 24 | 24 | 2,048 | 5,120 | 32 | 64 | No |
| EVA2.0_Base | 300M | 12 | 12 | 768 | 3,072 | 12 | 64 | Yes |
| EVA2.0_Large | 970M | 24 | 24 | 1,024 | 4,096 | 16 | 64 | Yes |
| EVA2.0_xLarge | 2.8B | 24 | 24 | 2,048 | 5,120 | 32 | 64 | Yes |
## How to use
Please refer to our GitHub [repository](https://github.com/thu-coai/EVA/tree/huggingface).
## Performance
We use the KdConv dataset to finetune and evaluate the model. Under the default hyperparameters in the scripts, we get the following results on the test set:
| | | | | |
| ---- | ---- | ---- | ---- | ---- |
| | loss | BLEU-4 | Dist-4 | f1 |
| eva1.0 Before finetune | 3.49 | 0.64 | 85.94 | 13.24 |
| eva1.0 After finetune | 2.37 | 3.94 | 72.47 | 19.80 |
| eva2.0 Before finetune | 3.14 | 1.02 | 82.67 | 14.36 |
| eva2.0 After finetune | 2.09 | 4.69 | 73.03 | 22.91 |
We also use beam search to run the interactive inference of EVA2.0:
```[]
Usr >>> 你好
Sys >>> 你好,请问你是?
Usr >>> 我是小明
Sys >>> 好的,小明,我是新来的,请多关照
Usr >>> 你了解人工智能吗?
Sys >>> 不了解,我只知道我是机器人
Usr >>> 那你很聪明
Sys >>> 谢谢夸奖,我会继续努力的
Usr >>> 你是学什么专业的呀
Sys >>> 计算机科学与技术,你呢?
Usr >>> 我也是学计算机的
Sys >>> 哦,那你有什么想知道的,可以问我
Usr >>> 编程语言你学过哪些?
Sys >>> java,c,c+都学过
Usr >>> 你学的真多
Sys >>> 呵呵,还好吧,我比较喜欢写代码
Usr >>> 哈哈,我也喜欢写代码,以后可以多多交流
Sys >>> 嗯,好的
Usr >>> 拜拜喽
Sys >>> 拜~
```
**NOET**: Since different hardware may have different implementations of random functions, even if you use the same random seed as ours, you may not be able to reproduce this case. But the overall performance will not differ much.
## Disclaimer
The pre-trained models aim to facilitate the research for conversation generation. The model provided in this repository is trained on a large dataset collected from various sources. Although a rigorous cleaning and filtering process has been carried out to the data and the model output, there is no guarantee that all the inappropriate contents have been completely banned. All the contents generated by the model do not represent the authors' opinions. The decoding script provided in this repository is only for research purposes. We are not responsible for any content generated using our model.
## Citation
```
@article{coai2021eva,
title={EVA: An Open-Domain Chinese Dialogue System with Large-Scale Generative Pre-Training},
author={Zhou, Hao and Ke, Pei and Zhang, Zheng and Gu, Yuxian and Zheng, Yinhe and Zheng, Chujie and Wang, Yida and Wu, Chen Henry and Sun, Hao and Yang, Xiaocong and Wen, Bosi and Zhu, Xiaoyan and Huang, Minlie and Tang, Jie},
journal={arXiv preprint arXiv:2108.01547},
year={2021}
}
@article{coai2022eva2,
title={{EVA2.0}: Investigating Open-Domain Chinese Dialogue Systems with Large-Scale Pre-Training},
author={Gu, Yuxian and Wen, Jiaxin and Sun, Hao and Song, Yi and Ke, Pei and Zheng, Chujie and Zhang, Zheng and Yao, Jianzhu and Zhu, Xiaoyan and Tang, Jie and Huang, Minlie},
journal={arXiv preprint arXiv:2203.09313},
year={2022}
}
``` |
jhonparra18/bert-base-cased-cv-studio_name-medium | 807a3b8cf8129bdca64842896809d1901978da72 | 2022-07-14T22:17:03.000Z | [
"pytorch",
"bert",
"text-classification",
"transformers",
"generated_from_trainer",
"license:apache-2.0",
"model-index"
] | text-classification | false | jhonparra18 | null | jhonparra18/bert-base-cased-cv-studio_name-medium | 21 | null | transformers | 8,266 | ---
license: apache-2.0
tags:
- generated_from_trainer
metrics:
- accuracy
model-index:
- name: bert-base-cased-cv-studio_name-medium
results: []
widget:
- text: "Egresado de la carrera Ingeniería en Computación Conocimientos de lenguajes HTML, CSS, Javascript y MySQL. Experiencia trabajando en ámbitos de redes de pequeña y mediana escala. Inglés Hablado nivel básico, escrito nivel intermedio.HTML, CSS y JavaScript. Realidad aumentada. Lenguaje R. HTML5, JavaScript y Nodejs"
- text: "mi nombre es Ivan Ducales Marquez, hago de subpresidente en la republica de Colombia. tengo experiencia en seguir órdenes de mis patrocinadores y repartir los recursos del país a empresarios corruptos"
---
<!-- This model card has been generated automatically according to the information the Trainer had access to. You
should probably proofread and complete it, then remove this comment. -->
# bert-base-cased-cv-studio_name-medium
This model is a fine-tuned version of [bert-base-cased](https://huggingface.co/bert-base-cased) on the None dataset.
It achieves the following results on the evaluation set:
- Loss: 2.3310
- F1 Micro: 0.6388
- F1 Macro: 0.5001
## Model description
Predicts a studio name based on a CV text
### Training hyperparameters
The following hyperparameters were used during training:
- learning_rate: 5e-05
- train_batch_size: 16
- eval_batch_size: 8
- seed: 42
- optimizer: Adam with betas=(0.9,0.999) and epsilon=1e-08
- lr_scheduler_type: linear
- lr_scheduler_warmup_steps: 20
- num_epochs: 10
### Training results
| Training Loss | Epoch | Step | Validation Loss | Accuracy | F1 Micro | F1 Macro | Precision Micro | Recall Micro |
|:-------------:|:-----:|:-----:|:---------------:|:--------:|:--------:|:--------:|:---------------:|:------------:|
| 1.4139 | 0.98 | 1000 | 1.3831 | 0.6039 | 0.6039 | 0.4188 | 0.6039 | 0.6039 |
| 1.1561 | 1.96 | 2000 | 1.2386 | 0.6554 | 0.6554 | 0.4743 | 0.6554 | 0.6554 |
| 0.9183 | 2.93 | 3000 | 1.2201 | 0.6576 | 0.6576 | 0.5011 | 0.6576 | 0.6576 |
| 0.677 | 3.91 | 4000 | 1.3478 | 0.6442 | 0.6442 | 0.5206 | 0.6442 | 0.6442 |
| 0.4857 | 4.89 | 5000 | 1.4765 | 0.6393 | 0.6393 | 0.5215 | 0.6393 | 0.6393 |
| 0.3318 | 5.87 | 6000 | 1.6924 | 0.6442 | 0.6442 | 0.5024 | 0.6442 | 0.6442 |
| 0.2273 | 6.84 | 7000 | 1.8645 | 0.6444 | 0.6444 | 0.5060 | 0.6444 | 0.6444 |
| 0.1396 | 7.82 | 8000 | 2.1143 | 0.6381 | 0.6381 | 0.5181 | 0.6381 | 0.6381 |
| 0.0841 | 8.8 | 9000 | 2.2699 | 0.6359 | 0.6359 | 0.5065 | 0.6359 | 0.6359 |
| 0.0598 | 9.78 | 10000 | 2.3310 | 0.6388 | 0.6388 | 0.5001 | 0.6388 | 0.6388 |
### Framework versions
- Transformers 4.19.0
- Pytorch 1.8.2+cu111
- Datasets 1.6.2
- Tokenizers 0.12.1
|
jhonparra18/roberta-base-cv-studio_name-medium | 80b48b379ba1ca5778cf24671e900d3db006686f | 2022-07-16T02:43:03.000Z | [
"pytorch",
"roberta",
"text-classification",
"transformers",
"generated_from_trainer",
"license:apache-2.0",
"model-index"
] | text-classification | false | jhonparra18 | null | jhonparra18/roberta-base-cv-studio_name-medium | 21 | null | transformers | 8,267 | ---
license: apache-2.0
tags:
- generated_from_trainer
metrics:
- accuracy
model-index:
- name: roberta-base-cv-studio_name-medium
results: []
widget:
- text: "Egresado de la carrera Ingeniería en Computación Conocimientos de lenguajes HTML, CSS, Javascript y MySQL. Experiencia trabajando en ámbitos de redes de pequeña y mediana escala. Inglés Hablado nivel básico, escrito nivel intermedio.HTML, CSS y JavaScript. Realidad aumentada. Lenguaje R. HTML5, JavaScript y Nodejs"
---
<!-- This model card has been generated automatically according to the information the Trainer had access to. You
should probably proofread and complete it, then remove this comment. -->
# bert-base-cased-cv-studio_name-medium
This model is a fine-tuned version of [roberta-base](https://huggingface.co/roberta-base) on the None dataset.
## Model description
Predicts a studio name based on a CV text
### Training hyperparameters
The following hyperparameters were used during training:
- learning_rate: 5e-05
- train_batch_size: 16
- eval_batch_size: 8
- seed: 42
- optimizer: Adam with betas=(0.9,0.999) and epsilon=1e-08
- lr_scheduler_type: linear
- lr_scheduler_warmup_steps: 20
- num_epochs: 10
### Framework versions
- Transformers 4.19.0
- Pytorch 1.8.2+cu111
- Datasets 1.6.2
- Tokenizers 0.12.1
|
jinwooChoi/KDH_NER_ELECTRA | 598b8d4ebeb7fd5771ea0bad8b14b9d8d20e2476 | 2022-07-19T07:54:14.000Z | [
"pytorch",
"distilbert",
"token-classification",
"transformers",
"autotrain_compatible"
] | token-classification | false | jinwooChoi | null | jinwooChoi/KDH_NER_ELECTRA | 21 | null | transformers | 8,268 | Entry not found |
Evelyn18/distilbert-base-uncased-modelo-becas0 | dcc0ed2933940ac7d3dc6ce53b1be22a0e94e919 | 2022-07-15T22:56:08.000Z | [
"pytorch",
"tensorboard",
"distilbert",
"question-answering",
"dataset:becasv3",
"transformers",
"generated_from_trainer",
"license:apache-2.0",
"model-index",
"autotrain_compatible"
] | question-answering | false | Evelyn18 | null | Evelyn18/distilbert-base-uncased-modelo-becas0 | 21 | null | transformers | 8,269 | ---
license: apache-2.0
tags:
- generated_from_trainer
datasets:
- becasv3
model-index:
- name: distilbert-base-uncased-modelo-becas0
results: []
---
<!-- This model card has been generated automatically according to the information the Trainer had access to. You
should probably proofread and complete it, then remove this comment. -->
# distilbert-base-uncased-modelo-becas0
This model is a fine-tuned version of [distilbert-base-uncased](https://huggingface.co/distilbert-base-uncased) on the becasv3 dataset.
It achieves the following results on the evaluation set:
- Loss: 3.1182
## Model description
More information needed
## Intended uses & limitations
More information needed
## Training and evaluation data
More information needed
## Training procedure
### Training hyperparameters
The following hyperparameters were used during training:
- learning_rate: 2e-05
- train_batch_size: 16
- eval_batch_size: 16
- seed: 42
- optimizer: Adam with betas=(0.9,0.999) and epsilon=1e-08
- lr_scheduler_type: linear
- num_epochs: 10
### Training results
| Training Loss | Epoch | Step | Validation Loss |
|:-------------:|:-----:|:----:|:---------------:|
| No log | 1.0 | 5 | 5.5381 |
| No log | 2.0 | 10 | 4.9493 |
| No log | 3.0 | 15 | 4.4985 |
| No log | 4.0 | 20 | 4.1063 |
| No log | 5.0 | 25 | 3.7708 |
| No log | 6.0 | 30 | 3.5205 |
| No log | 7.0 | 35 | 3.3313 |
| No log | 8.0 | 40 | 3.2195 |
| No log | 9.0 | 45 | 3.1453 |
| No log | 10.0 | 50 | 3.1182 |
### Framework versions
- Transformers 4.20.1
- Pytorch 1.12.0+cu113
- Datasets 2.3.2
- Tokenizers 0.12.1
|
Unso/roberta-large-finetuned-sst5 | 9c6fbad1c3e478f68a524b1f844259990f8375f2 | 2022-07-20T07:05:57.000Z | [
"pytorch",
"tensorboard",
"roberta",
"text-classification",
"transformers"
] | text-classification | false | Unso | null | Unso/roberta-large-finetuned-sst5 | 21 | null | transformers | 8,270 | Entry not found |
RobertoFont/pegasus-large-samsum | e68697845c4da36861d535d2252b7d30961d0340 | 2022-07-16T15:12:09.000Z | [
"pytorch",
"tensorboard",
"pegasus",
"text2text-generation",
"dataset:samsum",
"transformers",
"generated_from_trainer",
"model-index",
"autotrain_compatible"
] | text2text-generation | false | RobertoFont | null | RobertoFont/pegasus-large-samsum | 21 | null | transformers | 8,271 | ---
tags:
- generated_from_trainer
datasets:
- samsum
metrics:
- rouge
model-index:
- name: pegasus-large-samsum
results:
- task:
name: Sequence-to-sequence Language Modeling
type: text2text-generation
dataset:
name: samsum
type: samsum
args: samsum
metrics:
- name: Rouge1
type: rouge
value: 48.0968
---
<!-- This model card has been generated automatically according to the information the Trainer had access to. You
should probably proofread and complete it, then remove this comment. -->
# pegasus-large-samsum
This model is a fine-tuned version of [google/pegasus-large](https://huggingface.co/google/pegasus-large) on the samsum dataset.
It achieves the following results on the evaluation set:
- Loss: 1.4109
- Rouge1: 48.0968
- Rouge2: 24.6663
- Rougel: 40.2569
- Rougelsum: 44.0137
## Model description
More information needed
## Intended uses & limitations
More information needed
## Training and evaluation data
More information needed
## Training procedure
### Training hyperparameters
The following hyperparameters were used during training:
- learning_rate: 2e-05
- train_batch_size: 1
- eval_batch_size: 1
- seed: 42
- gradient_accumulation_steps: 64
- total_train_batch_size: 64
- optimizer: Adam with betas=(0.9,0.999) and epsilon=1e-08
- lr_scheduler_type: linear
- num_epochs: 3
### Training results
| Training Loss | Epoch | Step | Validation Loss | Rouge1 | Rouge2 | Rougel | Rougelsum |
|:-------------:|:-----:|:----:|:---------------:|:-------:|:-------:|:-------:|:---------:|
| No log | 1.0 | 230 | 1.4646 | 45.0631 | 22.5567 | 38.0518 | 41.2694 |
| No log | 2.0 | 460 | 1.4203 | 47.4122 | 24.158 | 39.7414 | 43.3485 |
| 1.699 | 3.0 | 690 | 1.4109 | 48.0968 | 24.6663 | 40.2569 | 44.0137 |
### Framework versions
- Transformers 4.20.1
- Pytorch 1.12.0+cu113
- Datasets 2.3.2
- Tokenizers 0.12.1
|
UT/BRTW_MULICLASS | 9b891cec9165a2cb4bc7ef7d78b68b109b9d51bf | 2022-07-17T10:57:14.000Z | [
"pytorch",
"bert",
"text-classification",
"transformers"
] | text-classification | false | UT | null | UT/BRTW_MULICLASS | 21 | null | transformers | 8,272 | Entry not found |
uer/roberta-large-wwm-chinese-cluecorpussmall | 96862a51fef0b5cc9cc485007f06db0ddd2c2dab | 2022-07-18T05:56:53.000Z | [
"pytorch",
"bert",
"fill-mask",
"zh",
"dataset:CLUECorpusSmall",
"arxiv:1909.05658",
"arxiv:1908.08962",
"transformers",
"autotrain_compatible"
] | fill-mask | false | uer | null | uer/roberta-large-wwm-chinese-cluecorpussmall | 21 | null | transformers | 8,273 | ---
language: zh
datasets: CLUECorpusSmall
widget:
- text: "北京是[MASK]国的首都。"
---
# Chinese Whole Word Masking RoBERTa Miniatures
## Model description
This is the set of 6 Chinese Whole Word Masking RoBERTa models pre-trained by [UER-py](https://arxiv.org/abs/1909.05658).
[Turc et al.](https://arxiv.org/abs/1908.08962) have shown that the standard BERT recipe is effective on a wide range of model sizes. Following their paper, we released the 6 Chinese Whole Word Masking RoBERTa models. In order to facilitate users to reproduce the results, we used the publicly available corpus and word segmentation tool, and provided all training details.
You can download the 6 Chinese RoBERTa miniatures either from the [UER-py Github page](https://github.com/dbiir/UER-py/), or via HuggingFace from the links below:
| | Link |
| -------- | :-----------------------: |
| **Tiny** | [**2/128 (Tiny)**][2_128] |
| **Mini** | [**4/256 (Mini)**][4_256] |
| **Small** | [**4/512 (Small)**][4_512] |
| **Medium** | [**8/512 (Medium)**][8_512] |
| **Base** | [**12/768 (Base)**][12_768] |
| **Large** | [**24/1024 (Large)**][24_1024] |
Here are scores on the devlopment set of six Chinese tasks:
| Model | Score | douban | chnsenticorp | lcqmc | tnews(CLUE) | iflytek(CLUE) | ocnli(CLUE) |
| ------------------ | :---: | :----: | :----------: | :---: | :---------: | :-----------: | :---------: |
| RoBERTa-Tiny-WWM | 72.1 | 82.8 | 91.8 | 81.8 | 62.1 | 55.4 | 58.6 |
| RoBERTa-Mini-WWM | 76.1 | 84.9 | 93.0 | 86.8 | 64.4 | 58.7 | 68.8 |
| RoBERTa-Small-WWM | 77.3 | 86.8 | 93.8 | 87.2 | 65.2 | 59.6 | 71.4 |
| RoBERTa-Medium-WWM | 78.4 | 88.2 | 94.4 | 88.8 | 66.0 | 59.9 | 73.2 |
| RoBERTa-Base-WWM | 80.1 | 90.0 | 95.8 | 89.4 | 67.5 | 61.8 | 76.2 |
| RoBERTa-Large-WWM | 81.0 | 90.4 | 95.8 | 90.0 | 68.5 | 62.1 | 79.1 |
For each task, we selected the best fine-tuning hyperparameters from the lists below, and trained with the sequence length of 128:
- epochs: 3, 5, 8
- batch sizes: 32, 64
- learning rates: 3e-5, 1e-4, 3e-4
## How to use
You can use this model directly with a pipeline for masked language modeling:
```python
>>> from transformers import pipeline
>>> unmasker = pipeline('fill-mask', model='uer/roberta-tiny-wwm-chinese-cluecorpussmall')
>>> unmasker("北京是[MASK]国的首都。")
[
{'score': 0.294228732585907,
'token': 704,
'token_str': '中',
'sequence': '北 京 是 中 国 的 首 都 。'},
{'score': 0.19691626727581024,
'token': 1266,
'token_str': '北',
'sequence': '北 京 是 北 国 的 首 都 。'},
{'score': 0.1070084273815155,
'token': 7506,
'token_str': '韩',
'sequence': '北 京 是 韩 国 的 首 都 。'},
{'score': 0.031527262181043625,
'token': 2769,
'token_str': '我',
'sequence': '北 京 是 我 国 的 首 都 。'},
{'score': 0.023054633289575577,
'token': 1298,
'token_str': '南',
'sequence': '北 京 是 南 国 的 首 都 。'}
]
```
Here is how to use this model to get the features of a given text in PyTorch:
```python
from transformers import BertTokenizer, BertModel
tokenizer = BertTokenizer.from_pretrained('uer/roberta-base-wwm-chinese-cluecorpussmall')
model = BertModel.from_pretrained("uer/roberta-base-wwm-chinese-cluecorpussmall")
text = "用你喜欢的任何文本替换我。"
encoded_input = tokenizer(text, return_tensors='pt')
output = model(**encoded_input)
```
and in TensorFlow:
```python
from transformers import BertTokenizer, TFBertModel
tokenizer = BertTokenizer.from_pretrained('uer/roberta-base-wwm-chinese-cluecorpussmall')
model = TFBertModel.from_pretrained("uer/roberta-base-wwm-chinese-cluecorpussmall")
text = "用你喜欢的任何文本替换我。"
encoded_input = tokenizer(text, return_tensors='tf')
output = model(encoded_input)
```
## Training data
[CLUECorpusSmall](https://github.com/CLUEbenchmark/CLUECorpus2020/) is used as training data.
## Training procedure
Models are pre-trained by [UER-py](https://github.com/dbiir/UER-py/) on [Tencent Cloud](https://cloud.tencent.com/). We pre-train 1,000,000 steps with a sequence length of 128 and then pre-train 250,000 additional steps with a sequence length of 512. We use the same hyper-parameters on different model sizes.
[jieba](https://github.com/fxsjy/jieba) is used as word segmentation tool.
Taking the case of Whole Word Masking RoBERTa-Medium
Stage1:
```
python3 preprocess.py --corpus_path corpora/cluecorpussmall.txt \
--vocab_path models/google_zh_vocab.txt \
--dataset_path cluecorpussmall_seq128_dataset.pt \
--processes_num 32 --seq_length 128 \
--dynamic_masking --data_processor mlm
```
```
python3 pretrain.py --dataset_path cluecorpussmall_word_seq128_dataset.pt \
--vocab_path models/google_zh_vocab.txt \
--config_path models/bert/medium_config.json \
--output_model_path models/cluecorpussmall_wwm_roberta_medium_seq128_model.bin \
--world_size 8 --gpu_ranks 0 1 2 3 4 5 6 7 \
--total_steps 1000000 --save_checkpoint_steps 100000 --report_steps 50000 \
--learning_rate 1e-4 --batch_size 64 \
--whole_word_masking \
--data_processor mlm --target mlm
```
Stage2:
```
python3 preprocess.py --corpus_path corpora/cluecorpussmall.txt \
--vocab_path models/google_zh_vocab.txt \
--dataset_path cluecorpussmall_seq512_dataset.pt \
--processes_num 32 --seq_length 512 \
--dynamic_masking --data_processor mlm
```
```
python3 pretrain.py --dataset_path cluecorpussmall_seq512_dataset.pt \
--vocab_path models/google_zh_vocab.txt \
--pretrained_model_path models/cluecorpussmall_wwm_roberta_medium_seq128_model.bin-1000000 \
--config_path models/bert/medium_config.json \
--output_model_path models/cluecorpussmall_wwm_roberta_medium_seq512_model.bin \
--world_size 8 --gpu_ranks 0 1 2 3 4 5 6 7 \
--total_steps 250000 --save_checkpoint_steps 50000 --report_steps 10000 \
--learning_rate 5e-5 --batch_size 16 \
--whole_word_masking \
--data_processor mlm --target mlm
```
Finally, we convert the pre-trained model into Huggingface's format:
```
python3 scripts/convert_bert_from_uer_to_huggingface.py --input_model_path models/cluecorpussmall_wwm_roberta_medium_seq512_model.bin \
--output_model_path pytorch_model.bin \
--layers_num 8 --type mlm
```
### BibTeX entry and citation info
```
@article{zhao2019uer,
title={UER: An Open-Source Toolkit for Pre-training Models},
author={Zhao, Zhe and Chen, Hui and Zhang, Jinbin and Zhao, Xin and Liu, Tao and Lu, Wei and Chen, Xi and Deng, Haotang and Ju, Qi and Du, Xiaoyong},
journal={EMNLP-IJCNLP 2019},
pages={241},
year={2019}
}
```
[2_128]:https://huggingface.co/uer/roberta-tiny-wwm-chinese-cluecorpussmall
[4_256]:https://huggingface.co/uer/roberta-mini-wwm-chinese-cluecorpussmall
[4_512]:https://huggingface.co/uer/roberta-small-wwm-chinese-cluecorpussmall
[8_512]:https://huggingface.co/uer/roberta-medium-wwm-chinese-cluecorpussmall
[12_768]:https://huggingface.co/uer/roberta-base-wwm-chinese-cluecorpussmall
[24_1024]:https://huggingface.co/uer/roberta-large-wwm-chinese-cluecorpussmall |
gary109/ai-light-dance_singing3_ft_wav2vec2-large-xlsr-53-v1-5gram | 0c024b28259a5a1b51331c6972b8f4b9aa2982df | 2022-07-29T08:18:10.000Z | [
"pytorch",
"tensorboard",
"wav2vec2",
"automatic-speech-recognition",
"transformers",
"gary109/AI_Light_Dance",
"generated_from_trainer",
"license:apache-2.0",
"model-index"
] | automatic-speech-recognition | false | gary109 | null | gary109/ai-light-dance_singing3_ft_wav2vec2-large-xlsr-53-v1-5gram | 21 | null | transformers | 8,274 | ---
license: apache-2.0
tags:
- automatic-speech-recognition
- gary109/AI_Light_Dance
- generated_from_trainer
model-index:
- name: ai-light-dance_singing3_ft_wav2vec2-large-xlsr-53-v1-5gram
results: []
---
<!-- This model card has been generated automatically according to the information the Trainer had access to. You
should probably proofread and complete it, then remove this comment. -->
# ai-light-dance_singing3_ft_wav2vec2-large-xlsr-53-v1-5gram
This model is a fine-tuned version of [gary109/ai-light-dance_singing3_ft_wav2vec2-large-xlsr-53-v1-5gram](https://huggingface.co/gary109/ai-light-dance_singing3_ft_wav2vec2-large-xlsr-53-v1-5gram) on the GARY109/AI_LIGHT_DANCE - ONSET-SINGING3 dataset.
It achieves the following results on the evaluation set:
- Loss: 0.4693
- Wer: 0.2046
## Model description
More information needed
## Intended uses & limitations
More information needed
## Training and evaluation data
More information needed
## Training procedure
### Training hyperparameters
The following hyperparameters were used during training:
- learning_rate: 4e-06
- train_batch_size: 4
- eval_batch_size: 4
- seed: 42
- gradient_accumulation_steps: 4
- total_train_batch_size: 16
- optimizer: Adam with betas=(0.9,0.999) and epsilon=1e-08
- lr_scheduler_type: linear
- lr_scheduler_warmup_steps: 500
- num_epochs: 100.0
- mixed_precision_training: Native AMP
### Training results
| Training Loss | Epoch | Step | Validation Loss | Wer |
|:-------------:|:-----:|:-----:|:---------------:|:------:|
| 0.3028 | 1.0 | 288 | 0.4693 | 0.2046 |
| 0.2986 | 2.0 | 576 | 0.4828 | 0.2058 |
| 0.297 | 3.0 | 864 | 0.5020 | 0.2038 |
| 0.2863 | 4.0 | 1152 | 0.5216 | 0.2020 |
| 0.3036 | 5.0 | 1440 | 0.4963 | 0.2008 |
| 0.3141 | 6.0 | 1728 | 0.5005 | 0.2020 |
| 0.2898 | 7.0 | 2016 | 0.4962 | 0.2029 |
| 0.2922 | 8.0 | 2304 | 0.5073 | 0.2031 |
| 0.266 | 9.0 | 2592 | 0.5159 | 0.2024 |
| 0.2817 | 10.0 | 2880 | 0.5238 | 0.2011 |
| 0.2922 | 11.0 | 3168 | 0.5080 | 0.2011 |
| 0.2869 | 12.0 | 3456 | 0.4974 | 0.2027 |
| 0.284 | 13.0 | 3744 | 0.5104 | 0.2006 |
| 0.2911 | 14.0 | 4032 | 0.5026 | 0.2017 |
| 0.2864 | 15.0 | 4320 | 0.5065 | 0.2002 |
| 0.2779 | 16.0 | 4608 | 0.5024 | 0.2010 |
| 0.2766 | 17.0 | 4896 | 0.5078 | 0.1998 |
| 0.2872 | 18.0 | 5184 | 0.5114 | 0.1981 |
| 0.268 | 19.0 | 5472 | 0.5078 | 0.1980 |
| 0.2631 | 20.0 | 5760 | 0.5262 | 0.2021 |
| 0.2753 | 21.0 | 6048 | 0.5161 | 0.1991 |
| 0.2797 | 22.0 | 6336 | 0.5097 | 0.2009 |
| 0.2667 | 23.0 | 6624 | 0.5131 | 0.1995 |
| 0.2722 | 24.0 | 6912 | 0.5098 | 0.1990 |
| 0.3026 | 25.0 | 7200 | 0.5193 | 0.2006 |
| 0.2888 | 26.0 | 7488 | 0.4987 | 0.1986 |
| 0.2732 | 27.0 | 7776 | 0.5063 | 0.2007 |
| 0.2567 | 28.0 | 8064 | 0.5103 | 0.2015 |
| 0.2845 | 29.0 | 8352 | 0.5084 | 0.2020 |
| 0.2591 | 30.0 | 8640 | 0.5109 | 0.1989 |
| 0.2777 | 31.0 | 8928 | 0.5179 | 0.1994 |
| 0.2784 | 32.0 | 9216 | 0.5183 | 0.1989 |
| 0.2801 | 33.0 | 9504 | 0.5222 | 0.2003 |
| 0.2554 | 34.0 | 9792 | 0.5137 | 0.1990 |
| 0.2708 | 35.0 | 10080 | 0.5094 | 0.1964 |
| 0.27 | 36.0 | 10368 | 0.5076 | 0.1980 |
| 0.2706 | 37.0 | 10656 | 0.5179 | 0.1983 |
| 0.2791 | 38.0 | 10944 | 0.5154 | 0.1976 |
| 0.3148 | 39.0 | 11232 | 0.5082 | 0.1990 |
| 0.2834 | 40.0 | 11520 | 0.5107 | 0.1980 |
| 0.2739 | 41.0 | 11808 | 0.5009 | 0.1990 |
| 0.2687 | 42.0 | 12096 | 0.5232 | 0.2011 |
| 0.2696 | 43.0 | 12384 | 0.5108 | 0.1986 |
| 0.2729 | 44.0 | 12672 | 0.5159 | 0.1991 |
| 0.2579 | 45.0 | 12960 | 0.5162 | 0.1991 |
| 0.283 | 46.0 | 13248 | 0.5032 | 0.1982 |
| 0.282 | 47.0 | 13536 | 0.5107 | 0.1980 |
| 0.2708 | 48.0 | 13824 | 0.5128 | 0.1982 |
| 0.2562 | 49.0 | 14112 | 0.5163 | 0.1991 |
| 0.2675 | 50.0 | 14400 | 0.5062 | 0.1994 |
| 0.285 | 51.0 | 14688 | 0.4999 | 0.1988 |
| 0.2756 | 52.0 | 14976 | 0.5030 | 0.1986 |
| 0.2888 | 53.0 | 15264 | 0.5043 | 0.1975 |
| 0.2778 | 54.0 | 15552 | 0.5111 | 0.1980 |
| 0.2707 | 55.0 | 15840 | 0.5117 | 0.1995 |
| 0.2566 | 56.0 | 16128 | 0.5197 | 0.2002 |
| 0.2517 | 57.0 | 16416 | 0.5211 | 0.1977 |
| 0.2629 | 58.0 | 16704 | 0.5080 | 0.1986 |
| 0.2787 | 59.0 | 16992 | 0.5133 | 0.1980 |
| 0.269 | 60.0 | 17280 | 0.5156 | 0.1973 |
| 0.2664 | 61.0 | 17568 | 0.5192 | 0.1949 |
| 0.2605 | 62.0 | 17856 | 0.5095 | 0.1970 |
| 0.2649 | 63.0 | 18144 | 0.5149 | 0.1970 |
| 0.246 | 64.0 | 18432 | 0.5165 | 0.1975 |
| 0.2567 | 65.0 | 18720 | 0.5072 | 0.1981 |
| 0.2509 | 66.0 | 19008 | 0.5061 | 0.1978 |
| 0.289 | 67.0 | 19296 | 0.5087 | 0.1957 |
| 0.2511 | 68.0 | 19584 | 0.5168 | 0.1982 |
| 0.2623 | 69.0 | 19872 | 0.5110 | 0.1959 |
| 0.2762 | 70.0 | 20160 | 0.5123 | 0.1959 |
| 0.2704 | 71.0 | 20448 | 0.5118 | 0.1966 |
| 0.2854 | 72.0 | 20736 | 0.5128 | 0.1949 |
| 0.2602 | 73.0 | 21024 | 0.5094 | 0.1966 |
| 0.2675 | 74.0 | 21312 | 0.5058 | 0.1961 |
| 0.2519 | 75.0 | 21600 | 0.5216 | 0.1988 |
| 0.2666 | 76.0 | 21888 | 0.5117 | 0.1959 |
| 0.2637 | 77.0 | 22176 | 0.5058 | 0.1957 |
| 0.273 | 78.0 | 22464 | 0.5187 | 0.1966 |
| 0.2666 | 79.0 | 22752 | 0.5176 | 0.1958 |
| 0.2627 | 80.0 | 23040 | 0.5142 | 0.1950 |
| 0.2508 | 81.0 | 23328 | 0.5158 | 0.1961 |
| 0.2499 | 82.0 | 23616 | 0.5131 | 0.1970 |
| 0.2583 | 83.0 | 23904 | 0.5150 | 0.1975 |
| 0.246 | 84.0 | 24192 | 0.5097 | 0.1962 |
| 0.272 | 85.0 | 24480 | 0.5043 | 0.1950 |
| 0.2601 | 86.0 | 24768 | 0.5091 | 0.1961 |
| 0.2719 | 87.0 | 25056 | 0.5087 | 0.1975 |
| 0.269 | 88.0 | 25344 | 0.5126 | 0.1966 |
| 0.2863 | 89.0 | 25632 | 0.5174 | 0.1966 |
| 0.2581 | 90.0 | 25920 | 0.5159 | 0.1969 |
| 0.26 | 91.0 | 26208 | 0.5146 | 0.1969 |
| 0.2796 | 92.0 | 26496 | 0.5150 | 0.1966 |
| 0.2723 | 93.0 | 26784 | 0.5133 | 0.1971 |
| 0.249 | 94.0 | 27072 | 0.5096 | 0.1961 |
| 0.266 | 95.0 | 27360 | 0.5116 | 0.1964 |
| 0.2683 | 96.0 | 27648 | 0.5133 | 0.1967 |
| 0.2451 | 97.0 | 27936 | 0.5141 | 0.1965 |
| 0.2723 | 98.0 | 28224 | 0.5123 | 0.1962 |
| 0.2527 | 99.0 | 28512 | 0.5120 | 0.1966 |
| 0.2604 | 100.0 | 28800 | 0.5111 | 0.1961 |
### Framework versions
- Transformers 4.21.0.dev0
- Pytorch 1.9.1+cu102
- Datasets 2.3.3.dev0
- Tokenizers 0.12.1
|
xhyi/layoutlmv3_docvqa_t11c5000 | 0965ab663472459606e8c8c5ee126157725a3d9a | 2022-07-22T18:53:47.000Z | [
"pytorch",
"layoutlmv3",
"question-answering",
"transformers",
"autotrain_compatible"
] | question-answering | false | xhyi | null | xhyi/layoutlmv3_docvqa_t11c5000 | 21 | null | transformers | 8,275 |
# LayoutLMv3: DocVQA Replication WIP
See experiments code: <https://github.com/redthing1/layoutlm_experiments>
|
razhan/codeqmul | 2da18b55944a2b6d0ea73dc2c746a501bf611a02 | 2022-07-28T18:25:06.000Z | [
"pytorch",
"gpt2",
"text-generation",
"transformers"
] | text-generation | false | razhan | null | razhan/codeqmul | 21 | null | transformers | 8,276 | Entry not found |
luffycodes/luke-base-conll | 85ae436586c1f3944ba07950f4050dbf86bba7b2 | 2022-07-29T04:32:14.000Z | [
"pytorch",
"luke",
"allennlp"
] | null | false | luffycodes | null | luffycodes/luke-base-conll | 21 | null | allennlp | 8,277 | ---
tags:
- allennlp
---
# TODO: Fill this model card
---
tags:
- allennlp
---
# TODO: Fill this model card
|
Aastha/wav2vec2-large-xls-r-300m-tr-colab | 10ec5e7ec56346b18f5e3d9fbc189b390f03a62f | 2022-01-23T20:43:52.000Z | [
"pytorch",
"tensorboard",
"wav2vec2",
"automatic-speech-recognition",
"transformers"
] | automatic-speech-recognition | false | Aastha | null | Aastha/wav2vec2-large-xls-r-300m-tr-colab | 20 | null | transformers | 8,278 | Entry not found |
AndrewMcDowell/wav2vec2-xls-r-300m-japanese | 831b85ecd8b3adc91e8de38984f761bcce9bfef6 | 2022-03-23T18:34:20.000Z | [
"pytorch",
"wav2vec2",
"automatic-speech-recognition",
"ja",
"dataset:mozilla-foundation/common_voice_8_0",
"transformers",
"generated_from_trainer",
"hf-asr-leaderboard",
"mozilla-foundation/common_voice_8_0",
"robust-speech-event",
"license:apache-2.0",
"model-index"
] | automatic-speech-recognition | false | AndrewMcDowell | null | AndrewMcDowell/wav2vec2-xls-r-300m-japanese | 20 | null | transformers | 8,279 | ---
language:
- ja
license: apache-2.0
tags:
- automatic-speech-recognition
- generated_from_trainer
- hf-asr-leaderboard
- ja
- mozilla-foundation/common_voice_8_0
- robust-speech-event
datasets:
- mozilla-foundation/common_voice_8_0
model-index:
- name: XLS-R-300-m
results:
- task:
name: Automatic Speech Recognition
type: automatic-speech-recognition
dataset:
name: Common Voice 8
type: mozilla-foundation/common_voice_8_0
args: ja
metrics:
- name: Test WER
type: wer
value: 95.82
- name: Test CER
type: cer
value: 23.64
- task:
name: Automatic Speech Recognition
type: automatic-speech-recognition
dataset:
name: Robust Speech Event - Dev Data
type: speech-recognition-community-v2/dev_data
args: de
metrics:
- name: Test WER
type: wer
value: 100.0
- name: Test CER
type: cer
value: 30.99
- task:
name: Automatic Speech Recognition
type: automatic-speech-recognition
dataset:
name: Robust Speech Event - Dev Data
type: speech-recognition-community-v2/dev_data
args: ja
metrics:
- name: Test CER
type: cer
value: 30.37
- task:
name: Automatic Speech Recognition
type: automatic-speech-recognition
dataset:
name: Robust Speech Event - Test Data
type: speech-recognition-community-v2/eval_data
args: ja
metrics:
- name: Test CER
type: cer
value: 34.42
---
<!-- This model card has been generated automatically according to the information the Trainer had access to. You
should probably proofread and complete it, then remove this comment. -->
#
This model is a fine-tuned version of [facebook/wav2vec2-xls-r-300m](https://huggingface.co/facebook/wav2vec2-xls-r-300m) on the MOZILLA-FOUNDATION/COMMON_VOICE_8_0 - JA dataset.
Kanji are converted into Hiragana using the [pykakasi](https://pykakasi.readthedocs.io/en/latest/index.html) library during training and evaluation. The model can output both Hiragana and Katakana characters. Since there is no spacing, WER is not a suitable metric for evaluating performance and CER is more suitable.
On mozilla-foundation/common_voice_8_0 it achieved:
- cer: 23.64%
On speech-recognition-community-v2/dev_data it achieved:
- cer: 30.99%
It achieves the following results on the evaluation set:
- Loss: 0.5212
- Wer: 1.3068
## Model description
More information needed
## Intended uses & limitations
More information needed
## Training and evaluation data
More information needed
## Training procedure
### Training hyperparameters
The following hyperparameters were used during training:
- learning_rate: 7.5e-05
- train_batch_size: 48
- eval_batch_size: 8
- seed: 42
- optimizer: Adam with betas=(0.9,0.999) and epsilon=1e-08
- lr_scheduler_type: linear
- lr_scheduler_warmup_steps: 2000
- num_epochs: 50.0
- mixed_precision_training: Native AMP
### Training results
| Training Loss | Epoch | Step | Validation Loss | Wer |
|:-------------:|:-----:|:-----:|:---------------:|:------:|
| 4.0974 | 4.72 | 1000 | 4.0178 | 1.9535 |
| 2.1276 | 9.43 | 2000 | 0.9301 | 1.2128 |
| 1.7622 | 14.15 | 3000 | 0.7103 | 1.5527 |
| 1.6397 | 18.87 | 4000 | 0.6729 | 1.4269 |
| 1.5468 | 23.58 | 5000 | 0.6087 | 1.2497 |
| 1.4885 | 28.3 | 6000 | 0.5786 | 1.3222 |
| 1.451 | 33.02 | 7000 | 0.5726 | 1.3768 |
| 1.3912 | 37.74 | 8000 | 0.5518 | 1.2497 |
| 1.3617 | 42.45 | 9000 | 0.5352 | 1.2694 |
| 1.3113 | 47.17 | 10000 | 0.5228 | 1.2781 |
### Framework versions
- Transformers 4.17.0.dev0
- Pytorch 1.10.2+cu102
- Datasets 1.18.2.dev0
- Tokenizers 0.11.0
#### Evaluation Commands
1. To evaluate on `mozilla-foundation/common_voice_8_0` with split `test`
```bash
python ./eval.py --model_id AndrewMcDowell/wav2vec2-xls-r-300m-japanese --dataset mozilla-foundation/common_voice_8_0 --config ja --split test --log_outputs
```
2. To evaluate on `mozilla-foundation/common_voice_8_0` with split `test`
```bash
python ./eval.py --model_id AndrewMcDowell/wav2vec2-xls-r-300m-japanese --dataset speech-recognition-community-v2/dev_data --config de --split validation --chunk_length_s 5.0 --stride_length_s 1.0
``` |
Andrija/M-bert-NER | c21a97f630f3ce9c97de7b2a5844d3d9101ca6c5 | 2021-08-13T09:46:42.000Z | [
"pytorch",
"bert",
"token-classification",
"hr",
"sr",
"dataset:hr500k",
"transformers",
"license:apache-2.0",
"autotrain_compatible"
] | token-classification | false | Andrija | null | Andrija/M-bert-NER | 20 | null | transformers | 8,280 | ---
datasets:
- hr500k
language:
- hr
- sr
widget:
- text: "Moje ime je Aleksandar i zivim u Beogradu pored Vlade Republike Srbije"
license: apache-2.0
---
Named Entity Recognition (Token Classification Head) for Serbian / Croatian languges.
Abbreviation|Description
-|-
O|Outside of a named entity
B-MIS |Beginning of a miscellaneous entity right after another miscellaneous entity
I-MIS | Miscellaneous entity
B-PER |Beginning of a person’s name right after another person’s name
B-DERIV-PER| Begginning derivative that describes relation to a person
I-PER |Person’s name
B-ORG |Beginning of an organization right after another organization
I-ORG |organization
B-LOC |Beginning of a location right after another location
I-LOC |Location |
Ayoola/cdial-yoruba-test | 9e6978cfe41814448ae39be633008abdd6d254a8 | 2021-12-12T09:21:21.000Z | [
"pytorch",
"wav2vec2",
"automatic-speech-recognition",
"transformers"
] | automatic-speech-recognition | false | Ayoola | null | Ayoola/cdial-yoruba-test | 20 | 1 | transformers | 8,281 | Entry not found |
BlindMan820/Sarcastic-News-Headlines | b499a7ba3786864ea571f8de1400d7e9a96fb674 | 2022-01-21T13:31:44.000Z | [
"pytorch",
"distilbert",
"text-classification",
"English",
"dataset:Kaggle Dataset",
"transformers",
"Text",
"Sequence-Classification",
"Sarcasm",
"DistilBert"
] | text-classification | false | BlindMan820 | null | BlindMan820/Sarcastic-News-Headlines | 20 | null | transformers | 8,282 | ---
language:
- English
tags:
- Text
- Sequence-Classification
- Sarcasm
- DistilBert
datasets:
- Kaggle Dataset
metrics :
- precision
- recall
- f1
---
Dataset Link - https://www.kaggle.com/rmisra/news-headlines-dataset-for-sarcasm-detection |
CenIA/bert-base-spanish-wwm-cased-finetuned-ner | 0cf7cc10bc005707fa8a70ba3739c7d1b50b2630 | 2022-01-06T20:06:50.000Z | [
"pytorch",
"bert",
"token-classification",
"transformers",
"autotrain_compatible"
] | token-classification | false | CenIA | null | CenIA/bert-base-spanish-wwm-cased-finetuned-ner | 20 | null | transformers | 8,283 | Entry not found |
DaNLP/da-xlmr-ned | 4a030b975894a7b9b17e9a9801dd18d1cd727d50 | 2021-09-17T12:10:46.000Z | [
"pytorch",
"tf",
"xlm-roberta",
"text-classification",
"da",
"dataset:DaNED",
"dataset:DaWikiNED",
"transformers",
"ned",
"license:cc-by-sa-4.0"
] | text-classification | false | DaNLP | null | DaNLP/da-xlmr-ned | 20 | null | transformers | 8,284 |
---
language:
- da
tags:
- ned
- xlm-roberta
- pytorch
- transformers
license: cc-by-sa-4.0
datasets:
- DaNED
- DaWikiNED
metrics:
- f1
---
# XLM-Roberta fine-tuned for Named Entity Disambiguation
Given a sentence and a knowledge graph context, the model detects whether a specific entity (represented by the knowledge graph context) is mentioned in the sentence (binary classification).
The base language model used is the [xlm-roberta-base](https://huggingface.co/xlm-roberta-base).
Here is how to use the model:
```python
from transformers import XLMRobertaTokenizer, XLMRobertaForSequenceClassification
model = XLMRobertaForSequenceClassification.from_pretrained("DaNLP/da-xlmr-ned")
tokenizer = XLMRobertaTokenizer.from_pretrained("DaNLP/da-xlmr-ned")
```
The tokenizer takes 2 strings has input: the sentence and the knowledge graph (KG) context.
Here is an example:
```python
sentence = "Karen Blixen vendte tilbage til Danmark, hvor hun boede resten af sit liv på Rungstedlund, som hun arvede efter sin mor i 1939"
kg_context = "udmærkelser modtaget Kritikerprisen udmærkelser modtaget Tagea Brandts Rejselegat udmærkelser modtaget Ingenio et arti udmærkelser modtaget Holbergmedaljen udmærkelser modtaget De Gyldne Laurbær mor Ingeborg Dinesen ægtefælle Bror von Blixen-Finecke køn kvinde Commons-kategori Karen Blixen LCAuth no95003722 VIAF 90663542 VIAF 121643918 GND-identifikator 118637878 ISNI 0000 0001 2096 6265 ISNI 0000 0003 6863 4408 ISNI 0000 0001 1891 0457 fødested Rungstedlund fødested Rungsted dødssted Rungstedlund dødssted København statsborgerskab Danmark NDL-nummer 00433530 dødsdato +1962-09-07T00:00:00Z dødsdato +1962-01-01T00:00:00Z fødselsdato +1885-04-17T00:00:00Z fødselsdato +1885-01-01T00:00:00Z AUT NKC jn20000600905 AUT NKC jo2015880827 AUT NKC xx0196181 emnets hovedkategori Kategori:Karen Blixen tilfælde af menneske billede Karen Blixen cropped from larger original.jpg IMDb-identifikationsnummer nm0227598 Freebase-ID /m/04ymd8w BNF 118857710 beskæftigelse skribent beskæftigelse selvbiograf beskæftigelse novelleforfatter ..."
```
A KG context, for a specific entity, can be generated from its Wikidata page.
In the previous example, the KG context is a string representation of the Wikidata page of [Karen Blixen (QID=Q182804)](https://www.wikidata.org/wiki/Q182804).
See the [DaNLP documentation](https://danlp-alexandra.readthedocs.io/en/latest/docs/tasks/ned.html#xlmr) for more details about how to generate a KG context.
## Training Data
The model has been trained on the [DaNED](https://danlp-alexandra.readthedocs.io/en/latest/docs/datasets.html#daned) and [DaWikiNED](https://danlp-alexandra.readthedocs.io/en/latest/docs/datasets.html#dawikined) datasets.
|
Darkrider/covidbert_medmarco | dcf299f1f7e791b63479cd7c5d3c10264592f62b | 2021-05-18T18:08:55.000Z | [
"pytorch",
"jax",
"bert",
"text-classification",
"arxiv:2010.05987",
"transformers"
] | text-classification | false | Darkrider | null | Darkrider/covidbert_medmarco | 20 | null | transformers | 8,285 | Fine-tuned CovidBERT on Med-Marco Dataset for passage ranking
# CovidBERT-MedNLI
This is the model **CovidBERT** trained by DeepSet on AllenAI's [CORD19 Dataset](https://pages.semanticscholar.org/coronavirus-research) of scientific articles about coronaviruses.
The model uses the original BERT wordpiece vocabulary and was subsequently fine-tuned on the [SNLI](https://nlp.stanford.edu/projects/snli/) and the [MultiNLI](https://www.nyu.edu/projects/bowman/multinli/) datasets using the [`sentence-transformers` library](https://github.com/UKPLab/sentence-transformers/) to produce universal sentence embeddings [1] using the **average pooling strategy** and a **softmax loss**.
It is further fine-tuned Med-Marco Dataset. MacAvaney et.al in their [paper](https://arxiv.org/abs/2010.05987) titled “SLEDGE-Z: A Zero-Shot Baseline for COVID-19 Literature Search” used MedSyn a lexicon of layperson and expert terminology for various medical conditions to filter for medical questions. One can also replace this by UMLs ontologies but the beauty of MedSyn is that the terms are more general human conversation lingo and not terms based on scientific literature.
Parameter details for the original training on CORD-19 are available on [DeepSet's MLFlow](https://public-mlflow.deepset.ai/#/experiments/2/runs/ba27d00c30044ef6a33b1d307b4a6cba)
**Base model**: `deepset/covid_bert_base` from HuggingFace's `AutoModel`.
|
Davlan/bert-base-multilingual-cased-masakhaner | b541a4b146aa178ddb6783638bbaa2ba86d9d349 | 2022-06-27T11:50:04.000Z | [
"pytorch",
"tf",
"bert",
"token-classification",
"ha",
"ig",
"rw",
"lg",
"luo",
"pcm",
"sw",
"wo",
"yo",
"multilingual",
"dataset:masakhaner",
"arxiv:2103.11811",
"transformers",
"autotrain_compatible"
] | token-classification | false | Davlan | null | Davlan/bert-base-multilingual-cased-masakhaner | 20 | null | transformers | 8,286 | Hugging Face's logo
---
language:
- ha
- ig
- rw
- lg
- luo
- pcm
- sw
- wo
- yo
- multilingual
datasets:
- masakhaner
---
# bert-base-multilingual-cased-masakhaner
## Model description
**bert-base-multilingual-cased-masakhaner** is the first **Named Entity Recognition** model for 9 African languages (Hausa, Igbo, Kinyarwanda, Luganda, Nigerian Pidgin, Swahilu, Wolof, and Yorùbá) based on a fine-tuned mBERT base model. It achieves the **state-of-the-art performance** for the NER task. It has been trained to recognize four types of entities: dates & times (DATE), location (LOC), organizations (ORG), and person (PER).
Specifically, this model is a *bert-base-multilingual-cased* model that was fine-tuned on an aggregation of African language datasets obtained from Masakhane [MasakhaNER](https://github.com/masakhane-io/masakhane-ner) dataset.
## Intended uses & limitations
#### How to use
You can use this model with Transformers *pipeline* for NER.
```python
from transformers import AutoTokenizer, AutoModelForTokenClassification
from transformers import pipeline
tokenizer = AutoTokenizer.from_pretrained("Davlan/bert-base-multilingual-cased-masakhaner")
model = AutoModelForTokenClassification.from_pretrained("Davlan/bert-base-multilingual-cased-masakhaner")
nlp = pipeline("ner", model=model, tokenizer=tokenizer)
example = "Emir of Kano turban Zhang wey don spend 18 years for Nigeria"
ner_results = nlp(example)
print(ner_results)
```
#### Limitations and bias
This model is limited by its training dataset of entity-annotated news articles from a specific span of time. This may not generalize well for all use cases in different domains.
## Training data
This model was fine-tuned on 9 African NER datasets (Hausa, Igbo, Kinyarwanda, Luganda, Nigerian Pidgin, Swahilu, Wolof, and Yorùbá) Masakhane [MasakhaNER](https://github.com/masakhane-io/masakhane-ner) dataset
The training dataset distinguishes between the beginning and continuation of an entity so that if there are back-to-back entities of the same type, the model can output where the second entity begins. As in the dataset, each token will be classified as one of the following classes:
Abbreviation|Description
-|-
O|Outside of a named entity
B-DATE |Beginning of a DATE entity right after another DATE entity
I-DATE |DATE entity
B-PER |Beginning of a person’s name right after another person’s name
I-PER |Person’s name
B-ORG |Beginning of an organisation right after another organisation
I-ORG |Organisation
B-LOC |Beginning of a location right after another location
I-LOC |Location
## Training procedure
This model was trained on a single NVIDIA V100 GPU with recommended hyperparameters from the [original MasakhaNER paper](https://arxiv.org/abs/2103.11811) which trained & evaluated the model on MasakhaNER corpus.
## Eval results on Test set (F-score)
language|F1-score
-|-
hau |88.66
ibo |85.72
kin |71.94
lug |81.73
luo |77.39
pcm |88.96
swa |88.23
wol |66.27
yor |80.09
### BibTeX entry and citation info
```
@article{adelani21tacl,
title = {Masakha{NER}: Named Entity Recognition for African Languages},
author = {David Ifeoluwa Adelani and Jade Abbott and Graham Neubig and Daniel D'souza and Julia Kreutzer and Constantine Lignos and Chester Palen-Michel and Happy Buzaaba and Shruti Rijhwani and Sebastian Ruder and Stephen Mayhew and Israel Abebe Azime and Shamsuddeen Muhammad and Chris Chinenye Emezue and Joyce Nakatumba-Nabende and Perez Ogayo and Anuoluwapo Aremu and Catherine Gitau and Derguene Mbaye and Jesujoba Alabi and Seid Muhie Yimam and Tajuddeen Gwadabe and Ignatius Ezeani and Rubungo Andre Niyongabo and Jonathan Mukiibi and Verrah Otiende and Iroro Orife and Davis David and Samba Ngom and Tosin Adewumi and Paul Rayson and Mofetoluwa Adeyemi and Gerald Muriuki and Emmanuel Anebi and Chiamaka Chukwuneke and Nkiruka Odu and Eric Peter Wairagala and Samuel Oyerinde and Clemencia Siro and Tobius Saul Bateesa and Temilola Oloyede and Yvonne Wambui and Victor Akinode and Deborah Nabagereka and Maurice Katusiime and Ayodele Awokoya and Mouhamadane MBOUP and Dibora Gebreyohannes and Henok Tilaye and Kelechi Nwaike and Degaga Wolde and Abdoulaye Faye and Blessing Sibanda and Orevaoghene Ahia and Bonaventure F. P. Dossou and Kelechi Ogueji and Thierno Ibrahima DIOP and Abdoulaye Diallo and Adewale Akinfaderin and Tendai Marengereke and Salomey Osei},
journal = {Transactions of the Association for Computational Linguistics (TACL)},
month = {},
url = {https://arxiv.org/abs/2103.11811},
year = {2021}
}
```
|
Geotrend/bert-base-zh-cased | 21847ddf72c2f6cfb6b2d214e04d5804fb8c2d12 | 2021-05-18T20:16:15.000Z | [
"pytorch",
"tf",
"jax",
"bert",
"fill-mask",
"zh",
"dataset:wikipedia",
"transformers",
"license:apache-2.0",
"autotrain_compatible"
] | fill-mask | false | Geotrend | null | Geotrend/bert-base-zh-cased | 20 | null | transformers | 8,287 | ---
language: zh
datasets: wikipedia
license: apache-2.0
---
# bert-base-zh-cased
We are sharing smaller versions of [bert-base-multilingual-cased](https://huggingface.co/bert-base-multilingual-cased) that handle a custom number of languages.
Unlike [distilbert-base-multilingual-cased](https://huggingface.co/distilbert-base-multilingual-cased), our versions give exactly the same representations produced by the original model which preserves the original accuracy.
For more information please visit our paper: [Load What You Need: Smaller Versions of Multilingual BERT](https://www.aclweb.org/anthology/2020.sustainlp-1.16.pdf).
## How to use
```python
from transformers import AutoTokenizer, AutoModel
tokenizer = AutoTokenizer.from_pretrained("Geotrend/bert-base-zh-cased")
model = AutoModel.from_pretrained("Geotrend/bert-base-zh-cased")
```
To generate other smaller versions of multilingual transformers please visit [our Github repo](https://github.com/Geotrend-research/smaller-transformers).
### How to cite
```bibtex
@inproceedings{smallermbert,
title={Load What You Need: Smaller Versions of Mutlilingual BERT},
author={Abdaoui, Amine and Pradel, Camille and Sigel, Grégoire},
booktitle={SustaiNLP / EMNLP},
year={2020}
}
```
## Contact
Please contact [email protected] for any question, feedback or request.
|
Helsinki-NLP/opus-mt-en-ts | 8093589efb39ad94f79db8e22c3dbabd6d598310 | 2021-09-09T21:40:13.000Z | [
"pytorch",
"marian",
"text2text-generation",
"en",
"ts",
"transformers",
"translation",
"license:apache-2.0",
"autotrain_compatible"
] | translation | false | Helsinki-NLP | null | Helsinki-NLP/opus-mt-en-ts | 20 | null | transformers | 8,288 | ---
tags:
- translation
license: apache-2.0
---
### opus-mt-en-ts
* source languages: en
* target languages: ts
* OPUS readme: [en-ts](https://github.com/Helsinki-NLP/OPUS-MT-train/blob/master/models/en-ts/README.md)
* dataset: opus
* model: transformer-align
* pre-processing: normalization + SentencePiece
* download original weights: [opus-2020-01-08.zip](https://object.pouta.csc.fi/OPUS-MT-models/en-ts/opus-2020-01-08.zip)
* test set translations: [opus-2020-01-08.test.txt](https://object.pouta.csc.fi/OPUS-MT-models/en-ts/opus-2020-01-08.test.txt)
* test set scores: [opus-2020-01-08.eval.txt](https://object.pouta.csc.fi/OPUS-MT-models/en-ts/opus-2020-01-08.eval.txt)
## Benchmarks
| testset | BLEU | chr-F |
|-----------------------|-------|-------|
| JW300.en.ts | 43.4 | 0.639 |
|
Helsinki-NLP/opus-mt-ja-sv | 1c70b4dc2dc82ada9a036ac3a4bc2cf552be3201 | 2021-09-10T13:53:27.000Z | [
"pytorch",
"marian",
"text2text-generation",
"ja",
"sv",
"transformers",
"translation",
"license:apache-2.0",
"autotrain_compatible"
] | translation | false | Helsinki-NLP | null | Helsinki-NLP/opus-mt-ja-sv | 20 | null | transformers | 8,289 | ---
tags:
- translation
license: apache-2.0
---
### opus-mt-ja-sv
* source languages: ja
* target languages: sv
* OPUS readme: [ja-sv](https://github.com/Helsinki-NLP/OPUS-MT-train/blob/master/models/ja-sv/README.md)
* dataset: opus
* model: transformer-align
* pre-processing: normalization + SentencePiece
* download original weights: [opus-2020-01-09.zip](https://object.pouta.csc.fi/OPUS-MT-models/ja-sv/opus-2020-01-09.zip)
* test set translations: [opus-2020-01-09.test.txt](https://object.pouta.csc.fi/OPUS-MT-models/ja-sv/opus-2020-01-09.test.txt)
* test set scores: [opus-2020-01-09.eval.txt](https://object.pouta.csc.fi/OPUS-MT-models/ja-sv/opus-2020-01-09.eval.txt)
## Benchmarks
| testset | BLEU | chr-F |
|-----------------------|-------|-------|
| Tatoeba.ja.sv | 26.1 | 0.445 |
|
Helsinki-NLP/opus-mt-kqn-en | 2fcb140432b927a8d8c726edda2fd73bf7d54378 | 2021-09-10T13:54:08.000Z | [
"pytorch",
"marian",
"text2text-generation",
"kqn",
"en",
"transformers",
"translation",
"license:apache-2.0",
"autotrain_compatible"
] | translation | false | Helsinki-NLP | null | Helsinki-NLP/opus-mt-kqn-en | 20 | null | transformers | 8,290 | ---
tags:
- translation
license: apache-2.0
---
### opus-mt-kqn-en
* source languages: kqn
* target languages: en
* OPUS readme: [kqn-en](https://github.com/Helsinki-NLP/OPUS-MT-train/blob/master/models/kqn-en/README.md)
* dataset: opus
* model: transformer-align
* pre-processing: normalization + SentencePiece
* download original weights: [opus-2020-01-09.zip](https://object.pouta.csc.fi/OPUS-MT-models/kqn-en/opus-2020-01-09.zip)
* test set translations: [opus-2020-01-09.test.txt](https://object.pouta.csc.fi/OPUS-MT-models/kqn-en/opus-2020-01-09.test.txt)
* test set scores: [opus-2020-01-09.eval.txt](https://object.pouta.csc.fi/OPUS-MT-models/kqn-en/opus-2020-01-09.eval.txt)
## Benchmarks
| testset | BLEU | chr-F |
|-----------------------|-------|-------|
| JW300.kqn.en | 32.6 | 0.480 |
|
Helsinki-NLP/opus-mt-loz-en | d975d0dcd374d47e05c15069ad5f63296d53a3c0 | 2021-09-10T13:55:15.000Z | [
"pytorch",
"marian",
"text2text-generation",
"loz",
"en",
"transformers",
"translation",
"license:apache-2.0",
"autotrain_compatible"
] | translation | false | Helsinki-NLP | null | Helsinki-NLP/opus-mt-loz-en | 20 | null | transformers | 8,291 | ---
tags:
- translation
license: apache-2.0
---
### opus-mt-loz-en
* source languages: loz
* target languages: en
* OPUS readme: [loz-en](https://github.com/Helsinki-NLP/OPUS-MT-train/blob/master/models/loz-en/README.md)
* dataset: opus
* model: transformer-align
* pre-processing: normalization + SentencePiece
* download original weights: [opus-2020-01-09.zip](https://object.pouta.csc.fi/OPUS-MT-models/loz-en/opus-2020-01-09.zip)
* test set translations: [opus-2020-01-09.test.txt](https://object.pouta.csc.fi/OPUS-MT-models/loz-en/opus-2020-01-09.test.txt)
* test set scores: [opus-2020-01-09.eval.txt](https://object.pouta.csc.fi/OPUS-MT-models/loz-en/opus-2020-01-09.eval.txt)
## Benchmarks
| testset | BLEU | chr-F |
|-----------------------|-------|-------|
| JW300.loz.en | 42.1 | 0.565 |
|
Helsinki-NLP/opus-mt-lun-en | 79da48c57f71876f29327472e09943a1cda155bf | 2021-09-10T13:56:41.000Z | [
"pytorch",
"marian",
"text2text-generation",
"lun",
"en",
"transformers",
"translation",
"license:apache-2.0",
"autotrain_compatible"
] | translation | false | Helsinki-NLP | null | Helsinki-NLP/opus-mt-lun-en | 20 | null | transformers | 8,292 | ---
tags:
- translation
license: apache-2.0
---
### opus-mt-lun-en
* source languages: lun
* target languages: en
* OPUS readme: [lun-en](https://github.com/Helsinki-NLP/OPUS-MT-train/blob/master/models/lun-en/README.md)
* dataset: opus
* model: transformer-align
* pre-processing: normalization + SentencePiece
* download original weights: [opus-2020-01-09.zip](https://object.pouta.csc.fi/OPUS-MT-models/lun-en/opus-2020-01-09.zip)
* test set translations: [opus-2020-01-09.test.txt](https://object.pouta.csc.fi/OPUS-MT-models/lun-en/opus-2020-01-09.test.txt)
* test set scores: [opus-2020-01-09.eval.txt](https://object.pouta.csc.fi/OPUS-MT-models/lun-en/opus-2020-01-09.eval.txt)
## Benchmarks
| testset | BLEU | chr-F |
|-----------------------|-------|-------|
| JW300.lun.en | 30.6 | 0.466 |
|
Helsinki-NLP/opus-mt-phi-en | e82e04a34f749e0f6a21beff9e7415dfa21d04d2 | 2020-08-21T14:42:48.000Z | [
"pytorch",
"marian",
"text2text-generation",
"phi",
"en",
"transformers",
"translation",
"license:apache-2.0",
"autotrain_compatible"
] | translation | false | Helsinki-NLP | null | Helsinki-NLP/opus-mt-phi-en | 20 | null | transformers | 8,293 | ---
language:
- phi
- en
tags:
- translation
license: apache-2.0
---
### phi-eng
* source group: Philippine languages
* target group: English
* OPUS readme: [phi-eng](https://github.com/Helsinki-NLP/Tatoeba-Challenge/tree/master/models/phi-eng/README.md)
* model: transformer
* source language(s): akl_Latn ceb hil ilo pag war
* target language(s): eng
* model: transformer
* pre-processing: normalization + SentencePiece (spm12k,spm12k)
* download original weights: [opus2m-2020-08-01.zip](https://object.pouta.csc.fi/Tatoeba-MT-models/phi-eng/opus2m-2020-08-01.zip)
* test set translations: [opus2m-2020-08-01.test.txt](https://object.pouta.csc.fi/Tatoeba-MT-models/phi-eng/opus2m-2020-08-01.test.txt)
* test set scores: [opus2m-2020-08-01.eval.txt](https://object.pouta.csc.fi/Tatoeba-MT-models/phi-eng/opus2m-2020-08-01.eval.txt)
## Benchmarks
| testset | BLEU | chr-F |
|-----------------------|-------|-------|
| Tatoeba-test.akl-eng.akl.eng | 11.6 | 0.321 |
| Tatoeba-test.ceb-eng.ceb.eng | 21.7 | 0.393 |
| Tatoeba-test.hil-eng.hil.eng | 17.6 | 0.371 |
| Tatoeba-test.ilo-eng.ilo.eng | 36.6 | 0.560 |
| Tatoeba-test.multi.eng | 21.5 | 0.391 |
| Tatoeba-test.pag-eng.pag.eng | 27.5 | 0.494 |
| Tatoeba-test.war-eng.war.eng | 17.3 | 0.380 |
### System Info:
- hf_name: phi-eng
- source_languages: phi
- target_languages: eng
- opus_readme_url: https://github.com/Helsinki-NLP/Tatoeba-Challenge/tree/master/models/phi-eng/README.md
- original_repo: Tatoeba-Challenge
- tags: ['translation']
- languages: ['phi', 'en']
- src_constituents: {'ilo', 'akl_Latn', 'war', 'hil', 'pag', 'ceb'}
- tgt_constituents: {'eng'}
- src_multilingual: True
- tgt_multilingual: False
- prepro: normalization + SentencePiece (spm12k,spm12k)
- url_model: https://object.pouta.csc.fi/Tatoeba-MT-models/phi-eng/opus2m-2020-08-01.zip
- url_test_set: https://object.pouta.csc.fi/Tatoeba-MT-models/phi-eng/opus2m-2020-08-01.test.txt
- src_alpha3: phi
- tgt_alpha3: eng
- short_pair: phi-en
- chrF2_score: 0.391
- bleu: 21.5
- brevity_penalty: 1.0
- ref_len: 2380.0
- src_name: Philippine languages
- tgt_name: English
- train_date: 2020-08-01
- src_alpha2: phi
- tgt_alpha2: en
- prefer_old: False
- long_pair: phi-eng
- helsinki_git_sha: 480fcbe0ee1bf4774bcbe6226ad9f58e63f6c535
- transformers_git_sha: 2207e5d8cb224e954a7cba69fa4ac2309e9ff30b
- port_machine: brutasse
- port_time: 2020-08-21-14:41 |
Helsinki-NLP/opus-mt-srn-en | 733852218fbe91aee17c1bc3a3cedf4737a3db6e | 2021-09-10T14:04:31.000Z | [
"pytorch",
"marian",
"text2text-generation",
"srn",
"en",
"transformers",
"translation",
"license:apache-2.0",
"autotrain_compatible"
] | translation | false | Helsinki-NLP | null | Helsinki-NLP/opus-mt-srn-en | 20 | null | transformers | 8,294 | ---
tags:
- translation
license: apache-2.0
---
### opus-mt-srn-en
* source languages: srn
* target languages: en
* OPUS readme: [srn-en](https://github.com/Helsinki-NLP/OPUS-MT-train/blob/master/models/srn-en/README.md)
* dataset: opus
* model: transformer-align
* pre-processing: normalization + SentencePiece
* download original weights: [opus-2020-01-21.zip](https://object.pouta.csc.fi/OPUS-MT-models/srn-en/opus-2020-01-21.zip)
* test set translations: [opus-2020-01-21.test.txt](https://object.pouta.csc.fi/OPUS-MT-models/srn-en/opus-2020-01-21.test.txt)
* test set scores: [opus-2020-01-21.eval.txt](https://object.pouta.csc.fi/OPUS-MT-models/srn-en/opus-2020-01-21.eval.txt)
## Benchmarks
| testset | BLEU | chr-F |
|-----------------------|-------|-------|
| JW300.srn.en | 40.3 | 0.555 |
|
Helsinki-NLP/opus-mt-taw-en | 3561d29d357076b0351253652cbed0d28f42b75d | 2020-08-21T14:42:50.000Z | [
"pytorch",
"marian",
"text2text-generation",
"lo",
"th",
"taw",
"en",
"transformers",
"translation",
"license:apache-2.0",
"autotrain_compatible"
] | translation | false | Helsinki-NLP | null | Helsinki-NLP/opus-mt-taw-en | 20 | null | transformers | 8,295 | ---
language:
- lo
- th
- taw
- en
tags:
- translation
license: apache-2.0
---
### taw-eng
* source group: Tai
* target group: English
* OPUS readme: [taw-eng](https://github.com/Helsinki-NLP/Tatoeba-Challenge/tree/master/models/taw-eng/README.md)
* model: transformer
* source language(s): lao tha
* target language(s): eng
* model: transformer
* pre-processing: normalization + SentencePiece (spm32k,spm32k)
* download original weights: [opus-2020-06-28.zip](https://object.pouta.csc.fi/Tatoeba-MT-models/taw-eng/opus-2020-06-28.zip)
* test set translations: [opus-2020-06-28.test.txt](https://object.pouta.csc.fi/Tatoeba-MT-models/taw-eng/opus-2020-06-28.test.txt)
* test set scores: [opus-2020-06-28.eval.txt](https://object.pouta.csc.fi/Tatoeba-MT-models/taw-eng/opus-2020-06-28.eval.txt)
## Benchmarks
| testset | BLEU | chr-F |
|-----------------------|-------|-------|
| Tatoeba-test.lao-eng.lao.eng | 1.1 | 0.133 |
| Tatoeba-test.multi.eng | 38.9 | 0.572 |
| Tatoeba-test.tha-eng.tha.eng | 40.6 | 0.588 |
### System Info:
- hf_name: taw-eng
- source_languages: taw
- target_languages: eng
- opus_readme_url: https://github.com/Helsinki-NLP/Tatoeba-Challenge/tree/master/models/taw-eng/README.md
- original_repo: Tatoeba-Challenge
- tags: ['translation']
- languages: ['lo', 'th', 'taw', 'en']
- src_constituents: {'lao', 'tha'}
- tgt_constituents: {'eng'}
- src_multilingual: True
- tgt_multilingual: False
- prepro: normalization + SentencePiece (spm32k,spm32k)
- url_model: https://object.pouta.csc.fi/Tatoeba-MT-models/taw-eng/opus-2020-06-28.zip
- url_test_set: https://object.pouta.csc.fi/Tatoeba-MT-models/taw-eng/opus-2020-06-28.test.txt
- src_alpha3: taw
- tgt_alpha3: eng
- short_pair: taw-en
- chrF2_score: 0.5720000000000001
- bleu: 38.9
- brevity_penalty: 1.0
- ref_len: 7630.0
- src_name: Tai
- tgt_name: English
- train_date: 2020-06-28
- src_alpha2: taw
- tgt_alpha2: en
- prefer_old: False
- long_pair: taw-eng
- helsinki_git_sha: 480fcbe0ee1bf4774bcbe6226ad9f58e63f6c535
- transformers_git_sha: 2207e5d8cb224e954a7cba69fa4ac2309e9ff30b
- port_machine: brutasse
- port_time: 2020-08-21-14:41 |
Helsinki-NLP/opus-mt-ts-en | 62fafde175b8164b5fc1cb28511184adf259ff94 | 2021-09-11T10:49:49.000Z | [
"pytorch",
"marian",
"text2text-generation",
"ts",
"en",
"transformers",
"translation",
"license:apache-2.0",
"autotrain_compatible"
] | translation | false | Helsinki-NLP | null | Helsinki-NLP/opus-mt-ts-en | 20 | null | transformers | 8,296 | ---
tags:
- translation
license: apache-2.0
---
### opus-mt-ts-en
* source languages: ts
* target languages: en
* OPUS readme: [ts-en](https://github.com/Helsinki-NLP/OPUS-MT-train/blob/master/models/ts-en/README.md)
* dataset: opus
* model: transformer-align
* pre-processing: normalization + SentencePiece
* download original weights: [opus-2020-01-16.zip](https://object.pouta.csc.fi/OPUS-MT-models/ts-en/opus-2020-01-16.zip)
* test set translations: [opus-2020-01-16.test.txt](https://object.pouta.csc.fi/OPUS-MT-models/ts-en/opus-2020-01-16.test.txt)
* test set scores: [opus-2020-01-16.eval.txt](https://object.pouta.csc.fi/OPUS-MT-models/ts-en/opus-2020-01-16.eval.txt)
## Benchmarks
| testset | BLEU | chr-F |
|-----------------------|-------|-------|
| JW300.ts.en | 44.0 | 0.590 |
|
Helsinki-NLP/opus-mt-zne-sv | 80dea0c78d7a8eb2647fc6e2aa17aeef46c682a6 | 2021-09-11T10:53:18.000Z | [
"pytorch",
"marian",
"text2text-generation",
"zne",
"sv",
"transformers",
"translation",
"license:apache-2.0",
"autotrain_compatible"
] | translation | false | Helsinki-NLP | null | Helsinki-NLP/opus-mt-zne-sv | 20 | null | transformers | 8,297 | ---
tags:
- translation
license: apache-2.0
---
### opus-mt-zne-sv
* source languages: zne
* target languages: sv
* OPUS readme: [zne-sv](https://github.com/Helsinki-NLP/OPUS-MT-train/blob/master/models/zne-sv/README.md)
* dataset: opus
* model: transformer-align
* pre-processing: normalization + SentencePiece
* download original weights: [opus-2020-01-16.zip](https://object.pouta.csc.fi/OPUS-MT-models/zne-sv/opus-2020-01-16.zip)
* test set translations: [opus-2020-01-16.test.txt](https://object.pouta.csc.fi/OPUS-MT-models/zne-sv/opus-2020-01-16.test.txt)
* test set scores: [opus-2020-01-16.eval.txt](https://object.pouta.csc.fi/OPUS-MT-models/zne-sv/opus-2020-01-16.eval.txt)
## Benchmarks
| testset | BLEU | chr-F |
|-----------------------|-------|-------|
| JW300.zne.sv | 25.2 | 0.425 |
|
IlyaGusev/gen_title_tg_bottleneck_encoder | 65142ed360a292834364ffbddf12648956c35401 | 2021-05-18T21:08:31.000Z | [
"pytorch",
"jax",
"bert",
"feature-extraction",
"transformers"
] | feature-extraction | false | IlyaGusev | null | IlyaGusev/gen_title_tg_bottleneck_encoder | 20 | null | transformers | 8,298 | Entry not found |
Jeska/VaccinChatSentenceClassifierDutch_fromBERTje2_DAdialogQonly09 | 6a55108f19f038fbde9fb430d0da009669698bbf | 2021-12-15T16:50:47.000Z | [
"pytorch",
"tensorboard",
"bert",
"text-classification",
"transformers",
"generated_from_trainer",
"model-index"
] | text-classification | false | Jeska | null | Jeska/VaccinChatSentenceClassifierDutch_fromBERTje2_DAdialogQonly09 | 20 | null | transformers | 8,299 | ---
tags:
- generated_from_trainer
metrics:
- accuracy
model-index:
- name: VaccinChatSentenceClassifierDutch_fromBERTje2_DAdialogQonly09
results: []
---
<!-- This model card has been generated automatically according to the information the Trainer had access to. You
should probably proofread and complete it, then remove this comment. -->
# VaccinChatSentenceClassifierDutch_fromBERTje2_DAdialogQonly09
This model is a fine-tuned version of [outputDAQonly09/](https://huggingface.co/outputDAQonly09/) on an unknown dataset.
It achieves the following results on the evaluation set:
- Loss: 0.4978
- Accuracy: 0.9031
## Model description
More information needed
## Intended uses & limitations
More information needed
## Training and evaluation data
More information needed
## Training procedure
### Training hyperparameters
The following hyperparameters were used during training:
- learning_rate: 1e-05
- train_batch_size: 32
- eval_batch_size: 8
- seed: 42
- optimizer: Adam with betas=(0.9,0.999) and epsilon=1e-08
- lr_scheduler_type: linear
- num_epochs: 30.0
### Training results
| Training Loss | Epoch | Step | Validation Loss | Accuracy |
|:-------------:|:-----:|:----:|:---------------:|:--------:|
| No log | 1.0 | 330 | 3.9692 | 0.2249 |
| 4.3672 | 2.0 | 660 | 3.1312 | 0.4031 |
| 4.3672 | 3.0 | 990 | 2.5068 | 0.5658 |
| 3.1495 | 4.0 | 1320 | 2.0300 | 0.6600 |
| 2.2491 | 5.0 | 1650 | 1.6517 | 0.7450 |
| 2.2491 | 6.0 | 1980 | 1.3604 | 0.7943 |
| 1.622 | 7.0 | 2310 | 1.1328 | 0.8327 |
| 1.1252 | 8.0 | 2640 | 0.9484 | 0.8611 |
| 1.1252 | 9.0 | 2970 | 0.8212 | 0.8757 |
| 0.7969 | 10.0 | 3300 | 0.7243 | 0.8830 |
| 0.5348 | 11.0 | 3630 | 0.6597 | 0.8867 |
| 0.5348 | 12.0 | 3960 | 0.5983 | 0.8857 |
| 0.3744 | 13.0 | 4290 | 0.5635 | 0.8976 |
| 0.2564 | 14.0 | 4620 | 0.5437 | 0.8985 |
| 0.2564 | 15.0 | 4950 | 0.5124 | 0.9013 |
| 0.1862 | 16.0 | 5280 | 0.5074 | 0.9022 |
| 0.1349 | 17.0 | 5610 | 0.5028 | 0.9049 |
| 0.1349 | 18.0 | 5940 | 0.4876 | 0.9077 |
| 0.0979 | 19.0 | 6270 | 0.4971 | 0.9049 |
| 0.0763 | 20.0 | 6600 | 0.4941 | 0.9022 |
| 0.0763 | 21.0 | 6930 | 0.4957 | 0.9049 |
| 0.0602 | 22.0 | 7260 | 0.4989 | 0.9049 |
| 0.0504 | 23.0 | 7590 | 0.4959 | 0.9040 |
| 0.0504 | 24.0 | 7920 | 0.4944 | 0.9031 |
| 0.0422 | 25.0 | 8250 | 0.4985 | 0.9040 |
| 0.0379 | 26.0 | 8580 | 0.4970 | 0.9049 |
| 0.0379 | 27.0 | 8910 | 0.4949 | 0.9040 |
| 0.0351 | 28.0 | 9240 | 0.4971 | 0.9040 |
| 0.0321 | 29.0 | 9570 | 0.4967 | 0.9031 |
| 0.0321 | 30.0 | 9900 | 0.4978 | 0.9031 |
### Framework versions
- Transformers 4.13.0.dev0
- Pytorch 1.10.0
- Datasets 1.16.1
- Tokenizers 0.10.3
|
Subsets and Splits
No community queries yet
The top public SQL queries from the community will appear here once available.