modelId
stringlengths 4
112
| sha
stringlengths 40
40
| lastModified
stringlengths 24
24
| tags
sequence | pipeline_tag
stringclasses 29
values | private
bool 1
class | author
stringlengths 2
38
β | config
null | id
stringlengths 4
112
| downloads
float64 0
36.8M
β | likes
float64 0
712
β | library_name
stringclasses 17
values | __index_level_0__
int64 0
38.5k
| readme
stringlengths 0
186k
|
---|---|---|---|---|---|---|---|---|---|---|---|---|---|
neuralmagic/oBERT-6-downstream-pruned-block4-80-squadv1 | dfdb9017e17ca2025f9814ad01170e4eb854fec2 | 2022-06-20T11:36:52.000Z | [
"pytorch",
"en",
"dataset:squad",
"arxiv:2203.07259",
"bert",
"oBERT",
"sparsity",
"pruning",
"compression"
] | null | false | neuralmagic | null | neuralmagic/oBERT-6-downstream-pruned-block4-80-squadv1 | 0 | null | null | 37,700 | ---
tags:
- bert
- oBERT
- sparsity
- pruning
- compression
language: en
datasets: squad
---
# oBERT-6-downstream-pruned-block4-80-squadv1
This model is obtained with [The Optimal BERT Surgeon: Scalable and Accurate Second-Order Pruning for Large Language Models](https://arxiv.org/abs/2203.07259).
It corresponds to the model presented in the `Table 3 - 6 Layers - Sparsity 80% - 4-block`.
```
Pruning method: oBERT downstream block-4
Paper: https://arxiv.org/abs/2203.07259
Dataset: SQuADv1
Sparsity: 80%
Number of layers: 6
```
The dev-set performance of this model:
```
EM = 79.55
F1 = 87.00
```
Code: _coming soon_
## BibTeX entry and citation info
```bibtex
@article{kurtic2022optimal,
title={The Optimal BERT Surgeon: Scalable and Accurate Second-Order Pruning for Large Language Models},
author={Kurtic, Eldar and Campos, Daniel and Nguyen, Tuan and Frantar, Elias and Kurtz, Mark and Fineran, Benjamin and Goin, Michael and Alistarh, Dan},
journal={arXiv preprint arXiv:2203.07259},
year={2022}
}
``` |
neuralmagic/oBERT-6-downstream-pruned-block4-90-squadv1 | 246146812f81943ea0f740481620da520453c814 | 2022-06-20T11:36:52.000Z | [
"pytorch",
"en",
"dataset:squad",
"arxiv:2203.07259",
"bert",
"oBERT",
"sparsity",
"pruning",
"compression"
] | null | false | neuralmagic | null | neuralmagic/oBERT-6-downstream-pruned-block4-90-squadv1 | 0 | null | null | 37,701 | ---
tags:
- bert
- oBERT
- sparsity
- pruning
- compression
language: en
datasets: squad
---
# oBERT-6-downstream-pruned-block4-90-squadv1
This model is obtained with [The Optimal BERT Surgeon: Scalable and Accurate Second-Order Pruning for Large Language Models](https://arxiv.org/abs/2203.07259).
It corresponds to the model presented in the `Table 3 - 6 Layers - Sparsity 90% - 4-block`.
```
Pruning method: oBERT downstream block-4
Paper: https://arxiv.org/abs/2203.07259
Dataset: SQuADv1
Sparsity: 90%
Number of layers: 6
```
The dev-set performance of this model:
```
EM = 77.65
F1 = 85.34
```
Code: _coming soon_
## BibTeX entry and citation info
```bibtex
@article{kurtic2022optimal,
title={The Optimal BERT Surgeon: Scalable and Accurate Second-Order Pruning for Large Language Models},
author={Kurtic, Eldar and Campos, Daniel and Nguyen, Tuan and Frantar, Elias and Kurtz, Mark and Fineran, Benjamin and Goin, Michael and Alistarh, Dan},
journal={arXiv preprint arXiv:2203.07259},
year={2022}
}
``` |
neuralmagic/oBERT-3-downstream-pruned-unstructured-80-squadv1 | b7f58099aa37f777c058816432e7ecd8c658472c | 2022-06-20T11:36:52.000Z | [
"pytorch",
"en",
"dataset:squad",
"arxiv:2203.07259",
"bert",
"oBERT",
"sparsity",
"pruning",
"compression"
] | null | false | neuralmagic | null | neuralmagic/oBERT-3-downstream-pruned-unstructured-80-squadv1 | 0 | null | null | 37,702 | ---
tags:
- bert
- oBERT
- sparsity
- pruning
- compression
language: en
datasets: squad
---
# oBERT-3-downstream-pruned-unstructured-80-squadv1
This model is obtained with [The Optimal BERT Surgeon: Scalable and Accurate Second-Order Pruning for Large Language Models](https://arxiv.org/abs/2203.07259).
It corresponds to the model presented in the `Table 3 - 3 Layers - Sparsity 80% - unstructured`.
```
Pruning method: oBERT downstream unstructured
Paper: https://arxiv.org/abs/2203.07259
Dataset: SQuADv1
Sparsity: 80%
Number of layers: 3
```
The dev-set performance of this model:
```
EM = 75.62
F1 = 84.08
```
Code: _coming soon_
## BibTeX entry and citation info
```bibtex
@article{kurtic2022optimal,
title={The Optimal BERT Surgeon: Scalable and Accurate Second-Order Pruning for Large Language Models},
author={Kurtic, Eldar and Campos, Daniel and Nguyen, Tuan and Frantar, Elias and Kurtz, Mark and Fineran, Benjamin and Goin, Michael and Alistarh, Dan},
journal={arXiv preprint arXiv:2203.07259},
year={2022}
}
``` |
neuralmagic/oBERT-3-downstream-pruned-unstructured-90-squadv1 | 24cf2937208d5e0c901bd688d61c606fa31f91d7 | 2022-06-20T11:36:52.000Z | [
"pytorch",
"en",
"dataset:squad",
"arxiv:2203.07259",
"bert",
"oBERT",
"sparsity",
"pruning",
"compression"
] | null | false | neuralmagic | null | neuralmagic/oBERT-3-downstream-pruned-unstructured-90-squadv1 | 0 | null | null | 37,703 | ---
tags:
- bert
- oBERT
- sparsity
- pruning
- compression
language: en
datasets: squad
---
# oBERT-3-downstream-pruned-unstructured-90-squadv1
This model is obtained with [The Optimal BERT Surgeon: Scalable and Accurate Second-Order Pruning for Large Language Models](https://arxiv.org/abs/2203.07259).
It corresponds to the model presented in the `Table 3 - 3 Layers - Sparsity 90% - unstructured`.
```
Pruning method: oBERT downstream unstructured
Paper: https://arxiv.org/abs/2203.07259
Dataset: SQuADv1
Sparsity: 90%
Number of layers: 3
```
The dev-set performance of this model:
```
EM = 73.61
F1 = 82.50
```
Code: _coming soon_
## BibTeX entry and citation info
```bibtex
@article{kurtic2022optimal,
title={The Optimal BERT Surgeon: Scalable and Accurate Second-Order Pruning for Large Language Models},
author={Kurtic, Eldar and Campos, Daniel and Nguyen, Tuan and Frantar, Elias and Kurtz, Mark and Fineran, Benjamin and Goin, Michael and Alistarh, Dan},
journal={arXiv preprint arXiv:2203.07259},
year={2022}
}
``` |
neuralmagic/oBERT-3-downstream-pruned-block4-80-squadv1 | 95f9815e4865c43d51bf95432f633bfaab4f44d2 | 2022-06-20T11:36:51.000Z | [
"pytorch",
"en",
"dataset:squad",
"arxiv:2203.07259",
"bert",
"oBERT",
"sparsity",
"pruning",
"compression"
] | null | false | neuralmagic | null | neuralmagic/oBERT-3-downstream-pruned-block4-80-squadv1 | 0 | null | null | 37,704 | ---
tags:
- bert
- oBERT
- sparsity
- pruning
- compression
language: en
datasets: squad
---
# oBERT-3-downstream-pruned-block4-80-squadv1
This model is obtained with [The Optimal BERT Surgeon: Scalable and Accurate Second-Order Pruning for Large Language Models](https://arxiv.org/abs/2203.07259).
It corresponds to the model presented in the `Table 3 - 3 Layers - Sparsity 80% - 4-block`.
```
Pruning method: oBERT downstream block-4
Paper: https://arxiv.org/abs/2203.07259
Dataset: SQuADv1
Sparsity: 80%
Number of layers: 3
```
The dev-set performance of this model:
```
EM = 74.07
F1 = 82.79
```
Code: _coming soon_
## BibTeX entry and citation info
```bibtex
@article{kurtic2022optimal,
title={The Optimal BERT Surgeon: Scalable and Accurate Second-Order Pruning for Large Language Models},
author={Kurtic, Eldar and Campos, Daniel and Nguyen, Tuan and Frantar, Elias and Kurtz, Mark and Fineran, Benjamin and Goin, Michael and Alistarh, Dan},
journal={arXiv preprint arXiv:2203.07259},
year={2022}
}
``` |
neuralmagic/oBERT-3-downstream-pruned-block4-90-squadv1 | 3bc01a4cd481fb99ff709d3eaf3b7c75615c570c | 2022-06-20T11:36:52.000Z | [
"pytorch",
"en",
"dataset:squad",
"arxiv:2203.07259",
"bert",
"oBERT",
"sparsity",
"pruning",
"compression"
] | null | false | neuralmagic | null | neuralmagic/oBERT-3-downstream-pruned-block4-90-squadv1 | 0 | null | null | 37,705 | ---
tags:
- bert
- oBERT
- sparsity
- pruning
- compression
language: en
datasets: squad
---
# oBERT-3-downstream-pruned-block4-90-squadv1
This model is obtained with [The Optimal BERT Surgeon: Scalable and Accurate Second-Order Pruning for Large Language Models](https://arxiv.org/abs/2203.07259).
It corresponds to the model presented in the `Table 3 - 3 Layers - Sparsity 90% - 4-block`.
```
Pruning method: oBERT downstream block-4
Paper: https://arxiv.org/abs/2203.07259
Dataset: SQuADv1
Sparsity: 90%
Number of layers: 3
```
The dev-set performance of this model:
```
EM = 71.36
F1 = 80.69
```
Code: _coming soon_
## BibTeX entry and citation info
```bibtex
@article{kurtic2022optimal,
title={The Optimal BERT Surgeon: Scalable and Accurate Second-Order Pruning for Large Language Models},
author={Kurtic, Eldar and Campos, Daniel and Nguyen, Tuan and Frantar, Elias and Kurtz, Mark and Fineran, Benjamin and Goin, Michael and Alistarh, Dan},
journal={arXiv preprint arXiv:2203.07259},
year={2022}
}
``` |
huggingtweets/sickziii | b7e4ff5bd09f12e5045d3ef5059c750319b4732f | 2022-05-25T16:18:04.000Z | [
"pytorch",
"gpt2",
"text-generation",
"en",
"transformers",
"huggingtweets"
] | text-generation | false | huggingtweets | null | huggingtweets/sickziii | 0 | null | transformers | 37,706 | ---
language: en
thumbnail: https://github.com/borisdayma/huggingtweets/blob/master/img/logo.png?raw=true
tags:
- huggingtweets
widget:
- text: "My dream is"
---
<div class="inline-flex flex-col" style="line-height: 1.5;">
<div class="flex">
<div
style="display:inherit; margin-left: 4px; margin-right: 4px; width: 92px; height:92px; border-radius: 50%; background-size: cover; background-image: url('https://pbs.twimg.com/profile_images/701052820754190336/OwxAZ9ES_400x400.jpg')">
</div>
<div
style="display:none; margin-left: 4px; margin-right: 4px; width: 92px; height:92px; border-radius: 50%; background-size: cover; background-image: url('')">
</div>
<div
style="display:none; margin-left: 4px; margin-right: 4px; width: 92px; height:92px; border-radius: 50%; background-size: cover; background-image: url('')">
</div>
</div>
<div style="text-align: center; margin-top: 3px; font-size: 16px; font-weight: 800">π€ AI BOT π€</div>
<div style="text-align: center; font-size: 16px; font-weight: 800">sickzee</div>
<div style="text-align: center; font-size: 14px;">@sickziii</div>
</div>
I was made with [huggingtweets](https://github.com/borisdayma/huggingtweets).
Create your own bot based on your favorite user with [the demo](https://colab.research.google.com/github/borisdayma/huggingtweets/blob/master/huggingtweets-demo.ipynb)!
## How does it work?
The model uses the following pipeline.
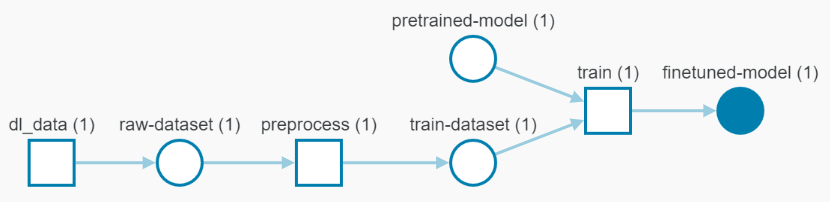
To understand how the model was developed, check the [W&B report](https://wandb.ai/wandb/huggingtweets/reports/HuggingTweets-Train-a-Model-to-Generate-Tweets--VmlldzoxMTY5MjI).
## Training data
The model was trained on tweets from sickzee.
| Data | sickzee |
| --- | --- |
| Tweets downloaded | 3214 |
| Retweets | 2499 |
| Short tweets | 224 |
| Tweets kept | 491 |
[Explore the data](https://wandb.ai/wandb/huggingtweets/runs/2hmehe5f/artifacts), which is tracked with [W&B artifacts](https://docs.wandb.com/artifacts) at every step of the pipeline.
## Training procedure
The model is based on a pre-trained [GPT-2](https://huggingface.co/gpt2) which is fine-tuned on @sickziii's tweets.
Hyperparameters and metrics are recorded in the [W&B training run](https://wandb.ai/wandb/huggingtweets/runs/drajr5oy) for full transparency and reproducibility.
At the end of training, [the final model](https://wandb.ai/wandb/huggingtweets/runs/drajr5oy/artifacts) is logged and versioned.
## How to use
You can use this model directly with a pipeline for text generation:
```python
from transformers import pipeline
generator = pipeline('text-generation',
model='huggingtweets/sickziii')
generator("My dream is", num_return_sequences=5)
```
## Limitations and bias
The model suffers from [the same limitations and bias as GPT-2](https://huggingface.co/gpt2#limitations-and-bias).
In addition, the data present in the user's tweets further affects the text generated by the model.
## About
*Built by Boris Dayma*
[](https://twitter.com/intent/follow?screen_name=borisdayma)
For more details, visit the project repository.
[](https://github.com/borisdayma/huggingtweets)
|
meetyildiz/TurQA-bert-base-turkish-cased-finetuned-toqad | a2fca4213ac8f0b0b4e9a5df236a3273c9e51e8f | 2022-06-02T22:41:29.000Z | [
"pytorch",
"tensorboard",
"bert",
"feature-extraction",
"dataset:squad",
"transformers",
"generated_from_trainer",
"license:mit",
"model-index"
] | feature-extraction | false | meetyildiz | null | meetyildiz/TurQA-bert-base-turkish-cased-finetuned-toqad | 0 | null | transformers | 37,707 | ---
license: mit
tags:
- generated_from_trainer
datasets:
- squad
model-index:
- name: TurQA-bert-base-turkish-cased-finetuned-toqad
results: []
---
<!-- This model card has been generated automatically according to the information the Trainer had access to. You
should probably proofread and complete it, then remove this comment. -->
# TurQA-bert-base-turkish-cased-finetuned-toqad
This model is a fine-tuned version of [dbmdz/bert-base-turkish-cased](https://huggingface.co/dbmdz/bert-base-turkish-cased) on the squad dataset.
It achieves the following results on the evaluation set:
- Loss: 2.9711
## Model description
More information needed
## Intended uses & limitations
More information needed
## Training and evaluation data
More information needed
## Training procedure
### Training hyperparameters
The following hyperparameters were used during training:
- learning_rate: 2e-05
- train_batch_size: 16
- eval_batch_size: 16
- seed: 42
- optimizer: Adam with betas=(0.9,0.999) and epsilon=1e-08
- lr_scheduler_type: linear
- num_epochs: 3
### Training results
| Training Loss | Epoch | Step | Validation Loss |
|:-------------:|:-----:|:----:|:---------------:|
| 2.7191 | 1.0 | 800 | 3.0920 |
| 1.6875 | 2.0 | 1600 | 2.9778 |
| 1.4582 | 3.0 | 2400 | 2.9711 |
### Framework versions
- Transformers 4.19.2
- Pytorch 1.11.0+cu113
- Datasets 2.2.2
- Tokenizers 0.12.1
|
theojolliffe/bart-cnn-pubmed-arxiv-pubmed-arxiv-arxiv-v3-e2 | 152c2f41dc5a640cf371770d9330b9d8a1dfe445 | 2022-05-25T18:51:56.000Z | [
"pytorch",
"tensorboard",
"bart",
"text2text-generation",
"transformers",
"generated_from_trainer",
"license:mit",
"model-index",
"autotrain_compatible"
] | text2text-generation | false | theojolliffe | null | theojolliffe/bart-cnn-pubmed-arxiv-pubmed-arxiv-arxiv-v3-e2 | 0 | null | transformers | 37,708 | ---
license: mit
tags:
- generated_from_trainer
metrics:
- rouge
model-index:
- name: bart-cnn-pubmed-arxiv-pubmed-arxiv-arxiv-v3-e2
results: []
---
<!-- This model card has been generated automatically according to the information the Trainer had access to. You
should probably proofread and complete it, then remove this comment. -->
# bart-cnn-pubmed-arxiv-pubmed-arxiv-arxiv-v3-e2
This model is a fine-tuned version of [theojolliffe/bart-cnn-pubmed-arxiv-pubmed-arxiv-arxiv](https://huggingface.co/theojolliffe/bart-cnn-pubmed-arxiv-pubmed-arxiv-arxiv) on an unknown dataset.
It achieves the following results on the evaluation set:
- Loss: 0.8604
- Rouge1: 53.7901
- Rouge2: 34.5052
- Rougel: 36.6399
- Rougelsum: 51.2331
- Gen Len: 141.7593
## Model description
More information needed
## Intended uses & limitations
More information needed
## Training and evaluation data
More information needed
## Training procedure
### Training hyperparameters
The following hyperparameters were used during training:
- learning_rate: 2e-05
- train_batch_size: 2
- eval_batch_size: 2
- seed: 42
- optimizer: Adam with betas=(0.9,0.999) and epsilon=1e-08
- lr_scheduler_type: linear
- num_epochs: 2
- mixed_precision_training: Native AMP
### Training results
| Training Loss | Epoch | Step | Validation Loss | Rouge1 | Rouge2 | Rougel | Rougelsum | Gen Len |
|:-------------:|:-----:|:----:|:---------------:|:-------:|:-------:|:-------:|:---------:|:--------:|
| No log | 1.0 | 398 | 0.8776 | 53.3731 | 34.1946 | 36.4438 | 50.7369 | 142.0 |
| 0.8266 | 2.0 | 796 | 0.8604 | 53.7901 | 34.5052 | 36.6399 | 51.2331 | 141.7593 |
### Framework versions
- Transformers 4.19.2
- Pytorch 1.11.0+cu113
- Datasets 2.2.2
- Tokenizers 0.12.1
|
theojolliffe/bart-cnn-pubmed-arxiv-pubmed-arxiv-arxiv-v3-e1 | 8f5dc6c3cd4fd0e86450e72bce47207d131b337e | 2022-05-25T18:43:19.000Z | [
"pytorch",
"tensorboard",
"bart",
"text2text-generation",
"transformers",
"generated_from_trainer",
"license:mit",
"model-index",
"autotrain_compatible"
] | text2text-generation | false | theojolliffe | null | theojolliffe/bart-cnn-pubmed-arxiv-pubmed-arxiv-arxiv-v3-e1 | 0 | null | transformers | 37,709 | ---
license: mit
tags:
- generated_from_trainer
metrics:
- rouge
model-index:
- name: bart-cnn-pubmed-arxiv-pubmed-arxiv-arxiv-v3-e1
results: []
---
<!-- This model card has been generated automatically according to the information the Trainer had access to. You
should probably proofread and complete it, then remove this comment. -->
# bart-cnn-pubmed-arxiv-pubmed-arxiv-arxiv-v3-e1
This model is a fine-tuned version of [theojolliffe/bart-cnn-pubmed-arxiv-pubmed-arxiv-arxiv](https://huggingface.co/theojolliffe/bart-cnn-pubmed-arxiv-pubmed-arxiv-arxiv) on an unknown dataset.
It achieves the following results on the evaluation set:
- Loss: 0.8952
- Rouge1: 53.0722
- Rouge2: 32.4229
- Rougel: 34.8749
- Rougelsum: 50.1772
- Gen Len: 142.0
## Model description
More information needed
## Intended uses & limitations
More information needed
## Training and evaluation data
More information needed
## Training procedure
### Training hyperparameters
The following hyperparameters were used during training:
- learning_rate: 2e-05
- train_batch_size: 1
- eval_batch_size: 1
- seed: 42
- optimizer: Adam with betas=(0.9,0.999) and epsilon=1e-08
- lr_scheduler_type: linear
- num_epochs: 1
- mixed_precision_training: Native AMP
### Training results
| Training Loss | Epoch | Step | Validation Loss | Rouge1 | Rouge2 | Rougel | Rougelsum | Gen Len |
|:-------------:|:-----:|:----:|:---------------:|:-------:|:-------:|:-------:|:---------:|:-------:|
| 0.686 | 1.0 | 795 | 0.8952 | 53.0722 | 32.4229 | 34.8749 | 50.1772 | 142.0 |
### Framework versions
- Transformers 4.19.2
- Pytorch 1.11.0+cu113
- Datasets 2.2.2
- Tokenizers 0.12.1
|
theojolliffe/bart-cnn-pubmed-arxiv-pubmed-arxiv-arxiv-v3-e4 | 88ff4eb4529f2f3c63c4975ddc0b0e0c73f81e31 | 2022-05-25T20:20:53.000Z | [
"pytorch",
"tensorboard",
"bart",
"text2text-generation",
"transformers",
"generated_from_trainer",
"license:mit",
"model-index",
"autotrain_compatible"
] | text2text-generation | false | theojolliffe | null | theojolliffe/bart-cnn-pubmed-arxiv-pubmed-arxiv-arxiv-v3-e4 | 0 | null | transformers | 37,710 | ---
license: mit
tags:
- generated_from_trainer
metrics:
- rouge
model-index:
- name: bart-cnn-pubmed-arxiv-pubmed-arxiv-arxiv-v3-e4
results: []
---
<!-- This model card has been generated automatically according to the information the Trainer had access to. You
should probably proofread and complete it, then remove this comment. -->
# bart-cnn-pubmed-arxiv-pubmed-arxiv-arxiv-v3-e4
This model is a fine-tuned version of [theojolliffe/bart-cnn-pubmed-arxiv-pubmed-arxiv-arxiv](https://huggingface.co/theojolliffe/bart-cnn-pubmed-arxiv-pubmed-arxiv-arxiv) on an unknown dataset.
It achieves the following results on the evaluation set:
- Loss: 0.8121
- Rouge1: 53.9237
- Rouge2: 34.5683
- Rougel: 36.5547
- Rougelsum: 51.0273
- Gen Len: 142.0
## Model description
More information needed
## Intended uses & limitations
More information needed
## Training and evaluation data
More information needed
## Training procedure
### Training hyperparameters
The following hyperparameters were used during training:
- learning_rate: 2e-05
- train_batch_size: 2
- eval_batch_size: 2
- seed: 42
- optimizer: Adam with betas=(0.9,0.999) and epsilon=1e-08
- lr_scheduler_type: linear
- num_epochs: 4
- mixed_precision_training: Native AMP
### Training results
| Training Loss | Epoch | Step | Validation Loss | Rouge1 | Rouge2 | Rougel | Rougelsum | Gen Len |
|:-------------:|:-----:|:----:|:---------------:|:-------:|:-------:|:-------:|:---------:|:-------:|
| No log | 1.0 | 398 | 0.8673 | 53.562 | 34.4013 | 36.5393 | 50.7868 | 142.0 |
| 0.826 | 2.0 | 796 | 0.8119 | 55.0909 | 36.5216 | 38.6034 | 52.718 | 142.0 |
| 0.5377 | 3.0 | 1194 | 0.8268 | 54.0198 | 35.9154 | 38.1218 | 51.2782 | 142.0 |
| 0.3817 | 4.0 | 1592 | 0.8121 | 53.9237 | 34.5683 | 36.5547 | 51.0273 | 142.0 |
### Framework versions
- Transformers 4.19.2
- Pytorch 1.11.0+cu113
- Datasets 2.2.2
- Tokenizers 0.12.1
|
theojolliffe/bart-cnn-pubmed-arxiv-pubmed-arxiv-arxiv-v3-e8 | ff8de15b3925d9ff2ff9d8aebe6ab72d3f1d3f1e | 2022-05-25T20:10:05.000Z | [
"pytorch",
"tensorboard",
"bart",
"text2text-generation",
"transformers",
"generated_from_trainer",
"license:mit",
"model-index",
"autotrain_compatible"
] | text2text-generation | false | theojolliffe | null | theojolliffe/bart-cnn-pubmed-arxiv-pubmed-arxiv-arxiv-v3-e8 | 0 | null | transformers | 37,711 | ---
license: mit
tags:
- generated_from_trainer
metrics:
- rouge
model-index:
- name: bart-cnn-pubmed-arxiv-pubmed-arxiv-arxiv-v3-e8
results: []
---
<!-- This model card has been generated automatically according to the information the Trainer had access to. You
should probably proofread and complete it, then remove this comment. -->
# bart-cnn-pubmed-arxiv-pubmed-arxiv-arxiv-v3-e8
This model is a fine-tuned version of [theojolliffe/bart-cnn-pubmed-arxiv-pubmed-arxiv-arxiv](https://huggingface.co/theojolliffe/bart-cnn-pubmed-arxiv-pubmed-arxiv-arxiv) on an unknown dataset.
It achieves the following results on the evaluation set:
- Loss: 0.8063
- Rouge1: 54.9922
- Rouge2: 38.7265
- Rougel: 41.9288
- Rougelsum: 52.8766
- Gen Len: 142.0
## Model description
More information needed
## Intended uses & limitations
More information needed
## Training and evaluation data
More information needed
## Training procedure
### Training hyperparameters
The following hyperparameters were used during training:
- learning_rate: 2e-05
- train_batch_size: 2
- eval_batch_size: 2
- seed: 42
- optimizer: Adam with betas=(0.9,0.999) and epsilon=1e-08
- lr_scheduler_type: linear
- num_epochs: 8
- mixed_precision_training: Native AMP
### Training results
| Training Loss | Epoch | Step | Validation Loss | Rouge1 | Rouge2 | Rougel | Rougelsum | Gen Len |
|:-------------:|:-----:|:----:|:---------------:|:-------:|:-------:|:-------:|:---------:|:--------:|
| No log | 1.0 | 398 | 0.8651 | 53.3185 | 33.3722 | 35.8852 | 50.5929 | 142.0 |
| 0.8268 | 2.0 | 796 | 0.8063 | 53.5267 | 34.3205 | 36.9783 | 51.0289 | 142.0 |
| 0.5331 | 3.0 | 1194 | 0.8155 | 53.5409 | 34.9962 | 38.078 | 51.2038 | 142.0 |
| 0.3588 | 4.0 | 1592 | 0.7883 | 53.7055 | 35.0869 | 38.1521 | 51.3094 | 141.4815 |
| 0.3588 | 5.0 | 1990 | 0.7770 | 54.4542 | 37.5817 | 39.8734 | 52.1947 | 141.7778 |
| 0.2447 | 6.0 | 2388 | 0.7929 | 55.1571 | 38.8425 | 41.4301 | 53.3049 | 141.4444 |
| 0.1765 | 7.0 | 2786 | 0.7909 | 55.5838 | 38.6226 | 42.0453 | 53.543 | 142.0 |
| 0.13 | 8.0 | 3184 | 0.8063 | 54.9922 | 38.7265 | 41.9288 | 52.8766 | 142.0 |
### Framework versions
- Transformers 4.19.2
- Pytorch 1.11.0+cu113
- Datasets 2.2.2
- Tokenizers 0.12.1
|
neuralmagic/oBERT-12-downstream-dense-QAT-squadv1 | 9db9bbf23407a11dccb768eeb2d9ada52cb769d7 | 2022-06-20T11:36:48.000Z | [
"pytorch",
"en",
"dataset:squad",
"arxiv:2203.07259",
"bert",
"oBERT",
"sparsity",
"pruning",
"compression"
] | null | false | neuralmagic | null | neuralmagic/oBERT-12-downstream-dense-QAT-squadv1 | 0 | null | null | 37,712 | ---
tags:
- bert
- oBERT
- sparsity
- pruning
- compression
language: en
datasets: squad
---
# oBERT-12-downstream-dense-QAT-squadv1
This model is obtained with [The Optimal BERT Surgeon: Scalable and Accurate Second-Order Pruning for Large Language Models](https://arxiv.org/abs/2203.07259).
It corresponds to the model presented in the `Table 3 - 12 Layers - 0% Sparsity - QAT`, and it represents an upper bound for performance of the corresponding pruned and quantized models:
- 80% unstructured QAT: `neuralmagic/oBERT-12-downstream-pruned-unstructured-80-QAT-squadv1`
- 80% block-4 QAT: `neuralmagic/oBERT-12-downstream-pruned-block4-80-QAT-squadv1`
- 90% unstructured QAT: `neuralmagic/oBERT-12-downstream-pruned-unstructured-90-QAT-squadv1`
- 90% block-4 QAT: `neuralmagic/oBERT-12-downstream-pruned-block4-90-QAT-squadv1`
SQuADv1 dev-set:
```
EM = 81.99
F1 = 89.06
```
Code: _coming soon_
## BibTeX entry and citation info
```bibtex
@article{kurtic2022optimal,
title={The Optimal BERT Surgeon: Scalable and Accurate Second-Order Pruning for Large Language Models},
author={Kurtic, Eldar and Campos, Daniel and Nguyen, Tuan and Frantar, Elias and Kurtz, Mark and Fineran, Benjamin and Goin, Michael and Alistarh, Dan},
journal={arXiv preprint arXiv:2203.07259},
year={2022}
}
``` |
neuralmagic/oBERT-12-downstream-pruned-block4-80-QAT-squadv1 | d3dc8fef050627bdd2b0cb541277990206742d5b | 2022-06-20T11:36:49.000Z | [
"pytorch",
"en",
"dataset:squad",
"arxiv:2203.07259",
"bert",
"oBERT",
"sparsity",
"pruning",
"compression"
] | null | false | neuralmagic | null | neuralmagic/oBERT-12-downstream-pruned-block4-80-QAT-squadv1 | 0 | null | null | 37,713 | ---
tags:
- bert
- oBERT
- sparsity
- pruning
- compression
language: en
datasets: squad
---
# oBERT-12-downstream-pruned-block4-80-QAT-squadv1
This model is obtained with [The Optimal BERT Surgeon: Scalable and Accurate Second-Order Pruning for Large Language Models](https://arxiv.org/abs/2203.07259).
It corresponds to the model presented in the `Table 3 - 12 Layers - Sparsity 80% - 4-block + QAT`.
```
Pruning method: oBERT downstream block-4 + QAT
Paper: https://arxiv.org/abs/2203.07259
Dataset: SQuADv1
Sparsity: 80%
Number of layers: 12
```
The dev-set performance of this model:
```
EM = 80.58
F1 = 87.89
```
Code: _coming soon_
## BibTeX entry and citation info
```bibtex
@article{kurtic2022optimal,
title={The Optimal BERT Surgeon: Scalable and Accurate Second-Order Pruning for Large Language Models},
author={Kurtic, Eldar and Campos, Daniel and Nguyen, Tuan and Frantar, Elias and Kurtz, Mark and Fineran, Benjamin and Goin, Michael and Alistarh, Dan},
journal={arXiv preprint arXiv:2203.07259},
year={2022}
}
``` |
neuralmagic/oBERT-12-downstream-pruned-block4-90-QAT-squadv1 | 8c7fd8995e983bf9365a9a798caf2a28684b452b | 2022-06-20T11:36:49.000Z | [
"pytorch",
"en",
"dataset:squad",
"arxiv:2203.07259",
"bert",
"oBERT",
"sparsity",
"pruning",
"compression"
] | null | false | neuralmagic | null | neuralmagic/oBERT-12-downstream-pruned-block4-90-QAT-squadv1 | 0 | null | null | 37,714 | ---
tags:
- bert
- oBERT
- sparsity
- pruning
- compression
language: en
datasets: squad
---
# oBERT-12-downstream-pruned-block4-90-QAT-squadv1
This model is obtained with [The Optimal BERT Surgeon: Scalable and Accurate Second-Order Pruning for Large Language Models](https://arxiv.org/abs/2203.07259).
It corresponds to the model presented in the `Table 3 - 12 Layers - Sparsity 90% - 4-block + QAT`.
```
Pruning method: oBERT downstream block-4 + QAT
Paper: https://arxiv.org/abs/2203.07259
Dataset: SQuADv1
Sparsity: 90%
Number of layers: 12
```
The dev-set performance of this model:
```
EM = 78.84
F1 = 86.68
```
Code: _coming soon_
## BibTeX entry and citation info
```bibtex
@article{kurtic2022optimal,
title={The Optimal BERT Surgeon: Scalable and Accurate Second-Order Pruning for Large Language Models},
author={Kurtic, Eldar and Campos, Daniel and Nguyen, Tuan and Frantar, Elias and Kurtz, Mark and Fineran, Benjamin and Goin, Michael and Alistarh, Dan},
journal={arXiv preprint arXiv:2203.07259},
year={2022}
}
``` |
neuralmagic/oBERT-6-downstream-dense-QAT-squadv1 | 9ad67eb4b4e5f0fcfc193f7c9e5c2b9ff255be0c | 2022-06-20T11:36:52.000Z | [
"pytorch",
"en",
"dataset:squad",
"arxiv:2203.07259",
"bert",
"oBERT",
"sparsity",
"pruning",
"compression"
] | null | false | neuralmagic | null | neuralmagic/oBERT-6-downstream-dense-QAT-squadv1 | 0 | null | null | 37,715 | ---
tags:
- bert
- oBERT
- sparsity
- pruning
- compression
language: en
datasets: squad
---
# oBERT-6-downstream-dense-QAT-squadv1
This model is obtained with [The Optimal BERT Surgeon: Scalable and Accurate Second-Order Pruning for Large Language Models](https://arxiv.org/abs/2203.07259).
It corresponds to the model presented in the `Table 3 - 6 Layers - 0% Sparsity - QAT`, and it represents an upper bound for performance of the corresponding pruned and quantized models:
- 80% unstructured QAT: `neuralmagic/oBERT-6-downstream-pruned-unstructured-80-QAT-squadv1`
- 80% block-4 QAT: `neuralmagic/oBERT-6-downstream-pruned-block4-80-QAT-squadv1`
- 90% unstructured QAT: `neuralmagic/oBERT-6-downstream-pruned-unstructured-90-QAT-squadv1`
- 90% block-4 QAT: `neuralmagic/oBERT-6-downstream-pruned-block4-90-QAT-squadv1`
SQuADv1 dev-set:
```
EM = 80.85
F1 = 87.94
```
Code: _coming soon_
## BibTeX entry and citation info
```bibtex
@article{kurtic2022optimal,
title={The Optimal BERT Surgeon: Scalable and Accurate Second-Order Pruning for Large Language Models},
author={Kurtic, Eldar and Campos, Daniel and Nguyen, Tuan and Frantar, Elias and Kurtz, Mark and Fineran, Benjamin and Goin, Michael and Alistarh, Dan},
journal={arXiv preprint arXiv:2203.07259},
year={2022}
}
``` |
neuralmagic/oBERT-6-downstream-pruned-block4-80-QAT-squadv1 | b6161a2782d8b8955755402c43defcf55ad0bae9 | 2022-06-20T11:36:52.000Z | [
"pytorch",
"en",
"dataset:squad",
"arxiv:2203.07259",
"bert",
"oBERT",
"sparsity",
"pruning",
"compression"
] | null | false | neuralmagic | null | neuralmagic/oBERT-6-downstream-pruned-block4-80-QAT-squadv1 | 0 | null | null | 37,716 | ---
tags:
- bert
- oBERT
- sparsity
- pruning
- compression
language: en
datasets: squad
---
# oBERT-6-downstream-pruned-block4-80-QAT-squadv1
This model is obtained with [The Optimal BERT Surgeon: Scalable and Accurate Second-Order Pruning for Large Language Models](https://arxiv.org/abs/2203.07259).
It corresponds to the model presented in the `Table 3 - 6 Layers - Sparsity 80% - 4-block + QAT`.
```
Pruning method: oBERT downstream block-4 + QAT
Paper: https://arxiv.org/abs/2203.07259
Dataset: SQuADv1
Sparsity: 80%
Number of layers: 6
```
The dev-set performance of this model:
```
EM = 78.28
F1 = 86.10
```
Code: _coming soon_
## BibTeX entry and citation info
```bibtex
@article{kurtic2022optimal,
title={The Optimal BERT Surgeon: Scalable and Accurate Second-Order Pruning for Large Language Models},
author={Kurtic, Eldar and Campos, Daniel and Nguyen, Tuan and Frantar, Elias and Kurtz, Mark and Fineran, Benjamin and Goin, Michael and Alistarh, Dan},
journal={arXiv preprint arXiv:2203.07259},
year={2022}
}
``` |
neuralmagic/oBERT-6-downstream-pruned-block4-90-QAT-squadv1 | 6a387f746d8b5430a9f50e98efeee72f04f8c895 | 2022-06-20T11:36:52.000Z | [
"pytorch",
"en",
"dataset:squad",
"arxiv:2203.07259",
"bert",
"oBERT",
"sparsity",
"pruning",
"compression"
] | null | false | neuralmagic | null | neuralmagic/oBERT-6-downstream-pruned-block4-90-QAT-squadv1 | 0 | null | null | 37,717 | ---
tags:
- bert
- oBERT
- sparsity
- pruning
- compression
language: en
datasets: squad
---
# oBERT-6-downstream-pruned-block4-90-QAT-squadv1
This model is obtained with [The Optimal BERT Surgeon: Scalable and Accurate Second-Order Pruning for Large Language Models](https://arxiv.org/abs/2203.07259).
It corresponds to the model presented in the `Table 3 - 6 Layers - Sparsity 90% - 4-block + QAT`.
```
Pruning method: oBERT downstream block-4 + QAT
Paper: https://arxiv.org/abs/2203.07259
Dataset: SQuADv1
Sparsity: 90%
Number of layers: 6
```
The dev-set performance of this model:
```
EM = 76.56
F1 = 84.59
```
Code: _coming soon_
## BibTeX entry and citation info
```bibtex
@article{kurtic2022optimal,
title={The Optimal BERT Surgeon: Scalable and Accurate Second-Order Pruning for Large Language Models},
author={Kurtic, Eldar and Campos, Daniel and Nguyen, Tuan and Frantar, Elias and Kurtz, Mark and Fineran, Benjamin and Goin, Michael and Alistarh, Dan},
journal={arXiv preprint arXiv:2203.07259},
year={2022}
}
``` |
neuralmagic/oBERT-3-downstream-dense-QAT-squadv1 | 28598d52dc6138baeb8886cec21c7b141b775163 | 2022-06-20T11:36:51.000Z | [
"pytorch",
"en",
"dataset:squad",
"arxiv:2203.07259",
"bert",
"oBERT",
"sparsity",
"pruning",
"compression"
] | null | false | neuralmagic | null | neuralmagic/oBERT-3-downstream-dense-QAT-squadv1 | 0 | null | null | 37,718 | ---
tags:
- bert
- oBERT
- sparsity
- pruning
- compression
language: en
datasets: squad
---
# oBERT-3-downstream-dense-QAT-squadv1
This model is obtained with [The Optimal BERT Surgeon: Scalable and Accurate Second-Order Pruning for Large Language Models](https://arxiv.org/abs/2203.07259).
It corresponds to the model presented in the `Table 3 - 3 Layers - 0% Sparsity - QAT`, and it represents an upper bound for performance of the corresponding pruned and quantized models:
- 80% unstructured QAT: `neuralmagic/oBERT-3-downstream-pruned-unstructured-80-QAT-squadv1`
- 80% block-4 QAT: `neuralmagic/oBERT-3-downstream-pruned-block4-80-QAT-squadv1`
- 90% unstructured QAT: `neuralmagic/oBERT-3-downstream-pruned-unstructured-90-QAT-squadv1`
- 90% block-4 QAT: `neuralmagic/oBERT-3-downstream-pruned-block4-90-QAT-squadv1`
SQuADv1 dev-set:
```
EM = 76.06
F1 = 84.25
```
Code: _coming soon_
## BibTeX entry and citation info
```bibtex
@article{kurtic2022optimal,
title={The Optimal BERT Surgeon: Scalable and Accurate Second-Order Pruning for Large Language Models},
author={Kurtic, Eldar and Campos, Daniel and Nguyen, Tuan and Frantar, Elias and Kurtz, Mark and Fineran, Benjamin and Goin, Michael and Alistarh, Dan},
journal={arXiv preprint arXiv:2203.07259},
year={2022}
}
``` |
neuralmagic/oBERT-3-downstream-pruned-block4-80-QAT-squadv1 | c8db32e67ca9b8f88448146e6011a9afb390fd3c | 2022-06-20T11:36:51.000Z | [
"pytorch",
"en",
"dataset:squad",
"arxiv:2203.07259",
"bert",
"oBERT",
"sparsity",
"pruning",
"compression"
] | null | false | neuralmagic | null | neuralmagic/oBERT-3-downstream-pruned-block4-80-QAT-squadv1 | 0 | null | null | 37,719 | ---
tags:
- bert
- oBERT
- sparsity
- pruning
- compression
language: en
datasets: squad
---
# oBERT-3-downstream-pruned-block4-80-QAT-squadv1
This model is obtained with [The Optimal BERT Surgeon: Scalable and Accurate Second-Order Pruning for Large Language Models](https://arxiv.org/abs/2203.07259).
It corresponds to the model presented in the `Table 3 - 3 Layers - Sparsity 80% - 4-block + QAT`.
```
Pruning method: oBERT downstream block-4 + QAT
Paper: https://arxiv.org/abs/2203.07259
Dataset: SQuADv1
Sparsity: 80%
Number of layers: 3
```
The dev-set performance of this model:
```
EM = 72.70
F1 = 82.04
```
Code: _coming soon_
## BibTeX entry and citation info
```bibtex
@article{kurtic2022optimal,
title={The Optimal BERT Surgeon: Scalable and Accurate Second-Order Pruning for Large Language Models},
author={Kurtic, Eldar and Campos, Daniel and Nguyen, Tuan and Frantar, Elias and Kurtz, Mark and Fineran, Benjamin and Goin, Michael and Alistarh, Dan},
journal={arXiv preprint arXiv:2203.07259},
year={2022}
}
``` |
neuralmagic/oBERT-3-downstream-pruned-block4-90-QAT-squadv1 | a9c7a514da17e9bf2fa7dc264f5cf79796a8a35c | 2022-06-20T11:36:51.000Z | [
"pytorch",
"en",
"dataset:squad",
"arxiv:2203.07259",
"bert",
"oBERT",
"sparsity",
"pruning",
"compression"
] | null | false | neuralmagic | null | neuralmagic/oBERT-3-downstream-pruned-block4-90-QAT-squadv1 | 0 | null | null | 37,720 | ---
tags:
- bert
- oBERT
- sparsity
- pruning
- compression
language: en
datasets: squad
---
# oBERT-3-downstream-pruned-block4-90-QAT-squadv1
This model is obtained with [The Optimal BERT Surgeon: Scalable and Accurate Second-Order Pruning for Large Language Models](https://arxiv.org/abs/2203.07259).
It corresponds to the model presented in the `Table 3 - 3 Layers - Sparsity 90% - 4-block + QAT`.
```
Pruning method: oBERT downstream block-4 + QAT
Paper: https://arxiv.org/abs/2203.07259
Dataset: SQuADv1
Sparsity: 90%
Number of layers: 3
```
The dev-set performance of this model:
```
EM = 70.00
F1 = 79.66
```
Code: _coming soon_
## BibTeX entry and citation info
```bibtex
@article{kurtic2022optimal,
title={The Optimal BERT Surgeon: Scalable and Accurate Second-Order Pruning for Large Language Models},
author={Kurtic, Eldar and Campos, Daniel and Nguyen, Tuan and Frantar, Elias and Kurtz, Mark and Fineran, Benjamin and Goin, Michael and Alistarh, Dan},
journal={arXiv preprint arXiv:2203.07259},
year={2022}
}
``` |
aioxlabs/dvoice-darija | aa2cfbaf722e02482e55e1cf33ba2c71dbce619e | 2022-05-28T08:21:44.000Z | [
"wav2vec2",
"feature-extraction",
"dar",
"dataset:commonvoice",
"speechbrain",
"CTC",
"pytorch",
"Transformer",
"license:apache-2.0",
"automatic-speech-recognition"
] | automatic-speech-recognition | false | aioxlabs | null | aioxlabs/dvoice-darija | 0 | null | speechbrain | 37,721 | ---
language: "dar"
thumbnail:
pipeline_tag: automatic-speech-recognition
tags:
- CTC
- pytorch
- speechbrain
- Transformer
license: "apache-2.0"
datasets:
- commonvoice
metrics:
- wer
- cer
---
<iframe src="https://ghbtns.com/github-btn.html?user=speechbrain&repo=speechbrain&type=star&count=true&size=large&v=2" frameborder="0" scrolling="0" width="170" height="30" title="GitHub"></iframe>
<br/><br/>
# wav2vec 2.0 with CTC/Attention trained on DVoice Darija (No LM)
This repository provides all the necessary tools to perform automatic speech
recognition from an end-to-end system pretrained on a [DVoice](https://zenodo.org/record/6342622) Darija dataset within
SpeechBrain. For a better experience, we encourage you to learn more about
[SpeechBrain](https://speechbrain.github.io).
| DVoice Release | Val. CER | Val. WER | Test CER | Test WER |
|:-------------:|:---------------------------:| -----:| -----:| -----:|
| v2.0 | 5.51 | 18.46 | 5.85 | 18.28 |
# Pipeline description
This ASR system is composed of 2 different but linked blocks:
- Tokenizer (unigram) that transforms words into subword units and trained with
the train transcriptions.
- Acoustic model (wav2vec2.0 + CTC). A pretrained wav2vec 2.0 model ([facebook/wav2vec2-large-xlsr-53](https://huggingface.co/facebook/wav2vec2-large-xlsr-53)) is combined with two DNN layers and finetuned on the Darija dataset.
The obtained final acoustic representation is given to the CTC greedy decoder.
The system is trained with recordings sampled at 16kHz (single channel).
The code will automatically normalize your audio (i.e., resampling + mono channel selection) when calling *transcribe_file* if needed.
# Install SpeechBrain
First of all, please install tranformers and SpeechBrain with the following command:
```
pip install speechbrain transformers
```
Please notice that we encourage you to read the SpeechBrain tutorials and learn more about
[SpeechBrain](https://speechbrain.github.io).
# Transcribing your own audio files (in Darija)
```python
from speechbrain.pretrained import EncoderASR
asr_model = EncoderASR.from_hparams(source="aioxlabs/dvoice-darija", savedir="pretrained_models/asr-wav2vec2-dvoice-dar")
asr_model.transcribe_file('./the_path_to_your_audio_file')
```
# Inference on GPU
To perform inference on the GPU, add `run_opts={"device":"cuda"}` when calling the `from_hparams` method.
# Training
To train the model from scratch, please see our GitHub tutorial [here](https://github.com/AIOXLABS/DVoice).
# Limitations
The SpeechBrain team does not provide any warranty on the performance achieved by this model when used on other datasets.
# Referencing SpeechBrain
```
@misc{SB2021,
author = {Ravanelli, Mirco and Parcollet, Titouan and Rouhe, Aku and Plantinga, Peter and Rastorgueva, Elena and Lugosch, Loren and Dawalatabad, Nauman and Ju-Chieh, Chou and Heba, Abdel and Grondin, Francois and Aris, William and Liao, Chien-Feng and Cornell, Samuele and Yeh, Sung-Lin and Na, Hwidong and Gao, Yan and Fu, Szu-Wei and Subakan, Cem and De Mori, Renato and Bengio, Yoshua },
title = {SpeechBrain},
year = {2021},
publisher = {GitHub},
journal = {GitHub repository},
howpublished = {\\\\url{https://github.com/speechbrain/speechbrain}},
}
```
# About DVoice
DVoice is a community initiative that aims to provide Africa low resources languages with data and models to facilitate their use of voice technologies. The lack of data on these languages makes it necessary to collect data using methods that are specific to each one. Two different approaches are currently used: the DVoice platforms ([https://dvoice.ma](https://dvoice.ma) and [https://dvoice.sn](https://dvoice.sn)), which are based on Mozilla Common Voice, for collecting authentic recordings from the community, and transfer learning techniques for automatically labeling recordings that are retrived from social medias. The DVoice platform currently manages 7 languages including Darija (Moroccan Arabic dialect) whose dataset appears on this version, Wolof, Mandingo, Serere, Pular, Diola and Soninke.
For this project, AIOX Labs the SI2M Laboratory are joining forces to build the future of technologies together.
# About AIOX Labs
Based in Rabat, London and Paris, AIOX-Labs mobilizes artificial intelligence technologies to meet the business needs and data projects of companies.
- He is at the service of the growth of groups, the optimization of processes or the improvement of the customer experience.
- AIOX-Labs is multi-sector, from fintech to industry, including retail and consumer goods.
- Business ready data products with a solid algorithmic base and adaptability for the specific needs of each client.
- A complementary team made up of doctors in AI and business experts with a solid scientific base and international publications.
Website: [https://www.aiox-labs.com/](https://www.aiox-labs.com/)
# SI2M Laboratory
The Information Systems, Intelligent Systems and Mathematical Modeling Research Laboratory (SI2M) is an academic research laboratory of the National Institute of Statistics and Applied Economics (INSEA). The research areas of the laboratories are Information Systems, Intelligent Systems, Artificial Intelligence, Decision Support, Network and System Security, Mathematical Modelling.
Website: [SI2M Laboratory](https://insea.ac.ma/index.php/pole-recherche/equipe-de-recherche/150-laboratoire-de-recherche-en-systemes-d-information-systemes-intelligents-et-modelisation-mathematique)
# About SpeechBrain
SpeechBrain is an open-source and all-in-one speech toolkit. It is designed to be simple, extremely flexible, and user-friendly. Competitive or state-of-the-art performance is obtained in various domains.
Website: https://speechbrain.github.io/
GitHub: https://github.com/speechbrain/speechbrain
# Referencing SpeechBrain
```
@misc{SB2021,
author = {Ravanelli, Mirco and Parcollet, Titouan and Rouhe, Aku and Plantinga, Peter and Rastorgueva, Elena and Lugosch, Loren and Dawalatabad, Nauman and Ju-Chieh, Chou and Heba, Abdel and Grondin, Francois and Aris, William and Liao, Chien-Feng and Cornell, Samuele and Yeh, Sung-Lin and Na, Hwidong and Gao, Yan and Fu, Szu-Wei and Subakan, Cem and De Mori, Renato and Bengio, Yoshua },
title = {SpeechBrain},
year = {2021},
publisher = {GitHub},
journal = {GitHub repository},
howpublished = {\\\\url{https://github.com/speechbrain/speechbrain}},
}
```
# Acknowledgements
This research was supported through computational resources of HPC-MARWAN (www.marwan.ma/hpc) provided by CNRST, Rabat, Morocco. We deeply thank this institution. |
oyousuf/afriberta_so1 | ad5308348a47c2bb42a3ca8a1c398e9ef3323ad1 | 2022-05-25T21:55:20.000Z | [
"pytorch",
"roberta",
"transformers"
] | null | false | oyousuf | null | oyousuf/afriberta_so1 | 0 | null | transformers | 37,722 | Entry not found |
stevemobs/deberta-base-combined-squad1-aqa-newsqa | 209424173fd1d79128f7a40f3d2e1da3db7a52ed | 2022-05-28T00:45:46.000Z | [
"pytorch",
"tensorboard",
"deberta",
"question-answering",
"transformers",
"generated_from_trainer",
"license:mit",
"model-index",
"autotrain_compatible"
] | question-answering | false | stevemobs | null | stevemobs/deberta-base-combined-squad1-aqa-newsqa | 0 | null | transformers | 37,723 | ---
license: mit
tags:
- generated_from_trainer
model-index:
- name: deberta-base-combined-squad1-aqa-newsqa
results: []
---
<!-- This model card has been generated automatically according to the information the Trainer had access to. You
should probably proofread and complete it, then remove this comment. -->
# deberta-base-combined-squad1-aqa-newsqa
This model is a fine-tuned version of [microsoft/deberta-base](https://huggingface.co/microsoft/deberta-base) on the None dataset.
It achieves the following results on the evaluation set:
- Loss: 0.8860
## Model description
More information needed
## Intended uses & limitations
More information needed
## Training and evaluation data
More information needed
## Training procedure
### Training hyperparameters
The following hyperparameters were used during training:
- learning_rate: 2e-05
- train_batch_size: 8
- eval_batch_size: 8
- seed: 42
- optimizer: Adam with betas=(0.9,0.999) and epsilon=1e-08
- lr_scheduler_type: linear
- num_epochs: 2
### Training results
| Training Loss | Epoch | Step | Validation Loss |
|:-------------:|:-----:|:-----:|:---------------:|
| 0.8812 | 1.0 | 40819 | 0.8762 |
| 0.6043 | 2.0 | 81638 | 0.8860 |
### Framework versions
- Transformers 4.19.2
- Pytorch 1.11.0+cu113
- Datasets 2.2.2
- Tokenizers 0.12.1
|
morahil/wav2vec2-hindi-new-4 | 614b3bb98d2d414bcf320ae847f89924e6d24861 | 2022-05-26T08:11:25.000Z | [
"pytorch",
"tensorboard",
"wav2vec2",
"automatic-speech-recognition",
"transformers",
"generated_from_trainer",
"license:apache-2.0",
"model-index"
] | automatic-speech-recognition | false | morahil | null | morahil/wav2vec2-hindi-new-4 | 0 | null | transformers | 37,724 | ---
license: apache-2.0
tags:
- generated_from_trainer
model-index:
- name: wav2vec2-hindi-new-4
results: []
---
<!-- This model card has been generated automatically according to the information the Trainer had access to. You
should probably proofread and complete it, then remove this comment. -->
# wav2vec2-hindi-new-4
This model is a fine-tuned version of [facebook/wav2vec2-large-xlsr-53](https://huggingface.co/facebook/wav2vec2-large-xlsr-53) on the None dataset.
It achieves the following results on the evaluation set:
- Loss: 2.3743
- Wer: 0.8926
## Model description
More information needed
## Intended uses & limitations
More information needed
## Training and evaluation data
More information needed
## Training procedure
### Training hyperparameters
The following hyperparameters were used during training:
- learning_rate: 0.0001
- train_batch_size: 16
- eval_batch_size: 8
- seed: 42
- gradient_accumulation_steps: 2
- total_train_batch_size: 32
- optimizer: Adam with betas=(0.9,0.999) and epsilon=1e-08
- lr_scheduler_type: linear
- lr_scheduler_warmup_steps: 500
- num_epochs: 40
- mixed_precision_training: Native AMP
### Training results
| Training Loss | Epoch | Step | Validation Loss | Wer |
|:-------------:|:-----:|:----:|:---------------:|:------:|
| 8.0662 | 6.45 | 400 | 3.6328 | 1.0 |
| 2.771 | 12.9 | 800 | 1.5110 | 0.8819 |
| 0.5131 | 19.35 | 1200 | 1.9369 | 0.8989 |
| 0.1955 | 25.8 | 1600 | 2.1664 | 0.8562 |
| 0.1264 | 32.26 | 2000 | 2.3343 | 0.8985 |
| 0.0992 | 38.7 | 2400 | 2.3743 | 0.8926 |
### Framework versions
- Transformers 4.20.0.dev0
- Pytorch 1.11.0+cu113
- Datasets 2.2.3.dev0
- Tokenizers 0.12.1
|
clisi2000/flairmodel | 714fc8eb1e0d2d60011c1ce42d5b99078ff4c2f2 | 2022-05-26T05:58:03.000Z | [
"pytorch",
"flair",
"token-classification"
] | token-classification | false | clisi2000 | null | clisi2000/flairmodel | 0 | null | flair | 37,725 | ---
tags:
- flair
- token-classification
widget:
- text: "does this work"
--- |
meetyildiz/TurQA-bert-base-turkish-uncased-finetuned-toqad | 5236f5439731bf539930e06e018a6b2732bab9b7 | 2022-06-02T22:50:29.000Z | [
"pytorch",
"tensorboard",
"bert",
"feature-extraction",
"dataset:squad",
"transformers",
"generated_from_trainer",
"license:mit",
"model-index"
] | feature-extraction | false | meetyildiz | null | meetyildiz/TurQA-bert-base-turkish-uncased-finetuned-toqad | 0 | null | transformers | 37,726 | ---
license: mit
tags:
- generated_from_trainer
datasets:
- squad
model-index:
- name: TurQA-bert-base-turkish-uncased-finetuned-toqad
results: []
---
<!-- This model card has been generated automatically according to the information the Trainer had access to. You
should probably proofread and complete it, then remove this comment. -->
# TurQA-bert-base-turkish-uncased-finetuned-toqad
This model is a fine-tuned version of [dbmdz/bert-base-turkish-uncased](https://huggingface.co/dbmdz/bert-base-turkish-uncased) on the squad dataset.
It achieves the following results on the evaluation set:
- Loss: 5.9506
## Model description
More information needed
## Intended uses & limitations
More information needed
## Training and evaluation data
More information needed
## Training procedure
### Training hyperparameters
The following hyperparameters were used during training:
- learning_rate: 0.002
- train_batch_size: 16
- eval_batch_size: 16
- seed: 42
- optimizer: Adam with betas=(0.9,0.999) and epsilon=1e-08
- lr_scheduler_type: linear
- num_epochs: 5
### Training results
| Training Loss | Epoch | Step | Validation Loss |
|:-------------:|:-----:|:----:|:---------------:|
| 5.9774 | 1.0 | 717 | 5.9506 |
| 5.9675 | 2.0 | 1434 | 5.9506 |
| 5.9584 | 3.0 | 2151 | 5.9506 |
| 5.957 | 4.0 | 2868 | 5.9506 |
| 5.9561 | 5.0 | 3585 | 5.9506 |
### Framework versions
- Transformers 4.19.2
- Pytorch 1.11.0+cu113
- Datasets 2.2.2
- Tokenizers 0.12.1
|
theojolliffe/bart-cnn-pubmed-arxiv-pubmed-arxiv-arxiv-v3-e10 | 8dce8e2d0d9ac63ac696654335383df6822d78d2 | 2022-05-26T09:31:55.000Z | [
"pytorch",
"tensorboard",
"bart",
"text2text-generation",
"transformers",
"generated_from_trainer",
"license:mit",
"model-index",
"autotrain_compatible"
] | text2text-generation | false | theojolliffe | null | theojolliffe/bart-cnn-pubmed-arxiv-pubmed-arxiv-arxiv-v3-e10 | 0 | null | transformers | 37,727 | ---
license: mit
tags:
- generated_from_trainer
metrics:
- rouge
model-index:
- name: bart-cnn-pubmed-arxiv-pubmed-arxiv-arxiv-v3-e10
results: []
---
<!-- This model card has been generated automatically according to the information the Trainer had access to. You
should probably proofread and complete it, then remove this comment. -->
# bart-cnn-pubmed-arxiv-pubmed-arxiv-arxiv-v3-e10
This model is a fine-tuned version of [theojolliffe/bart-cnn-pubmed-arxiv-pubmed-arxiv-arxiv](https://huggingface.co/theojolliffe/bart-cnn-pubmed-arxiv-pubmed-arxiv-arxiv) on an unknown dataset.
It achieves the following results on the evaluation set:
- Loss: 0.8234
- Rouge1: 55.5793
- Rouge2: 40.0855
- Rougel: 42.0964
- Rougelsum: 53.6353
- Gen Len: 142.0
## Model description
More information needed
## Intended uses & limitations
More information needed
## Training and evaluation data
More information needed
## Training procedure
### Training hyperparameters
The following hyperparameters were used during training:
- learning_rate: 2e-05
- train_batch_size: 2
- eval_batch_size: 2
- seed: 42
- optimizer: Adam with betas=(0.9,0.999) and epsilon=1e-08
- lr_scheduler_type: linear
- num_epochs: 10
- mixed_precision_training: Native AMP
### Training results
| Training Loss | Epoch | Step | Validation Loss | Rouge1 | Rouge2 | Rougel | Rougelsum | Gen Len |
|:-------------:|:-----:|:----:|:---------------:|:-------:|:-------:|:-------:|:---------:|:--------:|
| No log | 1.0 | 398 | 0.8670 | 53.2875 | 33.7336 | 36.1194 | 50.6842 | 142.0 |
| 0.8268 | 2.0 | 796 | 0.8041 | 53.8106 | 34.5241 | 37.4362 | 51.2786 | 142.0 |
| 0.5316 | 3.0 | 1194 | 0.8188 | 53.28 | 33.6 | 36.5483 | 50.6643 | 142.0 |
| 0.3572 | 4.0 | 1592 | 0.7821 | 53.9262 | 35.1924 | 37.8367 | 51.6176 | 141.7778 |
| 0.3572 | 5.0 | 1990 | 0.7837 | 55.35 | 37.6648 | 40.6764 | 52.5981 | 142.0 |
| 0.2426 | 6.0 | 2388 | 0.7760 | 55.4524 | 39.1414 | 42.4299 | 53.2113 | 141.9815 |
| 0.1698 | 7.0 | 2786 | 0.7921 | 56.7694 | 40.3148 | 43.3934 | 54.7093 | 142.0 |
| 0.1192 | 8.0 | 3184 | 0.8013 | 54.4313 | 37.6505 | 39.743 | 52.1465 | 142.0 |
| 0.1 | 9.0 | 3582 | 0.8139 | 55.6947 | 40.2425 | 42.7441 | 53.7018 | 142.0 |
| 0.1 | 10.0 | 3980 | 0.8234 | 55.5793 | 40.0855 | 42.0964 | 53.6353 | 142.0 |
### Framework versions
- Transformers 4.19.2
- Pytorch 1.11.0+cu113
- Datasets 2.2.2
- Tokenizers 0.12.1
|
theojolliffe/bart-cnn-pubmed-arxiv-pubmed-arxiv-arxiv-v3-e12 | 03aa2ce150eba9f44206fb6a5a9a3e115b76c409 | 2022-05-26T11:59:07.000Z | [
"pytorch",
"tensorboard",
"bart",
"text2text-generation",
"transformers",
"generated_from_trainer",
"license:mit",
"model-index",
"autotrain_compatible"
] | text2text-generation | false | theojolliffe | null | theojolliffe/bart-cnn-pubmed-arxiv-pubmed-arxiv-arxiv-v3-e12 | 0 | null | transformers | 37,728 | ---
license: mit
tags:
- generated_from_trainer
metrics:
- rouge
model-index:
- name: bart-cnn-pubmed-arxiv-pubmed-arxiv-arxiv-v3-e12
results: []
---
<!-- This model card has been generated automatically according to the information the Trainer had access to. You
should probably proofread and complete it, then remove this comment. -->
# bart-cnn-pubmed-arxiv-pubmed-arxiv-arxiv-v3-e12
This model is a fine-tuned version of [theojolliffe/bart-cnn-pubmed-arxiv-pubmed-arxiv-arxiv](https://huggingface.co/theojolliffe/bart-cnn-pubmed-arxiv-pubmed-arxiv-arxiv) on an unknown dataset.
It achieves the following results on the evaluation set:
- Loss: 0.8501
- Rouge1: 56.1453
- Rouge2: 40.018
- Rougel: 43.5586
- Rougelsum: 54.4271
- Gen Len: 142.0
## Model description
More information needed
## Intended uses & limitations
More information needed
## Training and evaluation data
More information needed
## Training procedure
### Training hyperparameters
The following hyperparameters were used during training:
- learning_rate: 2e-05
- train_batch_size: 2
- eval_batch_size: 2
- seed: 42
- optimizer: Adam with betas=(0.9,0.999) and epsilon=1e-08
- lr_scheduler_type: linear
- num_epochs: 12
- mixed_precision_training: Native AMP
### Training results
| Training Loss | Epoch | Step | Validation Loss | Rouge1 | Rouge2 | Rougel | Rougelsum | Gen Len |
|:-------------:|:-----:|:----:|:---------------:|:-------:|:-------:|:-------:|:---------:|:-------:|
| No log | 1.0 | 398 | 0.8670 | 54.4613 | 34.7958 | 36.5841 | 51.9208 | 142.0 |
| 0.8276 | 2.0 | 796 | 0.8061 | 53.5804 | 34.5801 | 37.4643 | 51.1494 | 142.0 |
| 0.5318 | 3.0 | 1194 | 0.8146 | 53.7541 | 34.2446 | 37.5488 | 51.2475 | 142.0 |
| 0.3541 | 4.0 | 1592 | 0.7578 | 53.7645 | 34.874 | 38.3958 | 51.3075 | 142.0 |
| 0.3541 | 5.0 | 1990 | 0.7778 | 55.2787 | 37.5539 | 40.5489 | 52.8514 | 142.0 |
| 0.2386 | 6.0 | 2388 | 0.7810 | 55.2487 | 38.6522 | 41.466 | 53.379 | 142.0 |
| 0.1652 | 7.0 | 2786 | 0.7905 | 54.3618 | 37.4987 | 40.7348 | 52.2938 | 142.0 |
| 0.1152 | 8.0 | 3184 | 0.7934 | 54.4888 | 37.649 | 40.3582 | 52.3451 | 142.0 |
| 0.0942 | 9.0 | 3582 | 0.8220 | 55.5489 | 39.8493 | 42.2318 | 53.727 | 142.0 |
| 0.0942 | 10.0 | 3980 | 0.8331 | 55.7509 | 39.9491 | 43.2336 | 53.9748 | 142.0 |
| 0.0669 | 11.0 | 4378 | 0.8298 | 57.3881 | 42.6588 | 45.4694 | 55.8334 | 142.0 |
| 0.0531 | 12.0 | 4776 | 0.8501 | 56.1453 | 40.018 | 43.5586 | 54.4271 | 142.0 |
### Framework versions
- Transformers 4.19.2
- Pytorch 1.11.0+cu113
- Datasets 2.2.2
- Tokenizers 0.12.1
|
peter2000/wav2vec2-large-xls-r-300m-kinyarwanda-colab | 6d5abc01cc936d85bf89ea9b7ba25a9489263575 | 2022-05-30T17:37:25.000Z | [
"pytorch",
"tensorboard",
"wav2vec2",
"automatic-speech-recognition",
"dataset:common_voice",
"transformers",
"generated_from_trainer",
"license:apache-2.0",
"model-index"
] | automatic-speech-recognition | false | peter2000 | null | peter2000/wav2vec2-large-xls-r-300m-kinyarwanda-colab | 0 | null | transformers | 37,729 | ---
license: apache-2.0
tags:
- generated_from_trainer
datasets:
- common_voice
model-index:
- name: wav2vec2-large-xls-r-300m-kinyarwanda-colab
results: []
---
<!-- This model card has been generated automatically according to the information the Trainer had access to. You
should probably proofread and complete it, then remove this comment. -->
# wav2vec2-large-xls-r-300m-kinyarwanda-colab
This model is a fine-tuned version of [facebook/wav2vec2-xls-r-300m](https://huggingface.co/facebook/wav2vec2-xls-r-300m) on the common_voice dataset.
It achieves the following results on the evaluation set:
- eval_loss: 0.6856
- eval_wer: 0.5693
- eval_runtime: 716.6268
- eval_samples_per_second: 6.584
- eval_steps_per_second: 0.823
- epoch: 2.98
- step: 2800
## Model description
More information needed
## Intended uses & limitations
More information needed
## Training and evaluation data
More information needed
## Training procedure
### Training hyperparameters
The following hyperparameters were used during training:
- learning_rate: 0.0003
- train_batch_size: 8
- eval_batch_size: 8
- seed: 42
- gradient_accumulation_steps: 2
- total_train_batch_size: 16
- optimizer: Adam with betas=(0.9,0.999) and epsilon=1e-08
- lr_scheduler_type: linear
- lr_scheduler_warmup_steps: 500
- num_epochs: 5
- mixed_precision_training: Native AMP
### Framework versions
- Transformers 4.11.3
- Pytorch 1.10.0+cu113
- Datasets 1.18.3
- Tokenizers 0.10.3
|
theojolliffe/bart-cnn-pubmed-arxiv-pubmed-arxiv-arxiv-v3-e3 | 62a83d3c888c8d57369d0b1ae00c5eb6ed6b0594 | 2022-05-26T13:07:26.000Z | [
"pytorch",
"tensorboard",
"bart",
"text2text-generation",
"transformers",
"generated_from_trainer",
"license:mit",
"model-index",
"autotrain_compatible"
] | text2text-generation | false | theojolliffe | null | theojolliffe/bart-cnn-pubmed-arxiv-pubmed-arxiv-arxiv-v3-e3 | 0 | null | transformers | 37,730 | ---
license: mit
tags:
- generated_from_trainer
metrics:
- rouge
model-index:
- name: bart-cnn-pubmed-arxiv-pubmed-arxiv-arxiv-v3-e3
results: []
---
<!-- This model card has been generated automatically according to the information the Trainer had access to. You
should probably proofread and complete it, then remove this comment. -->
# bart-cnn-pubmed-arxiv-pubmed-arxiv-arxiv-v3-e3
This model is a fine-tuned version of [theojolliffe/bart-cnn-pubmed-arxiv-pubmed-arxiv-arxiv](https://huggingface.co/theojolliffe/bart-cnn-pubmed-arxiv-pubmed-arxiv-arxiv) on an unknown dataset.
It achieves the following results on the evaluation set:
- Loss: 0.8311
- Rouge1: 53.458
- Rouge2: 34.076
- Rougel: 37.3287
- Rougelsum: 50.7849
- Gen Len: 142.0
## Model description
More information needed
## Intended uses & limitations
More information needed
## Training and evaluation data
More information needed
## Training procedure
### Training hyperparameters
The following hyperparameters were used during training:
- learning_rate: 2e-05
- train_batch_size: 2
- eval_batch_size: 2
- seed: 42
- optimizer: Adam with betas=(0.9,0.999) and epsilon=1e-08
- lr_scheduler_type: linear
- num_epochs: 3
- mixed_precision_training: Native AMP
### Training results
| Training Loss | Epoch | Step | Validation Loss | Rouge1 | Rouge2 | Rougel | Rougelsum | Gen Len |
|:-------------:|:-----:|:----:|:---------------:|:-------:|:-------:|:-------:|:---------:|:--------:|
| No log | 1.0 | 398 | 0.8697 | 52.6579 | 33.307 | 35.8099 | 49.9687 | 142.0 |
| 0.8264 | 2.0 | 796 | 0.8293 | 52.6738 | 33.7202 | 36.1502 | 50.0501 | 141.9815 |
| 0.5471 | 3.0 | 1194 | 0.8311 | 53.458 | 34.076 | 37.3287 | 50.7849 | 142.0 |
### Framework versions
- Transformers 4.19.2
- Pytorch 1.11.0+cu113
- Datasets 2.2.2
- Tokenizers 0.12.1
|
redcy/FrasierBotv1 | 11bed3a040d18982381ab16e901b278b5fce7c4b | 2022-05-26T12:25:09.000Z | [
"pytorch",
"gpt2",
"text-generation",
"transformers",
"conversational",
"license:afl-3.0"
] | conversational | false | redcy | null | redcy/FrasierBotv1 | 0 | null | transformers | 37,731 | ---
tags:
- conversational
license: afl-3.0
--- |
ruselkomp/deeppavlov-framebank-1x6size | 50f89db0c5c01b43453a43ac23cff62da6577aec | 2022-05-27T14:05:22.000Z | [
"pytorch",
"tensorboard",
"bert",
"question-answering",
"transformers",
"autotrain_compatible"
] | question-answering | false | ruselkomp | null | ruselkomp/deeppavlov-framebank-1x6size | 0 | null | transformers | 37,732 | Entry not found |
meetyildiz/TurQA-bert-base-turkish-128k-cased-finetuned-toqad | 42d2bb49eddfb6d77044d2c9a5b10e85c7868c29 | 2022-06-02T23:06:46.000Z | [
"pytorch",
"bert",
"feature-extraction",
"transformers"
] | feature-extraction | false | meetyildiz | null | meetyildiz/TurQA-bert-base-turkish-128k-cased-finetuned-toqad | 0 | null | transformers | 37,733 | Entry not found |
meetyildiz/TurQA-convbert-base-turkish-cased-finetuned-toqad | 1cae94340a47808eef67a227071c5b20e93543b8 | 2022-06-02T22:56:50.000Z | [
"pytorch",
"convbert",
"feature-extraction",
"transformers"
] | feature-extraction | false | meetyildiz | null | meetyildiz/TurQA-convbert-base-turkish-cased-finetuned-toqad | 0 | null | transformers | 37,734 | Entry not found |
zoha/wav2vec2-base-common-voice-persian-colab | 6267762ef6345f5e673123a26c873a4e340c08e2 | 2022-05-28T15:38:48.000Z | [
"pytorch",
"tensorboard",
"wav2vec2",
"automatic-speech-recognition",
"transformers",
"generated_from_trainer",
"license:apache-2.0",
"model-index"
] | automatic-speech-recognition | false | zoha | null | zoha/wav2vec2-base-common-voice-persian-colab | 0 | null | transformers | 37,735 | ---
license: apache-2.0
tags:
- generated_from_trainer
model-index:
- name: wav2vec2-base-common-voice-persian-colab
results: []
---
<!-- This model card has been generated automatically according to the information the Trainer had access to. You
should probably proofread and complete it, then remove this comment. -->
# wav2vec2-base-common-voice-persian-colab
This model is a fine-tuned version of [facebook/wav2vec2-base](https://huggingface.co/facebook/wav2vec2-base) on the None dataset.
It achieves the following results on the evaluation set:
- Loss: 1.1446
- Wer: 0.6911
## Model description
More information needed
## Intended uses & limitations
More information needed
## Training and evaluation data
More information needed
## Training procedure
### Training hyperparameters
The following hyperparameters were used during training:
- learning_rate: 0.0001
- train_batch_size: 16
- eval_batch_size: 8
- seed: 42
- optimizer: Adam with betas=(0.9,0.999) and epsilon=1e-08
- lr_scheduler_type: linear
- lr_scheduler_warmup_steps: 500
- num_epochs: 30
- mixed_precision_training: Native AMP
### Training results
| Training Loss | Epoch | Step | Validation Loss | Wer |
|:-------------:|:-----:|:----:|:---------------:|:------:|
| No log | 1.26 | 300 | 3.0670 | 1.0 |
| 3.3475 | 2.52 | 600 | 2.5530 | 1.0 |
| 3.3475 | 3.78 | 900 | 1.4598 | 0.9555 |
| 2.0348 | 5.04 | 1200 | 1.2189 | 0.8797 |
| 1.0817 | 6.3 | 1500 | 1.1242 | 0.8268 |
| 1.0817 | 7.56 | 1800 | 1.0764 | 0.7957 |
| 0.7973 | 8.82 | 2100 | 1.1023 | 0.7863 |
| 0.7973 | 10.08 | 2400 | 1.0583 | 0.7785 |
| 0.6514 | 11.34 | 2700 | 1.0963 | 0.7512 |
| 0.5878 | 12.61 | 3000 | 1.1200 | 0.7494 |
| 0.5878 | 13.87 | 3300 | 1.0396 | 0.7402 |
| 0.484 | 15.13 | 3600 | 1.1407 | 0.7340 |
| 0.484 | 16.39 | 3900 | 1.1534 | 0.7584 |
| 0.4384 | 17.65 | 4200 | 1.0973 | 0.7236 |
| 0.3966 | 18.91 | 4500 | 1.0623 | 0.7358 |
| 0.3966 | 20.17 | 4800 | 1.1655 | 0.7112 |
| 0.3408 | 21.43 | 5100 | 1.1825 | 0.7084 |
| 0.3408 | 22.69 | 5400 | 1.1436 | 0.7029 |
| 0.3274 | 23.95 | 5700 | 1.1077 | 0.6988 |
| 0.2948 | 25.21 | 6000 | 1.1454 | 0.7066 |
| 0.2948 | 26.47 | 6300 | 1.1411 | 0.6956 |
| 0.2545 | 27.73 | 6600 | 1.0952 | 0.6918 |
| 0.2545 | 28.99 | 6900 | 1.1446 | 0.6911 |
### Framework versions
- Transformers 4.11.3
- Pytorch 1.10.0+cu113
- Datasets 1.18.3
- Tokenizers 0.10.3
|
meetyildiz/TurQA-distilbert-base-turkish-cased-finetuned-toqad | de6adf3f17e6bdd824dbdc6a49c538085d888d3a | 2022-06-02T23:02:49.000Z | [
"pytorch",
"distilbert",
"feature-extraction",
"transformers"
] | feature-extraction | false | meetyildiz | null | meetyildiz/TurQA-distilbert-base-turkish-cased-finetuned-toqad | 0 | null | transformers | 37,736 | Entry not found |
inessilva/bert-base-portuguese-cased-finetuned-oparticles | 153c1298b8af92e6e7696a77c01281cc44e96d87 | 2022-05-26T16:53:43.000Z | [
"pytorch",
"tensorboard",
"bert",
"fill-mask",
"transformers",
"generated_from_trainer",
"license:mit",
"model-index",
"autotrain_compatible"
] | fill-mask | false | inessilva | null | inessilva/bert-base-portuguese-cased-finetuned-oparticles | 0 | null | transformers | 37,737 | ---
license: mit
tags:
- generated_from_trainer
model-index:
- name: bert-base-portuguese-cased-finetuned-oparticles
results: []
---
<!-- This model card has been generated automatically according to the information the Trainer had access to. You
should probably proofread and complete it, then remove this comment. -->
# bert-base-portuguese-cased-finetuned-oparticles
This model is a fine-tuned version of [neuralmind/bert-base-portuguese-cased](https://huggingface.co/neuralmind/bert-base-portuguese-cased) on the None dataset.
It achieves the following results on the evaluation set:
- Loss: 2.2012
## Model description
More information needed
## Intended uses & limitations
More information needed
## Training and evaluation data
More information needed
## Training procedure
### Training hyperparameters
The following hyperparameters were used during training:
- learning_rate: 2e-05
- train_batch_size: 16
- eval_batch_size: 16
- seed: 42
- optimizer: Adam with betas=(0.9,0.999) and epsilon=1e-08
- lr_scheduler_type: linear
- num_epochs: 3.0
- mixed_precision_training: Native AMP
### Training results
| Training Loss | Epoch | Step | Validation Loss |
|:-------------:|:-----:|:----:|:---------------:|
| 2.6294 | 1.0 | 85 | 2.2813 |
| 2.3647 | 2.0 | 170 | 2.2857 |
| 2.3189 | 3.0 | 255 | 2.3030 |
### Framework versions
- Transformers 4.19.2
- Pytorch 1.11.0+cu113
- Datasets 2.2.2
- Tokenizers 0.12.1
|
meetyildiz/TurQA-electra-base-turkish-cased-discriminator-finetuned-toqad | 8add4fd6a091b5f8a375984aba4684fc4f68fd5d | 2022-06-05T12:56:55.000Z | [
"pytorch",
"electra",
"question-answering",
"transformers",
"autotrain_compatible"
] | question-answering | false | meetyildiz | null | meetyildiz/TurQA-electra-base-turkish-cased-discriminator-finetuned-toqad | 0 | null | transformers | 37,738 | Entry not found |
ElMuchoDingDong/DialoGPT-medium-AudreyHepburn | 4b45f88ad85e93938ef3e05d8c31ae5e9218710b | 2022-05-26T18:24:51.000Z | [
"pytorch",
"gpt2",
"text-generation",
"transformers",
"conversational"
] | conversational | false | ElMuchoDingDong | null | ElMuchoDingDong/DialoGPT-medium-AudreyHepburn | 0 | null | transformers | 37,739 | ---
tags:
- conversational
---
#Audrey Hepburn DialoGPT Model |
tmills/clinical_tempeval_pubmedbert | 2109b929f1caa4c9437d3436835b36779288ece0 | 2022-05-26T23:12:50.000Z | [
"pytorch",
"cnlpt",
"transformers",
"license:apache-2.0"
] | null | false | tmills | null | tmills/clinical_tempeval_pubmedbert | 0 | null | transformers | 37,740 | ---
license: apache-2.0
---
|
ElMuchoDingDong/DialoGPT-medium-AudreyHepburn_v3 | eaa1c8caba34e7c291971c454fc533f46836afbe | 2022-05-27T02:46:59.000Z | [
"pytorch",
"gpt2",
"text-generation",
"transformers",
"conversational"
] | conversational | false | ElMuchoDingDong | null | ElMuchoDingDong/DialoGPT-medium-AudreyHepburn_v3 | 0 | null | transformers | 37,741 | ---
tags:
- conversational
---
#Audrey Hepburn DialoGPT Model |
kurapy/t5-small-finetuned-xsum | 81e5bf79a7250c891d68f6b3cd1d4dd6459f0784 | 2022-05-27T07:08:49.000Z | [
"pytorch",
"tensorboard",
"t5",
"text2text-generation",
"dataset:xsum",
"transformers",
"generated_from_trainer",
"license:apache-2.0",
"model-index",
"autotrain_compatible"
] | text2text-generation | false | kurapy | null | kurapy/t5-small-finetuned-xsum | 0 | null | transformers | 37,742 | ---
license: apache-2.0
tags:
- generated_from_trainer
datasets:
- xsum
metrics:
- rouge
model-index:
- name: t5-small-finetuned-xsum
results:
- task:
name: Sequence-to-sequence Language Modeling
type: text2text-generation
dataset:
name: xsum
type: xsum
args: default
metrics:
- name: Rouge1
type: rouge
value: 28.2621
---
<!-- This model card has been generated automatically according to the information the Trainer had access to. You
should probably proofread and complete it, then remove this comment. -->
# t5-small-finetuned-xsum
This model is a fine-tuned version of [t5-small](https://huggingface.co/t5-small) on the xsum dataset.
It achieves the following results on the evaluation set:
- Loss: 2.4782
- Rouge1: 28.2621
- Rouge2: 7.6583
- Rougel: 22.1971
- Rougelsum: 22.2
- Gen Len: 18.8243
## Model description
More information needed
## Intended uses & limitations
More information needed
## Training and evaluation data
More information needed
## Training procedure
### Training hyperparameters
The following hyperparameters were used during training:
- learning_rate: 2e-05
- train_batch_size: 16
- eval_batch_size: 16
- seed: 42
- optimizer: Adam with betas=(0.9,0.999) and epsilon=1e-08
- lr_scheduler_type: linear
- num_epochs: 1
- mixed_precision_training: Native AMP
### Training results
| Training Loss | Epoch | Step | Validation Loss | Rouge1 | Rouge2 | Rougel | Rougelsum | Gen Len |
|:-------------:|:-----:|:-----:|:---------------:|:-------:|:------:|:-------:|:---------:|:-------:|
| 2.7138 | 1.0 | 12753 | 2.4782 | 28.2621 | 7.6583 | 22.1971 | 22.2 | 18.8243 |
### Framework versions
- Transformers 4.19.2
- Pytorch 1.11.0+cu113
- Datasets 2.2.2
- Tokenizers 0.12.1
|
vai6hav/wav2vec2-large-xls-r-300m-hindi1-colab | 8bcca0c8510e17660a4824365c3c8e9128362c4d | 2022-05-27T06:46:58.000Z | [
"pytorch",
"tensorboard",
"wav2vec2",
"automatic-speech-recognition",
"dataset:common_voice",
"transformers",
"generated_from_trainer",
"license:apache-2.0",
"model-index"
] | automatic-speech-recognition | false | vai6hav | null | vai6hav/wav2vec2-large-xls-r-300m-hindi1-colab | 0 | null | transformers | 37,743 | ---
license: apache-2.0
tags:
- generated_from_trainer
datasets:
- common_voice
model-index:
- name: wav2vec2-large-xls-r-300m-hindi1-colab
results: []
---
<!-- This model card has been generated automatically according to the information the Trainer had access to. You
should probably proofread and complete it, then remove this comment. -->
# wav2vec2-large-xls-r-300m-hindi1-colab
This model is a fine-tuned version of [facebook/wav2vec2-xls-r-300m](https://huggingface.co/facebook/wav2vec2-xls-r-300m) on the common_voice dataset.
## Model description
More information needed
## Intended uses & limitations
More information needed
## Training and evaluation data
More information needed
## Training procedure
### Training hyperparameters
The following hyperparameters were used during training:
- learning_rate: 0.0003
- train_batch_size: 16
- eval_batch_size: 8
- seed: 42
- gradient_accumulation_steps: 2
- total_train_batch_size: 32
- optimizer: Adam with betas=(0.9,0.999) and epsilon=1e-08
- lr_scheduler_type: linear
- lr_scheduler_warmup_steps: 500
- num_epochs: 30
- mixed_precision_training: Native AMP
### Training results
### Framework versions
- Transformers 4.11.3
- Pytorch 1.10.0+cu113
- Datasets 1.18.3
- Tokenizers 0.10.3
|
tanviraumi/bert-base-uncased-issues-128 | e686a50bddcfd989c20ccaf1ddffca68d5bc246a | 2022-05-27T06:26:04.000Z | [
"pytorch",
"bert",
"fill-mask",
"transformers",
"generated_from_trainer",
"license:apache-2.0",
"model-index",
"autotrain_compatible"
] | fill-mask | false | tanviraumi | null | tanviraumi/bert-base-uncased-issues-128 | 0 | null | transformers | 37,744 | ---
license: apache-2.0
tags:
- generated_from_trainer
model-index:
- name: bert-base-uncased-issues-128
results: []
---
<!-- This model card has been generated automatically according to the information the Trainer had access to. You
should probably proofread and complete it, then remove this comment. -->
# bert-base-uncased-issues-128
This model is a fine-tuned version of [bert-base-uncased](https://huggingface.co/bert-base-uncased) on the None dataset.
It achieves the following results on the evaluation set:
- Loss: 1.2337
## Model description
More information needed
## Intended uses & limitations
More information needed
## Training and evaluation data
More information needed
## Training procedure
### Training hyperparameters
The following hyperparameters were used during training:
- learning_rate: 5e-05
- train_batch_size: 128
- eval_batch_size: 32
- seed: 42
- optimizer: Adam with betas=(0.9,0.999) and epsilon=1e-08
- lr_scheduler_type: linear
- num_epochs: 16
### Training results
| Training Loss | Epoch | Step | Validation Loss |
|:-------------:|:-----:|:----:|:---------------:|
| 2.3389 | 1.0 | 73 | 1.7400 |
| 1.8014 | 2.0 | 146 | 1.4690 |
| 1.634 | 3.0 | 219 | 1.4783 |
| 1.5461 | 4.0 | 292 | 1.3912 |
| 1.4706 | 5.0 | 365 | 1.3109 |
| 1.4161 | 6.0 | 438 | 1.3405 |
| 1.3664 | 7.0 | 511 | 1.3459 |
| 1.332 | 8.0 | 584 | 1.2745 |
| 1.3029 | 9.0 | 657 | 1.2633 |
| 1.2871 | 10.0 | 730 | 1.2336 |
| 1.2807 | 11.0 | 803 | 1.2966 |
| 1.2569 | 12.0 | 876 | 1.1508 |
| 1.2392 | 13.0 | 949 | 1.2530 |
| 1.237 | 14.0 | 1022 | 1.2485 |
| 1.2169 | 15.0 | 1095 | 1.2592 |
| 1.2272 | 16.0 | 1168 | 1.2337 |
### Framework versions
- Transformers 4.19.1
- Pytorch 1.12.0.dev20220513+cu113
- Datasets 2.2.1
- Tokenizers 0.12.1
|
Splend1dchan/t5small-squad-extractive | 2d10400e2ffa79a42fe172961cbec8433f9eac6c | 2022-05-27T07:48:00.000Z | [
"pytorch",
"tensorboard",
"dataset:squad",
"generated_from_trainer",
"license:apache-2.0",
"model-index"
] | null | false | Splend1dchan | null | Splend1dchan/t5small-squad-extractive | 0 | null | null | 37,745 | ---
license: apache-2.0
tags:
- generated_from_trainer
datasets:
- squad
model-index:
- name: t5_squad
results: []
---
<!-- This model card has been generated automatically according to the information the Trainer had access to. You
should probably proofread and complete it, then remove this comment. -->
# t5_squad
This model is a fine-tuned version of [t5-small](https://huggingface.co/t5-small) on the squad dataset, using the extractive method by isolating the encoder only.
## Model description
More information needed
## Intended uses & limitations
More information needed
## Training and evaluation data
More information needed
## Training procedure
### Training hyperparameters
The following hyperparameters were used during training:
- learning_rate: 3e-05
- train_batch_size: 12
- eval_batch_size: 8
- seed: 42
- optimizer: Adam with betas=(0.9,0.999) and epsilon=1e-08
- lr_scheduler_type: linear
- num_epochs: 3.0
### Training results
{
"epoch": 3.0,
"eval_exact_match": 70.06622516556291,
"eval_f1": 80.02993815400357,
"eval_samples": 10659
}
### Framework versions
- Transformers 4.20.0.dev0
- Pytorch 1.9.0+cu111
- Datasets 1.18.4
- Tokenizers 0.11.6
|
huggingtweets/terrybroad | 491b260dd73ea3b1246daafe79ac66b689464b2c | 2022-05-27T08:46:44.000Z | [
"pytorch",
"gpt2",
"text-generation",
"en",
"transformers",
"huggingtweets"
] | text-generation | false | huggingtweets | null | huggingtweets/terrybroad | 0 | null | transformers | 37,746 | ---
language: en
thumbnail: http://www.huggingtweets.com/terrybroad/1653641199493/predictions.png
tags:
- huggingtweets
widget:
- text: "My dream is"
---
<div class="inline-flex flex-col" style="line-height: 1.5;">
<div class="flex">
<div
style="display:inherit; margin-left: 4px; margin-right: 4px; width: 92px; height:92px; border-radius: 50%; background-size: cover; background-image: url('https://pbs.twimg.com/profile_images/1445695092325380098/Zk0H0J37_400x400.jpg')">
</div>
<div
style="display:none; margin-left: 4px; margin-right: 4px; width: 92px; height:92px; border-radius: 50%; background-size: cover; background-image: url('')">
</div>
<div
style="display:none; margin-left: 4px; margin-right: 4px; width: 92px; height:92px; border-radius: 50%; background-size: cover; background-image: url('')">
</div>
</div>
<div style="text-align: center; margin-top: 3px; font-size: 16px; font-weight: 800">π€ AI BOT π€</div>
<div style="text-align: center; font-size: 16px; font-weight: 800">Terence Broad</div>
<div style="text-align: center; font-size: 14px;">@terrybroad</div>
</div>
I was made with [huggingtweets](https://github.com/borisdayma/huggingtweets).
Create your own bot based on your favorite user with [the demo](https://colab.research.google.com/github/borisdayma/huggingtweets/blob/master/huggingtweets-demo.ipynb)!
## How does it work?
The model uses the following pipeline.
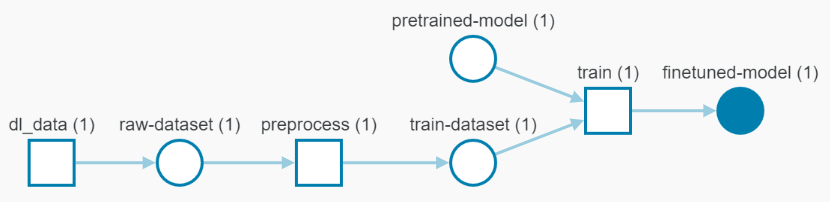
To understand how the model was developed, check the [W&B report](https://wandb.ai/wandb/huggingtweets/reports/HuggingTweets-Train-a-Model-to-Generate-Tweets--VmlldzoxMTY5MjI).
## Training data
The model was trained on tweets from Terence Broad.
| Data | Terence Broad |
| --- | --- |
| Tweets downloaded | 2248 |
| Retweets | 1230 |
| Short tweets | 231 |
| Tweets kept | 787 |
[Explore the data](https://wandb.ai/wandb/huggingtweets/runs/2v3f7i92/artifacts), which is tracked with [W&B artifacts](https://docs.wandb.com/artifacts) at every step of the pipeline.
## Training procedure
The model is based on a pre-trained [GPT-2](https://huggingface.co/gpt2) which is fine-tuned on @terrybroad's tweets.
Hyperparameters and metrics are recorded in the [W&B training run](https://wandb.ai/wandb/huggingtweets/runs/3fxvoi41) for full transparency and reproducibility.
At the end of training, [the final model](https://wandb.ai/wandb/huggingtweets/runs/3fxvoi41/artifacts) is logged and versioned.
## How to use
You can use this model directly with a pipeline for text generation:
```python
from transformers import pipeline
generator = pipeline('text-generation',
model='huggingtweets/terrybroad')
generator("My dream is", num_return_sequences=5)
```
## Limitations and bias
The model suffers from [the same limitations and bias as GPT-2](https://huggingface.co/gpt2#limitations-and-bias).
In addition, the data present in the user's tweets further affects the text generated by the model.
## About
*Built by Boris Dayma*
[](https://twitter.com/intent/follow?screen_name=borisdayma)
For more details, visit the project repository.
[](https://github.com/borisdayma/huggingtweets)
|
huggingtweets/mit_istnews | 44f043ee4253cfe5a669d4c5ee73942ecd29eeff | 2022-05-27T09:11:24.000Z | [
"pytorch",
"gpt2",
"text-generation",
"en",
"transformers",
"huggingtweets"
] | text-generation | false | huggingtweets | null | huggingtweets/mit_istnews | 0 | null | transformers | 37,747 | ---
language: en
thumbnail: http://www.huggingtweets.com/mit_istnews/1653642679545/predictions.png
tags:
- huggingtweets
widget:
- text: "My dream is"
---
<div class="inline-flex flex-col" style="line-height: 1.5;">
<div class="flex">
<div
style="display:inherit; margin-left: 4px; margin-right: 4px; width: 92px; height:92px; border-radius: 50%; background-size: cover; background-image: url('https://pbs.twimg.com/profile_images/875463526583857156/mxYzB8tm_400x400.jpg')">
</div>
<div
style="display:none; margin-left: 4px; margin-right: 4px; width: 92px; height:92px; border-radius: 50%; background-size: cover; background-image: url('')">
</div>
<div
style="display:none; margin-left: 4px; margin-right: 4px; width: 92px; height:92px; border-radius: 50%; background-size: cover; background-image: url('')">
</div>
</div>
<div style="text-align: center; margin-top: 3px; font-size: 16px; font-weight: 800">π€ AI BOT π€</div>
<div style="text-align: center; font-size: 16px; font-weight: 800">MIT IS&T</div>
<div style="text-align: center; font-size: 14px;">@mit_istnews</div>
</div>
I was made with [huggingtweets](https://github.com/borisdayma/huggingtweets).
Create your own bot based on your favorite user with [the demo](https://colab.research.google.com/github/borisdayma/huggingtweets/blob/master/huggingtweets-demo.ipynb)!
## How does it work?
The model uses the following pipeline.
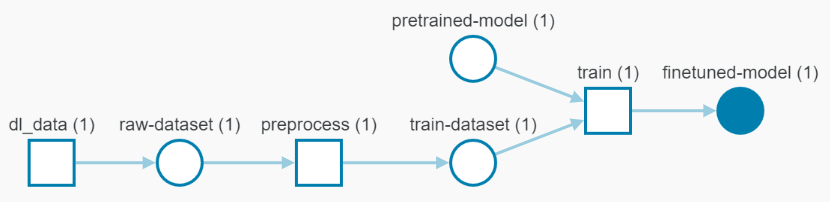
To understand how the model was developed, check the [W&B report](https://wandb.ai/wandb/huggingtweets/reports/HuggingTweets-Train-a-Model-to-Generate-Tweets--VmlldzoxMTY5MjI).
## Training data
The model was trained on tweets from MIT IS&T.
| Data | MIT IS&T |
| --- | --- |
| Tweets downloaded | 3250 |
| Retweets | 20 |
| Short tweets | 132 |
| Tweets kept | 3098 |
[Explore the data](https://wandb.ai/wandb/huggingtweets/runs/1b2tikho/artifacts), which is tracked with [W&B artifacts](https://docs.wandb.com/artifacts) at every step of the pipeline.
## Training procedure
The model is based on a pre-trained [GPT-2](https://huggingface.co/gpt2) which is fine-tuned on @mit_istnews's tweets.
Hyperparameters and metrics are recorded in the [W&B training run](https://wandb.ai/wandb/huggingtweets/runs/15k3tyvf) for full transparency and reproducibility.
At the end of training, [the final model](https://wandb.ai/wandb/huggingtweets/runs/15k3tyvf/artifacts) is logged and versioned.
## How to use
You can use this model directly with a pipeline for text generation:
```python
from transformers import pipeline
generator = pipeline('text-generation',
model='huggingtweets/mit_istnews')
generator("My dream is", num_return_sequences=5)
```
## Limitations and bias
The model suffers from [the same limitations and bias as GPT-2](https://huggingface.co/gpt2#limitations-and-bias).
In addition, the data present in the user's tweets further affects the text generated by the model.
## About
*Built by Boris Dayma*
[](https://twitter.com/intent/follow?screen_name=borisdayma)
For more details, visit the project repository.
[](https://github.com/borisdayma/huggingtweets)
|
huggingtweets/isaac_a_arthur | 95e007587641702cc757a24aa8a29d8743d98b58 | 2022-05-27T11:00:36.000Z | [
"pytorch",
"gpt2",
"text-generation",
"en",
"transformers",
"huggingtweets"
] | text-generation | false | huggingtweets | null | huggingtweets/isaac_a_arthur | 0 | null | transformers | 37,748 | ---
language: en
thumbnail: http://www.huggingtweets.com/isaac_a_arthur/1653649231789/predictions.png
tags:
- huggingtweets
widget:
- text: "My dream is"
---
<div class="inline-flex flex-col" style="line-height: 1.5;">
<div class="flex">
<div
style="display:inherit; margin-left: 4px; margin-right: 4px; width: 92px; height:92px; border-radius: 50%; background-size: cover; background-image: url('https://pbs.twimg.com/profile_images/1301946586331836421/at9dHQeU_400x400.jpg')">
</div>
<div
style="display:none; margin-left: 4px; margin-right: 4px; width: 92px; height:92px; border-radius: 50%; background-size: cover; background-image: url('')">
</div>
<div
style="display:none; margin-left: 4px; margin-right: 4px; width: 92px; height:92px; border-radius: 50%; background-size: cover; background-image: url('')">
</div>
</div>
<div style="text-align: center; margin-top: 3px; font-size: 16px; font-weight: 800">π€ AI BOT π€</div>
<div style="text-align: center; font-size: 16px; font-weight: 800">Isaac Arthur</div>
<div style="text-align: center; font-size: 14px;">@isaac_a_arthur</div>
</div>
I was made with [huggingtweets](https://github.com/borisdayma/huggingtweets).
Create your own bot based on your favorite user with [the demo](https://colab.research.google.com/github/borisdayma/huggingtweets/blob/master/huggingtweets-demo.ipynb)!
## How does it work?
The model uses the following pipeline.
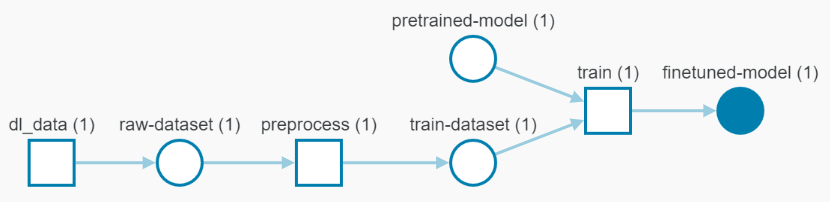
To understand how the model was developed, check the [W&B report](https://wandb.ai/wandb/huggingtweets/reports/HuggingTweets-Train-a-Model-to-Generate-Tweets--VmlldzoxMTY5MjI).
## Training data
The model was trained on tweets from Isaac Arthur.
| Data | Isaac Arthur |
| --- | --- |
| Tweets downloaded | 2697 |
| Retweets | 212 |
| Short tweets | 26 |
| Tweets kept | 2459 |
[Explore the data](https://wandb.ai/wandb/huggingtweets/runs/24wggcyw/artifacts), which is tracked with [W&B artifacts](https://docs.wandb.com/artifacts) at every step of the pipeline.
## Training procedure
The model is based on a pre-trained [GPT-2](https://huggingface.co/gpt2) which is fine-tuned on @isaac_a_arthur's tweets.
Hyperparameters and metrics are recorded in the [W&B training run](https://wandb.ai/wandb/huggingtweets/runs/2yxg71s3) for full transparency and reproducibility.
At the end of training, [the final model](https://wandb.ai/wandb/huggingtweets/runs/2yxg71s3/artifacts) is logged and versioned.
## How to use
You can use this model directly with a pipeline for text generation:
```python
from transformers import pipeline
generator = pipeline('text-generation',
model='huggingtweets/isaac_a_arthur')
generator("My dream is", num_return_sequences=5)
```
## Limitations and bias
The model suffers from [the same limitations and bias as GPT-2](https://huggingface.co/gpt2#limitations-and-bias).
In addition, the data present in the user's tweets further affects the text generated by the model.
## About
*Built by Boris Dayma*
[](https://twitter.com/intent/follow?screen_name=borisdayma)
For more details, visit the project repository.
[](https://github.com/borisdayma/huggingtweets)
|
huggingtweets/campbellclaret | 58904fd77558ec49596b713de0727b8a343deda5 | 2022-05-27T10:33:36.000Z | [
"pytorch",
"gpt2",
"text-generation",
"en",
"transformers",
"huggingtweets"
] | text-generation | false | huggingtweets | null | huggingtweets/campbellclaret | 0 | null | transformers | 37,749 | ---
language: en
thumbnail: http://www.huggingtweets.com/campbellclaret/1653647611538/predictions.png
tags:
- huggingtweets
widget:
- text: "My dream is"
---
<div class="inline-flex flex-col" style="line-height: 1.5;">
<div class="flex">
<div
style="display:inherit; margin-left: 4px; margin-right: 4px; width: 92px; height:92px; border-radius: 50%; background-size: cover; background-image: url('https://pbs.twimg.com/profile_images/1441638351052881920/13PTOAD0_400x400.jpg')">
</div>
<div
style="display:none; margin-left: 4px; margin-right: 4px; width: 92px; height:92px; border-radius: 50%; background-size: cover; background-image: url('')">
</div>
<div
style="display:none; margin-left: 4px; margin-right: 4px; width: 92px; height:92px; border-radius: 50%; background-size: cover; background-image: url('')">
</div>
</div>
<div style="text-align: center; margin-top: 3px; font-size: 16px; font-weight: 800">π€ AI BOT π€</div>
<div style="text-align: center; font-size: 16px; font-weight: 800">ALASTAIR CAMPBELL</div>
<div style="text-align: center; font-size: 14px;">@campbellclaret</div>
</div>
I was made with [huggingtweets](https://github.com/borisdayma/huggingtweets).
Create your own bot based on your favorite user with [the demo](https://colab.research.google.com/github/borisdayma/huggingtweets/blob/master/huggingtweets-demo.ipynb)!
## How does it work?
The model uses the following pipeline.
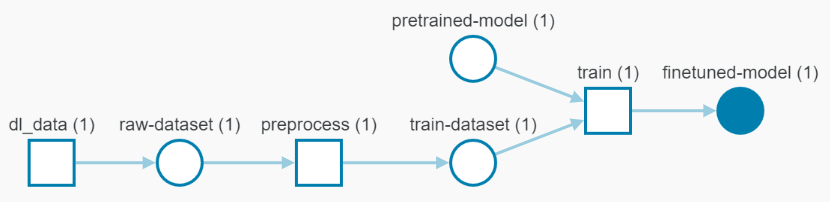
To understand how the model was developed, check the [W&B report](https://wandb.ai/wandb/huggingtweets/reports/HuggingTweets-Train-a-Model-to-Generate-Tweets--VmlldzoxMTY5MjI).
## Training data
The model was trained on tweets from ALASTAIR CAMPBELL.
| Data | ALASTAIR CAMPBELL |
| --- | --- |
| Tweets downloaded | 3239 |
| Retweets | 1921 |
| Short tweets | 112 |
| Tweets kept | 1206 |
[Explore the data](https://wandb.ai/wandb/huggingtweets/runs/1psic63j/artifacts), which is tracked with [W&B artifacts](https://docs.wandb.com/artifacts) at every step of the pipeline.
## Training procedure
The model is based on a pre-trained [GPT-2](https://huggingface.co/gpt2) which is fine-tuned on @campbellclaret's tweets.
Hyperparameters and metrics are recorded in the [W&B training run](https://wandb.ai/wandb/huggingtweets/runs/2bq64fuz) for full transparency and reproducibility.
At the end of training, [the final model](https://wandb.ai/wandb/huggingtweets/runs/2bq64fuz/artifacts) is logged and versioned.
## How to use
You can use this model directly with a pipeline for text generation:
```python
from transformers import pipeline
generator = pipeline('text-generation',
model='huggingtweets/campbellclaret')
generator("My dream is", num_return_sequences=5)
```
## Limitations and bias
The model suffers from [the same limitations and bias as GPT-2](https://huggingface.co/gpt2#limitations-and-bias).
In addition, the data present in the user's tweets further affects the text generated by the model.
## About
*Built by Boris Dayma*
[](https://twitter.com/intent/follow?screen_name=borisdayma)
For more details, visit the project repository.
[](https://github.com/borisdayma/huggingtweets)
|
Hemanth045/wav2vec2-large-xls-r-300m-hindi-colab | 77b4e45dcc8d19d97419f65a1bc7f963cf633d4a | 2022-05-30T18:13:35.000Z | [
"pytorch",
"tensorboard",
"wav2vec2",
"automatic-speech-recognition",
"dataset:common_voice",
"transformers",
"generated_from_trainer",
"license:apache-2.0",
"model-index"
] | automatic-speech-recognition | false | Hemanth045 | null | Hemanth045/wav2vec2-large-xls-r-300m-hindi-colab | 0 | null | transformers | 37,750 | ---
license: apache-2.0
tags:
- generated_from_trainer
datasets:
- common_voice
model-index:
- name: wav2vec2-large-xls-r-300m-hindi-colab
results: []
---
<!-- This model card has been generated automatically according to the information the Trainer had access to. You
should probably proofread and complete it, then remove this comment. -->
# wav2vec2-large-xls-r-300m-hindi-colab
This model is a fine-tuned version of [facebook/wav2vec2-xls-r-300m](https://huggingface.co/facebook/wav2vec2-xls-r-300m) on the common_voice dataset.
It achieves the following results on the evaluation set:
- Loss: 2.3273
- Wer: 0.9698
## Model description
More information needed
## Intended uses & limitations
More information needed
## Training and evaluation data
More information needed
## Training procedure
### Training hyperparameters
The following hyperparameters were used during training:
- learning_rate: 0.0003
- train_batch_size: 16
- eval_batch_size: 8
- seed: 42
- gradient_accumulation_steps: 2
- total_train_batch_size: 32
- optimizer: Adam with betas=(0.9,0.999) and epsilon=1e-08
- lr_scheduler_type: linear
- lr_scheduler_warmup_steps: 500
- num_epochs: 60
- mixed_precision_training: Native AMP
### Training results
| Training Loss | Epoch | Step | Validation Loss | Wer |
|:-------------:|:-----:|:----:|:---------------:|:------:|
| 6.6006 | 44.42 | 400 | 2.3273 | 0.9698 |
### Framework versions
- Transformers 4.11.3
- Pytorch 1.10.0+cu113
- Datasets 1.18.3
- Tokenizers 0.10.3
|
Splend1dchan/wav2vec2-large-lv60_t5lephone-small_speechfix_forExtractiveNMSQA | 20d9de2975c4f82938854b690923893fa3dfcd9d | 2022-06-07T19:44:13.000Z | [
"pytorch"
] | null | false | Splend1dchan | null | Splend1dchan/wav2vec2-large-lv60_t5lephone-small_speechfix_forExtractiveNMSQA | 0 | null | null | 37,751 | Entry not found |
huggingtweets/meliksahtas | 3375846b0a02ac76c5300f3ff69effa5497981a9 | 2022-05-27T11:01:12.000Z | [
"pytorch",
"gpt2",
"text-generation",
"en",
"transformers",
"huggingtweets"
] | text-generation | false | huggingtweets | null | huggingtweets/meliksahtas | 0 | null | transformers | 37,752 | ---
language: en
thumbnail: http://www.huggingtweets.com/meliksahtas/1653649268087/predictions.png
tags:
- huggingtweets
widget:
- text: "My dream is"
---
<div class="inline-flex flex-col" style="line-height: 1.5;">
<div class="flex">
<div
style="display:inherit; margin-left: 4px; margin-right: 4px; width: 92px; height:92px; border-radius: 50%; background-size: cover; background-image: url('https://pbs.twimg.com/profile_images/1229167506386014212/FKKauJpF_400x400.jpg')">
</div>
<div
style="display:none; margin-left: 4px; margin-right: 4px; width: 92px; height:92px; border-radius: 50%; background-size: cover; background-image: url('')">
</div>
<div
style="display:none; margin-left: 4px; margin-right: 4px; width: 92px; height:92px; border-radius: 50%; background-size: cover; background-image: url('')">
</div>
</div>
<div style="text-align: center; margin-top: 3px; font-size: 16px; font-weight: 800">π€ AI BOT π€</div>
<div style="text-align: center; font-size: 16px; font-weight: 800">meliksahtas</div>
<div style="text-align: center; font-size: 14px;">@meliksahtas</div>
</div>
I was made with [huggingtweets](https://github.com/borisdayma/huggingtweets).
Create your own bot based on your favorite user with [the demo](https://colab.research.google.com/github/borisdayma/huggingtweets/blob/master/huggingtweets-demo.ipynb)!
## How does it work?
The model uses the following pipeline.
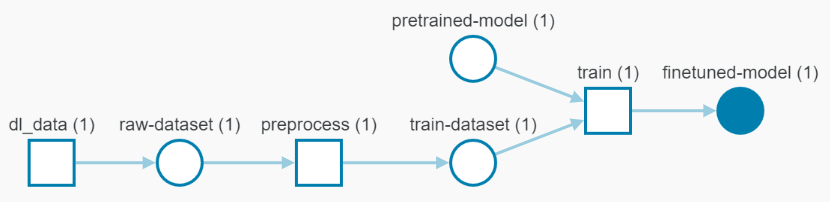
To understand how the model was developed, check the [W&B report](https://wandb.ai/wandb/huggingtweets/reports/HuggingTweets-Train-a-Model-to-Generate-Tweets--VmlldzoxMTY5MjI).
## Training data
The model was trained on tweets from meliksahtas.
| Data | meliksahtas |
| --- | --- |
| Tweets downloaded | 3247 |
| Retweets | 154 |
| Short tweets | 202 |
| Tweets kept | 2891 |
[Explore the data](https://wandb.ai/wandb/huggingtweets/runs/1ibkvi4w/artifacts), which is tracked with [W&B artifacts](https://docs.wandb.com/artifacts) at every step of the pipeline.
## Training procedure
The model is based on a pre-trained [GPT-2](https://huggingface.co/gpt2) which is fine-tuned on @meliksahtas's tweets.
Hyperparameters and metrics are recorded in the [W&B training run](https://wandb.ai/wandb/huggingtweets/runs/6flysmzm) for full transparency and reproducibility.
At the end of training, [the final model](https://wandb.ai/wandb/huggingtweets/runs/6flysmzm/artifacts) is logged and versioned.
## How to use
You can use this model directly with a pipeline for text generation:
```python
from transformers import pipeline
generator = pipeline('text-generation',
model='huggingtweets/meliksahtas')
generator("My dream is", num_return_sequences=5)
```
## Limitations and bias
The model suffers from [the same limitations and bias as GPT-2](https://huggingface.co/gpt2#limitations-and-bias).
In addition, the data present in the user's tweets further affects the text generated by the model.
## About
*Built by Boris Dayma*
[](https://twitter.com/intent/follow?screen_name=borisdayma)
For more details, visit the project repository.
[](https://github.com/borisdayma/huggingtweets)
|
huggingtweets/donhertzfeldt | b0745e5e35fb7bfb9891c44c3a090185cad8035c | 2022-05-27T11:02:23.000Z | [
"pytorch",
"gpt2",
"text-generation",
"en",
"transformers",
"huggingtweets"
] | text-generation | false | huggingtweets | null | huggingtweets/donhertzfeldt | 0 | null | transformers | 37,753 | ---
language: en
thumbnail: http://www.huggingtweets.com/donhertzfeldt/1653649338459/predictions.png
tags:
- huggingtweets
widget:
- text: "My dream is"
---
<div class="inline-flex flex-col" style="line-height: 1.5;">
<div class="flex">
<div
style="display:inherit; margin-left: 4px; margin-right: 4px; width: 92px; height:92px; border-radius: 50%; background-size: cover; background-image: url('https://pbs.twimg.com/profile_images/1617966805/star-avatar_400x400.jpg')">
</div>
<div
style="display:none; margin-left: 4px; margin-right: 4px; width: 92px; height:92px; border-radius: 50%; background-size: cover; background-image: url('')">
</div>
<div
style="display:none; margin-left: 4px; margin-right: 4px; width: 92px; height:92px; border-radius: 50%; background-size: cover; background-image: url('')">
</div>
</div>
<div style="text-align: center; margin-top: 3px; font-size: 16px; font-weight: 800">π€ AI BOT π€</div>
<div style="text-align: center; font-size: 16px; font-weight: 800">don hertzfeldt</div>
<div style="text-align: center; font-size: 14px;">@donhertzfeldt</div>
</div>
I was made with [huggingtweets](https://github.com/borisdayma/huggingtweets).
Create your own bot based on your favorite user with [the demo](https://colab.research.google.com/github/borisdayma/huggingtweets/blob/master/huggingtweets-demo.ipynb)!
## How does it work?
The model uses the following pipeline.
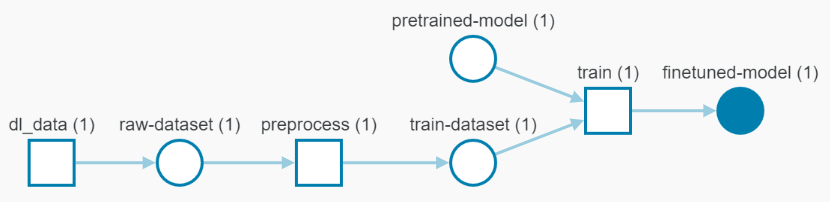
To understand how the model was developed, check the [W&B report](https://wandb.ai/wandb/huggingtweets/reports/HuggingTweets-Train-a-Model-to-Generate-Tweets--VmlldzoxMTY5MjI).
## Training data
The model was trained on tweets from don hertzfeldt.
| Data | don hertzfeldt |
| --- | --- |
| Tweets downloaded | 2513 |
| Retweets | 707 |
| Short tweets | 406 |
| Tweets kept | 1400 |
[Explore the data](https://wandb.ai/wandb/huggingtweets/runs/258eoxxi/artifacts), which is tracked with [W&B artifacts](https://docs.wandb.com/artifacts) at every step of the pipeline.
## Training procedure
The model is based on a pre-trained [GPT-2](https://huggingface.co/gpt2) which is fine-tuned on @donhertzfeldt's tweets.
Hyperparameters and metrics are recorded in the [W&B training run](https://wandb.ai/wandb/huggingtweets/runs/wxdijpch) for full transparency and reproducibility.
At the end of training, [the final model](https://wandb.ai/wandb/huggingtweets/runs/wxdijpch/artifacts) is logged and versioned.
## How to use
You can use this model directly with a pipeline for text generation:
```python
from transformers import pipeline
generator = pipeline('text-generation',
model='huggingtweets/donhertzfeldt')
generator("My dream is", num_return_sequences=5)
```
## Limitations and bias
The model suffers from [the same limitations and bias as GPT-2](https://huggingface.co/gpt2#limitations-and-bias).
In addition, the data present in the user's tweets further affects the text generated by the model.
## About
*Built by Boris Dayma*
[](https://twitter.com/intent/follow?screen_name=borisdayma)
For more details, visit the project repository.
[](https://github.com/borisdayma/huggingtweets)
|
huggingtweets/ancientorigins | f79ab280353c3d25edf61f898d446665d587f55d | 2022-05-27T11:03:39.000Z | [
"pytorch",
"gpt2",
"text-generation",
"en",
"transformers",
"huggingtweets"
] | text-generation | false | huggingtweets | null | huggingtweets/ancientorigins | 0 | null | transformers | 37,754 | ---
language: en
thumbnail: http://www.huggingtweets.com/ancientorigins/1653649414414/predictions.png
tags:
- huggingtweets
widget:
- text: "My dream is"
---
<div class="inline-flex flex-col" style="line-height: 1.5;">
<div class="flex">
<div
style="display:inherit; margin-left: 4px; margin-right: 4px; width: 92px; height:92px; border-radius: 50%; background-size: cover; background-image: url('https://pbs.twimg.com/profile_images/862334074702180352/Fjv-Np86_400x400.jpg')">
</div>
<div
style="display:none; margin-left: 4px; margin-right: 4px; width: 92px; height:92px; border-radius: 50%; background-size: cover; background-image: url('')">
</div>
<div
style="display:none; margin-left: 4px; margin-right: 4px; width: 92px; height:92px; border-radius: 50%; background-size: cover; background-image: url('')">
</div>
</div>
<div style="text-align: center; margin-top: 3px; font-size: 16px; font-weight: 800">π€ AI BOT π€</div>
<div style="text-align: center; font-size: 16px; font-weight: 800">Ancient Origins</div>
<div style="text-align: center; font-size: 14px;">@ancientorigins</div>
</div>
I was made with [huggingtweets](https://github.com/borisdayma/huggingtweets).
Create your own bot based on your favorite user with [the demo](https://colab.research.google.com/github/borisdayma/huggingtweets/blob/master/huggingtweets-demo.ipynb)!
## How does it work?
The model uses the following pipeline.
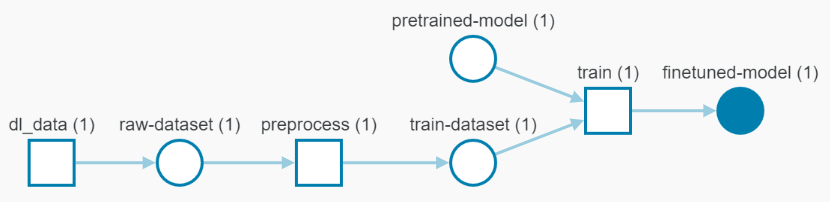
To understand how the model was developed, check the [W&B report](https://wandb.ai/wandb/huggingtweets/reports/HuggingTweets-Train-a-Model-to-Generate-Tweets--VmlldzoxMTY5MjI).
## Training data
The model was trained on tweets from Ancient Origins.
| Data | Ancient Origins |
| --- | --- |
| Tweets downloaded | 3250 |
| Retweets | 0 |
| Short tweets | 145 |
| Tweets kept | 3105 |
[Explore the data](https://wandb.ai/wandb/huggingtweets/runs/1ec3pwlj/artifacts), which is tracked with [W&B artifacts](https://docs.wandb.com/artifacts) at every step of the pipeline.
## Training procedure
The model is based on a pre-trained [GPT-2](https://huggingface.co/gpt2) which is fine-tuned on @ancientorigins's tweets.
Hyperparameters and metrics are recorded in the [W&B training run](https://wandb.ai/wandb/huggingtweets/runs/2ud8iwl7) for full transparency and reproducibility.
At the end of training, [the final model](https://wandb.ai/wandb/huggingtweets/runs/2ud8iwl7/artifacts) is logged and versioned.
## How to use
You can use this model directly with a pipeline for text generation:
```python
from transformers import pipeline
generator = pipeline('text-generation',
model='huggingtweets/ancientorigins')
generator("My dream is", num_return_sequences=5)
```
## Limitations and bias
The model suffers from [the same limitations and bias as GPT-2](https://huggingface.co/gpt2#limitations-and-bias).
In addition, the data present in the user's tweets further affects the text generated by the model.
## About
*Built by Boris Dayma*
[](https://twitter.com/intent/follow?screen_name=borisdayma)
For more details, visit the project repository.
[](https://github.com/borisdayma/huggingtweets)
|
huggingtweets/lolesports | fb9d7fc9c3b352bc466a472b7859ec2e480d45ee | 2022-05-27T11:10:55.000Z | [
"pytorch",
"gpt2",
"text-generation",
"en",
"transformers",
"huggingtweets"
] | text-generation | false | huggingtweets | null | huggingtweets/lolesports | 0 | null | transformers | 37,755 | ---
language: en
thumbnail: http://www.huggingtweets.com/lolesports/1653649850984/predictions.png
tags:
- huggingtweets
widget:
- text: "My dream is"
---
<div class="inline-flex flex-col" style="line-height: 1.5;">
<div class="flex">
<div
style="display:inherit; margin-left: 4px; margin-right: 4px; width: 92px; height:92px; border-radius: 50%; background-size: cover; background-image: url('https://pbs.twimg.com/profile_images/1522560089290592257/5TZEqZ0e_400x400.jpg')">
</div>
<div
style="display:none; margin-left: 4px; margin-right: 4px; width: 92px; height:92px; border-radius: 50%; background-size: cover; background-image: url('')">
</div>
<div
style="display:none; margin-left: 4px; margin-right: 4px; width: 92px; height:92px; border-radius: 50%; background-size: cover; background-image: url('')">
</div>
</div>
<div style="text-align: center; margin-top: 3px; font-size: 16px; font-weight: 800">π€ AI BOT π€</div>
<div style="text-align: center; font-size: 16px; font-weight: 800">LoL Esports</div>
<div style="text-align: center; font-size: 14px;">@lolesports</div>
</div>
I was made with [huggingtweets](https://github.com/borisdayma/huggingtweets).
Create your own bot based on your favorite user with [the demo](https://colab.research.google.com/github/borisdayma/huggingtweets/blob/master/huggingtweets-demo.ipynb)!
## How does it work?
The model uses the following pipeline.
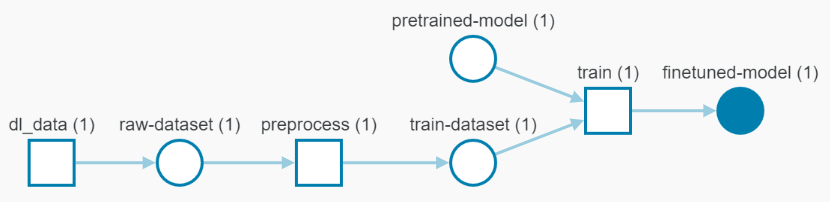
To understand how the model was developed, check the [W&B report](https://wandb.ai/wandb/huggingtweets/reports/HuggingTweets-Train-a-Model-to-Generate-Tweets--VmlldzoxMTY5MjI).
## Training data
The model was trained on tweets from LoL Esports.
| Data | LoL Esports |
| --- | --- |
| Tweets downloaded | 3250 |
| Retweets | 569 |
| Short tweets | 470 |
| Tweets kept | 2211 |
[Explore the data](https://wandb.ai/wandb/huggingtweets/runs/1lq68u80/artifacts), which is tracked with [W&B artifacts](https://docs.wandb.com/artifacts) at every step of the pipeline.
## Training procedure
The model is based on a pre-trained [GPT-2](https://huggingface.co/gpt2) which is fine-tuned on @lolesports's tweets.
Hyperparameters and metrics are recorded in the [W&B training run](https://wandb.ai/wandb/huggingtweets/runs/mskpd4dr) for full transparency and reproducibility.
At the end of training, [the final model](https://wandb.ai/wandb/huggingtweets/runs/mskpd4dr/artifacts) is logged and versioned.
## How to use
You can use this model directly with a pipeline for text generation:
```python
from transformers import pipeline
generator = pipeline('text-generation',
model='huggingtweets/lolesports')
generator("My dream is", num_return_sequences=5)
```
## Limitations and bias
The model suffers from [the same limitations and bias as GPT-2](https://huggingface.co/gpt2#limitations-and-bias).
In addition, the data present in the user's tweets further affects the text generated by the model.
## About
*Built by Boris Dayma*
[](https://twitter.com/intent/follow?screen_name=borisdayma)
For more details, visit the project repository.
[](https://github.com/borisdayma/huggingtweets)
|
huggingtweets/alejodorowsky | 642edb36bf797fc9ef8b42478a1d0f91e9b3063c | 2022-05-27T11:13:26.000Z | [
"pytorch",
"gpt2",
"text-generation",
"en",
"transformers",
"huggingtweets"
] | text-generation | false | huggingtweets | null | huggingtweets/alejodorowsky | 0 | null | transformers | 37,756 | ---
language: en
thumbnail: http://www.huggingtweets.com/alejodorowsky/1653650001771/predictions.png
tags:
- huggingtweets
widget:
- text: "My dream is"
---
<div class="inline-flex flex-col" style="line-height: 1.5;">
<div class="flex">
<div
style="display:inherit; margin-left: 4px; margin-right: 4px; width: 92px; height:92px; border-radius: 50%; background-size: cover; background-image: url('https://pbs.twimg.com/profile_images/784393032774873088/1x6o_3ws_400x400.jpg')">
</div>
<div
style="display:none; margin-left: 4px; margin-right: 4px; width: 92px; height:92px; border-radius: 50%; background-size: cover; background-image: url('')">
</div>
<div
style="display:none; margin-left: 4px; margin-right: 4px; width: 92px; height:92px; border-radius: 50%; background-size: cover; background-image: url('')">
</div>
</div>
<div style="text-align: center; margin-top: 3px; font-size: 16px; font-weight: 800">π€ AI BOT π€</div>
<div style="text-align: center; font-size: 16px; font-weight: 800">Alejandro Jodorowsky</div>
<div style="text-align: center; font-size: 14px;">@alejodorowsky</div>
</div>
I was made with [huggingtweets](https://github.com/borisdayma/huggingtweets).
Create your own bot based on your favorite user with [the demo](https://colab.research.google.com/github/borisdayma/huggingtweets/blob/master/huggingtweets-demo.ipynb)!
## How does it work?
The model uses the following pipeline.
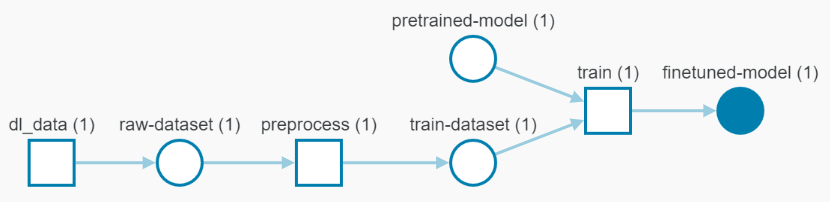
To understand how the model was developed, check the [W&B report](https://wandb.ai/wandb/huggingtweets/reports/HuggingTweets-Train-a-Model-to-Generate-Tweets--VmlldzoxMTY5MjI).
## Training data
The model was trained on tweets from Alejandro Jodorowsky.
| Data | Alejandro Jodorowsky |
| --- | --- |
| Tweets downloaded | 3245 |
| Retweets | 640 |
| Short tweets | 175 |
| Tweets kept | 2430 |
[Explore the data](https://wandb.ai/wandb/huggingtweets/runs/1vwsnx64/artifacts), which is tracked with [W&B artifacts](https://docs.wandb.com/artifacts) at every step of the pipeline.
## Training procedure
The model is based on a pre-trained [GPT-2](https://huggingface.co/gpt2) which is fine-tuned on @alejodorowsky's tweets.
Hyperparameters and metrics are recorded in the [W&B training run](https://wandb.ai/wandb/huggingtweets/runs/j8ai679x) for full transparency and reproducibility.
At the end of training, [the final model](https://wandb.ai/wandb/huggingtweets/runs/j8ai679x/artifacts) is logged and versioned.
## How to use
You can use this model directly with a pipeline for text generation:
```python
from transformers import pipeline
generator = pipeline('text-generation',
model='huggingtweets/alejodorowsky')
generator("My dream is", num_return_sequences=5)
```
## Limitations and bias
The model suffers from [the same limitations and bias as GPT-2](https://huggingface.co/gpt2#limitations-and-bias).
In addition, the data present in the user's tweets further affects the text generated by the model.
## About
*Built by Boris Dayma*
[](https://twitter.com/intent/follow?screen_name=borisdayma)
For more details, visit the project repository.
[](https://github.com/borisdayma/huggingtweets)
|
huggingtweets/mrbean | 103670f01465008ee0d3384d2f3b0f9b97a4b531 | 2022-05-27T11:30:30.000Z | [
"pytorch",
"gpt2",
"text-generation",
"en",
"transformers",
"huggingtweets"
] | text-generation | false | huggingtweets | null | huggingtweets/mrbean | 0 | null | transformers | 37,757 | ---
language: en
thumbnail: http://www.huggingtweets.com/mrbean/1653651025192/predictions.png
tags:
- huggingtweets
widget:
- text: "My dream is"
---
<div class="inline-flex flex-col" style="line-height: 1.5;">
<div class="flex">
<div
style="display:inherit; margin-left: 4px; margin-right: 4px; width: 92px; height:92px; border-radius: 50%; background-size: cover; background-image: url('https://pbs.twimg.com/profile_images/521655203011899392/pxOndDc7_400x400.png')">
</div>
<div
style="display:none; margin-left: 4px; margin-right: 4px; width: 92px; height:92px; border-radius: 50%; background-size: cover; background-image: url('')">
</div>
<div
style="display:none; margin-left: 4px; margin-right: 4px; width: 92px; height:92px; border-radius: 50%; background-size: cover; background-image: url('')">
</div>
</div>
<div style="text-align: center; margin-top: 3px; font-size: 16px; font-weight: 800">π€ AI BOT π€</div>
<div style="text-align: center; font-size: 16px; font-weight: 800">Mr Bean</div>
<div style="text-align: center; font-size: 14px;">@mrbean</div>
</div>
I was made with [huggingtweets](https://github.com/borisdayma/huggingtweets).
Create your own bot based on your favorite user with [the demo](https://colab.research.google.com/github/borisdayma/huggingtweets/blob/master/huggingtweets-demo.ipynb)!
## How does it work?
The model uses the following pipeline.
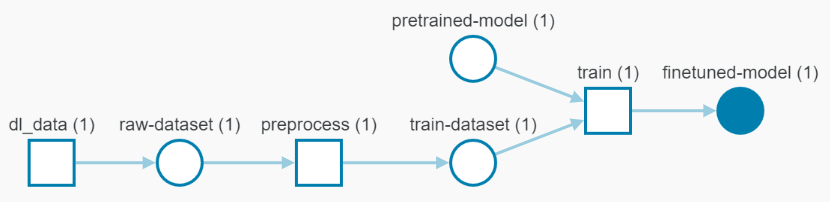
To understand how the model was developed, check the [W&B report](https://wandb.ai/wandb/huggingtweets/reports/HuggingTweets-Train-a-Model-to-Generate-Tweets--VmlldzoxMTY5MjI).
## Training data
The model was trained on tweets from Mr Bean.
| Data | Mr Bean |
| --- | --- |
| Tweets downloaded | 2324 |
| Retweets | 156 |
| Short tweets | 271 |
| Tweets kept | 1897 |
[Explore the data](https://wandb.ai/wandb/huggingtweets/runs/1nqdk593/artifacts), which is tracked with [W&B artifacts](https://docs.wandb.com/artifacts) at every step of the pipeline.
## Training procedure
The model is based on a pre-trained [GPT-2](https://huggingface.co/gpt2) which is fine-tuned on @mrbean's tweets.
Hyperparameters and metrics are recorded in the [W&B training run](https://wandb.ai/wandb/huggingtweets/runs/27zl3ib7) for full transparency and reproducibility.
At the end of training, [the final model](https://wandb.ai/wandb/huggingtweets/runs/27zl3ib7/artifacts) is logged and versioned.
## How to use
You can use this model directly with a pipeline for text generation:
```python
from transformers import pipeline
generator = pipeline('text-generation',
model='huggingtweets/mrbean')
generator("My dream is", num_return_sequences=5)
```
## Limitations and bias
The model suffers from [the same limitations and bias as GPT-2](https://huggingface.co/gpt2#limitations-and-bias).
In addition, the data present in the user's tweets further affects the text generated by the model.
## About
*Built by Boris Dayma*
[](https://twitter.com/intent/follow?screen_name=borisdayma)
For more details, visit the project repository.
[](https://github.com/borisdayma/huggingtweets)
|
huggingtweets/neinquarterly | 8100b3d4912f1e519bc5a3670fc88d108c9d4f42 | 2022-05-27T11:18:48.000Z | [
"pytorch",
"gpt2",
"text-generation",
"en",
"transformers",
"huggingtweets"
] | text-generation | false | huggingtweets | null | huggingtweets/neinquarterly | 0 | null | transformers | 37,758 | ---
language: en
thumbnail: http://www.huggingtweets.com/neinquarterly/1653650323364/predictions.png
tags:
- huggingtweets
widget:
- text: "My dream is"
---
<div class="inline-flex flex-col" style="line-height: 1.5;">
<div class="flex">
<div
style="display:inherit; margin-left: 4px; margin-right: 4px; width: 92px; height:92px; border-radius: 50%; background-size: cover; background-image: url('https://pbs.twimg.com/profile_images/702248569324093441/5HWfjcOQ_400x400.jpg')">
</div>
<div
style="display:none; margin-left: 4px; margin-right: 4px; width: 92px; height:92px; border-radius: 50%; background-size: cover; background-image: url('')">
</div>
<div
style="display:none; margin-left: 4px; margin-right: 4px; width: 92px; height:92px; border-radius: 50%; background-size: cover; background-image: url('')">
</div>
</div>
<div style="text-align: center; margin-top: 3px; font-size: 16px; font-weight: 800">π€ AI BOT π€</div>
<div style="text-align: center; font-size: 16px; font-weight: 800">Nein.</div>
<div style="text-align: center; font-size: 14px;">@neinquarterly</div>
</div>
I was made with [huggingtweets](https://github.com/borisdayma/huggingtweets).
Create your own bot based on your favorite user with [the demo](https://colab.research.google.com/github/borisdayma/huggingtweets/blob/master/huggingtweets-demo.ipynb)!
## How does it work?
The model uses the following pipeline.
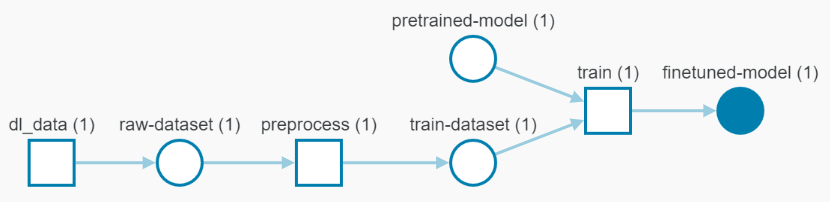
To understand how the model was developed, check the [W&B report](https://wandb.ai/wandb/huggingtweets/reports/HuggingTweets-Train-a-Model-to-Generate-Tweets--VmlldzoxMTY5MjI).
## Training data
The model was trained on tweets from Nein..
| Data | Nein. |
| --- | --- |
| Tweets downloaded | 3192 |
| Retweets | 156 |
| Short tweets | 117 |
| Tweets kept | 2919 |
[Explore the data](https://wandb.ai/wandb/huggingtweets/runs/3h1p4qh6/artifacts), which is tracked with [W&B artifacts](https://docs.wandb.com/artifacts) at every step of the pipeline.
## Training procedure
The model is based on a pre-trained [GPT-2](https://huggingface.co/gpt2) which is fine-tuned on @neinquarterly's tweets.
Hyperparameters and metrics are recorded in the [W&B training run](https://wandb.ai/wandb/huggingtweets/runs/35nwfk8z) for full transparency and reproducibility.
At the end of training, [the final model](https://wandb.ai/wandb/huggingtweets/runs/35nwfk8z/artifacts) is logged and versioned.
## How to use
You can use this model directly with a pipeline for text generation:
```python
from transformers import pipeline
generator = pipeline('text-generation',
model='huggingtweets/neinquarterly')
generator("My dream is", num_return_sequences=5)
```
## Limitations and bias
The model suffers from [the same limitations and bias as GPT-2](https://huggingface.co/gpt2#limitations-and-bias).
In addition, the data present in the user's tweets further affects the text generated by the model.
## About
*Built by Boris Dayma*
[](https://twitter.com/intent/follow?screen_name=borisdayma)
For more details, visit the project repository.
[](https://github.com/borisdayma/huggingtweets)
|
huggingtweets/emilythornberry | 2810568f75c52f7e170c3a2d2a4aec01760c0d36 | 2022-05-27T11:19:25.000Z | [
"pytorch",
"gpt2",
"text-generation",
"en",
"transformers",
"huggingtweets"
] | text-generation | false | huggingtweets | null | huggingtweets/emilythornberry | 0 | null | transformers | 37,759 | ---
language: en
thumbnail: https://github.com/borisdayma/huggingtweets/blob/master/img/logo.png?raw=true
tags:
- huggingtweets
widget:
- text: "My dream is"
---
<div class="inline-flex flex-col" style="line-height: 1.5;">
<div class="flex">
<div
style="display:inherit; margin-left: 4px; margin-right: 4px; width: 92px; height:92px; border-radius: 50%; background-size: cover; background-image: url('https://pbs.twimg.com/profile_images/1446231256052731905/octqXaR9_400x400.jpg')">
</div>
<div
style="display:none; margin-left: 4px; margin-right: 4px; width: 92px; height:92px; border-radius: 50%; background-size: cover; background-image: url('')">
</div>
<div
style="display:none; margin-left: 4px; margin-right: 4px; width: 92px; height:92px; border-radius: 50%; background-size: cover; background-image: url('')">
</div>
</div>
<div style="text-align: center; margin-top: 3px; font-size: 16px; font-weight: 800">π€ AI BOT π€</div>
<div style="text-align: center; font-size: 16px; font-weight: 800">Emily Thornberry</div>
<div style="text-align: center; font-size: 14px;">@emilythornberry</div>
</div>
I was made with [huggingtweets](https://github.com/borisdayma/huggingtweets).
Create your own bot based on your favorite user with [the demo](https://colab.research.google.com/github/borisdayma/huggingtweets/blob/master/huggingtweets-demo.ipynb)!
## How does it work?
The model uses the following pipeline.
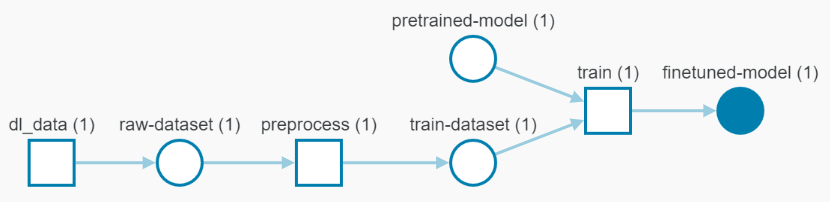
To understand how the model was developed, check the [W&B report](https://wandb.ai/wandb/huggingtweets/reports/HuggingTweets-Train-a-Model-to-Generate-Tweets--VmlldzoxMTY5MjI).
## Training data
The model was trained on tweets from Emily Thornberry.
| Data | Emily Thornberry |
| --- | --- |
| Tweets downloaded | 3234 |
| Retweets | 1153 |
| Short tweets | 274 |
| Tweets kept | 1807 |
[Explore the data](https://wandb.ai/wandb/huggingtweets/runs/gag2yg4r/artifacts), which is tracked with [W&B artifacts](https://docs.wandb.com/artifacts) at every step of the pipeline.
## Training procedure
The model is based on a pre-trained [GPT-2](https://huggingface.co/gpt2) which is fine-tuned on @emilythornberry's tweets.
Hyperparameters and metrics are recorded in the [W&B training run](https://wandb.ai/wandb/huggingtweets/runs/2zsqk4sk) for full transparency and reproducibility.
At the end of training, [the final model](https://wandb.ai/wandb/huggingtweets/runs/2zsqk4sk/artifacts) is logged and versioned.
## How to use
You can use this model directly with a pipeline for text generation:
```python
from transformers import pipeline
generator = pipeline('text-generation',
model='huggingtweets/emilythornberry')
generator("My dream is", num_return_sequences=5)
```
## Limitations and bias
The model suffers from [the same limitations and bias as GPT-2](https://huggingface.co/gpt2#limitations-and-bias).
In addition, the data present in the user's tweets further affects the text generated by the model.
## About
*Built by Boris Dayma*
[](https://twitter.com/intent/follow?screen_name=borisdayma)
For more details, visit the project repository.
[](https://github.com/borisdayma/huggingtweets)
|
huggingtweets/liwenliang | 9fbb3de3f9bacc0a9517ed5d1c873912b4c7a193 | 2022-05-27T11:26:23.000Z | [
"pytorch",
"gpt2",
"text-generation",
"en",
"transformers",
"huggingtweets"
] | text-generation | false | huggingtweets | null | huggingtweets/liwenliang | 0 | null | transformers | 37,760 | ---
language: en
thumbnail: http://www.huggingtweets.com/liwenliang/1653650598585/predictions.png
tags:
- huggingtweets
widget:
- text: "My dream is"
---
<div class="inline-flex flex-col" style="line-height: 1.5;">
<div class="flex">
<div
style="display:inherit; margin-left: 4px; margin-right: 4px; width: 92px; height:92px; border-radius: 50%; background-size: cover; background-image: url('https://pbs.twimg.com/profile_images/1197224526175784968/7n8Q3j05_400x400.jpg')">
</div>
<div
style="display:none; margin-left: 4px; margin-right: 4px; width: 92px; height:92px; border-radius: 50%; background-size: cover; background-image: url('')">
</div>
<div
style="display:none; margin-left: 4px; margin-right: 4px; width: 92px; height:92px; border-radius: 50%; background-size: cover; background-image: url('')">
</div>
</div>
<div style="text-align: center; margin-top: 3px; font-size: 16px; font-weight: 800">π€ AI BOT π€</div>
<div style="text-align: center; font-size: 16px; font-weight: 800">Kevin Li</div>
<div style="text-align: center; font-size: 14px;">@liwenliang</div>
</div>
I was made with [huggingtweets](https://github.com/borisdayma/huggingtweets).
Create your own bot based on your favorite user with [the demo](https://colab.research.google.com/github/borisdayma/huggingtweets/blob/master/huggingtweets-demo.ipynb)!
## How does it work?
The model uses the following pipeline.
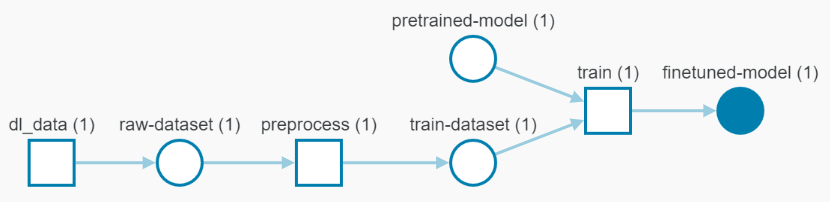
To understand how the model was developed, check the [W&B report](https://wandb.ai/wandb/huggingtweets/reports/HuggingTweets-Train-a-Model-to-Generate-Tweets--VmlldzoxMTY5MjI).
## Training data
The model was trained on tweets from Kevin Li.
| Data | Kevin Li |
| --- | --- |
| Tweets downloaded | 108 |
| Retweets | 21 |
| Short tweets | 5 |
| Tweets kept | 82 |
[Explore the data](https://wandb.ai/wandb/huggingtweets/runs/k8wvicoq/artifacts), which is tracked with [W&B artifacts](https://docs.wandb.com/artifacts) at every step of the pipeline.
## Training procedure
The model is based on a pre-trained [GPT-2](https://huggingface.co/gpt2) which is fine-tuned on @liwenliang's tweets.
Hyperparameters and metrics are recorded in the [W&B training run](https://wandb.ai/wandb/huggingtweets/runs/14q55e16) for full transparency and reproducibility.
At the end of training, [the final model](https://wandb.ai/wandb/huggingtweets/runs/14q55e16/artifacts) is logged and versioned.
## How to use
You can use this model directly with a pipeline for text generation:
```python
from transformers import pipeline
generator = pipeline('text-generation',
model='huggingtweets/liwenliang')
generator("My dream is", num_return_sequences=5)
```
## Limitations and bias
The model suffers from [the same limitations and bias as GPT-2](https://huggingface.co/gpt2#limitations-and-bias).
In addition, the data present in the user's tweets further affects the text generated by the model.
## About
*Built by Boris Dayma*
[](https://twitter.com/intent/follow?screen_name=borisdayma)
For more details, visit the project repository.
[](https://github.com/borisdayma/huggingtweets)
|
onewithnickelcoins/roberta-base-MLM | 384402c2d746ffe58ff045da1cbf967e22536a35 | 2022-05-27T11:57:24.000Z | [
"pytorch",
"roberta",
"fill-mask",
"transformers",
"generated_from_trainer",
"license:mit",
"model-index",
"autotrain_compatible"
] | fill-mask | false | onewithnickelcoins | null | onewithnickelcoins/roberta-base-MLM | 0 | null | transformers | 37,761 | ---
license: mit
tags:
- generated_from_trainer
metrics:
- accuracy
model-index:
- name: roberta-base-MLM
results: []
---
<!-- This model card has been generated automatically according to the information the Trainer had access to. You
should probably proofread and complete it, then remove this comment. -->
# roberta-base-MLM
This model is a fine-tuned version of [roberta-base](https://huggingface.co/roberta-base) on an unknown dataset.
It achieves the following results on the evaluation set:
- Loss: 2.0265
- Accuracy: 0.6009
## Model description
More information needed
## Intended uses & limitations
More information needed
## Training and evaluation data
More information needed
## Training procedure
### Training hyperparameters
The following hyperparameters were used during training:
- learning_rate: 5e-05
- train_batch_size: 8
- eval_batch_size: 8
- seed: 42
- distributed_type: tpu
- optimizer: Adam with betas=(0.9,0.999) and epsilon=1e-08
- lr_scheduler_type: linear
- num_epochs: 30.0
### Training results
### Framework versions
- Transformers 4.17.0
- Pytorch 1.10.2+cu113
- Datasets 1.18.4
- Tokenizers 0.11.6
|
peter2000/wav2vec2-large-xls-r-300m-kinyarwanda | 9a4464fb9c6bdfc1f1c454c55395363e1daaa32d | 2022-06-03T14:27:44.000Z | [
"pytorch",
"tensorboard",
"wav2vec2",
"automatic-speech-recognition",
"dataset:common_voice",
"transformers",
"generated_from_trainer",
"license:apache-2.0",
"model-index"
] | automatic-speech-recognition | false | peter2000 | null | peter2000/wav2vec2-large-xls-r-300m-kinyarwanda | 0 | null | transformers | 37,762 | ---
license: apache-2.0
tags:
- generated_from_trainer
datasets:
- common_voice
model-index:
- name: wav2vec2-large-xls-r-300m-kinyarwanda
results: []
---
<!-- This model card has been generated automatically according to the information the Trainer had access to. You
should probably proofread and complete it, then remove this comment. -->
# wav2vec2-large-xls-r-300m-kinyarwanda
This model is a fine-tuned version of [facebook/wav2vec2-xls-r-300m](https://huggingface.co/facebook/wav2vec2-xls-r-300m) on the common_voice dataset.
It achieves the following results on the evaluation set:
- Loss: 0.3917
- Wer: 0.3246
## Model description
More information needed
## Intended uses & limitations
More information needed
## Training and evaluation data
More information needed
## Training procedure
### Training hyperparameters
The following hyperparameters were used during training:
- learning_rate: 7e-05
- train_batch_size: 12
- eval_batch_size: 8
- seed: 42
- gradient_accumulation_steps: 2
- total_train_batch_size: 24
- optimizer: Adam with betas=(0.9,0.999) and epsilon=1e-08
- lr_scheduler_type: linear
- lr_scheduler_warmup_steps: 400
- num_epochs: 8
- mixed_precision_training: Native AMP
### Training results
| Training Loss | Epoch | Step | Validation Loss | Wer |
|:-------------:|:-----:|:-----:|:---------------:|:------:|
| 9.0634 | 0.12 | 400 | 3.0554 | 1.0 |
| 2.8009 | 0.24 | 800 | 1.5927 | 0.9554 |
| 0.9022 | 0.36 | 1200 | 0.7328 | 0.6445 |
| 0.6213 | 0.48 | 1600 | 0.6138 | 0.5510 |
| 0.5299 | 0.6 | 2000 | 0.6072 | 0.5223 |
| 0.4999 | 0.72 | 2400 | 0.5449 | 0.4969 |
| 0.4731 | 0.84 | 2800 | 0.5261 | 0.4828 |
| 0.458 | 0.96 | 3200 | 0.5058 | 0.4607 |
| 0.4158 | 1.09 | 3600 | 0.4892 | 0.4463 |
| 0.4037 | 1.21 | 4000 | 0.4759 | 0.4429 |
| 0.4021 | 1.33 | 4400 | 0.4615 | 0.4330 |
| 0.3934 | 1.45 | 4800 | 0.4593 | 0.4315 |
| 0.3808 | 1.57 | 5200 | 0.4736 | 0.4344 |
| 0.3838 | 1.69 | 5600 | 0.4569 | 0.4249 |
| 0.3726 | 1.81 | 6000 | 0.4473 | 0.4140 |
| 0.3623 | 1.93 | 6400 | 0.4403 | 0.4097 |
| 0.3517 | 2.05 | 6800 | 0.4389 | 0.4061 |
| 0.333 | 2.17 | 7200 | 0.4383 | 0.4104 |
| 0.3354 | 2.29 | 7600 | 0.4360 | 0.3955 |
| 0.3257 | 2.41 | 8000 | 0.4226 | 0.3942 |
| 0.3275 | 2.53 | 8400 | 0.4206 | 0.4040 |
| 0.3262 | 2.65 | 8800 | 0.4172 | 0.3875 |
| 0.3206 | 2.77 | 9200 | 0.4209 | 0.3877 |
| 0.323 | 2.89 | 9600 | 0.4177 | 0.3825 |
| 0.3099 | 3.01 | 10000 | 0.4101 | 0.3691 |
| 0.3008 | 3.14 | 10400 | 0.4055 | 0.3709 |
| 0.2918 | 3.26 | 10800 | 0.4085 | 0.3800 |
| 0.292 | 3.38 | 11200 | 0.4089 | 0.3713 |
| 0.292 | 3.5 | 11600 | 0.4092 | 0.3730 |
| 0.2785 | 3.62 | 12000 | 0.4151 | 0.3687 |
| 0.2941 | 3.74 | 12400 | 0.4004 | 0.3639 |
| 0.2838 | 3.86 | 12800 | 0.4108 | 0.3703 |
| 0.2854 | 3.98 | 13200 | 0.3911 | 0.3596 |
| 0.2683 | 4.1 | 13600 | 0.3944 | 0.3575 |
| 0.2647 | 4.22 | 14000 | 0.3836 | 0.3538 |
| 0.2704 | 4.34 | 14400 | 0.4006 | 0.3540 |
| 0.2664 | 4.46 | 14800 | 0.3974 | 0.3553 |
| 0.2662 | 4.58 | 15200 | 0.3890 | 0.3470 |
| 0.2615 | 4.7 | 15600 | 0.3856 | 0.3507 |
| 0.2553 | 4.82 | 16000 | 0.3814 | 0.3497 |
| 0.2587 | 4.94 | 16400 | 0.3837 | 0.3440 |
| 0.2522 | 5.06 | 16800 | 0.3834 | 0.3486 |
| 0.2451 | 5.19 | 17200 | 0.3897 | 0.3414 |
| 0.2423 | 5.31 | 17600 | 0.3864 | 0.3481 |
| 0.2434 | 5.43 | 18000 | 0.3808 | 0.3416 |
| 0.2525 | 5.55 | 18400 | 0.3795 | 0.3408 |
| 0.2427 | 5.67 | 18800 | 0.3841 | 0.3411 |
| 0.2411 | 5.79 | 19200 | 0.3804 | 0.3366 |
| 0.2404 | 5.91 | 19600 | 0.3800 | 0.3328 |
| 0.2372 | 6.03 | 20000 | 0.3749 | 0.3335 |
| 0.2244 | 6.15 | 20400 | 0.3820 | 0.3327 |
| 0.2381 | 6.27 | 20800 | 0.3789 | 0.3325 |
| 0.2294 | 6.39 | 21200 | 0.3867 | 0.3298 |
| 0.2378 | 6.51 | 21600 | 0.3843 | 0.3281 |
| 0.2312 | 6.63 | 22000 | 0.3813 | 0.3277 |
| 0.2411 | 6.75 | 22400 | 0.3780 | 0.3268 |
| 0.2315 | 6.87 | 22800 | 0.3790 | 0.3280 |
| 0.241 | 6.99 | 23200 | 0.3776 | 0.3281 |
| 0.2313 | 7.11 | 23600 | 0.3929 | 0.3283 |
| 0.2423 | 7.24 | 24000 | 0.3905 | 0.3280 |
| 0.2337 | 7.36 | 24400 | 0.3979 | 0.3249 |
| 0.2368 | 7.48 | 24800 | 0.3980 | 0.3257 |
| 0.2409 | 7.6 | 25200 | 0.3937 | 0.3229 |
| 0.2416 | 7.72 | 25600 | 0.3867 | 0.3237 |
| 0.2364 | 7.84 | 26000 | 0.3912 | 0.3253 |
| 0.234 | 7.96 | 26400 | 0.3917 | 0.3246 |
### Framework versions
- Transformers 4.11.3
- Pytorch 1.10.0+cu113
- Datasets 1.18.3
- Tokenizers 0.10.3
|
ruselkomp/deeppavlov-framebank-1x6size-2 | 9d07c83f37cba17c6b5787a620d29a7d63b72428 | 2022-05-27T19:53:48.000Z | [
"pytorch",
"tensorboard",
"bert",
"question-answering",
"transformers",
"generated_from_trainer",
"model-index",
"autotrain_compatible"
] | question-answering | false | ruselkomp | null | ruselkomp/deeppavlov-framebank-1x6size-2 | 0 | null | transformers | 37,763 | ---
tags:
- generated_from_trainer
model-index:
- name: deeppavlov-framebank-1x6size-2
results: []
---
<!-- This model card has been generated automatically according to the information the Trainer had access to. You
should probably proofread and complete it, then remove this comment. -->
# deeppavlov-framebank-1x6size-2
This model is a fine-tuned version of [DeepPavlov/rubert-base-cased](https://huggingface.co/DeepPavlov/rubert-base-cased) on the None dataset.
It achieves the following results on the evaluation set:
- Loss: 1.1085
## Model description
More information needed
## Intended uses & limitations
More information needed
## Training and evaluation data
More information needed
## Training procedure
### Training hyperparameters
The following hyperparameters were used during training:
- learning_rate: 2e-05
- train_batch_size: 16
- eval_batch_size: 16
- seed: 42
- optimizer: Adam with betas=(0.9,0.999) and epsilon=1e-08
- lr_scheduler_type: linear
- num_epochs: 3
### Training results
| Training Loss | Epoch | Step | Validation Loss |
|:-------------:|:-----:|:----:|:---------------:|
| 1.0723 | 1.0 | 2827 | 1.0127 |
| 0.7797 | 2.0 | 5654 | 1.0359 |
| 0.5878 | 3.0 | 8481 | 1.1085 |
### Framework versions
- Transformers 4.19.0.dev0
- Pytorch 1.11.0+cu113
- Datasets 2.2.3.dev0
- Tokenizers 0.12.1
|
coreybrady/coreyresults-smaller | 7fb64ecd27fef6b164b600012663f2faa5803567 | 2022-05-27T22:02:32.000Z | [
"pytorch",
"tensorboard",
"roberta",
"fill-mask",
"transformers",
"generated_from_trainer",
"license:apache-2.0",
"model-index",
"autotrain_compatible"
] | fill-mask | false | coreybrady | null | coreybrady/coreyresults-smaller | 0 | null | transformers | 37,764 | ---
license: apache-2.0
tags:
- generated_from_trainer
model-index:
- name: coreyresults-smaller
results: []
---
<!-- This model card has been generated automatically according to the information the Trainer had access to. You
should probably proofread and complete it, then remove this comment. -->
# coreyresults-smaller
This model is a fine-tuned version of [distilroberta-base](https://huggingface.co/distilroberta-base) on the None dataset.
## Model description
More information needed
## Intended uses & limitations
More information needed
## Training and evaluation data
More information needed
## Training procedure
### Training hyperparameters
The following hyperparameters were used during training:
- learning_rate: 2e-05
- train_batch_size: 4
- eval_batch_size: 4
- seed: 42
- optimizer: Adam with betas=(0.9,0.999) and epsilon=1e-08
- lr_scheduler_type: linear
- num_epochs: 3
### Training results
### Framework versions
- Transformers 4.19.2
- Pytorch 1.11.0+cu113
- Datasets 2.2.2
- Tokenizers 0.12.1
|
kenkaneki/bert-base-aeslc | 43f724f96126410351436c7148ff0947b2c2c2d0 | 2022-05-27T20:38:16.000Z | [
"pytorch",
"bert",
"fill-mask",
"transformers",
"autotrain_compatible"
] | fill-mask | false | kenkaneki | null | kenkaneki/bert-base-aeslc | 0 | null | transformers | 37,765 | Entry not found |
huggingtweets/0xgaut | e6f5eddc6e1a13d7f8d855db2231a94127866008 | 2022-05-27T22:31:37.000Z | [
"pytorch",
"gpt2",
"text-generation",
"en",
"transformers",
"huggingtweets"
] | text-generation | false | huggingtweets | null | huggingtweets/0xgaut | 0 | null | transformers | 37,766 | ---
language: en
thumbnail: http://www.huggingtweets.com/0xgaut/1653690692376/predictions.png
tags:
- huggingtweets
widget:
- text: "My dream is"
---
<div class="inline-flex flex-col" style="line-height: 1.5;">
<div class="flex">
<div
style="display:inherit; margin-left: 4px; margin-right: 4px; width: 92px; height:92px; border-radius: 50%; background-size: cover; background-image: url('https://pbs.twimg.com/profile_images/1497681806300168198/YO7feRFJ_400x400.jpg')">
</div>
<div
style="display:none; margin-left: 4px; margin-right: 4px; width: 92px; height:92px; border-radius: 50%; background-size: cover; background-image: url('')">
</div>
<div
style="display:none; margin-left: 4px; margin-right: 4px; width: 92px; height:92px; border-radius: 50%; background-size: cover; background-image: url('')">
</div>
</div>
<div style="text-align: center; margin-top: 3px; font-size: 16px; font-weight: 800">π€ AI BOT π€</div>
<div style="text-align: center; font-size: 16px; font-weight: 800">gaut</div>
<div style="text-align: center; font-size: 14px;">@0xgaut</div>
</div>
I was made with [huggingtweets](https://github.com/borisdayma/huggingtweets).
Create your own bot based on your favorite user with [the demo](https://colab.research.google.com/github/borisdayma/huggingtweets/blob/master/huggingtweets-demo.ipynb)!
## How does it work?
The model uses the following pipeline.
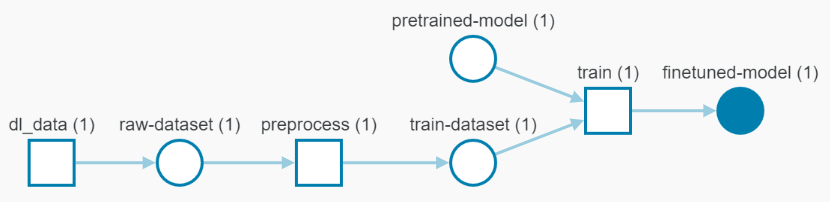
To understand how the model was developed, check the [W&B report](https://wandb.ai/wandb/huggingtweets/reports/HuggingTweets-Train-a-Model-to-Generate-Tweets--VmlldzoxMTY5MjI).
## Training data
The model was trained on tweets from gaut.
| Data | gaut |
| --- | --- |
| Tweets downloaded | 3247 |
| Retweets | 55 |
| Short tweets | 1155 |
| Tweets kept | 2037 |
[Explore the data](https://wandb.ai/wandb/huggingtweets/runs/1zfws042/artifacts), which is tracked with [W&B artifacts](https://docs.wandb.com/artifacts) at every step of the pipeline.
## Training procedure
The model is based on a pre-trained [GPT-2](https://huggingface.co/gpt2) which is fine-tuned on @0xgaut's tweets.
Hyperparameters and metrics are recorded in the [W&B training run](https://wandb.ai/wandb/huggingtweets/runs/1ds9xc41) for full transparency and reproducibility.
At the end of training, [the final model](https://wandb.ai/wandb/huggingtweets/runs/1ds9xc41/artifacts) is logged and versioned.
## How to use
You can use this model directly with a pipeline for text generation:
```python
from transformers import pipeline
generator = pipeline('text-generation',
model='huggingtweets/0xgaut')
generator("My dream is", num_return_sequences=5)
```
## Limitations and bias
The model suffers from [the same limitations and bias as GPT-2](https://huggingface.co/gpt2#limitations-and-bias).
In addition, the data present in the user's tweets further affects the text generated by the model.
## About
*Built by Boris Dayma*
[](https://twitter.com/intent/follow?screen_name=borisdayma)
For more details, visit the project repository.
[](https://github.com/borisdayma/huggingtweets)
|
reemalyami/AraRoBERTa_Poem | 2e66caa86ee749ebbe7e2d059d9210f050d79164 | 2022-05-28T01:50:48.000Z | [
"pytorch",
"roberta",
"fill-mask",
"transformers",
"autotrain_compatible"
] | fill-mask | false | reemalyami | null | reemalyami/AraRoBERTa_Poem | 0 | null | transformers | 37,767 | Entry not found |
sanbohork/t5 | 60b7b5a9b9c46f5ffd39f0aae5876b9e3b5f3ecb | 2022-05-28T12:26:51.000Z | [
"pytorch",
"t5",
"text2text-generation",
"transformers",
"license:afl-3.0",
"autotrain_compatible"
] | text2text-generation | false | sanbohork | null | sanbohork/t5 | 0 | null | transformers | 37,768 | ---
license: afl-3.0
---
Este modelo busca generar el titulo de un texto, se tomo como base el articulo:
https://medium.com/nlplanet/a-full-guide-to-finetuning-t5-for-text2text-and-building-a-demo-with-streamlit-c72009631887
Se entreno el modelo con 500 elementos del dataset
Genera el titulo del texto |
sriiikar/wav2vec2-hindi | 79b5cd0d45de9d408539eaa5e516f9152ed6b8dc | 2022-05-28T11:25:59.000Z | [
"pytorch",
"tensorboard",
"wav2vec2",
"automatic-speech-recognition",
"transformers",
"generated_from_trainer",
"license:apache-2.0",
"model-index"
] | automatic-speech-recognition | false | sriiikar | null | sriiikar/wav2vec2-hindi | 0 | null | transformers | 37,769 | ---
license: apache-2.0
tags:
- generated_from_trainer
model-index:
- name: wav2vec2-hindi
results: []
---
<!-- This model card has been generated automatically according to the information the Trainer had access to. You
should probably proofread and complete it, then remove this comment. -->
# wav2vec2-hindi
This model is a fine-tuned version of [facebook/wav2vec2-large-xlsr-53](https://huggingface.co/facebook/wav2vec2-large-xlsr-53) on the None dataset.
It achieves the following results on the evaluation set:
- Loss: 2.8814
- Wer: 1.0
## Model description
More information needed
## Intended uses & limitations
More information needed
## Training and evaluation data
More information needed
## Training procedure
### Training hyperparameters
The following hyperparameters were used during training:
- learning_rate: 0.0001
- train_batch_size: 16
- eval_batch_size: 8
- seed: 42
- gradient_accumulation_steps: 2
- total_train_batch_size: 32
- optimizer: Adam with betas=(0.9,0.999) and epsilon=1e-08
- lr_scheduler_type: linear
- lr_scheduler_warmup_steps: 500
- num_epochs: 40
- mixed_precision_training: Native AMP
### Training results
| Training Loss | Epoch | Step | Validation Loss | Wer |
|:-------------:|:-----:|:----:|:---------------:|:---:|
| 23.6834 | 6.25 | 100 | 13.5748 | 1.0 |
| 8.2358 | 12.5 | 200 | 3.9834 | 1.0 |
| 3.6953 | 18.75 | 300 | 3.7861 | 1.0 |
| 3.4186 | 25.0 | 400 | 3.8232 | 1.0 |
| 3.2462 | 31.25 | 500 | 3.4688 | 1.0 |
| 2.8108 | 37.5 | 600 | 2.8814 | 1.0 |
### Framework versions
- Transformers 4.20.0.dev0
- Pytorch 1.11.0+cu113
- Datasets 2.2.3.dev0
- Tokenizers 0.12.1
|
huggingtweets/vox_akuma | dc42437b1ae38ef34551487827bbfcc5631fbc30 | 2022-06-19T03:26:08.000Z | [
"pytorch",
"gpt2",
"text-generation",
"en",
"transformers",
"huggingtweets"
] | text-generation | false | huggingtweets | null | huggingtweets/vox_akuma | 0 | null | transformers | 37,770 | ---
language: en
thumbnail: http://www.huggingtweets.com/vox_akuma/1655609164156/predictions.png
tags:
- huggingtweets
widget:
- text: "My dream is"
---
<div class="inline-flex flex-col" style="line-height: 1.5;">
<div class="flex">
<div
style="display:inherit; margin-left: 4px; margin-right: 4px; width: 92px; height:92px; border-radius: 50%; background-size: cover; background-image: url('https://pbs.twimg.com/profile_images/1509960920449093633/c0in4uvf_400x400.jpg')">
</div>
<div
style="display:none; margin-left: 4px; margin-right: 4px; width: 92px; height:92px; border-radius: 50%; background-size: cover; background-image: url('')">
</div>
<div
style="display:none; margin-left: 4px; margin-right: 4px; width: 92px; height:92px; border-radius: 50%; background-size: cover; background-image: url('')">
</div>
</div>
<div style="text-align: center; margin-top: 3px; font-size: 16px; font-weight: 800">π€ AI BOT π€</div>
<div style="text-align: center; font-size: 16px; font-weight: 800">Vox Akuma πΉπ§§ NIJISANJI EN</div>
<div style="text-align: center; font-size: 14px;">@vox_akuma</div>
</div>
I was made with [huggingtweets](https://github.com/borisdayma/huggingtweets).
Create your own bot based on your favorite user with [the demo](https://colab.research.google.com/github/borisdayma/huggingtweets/blob/master/huggingtweets-demo.ipynb)!
## How does it work?
The model uses the following pipeline.
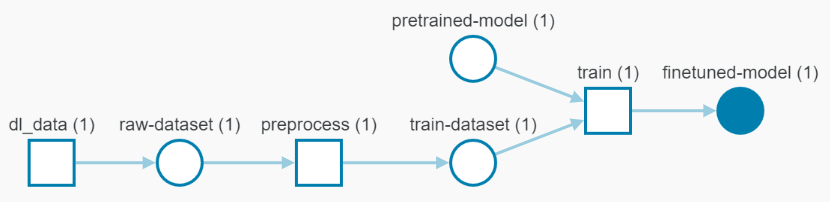
To understand how the model was developed, check the [W&B report](https://wandb.ai/wandb/huggingtweets/reports/HuggingTweets-Train-a-Model-to-Generate-Tweets--VmlldzoxMTY5MjI).
## Training data
The model was trained on tweets from Vox Akuma πΉπ§§ NIJISANJI EN.
| Data | Vox Akuma πΉπ§§ NIJISANJI EN |
| --- | --- |
| Tweets downloaded | 3149 |
| Retweets | 948 |
| Short tweets | 465 |
| Tweets kept | 1736 |
[Explore the data](https://wandb.ai/wandb/huggingtweets/runs/2g4om0wh/artifacts), which is tracked with [W&B artifacts](https://docs.wandb.com/artifacts) at every step of the pipeline.
## Training procedure
The model is based on a pre-trained [GPT-2](https://huggingface.co/gpt2) which is fine-tuned on @vox_akuma's tweets.
Hyperparameters and metrics are recorded in the [W&B training run](https://wandb.ai/wandb/huggingtweets/runs/qy49fjem) for full transparency and reproducibility.
At the end of training, [the final model](https://wandb.ai/wandb/huggingtweets/runs/qy49fjem/artifacts) is logged and versioned.
## How to use
You can use this model directly with a pipeline for text generation:
```python
from transformers import pipeline
generator = pipeline('text-generation',
model='huggingtweets/vox_akuma')
generator("My dream is", num_return_sequences=5)
```
## Limitations and bias
The model suffers from [the same limitations and bias as GPT-2](https://huggingface.co/gpt2#limitations-and-bias).
In addition, the data present in the user's tweets further affects the text generated by the model.
## About
*Built by Boris Dayma*
[](https://twitter.com/intent/follow?screen_name=borisdayma)
For more details, visit the project repository.
[](https://github.com/borisdayma/huggingtweets)
|
huggingtweets/protectandwag | 3aab96c18ec30609aa204eae301f78f2f3486df0 | 2022-05-28T19:21:17.000Z | [
"pytorch",
"gpt2",
"text-generation",
"en",
"transformers",
"huggingtweets"
] | text-generation | false | huggingtweets | null | huggingtweets/protectandwag | 0 | null | transformers | 37,771 | ---
language: en
thumbnail: http://www.huggingtweets.com/protectandwag/1653765651734/predictions.png
tags:
- huggingtweets
widget:
- text: "My dream is"
---
<div class="inline-flex flex-col" style="line-height: 1.5;">
<div class="flex">
<div
style="display:inherit; margin-left: 4px; margin-right: 4px; width: 92px; height:92px; border-radius: 50%; background-size: cover; background-image: url('https://pbs.twimg.com/profile_images/1530322632557592576/riUHOeVY_400x400.jpg')">
</div>
<div
style="display:none; margin-left: 4px; margin-right: 4px; width: 92px; height:92px; border-radius: 50%; background-size: cover; background-image: url('')">
</div>
<div
style="display:none; margin-left: 4px; margin-right: 4px; width: 92px; height:92px; border-radius: 50%; background-size: cover; background-image: url('')">
</div>
</div>
<div style="text-align: center; margin-top: 3px; font-size: 16px; font-weight: 800">π€ AI BOT π€</div>
<div style="text-align: center; font-size: 16px; font-weight: 800">soppy WHAT π΅βπ«</div>
<div style="text-align: center; font-size: 14px;">@protectandwag</div>
</div>
I was made with [huggingtweets](https://github.com/borisdayma/huggingtweets).
Create your own bot based on your favorite user with [the demo](https://colab.research.google.com/github/borisdayma/huggingtweets/blob/master/huggingtweets-demo.ipynb)!
## How does it work?
The model uses the following pipeline.
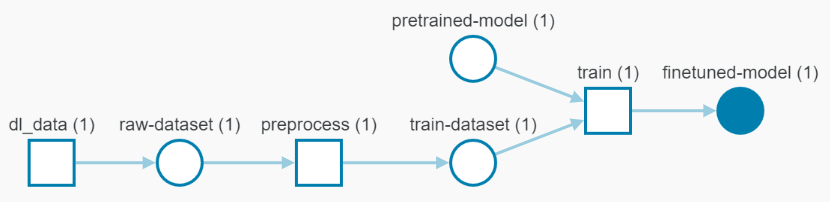
To understand how the model was developed, check the [W&B report](https://wandb.ai/wandb/huggingtweets/reports/HuggingTweets-Train-a-Model-to-Generate-Tweets--VmlldzoxMTY5MjI).
## Training data
The model was trained on tweets from soppy WHAT π΅βπ«.
| Data | soppy WHAT π΅βπ« |
| --- | --- |
| Tweets downloaded | 973 |
| Retweets | 34 |
| Short tweets | 217 |
| Tweets kept | 722 |
[Explore the data](https://wandb.ai/wandb/huggingtweets/runs/3ipwebzp/artifacts), which is tracked with [W&B artifacts](https://docs.wandb.com/artifacts) at every step of the pipeline.
## Training procedure
The model is based on a pre-trained [GPT-2](https://huggingface.co/gpt2) which is fine-tuned on @protectandwag's tweets.
Hyperparameters and metrics are recorded in the [W&B training run](https://wandb.ai/wandb/huggingtweets/runs/1a3jvx5q) for full transparency and reproducibility.
At the end of training, [the final model](https://wandb.ai/wandb/huggingtweets/runs/1a3jvx5q/artifacts) is logged and versioned.
## How to use
You can use this model directly with a pipeline for text generation:
```python
from transformers import pipeline
generator = pipeline('text-generation',
model='huggingtweets/protectandwag')
generator("My dream is", num_return_sequences=5)
```
## Limitations and bias
The model suffers from [the same limitations and bias as GPT-2](https://huggingface.co/gpt2#limitations-and-bias).
In addition, the data present in the user's tweets further affects the text generated by the model.
## About
*Built by Boris Dayma*
[](https://twitter.com/intent/follow?screen_name=borisdayma)
For more details, visit the project repository.
[](https://github.com/borisdayma/huggingtweets)
|
thanhchauns2/DialoGPT-medium-Luna | 61d5653832219c6ebf1cf6334484aad30d27190a | 2022-05-28T23:27:51.000Z | [
"pytorch",
"gpt2",
"text-generation",
"transformers",
"conversational"
] | conversational | false | thanhchauns2 | null | thanhchauns2/DialoGPT-medium-Luna | 0 | null | transformers | 37,772 | ---
tags:
- conversational
---
# My Awesome Model |
tclong/wav2vec2-base-vios-google-colab | d585d6da67fb98e656517a15f5e0b832871aab22 | 2022-06-11T13:26:15.000Z | [
"pytorch",
"tensorboard",
"wav2vec2",
"automatic-speech-recognition",
"transformers",
"generated_from_trainer",
"license:apache-2.0",
"model-index"
] | automatic-speech-recognition | false | tclong | null | tclong/wav2vec2-base-vios-google-colab | 0 | null | transformers | 37,773 | ---
license: apache-2.0
tags:
- generated_from_trainer
model-index:
- name: wav2vec2-base-vios-google-colab
results: []
---
<!-- This model card has been generated automatically according to the information the Trainer had access to. You
should probably proofread and complete it, then remove this comment. -->
# wav2vec2-base-vios-google-colab
This model is a fine-tuned version of [facebook/wav2vec2-large-xlsr-53](https://huggingface.co/facebook/wav2vec2-large-xlsr-53) on the None dataset.
It achieves the following results on the evaluation set:
- Loss: 0.5647
- Wer: 0.4970
## Model description
More information needed
## Intended uses & limitations
More information needed
## Training and evaluation data
More information needed
## Training procedure
### Training hyperparameters
The following hyperparameters were used during training:
- learning_rate: 0.0001
- train_batch_size: 16
- eval_batch_size: 8
- seed: 42
- gradient_accumulation_steps: 2
- total_train_batch_size: 32
- optimizer: Adam with betas=(0.9,0.999) and epsilon=1e-08
- lr_scheduler_type: linear
- lr_scheduler_warmup_steps: 1000
- num_epochs: 30
- mixed_precision_training: Native AMP
### Training results
| Training Loss | Epoch | Step | Validation Loss | Wer |
|:-------------:|:-----:|:----:|:---------------:|:------:|
| 7.7292 | 2.0 | 500 | 3.4159 | 1.0 |
| 3.0762 | 4.0 | 1000 | 1.3005 | 0.9615 |
| 0.8812 | 6.0 | 1500 | 0.4664 | 0.4740 |
| 0.5076 | 8.0 | 2000 | 0.4101 | 0.4180 |
| 0.4075 | 10.0 | 2500 | 0.3815 | 0.3802 |
| 0.3724 | 12.0 | 3000 | 0.3785 | 0.3741 |
| 0.3762 | 14.0 | 3500 | 0.4404 | 0.3766 |
| 0.4541 | 16.0 | 4000 | 0.4671 | 0.3822 |
| 0.6391 | 18.0 | 4500 | 0.5643 | 0.4200 |
| 0.7681 | 20.0 | 5000 | 0.6564 | 0.5214 |
| 0.8131 | 22.0 | 5500 | 0.5786 | 0.4934 |
| 0.7448 | 24.0 | 6000 | 0.5561 | 0.4920 |
| 0.7337 | 26.0 | 6500 | 0.5631 | 0.4964 |
| 0.7359 | 28.0 | 7000 | 0.5647 | 0.4968 |
| 0.7397 | 30.0 | 7500 | 0.5647 | 0.4970 |
### Framework versions
- Transformers 4.17.0
- Pytorch 1.11.0+cu113
- Datasets 1.18.3
- Tokenizers 0.12.1
|
vai6hav/wav2vec2-large-xls-r-300m-hindi-epochs40-colab | 18e9ddcbd5983741a9662e335a846a7ea9e5fd44 | 2022-05-29T10:06:38.000Z | [
"pytorch",
"tensorboard",
"wav2vec2",
"automatic-speech-recognition",
"dataset:common_voice",
"transformers",
"generated_from_trainer",
"license:apache-2.0",
"model-index"
] | automatic-speech-recognition | false | vai6hav | null | vai6hav/wav2vec2-large-xls-r-300m-hindi-epochs40-colab | 0 | null | transformers | 37,774 | ---
license: apache-2.0
tags:
- generated_from_trainer
datasets:
- common_voice
model-index:
- name: wav2vec2-large-xls-r-300m-hindi-epochs40-colab
results: []
---
<!-- This model card has been generated automatically according to the information the Trainer had access to. You
should probably proofread and complete it, then remove this comment. -->
# wav2vec2-large-xls-r-300m-hindi-epochs40-colab
This model is a fine-tuned version of [facebook/wav2vec2-xls-r-300m](https://huggingface.co/facebook/wav2vec2-xls-r-300m) on the common_voice dataset.
## Model description
More information needed
## Intended uses & limitations
More information needed
## Training and evaluation data
More information needed
## Training procedure
### Training hyperparameters
The following hyperparameters were used during training:
- learning_rate: 0.0003
- train_batch_size: 16
- eval_batch_size: 8
- seed: 42
- gradient_accumulation_steps: 2
- total_train_batch_size: 32
- optimizer: Adam with betas=(0.9,0.999) and epsilon=1e-08
- lr_scheduler_type: linear
- lr_scheduler_warmup_steps: 500
- num_epochs: 40
- mixed_precision_training: Native AMP
### Training results
### Framework versions
- Transformers 4.11.3
- Pytorch 1.10.0+cu113
- Datasets 1.18.3
- Tokenizers 0.10.3
|
stevemobs/deberta-base-finetuned-aqa-newsqa | e3967610b34f26891c86d7548b9b492f747785eb | 2022-05-29T17:36:20.000Z | [
"pytorch",
"tensorboard",
"deberta",
"question-answering",
"transformers",
"generated_from_trainer",
"license:mit",
"model-index",
"autotrain_compatible"
] | question-answering | false | stevemobs | null | stevemobs/deberta-base-finetuned-aqa-newsqa | 0 | null | transformers | 37,775 | ---
license: mit
tags:
- generated_from_trainer
model-index:
- name: deberta-base-finetuned-aqa-newsqa
results: []
---
<!-- This model card has been generated automatically according to the information the Trainer had access to. You
should probably proofread and complete it, then remove this comment. -->
# deberta-base-finetuned-aqa-newsqa
This model is a fine-tuned version of [stevemobs/deberta-base-finetuned-aqa](https://huggingface.co/stevemobs/deberta-base-finetuned-aqa) on the None dataset.
It achieves the following results on the evaluation set:
- Loss: 0.7657
## Model description
More information needed
## Intended uses & limitations
More information needed
## Training and evaluation data
More information needed
## Training procedure
### Training hyperparameters
The following hyperparameters were used during training:
- learning_rate: 2e-05
- train_batch_size: 12
- eval_batch_size: 12
- seed: 42
- optimizer: Adam with betas=(0.9,0.999) and epsilon=1e-08
- lr_scheduler_type: linear
- num_epochs: 2
### Training results
| Training Loss | Epoch | Step | Validation Loss |
|:-------------:|:-----:|:-----:|:---------------:|
| 0.6883 | 1.0 | 17307 | 0.7325 |
| 0.4807 | 2.0 | 34614 | 0.7657 |
### Framework versions
- Transformers 4.19.2
- Pytorch 1.11.0+cu113
- Datasets 2.2.2
- Tokenizers 0.12.1
|
stevemobs/deberta-base-combined-squad1-aqa-newsqa-and-newsqa | 221030e3ef9995c735502a511a87a87a6a691347 | 2022-05-29T15:10:56.000Z | [
"pytorch",
"tensorboard",
"deberta",
"question-answering",
"transformers",
"generated_from_trainer",
"license:mit",
"model-index",
"autotrain_compatible"
] | question-answering | false | stevemobs | null | stevemobs/deberta-base-combined-squad1-aqa-newsqa-and-newsqa | 0 | null | transformers | 37,776 | ---
license: mit
tags:
- generated_from_trainer
model-index:
- name: deberta-base-combined-squad1-aqa-newsqa-and-newsqa
results: []
---
<!-- This model card has been generated automatically according to the information the Trainer had access to. You
should probably proofread and complete it, then remove this comment. -->
# deberta-base-combined-squad1-aqa-newsqa-and-newsqa
This model is a fine-tuned version of [stevemobs/deberta-base-combined-squad1-aqa-newsqa](https://huggingface.co/stevemobs/deberta-base-combined-squad1-aqa-newsqa) on the None dataset.
It achieves the following results on the evaluation set:
- Loss: 0.9874
## Model description
More information needed
## Intended uses & limitations
More information needed
## Training and evaluation data
More information needed
## Training procedure
### Training hyperparameters
The following hyperparameters were used during training:
- learning_rate: 2e-05
- train_batch_size: 12
- eval_batch_size: 12
- seed: 42
- optimizer: Adam with betas=(0.9,0.999) and epsilon=1e-08
- lr_scheduler_type: linear
- num_epochs: 2
### Training results
| Training Loss | Epoch | Step | Validation Loss |
|:-------------:|:-----:|:-----:|:---------------:|
| 0.491 | 1.0 | 17307 | 0.8047 |
| 0.3064 | 2.0 | 34614 | 0.9874 |
### Framework versions
- Transformers 4.18.0
- Pytorch 1.11.0
- Datasets 2.1.0
- Tokenizers 0.12.1
|
lenses/distilroberta-base-finetuned-assignment2 | b413e49832f92238bc4aace51262d4570147f6ae | 2022-05-29T13:36:46.000Z | [
"pytorch",
"tensorboard",
"roberta",
"fill-mask",
"transformers",
"generated_from_trainer",
"license:apache-2.0",
"model-index",
"autotrain_compatible"
] | fill-mask | false | lenses | null | lenses/distilroberta-base-finetuned-assignment2 | 0 | null | transformers | 37,777 | ---
license: apache-2.0
tags:
- generated_from_trainer
model-index:
- name: distilroberta-base-finetuned-assignment2
results: []
---
<!-- This model card has been generated automatically according to the information the Trainer had access to. You
should probably proofread and complete it, then remove this comment. -->
# distilroberta-base-finetuned-assignment2
This model is a fine-tuned version of [distilroberta-base](https://huggingface.co/distilroberta-base) on the None dataset.
It achieves the following results on the evaluation set:
- Loss: 0.5976
## Model description
More information needed
## Intended uses & limitations
More information needed
## Training and evaluation data
More information needed
## Training procedure
### Training hyperparameters
The following hyperparameters were used during training:
- learning_rate: 2e-05
- train_batch_size: 8
- eval_batch_size: 8
- seed: 42
- optimizer: Adam with betas=(0.9,0.999) and epsilon=1e-08
- lr_scheduler_type: linear
- num_epochs: 3.0
### Training results
| Training Loss | Epoch | Step | Validation Loss |
|:-------------:|:-----:|:----:|:---------------:|
| No log | 1.0 | 52 | 0.6602 |
| No log | 2.0 | 104 | 0.5939 |
| No log | 3.0 | 156 | 0.6450 |
### Framework versions
- Transformers 4.19.2
- Pytorch 1.11.0+cu113
- Datasets 2.2.2
- Tokenizers 0.12.1
|
hw0724/wmt16-en-ro | 83fca26decf5a046e19696e4713df5f25747a989 | 2022-05-29T11:45:06.000Z | [
"pytorch",
"marian",
"text2text-generation",
"transformers",
"license:apache-2.0",
"autotrain_compatible"
] | text2text-generation | false | hw0724 | null | hw0724/wmt16-en-ro | 0 | null | transformers | 37,778 | ---
license: apache-2.0
---
|
vai6hav/wav2vec2-large-xls-r-300m-hindi-epochs35-colab | 10c2f82db919294b3771022e4d78007ff7b0dcb9 | 2022-05-29T12:48:35.000Z | [
"pytorch",
"tensorboard",
"wav2vec2",
"automatic-speech-recognition",
"dataset:common_voice",
"transformers",
"generated_from_trainer",
"license:apache-2.0",
"model-index"
] | automatic-speech-recognition | false | vai6hav | null | vai6hav/wav2vec2-large-xls-r-300m-hindi-epochs35-colab | 0 | null | transformers | 37,779 | ---
license: apache-2.0
tags:
- generated_from_trainer
datasets:
- common_voice
model-index:
- name: wav2vec2-large-xls-r-300m-hindi-epochs35-colab
results: []
---
<!-- This model card has been generated automatically according to the information the Trainer had access to. You
should probably proofread and complete it, then remove this comment. -->
# wav2vec2-large-xls-r-300m-hindi-epochs35-colab
This model is a fine-tuned version of [facebook/wav2vec2-xls-r-300m](https://huggingface.co/facebook/wav2vec2-xls-r-300m) on the common_voice dataset.
## Model description
More information needed
## Intended uses & limitations
More information needed
## Training and evaluation data
More information needed
## Training procedure
### Training hyperparameters
The following hyperparameters were used during training:
- learning_rate: 0.0003
- train_batch_size: 16
- eval_batch_size: 8
- seed: 42
- gradient_accumulation_steps: 2
- total_train_batch_size: 32
- optimizer: Adam with betas=(0.9,0.999) and epsilon=1e-08
- lr_scheduler_type: linear
- lr_scheduler_warmup_steps: 500
- num_epochs: 35
- mixed_precision_training: Native AMP
### Training results
### Framework versions
- Transformers 4.11.3
- Pytorch 1.10.0+cu113
- Datasets 1.18.3
- Tokenizers 0.10.3
|
vai6hav/wav2vec2-large-xls-r-300m-hindi-epochs60-colab | 2567665a85d4ff0ff5b2379e02fe3e170969f093 | 2022-05-29T15:04:50.000Z | [
"pytorch",
"tensorboard",
"wav2vec2",
"automatic-speech-recognition",
"dataset:common_voice",
"transformers",
"generated_from_trainer",
"license:apache-2.0",
"model-index"
] | automatic-speech-recognition | false | vai6hav | null | vai6hav/wav2vec2-large-xls-r-300m-hindi-epochs60-colab | 0 | null | transformers | 37,780 | ---
license: apache-2.0
tags:
- generated_from_trainer
datasets:
- common_voice
model-index:
- name: wav2vec2-large-xls-r-300m-hindi-epochs60-colab
results: []
---
<!-- This model card has been generated automatically according to the information the Trainer had access to. You
should probably proofread and complete it, then remove this comment. -->
# wav2vec2-large-xls-r-300m-hindi-epochs60-colab
This model is a fine-tuned version of [facebook/wav2vec2-xls-r-300m](https://huggingface.co/facebook/wav2vec2-xls-r-300m) on the common_voice dataset.
It achieves the following results on the evaluation set:
- Loss: 1.7322
- Wer: 0.9188
## Model description
More information needed
## Intended uses & limitations
More information needed
## Training and evaluation data
More information needed
## Training procedure
### Training hyperparameters
The following hyperparameters were used during training:
- learning_rate: 0.0003
- train_batch_size: 16
- eval_batch_size: 8
- seed: 42
- gradient_accumulation_steps: 2
- total_train_batch_size: 32
- optimizer: Adam with betas=(0.9,0.999) and epsilon=1e-08
- lr_scheduler_type: linear
- lr_scheduler_warmup_steps: 500
- num_epochs: 60
### Training results
| Training Loss | Epoch | Step | Validation Loss | Wer |
|:-------------:|:-----:|:----:|:---------------:|:------:|
| 6.2832 | 44.42 | 400 | 1.7322 | 0.9188 |
### Framework versions
- Transformers 4.11.3
- Pytorch 1.10.0+cu113
- Datasets 1.18.3
- Tokenizers 0.10.3
|
tclong/wav2vec2-dataset-vios | 935c98b450153f4aeb859c20feff54c70d611ebb | 2022-05-30T17:12:49.000Z | [
"pytorch",
"tensorboard",
"wav2vec2",
"automatic-speech-recognition",
"dataset:vivos_dataset",
"transformers",
"generated_from_trainer",
"license:apache-2.0",
"model-index"
] | automatic-speech-recognition | false | tclong | null | tclong/wav2vec2-dataset-vios | 0 | null | transformers | 37,781 | ---
license: apache-2.0
tags:
- generated_from_trainer
datasets:
- vivos_dataset
model-index:
- name: wav2vec2-dataset-vios
results: []
---
<!-- This model card has been generated automatically according to the information the Trainer had access to. You
should probably proofread and complete it, then remove this comment. -->
# wav2vec2-dataset-vios
This model is a fine-tuned version of [facebook/wav2vec2-xls-r-300m](https://huggingface.co/facebook/wav2vec2-xls-r-300m) on the vivos_dataset dataset.
It achieves the following results on the evaluation set:
- Loss: 0.5423
- Wer: 0.4075
## Model description
More information needed
## Intended uses & limitations
More information needed
## Training and evaluation data
More information needed
## Training procedure
### Training hyperparameters
The following hyperparameters were used during training:
- learning_rate: 0.0003
- train_batch_size: 16
- eval_batch_size: 8
- seed: 42
- gradient_accumulation_steps: 2
- total_train_batch_size: 32
- optimizer: Adam with betas=(0.9,0.999) and epsilon=1e-08
- lr_scheduler_type: linear
- lr_scheduler_warmup_steps: 500
- num_epochs: 30
- mixed_precision_training: Native AMP
### Training results
| Training Loss | Epoch | Step | Validation Loss | Wer |
|:-------------:|:-----:|:-----:|:---------------:|:------:|
| 5.0963 | 1.1 | 400 | 1.1336 | 0.7374 |
| 0.6576 | 2.2 | 800 | 0.4716 | 0.3727 |
| 0.4099 | 3.3 | 1200 | 0.3907 | 0.3100 |
| 0.3332 | 4.4 | 1600 | 0.3735 | 0.2766 |
| 0.2976 | 5.49 | 2000 | 0.3932 | 0.2801 |
| 0.2645 | 6.59 | 2400 | 0.3628 | 0.2542 |
| 0.2395 | 7.69 | 2800 | 0.3702 | 0.2734 |
| 0.2208 | 8.79 | 3200 | 0.3667 | 0.2467 |
| 0.1974 | 9.89 | 3600 | 0.3688 | 0.2398 |
| 0.1772 | 10.99 | 4000 | 0.3819 | 0.2457 |
| 0.1695 | 12.09 | 4400 | 0.3840 | 0.2451 |
| 0.319 | 13.19 | 4800 | 0.6531 | 0.4084 |
| 0.7305 | 14.29 | 5200 | 0.9883 | 0.6348 |
| 0.5787 | 15.38 | 5600 | 0.5260 | 0.3063 |
| 0.8558 | 16.48 | 6000 | 1.2870 | 0.7692 |
| 1.155 | 17.58 | 6400 | 1.0568 | 0.6353 |
| 0.8393 | 18.68 | 6800 | 0.7360 | 0.4486 |
| 0.6094 | 19.78 | 7200 | 0.6072 | 0.4108 |
| 0.5346 | 20.88 | 7600 | 0.5749 | 0.4095 |
| 0.5073 | 21.98 | 8000 | 0.5588 | 0.4056 |
| 0.4859 | 23.08 | 8400 | 0.5475 | 0.4015 |
| 0.475 | 24.18 | 8800 | 0.5430 | 0.4011 |
| 0.4683 | 25.27 | 9200 | 0.5400 | 0.3990 |
| 0.4673 | 26.37 | 9600 | 0.5407 | 0.4011 |
| 0.4665 | 27.47 | 10000 | 0.5408 | 0.3992 |
| 0.4703 | 28.57 | 10400 | 0.5420 | 0.4070 |
| 0.4709 | 29.67 | 10800 | 0.5423 | 0.4075 |
### Framework versions
- Transformers 4.11.3
- Pytorch 1.10.0+cu113
- Datasets 1.18.3
- Tokenizers 0.10.3
|
pparkji/wav2vec2_timit | d6a94e68a338fe41e585f09e78089dc45a60ce3c | 2022-05-29T15:10:06.000Z | [
"pytorch",
"wav2vec2",
"automatic-speech-recognition",
"transformers"
] | automatic-speech-recognition | false | pparkji | null | pparkji/wav2vec2_timit | 0 | null | transformers | 37,782 | Entry not found |
stevemobs/deberta-base-combined-squad1-aqa-newsqa-and-newsqa-1epoch | 471f5bd14f37a4fface9b753fb1a36d5007096ba | 2022-05-29T17:25:24.000Z | [
"pytorch",
"tensorboard",
"deberta",
"question-answering",
"transformers",
"generated_from_trainer",
"license:mit",
"model-index",
"autotrain_compatible"
] | question-answering | false | stevemobs | null | stevemobs/deberta-base-combined-squad1-aqa-newsqa-and-newsqa-1epoch | 0 | null | transformers | 37,783 | ---
license: mit
tags:
- generated_from_trainer
model-index:
- name: deberta-base-combined-squad1-aqa-newsqa-and-newsqa-1epoch
results: []
---
<!-- This model card has been generated automatically according to the information the Trainer had access to. You
should probably proofread and complete it, then remove this comment. -->
# deberta-base-combined-squad1-aqa-newsqa-and-newsqa-1epoch
This model is a fine-tuned version of [stevemobs/deberta-base-combined-squad1-aqa-newsqa](https://huggingface.co/stevemobs/deberta-base-combined-squad1-aqa-newsqa) on the None dataset.
It achieves the following results on the evaluation set:
- Loss: 0.7915
## Model description
More information needed
## Intended uses & limitations
More information needed
## Training and evaluation data
More information needed
## Training procedure
### Training hyperparameters
The following hyperparameters were used during training:
- learning_rate: 2e-05
- train_batch_size: 12
- eval_batch_size: 12
- seed: 42
- optimizer: Adam with betas=(0.9,0.999) and epsilon=1e-08
- lr_scheduler_type: linear
- num_epochs: 1
### Training results
| Training Loss | Epoch | Step | Validation Loss |
|:-------------:|:-----:|:-----:|:---------------:|
| 0.4499 | 1.0 | 17307 | 0.7915 |
### Framework versions
- Transformers 4.18.0
- Pytorch 1.11.0
- Datasets 2.1.0
- Tokenizers 0.12.1
|
uygarkurt/gpt2-poet | 489e6706780caa2328f2e50c758641475633c394 | 2022-05-29T17:09:19.000Z | [
"pytorch",
"tensorboard",
"gpt2",
"text-generation",
"transformers",
"generated_from_trainer",
"license:mit",
"model-index"
] | text-generation | false | uygarkurt | null | uygarkurt/gpt2-poet | 0 | null | transformers | 37,784 | ---
license: mit
tags:
- generated_from_trainer
model-index:
- name: gpt2-poet
results: []
---
<!-- This model card has been generated automatically according to the information the Trainer had access to. You
should probably proofread and complete it, then remove this comment. -->
# gpt2-poet
This model is a fine-tuned version of [gpt2](https://huggingface.co/gpt2) on an unknown dataset.
It achieves the following results on the evaluation set:
- Loss: 5.2026
## Model description
More information needed
## Intended uses & limitations
More information needed
## Training and evaluation data
More information needed
## Training procedure
### Training hyperparameters
The following hyperparameters were used during training:
- learning_rate: 5e-05
- train_batch_size: 32
- eval_batch_size: 32
- seed: 42
- optimizer: Adam with betas=(0.9,0.999) and epsilon=1e-08
- lr_scheduler_type: linear
- lr_scheduler_warmup_steps: 500
- num_epochs: 3
### Training results
| Training Loss | Epoch | Step | Validation Loss |
|:-------------:|:-----:|:----:|:---------------:|
| 4.9797 | 1.0 | 69 | 5.1736 |
| 4.8746 | 2.0 | 138 | 5.1852 |
| 4.7168 | 3.0 | 207 | 5.2026 |
### Framework versions
- Transformers 4.19.2
- Pytorch 1.11.0+cu113
- Datasets 2.2.2
- Tokenizers 0.12.1
|
jadkheirallah/DialoGPT-med-wie | d14b29a82fc3d137082cea51a3a95d4120fb124a | 2022-05-31T17:47:25.000Z | [
"pytorch",
"gpt2",
"text-generation",
"transformers"
] | text-generation | false | jadkheirallah | null | jadkheirallah/DialoGPT-med-wie | 0 | null | transformers | 37,785 | Entry not found |
pgilles/wav2vec2-large-xls-r-LUXEMBOURGISH | 49c67e4bf5b71c54084a15e268499a619554d365 | 2022-06-02T12:56:09.000Z | [
"pytorch",
"wav2vec2",
"automatic-speech-recognition",
"lb",
"ltz",
"dataset:Wav2Vec2-XLS-R-300M",
"transformers",
"ASR",
"Automatic Speech Recognition",
"speech",
"license:mit"
] | automatic-speech-recognition | false | pgilles | null | pgilles/wav2vec2-large-xls-r-LUXEMBOURGISH | 0 | 1 | transformers | 37,786 | ---
language:
- lb
- ltz
#thumbnail: "url to a thumbnail used in social sharing"
tags:
- ASR
- Automatic Speech Recognition
- speech
- wav2vec2
license: "mit"
datasets:
- "Wav2Vec2-XLS-R-300M"
#- "trained on custom data set (~8 hours)"
metrics:
- type: wer
- value: 0.188285
widget:
- src: https://luxappsdata.uni.lu/schnessen/media/recording_recordings/2021-10-13/pg_71_4629_rdc1j.wav
example_title: Example 1
- src: https://luxappsdata.uni.lu/schnessen/media/recording_recordings/2021-08-20/pg_73_6801_3j86c.wav
example_title: Example 2
- src: https://luxappsdata.uni.lu/schnessen/media/recording_recordings/2018-11-29/pg_347_1313_kolin.wav
example_title: Example 3
- src: https://luxappsdata.uni.lu/schnessen/media/recording_recordings/2018-08-05/pg_192_3299_1zqrn.wav
example_title: Example 4
- src: https://huggingface.co/pgilles/wav2vec2-large-xls-r-LUXEMBOURGISH/resolve/main/Chamber_2020_10_13.wav
example_title: Example 5
---
# First Automatic Speech Recognition Model for Luxembourgish
Training pipeline based on the tutorials:
- [Fine-tuning XLS-R for Multi-Lingual ASR with π€ Transformers](https://colab.research.google.com/github/patrickvonplaten/notebooks/blob/master/Fine_Tune_XLSR_Wav2Vec2_on_Turkish_ASR_with_%F0%9F%A4%97_Transformers.ipynb)
- [Fine-tuning XLSR-Wav2Vec2 for WOLOF ASR with π€](https://www.kaggle.com/kingabzpro/fine-tuning-xlsr-wav2vec2-for-wolof-asr-with/notebook)
Trained on custom data set (~8 hours of Luxembourgish audio+transcript data)
This a first shot and purely experimental. The inferences are thus based only on the trained acoustic model. No language model has been used yet.
WER: 0.18. |
jppaolim/v36_Naive | 5318e01ca885ba05f25df040fe4489912c60ee9f | 2022-05-29T20:16:05.000Z | [
"pytorch",
"gpt2",
"text-generation",
"transformers"
] | text-generation | false | jppaolim | null | jppaolim/v36_Naive | 0 | null | transformers | 37,787 | Entry not found |
jayklaws0606/dgpt-small-jaybot | 5b525ebf7345647ba149c735bc0c61087b68658f | 2022-05-29T22:23:43.000Z | [
"pytorch",
"gpt2",
"text-generation",
"transformers",
"conversational"
] | conversational | false | jayklaws0606 | null | jayklaws0606/dgpt-small-jaybot | 0 | null | transformers | 37,788 | ---
tags:
- conversational
---
#jaybot 2.0 |
stevemobs/deberta-base-combined-squad1-aqa-1epoch | 0304a48cbe6e1e82a53ceb8909f67bad79ede785 | 2022-05-30T02:38:48.000Z | [
"pytorch",
"tensorboard",
"deberta",
"question-answering",
"transformers",
"generated_from_trainer",
"license:mit",
"model-index",
"autotrain_compatible"
] | question-answering | false | stevemobs | null | stevemobs/deberta-base-combined-squad1-aqa-1epoch | 0 | null | transformers | 37,789 | ---
license: mit
tags:
- generated_from_trainer
model-index:
- name: deberta-base-combined-squad1-aqa-1epoch
results: []
---
<!-- This model card has been generated automatically according to the information the Trainer had access to. You
should probably proofread and complete it, then remove this comment. -->
# deberta-base-combined-squad1-aqa-1epoch
This model is a fine-tuned version of [microsoft/deberta-base](https://huggingface.co/microsoft/deberta-base) on the None dataset.
It achieves the following results on the evaluation set:
- Loss: 0.9431
## Model description
More information needed
## Intended uses & limitations
More information needed
## Training and evaluation data
More information needed
## Training procedure
### Training hyperparameters
The following hyperparameters were used during training:
- learning_rate: 2e-05
- train_batch_size: 12
- eval_batch_size: 12
- seed: 42
- optimizer: Adam with betas=(0.9,0.999) and epsilon=1e-08
- lr_scheduler_type: linear
- num_epochs: 1
### Training results
| Training Loss | Epoch | Step | Validation Loss |
|:-------------:|:-----:|:----:|:---------------:|
| 1.0971 | 1.0 | 9906 | 0.9431 |
### Framework versions
- Transformers 4.18.0
- Pytorch 1.11.0
- Datasets 2.1.0
- Tokenizers 0.12.1
|
imamnurby/rob2rand_chen_w_prefix_c_fc | 5910bc63da52ee280a4435c3ffa6773f885e7140 | 2022-05-30T02:25:04.000Z | [
"pytorch",
"encoder-decoder",
"text2text-generation",
"transformers",
"generated_from_trainer",
"model-index",
"autotrain_compatible"
] | text2text-generation | false | imamnurby | null | imamnurby/rob2rand_chen_w_prefix_c_fc | 0 | null | transformers | 37,790 | ---
tags:
- generated_from_trainer
model-index:
- name: rob2rand_chen_w_prefix_c_fc
results: []
---
<!-- This model card has been generated automatically according to the information the Trainer had access to. You
should probably proofread and complete it, then remove this comment. -->
# rob2rand_chen_w_prefix_c_fc
This model was trained from scratch on the None dataset.
It achieves the following results on the evaluation set:
- eval_loss: 0.0939
- eval_bleu: 84.4530
- eval_em: 52.0156
- eval_bleu_em: 68.2343
- eval_runtime: 21.0016
- eval_samples_per_second: 36.616
- eval_steps_per_second: 0.619
- step: 0
## Model description
More information needed
## Intended uses & limitations
More information needed
## Training and evaluation data
More information needed
## Training procedure
### Training hyperparameters
The following hyperparameters were used during training:
- learning_rate: 5e-06
- train_batch_size: 64
- eval_batch_size: 64
- seed: 42
- optimizer: Adam with betas=(0.9,0.999) and epsilon=1e-08
- lr_scheduler_type: linear
- lr_scheduler_warmup_steps: 1000
- num_epochs: 3
- mixed_precision_training: Native AMP
### Framework versions
- Transformers 4.18.0
- Pytorch 1.7.1
- Datasets 2.1.0
- Tokenizers 0.12.1
|
knurm/my-finetuned-xml-roberta4 | e1d03025c2c814f842183c0c851fd29a6b02bbfb | 2022-05-30T16:14:33.000Z | [
"pytorch",
"tensorboard",
"xlm-roberta",
"question-answering",
"transformers",
"generated_from_trainer",
"license:mit",
"model-index",
"autotrain_compatible"
] | question-answering | false | knurm | null | knurm/my-finetuned-xml-roberta4 | 0 | null | transformers | 37,791 | ---
license: mit
tags:
- generated_from_trainer
model-index:
- name: my-finetuned-xml-roberta4
results: []
---
<!-- This model card has been generated automatically according to the information the Trainer had access to. You
should probably proofread and complete it, then remove this comment. -->
# my-finetuned-xml-roberta4
This model is a fine-tuned version of [knurm/xlm-roberta-base-finetuned-est](https://huggingface.co/knurm/xlm-roberta-base-finetuned-est) on an unknown dataset.
It achieves the following results on the evaluation set:
- Loss: 3.7709
## Model description
More information needed
## Intended uses & limitations
More information needed
## Training and evaluation data
More information needed
## Training procedure
### Training hyperparameters
The following hyperparameters were used during training:
- learning_rate: 2e-05
- train_batch_size: 16
- eval_batch_size: 16
- seed: 42
- optimizer: Adam with betas=(0.9,0.999) and epsilon=1e-08
- lr_scheduler_type: linear
- num_epochs: 7
### Training results
| Training Loss | Epoch | Step | Validation Loss |
|:-------------:|:-----:|:-----:|:---------------:|
| 3.4629 | 1.0 | 5652 | 3.3367 |
| 3.1814 | 2.0 | 11304 | 3.2952 |
| 2.9718 | 3.0 | 16956 | 3.2592 |
| 2.7442 | 4.0 | 22608 | 3.3133 |
| 2.5991 | 5.0 | 28260 | 3.4292 |
| 2.4221 | 6.0 | 33912 | 3.5928 |
| 2.3259 | 7.0 | 39564 | 3.7709 |
### Framework versions
- Transformers 4.19.1
- Pytorch 1.11.0+cu113
- Datasets 2.2.1
- Tokenizers 0.12.1
|
melnikoff-oleg/distilbart-sep-mask-all | 3dd3ec2e4c1506d632060c22a06495c9a3ffbf3e | 2022-05-30T08:12:43.000Z | [
"pytorch",
"bart",
"text2text-generation",
"transformers",
"autotrain_compatible"
] | text2text-generation | false | melnikoff-oleg | null | melnikoff-oleg/distilbart-sep-mask-all | 0 | null | transformers | 37,792 | Entry not found |
kabelomalapane/en_tn_ukuxhumana_model2 | baf690096c944f6e29b6fe65fdffc601a3402252 | 2022-05-31T16:59:22.000Z | [
"pytorch",
"tensorboard",
"marian",
"text2text-generation",
"transformers",
"translation",
"generated_from_trainer",
"license:apache-2.0",
"model-index",
"autotrain_compatible"
] | translation | false | kabelomalapane | null | kabelomalapane/en_tn_ukuxhumana_model2 | 0 | null | transformers | 37,793 | ---
license: apache-2.0
tags:
- translation
- generated_from_trainer
metrics:
- bleu
model-index:
- name: en_tn_ukuxhumana_model2
results: []
---
<!-- This model card has been generated automatically according to the information the Trainer had access to. You
should probably proofread and complete it, then remove this comment. -->
# en_tn_ukuxhumana_model2
This model is a fine-tuned version of [Helsinki-NLP/opus-mt-en-tn](https://huggingface.co/Helsinki-NLP/opus-mt-en-tn) on the ukuxhumana dataset.
- Train_data = 12080
- Dev_data = 3000
It achieves the following results on the evaluation set:
After training:
- Loss: 2.6466
- Bleu: 21.8204
## Model description
More information needed
## Intended uses & limitations
More information needed
## Training and evaluation data
More information needed
## Training procedure
### Training hyperparameters
The following hyperparameters were used during training:
- learning_rate: 2e-05
- train_batch_size: 32
- eval_batch_size: 64
- seed: 42
- optimizer: Adam with betas=(0.9,0.999) and epsilon=1e-08
- lr_scheduler_type: linear
- num_epochs: 3
### Training results
### Framework versions
- Transformers 4.19.2
- Pytorch 1.11.0+cu113
- Datasets 2.2.2
- Tokenizers 0.12.1
|
huggingtweets/erinkhoo | 7635c8aa2dec2c15213bce2f91a56b609e489d49 | 2022-05-30T16:48:54.000Z | [
"pytorch",
"gpt2",
"text-generation",
"en",
"transformers",
"huggingtweets"
] | text-generation | false | huggingtweets | null | huggingtweets/erinkhoo | 0 | null | transformers | 37,794 | ---
language: en
thumbnail: https://github.com/borisdayma/huggingtweets/blob/master/img/logo.png?raw=true
tags:
- huggingtweets
widget:
- text: "My dream is"
---
<div class="inline-flex flex-col" style="line-height: 1.5;">
<div class="flex">
<div
style="display:inherit; margin-left: 4px; margin-right: 4px; width: 92px; height:92px; border-radius: 50%; background-size: cover; background-image: url('https://pbs.twimg.com/profile_images/1362800111118659591/O6gxa7NN_400x400.jpg')">
</div>
<div
style="display:none; margin-left: 4px; margin-right: 4px; width: 92px; height:92px; border-radius: 50%; background-size: cover; background-image: url('')">
</div>
<div
style="display:none; margin-left: 4px; margin-right: 4px; width: 92px; height:92px; border-radius: 50%; background-size: cover; background-image: url('')">
</div>
</div>
<div style="text-align: center; margin-top: 3px; font-size: 16px; font-weight: 800">π€ AI BOT π€</div>
<div style="text-align: center; font-size: 16px; font-weight: 800">erinkhoo.x</div>
<div style="text-align: center; font-size: 14px;">@erinkhoo</div>
</div>
I was made with [huggingtweets](https://github.com/borisdayma/huggingtweets).
Create your own bot based on your favorite user with [the demo](https://colab.research.google.com/github/borisdayma/huggingtweets/blob/master/huggingtweets-demo.ipynb)!
## How does it work?
The model uses the following pipeline.
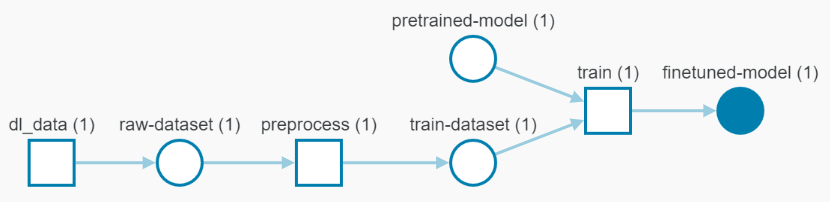
To understand how the model was developed, check the [W&B report](https://wandb.ai/wandb/huggingtweets/reports/HuggingTweets-Train-a-Model-to-Generate-Tweets--VmlldzoxMTY5MjI).
## Training data
The model was trained on tweets from erinkhoo.x.
| Data | erinkhoo.x |
| --- | --- |
| Tweets downloaded | 3216 |
| Retweets | 1795 |
| Short tweets | 181 |
| Tweets kept | 1240 |
[Explore the data](https://wandb.ai/wandb/huggingtweets/runs/navmzjcl/artifacts), which is tracked with [W&B artifacts](https://docs.wandb.com/artifacts) at every step of the pipeline.
## Training procedure
The model is based on a pre-trained [GPT-2](https://huggingface.co/gpt2) which is fine-tuned on @erinkhoo's tweets.
Hyperparameters and metrics are recorded in the [W&B training run](https://wandb.ai/wandb/huggingtweets/runs/3uoi8z43) for full transparency and reproducibility.
At the end of training, [the final model](https://wandb.ai/wandb/huggingtweets/runs/3uoi8z43/artifacts) is logged and versioned.
## How to use
You can use this model directly with a pipeline for text generation:
```python
from transformers import pipeline
generator = pipeline('text-generation',
model='huggingtweets/erinkhoo')
generator("My dream is", num_return_sequences=5)
```
## Limitations and bias
The model suffers from [the same limitations and bias as GPT-2](https://huggingface.co/gpt2#limitations-and-bias).
In addition, the data present in the user's tweets further affects the text generated by the model.
## About
*Built by Boris Dayma*
[](https://twitter.com/intent/follow?screen_name=borisdayma)
For more details, visit the project repository.
[](https://github.com/borisdayma/huggingtweets)
|
income/bpr-base-msmarco-distilbert-tas-b | 7aa5c436509e30e95fe7bca6a5a541636f68487b | 2022-05-30T21:23:31.000Z | [
"pytorch",
"distilbert",
"feature-extraction",
"transformers",
"license:apache-2.0"
] | feature-extraction | false | income | null | income/bpr-base-msmarco-distilbert-tas-b | 0 | null | transformers | 37,795 | ---
license: apache-2.0
--- |
jppaolim/v37_Best2Epoch | 8df5e40b9b62ec1930b237a2c078fb8db2c99847 | 2022-05-30T21:44:18.000Z | [
"pytorch",
"gpt2",
"text-generation",
"transformers"
] | text-generation | false | jppaolim | null | jppaolim/v37_Best2Epoch | 0 | null | transformers | 37,796 | # My Story model
Arthur goes to the beach. Arthur wanted to go to the beach with his friends. He found out he couldn't swim. Arthur went to the doctor. The doctor said he could not swim. Arthur decides to stay at home and get his prescription.
Arthur goes to the beach. Arthur went to the beach one day. He was excited to see the ocean. He decided to go out on a boat and get lunch. After eating lunch, Arthur realized he was hungry. He decided to eat more fruit.
Arthur goes to the beach. Arthur has a date at the beach. He is nervous and asks his date if he can go with him. His date says yes and they walk down the beach. Arthur is so excited that he decides to try out for the beach. Arthur loves his new friends at the beach.
Arthur goes to the beach. Arthur decides to take a nap in the sand. He gets up early and goes to work. Arthur is very tired at work. Arthur wakes up the next morning feeling very tired. Arthur sleeps for hours.
Arthur goes to the beach. Arthur had been looking forward to going to the beach with his friends for a few days. He was excited to go swimming at the beach. However, he saw that it was raining hard. Arthur decided not to go swimming in the rainy. He decided to just wait until the rainy cleared.
Arthur goes to the beach. Arthur was going to go to the beach with his family. He packed up all of his stuff and headed out. He arrived at the beach on time. Arthur's dad came over to help him. Arthur was very happy that he had been able to go to the beach.
Arthur goes to the beach. Arthur loves to go to the beach. He loves to swim and swims. One day, Arthur is out on a date with a girl. He doesn't know her well enough to ask her out. He decides that he will never ask her out again.
Arthur goes to the beach. Arthur decides he wants to go on a cruise. He buys a ticket to the beach and goes out. The sun goes down and he feels great. He gets to the beach and relaxes. Arthur is glad he took the time to spend with his family.
Arthur goes to the beach. Arthur went to the beach. He wanted to play with his friends. But they all had a bad day. They all had a bad day. Arthur was happy that he got to go to the beach.
Arthur goes to the beach. Arthur loves going to the ocean. He has never been to a beach before. He decides he wants to go to the beach. He buys a surfboard and rides the waves. Arthur is happy that he went to the beach.
Arthur goes to the beach. Arthur is going to the beach with his family. He has never been to a beach before. He decides he needs to get in shape. He begins to swim everyday. Arthur feels much better after swimming.
Arthur goes to the beach. Arthur is at the beach with his friends. He is playing in a pool. Suddenly he feels a tug on his shirt. He pulls out his shirt and tries to swim away. Arthur is able get back on the water safely.
Arthur goes to the beach. Arthur was going to the beach with his friends. He had never been to a beach before. They went out to the sand and sat on the sand. Arthur felt very relaxed after he finished. He decided to go back to the beach later that day.
Arthur goes to the beach. Arthur was going to the beach with his family. He had never been to a beach before. He went up to the beach and looked around. He saw a huge wave. Arthur decided he would go back to the beach after.
Arthur goes to the beach. Arthur is going to the beach with his family. He has been saving up for months to buy a new car. When he gets there he is very excited. He checks out the car and it is amazing. He loves his new car.
|
huggingtweets/sun_soony-unjaded_jade-veganhollyg | bb7fc8ad5fc47e9dd1a3c9d12403866ebf7c3057 | 2022-06-08T21:45:56.000Z | [
"pytorch",
"gpt2",
"text-generation",
"en",
"transformers",
"huggingtweets"
] | text-generation | false | huggingtweets | null | huggingtweets/sun_soony-unjaded_jade-veganhollyg | 0 | null | transformers | 37,797 | ---
language: en
thumbnail: http://www.huggingtweets.com/sun_soony-unjaded_jade-veganhollyg/1654724750416/predictions.png
tags:
- huggingtweets
widget:
- text: "My dream is"
---
<div class="inline-flex flex-col" style="line-height: 1.5;">
<div class="flex">
<div
style="display:inherit; margin-left: 4px; margin-right: 4px; width: 92px; height:92px; border-radius: 50%; background-size: cover; background-image: url('https://pbs.twimg.com/profile_images/1105554414427885569/XkyfcoMJ_400x400.jpg')">
</div>
<div
style="display:inherit; margin-left: 4px; margin-right: 4px; width: 92px; height:92px; border-radius: 50%; background-size: cover; background-image: url('https://pbs.twimg.com/profile_images/1290809762637131776/uwGH2mYu_400x400.jpg')">
</div>
<div
style="display:inherit; margin-left: 4px; margin-right: 4px; width: 92px; height:92px; border-radius: 50%; background-size: cover; background-image: url('https://pbs.twimg.com/profile_images/900359049061036032/LYf3Ouv__400x400.jpg')">
</div>
</div>
<div style="text-align: center; margin-top: 3px; font-size: 16px; font-weight: 800">π€ AI CYBORG π€</div>
<div style="text-align: center; font-size: 16px; font-weight: 800">Jade Bowler & soony & Holly Gabrielle</div>
<div style="text-align: center; font-size: 14px;">@sun_soony-unjaded_jade-veganhollyg</div>
</div>
I was made with [huggingtweets](https://github.com/borisdayma/huggingtweets).
Create your own bot based on your favorite user with [the demo](https://colab.research.google.com/github/borisdayma/huggingtweets/blob/master/huggingtweets-demo.ipynb)!
## How does it work?
The model uses the following pipeline.
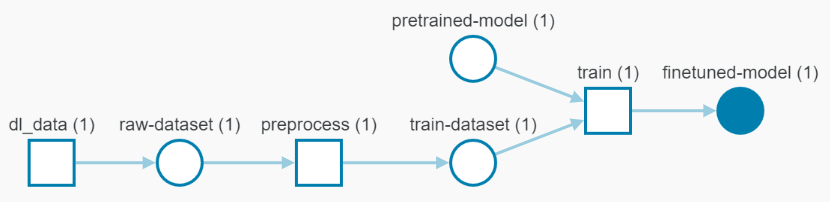
To understand how the model was developed, check the [W&B report](https://wandb.ai/wandb/huggingtweets/reports/HuggingTweets-Train-a-Model-to-Generate-Tweets--VmlldzoxMTY5MjI).
## Training data
The model was trained on tweets from Jade Bowler & soony & Holly Gabrielle.
| Data | Jade Bowler | soony | Holly Gabrielle |
| --- | --- | --- | --- |
| Tweets downloaded | 3170 | 815 | 1802 |
| Retweets | 121 | 260 | 276 |
| Short tweets | 120 | 47 | 253 |
| Tweets kept | 2929 | 508 | 1273 |
[Explore the data](https://wandb.ai/wandb/huggingtweets/runs/afi2j4p2/artifacts), which is tracked with [W&B artifacts](https://docs.wandb.com/artifacts) at every step of the pipeline.
## Training procedure
The model is based on a pre-trained [GPT-2](https://huggingface.co/gpt2) which is fine-tuned on @sun_soony-unjaded_jade-veganhollyg's tweets.
Hyperparameters and metrics are recorded in the [W&B training run](https://wandb.ai/wandb/huggingtweets/runs/3uiqxuec) for full transparency and reproducibility.
At the end of training, [the final model](https://wandb.ai/wandb/huggingtweets/runs/3uiqxuec/artifacts) is logged and versioned.
## How to use
You can use this model directly with a pipeline for text generation:
```python
from transformers import pipeline
generator = pipeline('text-generation',
model='huggingtweets/sun_soony-unjaded_jade-veganhollyg')
generator("My dream is", num_return_sequences=5)
```
## Limitations and bias
The model suffers from [the same limitations and bias as GPT-2](https://huggingface.co/gpt2#limitations-and-bias).
In addition, the data present in the user's tweets further affects the text generated by the model.
## About
*Built by Boris Dayma*
[](https://twitter.com/intent/follow?screen_name=borisdayma)
For more details, visit the project repository.
[](https://github.com/borisdayma/huggingtweets)
|
roshnir/bert-multi-uncased-trained-squadv2 | 90c6f0897f042f1733964d76a2ad29ee28dd3423 | 2022-06-02T20:15:14.000Z | [
"pytorch",
"bert",
"question-answering",
"transformers",
"autotrain_compatible"
] | question-answering | false | roshnir | null | roshnir/bert-multi-uncased-trained-squadv2 | 0 | null | transformers | 37,798 | mBERT base uncased model trained on 50% SQUAD data. This model can further be used to fine-tune using dev data for QA system on a specific language. The process is similar to what was followed in MLQA paper[https://aclanthology.org/2020.acl-main.421.pdf].
|
stfuowned/rickfinal | 9867253d39276ac1cb75c3f5d6c074f7f3768070 | 2022-05-31T00:14:42.000Z | [
"pytorch",
"gpt2",
"text-generation",
"transformers",
"conversational"
] | conversational | false | stfuowned | null | stfuowned/rickfinal | 0 | null | transformers | 37,799 | ---
tags:
- conversational
---
#rickfinal |
Subsets and Splits
No community queries yet
The top public SQL queries from the community will appear here once available.