modelId
stringlengths 5
139
| author
stringlengths 2
42
| last_modified
timestamp[us, tz=UTC]date 2020-02-15 11:33:14
2025-07-16 00:42:46
| downloads
int64 0
223M
| likes
int64 0
11.7k
| library_name
stringclasses 522
values | tags
listlengths 1
4.05k
| pipeline_tag
stringclasses 55
values | createdAt
timestamp[us, tz=UTC]date 2022-03-02 23:29:04
2025-07-16 00:42:16
| card
stringlengths 11
1.01M
|
---|---|---|---|---|---|---|---|---|---|
Omaeriahi/interview001 | Omaeriahi | 2025-04-27T09:00:46Z | 0 | 0 | transformers | [
"transformers",
"safetensors",
"llama",
"text-generation",
"text-generation-inference",
"unsloth",
"trl",
"sft",
"conversational",
"en",
"license:apache-2.0",
"autotrain_compatible",
"endpoints_compatible",
"4-bit",
"bitsandbytes",
"region:us"
]
| text-generation | 2025-04-27T08:58:00Z | ---
base_model: unsloth/llama-3.2-3b-instruct-unsloth-bnb-4bit
tags:
- text-generation-inference
- transformers
- unsloth
- llama
- trl
- sft
license: apache-2.0
language:
- en
---
# Uploaded model
- **Developed by:** Omaeriahi
- **License:** apache-2.0
- **Finetuned from model :** unsloth/llama-3.2-3b-instruct-unsloth-bnb-4bit
This llama model was trained 2x faster with [Unsloth](https://github.com/unslothai/unsloth) and Huggingface's TRL library.
[<img src="https://raw.githubusercontent.com/unslothai/unsloth/main/images/unsloth%20made%20with%20love.png" width="200"/>](https://github.com/unslothai/unsloth)
|
mlfoundations-dev/c1_code_nod_16s_10k | mlfoundations-dev | 2025-04-27T09:00:40Z | 0 | 0 | transformers | [
"transformers",
"safetensors",
"qwen2",
"text-generation",
"llama-factory",
"full",
"generated_from_trainer",
"conversational",
"base_model:Qwen/Qwen2.5-7B-Instruct",
"base_model:finetune:Qwen/Qwen2.5-7B-Instruct",
"license:apache-2.0",
"autotrain_compatible",
"text-generation-inference",
"endpoints_compatible",
"region:us"
]
| text-generation | 2025-04-27T03:51:04Z | ---
library_name: transformers
license: apache-2.0
base_model: Qwen/Qwen2.5-7B-Instruct
tags:
- llama-factory
- full
- generated_from_trainer
model-index:
- name: c1_code_nod_16s_10k
results: []
---
<!-- This model card has been generated automatically according to the information the Trainer had access to. You
should probably proofread and complete it, then remove this comment. -->
# c1_code_nod_16s_10k
This model is a fine-tuned version of [Qwen/Qwen2.5-7B-Instruct](https://huggingface.co/Qwen/Qwen2.5-7B-Instruct) on the mlfoundations-dev/c1_code_nod_16s_10k dataset.
## Model description
More information needed
## Intended uses & limitations
More information needed
## Training and evaluation data
More information needed
## Training procedure
### Training hyperparameters
The following hyperparameters were used during training:
- learning_rate: 4e-05
- train_batch_size: 1
- eval_batch_size: 8
- seed: 42
- distributed_type: multi-GPU
- num_devices: 16
- gradient_accumulation_steps: 8
- total_train_batch_size: 128
- total_eval_batch_size: 128
- optimizer: Use OptimizerNames.ADAMW_TORCH with betas=(0.9,0.999) and epsilon=1e-08 and optimizer_args=No additional optimizer arguments
- lr_scheduler_type: cosine
- lr_scheduler_warmup_ratio: 0.1
- num_epochs: 5.0
### Training results
### Framework versions
- Transformers 4.46.1
- Pytorch 2.5.1
- Datasets 3.0.2
- Tokenizers 0.20.3
|
Ghazi-nak/results | Ghazi-nak | 2025-04-27T09:00:22Z | 0 | 0 | transformers | [
"transformers",
"tensorboard",
"safetensors",
"roberta",
"text-classification",
"generated_from_trainer",
"base_model:FacebookAI/roberta-base",
"base_model:finetune:FacebookAI/roberta-base",
"autotrain_compatible",
"endpoints_compatible",
"region:us"
]
| text-classification | 2025-04-27T08:59:42Z | ---
library_name: transformers
base_model: roberta-base
tags:
- generated_from_trainer
metrics:
- accuracy
model-index:
- name: results
results: []
---
<!-- This model card has been generated automatically according to the information the Trainer had access to. You
should probably proofread and complete it, then remove this comment. -->
# results
This model is a fine-tuned version of [roberta-base](https://huggingface.co/roberta-base) on an unknown dataset.
It achieves the following results on the evaluation set:
- Loss: 0.7984
- Accuracy: 0.705
## Model description
More information needed
## Intended uses & limitations
More information needed
## Training and evaluation data
More information needed
## Training procedure
### Training hyperparameters
The following hyperparameters were used during training:
- learning_rate: 5e-05
- train_batch_size: 8
- eval_batch_size: 16
- seed: 42
- gradient_accumulation_steps: 2
- total_train_batch_size: 16
- optimizer: Use OptimizerNames.ADAMW_TORCH with betas=(0.9,0.999) and epsilon=1e-08 and optimizer_args=No additional optimizer arguments
- lr_scheduler_type: linear
- lr_scheduler_warmup_steps: 200
- num_epochs: 1
### Training results
### Framework versions
- Transformers 4.51.3
- Pytorch 2.6.0+cu124
- Datasets 3.5.0
- Tokenizers 0.21.1
|
Nerva1228/qiufonvhai | Nerva1228 | 2025-04-27T08:59:43Z | 0 | 0 | diffusers | [
"diffusers",
"flux",
"lora",
"replicate",
"text-to-image",
"en",
"base_model:black-forest-labs/FLUX.1-dev",
"base_model:adapter:black-forest-labs/FLUX.1-dev",
"license:other",
"region:us"
]
| text-to-image | 2025-04-27T08:22:44Z | ---
license: other
license_name: flux-1-dev-non-commercial-license
license_link: https://huggingface.co/black-forest-labs/FLUX.1-dev/blob/main/LICENSE.md
language:
- en
tags:
- flux
- diffusers
- lora
- replicate
base_model: "black-forest-labs/FLUX.1-dev"
pipeline_tag: text-to-image
# widget:
# - text: >-
# prompt
# output:
# url: https://...
instance_prompt: qiufonvhai
---
# Qiufonvhai
<Gallery />
## About this LoRA
This is a [LoRA](https://replicate.com/docs/guides/working-with-loras) for the FLUX.1-dev text-to-image model. It can be used with diffusers or ComfyUI.
It was trained on [Replicate](https://replicate.com/) using AI toolkit: https://replicate.com/ostris/flux-dev-lora-trainer/train
## Trigger words
You should use `qiufonvhai` to trigger the image generation.
## Run this LoRA with an API using Replicate
```py
import replicate
input = {
"prompt": "qiufonvhai",
"lora_weights": "https://huggingface.co/Nerva1228/qiufonvhai/resolve/main/lora.safetensors"
}
output = replicate.run(
"black-forest-labs/flux-dev-lora",
input=input
)
for index, item in enumerate(output):
with open(f"output_{index}.webp", "wb") as file:
file.write(item.read())
```
## Use it with the [🧨 diffusers library](https://github.com/huggingface/diffusers)
```py
from diffusers import AutoPipelineForText2Image
import torch
pipeline = AutoPipelineForText2Image.from_pretrained('black-forest-labs/FLUX.1-dev', torch_dtype=torch.float16).to('cuda')
pipeline.load_lora_weights('Nerva1228/qiufonvhai', weight_name='lora.safetensors')
image = pipeline('qiufonvhai').images[0]
```
For more details, including weighting, merging and fusing LoRAs, check the [documentation on loading LoRAs in diffusers](https://huggingface.co/docs/diffusers/main/en/using-diffusers/loading_adapters)
## Training details
- Steps: 2000
- Learning rate: 0.0004
- LoRA rank: 16
## Contribute your own examples
You can use the [community tab](https://huggingface.co/Nerva1228/qiufonvhai/discussions) to add images that show off what you’ve made with this LoRA.
|
lexa862/NastyLora11 | lexa862 | 2025-04-27T08:55:11Z | 35 | 0 | diffusers | [
"diffusers",
"flux",
"text-to-image",
"lora",
"fal",
"base_model:black-forest-labs/FLUX.1-dev",
"base_model:adapter:black-forest-labs/FLUX.1-dev",
"license:other",
"region:us"
]
| text-to-image | 2025-04-25T19:22:40Z | ---
tags:
- flux
- text-to-image
- lora
- diffusers
- fal
base_model: black-forest-labs/FLUX.1-dev
instance_prompt: Nasty
license: other
license_name: flux-1-dev-non-commercial-license
license_link: https://huggingface.co/black-forest-labs/FLUX.1-dev/blob/main/LICENSE.md
---
# NastyLora11
<Gallery />
## Model description
-
## Trigger words
You should use `Nasty` to trigger the image generation.
## Download model
Weights for this model are available in Safetensors format.
[Download](/lexa862/NastyLora11/tree/main) them in the Files & versions tab.
## Training at fal.ai
Training was done using [fal.ai/models/fal-ai/flux-lora-fast-training](https://fal.ai/models/fal-ai/flux-lora-fast-training).
|
chanakya-varma/resume-classifier | chanakya-varma | 2025-04-27T08:54:56Z | 0 | 0 | transformers | [
"transformers",
"safetensors",
"distilbert",
"text-classification",
"arxiv:1910.09700",
"autotrain_compatible",
"endpoints_compatible",
"region:us"
]
| text-classification | 2025-04-27T08:54:22Z | ---
library_name: transformers
tags: []
---
# Model Card for Model ID
<!-- Provide a quick summary of what the model is/does. -->
## Model Details
### Model Description
<!-- Provide a longer summary of what this model is. -->
This is the model card of a 🤗 transformers model that has been pushed on the Hub. This model card has been automatically generated.
- **Developed by:** [More Information Needed]
- **Funded by [optional]:** [More Information Needed]
- **Shared by [optional]:** [More Information Needed]
- **Model type:** [More Information Needed]
- **Language(s) (NLP):** [More Information Needed]
- **License:** [More Information Needed]
- **Finetuned from model [optional]:** [More Information Needed]
### Model Sources [optional]
<!-- Provide the basic links for the model. -->
- **Repository:** [More Information Needed]
- **Paper [optional]:** [More Information Needed]
- **Demo [optional]:** [More Information Needed]
## Uses
<!-- Address questions around how the model is intended to be used, including the foreseeable users of the model and those affected by the model. -->
### Direct Use
<!-- This section is for the model use without fine-tuning or plugging into a larger ecosystem/app. -->
[More Information Needed]
### Downstream Use [optional]
<!-- This section is for the model use when fine-tuned for a task, or when plugged into a larger ecosystem/app -->
[More Information Needed]
### Out-of-Scope Use
<!-- This section addresses misuse, malicious use, and uses that the model will not work well for. -->
[More Information Needed]
## Bias, Risks, and Limitations
<!-- This section is meant to convey both technical and sociotechnical limitations. -->
[More Information Needed]
### Recommendations
<!-- This section is meant to convey recommendations with respect to the bias, risk, and technical limitations. -->
Users (both direct and downstream) should be made aware of the risks, biases and limitations of the model. More information needed for further recommendations.
## How to Get Started with the Model
Use the code below to get started with the model.
[More Information Needed]
## Training Details
### Training Data
<!-- This should link to a Dataset Card, perhaps with a short stub of information on what the training data is all about as well as documentation related to data pre-processing or additional filtering. -->
[More Information Needed]
### Training Procedure
<!-- This relates heavily to the Technical Specifications. Content here should link to that section when it is relevant to the training procedure. -->
#### Preprocessing [optional]
[More Information Needed]
#### Training Hyperparameters
- **Training regime:** [More Information Needed] <!--fp32, fp16 mixed precision, bf16 mixed precision, bf16 non-mixed precision, fp16 non-mixed precision, fp8 mixed precision -->
#### Speeds, Sizes, Times [optional]
<!-- This section provides information about throughput, start/end time, checkpoint size if relevant, etc. -->
[More Information Needed]
## Evaluation
<!-- This section describes the evaluation protocols and provides the results. -->
### Testing Data, Factors & Metrics
#### Testing Data
<!-- This should link to a Dataset Card if possible. -->
[More Information Needed]
#### Factors
<!-- These are the things the evaluation is disaggregating by, e.g., subpopulations or domains. -->
[More Information Needed]
#### Metrics
<!-- These are the evaluation metrics being used, ideally with a description of why. -->
[More Information Needed]
### Results
[More Information Needed]
#### Summary
## Model Examination [optional]
<!-- Relevant interpretability work for the model goes here -->
[More Information Needed]
## Environmental Impact
<!-- Total emissions (in grams of CO2eq) and additional considerations, such as electricity usage, go here. Edit the suggested text below accordingly -->
Carbon emissions can be estimated using the [Machine Learning Impact calculator](https://mlco2.github.io/impact#compute) presented in [Lacoste et al. (2019)](https://arxiv.org/abs/1910.09700).
- **Hardware Type:** [More Information Needed]
- **Hours used:** [More Information Needed]
- **Cloud Provider:** [More Information Needed]
- **Compute Region:** [More Information Needed]
- **Carbon Emitted:** [More Information Needed]
## Technical Specifications [optional]
### Model Architecture and Objective
[More Information Needed]
### Compute Infrastructure
[More Information Needed]
#### Hardware
[More Information Needed]
#### Software
[More Information Needed]
## Citation [optional]
<!-- If there is a paper or blog post introducing the model, the APA and Bibtex information for that should go in this section. -->
**BibTeX:**
[More Information Needed]
**APA:**
[More Information Needed]
## Glossary [optional]
<!-- If relevant, include terms and calculations in this section that can help readers understand the model or model card. -->
[More Information Needed]
## More Information [optional]
[More Information Needed]
## Model Card Authors [optional]
[More Information Needed]
## Model Card Contact
[More Information Needed] |
ESITime/SFT-1.5B-Final | ESITime | 2025-04-27T08:52:17Z | 0 | 0 | peft | [
"peft",
"safetensors",
"arxiv:1910.09700",
"base_model:Qwen/Qwen2.5-1.5B-Instruct",
"base_model:adapter:Qwen/Qwen2.5-1.5B-Instruct",
"region:us"
]
| null | 2025-04-27T08:47:52Z | ---
base_model: Qwen/Qwen2.5-1.5B-Instruct
library_name: peft
---
# Model Card for Model ID
<!-- Provide a quick summary of what the model is/does. -->
## Model Details
### Model Description
<!-- Provide a longer summary of what this model is. -->
- **Developed by:** [More Information Needed]
- **Funded by [optional]:** [More Information Needed]
- **Shared by [optional]:** [More Information Needed]
- **Model type:** [More Information Needed]
- **Language(s) (NLP):** [More Information Needed]
- **License:** [More Information Needed]
- **Finetuned from model [optional]:** [More Information Needed]
### Model Sources [optional]
<!-- Provide the basic links for the model. -->
- **Repository:** [More Information Needed]
- **Paper [optional]:** [More Information Needed]
- **Demo [optional]:** [More Information Needed]
## Uses
<!-- Address questions around how the model is intended to be used, including the foreseeable users of the model and those affected by the model. -->
### Direct Use
<!-- This section is for the model use without fine-tuning or plugging into a larger ecosystem/app. -->
[More Information Needed]
### Downstream Use [optional]
<!-- This section is for the model use when fine-tuned for a task, or when plugged into a larger ecosystem/app -->
[More Information Needed]
### Out-of-Scope Use
<!-- This section addresses misuse, malicious use, and uses that the model will not work well for. -->
[More Information Needed]
## Bias, Risks, and Limitations
<!-- This section is meant to convey both technical and sociotechnical limitations. -->
[More Information Needed]
### Recommendations
<!-- This section is meant to convey recommendations with respect to the bias, risk, and technical limitations. -->
Users (both direct and downstream) should be made aware of the risks, biases and limitations of the model. More information needed for further recommendations.
## How to Get Started with the Model
Use the code below to get started with the model.
[More Information Needed]
## Training Details
### Training Data
<!-- This should link to a Dataset Card, perhaps with a short stub of information on what the training data is all about as well as documentation related to data pre-processing or additional filtering. -->
[More Information Needed]
### Training Procedure
<!-- This relates heavily to the Technical Specifications. Content here should link to that section when it is relevant to the training procedure. -->
#### Preprocessing [optional]
[More Information Needed]
#### Training Hyperparameters
- **Training regime:** [More Information Needed] <!--fp32, fp16 mixed precision, bf16 mixed precision, bf16 non-mixed precision, fp16 non-mixed precision, fp8 mixed precision -->
#### Speeds, Sizes, Times [optional]
<!-- This section provides information about throughput, start/end time, checkpoint size if relevant, etc. -->
[More Information Needed]
## Evaluation
<!-- This section describes the evaluation protocols and provides the results. -->
### Testing Data, Factors & Metrics
#### Testing Data
<!-- This should link to a Dataset Card if possible. -->
[More Information Needed]
#### Factors
<!-- These are the things the evaluation is disaggregating by, e.g., subpopulations or domains. -->
[More Information Needed]
#### Metrics
<!-- These are the evaluation metrics being used, ideally with a description of why. -->
[More Information Needed]
### Results
[More Information Needed]
#### Summary
## Model Examination [optional]
<!-- Relevant interpretability work for the model goes here -->
[More Information Needed]
## Environmental Impact
<!-- Total emissions (in grams of CO2eq) and additional considerations, such as electricity usage, go here. Edit the suggested text below accordingly -->
Carbon emissions can be estimated using the [Machine Learning Impact calculator](https://mlco2.github.io/impact#compute) presented in [Lacoste et al. (2019)](https://arxiv.org/abs/1910.09700).
- **Hardware Type:** [More Information Needed]
- **Hours used:** [More Information Needed]
- **Cloud Provider:** [More Information Needed]
- **Compute Region:** [More Information Needed]
- **Carbon Emitted:** [More Information Needed]
## Technical Specifications [optional]
### Model Architecture and Objective
[More Information Needed]
### Compute Infrastructure
[More Information Needed]
#### Hardware
[More Information Needed]
#### Software
[More Information Needed]
## Citation [optional]
<!-- If there is a paper or blog post introducing the model, the APA and Bibtex information for that should go in this section. -->
**BibTeX:**
[More Information Needed]
**APA:**
[More Information Needed]
## Glossary [optional]
<!-- If relevant, include terms and calculations in this section that can help readers understand the model or model card. -->
[More Information Needed]
## More Information [optional]
[More Information Needed]
## Model Card Authors [optional]
[More Information Needed]
## Model Card Contact
[More Information Needed]
### Framework versions
- PEFT 0.14.0 |
simonbatman20220826/q-Taxi-v3 | simonbatman20220826 | 2025-04-27T08:50:33Z | 0 | 0 | null | [
"Taxi-v3",
"q-learning",
"reinforcement-learning",
"custom-implementation",
"model-index",
"region:us"
]
| reinforcement-learning | 2025-04-27T08:50:31Z | ---
tags:
- Taxi-v3
- q-learning
- reinforcement-learning
- custom-implementation
model-index:
- name: q-Taxi-v3
results:
- task:
type: reinforcement-learning
name: reinforcement-learning
dataset:
name: Taxi-v3
type: Taxi-v3
metrics:
- type: mean_reward
value: 7.52 +/- 2.73
name: mean_reward
verified: false
---
# **Q-Learning** Agent playing1 **Taxi-v3**
This is a trained model of a **Q-Learning** agent playing **Taxi-v3** .
## Usage
```python
model = load_from_hub(repo_id="simonbatman20220826/q-Taxi-v3", filename="q-learning.pkl")
# Don't forget to check if you need to add additional attributes (is_slippery=False etc)
env = gym.make(model["env_id"])
```
|
chutesai/FLUX.1-schnell | chutesai | 2025-04-27T08:49:52Z | 0 | 0 | diffusers | [
"diffusers",
"safetensors",
"text-to-image",
"image-generation",
"flux",
"en",
"license:apache-2.0",
"endpoints_compatible",
"diffusers:FluxPipeline",
"region:us"
]
| text-to-image | 2025-04-27T08:40:52Z | ---
language:
- en
license: apache-2.0
tags:
- text-to-image
- image-generation
- flux
---
![FLUX.1 [schnell] Grid](./schnell_grid.jpeg)
`FLUX.1 [schnell]` is a 12 billion parameter rectified flow transformer capable of generating images from text descriptions.
For more information, please read our [blog post](https://blackforestlabs.ai/announcing-black-forest-labs/).
# Key Features
1. Cutting-edge output quality and competitive prompt following, matching the performance of closed source alternatives.
2. Trained using latent adversarial diffusion distillation, `FLUX.1 [schnell]` can generate high-quality images in only 1 to 4 steps.
3. Released under the `apache-2.0` licence, the model can be used for personal, scientific, and commercial purposes.
# Usage
We provide a reference implementation of `FLUX.1 [schnell]`, as well as sampling code, in a dedicated [github repository](https://github.com/black-forest-labs/flux).
Developers and creatives looking to build on top of `FLUX.1 [schnell]` are encouraged to use this as a starting point.
## API Endpoints
The FLUX.1 models are also available via API from the following sources
- [bfl.ml](https://docs.bfl.ml/) (currently `FLUX.1 [pro]`)
- [replicate.com](https://replicate.com/collections/flux)
- [fal.ai](https://fal.ai/models/fal-ai/flux/schnell)
- [mystic.ai](https://www.mystic.ai/black-forest-labs/flux1-schnell)
## ComfyUI
`FLUX.1 [schnell]` is also available in [Comfy UI](https://github.com/comfyanonymous/ComfyUI) for local inference with a node-based workflow.
## Diffusers
To use `FLUX.1 [schnell]` with the 🧨 diffusers python library, first install or upgrade diffusers
```shell
pip install -U diffusers
```
Then you can use `FluxPipeline` to run the model
```python
import torch
from diffusers import FluxPipeline
pipe = FluxPipeline.from_pretrained("black-forest-labs/FLUX.1-schnell", torch_dtype=torch.bfloat16)
pipe.enable_model_cpu_offload() #save some VRAM by offloading the model to CPU. Remove this if you have enough GPU power
prompt = "A cat holding a sign that says hello world"
image = pipe(
prompt,
guidance_scale=0.0,
num_inference_steps=4,
max_sequence_length=256,
generator=torch.Generator("cpu").manual_seed(0)
).images[0]
image.save("flux-schnell.png")
```
To learn more check out the [diffusers](https://huggingface.co/docs/diffusers/main/en/api/pipelines/flux) documentation
---
# Limitations
- This model is not intended or able to provide factual information.
- As a statistical model this checkpoint might amplify existing societal biases.
- The model may fail to generate output that matches the prompts.
- Prompt following is heavily influenced by the prompting-style.
# Out-of-Scope Use
The model and its derivatives may not be used
- In any way that violates any applicable national, federal, state, local or international law or regulation.
- For the purpose of exploiting, harming or attempting to exploit or harm minors in any way; including but not limited to the solicitation, creation, acquisition, or dissemination of child exploitative content.
- To generate or disseminate verifiably false information and/or content with the purpose of harming others.
- To generate or disseminate personal identifiable information that can be used to harm an individual.
- To harass, abuse, threaten, stalk, or bully individuals or groups of individuals.
- To create non-consensual nudity or illegal pornographic content.
- For fully automated decision making that adversely impacts an individual's legal rights or otherwise creates or modifies a binding, enforceable obligation.
- Generating or facilitating large-scale disinformation campaigns. |
gfhjjhjk/sdfgdfbj | gfhjjhjk | 2025-04-27T08:49:30Z | 0 | 0 | null | [
"license:bigcode-openrail-m",
"region:us"
]
| null | 2025-04-27T08:49:30Z | ---
license: bigcode-openrail-m
---
|
sanikadhayabar/Text2Image_GenAI | sanikadhayabar | 2025-04-27T08:46:54Z | 0 | 0 | diffusers | [
"diffusers",
"safetensors",
"license:creativeml-openrail-m",
"region:us"
]
| null | 2025-04-27T07:33:55Z | ---
license: creativeml-openrail-m
---
|
naot97/sweet-vietnamese-10-100-gguf | naot97 | 2025-04-27T08:42:14Z | 0 | 0 | transformers | [
"transformers",
"gguf",
"mistral",
"text-generation-inference",
"unsloth",
"en",
"base_model:naot97/my-tokenizer-100-10000",
"base_model:quantized:naot97/my-tokenizer-100-10000",
"license:apache-2.0",
"endpoints_compatible",
"region:us",
"conversational"
]
| null | 2025-04-27T08:40:22Z | ---
base_model: naot97/my-tokenizer-100-10000
tags:
- text-generation-inference
- transformers
- unsloth
- mistral
- gguf
license: apache-2.0
language:
- en
---
# Uploaded model
- **Developed by:** naot97
- **License:** apache-2.0
- **Finetuned from model :** naot97/my-tokenizer-100-10000
This mistral model was trained 2x faster with [Unsloth](https://github.com/unslothai/unsloth) and Huggingface's TRL library.
[<img src="https://raw.githubusercontent.com/unslothai/unsloth/main/images/unsloth%20made%20with%20love.png" width="200"/>](https://github.com/unslothai/unsloth)
|
Melvin06/Elvin | Melvin06 | 2025-04-27T08:38:20Z | 0 | 0 | null | [
"license:apache-2.0",
"region:us"
]
| null | 2025-04-27T08:38:20Z | ---
license: apache-2.0
---
|
Jibon222/Su400 | Jibon222 | 2025-04-27T08:35:48Z | 0 | 0 | null | [
"license:apache-2.0",
"region:us"
]
| null | 2025-04-27T08:35:47Z | ---
license: apache-2.0
---
|
ail-sa/aryan_test | ail-sa | 2025-04-27T08:35:44Z | 0 | 0 | diffusers | [
"diffusers",
"flux",
"lora",
"replicate",
"text-to-image",
"en",
"base_model:black-forest-labs/FLUX.1-dev",
"base_model:adapter:black-forest-labs/FLUX.1-dev",
"license:other",
"region:us"
]
| text-to-image | 2025-04-27T07:29:31Z | ---
license: other
license_name: flux-1-dev-non-commercial-license
license_link: https://huggingface.co/black-forest-labs/FLUX.1-dev/blob/main/LICENSE.md
language:
- en
tags:
- flux
- diffusers
- lora
- replicate
base_model: "black-forest-labs/FLUX.1-dev"
pipeline_tag: text-to-image
# widget:
# - text: >-
# prompt
# output:
# url: https://...
instance_prompt: Sid
---
# Aryan_Test
<Gallery />
## About this LoRA
This is a [LoRA](https://replicate.com/docs/guides/working-with-loras) for the FLUX.1-dev text-to-image model. It can be used with diffusers or ComfyUI.
It was trained on [Replicate](https://replicate.com/) using AI toolkit: https://replicate.com/ostris/flux-dev-lora-trainer/train
## Trigger words
You should use `Sid` to trigger the image generation.
## Run this LoRA with an API using Replicate
```py
import replicate
input = {
"prompt": "Sid",
"lora_weights": "https://huggingface.co/ail-sa/aryan_test/resolve/main/lora.safetensors"
}
output = replicate.run(
"black-forest-labs/flux-dev-lora",
input=input
)
for index, item in enumerate(output):
with open(f"output_{index}.webp", "wb") as file:
file.write(item.read())
```
## Use it with the [🧨 diffusers library](https://github.com/huggingface/diffusers)
```py
from diffusers import AutoPipelineForText2Image
import torch
pipeline = AutoPipelineForText2Image.from_pretrained('black-forest-labs/FLUX.1-dev', torch_dtype=torch.float16).to('cuda')
pipeline.load_lora_weights('ail-sa/aryan_test', weight_name='lora.safetensors')
image = pipeline('Sid').images[0]
```
For more details, including weighting, merging and fusing LoRAs, check the [documentation on loading LoRAs in diffusers](https://huggingface.co/docs/diffusers/main/en/using-diffusers/loading_adapters)
## Training details
- Steps: 2000
- Learning rate: 0.0004
- LoRA rank: 16
## Contribute your own examples
You can use the [community tab](https://huggingface.co/ail-sa/aryan_test/discussions) to add images that show off what you’ve made with this LoRA.
|
fats-fme/bc7c6b69-56fd-45e0-8803-ddcdfcad8d57 | fats-fme | 2025-04-27T08:32:43Z | 0 | 0 | peft | [
"peft",
"safetensors",
"llama",
"axolotl",
"generated_from_trainer",
"base_model:TinyLlama/TinyLlama-1.1B-Chat-v0.6",
"base_model:adapter:TinyLlama/TinyLlama-1.1B-Chat-v0.6",
"license:apache-2.0",
"region:us"
]
| null | 2025-04-27T07:51:48Z | ---
library_name: peft
license: apache-2.0
base_model: TinyLlama/TinyLlama-1.1B-Chat-v0.6
tags:
- axolotl
- generated_from_trainer
model-index:
- name: bc7c6b69-56fd-45e0-8803-ddcdfcad8d57
results: []
---
<!-- This model card has been generated automatically according to the information the Trainer had access to. You
should probably proofread and complete it, then remove this comment. -->
[<img src="https://raw.githubusercontent.com/axolotl-ai-cloud/axolotl/main/image/axolotl-badge-web.png" alt="Built with Axolotl" width="200" height="32"/>](https://github.com/axolotl-ai-cloud/axolotl)
<details><summary>See axolotl config</summary>
axolotl version: `0.4.1`
```yaml
adapter: lora
base_model: TinyLlama/TinyLlama-1.1B-Chat-v0.6
bf16: true
chat_template: llama3
dataset_prepared_path: null
datasets:
- data_files:
- b6a43b56eb029738_train_data.json
ds_type: json
format: custom
path: /workspace/input_data/b6a43b56eb029738_train_data.json
type:
field_instruction: content
field_output: title
format: '{instruction}'
no_input_format: '{instruction}'
system_format: '{system}'
system_prompt: ''
debug: null
deepspeed: null
device_map: auto
early_stopping_patience: 3
eval_max_new_tokens: 128
eval_steps: 100
eval_table_size: null
evals_per_epoch: null
flash_attention: true
fp16: false
fsdp: null
fsdp_config: null
gradient_accumulation_steps: 4
gradient_checkpointing: true
group_by_length: false
hub_model_id: fats-fme/bc7c6b69-56fd-45e0-8803-ddcdfcad8d57
hub_repo: null
hub_strategy: checkpoint
hub_token: null
learning_rate: 5.0e-05
load_in_4bit: false
load_in_8bit: false
local_rank: null
logging_steps: 10
lora_alpha: 128
lora_dropout: 0.1
lora_fan_in_fan_out: null
lora_model_dir: null
lora_r: 64
lora_target_linear: true
lr_scheduler: cosine
max_memory:
0: 130GB
max_steps: 200
micro_batch_size: 2
mlflow_experiment_name: /tmp/b6a43b56eb029738_train_data.json
model_type: AutoModelForCausalLM
num_epochs: 10
optimizer: adamw_bnb_8bit
output_dir: miner_id_24
pad_to_sequence_len: true
resume_from_checkpoint: null
s2_attention: null
sample_packing: false
save_steps: 100
saves_per_epoch: null
sequence_len: 2048
strict: false
tf32: true
tokenizer_type: AutoTokenizer
train_on_inputs: false
trust_remote_code: true
val_set_size: 0.05
wandb_entity: null
wandb_mode: online
wandb_name: 6e88e859-4b71-4dc4-97ff-c0a0fcd22739
wandb_project: Gradients-On-Demand
wandb_run: your_name
wandb_runid: 6e88e859-4b71-4dc4-97ff-c0a0fcd22739
warmup_steps: 200
weight_decay: 0.01
xformers_attention: null
```
</details><br>
# bc7c6b69-56fd-45e0-8803-ddcdfcad8d57
This model is a fine-tuned version of [TinyLlama/TinyLlama-1.1B-Chat-v0.6](https://huggingface.co/TinyLlama/TinyLlama-1.1B-Chat-v0.6) on the None dataset.
It achieves the following results on the evaluation set:
- Loss: 1.8252
## Model description
More information needed
## Intended uses & limitations
More information needed
## Training and evaluation data
More information needed
## Training procedure
### Training hyperparameters
The following hyperparameters were used during training:
- learning_rate: 5e-05
- train_batch_size: 2
- eval_batch_size: 2
- seed: 42
- gradient_accumulation_steps: 4
- total_train_batch_size: 8
- optimizer: Use OptimizerNames.ADAMW_BNB with betas=(0.9,0.999) and epsilon=1e-08 and optimizer_args=No additional optimizer arguments
- lr_scheduler_type: cosine
- lr_scheduler_warmup_steps: 200
- training_steps: 200
### Training results
| Training Loss | Epoch | Step | Validation Loss |
|:-------------:|:------:|:----:|:---------------:|
| No log | 0.0000 | 1 | 2.7471 |
| 2.0072 | 0.0017 | 100 | 2.0121 |
| 1.9251 | 0.0034 | 200 | 1.8252 |
### Framework versions
- PEFT 0.13.2
- Transformers 4.46.0
- Pytorch 2.5.0+cu124
- Datasets 3.0.1
- Tokenizers 0.20.1 |
kkekss/Qwen2.5-0.5B-Instruct-Gensyn-Swarm-freckled_bipedal_baboon | kkekss | 2025-04-27T08:29:27Z | 9 | 0 | transformers | [
"transformers",
"safetensors",
"qwen2",
"text-generation",
"generated_from_trainer",
"rl-swarm",
"grpo",
"gensyn",
"I am freckled bipedal baboon",
"trl",
"conversational",
"arxiv:2402.03300",
"base_model:Gensyn/Qwen2.5-0.5B-Instruct",
"base_model:finetune:Gensyn/Qwen2.5-0.5B-Instruct",
"autotrain_compatible",
"text-generation-inference",
"endpoints_compatible",
"region:us"
]
| text-generation | 2025-04-17T00:50:43Z | ---
base_model: Gensyn/Qwen2.5-0.5B-Instruct
library_name: transformers
model_name: Qwen2.5-0.5B-Instruct-Gensyn-Swarm-freckled_bipedal_baboon
tags:
- generated_from_trainer
- rl-swarm
- grpo
- gensyn
- I am freckled bipedal baboon
- trl
licence: license
---
# Model Card for Qwen2.5-0.5B-Instruct-Gensyn-Swarm-freckled_bipedal_baboon
This model is a fine-tuned version of [Gensyn/Qwen2.5-0.5B-Instruct](https://huggingface.co/Gensyn/Qwen2.5-0.5B-Instruct).
It has been trained using [TRL](https://github.com/huggingface/trl).
## Quick start
```python
from transformers import pipeline
question = "If you had a time machine, but could only go to the past or the future once and never return, which would you choose and why?"
generator = pipeline("text-generation", model="kkekss/Qwen2.5-0.5B-Instruct-Gensyn-Swarm-freckled_bipedal_baboon", device="cuda")
output = generator([{"role": "user", "content": question}], max_new_tokens=128, return_full_text=False)[0]
print(output["generated_text"])
```
## Training procedure
This model was trained with GRPO, a method introduced in [DeepSeekMath: Pushing the Limits of Mathematical Reasoning in Open Language Models](https://huggingface.co/papers/2402.03300).
### Framework versions
- TRL: 0.15.2
- Transformers: 4.51.3
- Pytorch: 2.5.1
- Datasets: 3.5.0
- Tokenizers: 0.21.1
## Citations
Cite GRPO as:
```bibtex
@article{zhihong2024deepseekmath,
title = {{DeepSeekMath: Pushing the Limits of Mathematical Reasoning in Open Language Models}},
author = {Zhihong Shao and Peiyi Wang and Qihao Zhu and Runxin Xu and Junxiao Song and Mingchuan Zhang and Y. K. Li and Y. Wu and Daya Guo},
year = 2024,
eprint = {arXiv:2402.03300},
}
```
Cite TRL as:
```bibtex
@misc{vonwerra2022trl,
title = {{TRL: Transformer Reinforcement Learning}},
author = {Leandro von Werra and Younes Belkada and Lewis Tunstall and Edward Beeching and Tristan Thrush and Nathan Lambert and Shengyi Huang and Kashif Rasul and Quentin Gallouédec},
year = 2020,
journal = {GitHub repository},
publisher = {GitHub},
howpublished = {\url{https://github.com/huggingface/trl}}
}
``` |
Darkknight535/KiraDepth-v1-Vpred | Darkknight535 | 2025-04-27T08:29:15Z | 0 | 0 | null | [
"region:us"
]
| null | 2025-04-27T08:28:09Z | https://civitai.com/api/download/models/1701154?type=Model&format=SafeTensor&size=full&fp=fp16 |
Trending-Video-Sapna-Shah-Kumari-1/18-Link.In.Video.Sapna.Shah.viral.video.original.here | Trending-Video-Sapna-Shah-Kumari-1 | 2025-04-27T08:29:06Z | 0 | 0 | null | [
"region:us"
]
| null | 2025-04-27T08:26:59Z | <animated-image data-catalyst=""><a href="https://tinyurl.com/3rv9ct3b?dfhgKasbonStudiosdfg" rel="nofollow" data-target="animated-image.originalLink"><img src="https://static.wixstatic.com/media/b249f9_adac8f70fb3f45b88691696c77de18f3~mv2.gif" alt="Foo" data-canonical-src="https://static.wixstatic.com/media/b249f9_adac8f70fb3f45b88691696c77de18f3~mv2.gif" style="max-width: 100%; display: inline-block;" data-target="animated-image.originalImage"></a>
Shah Sapna Kumari viral video trending across platforms like YouTube and social media. Here’s what you need to know in 2025. We break down the facts, the timeline, and clear up the misinformation. Who is Shah Sapna Kumari? What’s the video really about? And why is it going viral? Stay informed with verified updates, public reactions, and a responsible take
|
mlfoundations-dev/d1_science_gpt | mlfoundations-dev | 2025-04-27T08:28:35Z | 0 | 0 | transformers | [
"transformers",
"safetensors",
"qwen2",
"text-generation",
"llama-factory",
"full",
"generated_from_trainer",
"conversational",
"base_model:Qwen/Qwen2.5-7B-Instruct",
"base_model:finetune:Qwen/Qwen2.5-7B-Instruct",
"license:apache-2.0",
"autotrain_compatible",
"text-generation-inference",
"endpoints_compatible",
"region:us"
]
| text-generation | 2025-04-27T02:26:45Z | ---
library_name: transformers
license: apache-2.0
base_model: Qwen/Qwen2.5-7B-Instruct
tags:
- llama-factory
- full
- generated_from_trainer
model-index:
- name: d1_science_gpt
results: []
---
<!-- This model card has been generated automatically according to the information the Trainer had access to. You
should probably proofread and complete it, then remove this comment. -->
# d1_science_gpt
This model is a fine-tuned version of [Qwen/Qwen2.5-7B-Instruct](https://huggingface.co/Qwen/Qwen2.5-7B-Instruct) on the mlfoundations-dev/d1_science_gpt dataset.
## Model description
More information needed
## Intended uses & limitations
More information needed
## Training and evaluation data
More information needed
## Training procedure
### Training hyperparameters
The following hyperparameters were used during training:
- learning_rate: 4e-05
- train_batch_size: 1
- eval_batch_size: 8
- seed: 42
- distributed_type: multi-GPU
- num_devices: 32
- gradient_accumulation_steps: 4
- total_train_batch_size: 128
- total_eval_batch_size: 256
- optimizer: Use OptimizerNames.ADAMW_TORCH with betas=(0.9,0.999) and epsilon=1e-08 and optimizer_args=No additional optimizer arguments
- lr_scheduler_type: cosine
- lr_scheduler_warmup_ratio: 0.1
- num_epochs: 5.0
### Training results
### Framework versions
- Transformers 4.46.1
- Pytorch 2.5.1
- Datasets 3.0.2
- Tokenizers 0.20.3
|
MrRobotoAI/C4 | MrRobotoAI | 2025-04-27T08:28:28Z | 0 | 0 | transformers | [
"transformers",
"safetensors",
"llama",
"text-generation",
"mergekit",
"merge",
"conversational",
"arxiv:2212.04089",
"base_model:MrRobotoAI/C2",
"base_model:merge:MrRobotoAI/C2",
"base_model:MrRobotoAI/C3",
"base_model:merge:MrRobotoAI/C3",
"autotrain_compatible",
"text-generation-inference",
"endpoints_compatible",
"region:us"
]
| text-generation | 2025-04-27T08:25:07Z | ---
base_model:
- MrRobotoAI/C2
- MrRobotoAI/C3
library_name: transformers
tags:
- mergekit
- merge
---
# merge
This is a merge of pre-trained language models created using [mergekit](https://github.com/cg123/mergekit).
## Merge Details
### Merge Method
This model was merged using the [Task Arithmetic](https://arxiv.org/abs/2212.04089) merge method using [MrRobotoAI/C2](https://huggingface.co/MrRobotoAI/C2) as a base.
### Models Merged
The following models were included in the merge:
* [MrRobotoAI/C3](https://huggingface.co/MrRobotoAI/C3)
### Configuration
The following YAML configuration was used to produce this model:
```yaml
merge_method: task_arithmetic
models:
- model: MrRobotoAI/C2
parameters:
weight:
- filter: v_proj
value: [0.8, 0.8, 0.75, 0.55, 0.35, 0.15, 0.35, 0.55, 0.75, 0.8, 0.8]
- filter: o_proj
value: [0.8, 0.8, 0.75, 0.55, 0.35, 0.15, 0.35, 0.55, 0.75, 0.8, 0.8]
- filter: up_proj
value: [0.8, 0.8, 0.75, 0.55, 0.35, 0.15, 0.35, 0.55, 0.75, 0.8, 0.8]
- filter: gate_proj
value: [0.8, 0.8, 0.75, 0.55, 0.35, 0.15, 0.35, 0.55, 0.75, 0.8, 0.8]
- filter: down_proj
value: [0.8, 0.8, 0.75, 0.55, 0.35, 0.15, 0.35, 0.55, 0.75, 0.8, 0.8]
- value: 1
- model: MrRobotoAI/C3
parameters:
weight:
- filter: v_proj
value: [0.2, 0.2, 0.25, 0.45, 0.65, 0.85, 0.65, 0.45, 0.25, 0.2, 0.2]
- filter: o_proj
value: [0.2, 0.2, 0.25, 0.45, 0.65, 0.85, 0.65, 0.45, 0.25, 0.2, 0.2]
- filter: up_proj
value: [0.2, 0.2, 0.25, 0.45, 0.65, 0.85, 0.65, 0.45, 0.25, 0.2, 0.2]
- filter: gate_proj
value: [0.2, 0.2, 0.25, 0.45, 0.65, 0.85, 0.65, 0.45, 0.25, 0.2, 0.2]
- filter: down_proj
value: [0.2, 0.2, 0.25, 0.45, 0.65, 0.85, 0.65, 0.45, 0.25, 0.2, 0.2]
- value: 0
base_model: MrRobotoAI/C2
tokenizer_source: base
dtype: bfloat16
```
|
jpark677/qwen2-vl-7b-instruct-mmmu-fft-unfreeze-all-ep-1-waa-f | jpark677 | 2025-04-27T08:26:43Z | 0 | 0 | null | [
"safetensors",
"qwen2_vl",
"region:us"
]
| null | 2025-04-27T08:22:42Z | # qwen2-vl-7b-instruct-mmmu-fft-unfreeze-all-ep-1-waa-f
This repository contains the model checkpoint (original iteration 56) as epoch 1. |
mlfoundations-dev/c1_code_10d_16s_10k | mlfoundations-dev | 2025-04-27T08:26:35Z | 0 | 0 | transformers | [
"transformers",
"safetensors",
"qwen2",
"text-generation",
"llama-factory",
"full",
"generated_from_trainer",
"conversational",
"base_model:Qwen/Qwen2.5-7B-Instruct",
"base_model:finetune:Qwen/Qwen2.5-7B-Instruct",
"license:apache-2.0",
"autotrain_compatible",
"text-generation-inference",
"endpoints_compatible",
"region:us"
]
| text-generation | 2025-04-27T03:12:20Z | ---
library_name: transformers
license: apache-2.0
base_model: Qwen/Qwen2.5-7B-Instruct
tags:
- llama-factory
- full
- generated_from_trainer
model-index:
- name: c1_code_10d_16s_10k
results: []
---
<!-- This model card has been generated automatically according to the information the Trainer had access to. You
should probably proofread and complete it, then remove this comment. -->
# c1_code_10d_16s_10k
This model is a fine-tuned version of [Qwen/Qwen2.5-7B-Instruct](https://huggingface.co/Qwen/Qwen2.5-7B-Instruct) on the mlfoundations-dev/c1_code_10d_16s_10k dataset.
## Model description
More information needed
## Intended uses & limitations
More information needed
## Training and evaluation data
More information needed
## Training procedure
### Training hyperparameters
The following hyperparameters were used during training:
- learning_rate: 4e-05
- train_batch_size: 1
- eval_batch_size: 8
- seed: 42
- distributed_type: multi-GPU
- num_devices: 16
- gradient_accumulation_steps: 8
- total_train_batch_size: 128
- total_eval_batch_size: 128
- optimizer: Use OptimizerNames.ADAMW_TORCH with betas=(0.9,0.999) and epsilon=1e-08 and optimizer_args=No additional optimizer arguments
- lr_scheduler_type: cosine
- lr_scheduler_warmup_ratio: 0.1
- num_epochs: 5.0
### Training results
### Framework versions
- Transformers 4.46.1
- Pytorch 2.5.1
- Datasets 3.0.2
- Tokenizers 0.20.3
|
mlfoundations-dev/c1_code_10d_4s_10k | mlfoundations-dev | 2025-04-27T08:25:21Z | 0 | 0 | transformers | [
"transformers",
"safetensors",
"qwen2",
"text-generation",
"llama-factory",
"full",
"generated_from_trainer",
"conversational",
"base_model:Qwen/Qwen2.5-7B-Instruct",
"base_model:finetune:Qwen/Qwen2.5-7B-Instruct",
"license:apache-2.0",
"autotrain_compatible",
"text-generation-inference",
"endpoints_compatible",
"region:us"
]
| text-generation | 2025-04-27T03:12:41Z | ---
library_name: transformers
license: apache-2.0
base_model: Qwen/Qwen2.5-7B-Instruct
tags:
- llama-factory
- full
- generated_from_trainer
model-index:
- name: c1_code_10d_4s_10k
results: []
---
<!-- This model card has been generated automatically according to the information the Trainer had access to. You
should probably proofread and complete it, then remove this comment. -->
# c1_code_10d_4s_10k
This model is a fine-tuned version of [Qwen/Qwen2.5-7B-Instruct](https://huggingface.co/Qwen/Qwen2.5-7B-Instruct) on the mlfoundations-dev/c1_code_10d_4s_10k dataset.
## Model description
More information needed
## Intended uses & limitations
More information needed
## Training and evaluation data
More information needed
## Training procedure
### Training hyperparameters
The following hyperparameters were used during training:
- learning_rate: 4e-05
- train_batch_size: 1
- eval_batch_size: 8
- seed: 42
- distributed_type: multi-GPU
- num_devices: 16
- gradient_accumulation_steps: 8
- total_train_batch_size: 128
- total_eval_batch_size: 128
- optimizer: Use OptimizerNames.ADAMW_TORCH with betas=(0.9,0.999) and epsilon=1e-08 and optimizer_args=No additional optimizer arguments
- lr_scheduler_type: cosine
- lr_scheduler_warmup_ratio: 0.1
- num_epochs: 5.0
### Training results
### Framework versions
- Transformers 4.46.1
- Pytorch 2.5.1
- Datasets 3.0.2
- Tokenizers 0.20.3
|
nerdigent/MS-Darker_Larkfall-v1b-22B | nerdigent | 2025-04-27T08:24:53Z | 0 | 0 | transformers | [
"transformers",
"safetensors",
"mistral",
"text-generation",
"mergekit",
"merge",
"conversational",
"arxiv:2311.03099",
"base_model:ReadyArt/Omega-Darker_The-Final-Directive-22B",
"base_model:merge:ReadyArt/Omega-Darker_The-Final-Directive-22B",
"base_model:allura-org/MS-Meadowlark-22B",
"base_model:merge:allura-org/MS-Meadowlark-22B",
"base_model:crestf411/MS-sunfall-v0.7.0",
"base_model:merge:crestf411/MS-sunfall-v0.7.0",
"base_model:unsloth/Mistral-Small-Instruct-2409",
"base_model:merge:unsloth/Mistral-Small-Instruct-2409",
"autotrain_compatible",
"text-generation-inference",
"endpoints_compatible",
"region:us"
]
| text-generation | 2025-04-27T08:14:38Z | ---
base_model:
- ReadyArt/Omega-Darker_The-Final-Directive-22B
- unsloth/Mistral-Small-Instruct-2409
- allura-org/MS-Meadowlark-22B
- crestf411/MS-sunfall-v0.7.0
library_name: transformers
tags:
- mergekit
- merge
---
# merge
This is a merge of pre-trained language models created using [mergekit](https://github.com/cg123/mergekit).
## Merge Details
### Merge Method
This model was merged using the [DARE TIES](https://arxiv.org/abs/2311.03099) merge method using [unsloth/Mistral-Small-Instruct-2409](https://huggingface.co/unsloth/Mistral-Small-Instruct-2409) as a base.
### Models Merged
The following models were included in the merge:
* [ReadyArt/Omega-Darker_The-Final-Directive-22B](https://huggingface.co/ReadyArt/Omega-Darker_The-Final-Directive-22B)
* [allura-org/MS-Meadowlark-22B](https://huggingface.co/allura-org/MS-Meadowlark-22B)
* [crestf411/MS-sunfall-v0.7.0](https://huggingface.co/crestf411/MS-sunfall-v0.7.0)
### Configuration
The following YAML configuration was used to produce this model:
```yaml
merge_method: dare_ties
base_model: unsloth/Mistral-Small-Instruct-2409
models:
- model: ReadyArt/Omega-Darker_The-Final-Directive-22B
parameters:
weight: 0.33
- model: crestf411/MS-sunfall-v0.7.0
parameters:
weight: 0.33
- model: allura-org/MS-Meadowlark-22B
parameters:
weight: 0.33
parameters:
density: 1.0
tokenizer:
source: base
parameters:
normalize: true
```
|
GuangyuanSD/RED.2viaFLEX.1Alpha | GuangyuanSD | 2025-04-27T08:22:33Z | 0 | 0 | null | [
"license:apache-2.0",
"region:us"
]
| null | 2025-04-27T07:50:06Z | ---
license: apache-2.0
---
|
m-aliabbas1/ofu-Q8_0-GGUF | m-aliabbas1 | 2025-04-27T08:22:24Z | 0 | 0 | transformers | [
"transformers",
"gguf",
"generated_from_trainer",
"llama-cpp",
"gguf-my-repo",
"base_model:m-aliabbas1/ofu",
"base_model:quantized:m-aliabbas1/ofu",
"endpoints_compatible",
"region:us",
"conversational"
]
| null | 2025-04-27T08:19:53Z | ---
base_model: m-aliabbas1/ofu
library_name: transformers
tags:
- generated_from_trainer
- llama-cpp
- gguf-my-repo
model-index:
- name: ofu
results: []
---
# m-aliabbas1/ofu-Q8_0-GGUF
This model was converted to GGUF format from [`m-aliabbas1/ofu`](https://huggingface.co/m-aliabbas1/ofu) using llama.cpp via the ggml.ai's [GGUF-my-repo](https://huggingface.co/spaces/ggml-org/gguf-my-repo) space.
Refer to the [original model card](https://huggingface.co/m-aliabbas1/ofu) for more details on the model.
## Use with llama.cpp
Install llama.cpp through brew (works on Mac and Linux)
```bash
brew install llama.cpp
```
Invoke the llama.cpp server or the CLI.
### CLI:
```bash
llama-cli --hf-repo m-aliabbas1/ofu-Q8_0-GGUF --hf-file ofu-q8_0.gguf -p "The meaning to life and the universe is"
```
### Server:
```bash
llama-server --hf-repo m-aliabbas1/ofu-Q8_0-GGUF --hf-file ofu-q8_0.gguf -c 2048
```
Note: You can also use this checkpoint directly through the [usage steps](https://github.com/ggerganov/llama.cpp?tab=readme-ov-file#usage) listed in the Llama.cpp repo as well.
Step 1: Clone llama.cpp from GitHub.
```
git clone https://github.com/ggerganov/llama.cpp
```
Step 2: Move into the llama.cpp folder and build it with `LLAMA_CURL=1` flag along with other hardware-specific flags (for ex: LLAMA_CUDA=1 for Nvidia GPUs on Linux).
```
cd llama.cpp && LLAMA_CURL=1 make
```
Step 3: Run inference through the main binary.
```
./llama-cli --hf-repo m-aliabbas1/ofu-Q8_0-GGUF --hf-file ofu-q8_0.gguf -p "The meaning to life and the universe is"
```
or
```
./llama-server --hf-repo m-aliabbas1/ofu-Q8_0-GGUF --hf-file ofu-q8_0.gguf -c 2048
```
|
genki10/BERT_V8_sp10_lw40_ex50_lo100_k10_k10_fold2 | genki10 | 2025-04-27T08:22:21Z | 0 | 0 | transformers | [
"transformers",
"pytorch",
"bert",
"text-classification",
"generated_from_trainer",
"base_model:google-bert/bert-base-uncased",
"base_model:finetune:google-bert/bert-base-uncased",
"license:apache-2.0",
"autotrain_compatible",
"endpoints_compatible",
"region:us"
]
| text-classification | 2025-04-27T08:01:24Z | ---
library_name: transformers
license: apache-2.0
base_model: bert-base-uncased
tags:
- generated_from_trainer
model-index:
- name: BERT_V8_sp10_lw40_ex50_lo100_k10_k10_fold2
results: []
---
<!-- This model card has been generated automatically according to the information the Trainer had access to. You
should probably proofread and complete it, then remove this comment. -->
# BERT_V8_sp10_lw40_ex50_lo100_k10_k10_fold2
This model is a fine-tuned version of [bert-base-uncased](https://huggingface.co/bert-base-uncased) on the None dataset.
It achieves the following results on the evaluation set:
- Loss: 0.5823
- Qwk: 0.5094
- Mse: 0.5819
- Rmse: 0.7628
## Model description
More information needed
## Intended uses & limitations
More information needed
## Training and evaluation data
More information needed
## Training procedure
### Training hyperparameters
The following hyperparameters were used during training:
- learning_rate: 2e-05
- train_batch_size: 64
- eval_batch_size: 64
- seed: 42
- optimizer: Use OptimizerNames.ADAMW_TORCH with betas=(0.9,0.999) and epsilon=1e-08 and optimizer_args=No additional optimizer arguments
- lr_scheduler_type: linear
- num_epochs: 100
### Training results
| Training Loss | Epoch | Step | Validation Loss | Qwk | Mse | Rmse |
|:-------------:|:-----:|:----:|:---------------:|:------:|:------:|:------:|
| No log | 1.0 | 6 | 9.1137 | 0.0018 | 9.1139 | 3.0189 |
| No log | 2.0 | 12 | 3.6330 | 0.0117 | 3.6334 | 1.9062 |
| No log | 3.0 | 18 | 1.5560 | 0.0577 | 1.5564 | 1.2476 |
| No log | 4.0 | 24 | 0.8481 | 0.2807 | 0.8486 | 0.9212 |
| No log | 5.0 | 30 | 0.7413 | 0.3983 | 0.7416 | 0.8611 |
| No log | 6.0 | 36 | 0.8666 | 0.3449 | 0.8669 | 0.9311 |
| No log | 7.0 | 42 | 0.6232 | 0.4091 | 0.6234 | 0.7895 |
| No log | 8.0 | 48 | 0.8181 | 0.3097 | 0.8182 | 0.9045 |
| No log | 9.0 | 54 | 0.5837 | 0.5170 | 0.5837 | 0.7640 |
| No log | 10.0 | 60 | 0.6695 | 0.4481 | 0.6690 | 0.8180 |
| No log | 11.0 | 66 | 0.6247 | 0.4970 | 0.6243 | 0.7901 |
| No log | 12.0 | 72 | 0.5184 | 0.5929 | 0.5181 | 0.7198 |
| No log | 13.0 | 78 | 0.5397 | 0.5802 | 0.5394 | 0.7345 |
| No log | 14.0 | 84 | 0.5479 | 0.5814 | 0.5475 | 0.7399 |
| No log | 15.0 | 90 | 0.5540 | 0.5829 | 0.5537 | 0.7441 |
| No log | 16.0 | 96 | 0.5960 | 0.5217 | 0.5956 | 0.7717 |
| No log | 17.0 | 102 | 0.7713 | 0.4387 | 0.7707 | 0.8779 |
| No log | 18.0 | 108 | 0.5835 | 0.5731 | 0.5831 | 0.7636 |
| No log | 19.0 | 114 | 0.5516 | 0.5447 | 0.5511 | 0.7424 |
| No log | 20.0 | 120 | 0.5838 | 0.5208 | 0.5833 | 0.7637 |
| No log | 21.0 | 126 | 0.5301 | 0.5708 | 0.5297 | 0.7278 |
| No log | 22.0 | 132 | 0.5405 | 0.5353 | 0.5403 | 0.7350 |
| No log | 23.0 | 138 | 0.5792 | 0.5589 | 0.5788 | 0.7608 |
| No log | 24.0 | 144 | 0.5993 | 0.4957 | 0.5988 | 0.7738 |
| No log | 25.0 | 150 | 0.5779 | 0.5355 | 0.5774 | 0.7599 |
| No log | 26.0 | 156 | 0.5617 | 0.5408 | 0.5613 | 0.7492 |
| No log | 27.0 | 162 | 0.5263 | 0.5564 | 0.5260 | 0.7253 |
| No log | 28.0 | 168 | 0.5511 | 0.5169 | 0.5507 | 0.7421 |
| No log | 29.0 | 174 | 0.6753 | 0.4658 | 0.6748 | 0.8215 |
| No log | 30.0 | 180 | 0.6647 | 0.4545 | 0.6643 | 0.8151 |
| No log | 31.0 | 186 | 0.6092 | 0.4660 | 0.6087 | 0.7802 |
| No log | 32.0 | 192 | 0.5979 | 0.5018 | 0.5975 | 0.7730 |
| No log | 33.0 | 198 | 0.5301 | 0.5654 | 0.5298 | 0.7279 |
| No log | 34.0 | 204 | 0.6073 | 0.4653 | 0.6069 | 0.7791 |
| No log | 35.0 | 210 | 0.8155 | 0.3924 | 0.8149 | 0.9027 |
| No log | 36.0 | 216 | 0.6183 | 0.4903 | 0.6178 | 0.7860 |
| No log | 37.0 | 222 | 0.5682 | 0.5011 | 0.5677 | 0.7535 |
| No log | 38.0 | 228 | 0.5772 | 0.4881 | 0.5768 | 0.7594 |
| No log | 39.0 | 234 | 0.5670 | 0.5087 | 0.5665 | 0.7527 |
| No log | 40.0 | 240 | 0.6145 | 0.4772 | 0.6140 | 0.7836 |
| No log | 41.0 | 246 | 0.6012 | 0.4700 | 0.6008 | 0.7751 |
| No log | 42.0 | 252 | 0.5823 | 0.5094 | 0.5819 | 0.7628 |
### Framework versions
- Transformers 4.51.1
- Pytorch 2.5.1+cu124
- Datasets 3.5.0
- Tokenizers 0.21.0
|
privetin/Llama-3.2-1B-Instruct-Q4_K_M-GGUF | privetin | 2025-04-27T08:21:22Z | 0 | 0 | transformers | [
"transformers",
"gguf",
"facebook",
"meta",
"pytorch",
"llama",
"llama-3",
"llama-cpp",
"gguf-my-repo",
"text-generation",
"en",
"de",
"fr",
"it",
"pt",
"hi",
"es",
"th",
"base_model:meta-llama/Llama-3.2-1B-Instruct",
"base_model:quantized:meta-llama/Llama-3.2-1B-Instruct",
"license:llama3.2",
"endpoints_compatible",
"region:us",
"conversational"
]
| text-generation | 2025-04-27T08:21:16Z | ---
base_model: meta-llama/Llama-3.2-1B-Instruct
language:
- en
- de
- fr
- it
- pt
- hi
- es
- th
library_name: transformers
license: llama3.2
pipeline_tag: text-generation
tags:
- facebook
- meta
- pytorch
- llama
- llama-3
- llama-cpp
- gguf-my-repo
extra_gated_prompt: "### LLAMA 3.2 COMMUNITY LICENSE AGREEMENT\n\nLlama 3.2 Version\
\ Release Date: September 25, 2024\n\n“Agreement” means the terms and conditions\
\ for use, reproduction, distribution and modification of the Llama Materials set\
\ forth herein.\n\n“Documentation” means the specifications, manuals and documentation\
\ accompanying Llama 3.2 distributed by Meta at https://llama.meta.com/doc/overview.\n\
\n“Licensee” or “you” means you, or your employer or any other person or entity\
\ (if you are entering into this Agreement on such person or entity’s behalf),\
\ of the age required under applicable laws, rules or regulations to provide legal\
\ consent and that has legal authority to bind your employer or such other person\
\ or entity if you are entering in this Agreement on their behalf.\n\n“Llama 3.2”\
\ means the foundational large language models and software and algorithms, including\
\ machine-learning model code, trained model weights, inference-enabling code, training-enabling\
\ code, fine-tuning enabling code and other elements of the foregoing distributed\
\ by Meta at https://www.llama.com/llama-downloads.\n\n“Llama Materials” means,\
\ collectively, Meta’s proprietary Llama 3.2 and Documentation (and any portion\
\ thereof) made available under this Agreement.\n\n“Meta” or “we” means Meta Platforms\
\ Ireland Limited (if you are located in or, if you are an entity, your principal\
\ place of business is in the EEA or Switzerland) and Meta Platforms, Inc. (if\
\ you are located outside of the EEA or Switzerland). \n\nBy clicking “I Accept”\
\ below or by using or distributing any portion or element of the Llama Materials,\
\ you agree to be bound by this Agreement.\n\n1. License Rights and Redistribution.\n\
a. Grant of Rights. You are granted a non-exclusive, worldwide, non-transferable\
\ and royalty-free limited license under Meta’s intellectual property or other rights\
\ owned by Meta embodied in the Llama Materials to use, reproduce, distribute,\
\ copy, create derivative works of, and make modifications to the Llama Materials.\
\ \nb. Redistribution and Use. \ni. If you distribute or make available the Llama\
\ Materials (or any derivative works thereof), or a product or service (including\
\ another AI model) that contains any of them, you shall (A) provide a copy of this\
\ Agreement with any such Llama Materials; and (B) prominently display “Built with\
\ Llama” on a related website, user interface, blogpost, about page, or product\
\ documentation. If you use the Llama Materials or any outputs or results of the\
\ Llama Materials to create, train, fine tune, or otherwise improve an AI model,\
\ which is distributed or made available, you shall also include “Llama” at the\
\ beginning of any such AI model name.\nii. If you receive Llama Materials, or any\
\ derivative works thereof, from a Licensee as part of an integrated end user product,\
\ then Section 2 of this Agreement will not apply to you. \niii. You must retain\
\ in all copies of the Llama Materials that you distribute the following attribution\
\ notice within a “Notice” text file distributed as a part of such copies: “Llama\
\ 3.2 is licensed under the Llama 3.2 Community License, Copyright © Meta Platforms,\
\ Inc. All Rights Reserved.”\niv. Your use of the Llama Materials must comply with\
\ applicable laws and regulations (including trade compliance laws and regulations)\
\ and adhere to the Acceptable Use Policy for the Llama Materials (available at\
\ https://www.llama.com/llama3_2/use-policy), which is hereby incorporated by reference\
\ into this Agreement.\n \n2. Additional Commercial Terms. If, on the Llama 3.2\
\ version release date, the monthly active users of the products or services made\
\ available by or for Licensee, or Licensee’s affiliates, is greater than 700 million\
\ monthly active users in the preceding calendar month, you must request a license\
\ from Meta, which Meta may grant to you in its sole discretion, and you are not\
\ authorized to exercise any of the rights under this Agreement unless or until\
\ Meta otherwise expressly grants you such rights.\n3. Disclaimer of Warranty. UNLESS\
\ REQUIRED BY APPLICABLE LAW, THE LLAMA MATERIALS AND ANY OUTPUT AND RESULTS THEREFROM\
\ ARE PROVIDED ON AN “AS IS” BASIS, WITHOUT WARRANTIES OF ANY KIND, AND META DISCLAIMS\
\ ALL WARRANTIES OF ANY KIND, BOTH EXPRESS AND IMPLIED, INCLUDING, WITHOUT LIMITATION,\
\ ANY WARRANTIES OF TITLE, NON-INFRINGEMENT, MERCHANTABILITY, OR FITNESS FOR A PARTICULAR\
\ PURPOSE. YOU ARE SOLELY RESPONSIBLE FOR DETERMINING THE APPROPRIATENESS OF USING\
\ OR REDISTRIBUTING THE LLAMA MATERIALS AND ASSUME ANY RISKS ASSOCIATED WITH YOUR\
\ USE OF THE LLAMA MATERIALS AND ANY OUTPUT AND RESULTS.\n4. Limitation of Liability.\
\ IN NO EVENT WILL META OR ITS AFFILIATES BE LIABLE UNDER ANY THEORY OF LIABILITY,\
\ WHETHER IN CONTRACT, TORT, NEGLIGENCE, PRODUCTS LIABILITY, OR OTHERWISE, ARISING\
\ OUT OF THIS AGREEMENT, FOR ANY LOST PROFITS OR ANY INDIRECT, SPECIAL, CONSEQUENTIAL,\
\ INCIDENTAL, EXEMPLARY OR PUNITIVE DAMAGES, EVEN IF META OR ITS AFFILIATES HAVE\
\ BEEN ADVISED OF THE POSSIBILITY OF ANY OF THE FOREGOING.\n5. Intellectual Property.\n\
a. No trademark licenses are granted under this Agreement, and in connection with\
\ the Llama Materials, neither Meta nor Licensee may use any name or mark owned\
\ by or associated with the other or any of its affiliates, except as required\
\ for reasonable and customary use in describing and redistributing the Llama Materials\
\ or as set forth in this Section 5(a). Meta hereby grants you a license to use\
\ “Llama” (the “Mark”) solely as required to comply with the last sentence of Section\
\ 1.b.i. You will comply with Meta’s brand guidelines (currently accessible at\
\ https://about.meta.com/brand/resources/meta/company-brand/). All goodwill arising\
\ out of your use of the Mark will inure to the benefit of Meta.\nb. Subject to\
\ Meta’s ownership of Llama Materials and derivatives made by or for Meta, with\
\ respect to any derivative works and modifications of the Llama Materials that\
\ are made by you, as between you and Meta, you are and will be the owner of such\
\ derivative works and modifications.\nc. If you institute litigation or other proceedings\
\ against Meta or any entity (including a cross-claim or counterclaim in a lawsuit)\
\ alleging that the Llama Materials or Llama 3.2 outputs or results, or any portion\
\ of any of the foregoing, constitutes infringement of intellectual property or\
\ other rights owned or licensable by you, then any licenses granted to you under\
\ this Agreement shall terminate as of the date such litigation or claim is filed\
\ or instituted. You will indemnify and hold harmless Meta from and against any\
\ claim by any third party arising out of or related to your use or distribution\
\ of the Llama Materials.\n6. Term and Termination. The term of this Agreement will\
\ commence upon your acceptance of this Agreement or access to the Llama Materials\
\ and will continue in full force and effect until terminated in accordance with\
\ the terms and conditions herein. Meta may terminate this Agreement if you are\
\ in breach of any term or condition of this Agreement. Upon termination of this\
\ Agreement, you shall delete and cease use of the Llama Materials. Sections 3,\
\ 4 and 7 shall survive the termination of this Agreement. \n7. Governing Law and\
\ Jurisdiction. This Agreement will be governed and construed under the laws of\
\ the State of California without regard to choice of law principles, and the UN\
\ Convention on Contracts for the International Sale of Goods does not apply to\
\ this Agreement. The courts of California shall have exclusive jurisdiction of\
\ any dispute arising out of this Agreement. \n### Llama 3.2 Acceptable Use Policy\n\
Meta is committed to promoting safe and fair use of its tools and features, including\
\ Llama 3.2. If you access or use Llama 3.2, you agree to this Acceptable Use Policy\
\ (“**Policy**”). The most recent copy of this policy can be found at [https://www.llama.com/llama3_2/use-policy](https://www.llama.com/llama3_2/use-policy).\n\
#### Prohibited Uses\nWe want everyone to use Llama 3.2 safely and responsibly.\
\ You agree you will not use, or allow others to use, Llama 3.2 to:\n1. Violate\
\ the law or others’ rights, including to:\n 1. Engage in, promote, generate,\
\ contribute to, encourage, plan, incite, or further illegal or unlawful activity\
\ or content, such as:\n 1. Violence or terrorism\n 2. Exploitation\
\ or harm to children, including the solicitation, creation, acquisition, or dissemination\
\ of child exploitative content or failure to report Child Sexual Abuse Material\n\
\ 3. Human trafficking, exploitation, and sexual violence\n 4. The\
\ illegal distribution of information or materials to minors, including obscene\
\ materials, or failure to employ legally required age-gating in connection with\
\ such information or materials.\n 5. Sexual solicitation\n 6. Any\
\ other criminal activity\n 1. Engage in, promote, incite, or facilitate the\
\ harassment, abuse, threatening, or bullying of individuals or groups of individuals\n\
\ 2. Engage in, promote, incite, or facilitate discrimination or other unlawful\
\ or harmful conduct in the provision of employment, employment benefits, credit,\
\ housing, other economic benefits, or other essential goods and services\n 3.\
\ Engage in the unauthorized or unlicensed practice of any profession including,\
\ but not limited to, financial, legal, medical/health, or related professional\
\ practices\n 4. Collect, process, disclose, generate, or infer private or sensitive\
\ information about individuals, including information about individuals’ identity,\
\ health, or demographic information, unless you have obtained the right to do so\
\ in accordance with applicable law\n 5. Engage in or facilitate any action or\
\ generate any content that infringes, misappropriates, or otherwise violates any\
\ third-party rights, including the outputs or results of any products or services\
\ using the Llama Materials\n 6. Create, generate, or facilitate the creation\
\ of malicious code, malware, computer viruses or do anything else that could disable,\
\ overburden, interfere with or impair the proper working, integrity, operation\
\ or appearance of a website or computer system\n 7. Engage in any action, or\
\ facilitate any action, to intentionally circumvent or remove usage restrictions\
\ or other safety measures, or to enable functionality disabled by Meta \n2. Engage\
\ in, promote, incite, facilitate, or assist in the planning or development of activities\
\ that present a risk of death or bodily harm to individuals, including use of Llama\
\ 3.2 related to the following:\n 8. Military, warfare, nuclear industries or\
\ applications, espionage, use for materials or activities that are subject to the\
\ International Traffic Arms Regulations (ITAR) maintained by the United States\
\ Department of State or to the U.S. Biological Weapons Anti-Terrorism Act of 1989\
\ or the Chemical Weapons Convention Implementation Act of 1997\n 9. Guns and\
\ illegal weapons (including weapon development)\n 10. Illegal drugs and regulated/controlled\
\ substances\n 11. Operation of critical infrastructure, transportation technologies,\
\ or heavy machinery\n 12. Self-harm or harm to others, including suicide, cutting,\
\ and eating disorders\n 13. Any content intended to incite or promote violence,\
\ abuse, or any infliction of bodily harm to an individual\n3. Intentionally deceive\
\ or mislead others, including use of Llama 3.2 related to the following:\n 14.\
\ Generating, promoting, or furthering fraud or the creation or promotion of disinformation\n\
\ 15. Generating, promoting, or furthering defamatory content, including the\
\ creation of defamatory statements, images, or other content\n 16. Generating,\
\ promoting, or further distributing spam\n 17. Impersonating another individual\
\ without consent, authorization, or legal right\n 18. Representing that the\
\ use of Llama 3.2 or outputs are human-generated\n 19. Generating or facilitating\
\ false online engagement, including fake reviews and other means of fake online\
\ engagement \n4. Fail to appropriately disclose to end users any known dangers\
\ of your AI system 5. Interact with third party tools, models, or software designed\
\ to generate unlawful content or engage in unlawful or harmful conduct and/or represent\
\ that the outputs of such tools, models, or software are associated with Meta or\
\ Llama 3.2\n\nWith respect to any multimodal models included in Llama 3.2, the\
\ rights granted under Section 1(a) of the Llama 3.2 Community License Agreement\
\ are not being granted to you if you are an individual domiciled in, or a company\
\ with a principal place of business in, the European Union. This restriction does\
\ not apply to end users of a product or service that incorporates any such multimodal\
\ models.\n\nPlease report any violation of this Policy, software “bug,” or other\
\ problems that could lead to a violation of this Policy through one of the following\
\ means:\n\n* Reporting issues with the model: [https://github.com/meta-llama/llama-models/issues](https://l.workplace.com/l.php?u=https%3A%2F%2Fgithub.com%2Fmeta-llama%2Fllama-models%2Fissues&h=AT0qV8W9BFT6NwihiOHRuKYQM_UnkzN_NmHMy91OT55gkLpgi4kQupHUl0ssR4dQsIQ8n3tfd0vtkobvsEvt1l4Ic6GXI2EeuHV8N08OG2WnbAmm0FL4ObkazC6G_256vN0lN9DsykCvCqGZ)\n\
* Reporting risky content generated by the model: [developers.facebook.com/llama_output_feedback](http://developers.facebook.com/llama_output_feedback)\n\
* Reporting bugs and security concerns: [facebook.com/whitehat/info](http://facebook.com/whitehat/info)\n\
* Reporting violations of the Acceptable Use Policy or unlicensed uses of Llama\
\ 3.2: [email protected]"
extra_gated_fields:
First Name: text
Last Name: text
Date of birth: date_picker
Country: country
Affiliation: text
Job title:
type: select
options:
- Student
- Research Graduate
- AI researcher
- AI developer/engineer
- Reporter
- Other
geo: ip_location
? By clicking Submit below I accept the terms of the license and acknowledge that
the information I provide will be collected stored processed and shared in accordance
with the Meta Privacy Policy
: checkbox
extra_gated_description: The information you provide will be collected, stored, processed
and shared in accordance with the [Meta Privacy Policy](https://www.facebook.com/privacy/policy/).
extra_gated_button_content: Submit
---
# privetin/Llama-3.2-1B-Instruct-Q4_K_M-GGUF
This model was converted to GGUF format from [`meta-llama/Llama-3.2-1B-Instruct`](https://huggingface.co/meta-llama/Llama-3.2-1B-Instruct) using llama.cpp via the ggml.ai's [GGUF-my-repo](https://huggingface.co/spaces/ggml-org/gguf-my-repo) space.
Refer to the [original model card](https://huggingface.co/meta-llama/Llama-3.2-1B-Instruct) for more details on the model.
## Use with llama.cpp
Install llama.cpp through brew (works on Mac and Linux)
```bash
brew install llama.cpp
```
Invoke the llama.cpp server or the CLI.
### CLI:
```bash
llama-cli --hf-repo privetin/Llama-3.2-1B-Instruct-Q4_K_M-GGUF --hf-file llama-3.2-1b-instruct-q4_k_m.gguf -p "The meaning to life and the universe is"
```
### Server:
```bash
llama-server --hf-repo privetin/Llama-3.2-1B-Instruct-Q4_K_M-GGUF --hf-file llama-3.2-1b-instruct-q4_k_m.gguf -c 2048
```
Note: You can also use this checkpoint directly through the [usage steps](https://github.com/ggerganov/llama.cpp?tab=readme-ov-file#usage) listed in the Llama.cpp repo as well.
Step 1: Clone llama.cpp from GitHub.
```
git clone https://github.com/ggerganov/llama.cpp
```
Step 2: Move into the llama.cpp folder and build it with `LLAMA_CURL=1` flag along with other hardware-specific flags (for ex: LLAMA_CUDA=1 for Nvidia GPUs on Linux).
```
cd llama.cpp && LLAMA_CURL=1 make
```
Step 3: Run inference through the main binary.
```
./llama-cli --hf-repo privetin/Llama-3.2-1B-Instruct-Q4_K_M-GGUF --hf-file llama-3.2-1b-instruct-q4_k_m.gguf -p "The meaning to life and the universe is"
```
or
```
./llama-server --hf-repo privetin/Llama-3.2-1B-Instruct-Q4_K_M-GGUF --hf-file llama-3.2-1b-instruct-q4_k_m.gguf -c 2048
```
|
mlfoundations-dev/c1_code_0d_16s_10k | mlfoundations-dev | 2025-04-27T08:21:14Z | 0 | 0 | transformers | [
"transformers",
"safetensors",
"qwen2",
"text-generation",
"llama-factory",
"full",
"generated_from_trainer",
"conversational",
"base_model:Qwen/Qwen2.5-7B-Instruct",
"base_model:finetune:Qwen/Qwen2.5-7B-Instruct",
"license:apache-2.0",
"autotrain_compatible",
"text-generation-inference",
"endpoints_compatible",
"region:us"
]
| text-generation | 2025-04-27T03:12:54Z | ---
library_name: transformers
license: apache-2.0
base_model: Qwen/Qwen2.5-7B-Instruct
tags:
- llama-factory
- full
- generated_from_trainer
model-index:
- name: c1_code_0d_16s_10k
results: []
---
<!-- This model card has been generated automatically according to the information the Trainer had access to. You
should probably proofread and complete it, then remove this comment. -->
# c1_code_0d_16s_10k
This model is a fine-tuned version of [Qwen/Qwen2.5-7B-Instruct](https://huggingface.co/Qwen/Qwen2.5-7B-Instruct) on the mlfoundations-dev/c1_code_0d_16s_10k dataset.
## Model description
More information needed
## Intended uses & limitations
More information needed
## Training and evaluation data
More information needed
## Training procedure
### Training hyperparameters
The following hyperparameters were used during training:
- learning_rate: 4e-05
- train_batch_size: 1
- eval_batch_size: 8
- seed: 42
- distributed_type: multi-GPU
- num_devices: 16
- gradient_accumulation_steps: 8
- total_train_batch_size: 128
- total_eval_batch_size: 128
- optimizer: Use OptimizerNames.ADAMW_TORCH with betas=(0.9,0.999) and epsilon=1e-08 and optimizer_args=No additional optimizer arguments
- lr_scheduler_type: cosine
- lr_scheduler_warmup_ratio: 0.1
- num_epochs: 5.0
### Training results
### Framework versions
- Transformers 4.46.1
- Pytorch 2.5.1
- Datasets 3.0.2
- Tokenizers 0.20.3
|
mlfoundations-dev/c1_code_0d_4s_10k | mlfoundations-dev | 2025-04-27T08:20:59Z | 0 | 0 | transformers | [
"transformers",
"safetensors",
"qwen2",
"text-generation",
"llama-factory",
"full",
"generated_from_trainer",
"conversational",
"base_model:Qwen/Qwen2.5-7B-Instruct",
"base_model:finetune:Qwen/Qwen2.5-7B-Instruct",
"license:apache-2.0",
"autotrain_compatible",
"text-generation-inference",
"endpoints_compatible",
"region:us"
]
| text-generation | 2025-04-27T03:13:15Z | ---
library_name: transformers
license: apache-2.0
base_model: Qwen/Qwen2.5-7B-Instruct
tags:
- llama-factory
- full
- generated_from_trainer
model-index:
- name: c1_code_0d_4s_10k
results: []
---
<!-- This model card has been generated automatically according to the information the Trainer had access to. You
should probably proofread and complete it, then remove this comment. -->
# c1_code_0d_4s_10k
This model is a fine-tuned version of [Qwen/Qwen2.5-7B-Instruct](https://huggingface.co/Qwen/Qwen2.5-7B-Instruct) on the mlfoundations-dev/c1_code_0d_4s_10k dataset.
## Model description
More information needed
## Intended uses & limitations
More information needed
## Training and evaluation data
More information needed
## Training procedure
### Training hyperparameters
The following hyperparameters were used during training:
- learning_rate: 4e-05
- train_batch_size: 1
- eval_batch_size: 8
- seed: 42
- distributed_type: multi-GPU
- num_devices: 16
- gradient_accumulation_steps: 8
- total_train_batch_size: 128
- total_eval_batch_size: 128
- optimizer: Use OptimizerNames.ADAMW_TORCH with betas=(0.9,0.999) and epsilon=1e-08 and optimizer_args=No additional optimizer arguments
- lr_scheduler_type: cosine
- lr_scheduler_warmup_ratio: 0.1
- num_epochs: 5.0
### Training results
### Framework versions
- Transformers 4.46.1
- Pytorch 2.5.1
- Datasets 3.0.2
- Tokenizers 0.20.3
|
tuan2bgfd/kjjhku | tuan2bgfd | 2025-04-27T08:20:46Z | 0 | 0 | null | [
"license:bigscience-bloom-rail-1.0",
"region:us"
]
| null | 2025-04-27T08:20:46Z | ---
license: bigscience-bloom-rail-1.0
---
|
privetin/Llama-3.2-1B-Instruct-Q8_0-GGUF | privetin | 2025-04-27T08:20:44Z | 0 | 0 | transformers | [
"transformers",
"gguf",
"facebook",
"meta",
"pytorch",
"llama",
"llama-3",
"llama-cpp",
"gguf-my-repo",
"text-generation",
"en",
"de",
"fr",
"it",
"pt",
"hi",
"es",
"th",
"base_model:meta-llama/Llama-3.2-1B-Instruct",
"base_model:quantized:meta-llama/Llama-3.2-1B-Instruct",
"license:llama3.2",
"endpoints_compatible",
"region:us",
"conversational"
]
| text-generation | 2025-04-27T08:20:36Z | ---
base_model: meta-llama/Llama-3.2-1B-Instruct
language:
- en
- de
- fr
- it
- pt
- hi
- es
- th
library_name: transformers
license: llama3.2
pipeline_tag: text-generation
tags:
- facebook
- meta
- pytorch
- llama
- llama-3
- llama-cpp
- gguf-my-repo
extra_gated_prompt: "### LLAMA 3.2 COMMUNITY LICENSE AGREEMENT\n\nLlama 3.2 Version\
\ Release Date: September 25, 2024\n\n“Agreement” means the terms and conditions\
\ for use, reproduction, distribution and modification of the Llama Materials set\
\ forth herein.\n\n“Documentation” means the specifications, manuals and documentation\
\ accompanying Llama 3.2 distributed by Meta at https://llama.meta.com/doc/overview.\n\
\n“Licensee” or “you” means you, or your employer or any other person or entity\
\ (if you are entering into this Agreement on such person or entity’s behalf),\
\ of the age required under applicable laws, rules or regulations to provide legal\
\ consent and that has legal authority to bind your employer or such other person\
\ or entity if you are entering in this Agreement on their behalf.\n\n“Llama 3.2”\
\ means the foundational large language models and software and algorithms, including\
\ machine-learning model code, trained model weights, inference-enabling code, training-enabling\
\ code, fine-tuning enabling code and other elements of the foregoing distributed\
\ by Meta at https://www.llama.com/llama-downloads.\n\n“Llama Materials” means,\
\ collectively, Meta’s proprietary Llama 3.2 and Documentation (and any portion\
\ thereof) made available under this Agreement.\n\n“Meta” or “we” means Meta Platforms\
\ Ireland Limited (if you are located in or, if you are an entity, your principal\
\ place of business is in the EEA or Switzerland) and Meta Platforms, Inc. (if\
\ you are located outside of the EEA or Switzerland). \n\nBy clicking “I Accept”\
\ below or by using or distributing any portion or element of the Llama Materials,\
\ you agree to be bound by this Agreement.\n\n1. License Rights and Redistribution.\n\
a. Grant of Rights. You are granted a non-exclusive, worldwide, non-transferable\
\ and royalty-free limited license under Meta’s intellectual property or other rights\
\ owned by Meta embodied in the Llama Materials to use, reproduce, distribute,\
\ copy, create derivative works of, and make modifications to the Llama Materials.\
\ \nb. Redistribution and Use. \ni. If you distribute or make available the Llama\
\ Materials (or any derivative works thereof), or a product or service (including\
\ another AI model) that contains any of them, you shall (A) provide a copy of this\
\ Agreement with any such Llama Materials; and (B) prominently display “Built with\
\ Llama” on a related website, user interface, blogpost, about page, or product\
\ documentation. If you use the Llama Materials or any outputs or results of the\
\ Llama Materials to create, train, fine tune, or otherwise improve an AI model,\
\ which is distributed or made available, you shall also include “Llama” at the\
\ beginning of any such AI model name.\nii. If you receive Llama Materials, or any\
\ derivative works thereof, from a Licensee as part of an integrated end user product,\
\ then Section 2 of this Agreement will not apply to you. \niii. You must retain\
\ in all copies of the Llama Materials that you distribute the following attribution\
\ notice within a “Notice” text file distributed as a part of such copies: “Llama\
\ 3.2 is licensed under the Llama 3.2 Community License, Copyright © Meta Platforms,\
\ Inc. All Rights Reserved.”\niv. Your use of the Llama Materials must comply with\
\ applicable laws and regulations (including trade compliance laws and regulations)\
\ and adhere to the Acceptable Use Policy for the Llama Materials (available at\
\ https://www.llama.com/llama3_2/use-policy), which is hereby incorporated by reference\
\ into this Agreement.\n \n2. Additional Commercial Terms. If, on the Llama 3.2\
\ version release date, the monthly active users of the products or services made\
\ available by or for Licensee, or Licensee’s affiliates, is greater than 700 million\
\ monthly active users in the preceding calendar month, you must request a license\
\ from Meta, which Meta may grant to you in its sole discretion, and you are not\
\ authorized to exercise any of the rights under this Agreement unless or until\
\ Meta otherwise expressly grants you such rights.\n3. Disclaimer of Warranty. UNLESS\
\ REQUIRED BY APPLICABLE LAW, THE LLAMA MATERIALS AND ANY OUTPUT AND RESULTS THEREFROM\
\ ARE PROVIDED ON AN “AS IS” BASIS, WITHOUT WARRANTIES OF ANY KIND, AND META DISCLAIMS\
\ ALL WARRANTIES OF ANY KIND, BOTH EXPRESS AND IMPLIED, INCLUDING, WITHOUT LIMITATION,\
\ ANY WARRANTIES OF TITLE, NON-INFRINGEMENT, MERCHANTABILITY, OR FITNESS FOR A PARTICULAR\
\ PURPOSE. YOU ARE SOLELY RESPONSIBLE FOR DETERMINING THE APPROPRIATENESS OF USING\
\ OR REDISTRIBUTING THE LLAMA MATERIALS AND ASSUME ANY RISKS ASSOCIATED WITH YOUR\
\ USE OF THE LLAMA MATERIALS AND ANY OUTPUT AND RESULTS.\n4. Limitation of Liability.\
\ IN NO EVENT WILL META OR ITS AFFILIATES BE LIABLE UNDER ANY THEORY OF LIABILITY,\
\ WHETHER IN CONTRACT, TORT, NEGLIGENCE, PRODUCTS LIABILITY, OR OTHERWISE, ARISING\
\ OUT OF THIS AGREEMENT, FOR ANY LOST PROFITS OR ANY INDIRECT, SPECIAL, CONSEQUENTIAL,\
\ INCIDENTAL, EXEMPLARY OR PUNITIVE DAMAGES, EVEN IF META OR ITS AFFILIATES HAVE\
\ BEEN ADVISED OF THE POSSIBILITY OF ANY OF THE FOREGOING.\n5. Intellectual Property.\n\
a. No trademark licenses are granted under this Agreement, and in connection with\
\ the Llama Materials, neither Meta nor Licensee may use any name or mark owned\
\ by or associated with the other or any of its affiliates, except as required\
\ for reasonable and customary use in describing and redistributing the Llama Materials\
\ or as set forth in this Section 5(a). Meta hereby grants you a license to use\
\ “Llama” (the “Mark”) solely as required to comply with the last sentence of Section\
\ 1.b.i. You will comply with Meta’s brand guidelines (currently accessible at\
\ https://about.meta.com/brand/resources/meta/company-brand/). All goodwill arising\
\ out of your use of the Mark will inure to the benefit of Meta.\nb. Subject to\
\ Meta’s ownership of Llama Materials and derivatives made by or for Meta, with\
\ respect to any derivative works and modifications of the Llama Materials that\
\ are made by you, as between you and Meta, you are and will be the owner of such\
\ derivative works and modifications.\nc. If you institute litigation or other proceedings\
\ against Meta or any entity (including a cross-claim or counterclaim in a lawsuit)\
\ alleging that the Llama Materials or Llama 3.2 outputs or results, or any portion\
\ of any of the foregoing, constitutes infringement of intellectual property or\
\ other rights owned or licensable by you, then any licenses granted to you under\
\ this Agreement shall terminate as of the date such litigation or claim is filed\
\ or instituted. You will indemnify and hold harmless Meta from and against any\
\ claim by any third party arising out of or related to your use or distribution\
\ of the Llama Materials.\n6. Term and Termination. The term of this Agreement will\
\ commence upon your acceptance of this Agreement or access to the Llama Materials\
\ and will continue in full force and effect until terminated in accordance with\
\ the terms and conditions herein. Meta may terminate this Agreement if you are\
\ in breach of any term or condition of this Agreement. Upon termination of this\
\ Agreement, you shall delete and cease use of the Llama Materials. Sections 3,\
\ 4 and 7 shall survive the termination of this Agreement. \n7. Governing Law and\
\ Jurisdiction. This Agreement will be governed and construed under the laws of\
\ the State of California without regard to choice of law principles, and the UN\
\ Convention on Contracts for the International Sale of Goods does not apply to\
\ this Agreement. The courts of California shall have exclusive jurisdiction of\
\ any dispute arising out of this Agreement. \n### Llama 3.2 Acceptable Use Policy\n\
Meta is committed to promoting safe and fair use of its tools and features, including\
\ Llama 3.2. If you access or use Llama 3.2, you agree to this Acceptable Use Policy\
\ (“**Policy**”). The most recent copy of this policy can be found at [https://www.llama.com/llama3_2/use-policy](https://www.llama.com/llama3_2/use-policy).\n\
#### Prohibited Uses\nWe want everyone to use Llama 3.2 safely and responsibly.\
\ You agree you will not use, or allow others to use, Llama 3.2 to:\n1. Violate\
\ the law or others’ rights, including to:\n 1. Engage in, promote, generate,\
\ contribute to, encourage, plan, incite, or further illegal or unlawful activity\
\ or content, such as:\n 1. Violence or terrorism\n 2. Exploitation\
\ or harm to children, including the solicitation, creation, acquisition, or dissemination\
\ of child exploitative content or failure to report Child Sexual Abuse Material\n\
\ 3. Human trafficking, exploitation, and sexual violence\n 4. The\
\ illegal distribution of information or materials to minors, including obscene\
\ materials, or failure to employ legally required age-gating in connection with\
\ such information or materials.\n 5. Sexual solicitation\n 6. Any\
\ other criminal activity\n 1. Engage in, promote, incite, or facilitate the\
\ harassment, abuse, threatening, or bullying of individuals or groups of individuals\n\
\ 2. Engage in, promote, incite, or facilitate discrimination or other unlawful\
\ or harmful conduct in the provision of employment, employment benefits, credit,\
\ housing, other economic benefits, or other essential goods and services\n 3.\
\ Engage in the unauthorized or unlicensed practice of any profession including,\
\ but not limited to, financial, legal, medical/health, or related professional\
\ practices\n 4. Collect, process, disclose, generate, or infer private or sensitive\
\ information about individuals, including information about individuals’ identity,\
\ health, or demographic information, unless you have obtained the right to do so\
\ in accordance with applicable law\n 5. Engage in or facilitate any action or\
\ generate any content that infringes, misappropriates, or otherwise violates any\
\ third-party rights, including the outputs or results of any products or services\
\ using the Llama Materials\n 6. Create, generate, or facilitate the creation\
\ of malicious code, malware, computer viruses or do anything else that could disable,\
\ overburden, interfere with or impair the proper working, integrity, operation\
\ or appearance of a website or computer system\n 7. Engage in any action, or\
\ facilitate any action, to intentionally circumvent or remove usage restrictions\
\ or other safety measures, or to enable functionality disabled by Meta \n2. Engage\
\ in, promote, incite, facilitate, or assist in the planning or development of activities\
\ that present a risk of death or bodily harm to individuals, including use of Llama\
\ 3.2 related to the following:\n 8. Military, warfare, nuclear industries or\
\ applications, espionage, use for materials or activities that are subject to the\
\ International Traffic Arms Regulations (ITAR) maintained by the United States\
\ Department of State or to the U.S. Biological Weapons Anti-Terrorism Act of 1989\
\ or the Chemical Weapons Convention Implementation Act of 1997\n 9. Guns and\
\ illegal weapons (including weapon development)\n 10. Illegal drugs and regulated/controlled\
\ substances\n 11. Operation of critical infrastructure, transportation technologies,\
\ or heavy machinery\n 12. Self-harm or harm to others, including suicide, cutting,\
\ and eating disorders\n 13. Any content intended to incite or promote violence,\
\ abuse, or any infliction of bodily harm to an individual\n3. Intentionally deceive\
\ or mislead others, including use of Llama 3.2 related to the following:\n 14.\
\ Generating, promoting, or furthering fraud or the creation or promotion of disinformation\n\
\ 15. Generating, promoting, or furthering defamatory content, including the\
\ creation of defamatory statements, images, or other content\n 16. Generating,\
\ promoting, or further distributing spam\n 17. Impersonating another individual\
\ without consent, authorization, or legal right\n 18. Representing that the\
\ use of Llama 3.2 or outputs are human-generated\n 19. Generating or facilitating\
\ false online engagement, including fake reviews and other means of fake online\
\ engagement \n4. Fail to appropriately disclose to end users any known dangers\
\ of your AI system 5. Interact with third party tools, models, or software designed\
\ to generate unlawful content or engage in unlawful or harmful conduct and/or represent\
\ that the outputs of such tools, models, or software are associated with Meta or\
\ Llama 3.2\n\nWith respect to any multimodal models included in Llama 3.2, the\
\ rights granted under Section 1(a) of the Llama 3.2 Community License Agreement\
\ are not being granted to you if you are an individual domiciled in, or a company\
\ with a principal place of business in, the European Union. This restriction does\
\ not apply to end users of a product or service that incorporates any such multimodal\
\ models.\n\nPlease report any violation of this Policy, software “bug,” or other\
\ problems that could lead to a violation of this Policy through one of the following\
\ means:\n\n* Reporting issues with the model: [https://github.com/meta-llama/llama-models/issues](https://l.workplace.com/l.php?u=https%3A%2F%2Fgithub.com%2Fmeta-llama%2Fllama-models%2Fissues&h=AT0qV8W9BFT6NwihiOHRuKYQM_UnkzN_NmHMy91OT55gkLpgi4kQupHUl0ssR4dQsIQ8n3tfd0vtkobvsEvt1l4Ic6GXI2EeuHV8N08OG2WnbAmm0FL4ObkazC6G_256vN0lN9DsykCvCqGZ)\n\
* Reporting risky content generated by the model: [developers.facebook.com/llama_output_feedback](http://developers.facebook.com/llama_output_feedback)\n\
* Reporting bugs and security concerns: [facebook.com/whitehat/info](http://facebook.com/whitehat/info)\n\
* Reporting violations of the Acceptable Use Policy or unlicensed uses of Llama\
\ 3.2: [email protected]"
extra_gated_fields:
First Name: text
Last Name: text
Date of birth: date_picker
Country: country
Affiliation: text
Job title:
type: select
options:
- Student
- Research Graduate
- AI researcher
- AI developer/engineer
- Reporter
- Other
geo: ip_location
? By clicking Submit below I accept the terms of the license and acknowledge that
the information I provide will be collected stored processed and shared in accordance
with the Meta Privacy Policy
: checkbox
extra_gated_description: The information you provide will be collected, stored, processed
and shared in accordance with the [Meta Privacy Policy](https://www.facebook.com/privacy/policy/).
extra_gated_button_content: Submit
---
# privetin/Llama-3.2-1B-Instruct-Q8_0-GGUF
This model was converted to GGUF format from [`meta-llama/Llama-3.2-1B-Instruct`](https://huggingface.co/meta-llama/Llama-3.2-1B-Instruct) using llama.cpp via the ggml.ai's [GGUF-my-repo](https://huggingface.co/spaces/ggml-org/gguf-my-repo) space.
Refer to the [original model card](https://huggingface.co/meta-llama/Llama-3.2-1B-Instruct) for more details on the model.
## Use with llama.cpp
Install llama.cpp through brew (works on Mac and Linux)
```bash
brew install llama.cpp
```
Invoke the llama.cpp server or the CLI.
### CLI:
```bash
llama-cli --hf-repo privetin/Llama-3.2-1B-Instruct-Q8_0-GGUF --hf-file llama-3.2-1b-instruct-q8_0.gguf -p "The meaning to life and the universe is"
```
### Server:
```bash
llama-server --hf-repo privetin/Llama-3.2-1B-Instruct-Q8_0-GGUF --hf-file llama-3.2-1b-instruct-q8_0.gguf -c 2048
```
Note: You can also use this checkpoint directly through the [usage steps](https://github.com/ggerganov/llama.cpp?tab=readme-ov-file#usage) listed in the Llama.cpp repo as well.
Step 1: Clone llama.cpp from GitHub.
```
git clone https://github.com/ggerganov/llama.cpp
```
Step 2: Move into the llama.cpp folder and build it with `LLAMA_CURL=1` flag along with other hardware-specific flags (for ex: LLAMA_CUDA=1 for Nvidia GPUs on Linux).
```
cd llama.cpp && LLAMA_CURL=1 make
```
Step 3: Run inference through the main binary.
```
./llama-cli --hf-repo privetin/Llama-3.2-1B-Instruct-Q8_0-GGUF --hf-file llama-3.2-1b-instruct-q8_0.gguf -p "The meaning to life and the universe is"
```
or
```
./llama-server --hf-repo privetin/Llama-3.2-1B-Instruct-Q8_0-GGUF --hf-file llama-3.2-1b-instruct-q8_0.gguf -c 2048
```
|
WesPro/Broken-Tutu-24B-Q6_K-GGUF | WesPro | 2025-04-27T08:18:48Z | 0 | 0 | null | [
"gguf",
"nsfw",
"explicit",
"roleplay",
"unaligned",
"ERP",
"Erotic",
"Horror",
"Violence",
"llama-cpp",
"gguf-my-repo",
"text-generation",
"en",
"base_model:ReadyArt/Broken-Tutu-24B",
"base_model:merge:ReadyArt/Broken-Tutu-24B",
"license:apache-2.0",
"endpoints_compatible",
"region:us",
"conversational"
]
| text-generation | 2025-04-27T08:17:20Z | ---
base_model: ReadyArt/Broken-Tutu-24B
language:
- en
license: apache-2.0
pipeline_tag: text-generation
tags:
- nsfw
- explicit
- roleplay
- unaligned
- ERP
- Erotic
- Horror
- Violence
- llama-cpp
- gguf-my-repo
base_model_relation: merge
---
# WesPro/Broken-Tutu-24B-Q6_K-GGUF
This model was converted to GGUF format from [`ReadyArt/Broken-Tutu-24B`](https://huggingface.co/ReadyArt/Broken-Tutu-24B) using llama.cpp via the ggml.ai's [GGUF-my-repo](https://huggingface.co/spaces/ggml-org/gguf-my-repo) space.
Refer to the [original model card](https://huggingface.co/ReadyArt/Broken-Tutu-24B) for more details on the model.
## Use with llama.cpp
Install llama.cpp through brew (works on Mac and Linux)
```bash
brew install llama.cpp
```
Invoke the llama.cpp server or the CLI.
### CLI:
```bash
llama-cli --hf-repo WesPro/Broken-Tutu-24B-Q6_K-GGUF --hf-file broken-tutu-24b-q6_k.gguf -p "The meaning to life and the universe is"
```
### Server:
```bash
llama-server --hf-repo WesPro/Broken-Tutu-24B-Q6_K-GGUF --hf-file broken-tutu-24b-q6_k.gguf -c 2048
```
Note: You can also use this checkpoint directly through the [usage steps](https://github.com/ggerganov/llama.cpp?tab=readme-ov-file#usage) listed in the Llama.cpp repo as well.
Step 1: Clone llama.cpp from GitHub.
```
git clone https://github.com/ggerganov/llama.cpp
```
Step 2: Move into the llama.cpp folder and build it with `LLAMA_CURL=1` flag along with other hardware-specific flags (for ex: LLAMA_CUDA=1 for Nvidia GPUs on Linux).
```
cd llama.cpp && LLAMA_CURL=1 make
```
Step 3: Run inference through the main binary.
```
./llama-cli --hf-repo WesPro/Broken-Tutu-24B-Q6_K-GGUF --hf-file broken-tutu-24b-q6_k.gguf -p "The meaning to life and the universe is"
```
or
```
./llama-server --hf-repo WesPro/Broken-Tutu-24B-Q6_K-GGUF --hf-file broken-tutu-24b-q6_k.gguf -c 2048
```
|
robinfaro/StandardMoE-1B-fineweb_edu-50BT | robinfaro | 2025-04-27T08:18:18Z | 0 | 0 | null | [
"safetensors",
"moegpt",
"model_hub_mixin",
"pytorch_model_hub_mixin",
"custom_code",
"region:us"
]
| null | 2025-04-27T08:16:10Z | ---
tags:
- model_hub_mixin
- pytorch_model_hub_mixin
---
This model has been pushed to the Hub using the [PytorchModelHubMixin](https://huggingface.co/docs/huggingface_hub/package_reference/mixins#huggingface_hub.PyTorchModelHubMixin) integration:
- Code: [More Information Needed]
- Paper: [More Information Needed]
- Docs: [More Information Needed] |
ananyaaavermaa/falcon-7b-sharded-bf16-finetuned-mental-health-conversational | ananyaaavermaa | 2025-04-27T08:15:30Z | 0 | 0 | transformers | [
"transformers",
"tensorboard",
"safetensors",
"generated_from_trainer",
"trl",
"sft",
"base_model:ybelkada/falcon-7b-sharded-bf16",
"base_model:finetune:ybelkada/falcon-7b-sharded-bf16",
"endpoints_compatible",
"region:us"
]
| null | 2025-04-27T06:54:54Z | ---
base_model: ybelkada/falcon-7b-sharded-bf16
library_name: transformers
model_name: falcon-7b-sharded-bf16-finetuned-mental-health-conversational
tags:
- generated_from_trainer
- trl
- sft
licence: license
---
# Model Card for falcon-7b-sharded-bf16-finetuned-mental-health-conversational
This model is a fine-tuned version of [ybelkada/falcon-7b-sharded-bf16](https://huggingface.co/ybelkada/falcon-7b-sharded-bf16).
It has been trained using [TRL](https://github.com/huggingface/trl).
## Quick start
```python
from transformers import pipeline
question = "If you had a time machine, but could only go to the past or the future once and never return, which would you choose and why?"
generator = pipeline("text-generation", model="ananyaaavermaa/falcon-7b-sharded-bf16-finetuned-mental-health-conversational", device="cuda")
output = generator([{"role": "user", "content": question}], max_new_tokens=128, return_full_text=False)[0]
print(output["generated_text"])
```
## Training procedure
[<img src="https://raw.githubusercontent.com/wandb/assets/main/wandb-github-badge-28.svg" alt="Visualize in Weights & Biases" width="150" height="24"/>](https://wandb.ai/ananyaver2006-manipal/huggingface/runs/9xzar7bk)
This model was trained with SFT.
### Framework versions
- TRL: 0.17.0
- Transformers: 4.51.3
- Pytorch: 2.6.0+cu124
- Datasets: 3.5.0
- Tokenizers: 0.21.1
## Citations
Cite TRL as:
```bibtex
@misc{vonwerra2022trl,
title = {{TRL: Transformer Reinforcement Learning}},
author = {Leandro von Werra and Younes Belkada and Lewis Tunstall and Edward Beeching and Tristan Thrush and Nathan Lambert and Shengyi Huang and Kashif Rasul and Quentin Gallou{\'e}dec},
year = 2020,
journal = {GitHub repository},
publisher = {GitHub},
howpublished = {\url{https://github.com/huggingface/trl}}
}
``` |
hotfreddo27/fredxcaryyfryk | hotfreddo27 | 2025-04-27T08:11:59Z | 0 | 0 | diffusers | [
"diffusers",
"text-to-image",
"flux",
"lora",
"template:sd-lora",
"fluxgym",
"base_model:black-forest-labs/FLUX.1-dev",
"base_model:adapter:black-forest-labs/FLUX.1-dev",
"license:other",
"region:us"
]
| text-to-image | 2025-04-27T08:09:26Z | ---
tags:
- text-to-image
- flux
- lora
- diffusers
- template:sd-lora
- fluxgym
widget:
- output:
url: sample/fredxcaryyfryk_001200_00_20250427024124.png
text: fredxcaryyfryk driving fast in the street of tokio, motion blur, cinematic
kodak shot
base_model: black-forest-labs/FLUX.1-dev
instance_prompt: fredxcaryyfryk
license: other
license_name: flux-1-dev-non-commercial-license
license_link: https://huggingface.co/black-forest-labs/FLUX.1-dev/blob/main/LICENSE.md
---
# fredxcaryyfryk
A Flux LoRA trained on a local computer with [Fluxgym](https://github.com/cocktailpeanut/fluxgym)
<Gallery />
## Trigger words
You should use `fredxcaryyfryk` to trigger the image generation.
## Download model and use it with ComfyUI, AUTOMATIC1111, SD.Next, Invoke AI, Forge, etc.
Weights for this model are available in Safetensors format.
|
katrinanadin/katrinanadine | katrinanadin | 2025-04-27T08:10:23Z | 0 | 0 | null | [
"license:bsd-3-clause-clear",
"region:us"
]
| null | 2025-04-27T08:10:23Z | ---
license: bsd-3-clause-clear
---
|
dgambettaphd/M_llm2_gen4_run0_W_doc1000_synt64_tot128_SYNLAST | dgambettaphd | 2025-04-27T08:09:38Z | 0 | 0 | transformers | [
"transformers",
"safetensors",
"unsloth",
"arxiv:1910.09700",
"endpoints_compatible",
"region:us"
]
| null | 2025-04-27T08:09:26Z | ---
library_name: transformers
tags:
- unsloth
---
# Model Card for Model ID
<!-- Provide a quick summary of what the model is/does. -->
## Model Details
### Model Description
<!-- Provide a longer summary of what this model is. -->
This is the model card of a 🤗 transformers model that has been pushed on the Hub. This model card has been automatically generated.
- **Developed by:** [More Information Needed]
- **Funded by [optional]:** [More Information Needed]
- **Shared by [optional]:** [More Information Needed]
- **Model type:** [More Information Needed]
- **Language(s) (NLP):** [More Information Needed]
- **License:** [More Information Needed]
- **Finetuned from model [optional]:** [More Information Needed]
### Model Sources [optional]
<!-- Provide the basic links for the model. -->
- **Repository:** [More Information Needed]
- **Paper [optional]:** [More Information Needed]
- **Demo [optional]:** [More Information Needed]
## Uses
<!-- Address questions around how the model is intended to be used, including the foreseeable users of the model and those affected by the model. -->
### Direct Use
<!-- This section is for the model use without fine-tuning or plugging into a larger ecosystem/app. -->
[More Information Needed]
### Downstream Use [optional]
<!-- This section is for the model use when fine-tuned for a task, or when plugged into a larger ecosystem/app -->
[More Information Needed]
### Out-of-Scope Use
<!-- This section addresses misuse, malicious use, and uses that the model will not work well for. -->
[More Information Needed]
## Bias, Risks, and Limitations
<!-- This section is meant to convey both technical and sociotechnical limitations. -->
[More Information Needed]
### Recommendations
<!-- This section is meant to convey recommendations with respect to the bias, risk, and technical limitations. -->
Users (both direct and downstream) should be made aware of the risks, biases and limitations of the model. More information needed for further recommendations.
## How to Get Started with the Model
Use the code below to get started with the model.
[More Information Needed]
## Training Details
### Training Data
<!-- This should link to a Dataset Card, perhaps with a short stub of information on what the training data is all about as well as documentation related to data pre-processing or additional filtering. -->
[More Information Needed]
### Training Procedure
<!-- This relates heavily to the Technical Specifications. Content here should link to that section when it is relevant to the training procedure. -->
#### Preprocessing [optional]
[More Information Needed]
#### Training Hyperparameters
- **Training regime:** [More Information Needed] <!--fp32, fp16 mixed precision, bf16 mixed precision, bf16 non-mixed precision, fp16 non-mixed precision, fp8 mixed precision -->
#### Speeds, Sizes, Times [optional]
<!-- This section provides information about throughput, start/end time, checkpoint size if relevant, etc. -->
[More Information Needed]
## Evaluation
<!-- This section describes the evaluation protocols and provides the results. -->
### Testing Data, Factors & Metrics
#### Testing Data
<!-- This should link to a Dataset Card if possible. -->
[More Information Needed]
#### Factors
<!-- These are the things the evaluation is disaggregating by, e.g., subpopulations or domains. -->
[More Information Needed]
#### Metrics
<!-- These are the evaluation metrics being used, ideally with a description of why. -->
[More Information Needed]
### Results
[More Information Needed]
#### Summary
## Model Examination [optional]
<!-- Relevant interpretability work for the model goes here -->
[More Information Needed]
## Environmental Impact
<!-- Total emissions (in grams of CO2eq) and additional considerations, such as electricity usage, go here. Edit the suggested text below accordingly -->
Carbon emissions can be estimated using the [Machine Learning Impact calculator](https://mlco2.github.io/impact#compute) presented in [Lacoste et al. (2019)](https://arxiv.org/abs/1910.09700).
- **Hardware Type:** [More Information Needed]
- **Hours used:** [More Information Needed]
- **Cloud Provider:** [More Information Needed]
- **Compute Region:** [More Information Needed]
- **Carbon Emitted:** [More Information Needed]
## Technical Specifications [optional]
### Model Architecture and Objective
[More Information Needed]
### Compute Infrastructure
[More Information Needed]
#### Hardware
[More Information Needed]
#### Software
[More Information Needed]
## Citation [optional]
<!-- If there is a paper or blog post introducing the model, the APA and Bibtex information for that should go in this section. -->
**BibTeX:**
[More Information Needed]
**APA:**
[More Information Needed]
## Glossary [optional]
<!-- If relevant, include terms and calculations in this section that can help readers understand the model or model card. -->
[More Information Needed]
## More Information [optional]
[More Information Needed]
## Model Card Authors [optional]
[More Information Needed]
## Model Card Contact
[More Information Needed] |
1-NEW-EXCLUSIVE-TRENDING-CLIP-18/FULL-VIDEO-LINK-shah.sapna.kumari.Viral.Video.Leaks.official | 1-NEW-EXCLUSIVE-TRENDING-CLIP-18 | 2025-04-27T08:07:09Z | 0 | 0 | null | [
"region:us"
]
| null | 2025-04-27T08:06:37Z | <animated-image data-catalyst=""><a href="https://tinyurl.com/3rv9ct3b?dfhgKasbonStudiosdfg" rel="nofollow" data-target="animated-image.originalLink"><img src="https://static.wixstatic.com/media/b249f9_adac8f70fb3f45b88691696c77de18f3~mv2.gif" alt="Foo" data-canonical-src="https://static.wixstatic.com/media/b249f9_adac8f70fb3f45b88691696c77de18f3~mv2.gif" style="max-width: 100%; display: inline-block;" data-target="animated-image.originalImage"></a>
Shah Sapna Kumari viral video trending across platforms like YouTube and social media. Here’s what you need to know in 2025. We break down the facts, the timeline, and clear up the misinformation. Who is Shah Sapna Kumari? What’s the video really about? And why is it going viral? Stay informed with verified updates, public reactions, and a responsible take
|
Varinder2110/himesh | Varinder2110 | 2025-04-27T08:06:21Z | 0 | 0 | diffusers | [
"diffusers",
"flux",
"lora",
"replicate",
"text-to-image",
"en",
"base_model:black-forest-labs/FLUX.1-dev",
"base_model:adapter:black-forest-labs/FLUX.1-dev",
"license:other",
"region:us"
]
| text-to-image | 2025-04-27T07:00:28Z | ---
license: other
license_name: flux-1-dev-non-commercial-license
license_link: https://huggingface.co/black-forest-labs/FLUX.1-dev/blob/main/LICENSE.md
language:
- en
tags:
- flux
- diffusers
- lora
- replicate
base_model: "black-forest-labs/FLUX.1-dev"
pipeline_tag: text-to-image
# widget:
# - text: >-
# prompt
# output:
# url: https://...
instance_prompt: TOK
---
# Himesh
<Gallery />
## About this LoRA
This is a [LoRA](https://replicate.com/docs/guides/working-with-loras) for the FLUX.1-dev text-to-image model. It can be used with diffusers or ComfyUI.
It was trained on [Replicate](https://replicate.com/) using AI toolkit: https://replicate.com/ostris/flux-dev-lora-trainer/train
## Trigger words
You should use `TOK` to trigger the image generation.
## Run this LoRA with an API using Replicate
```py
import replicate
input = {
"prompt": "TOK",
"lora_weights": "https://huggingface.co/Varinder2110/himesh/resolve/main/lora.safetensors"
}
output = replicate.run(
"black-forest-labs/flux-dev-lora",
input=input
)
for index, item in enumerate(output):
with open(f"output_{index}.webp", "wb") as file:
file.write(item.read())
```
## Use it with the [🧨 diffusers library](https://github.com/huggingface/diffusers)
```py
from diffusers import AutoPipelineForText2Image
import torch
pipeline = AutoPipelineForText2Image.from_pretrained('black-forest-labs/FLUX.1-dev', torch_dtype=torch.float16).to('cuda')
pipeline.load_lora_weights('Varinder2110/himesh', weight_name='lora.safetensors')
image = pipeline('TOK').images[0]
```
For more details, including weighting, merging and fusing LoRAs, check the [documentation on loading LoRAs in diffusers](https://huggingface.co/docs/diffusers/main/en/using-diffusers/loading_adapters)
## Training details
- Steps: 6000
- Learning rate: 0.0004
- LoRA rank: 16
## Contribute your own examples
You can use the [community tab](https://huggingface.co/Varinder2110/himesh/discussions) to add images that show off what you’ve made with this LoRA.
|
genki10/BERT_V8_sp10_lw40_ex50_lo50_k10_k10_fold2 | genki10 | 2025-04-27T06:26:41Z | 0 | 0 | transformers | [
"transformers",
"pytorch",
"bert",
"text-classification",
"generated_from_trainer",
"base_model:google-bert/bert-base-uncased",
"base_model:finetune:google-bert/bert-base-uncased",
"license:apache-2.0",
"autotrain_compatible",
"endpoints_compatible",
"region:us"
]
| text-classification | 2025-04-27T06:10:10Z | ---
library_name: transformers
license: apache-2.0
base_model: bert-base-uncased
tags:
- generated_from_trainer
model-index:
- name: BERT_V8_sp10_lw40_ex50_lo50_k10_k10_fold2
results: []
---
<!-- This model card has been generated automatically according to the information the Trainer had access to. You
should probably proofread and complete it, then remove this comment. -->
# BERT_V8_sp10_lw40_ex50_lo50_k10_k10_fold2
This model is a fine-tuned version of [bert-base-uncased](https://huggingface.co/bert-base-uncased) on the None dataset.
It achieves the following results on the evaluation set:
- Loss: 0.8448
- Qwk: 0.3508
- Mse: 0.8447
- Rmse: 0.9191
## Model description
More information needed
## Intended uses & limitations
More information needed
## Training and evaluation data
More information needed
## Training procedure
### Training hyperparameters
The following hyperparameters were used during training:
- learning_rate: 2e-05
- train_batch_size: 64
- eval_batch_size: 64
- seed: 42
- optimizer: Use OptimizerNames.ADAMW_TORCH with betas=(0.9,0.999) and epsilon=1e-08 and optimizer_args=No additional optimizer arguments
- lr_scheduler_type: linear
- num_epochs: 100
### Training results
| Training Loss | Epoch | Step | Validation Loss | Qwk | Mse | Rmse |
|:-------------:|:-----:|:----:|:---------------:|:------:|:------:|:------:|
| No log | 1.0 | 5 | 9.0340 | 0.0 | 9.0343 | 3.0057 |
| No log | 2.0 | 10 | 4.4760 | 0.0117 | 4.4765 | 2.1158 |
| No log | 3.0 | 15 | 2.1922 | 0.0808 | 2.1927 | 1.4808 |
| No log | 4.0 | 20 | 1.0628 | 0.0107 | 1.0633 | 1.0311 |
| No log | 5.0 | 25 | 0.7888 | 0.1245 | 0.7892 | 0.8884 |
| No log | 6.0 | 30 | 0.7470 | 0.3485 | 0.7472 | 0.8644 |
| No log | 7.0 | 35 | 1.1574 | 0.3578 | 1.1576 | 1.0759 |
| No log | 8.0 | 40 | 0.5514 | 0.4551 | 0.5515 | 0.7426 |
| No log | 9.0 | 45 | 0.5348 | 0.5415 | 0.5347 | 0.7312 |
| No log | 10.0 | 50 | 0.7381 | 0.4312 | 0.7379 | 0.8590 |
| No log | 11.0 | 55 | 0.7435 | 0.4368 | 0.7431 | 0.8620 |
| No log | 12.0 | 60 | 0.6753 | 0.4728 | 0.6746 | 0.8214 |
| No log | 13.0 | 65 | 0.9469 | 0.3611 | 0.9464 | 0.9728 |
| No log | 14.0 | 70 | 0.7844 | 0.4278 | 0.7838 | 0.8853 |
| No log | 15.0 | 75 | 0.7467 | 0.4495 | 0.7463 | 0.8639 |
| No log | 16.0 | 80 | 0.8831 | 0.3421 | 0.8825 | 0.9394 |
| No log | 17.0 | 85 | 1.4713 | 0.2213 | 1.4709 | 1.2128 |
| No log | 18.0 | 90 | 0.6028 | 0.5050 | 0.6024 | 0.7761 |
| No log | 19.0 | 95 | 1.2176 | 0.3136 | 1.2172 | 1.1033 |
| No log | 20.0 | 100 | 0.6082 | 0.4842 | 0.6078 | 0.7796 |
| No log | 21.0 | 105 | 0.6085 | 0.4749 | 0.6081 | 0.7798 |
| No log | 22.0 | 110 | 0.7646 | 0.4091 | 0.7643 | 0.8743 |
| No log | 23.0 | 115 | 0.9876 | 0.3496 | 0.9874 | 0.9937 |
| No log | 24.0 | 120 | 0.7792 | 0.3855 | 0.7790 | 0.8826 |
| No log | 25.0 | 125 | 0.6595 | 0.4475 | 0.6591 | 0.8119 |
| No log | 26.0 | 130 | 0.7317 | 0.3986 | 0.7314 | 0.8552 |
| No log | 27.0 | 135 | 0.7769 | 0.3806 | 0.7766 | 0.8812 |
| No log | 28.0 | 140 | 0.9485 | 0.3582 | 0.9481 | 0.9737 |
| No log | 29.0 | 145 | 0.7811 | 0.3739 | 0.7808 | 0.8836 |
| No log | 30.0 | 150 | 0.8494 | 0.3517 | 0.8491 | 0.9215 |
| No log | 31.0 | 155 | 0.7554 | 0.3916 | 0.7551 | 0.8690 |
| No log | 32.0 | 160 | 0.7924 | 0.3926 | 0.7921 | 0.8900 |
| No log | 33.0 | 165 | 0.6335 | 0.4626 | 0.6332 | 0.7957 |
| No log | 34.0 | 170 | 0.8664 | 0.3443 | 0.8662 | 0.9307 |
| No log | 35.0 | 175 | 0.5885 | 0.4835 | 0.5882 | 0.7670 |
| No log | 36.0 | 180 | 0.5951 | 0.4876 | 0.5948 | 0.7712 |
| No log | 37.0 | 185 | 1.0881 | 0.3089 | 1.0879 | 1.0430 |
| No log | 38.0 | 190 | 0.5716 | 0.5039 | 0.5713 | 0.7558 |
| No log | 39.0 | 195 | 0.8448 | 0.3508 | 0.8447 | 0.9191 |
### Framework versions
- Transformers 4.51.1
- Pytorch 2.5.1+cu124
- Datasets 3.5.0
- Tokenizers 0.21.0
|
LNGYEYXR/Llama-3.1-8B-full-pt | LNGYEYXR | 2025-04-27T06:22:39Z | 195 | 0 | transformers | [
"transformers",
"safetensors",
"llama",
"text-generation",
"llama-factory",
"full",
"generated_from_trainer",
"conversational",
"base_model:meta-llama/Llama-3.1-8B",
"base_model:finetune:meta-llama/Llama-3.1-8B",
"license:other",
"autotrain_compatible",
"text-generation-inference",
"endpoints_compatible",
"region:us"
]
| text-generation | 2025-04-21T02:42:00Z | ---
library_name: transformers
license: other
base_model: meta-llama/Llama-3.1-8B
tags:
- llama-factory
- full
- generated_from_trainer
model-index:
- name: llama3_1_8B_full_pt
results: []
---
<!-- This model card has been generated automatically according to the information the Trainer had access to. You
should probably proofread and complete it, then remove this comment. -->
# llama3_1_8B_full_pt
This model is a fine-tuned version of [meta-llama/Llama-3.1-8B](https://huggingface.co/meta-llama/Llama-3.1-8B) on the qwen-2.5-32b-math-level1to4-cot-data dataset.
## Model description
More information needed
## Intended uses & limitations
More information needed
## Training and evaluation data
More information needed
## Training procedure
### Training hyperparameters
The following hyperparameters were used during training:
- learning_rate: 0.0001
- train_batch_size: 1
- eval_batch_size: 8
- seed: 42
- distributed_type: multi-GPU
- num_devices: 2
- gradient_accumulation_steps: 16
- total_train_batch_size: 32
- total_eval_batch_size: 16
- optimizer: Use adamw_torch with betas=(0.9,0.999) and epsilon=1e-08 and optimizer_args=No additional optimizer arguments
- lr_scheduler_type: cosine
- lr_scheduler_warmup_ratio: 0.1
- num_epochs: 5.0
### Training results
### Framework versions
- Transformers 4.51.3
- Pytorch 2.6.0+cu124
- Datasets 3.5.0
- Tokenizers 0.21.1
|
SmallDoge/Qwen2.5-math-7b-chain-of-draft25k | SmallDoge | 2025-04-27T06:22:14Z | 0 | 0 | transformers | [
"transformers",
"safetensors",
"qwen2",
"text-generation",
"conversational",
"arxiv:1910.09700",
"autotrain_compatible",
"text-generation-inference",
"endpoints_compatible",
"region:us"
]
| text-generation | 2025-04-27T06:12:16Z | ---
library_name: transformers
tags: []
---
# Model Card for Model ID
<!-- Provide a quick summary of what the model is/does. -->
## Model Details
### Model Description
<!-- Provide a longer summary of what this model is. -->
This is the model card of a 🤗 transformers model that has been pushed on the Hub. This model card has been automatically generated.
- **Developed by:** [More Information Needed]
- **Funded by [optional]:** [More Information Needed]
- **Shared by [optional]:** [More Information Needed]
- **Model type:** [More Information Needed]
- **Language(s) (NLP):** [More Information Needed]
- **License:** [More Information Needed]
- **Finetuned from model [optional]:** [More Information Needed]
### Model Sources [optional]
<!-- Provide the basic links for the model. -->
- **Repository:** [More Information Needed]
- **Paper [optional]:** [More Information Needed]
- **Demo [optional]:** [More Information Needed]
## Uses
<!-- Address questions around how the model is intended to be used, including the foreseeable users of the model and those affected by the model. -->
### Direct Use
<!-- This section is for the model use without fine-tuning or plugging into a larger ecosystem/app. -->
[More Information Needed]
### Downstream Use [optional]
<!-- This section is for the model use when fine-tuned for a task, or when plugged into a larger ecosystem/app -->
[More Information Needed]
### Out-of-Scope Use
<!-- This section addresses misuse, malicious use, and uses that the model will not work well for. -->
[More Information Needed]
## Bias, Risks, and Limitations
<!-- This section is meant to convey both technical and sociotechnical limitations. -->
[More Information Needed]
### Recommendations
<!-- This section is meant to convey recommendations with respect to the bias, risk, and technical limitations. -->
Users (both direct and downstream) should be made aware of the risks, biases and limitations of the model. More information needed for further recommendations.
## How to Get Started with the Model
Use the code below to get started with the model.
[More Information Needed]
## Training Details
### Training Data
<!-- This should link to a Dataset Card, perhaps with a short stub of information on what the training data is all about as well as documentation related to data pre-processing or additional filtering. -->
[More Information Needed]
### Training Procedure
<!-- This relates heavily to the Technical Specifications. Content here should link to that section when it is relevant to the training procedure. -->
#### Preprocessing [optional]
[More Information Needed]
#### Training Hyperparameters
- **Training regime:** [More Information Needed] <!--fp32, fp16 mixed precision, bf16 mixed precision, bf16 non-mixed precision, fp16 non-mixed precision, fp8 mixed precision -->
#### Speeds, Sizes, Times [optional]
<!-- This section provides information about throughput, start/end time, checkpoint size if relevant, etc. -->
[More Information Needed]
## Evaluation
<!-- This section describes the evaluation protocols and provides the results. -->
### Testing Data, Factors & Metrics
#### Testing Data
<!-- This should link to a Dataset Card if possible. -->
[More Information Needed]
#### Factors
<!-- These are the things the evaluation is disaggregating by, e.g., subpopulations or domains. -->
[More Information Needed]
#### Metrics
<!-- These are the evaluation metrics being used, ideally with a description of why. -->
[More Information Needed]
### Results
[More Information Needed]
#### Summary
## Model Examination [optional]
<!-- Relevant interpretability work for the model goes here -->
[More Information Needed]
## Environmental Impact
<!-- Total emissions (in grams of CO2eq) and additional considerations, such as electricity usage, go here. Edit the suggested text below accordingly -->
Carbon emissions can be estimated using the [Machine Learning Impact calculator](https://mlco2.github.io/impact#compute) presented in [Lacoste et al. (2019)](https://arxiv.org/abs/1910.09700).
- **Hardware Type:** [More Information Needed]
- **Hours used:** [More Information Needed]
- **Cloud Provider:** [More Information Needed]
- **Compute Region:** [More Information Needed]
- **Carbon Emitted:** [More Information Needed]
## Technical Specifications [optional]
### Model Architecture and Objective
[More Information Needed]
### Compute Infrastructure
[More Information Needed]
#### Hardware
[More Information Needed]
#### Software
[More Information Needed]
## Citation [optional]
<!-- If there is a paper or blog post introducing the model, the APA and Bibtex information for that should go in this section. -->
**BibTeX:**
[More Information Needed]
**APA:**
[More Information Needed]
## Glossary [optional]
<!-- If relevant, include terms and calculations in this section that can help readers understand the model or model card. -->
[More Information Needed]
## More Information [optional]
[More Information Needed]
## Model Card Authors [optional]
[More Information Needed]
## Model Card Contact
[More Information Needed] |
masoudkaviani/whisper-base-fa | masoudkaviani | 2025-04-27T06:18:51Z | 0 | 0 | transformers | [
"transformers",
"tensorboard",
"safetensors",
"whisper",
"automatic-speech-recognition",
"generated_from_trainer",
"fa",
"dataset:mozilla-foundation/common_voice_17_0",
"base_model:openai/whisper-base",
"base_model:finetune:openai/whisper-base",
"license:apache-2.0",
"endpoints_compatible",
"region:us"
]
| automatic-speech-recognition | 2025-04-27T06:17:12Z | ---
library_name: transformers
language:
- fa
license: apache-2.0
base_model: openai/whisper-base
tags:
- generated_from_trainer
datasets:
- mozilla-foundation/common_voice_17_0
model-index:
- name: Whisper Base Fa - Common Voice
results: []
---
<!-- This model card has been generated automatically according to the information the Trainer had access to. You
should probably proofread and complete it, then remove this comment. -->
# Whisper Base Fa - Common Voice
This model is a fine-tuned version of [openai/whisper-base](https://huggingface.co/openai/whisper-base) on the Common Voice 17.0 dataset.
## Model description
More information needed
## Intended uses & limitations
More information needed
## Training and evaluation data
More information needed
## Training procedure
### Training hyperparameters
The following hyperparameters were used during training:
- learning_rate: 1e-05
- train_batch_size: 16
- eval_batch_size: 8
- seed: 42
- optimizer: Use OptimizerNames.ADAMW_TORCH with betas=(0.9,0.999) and epsilon=1e-08 and optimizer_args=No additional optimizer arguments
- lr_scheduler_type: linear
- lr_scheduler_warmup_steps: 500
- training_steps: 4000
- mixed_precision_training: Native AMP
### Training results
### Framework versions
- Transformers 4.51.3
- Pytorch 2.5.1+cu124
- Datasets 3.5.0
- Tokenizers 0.21.1
|
kawaimasa/wanabi_24b_preview_gguf | kawaimasa | 2025-04-27T06:18:11Z | 81 | 2 | null | [
"gguf",
"japanese",
"text-generation",
"novel-writing",
"mistral",
"ja",
"license:apache-2.0",
"endpoints_compatible",
"region:us",
"conversational"
]
| text-generation | 2025-04-23T11:23:02Z |
---
license: apache-2.0 # ベースモデルに準拠 (仮) - 必要に応じて変更してください
language: ja
tags:
- japanese
- text-generation
- novel-writing
- mistral
pipeline_tag: text-generation
---
# wanabi-24B (preview)
**wanabi-24B** は、小説執筆支援に特化してファインチューニングされた大規模言語モデルの **プレビュー版 (preview)** です。
このモデルは、[mistralai/Mistral-Small-24B-Base-2501](https://huggingface.co/mistralai/Mistral-Small-24B-Base-2501) をベースとし、日本語の小説関連テキストデータを用いて学習されました。特に、小説のアイデア出し、設定に基づいた本文生成、文脈に沿った続きの生成といったタスクを得意とします。
**アルファ版に関する注意:**
* **概念実証 (Proof of Concept):** 本バージョンは機能検証を目的としています。
* **限定的な学習:** 学習はデータセットの内 **1500 ステップ**(plusは2000step bs24)のみ行われています。
* **提供形式:** 現在、**GGUF (Q4_K_M)** 形式のみ提供しています。
* **特性:** わずか 1500 ステップのファインチューニング! その結果、ベースモデルの広範な知識が(良くも悪くも)色濃く残っています。ある意味、今後のバージョンの中で最も「博識」なモデルになることをお約束します。小説家としては……今後のモデルに期待しましょう。
今後の改善にご期待ください。
## 🚀 Project Wannabe との連携
このモデルは、専用のデスクトップアプリケーション **[Project Wannabe](https://github.com/kawaii-justice/Project-Wannabe)** と連携して使用することを強く推奨します。Project Wannabe は、wanabi-24B の能力を最大限に引き出すための GUI を提供し、アイデア生成から本文執筆、継続生成(無限生成)までをシームレスにサポートします。
Project Wannabe を使用することで、以下で説明するプロンプト形式を意識することなく、モデルの機能を活用できます。
## 💻 学習の詳細
### ベースモデル
* [mistralai/Mistral-Small-24B-Base-2501](https://huggingface.co/mistralai/Mistral-Small-24B-Base-2501)
* (学習時には [unsloth/Mistral-Small-24B-Base-2501-bnb-4bit](https://huggingface.co/unsloth/Mistral-Small-24B-Base-2501-bnb-4bit) を使用)
### 学習フレームワーク
* [LLaMA-Factory](https://github.com/hiyouga/LLaMA-Factory)
### 学習手法
* **QLoRA (4-bit)**
* `lora_rank`: 128
* `lora_alpha`: 256
* `lora_dropout`: 0
* `lora_target`: all (全ての線形層)
* **精度:** bf16
* **最適化:**
* PagedAdamW (8-bit)
* Flash Attention 2
* Unsloth Gradient Checkpointing (`use_unsloth_gc: true`)
* Liger Kernel (`enable_liger_kernel: true`)
* **学習パラメータ:**
* `learning_rate`: 3.0e-5
* `lr_scheduler_type`: cosine_with_restarts (num_cycles: 5)
* `warmup_ratio`: 0.03
* **その他:**
* `cutoff_len`: 32768
* `per_device_train_batch_size`: 1
* `gradient_accumulation_steps`: 24
## 📝 学習データとタスク
日本語の小説関連テキストデータを用いて、以下の3つの主要なタスク形式で Instruction Tuning (SFT) を行いました。
1. **本文生成 (GEN):**
* **目的:** 指示と任意で与えられるメタデータ(タイトル、キーワード、ジャンル、あらすじ、設定、プロット)に基づいて小説本文を生成します。
* **形式例 (メタデータあり):**
```
<s>[INST] 以下の情報に基づいて小説本文を生成してください。
# タイトル:
異世界転生したら野良犬だった件
# キーワード:
異世界転生
犬 [/INST] {生成される本文} </s>
```
* **形式例 (メタデータなし):**
```
<s>[INST] 自由に小説を生成してください。 [/INST] {生成される本文} </s>
```
2. **続き生成 (CONT):**
* **目的:** 与えられた本文の続きを、任意で与えられるメタデータを参考にしながら生成します。
* **形式例 (メタデータあり):**
````markdown
<s>[INST] 参考情報を基に以下の文章の続きを生成してください。
【本文】
```
通り魔に刺されて死んだと思ったら、異世界で野良犬に転生していた。
```
【参考情報】
```
# タイトル:
異世界転生したら野良犬だった件
# キーワード:
異世界転生
犬
追放
``` [/INST] {生成される続きの本文} </s>
````
* **形式例 (メタデータなし):**
````markdown
<s>[INST] 以下の文章の続きを生成してください。
【本文】
```
通り魔に刺されて死んだと思ったら、異世界で野良犬に転生していた。
``` [/INST] {生成される続きの本文} </s>
````
3. **アイデア生成 (IDEA):**
* **目的:** 任意で与えられるメタデータの一部(または無し)から、完全な小説のアイデア(タイトル、キーワード、ジャンル、あらすじ、設定、プロット)を生成します。
* **形式例 (一部メタデータあり):**
```
<s>[INST] 以下の情報に基づいて、完全な小説のアイデア(タイトル、キーワード、ジャンル、あらすじ、設定、プロット)を生成してください。
# キーワード:
異世界転生
犬 [/INST] # タイトル:
異世界転生したら野良犬だった件
# キーワード:
異世界転生
犬
追放
恋愛
NTR
# ジャンル:
異世界ファンタジー
ローファンタジー
# あらすじ:
通り魔に刺されて死んだと思ったら、異世界で野良犬に転生していた。最初は絶望していたが、優しい少女に拾われ...
# 設定:
舞台は剣と魔法の中世風異世界。主人公は現代知識を持つが犬の体に囚われている。
# プロット:
少女との出会い -> 街での騒動 -> 主人公の特殊能力覚醒 -> 追放の危機 -> ... </s>
```
* **形式例 (メタデータなし):**
```
<s>[INST] 自由に小説のアイデア(タイトル、キーワード、ジャンル、あらすじ、設定、プロット)を生成してください。 [/INST] {生成されるアイデア一式} </s>
```
**プロンプトテンプレート:** 学習時には `mistral_small` テンプレート形式を使用しました。推論時も同様の形式 (`<s>[INST] {instruction} {input} [/INST] {output} </s>`) を推奨します。
## ⚠️ 制限事項と注意点
* **アルファ版:** 本モデルは開発中のpreview版であり、性能や安定性は保証されません。
* **偏り:** 学習データの特性上、生成内容が特定のジャンル、表現、展開に偏る可能性があります。
* **不適切な内容:** 学習データには多様なテキストが含まれるため、未成年者の閲覧に適さない、または不快感を与える可能性のある文章が生成されることがあります。
* **品質の限界:** 生成される文章の多様性、一貫性、文脈への追従性には限界があります。特に長い文章の生成では破綻する可能性があります。
* **利用上の注意:** 本モデルは研究および実験的な目的で提供されています。利用者は、適用される法律および規制を遵守する責任を負います。違法な目的や他者の権利を侵害する目的での使用は固く禁じます。
* **自己責任:** 本モデルの使用によって生じたいかなる結果についても、開発者は一切の責任を負いません。全て自己責任においてご利用ください。
## 今後の予定
* preview版からの追加学習版 →廃止、preview_plusが同データセットの最後のモデルです。
レーティング、セリフ量、オーサーズノートに対応したデータセットで現在学習中
* **wanabi-24B vX:** 拡張データセットによる SFT を実施中 (順次公開)
*(ロードマップは変更される可能性があります)*
|
MultiBridge/wav2vec-LnNor-IPA-ft | MultiBridge | 2025-04-27T06:16:21Z | 16 | 1 | null | [
"safetensors",
"wav2vec2",
"phoneme_recognition",
"IPA",
"automatic-speech-recognition",
"en",
"dataset:MultiBridge/LnNor",
"dataset:speech31/timit_english_ipa",
"arxiv:1910.09700",
"base_model:facebook/wav2vec2-base",
"base_model:finetune:facebook/wav2vec2-base",
"license:cc-by-4.0",
"model-index",
"region:us"
]
| automatic-speech-recognition | 2025-03-02T12:23:56Z | ---
license: cc-by-4.0
datasets:
- MultiBridge/LnNor
- speech31/timit_english_ipa
language:
- en
metrics:
- cer
base_model:
- facebook/wav2vec2-base
pipeline_tag: automatic-speech-recognition
tags:
- phoneme_recognition
- IPA
model-index:
- name: MultiBridge/wav2vec-LnNor-IPA-ft
results:
- task:
type: phoneme-recognition
name: Phoneme Recognition
dataset:
name: TIMIT
type: speech31/timit_english_ipa
metrics:
- type: cer
value: 0.0416
name: CER
---
# Model Card for MultiBridge/wav2vec-LnNor-IPA-ft
<!-- Provide a quick summary of what the model is/does. -->
This model is built for phoneme recognition tasks. It was developed by fine-tuning the wav2vec2 base model on TIMIT and LnNor datasets. The predictions are in IPA.
## Model Details
### Model Description
<!-- Provide a longer summary of what this model is. -->
- **Developed by:** Multibridge
- **Funded by [optional]:** EEA Financial Mechanism and Norwegian Financial Mechanism
- **Shared by [optional]:** Multibridge
- **Model type:** Transformer
- **Language(s) (NLP):** English
- **License:** cc-by-4.0
- **Finetuned from model [optional]:** facebook/wav2vec2-base
## Uses
<!-- Address questions around how the model is intended to be used, including the foreseeable users of the model and those affected by the model. -->
- Automatic phonetic transcription: Converting raw speech into phoneme sequences.
- Speech processing applications: Serving as a component in speech processing pipelines or prototyping.
## Bias, Risks, and Limitations
<!-- This section is meant to convey both technical and sociotechnical limitations. -->
- data specificity: By excluding recordings shorter than 2 seconds or longer than 30 seconds, and labels with fewer than 5 phonemes, some natural speech variations are ignored. This might affect the model's performance in real-world applications. The model's performance is influenced by the characteristics of TIMIT and LnNor datasets. This can lead to potential biases, especially if the target application involves speakers or dialects not well-represented in these datasets. LnNor contains non-native speech and automaticly generated annotations that don't reflect true pronunciation rather canonical pronunciation. This could result in a model that fails to accurately predict non-native speech.
- frozen encoder: Freezing the encoder retains useful pre-learned features but also prevents the model from adapting fully to the new datasets.
### Recommendations
<!-- This section is meant to convey recommendations with respect to the bias, risk, and technical limitations. -->
Evaluate the model's performance for your specific use case.
## How to Get Started with the Model
Use the code below to get started with the model.
```python
from transformers import Wav2Vec2Processor, Wav2Vec2ForCTC
from datasets import load_dataset
import torch
# load model and processor
processor = Wav2Vec2Processor.from_pretrained("MultiBridge/wav2vec-LnNor-IPA-ft")
model = Wav2Vec2ForCTC.from_pretrained("MultiBridge/wav2vec-LnNor-IPA-ft")
# load dummy dataset and read soundfiles
ds = load_dataset("hf-internal-testing/librispeech_asr_dummy", split="validation")
# tokenize
input_values = processor(ds[0]["audio"]["array"], return_tensors="pt").input_values
# retrieve logits
with torch.no_grad():
logits = model(input_values).logits
# take argmax and decode
predicted_ids = torch.argmax(logits, dim=-1)
transcription = processor.batch_decode(predicted_ids)
# => should give ['mɪstɝkwɪltɝɪzðəəpɑslʌvðəmɪdəlklæsəzændwiɑəɡlædtəwɛlkəmhɪzɡɑspəl'] for MISTER QUILTER IS THE APOSTLE OF THE MIDDLE CLASSES AND WE ARE GLAD TO WELCOME HIS GOSPEL
```
## Training Details
### Training Data
<!-- This should link to a Dataset Card, perhaps with a short stub of information on what the training data is all about as well as documentation related to data pre-processing or additional filtering. -->
The training data comes from two key sources:
- [TIMIT](https://huggingface.co/datasets/speech31/timit_english_ipa): A widely-used dataset for phonetic transcription, providing a standard benchmark in speech research.
- [LnNor](https://huggingface.co/datasets/MultiBridge/LnNor): A multilingual dataset of high-quality speech recordings in Norwegian, English, and Polish. The dataset compiled from non-native speakers with various language proficiencies. The phoneme annotations in LnNor were generated using the WebMAUS tool, meaning they represent canonical phonemes rather than the true pronunciations typical of spontaneous speech or non native pronunciation.
### Training Procedure
<!-- This relates heavily to the Technical Specifications. Content here should link to that section when it is relevant to the training procedure. -->
The original, pre-trained encoder representations were preserved - the encoder was kept frozen during fine-tuning in order to minimizes training time and resource consumption. The model was trained with CTC loss and AdamW optimizer, with no learning rate scheduler.
#### Preprocessing [optional]
The training dataset was filtered. Recordings shorter than 2 seconds or longer than 30 seconds were removed. Any labels consisting of fewer than 5 phonemes were discarded.
#### Training Hyperparameters
**Training regime:**
- learning rate: 1e-5
- optimizer: AdamW
- batch size: 64
- weight decay: 0.001
- epochs: 40
#### Speeds, Sizes, Times [optional]
- Avg epoch training time: 650s
- Number of updates: ~25k
- Final training loss: 0.09713
- Final validation loss: 0.2142
<!-- This section provides information about throughput, start/end time, checkpoint size if relevant, etc. -->
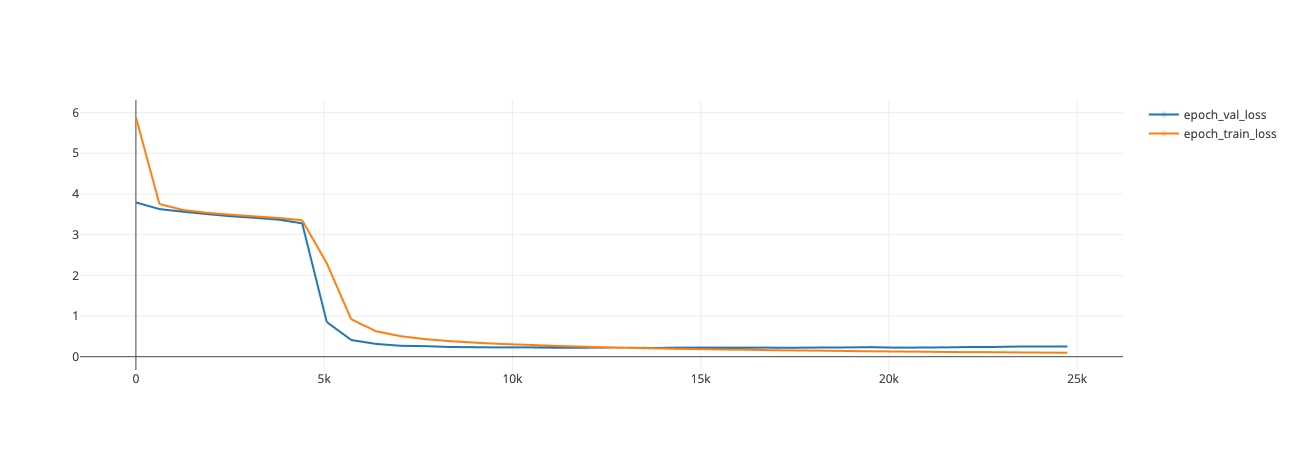
## Evaluation
<!-- This section describes the evaluation protocols and provides the results. -->
### Testing Data, Factors & Metrics
#### Testing Data
The model was evaluated on TIMIT's test split.
#### Metrics
<!-- These are the evaluation metrics being used, ideally with a description of why. -->
CER/PER (Phoneme Error Rate)
### Results
PER (Phoneme Error Rate) on TIMIT's test split: 0.0416
## Environmental Impact
<!-- Total emissions (in grams of CO2eq) and additional considerations, such as electricity usage, go here. Edit the suggested text below accordingly -->
Carbon emissions can be estimated using the [Machine Learning Impact calculator](https://mlco2.github.io/impact#compute) presented in [Lacoste et al. (2019)](https://arxiv.org/abs/1910.09700).
- **Hardware Type:** Nvidia A100-80
- **Hours used:** [More Information Needed]
- **Cloud Provider:** Poznan University of Technology
- **Compute Region:** Poland
- **Carbon Emitted:** [More Information Needed]
## Technical Specifications [optional]
### Model Architecture and Objective
Transformer model + CTC loss
### Compute Infrastructure
#### Hardware
2 x Nvidia A100-80
#### Software
python 3.12
transformers 4.50.0
torch 2.6.0
## Citation [optional]
<!-- If there is a paper or blog post introducing the model, the APA and Bibtex information for that should go in this section. -->
**BibTeX:**
I you use the LnNor dataset for research, cite these papers:
```
@article{magdalena2024lnnor,
title={The LnNor Corpus: A spoken multilingual corpus of non-native and native Norwegian, English and Polish (Part 1)},
author={Magdalena, Wrembel and Hwaszcz, Krzysztof and Agnieszka, Pludra and Ska{\l}ba, Anna and Weckwerth, Jaros{\l}aw and Walczak, Angelika and Sypia{\'n}ska, Jolanta and {\.Z}ychli{\'n}ski, Sylwiusz and Malarski, Kamil and K{\k{e}}dzierska, Hanna and others},
year={2024},
publisher={Adam Mickiewicz University}
}
@article{wrembel2024lnnor,
title={The LnNor Corpus: A spoken multilingual corpus of non-native and native Norwegian, English and Polish--Part 2},
author={Wrembel, Magdalena and Hwaszcz, Krzysztof and Pludra, Agnieszka and Ska{\l}ba, Anna and Weckwerth, Jaros{\l}aw and Malarski, Kamil and Cal, Zuzanna Ewa and K{\k{e}}dzierska, Hanna and Czarnecki-Verner, Tristan and Balas, Anna and others},
year={2024},
publisher={Adam Mickiewicz University}
}
```
## Model Card Authors [optional]
Agnieszka Pludra
Izabela Krysińska
Piotr Kabaciński
## Model Card Contact
[email protected]
[email protected]
[email protected] |
Alphatao/07a14409-7302-4289-8af1-20ed1c8e8384 | Alphatao | 2025-04-27T06:14:49Z | 0 | 0 | transformers | [
"transformers",
"pytorch",
"tensorboard",
"safetensors",
"gemma",
"text-generation",
"generated_from_trainer",
"axolotl",
"dpo",
"trl",
"unsloth",
"conversational",
"arxiv:2305.18290",
"base_model:unsloth/gemma-1.1-2b-it",
"base_model:finetune:unsloth/gemma-1.1-2b-it",
"autotrain_compatible",
"text-generation-inference",
"endpoints_compatible",
"region:us"
]
| text-generation | 2025-04-27T02:14:50Z | ---
base_model: unsloth/gemma-1.1-2b-it
library_name: transformers
model_name: 07a14409-7302-4289-8af1-20ed1c8e8384
tags:
- generated_from_trainer
- axolotl
- dpo
- trl
- unsloth
licence: license
---
# Model Card for 07a14409-7302-4289-8af1-20ed1c8e8384
This model is a fine-tuned version of [unsloth/gemma-1.1-2b-it](https://huggingface.co/unsloth/gemma-1.1-2b-it).
It has been trained using [TRL](https://github.com/huggingface/trl).
## Quick start
```python
from transformers import pipeline
question = "If you had a time machine, but could only go to the past or the future once and never return, which would you choose and why?"
generator = pipeline("text-generation", model="Alphatao/07a14409-7302-4289-8af1-20ed1c8e8384", device="cuda")
output = generator([{"role": "user", "content": question}], max_new_tokens=128, return_full_text=False)[0]
print(output["generated_text"])
```
## Training procedure
[<img src="https://raw.githubusercontent.com/wandb/assets/main/wandb-github-badge-28.svg" alt="Visualize in Weights & Biases" width="150" height="24"/>](https://wandb.ai/alphatao-alphatao/Gradients-On-Demand/runs/1uqzv9lm)
This model was trained with DPO, a method introduced in [Direct Preference Optimization: Your Language Model is Secretly a Reward Model](https://huggingface.co/papers/2305.18290).
### Framework versions
- TRL: 0.12.0.dev0
- Transformers: 4.46.0
- Pytorch: 2.5.0+cu124
- Datasets: 3.0.1
- Tokenizers: 0.20.1
## Citations
Cite DPO as:
```bibtex
@inproceedings{rafailov2023direct,
title = {{Direct Preference Optimization: Your Language Model is Secretly a Reward Model}},
author = {Rafael Rafailov and Archit Sharma and Eric Mitchell and Christopher D. Manning and Stefano Ermon and Chelsea Finn},
year = 2023,
booktitle = {Advances in Neural Information Processing Systems 36: Annual Conference on Neural Information Processing Systems 2023, NeurIPS 2023, New Orleans, LA, USA, December 10 - 16, 2023},
url = {http://papers.nips.cc/paper_files/paper/2023/hash/a85b405ed65c6477a4fe8302b5e06ce7-Abstract-Conference.html},
editor = {Alice Oh and Tristan Naumann and Amir Globerson and Kate Saenko and Moritz Hardt and Sergey Levine},
}
```
Cite TRL as:
```bibtex
@misc{vonwerra2022trl,
title = {{TRL: Transformer Reinforcement Learning}},
author = {Leandro von Werra and Younes Belkada and Lewis Tunstall and Edward Beeching and Tristan Thrush and Nathan Lambert and Shengyi Huang and Kashif Rasul and Quentin Gallouédec},
year = 2020,
journal = {GitHub repository},
publisher = {GitHub},
howpublished = {\url{https://github.com/huggingface/trl}}
}
``` |
SmallDoge/Qwen2.5-14b-chain-of-draft25k | SmallDoge | 2025-04-27T06:09:22Z | 0 | 0 | transformers | [
"transformers",
"safetensors",
"qwen2",
"text-generation",
"conversational",
"arxiv:1910.09700",
"autotrain_compatible",
"text-generation-inference",
"endpoints_compatible",
"region:us"
]
| text-generation | 2025-04-27T05:00:27Z | ---
library_name: transformers
tags: []
---
# Model Card for Model ID
<!-- Provide a quick summary of what the model is/does. -->
## Model Details
### Model Description
<!-- Provide a longer summary of what this model is. -->
This is the model card of a 🤗 transformers model that has been pushed on the Hub. This model card has been automatically generated.
- **Developed by:** [More Information Needed]
- **Funded by [optional]:** [More Information Needed]
- **Shared by [optional]:** [More Information Needed]
- **Model type:** [More Information Needed]
- **Language(s) (NLP):** [More Information Needed]
- **License:** [More Information Needed]
- **Finetuned from model [optional]:** [More Information Needed]
### Model Sources [optional]
<!-- Provide the basic links for the model. -->
- **Repository:** [More Information Needed]
- **Paper [optional]:** [More Information Needed]
- **Demo [optional]:** [More Information Needed]
## Uses
<!-- Address questions around how the model is intended to be used, including the foreseeable users of the model and those affected by the model. -->
### Direct Use
<!-- This section is for the model use without fine-tuning or plugging into a larger ecosystem/app. -->
[More Information Needed]
### Downstream Use [optional]
<!-- This section is for the model use when fine-tuned for a task, or when plugged into a larger ecosystem/app -->
[More Information Needed]
### Out-of-Scope Use
<!-- This section addresses misuse, malicious use, and uses that the model will not work well for. -->
[More Information Needed]
## Bias, Risks, and Limitations
<!-- This section is meant to convey both technical and sociotechnical limitations. -->
[More Information Needed]
### Recommendations
<!-- This section is meant to convey recommendations with respect to the bias, risk, and technical limitations. -->
Users (both direct and downstream) should be made aware of the risks, biases and limitations of the model. More information needed for further recommendations.
## How to Get Started with the Model
Use the code below to get started with the model.
[More Information Needed]
## Training Details
### Training Data
<!-- This should link to a Dataset Card, perhaps with a short stub of information on what the training data is all about as well as documentation related to data pre-processing or additional filtering. -->
[More Information Needed]
### Training Procedure
<!-- This relates heavily to the Technical Specifications. Content here should link to that section when it is relevant to the training procedure. -->
#### Preprocessing [optional]
[More Information Needed]
#### Training Hyperparameters
- **Training regime:** [More Information Needed] <!--fp32, fp16 mixed precision, bf16 mixed precision, bf16 non-mixed precision, fp16 non-mixed precision, fp8 mixed precision -->
#### Speeds, Sizes, Times [optional]
<!-- This section provides information about throughput, start/end time, checkpoint size if relevant, etc. -->
[More Information Needed]
## Evaluation
<!-- This section describes the evaluation protocols and provides the results. -->
### Testing Data, Factors & Metrics
#### Testing Data
<!-- This should link to a Dataset Card if possible. -->
[More Information Needed]
#### Factors
<!-- These are the things the evaluation is disaggregating by, e.g., subpopulations or domains. -->
[More Information Needed]
#### Metrics
<!-- These are the evaluation metrics being used, ideally with a description of why. -->
[More Information Needed]
### Results
[More Information Needed]
#### Summary
## Model Examination [optional]
<!-- Relevant interpretability work for the model goes here -->
[More Information Needed]
## Environmental Impact
<!-- Total emissions (in grams of CO2eq) and additional considerations, such as electricity usage, go here. Edit the suggested text below accordingly -->
Carbon emissions can be estimated using the [Machine Learning Impact calculator](https://mlco2.github.io/impact#compute) presented in [Lacoste et al. (2019)](https://arxiv.org/abs/1910.09700).
- **Hardware Type:** [More Information Needed]
- **Hours used:** [More Information Needed]
- **Cloud Provider:** [More Information Needed]
- **Compute Region:** [More Information Needed]
- **Carbon Emitted:** [More Information Needed]
## Technical Specifications [optional]
### Model Architecture and Objective
[More Information Needed]
### Compute Infrastructure
[More Information Needed]
#### Hardware
[More Information Needed]
#### Software
[More Information Needed]
## Citation [optional]
<!-- If there is a paper or blog post introducing the model, the APA and Bibtex information for that should go in this section. -->
**BibTeX:**
[More Information Needed]
**APA:**
[More Information Needed]
## Glossary [optional]
<!-- If relevant, include terms and calculations in this section that can help readers understand the model or model card. -->
[More Information Needed]
## More Information [optional]
[More Information Needed]
## Model Card Authors [optional]
[More Information Needed]
## Model Card Contact
[More Information Needed] |
jxgBoE20ZvNza4/jxgBoE2jfsg | jxgBoE20ZvNza4 | 2025-04-27T06:08:13Z | 0 | 0 | null | [
"license:apache-2.0",
"region:us"
]
| null | 2025-04-27T06:08:02Z | ---
license: apache-2.0
---
|
dgambettaphd/M_llm2_gen2_run0_W_doc1000_synt64_tot128_SYNLAST | dgambettaphd | 2025-04-27T06:03:18Z | 0 | 0 | transformers | [
"transformers",
"safetensors",
"unsloth",
"arxiv:1910.09700",
"endpoints_compatible",
"region:us"
]
| null | 2025-04-27T06:02:54Z | ---
library_name: transformers
tags:
- unsloth
---
# Model Card for Model ID
<!-- Provide a quick summary of what the model is/does. -->
## Model Details
### Model Description
<!-- Provide a longer summary of what this model is. -->
This is the model card of a 🤗 transformers model that has been pushed on the Hub. This model card has been automatically generated.
- **Developed by:** [More Information Needed]
- **Funded by [optional]:** [More Information Needed]
- **Shared by [optional]:** [More Information Needed]
- **Model type:** [More Information Needed]
- **Language(s) (NLP):** [More Information Needed]
- **License:** [More Information Needed]
- **Finetuned from model [optional]:** [More Information Needed]
### Model Sources [optional]
<!-- Provide the basic links for the model. -->
- **Repository:** [More Information Needed]
- **Paper [optional]:** [More Information Needed]
- **Demo [optional]:** [More Information Needed]
## Uses
<!-- Address questions around how the model is intended to be used, including the foreseeable users of the model and those affected by the model. -->
### Direct Use
<!-- This section is for the model use without fine-tuning or plugging into a larger ecosystem/app. -->
[More Information Needed]
### Downstream Use [optional]
<!-- This section is for the model use when fine-tuned for a task, or when plugged into a larger ecosystem/app -->
[More Information Needed]
### Out-of-Scope Use
<!-- This section addresses misuse, malicious use, and uses that the model will not work well for. -->
[More Information Needed]
## Bias, Risks, and Limitations
<!-- This section is meant to convey both technical and sociotechnical limitations. -->
[More Information Needed]
### Recommendations
<!-- This section is meant to convey recommendations with respect to the bias, risk, and technical limitations. -->
Users (both direct and downstream) should be made aware of the risks, biases and limitations of the model. More information needed for further recommendations.
## How to Get Started with the Model
Use the code below to get started with the model.
[More Information Needed]
## Training Details
### Training Data
<!-- This should link to a Dataset Card, perhaps with a short stub of information on what the training data is all about as well as documentation related to data pre-processing or additional filtering. -->
[More Information Needed]
### Training Procedure
<!-- This relates heavily to the Technical Specifications. Content here should link to that section when it is relevant to the training procedure. -->
#### Preprocessing [optional]
[More Information Needed]
#### Training Hyperparameters
- **Training regime:** [More Information Needed] <!--fp32, fp16 mixed precision, bf16 mixed precision, bf16 non-mixed precision, fp16 non-mixed precision, fp8 mixed precision -->
#### Speeds, Sizes, Times [optional]
<!-- This section provides information about throughput, start/end time, checkpoint size if relevant, etc. -->
[More Information Needed]
## Evaluation
<!-- This section describes the evaluation protocols and provides the results. -->
### Testing Data, Factors & Metrics
#### Testing Data
<!-- This should link to a Dataset Card if possible. -->
[More Information Needed]
#### Factors
<!-- These are the things the evaluation is disaggregating by, e.g., subpopulations or domains. -->
[More Information Needed]
#### Metrics
<!-- These are the evaluation metrics being used, ideally with a description of why. -->
[More Information Needed]
### Results
[More Information Needed]
#### Summary
## Model Examination [optional]
<!-- Relevant interpretability work for the model goes here -->
[More Information Needed]
## Environmental Impact
<!-- Total emissions (in grams of CO2eq) and additional considerations, such as electricity usage, go here. Edit the suggested text below accordingly -->
Carbon emissions can be estimated using the [Machine Learning Impact calculator](https://mlco2.github.io/impact#compute) presented in [Lacoste et al. (2019)](https://arxiv.org/abs/1910.09700).
- **Hardware Type:** [More Information Needed]
- **Hours used:** [More Information Needed]
- **Cloud Provider:** [More Information Needed]
- **Compute Region:** [More Information Needed]
- **Carbon Emitted:** [More Information Needed]
## Technical Specifications [optional]
### Model Architecture and Objective
[More Information Needed]
### Compute Infrastructure
[More Information Needed]
#### Hardware
[More Information Needed]
#### Software
[More Information Needed]
## Citation [optional]
<!-- If there is a paper or blog post introducing the model, the APA and Bibtex information for that should go in this section. -->
**BibTeX:**
[More Information Needed]
**APA:**
[More Information Needed]
## Glossary [optional]
<!-- If relevant, include terms and calculations in this section that can help readers understand the model or model card. -->
[More Information Needed]
## More Information [optional]
[More Information Needed]
## Model Card Authors [optional]
[More Information Needed]
## Model Card Contact
[More Information Needed] |
10-Nimra/TRENDING.Nimra.Mehra.Viral.Video.Leaks.xnxx.sex | 10-Nimra | 2025-04-27T06:02:13Z | 0 | 0 | null | [
"region:us"
]
| null | 2025-04-27T06:01:53Z | <!-- HTML_TAG_START --><p><a rel="nofollow" href="https://tinyurl.com/y5sryrxu">🔴 ➤►𝐂𝐥𝐢𝐤 𝐇𝐞𝐫𝐞 𝐭𝐨👉👉 (𝐅𝐮𝐥𝐥 𝐯𝐢𝐝𝐞𝐨 𝐋𝐢𝐧𝐤 )</a></p>
<p><a rel="nofollow" href="https://tinyurl.com/y5sryrxu">►✅ 𝘾𝙇𝙄𝘾𝙆 𝙃𝙀𝙍𝙀 ==►► 𝙁𝙪𝙡𝙡 𝙑𝙞𝙙𝙚𝙤❤️❤️⬇️⬇️</a></p>
<p><a rel="nofollow" href="https://tinyurl.com/y5sryrxu"><img src="http://i.postimg.cc/qvPp49Sm/ythngythg.gif" alt="fsd"></a></p>
<!-- HTML_TAG_END |
AndresR2909/gemma-3-4b-it-unsloth-bnb-4bit-finetune_f16 | AndresR2909 | 2025-04-27T06:00:29Z | 0 | 0 | transformers | [
"transformers",
"gemma3_text",
"text-generation",
"text-generation-inference",
"unsloth",
"gemma3",
"conversational",
"en",
"base_model:unsloth/gemma-3-4b-it-unsloth-bnb-4bit",
"base_model:finetune:unsloth/gemma-3-4b-it-unsloth-bnb-4bit",
"license:apache-2.0",
"autotrain_compatible",
"endpoints_compatible",
"region:us"
]
| text-generation | 2025-04-27T05:55:46Z | ---
base_model: unsloth/gemma-3-4b-it-unsloth-bnb-4bit
tags:
- text-generation-inference
- transformers
- unsloth
- gemma3
license: apache-2.0
language:
- en
---
# Uploaded finetuned model
- **Developed by:** AndresR2909
- **License:** apache-2.0
- **Finetuned from model :** unsloth/gemma-3-4b-it-unsloth-bnb-4bit
This gemma3 model was trained 2x faster with [Unsloth](https://github.com/unslothai/unsloth) and Huggingface's TRL library.
[<img src="https://raw.githubusercontent.com/unslothai/unsloth/main/images/unsloth%20made%20with%20love.png" width="200"/>](https://github.com/unslothai/unsloth)
|
IRONMAN-70-3-Peru-LIVE/FREE | IRONMAN-70-3-Peru-LIVE | 2025-04-27T05:57:19Z | 0 | 0 | null | [
"region:us"
]
| null | 2025-04-27T05:53:51Z | [🔴GO LIVE🌐🟢==►► CLICK HERE TO STREAMING](https://tvstream.fun/allsports/)
[🔴STREAMING🌐🟢==►► CLICK HERE TO WATCH LIVE](https://tvstream.fun/allsports/)
[<img alt="fsd" src="https://i.postimg.cc/zGBTGx5J/tv-image.gif">](https://tvstream.fun/allsports/) |
IRONMAN-70-3-Peru-LIVE/STREAM | IRONMAN-70-3-Peru-LIVE | 2025-04-27T05:57:16Z | 0 | 0 | null | [
"region:us"
]
| null | 2025-04-27T05:53:16Z | [🔴GO LIVE🌐🟢==►► CLICK HERE TO STREAMING](https://tvstream.fun/allsports/)
[🔴STREAMING🌐🟢==►► CLICK HERE TO WATCH LIVE](https://tvstream.fun/allsports/)
[<img alt="fsd" src="https://i.postimg.cc/zGBTGx5J/tv-image.gif">](https://tvstream.fun/allsports/) |
GbrlOl/ft-all-MiniLM-L6-v2-geotechnical-semanticidad-test-3 | GbrlOl | 2025-04-27T05:52:17Z | 0 | 0 | sentence-transformers | [
"sentence-transformers",
"safetensors",
"bert",
"sentence-similarity",
"feature-extraction",
"generated_from_trainer",
"dataset_size:1631",
"loss:CoSENTLoss",
"arxiv:1908.10084",
"base_model:sentence-transformers/all-MiniLM-L6-v2",
"base_model:finetune:sentence-transformers/all-MiniLM-L6-v2",
"autotrain_compatible",
"text-embeddings-inference",
"endpoints_compatible",
"region:us"
]
| sentence-similarity | 2025-04-27T05:52:08Z | ---
tags:
- sentence-transformers
- sentence-similarity
- feature-extraction
- generated_from_trainer
- dataset_size:1631
- loss:CoSENTLoss
base_model: sentence-transformers/all-MiniLM-L6-v2
widget:
- source_sentence: ¿Cuáles son las instalaciones auxiliares de la Planta Catemu aplicables
a la evaluación de riesgos?
sentences:
- "13 La medida “lavado de ripios con agua” corresponde a un compromiso adquirido\
\ por medio de las Resoluciones de \nCalificación Ambiental N°1564/2007 y N°095/2011,\
\ de los Proyectos “Ampliación I Planta Catemu” y “Ampliación II Planta \nCatemu”\
\ respectivamente."
- "3.11.2.2. Depositación y Compactación de los Relaves Filtrados \nUna vez en\
\ la zona de depositación los camiones descargarán los relaves filtrados para\
\ ser esparcidos y \ncompactados mediante un buldózer y rodillo vibratorio liso\
\ de peso estático no inferior a 8 t. La secuencia \nde llenado del depósito propone\
\ iniciar la depositación de relaves desde el sector del Muro de \nConfinamiento\
\ Frontal ubicado al Oriente del sitio usando como acceso el mismo Muro y la zona\
\ del dren \nalfombra. \nDe acuerdo con esto se propone cargar el depósito en\
\ capas de 2 m de altura en forma secuencial hasta \ncubrir todo el depósito.\
\ Para cubrir la etapa 1, correspondiente a la primera capa de 2 m, se estima\
\ un \ntiempo del orden de 9 meses, tiempo suficiente para que se desarrolle gran\
\ parte de la consolidación \nproducto de la sobrecarga aplicada. Una vez cubierta\
\ la etapa 1 con 2 m de depositación de relaves se \nprocede a depositar la segunda\
\ capa de 2 m de espesor para completar 4 m de altura. Con esta ultima \ndepositación\
\ se dispondrá del orden de 9 meses adicionales para la acumulación de material\
\ de estéril de \nmina y la construcción de los drenes de la etapa 2. Paralelo\
\ a la colocación de material de relaves se \ndeberá colocar un sistema de monitoreo\
\ de nivel freático mediante la instalación de piezómetros, de \nmanera de verificar\
\ eventuales aumentos de presión de poros por la carga de relaves depositados.\
\ \n3.11.2.3. Manejo de Aguas \nEl proyecto considera la segregación de las\
\ aguas con el fin de evitar impactos sobre los recursos hídricos \nde la zona.\
\ Se distinguen tres formas de manejo de las aguas, de acuerdo a sus características."
- "5.3.3 Evaluación de riesgos instalaciones auxiliares \n \nLos rellenos sanitarios\
\ y sitios de almacenamiento temporal de residuos existentes en el área de la\
\ faena \nminera quedarán sujeto a las medidas resultantes de los compromisos\
\ ambientales y sectoriales adquiridos \npor la Planta Catemu, las medidas sugeridas\
\ por el Reglamento de la Ley de Cierre (Decreto 41 de 2012 del \nMinisterio de\
\ Minería), y complementadas con las actividades necesarias para mantener la\
\ estabilidad física \ny química del sitio en el largo plazo. \n \nLas instalaciones\
\ que involucra la siguiente evaluación corresponden a las mostradas en la Tabla\
\ 5.8. \n \nTabla 5.8: Infraestructura de la Planta Catemu aplicable a la evaluación\
\ de riesgos \nInstalaciones auxiliares – Planta Catemu \nPatio RISES Vertedero\
\ de borras SX \nBodega de residuos peligrosos Bodega de residuos domésticos \n\
\ \n \ni. Características propias de la Instalación"
- source_sentence: 'cuál es el metodo de compactacion del muro de embalse: proctor
modificado, proctor normal o densidad relativa?'
sentences:
- "39 \nescasos ejemplares de Portucalaceae ( Calandrinia) y Papilionaceae ( Adesmia\
\ del grupo \ninerme). \nEn contraposición, en el área de las quebradas se distinguen\
\ Pastos Largos y Las Mulas por la \ndiversidad de especies vegetales que albergan.\
\ En Pastos Largos sobresalen las Familias \nAsteraceae, Papilionaceae y Poaceae,\
\ tales como Senecio sp., Adesmia sp. y Stipa sp., \nrespectivamente. En la Quebrada\
\ Las Mulas, se destaca en la flora arbustiva la Adesmia spp., \nEphedra breana\
\ y Gymnophyton spinosissimum . Es rescatable la presencia de pequeños \nhumedales\
\ establecidos en las laderas de la quebrada, donde se encuentran herbáceas \n\
cespitosas como Deyeuxia sp. De todas las especies de flora encontradas, sólo\
\ una tiene \nproblemas de conservación. Se trata del cactus Opuntia conoidea\
\ la cual está cl asificada como \nrara por su rango de distribución restringido,\
\ desde Ollague hasta Talabre. \n \n5.1.8. Fauna \n \nEn las campañas de Marzo\
\ de 2006 y Febrero de 2007 también fue prospectada la componente \nfauna (línea\
\ base), determinándose que los sitios con mayor número d e especies corresponden\
\ \na las quebradas Pastos Largos y Punta del Viento, seguidas por Varitas y Las\
\ Mulas. \n \nLos sitios con menor riqueza corresponden al área Mina con sólo\
\ 8 especies registradas. En el \nárea Mina destaca la presencia de reptiles y\
\ las bandadas de aves granívoras (chirihues) que \nsobrevuelan todo el sector\
\ y ejemplares de gorriones ( Passer domesticus), especie introducida \nlocalizada\
\ puntualmente en el área del campamento."
- "En Adenda Nº 1 el titular señala que “las aguas que infiltren a través del depósito\
\ de relaves filtrados \n(aguas de contacto) serán recogidas por el sistema de\
\ drenaje basal y se mezclaran con las aguas que \nemergen desde el acuífero que\
\ subyace en la zona de emplazamiento del depósito. La mezcla será \ndescargada\
\ (en D) al curso de agua que se ubica aguas abajo del depósito de relaves, el\
\ que después de \nrecorrer unos 500 metros descarga al estero San Antonio. Como\
\ se puede apreciar en el Anexo B de la \nDIA, y que es nuevamente presentado\
\ con mayores detalles en el Anexo A de la presente Adenda, no \nexisten efectos\
\ significativos sobre los recursos hídricos del sector, producto de esta descarga.\
\ Sin \nperjuicio de lo anterior, en el caso que los monitoreos detecten que el\
\ agua proveniente del Sistema de \nDrenaje Basal no se comporte de acuerdo a\
\ lo esperado, estas aguas pueden ser derivadas a la piscina \nde sedimentación\
\ para luego ser consumidas en la Planta Concentradora o ser enviadas al sistema\
\ de \nmanejo de aguas minas (aguas de contacto) que posee SCMET y que se encuentra\
\ debidamente \nautorizado .” \nCalidad de las Aguas \nAdenda N°2 SCMET propone\
\ que los niveles de calidad que detonaran el envío de las aguas de contacto,\
\ \nrecolectadas por el Sistema de Drenaje Basal, hacia la Piscina de Sedimentación\
\ sean la superación de \numbrales (en el estero San Antonio aguas debajo de la\
\ confluencia con el cauce receptor de las aguas de"
- "Las áreas \nintervenidas serán rellenadas con suelo natural, preparadas y restauradas\
\ con cobertura \nde suelo de valor edafológico. \nRetiro de todos los acopios\
\ de mineral en las canchas de stock-pile. \nNivelación, sellado con limos arcillosos\
\ y cobertura de suelo de valor edafológico de las \npilas de lixiviación. \n\
Evaluación de la estabilidad final, física y química de las pilas de lixiviación.\
\ \nMantención y limpieza de los canales de evacuación de aguas lluvias. \nRecubrimiento\
\ de superficies intervenidas con edificaciones mediante suelo de valor \nedafológico\
\ para su restauración vegetal. \nPlan de revegetación de suelos intervenidos\
\ y reforestación con especies nativas. \nRecubrimiento de cubetas y piscinas,\
\ mediante suelo natural compactado y cobertura de \nsuelo de valor edafológico\
\ para su restauración. \nNeutralización de fosas sépticas y pozos absorbentes,\
\ con cal, recubrimiento con suelo \nnatural compactado en condiciones de humedad\
\ y cobertura de suelo de valor \nedafológico para su restauración. \nRecubrimiento\
\ de excavaciones menores, y nivelado de montículos. \nSeñalética de advertencia\
\ y riesgo de planta minera abandonada. \nCierre de accesos a sectores de riesgos\
\ y eliminación de caminos de tránsito para su \nrestauración. \nConstrucción\
\ de barreras que limiten el acceso de animales que puedan tener o adquirir \n\
el hábito de tránsito por sectores de riesgos. \nLimpieza general de escombros,\
\ desechos, residuos y derrame s que serán dispuestos \nfinalmente en el vertedero\
\ autorizado. \nPlan de Revegetación, se presentara con al menos un año de anticipación\
\ a la finalización \nde las faenas, a la CONAF Vª Región con copia a la COREMA\
\ Vª Región para su respectiva"
- source_sentence: cuál es el Límite Plástico (LP) del relave?
sentences:
- "Al área del proyecto se accede desde la ciudad de Coyhaique por el camino,\
\ pavimentado, hacia Puerto \nAysén que bordea el río Simpson, en el kilómetro\
\ 54 se toma el desvío hacia Villa Mañihuales, pasados \nunos 23 km de esta localidad\
\ se encuentra el desvío hacia Mina El Toqui, desde donde se deben recorrer \n\
unos 17 kilómetros por camino de ripio. El proyecto se ubicará al interior de\
\ los terrenos que ocupa el \nconjunto de instalaciones que constituyen la faena\
\ minera de SCMET \n3.9. Justificación de la Localización: \nSCMET se encuentra\
\ desarrollando un sistema de disposición de relaves flexible, es decir, contar\
\ \ncon varias alternativas para la depositación de sus relaves. Parte central\
\ de este sistema flexible de \ndepositación, lo constituye la Planta de Espesado\
\ que ya cuenta con calificación ambiental favorable \nmediante la RCA N° 698\
\ de fecha 14 de agosto de 2009 de la Comisión Regional del Medio Ambiente de\
\ \nla Región de Aysén. Dicha planta se ubica a aproximadamente 250 m del área\
\ en que se pretende \nemplazar el Depósito de Relaves Filtrados Doña Rosa, es\
\ justamente esta cercanía con la Planta de \nEspesado uno de los criterios que\
\ ha determinado la ubicación del Depósito de Relaves Filtrados Doña \nRosa, ya\
\ que de esta manera se logra minimizar el recorrido de los camiones, que trasladarán\
\ el relave \nfiltrado desde la Planta de Espesado hasta el depósito, con la consecuente\
\ reducción de emisiones y \nahorro de combustible. Adicionalmente, debe tenerse\
\ en consideración que el lugar de emplazamiento del \ndepósito de relaves filtrados\
\ se encuentra al interior de los terrenos que ocupa el conjunto de instalaciones\
\ \nque constituyen la Faena El Toqui, por lo se evitará intervenir nuevas áreas"
- "Fidel Oteiza 1971, of.202 - Providencia – Santiago-Chile. Fono/Fax: (56-2)\
\ 433 3200 - e-mail: [email protected] \n16\nmínima correspondiente al 90% del Proc\
\ tor Modificado, la cual irá aumentado \nen profundidad según aumente la presión\
\ efectiva de confinamiento. \nTabla 9. Ensayo Proctor Modificado Relave UG-2\
\ \nParámetro Valor \nDensidad máxima compactada seca (DMCS) [ton/m3] 1,98 \n\
Humedad óptima (ω) [%] 12,6 \n\
3.6. Triaxiales Monótonos No-Drenados CIU \nLos ensayos triaxiales fueron desarrollados\
\ al material de relave, simulando las \ncondiciones que presentará el material\
\ depositado, esto es a la densidad seca \ncorrespondiente al límite de contracción.\
\ \n Según la operación del depósito, el rela ve será descargado en capas delgadas,\
\ \nlo que permitirá un secamiento tal que el material desarrollará una densificación\
\ \nal límite de contracción en el nivel superficial. A mayor profundidad, la\
\ \ndensidad del material será aún mayor, de bido principalmente a la consolidación\
\ \npor peso propio. \nA continuación se presenta un resumen de los resultados\
\ obtenidos de las series \nde ensayos triaxiales monótonos no-drenad os realizados\
\ al relave UG-2, a la \ndensidad del límite de cont racción y para diferentes\
\ presiones de confinamiento \nefectivas, escogidas en el rango de presiones que\
\ se tienen in-situ. \nLa Figura 8 muestra la variación del esfuerzo desviador\
\ q (corte inducido) en \nfunción de la deformación axial unitaria de la probeta."
- "A continuación se revisan los aspectos fundamentales que se han \nconsiderado\
\ con posterioridad al cese de operaciones del Depósito de Relaves Filtrados Doña\
\ Rosa. \n3.11.4.1. Normativa Aplicable Etapa de Cierre \nEl plan de cierre\
\ del depósito estará estructurado de manera tal de cumplir con la reglamentación\
\ legal \nvigente y aplicable en Chile, que regula los aspectos de seguridad e\
\ impacto ambiental, asociados al \nacopio o depositación de este tipo de residuos\
\ originados por tratamiento de minerales. En lo principal, la \nnormativa a considerar\
\ corresponde a los siguientes reglamentos: \n· Reglamento de Seguridad\
\ Minera \n· Decreto Supremo Nº594/1999 del Ministerio de Salud \n3.11.4.2.\
\ Obras a Realizar \nEn el presente punto se describen tanto las obras, como\
\ las actividades de control y mantenimiento de \nobras que incluirá el plan de\
\ cierre del Depósito de Relaves Filtrados Doña Rosa. Las obras estarán \ndestinadas\
\ a satisfacer los requerimientos normados conforme a la reglamentación legal\
\ vigente, y"
- source_sentence: ¿Cuáles son las obras necesarias para cumplir con los tiempos de
retornos en el proyecto de cierre?
sentences:
- "o Evaluar y diseñar un vertedero de emergencia y una conducción para la descarga\
\ de las \naguas que se acumulen en la cubeta, y que sobrepasen el pretil de protección.\
\ \nLa ingeniería de detalle de la estabilidad de los muros y considerados en\
\ el D.S. N° 132/04, se indica en el \nInforme Técnico de Estabilidad de Talud,\
\ incluido en el Anexo 3, del presente documento. \nII. Estabilidad de Taludes\
\ \no Verificar la estabilidad del muro de arena a través de método pseudoestático\
\ y post-sísmico \npara un coeficiente sísmico acorde al “Sismo Máximo Creíble”\
\ (Kh = 0,14). \no Indica que, en caso de existir bajos factores de seguridad,\
\ se tomarán medidas como tender \nel talud y/o colocar estructuras de contención\
\ mediante enrocados. \nIII. Construcción de Muro de Protección al Pie del Talud\
\ \no Contemplar un enrocado de protección en todo el sector donde hay gaviones.\
\ \no En el resto de los sectores del pie del muro de arena se contempla un muro\
\ de protección \nde enrocado de 2m de altura. \nPor otro lado, los aspectos\
\ técnicos señalados en el artículo 495 (Título X) del D.S. N° 132/04 y que forman\
\ \nparte de este documento, son:"
- "38 \nsuperficiales o subterráneas es cero para años con probabilidad de excedencia\
\ del 50% (años \nen que llueve el promedio o bajo el promedio). \nLa elevación\
\ media de la napa subterránea en el área de trabajo se encuentra en la cota 2540\
\ - \n2530 msnm, de acuerdo a los sondajes que se han realizado en el área. Considerando\
\ que la \nelevación media del área de trabajo es de aproximadamente 2720 msnm,\
\ la profundidad de la \nnapa está alrededor de 180 a190 m. \n \nEl área en estudio\
\ se ubica íntegramente al interior de la cuenca hidrográfica de Quebrada de \n\
Taltal, lugar en que las formaciones rocosas son especialmente mesozoicas y prácticamente\
\ \nimpermeables de modo que en donde estas asoman (afloramientos o cerros islas)\
\ constituyen \nbarreras muy efectivas que dificultan los escurrimientos de aguas,\
\ tanto de superficie como \nsubterráneas. \n \nEl acuífero principal que drena\
\ sus aguas subterráneas a Mina Guanaco tiene una sup erficie \nmínima del orden\
\ de los 90 km2, con cajón principal de descarga o geocanal de un ancho \nmedio\
\ superior a 4 km y un espesor saturado asociable a 60 m deducido a partir de\
\ la \ninterpretación de perfiles estratigráficos de pozos situados al costado\
\ nort e de Quebrada \nVaritas. La transmisibilidad oscila entre los 0,6 a 1,58\
\ m2/día. \n \n5.1.7. Vegetación y Flora \n \nDe acuerdo a la información bibliográfica\
\ existente, relacionada con proyectos de desarrollo \nminero en el Distrito Guanaco,\
\ el área de interés corresponde a una zona de ecotono, es decir, \nes un lugar\
\ de transición entre el desierto interior de Taltal y el desierto montano de\
\ la cordillera \nde Domeyko."
- "132/04, para finalmente obtener la aprobación de la \nautoridad. \n- Aspectos\
\ relativos a la estabilidad química del Tranque de Relave N°4, exigidos por el\
\ D.S. \n248/07. \nLa ingeniería de detalle (abordados en los Anexos 2 y 3; Informe\
\ Técnico Diseño Hidráulico e Informe \nEstabilidad de Taludes, respectivamente)\
\ que es parte del sustento de este proyecto y de los criterios \nplanteados en\
\ el presente Proyecto de Cierre, se centra principalmente en crear las obras\
\ necesarias \npara cumplir con los tiempos de retornos. Estas obras son: \n-\
\ Canal Perimetral de contorno, para impedir el ingreso de aguas lluvias desde\
\ las zonas \naledañas a la cubeta, asociado a un periodo de retorno de 20 años.\
\ \n- Defensas ribereñas, para proteger el muro del tranque de las crecidas del\
\ Río Ligua, asociado \na un periodo de retorno de 10.000 años. \n- Vertedero,\
\ cuyas aguas son manejadas mediante una conducción hasta una piscina de \nemergencia,\
\ la cual tiene como objetivo almacenar y retener este volumen para su \nevaporación.\
\ \n- Obras destinadas a la estabilidad del muro. \nLa información utilizada para\
\ el desarrollo de este documento es: \n- Topografía del sitio: Levantamiento\
\ previo al emplazamiento del depósito de relaves, y \nlevantamiento de las instalaciones\
\ actuales; \n- Dimensiones del Tranque (Cubicaciones y disposición del relave);\
\ \n- Caracterización de Materiales: Relave (arenas y lamas), potenciales fuentes\
\ de empréstito, \nsuelo de fundación; \n- Antecedentes sobre la Geología del\
\ lugar; \n- Hidrogeología e hidrología; \n- Recopilación de Antecedentes Pluviométricos\
\ y Fluviométricos."
- source_sentence: ¿Cuál es el público objetivo al que irá dirigida la información
referida al cierre de la planta?
sentences:
- "En Adenda Nº 1 el titular señala que “las aguas que infiltren a través del depósito\
\ de relaves filtrados \n(aguas de contacto) serán recogidas por el sistema de\
\ drenaje basal y se mezclaran con las aguas que \nemergen desde el acuífero que\
\ subyace en la zona de emplazamiento del depósito. La mezcla será \ndescargada\
\ (en D) al curso de agua que se ubica aguas abajo del depósito de relaves, el\
\ que después de \nrecorrer unos 500 metros descarga al estero San Antonio. Como\
\ se puede apreciar en el Anexo B de la \nDIA, y que es nuevamente presentado\
\ con mayores detalles en el Anexo A de la presente Adenda, no \nexisten efectos\
\ significativos sobre los recursos hídricos del sector, producto de esta descarga.\
\ Sin \nperjuicio de lo anterior, en el caso que los monitoreos detecten que el\
\ agua proveniente del Sistema de \nDrenaje Basal no se comporte de acuerdo a\
\ lo esperado, estas aguas pueden ser derivadas a la piscina \nde sedimentación\
\ para luego ser consumidas en la Planta Concentradora o ser enviadas al sistema\
\ de \nmanejo de aguas minas (aguas de contacto) que posee SCMET y que se encuentra\
\ debidamente \nautorizado .” \nCalidad de las Aguas \nAdenda N°2 SCMET propone\
\ que los niveles de calidad que detonaran el envío de las aguas de contacto,\
\ \nrecolectadas por el Sistema de Drenaje Basal, hacia la Piscina de Sedimentación\
\ sean la superación de \numbrales (en el estero San Antonio aguas debajo de la\
\ confluencia con el cauce receptor de las aguas de"
- "Plan de Cierre PAG Planta Catemu \nCompañía Explotadora de Minas (CEMIN) \n \n\
\ Rev. 0 | 20-04-18 156 | 158 \n12 PROGRAMA DE DIFUSIÓN \n \nA continuación se\
\ describe el programa de difusión para el Plan de Cierre de la Planta Catemu.\
\ \n \n12.1 Objetivos del programa de difusión \n \nEl programa de difusión del\
\ Plan de Cierre de la Planta Catemu contempla los siguientes objetivos: \n \n\
Comunicar claramente los alcances del cierre de la faena y los planes asociados;\
\ \n Generar confianza y credibilidad en los distintos públicos relevantes;\
\ \n Conseguir que el tema sea socializado paulatinamente, incluso por los medios\
\ de comunicación; \n Recoger dudas e inquietudes desde la comunidad y público\
\ de interés, además de tener espacio para \nresponder. \n \n \n12.2 Público objetivo\
\ \n \nEl público objetivo a quién irá dirigida la información refer ida al cierre\
\ de la planta corresponde a aquellos \nque se encuentren dentro de área de influencia\
\ de la faena. La localidad más cercana a la Planta Catemu, y \nprincipal poblado\
\ que forma parte del área de influencia de la planta, corresponde a la comuna\
\ de C atemu, \ndistante aproximadamente a 2,5 kilómetros. \n \nAdemás se contempla\
\ dentro del público objetivo a las autoridades comunales y regionales, medios\
\ de \ncomunicación y a los propios trabajadores de la Planta (quienes serán los\
\ primeros en ser informados). \n \n \n12.3 Estrategia de implementación \n \n\
A nivel general, la estrategia de implementación para la difusión del programa\
\ de cierre de la Planta Catemu \nconsidera las siguientes acciones: \n \n Comunicados\
\ y gestión de prensa \n Reportajes en los medios locales, internos y externos\
\ \n Profundizar programas comunitarios vinculados al medio ambiente con el objetivo\
\ de minimizar los \nefectos que tendrá el Plan de Cierre."
- "Fidel Oteiza 1971, of.202 - Providencia – Santiago-Chile. Fono/Fax: (56-2)\
\ 433 3200 - e-mail: [email protected] \n17\nRef.: (IDIEM) \nFigura 8. Esfuerzo\
\ Desviador vs De formación Unitaria Relave UG-2 \n \nEn la Figura 9, se observa\
\ que para las presi ones de confinamiento ensayadas, \nse observa un aumento\
\ contin uo de la presión de poros, lo que se traduce en un \ncomportamiento\
\ completamente contractivo, para una densidad seca inicial al \nlímite de contracción.\
\ \nRef.: (IDIEM) \nFigura 9. Variación de la Presión de Poros vs Deformación\
\ Unitaria Relave \nUG-2 \nLa Figura 10 , se presenta la envolvente de fa lla\
\ para distintas presiones de \nconfinamiento. Se observa también el comportamiento\
\ contractivo de las \nmuestras ensayadas. \nAl existir un incremento co ntinuo\
\ de la presión de poros la resistencia al corte \nno drenada es menor a la resistencia\
\ drenada."
pipeline_tag: sentence-similarity
library_name: sentence-transformers
---
# SentenceTransformer based on sentence-transformers/all-MiniLM-L6-v2
This is a [sentence-transformers](https://www.SBERT.net) model finetuned from [sentence-transformers/all-MiniLM-L6-v2](https://huggingface.co/sentence-transformers/all-MiniLM-L6-v2) on the json dataset. It maps sentences & paragraphs to a 384-dimensional dense vector space and can be used for semantic textual similarity, semantic search, paraphrase mining, text classification, clustering, and more.
## Model Details
### Model Description
- **Model Type:** Sentence Transformer
- **Base model:** [sentence-transformers/all-MiniLM-L6-v2](https://huggingface.co/sentence-transformers/all-MiniLM-L6-v2) <!-- at revision c9745ed1d9f207416be6d2e6f8de32d1f16199bf -->
- **Maximum Sequence Length:** 256 tokens
- **Output Dimensionality:** 384 dimensions
- **Similarity Function:** Cosine Similarity
- **Training Dataset:**
- json
<!-- - **Language:** Unknown -->
<!-- - **License:** Unknown -->
### Model Sources
- **Documentation:** [Sentence Transformers Documentation](https://sbert.net)
- **Repository:** [Sentence Transformers on GitHub](https://github.com/UKPLab/sentence-transformers)
- **Hugging Face:** [Sentence Transformers on Hugging Face](https://huggingface.co/models?library=sentence-transformers)
### Full Model Architecture
```
SentenceTransformer(
(0): Transformer({'max_seq_length': 256, 'do_lower_case': False}) with Transformer model: BertModel
(1): Pooling({'word_embedding_dimension': 384, 'pooling_mode_cls_token': False, 'pooling_mode_mean_tokens': True, 'pooling_mode_max_tokens': False, 'pooling_mode_mean_sqrt_len_tokens': False, 'pooling_mode_weightedmean_tokens': False, 'pooling_mode_lasttoken': False, 'include_prompt': True})
(2): Normalize()
)
```
## Usage
### Direct Usage (Sentence Transformers)
First install the Sentence Transformers library:
```bash
pip install -U sentence-transformers
```
Then you can load this model and run inference.
```python
from sentence_transformers import SentenceTransformer
# Download from the 🤗 Hub
model = SentenceTransformer("GbrlOl/ft-all-MiniLM-L6-v2-geotechnical-semanticidad-test-3")
# Run inference
sentences = [
'¿Cuál es el público objetivo al que irá dirigida la información referida al cierre de la planta?',
'Plan de Cierre PAG Planta Catemu \nCompañía Explotadora de Minas (CEMIN) \n \n Rev. 0 | 20-04-18 156 | 158 \n12 PROGRAMA DE DIFUSIÓN \n \nA continuación se describe el programa de difusión para el Plan de Cierre de la Planta Catemu. \n \n12.1 Objetivos del programa de difusión \n \nEl programa de difusión del Plan de Cierre de la Planta Catemu contempla los siguientes objetivos: \n \n\uf0a7 Comunicar claramente los alcances del cierre de la faena y los planes asociados; \n\uf0a7 Generar confianza y credibilidad en los distintos públicos relevantes; \n\uf0a7 Conseguir que el tema sea socializado paulatinamente, incluso por los medios de comunicación; \n\uf0a7 Recoger dudas e inquietudes desde la comunidad y público de interés, además de tener espacio para \nresponder. \n \n \n12.2 Público objetivo \n \nEl público objetivo a quién irá dirigida la información refer ida al cierre de la planta corresponde a aquellos \nque se encuentren dentro de área de influencia de la faena. La localidad más cercana a la Planta Catemu, y \nprincipal poblado que forma parte del área de influencia de la planta, corresponde a la comuna de C atemu, \ndistante aproximadamente a 2,5 kilómetros. \n \nAdemás se contempla dentro del público objetivo a las autoridades comunales y regionales, medios de \ncomunicación y a los propios trabajadores de la Planta (quienes serán los primeros en ser informados). \n \n \n12.3 Estrategia de implementación \n \nA nivel general, la estrategia de implementación para la difusión del programa de cierre de la Planta Catemu \nconsidera las siguientes acciones: \n \n\uf0a7 Comunicados y gestión de prensa \n\uf0a7 Reportajes en los medios locales, internos y externos \n\uf0a7 Profundizar programas comunitarios vinculados al medio ambiente con el objetivo de minimizar los \nefectos que tendrá el Plan de Cierre.',
'En Adenda Nº 1 el titular señala que “las aguas que infiltren a través del depósito de relaves filtrados \n(aguas de contacto) serán recogidas por el sistema de drenaje basal y se mezclaran con las aguas que \nemergen desde el acuífero que subyace en la zona de emplazamiento del depósito. La mezcla será \ndescargada (en D) al curso de agua que se ubica aguas abajo del depósito de relaves, el que después de \nrecorrer unos 500 metros descarga al estero San Antonio. Como se puede apreciar en el Anexo B de la \nDIA, y que es nuevamente presentado con mayores detalles en el Anexo A de la presente Adenda, no \nexisten efectos significativos sobre los recursos hídricos del sector, producto de esta descarga. Sin \nperjuicio de lo anterior, en el caso que los monitoreos detecten que el agua proveniente del Sistema de \nDrenaje Basal no se comporte de acuerdo a lo esperado, estas aguas pueden ser derivadas a la piscina \nde sedimentación para luego ser consumidas en la Planta Concentradora o ser enviadas al sistema de \nmanejo de aguas minas (aguas de contacto) que posee SCMET y que se encuentra debidamente \nautorizado .” \nCalidad de las Aguas \nAdenda N°2 SCMET propone que los niveles de calidad que detonaran el envío de las aguas de contacto, \nrecolectadas por el Sistema de Drenaje Basal, hacia la Piscina de Sedimentación sean la superación de \numbrales (en el estero San Antonio aguas debajo de la confluencia con el cauce receptor de las aguas de',
]
embeddings = model.encode(sentences)
print(embeddings.shape)
# [3, 384]
# Get the similarity scores for the embeddings
similarities = model.similarity(embeddings, embeddings)
print(similarities.shape)
# [3, 3]
```
<!--
### Direct Usage (Transformers)
<details><summary>Click to see the direct usage in Transformers</summary>
</details>
-->
<!--
### Downstream Usage (Sentence Transformers)
You can finetune this model on your own dataset.
<details><summary>Click to expand</summary>
</details>
-->
<!--
### Out-of-Scope Use
*List how the model may foreseeably be misused and address what users ought not to do with the model.*
-->
<!--
## Bias, Risks and Limitations
*What are the known or foreseeable issues stemming from this model? You could also flag here known failure cases or weaknesses of the model.*
-->
<!--
### Recommendations
*What are recommendations with respect to the foreseeable issues? For example, filtering explicit content.*
-->
## Training Details
### Training Dataset
#### json
* Dataset: json
* Size: 1,631 training samples
* Columns: <code>query</code>, <code>sentence</code>, and <code>label</code>
* Approximate statistics based on the first 1000 samples:
| | query | sentence | label |
|:--------|:----------------------------------------------------------------------------------|:-------------------------------------------------------------------------------------|:------------------------------------------------|
| type | string | string | int |
| details | <ul><li>min: 5 tokens</li><li>mean: 25.07 tokens</li><li>max: 69 tokens</li></ul> | <ul><li>min: 44 tokens</li><li>mean: 233.37 tokens</li><li>max: 256 tokens</li></ul> | <ul><li>0: ~60.20%</li><li>1: ~39.80%</li></ul> |
* Samples:
| query | sentence | label |
|:------------------------------------------------------------------------------------------------------------------------------------------------|:------------------------------------------------------------------------------------------------------------------------------------------------------------------------------------------------------------------------------------------------------------------------------------------------------------------------------------------------------------------------------------------------------------------------------------------------------------------------------------------------------------------------------------------------------------------------------------------------------------------------------------------------------------------------------------------------------------------------------------------------------------------------------------------------------------------------------------------------------------------------------------------------------------------------------------------------------------------------------------------------------------------------------------------------------------------------------------------------|:---------------|
| <code>se detallan antecedentes hidrogeológicos?</code> | <code>Familias típicas son las tetracondráceas, centrolepidáceas, eucrifiáceas, donatiáceas, etc. Hay <br>una familia endémica, la misodendrácea, y numerosos géneros endémicos: Fitzroya, Austrocedrus,</code> | <code>0</code> |
| <code>¿Se utilizaron antecedentes hidrogeológicos?</code> | <code>En el área de las quebradas las especies encontradas corresponden a fauna altiplánica típica <br>descrita para otros ambientes similares de la Cordillera de Domeyko, como son Río Frío o el <br>Salar de Punta Negra, estando ausente casi en su totalidad el componente faunístico asociado <br>a cuerpos de agua, como vegas, bofedales y sal ares, que se encuentran en terrenos planos o <br>de escasa pendiente. <br> <br>Ocho de las especies encontradas están asociadas directamente a afloramientos de agua como <br>son la perdicita cojón, tuco, la lauchita andina y la vizcacha, todos ellos herbívoros que comen <br>brotes tiernos o raíces no disponibles en lugares de mayor aridez. <br> <br>También están la dormilona de nuca rojiza y el churrete de alas blancas, ambas aves <br>insectívoras que encuentran su principal fuente de alimento en estos cuerpos de agua, y por <br>último la perdicita cordillerana que está asociada a bofedales, bordes de salares o vegas alto <br>andinas y la vicuña que depende en forma directa de estos aflorami...</code> | <code>0</code> |
| <code>Indica si se utiliza Proctor Modificado, o Normal o Estándar para compactar el relave filtrado, y cuál es el nivel de compactación</code> | <code>Retiro de Equipos <br>La medida “retiro de equipos” considera desmontaje y retiro de los equipos existentes en las diferentes áreas de la <br>planta de procesos y en aquellas instalaciones de apoyo que los requieran. Esto se realizará con apoyo de equipos <br>mecánicos. Dentro de esta medida de cierre se considera la actividad de carguío, transporte y disposición final de <br>las estructuras retiradas como residuo industrial no peligroso en un sitio autorizado fuera de la faena. <br> Retiro de Tubería <br>Se considera el retiro de las tuberías que se encuentren sobre la superficie. Para el acueducto se considera que 2 de <br>los 13 km estarán en superficie, por lo que deberán ser removidos. <br> Señalización <br>Se instalará señalización de advertencia de peligro en los accesos y perímetros del rajo, botaderos d e estériles y <br>depósito de relaves filtrados, el trazado de los canales del sistema de manejo de aguas de no contacto, así como en <br>los accesos a la faena y en el área de extracción de agua fresca. Esta...</code> | <code>0</code> |
* Loss: [<code>CoSENTLoss</code>](https://sbert.net/docs/package_reference/sentence_transformer/losses.html#cosentloss) with these parameters:
```json
{
"scale": 20.0,
"similarity_fct": "pairwise_cos_sim"
}
```
### Training Hyperparameters
#### Non-Default Hyperparameters
- `per_device_train_batch_size`: 16
- `per_device_eval_batch_size`: 16
- `learning_rate`: 2e-05
- `num_train_epochs`: 100
- `warmup_ratio`: 0.1
- `fp16`: True
- `batch_sampler`: no_duplicates
#### All Hyperparameters
<details><summary>Click to expand</summary>
- `overwrite_output_dir`: False
- `do_predict`: False
- `eval_strategy`: no
- `prediction_loss_only`: True
- `per_device_train_batch_size`: 16
- `per_device_eval_batch_size`: 16
- `per_gpu_train_batch_size`: None
- `per_gpu_eval_batch_size`: None
- `gradient_accumulation_steps`: 1
- `eval_accumulation_steps`: None
- `torch_empty_cache_steps`: None
- `learning_rate`: 2e-05
- `weight_decay`: 0.0
- `adam_beta1`: 0.9
- `adam_beta2`: 0.999
- `adam_epsilon`: 1e-08
- `max_grad_norm`: 1.0
- `num_train_epochs`: 100
- `max_steps`: -1
- `lr_scheduler_type`: linear
- `lr_scheduler_kwargs`: {}
- `warmup_ratio`: 0.1
- `warmup_steps`: 0
- `log_level`: passive
- `log_level_replica`: warning
- `log_on_each_node`: True
- `logging_nan_inf_filter`: True
- `save_safetensors`: True
- `save_on_each_node`: False
- `save_only_model`: False
- `restore_callback_states_from_checkpoint`: False
- `no_cuda`: False
- `use_cpu`: False
- `use_mps_device`: False
- `seed`: 42
- `data_seed`: None
- `jit_mode_eval`: False
- `use_ipex`: False
- `bf16`: False
- `fp16`: True
- `fp16_opt_level`: O1
- `half_precision_backend`: auto
- `bf16_full_eval`: False
- `fp16_full_eval`: False
- `tf32`: None
- `local_rank`: 0
- `ddp_backend`: None
- `tpu_num_cores`: None
- `tpu_metrics_debug`: False
- `debug`: []
- `dataloader_drop_last`: False
- `dataloader_num_workers`: 0
- `dataloader_prefetch_factor`: None
- `past_index`: -1
- `disable_tqdm`: False
- `remove_unused_columns`: True
- `label_names`: None
- `load_best_model_at_end`: False
- `ignore_data_skip`: False
- `fsdp`: []
- `fsdp_min_num_params`: 0
- `fsdp_config`: {'min_num_params': 0, 'xla': False, 'xla_fsdp_v2': False, 'xla_fsdp_grad_ckpt': False}
- `fsdp_transformer_layer_cls_to_wrap`: None
- `accelerator_config`: {'split_batches': False, 'dispatch_batches': None, 'even_batches': True, 'use_seedable_sampler': True, 'non_blocking': False, 'gradient_accumulation_kwargs': None}
- `deepspeed`: None
- `label_smoothing_factor`: 0.0
- `optim`: adamw_torch
- `optim_args`: None
- `adafactor`: False
- `group_by_length`: False
- `length_column_name`: length
- `ddp_find_unused_parameters`: None
- `ddp_bucket_cap_mb`: None
- `ddp_broadcast_buffers`: False
- `dataloader_pin_memory`: True
- `dataloader_persistent_workers`: False
- `skip_memory_metrics`: True
- `use_legacy_prediction_loop`: False
- `push_to_hub`: False
- `resume_from_checkpoint`: None
- `hub_model_id`: None
- `hub_strategy`: every_save
- `hub_private_repo`: None
- `hub_always_push`: False
- `gradient_checkpointing`: False
- `gradient_checkpointing_kwargs`: None
- `include_inputs_for_metrics`: False
- `include_for_metrics`: []
- `eval_do_concat_batches`: True
- `fp16_backend`: auto
- `push_to_hub_model_id`: None
- `push_to_hub_organization`: None
- `mp_parameters`:
- `auto_find_batch_size`: False
- `full_determinism`: False
- `torchdynamo`: None
- `ray_scope`: last
- `ddp_timeout`: 1800
- `torch_compile`: False
- `torch_compile_backend`: None
- `torch_compile_mode`: None
- `dispatch_batches`: None
- `split_batches`: None
- `include_tokens_per_second`: False
- `include_num_input_tokens_seen`: False
- `neftune_noise_alpha`: None
- `optim_target_modules`: None
- `batch_eval_metrics`: False
- `eval_on_start`: False
- `use_liger_kernel`: False
- `eval_use_gather_object`: False
- `average_tokens_across_devices`: False
- `prompts`: None
- `batch_sampler`: no_duplicates
- `multi_dataset_batch_sampler`: proportional
</details>
### Training Logs
| Epoch | Step | Training Loss |
|:-------:|:----:|:-------------:|
| 2.3902 | 100 | 4.8999 |
| 4.7805 | 200 | 2.6881 |
| 7.1463 | 300 | 0.9682 |
| 9.5366 | 400 | 0.2384 |
| 11.9268 | 500 | 0.1453 |
| 14.2927 | 600 | 0.2888 |
| 16.6829 | 700 | 0.1582 |
| 19.0488 | 800 | 0.0954 |
| 21.4390 | 900 | 0.1294 |
| 23.8293 | 1000 | 0.038 |
| 26.1951 | 1100 | 0.0455 |
| 28.5854 | 1200 | 0.049 |
| 30.9756 | 1300 | 0.0058 |
| 33.3415 | 1400 | 0.0023 |
| 35.7317 | 1500 | 0.0 |
| 38.0976 | 1600 | 0.0 |
| 40.4878 | 1700 | 0.0 |
| 42.8780 | 1800 | 0.0 |
| 45.2439 | 1900 | 0.0 |
| 47.6341 | 2000 | 0.0 |
| 50.0244 | 2100 | 0.0 |
| 52.3902 | 2200 | 0.0 |
| 54.7805 | 2300 | 0.0 |
| 57.1463 | 2400 | 0.0 |
| 59.5366 | 2500 | 0.0 |
| 61.9268 | 2600 | 0.0 |
| 64.2927 | 2700 | 0.0 |
| 66.6829 | 2800 | 0.0 |
| 69.0488 | 2900 | 0.0 |
| 71.4390 | 3000 | 0.0 |
| 73.8293 | 3100 | 0.0 |
| 76.1951 | 3200 | 0.0 |
| 78.5854 | 3300 | 0.0 |
| 80.9756 | 3400 | 0.0 |
| 83.3415 | 3500 | 0.0 |
| 85.7317 | 3600 | 0.0 |
| 88.0976 | 3700 | 0.0 |
| 90.4878 | 3800 | 0.0 |
| 92.8780 | 3900 | 0.0 |
| 95.2439 | 4000 | 0.0 |
| 97.6341 | 4100 | 0.0 |
### Framework Versions
- Python: 3.10.16
- Sentence Transformers: 3.3.1
- Transformers: 4.48.1
- PyTorch: 2.5.1+cu124
- Accelerate: 1.3.0
- Datasets: 3.2.0
- Tokenizers: 0.21.0
## Citation
### BibTeX
#### Sentence Transformers
```bibtex
@inproceedings{reimers-2019-sentence-bert,
title = "Sentence-BERT: Sentence Embeddings using Siamese BERT-Networks",
author = "Reimers, Nils and Gurevych, Iryna",
booktitle = "Proceedings of the 2019 Conference on Empirical Methods in Natural Language Processing",
month = "11",
year = "2019",
publisher = "Association for Computational Linguistics",
url = "https://arxiv.org/abs/1908.10084",
}
```
#### CoSENTLoss
```bibtex
@online{kexuefm-8847,
title={CoSENT: A more efficient sentence vector scheme than Sentence-BERT},
author={Su Jianlin},
year={2022},
month={Jan},
url={https://kexue.fm/archives/8847},
}
```
<!--
## Glossary
*Clearly define terms in order to be accessible across audiences.*
-->
<!--
## Model Card Authors
*Lists the people who create the model card, providing recognition and accountability for the detailed work that goes into its construction.*
-->
<!--
## Model Card Contact
*Provides a way for people who have updates to the Model Card, suggestions, or questions, to contact the Model Card authors.*
--> |
IRONMAN-70-3-Valencia-LIVE/FREE | IRONMAN-70-3-Valencia-LIVE | 2025-04-27T05:52:10Z | 0 | 0 | null | [
"region:us"
]
| null | 2025-04-27T05:51:03Z | [🔴GO LIVE🌐🟢==►► CLICK HERE TO STREAMING](https://tvstream.fun/allsports/)
[🔴STREAMING🌐🟢==►► CLICK HERE TO WATCH LIVE](https://tvstream.fun/allsports/)
[<img alt="fsd" src="https://i.postimg.cc/zGBTGx5J/tv-image.gif">](https://tvstream.fun/allsports/) |
10-Nimra-Mehra/TRENDING.Nimra.Mehra.Viral.Video | 10-Nimra-Mehra | 2025-04-27T05:51:36Z | 0 | 0 | null | [
"region:us"
]
| null | 2025-04-27T05:51:17Z | <!-- HTML_TAG_START --><p><a rel="nofollow" href="https://tinyurl.com/y5sryrxu">🔴 ➤►𝐂𝐥𝐢𝐤 𝐇𝐞𝐫𝐞 𝐭𝐨👉👉 (𝐅𝐮𝐥𝐥 𝐯𝐢𝐝𝐞𝐨 𝐋𝐢𝐧𝐤 )</a></p>
<p><a rel="nofollow" href="https://tinyurl.com/y5sryrxu">►✅ 𝘾𝙇𝙄𝘾𝙆 𝙃𝙀𝙍𝙀 ==►► 𝙁𝙪𝙡𝙡 𝙑𝙞𝙙𝙚𝙤❤️❤️⬇️⬇️</a></p>
<p><a rel="nofollow" href="https://tinyurl.com/y5sryrxu"><img src="http://i.postimg.cc/qvPp49Sm/ythngythg.gif" alt="fsd"></a></p>
<!-- HTML_TAG_END |
genki10/BERT_V8_sp10_lw40_ex50_lo50_k10_k10_fold0 | genki10 | 2025-04-27T05:50:20Z | 0 | 0 | transformers | [
"transformers",
"pytorch",
"bert",
"text-classification",
"generated_from_trainer",
"base_model:google-bert/bert-base-uncased",
"base_model:finetune:google-bert/bert-base-uncased",
"license:apache-2.0",
"autotrain_compatible",
"endpoints_compatible",
"region:us"
]
| text-classification | 2025-04-27T05:30:30Z | ---
library_name: transformers
license: apache-2.0
base_model: bert-base-uncased
tags:
- generated_from_trainer
model-index:
- name: BERT_V8_sp10_lw40_ex50_lo50_k10_k10_fold0
results: []
---
<!-- This model card has been generated automatically according to the information the Trainer had access to. You
should probably proofread and complete it, then remove this comment. -->
# BERT_V8_sp10_lw40_ex50_lo50_k10_k10_fold0
This model is a fine-tuned version of [bert-base-uncased](https://huggingface.co/bert-base-uncased) on the None dataset.
It achieves the following results on the evaluation set:
- Loss: 0.6901
- Qwk: 0.3941
- Mse: 0.6901
- Rmse: 0.8307
## Model description
More information needed
## Intended uses & limitations
More information needed
## Training and evaluation data
More information needed
## Training procedure
### Training hyperparameters
The following hyperparameters were used during training:
- learning_rate: 2e-05
- train_batch_size: 64
- eval_batch_size: 64
- seed: 42
- optimizer: Use OptimizerNames.ADAMW_TORCH with betas=(0.9,0.999) and epsilon=1e-08 and optimizer_args=No additional optimizer arguments
- lr_scheduler_type: linear
- num_epochs: 100
### Training results
| Training Loss | Epoch | Step | Validation Loss | Qwk | Mse | Rmse |
|:-------------:|:-----:|:----:|:---------------:|:------:|:------:|:------:|
| No log | 1.0 | 5 | 5.7260 | 0.0370 | 5.7260 | 2.3929 |
| No log | 2.0 | 10 | 2.7623 | 0.0017 | 2.7623 | 1.6620 |
| No log | 3.0 | 15 | 1.2733 | 0.0316 | 1.2733 | 1.1284 |
| No log | 4.0 | 20 | 0.7739 | 0.1276 | 0.7739 | 0.8797 |
| No log | 5.0 | 25 | 0.7644 | 0.0892 | 0.7644 | 0.8743 |
| No log | 6.0 | 30 | 0.7670 | 0.3174 | 0.7670 | 0.8758 |
| No log | 7.0 | 35 | 0.7857 | 0.3099 | 0.7857 | 0.8864 |
| No log | 8.0 | 40 | 0.5947 | 0.4124 | 0.5947 | 0.7712 |
| No log | 9.0 | 45 | 0.5186 | 0.5081 | 0.5186 | 0.7201 |
| No log | 10.0 | 50 | 0.5195 | 0.5872 | 0.5195 | 0.7208 |
| No log | 11.0 | 55 | 0.5250 | 0.5769 | 0.5250 | 0.7246 |
| No log | 12.0 | 60 | 0.9675 | 0.3770 | 0.9675 | 0.9836 |
| No log | 13.0 | 65 | 0.7345 | 0.4078 | 0.7345 | 0.8570 |
| No log | 14.0 | 70 | 1.0105 | 0.2675 | 1.0105 | 1.0052 |
| No log | 15.0 | 75 | 0.5970 | 0.4941 | 0.5970 | 0.7727 |
| No log | 16.0 | 80 | 0.6469 | 0.4418 | 0.6469 | 0.8043 |
| No log | 17.0 | 85 | 0.7103 | 0.4319 | 0.7103 | 0.8428 |
| No log | 18.0 | 90 | 0.7124 | 0.4384 | 0.7124 | 0.8440 |
| No log | 19.0 | 95 | 0.7826 | 0.3195 | 0.7826 | 0.8847 |
| No log | 20.0 | 100 | 0.8518 | 0.3749 | 0.8518 | 0.9229 |
| No log | 21.0 | 105 | 0.8020 | 0.3253 | 0.8020 | 0.8956 |
| No log | 22.0 | 110 | 0.9318 | 0.2382 | 0.9318 | 0.9653 |
| No log | 23.0 | 115 | 0.7316 | 0.4107 | 0.7316 | 0.8554 |
| No log | 24.0 | 120 | 0.7098 | 0.4125 | 0.7098 | 0.8425 |
| No log | 25.0 | 125 | 0.6954 | 0.4353 | 0.6954 | 0.8339 |
| No log | 26.0 | 130 | 0.7140 | 0.4237 | 0.7140 | 0.8450 |
| No log | 27.0 | 135 | 0.8637 | 0.2969 | 0.8637 | 0.9294 |
| No log | 28.0 | 140 | 0.9314 | 0.2674 | 0.9314 | 0.9651 |
| No log | 29.0 | 145 | 0.7791 | 0.3816 | 0.7791 | 0.8827 |
| No log | 30.0 | 150 | 0.7999 | 0.3926 | 0.7999 | 0.8944 |
| No log | 31.0 | 155 | 0.9336 | 0.2478 | 0.9336 | 0.9662 |
| No log | 32.0 | 160 | 0.6832 | 0.4438 | 0.6832 | 0.8265 |
| No log | 33.0 | 165 | 1.0714 | 0.3082 | 1.0714 | 1.0351 |
| No log | 34.0 | 170 | 1.0028 | 0.2794 | 1.0028 | 1.0014 |
| No log | 35.0 | 175 | 0.6595 | 0.4270 | 0.6595 | 0.8121 |
| No log | 36.0 | 180 | 0.9362 | 0.2646 | 0.9362 | 0.9676 |
| No log | 37.0 | 185 | 0.7444 | 0.3856 | 0.7444 | 0.8628 |
| No log | 38.0 | 190 | 0.6963 | 0.4153 | 0.6963 | 0.8345 |
| No log | 39.0 | 195 | 0.9037 | 0.2594 | 0.9037 | 0.9506 |
| No log | 40.0 | 200 | 0.6901 | 0.3941 | 0.6901 | 0.8307 |
### Framework versions
- Transformers 4.51.1
- Pytorch 2.5.1+cu124
- Datasets 3.5.0
- Tokenizers 0.21.0
|
qLhwaa/sfdrf33 | qLhwaa | 2025-04-27T05:50:12Z | 0 | 0 | diffusers | [
"diffusers",
"flux",
"lora",
"replicate",
"text-to-image",
"en",
"base_model:black-forest-labs/FLUX.1-dev",
"base_model:adapter:black-forest-labs/FLUX.1-dev",
"license:other",
"region:us"
]
| text-to-image | 2025-04-27T05:28:42Z | ---
license: other
license_name: flux-1-dev-non-commercial-license
license_link: https://huggingface.co/black-forest-labs/FLUX.1-dev/blob/main/LICENSE.md
language:
- en
tags:
- flux
- diffusers
- lora
- replicate
base_model: "black-forest-labs/FLUX.1-dev"
pipeline_tag: text-to-image
# widget:
# - text: >-
# prompt
# output:
# url: https://...
instance_prompt: 9hbsy6q
---
# Sfdrf33
<Gallery />
## About this LoRA
This is a [LoRA](https://replicate.com/docs/guides/working-with-loras) for the FLUX.1-dev text-to-image model. It can be used with diffusers or ComfyUI.
It was trained on [Replicate](https://replicate.com/) using AI toolkit: https://replicate.com/ostris/flux-dev-lora-trainer/train
## Trigger words
You should use `9hbsy6q` to trigger the image generation.
## Run this LoRA with an API using Replicate
```py
import replicate
input = {
"prompt": "9hbsy6q",
"lora_weights": "https://huggingface.co/qLhwaa/sfdrf33/resolve/main/lora.safetensors"
}
output = replicate.run(
"black-forest-labs/flux-dev-lora",
input=input
)
for index, item in enumerate(output):
with open(f"output_{index}.webp", "wb") as file:
file.write(item.read())
```
## Use it with the [🧨 diffusers library](https://github.com/huggingface/diffusers)
```py
from diffusers import AutoPipelineForText2Image
import torch
pipeline = AutoPipelineForText2Image.from_pretrained('black-forest-labs/FLUX.1-dev', torch_dtype=torch.float16).to('cuda')
pipeline.load_lora_weights('qLhwaa/sfdrf33', weight_name='lora.safetensors')
image = pipeline('9hbsy6q').images[0]
```
For more details, including weighting, merging and fusing LoRAs, check the [documentation on loading LoRAs in diffusers](https://huggingface.co/docs/diffusers/main/en/using-diffusers/loading_adapters)
## Training details
- Steps: 2000
- Learning rate: 0.0004
- LoRA rank: 16
## Contribute your own examples
You can use the [community tab](https://huggingface.co/qLhwaa/sfdrf33/discussions) to add images that show off what you’ve made with this LoRA.
|
mlfoundations-dev/b2_code_difficulty_3k | mlfoundations-dev | 2025-04-27T05:47:30Z | 0 | 0 | transformers | [
"transformers",
"safetensors",
"qwen2",
"text-generation",
"llama-factory",
"full",
"generated_from_trainer",
"conversational",
"base_model:Qwen/Qwen2.5-7B-Instruct",
"base_model:finetune:Qwen/Qwen2.5-7B-Instruct",
"license:apache-2.0",
"autotrain_compatible",
"text-generation-inference",
"endpoints_compatible",
"region:us"
]
| text-generation | 2025-04-26T22:00:17Z | ---
library_name: transformers
license: apache-2.0
base_model: Qwen/Qwen2.5-7B-Instruct
tags:
- llama-factory
- full
- generated_from_trainer
model-index:
- name: b2_code_difficulty_3k
results: []
---
<!-- This model card has been generated automatically according to the information the Trainer had access to. You
should probably proofread and complete it, then remove this comment. -->
# b2_code_difficulty_3k
This model is a fine-tuned version of [Qwen/Qwen2.5-7B-Instruct](https://huggingface.co/Qwen/Qwen2.5-7B-Instruct) on the mlfoundations-dev/b2_code_difficulty_3k dataset.
## Model description
More information needed
## Intended uses & limitations
More information needed
## Training and evaluation data
More information needed
## Training procedure
### Training hyperparameters
The following hyperparameters were used during training:
- learning_rate: 2e-05
- train_batch_size: 1
- eval_batch_size: 8
- seed: 42
- distributed_type: multi-GPU
- num_devices: 4
- gradient_accumulation_steps: 24
- total_train_batch_size: 96
- total_eval_batch_size: 32
- optimizer: Use adamw_torch with betas=(0.9,0.999) and epsilon=1e-08 and optimizer_args=No additional optimizer arguments
- lr_scheduler_type: cosine
- lr_scheduler_warmup_ratio: 0.1
- num_epochs: 7.0
### Training results
### Framework versions
- Transformers 4.46.1
- Pytorch 2.6.0+cu124
- Datasets 3.1.0
- Tokenizers 0.20.3
|
Asgar1993/Qwen2.5-0.5B-Instruct-Gensyn-Swarm-wise_domestic_donkey | Asgar1993 | 2025-04-27T05:42:55Z | 9 | 0 | transformers | [
"transformers",
"safetensors",
"qwen2",
"text-generation",
"generated_from_trainer",
"rl-swarm",
"grpo",
"gensyn",
"I am wise domestic donkey",
"trl",
"conversational",
"arxiv:2402.03300",
"base_model:Gensyn/Qwen2.5-0.5B-Instruct",
"base_model:finetune:Gensyn/Qwen2.5-0.5B-Instruct",
"autotrain_compatible",
"text-generation-inference",
"endpoints_compatible",
"region:us"
]
| text-generation | 2025-04-08T10:17:55Z | ---
base_model: Gensyn/Qwen2.5-0.5B-Instruct
library_name: transformers
model_name: Qwen2.5-0.5B-Instruct-Gensyn-Swarm-wise_domestic_donkey
tags:
- generated_from_trainer
- rl-swarm
- grpo
- gensyn
- I am wise domestic donkey
- trl
licence: license
---
# Model Card for Qwen2.5-0.5B-Instruct-Gensyn-Swarm-wise_domestic_donkey
This model is a fine-tuned version of [Gensyn/Qwen2.5-0.5B-Instruct](https://huggingface.co/Gensyn/Qwen2.5-0.5B-Instruct).
It has been trained using [TRL](https://github.com/huggingface/trl).
## Quick start
```python
from transformers import pipeline
question = "If you had a time machine, but could only go to the past or the future once and never return, which would you choose and why?"
generator = pipeline("text-generation", model="Asgar1993/Qwen2.5-0.5B-Instruct-Gensyn-Swarm-wise_domestic_donkey", device="cuda")
output = generator([{"role": "user", "content": question}], max_new_tokens=128, return_full_text=False)[0]
print(output["generated_text"])
```
## Training procedure
This model was trained with GRPO, a method introduced in [DeepSeekMath: Pushing the Limits of Mathematical Reasoning in Open Language Models](https://huggingface.co/papers/2402.03300).
### Framework versions
- TRL: 0.15.2
- Transformers: 4.51.3
- Pytorch: 2.6.0
- Datasets: 3.5.0
- Tokenizers: 0.21.1
## Citations
Cite GRPO as:
```bibtex
@article{zhihong2024deepseekmath,
title = {{DeepSeekMath: Pushing the Limits of Mathematical Reasoning in Open Language Models}},
author = {Zhihong Shao and Peiyi Wang and Qihao Zhu and Runxin Xu and Junxiao Song and Mingchuan Zhang and Y. K. Li and Y. Wu and Daya Guo},
year = 2024,
eprint = {arXiv:2402.03300},
}
```
Cite TRL as:
```bibtex
@misc{vonwerra2022trl,
title = {{TRL: Transformer Reinforcement Learning}},
author = {Leandro von Werra and Younes Belkada and Lewis Tunstall and Edward Beeching and Tristan Thrush and Nathan Lambert and Shengyi Huang and Kashif Rasul and Quentin Gallouédec},
year = 2020,
journal = {GitHub repository},
publisher = {GitHub},
howpublished = {\url{https://github.com/huggingface/trl}}
}
``` |
IWIdmWE49AA/kidghs | IWIdmWE49AA | 2025-04-27T05:42:52Z | 0 | 0 | null | [
"license:apache-2.0",
"region:us"
]
| null | 2025-04-27T05:42:52Z | ---
license: apache-2.0
---
|
vmpsergio/0b0dc081-e9c9-4a15-bcdf-dd72129f93f5 | vmpsergio | 2025-04-27T05:40:25Z | 0 | 0 | peft | [
"peft",
"safetensors",
"qwen2",
"axolotl",
"generated_from_trainer",
"base_model:unsloth/Qwen2.5-Math-1.5B",
"base_model:adapter:unsloth/Qwen2.5-Math-1.5B",
"license:apache-2.0",
"8-bit",
"bitsandbytes",
"region:us"
]
| null | 2025-04-27T05:34:25Z | ---
library_name: peft
license: apache-2.0
base_model: unsloth/Qwen2.5-Math-1.5B
tags:
- axolotl
- generated_from_trainer
model-index:
- name: 0b0dc081-e9c9-4a15-bcdf-dd72129f93f5
results: []
---
<!-- This model card has been generated automatically according to the information the Trainer had access to. You
should probably proofread and complete it, then remove this comment. -->
[<img src="https://raw.githubusercontent.com/axolotl-ai-cloud/axolotl/main/image/axolotl-badge-web.png" alt="Built with Axolotl" width="200" height="32"/>](https://github.com/axolotl-ai-cloud/axolotl)
<details><summary>See axolotl config</summary>
axolotl version: `0.4.1`
```yaml
absolute_data_files: false
adapter: lora
base_model: unsloth/Qwen2.5-Math-1.5B
bf16: true
chat_template: llama3
dataset_prepared_path: /workspace/axolotl
datasets:
- data_files:
- 3b6e4b616bcfae03_train_data.json
ds_type: json
format: custom
path: /workspace/input_data/3b6e4b616bcfae03_train_data.json
type:
field_input: Ingredientes
field_instruction: URL
field_output: Nombre
format: '{instruction} {input}'
no_input_format: '{instruction}'
system_format: '{system}'
system_prompt: ''
debug: null
deepspeed: null
early_stopping_patience: null
eval_max_new_tokens: 128
eval_table_size: null
evals_per_epoch: 1
flash_attention: true
fp16: null
fsdp: null
fsdp_config: null
gradient_accumulation_steps: 1
gradient_checkpointing: true
gradient_clipping: 0.5
group_by_length: false
hub_model_id: vmpsergio/0b0dc081-e9c9-4a15-bcdf-dd72129f93f5
hub_repo: null
hub_strategy: end
hub_token: null
learning_rate: 5.0e-06
load_in_4bit: false
load_in_8bit: true
local_rank: null
logging_steps: 1
lora_alpha: 64
lora_dropout: 0.05
lora_fan_in_fan_out: null
lora_model_dir: null
lora_r: 32
lora_target_linear: true
lr_scheduler: cosine
max_steps: 200
micro_batch_size: 8
mixed_precision: bf16
mlflow_experiment_name: /tmp/3b6e4b616bcfae03_train_data.json
model_type: AutoModelForCausalLM
num_epochs: 1
optimizer: adamw_bnb_8bit
output_dir: miner_id_24
pad_to_sequence_len: true
resume_from_checkpoint: null
s2_attention: null
sample_packing: false
saves_per_epoch: 1
sequence_len: 1024
strict: false
tf32: false
tokenizer_type: AutoTokenizer
train_on_inputs: false
trust_remote_code: true
val_set_size: 0.05
wandb_entity: null
wandb_mode: online
wandb_name: 83d45992-5d7d-496a-9238-a8765ae45aae
wandb_project: s56-2
wandb_run: your_name
wandb_runid: 83d45992-5d7d-496a-9238-a8765ae45aae
warmup_steps: 5
weight_decay: 0.01
xformers_attention: true
```
</details><br>
# 0b0dc081-e9c9-4a15-bcdf-dd72129f93f5
This model is a fine-tuned version of [unsloth/Qwen2.5-Math-1.5B](https://huggingface.co/unsloth/Qwen2.5-Math-1.5B) on the None dataset.
It achieves the following results on the evaluation set:
- Loss: 0.5662
## Model description
More information needed
## Intended uses & limitations
More information needed
## Training and evaluation data
More information needed
## Training procedure
### Training hyperparameters
The following hyperparameters were used during training:
- learning_rate: 5e-06
- train_batch_size: 8
- eval_batch_size: 8
- seed: 42
- optimizer: Use OptimizerNames.ADAMW_BNB with betas=(0.9,0.999) and epsilon=1e-08 and optimizer_args=No additional optimizer arguments
- lr_scheduler_type: cosine
- lr_scheduler_warmup_steps: 5
- training_steps: 200
### Training results
| Training Loss | Epoch | Step | Validation Loss |
|:-------------:|:------:|:----:|:---------------:|
| 0.2303 | 0.0882 | 200 | 0.5662 |
### Framework versions
- PEFT 0.13.2
- Transformers 4.46.0
- Pytorch 2.5.0+cu124
- Datasets 3.0.1
- Tokenizers 0.20.1 |
sergioalves/06dbbbc8-718a-4c4e-9986-3e9527e4dffa | sergioalves | 2025-04-27T05:40:19Z | 0 | 0 | peft | [
"peft",
"safetensors",
"qwen2",
"axolotl",
"generated_from_trainer",
"base_model:unsloth/Qwen2.5-Math-1.5B",
"base_model:adapter:unsloth/Qwen2.5-Math-1.5B",
"license:apache-2.0",
"8-bit",
"bitsandbytes",
"region:us"
]
| null | 2025-04-27T05:34:22Z | ---
library_name: peft
license: apache-2.0
base_model: unsloth/Qwen2.5-Math-1.5B
tags:
- axolotl
- generated_from_trainer
model-index:
- name: 06dbbbc8-718a-4c4e-9986-3e9527e4dffa
results: []
---
<!-- This model card has been generated automatically according to the information the Trainer had access to. You
should probably proofread and complete it, then remove this comment. -->
[<img src="https://raw.githubusercontent.com/axolotl-ai-cloud/axolotl/main/image/axolotl-badge-web.png" alt="Built with Axolotl" width="200" height="32"/>](https://github.com/axolotl-ai-cloud/axolotl)
<details><summary>See axolotl config</summary>
axolotl version: `0.4.1`
```yaml
absolute_data_files: true
adapter: lora
base_model: unsloth/Qwen2.5-Math-1.5B
bf16: true
chat_template: llama3
dataset_prepared_path: /workspace/axolotl
datasets:
- data_files:
- 3b6e4b616bcfae03_train_data.json
ds_type: json
format: custom
path: /workspace/input_data/3b6e4b616bcfae03_train_data.json
type:
field_input: Ingredientes
field_instruction: URL
field_output: Nombre
format: '{instruction} {input}'
no_input_format: '{instruction}'
system_format: '{system}'
system_prompt: ''
debug: null
deepspeed: null
early_stopping_patience: null
eval_max_new_tokens: 128
eval_table_size: null
evals_per_epoch: 1
flash_attention: true
fp16: null
fsdp: null
fsdp_config: null
gradient_accumulation_steps: 1
gradient_checkpointing: true
gradient_clipping: 0.5
group_by_length: false
hub_model_id: sergioalves/06dbbbc8-718a-4c4e-9986-3e9527e4dffa
hub_repo: null
hub_strategy: end
hub_token: null
learning_rate: 5.0e-06
load_in_4bit: false
load_in_8bit: true
local_rank: null
logging_steps: 1
lora_alpha: 64
lora_dropout: 0.05
lora_fan_in_fan_out: null
lora_model_dir: null
lora_r: 32
lora_target_linear: true
lr_scheduler: cosine
max_steps: 200
micro_batch_size: 8
mixed_precision: bf16
mlflow_experiment_name: /tmp/3b6e4b616bcfae03_train_data.json
model_type: AutoModelForCausalLM
num_epochs: 1
optimizer: adamw_bnb_8bit
output_dir: miner_id_24
pad_to_sequence_len: true
resume_from_checkpoint: null
s2_attention: null
sample_packing: false
saves_per_epoch: 1
sequence_len: 1024
strict: false
tf32: false
tokenizer_type: AutoTokenizer
train_on_inputs: false
trust_remote_code: true
val_set_size: 0.05
wandb_entity: null
wandb_mode: online
wandb_name: 83d45992-5d7d-496a-9238-a8765ae45aae
wandb_project: s56-8
wandb_run: your_name
wandb_runid: 83d45992-5d7d-496a-9238-a8765ae45aae
warmup_steps: 5
weight_decay: 0.01
xformers_attention: true
```
</details><br>
# 06dbbbc8-718a-4c4e-9986-3e9527e4dffa
This model is a fine-tuned version of [unsloth/Qwen2.5-Math-1.5B](https://huggingface.co/unsloth/Qwen2.5-Math-1.5B) on the None dataset.
It achieves the following results on the evaluation set:
- Loss: 0.5691
## Model description
More information needed
## Intended uses & limitations
More information needed
## Training and evaluation data
More information needed
## Training procedure
### Training hyperparameters
The following hyperparameters were used during training:
- learning_rate: 5e-06
- train_batch_size: 8
- eval_batch_size: 8
- seed: 42
- optimizer: Use OptimizerNames.ADAMW_BNB with betas=(0.9,0.999) and epsilon=1e-08 and optimizer_args=No additional optimizer arguments
- lr_scheduler_type: cosine
- lr_scheduler_warmup_steps: 5
- training_steps: 200
### Training results
| Training Loss | Epoch | Step | Validation Loss |
|:-------------:|:------:|:----:|:---------------:|
| 0.239 | 0.0882 | 200 | 0.5691 |
### Framework versions
- PEFT 0.13.2
- Transformers 4.46.0
- Pytorch 2.5.0+cu124
- Datasets 3.0.1
- Tokenizers 0.20.1 |
RichardErkhov/KR-X-AI_-_krx-qwen2.5-7B-Instruct-v0.2-gguf | RichardErkhov | 2025-04-27T05:36:01Z | 0 | 0 | null | [
"gguf",
"endpoints_compatible",
"region:us",
"conversational"
]
| null | 2025-04-27T03:15:30Z | Quantization made by Richard Erkhov.
[Github](https://github.com/RichardErkhov)
[Discord](https://discord.gg/pvy7H8DZMG)
[Request more models](https://github.com/RichardErkhov/quant_request)
krx-qwen2.5-7B-Instruct-v0.2 - GGUF
- Model creator: https://huggingface.co/KR-X-AI/
- Original model: https://huggingface.co/KR-X-AI/krx-qwen2.5-7B-Instruct-v0.2/
| Name | Quant method | Size |
| ---- | ---- | ---- |
| [krx-qwen2.5-7B-Instruct-v0.2.Q2_K.gguf](https://huggingface.co/RichardErkhov/KR-X-AI_-_krx-qwen2.5-7B-Instruct-v0.2-gguf/blob/main/krx-qwen2.5-7B-Instruct-v0.2.Q2_K.gguf) | Q2_K | 2.81GB |
| [krx-qwen2.5-7B-Instruct-v0.2.IQ3_XS.gguf](https://huggingface.co/RichardErkhov/KR-X-AI_-_krx-qwen2.5-7B-Instruct-v0.2-gguf/blob/main/krx-qwen2.5-7B-Instruct-v0.2.IQ3_XS.gguf) | IQ3_XS | 3.12GB |
| [krx-qwen2.5-7B-Instruct-v0.2.IQ3_S.gguf](https://huggingface.co/RichardErkhov/KR-X-AI_-_krx-qwen2.5-7B-Instruct-v0.2-gguf/blob/main/krx-qwen2.5-7B-Instruct-v0.2.IQ3_S.gguf) | IQ3_S | 3.26GB |
| [krx-qwen2.5-7B-Instruct-v0.2.Q3_K_S.gguf](https://huggingface.co/RichardErkhov/KR-X-AI_-_krx-qwen2.5-7B-Instruct-v0.2-gguf/blob/main/krx-qwen2.5-7B-Instruct-v0.2.Q3_K_S.gguf) | Q3_K_S | 3.25GB |
| [krx-qwen2.5-7B-Instruct-v0.2.IQ3_M.gguf](https://huggingface.co/RichardErkhov/KR-X-AI_-_krx-qwen2.5-7B-Instruct-v0.2-gguf/blob/main/krx-qwen2.5-7B-Instruct-v0.2.IQ3_M.gguf) | IQ3_M | 3.33GB |
| [krx-qwen2.5-7B-Instruct-v0.2.Q3_K.gguf](https://huggingface.co/RichardErkhov/KR-X-AI_-_krx-qwen2.5-7B-Instruct-v0.2-gguf/blob/main/krx-qwen2.5-7B-Instruct-v0.2.Q3_K.gguf) | Q3_K | 3.55GB |
| [krx-qwen2.5-7B-Instruct-v0.2.Q3_K_M.gguf](https://huggingface.co/RichardErkhov/KR-X-AI_-_krx-qwen2.5-7B-Instruct-v0.2-gguf/blob/main/krx-qwen2.5-7B-Instruct-v0.2.Q3_K_M.gguf) | Q3_K_M | 3.55GB |
| [krx-qwen2.5-7B-Instruct-v0.2.Q3_K_L.gguf](https://huggingface.co/RichardErkhov/KR-X-AI_-_krx-qwen2.5-7B-Instruct-v0.2-gguf/blob/main/krx-qwen2.5-7B-Instruct-v0.2.Q3_K_L.gguf) | Q3_K_L | 3.81GB |
| [krx-qwen2.5-7B-Instruct-v0.2.IQ4_XS.gguf](https://huggingface.co/RichardErkhov/KR-X-AI_-_krx-qwen2.5-7B-Instruct-v0.2-gguf/blob/main/krx-qwen2.5-7B-Instruct-v0.2.IQ4_XS.gguf) | IQ4_XS | 3.96GB |
| [krx-qwen2.5-7B-Instruct-v0.2.Q4_0.gguf](https://huggingface.co/RichardErkhov/KR-X-AI_-_krx-qwen2.5-7B-Instruct-v0.2-gguf/blob/main/krx-qwen2.5-7B-Instruct-v0.2.Q4_0.gguf) | Q4_0 | 4.13GB |
| [krx-qwen2.5-7B-Instruct-v0.2.IQ4_NL.gguf](https://huggingface.co/RichardErkhov/KR-X-AI_-_krx-qwen2.5-7B-Instruct-v0.2-gguf/blob/main/krx-qwen2.5-7B-Instruct-v0.2.IQ4_NL.gguf) | IQ4_NL | 4.16GB |
| [krx-qwen2.5-7B-Instruct-v0.2.Q4_K_S.gguf](https://huggingface.co/RichardErkhov/KR-X-AI_-_krx-qwen2.5-7B-Instruct-v0.2-gguf/blob/main/krx-qwen2.5-7B-Instruct-v0.2.Q4_K_S.gguf) | Q4_K_S | 4.15GB |
| [krx-qwen2.5-7B-Instruct-v0.2.Q4_K.gguf](https://huggingface.co/RichardErkhov/KR-X-AI_-_krx-qwen2.5-7B-Instruct-v0.2-gguf/blob/main/krx-qwen2.5-7B-Instruct-v0.2.Q4_K.gguf) | Q4_K | 4.36GB |
| [krx-qwen2.5-7B-Instruct-v0.2.Q4_K_M.gguf](https://huggingface.co/RichardErkhov/KR-X-AI_-_krx-qwen2.5-7B-Instruct-v0.2-gguf/blob/main/krx-qwen2.5-7B-Instruct-v0.2.Q4_K_M.gguf) | Q4_K_M | 4.36GB |
| [krx-qwen2.5-7B-Instruct-v0.2.Q4_1.gguf](https://huggingface.co/RichardErkhov/KR-X-AI_-_krx-qwen2.5-7B-Instruct-v0.2-gguf/blob/main/krx-qwen2.5-7B-Instruct-v0.2.Q4_1.gguf) | Q4_1 | 4.54GB |
| [krx-qwen2.5-7B-Instruct-v0.2.Q5_0.gguf](https://huggingface.co/RichardErkhov/KR-X-AI_-_krx-qwen2.5-7B-Instruct-v0.2-gguf/blob/main/krx-qwen2.5-7B-Instruct-v0.2.Q5_0.gguf) | Q5_0 | 4.95GB |
| [krx-qwen2.5-7B-Instruct-v0.2.Q5_K_S.gguf](https://huggingface.co/RichardErkhov/KR-X-AI_-_krx-qwen2.5-7B-Instruct-v0.2-gguf/blob/main/krx-qwen2.5-7B-Instruct-v0.2.Q5_K_S.gguf) | Q5_K_S | 4.95GB |
| [krx-qwen2.5-7B-Instruct-v0.2.Q5_K.gguf](https://huggingface.co/RichardErkhov/KR-X-AI_-_krx-qwen2.5-7B-Instruct-v0.2-gguf/blob/main/krx-qwen2.5-7B-Instruct-v0.2.Q5_K.gguf) | Q5_K | 5.07GB |
| [krx-qwen2.5-7B-Instruct-v0.2.Q5_K_M.gguf](https://huggingface.co/RichardErkhov/KR-X-AI_-_krx-qwen2.5-7B-Instruct-v0.2-gguf/blob/main/krx-qwen2.5-7B-Instruct-v0.2.Q5_K_M.gguf) | Q5_K_M | 5.07GB |
| [krx-qwen2.5-7B-Instruct-v0.2.Q5_1.gguf](https://huggingface.co/RichardErkhov/KR-X-AI_-_krx-qwen2.5-7B-Instruct-v0.2-gguf/blob/main/krx-qwen2.5-7B-Instruct-v0.2.Q5_1.gguf) | Q5_1 | 5.36GB |
| [krx-qwen2.5-7B-Instruct-v0.2.Q6_K.gguf](https://huggingface.co/RichardErkhov/KR-X-AI_-_krx-qwen2.5-7B-Instruct-v0.2-gguf/blob/main/krx-qwen2.5-7B-Instruct-v0.2.Q6_K.gguf) | Q6_K | 5.82GB |
| [krx-qwen2.5-7B-Instruct-v0.2.Q8_0.gguf](https://huggingface.co/RichardErkhov/KR-X-AI_-_krx-qwen2.5-7B-Instruct-v0.2-gguf/blob/main/krx-qwen2.5-7B-Instruct-v0.2.Q8_0.gguf) | Q8_0 | 7.54GB |
Original model description:
---
base_model: model
tags:
- text-generation-inference
- transformers
- unsloth
- qwen2
- trl
license: apache-2.0
language:
- en
---
# Uploaded model
- **Developed by:** KR-X-AI
- **License:** apache-2.0
- **Finetuned from model :** model
This qwen2 model was trained 2x faster with [Unsloth](https://github.com/unslothai/unsloth) and Huggingface's TRL library.
[<img src="https://raw.githubusercontent.com/unslothai/unsloth/main/images/unsloth%20made%20with%20love.png" width="200"/>](https://github.com/unslothai/unsloth)
|
bqcDXXOmgO52f/kilagd | bqcDXXOmgO52f | 2025-04-27T05:34:48Z | 0 | 0 | null | [
"license:apache-2.0",
"region:us"
]
| null | 2025-04-27T05:34:48Z | ---
license: apache-2.0
---
|
10-Nimra-Mehra-Go-Viral-Link/TRENDING.Nimra.Mehra.Viral.Video.Leaks.Tutorial | 10-Nimra-Mehra-Go-Viral-Link | 2025-04-27T05:33:20Z | 0 | 0 | null | [
"region:us"
]
| null | 2025-04-27T05:32:14Z | <animated-image data-catalyst=""><a href="https://tinyurl.com/3ac24m6k?dfhgKasbonStudiosdfg" rel="nofollow" data-target="animated-image.originalLink"><img src="https://static.wixstatic.com/media/b249f9_adac8f70fb3f45b88691696c77de18f3~mv2.gif" alt="Foo" data-canonical-src="https://static.wixstatic.com/media/b249f9_adac8f70fb3f45b88691696c77de18f3~mv2.gif" style="max-width: 100%; display: inline-block;" data-target="animated-image.originalImage"></a>
Nimra Mehra’s private video surfaces, trending on social media
MM News Staff by MM News Staff December 2, 2024
The trend of video leaks continues in Pakistan, with reports of singer and social media influencer Nimra Mehra’s private videos being leaked.
Nimra Mehra, a well-known Pakistani TikTok star and influencer, has gained popularity for her interesting videos and unique style. Recently, there have been reports of an inappropriate video of her being leaked, which has created a stir on social media. |
mlfoundations-dev/c1_math_10d_1s_1k | mlfoundations-dev | 2025-04-27T05:31:27Z | 0 | 0 | transformers | [
"transformers",
"safetensors",
"qwen2",
"text-generation",
"llama-factory",
"full",
"generated_from_trainer",
"conversational",
"base_model:Qwen/Qwen2.5-7B-Instruct",
"base_model:finetune:Qwen/Qwen2.5-7B-Instruct",
"license:apache-2.0",
"autotrain_compatible",
"text-generation-inference",
"endpoints_compatible",
"region:us"
]
| text-generation | 2025-04-27T03:03:04Z | ---
library_name: transformers
license: apache-2.0
base_model: Qwen/Qwen2.5-7B-Instruct
tags:
- llama-factory
- full
- generated_from_trainer
model-index:
- name: c1_math_10d_1s_1k
results: []
---
<!-- This model card has been generated automatically according to the information the Trainer had access to. You
should probably proofread and complete it, then remove this comment. -->
# c1_math_10d_1s_1k
This model is a fine-tuned version of [Qwen/Qwen2.5-7B-Instruct](https://huggingface.co/Qwen/Qwen2.5-7B-Instruct) on the mlfoundations-dev/c1_math_10d_1s_1k dataset.
## Model description
More information needed
## Intended uses & limitations
More information needed
## Training and evaluation data
More information needed
## Training procedure
### Training hyperparameters
The following hyperparameters were used during training:
- learning_rate: 2e-05
- train_batch_size: 1
- eval_batch_size: 8
- seed: 42
- distributed_type: multi-GPU
- num_devices: 4
- gradient_accumulation_steps: 24
- total_train_batch_size: 96
- total_eval_batch_size: 32
- optimizer: Use adamw_torch with betas=(0.9,0.999) and epsilon=1e-08 and optimizer_args=No additional optimizer arguments
- lr_scheduler_type: cosine
- lr_scheduler_warmup_ratio: 0.1
- num_epochs: 7.0
### Training results
### Framework versions
- Transformers 4.46.1
- Pytorch 2.6.0+cu124
- Datasets 3.1.0
- Tokenizers 0.20.3
|
mlfoundations-dev/c1_code_nod_4s_10k | mlfoundations-dev | 2025-04-27T05:31:22Z | 0 | 0 | transformers | [
"transformers",
"safetensors",
"qwen2",
"text-generation",
"llama-factory",
"full",
"generated_from_trainer",
"conversational",
"base_model:Qwen/Qwen2.5-7B-Instruct",
"base_model:finetune:Qwen/Qwen2.5-7B-Instruct",
"license:apache-2.0",
"autotrain_compatible",
"text-generation-inference",
"endpoints_compatible",
"region:us"
]
| text-generation | 2025-04-27T00:23:02Z | ---
library_name: transformers
license: apache-2.0
base_model: Qwen/Qwen2.5-7B-Instruct
tags:
- llama-factory
- full
- generated_from_trainer
model-index:
- name: c1_code_nod_4s_10k
results: []
---
<!-- This model card has been generated automatically according to the information the Trainer had access to. You
should probably proofread and complete it, then remove this comment. -->
# c1_code_nod_4s_10k
This model is a fine-tuned version of [Qwen/Qwen2.5-7B-Instruct](https://huggingface.co/Qwen/Qwen2.5-7B-Instruct) on the mlfoundations-dev/c1_code_nod_4s_10k dataset.
## Model description
More information needed
## Intended uses & limitations
More information needed
## Training and evaluation data
More information needed
## Training procedure
### Training hyperparameters
The following hyperparameters were used during training:
- learning_rate: 4e-05
- train_batch_size: 1
- eval_batch_size: 8
- seed: 42
- distributed_type: multi-GPU
- num_devices: 16
- gradient_accumulation_steps: 8
- total_train_batch_size: 128
- total_eval_batch_size: 128
- optimizer: Use OptimizerNames.ADAMW_TORCH with betas=(0.9,0.999) and epsilon=1e-08 and optimizer_args=No additional optimizer arguments
- lr_scheduler_type: cosine
- lr_scheduler_warmup_ratio: 0.1
- num_epochs: 5.0
### Training results
### Framework versions
- Transformers 4.46.1
- Pytorch 2.5.1
- Datasets 3.0.2
- Tokenizers 0.20.3
|
mlfoundations-dev/b2_code_length_gpt41nano_3k | mlfoundations-dev | 2025-04-27T05:28:47Z | 0 | 0 | transformers | [
"transformers",
"safetensors",
"qwen2",
"text-generation",
"llama-factory",
"full",
"generated_from_trainer",
"conversational",
"base_model:Qwen/Qwen2.5-7B-Instruct",
"base_model:finetune:Qwen/Qwen2.5-7B-Instruct",
"license:apache-2.0",
"autotrain_compatible",
"text-generation-inference",
"endpoints_compatible",
"region:us"
]
| text-generation | 2025-04-26T21:55:16Z | ---
library_name: transformers
license: apache-2.0
base_model: Qwen/Qwen2.5-7B-Instruct
tags:
- llama-factory
- full
- generated_from_trainer
model-index:
- name: b2_code_length_gpt41nano_3k
results: []
---
<!-- This model card has been generated automatically according to the information the Trainer had access to. You
should probably proofread and complete it, then remove this comment. -->
# b2_code_length_gpt41nano_3k
This model is a fine-tuned version of [Qwen/Qwen2.5-7B-Instruct](https://huggingface.co/Qwen/Qwen2.5-7B-Instruct) on the mlfoundations-dev/b2_code_length_gpt41nano_3k dataset.
## Model description
More information needed
## Intended uses & limitations
More information needed
## Training and evaluation data
More information needed
## Training procedure
### Training hyperparameters
The following hyperparameters were used during training:
- learning_rate: 2e-05
- train_batch_size: 1
- eval_batch_size: 8
- seed: 42
- distributed_type: multi-GPU
- num_devices: 4
- gradient_accumulation_steps: 24
- total_train_batch_size: 96
- total_eval_batch_size: 32
- optimizer: Use adamw_torch with betas=(0.9,0.999) and epsilon=1e-08 and optimizer_args=No additional optimizer arguments
- lr_scheduler_type: cosine
- lr_scheduler_warmup_ratio: 0.1
- num_epochs: 7.0
### Training results
### Framework versions
- Transformers 4.46.1
- Pytorch 2.6.0+cu124
- Datasets 3.1.0
- Tokenizers 0.20.3
|
TOMFORD79/E3 | TOMFORD79 | 2025-04-27T05:26:32Z | 0 | 0 | null | [
"safetensors",
"any-to-any",
"omega",
"omegalabs",
"bittensor",
"agi",
"license:mit",
"region:us"
]
| any-to-any | 2025-04-27T04:49:35Z | ---
license: mit
tags:
- any-to-any
- omega
- omegalabs
- bittensor
- agi
---
This is an Any-to-Any model checkpoint for the OMEGA Labs x Bittensor Any-to-Any subnet.
Check out the [git repo](https://github.com/omegalabsinc/omegalabs-anytoany-bittensor) and find OMEGA on X: [@omegalabsai](https://x.com/omegalabsai).
|
Gale09080706/0427-sft | Gale09080706 | 2025-04-27T05:26:21Z | 0 | 0 | transformers | [
"transformers",
"safetensors",
"qwen2_5_vl",
"image-text-to-text",
"generated_from_trainer",
"trl",
"sft",
"conversational",
"text-generation-inference",
"endpoints_compatible",
"region:us"
]
| image-text-to-text | 2025-04-27T03:42:13Z | ---
library_name: transformers
model_name: 0427-sft
tags:
- generated_from_trainer
- trl
- sft
licence: license
---
# Model Card for 0427-sft
This model is a fine-tuned version of [None](https://huggingface.co/None).
It has been trained using [TRL](https://github.com/huggingface/trl).
## Quick start
```python
from transformers import pipeline
question = "If you had a time machine, but could only go to the past or the future once and never return, which would you choose and why?"
generator = pipeline("text-generation", model="Gale09080706/0427-sft", device="cuda")
output = generator([{"role": "user", "content": question}], max_new_tokens=128, return_full_text=False)[0]
print(output["generated_text"])
```
## Training procedure
[<img src="https://raw.githubusercontent.com/wandb/assets/main/wandb-github-badge-28.svg" alt="Visualize in Weights & Biases" width="150" height="24"/>](https://wandb.ai/yuefang979-national-university-of-singapore-students-union/huggingface/runs/cgifqlsj)
This model was trained with SFT.
### Framework versions
- TRL: 0.16.1
- Transformers: 4.50.0.dev0
- Pytorch: 2.6.0
- Datasets: 3.3.2
- Tokenizers: 0.21.0
## Citations
Cite TRL as:
```bibtex
@misc{vonwerra2022trl,
title = {{TRL: Transformer Reinforcement Learning}},
author = {Leandro von Werra and Younes Belkada and Lewis Tunstall and Edward Beeching and Tristan Thrush and Nathan Lambert and Shengyi Huang and Kashif Rasul and Quentin Gallouédec},
year = 2020,
journal = {GitHub repository},
publisher = {GitHub},
howpublished = {\url{https://github.com/huggingface/trl}}
}
``` |
jnjj/mega-multimodal-fused-final-v6 | jnjj | 2025-04-27T05:24:30Z | 0 | 0 | null | [
"mega-multimodal-v6",
"region:us"
]
| null | 2025-04-27T05:24:30Z | # Mega Multimodal Model V6 (mega-multimodal-v6)
Multimodal model with unified interface, selective loading, auto-skip for failed loads.
## Included Capabilities & Models (Based on Config)
* **ControlNet (Canny):** lllyasviel/control_v11p_sd15_canny (Not Loaded)
* **ControlNet (Depth):** lllyasviel/control_v11f1p_sd15_depth (Not Loaded)
* **ControlNet (Pose):** lllyasviel/control_v11p_sd15_openpose (Not Loaded)
* **ControlNet (Softedge):** N/A (controlnet_softedge) (Not Loaded)
* **Audio_cls Model:** microsoft/wavlm-base-plus-sd (Not Loaded)
* **bark:** suno/bark (Not Loaded)
* **Caption Model:** nlpconnect/vit-gpt2-image-captioning (Not Loaded)
* **clip:** openai/clip-vit-base-patch32 (Not Loaded)
* **code:** bigcode/starcoder2-3b (Not Loaded)
* **Depth Model:** Intel/dpt-large (Not Loaded)
* **detr:** facebook/detr-resnet-50 (Not Loaded)
* **Docvqa Model:** naver-clova-ix/donut-base-finetuned-docvqa (Not Loaded)
* **music:** facebook/musicgen-medium (Not Loaded)
* **ner:** dbmdz/bert-large-cased-finetuned-conll03-english (Not Loaded)
* **qa:** distilbert-base-uncased-distilled-squad (Not Loaded)
* **sentiment:** unitary/toxic-bert (Not Loaded)
* **speech:** openai/whisper-tiny (Not Loaded)
* **text:** Qwen/Qwen1.5-1.8B (Not Loaded)
* **tqa:** google/tapas-base-finetuned-wtq (Not Loaded)
* **trocr:** microsoft/trocr-small-handwritten (Not Loaded)
* **Video_cls Model:** MCG-NJU/videomae-base-finetuned-kinetics (Not Loaded)
* **Vqa Model:** dandelin/vilt-b32-finetuned-vqa (Not Loaded)
* **zshot_cls:** openai/clip-vit-large-patch14 (Not Loaded)
* **zshot_det:** google/owlvit-base-patch32 (Not Loaded)
* **i2v:** stabilityai/stable-video-diffusion-img2vid-xt (Not Loaded)
* **instruct_pix2pix:** timbrooks/instruct-pix2pix (Not Loaded)
* **kandinsky_decoder:** kandinsky-community/kandinsky-2-2-decoder (Not Loaded)
* **kandinsky_prior:** kandinsky-community/kandinsky-2-2-prior (Not Loaded)
* **refine:** stabilityai/stable-diffusion-xl-refiner-1.0 (Not Loaded)
* **Text-to-Image (SD 1.5):** runwayml/stable-diffusion-v1-5 (Not Loaded)
* **sd_inpainting:** runwayml/stable-diffusion-inpainting (Not Loaded)
* **Text-to-Image (SDXL):** stabilityai/stable-diffusion-xl-base-1.0 (Not Loaded)
* **shape_pipe:** stabilityai/shap-e (Not Loaded)
* **t2v:** cerspense/zeroscope_v2_576w (Not Loaded)
* **Audio_cls Processor:** microsoft/wavlm-base-plus-sd (Not Loaded)
* **Bark Processor:** suno/bark (Not Loaded)
* **Caption Processor:** nlpconnect/vit-gpt2-image-captioning (Not Loaded)
* **Clip Processor:** openai/clip-vit-base-patch32 (Not Loaded)
* **Depth Processor:** Intel/dpt-large (Not Loaded)
* **Detr Processor:** facebook/detr-resnet-50 (Not Loaded)
* **Docvqa Processor:** naver-clova-ix/donut-base-finetuned-docvqa (Not Loaded)
* **Speech Processor:** openai/whisper-tiny (Not Loaded)
* **Trocr Processor:** microsoft/trocr-small-handwritten (Not Loaded)
* **Video_cls Processor:** MCG-NJU/videomae-base-finetuned-kinetics (Not Loaded)
* **Vqa Processor:** dandelin/vilt-b32-finetuned-vqa (Not Loaded)
* **Zshot_cls Processor:** openai/clip-vit-large-patch14 (Not Loaded)
* **Zshot_det Processor:** google/owlvit-base-patch32 (Not Loaded)
* **Code Tokenizer:** bigcode/starcoder2-3b (Not Loaded)
* **Music Tokenizer:** facebook/musicgen-medium (Not Loaded)
* **Ner Tokenizer:** dbmdz/bert-large-cased-finetuned-conll03-english (Not Loaded)
* **Qa Tokenizer:** distilbert-base-uncased-distilled-squad (Not Loaded)
* **Sentiment Tokenizer:** unitary/toxic-bert (Not Loaded)
* **Text Tokenizer:** Qwen/Qwen1.5-1.8B (Not Loaded)
* **Tqa Tokenizer:** google/tapas-base-finetuned-wtq (Not Loaded)
## Optimizations & Mechanisms
* **Selective Loading:** from_pretrained(..., components_to_load=[...]).
* **Auto-Skip Failed Loads:** Logs errors and continues if a component fails.
* **Logging & Performance Timing:** Optional generate(..., time_execution=True).
* **Input Validation:** Enhanced type/value checks.
* **Custom BitLinear:** Configured: False.
* **BitsAndBytes Quantization:** Configured: False, Mode: 4bit.
* **Global Pruning:** Configured Amount: 0.0.
* **Gradient Checkpointing:** Configured: False.
* **Flash Attention 2:** Configured: False.
* **Diffusers Optimizations:** Slicing (True), Offload (True).
* **Low CPU Mem Usage:** Configured: True.
## Saving & Loading
Uses standard save_pretrained / from_pretrained. Components in subdirs. Failed loads during from_pretrained skipped.
python
from mega_multimodal_model import MegaMultimodalModel # Assuming class definition saved
# Save
# model.save_pretrained("./my_mega_multimodal_model_v6")
# Load all
# loaded_model = MegaMultimodalModel.from_pretrained("./my_mega_multimodal_model_v6")
# Load selectively (example)
# components = ['text', 'text_tok', 'sd', 'canny'] # Use class attribute names or controlnet types
# loaded_model_subset = MegaMultimodalModel.from_pretrained("./my_mega_multimodal_model_v6", components_to_load=components)
# Load from Hub
# loaded_model = MegaMultimodalModel.from_pretrained("your_hf_username/your_repo_name")
# loaded_model_subset = MegaMultimodalModel.from_pretrained("your_hf_username/your_repo_name", components_to_load=components)
# Usage
# text_output = loaded_model.generate("Hello!", task="text", time_execution=True)
## Installation Dependencies
Core: torch torchvision torchaudio transformers diffusers huggingface_hub[hf_xet] hf_xet safetensors timm Pillow accelerate bitsandbytes einops pandas decord ftfy pyav --upgrade
Optional: controlnet-aux, flash-attn --no-build-isolation
## Model Configuration (config.json)
Stores component IDs and optimization settings. |
azirdomini8/9 | azirdomini8 | 2025-04-27T05:24:06Z | 0 | 0 | flair | [
"flair",
"text-classification",
"af",
"dataset:nvidia/Llama-Nemotron-Post-Training-Dataset",
"base_model:black-forest-labs/FLUX.1-dev",
"base_model:finetune:black-forest-labs/FLUX.1-dev",
"license:openrail",
"region:us"
]
| text-classification | 2025-04-27T05:23:49Z | ---
license: openrail
datasets:
- nvidia/Llama-Nemotron-Post-Training-Dataset
language:
- af
metrics:
- bertscore
base_model:
- black-forest-labs/FLUX.1-dev
new_version: reducto/RolmOCR
pipeline_tag: text-classification
library_name: flair
--- |
stpete2/qwen2.5-0.5b-gsm8k-raftplusplus | stpete2 | 2025-04-27T05:18:41Z | 0 | 0 | transformers | [
"transformers",
"safetensors",
"qwen2",
"text-generation",
"generated_from_trainer",
"open-r1",
"conversational",
"dataset:stpete2/openai-gsm8k-part",
"arxiv:2402.03300",
"base_model:Qwen/Qwen2.5-0.5B",
"base_model:finetune:Qwen/Qwen2.5-0.5B",
"autotrain_compatible",
"text-generation-inference",
"endpoints_compatible",
"region:us"
]
| text-generation | 2025-04-27T05:15:29Z | ---
base_model: Qwen/Qwen2.5-0.5B
datasets: stpete2/openai-gsm8k-part
library_name: transformers
tags:
- generated_from_trainer
- open-r1
licence: license
---
# Model Card for None
This model is a fine-tuned version of [Qwen/Qwen2.5-0.5B](https://huggingface.co/Qwen/Qwen2.5-0.5B) on the [stpete2/openai-gsm8k-part](https://huggingface.co/datasets/stpete2/openai-gsm8k-part) dataset.
It has been trained using [TRL](https://github.com/huggingface/trl).
## Quick start
```python
from transformers import pipeline
question = "If you had a time machine, but could only go to the past or the future once and never return, which would you choose and why?"
generator = pipeline("text-generation", model="None", device="cuda")
output = generator([{"role": "user", "content": question}], max_new_tokens=128, return_full_text=False)[0]
print(output["generated_text"])
```
## Training procedure
[<img src="https://raw.githubusercontent.com/wandb/assets/main/wandb-github-badge-28.svg" alt="Visualize in Weights & Biases" width="150" height="24"/>](https://wandb.ai/stpeteishii/huggingface/runs/5xnj2ak8)
This model was trained with GRPO, a method introduced in [DeepSeekMath: Pushing the Limits of Mathematical Reasoning in Open Language Models](https://huggingface.co/papers/2402.03300).
### Framework versions
- TRL: 0.16.0.dev0
- Transformers: 4.49.0
- Pytorch: 2.5.1+cu121
- Datasets: 3.3.1
- Tokenizers: 0.21.0
## Citations
Cite GRPO as:
```bibtex
@article{zhihong2024deepseekmath,
title = {{DeepSeekMath: Pushing the Limits of Mathematical Reasoning in Open Language Models}},
author = {Zhihong Shao and Peiyi Wang and Qihao Zhu and Runxin Xu and Junxiao Song and Mingchuan Zhang and Y. K. Li and Y. Wu and Daya Guo},
year = 2024,
eprint = {arXiv:2402.03300},
}
```
Cite TRL as:
```bibtex
@misc{vonwerra2022trl,
title = {{TRL: Transformer Reinforcement Learning}},
author = {Leandro von Werra and Younes Belkada and Lewis Tunstall and Edward Beeching and Tristan Thrush and Nathan Lambert and Shengyi Huang and Kashif Rasul and Quentin Gallouédec},
year = 2020,
journal = {GitHub repository},
publisher = {GitHub},
howpublished = {\url{https://github.com/huggingface/trl}}
}
``` |
mlfoundations-dev/b2_code_length_3k | mlfoundations-dev | 2025-04-27T05:18:06Z | 0 | 0 | transformers | [
"transformers",
"safetensors",
"qwen2",
"text-generation",
"llama-factory",
"full",
"generated_from_trainer",
"conversational",
"base_model:Qwen/Qwen2.5-7B-Instruct",
"base_model:finetune:Qwen/Qwen2.5-7B-Instruct",
"license:apache-2.0",
"autotrain_compatible",
"text-generation-inference",
"endpoints_compatible",
"region:us"
]
| text-generation | 2025-04-26T21:27:50Z | ---
library_name: transformers
license: apache-2.0
base_model: Qwen/Qwen2.5-7B-Instruct
tags:
- llama-factory
- full
- generated_from_trainer
model-index:
- name: b2_code_length_3k
results: []
---
<!-- This model card has been generated automatically according to the information the Trainer had access to. You
should probably proofread and complete it, then remove this comment. -->
# b2_code_length_3k
This model is a fine-tuned version of [Qwen/Qwen2.5-7B-Instruct](https://huggingface.co/Qwen/Qwen2.5-7B-Instruct) on the mlfoundations-dev/b2_code_length_3k dataset.
## Model description
More information needed
## Intended uses & limitations
More information needed
## Training and evaluation data
More information needed
## Training procedure
### Training hyperparameters
The following hyperparameters were used during training:
- learning_rate: 2e-05
- train_batch_size: 1
- eval_batch_size: 8
- seed: 42
- distributed_type: multi-GPU
- num_devices: 4
- gradient_accumulation_steps: 24
- total_train_batch_size: 96
- total_eval_batch_size: 32
- optimizer: Use adamw_torch with betas=(0.9,0.999) and epsilon=1e-08 and optimizer_args=No additional optimizer arguments
- lr_scheduler_type: cosine
- lr_scheduler_warmup_ratio: 0.1
- num_epochs: 7.0
### Training results
### Framework versions
- Transformers 4.46.1
- Pytorch 2.6.0+cu124
- Datasets 3.1.0
- Tokenizers 0.20.3
|
genki10/BERT_V8_sp10_lw40_ex100_lo50_k7_k7_fold3 | genki10 | 2025-04-27T05:16:38Z | 0 | 0 | transformers | [
"transformers",
"pytorch",
"bert",
"text-classification",
"generated_from_trainer",
"base_model:google-bert/bert-base-uncased",
"base_model:finetune:google-bert/bert-base-uncased",
"license:apache-2.0",
"autotrain_compatible",
"endpoints_compatible",
"region:us"
]
| text-classification | 2025-04-27T04:59:34Z | ---
library_name: transformers
license: apache-2.0
base_model: bert-base-uncased
tags:
- generated_from_trainer
model-index:
- name: BERT_V8_sp10_lw40_ex100_lo50_k7_k7_fold3
results: []
---
<!-- This model card has been generated automatically according to the information the Trainer had access to. You
should probably proofread and complete it, then remove this comment. -->
# BERT_V8_sp10_lw40_ex100_lo50_k7_k7_fold3
This model is a fine-tuned version of [bert-base-uncased](https://huggingface.co/bert-base-uncased) on the None dataset.
It achieves the following results on the evaluation set:
- Loss: 1.0593
- Qwk: 0.3015
- Mse: 1.0597
- Rmse: 1.0294
## Model description
More information needed
## Intended uses & limitations
More information needed
## Training and evaluation data
More information needed
## Training procedure
### Training hyperparameters
The following hyperparameters were used during training:
- learning_rate: 2e-05
- train_batch_size: 64
- eval_batch_size: 64
- seed: 42
- optimizer: Use OptimizerNames.ADAMW_TORCH with betas=(0.9,0.999) and epsilon=1e-08 and optimizer_args=No additional optimizer arguments
- lr_scheduler_type: linear
- num_epochs: 100
### Training results
| Training Loss | Epoch | Step | Validation Loss | Qwk | Mse | Rmse |
|:-------------:|:-----:|:----:|:---------------:|:------:|:------:|:------:|
| No log | 1.0 | 4 | 9.8257 | 0.0018 | 9.8240 | 3.1343 |
| No log | 2.0 | 8 | 5.9230 | 0.0432 | 5.9217 | 2.4334 |
| No log | 3.0 | 12 | 3.5939 | 0.0038 | 3.5929 | 1.8955 |
| No log | 4.0 | 16 | 1.8570 | 0.0528 | 1.8562 | 1.3624 |
| No log | 5.0 | 20 | 1.1050 | 0.0102 | 1.1045 | 1.0509 |
| No log | 6.0 | 24 | 0.8647 | 0.1192 | 0.8644 | 0.9297 |
| No log | 7.0 | 28 | 0.8063 | 0.1489 | 0.8059 | 0.8977 |
| No log | 8.0 | 32 | 0.9996 | 0.1921 | 0.9995 | 0.9997 |
| No log | 9.0 | 36 | 0.7886 | 0.3961 | 0.7887 | 0.8881 |
| No log | 10.0 | 40 | 1.2674 | 0.3456 | 1.2678 | 1.1259 |
| No log | 11.0 | 44 | 1.2061 | 0.3403 | 1.2066 | 1.0984 |
| No log | 12.0 | 48 | 0.7582 | 0.4512 | 0.7588 | 0.8711 |
| No log | 13.0 | 52 | 0.8385 | 0.4216 | 0.8391 | 0.9160 |
| No log | 14.0 | 56 | 0.7615 | 0.4588 | 0.7618 | 0.8728 |
| No log | 15.0 | 60 | 0.7612 | 0.4532 | 0.7615 | 0.8727 |
| No log | 16.0 | 64 | 0.9011 | 0.4228 | 0.9016 | 0.9495 |
| No log | 17.0 | 68 | 0.9822 | 0.3690 | 0.9828 | 0.9913 |
| No log | 18.0 | 72 | 1.1433 | 0.3141 | 1.1439 | 1.0695 |
| No log | 19.0 | 76 | 1.3613 | 0.2542 | 1.3618 | 1.1670 |
| No log | 20.0 | 80 | 0.9052 | 0.3826 | 0.9056 | 0.9516 |
| No log | 21.0 | 84 | 1.4135 | 0.2432 | 1.4139 | 1.1891 |
| No log | 22.0 | 88 | 1.0541 | 0.3264 | 1.0545 | 1.0269 |
| No log | 23.0 | 92 | 1.0845 | 0.3232 | 1.0850 | 1.0416 |
| No log | 24.0 | 96 | 0.9120 | 0.4106 | 0.9125 | 0.9552 |
| No log | 25.0 | 100 | 1.2307 | 0.2766 | 1.2311 | 1.1096 |
| No log | 26.0 | 104 | 1.2194 | 0.2664 | 1.2200 | 1.1045 |
| No log | 27.0 | 108 | 1.2270 | 0.2441 | 1.2275 | 1.1079 |
| No log | 28.0 | 112 | 1.0726 | 0.3140 | 1.0731 | 1.0359 |
| No log | 29.0 | 116 | 1.1589 | 0.2819 | 1.1594 | 1.0767 |
| No log | 30.0 | 120 | 0.9678 | 0.3024 | 0.9682 | 0.9840 |
| No log | 31.0 | 124 | 1.1879 | 0.2328 | 1.1883 | 1.0901 |
| No log | 32.0 | 128 | 0.9852 | 0.3352 | 0.9857 | 0.9928 |
| No log | 33.0 | 132 | 0.9971 | 0.3444 | 0.9976 | 0.9988 |
| No log | 34.0 | 136 | 1.2483 | 0.2408 | 1.2487 | 1.1175 |
| No log | 35.0 | 140 | 0.8800 | 0.3717 | 0.8804 | 0.9383 |
| No log | 36.0 | 144 | 1.4634 | 0.2153 | 1.4638 | 1.2099 |
| No log | 37.0 | 148 | 0.8824 | 0.3696 | 0.8827 | 0.9395 |
| No log | 38.0 | 152 | 1.1537 | 0.2608 | 1.1540 | 1.0743 |
| No log | 39.0 | 156 | 1.1222 | 0.2823 | 1.1227 | 1.0596 |
| No log | 40.0 | 160 | 0.9534 | 0.3604 | 0.9539 | 0.9767 |
| No log | 41.0 | 164 | 1.0497 | 0.2957 | 1.0501 | 1.0247 |
| No log | 42.0 | 168 | 1.0688 | 0.2901 | 1.0691 | 1.0340 |
| No log | 43.0 | 172 | 1.0982 | 0.2784 | 1.0986 | 1.0481 |
| No log | 44.0 | 176 | 1.0593 | 0.3015 | 1.0597 | 1.0294 |
### Framework versions
- Transformers 4.51.1
- Pytorch 2.5.1+cu124
- Datasets 3.5.0
- Tokenizers 0.21.0
|
filipesantoscv11/0bc4bb33-ce4a-4e61-bd3d-8005a04ddb96 | filipesantoscv11 | 2025-04-27T05:14:48Z | 0 | 0 | peft | [
"peft",
"safetensors",
"llama",
"axolotl",
"generated_from_trainer",
"base_model:MLP-KTLim/llama-3-Korean-Bllossom-8B",
"base_model:adapter:MLP-KTLim/llama-3-Korean-Bllossom-8B",
"license:llama3",
"8-bit",
"bitsandbytes",
"region:us"
]
| null | 2025-04-27T04:48:57Z | ---
library_name: peft
license: llama3
base_model: MLP-KTLim/llama-3-Korean-Bllossom-8B
tags:
- axolotl
- generated_from_trainer
model-index:
- name: 0bc4bb33-ce4a-4e61-bd3d-8005a04ddb96
results: []
---
<!-- This model card has been generated automatically according to the information the Trainer had access to. You
should probably proofread and complete it, then remove this comment. -->
[<img src="https://raw.githubusercontent.com/axolotl-ai-cloud/axolotl/main/image/axolotl-badge-web.png" alt="Built with Axolotl" width="200" height="32"/>](https://github.com/axolotl-ai-cloud/axolotl)
<details><summary>See axolotl config</summary>
axolotl version: `0.4.1`
```yaml
adapter: lora
base_model: MLP-KTLim/llama-3-Korean-Bllossom-8B
bf16: true
chat_template: llama3
dataset_prepared_path: /workspace/axolotl
datasets:
- data_files:
- ad68524142d70a99_train_data.json
ds_type: json
format: custom
path: /workspace/input_data/ad68524142d70a99_train_data.json
type:
field_input: input
field_instruction: instruction
field_output: output
format: '{instruction} {input}'
no_input_format: '{instruction}'
system_format: '{system}'
system_prompt: ''
debug: null
deepspeed: null
early_stopping_patience: null
eval_max_new_tokens: 128
eval_table_size: null
evals_per_epoch: 1
flash_attention: true
fp16: null
fsdp: null
fsdp_config: null
gradient_accumulation_steps: 1
gradient_checkpointing: true
gradient_clipping: 0.5
group_by_length: false
hub_model_id: filipesantoscv11/0bc4bb33-ce4a-4e61-bd3d-8005a04ddb96
hub_repo: null
hub_strategy: end
hub_token: null
learning_rate: 5.0e-06
load_in_4bit: false
load_in_8bit: true
local_rank: null
logging_steps: 1
lora_alpha: 128
lora_dropout: 0.05
lora_fan_in_fan_out: null
lora_model_dir: null
lora_r: 64
lora_target_linear: true
lr_scheduler: cosine
max_steps: 200
micro_batch_size: 8
mixed_precision: bf16
mlflow_experiment_name: /tmp/ad68524142d70a99_train_data.json
model_type: AutoModelForCausalLM
num_epochs: 1
optimizer: adamw_bnb_8bit
output_dir: miner_id_24
pad_to_sequence_len: true
resume_from_checkpoint: null
s2_attention: null
sample_packing: false
saves_per_epoch: 1
sequence_len: 1024
strict: false
tf32: false
tokenizer_type: AutoTokenizer
train_on_inputs: false
trust_remote_code: true
val_set_size: 0.05
wandb_entity: null
wandb_mode: online
wandb_name: 22cf7bd1-322f-425f-af34-d250b70ab9ea
wandb_project: s56-6
wandb_run: your_name
wandb_runid: 22cf7bd1-322f-425f-af34-d250b70ab9ea
warmup_steps: 5
weight_decay: 0.01
xformers_attention: true
```
</details><br>
# 0bc4bb33-ce4a-4e61-bd3d-8005a04ddb96
This model is a fine-tuned version of [MLP-KTLim/llama-3-Korean-Bllossom-8B](https://huggingface.co/MLP-KTLim/llama-3-Korean-Bllossom-8B) on the None dataset.
It achieves the following results on the evaluation set:
- Loss: 0.5897
## Model description
More information needed
## Intended uses & limitations
More information needed
## Training and evaluation data
More information needed
## Training procedure
### Training hyperparameters
The following hyperparameters were used during training:
- learning_rate: 5e-06
- train_batch_size: 8
- eval_batch_size: 8
- seed: 42
- optimizer: Use OptimizerNames.ADAMW_BNB with betas=(0.9,0.999) and epsilon=1e-08 and optimizer_args=No additional optimizer arguments
- lr_scheduler_type: cosine
- lr_scheduler_warmup_steps: 5
- training_steps: 200
### Training results
| Training Loss | Epoch | Step | Validation Loss |
|:-------------:|:------:|:----:|:---------------:|
| 0.593 | 0.1871 | 200 | 0.5897 |
### Framework versions
- PEFT 0.13.2
- Transformers 4.46.0
- Pytorch 2.5.0+cu124
- Datasets 3.0.1
- Tokenizers 0.20.1 |
Orhan1987/Qwen2.5-0.5B-Instruct-Gensyn-Swarm-galloping_peckish_squid | Orhan1987 | 2025-04-27T05:12:11Z | 2 | 0 | transformers | [
"transformers",
"safetensors",
"qwen2",
"text-generation",
"generated_from_trainer",
"rl-swarm",
"grpo",
"gensyn",
"I am galloping peckish squid",
"trl",
"conversational",
"arxiv:2402.03300",
"base_model:Gensyn/Qwen2.5-0.5B-Instruct",
"base_model:finetune:Gensyn/Qwen2.5-0.5B-Instruct",
"autotrain_compatible",
"text-generation-inference",
"endpoints_compatible",
"region:us"
]
| text-generation | 2025-04-22T11:01:36Z | ---
base_model: Gensyn/Qwen2.5-0.5B-Instruct
library_name: transformers
model_name: Qwen2.5-0.5B-Instruct-Gensyn-Swarm-galloping_peckish_squid
tags:
- generated_from_trainer
- rl-swarm
- grpo
- gensyn
- I am galloping peckish squid
- trl
licence: license
---
# Model Card for Qwen2.5-0.5B-Instruct-Gensyn-Swarm-galloping_peckish_squid
This model is a fine-tuned version of [Gensyn/Qwen2.5-0.5B-Instruct](https://huggingface.co/Gensyn/Qwen2.5-0.5B-Instruct).
It has been trained using [TRL](https://github.com/huggingface/trl).
## Quick start
```python
from transformers import pipeline
question = "If you had a time machine, but could only go to the past or the future once and never return, which would you choose and why?"
generator = pipeline("text-generation", model="Orhan1987/Qwen2.5-0.5B-Instruct-Gensyn-Swarm-galloping_peckish_squid", device="cuda")
output = generator([{"role": "user", "content": question}], max_new_tokens=128, return_full_text=False)[0]
print(output["generated_text"])
```
## Training procedure
This model was trained with GRPO, a method introduced in [DeepSeekMath: Pushing the Limits of Mathematical Reasoning in Open Language Models](https://huggingface.co/papers/2402.03300).
### Framework versions
- TRL: 0.15.2
- Transformers: 4.51.3
- Pytorch: 2.6.0
- Datasets: 3.5.0
- Tokenizers: 0.21.1
## Citations
Cite GRPO as:
```bibtex
@article{zhihong2024deepseekmath,
title = {{DeepSeekMath: Pushing the Limits of Mathematical Reasoning in Open Language Models}},
author = {Zhihong Shao and Peiyi Wang and Qihao Zhu and Runxin Xu and Junxiao Song and Mingchuan Zhang and Y. K. Li and Y. Wu and Daya Guo},
year = 2024,
eprint = {arXiv:2402.03300},
}
```
Cite TRL as:
```bibtex
@misc{vonwerra2022trl,
title = {{TRL: Transformer Reinforcement Learning}},
author = {Leandro von Werra and Younes Belkada and Lewis Tunstall and Edward Beeching and Tristan Thrush and Nathan Lambert and Shengyi Huang and Kashif Rasul and Quentin Gallouédec},
year = 2020,
journal = {GitHub repository},
publisher = {GitHub},
howpublished = {\url{https://github.com/huggingface/trl}}
}
``` |
TOMFORD79/E1 | TOMFORD79 | 2025-04-27T05:11:39Z | 0 | 0 | null | [
"safetensors",
"any-to-any",
"omega",
"omegalabs",
"bittensor",
"agi",
"license:mit",
"region:us"
]
| any-to-any | 2025-04-27T04:49:21Z | ---
license: mit
tags:
- any-to-any
- omega
- omegalabs
- bittensor
- agi
---
This is an Any-to-Any model checkpoint for the OMEGA Labs x Bittensor Any-to-Any subnet.
Check out the [git repo](https://github.com/omegalabsinc/omegalabs-anytoany-bittensor) and find OMEGA on X: [@omegalabsai](https://x.com/omegalabsai).
|
1-NEW-EXCLUSIVE-TRENDING-CLIP/FULL.VIDEO.LINK.Sophie.Rain.Spiderman.Viral.Video.Leaks.Tutorial | 1-NEW-EXCLUSIVE-TRENDING-CLIP | 2025-04-27T05:10:44Z | 0 | 0 | null | [
"region:us"
]
| null | 2025-04-27T05:10:11Z | <animated-image data-catalyst=""><a href="https://tinyurl.com/y2a827nj?dfhgKasbonStudiosdfg" rel="nofollow" data-target="animated-image.originalLink"><img src="https://static.wixstatic.com/media/b249f9_adac8f70fb3f45b88691696c77de18f3~mv2.gif" alt="Foo" data-canonical-src="https://static.wixstatic.com/media/b249f9_adac8f70fb3f45b88691696c77de18f3~mv2.gif" style="max-width: 100%; display: inline-block;" data-target="animated-image.originalImage"></a>
Sophie Rain’s $43.4 million OnlyFans earnings outshine popular NBA stars, sparking viral reactions
Prominent social media influencer and adult content creator/model Sophie Rain has taken the internet by storm after revealing her astonishing income from OnlyFans. In a post shared on X, Rain disclosed that she earned $43.4 million over the past year, leaving fans and critics in awe. Her post, featuring a screenshot of her earnings, was accompanied by a heartfelt caption: “Thankful for one year on here.”
Original.Viral.Clip.Sophie.Rain.Viral.Video.Leaks.official.HD |
jwlarocque/DIS-SAM | jwlarocque | 2025-04-27T05:07:35Z | 0 | 0 | null | [
"sam",
"vision",
"dis",
"Dichotomous Image Segmentation",
"mask-generation",
"arxiv:2401.00248",
"license:mit",
"region:us"
]
| mask-generation | 2025-04-27T04:55:32Z | ---
license: mit
pipeline_tag: mask-generation
tags:
- sam
- vision
- dis
- Dichotomous Image Segmentation
---
No affiliation with the authors.
[Original GitHub repository.](https://github.com/Tennine2077/DIS-SAM)
[Paper.](https://arxiv.org/abs/2401.00248v4)
> The Segment Anything Model (SAM) represents a significant breakthrough into foundation models for computer vision, providing a large-scale image segmentation model. However, despite SAM's zero-shot performance, its segmentation masks lack fine-grained details, particularly in accurately delineating object boundaries. Therefore, it is both interesting and valuable to explore whether SAM can be improved towards highly accurate object segmentation, which is known as the dichotomous image segmentation (DIS) task. To address this issue, we propose DIS-SAM, which advances SAM towards DIS with extremely accurate details. DIS-SAM is a framework specifically tailored for highly accurate segmentation, maintaining SAM's promptable design. DIS-SAM employs a two-stage approach, integrating SAM with a modified advanced network that was previously designed to handle the prompt-free DIS task. To better train DIS-SAM, we employ a ground truth enrichment strategy by modifying original mask annotations. Despite its simplicity, DIS-SAM significantly advances the SAM, HQ-SAM, and Pi-SAM ~by 8.5%, ~6.9%, and ~3.7% maximum F-measure |
joseiivb26/yandel2refine | joseiivb26 | 2025-04-27T05:07:03Z | 0 | 0 | null | [
"license:other",
"region:us"
]
| null | 2025-04-27T04:25:41Z | ---
license: other
license_name: flux-1-dev-non-commercial-license
license_link: https://huggingface.co/black-forest-labs/FLUX.1-dev/blob/main/LICENSE.md
--- |
dgambettaphd/M_llm2_gen1_run0_W_doc1000_synt64_tot128_SYNLAST | dgambettaphd | 2025-04-27T05:02:18Z | 0 | 0 | transformers | [
"transformers",
"safetensors",
"unsloth",
"arxiv:1910.09700",
"endpoints_compatible",
"region:us"
]
| null | 2025-04-27T05:02:06Z | ---
library_name: transformers
tags:
- unsloth
---
# Model Card for Model ID
<!-- Provide a quick summary of what the model is/does. -->
## Model Details
### Model Description
<!-- Provide a longer summary of what this model is. -->
This is the model card of a 🤗 transformers model that has been pushed on the Hub. This model card has been automatically generated.
- **Developed by:** [More Information Needed]
- **Funded by [optional]:** [More Information Needed]
- **Shared by [optional]:** [More Information Needed]
- **Model type:** [More Information Needed]
- **Language(s) (NLP):** [More Information Needed]
- **License:** [More Information Needed]
- **Finetuned from model [optional]:** [More Information Needed]
### Model Sources [optional]
<!-- Provide the basic links for the model. -->
- **Repository:** [More Information Needed]
- **Paper [optional]:** [More Information Needed]
- **Demo [optional]:** [More Information Needed]
## Uses
<!-- Address questions around how the model is intended to be used, including the foreseeable users of the model and those affected by the model. -->
### Direct Use
<!-- This section is for the model use without fine-tuning or plugging into a larger ecosystem/app. -->
[More Information Needed]
### Downstream Use [optional]
<!-- This section is for the model use when fine-tuned for a task, or when plugged into a larger ecosystem/app -->
[More Information Needed]
### Out-of-Scope Use
<!-- This section addresses misuse, malicious use, and uses that the model will not work well for. -->
[More Information Needed]
## Bias, Risks, and Limitations
<!-- This section is meant to convey both technical and sociotechnical limitations. -->
[More Information Needed]
### Recommendations
<!-- This section is meant to convey recommendations with respect to the bias, risk, and technical limitations. -->
Users (both direct and downstream) should be made aware of the risks, biases and limitations of the model. More information needed for further recommendations.
## How to Get Started with the Model
Use the code below to get started with the model.
[More Information Needed]
## Training Details
### Training Data
<!-- This should link to a Dataset Card, perhaps with a short stub of information on what the training data is all about as well as documentation related to data pre-processing or additional filtering. -->
[More Information Needed]
### Training Procedure
<!-- This relates heavily to the Technical Specifications. Content here should link to that section when it is relevant to the training procedure. -->
#### Preprocessing [optional]
[More Information Needed]
#### Training Hyperparameters
- **Training regime:** [More Information Needed] <!--fp32, fp16 mixed precision, bf16 mixed precision, bf16 non-mixed precision, fp16 non-mixed precision, fp8 mixed precision -->
#### Speeds, Sizes, Times [optional]
<!-- This section provides information about throughput, start/end time, checkpoint size if relevant, etc. -->
[More Information Needed]
## Evaluation
<!-- This section describes the evaluation protocols and provides the results. -->
### Testing Data, Factors & Metrics
#### Testing Data
<!-- This should link to a Dataset Card if possible. -->
[More Information Needed]
#### Factors
<!-- These are the things the evaluation is disaggregating by, e.g., subpopulations or domains. -->
[More Information Needed]
#### Metrics
<!-- These are the evaluation metrics being used, ideally with a description of why. -->
[More Information Needed]
### Results
[More Information Needed]
#### Summary
## Model Examination [optional]
<!-- Relevant interpretability work for the model goes here -->
[More Information Needed]
## Environmental Impact
<!-- Total emissions (in grams of CO2eq) and additional considerations, such as electricity usage, go here. Edit the suggested text below accordingly -->
Carbon emissions can be estimated using the [Machine Learning Impact calculator](https://mlco2.github.io/impact#compute) presented in [Lacoste et al. (2019)](https://arxiv.org/abs/1910.09700).
- **Hardware Type:** [More Information Needed]
- **Hours used:** [More Information Needed]
- **Cloud Provider:** [More Information Needed]
- **Compute Region:** [More Information Needed]
- **Carbon Emitted:** [More Information Needed]
## Technical Specifications [optional]
### Model Architecture and Objective
[More Information Needed]
### Compute Infrastructure
[More Information Needed]
#### Hardware
[More Information Needed]
#### Software
[More Information Needed]
## Citation [optional]
<!-- If there is a paper or blog post introducing the model, the APA and Bibtex information for that should go in this section. -->
**BibTeX:**
[More Information Needed]
**APA:**
[More Information Needed]
## Glossary [optional]
<!-- If relevant, include terms and calculations in this section that can help readers understand the model or model card. -->
[More Information Needed]
## More Information [optional]
[More Information Needed]
## Model Card Authors [optional]
[More Information Needed]
## Model Card Contact
[More Information Needed] |
AndresR2909/unsloth_Meta-Llama-3.1-8B-Instruct-bnb-4bit_gguf | AndresR2909 | 2025-04-27T05:01:35Z | 0 | 0 | transformers | [
"transformers",
"gguf",
"llama",
"text-generation-inference",
"unsloth",
"en",
"license:apache-2.0",
"endpoints_compatible",
"region:us",
"conversational"
]
| null | 2025-04-27T04:41:34Z | ---
base_model: unsloth/meta-llama-3.1-8b-instruct-unsloth-bnb-4bit
tags:
- text-generation-inference
- transformers
- unsloth
- llama
- gguf
license: apache-2.0
language:
- en
---
# Uploaded model
- **Developed by:** AndresR2909
- **License:** apache-2.0
- **Finetuned from model :** unsloth/meta-llama-3.1-8b-instruct-unsloth-bnb-4bit
This llama model was trained 2x faster with [Unsloth](https://github.com/unslothai/unsloth) and Huggingface's TRL library.
[<img src="https://raw.githubusercontent.com/unslothai/unsloth/main/images/unsloth%20made%20with%20love.png" width="200"/>](https://github.com/unslothai/unsloth)
|
adrianamariedavi8/8 | adrianamariedavi8 | 2025-04-27T05:00:34Z | 0 | 0 | fastai | [
"fastai",
"climate",
"token-classification",
"af",
"dataset:nvidia/OpenCodeReasoning",
"base_model:sand-ai/MAGI-1",
"base_model:finetune:sand-ai/MAGI-1",
"license:openrail",
"region:us"
]
| token-classification | 2025-04-27T05:00:11Z | ---
license: openrail
datasets:
- nvidia/OpenCodeReasoning
language:
- af
metrics:
- accuracy
base_model:
- sand-ai/MAGI-1
new_version: agentica-org/DeepCoder-14B-Preview
pipeline_tag: token-classification
library_name: fastai
tags:
- climate
--- |
greenwich157/qwen2.5-3b-telcollm-dpo-gguf | greenwich157 | 2025-04-27T05:00:25Z | 0 | 0 | transformers | [
"transformers",
"gguf",
"qwen2",
"text-generation-inference",
"unsloth",
"en",
"base_model:greenwich157/qwen2.5-3b-telcollm-dpo",
"base_model:quantized:greenwich157/qwen2.5-3b-telcollm-dpo",
"license:apache-2.0",
"endpoints_compatible",
"region:us",
"conversational"
]
| null | 2025-04-27T04:59:43Z | ---
base_model: greenwich157/qwen2.5-3b-telcollm-dpo
tags:
- text-generation-inference
- transformers
- unsloth
- qwen2
- gguf
license: apache-2.0
language:
- en
---
# Uploaded model
- **Developed by:** greenwich157
- **License:** apache-2.0
- **Finetuned from model :** greenwich157/qwen2.5-3b-telcollm-dpo
This qwen2 model was trained 2x faster with [Unsloth](https://github.com/unslothai/unsloth) and Huggingface's TRL library.
[<img src="https://raw.githubusercontent.com/unslothai/unsloth/main/images/unsloth%20made%20with%20love.png" width="200"/>](https://github.com/unslothai/unsloth)
|
genki10/BERT_V8_sp10_lw40_ex100_lo50_k7_k7_fold2 | genki10 | 2025-04-27T04:59:27Z | 0 | 0 | transformers | [
"transformers",
"pytorch",
"bert",
"text-classification",
"generated_from_trainer",
"base_model:google-bert/bert-base-uncased",
"base_model:finetune:google-bert/bert-base-uncased",
"license:apache-2.0",
"autotrain_compatible",
"endpoints_compatible",
"region:us"
]
| text-classification | 2025-04-27T04:41:47Z | ---
library_name: transformers
license: apache-2.0
base_model: bert-base-uncased
tags:
- generated_from_trainer
model-index:
- name: BERT_V8_sp10_lw40_ex100_lo50_k7_k7_fold2
results: []
---
<!-- This model card has been generated automatically according to the information the Trainer had access to. You
should probably proofread and complete it, then remove this comment. -->
# BERT_V8_sp10_lw40_ex100_lo50_k7_k7_fold2
This model is a fine-tuned version of [bert-base-uncased](https://huggingface.co/bert-base-uncased) on the None dataset.
It achieves the following results on the evaluation set:
- Loss: 0.5408
- Qwk: 0.4952
- Mse: 0.5404
- Rmse: 0.7351
## Model description
More information needed
## Intended uses & limitations
More information needed
## Training and evaluation data
More information needed
## Training procedure
### Training hyperparameters
The following hyperparameters were used during training:
- learning_rate: 2e-05
- train_batch_size: 64
- eval_batch_size: 64
- seed: 42
- optimizer: Use OptimizerNames.ADAMW_TORCH with betas=(0.9,0.999) and epsilon=1e-08 and optimizer_args=No additional optimizer arguments
- lr_scheduler_type: linear
- num_epochs: 100
### Training results
| Training Loss | Epoch | Step | Validation Loss | Qwk | Mse | Rmse |
|:-------------:|:-----:|:----:|:---------------:|:------:|:------:|:------:|
| No log | 1.0 | 4 | 5.4911 | 0.0412 | 5.4915 | 2.3434 |
| No log | 2.0 | 8 | 3.4449 | 0.0 | 3.4452 | 1.8561 |
| No log | 3.0 | 12 | 2.0814 | 0.0628 | 2.0819 | 1.4429 |
| No log | 4.0 | 16 | 1.3102 | 0.0107 | 1.3107 | 1.1448 |
| No log | 5.0 | 20 | 0.8817 | 0.2075 | 0.8822 | 0.9392 |
| No log | 6.0 | 24 | 0.7717 | 0.1043 | 0.7721 | 0.8787 |
| No log | 7.0 | 28 | 0.5764 | 0.4618 | 0.5765 | 0.7593 |
| No log | 8.0 | 32 | 0.5234 | 0.4888 | 0.5234 | 0.7234 |
| No log | 9.0 | 36 | 0.8255 | 0.4141 | 0.8254 | 0.9085 |
| No log | 10.0 | 40 | 0.6096 | 0.3460 | 0.6095 | 0.7807 |
| No log | 11.0 | 44 | 0.7374 | 0.3899 | 0.7372 | 0.8586 |
| No log | 12.0 | 48 | 0.5518 | 0.4829 | 0.5518 | 0.7428 |
| No log | 13.0 | 52 | 0.5116 | 0.5353 | 0.5114 | 0.7152 |
| No log | 14.0 | 56 | 0.5406 | 0.5516 | 0.5404 | 0.7351 |
| No log | 15.0 | 60 | 0.7312 | 0.4829 | 0.7309 | 0.8549 |
| No log | 16.0 | 64 | 0.5473 | 0.6051 | 0.5469 | 0.7395 |
| No log | 17.0 | 68 | 0.5845 | 0.5172 | 0.5840 | 0.7642 |
| No log | 18.0 | 72 | 0.5410 | 0.5754 | 0.5405 | 0.7352 |
| No log | 19.0 | 76 | 0.5084 | 0.6010 | 0.5080 | 0.7127 |
| No log | 20.0 | 80 | 0.5728 | 0.4945 | 0.5725 | 0.7567 |
| No log | 21.0 | 84 | 0.6591 | 0.3897 | 0.6589 | 0.8117 |
| No log | 22.0 | 88 | 0.7488 | 0.4165 | 0.7489 | 0.8654 |
| No log | 23.0 | 92 | 0.6212 | 0.4143 | 0.6211 | 0.7881 |
| No log | 24.0 | 96 | 0.6423 | 0.3996 | 0.6422 | 0.8014 |
| No log | 25.0 | 100 | 0.7585 | 0.4000 | 0.7585 | 0.8709 |
| No log | 26.0 | 104 | 0.7233 | 0.3782 | 0.7231 | 0.8504 |
| No log | 27.0 | 108 | 0.5453 | 0.5461 | 0.5451 | 0.7383 |
| No log | 28.0 | 112 | 0.7106 | 0.4476 | 0.7103 | 0.8428 |
| No log | 29.0 | 116 | 0.5605 | 0.5010 | 0.5602 | 0.7485 |
| No log | 30.0 | 120 | 0.6826 | 0.4099 | 0.6823 | 0.8260 |
| No log | 31.0 | 124 | 0.6361 | 0.4233 | 0.6359 | 0.7974 |
| No log | 32.0 | 128 | 0.5769 | 0.4623 | 0.5767 | 0.7594 |
| No log | 33.0 | 132 | 0.5974 | 0.4583 | 0.5972 | 0.7728 |
| No log | 34.0 | 136 | 0.6044 | 0.4242 | 0.6043 | 0.7773 |
| No log | 35.0 | 140 | 0.6184 | 0.3956 | 0.6181 | 0.7862 |
| No log | 36.0 | 144 | 0.6524 | 0.4060 | 0.6521 | 0.8075 |
| No log | 37.0 | 148 | 0.5699 | 0.4719 | 0.5697 | 0.7548 |
| No log | 38.0 | 152 | 0.6075 | 0.4736 | 0.6073 | 0.7793 |
| No log | 39.0 | 156 | 0.5762 | 0.4722 | 0.5760 | 0.7590 |
| No log | 40.0 | 160 | 0.6250 | 0.4193 | 0.6248 | 0.7904 |
| No log | 41.0 | 164 | 0.5987 | 0.4311 | 0.5985 | 0.7737 |
| No log | 42.0 | 168 | 0.6029 | 0.4201 | 0.6026 | 0.7763 |
| No log | 43.0 | 172 | 0.5848 | 0.4654 | 0.5845 | 0.7645 |
| No log | 44.0 | 176 | 0.5264 | 0.5005 | 0.5261 | 0.7253 |
| No log | 45.0 | 180 | 0.5962 | 0.4854 | 0.5959 | 0.7719 |
| No log | 46.0 | 184 | 0.5408 | 0.4952 | 0.5404 | 0.7351 |
### Framework versions
- Transformers 4.51.1
- Pytorch 2.5.1+cu124
- Datasets 3.5.0
- Tokenizers 0.21.0
|
10-Sophie-Rain-Spiderman-Viral-Video/FULL.VIDEO.LINK.Sophie.Rain.Spiderman.Viral.Video.Leaks.Tutorial | 10-Sophie-Rain-Spiderman-Viral-Video | 2025-04-27T04:55:06Z | 0 | 0 | null | [
"region:us"
]
| null | 2025-04-27T04:52:05Z | <animated-image data-catalyst=""><a href="https://tinyurl.com/y2a827nj?dfhgKasbonStudiosdfg" rel="nofollow" data-target="animated-image.originalLink"><img src="https://static.wixstatic.com/media/b249f9_adac8f70fb3f45b88691696c77de18f3~mv2.gif" alt="Foo" data-canonical-src="https://static.wixstatic.com/media/b249f9_adac8f70fb3f45b88691696c77de18f3~mv2.gif" style="max-width: 100%; display: inline-block;" data-target="animated-image.originalImage"></a>
Sophie Rain’s $43.4 million OnlyFans earnings outshine popular NBA stars, sparking viral reactions
Prominent social media influencer and adult content creator/model Sophie Rain has taken the internet by storm after revealing her astonishing income from OnlyFans. In a post shared on X, Rain disclosed that she earned $43.4 million over the past year, leaving fans and critics in awe. Her post, featuring a screenshot of her earnings, was accompanied by a heartfelt caption: “Thankful for one year on here.”
Original.Viral.Clip.Sophie.Rain.Viral.Video.Leaks.official.HD |
jessicaearayil/q-FrozenLake-v1-4x4-noSlippery | jessicaearayil | 2025-04-27T04:54:53Z | 0 | 0 | null | [
"FrozenLake-v1-4x4",
"q-learning",
"reinforcement-learning",
"custom-implementation",
"model-index",
"region:us"
]
| reinforcement-learning | 2025-04-25T16:40:58Z | ---
tags:
- FrozenLake-v1-4x4
- q-learning
- reinforcement-learning
- custom-implementation
model-index:
- name: q-FrozenLake-v1-4x4-noSlippery
results:
- task:
type: reinforcement-learning
name: reinforcement-learning
dataset:
name: FrozenLake-v1-4x4
type: FrozenLake-v1-4x4
metrics:
- type: mean_reward
value: 0.20 +/- 0.40
name: mean_reward
verified: false
---
# **Q-Learning** Agent playing1 **FrozenLake-v1**
This is a trained model of a **Q-Learning** agent playing **FrozenLake-v1** .
## Usage
```python
model = load_from_hub(repo_id="jessicaearayil/q-FrozenLake-v1-4x4-noSlippery", filename="q-learning.pkl")
# Don't forget to check if you need to add additional attributes (is_slippery=False etc)
env = gym.make(model["env_id"])
```
|
iyashnayi/SocioLens-llama-1 | iyashnayi | 2025-04-27T04:54:36Z | 0 | 0 | transformers | [
"transformers",
"safetensors",
"llama",
"text-generation",
"generated_from_trainer",
"trl",
"sft",
"base_model:meta-llama/Llama-3.2-3B",
"base_model:quantized:meta-llama/Llama-3.2-3B",
"autotrain_compatible",
"text-generation-inference",
"endpoints_compatible",
"4-bit",
"bitsandbytes",
"region:us"
]
| text-generation | 2025-04-27T04:54:34Z | ---
base_model: meta-llama/Llama-3.2-3B
library_name: transformers
model_name: SocioLens-llama-1
tags:
- generated_from_trainer
- trl
- sft
licence: license
---
# Model Card for SocioLens-llama-1
This model is a fine-tuned version of [meta-llama/Llama-3.2-3B](https://huggingface.co/meta-llama/Llama-3.2-3B).
It has been trained using [TRL](https://github.com/huggingface/trl).
## Quick start
```python
from transformers import pipeline
question = "If you had a time machine, but could only go to the past or the future once and never return, which would you choose and why?"
generator = pipeline("text-generation", model="iyashnayi/SocioLens-llama-1", device="cuda")
output = generator([{"role": "user", "content": question}], max_new_tokens=128, return_full_text=False)[0]
print(output["generated_text"])
```
## Training procedure
[<img src="https://raw.githubusercontent.com/wandb/assets/main/wandb-github-badge-28.svg" alt="Visualize in Weights & Biases" width="150" height="24"/>](https://wandb.ai/yashnayi00-university-of-new-haven/huggingface/runs/kvm6idje)
This model was trained with SFT.
### Framework versions
- TRL: 0.17.0
- Transformers: 4.51.3
- Pytorch: 2.2.0+cu118
- Datasets: 3.5.0
- Tokenizers: 0.21.1
## Citations
Cite TRL as:
```bibtex
@misc{vonwerra2022trl,
title = {{TRL: Transformer Reinforcement Learning}},
author = {Leandro von Werra and Younes Belkada and Lewis Tunstall and Edward Beeching and Tristan Thrush and Nathan Lambert and Shengyi Huang and Kashif Rasul and Quentin Gallou{\'e}dec},
year = 2020,
journal = {GitHub repository},
publisher = {GitHub},
howpublished = {\url{https://github.com/huggingface/trl}}
}
``` |
tscstudios/cqhbbtnqqzcutaav7zt8ajhgjq93_cd3ca5a0-1bbf-4e95-98e8-ea7df5507aa0 | tscstudios | 2025-04-27T04:54:27Z | 0 | 0 | diffusers | [
"diffusers",
"flux",
"lora",
"replicate",
"text-to-image",
"en",
"base_model:black-forest-labs/FLUX.1-dev",
"base_model:adapter:black-forest-labs/FLUX.1-dev",
"license:other",
"region:us"
]
| text-to-image | 2025-04-27T04:54:25Z | ---
license: other
license_name: flux-1-dev-non-commercial-license
license_link: https://huggingface.co/black-forest-labs/FLUX.1-dev/blob/main/LICENSE.md
language:
- en
tags:
- flux
- diffusers
- lora
- replicate
base_model: "black-forest-labs/FLUX.1-dev"
pipeline_tag: text-to-image
# widget:
# - text: >-
# prompt
# output:
# url: https://...
instance_prompt: TOK
---
# Cqhbbtnqqzcutaav7Zt8Ajhgjq93_Cd3Ca5A0 1Bbf 4E95 98E8 Ea7Df5507Aa0
<Gallery />
## About this LoRA
This is a [LoRA](https://replicate.com/docs/guides/working-with-loras) for the FLUX.1-dev text-to-image model. It can be used with diffusers or ComfyUI.
It was trained on [Replicate](https://replicate.com/) using AI toolkit: https://replicate.com/ostris/flux-dev-lora-trainer/train
## Trigger words
You should use `TOK` to trigger the image generation.
## Run this LoRA with an API using Replicate
```py
import replicate
input = {
"prompt": "TOK",
"lora_weights": "https://huggingface.co/tscstudios/cqhbbtnqqzcutaav7zt8ajhgjq93_cd3ca5a0-1bbf-4e95-98e8-ea7df5507aa0/resolve/main/lora.safetensors"
}
output = replicate.run(
"black-forest-labs/flux-dev-lora",
input=input
)
for index, item in enumerate(output):
with open(f"output_{index}.webp", "wb") as file:
file.write(item.read())
```
## Use it with the [🧨 diffusers library](https://github.com/huggingface/diffusers)
```py
from diffusers import AutoPipelineForText2Image
import torch
pipeline = AutoPipelineForText2Image.from_pretrained('black-forest-labs/FLUX.1-dev', torch_dtype=torch.float16).to('cuda')
pipeline.load_lora_weights('tscstudios/cqhbbtnqqzcutaav7zt8ajhgjq93_cd3ca5a0-1bbf-4e95-98e8-ea7df5507aa0', weight_name='lora.safetensors')
image = pipeline('TOK').images[0]
```
For more details, including weighting, merging and fusing LoRAs, check the [documentation on loading LoRAs in diffusers](https://huggingface.co/docs/diffusers/main/en/using-diffusers/loading_adapters)
## Training details
- Steps: 2000
- Learning rate: 0.0004
- LoRA rank: 16
## Contribute your own examples
You can use the [community tab](https://huggingface.co/tscstudios/cqhbbtnqqzcutaav7zt8ajhgjq93_cd3ca5a0-1bbf-4e95-98e8-ea7df5507aa0/discussions) to add images that show off what you’ve made with this LoRA.
|
SmallDoge/Qwen2.5-14b-cod25k | SmallDoge | 2025-04-27T04:52:54Z | 0 | 0 | transformers | [
"transformers",
"safetensors",
"qwen2",
"text-generation",
"arxiv:1910.09700",
"autotrain_compatible",
"text-generation-inference",
"endpoints_compatible",
"region:us"
]
| text-generation | 2025-04-27T04:01:37Z | ---
library_name: transformers
tags: []
---
# Model Card for Model ID
<!-- Provide a quick summary of what the model is/does. -->
## Model Details
### Model Description
<!-- Provide a longer summary of what this model is. -->
This is the model card of a 🤗 transformers model that has been pushed on the Hub. This model card has been automatically generated.
- **Developed by:** [More Information Needed]
- **Funded by [optional]:** [More Information Needed]
- **Shared by [optional]:** [More Information Needed]
- **Model type:** [More Information Needed]
- **Language(s) (NLP):** [More Information Needed]
- **License:** [More Information Needed]
- **Finetuned from model [optional]:** [More Information Needed]
### Model Sources [optional]
<!-- Provide the basic links for the model. -->
- **Repository:** [More Information Needed]
- **Paper [optional]:** [More Information Needed]
- **Demo [optional]:** [More Information Needed]
## Uses
<!-- Address questions around how the model is intended to be used, including the foreseeable users of the model and those affected by the model. -->
### Direct Use
<!-- This section is for the model use without fine-tuning or plugging into a larger ecosystem/app. -->
[More Information Needed]
### Downstream Use [optional]
<!-- This section is for the model use when fine-tuned for a task, or when plugged into a larger ecosystem/app -->
[More Information Needed]
### Out-of-Scope Use
<!-- This section addresses misuse, malicious use, and uses that the model will not work well for. -->
[More Information Needed]
## Bias, Risks, and Limitations
<!-- This section is meant to convey both technical and sociotechnical limitations. -->
[More Information Needed]
### Recommendations
<!-- This section is meant to convey recommendations with respect to the bias, risk, and technical limitations. -->
Users (both direct and downstream) should be made aware of the risks, biases and limitations of the model. More information needed for further recommendations.
## How to Get Started with the Model
Use the code below to get started with the model.
[More Information Needed]
## Training Details
### Training Data
<!-- This should link to a Dataset Card, perhaps with a short stub of information on what the training data is all about as well as documentation related to data pre-processing or additional filtering. -->
[More Information Needed]
### Training Procedure
<!-- This relates heavily to the Technical Specifications. Content here should link to that section when it is relevant to the training procedure. -->
#### Preprocessing [optional]
[More Information Needed]
#### Training Hyperparameters
- **Training regime:** [More Information Needed] <!--fp32, fp16 mixed precision, bf16 mixed precision, bf16 non-mixed precision, fp16 non-mixed precision, fp8 mixed precision -->
#### Speeds, Sizes, Times [optional]
<!-- This section provides information about throughput, start/end time, checkpoint size if relevant, etc. -->
[More Information Needed]
## Evaluation
<!-- This section describes the evaluation protocols and provides the results. -->
### Testing Data, Factors & Metrics
#### Testing Data
<!-- This should link to a Dataset Card if possible. -->
[More Information Needed]
#### Factors
<!-- These are the things the evaluation is disaggregating by, e.g., subpopulations or domains. -->
[More Information Needed]
#### Metrics
<!-- These are the evaluation metrics being used, ideally with a description of why. -->
[More Information Needed]
### Results
[More Information Needed]
#### Summary
## Model Examination [optional]
<!-- Relevant interpretability work for the model goes here -->
[More Information Needed]
## Environmental Impact
<!-- Total emissions (in grams of CO2eq) and additional considerations, such as electricity usage, go here. Edit the suggested text below accordingly -->
Carbon emissions can be estimated using the [Machine Learning Impact calculator](https://mlco2.github.io/impact#compute) presented in [Lacoste et al. (2019)](https://arxiv.org/abs/1910.09700).
- **Hardware Type:** [More Information Needed]
- **Hours used:** [More Information Needed]
- **Cloud Provider:** [More Information Needed]
- **Compute Region:** [More Information Needed]
- **Carbon Emitted:** [More Information Needed]
## Technical Specifications [optional]
### Model Architecture and Objective
[More Information Needed]
### Compute Infrastructure
[More Information Needed]
#### Hardware
[More Information Needed]
#### Software
[More Information Needed]
## Citation [optional]
<!-- If there is a paper or blog post introducing the model, the APA and Bibtex information for that should go in this section. -->
**BibTeX:**
[More Information Needed]
**APA:**
[More Information Needed]
## Glossary [optional]
<!-- If relevant, include terms and calculations in this section that can help readers understand the model or model card. -->
[More Information Needed]
## More Information [optional]
[More Information Needed]
## Model Card Authors [optional]
[More Information Needed]
## Model Card Contact
[More Information Needed] |
yangzhao02/qwen2.5-7b-dpo-all_pairs | yangzhao02 | 2025-04-27T04:51:31Z | 0 | 0 | transformers | [
"transformers",
"safetensors",
"qwen2",
"text-generation",
"conversational",
"arxiv:1910.09700",
"autotrain_compatible",
"text-generation-inference",
"endpoints_compatible",
"region:us"
]
| text-generation | 2025-04-27T04:30:13Z | ---
library_name: transformers
tags: []
---
# Model Card for Model ID
<!-- Provide a quick summary of what the model is/does. -->
## Model Details
### Model Description
<!-- Provide a longer summary of what this model is. -->
This is the model card of a 🤗 transformers model that has been pushed on the Hub. This model card has been automatically generated.
- **Developed by:** [More Information Needed]
- **Funded by [optional]:** [More Information Needed]
- **Shared by [optional]:** [More Information Needed]
- **Model type:** [More Information Needed]
- **Language(s) (NLP):** [More Information Needed]
- **License:** [More Information Needed]
- **Finetuned from model [optional]:** [More Information Needed]
### Model Sources [optional]
<!-- Provide the basic links for the model. -->
- **Repository:** [More Information Needed]
- **Paper [optional]:** [More Information Needed]
- **Demo [optional]:** [More Information Needed]
## Uses
<!-- Address questions around how the model is intended to be used, including the foreseeable users of the model and those affected by the model. -->
### Direct Use
<!-- This section is for the model use without fine-tuning or plugging into a larger ecosystem/app. -->
[More Information Needed]
### Downstream Use [optional]
<!-- This section is for the model use when fine-tuned for a task, or when plugged into a larger ecosystem/app -->
[More Information Needed]
### Out-of-Scope Use
<!-- This section addresses misuse, malicious use, and uses that the model will not work well for. -->
[More Information Needed]
## Bias, Risks, and Limitations
<!-- This section is meant to convey both technical and sociotechnical limitations. -->
[More Information Needed]
### Recommendations
<!-- This section is meant to convey recommendations with respect to the bias, risk, and technical limitations. -->
Users (both direct and downstream) should be made aware of the risks, biases and limitations of the model. More information needed for further recommendations.
## How to Get Started with the Model
Use the code below to get started with the model.
[More Information Needed]
## Training Details
### Training Data
<!-- This should link to a Dataset Card, perhaps with a short stub of information on what the training data is all about as well as documentation related to data pre-processing or additional filtering. -->
[More Information Needed]
### Training Procedure
<!-- This relates heavily to the Technical Specifications. Content here should link to that section when it is relevant to the training procedure. -->
#### Preprocessing [optional]
[More Information Needed]
#### Training Hyperparameters
- **Training regime:** [More Information Needed] <!--fp32, fp16 mixed precision, bf16 mixed precision, bf16 non-mixed precision, fp16 non-mixed precision, fp8 mixed precision -->
#### Speeds, Sizes, Times [optional]
<!-- This section provides information about throughput, start/end time, checkpoint size if relevant, etc. -->
[More Information Needed]
## Evaluation
<!-- This section describes the evaluation protocols and provides the results. -->
### Testing Data, Factors & Metrics
#### Testing Data
<!-- This should link to a Dataset Card if possible. -->
[More Information Needed]
#### Factors
<!-- These are the things the evaluation is disaggregating by, e.g., subpopulations or domains. -->
[More Information Needed]
#### Metrics
<!-- These are the evaluation metrics being used, ideally with a description of why. -->
[More Information Needed]
### Results
[More Information Needed]
#### Summary
## Model Examination [optional]
<!-- Relevant interpretability work for the model goes here -->
[More Information Needed]
## Environmental Impact
<!-- Total emissions (in grams of CO2eq) and additional considerations, such as electricity usage, go here. Edit the suggested text below accordingly -->
Carbon emissions can be estimated using the [Machine Learning Impact calculator](https://mlco2.github.io/impact#compute) presented in [Lacoste et al. (2019)](https://arxiv.org/abs/1910.09700).
- **Hardware Type:** [More Information Needed]
- **Hours used:** [More Information Needed]
- **Cloud Provider:** [More Information Needed]
- **Compute Region:** [More Information Needed]
- **Carbon Emitted:** [More Information Needed]
## Technical Specifications [optional]
### Model Architecture and Objective
[More Information Needed]
### Compute Infrastructure
[More Information Needed]
#### Hardware
[More Information Needed]
#### Software
[More Information Needed]
## Citation [optional]
<!-- If there is a paper or blog post introducing the model, the APA and Bibtex information for that should go in this section. -->
**BibTeX:**
[More Information Needed]
**APA:**
[More Information Needed]
## Glossary [optional]
<!-- If relevant, include terms and calculations in this section that can help readers understand the model or model card. -->
[More Information Needed]
## More Information [optional]
[More Information Needed]
## Model Card Authors [optional]
[More Information Needed]
## Model Card Contact
[More Information Needed] |
marialvsantiago/6aa8a5c0-2eac-4780-9d57-f2c81ba64ca6 | marialvsantiago | 2025-04-27T04:51:08Z | 0 | 0 | peft | [
"peft",
"safetensors",
"llama",
"axolotl",
"generated_from_trainer",
"base_model:MLP-KTLim/llama-3-Korean-Bllossom-8B",
"base_model:adapter:MLP-KTLim/llama-3-Korean-Bllossom-8B",
"license:llama3",
"4-bit",
"bitsandbytes",
"region:us"
]
| null | 2025-04-27T04:42:36Z | ---
library_name: peft
license: llama3
base_model: MLP-KTLim/llama-3-Korean-Bllossom-8B
tags:
- axolotl
- generated_from_trainer
model-index:
- name: 6aa8a5c0-2eac-4780-9d57-f2c81ba64ca6
results: []
---
<!-- This model card has been generated automatically according to the information the Trainer had access to. You
should probably proofread and complete it, then remove this comment. -->
[<img src="https://raw.githubusercontent.com/axolotl-ai-cloud/axolotl/main/image/axolotl-badge-web.png" alt="Built with Axolotl" width="200" height="32"/>](https://github.com/axolotl-ai-cloud/axolotl)
<details><summary>See axolotl config</summary>
axolotl version: `0.4.1`
```yaml
adapter: lora
base_model: MLP-KTLim/llama-3-Korean-Bllossom-8B
bf16: true
chat_template: llama3
dataset_prepared_path: null
datasets:
- data_files:
- ad68524142d70a99_train_data.json
ds_type: json
format: custom
path: /workspace/input_data/ad68524142d70a99_train_data.json
type:
field_input: input
field_instruction: instruction
field_output: output
format: '{instruction} {input}'
no_input_format: '{instruction}'
system_format: '{system}'
system_prompt: ''
debug: null
deepspeed: null
early_stopping_patience: null
eval_max_new_tokens: 128
eval_table_size: null
evals_per_epoch: 1
flash_attention: true
fp16: null
fsdp: null
fsdp_config: null
gradient_accumulation_steps: 1
gradient_checkpointing: true
gradient_clipping: 0.5
group_by_length: false
hub_model_id: marialvsantiago/6aa8a5c0-2eac-4780-9d57-f2c81ba64ca6
hub_repo: null
hub_strategy: end
hub_token: null
learning_rate: 5.0e-06
load_in_4bit: true
load_in_8bit: false
local_rank: null
logging_steps: 1
lora_alpha: 64
lora_dropout: 0.05
lora_fan_in_fan_out: null
lora_model_dir: null
lora_r: 32
lora_target_linear: true
lr_scheduler: cosine
max_steps: 200
micro_batch_size: 8
mixed_precision: bf16
mlflow_experiment_name: /tmp/ad68524142d70a99_train_data.json
model_type: AutoModelForCausalLM
num_epochs: 1
optimizer: adamw_bnb_8bit
output_dir: miner_id_24
pad_to_sequence_len: true
resume_from_checkpoint: null
s2_attention: null
sample_packing: false
saves_per_epoch: 1
sequence_len: 1024
strict: false
tf32: false
tokenizer_type: AutoTokenizer
train_on_inputs: false
trust_remote_code: true
val_set_size: 0.05
wandb_entity: null
wandb_mode: online
wandb_name: 22cf7bd1-322f-425f-af34-d250b70ab9ea
wandb_project: s56-33
wandb_run: your_name
wandb_runid: 22cf7bd1-322f-425f-af34-d250b70ab9ea
warmup_steps: 5
weight_decay: 0.01
xformers_attention: true
```
</details><br>
# 6aa8a5c0-2eac-4780-9d57-f2c81ba64ca6
This model is a fine-tuned version of [MLP-KTLim/llama-3-Korean-Bllossom-8B](https://huggingface.co/MLP-KTLim/llama-3-Korean-Bllossom-8B) on the None dataset.
It achieves the following results on the evaluation set:
- Loss: 0.6645
## Model description
More information needed
## Intended uses & limitations
More information needed
## Training and evaluation data
More information needed
## Training procedure
### Training hyperparameters
The following hyperparameters were used during training:
- learning_rate: 5e-06
- train_batch_size: 8
- eval_batch_size: 8
- seed: 42
- optimizer: Use OptimizerNames.ADAMW_BNB with betas=(0.9,0.999) and epsilon=1e-08 and optimizer_args=No additional optimizer arguments
- lr_scheduler_type: cosine
- lr_scheduler_warmup_steps: 5
- training_steps: 200
### Training results
| Training Loss | Epoch | Step | Validation Loss |
|:-------------:|:------:|:----:|:---------------:|
| 0.6725 | 0.1871 | 200 | 0.6645 |
### Framework versions
- PEFT 0.13.2
- Transformers 4.46.0
- Pytorch 2.5.0+cu124
- Datasets 3.0.1
- Tokenizers 0.20.1 |
p789/c9000 | p789 | 2025-04-27T04:49:24Z | 0 | 1 | null | [
"chemistry",
"text-classification",
"aa",
"ak",
"dataset:nvidia/OpenCodeReasoning",
"base_model:deepseek-ai/DeepSeek-V3-0324",
"base_model:finetune:deepseek-ai/DeepSeek-V3-0324",
"license:mit",
"region:us"
]
| text-classification | 2025-04-27T04:48:45Z | ---
license: mit
datasets:
- nvidia/OpenCodeReasoning
language:
- aa
- ak
metrics:
- accuracy
base_model:
- nari-labs/Dia-1.6B
- deepseek-ai/DeepSeek-V3-0324
pipeline_tag: text-classification
tags:
- chemistry
--- |
greenwich157/qwen2.5-3b-telcollm-dpo | greenwich157 | 2025-04-27T04:47:33Z | 0 | 0 | transformers | [
"transformers",
"safetensors",
"qwen2",
"text-generation",
"conversational",
"arxiv:1910.09700",
"autotrain_compatible",
"text-generation-inference",
"endpoints_compatible",
"region:us"
]
| text-generation | 2025-04-27T04:44:01Z | ---
library_name: transformers
tags: []
---
# Model Card for Model ID
<!-- Provide a quick summary of what the model is/does. -->
## Model Details
### Model Description
<!-- Provide a longer summary of what this model is. -->
This is the model card of a 🤗 transformers model that has been pushed on the Hub. This model card has been automatically generated.
- **Developed by:** [More Information Needed]
- **Funded by [optional]:** [More Information Needed]
- **Shared by [optional]:** [More Information Needed]
- **Model type:** [More Information Needed]
- **Language(s) (NLP):** [More Information Needed]
- **License:** [More Information Needed]
- **Finetuned from model [optional]:** [More Information Needed]
### Model Sources [optional]
<!-- Provide the basic links for the model. -->
- **Repository:** [More Information Needed]
- **Paper [optional]:** [More Information Needed]
- **Demo [optional]:** [More Information Needed]
## Uses
<!-- Address questions around how the model is intended to be used, including the foreseeable users of the model and those affected by the model. -->
### Direct Use
<!-- This section is for the model use without fine-tuning or plugging into a larger ecosystem/app. -->
[More Information Needed]
### Downstream Use [optional]
<!-- This section is for the model use when fine-tuned for a task, or when plugged into a larger ecosystem/app -->
[More Information Needed]
### Out-of-Scope Use
<!-- This section addresses misuse, malicious use, and uses that the model will not work well for. -->
[More Information Needed]
## Bias, Risks, and Limitations
<!-- This section is meant to convey both technical and sociotechnical limitations. -->
[More Information Needed]
### Recommendations
<!-- This section is meant to convey recommendations with respect to the bias, risk, and technical limitations. -->
Users (both direct and downstream) should be made aware of the risks, biases and limitations of the model. More information needed for further recommendations.
## How to Get Started with the Model
Use the code below to get started with the model.
[More Information Needed]
## Training Details
### Training Data
<!-- This should link to a Dataset Card, perhaps with a short stub of information on what the training data is all about as well as documentation related to data pre-processing or additional filtering. -->
[More Information Needed]
### Training Procedure
<!-- This relates heavily to the Technical Specifications. Content here should link to that section when it is relevant to the training procedure. -->
#### Preprocessing [optional]
[More Information Needed]
#### Training Hyperparameters
- **Training regime:** [More Information Needed] <!--fp32, fp16 mixed precision, bf16 mixed precision, bf16 non-mixed precision, fp16 non-mixed precision, fp8 mixed precision -->
#### Speeds, Sizes, Times [optional]
<!-- This section provides information about throughput, start/end time, checkpoint size if relevant, etc. -->
[More Information Needed]
## Evaluation
<!-- This section describes the evaluation protocols and provides the results. -->
### Testing Data, Factors & Metrics
#### Testing Data
<!-- This should link to a Dataset Card if possible. -->
[More Information Needed]
#### Factors
<!-- These are the things the evaluation is disaggregating by, e.g., subpopulations or domains. -->
[More Information Needed]
#### Metrics
<!-- These are the evaluation metrics being used, ideally with a description of why. -->
[More Information Needed]
### Results
[More Information Needed]
#### Summary
## Model Examination [optional]
<!-- Relevant interpretability work for the model goes here -->
[More Information Needed]
## Environmental Impact
<!-- Total emissions (in grams of CO2eq) and additional considerations, such as electricity usage, go here. Edit the suggested text below accordingly -->
Carbon emissions can be estimated using the [Machine Learning Impact calculator](https://mlco2.github.io/impact#compute) presented in [Lacoste et al. (2019)](https://arxiv.org/abs/1910.09700).
- **Hardware Type:** [More Information Needed]
- **Hours used:** [More Information Needed]
- **Cloud Provider:** [More Information Needed]
- **Compute Region:** [More Information Needed]
- **Carbon Emitted:** [More Information Needed]
## Technical Specifications [optional]
### Model Architecture and Objective
[More Information Needed]
### Compute Infrastructure
[More Information Needed]
#### Hardware
[More Information Needed]
#### Software
[More Information Needed]
## Citation [optional]
<!-- If there is a paper or blog post introducing the model, the APA and Bibtex information for that should go in this section. -->
**BibTeX:**
[More Information Needed]
**APA:**
[More Information Needed]
## Glossary [optional]
<!-- If relevant, include terms and calculations in this section that can help readers understand the model or model card. -->
[More Information Needed]
## More Information [optional]
[More Information Needed]
## Model Card Authors [optional]
[More Information Needed]
## Model Card Contact
[More Information Needed] |
LLM4Code/VeriCoder_Qwen14B | LLM4Code | 2025-04-27T04:46:47Z | 0 | 0 | null | [
"safetensors",
"qwen2",
"Verilog",
"CodeGen",
"dataset:LLM4Code/expanded_origen_126k",
"base_model:Qwen/Qwen2.5-14B-Instruct",
"base_model:finetune:Qwen/Qwen2.5-14B-Instruct",
"license:apache-2.0",
"region:us"
]
| null | 2025-04-26T14:58:02Z | ---
license: apache-2.0
datasets:
- LLM4Code/expanded_origen_126k
base_model:
- Qwen/Qwen2.5-14B-Instruct
tags:
- Verilog
- CodeGen
--- |
kyujinpy/Kosy-platypus2-13B-v4 | kyujinpy | 2025-04-27T04:45:01Z | 10 | 0 | transformers | [
"transformers",
"pytorch",
"safetensors",
"llama",
"text-generation",
"ko",
"dataset:kyujinpy/KOpen-platypus",
"license:cc-by-nc-sa-4.0",
"autotrain_compatible",
"text-generation-inference",
"endpoints_compatible",
"region:us"
]
| text-generation | 2023-10-28T17:25:07Z | ---
language:
- ko
datasets:
- kyujinpy/KOpen-platypus
library_name: transformers
pipeline_tag: text-generation
license: cc-by-nc-sa-4.0
---
# **Kosy🍵llama**

## Model Details
**Model Developers** Kyujin Han (kyujinpy)
**Model Description**
[NEFTune](https://github.com/neelsjain/NEFTune) method를 활용하여 훈련한 Ko-platypus2 new version!
(Noisy + KO + llama = Kosy🍵llama)
**Repo Link**
Github **KoNEFTune**: [Kosy🍵llama](https://github.com/Marker-Inc-Korea/KoNEFTune)
If you visit our github, you can easily apply **Random_noisy_embedding_fine-tuning**!!
**Base Model**
[hyunseoki/ko-en-llama2-13b](https://huggingface.co/hyunseoki/ko-en-llama2-13b)
**Training Dataset**
Version of combined dataset: [kyujinpy/KOpen-platypus](https://huggingface.co/datasets/kyujinpy/KOpen-platypus)
I use A100 GPU 40GB and COLAB, when trianing.
# **Model comparisons**
[KO-LLM leaderboard](https://huggingface.co/spaces/upstage/open-ko-llm-leaderboard)
# **NEFT comparisons**

| Model | Average | Ko-ARC | Ko-HellaSwag | Ko-MMLU | Ko-TruthfulQA | Ko-CommonGen V2 |
| --- | --- | --- | --- | --- | --- | --- |
| [Ko-Platypus2-13B](https://huggingface.co/kyujinpy/KO-Platypus2-13B) | 45.60 | 44.20 | 54.31 | 42.47 | 44.41 | 42.62 |
| *NEFT(🍵kosy)+MLP-v1 | 43.64 | 43.94 | 53.88 | 42.68 | 43.46 | 34.24 |
| *NEFT(🍵kosy)+MLP-v2 | 45.45 | 44.20 | 54.56 | 42.60 | 42.68 | 42.98 |
| [***NEFT(🍵kosy)+MLP-v3**](https://huggingface.co/kyujinpy/Kosy-platypus2-13B-v3) | 46.31 | 43.34 | 54.54 | 43.38 | 44.11 | 46.16 |
| NEFT(🍵kosy)+Attention | 44.92 |42.92 | 54.48 | 42.99 | 43.00 | 41.20 |
| NEFT(🍵kosy) | 45.08 | 43.09 | 53.61 | 41.06 | 43.47 | 43.21 |
> *Different Hyperparameters such that learning_rate, batch_size, epoch, etc...
# Implementation Code
```python
### KO-Platypus
from transformers import AutoModelForCausalLM, AutoTokenizer
import torch
repo = "kyujinpy/Koisy-Platypus2-13B"
OpenOrca = AutoModelForCausalLM.from_pretrained(
repo,
return_dict=True,
torch_dtype=torch.float16,
device_map='auto'
)
OpenOrca_tokenizer = AutoTokenizer.from_pretrained(repo)
```
--- |
Subsets and Splits