modelId
stringlengths 4
112
| sha
stringlengths 40
40
| lastModified
stringlengths 24
24
| tags
sequence | pipeline_tag
stringclasses 29
values | private
bool 1
class | author
stringlengths 2
38
⌀ | config
null | id
stringlengths 4
112
| downloads
float64 0
36.8M
⌀ | likes
float64 0
712
⌀ | library_name
stringclasses 17
values | __index_level_0__
int64 0
38.5k
| readme
stringlengths 0
186k
|
---|---|---|---|---|---|---|---|---|---|---|---|---|---|
tner/xlm-roberta-base-panx-dataset-ru | e70931cf283091559ff1606d640c91326730986c | 2021-02-13T00:08:30.000Z | [
"pytorch",
"xlm-roberta",
"token-classification",
"transformers",
"autotrain_compatible"
] | token-classification | false | tner | null | tner/xlm-roberta-base-panx-dataset-ru | 0 | null | transformers | 33,600 | # XLM-RoBERTa for NER
XLM-RoBERTa finetuned on NER. Check more detail at [TNER repository](https://github.com/asahi417/tner).
## Usage
```
from transformers import AutoTokenizer, AutoModelForTokenClassification
tokenizer = AutoTokenizer.from_pretrained("asahi417/tner-xlm-roberta-base-panx-dataset-ru")
model = AutoModelForTokenClassification.from_pretrained("asahi417/tner-xlm-roberta-base-panx-dataset-ru")
``` |
tner/xlm-roberta-base-uncased-bc5cdr | a94f829854c121c128531f628b068547b7528a63 | 2021-02-13T00:08:23.000Z | [
"pytorch",
"xlm-roberta",
"token-classification",
"transformers",
"autotrain_compatible"
] | token-classification | false | tner | null | tner/xlm-roberta-base-uncased-bc5cdr | 0 | null | transformers | 33,601 | # XLM-RoBERTa for NER
XLM-RoBERTa finetuned on NER. Check more detail at [TNER repository](https://github.com/asahi417/tner).
## Usage
```
from transformers import AutoTokenizer, AutoModelForTokenClassification
tokenizer = AutoTokenizer.from_pretrained("asahi417/tner-xlm-roberta-base-uncased-bc5cdr")
model = AutoModelForTokenClassification.from_pretrained("asahi417/tner-xlm-roberta-base-uncased-bc5cdr")
``` |
tner/xlm-roberta-large-panx-dataset-en | 5a9a00b3df08682bd91282e17af7e7ffd8875529 | 2021-02-13T00:11:22.000Z | [
"pytorch",
"xlm-roberta",
"token-classification",
"transformers",
"autotrain_compatible"
] | token-classification | false | tner | null | tner/xlm-roberta-large-panx-dataset-en | 0 | null | transformers | 33,602 | # XLM-RoBERTa for NER
XLM-RoBERTa finetuned on NER. Check more detail at [TNER repository](https://github.com/asahi417/tner).
## Usage
```
from transformers import AutoTokenizer, AutoModelForTokenClassification
tokenizer = AutoTokenizer.from_pretrained("asahi417/tner-xlm-roberta-large-panx-dataset-en")
model = AutoModelForTokenClassification.from_pretrained("asahi417/tner-xlm-roberta-large-panx-dataset-en")
``` |
tner/xlm-roberta-large-panx-dataset-ru | c928186c245847850aa748400bddd6893995eeec | 2021-02-13T00:11:34.000Z | [
"pytorch",
"xlm-roberta",
"token-classification",
"transformers",
"autotrain_compatible"
] | token-classification | false | tner | null | tner/xlm-roberta-large-panx-dataset-ru | 0 | null | transformers | 33,603 | # XLM-RoBERTa for NER
XLM-RoBERTa finetuned on NER. Check more detail at [TNER repository](https://github.com/asahi417/tner).
## Usage
```
from transformers import AutoTokenizer, AutoModelForTokenClassification
tokenizer = AutoTokenizer.from_pretrained("asahi417/tner-xlm-roberta-large-panx-dataset-ru")
model = AutoModelForTokenClassification.from_pretrained("asahi417/tner-xlm-roberta-large-panx-dataset-ru")
``` |
tner/xlm-roberta-large-uncased-bc5cdr | 973e4f0fac04e6e73374ec5023a3a2505406fd13 | 2021-02-13T00:11:43.000Z | [
"pytorch",
"xlm-roberta",
"token-classification",
"transformers",
"autotrain_compatible"
] | token-classification | false | tner | null | tner/xlm-roberta-large-uncased-bc5cdr | 0 | null | transformers | 33,604 | # XLM-RoBERTa for NER
XLM-RoBERTa finetuned on NER. Check more detail at [TNER repository](https://github.com/asahi417/tner).
## Usage
```
from transformers import AutoTokenizer, AutoModelForTokenClassification
tokenizer = AutoTokenizer.from_pretrained("asahi417/tner-xlm-roberta-large-uncased-bc5cdr")
model = AutoModelForTokenClassification.from_pretrained("asahi417/tner-xlm-roberta-large-uncased-bc5cdr")
``` |
tner/xlm-roberta-large-uncased-fin | 92eb88f13a760c1524fe6c6faacc95e7a30aead0 | 2021-02-13T00:05:55.000Z | [
"pytorch",
"xlm-roberta",
"token-classification",
"transformers",
"autotrain_compatible"
] | token-classification | false | tner | null | tner/xlm-roberta-large-uncased-fin | 0 | null | transformers | 33,605 | # XLM-RoBERTa for NER
XLM-RoBERTa finetuned on NER. Check more detail at [TNER repository](https://github.com/asahi417/tner).
## Usage
```
from transformers import AutoTokenizer, AutoModelForTokenClassification
tokenizer = AutoTokenizer.from_pretrained("asahi417/tner-xlm-roberta-large-uncased-fin")
model = AutoModelForTokenClassification.from_pretrained("asahi417/tner-xlm-roberta-large-uncased-fin")
``` |
bgfruna/double-bart-ensemble-squad2 | 1bb9a0f44dd99ec7c27738cd70112647badc8716 | 2021-07-21T22:47:12.000Z | [
"pytorch",
"en",
"dataset:squad_v2",
"dataset:squad2",
"question-answering",
"license:cc-by-4.0"
] | question-answering | false | bgfruna | null | bgfruna/double-bart-ensemble-squad2 | 0 | null | null | 33,606 | ---
language: en
tags:
- pytorch
- question-answering
datasets:
- squad_v2
- squad2
license: cc-by-4.0
metrics:
- squad_v2
- exact
- f1
widget:
- text: "By what main attribute are computational problems classified utilizing computational complexity theory?"
context: "Computational complexity theory is a branch of the theory of computation in theoretical computer science that focuses on classifying computational problems according to their inherent difficulty, and relating those classes to each other. A computational problem is understood to be a task that is in principle amenable to being solved by a computer, which is equivalent to stating that the problem may be solved by mechanical application of mathematical steps, such as an algorithm."
---
# Performance
This ensemble was evaluated on [SQuAD 2.0](https://huggingface.co/datasets/squad_v2) with the following results:
```
{'HasAns_exact': 52.5472334682861,
'HasAns_f1': 67.94939813758602,
'HasAns_total': 5928,
'NoAns_exact': 91.75777964676199,
'NoAns_f1': 91.75777964676199,
'NoAns_total': 5945,
'best_exact': 72.16373283921503,
'best_exact_thresh': 0.0,
'best_f1': 79.85378860941708,
'best_f1_thresh': 0.0,
'exact': 72.1805777815211,
'f1': 79.87063355172326,
'total': 11873
}
``` |
bluebalam/paper-rec | 8ed91678a65abd9e178f930150cfb2c7136b8f5f | 2022-02-04T21:37:35.000Z | [
"en",
"arxiv:2109.03955",
"arxiv:1908.10084",
"recsys",
"pytorch",
"sentence_transformers",
"license:mit"
] | null | false | bluebalam | null | bluebalam/paper-rec | 0 | 3 | null | 33,607 | ---
language:
- en
license: mit
tags:
- recsys
- pytorch
- sentence_transformers
#datasets:
#- {dataset_0} # Example: common_voice. Use dataset id from https://hf.co/datasets
#metrics:
#- {metric_0} # Example: wer. Use metric id from https://hf.co/metrics
---
# `paper-rec` Model Card
Last updated: 2022-02-04
## Model Details
`paper-rec` goal is to recommend users what scientific papers to read next based on their preferences. This is a test model used to explore Hugging Face Hub capabilities and identify requirements to enable support for recommendation task in the ecosystem.
### Model date
2022-02-04
### Model type
Recommender System model with support of a Language Model for feature extraction.
### Paper & samples
The overall idea for `paper-rec` test model is inspired by this work: [NU:BRIEF – A Privacy-aware Newsletter Personalization Engine for Publishers](https://arxiv.org/abs/2109.03955).
However, for `paper-rec`, we use a different language model more suitable for longer text, namely *Sentence Transformers*: [Sentence-BERT: Sentence Embeddings using Siamese BERT-Networks](https://arxiv.org/abs/1908.10084), in particular: [sentence-transformers/all-MiniLM-L6-v2](https://huggingface.co/sentence-transformers/all-MiniLM-L6-v2).
## Model Use
The intended direct users are recommender systems' practitioners and enthusiasts that would like to experiment with the task of scientific paper recommendation.
## Data, Performance, and Limitations
### Data
The data used for this model corresponds to the [RSS news feeds for arXiv updates](https://arxiv.org/help/rss) accessed on 2022-02-04. In particular to the ones related to Machine Learning and AI:
1. [Artificial Intelligence](http://arxiv.org/rss/cs.AI)
1. [Computation and Language](http://arxiv.org/rss/cs.CL)
1. [Computer Vision and Pattern Recognition](http://arxiv.org/rss/cs.CV)
1. [Information Retrieval](http://arxiv.org/rss/cs.IR)
1. [Machine Learning (cs)](http://arxiv.org/rss/cs.LG)
1. [Machine Learning (stat)](http://arxiv.org/rss/stat.ML)
### Performance
N/A
## Limitations
The model is limited to the papers fetched on 2022-02-04, that is, those papers are the only ones it can recommend.
|
cross/words | d2097c5faa2c8db9d4e905059369c9bc5edf30e8 | 2021-03-19T12:05:58.000Z | [
"pytorch"
] | null | false | cross | null | cross/words | 0 | null | null | 33,608 | Entry not found |
cyou/bert-base-jp1 | b2b0a3e484cc3ffc3a3c533aa8fe66312b61299d | 2021-11-01T21:15:25.000Z | [
"pytorch"
] | null | false | cyou | null | cyou/bert-base-jp1 | 0 | null | null | 33,609 | |
drcod/DagaareBERTa | 552f8cae48ba2ea1ebdaed209b58b40aa5cabd56 | 2021-08-24T22:23:45.000Z | [
"pytorch",
"tf",
"dataset:Bible",
"arxiv:1907.11692"
] | null | false | drcod | null | drcod/DagaareBERTa | 0 | null | null | 33,610 | ---
datasets:
- Bible
---
Pretrained model on Dagaare language using a masked language modeling (MLM) objective first introduced in
[this paper](https://arxiv.org/abs/1907.11692) and first released in
[this repository](https://github.com/pytorch/fairseq/tree/master/examples/roberta)\
|
facebook/wav2vec2-base-10k-voxpopuli-ft-de | 1cbaf198475e1af97cc479ca081ce4ddd2d6b5cf | 2021-07-06T01:48:44.000Z | [
"pytorch",
"wav2vec2",
"automatic-speech-recognition",
"de",
"arxiv:2101.00390",
"transformers",
"audio",
"voxpopuli",
"license:cc-by-nc-4.0"
] | automatic-speech-recognition | false | facebook | null | facebook/wav2vec2-base-10k-voxpopuli-ft-de | 0 | 1 | transformers | 33,611 | ---
language: de
tags:
- audio
- automatic-speech-recognition
- voxpopuli
license: cc-by-nc-4.0
---
# Wav2Vec2-Base-VoxPopuli-Finetuned
[Facebook's Wav2Vec2](https://ai.facebook.com/blog/wav2vec-20-learning-the-structure-of-speech-from-raw-audio/) base model pretrained on the 10K unlabeled subset of [VoxPopuli corpus](https://arxiv.org/abs/2101.00390) and fine-tuned on the transcribed data in de (refer to Table 1 of paper for more information).
**Paper**: *[VoxPopuli: A Large-Scale Multilingual Speech Corpus for Representation
Learning, Semi-Supervised Learning and Interpretation](https://arxiv.org/abs/2101.00390)*
**Authors**: *Changhan Wang, Morgane Riviere, Ann Lee, Anne Wu, Chaitanya Talnikar, Daniel Haziza, Mary Williamson, Juan Pino, Emmanuel Dupoux* from *Facebook AI*
See the official website for more information, [here](https://github.com/facebookresearch/voxpopuli/)
# Usage for inference
In the following it is shown how the model can be used in inference on a sample of the [Common Voice dataset](https://commonvoice.mozilla.org/en/datasets)
```python
#!/usr/bin/env python3
from transformers import Wav2Vec2Processor, Wav2Vec2ForCTC
from datasets import load_dataset
import torchaudio
import torch
# resample audio
# load model & processor
model = Wav2Vec2ForCTC.from_pretrained("facebook/wav2vec2-base-10k-voxpopuli-ft-de")
processor = Wav2Vec2Processor.from_pretrained("facebook/wav2vec2-base-10k-voxpopuli-ft-de")
# load dataset
ds = load_dataset("common_voice", "de", split="validation[:1%]")
# common voice does not match target sampling rate
common_voice_sample_rate = 48000
target_sample_rate = 16000
resampler = torchaudio.transforms.Resample(common_voice_sample_rate, target_sample_rate)
# define mapping fn to read in sound file and resample
def map_to_array(batch):
speech, _ = torchaudio.load(batch["path"])
speech = resampler(speech)
batch["speech"] = speech[0]
return batch
# load all audio files
ds = ds.map(map_to_array)
# run inference on the first 5 data samples
inputs = processor(ds[:5]["speech"], sampling_rate=target_sample_rate, return_tensors="pt", padding=True)
# inference
logits = model(**inputs).logits
predicted_ids = torch.argmax(logits, axis=-1)
print(processor.batch_decode(predicted_ids))
```
|
facebook/wav2vec2-base-10k-voxpopuli-ft-hu | fd70fe41f6e7b7dc71bc952a9cd4b4e23ed7e17f | 2021-07-06T01:50:56.000Z | [
"pytorch",
"wav2vec2",
"automatic-speech-recognition",
"hu",
"arxiv:2101.00390",
"transformers",
"audio",
"voxpopuli",
"license:cc-by-nc-4.0"
] | automatic-speech-recognition | false | facebook | null | facebook/wav2vec2-base-10k-voxpopuli-ft-hu | 0 | null | transformers | 33,612 | ---
language: hu
tags:
- audio
- automatic-speech-recognition
- voxpopuli
license: cc-by-nc-4.0
---
# Wav2Vec2-Base-VoxPopuli-Finetuned
[Facebook's Wav2Vec2](https://ai.facebook.com/blog/wav2vec-20-learning-the-structure-of-speech-from-raw-audio/) base model pretrained on the 10K unlabeled subset of [VoxPopuli corpus](https://arxiv.org/abs/2101.00390) and fine-tuned on the transcribed data in hu (refer to Table 1 of paper for more information).
**Paper**: *[VoxPopuli: A Large-Scale Multilingual Speech Corpus for Representation
Learning, Semi-Supervised Learning and Interpretation](https://arxiv.org/abs/2101.00390)*
**Authors**: *Changhan Wang, Morgane Riviere, Ann Lee, Anne Wu, Chaitanya Talnikar, Daniel Haziza, Mary Williamson, Juan Pino, Emmanuel Dupoux* from *Facebook AI*
See the official website for more information, [here](https://github.com/facebookresearch/voxpopuli/)
# Usage for inference
In the following it is shown how the model can be used in inference on a sample of the [Common Voice dataset](https://commonvoice.mozilla.org/en/datasets)
```python
#!/usr/bin/env python3
from transformers import Wav2Vec2Processor, Wav2Vec2ForCTC
from datasets import load_dataset
import torchaudio
import torch
# resample audio
# load model & processor
model = Wav2Vec2ForCTC.from_pretrained("facebook/wav2vec2-base-10k-voxpopuli-ft-hu")
processor = Wav2Vec2Processor.from_pretrained("facebook/wav2vec2-base-10k-voxpopuli-ft-hu")
# load dataset
ds = load_dataset("common_voice", "hu", split="validation[:1%]")
# common voice does not match target sampling rate
common_voice_sample_rate = 48000
target_sample_rate = 16000
resampler = torchaudio.transforms.Resample(common_voice_sample_rate, target_sample_rate)
# define mapping fn to read in sound file and resample
def map_to_array(batch):
speech, _ = torchaudio.load(batch["path"])
speech = resampler(speech)
batch["speech"] = speech[0]
return batch
# load all audio files
ds = ds.map(map_to_array)
# run inference on the first 5 data samples
inputs = processor(ds[:5]["speech"], sampling_rate=target_sample_rate, return_tensors="pt", padding=True)
# inference
logits = model(**inputs).logits
predicted_ids = torch.argmax(logits, axis=-1)
print(processor.batch_decode(predicted_ids))
```
|
facebook/wav2vec2-base-10k-voxpopuli-ft-it | 653b3c789c7d83134924bda6fabe0c6bb84f579c | 2021-07-06T01:51:18.000Z | [
"pytorch",
"wav2vec2",
"automatic-speech-recognition",
"it",
"arxiv:2101.00390",
"transformers",
"audio",
"voxpopuli",
"license:cc-by-nc-4.0"
] | automatic-speech-recognition | false | facebook | null | facebook/wav2vec2-base-10k-voxpopuli-ft-it | 0 | null | transformers | 33,613 | ---
language: it
tags:
- audio
- automatic-speech-recognition
- voxpopuli
license: cc-by-nc-4.0
---
# Wav2Vec2-Base-VoxPopuli-Finetuned
[Facebook's Wav2Vec2](https://ai.facebook.com/blog/wav2vec-20-learning-the-structure-of-speech-from-raw-audio/) base model pretrained on the 10K unlabeled subset of [VoxPopuli corpus](https://arxiv.org/abs/2101.00390) and fine-tuned on the transcribed data in it (refer to Table 1 of paper for more information).
**Paper**: *[VoxPopuli: A Large-Scale Multilingual Speech Corpus for Representation
Learning, Semi-Supervised Learning and Interpretation](https://arxiv.org/abs/2101.00390)*
**Authors**: *Changhan Wang, Morgane Riviere, Ann Lee, Anne Wu, Chaitanya Talnikar, Daniel Haziza, Mary Williamson, Juan Pino, Emmanuel Dupoux* from *Facebook AI*
See the official website for more information, [here](https://github.com/facebookresearch/voxpopuli/)
# Usage for inference
In the following it is shown how the model can be used in inference on a sample of the [Common Voice dataset](https://commonvoice.mozilla.org/en/datasets)
```python
#!/usr/bin/env python3
from transformers import Wav2Vec2Processor, Wav2Vec2ForCTC
from datasets import load_dataset
import torchaudio
import torch
# resample audio
# load model & processor
model = Wav2Vec2ForCTC.from_pretrained("facebook/wav2vec2-base-10k-voxpopuli-ft-it")
processor = Wav2Vec2Processor.from_pretrained("facebook/wav2vec2-base-10k-voxpopuli-ft-it")
# load dataset
ds = load_dataset("common_voice", "it", split="validation[:1%]")
# common voice does not match target sampling rate
common_voice_sample_rate = 48000
target_sample_rate = 16000
resampler = torchaudio.transforms.Resample(common_voice_sample_rate, target_sample_rate)
# define mapping fn to read in sound file and resample
def map_to_array(batch):
speech, _ = torchaudio.load(batch["path"])
speech = resampler(speech)
batch["speech"] = speech[0]
return batch
# load all audio files
ds = ds.map(map_to_array)
# run inference on the first 5 data samples
inputs = processor(ds[:5]["speech"], sampling_rate=target_sample_rate, return_tensors="pt", padding=True)
# inference
logits = model(**inputs).logits
predicted_ids = torch.argmax(logits, axis=-1)
print(processor.batch_decode(predicted_ids))
```
|
facebook/wav2vec2-xlsr-53-phon-cv-babel-ft | 2598051121aed794d856e0edac36df88613724ba | 2021-11-10T12:02:20.000Z | [
"pytorch",
"wav2vec2",
"automatic-speech-recognition",
"transformers"
] | automatic-speech-recognition | false | facebook | null | facebook/wav2vec2-xlsr-53-phon-cv-babel-ft | 0 | null | transformers | 33,614 | Entry not found |
fadhilarkan/t5_paw_global | de340fecbdad839e4cb967aad569361929509139 | 2021-08-23T15:42:54.000Z | [
"pytorch",
"t5",
"text2text-generation",
"transformers",
"autotrain_compatible"
] | text2text-generation | false | fadhilarkan | null | fadhilarkan/t5_paw_global | 0 | null | transformers | 33,615 | Entry not found |
fadhilarkan/tmpvqruuuz0 | 6477d617cef8553baa2eb1a4088c427cc16fc2f5 | 2021-08-23T17:06:39.000Z | [
"pytorch",
"t5",
"text2text-generation",
"transformers",
"autotrain_compatible"
] | text2text-generation | false | fadhilarkan | null | fadhilarkan/tmpvqruuuz0 | 0 | null | transformers | 33,616 | Entry not found |
faketermz/DialoGPT | 5c1c9ab8d18265c98d4ea73cc6b4f84a5bc5790c | 2021-12-05T13:02:46.000Z | [
"pytorch",
"gpt2",
"text-generation",
"transformers",
"conversational"
] | conversational | false | faketermz | null | faketermz/DialoGPT | 0 | null | transformers | 33,617 | ---
tags:
- conversational
---
# test DialoGPT Model |
famodde/optimizer-ner-fineTune-lst2021 | b7c5561849ab201f82e58b0442e954e82484ab71 | 2022-02-18T05:01:27.000Z | [
"pytorch",
"camembert",
"token-classification",
"transformers",
"autotrain_compatible"
] | token-classification | false | famodde | null | famodde/optimizer-ner-fineTune-lst2021 | 0 | null | transformers | 33,618 | Entry not found |
famodde/optimizer-ner-fineTune | 9711ce205f254d30f581a73fb57c7053b811ab1d | 2022-02-16T18:41:51.000Z | [
"pytorch",
"camembert",
"token-classification",
"transformers",
"autotrain_compatible"
] | token-classification | false | famodde | null | famodde/optimizer-ner-fineTune | 0 | null | transformers | 33,619 | Entry not found |
famodde/wangchanberta-ner-fineTune | 748a4cc18ff673826965a3b409d38de1fc0a4cf1 | 2022-02-16T08:08:19.000Z | [
"pytorch",
"camembert",
"token-classification",
"transformers",
"autotrain_compatible"
] | token-classification | false | famodde | null | famodde/wangchanberta-ner-fineTune | 0 | null | transformers | 33,620 | Entry not found |
fatemaMeem98/DialoGPT-medium-HermioneGrangerBot | ae7a18a3e2bdf2b41f27661d2cea38143e526ad7 | 2021-12-20T05:35:09.000Z | [
"pytorch",
"gpt2",
"text-generation",
"transformers",
"conversational"
] | conversational | false | fatemaMeem98 | null | fatemaMeem98/DialoGPT-medium-HermioneGrangerBot | 0 | null | transformers | 33,621 | ---
tags:
- conversational
---
# Hermione Granger DialoGPT Model |
fav-kky/FERNET-News_sk | 81e1bf2f8ad90f7f38a171a924f839276bae1217 | 2021-09-09T14:02:40.000Z | [
"pytorch",
"tf",
"roberta",
"fill-mask",
"sk",
"arxiv:2107.10042",
"transformers",
"Slovak",
"KKY",
"FAV",
"license:cc-by-nc-sa-4.0",
"autotrain_compatible"
] | fill-mask | false | fav-kky | null | fav-kky/FERNET-News_sk | 0 | null | transformers | 33,622 | ---
language: "sk"
tags:
- Slovak
- KKY
- FAV
license: "cc-by-nc-sa-4.0"
---
# FERNET-News_sk
FERNET-News_sk is a monolingual Slovak RoBERTa-base model pre-trained from 4.5GB of thoroughly cleaned Slovak news corpus.
It is a Slovak version of our Czech [FERNET-News](https://huggingface.co/fav-kky/FERNET-News) model.
Preprint of our paper is available at https://arxiv.org/abs/2107.10042. |
felixai/distilmbart-9-3 | a4499e41be9451ef8c471f44ab5c51875acefe25 | 2021-07-16T14:41:20.000Z | [
"pytorch",
"mbart",
"text2text-generation",
"transformers",
"autotrain_compatible"
] | text2text-generation | false | felixai | null | felixai/distilmbart-9-3 | 0 | null | transformers | 33,623 | # mbart for 9-3
|
ffrmns/t5-small_XSum-finetuned | 9f783d62ef4b9eafd0a82deae62cbef11da85cee | 2021-04-20T00:59:58.000Z | [
"pytorch",
"t5",
"text2text-generation",
"transformers",
"autotrain_compatible"
] | text2text-generation | false | ffrmns | null | ffrmns/t5-small_XSum-finetuned | 0 | null | transformers | 33,624 | Entry not found |
ffsouza/tiny-mbart-length-96-learning_rate-2e-05-weight_decay-0.005-finetuned-en-to-ro | 119229529e68cec6e0bd457227c9d1d64fca142a | 2021-11-30T19:57:36.000Z | [
"pytorch",
"tensorboard",
"mbart",
"text2text-generation",
"dataset:wmt16_en_ro_pre_processed",
"transformers",
"generated_from_trainer",
"model-index",
"autotrain_compatible"
] | text2text-generation | false | ffsouza | null | ffsouza/tiny-mbart-length-96-learning_rate-2e-05-weight_decay-0.005-finetuned-en-to-ro | 0 | null | transformers | 33,625 | ---
tags:
- generated_from_trainer
datasets:
- wmt16_en_ro_pre_processed
metrics:
- bleu
model-index:
- name: tiny-mbart-length-96-learning_rate-2e-05-weight_decay-0.005-finetuned-en-to-ro
results:
- task:
name: Sequence-to-sequence Language Modeling
type: text2text-generation
dataset:
name: wmt16_en_ro_pre_processed
type: wmt16_en_ro_pre_processed
args: enro
metrics:
- name: Bleu
type: bleu
value: 0.0
---
<!-- This model card has been generated automatically according to the information the Trainer had access to. You
should probably proofread and complete it, then remove this comment. -->
# tiny-mbart-length-96-learning_rate-2e-05-weight_decay-0.005-finetuned-en-to-ro
This model is a fine-tuned version of [sshleifer/tiny-mbart](https://huggingface.co/sshleifer/tiny-mbart) on the wmt16_en_ro_pre_processed dataset.
It achieves the following results on the evaluation set:
- Loss: 8.5983
- Bleu: 0.0
- Gen Len: 20.0
## Model description
More information needed
## Intended uses & limitations
More information needed
## Training and evaluation data
More information needed
## Training procedure
### Training hyperparameters
The following hyperparameters were used during training:
- learning_rate: 2e-05
- train_batch_size: 8
- eval_batch_size: 8
- seed: 42
- optimizer: Adam with betas=(0.9,0.999) and epsilon=1e-08
- lr_scheduler_type: linear
- num_epochs: 1
- mixed_precision_training: Native AMP
### Training results
| Training Loss | Epoch | Step | Validation Loss | Bleu | Gen Len |
|:-------------:|:-----:|:-----:|:---------------:|:----:|:-------:|
| 8.3753 | 1.0 | 76290 | 8.5983 | 0.0 | 20.0 |
### Framework versions
- Transformers 4.12.5
- Pytorch 1.10.0+cu102
- Datasets 1.15.1
- Tokenizers 0.10.3
|
ffsouza/tiny-mbart-length-96-learning_rate-2e-05-weight_decay-0.02-finetuned-en-to-ro | 6da962a68f0deab4ea27c59dbbaaefd973af78fb | 2021-11-30T20:10:38.000Z | [
"pytorch",
"tensorboard",
"mbart",
"text2text-generation",
"transformers",
"autotrain_compatible"
] | text2text-generation | false | ffsouza | null | ffsouza/tiny-mbart-length-96-learning_rate-2e-05-weight_decay-0.02-finetuned-en-to-ro | 0 | null | transformers | 33,626 | Entry not found |
fgaim/t5-small-squad-v2 | dcbbdbea810d304e270c8a6ab12c475bc0b1f151 | 2022-01-30T21:35:54.000Z | [
"pytorch",
"t5",
"text2text-generation",
"en",
"dataset:c4",
"dataset:squad",
"transformers",
"license:apache-2.0",
"autotrain_compatible"
] | text2text-generation | false | fgaim | null | fgaim/t5-small-squad-v2 | 0 | null | transformers | 33,627 | ---
language:
- en
datasets:
- c4
- squad
tags:
- text2text-generation
widget:
- text: "question: What is the atomic number for oxygen? context: Oxygen is a chemical element with symbol O and atomic number 8."
- text: "question: What is the chemical symbol of Oxygen? context: Oxygen is a chemical element with symbol O and atomic number 8."
license: apache-2.0
---
T5-small for QA
---
[Google's T5-small](https://ai.googleblog.com/2020/02/exploring-transfer-learning-with-t5.html) pre-trained on the [C4](https://huggingface.co/datasets/c4) dataset, fine-tuned for Question-Answering on [SQuAD v2](https://huggingface.co/datasets/squad_v2) with the following hyperparameters:
```
optimizer=adamw_hf
learning_rate=3e-5
adam_beta1=0.9
adam_beta2=0.999
adam_epsilon=1e-08
num_train_epochs=2
per_device_train_batch_size=12
```
Usage
---
The input [context and question] has to be prepared in a specific way as follows:
```python
from transformers import pipeline
def prep_input(_context, _question):
return " ".join(["question:", _question.strip(), "context:", _context.strip()])
t5qa = pipeline("text2text-generation", "fgaim/t5-small-squad-v2")
context = """
Oxygen is a chemical element with symbol O and atomic number 8. It is a member of the chalcogen group on the periodic table and is a highly reactive nonmetal and oxidizing agent that readily forms compounds (notably oxides) with most elements. By mass, oxygen is the third-most abundant element in the universe, after hydrogen and helium. At standard temperature and pressure, two atoms of the element bind to form dioxygen, a colorless and odorless diatomic gas with the formula O.
"""
t5qa(prep_input(context, "How many atoms combine to form dioxygen?"))
# [{'generated_text': 'two'}]
t5qa(prep_input(context, "What element makes up almost half of the earth's crust by mass?"))
# [{'generated_text': 'oxygen'}]
t5qa(prep_input(context, "What are the most abundent elements of the universe by mass?"))
# [{'generated_text': 'hydrogen and helium'}]
```
|
fgua/bert-base-uncased-wikitext2 | 95764d9cfa4dc9ae7291451ddd4e690de53b3b22 | 2022-04-26T15:04:36.000Z | [
"pytorch",
"bert",
"fill-mask",
"transformers",
"autotrain_compatible"
] | fill-mask | false | fgua | null | fgua/bert-base-uncased-wikitext2 | 0 | null | transformers | 33,628 | Entry not found |
fibruh/DialoGPT-small-harrypotter | 46acbb31b70e79dc53b646daee1714fb41bcdc3f | 2021-12-15T04:18:11.000Z | [
"pytorch",
"gpt2",
"text-generation",
"transformers",
"conversational"
] | conversational | false | fibruh | null | fibruh/DialoGPT-small-harrypotter | 0 | null | transformers | 33,629 | ---
tags:
- conversational
---
# Fibruh Bot Model |
finiteautomata/bert-non-contextualized-hate-category-es | 09ecb41256785c2c7cfe8706431c77c54598959b | 2021-05-19T16:51:52.000Z | [
"pytorch",
"bert",
"transformers"
] | null | false | finiteautomata | null | finiteautomata/bert-non-contextualized-hate-category-es | 0 | null | transformers | 33,630 | Entry not found |
finiteautomata/beto-fine-grained-hatespeech-news | f4a730bd8173344c79513e47a92ac20cc3cc9d6d | 2021-06-24T14:54:12.000Z | [
"pytorch",
"bert",
"transformers"
] | null | false | finiteautomata | null | finiteautomata/beto-fine-grained-hatespeech-news | 0 | null | transformers | 33,631 | ## Fine Grained Hate Speech in News
### WARNING: Work in progress
Model trained on news comments. |
finiteautomata/betonews-bodycontext | efccfffb07da8ad1143d183e8062b37bc39c6cdb | 2021-10-08T14:53:18.000Z | [
"pytorch",
"bert",
"fill-mask",
"transformers",
"autotrain_compatible"
] | fill-mask | false | finiteautomata | null | finiteautomata/betonews-bodycontext | 0 | null | transformers | 33,632 | Entry not found |
finiteautomata/betonews-nonecontext | 444990c470e160583943cabe30d304ddcee642f4 | 2021-10-01T21:32:37.000Z | [
"pytorch",
"bert",
"fill-mask",
"transformers",
"autotrain_compatible"
] | fill-mask | false | finiteautomata | null | finiteautomata/betonews-nonecontext | 0 | null | transformers | 33,633 | Entry not found |
fkHug/modelFromWav2vec | d778372ac925e17981e14547b93dc47b313c3075 | 2021-12-03T17:35:19.000Z | [
"pytorch",
"wav2vec2",
"automatic-speech-recognition",
"transformers"
] | automatic-speech-recognition | false | fkHug | null | fkHug/modelFromWav2vec | 0 | null | transformers | 33,634 | this is my model card
|
flairbook/flairmodel | 5966905c3b083679ba6d327261d1ce73074e6341 | 2022-01-08T18:06:53.000Z | [
"pytorch",
"flair",
"token-classification"
] | token-classification | false | flairbook | null | flairbook/flairmodel | 0 | null | flair | 33,635 | ---
tags:
- flair
- token-classification
widget:
- text: "does this work"
---
## Test model README
Some test README description |
flakje/DialoGPT-small-Marty | 2e8d61849608dda317dc7f069914b2b4ef30a599 | 2021-11-20T18:34:54.000Z | [
"pytorch",
"gpt2",
"text-generation",
"transformers",
"conversational"
] | conversational | false | flakje | null | flakje/DialoGPT-small-Marty | 0 | null | transformers | 33,636 | ---
tags:
- conversational
---
# Marty DialoGPT Model |
flavio-nakasato/deeppolicytracker_500k | aa3c41c375fe846894cd19ffd4088876788b3c5b | 2021-08-14T22:14:07.000Z | [
"pytorch",
"tensorboard",
"roberta",
"fill-mask",
"transformers",
"autotrain_compatible"
] | fill-mask | false | flavio-nakasato | null | flavio-nakasato/deeppolicytracker_500k | 0 | null | transformers | 33,637 | RoBERTa model pretrained on the Brazilian Federal Official Gazette (500k instances).
|
flax-community/gpt-code-clippy-125M-bs2048-raw | 39fa8caa315e1524b9302e094262888e342b9188 | 2021-07-16T10:29:45.000Z | [
"pytorch",
"jax",
"gpt_neo",
"text-generation",
"transformers"
] | text-generation | false | flax-community | null | flax-community/gpt-code-clippy-125M-bs2048-raw | 0 | null | transformers | 33,638 | Entry not found |
flax-community/gpt-neo-1.3B-resized-embed | b75d14484ef2e569ef6ef10dd60f6a4e8829b674 | 2021-07-16T11:25:41.000Z | [
"pytorch",
"jax",
"gpt_neo",
"text-generation",
"transformers"
] | text-generation | false | flax-community | null | flax-community/gpt-neo-1.3B-resized-embed | 0 | null | transformers | 33,639 | Entry not found |
flax-sentence-embeddings/all_datasets_v4_MiniLM-L12 | 70c1ce7853189f5b4cb094ff364dc1f0869c11be | 2021-07-23T16:01:01.000Z | [
"pytorch",
"bert",
"en",
"arxiv:2104.08727",
"arxiv:1810.09305",
"arxiv:2102.07033",
"arxiv:1904.06472",
"sentence-transformers",
"feature-extraction",
"sentence-similarity"
] | sentence-similarity | false | flax-sentence-embeddings | null | flax-sentence-embeddings/all_datasets_v4_MiniLM-L12 | 0 | 2 | sentence-transformers | 33,640 | ---
pipeline_tag: sentence-similarity
tags:
- sentence-transformers
- feature-extraction
- sentence-similarity
language: en
---
# Model description
The project aims to train sentence embedding models on very large sentence level datasets using a self-supervised
contrastive learning objective. We used the pretrained ['MiniLM-L12'](https://huggingface.co/microsoft/MiniLM-L12-H384-uncased) model and fine-tuned in on a
1B sentence pairs dataset. We use a contrastive learning objective: given a sentence from the pair, the model should predict which out of a set of randomly sampled other sentences, was actually paired with it in our dataset.
We developped this model during the
[Community week using JAX/Flax for NLP & CV](https://discuss.huggingface.co/t/open-to-the-community-community-week-using-jax-flax-for-nlp-cv/7104),
organized by Hugging Face. We developped this model as part of the project:
[Train the Best Sentence Embedding Model Ever with 1B Training Pairs](https://discuss.huggingface.co/t/train-the-best-sentence-embedding-model-ever-with-1b-training-pairs/7354). We benefited from efficient hardware infrastructure to run the project: 7 TPUs v3-8, as well
as intervention from Google’s Flax, JAX, and Cloud team member about efficient deep learning frameworks.
## Intended uses
Our model is intented to be used as a sentence encoder. Given an input sentence, it ouptuts a vector which captures
the sentence semantic information. The sentence vector may be used for information retrieval, clustering or sentence
similarity tasks.
## How to use
Here is how to use this model to get the features of a given text using [SentenceTransformers](https://github.com/UKPLab/sentence-transformers) library:
```python
from sentence_transformers import SentenceTransformer
model = SentenceTransformer('flax-sentence-embeddings/all_datasets_v4_MiniLM-L12')
text = "Replace me by any text you'd like."
text_embbedding = model.encode(text)
# array([-0.01559514, 0.04046123, 0.1317083 , 0.00085931, 0.04585106,
# -0.05607086, 0.0138078 , 0.03569756, 0.01420381, 0.04266302 ...],
# dtype=float32)
```
# Training procedure
## Pre-training
We use the pretrained ['MiniLM-L12'](https://huggingface.co/microsoft/MiniLM-L12-H384-uncased).
Please refer to the model card for more detailed information about the pre-training procedure.
## Fine-tuning
We fine-tune the model using a contrastive objective. Formally, we compute the cosine similarity from each possible sentence pairs from the batch.
We then apply the cross entropy loss by comparing with true pairs.
### Hyper parameters
We trained ou model on a TPU v3-8. We train the model during 540k steps using a batch size of 1024 (128 per TPU core).
We use a learning rate warm up of 500. The sequence length was limited to 128 tokens. We used the AdamW optimizer with
a 2e-5 learning rate. The full training script is accessible in this current repository.
### Training data
We use the concatenation from multiple datasets to fine-tune our model. The total number of sentence pairs is above 1 billion sentences.
We sampled each dataset given a weighted probability which configuration is detailed in the `data_config.json` file.
| Dataset | Paper | Number of training tuples |
|:--------------------------------------------------------:|:----------------------------------------:|:--------------------------:|
| [GOOAQ: Open Question Answering with Diverse Answer Types](https://github.com/allenai/gooaq) | [paper](https://arxiv.org/pdf/2104.08727.pdf) | 3,012,496 |
| [Stack Exchange](https://huggingface.co/datasets/flax-sentence-embeddings/stackexchange_title_body_jsonl) | - | 364,001 |
| [Flickr 30k](https://shannon.cs.illinois.edu/DenotationGraph/) | [paper](https://transacl.org/ojs/index.php/tacl/article/view/229/33) | 317,695 |
| [COCO 2020](COCO 2020) | [paper](https://link.springer.com/chapter/10.1007%2F978-3-319-10602-1_48) | 828,395|
| [Code Search](https://huggingface.co/datasets/code_search_net) | - | 1,151,414 |
| [TriviaqQA](https://huggingface.co/datasets/trivia_qa) | - | 73,346 |
| [SQuAD2.0](https://rajpurkar.github.io/SQuAD-explorer/) | [paper](https://aclanthology.org/P18-2124.pdf) | 87,599 |
| [Natural Questions (NQ)](https://ai.google.com/research/NaturalQuestions) | [paper](https://transacl.org/ojs/index.php/tacl/article/view/1455) | 100,231 |
| [Simple Wikipedia](https://cs.pomona.edu/~dkauchak/simplification/) | [paper](https://www.aclweb.org/anthology/P11-2117/) | 102,225 |
| [Quora Question Pairs](https://quoradata.quora.com/First-Quora-Dataset-Release-Question-Pairs) | - | 103,663 |
| [Altlex](https://github.com/chridey/altlex/) | [paper](https://aclanthology.org/P16-1135.pdf) | 112,696 |
| [Wikihow](https://github.com/pvl/wikihow_pairs_dataset) | [paper](https://arxiv.org/abs/1810.09305) | 128,542 |
| [Sentence Compression](https://github.com/google-research-datasets/sentence-compression) | [paper](https://www.aclweb.org/anthology/D13-1155/) | 180,000 |
| AllNLI ([SNLI](https://nlp.stanford.edu/projects/snli/) and [MultiNLI](https://cims.nyu.edu/~sbowman/multinli/) | [paper SNLI](https://doi.org/10.18653/v1/d15-1075), [paper MultiNLI](https://doi.org/10.18653/v1/n18-1101) | 277,230 |
| [Eli5](https://huggingface.co/datasets/eli5) | [paper](https://doi.org/10.18653/v1/p19-1346) | 325,475 |
| [SPECTER](https://github.com/allenai/specter) | [paper](https://doi.org/10.18653/v1/2020.acl-main.207) | 684,100 |
| [S2ORC](https://github.com/allenai/s2orc) Title/Abstract | [paper](https://aclanthology.org/2020.acl-main.447/) | 41,769,185 |
| [S2ORC](https://github.com/allenai/s2orc) Citation/Citation | [paper](https://aclanthology.org/2020.acl-main.447/) | 52,603,982 |
| [S2ORC](https://github.com/allenai/s2orc) Citation/Abstract | [paper](https://aclanthology.org/2020.acl-main.447/) | 116,288,806 |
| [PAQ](https://github.com/facebookresearch/PAQ) | [paper](https://arxiv.org/abs/2102.07033) | 64,371,441 |
| [WikiAnswers](https://github.com/afader/oqa#wikianswers-corpus) | [paper](https://doi.org/10.1145/2623330.2623677) | 77,427,422 |
| SearchQA | - | 582,261 |
| [Yahoo Answers](https://www.kaggle.com/soumikrakshit/yahoo-answers-dataset) Title/Answer | [paper](https://proceedings.neurips.cc/paper/2015/hash/250cf8b51c773f3f8dc8b4be867a9a02-Abstract.html) | 1,198,260 |
| [Yahoo Answers](https://www.kaggle.com/soumikrakshit/yahoo-answers-dataset) Title/Question | [paper](https://proceedings.neurips.cc/paper/2015/hash/250cf8b51c773f3f8dc8b4be867a9a02-Abstract.html) | 659,896 |
| [Yahoo Answers](https://www.kaggle.com/soumikrakshit/yahoo-answers-dataset) Question/Answer | [paper](https://proceedings.neurips.cc/paper/2015/hash/250cf8b51c773f3f8dc8b4be867a9a02-Abstract.html) | 681,164 |
| [MS MARCO](https://microsoft.github.io/msmarco/) | [paper](https://doi.org/10.1145/3404835.3462804) | 9,144,553 |
| [Reddit conversationnal](https://github.com/PolyAI-LDN/conversational-datasets/tree/master/reddit) | [paper](https://arxiv.org/abs/1904.06472) | 726,484,430 |
| total | | 1,097,953,922 |
|
flboehm/reddit-bert-text2 | e65bd2cef8e20a463711d1ae957444761012de89 | 2021-12-07T14:45:38.000Z | [
"pytorch",
"tensorboard",
"bert",
"fill-mask",
"transformers",
"generated_from_trainer",
"license:apache-2.0",
"model-index",
"autotrain_compatible"
] | fill-mask | false | flboehm | null | flboehm/reddit-bert-text2 | 0 | null | transformers | 33,641 | ---
license: apache-2.0
tags:
- generated_from_trainer
model-index:
- name: reddit-bert-text2
results: []
---
<!-- This model card has been generated automatically according to the information the Trainer had access to. You
should probably proofread and complete it, then remove this comment. -->
# reddit-bert-text2
This model is a fine-tuned version of [bert-base-uncased](https://huggingface.co/bert-base-uncased) on an unknown dataset.
It achieves the following results on the evaluation set:
- Loss: 2.4969
- Perplexity: 12.14
## Model description
More information needed
## Intended uses & limitations
More information needed
## Training and evaluation data
More information needed
## Training procedure
### Training hyperparameters
The following hyperparameters were used during training:
- learning_rate: 2e-05
- train_batch_size: 8
- eval_batch_size: 8
- seed: 42
- optimizer: Adam with betas=(0.9,0.999) and epsilon=1e-08
- lr_scheduler_type: linear
- num_epochs: 3.0
### Training results
| Training Loss | Epoch | Step | Validation Loss |
|:-------------:|:-----:|:----:|:---------------:|
| 2.8378 | 1.0 | 1007 | 2.6379 |
| 2.6493 | 2.0 | 2014 | 2.5655 |
| 2.5561 | 3.0 | 3021 | 2.5382 |
### Framework versions
- Transformers 4.12.5
- Pytorch 1.10.0+cu113
- Datasets 1.16.1
- Tokenizers 0.10.3
|
flboehm/reddit-bert-text3 | ad988e5ed57aa5368c34d6c5202aeddf458b7e78 | 2021-12-08T15:32:43.000Z | [
"pytorch",
"tensorboard",
"bert",
"fill-mask",
"transformers",
"generated_from_trainer",
"license:apache-2.0",
"model-index",
"autotrain_compatible"
] | fill-mask | false | flboehm | null | flboehm/reddit-bert-text3 | 0 | null | transformers | 33,642 | ---
license: apache-2.0
tags:
- generated_from_trainer
model-index:
- name: reddit-bert-text3
results: []
---
<!-- This model card has been generated automatically according to the information the Trainer had access to. You
should probably proofread and complete it, then remove this comment. -->
# reddit-bert-text3
This model is a fine-tuned version of [bert-base-uncased](https://huggingface.co/bert-base-uncased) on an unknown dataset.
It achieves the following results on the evaluation set:
- Loss: 2.5346
## Model description
More information needed
## Intended uses & limitations
More information needed
## Training and evaluation data
More information needed
## Training procedure
### Training hyperparameters
The following hyperparameters were used during training:
- learning_rate: 2e-05
- train_batch_size: 8
- eval_batch_size: 8
- seed: 42
- optimizer: Adam with betas=(0.9,0.999) and epsilon=1e-08
- lr_scheduler_type: linear
- num_epochs: 3.0
### Training results
| Training Loss | Epoch | Step | Validation Loss |
|:-------------:|:-----:|:----:|:---------------:|
| 3.1924 | 1.0 | 981 | 2.6541 |
| 2.7158 | 2.0 | 1962 | 2.5480 |
| 2.6583 | 3.0 | 2943 | 2.5072 |
### Framework versions
- Transformers 4.12.5
- Pytorch 1.10.0+cu113
- Datasets 1.16.1
- Tokenizers 0.10.3
|
flboehm/reddit-bert-text4 | 51b7eff9331ea63931d73c1b68120bf5269403c3 | 2021-12-15T08:41:48.000Z | [
"pytorch",
"tensorboard",
"bert",
"fill-mask",
"transformers",
"generated_from_trainer",
"license:apache-2.0",
"model-index",
"autotrain_compatible"
] | fill-mask | false | flboehm | null | flboehm/reddit-bert-text4 | 0 | null | transformers | 33,643 | ---
license: apache-2.0
tags:
- generated_from_trainer
model-index:
- name: reddit-bert-text4
results: []
---
<!-- This model card has been generated automatically according to the information the Trainer had access to. You
should probably proofread and complete it, then remove this comment. -->
# reddit-bert-text4
This model is a fine-tuned version of [bert-base-uncased](https://huggingface.co/bert-base-uncased) on an unknown dataset.
It achieves the following results on the evaluation set:
- Loss: 2.4763
## Model description
More information needed
## Intended uses & limitations
More information needed
## Training and evaluation data
More information needed
## Training procedure
### Training hyperparameters
The following hyperparameters were used during training:
- learning_rate: 2e-05
- train_batch_size: 8
- eval_batch_size: 8
- seed: 42
- optimizer: Adam with betas=(0.9,0.999) and epsilon=1e-08
- lr_scheduler_type: linear
- num_epochs: 3.0
### Training results
| Training Loss | Epoch | Step | Validation Loss |
|:-------------:|:-----:|:----:|:---------------:|
| 3.1071 | 1.0 | 978 | 2.6170 |
| 2.6788 | 2.0 | 1956 | 2.5332 |
| 2.6112 | 3.0 | 2934 | 2.4844 |
### Framework versions
- Transformers 4.13.0
- Pytorch 1.10.0+cu113
- Datasets 1.16.1
- Tokenizers 0.10.3
|
flymushroom/model1 | 3e012e3c0682fd818e16f8b9255fac04851d9539 | 2021-11-02T05:07:30.000Z | [
"pytorch",
"bert",
"fill-mask",
"transformers",
"autotrain_compatible"
] | fill-mask | false | flymushroom | null | flymushroom/model1 | 0 | null | transformers | 33,644 | Entry not found |
formermagic/codet5-small | 770eff8c18ee77115cfade8021030072dfe495ab | 2021-09-21T23:20:48.000Z | [
"pytorch",
"jax",
"tensorboard",
"t5",
"text2text-generation",
"transformers",
"autotrain_compatible"
] | text2text-generation | false | formermagic | null | formermagic/codet5-small | 0 | 1 | transformers | 33,645 | Entry not found |
formermagic/codet5x-small | 921f2c65cd0919588882387818dc57e9073659f9 | 2021-10-08T02:51:31.000Z | [
"pytorch",
"jax",
"t5",
"text2text-generation",
"transformers",
"autotrain_compatible"
] | text2text-generation | false | formermagic | null | formermagic/codet5x-small | 0 | null | transformers | 33,646 | Entry not found |
francoMG/sara-qa | 2baf25628af770dc41eeca1c0dbd252aa5faf954 | 2021-10-08T02:45:39.000Z | [
"pytorch",
"bert",
"question-answering",
"transformers",
"autotrain_compatible"
] | question-answering | false | francoMG | null | francoMG/sara-qa | 0 | null | transformers | 33,647 | Entry not found |
fznmhmmd/gpt2-wikitext2 | 848acc2279df64fd4d77f0b21cc88727b0312d66 | 2022-02-09T15:44:05.000Z | [
"pytorch",
"tensorboard",
"gpt2",
"text-generation",
"transformers",
"generated_from_trainer",
"license:mit",
"model-index"
] | text-generation | false | fznmhmmd | null | fznmhmmd/gpt2-wikitext2 | 0 | null | transformers | 33,648 | ---
license: mit
tags:
- generated_from_trainer
model-index:
- name: gpt2-wikitext2
results: []
---
<!-- This model card has been generated automatically according to the information the Trainer had access to. You
should probably proofread and complete it, then remove this comment. -->
# gpt2-wikitext2
This model is a fine-tuned version of [gpt2](https://huggingface.co/gpt2) on the None dataset.
It achieves the following results on the evaluation set:
- Loss: 6.1112
## Model description
More information needed
## Intended uses & limitations
More information needed
## Training and evaluation data
More information needed
## Training procedure
### Training hyperparameters
The following hyperparameters were used during training:
- learning_rate: 2e-05
- train_batch_size: 8
- eval_batch_size: 8
- seed: 42
- optimizer: Adam with betas=(0.9,0.999) and epsilon=1e-08
- lr_scheduler_type: linear
- num_epochs: 3.0
### Training results
| Training Loss | Epoch | Step | Validation Loss |
|:-------------:|:-----:|:----:|:---------------:|
| 6.5571 | 1.0 | 2249 | 6.4684 |
| 6.1921 | 2.0 | 4498 | 6.1984 |
| 6.0016 | 3.0 | 6747 | 6.1112 |
### Framework versions
- Transformers 4.16.2
- Pytorch 1.10.0+cu111
- Datasets 1.18.3
- Tokenizers 0.11.0
|
gabtan99/dialogpt-tagalog-medium-10 | 62ec78049ea2dcc6442ed4e487c3b9e872f85c45 | 2021-07-26T10:19:09.000Z | [
"pytorch",
"gpt2",
"text-generation",
"tl",
"transformers",
"conversational",
"tagalog",
"filipino"
] | conversational | false | gabtan99 | null | gabtan99/dialogpt-tagalog-medium-10 | 0 | null | transformers | 33,649 | ---
tags:
- conversational
- tagalog
- filipino
language:
- tl
---
# Tagalog DialoGPT
This is an extension of the base Tagalog DialoGPT model (https://huggingface.co/gabtan99/dialogpt-tagalog-medium).
This model is trained on 52K original conversations and 52K synthetic conversations, where 10% of tokens in each utterance in the synthetic conversation are machine-generated tokens.
|
gabtan99/dialogpt-tagalog-medium-20 | 1c1c4a011e106f4b37d3811a8be49d7c064bbcfa | 2021-08-18T03:04:51.000Z | [
"pytorch",
"gpt2",
"text-generation",
"tl",
"transformers",
"conversational",
"tagalog",
"filipino"
] | conversational | false | gabtan99 | null | gabtan99/dialogpt-tagalog-medium-20 | 0 | null | transformers | 33,650 | ---
tags:
- conversational
- tagalog
- filipino
inference: false
language:
- tl
---
# Tagalog DialoGPT
This is an extension of the base Tagalog DialoGPT model (https://huggingface.co/gabtan99/dialogpt-tagalog-medium).
This model is trained on 52K original conversations and 52K synthetic conversations, where 20% of tokens in each utterance in the synthetic conversation are machine-generated tokens.
|
gabtan99/dialogpt-tagalog-medium-30 | a40da3ce9ff019535b8ae2da4e97c24644b28f28 | 2021-08-18T03:05:00.000Z | [
"pytorch",
"gpt2",
"text-generation",
"tl",
"transformers",
"conversational",
"tagalog",
"filipino"
] | conversational | false | gabtan99 | null | gabtan99/dialogpt-tagalog-medium-30 | 0 | null | transformers | 33,651 | ---
tags:
- conversational
- tagalog
- filipino
inference: false
language:
- tl
---
# Tagalog DialoGPT
This is an extension of the base Tagalog DialoGPT model (https://huggingface.co/gabtan99/dialogpt-tagalog-medium).
This model is trained on 52K original conversations and 52K synthetic conversations, where 30% of tokens in each utterance in the synthetic conversation are machine-generated tokens.
|
gagan3012/wav2vec2-xlsr-chuvash | d08849c6cfdee971e326ced6e4d35ad52369f8ee | 2021-07-06T03:45:55.000Z | [
"pytorch",
"jax",
"wav2vec2",
"automatic-speech-recognition",
"cv",
"dataset:common_voice",
"transformers",
"audio",
"speech",
"xlsr-fine-tuning-week",
"license:apache-2.0",
"model-index"
] | automatic-speech-recognition | false | gagan3012 | null | gagan3012/wav2vec2-xlsr-chuvash | 0 | null | transformers | 33,652 | ---
language: cv
datasets:
- common_voice
metrics:
- wer
tags:
- audio
- automatic-speech-recognition
- speech
- xlsr-fine-tuning-week
license: apache-2.0
model-index:
- name: wav2vec2-xlsr-chuvash by Gagan Bhatia
results:
- task:
name: Speech Recognition
type: automatic-speech-recognition
dataset:
name: Common Voice cv
type: common_voice
args: cv
metrics:
- name: Test WER
type: wer
value: 48.40
---
# Wav2Vec2-Large-XLSR-53-Chuvash
Fine-tuned [facebook/wav2vec2-large-xlsr-53](https://huggingface.co/facebook/wav2vec2-large-xlsr-53) on Chuvash using the [Common Voice](https://huggingface.co/datasets/common_voice)
When using this model, make sure that your speech input is sampled at 16kHz.
## Usage
The model can be used directly (without a language model) as follows:
```python
import torch
import torchaudio
from datasets import load_dataset
from transformers import Wav2Vec2ForCTC, Wav2Vec2Processor
test_dataset = load_dataset("common_voice", "cv", split="test")
processor = Wav2Vec2Processor.from_pretrained("gagan3012/wav2vec2-xlsr-chuvash")
model = Wav2Vec2ForCTC.from_pretrained("gagan3012/wav2vec2-xlsr-chuvash")
resampler = torchaudio.transforms.Resample(48_000, 16_000)
# Preprocessing the datasets.
# We need to read the aduio files as arrays
def speech_file_to_array_fn(batch):
\\tspeech_array, sampling_rate = torchaudio.load(batch["path"])
\\tbatch["speech"] = resampler(speech_array).squeeze().numpy()
\\treturn batch
test_dataset = test_dataset.map(speech_file_to_array_fn)
inputs = processor(test_dataset["speech"][:2], sampling_rate=16_000, return_tensors="pt", padding=True)
with torch.no_grad():
\\tlogits = model(inputs.input_values, attention_mask=inputs.attention_mask).logits
predicted_ids = torch.argmax(logits, dim=-1)
print("Prediction:", processor.batch_decode(predicted_ids))
print("Reference:", test_dataset["sentence"][:2])
```
#### Results:
Prediction: ['проектпа килӗшӳллӗн тӗлӗ мероприяти иртермелле', 'твăра çак планета минтӗ пуяни калленнана']
Reference: ['Проектпа килӗшӳллӗн, тӗрлӗ мероприяти ирттермелле.', 'Çак планета питĕ пуян иккен.']
## Evaluation
The model can be evaluated as follows on the Chuvash test data of Common Voice.
```python
import torch
import torchaudio
from datasets import load_dataset, load_metric
from transformers import Wav2Vec2ForCTC, Wav2Vec2Processor
import re
!mkdir cer
!wget -O cer/cer.py https://huggingface.co/ctl/wav2vec2-large-xlsr-cantonese/raw/main/cer.py
test_dataset = load_dataset("common_voice", "cv", split="test") #TODO: replace {lang_id} in your language code here. Make sure the code is one of the *ISO codes* of [this](https://huggingface.co/languages) site.
wer = load_metric("wer")
cer = load_metric("cer")
processor = Wav2Vec2Processor.from_pretrained("gagan3012/wav2vec2-xlsr-chuvash")
model = Wav2Vec2ForCTC.from_pretrained("gagan3012/wav2vec2-xlsr-chuvash")
model.to("cuda")
chars_to_ignore_regex = '[\\\\,\\\\?\\\\.\\\\!\\\\-\\\\;\\\\:\\\\"\\\\“]' # TODO: adapt this list to include all special characters you removed from the data
resampler = torchaudio.transforms.Resample(48_000, 16_000)
# Preprocessing the datasets.
# We need to read the aduio files as arrays
def speech_file_to_array_fn(batch):
\\tbatch["sentence"] = re.sub(chars_to_ignore_regex, '', batch["sentence"]).lower()
\\tspeech_array, sampling_rate = torchaudio.load(batch["path"])
\\tbatch["speech"] = resampler(speech_array).squeeze().numpy()
\\treturn batch
test_dataset = test_dataset.map(speech_file_to_array_fn)
# Preprocessing the datasets.
# We need to read the aduio files as arrays
def evaluate(batch):
\\tinputs = processor(batch["speech"], sampling_rate=16_000, return_tensors="pt", padding=True)
\\twith torch.no_grad():
\\t\\tlogits = model(inputs.input_values.to("cuda"), attention_mask=inputs.attention_mask.to("cuda")).logits
\\tpred_ids = torch.argmax(logits, dim=-1)
\\tbatch["pred_strings"] = processor.batch_decode(pred_ids)
\\treturn batch
result = test_dataset.map(evaluate, batched=True, batch_size=8)
print("WER: {:2f}".format(100 * wer.compute(predictions=result["pred_strings"], references=result["sentence"])))
print("CER: {:2f}".format(100 * cer.compute(predictions=result["pred_strings"], references=result["sentence"])))
```
**Test Result**: 48.40 %
## Training
The script used for training can be found [here](https://colab.research.google.com/drive/1A7Y20c1QkSHfdOmLXPMiOEpwlTjDZ7m5?usp=sharing) |
gagan3012/wav2vec2-xlsr-punjabi | d37bc866ee1867595d13bbb20b4031cda0adf98a | 2021-07-06T04:21:10.000Z | [
"pytorch",
"jax",
"wav2vec2",
"automatic-speech-recognition",
"pa-IN",
"dataset:common_voice",
"transformers",
"audio",
"speech",
"xlsr-fine-tuning-week",
"license:apache-2.0",
"model-index"
] | automatic-speech-recognition | false | gagan3012 | null | gagan3012/wav2vec2-xlsr-punjabi | 0 | null | transformers | 33,653 | ---
language: pa-IN
datasets:
- common_voice
metrics:
- wer
tags:
- audio
- automatic-speech-recognition
- speech
- xlsr-fine-tuning-week
license: apache-2.0
model-index:
- name: wav2vec2-xlsr-punjabi
results:
- task:
name: Speech Recognition
type: automatic-speech-recognition
dataset:
name: Common Voice pa
type: common_voice
args: pa-IN
metrics:
- name: Test WER
type: wer
value: 58.06
---
# Wav2Vec2-Large-XLSR-53-Punjabi
Fine-tuned [facebook/wav2vec2-large-xlsr-53](https://huggingface.co/facebook/wav2vec2-large-xlsr-53) on Punjabi using the [Common Voice](https://huggingface.co/datasets/common_voice)
When using this model, make sure that your speech input is sampled at 16kHz.
## Usage
The model can be used directly (without a language model) as follows:
```python
import torch
import torchaudio
from datasets import load_dataset
from transformers import Wav2Vec2ForCTC, Wav2Vec2Processor
test_dataset = load_dataset("common_voice", "pa-IN", split="test")
processor = Wav2Vec2Processor.from_pretrained("gagan3012/wav2vec2-xlsr-punjabi")
model = Wav2Vec2ForCTC.from_pretrained("gagan3012/wav2vec2-xlsr-punjabi")
resampler = torchaudio.transforms.Resample(48_000, 16_000)
# Preprocessing the datasets.
# We need to read the aduio files as arrays
def speech_file_to_array_fn(batch):
\\\\tspeech_array, sampling_rate = torchaudio.load(batch["path"])
\\\\tbatch["speech"] = resampler(speech_array).squeeze().numpy()
\\\\treturn batch
test_dataset = test_dataset.map(speech_file_to_array_fn)
inputs = processor(test_dataset["speech"][:2], sampling_rate=16_000, return_tensors="pt", padding=True)
with torch.no_grad():
\\\\tlogits = model(inputs.input_values, attention_mask=inputs.attention_mask).logits
predicted_ids = torch.argmax(logits, dim=-1)
print("Prediction:", processor.batch_decode(predicted_ids))
print("Reference:", test_dataset["sentence"][:2])
```
#### Results:
Prediction: ['ਹਵਾ ਲਾਤ ਵਿੱਚ ਪੰਦ ਛੇ ਇਖਲਾਟਕੀ ਮੁਜਰਮ ਸਨ', 'ਮੈ ਇ ਹਾ ਪੈਸੇ ਲੇਹੜ ਨਹੀਂ ਸੀ ਚੌਨਾ']
Reference: ['ਹਵਾਲਾਤ ਵਿੱਚ ਪੰਜ ਛੇ ਇਖ਼ਲਾਕੀ ਮੁਜਰਮ ਸਨ', 'ਮੈਂ ਇਹ ਪੈਸੇ ਲੈਣੇ ਨਹੀਂ ਸੀ ਚਾਹੁੰਦਾ']
## Evaluation
The model can be evaluated as follows on the {language} test data of Common Voice. # TODO: replace #TODO: replace language with your {language}, *e.g.* French
```python
import torch
import torchaudio
from datasets import load_dataset, load_metric
from transformers import Wav2Vec2ForCTC, Wav2Vec2Processor
import re
test_dataset = load_dataset("common_voice", "pa-IN", split="test") #TODO: replace {lang_id} in your language code here. Make sure the code is one of the *ISO codes* of [this](https://huggingface.co/languages) site.
wer = load_metric("wer")
processor = Wav2Vec2Processor.from_pretrained("gagan3012/wav2vec2-xlsr-punjabi")
model = Wav2Vec2ForCTC.from_pretrained("gagan3012/wav2vec2-xlsr-punjabi")
model.to("cuda")
chars_to_ignore_regex = '[\\\\\\\\\\\\\\\\,\\\\\\\\\\\\\\\\?\\\\\\\\\\\\\\\\.\\\\\\\\\\\\\\\\!\\\\\\\\\\\\\\\\-\\\\\\\\\\\\\\\\;\\\\\\\\\\\\\\\\:\\\\\\\\\\\\\\\\"\\\\\\\\\\\\\\\\“]' # TODO: adapt this list to include all special characters you removed from the data
resampler = torchaudio.transforms.Resample(48_000, 16_000)
# Preprocessing the datasets.
# We need to read the aduio files as arrays
def speech_file_to_array_fn(batch):
\\\\\\\\tbatch["sentence"] = re.sub(chars_to_ignore_regex, '', batch["sentence"]).lower()
\\\\\\\\tspeech_array, sampling_rate = torchaudio.load(batch["path"])
\\\\\\\\tbatch["speech"] = resampler(speech_array).squeeze().numpy()
\\\\\\\\treturn batch
test_dataset = test_dataset.map(speech_file_to_array_fn)
# Preprocessing the datasets.
# We need to read the aduio files as arrays
def evaluate(batch):
\\\\\\\\tinputs = processor(batch["speech"], sampling_rate=16_000, return_tensors="pt", padding=True)
\\\\\\\\twith torch.no_grad():
\\\\\\\\t\\\\\\\\tlogits = model(inputs.input_values.to("cuda"), attention_mask=inputs.attention_mask.to("cuda")).logits
\\\\\\\\tpred_ids = torch.argmax(logits, dim=-1)
\\\\\\\\tbatch["pred_strings"] = processor.batch_decode(pred_ids)
\\\\\\\\treturn batch
result = test_dataset.map(evaluate, batched=True, batch_size=8)
print("WER: {:2f}".format(100 * wer.compute(predictions=result["pred_strings"], references=result["sentence"])))
```
**Test Result**: 58.05 %
## Training
The script used for training can be found [here](https://colab.research.google.com/drive/1A7Y20c1QkSHfdOmLXPMiOEpwlTjDZ7m5?usp=sharing) |
gagan3012/xls-r-300m-pa | cf684782f31901700be698d8649c8b26608251e0 | 2022-01-31T15:27:47.000Z | [
"pytorch",
"wav2vec2",
"automatic-speech-recognition",
"pa-IN",
"dataset:common_voice",
"transformers",
"mozilla-foundation/common_voice_8_0",
"generated_from_trainer",
"license:apache-2.0",
"model-index"
] | automatic-speech-recognition | false | gagan3012 | null | gagan3012/xls-r-300m-pa | 0 | null | transformers | 33,654 | ---
language:
- pa-IN
license: apache-2.0
tags:
- automatic-speech-recognition
- mozilla-foundation/common_voice_8_0
- generated_from_trainer
datasets:
- common_voice
model-index:
- name: xls-r-300m-pa
results: []
---
<!-- This model card has been generated automatically according to the information the Trainer had access to. You
should probably proofread and complete it, then remove this comment. -->
# xls-r-300m-pa
This model is a fine-tuned version of [facebook/wav2vec2-xls-r-300m](https://huggingface.co/facebook/wav2vec2-xls-r-300m) on the MOZILLA-FOUNDATION/COMMON_VOICE_8_0 - PA-IN dataset.
It achieves the following results on the evaluation set:
- Loss: 1.0443
- Wer: 0.5715
## Model description
More information needed
## Intended uses & limitations
More information needed
## Training and evaluation data
More information needed
## Training procedure
### Training hyperparameters
The following hyperparameters were used during training:
- learning_rate: 7.5e-05
- train_batch_size: 8
- eval_batch_size: 8
- seed: 42
- gradient_accumulation_steps: 4
- total_train_batch_size: 32
- optimizer: Adam with betas=(0.9,0.999) and epsilon=1e-08
- lr_scheduler_type: linear
- lr_scheduler_warmup_steps: 2000
- num_epochs: 500.0
- mixed_precision_training: Native AMP
### Training results
| Training Loss | Epoch | Step | Validation Loss | Wer |
|:-------------:|:------:|:-----:|:---------------:|:------:|
| 4.6694 | 19.22 | 500 | 4.0455 | 1.0 |
| 3.3907 | 38.45 | 1000 | 3.2836 | 1.0 |
| 2.0866 | 57.67 | 1500 | 1.2788 | 0.7715 |
| 1.4106 | 76.9 | 2000 | 0.7866 | 0.6891 |
| 1.1711 | 96.15 | 2500 | 0.6556 | 0.6272 |
| 1.038 | 115.37 | 3000 | 0.6195 | 0.5680 |
| 0.8989 | 134.6 | 3500 | 0.6563 | 0.5602 |
| 0.8021 | 153.82 | 4000 | 0.6644 | 0.5327 |
| 0.7161 | 173.07 | 4500 | 0.6844 | 0.5253 |
| 0.6449 | 192.3 | 5000 | 0.7018 | 0.5331 |
| 0.5659 | 211.52 | 5500 | 0.7451 | 0.5465 |
| 0.5118 | 230.75 | 6000 | 0.7857 | 0.5386 |
| 0.4385 | 249.97 | 6500 | 0.8062 | 0.5382 |
| 0.3984 | 269.22 | 7000 | 0.8316 | 0.5621 |
| 0.3666 | 288.45 | 7500 | 0.8736 | 0.5504 |
| 0.3256 | 307.67 | 8000 | 0.9133 | 0.5688 |
| 0.289 | 326.9 | 8500 | 0.9556 | 0.5684 |
| 0.2663 | 346.15 | 9000 | 0.9344 | 0.5708 |
| 0.2445 | 365.37 | 9500 | 0.9472 | 0.5590 |
| 0.2289 | 384.6 | 10000 | 0.9713 | 0.5672 |
| 0.2048 | 403.82 | 10500 | 0.9978 | 0.5762 |
| 0.1857 | 423.07 | 11000 | 1.0230 | 0.5798 |
| 0.1751 | 442.3 | 11500 | 1.0409 | 0.5755 |
| 0.1688 | 461.52 | 12000 | 1.0445 | 0.5727 |
| 0.1633 | 480.75 | 12500 | 1.0484 | 0.5739 |
| 0.1488 | 499.97 | 13000 | 1.0443 | 0.5715 |
### Framework versions
- Transformers 4.17.0.dev0
- Pytorch 1.10.2+cu102
- Datasets 1.18.2.dev0
- Tokenizers 0.11.0
|
gaotianyu1350/unsup-simcse-bert-large-uncased | c4294b4e7c593ed61814accee7e4837045ea7474 | 2021-05-19T17:11:09.000Z | [
"pytorch",
"jax",
"bert",
"feature-extraction",
"transformers"
] | feature-extraction | false | gaotianyu1350 | null | gaotianyu1350/unsup-simcse-bert-large-uncased | 0 | null | transformers | 33,655 | Entry not found |
gaotianyu1350/unsup-simcse-roberta-large | c0abe3578e9554542f25b4a0da9ed19188ec7a51 | 2021-05-20T16:29:43.000Z | [
"pytorch",
"jax",
"roberta",
"feature-extraction",
"transformers"
] | feature-extraction | false | gaotianyu1350 | null | gaotianyu1350/unsup-simcse-roberta-large | 0 | null | transformers | 33,656 | Entry not found |
gaussfer/test_simcse_new | d44790f8bce80e98087ce050bcfcf5d84f5791b1 | 2022-01-05T09:03:36.000Z | [
"pytorch",
"bert",
"feature-extraction",
"sentence-transformers",
"sentence-similarity",
"transformers"
] | sentence-similarity | false | gaussfer | null | gaussfer/test_simcse_new | 0 | null | sentence-transformers | 33,657 | ---
pipeline_tag: sentence-similarity
tags:
- sentence-transformers
- feature-extraction
- sentence-similarity
- transformers
---
# gaussfer/test_simcse_new
This is a [sentence-transformers](https://www.SBERT.net) model: It maps sentences & paragraphs to a 768 dimensional dense vector space and can be used for tasks like clustering or semantic search.
<!--- Describe your model here -->
## Usage (Sentence-Transformers)
Using this model becomes easy when you have [sentence-transformers](https://www.SBERT.net) installed:
```
pip install -U sentence-transformers
```
Then you can use the model like this:
```python
from sentence_transformers import SentenceTransformer
sentences = ["This is an example sentence", "Each sentence is converted"]
model = SentenceTransformer('gaussfer/test_simcse_new')
embeddings = model.encode(sentences)
print(embeddings)
```
## Usage (HuggingFace Transformers)
Without [sentence-transformers](https://www.SBERT.net), you can use the model like this: First, you pass your input through the transformer model, then you have to apply the right pooling-operation on-top of the contextualized word embeddings.
```python
from transformers import AutoTokenizer, AutoModel
import torch
#Mean Pooling - Take attention mask into account for correct averaging
def mean_pooling(model_output, attention_mask):
token_embeddings = model_output[0] #First element of model_output contains all token embeddings
input_mask_expanded = attention_mask.unsqueeze(-1).expand(token_embeddings.size()).float()
return torch.sum(token_embeddings * input_mask_expanded, 1) / torch.clamp(input_mask_expanded.sum(1), min=1e-9)
# Sentences we want sentence embeddings for
sentences = ['This is an example sentence', 'Each sentence is converted']
# Load model from HuggingFace Hub
tokenizer = AutoTokenizer.from_pretrained('gaussfer/test_simcse_new')
model = AutoModel.from_pretrained('gaussfer/test_simcse_new')
# Tokenize sentences
encoded_input = tokenizer(sentences, padding=True, truncation=True, return_tensors='pt')
# Compute token embeddings
with torch.no_grad():
model_output = model(**encoded_input)
# Perform pooling. In this case, mean pooling.
sentence_embeddings = mean_pooling(model_output, encoded_input['attention_mask'])
print("Sentence embeddings:")
print(sentence_embeddings)
```
## Evaluation Results
<!--- Describe how your model was evaluated -->
For an automated evaluation of this model, see the *Sentence Embeddings Benchmark*: [https://seb.sbert.net](https://seb.sbert.net?model_name=gaussfer/test_simcse_new)
## Training
The model was trained with the parameters:
**DataLoader**:
`torch.utils.data.dataloader.DataLoader` of length 875 with parameters:
```
{'batch_size': 16, 'sampler': 'torch.utils.data.sampler.RandomSampler', 'batch_sampler': 'torch.utils.data.sampler.BatchSampler'}
```
**Loss**:
`sentence_transformers.losses.MultipleNegativesRankingLoss.MultipleNegativesRankingLoss` with parameters:
```
{'scale': 20.0, 'similarity_fct': 'cos_sim'}
```
Parameters of the fit()-Method:
```
{
"epochs": 40,
"evaluation_steps": 0,
"evaluator": "NoneType",
"max_grad_norm": 1,
"optimizer_class": "<class 'transformers.optimization.AdamW'>",
"optimizer_params": {
"lr": 2e-05
},
"scheduler": "WarmupLinear",
"steps_per_epoch": null,
"warmup_steps": 10000,
"weight_decay": 0.01
}
```
## Full Model Architecture
```
SentenceTransformer(
(0): Transformer({'max_seq_length': 512, 'do_lower_case': False}) with Transformer model: BertModel
(1): Pooling({'word_embedding_dimension': 768, 'pooling_mode_cls_token': False, 'pooling_mode_mean_tokens': True, 'pooling_mode_max_tokens': False, 'pooling_mode_mean_sqrt_len_tokens': False})
)
```
## Citing & Authors
<!--- Describe where people can find more information --> |
gayanin/bart-mlm-pubmed-15 | f8380e42baed492b6960f730b5d9b88f1030e8d1 | 2021-11-22T20:33:06.000Z | [
"pytorch",
"tensorboard",
"bart",
"text2text-generation",
"transformers",
"generated_from_trainer",
"license:apache-2.0",
"model-index",
"autotrain_compatible"
] | text2text-generation | false | gayanin | null | gayanin/bart-mlm-pubmed-15 | 0 | null | transformers | 33,658 | ---
license: apache-2.0
tags:
- generated_from_trainer
model-index:
- name: bart-mlm-pubmed-15
results: []
---
<!-- This model card has been generated automatically according to the information the Trainer had access to. You
should probably proofread and complete it, then remove this comment. -->
# bart-mlm-pubmed-15
This model is a fine-tuned version of [facebook/bart-base](https://huggingface.co/facebook/bart-base) on an unknown dataset.
It achieves the following results on the evaluation set:
- Loss: 0.4822
- Rouge2 Precision: 0.7578
- Rouge2 Recall: 0.5933
- Rouge2 Fmeasure: 0.6511
## Model description
More information needed
## Intended uses & limitations
More information needed
## Training and evaluation data
More information needed
## Training procedure
### Training hyperparameters
The following hyperparameters were used during training:
- learning_rate: 2e-05
- train_batch_size: 16
- eval_batch_size: 16
- seed: 42
- optimizer: Adam with betas=(0.9,0.999) and epsilon=1e-08
- lr_scheduler_type: linear
- num_epochs: 10
- mixed_precision_training: Native AMP
### Training results
| Training Loss | Epoch | Step | Validation Loss | Rouge2 Precision | Rouge2 Recall | Rouge2 Fmeasure |
|:-------------:|:-----:|:----:|:---------------:|:----------------:|:-------------:|:---------------:|
| 0.7006 | 1.0 | 663 | 0.5062 | 0.7492 | 0.5855 | 0.6434 |
| 0.5709 | 2.0 | 1326 | 0.4811 | 0.7487 | 0.5879 | 0.6447 |
| 0.5011 | 3.0 | 1989 | 0.4734 | 0.7541 | 0.5906 | 0.6483 |
| 0.4164 | 4.0 | 2652 | 0.4705 | 0.7515 | 0.5876 | 0.6452 |
| 0.3888 | 5.0 | 3315 | 0.4703 | 0.7555 | 0.5946 | 0.6515 |
| 0.3655 | 6.0 | 3978 | 0.4725 | 0.7572 | 0.5943 | 0.6516 |
| 0.319 | 7.0 | 4641 | 0.4733 | 0.7557 | 0.5911 | 0.6491 |
| 0.3089 | 8.0 | 5304 | 0.4792 | 0.7577 | 0.5936 | 0.6513 |
| 0.2907 | 9.0 | 5967 | 0.4799 | 0.7577 | 0.5931 | 0.6509 |
| 0.275 | 10.0 | 6630 | 0.4822 | 0.7578 | 0.5933 | 0.6511 |
### Framework versions
- Transformers 4.12.5
- Pytorch 1.10.0+cu111
- Datasets 1.15.1
- Tokenizers 0.10.3
|
gayanin/bart-mlm-pubmed-35 | 429403835be043886f4d3c8bf2afc4ead6191f96 | 2021-11-22T21:16:10.000Z | [
"pytorch",
"tensorboard",
"bart",
"text2text-generation",
"transformers",
"generated_from_trainer",
"license:apache-2.0",
"model-index",
"autotrain_compatible"
] | text2text-generation | false | gayanin | null | gayanin/bart-mlm-pubmed-35 | 0 | null | transformers | 33,659 | ---
license: apache-2.0
tags:
- generated_from_trainer
model-index:
- name: bart-mlm-pubmed-35
results: []
---
<!-- This model card has been generated automatically according to the information the Trainer had access to. You
should probably proofread and complete it, then remove this comment. -->
# bart-mlm-pubmed-35
This model is a fine-tuned version of [facebook/bart-base](https://huggingface.co/facebook/bart-base) on an unknown dataset.
It achieves the following results on the evaluation set:
- Loss: 0.9359
- Rouge2 Precision: 0.5451
- Rouge2 Recall: 0.4232
- Rouge2 Fmeasure: 0.4666
## Model description
More information needed
## Intended uses & limitations
More information needed
## Training and evaluation data
More information needed
## Training procedure
### Training hyperparameters
The following hyperparameters were used during training:
- learning_rate: 2e-05
- train_batch_size: 16
- eval_batch_size: 16
- seed: 42
- optimizer: Adam with betas=(0.9,0.999) and epsilon=1e-08
- lr_scheduler_type: linear
- num_epochs: 10
- mixed_precision_training: Native AMP
### Training results
| Training Loss | Epoch | Step | Validation Loss | Rouge2 Precision | Rouge2 Recall | Rouge2 Fmeasure |
|:-------------:|:-----:|:----:|:---------------:|:----------------:|:-------------:|:---------------:|
| 1.4156 | 1.0 | 663 | 1.0366 | 0.5165 | 0.3967 | 0.4394 |
| 1.1773 | 2.0 | 1326 | 0.9841 | 0.5354 | 0.4168 | 0.4589 |
| 1.0894 | 3.0 | 1989 | 0.9554 | 0.5346 | 0.4133 | 0.4563 |
| 0.9359 | 4.0 | 2652 | 0.9440 | 0.5357 | 0.4163 | 0.4587 |
| 0.8758 | 5.0 | 3315 | 0.9340 | 0.5428 | 0.4226 | 0.465 |
| 0.8549 | 6.0 | 3978 | 0.9337 | 0.5385 | 0.422 | 0.4634 |
| 0.7743 | 7.0 | 4641 | 0.9330 | 0.542 | 0.422 | 0.4647 |
| 0.7465 | 8.0 | 5304 | 0.9315 | 0.5428 | 0.4231 | 0.4654 |
| 0.7348 | 9.0 | 5967 | 0.9344 | 0.5462 | 0.4244 | 0.4674 |
| 0.7062 | 10.0 | 6630 | 0.9359 | 0.5451 | 0.4232 | 0.4666 |
### Framework versions
- Transformers 4.12.5
- Pytorch 1.10.0+cu111
- Datasets 1.15.1
- Tokenizers 0.10.3
|
gayanin/bart-mlm-pubmed-45 | 92b87fe381d769a93361abedaaedf33e4cdfdea5 | 2021-11-22T21:54:14.000Z | [
"pytorch",
"tensorboard",
"bart",
"text2text-generation",
"transformers",
"generated_from_trainer",
"license:apache-2.0",
"model-index",
"autotrain_compatible"
] | text2text-generation | false | gayanin | null | gayanin/bart-mlm-pubmed-45 | 0 | null | transformers | 33,660 | ---
license: apache-2.0
tags:
- generated_from_trainer
model-index:
- name: bart-mlm-pubmed-45
results: []
---
<!-- This model card has been generated automatically according to the information the Trainer had access to. You
should probably proofread and complete it, then remove this comment. -->
# bart-mlm-pubmed-45
This model is a fine-tuned version of [facebook/bart-base](https://huggingface.co/facebook/bart-base) on an unknown dataset.
It achieves the following results on the evaluation set:
- Loss: 1.1797
- Rouge2 Precision: 0.4333
- Rouge2 Recall: 0.3331
- Rouge2 Fmeasure: 0.3684
## Model description
More information needed
## Intended uses & limitations
More information needed
## Training and evaluation data
More information needed
## Training procedure
### Training hyperparameters
The following hyperparameters were used during training:
- learning_rate: 2e-05
- train_batch_size: 16
- eval_batch_size: 16
- seed: 42
- optimizer: Adam with betas=(0.9,0.999) and epsilon=1e-08
- lr_scheduler_type: linear
- num_epochs: 10
- mixed_precision_training: Native AMP
### Training results
| Training Loss | Epoch | Step | Validation Loss | Rouge2 Precision | Rouge2 Recall | Rouge2 Fmeasure |
|:-------------:|:-----:|:----:|:---------------:|:----------------:|:-------------:|:---------------:|
| 1.7989 | 1.0 | 663 | 1.3385 | 0.4097 | 0.3086 | 0.3444 |
| 1.5072 | 2.0 | 1326 | 1.2582 | 0.4218 | 0.3213 | 0.3569 |
| 1.4023 | 3.0 | 1989 | 1.2236 | 0.4207 | 0.3211 | 0.3562 |
| 1.2205 | 4.0 | 2652 | 1.2025 | 0.4359 | 0.3331 | 0.3696 |
| 1.1584 | 5.0 | 3315 | 1.1910 | 0.4304 | 0.3307 | 0.3658 |
| 1.1239 | 6.0 | 3978 | 1.1830 | 0.4247 | 0.3279 | 0.3618 |
| 1.0384 | 7.0 | 4641 | 1.1761 | 0.4308 | 0.3325 | 0.367 |
| 1.0168 | 8.0 | 5304 | 1.1762 | 0.4314 | 0.3336 | 0.368 |
| 0.9966 | 9.0 | 5967 | 1.1773 | 0.4335 | 0.3341 | 0.369 |
| 0.961 | 10.0 | 6630 | 1.1797 | 0.4333 | 0.3331 | 0.3684 |
### Framework versions
- Transformers 4.12.5
- Pytorch 1.10.0+cu111
- Datasets 1.15.1
- Tokenizers 0.10.3
|
gayanin/t5-small-finetuned-pubmed | 7d519c1f7334e135a9b7877b02322b6d9dccb294 | 2021-11-04T03:22:48.000Z | [
"pytorch",
"tensorboard",
"t5",
"text2text-generation",
"transformers",
"generated_from_trainer",
"license:apache-2.0",
"model-index",
"autotrain_compatible"
] | text2text-generation | false | gayanin | null | gayanin/t5-small-finetuned-pubmed | 0 | null | transformers | 33,661 | ---
license: apache-2.0
tags:
- generated_from_trainer
model-index:
- name: t5-small-finetuned-pubmed
results: []
---
<!-- This model card has been generated automatically according to the information the Trainer had access to. You
should probably proofread and complete it, then remove this comment. -->
# t5-small-finetuned-pubmed
This model is a fine-tuned version of [t5-small](https://huggingface.co/t5-small) on an unknown dataset.
It achieves the following results on the evaluation set:
- Loss: 1.6131
- Rouge2 Precision: 0.3
- Rouge2 Recall: 0.2152
- Rouge2 Fmeasure: 0.2379
## Model description
More information needed
## Intended uses & limitations
More information needed
## Training and evaluation data
More information needed
## Training procedure
### Training hyperparameters
The following hyperparameters were used during training:
- learning_rate: 2e-05
- train_batch_size: 16
- eval_batch_size: 16
- seed: 42
- optimizer: Adam with betas=(0.9,0.999) and epsilon=1e-08
- lr_scheduler_type: linear
- num_epochs: 10
- mixed_precision_training: Native AMP
### Training results
| Training Loss | Epoch | Step | Validation Loss | Rouge2 Precision | Rouge2 Recall | Rouge2 Fmeasure |
|:-------------:|:-----:|:----:|:---------------:|:----------------:|:-------------:|:---------------:|
| 2.1335 | 1.0 | 563 | 1.7632 | 0.2716 | 0.1936 | 0.2135 |
| 1.9373 | 2.0 | 1126 | 1.7037 | 0.2839 | 0.2068 | 0.2265 |
| 1.8827 | 3.0 | 1689 | 1.6723 | 0.2901 | 0.2118 | 0.2316 |
| 1.8257 | 4.0 | 2252 | 1.6503 | 0.2938 | 0.2115 | 0.2332 |
| 1.8152 | 5.0 | 2815 | 1.6386 | 0.2962 | 0.2139 | 0.2357 |
| 1.7939 | 6.0 | 3378 | 1.6284 | 0.2976 | 0.212 | 0.2354 |
| 1.7845 | 7.0 | 3941 | 1.6211 | 0.2991 | 0.2155 | 0.2383 |
| 1.7468 | 8.0 | 4504 | 1.6167 | 0.2994 | 0.217 | 0.239 |
| 1.7464 | 9.0 | 5067 | 1.6137 | 0.3007 | 0.2154 | 0.2382 |
| 1.744 | 10.0 | 5630 | 1.6131 | 0.3 | 0.2152 | 0.2379 |
### Framework versions
- Transformers 4.12.3
- Pytorch 1.9.0+cu111
- Datasets 1.15.1
- Tokenizers 0.10.3
|
gayanin/t5-small-mlm-pubmed-15 | f070f21ba96b8e9012919dc3d6ac42ca61777939 | 2021-11-22T21:10:30.000Z | [
"pytorch",
"tensorboard",
"t5",
"text2text-generation",
"transformers",
"generated_from_trainer",
"license:apache-2.0",
"model-index",
"autotrain_compatible"
] | text2text-generation | false | gayanin | null | gayanin/t5-small-mlm-pubmed-15 | 0 | null | transformers | 33,662 | ---
license: apache-2.0
tags:
- generated_from_trainer
model-index:
- name: t5-small-mlm-pubmed-15
results: []
---
<!-- This model card has been generated automatically according to the information the Trainer had access to. You
should probably proofread and complete it, then remove this comment. -->
# t5-small-mlm-pubmed-15
This model is a fine-tuned version of [t5-small](https://huggingface.co/t5-small) on an unknown dataset.
It achieves the following results on the evaluation set:
- Loss: 0.5389
- Rouge2 Precision: 0.7165
- Rouge2 Recall: 0.5375
- Rouge2 Fmeasure: 0.5981
## Model description
More information needed
## Intended uses & limitations
More information needed
## Training and evaluation data
More information needed
## Training procedure
### Training hyperparameters
The following hyperparameters were used during training:
- learning_rate: 2e-05
- train_batch_size: 16
- eval_batch_size: 16
- seed: 42
- optimizer: Adam with betas=(0.9,0.999) and epsilon=1e-08
- lr_scheduler_type: linear
- num_epochs: 40
- mixed_precision_training: Native AMP
### Training results
| Training Loss | Epoch | Step | Validation Loss | Rouge2 Precision | Rouge2 Recall | Rouge2 Fmeasure |
|:-------------:|:-----:|:-----:|:---------------:|:----------------:|:-------------:|:---------------:|
| 1.1024 | 0.75 | 500 | 0.7890 | 0.6854 | 0.4813 | 0.5502 |
| 0.8788 | 1.51 | 1000 | 0.7176 | 0.6906 | 0.4989 | 0.5638 |
| 0.8086 | 2.26 | 1500 | 0.6830 | 0.6872 | 0.5052 | 0.5663 |
| 0.7818 | 3.02 | 2000 | 0.6650 | 0.6912 | 0.5104 | 0.5711 |
| 0.7466 | 3.77 | 2500 | 0.6458 | 0.6965 | 0.5167 | 0.5774 |
| 0.731 | 4.52 | 3000 | 0.6355 | 0.6955 | 0.5161 | 0.5763 |
| 0.7126 | 5.28 | 3500 | 0.6249 | 0.6924 | 0.517 | 0.576 |
| 0.6998 | 6.03 | 4000 | 0.6166 | 0.6995 | 0.5207 | 0.5809 |
| 0.6855 | 6.79 | 4500 | 0.6076 | 0.6981 | 0.5215 | 0.5813 |
| 0.676 | 7.54 | 5000 | 0.6015 | 0.7003 | 0.5242 | 0.5836 |
| 0.6688 | 8.3 | 5500 | 0.5962 | 0.7004 | 0.5235 | 0.583 |
| 0.6569 | 9.05 | 6000 | 0.5900 | 0.6997 | 0.5234 | 0.5827 |
| 0.6503 | 9.8 | 6500 | 0.5880 | 0.703 | 0.5257 | 0.5856 |
| 0.6455 | 10.56 | 7000 | 0.5818 | 0.7008 | 0.5259 | 0.5849 |
| 0.635 | 11.31 | 7500 | 0.5796 | 0.7017 | 0.5271 | 0.5861 |
| 0.6323 | 12.07 | 8000 | 0.5769 | 0.7053 | 0.5276 | 0.5877 |
| 0.6241 | 12.82 | 8500 | 0.5730 | 0.7011 | 0.5243 | 0.5838 |
| 0.6224 | 13.57 | 9000 | 0.5696 | 0.7046 | 0.5286 | 0.5879 |
| 0.6139 | 14.33 | 9500 | 0.5685 | 0.7047 | 0.5295 | 0.5886 |
| 0.6118 | 15.08 | 10000 | 0.5653 | 0.704 | 0.5297 | 0.5886 |
| 0.6089 | 15.84 | 10500 | 0.5633 | 0.703 | 0.5272 | 0.5865 |
| 0.598 | 16.59 | 11000 | 0.5613 | 0.7059 | 0.5293 | 0.5889 |
| 0.6003 | 17.35 | 11500 | 0.5602 | 0.7085 | 0.532 | 0.5918 |
| 0.5981 | 18.1 | 12000 | 0.5587 | 0.7106 | 0.5339 | 0.5938 |
| 0.5919 | 18.85 | 12500 | 0.5556 | 0.708 | 0.5319 | 0.5914 |
| 0.5897 | 19.61 | 13000 | 0.5556 | 0.7106 | 0.5327 | 0.5931 |
| 0.5899 | 20.36 | 13500 | 0.5526 | 0.7114 | 0.534 | 0.5939 |
| 0.5804 | 21.12 | 14000 | 0.5521 | 0.7105 | 0.5328 | 0.5928 |
| 0.5764 | 21.87 | 14500 | 0.5520 | 0.715 | 0.537 | 0.5976 |
| 0.5793 | 22.62 | 15000 | 0.5506 | 0.713 | 0.5346 | 0.5951 |
| 0.5796 | 23.38 | 15500 | 0.5492 | 0.7124 | 0.5352 | 0.5952 |
| 0.5672 | 24.13 | 16000 | 0.5482 | 0.7124 | 0.5346 | 0.5948 |
| 0.5737 | 24.89 | 16500 | 0.5470 | 0.7134 | 0.5352 | 0.5956 |
| 0.5685 | 25.64 | 17000 | 0.5463 | 0.7117 | 0.5346 | 0.5946 |
| 0.5658 | 26.4 | 17500 | 0.5457 | 0.7145 | 0.5359 | 0.5965 |
| 0.5657 | 27.15 | 18000 | 0.5447 | 0.7145 | 0.5367 | 0.597 |
| 0.5645 | 27.9 | 18500 | 0.5441 | 0.7141 | 0.5362 | 0.5964 |
| 0.565 | 28.66 | 19000 | 0.5436 | 0.7151 | 0.5367 | 0.5972 |
| 0.5579 | 29.41 | 19500 | 0.5426 | 0.7162 | 0.5378 | 0.5982 |
| 0.563 | 30.17 | 20000 | 0.5424 | 0.7155 | 0.5373 | 0.5977 |
| 0.556 | 30.92 | 20500 | 0.5418 | 0.7148 | 0.536 | 0.5966 |
| 0.5576 | 31.67 | 21000 | 0.5411 | 0.7141 | 0.5356 | 0.5961 |
| 0.5546 | 32.43 | 21500 | 0.5409 | 0.7149 | 0.5364 | 0.5967 |
| 0.556 | 33.18 | 22000 | 0.5405 | 0.7143 | 0.5356 | 0.596 |
| 0.5536 | 33.94 | 22500 | 0.5401 | 0.7165 | 0.5377 | 0.5982 |
| 0.5527 | 34.69 | 23000 | 0.5397 | 0.7188 | 0.5389 | 0.5999 |
| 0.5531 | 35.44 | 23500 | 0.5395 | 0.7172 | 0.538 | 0.5989 |
| 0.5508 | 36.2 | 24000 | 0.5392 | 0.7166 | 0.538 | 0.5985 |
| 0.5495 | 36.95 | 24500 | 0.5391 | 0.7176 | 0.5387 | 0.5993 |
| 0.5539 | 37.71 | 25000 | 0.5391 | 0.7169 | 0.5372 | 0.598 |
| 0.5452 | 38.46 | 25500 | 0.5390 | 0.7179 | 0.5384 | 0.5991 |
| 0.5513 | 39.22 | 26000 | 0.5390 | 0.717 | 0.5377 | 0.5984 |
| 0.5506 | 39.97 | 26500 | 0.5389 | 0.7165 | 0.5375 | 0.5981 |
### Framework versions
- Transformers 4.12.5
- Pytorch 1.10.0+cu111
- Datasets 1.15.1
- Tokenizers 0.10.3
|
gayanin/t5-small-mlm-pubmed-35 | 65b895e8e4aee48cc576ca045ff4f6be27029654 | 2021-11-22T22:24:30.000Z | [
"pytorch",
"tensorboard",
"t5",
"text2text-generation",
"transformers",
"generated_from_trainer",
"license:apache-2.0",
"model-index",
"autotrain_compatible"
] | text2text-generation | false | gayanin | null | gayanin/t5-small-mlm-pubmed-35 | 0 | null | transformers | 33,663 | ---
license: apache-2.0
tags:
- generated_from_trainer
model-index:
- name: t5-small-mlm-pubmed-35
results: []
---
<!-- This model card has been generated automatically according to the information the Trainer had access to. You
should probably proofread and complete it, then remove this comment. -->
# t5-small-mlm-pubmed-35
This model is a fine-tuned version of [t5-small](https://huggingface.co/t5-small) on an unknown dataset.
It achieves the following results on the evaluation set:
- Loss: 1.1101
- Rouge2 Precision: 0.4758
- Rouge2 Recall: 0.3498
- Rouge2 Fmeasure: 0.3927
## Model description
More information needed
## Intended uses & limitations
More information needed
## Training and evaluation data
More information needed
## Training procedure
### Training hyperparameters
The following hyperparameters were used during training:
- learning_rate: 2e-05
- train_batch_size: 16
- eval_batch_size: 16
- seed: 42
- optimizer: Adam with betas=(0.9,0.999) and epsilon=1e-08
- lr_scheduler_type: linear
- num_epochs: 40
- mixed_precision_training: Native AMP
### Training results
| Training Loss | Epoch | Step | Validation Loss | Rouge2 Precision | Rouge2 Recall | Rouge2 Fmeasure |
|:-------------:|:-----:|:-----:|:---------------:|:----------------:|:-------------:|:---------------:|
| 1.8404 | 0.75 | 500 | 1.5005 | 0.4265 | 0.2786 | 0.3273 |
| 1.6858 | 1.51 | 1000 | 1.4216 | 0.4318 | 0.2946 | 0.3404 |
| 1.6071 | 2.26 | 1500 | 1.3777 | 0.4472 | 0.3148 | 0.3598 |
| 1.5551 | 3.02 | 2000 | 1.3360 | 0.4406 | 0.3168 | 0.3586 |
| 1.5116 | 3.77 | 2500 | 1.3128 | 0.4523 | 0.3234 | 0.3671 |
| 1.4837 | 4.52 | 3000 | 1.2937 | 0.4477 | 0.3215 | 0.3645 |
| 1.4513 | 5.28 | 3500 | 1.2766 | 0.4511 | 0.3262 | 0.3689 |
| 1.4336 | 6.03 | 4000 | 1.2626 | 0.4548 | 0.3283 | 0.3718 |
| 1.4149 | 6.79 | 4500 | 1.2449 | 0.4495 | 0.3274 | 0.3687 |
| 1.3977 | 7.54 | 5000 | 1.2349 | 0.4507 | 0.3305 | 0.3712 |
| 1.3763 | 8.3 | 5500 | 1.2239 | 0.4519 | 0.3266 | 0.3688 |
| 1.371 | 9.05 | 6000 | 1.2171 | 0.4546 | 0.3305 | 0.3727 |
| 1.3501 | 9.8 | 6500 | 1.2080 | 0.4575 | 0.3329 | 0.3755 |
| 1.3443 | 10.56 | 7000 | 1.2017 | 0.4576 | 0.3314 | 0.3742 |
| 1.326 | 11.31 | 7500 | 1.1926 | 0.4578 | 0.333 | 0.3757 |
| 1.3231 | 12.07 | 8000 | 1.1866 | 0.4606 | 0.3357 | 0.3782 |
| 1.3089 | 12.82 | 8500 | 1.1816 | 0.4591 | 0.3338 | 0.3765 |
| 1.3007 | 13.57 | 9000 | 1.1764 | 0.4589 | 0.3361 | 0.3777 |
| 1.2943 | 14.33 | 9500 | 1.1717 | 0.4641 | 0.3382 | 0.3811 |
| 1.2854 | 15.08 | 10000 | 1.1655 | 0.4617 | 0.3378 | 0.38 |
| 1.2777 | 15.84 | 10500 | 1.1612 | 0.464 | 0.3401 | 0.3823 |
| 1.2684 | 16.59 | 11000 | 1.1581 | 0.4608 | 0.3367 | 0.3789 |
| 1.2612 | 17.35 | 11500 | 1.1554 | 0.4623 | 0.3402 | 0.3818 |
| 1.2625 | 18.1 | 12000 | 1.1497 | 0.4613 | 0.3381 | 0.3802 |
| 1.2529 | 18.85 | 12500 | 1.1465 | 0.4671 | 0.3419 | 0.3848 |
| 1.2461 | 19.61 | 13000 | 1.1431 | 0.4646 | 0.3399 | 0.3824 |
| 1.2415 | 20.36 | 13500 | 1.1419 | 0.4659 | 0.341 | 0.3835 |
| 1.2375 | 21.12 | 14000 | 1.1377 | 0.4693 | 0.3447 | 0.3873 |
| 1.2315 | 21.87 | 14500 | 1.1353 | 0.4672 | 0.3433 | 0.3855 |
| 1.2263 | 22.62 | 15000 | 1.1333 | 0.467 | 0.3433 | 0.3854 |
| 1.2214 | 23.38 | 15500 | 1.1305 | 0.4682 | 0.3446 | 0.3869 |
| 1.2202 | 24.13 | 16000 | 1.1291 | 0.4703 | 0.3465 | 0.3888 |
| 1.2155 | 24.89 | 16500 | 1.1270 | 0.472 | 0.348 | 0.3903 |
| 1.2064 | 25.64 | 17000 | 1.1261 | 0.4724 | 0.3479 | 0.3905 |
| 1.2173 | 26.4 | 17500 | 1.1236 | 0.4734 | 0.3485 | 0.3912 |
| 1.1994 | 27.15 | 18000 | 1.1220 | 0.4739 | 0.3486 | 0.3915 |
| 1.2018 | 27.9 | 18500 | 1.1217 | 0.4747 | 0.3489 | 0.3921 |
| 1.2045 | 28.66 | 19000 | 1.1194 | 0.4735 | 0.3488 | 0.3916 |
| 1.1949 | 29.41 | 19500 | 1.1182 | 0.4732 | 0.3484 | 0.3911 |
| 1.19 | 30.17 | 20000 | 1.1166 | 0.4724 | 0.3479 | 0.3904 |
| 1.1932 | 30.92 | 20500 | 1.1164 | 0.4753 | 0.3494 | 0.3924 |
| 1.1952 | 31.67 | 21000 | 1.1147 | 0.4733 | 0.3485 | 0.3911 |
| 1.1922 | 32.43 | 21500 | 1.1146 | 0.475 | 0.3494 | 0.3923 |
| 1.1889 | 33.18 | 22000 | 1.1132 | 0.4765 | 0.3499 | 0.3933 |
| 1.1836 | 33.94 | 22500 | 1.1131 | 0.4768 | 0.351 | 0.3939 |
| 1.191 | 34.69 | 23000 | 1.1127 | 0.4755 | 0.3495 | 0.3926 |
| 1.1811 | 35.44 | 23500 | 1.1113 | 0.4748 | 0.349 | 0.3919 |
| 1.1864 | 36.2 | 24000 | 1.1107 | 0.4751 | 0.3494 | 0.3921 |
| 1.1789 | 36.95 | 24500 | 1.1103 | 0.4756 | 0.3499 | 0.3927 |
| 1.1819 | 37.71 | 25000 | 1.1101 | 0.4758 | 0.35 | 0.3932 |
| 1.1862 | 38.46 | 25500 | 1.1099 | 0.4755 | 0.3497 | 0.3926 |
| 1.1764 | 39.22 | 26000 | 1.1101 | 0.4759 | 0.3498 | 0.3928 |
| 1.1819 | 39.97 | 26500 | 1.1101 | 0.4758 | 0.3498 | 0.3927 |
### Framework versions
- Transformers 4.12.5
- Pytorch 1.10.0+cu111
- Datasets 1.15.1
- Tokenizers 0.10.3
|
gchhablani/wav2vec2-large-xlsr-or | bdeb0c534df834da1ba679b1dbacef9a1bc6042c | 2021-07-06T05:17:20.000Z | [
"pytorch",
"jax",
"wav2vec2",
"automatic-speech-recognition",
"or",
"dataset:common_voice",
"transformers",
"audio",
"speech",
"xlsr-fine-tuning-week",
"license:apache-2.0",
"model-index"
] | automatic-speech-recognition | false | gchhablani | null | gchhablani/wav2vec2-large-xlsr-or | 0 | null | transformers | 33,664 | ---
language: or
datasets:
- common_voice
metrics:
- wer
tags:
- audio
- automatic-speech-recognition
- speech
- xlsr-fine-tuning-week
license: apache-2.0
model-index:
- name: XLSR Wav2Vec2 Large 53 Odia by Gunjan Chhablani
results:
- task:
name: Speech Recognition
type: automatic-speech-recognition
dataset:
name: Common Voice or
type: common_voice
args: or
metrics:
- name: Test WER
type: wer
value: 52.64
---
# Wav2Vec2-Large-XLSR-53-Odia
Fine-tuned [facebook/wav2vec2-large-xlsr-53](https://huggingface.co/facebook/wav2vec2-large-xlsr-53) on Odia using the [Common Voice](https://huggingface.co/datasets/common_voice).
When using this model, make sure that your speech input is sampled at 16kHz.
## Usage
The model can be used directly (without a language model) as follows:
```python
import torch
import torchaudio
from datasets import load_dataset
from transformers import Wav2Vec2ForCTC, Wav2Vec2Processor
test_dataset = load_dataset("common_voice", "or", split="test[:2%]")
processor = Wav2Vec2Processor.from_pretrained("gchhablani/wav2vec2-large-xlsr-or")
model = Wav2Vec2ForCTC.from_pretrained("gchhablani/wav2vec2-large-xlsr-or")
resampler = torchaudio.transforms.Resample(48_000, 16_000)
# Preprocessing the datasets.
# We need to read the aduio files as arrays
def speech_file_to_array_fn(batch):
speech_array, sampling_rate = torchaudio.load(batch["path"])
batch["speech"] = resampler(speech_array).squeeze().numpy()
return batch
test_dataset = test_dataset.map(speech_file_to_array_fn)
inputs = processor(test_dataset["speech"][:2], sampling_rate=16_000, return_tensors="pt", padding=True)
with torch.no_grad():
logits = model(inputs.input_values, attention_mask=inputs.attention_mask).logits
predicted_ids = torch.argmax(logits, dim=-1)
print("Prediction:", processor.batch_decode(predicted_ids))
print("Reference:", test_dataset["sentence"][:2])
```
## Evaluation
The model can be evaluated as follows on the Odia test data of Common Voice.
```python
import torch
import torchaudio
from datasets import load_dataset, load_metric
from transformers import Wav2Vec2ForCTC, Wav2Vec2Processor
import re
test_dataset = load_dataset("common_voice", "or", split="test")
wer = load_metric("wer")
processor = Wav2Vec2Processor.from_pretrained("gchhablani/wav2vec2-large-xlsr-or")
model = Wav2Vec2ForCTC.from_pretrained("gchhablani/wav2vec2-large-xlsr-or")
model.to("cuda")
chars_to_ignore_regex = '[\,\?\.\!\-\;\:\"\“\%\‘\”\�\–\…\'\_\’\।\|]'
resampler = torchaudio.transforms.Resample(48_000, 16_000)
# Preprocessing the datasets.
# We need to read the aduio files as arrays
def speech_file_to_array_fn(batch):
batch["sentence"] = re.sub(chars_to_ignore_regex, '', batch["sentence"]).lower()
speech_array, sampling_rate = torchaudio.load(batch["path"])
batch["speech"] = resampler(speech_array).squeeze().numpy()
return batch
test_dataset = test_dataset.map(speech_file_to_array_fn)
# Preprocessing the datasets.
# We need to read the aduio files as arrays
def evaluate(batch):
inputs = processor(batch["speech"], sampling_rate=16_000, return_tensors="pt", padding=True)
with torch.no_grad():
logits = model(inputs.input_values.to("cuda"), attention_mask=inputs.attention_mask.to("cuda")).logits
pred_ids = torch.argmax(logits, dim=-1)
batch["pred_strings"] = processor.batch_decode(pred_ids)
return batch
result = test_dataset.map(evaluate, batched=True, batch_size=8)
print("WER: {:2f}".format(100 * wer.compute(predictions=result["pred_strings"], references=result["sentence"])))
```
**Test Result**: 52.64 %
## Training
The Common Voice `train` and `validation` datasets were used for training.The colab notebook used can be found [here](https://colab.research.google.com/drive/1s8DrwgB5y4Z7xXIrPXo1rQA5_1OZ8WD5?usp=sharing). |
gchhablani/wav2vec2-large-xlsr-rm-sursilv | 5a7ae7b5e63a5c837829a986345481e225591929 | 2021-07-06T05:27:40.000Z | [
"pytorch",
"jax",
"wav2vec2",
"automatic-speech-recognition",
"rm-sursilv",
"dataset:common_voice",
"transformers",
"audio",
"speech",
"xlsr-fine-tuning-week",
"license:apache-2.0",
"model-index"
] | automatic-speech-recognition | false | gchhablani | null | gchhablani/wav2vec2-large-xlsr-rm-sursilv | 0 | null | transformers | 33,665 | ---
language: rm-sursilv
datasets:
- common_voice
metrics:
- wer
tags:
- audio
- automatic-speech-recognition
- speech
- xlsr-fine-tuning-week
license: apache-2.0
model-index:
- name: Wav2Vec2 Large 53 Romansh Sursilvan by Gunjan Chhablani
results:
- task:
name: Speech Recognition
type: automatic-speech-recognition
dataset:
name: Common Voice rm-sursilv
type: common_voice
args: rm-sursilv
metrics:
- name: Test WER
type: wer
value: 25.16
---
# Wav2Vec2-Large-XLSR-53-Romansh-Sursilvan
Fine-tuned [facebook/wav2vec2-large-xlsr-53](https://huggingface.co/facebook/wav2vec2-large-xlsr-53) on Romansh Sursilvan using the [Common Voice](https://huggingface.co/datasets/common_voice) dataset.
When using this model, make sure that your speech input is sampled at 16kHz.
## Usage
The model can be used directly (without a language model) as follows:
```python
import torch
import torchaudio
from datasets import load_dataset
from transformers import Wav2Vec2ForCTC, Wav2Vec2Processor
test_dataset = load_dataset("common_voice", "rm-sursilv", split="test[:2%]")
processor = Wav2Vec2Processor.from_pretrained("gchhablani/wav2vec2-large-xlsr-rm-sursilv")
model = Wav2Vec2ForCTC.from_pretrained("gchhablani/wav2vec2-large-xlsr-rm-sursilv")
resampler = torchaudio.transforms.Resample(48_000, 16_000)
# Preprocessing the datasets.
# We need to read the aduio files as arrays
def speech_file_to_array_fn(batch):
speech_array, sampling_rate = torchaudio.load(batch["path"])
batch["speech"] = resampler(speech_array).squeeze().numpy()
return batch
test_dataset = test_dataset.map(speech_file_to_array_fn)
inputs = processor(test_dataset["speech"][:2], sampling_rate=16_000, return_tensors="pt", padding=True)
with torch.no_grad():
logits = model(inputs.input_values, attention_mask=inputs.attention_mask).logits
predicted_ids = torch.argmax(logits, dim=-1)
print("Prediction:", processor.batch_decode(predicted_ids))
print("Reference:", test_dataset["sentence"][:2])
```
## Evaluation
The model can be evaluated as follows on the Portuguese test data of Common Voice.
```python
import torch
import torchaudio
from datasets import load_dataset, load_metric
from transformers import Wav2Vec2ForCTC, Wav2Vec2Processor
import re
test_dataset = load_dataset("common_voice", "rm-sursilv", split="test")
wer = load_metric("wer")
processor = Wav2Vec2Processor.from_pretrained("gchhablani/wav2vec2-large-xlsr-rm-sursilv")
model = Wav2Vec2ForCTC.from_pretrained("gchhablani/wav2vec2-large-xlsr-rm-sursilv")
model.to("cuda")
chars_to_ignore_regex = '[\\,\\?\\.\\!\\-\\;\\:\\"\\“\\%\\‘\\”\\�\\…\\«\\»\\–]'
resampler = torchaudio.transforms.Resample(48_000, 16_000)
# Preprocessing the datasets.
# We need to read the aduio files as arrays
def speech_file_to_array_fn(batch):
batch["sentence"] = re.sub(chars_to_ignore_regex, '', batch["sentence"]).lower()
speech_array, sampling_rate = torchaudio.load(batch["path"])
batch["speech"] = resampler(speech_array).squeeze().numpy()
return batch
test_dataset = test_dataset.map(speech_file_to_array_fn)
# Preprocessing the datasets.
# We need to read the aduio files as arrays
def evaluate(batch):
inputs = processor(batch["speech"], sampling_rate=16_000, return_tensors="pt", padding=True)
with torch.no_grad():
logits = model(inputs.input_values.to("cuda"), attention_mask=inputs.attention_mask.to("cuda")).logits
pred_ids = torch.argmax(logits, dim=-1)
batch["pred_strings"] = processor.batch_decode(pred_ids)
return batch
result = test_dataset.map(evaluate, batched=True, batch_size=8)
print("WER: {:2f}".format(100 * wer.compute(predictions=result["pred_strings"], references=result["sentence"])))
```
**Test Result**: 25.16 %
## Training
The Common Voice `train` and `validation` datasets were used for training. The code can be found [here](https://colab.research.google.com/drive/1dpZr_GzRowCciUbzM3GnW04TNKnB7vrP?usp=sharing). |
gfdream/dialogpt-small-familyguy | a39cb4a7dd8581c0e61a64c3d376c88251be86ea | 2021-09-14T23:33:22.000Z | [
"pytorch",
"gpt2",
"text-generation",
"transformers",
"conversational"
] | conversational | false | gfdream | null | gfdream/dialogpt-small-familyguy | 0 | null | transformers | 33,666 | ---
tags:
- conversational
---
# Family Guy (Peter) DialoGPT Model |
ggosline/t5-small-herblabels | 3101a2ebbeb2ea824a7d70c9151074311354bb3d | 2021-12-08T00:16:57.000Z | [
"pytorch",
"t5",
"text2text-generation",
"transformers",
"generated_from_trainer",
"license:apache-2.0",
"model-index",
"autotrain_compatible"
] | text2text-generation | false | ggosline | null | ggosline/t5-small-herblabels | 0 | null | transformers | 33,667 | ---
license: apache-2.0
tags:
- generated_from_trainer
metrics:
- rouge
model-index:
- name: t5-small-herblabels
results: []
---
<!-- This model card has been generated automatically according to the information the Trainer had access to. You
should probably proofread and complete it, then remove this comment. -->
# t5-small-herblabels
This model is a fine-tuned version of [t5-small](https://huggingface.co/t5-small) on an unknown dataset.
It achieves the following results on the evaluation set:
- Loss: 0.4823
- Rouge1: 3.0759
- Rouge2: 1.0495
- Rougel: 3.0758
- Rougelsum: 3.0431
- Gen Len: 18.9716
## Model description
More information needed
## Intended uses & limitations
More information needed
## Training and evaluation data
More information needed
## Training procedure
### Training hyperparameters
The following hyperparameters were used during training:
- learning_rate: 2e-05
- train_batch_size: 8
- eval_batch_size: 8
- seed: 42
- optimizer: Adam with betas=(0.9,0.999) and epsilon=1e-08
- lr_scheduler_type: linear
- num_epochs: 10
- mixed_precision_training: Native AMP
### Training results
| Training Loss | Epoch | Step | Validation Loss | Rouge1 | Rouge2 | Rougel | Rougelsum | Gen Len |
|:-------------:|:-----:|:----:|:---------------:|:------:|:------:|:------:|:---------:|:-------:|
| No log | 1.0 | 264 | 1.6010 | 2.4276 | 0.5658 | 2.3546 | 2.3099 | 18.9091 |
| 2.5052 | 2.0 | 528 | 1.0237 | 2.9016 | 0.3395 | 2.8221 | 2.783 | 18.9673 |
| 2.5052 | 3.0 | 792 | 0.7793 | 2.962 | 0.3091 | 2.9375 | 2.8786 | 18.9588 |
| 1.1552 | 4.0 | 1056 | 0.6530 | 2.98 | 0.4375 | 2.9584 | 2.8711 | 18.9588 |
| 1.1552 | 5.0 | 1320 | 0.5863 | 3.0023 | 0.5882 | 2.987 | 2.9155 | 18.9588 |
| 0.8659 | 6.0 | 1584 | 0.5428 | 3.0576 | 0.8019 | 3.0494 | 2.9989 | 18.9716 |
| 0.8659 | 7.0 | 1848 | 0.5145 | 3.0808 | 0.9476 | 3.0719 | 3.0237 | 18.9716 |
| 0.747 | 8.0 | 2112 | 0.4962 | 3.0748 | 1.0032 | 3.0683 | 3.0359 | 18.9716 |
| 0.747 | 9.0 | 2376 | 0.4856 | 3.0702 | 1.0196 | 3.0665 | 3.0328 | 18.9716 |
| 0.6987 | 10.0 | 2640 | 0.4823 | 3.0759 | 1.0495 | 3.0758 | 3.0431 | 18.9716 |
### Framework versions
- Transformers 4.12.5
- Pytorch 1.10.0
- Datasets 1.16.1
- Tokenizers 0.10.3
|
ghadeermobasher/BC5CDR-Chemical_Modified_BioM-ELECTRA-Base-Discriminator | 7b9cd74e3b0ba39ce6adf12024f57805d29b2be3 | 2022-01-22T23:47:01.000Z | [
"pytorch",
"electra",
"token-classification",
"transformers",
"autotrain_compatible"
] | token-classification | false | ghadeermobasher | null | ghadeermobasher/BC5CDR-Chemical_Modified_BioM-ELECTRA-Base-Discriminator | 0 | null | transformers | 33,668 | Entry not found |
ghadeermobasher/CRAFT-Chem_ImbalancedBioM-ELECTRA-Base-Discriminator | c8ed19fd0bef8890cf8f49a6cc19d44fe2a728b5 | 2022-01-23T01:55:20.000Z | [
"pytorch",
"electra",
"token-classification",
"transformers",
"autotrain_compatible"
] | token-classification | false | ghadeermobasher | null | ghadeermobasher/CRAFT-Chem_ImbalancedBioM-ELECTRA-Base-Discriminator | 0 | null | transformers | 33,669 | Entry not found |
ghazikhanihamed/TCDB-BERT | 36cf782d1496a5b40aaa4113fcf89af0d615679b | 2022-02-19T16:20:12.000Z | [
"pytorch",
"bert",
"fill-mask",
"transformers",
"autotrain_compatible"
] | fill-mask | false | ghazikhanihamed | null | ghazikhanihamed/TCDB-BERT | 0 | null | transformers | 33,670 | Entry not found |
ghazikhanihamed/TransportersBERT | 84488b26da5828cd5d9a94154e8d3419bc471a01 | 2022-02-18T11:39:32.000Z | [
"pytorch",
"bert",
"transformers"
] | null | false | ghazikhanihamed | null | ghazikhanihamed/TransportersBERT | 0 | null | transformers | 33,671 | This repository belongs to TransportersBERT from ActTrans publication.
Taju, Semmy Wellem, Syed Muazzam Ali Shah, and Yu-Yen Ou. “ActTRANS: Functional Classification in Active Transport Proteins Based on Transfer Learning and Contextual Representations.” Computational Biology and Chemistry 93 (August 1, 2021): 107537. https://doi.org/10.1016/j.compbiolchem.2021.107537.
|
ghhostboy/DialoGPT-medium-connorDBH3-21 | 5ba7b65a620fd29bb50f65d8fbef67f26cb2841e | 2021-11-27T04:29:16.000Z | [
"pytorch",
"gpt2",
"text-generation",
"transformers",
"conversational"
] | conversational | false | ghhostboy | null | ghhostboy/DialoGPT-medium-connorDBH3-21 | 0 | null | transformers | 33,672 | ---
tags:
- conversational
---
# Connor |
giacomomiolo/electramed_base_scivocab_750 | aac2aa9460031bb894b52caa39d6529de28bdcf8 | 2020-09-30T11:47:57.000Z | [
"pytorch",
"tf",
"electra",
"pretraining",
"transformers"
] | null | false | giacomomiolo | null | giacomomiolo/electramed_base_scivocab_750 | 0 | null | transformers | 33,673 | Entry not found |
gizmo-dev/DialoGPT-small-jake | b3885bdae8312001dcd5da5b40576656d7b7c8d7 | 2021-08-28T10:16:41.000Z | [
"pytorch",
"gpt2",
"text-generation",
"transformers",
"conversational"
] | conversational | false | gizmo-dev | null | gizmo-dev/DialoGPT-small-jake | 0 | null | transformers | 33,674 | ---
tags:
- conversational
---
# Jake Peralta DialoGPT model |
glasses/deit_base_patch16_224 | 8c4d6fb2a2506f481d7273e69d8f6e43dc5efdab | 2021-04-22T18:44:42.000Z | [
"pytorch",
"arxiv:2010.11929",
"transformers"
] | null | false | glasses | null | glasses/deit_base_patch16_224 | 0 | null | transformers | 33,675 | # deit_base_patch16_224
Implementation of DeiT proposed in [Training data-efficient image
transformers & distillation through
attention](https://arxiv.org/pdf/2010.11929.pdf)
An attention based distillation is proposed where a new token is added
to the model, the [dist]{.title-ref} token.
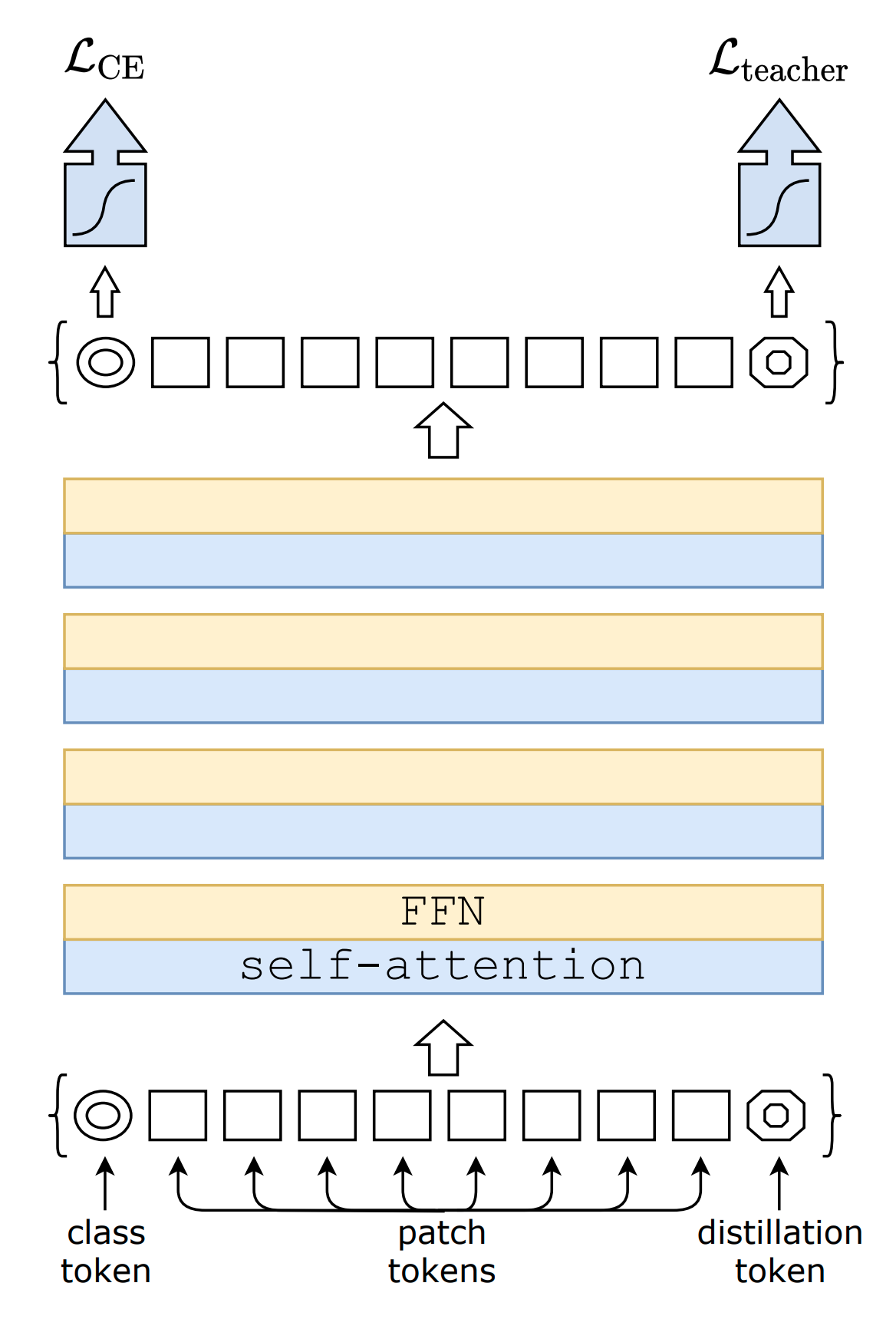
``` {.sourceCode .}
DeiT.deit_tiny_patch16_224()
DeiT.deit_small_patch16_224()
DeiT.deit_base_patch16_224()
DeiT.deit_base_patch16_384()
```
|
glasses/deit_base_patch16_384 | a160baa533a2c7b7b87d14f6ea97f4626da8639c | 2021-04-22T18:44:58.000Z | [
"pytorch",
"arxiv:2010.11929",
"transformers"
] | null | false | glasses | null | glasses/deit_base_patch16_384 | 0 | null | transformers | 33,676 | # deit_base_patch16_384
Implementation of DeiT proposed in [Training data-efficient image
transformers & distillation through
attention](https://arxiv.org/pdf/2010.11929.pdf)
An attention based distillation is proposed where a new token is added
to the model, the [dist]{.title-ref} token.
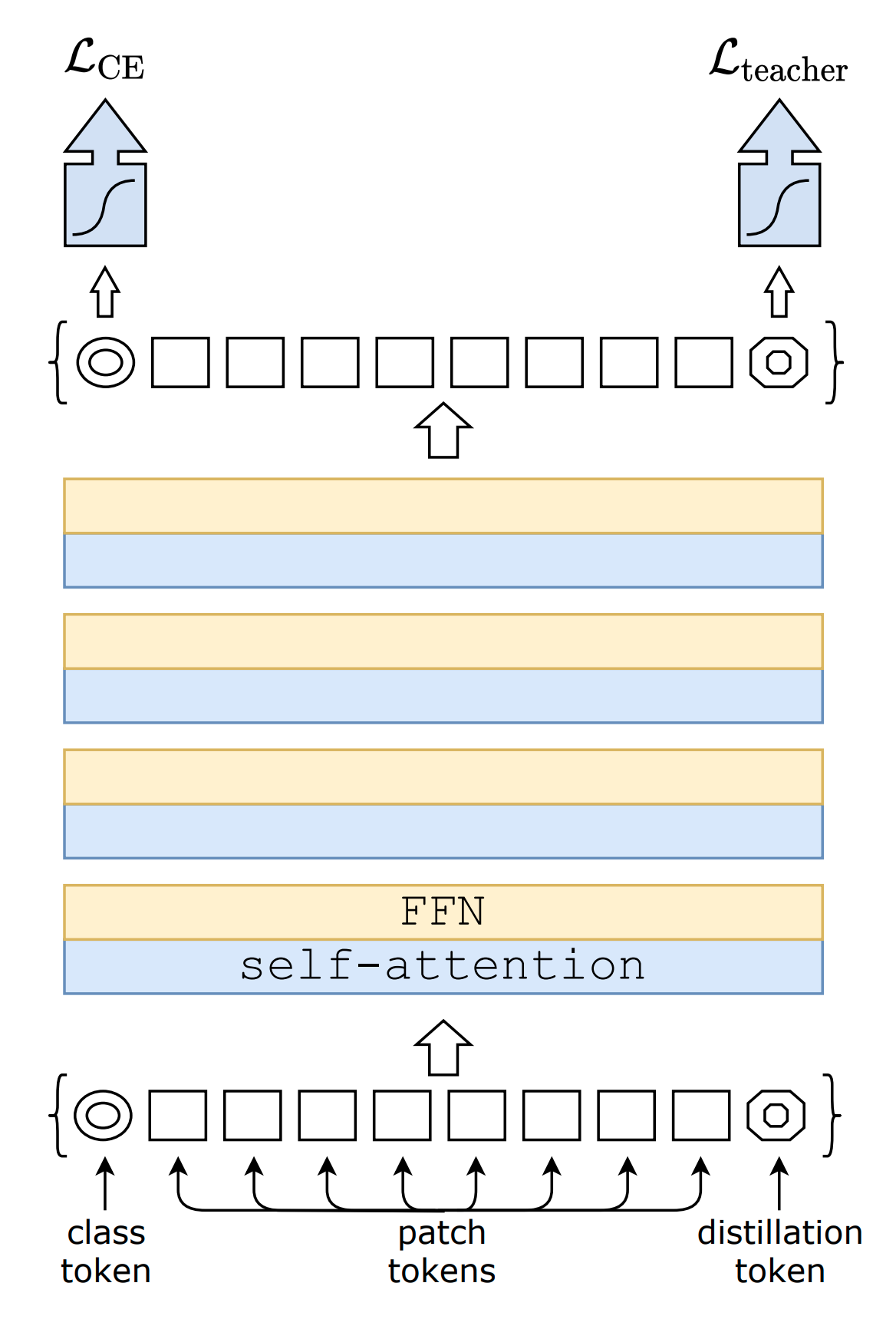
``` {.sourceCode .}
DeiT.deit_tiny_patch16_224()
DeiT.deit_small_patch16_224()
DeiT.deit_base_patch16_224()
DeiT.deit_base_patch16_384()
```
|
glasses/deit_small_patch16_224 | a2b6964107f0eb4afaee873969f7fe0f1ee79ea4 | 2021-04-22T18:44:25.000Z | [
"pytorch",
"arxiv:2010.11929",
"transformers"
] | null | false | glasses | null | glasses/deit_small_patch16_224 | 0 | null | transformers | 33,677 | # deit_small_patch16_224
Implementation of DeiT proposed in [Training data-efficient image
transformers & distillation through
attention](https://arxiv.org/pdf/2010.11929.pdf)
An attention based distillation is proposed where a new token is added
to the model, the [dist]{.title-ref} token.
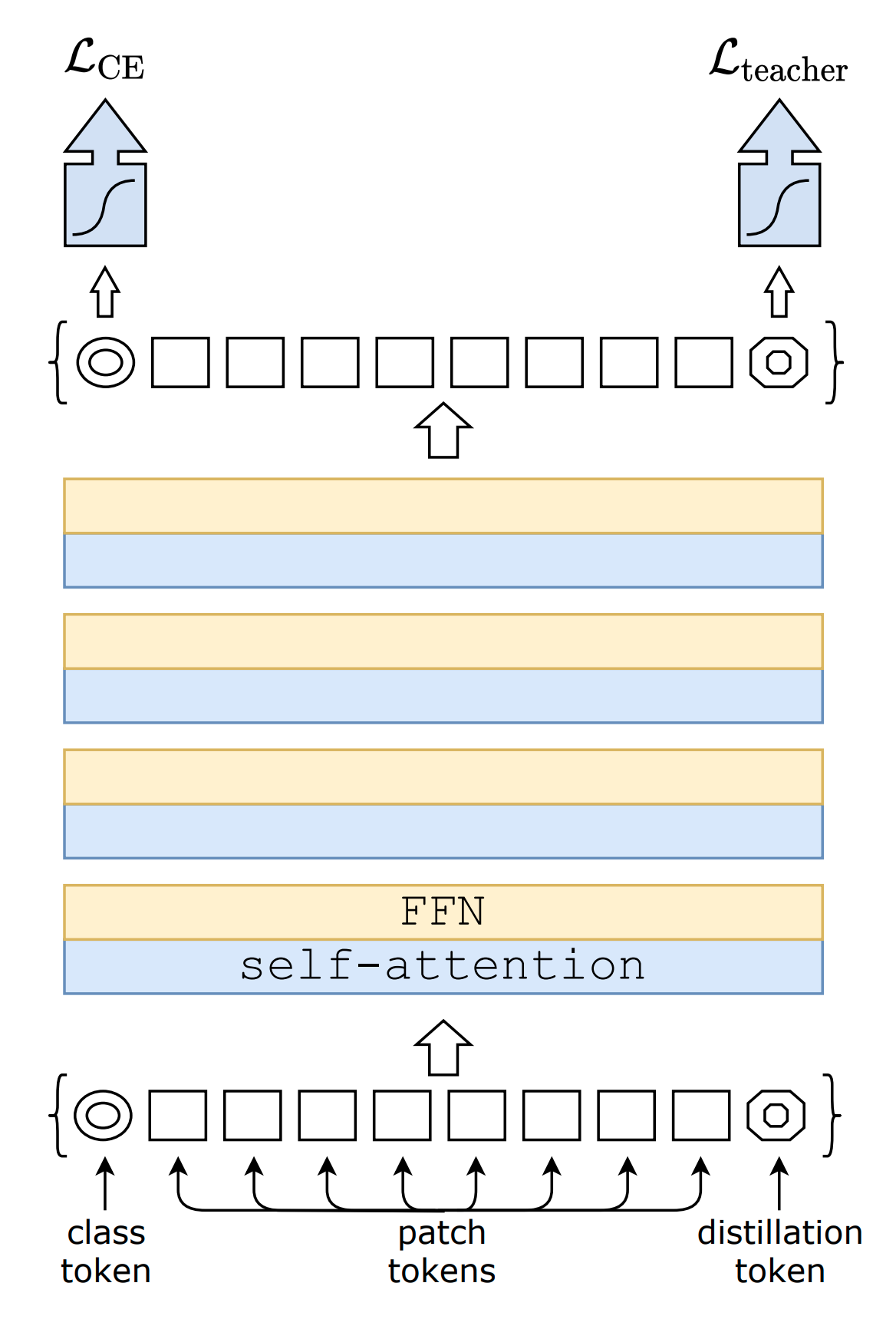
``` {.sourceCode .}
DeiT.deit_tiny_patch16_224()
DeiT.deit_small_patch16_224()
DeiT.deit_base_patch16_224()
DeiT.deit_base_patch16_384()
```
|
glasses/eca_resnet50d | 13bd25b5877737d0f1695d112cdd9d4c98893bae | 2021-11-30T20:23:56.000Z | [
"pytorch",
"transformers"
] | null | false | glasses | null | glasses/eca_resnet50d | 0 | null | transformers | 33,678 | Entry not found |
glasses/regnetx_016 | cf66d97a7ad317998015fbb985143e1546832d53 | 2021-11-30T20:26:57.000Z | [
"pytorch",
"arxiv:2003.13678",
"transformers"
] | null | false | glasses | null | glasses/regnetx_016 | 0 | null | transformers | 33,679 | # regnetx_016
Implementation of RegNet proposed in [Designing Network Design
Spaces](https://arxiv.org/abs/2003.13678)
The main idea is to start with a high dimensional search space and
iteratively reduce the search space by empirically apply constrains
based on the best performing models sampled by the current search
space.
The resulting models are light, accurate, and faster than
EfficientNets (up to 5x times!)
For example, to go from $AnyNet_A$ to $AnyNet_B$ they fixed the
bottleneck ratio $b_i$ for all stage $i$. The following table shows
all the restrictions applied from one search space to the next one.
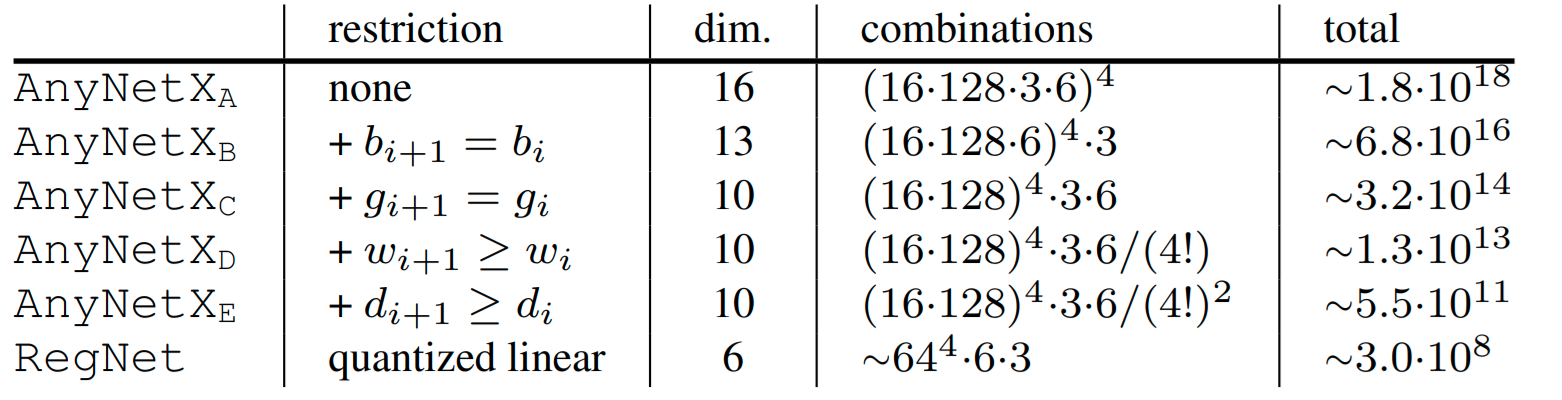
The paper is really well written and very interesting, I highly
recommended read it.
``` python
ResNet.regnetx_002()
ResNet.regnetx_004()
ResNet.regnetx_006()
ResNet.regnetx_008()
ResNet.regnetx_016()
ResNet.regnetx_040()
ResNet.regnetx_064()
ResNet.regnetx_080()
ResNet.regnetx_120()
ResNet.regnetx_160()
ResNet.regnetx_320()
# Y variants (with SE)
ResNet.regnety_002()
# ...
ResNet.regnetx_320()
You can easily customize your model
```
Examples:
``` python
# change activation
RegNet.regnetx_004(activation = nn.SELU)
# change number of classes (default is 1000 )
RegNet.regnetx_004(n_classes=100)
# pass a different block
RegNet.regnetx_004(block=RegNetYBotteneckBlock)
# change the steam
model = RegNet.regnetx_004(stem=ResNetStemC)
change shortcut
model = RegNet.regnetx_004(block=partial(RegNetYBotteneckBlock, shortcut=ResNetShorcutD))
# store each feature
x = torch.rand((1, 3, 224, 224))
# get features
model = RegNet.regnetx_004()
# first call .features, this will activate the forward hooks and tells the model you'll like to get the features
model.encoder.features
model(torch.randn((1,3,224,224)))
# get the features from the encoder
features = model.encoder.features
print([x.shape for x in features])
#[torch.Size([1, 32, 112, 112]), torch.Size([1, 32, 56, 56]), torch.Size([1, 64, 28, 28]), torch.Size([1, 160, 14, 14])]
```
|
glasses/regnety_006 | 0a70fe056933af6649c2a22731b5aaf43012b896 | 2021-12-01T07:46:05.000Z | [
"pytorch",
"arxiv:2003.13678",
"transformers"
] | null | false | glasses | null | glasses/regnety_006 | 0 | null | transformers | 33,680 | # regnety_006
Implementation of RegNet proposed in [Designing Network Design
Spaces](https://arxiv.org/abs/2003.13678)
The main idea is to start with a high dimensional search space and
iteratively reduce the search space by empirically apply constrains
based on the best performing models sampled by the current search
space.
The resulting models are light, accurate, and faster than
EfficientNets (up to 5x times!)
For example, to go from $AnyNet_A$ to $AnyNet_B$ they fixed the
bottleneck ratio $b_i$ for all stage $i$. The following table shows
all the restrictions applied from one search space to the next one.
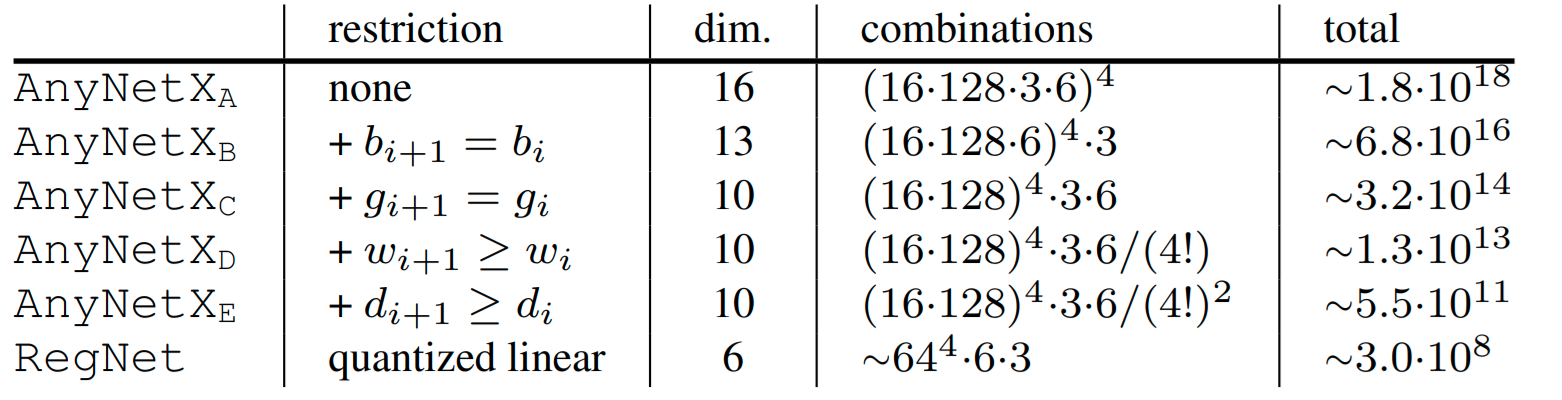
The paper is really well written and very interesting, I highly
recommended read it.
``` python
ResNet.regnetx_002()
ResNet.regnetx_004()
ResNet.regnetx_006()
ResNet.regnetx_008()
ResNet.regnetx_016()
ResNet.regnetx_040()
ResNet.regnetx_064()
ResNet.regnetx_080()
ResNet.regnetx_120()
ResNet.regnetx_160()
ResNet.regnetx_320()
# Y variants (with SE)
ResNet.regnety_002()
# ...
ResNet.regnetx_320()
You can easily customize your model
```
Examples:
``` python
# change activation
RegNet.regnetx_004(activation = nn.SELU)
# change number of classes (default is 1000 )
RegNet.regnetx_004(n_classes=100)
# pass a different block
RegNet.regnetx_004(block=RegNetYBotteneckBlock)
# change the steam
model = RegNet.regnetx_004(stem=ResNetStemC)
change shortcut
model = RegNet.regnetx_004(block=partial(RegNetYBotteneckBlock, shortcut=ResNetShorcutD))
# store each feature
x = torch.rand((1, 3, 224, 224))
# get features
model = RegNet.regnetx_004()
# first call .features, this will activate the forward hooks and tells the model you'll like to get the features
model.encoder.features
model(torch.randn((1,3,224,224)))
# get the features from the encoder
features = model.encoder.features
print([x.shape for x in features])
#[torch.Size([1, 32, 112, 112]), torch.Size([1, 32, 56, 56]), torch.Size([1, 64, 28, 28]), torch.Size([1, 160, 14, 14])]
```
|
glasses/resnext101_32x8d | 72729573dbbeaf1bd692695ac1d1f4102de02ad1 | 2021-11-30T20:15:04.000Z | [
"pytorch",
"arxiv:1611.05431",
"transformers"
] | null | false | glasses | null | glasses/resnext101_32x8d | 0 | null | transformers | 33,681 | # resnext101_32x8d
Implementation of ResNetXt proposed in [\"Aggregated Residual
Transformation for Deep Neural
Networks\"](https://arxiv.org/pdf/1611.05431.pdf)
Create a default model
``` python
ResNetXt.resnext50_32x4d()
ResNetXt.resnext101_32x8d()
# create a resnetxt18_32x4d
ResNetXt.resnet18(block=ResNetXtBottleNeckBlock, groups=32, base_width=4)
```
Examples:
: ``` python
# change activation
ResNetXt.resnext50_32x4d(activation = nn.SELU)
# change number of classes (default is 1000 )
ResNetXt.resnext50_32x4d(n_classes=100)
# pass a different block
ResNetXt.resnext50_32x4d(block=SENetBasicBlock)
# change the initial convolution
model = ResNetXt.resnext50_32x4d
model.encoder.gate.conv1 = nn.Conv2d(3, 64, kernel_size=3)
# store each feature
x = torch.rand((1, 3, 224, 224))
model = ResNetXt.resnext50_32x4d()
# first call .features, this will activate the forward hooks and tells the model you'll like to get the features
model.encoder.features
model(torch.randn((1,3,224,224)))
# get the features from the encoder
features = model.encoder.features
print([x.shape for x in features])
#[torch.Size([1, 64, 112, 112]), torch.Size([1, 64, 56, 56]), torch.Size([1, 128, 28, 28]), torch.Size([1, 256, 14, 14])]
```
|
glasses/vgg13_bn | e09a0a7152c3925e6fafbabfaeb3839019a3be0b | 2021-12-01T08:02:05.000Z | [
"pytorch",
"transformers"
] | null | false | glasses | null | glasses/vgg13_bn | 0 | null | transformers | 33,682 | # vgg13_bn
Implementation of VGG proposed in [Very Deep Convolutional Networks For
Large-Scale Image Recognition](https://arxiv.org/pdf/1409.1556.pdf)
``` python
VGG.vgg11()
VGG.vgg13()
VGG.vgg16()
VGG.vgg19()
VGG.vgg11_bn()
VGG.vgg13_bn()
VGG.vgg16_bn()
VGG.vgg19_bn()
```
Please be aware that the [bn]{.title-ref} models uses BatchNorm but
they are very old and people back then don\'t know the bias is
superfluous in a conv followed by a batchnorm.
Examples:
``` python
# change activation
VGG.vgg11(activation = nn.SELU)
# change number of classes (default is 1000 )
VGG.vgg11(n_classes=100)
# pass a different block
from nn.models.classification.senet import SENetBasicBlock
VGG.vgg11(block=SENetBasicBlock)
# store the features tensor after every block
```
|
glasses/vit_base_patch16_384 | eed350bba31b52e44b7d19bc64d546ec824a823a | 2021-12-01T08:26:46.000Z | [
"pytorch",
"arxiv:2010.11929",
"transformers"
] | null | false | glasses | null | glasses/vit_base_patch16_384 | 0 | null | transformers | 33,683 | # vit_base_patch16_384
Implementation of Vision Transformer (ViT) proposed in [An Image Is
Worth 16x16 Words: Transformers For Image Recognition At
Scale](https://arxiv.org/pdf/2010.11929.pdf)
The following image from the authors shows the architecture.

``` python
ViT.vit_small_patch16_224()
ViT.vit_base_patch16_224()
ViT.vit_base_patch16_384()
ViT.vit_base_patch32_384()
ViT.vit_huge_patch16_224()
ViT.vit_huge_patch32_384()
ViT.vit_large_patch16_224()
ViT.vit_large_patch16_384()
ViT.vit_large_patch32_384()
```
Examples:
``` python
# change activation
ViT.vit_base_patch16_224(activation = nn.SELU)
# change number of classes (default is 1000 )
ViT.vit_base_patch16_224(n_classes=100)
# pass a different block, default is TransformerEncoderBlock
ViT.vit_base_patch16_224(block=MyCoolTransformerBlock)
# get features
model = ViT.vit_base_patch16_224
# first call .features, this will activate the forward hooks and tells the model you'll like to get the features
model.encoder.features
model(torch.randn((1,3,224,224)))
# get the features from the encoder
features = model.encoder.features
print([x.shape for x in features])
#[[torch.Size([1, 197, 768]), torch.Size([1, 197, 768]), ...]
# change the tokens, you have to subclass ViTTokens
class MyTokens(ViTTokens):
def __init__(self, emb_size: int):
super().__init__(emb_size)
self.my_new_token = nn.Parameter(torch.randn(1, 1, emb_size))
ViT(tokens=MyTokens)
```
|
glasses/vit_large_patch16_224 | a975743fb7b441be565d7532b9fd2fda8635e4bd | 2021-04-22T18:42:35.000Z | [
"pytorch",
"arxiv:2010.11929",
"transformers"
] | null | false | glasses | null | glasses/vit_large_patch16_224 | 0 | null | transformers | 33,684 | # vit_large_patch16_224
Implementation of Vision Transformer (ViT) proposed in [An Image Is
Worth 16x16 Words: Transformers For Image Recognition At
Scale](https://arxiv.org/pdf/2010.11929.pdf)
The following image from the authors shows the architecture.

``` python
ViT.vit_small_patch16_224()
ViT.vit_base_patch16_224()
ViT.vit_base_patch16_384()
ViT.vit_base_patch32_384()
ViT.vit_huge_patch16_224()
ViT.vit_huge_patch32_384()
ViT.vit_large_patch16_224()
ViT.vit_large_patch16_384()
ViT.vit_large_patch32_384()
```
Examples:
``` python
# change activation
ViT.vit_base_patch16_224(activation = nn.SELU)
# change number of classes (default is 1000 )
ViT.vit_base_patch16_224(n_classes=100)
# pass a different block, default is TransformerEncoderBlock
ViT.vit_base_patch16_224(block=MyCoolTransformerBlock)
# get features
model = ViT.vit_base_patch16_224
# first call .features, this will activate the forward hooks and tells the model you'll like to get the features
model.encoder.features
model(torch.randn((1,3,224,224)))
# get the features from the encoder
features = model.encoder.features
print([x.shape for x in features])
#[[torch.Size([1, 197, 768]), torch.Size([1, 197, 768]), ...]
# change the tokens, you have to subclass ViTTokens
class MyTokens(ViTTokens):
def __init__(self, emb_size: int):
super().__init__(emb_size)
self.my_new_token = nn.Parameter(torch.randn(1, 1, emb_size))
ViT(tokens=MyTokens)
```
|
glasses/vit_large_patch16_384 | 193c63a7c3aeff06b8a47495faad33a83ad1e364 | 2021-04-22T18:43:25.000Z | [
"pytorch",
"arxiv:2010.11929",
"transformers"
] | null | false | glasses | null | glasses/vit_large_patch16_384 | 0 | null | transformers | 33,685 | # vit_large_patch16_384
Implementation of Vision Transformer (ViT) proposed in [An Image Is
Worth 16x16 Words: Transformers For Image Recognition At
Scale](https://arxiv.org/pdf/2010.11929.pdf)
The following image from the authors shows the architecture.

``` python
ViT.vit_small_patch16_224()
ViT.vit_base_patch16_224()
ViT.vit_base_patch16_384()
ViT.vit_base_patch32_384()
ViT.vit_huge_patch16_224()
ViT.vit_huge_patch32_384()
ViT.vit_large_patch16_224()
ViT.vit_large_patch16_384()
ViT.vit_large_patch32_384()
```
Examples:
``` python
# change activation
ViT.vit_base_patch16_224(activation = nn.SELU)
# change number of classes (default is 1000 )
ViT.vit_base_patch16_224(n_classes=100)
# pass a different block, default is TransformerEncoderBlock
ViT.vit_base_patch16_224(block=MyCoolTransformerBlock)
# get features
model = ViT.vit_base_patch16_224
# first call .features, this will activate the forward hooks and tells the model you'll like to get the features
model.encoder.features
model(torch.randn((1,3,224,224)))
# get the features from the encoder
features = model.encoder.features
print([x.shape for x in features])
#[[torch.Size([1, 197, 768]), torch.Size([1, 197, 768]), ...]
# change the tokens, you have to subclass ViTTokens
class MyTokens(ViTTokens):
def __init__(self, emb_size: int):
super().__init__(emb_size)
self.my_new_token = nn.Parameter(torch.randn(1, 1, emb_size))
ViT(tokens=MyTokens)
```
|
glob-asr/test-asr-sp-model | 06a12ac26c85f73aaf1e2e8e93ef0ee25604aed5 | 2022-01-28T20:46:59.000Z | [
"pytorch",
"wav2vec2",
"feature-extraction",
"transformers"
] | feature-extraction | false | glob-asr | null | glob-asr/test-asr-sp-model | 0 | null | transformers | 33,686 | Entry not found |
gngpostalsrvc/BERiTmodel2 | 4b0d0418776894a2483ba5b7534322a10b3da95b | 2021-12-22T17:25:25.000Z | [
"pytorch",
"tensorboard",
"roberta",
"fill-mask",
"transformers",
"generated_from_trainer",
"license:mit",
"model-index",
"autotrain_compatible"
] | fill-mask | false | gngpostalsrvc | null | gngpostalsrvc/BERiTmodel2 | 0 | null | transformers | 33,687 | ---
license: mit
tags:
- generated_from_trainer
model-index:
- name: BERiTmodel2
results: []
---
<!-- This model card has been generated automatically according to the information the Trainer had access to. You
should probably proofread and complete it, then remove this comment. -->
# BERiTmodel2
This model is a fine-tuned version of [roberta-base](https://huggingface.co/roberta-base) on an unknown dataset.
It achieves the following results on the evaluation set:
- Loss: 3.1508
## Model description
More information needed
## Intended uses & limitations
More information needed
## Training and evaluation data
More information needed
## Training procedure
### Training hyperparameters
The following hyperparameters were used during training:
- learning_rate: 5e-05
- train_batch_size: 8
- eval_batch_size: 8
- seed: 42
- optimizer: Adam with betas=(0.9,0.999) and epsilon=1e-08
- lr_scheduler_type: cosine
- lr_scheduler_warmup_steps: 280
- num_epochs: 10
### Training results
| Training Loss | Epoch | Step | Validation Loss |
|:-------------:|:-----:|:-----:|:---------------:|
| 3.1924 | 1.0 | 2854 | 3.4329 |
| 3.0936 | 2.0 | 5708 | 3.5036 |
| 2.9998 | 3.0 | 8562 | 3.1906 |
| 2.9064 | 4.0 | 11416 | 3.4867 |
| 2.8493 | 5.0 | 14270 | 3.2027 |
| 2.7538 | 6.0 | 17124 | 2.9772 |
| 2.7273 | 7.0 | 19978 | 2.9950 |
| 2.7399 | 8.0 | 22832 | 2.9690 |
| 2.67 | 9.0 | 25686 | 3.0311 |
| 2.6388 | 10.0 | 28540 | 3.1508 |
### Framework versions
- Transformers 4.14.1
- Pytorch 1.10.0+cu111
- Datasets 1.17.0
- Tokenizers 0.10.3
|
gokulkarthik/distilbert-base-uncased-finetuned-squad | 8de82646b339fc7f3a67bc032ff0c1467e145dc0 | 2021-09-29T15:13:52.000Z | [
"pytorch",
"tensorboard",
"distilbert",
"question-answering",
"dataset:squad",
"transformers",
"generated_from_trainer",
"license:apache-2.0",
"model-index",
"autotrain_compatible"
] | question-answering | false | gokulkarthik | null | gokulkarthik/distilbert-base-uncased-finetuned-squad | 0 | null | transformers | 33,688 | ---
license: apache-2.0
tags:
- generated_from_trainer
datasets:
- squad
model-index:
- name: distilbert-base-uncased-finetuned-squad
results: []
---
<!-- This model card has been generated automatically according to the information the Trainer had access to. You
should probably proofread and complete it, then remove this comment. -->
# distilbert-base-uncased-finetuned-squad
This model is a fine-tuned version of [distilbert-base-uncased](https://huggingface.co/distilbert-base-uncased) on the squad dataset.
## Model description
More information needed
## Intended uses & limitations
More information needed
## Training and evaluation data
More information needed
## Training procedure
### Training hyperparameters
The following hyperparameters were used during training:
- learning_rate: 2e-05
- train_batch_size: 16
- eval_batch_size: 16
- seed: 42
- optimizer: Adam with betas=(0.9,0.999) and epsilon=1e-08
- lr_scheduler_type: linear
- num_epochs: 3
### Framework versions
- Transformers 4.11.0
- Pytorch 1.9.0+cu102
- Datasets 1.12.1
- Tokenizers 0.10.3
|
goodjw/klue-bert-mlm | 451afde04a32165ed465df79fdbed0f5c75473c9 | 2021-10-02T05:18:01.000Z | [
"pytorch",
"bert",
"fill-mask",
"transformers",
"autotrain_compatible"
] | fill-mask | false | goodjw | null | goodjw/klue-bert-mlm | 0 | 1 | transformers | 33,689 | Entry not found |
goodjw/klue-roberta-large-tapt | 4311c1c3cd555818ec2b84a4a30fe3ec7cb3ca45 | 2021-10-06T06:22:40.000Z | [
"pytorch",
"roberta",
"fill-mask",
"transformers",
"autotrain_compatible"
] | fill-mask | false | goodjw | null | goodjw/klue-roberta-large-tapt | 0 | null | transformers | 33,690 | Entry not found |
goodjw/klue-roberta-mlm | af64935da25fab9997064cdd9b4f18e9baa60400 | 2021-10-05T04:17:02.000Z | [
"pytorch",
"roberta",
"fill-mask",
"transformers",
"autotrain_compatible"
] | fill-mask | false | goodjw | null | goodjw/klue-roberta-mlm | 0 | 1 | transformers | 33,691 | Entry not found |
goodjw/koelectra-mlm | 1668a75be7d81a60f132293f5d1764be51cdd9f6 | 2021-10-06T01:17:58.000Z | [
"pytorch",
"electra",
"fill-mask",
"transformers",
"autotrain_compatible"
] | fill-mask | false | goodjw | null | goodjw/koelectra-mlm | 0 | null | transformers | 33,692 | Entry not found |
google/multiberts-seed_0-step_120k | 0cf4a460cb80f7054f426a34f1e430a9de19a9cc | 2021-11-05T23:47:25.000Z | [
"pytorch",
"tf",
"bert",
"pretraining",
"en",
"arxiv:2106.16163",
"arxiv:1908.08962",
"transformers",
"multiberts",
"multiberts-seed_0",
"multiberts-seed_0-step_120k",
"license:apache-2.0"
] | null | false | google | null | google/multiberts-seed_0-step_120k | 0 | null | transformers | 33,693 | ---
language: en
tags:
- multiberts
- multiberts-seed_0
- multiberts-seed_0-step_120k
license: apache-2.0
---
# MultiBERTs, Intermediate Checkpoint - Seed 0, Step 120k
MultiBERTs is a collection of checkpoints and a statistical library to support
robust research on BERT. We provide 25 BERT-base models trained with
similar hyper-parameters as
[the original BERT model](https://github.com/google-research/bert) but
with different random seeds, which causes variations in the initial weights and order of
training instances. The aim is to distinguish findings that apply to a specific
artifact (i.e., a particular instance of the model) from those that apply to the
more general procedure.
We also provide 140 intermediate checkpoints captured
during the course of pre-training (we saved 28 checkpoints for the first 5 runs).
The models were originally released through
[http://goo.gle/multiberts](http://goo.gle/multiberts). We describe them in our
paper
[The MultiBERTs: BERT Reproductions for Robustness Analysis](https://arxiv.org/abs/2106.16163).
This is model #0, captured at step 120k (max: 2000k, i.e., 2M steps).
## Model Description
This model was captured during a reproduction of
[BERT-base uncased](https://github.com/google-research/bert), for English: it
is a Transformers model pretrained on a large corpus of English data, using the
Masked Language Modelling (MLM) and the Next Sentence Prediction (NSP)
objectives.
The intended uses, limitations, training data and training procedure for the fully trained model are similar
to [BERT-base uncased](https://github.com/google-research/bert). Two major
differences with the original model:
* We pre-trained the MultiBERTs models for 2 million steps using sequence
length 512 (instead of 1 million steps using sequence length 128 then 512).
* We used an alternative version of Wikipedia and Books Corpus, initially
collected for [Turc et al., 2019](https://arxiv.org/abs/1908.08962).
This is a best-effort reproduction, and so it is probable that differences with
the original model have gone unnoticed. The performance of MultiBERTs on GLUE after full training is oftentimes comparable to that of original
BERT, but we found significant differences on the dev set of SQuAD (MultiBERTs outperforms original BERT).
See our [technical report](https://arxiv.org/abs/2106.16163) for more details.
### How to use
Using code from
[BERT-base uncased](https://huggingface.co/bert-base-uncased), here is an example based on
Tensorflow:
```
from transformers import BertTokenizer, TFBertModel
tokenizer = BertTokenizer.from_pretrained('google/multiberts-seed_0-step_120k')
model = TFBertModel.from_pretrained("google/multiberts-seed_0-step_120k")
text = "Replace me by any text you'd like."
encoded_input = tokenizer(text, return_tensors='tf')
output = model(encoded_input)
```
PyTorch version:
```
from transformers import BertTokenizer, BertModel
tokenizer = BertTokenizer.from_pretrained('google/multiberts-seed_0-step_120k')
model = BertModel.from_pretrained("google/multiberts-seed_0-step_120k")
text = "Replace me by any text you'd like."
encoded_input = tokenizer(text, return_tensors='pt')
output = model(**encoded_input)
```
## Citation info
```bibtex
@article{sellam2021multiberts,
title={The MultiBERTs: BERT Reproductions for Robustness Analysis},
author={Thibault Sellam and Steve Yadlowsky and Jason Wei and Naomi Saphra and Alexander D'Amour and Tal Linzen and Jasmijn Bastings and Iulia Turc and Jacob Eisenstein and Dipanjan Das and Ian Tenney and Ellie Pavlick},
journal={arXiv preprint arXiv:2106.16163},
year={2021}
}
```
|
google/multiberts-seed_0-step_140k | e6f8f170bf6295091ff2cfce1723b3b3329b9fec | 2021-11-05T23:49:02.000Z | [
"pytorch",
"tf",
"bert",
"pretraining",
"en",
"arxiv:2106.16163",
"arxiv:1908.08962",
"transformers",
"multiberts",
"multiberts-seed_0",
"multiberts-seed_0-step_140k",
"license:apache-2.0"
] | null | false | google | null | google/multiberts-seed_0-step_140k | 0 | null | transformers | 33,694 | ---
language: en
tags:
- multiberts
- multiberts-seed_0
- multiberts-seed_0-step_140k
license: apache-2.0
---
# MultiBERTs, Intermediate Checkpoint - Seed 0, Step 140k
MultiBERTs is a collection of checkpoints and a statistical library to support
robust research on BERT. We provide 25 BERT-base models trained with
similar hyper-parameters as
[the original BERT model](https://github.com/google-research/bert) but
with different random seeds, which causes variations in the initial weights and order of
training instances. The aim is to distinguish findings that apply to a specific
artifact (i.e., a particular instance of the model) from those that apply to the
more general procedure.
We also provide 140 intermediate checkpoints captured
during the course of pre-training (we saved 28 checkpoints for the first 5 runs).
The models were originally released through
[http://goo.gle/multiberts](http://goo.gle/multiberts). We describe them in our
paper
[The MultiBERTs: BERT Reproductions for Robustness Analysis](https://arxiv.org/abs/2106.16163).
This is model #0, captured at step 140k (max: 2000k, i.e., 2M steps).
## Model Description
This model was captured during a reproduction of
[BERT-base uncased](https://github.com/google-research/bert), for English: it
is a Transformers model pretrained on a large corpus of English data, using the
Masked Language Modelling (MLM) and the Next Sentence Prediction (NSP)
objectives.
The intended uses, limitations, training data and training procedure for the fully trained model are similar
to [BERT-base uncased](https://github.com/google-research/bert). Two major
differences with the original model:
* We pre-trained the MultiBERTs models for 2 million steps using sequence
length 512 (instead of 1 million steps using sequence length 128 then 512).
* We used an alternative version of Wikipedia and Books Corpus, initially
collected for [Turc et al., 2019](https://arxiv.org/abs/1908.08962).
This is a best-effort reproduction, and so it is probable that differences with
the original model have gone unnoticed. The performance of MultiBERTs on GLUE after full training is oftentimes comparable to that of original
BERT, but we found significant differences on the dev set of SQuAD (MultiBERTs outperforms original BERT).
See our [technical report](https://arxiv.org/abs/2106.16163) for more details.
### How to use
Using code from
[BERT-base uncased](https://huggingface.co/bert-base-uncased), here is an example based on
Tensorflow:
```
from transformers import BertTokenizer, TFBertModel
tokenizer = BertTokenizer.from_pretrained('google/multiberts-seed_0-step_140k')
model = TFBertModel.from_pretrained("google/multiberts-seed_0-step_140k")
text = "Replace me by any text you'd like."
encoded_input = tokenizer(text, return_tensors='tf')
output = model(encoded_input)
```
PyTorch version:
```
from transformers import BertTokenizer, BertModel
tokenizer = BertTokenizer.from_pretrained('google/multiberts-seed_0-step_140k')
model = BertModel.from_pretrained("google/multiberts-seed_0-step_140k")
text = "Replace me by any text you'd like."
encoded_input = tokenizer(text, return_tensors='pt')
output = model(**encoded_input)
```
## Citation info
```bibtex
@article{sellam2021multiberts,
title={The MultiBERTs: BERT Reproductions for Robustness Analysis},
author={Thibault Sellam and Steve Yadlowsky and Jason Wei and Naomi Saphra and Alexander D'Amour and Tal Linzen and Jasmijn Bastings and Iulia Turc and Jacob Eisenstein and Dipanjan Das and Ian Tenney and Ellie Pavlick},
journal={arXiv preprint arXiv:2106.16163},
year={2021}
}
```
|
google/multiberts-seed_0-step_1500k | 1aac894fd46ed128076db103a331475420a04767 | 2021-11-06T00:16:49.000Z | [
"pytorch",
"tf",
"bert",
"pretraining",
"en",
"arxiv:2106.16163",
"arxiv:1908.08962",
"transformers",
"multiberts",
"multiberts-seed_0",
"multiberts-seed_0-step_1500k",
"license:apache-2.0"
] | null | false | google | null | google/multiberts-seed_0-step_1500k | 0 | null | transformers | 33,695 | ---
language: en
tags:
- multiberts
- multiberts-seed_0
- multiberts-seed_0-step_1500k
license: apache-2.0
---
# MultiBERTs, Intermediate Checkpoint - Seed 0, Step 1500k
MultiBERTs is a collection of checkpoints and a statistical library to support
robust research on BERT. We provide 25 BERT-base models trained with
similar hyper-parameters as
[the original BERT model](https://github.com/google-research/bert) but
with different random seeds, which causes variations in the initial weights and order of
training instances. The aim is to distinguish findings that apply to a specific
artifact (i.e., a particular instance of the model) from those that apply to the
more general procedure.
We also provide 140 intermediate checkpoints captured
during the course of pre-training (we saved 28 checkpoints for the first 5 runs).
The models were originally released through
[http://goo.gle/multiberts](http://goo.gle/multiberts). We describe them in our
paper
[The MultiBERTs: BERT Reproductions for Robustness Analysis](https://arxiv.org/abs/2106.16163).
This is model #0, captured at step 1500k (max: 2000k, i.e., 2M steps).
## Model Description
This model was captured during a reproduction of
[BERT-base uncased](https://github.com/google-research/bert), for English: it
is a Transformers model pretrained on a large corpus of English data, using the
Masked Language Modelling (MLM) and the Next Sentence Prediction (NSP)
objectives.
The intended uses, limitations, training data and training procedure for the fully trained model are similar
to [BERT-base uncased](https://github.com/google-research/bert). Two major
differences with the original model:
* We pre-trained the MultiBERTs models for 2 million steps using sequence
length 512 (instead of 1 million steps using sequence length 128 then 512).
* We used an alternative version of Wikipedia and Books Corpus, initially
collected for [Turc et al., 2019](https://arxiv.org/abs/1908.08962).
This is a best-effort reproduction, and so it is probable that differences with
the original model have gone unnoticed. The performance of MultiBERTs on GLUE after full training is oftentimes comparable to that of original
BERT, but we found significant differences on the dev set of SQuAD (MultiBERTs outperforms original BERT).
See our [technical report](https://arxiv.org/abs/2106.16163) for more details.
### How to use
Using code from
[BERT-base uncased](https://huggingface.co/bert-base-uncased), here is an example based on
Tensorflow:
```
from transformers import BertTokenizer, TFBertModel
tokenizer = BertTokenizer.from_pretrained('google/multiberts-seed_0-step_1500k')
model = TFBertModel.from_pretrained("google/multiberts-seed_0-step_1500k")
text = "Replace me by any text you'd like."
encoded_input = tokenizer(text, return_tensors='tf')
output = model(encoded_input)
```
PyTorch version:
```
from transformers import BertTokenizer, BertModel
tokenizer = BertTokenizer.from_pretrained('google/multiberts-seed_0-step_1500k')
model = BertModel.from_pretrained("google/multiberts-seed_0-step_1500k")
text = "Replace me by any text you'd like."
encoded_input = tokenizer(text, return_tensors='pt')
output = model(**encoded_input)
```
## Citation info
```bibtex
@article{sellam2021multiberts,
title={The MultiBERTs: BERT Reproductions for Robustness Analysis},
author={Thibault Sellam and Steve Yadlowsky and Jason Wei and Naomi Saphra and Alexander D'Amour and Tal Linzen and Jasmijn Bastings and Iulia Turc and Jacob Eisenstein and Dipanjan Das and Ian Tenney and Ellie Pavlick},
journal={arXiv preprint arXiv:2106.16163},
year={2021}
}
```
|
google/multiberts-seed_0-step_300k | ba194e26ef19c2ac7a22c2c1142ed8c2b2f271c5 | 2021-11-05T23:56:05.000Z | [
"pytorch",
"tf",
"bert",
"pretraining",
"en",
"arxiv:2106.16163",
"arxiv:1908.08962",
"transformers",
"multiberts",
"multiberts-seed_0",
"multiberts-seed_0-step_300k",
"license:apache-2.0"
] | null | false | google | null | google/multiberts-seed_0-step_300k | 0 | null | transformers | 33,696 | ---
language: en
tags:
- multiberts
- multiberts-seed_0
- multiberts-seed_0-step_300k
license: apache-2.0
---
# MultiBERTs, Intermediate Checkpoint - Seed 0, Step 300k
MultiBERTs is a collection of checkpoints and a statistical library to support
robust research on BERT. We provide 25 BERT-base models trained with
similar hyper-parameters as
[the original BERT model](https://github.com/google-research/bert) but
with different random seeds, which causes variations in the initial weights and order of
training instances. The aim is to distinguish findings that apply to a specific
artifact (i.e., a particular instance of the model) from those that apply to the
more general procedure.
We also provide 140 intermediate checkpoints captured
during the course of pre-training (we saved 28 checkpoints for the first 5 runs).
The models were originally released through
[http://goo.gle/multiberts](http://goo.gle/multiberts). We describe them in our
paper
[The MultiBERTs: BERT Reproductions for Robustness Analysis](https://arxiv.org/abs/2106.16163).
This is model #0, captured at step 300k (max: 2000k, i.e., 2M steps).
## Model Description
This model was captured during a reproduction of
[BERT-base uncased](https://github.com/google-research/bert), for English: it
is a Transformers model pretrained on a large corpus of English data, using the
Masked Language Modelling (MLM) and the Next Sentence Prediction (NSP)
objectives.
The intended uses, limitations, training data and training procedure for the fully trained model are similar
to [BERT-base uncased](https://github.com/google-research/bert). Two major
differences with the original model:
* We pre-trained the MultiBERTs models for 2 million steps using sequence
length 512 (instead of 1 million steps using sequence length 128 then 512).
* We used an alternative version of Wikipedia and Books Corpus, initially
collected for [Turc et al., 2019](https://arxiv.org/abs/1908.08962).
This is a best-effort reproduction, and so it is probable that differences with
the original model have gone unnoticed. The performance of MultiBERTs on GLUE after full training is oftentimes comparable to that of original
BERT, but we found significant differences on the dev set of SQuAD (MultiBERTs outperforms original BERT).
See our [technical report](https://arxiv.org/abs/2106.16163) for more details.
### How to use
Using code from
[BERT-base uncased](https://huggingface.co/bert-base-uncased), here is an example based on
Tensorflow:
```
from transformers import BertTokenizer, TFBertModel
tokenizer = BertTokenizer.from_pretrained('google/multiberts-seed_0-step_300k')
model = TFBertModel.from_pretrained("google/multiberts-seed_0-step_300k")
text = "Replace me by any text you'd like."
encoded_input = tokenizer(text, return_tensors='tf')
output = model(encoded_input)
```
PyTorch version:
```
from transformers import BertTokenizer, BertModel
tokenizer = BertTokenizer.from_pretrained('google/multiberts-seed_0-step_300k')
model = BertModel.from_pretrained("google/multiberts-seed_0-step_300k")
text = "Replace me by any text you'd like."
encoded_input = tokenizer(text, return_tensors='pt')
output = model(**encoded_input)
```
## Citation info
```bibtex
@article{sellam2021multiberts,
title={The MultiBERTs: BERT Reproductions for Robustness Analysis},
author={Thibault Sellam and Steve Yadlowsky and Jason Wei and Naomi Saphra and Alexander D'Amour and Tal Linzen and Jasmijn Bastings and Iulia Turc and Jacob Eisenstein and Dipanjan Das and Ian Tenney and Ellie Pavlick},
journal={arXiv preprint arXiv:2106.16163},
year={2021}
}
```
|
google/multiberts-seed_0-step_400k | 5e9c41a7458165ad3230c44bb53efdfc79796c29 | 2021-11-05T23:57:51.000Z | [
"pytorch",
"tf",
"bert",
"pretraining",
"en",
"arxiv:2106.16163",
"arxiv:1908.08962",
"transformers",
"multiberts",
"multiberts-seed_0",
"multiberts-seed_0-step_400k",
"license:apache-2.0"
] | null | false | google | null | google/multiberts-seed_0-step_400k | 0 | null | transformers | 33,697 | ---
language: en
tags:
- multiberts
- multiberts-seed_0
- multiberts-seed_0-step_400k
license: apache-2.0
---
# MultiBERTs, Intermediate Checkpoint - Seed 0, Step 400k
MultiBERTs is a collection of checkpoints and a statistical library to support
robust research on BERT. We provide 25 BERT-base models trained with
similar hyper-parameters as
[the original BERT model](https://github.com/google-research/bert) but
with different random seeds, which causes variations in the initial weights and order of
training instances. The aim is to distinguish findings that apply to a specific
artifact (i.e., a particular instance of the model) from those that apply to the
more general procedure.
We also provide 140 intermediate checkpoints captured
during the course of pre-training (we saved 28 checkpoints for the first 5 runs).
The models were originally released through
[http://goo.gle/multiberts](http://goo.gle/multiberts). We describe them in our
paper
[The MultiBERTs: BERT Reproductions for Robustness Analysis](https://arxiv.org/abs/2106.16163).
This is model #0, captured at step 400k (max: 2000k, i.e., 2M steps).
## Model Description
This model was captured during a reproduction of
[BERT-base uncased](https://github.com/google-research/bert), for English: it
is a Transformers model pretrained on a large corpus of English data, using the
Masked Language Modelling (MLM) and the Next Sentence Prediction (NSP)
objectives.
The intended uses, limitations, training data and training procedure for the fully trained model are similar
to [BERT-base uncased](https://github.com/google-research/bert). Two major
differences with the original model:
* We pre-trained the MultiBERTs models for 2 million steps using sequence
length 512 (instead of 1 million steps using sequence length 128 then 512).
* We used an alternative version of Wikipedia and Books Corpus, initially
collected for [Turc et al., 2019](https://arxiv.org/abs/1908.08962).
This is a best-effort reproduction, and so it is probable that differences with
the original model have gone unnoticed. The performance of MultiBERTs on GLUE after full training is oftentimes comparable to that of original
BERT, but we found significant differences on the dev set of SQuAD (MultiBERTs outperforms original BERT).
See our [technical report](https://arxiv.org/abs/2106.16163) for more details.
### How to use
Using code from
[BERT-base uncased](https://huggingface.co/bert-base-uncased), here is an example based on
Tensorflow:
```
from transformers import BertTokenizer, TFBertModel
tokenizer = BertTokenizer.from_pretrained('google/multiberts-seed_0-step_400k')
model = TFBertModel.from_pretrained("google/multiberts-seed_0-step_400k")
text = "Replace me by any text you'd like."
encoded_input = tokenizer(text, return_tensors='tf')
output = model(encoded_input)
```
PyTorch version:
```
from transformers import BertTokenizer, BertModel
tokenizer = BertTokenizer.from_pretrained('google/multiberts-seed_0-step_400k')
model = BertModel.from_pretrained("google/multiberts-seed_0-step_400k")
text = "Replace me by any text you'd like."
encoded_input = tokenizer(text, return_tensors='pt')
output = model(**encoded_input)
```
## Citation info
```bibtex
@article{sellam2021multiberts,
title={The MultiBERTs: BERT Reproductions for Robustness Analysis},
author={Thibault Sellam and Steve Yadlowsky and Jason Wei and Naomi Saphra and Alexander D'Amour and Tal Linzen and Jasmijn Bastings and Iulia Turc and Jacob Eisenstein and Dipanjan Das and Ian Tenney and Ellie Pavlick},
journal={arXiv preprint arXiv:2106.16163},
year={2021}
}
```
|
google/multiberts-seed_1-step_1100k | 4c6ea4453f497729d2308ff565d41dd64e2276c2 | 2021-11-06T01:05:05.000Z | [
"pytorch",
"tf",
"bert",
"pretraining",
"en",
"arxiv:2106.16163",
"arxiv:1908.08962",
"transformers",
"multiberts",
"multiberts-seed_1",
"multiberts-seed_1-step_1100k",
"license:apache-2.0"
] | null | false | google | null | google/multiberts-seed_1-step_1100k | 0 | null | transformers | 33,698 | ---
language: en
tags:
- multiberts
- multiberts-seed_1
- multiberts-seed_1-step_1100k
license: apache-2.0
---
# MultiBERTs, Intermediate Checkpoint - Seed 1, Step 1100k
MultiBERTs is a collection of checkpoints and a statistical library to support
robust research on BERT. We provide 25 BERT-base models trained with
similar hyper-parameters as
[the original BERT model](https://github.com/google-research/bert) but
with different random seeds, which causes variations in the initial weights and order of
training instances. The aim is to distinguish findings that apply to a specific
artifact (i.e., a particular instance of the model) from those that apply to the
more general procedure.
We also provide 140 intermediate checkpoints captured
during the course of pre-training (we saved 28 checkpoints for the first 5 runs).
The models were originally released through
[http://goo.gle/multiberts](http://goo.gle/multiberts). We describe them in our
paper
[The MultiBERTs: BERT Reproductions for Robustness Analysis](https://arxiv.org/abs/2106.16163).
This is model #1, captured at step 1100k (max: 2000k, i.e., 2M steps).
## Model Description
This model was captured during a reproduction of
[BERT-base uncased](https://github.com/google-research/bert), for English: it
is a Transformers model pretrained on a large corpus of English data, using the
Masked Language Modelling (MLM) and the Next Sentence Prediction (NSP)
objectives.
The intended uses, limitations, training data and training procedure for the fully trained model are similar
to [BERT-base uncased](https://github.com/google-research/bert). Two major
differences with the original model:
* We pre-trained the MultiBERTs models for 2 million steps using sequence
length 512 (instead of 1 million steps using sequence length 128 then 512).
* We used an alternative version of Wikipedia and Books Corpus, initially
collected for [Turc et al., 2019](https://arxiv.org/abs/1908.08962).
This is a best-effort reproduction, and so it is probable that differences with
the original model have gone unnoticed. The performance of MultiBERTs on GLUE after full training is oftentimes comparable to that of original
BERT, but we found significant differences on the dev set of SQuAD (MultiBERTs outperforms original BERT).
See our [technical report](https://arxiv.org/abs/2106.16163) for more details.
### How to use
Using code from
[BERT-base uncased](https://huggingface.co/bert-base-uncased), here is an example based on
Tensorflow:
```
from transformers import BertTokenizer, TFBertModel
tokenizer = BertTokenizer.from_pretrained('google/multiberts-seed_1-step_1100k')
model = TFBertModel.from_pretrained("google/multiberts-seed_1-step_1100k")
text = "Replace me by any text you'd like."
encoded_input = tokenizer(text, return_tensors='tf')
output = model(encoded_input)
```
PyTorch version:
```
from transformers import BertTokenizer, BertModel
tokenizer = BertTokenizer.from_pretrained('google/multiberts-seed_1-step_1100k')
model = BertModel.from_pretrained("google/multiberts-seed_1-step_1100k")
text = "Replace me by any text you'd like."
encoded_input = tokenizer(text, return_tensors='pt')
output = model(**encoded_input)
```
## Citation info
```bibtex
@article{sellam2021multiberts,
title={The MultiBERTs: BERT Reproductions for Robustness Analysis},
author={Thibault Sellam and Steve Yadlowsky and Jason Wei and Naomi Saphra and Alexander D'Amour and Tal Linzen and Jasmijn Bastings and Iulia Turc and Jacob Eisenstein and Dipanjan Das and Ian Tenney and Ellie Pavlick},
journal={arXiv preprint arXiv:2106.16163},
year={2021}
}
```
|
google/multiberts-seed_1-step_120k | 7a41b87e6d9246dcd586b59f83cd151f06552b77 | 2021-11-06T00:43:27.000Z | [
"pytorch",
"tf",
"bert",
"pretraining",
"en",
"arxiv:2106.16163",
"arxiv:1908.08962",
"transformers",
"multiberts",
"multiberts-seed_1",
"multiberts-seed_1-step_120k",
"license:apache-2.0"
] | null | false | google | null | google/multiberts-seed_1-step_120k | 0 | null | transformers | 33,699 | ---
language: en
tags:
- multiberts
- multiberts-seed_1
- multiberts-seed_1-step_120k
license: apache-2.0
---
# MultiBERTs, Intermediate Checkpoint - Seed 1, Step 120k
MultiBERTs is a collection of checkpoints and a statistical library to support
robust research on BERT. We provide 25 BERT-base models trained with
similar hyper-parameters as
[the original BERT model](https://github.com/google-research/bert) but
with different random seeds, which causes variations in the initial weights and order of
training instances. The aim is to distinguish findings that apply to a specific
artifact (i.e., a particular instance of the model) from those that apply to the
more general procedure.
We also provide 140 intermediate checkpoints captured
during the course of pre-training (we saved 28 checkpoints for the first 5 runs).
The models were originally released through
[http://goo.gle/multiberts](http://goo.gle/multiberts). We describe them in our
paper
[The MultiBERTs: BERT Reproductions for Robustness Analysis](https://arxiv.org/abs/2106.16163).
This is model #1, captured at step 120k (max: 2000k, i.e., 2M steps).
## Model Description
This model was captured during a reproduction of
[BERT-base uncased](https://github.com/google-research/bert), for English: it
is a Transformers model pretrained on a large corpus of English data, using the
Masked Language Modelling (MLM) and the Next Sentence Prediction (NSP)
objectives.
The intended uses, limitations, training data and training procedure for the fully trained model are similar
to [BERT-base uncased](https://github.com/google-research/bert). Two major
differences with the original model:
* We pre-trained the MultiBERTs models for 2 million steps using sequence
length 512 (instead of 1 million steps using sequence length 128 then 512).
* We used an alternative version of Wikipedia and Books Corpus, initially
collected for [Turc et al., 2019](https://arxiv.org/abs/1908.08962).
This is a best-effort reproduction, and so it is probable that differences with
the original model have gone unnoticed. The performance of MultiBERTs on GLUE after full training is oftentimes comparable to that of original
BERT, but we found significant differences on the dev set of SQuAD (MultiBERTs outperforms original BERT).
See our [technical report](https://arxiv.org/abs/2106.16163) for more details.
### How to use
Using code from
[BERT-base uncased](https://huggingface.co/bert-base-uncased), here is an example based on
Tensorflow:
```
from transformers import BertTokenizer, TFBertModel
tokenizer = BertTokenizer.from_pretrained('google/multiberts-seed_1-step_120k')
model = TFBertModel.from_pretrained("google/multiberts-seed_1-step_120k")
text = "Replace me by any text you'd like."
encoded_input = tokenizer(text, return_tensors='tf')
output = model(encoded_input)
```
PyTorch version:
```
from transformers import BertTokenizer, BertModel
tokenizer = BertTokenizer.from_pretrained('google/multiberts-seed_1-step_120k')
model = BertModel.from_pretrained("google/multiberts-seed_1-step_120k")
text = "Replace me by any text you'd like."
encoded_input = tokenizer(text, return_tensors='pt')
output = model(**encoded_input)
```
## Citation info
```bibtex
@article{sellam2021multiberts,
title={The MultiBERTs: BERT Reproductions for Robustness Analysis},
author={Thibault Sellam and Steve Yadlowsky and Jason Wei and Naomi Saphra and Alexander D'Amour and Tal Linzen and Jasmijn Bastings and Iulia Turc and Jacob Eisenstein and Dipanjan Das and Ian Tenney and Ellie Pavlick},
journal={arXiv preprint arXiv:2106.16163},
year={2021}
}
```
|
Subsets and Splits
No saved queries yet
Save your SQL queries to embed, download, and access them later. Queries will appear here once saved.