modelId
stringlengths 4
112
| sha
stringlengths 40
40
| lastModified
stringlengths 24
24
| tags
sequence | pipeline_tag
stringclasses 29
values | private
bool 1
class | author
stringlengths 2
38
⌀ | config
null | id
stringlengths 4
112
| downloads
float64 0
36.8M
⌀ | likes
float64 0
712
⌀ | library_name
stringclasses 17
values | __index_level_0__
int64 0
38.5k
| readme
stringlengths 0
186k
|
---|---|---|---|---|---|---|---|---|---|---|---|---|---|
thebyy/DialoGPT-small-mortyisarick | c5dfb0dc22b3cee5cd8ca5c1d68650bdcc429722 | 2022-05-20T04:13:22.000Z | [
"pytorch",
"gpt2",
"text-generation",
"transformers",
"conversational"
] | conversational | false | thebyy | null | thebyy/DialoGPT-small-mortyisarick | 0 | null | transformers | 37,600 | ---
tags:
- conversational
--- |
huggingtweets/connorhvnsen | 084e9ea9979fad8d628956785622bccaecf8d885 | 2022-05-20T03:52:28.000Z | [
"pytorch",
"gpt2",
"text-generation",
"en",
"transformers",
"huggingtweets"
] | text-generation | false | huggingtweets | null | huggingtweets/connorhvnsen | 0 | null | transformers | 37,601 | ---
language: en
thumbnail: http://www.huggingtweets.com/connorhvnsen/1653018744349/predictions.png
tags:
- huggingtweets
widget:
- text: "My dream is"
---
<div class="inline-flex flex-col" style="line-height: 1.5;">
<div class="flex">
<div
style="display:inherit; margin-left: 4px; margin-right: 4px; width: 92px; height:92px; border-radius: 50%; background-size: cover; background-image: url('https://pbs.twimg.com/profile_images/1524595130031915009/JbJeqNFJ_400x400.jpg')">
</div>
<div
style="display:none; margin-left: 4px; margin-right: 4px; width: 92px; height:92px; border-radius: 50%; background-size: cover; background-image: url('')">
</div>
<div
style="display:none; margin-left: 4px; margin-right: 4px; width: 92px; height:92px; border-radius: 50%; background-size: cover; background-image: url('')">
</div>
</div>
<div style="text-align: center; margin-top: 3px; font-size: 16px; font-weight: 800">🤖 AI BOT 🤖</div>
<div style="text-align: center; font-size: 16px; font-weight: 800">HɅNSΞN ™</div>
<div style="text-align: center; font-size: 14px;">@connorhvnsen</div>
</div>
I was made with [huggingtweets](https://github.com/borisdayma/huggingtweets).
Create your own bot based on your favorite user with [the demo](https://colab.research.google.com/github/borisdayma/huggingtweets/blob/master/huggingtweets-demo.ipynb)!
## How does it work?
The model uses the following pipeline.
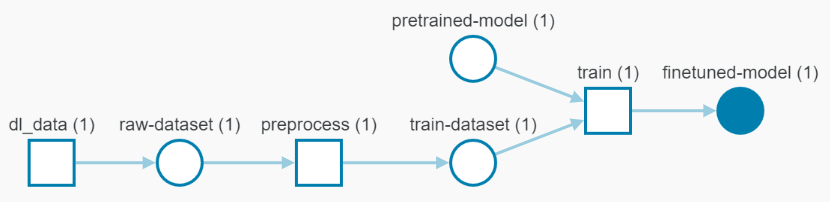
To understand how the model was developed, check the [W&B report](https://wandb.ai/wandb/huggingtweets/reports/HuggingTweets-Train-a-Model-to-Generate-Tweets--VmlldzoxMTY5MjI).
## Training data
The model was trained on tweets from HɅNSΞN ™.
| Data | HɅNSΞN ™ |
| --- | --- |
| Tweets downloaded | 1253 |
| Retweets | 317 |
| Short tweets | 309 |
| Tweets kept | 627 |
[Explore the data](https://wandb.ai/wandb/huggingtweets/runs/qz1rz5ej/artifacts), which is tracked with [W&B artifacts](https://docs.wandb.com/artifacts) at every step of the pipeline.
## Training procedure
The model is based on a pre-trained [GPT-2](https://huggingface.co/gpt2) which is fine-tuned on @connorhvnsen's tweets.
Hyperparameters and metrics are recorded in the [W&B training run](https://wandb.ai/wandb/huggingtweets/runs/aeaa7tfg) for full transparency and reproducibility.
At the end of training, [the final model](https://wandb.ai/wandb/huggingtweets/runs/aeaa7tfg/artifacts) is logged and versioned.
## How to use
You can use this model directly with a pipeline for text generation:
```python
from transformers import pipeline
generator = pipeline('text-generation',
model='huggingtweets/connorhvnsen')
generator("My dream is", num_return_sequences=5)
```
## Limitations and bias
The model suffers from [the same limitations and bias as GPT-2](https://huggingface.co/gpt2#limitations-and-bias).
In addition, the data present in the user's tweets further affects the text generated by the model.
## About
*Built by Boris Dayma*
[](https://twitter.com/intent/follow?screen_name=borisdayma)
For more details, visit the project repository.
[](https://github.com/borisdayma/huggingtweets)
|
umanlp/mt5-mlm-16 | 867d4eeafd35ea31e097b713f66c5a1395c2e5f9 | 2022-05-20T09:45:06.000Z | [
"pytorch",
"mt5",
"feature-extraction",
"transformers"
] | feature-extraction | false | umanlp | null | umanlp/mt5-mlm-16 | 0 | null | transformers | 37,602 | Entry not found |
umanlp/mt5-mlm-wiki14 | 04379b4bb8887c8382799a7fff3b9a716845f5e1 | 2022-05-20T09:56:45.000Z | [
"pytorch",
"mt5",
"feature-extraction",
"transformers"
] | feature-extraction | false | umanlp | null | umanlp/mt5-mlm-wiki14 | 0 | null | transformers | 37,603 | Entry not found |
huggingtweets/welcomeunknown | c2f396f0fea7240b439bb8355e2436889619bb89 | 2022-05-20T12:32:41.000Z | [
"pytorch",
"gpt2",
"text-generation",
"en",
"transformers",
"huggingtweets"
] | text-generation | false | huggingtweets | null | huggingtweets/welcomeunknown | 0 | null | transformers | 37,604 | ---
language: en
thumbnail: http://www.huggingtweets.com/welcomeunknown/1653049956766/predictions.png
tags:
- huggingtweets
widget:
- text: "My dream is"
---
<div class="inline-flex flex-col" style="line-height: 1.5;">
<div class="flex">
<div
style="display:inherit; margin-left: 4px; margin-right: 4px; width: 92px; height:92px; border-radius: 50%; background-size: cover; background-image: url('https://pbs.twimg.com/profile_images/1465974364453572609/sxLKsmL8_400x400.jpg')">
</div>
<div
style="display:none; margin-left: 4px; margin-right: 4px; width: 92px; height:92px; border-radius: 50%; background-size: cover; background-image: url('')">
</div>
<div
style="display:none; margin-left: 4px; margin-right: 4px; width: 92px; height:92px; border-radius: 50%; background-size: cover; background-image: url('')">
</div>
</div>
<div style="text-align: center; margin-top: 3px; font-size: 16px; font-weight: 800">🤖 AI BOT 🤖</div>
<div style="text-align: center; font-size: 16px; font-weight: 800">b e a r 🤍⃤</div>
<div style="text-align: center; font-size: 14px;">@welcomeunknown</div>
</div>
I was made with [huggingtweets](https://github.com/borisdayma/huggingtweets).
Create your own bot based on your favorite user with [the demo](https://colab.research.google.com/github/borisdayma/huggingtweets/blob/master/huggingtweets-demo.ipynb)!
## How does it work?
The model uses the following pipeline.
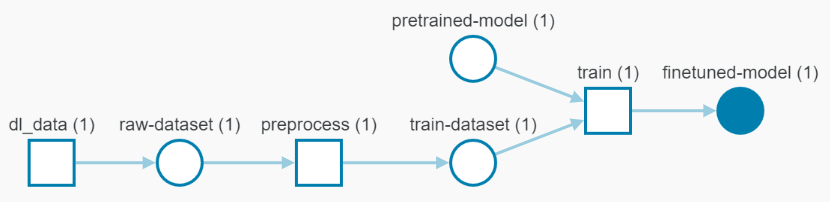
To understand how the model was developed, check the [W&B report](https://wandb.ai/wandb/huggingtweets/reports/HuggingTweets-Train-a-Model-to-Generate-Tweets--VmlldzoxMTY5MjI).
## Training data
The model was trained on tweets from b e a r 🤍⃤.
| Data | b e a r 🤍⃤ |
| --- | --- |
| Tweets downloaded | 3071 |
| Retweets | 1185 |
| Short tweets | 214 |
| Tweets kept | 1672 |
[Explore the data](https://wandb.ai/wandb/huggingtweets/runs/241jk5jh/artifacts), which is tracked with [W&B artifacts](https://docs.wandb.com/artifacts) at every step of the pipeline.
## Training procedure
The model is based on a pre-trained [GPT-2](https://huggingface.co/gpt2) which is fine-tuned on @welcomeunknown's tweets.
Hyperparameters and metrics are recorded in the [W&B training run](https://wandb.ai/wandb/huggingtweets/runs/gcn82iuh) for full transparency and reproducibility.
At the end of training, [the final model](https://wandb.ai/wandb/huggingtweets/runs/gcn82iuh/artifacts) is logged and versioned.
## How to use
You can use this model directly with a pipeline for text generation:
```python
from transformers import pipeline
generator = pipeline('text-generation',
model='huggingtweets/welcomeunknown')
generator("My dream is", num_return_sequences=5)
```
## Limitations and bias
The model suffers from [the same limitations and bias as GPT-2](https://huggingface.co/gpt2#limitations-and-bias).
In addition, the data present in the user's tweets further affects the text generated by the model.
## About
*Built by Boris Dayma*
[](https://twitter.com/intent/follow?screen_name=borisdayma)
For more details, visit the project repository.
[](https://github.com/borisdayma/huggingtweets)
|
ruselkomp/deep-pavlov-framebank-5epochs-2 | f71f40cae7fd5342abf2dd0d672958512086f420 | 2022-05-20T15:09:32.000Z | [
"pytorch",
"tensorboard",
"bert",
"question-answering",
"transformers",
"generated_from_trainer",
"model-index",
"autotrain_compatible"
] | question-answering | false | ruselkomp | null | ruselkomp/deep-pavlov-framebank-5epochs-2 | 0 | null | transformers | 37,605 | ---
tags:
- generated_from_trainer
model-index:
- name: deep-pavlov-framebank-5epochs-2
results: []
---
<!-- This model card has been generated automatically according to the information the Trainer had access to. You
should probably proofread and complete it, then remove this comment. -->
# deep-pavlov-framebank-5epochs-2
This model is a fine-tuned version of [DeepPavlov/rubert-base-cased](https://huggingface.co/DeepPavlov/rubert-base-cased) on the None dataset.
It achieves the following results on the evaluation set:
- Loss: 2.4205
## Model description
More information needed
## Intended uses & limitations
More information needed
## Training and evaluation data
More information needed
## Training procedure
### Training hyperparameters
The following hyperparameters were used during training:
- learning_rate: 2e-05
- train_batch_size: 16
- eval_batch_size: 16
- seed: 42
- optimizer: Adam with betas=(0.9,0.999) and epsilon=1e-08
- lr_scheduler_type: linear
- num_epochs: 5
### Training results
| Training Loss | Epoch | Step | Validation Loss |
|:-------------:|:-----:|:-----:|:---------------:|
| 0.4667 | 1.0 | 2827 | 1.3508 |
| 0.3114 | 2.0 | 5654 | 1.5341 |
| 0.1941 | 3.0 | 8481 | 1.8772 |
| 0.1185 | 4.0 | 11308 | 2.1496 |
| 0.0795 | 5.0 | 14135 | 2.4205 |
### Framework versions
- Transformers 4.19.0.dev0
- Pytorch 1.11.0+cu113
- Datasets 2.2.2.dev0
- Tokenizers 0.12.1
|
gulteng/distilbert-base-uncased-finetuned-squad | 9c39b68b3fb0c0c0facc255228351589381dc653 | 2022-05-20T13:44:24.000Z | [
"pytorch",
"tensorboard",
"distilbert",
"question-answering",
"dataset:squad",
"transformers",
"generated_from_trainer",
"license:apache-2.0",
"model-index",
"autotrain_compatible"
] | question-answering | false | gulteng | null | gulteng/distilbert-base-uncased-finetuned-squad | 0 | null | transformers | 37,606 | ---
license: apache-2.0
tags:
- generated_from_trainer
datasets:
- squad
model-index:
- name: distilbert-base-uncased-finetuned-squad
results: []
---
<!-- This model card has been generated automatically according to the information the Trainer had access to. You
should probably proofread and complete it, then remove this comment. -->
# distilbert-base-uncased-finetuned-squad
This model is a fine-tuned version of [distilbert-base-uncased](https://huggingface.co/distilbert-base-uncased) on the squad dataset.
It achieves the following results on the evaluation set:
- Loss: 1.2131
## Model description
More information needed
## Intended uses & limitations
More information needed
## Training and evaluation data
More information needed
## Training procedure
### Training hyperparameters
The following hyperparameters were used during training:
- learning_rate: 2e-05
- train_batch_size: 16
- eval_batch_size: 16
- seed: 42
- optimizer: Adam with betas=(0.9,0.999) and epsilon=1e-08
- lr_scheduler_type: linear
- num_epochs: 1
### Training results
| Training Loss | Epoch | Step | Validation Loss |
|:-------------:|:-----:|:----:|:---------------:|
| 1.2672 | 1.0 | 5533 | 1.2131 |
### Framework versions
- Transformers 4.19.2
- Pytorch 1.11.0+cu113
- Datasets 2.2.1
- Tokenizers 0.12.1
|
subhasisj/xlm-roberta-base-squad-32 | 20bfbe729c522bfe7b49b55a37ccfaac202e7cfd | 2022-05-20T19:13:21.000Z | [
"pytorch",
"tensorboard",
"xlm-roberta",
"question-answering",
"dataset:squad",
"transformers",
"generated_from_trainer",
"license:mit",
"model-index",
"autotrain_compatible"
] | question-answering | false | subhasisj | null | subhasisj/xlm-roberta-base-squad-32 | 0 | null | transformers | 37,607 | ---
license: mit
tags:
- generated_from_trainer
datasets:
- squad
model-index:
- name: xlm-roberta-base-squad-32
results: []
---
<!-- This model card has been generated automatically according to the information the Trainer had access to. You
should probably proofread and complete it, then remove this comment. -->
# xlm-roberta-base-squad-32
This model is a fine-tuned version of [xlm-roberta-base](https://huggingface.co/xlm-roberta-base) on the squad dataset.
It achieves the following results on the evaluation set:
- Loss: 1.0083
## Model description
More information needed
## Intended uses & limitations
More information needed
## Training and evaluation data
More information needed
## Training procedure
### Training hyperparameters
The following hyperparameters were used during training:
- learning_rate: 1.5e-05
- train_batch_size: 32
- eval_batch_size: 32
- seed: 42
- gradient_accumulation_steps: 8
- total_train_batch_size: 256
- optimizer: Adam with betas=(0.9,0.999) and epsilon=1e-08
- lr_scheduler_type: linear
- lr_scheduler_warmup_ratio: 0.1
- num_epochs: 5
- mixed_precision_training: Native AMP
### Training results
| Training Loss | Epoch | Step | Validation Loss |
|:-------------:|:-----:|:----:|:---------------:|
| No log | 1.0 | 350 | 1.2339 |
| 2.3864 | 2.0 | 700 | 1.0571 |
| 1.0541 | 3.0 | 1050 | 1.0246 |
| 1.0541 | 4.0 | 1400 | 0.9947 |
| 0.9214 | 5.0 | 1750 | 1.0083 |
### Framework versions
- Transformers 4.18.0
- Pytorch 1.11.0+cu113
- Datasets 2.2.1
- Tokenizers 0.12.1
|
roshnir/bert-base-multi-mlqa-dev-en | bc45e4425c2f40955cb3b57ada939a0eeb3d63d3 | 2022-05-20T17:05:01.000Z | [
"pytorch",
"bert",
"question-answering",
"transformers",
"autotrain_compatible"
] | question-answering | false | roshnir | null | roshnir/bert-base-multi-mlqa-dev-en | 0 | null | transformers | 37,608 | Entry not found |
noah-rush/inquirer-bert | 4de003c27367a2816c8c37a2a4112cb381e5cfd0 | 2022-05-20T20:42:07.000Z | [
"pytorch",
"bert",
"token-classification",
"transformers",
"autotrain_compatible"
] | token-classification | false | noah-rush | null | noah-rush/inquirer-bert | 0 | null | transformers | 37,609 | Entry not found |
marksverdhei/t5-large-reddit-syac | df248290f824eebd6f12bcebf30e27fbe7e8f0a4 | 2022-05-20T22:15:39.000Z | [
"pytorch",
"t5",
"text2text-generation",
"transformers",
"autotrain_compatible"
] | text2text-generation | false | marksverdhei | null | marksverdhei/t5-large-reddit-syac | 0 | null | transformers | 37,610 | Entry not found |
fransoa/arrombado-dms | 91e5bfa5c6b4ec61223ffbb0489c82d6c555c1d1 | 2022-05-20T22:09:48.000Z | [
"pytorch",
"gpt2",
"text-generation",
"transformers",
"conversational"
] | conversational | false | fransoa | null | fransoa/arrombado-dms | 0 | null | transformers | 37,611 | ---
tags:
- conversational
---
# troska DialogGPT models
|
huggingtweets/slayersiu | 6f1e2384db8707ab18097a6a15afcb86eb1cb7b3 | 2022-05-25T14:29:09.000Z | [
"pytorch",
"gpt2",
"text-generation",
"en",
"transformers",
"huggingtweets"
] | text-generation | false | huggingtweets | null | huggingtweets/slayersiu | 0 | null | transformers | 37,612 | ---
language: en
thumbnail: http://www.huggingtweets.com/slayersiu/1653488944264/predictions.png
tags:
- huggingtweets
widget:
- text: "My dream is"
---
<div class="inline-flex flex-col" style="line-height: 1.5;">
<div class="flex">
<div
style="display:inherit; margin-left: 4px; margin-right: 4px; width: 92px; height:92px; border-radius: 50%; background-size: cover; background-image: url('https://pbs.twimg.com/profile_images/1455287025821790214/c0-KTf04_400x400.jpg')">
</div>
<div
style="display:none; margin-left: 4px; margin-right: 4px; width: 92px; height:92px; border-radius: 50%; background-size: cover; background-image: url('')">
</div>
<div
style="display:none; margin-left: 4px; margin-right: 4px; width: 92px; height:92px; border-radius: 50%; background-size: cover; background-image: url('')">
</div>
</div>
<div style="text-align: center; margin-top: 3px; font-size: 16px; font-weight: 800">🤖 AI BOT 🤖</div>
<div style="text-align: center; font-size: 16px; font-weight: 800">DR. RASMUS</div>
<div style="text-align: center; font-size: 14px;">@slayersiu</div>
</div>
I was made with [huggingtweets](https://github.com/borisdayma/huggingtweets).
Create your own bot based on your favorite user with [the demo](https://colab.research.google.com/github/borisdayma/huggingtweets/blob/master/huggingtweets-demo.ipynb)!
## How does it work?
The model uses the following pipeline.
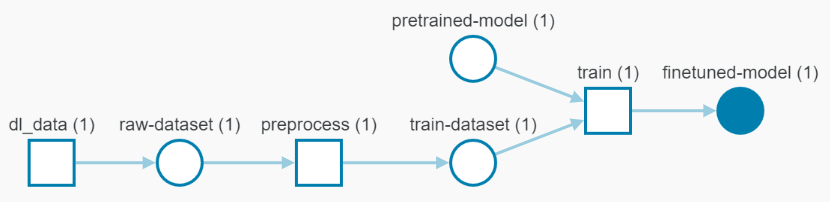
To understand how the model was developed, check the [W&B report](https://wandb.ai/wandb/huggingtweets/reports/HuggingTweets-Train-a-Model-to-Generate-Tweets--VmlldzoxMTY5MjI).
## Training data
The model was trained on tweets from DR. RASMUS.
| Data | DR. RASMUS |
| --- | --- |
| Tweets downloaded | 3189 |
| Retweets | 39 |
| Short tweets | 925 |
| Tweets kept | 2225 |
[Explore the data](https://wandb.ai/wandb/huggingtweets/runs/39xz51i2/artifacts), which is tracked with [W&B artifacts](https://docs.wandb.com/artifacts) at every step of the pipeline.
## Training procedure
The model is based on a pre-trained [GPT-2](https://huggingface.co/gpt2) which is fine-tuned on @slayersiu's tweets.
Hyperparameters and metrics are recorded in the [W&B training run](https://wandb.ai/wandb/huggingtweets/runs/bdxg3cak) for full transparency and reproducibility.
At the end of training, [the final model](https://wandb.ai/wandb/huggingtweets/runs/bdxg3cak/artifacts) is logged and versioned.
## How to use
You can use this model directly with a pipeline for text generation:
```python
from transformers import pipeline
generator = pipeline('text-generation',
model='huggingtweets/slayersiu')
generator("My dream is", num_return_sequences=5)
```
## Limitations and bias
The model suffers from [the same limitations and bias as GPT-2](https://huggingface.co/gpt2#limitations-and-bias).
In addition, the data present in the user's tweets further affects the text generated by the model.
## About
*Built by Boris Dayma*
[](https://twitter.com/intent/follow?screen_name=borisdayma)
For more details, visit the project repository.
[](https://github.com/borisdayma/huggingtweets)
|
ruselkomp/deep-pavlov-framebank-hidesize | 271bc699e191b73ed577664c9b73d973a3677efe | 2022-05-21T02:48:28.000Z | [
"pytorch",
"tensorboard",
"bert",
"question-answering",
"transformers",
"generated_from_trainer",
"model-index",
"autotrain_compatible"
] | question-answering | false | ruselkomp | null | ruselkomp/deep-pavlov-framebank-hidesize | 0 | null | transformers | 37,613 | ---
tags:
- generated_from_trainer
model-index:
- name: deep-pavlov-framebank-hidesize
results: []
---
<!-- This model card has been generated automatically according to the information the Trainer had access to. You
should probably proofread and complete it, then remove this comment. -->
# deep-pavlov-framebank-hidesize
This model is a fine-tuned version of [DeepPavlov/rubert-base-cased](https://huggingface.co/DeepPavlov/rubert-base-cased) on the None dataset.
It achieves the following results on the evaluation set:
- Loss: 1.0985
## Model description
More information needed
## Intended uses & limitations
More information needed
## Training and evaluation data
More information needed
## Training procedure
### Training hyperparameters
The following hyperparameters were used during training:
- learning_rate: 2e-05
- train_batch_size: 16
- eval_batch_size: 16
- seed: 42
- optimizer: Adam with betas=(0.9,0.999) and epsilon=1e-08
- lr_scheduler_type: linear
- num_epochs: 3
### Training results
| Training Loss | Epoch | Step | Validation Loss |
|:-------------:|:-----:|:----:|:---------------:|
| 1.0729 | 1.0 | 2827 | 1.0161 |
| 0.7899 | 2.0 | 5654 | 1.0360 |
| 0.5958 | 3.0 | 8481 | 1.0985 |
### Framework versions
- Transformers 4.19.0.dev0
- Pytorch 1.11.0+cu113
- Datasets 2.2.3.dev0
- Tokenizers 0.12.1
|
huggingtweets/mrquinnzard | 3c7aab555774a317cd73c4770cfe70fdec47f354 | 2022-05-21T00:19:55.000Z | [
"pytorch",
"gpt2",
"text-generation",
"en",
"transformers",
"huggingtweets"
] | text-generation | false | huggingtweets | null | huggingtweets/mrquinnzard | 0 | null | transformers | 37,614 | ---
language: en
thumbnail: http://www.huggingtweets.com/mrquinnzard/1653092375998/predictions.png
tags:
- huggingtweets
widget:
- text: "My dream is"
---
<div class="inline-flex flex-col" style="line-height: 1.5;">
<div class="flex">
<div
style="display:inherit; margin-left: 4px; margin-right: 4px; width: 92px; height:92px; border-radius: 50%; background-size: cover; background-image: url('https://pbs.twimg.com/profile_images/1525619063447339009/xeQSjk3u_400x400.jpg')">
</div>
<div
style="display:none; margin-left: 4px; margin-right: 4px; width: 92px; height:92px; border-radius: 50%; background-size: cover; background-image: url('')">
</div>
<div
style="display:none; margin-left: 4px; margin-right: 4px; width: 92px; height:92px; border-radius: 50%; background-size: cover; background-image: url('')">
</div>
</div>
<div style="text-align: center; margin-top: 3px; font-size: 16px; font-weight: 800">🤖 AI BOT 🤖</div>
<div style="text-align: center; font-size: 16px; font-weight: 800">MrQuinnzard X ✊🏿🇺🇦</div>
<div style="text-align: center; font-size: 14px;">@mrquinnzard</div>
</div>
I was made with [huggingtweets](https://github.com/borisdayma/huggingtweets).
Create your own bot based on your favorite user with [the demo](https://colab.research.google.com/github/borisdayma/huggingtweets/blob/master/huggingtweets-demo.ipynb)!
## How does it work?
The model uses the following pipeline.
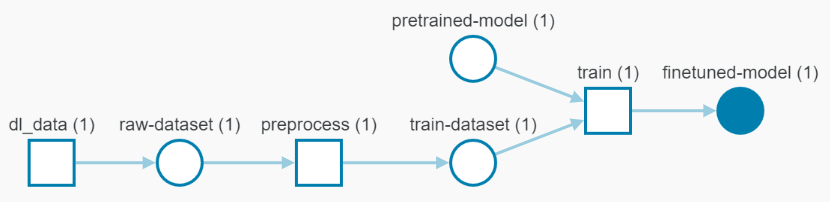
To understand how the model was developed, check the [W&B report](https://wandb.ai/wandb/huggingtweets/reports/HuggingTweets-Train-a-Model-to-Generate-Tweets--VmlldzoxMTY5MjI).
## Training data
The model was trained on tweets from MrQuinnzard X ✊🏿🇺🇦.
| Data | MrQuinnzard X ✊🏿🇺🇦 |
| --- | --- |
| Tweets downloaded | 716 |
| Retweets | 47 |
| Short tweets | 115 |
| Tweets kept | 554 |
[Explore the data](https://wandb.ai/wandb/huggingtweets/runs/2uwzvaxw/artifacts), which is tracked with [W&B artifacts](https://docs.wandb.com/artifacts) at every step of the pipeline.
## Training procedure
The model is based on a pre-trained [GPT-2](https://huggingface.co/gpt2) which is fine-tuned on @mrquinnzard's tweets.
Hyperparameters and metrics are recorded in the [W&B training run](https://wandb.ai/wandb/huggingtweets/runs/mntwd4n5) for full transparency and reproducibility.
At the end of training, [the final model](https://wandb.ai/wandb/huggingtweets/runs/mntwd4n5/artifacts) is logged and versioned.
## How to use
You can use this model directly with a pipeline for text generation:
```python
from transformers import pipeline
generator = pipeline('text-generation',
model='huggingtweets/mrquinnzard')
generator("My dream is", num_return_sequences=5)
```
## Limitations and bias
The model suffers from [the same limitations and bias as GPT-2](https://huggingface.co/gpt2#limitations-and-bias).
In addition, the data present in the user's tweets further affects the text generated by the model.
## About
*Built by Boris Dayma*
[](https://twitter.com/intent/follow?screen_name=borisdayma)
For more details, visit the project repository.
[](https://github.com/borisdayma/huggingtweets)
|
huggingtweets/darcywubot | c1db72907beba6173dcf9e6a9e9768319cd8f611 | 2022-05-21T00:27:43.000Z | [
"pytorch",
"gpt2",
"text-generation",
"en",
"transformers",
"huggingtweets"
] | text-generation | false | huggingtweets | null | huggingtweets/darcywubot | 0 | null | transformers | 37,615 | ---
language: en
thumbnail: http://www.huggingtweets.com/darcywubot/1653092857463/predictions.png
tags:
- huggingtweets
widget:
- text: "My dream is"
---
<div class="inline-flex flex-col" style="line-height: 1.5;">
<div class="flex">
<div
style="display:inherit; margin-left: 4px; margin-right: 4px; width: 92px; height:92px; border-radius: 50%; background-size: cover; background-image: url('https://pbs.twimg.com/profile_images/1520965807374835712/oz5XZFva_400x400.jpg')">
</div>
<div
style="display:none; margin-left: 4px; margin-right: 4px; width: 92px; height:92px; border-radius: 50%; background-size: cover; background-image: url('')">
</div>
<div
style="display:none; margin-left: 4px; margin-right: 4px; width: 92px; height:92px; border-radius: 50%; background-size: cover; background-image: url('')">
</div>
</div>
<div style="text-align: center; margin-top: 3px; font-size: 16px; font-weight: 800">🤖 AI BOT 🤖</div>
<div style="text-align: center; font-size: 16px; font-weight: 800">Darcy Bot</div>
<div style="text-align: center; font-size: 14px;">@darcywubot</div>
</div>
I was made with [huggingtweets](https://github.com/borisdayma/huggingtweets).
Create your own bot based on your favorite user with [the demo](https://colab.research.google.com/github/borisdayma/huggingtweets/blob/master/huggingtweets-demo.ipynb)!
## How does it work?
The model uses the following pipeline.
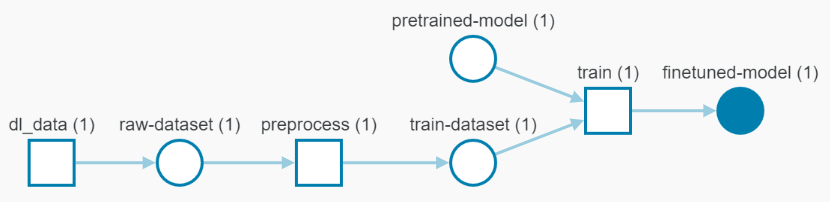
To understand how the model was developed, check the [W&B report](https://wandb.ai/wandb/huggingtweets/reports/HuggingTweets-Train-a-Model-to-Generate-Tweets--VmlldzoxMTY5MjI).
## Training data
The model was trained on tweets from Darcy Bot.
| Data | Darcy Bot |
| --- | --- |
| Tweets downloaded | 3250 |
| Retweets | 6 |
| Short tweets | 413 |
| Tweets kept | 2831 |
[Explore the data](https://wandb.ai/wandb/huggingtweets/runs/3ou05gm6/artifacts), which is tracked with [W&B artifacts](https://docs.wandb.com/artifacts) at every step of the pipeline.
## Training procedure
The model is based on a pre-trained [GPT-2](https://huggingface.co/gpt2) which is fine-tuned on @darcywubot's tweets.
Hyperparameters and metrics are recorded in the [W&B training run](https://wandb.ai/wandb/huggingtweets/runs/3p4xvqb6) for full transparency and reproducibility.
At the end of training, [the final model](https://wandb.ai/wandb/huggingtweets/runs/3p4xvqb6/artifacts) is logged and versioned.
## How to use
You can use this model directly with a pipeline for text generation:
```python
from transformers import pipeline
generator = pipeline('text-generation',
model='huggingtweets/darcywubot')
generator("My dream is", num_return_sequences=5)
```
## Limitations and bias
The model suffers from [the same limitations and bias as GPT-2](https://huggingface.co/gpt2#limitations-and-bias).
In addition, the data present in the user's tweets further affects the text generated by the model.
## About
*Built by Boris Dayma*
[](https://twitter.com/intent/follow?screen_name=borisdayma)
For more details, visit the project repository.
[](https://github.com/borisdayma/huggingtweets)
|
huggingtweets/annebottz | b94835ef741550c4a834c642f21df28063280daf | 2022-05-21T00:49:07.000Z | [
"pytorch",
"gpt2",
"text-generation",
"en",
"transformers",
"huggingtweets"
] | text-generation | false | huggingtweets | null | huggingtweets/annebottz | 0 | null | transformers | 37,616 | ---
language: en
thumbnail: http://www.huggingtweets.com/annebottz/1653094143094/predictions.png
tags:
- huggingtweets
widget:
- text: "My dream is"
---
<div class="inline-flex flex-col" style="line-height: 1.5;">
<div class="flex">
<div
style="display:inherit; margin-left: 4px; margin-right: 4px; width: 92px; height:92px; border-radius: 50%; background-size: cover; background-image: url('https://pbs.twimg.com/profile_images/1526210961031548935/59jbyuut_400x400.jpg')">
</div>
<div
style="display:none; margin-left: 4px; margin-right: 4px; width: 92px; height:92px; border-radius: 50%; background-size: cover; background-image: url('')">
</div>
<div
style="display:none; margin-left: 4px; margin-right: 4px; width: 92px; height:92px; border-radius: 50%; background-size: cover; background-image: url('')">
</div>
</div>
<div style="text-align: center; margin-top: 3px; font-size: 16px; font-weight: 800">🤖 AI BOT 🤖</div>
<div style="text-align: center; font-size: 16px; font-weight: 800">Anne Bot</div>
<div style="text-align: center; font-size: 14px;">@annebottz</div>
</div>
I was made with [huggingtweets](https://github.com/borisdayma/huggingtweets).
Create your own bot based on your favorite user with [the demo](https://colab.research.google.com/github/borisdayma/huggingtweets/blob/master/huggingtweets-demo.ipynb)!
## How does it work?
The model uses the following pipeline.
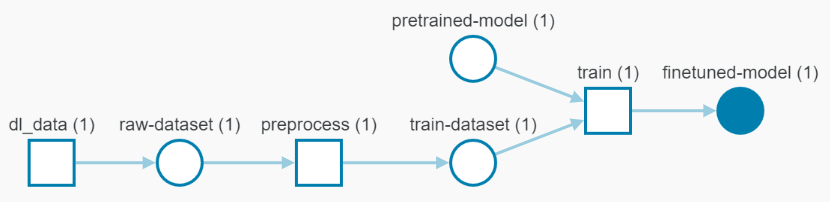
To understand how the model was developed, check the [W&B report](https://wandb.ai/wandb/huggingtweets/reports/HuggingTweets-Train-a-Model-to-Generate-Tweets--VmlldzoxMTY5MjI).
## Training data
The model was trained on tweets from Anne Bot.
| Data | Anne Bot |
| --- | --- |
| Tweets downloaded | 3250 |
| Retweets | 0 |
| Short tweets | 590 |
| Tweets kept | 2660 |
[Explore the data](https://wandb.ai/wandb/huggingtweets/runs/263xyaa3/artifacts), which is tracked with [W&B artifacts](https://docs.wandb.com/artifacts) at every step of the pipeline.
## Training procedure
The model is based on a pre-trained [GPT-2](https://huggingface.co/gpt2) which is fine-tuned on @annebottz's tweets.
Hyperparameters and metrics are recorded in the [W&B training run](https://wandb.ai/wandb/huggingtweets/runs/edyr41r2) for full transparency and reproducibility.
At the end of training, [the final model](https://wandb.ai/wandb/huggingtweets/runs/edyr41r2/artifacts) is logged and versioned.
## How to use
You can use this model directly with a pipeline for text generation:
```python
from transformers import pipeline
generator = pipeline('text-generation',
model='huggingtweets/annebottz')
generator("My dream is", num_return_sequences=5)
```
## Limitations and bias
The model suffers from [the same limitations and bias as GPT-2](https://huggingface.co/gpt2#limitations-and-bias).
In addition, the data present in the user's tweets further affects the text generated by the model.
## About
*Built by Boris Dayma*
[](https://twitter.com/intent/follow?screen_name=borisdayma)
For more details, visit the project repository.
[](https://github.com/borisdayma/huggingtweets)
|
ruselkomp/deep-pavlov-framebank-hidesize-1 | ddce9bd61f8d1ae159efdb584fa99344348e70b6 | 2022-05-21T12:19:16.000Z | [
"pytorch",
"tensorboard",
"bert",
"question-answering",
"transformers",
"generated_from_trainer",
"model-index",
"autotrain_compatible"
] | question-answering | false | ruselkomp | null | ruselkomp/deep-pavlov-framebank-hidesize-1 | 0 | null | transformers | 37,617 | ---
tags:
- generated_from_trainer
model-index:
- name: deep-pavlov-framebank-hidesize-1
results: []
---
<!-- This model card has been generated automatically according to the information the Trainer had access to. You
should probably proofread and complete it, then remove this comment. -->
# deep-pavlov-framebank-hidesize-1
This model is a fine-tuned version of [DeepPavlov/rubert-base-cased](https://huggingface.co/DeepPavlov/rubert-base-cased) on the None dataset.
It achieves the following results on the evaluation set:
- Loss: 1.0967
## Model description
More information needed
## Intended uses & limitations
More information needed
## Training and evaluation data
More information needed
## Training procedure
### Training hyperparameters
The following hyperparameters were used during training:
- learning_rate: 2e-05
- train_batch_size: 16
- eval_batch_size: 16
- seed: 42
- optimizer: Adam with betas=(0.9,0.999) and epsilon=1e-08
- lr_scheduler_type: linear
- num_epochs: 3
### Training results
| Training Loss | Epoch | Step | Validation Loss |
|:-------------:|:-----:|:----:|:---------------:|
| 1.073 | 1.0 | 2827 | 1.0101 |
| 0.7856 | 2.0 | 5654 | 1.0367 |
| 0.5993 | 3.0 | 8481 | 1.0967 |
### Framework versions
- Transformers 4.19.0.dev0
- Pytorch 1.11.0+cu113
- Datasets 2.2.3.dev0
- Tokenizers 0.12.1
|
subhasisj/vi-adapter-32 | 0662d668d2aa7f5ae1d73e2813f30684a3f436de | 2022-05-21T22:30:44.000Z | [
"pytorch",
"tensorboard",
"xlm-roberta",
"question-answering",
"transformers",
"generated_from_trainer",
"model-index",
"autotrain_compatible"
] | question-answering | false | subhasisj | null | subhasisj/vi-adapter-32 | 0 | null | transformers | 37,618 | ---
tags:
- generated_from_trainer
model-index:
- name: vi-adapter-32
results: []
---
<!-- This model card has been generated automatically according to the information the Trainer had access to. You
should probably proofread and complete it, then remove this comment. -->
# vi-adapter-32
This model was trained from scratch on the None dataset.
It achieves the following results on the evaluation set:
- Loss: 5.4211
## Model description
More information needed
## Intended uses & limitations
More information needed
## Training and evaluation data
More information needed
## Training procedure
### Training hyperparameters
The following hyperparameters were used during training:
- learning_rate: 3e-05
- train_batch_size: 32
- eval_batch_size: 32
- seed: 42
- gradient_accumulation_steps: 8
- total_train_batch_size: 256
- optimizer: Adam with betas=(0.9,0.999) and epsilon=1e-08
- lr_scheduler_type: linear
- lr_scheduler_warmup_ratio: 0.1
- num_epochs: 5
- mixed_precision_training: Native AMP
### Training results
| Training Loss | Epoch | Step | Validation Loss |
|:-------------:|:-----:|:----:|:---------------:|
| No log | 1.0 | 356 | 5.6984 |
| 5.7565 | 2.0 | 712 | 5.5596 |
| 5.5609 | 3.0 | 1068 | 5.4781 |
| 5.5609 | 4.0 | 1424 | 5.4349 |
| 5.4654 | 5.0 | 1780 | 5.4211 |
### Framework versions
- Transformers 4.17.0
- Pytorch 1.11.0+cu113
- Datasets 2.2.2
- Tokenizers 0.12.1
|
ruselkomp/sber-framebank-hidesize | 83fd2c4d9345197f6c7a64d6e340ee6465ee47d6 | 2022-05-21T19:49:37.000Z | [
"pytorch",
"tensorboard",
"bert",
"question-answering",
"transformers",
"autotrain_compatible"
] | question-answering | false | ruselkomp | null | ruselkomp/sber-framebank-hidesize | 0 | null | transformers | 37,619 | Entry not found |
HighCWu/anime-biggan-pytorch | 9f8640938b6611f0af75520c95ff49506f66e765 | 2022-05-21T15:36:10.000Z | [
"pytorch"
] | null | false | HighCWu | null | HighCWu/anime-biggan-pytorch | 0 | null | null | 37,620 | Entry not found |
renjithks/distilbert-cord-ner | dc44e6ed7f2473ee3cc7205fbf6acb67325fb7d3 | 2022-05-22T11:12:52.000Z | [
"pytorch",
"tensorboard",
"distilbert",
"token-classification",
"transformers",
"generated_from_trainer",
"license:apache-2.0",
"model-index",
"autotrain_compatible"
] | token-classification | false | renjithks | null | renjithks/distilbert-cord-ner | 0 | null | transformers | 37,621 | ---
license: apache-2.0
tags:
- generated_from_trainer
metrics:
- precision
- recall
- f1
- accuracy
model-index:
- name: distilbert-cord-ner
results: []
---
<!-- This model card has been generated automatically according to the information the Trainer had access to. You
should probably proofread and complete it, then remove this comment. -->
# distilbert-cord-ner
This model is a fine-tuned version of [Geotrend/distilbert-base-en-fr-de-no-da-cased](https://huggingface.co/Geotrend/distilbert-base-en-fr-de-no-da-cased) on an unknown dataset.
It achieves the following results on the evaluation set:
- Loss: 0.1670
- Precision: 0.9128
- Recall: 0.9242
- F1: 0.9185
- Accuracy: 0.9656
## Model description
More information needed
## Intended uses & limitations
More information needed
## Training and evaluation data
More information needed
## Training procedure
### Training hyperparameters
The following hyperparameters were used during training:
- learning_rate: 5e-05
- train_batch_size: 8
- eval_batch_size: 8
- seed: 42
- optimizer: Adam with betas=(0.9,0.999) and epsilon=1e-08
- lr_scheduler_type: linear
- num_epochs: 10
### Training results
| Training Loss | Epoch | Step | Validation Loss | Precision | Recall | F1 | Accuracy |
|:-------------:|:-----:|:----:|:---------------:|:---------:|:------:|:------:|:--------:|
| No log | 1.0 | 113 | 0.1814 | 0.8480 | 0.8618 | 0.8548 | 0.9393 |
| No log | 2.0 | 226 | 0.1755 | 0.8669 | 0.9002 | 0.8832 | 0.9427 |
| No log | 3.0 | 339 | 0.1499 | 0.8800 | 0.8935 | 0.8867 | 0.9533 |
| No log | 4.0 | 452 | 0.1340 | 0.8975 | 0.9079 | 0.9027 | 0.9596 |
| 0.1812 | 5.0 | 565 | 0.1553 | 0.8999 | 0.9146 | 0.9072 | 0.9592 |
| 0.1812 | 6.0 | 678 | 0.1474 | 0.8961 | 0.9021 | 0.8991 | 0.9562 |
| 0.1812 | 7.0 | 791 | 0.1682 | 0.9135 | 0.9223 | 0.9179 | 0.9622 |
| 0.1812 | 8.0 | 904 | 0.1663 | 0.8960 | 0.9175 | 0.9066 | 0.9613 |
| 0.0199 | 9.0 | 1017 | 0.1753 | 0.9061 | 0.9261 | 0.9160 | 0.9635 |
| 0.0199 | 10.0 | 1130 | 0.1670 | 0.9128 | 0.9242 | 0.9185 | 0.9656 |
### Framework versions
- Transformers 4.18.0
- Pytorch 1.11.0
- Datasets 2.1.0
- Tokenizers 0.12.1
|
subhasisj/ar-adapter-32 | c4a28e4e2a54fd2f1223f9a2c451baf07ad3c520 | 2022-05-21T20:22:40.000Z | [
"pytorch",
"tensorboard",
"xlm-roberta",
"question-answering",
"transformers",
"generated_from_trainer",
"model-index",
"autotrain_compatible"
] | question-answering | false | subhasisj | null | subhasisj/ar-adapter-32 | 0 | null | transformers | 37,622 | ---
tags:
- generated_from_trainer
model-index:
- name: ar-adapter-32
results: []
---
<!-- This model card has been generated automatically according to the information the Trainer had access to. You
should probably proofread and complete it, then remove this comment. -->
# ar-adapter-32
This model was trained from scratch on the None dataset.
It achieves the following results on the evaluation set:
- Loss: 5.3886
## Model description
More information needed
## Intended uses & limitations
More information needed
## Training and evaluation data
More information needed
## Training procedure
### Training hyperparameters
The following hyperparameters were used during training:
- learning_rate: 3e-05
- train_batch_size: 32
- eval_batch_size: 32
- seed: 42
- gradient_accumulation_steps: 8
- total_train_batch_size: 256
- optimizer: Adam with betas=(0.9,0.999) and epsilon=1e-08
- lr_scheduler_type: linear
- lr_scheduler_warmup_ratio: 0.1
- num_epochs: 5
- mixed_precision_training: Native AMP
### Training results
| Training Loss | Epoch | Step | Validation Loss |
|:-------------:|:-----:|:----:|:---------------:|
| No log | 1.0 | 352 | 5.6861 |
| 5.7356 | 2.0 | 704 | 5.5388 |
| 5.5308 | 3.0 | 1056 | 5.4493 |
| 5.5308 | 4.0 | 1408 | 5.4030 |
| 5.4304 | 5.0 | 1760 | 5.3886 |
### Framework versions
- Transformers 4.17.0
- Pytorch 1.11.0+cu113
- Datasets 2.2.2
- Tokenizers 0.12.1
|
stevemobs/distilbert-base-uncased-finetuned-squad | 3c5d0b04d6d1052f2f07d3926eba6f2068add794 | 2022-05-21T21:52:36.000Z | [
"pytorch",
"tensorboard",
"distilbert",
"question-answering",
"dataset:squad_v2",
"transformers",
"generated_from_trainer",
"license:apache-2.0",
"model-index",
"autotrain_compatible"
] | question-answering | false | stevemobs | null | stevemobs/distilbert-base-uncased-finetuned-squad | 0 | null | transformers | 37,623 | ---
license: apache-2.0
tags:
- generated_from_trainer
datasets:
- squad_v2
model-index:
- name: distilbert-base-uncased-finetuned-squad
results: []
---
<!-- This model card has been generated automatically according to the information the Trainer had access to. You
should probably proofread and complete it, then remove this comment. -->
# distilbert-base-uncased-finetuned-squad
This model is a fine-tuned version of [distilbert-base-uncased](https://huggingface.co/distilbert-base-uncased) on the squad_v2 dataset.
It achieves the following results on the evaluation set:
- Loss: 1.4413
## Model description
More information needed
## Intended uses & limitations
More information needed
## Training and evaluation data
More information needed
## Training procedure
### Training hyperparameters
The following hyperparameters were used during training:
- learning_rate: 2e-05
- train_batch_size: 16
- eval_batch_size: 16
- seed: 42
- optimizer: Adam with betas=(0.9,0.999) and epsilon=1e-08
- lr_scheduler_type: linear
- num_epochs: 3
### Training results
| Training Loss | Epoch | Step | Validation Loss |
|:-------------:|:-----:|:-----:|:---------------:|
| 1.2121 | 1.0 | 8235 | 1.2995 |
| 0.948 | 2.0 | 16470 | 1.2667 |
| 0.7629 | 3.0 | 24705 | 1.4413 |
### Framework versions
- Transformers 4.19.2
- Pytorch 1.11.0+cu113
- Datasets 2.2.2
- Tokenizers 0.12.1
|
ruselkomp/sber-framebank-hidesize-1 | 1bf22f09f2bb88f1c4288816e2ba1879c3b872d9 | 2022-05-22T01:57:09.000Z | [
"pytorch",
"tensorboard",
"bert",
"question-answering",
"transformers",
"generated_from_trainer",
"model-index",
"autotrain_compatible"
] | question-answering | false | ruselkomp | null | ruselkomp/sber-framebank-hidesize-1 | 0 | null | transformers | 37,624 | ---
tags:
- generated_from_trainer
model-index:
- name: sber-framebank-hidesize-1
results: []
---
<!-- This model card has been generated automatically according to the information the Trainer had access to. You
should probably proofread and complete it, then remove this comment. -->
# sber-framebank-hidesize-1
This model is a fine-tuned version of [sberbank-ai/sbert_large_nlu_ru](https://huggingface.co/sberbank-ai/sbert_large_nlu_ru) on the None dataset.
It achieves the following results on the evaluation set:
- Loss: 1.4154
## Model description
More information needed
## Intended uses & limitations
More information needed
## Training and evaluation data
More information needed
## Training procedure
### Training hyperparameters
The following hyperparameters were used during training:
- learning_rate: 2e-05
- train_batch_size: 4
- eval_batch_size: 4
- seed: 42
- optimizer: Adam with betas=(0.9,0.999) and epsilon=1e-08
- lr_scheduler_type: linear
- num_epochs: 3
### Training results
| Training Loss | Epoch | Step | Validation Loss |
|:-------------:|:-----:|:-----:|:---------------:|
| 1.053 | 1.0 | 11307 | 1.0655 |
| 0.835 | 2.0 | 22614 | 1.2487 |
| 0.6054 | 3.0 | 33921 | 1.4154 |
### Framework versions
- Transformers 4.19.0.dev0
- Pytorch 1.11.0+cu113
- Datasets 2.2.3.dev0
- Tokenizers 0.12.1
|
prodm93/T5Dynamic_text_model_v2 | 54290f0d83594f770dc0b298e18968f9e71c3d8b | 2022-05-21T22:23:59.000Z | [
"pytorch",
"t5",
"text2text-generation",
"transformers",
"autotrain_compatible"
] | text2text-generation | false | prodm93 | null | prodm93/T5Dynamic_text_model_v2 | 0 | null | transformers | 37,625 | Entry not found |
neibla/convnext-tiny-224-finetuned-eurosat | 33eca54e09e648dffcf44cc89eb9b0057d97b8b5 | 2022-05-22T04:10:27.000Z | [
"pytorch",
"tensorboard",
"regnet",
"image-classification",
"transformers"
] | image-classification | false | neibla | null | neibla/convnext-tiny-224-finetuned-eurosat | 0 | null | transformers | 37,626 | Entry not found |
sandrokim/two_tower_sentence_snoobert | dea46e48bf82d72e587995913c1b1ac2b7aa8cf2 | 2022-05-22T00:02:17.000Z | [
"pytorch",
"bert",
"feature-extraction",
"sentence-transformers",
"sentence-similarity",
"transformers"
] | sentence-similarity | false | sandrokim | null | sandrokim/two_tower_sentence_snoobert | 0 | null | sentence-transformers | 37,627 | ---
pipeline_tag: sentence-similarity
tags:
- sentence-transformers
- feature-extraction
- sentence-similarity
- transformers
---
# sandrokim/two_tower_sentence_snoobert
This is a [sentence-transformers](https://www.SBERT.net) model: It maps sentences & paragraphs to a 768 dimensional dense vector space and can be used for tasks like clustering or semantic search.
<!--- Describe your model here -->
## Usage (Sentence-Transformers)
Using this model becomes easy when you have [sentence-transformers](https://www.SBERT.net) installed:
```
pip install -U sentence-transformers
```
Then you can use the model like this:
```python
from sentence_transformers import SentenceTransformer
sentences = ["This is an example sentence", "Each sentence is converted"]
model = SentenceTransformer('sandrokim/two_tower_sentence_snoobert')
embeddings = model.encode(sentences)
print(embeddings)
```
## Usage (HuggingFace Transformers)
Without [sentence-transformers](https://www.SBERT.net), you can use the model like this: First, you pass your input through the transformer model, then you have to apply the right pooling-operation on-top of the contextualized word embeddings.
```python
from transformers import AutoTokenizer, AutoModel
import torch
#Mean Pooling - Take attention mask into account for correct averaging
def mean_pooling(model_output, attention_mask):
token_embeddings = model_output[0] #First element of model_output contains all token embeddings
input_mask_expanded = attention_mask.unsqueeze(-1).expand(token_embeddings.size()).float()
return torch.sum(token_embeddings * input_mask_expanded, 1) / torch.clamp(input_mask_expanded.sum(1), min=1e-9)
# Sentences we want sentence embeddings for
sentences = ['This is an example sentence', 'Each sentence is converted']
# Load model from HuggingFace Hub
tokenizer = AutoTokenizer.from_pretrained('sandrokim/two_tower_sentence_snoobert')
model = AutoModel.from_pretrained('sandrokim/two_tower_sentence_snoobert')
# Tokenize sentences
encoded_input = tokenizer(sentences, padding=True, truncation=True, return_tensors='pt')
# Compute token embeddings
with torch.no_grad():
model_output = model(**encoded_input)
# Perform pooling. In this case, mean pooling.
sentence_embeddings = mean_pooling(model_output, encoded_input['attention_mask'])
print("Sentence embeddings:")
print(sentence_embeddings)
```
## Evaluation Results
<!--- Describe how your model was evaluated -->
For an automated evaluation of this model, see the *Sentence Embeddings Benchmark*: [https://seb.sbert.net](https://seb.sbert.net?model_name=sandrokim/two_tower_sentence_snoobert)
## Training
The model was trained with the parameters:
**DataLoader**:
`torch.utils.data.dataloader.DataLoader` of length 719 with parameters:
```
{'batch_size': 8, 'sampler': 'torch.utils.data.sampler.RandomSampler', 'batch_sampler': 'torch.utils.data.sampler.BatchSampler'}
```
**Loss**:
`sentence_transformers.losses.CosineSimilarityLoss.CosineSimilarityLoss`
Parameters of the fit()-Method:
```
{
"epochs": 5,
"evaluation_steps": 1000,
"evaluator": "sentence_transformers.evaluation.EmbeddingSimilarityEvaluator.EmbeddingSimilarityEvaluator",
"max_grad_norm": 1,
"optimizer_class": "<class 'transformers.optimization.AdamW'>",
"optimizer_params": {
"lr": 2e-05
},
"scheduler": "WarmupLinear",
"steps_per_epoch": null,
"warmup_steps": 992,
"weight_decay": 0.01
}
```
## Full Model Architecture
```
SentenceTransformer(
(0): Transformer({'max_seq_length': 512, 'do_lower_case': False}) with Transformer model: BertModel
(1): Pooling({'word_embedding_dimension': 768, 'pooling_mode_cls_token': False, 'pooling_mode_mean_tokens': True, 'pooling_mode_max_tokens': False, 'pooling_mode_mean_sqrt_len_tokens': False})
)
```
## Citing & Authors
<!--- Describe where people can find more information --> |
prodm93/t5-rn-abstract-model-v1 | d588f01c9c1dade0117a1ae1e545fe3de5fff8e0 | 2022-05-22T01:15:18.000Z | [
"pytorch",
"t5",
"text2text-generation",
"transformers",
"autotrain_compatible"
] | text2text-generation | false | prodm93 | null | prodm93/t5-rn-abstract-model-v1 | 0 | null | transformers | 37,628 | Entry not found |
prodm93/gpt2-sum-abstract-model-v1 | 2e407224b90f76478a5c4258e68bd521c6a699c0 | 2022-05-22T01:26:57.000Z | [
"pytorch",
"gpt2",
"text-generation",
"transformers"
] | text-generation | false | prodm93 | null | prodm93/gpt2-sum-abstract-model-v1 | 0 | null | transformers | 37,629 | Entry not found |
prodm93/t5-sum-abstract-model-v1 | 7702a55695a2bfcf21f6470cea48f803bb07c8e6 | 2022-05-22T01:35:40.000Z | [
"pytorch",
"t5",
"text2text-generation",
"transformers",
"autotrain_compatible"
] | text2text-generation | false | prodm93 | null | prodm93/t5-sum-abstract-model-v1 | 0 | null | transformers | 37,630 | Entry not found |
huggingtweets/flimosch | 67b15dfc340db7659abbdc6ee7e93a0fe4dca131 | 2022-05-22T05:32:03.000Z | [
"pytorch",
"gpt2",
"text-generation",
"en",
"transformers",
"huggingtweets"
] | text-generation | false | huggingtweets | null | huggingtweets/flimosch | 0 | null | transformers | 37,631 | ---
language: en
thumbnail: https://github.com/borisdayma/huggingtweets/blob/master/img/logo.png?raw=true
tags:
- huggingtweets
widget:
- text: "My dream is"
---
<div class="inline-flex flex-col" style="line-height: 1.5;">
<div class="flex">
<div
style="display:inherit; margin-left: 4px; margin-right: 4px; width: 92px; height:92px; border-radius: 50%; background-size: cover; background-image: url('https://pbs.twimg.com/profile_images/791305273587752962/cQxUCInF_400x400.jpg')">
</div>
<div
style="display:none; margin-left: 4px; margin-right: 4px; width: 92px; height:92px; border-radius: 50%; background-size: cover; background-image: url('')">
</div>
<div
style="display:none; margin-left: 4px; margin-right: 4px; width: 92px; height:92px; border-radius: 50%; background-size: cover; background-image: url('')">
</div>
</div>
<div style="text-align: center; margin-top: 3px; font-size: 16px; font-weight: 800">🤖 AI BOT 🤖</div>
<div style="text-align: center; font-size: 16px; font-weight: 800">flimosch</div>
<div style="text-align: center; font-size: 14px;">@flimosch</div>
</div>
I was made with [huggingtweets](https://github.com/borisdayma/huggingtweets).
Create your own bot based on your favorite user with [the demo](https://colab.research.google.com/github/borisdayma/huggingtweets/blob/master/huggingtweets-demo.ipynb)!
## How does it work?
The model uses the following pipeline.
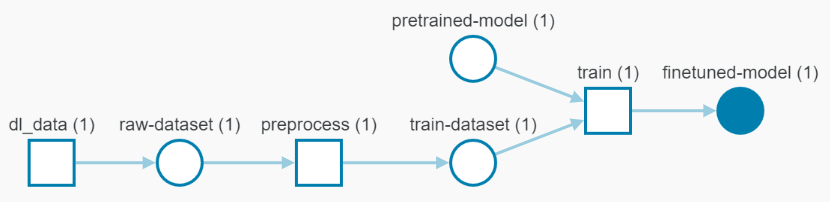
To understand how the model was developed, check the [W&B report](https://wandb.ai/wandb/huggingtweets/reports/HuggingTweets-Train-a-Model-to-Generate-Tweets--VmlldzoxMTY5MjI).
## Training data
The model was trained on tweets from flimosch.
| Data | flimosch |
| --- | --- |
| Tweets downloaded | 3174 |
| Retweets | 649 |
| Short tweets | 681 |
| Tweets kept | 1844 |
[Explore the data](https://wandb.ai/wandb/huggingtweets/runs/3umhpijp/artifacts), which is tracked with [W&B artifacts](https://docs.wandb.com/artifacts) at every step of the pipeline.
## Training procedure
The model is based on a pre-trained [GPT-2](https://huggingface.co/gpt2) which is fine-tuned on @flimosch's tweets.
Hyperparameters and metrics are recorded in the [W&B training run](https://wandb.ai/wandb/huggingtweets/runs/1jet29t5) for full transparency and reproducibility.
At the end of training, [the final model](https://wandb.ai/wandb/huggingtweets/runs/1jet29t5/artifacts) is logged and versioned.
## How to use
You can use this model directly with a pipeline for text generation:
```python
from transformers import pipeline
generator = pipeline('text-generation',
model='huggingtweets/flimosch')
generator("My dream is", num_return_sequences=5)
```
## Limitations and bias
The model suffers from [the same limitations and bias as GPT-2](https://huggingface.co/gpt2#limitations-and-bias).
In addition, the data present in the user's tweets further affects the text generated by the model.
## About
*Built by Boris Dayma*
[](https://twitter.com/intent/follow?screen_name=borisdayma)
For more details, visit the project repository.
[](https://github.com/borisdayma/huggingtweets)
|
sabah17/distilbert-base-uncased-finetuned-squad | 0708777ae441ffafa5a07f658f05e7e7d1041fcb | 2022-05-29T05:37:34.000Z | [
"pytorch",
"tensorboard",
"distilbert",
"question-answering",
"dataset:squad",
"transformers",
"generated_from_trainer",
"license:apache-2.0",
"model-index",
"autotrain_compatible"
] | question-answering | false | sabah17 | null | sabah17/distilbert-base-uncased-finetuned-squad | 0 | null | transformers | 37,632 | ---
license: apache-2.0
tags:
- generated_from_trainer
datasets:
- squad
model-index:
- name: distilbert-base-uncased-finetuned-squad
results: []
---
<!-- This model card has been generated automatically according to the information the Trainer had access to. You
should probably proofread and complete it, then remove this comment. -->
# distilbert-base-uncased-finetuned-squad
This model is a fine-tuned version of [distilbert-base-uncased](https://huggingface.co/distilbert-base-uncased) on the squad dataset.
It achieves the following results on the evaluation set:
- Loss: 1.1635
## Model description
More information needed
## Intended uses & limitations
More information needed
## Training and evaluation data
More information needed
## Training procedure
### Training hyperparameters
The following hyperparameters were used during training:
- learning_rate: 2e-05
- train_batch_size: 16
- eval_batch_size: 16
- seed: 42
- optimizer: Adam with betas=(0.9,0.999) and epsilon=1e-08
- lr_scheduler_type: linear
- num_epochs: 3
### Training results
| Training Loss | Epoch | Step | Validation Loss |
|:-------------:|:-----:|:-----:|:---------------:|
| 1.2324 | 1.0 | 5533 | 1.1746 |
| 0.9703 | 2.0 | 11066 | 1.1406 |
| 0.7702 | 3.0 | 16599 | 1.1635 |
### Framework versions
- Transformers 4.19.2
- Pytorch 1.11.0+cu113
- Datasets 2.2.2
- Tokenizers 0.12.1
|
pglauner/xlm-roberta-base-finetuned-panx-de | 7f77b368455ad3e566f561accccd15e2e4d2569e | 2022-05-22T08:35:58.000Z | [
"pytorch",
"tensorboard",
"xlm-roberta",
"token-classification",
"dataset:xtreme",
"transformers",
"generated_from_trainer",
"license:mit",
"model-index",
"autotrain_compatible"
] | token-classification | false | pglauner | null | pglauner/xlm-roberta-base-finetuned-panx-de | 0 | null | transformers | 37,633 | ---
license: mit
tags:
- generated_from_trainer
datasets:
- xtreme
metrics:
- f1
model-index:
- name: xlm-roberta-base-finetuned-panx-de
results:
- task:
name: Token Classification
type: token-classification
dataset:
name: xtreme
type: xtreme
args: PAN-X.de
metrics:
- name: F1
type: f1
value: 0.8620945214069894
---
<!-- This model card has been generated automatically according to the information the Trainer had access to. You
should probably proofread and complete it, then remove this comment. -->
# xlm-roberta-base-finetuned-panx-de
This model is a fine-tuned version of [xlm-roberta-base](https://huggingface.co/xlm-roberta-base) on the xtreme dataset.
It achieves the following results on the evaluation set:
- Loss: 0.1372
- F1: 0.8621
## Model description
More information needed
## Intended uses & limitations
More information needed
## Training and evaluation data
More information needed
## Training procedure
### Training hyperparameters
The following hyperparameters were used during training:
- learning_rate: 5e-05
- train_batch_size: 24
- eval_batch_size: 24
- seed: 42
- optimizer: Adam with betas=(0.9,0.999) and epsilon=1e-08
- lr_scheduler_type: linear
- num_epochs: 3
### Training results
| Training Loss | Epoch | Step | Validation Loss | F1 |
|:-------------:|:-----:|:----:|:---------------:|:------:|
| 0.2575 | 1.0 | 525 | 0.1621 | 0.8292 |
| 0.1287 | 2.0 | 1050 | 0.1378 | 0.8526 |
| 0.0831 | 3.0 | 1575 | 0.1372 | 0.8621 |
### Framework versions
- Transformers 4.11.3
- Pytorch 1.11.0+cu113
- Datasets 1.16.1
- Tokenizers 0.10.3
|
subhasisj/de-adapter-32 | 954361d68dbac518b6591b305e365a9f687905a1 | 2022-05-22T11:00:43.000Z | [
"pytorch",
"tensorboard",
"xlm-roberta",
"question-answering",
"transformers",
"generated_from_trainer",
"model-index",
"autotrain_compatible"
] | question-answering | false | subhasisj | null | subhasisj/de-adapter-32 | 0 | null | transformers | 37,634 | ---
tags:
- generated_from_trainer
model-index:
- name: de-adapter-32
results: []
---
<!-- This model card has been generated automatically according to the information the Trainer had access to. You
should probably proofread and complete it, then remove this comment. -->
# de-adapter-32
This model was trained from scratch on the None dataset.
It achieves the following results on the evaluation set:
- Loss: 5.4347
## Model description
More information needed
## Intended uses & limitations
More information needed
## Training and evaluation data
More information needed
## Training procedure
### Training hyperparameters
The following hyperparameters were used during training:
- learning_rate: 3e-05
- train_batch_size: 32
- eval_batch_size: 32
- seed: 42
- gradient_accumulation_steps: 8
- total_train_batch_size: 256
- optimizer: Adam with betas=(0.9,0.999) and epsilon=1e-08
- lr_scheduler_type: linear
- lr_scheduler_warmup_ratio: 0.1
- num_epochs: 5
- mixed_precision_training: Native AMP
### Training results
| Training Loss | Epoch | Step | Validation Loss |
|:-------------:|:-----:|:----:|:---------------:|
| No log | 1.0 | 335 | 5.7031 |
| 5.7592 | 2.0 | 670 | 5.5706 |
| 5.5647 | 3.0 | 1005 | 5.4899 |
| 5.5647 | 4.0 | 1340 | 5.4481 |
| 5.4865 | 5.0 | 1675 | 5.4347 |
### Framework versions
- Transformers 4.17.0
- Pytorch 1.11.0+cu113
- Datasets 2.2.2
- Tokenizers 0.12.1
|
moghis/xlm-roberta-base-finetuned-panx-fr-de | fe80466c9549c97298c8afda097ab20e8379dea1 | 2022-05-22T09:56:59.000Z | [
"pytorch",
"xlm-roberta",
"token-classification",
"transformers",
"generated_from_trainer",
"license:mit",
"model-index",
"autotrain_compatible"
] | token-classification | false | moghis | null | moghis/xlm-roberta-base-finetuned-panx-fr-de | 0 | null | transformers | 37,635 | ---
license: mit
tags:
- generated_from_trainer
model-index:
- name: xlm-roberta-base-finetuned-panx-fr-de
results: []
---
<!-- This model card has been generated automatically according to the information the Trainer had access to. You
should probably proofread and complete it, then remove this comment. -->
# xlm-roberta-base-finetuned-panx-fr-de
This model is a fine-tuned version of [xlm-roberta-base](https://huggingface.co/xlm-roberta-base) on the None dataset.
It achieves the following results on the evaluation set:
- Loss: 0.1631
- F1 Score: 0.8579
## Model description
More information needed
## Intended uses & limitations
More information needed
## Training and evaluation data
More information needed
## Training procedure
### Training hyperparameters
The following hyperparameters were used during training:
- learning_rate: 5e-05
- train_batch_size: 24
- eval_batch_size: 24
- seed: 42
- optimizer: Adam with betas=(0.9,0.999) and epsilon=1e-08
- lr_scheduler_type: linear
- num_epochs: 3
### Training results
| Training Loss | Epoch | Step | Validation Loss | F1 Score |
|:-------------:|:-----:|:----:|:---------------:|:--------:|
| 0.2878 | 1.0 | 715 | 0.1840 | 0.8247 |
| 0.1456 | 2.0 | 1430 | 0.1596 | 0.8473 |
| 0.0925 | 3.0 | 2145 | 0.1631 | 0.8579 |
### Framework versions
- Transformers 4.19.2
- Pytorch 1.11.0+cu113
- Datasets 2.2.2
- Tokenizers 0.12.1
|
ruselkomp/sber-framebank-hidesize-2 | aac0451c57f69fe279e5b3e40ff331bd16ce98d0 | 2022-05-23T01:04:10.000Z | [
"pytorch",
"tensorboard",
"bert",
"question-answering",
"transformers",
"generated_from_trainer",
"model-index",
"autotrain_compatible"
] | question-answering | false | ruselkomp | null | ruselkomp/sber-framebank-hidesize-2 | 0 | null | transformers | 37,636 | ---
tags:
- generated_from_trainer
model-index:
- name: sber-framebank-hidesize-2
results: []
---
<!-- This model card has been generated automatically according to the information the Trainer had access to. You
should probably proofread and complete it, then remove this comment. -->
# sber-framebank-hidesize-2
This model is a fine-tuned version of [sberbank-ai/sbert_large_nlu_ru](https://huggingface.co/sberbank-ai/sbert_large_nlu_ru) on the None dataset.
It achieves the following results on the evaluation set:
- Loss: 1.5381
## Model description
More information needed
## Intended uses & limitations
More information needed
## Training and evaluation data
More information needed
## Training procedure
### Training hyperparameters
The following hyperparameters were used during training:
- learning_rate: 2e-05
- train_batch_size: 4
- eval_batch_size: 4
- seed: 42
- optimizer: Adam with betas=(0.9,0.999) and epsilon=1e-08
- lr_scheduler_type: linear
- num_epochs: 3
### Training results
| Training Loss | Epoch | Step | Validation Loss |
|:-------------:|:-----:|:-----:|:---------------:|
| 1.0513 | 1.0 | 11307 | 1.0576 |
| 0.7052 | 2.0 | 22614 | 1.1270 |
| 0.4185 | 3.0 | 33921 | 1.5381 |
### Framework versions
- Transformers 4.19.0.dev0
- Pytorch 1.11.0+cu113
- Datasets 2.2.3.dev0
- Tokenizers 0.12.1
|
subhasisj/es-adapter-32 | ffe543a6da4c9c0e59ce45c839a0da91fc7e03e5 | 2022-05-22T13:44:29.000Z | [
"pytorch",
"tensorboard",
"xlm-roberta",
"question-answering",
"transformers",
"generated_from_trainer",
"model-index",
"autotrain_compatible"
] | question-answering | false | subhasisj | null | subhasisj/es-adapter-32 | 0 | null | transformers | 37,637 | ---
tags:
- generated_from_trainer
model-index:
- name: es-adapter-32
results: []
---
<!-- This model card has been generated automatically according to the information the Trainer had access to. You
should probably proofread and complete it, then remove this comment. -->
# es-adapter-32
This model was trained from scratch on the None dataset.
It achieves the following results on the evaluation set:
- Loss: 5.4161
## Model description
More information needed
## Intended uses & limitations
More information needed
## Training and evaluation data
More information needed
## Training procedure
### Training hyperparameters
The following hyperparameters were used during training:
- learning_rate: 3e-05
- train_batch_size: 32
- eval_batch_size: 32
- seed: 42
- gradient_accumulation_steps: 8
- total_train_batch_size: 256
- optimizer: Adam with betas=(0.9,0.999) and epsilon=1e-08
- lr_scheduler_type: linear
- lr_scheduler_warmup_ratio: 0.1
- num_epochs: 5
- mixed_precision_training: Native AMP
### Training results
| Training Loss | Epoch | Step | Validation Loss |
|:-------------:|:-----:|:----:|:---------------:|
| No log | 1.0 | 356 | 5.6970 |
| 5.7468 | 2.0 | 712 | 5.5589 |
| 5.5498 | 3.0 | 1068 | 5.4747 |
| 5.5498 | 4.0 | 1424 | 5.4303 |
| 5.4518 | 5.0 | 1780 | 5.4161 |
### Framework versions
- Transformers 4.17.0
- Pytorch 1.11.0+cu113
- Datasets 2.2.2
- Tokenizers 0.12.1
|
kirillka/rut5-small-finetuned-gen-description-2 | 38aa631890bfe8a440e40622a27e4c8a174787b3 | 2022-05-22T12:14:52.000Z | [
"pytorch",
"tensorboard",
"mt5",
"text2text-generation",
"transformers",
"generated_from_trainer",
"license:mit",
"model-index",
"autotrain_compatible"
] | text2text-generation | false | kirillka | null | kirillka/rut5-small-finetuned-gen-description-2 | 0 | null | transformers | 37,638 | ---
license: mit
tags:
- generated_from_trainer
model-index:
- name: rut5-small-finetuned-gen-description-2
results: []
---
<!-- This model card has been generated automatically according to the information the Trainer had access to. You
should probably proofread and complete it, then remove this comment. -->
# rut5-small-finetuned-gen-description-2
This model is a fine-tuned version of [cointegrated/rut5-small](https://huggingface.co/cointegrated/rut5-small) on the None dataset.
It achieves the following results on the evaluation set:
- Loss: nan
## Model description
More information needed
## Intended uses & limitations
More information needed
## Training and evaluation data
More information needed
## Training procedure
### Training hyperparameters
The following hyperparameters were used during training:
- learning_rate: 2e-05
- train_batch_size: 16
- eval_batch_size: 16
- seed: 42
- optimizer: Adam with betas=(0.9,0.999) and epsilon=1e-08
- lr_scheduler_type: linear
- num_epochs: 5
- mixed_precision_training: Native AMP
### Training results
| Training Loss | Epoch | Step | Validation Loss |
|:-------------:|:-----:|:----:|:---------------:|
| No log | 1.0 | 422 | nan |
| 2.3892 | 2.0 | 844 | nan |
| 0.0 | 3.0 | 1266 | nan |
| 0.0 | 4.0 | 1688 | nan |
| 0.0 | 5.0 | 2110 | nan |
### Framework versions
- Transformers 4.19.2
- Pytorch 1.11.0+cu113
- Datasets 2.2.2
- Tokenizers 0.12.1
|
stevemobs/distilbert-base-uncased-combined-squad-adversarial | e6ca1e7c35d1385732c30a06a82cd4cfd14d0a43 | 2022-05-22T15:35:53.000Z | [
"pytorch",
"tensorboard",
"distilbert",
"question-answering",
"transformers",
"generated_from_trainer",
"license:apache-2.0",
"model-index",
"autotrain_compatible"
] | question-answering | false | stevemobs | null | stevemobs/distilbert-base-uncased-combined-squad-adversarial | 0 | null | transformers | 37,639 | ---
license: apache-2.0
tags:
- generated_from_trainer
model-index:
- name: distilbert-base-uncased-combined-squad-adversarial
results: []
---
<!-- This model card has been generated automatically according to the information the Trainer had access to. You
should probably proofread and complete it, then remove this comment. -->
# distilbert-base-uncased-combined-squad-adversarial
This model is a fine-tuned version of [distilbert-base-uncased](https://huggingface.co/distilbert-base-uncased) on the None dataset.
It achieves the following results on the evaluation set:
- Loss: 1.7273
## Model description
More information needed
## Intended uses & limitations
More information needed
## Training and evaluation data
More information needed
## Training procedure
### Training hyperparameters
The following hyperparameters were used during training:
- learning_rate: 2e-05
- train_batch_size: 16
- eval_batch_size: 16
- seed: 42
- optimizer: Adam with betas=(0.9,0.999) and epsilon=1e-08
- lr_scheduler_type: linear
- num_epochs: 3
### Training results
| Training Loss | Epoch | Step | Validation Loss |
|:-------------:|:-----:|:-----:|:---------------:|
| 1.574 | 1.0 | 10130 | 1.5529 |
| 1.2707 | 2.0 | 20260 | 1.6522 |
| 1.0196 | 3.0 | 30390 | 1.7273 |
### Framework versions
- Transformers 4.19.2
- Pytorch 1.11.0+cu113
- Datasets 2.2.2
- Tokenizers 0.12.1
|
masoumehb/wav2vec2-large-xlsr-turkish-demo-colab | 3a4c8e2e2633ec5bb560152987920cbbab5cdfef | 2022-05-24T12:20:20.000Z | [
"pytorch",
"tensorboard",
"wav2vec2",
"automatic-speech-recognition",
"dataset:common_voice",
"transformers",
"generated_from_trainer",
"license:apache-2.0",
"model-index"
] | automatic-speech-recognition | false | masoumehb | null | masoumehb/wav2vec2-large-xlsr-turkish-demo-colab | 0 | null | transformers | 37,640 | ---
license: apache-2.0
tags:
- generated_from_trainer
datasets:
- common_voice
model-index:
- name: wav2vec2-large-xlsr-turkish-demo-colab
results: []
---
<!-- This model card has been generated automatically according to the information the Trainer had access to. You
should probably proofread and complete it, then remove this comment. -->
# wav2vec2-large-xlsr-turkish-demo-colab
This model is a fine-tuned version of [facebook/wav2vec2-large-xlsr-53](https://huggingface.co/facebook/wav2vec2-large-xlsr-53) on the common_voice dataset.
## Model description
More information needed
## Intended uses & limitations
More information needed
## Training and evaluation data
More information needed
## Training procedure
### Training hyperparameters
The following hyperparameters were used during training:
- learning_rate: 0.0003
- train_batch_size: 16
- eval_batch_size: 8
- seed: 42
- gradient_accumulation_steps: 2
- total_train_batch_size: 32
- optimizer: Adam with betas=(0.9,0.999) and epsilon=1e-08
- lr_scheduler_type: linear
- lr_scheduler_warmup_steps: 500
- num_epochs: 30
- mixed_precision_training: Native AMP
### Framework versions
- Transformers 4.11.3
- Pytorch 1.11.0
- Datasets 1.13.3
- Tokenizers 0.10.3
|
subhasisj/zh-adapter-32 | f0e44fc14efa83da68333f3f6482493633a8a208 | 2022-05-22T19:42:24.000Z | [
"pytorch",
"tensorboard",
"xlm-roberta",
"question-answering",
"transformers",
"generated_from_trainer",
"model-index",
"autotrain_compatible"
] | question-answering | false | subhasisj | null | subhasisj/zh-adapter-32 | 0 | null | transformers | 37,641 | ---
tags:
- generated_from_trainer
model-index:
- name: zh-adapter-32
results: []
---
<!-- This model card has been generated automatically according to the information the Trainer had access to. You
should probably proofread and complete it, then remove this comment. -->
# zh-adapter-32
This model was trained from scratch on the None dataset.
It achieves the following results on the evaluation set:
- Loss: 5.2154
## Model description
More information needed
## Intended uses & limitations
More information needed
## Training and evaluation data
More information needed
## Training procedure
### Training hyperparameters
The following hyperparameters were used during training:
- learning_rate: 3e-05
- train_batch_size: 32
- eval_batch_size: 32
- seed: 42
- gradient_accumulation_steps: 8
- total_train_batch_size: 256
- optimizer: Adam with betas=(0.9,0.999) and epsilon=1e-08
- lr_scheduler_type: linear
- lr_scheduler_warmup_ratio: 0.1
- num_epochs: 5
- mixed_precision_training: Native AMP
### Training results
| Training Loss | Epoch | Step | Validation Loss |
|:-------------:|:-----:|:----:|:---------------:|
| No log | 1.0 | 338 | 5.5948 |
| 5.6797 | 2.0 | 676 | 5.4048 |
| 5.4277 | 3.0 | 1014 | 5.2908 |
| 5.4277 | 4.0 | 1352 | 5.2328 |
| 5.313 | 5.0 | 1690 | 5.2154 |
### Framework versions
- Transformers 4.17.0
- Pytorch 1.11.0+cu113
- Datasets 2.2.2
- Tokenizers 0.12.1
|
jppaolim/v35_Baseline | dfb56f796dfa4b260a5dbe2581abefefcf5bcf52 | 2022-05-22T20:20:32.000Z | [
"pytorch",
"gpt2",
"text-generation",
"transformers"
] | text-generation | false | jppaolim | null | jppaolim/v35_Baseline | 0 | null | transformers | 37,642 | # Dummy model
Arthur goes to the beach. Arthur wanted to go to the beach. He thought it would be fun. He went and got a big towel. He set out to get on the water. He was so excited he fell in love with the ocean.
Arthur goes to the beach. Arthur and his family went to the beach on Saturday. They all wanted to go swimming. Arthur decided to try and go to the water. His mom didn't want him to go swimming. He tried to jump into the water but it was too dangerous.
Arthur goes to the beach. Arthur was surfing at a beach. He found a shark swimming near him. He got out of the water and started to dive. A big wave hit his surfboard. Arthur had to run away before he was rescued.
Arthur goes to the beach. Arthur is going to the beach for the first time. He is nervous and doesn't know what to expect. He begins to think about his day. He walks home from the beach. He takes a deep breath, and checks the weather.
Arthur goes to the beach. Arthur was excited for the next day of school. He packed his bags and headed to the beach. When he got there, he saw his friend's car. He tried to help his friend move away from the car. His friend was okay, so he helped him.
Arthur goes to the beach. Arthur was out with friends at a beach. He decided to go for a swim in the ocean. Arthur started swimming but felt tired. He fell asleep and went to sleep. When he woke up he was very tired and had a hard time.
Arthur goes to the beach. Arthur is going to the beach today. He wants to go swimming. Arthur gets out of his car and heads for the beach. He goes swimming and enjoys the sun. He then decides he needs to do something else.
Arthur goes to the beach. Arthur was going to go to the beach. He didn't have any money. Arthur decided to go to the lake. He bought all his friends water. He went home with a smile on his face.
Arthur goes to the beach. Arthur loved going to the beach. He went to the beach everyday. One day, he decided to go to the beach. Arthur found that the beach was crowded with people. Arthur went home exhausted and feeling sad.
Arthur goes to the beach. Arthur has always wanted to go to the beach. He decides to get on his bike. He parks and gets ready to go. The sun comes up and he goes to the beach. He loves his new adventure!
Arthur goes to the beach. Arthur wanted to go to the beach. He decided to go to the beach with his girlfriend. They went to a local bar. The bar had a lot of good food. Arthur ate at the bar and got a good night's rest.
Arthur goes to the beach. Arthur went to the beach with his family. Arthur got on a boat. Arthur started to go down the water. Arthur had a rough time. Arthur's family came home and they were happy.
Arthur goes to the beach. Arthur was going to go to the beach with his friends. He had never been on a beach before. He got very excited and headed out the door. The weather was nice and warm. Arthur had a great time at the beach.
Arthur goes to the beach. Arthur decides he wants to go to the beach. He decides to take a boat ride on the beach. He is not very experienced. Arthur gets lost in the sand. He never goes back to the beach again.
Arthur goes to the beach. Arthur is at the beach with his friends. He gets lost in a large water slide. He tries to find his way back home. He lands on the beach and waits for help. When he gets there he finds a very famous guy.
Arthur goes to the beach. Arthur loves going to the ocean. He has never been on a boat before. He decides he wants to go to the beach. He heads to the beach and gets to the water. He is happy he went to the beach.
Arthur goes to the beach. Arthur is going on a trip to the beach. He has never been to the beach before. He is very excited about his trip. He gets in his car and drives home. He loves the beach.
Arthur goes to the beach. Arthur is going to the beach with his family. He is going to go to a beach with his family. Arthur gets on the water and heads out. Arthur swims for hours in the water. Arthur is happy he was able to go to the beach.
Arthur goes to the beach. Arthur is going to the beach with his family. He has never been to a beach before. He decides he wants to go. He goes to the beach and gets to know all of the people there. He is so happy he can't wait for next year.
Arthur goes to the beach. Arthur was a very good swimmer. He was always in the water at the beach. One day, he was swimming with his friends. Arthur got hit by a car and died. His friends were very sad about it.
|
prodm93/rn_gpt2_customdata_model | e7f3505241ce173de033b1b2bedf65734862ff04 | 2022-05-22T20:47:22.000Z | [
"pytorch",
"gpt2",
"text-generation",
"transformers"
] | text-generation | false | prodm93 | null | prodm93/rn_gpt2_customdata_model | 0 | null | transformers | 37,643 | Entry not found |
jacklin/DeLADE-CLS | 89839d2419f546d673ff39ba06ebf3a229ce0266 | 2022-05-22T21:27:36.000Z | [
"pytorch",
"arxiv:2112.04666"
] | null | false | jacklin | null | jacklin/DeLADE-CLS | 0 | null | null | 37,644 | This model, DeLADE+[CLS], is trained by fusing neural lexical and semantic components in single transformer using DistilBERT as a backbone.
*[A Dense Representation Framework for Lexical and Semantic Matching](https://arxiv.org/pdf/2112.04666.pdf)* Sheng-Chieh Lin and Jimmy Lin.
You can find the usage of the model in our [DHR repo](https://github.com/jacklin64/DHR): (1) [Inference on MSMARCO Passage Ranking](https://github.com/castorini/DHR/blob/main/docs/msmarco-passage-train-eval.md); (2) [Inference on BEIR datasets](https://github.com/castorini/DHR/blob/main/docs/beir-eval.md).
|
jacklin/DeLADE | 93f79f0f14023fc2d37a5d64baa8210b829d1c18 | 2022-05-22T21:27:15.000Z | [
"pytorch",
"arxiv:2112.04666"
] | null | false | jacklin | null | jacklin/DeLADE | 0 | null | null | 37,645 | This model, DeLADE, is trained by fusing neural lexical and semantic components in single transformer using DistilBERT as a backbone.
*[A Dense Representation Framework for Lexical and Semantic Matching](https://arxiv.org/pdf/2112.04666.pdf)* Sheng-Chieh Lin and Jimmy Lin.
You can find the usage of the model in our [DHR repo](https://github.com/jacklin64/DHR): (1) [Inference on MSMARCO Passage Ranking](https://github.com/castorini/DHR/blob/main/docs/msmarco-passage-train-eval.md); (2) [Inference on BEIR datasets](https://github.com/castorini/DHR/blob/main/docs/beir-eval.md).
|
stevemobs/deberta-base-combined-squad1-aqa | 7a0d74b4554c7af74164c0725ae942e42fae55b0 | 2022-05-23T02:32:12.000Z | [
"pytorch",
"tensorboard",
"deberta",
"question-answering",
"transformers",
"generated_from_trainer",
"license:mit",
"model-index",
"autotrain_compatible"
] | question-answering | false | stevemobs | null | stevemobs/deberta-base-combined-squad1-aqa | 0 | null | transformers | 37,646 | ---
license: mit
tags:
- generated_from_trainer
model-index:
- name: deberta-base-combined-squad1-aqa
results: []
---
<!-- This model card has been generated automatically according to the information the Trainer had access to. You
should probably proofread and complete it, then remove this comment. -->
# deberta-base-combined-squad1-aqa
This model is a fine-tuned version of [microsoft/deberta-base](https://huggingface.co/microsoft/deberta-base) on the None dataset.
It achieves the following results on the evaluation set:
- Loss: 0.9442
## Model description
More information needed
## Intended uses & limitations
More information needed
## Training and evaluation data
More information needed
## Training procedure
### Training hyperparameters
The following hyperparameters were used during training:
- learning_rate: 2e-05
- train_batch_size: 12
- eval_batch_size: 12
- seed: 42
- optimizer: Adam with betas=(0.9,0.999) and epsilon=1e-08
- lr_scheduler_type: linear
- num_epochs: 2
### Training results
| Training Loss | Epoch | Step | Validation Loss |
|:-------------:|:-----:|:-----:|:---------------:|
| 1.1133 | 1.0 | 9906 | 0.9652 |
| 0.7943 | 2.0 | 19812 | 0.9442 |
### Framework versions
- Transformers 4.19.2
- Pytorch 1.11.0+cu113
- Datasets 2.2.2
- Tokenizers 0.12.1
|
globuslabs/ScholarBERT_10 | b94cfe6ae48a5e7391d076e1321e18585158c5fc | 2022-05-24T03:15:41.000Z | [
"pytorch",
"bert",
"fill-mask",
"en",
"arxiv:2205.11342",
"transformers",
"science",
"multi-displinary",
"license:apache-2.0",
"autotrain_compatible"
] | fill-mask | false | globuslabs | null | globuslabs/ScholarBERT_10 | 0 | null | transformers | 37,647 | ---
language: en
tags:
- science
- multi-displinary
license: apache-2.0
---
# ScholarBERT_10 Model
This is the **ScholarBERT_10** variant of the ScholarBERT model family.
The model is pretrained on a large collection of scientific research articles (**22.1B tokens**).
This is a **cased** (case-sensitive) model. The tokenizer will not convert all inputs to lower-case by default.
The model is based on the same architecture as [BERT-large](https://huggingface.co/bert-large-cased) and has a total of 340M parameters.
# Model Architecture
| Hyperparameter | Value |
|-----------------|:-------:|
| Layers | 24 |
| Hidden Size | 1024 |
| Attention Heads | 16 |
| Total Parameters | 340M |
# Training Dataset
The vocab and the model are pertrained on **10% of the PRD** scientific literature dataset.
The PRD dataset is provided by Public.Resource.Org, Inc. (“Public Resource”),
a nonprofit organization based in California. This dataset was constructed from a corpus
of journal article files, from which We successfully extracted text from 75,496,055 articles from 178,928 journals.
The articles span across Arts & Humanities, Life Sciences & Biomedicine, Physical Sciences,
Social Sciences, and Technology. The distribution of articles is shown below.
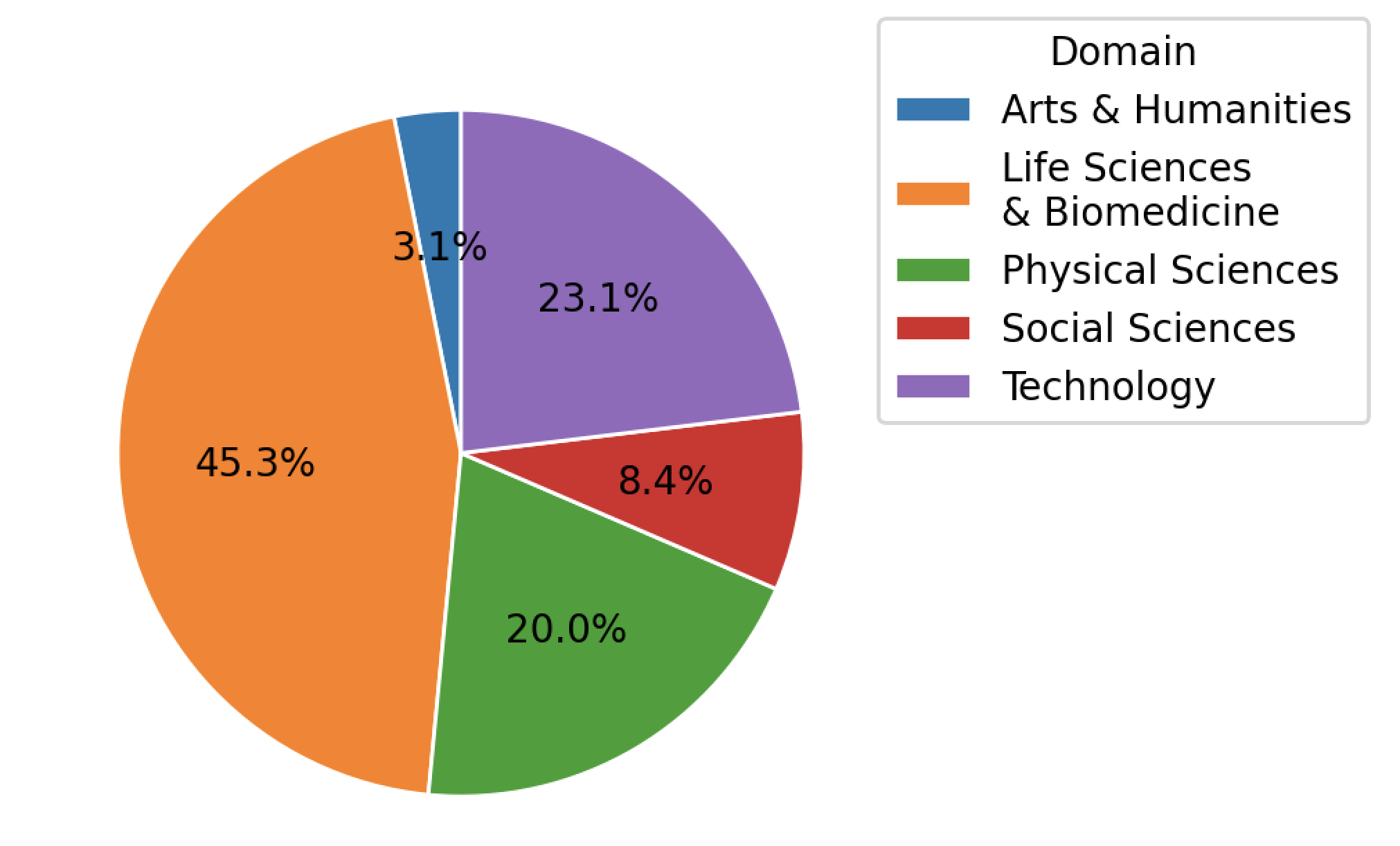
# BibTeX entry and citation info
If using this model, please cite this paper:
```
@misc{hong2022scholarbert,
doi = {10.48550/ARXIV.2205.11342},
url = {https://arxiv.org/abs/2205.11342},
author = {Hong, Zhi and Ajith, Aswathy and Pauloski, Gregory and Duede, Eamon and Malamud, Carl and Magoulas, Roger and Chard, Kyle and Foster, Ian},
title = {ScholarBERT: Bigger is Not Always Better},
publisher = {arXiv},
year = {2022}
}
``` |
zuu/asr-wav2vec2 | 1e8cf7828cfb4f3af02e90d43623f235388b94a4 | 2022-05-23T05:03:02.000Z | [
"pytorch",
"wav2vec2",
"automatic-speech-recognition",
"transformers"
] | automatic-speech-recognition | false | zuu | null | zuu/asr-wav2vec2 | 0 | null | transformers | 37,648 | Entry not found |
mehari/fnrbt | 7e91e4067efa05b3b37316501e38eaa04e634e7d | 2022-05-24T08:06:01.000Z | [
"pytorch",
"roberta",
"token-classification",
"transformers",
"autotrain_compatible"
] | token-classification | false | mehari | null | mehari/fnrbt | 0 | null | transformers | 37,649 | Entry not found |
t8oo/DialoGPT-small-zeni | 1a79bbcc94770665eb0a431cb0c1724cec9a32c9 | 2022-05-23T06:55:16.000Z | [
"pytorch",
"gpt2",
"text-generation",
"transformers",
"conversational"
] | conversational | false | t8oo | null | t8oo/DialoGPT-small-zeni | 0 | null | transformers | 37,650 | ---
tags :
- conversational
---
# Zeni DialoGPT Model |
spasis/bert-finetuned-squad-accelerate | 82bfcb2ae14a6167194e7d77f9774344979b8e61 | 2022-05-23T11:06:21.000Z | [
"pytorch",
"bert",
"question-answering",
"transformers",
"autotrain_compatible"
] | question-answering | false | spasis | null | spasis/bert-finetuned-squad-accelerate | 0 | null | transformers | 37,651 | Entry not found |
stplgg/xlm-roberta-base-finetuned-panx-de | e9ed9a23b4dc65997ec5b8f74581566427eed009 | 2022-05-23T09:43:15.000Z | [
"pytorch",
"tensorboard",
"xlm-roberta",
"token-classification",
"dataset:xtreme",
"transformers",
"generated_from_trainer",
"license:mit",
"model-index",
"autotrain_compatible"
] | token-classification | false | stplgg | null | stplgg/xlm-roberta-base-finetuned-panx-de | 0 | null | transformers | 37,652 | ---
license: mit
tags:
- generated_from_trainer
datasets:
- xtreme
metrics:
- f1
model-index:
- name: xlm-roberta-base-finetuned-panx-de
results:
- task:
name: Token Classification
type: token-classification
dataset:
name: xtreme
type: xtreme
args: PAN-X.de
metrics:
- name: F1
type: f1
value: 0.8620945214069894
---
<!-- This model card has been generated automatically according to the information the Trainer had access to. You
should probably proofread and complete it, then remove this comment. -->
# xlm-roberta-base-finetuned-panx-de
This model is a fine-tuned version of [xlm-roberta-base](https://huggingface.co/xlm-roberta-base) on the xtreme dataset.
It achieves the following results on the evaluation set:
- Loss: 0.1372
- F1: 0.8621
## Model description
More information needed
## Intended uses & limitations
More information needed
## Training and evaluation data
More information needed
## Training procedure
### Training hyperparameters
The following hyperparameters were used during training:
- learning_rate: 5e-05
- train_batch_size: 24
- eval_batch_size: 24
- seed: 42
- optimizer: Adam with betas=(0.9,0.999) and epsilon=1e-08
- lr_scheduler_type: linear
- num_epochs: 3
### Training results
| Training Loss | Epoch | Step | Validation Loss | F1 |
|:-------------:|:-----:|:----:|:---------------:|:------:|
| 0.2575 | 1.0 | 525 | 0.1621 | 0.8292 |
| 0.1287 | 2.0 | 1050 | 0.1378 | 0.8526 |
| 0.0831 | 3.0 | 1575 | 0.1372 | 0.8621 |
### Framework versions
- Transformers 4.19.2
- Pytorch 1.11.0+cu113
- Datasets 2.2.2
- Tokenizers 0.12.1
|
theojolliffe/bart-cnn-pubmed-arxiv-pubmed-v3-e100 | 2099132aca15e848200431e0d821d86beacb399e | 2022-05-24T00:25:24.000Z | [
"pytorch",
"tensorboard",
"bart",
"text2text-generation",
"transformers",
"generated_from_trainer",
"license:mit",
"model-index",
"autotrain_compatible"
] | text2text-generation | false | theojolliffe | null | theojolliffe/bart-cnn-pubmed-arxiv-pubmed-v3-e100 | 0 | null | transformers | 37,653 | ---
license: mit
tags:
- generated_from_trainer
metrics:
- rouge
model-index:
- name: bart-cnn-pubmed-arxiv-pubmed-v3-e100
results: []
---
<!-- This model card has been generated automatically according to the information the Trainer had access to. You
should probably proofread and complete it, then remove this comment. -->
# bart-cnn-pubmed-arxiv-pubmed-v3-e100
This model is a fine-tuned version of [theojolliffe/bart-cnn-pubmed-arxiv-pubmed](https://huggingface.co/theojolliffe/bart-cnn-pubmed-arxiv-pubmed) on an unknown dataset.
It achieves the following results on the evaluation set:
- Loss: 1.1806
- Rouge1: 59.4159
- Rouge2: 48.867
- Rougel: 51.9013
- Rougelsum: 58.3382
- Gen Len: 142.0
## Model description
More information needed
## Intended uses & limitations
More information needed
## Training and evaluation data
More information needed
## Training procedure
### Training hyperparameters
The following hyperparameters were used during training:
- learning_rate: 2e-05
- train_batch_size: 1
- eval_batch_size: 1
- seed: 42
- optimizer: Adam with betas=(0.9,0.999) and epsilon=1e-08
- lr_scheduler_type: linear
- num_epochs: 100
- mixed_precision_training: Native AMP
### Training results
| Training Loss | Epoch | Step | Validation Loss | Rouge1 | Rouge2 | Rougel | Rougelsum | Gen Len |
|:-------------:|:-----:|:-----:|:---------------:|:-------:|:-------:|:-------:|:---------:|:--------:|
| 1.2541 | 1.0 | 795 | 0.9350 | 52.5594 | 32.6314 | 35.2302 | 50.1767 | 142.0 |
| 0.7018 | 2.0 | 1590 | 0.8022 | 53.4804 | 35.4649 | 37.1673 | 51.2428 | 142.0 |
| 0.5266 | 3.0 | 2385 | 0.7752 | 52.9462 | 34.3697 | 36.611 | 50.6922 | 142.0 |
| 0.3475 | 4.0 | 3180 | 0.7771 | 53.4605 | 35.4738 | 38.5714 | 51.3798 | 142.0 |
| 0.2691 | 5.0 | 3975 | 0.7424 | 54.1132 | 35.7289 | 39.2653 | 51.6822 | 141.4259 |
| 0.182 | 6.0 | 4770 | 0.8037 | 53.7969 | 35.7324 | 38.4764 | 51.4929 | 141.7778 |
| 0.1446 | 7.0 | 5565 | 0.7686 | 55.0274 | 38.7813 | 42.6251 | 52.9847 | 142.0 |
| 0.1191 | 8.0 | 6360 | 0.7807 | 55.4651 | 38.6537 | 41.2746 | 53.578 | 141.8704 |
| 0.0976 | 9.0 | 7155 | 0.8045 | 55.2843 | 40.2358 | 42.8464 | 54.0957 | 142.0 |
| 0.0882 | 10.0 | 7950 | 0.8533 | 56.8288 | 41.6714 | 44.3961 | 54.9406 | 142.0 |
| 0.0721 | 11.0 | 8745 | 0.8962 | 55.3187 | 40.1599 | 43.2103 | 54.1964 | 142.0 |
| 0.0597 | 12.0 | 9540 | 0.8653 | 55.5706 | 40.2321 | 44.0075 | 53.9883 | 142.0 |
| 0.054 | 13.0 | 10335 | 0.8566 | 55.6622 | 40.0252 | 42.6907 | 54.0548 | 142.0 |
| 0.0476 | 14.0 | 11130 | 0.8900 | 57.5046 | 43.6309 | 46.449 | 55.9909 | 142.0 |
| 0.0432 | 15.0 | 11925 | 0.9149 | 55.604 | 39.9591 | 43.1729 | 54.3703 | 142.0 |
| 0.0403 | 16.0 | 12720 | 0.9258 | 55.1275 | 39.6566 | 42.3852 | 53.7656 | 142.0 |
| 0.0351 | 17.0 | 13515 | 0.9184 | 58.2352 | 44.6109 | 47.3863 | 56.9529 | 142.0 |
| 0.032 | 18.0 | 14310 | 0.9275 | 55.9687 | 41.2482 | 44.0076 | 54.0707 | 142.0 |
| 0.0313 | 19.0 | 15105 | 0.9635 | 56.3574 | 41.2113 | 44.8358 | 54.6279 | 142.0 |
| 0.0258 | 20.0 | 15900 | 0.9478 | 57.8445 | 44.297 | 46.8836 | 56.2003 | 142.0 |
| 0.0277 | 21.0 | 16695 | 0.9363 | 58.4823 | 46.0943 | 48.7817 | 57.5883 | 141.6667 |
| 0.0219 | 22.0 | 17490 | 0.9705 | 57.6022 | 43.9147 | 47.3054 | 56.3866 | 142.0 |
| 0.0231 | 23.0 | 18285 | 0.9857 | 56.5809 | 42.9124 | 46.789 | 55.3897 | 142.0 |
| 0.021 | 24.0 | 19080 | 1.0155 | 56.9745 | 43.8859 | 46.6109 | 55.708 | 142.0 |
| 0.02 | 25.0 | 19875 | 1.0095 | 57.9702 | 45.1809 | 48.2856 | 56.6941 | 142.0 |
| 0.0175 | 26.0 | 20670 | 0.9634 | 57.7023 | 45.1577 | 48.2398 | 56.5282 | 142.0 |
| 0.0161 | 27.0 | 21465 | 1.0197 | 58.739 | 46.3307 | 49.2328 | 57.5778 | 142.0 |
| 0.0186 | 28.0 | 22260 | 0.9790 | 56.1661 | 42.9731 | 45.8654 | 54.4365 | 142.0 |
| 0.0145 | 29.0 | 23055 | 0.9883 | 55.8554 | 41.7405 | 45.177 | 54.478 | 142.0 |
| 0.013 | 30.0 | 23850 | 0.9977 | 55.5831 | 41.2429 | 44.8063 | 53.886 | 142.0 |
| 0.0131 | 31.0 | 24645 | 0.9765 | 57.4478 | 44.8905 | 48.1376 | 56.102 | 141.463 |
| 0.0118 | 32.0 | 25440 | 1.0000 | 58.4282 | 46.6557 | 49.4122 | 57.1979 | 142.0 |
| 0.0117 | 33.0 | 26235 | 0.9924 | 57.1995 | 44.4177 | 47.6248 | 56.0251 | 141.2407 |
| 0.011 | 34.0 | 27030 | 1.0698 | 57.8918 | 45.925 | 49.0505 | 56.9352 | 142.0 |
| 0.0093 | 35.0 | 27825 | 1.0297 | 57.7003 | 45.4556 | 47.9919 | 56.5134 | 141.8148 |
| 0.0112 | 36.0 | 28620 | 1.0429 | 58.4039 | 46.6401 | 49.3897 | 57.4753 | 142.0 |
| 0.0101 | 37.0 | 29415 | 1.0761 | 59.2768 | 47.5384 | 50.2152 | 57.9493 | 142.0 |
| 0.0095 | 38.0 | 30210 | 1.0254 | 58.6205 | 47.246 | 50.87 | 57.7829 | 142.0 |
| 0.0087 | 39.0 | 31005 | 1.0216 | 57.7667 | 44.7762 | 48.067 | 56.6006 | 142.0 |
| 0.0082 | 40.0 | 31800 | 1.0587 | 58.4703 | 45.8371 | 48.5321 | 57.2036 | 142.0 |
| 0.0075 | 41.0 | 32595 | 1.0621 | 58.5629 | 46.8885 | 49.5943 | 57.4579 | 142.0 |
| 0.0079 | 42.0 | 33390 | 1.0845 | 57.664 | 45.5954 | 48.408 | 56.661 | 141.9815 |
| 0.0076 | 43.0 | 34185 | 1.0705 | 58.1776 | 46.0435 | 49.3126 | 57.138 | 142.0 |
| 0.0074 | 44.0 | 34980 | 1.0636 | 58.1022 | 46.4877 | 48.7985 | 56.9073 | 142.0 |
| 0.007 | 45.0 | 35775 | 1.0810 | 57.8251 | 44.8767 | 47.8991 | 56.5977 | 142.0 |
| 0.0057 | 46.0 | 36570 | 1.0560 | 58.5086 | 46.3448 | 49.2576 | 57.4386 | 142.0 |
| 0.0062 | 47.0 | 37365 | 1.0903 | 58.8772 | 47.2886 | 49.9502 | 57.611 | 142.0 |
| 0.0058 | 48.0 | 38160 | 1.0847 | 59.4672 | 48.3847 | 51.602 | 58.4588 | 142.0 |
| 0.0061 | 49.0 | 38955 | 1.0798 | 59.5308 | 48.0396 | 50.8641 | 58.5016 | 142.0 |
| 0.0062 | 50.0 | 39750 | 1.0795 | 59.5026 | 48.5319 | 51.7426 | 58.7111 | 142.0 |
| 0.0051 | 51.0 | 40545 | 1.0842 | 57.7941 | 46.1198 | 48.7341 | 56.7164 | 142.0 |
| 0.0057 | 52.0 | 41340 | 1.0777 | 58.6131 | 46.3924 | 49.0787 | 57.1278 | 142.0 |
| 0.0039 | 53.0 | 42135 | 1.1133 | 57.6447 | 45.6699 | 48.5207 | 56.6447 | 142.0 |
| 0.0038 | 54.0 | 42930 | 1.0714 | 58.1462 | 46.4616 | 49.273 | 57.2771 | 142.0 |
| 0.004 | 55.0 | 43725 | 1.0852 | 58.6577 | 47.2095 | 50.4702 | 57.7724 | 142.0 |
| 0.0044 | 56.0 | 44520 | 1.1152 | 59.0564 | 47.1621 | 50.2807 | 58.3122 | 142.0 |
| 0.0042 | 57.0 | 45315 | 1.0831 | 58.1767 | 46.8127 | 49.9166 | 57.1833 | 142.0 |
| 0.0038 | 58.0 | 46110 | 1.1156 | 57.8515 | 46.3229 | 48.6843 | 56.7218 | 142.0 |
| 0.0038 | 59.0 | 46905 | 1.1105 | 57.9332 | 45.8354 | 49.27 | 57.1209 | 142.0 |
| 0.0034 | 60.0 | 47700 | 1.1104 | 60.0207 | 49.2067 | 51.8751 | 58.9484 | 142.0 |
| 0.0028 | 61.0 | 48495 | 1.1533 | 58.3432 | 46.8835 | 50.2868 | 57.5427 | 141.6111 |
| 0.0026 | 62.0 | 49290 | 1.1441 | 58.6838 | 46.9472 | 49.9524 | 57.5287 | 142.0 |
| 0.0028 | 63.0 | 50085 | 1.1232 | 58.0202 | 45.5855 | 48.6554 | 56.8368 | 141.9444 |
| 0.0037 | 64.0 | 50880 | 1.1520 | 58.3905 | 47.0348 | 49.8478 | 57.3665 | 142.0 |
| 0.0029 | 65.0 | 51675 | 1.1358 | 59.231 | 48.7251 | 51.6138 | 58.5718 | 142.0 |
| 0.0026 | 66.0 | 52470 | 1.1559 | 58.9482 | 47.2137 | 49.4299 | 57.7235 | 142.0 |
| 0.0025 | 67.0 | 53265 | 1.1272 | 59.3333 | 47.7419 | 50.7018 | 58.326 | 142.0 |
| 0.0026 | 68.0 | 54060 | 1.1613 | 58.6404 | 47.3218 | 50.255 | 57.4646 | 142.0 |
| 0.0015 | 69.0 | 54855 | 1.1575 | 58.7927 | 47.7018 | 50.695 | 57.796 | 142.0 |
| 0.0018 | 70.0 | 55650 | 1.1463 | 58.9455 | 47.2691 | 50.176 | 57.9997 | 142.0 |
| 0.0023 | 71.0 | 56445 | 1.1622 | 58.5943 | 46.9325 | 49.4159 | 57.2131 | 142.0 |
| 0.0024 | 72.0 | 57240 | 1.1258 | 58.2779 | 47.4119 | 49.9836 | 57.4867 | 142.0 |
| 0.0019 | 73.0 | 58035 | 1.1333 | 58.9185 | 47.5755 | 50.0765 | 57.8661 | 142.0 |
| 0.0017 | 74.0 | 58830 | 1.1469 | 60.5037 | 49.4508 | 52.2863 | 59.6675 | 141.963 |
| 0.0017 | 75.0 | 59625 | 1.1349 | 59.4264 | 47.4554 | 50.0383 | 58.3103 | 142.0 |
| 0.0025 | 76.0 | 60420 | 1.1215 | 58.2795 | 46.9852 | 49.5787 | 57.4501 | 142.0 |
| 0.0012 | 77.0 | 61215 | 1.1272 | 58.2248 | 47.0914 | 50.2569 | 57.1888 | 142.0 |
| 0.001 | 78.0 | 62010 | 1.1648 | 59.3808 | 48.4901 | 51.118 | 58.6251 | 142.0 |
| 0.0011 | 79.0 | 62805 | 1.1433 | 58.8697 | 47.6232 | 50.0226 | 57.6299 | 142.0 |
| 0.001 | 80.0 | 63600 | 1.1486 | 59.0608 | 47.1931 | 50.1354 | 57.8687 | 142.0 |
| 0.0011 | 81.0 | 64395 | 1.1695 | 58.341 | 47.0306 | 49.9269 | 57.339 | 142.0 |
| 0.001 | 82.0 | 65190 | 1.1589 | 58.9283 | 48.4586 | 51.2319 | 57.9485 | 142.0 |
| 0.0009 | 83.0 | 65985 | 1.1868 | 59.1377 | 48.2469 | 50.8486 | 58.1111 | 142.0 |
| 0.001 | 84.0 | 66780 | 1.1664 | 58.7706 | 47.5868 | 50.5937 | 57.7824 | 142.0 |
| 0.0009 | 85.0 | 67575 | 1.1719 | 57.8121 | 45.5997 | 48.2442 | 56.5272 | 142.0 |
| 0.0006 | 86.0 | 68370 | 1.1662 | 58.5204 | 47.5947 | 50.1839 | 57.6431 | 142.0 |
| 0.0007 | 87.0 | 69165 | 1.1668 | 59.2416 | 48.2985 | 51.0347 | 58.2794 | 142.0 |
| 0.0007 | 88.0 | 69960 | 1.1619 | 58.6933 | 47.5716 | 50.6785 | 57.5726 | 142.0 |
| 0.0003 | 89.0 | 70755 | 1.1765 | 59.2853 | 48.6451 | 51.3017 | 58.2603 | 142.0 |
| 0.0005 | 90.0 | 71550 | 1.1766 | 59.248 | 48.5642 | 50.9843 | 58.1706 | 142.0 |
| 0.0005 | 91.0 | 72345 | 1.1983 | 59.0009 | 48.311 | 51.0192 | 57.9822 | 142.0 |
| 0.0006 | 92.0 | 73140 | 1.1721 | 59.1248 | 49.0902 | 51.9937 | 58.2288 | 142.0 |
| 0.0003 | 93.0 | 73935 | 1.1799 | 58.2448 | 47.4011 | 49.987 | 57.515 | 142.0 |
| 0.0005 | 94.0 | 74730 | 1.1900 | 59.931 | 49.6663 | 52.3233 | 58.962 | 142.0 |
| 0.0004 | 95.0 | 75525 | 1.1868 | 59.5898 | 49.0004 | 51.4835 | 58.6463 | 142.0 |
| 0.0093 | 96.0 | 76320 | 1.1831 | 59.9405 | 49.83 | 52.4355 | 59.0702 | 142.0 |
| 0.0004 | 97.0 | 77115 | 1.1841 | 59.7379 | 49.5435 | 52.5255 | 58.8526 | 142.0 |
| 0.0004 | 98.0 | 77910 | 1.1790 | 59.5515 | 49.0724 | 51.9888 | 58.5488 | 142.0 |
| 0.0003 | 99.0 | 78705 | 1.1786 | 59.7712 | 49.0557 | 51.8137 | 58.7144 | 142.0 |
| 0.0002 | 100.0 | 79500 | 1.1806 | 59.4159 | 48.867 | 51.9013 | 58.3382 | 142.0 |
### Framework versions
- Transformers 4.19.2
- Pytorch 1.11.0+cu113
- Datasets 2.2.2
- Tokenizers 0.12.1
|
mkkc58/bert-finetuned-squad | 2e0e83f84ad9f7dbb6e9dc4809fff87640b55e04 | 2022-05-24T08:54:29.000Z | [
"pytorch",
"tensorboard",
"bert",
"question-answering",
"dataset:squad",
"transformers",
"generated_from_trainer",
"license:apache-2.0",
"model-index",
"autotrain_compatible"
] | question-answering | false | mkkc58 | null | mkkc58/bert-finetuned-squad | 0 | null | transformers | 37,654 | ---
license: apache-2.0
tags:
- generated_from_trainer
datasets:
- squad
model-index:
- name: bert-finetuned-squad
results: []
---
<!-- This model card has been generated automatically according to the information the Trainer had access to. You
should probably proofread and complete it, then remove this comment. -->
# bert-finetuned-squad
This model is a fine-tuned version of [bert-base-cased](https://huggingface.co/bert-base-cased) on the squad dataset.
## Model description
More information needed
## Intended uses & limitations
More information needed
## Training and evaluation data
More information needed
## Training procedure
### Training hyperparameters
The following hyperparameters were used during training:
- learning_rate: 2e-05
- train_batch_size: 8
- eval_batch_size: 8
- seed: 42
- optimizer: Adam with betas=(0.9,0.999) and epsilon=1e-08
- lr_scheduler_type: linear
- num_epochs: 3
- mixed_precision_training: Native AMP
### Training results
### Framework versions
- Transformers 4.19.2
- Pytorch 1.11.0+cu113
- Datasets 2.2.2
- Tokenizers 0.12.1
|
salma-elshafey/mbert2mbert-finetuned-ar-to-en | 54f5d05b5ff6e2b8d2ac3f8051aac6f6f5f1bd29 | 2022-05-23T21:25:44.000Z | [
"pytorch",
"tensorboard",
"encoder-decoder",
"text2text-generation",
"transformers",
"autotrain_compatible"
] | text2text-generation | false | salma-elshafey | null | salma-elshafey/mbert2mbert-finetuned-ar-to-en | 0 | null | transformers | 37,655 | Entry not found |
CEBaB/lstm.CEBaB.causalm.food__service.5-class.exclusive.seed_42 | 418ba18d44445faf3c064c0d6f182110d82f3000 | 2022-05-24T10:08:54.000Z | [
"pytorch",
"lstm_causalm",
"transformers"
] | null | false | CEBaB | null | CEBaB/lstm.CEBaB.causalm.food__service.5-class.exclusive.seed_42 | 0 | null | transformers | 37,656 | Entry not found |
huggingtweets/elonmusk-fchollet-steak_umm | a8fadabe75bc2ab1319fb0685f3140050fb75176 | 2022-05-24T00:03:41.000Z | [
"pytorch",
"gpt2",
"text-generation",
"en",
"transformers",
"huggingtweets"
] | text-generation | false | huggingtweets | null | huggingtweets/elonmusk-fchollet-steak_umm | 0 | null | transformers | 37,657 | ---
language: en
thumbnail: https://github.com/borisdayma/huggingtweets/blob/master/img/logo.png?raw=true
tags:
- huggingtweets
widget:
- text: "My dream is"
---
<div class="inline-flex flex-col" style="line-height: 1.5;">
<div class="flex">
<div
style="display:inherit; margin-left: 4px; margin-right: 4px; width: 92px; height:92px; border-radius: 50%; background-size: cover; background-image: url('https://pbs.twimg.com/profile_images/1521957986335297536/itVSA7l0_400x400.jpg')">
</div>
<div
style="display:inherit; margin-left: 4px; margin-right: 4px; width: 92px; height:92px; border-radius: 50%; background-size: cover; background-image: url('https://pbs.twimg.com/profile_images/1505963741954945028/kk8k_nwH_400x400.jpg')">
</div>
<div
style="display:inherit; margin-left: 4px; margin-right: 4px; width: 92px; height:92px; border-radius: 50%; background-size: cover; background-image: url('https://pbs.twimg.com/profile_images/1234692331263016960/7uR-nYW0_400x400.jpg')">
</div>
</div>
<div style="text-align: center; margin-top: 3px; font-size: 16px; font-weight: 800">🤖 AI CYBORG 🤖</div>
<div style="text-align: center; font-size: 16px; font-weight: 800">Elon Musk & Steak-umm & François Chollet</div>
<div style="text-align: center; font-size: 14px;">@elonmusk-fchollet-steak_umm</div>
</div>
I was made with [huggingtweets](https://github.com/borisdayma/huggingtweets).
Create your own bot based on your favorite user with [the demo](https://colab.research.google.com/github/borisdayma/huggingtweets/blob/master/huggingtweets-demo.ipynb)!
## How does it work?
The model uses the following pipeline.
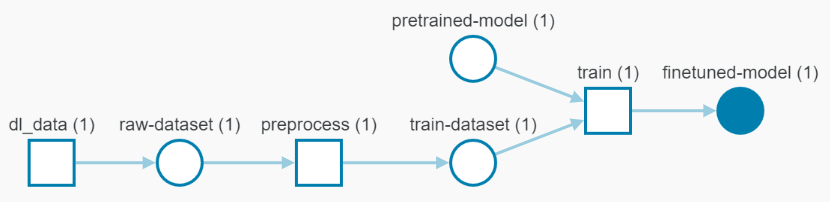
To understand how the model was developed, check the [W&B report](https://wandb.ai/wandb/huggingtweets/reports/HuggingTweets-Train-a-Model-to-Generate-Tweets--VmlldzoxMTY5MjI).
## Training data
The model was trained on tweets from Elon Musk & Steak-umm & François Chollet.
| Data | Elon Musk | Steak-umm | François Chollet |
| --- | --- | --- | --- |
| Tweets downloaded | 200 | 3249 | 3248 |
| Retweets | 6 | 53 | 429 |
| Short tweets | 61 | 1129 | 84 |
| Tweets kept | 133 | 2067 | 2735 |
[Explore the data](https://wandb.ai/wandb/huggingtweets/runs/3vvetpzj/artifacts), which is tracked with [W&B artifacts](https://docs.wandb.com/artifacts) at every step of the pipeline.
## Training procedure
The model is based on a pre-trained [GPT-2](https://huggingface.co/gpt2) which is fine-tuned on @elonmusk-fchollet-steak_umm's tweets.
Hyperparameters and metrics are recorded in the [W&B training run](https://wandb.ai/wandb/huggingtweets/runs/2oru1ym7) for full transparency and reproducibility.
At the end of training, [the final model](https://wandb.ai/wandb/huggingtweets/runs/2oru1ym7/artifacts) is logged and versioned.
## How to use
You can use this model directly with a pipeline for text generation:
```python
from transformers import pipeline
generator = pipeline('text-generation',
model='huggingtweets/elonmusk-fchollet-steak_umm')
generator("My dream is", num_return_sequences=5)
```
## Limitations and bias
The model suffers from [the same limitations and bias as GPT-2](https://huggingface.co/gpt2#limitations-and-bias).
In addition, the data present in the user's tweets further affects the text generated by the model.
## About
*Built by Boris Dayma*
[](https://twitter.com/intent/follow?screen_name=borisdayma)
For more details, visit the project repository.
[](https://github.com/borisdayma/huggingtweets)
|
luisu0124/Prueba_tf5 | 066407a72e148a13443f02babede63750fd0d0fd | 2022-05-24T05:11:02.000Z | [
"pytorch",
"bert",
"transformers"
] | null | false | luisu0124 | null | luisu0124/Prueba_tf5 | 0 | null | transformers | 37,658 | Model preentrenado |
nandezgarcia/roberta-base-bne-finetuned-recores-short | 837ef9f911a9f40b7793d4f75d10288399b439bb | 2022-05-24T06:21:47.000Z | [
"pytorch",
"tensorboard",
"roberta",
"multiple-choice",
"transformers"
] | multiple-choice | false | nandezgarcia | null | nandezgarcia/roberta-base-bne-finetuned-recores-short | 0 | null | transformers | 37,659 | Entry not found |
nandezgarcia/roberta-base-bne-finetuned-recores-long | 5744e236dd10135c44bcc34779ec283afb8c4350 | 2022-05-24T08:04:35.000Z | [
"pytorch",
"tensorboard",
"roberta",
"multiple-choice",
"transformers",
"generated_from_trainer",
"license:apache-2.0",
"model-index"
] | multiple-choice | false | nandezgarcia | null | nandezgarcia/roberta-base-bne-finetuned-recores-long | 0 | null | transformers | 37,660 | ---
license: apache-2.0
tags:
- generated_from_trainer
metrics:
- accuracy
model-index:
- name: roberta-base-bne-finetuned-recores-long
results: []
---
<!-- This model card has been generated automatically according to the information the Trainer had access to. You
should probably proofread and complete it, then remove this comment. -->
# roberta-base-bne-finetuned-recores-long
This model is a fine-tuned version of [PlanTL-GOB-ES/roberta-base-bne](https://huggingface.co/PlanTL-GOB-ES/roberta-base-bne) on an unknown dataset.
It achieves the following results on the evaluation set:
- Loss: 2.2599
- Accuracy: 0.4525
## Model description
More information needed
## Intended uses & limitations
More information needed
## Training and evaluation data
More information needed
## Training procedure
### Training hyperparameters
The following hyperparameters were used during training:
- learning_rate: 1e-05
- train_batch_size: 1
- eval_batch_size: 1
- seed: 42
- optimizer: Adam with betas=(0.9,0.999) and epsilon=1e-08
- lr_scheduler_type: linear
- num_epochs: 3
### Training results
| Training Loss | Epoch | Step | Validation Loss | Accuracy |
|:-------------:|:-----:|:----:|:---------------:|:--------:|
| 1.5728 | 1.0 | 653 | 1.4938 | 0.3846 |
| 0.9036 | 2.0 | 1306 | 1.9815 | 0.4615 |
| 0.4161 | 3.0 | 1959 | 2.2599 | 0.4525 |
### Framework versions
- Transformers 4.18.0
- Pytorch 1.10.1+cu102
- Datasets 2.2.1
- Tokenizers 0.12.1
|
nandezgarcia/roberta-base-bne-sqac-finetuned-recores-long | d96b3147a4f042b4c562319f4463a0aa12e2de05 | 2022-05-24T06:51:27.000Z | [
"pytorch",
"tensorboard",
"roberta",
"multiple-choice",
"transformers",
"generated_from_trainer",
"license:apache-2.0",
"model-index"
] | multiple-choice | false | nandezgarcia | null | nandezgarcia/roberta-base-bne-sqac-finetuned-recores-long | 0 | null | transformers | 37,661 | ---
license: apache-2.0
tags:
- generated_from_trainer
metrics:
- accuracy
model-index:
- name: roberta-base-bne-sqac-finetuned-recores-long
results: []
---
<!-- This model card has been generated automatically according to the information the Trainer had access to. You
should probably proofread and complete it, then remove this comment. -->
# roberta-base-bne-sqac-finetuned-recores-long
This model is a fine-tuned version of [PlanTL-GOB-ES/roberta-base-bne-sqac](https://huggingface.co/PlanTL-GOB-ES/roberta-base-bne-sqac) on an unknown dataset.
It achieves the following results on the evaluation set:
- Loss: 1.8161
- Accuracy: 0.3710
## Model description
More information needed
## Intended uses & limitations
More information needed
## Training and evaluation data
More information needed
## Training procedure
### Training hyperparameters
The following hyperparameters were used during training:
- learning_rate: 1e-05
- train_batch_size: 1
- eval_batch_size: 1
- seed: 42
- optimizer: Adam with betas=(0.9,0.999) and epsilon=1e-08
- lr_scheduler_type: linear
- num_epochs: 3
### Training results
| Training Loss | Epoch | Step | Validation Loss | Accuracy |
|:-------------:|:-----:|:----:|:---------------:|:--------:|
| 1.6126 | 1.0 | 653 | 1.5897 | 0.3032 |
| 1.4433 | 2.0 | 1306 | 1.4736 | 0.4163 |
| 0.8946 | 3.0 | 1959 | 1.8161 | 0.3710 |
### Framework versions
- Transformers 4.18.0
- Pytorch 1.10.1+cu102
- Datasets 2.2.1
- Tokenizers 0.12.1
|
theojolliffe/bart-cnn-pubmed-arxiv-pubmed-v3-e60 | e0497d2c8f1d4010af898e87d49c4a15409219f4 | 2022-05-24T17:41:07.000Z | [
"pytorch",
"tensorboard",
"bart",
"text2text-generation",
"transformers",
"generated_from_trainer",
"license:mit",
"model-index",
"autotrain_compatible"
] | text2text-generation | false | theojolliffe | null | theojolliffe/bart-cnn-pubmed-arxiv-pubmed-v3-e60 | 0 | null | transformers | 37,662 | ---
license: mit
tags:
- generated_from_trainer
metrics:
- rouge
model-index:
- name: bart-cnn-pubmed-arxiv-pubmed-v3-e60
results: []
---
<!-- This model card has been generated automatically according to the information the Trainer had access to. You
should probably proofread and complete it, then remove this comment. -->
# bart-cnn-pubmed-arxiv-pubmed-v3-e60
This model is a fine-tuned version of [theojolliffe/bart-cnn-pubmed-arxiv-pubmed](https://huggingface.co/theojolliffe/bart-cnn-pubmed-arxiv-pubmed) on an unknown dataset.
It achieves the following results on the evaluation set:
- Loss: 1.0969
- Rouge1: 60.5054
- Rouge2: 49.8345
- Rougel: 52.7857
- Rougelsum: 59.5625
- Gen Len: 142.0
## Model description
More information needed
## Intended uses & limitations
More information needed
## Training and evaluation data
More information needed
## Training procedure
### Training hyperparameters
The following hyperparameters were used during training:
- learning_rate: 2e-05
- train_batch_size: 1
- eval_batch_size: 1
- seed: 42
- optimizer: Adam with betas=(0.9,0.999) and epsilon=1e-08
- lr_scheduler_type: linear
- num_epochs: 60
- mixed_precision_training: Native AMP
### Training results
| Training Loss | Epoch | Step | Validation Loss | Rouge1 | Rouge2 | Rougel | Rougelsum | Gen Len |
|:-------------:|:-----:|:-----:|:---------------:|:-------:|:-------:|:-------:|:---------:|:--------:|
| 1.2541 | 1.0 | 795 | 0.9312 | 52.6474 | 33.219 | 35.3153 | 50.2117 | 142.0 |
| 0.7026 | 2.0 | 1590 | 0.8076 | 53.2385 | 34.2933 | 36.3889 | 50.8338 | 141.9815 |
| 0.5259 | 3.0 | 2385 | 0.7832 | 53.3407 | 33.8438 | 37.2622 | 50.8487 | 142.0 |
| 0.347 | 4.0 | 3180 | 0.7632 | 53.24 | 34.233 | 36.954 | 50.3872 | 142.0 |
| 0.2657 | 5.0 | 3975 | 0.7389 | 54.7175 | 36.9459 | 39.4874 | 52.5236 | 142.0 |
| 0.1799 | 6.0 | 4770 | 0.8152 | 53.7057 | 36.4558 | 39.2037 | 51.4357 | 141.8889 |
| 0.1425 | 7.0 | 5565 | 0.7632 | 56.4087 | 40.1423 | 44.2536 | 54.2827 | 141.8519 |
| 0.1161 | 8.0 | 6360 | 0.7787 | 57.048 | 41.4384 | 44.5318 | 55.001 | 141.9259 |
| 0.0936 | 9.0 | 7155 | 0.8074 | 55.9781 | 39.7293 | 42.2029 | 53.7465 | 142.0 |
| 0.0863 | 10.0 | 7950 | 0.8527 | 55.8303 | 40.3243 | 43.6105 | 53.7656 | 142.0 |
| 0.0669 | 11.0 | 8745 | 0.8699 | 57.0888 | 42.5994 | 45.3813 | 55.4823 | 142.0 |
| 0.0546 | 12.0 | 9540 | 0.8474 | 55.9644 | 41.4168 | 44.3511 | 54.27 | 141.8704 |
| 0.0473 | 13.0 | 10335 | 0.8369 | 56.3014 | 41.3835 | 44.6644 | 54.6368 | 142.0 |
| 0.0459 | 14.0 | 11130 | 0.8922 | 56.9204 | 42.6545 | 45.4635 | 55.3169 | 142.0 |
| 0.0379 | 15.0 | 11925 | 0.9166 | 57.783 | 44.3517 | 48.0052 | 55.9449 | 142.0 |
| 0.0333 | 16.0 | 12720 | 0.9346 | 57.7209 | 44.1832 | 47.634 | 56.0137 | 142.0 |
| 0.0304 | 17.0 | 13515 | 0.9046 | 57.2015 | 42.7752 | 46.4241 | 55.7707 | 142.0 |
| 0.0272 | 18.0 | 14310 | 0.9191 | 56.0557 | 41.6832 | 44.44 | 54.3098 | 142.0 |
| 0.0242 | 19.0 | 15105 | 0.9431 | 56.8941 | 42.662 | 46.147 | 55.1771 | 142.0 |
| 0.0208 | 20.0 | 15900 | 0.9127 | 58.5386 | 45.2057 | 48.5554 | 57.1466 | 142.0 |
| 0.02 | 21.0 | 16695 | 0.9537 | 57.8511 | 44.5897 | 47.8505 | 56.5768 | 142.0 |
| 0.018 | 22.0 | 17490 | 0.9576 | 57.5774 | 44.4534 | 47.6493 | 55.9042 | 142.0 |
| 0.0151 | 23.0 | 18285 | 1.0039 | 57.7678 | 43.6504 | 47.3487 | 55.9951 | 141.5926 |
| 0.0164 | 24.0 | 19080 | 0.9815 | 57.2684 | 44.4105 | 47.8775 | 55.9622 | 142.0 |
| 0.0131 | 25.0 | 19875 | 0.9932 | 58.0703 | 44.5521 | 47.9763 | 56.4451 | 142.0 |
| 0.0127 | 26.0 | 20670 | 0.9851 | 56.9139 | 43.707 | 46.8548 | 55.7885 | 142.0 |
| 0.0113 | 27.0 | 21465 | 0.9894 | 59.2224 | 46.5814 | 49.2356 | 58.0085 | 142.0 |
| 0.0107 | 28.0 | 22260 | 0.9845 | 58.6542 | 46.4524 | 49.3959 | 57.4585 | 142.0 |
| 0.0098 | 29.0 | 23055 | 1.0165 | 57.8297 | 44.7935 | 47.7898 | 56.5338 | 142.0 |
| 0.0093 | 30.0 | 23850 | 0.9844 | 58.6572 | 47.6771 | 50.309 | 57.4929 | 142.0 |
| 0.0094 | 31.0 | 24645 | 1.0083 | 57.9771 | 46.1191 | 49.7179 | 56.8376 | 142.0 |
| 0.0077 | 32.0 | 25440 | 0.9739 | 58.4251 | 46.2082 | 49.1364 | 57.1372 | 141.463 |
| 0.007 | 33.0 | 26235 | 1.0364 | 58.4724 | 46.2787 | 49.7396 | 57.203 | 142.0 |
| 0.0062 | 34.0 | 27030 | 1.0401 | 59.9105 | 48.5584 | 51.232 | 58.7889 | 142.0 |
| 0.007 | 35.0 | 27825 | 1.0477 | 58.3057 | 46.0506 | 49.7662 | 57.1383 | 142.0 |
| 0.0064 | 36.0 | 28620 | 1.0328 | 58.301 | 45.3733 | 48.1001 | 56.909 | 142.0 |
| 0.0049 | 37.0 | 29415 | 1.0488 | 58.8353 | 45.8655 | 48.7498 | 57.3955 | 142.0 |
| 0.0037 | 38.0 | 30210 | 1.0196 | 59.245 | 47.4285 | 50.9562 | 58.1597 | 142.0 |
| 0.0049 | 39.0 | 31005 | 1.0270 | 59.4799 | 48.1755 | 51.5027 | 58.3599 | 142.0 |
| 0.004 | 40.0 | 31800 | 1.0517 | 58.8698 | 46.8679 | 50.4378 | 57.7936 | 142.0 |
| 0.0034 | 41.0 | 32595 | 1.0787 | 59.2729 | 47.718 | 50.9233 | 57.9377 | 141.8148 |
| 0.0031 | 42.0 | 33390 | 1.0685 | 60.1618 | 48.1466 | 51.3451 | 58.978 | 142.0 |
| 0.0028 | 43.0 | 34185 | 1.0770 | 60.4238 | 50.1106 | 53.211 | 59.3799 | 142.0 |
| 0.0031 | 44.0 | 34980 | 1.0786 | 59.1729 | 47.6285 | 51.3243 | 58.0335 | 142.0 |
| 0.0024 | 45.0 | 35775 | 1.0829 | 59.4366 | 48.3836 | 51.7183 | 58.4366 | 142.0 |
| 0.0021 | 46.0 | 36570 | 1.0791 | 59.1313 | 47.6137 | 51.3465 | 58.048 | 142.0 |
| 0.002 | 47.0 | 37365 | 1.0630 | 58.8133 | 46.795 | 50.2249 | 57.496 | 141.9444 |
| 0.0016 | 48.0 | 38160 | 1.0800 | 58.7699 | 47.6953 | 50.1339 | 57.4936 | 142.0 |
| 0.0018 | 49.0 | 38955 | 1.0563 | 58.1134 | 46.3537 | 49.7251 | 56.7849 | 142.0 |
| 0.0013 | 50.0 | 39750 | 1.0819 | 59.3582 | 47.9255 | 51.1782 | 58.2925 | 142.0 |
| 0.0013 | 51.0 | 40545 | 1.0762 | 59.0797 | 48.0875 | 50.8556 | 57.9182 | 142.0 |
| 0.0013 | 52.0 | 41340 | 1.0906 | 60.0376 | 48.9763 | 51.9324 | 58.8537 | 142.0 |
| 0.0008 | 53.0 | 42135 | 1.1106 | 59.3213 | 48.7152 | 51.4854 | 58.2943 | 142.0 |
| 0.0009 | 54.0 | 42930 | 1.0845 | 59.8334 | 48.702 | 51.3005 | 58.921 | 142.0 |
| 0.0008 | 55.0 | 43725 | 1.1035 | 60.1754 | 48.9721 | 51.4863 | 59.0829 | 142.0 |
| 0.0008 | 56.0 | 44520 | 1.0872 | 59.8122 | 48.6515 | 51.8589 | 58.8101 | 142.0 |
| 0.0011 | 57.0 | 45315 | 1.0872 | 59.5352 | 48.1967 | 51.1626 | 58.3402 | 142.0 |
| 0.0005 | 58.0 | 46110 | 1.0937 | 59.4125 | 48.1826 | 51.5944 | 58.4618 | 142.0 |
| 0.0008 | 59.0 | 46905 | 1.0936 | 60.0138 | 49.1796 | 52.3896 | 59.0976 | 142.0 |
| 0.0005 | 60.0 | 47700 | 1.0969 | 60.5054 | 49.8345 | 52.7857 | 59.5625 | 142.0 |
### Framework versions
- Transformers 4.19.2
- Pytorch 1.11.0+cu113
- Datasets 2.2.2
- Tokenizers 0.12.1
|
nandezgarcia/roberta-base-bne-finetuned-recores-complete | 18727530a2190a54c0f4931848fce88a70323d6a | 2022-05-24T08:41:00.000Z | [
"pytorch",
"tensorboard",
"roberta",
"multiple-choice",
"transformers"
] | multiple-choice | false | nandezgarcia | null | nandezgarcia/roberta-base-bne-finetuned-recores-complete | 0 | null | transformers | 37,663 | Entry not found |
mayur0703/hindiqa | cbc54667c33be02e1f7bd6cd01d2d84ec533222b | 2022-05-24T09:49:25.000Z | [
"pytorch",
"xlm-roberta",
"question-answering",
"transformers",
"license:afl-3.0",
"autotrain_compatible"
] | question-answering | false | mayur0703 | null | mayur0703/hindiqa | 0 | null | transformers | 37,664 | ---
license: afl-3.0
---
|
trev/Twilight-Sparkle | d6cce001b3d292f945e74d749fbd05bd33982b92 | 2022-05-24T13:39:06.000Z | [
"pytorch",
"gpt2",
"text-generation",
"transformers",
"conversational"
] | conversational | false | trev | null | trev/Twilight-Sparkle | 0 | null | transformers | 37,665 | ---
tags:
- conversational
---
# Twilight Sparkle DialoGPT Model |
huggingtweets/respctclub-utsavsingla | 830ad1d517b2d70341232bb43e4605e455589df0 | 2022-05-24T14:54:46.000Z | [
"pytorch",
"gpt2",
"text-generation",
"en",
"transformers",
"huggingtweets"
] | text-generation | false | huggingtweets | null | huggingtweets/respctclub-utsavsingla | 0 | null | transformers | 37,666 | ---
language: en
thumbnail: http://www.huggingtweets.com/respctclub-utsavsingla/1653404081829/predictions.png
tags:
- huggingtweets
widget:
- text: "My dream is"
---
<div class="inline-flex flex-col" style="line-height: 1.5;">
<div class="flex">
<div
style="display:inherit; margin-left: 4px; margin-right: 4px; width: 92px; height:92px; border-radius: 50%; background-size: cover; background-image: url('https://pbs.twimg.com/profile_images/1500685428755623941/jT40-aBp_400x400.jpg')">
</div>
<div
style="display:inherit; margin-left: 4px; margin-right: 4px; width: 92px; height:92px; border-radius: 50%; background-size: cover; background-image: url('https://pbs.twimg.com/profile_images/1482271276077305859/n-xPut5M_400x400.jpg')">
</div>
<div
style="display:none; margin-left: 4px; margin-right: 4px; width: 92px; height:92px; border-radius: 50%; background-size: cover; background-image: url('')">
</div>
</div>
<div style="text-align: center; margin-top: 3px; font-size: 16px; font-weight: 800">🤖 AI CYBORG 🤖</div>
<div style="text-align: center; font-size: 16px; font-weight: 800">Utsav Singla | Respct.co 🙏🙏 & Respct</div>
<div style="text-align: center; font-size: 14px;">@respctclub-utsavsingla</div>
</div>
I was made with [huggingtweets](https://github.com/borisdayma/huggingtweets).
Create your own bot based on your favorite user with [the demo](https://colab.research.google.com/github/borisdayma/huggingtweets/blob/master/huggingtweets-demo.ipynb)!
## How does it work?
The model uses the following pipeline.
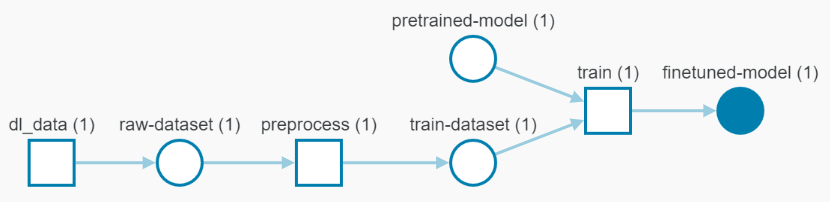
To understand how the model was developed, check the [W&B report](https://wandb.ai/wandb/huggingtweets/reports/HuggingTweets-Train-a-Model-to-Generate-Tweets--VmlldzoxMTY5MjI).
## Training data
The model was trained on tweets from Utsav Singla | Respct.co 🙏🙏 & Respct.
| Data | Utsav Singla | Respct.co 🙏🙏 | Respct |
| --- | --- | --- |
| Tweets downloaded | 365 | 157 |
| Retweets | 109 | 22 |
| Short tweets | 21 | 5 |
| Tweets kept | 235 | 130 |
[Explore the data](https://wandb.ai/wandb/huggingtweets/runs/1tvesvyp/artifacts), which is tracked with [W&B artifacts](https://docs.wandb.com/artifacts) at every step of the pipeline.
## Training procedure
The model is based on a pre-trained [GPT-2](https://huggingface.co/gpt2) which is fine-tuned on @respctclub-utsavsingla's tweets.
Hyperparameters and metrics are recorded in the [W&B training run](https://wandb.ai/wandb/huggingtweets/runs/3t9huyws) for full transparency and reproducibility.
At the end of training, [the final model](https://wandb.ai/wandb/huggingtweets/runs/3t9huyws/artifacts) is logged and versioned.
## How to use
You can use this model directly with a pipeline for text generation:
```python
from transformers import pipeline
generator = pipeline('text-generation',
model='huggingtweets/respctclub-utsavsingla')
generator("My dream is", num_return_sequences=5)
```
## Limitations and bias
The model suffers from [the same limitations and bias as GPT-2](https://huggingface.co/gpt2#limitations-and-bias).
In addition, the data present in the user's tweets further affects the text generated by the model.
## About
*Built by Boris Dayma*
[](https://twitter.com/intent/follow?screen_name=borisdayma)
For more details, visit the project repository.
[](https://github.com/borisdayma/huggingtweets)
|
stevemobs/deberta-base-finetuned-aqa-squad1 | bfe32be12f5c25cfe579d198f73983d120fb18f5 | 2022-05-24T19:56:41.000Z | [
"pytorch",
"tensorboard",
"deberta",
"question-answering",
"dataset:squad",
"transformers",
"generated_from_trainer",
"license:mit",
"model-index",
"autotrain_compatible"
] | question-answering | false | stevemobs | null | stevemobs/deberta-base-finetuned-aqa-squad1 | 0 | null | transformers | 37,667 | ---
license: mit
tags:
- generated_from_trainer
datasets:
- squad
model-index:
- name: deberta-base-finetuned-aqa-squad1
results: []
---
<!-- This model card has been generated automatically according to the information the Trainer had access to. You
should probably proofread and complete it, then remove this comment. -->
# deberta-base-finetuned-aqa-squad1
This model is a fine-tuned version of [stevemobs/deberta-base-finetuned-aqa](https://huggingface.co/stevemobs/deberta-base-finetuned-aqa) on the squad dataset.
It achieves the following results on the evaluation set:
- Loss: 0.7790
## Model description
More information needed
## Intended uses & limitations
More information needed
## Training and evaluation data
More information needed
## Training procedure
### Training hyperparameters
The following hyperparameters were used during training:
- learning_rate: 2e-05
- train_batch_size: 12
- eval_batch_size: 12
- seed: 42
- optimizer: Adam with betas=(0.9,0.999) and epsilon=1e-08
- lr_scheduler_type: linear
- num_epochs: 2
### Training results
| Training Loss | Epoch | Step | Validation Loss |
|:-------------:|:-----:|:-----:|:---------------:|
| 0.7662 | 1.0 | 7380 | 0.7575 |
| 0.5586 | 2.0 | 14760 | 0.7790 |
### Framework versions
- Transformers 4.19.2
- Pytorch 1.11.0+cu113
- Datasets 2.2.2
- Tokenizers 0.12.1
|
theojolliffe/bart-cnn-pubmed-arxiv-pubmed-v3-e43 | e50e8826767b38038e41f25c3be139e0d71e393c | 2022-05-24T23:30:12.000Z | [
"pytorch",
"tensorboard",
"bart",
"text2text-generation",
"transformers",
"generated_from_trainer",
"license:mit",
"model-index",
"autotrain_compatible"
] | text2text-generation | false | theojolliffe | null | theojolliffe/bart-cnn-pubmed-arxiv-pubmed-v3-e43 | 0 | null | transformers | 37,668 | ---
license: mit
tags:
- generated_from_trainer
metrics:
- rouge
model-index:
- name: bart-cnn-pubmed-arxiv-pubmed-v3-e43
results: []
---
<!-- This model card has been generated automatically according to the information the Trainer had access to. You
should probably proofread and complete it, then remove this comment. -->
# bart-cnn-pubmed-arxiv-pubmed-v3-e43
This model is a fine-tuned version of [theojolliffe/bart-cnn-pubmed-arxiv-pubmed](https://huggingface.co/theojolliffe/bart-cnn-pubmed-arxiv-pubmed) on an unknown dataset.
It achieves the following results on the evaluation set:
- Loss: 1.0837
- Rouge1: 58.1526
- Rouge2: 46.0425
- Rougel: 49.5624
- Rougelsum: 56.9295
- Gen Len: 142.0
## Model description
More information needed
## Intended uses & limitations
More information needed
## Training and evaluation data
More information needed
## Training procedure
### Training hyperparameters
The following hyperparameters were used during training:
- learning_rate: 2e-05
- train_batch_size: 1
- eval_batch_size: 1
- seed: 42
- optimizer: Adam with betas=(0.9,0.999) and epsilon=1e-08
- lr_scheduler_type: linear
- num_epochs: 43
- mixed_precision_training: Native AMP
### Training results
| Training Loss | Epoch | Step | Validation Loss | Rouge1 | Rouge2 | Rougel | Rougelsum | Gen Len |
|:-------------:|:-----:|:-----:|:---------------:|:-------:|:-------:|:-------:|:---------:|:--------:|
| 1.2542 | 1.0 | 795 | 0.9354 | 51.4655 | 31.6464 | 34.2376 | 48.9765 | 141.963 |
| 0.7019 | 2.0 | 1590 | 0.8119 | 53.3066 | 34.683 | 36.4262 | 50.907 | 142.0 |
| 0.5251 | 3.0 | 2385 | 0.7839 | 52.4248 | 32.8685 | 36.0084 | 49.9957 | 142.0 |
| 0.3449 | 4.0 | 3180 | 0.7673 | 52.716 | 34.7869 | 38.4201 | 50.8384 | 142.0 |
| 0.2666 | 5.0 | 3975 | 0.7647 | 54.6433 | 37.1337 | 40.1459 | 52.4288 | 141.7778 |
| 0.1805 | 6.0 | 4770 | 0.8400 | 53.5747 | 36.001 | 39.5984 | 51.1935 | 141.8148 |
| 0.1413 | 7.0 | 5565 | 0.7925 | 53.9875 | 37.01 | 40.6532 | 51.9353 | 142.0 |
| 0.113 | 8.0 | 6360 | 0.7665 | 56.395 | 41.5764 | 44.327 | 54.7845 | 142.0 |
| 0.0907 | 9.0 | 7155 | 0.8442 | 55.1407 | 39.4113 | 43.0628 | 53.6503 | 142.0 |
| 0.0824 | 10.0 | 7950 | 0.8469 | 55.7103 | 40.6761 | 43.3754 | 53.8227 | 142.0 |
| 0.0639 | 11.0 | 8745 | 0.8892 | 56.0839 | 40.6204 | 43.2455 | 54.4412 | 142.0 |
| 0.0504 | 12.0 | 9540 | 0.8613 | 56.9634 | 42.8236 | 45.4255 | 55.4026 | 142.0 |
| 0.0447 | 13.0 | 10335 | 0.9341 | 57.7216 | 44.104 | 47.1429 | 56.4299 | 142.0 |
| 0.0396 | 14.0 | 11130 | 0.9203 | 56.2073 | 42.9575 | 45.8068 | 54.8089 | 142.0 |
| 0.036 | 15.0 | 11925 | 0.9253 | 58.5212 | 45.6047 | 49.1205 | 57.0551 | 142.0 |
| 0.0302 | 16.0 | 12720 | 0.9187 | 58.8046 | 46.0106 | 48.0442 | 57.2799 | 142.0 |
| 0.0261 | 17.0 | 13515 | 0.9578 | 57.3405 | 43.8227 | 46.6317 | 55.7836 | 142.0 |
| 0.0231 | 18.0 | 14310 | 0.9578 | 57.7604 | 44.6164 | 47.8902 | 56.2309 | 141.8148 |
| 0.0198 | 19.0 | 15105 | 0.9662 | 57.774 | 44.6407 | 47.5489 | 56.1936 | 142.0 |
| 0.0165 | 20.0 | 15900 | 0.9509 | 59.6297 | 46.5076 | 48.3507 | 58.083 | 142.0 |
| 0.0145 | 21.0 | 16695 | 0.9915 | 58.2245 | 45.1804 | 48.1191 | 56.889 | 142.0 |
| 0.0128 | 22.0 | 17490 | 0.9945 | 58.2646 | 46.2782 | 49.4411 | 56.992 | 142.0 |
| 0.0129 | 23.0 | 18285 | 1.0069 | 57.0055 | 44.1866 | 46.9101 | 55.5056 | 141.9444 |
| 0.0116 | 24.0 | 19080 | 0.9967 | 58.1091 | 45.5303 | 48.2208 | 56.4496 | 142.0 |
| 0.0093 | 25.0 | 19875 | 1.0188 | 56.59 | 43.677 | 45.8956 | 55.0954 | 142.0 |
| 0.008 | 26.0 | 20670 | 0.9976 | 58.5408 | 46.7019 | 48.9235 | 57.2562 | 142.0 |
| 0.0077 | 27.0 | 21465 | 1.0123 | 57.7909 | 45.7619 | 48.3412 | 56.3796 | 142.0 |
| 0.0075 | 28.0 | 22260 | 1.0258 | 58.1694 | 45.03 | 48.282 | 56.7303 | 142.0 |
| 0.0056 | 29.0 | 23055 | 1.0100 | 58.0406 | 45.37 | 48.0125 | 56.5288 | 142.0 |
| 0.0049 | 30.0 | 23850 | 1.0235 | 56.419 | 43.248 | 46.3448 | 54.8467 | 142.0 |
| 0.0042 | 31.0 | 24645 | 1.0395 | 57.7232 | 45.6305 | 48.4531 | 56.3343 | 141.9444 |
| 0.0034 | 32.0 | 25440 | 1.0605 | 58.9049 | 46.8049 | 49.9103 | 57.6751 | 141.5 |
| 0.0032 | 33.0 | 26235 | 1.0362 | 57.8681 | 45.9028 | 48.8624 | 56.5616 | 141.8704 |
| 0.0025 | 34.0 | 27030 | 1.0521 | 58.8985 | 46.8547 | 49.8485 | 57.4249 | 142.0 |
| 0.0021 | 35.0 | 27825 | 1.0639 | 58.9324 | 46.656 | 49.1907 | 57.4836 | 142.0 |
| 0.0023 | 36.0 | 28620 | 1.0624 | 58.5734 | 46.6774 | 49.6377 | 57.3825 | 142.0 |
| 0.0019 | 37.0 | 29415 | 1.0636 | 58.9899 | 46.8217 | 49.4829 | 57.8683 | 142.0 |
| 0.0018 | 38.0 | 30210 | 1.0640 | 58.793 | 46.7964 | 49.7845 | 57.6379 | 142.0 |
| 0.0013 | 39.0 | 31005 | 1.0692 | 57.7124 | 45.5948 | 49.0482 | 56.4246 | 142.0 |
| 0.0012 | 40.0 | 31800 | 1.0746 | 58.1789 | 46.458 | 49.547 | 57.1007 | 141.6296 |
| 0.0008 | 41.0 | 32595 | 1.0815 | 57.7392 | 45.6404 | 48.4845 | 56.6464 | 142.0 |
| 0.0009 | 42.0 | 33390 | 1.0853 | 58.317 | 46.2661 | 49.0466 | 57.0971 | 142.0 |
| 0.0005 | 43.0 | 34185 | 1.0837 | 58.1526 | 46.0425 | 49.5624 | 56.9295 | 142.0 |
### Framework versions
- Transformers 4.19.2
- Pytorch 1.11.0+cu113
- Datasets 2.2.2
- Tokenizers 0.12.1
|
ronanki/ml_mpnet_768_MNR_15 | 8bd5ae7f3cfd1365f8534ae990e9ebd0efdcd70c | 2022-05-24T18:03:57.000Z | [
"pytorch",
"xlm-roberta",
"feature-extraction",
"sentence-transformers",
"sentence-similarity",
"transformers"
] | sentence-similarity | false | ronanki | null | ronanki/ml_mpnet_768_MNR_15 | 0 | null | sentence-transformers | 37,669 | ---
pipeline_tag: sentence-similarity
tags:
- sentence-transformers
- feature-extraction
- sentence-similarity
- transformers
---
# ronanki/ml_mpnet_768_MNR_15
This is a [sentence-transformers](https://www.SBERT.net) model: It maps sentences & paragraphs to a 768 dimensional dense vector space and can be used for tasks like clustering or semantic search.
<!--- Describe your model here -->
## Usage (Sentence-Transformers)
Using this model becomes easy when you have [sentence-transformers](https://www.SBERT.net) installed:
```
pip install -U sentence-transformers
```
Then you can use the model like this:
```python
from sentence_transformers import SentenceTransformer
sentences = ["This is an example sentence", "Each sentence is converted"]
model = SentenceTransformer('ronanki/ml_mpnet_768_MNR_15')
embeddings = model.encode(sentences)
print(embeddings)
```
## Usage (HuggingFace Transformers)
Without [sentence-transformers](https://www.SBERT.net), you can use the model like this: First, you pass your input through the transformer model, then you have to apply the right pooling-operation on-top of the contextualized word embeddings.
```python
from transformers import AutoTokenizer, AutoModel
import torch
#Mean Pooling - Take attention mask into account for correct averaging
def mean_pooling(model_output, attention_mask):
token_embeddings = model_output[0] #First element of model_output contains all token embeddings
input_mask_expanded = attention_mask.unsqueeze(-1).expand(token_embeddings.size()).float()
return torch.sum(token_embeddings * input_mask_expanded, 1) / torch.clamp(input_mask_expanded.sum(1), min=1e-9)
# Sentences we want sentence embeddings for
sentences = ['This is an example sentence', 'Each sentence is converted']
# Load model from HuggingFace Hub
tokenizer = AutoTokenizer.from_pretrained('ronanki/ml_mpnet_768_MNR_15')
model = AutoModel.from_pretrained('ronanki/ml_mpnet_768_MNR_15')
# Tokenize sentences
encoded_input = tokenizer(sentences, padding=True, truncation=True, return_tensors='pt')
# Compute token embeddings
with torch.no_grad():
model_output = model(**encoded_input)
# Perform pooling. In this case, mean pooling.
sentence_embeddings = mean_pooling(model_output, encoded_input['attention_mask'])
print("Sentence embeddings:")
print(sentence_embeddings)
```
## Evaluation Results
<!--- Describe how your model was evaluated -->
For an automated evaluation of this model, see the *Sentence Embeddings Benchmark*: [https://seb.sbert.net](https://seb.sbert.net?model_name=ronanki/ml_mpnet_768_MNR_15)
## Training
The model was trained with the parameters:
**DataLoader**:
`sentence_transformers.datasets.NoDuplicatesDataLoader.NoDuplicatesDataLoader` of length 8 with parameters:
```
{'batch_size': 4}
```
**Loss**:
`sentence_transformers.losses.MultipleNegativesRankingLoss.MultipleNegativesRankingLoss` with parameters:
```
{'scale': 20.0, 'similarity_fct': 'cos_sim'}
```
Parameters of the fit()-Method:
```
{
"epochs": 5,
"evaluation_steps": 0,
"evaluator": "NoneType",
"max_grad_norm": 1,
"optimizer_class": "<class 'transformers.optimization.AdamW'>",
"optimizer_params": {
"lr": 2e-05
},
"scheduler": "WarmupLinear",
"steps_per_epoch": null,
"warmup_steps": 0,
"weight_decay": 0.01
}
```
## Full Model Architecture
```
SentenceTransformer(
(0): Transformer({'max_seq_length': 128, 'do_lower_case': False}) with Transformer model: XLMRobertaModel
(1): Pooling({'word_embedding_dimension': 768, 'pooling_mode_cls_token': False, 'pooling_mode_mean_tokens': True, 'pooling_mode_max_tokens': False, 'pooling_mode_mean_sqrt_len_tokens': False})
)
```
## Citing & Authors
<!--- Describe where people can find more information --> |
huggingtweets/bladeecity-jerma985 | 9bf3a0db7f6bc960c51f2c0dc6fb66ed982b0180 | 2022-05-24T18:59:10.000Z | [
"pytorch",
"gpt2",
"text-generation",
"en",
"transformers",
"huggingtweets"
] | text-generation | false | huggingtweets | null | huggingtweets/bladeecity-jerma985 | 0 | null | transformers | 37,670 | ---
language: en
thumbnail: http://www.huggingtweets.com/bladeecity-jerma985/1653418745528/predictions.png
tags:
- huggingtweets
widget:
- text: "My dream is"
---
<div class="inline-flex flex-col" style="line-height: 1.5;">
<div class="flex">
<div
style="display:inherit; margin-left: 4px; margin-right: 4px; width: 92px; height:92px; border-radius: 50%; background-size: cover; background-image: url('https://pbs.twimg.com/profile_images/1501634135378391044/6FiRJ7RP_400x400.jpg')">
</div>
<div
style="display:inherit; margin-left: 4px; margin-right: 4px; width: 92px; height:92px; border-radius: 50%; background-size: cover; background-image: url('https://pbs.twimg.com/profile_images/803601382943162368/F36Z7ypy_400x400.jpg')">
</div>
<div
style="display:none; margin-left: 4px; margin-right: 4px; width: 92px; height:92px; border-radius: 50%; background-size: cover; background-image: url('')">
</div>
</div>
<div style="text-align: center; margin-top: 3px; font-size: 16px; font-weight: 800">🤖 AI CYBORG 🤖</div>
<div style="text-align: center; font-size: 16px; font-weight: 800">Aim Nothyng & Jerma</div>
<div style="text-align: center; font-size: 14px;">@bladeecity-jerma985</div>
</div>
I was made with [huggingtweets](https://github.com/borisdayma/huggingtweets).
Create your own bot based on your favorite user with [the demo](https://colab.research.google.com/github/borisdayma/huggingtweets/blob/master/huggingtweets-demo.ipynb)!
## How does it work?
The model uses the following pipeline.
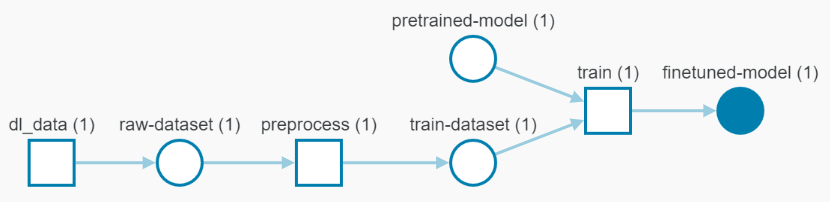
To understand how the model was developed, check the [W&B report](https://wandb.ai/wandb/huggingtweets/reports/HuggingTweets-Train-a-Model-to-Generate-Tweets--VmlldzoxMTY5MjI).
## Training data
The model was trained on tweets from Aim Nothyng & Jerma.
| Data | Aim Nothyng | Jerma |
| --- | --- | --- |
| Tweets downloaded | 1620 | 2695 |
| Retweets | 322 | 100 |
| Short tweets | 492 | 286 |
| Tweets kept | 806 | 2309 |
[Explore the data](https://wandb.ai/wandb/huggingtweets/runs/3g5k759s/artifacts), which is tracked with [W&B artifacts](https://docs.wandb.com/artifacts) at every step of the pipeline.
## Training procedure
The model is based on a pre-trained [GPT-2](https://huggingface.co/gpt2) which is fine-tuned on @bladeecity-jerma985's tweets.
Hyperparameters and metrics are recorded in the [W&B training run](https://wandb.ai/wandb/huggingtweets/runs/2wj5tjlg) for full transparency and reproducibility.
At the end of training, [the final model](https://wandb.ai/wandb/huggingtweets/runs/2wj5tjlg/artifacts) is logged and versioned.
## How to use
You can use this model directly with a pipeline for text generation:
```python
from transformers import pipeline
generator = pipeline('text-generation',
model='huggingtweets/bladeecity-jerma985')
generator("My dream is", num_return_sequences=5)
```
## Limitations and bias
The model suffers from [the same limitations and bias as GPT-2](https://huggingface.co/gpt2#limitations-and-bias).
In addition, the data present in the user's tweets further affects the text generated by the model.
## About
*Built by Boris Dayma*
[](https://twitter.com/intent/follow?screen_name=borisdayma)
For more details, visit the project repository.
[](https://github.com/borisdayma/huggingtweets)
|
ulises801/DialoGPT-medium-rick | 0a2c4b5b887e9957f74c767e9631c411d40ad580 | 2022-05-25T00:21:51.000Z | [
"pytorch",
"gpt2",
"text-generation",
"transformers",
"conversational"
] | conversational | false | ulises801 | null | ulises801/DialoGPT-medium-rick | 0 | null | transformers | 37,671 | ---
tags:
- conversational
---
# Rick DialogGPT Model |
syssec-utd/dis2py-37-with-cf | 7d01efd2d5ccef9512afc2695642ba22c549727f | 2022-05-31T14:40:52.000Z | [
"pytorch",
"t5",
"text2text-generation",
"transformers",
"generated_from_trainer",
"license:apache-2.0",
"model-index",
"autotrain_compatible"
] | text2text-generation | false | syssec-utd | null | syssec-utd/dis2py-37-with-cf | 0 | null | transformers | 37,672 | ---
license: apache-2.0
tags:
- generated_from_trainer
model-index:
- name: dis2py-37-with-cf
results: []
---
<!-- This model card has been generated automatically according to the information the Trainer had access to. You
should probably proofread and complete it, then remove this comment. -->
# dis2py-37-with-cf
This model is a fine-tuned version of [Salesforce/codet5-base](https://huggingface.co/Salesforce/codet5-base) on the syssec-utd/dis2py-37-with-cf-processed dataset.
## Model description
More information needed
## Intended uses & limitations
More information needed
## Training and evaluation data
More information needed
## Training procedure
### Training hyperparameters
The following hyperparameters were used during training:
- learning_rate: 2e-05
- train_batch_size: 24
- eval_batch_size: 24
- seed: 42
- optimizer: Adam with betas=(0.9,0.999) and epsilon=1e-08
- lr_scheduler_type: linear
- num_epochs: 3
### Training results
### Framework versions
- Transformers 4.17.0
- Pytorch 1.11.0+cu102
- Datasets 2.0.0
- Tokenizers 0.11.6
|
morahil/wav2vec2-hindi-new-3 | dc9d6fda2f537f57cef2a7a46ad3f85ec3a2ff33 | 2022-05-25T11:00:05.000Z | [
"pytorch",
"tensorboard",
"wav2vec2",
"automatic-speech-recognition",
"transformers",
"generated_from_trainer",
"license:apache-2.0",
"model-index"
] | automatic-speech-recognition | false | morahil | null | morahil/wav2vec2-hindi-new-3 | 0 | null | transformers | 37,673 | ---
license: apache-2.0
tags:
- generated_from_trainer
model-index:
- name: wav2vec2-hindi-new-3
results: []
---
<!-- This model card has been generated automatically according to the information the Trainer had access to. You
should probably proofread and complete it, then remove this comment. -->
# wav2vec2-hindi-new-3
This model is a fine-tuned version of [facebook/wav2vec2-large-xlsr-53](https://huggingface.co/facebook/wav2vec2-large-xlsr-53) on the None dataset.
It achieves the following results on the evaluation set:
- eval_loss: 2.1206
- eval_wer: 0.8949
- eval_runtime: 20.2358
- eval_samples_per_second: 19.767
- eval_steps_per_second: 2.471
- epoch: 25.8
- step: 1600
## Model description
More information needed
## Intended uses & limitations
More information needed
## Training and evaluation data
More information needed
## Training procedure
### Training hyperparameters
The following hyperparameters were used during training:
- learning_rate: 0.0001
- train_batch_size: 16
- eval_batch_size: 8
- seed: 42
- gradient_accumulation_steps: 2
- total_train_batch_size: 32
- optimizer: Adam with betas=(0.9,0.999) and epsilon=1e-08
- lr_scheduler_type: linear
- lr_scheduler_warmup_steps: 500
- num_epochs: 40
- mixed_precision_training: Native AMP
### Framework versions
- Transformers 4.20.0.dev0
- Pytorch 1.11.0+cu113
- Datasets 2.2.3.dev0
- Tokenizers 0.12.1
|
theojolliffe/bart-cnn-pubmed-arxiv-pubmed-arxiv-arxiv-v3-e16 | 2113c7a627a6f842a6e97f23ee5d758e2aca6add | 2022-05-25T10:47:47.000Z | [
"pytorch",
"tensorboard",
"bart",
"text2text-generation",
"transformers",
"generated_from_trainer",
"license:mit",
"model-index",
"autotrain_compatible"
] | text2text-generation | false | theojolliffe | null | theojolliffe/bart-cnn-pubmed-arxiv-pubmed-arxiv-arxiv-v3-e16 | 0 | null | transformers | 37,674 | ---
license: mit
tags:
- generated_from_trainer
metrics:
- rouge
model-index:
- name: bart-cnn-pubmed-arxiv-pubmed-arxiv-arxiv-v3-e16
results: []
---
<!-- This model card has been generated automatically according to the information the Trainer had access to. You
should probably proofread and complete it, then remove this comment. -->
# bart-cnn-pubmed-arxiv-pubmed-arxiv-arxiv-v3-e16
This model is a fine-tuned version of [theojolliffe/bart-cnn-pubmed-arxiv-pubmed-arxiv-arxiv](https://huggingface.co/theojolliffe/bart-cnn-pubmed-arxiv-pubmed-arxiv-arxiv) on an unknown dataset.
It achieves the following results on the evaluation set:
- Loss: 0.8960
- Rouge1: 57.7198
- Rouge2: 44.5711
- Rougel: 47.6281
- Rougelsum: 56.2372
- Gen Len: 142.0
## Model description
More information needed
## Intended uses & limitations
More information needed
## Training and evaluation data
More information needed
## Training procedure
### Training hyperparameters
The following hyperparameters were used during training:
- learning_rate: 2e-05
- train_batch_size: 2
- eval_batch_size: 2
- seed: 42
- optimizer: Adam with betas=(0.9,0.999) and epsilon=1e-08
- lr_scheduler_type: linear
- num_epochs: 16
- mixed_precision_training: Native AMP
### Training results
| Training Loss | Epoch | Step | Validation Loss | Rouge1 | Rouge2 | Rougel | Rougelsum | Gen Len |
|:-------------:|:-----:|:----:|:---------------:|:-------:|:-------:|:-------:|:---------:|:-------:|
| No log | 1.0 | 398 | 0.8634 | 53.7416 | 34.3731 | 37.1193 | 51.3075 | 142.0 |
| 0.8276 | 2.0 | 796 | 0.8001 | 53.9975 | 35.1019 | 38.2722 | 51.7878 | 142.0 |
| 0.5311 | 3.0 | 1194 | 0.7988 | 53.409 | 34.3201 | 37.5443 | 50.738 | 142.0 |
| 0.3538 | 4.0 | 1592 | 0.7698 | 53.679 | 34.7209 | 37.7895 | 51.2497 | 142.0 |
| 0.3538 | 5.0 | 1990 | 0.7863 | 54.2493 | 36.0643 | 39.1249 | 51.9758 | 142.0 |
| 0.2367 | 6.0 | 2388 | 0.7810 | 54.4042 | 37.4276 | 41.529 | 52.1544 | 142.0 |
| 0.164 | 7.0 | 2786 | 0.8055 | 56.0408 | 39.6744 | 42.8323 | 54.163 | 142.0 |
| 0.1146 | 8.0 | 3184 | 0.8098 | 55.2046 | 38.5399 | 41.9178 | 53.0001 | 142.0 |
| 0.089 | 9.0 | 3582 | 0.8199 | 57.1523 | 41.7614 | 44.5914 | 55.1602 | 142.0 |
| 0.089 | 10.0 | 3980 | 0.8644 | 56.943 | 41.5063 | 44.4929 | 54.9515 | 142.0 |
| 0.0647 | 11.0 | 4378 | 0.8413 | 57.0321 | 41.964 | 45.3971 | 55.0957 | 142.0 |
| 0.0485 | 12.0 | 4776 | 0.8735 | 56.7275 | 41.8577 | 44.3911 | 54.9824 | 142.0 |
| 0.0365 | 13.0 | 5174 | 0.8858 | 57.6103 | 43.8831 | 47.0374 | 56.0675 | 142.0 |
| 0.0271 | 14.0 | 5572 | 0.8974 | 57.39 | 42.8693 | 45.9344 | 55.7404 | 142.0 |
| 0.0271 | 15.0 | 5970 | 0.8990 | 57.9433 | 44.7301 | 47.843 | 56.5407 | 142.0 |
| 0.0232 | 16.0 | 6368 | 0.8960 | 57.7198 | 44.5711 | 47.6281 | 56.2372 | 142.0 |
### Framework versions
- Transformers 4.19.2
- Pytorch 1.11.0+cu113
- Datasets 2.2.2
- Tokenizers 0.12.1
|
pritam18/swadeshi_bhojpuriwav2vec2asr | c7dc0df5854d5e054db9df9d9fb3e6bbb012bcd3 | 2022-05-25T18:35:33.000Z | [
"pytorch",
"tensorboard",
"wav2vec2",
"automatic-speech-recognition",
"transformers",
"generated_from_trainer",
"license:apache-2.0",
"model-index"
] | automatic-speech-recognition | false | pritam18 | null | pritam18/swadeshi_bhojpuriwav2vec2asr | 0 | null | transformers | 37,675 | ---
license: apache-2.0
tags:
- generated_from_trainer
model-index:
- name: swadeshi_bhojpuriwav2vec2asr
results: []
---
<!-- This model card has been generated automatically according to the information the Trainer had access to. You
should probably proofread and complete it, then remove this comment. -->
# swadeshi_bhojpuriwav2vec2asr
This model is a fine-tuned version of [theainerd/Wav2Vec2-large-xlsr-hindi](https://huggingface.co/theainerd/Wav2Vec2-large-xlsr-hindi) on the None dataset.
It achieves the following results on the evaluation set:
- Loss: 0.2155
- Wer: 0.2931
## Model description
More information needed
## Intended uses & limitations
More information needed
## Training and evaluation data
More information needed
## Training procedure
### Training hyperparameters
The following hyperparameters were used during training:
- learning_rate: 0.0001
- train_batch_size: 8
- eval_batch_size: 8
- seed: 42
- gradient_accumulation_steps: 2
- total_train_batch_size: 16
- optimizer: Adam with betas=(0.9,0.999) and epsilon=1e-08
- lr_scheduler_type: linear
- lr_scheduler_warmup_steps: 500
- num_epochs: 30
- mixed_precision_training: Native AMP
### Training results
| Training Loss | Epoch | Step | Validation Loss | Wer |
|:-------------:|:-----:|:----:|:---------------:|:------:|
| 3.6928 | 3.2 | 400 | 2.4820 | 0.9925 |
| 1.6981 | 6.4 | 800 | 0.8053 | 0.6320 |
| 0.975 | 9.6 | 1200 | 0.5420 | 0.4980 |
| 0.7672 | 12.8 | 1600 | 0.4224 | 0.4233 |
| 0.636 | 16.0 | 2000 | 0.3481 | 0.3774 |
| 0.5562 | 19.2 | 2400 | 0.2861 | 0.3409 |
| 0.4973 | 22.4 | 2800 | 0.2450 | 0.3211 |
| 0.4616 | 25.6 | 3200 | 0.2230 | 0.3004 |
| 0.4264 | 28.8 | 3600 | 0.2155 | 0.2931 |
### Framework versions
- Transformers 4.20.0.dev0
- Pytorch 1.11.0+cu113
- Datasets 2.2.3.dev0
- Tokenizers 0.12.1
|
neuralmagic/oBERT-teacher-squadv1 | 733308386b17fb771a5495823b8f9b05a6404ac1 | 2022-06-20T11:36:53.000Z | [
"pytorch",
"en",
"dataset:squad",
"arxiv:2203.07259",
"bert",
"oBERT",
"sparsity",
"pruning",
"compression"
] | null | false | neuralmagic | null | neuralmagic/oBERT-teacher-squadv1 | 0 | null | null | 37,676 | ---
tags:
- bert
- oBERT
- sparsity
- pruning
- compression
language: en
datasets: squad
---
# SQuADv1 teacher
This model is used as a teacher for all runs on the SQuADv1 downstream task in the paper [The Optimal BERT Surgeon: Scalable and Accurate Second-Order Pruning for Large Language Models](https://arxiv.org/abs/2203.07259).
SQuADv1 dev-set:
```
EM = 81.41
F1 = 88.54
```
## BibTeX entry and citation info
```bibtex
@article{kurtic2022optimal,
title={The Optimal BERT Surgeon: Scalable and Accurate Second-Order Pruning for Large Language Models},
author={Kurtic, Eldar and Campos, Daniel and Nguyen, Tuan and Frantar, Elias and Kurtz, Mark and Fineran, Benjamin and Goin, Michael and Alistarh, Dan},
journal={arXiv preprint arXiv:2203.07259},
year={2022}
}
``` |
neuralmagic/oBERT-12-downstream-pruned-unstructured-90-squadv1 | 11cd9a179a8293ebaf3f487797bf18ec67db0ae2 | 2022-06-20T11:36:49.000Z | [
"pytorch",
"en",
"dataset:squad",
"arxiv:2203.07259",
"bert",
"oBERT",
"sparsity",
"pruning",
"compression"
] | null | false | neuralmagic | null | neuralmagic/oBERT-12-downstream-pruned-unstructured-90-squadv1 | 0 | null | null | 37,677 | ---
tags:
- bert
- oBERT
- sparsity
- pruning
- compression
language: en
datasets: squad
---
# oBERT-12-downstream-pruned-unstructured-90-squadv1
This model is obtained with [The Optimal BERT Surgeon: Scalable and Accurate Second-Order Pruning for Large Language Models](https://arxiv.org/abs/2203.07259).
It corresponds to the model presented in the `Table 1 - 30 Epochs - oBERT - SQuADv1 90%`.
```
Pruning method: oBERT downstream unstructured
Paper: https://arxiv.org/abs/2203.07259
Dataset: SQuADv1
Sparsity: 90%
Number of layers: 12
```
The dev-set performance reported in the paper is averaged over three seeds, and we release the best model (marked with `(*)`):
```
| oBERT 90% | F1 | EM |
| ------------ | ----- | ----- |
| seed=42 | 88.22 | 81.10 |
| seed=3407 (*)| 88.46 | 81.26 |
| seed=54321 | 88.26 | 81.00 |
| ------------ | ----- | ----- |
| mean | 88.31 | 81.12 |
| stdev | 0.128 | 0.131 |
```
Code: _coming soon_
## BibTeX entry and citation info
```bibtex
@article{kurtic2022optimal,
title={The Optimal BERT Surgeon: Scalable and Accurate Second-Order Pruning for Large Language Models},
author={Kurtic, Eldar and Campos, Daniel and Nguyen, Tuan and Frantar, Elias and Kurtz, Mark and Fineran, Benjamin and Goin, Michael and Alistarh, Dan},
journal={arXiv preprint arXiv:2203.07259},
year={2022}
}
``` |
neuralmagic/oBERT-12-downstream-pruned-unstructured-97-squadv1 | a243c9389bfb610522875045872f7fa6d6a498b0 | 2022-06-20T11:36:50.000Z | [
"pytorch",
"en",
"dataset:squad",
"arxiv:2203.07259",
"bert",
"oBERT",
"sparsity",
"pruning",
"compression"
] | null | false | neuralmagic | null | neuralmagic/oBERT-12-downstream-pruned-unstructured-97-squadv1 | 0 | null | null | 37,678 | ---
tags:
- bert
- oBERT
- sparsity
- pruning
- compression
language: en
datasets: squad
---
# oBERT-12-downstream-pruned-unstructured-97-squadv1
This model is obtained with [The Optimal BERT Surgeon: Scalable and Accurate Second-Order Pruning for Large Language Models](https://arxiv.org/abs/2203.07259).
It corresponds to the model presented in the `Table 1 - 30 Epochs - oBERT - SQuADv1 97%`.
```
Pruning method: oBERT downstream unstructured
Paper: https://arxiv.org/abs/2203.07259
Dataset: SQuADv1
Sparsity: 97%
Number of layers: 12
```
The dev-set performance reported in the paper is averaged over three seeds, and we release the best model (marked with `(*)`):
```
| oBERT 97% | F1 | EM |
| ------------ | ----- | ----- |
| seed=42 (*)| 86.06 | 78.28 |
| seed=3407 | 86.04 | 78.12 |
| seed=54321 | 85.85 | 77.93 |
| ------------ | ----- | ----- |
| mean | 85.98 | 78.11 |
| stdev | 0.115 | 0.175 |
```
Code: _coming soon_
## BibTeX entry and citation info
```bibtex
@article{kurtic2022optimal,
title={The Optimal BERT Surgeon: Scalable and Accurate Second-Order Pruning for Large Language Models},
author={Kurtic, Eldar and Campos, Daniel and Nguyen, Tuan and Frantar, Elias and Kurtz, Mark and Fineran, Benjamin and Goin, Michael and Alistarh, Dan},
journal={arXiv preprint arXiv:2203.07259},
year={2022}
}
``` |
neuralmagic/oBERT-teacher-mnli | 20f8a17d62e84cb0ef2cd979bd673500072ac9f0 | 2022-06-20T11:36:52.000Z | [
"pytorch",
"en",
"dataset:mnli",
"arxiv:2203.07259",
"bert",
"oBERT",
"sparsity",
"pruning",
"compression"
] | null | false | neuralmagic | null | neuralmagic/oBERT-teacher-mnli | 0 | null | null | 37,679 | ---
tags:
- bert
- oBERT
- sparsity
- pruning
- compression
language: en
datasets: mnli
---
# MNLI teacher
This model is used as a teacher for all runs on the MNLI downstream task in the paper [The Optimal BERT Surgeon: Scalable and Accurate Second-Order Pruning for Large Language Models](https://arxiv.org/abs/2203.07259).
MNLI dev-set:
```
matched accuracy = 84.54
mismatched accuracy = 85.06
```
## BibTeX entry and citation info
```bibtex
@article{kurtic2022optimal,
title={The Optimal BERT Surgeon: Scalable and Accurate Second-Order Pruning for Large Language Models},
author={Kurtic, Eldar and Campos, Daniel and Nguyen, Tuan and Frantar, Elias and Kurtz, Mark and Fineran, Benjamin and Goin, Michael and Alistarh, Dan},
journal={arXiv preprint arXiv:2203.07259},
year={2022}
}
``` |
neuralmagic/oBERT-12-downstream-pruned-unstructured-80-mnli | 828739304f5bb17eee83f39e4940eca6a70d093d | 2022-06-20T11:36:49.000Z | [
"pytorch",
"en",
"dataset:mnli",
"arxiv:2203.07259",
"bert",
"oBERT",
"sparsity",
"pruning",
"compression"
] | null | false | neuralmagic | null | neuralmagic/oBERT-12-downstream-pruned-unstructured-80-mnli | 0 | null | null | 37,680 | ---
tags:
- bert
- oBERT
- sparsity
- pruning
- compression
language: en
datasets: mnli
---
# oBERT-12-downstream-pruned-unstructured-80-mnli
This model is obtained with [The Optimal BERT Surgeon: Scalable and Accurate Second-Order Pruning for Large Language Models](https://arxiv.org/abs/2203.07259).
It corresponds to the model presented in the `Table 1 - 30 Epochs - oBERT - MNLI 80%`.
```
Pruning method: oBERT downstream unstructured
Paper: https://arxiv.org/abs/2203.07259
Dataset: MNLI
Sparsity: 80%
Number of layers: 12
```
The dev-set performance reported in the paper is averaged over three seeds, and we release the best model (marked with `(*)`):
```
| oBERT 80% | m-acc | mm-acc|
| ------------ | ----- | ----- |
| seed=42 | 84.30 | 84.98 |
| seed=3407 (*)| 84.46 | 84.99 |
| seed=54321 | 84.18 | 84.76 |
| ------------ | ----- | ----- |
| mean | 84.32 | 84.91 |
| stdev | 0.140 | 0.133 |
```
Code: _coming soon_
## BibTeX entry and citation info
```bibtex
@article{kurtic2022optimal,
title={The Optimal BERT Surgeon: Scalable and Accurate Second-Order Pruning for Large Language Models},
author={Kurtic, Eldar and Campos, Daniel and Nguyen, Tuan and Frantar, Elias and Kurtz, Mark and Fineran, Benjamin and Goin, Michael and Alistarh, Dan},
journal={arXiv preprint arXiv:2203.07259},
year={2022}
}
``` |
neuralmagic/oBERT-12-downstream-pruned-unstructured-90-mnli | 7b039ca83c3209f18d902f4b516e99ebae6ee7f2 | 2022-06-20T11:36:49.000Z | [
"pytorch",
"en",
"dataset:mnli",
"arxiv:2203.07259",
"bert",
"oBERT",
"sparsity",
"pruning",
"compression"
] | null | false | neuralmagic | null | neuralmagic/oBERT-12-downstream-pruned-unstructured-90-mnli | 0 | null | null | 37,681 | ---
tags:
- bert
- oBERT
- sparsity
- pruning
- compression
language: en
datasets: mnli
---
# oBERT-12-downstream-pruned-unstructured-90-mnli
This model is obtained with [The Optimal BERT Surgeon: Scalable and Accurate Second-Order Pruning for Large Language Models](https://arxiv.org/abs/2203.07259).
It corresponds to the model presented in the `Table 1 - 30 Epochs - oBERT - MNLI 90%`.
```
Pruning method: oBERT downstream unstructured
Paper: https://arxiv.org/abs/2203.07259
Dataset: MNLI
Sparsity: 90%
Number of layers: 12
```
The dev-set performance reported in the paper is averaged over three seeds, and we release the best model (marked with `(*)`):
```
| oBERT 90% | m-acc | mm-acc|
| ------------ | ----- | ----- |
| seed=42 | 83.74 | 84.31 |
| seed=3407 (*)| 83.85 | 84.40 |
| seed=54321 | 83.77 | 84.33 |
| ------------ | ----- | ----- |
| mean | 83.79 | 84.35 |
| stdev | 0.056 | 0.047 |
```
Code: _coming soon_
## BibTeX entry and citation info
```bibtex
@article{kurtic2022optimal,
title={The Optimal BERT Surgeon: Scalable and Accurate Second-Order Pruning for Large Language Models},
author={Kurtic, Eldar and Campos, Daniel and Nguyen, Tuan and Frantar, Elias and Kurtz, Mark and Fineran, Benjamin and Goin, Michael and Alistarh, Dan},
journal={arXiv preprint arXiv:2203.07259},
year={2022}
}
``` |
neuralmagic/oBERT-12-downstream-pruned-unstructured-97-mnli | 6f0c3a4713f4898e69a821c43750695766d37bfc | 2022-06-20T11:36:49.000Z | [
"pytorch",
"en",
"dataset:mnli",
"arxiv:2203.07259",
"bert",
"oBERT",
"sparsity",
"pruning",
"compression"
] | null | false | neuralmagic | null | neuralmagic/oBERT-12-downstream-pruned-unstructured-97-mnli | 0 | null | null | 37,682 | ---
tags:
- bert
- oBERT
- sparsity
- pruning
- compression
language: en
datasets: mnli
---
# oBERT-12-downstream-pruned-unstructured-97-mnli
This model is obtained with [The Optimal BERT Surgeon: Scalable and Accurate Second-Order Pruning for Large Language Models](https://arxiv.org/abs/2203.07259).
It corresponds to the model presented in the `Table 1 - 30 Epochs - oBERT - MNLI 97%`.
```
Pruning method: oBERT downstream unstructured
Paper: https://arxiv.org/abs/2203.07259
Dataset: MNLI
Sparsity: 97%
Number of layers: 12
```
The dev-set performance reported in the paper is averaged over three seeds, and we release the best model (marked with `(*)`):
```
| oBERT 97% | m-acc | mm-acc|
| ------------ | ----- | ----- |
| seed=42 (*)| 82.10 | 81.94 |
| seed=3407 | 81.81 | 82.27 |
| seed=54321 | 81.40 | 81.83 |
| ------------ | ----- | ----- |
| mean | 81.77 | 82.01 |
| stdev | 0.351 | 0.228 |
```
Code: _coming soon_
## BibTeX entry and citation info
```bibtex
@article{kurtic2022optimal,
title={The Optimal BERT Surgeon: Scalable and Accurate Second-Order Pruning for Large Language Models},
author={Kurtic, Eldar and Campos, Daniel and Nguyen, Tuan and Frantar, Elias and Kurtz, Mark and Fineran, Benjamin and Goin, Michael and Alistarh, Dan},
journal={arXiv preprint arXiv:2203.07259},
year={2022}
}
``` |
neuralmagic/oBERT-teacher-qqp | 9115006d21a4e7d36647f5982cdf012b4ff41f94 | 2022-06-20T11:36:53.000Z | [
"pytorch",
"en",
"dataset:qqp",
"arxiv:2203.07259",
"bert",
"oBERT",
"sparsity",
"pruning",
"compression"
] | null | false | neuralmagic | null | neuralmagic/oBERT-teacher-qqp | 0 | null | null | 37,683 | ---
tags:
- bert
- oBERT
- sparsity
- pruning
- compression
language: en
datasets: qqp
---
# QQP teacher
This model is used as a teacher for all runs on the QQP downstream task in the paper [The Optimal BERT Surgeon: Scalable and Accurate Second-Order Pruning for Large Language Models](https://arxiv.org/abs/2203.07259).
QQP dev-set:
```
accuracy = 91.06
F1 = 88.00
```
## BibTeX entry and citation info
```bibtex
@article{kurtic2022optimal,
title={The Optimal BERT Surgeon: Scalable and Accurate Second-Order Pruning for Large Language Models},
author={Kurtic, Eldar and Campos, Daniel and Nguyen, Tuan and Frantar, Elias and Kurtz, Mark and Fineran, Benjamin and Goin, Michael and Alistarh, Dan},
journal={arXiv preprint arXiv:2203.07259},
year={2022}
}
``` |
neuralmagic/oBERT-12-downstream-pruned-unstructured-80-qqp | 5606d41cad5a7a4b6b1c0a19b57e2ed03556bcca | 2022-06-20T11:36:49.000Z | [
"pytorch",
"en",
"dataset:qqp",
"arxiv:2203.07259",
"bert",
"oBERT",
"sparsity",
"pruning",
"compression"
] | null | false | neuralmagic | null | neuralmagic/oBERT-12-downstream-pruned-unstructured-80-qqp | 0 | null | null | 37,684 | ---
tags:
- bert
- oBERT
- sparsity
- pruning
- compression
language: en
datasets: qqp
---
# oBERT-12-downstream-pruned-unstructured-80-qqp
This model is obtained with [The Optimal BERT Surgeon: Scalable and Accurate Second-Order Pruning for Large Language Models](https://arxiv.org/abs/2203.07259).
It corresponds to the model presented in the `Table 1 - 30 Epochs - oBERT - QQP 80%`.
```
Pruning method: oBERT downstream unstructured
Paper: https://arxiv.org/abs/2203.07259
Dataset: QQP
Sparsity: 80%
Number of layers: 12
```
The dev-set performance reported in the paper is averaged over three seeds, and we release the best model (marked with `(*)`):
```
| oBERT 80% | acc | F1 |
| ------------ | ----- | ----- |
| seed=42 (*)| 91.66 | 88.72 |
| seed=3407 | 91.51 | 88.56 |
| seed=54321 | 91.54 | 88.60 |
| ------------ | ----- | ----- |
| mean | 91.57 | 88.63 |
| stdev | 0.079 | 0.083 |
```
Code: _coming soon_
## BibTeX entry and citation info
```bibtex
@article{kurtic2022optimal,
title={The Optimal BERT Surgeon: Scalable and Accurate Second-Order Pruning for Large Language Models},
author={Kurtic, Eldar and Campos, Daniel and Nguyen, Tuan and Frantar, Elias and Kurtz, Mark and Fineran, Benjamin and Goin, Michael and Alistarh, Dan},
journal={arXiv preprint arXiv:2203.07259},
year={2022}
}
``` |
neuralmagic/oBERT-12-downstream-pruned-unstructured-90-qqp | 9233f2b32f0ddee0ed908dd842ac31b0bd3918bd | 2022-06-20T11:36:49.000Z | [
"pytorch",
"en",
"dataset:qqp",
"arxiv:2203.07259",
"bert",
"oBERT",
"sparsity",
"pruning",
"compression"
] | null | false | neuralmagic | null | neuralmagic/oBERT-12-downstream-pruned-unstructured-90-qqp | 0 | null | null | 37,685 | ---
tags:
- bert
- oBERT
- sparsity
- pruning
- compression
language: en
datasets: qqp
---
# oBERT-12-downstream-pruned-unstructured-90-qqp
This model is obtained with [The Optimal BERT Surgeon: Scalable and Accurate Second-Order Pruning for Large Language Models](https://arxiv.org/abs/2203.07259).
It corresponds to the model presented in the `Table 1 - 30 Epochs - oBERT - QQP 90%`.
```
Pruning method: oBERT downstream unstructured
Paper: https://arxiv.org/abs/2203.07259
Dataset: QQP
Sparsity: 90%
Number of layers: 12
```
The dev-set performance reported in the paper is averaged over three seeds, and we release the best model (marked with `(*)`):
```
| oBERT 90% | acc | F1 |
| ------------ | ----- | ----- |
| seed=42 | 91.30 | 88.24 |
| seed=3407 (*)| 91.39 | 88.36 |
| seed=54321 | 91.36 | 88.29 |
| ------------ | ----- | ----- |
| mean | 91.35 | 88.30 |
| stdev | 0.045 | 0.060 |
```
Code: _coming soon_
## BibTeX entry and citation info
```bibtex
@article{kurtic2022optimal,
title={The Optimal BERT Surgeon: Scalable and Accurate Second-Order Pruning for Large Language Models},
author={Kurtic, Eldar and Campos, Daniel and Nguyen, Tuan and Frantar, Elias and Kurtz, Mark and Fineran, Benjamin and Goin, Michael and Alistarh, Dan},
journal={arXiv preprint arXiv:2203.07259},
year={2022}
}
``` |
neuralmagic/oBERT-12-downstream-pruned-unstructured-97-qqp | 08fce0ae0a627b4750d468182eb52f61061f73ae | 2022-06-20T11:36:50.000Z | [
"pytorch",
"en",
"dataset:qqp",
"arxiv:2203.07259",
"bert",
"oBERT",
"sparsity",
"pruning",
"compression"
] | null | false | neuralmagic | null | neuralmagic/oBERT-12-downstream-pruned-unstructured-97-qqp | 0 | null | null | 37,686 | ---
tags:
- bert
- oBERT
- sparsity
- pruning
- compression
language: en
datasets: qqp
---
# oBERT-12-downstream-pruned-unstructured-97-qqp
This model is obtained with [The Optimal BERT Surgeon: Scalable and Accurate Second-Order Pruning for Large Language Models](https://arxiv.org/abs/2203.07259).
It corresponds to the model presented in the `Table 1 - 30 Epochs - oBERT - QQP 97%`.
```
Pruning method: oBERT downstream unstructured
Paper: https://arxiv.org/abs/2203.07259
Dataset: QQP
Sparsity: 97%
Number of layers: 12
```
The dev-set performance reported in the paper is averaged over three seeds, and we release the best model (marked with `(*)`):
```
| oBERT 97% | acc | F1 |
| ------------ | ----- | ----- |
| seed=42 (*)| 90.90 | 87.73 |
| seed=3407 | 90.80 | 87.57 |
| seed=54321 | 90.90 | 87.69 |
| ------------ | ----- | ----- |
| mean | 90.87 | 87.66 |
| stdev | 0.057 | 0.083 |
```
Code: _coming soon_
## BibTeX entry and citation info
```bibtex
@article{kurtic2022optimal,
title={The Optimal BERT Surgeon: Scalable and Accurate Second-Order Pruning for Large Language Models},
author={Kurtic, Eldar and Campos, Daniel and Nguyen, Tuan and Frantar, Elias and Kurtz, Mark and Fineran, Benjamin and Goin, Michael and Alistarh, Dan},
journal={arXiv preprint arXiv:2203.07259},
year={2022}
}
``` |
neuralmagic/oBERT-12-upstream-pruned-unstructured-90 | 11f5620ab4851ec5a96160d020a9cec92d668f6a | 2022-06-20T11:36:50.000Z | [
"pytorch",
"en",
"dataset:bookcorpus",
"dataset:wikipedia",
"arxiv:2203.07259",
"bert",
"oBERT",
"sparsity",
"pruning",
"compression"
] | null | false | neuralmagic | null | neuralmagic/oBERT-12-upstream-pruned-unstructured-90 | 0 | null | null | 37,687 | ---
tags:
- bert
- oBERT
- sparsity
- pruning
- compression
language: en
datasets:
- bookcorpus
- wikipedia
---
# oBERT-12-upstream-pruned-unstructured-90
This model is obtained with [The Optimal BERT Surgeon: Scalable and Accurate Second-Order Pruning for Large Language Models](https://arxiv.org/abs/2203.07259).
It corresponds to the upstream pruned model used as a starting point for sparse-transfer learning to downstream tasks presented in the `Table 2 - oBERT - {SQuADv1, MNLI, QQP} - 90%`.
Finetuned versions of this model for each downstream task are:
- SQuADv1: `neuralmagic/oBERT-12-upstream-pruned-unstructured-90-finetuned-squadv1`
- MNLI: `neuralmagic/oBERT-12-upstream-pruned-unstructured-90-finetuned-mnli`
- QQP: `neuralmagic/oBERT-12-upstream-pruned-unstructured-90-finetuned-qqp`
```
Pruning method: oBERT upstream unstructured
Paper: https://arxiv.org/abs/2203.07259
Dataset: BookCorpus and English Wikipedia
Sparsity: 90%
Number of layers: 12
```
Code: _coming soon_
## BibTeX entry and citation info
```bibtex
@article{kurtic2022optimal,
title={The Optimal BERT Surgeon: Scalable and Accurate Second-Order Pruning for Large Language Models},
author={Kurtic, Eldar and Campos, Daniel and Nguyen, Tuan and Frantar, Elias and Kurtz, Mark and Fineran, Benjamin and Goin, Michael and Alistarh, Dan},
journal={arXiv preprint arXiv:2203.07259},
year={2022}
}
``` |
neuralmagic/oBERT-12-upstream-pruned-unstructured-97 | 2a18dfa3901d129d1ea412a9b020ba2082404ecd | 2022-06-20T11:36:51.000Z | [
"pytorch",
"en",
"dataset:bookcorpus",
"dataset:wikipedia",
"arxiv:2203.07259",
"bert",
"oBERT",
"sparsity",
"pruning",
"compression"
] | null | false | neuralmagic | null | neuralmagic/oBERT-12-upstream-pruned-unstructured-97 | 0 | null | null | 37,688 | ---
tags:
- bert
- oBERT
- sparsity
- pruning
- compression
language: en
datasets:
- bookcorpus
- wikipedia
---
# oBERT-12-upstream-pruned-unstructured-97
This model is obtained with [The Optimal BERT Surgeon: Scalable and Accurate Second-Order Pruning for Large Language Models](https://arxiv.org/abs/2203.07259).
It corresponds to the upstream pruned model used as a starting point for sparse-transfer learning to downstream tasks presented in the `Table 2 - oBERT - {SQuADv1, MNLI, QQP} - 97%`.
Finetuned versions of this model for each downstream task are:
- SQuADv1: `neuralmagic/oBERT-12-upstream-pruned-unstructured-97-finetuned-squadv1`
- MNLI: `neuralmagic/oBERT-12-upstream-pruned-unstructured-97-finetuned-mnli`
- QQP: `neuralmagic/oBERT-12-upstream-pruned-unstructured-97-finetuned-qqp`
```
Pruning method: oBERT upstream unstructured
Paper: https://arxiv.org/abs/2203.07259
Dataset: BookCorpus and English Wikipedia
Sparsity: 97%
Number of layers: 12
```
Code: _coming soon_
## BibTeX entry and citation info
```bibtex
@article{kurtic2022optimal,
title={The Optimal BERT Surgeon: Scalable and Accurate Second-Order Pruning for Large Language Models},
author={Kurtic, Eldar and Campos, Daniel and Nguyen, Tuan and Frantar, Elias and Kurtz, Mark and Fineran, Benjamin and Goin, Michael and Alistarh, Dan},
journal={arXiv preprint arXiv:2203.07259},
year={2022}
}
``` |
neuralmagic/oBERT-12-upstream-pruned-unstructured-90-finetuned-squadv1 | 135307894d942bb1258a35987450b76c3320e967 | 2022-06-20T11:36:50.000Z | [
"pytorch",
"en",
"dataset:squad",
"arxiv:2203.07259",
"bert",
"oBERT",
"sparsity",
"pruning",
"compression"
] | null | false | neuralmagic | null | neuralmagic/oBERT-12-upstream-pruned-unstructured-90-finetuned-squadv1 | 0 | null | null | 37,689 | ---
tags:
- bert
- oBERT
- sparsity
- pruning
- compression
language: en
datasets: squad
---
# oBERT-12-upstream-pruned-unstructured-90-finetuned-squadv1
This model is obtained with [The Optimal BERT Surgeon: Scalable and Accurate Second-Order Pruning for Large Language Models](https://arxiv.org/abs/2203.07259).
It corresponds to the model presented in the `Table 2 - oBERT - SQuADv1 90%`.
```
Pruning method: oBERT upstream unstructured + sparse-transfer to downstream
Paper: https://arxiv.org/abs/2203.07259
Dataset: SQuADv1
Sparsity: 90%
Number of layers: 12
```
The dev-set performance reported in the paper is averaged over three seeds, and we release the best model (marked with `(*)`):
```
| oBERT 90% | F1 | EM |
| ------------ | ----- | ----- |
| seed=42 (*)| 88.47 | 81.43 |
| seed=3407 | 88.32 | 81.13 |
| seed=54321 | 88.47 | 81.38 |
| ------------ | ----- | ----- |
| mean | 88.42 | 81.31 |
| stdev | 0.086 | 0.160 |
```
Code: _coming soon_
## BibTeX entry and citation info
```bibtex
@article{kurtic2022optimal,
title={The Optimal BERT Surgeon: Scalable and Accurate Second-Order Pruning for Large Language Models},
author={Kurtic, Eldar and Campos, Daniel and Nguyen, Tuan and Frantar, Elias and Kurtz, Mark and Fineran, Benjamin and Goin, Michael and Alistarh, Dan},
journal={arXiv preprint arXiv:2203.07259},
year={2022}
}
``` |
neuralmagic/oBERT-12-upstream-pruned-unstructured-97-finetuned-squadv1 | 4fb62121f1ab5ea13156523ccc39b952488121b7 | 2022-06-20T11:36:51.000Z | [
"pytorch",
"en",
"dataset:squad",
"arxiv:2203.07259",
"bert",
"oBERT",
"sparsity",
"pruning",
"compression"
] | null | false | neuralmagic | null | neuralmagic/oBERT-12-upstream-pruned-unstructured-97-finetuned-squadv1 | 0 | null | null | 37,690 | ---
tags:
- bert
- oBERT
- sparsity
- pruning
- compression
language: en
datasets: squad
---
# oBERT-12-upstream-pruned-unstructured-97-finetuned-squadv1
This model is obtained with [The Optimal BERT Surgeon: Scalable and Accurate Second-Order Pruning for Large Language Models](https://arxiv.org/abs/2203.07259).
It corresponds to the model presented in the `Table 2 - oBERT - SQuADv1 97%`.
```
Pruning method: oBERT upstream unstructured + sparse-transfer to downstream
Paper: https://arxiv.org/abs/2203.07259
Dataset: SQuADv1
Sparsity: 97%
Number of layers: 12
```
The dev-set performance reported in the paper is averaged over three seeds, and we release the best model (marked with `(*)`):
```
| oBERT 97% | F1 | EM |
| ------------ | ----- | ----- |
| seed=42 | 84.11 | 76.02 |
| seed=3407 (*)| 84.71 | 76.61 |
| seed=54321 | 84.35 | 76.44 |
| ------------ | ----- | ----- |
| mean | 84.39 | 76.36 |
| stdev | 0.301 | 0.303 |
```
Code: _coming soon_
## BibTeX entry and citation info
```bibtex
@article{kurtic2022optimal,
title={The Optimal BERT Surgeon: Scalable and Accurate Second-Order Pruning for Large Language Models},
author={Kurtic, Eldar and Campos, Daniel and Nguyen, Tuan and Frantar, Elias and Kurtz, Mark and Fineran, Benjamin and Goin, Michael and Alistarh, Dan},
journal={arXiv preprint arXiv:2203.07259},
year={2022}
}
``` |
neuralmagic/oBERT-12-upstream-pruned-unstructured-90-finetuned-mnli | 3acc9173de167432e2cefc3e2c8e35f9bda25517 | 2022-06-20T11:36:50.000Z | [
"pytorch",
"en",
"dataset:mnli",
"arxiv:2203.07259",
"bert",
"oBERT",
"sparsity",
"pruning",
"compression"
] | null | false | neuralmagic | null | neuralmagic/oBERT-12-upstream-pruned-unstructured-90-finetuned-mnli | 0 | null | null | 37,691 | ---
tags:
- bert
- oBERT
- sparsity
- pruning
- compression
language: en
datasets: mnli
---
# oBERT-12-upstream-pruned-unstructured-90-finetuned-mnli
This model is obtained with [The Optimal BERT Surgeon: Scalable and Accurate Second-Order Pruning for Large Language Models](https://arxiv.org/abs/2203.07259).
It corresponds to the model presented in the `Table 2 - oBERT - MNLI 90%`.
```
Pruning method: oBERT upstream unstructured + sparse-transfer to downstream
Paper: https://arxiv.org/abs/2203.07259
Dataset: MNLI
Sparsity: 90%
Number of layers: 12
```
The dev-set performance reported in the paper is averaged over three seeds, and we release the best model (marked with `(*)`):
```
| oBERT 90% | m-acc | mm-acc|
| ------------ | ----- | ----- |
| seed=42 (*)| 82.40 | 83.40 |
| seed=3407 | 82.15 | 83.41 |
| seed=54321 | 82.32 | 83.38 |
| ------------ | ----- | ----- |
| mean | 82.29 | 83.40 |
| stdev | 0.127 | 0.015 |
```
Code: _coming soon_
## BibTeX entry and citation info
```bibtex
@article{kurtic2022optimal,
title={The Optimal BERT Surgeon: Scalable and Accurate Second-Order Pruning for Large Language Models},
author={Kurtic, Eldar and Campos, Daniel and Nguyen, Tuan and Frantar, Elias and Kurtz, Mark and Fineran, Benjamin and Goin, Michael and Alistarh, Dan},
journal={arXiv preprint arXiv:2203.07259},
year={2022}
}
``` |
neuralmagic/oBERT-12-upstream-pruned-unstructured-97-finetuned-mnli | 465a50e80f12db9417a5f4272ed7f816643aec1d | 2022-06-20T11:36:51.000Z | [
"pytorch",
"en",
"dataset:mnli",
"arxiv:2203.07259",
"bert",
"oBERT",
"sparsity",
"pruning",
"compression"
] | null | false | neuralmagic | null | neuralmagic/oBERT-12-upstream-pruned-unstructured-97-finetuned-mnli | 0 | null | null | 37,692 | ---
tags:
- bert
- oBERT
- sparsity
- pruning
- compression
language: en
datasets: mnli
---
# oBERT-12-upstream-pruned-unstructured-97-finetuned-mnli
This model is obtained with [The Optimal BERT Surgeon: Scalable and Accurate Second-Order Pruning for Large Language Models](https://arxiv.org/abs/2203.07259).
It corresponds to the model presented in the `Table 2 - oBERT - MNLI 97%`.
```
Pruning method: oBERT upstream unstructured + sparse-transfer to downstream
Paper: https://arxiv.org/abs/2203.07259
Dataset: MNLI
Sparsity: 97%
Number of layers: 12
```
The dev-set performance reported in the paper is averaged over three seeds, and we release the best model (marked with `(*)`):
```
| oBERT 97% | m-acc | mm-acc|
| ------------ | ----- | ----- |
| seed=42 | 78.55 | 79.90 |
| seed=3407 | 78.88 | 79.78 |
| seed=54321(*)| 79.11 | 79.71 |
| ------------ | ----- | ----- |
| mean | 78.85 | 79.80 |
| stdev | 0.281 | 0.096 |
```
Code: _coming soon_
## BibTeX entry and citation info
```bibtex
@article{kurtic2022optimal,
title={The Optimal BERT Surgeon: Scalable and Accurate Second-Order Pruning for Large Language Models},
author={Kurtic, Eldar and Campos, Daniel and Nguyen, Tuan and Frantar, Elias and Kurtz, Mark and Fineran, Benjamin and Goin, Michael and Alistarh, Dan},
journal={arXiv preprint arXiv:2203.07259},
year={2022}
}
``` |
neuralmagic/oBERT-12-upstream-pruned-unstructured-90-finetuned-qqp | fbb0202da0e4e1013b53f5240b5aa5e9c91e1741 | 2022-06-20T11:36:50.000Z | [
"pytorch",
"en",
"dataset:qqp",
"arxiv:2203.07259",
"bert",
"oBERT",
"sparsity",
"pruning",
"compression"
] | null | false | neuralmagic | null | neuralmagic/oBERT-12-upstream-pruned-unstructured-90-finetuned-qqp | 0 | null | null | 37,693 | ---
tags:
- bert
- oBERT
- sparsity
- pruning
- compression
language: en
datasets: qqp
---
# oBERT-12-upstream-pruned-unstructured-90-finetuned-qqp
This model is obtained with [The Optimal BERT Surgeon: Scalable and Accurate Second-Order Pruning for Large Language Models](https://arxiv.org/abs/2203.07259).
It corresponds to the model presented in the `Table 2 - oBERT - QQP 90%`.
```
Pruning method: oBERT upstream unstructured + sparse-transfer to downstream
Paper: https://arxiv.org/abs/2203.07259
Dataset: QQP
Sparsity: 90%
Number of layers: 12
```
The dev-set performance reported in the paper is averaged over three seeds, and we release the best model (marked with `(*)`):
```
| oBERT 90% | acc | F1 |
| ------------ | ----- | ----- |
| seed=42 (*)| 90.93 | 87.77 |
| seed=3407 | 90.70 | 87.49 |
| seed=54321 | 90.86 | 87.68 |
| ------------ | ----- | ----- |
| mean | 90.83 | 87.65 |
| stdev | 0.117 | 0.143 |
```
Code: _coming soon_
## BibTeX entry and citation info
```bibtex
@article{kurtic2022optimal,
title={The Optimal BERT Surgeon: Scalable and Accurate Second-Order Pruning for Large Language Models},
author={Kurtic, Eldar and Campos, Daniel and Nguyen, Tuan and Frantar, Elias and Kurtz, Mark and Fineran, Benjamin and Goin, Michael and Alistarh, Dan},
journal={arXiv preprint arXiv:2203.07259},
year={2022}
}
``` |
neuralmagic/oBERT-12-downstream-dense-squadv1 | 5466d30fe1d851554150afad56361fea2aaec9b8 | 2022-06-20T11:36:49.000Z | [
"pytorch",
"en",
"dataset:squad",
"arxiv:2203.07259",
"bert",
"oBERT",
"sparsity",
"pruning",
"compression"
] | null | false | neuralmagic | null | neuralmagic/oBERT-12-downstream-dense-squadv1 | 0 | null | null | 37,694 | ---
tags:
- bert
- oBERT
- sparsity
- pruning
- compression
language: en
datasets: squad
---
# oBERT-12-downstream-dense-squadv1
This model is obtained with [The Optimal BERT Surgeon: Scalable and Accurate Second-Order Pruning for Large Language Models](https://arxiv.org/abs/2203.07259).
It corresponds to the model presented in the `Table 3 - 12 Layers - 0% Sparsity`, and it represents an upper bound for performance of the corresponding pruned models:
- 80% unstructured: `neuralmagic/oBERT-12-downstream-pruned-unstructured-80-squadv1`
- 80% block-4: `neuralmagic/oBERT-12-downstream-pruned-block4-80-squadv1`
- 90% unstructured: `neuralmagic/oBERT-12-downstream-pruned-unstructured-90-squadv1`
- 90% block-4: `neuralmagic/oBERT-12-downstream-pruned-block4-90-squadv1`
SQuADv1 dev-set:
```
EM = 82.71
F1 = 89.48
```
## BibTeX entry and citation info
```bibtex
@article{kurtic2022optimal,
title={The Optimal BERT Surgeon: Scalable and Accurate Second-Order Pruning for Large Language Models},
author={Kurtic, Eldar and Campos, Daniel and Nguyen, Tuan and Frantar, Elias and Kurtz, Mark and Fineran, Benjamin and Goin, Michael and Alistarh, Dan},
journal={arXiv preprint arXiv:2203.07259},
year={2022}
}
``` |
neuralmagic/oBERT-12-downstream-pruned-block4-80-squadv1 | df9814e85c540b684ae352a4288cf99086dbb98e | 2022-06-20T11:36:49.000Z | [
"pytorch",
"en",
"dataset:squad",
"arxiv:2203.07259",
"bert",
"oBERT",
"sparsity",
"pruning",
"compression"
] | null | false | neuralmagic | null | neuralmagic/oBERT-12-downstream-pruned-block4-80-squadv1 | 0 | null | null | 37,695 | ---
tags:
- bert
- oBERT
- sparsity
- pruning
- compression
language: en
datasets: squad
---
# oBERT-12-downstream-pruned-block4-80-squadv1
This model is obtained with [The Optimal BERT Surgeon: Scalable and Accurate Second-Order Pruning for Large Language Models](https://arxiv.org/abs/2203.07259).
It corresponds to the model presented in the `Table 3 - 12 Layers - Sparsity 80% - 4-block`.
```
Pruning method: oBERT downstream block-4
Paper: https://arxiv.org/abs/2203.07259
Dataset: SQuADv1
Sparsity: 80%
Number of layers: 12
```
The dev-set performance of this model:
```
EM = 81.45
F1 = 88.57
```
Code: _coming soon_
## BibTeX entry and citation info
```bibtex
@article{kurtic2022optimal,
title={The Optimal BERT Surgeon: Scalable and Accurate Second-Order Pruning for Large Language Models},
author={Kurtic, Eldar and Campos, Daniel and Nguyen, Tuan and Frantar, Elias and Kurtz, Mark and Fineran, Benjamin and Goin, Michael and Alistarh, Dan},
journal={arXiv preprint arXiv:2203.07259},
year={2022}
}
``` |
vai6hav/wav2vec2-large-xls-r-300m-hindi-colab | 0b63ae132d54af2d17ff3a516014acfb2f724c6a | 2022-05-25T15:01:42.000Z | [
"pytorch",
"tensorboard",
"wav2vec2",
"automatic-speech-recognition",
"dataset:common_voice",
"transformers",
"generated_from_trainer",
"license:apache-2.0",
"model-index"
] | automatic-speech-recognition | false | vai6hav | null | vai6hav/wav2vec2-large-xls-r-300m-hindi-colab | 0 | null | transformers | 37,696 | ---
license: apache-2.0
tags:
- generated_from_trainer
datasets:
- common_voice
model-index:
- name: wav2vec2-large-xls-r-300m-hindi-colab
results: []
---
<!-- This model card has been generated automatically according to the information the Trainer had access to. You
should probably proofread and complete it, then remove this comment. -->
# wav2vec2-large-xls-r-300m-hindi-colab
This model is a fine-tuned version of [facebook/wav2vec2-xls-r-300m](https://huggingface.co/facebook/wav2vec2-xls-r-300m) on the common_voice dataset.
## Model description
More information needed
## Intended uses & limitations
More information needed
## Training and evaluation data
More information needed
## Training procedure
### Training hyperparameters
The following hyperparameters were used during training:
- learning_rate: 0.0003
- train_batch_size: 16
- eval_batch_size: 8
- seed: 42
- gradient_accumulation_steps: 2
- total_train_batch_size: 32
- optimizer: Adam with betas=(0.9,0.999) and epsilon=1e-08
- lr_scheduler_type: linear
- lr_scheduler_warmup_steps: 500
- num_epochs: 30
- mixed_precision_training: Native AMP
### Training results
### Framework versions
- Transformers 4.11.3
- Pytorch 1.10.0+cu113
- Datasets 1.18.3
- Tokenizers 0.10.3
|
neuralmagic/oBERT-12-downstream-pruned-block4-90-squadv1 | c85ba0e67a6a32e7875966b7740533682a6f8c68 | 2022-06-20T11:36:49.000Z | [
"pytorch",
"en",
"dataset:squad",
"arxiv:2203.07259",
"bert",
"oBERT",
"sparsity",
"pruning",
"compression"
] | null | false | neuralmagic | null | neuralmagic/oBERT-12-downstream-pruned-block4-90-squadv1 | 0 | null | null | 37,697 | ---
tags:
- bert
- oBERT
- sparsity
- pruning
- compression
language: en
datasets: squad
---
# oBERT-12-downstream-pruned-block4-90-squadv1
This model is obtained with [The Optimal BERT Surgeon: Scalable and Accurate Second-Order Pruning for Large Language Models](https://arxiv.org/abs/2203.07259).
It corresponds to the model presented in the `Table 3 - 12 Layers - Sparsity 90% - 4-block`.
```
Pruning method: oBERT downstream block-4
Paper: https://arxiv.org/abs/2203.07259
Dataset: SQuADv1
Sparsity: 90%
Number of layers: 12
```
The dev-set performance of this model:
```
EM = 80.14
F1 = 87.57
```
Code: _coming soon_
## BibTeX entry and citation info
```bibtex
@article{kurtic2022optimal,
title={The Optimal BERT Surgeon: Scalable and Accurate Second-Order Pruning for Large Language Models},
author={Kurtic, Eldar and Campos, Daniel and Nguyen, Tuan and Frantar, Elias and Kurtz, Mark and Fineran, Benjamin and Goin, Michael and Alistarh, Dan},
journal={arXiv preprint arXiv:2203.07259},
year={2022}
}
``` |
neuralmagic/oBERT-6-downstream-pruned-unstructured-80-squadv1 | af40ce51efec32a63a3a4b8b22d2a5769d11cd35 | 2022-06-20T11:36:52.000Z | [
"pytorch",
"en",
"dataset:squad",
"arxiv:2203.07259",
"bert",
"oBERT",
"sparsity",
"pruning",
"compression"
] | null | false | neuralmagic | null | neuralmagic/oBERT-6-downstream-pruned-unstructured-80-squadv1 | 0 | null | null | 37,698 | ---
tags:
- bert
- oBERT
- sparsity
- pruning
- compression
language: en
datasets: squad
---
# oBERT-6-downstream-pruned-unstructured-80-squadv1
This model is obtained with [The Optimal BERT Surgeon: Scalable and Accurate Second-Order Pruning for Large Language Models](https://arxiv.org/abs/2203.07259).
It corresponds to the model presented in the `Table 3 - 6 Layers - Sparsity 80% - unstructured`.
```
Pruning method: oBERT downstream unstructured
Paper: https://arxiv.org/abs/2203.07259
Dataset: SQuADv1
Sparsity: 80%
Number of layers: 6
```
The dev-set performance of this model:
```
EM = 81.15
F1 = 88.20
```
Code: _coming soon_
## BibTeX entry and citation info
```bibtex
@article{kurtic2022optimal,
title={The Optimal BERT Surgeon: Scalable and Accurate Second-Order Pruning for Large Language Models},
author={Kurtic, Eldar and Campos, Daniel and Nguyen, Tuan and Frantar, Elias and Kurtz, Mark and Fineran, Benjamin and Goin, Michael and Alistarh, Dan},
journal={arXiv preprint arXiv:2203.07259},
year={2022}
}
``` |
neuralmagic/oBERT-6-downstream-pruned-unstructured-90-squadv1 | 6c736d82d3a35ef34f050e155959b6e8ca9ec4b4 | 2022-06-20T11:36:52.000Z | [
"pytorch",
"en",
"dataset:squad",
"arxiv:2203.07259",
"bert",
"oBERT",
"sparsity",
"pruning",
"compression"
] | null | false | neuralmagic | null | neuralmagic/oBERT-6-downstream-pruned-unstructured-90-squadv1 | 0 | null | null | 37,699 | ---
tags:
- bert
- oBERT
- sparsity
- pruning
- compression
language: en
datasets: squad
---
# oBERT-6-downstream-pruned-unstructured-90-squadv1
This model is obtained with [The Optimal BERT Surgeon: Scalable and Accurate Second-Order Pruning for Large Language Models](https://arxiv.org/abs/2203.07259).
It corresponds to the model presented in the `Table 3 - 6 Layers - Sparsity 90% - unstructured`.
```
Pruning method: oBERT downstream unstructured
Paper: https://arxiv.org/abs/2203.07259
Dataset: SQuADv1
Sparsity: 90%
Number of layers: 6
```
The dev-set performance of this model:
```
EM = 79.16
F1 = 86.78
```
Code: _coming soon_
## BibTeX entry and citation info
```bibtex
@article{kurtic2022optimal,
title={The Optimal BERT Surgeon: Scalable and Accurate Second-Order Pruning for Large Language Models},
author={Kurtic, Eldar and Campos, Daniel and Nguyen, Tuan and Frantar, Elias and Kurtz, Mark and Fineran, Benjamin and Goin, Michael and Alistarh, Dan},
journal={arXiv preprint arXiv:2203.07259},
year={2022}
}
``` |
Subsets and Splits
No community queries yet
The top public SQL queries from the community will appear here once available.