modelId
stringlengths 4
112
| sha
stringlengths 40
40
| lastModified
stringlengths 24
24
| tags
sequence | pipeline_tag
stringclasses 29
values | private
bool 1
class | author
stringlengths 2
38
⌀ | config
null | id
stringlengths 4
112
| downloads
float64 0
36.8M
⌀ | likes
float64 0
712
⌀ | library_name
stringclasses 17
values | __index_level_0__
int64 0
38.5k
| readme
stringlengths 0
186k
|
---|---|---|---|---|---|---|---|---|---|---|---|---|---|
PSW/last-ut-pred-pre-train | ee1ffa8307e3eaffd85224088a2673876ed8bfcb | 2022-04-18T03:11:33.000Z | [
"pytorch",
"bart",
"text2text-generation",
"transformers",
"autotrain_compatible"
] | text2text-generation | false | PSW | null | PSW/last-ut-pred-pre-train | 1 | null | transformers | 31,300 | Entry not found |
csikasote/xls-r-300m-bemba-10hrs | 7ac202baf8c32cf17a60e51c71eab8e7c1fbacc6 | 2022-04-18T15:05:29.000Z | [
"pytorch",
"tensorboard",
"wav2vec2",
"automatic-speech-recognition",
"transformers",
"generated_from_trainer",
"license:apache-2.0",
"model-index"
] | automatic-speech-recognition | false | csikasote | null | csikasote/xls-r-300m-bemba-10hrs | 1 | null | transformers | 31,301 | ---
license: apache-2.0
tags:
- generated_from_trainer
model-index:
- name: xls-r-300m-bemba-10hrs
results: []
---
<!-- This model card has been generated automatically according to the information the Trainer had access to. You
should probably proofread and complete it, then remove this comment. -->
# xls-r-300m-bemba-10hrs
This model is a fine-tuned version of [facebook/wav2vec2-xls-r-300m](https://huggingface.co/facebook/wav2vec2-xls-r-300m) on an unknown dataset.
It achieves the following results on the evaluation set:
- Loss: 0.3022
- Wer: 0.3976
## Model description
More information needed
## Intended uses & limitations
More information needed
## Training and evaluation data
More information needed
## Training procedure
### Training hyperparameters
The following hyperparameters were used during training:
- learning_rate: 0.0003
- train_batch_size: 8
- eval_batch_size: 8
- seed: 42
- gradient_accumulation_steps: 2
- total_train_batch_size: 16
- optimizer: Adam with betas=(0.9,0.999) and epsilon=1e-08
- lr_scheduler_type: linear
- lr_scheduler_warmup_steps: 500
- num_epochs: 10
- mixed_precision_training: Native AMP
### Training results
| Training Loss | Epoch | Step | Validation Loss | Wer |
|:-------------:|:-----:|:----:|:---------------:|:------:|
| 3.4544 | 1.07 | 400 | 0.4912 | 0.6813 |
| 0.662 | 2.14 | 800 | 0.3667 | 0.5690 |
| 0.4601 | 3.22 | 1200 | 0.2792 | 0.4819 |
| 0.3816 | 4.29 | 1600 | 0.2828 | 0.4608 |
| 0.3012 | 5.36 | 2000 | 0.2881 | 0.4651 |
| 0.2427 | 6.43 | 2400 | 0.2758 | 0.4219 |
| 0.1888 | 7.51 | 2800 | 0.2743 | 0.4094 |
| 0.1559 | 8.58 | 3200 | 0.2893 | 0.4021 |
| 0.1203 | 9.65 | 3600 | 0.3022 | 0.3976 |
### Framework versions
- Transformers 4.19.0.dev0
- Pytorch 1.10.0+cu111
- Datasets 2.1.0
- Tokenizers 0.12.1
|
ajaypyatha/sdsqna | 7cb0f8c1595708e0c1c92ed0b2e322604d0586d6 | 2022-04-27T04:24:57.000Z | [
"pytorch",
"distilbert",
"question-answering",
"transformers",
"license:afl-3.0",
"autotrain_compatible"
] | question-answering | false | ajaypyatha | null | ajaypyatha/sdsqna | 1 | null | transformers | 31,302 | ---
license: afl-3.0
---
|
eslamxm/AraBART-finetuned-ar-wikilingua | d392f1b9ba7ee8a34dde221d2e4ec7a4a02933b6 | 2022-04-18T10:01:00.000Z | [
"pytorch",
"mbart",
"text2text-generation",
"dataset:wiki_lingua",
"transformers",
"summarization",
"generated_from_trainer",
"license:apache-2.0",
"model-index",
"autotrain_compatible"
] | summarization | false | eslamxm | null | eslamxm/AraBART-finetuned-ar-wikilingua | 1 | null | transformers | 31,303 | ---
license: apache-2.0
tags:
- summarization
- generated_from_trainer
datasets:
- wiki_lingua
model-index:
- name: AraBART-finetuned-ar-wikilingua
results: []
---
<!-- This model card has been generated automatically according to the information the Trainer had access to. You
should probably proofread and complete it, then remove this comment. -->
# AraBART-finetuned-ar-wikilingua
This model is a fine-tuned version of [moussaKam/AraBART](https://huggingface.co/moussaKam/AraBART) on the wiki_lingua dataset.
It achieves the following results on the evaluation set:
- Loss: 3.9990
- Rouge-1: 23.82
- Rouge-2: 8.97
- Rouge-l: 21.05
- Gen Len: 19.06
- Bertscore: 72.08
## Model description
More information needed
## Intended uses & limitations
More information needed
## Training and evaluation data
More information needed
## Training procedure
### Training hyperparameters
The following hyperparameters were used during training:
- learning_rate: 5e-05
- train_batch_size: 4
- eval_batch_size: 4
- seed: 42
- optimizer: Adam with betas=(0.9,0.999) and epsilon=1e-08
- lr_scheduler_type: linear
- lr_scheduler_warmup_steps: 250
- num_epochs: 8
- label_smoothing_factor: 0.1
### Training results
| Training Loss | Epoch | Step | Validation Loss | Rouge-1 | Rouge-2 | Rouge-l | Gen Len | Bertscore |
|:-------------:|:-----:|:-----:|:---------------:|:-------:|:-------:|:-------:|:-------:|:---------:|
| 4.2331 | 1.0 | 5111 | 4.0713 | 21.42 | 7.69 | 19.08 | 18.79 | 71.22 |
| 3.9438 | 2.0 | 10222 | 4.0251 | 23.1 | 8.63 | 20.59 | 18.41 | 71.86 |
| 3.7372 | 3.0 | 15333 | 3.9744 | 22.98 | 8.47 | 20.3 | 19.2 | 71.74 |
| 3.5782 | 4.0 | 20444 | 3.9680 | 23.37 | 8.67 | 20.79 | 18.93 | 71.85 |
| 3.4509 | 5.0 | 25555 | 3.9643 | 23.42 | 8.85 | 20.71 | 19.33 | 71.88 |
| 3.3471 | 6.0 | 30666 | 3.9831 | 23.41 | 8.75 | 20.69 | 19.18 | 71.97 |
| 3.2673 | 7.0 | 35777 | 3.9917 | 23.93 | 9.13 | 21.16 | 19.0 | 72.11 |
| 3.214 | 8.0 | 40888 | 3.9990 | 23.94 | 9.1 | 21.21 | 19.13 | 72.11 |
### Framework versions
- Transformers 4.18.0
- Pytorch 1.10.0+cu111
- Datasets 2.1.0
- Tokenizers 0.12.1
|
BFMeriem/model | 337427975d539d95c1fd7ada5bcb7aea797745e8 | 2022-04-18T04:46:51.000Z | [
"pytorch",
"gpt2",
"text-generation",
"transformers",
"conversational"
] | conversational | false | BFMeriem | null | BFMeriem/model | 1 | null | transformers | 31,304 | ---
tags:
- conversational
---
#Michael Scott Chatbot |
huggingtweets/buckeshot-onlinepete | 806d8446dadb2aa262a0a6e42dc0256fa0518734 | 2022-04-18T07:25:28.000Z | [
"pytorch",
"gpt2",
"text-generation",
"en",
"transformers",
"huggingtweets"
] | text-generation | false | huggingtweets | null | huggingtweets/buckeshot-onlinepete | 1 | null | transformers | 31,305 | ---
language: en
thumbnail: https://github.com/borisdayma/huggingtweets/blob/master/img/logo.png?raw=true
tags:
- huggingtweets
widget:
- text: "My dream is"
---
<div class="inline-flex flex-col" style="line-height: 1.5;">
<div class="flex">
<div
style="display:inherit; margin-left: 4px; margin-right: 4px; width: 92px; height:92px; border-radius: 50%; background-size: cover; background-image: url('https://pbs.twimg.com/profile_images/456958582731603969/QZKpv6eI_400x400.jpeg')">
</div>
<div
style="display:inherit; margin-left: 4px; margin-right: 4px; width: 92px; height:92px; border-radius: 50%; background-size: cover; background-image: url('https://pbs.twimg.com/profile_images/1492494175849353223/nhm3MajO_400x400.jpg')">
</div>
<div
style="display:none; margin-left: 4px; margin-right: 4px; width: 92px; height:92px; border-radius: 50%; background-size: cover; background-image: url('')">
</div>
</div>
<div style="text-align: center; margin-top: 3px; font-size: 16px; font-weight: 800">🤖 AI CYBORG 🤖</div>
<div style="text-align: center; font-size: 16px; font-weight: 800">im pete online & BUCKSHOT</div>
<div style="text-align: center; font-size: 14px;">@buckeshot-onlinepete</div>
</div>
I was made with [huggingtweets](https://github.com/borisdayma/huggingtweets).
Create your own bot based on your favorite user with [the demo](https://colab.research.google.com/github/borisdayma/huggingtweets/blob/master/huggingtweets-demo.ipynb)!
## How does it work?
The model uses the following pipeline.
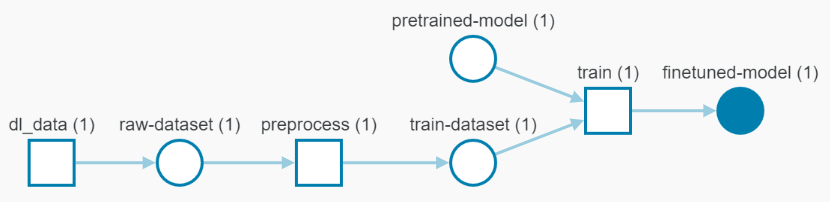
To understand how the model was developed, check the [W&B report](https://wandb.ai/wandb/huggingtweets/reports/HuggingTweets-Train-a-Model-to-Generate-Tweets--VmlldzoxMTY5MjI).
## Training data
The model was trained on tweets from im pete online & BUCKSHOT.
| Data | im pete online | BUCKSHOT |
| --- | --- | --- |
| Tweets downloaded | 3190 | 211 |
| Retweets | 94 | 52 |
| Short tweets | 1003 | 28 |
| Tweets kept | 2093 | 131 |
[Explore the data](https://wandb.ai/wandb/huggingtweets/runs/my5myk60/artifacts), which is tracked with [W&B artifacts](https://docs.wandb.com/artifacts) at every step of the pipeline.
## Training procedure
The model is based on a pre-trained [GPT-2](https://huggingface.co/gpt2) which is fine-tuned on @buckeshot-onlinepete's tweets.
Hyperparameters and metrics are recorded in the [W&B training run](https://wandb.ai/wandb/huggingtweets/runs/b9ea5prx) for full transparency and reproducibility.
At the end of training, [the final model](https://wandb.ai/wandb/huggingtweets/runs/b9ea5prx/artifacts) is logged and versioned.
## How to use
You can use this model directly with a pipeline for text generation:
```python
from transformers import pipeline
generator = pipeline('text-generation',
model='huggingtweets/buckeshot-onlinepete')
generator("My dream is", num_return_sequences=5)
```
## Limitations and bias
The model suffers from [the same limitations and bias as GPT-2](https://huggingface.co/gpt2#limitations-and-bias).
In addition, the data present in the user's tweets further affects the text generated by the model.
## About
*Built by Boris Dayma*
[](https://twitter.com/intent/follow?screen_name=borisdayma)
For more details, visit the project repository.
[](https://github.com/borisdayma/huggingtweets)
|
PrajwalS/wav2vec2-large-960h-lv60-self-timit-fine-tuned | 9d11d10da6b1bbf0ea19bbb8df1cc385209ed8c2 | 2022-04-21T07:17:16.000Z | [
"pytorch",
"wav2vec2",
"automatic-speech-recognition",
"transformers"
] | automatic-speech-recognition | false | PrajwalS | null | PrajwalS/wav2vec2-large-960h-lv60-self-timit-fine-tuned | 1 | null | transformers | 31,306 | Entry not found |
rmihaylov/roberta-base-use-qa-bg | d141e8bbfce0d35906764659cd559659e92e9f44 | 2022-04-18T09:10:52.000Z | [
"pytorch",
"xlm-roberta",
"feature-extraction",
"bg",
"dataset:oscar",
"dataset:chitanka",
"dataset:wikipedia",
"arxiv:2004.09813",
"transformers",
"torch",
"license:mit",
"sentence-similarity"
] | sentence-similarity | false | rmihaylov | null | rmihaylov/roberta-base-use-qa-bg | 1 | null | transformers | 31,307 | ---
inference: false
pipeline_tag: sentence-similarity
language:
- bg
license: mit
datasets:
- oscar
- chitanka
- wikipedia
tags:
- torch
---
# ROBERTA BASE (cased) trained on private Bulgarian-English parallel data
This is a Multilingual Roberta model. It could be used for creating embeddings of Bulgarian sentences.
Using the ideas from [Sentence-BERT](https://arxiv.org/abs/2004.09813), the training is based on the idea that a translated sentence should be mapped to the same location in the vector space as the original sentence.
The teacher model is the [USE model by Google](https://aclanthology.org/D18-2029/).
This model is cased: it does make a difference between bulgarian and Bulgarian.
It was trained on private Bulgarian-English parallel data.
### How to use
Here is how to use this model in PyTorch:
```python
>>> import scipy
>>> import torch
>>> from transformers import AutoModel, AutoTokenizer
>>>
>>> model = AutoModel.from_pretrained('rmihaylov/roberta-base-use-qa-bg')
>>> tokenizer = AutoTokenizer.from_pretrained('rmihaylov/roberta-base-use-qa-bg')
>>>
>>> query = "Какви са съставките на бисквитките?"
>>>
>>> answers = [
>>> "Бисквитката е печена или варена храна, която обикновено е малка, плоска и сладка.",
>>> "Бисквитките обикновено съдържат брашно, захар и някакъв вид масло или мазнини. Те могат да включват други съставки като стафиди, овес, шоколадов чипс, ядки и др.",
>>> "В повечето англоговорящи страни, с изключение на САЩ и Канада, хрупкавите бисквитки се наричат бисквити.",
>>> "Бисквитите Chewier понякога се наричат бисквитки дори в Обединеното кралство. Някои бисквитки могат също да бъдат назовавани според формата им, като квадратчета с дата или барове.",
>>> "Бисквитките или бисквитите могат да се произвеждат масово във фабрики, направени в малки пекарни или домашно приготвени.",
>>> "Вариантите за бисквити или бисквити включват сандвич бисквити, като крем крем, Jammie Dodgers, Bourbons и Oreos, с пълнеж от ружа или конфитюр и понякога потопени в шоколад или друго сладко покритие.",
>>> "Бисквитките често се сервират с напитки като мляко, кафе или чай.",
>>> "Фабричните бисквитки се продават в магазини за хранителни стоки, магазини за удобство и автомати.",
>>> "Американската употреба произлиза от холандското koekje „малка торта“, което е умалително от „koek“ („торта“), което произлиза от средно холандската дума „koke“.",
>>> "Cookie Monster е Muppet в дългогодишното детско телевизионно шоу Sesame Street, който е най-известен с ненаситния си апетит към бисквитките и известните си фрази за ядене, като „Me want cookie!“, „Me eat cookie!“ (или просто „COOKIE!“) и „Om nom nom nom“ (казано през уста, пълна с храна).",
>>> "Домашните бисквитки обикновено се правят от тесто, оформено на малки топчета и пуснато върху лист с бисквитки. След това се пекат във фурна за 5 до 15 минути, в зависимост от рецептата. Температурата на фурната варира от 250 до 350 градуса.",
>>> "Повечето бисквитки със среден размер, ако са направени със захар, брашно и скъсяване, ще съдържат между 100 и 200 калории.",
>>> ]
>>>
>>> query_embedding = model.question(**tokenizer.encode_plus(query, return_tensors='pt')).detach().numpy()[0]
>>>
>>> corpus, corpus_embeddings = [], []
>>> for answer in answers:
>>> value_inputs = tokenizer.encode_plus(answer, answer, return_tensors='pt')
>>> embedding = model.answer(**value_inputs).detach().numpy()[0]
>>> corpus.append(answer)
>>> corpus_embeddings.append(embedding)
>>>
>>> distances = scipy.spatial.distance.cdist([query_embedding], corpus_embeddings, "cosine")[0]
>>>
>>> results = zip(range(len(distances)), distances)
>>> results = sorted(results, key=lambda x: x[1])
>>>
>>> print([[corpus[idx].strip(), (1.0 - distance)] for idx, distance in results])
[['Бисквитките обикновено съдържат брашно, захар и някакъв вид масло или мазнини. Те могат да включват други съставки като стафиди, овес, шоколадов чипс, ядки и др.',
0.620301064877746],
['Бисквитката е печена или варена храна, която обикновено е малка, плоска и сладка.',
0.5696434424179133],
['Повечето бисквитки със среден размер, ако са направени със захар, брашно и скъсяване, ще съдържат между 100 и 200 калории.',
0.5496458499598336],
['Бисквитките или бисквитите могат да се произвеждат масово във фабрики, направени в малки пекарни или домашно приготвени.',
0.5365738121336622],
['Бисквитите Chewier понякога се наричат \u200b\u200bбисквитки дори в Обединеното кралство. Някои бисквитки могат също да бъдат назовавани според формата им, като квадратчета с дата или барове.',
0.5278547550921155],
['Вариантите за бисквити или бисквити включват сандвич бисквити, като крем крем, Jammie Dodgers, Bourbons и Oreos, с пълнеж от ружа или конфитюр и понякога потопени в шоколад или друго сладко покритие.',
0.5231947553588652],
['Фабричните бисквитки се продават в магазини за хранителни стоки, магазини за удобство и автомати.',
0.5222493948012543],
['В повечето англоговорящи страни, с изключение на САЩ и Канада, хрупкавите бисквитки се наричат \u200b\u200bбисквити.',
0.5185776999549867],
['Домашните бисквитки обикновено се правят от тесто, оформено на малки топчета и пуснато върху лист с бисквитки. След това се пекат във фурна за 5 до 15 минути, в зависимост от рецептата. Температурата на фурната варира от 250 до 350 градуса.',
0.5113299248563532],
['Cookie Monster е Muppet в дългогодишното детско телевизионно шоу Sesame Street, който е най-известен с ненаситния си апетит към бисквитките и известните си фрази за ядене, като „Me want cookie!“, „Me eat cookie!“ (или просто „COOKIE!“) и „Om nom nom nom“ (казано през уста, пълна с храна).',
0.4642001162793412],
['Бисквитките често се сервират с напитки като мляко, кафе или чай.',
0.44902199326988135],
['Американската употреба произлиза от холандското koekje „малка торта“, което е умалително от „koek“ („торта“), което произлиза от средно холандската дума „koke“.',
0.25256183690274214]]
```
|
orendar/en_he_roberta_shared | 4b32ac5aa9f567c3dbab8a44441044a3c0c704af | 2022-04-18T12:58:23.000Z | [
"pytorch",
"encoder-decoder",
"text2text-generation",
"transformers",
"autotrain_compatible"
] | text2text-generation | false | orendar | null | orendar/en_he_roberta_shared | 1 | null | transformers | 31,308 | Entry not found |
4m1g0/wav2vec2-large-xls-r-300m-gl-jupyter3 | 14a99f176151d42535d8f315bf5359be495765f5 | 2022-04-18T12:10:03.000Z | [
"pytorch",
"tensorboard",
"wav2vec2",
"automatic-speech-recognition",
"transformers"
] | automatic-speech-recognition | false | 4m1g0 | null | 4m1g0/wav2vec2-large-xls-r-300m-gl-jupyter3 | 1 | null | transformers | 31,309 | Entry not found |
npleshkanov/dannysmirnov_toxicity_model | 1b284645f954e98f713457117a943b735e06581d | 2022-04-18T12:54:20.000Z | [
"pytorch",
"bert",
"transformers"
] | null | false | npleshkanov | null | npleshkanov/dannysmirnov_toxicity_model | 1 | null | transformers | 31,310 | Entry not found |
ucabqfe/bigBird_AAE_bio | a5dde8c43f5122e0ebdc66cbb59a1986e753468d | 2022-04-18T15:32:25.000Z | [
"pytorch",
"big_bird",
"token-classification",
"transformers",
"autotrain_compatible"
] | token-classification | false | ucabqfe | null | ucabqfe/bigBird_AAE_bio | 1 | null | transformers | 31,311 | Entry not found |
Tianle/bert-base-uncased-finetuned-squad | 1b8b1c2456b7270049c5517b0fa89c54a0607e9a | 2022-04-18T20:25:14.000Z | [
"pytorch",
"tensorboard",
"bert",
"question-answering",
"dataset:squad",
"transformers",
"generated_from_trainer",
"license:apache-2.0",
"model-index",
"autotrain_compatible"
] | question-answering | false | Tianle | null | Tianle/bert-base-uncased-finetuned-squad | 1 | null | transformers | 31,312 | ---
license: apache-2.0
tags:
- generated_from_trainer
datasets:
- squad
model-index:
- name: bert-base-uncased-finetuned-squad
results: []
---
<!-- This model card has been generated automatically according to the information the Trainer had access to. You
should probably proofread and complete it, then remove this comment. -->
# bert-base-uncased-finetuned-squad
This model is a fine-tuned version of [bert-base-uncased](https://huggingface.co/bert-base-uncased) on the squad dataset.
It achieves the following results on the evaluation set:
- Loss: 1.1006
## Model description
More information needed
## Intended uses & limitations
More information needed
## Training and evaluation data
More information needed
## Training procedure
### Training hyperparameters
The following hyperparameters were used during training:
- learning_rate: 2e-05
- train_batch_size: 16
- eval_batch_size: 16
- seed: 42
- optimizer: Adam with betas=(0.9,0.999) and epsilon=1e-08
- lr_scheduler_type: linear
- num_epochs: 1
### Training results
| Training Loss | Epoch | Step | Validation Loss |
|:-------------:|:-----:|:----:|:---------------:|
| 1.0275 | 1.0 | 5533 | 1.1006 |
### Framework versions
- Transformers 4.18.0
- Pytorch 1.10.0+cu111
- Datasets 2.1.0
- Tokenizers 0.12.1
|
StringCheese/Dialog-small-bigbang | 1a38cef9485f666d45ff44f1745209dde5434c8b | 2022-04-18T17:59:21.000Z | [
"pytorch",
"gpt2",
"text-generation",
"transformers",
"conversational"
] | conversational | false | StringCheese | null | StringCheese/Dialog-small-bigbang | 1 | null | transformers | 31,313 | ---
tags:
- conversational
---
# Big Bang Theory Dialog Model |
ucabqfe/bigBird_AAE_bieo | 6721406fb275217d976fe8dc60782045e6a6c4c2 | 2022-04-18T18:09:50.000Z | [
"pytorch",
"big_bird",
"token-classification",
"transformers",
"autotrain_compatible"
] | token-classification | false | ucabqfe | null | ucabqfe/bigBird_AAE_bieo | 1 | null | transformers | 31,314 | Entry not found |
ucabqfe/bigBird_AAE_io | bbb7a271f992583d2e89ea76954a09409937a394 | 2022-04-18T18:11:18.000Z | [
"pytorch",
"big_bird",
"token-classification",
"transformers",
"autotrain_compatible"
] | token-classification | false | ucabqfe | null | ucabqfe/bigBird_AAE_io | 1 | null | transformers | 31,315 | Entry not found |
ucabqfe/bigBird_PER_bieo | 2d7c2b7ccf945de57394dac10bf1589e12bf2fb8 | 2022-04-18T18:16:30.000Z | [
"pytorch",
"big_bird",
"token-classification",
"transformers",
"autotrain_compatible"
] | token-classification | false | ucabqfe | null | ucabqfe/bigBird_PER_bieo | 1 | null | transformers | 31,316 | Entry not found |
zoha/wav2vec2-base-common-voice-fa-demo-colab | 399f053bb802d6413c5af61d0350ac4912263c4d | 2022-04-29T21:09:20.000Z | [
"pytorch",
"tensorboard",
"wav2vec2",
"automatic-speech-recognition",
"transformers",
"generated_from_trainer",
"license:apache-2.0",
"model-index"
] | automatic-speech-recognition | false | zoha | null | zoha/wav2vec2-base-common-voice-fa-demo-colab | 1 | null | transformers | 31,317 | ---
license: apache-2.0
tags:
- generated_from_trainer
model-index:
- name: wav2vec2-base-common-voice-fa-demo-colab
results: []
---
<!-- This model card has been generated automatically according to the information the Trainer had access to. You
should probably proofread and complete it, then remove this comment. -->
# wav2vec2-base-common-voice-fa-demo-colab
This model is a fine-tuned version of [facebook/wav2vec2-base](https://huggingface.co/facebook/wav2vec2-base) on the None dataset.
It achieves the following results on the evaluation set:
- Loss: 3.0558
- Wer: 1.0
## Model description
More information needed
## Intended uses & limitations
More information needed
## Training and evaluation data
More information needed
## Training procedure
### Training hyperparameters
The following hyperparameters were used during training:
- learning_rate: 0.0001
- train_batch_size: 16
- eval_batch_size: 8
- seed: 42
- optimizer: Adam with betas=(0.9,0.999) and epsilon=1e-08
- lr_scheduler_type: linear
- lr_scheduler_warmup_steps: 1000
- num_epochs: 3
- mixed_precision_training: Native AMP
### Training results
| Training Loss | Epoch | Step | Validation Loss | Wer |
|:-------------:|:-----:|:----:|:---------------:|:---:|
| 5.1626 | 0.3 | 100 | 4.0692 | 1.0 |
| 5.1776 | 0.6 | 200 | 3.6640 | 1.0 |
| 3.6628 | 0.9 | 300 | 3.3832 | 1.0 |
| 3.2022 | 1.2 | 400 | 3.3492 | 1.0 |
| 3.1714 | 1.5 | 500 | 3.3215 | 1.0 |
| 3.0689 | 1.8 | 600 | 3.0806 | 1.0 |
| 3.1478 | 2.1 | 700 | 3.0624 | 1.0 |
| 3.1818 | 2.4 | 800 | 3.0777 | 1.0 |
| 3.159 | 2.7 | 900 | 3.0558 | 1.0 |
### Framework versions
- Transformers 4.11.3
- Pytorch 1.10.0+cu113
- Datasets 1.18.3
- Tokenizers 0.10.3
|
TJKlein/distilbert-base-uncased-finetuned-ner | 647ec4a158c4d2745ad4df4da6d76cd91687c8a6 | 2022-04-18T23:32:29.000Z | [
"pytorch",
"tensorboard",
"distilbert",
"token-classification",
"transformers",
"autotrain_compatible"
] | token-classification | false | TJKlein | null | TJKlein/distilbert-base-uncased-finetuned-ner | 1 | null | transformers | 31,318 | Entry not found |
samwell/marian-finetuned-kde4-en-to-fr | d752c764a2adc4f77d44488cdf2550f8bc9d2448 | 2022-04-18T23:53:11.000Z | [
"pytorch",
"tensorboard",
"marian",
"text2text-generation",
"transformers",
"translation",
"generated_from_trainer",
"license:apache-2.0",
"model-index",
"autotrain_compatible"
] | translation | false | samwell | null | samwell/marian-finetuned-kde4-en-to-fr | 1 | null | transformers | 31,319 | ---
license: apache-2.0
tags:
- translation
- generated_from_trainer
metrics:
- bleu
model-index:
- name: marian-finetuned-kde4-en-to-fr
results: []
---
<!-- This model card has been generated automatically according to the information the Trainer had access to. You
should probably proofread and complete it, then remove this comment. -->
# marian-finetuned-kde4-en-to-fr
This model is a fine-tuned version of [Helsinki-NLP/opus-mt-en-fr](https://huggingface.co/Helsinki-NLP/opus-mt-en-fr) on an unknown dataset.
It achieves the following results on the evaluation set:
- Loss: 2.2663
- Bleu: 0.0
## Model description
More information needed
## Intended uses & limitations
More information needed
## Training and evaluation data
More information needed
## Training procedure
### Training hyperparameters
The following hyperparameters were used during training:
- learning_rate: 2e-05
- train_batch_size: 32
- eval_batch_size: 64
- seed: 42
- optimizer: Adam with betas=(0.9,0.999) and epsilon=1e-08
- lr_scheduler_type: linear
- num_epochs: 3
### Training results
### Framework versions
- Transformers 4.18.0
- Pytorch 1.10.0+cu111
- Datasets 2.1.0
- Tokenizers 0.12.1
|
KevinChoi/bert-finetuned-squad | 74b32411460f85ad338f740b5e3dd4a987e800be | 2022-04-19T09:27:21.000Z | [
"pytorch",
"bert",
"question-answering",
"transformers",
"autotrain_compatible"
] | question-answering | false | KevinChoi | null | KevinChoi/bert-finetuned-squad | 1 | null | transformers | 31,320 | Entry not found |
KevinChoi/bert-finetuned-squad-accelerate | f356e0224a4546bc42e3521e2e5e5015b74b288f | 2022-04-19T13:05:47.000Z | [
"pytorch",
"bert",
"question-answering",
"transformers",
"autotrain_compatible"
] | question-answering | false | KevinChoi | null | KevinChoi/bert-finetuned-squad-accelerate | 1 | null | transformers | 31,321 | Entry not found |
PSW/max_sim_del | fb4ed4960def1b150d503daf54f6c66bca7846ef | 2022-04-19T12:27:14.000Z | [
"pytorch",
"bart",
"text2text-generation",
"transformers",
"autotrain_compatible"
] | text2text-generation | false | PSW | null | PSW/max_sim_del | 1 | null | transformers | 31,322 | Entry not found |
rmihaylov/pegasus-base-qag-bg | 04c853ec47297b4a62504caa267c7160713ddea6 | 2022-04-19T14:54:31.000Z | [
"pytorch",
"pegasus",
"text2text-generation",
"bg",
"dataset:oscar",
"dataset:chitanka",
"dataset:wikipedia",
"arxiv:1912.08777",
"transformers",
"torch",
"license:mit",
"autotrain_compatible"
] | text2text-generation | false | rmihaylov | null | rmihaylov/pegasus-base-qag-bg | 1 | null | transformers | 31,323 | ---
inference: false
language:
- bg
license: mit
datasets:
- oscar
- chitanka
- wikipedia
tags:
- torch
---
# PEGASUS BASE
This model was pretrained on Bulgarian language. It was intorduced in [this paper](https://arxiv.org/pdf/1912.08777.pdf).
## Model description
The training data is private Bulgarian squad data.
## Intended uses & limitations
You can use the raw model for generation of question-answer pairs related with given Bulgarian text.
### How to use
Here is how to use this model in PyTorch:
```python
>>> from transformers import PegasusForConditionalGeneration, AlbertTokenizer
>>>
>>> model_id = "rmihaylov/pegasus-base-qag-bg"
>>> model = PegasusForConditionalGeneration.from_pretrained(model_id)
>>> tokenizer = AlbertTokenizer.from_pretrained(model_id)
>>>
>>> text = """Това, че някой може да заяви на най-силен глас исканията си, не означава те да бъдат удовлетворени, заяви Костадин Ангелов.
Той допълни, че приоритетите на властите са здравето, образование и спорта, давайки знак, че се търси разхлабване на мерките в болничните заведения, връщането на учениците в класните стаи и отварянето на обектите за масов спорт.
"""
>>>
>>> inputs = tokenizer.encode_plus(
>>> text,
>>> return_tensors='pt',
>>> truncation=True,
>>> max_length=512,
>>> return_token_type_ids=False,
>>> return_attention_mask=True)
>>>
>>> outputs = model.generate(**inputs,
>>> max_length=150,
>>> top_p=0.95,
>>> top_k=20,
>>> do_sample=True,
>>> num_return_sequences=10,
>>> num_beams=1,
>>> eos_token_id=50259,
>>> decoder_start_token_id=50257,
>>> return_dict_in_generate=True,
>>> output_scores=True)
>>>
>>> for g in outputs.sequences:
>>> text_gen = tokenizer.decode(g, skip_special_tokens=False)
>>>
>>> if ('[SEP]' not in text_gen) or ('[MASK]' not in text_gen) or ('[CLS]' not in text_gen):
>>> continue
>>>
>>> question, answer = text_gen.replace('[CLS]', '').strip().split('[SEP]')
>>> answer = answer.split('[MASK]')[0].strip()
>>>
>>> if (not answer) or (answer not in text) or (len(answer) <= 1):
>>> continue
>>>
>>> print(f'{question.strip()}\n{answer.strip()}', '\n\n')
Какво трябва да се предприеме, за да се случи?
разхлабване
Какви са приоритетите на управляващите?
здравето, образование и спорта,
Какви усилия има правителството за стимулиране на раждаемостта?
разхлабване на мерките
Какъв е основният проблем, който може да реши?
образование
```
|
PSW/min_sim_del | d00227f9c376e5986ea008ecbe6be72df5bf6296 | 2022-04-19T13:18:08.000Z | [
"pytorch",
"bart",
"text2text-generation",
"transformers",
"autotrain_compatible"
] | text2text-generation | false | PSW | null | PSW/min_sim_del | 1 | null | transformers | 31,324 | Entry not found |
jamie613/xlm-roberta-base-finetuned-panx-de | 485545e8ecc330c743750418467b2a433c02e8a8 | 2022-05-13T07:27:12.000Z | [
"pytorch",
"tensorboard",
"xlm-roberta",
"token-classification",
"dataset:xtreme",
"transformers",
"generated_from_trainer",
"license:mit",
"model-index",
"autotrain_compatible"
] | token-classification | false | jamie613 | null | jamie613/xlm-roberta-base-finetuned-panx-de | 1 | null | transformers | 31,325 | ---
license: mit
tags:
- generated_from_trainer
datasets:
- xtreme
metrics:
- f1
model-index:
- name: xlm-roberta-base-finetuned-panx-de
results:
- task:
name: Token Classification
type: token-classification
dataset:
name: xtreme
type: xtreme
args: PAN-X.de
metrics:
- name: F1
type: f1
value: 0.8654425558524246
---
<!-- This model card has been generated automatically according to the information the Trainer had access to. You
should probably proofread and complete it, then remove this comment. -->
# xlm-roberta-base-finetuned-panx-de
This model is a fine-tuned version of [xlm-roberta-base](https://huggingface.co/xlm-roberta-base) on the xtreme dataset.
It achieves the following results on the evaluation set:
- Loss: 0.1334
- F1: 0.8654
## Model description
More information needed
## Intended uses & limitations
More information needed
## Training and evaluation data
More information needed
## Training procedure
### Training hyperparameters
The following hyperparameters were used during training:
- learning_rate: 5e-05
- train_batch_size: 24
- eval_batch_size: 24
- seed: 42
- optimizer: Adam with betas=(0.9,0.999) and epsilon=1e-08
- lr_scheduler_type: linear
- num_epochs: 3
### Training results
| Training Loss | Epoch | Step | Validation Loss | F1 |
|:-------------:|:-----:|:----:|:---------------:|:------:|
| 0.2541 | 1.0 | 525 | 0.1596 | 0.8242 |
| 0.1284 | 2.0 | 1050 | 0.1360 | 0.8499 |
| 0.0827 | 3.0 | 1575 | 0.1334 | 0.8654 |
### Framework versions
- Transformers 4.11.3
- Pytorch 1.11.0+cu113
- Datasets 1.16.1
- Tokenizers 0.10.3
|
PBusienei/Nashville_Analytics_Summit_conference_helper | b6b7060dc673b117920eac21a6be1d94832f4119 | 2022-04-19T13:58:57.000Z | [
"pytorch",
"bert",
"feature-extraction",
"sentence-transformers",
"sentence-similarity"
] | sentence-similarity | false | PBusienei | null | PBusienei/Nashville_Analytics_Summit_conference_helper | 1 | null | sentence-transformers | 31,326 | ---
pipeline_tag: sentence-similarity
tags:
- sentence-transformers
- feature-extraction
- sentence-similarity
---
# Conference Helper
This is a [sentence-transformers](https://www.SBERT.net) model: It maps sentences & paragraphs to a 384 dimensional dense vector space and was designed for **semantic search**. It has been trained on 215M (question, answer) pairs from diverse sources.
## Usage (Sentence-Transformers)
The usage of this model is easy when you have [sentence-transformers](https://www.SBERT.net) installed:
```
pip install -U sentence-transformers
```
Thus the model can be used as:
```python
from sentence_transformers import SentenceTransformer, util
query = "Health Analytics?"
docs = ["The output is 3 top most similar sessions from the summit"]
#Load the model
model = SentenceTransformer('sentence-transformers/multi-qa-MiniLM-L6-cos-v1')
#Encode query and documents
query_emb = model.encode(query)
doc_emb = model.encode(docs)
#Compute dot score between query and all document embeddings
scores = util.dot_score(query_emb, doc_emb)[0].cpu().tolist()
#Combine docs & scores
doc_score_pairs = list(zip(docs, scores))
#Sort by decreasing score
doc_score_pairs = sorted(doc_score_pairs, key=lambda x: x[1], reverse=True)
#Output passages & scores
for doc, score in doc_score_pairs:
print(score, doc)
```
## Usage (HuggingFace Transformers)
Without [sentence-transformers](https://www.SBERT.net), you can take the following steps:
1. Pass input through the transformer model,
2. Apply the correct pooling-operation on-top of the contextualized word embeddings.
```python
from transformers import AutoTokenizer, AutoModel
import torch
import torch.nn.functional as F
#Mean Pooling - Take average of all tokens
def mean_pooling(model_output, attention_mask):
token_embeddings = model_output.last_hidden_state #The first element of model_output containing all token embeddings
input_mask_expanded = attention_mask.unsqueeze(-1).expand(token_embeddings.size()).float()
return torch.sum(token_embeddings * input_mask_expanded, 1) / torch.clamp(input_mask_expanded.sum(1), min=1e-9)
#Encode text
def encode(texts):
# Tokenize sentences
encoded_input = tokenizer(texts, padding=True, truncation=True, return_tensors='pt')
# Compute token embeddings
with torch.no_grad():
model_output = model(**encoded_input, return_dict=True)
# Perform pooling
embeddings = mean_pooling(model_output, encoded_input['attention_mask'])
# Normalize embeddings
embeddings = F.normalize(embeddings, p=2, dim=1)
return embeddings
# Sentences we want sentence embeddings for
query = "Health Analytics?"
docs = ["The output is 3 top most similar sessions from the summit"]
# Load model from HuggingFace Hub
tokenizer = AutoTokenizer.from_pretrained("sentence-transformers/multi-qa-MiniLM-L6-cos-v1")
model = AutoModel.from_pretrained("sentence-transformers/multi-qa-MiniLM-L6-cos-v1")
#Encode query and docs
query_emb = encode(query)
doc_emb = encode(docs)
#Compute dot score between query and all document embeddings
scores = torch.mm(query_emb, doc_emb.transpose(0, 1))[0].cpu().tolist()
#Combine docs & scores
doc_score_pairs = list(zip(docs, scores))
#Sort by decreasing score
doc_score_pairs = sorted(doc_score_pairs, key=lambda x: x[1], reverse=True)
#Output passages & scores
for doc, score in doc_score_pairs:
print(score, doc)
```
## Technical Details
In the following some technical details how this model must be used:
| Setting | Value |
| --- | :---: |
| Dimensions | 384 |
| Produces normalized embeddings | Yes |
| Pooling-Method | Mean pooling |
| Suitable score functions | dot-product (`util.dot_score`), cosine-similarity (`util.cos_sim`), or euclidean distance |
Note: When loaded with `sentence-transformers`, this model produces normalized embeddings with length 1. In that case, dot-product and cosine-similarity are equivalent. dot-product is preferred as it is faster. Euclidean distance is proportional to dot-product and can also be used.
----
## Background
The project aims to train sentence embedding models on very large sentence level datasets using a self-supervised
contrastive learning objective. We use a contrastive learning objective: given a sentence from the pair, the model should predict which out of a set of randomly sampled other sentences, was actually paired with it in our dataset.
## Intended uses
The model is intended to be used for semantic search at Nashville Analytics Summit: It encodes queries / questions and text paragraphs in a dense vector space. It finds relevant documents for the given passages.
Note that there is a limit of 512 word pieces: Text longer than that will be truncated. Further note that the model was just trained on input text up to 250 word pieces. It might not work well for longer text.
## Training procedure
The full training script is accessible in: `train_script.py`.
### Pre-training
The pretrained [`nreimers/MiniLM-L6-H384-uncased`](https://huggingface.co/nreimers/MiniLM-L6-H384-uncased) model.
#### Training
We use the concatenation from multiple datasets to fine-tune our model. In total we have about 215M (question, answer) pairs.
We sampled each dataset given a weighted probability which configuration is detailed in the `data_config.json` file.
The model was trained with [MultipleNegativesRankingLoss](https://www.sbert.net/docs/package_reference/losses.html#multiplenegativesrankingloss) using Mean-pooling, cosine-similarity as similarity function, and a scale of 20.
|
tartuNLP/est-roberta-hist-ner | 7cfffaf8114f34025ffd4e8b4dc143c2a66098ce | 2022-06-29T08:48:58.000Z | [
"pytorch",
"camembert",
"token-classification",
"et",
"transformers",
"license:cc-by-sa-4.0",
"autotrain_compatible"
] | token-classification | false | tartuNLP | null | tartuNLP/est-roberta-hist-ner | 1 | null | transformers | 31,327 | ---
language: et
license: cc-by-sa-4.0
inference: false
---
# est-roberta-hist-ner
## Model description
est-roberta-hist-ner is an [Est-RoBERTa](https://huggingface.co/EMBEDDIA/est-roberta) based model fine-tuned for named entity recognition in Estonian 19th century parish court records (for details, see [this repository](https://github.com/soras/vk_ner_lrec_2022)).
The following types of entities are recognized: person names (PER), ambiguous locations-organizations (LOC_ORG), locations (LOC), organizations (ORG) and MISC (miscellaneous names).
## How to use
Recommended usage of the model is with approriate pre- and postprocessing by EstNLTK.
For an usage example, see this tutorial: [https://github.com/soras/vk\_ner\_lrec\_2022/blob/main/using\_bert\_ner\_tagger.ipynb](https://github.com/soras/vk_ner_lrec_2022/blob/main/using_bert_ner_tagger.ipynb)
## Citation
If you use this model in your work, please cite us as follows:
@InProceedings{orasmaa-EtAl:2022:LREC,
author = {Orasmaa, Siim and Muischnek, Kadri and Poska, Kristjan and Edela, Anna},
title = {Named Entity Recognition in Estonian 19th Century Parish Court Records},
booktitle = {Proceedings of the Language Resources and Evaluation Conference},
month = {June},
year = {2022},
address = {Marseille, France},
publisher = {European Language Resources Association},
pages = {5304--5313},
url = {https://aclanthology.org/2022.lrec-1.568}
}
|
PSW/min_sim_del_seed1 | 3c88c1269c1c0e60a2af1b1a5167938303d933eb | 2022-04-19T14:15:07.000Z | [
"pytorch",
"bart",
"text2text-generation",
"transformers",
"autotrain_compatible"
] | text2text-generation | false | PSW | null | PSW/min_sim_del_seed1 | 1 | null | transformers | 31,328 | Entry not found |
GPL/newsqa-msmarco-distilbert-gpl | 9322db85ca404ea49623675d4b50ba832fdbf0a0 | 2022-04-19T15:14:23.000Z | [
"pytorch",
"distilbert",
"feature-extraction",
"sentence-transformers",
"sentence-similarity",
"transformers"
] | sentence-similarity | false | GPL | null | GPL/newsqa-msmarco-distilbert-gpl | 1 | null | sentence-transformers | 31,329 | ---
pipeline_tag: sentence-similarity
tags:
- sentence-transformers
- feature-extraction
- sentence-similarity
- transformers
---
# {MODEL_NAME}
This is a [sentence-transformers](https://www.SBERT.net) model: It maps sentences & paragraphs to a 768 dimensional dense vector space and can be used for tasks like clustering or semantic search.
<!--- Describe your model here -->
## Usage (Sentence-Transformers)
Using this model becomes easy when you have [sentence-transformers](https://www.SBERT.net) installed:
```
pip install -U sentence-transformers
```
Then you can use the model like this:
```python
from sentence_transformers import SentenceTransformer
sentences = ["This is an example sentence", "Each sentence is converted"]
model = SentenceTransformer('{MODEL_NAME}')
embeddings = model.encode(sentences)
print(embeddings)
```
## Usage (HuggingFace Transformers)
Without [sentence-transformers](https://www.SBERT.net), you can use the model like this: First, you pass your input through the transformer model, then you have to apply the right pooling-operation on-top of the contextualized word embeddings.
```python
from transformers import AutoTokenizer, AutoModel
import torch
#Mean Pooling - Take attention mask into account for correct averaging
def mean_pooling(model_output, attention_mask):
token_embeddings = model_output[0] #First element of model_output contains all token embeddings
input_mask_expanded = attention_mask.unsqueeze(-1).expand(token_embeddings.size()).float()
return torch.sum(token_embeddings * input_mask_expanded, 1) / torch.clamp(input_mask_expanded.sum(1), min=1e-9)
# Sentences we want sentence embeddings for
sentences = ['This is an example sentence', 'Each sentence is converted']
# Load model from HuggingFace Hub
tokenizer = AutoTokenizer.from_pretrained('{MODEL_NAME}')
model = AutoModel.from_pretrained('{MODEL_NAME}')
# Tokenize sentences
encoded_input = tokenizer(sentences, padding=True, truncation=True, return_tensors='pt')
# Compute token embeddings
with torch.no_grad():
model_output = model(**encoded_input)
# Perform pooling. In this case, mean pooling.
sentence_embeddings = mean_pooling(model_output, encoded_input['attention_mask'])
print("Sentence embeddings:")
print(sentence_embeddings)
```
## Evaluation Results
<!--- Describe how your model was evaluated -->
For an automated evaluation of this model, see the *Sentence Embeddings Benchmark*: [https://seb.sbert.net](https://seb.sbert.net?model_name={MODEL_NAME})
## Training
The model was trained with the parameters:
**DataLoader**:
`torch.utils.data.dataloader.DataLoader` of length 140000 with parameters:
```
{'batch_size': 32, 'sampler': 'torch.utils.data.sampler.SequentialSampler', 'batch_sampler': 'torch.utils.data.sampler.BatchSampler'}
```
**Loss**:
`gpl.toolkit.loss.MarginDistillationLoss`
Parameters of the fit()-Method:
```
{
"epochs": 1,
"evaluation_steps": 0,
"evaluator": "NoneType",
"max_grad_norm": 1,
"optimizer_class": "<class 'transformers.optimization.AdamW'>",
"optimizer_params": {
"lr": 2e-05
},
"scheduler": "WarmupLinear",
"steps_per_epoch": 140000,
"warmup_steps": 1000,
"weight_decay": 0.01
}
```
## Full Model Architecture
```
SentenceTransformer(
(0): Transformer({'max_seq_length': 350, 'do_lower_case': False}) with Transformer model: DistilBertModel
(1): Pooling({'word_embedding_dimension': 768, 'pooling_mode_cls_token': False, 'pooling_mode_mean_tokens': True, 'pooling_mode_max_tokens': False, 'pooling_mode_mean_sqrt_len_tokens': False})
)
```
## Citing & Authors
<!--- Describe where people can find more information --> |
GPL/nq-msmarco-distilbert-gpl | 50f21a6219c7565c1323b7ef1d95f084b7761ae7 | 2022-04-19T15:15:00.000Z | [
"pytorch",
"distilbert",
"feature-extraction",
"sentence-transformers",
"sentence-similarity",
"transformers"
] | sentence-similarity | false | GPL | null | GPL/nq-msmarco-distilbert-gpl | 1 | null | sentence-transformers | 31,330 | ---
pipeline_tag: sentence-similarity
tags:
- sentence-transformers
- feature-extraction
- sentence-similarity
- transformers
---
# {MODEL_NAME}
This is a [sentence-transformers](https://www.SBERT.net) model: It maps sentences & paragraphs to a 768 dimensional dense vector space and can be used for tasks like clustering or semantic search.
<!--- Describe your model here -->
## Usage (Sentence-Transformers)
Using this model becomes easy when you have [sentence-transformers](https://www.SBERT.net) installed:
```
pip install -U sentence-transformers
```
Then you can use the model like this:
```python
from sentence_transformers import SentenceTransformer
sentences = ["This is an example sentence", "Each sentence is converted"]
model = SentenceTransformer('{MODEL_NAME}')
embeddings = model.encode(sentences)
print(embeddings)
```
## Usage (HuggingFace Transformers)
Without [sentence-transformers](https://www.SBERT.net), you can use the model like this: First, you pass your input through the transformer model, then you have to apply the right pooling-operation on-top of the contextualized word embeddings.
```python
from transformers import AutoTokenizer, AutoModel
import torch
#Mean Pooling - Take attention mask into account for correct averaging
def mean_pooling(model_output, attention_mask):
token_embeddings = model_output[0] #First element of model_output contains all token embeddings
input_mask_expanded = attention_mask.unsqueeze(-1).expand(token_embeddings.size()).float()
return torch.sum(token_embeddings * input_mask_expanded, 1) / torch.clamp(input_mask_expanded.sum(1), min=1e-9)
# Sentences we want sentence embeddings for
sentences = ['This is an example sentence', 'Each sentence is converted']
# Load model from HuggingFace Hub
tokenizer = AutoTokenizer.from_pretrained('{MODEL_NAME}')
model = AutoModel.from_pretrained('{MODEL_NAME}')
# Tokenize sentences
encoded_input = tokenizer(sentences, padding=True, truncation=True, return_tensors='pt')
# Compute token embeddings
with torch.no_grad():
model_output = model(**encoded_input)
# Perform pooling. In this case, mean pooling.
sentence_embeddings = mean_pooling(model_output, encoded_input['attention_mask'])
print("Sentence embeddings:")
print(sentence_embeddings)
```
## Evaluation Results
<!--- Describe how your model was evaluated -->
For an automated evaluation of this model, see the *Sentence Embeddings Benchmark*: [https://seb.sbert.net](https://seb.sbert.net?model_name={MODEL_NAME})
## Training
The model was trained with the parameters:
**DataLoader**:
`torch.utils.data.dataloader.DataLoader` of length 140000 with parameters:
```
{'batch_size': 32, 'sampler': 'torch.utils.data.sampler.SequentialSampler', 'batch_sampler': 'torch.utils.data.sampler.BatchSampler'}
```
**Loss**:
`gpl.toolkit.loss.MarginDistillationLoss`
Parameters of the fit()-Method:
```
{
"epochs": 1,
"evaluation_steps": 0,
"evaluator": "NoneType",
"max_grad_norm": 1,
"optimizer_class": "<class 'transformers.optimization.AdamW'>",
"optimizer_params": {
"lr": 2e-05
},
"scheduler": "WarmupLinear",
"steps_per_epoch": 140000,
"warmup_steps": 1000,
"weight_decay": 0.01
}
```
## Full Model Architecture
```
SentenceTransformer(
(0): Transformer({'max_seq_length': 350, 'do_lower_case': False}) with Transformer model: DistilBertModel
(1): Pooling({'word_embedding_dimension': 768, 'pooling_mode_cls_token': False, 'pooling_mode_mean_tokens': True, 'pooling_mode_max_tokens': False, 'pooling_mode_mean_sqrt_len_tokens': False})
)
```
## Citing & Authors
<!--- Describe where people can find more information --> |
GPL/signal1m-msmarco-distilbert-gpl | be2a6c5783a9afc0dfdf4b2308a8ec21cad151fc | 2022-04-19T15:15:37.000Z | [
"pytorch",
"distilbert",
"feature-extraction",
"sentence-transformers",
"sentence-similarity",
"transformers"
] | sentence-similarity | false | GPL | null | GPL/signal1m-msmarco-distilbert-gpl | 1 | null | sentence-transformers | 31,331 | ---
pipeline_tag: sentence-similarity
tags:
- sentence-transformers
- feature-extraction
- sentence-similarity
- transformers
---
# {MODEL_NAME}
This is a [sentence-transformers](https://www.SBERT.net) model: It maps sentences & paragraphs to a 768 dimensional dense vector space and can be used for tasks like clustering or semantic search.
<!--- Describe your model here -->
## Usage (Sentence-Transformers)
Using this model becomes easy when you have [sentence-transformers](https://www.SBERT.net) installed:
```
pip install -U sentence-transformers
```
Then you can use the model like this:
```python
from sentence_transformers import SentenceTransformer
sentences = ["This is an example sentence", "Each sentence is converted"]
model = SentenceTransformer('{MODEL_NAME}')
embeddings = model.encode(sentences)
print(embeddings)
```
## Usage (HuggingFace Transformers)
Without [sentence-transformers](https://www.SBERT.net), you can use the model like this: First, you pass your input through the transformer model, then you have to apply the right pooling-operation on-top of the contextualized word embeddings.
```python
from transformers import AutoTokenizer, AutoModel
import torch
#Mean Pooling - Take attention mask into account for correct averaging
def mean_pooling(model_output, attention_mask):
token_embeddings = model_output[0] #First element of model_output contains all token embeddings
input_mask_expanded = attention_mask.unsqueeze(-1).expand(token_embeddings.size()).float()
return torch.sum(token_embeddings * input_mask_expanded, 1) / torch.clamp(input_mask_expanded.sum(1), min=1e-9)
# Sentences we want sentence embeddings for
sentences = ['This is an example sentence', 'Each sentence is converted']
# Load model from HuggingFace Hub
tokenizer = AutoTokenizer.from_pretrained('{MODEL_NAME}')
model = AutoModel.from_pretrained('{MODEL_NAME}')
# Tokenize sentences
encoded_input = tokenizer(sentences, padding=True, truncation=True, return_tensors='pt')
# Compute token embeddings
with torch.no_grad():
model_output = model(**encoded_input)
# Perform pooling. In this case, mean pooling.
sentence_embeddings = mean_pooling(model_output, encoded_input['attention_mask'])
print("Sentence embeddings:")
print(sentence_embeddings)
```
## Evaluation Results
<!--- Describe how your model was evaluated -->
For an automated evaluation of this model, see the *Sentence Embeddings Benchmark*: [https://seb.sbert.net](https://seb.sbert.net?model_name={MODEL_NAME})
## Training
The model was trained with the parameters:
**DataLoader**:
`torch.utils.data.dataloader.DataLoader` of length 140000 with parameters:
```
{'batch_size': 32, 'sampler': 'torch.utils.data.sampler.SequentialSampler', 'batch_sampler': 'torch.utils.data.sampler.BatchSampler'}
```
**Loss**:
`gpl.toolkit.loss.MarginDistillationLoss`
Parameters of the fit()-Method:
```
{
"epochs": 1,
"evaluation_steps": 0,
"evaluator": "NoneType",
"max_grad_norm": 1,
"optimizer_class": "<class 'transformers.optimization.AdamW'>",
"optimizer_params": {
"lr": 2e-05
},
"scheduler": "WarmupLinear",
"steps_per_epoch": 140000,
"warmup_steps": 1000,
"weight_decay": 0.01
}
```
## Full Model Architecture
```
SentenceTransformer(
(0): Transformer({'max_seq_length': 350, 'do_lower_case': False}) with Transformer model: DistilBertModel
(1): Pooling({'word_embedding_dimension': 768, 'pooling_mode_cls_token': False, 'pooling_mode_mean_tokens': True, 'pooling_mode_max_tokens': False, 'pooling_mode_mean_sqrt_len_tokens': False})
)
```
## Citing & Authors
<!--- Describe where people can find more information --> |
GPL/scidocs-msmarco-distilbert-gpl | 9ed45cb4912339b5dad97c0a022f9a8d234b822b | 2022-04-19T15:16:34.000Z | [
"pytorch",
"distilbert",
"feature-extraction",
"sentence-transformers",
"sentence-similarity",
"transformers"
] | sentence-similarity | false | GPL | null | GPL/scidocs-msmarco-distilbert-gpl | 1 | null | sentence-transformers | 31,332 | ---
pipeline_tag: sentence-similarity
tags:
- sentence-transformers
- feature-extraction
- sentence-similarity
- transformers
---
# {MODEL_NAME}
This is a [sentence-transformers](https://www.SBERT.net) model: It maps sentences & paragraphs to a 768 dimensional dense vector space and can be used for tasks like clustering or semantic search.
<!--- Describe your model here -->
## Usage (Sentence-Transformers)
Using this model becomes easy when you have [sentence-transformers](https://www.SBERT.net) installed:
```
pip install -U sentence-transformers
```
Then you can use the model like this:
```python
from sentence_transformers import SentenceTransformer
sentences = ["This is an example sentence", "Each sentence is converted"]
model = SentenceTransformer('{MODEL_NAME}')
embeddings = model.encode(sentences)
print(embeddings)
```
## Usage (HuggingFace Transformers)
Without [sentence-transformers](https://www.SBERT.net), you can use the model like this: First, you pass your input through the transformer model, then you have to apply the right pooling-operation on-top of the contextualized word embeddings.
```python
from transformers import AutoTokenizer, AutoModel
import torch
#Mean Pooling - Take attention mask into account for correct averaging
def mean_pooling(model_output, attention_mask):
token_embeddings = model_output[0] #First element of model_output contains all token embeddings
input_mask_expanded = attention_mask.unsqueeze(-1).expand(token_embeddings.size()).float()
return torch.sum(token_embeddings * input_mask_expanded, 1) / torch.clamp(input_mask_expanded.sum(1), min=1e-9)
# Sentences we want sentence embeddings for
sentences = ['This is an example sentence', 'Each sentence is converted']
# Load model from HuggingFace Hub
tokenizer = AutoTokenizer.from_pretrained('{MODEL_NAME}')
model = AutoModel.from_pretrained('{MODEL_NAME}')
# Tokenize sentences
encoded_input = tokenizer(sentences, padding=True, truncation=True, return_tensors='pt')
# Compute token embeddings
with torch.no_grad():
model_output = model(**encoded_input)
# Perform pooling. In this case, mean pooling.
sentence_embeddings = mean_pooling(model_output, encoded_input['attention_mask'])
print("Sentence embeddings:")
print(sentence_embeddings)
```
## Evaluation Results
<!--- Describe how your model was evaluated -->
For an automated evaluation of this model, see the *Sentence Embeddings Benchmark*: [https://seb.sbert.net](https://seb.sbert.net?model_name={MODEL_NAME})
## Training
The model was trained with the parameters:
**DataLoader**:
`torch.utils.data.dataloader.DataLoader` of length 140000 with parameters:
```
{'batch_size': 32, 'sampler': 'torch.utils.data.sampler.SequentialSampler', 'batch_sampler': 'torch.utils.data.sampler.BatchSampler'}
```
**Loss**:
`gpl.toolkit.loss.MarginDistillationLoss`
Parameters of the fit()-Method:
```
{
"epochs": 1,
"evaluation_steps": 0,
"evaluator": "NoneType",
"max_grad_norm": 1,
"optimizer_class": "<class 'transformers.optimization.AdamW'>",
"optimizer_params": {
"lr": 2e-05
},
"scheduler": "WarmupLinear",
"steps_per_epoch": 140000,
"warmup_steps": 1000,
"weight_decay": 0.01
}
```
## Full Model Architecture
```
SentenceTransformer(
(0): Transformer({'max_seq_length': 350, 'do_lower_case': False}) with Transformer model: DistilBertModel
(1): Pooling({'word_embedding_dimension': 768, 'pooling_mode_cls_token': False, 'pooling_mode_mean_tokens': True, 'pooling_mode_max_tokens': False, 'pooling_mode_mean_sqrt_len_tokens': False})
)
```
## Citing & Authors
<!--- Describe where people can find more information --> |
GPL/dbpedia-entity-tsdae-msmarco-distilbert-margin-mse | d6124db76758ea4c9de6547c8a22270989cf057f | 2022-04-19T16:43:18.000Z | [
"pytorch",
"distilbert",
"feature-extraction",
"transformers"
] | feature-extraction | false | GPL | null | GPL/dbpedia-entity-tsdae-msmarco-distilbert-margin-mse | 1 | null | transformers | 31,333 | Entry not found |
GPL/nq-tsdae-msmarco-distilbert-margin-mse | 44ae3ed343790853afcb68efe3d9e858164e60ea | 2022-04-19T16:44:50.000Z | [
"pytorch",
"distilbert",
"feature-extraction",
"transformers"
] | feature-extraction | false | GPL | null | GPL/nq-tsdae-msmarco-distilbert-margin-mse | 1 | null | transformers | 31,334 | Entry not found |
GPL/signal1m-tsdae-msmarco-distilbert-margin-mse | fe18a5eed63f0903399e2e06e9ae98ecb7f6b755 | 2022-04-19T16:46:08.000Z | [
"pytorch",
"distilbert",
"feature-extraction",
"transformers"
] | feature-extraction | false | GPL | null | GPL/signal1m-tsdae-msmarco-distilbert-margin-mse | 1 | null | transformers | 31,335 | Entry not found |
GPL/bioasq-tsdae-msmarco-distilbert-margin-mse | eafe1fb1ac2d6c35da757addc3443a28c8e0e75a | 2022-04-19T16:48:37.000Z | [
"pytorch",
"distilbert",
"feature-extraction",
"transformers"
] | feature-extraction | false | GPL | null | GPL/bioasq-tsdae-msmarco-distilbert-margin-mse | 1 | null | transformers | 31,336 | Entry not found |
robkayinto/xlm-roberta-base-finetuned-panx-de-fr | b9b5e946b7808e1589e6db297d68f8c9b2d5e9f8 | 2022-07-13T17:45:25.000Z | [
"pytorch",
"xlm-roberta",
"token-classification",
"transformers",
"generated_from_trainer",
"license:mit",
"model-index",
"autotrain_compatible"
] | token-classification | false | robkayinto | null | robkayinto/xlm-roberta-base-finetuned-panx-de-fr | 1 | null | transformers | 31,337 | ---
license: mit
tags:
- generated_from_trainer
metrics:
- f1
model-index:
- name: xlm-roberta-base-finetuned-panx-de-fr
results: []
---
<!-- This model card has been generated automatically according to the information the Trainer had access to. You
should probably proofread and complete it, then remove this comment. -->
# xlm-roberta-base-finetuned-panx-de-fr
This model is a fine-tuned version of [xlm-roberta-base](https://huggingface.co/xlm-roberta-base) on the None dataset.
It achieves the following results on the evaluation set:
- Loss: 0.1654
- F1: 0.8590
## Model description
More information needed
## Intended uses & limitations
More information needed
## Training and evaluation data
More information needed
## Training procedure
### Training hyperparameters
The following hyperparameters were used during training:
- learning_rate: 5e-05
- train_batch_size: 24
- eval_batch_size: 24
- seed: 42
- optimizer: Adam with betas=(0.9,0.999) and epsilon=1e-08
- lr_scheduler_type: linear
- num_epochs: 3
### Training results
| Training Loss | Epoch | Step | Validation Loss | F1 |
|:-------------:|:-----:|:----:|:---------------:|:------:|
| 0.2845 | 1.0 | 715 | 0.1831 | 0.8249 |
| 0.1449 | 2.0 | 1430 | 0.1643 | 0.8479 |
| 0.0929 | 3.0 | 2145 | 0.1654 | 0.8590 |
### Framework versions
- Transformers 4.11.3
- Pytorch 1.12.0+cu113
- Datasets 1.16.1
- Tokenizers 0.10.3
|
crystina-z/mdpr-tied-nq | 6d2f749155429e4738afd191b86bb4594e1528cb | 2022-04-19T18:39:42.000Z | [
"pytorch",
"bert",
"feature-extraction",
"transformers"
] | feature-extraction | false | crystina-z | null | crystina-z/mdpr-tied-nq | 1 | null | transformers | 31,338 | Entry not found |
kniemiec/test | b29d144181bc8eb1e28bbb0969e8344c7d1c6beb | 2022-04-19T20:39:56.000Z | [
"pytorch",
"segformer",
"transformers"
] | null | false | kniemiec | null | kniemiec/test | 1 | null | transformers | 31,339 | Entry not found |
jqsl2012/layoutlmv2-cord-test | 591fac28f32b9f7cb551ec9a344218b7b8a4bc50 | 2022-04-20T07:00:26.000Z | [
"pytorch",
"layoutlmv2",
"token-classification",
"transformers",
"license:apache-2.0",
"autotrain_compatible"
] | token-classification | false | jqsl2012 | null | jqsl2012/layoutlmv2-cord-test | 1 | null | transformers | 31,340 | ---
license: apache-2.0
---
|
PSW/max_sim_del_seed1 | 6eb9168411cbfdb5a289175965dec87f32cfcfd9 | 2022-04-20T03:58:25.000Z | [
"pytorch",
"bart",
"text2text-generation",
"transformers",
"autotrain_compatible"
] | text2text-generation | false | PSW | null | PSW/max_sim_del_seed1 | 1 | null | transformers | 31,341 | Entry not found |
scasutt/wav2vec2-large-xlsr-53_toy_train_fast_masked_augment_random_noise_slow_fast_high_low | 7f07aae8f7f98ffc25a607c44960a79b46fe5730 | 2022-04-20T13:35:58.000Z | [
"pytorch",
"wav2vec2",
"automatic-speech-recognition",
"transformers"
] | automatic-speech-recognition | false | scasutt | null | scasutt/wav2vec2-large-xlsr-53_toy_train_fast_masked_augment_random_noise_slow_fast_high_low | 1 | null | transformers | 31,342 | Entry not found |
PSW/half_sim_del_seed1 | 0dacc106ac56a88fa943d708bb01fa81d60d0617 | 2022-04-20T06:55:56.000Z | [
"pytorch",
"bart",
"text2text-generation",
"transformers",
"autotrain_compatible"
] | text2text-generation | false | PSW | null | PSW/half_sim_del_seed1 | 1 | null | transformers | 31,343 | Entry not found |
PSW/half_sim_del | 1b85bebfa6b2ec254432ea7d39b22e530cf3e84b | 2022-04-20T08:28:34.000Z | [
"pytorch",
"bart",
"text2text-generation",
"transformers",
"autotrain_compatible"
] | text2text-generation | false | PSW | null | PSW/half_sim_del | 1 | null | transformers | 31,344 | Entry not found |
DongHyoungLee/bluebert-base-uncased-tokenclassification-2layers | 43fe96c8b244690509b069e7223b937d9a6c2e24 | 2022-04-20T08:25:49.000Z | [
"pytorch",
"bert",
"transformers"
] | null | false | DongHyoungLee | null | DongHyoungLee/bluebert-base-uncased-tokenclassification-2layers | 1 | null | transformers | 31,345 | Entry not found |
MeshalAlamr/wav2vec2-large-xls-r-300m-ar-2 | 0d207a74c3302e149492fdf26af2d451b740afb1 | 2022-04-21T06:54:05.000Z | [
"pytorch",
"wav2vec2",
"automatic-speech-recognition",
"dataset:common_voice",
"transformers",
"generated_from_trainer",
"license:apache-2.0",
"model-index"
] | automatic-speech-recognition | false | MeshalAlamr | null | MeshalAlamr/wav2vec2-large-xls-r-300m-ar-2 | 1 | null | transformers | 31,346 | ---
license: apache-2.0
tags:
- generated_from_trainer
datasets:
- common_voice
model-index:
- name: wav2vec2-large-xls-r-300m-ar-2
results: []
---
<!-- This model card has been generated automatically according to the information the Trainer had access to. You
should probably proofread and complete it, then remove this comment. -->
# wav2vec2-large-xls-r-300m-ar-2
This model is a fine-tuned version of [facebook/wav2vec2-xls-r-300m](https://huggingface.co/facebook/wav2vec2-xls-r-300m) on the common_voice dataset.
It achieves the following results on the evaluation set:
- Loss: 0.4764
- Wer: 0.3073
## Model description
More information needed
## Intended uses & limitations
More information needed
## Training and evaluation data
More information needed
## Training procedure
### Training hyperparameters
The following hyperparameters were used during training:
- learning_rate: 0.001
- train_batch_size: 32
- eval_batch_size: 16
- seed: 42
- gradient_accumulation_steps: 2
- total_train_batch_size: 64
- optimizer: Adam with betas=(0.9,0.999) and epsilon=1e-08
- lr_scheduler_type: linear
- lr_scheduler_warmup_steps: 500
- num_epochs: 30
- mixed_precision_training: Native AMP
### Training results
| Training Loss | Epoch | Step | Validation Loss | Wer |
|:-------------:|:-----:|:-----:|:---------------:|:------:|
| 1.0851 | 1.18 | 400 | 0.5614 | 0.4888 |
| 0.691 | 2.35 | 800 | 0.6557 | 0.5558 |
| 0.6128 | 3.53 | 1200 | 0.5852 | 0.5070 |
| 0.543 | 4.71 | 1600 | 0.5591 | 0.4838 |
| 0.5185 | 5.88 | 2000 | 0.6649 | 0.5514 |
| 0.4816 | 7.06 | 2400 | 0.5598 | 0.4689 |
| 0.4336 | 8.24 | 2800 | 0.5384 | 0.4515 |
| 0.405 | 9.41 | 3200 | 0.4987 | 0.4138 |
| 0.3811 | 10.59 | 3600 | 0.5427 | 0.4644 |
| 0.3539 | 11.76 | 4000 | 0.4881 | 0.4159 |
| 0.3299 | 12.94 | 4400 | 0.5160 | 0.4198 |
| 0.3096 | 14.12 | 4800 | 0.5019 | 0.4077 |
| 0.2881 | 15.29 | 5200 | 0.5146 | 0.4140 |
| 0.2894 | 16.47 | 5600 | 0.4861 | 0.4026 |
| 0.2461 | 17.65 | 6000 | 0.4765 | 0.3742 |
| 0.2371 | 18.82 | 6400 | 0.4679 | 0.3672 |
| 0.2182 | 20.0 | 6800 | 0.4699 | 0.3603 |
| 0.1942 | 21.18 | 7200 | 0.4769 | 0.3519 |
| 0.1823 | 22.35 | 7600 | 0.4719 | 0.3497 |
| 0.1682 | 23.53 | 8000 | 0.4876 | 0.3456 |
| 0.1526 | 24.71 | 8400 | 0.4591 | 0.3300 |
| 0.137 | 25.88 | 8800 | 0.4819 | 0.3314 |
| 0.1283 | 27.06 | 9200 | 0.4823 | 0.3213 |
| 0.1174 | 28.24 | 9600 | 0.4879 | 0.3174 |
| 0.1104 | 29.41 | 10000 | 0.4764 | 0.3073 |
### Framework versions
- Transformers 4.17.0
- Pytorch 1.11.0
- Datasets 1.18.4
- Tokenizers 0.11.6
|
4m1g0/wav2vec2-large-xls-r-53m-gl-jupyter2 | d1e75f1fb17582e064d240fc1d7aecfa112d00bf | 2022-04-20T16:27:03.000Z | [
"pytorch",
"tensorboard",
"wav2vec2",
"automatic-speech-recognition",
"transformers",
"generated_from_trainer",
"license:apache-2.0",
"model-index"
] | automatic-speech-recognition | false | 4m1g0 | null | 4m1g0/wav2vec2-large-xls-r-53m-gl-jupyter2 | 1 | null | transformers | 31,347 | ---
license: apache-2.0
tags:
- generated_from_trainer
model-index:
- name: wav2vec2-large-xls-r-53m-gl-jupyter2
results: []
---
<!-- This model card has been generated automatically according to the information the Trainer had access to. You
should probably proofread and complete it, then remove this comment. -->
# wav2vec2-large-xls-r-53m-gl-jupyter2
This model is a fine-tuned version of [facebook/wav2vec2-large-xlsr-53](https://huggingface.co/facebook/wav2vec2-large-xlsr-53) on the None dataset.
It achieves the following results on the evaluation set:
- Loss: 0.0941
- Wer: 0.0615
## Model description
More information needed
## Intended uses & limitations
More information needed
## Training and evaluation data
More information needed
## Training procedure
### Training hyperparameters
The following hyperparameters were used during training:
- learning_rate: 0.0003
- train_batch_size: 16
- eval_batch_size: 8
- seed: 42
- gradient_accumulation_steps: 2
- total_train_batch_size: 32
- optimizer: Adam with betas=(0.9,0.999) and epsilon=1e-08
- lr_scheduler_type: linear
- lr_scheduler_warmup_steps: 500
- num_epochs: 45
- mixed_precision_training: Native AMP
### Training results
| Training Loss | Epoch | Step | Validation Loss | Wer |
|:-------------:|:-----:|:----:|:---------------:|:------:|
| 3.7298 | 3.36 | 400 | 0.2477 | 0.2493 |
| 0.1507 | 6.72 | 800 | 0.1294 | 0.1264 |
| 0.066 | 10.08 | 1200 | 0.1235 | 0.1161 |
| 0.0456 | 13.44 | 1600 | 0.1011 | 0.1001 |
| 0.0347 | 16.8 | 2000 | 0.1033 | 0.0909 |
| 0.0284 | 20.17 | 2400 | 0.1083 | 0.0861 |
| 0.0221 | 23.53 | 2800 | 0.1010 | 0.0761 |
| 0.0199 | 26.89 | 3200 | 0.0911 | 0.0754 |
| 0.0155 | 30.25 | 3600 | 0.1026 | 0.0743 |
| 0.0142 | 33.61 | 4000 | 0.1024 | 0.0719 |
| 0.0125 | 36.97 | 4400 | 0.0977 | 0.0676 |
| 0.0104 | 40.33 | 4800 | 0.0945 | 0.0664 |
| 0.0089 | 43.69 | 5200 | 0.0941 | 0.0615 |
### Framework versions
- Transformers 4.11.3
- Pytorch 1.9.1+cu111
- Datasets 1.18.3
- Tokenizers 0.10.3
|
masakhane/m2m100_418M_fr_wol_rel_news | d7e435de479c282d4f6913d66110e4b5114344ec | 2022-04-20T17:34:57.000Z | [
"pytorch",
"m2m_100",
"text2text-generation",
"transformers",
"license:afl-3.0",
"autotrain_compatible"
] | text2text-generation | false | masakhane | null | masakhane/m2m100_418M_fr_wol_rel_news | 1 | null | transformers | 31,348 | ---
license: afl-3.0
---
|
masakhane/m2m100_418M_wol_fr_rel_news_ft | 4e48e6b372fcbdb30f540e0545d66dec1ffa62c2 | 2022-04-20T18:36:02.000Z | [
"pytorch",
"m2m_100",
"text2text-generation",
"transformers",
"license:afl-3.0",
"autotrain_compatible"
] | text2text-generation | false | masakhane | null | masakhane/m2m100_418M_wol_fr_rel_news_ft | 1 | null | transformers | 31,349 | ---
license: afl-3.0
---
|
masakhane/m2m100_418M_wol_fr_rel | 4fc87c6fcc66c748db7504fedccd57989de3126a | 2022-04-20T19:20:17.000Z | [
"pytorch",
"m2m_100",
"text2text-generation",
"transformers",
"license:afl-3.0",
"autotrain_compatible"
] | text2text-generation | false | masakhane | null | masakhane/m2m100_418M_wol_fr_rel | 1 | null | transformers | 31,350 | ---
license: afl-3.0
---
|
4m1g0/wav2vec2-large-xls-r-300m-gl-jupyter5 | d0b687219d144d1ad81188b20566865d3345e014 | 2022-04-20T16:10:50.000Z | [
"pytorch",
"tensorboard",
"wav2vec2",
"automatic-speech-recognition",
"transformers"
] | automatic-speech-recognition | false | 4m1g0 | null | 4m1g0/wav2vec2-large-xls-r-300m-gl-jupyter5 | 1 | null | transformers | 31,351 | Entry not found |
frozenwalker/T5_pubmedqa_question_generation_preTrained_MedQuad_modified | 9bedc2473cb94487ec94274f6be3ea72fddeb12c | 2022-04-20T13:48:46.000Z | [
"pytorch",
"t5",
"text2text-generation",
"transformers",
"autotrain_compatible"
] | text2text-generation | false | frozenwalker | null | frozenwalker/T5_pubmedqa_question_generation_preTrained_MedQuad_modified | 1 | null | transformers | 31,352 | Entry not found |
csikasote/xls-r-1b-bemba-15hrs | e02444e784111effb0cb94b61b191379c9d883db | 2022-04-24T17:47:51.000Z | [
"pytorch",
"tensorboard",
"wav2vec2",
"automatic-speech-recognition",
"transformers",
"generated_from_trainer",
"license:apache-2.0",
"model-index"
] | automatic-speech-recognition | false | csikasote | null | csikasote/xls-r-1b-bemba-15hrs | 1 | null | transformers | 31,353 | ---
license: apache-2.0
tags:
- generated_from_trainer
model-index:
- name: xls-r-1b-bemba-15hrs
results: []
---
<!-- This model card has been generated automatically according to the information the Trainer had access to. You
should probably proofread and complete it, then remove this comment. -->
# xls-r-1b-bemba-15hrs
This model is a fine-tuned version of [facebook/wav2vec2-xls-r-1b](https://huggingface.co/facebook/wav2vec2-xls-r-1b) on an unknown dataset.
It achieves the following results on the evaluation set:
- Loss: 0.2134
- Wer: 0.3485
## Model description
More information needed
## Intended uses & limitations
More information needed
## Training and evaluation data
More information needed
## Training procedure
### Training hyperparameters
The following hyperparameters were used during training:
- learning_rate: 3e-05
- train_batch_size: 4
- eval_batch_size: 8
- seed: 42
- gradient_accumulation_steps: 2
- total_train_batch_size: 8
- optimizer: Adam with betas=(0.9,0.999) and epsilon=1e-08
- lr_scheduler_type: linear
- lr_scheduler_warmup_steps: 400
- num_epochs: 10
- mixed_precision_training: Native AMP
### Training results
| Training Loss | Epoch | Step | Validation Loss | Wer |
|:-------------:|:-----:|:----:|:---------------:|:------:|
| 2.3016 | 0.36 | 400 | 0.6032 | 0.9932 |
| 0.5196 | 0.71 | 800 | 0.3089 | 0.5020 |
| 0.4397 | 1.07 | 1200 | 0.2562 | 0.4223 |
| 0.3617 | 1.43 | 1600 | 0.2269 | 0.4009 |
| 0.36 | 1.79 | 2000 | 0.2106 | 0.3896 |
| 0.3404 | 2.14 | 2400 | 0.2079 | 0.3681 |
| 0.2915 | 2.5 | 2800 | 0.2024 | 0.3488 |
| 0.2869 | 2.86 | 3200 | 0.2068 | 0.3550 |
| 0.2492 | 3.22 | 3600 | 0.1925 | 0.3273 |
| 0.2542 | 3.57 | 4000 | 0.2041 | 0.3446 |
| 0.2333 | 3.93 | 4400 | 0.1985 | 0.3386 |
| 0.2023 | 4.29 | 4800 | 0.2134 | 0.3485 |
### Framework versions
- Transformers 4.19.0.dev0
- Pytorch 1.10.0+cu111
- Datasets 2.1.0
- Tokenizers 0.12.1
|
mnazari/delete_this_later | 8990fad660ac092682fbad422a8fcc30cd04407f | 2022-04-23T00:06:41.000Z | [
"pytorch",
"wav2vec2",
"automatic-speech-recognition",
"transformers"
] | automatic-speech-recognition | false | mnazari | null | mnazari/delete_this_later | 1 | null | transformers | 31,354 | Entry not found |
shkim/distilbert-base-uncased-finetuned-imdb-accelerate | 068bdae154b14a10ab16df4c96c8de3eaae532eb | 2022-04-20T14:35:34.000Z | [
"pytorch",
"distilbert",
"fill-mask",
"transformers",
"autotrain_compatible"
] | fill-mask | false | shkim | null | shkim/distilbert-base-uncased-finetuned-imdb-accelerate | 1 | null | transformers | 31,355 | Entry not found |
ffalcao/pegasus-samsum | 21441de6cb3408c7031cff2281026e0f9e04b18e | 2022-04-27T13:09:17.000Z | [
"pytorch",
"tensorboard",
"pegasus",
"text2text-generation",
"dataset:samsum",
"transformers",
"generated_from_trainer",
"model-index",
"autotrain_compatible"
] | text2text-generation | false | ffalcao | null | ffalcao/pegasus-samsum | 1 | null | transformers | 31,356 | ---
tags:
- generated_from_trainer
datasets:
- samsum
model-index:
- name: pegasus-samsum
results: []
---
<!-- This model card has been generated automatically according to the information the Trainer had access to. You
should probably proofread and complete it, then remove this comment. -->
# pegasus-samsum
This model is a fine-tuned version of [google/pegasus-cnn_dailymail](https://huggingface.co/google/pegasus-cnn_dailymail) on the samsum dataset.
It achieves the following results on the evaluation set:
- Loss: 1.4874
## Model description
More information needed
## Intended uses & limitations
More information needed
## Training and evaluation data
More information needed
## Training procedure
### Training hyperparameters
The following hyperparameters were used during training:
- learning_rate: 5e-05
- train_batch_size: 1
- eval_batch_size: 1
- seed: 42
- gradient_accumulation_steps: 16
- total_train_batch_size: 16
- optimizer: Adam with betas=(0.9,0.999) and epsilon=1e-08
- lr_scheduler_type: linear
- lr_scheduler_warmup_steps: 500
- num_epochs: 1
### Training results
| Training Loss | Epoch | Step | Validation Loss |
|:-------------:|:-----:|:----:|:---------------:|
| 1.702 | 0.54 | 500 | 1.4874 |
### Framework versions
- Transformers 4.11.3
- Pytorch 1.9.1
- Datasets 1.16.1
- Tokenizers 0.10.3
|
4m1g0/wav2vec2-large-xls-r-53m-gl-jupyter3 | 8ab722626d9233219beb0cc6689dd316962b312a | 2022-04-20T17:05:08.000Z | [
"pytorch",
"tensorboard",
"wav2vec2",
"automatic-speech-recognition",
"transformers"
] | automatic-speech-recognition | false | 4m1g0 | null | 4m1g0/wav2vec2-large-xls-r-53m-gl-jupyter3 | 1 | null | transformers | 31,357 | Entry not found |
Tejas21/Totto_t5_base_BERT_Score_20k_steps | 990c260dcc98a5fb8a669574f128c7a6d8aee127 | 2022-04-21T18:47:18.000Z | [
"pytorch",
"t5",
"text2text-generation",
"transformers",
"license:apache-2.0",
"autotrain_compatible"
] | text2text-generation | false | Tejas21 | null | Tejas21/Totto_t5_base_BERT_Score_20k_steps | 1 | null | transformers | 31,358 | ---
license: apache-2.0
---
language:
- en
tags:
- Table to text
- Data to text
## Dataset:
- [ToTTo](https://github.com/google-research-datasets/ToTTo)
A Controlled Table-to-Text Dataset. Totto is an open-source table-to-text dataset with over 1,20,000 examples in the English language. It defines a controlled generation task as: given a Wikipedia table and a set of highlighted cells, generate a one-sentence description.
## Base Model - T5-Base
[Google's T5](https://ai.googleblog.com/2020/02/exploring-transfer-learning-with-t5.html)
The T5 was built by the Google team in order to create a general-purpose model that can understand the text. The basic idea behind t5 was to deal with the text processing problem as a “text-to-text” problem, i.e. taking the text as input and producing new text as output.
## Baseline Preprocessing
[Baseline Preprocessing](https://github.com/google-research/language/tree/master/language/totto)
This code repository serves as a supplementary for the main repository, which can be used to do basic preprocessing of the Totto dataset.
## Fine-tuning
On the Totto dataset, we used the T5 for the conditional generation model and fine-tuned it with 10000 steps BLEU and then 20000 steps [BERT-SCORE](https://github.com/Tiiiger/bert_score) as a metric.
|
negfir/bert_uncased_L-2_H-768_A-12wiki103 | 11391e8e5dcc155f48612a6514a3ed63da7e3c30 | 2022-04-20T20:53:22.000Z | [
"pytorch",
"bert",
"fill-mask",
"transformers",
"autotrain_compatible"
] | fill-mask | false | negfir | null | negfir/bert_uncased_L-2_H-768_A-12wiki103 | 1 | null | transformers | 31,359 | Entry not found |
dlu66061/wav2vec2-base-timit-demo | b79a0a94975f78c4af0290a810b04d51e62cc80f | 2022-04-21T03:16:11.000Z | [
"pytorch",
"wav2vec2",
"automatic-speech-recognition",
"transformers",
"generated_from_trainer",
"license:apache-2.0",
"model-index"
] | automatic-speech-recognition | false | dlu66061 | null | dlu66061/wav2vec2-base-timit-demo | 1 | null | transformers | 31,360 | ---
license: apache-2.0
tags:
- generated_from_trainer
model-index:
- name: wav2vec2-base-timit-demo
results: []
---
<!-- This model card has been generated automatically according to the information the Trainer had access to. You
should probably proofread and complete it, then remove this comment. -->
# wav2vec2-base-timit-demo
This model is a fine-tuned version of [facebook/wav2vec2-base](https://huggingface.co/facebook/wav2vec2-base) on the None dataset.
It achieves the following results on the evaluation set:
- Loss: 0.4094
- Wer: 0.2825
## Model description
More information needed
## Intended uses & limitations
More information needed
## Training and evaluation data
More information needed
## Training procedure
### Training hyperparameters
The following hyperparameters were used during training:
- learning_rate: 0.0001
- train_batch_size: 32
- eval_batch_size: 8
- seed: 42
- optimizer: Adam with betas=(0.9,0.999) and epsilon=1e-08
- lr_scheduler_type: linear
- lr_scheduler_warmup_steps: 1000
- num_epochs: 30
- mixed_precision_training: Native AMP
### Training results
| Training Loss | Epoch | Step | Validation Loss | Wer |
|:-------------:|:-----:|:----:|:---------------:|:------:|
| 3.5419 | 3.45 | 500 | 1.2376 | 0.8772 |
| 0.5393 | 6.9 | 1000 | 0.4489 | 0.3894 |
| 0.1916 | 10.34 | 1500 | 0.3777 | 0.3185 |
| 0.1139 | 13.79 | 2000 | 0.4041 | 0.3058 |
| 0.0798 | 17.24 | 2500 | 0.3742 | 0.2988 |
| 0.0602 | 20.69 | 3000 | 0.3751 | 0.2897 |
| 0.0463 | 24.14 | 3500 | 0.4067 | 0.2865 |
| 0.0388 | 27.59 | 4000 | 0.4094 | 0.2825 |
### Framework versions
- Transformers 4.18.0
- Pytorch 1.11.0+cu102
- Datasets 1.18.3
- Tokenizers 0.11.6
|
4m1g0/wav2vec2-large-xls-r-53m-gl-jupyter5 | db41901ca72856f504d71d566dc4c7aacebbeb59 | 2022-04-21T05:58:07.000Z | [
"pytorch",
"tensorboard",
"wav2vec2",
"automatic-speech-recognition",
"transformers",
"generated_from_trainer",
"license:apache-2.0",
"model-index"
] | automatic-speech-recognition | false | 4m1g0 | null | 4m1g0/wav2vec2-large-xls-r-53m-gl-jupyter5 | 1 | null | transformers | 31,361 | ---
license: apache-2.0
tags:
- generated_from_trainer
model-index:
- name: wav2vec2-large-xls-r-53m-gl-jupyter5
results: []
---
<!-- This model card has been generated automatically according to the information the Trainer had access to. You
should probably proofread and complete it, then remove this comment. -->
# wav2vec2-large-xls-r-53m-gl-jupyter5
This model is a fine-tuned version of [facebook/wav2vec2-large-xlsr-53](https://huggingface.co/facebook/wav2vec2-large-xlsr-53) on the None dataset.
It achieves the following results on the evaluation set:
- Loss: 0.1025
- Wer: 0.0625
## Model description
More information needed
## Intended uses & limitations
More information needed
## Training and evaluation data
More information needed
## Training procedure
### Training hyperparameters
The following hyperparameters were used during training:
- learning_rate: 0.0003
- train_batch_size: 16
- eval_batch_size: 8
- seed: 42
- gradient_accumulation_steps: 2
- total_train_batch_size: 32
- optimizer: Adam with betas=(0.9,0.999) and epsilon=1e-08
- lr_scheduler_type: linear
- lr_scheduler_warmup_steps: 500
- num_epochs: 60
- mixed_precision_training: Native AMP
### Training results
| Training Loss | Epoch | Step | Validation Loss | Wer |
|:-------------:|:-----:|:----:|:---------------:|:------:|
| 3.6862 | 3.36 | 400 | 0.2455 | 0.2344 |
| 0.1517 | 6.72 | 800 | 0.1195 | 0.1233 |
| 0.0772 | 10.08 | 1200 | 0.1219 | 0.1155 |
| 0.0472 | 13.44 | 1600 | 0.1162 | 0.1034 |
| 0.0357 | 16.8 | 2000 | 0.1070 | 0.1006 |
| 0.0307 | 20.17 | 2400 | 0.1131 | 0.1013 |
| 0.0258 | 23.53 | 2800 | 0.1163 | 0.0847 |
| 0.0229 | 26.89 | 3200 | 0.1100 | 0.0858 |
| 0.0183 | 30.25 | 3600 | 0.1062 | 0.0810 |
| 0.0182 | 33.61 | 4000 | 0.1068 | 0.0800 |
| 0.0151 | 36.97 | 4400 | 0.1088 | 0.0780 |
| 0.0138 | 40.33 | 4800 | 0.1062 | 0.0737 |
| 0.0121 | 43.69 | 5200 | 0.1061 | 0.0722 |
| 0.0088 | 47.06 | 5600 | 0.1055 | 0.0670 |
| 0.008 | 50.42 | 6000 | 0.1059 | 0.0646 |
| 0.007 | 53.78 | 6400 | 0.1020 | 0.0634 |
| 0.0065 | 57.14 | 6800 | 0.1025 | 0.0625 |
### Framework versions
- Transformers 4.11.3
- Pytorch 1.9.1+cu111
- Datasets 1.18.3
- Tokenizers 0.10.3
|
negfir/bert_uncased_L-2_H-512_A-8wiki103 | 622d7913e9067bbdac4663c92587433f3f25fe2a | 2022-04-21T01:17:41.000Z | [
"pytorch",
"bert",
"fill-mask",
"transformers",
"autotrain_compatible"
] | fill-mask | false | negfir | null | negfir/bert_uncased_L-2_H-512_A-8wiki103 | 1 | null | transformers | 31,362 | Entry not found |
obokkkk/wav2vec2-base-timit-demo-colab3 | 3946e18144660bc1cd65c5cbd7231a5fab503ce9 | 2022-04-21T04:10:35.000Z | [
"pytorch",
"tensorboard",
"wav2vec2",
"automatic-speech-recognition",
"transformers",
"generated_from_trainer",
"license:apache-2.0",
"model-index"
] | automatic-speech-recognition | false | obokkkk | null | obokkkk/wav2vec2-base-timit-demo-colab3 | 1 | null | transformers | 31,363 | ---
license: apache-2.0
tags:
- generated_from_trainer
model-index:
- name: wav2vec2-base-timit-demo-colab3
results: []
---
<!-- This model card has been generated automatically according to the information the Trainer had access to. You
should probably proofread and complete it, then remove this comment. -->
# wav2vec2-base-timit-demo-colab3
This model is a fine-tuned version of [facebook/wav2vec2-base](https://huggingface.co/facebook/wav2vec2-base) on the None dataset.
It achieves the following results on the evaluation set:
- Loss: 0.4832
- Wer: 0.3419
## Model description
More information needed
## Intended uses & limitations
More information needed
## Training and evaluation data
More information needed
## Training procedure
### Training hyperparameters
The following hyperparameters were used during training:
- learning_rate: 0.0001
- train_batch_size: 32
- eval_batch_size: 8
- seed: 42
- optimizer: Adam with betas=(0.9,0.999) and epsilon=1e-08
- lr_scheduler_type: linear
- lr_scheduler_warmup_steps: 1000
- num_epochs: 30
- mixed_precision_training: Native AMP
### Training results
| Training Loss | Epoch | Step | Validation Loss | Wer |
|:-------------:|:-----:|:----:|:---------------:|:------:|
| 2.292 | 4.0 | 500 | 0.7903 | 0.6305 |
| 0.5022 | 8.0 | 1000 | 0.4497 | 0.4332 |
| 0.2129 | 12.0 | 1500 | 0.4998 | 0.3940 |
| 0.1251 | 16.0 | 2000 | 0.4728 | 0.3667 |
| 0.0861 | 20.0 | 2500 | 0.4663 | 0.3644 |
| 0.0594 | 24.0 | 3000 | 0.4773 | 0.3497 |
| 0.0446 | 28.0 | 3500 | 0.4832 | 0.3419 |
### Framework versions
- Transformers 4.11.3
- Pytorch 1.10.0+cu111
- Datasets 1.18.3
- Tokenizers 0.10.3
|
ToToKr/wav2vec2-base-timit-demo-colab | 447fde3d3f13e82aa47ba51c81c62255fc7945d7 | 2022-04-27T07:50:31.000Z | [
"pytorch",
"tensorboard",
"wav2vec2",
"automatic-speech-recognition",
"transformers",
"generated_from_trainer",
"license:apache-2.0",
"model-index"
] | automatic-speech-recognition | false | ToToKr | null | ToToKr/wav2vec2-base-timit-demo-colab | 1 | null | transformers | 31,364 | ---
license: apache-2.0
tags:
- generated_from_trainer
model-index:
- name: wav2vec2-base-timit-demo-colab
results: []
---
<!-- This model card has been generated automatically according to the information the Trainer had access to. You
should probably proofread and complete it, then remove this comment. -->
# wav2vec2-base-timit-demo-colab
This model is a fine-tuned version of [facebook/wav2vec2-base](https://huggingface.co/facebook/wav2vec2-base) on the None dataset.
It achieves the following results on the evaluation set:
- Loss: 0.4520
- Wer: 0.2286
## Model description
More information needed
## Intended uses & limitations
More information needed
## Training and evaluation data
More information needed
## Training procedure
### Training hyperparameters
The following hyperparameters were used during training:
- learning_rate: 0.0001
- train_batch_size: 32
- eval_batch_size: 8
- seed: 42
- optimizer: Adam with betas=(0.9,0.999) and epsilon=1e-08
- lr_scheduler_type: linear
- lr_scheduler_warmup_steps: 1000
- num_epochs: 30
- mixed_precision_training: Native AMP
### Training results
| Training Loss | Epoch | Step | Validation Loss | Wer |
|:-------------:|:-----:|:----:|:---------------:|:------:|
| 3.3811 | 4.0 | 500 | 1.1887 | 0.8528 |
| 0.5798 | 8.0 | 1000 | 0.4544 | 0.3357 |
| 0.2197 | 12.0 | 1500 | 0.4424 | 0.2699 |
| 0.1279 | 16.0 | 2000 | 0.4388 | 0.2559 |
| 0.0855 | 20.0 | 2500 | 0.4572 | 0.2450 |
| 0.062 | 24.0 | 3000 | 0.4385 | 0.2353 |
| 0.0469 | 28.0 | 3500 | 0.4520 | 0.2286 |
### Framework versions
- Transformers 4.11.3
- Pytorch 1.10.0+cu111
- Datasets 1.18.3
- Tokenizers 0.10.3
|
negfir/bert_uncased_L-2_H-256_A-4wiki103 | da9f334409f11bbc38fb634b81077f23475896bd | 2022-04-21T02:25:12.000Z | [
"pytorch",
"bert",
"fill-mask",
"transformers",
"autotrain_compatible"
] | fill-mask | false | negfir | null | negfir/bert_uncased_L-2_H-256_A-4wiki103 | 1 | null | transformers | 31,365 | Entry not found |
negfir/bert_uncased_L-2_H-128_A-2wiki103 | d84479ebc3280b0a3631d1bab1464790b94243dd | 2022-04-21T03:14:53.000Z | [
"pytorch",
"bert",
"fill-mask",
"transformers",
"autotrain_compatible"
] | fill-mask | false | negfir | null | negfir/bert_uncased_L-2_H-128_A-2wiki103 | 1 | null | transformers | 31,366 | Entry not found |
DongHyoungLee/oubiobert-tokenclassification-2layers-init | 753c98c096738130156a7d617b9e8f27fe594a1b | 2022-04-21T08:55:38.000Z | [
"pytorch",
"bert",
"transformers"
] | null | false | DongHyoungLee | null | DongHyoungLee/oubiobert-tokenclassification-2layers-init | 1 | null | transformers | 31,367 | Entry not found |
umanlp/TOD-XLMR | 2aaeb95e444bc679dd922502996f8fff8eae9a65 | 2022-05-02T14:16:51.000Z | [
"pytorch",
"xlm-roberta",
"fill-mask",
"multilingual",
"transformers",
"exbert",
"license:mit",
"autotrain_compatible"
] | fill-mask | false | umanlp | null | umanlp/TOD-XLMR | 1 | 2 | transformers | 31,368 | ---
tags:
- exbert
language: multilingual
license: mit
---
# TOD-XLMR
TOD-XLMR is a conversationally specialized multilingual version based on [XLM-RoBERTa](https://huggingface.co/xlm-roberta-base). It is pre-trained on English conversational corpora consisting of nine human-to-human multi-turn task-oriented dialog (TOD) datasets as proposed in the paper [TOD-BERT: Pre-trained Natural Language Understanding for Task-Oriented Dialogue](https://aclanthology.org/2020.emnlp-main.66.pdf) by Wu et al. and first released in [this repository](https://huggingface.co/TODBERT).
The model is jointly trained with two objectives as proposed in TOD-BERT, including masked language modeling (MLM) and response contrastive loss (RCL). Masked language modeling is a common pretraining strategy utilized for BERT-based architectures, where a random sample of tokens in the input sequence is replaced with the special token [MASK] for predicting the original masked tokens. To further encourage the model to capture dialogic structure (i.e., dialog sequential order), response contrastive loss is implemented by using in-batch negative training with contrastive learning.
### How to use
Here is how to use this model to get the features of a given text in PyTorch:
```
from transformers import AutoTokenizer, AutoModelForMaskedLM
tokenizer = AutoTokenizer.from_pretrained("umanlp/TOD-XLMR")
model = AutoModelForMaskedLM.from_pretrained("umanlp/TOD-XLMR")
# prepare input
text = "Replace me by any text you'd like."
encoded_input = tokenizer(text, return_tensors='pt')
# forward pass
output = model(**encoded_input)
```
Or you can also use `AutoModel` to load the pretrained model and further apply to downstream tasks:
```
from transformers import AutoTokenizer, AutoModel
tokenizer = AutoTokenizer.from_pretrained("umanlp/TOD-XLMR")
model = AutoModel("umanlp/TOD-XLMR")
# prepare input
text = "Replace me by any text you'd like."
encoded_input = tokenizer(text, return_tensors='pt')
# forward pass
output = model(**encoded_input)
```
|
lamyae/distilroberta-base-finetuned-wikitext2 | afd343178237cef73d24917d7180d843b11e2219 | 2022-04-21T12:48:59.000Z | [
"pytorch",
"tensorboard",
"roberta",
"fill-mask",
"transformers",
"generated_from_trainer",
"license:apache-2.0",
"model-index",
"autotrain_compatible"
] | fill-mask | false | lamyae | null | lamyae/distilroberta-base-finetuned-wikitext2 | 1 | null | transformers | 31,369 | ---
license: apache-2.0
tags:
- generated_from_trainer
model-index:
- name: distilroberta-base-finetuned-wikitext2
results: []
---
<!-- This model card has been generated automatically according to the information the Trainer had access to. You
should probably proofread and complete it, then remove this comment. -->
# distilroberta-base-finetuned-wikitext2
This model is a fine-tuned version of [distilroberta-base](https://huggingface.co/distilroberta-base) on the None dataset.
It achieves the following results on the evaluation set:
- Loss: 3.0917
## Model description
More information needed
## Intended uses & limitations
More information needed
## Training and evaluation data
More information needed
## Training procedure
### Training hyperparameters
The following hyperparameters were used during training:
- learning_rate: 2e-05
- train_batch_size: 8
- eval_batch_size: 8
- seed: 42
- optimizer: Adam with betas=(0.9,0.999) and epsilon=1e-08
- lr_scheduler_type: linear
- num_epochs: 3.0
### Training results
| Training Loss | Epoch | Step | Validation Loss |
|:-------------:|:-----:|:----:|:---------------:|
| No log | 1.0 | 9 | 3.3324 |
| No log | 2.0 | 18 | 3.1066 |
| No log | 3.0 | 27 | 3.2930 |
### Framework versions
- Transformers 4.18.0
- Pytorch 1.10.0+cu111
- Datasets 2.1.0
- Tokenizers 0.12.1
|
huggingtweets/kfc_uki | 63587bae2bf8e940be0dfa91a268ee140cda6ff1 | 2022-04-21T13:52:15.000Z | [
"pytorch",
"gpt2",
"text-generation",
"en",
"transformers",
"huggingtweets"
] | text-generation | false | huggingtweets | null | huggingtweets/kfc_uki | 1 | null | transformers | 31,370 | ---
language: en
thumbnail: http://www.huggingtweets.com/kfc_uki/1650549131420/predictions.png
tags:
- huggingtweets
widget:
- text: "My dream is"
---
<div class="inline-flex flex-col" style="line-height: 1.5;">
<div class="flex">
<div
style="display:inherit; margin-left: 4px; margin-right: 4px; width: 92px; height:92px; border-radius: 50%; background-size: cover; background-image: url('https://pbs.twimg.com/profile_images/1062716172418699265/ObupAaDb_400x400.jpg')">
</div>
<div
style="display:none; margin-left: 4px; margin-right: 4px; width: 92px; height:92px; border-radius: 50%; background-size: cover; background-image: url('')">
</div>
<div
style="display:none; margin-left: 4px; margin-right: 4px; width: 92px; height:92px; border-radius: 50%; background-size: cover; background-image: url('')">
</div>
</div>
<div style="text-align: center; margin-top: 3px; font-size: 16px; font-weight: 800">🤖 AI BOT 🤖</div>
<div style="text-align: center; font-size: 16px; font-weight: 800">KFC UK</div>
<div style="text-align: center; font-size: 14px;">@kfc_uki</div>
</div>
I was made with [huggingtweets](https://github.com/borisdayma/huggingtweets).
Create your own bot based on your favorite user with [the demo](https://colab.research.google.com/github/borisdayma/huggingtweets/blob/master/huggingtweets-demo.ipynb)!
## How does it work?
The model uses the following pipeline.
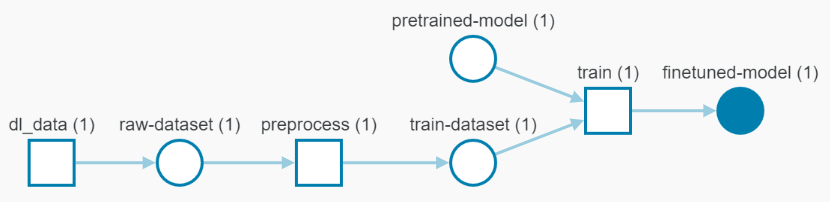
To understand how the model was developed, check the [W&B report](https://wandb.ai/wandb/huggingtweets/reports/HuggingTweets-Train-a-Model-to-Generate-Tweets--VmlldzoxMTY5MjI).
## Training data
The model was trained on tweets from KFC UK.
| Data | KFC UK |
| --- | --- |
| Tweets downloaded | 3250 |
| Retweets | 4 |
| Short tweets | 596 |
| Tweets kept | 2650 |
[Explore the data](https://wandb.ai/wandb/huggingtweets/runs/1x91e62j/artifacts), which is tracked with [W&B artifacts](https://docs.wandb.com/artifacts) at every step of the pipeline.
## Training procedure
The model is based on a pre-trained [GPT-2](https://huggingface.co/gpt2) which is fine-tuned on @kfc_uki's tweets.
Hyperparameters and metrics are recorded in the [W&B training run](https://wandb.ai/wandb/huggingtweets/runs/3auxmk8k) for full transparency and reproducibility.
At the end of training, [the final model](https://wandb.ai/wandb/huggingtweets/runs/3auxmk8k/artifacts) is logged and versioned.
## How to use
You can use this model directly with a pipeline for text generation:
```python
from transformers import pipeline
generator = pipeline('text-generation',
model='huggingtweets/kfc_uki')
generator("My dream is", num_return_sequences=5)
```
## Limitations and bias
The model suffers from [the same limitations and bias as GPT-2](https://huggingface.co/gpt2#limitations-and-bias).
In addition, the data present in the user's tweets further affects the text generated by the model.
## About
*Built by Boris Dayma*
[](https://twitter.com/intent/follow?screen_name=borisdayma)
For more details, visit the project repository.
[](https://github.com/borisdayma/huggingtweets)
|
Onlydrinkwater/T5-small-de-en | 687360587c344dd24f160b7c89ee11bc0ef4bab7 | 2022-04-21T16:52:00.000Z | [
"pytorch",
"t5",
"text2text-generation",
"transformers",
"autotrain_compatible"
] | text2text-generation | false | Onlydrinkwater | null | Onlydrinkwater/T5-small-de-en | 1 | null | transformers | 31,371 | Entry not found |
negfir/bert_uncased_L-8_H-768_A-12wiki103 | 5b08990331ddecb50e381a466491768ed1033672 | 2022-04-21T17:24:12.000Z | [
"pytorch",
"bert",
"fill-mask",
"transformers",
"autotrain_compatible"
] | fill-mask | false | negfir | null | negfir/bert_uncased_L-8_H-768_A-12wiki103 | 1 | null | transformers | 31,372 | Entry not found |
negfir/bert_uncased_L-8_H-512_A-8wiki103 | 637979d201a719dff8e79253ca35e5d266e001f2 | 2022-04-21T20:07:07.000Z | [
"pytorch",
"bert",
"fill-mask",
"transformers",
"autotrain_compatible"
] | fill-mask | false | negfir | null | negfir/bert_uncased_L-8_H-512_A-8wiki103 | 1 | null | transformers | 31,373 | Entry not found |
surajnair/r3m-34 | ae6d653f2ab737c79be68f705c30f4fbd645d782 | 2022-04-21T20:32:46.000Z | [
"pytorch",
"r3m",
"transformers"
] | null | false | surajnair | null | surajnair/r3m-34 | 1 | null | transformers | 31,374 | This model contains the pre-trained ResNet34 R3M model from the paper "R3M: A Universal Visual Representation for Robot Manipulation" (Nair et al.) The model is trained on the Ego4D dataset using time-contrastive learning, video-language alignment, and sparsity objectives. It is used for efficient downstream robotic learning.
|
surajnair/r3m-18 | 1a4f077fe01db52c8f5d9f8d6641b6e03f688420 | 2022-04-21T20:32:32.000Z | [
"pytorch",
"r3m",
"transformers"
] | null | false | surajnair | null | surajnair/r3m-18 | 1 | null | transformers | 31,375 | This model contains the pre-trained ResNet18 R3M model from the paper "R3M: A Universal Visual Representation for Robot Manipulation" (Nair et al.) The model is trained on the Ego4D dataset using time-contrastive learning, video-language alignment, and sparsity objectives. It is used for efficient downstream robotic learning.
|
masakhane/afrimt5_en_ibo_news | fa320c776ce021828967c899ba731132186be989 | 2022-04-22T09:40:53.000Z | [
"pytorch",
"mt5",
"text2text-generation",
"transformers",
"license:afl-3.0",
"autotrain_compatible"
] | text2text-generation | false | masakhane | null | masakhane/afrimt5_en_ibo_news | 1 | null | transformers | 31,376 | ---
license: afl-3.0
---
|
masakhane/afrimt5_ibo_en_news | acb7de78a9e450ee8736ad2c23a2d889467e308e | 2022-04-22T09:40:56.000Z | [
"pytorch",
"mt5",
"text2text-generation",
"transformers",
"license:afl-3.0",
"autotrain_compatible"
] | text2text-generation | false | masakhane | null | masakhane/afrimt5_ibo_en_news | 1 | null | transformers | 31,377 | ---
license: afl-3.0
---
|
masakhane/afribyt5_en_ibo_news | 4c77ffe12790ef25dfba38f97fd90ed1546b12ee | 2022-04-22T10:50:19.000Z | [
"pytorch",
"t5",
"text2text-generation",
"transformers",
"license:afl-3.0",
"autotrain_compatible"
] | text2text-generation | false | masakhane | null | masakhane/afribyt5_en_ibo_news | 1 | null | transformers | 31,378 | ---
license: afl-3.0
---
|
masakhane/mbart50_en_ibo_news | 042cd9a8119f24a5f037409ed712543b2e04a009 | 2022-04-22T10:50:25.000Z | [
"pytorch",
"mbart",
"text2text-generation",
"transformers",
"license:afl-3.0",
"autotrain_compatible"
] | text2text-generation | false | masakhane | null | masakhane/mbart50_en_ibo_news | 1 | null | transformers | 31,379 | ---
license: afl-3.0
---
|
masakhane/m2m100_418M_ibo_en_news | 51ace50ecd1d8312b727294687016bdd8ba0682a | 2022-04-22T12:45:19.000Z | [
"pytorch",
"m2m_100",
"text2text-generation",
"transformers",
"license:afl-3.0",
"autotrain_compatible"
] | text2text-generation | false | masakhane | null | masakhane/m2m100_418M_ibo_en_news | 1 | null | transformers | 31,380 | ---
license: afl-3.0
---
|
masakhane/m2m100_418M_en_ibo_rel_news_ft | 48c2e9917fc68ed89ac131eb59b720d2bcec176c | 2022-04-22T13:49:32.000Z | [
"pytorch",
"m2m_100",
"text2text-generation",
"transformers",
"license:afl-3.0",
"autotrain_compatible"
] | text2text-generation | false | masakhane | null | masakhane/m2m100_418M_en_ibo_rel_news_ft | 1 | null | transformers | 31,381 | ---
license: afl-3.0
---
|
masakhane/m2m100_418M_ibo_en_rel_ft | db01073cdb7a9cbf2451b4d7f45e67d2a8e1bb86 | 2022-04-22T13:49:24.000Z | [
"pytorch",
"m2m_100",
"text2text-generation",
"transformers",
"license:afl-3.0",
"autotrain_compatible"
] | text2text-generation | false | masakhane | null | masakhane/m2m100_418M_ibo_en_rel_ft | 1 | null | transformers | 31,382 | ---
license: afl-3.0
---
|
jjezabek/roberta-base-imdb | f93ff302737e204616b7b2020821bd61cc9ca417 | 2022-04-21T23:02:27.000Z | [
"pytorch"
] | null | false | jjezabek | null | jjezabek/roberta-base-imdb | 1 | null | null | 31,383 | Entry not found |
negfir/bert_uncased_L-8_H-128_A-2wiki103 | 64ab85572447822611637d3b7ad19591156efb65 | 2022-04-21T23:06:20.000Z | [
"pytorch",
"bert",
"fill-mask",
"transformers",
"autotrain_compatible"
] | fill-mask | false | negfir | null | negfir/bert_uncased_L-8_H-128_A-2wiki103 | 1 | null | transformers | 31,384 | Entry not found |
Scaprod/DialoGPT-small-arbiter | 94130be2e3403f7c5fbd6d8a28665535825f1ff6 | 2022-04-23T23:18:21.000Z | [
"pytorch",
"gpt2",
"text-generation",
"transformers",
"conversational"
] | conversational | false | Scaprod | null | Scaprod/DialoGPT-small-arbiter | 1 | null | transformers | 31,385 | ---
tags:
- conversational
---
# Arbiter DialoGPT Model |
obokkkk/wav2vec2-base-960h-timit-demo-colab | e0d91c4ba9bf9444ad4ec98db98b809b29580328 | 2022-04-22T04:45:54.000Z | [
"pytorch",
"tensorboard",
"wav2vec2",
"automatic-speech-recognition",
"transformers",
"generated_from_trainer",
"license:apache-2.0",
"model-index"
] | automatic-speech-recognition | false | obokkkk | null | obokkkk/wav2vec2-base-960h-timit-demo-colab | 1 | 1 | transformers | 31,386 | ---
license: apache-2.0
tags:
- generated_from_trainer
model-index:
- name: wav2vec2-base-960h-timit-demo-colab
results: []
---
<!-- This model card has been generated automatically according to the information the Trainer had access to. You
should probably proofread and complete it, then remove this comment. -->
# wav2vec2-base-960h-timit-demo-colab
This model is a fine-tuned version of [facebook/wav2vec2-base-960h](https://huggingface.co/facebook/wav2vec2-base-960h) on the None dataset.
It achieves the following results on the evaluation set:
- Loss: 0.2002
- Wer: 0.2160
## Model description
More information needed
## Intended uses & limitations
More information needed
## Training and evaluation data
More information needed
## Training procedure
### Training hyperparameters
The following hyperparameters were used during training:
- learning_rate: 0.0001
- train_batch_size: 32
- eval_batch_size: 8
- seed: 42
- optimizer: Adam with betas=(0.9,0.999) and epsilon=1e-08
- lr_scheduler_type: linear
- lr_scheduler_warmup_steps: 1000
- num_epochs: 30
- mixed_precision_training: Native AMP
### Training results
| Training Loss | Epoch | Step | Validation Loss | Wer |
|:-------------:|:-----:|:----:|:---------------:|:------:|
| 5.7805 | 4.0 | 500 | 3.0558 | 1.0 |
| 2.2936 | 8.0 | 1000 | 0.2937 | 0.3479 |
| 0.4155 | 12.0 | 1500 | 0.2108 | 0.2473 |
| 0.2439 | 16.0 | 2000 | 0.2313 | 0.2391 |
| 0.1617 | 20.0 | 2500 | 0.2003 | 0.2255 |
| 0.1443 | 24.0 | 3000 | 0.2175 | 0.2207 |
| 0.119 | 28.0 | 3500 | 0.2002 | 0.2160 |
### Framework versions
- Transformers 4.11.3
- Pytorch 1.10.0+cu111
- Datasets 1.18.3
- Tokenizers 0.10.3
|
obokkkk/hubert-large-ls960-ft-timit | b0ee8ea5cdf2aaf82bfa02dedb9c86fcf0dec4f2 | 2022-04-22T08:44:25.000Z | [
"pytorch",
"tensorboard",
"hubert",
"automatic-speech-recognition",
"transformers",
"generated_from_trainer",
"license:apache-2.0",
"model-index"
] | automatic-speech-recognition | false | obokkkk | null | obokkkk/hubert-large-ls960-ft-timit | 1 | null | transformers | 31,387 | ---
license: apache-2.0
tags:
- generated_from_trainer
model-index:
- name: hubert-large-ls960-ft-timit
results: []
---
<!-- This model card has been generated automatically according to the information the Trainer had access to. You
should probably proofread and complete it, then remove this comment. -->
# hubert-large-ls960-ft-timit
This model is a fine-tuned version of [facebook/hubert-large-ls960-ft](https://huggingface.co/facebook/hubert-large-ls960-ft) on the None dataset.
It achieves the following results on the evaluation set:
- Loss: 0.1074
- Wer: 0.1708
## Model description
More information needed
## Intended uses & limitations
More information needed
## Training and evaluation data
More information needed
## Training procedure
### Training hyperparameters
The following hyperparameters were used during training:
- learning_rate: 0.0001
- train_batch_size: 32
- eval_batch_size: 8
- seed: 42
- optimizer: Adam with betas=(0.9,0.999) and epsilon=1e-08
- lr_scheduler_type: linear
- lr_scheduler_warmup_steps: 1000
- num_epochs: 30
- mixed_precision_training: Native AMP
### Training results
| Training Loss | Epoch | Step | Validation Loss | Wer |
|:-------------:|:-----:|:----:|:---------------:|:------:|
| 6.2278 | 4.0 | 500 | 2.6282 | 0.9999 |
| 0.9389 | 8.0 | 1000 | 0.1154 | 0.2096 |
| 0.2005 | 12.0 | 1500 | 0.0951 | 0.1732 |
| 0.1985 | 16.0 | 2000 | 0.0974 | 0.1759 |
| 0.124 | 20.0 | 2500 | 0.0951 | 0.1728 |
| 0.0797 | 24.0 | 3000 | 0.1064 | 0.1713 |
| 0.1047 | 28.0 | 3500 | 0.1074 | 0.1708 |
### Framework versions
- Transformers 4.11.3
- Pytorch 1.10.0+cu111
- Datasets 1.18.3
- Tokenizers 0.10.3
|
proseph/ctrlv-speechrecognition-model | 094e5a53c778f4777d41da7fcee4e785b60fb9b1 | 2022-05-19T09:59:57.000Z | [
"pytorch",
"tensorboard",
"wav2vec2",
"automatic-speech-recognition",
"transformers",
"generated_from_trainer",
"license:apache-2.0",
"model-index"
] | automatic-speech-recognition | false | proseph | null | proseph/ctrlv-speechrecognition-model | 1 | 1 | transformers | 31,388 | ---
license: apache-2.0
tags:
- generated_from_trainer
model-index:
- name: ctrlv-speechrecognition-model
results: []
---
<!-- This model card has been generated automatically according to the information the Trainer had access to. You
should probably proofread and complete it, then remove this comment. -->
# ctrlv-speechrecognition-model
This model is a fine-tuned version of [facebook/wav2vec2-base](https://huggingface.co/facebook/wav2vec2-base) on the TIMIT dataset.
It achieves the following results on the evaluation set:
- Loss: 0.4730
- Wer: 0.3031
## Test WER in TIMIT dataset
- Wer: 0.189
[Google Colab Notebook](https://colab.research.google.com/drive/1M9ZbqvoRqshEccIlpTQGsgptpiGVgauH)
## Model description
More information needed
## Intended uses & limitations
More information needed
## Training and evaluation data
More information needed
## Training procedure
### Training hyperparameters
The following hyperparameters were used during training:
- learning_rate: 0.0001
- train_batch_size: 32
- eval_batch_size: 8
- seed: 42
- optimizer: Adam with betas=(0.9,0.999) and epsilon=1e-08
- lr_scheduler_type: linear
- lr_scheduler_warmup_steps: 1000
- num_epochs: 60
- mixed_precision_training: Native AMP
### Training results
| Training Loss | Epoch | Step | Validation Loss | Wer |
|:-------------:|:-----:|:----:|:---------------:|:------:|
| 3.53 | 3.45 | 500 | 1.4021 | 0.9307 |
| 0.6077 | 6.9 | 1000 | 0.4255 | 0.4353 |
| 0.2331 | 10.34 | 1500 | 0.3887 | 0.3650 |
| 0.1436 | 13.79 | 2000 | 0.3579 | 0.3393 |
| 0.1021 | 17.24 | 2500 | 0.4447 | 0.3440 |
| 0.0797 | 20.69 | 3000 | 0.4041 | 0.3291 |
| 0.0657 | 24.14 | 3500 | 0.4262 | 0.3368 |
| 0.0525 | 27.59 | 4000 | 0.4937 | 0.3429 |
| 0.0454 | 31.03 | 4500 | 0.4449 | 0.3244 |
| 0.0373 | 34.48 | 5000 | 0.4363 | 0.3288 |
| 0.0321 | 37.93 | 5500 | 0.4519 | 0.3204 |
| 0.0288 | 41.38 | 6000 | 0.4440 | 0.3145 |
| 0.0259 | 44.83 | 6500 | 0.4691 | 0.3182 |
| 0.0203 | 48.28 | 7000 | 0.5062 | 0.3162 |
| 0.0171 | 51.72 | 7500 | 0.4762 | 0.3129 |
| 0.0166 | 55.17 | 8000 | 0.4772 | 0.3090 |
| 0.0147 | 58.62 | 8500 | 0.4730 | 0.3031 |
### Framework versions
- Transformers 4.11.3
- Pytorch 1.10.0+cu111
- Datasets 1.18.3
- Tokenizers 0.10.3 |
Khalsuu/filipino-wav2vec2-l-xls-r-300m-test | 024952b9b990b6609be5bd85bb9cfbe6e37019c4 | 2022-04-23T08:27:45.000Z | [
"pytorch",
"tensorboard",
"wav2vec2",
"automatic-speech-recognition",
"dataset:filipino_voice",
"transformers",
"generated_from_trainer",
"license:apache-2.0",
"model-index"
] | automatic-speech-recognition | false | Khalsuu | null | Khalsuu/filipino-wav2vec2-l-xls-r-300m-test | 1 | null | transformers | 31,389 | ---
license: apache-2.0
tags:
- generated_from_trainer
datasets:
- filipino_voice
model-index:
- name: filipino-wav2vec2-l-xls-r-300m-test
results: []
---
<!-- This model card has been generated automatically according to the information the Trainer had access to. You
should probably proofread and complete it, then remove this comment. -->
# filipino-wav2vec2-l-xls-r-300m-test
This model is a fine-tuned version of [facebook/wav2vec2-xls-r-300m](https://huggingface.co/facebook/wav2vec2-xls-r-300m) on the filipino_voice dataset.
It achieves the following results on the evaluation set:
- Loss: 0.7753
- Wer: 0.4831
## Model description
More information needed
## Intended uses & limitations
More information needed
## Training and evaluation data
More information needed
## Training procedure
### Training hyperparameters
The following hyperparameters were used during training:
- learning_rate: 0.001
- train_batch_size: 8
- eval_batch_size: 8
- seed: 42
- gradient_accumulation_steps: 2
- total_train_batch_size: 16
- optimizer: Adam with betas=(0.9,0.999) and epsilon=1e-08
- lr_scheduler_type: linear
- lr_scheduler_warmup_steps: 500
- num_epochs: 20
- mixed_precision_training: Native AMP
### Training results
| Training Loss | Epoch | Step | Validation Loss | Wer |
|:-------------:|:-----:|:----:|:---------------:|:------:|
| 2.7314 | 2.09 | 400 | 0.7541 | 0.7262 |
| 0.6065 | 4.19 | 800 | 0.6738 | 0.6314 |
| 0.4063 | 6.28 | 1200 | 0.6310 | 0.5992 |
| 0.2986 | 8.38 | 1600 | 0.6301 | 0.5340 |
| 0.2263 | 10.47 | 2000 | 0.6598 | 0.5391 |
| 0.1714 | 12.57 | 2400 | 0.7778 | 0.5593 |
| 0.1303 | 14.66 | 2800 | 0.7231 | 0.4907 |
| 0.1056 | 16.75 | 3200 | 0.8031 | 0.4885 |
| 0.0851 | 18.85 | 3600 | 0.7753 | 0.4831 |
### Framework versions
- Transformers 4.11.3
- Pytorch 1.10.0+cu111
- Datasets 1.18.3
- Tokenizers 0.10.3
|
stevems1/bert-base-uncased-Ganesh123 | fcea1a7026eb341123610d0c1bbfa2a494fb4006 | 2022-04-22T07:46:54.000Z | [
"pytorch",
"tensorboard",
"bert",
"fill-mask",
"transformers",
"generated_from_trainer",
"model-index",
"autotrain_compatible"
] | fill-mask | false | stevems1 | null | stevems1/bert-base-uncased-Ganesh123 | 1 | null | transformers | 31,390 | ---
tags:
- generated_from_trainer
model-index:
- name: bert-base-uncased-Ganesh123
results: []
---
<!-- This model card has been generated automatically according to the information the Trainer had access to. You
should probably proofread and complete it, then remove this comment. -->
# bert-base-uncased-Ganesh123
This model is a fine-tuned version of [](https://huggingface.co/) on an unknown dataset.
## Model description
More information needed
## Intended uses & limitations
More information needed
## Training and evaluation data
More information needed
## Training procedure
### Training hyperparameters
The following hyperparameters were used during training:
- learning_rate: 5e-05
- train_batch_size: 32
- eval_batch_size: 8
- seed: 42
- optimizer: Adam with betas=(0.9,0.999) and epsilon=1e-08
- lr_scheduler_type: linear
- num_epochs: 1
### Training results
### Framework versions
- Transformers 4.18.0
- Pytorch 1.10.0+cu111
- Datasets 2.1.0
- Tokenizers 0.12.1
|
Vishfeb27/wav2vec2-base-timit-demo-colab | 843bf4520279d9036d0df3917a1e0d1924f8e49d | 2022-04-22T11:31:09.000Z | [
"pytorch",
"tensorboard",
"wav2vec2",
"automatic-speech-recognition",
"transformers",
"generated_from_trainer",
"license:apache-2.0",
"model-index"
] | automatic-speech-recognition | false | Vishfeb27 | null | Vishfeb27/wav2vec2-base-timit-demo-colab | 1 | null | transformers | 31,391 | ---
license: apache-2.0
tags:
- generated_from_trainer
model-index:
- name: wav2vec2-base-timit-demo-colab
results: []
---
<!-- This model card has been generated automatically according to the information the Trainer had access to. You
should probably proofread and complete it, then remove this comment. -->
# wav2vec2-base-timit-demo-colab
This model is a fine-tuned version of [facebook/wav2vec2-base](https://huggingface.co/facebook/wav2vec2-base) on the None dataset.
## Model description
More information needed
## Intended uses & limitations
More information needed
## Training and evaluation data
More information needed
## Training procedure
### Training hyperparameters
The following hyperparameters were used during training:
- learning_rate: 0.0001
- train_batch_size: 32
- eval_batch_size: 8
- seed: 42
- optimizer: Adam with betas=(0.9,0.999) and epsilon=1e-08
- lr_scheduler_type: linear
- lr_scheduler_warmup_steps: 1000
- num_epochs: 1
- mixed_precision_training: Native AMP
### Training results
### Framework versions
- Transformers 4.11.3
- Pytorch 1.10.0+cu111
- Datasets 1.18.3
- Tokenizers 0.10.3
|
spuun/kekbot-beta-1 | c710f76dfbef650e1e32989b36b29d8ad5791379 | 2022-04-22T14:32:20.000Z | [
"pytorch",
"gpt2",
"text-generation",
"transformers",
"conversational",
"license:cc-by-nc-sa-4.0"
] | conversational | false | spuun | null | spuun/kekbot-beta-1 | 1 | null | transformers | 31,392 | ---
tags:
- conversational
license: cc-by-nc-sa-4.0
---
|
alifabdulR/nn | 9d3edf6c053d57e63d349f409088dca125e72a15 | 2022-04-22T15:48:52.000Z | [
"pytorch",
"wav2vec2",
"automatic-speech-recognition",
"transformers"
] | automatic-speech-recognition | false | alifabdulR | null | alifabdulR/nn | 1 | null | transformers | 31,393 | Entry not found |
mimicheng/codeparrot-ds-sample-2ep-batchsize32 | 9ae34042702f9975f8688a91d6d000ddff5c2b2a | 2022-04-23T01:54:26.000Z | [
"pytorch",
"tensorboard",
"gpt2",
"text-generation",
"transformers",
"generated_from_trainer",
"license:mit",
"model-index"
] | text-generation | false | mimicheng | null | mimicheng/codeparrot-ds-sample-2ep-batchsize32 | 1 | null | transformers | 31,394 | ---
license: mit
tags:
- generated_from_trainer
model-index:
- name: codeparrot-ds-sample-2ep-batchsize32
results: []
---
<!-- This model card has been generated automatically according to the information the Trainer had access to. You
should probably proofread and complete it, then remove this comment. -->
# codeparrot-ds-sample-2ep-batchsize32
This model is a fine-tuned version of [gpt2](https://huggingface.co/gpt2) on an unknown dataset.
It achieves the following results on the evaluation set:
- Loss: 1.5721
## Model description
More information needed
## Intended uses & limitations
More information needed
## Training and evaluation data
More information needed
## Training procedure
### Training hyperparameters
The following hyperparameters were used during training:
- learning_rate: 0.0005
- train_batch_size: 32
- eval_batch_size: 32
- seed: 42
- distributed_type: tpu
- gradient_accumulation_steps: 8
- total_train_batch_size: 256
- optimizer: Adam with betas=(0.9,0.999) and epsilon=1e-08
- lr_scheduler_type: cosine
- lr_scheduler_warmup_steps: 1000
- num_epochs: 2
### Training results
| Training Loss | Epoch | Step | Validation Loss |
|:-------------:|:-----:|:-----:|:---------------:|
| 4.3529 | 0.19 | 1000 | 2.8073 |
| 2.4602 | 0.37 | 2000 | 2.2907 |
| 2.1127 | 0.56 | 3000 | 2.0745 |
| 1.9187 | 0.74 | 4000 | 1.9287 |
| 1.782 | 0.93 | 5000 | 1.8234 |
| 1.639 | 1.11 | 6000 | 1.7456 |
| 1.5519 | 1.3 | 7000 | 1.6738 |
| 1.489 | 1.49 | 8000 | 1.6235 |
| 1.4372 | 1.67 | 9000 | 1.5874 |
| 1.4122 | 1.86 | 10000 | 1.5721 |
### Framework versions
- Transformers 4.18.0
- Pytorch 1.11.0+cu102
- Datasets 2.1.0
- Tokenizers 0.12.1
|
negfir/bert_uncased_L-6_H-768_A-12wiki103 | 8c800f03dc1a873d62c4eeade3398246b14fbb98 | 2022-04-22T18:11:42.000Z | [
"pytorch",
"bert",
"fill-mask",
"transformers",
"autotrain_compatible"
] | fill-mask | false | negfir | null | negfir/bert_uncased_L-6_H-768_A-12wiki103 | 1 | null | transformers | 31,395 | Entry not found |
princeton-nlp/efficient_mlm_m0.15 | f0bc11138f73eccefadb049194e01744836e6a5c | 2022-04-27T18:54:34.000Z | [
"pytorch",
"roberta",
"fill-mask",
"arxiv:2202.08005",
"transformers",
"autotrain_compatible"
] | fill-mask | false | princeton-nlp | null | princeton-nlp/efficient_mlm_m0.15 | 1 | null | transformers | 31,396 | ---
inference: false
---
This is a model checkpoint for ["Should You Mask 15% in Masked Language Modeling"](https://arxiv.org/abs/2202.08005) [(code)](https://github.com/princeton-nlp/DinkyTrain.git). We use pre layer norm, which is not supported by HuggingFace. To use our model, go to our [github repo](https://github.com/princeton-nlp/DinkyTrain.git), download our code, and import the RoBERTa class from `huggingface/modeling_roberta_prelayernorm.py`. For example,
``` bash
from huggingface.modeling_roberta_prelayernorm import RobertaForMaskedLM, RobertaForSequenceClassification
``` |
princeton-nlp/efficient_mlm_m0.40 | 08e44702760ae2ed21cf92d100a82bce4f72f13b | 2022-04-27T18:54:13.000Z | [
"pytorch",
"roberta",
"fill-mask",
"arxiv:2202.08005",
"transformers",
"autotrain_compatible"
] | fill-mask | false | princeton-nlp | null | princeton-nlp/efficient_mlm_m0.40 | 1 | null | transformers | 31,397 | ---
inference: false
---
This is a model checkpoint for ["Should You Mask 15% in Masked Language Modeling"](https://arxiv.org/abs/2202.08005) [(code)](https://github.com/princeton-nlp/DinkyTrain.git). We use pre layer norm, which is not supported by HuggingFace. To use our model, go to our [github repo](https://github.com/princeton-nlp/DinkyTrain.git), download our code, and import the RoBERTa class from `huggingface/modeling_roberta_prelayernorm.py`. For example,
``` bash
from huggingface.modeling_roberta_prelayernorm import RobertaForMaskedLM, RobertaForSequenceClassification
``` |
princeton-nlp/efficient_mlm_m0.15-801010 | 490aba78955a05e7139921646d2bfc48cad555bc | 2022-04-27T18:54:45.000Z | [
"pytorch",
"roberta",
"fill-mask",
"arxiv:2202.08005",
"transformers",
"autotrain_compatible"
] | fill-mask | false | princeton-nlp | null | princeton-nlp/efficient_mlm_m0.15-801010 | 1 | null | transformers | 31,398 | ---
inference: false
---
This is a model checkpoint for ["Should You Mask 15% in Masked Language Modeling"](https://arxiv.org/abs/2202.08005) [(code)](https://github.com/princeton-nlp/DinkyTrain.git). We use pre layer norm, which is not supported by HuggingFace. To use our model, go to our [github repo](https://github.com/princeton-nlp/DinkyTrain.git), download our code, and import the RoBERTa class from `huggingface/modeling_roberta_prelayernorm.py`. For example,
``` bash
from huggingface.modeling_roberta_prelayernorm import RobertaForMaskedLM, RobertaForSequenceClassification
``` |
AntoDono/DialoGPT-Bopy | 83d24c33c97f89a0f4c4e3bb1eeb7659d8e980d0 | 2022-04-22T19:02:06.000Z | [
"pytorch",
"gpt2",
"text-generation",
"transformers"
] | text-generation | false | AntoDono | null | AntoDono/DialoGPT-Bopy | 1 | null | transformers | 31,399 | Entry not found |
Subsets and Splits
No community queries yet
The top public SQL queries from the community will appear here once available.