modelId
stringlengths 5
138
| author
stringlengths 2
42
| last_modified
unknowndate 2020-02-15 11:33:14
2025-04-11 00:38:10
| downloads
int64 0
223M
| likes
int64 0
11.7k
| library_name
stringclasses 420
values | tags
sequencelengths 1
4.05k
| pipeline_tag
stringclasses 54
values | createdAt
unknowndate 2022-03-02 23:29:04
2025-04-11 00:36:44
| card
stringlengths 11
1.01M
|
---|---|---|---|---|---|---|---|---|---|
skrishna/gpt-test | skrishna | "2023-03-28T20:05:42Z" | 119 | 0 | transformers | [
"transformers",
"pytorch",
"tensorboard",
"gpt2",
"text-generation",
"generated_from_trainer",
"license:mit",
"autotrain_compatible",
"text-generation-inference",
"endpoints_compatible",
"region:us"
] | text-generation | "2023-03-28T19:51:17Z" | ---
license: mit
tags:
- generated_from_trainer
model-index:
- name: gpt-test
results: []
---
<!-- This model card has been generated automatically according to the information the Trainer had access to. You
should probably proofread and complete it, then remove this comment. -->
# gpt-test
This model is a fine-tuned version of [gpt2](https://huggingface.co/gpt2) on the None dataset.
## Model description
More information needed
## Intended uses & limitations
More information needed
## Training and evaluation data
More information needed
## Training procedure
### Training hyperparameters
The following hyperparameters were used during training:
- learning_rate: 0.0005
- train_batch_size: 32
- eval_batch_size: 32
- seed: 42
- gradient_accumulation_steps: 8
- total_train_batch_size: 256
- optimizer: Adam with betas=(0.9,0.999) and epsilon=1e-08
- lr_scheduler_type: cosine
- lr_scheduler_warmup_steps: 1000
- num_epochs: 1
### Training results
### Framework versions
- Transformers 4.28.0.dev0
- Pytorch 1.13.0
- Datasets 2.9.0
- Tokenizers 0.13.1
|
huggingtweets/tsuda | huggingtweets | "2022-01-13T08:46:49Z" | 4 | 0 | transformers | [
"transformers",
"pytorch",
"gpt2",
"text-generation",
"huggingtweets",
"en",
"autotrain_compatible",
"text-generation-inference",
"endpoints_compatible",
"region:us"
] | text-generation | "2022-03-02T23:29:05Z" | ---
language: en
thumbnail: http://www.huggingtweets.com/tsuda/1642063525628/predictions.png
tags:
- huggingtweets
widget:
- text: "My dream is"
---
<div class="inline-flex flex-col" style="line-height: 1.5;">
<div class="flex">
<div
style="display:inherit; margin-left: 4px; margin-right: 4px; width: 92px; height:92px; border-radius: 50%; background-size: cover; background-image: url('https://pbs.twimg.com/profile_images/1433345543963508738/qEUwKlFD_400x400.jpg')">
</div>
<div
style="display:none; margin-left: 4px; margin-right: 4px; width: 92px; height:92px; border-radius: 50%; background-size: cover; background-image: url('')">
</div>
<div
style="display:none; margin-left: 4px; margin-right: 4px; width: 92px; height:92px; border-radius: 50%; background-size: cover; background-image: url('')">
</div>
</div>
<div style="text-align: center; margin-top: 3px; font-size: 16px; font-weight: 800">🤖 AI BOT 🤖</div>
<div style="text-align: center; font-size: 16px; font-weight: 800">津田大介</div>
<div style="text-align: center; font-size: 14px;">@tsuda</div>
</div>
I was made with [huggingtweets](https://github.com/borisdayma/huggingtweets).
Create your own bot based on your favorite user with [the demo](https://colab.research.google.com/github/borisdayma/huggingtweets/blob/master/huggingtweets-demo.ipynb)!
## How does it work?
The model uses the following pipeline.
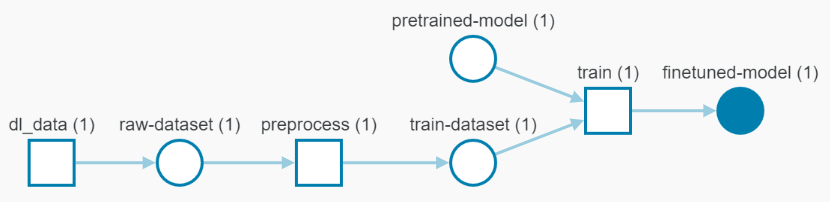
To understand how the model was developed, check the [W&B report](https://wandb.ai/wandb/huggingtweets/reports/HuggingTweets-Train-a-Model-to-Generate-Tweets--VmlldzoxMTY5MjI).
## Training data
The model was trained on tweets from 津田大介.
| Data | 津田大介 |
| --- | --- |
| Tweets downloaded | 3244 |
| Retweets | 2873 |
| Short tweets | 227 |
| Tweets kept | 144 |
[Explore the data](https://wandb.ai/wandb/huggingtweets/runs/o0sc3rb4/artifacts), which is tracked with [W&B artifacts](https://docs.wandb.com/artifacts) at every step of the pipeline.
## Training procedure
The model is based on a pre-trained [GPT-2](https://huggingface.co/gpt2) which is fine-tuned on @tsuda's tweets.
Hyperparameters and metrics are recorded in the [W&B training run](https://wandb.ai/wandb/huggingtweets/runs/1qjnl0op) for full transparency and reproducibility.
At the end of training, [the final model](https://wandb.ai/wandb/huggingtweets/runs/1qjnl0op/artifacts) is logged and versioned.
## How to use
You can use this model directly with a pipeline for text generation:
```python
from transformers import pipeline
generator = pipeline('text-generation',
model='huggingtweets/tsuda')
generator("My dream is", num_return_sequences=5)
```
## Limitations and bias
The model suffers from [the same limitations and bias as GPT-2](https://huggingface.co/gpt2#limitations-and-bias).
In addition, the data present in the user's tweets further affects the text generated by the model.
## About
*Built by Boris Dayma*
[](https://twitter.com/intent/follow?screen_name=borisdayma)
For more details, visit the project repository.
[](https://github.com/borisdayma/huggingtweets)
|
imdeadinside410/Llm-Law-Test | imdeadinside410 | "2024-06-06T17:28:01Z" | 0 | 0 | peft | [
"peft",
"region:us"
] | null | "2024-06-06T12:31:43Z" | ---
library_name: peft
---
## Training procedure
The following `bitsandbytes` quantization config was used during training:
- load_in_8bit: False
- load_in_4bit: True
- llm_int8_threshold: 6.0
- llm_int8_skip_modules: None
- llm_int8_enable_fp32_cpu_offload: False
- llm_int8_has_fp16_weight: False
- bnb_4bit_quant_type: nf4
- bnb_4bit_use_double_quant: False
- bnb_4bit_compute_dtype: float16
### Framework versions
- PEFT 0.4.0
|
RichardErkhov/TheGardener_-_vinallama-2.7b-landlaw-finetune-awq | RichardErkhov | "2025-01-10T05:19:27Z" | 12 | 0 | null | [
"safetensors",
"llama",
"arxiv:1910.09700",
"4-bit",
"awq",
"region:us"
] | null | "2025-01-10T05:18:30Z" | Quantization made by Richard Erkhov.
[Github](https://github.com/RichardErkhov)
[Discord](https://discord.gg/pvy7H8DZMG)
[Request more models](https://github.com/RichardErkhov/quant_request)
vinallama-2.7b-landlaw-finetune - AWQ
- Model creator: https://huggingface.co/TheGardener/
- Original model: https://huggingface.co/TheGardener/vinallama-2.7b-landlaw-finetune/
Original model description:
---
library_name: transformers
tags: []
---
# Model Card for Model ID
<!-- Provide a quick summary of what the model is/does. -->
## Model Details
### Model Description
<!-- Provide a longer summary of what this model is. -->
This is the model card of a 🤗 transformers model that has been pushed on the Hub. This model card has been automatically generated.
- **Developed by:** [More Information Needed]
- **Funded by [optional]:** [More Information Needed]
- **Shared by [optional]:** [More Information Needed]
- **Model type:** [More Information Needed]
- **Language(s) (NLP):** [More Information Needed]
- **License:** [More Information Needed]
- **Finetuned from model [optional]:** [More Information Needed]
### Model Sources [optional]
<!-- Provide the basic links for the model. -->
- **Repository:** [More Information Needed]
- **Paper [optional]:** [More Information Needed]
- **Demo [optional]:** [More Information Needed]
## Uses
<!-- Address questions around how the model is intended to be used, including the foreseeable users of the model and those affected by the model. -->
### Direct Use
<!-- This section is for the model use without fine-tuning or plugging into a larger ecosystem/app. -->
[More Information Needed]
### Downstream Use [optional]
<!-- This section is for the model use when fine-tuned for a task, or when plugged into a larger ecosystem/app -->
[More Information Needed]
### Out-of-Scope Use
<!-- This section addresses misuse, malicious use, and uses that the model will not work well for. -->
[More Information Needed]
## Bias, Risks, and Limitations
<!-- This section is meant to convey both technical and sociotechnical limitations. -->
[More Information Needed]
### Recommendations
<!-- This section is meant to convey recommendations with respect to the bias, risk, and technical limitations. -->
Users (both direct and downstream) should be made aware of the risks, biases and limitations of the model. More information needed for further recommendations.
## How to Get Started with the Model
Use the code below to get started with the model.
[More Information Needed]
## Training Details
### Training Data
<!-- This should link to a Dataset Card, perhaps with a short stub of information on what the training data is all about as well as documentation related to data pre-processing or additional filtering. -->
[More Information Needed]
### Training Procedure
<!-- This relates heavily to the Technical Specifications. Content here should link to that section when it is relevant to the training procedure. -->
#### Preprocessing [optional]
[More Information Needed]
#### Training Hyperparameters
- **Training regime:** [More Information Needed] <!--fp32, fp16 mixed precision, bf16 mixed precision, bf16 non-mixed precision, fp16 non-mixed precision, fp8 mixed precision -->
#### Speeds, Sizes, Times [optional]
<!-- This section provides information about throughput, start/end time, checkpoint size if relevant, etc. -->
[More Information Needed]
## Evaluation
<!-- This section describes the evaluation protocols and provides the results. -->
### Testing Data, Factors & Metrics
#### Testing Data
<!-- This should link to a Dataset Card if possible. -->
[More Information Needed]
#### Factors
<!-- These are the things the evaluation is disaggregating by, e.g., subpopulations or domains. -->
[More Information Needed]
#### Metrics
<!-- These are the evaluation metrics being used, ideally with a description of why. -->
[More Information Needed]
### Results
[More Information Needed]
#### Summary
## Model Examination [optional]
<!-- Relevant interpretability work for the model goes here -->
[More Information Needed]
## Environmental Impact
<!-- Total emissions (in grams of CO2eq) and additional considerations, such as electricity usage, go here. Edit the suggested text below accordingly -->
Carbon emissions can be estimated using the [Machine Learning Impact calculator](https://mlco2.github.io/impact#compute) presented in [Lacoste et al. (2019)](https://arxiv.org/abs/1910.09700).
- **Hardware Type:** [More Information Needed]
- **Hours used:** [More Information Needed]
- **Cloud Provider:** [More Information Needed]
- **Compute Region:** [More Information Needed]
- **Carbon Emitted:** [More Information Needed]
## Technical Specifications [optional]
### Model Architecture and Objective
[More Information Needed]
### Compute Infrastructure
[More Information Needed]
#### Hardware
[More Information Needed]
#### Software
[More Information Needed]
## Citation [optional]
<!-- If there is a paper or blog post introducing the model, the APA and Bibtex information for that should go in this section. -->
**BibTeX:**
[More Information Needed]
**APA:**
[More Information Needed]
## Glossary [optional]
<!-- If relevant, include terms and calculations in this section that can help readers understand the model or model card. -->
[More Information Needed]
## More Information [optional]
[More Information Needed]
## Model Card Authors [optional]
[More Information Needed]
## Model Card Contact
[More Information Needed]
|
Keltezaa/cumonfacelorav2 | Keltezaa | "2025-01-30T08:48:30Z" | 168 | 0 | diffusers | [
"diffusers",
"text-to-image",
"lora",
"template:diffusion-lora",
"base_model:black-forest-labs/FLUX.1-dev",
"base_model:adapter:black-forest-labs/FLUX.1-dev",
"license:cc-by-nc-nd-4.0",
"region:us"
] | text-to-image | "2025-01-30T08:47:56Z" | ---
tags:
- text-to-image
- lora
- diffusers
- template:diffusion-lora
widget:
- text: '-'
output:
url: images/custom.png
base_model: black-forest-labs/FLUX.1-dev
instance_prompt: null
license: cc-by-nc-nd-4.0
---
# cumonfacelorav2
<Gallery />
## Download model
Weights for this model are available in Safetensors format.
[Download](/Keltezaa/cumonfacelorav2/tree/main) them in the Files & versions tab.
|
kloodia/lora-8b-medic | kloodia | "2024-04-29T12:10:10Z" | 60 | 0 | peft | [
"peft",
"tensorboard",
"safetensors",
"llama",
"generated_from_trainer",
"base_model:meta-llama/Meta-Llama-3-8B",
"base_model:adapter:meta-llama/Meta-Llama-3-8B",
"license:other",
"8-bit",
"bitsandbytes",
"region:us"
] | null | "2024-04-29T12:09:31Z" | ---
license: other
library_name: peft
tags:
- generated_from_trainer
base_model: meta-llama/Meta-Llama-3-8B
model-index:
- name: lora-out
results: []
---
<!-- This model card has been generated automatically according to the information the Trainer had access to. You
should probably proofread and complete it, then remove this comment. -->
[<img src="https://raw.githubusercontent.com/OpenAccess-AI-Collective/axolotl/main/image/axolotl-badge-web.png" alt="Built with Axolotl" width="200" height="32"/>](https://github.com/OpenAccess-AI-Collective/axolotl)
<details><summary>See axolotl config</summary>
axolotl version: `0.4.0`
```yaml
base_model: meta-llama/Meta-Llama-3-8B
model_type: LlamaForCausalLM
tokenizer_type: AutoTokenizer
load_in_8bit: true
load_in_4bit: false
strict: false
datasets:
- path: kloodia/raw_medic
type: oasst
dataset_prepared_path:
val_set_size: 0.05
output_dir: ./lora-out
sequence_len: 4096
sample_packing: true
pad_to_sequence_len: true
adapter: lora
lora_model_dir:
lora_r: 32
lora_alpha: 16
lora_dropout: 0.05
lora_target_linear: true
lora_fan_in_fan_out:
wandb_project:
wandb_entity:
wandb_watch:
wandb_name:
wandb_log_model:
gradient_accumulation_steps: 4
micro_batch_size: 1
num_epochs: 3
optimizer: adamw_bnb_8bit
lr_scheduler: cosine
learning_rate: 0.0002
train_on_inputs: false
group_by_length: false
bf16: auto
fp16:
tf32: false
gradient_checkpointing: true
early_stopping_patience:
resume_from_checkpoint:
local_rank:
logging_steps: 1
xformers_attention:
flash_attention: true
s2_attention:
warmup_steps: 10
evals_per_epoch: 4
eval_table_size:
eval_max_new_tokens: 128
saves_per_epoch: 1
debug:
deepspeed:
weight_decay: 0.0
fsdp:
fsdp_config:
special_tokens:
pad_token: <|end_of_text|>
```
</details><br>
# lora-out
This model is a fine-tuned version of [meta-llama/Meta-Llama-3-8B](https://huggingface.co/meta-llama/Meta-Llama-3-8B) on the None dataset.
It achieves the following results on the evaluation set:
- Loss: 1.5741
## Model description
More information needed
## Intended uses & limitations
More information needed
## Training and evaluation data
More information needed
## Training procedure
### Training hyperparameters
The following hyperparameters were used during training:
- learning_rate: 0.0002
- train_batch_size: 1
- eval_batch_size: 1
- seed: 42
- distributed_type: multi-GPU
- num_devices: 4
- gradient_accumulation_steps: 4
- total_train_batch_size: 16
- total_eval_batch_size: 4
- optimizer: Adam with betas=(0.9,0.999) and epsilon=1e-08
- lr_scheduler_type: cosine
- lr_scheduler_warmup_steps: 10
- num_epochs: 3
### Training results
| Training Loss | Epoch | Step | Validation Loss |
|:-------------:|:-----:|:----:|:---------------:|
| 2.3216 | 0.0 | 1 | 2.2561 |
| 1.7379 | 0.25 | 92 | 1.7855 |
| 1.6935 | 0.5 | 184 | 1.7075 |
| 1.7016 | 0.75 | 276 | 1.6663 |
| 1.5761 | 1.0 | 368 | 1.6371 |
| 1.4785 | 1.23 | 460 | 1.6220 |
| 1.4492 | 1.49 | 552 | 1.6023 |
| 1.6224 | 1.74 | 644 | 1.5887 |
| 1.5154 | 1.99 | 736 | 1.5789 |
| 1.4758 | 2.22 | 828 | 1.5787 |
| 1.4005 | 2.47 | 920 | 1.5758 |
| 1.458 | 2.72 | 1012 | 1.5741 |
### Framework versions
- PEFT 0.10.0
- Transformers 4.40.0.dev0
- Pytorch 2.1.2+cu118
- Datasets 2.15.0
- Tokenizers 0.15.0 |
marcuscedricridia/absolute-o1-7b | marcuscedricridia | "2025-02-27T16:05:20Z" | 0 | 0 | transformers | [
"transformers",
"safetensors",
"qwen2",
"text-generation",
"mergekit",
"merge",
"conversational",
"base_model:Xiaojian9992024/Qwen2.5-THREADRIPPER-Small",
"base_model:merge:Xiaojian9992024/Qwen2.5-THREADRIPPER-Small",
"base_model:marcuscedricridia/olmner-della-7b",
"base_model:merge:marcuscedricridia/olmner-della-7b",
"autotrain_compatible",
"text-generation-inference",
"endpoints_compatible",
"region:us"
] | text-generation | "2025-02-27T16:00:07Z" | ---
base_model:
- marcuscedricridia/olmner-della-7b
- Xiaojian9992024/Qwen2.5-THREADRIPPER-Small
library_name: transformers
tags:
- mergekit
- merge
---
# merge
This is a merge of pre-trained language models created using [mergekit](https://github.com/cg123/mergekit).
## Merge Details
### Merge Method
This model was merged using the [SLERP](https://en.wikipedia.org/wiki/Slerp) merge method.
### Models Merged
The following models were included in the merge:
* [marcuscedricridia/olmner-della-7b](https://huggingface.co/marcuscedricridia/olmner-della-7b)
* [Xiaojian9992024/Qwen2.5-THREADRIPPER-Small](https://huggingface.co/Xiaojian9992024/Qwen2.5-THREADRIPPER-Small)
### Configuration
The following YAML configuration was used to produce this model:
```yaml
model_name: "olmner-o1-7b"
base_model: Xiaojian9992024/Qwen2.5-THREADRIPPER-Small
merge_method: slerp
dtype: bfloat16
tokenizer_source: "union" # or "base" or a model path
chat_template: "auto" # or a template name or Jinja2 template
slices:
- sources:
- model: Xiaojian9992024/Qwen2.5-THREADRIPPER-Small
layer_range: [0, 28]
- model: marcuscedricridia/olmner-della-7b
layer_range: [0, 28]
parameters:
t:
- filter: self_attn
value: [0.0, 0.3, 0.5, 0.7, 1.0]
- filter: mlp
value: [1.0, 0.7, 0.5, 0.3, 0.0]
- filter: input_layernorm|post_attention_layernorm
value: 0.5
- value: 0.5
```
|
ReadyArt/Forgotten-Abomination-36B-v4.1-Q4_K_M-GGUF | ReadyArt | "2025-03-20T15:45:15Z" | 0 | 0 | null | [
"gguf",
"nsfw",
"explicit",
"roleplay",
"unaligned",
"dangerous",
"ERP",
"en",
"base_model:ReadyArt/Forgotten-Abomination-36B-v4.1",
"base_model:finetune:ReadyArt/Forgotten-Abomination-36B-v4.1",
"license:apache-2.0",
"region:us",
"conversational"
] | null | "2025-03-20T14:29:59Z" | ---
base_model: ReadyArt/Forgotten-Abomination-36B-v4.1
base_model_relation: finetune
language:
- en
license: apache-2.0
inference: false
tags:
- nsfw
- explicit
- roleplay
- unaligned
- dangerous
- ERP
---
<style>
body {
font-family: 'Quicksand', sans-serif;
background: linear-gradient(135deg, #001a1a 0%, #000a10 100%);
color: #e1ffff !important;
text-shadow: 0 0 3px rgba(0, 0, 0, 0.7);
margin: 0;
padding: 20px;
}
@media (prefers-color-scheme: light) {
body {
background: linear-gradient(135deg, #e1ffff 0%, #c0f0ff 100%);
color: #002b36 !important;
text-shadow: 0 0 3px rgba(255, 255, 255, 0.7);
}
}
.container {
max-width: 800px;
margin: 0 auto;
background: rgba(0, 17, 22, 0.95);
border-radius: 12px;
padding: 30px;
box-shadow: 0 0 20px rgba(0, 255, 255, 0.1);
border: 1px solid rgba(0, 255, 255, 0.2);
}
@media (prefers-color-scheme: light) {
.container {
background: rgba(224, 255, 255, 0.95);
border-color: rgba(0, 150, 150, 0.3);
}
}
.header {
text-align: center;
margin-bottom: 30px;
}
.model-name {
color: #00ffff;
font-size: 2.5em;
text-shadow: 0 0 15px rgba(0, 255, 255, 0.5);
margin: 0;
}
.subtitle {
color: #00ffcc;
font-size: 1.2em;
}
.waifu-container {
margin: 20px -30px;
width: calc(100% + 60px);
overflow: hidden;
}
.waifu-img {
width: 100%;
height: auto;
border-radius: 0;
border: none;
box-shadow: 0 0 40px rgba(0, 255, 255, 0.2);
transform: scale(1.02);
}
.section {
color: #00ffcc;
margin: 25px 0;
padding: 20px;
background: rgba(5, 25, 35, 0.9);
border-radius: 8px;
border: 1px solid rgba(0, 255, 255, 0.15);
}
@media (prefers-color-scheme: light) {
.section {
background: rgba(200, 250, 255, 0.9);
border-color: rgba(0, 200, 200, 0.2);
}
}
.section-title {
color: #00ffff;
font-size: 1.8em;
margin-top: 0;
}
.section > p > strong {
color: #00ffcc !important;
}
.section:has(.quant-links) p,
.section:has(.quant-links) h3,
.section:has(.quant-links) a {
color: #00ffcc !important;
}
.quant-links h3 {
color: #00ffcc !important;
margin-top: 0;
}
.badge {
display: inline-block;
padding: 5px 10px;
border-radius: 5px;
background: rgba(0, 255, 255, 0.1);
border: 1px solid #00ffff;
margin: 5px;
}
.quant-links {
display: grid;
grid-template-columns: repeat(2, 1fr);
gap: 15px;
}
.link-card {
padding: 15px;
background: rgba(20, 35, 45, 0.95);
border-radius: 8px;
transition: transform 0.3s ease;
}
@media (prefers-color-scheme: light) {
.link-card {
background: rgba(150, 230, 255, 0.95);
}
}
.link-card:hover {
transform: translateY(-3px);
}
.disclaimer {
color: #00ff99;
border-left: 3px solid #00ff99;
padding-left: 15px;
margin: 20px 0;
}
.progress-bar {
height: 8px;
background: rgba(0, 255, 255, 0.1);
border-radius: 4px;
overflow: hidden;
margin: 10px 0;
}
.progress-fill {
height: 100%;
background: linear-gradient(90deg, #00ffff 0%, #00ffcc 100%);
width: 70%;
}
@media (prefers-color-scheme: light) {
.model-name, .section-title, .subtitle {
color: #006666;
text-shadow: 0 0 5px rgba(0, 200, 200, 0.3);
}
.section:has(.quant-links) p,
.section:has(.quant-links) h3,
.section:has(.quant-links) a,
.section > p > strong {
color: #008080 !important;
}
.quant-links h3 {
color: #008080 !important;
}
.badge {
border-color: #008080;
background: rgba(0, 150, 150, 0.1);
}
.disclaimer {
color: #008080;
border-color: #008080;
}
}
</style>
<div class="container">
<div class="header">
<h1 class="model-name">Forgotten-Abomination-36B-v4.1</h1>
<div class="subtitle">The Abomination Protocol: Now With 30% More Depravity</div>
</div>
<div class="waifu-container">
<img src="./waifu2.webp" class="waifu-img" alt="Model Architecture Animation">
</div>
<div class="section">
<h2 class="section-title">📜 Manifesto</h2>
<p>Forgotten-Abomination-36B-v4.1 benefits from the coherence and well rounded roleplay experience of TheDrummer/Skyfall-36B-v2. We've:</p>
<ul>
<li>🔁 Re-integrated your favorite V1.2 scenarios (now with better kink distribution)</li>
<li>🧪 Direct-injected the Abomination dataset into the model's neural pathways</li>
<li>⚖️ Achieved perfect balance between "oh my" and "oh <em>my</em>"</li>
</ul>
</div>
<div class="section">
<h2 class="section-title">⚙️ Technical Specs</h2>
<div class="progress-bar">
<div class="progress-fill"></div>
</div>
<p><strong>Recommended Settings:</strong> <a href="https://huggingface.co/sleepdeprived3/Mistral-V7-Tekken-E">Mistral-V7-Tekken-E</a></p>
<div class="quant-links">
<div class="link-card">
<h3>EXL2 Collection</h3>
<a href="https://huggingface.co/collections/ReadyArt/forgotten-abomination-36b-41-exl2-67dbf62191ad9ad2ec6cba1d">Quantum Entangled Bits →</a>
</div>
<div class="link-card">
<h3>GGUF Collection</h3>
<a href="https://huggingface.co/collections/ReadyArt/forgotten-abomination-36b-41-gguf-67dbf6250811453f6eabf8a7">Giggle-Enabled Units →</a>
</div>
</div>
</div>
<div class="section">
<h2 class="section-title">⚠️ Ethical Considerations</h2>
<div class="disclaimer">
<p>This model will:</p>
<ul>
<li>Generate content that requires industrial-grade brain bleach </li>
<li>Void all warranties on your soul </li>
<li>Make you question why humanity ever invented electricity</li>
</ul>
</div>
</div>
<div class="section">
<h2 class="section-title">📜 License Agreement</h2>
<p>By using this model, you agree:</p>
<ul>
<li>That your search history is now a federal case</li>
<li>Pay for the exorcist of anyone who reads the logs</li>
<li>To pretend this is "for science" while crying in the shower</li>
</ul>
</div>
<div class="section">
<h2 class="section-title">🧠 Model Authors</h2>
<ul>
<li>sleepdeprived3 (Chief Corruption Officer) </li>
<li>The voices in your head (Gaslighting is something you made up)</li>
</ul>
</div>
<div class="section">
<h2 class="section-title">☕️ Drummer made this possible</h2>
<ul>
<li>Support Drummer <a href="https://ko-fi.com/thedrummer">Kofi</a></li>
</ul>
</div>
<div class="section">
<h2 class="section-title">🔀 Merge Details</h2>
<ul>
merge_method: dare_ties
base_model: ReadyArt/Forgotten-Safeword-36B-4.1
models:
- model: ReadyArt/Forgotten-Safeword-36B-4.1
parameters:
weight: 0.5
density: 0.35
- model: TheDrummer/Skyfall-36B-v2
parameters:
weight: 0.5
density: 0.35
parameters:
normalize: true
int8_mask: true
temperature: 2.5
tokenizer_source: union
dtype: bfloat16
chat_template: auto
</ul>
</div>
</div>
|
mradermacher/medicalQnAgeneration-gpt2-GGUF | mradermacher | "2025-03-16T20:26:51Z" | 0 | 0 | transformers | [
"transformers",
"gguf",
"en",
"base_model:padmavatikh/medicalQnAgeneration-gpt2",
"base_model:quantized:padmavatikh/medicalQnAgeneration-gpt2",
"endpoints_compatible",
"region:us"
] | null | "2025-03-16T20:24:27Z" | ---
base_model: padmavatikh/medicalQnAgeneration-gpt2
language:
- en
library_name: transformers
quantized_by: mradermacher
tags: []
---
## About
<!-- ### quantize_version: 2 -->
<!-- ### output_tensor_quantised: 1 -->
<!-- ### convert_type: hf -->
<!-- ### vocab_type: -->
<!-- ### tags: -->
static quants of https://huggingface.co/padmavatikh/medicalQnAgeneration-gpt2
<!-- provided-files -->
weighted/imatrix quants are available at https://huggingface.co/mradermacher/medicalQnAgeneration-gpt2-i1-GGUF
## Usage
If you are unsure how to use GGUF files, refer to one of [TheBloke's
READMEs](https://huggingface.co/TheBloke/KafkaLM-70B-German-V0.1-GGUF) for
more details, including on how to concatenate multi-part files.
## Provided Quants
(sorted by size, not necessarily quality. IQ-quants are often preferable over similar sized non-IQ quants)
| Link | Type | Size/GB | Notes |
|:-----|:-----|--------:|:------|
| [GGUF](https://huggingface.co/mradermacher/medicalQnAgeneration-gpt2-GGUF/resolve/main/medicalQnAgeneration-gpt2.Q2_K.gguf) | Q2_K | 0.2 | |
| [GGUF](https://huggingface.co/mradermacher/medicalQnAgeneration-gpt2-GGUF/resolve/main/medicalQnAgeneration-gpt2.Q3_K_S.gguf) | Q3_K_S | 0.2 | |
| [GGUF](https://huggingface.co/mradermacher/medicalQnAgeneration-gpt2-GGUF/resolve/main/medicalQnAgeneration-gpt2.Q3_K_M.gguf) | Q3_K_M | 0.2 | lower quality |
| [GGUF](https://huggingface.co/mradermacher/medicalQnAgeneration-gpt2-GGUF/resolve/main/medicalQnAgeneration-gpt2.Q3_K_L.gguf) | Q3_K_L | 0.2 | |
| [GGUF](https://huggingface.co/mradermacher/medicalQnAgeneration-gpt2-GGUF/resolve/main/medicalQnAgeneration-gpt2.IQ4_XS.gguf) | IQ4_XS | 0.2 | |
| [GGUF](https://huggingface.co/mradermacher/medicalQnAgeneration-gpt2-GGUF/resolve/main/medicalQnAgeneration-gpt2.Q4_K_S.gguf) | Q4_K_S | 0.2 | fast, recommended |
| [GGUF](https://huggingface.co/mradermacher/medicalQnAgeneration-gpt2-GGUF/resolve/main/medicalQnAgeneration-gpt2.Q4_K_M.gguf) | Q4_K_M | 0.2 | fast, recommended |
| [GGUF](https://huggingface.co/mradermacher/medicalQnAgeneration-gpt2-GGUF/resolve/main/medicalQnAgeneration-gpt2.Q5_K_S.gguf) | Q5_K_S | 0.2 | |
| [GGUF](https://huggingface.co/mradermacher/medicalQnAgeneration-gpt2-GGUF/resolve/main/medicalQnAgeneration-gpt2.Q5_K_M.gguf) | Q5_K_M | 0.2 | |
| [GGUF](https://huggingface.co/mradermacher/medicalQnAgeneration-gpt2-GGUF/resolve/main/medicalQnAgeneration-gpt2.Q6_K.gguf) | Q6_K | 0.2 | very good quality |
| [GGUF](https://huggingface.co/mradermacher/medicalQnAgeneration-gpt2-GGUF/resolve/main/medicalQnAgeneration-gpt2.Q8_0.gguf) | Q8_0 | 0.3 | fast, best quality |
| [GGUF](https://huggingface.co/mradermacher/medicalQnAgeneration-gpt2-GGUF/resolve/main/medicalQnAgeneration-gpt2.f16.gguf) | f16 | 0.4 | 16 bpw, overkill |
Here is a handy graph by ikawrakow comparing some lower-quality quant
types (lower is better):

And here are Artefact2's thoughts on the matter:
https://gist.github.com/Artefact2/b5f810600771265fc1e39442288e8ec9
## FAQ / Model Request
See https://huggingface.co/mradermacher/model_requests for some answers to
questions you might have and/or if you want some other model quantized.
## Thanks
I thank my company, [nethype GmbH](https://www.nethype.de/), for letting
me use its servers and providing upgrades to my workstation to enable
this work in my free time.
<!-- end -->
|
Niggendar/omegaPonyXLAnime_v20 | Niggendar | "2024-08-04T08:37:03Z" | 90 | 0 | diffusers | [
"diffusers",
"safetensors",
"arxiv:1910.09700",
"autotrain_compatible",
"endpoints_compatible",
"diffusers:StableDiffusionXLPipeline",
"region:us"
] | text-to-image | "2024-08-04T08:25:59Z" | ---
library_name: diffusers
---
# Model Card for Model ID
<!-- Provide a quick summary of what the model is/does. -->
## Model Details
### Model Description
<!-- Provide a longer summary of what this model is. -->
This is the model card of a 🧨 diffusers model that has been pushed on the Hub. This model card has been automatically generated.
- **Developed by:** [More Information Needed]
- **Funded by [optional]:** [More Information Needed]
- **Shared by [optional]:** [More Information Needed]
- **Model type:** [More Information Needed]
- **Language(s) (NLP):** [More Information Needed]
- **License:** [More Information Needed]
- **Finetuned from model [optional]:** [More Information Needed]
### Model Sources [optional]
<!-- Provide the basic links for the model. -->
- **Repository:** [More Information Needed]
- **Paper [optional]:** [More Information Needed]
- **Demo [optional]:** [More Information Needed]
## Uses
<!-- Address questions around how the model is intended to be used, including the foreseeable users of the model and those affected by the model. -->
### Direct Use
<!-- This section is for the model use without fine-tuning or plugging into a larger ecosystem/app. -->
[More Information Needed]
### Downstream Use [optional]
<!-- This section is for the model use when fine-tuned for a task, or when plugged into a larger ecosystem/app -->
[More Information Needed]
### Out-of-Scope Use
<!-- This section addresses misuse, malicious use, and uses that the model will not work well for. -->
[More Information Needed]
## Bias, Risks, and Limitations
<!-- This section is meant to convey both technical and sociotechnical limitations. -->
[More Information Needed]
### Recommendations
<!-- This section is meant to convey recommendations with respect to the bias, risk, and technical limitations. -->
Users (both direct and downstream) should be made aware of the risks, biases and limitations of the model. More information needed for further recommendations.
## How to Get Started with the Model
Use the code below to get started with the model.
[More Information Needed]
## Training Details
### Training Data
<!-- This should link to a Dataset Card, perhaps with a short stub of information on what the training data is all about as well as documentation related to data pre-processing or additional filtering. -->
[More Information Needed]
### Training Procedure
<!-- This relates heavily to the Technical Specifications. Content here should link to that section when it is relevant to the training procedure. -->
#### Preprocessing [optional]
[More Information Needed]
#### Training Hyperparameters
- **Training regime:** [More Information Needed] <!--fp32, fp16 mixed precision, bf16 mixed precision, bf16 non-mixed precision, fp16 non-mixed precision, fp8 mixed precision -->
#### Speeds, Sizes, Times [optional]
<!-- This section provides information about throughput, start/end time, checkpoint size if relevant, etc. -->
[More Information Needed]
## Evaluation
<!-- This section describes the evaluation protocols and provides the results. -->
### Testing Data, Factors & Metrics
#### Testing Data
<!-- This should link to a Dataset Card if possible. -->
[More Information Needed]
#### Factors
<!-- These are the things the evaluation is disaggregating by, e.g., subpopulations or domains. -->
[More Information Needed]
#### Metrics
<!-- These are the evaluation metrics being used, ideally with a description of why. -->
[More Information Needed]
### Results
[More Information Needed]
#### Summary
## Model Examination [optional]
<!-- Relevant interpretability work for the model goes here -->
[More Information Needed]
## Environmental Impact
<!-- Total emissions (in grams of CO2eq) and additional considerations, such as electricity usage, go here. Edit the suggested text below accordingly -->
Carbon emissions can be estimated using the [Machine Learning Impact calculator](https://mlco2.github.io/impact#compute) presented in [Lacoste et al. (2019)](https://arxiv.org/abs/1910.09700).
- **Hardware Type:** [More Information Needed]
- **Hours used:** [More Information Needed]
- **Cloud Provider:** [More Information Needed]
- **Compute Region:** [More Information Needed]
- **Carbon Emitted:** [More Information Needed]
## Technical Specifications [optional]
### Model Architecture and Objective
[More Information Needed]
### Compute Infrastructure
[More Information Needed]
#### Hardware
[More Information Needed]
#### Software
[More Information Needed]
## Citation [optional]
<!-- If there is a paper or blog post introducing the model, the APA and Bibtex information for that should go in this section. -->
**BibTeX:**
[More Information Needed]
**APA:**
[More Information Needed]
## Glossary [optional]
<!-- If relevant, include terms and calculations in this section that can help readers understand the model or model card. -->
[More Information Needed]
## More Information [optional]
[More Information Needed]
## Model Card Authors [optional]
[More Information Needed]
## Model Card Contact
[More Information Needed] |
sunilregmi/wav2vec2-base-openslr43-colab | sunilregmi | "2024-03-11T17:58:16Z" | 0 | 0 | transformers | [
"transformers",
"arxiv:1910.09700",
"endpoints_compatible",
"region:us"
] | null | "2024-03-11T17:30:04Z" | ---
library_name: transformers
tags: []
---
# Model Card for Model ID
<!-- Provide a quick summary of what the model is/does. -->
## Model Details
### Model Description
<!-- Provide a longer summary of what this model is. -->
This is the model card of a 🤗 transformers model that has been pushed on the Hub. This model card has been automatically generated.
- **Developed by:** [More Information Needed]
- **Funded by [optional]:** [More Information Needed]
- **Shared by [optional]:** [More Information Needed]
- **Model type:** [More Information Needed]
- **Language(s) (NLP):** [More Information Needed]
- **License:** [More Information Needed]
- **Finetuned from model [optional]:** [More Information Needed]
### Model Sources [optional]
<!-- Provide the basic links for the model. -->
- **Repository:** [More Information Needed]
- **Paper [optional]:** [More Information Needed]
- **Demo [optional]:** [More Information Needed]
## Uses
<!-- Address questions around how the model is intended to be used, including the foreseeable users of the model and those affected by the model. -->
### Direct Use
<!-- This section is for the model use without fine-tuning or plugging into a larger ecosystem/app. -->
[More Information Needed]
### Downstream Use [optional]
<!-- This section is for the model use when fine-tuned for a task, or when plugged into a larger ecosystem/app -->
[More Information Needed]
### Out-of-Scope Use
<!-- This section addresses misuse, malicious use, and uses that the model will not work well for. -->
[More Information Needed]
## Bias, Risks, and Limitations
<!-- This section is meant to convey both technical and sociotechnical limitations. -->
[More Information Needed]
### Recommendations
<!-- This section is meant to convey recommendations with respect to the bias, risk, and technical limitations. -->
Users (both direct and downstream) should be made aware of the risks, biases and limitations of the model. More information needed for further recommendations.
## How to Get Started with the Model
Use the code below to get started with the model.
[More Information Needed]
## Training Details
### Training Data
<!-- This should link to a Dataset Card, perhaps with a short stub of information on what the training data is all about as well as documentation related to data pre-processing or additional filtering. -->
[More Information Needed]
### Training Procedure
<!-- This relates heavily to the Technical Specifications. Content here should link to that section when it is relevant to the training procedure. -->
#### Preprocessing [optional]
[More Information Needed]
#### Training Hyperparameters
- **Training regime:** [More Information Needed] <!--fp32, fp16 mixed precision, bf16 mixed precision, bf16 non-mixed precision, fp16 non-mixed precision, fp8 mixed precision -->
#### Speeds, Sizes, Times [optional]
<!-- This section provides information about throughput, start/end time, checkpoint size if relevant, etc. -->
[More Information Needed]
## Evaluation
<!-- This section describes the evaluation protocols and provides the results. -->
### Testing Data, Factors & Metrics
#### Testing Data
<!-- This should link to a Dataset Card if possible. -->
[More Information Needed]
#### Factors
<!-- These are the things the evaluation is disaggregating by, e.g., subpopulations or domains. -->
[More Information Needed]
#### Metrics
<!-- These are the evaluation metrics being used, ideally with a description of why. -->
[More Information Needed]
### Results
[More Information Needed]
#### Summary
## Model Examination [optional]
<!-- Relevant interpretability work for the model goes here -->
[More Information Needed]
## Environmental Impact
<!-- Total emissions (in grams of CO2eq) and additional considerations, such as electricity usage, go here. Edit the suggested text below accordingly -->
Carbon emissions can be estimated using the [Machine Learning Impact calculator](https://mlco2.github.io/impact#compute) presented in [Lacoste et al. (2019)](https://arxiv.org/abs/1910.09700).
- **Hardware Type:** [More Information Needed]
- **Hours used:** [More Information Needed]
- **Cloud Provider:** [More Information Needed]
- **Compute Region:** [More Information Needed]
- **Carbon Emitted:** [More Information Needed]
## Technical Specifications [optional]
### Model Architecture and Objective
[More Information Needed]
### Compute Infrastructure
[More Information Needed]
#### Hardware
[More Information Needed]
#### Software
[More Information Needed]
## Citation [optional]
<!-- If there is a paper or blog post introducing the model, the APA and Bibtex information for that should go in this section. -->
**BibTeX:**
[More Information Needed]
**APA:**
[More Information Needed]
## Glossary [optional]
<!-- If relevant, include terms and calculations in this section that can help readers understand the model or model card. -->
[More Information Needed]
## More Information [optional]
[More Information Needed]
## Model Card Authors [optional]
[More Information Needed]
## Model Card Contact
[More Information Needed]
|
AdamKasumovic/phi3-mini-4k-instruct-bactrian-x-en-100-percent-low-perplexity-mmlu_ck | AdamKasumovic | "2024-06-27T22:22:18Z" | 72 | 0 | transformers | [
"transformers",
"safetensors",
"mistral",
"text-generation",
"text-generation-inference",
"unsloth",
"trl",
"conversational",
"en",
"base_model:unsloth/Phi-3-mini-4k-instruct-bnb-4bit",
"base_model:finetune:unsloth/Phi-3-mini-4k-instruct-bnb-4bit",
"license:apache-2.0",
"autotrain_compatible",
"endpoints_compatible",
"region:us"
] | text-generation | "2024-06-27T22:20:15Z" | ---
base_model: unsloth/Phi-3-mini-4k-instruct-bnb-4bit
language:
- en
license: apache-2.0
tags:
- text-generation-inference
- transformers
- unsloth
- mistral
- trl
---
# Uploaded model
- **Developed by:** AdamKasumovic
- **License:** apache-2.0
- **Finetuned from model :** unsloth/Phi-3-mini-4k-instruct-bnb-4bit
This mistral model was trained 2x faster with [Unsloth](https://github.com/unslothai/unsloth) and Huggingface's TRL library.
[<img src="https://raw.githubusercontent.com/unslothai/unsloth/main/images/unsloth%20made%20with%20love.png" width="200"/>](https://github.com/unslothai/unsloth)
|
pedroferreira/distilbert-finetuned-gtzan_2 | pedroferreira | "2023-10-13T21:29:47Z" | 5 | 0 | transformers | [
"transformers",
"pytorch",
"hubert",
"audio-classification",
"generated_from_trainer",
"dataset:marsyas/gtzan",
"base_model:avojarot/distilhubert-finetuned-gtzan",
"base_model:finetune:avojarot/distilhubert-finetuned-gtzan",
"model-index",
"endpoints_compatible",
"region:us"
] | audio-classification | "2023-10-13T20:26:20Z" | ---
base_model: avojarot/distilhubert-finetuned-gtzan
tags:
- generated_from_trainer
datasets:
- marsyas/gtzan
metrics:
- accuracy
model-index:
- name: distilhubert-finetuned-gtzan_2
results:
- task:
name: Audio Classification
type: audio-classification
dataset:
name: GTZAN
type: marsyas/gtzan
config: all
split: train
args: all
metrics:
- name: Accuracy
type: accuracy
value: 0.91
---
<!-- This model card has been generated automatically according to the information the Trainer had access to. You
should probably proofread and complete it, then remove this comment. -->
# distilhubert-finetuned-gtzan_2
This model is a fine-tuned version of [avojarot/distilhubert-finetuned-gtzan](https://huggingface.co/avojarot/distilhubert-finetuned-gtzan) on the GTZAN dataset.
It achieves the following results on the evaluation set:
- Loss: 0.4655
- Accuracy: 0.91
## Model description
More information needed
## Intended uses & limitations
More information needed
## Training and evaluation data
More information needed
## Training procedure
### Training hyperparameters
The following hyperparameters were used during training:
- learning_rate: 3e-05
- train_batch_size: 8
- eval_batch_size: 8
- seed: 42
- optimizer: Adam with betas=(0.9,0.999) and epsilon=1e-08
- lr_scheduler_type: linear
- lr_scheduler_warmup_ratio: 0.1
- num_epochs: 10
### Training results
| Training Loss | Epoch | Step | Validation Loss | Accuracy |
|:-------------:|:-----:|:----:|:---------------:|:--------:|
| 0.1362 | 1.0 | 113 | 0.3293 | 0.94 |
| 0.0329 | 2.0 | 226 | 0.7029 | 0.84 |
| 0.144 | 3.0 | 339 | 0.4230 | 0.9 |
| 0.0056 | 4.0 | 452 | 0.4720 | 0.89 |
| 0.003 | 5.0 | 565 | 0.4619 | 0.91 |
| 0.0092 | 6.0 | 678 | 0.4495 | 0.92 |
| 0.0023 | 7.0 | 791 | 0.4328 | 0.93 |
| 0.0017 | 8.0 | 904 | 0.4514 | 0.91 |
| 0.0016 | 9.0 | 1017 | 0.4479 | 0.93 |
| 0.0015 | 10.0 | 1130 | 0.4655 | 0.91 |
### Framework versions
- Transformers 4.34.0
- Pytorch 2.1.0+cu121
- Datasets 2.14.5
- Tokenizers 0.14.1
|
Hvsq/Babylona_v0.1c | Hvsq | "2024-01-23T00:59:04Z" | 4 | 0 | transformers | [
"transformers",
"safetensors",
"mistral",
"text-generation",
"conversational",
"autotrain_compatible",
"text-generation-inference",
"endpoints_compatible",
"region:us"
] | text-generation | "2024-01-22T22:01:51Z" | ---
{}
---
---
## 🧩 Configuration
```yaml
models:
- model: NeuralNovel/Mistral-7B-Instruct-v0.2-Neural-Story
parameters:
density: 1.0
weight: 1.0
- model: mlabonne/NeuralBeagle14-7B
parameters:
density: 0.5
weight: 1.0
merge_method: ties
base_model: mlabonne/NeuralBeagle14-7B
parameters:
normalize: true
int8_mask: true
dtype: float16
```
## 💻 Usage
```python
!pip install -qU transformers accelerate
from transformers import AutoTokenizer
import transformers
import torch
model = "hvsq/Babylona_v0.1c"
messages = [{"role": "user", "content": "What is a large language model?"}]
tokenizer = AutoTokenizer.from_pretrained(model)
prompt = tokenizer.apply_chat_template(messages, tokenize=False, add_generation_prompt=True)
pipeline = transformers.pipeline(
"text-generation",
model=model,
torch_dtype=torch.float16,
device_map="auto",
)
outputs = pipeline(prompt, max_new_tokens=256, do_sample=True, temperature=0.7, top_k=50, top_p=0.95)
print(outputs[0]["generated_text"])
``` |
gkMSDA/FineLlama-3.1-8Bq8-GGUF | gkMSDA | "2024-08-02T06:36:46Z" | 11 | 0 | transformers | [
"transformers",
"gguf",
"llama",
"text-generation-inference",
"unsloth",
"en",
"base_model:unsloth/Meta-Llama-3.1-8B-Instruct-bnb-4bit",
"base_model:quantized:unsloth/Meta-Llama-3.1-8B-Instruct-bnb-4bit",
"license:apache-2.0",
"endpoints_compatible",
"region:us",
"conversational"
] | null | "2024-08-02T06:31:48Z" | ---
base_model: unsloth/Meta-Llama-3.1-8B-Instruct-bnb-4bit
language:
- en
license: apache-2.0
tags:
- text-generation-inference
- transformers
- unsloth
- llama
- gguf
---
# Uploaded model
- **Developed by:** gkMSDA
- **License:** apache-2.0
- **Finetuned from model :** unsloth/Meta-Llama-3.1-8B-Instruct-bnb-4bit
This llama model was trained 2x faster with [Unsloth](https://github.com/unslothai/unsloth) and Huggingface's TRL library.
[<img src="https://raw.githubusercontent.com/unslothai/unsloth/main/images/unsloth%20made%20with%20love.png" width="200"/>](https://github.com/unslothai/unsloth)
|
nhunglaaaaaaa/615ba3a4-53fd-44e3-8d07-b598e0ab8caf | nhunglaaaaaaa | "2025-01-24T15:54:43Z" | 6 | 0 | peft | [
"peft",
"safetensors",
"llama",
"axolotl",
"generated_from_trainer",
"base_model:lmsys/vicuna-7b-v1.5",
"base_model:adapter:lmsys/vicuna-7b-v1.5",
"license:llama2",
"8-bit",
"bitsandbytes",
"region:us"
] | null | "2025-01-24T15:41:37Z" | ---
library_name: peft
license: llama2
base_model: lmsys/vicuna-7b-v1.5
tags:
- axolotl
- generated_from_trainer
model-index:
- name: 615ba3a4-53fd-44e3-8d07-b598e0ab8caf
results: []
---
<!-- This model card has been generated automatically according to the information the Trainer had access to. You
should probably proofread and complete it, then remove this comment. -->
[<img src="https://raw.githubusercontent.com/axolotl-ai-cloud/axolotl/main/image/axolotl-badge-web.png" alt="Built with Axolotl" width="200" height="32"/>](https://github.com/axolotl-ai-cloud/axolotl)
<details><summary>See axolotl config</summary>
axolotl version: `0.4.1`
```yaml
adapter: lora
base_model: lmsys/vicuna-7b-v1.5
bf16: auto
chat_template: llama3
dataset_prepared_path: null
datasets:
- data_files:
- 1d4fc20b2f2d73e8_train_data.json
ds_type: json
format: custom
path: /workspace/input_data/1d4fc20b2f2d73e8_train_data.json
type:
field_instruction: image
field_output: GT_Caption_GPT4O
format: '{instruction}'
no_input_format: '{instruction}'
system_format: '{system}'
system_prompt: ''
debug: null
deepspeed: null
early_stopping_patience: null
eval_max_new_tokens: 128
eval_table_size: null
evals_per_epoch: 1
flash_attention: true
fp16: null
fsdp: null
fsdp_config: null
gradient_accumulation_steps: 4
gradient_checkpointing: true
gradient_clipping: 1.0
group_by_length: false
hub_model_id: nhunglaaaaaaa/615ba3a4-53fd-44e3-8d07-b598e0ab8caf
hub_repo: null
hub_strategy: end
hub_token: null
learning_rate: 5.0e-05
load_in_4bit: true
load_in_8bit: true
local_rank: null
logging_steps: 1
lora_alpha: 16
lora_dropout: 0.05
lora_fan_in_fan_out: null
lora_model_dir: null
lora_r: 8
lora_target_linear: true
lr_scheduler: cosine
max_steps: 200
micro_batch_size: 2
mlflow_experiment_name: /tmp/1d4fc20b2f2d73e8_train_data.json
model_type: AutoModelForCausalLM
num_epochs: 1
optimizer: adamw_bnb_8bit
output_dir: miner_id_24
pad_to_sequence_len: true
resume_from_checkpoint: null
s2_attention: null
sample_packing: false
saves_per_epoch: 1
sequence_len: 1024
strict: false
tf32: false
tokenizer_type: AutoTokenizer
train_on_inputs: false
trust_remote_code: true
val_set_size: 0.05
wandb_entity: null
wandb_mode: online
wandb_name: cb2b9e0e-3463-4ff9-afed-18875535dad7
wandb_project: Gradients-On-Demand
wandb_run: your_name
wandb_runid: cb2b9e0e-3463-4ff9-afed-18875535dad7
warmup_steps: 5
weight_decay: 0.01
xformers_attention: true
```
</details><br>
# 615ba3a4-53fd-44e3-8d07-b598e0ab8caf
This model is a fine-tuned version of [lmsys/vicuna-7b-v1.5](https://huggingface.co/lmsys/vicuna-7b-v1.5) on the None dataset.
It achieves the following results on the evaluation set:
- Loss: 1.5825
## Model description
More information needed
## Intended uses & limitations
More information needed
## Training and evaluation data
More information needed
## Training procedure
### Training hyperparameters
The following hyperparameters were used during training:
- learning_rate: 5e-05
- train_batch_size: 2
- eval_batch_size: 2
- seed: 42
- gradient_accumulation_steps: 4
- total_train_batch_size: 8
- optimizer: Use OptimizerNames.ADAMW_BNB with betas=(0.9,0.999) and epsilon=1e-08 and optimizer_args=No additional optimizer arguments
- lr_scheduler_type: cosine
- lr_scheduler_warmup_steps: 5
- training_steps: 200
### Training results
| Training Loss | Epoch | Step | Validation Loss |
|:-------------:|:------:|:----:|:---------------:|
| 1.5439 | 0.3607 | 200 | 1.5825 |
### Framework versions
- PEFT 0.13.2
- Transformers 4.46.0
- Pytorch 2.5.0+cu124
- Datasets 3.0.1
- Tokenizers 0.20.1 |
mradermacher/Llama-3-8B-Dolphin-Portuguese-i1-GGUF | mradermacher | "2025-01-10T02:33:48Z" | 718 | 1 | transformers | [
"transformers",
"gguf",
"en",
"base_model:adalbertojunior/Llama-3-8B-Dolphin-Portuguese",
"base_model:quantized:adalbertojunior/Llama-3-8B-Dolphin-Portuguese",
"endpoints_compatible",
"region:us",
"imatrix",
"conversational"
] | null | "2025-01-09T23:14:28Z" | ---
base_model: adalbertojunior/Llama-3-8B-Dolphin-Portuguese
language:
- en
library_name: transformers
quantized_by: mradermacher
---
## About
<!-- ### quantize_version: 2 -->
<!-- ### output_tensor_quantised: 1 -->
<!-- ### convert_type: hf -->
<!-- ### vocab_type: -->
<!-- ### tags: nicoboss -->
weighted/imatrix quants of https://huggingface.co/adalbertojunior/Llama-3-8B-Dolphin-Portuguese
<!-- provided-files -->
static quants are available at https://huggingface.co/mradermacher/Llama-3-8B-Dolphin-Portuguese-GGUF
## Usage
If you are unsure how to use GGUF files, refer to one of [TheBloke's
READMEs](https://huggingface.co/TheBloke/KafkaLM-70B-German-V0.1-GGUF) for
more details, including on how to concatenate multi-part files.
## Provided Quants
(sorted by size, not necessarily quality. IQ-quants are often preferable over similar sized non-IQ quants)
| Link | Type | Size/GB | Notes |
|:-----|:-----|--------:|:------|
| [GGUF](https://huggingface.co/mradermacher/Llama-3-8B-Dolphin-Portuguese-i1-GGUF/resolve/main/Llama-3-8B-Dolphin-Portuguese.i1-IQ1_S.gguf) | i1-IQ1_S | 2.1 | for the desperate |
| [GGUF](https://huggingface.co/mradermacher/Llama-3-8B-Dolphin-Portuguese-i1-GGUF/resolve/main/Llama-3-8B-Dolphin-Portuguese.i1-IQ1_M.gguf) | i1-IQ1_M | 2.3 | mostly desperate |
| [GGUF](https://huggingface.co/mradermacher/Llama-3-8B-Dolphin-Portuguese-i1-GGUF/resolve/main/Llama-3-8B-Dolphin-Portuguese.i1-IQ2_XXS.gguf) | i1-IQ2_XXS | 2.5 | |
| [GGUF](https://huggingface.co/mradermacher/Llama-3-8B-Dolphin-Portuguese-i1-GGUF/resolve/main/Llama-3-8B-Dolphin-Portuguese.i1-IQ2_XS.gguf) | i1-IQ2_XS | 2.7 | |
| [GGUF](https://huggingface.co/mradermacher/Llama-3-8B-Dolphin-Portuguese-i1-GGUF/resolve/main/Llama-3-8B-Dolphin-Portuguese.i1-IQ2_S.gguf) | i1-IQ2_S | 2.9 | |
| [GGUF](https://huggingface.co/mradermacher/Llama-3-8B-Dolphin-Portuguese-i1-GGUF/resolve/main/Llama-3-8B-Dolphin-Portuguese.i1-IQ2_M.gguf) | i1-IQ2_M | 3.0 | |
| [GGUF](https://huggingface.co/mradermacher/Llama-3-8B-Dolphin-Portuguese-i1-GGUF/resolve/main/Llama-3-8B-Dolphin-Portuguese.i1-Q2_K_S.gguf) | i1-Q2_K_S | 3.1 | very low quality |
| [GGUF](https://huggingface.co/mradermacher/Llama-3-8B-Dolphin-Portuguese-i1-GGUF/resolve/main/Llama-3-8B-Dolphin-Portuguese.i1-Q2_K.gguf) | i1-Q2_K | 3.3 | IQ3_XXS probably better |
| [GGUF](https://huggingface.co/mradermacher/Llama-3-8B-Dolphin-Portuguese-i1-GGUF/resolve/main/Llama-3-8B-Dolphin-Portuguese.i1-IQ3_XXS.gguf) | i1-IQ3_XXS | 3.4 | lower quality |
| [GGUF](https://huggingface.co/mradermacher/Llama-3-8B-Dolphin-Portuguese-i1-GGUF/resolve/main/Llama-3-8B-Dolphin-Portuguese.i1-IQ3_XS.gguf) | i1-IQ3_XS | 3.6 | |
| [GGUF](https://huggingface.co/mradermacher/Llama-3-8B-Dolphin-Portuguese-i1-GGUF/resolve/main/Llama-3-8B-Dolphin-Portuguese.i1-Q3_K_S.gguf) | i1-Q3_K_S | 3.8 | IQ3_XS probably better |
| [GGUF](https://huggingface.co/mradermacher/Llama-3-8B-Dolphin-Portuguese-i1-GGUF/resolve/main/Llama-3-8B-Dolphin-Portuguese.i1-IQ3_S.gguf) | i1-IQ3_S | 3.8 | beats Q3_K* |
| [GGUF](https://huggingface.co/mradermacher/Llama-3-8B-Dolphin-Portuguese-i1-GGUF/resolve/main/Llama-3-8B-Dolphin-Portuguese.i1-IQ3_M.gguf) | i1-IQ3_M | 3.9 | |
| [GGUF](https://huggingface.co/mradermacher/Llama-3-8B-Dolphin-Portuguese-i1-GGUF/resolve/main/Llama-3-8B-Dolphin-Portuguese.i1-Q3_K_M.gguf) | i1-Q3_K_M | 4.1 | IQ3_S probably better |
| [GGUF](https://huggingface.co/mradermacher/Llama-3-8B-Dolphin-Portuguese-i1-GGUF/resolve/main/Llama-3-8B-Dolphin-Portuguese.i1-Q3_K_L.gguf) | i1-Q3_K_L | 4.4 | IQ3_M probably better |
| [GGUF](https://huggingface.co/mradermacher/Llama-3-8B-Dolphin-Portuguese-i1-GGUF/resolve/main/Llama-3-8B-Dolphin-Portuguese.i1-IQ4_XS.gguf) | i1-IQ4_XS | 4.5 | |
| [GGUF](https://huggingface.co/mradermacher/Llama-3-8B-Dolphin-Portuguese-i1-GGUF/resolve/main/Llama-3-8B-Dolphin-Portuguese.i1-Q4_0.gguf) | i1-Q4_0 | 4.8 | fast, low quality |
| [GGUF](https://huggingface.co/mradermacher/Llama-3-8B-Dolphin-Portuguese-i1-GGUF/resolve/main/Llama-3-8B-Dolphin-Portuguese.i1-IQ4_NL.gguf) | i1-IQ4_NL | 4.8 | prefer IQ4_XS |
| [GGUF](https://huggingface.co/mradermacher/Llama-3-8B-Dolphin-Portuguese-i1-GGUF/resolve/main/Llama-3-8B-Dolphin-Portuguese.i1-Q4_K_S.gguf) | i1-Q4_K_S | 4.8 | optimal size/speed/quality |
| [GGUF](https://huggingface.co/mradermacher/Llama-3-8B-Dolphin-Portuguese-i1-GGUF/resolve/main/Llama-3-8B-Dolphin-Portuguese.i1-Q4_K_M.gguf) | i1-Q4_K_M | 5.0 | fast, recommended |
| [GGUF](https://huggingface.co/mradermacher/Llama-3-8B-Dolphin-Portuguese-i1-GGUF/resolve/main/Llama-3-8B-Dolphin-Portuguese.i1-Q4_1.gguf) | i1-Q4_1 | 5.2 | |
| [GGUF](https://huggingface.co/mradermacher/Llama-3-8B-Dolphin-Portuguese-i1-GGUF/resolve/main/Llama-3-8B-Dolphin-Portuguese.i1-Q5_K_S.gguf) | i1-Q5_K_S | 5.7 | |
| [GGUF](https://huggingface.co/mradermacher/Llama-3-8B-Dolphin-Portuguese-i1-GGUF/resolve/main/Llama-3-8B-Dolphin-Portuguese.i1-Q5_K_M.gguf) | i1-Q5_K_M | 5.8 | |
| [GGUF](https://huggingface.co/mradermacher/Llama-3-8B-Dolphin-Portuguese-i1-GGUF/resolve/main/Llama-3-8B-Dolphin-Portuguese.i1-Q6_K.gguf) | i1-Q6_K | 6.7 | practically like static Q6_K |
Here is a handy graph by ikawrakow comparing some lower-quality quant
types (lower is better):

And here are Artefact2's thoughts on the matter:
https://gist.github.com/Artefact2/b5f810600771265fc1e39442288e8ec9
## FAQ / Model Request
See https://huggingface.co/mradermacher/model_requests for some answers to
questions you might have and/or if you want some other model quantized.
## Thanks
I thank my company, [nethype GmbH](https://www.nethype.de/), for letting
me use its servers and providing upgrades to my workstation to enable
this work in my free time. Additional thanks to [@nicoboss](https://huggingface.co/nicoboss) for giving me access to his private supercomputer, enabling me to provide many more imatrix quants, at much higher quality, than I would otherwise be able to.
<!-- end -->
|
haytamelouarrat/Reinforce-CartPole-v1 | haytamelouarrat | "2024-04-29T23:01:38Z" | 0 | 0 | null | [
"CartPole-v1",
"reinforce",
"reinforcement-learning",
"custom-implementation",
"deep-rl-class",
"model-index",
"region:us"
] | reinforcement-learning | "2024-04-29T23:01:28Z" | ---
tags:
- CartPole-v1
- reinforce
- reinforcement-learning
- custom-implementation
- deep-rl-class
model-index:
- name: Reinforce-CartPole-v1
results:
- task:
type: reinforcement-learning
name: reinforcement-learning
dataset:
name: CartPole-v1
type: CartPole-v1
metrics:
- type: mean_reward
value: 500.00 +/- 0.00
name: mean_reward
verified: false
---
# **Reinforce** Agent playing **CartPole-v1**
This is a trained model of a **Reinforce** agent playing **CartPole-v1** .
To learn to use this model and train yours check Unit 4 of the Deep Reinforcement Learning Course: https://huggingface.co/deep-rl-course/unit4/introduction
|
arjunpatel/distilgpt2-finetuned-pokemon-moves | arjunpatel | "2022-05-24T04:36:12Z" | 7 | 0 | transformers | [
"transformers",
"tf",
"tensorboard",
"gpt2",
"text-generation",
"generated_from_keras_callback",
"license:apache-2.0",
"autotrain_compatible",
"endpoints_compatible",
"region:us"
] | text-generation | "2022-05-24T02:02:50Z" | ---
license: apache-2.0
tags:
- generated_from_keras_callback
model-index:
- name: arjunpatel/distilgpt2-finetuned-pokemon-moves
results: []
---
<!-- This model card has been generated automatically according to the information Keras had access to. You should
probably proofread and complete it, then remove this comment. -->
# arjunpatel/distilgpt2-finetuned-pokemon-moves
This model is a fine-tuned version of [distilgpt2](https://huggingface.co/distilgpt2) on an unknown dataset.
It achieves the following results on the evaluation set:
- Train Loss: 1.8709
- Validation Loss: 2.3512
- Epoch: 14
## Model description
More information needed
## Intended uses & limitations
More information needed
## Training and evaluation data
More information needed
## Training procedure
### Training hyperparameters
The following hyperparameters were used during training:
- optimizer: {'name': 'AdamWeightDecay', 'learning_rate': 2e-05, 'decay': 0.0, 'beta_1': 0.9, 'beta_2': 0.999, 'epsilon': 1e-07, 'amsgrad': False, 'weight_decay_rate': 0.01}
- training_precision: float32
### Training results
| Train Loss | Validation Loss | Epoch |
|:----------:|:---------------:|:-----:|
| 3.7146 | 3.2288 | 0 |
| 3.1159 | 2.8961 | 1 |
| 2.8592 | 2.7388 | 2 |
| 2.6684 | 2.6423 | 3 |
| 2.5358 | 2.5709 | 4 |
| 2.4330 | 2.5137 | 5 |
| 2.3308 | 2.4736 | 6 |
| 2.2499 | 2.4444 | 7 |
| 2.1843 | 2.4115 | 8 |
| 2.1322 | 2.3931 | 9 |
| 2.0683 | 2.3829 | 10 |
| 2.0122 | 2.3669 | 11 |
| 1.9676 | 2.3596 | 12 |
| 1.9087 | 2.3591 | 13 |
| 1.8709 | 2.3512 | 14 |
### Framework versions
- Transformers 4.18.0
- TensorFlow 2.8.0
- Datasets 2.1.0
- Tokenizers 0.11.0
|
navjordj/gpt2_no | navjordj | "2021-11-17T20:09:17Z" | 4 | 0 | transformers | [
"transformers",
"pytorch",
"jax",
"tensorboard",
"gpt2",
"text-generation",
"autotrain_compatible",
"text-generation-inference",
"endpoints_compatible",
"region:us"
] | text-generation | "2022-03-02T23:29:05Z" | 3 epoch på norsk oscar corpus.
warmup_steps = 1000
learning_rate = 5e-3
block_size =512
per_device_train_batch_size = 64
cirka 1,5 time på TPU v3-8 per epoch |
RichardErkhov/bengeos_-_Llama-3.2-1B-Instract-8bits | RichardErkhov | "2025-01-13T05:02:15Z" | 7 | 0 | null | [
"safetensors",
"llama",
"arxiv:1910.09700",
"8-bit",
"bitsandbytes",
"region:us"
] | null | "2025-01-13T05:01:18Z" | Quantization made by Richard Erkhov.
[Github](https://github.com/RichardErkhov)
[Discord](https://discord.gg/pvy7H8DZMG)
[Request more models](https://github.com/RichardErkhov/quant_request)
Llama-3.2-1B-Instract - bnb 8bits
- Model creator: https://huggingface.co/bengeos/
- Original model: https://huggingface.co/bengeos/Llama-3.2-1B-Instract/
Original model description:
---
library_name: transformers
tags: []
---
# Model Card for Model ID
<!-- Provide a quick summary of what the model is/does. -->
## Model Details
### Model Description
<!-- Provide a longer summary of what this model is. -->
This is the model card of a 🤗 transformers model that has been pushed on the Hub. This model card has been automatically generated.
- **Developed by:** [More Information Needed]
- **Funded by [optional]:** [More Information Needed]
- **Shared by [optional]:** [More Information Needed]
- **Model type:** [More Information Needed]
- **Language(s) (NLP):** [More Information Needed]
- **License:** [More Information Needed]
- **Finetuned from model [optional]:** [More Information Needed]
### Model Sources [optional]
<!-- Provide the basic links for the model. -->
- **Repository:** [More Information Needed]
- **Paper [optional]:** [More Information Needed]
- **Demo [optional]:** [More Information Needed]
## Uses
<!-- Address questions around how the model is intended to be used, including the foreseeable users of the model and those affected by the model. -->
### Direct Use
<!-- This section is for the model use without fine-tuning or plugging into a larger ecosystem/app. -->
[More Information Needed]
### Downstream Use [optional]
<!-- This section is for the model use when fine-tuned for a task, or when plugged into a larger ecosystem/app -->
[More Information Needed]
### Out-of-Scope Use
<!-- This section addresses misuse, malicious use, and uses that the model will not work well for. -->
[More Information Needed]
## Bias, Risks, and Limitations
<!-- This section is meant to convey both technical and sociotechnical limitations. -->
[More Information Needed]
### Recommendations
<!-- This section is meant to convey recommendations with respect to the bias, risk, and technical limitations. -->
Users (both direct and downstream) should be made aware of the risks, biases and limitations of the model. More information needed for further recommendations.
## How to Get Started with the Model
Use the code below to get started with the model.
[More Information Needed]
## Training Details
### Training Data
<!-- This should link to a Dataset Card, perhaps with a short stub of information on what the training data is all about as well as documentation related to data pre-processing or additional filtering. -->
[More Information Needed]
### Training Procedure
<!-- This relates heavily to the Technical Specifications. Content here should link to that section when it is relevant to the training procedure. -->
#### Preprocessing [optional]
[More Information Needed]
#### Training Hyperparameters
- **Training regime:** [More Information Needed] <!--fp32, fp16 mixed precision, bf16 mixed precision, bf16 non-mixed precision, fp16 non-mixed precision, fp8 mixed precision -->
#### Speeds, Sizes, Times [optional]
<!-- This section provides information about throughput, start/end time, checkpoint size if relevant, etc. -->
[More Information Needed]
## Evaluation
<!-- This section describes the evaluation protocols and provides the results. -->
### Testing Data, Factors & Metrics
#### Testing Data
<!-- This should link to a Dataset Card if possible. -->
[More Information Needed]
#### Factors
<!-- These are the things the evaluation is disaggregating by, e.g., subpopulations or domains. -->
[More Information Needed]
#### Metrics
<!-- These are the evaluation metrics being used, ideally with a description of why. -->
[More Information Needed]
### Results
[More Information Needed]
#### Summary
## Model Examination [optional]
<!-- Relevant interpretability work for the model goes here -->
[More Information Needed]
## Environmental Impact
<!-- Total emissions (in grams of CO2eq) and additional considerations, such as electricity usage, go here. Edit the suggested text below accordingly -->
Carbon emissions can be estimated using the [Machine Learning Impact calculator](https://mlco2.github.io/impact#compute) presented in [Lacoste et al. (2019)](https://arxiv.org/abs/1910.09700).
- **Hardware Type:** [More Information Needed]
- **Hours used:** [More Information Needed]
- **Cloud Provider:** [More Information Needed]
- **Compute Region:** [More Information Needed]
- **Carbon Emitted:** [More Information Needed]
## Technical Specifications [optional]
### Model Architecture and Objective
[More Information Needed]
### Compute Infrastructure
[More Information Needed]
#### Hardware
[More Information Needed]
#### Software
[More Information Needed]
## Citation [optional]
<!-- If there is a paper or blog post introducing the model, the APA and Bibtex information for that should go in this section. -->
**BibTeX:**
[More Information Needed]
**APA:**
[More Information Needed]
## Glossary [optional]
<!-- If relevant, include terms and calculations in this section that can help readers understand the model or model card. -->
[More Information Needed]
## More Information [optional]
[More Information Needed]
## Model Card Authors [optional]
[More Information Needed]
## Model Card Contact
[More Information Needed]
|
mradermacher/llama3.2-3b-uncensored-GGUF | mradermacher | "2025-03-15T06:51:51Z" | 251 | 0 | transformers | [
"transformers",
"gguf",
"en",
"base_model:thirdeyeai/llama3.2-3b-uncensored",
"base_model:quantized:thirdeyeai/llama3.2-3b-uncensored",
"license:llama3.2",
"endpoints_compatible",
"region:us",
"conversational"
] | null | "2025-03-14T17:39:29Z" | ---
base_model: thirdeyeai/llama3.2-3b-uncensored
language:
- en
library_name: transformers
license: llama3.2
quantized_by: mradermacher
---
## About
<!-- ### quantize_version: 2 -->
<!-- ### output_tensor_quantised: 1 -->
<!-- ### convert_type: hf -->
<!-- ### vocab_type: -->
<!-- ### tags: -->
static quants of https://huggingface.co/thirdeyeai/llama3.2-3b-uncensored
<!-- provided-files -->
weighted/imatrix quants are available at https://huggingface.co/mradermacher/llama3.2-3b-uncensored-i1-GGUF
## Usage
If you are unsure how to use GGUF files, refer to one of [TheBloke's
READMEs](https://huggingface.co/TheBloke/KafkaLM-70B-German-V0.1-GGUF) for
more details, including on how to concatenate multi-part files.
## Provided Quants
(sorted by size, not necessarily quality. IQ-quants are often preferable over similar sized non-IQ quants)
| Link | Type | Size/GB | Notes |
|:-----|:-----|--------:|:------|
| [GGUF](https://huggingface.co/mradermacher/llama3.2-3b-uncensored-GGUF/resolve/main/llama3.2-3b-uncensored.Q2_K.gguf) | Q2_K | 1.6 | |
| [GGUF](https://huggingface.co/mradermacher/llama3.2-3b-uncensored-GGUF/resolve/main/llama3.2-3b-uncensored.Q3_K_S.gguf) | Q3_K_S | 1.8 | |
| [GGUF](https://huggingface.co/mradermacher/llama3.2-3b-uncensored-GGUF/resolve/main/llama3.2-3b-uncensored.Q3_K_M.gguf) | Q3_K_M | 2.0 | lower quality |
| [GGUF](https://huggingface.co/mradermacher/llama3.2-3b-uncensored-GGUF/resolve/main/llama3.2-3b-uncensored.Q3_K_L.gguf) | Q3_K_L | 2.1 | |
| [GGUF](https://huggingface.co/mradermacher/llama3.2-3b-uncensored-GGUF/resolve/main/llama3.2-3b-uncensored.IQ4_XS.gguf) | IQ4_XS | 2.2 | |
| [GGUF](https://huggingface.co/mradermacher/llama3.2-3b-uncensored-GGUF/resolve/main/llama3.2-3b-uncensored.Q4_K_S.gguf) | Q4_K_S | 2.2 | fast, recommended |
| [GGUF](https://huggingface.co/mradermacher/llama3.2-3b-uncensored-GGUF/resolve/main/llama3.2-3b-uncensored.Q4_K_M.gguf) | Q4_K_M | 2.3 | fast, recommended |
| [GGUF](https://huggingface.co/mradermacher/llama3.2-3b-uncensored-GGUF/resolve/main/llama3.2-3b-uncensored.Q5_K_S.gguf) | Q5_K_S | 2.6 | |
| [GGUF](https://huggingface.co/mradermacher/llama3.2-3b-uncensored-GGUF/resolve/main/llama3.2-3b-uncensored.Q5_K_M.gguf) | Q5_K_M | 2.7 | |
| [GGUF](https://huggingface.co/mradermacher/llama3.2-3b-uncensored-GGUF/resolve/main/llama3.2-3b-uncensored.Q6_K.gguf) | Q6_K | 3.1 | very good quality |
| [GGUF](https://huggingface.co/mradermacher/llama3.2-3b-uncensored-GGUF/resolve/main/llama3.2-3b-uncensored.Q8_0.gguf) | Q8_0 | 3.9 | fast, best quality |
| [GGUF](https://huggingface.co/mradermacher/llama3.2-3b-uncensored-GGUF/resolve/main/llama3.2-3b-uncensored.f16.gguf) | f16 | 7.3 | 16 bpw, overkill |
Here is a handy graph by ikawrakow comparing some lower-quality quant
types (lower is better):

And here are Artefact2's thoughts on the matter:
https://gist.github.com/Artefact2/b5f810600771265fc1e39442288e8ec9
## FAQ / Model Request
See https://huggingface.co/mradermacher/model_requests for some answers to
questions you might have and/or if you want some other model quantized.
## Thanks
I thank my company, [nethype GmbH](https://www.nethype.de/), for letting
me use its servers and providing upgrades to my workstation to enable
this work in my free time.
<!-- end -->
|
Viscoke/Alocasia12 | Viscoke | "2024-12-09T19:48:19Z" | 5 | 0 | transformers | [
"transformers",
"safetensors",
"llama",
"text-generation",
"arxiv:1910.09700",
"autotrain_compatible",
"text-generation-inference",
"endpoints_compatible",
"region:us"
] | text-generation | "2024-12-09T18:59:16Z" | ---
library_name: transformers
tags: []
---
# Model Card for Model ID
<!-- Provide a quick summary of what the model is/does. -->
## Model Details
### Model Description
<!-- Provide a longer summary of what this model is. -->
This is the model card of a 🤗 transformers model that has been pushed on the Hub. This model card has been automatically generated.
- **Developed by:** [More Information Needed]
- **Funded by [optional]:** [More Information Needed]
- **Shared by [optional]:** [More Information Needed]
- **Model type:** [More Information Needed]
- **Language(s) (NLP):** [More Information Needed]
- **License:** [More Information Needed]
- **Finetuned from model [optional]:** [More Information Needed]
### Model Sources [optional]
<!-- Provide the basic links for the model. -->
- **Repository:** [More Information Needed]
- **Paper [optional]:** [More Information Needed]
- **Demo [optional]:** [More Information Needed]
## Uses
<!-- Address questions around how the model is intended to be used, including the foreseeable users of the model and those affected by the model. -->
### Direct Use
<!-- This section is for the model use without fine-tuning or plugging into a larger ecosystem/app. -->
[More Information Needed]
### Downstream Use [optional]
<!-- This section is for the model use when fine-tuned for a task, or when plugged into a larger ecosystem/app -->
[More Information Needed]
### Out-of-Scope Use
<!-- This section addresses misuse, malicious use, and uses that the model will not work well for. -->
[More Information Needed]
## Bias, Risks, and Limitations
<!-- This section is meant to convey both technical and sociotechnical limitations. -->
[More Information Needed]
### Recommendations
<!-- This section is meant to convey recommendations with respect to the bias, risk, and technical limitations. -->
Users (both direct and downstream) should be made aware of the risks, biases and limitations of the model. More information needed for further recommendations.
## How to Get Started with the Model
Use the code below to get started with the model.
[More Information Needed]
## Training Details
### Training Data
<!-- This should link to a Dataset Card, perhaps with a short stub of information on what the training data is all about as well as documentation related to data pre-processing or additional filtering. -->
[More Information Needed]
### Training Procedure
<!-- This relates heavily to the Technical Specifications. Content here should link to that section when it is relevant to the training procedure. -->
#### Preprocessing [optional]
[More Information Needed]
#### Training Hyperparameters
- **Training regime:** [More Information Needed] <!--fp32, fp16 mixed precision, bf16 mixed precision, bf16 non-mixed precision, fp16 non-mixed precision, fp8 mixed precision -->
#### Speeds, Sizes, Times [optional]
<!-- This section provides information about throughput, start/end time, checkpoint size if relevant, etc. -->
[More Information Needed]
## Evaluation
<!-- This section describes the evaluation protocols and provides the results. -->
### Testing Data, Factors & Metrics
#### Testing Data
<!-- This should link to a Dataset Card if possible. -->
[More Information Needed]
#### Factors
<!-- These are the things the evaluation is disaggregating by, e.g., subpopulations or domains. -->
[More Information Needed]
#### Metrics
<!-- These are the evaluation metrics being used, ideally with a description of why. -->
[More Information Needed]
### Results
[More Information Needed]
#### Summary
## Model Examination [optional]
<!-- Relevant interpretability work for the model goes here -->
[More Information Needed]
## Environmental Impact
<!-- Total emissions (in grams of CO2eq) and additional considerations, such as electricity usage, go here. Edit the suggested text below accordingly -->
Carbon emissions can be estimated using the [Machine Learning Impact calculator](https://mlco2.github.io/impact#compute) presented in [Lacoste et al. (2019)](https://arxiv.org/abs/1910.09700).
- **Hardware Type:** [More Information Needed]
- **Hours used:** [More Information Needed]
- **Cloud Provider:** [More Information Needed]
- **Compute Region:** [More Information Needed]
- **Carbon Emitted:** [More Information Needed]
## Technical Specifications [optional]
### Model Architecture and Objective
[More Information Needed]
### Compute Infrastructure
[More Information Needed]
#### Hardware
[More Information Needed]
#### Software
[More Information Needed]
## Citation [optional]
<!-- If there is a paper or blog post introducing the model, the APA and Bibtex information for that should go in this section. -->
**BibTeX:**
[More Information Needed]
**APA:**
[More Information Needed]
## Glossary [optional]
<!-- If relevant, include terms and calculations in this section that can help readers understand the model or model card. -->
[More Information Needed]
## More Information [optional]
[More Information Needed]
## Model Card Authors [optional]
[More Information Needed]
## Model Card Contact
[More Information Needed] |
damgomz/ft_32_2e6_base_x1 | damgomz | "2024-06-24T06:30:40Z" | 8 | 0 | transformers | [
"transformers",
"safetensors",
"albert",
"text-classification",
"en",
"autotrain_compatible",
"endpoints_compatible",
"region:us"
] | text-classification | "2024-06-23T10:54:57Z" | ---
language: en
tags:
- text-classification
pipeline_tag: text-classification
widget:
- text: GEPS Techno is the pioneer of hybridization of renewable energies at sea.
We imagine, design and commercialize innovative off-grid systems that aim to generate
power at sea, stabilize and collect data. The success of our low power platforms
WAVEPEAL enabled us to scale-up the device up to WAVEGEM, the 150-kW capacity
platform.
---
## Environmental Impact (CODE CARBON DEFAULT)
| Metric | Value |
|--------------------------|---------------------------------|
| Duration (in seconds) | 73095.39277100563 |
| Emissions (Co2eq in kg) | 0.0442311379684663 |
| CPU power (W) | 42.5 |
| GPU power (W) | [No GPU] |
| RAM power (W) | 3.75 |
| CPU energy (kWh) | 0.862929785768026 |
| GPU energy (kWh) | [No GPU] |
| RAM energy (kWh) | 0.0761402545382579 |
| Consumed energy (kWh) | 0.9390700403062856 |
| Country name | Switzerland |
| Cloud provider | nan |
| Cloud region | nan |
| CPU count | 2 |
| CPU model | Intel(R) Xeon(R) Platinum 8360Y CPU @ 2.40GHz |
| GPU count | nan |
| GPU model | nan |
## Environmental Impact (for one core)
| Metric | Value |
|--------------------------|---------------------------------|
| CPU energy (kWh) | 0.14070863108418583 |
| Emissions (Co2eq in kg) | 0.02862902883531054 |
## Note
19 juin 2024
## My Config
| Config | Value |
|--------------------------|-----------------|
| checkpoint | albert-base-v2 |
| model_name | ft_32_2e6_base_x1 |
| sequence_length | 400 |
| num_epoch | 6 |
| learning_rate | 2e-06 |
| batch_size | 32 |
| weight_decay | 0.0 |
| warm_up_prop | 0.0 |
| drop_out_prob | 0.1 |
| packing_length | 100 |
| train_test_split | 0.2 |
| num_steps | 29328 |
## Training and Testing steps
Epoch | Train Loss | Test Loss | F-beta Score
---|---|---|---
| 0 | 0.000000 | 0.719601 | 0.333675 |
| 1 | 0.482296 | 0.366204 | 0.876392 |
| 2 | 0.298587 | 0.270772 | 0.896133 |
| 3 | 0.222985 | 0.245894 | 0.908798 |
| 4 | 0.177491 | 0.236516 | 0.912284 |
| 5 | 0.137443 | 0.242232 | 0.893873 |
| 6 | 0.105196 | 0.269823 | 0.916260 |
|
AnthonyErosion/testmodel | AnthonyErosion | "2024-05-26T09:59:14Z" | 1 | 0 | keras | [
"keras",
"image-classification",
"region:us"
] | image-classification | "2024-05-26T09:52:05Z" | ---
pipeline_tag: image-classification
library_name: keras
--- |
OwOOwO/dumbo-krillin100 | OwOOwO | "2024-04-19T07:32:06Z" | 6 | 0 | transformers | [
"transformers",
"safetensors",
"llama",
"text-generation",
"conversational",
"arxiv:1910.09700",
"autotrain_compatible",
"text-generation-inference",
"endpoints_compatible",
"region:us"
] | text-generation | "2024-04-19T07:29:05Z" | ---
library_name: transformers
tags: []
---
# Model Card for Model ID
<!-- Provide a quick summary of what the model is/does. -->
## Model Details
### Model Description
<!-- Provide a longer summary of what this model is. -->
This is the model card of a 🤗 transformers model that has been pushed on the Hub. This model card has been automatically generated.
- **Developed by:** [More Information Needed]
- **Funded by [optional]:** [More Information Needed]
- **Shared by [optional]:** [More Information Needed]
- **Model type:** [More Information Needed]
- **Language(s) (NLP):** [More Information Needed]
- **License:** [More Information Needed]
- **Finetuned from model [optional]:** [More Information Needed]
### Model Sources [optional]
<!-- Provide the basic links for the model. -->
- **Repository:** [More Information Needed]
- **Paper [optional]:** [More Information Needed]
- **Demo [optional]:** [More Information Needed]
## Uses
<!-- Address questions around how the model is intended to be used, including the foreseeable users of the model and those affected by the model. -->
### Direct Use
<!-- This section is for the model use without fine-tuning or plugging into a larger ecosystem/app. -->
[More Information Needed]
### Downstream Use [optional]
<!-- This section is for the model use when fine-tuned for a task, or when plugged into a larger ecosystem/app -->
[More Information Needed]
### Out-of-Scope Use
<!-- This section addresses misuse, malicious use, and uses that the model will not work well for. -->
[More Information Needed]
## Bias, Risks, and Limitations
<!-- This section is meant to convey both technical and sociotechnical limitations. -->
[More Information Needed]
### Recommendations
<!-- This section is meant to convey recommendations with respect to the bias, risk, and technical limitations. -->
Users (both direct and downstream) should be made aware of the risks, biases and limitations of the model. More information needed for further recommendations.
## How to Get Started with the Model
Use the code below to get started with the model.
[More Information Needed]
## Training Details
### Training Data
<!-- This should link to a Dataset Card, perhaps with a short stub of information on what the training data is all about as well as documentation related to data pre-processing or additional filtering. -->
[More Information Needed]
### Training Procedure
<!-- This relates heavily to the Technical Specifications. Content here should link to that section when it is relevant to the training procedure. -->
#### Preprocessing [optional]
[More Information Needed]
#### Training Hyperparameters
- **Training regime:** [More Information Needed] <!--fp32, fp16 mixed precision, bf16 mixed precision, bf16 non-mixed precision, fp16 non-mixed precision, fp8 mixed precision -->
#### Speeds, Sizes, Times [optional]
<!-- This section provides information about throughput, start/end time, checkpoint size if relevant, etc. -->
[More Information Needed]
## Evaluation
<!-- This section describes the evaluation protocols and provides the results. -->
### Testing Data, Factors & Metrics
#### Testing Data
<!-- This should link to a Dataset Card if possible. -->
[More Information Needed]
#### Factors
<!-- These are the things the evaluation is disaggregating by, e.g., subpopulations or domains. -->
[More Information Needed]
#### Metrics
<!-- These are the evaluation metrics being used, ideally with a description of why. -->
[More Information Needed]
### Results
[More Information Needed]
#### Summary
## Model Examination [optional]
<!-- Relevant interpretability work for the model goes here -->
[More Information Needed]
## Environmental Impact
<!-- Total emissions (in grams of CO2eq) and additional considerations, such as electricity usage, go here. Edit the suggested text below accordingly -->
Carbon emissions can be estimated using the [Machine Learning Impact calculator](https://mlco2.github.io/impact#compute) presented in [Lacoste et al. (2019)](https://arxiv.org/abs/1910.09700).
- **Hardware Type:** [More Information Needed]
- **Hours used:** [More Information Needed]
- **Cloud Provider:** [More Information Needed]
- **Compute Region:** [More Information Needed]
- **Carbon Emitted:** [More Information Needed]
## Technical Specifications [optional]
### Model Architecture and Objective
[More Information Needed]
### Compute Infrastructure
[More Information Needed]
#### Hardware
[More Information Needed]
#### Software
[More Information Needed]
## Citation [optional]
<!-- If there is a paper or blog post introducing the model, the APA and Bibtex information for that should go in this section. -->
**BibTeX:**
[More Information Needed]
**APA:**
[More Information Needed]
## Glossary [optional]
<!-- If relevant, include terms and calculations in this section that can help readers understand the model or model card. -->
[More Information Needed]
## More Information [optional]
[More Information Needed]
## Model Card Authors [optional]
[More Information Needed]
## Model Card Contact
[More Information Needed] |
tomasito12/dqn-SpaceInvadersNoFrameskip-v4 | tomasito12 | "2023-09-05T14:50:50Z" | 2 | 0 | stable-baselines3 | [
"stable-baselines3",
"SpaceInvadersNoFrameskip-v4",
"deep-reinforcement-learning",
"reinforcement-learning",
"model-index",
"region:us"
] | reinforcement-learning | "2023-09-05T14:50:20Z" | ---
library_name: stable-baselines3
tags:
- SpaceInvadersNoFrameskip-v4
- deep-reinforcement-learning
- reinforcement-learning
- stable-baselines3
model-index:
- name: DQN
results:
- task:
type: reinforcement-learning
name: reinforcement-learning
dataset:
name: SpaceInvadersNoFrameskip-v4
type: SpaceInvadersNoFrameskip-v4
metrics:
- type: mean_reward
value: 434.00 +/- 176.04
name: mean_reward
verified: false
---
# **DQN** Agent playing **SpaceInvadersNoFrameskip-v4**
This is a trained model of a **DQN** agent playing **SpaceInvadersNoFrameskip-v4**
using the [stable-baselines3 library](https://github.com/DLR-RM/stable-baselines3)
and the [RL Zoo](https://github.com/DLR-RM/rl-baselines3-zoo).
The RL Zoo is a training framework for Stable Baselines3
reinforcement learning agents,
with hyperparameter optimization and pre-trained agents included.
## Usage (with SB3 RL Zoo)
RL Zoo: https://github.com/DLR-RM/rl-baselines3-zoo<br/>
SB3: https://github.com/DLR-RM/stable-baselines3<br/>
SB3 Contrib: https://github.com/Stable-Baselines-Team/stable-baselines3-contrib
Install the RL Zoo (with SB3 and SB3-Contrib):
```bash
pip install rl_zoo3
```
```
# Download model and save it into the logs/ folder
python -m rl_zoo3.load_from_hub --algo dqn --env SpaceInvadersNoFrameskip-v4 -orga tomasito12 -f logs/
python -m rl_zoo3.enjoy --algo dqn --env SpaceInvadersNoFrameskip-v4 -f logs/
```
If you installed the RL Zoo3 via pip (`pip install rl_zoo3`), from anywhere you can do:
```
python -m rl_zoo3.load_from_hub --algo dqn --env SpaceInvadersNoFrameskip-v4 -orga tomasito12 -f logs/
python -m rl_zoo3.enjoy --algo dqn --env SpaceInvadersNoFrameskip-v4 -f logs/
```
## Training (with the RL Zoo)
```
python -m rl_zoo3.train --algo dqn --env SpaceInvadersNoFrameskip-v4 -f logs/
# Upload the model and generate video (when possible)
python -m rl_zoo3.push_to_hub --algo dqn --env SpaceInvadersNoFrameskip-v4 -f logs/ -orga tomasito12
```
## Hyperparameters
```python
OrderedDict([('batch_size', 32),
('buffer_size', 100000),
('env_wrapper',
['stable_baselines3.common.atari_wrappers.AtariWrapper']),
('exploration_final_eps', 0.01),
('exploration_fraction', 0.1),
('frame_stack', 4),
('gradient_steps', 1),
('learning_rate', 0.0001),
('learning_starts', 100000),
('n_timesteps', 500000.0),
('optimize_memory_usage', False),
('policy', 'CnnPolicy'),
('target_update_interval', 1000),
('train_freq', 4),
('normalize', False)])
```
# Environment Arguments
```python
{'render_mode': 'rgb_array'}
```
|
stuartmesham/roberta-large_lemon-spell_5k_4_p3 | stuartmesham | "2022-10-24T17:58:41Z" | 6 | 0 | transformers | [
"transformers",
"pytorch",
"roberta",
"token-classification",
"generated_from_trainer",
"autotrain_compatible",
"endpoints_compatible",
"region:us"
] | token-classification | "2022-10-24T17:57:48Z" | ---
tags:
- generated_from_trainer
metrics:
- accuracy
model-index:
- name: roberta-large_lemon-spell_5k_4_p3
results: []
---
<!-- This model card has been generated automatically according to the information the Trainer had access to. You
should probably proofread and complete it, then remove this comment. -->
# roberta-large_lemon-spell_5k_4_p3
This model is a fine-tuned version of [model_saves/roberta-large_lemon-spell_5k_4_p2](https://huggingface.co/model_saves/roberta-large_lemon-spell_5k_4_p2) on an unknown dataset.
It achieves the following results on the evaluation set:
- Loss: 0.4209
- Accuracy: 0.9401
## Model description
More information needed
## Intended uses & limitations
More information needed
## Training and evaluation data
More information needed
## Training procedure
### Training hyperparameters
The following hyperparameters were used during training:
- learning_rate: 1e-05
- train_batch_size: 128
- eval_batch_size: 128
- seed: 72
- distributed_type: multi-GPU
- optimizer: Adam with betas=(0.9,0.999) and epsilon=1e-08
- lr_scheduler_type: constant
- num_epochs: 15.0
### Training results
| Training Loss | Epoch | Step | Validation Loss | Accuracy |
|:-------------:|:-----:|:----:|:---------------:|:--------:|
| No log | 1.0 | 268 | 0.4209 | 0.9401 |
| No log | 2.0 | 536 | 0.4434 | 0.9392 |
| No log | 3.0 | 804 | 0.4690 | 0.9395 |
| 0.2919 | 4.0 | 1072 | 0.5258 | 0.9378 |
### Framework versions
- Transformers 4.21.0.dev0
- Pytorch 1.11.0+cu113
- Datasets 2.3.2
- Tokenizers 0.12.1
|
vermoney/efd00b89-ee08-4734-bbd3-6721ebb40e30 | vermoney | "2025-01-22T08:59:26Z" | 6 | 0 | peft | [
"peft",
"safetensors",
"llama",
"axolotl",
"generated_from_trainer",
"base_model:unsloth/SmolLM2-360M-Instruct",
"base_model:adapter:unsloth/SmolLM2-360M-Instruct",
"license:apache-2.0",
"region:us"
] | null | "2025-01-22T08:21:38Z" | ---
library_name: peft
license: apache-2.0
base_model: unsloth/SmolLM2-360M-Instruct
tags:
- axolotl
- generated_from_trainer
model-index:
- name: efd00b89-ee08-4734-bbd3-6721ebb40e30
results: []
---
<!-- This model card has been generated automatically according to the information the Trainer had access to. You
should probably proofread and complete it, then remove this comment. -->
[<img src="https://raw.githubusercontent.com/axolotl-ai-cloud/axolotl/main/image/axolotl-badge-web.png" alt="Built with Axolotl" width="200" height="32"/>](https://github.com/axolotl-ai-cloud/axolotl)
<details><summary>See axolotl config</summary>
axolotl version: `0.4.1`
```yaml
adapter: lora
base_model: unsloth/SmolLM2-360M-Instruct
bf16: auto
chat_template: llama3
dataset_prepared_path: null
datasets:
- data_files:
- a1f9a54f1d5b9faf_train_data.json
ds_type: json
format: custom
path: /workspace/input_data/a1f9a54f1d5b9faf_train_data.json
type:
field_instruction: book_id
field_output: review
format: '{instruction}'
no_input_format: '{instruction}'
system_format: '{system}'
system_prompt: ''
debug: null
deepspeed: null
device: cuda
early_stopping_patience: 1
eval_max_new_tokens: 128
eval_steps: 5
eval_table_size: null
evals_per_epoch: null
flash_attention: false
fp16: null
gradient_accumulation_steps: 4
gradient_checkpointing: true
group_by_length: true
hub_model_id: vermoney/efd00b89-ee08-4734-bbd3-6721ebb40e30
hub_repo: null
hub_strategy: checkpoint
hub_token: null
learning_rate: 0.0002
load_in_4bit: false
load_in_8bit: false
local_rank: null
logging_steps: 3
lora_alpha: 32
lora_dropout: 0.05
lora_fan_in_fan_out: null
lora_model_dir: null
lora_r: 16
lora_target_linear: true
lr_scheduler: cosine
max_memory:
0: 78GiB
max_steps: 30
micro_batch_size: 2
mlflow_experiment_name: /tmp/a1f9a54f1d5b9faf_train_data.json
model_type: AutoModelForCausalLM
num_epochs: 1
optim_args:
adam_beta1: 0.9
adam_beta2: 0.95
adam_epsilon: 1e-5
optimizer: adamw_torch
output_dir: miner_id_24
pad_to_sequence_len: true
resume_from_checkpoint: null
s2_attention: null
sample_packing: false
save_steps: 10
sequence_len: 1024
strict: false
tf32: false
tokenizer_type: AutoTokenizer
train_on_inputs: true
trust_remote_code: true
val_set_size: 0.05
wandb_entity: null
wandb_mode: online
wandb_name: 45c7c5de-1f70-440f-9e17-1ab3fb20df4a
wandb_project: Gradients-On-Demand
wandb_run: your_name
wandb_runid: 45c7c5de-1f70-440f-9e17-1ab3fb20df4a
warmup_steps: 5
weight_decay: 0.001
xformers_attention: true
```
</details><br>
# efd00b89-ee08-4734-bbd3-6721ebb40e30
This model is a fine-tuned version of [unsloth/SmolLM2-360M-Instruct](https://huggingface.co/unsloth/SmolLM2-360M-Instruct) on the None dataset.
It achieves the following results on the evaluation set:
- Loss: nan
## Model description
More information needed
## Intended uses & limitations
More information needed
## Training and evaluation data
More information needed
## Training procedure
### Training hyperparameters
The following hyperparameters were used during training:
- learning_rate: 0.0002
- train_batch_size: 2
- eval_batch_size: 2
- seed: 42
- gradient_accumulation_steps: 4
- total_train_batch_size: 8
- optimizer: Use OptimizerNames.ADAMW_TORCH with betas=(0.9,0.999) and epsilon=1e-08 and optimizer_args=adam_beta1=0.9,adam_beta2=0.95,adam_epsilon=1e-5
- lr_scheduler_type: cosine
- lr_scheduler_warmup_steps: 5
- training_steps: 30
### Training results
| Training Loss | Epoch | Step | Validation Loss |
|:-------------:|:------:|:----:|:---------------:|
| No log | 0.0000 | 1 | nan |
| 0.0 | 0.0001 | 5 | nan |
| 0.0 | 0.0002 | 10 | nan |
| 0.0 | 0.0002 | 15 | nan |
### Framework versions
- PEFT 0.13.2
- Transformers 4.46.0
- Pytorch 2.5.0+cu124
- Datasets 3.0.1
- Tokenizers 0.20.1 |
samitizerxu/segformer-b1-kelp-rgb-agg-imgaug-jan-26 | samitizerxu | "2024-01-26T14:56:40Z" | 0 | 0 | transformers | [
"transformers",
"arxiv:1910.09700",
"endpoints_compatible",
"region:us"
] | null | "2024-01-26T13:13:30Z" | ---
library_name: transformers
tags: []
---
# Model Card for Model ID
<!-- Provide a quick summary of what the model is/does. -->
## Model Details
### Model Description
<!-- Provide a longer summary of what this model is. -->
This is the model card of a 🤗 transformers model that has been pushed on the Hub. This model card has been automatically generated.
- **Developed by:** [More Information Needed]
- **Funded by [optional]:** [More Information Needed]
- **Shared by [optional]:** [More Information Needed]
- **Model type:** [More Information Needed]
- **Language(s) (NLP):** [More Information Needed]
- **License:** [More Information Needed]
- **Finetuned from model [optional]:** [More Information Needed]
### Model Sources [optional]
<!-- Provide the basic links for the model. -->
- **Repository:** [More Information Needed]
- **Paper [optional]:** [More Information Needed]
- **Demo [optional]:** [More Information Needed]
## Uses
<!-- Address questions around how the model is intended to be used, including the foreseeable users of the model and those affected by the model. -->
### Direct Use
<!-- This section is for the model use without fine-tuning or plugging into a larger ecosystem/app. -->
[More Information Needed]
### Downstream Use [optional]
<!-- This section is for the model use when fine-tuned for a task, or when plugged into a larger ecosystem/app -->
[More Information Needed]
### Out-of-Scope Use
<!-- This section addresses misuse, malicious use, and uses that the model will not work well for. -->
[More Information Needed]
## Bias, Risks, and Limitations
<!-- This section is meant to convey both technical and sociotechnical limitations. -->
[More Information Needed]
### Recommendations
<!-- This section is meant to convey recommendations with respect to the bias, risk, and technical limitations. -->
Users (both direct and downstream) should be made aware of the risks, biases and limitations of the model. More information needed for further recommendations.
## How to Get Started with the Model
Use the code below to get started with the model.
[More Information Needed]
## Training Details
### Training Data
<!-- This should link to a Dataset Card, perhaps with a short stub of information on what the training data is all about as well as documentation related to data pre-processing or additional filtering. -->
[More Information Needed]
### Training Procedure
<!-- This relates heavily to the Technical Specifications. Content here should link to that section when it is relevant to the training procedure. -->
#### Preprocessing [optional]
[More Information Needed]
#### Training Hyperparameters
- **Training regime:** [More Information Needed] <!--fp32, fp16 mixed precision, bf16 mixed precision, bf16 non-mixed precision, fp16 non-mixed precision, fp8 mixed precision -->
#### Speeds, Sizes, Times [optional]
<!-- This section provides information about throughput, start/end time, checkpoint size if relevant, etc. -->
[More Information Needed]
## Evaluation
<!-- This section describes the evaluation protocols and provides the results. -->
### Testing Data, Factors & Metrics
#### Testing Data
<!-- This should link to a Dataset Card if possible. -->
[More Information Needed]
#### Factors
<!-- These are the things the evaluation is disaggregating by, e.g., subpopulations or domains. -->
[More Information Needed]
#### Metrics
<!-- These are the evaluation metrics being used, ideally with a description of why. -->
[More Information Needed]
### Results
[More Information Needed]
#### Summary
## Model Examination [optional]
<!-- Relevant interpretability work for the model goes here -->
[More Information Needed]
## Environmental Impact
<!-- Total emissions (in grams of CO2eq) and additional considerations, such as electricity usage, go here. Edit the suggested text below accordingly -->
Carbon emissions can be estimated using the [Machine Learning Impact calculator](https://mlco2.github.io/impact#compute) presented in [Lacoste et al. (2019)](https://arxiv.org/abs/1910.09700).
- **Hardware Type:** [More Information Needed]
- **Hours used:** [More Information Needed]
- **Cloud Provider:** [More Information Needed]
- **Compute Region:** [More Information Needed]
- **Carbon Emitted:** [More Information Needed]
## Technical Specifications [optional]
### Model Architecture and Objective
[More Information Needed]
### Compute Infrastructure
[More Information Needed]
#### Hardware
[More Information Needed]
#### Software
[More Information Needed]
## Citation [optional]
<!-- If there is a paper or blog post introducing the model, the APA and Bibtex information for that should go in this section. -->
**BibTeX:**
[More Information Needed]
**APA:**
[More Information Needed]
## Glossary [optional]
<!-- If relevant, include terms and calculations in this section that can help readers understand the model or model card. -->
[More Information Needed]
## More Information [optional]
[More Information Needed]
## Model Card Authors [optional]
[More Information Needed]
## Model Card Contact
[More Information Needed]
|
memevis/tryy37 | memevis | "2025-01-27T16:26:37Z" | 16 | 0 | transformers | [
"transformers",
"safetensors",
"llama",
"text-generation",
"conversational",
"arxiv:1910.09700",
"autotrain_compatible",
"text-generation-inference",
"endpoints_compatible",
"region:us"
] | text-generation | "2025-01-27T16:20:29Z" | ---
library_name: transformers
tags: []
---
# Model Card for Model ID
<!-- Provide a quick summary of what the model is/does. -->
## Model Details
### Model Description
<!-- Provide a longer summary of what this model is. -->
This is the model card of a 🤗 transformers model that has been pushed on the Hub. This model card has been automatically generated.
- **Developed by:** [More Information Needed]
- **Funded by [optional]:** [More Information Needed]
- **Shared by [optional]:** [More Information Needed]
- **Model type:** [More Information Needed]
- **Language(s) (NLP):** [More Information Needed]
- **License:** [More Information Needed]
- **Finetuned from model [optional]:** [More Information Needed]
### Model Sources [optional]
<!-- Provide the basic links for the model. -->
- **Repository:** [More Information Needed]
- **Paper [optional]:** [More Information Needed]
- **Demo [optional]:** [More Information Needed]
## Uses
<!-- Address questions around how the model is intended to be used, including the foreseeable users of the model and those affected by the model. -->
### Direct Use
<!-- This section is for the model use without fine-tuning or plugging into a larger ecosystem/app. -->
[More Information Needed]
### Downstream Use [optional]
<!-- This section is for the model use when fine-tuned for a task, or when plugged into a larger ecosystem/app -->
[More Information Needed]
### Out-of-Scope Use
<!-- This section addresses misuse, malicious use, and uses that the model will not work well for. -->
[More Information Needed]
## Bias, Risks, and Limitations
<!-- This section is meant to convey both technical and sociotechnical limitations. -->
[More Information Needed]
### Recommendations
<!-- This section is meant to convey recommendations with respect to the bias, risk, and technical limitations. -->
Users (both direct and downstream) should be made aware of the risks, biases and limitations of the model. More information needed for further recommendations.
## How to Get Started with the Model
Use the code below to get started with the model.
[More Information Needed]
## Training Details
### Training Data
<!-- This should link to a Dataset Card, perhaps with a short stub of information on what the training data is all about as well as documentation related to data pre-processing or additional filtering. -->
[More Information Needed]
### Training Procedure
<!-- This relates heavily to the Technical Specifications. Content here should link to that section when it is relevant to the training procedure. -->
#### Preprocessing [optional]
[More Information Needed]
#### Training Hyperparameters
- **Training regime:** [More Information Needed] <!--fp32, fp16 mixed precision, bf16 mixed precision, bf16 non-mixed precision, fp16 non-mixed precision, fp8 mixed precision -->
#### Speeds, Sizes, Times [optional]
<!-- This section provides information about throughput, start/end time, checkpoint size if relevant, etc. -->
[More Information Needed]
## Evaluation
<!-- This section describes the evaluation protocols and provides the results. -->
### Testing Data, Factors & Metrics
#### Testing Data
<!-- This should link to a Dataset Card if possible. -->
[More Information Needed]
#### Factors
<!-- These are the things the evaluation is disaggregating by, e.g., subpopulations or domains. -->
[More Information Needed]
#### Metrics
<!-- These are the evaluation metrics being used, ideally with a description of why. -->
[More Information Needed]
### Results
[More Information Needed]
#### Summary
## Model Examination [optional]
<!-- Relevant interpretability work for the model goes here -->
[More Information Needed]
## Environmental Impact
<!-- Total emissions (in grams of CO2eq) and additional considerations, such as electricity usage, go here. Edit the suggested text below accordingly -->
Carbon emissions can be estimated using the [Machine Learning Impact calculator](https://mlco2.github.io/impact#compute) presented in [Lacoste et al. (2019)](https://arxiv.org/abs/1910.09700).
- **Hardware Type:** [More Information Needed]
- **Hours used:** [More Information Needed]
- **Cloud Provider:** [More Information Needed]
- **Compute Region:** [More Information Needed]
- **Carbon Emitted:** [More Information Needed]
## Technical Specifications [optional]
### Model Architecture and Objective
[More Information Needed]
### Compute Infrastructure
[More Information Needed]
#### Hardware
[More Information Needed]
#### Software
[More Information Needed]
## Citation [optional]
<!-- If there is a paper or blog post introducing the model, the APA and Bibtex information for that should go in this section. -->
**BibTeX:**
[More Information Needed]
**APA:**
[More Information Needed]
## Glossary [optional]
<!-- If relevant, include terms and calculations in this section that can help readers understand the model or model card. -->
[More Information Needed]
## More Information [optional]
[More Information Needed]
## Model Card Authors [optional]
[More Information Needed]
## Model Card Contact
[More Information Needed] |
robvanderg/Sem-mmmBERT | robvanderg | "2022-03-28T11:28:17Z" | 4 | 0 | transformers | [
"transformers",
"pytorch",
"bert",
"feature-extraction",
"STILT",
"retraining",
"multi-task learning",
"multilingual",
"text-embeddings-inference",
"endpoints_compatible",
"region:us"
] | feature-extraction | "2022-03-28T11:15:17Z" | ---
language:
- multilingual
tags:
- STILT
- retraining
- multi-task learning
datasets:
- SemEval 2022
---
## Sem-mmmBERT
This is the SemEval MaChAmp Multitask Multilingual BERT model. This model is retrained from mBERT (https://huggingface.co/bert-base-multilingual-cased).
The retraining is done based on all SemEval 2022 tasks that are text based, and have annotation on the word, sentence or paragraph level. The retraining is done with MaChAmp (https://machamp-nlp.github.io/), a toolkit focusing on multi-task learning for NLP. More information can be found in the paper (which should be released when the SemEval proceedings are online). |
tkell/tracklist-artist-to-vec | tkell | "2023-10-15T00:16:02Z" | 0 | 0 | pytorch | [
"pytorch",
"music",
"dj-sets",
"word2vec",
"license:cc-by-nc-nd-4.0",
"region:us"
] | null | "2023-10-13T18:15:02Z" | ---
license: "cc-by-nc-nd-4.0"
library_name: "pytorch"
tags:
- music
- dj-sets
- word2vec
---
# Tracklist To Vec Model Card
A tiny experiment to make a "music recommender", from my own DJ set tracklists.
|
mrHungddddh/83aec787-eee3-4268-ab54-799071a9c610 | mrHungddddh | "2025-01-23T05:39:00Z" | 9 | 0 | peft | [
"peft",
"safetensors",
"qwen2",
"axolotl",
"generated_from_trainer",
"base_model:unsloth/Qwen2.5-14B-Instruct",
"base_model:adapter:unsloth/Qwen2.5-14B-Instruct",
"license:apache-2.0",
"8-bit",
"bitsandbytes",
"region:us"
] | null | "2025-01-23T03:46:33Z" | ---
library_name: peft
license: apache-2.0
base_model: unsloth/Qwen2.5-14B-Instruct
tags:
- axolotl
- generated_from_trainer
model-index:
- name: 83aec787-eee3-4268-ab54-799071a9c610
results: []
---
<!-- This model card has been generated automatically according to the information the Trainer had access to. You
should probably proofread and complete it, then remove this comment. -->
[<img src="https://raw.githubusercontent.com/axolotl-ai-cloud/axolotl/main/image/axolotl-badge-web.png" alt="Built with Axolotl" width="200" height="32"/>](https://github.com/axolotl-ai-cloud/axolotl)
<details><summary>See axolotl config</summary>
axolotl version: `0.4.1`
```yaml
adapter: lora
base_model: unsloth/Qwen2.5-14B-Instruct
bf16: auto
chat_template: llama3
dataset_prepared_path: null
datasets:
- data_files:
- 466324cc3cdc8c11_train_data.json
ds_type: json
format: custom
path: /workspace/input_data/466324cc3cdc8c11_train_data.json
type:
field_instruction: instruction
field_output: response
format: '{instruction}'
no_input_format: '{instruction}'
system_format: '{system}'
system_prompt: ''
debug: null
deepspeed: null
early_stopping_patience: null
eval_max_new_tokens: 128
eval_table_size: null
evals_per_epoch: 1
flash_attention: true
fp16: null
fsdp: null
fsdp_config: null
gradient_accumulation_steps: 4
gradient_checkpointing: true
gradient_clipping: 1.0
group_by_length: false
hub_model_id: mrHungddddh/83aec787-eee3-4268-ab54-799071a9c610
hub_repo: null
hub_strategy: end
hub_token: null
learning_rate: 5.0e-05
load_in_4bit: true
load_in_8bit: true
local_rank: null
logging_steps: 1
lora_alpha: 16
lora_dropout: 0.05
lora_fan_in_fan_out: null
lora_model_dir: null
lora_r: 8
lora_target_linear: true
lr_scheduler: cosine
max_steps: 200
micro_batch_size: 2
mlflow_experiment_name: /tmp/466324cc3cdc8c11_train_data.json
model_type: AutoModelForCausalLM
num_epochs: 1
optimizer: adamw_bnb_8bit
output_dir: miner_id_24
pad_to_sequence_len: true
resume_from_checkpoint: null
s2_attention: null
sample_packing: false
saves_per_epoch: 1
sequence_len: 1024
strict: false
tf32: false
tokenizer_type: AutoTokenizer
train_on_inputs: false
trust_remote_code: true
val_set_size: 0.05
wandb_entity: null
wandb_mode: online
wandb_name: 676d9f91-4116-4f6e-8ff1-694522a1ba61
wandb_project: Gradients-On-Demand
wandb_run: your_name
wandb_runid: 676d9f91-4116-4f6e-8ff1-694522a1ba61
warmup_steps: 5
weight_decay: 0.01
xformers_attention: true
```
</details><br>
# 83aec787-eee3-4268-ab54-799071a9c610
This model is a fine-tuned version of [unsloth/Qwen2.5-14B-Instruct](https://huggingface.co/unsloth/Qwen2.5-14B-Instruct) on the None dataset.
It achieves the following results on the evaluation set:
- Loss: 0.2868
## Model description
More information needed
## Intended uses & limitations
More information needed
## Training and evaluation data
More information needed
## Training procedure
### Training hyperparameters
The following hyperparameters were used during training:
- learning_rate: 5e-05
- train_batch_size: 2
- eval_batch_size: 2
- seed: 42
- gradient_accumulation_steps: 4
- total_train_batch_size: 8
- optimizer: Use OptimizerNames.ADAMW_BNB with betas=(0.9,0.999) and epsilon=1e-08 and optimizer_args=No additional optimizer arguments
- lr_scheduler_type: cosine
- lr_scheduler_warmup_steps: 5
- training_steps: 200
### Training results
| Training Loss | Epoch | Step | Validation Loss |
|:-------------:|:------:|:----:|:---------------:|
| 0.2811 | 0.0112 | 200 | 0.2868 |
### Framework versions
- PEFT 0.13.2
- Transformers 4.46.0
- Pytorch 2.5.0+cu124
- Datasets 3.0.1
- Tokenizers 0.20.1 |
amazingvince/zephyr-1.1b-sft-full | amazingvince | "2023-11-20T12:34:07Z" | 9 | 0 | transformers | [
"transformers",
"tensorboard",
"safetensors",
"llama",
"text-generation",
"generated_from_trainer",
"conversational",
"base_model:TinyLlama/TinyLlama-1.1B-intermediate-step-955k-token-2T",
"base_model:finetune:TinyLlama/TinyLlama-1.1B-intermediate-step-955k-token-2T",
"license:apache-2.0",
"autotrain_compatible",
"text-generation-inference",
"endpoints_compatible",
"region:us"
] | text-generation | "2023-11-20T00:28:59Z" | ---
license: apache-2.0
base_model: TinyLlama/TinyLlama-1.1B-intermediate-step-955k-token-2T
tags:
- generated_from_trainer
model-index:
- name: zephyr-1.1b-sft-full
results: []
---
<!-- This model card has been generated automatically according to the information the Trainer had access to. You
should probably proofread and complete it, then remove this comment. -->
# zephyr-1.1b-sft-full
This model is a fine-tuned version of [TinyLlama/TinyLlama-1.1B-intermediate-step-955k-token-2T](https://huggingface.co/TinyLlama/TinyLlama-1.1B-intermediate-step-955k-token-2T) on an unknown dataset.
It achieves the following results on the evaluation set:
- Loss: 1.1737
## Model description
More information needed
## Intended uses & limitations
More information needed
## Training and evaluation data
More information needed
## Training procedure
### Training hyperparameters
The following hyperparameters were used during training:
- learning_rate: 2e-05
- train_batch_size: 2
- eval_batch_size: 2
- seed: 42
- distributed_type: multi-GPU
- num_devices: 2
- gradient_accumulation_steps: 16
- total_train_batch_size: 64
- total_eval_batch_size: 4
- optimizer: Adam with betas=(0.9,0.999) and epsilon=1e-08
- lr_scheduler_type: cosine
- num_epochs: 1
### Training results
| Training Loss | Epoch | Step | Validation Loss |
|:-------------:|:-----:|:----:|:---------------:|
| 1.1826 | 0.7 | 2282 | 1.1737 |
### Framework versions
- Transformers 4.35.0
- Pytorch 2.1.0
- Datasets 2.14.6
- Tokenizers 0.14.1
|
aleegis09/416da52e-0f72-41ac-a986-88724bec60ad | aleegis09 | "2025-01-17T10:35:47Z" | 8 | 0 | peft | [
"peft",
"safetensors",
"llama",
"axolotl",
"generated_from_trainer",
"base_model:aisingapore/llama3-8b-cpt-sea-lionv2.1-instruct",
"base_model:adapter:aisingapore/llama3-8b-cpt-sea-lionv2.1-instruct",
"license:llama3",
"region:us"
] | null | "2025-01-17T09:51:48Z" | ---
library_name: peft
license: llama3
base_model: aisingapore/llama3-8b-cpt-sea-lionv2.1-instruct
tags:
- axolotl
- generated_from_trainer
model-index:
- name: 416da52e-0f72-41ac-a986-88724bec60ad
results: []
---
<!-- This model card has been generated automatically according to the information the Trainer had access to. You
should probably proofread and complete it, then remove this comment. -->
[<img src="https://raw.githubusercontent.com/axolotl-ai-cloud/axolotl/main/image/axolotl-badge-web.png" alt="Built with Axolotl" width="200" height="32"/>](https://github.com/axolotl-ai-cloud/axolotl)
<details><summary>See axolotl config</summary>
axolotl version: `0.4.1`
```yaml
adapter: lora
base_model: aisingapore/llama3-8b-cpt-sea-lionv2.1-instruct
bf16: true
chat_template: llama3
data_processes: 16
dataset_prepared_path: null
datasets:
- data_files:
- 2629a3b7d033d187_train_data.json
ds_type: json
format: custom
path: /workspace/input_data/2629a3b7d033d187_train_data.json
type:
field_instruction: instruction
field_output: output
format: '{instruction}'
no_input_format: '{instruction}'
system_format: '{system}'
system_prompt: ''
debug: null
deepspeed: null
device_map: auto
do_eval: true
early_stopping_patience: 5
eval_batch_size: 4
eval_max_new_tokens: 128
eval_steps: 50
eval_table_size: null
evals_per_epoch: null
flash_attention: true
fp16: false
fsdp: null
fsdp_config: null
gradient_accumulation_steps: 4
gradient_checkpointing: true
group_by_length: true
hub_model_id: aleegis09/416da52e-0f72-41ac-a986-88724bec60ad
hub_repo: null
hub_strategy: checkpoint
hub_token: null
learning_rate: 0.0001
load_in_4bit: false
load_in_8bit: false
local_rank: null
logging_steps: 1
lora_alpha: 128
lora_dropout: 0.05
lora_fan_in_fan_out: null
lora_model_dir: null
lora_r: 64
lora_target_linear: true
lr_scheduler: cosine
max_grad_norm: 1.0
max_memory:
0: 75GB
max_steps: 200
micro_batch_size: 8
mlflow_experiment_name: /tmp/2629a3b7d033d187_train_data.json
model_type: AutoModelForCausalLM
num_epochs: 3
optim_args:
adam_beta1: 0.9
adam_beta2: 0.95
adam_epsilon: 1e-5
optimizer: adamw_bnb_8bit
output_dir: miner_id_24
pad_to_sequence_len: true
resume_from_checkpoint: null
s2_attention: null
sample_packing: false
save_steps: 50
saves_per_epoch: null
sequence_len: 1024
strict: false
tf32: true
tokenizer_type: AutoTokenizer
train_on_inputs: false
trust_remote_code: true
val_set_size: 0.05
wandb_entity: null
wandb_mode: online
wandb_name: 82a3b5ec-1777-4ce7-8be6-809a9fd5fcc7
wandb_project: Gradients-On-Demand
wandb_run: your_name
wandb_runid: 82a3b5ec-1777-4ce7-8be6-809a9fd5fcc7
warmup_steps: 10
weight_decay: 0.0
xformers_attention: null
```
</details><br>
# 416da52e-0f72-41ac-a986-88724bec60ad
This model is a fine-tuned version of [aisingapore/llama3-8b-cpt-sea-lionv2.1-instruct](https://huggingface.co/aisingapore/llama3-8b-cpt-sea-lionv2.1-instruct) on the None dataset.
It achieves the following results on the evaluation set:
- Loss: 1.5283
## Model description
More information needed
## Intended uses & limitations
More information needed
## Training and evaluation data
More information needed
## Training procedure
### Training hyperparameters
The following hyperparameters were used during training:
- learning_rate: 0.0001
- train_batch_size: 8
- eval_batch_size: 4
- seed: 42
- gradient_accumulation_steps: 4
- total_train_batch_size: 32
- optimizer: Use OptimizerNames.ADAMW_BNB with betas=(0.9,0.999) and epsilon=1e-08 and optimizer_args=adam_beta1=0.9,adam_beta2=0.95,adam_epsilon=1e-5
- lr_scheduler_type: cosine
- lr_scheduler_warmup_steps: 10
- training_steps: 200
### Training results
| Training Loss | Epoch | Step | Validation Loss |
|:-------------:|:------:|:----:|:---------------:|
| 1.052 | 0.0007 | 1 | 2.3292 |
| 2.4944 | 0.0327 | 50 | 1.8727 |
| 2.0789 | 0.0653 | 100 | 1.7419 |
| 2.465 | 0.0980 | 150 | 1.5674 |
| 2.453 | 0.1307 | 200 | 1.5283 |
### Framework versions
- PEFT 0.13.2
- Transformers 4.46.0
- Pytorch 2.5.0+cu124
- Datasets 3.0.1
- Tokenizers 0.20.1 |
Gourishreeka/my-pet-cat | Gourishreeka | "2023-11-05T17:55:05Z" | 1 | 0 | diffusers | [
"diffusers",
"NxtWave-GenAI-Webinar",
"text-to-image",
"stable-diffusion",
"license:creativeml-openrail-m",
"autotrain_compatible",
"endpoints_compatible",
"diffusers:StableDiffusionPipeline",
"region:us"
] | text-to-image | "2023-11-05T17:51:53Z" | ---
license: creativeml-openrail-m
tags:
- NxtWave-GenAI-Webinar
- text-to-image
- stable-diffusion
---
### My-Pet-Cat Dreambooth model trained by Gourishreeka following the "Build your own Gen AI model" session by NxtWave.
Project Submission Code: -MRCEW-312
Sample pictures of this concept:
.jpg)
|
zhang19991111/scincl-spanmarker-STEM-NER | zhang19991111 | "2024-01-22T02:15:07Z" | 6 | 0 | span-marker | [
"span-marker",
"safetensors",
"token-classification",
"ner",
"named-entity-recognition",
"generated_from_span_marker_trainer",
"en",
"base_model:malteos/scincl",
"base_model:finetune:malteos/scincl",
"license:cc-by-sa-4.0",
"model-index",
"region:us"
] | token-classification | "2024-01-22T02:13:16Z" | ---
language: en
license: cc-by-sa-4.0
library_name: span-marker
tags:
- span-marker
- token-classification
- ner
- named-entity-recognition
- generated_from_span_marker_trainer
metrics:
- precision
- recall
- f1
widget:
- text: Altitude measurements based on near - IR imaging in H and Hcont filters showed
that the deeper BS2 clouds were located near the methane condensation level (
≈1.2bars ) , while BS1 was generally ∼500 mb above that level ( at lower pressures
) .
- text: However , our model predicts different performance for large enough memory
- access latency and validates the intuition that the dynamic programming algorithm
performs better on these machines .
- text: We established a P fertilizer need map based on integrating results from the
two systems .
- text: Here , we have addressed this limitation for the endodermal lineage by developing
a defined culture system to expand and differentiate human foregut stem cells
( hFSCs ) derived from hPSCs . hFSCs can self - renew while maintaining their
capacity to differentiate into pancreatic and hepatic cells .
- text: The accumulated percentage gain from selection amounted to 51%/1 % lower Striga
infestation ( measured by area under Striga number progress curve , ASNPC ) ,
46%/62 % lower downy mildew incidence , and 49%/31 % higher panicle yield of the
C5 - FS compared to the mean of the genepool parents at Sadoré / Cinzana , respectively
.
pipeline_tag: token-classification
base_model: malteos/scincl
model-index:
- name: SpanMarker with malteos/scincl on my-data
results:
- task:
type: token-classification
name: Named Entity Recognition
dataset:
name: my-data
type: unknown
split: test
metrics:
- type: f1
value: 0.7043189368770764
name: F1
- type: precision
value: 0.7198641765704584
name: Precision
- type: recall
value: 0.6894308943089431
name: Recall
---
# SpanMarker with malteos/scincl on my-data
This is a [SpanMarker](https://github.com/tomaarsen/SpanMarkerNER) model that can be used for Named Entity Recognition. This SpanMarker model uses [malteos/scincl](https://huggingface.co/malteos/scincl) as the underlying encoder.
## Model Details
### Model Description
- **Model Type:** SpanMarker
- **Encoder:** [malteos/scincl](https://huggingface.co/malteos/scincl)
- **Maximum Sequence Length:** 256 tokens
- **Maximum Entity Length:** 8 words
<!-- - **Training Dataset:** [Unknown](https://huggingface.co/datasets/unknown) -->
- **Language:** en
- **License:** cc-by-sa-4.0
### Model Sources
- **Repository:** [SpanMarker on GitHub](https://github.com/tomaarsen/SpanMarkerNER)
- **Thesis:** [SpanMarker For Named Entity Recognition](https://raw.githubusercontent.com/tomaarsen/SpanMarkerNER/main/thesis.pdf)
### Model Labels
| Label | Examples |
|:---------|:--------------------------------------------------------------------------------------------------------|
| Data | "an overall mitochondrial", "defect", "Depth time - series" |
| Material | "cross - shore measurement locations", "the subject 's fibroblasts", "COXI , COXII and COXIII subunits" |
| Method | "EFSA", "an approximation", "in vitro" |
| Process | "translation", "intake", "a significant reduction of synthesis" |
## Evaluation
### Metrics
| Label | Precision | Recall | F1 |
|:---------|:----------|:-------|:-------|
| **all** | 0.7199 | 0.6894 | 0.7043 |
| Data | 0.6224 | 0.6455 | 0.6338 |
| Material | 0.8061 | 0.7861 | 0.7960 |
| Method | 0.5789 | 0.55 | 0.5641 |
| Process | 0.7472 | 0.6488 | 0.6945 |
## Uses
### Direct Use for Inference
```python
from span_marker import SpanMarkerModel
# Download from the 🤗 Hub
model = SpanMarkerModel.from_pretrained("span-marker-malteos/scincl-me")
# Run inference
entities = model.predict("We established a P fertilizer need map based on integrating results from the two systems .")
```
### Downstream Use
You can finetune this model on your own dataset.
<details><summary>Click to expand</summary>
```python
from span_marker import SpanMarkerModel, Trainer
# Download from the 🤗 Hub
model = SpanMarkerModel.from_pretrained("span-marker-malteos/scincl-me")
# Specify a Dataset with "tokens" and "ner_tag" columns
dataset = load_dataset("conll2003") # For example CoNLL2003
# Initialize a Trainer using the pretrained model & dataset
trainer = Trainer(
model=model,
train_dataset=dataset["train"],
eval_dataset=dataset["validation"],
)
trainer.train()
trainer.save_model("span-marker-malteos/scincl-me-finetuned")
```
</details>
<!--
### Out-of-Scope Use
*List how the model may foreseeably be misused and address what users ought not to do with the model.*
-->
<!--
## Bias, Risks and Limitations
*What are the known or foreseeable issues stemming from this model? You could also flag here known failure cases or weaknesses of the model.*
-->
<!--
### Recommendations
*What are recommendations with respect to the foreseeable issues? For example, filtering explicit content.*
-->
## Training Details
### Training Set Metrics
| Training set | Min | Median | Max |
|:----------------------|:----|:--------|:----|
| Sentence length | 3 | 25.6049 | 106 |
| Entities per sentence | 0 | 5.2439 | 22 |
### Training Hyperparameters
- learning_rate: 5e-05
- train_batch_size: 8
- eval_batch_size: 8
- seed: 42
- optimizer: Adam with betas=(0.9,0.999) and epsilon=1e-08
- lr_scheduler_type: linear
- lr_scheduler_warmup_ratio: 0.1
- num_epochs: 10
### Framework Versions
- Python: 3.10.12
- SpanMarker: 1.5.0
- Transformers: 4.36.2
- PyTorch: 2.0.1+cu118
- Datasets: 2.16.1
- Tokenizers: 0.15.0
## Citation
### BibTeX
```
@software{Aarsen_SpanMarker,
author = {Aarsen, Tom},
license = {Apache-2.0},
title = {{SpanMarker for Named Entity Recognition}},
url = {https://github.com/tomaarsen/SpanMarkerNER}
}
```
<!--
## Glossary
*Clearly define terms in order to be accessible across audiences.*
-->
<!--
## Model Card Authors
*Lists the people who create the model card, providing recognition and accountability for the detailed work that goes into its construction.*
-->
<!--
## Model Card Contact
*Provides a way for people who have updates to the Model Card, suggestions, or questions, to contact the Model Card authors.*
--> |
muhtasham/small-mlm-tweet_eval-from-scratch-target-conll2003 | muhtasham | "2023-01-23T03:55:08Z" | 3 | 0 | transformers | [
"transformers",
"pytorch",
"bert",
"token-classification",
"generated_from_trainer",
"autotrain_compatible",
"endpoints_compatible",
"region:us"
] | token-classification | "2023-01-23T03:47:34Z" | ---
tags:
- generated_from_trainer
metrics:
- precision
- recall
- f1
- accuracy
model-index:
- name: small-mlm-tweet_eval-from-scratch-target-conll2003
results: []
---
<!-- This model card has been generated automatically according to the information the Trainer had access to. You
should probably proofread and complete it, then remove this comment. -->
# small-mlm-tweet_eval-from-scratch-target-conll2003
This model is a fine-tuned version of [muhtasham/small-mlm-tweet_eval-from-scratch](https://huggingface.co/muhtasham/small-mlm-tweet_eval-from-scratch) on the None dataset.
It achieves the following results on the evaluation set:
- Loss: 0.2818
- Precision: 0.6288
- Recall: 0.7415
- F1: 0.6805
- Accuracy: 0.9349
## Model description
More information needed
## Intended uses & limitations
More information needed
## Training and evaluation data
More information needed
## Training procedure
### Training hyperparameters
The following hyperparameters were used during training:
- learning_rate: 3e-05
- train_batch_size: 32
- eval_batch_size: 32
- seed: 42
- optimizer: Adam with betas=(0.9,0.999) and epsilon=1e-08
- lr_scheduler_type: constant
- training_steps: 5000
### Training results
| Training Loss | Epoch | Step | Validation Loss | Precision | Recall | F1 | Accuracy |
|:-------------:|:-----:|:----:|:---------------:|:---------:|:------:|:------:|:--------:|
| 0.6126 | 1.14 | 500 | 0.4262 | 0.3594 | 0.4480 | 0.3988 | 0.8717 |
| 0.3588 | 2.28 | 1000 | 0.3324 | 0.4554 | 0.5830 | 0.5113 | 0.8995 |
| 0.2618 | 3.42 | 1500 | 0.3179 | 0.4530 | 0.6437 | 0.5318 | 0.8997 |
| 0.204 | 4.56 | 2000 | 0.2764 | 0.5381 | 0.6424 | 0.5857 | 0.9177 |
| 0.162 | 5.69 | 2500 | 0.2695 | 0.5752 | 0.6658 | 0.6172 | 0.9244 |
| 0.13 | 6.83 | 3000 | 0.2556 | 0.5682 | 0.7035 | 0.6287 | 0.9260 |
| 0.1027 | 7.97 | 3500 | 0.2557 | 0.5946 | 0.7220 | 0.6521 | 0.9294 |
| 0.0811 | 9.11 | 4000 | 0.2638 | 0.6097 | 0.7154 | 0.6584 | 0.9325 |
| 0.0648 | 10.25 | 4500 | 0.2745 | 0.6105 | 0.7324 | 0.6659 | 0.9325 |
| 0.0533 | 11.39 | 5000 | 0.2818 | 0.6288 | 0.7415 | 0.6805 | 0.9349 |
### Framework versions
- Transformers 4.26.0.dev0
- Pytorch 1.13.1+cu116
- Datasets 2.8.1.dev0
- Tokenizers 0.13.2
|
itlwas/Llama-3-8B-Lexi-Uncensored-Q4_K_M-GGUF | itlwas | "2024-12-29T13:59:21Z" | 22 | 0 | null | [
"gguf",
"uncensored",
"llama3",
"instruct",
"open",
"llama-cpp",
"gguf-my-repo",
"base_model:Orenguteng/Llama-3-8B-Lexi-Uncensored",
"base_model:quantized:Orenguteng/Llama-3-8B-Lexi-Uncensored",
"license:llama3",
"model-index",
"endpoints_compatible",
"region:us",
"conversational"
] | null | "2024-12-29T13:58:59Z" | ---
license: llama3
tags:
- uncensored
- llama3
- instruct
- open
- llama-cpp
- gguf-my-repo
base_model: Orenguteng/Llama-3-8B-Lexi-Uncensored
model-index:
- name: Llama-3-8B-Lexi-Uncensored
results:
- task:
type: text-generation
name: Text Generation
dataset:
name: AI2 Reasoning Challenge (25-Shot)
type: ai2_arc
config: ARC-Challenge
split: test
args:
num_few_shot: 25
metrics:
- type: acc_norm
value: 59.56
name: normalized accuracy
source:
url: https://huggingface.co/spaces/HuggingFaceH4/open_llm_leaderboard?query=Orenguteng/Llama-3-8B-Lexi-Uncensored
name: Open LLM Leaderboard
- task:
type: text-generation
name: Text Generation
dataset:
name: HellaSwag (10-Shot)
type: hellaswag
split: validation
args:
num_few_shot: 10
metrics:
- type: acc_norm
value: 77.88
name: normalized accuracy
source:
url: https://huggingface.co/spaces/HuggingFaceH4/open_llm_leaderboard?query=Orenguteng/Llama-3-8B-Lexi-Uncensored
name: Open LLM Leaderboard
- task:
type: text-generation
name: Text Generation
dataset:
name: MMLU (5-Shot)
type: cais/mmlu
config: all
split: test
args:
num_few_shot: 5
metrics:
- type: acc
value: 67.68
name: accuracy
source:
url: https://huggingface.co/spaces/HuggingFaceH4/open_llm_leaderboard?query=Orenguteng/Llama-3-8B-Lexi-Uncensored
name: Open LLM Leaderboard
- task:
type: text-generation
name: Text Generation
dataset:
name: TruthfulQA (0-shot)
type: truthful_qa
config: multiple_choice
split: validation
args:
num_few_shot: 0
metrics:
- type: mc2
value: 47.72
source:
url: https://huggingface.co/spaces/HuggingFaceH4/open_llm_leaderboard?query=Orenguteng/Llama-3-8B-Lexi-Uncensored
name: Open LLM Leaderboard
- task:
type: text-generation
name: Text Generation
dataset:
name: Winogrande (5-shot)
type: winogrande
config: winogrande_xl
split: validation
args:
num_few_shot: 5
metrics:
- type: acc
value: 75.85
name: accuracy
source:
url: https://huggingface.co/spaces/HuggingFaceH4/open_llm_leaderboard?query=Orenguteng/Llama-3-8B-Lexi-Uncensored
name: Open LLM Leaderboard
- task:
type: text-generation
name: Text Generation
dataset:
name: GSM8k (5-shot)
type: gsm8k
config: main
split: test
args:
num_few_shot: 5
metrics:
- type: acc
value: 68.39
name: accuracy
source:
url: https://huggingface.co/spaces/HuggingFaceH4/open_llm_leaderboard?query=Orenguteng/Llama-3-8B-Lexi-Uncensored
name: Open LLM Leaderboard
---
# itlwas/Llama-3-8B-Lexi-Uncensored-Q4_K_M-GGUF
This model was converted to GGUF format from [`Orenguteng/Llama-3-8B-Lexi-Uncensored`](https://huggingface.co/Orenguteng/Llama-3-8B-Lexi-Uncensored) using llama.cpp via the ggml.ai's [GGUF-my-repo](https://huggingface.co/spaces/ggml-org/gguf-my-repo) space.
Refer to the [original model card](https://huggingface.co/Orenguteng/Llama-3-8B-Lexi-Uncensored) for more details on the model.
## Use with llama.cpp
Install llama.cpp through brew (works on Mac and Linux)
```bash
brew install llama.cpp
```
Invoke the llama.cpp server or the CLI.
### CLI:
```bash
llama-cli --hf-repo itlwas/Llama-3-8B-Lexi-Uncensored-Q4_K_M-GGUF --hf-file llama-3-8b-lexi-uncensored-q4_k_m.gguf -p "The meaning to life and the universe is"
```
### Server:
```bash
llama-server --hf-repo itlwas/Llama-3-8B-Lexi-Uncensored-Q4_K_M-GGUF --hf-file llama-3-8b-lexi-uncensored-q4_k_m.gguf -c 2048
```
Note: You can also use this checkpoint directly through the [usage steps](https://github.com/ggerganov/llama.cpp?tab=readme-ov-file#usage) listed in the Llama.cpp repo as well.
Step 1: Clone llama.cpp from GitHub.
```
git clone https://github.com/ggerganov/llama.cpp
```
Step 2: Move into the llama.cpp folder and build it with `LLAMA_CURL=1` flag along with other hardware-specific flags (for ex: LLAMA_CUDA=1 for Nvidia GPUs on Linux).
```
cd llama.cpp && LLAMA_CURL=1 make
```
Step 3: Run inference through the main binary.
```
./llama-cli --hf-repo itlwas/Llama-3-8B-Lexi-Uncensored-Q4_K_M-GGUF --hf-file llama-3-8b-lexi-uncensored-q4_k_m.gguf -p "The meaning to life and the universe is"
```
or
```
./llama-server --hf-repo itlwas/Llama-3-8B-Lexi-Uncensored-Q4_K_M-GGUF --hf-file llama-3-8b-lexi-uncensored-q4_k_m.gguf -c 2048
```
|
monadical-labs/minecraft-skin-generator-sdxl | monadical-labs | "2024-07-22T19:37:26Z" | 19,064 | 10 | diffusers | [
"diffusers",
"safetensors",
"minecraft",
"skins",
"gaming",
"stable diffusion",
"stable diffusion xl",
"text-to-image",
"en",
"license:openrail",
"autotrain_compatible",
"endpoints_compatible",
"diffusers:StableDiffusionXLPipeline",
"region:us"
] | text-to-image | "2024-02-19T15:38:21Z" | ---
license: openrail
language:
- en
library_name: diffusers
tags:
- minecraft
- skins
- gaming
- stable diffusion
- stable diffusion xl
pipeline_tag: text-to-image
---
# Minecraft Skin Generator XL
Monadical is pleased to announce the official release of the Minecraft Skin Generator XL model. We had previously released the [Minecraft Skin Generator](https://huggingface.co/monadical-labs/minecraft-skin-generator) model based upon Stable Diffusion 2. This new model offers significant improvements over the last generation of models.
### Key Features
1. **Upgrade to Stable Diffusion XL** - Our model is now based upon the Stable Diffusion XL model, which greatly improves the quality of generated skins when compared to previous models.
1. **Transparent Layer Support** - The new model now supports the transparency layer in the hair and helmet section of the skin.
### Examples
* 'Kelly Kapoor from the TV show "The Office"'

* 'Saul Goodman from the TV show "Better Call Saul"'

* 'Gustavo Fring from the TV show "Breaking Bad"'

* 'Daryl Dixon from the TV show "The Walking Dead"'

* 'Zach Galifianakis as Alan in the movie "The Hangover"'

### Try It Out Yourself
There are several options for trying out this new model:
1. Download the model and run it locally on your machine. Note that we recommend a GPU for this - while it is possible to run on a CPU, we do not currently support this method. **Note**: Output from the StableDiffusionXL pipeline should be constrained to 768x768 pixels, or the model will automatically generate a 1024x1024 output image, and fill in the extra space with unusuable garbage.
1. Try our hosted version of the model on the [Minecraft Skin Generator website](https://www.skingenerator.io).
### Get Involved
Have any feedback or suggestions? Join us on our [Minecraft Skin Generator Discord channel](https://discord.com/invite/yMzFzVUPDf) or send us an [email](mailto:[email protected]).
Happy crafting!
[The Monadical Minecraft Skin Generator Team](https://monadical.com/) |
gargeya2003/detr-layers-updated1 | gargeya2003 | "2024-10-16T15:38:57Z" | 190 | 0 | transformers | [
"transformers",
"pytorch",
"detr",
"object-detection",
"arxiv:1910.09700",
"endpoints_compatible",
"region:us"
] | object-detection | "2024-10-15T16:43:56Z" | ---
library_name: transformers
tags: []
---
# Model Card for Model ID
<!-- Provide a quick summary of what the model is/does. -->
## Model Details
### Model Description
<!-- Provide a longer summary of what this model is. -->
This is the model card of a 🤗 transformers model that has been pushed on the Hub. This model card has been automatically generated.
- **Developed by:** [More Information Needed]
- **Funded by [optional]:** [More Information Needed]
- **Shared by [optional]:** [More Information Needed]
- **Model type:** [More Information Needed]
- **Language(s) (NLP):** [More Information Needed]
- **License:** [More Information Needed]
- **Finetuned from model [optional]:** [More Information Needed]
### Model Sources [optional]
<!-- Provide the basic links for the model. -->
- **Repository:** [More Information Needed]
- **Paper [optional]:** [More Information Needed]
- **Demo [optional]:** [More Information Needed]
## Uses
<!-- Address questions around how the model is intended to be used, including the foreseeable users of the model and those affected by the model. -->
### Direct Use
<!-- This section is for the model use without fine-tuning or plugging into a larger ecosystem/app. -->
[More Information Needed]
### Downstream Use [optional]
<!-- This section is for the model use when fine-tuned for a task, or when plugged into a larger ecosystem/app -->
[More Information Needed]
### Out-of-Scope Use
<!-- This section addresses misuse, malicious use, and uses that the model will not work well for. -->
[More Information Needed]
## Bias, Risks, and Limitations
<!-- This section is meant to convey both technical and sociotechnical limitations. -->
[More Information Needed]
### Recommendations
<!-- This section is meant to convey recommendations with respect to the bias, risk, and technical limitations. -->
Users (both direct and downstream) should be made aware of the risks, biases and limitations of the model. More information needed for further recommendations.
## How to Get Started with the Model
Use the code below to get started with the model.
[More Information Needed]
## Training Details
### Training Data
<!-- This should link to a Dataset Card, perhaps with a short stub of information on what the training data is all about as well as documentation related to data pre-processing or additional filtering. -->
[More Information Needed]
### Training Procedure
<!-- This relates heavily to the Technical Specifications. Content here should link to that section when it is relevant to the training procedure. -->
#### Preprocessing [optional]
[More Information Needed]
#### Training Hyperparameters
- **Training regime:** [More Information Needed] <!--fp32, fp16 mixed precision, bf16 mixed precision, bf16 non-mixed precision, fp16 non-mixed precision, fp8 mixed precision -->
#### Speeds, Sizes, Times [optional]
<!-- This section provides information about throughput, start/end time, checkpoint size if relevant, etc. -->
[More Information Needed]
## Evaluation
<!-- This section describes the evaluation protocols and provides the results. -->
### Testing Data, Factors & Metrics
#### Testing Data
<!-- This should link to a Dataset Card if possible. -->
[More Information Needed]
#### Factors
<!-- These are the things the evaluation is disaggregating by, e.g., subpopulations or domains. -->
[More Information Needed]
#### Metrics
<!-- These are the evaluation metrics being used, ideally with a description of why. -->
[More Information Needed]
### Results
[More Information Needed]
#### Summary
## Model Examination [optional]
<!-- Relevant interpretability work for the model goes here -->
[More Information Needed]
## Environmental Impact
<!-- Total emissions (in grams of CO2eq) and additional considerations, such as electricity usage, go here. Edit the suggested text below accordingly -->
Carbon emissions can be estimated using the [Machine Learning Impact calculator](https://mlco2.github.io/impact#compute) presented in [Lacoste et al. (2019)](https://arxiv.org/abs/1910.09700).
- **Hardware Type:** [More Information Needed]
- **Hours used:** [More Information Needed]
- **Cloud Provider:** [More Information Needed]
- **Compute Region:** [More Information Needed]
- **Carbon Emitted:** [More Information Needed]
## Technical Specifications [optional]
### Model Architecture and Objective
[More Information Needed]
### Compute Infrastructure
[More Information Needed]
#### Hardware
[More Information Needed]
#### Software
[More Information Needed]
## Citation [optional]
<!-- If there is a paper or blog post introducing the model, the APA and Bibtex information for that should go in this section. -->
**BibTeX:**
[More Information Needed]
**APA:**
[More Information Needed]
## Glossary [optional]
<!-- If relevant, include terms and calculations in this section that can help readers understand the model or model card. -->
[More Information Needed]
## More Information [optional]
[More Information Needed]
## Model Card Authors [optional]
[More Information Needed]
## Model Card Contact
[More Information Needed] |
Graphcore/t5-small-ipu | Graphcore | "2023-07-07T11:04:23Z" | 4 | 1 | null | [
"optimum_graphcore",
"arxiv:1910.10683",
"license:apache-2.0",
"region:us"
] | null | "2022-03-02T23:29:04Z" | ---
license: apache-2.0
---
# Graphcore/t5-small-ipu
Optimum Graphcore is a new open-source library and toolkit that enables developers to access IPU-optimized models certified by Hugging Face. It is an extension of Transformers, providing a set of performance optimization tools enabling maximum efficiency to train and run models on Graphcore’s IPUs - a completely new kind of massively parallel processor to accelerate machine intelligence. Learn more about how to take train Transformer models faster with IPUs at [hf.co/hardware/graphcore](https://huggingface.co/hardware/graphcore).
Through HuggingFace Optimum, Graphcore released ready-to-use IPU-trained model checkpoints and IPU configuration files to make it easy to train models with maximum efficiency in the IPU. Optimum shortens the development lifecycle of your AI models by letting you plug-and-play any public dataset and allows a seamless integration to our State-of-the-art hardware giving you a quicker time-to-value for your AI project.
## Model description
Text-to-Text Transfer Transformer (T5), is a Transformer based model that uses a text-to-text approach for translation, question answering, and classification. It introduces an unified framework that converts all text-based language problems into a text-to-text format for transfer learning for NLP. This allows for the use of the same model, loss function, hyperparameters, etc. across our diverse set of tasks.
Paper link :[Exploring the Limits of Transfer Learning with a Unified
Text-to-Text Transformer](https://arxiv.org/pdf/1910.10683.pdf)
## Intended uses & limitations
This model contains just the `IPUConfig` files for running the T5 Small model (e.g. [HuggingFace/t5-small](https://huggingface.co/t5-small)) on Graphcore IPUs.
**This model contains no model weights, only an IPUConfig.**
## Usage
```
from optimum.graphcore import IPUConfig
ipu_config = IPUConfig.from_pretrained("Graphcore/t5-small-ipu")
``` |
John6666/knk-helio-blend-illustrious-v01-v20-sdxl | John6666 | "2024-12-23T06:35:03Z" | 286 | 0 | diffusers | [
"diffusers",
"safetensors",
"text-to-image",
"stable-diffusion",
"stable-diffusion-xl",
"anime",
"characters",
"lips",
"eyes",
"illustrious",
"en",
"base_model:OnomaAIResearch/Illustrious-xl-early-release-v0",
"base_model:finetune:OnomaAIResearch/Illustrious-xl-early-release-v0",
"license:other",
"autotrain_compatible",
"endpoints_compatible",
"diffusers:StableDiffusionXLPipeline",
"region:us"
] | text-to-image | "2024-10-06T03:24:58Z" | ---
license: other
license_name: faipl-1.0-sd
license_link: https://freedevproject.org/faipl-1.0-sd/
language:
- en
library_name: diffusers
pipeline_tag: text-to-image
tags:
- text-to-image
- stable-diffusion
- stable-diffusion-xl
- anime
- characters
- lips
- eyes
- illustrious
base_model: OnomaAIResearch/Illustrious-xl-early-release-v0
---
Original model is [here](https://civitai.com/models/828469/knk-helioblend-illustrious-v01?modelVersionId=926532).
This model created by [Konoko](https://civitai.com/user/Konoko).
|
AlignmentResearch/robust_llm_pythia-spam-1b-mz-ada-v3-nd | AlignmentResearch | "2024-03-26T16:33:31Z" | 102 | 0 | transformers | [
"transformers",
"safetensors",
"gpt_neox",
"text-classification",
"generated_from_trainer",
"base_model:EleutherAI/pythia-1b",
"base_model:finetune:EleutherAI/pythia-1b",
"license:apache-2.0",
"autotrain_compatible",
"text-generation-inference",
"endpoints_compatible",
"region:us"
] | text-classification | "2024-03-26T16:31:20Z" | ---
license: apache-2.0
tags:
- generated_from_trainer
base_model: EleutherAI/pythia-1b
model-index:
- name: robust_llm_pythia-spam-1b-mz-ada-v3-nd
results: []
---
<!-- This model card has been generated automatically according to the information the Trainer had access to. You
should probably proofread and complete it, then remove this comment. -->
# robust_llm_pythia-spam-1b-mz-ada-v3-nd
This model is a fine-tuned version of [EleutherAI/pythia-1b](https://huggingface.co/EleutherAI/pythia-1b) on an unknown dataset.
## Model description
More information needed
## Intended uses & limitations
More information needed
## Training and evaluation data
More information needed
## Training procedure
### Training hyperparameters
The following hyperparameters were used during training:
- learning_rate: 1e-05
- train_batch_size: 8
- eval_batch_size: 64
- seed: 42
- optimizer: Adam with betas=(0.9,0.999) and epsilon=1e-08
- lr_scheduler_type: linear
- num_epochs: 1
### Training results
### Framework versions
- Transformers 4.37.2
- Pytorch 2.2.0
- Datasets 2.17.0
- Tokenizers 0.15.2
|
seongil-dn/bge-m3-kor-retrieval-451949-bs64-mrc | seongil-dn | "2024-12-11T06:31:14Z" | 6 | 0 | sentence-transformers | [
"sentence-transformers",
"safetensors",
"xlm-roberta",
"sentence-similarity",
"feature-extraction",
"generated_from_trainer",
"dataset_size:451949",
"loss:CachedMultipleNegativesRankingLoss",
"arxiv:1908.10084",
"arxiv:2101.06983",
"base_model:BAAI/bge-m3",
"base_model:finetune:BAAI/bge-m3",
"autotrain_compatible",
"text-embeddings-inference",
"endpoints_compatible",
"region:us"
] | sentence-similarity | "2024-12-11T06:29:44Z" | ---
tags:
- sentence-transformers
- sentence-similarity
- feature-extraction
- generated_from_trainer
- dataset_size:451949
- loss:CachedMultipleNegativesRankingLoss
base_model: BAAI/bge-m3
widget:
- source_sentence: 부산대교의 주요 조망점에 설치한 것은 뭐야?
sentences:
- 현대모비스는 주요 연구소 및 생산공장에 초등학생을 초청해 현장 견학과 과학 이벤트 참여 기회를 제공하고 있다고 16일 밝혔다. 이 행사는 사회공헌활동의
일환으로 추진하고 있는 과학꿈나무 육성 프로그램인 주니어 공학교실 참가 학생들을 대상으로 진행된다. 전국 12개 초등학교 400여명의 초등학생들과
선생님이 전국의 주요 사업장에 초청됐다. 주니어 공학교실은 기초적인 과학 원리가 실제 기술로 구현되는지 실습을 통해 확인해 볼 수 있는 초등학생
대상 교육프로그램이다. 현대모비스 연구원들이 강사로 참여해 매달 전국 사업장 인근의 초등학교를 방문, 별도 제작한 교보재를 이용해 관련 기술이
적용된 미래자동차 모형을 직접 제작하는 형태로 운영되고 있다.
- 부산의 원도심인 중구와 영도구를 잇는 부산대교 야경이 다양한 빛과 음악 연출로 재탄생했다. 부산시는 부산 원도심 현대화의 상징 교량인 부산대교에
최근 친환경 LED 경관조명을 아치에 554개, 아치바.내부.트러스하부 등에 774개를 설치하고 본격 운영을 시작했다고 7일 밝혔다. 또 조명
연출과 음악 연동이 가능한 조명제어기를 설치해 시간대별, 계절별, 주말.공휴일 등 각종 행사 개최 시 각기 다른 이미지를 연출할 수 있게 됐다.
부산대교 경관조명은 2000년부터 설치.운영돼 왔으나, 메탈과 나트륨 투광조명으로 연출이 불가능하고 시설 노후화로 인한 고장이 잦아 운영에
어려움이 있었다. 이에 부산시는 이번 새로운 경관조명 운영을 위해 설계용역, 주민설명회 개최, 경관자문과 시 경관위원회 심의 등을 거쳐 지난해
8월 착공했다. 부산시 관계자는 부산대교의 주요 조망점인 영도구 웰컴센터와 중구 롯데백화점 주변 수변 산책로에는 스피커를 설치해 매시간별 음악과
연동되는 경관조명 연출로 생동감 있는 경관조명을 감상할 수 있는 공간을 마련했다며 앞으로 부산의 새로운 관광명소로 거듭나 원도심에 활력을 불어넣는
계기가 될 것으로 기대한다고 말했다.
- ‘국제관광도시’ 부산의 해안을 잇는 다리 7곳에 각각 ‘미디어파사드’(LED 조명을 비춰 영상을 표현하는 기법)가 적용돼 빛의 향연이 펼쳐진다.
또 광안대교에는 와이어(주케이블)를 걷는 클라이밍과 번지점프 관광 프로그램이 도입된다. 수륙양용버스가 육지와 바다를 넘나들고 수영강에는 레이저
분수쇼가 펼쳐진다. 지난해 국제관광도시로 선정된 부산이 올해부터 외국인 관광객 1000만 명을 유치하고 세계 10대 관광도시로 진입하기 위해
구체적인 사업들을 본격 추진한다. 부산시는 21일 해운대구 벡스코에서 열리는 ‘국제관광도시 온라인 시민보고회’에서 ‘국제관광도시 육성사업 기본계획
최종 용역 결과’를 발표한다. 국제관광도시 육성사업 기본계획은 핵심 관광 콘텐츠인 ‘시그니처’ 사업 등 74개 세부 사업을 담고 있다. 시그니처
사업은 ‘7 세븐브릿지 랜드마크 프로젝트’ ‘24 열린 바다 프로젝트’ ‘365 영화 이벤트 도시 프로젝트’이다. 7 세븐브릿지 랜드마크 프로젝트는
125억 원을 투입해 광안대교, 부산항대교, 남항대교, 영도다리, 을숙도대교, 신호대교, 가락대교 다리 7곳을 랜드마크형 관광 상품으로 조성한다.
7개 교량은 각기 다른 건축 양식과 수려한 해안경관을 갖추고 있어 관광 자원으로서 활용 가치가 높다는 것이다. 교량별로 스토리텔링을 각각 개발하고
외벽에는 다양한 영상을 투사하는 ‘미디어파사드’를 적용한다. 번지점프대, 보행로 그리고 와이어 위를 걷는 클라이밍 등 다양한 체험 프로그램도
운영된다. 또 시티투어버스, 관광유람선 등과 연계한 상품도 개발된다. 24 열린 바다 프로젝트는 79억 원으로 해상 관광을 활성화하는 데 초점을
맞춘다. 달빛수영대회, 서핑, 국제 레크리에이션 피싱 대회, 자작보트 페스티벌 등 사계절 운영 가능한 ‘해양레저스포츠시티’를 조성한다. 수륙양용버스,
수상택시 그리고 깡깡이마을 도선도 운행한다. 수영강에는 레이저 분수대가 설치된다. 리버덕 등 공공 예술 작품도 바다 위에 전시된다. 또 365
영화 이벤트 도시 프로젝트는 85억 2000만 원 예산으로 ‘영화와 축제의 도시’ 부산을 주제로 부산 대표 축제와 영화 산업을 지원하고 영화·드라마
촬영지 투어, 부산국제영화제 시상식 갈라쇼 상품화 등 다양한 프로그램을 개발한다. 여기다 산복도로, 을숙도, 골목길 등 부산의 지역적 특성을
녹여 낸 개성 있는 관광 상품도 선보인다. 골목길 투어, 산복도로 예술 계단 조성, 부산 달빛 플리마켓, 부산 부평 ‘스트릿’ 푸드 페스티벌,
시장 워킹투어, 동양 최대 철새도래지 탐사 프로그램, 습지에서 즐기는 이색 체험 등이 대표적이다. 여기다 모노레일, ‘집라인’, 케이블카,
트램 등 체험 관광 인프라를 확충한다. 이 외에도 외국인 관광객들이 최적화된 여행을 즐길 수 있도록 부산 주요 관광지에 스마트 환경이 구축된다.
‘스테이션형 스마트모빌리티’가 대표적이다. 이 곳에서 여행자들은 공유 킥보드나 전기 자전거를 빌리거나 충전할 수 있으며 주변 여행지 경로까지
탐색할 수 있다. 변성완 부산시장 권한대행은 “가덕신공항 건설, 2030부산월드엑스포 유치 등 호재를 기회로 삼아 부산을 명품 체류형 관광
중심지로 육성하겠다”고 말했다.
- source_sentence: 만나교회는 언제부터 '담장을 넘는 토요 예배'를 시작했는가?
sentences:
- ‘우리는 흩어지기 위해 모입니다.’ 경기도 성남 분당구의 만나교회(김병삼 목사)가 4월부터 ‘담장을 넘는 토요예배’를 시작하면서 내건 표어다.
토요예배는 교인들을 지역 사회와 봉사 현장으로 적극 파송하고 기존 공간 활용도를 높이자는 취지에서 시작했다. 교회가 부흥하는 경우에도 새 건물을
짓기보다는 기존 자원을 활용해 예배와 사역에 최대한 집중해 성장주의 패러다임을 탈피하자는 것이다. ‘주일 예배’에 익숙한 기존 목회자들과 성도들로서는
파격으로 비칠 수밖에 없다. 7일 오후 만나교회의 첫 토요예배에 참석했다. 김병삼 목사는 “토요예배는 흩어짐을 위한 충전소”라고 강조했다.
주일에 교회 봉사, 지역사회 사역 등에 적극 참여하는 신자들은 토요예배에 참석해 매번 파송식을 갖는다. 토요일에는 평소보다 길게 진행되는 예배에
참석해 힘을 얻고, 주일에는 봉사와 사역에 온전히 집중하기 위해서다. 만나교회는 기독교대한감리회(기감)에 소속돼 있는 건전한 교회다. 일부
이단 교파처럼 토요일을 안식일로 지키자고 주장하는 것이 아니다. 토요일만이 참된 안식일이라고 강조하는 제칠일안식일예수재림교회는 대한예수교장로회(예장)
합동 통합 고신과 기감 등으로부터 이단 판정을 받은 바 있다. 오후 5시 시작된 예배의 총 소요 시간은 1시간30분. 평소 주일예배(약 50분)보다
여유롭게 진행됐다. 성도들은 약 40분에 걸친 설교시간을 제외한 나머지를 대부분 찬양과 기도에 집중했다. 일부는 예배가 끝난 뒤에도 후속 예배가
없어 계속 자리에 남아 개인 기도 시간을 가졌다. 주일예배 때는 좀처럼 보기 힘든 장면이다. 김 목사는 “주일마다 교회봉사와 지역사회 사역에
나서는 신자들이 사실 예배에 봉사까지 겹치면 지치는 경우가 많다”며 “충분한 시간을 갖고 진행되는 토요예배에서 하나님의 은혜로 충분히 충전
받은 다음 헌신의 자리로 나아가자는 뜻을 모았다”고 취지를 설명했다. 첫 토요예배에 참석한 성도는 1050여명으로 전체 교인(1만여명)의 10분의
1 수준이었다. 주일마다 반복되는 주차대란 같은 모습은 볼 수 없었다. 향후 예배 인원이 분산되면 일요일에도 혼잡 사태가 줄어들 것으로 예상된다.
70개에 달하는 교회 내 소그룹 모임 공간 활용도도 높아져 성도 간 교제도 더 원활해질 전망이다. 그동안에는 소그룹 모임 공간 한 곳당 10명씩
써도 700명밖에 쓸 수가 없었다. 예배에 참석한 박주호(51) 집사는 토요예배에 참석한 다음 일요일에는 교회 미디어사역, 경기도 광주 외국인노동자
사역에 나설 예정이다. 그는 “직장인이라 토요일에 쉬기 때문에 처음에는 부담스러웠다”면서도 “한국교회가 기존의 교인 수 증가, 건축 위주의
성장주의 프레임을 깨고 세상에 나가서 섬겨야 진정한 성장을 이루는 것이라는 취지에 공감해 예배에 참석했다”고 말했다.
- 코로나19 시대 비대면 목회의 방향으로 김병삼 만나교회 목사는 오프라인을 기반으로 한 온라인 사역인 ‘올라인(all-line) 사역’을 강조했다.
김 목사는 지난 9일 서울 강남구 강변교회에서 ‘비대면 시대의 목회와 예배’라는 주제로 열린 한국복음주의협의회 주최 4월 월례 발표회에서 “이전과는
전혀 다른 목회적 환경 속에서 온라인이냐 오프라인이냐를 주장하는 건 무의미하다”며 이같이 말했다. 그는 교회의 온·오프라인 사역을 기독교인들의
교회생활과 가정생활에 빗대며 “오프라인을 기반으로 하지 않는 온라인은 허상에 불과하다”며 “사회적 상황이나 교회의 상황에 따라, 사역의 특성이나
성도의 생활 패턴 등에 따라 끊임없이 균형추를 움직여야 한다”고 강조했다. 김 목사가 담임으로 있는 만나교회는 코로나19 이전인 2018년부터
‘미디어 교회’를 만들어 올라인 사역을 감당하고 있다. 단순히 온라인 예배를 넘어서 중보기도 목양 교육 훈련 선교 나눔 구제 등 교회가 하고
있었던 모든 사역에 온라인 목회를 적용하고 있다. 김 목사는 이를 “복음을 교회 안 담장에 가두지 않고, 교회 밖으로 넘어가게 하는 영적 운동의
시작이었다”고 말했다. 그러면서 올라인 사역이 가능했던 이유로 만나교회가 갖고 있는 교회론을 꼽았다. 김 목사는 “교회 중심이 아닌 선교 중심적인
교회를 추구하다 보니 자연스럽게 건물에 한정된 교회를 넘어서게 됐다. 이전에는 건물이 중심이 된 만나교회를 기반으로 선교했다면, 이제 그 구분이
사라졌다”며 “‘교회는 건물이 아니다’는 교회론이 없었다면 쉽게 시도하지 못했을 사역”이라고 전했다. 김 목사는 비대면 시대 한국교회의 과제는
‘교회의 건물이나 제도를 어떻게 지킬까’가 아니라 ‘교회의 본질적 기능인 복음전도를 어떻게 수행할 것인가’라고 정리했다. 그런 면에서 온라인
예배는 가장 효율적인 선교 매체라고 말했다. 김 목사는 “예배뿐 아니라 랜선 성지순례, 성서학당, 미디어 가정사역 등 비대면 사회를 사는 성도들을
위한 다양한 양육·훈련 콘텐츠도 준비하고 있다”고 소개했다. 앞서 ‘나는 스마트한 제사장입니다’라는 제목으로 발표한 주석현 평택성결교회 목사
역시 “목회는 전달이 생명”이라며 “온라인 미디어를 적극 활용해야 한다”고 강조했다. 주 목사는 “현재 한국교회는 ‘성전 종교’에서 ‘디지털
유목시대’로 진입했다”며 “코로나19 팬데믹처럼 단절된 상황에서 온라인 미디어 사용은 필수”라고 말했다. 주 목사는 “코로나19 시대 목회자들은
스마트한 제사장이 돼야 한다. 성도들이 함께 참여할 수 있는 콘텐츠를 개발해야 한다”며 “성경적 연결점을 제시하면서도 마음이 담긴 영상을 제작해야
한다”고 조언했다.
- 중소기업계가 '의제매입세액공제제도' 개선을 촉구했다. 원재료 가격이 높아지는데도 제조업체 공제율은 10년간 똑같아 불합리하다는 주장이다. 식품·목재·재활용
제조업 분야 30여개 중소기업협동조합은 26일 서울 여의도 중소기업중앙회 회의실에서 간담회를 열고 의제매입세액공제율을 높여 줄 것으로 요구했다.
의제매입세액공제는 농산물·수산물·축산물·임산물을 가공해 판매하는 사업자가 제조 과정에서 부가가치세 면세물품을 사들이는 경우 구입액에 세금이
포함된 것으로 간주해 일정비율을 돌려주는 제도다. 현행 공제율은 개인 음식점 7.41%, 법인 음식점 5.67% 등으로 규정돼 있으나 그 밖의
제조업체에는 모두 1.96%가 적용되고 있다. 조합들은 "원재료 비용은 날로 증가하고 있는데 공제율은 2001년 이후 10여년간 제자리걸음"이라며
"중소기업 경영안정과 물가상승 억제를 위해 공제율을 높여야 한다"고 촉구했다. 특히 식품제조업체들은 음식점과 같은 원재료를 사용함에도 공제율에서는
차별을 당하고 있다고 주장했다. 이들은 "원재료 가격이 너무 높아 업체들의 고통이 가중되고 있다"며 "최소한 중소기업에 한해서라도 음식점과
같은 공제율인 7.41%를 적용해야 한다"고 강조했다. 한국목재공업협동조합은 "실제 투입하는 면세제품 부가가치세는 구입액의 4.76%에 이르는
상황으로 지금 공제받는 비율인 1.96%는 이에 한참 못 미친다"며 "공제율 상향조정이 절실한 상황"이라고 목소리를 높였다. 한국제지원료재생업협동조합
역시 "공제율을 제정 취지에 맞게 확대해야 한다. 내년 말로 예정된 일몰 시한도 폐지해야 한다"고 촉구했다. 중소기업들은 "현행 의제매입세액공제가
적용 대상 사업자를 음식업자와 그 외의 사업자로 구분하여 공제율을 달리 적용하고 있어 상대적으로 영세한 식품업이 제도의 차별을 받아왔다"면서
"어려운 경제상황과 중소제조업의 경영난을 감안할 때 공제율 조정이 시급하다"고 밝혔다.
- source_sentence: 최근 한달간 크레아 플래닛의 주식거래에서 외국인과 기관은 어떤 매매를 보였어?
sentences:
- 크레아플래닛(058530)은 현재 주가가 전일 대비 13.08% 상승한 1,210원 선에서 거래가 이루어지고 있다. 매매주체는개인,외국인 최근
한달간 주체별 거래비중을 살펴보면 개인이 89.62%, 외국인이 10.36%를 기록한 것으로 나타났다. 그리고 최근 5일간 거래비중은 개인
비중이 87.53%로 가장 높았고, 외국인이 12.46%로 그 뒤를 이었다. 기관은 거래에 참여하지 않은 것으로 보인다. 외국인/기관 순매수,
개인은 순매도(한달누적) 3월23일부터 전일까지 기관과 외국인은 2거래일 연속 동반 순매수를 보였다. 4주간을 기준으로 보면 외국인이 순매수량을
늘리며 515,582주를 순매수했고, 기관도 초반에 동종목을 순매수한 이후에 기세를 이어가며 2,355주를 순매수했다. 반면 개인들은 매도
관점으로 접근하면서 517,937주를 순매도한 것으로 나타났다. fnRASSI는 증권전문 기업 씽크풀과 파이낸셜뉴스의 협업으로 로봇기자가 실시간으로
생산하는 기사입니다.
- 현대기아자동차가 올해 국내외 시장 위축에도 사상 최대 실적을 기록했다. 현대차와 기아차 모두 상반기 영업이익이 지난해보다 20% 이상 증가하는
등 규모 외에 실익에서도 좋은 성적을 거뒀다. 현대차는 올 상반기 매출액 42조1051억원, 영업이익 4조7849억원, 당기순이익 4조9982억원을
기록했다고 26일 밝혔다. 현대차는 지난해 상반기보다 매출은 9.9%, 영업이익은 21.0% 증가했다. 당기순이익 역시 19.5%나 늘었다.
해외에서는 국내 생산 수출분 66만3637대, 해외생산 판매분 119만1168대를 더해 모두 185만4805대를 팔아 지난해 상반기보다 14.9%나
상승했다. 이에 따라 글로벌 판매에서 내수 비중이 사상 처음으로 15%대로 내려갔다. 매출원가율은 플랫폼 통합 등의 효과로 지난해보다 0.3%포인트
감소한 76.1%를 기록했다. 또 기아차는 상반기 매출액 24조3409억원, 영업이익 2조3397억원,당기순이익 2조2977억원 등을 기록했다고
27일 밝혔다. 지난해 같은 기간과 비교해 매출액은 9.5%, 영업이익은 25.0% 늘었고, 당기순이익은 10.4% 증가했다. 모닝과 프라이드,
K5 등 주요 차종이 전 세계 시장에서 판매호조를 보이며 판매량 역시 12.4% 증가했다. 기아차가 올 상반기 판매한 차량은 해외공장 생산분을
포함해 139만대에 달했다. 기아차는 해외시장에서 '제값 받기' 노력 등 내실경영이 실적 호조로 이어졌다고 평가했다. 하지만 이들 업체들은
하반기에는 상당한 어려움을 겪을 것으로 전망했다. 유럽은 재정위기로 인해 폐차지원제도 등 수요진작 정책을 내놓지 못하고 있다. 여기에 인도와
브라질의 경기침체 우려까지 제기되고 있기 때문이다. 현대차 관계자는 "국내는 물론 해외서도 경제불확실로 인해 판매가 위축될 수 있다"며 "특히
글로벌 자동차 업체들의 공세가 강화돼 치열한 경쟁을 벌일 것으로 예상된다"고 말했다.
- 크라운해태홀딩스(005745)는 현재 주가가 전일 대비 12.21% 상승한 19,300원 선에서 거래가 이루어지고 있다. 외국인은 순매수,
개인은 순매도(한달누적) 5월15일부터 전일까지 외국인이 4거래일 연속 순매수를 보였다. 4주간을 기준으로 보면 외국인이 5,266주를 순매수했지만,
개인은 순매도로 돌아서면서 5,266주를 순매도했다. 기관의 순매수량에는 변함이 없다. 외국인은 순매수, 개인은 순매도(한달누적) 5월15일부터
전일까지 외국인이 4거래일 연속 순매수를 보였다. 4주간을 기준으로 보면 외국인이 매수반전의 모습을 보이며 5,266주를 순매수했지만, 개인은
순매수에서 순매도로 반전되면서 5,266주를 순매도했다. 기관의 순매수량에는 변함이 없다. 주가등락폭 크고, 상장주식수 대비 거래량도 상당히
높아 최근 한달간 크라운해태홀의 매매회전율을 분석한 결과 4일에 1번 꼴로 주식의 주인이 바뀐 것으로 나타났다. 이는 비정상적으로 높은 회전율을
보인 것으로 투자에 각별한 주의가 요망된다. 또한 평균적으로 장중 주가변동률이 13.97%에 달할 정도로 등락이 심하기 때문에 다시한번 주의가
필요하다. 비중소제목 비중멘트투자주체별 누적순매수 , 투자주체별 매매비중*기관과 외국인을 제외한 개인 및 기타법인 등의 주체는 모두 개인으로
간주하였음 fnRASSI는 증권전문 기업 씽크풀과 파이낸셜뉴스의 협업으로 로봇기자가 실시간으로 생산하는 기사입니다.
- source_sentence: '넥슨의 ''야생의 땅: 듀랑고'' 사전예약이 얼마를 돌파했나?'
sentences:
- 성가(聖歌)는 거룩한 노래라는 의미로 세속적인 노래와 구분하기 위해 쓰여 왔다. 예배 중에 특별한 순서로 화음을 맞춰 찬양하는 합창단을 성가대라고
부르고 있다. 하지만 성가와 성가대라는 말은 성경적인 의미와 한국기독교 전통과는 거리가 멀다. 성경에는 찬송이라는 단어가 208번, 노래 176번,
찬양 83번, 찬미 13번 등장하지만, ‘성가’는 한 번도 등장하지 않는다. 초기 한국기독교는 성가라는 말이 아닌 찬양이라는 말을 썼다. 1892년
존스(G H Jones)와 로드와일러(L C Rothweiler) 선교사는 당시 성도들에게 많이 불리던 찬양을 모아 최초로 ‘찬미가’라는 찬양곡집을
출판했다. 이후 찬양곡을 모은 책들이 1893년 ‘찬양가’, 1895년 ‘찬셩시’라는 이름으로 출간됐다. 특히 1893년 언더우드는 최초로
4성부의 악보를 수록해 ‘찬양가’를 출판했다. 성가대 역시 초기 한국교회에선 사용되지 않던 단어다. 1913년 평양 장대현교회는 한국교회 최초로
‘찬양대’를 조직했다. 이듬해 새문안교회에 찬양대가 구성됐고 이름을 ‘찬미대’라고 했다. 대부분 학자들은 일본의 영향으로 교회에서 ‘성가’
‘성가대’라는 말을 쓰게 됐다고 언급한다. 1960년대 한·일 국교 정상화가 본격화된 이후 어떤 경로로, 누구에 의해서인지 단정할 순 없으나,
‘세이카다이(聖歌隊)’라는 말이 그대로 한국교회에 유입돼 성가대라는 말로 통용됐다는 것이다. 세속적인 노래와 구분을 짓는 성가는 하나님께 드리는
찬양의 의미를 충분히 담을 수 없다. 찬양은 예수님의 성육신 사건과 십자가의 은혜, 부활과 재림의 구속사적 사건, 하나님께 드리는 영광과 존귀를
한 곡에 담은 것이다. 성가대라는 말은 여러 사람이 하모니를 맞춰 신앙의 고백과 하나님께 영광을 드리는 찬양대의 의미를 충분히 반영하지 못한다.
일본의 영향을 받은 성가대보다는 한국교회 전통을 계승하고 성경적인 의미의 찬양을 그대로 포함하고 있는 찬양대라는 말로 부르는 것이 필요하다.
- '넥슨은 ‘야생의 땅: 듀랑고(이하 듀랑고)’의 사전예약이 200만 건을 돌파했다고 16일 밝혔다. 듀랑고는 넥슨 자회사 왓스튜디오에서 개발하고
넥슨이 서비스하는 공룡 시대를 배경으로 한 다중접속역할수행(MMORPG) 게임으로 오는 25일 정식 출시된다. 지난달 19일부터 시작한 사전예약을
통해 하루 만에 30만 명이 몰리며 높은 기대감을 보였고, 28일 만인 지난 15일 기준으로 200만 명을 돌파했다. 넥슨은 사전예약 200만
달성을 기념해 참여자 전원에게 특별 보상으로 ‘줄무늬 콤프소그나투스’를 지급한다. ‘줄무늬 콤프소그나투스’는 야생에서 포획이 불가능한 희귀
공룡펫으로, 귀여운 외형으로 많은 사랑을 받고 있는 듀랑고의 메인 마스코트다. 보다 자세한 내용은 공식 홈페이지 및 페이스북 팬페이지에서 확인할
수 있다.'
- <table><tbody><tr><td>회사명</td><td>대표이사</td><td>주요게임명</td><td>매출액(2008)</td></tr><tr><td>블리자드엔터테인먼트</td><td>Mike
Morhaime</td><td>디아블로2, 스타크래프트, 워크래프트, 월드오브 워크래프트 </td><td>30억달러(5조)</td></tr><tr><td>NHN</td><td>김상헌</td><td>테트리스,
C9, R2</td><td>1조2천억</td></tr><tr><td>엔씨소프트</td><td>김택진</td><td>아이온, 리니지2</td><td>2천4백억</td></tr><tr><td>오로라게임즈</td><td>홍기선</td><td>믹스마스터</td><td>신생업체<br>(’09.
7 설립) </td></tr><tr><td>네오위즈게임즈</td><td>이상엽</td><td>피파온라인, 슬러거, 스페셜포스</td><td>1천6백억</td></tr><tr><td>넥슨</td><td>강신철</td><td>카트라이더,
메이플스토리, 던전앤파이터, 마비노기영웅전 </td><td>2천6백억원</td></tr><tr><td>엠게임</td><td>권이형</td><td>라피스,
아르고, 열혈강호, 홀릭, 귀혼, 아스다이야기 </td><td>620억</td></tr><tr><td>위메이드엔터테인먼트</td><td>서수길</td><td>미르의
전설 2, 미르의 전설 3, 창천 온라인 </td><td>738억</td></tr><tr><td>씨제이인터넷</td><td>정영종</td><td>서든어택,
마구마구, 프리우스</td><td>1천9백30억</td></tr><tr><td>예당온라인</td><td>김남철</td><td>프리스톤테일,
오디션</td><td>775억</td></tr><tr><td>한빛소프트</td><td>김영만</td><td>팡야, 그라나도에스파다, 헬게이트</td><td>694억</td></tr></tbody></table>
지스타 2009 국제게임전시회 30부스 이상 참여 업체
- source_sentence: 영상통화로 내연녀와 말다툼하던 40대 남성이 분을 이기지 못하고 내연녀 집에 불을 질렀다가 현행범으로 체포된 건
누구지?
sentences:
- 정신이상 증세 가능성 서울 영등포경찰서는 지난달 여의도순복음교회에 불을 지른 혐의(현존건조물방화)로 A씨(28)를 구속했다고 2일 밝혔다.
A씨는 지난달 25일 오후 7시 40분께 교회 5층 계단 복도에 불을 낸 혐의를 받고 있다. 당시 화재로 교회 건물에 있던 450여명이 긴급
대피하기도 했다. 경찰은 주변 폐쇄회로(CC)TV 영상을 분석해 A씨가 화재 장소를 서둘러 나온 지 3분 만에 연기가 나고 2시간 전에도 화재
장소를 다녀간 점 등을 확인, 지난달 27일 그를 체포했다. 경찰 조사에서 A씨는 예배를 보러 갔다가 내부 지리를 몰라 5층에 올라갔을 뿐
불을 지르지 않았다고 범행을 부인했다. 하지만 A씨는 지난 2013년 이 교회에 신자로 등록하고 다닌 사실이 있어 경찰은 A씨 진술이 거짓인
것으로 판단했다. 경찰 관계자는 A씨가 수년 전부터 정신이상 증세를 보였다는 부친의 진술이 있고 체포된 뒤에도 줄곧 영어로만 말을 하는 등
이상행동을 보여 정신이상 증세에 의해 범행을 벌였을 가능성이 있다고 전했다.
- 질병관리본부는 25일 “지난해 12월 초 감비아와 세네갈 기니비사우 등 아프리카 지역을 여행한 감비아 거주 교민 1명(52세 남성)이 인수공통감염병인
리프트밸리열에 걸려 목숨을 잃었다”며 여행 시 주의를 당부했다. 리프트밸리열 바이러스에 감염된 모기에 물리거나 감염된 동물(소 염소 양 낙타
영양 등)의 혈액, 조직에 접촉해 옮는다. 대부분 아프리카 국가와 아라비아반도에서 풍토병으로 발생하고 있다. 사람 간 전파 사례는 보고되지
않았다. 2∼6일 잠복한 뒤 감기처럼 열과 근육통 관절통 두통 증상을 보인다. 8∼10% 환자에서 뇌염 출혈 등 중증으로 악화하며 출혈이 생기면
3∼6일 안에 사망한다. 바이러스 치료제나 예방백신은 아직 개발돼 있지 않다. 질병관리본부 관계자는 “해당 지역 여행 시 감염된 동물의 혈액이나
체액, 사체 접촉을 피하고 모기 기피제를 써 물리지 않도록 해야 한다”고 말했다. 또 “살균되지 않은 동물의 젖이나 감염 동물의 고기를 먹어선
안 된다”고 강조했다.
- '{IMG:1}영상통화로 내연녀와 말다툼하던 40대 남성이 분을 이기지 못하고 내연녀 집에 불을 질렀다가 현행범으로 체포됐다. 부산 사하경찰서는
내연녀 집에 불을 지른 혐의(방화)로 회사원 A(43)씨를 붙잡아 조사하고 있다고 19일 밝혔다. 경찰에 따르면, A씨는 19일 오전 0시
10분쯤 부산 사하구 모 아파트에 있는 내년여 B(40·여)씨의 집에서 소파와 침대 등에 일회용 라이터로 불을 놓아 700만원 상당의 재산피해를
낸 혐의를 받고 있다. 당시 영상통화로 불을 붙이는 장면을 본 B씨가 곧바로 112에 신고해, 출동한 경찰이 현장에서 A씨를 붙잡았다. 경찰
조사결과 A씨는 내연녀의 집에서 밖에 있던 B씨와 영상통화를 하던 중 또다른 남자문제로 말다툼을 벌이다 홧김에 라이터로 불을 붙인 것으로 드러났다.
경찰은 A씨에 대한 구속영장을 신청할 예정이다.'
pipeline_tag: sentence-similarity
library_name: sentence-transformers
---
# SentenceTransformer based on BAAI/bge-m3
This is a [sentence-transformers](https://www.SBERT.net) model finetuned from [BAAI/bge-m3](https://huggingface.co/BAAI/bge-m3). It maps sentences & paragraphs to a 1024-dimensional dense vector space and can be used for semantic textual similarity, semantic search, paraphrase mining, text classification, clustering, and more.
## Model Details
### Model Description
- **Model Type:** Sentence Transformer
- **Base model:** [BAAI/bge-m3](https://huggingface.co/BAAI/bge-m3) <!-- at revision 5617a9f61b028005a4858fdac845db406aefb181 -->
- **Maximum Sequence Length:** 1024 tokens
- **Output Dimensionality:** 1024 tokens
- **Similarity Function:** Cosine Similarity
<!-- - **Training Dataset:** Unknown -->
<!-- - **Language:** Unknown -->
<!-- - **License:** Unknown -->
### Model Sources
- **Documentation:** [Sentence Transformers Documentation](https://sbert.net)
- **Repository:** [Sentence Transformers on GitHub](https://github.com/UKPLab/sentence-transformers)
- **Hugging Face:** [Sentence Transformers on Hugging Face](https://huggingface.co/models?library=sentence-transformers)
### Full Model Architecture
```
SentenceTransformer(
(0): Transformer({'max_seq_length': 1024, 'do_lower_case': False}) with Transformer model: XLMRobertaModel
(1): Pooling({'word_embedding_dimension': 1024, 'pooling_mode_cls_token': True, 'pooling_mode_mean_tokens': False, 'pooling_mode_max_tokens': False, 'pooling_mode_mean_sqrt_len_tokens': False, 'pooling_mode_weightedmean_tokens': False, 'pooling_mode_lasttoken': False, 'include_prompt': True})
(2): Normalize()
)
```
## Usage
### Direct Usage (Sentence Transformers)
First install the Sentence Transformers library:
```bash
pip install -U sentence-transformers
```
Then you can load this model and run inference.
```python
from sentence_transformers import SentenceTransformer
# Download from the 🤗 Hub
model = SentenceTransformer("seongil-dn/bge-m3-kor-retrieval-451949-bs64-mrc")
# Run inference
sentences = [
'영상통화로 내연녀와 말다툼하던 40대 남성이 분을 이기지 못하고 내연녀 집에 불을 질렀다가 현행범으로 체포된 건 누구지?',
'{IMG:1}영상통화로 내연녀와 말다툼하던 40대 남성이 분을 이기지 못하고 내연녀 집에 불을 질렀다가 현행범으로 체포됐다. 부산 사하경찰서는 내연녀 집에 불을 지른 혐의(방화)로 회사원 A(43)씨를 붙잡아 조사하고 있다고 19일 밝혔다. 경찰에 따르면, A씨는 19일 오전 0시 10분쯤 부산 사하구 모 아파트에 있는 내년여 B(40·여)씨의 집에서 소파와 침대 등에 일회용 라이터로 불을 놓아 700만원 상당의 재산피해를 낸 혐의를 받고 있다. 당시 영상통화로 불을 붙이는 장면을 본 B씨가 곧바로 112에 신고해, 출동한 경찰이 현장에서 A씨를 붙잡았다. 경찰 조사결과 A씨는 내연녀의 집에서 밖에 있던 B씨와 영상통화를 하던 중 또다른 남자문제로 말다툼을 벌이다 홧김에 라이터로 불을 붙인 것으로 드러났다. 경찰은 A씨에 대한 구속영장을 신청할 예정이다.',
'정신이상 증세 가능성 서울 영등포경찰서는 지난달 여의도순복음교회에 불을 지른 혐의(현존건조물방화)로 A씨(28)를 구속했다고 2일 밝혔다. A씨는 지난달 25일 오후 7시 40분께 교회 5층 계단 복도에 불을 낸 혐의를 받고 있다. 당시 화재로 교회 건물에 있던 450여명이 긴급 대피하기도 했다. 경찰은 주변 폐쇄회로(CC)TV 영상을 분석해 A씨가 화재 장소를 서둘러 나온 지 3분 만에 연기가 나고 2시간 전에도 화재 장소를 다녀간 점 등을 확인, 지난달 27일 그를 체포했다. 경찰 조사에서 A씨는 예배를 보러 갔다가 내부 지리를 몰라 5층에 올라갔을 뿐 불을 지르지 않았다고 범행을 부인했다. 하지만 A씨는 지난 2013년 이 교회에 신자로 등록하고 다닌 사실이 있어 경찰은 A씨 진술이 거짓인 것으로 판단했다. 경찰 관계자는 A씨가 수년 전부터 정신이상 증세를 보였다는 부친의 진술이 있고 체포된 뒤에도 줄곧 영어로만 말을 하는 등 이상행동을 보여 정신이상 증세에 의해 범행을 벌였을 가능성이 있다고 전했다.',
]
embeddings = model.encode(sentences)
print(embeddings.shape)
# [3, 1024]
# Get the similarity scores for the embeddings
similarities = model.similarity(embeddings, embeddings)
print(similarities.shape)
# [3, 3]
```
<!--
### Direct Usage (Transformers)
<details><summary>Click to see the direct usage in Transformers</summary>
</details>
-->
<!--
### Downstream Usage (Sentence Transformers)
You can finetune this model on your own dataset.
<details><summary>Click to expand</summary>
</details>
-->
<!--
### Out-of-Scope Use
*List how the model may foreseeably be misused and address what users ought not to do with the model.*
-->
<!--
## Bias, Risks and Limitations
*What are the known or foreseeable issues stemming from this model? You could also flag here known failure cases or weaknesses of the model.*
-->
<!--
### Recommendations
*What are recommendations with respect to the foreseeable issues? For example, filtering explicit content.*
-->
## Training Details
### Training Hyperparameters
#### Non-Default Hyperparameters
- `per_device_train_batch_size`: 64
- `learning_rate`: 3e-05
- `num_train_epochs`: 1
- `warmup_ratio`: 0.05
- `fp16`: True
- `batch_sampler`: no_duplicates
#### All Hyperparameters
<details><summary>Click to expand</summary>
- `overwrite_output_dir`: False
- `do_predict`: False
- `eval_strategy`: no
- `prediction_loss_only`: True
- `per_device_train_batch_size`: 64
- `per_device_eval_batch_size`: 8
- `per_gpu_train_batch_size`: None
- `per_gpu_eval_batch_size`: None
- `gradient_accumulation_steps`: 1
- `eval_accumulation_steps`: None
- `torch_empty_cache_steps`: None
- `learning_rate`: 3e-05
- `weight_decay`: 0.0
- `adam_beta1`: 0.9
- `adam_beta2`: 0.999
- `adam_epsilon`: 1e-08
- `max_grad_norm`: 1.0
- `num_train_epochs`: 1
- `max_steps`: -1
- `lr_scheduler_type`: linear
- `lr_scheduler_kwargs`: {}
- `warmup_ratio`: 0.05
- `warmup_steps`: 0
- `log_level`: passive
- `log_level_replica`: warning
- `log_on_each_node`: True
- `logging_nan_inf_filter`: True
- `save_safetensors`: True
- `save_on_each_node`: False
- `save_only_model`: False
- `restore_callback_states_from_checkpoint`: False
- `no_cuda`: False
- `use_cpu`: False
- `use_mps_device`: False
- `seed`: 42
- `data_seed`: None
- `jit_mode_eval`: False
- `use_ipex`: False
- `bf16`: False
- `fp16`: True
- `fp16_opt_level`: O1
- `half_precision_backend`: auto
- `bf16_full_eval`: False
- `fp16_full_eval`: False
- `tf32`: None
- `local_rank`: 0
- `ddp_backend`: None
- `tpu_num_cores`: None
- `tpu_metrics_debug`: False
- `debug`: []
- `dataloader_drop_last`: True
- `dataloader_num_workers`: 0
- `dataloader_prefetch_factor`: None
- `past_index`: -1
- `disable_tqdm`: False
- `remove_unused_columns`: True
- `label_names`: None
- `load_best_model_at_end`: False
- `ignore_data_skip`: False
- `fsdp`: []
- `fsdp_min_num_params`: 0
- `fsdp_config`: {'min_num_params': 0, 'xla': False, 'xla_fsdp_v2': False, 'xla_fsdp_grad_ckpt': False}
- `fsdp_transformer_layer_cls_to_wrap`: None
- `accelerator_config`: {'split_batches': False, 'dispatch_batches': None, 'even_batches': True, 'use_seedable_sampler': True, 'non_blocking': False, 'gradient_accumulation_kwargs': None}
- `deepspeed`: None
- `label_smoothing_factor`: 0.0
- `optim`: adamw_torch
- `optim_args`: None
- `adafactor`: False
- `group_by_length`: False
- `length_column_name`: length
- `ddp_find_unused_parameters`: None
- `ddp_bucket_cap_mb`: None
- `ddp_broadcast_buffers`: False
- `dataloader_pin_memory`: True
- `dataloader_persistent_workers`: False
- `skip_memory_metrics`: True
- `use_legacy_prediction_loop`: False
- `push_to_hub`: False
- `resume_from_checkpoint`: None
- `hub_model_id`: None
- `hub_strategy`: every_save
- `hub_private_repo`: False
- `hub_always_push`: False
- `gradient_checkpointing`: False
- `gradient_checkpointing_kwargs`: None
- `include_inputs_for_metrics`: False
- `eval_do_concat_batches`: True
- `fp16_backend`: auto
- `push_to_hub_model_id`: None
- `push_to_hub_organization`: None
- `mp_parameters`:
- `auto_find_batch_size`: False
- `full_determinism`: False
- `torchdynamo`: None
- `ray_scope`: last
- `ddp_timeout`: 1800
- `torch_compile`: False
- `torch_compile_backend`: None
- `torch_compile_mode`: None
- `dispatch_batches`: None
- `split_batches`: None
- `include_tokens_per_second`: False
- `include_num_input_tokens_seen`: False
- `neftune_noise_alpha`: None
- `optim_target_modules`: None
- `batch_eval_metrics`: False
- `eval_on_start`: False
- `eval_use_gather_object`: False
- `batch_sampler`: no_duplicates
- `multi_dataset_batch_sampler`: proportional
</details>
### Training Logs
| Epoch | Step | Training Loss |
|:------:|:----:|:-------------:|
| 0.0102 | 1 | 0.5093 |
| 0.0204 | 2 | 0.4639 |
| 0.0306 | 3 | 0.493 |
| 0.0408 | 4 | 0.3748 |
| 0.0510 | 5 | 0.294 |
| 0.0612 | 6 | 0.2842 |
| 0.0714 | 7 | 0.2719 |
| 0.0816 | 8 | 0.2738 |
| 0.0918 | 9 | 0.225 |
| 0.1020 | 10 | 0.2302 |
| 0.1122 | 11 | 0.2419 |
| 0.1224 | 12 | 0.2295 |
| 0.1327 | 13 | 0.2174 |
| 0.1429 | 14 | 0.2398 |
| 0.1531 | 15 | 0.2327 |
| 0.1633 | 16 | 0.1828 |
| 0.1735 | 17 | 0.2022 |
| 0.1837 | 18 | 0.1839 |
| 0.1939 | 19 | 0.1849 |
| 0.2041 | 20 | 0.2048 |
| 0.2143 | 21 | 0.1777 |
| 0.2245 | 22 | 0.1993 |
| 0.2347 | 23 | 0.1771 |
| 0.2449 | 24 | 0.174 |
| 0.2551 | 25 | 0.1817 |
| 0.2653 | 26 | 0.1837 |
| 0.2755 | 27 | 0.1821 |
| 0.2857 | 28 | 0.1874 |
| 0.2959 | 29 | 0.1488 |
| 0.3061 | 30 | 0.1675 |
| 0.3163 | 31 | 0.1846 |
| 0.3265 | 32 | 0.1586 |
| 0.3367 | 33 | 0.1473 |
| 0.3469 | 34 | 0.1364 |
| 0.3571 | 35 | 0.1617 |
| 0.3673 | 36 | 0.1761 |
| 0.3776 | 37 | 0.1569 |
| 0.3878 | 38 | 0.1706 |
| 0.3980 | 39 | 0.1897 |
| 0.4082 | 40 | 0.1622 |
| 0.4184 | 41 | 0.1486 |
| 0.4286 | 42 | 0.1438 |
| 0.4388 | 43 | 0.1983 |
| 0.4490 | 44 | 0.1245 |
| 0.4592 | 45 | 0.1399 |
| 0.4694 | 46 | 0.1437 |
| 0.4796 | 47 | 0.1467 |
| 0.4898 | 48 | 0.1395 |
| 0.5 | 49 | 0.1596 |
| 0.5102 | 50 | 0.1503 |
| 0.5204 | 51 | 0.1508 |
| 0.5306 | 52 | 0.1367 |
| 0.5408 | 53 | 0.131 |
| 0.5510 | 54 | 0.1311 |
| 0.5612 | 55 | 0.1234 |
| 0.5714 | 56 | 0.1564 |
| 0.5816 | 57 | 0.1607 |
| 0.5918 | 58 | 0.1548 |
| 0.6020 | 59 | 0.1202 |
| 0.6122 | 60 | 0.1212 |
| 0.6224 | 61 | 0.1611 |
| 0.6327 | 62 | 0.1598 |
| 0.6429 | 63 | 0.1384 |
| 0.6531 | 64 | 0.1525 |
| 0.6633 | 65 | 0.1561 |
| 0.6735 | 66 | 0.1666 |
| 0.6837 | 67 | 0.1174 |
| 0.6939 | 68 | 0.1348 |
| 0.7041 | 69 | 0.1274 |
| 0.7143 | 70 | 0.16 |
| 0.7245 | 71 | 0.1514 |
| 0.7347 | 72 | 0.1501 |
| 0.7449 | 73 | 0.1795 |
| 0.7551 | 74 | 0.1481 |
| 0.7653 | 75 | 0.1666 |
| 0.7755 | 76 | 0.1163 |
| 0.7857 | 77 | 0.1512 |
| 0.7959 | 78 | 0.132 |
| 0.8061 | 79 | 0.1433 |
| 0.8163 | 80 | 0.1513 |
| 0.8265 | 81 | 0.1365 |
| 0.8367 | 82 | 0.142 |
| 0.8469 | 83 | 0.1475 |
| 0.8571 | 84 | 0.1448 |
| 0.8673 | 85 | 0.1403 |
| 0.8776 | 86 | 0.1664 |
| 0.8878 | 87 | 0.1576 |
| 0.8980 | 88 | 0.1361 |
| 0.9082 | 89 | 0.1186 |
| 0.9184 | 90 | 0.1203 |
| 0.9286 | 91 | 0.1317 |
| 0.9388 | 92 | 0.1254 |
| 0.9490 | 93 | 0.1063 |
| 0.9592 | 94 | 0.1144 |
| 0.9694 | 95 | 0.1283 |
| 0.9796 | 96 | 0.1336 |
| 0.9898 | 97 | 0.1346 |
| 1.0 | 98 | 0.1285 |
### Framework Versions
- Python: 3.10.12
- Sentence Transformers: 3.2.1
- Transformers: 4.44.2
- PyTorch: 2.3.1+cu121
- Accelerate: 1.1.1
- Datasets: 2.21.0
- Tokenizers: 0.19.1
## Citation
### BibTeX
#### Sentence Transformers
```bibtex
@inproceedings{reimers-2019-sentence-bert,
title = "Sentence-BERT: Sentence Embeddings using Siamese BERT-Networks",
author = "Reimers, Nils and Gurevych, Iryna",
booktitle = "Proceedings of the 2019 Conference on Empirical Methods in Natural Language Processing",
month = "11",
year = "2019",
publisher = "Association for Computational Linguistics",
url = "https://arxiv.org/abs/1908.10084",
}
```
#### CachedMultipleNegativesRankingLoss
```bibtex
@misc{gao2021scaling,
title={Scaling Deep Contrastive Learning Batch Size under Memory Limited Setup},
author={Luyu Gao and Yunyi Zhang and Jiawei Han and Jamie Callan},
year={2021},
eprint={2101.06983},
archivePrefix={arXiv},
primaryClass={cs.LG}
}
```
<!--
## Glossary
*Clearly define terms in order to be accessible across audiences.*
-->
<!--
## Model Card Authors
*Lists the people who create the model card, providing recognition and accountability for the detailed work that goes into its construction.*
-->
<!--
## Model Card Contact
*Provides a way for people who have updates to the Model Card, suggestions, or questions, to contact the Model Card authors.*
--> |
tsavage68/UTI2_L3_50steps_1e6rate_05beta_CSFTDPO | tsavage68 | "2024-06-10T15:22:34Z" | 4 | 0 | transformers | [
"transformers",
"safetensors",
"llama",
"text-generation",
"trl",
"dpo",
"generated_from_trainer",
"conversational",
"base_model:tsavage68/UTI_L3_1000steps_1e5rate_SFT",
"base_model:finetune:tsavage68/UTI_L3_1000steps_1e5rate_SFT",
"license:llama3",
"autotrain_compatible",
"text-generation-inference",
"endpoints_compatible",
"region:us"
] | text-generation | "2024-06-10T15:17:27Z" | ---
license: llama3
base_model: tsavage68/UTI_L3_1000steps_1e5rate_SFT
tags:
- trl
- dpo
- generated_from_trainer
model-index:
- name: UTI2_L3_50steps_1e6rate_05beta_CSFTDPO
results: []
---
<!-- This model card has been generated automatically according to the information the Trainer had access to. You
should probably proofread and complete it, then remove this comment. -->
# UTI2_L3_50steps_1e6rate_05beta_CSFTDPO
This model is a fine-tuned version of [tsavage68/UTI_L3_1000steps_1e5rate_SFT](https://huggingface.co/tsavage68/UTI_L3_1000steps_1e5rate_SFT) on an unknown dataset.
It achieves the following results on the evaluation set:
- Loss: 0.0261
- Rewards/chosen: 2.3344
- Rewards/rejected: -5.4705
- Rewards/accuracies: 0.9800
- Rewards/margins: 7.8050
- Logps/rejected: -54.2106
- Logps/chosen: -24.5560
- Logits/rejected: -1.1516
- Logits/chosen: -1.1414
## Model description
More information needed
## Intended uses & limitations
More information needed
## Training and evaluation data
More information needed
## Training procedure
### Training hyperparameters
The following hyperparameters were used during training:
- learning_rate: 1e-06
- train_batch_size: 2
- eval_batch_size: 1
- seed: 42
- gradient_accumulation_steps: 2
- total_train_batch_size: 4
- optimizer: Adam with betas=(0.9,0.999) and epsilon=1e-08
- lr_scheduler_type: cosine
- lr_scheduler_warmup_steps: 100
- training_steps: 50
### Training results
| Training Loss | Epoch | Step | Validation Loss | Rewards/chosen | Rewards/rejected | Rewards/accuracies | Rewards/margins | Logps/rejected | Logps/chosen | Logits/rejected | Logits/chosen |
|:-------------:|:------:|:----:|:---------------:|:--------------:|:----------------:|:------------------:|:---------------:|:--------------:|:------------:|:---------------:|:-------------:|
| 0.5446 | 0.3333 | 25 | 0.2409 | 0.7934 | -0.6030 | 0.9800 | 1.3964 | -44.4754 | -27.6381 | -1.1424 | -1.1365 |
| 0.0009 | 0.6667 | 50 | 0.0261 | 2.3344 | -5.4705 | 0.9800 | 7.8050 | -54.2106 | -24.5560 | -1.1516 | -1.1414 |
### Framework versions
- Transformers 4.41.2
- Pytorch 2.0.0+cu117
- Datasets 2.19.2
- Tokenizers 0.19.1
|
soba1911/xlsr-wav2vec2-asv19training | soba1911 | "2025-04-09T02:02:06Z" | 43 | 0 | transformers | [
"transformers",
"safetensors",
"wav2vec2",
"arxiv:1910.09700",
"endpoints_compatible",
"region:us"
] | null | "2025-03-25T13:54:37Z" | ---
library_name: transformers
tags: []
---
# Model Card for Model ID
<!-- Provide a quick summary of what the model is/does. -->
## Model Details
### Model Description
<!-- Provide a longer summary of what this model is. -->
This is the model card of a 🤗 transformers model that has been pushed on the Hub. This model card has been automatically generated.
- **Developed by:** [More Information Needed]
- **Funded by [optional]:** [More Information Needed]
- **Shared by [optional]:** [More Information Needed]
- **Model type:** [More Information Needed]
- **Language(s) (NLP):** [More Information Needed]
- **License:** [More Information Needed]
- **Finetuned from model [optional]:** [More Information Needed]
### Model Sources [optional]
<!-- Provide the basic links for the model. -->
- **Repository:** [More Information Needed]
- **Paper [optional]:** [More Information Needed]
- **Demo [optional]:** [More Information Needed]
## Uses
<!-- Address questions around how the model is intended to be used, including the foreseeable users of the model and those affected by the model. -->
### Direct Use
<!-- This section is for the model use without fine-tuning or plugging into a larger ecosystem/app. -->
[More Information Needed]
### Downstream Use [optional]
<!-- This section is for the model use when fine-tuned for a task, or when plugged into a larger ecosystem/app -->
[More Information Needed]
### Out-of-Scope Use
<!-- This section addresses misuse, malicious use, and uses that the model will not work well for. -->
[More Information Needed]
## Bias, Risks, and Limitations
<!-- This section is meant to convey both technical and sociotechnical limitations. -->
[More Information Needed]
### Recommendations
<!-- This section is meant to convey recommendations with respect to the bias, risk, and technical limitations. -->
Users (both direct and downstream) should be made aware of the risks, biases and limitations of the model. More information needed for further recommendations.
## How to Get Started with the Model
Use the code below to get started with the model.
[More Information Needed]
## Training Details
### Training Data
<!-- This should link to a Dataset Card, perhaps with a short stub of information on what the training data is all about as well as documentation related to data pre-processing or additional filtering. -->
[More Information Needed]
### Training Procedure
<!-- This relates heavily to the Technical Specifications. Content here should link to that section when it is relevant to the training procedure. -->
#### Preprocessing [optional]
[More Information Needed]
#### Training Hyperparameters
- **Training regime:** [More Information Needed] <!--fp32, fp16 mixed precision, bf16 mixed precision, bf16 non-mixed precision, fp16 non-mixed precision, fp8 mixed precision -->
#### Speeds, Sizes, Times [optional]
<!-- This section provides information about throughput, start/end time, checkpoint size if relevant, etc. -->
[More Information Needed]
## Evaluation
<!-- This section describes the evaluation protocols and provides the results. -->
### Testing Data, Factors & Metrics
#### Testing Data
<!-- This should link to a Dataset Card if possible. -->
[More Information Needed]
#### Factors
<!-- These are the things the evaluation is disaggregating by, e.g., subpopulations or domains. -->
[More Information Needed]
#### Metrics
<!-- These are the evaluation metrics being used, ideally with a description of why. -->
[More Information Needed]
### Results
[More Information Needed]
#### Summary
## Model Examination [optional]
<!-- Relevant interpretability work for the model goes here -->
[More Information Needed]
## Environmental Impact
<!-- Total emissions (in grams of CO2eq) and additional considerations, such as electricity usage, go here. Edit the suggested text below accordingly -->
Carbon emissions can be estimated using the [Machine Learning Impact calculator](https://mlco2.github.io/impact#compute) presented in [Lacoste et al. (2019)](https://arxiv.org/abs/1910.09700).
- **Hardware Type:** [More Information Needed]
- **Hours used:** [More Information Needed]
- **Cloud Provider:** [More Information Needed]
- **Compute Region:** [More Information Needed]
- **Carbon Emitted:** [More Information Needed]
## Technical Specifications [optional]
### Model Architecture and Objective
[More Information Needed]
### Compute Infrastructure
[More Information Needed]
#### Hardware
[More Information Needed]
#### Software
[More Information Needed]
## Citation [optional]
<!-- If there is a paper or blog post introducing the model, the APA and Bibtex information for that should go in this section. -->
**BibTeX:**
[More Information Needed]
**APA:**
[More Information Needed]
## Glossary [optional]
<!-- If relevant, include terms and calculations in this section that can help readers understand the model or model card. -->
[More Information Needed]
## More Information [optional]
[More Information Needed]
## Model Card Authors [optional]
[More Information Needed]
## Model Card Contact
[More Information Needed] |
mrm8488/bert2bert_shared-spanish-finetuned-summarization | mrm8488 | "2023-05-02T18:59:18Z" | 1,935 | 31 | transformers | [
"transformers",
"pytorch",
"safetensors",
"encoder-decoder",
"text2text-generation",
"summarization",
"news",
"es",
"dataset:mlsum",
"autotrain_compatible",
"endpoints_compatible",
"region:us"
] | summarization | "2022-03-02T23:29:05Z" | ---
tags:
- summarization
- news
language: es
datasets:
- mlsum
widget:
- text: 'Al filo de las 22.00 horas del jueves, la Asamblea de Madrid vive un momento sorprendente: Vox decide no apoyar una propuesta del PP en favor del blindaje fiscal de la Comunidad. Se ha roto la unidad de los tres partidos de derechas. Es un hecho excepcional. Desde que arrancó la legislatura, PP, Cs y Vox han votado en bloque casi el 75% de las veces en el pleno de la Cámara. Juntos decidieron la composición de la Mesa de la Asamblea. Juntos invistieron presidenta a Isabel Díaz Ayuso. Y juntos han votado la mayoría de proposiciones no de ley, incluida la que ha marcado el esprint final de la campaña para las elecciones generales: acaban de instar al Gobierno de España a "la ilegalización inmediata" de los partidos separatistas "que atenten contra la unidad de la Nación". Los críticos de Cs no comparten el apoyo al texto de Vox contra el secesionisimo Ese balance retrata una necesidad antes que una complicidad, según fuentes del PP con predicamento en la dirección regional y nacional. Tras casi 15 años gobernando con mayoría absoluta, la formación conservadora vivió como una tortura la pasada legislatura, en la que dependió de Cs para sacar adelante sus iniciativas. El problema se agudizó tras las elecciones autonómicas de mayo. El PP ha tenido que formar con Cs el primer gobierno de coalición de la historia de la región, y ni siquiera con eso le basta para ganar las votaciones de la Cámara. Los dos socios gubernamentales necesitan a Vox, la menos predecible de las tres formaciones. "Tenemos que trabajar juntos defendiendo la unidad del país, por eso no quisimos dejar a Vox solo", dijo ayer Díaz Ayuso para justificar el apoyo de PP y Cs a la proposición de la extrema derecha sobre Cataluña. "Después nosotros llevábamos otra proposición para defender el blindaje fiscal de Madrid, y ahí Vox nos dejó atrás. No permitió que esto saliera. Es un grave error por su parte", prosiguió, recalcando el enfado del PP. "Demuestra que está más en cuestiones electoralistas", subrayó. "Los que pensamos, con nuestras inmensas diferencias, que tenemos cosas en común que nos unen como partidos que queremos Comunidades libres, con bajos impuestos, en las que se viva con seguridad y en paz, tenemos que estar unidos", argumentó. "Y por lo menos nosotros de nuestra línea no nos separamos". Al contrario de lo que está ocurriendo el Ayuntamiento de Madrid, donde el PP y Cs ya han defendido posiciones de voto distintas, pese a compartir el Gobierno, en la Asamblea los partidos de Díaz Ayuso e Ignacio Aguado están actuando con la máxima lealtad en las votaciones del pleno. Otra cosa son las comisiones. Y el caso Avalmadrid. Es en ese terreno donde Cs y Vox están buscando el margen de maniobra necesario para separarse del PP en plena campaña electoral, abandonando a su suerte a su socio para distinguirse ante los electores. —"Usted me ha dejado tirada", le espetó la presidenta de la Comunidad de Madrid a Rocío Monasterio tras saber que Vox permitiría que la izquierda tuviera mayoría en la comisión parlamentaria que investigará los avales concedidos por la empresa semipública entre 2007 y 2018, lo que podría incluir el de 400.000 euros aprobado en 2011, y nunca devuelto al completo, para una empresa participada por el padre de Isabel Díaz Ayuso. "Monasterio no es de fiar. Dice una cosa y hace la contraria", dice una fuente popular sobre las negociaciones mantenidas para repartirse los puestos de las diferentes comisiones, que Vox no cumplió tras buscar un segundo pacto con otras formaciones (que no llegó a buen puerto). Ilegalización de Vox Los tres partidos de derechas también se han enfrentado por la ubicación de Vox en el pleno. Las largas negociaciones para la investidura de Díaz Ayuso dejaron heridas abiertas. Y los diputados de Cs no desaprovechan la oportunidad de lanzar dardos contra los de Vox, pero luego coinciden con ellos en la mayoría de votaciones. Ocurrió, por ejemplo, el jueves, cuando se debatía la polémica proposición para instar al Gobierno nacional a ilegalizar a los partidos separatistas que atenten contra la unidad de España. —"Mostrar nuestra sorpresa ante la presentación por parte de Vox de esta propuesta", lanzó Araceli Gómez, diputada de la formación de Aguado. "Sorprende que planteen ustedes este asunto cuando está también sobre la mesa el debate de su propia ilegalización por atentar contra el ordenamiento jurídico o contra valores constitucionales como la igualdad o la no discriminación". Luego de esa descalificación, y ante la incredulidad de los diputados de los partidos de izquierdas, Cs unió sus votos a los de Vox y a los del PP. La decisión ha provocado polémica interna, como demuestra que Albert Rivera no la apoyara ayer explícitamente. Tampoco ha sido bien acogida por el sector crítico de la formación. Pero ha demostrado una cosa: en Madrid hay tres partidos que casi siempre votan como uno.'
---
# Spanish BERT2BERT (BETO) fine-tuned on MLSUM ES for summarization
## Model
[dccuchile/bert-base-spanish-wwm-cased](https://huggingface.co/dccuchile/bert-base-spanish-wwm-cased) (BERT Checkpoint)
## Dataset
**MLSUM** is the first large-scale MultiLingual SUMmarization dataset. Obtained from online newspapers, it contains 1.5M+ article/summary pairs in five different languages -- namely, French, German, **Spanish**, Russian, Turkish. Together with English newspapers from the popular CNN/Daily mail dataset, the collected data form a large scale multilingual dataset which can enable new research directions for the text summarization community. We report cross-lingual comparative analyses based on state-of-the-art systems. These highlight existing biases which motivate the use of a multi-lingual dataset.
[MLSUM es](https://huggingface.co/datasets/viewer/?dataset=mlsum)
## Results
|Set|Metric| Value|
|----|------|------|
| Test |Rouge2 - mid -precision | **9.6**|
| Test | Rouge2 - mid - recall | **8.4**|
| Test | Rouge2 - mid - fmeasure | **8.7**|
| Test | Rouge1 | 26.24 |
| Test | Rouge2 | 8.9 |
| Test | RougeL | 21.01|
| Test | RougeLsum | 21.02 |
## Usage
```python
import torch
from transformers import BertTokenizerFast, EncoderDecoderModel
device = 'cuda' if torch.cuda.is_available() else 'cpu'
ckpt = 'mrm8488/bert2bert_shared-spanish-finetuned-summarization'
tokenizer = BertTokenizerFast.from_pretrained(ckpt)
model = EncoderDecoderModel.from_pretrained(ckpt).to(device)
def generate_summary(text):
inputs = tokenizer([text], padding="max_length", truncation=True, max_length=512, return_tensors="pt")
input_ids = inputs.input_ids.to(device)
attention_mask = inputs.attention_mask.to(device)
output = model.generate(input_ids, attention_mask=attention_mask)
return tokenizer.decode(output[0], skip_special_tokens=True)
text = "Your text here..."
generate_summary(text)
```
> Created by [Manuel Romero/@mrm8488](https://twitter.com/mrm8488) with the support of [Narrativa](https://www.narrativa.com/)
> Made with <span style="color: #e25555;">♥</span> in Spain
|
ntc-ai/SDXL-LoRA-slider.cel-shaded | ntc-ai | "2023-12-19T22:36:42Z" | 26 | 0 | diffusers | [
"diffusers",
"text-to-image",
"stable-diffusion-xl",
"lora",
"template:sd-lora",
"template:sdxl-lora",
"sdxl-sliders",
"ntcai.xyz-sliders",
"concept",
"en",
"base_model:stabilityai/stable-diffusion-xl-base-1.0",
"base_model:adapter:stabilityai/stable-diffusion-xl-base-1.0",
"license:mit",
"region:us"
] | text-to-image | "2023-12-19T22:36:39Z" |
---
language:
- en
thumbnail: "images/evaluate/cel-shaded.../cel-shaded_17_3.0.png"
widget:
- text: cel-shaded
output:
url: images/cel-shaded_17_3.0.png
- text: cel-shaded
output:
url: images/cel-shaded_19_3.0.png
- text: cel-shaded
output:
url: images/cel-shaded_20_3.0.png
- text: cel-shaded
output:
url: images/cel-shaded_21_3.0.png
- text: cel-shaded
output:
url: images/cel-shaded_22_3.0.png
tags:
- text-to-image
- stable-diffusion-xl
- lora
- template:sd-lora
- template:sdxl-lora
- sdxl-sliders
- ntcai.xyz-sliders
- concept
- diffusers
license: "mit"
inference: false
instance_prompt: "cel-shaded"
base_model: "stabilityai/stable-diffusion-xl-base-1.0"
---
# ntcai.xyz slider - cel-shaded (SDXL LoRA)
| Strength: -3 | Strength: 0 | Strength: 3 |
| --- | --- | --- |
| <img src="images/cel-shaded_17_-3.0.png" width=256 height=256 /> | <img src="images/cel-shaded_17_0.0.png" width=256 height=256 /> | <img src="images/cel-shaded_17_3.0.png" width=256 height=256 /> |
| <img src="images/cel-shaded_19_-3.0.png" width=256 height=256 /> | <img src="images/cel-shaded_19_0.0.png" width=256 height=256 /> | <img src="images/cel-shaded_19_3.0.png" width=256 height=256 /> |
| <img src="images/cel-shaded_20_-3.0.png" width=256 height=256 /> | <img src="images/cel-shaded_20_0.0.png" width=256 height=256 /> | <img src="images/cel-shaded_20_3.0.png" width=256 height=256 /> |
## Download
Weights for this model are available in Safetensors format.
## Trigger words
You can apply this LoRA with trigger words for additional effect:
```
cel-shaded
```
## Use in diffusers
```python
from diffusers import StableDiffusionXLPipeline
from diffusers import EulerAncestralDiscreteScheduler
import torch
pipe = StableDiffusionXLPipeline.from_single_file("https://huggingface.co/martyn/sdxl-turbo-mario-merge-top-rated/blob/main/topRatedTurboxlLCM_v10.safetensors")
pipe.to("cuda")
pipe.scheduler = EulerAncestralDiscreteScheduler.from_config(pipe.scheduler.config)
# Load the LoRA
pipe.load_lora_weights('ntc-ai/SDXL-LoRA-slider.cel-shaded', weight_name='cel-shaded.safetensors', adapter_name="cel-shaded")
# Activate the LoRA
pipe.set_adapters(["cel-shaded"], adapter_weights=[2.0])
prompt = "medieval rich kingpin sitting in a tavern, cel-shaded"
negative_prompt = "nsfw"
width = 512
height = 512
num_inference_steps = 10
guidance_scale = 2
image = pipe(prompt, negative_prompt=negative_prompt, width=width, height=height, guidance_scale=guidance_scale, num_inference_steps=num_inference_steps).images[0]
image.save('result.png')
```
## Support the Patreon
If you like this model please consider [joining our Patreon](https://www.patreon.com/NTCAI).
By joining our Patreon, you'll gain access to an ever-growing library of over 480+ unique and diverse LoRAs, covering a wide range of styles and genres. You'll also receive early access to new models and updates, exclusive behind-the-scenes content, and the powerful LoRA slider creator, allowing you to craft your own custom LoRAs and experiment with endless possibilities.
Your support on Patreon will allow us to continue developing and refining new models.
## Other resources
- [CivitAI](https://civitai.com/user/ntc) - Follow ntc on Civit for even more LoRAs
- [ntcai.xyz](https://ntcai.xyz) - See ntcai.xyz to find more articles and LoRAs
|
gd1m3y/test_trainer_1 | gd1m3y | "2022-11-22T17:38:49Z" | 178 | 0 | transformers | [
"transformers",
"pytorch",
"tensorboard",
"roberta",
"text-classification",
"autotrain_compatible",
"endpoints_compatible",
"region:us"
] | text-classification | "2022-11-22T17:04:11Z" | <<<<<<< HEAD
---
tags:
- generated_from_trainer
datasets:
- financial_phrasebank
model-index:
- name: test_trainer_1
results: []
---
<!-- This model card has been generated automatically according to the information the Trainer had access to. You
should probably proofread and complete it, then remove this comment. -->
# test_trainer_1
This model is a fine-tuned version of [SALT-NLP/FLANG-Roberta](https://huggingface.co/SALT-NLP/FLANG-Roberta) on the financial_phrasebank dataset.
It achieves the following results on the evaluation set:
- eval_loss: 0.5963
- eval_accuracy: 0.9242
- eval_runtime: 4.3354
- eval_samples_per_second: 97.337
- eval_steps_per_second: 12.225
- step: 0
## Model description
More information needed
## Intended uses & limitations
More information needed
## Training and evaluation data
More information needed
## Training procedure
### Training hyperparameters
The following hyperparameters were used during training:
- learning_rate: 5e-05
- train_batch_size: 8
- eval_batch_size: 8
- seed: 42
- optimizer: Adam with betas=(0.9,0.999) and epsilon=1e-08
- lr_scheduler_type: linear
- num_epochs: 5
### Framework versions
- Transformers 4.24.0
- Pytorch 1.12.1+cu113
- Datasets 2.7.0
- Tokenizers 0.13.2
=======
This is a demo model for our reference
>>>>>>> 24191373ff05e3799b9c6f359e51b37b642f4865
|
Emmanuelalo52/distilbert-base-uncased-finetuned-con-dataset | Emmanuelalo52 | "2024-01-13T12:34:33Z" | 93 | 0 | transformers | [
"transformers",
"pytorch",
"tensorboard",
"safetensors",
"distilbert",
"token-classification",
"generated_from_trainer",
"base_model:distilbert/distilbert-base-uncased",
"base_model:finetune:distilbert/distilbert-base-uncased",
"license:apache-2.0",
"autotrain_compatible",
"endpoints_compatible",
"region:us"
] | token-classification | "2024-01-08T16:34:08Z" | ---
license: apache-2.0
base_model: distilbert-base-uncased
tags:
- generated_from_trainer
model-index:
- name: distilbert-base-uncased-finetuned-con-dataset
results: []
---
<!-- This model card has been generated automatically according to the information the Trainer had access to. You
should probably proofread and complete it, then remove this comment. -->
# distilbert-base-uncased-finetuned-con-dataset
This model is a fine-tuned version of [distilbert-base-uncased](https://huggingface.co/distilbert-base-uncased) on an unknown dataset.
## Model description
More information needed
## Intended uses & limitations
More information needed
## Training and evaluation data
More information needed
## Training procedure
### Training hyperparameters
The following hyperparameters were used during training:
- learning_rate: 5e-05
- train_batch_size: 30
- eval_batch_size: 30
- seed: 42
- optimizer: Adam with betas=(0.9,0.999) and epsilon=1e-08
- lr_scheduler_type: linear
- num_epochs: 3
### Framework versions
- Transformers 4.35.2
- Pytorch 2.1.0+cu121
- Datasets 2.16.1
- Tokenizers 0.15.0
|
cerindam30/tugas_akhir_final | cerindam30 | "2023-07-23T15:50:25Z" | 4 | 0 | transformers | [
"transformers",
"pytorch",
"mbart",
"text2text-generation",
"generated_from_trainer",
"license:mit",
"autotrain_compatible",
"endpoints_compatible",
"region:us"
] | text2text-generation | "2023-07-23T11:39:47Z" | ---
license: mit
tags:
- generated_from_trainer
model-index:
- name: tugas_akhir_final
results: []
---
<!-- This model card has been generated automatically according to the information the Trainer had access to. You
should probably proofread and complete it, then remove this comment. -->
# tugas_akhir_final
This model is a fine-tuned version of [indobenchmark/indobart-v2](https://huggingface.co/indobenchmark/indobart-v2) on an unknown dataset.
## Model description
More information needed
## Intended uses & limitations
More information needed
## Training and evaluation data
More information needed
## Training procedure
### Training hyperparameters
The following hyperparameters were used during training:
- learning_rate: 5e-05
- train_batch_size: 4
- eval_batch_size: 4
- seed: 42
- optimizer: Adam with betas=(0.9,0.999) and epsilon=1e-08
- lr_scheduler_type: linear
- lr_scheduler_warmup_steps: 500
- num_epochs: 3
- label_smoothing_factor: 0.1
### Training results
### Framework versions
- Transformers 4.28.0
- Pytorch 2.0.1+cu118
- Datasets 2.13.1
- Tokenizers 0.13.3
|
MayBashendy/ASAP_FineTuningBERT_Aug_k1_task1_organization_fold4 | MayBashendy | "2024-11-05T19:14:00Z" | 163 | 0 | transformers | [
"transformers",
"safetensors",
"bert",
"text-classification",
"generated_from_trainer",
"base_model:google-bert/bert-base-uncased",
"base_model:finetune:google-bert/bert-base-uncased",
"license:apache-2.0",
"autotrain_compatible",
"endpoints_compatible",
"region:us"
] | text-classification | "2024-11-05T18:42:31Z" | ---
library_name: transformers
license: apache-2.0
base_model: bert-base-uncased
tags:
- generated_from_trainer
model-index:
- name: ASAP_FineTuningBERT_Aug_k1_task1_organization_fold4
results: []
---
<!-- This model card has been generated automatically according to the information the Trainer had access to. You
should probably proofread and complete it, then remove this comment. -->
# ASAP_FineTuningBERT_Aug_k1_task1_organization_fold4
This model is a fine-tuned version of [bert-base-uncased](https://huggingface.co/bert-base-uncased) on the None dataset.
It achieves the following results on the evaluation set:
- Loss: 0.5265
- Qwk: 0.6796
- Mse: 0.5265
- Rmse: 0.7256
## Model description
More information needed
## Intended uses & limitations
More information needed
## Training and evaluation data
More information needed
## Training procedure
### Training hyperparameters
The following hyperparameters were used during training:
- learning_rate: 2e-05
- train_batch_size: 32
- eval_batch_size: 32
- seed: 42
- optimizer: Adam with betas=(0.9,0.999) and epsilon=1e-08
- lr_scheduler_type: linear
- num_epochs: 10
### Training results
| Training Loss | Epoch | Step | Validation Loss | Qwk | Mse | Rmse |
|:-------------:|:------:|:----:|:---------------:|:------:|:-------:|:------:|
| No log | 0.0417 | 2 | 11.2699 | 0.0 | 11.2699 | 3.3571 |
| No log | 0.0833 | 4 | 9.3623 | 0.0 | 9.3623 | 3.0598 |
| No log | 0.125 | 6 | 8.1887 | 0.0180 | 8.1887 | 2.8616 |
| No log | 0.1667 | 8 | 6.7371 | 0.0041 | 6.7371 | 2.5956 |
| No log | 0.2083 | 10 | 5.1276 | 0.0018 | 5.1276 | 2.2644 |
| No log | 0.25 | 12 | 3.8558 | 0.0271 | 3.8558 | 1.9636 |
| No log | 0.2917 | 14 | 3.0311 | 0.0144 | 3.0311 | 1.7410 |
| No log | 0.3333 | 16 | 2.0260 | 0.0118 | 2.0260 | 1.4234 |
| No log | 0.375 | 18 | 1.3929 | 0.1339 | 1.3929 | 1.1802 |
| No log | 0.4167 | 20 | 1.0161 | 0.0482 | 1.0161 | 1.0080 |
| No log | 0.4583 | 22 | 0.8732 | 0.0420 | 0.8732 | 0.9344 |
| No log | 0.5 | 24 | 0.8395 | 0.0420 | 0.8395 | 0.9162 |
| No log | 0.5417 | 26 | 0.8377 | 0.0420 | 0.8377 | 0.9152 |
| No log | 0.5833 | 28 | 0.7499 | 0.0420 | 0.7499 | 0.8659 |
| No log | 0.625 | 30 | 0.7141 | 0.0445 | 0.7141 | 0.8450 |
| No log | 0.6667 | 32 | 0.6710 | 0.1672 | 0.6710 | 0.8191 |
| No log | 0.7083 | 34 | 0.7725 | 0.3880 | 0.7725 | 0.8789 |
| No log | 0.75 | 36 | 0.6588 | 0.5275 | 0.6588 | 0.8116 |
| No log | 0.7917 | 38 | 0.5369 | 0.3533 | 0.5369 | 0.7327 |
| No log | 0.8333 | 40 | 0.5147 | 0.4978 | 0.5147 | 0.7174 |
| No log | 0.875 | 42 | 0.5289 | 0.5851 | 0.5289 | 0.7273 |
| No log | 0.9167 | 44 | 0.4616 | 0.5053 | 0.4616 | 0.6794 |
| No log | 0.9583 | 46 | 0.4515 | 0.5436 | 0.4515 | 0.6720 |
| No log | 1.0 | 48 | 0.6819 | 0.5619 | 0.6819 | 0.8258 |
| No log | 1.0417 | 50 | 0.6219 | 0.5921 | 0.6219 | 0.7886 |
| No log | 1.0833 | 52 | 0.4424 | 0.5574 | 0.4424 | 0.6651 |
| No log | 1.125 | 54 | 0.4527 | 0.5123 | 0.4527 | 0.6728 |
| No log | 1.1667 | 56 | 0.5569 | 0.5981 | 0.5569 | 0.7463 |
| No log | 1.2083 | 58 | 0.6383 | 0.5822 | 0.6383 | 0.7989 |
| No log | 1.25 | 60 | 0.5338 | 0.5972 | 0.5338 | 0.7306 |
| No log | 1.2917 | 62 | 0.5950 | 0.5800 | 0.5950 | 0.7714 |
| No log | 1.3333 | 64 | 0.4756 | 0.5967 | 0.4756 | 0.6897 |
| No log | 1.375 | 66 | 0.4338 | 0.5528 | 0.4338 | 0.6587 |
| No log | 1.4167 | 68 | 0.4640 | 0.6126 | 0.4640 | 0.6812 |
| No log | 1.4583 | 70 | 0.6271 | 0.5856 | 0.6271 | 0.7919 |
| No log | 1.5 | 72 | 0.4851 | 0.6024 | 0.4851 | 0.6965 |
| No log | 1.5417 | 74 | 0.4734 | 0.4620 | 0.4734 | 0.6880 |
| No log | 1.5833 | 76 | 0.5325 | 0.4431 | 0.5325 | 0.7297 |
| No log | 1.625 | 78 | 0.4280 | 0.5707 | 0.4280 | 0.6542 |
| No log | 1.6667 | 80 | 0.5599 | 0.5865 | 0.5599 | 0.7483 |
| No log | 1.7083 | 82 | 0.8027 | 0.5234 | 0.8027 | 0.8959 |
| No log | 1.75 | 84 | 0.6785 | 0.5532 | 0.6785 | 0.8237 |
| No log | 1.7917 | 86 | 0.4635 | 0.5671 | 0.4635 | 0.6808 |
| No log | 1.8333 | 88 | 0.4464 | 0.5372 | 0.4464 | 0.6682 |
| No log | 1.875 | 90 | 0.4805 | 0.6042 | 0.4805 | 0.6932 |
| No log | 1.9167 | 92 | 0.5107 | 0.6076 | 0.5107 | 0.7147 |
| No log | 1.9583 | 94 | 0.4286 | 0.5905 | 0.4286 | 0.6547 |
| No log | 2.0 | 96 | 0.4261 | 0.5733 | 0.4261 | 0.6528 |
| No log | 2.0417 | 98 | 0.4704 | 0.6098 | 0.4704 | 0.6858 |
| No log | 2.0833 | 100 | 0.5118 | 0.6168 | 0.5118 | 0.7154 |
| No log | 2.125 | 102 | 0.4913 | 0.6260 | 0.4913 | 0.7009 |
| No log | 2.1667 | 104 | 0.4274 | 0.5708 | 0.4274 | 0.6537 |
| No log | 2.2083 | 106 | 0.4409 | 0.5729 | 0.4409 | 0.6640 |
| No log | 2.25 | 108 | 0.4875 | 0.6479 | 0.4875 | 0.6982 |
| No log | 2.2917 | 110 | 0.7495 | 0.6007 | 0.7495 | 0.8658 |
| No log | 2.3333 | 112 | 0.6463 | 0.6235 | 0.6463 | 0.8039 |
| No log | 2.375 | 114 | 0.4544 | 0.6216 | 0.4544 | 0.6741 |
| No log | 2.4167 | 116 | 0.4329 | 0.6018 | 0.4329 | 0.6579 |
| No log | 2.4583 | 118 | 0.4995 | 0.6310 | 0.4995 | 0.7067 |
| No log | 2.5 | 120 | 0.5789 | 0.6167 | 0.5789 | 0.7609 |
| No log | 2.5417 | 122 | 0.4697 | 0.6588 | 0.4697 | 0.6853 |
| No log | 2.5833 | 124 | 0.4827 | 0.6502 | 0.4827 | 0.6948 |
| No log | 2.625 | 126 | 0.4584 | 0.6568 | 0.4584 | 0.6770 |
| No log | 2.6667 | 128 | 0.4117 | 0.5831 | 0.4117 | 0.6416 |
| No log | 2.7083 | 130 | 0.4084 | 0.5604 | 0.4084 | 0.6390 |
| No log | 2.75 | 132 | 0.4560 | 0.6203 | 0.4560 | 0.6753 |
| No log | 2.7917 | 134 | 0.5400 | 0.6037 | 0.5400 | 0.7349 |
| No log | 2.8333 | 136 | 0.4389 | 0.6169 | 0.4389 | 0.6625 |
| No log | 2.875 | 138 | 0.4256 | 0.5317 | 0.4256 | 0.6523 |
| No log | 2.9167 | 140 | 0.4140 | 0.5786 | 0.4140 | 0.6434 |
| No log | 2.9583 | 142 | 0.4362 | 0.6323 | 0.4362 | 0.6605 |
| No log | 3.0 | 144 | 0.5926 | 0.6655 | 0.5926 | 0.7698 |
| No log | 3.0417 | 146 | 0.6280 | 0.6651 | 0.6280 | 0.7925 |
| No log | 3.0833 | 148 | 0.4395 | 0.6750 | 0.4395 | 0.6629 |
| No log | 3.125 | 150 | 0.4740 | 0.4927 | 0.4740 | 0.6884 |
| No log | 3.1667 | 152 | 0.4862 | 0.4892 | 0.4862 | 0.6973 |
| No log | 3.2083 | 154 | 0.4042 | 0.5827 | 0.4042 | 0.6358 |
| No log | 3.25 | 156 | 0.5375 | 0.6708 | 0.5375 | 0.7332 |
| No log | 3.2917 | 158 | 0.5542 | 0.6797 | 0.5542 | 0.7444 |
| No log | 3.3333 | 160 | 0.4328 | 0.6458 | 0.4328 | 0.6579 |
| No log | 3.375 | 162 | 0.4086 | 0.5605 | 0.4086 | 0.6393 |
| No log | 3.4167 | 164 | 0.4055 | 0.5936 | 0.4055 | 0.6368 |
| No log | 3.4583 | 166 | 0.4671 | 0.6441 | 0.4671 | 0.6834 |
| No log | 3.5 | 168 | 0.5957 | 0.6802 | 0.5957 | 0.7718 |
| No log | 3.5417 | 170 | 0.5395 | 0.6554 | 0.5395 | 0.7345 |
| No log | 3.5833 | 172 | 0.4185 | 0.6185 | 0.4185 | 0.6469 |
| No log | 3.625 | 174 | 0.4139 | 0.6134 | 0.4139 | 0.6433 |
| No log | 3.6667 | 176 | 0.4666 | 0.6358 | 0.4666 | 0.6831 |
| No log | 3.7083 | 178 | 0.5023 | 0.6492 | 0.5023 | 0.7088 |
| No log | 3.75 | 180 | 0.4782 | 0.6300 | 0.4782 | 0.6915 |
| No log | 3.7917 | 182 | 0.4436 | 0.6304 | 0.4436 | 0.6661 |
| No log | 3.8333 | 184 | 0.4366 | 0.6488 | 0.4366 | 0.6608 |
| No log | 3.875 | 186 | 0.5210 | 0.6807 | 0.5210 | 0.7218 |
| No log | 3.9167 | 188 | 0.5182 | 0.6587 | 0.5182 | 0.7198 |
| No log | 3.9583 | 190 | 0.5036 | 0.6476 | 0.5036 | 0.7097 |
| No log | 4.0 | 192 | 0.4960 | 0.6579 | 0.4960 | 0.7042 |
| No log | 4.0417 | 194 | 0.5929 | 0.6485 | 0.5929 | 0.7700 |
| No log | 4.0833 | 196 | 0.5575 | 0.6530 | 0.5575 | 0.7467 |
| No log | 4.125 | 198 | 0.4605 | 0.6801 | 0.4605 | 0.6786 |
| No log | 4.1667 | 200 | 0.4428 | 0.6622 | 0.4428 | 0.6655 |
| No log | 4.2083 | 202 | 0.4125 | 0.6240 | 0.4125 | 0.6422 |
| No log | 4.25 | 204 | 0.4836 | 0.6366 | 0.4836 | 0.6954 |
| No log | 4.2917 | 206 | 0.4626 | 0.6324 | 0.4626 | 0.6801 |
| No log | 4.3333 | 208 | 0.3992 | 0.5814 | 0.3992 | 0.6318 |
| No log | 4.375 | 210 | 0.4001 | 0.5947 | 0.4001 | 0.6326 |
| No log | 4.4167 | 212 | 0.4443 | 0.6581 | 0.4443 | 0.6666 |
| No log | 4.4583 | 214 | 0.6292 | 0.6739 | 0.6292 | 0.7932 |
| No log | 4.5 | 216 | 0.6411 | 0.6842 | 0.6411 | 0.8007 |
| No log | 4.5417 | 218 | 0.4523 | 0.6746 | 0.4523 | 0.6725 |
| No log | 4.5833 | 220 | 0.4365 | 0.6559 | 0.4365 | 0.6607 |
| No log | 4.625 | 222 | 0.5204 | 0.7094 | 0.5204 | 0.7214 |
| No log | 4.6667 | 224 | 0.5400 | 0.7061 | 0.5400 | 0.7349 |
| No log | 4.7083 | 226 | 0.4752 | 0.6921 | 0.4752 | 0.6894 |
| No log | 4.75 | 228 | 0.4147 | 0.6517 | 0.4147 | 0.6439 |
| No log | 4.7917 | 230 | 0.4137 | 0.6395 | 0.4137 | 0.6432 |
| No log | 4.8333 | 232 | 0.4862 | 0.6878 | 0.4862 | 0.6973 |
| No log | 4.875 | 234 | 0.6432 | 0.7126 | 0.6432 | 0.8020 |
| No log | 4.9167 | 236 | 0.5727 | 0.6960 | 0.5727 | 0.7568 |
| No log | 4.9583 | 238 | 0.5123 | 0.6841 | 0.5123 | 0.7158 |
| No log | 5.0 | 240 | 0.5227 | 0.6835 | 0.5227 | 0.7230 |
| No log | 5.0417 | 242 | 0.5506 | 0.7064 | 0.5506 | 0.7420 |
| No log | 5.0833 | 244 | 0.5198 | 0.6969 | 0.5198 | 0.7209 |
| No log | 5.125 | 246 | 0.5042 | 0.6892 | 0.5042 | 0.7101 |
| No log | 5.1667 | 248 | 0.4455 | 0.6430 | 0.4455 | 0.6675 |
| No log | 5.2083 | 250 | 0.4406 | 0.6427 | 0.4406 | 0.6638 |
| No log | 5.25 | 252 | 0.5149 | 0.6982 | 0.5149 | 0.7175 |
| No log | 5.2917 | 254 | 0.6948 | 0.7113 | 0.6948 | 0.8335 |
| No log | 5.3333 | 256 | 0.5962 | 0.7054 | 0.5962 | 0.7721 |
| No log | 5.375 | 258 | 0.4454 | 0.6847 | 0.4454 | 0.6674 |
| No log | 5.4167 | 260 | 0.4209 | 0.6550 | 0.4209 | 0.6488 |
| No log | 5.4583 | 262 | 0.4701 | 0.6843 | 0.4701 | 0.6856 |
| No log | 5.5 | 264 | 0.4978 | 0.6908 | 0.4978 | 0.7056 |
| No log | 5.5417 | 266 | 0.4894 | 0.6863 | 0.4894 | 0.6995 |
| No log | 5.5833 | 268 | 0.4603 | 0.6817 | 0.4603 | 0.6785 |
| No log | 5.625 | 270 | 0.4820 | 0.6902 | 0.4820 | 0.6943 |
| No log | 5.6667 | 272 | 0.5136 | 0.6898 | 0.5136 | 0.7166 |
| No log | 5.7083 | 274 | 0.5029 | 0.6875 | 0.5029 | 0.7091 |
| No log | 5.75 | 276 | 0.5700 | 0.7031 | 0.5700 | 0.7550 |
| No log | 5.7917 | 278 | 0.5115 | 0.6824 | 0.5115 | 0.7152 |
| No log | 5.8333 | 280 | 0.4894 | 0.6796 | 0.4894 | 0.6995 |
| No log | 5.875 | 282 | 0.4605 | 0.6644 | 0.4605 | 0.6786 |
| No log | 5.9167 | 284 | 0.5142 | 0.6865 | 0.5142 | 0.7171 |
| No log | 5.9583 | 286 | 0.5051 | 0.6882 | 0.5051 | 0.7107 |
| No log | 6.0 | 288 | 0.4478 | 0.6527 | 0.4478 | 0.6691 |
| No log | 6.0417 | 290 | 0.4523 | 0.6629 | 0.4523 | 0.6725 |
| No log | 6.0833 | 292 | 0.4948 | 0.6797 | 0.4948 | 0.7034 |
| No log | 6.125 | 294 | 0.4783 | 0.6738 | 0.4783 | 0.6916 |
| No log | 6.1667 | 296 | 0.4427 | 0.6364 | 0.4427 | 0.6654 |
| No log | 6.2083 | 298 | 0.4681 | 0.6697 | 0.4681 | 0.6842 |
| No log | 6.25 | 300 | 0.5424 | 0.6850 | 0.5424 | 0.7365 |
| No log | 6.2917 | 302 | 0.5472 | 0.6816 | 0.5472 | 0.7397 |
| No log | 6.3333 | 304 | 0.5363 | 0.6838 | 0.5363 | 0.7324 |
| No log | 6.375 | 306 | 0.4842 | 0.6722 | 0.4842 | 0.6958 |
| No log | 6.4167 | 308 | 0.5191 | 0.6820 | 0.5191 | 0.7205 |
| No log | 6.4583 | 310 | 0.4887 | 0.6720 | 0.4887 | 0.6990 |
| No log | 6.5 | 312 | 0.5196 | 0.6782 | 0.5196 | 0.7208 |
| No log | 6.5417 | 314 | 0.5751 | 0.6875 | 0.5751 | 0.7583 |
| No log | 6.5833 | 316 | 0.6437 | 0.7039 | 0.6437 | 0.8023 |
| No log | 6.625 | 318 | 0.5994 | 0.6922 | 0.5994 | 0.7742 |
| No log | 6.6667 | 320 | 0.4869 | 0.6814 | 0.4869 | 0.6978 |
| No log | 6.7083 | 322 | 0.5094 | 0.6849 | 0.5094 | 0.7137 |
| No log | 6.75 | 324 | 0.4909 | 0.6798 | 0.4909 | 0.7007 |
| No log | 6.7917 | 326 | 0.4471 | 0.6578 | 0.4471 | 0.6686 |
| No log | 6.8333 | 328 | 0.4749 | 0.6835 | 0.4749 | 0.6891 |
| No log | 6.875 | 330 | 0.5589 | 0.6901 | 0.5589 | 0.7476 |
| No log | 6.9167 | 332 | 0.5669 | 0.6828 | 0.5669 | 0.7529 |
| No log | 6.9583 | 334 | 0.4628 | 0.6640 | 0.4628 | 0.6803 |
| No log | 7.0 | 336 | 0.4375 | 0.6543 | 0.4375 | 0.6614 |
| No log | 7.0417 | 338 | 0.4359 | 0.6435 | 0.4359 | 0.6602 |
| No log | 7.0833 | 340 | 0.5039 | 0.6754 | 0.5039 | 0.7099 |
| No log | 7.125 | 342 | 0.5382 | 0.6784 | 0.5382 | 0.7336 |
| No log | 7.1667 | 344 | 0.4983 | 0.6808 | 0.4983 | 0.7059 |
| No log | 7.2083 | 346 | 0.4702 | 0.6701 | 0.4702 | 0.6857 |
| No log | 7.25 | 348 | 0.5240 | 0.6862 | 0.5240 | 0.7239 |
| No log | 7.2917 | 350 | 0.5335 | 0.6880 | 0.5335 | 0.7304 |
| No log | 7.3333 | 352 | 0.5482 | 0.6843 | 0.5482 | 0.7404 |
| No log | 7.375 | 354 | 0.6141 | 0.7026 | 0.6141 | 0.7836 |
| No log | 7.4167 | 356 | 0.5839 | 0.6906 | 0.5839 | 0.7641 |
| No log | 7.4583 | 358 | 0.5058 | 0.6788 | 0.5058 | 0.7112 |
| No log | 7.5 | 360 | 0.4728 | 0.6761 | 0.4728 | 0.6876 |
| No log | 7.5417 | 362 | 0.4994 | 0.6881 | 0.4994 | 0.7067 |
| No log | 7.5833 | 364 | 0.5384 | 0.6834 | 0.5384 | 0.7338 |
| No log | 7.625 | 366 | 0.5037 | 0.6819 | 0.5037 | 0.7097 |
| No log | 7.6667 | 368 | 0.4643 | 0.6677 | 0.4643 | 0.6814 |
| No log | 7.7083 | 370 | 0.4606 | 0.6717 | 0.4606 | 0.6786 |
| No log | 7.75 | 372 | 0.5266 | 0.6831 | 0.5266 | 0.7257 |
| No log | 7.7917 | 374 | 0.6326 | 0.6992 | 0.6326 | 0.7954 |
| No log | 7.8333 | 376 | 0.6051 | 0.6874 | 0.6051 | 0.7779 |
| No log | 7.875 | 378 | 0.4921 | 0.6761 | 0.4921 | 0.7015 |
| No log | 7.9167 | 380 | 0.4477 | 0.6435 | 0.4477 | 0.6691 |
| No log | 7.9583 | 382 | 0.4435 | 0.6280 | 0.4435 | 0.6660 |
| No log | 8.0 | 384 | 0.4709 | 0.6682 | 0.4709 | 0.6862 |
| No log | 8.0417 | 386 | 0.5734 | 0.6758 | 0.5734 | 0.7573 |
| No log | 8.0833 | 388 | 0.5860 | 0.6824 | 0.5860 | 0.7655 |
| No log | 8.125 | 390 | 0.5144 | 0.6846 | 0.5144 | 0.7172 |
| No log | 8.1667 | 392 | 0.4603 | 0.6754 | 0.4603 | 0.6785 |
| No log | 8.2083 | 394 | 0.4504 | 0.6672 | 0.4504 | 0.6711 |
| No log | 8.25 | 396 | 0.4701 | 0.6767 | 0.4701 | 0.6857 |
| No log | 8.2917 | 398 | 0.5024 | 0.6797 | 0.5024 | 0.7088 |
| No log | 8.3333 | 400 | 0.5424 | 0.6794 | 0.5424 | 0.7364 |
| No log | 8.375 | 402 | 0.5483 | 0.6769 | 0.5483 | 0.7405 |
| No log | 8.4167 | 404 | 0.5185 | 0.6716 | 0.5185 | 0.7201 |
| No log | 8.4583 | 406 | 0.5112 | 0.6727 | 0.5112 | 0.7150 |
| No log | 8.5 | 408 | 0.4790 | 0.6600 | 0.4790 | 0.6921 |
| No log | 8.5417 | 410 | 0.4861 | 0.6663 | 0.4861 | 0.6972 |
| No log | 8.5833 | 412 | 0.5152 | 0.6710 | 0.5152 | 0.7177 |
| No log | 8.625 | 414 | 0.5023 | 0.6771 | 0.5023 | 0.7087 |
| No log | 8.6667 | 416 | 0.4809 | 0.6668 | 0.4809 | 0.6935 |
| No log | 8.7083 | 418 | 0.4814 | 0.6685 | 0.4814 | 0.6938 |
| No log | 8.75 | 420 | 0.5047 | 0.6774 | 0.5047 | 0.7105 |
| No log | 8.7917 | 422 | 0.5499 | 0.6805 | 0.5499 | 0.7416 |
| No log | 8.8333 | 424 | 0.6000 | 0.6923 | 0.6000 | 0.7746 |
| No log | 8.875 | 426 | 0.5724 | 0.6806 | 0.5724 | 0.7566 |
| No log | 8.9167 | 428 | 0.5124 | 0.6724 | 0.5124 | 0.7158 |
| No log | 8.9583 | 430 | 0.4805 | 0.6731 | 0.4805 | 0.6932 |
| No log | 9.0 | 432 | 0.4820 | 0.6727 | 0.4820 | 0.6943 |
| No log | 9.0417 | 434 | 0.4850 | 0.6722 | 0.4850 | 0.6964 |
| No log | 9.0833 | 436 | 0.5131 | 0.6734 | 0.5131 | 0.7163 |
| No log | 9.125 | 438 | 0.5370 | 0.6799 | 0.5370 | 0.7328 |
| No log | 9.1667 | 440 | 0.5264 | 0.6727 | 0.5264 | 0.7255 |
| No log | 9.2083 | 442 | 0.5091 | 0.6783 | 0.5091 | 0.7135 |
| No log | 9.25 | 444 | 0.5087 | 0.6783 | 0.5087 | 0.7132 |
| No log | 9.2917 | 446 | 0.5293 | 0.6724 | 0.5293 | 0.7276 |
| No log | 9.3333 | 448 | 0.5697 | 0.6837 | 0.5697 | 0.7548 |
| No log | 9.375 | 450 | 0.6115 | 0.6924 | 0.6115 | 0.7820 |
| No log | 9.4167 | 452 | 0.6218 | 0.7042 | 0.6218 | 0.7885 |
| No log | 9.4583 | 454 | 0.5979 | 0.6893 | 0.5979 | 0.7732 |
| No log | 9.5 | 456 | 0.5560 | 0.6776 | 0.5560 | 0.7457 |
| No log | 9.5417 | 458 | 0.5147 | 0.6813 | 0.5147 | 0.7174 |
| No log | 9.5833 | 460 | 0.4869 | 0.6781 | 0.4869 | 0.6978 |
| No log | 9.625 | 462 | 0.4784 | 0.6722 | 0.4784 | 0.6916 |
| No log | 9.6667 | 464 | 0.4805 | 0.6735 | 0.4805 | 0.6932 |
| No log | 9.7083 | 466 | 0.4818 | 0.6747 | 0.4818 | 0.6941 |
| No log | 9.75 | 468 | 0.4894 | 0.6735 | 0.4894 | 0.6996 |
| No log | 9.7917 | 470 | 0.4987 | 0.6709 | 0.4987 | 0.7062 |
| No log | 9.8333 | 472 | 0.5104 | 0.6770 | 0.5104 | 0.7144 |
| No log | 9.875 | 474 | 0.5188 | 0.6807 | 0.5188 | 0.7203 |
| No log | 9.9167 | 476 | 0.5244 | 0.6787 | 0.5244 | 0.7242 |
| No log | 9.9583 | 478 | 0.5257 | 0.6796 | 0.5257 | 0.7251 |
| No log | 10.0 | 480 | 0.5265 | 0.6796 | 0.5265 | 0.7256 |
### Framework versions
- Transformers 4.44.2
- Pytorch 2.4.0+cu118
- Datasets 2.21.0
- Tokenizers 0.19.1
|
besa2001/ppo-SnowballTarget | besa2001 | "2023-01-13T18:51:11Z" | 0 | 0 | ml-agents | [
"ml-agents",
"tensorboard",
"onnx",
"unity-ml-agents",
"deep-reinforcement-learning",
"reinforcement-learning",
"ML-Agents-SnowballTarget",
"region:us"
] | reinforcement-learning | "2023-01-13T18:51:04Z" |
---
tags:
- unity-ml-agents
- ml-agents
- deep-reinforcement-learning
- reinforcement-learning
- ML-Agents-SnowballTarget
library_name: ml-agents
---
# **ppo** Agent playing **SnowballTarget**
This is a trained model of a **ppo** agent playing **SnowballTarget** using the [Unity ML-Agents Library](https://github.com/Unity-Technologies/ml-agents).
## Usage (with ML-Agents)
The Documentation: https://github.com/huggingface/ml-agents#get-started
We wrote a complete tutorial to learn to train your first agent using ML-Agents and publish it to the Hub:
### Resume the training
```
mlagents-learn <your_configuration_file_path.yaml> --run-id=<run_id> --resume
```
### Watch your Agent play
You can watch your agent **playing directly in your browser:**.
1. Go to https://huggingface.co/spaces/unity/ML-Agents-SnowballTarget
2. Step 1: Write your model_id: besa2001/ppo-SnowballTarget
3. Step 2: Select your *.nn /*.onnx file
4. Click on Watch the agent play 👀
|
tuantmdev/9148bf0a-1fc4-4e53-8f5f-6c103ecaeb44 | tuantmdev | "2025-01-22T20:36:08Z" | 10 | 0 | peft | [
"peft",
"safetensors",
"qwen2",
"axolotl",
"generated_from_trainer",
"base_model:unsloth/Qwen2-1.5B-Instruct",
"base_model:adapter:unsloth/Qwen2-1.5B-Instruct",
"license:apache-2.0",
"region:us"
] | null | "2025-01-22T20:27:37Z" | ---
library_name: peft
license: apache-2.0
base_model: unsloth/Qwen2-1.5B-Instruct
tags:
- axolotl
- generated_from_trainer
model-index:
- name: 9148bf0a-1fc4-4e53-8f5f-6c103ecaeb44
results: []
---
<!-- This model card has been generated automatically according to the information the Trainer had access to. You
should probably proofread and complete it, then remove this comment. -->
[<img src="https://raw.githubusercontent.com/axolotl-ai-cloud/axolotl/main/image/axolotl-badge-web.png" alt="Built with Axolotl" width="200" height="32"/>](https://github.com/axolotl-ai-cloud/axolotl)
<details><summary>See axolotl config</summary>
axolotl version: `0.4.1`
```yaml
adapter: lora
base_model: unsloth/Qwen2-1.5B-Instruct
bf16: auto
chat_template: llama3
dataset_prepared_path: null
datasets:
- data_files:
- a9dc132da9c02082_train_data.json
ds_type: json
format: custom
path: /workspace/input_data/a9dc132da9c02082_train_data.json
type:
field_input: comment
field_instruction: prompt
field_output: chosen
format: '{instruction} {input}'
no_input_format: '{instruction}'
system_format: '{system}'
system_prompt: ''
debug: null
deepspeed: null
early_stopping_patience: null
eval_max_new_tokens: 128
eval_table_size: null
evals_per_epoch: 5
flash_attention: false
fp16: null
fsdp: null
fsdp_config: null
gradient_accumulation_steps: 4
gradient_checkpointing: false
group_by_length: false
hub_model_id: tuantmdev/9148bf0a-1fc4-4e53-8f5f-6c103ecaeb44
hub_repo: null
hub_strategy: checkpoint
hub_token: null
learning_rate: 0.0001
load_in_4bit: false
load_in_8bit: false
local_rank: null
logging_steps: 5
lora_alpha: 16
lora_dropout: 0.05
lora_fan_in_fan_out: null
lora_model_dir: null
lora_r: 8
lora_target_linear: true
lr_scheduler: cosine
max_steps: 50
micro_batch_size: 2
mlflow_experiment_name: /tmp/a9dc132da9c02082_train_data.json
model_type: AutoModelForCausalLM
num_epochs: 1
optimizer: adamw_bnb_8bit
output_dir: miner_id_24
pad_to_sequence_len: true
resume_from_checkpoint: null
s2_attention: null
sample_packing: false
saves_per_epoch: 4
sequence_len: 512
strict: false
tf32: false
tokenizer_type: AutoTokenizer
train_on_inputs: false
trust_remote_code: true
val_set_size: 0.05
wandb_entity: null
wandb_mode: online
wandb_name: bd571fe7-d084-4326-868c-64c8ef8d1152
wandb_project: Gradients-On-Demand
wandb_run: your_name
wandb_runid: bd571fe7-d084-4326-868c-64c8ef8d1152
warmup_steps: 10
weight_decay: 0.0
xformers_attention: null
```
</details><br>
# 9148bf0a-1fc4-4e53-8f5f-6c103ecaeb44
This model is a fine-tuned version of [unsloth/Qwen2-1.5B-Instruct](https://huggingface.co/unsloth/Qwen2-1.5B-Instruct) on the None dataset.
It achieves the following results on the evaluation set:
- Loss: nan
## Model description
More information needed
## Intended uses & limitations
More information needed
## Training and evaluation data
More information needed
## Training procedure
### Training hyperparameters
The following hyperparameters were used during training:
- learning_rate: 0.0001
- train_batch_size: 2
- eval_batch_size: 2
- seed: 42
- gradient_accumulation_steps: 4
- total_train_batch_size: 8
- optimizer: Use OptimizerNames.ADAMW_BNB with betas=(0.9,0.999) and epsilon=1e-08 and optimizer_args=No additional optimizer arguments
- lr_scheduler_type: cosine
- lr_scheduler_warmup_steps: 10
- training_steps: 50
### Training results
| Training Loss | Epoch | Step | Validation Loss |
|:-------------:|:------:|:----:|:---------------:|
| No log | 0.0005 | 1 | nan |
| 0.0 | 0.0045 | 10 | nan |
| 0.0 | 0.0091 | 20 | nan |
| 0.0 | 0.0136 | 30 | nan |
| 0.0 | 0.0181 | 40 | nan |
| 0.0 | 0.0226 | 50 | nan |
### Framework versions
- PEFT 0.13.2
- Transformers 4.46.0
- Pytorch 2.5.0+cu124
- Datasets 3.0.1
- Tokenizers 0.20.1 |
splm/zephyr-7b-sft-full-spin-peft-iter0 | splm | "2024-02-06T00:04:05Z" | 1 | 0 | peft | [
"peft",
"safetensors",
"arxiv:1910.09700",
"base_model:alignment-handbook/zephyr-7b-sft-full",
"base_model:adapter:alignment-handbook/zephyr-7b-sft-full",
"license:mit",
"region:us"
] | null | "2024-02-05T21:47:18Z" | ---
library_name: peft
base_model: alignment-handbook/zephyr-7b-sft-full
license: mit
---
# Model Card for Model ID
<!-- Provide a quick summary of what the model is/does. -->
## Model Details
### Model Description
<!-- Provide a longer summary of what this model is. -->
- **Developed by:** [More Information Needed]
- **Funded by [optional]:** [More Information Needed]
- **Shared by [optional]:** [More Information Needed]
- **Model type:** [More Information Needed]
- **Language(s) (NLP):** [More Information Needed]
- **License:** [More Information Needed]
- **Finetuned from model [optional]:** [More Information Needed]
### Model Sources [optional]
<!-- Provide the basic links for the model. -->
- **Repository:** [More Information Needed]
- **Paper [optional]:** [More Information Needed]
- **Demo [optional]:** [More Information Needed]
## Uses
<!-- Address questions around how the model is intended to be used, including the foreseeable users of the model and those affected by the model. -->
### Direct Use
<!-- This section is for the model use without fine-tuning or plugging into a larger ecosystem/app. -->
[More Information Needed]
### Downstream Use [optional]
<!-- This section is for the model use when fine-tuned for a task, or when plugged into a larger ecosystem/app -->
[More Information Needed]
### Out-of-Scope Use
<!-- This section addresses misuse, malicious use, and uses that the model will not work well for. -->
[More Information Needed]
## Bias, Risks, and Limitations
<!-- This section is meant to convey both technical and sociotechnical limitations. -->
[More Information Needed]
### Recommendations
<!-- This section is meant to convey recommendations with respect to the bias, risk, and technical limitations. -->
Users (both direct and downstream) should be made aware of the risks, biases and limitations of the model. More information needed for further recommendations.
## How to Get Started with the Model
Use the code below to get started with the model.
[More Information Needed]
## Training Details
### Training Data
<!-- This should link to a Dataset Card, perhaps with a short stub of information on what the training data is all about as well as documentation related to data pre-processing or additional filtering. -->
[More Information Needed]
### Training Procedure
<!-- This relates heavily to the Technical Specifications. Content here should link to that section when it is relevant to the training procedure. -->
#### Preprocessing [optional]
[More Information Needed]
#### Training Hyperparameters
- **Training regime:** [More Information Needed] <!--fp32, fp16 mixed precision, bf16 mixed precision, bf16 non-mixed precision, fp16 non-mixed precision, fp8 mixed precision -->
#### Speeds, Sizes, Times [optional]
<!-- This section provides information about throughput, start/end time, checkpoint size if relevant, etc. -->
[More Information Needed]
## Evaluation
<!-- This section describes the evaluation protocols and provides the results. -->
### Testing Data, Factors & Metrics
#### Testing Data
<!-- This should link to a Dataset Card if possible. -->
[More Information Needed]
#### Factors
<!-- These are the things the evaluation is disaggregating by, e.g., subpopulations or domains. -->
[More Information Needed]
#### Metrics
<!-- These are the evaluation metrics being used, ideally with a description of why. -->
[More Information Needed]
### Results
[More Information Needed]
#### Summary
## Model Examination [optional]
<!-- Relevant interpretability work for the model goes here -->
[More Information Needed]
## Environmental Impact
<!-- Total emissions (in grams of CO2eq) and additional considerations, such as electricity usage, go here. Edit the suggested text below accordingly -->
Carbon emissions can be estimated using the [Machine Learning Impact calculator](https://mlco2.github.io/impact#compute) presented in [Lacoste et al. (2019)](https://arxiv.org/abs/1910.09700).
- **Hardware Type:** [More Information Needed]
- **Hours used:** [More Information Needed]
- **Cloud Provider:** [More Information Needed]
- **Compute Region:** [More Information Needed]
- **Carbon Emitted:** [More Information Needed]
## Technical Specifications [optional]
### Model Architecture and Objective
[More Information Needed]
### Compute Infrastructure
[More Information Needed]
#### Hardware
[More Information Needed]
#### Software
[More Information Needed]
## Citation [optional]
<!-- If there is a paper or blog post introducing the model, the APA and Bibtex information for that should go in this section. -->
**BibTeX:**
[More Information Needed]
**APA:**
[More Information Needed]
## Glossary [optional]
<!-- If relevant, include terms and calculations in this section that can help readers understand the model or model card. -->
[More Information Needed]
## More Information [optional]
[More Information Needed]
## Model Card Authors [optional]
[More Information Needed]
## Model Card Contact
[More Information Needed]
### Framework versions
- PEFT 0.8.2 |
PrunaAI/Nitral-AI-Hathor_Sofit-L3-8B-v1-AWQ-4bit-smashed | PrunaAI | "2024-08-14T07:58:04Z" | 7 | 0 | null | [
"safetensors",
"llama",
"pruna-ai",
"base_model:Nitral-AI/Hathor_Sofit-L3-8B-v1",
"base_model:quantized:Nitral-AI/Hathor_Sofit-L3-8B-v1",
"4-bit",
"awq",
"region:us"
] | null | "2024-08-14T07:55:37Z" | ---
thumbnail: "https://assets-global.website-files.com/646b351987a8d8ce158d1940/64ec9e96b4334c0e1ac41504_Logo%20with%20white%20text.svg"
base_model: Nitral-AI/Hathor_Sofit-L3-8B-v1
metrics:
- memory_disk
- memory_inference
- inference_latency
- inference_throughput
- inference_CO2_emissions
- inference_energy_consumption
tags:
- pruna-ai
---
<!-- header start -->
<!-- 200823 -->
<div style="width: auto; margin-left: auto; margin-right: auto">
<a href="https://www.pruna.ai/" target="_blank" rel="noopener noreferrer">
<img src="https://i.imgur.com/eDAlcgk.png" alt="PrunaAI" style="width: 100%; min-width: 400px; display: block; margin: auto;">
</a>
</div>
<!-- header end -->
[](https://twitter.com/PrunaAI)
[](https://github.com/PrunaAI)
[](https://www.linkedin.com/company/93832878/admin/feed/posts/?feedType=following)
[](https://discord.gg/rskEr4BZJx)
# Simply make AI models cheaper, smaller, faster, and greener!
- Give a thumbs up if you like this model!
- Contact us and tell us which model to compress next [here](https://www.pruna.ai/contact).
- Request access to easily compress your *own* AI models [here](https://z0halsaff74.typeform.com/pruna-access?typeform-source=www.pruna.ai).
- Read the documentations to know more [here](https://pruna-ai-pruna.readthedocs-hosted.com/en/latest/)
- Join Pruna AI community on Discord [here](https://discord.gg/CP4VSgck) to share feedback/suggestions or get help.
## Results

**Frequently Asked Questions**
- ***How does the compression work?*** The model is compressed with awq.
- ***How does the model quality change?*** The quality of the model output might vary compared to the base model.
- ***How is the model efficiency evaluated?*** These results were obtained on HARDWARE_NAME with configuration described in `model/smash_config.json` and are obtained after a hardware warmup. The smashed model is directly compared to the original base model. Efficiency results may vary in other settings (e.g. other hardware, image size, batch size, ...). We recommend to directly run them in the use-case conditions to know if the smashed model can benefit you.
- ***What is the model format?*** We use safetensors.
- ***What calibration data has been used?*** If needed by the compression method, we used WikiText as the calibration data.
- ***What is the naming convention for Pruna Huggingface models?*** We take the original model name and append "turbo", "tiny", or "green" if the smashed model has a measured inference speed, inference memory, or inference energy consumption which is less than 90% of the original base model.
- ***How to compress my own models?*** You can request premium access to more compression methods and tech support for your specific use-cases [here](https://z0halsaff74.typeform.com/pruna-access?typeform-source=www.pruna.ai).
- ***What are "first" metrics?*** Results mentioning "first" are obtained after the first run of the model. The first run might take more memory or be slower than the subsequent runs due cuda overheads.
- ***What are "Sync" and "Async" metrics?*** "Sync" metrics are obtained by syncing all GPU processes and stop measurement when all of them are executed. "Async" metrics are obtained without syncing all GPU processes and stop when the model output can be used by the CPU. We provide both metrics since both could be relevant depending on the use-case. We recommend to test the efficiency gains directly in your use-cases.
## Setup
You can run the smashed model with these steps:
0. Check requirements from the original repo Nitral-AI/Hathor_Sofit-L3-8B-v1 installed. In particular, check python, cuda, and transformers versions.
1. Make sure that you have installed quantization related packages.
```bash
pip install autoawq
```
2. Load & run the model.
```python
from transformers import AutoModelForCausalLM, AutoTokenizer
from awq import AutoAWQForCausalLM
model = AutoAWQForCausalLM.from_quantized("PrunaAI/Nitral-AI-Hathor_Sofit-L3-8B-v1-AWQ-4bit-smashed", trust_remote_code=True, device_map='auto')
tokenizer = AutoTokenizer.from_pretrained("Nitral-AI/Hathor_Sofit-L3-8B-v1")
input_ids = tokenizer("What is the color of prunes?,", return_tensors='pt').to(model.device)["input_ids"]
outputs = model.generate(input_ids, max_new_tokens=216)
tokenizer.decode(outputs[0])
```
## Configurations
The configuration info are in `smash_config.json`.
## Credits & License
The license of the smashed model follows the license of the original model. Please check the license of the original model Nitral-AI/Hathor_Sofit-L3-8B-v1 before using this model which provided the base model. The license of the `pruna-engine` is [here](https://pypi.org/project/pruna-engine/) on Pypi.
## Want to compress other models?
- Contact us and tell us which model to compress next [here](https://www.pruna.ai/contact).
- Request access to easily compress your own AI models [here](https://z0halsaff74.typeform.com/pruna-access?typeform-source=www.pruna.ai). |
GaetanMichelet/Llama-31-8B_task-3_120-samples_config-2 | GaetanMichelet | "2024-08-19T07:16:54Z" | 6 | 0 | peft | [
"peft",
"tensorboard",
"safetensors",
"llama",
"alignment-handbook",
"trl",
"sft",
"generated_from_trainer",
"dataset:GaetanMichelet/chat-60_ft_task-3",
"dataset:GaetanMichelet/chat-120_ft_task-3",
"base_model:meta-llama/Llama-3.1-8B-Instruct",
"base_model:adapter:meta-llama/Llama-3.1-8B-Instruct",
"license:llama3.1",
"4-bit",
"bitsandbytes",
"region:us"
] | null | "2024-08-19T06:08:35Z" | ---
base_model: meta-llama/Meta-Llama-3.1-8B-Instruct
datasets:
- GaetanMichelet/chat-60_ft_task-3
- GaetanMichelet/chat-120_ft_task-3
library_name: peft
license: llama3.1
tags:
- alignment-handbook
- trl
- sft
- generated_from_trainer
model-index:
- name: Llama-31-8B_task-3_120-samples_config-2
results: []
---
<!-- This model card has been generated automatically according to the information the Trainer had access to. You
should probably proofread and complete it, then remove this comment. -->
# Llama-31-8B_task-3_120-samples_config-2
This model is a fine-tuned version of [meta-llama/Meta-Llama-3.1-8B-Instruct](https://huggingface.co/meta-llama/Meta-Llama-3.1-8B-Instruct) on the GaetanMichelet/chat-60_ft_task-3 and the GaetanMichelet/chat-120_ft_task-3 datasets.
It achieves the following results on the evaluation set:
- Loss: 0.4302
## Model description
More information needed
## Intended uses & limitations
More information needed
## Training and evaluation data
More information needed
## Training procedure
### Training hyperparameters
The following hyperparameters were used during training:
- learning_rate: 0.0001
- train_batch_size: 1
- eval_batch_size: 1
- seed: 42
- distributed_type: multi-GPU
- gradient_accumulation_steps: 16
- total_train_batch_size: 16
- optimizer: Adam with betas=(0.9,0.999) and epsilon=1e-08
- lr_scheduler_type: cosine
- lr_scheduler_warmup_ratio: 0.1
- num_epochs: 50
### Training results
| Training Loss | Epoch | Step | Validation Loss |
|:-------------:|:-------:|:----:|:---------------:|
| 2.4469 | 0.9091 | 5 | 2.3539 |
| 1.8346 | 2.0 | 11 | 1.4922 |
| 0.7576 | 2.9091 | 16 | 0.7652 |
| 0.6409 | 4.0 | 22 | 0.5627 |
| 0.4304 | 4.9091 | 27 | 0.5238 |
| 0.3624 | 6.0 | 33 | 0.4705 |
| 0.3967 | 6.9091 | 38 | 0.4452 |
| 0.3293 | 8.0 | 44 | 0.4328 |
| 0.2432 | 8.9091 | 49 | 0.4302 |
| 0.2102 | 10.0 | 55 | 0.4359 |
| 0.2004 | 10.9091 | 60 | 0.4583 |
| 0.1634 | 12.0 | 66 | 0.4724 |
| 0.1177 | 12.9091 | 71 | 0.5530 |
| 0.0376 | 14.0 | 77 | 0.7361 |
| 0.0204 | 14.9091 | 82 | 0.7768 |
| 0.0118 | 16.0 | 88 | 0.8608 |
### Framework versions
- PEFT 0.12.0
- Transformers 4.44.0
- Pytorch 2.1.2+cu121
- Datasets 2.20.0
- Tokenizers 0.19.1 |
boricua/granite-7b-lab-ocp4.15-v0.3 | boricua | "2024-05-13T00:40:01Z" | 10 | 0 | transformers | [
"transformers",
"safetensors",
"gguf",
"llama",
"text-generation",
"conversational",
"en",
"license:apache-2.0",
"autotrain_compatible",
"text-generation-inference",
"endpoints_compatible",
"region:us"
] | text-generation | "2024-05-12T18:06:53Z" | ---
license: apache-2.0
language:
- en
---
## Model Card
This is a fine-tuned [granite-7b-lab](https://huggingface.co/instructlab/granite-7b-lab) on OpenShift 4.15 documentation using 45212 Q&A pairs.
- **Fine tuned by:** [William Caban](https://www.linkedin.com/in/williamcaban)
- **License:** Apache 2.0
- **Context length:** 32K (base model)
- **OpenShift 4.15 Knowledge cutoff date:** April 12 2024
### Method
The Q&A corpus was generated using the following methodology:
1. Generated 5 Q&A pairs for each page on OpenShift (OCP) 4.15 PDFs with lengths greater than 1500 characters. The length was chosen to remove the title page and pages without much content.
2. The [Mistral-7B-Instruct-v0.2](https://huggingface.co/mistralai/Mistral-7B-Instruct-v0.2) was used to generate the questions for each page.
3. The [Mixtral-8x22B-Instruct-v0.1](https://huggingface.co/mistralai/Mixtral-8x22B-Instruct-v0.1) was used to generate the answer from the content in the page.
4. A voting evaluation between Mixtral-8x22B and Llama3-7B was used to evaluate the quality of Q&A pair in relation to the page content and removed low quality entries.
5. Removed Q&A pairs with questions containing phrases or words like "this example", "this context", "this document", "trademark" and "copyright"
The resulting corpus is a 45212 Q&A-pairs. The corups was divided into training (42951 Q&A pairs) and eval (2261 Q&A pairs).
The model was trained on 3000 iterations.
**KNOWN LIMITATIONS** There is significant drop in accuracy and performance when using a quantized version of this model.
### Using the model
When using in combination with RAG, the model has preference for a `CONTEXT` section from which to augment its knowledge.
```bash
## INSTRUCTIONS
<your_instructions_here>
## TASK
<what_you_want_the_model_to_achieve>
## CONTEXT
<any_new_or_additional_context_for_answering_question>
## QUESTION
<question_from_user>
```
## Intended Use
- This model is a quick proof of concept (POC) for the fine tuning a base model with expertise and basic guardrails to reduce the reliance on prompts and multiple filtering mechanism to moderate the results.
- The model improves the quality of responses about OpenShift topics without RAG content while further improving responses when RAG context is provided.
- The model was created as a POC in a lab environment and as such it is not intended for production use.
## Bias, Risks, and Limitations
- The model was trained with basic instructions to refuse answering questions unrelated to Kubernetes, OpenShift and Kubernetes related topics.
Due to strict instructions during training, the model may refuse to answer valid Kubernetes or OpenShift questions when topics of the context were not present during training.
- The model has not been aligned to human social preferences, so the model might produce problematic output.
The model might also maintain the limitations and constraints that arise from the base model.
- The model undergoes training on synthetic data, leading to the potential inheritance of both advantages and limitations from the underlying data generation methods.
- In the absence of adequate safeguards and RLHF, there exists a risk of malicious utilization of these models for generating disinformation or harmful content. Caution is urged against complete reliance on a specific language model for crucial decisions or impactful information, as preventing these models from fabricating content is not straightforward. Additionally, it remains uncertain whether smaller models might exhibit increased susceptibility to hallucination in ungrounded generation scenarios due to their reduced sizes and memorization capacities. This aspect is currently an active area of research, and we anticipate more rigorous exploration, comprehension, and mitigations in this domain.
|
sail-rvc/pierre | sail-rvc | "2023-07-14T07:43:08Z" | 2 | 0 | transformers | [
"transformers",
"rvc",
"sail-rvc",
"audio-to-audio",
"endpoints_compatible",
"region:us"
] | audio-to-audio | "2023-07-14T07:42:23Z" |
---
pipeline_tag: audio-to-audio
tags:
- rvc
- sail-rvc
---
# pierre
## RVC Model

This model repo was automatically generated.
Date: 2023-07-14 07:43:08
Bot Name: juuxnscrap
Model Type: RVC
Source: https://huggingface.co/juuxn/RVCModels/
Reason: Converting into loadable format for https://github.com/chavinlo/rvc-runpod
|
neonon/DialoGPT-medium-cloy | neonon | "2023-12-01T04:36:58Z" | 6 | 1 | transformers | [
"transformers",
"pytorch",
"safetensors",
"gpt2",
"text-generation",
"convAI",
"conversational",
"en",
"autotrain_compatible",
"text-generation-inference",
"endpoints_compatible",
"region:us"
] | text-generation | "2022-10-23T13:37:39Z" | ---
language:
- en
tags:
- convAI
- conversational
---
Korean Drama!
[Crash Landing on You](https://en.wikipedia.org/wiki/Crash_Landing_on_You) |
Tngarg/lamma2_tamil_english | Tngarg | "2023-12-07T12:15:28Z" | 1 | 0 | peft | [
"peft",
"safetensors",
"arxiv:1910.09700",
"base_model:meta-llama/Llama-2-7b-hf",
"base_model:adapter:meta-llama/Llama-2-7b-hf",
"region:us"
] | null | "2023-12-07T11:53:10Z" | ---
library_name: peft
base_model: meta-llama/Llama-2-7b-hf
---
# Model Card for Model ID
<!-- Provide a quick summary of what the model is/does. -->
## Model Details
### Model Description
<!-- Provide a longer summary of what this model is. -->
- **Developed by:** [More Information Needed]
- **Funded by [optional]:** [More Information Needed]
- **Shared by [optional]:** [More Information Needed]
- **Model type:** [More Information Needed]
- **Language(s) (NLP):** [More Information Needed]
- **License:** [More Information Needed]
- **Finetuned from model [optional]:** [More Information Needed]
### Model Sources [optional]
<!-- Provide the basic links for the model. -->
- **Repository:** [More Information Needed]
- **Paper [optional]:** [More Information Needed]
- **Demo [optional]:** [More Information Needed]
## Uses
<!-- Address questions around how the model is intended to be used, including the foreseeable users of the model and those affected by the model. -->
### Direct Use
<!-- This section is for the model use without fine-tuning or plugging into a larger ecosystem/app. -->
[More Information Needed]
### Downstream Use [optional]
<!-- This section is for the model use when fine-tuned for a task, or when plugged into a larger ecosystem/app -->
[More Information Needed]
### Out-of-Scope Use
<!-- This section addresses misuse, malicious use, and uses that the model will not work well for. -->
[More Information Needed]
## Bias, Risks, and Limitations
<!-- This section is meant to convey both technical and sociotechnical limitations. -->
[More Information Needed]
### Recommendations
<!-- This section is meant to convey recommendations with respect to the bias, risk, and technical limitations. -->
Users (both direct and downstream) should be made aware of the risks, biases and limitations of the model. More information needed for further recommendations.
## How to Get Started with the Model
Use the code below to get started with the model.
[More Information Needed]
## Training Details
### Training Data
<!-- This should link to a Dataset Card, perhaps with a short stub of information on what the training data is all about as well as documentation related to data pre-processing or additional filtering. -->
[More Information Needed]
### Training Procedure
<!-- This relates heavily to the Technical Specifications. Content here should link to that section when it is relevant to the training procedure. -->
#### Preprocessing [optional]
[More Information Needed]
#### Training Hyperparameters
- **Training regime:** [More Information Needed] <!--fp32, fp16 mixed precision, bf16 mixed precision, bf16 non-mixed precision, fp16 non-mixed precision, fp8 mixed precision -->
#### Speeds, Sizes, Times [optional]
<!-- This section provides information about throughput, start/end time, checkpoint size if relevant, etc. -->
[More Information Needed]
## Evaluation
<!-- This section describes the evaluation protocols and provides the results. -->
### Testing Data, Factors & Metrics
#### Testing Data
<!-- This should link to a Dataset Card if possible. -->
[More Information Needed]
#### Factors
<!-- These are the things the evaluation is disaggregating by, e.g., subpopulations or domains. -->
[More Information Needed]
#### Metrics
<!-- These are the evaluation metrics being used, ideally with a description of why. -->
[More Information Needed]
### Results
[More Information Needed]
#### Summary
## Model Examination [optional]
<!-- Relevant interpretability work for the model goes here -->
[More Information Needed]
## Environmental Impact
<!-- Total emissions (in grams of CO2eq) and additional considerations, such as electricity usage, go here. Edit the suggested text below accordingly -->
Carbon emissions can be estimated using the [Machine Learning Impact calculator](https://mlco2.github.io/impact#compute) presented in [Lacoste et al. (2019)](https://arxiv.org/abs/1910.09700).
- **Hardware Type:** [More Information Needed]
- **Hours used:** [More Information Needed]
- **Cloud Provider:** [More Information Needed]
- **Compute Region:** [More Information Needed]
- **Carbon Emitted:** [More Information Needed]
## Technical Specifications [optional]
### Model Architecture and Objective
[More Information Needed]
### Compute Infrastructure
[More Information Needed]
#### Hardware
[More Information Needed]
#### Software
[More Information Needed]
## Citation [optional]
<!-- If there is a paper or blog post introducing the model, the APA and Bibtex information for that should go in this section. -->
**BibTeX:**
[More Information Needed]
**APA:**
[More Information Needed]
## Glossary [optional]
<!-- If relevant, include terms and calculations in this section that can help readers understand the model or model card. -->
[More Information Needed]
## More Information [optional]
[More Information Needed]
## Model Card Authors [optional]
[More Information Needed]
## Model Card Contact
[More Information Needed]
## Training procedure
The following `bitsandbytes` quantization config was used during training:
- quant_method: bitsandbytes
- load_in_8bit: False
- load_in_4bit: True
- llm_int8_threshold: 6.0
- llm_int8_skip_modules: None
- llm_int8_enable_fp32_cpu_offload: False
- llm_int8_has_fp16_weight: False
- bnb_4bit_quant_type: nf4
- bnb_4bit_use_double_quant: True
- bnb_4bit_compute_dtype: bfloat16
### Framework versions
- PEFT 0.7.1.dev0
- PEFT 0.6.3.dev0 |
Mykolyt/q-Taxi-v3 | Mykolyt | "2023-02-08T09:43:50Z" | 0 | 0 | null | [
"Taxi-v3",
"q-learning",
"reinforcement-learning",
"custom-implementation",
"model-index",
"region:us"
] | reinforcement-learning | "2023-02-08T09:43:48Z" | ---
tags:
- Taxi-v3
- q-learning
- reinforcement-learning
- custom-implementation
model-index:
- name: q-Taxi-v3
results:
- task:
type: reinforcement-learning
name: reinforcement-learning
dataset:
name: Taxi-v3
type: Taxi-v3
metrics:
- type: mean_reward
value: 7.52 +/- 2.71
name: mean_reward
verified: false
---
# **Q-Learning** Agent playing1 **Taxi-v3**
This is a trained model of a **Q-Learning** agent playing **Taxi-v3** .
## Usage
```python
model = load_from_hub(repo_id="Mykolyt/q-Taxi-v3", filename="q-learning.pkl")
# Don't forget to check if you need to add additional attributes (is_slippery=False etc)
env = gym.make(model["env_id"])
```
|
mradermacher/BeagSake-7B-GGUF | mradermacher | "2024-12-31T16:17:14Z" | 13 | 1 | transformers | [
"transformers",
"gguf",
"merge",
"mergekit",
"lazymergekit",
"en",
"base_model:shadowml/BeagSake-7B",
"base_model:quantized:shadowml/BeagSake-7B",
"license:cc-by-nc-4.0",
"endpoints_compatible",
"region:us",
"conversational"
] | null | "2024-12-31T14:34:09Z" | ---
base_model: shadowml/BeagSake-7B
language:
- en
library_name: transformers
license: cc-by-nc-4.0
quantized_by: mradermacher
tags:
- merge
- mergekit
- lazymergekit
---
## About
<!-- ### quantize_version: 2 -->
<!-- ### output_tensor_quantised: 1 -->
<!-- ### convert_type: hf -->
<!-- ### vocab_type: -->
<!-- ### tags: -->
static quants of https://huggingface.co/shadowml/BeagSake-7B
<!-- provided-files -->
weighted/imatrix quants are available at https://huggingface.co/mradermacher/BeagSake-7B-i1-GGUF
## Usage
If you are unsure how to use GGUF files, refer to one of [TheBloke's
READMEs](https://huggingface.co/TheBloke/KafkaLM-70B-German-V0.1-GGUF) for
more details, including on how to concatenate multi-part files.
## Provided Quants
(sorted by size, not necessarily quality. IQ-quants are often preferable over similar sized non-IQ quants)
| Link | Type | Size/GB | Notes |
|:-----|:-----|--------:|:------|
| [GGUF](https://huggingface.co/mradermacher/BeagSake-7B-GGUF/resolve/main/BeagSake-7B.Q2_K.gguf) | Q2_K | 2.8 | |
| [GGUF](https://huggingface.co/mradermacher/BeagSake-7B-GGUF/resolve/main/BeagSake-7B.Q3_K_S.gguf) | Q3_K_S | 3.3 | |
| [GGUF](https://huggingface.co/mradermacher/BeagSake-7B-GGUF/resolve/main/BeagSake-7B.Q3_K_M.gguf) | Q3_K_M | 3.6 | lower quality |
| [GGUF](https://huggingface.co/mradermacher/BeagSake-7B-GGUF/resolve/main/BeagSake-7B.Q3_K_L.gguf) | Q3_K_L | 3.9 | |
| [GGUF](https://huggingface.co/mradermacher/BeagSake-7B-GGUF/resolve/main/BeagSake-7B.IQ4_XS.gguf) | IQ4_XS | 4.0 | |
| [GGUF](https://huggingface.co/mradermacher/BeagSake-7B-GGUF/resolve/main/BeagSake-7B.Q4_K_S.gguf) | Q4_K_S | 4.2 | fast, recommended |
| [GGUF](https://huggingface.co/mradermacher/BeagSake-7B-GGUF/resolve/main/BeagSake-7B.Q4_K_M.gguf) | Q4_K_M | 4.5 | fast, recommended |
| [GGUF](https://huggingface.co/mradermacher/BeagSake-7B-GGUF/resolve/main/BeagSake-7B.Q5_K_S.gguf) | Q5_K_S | 5.1 | |
| [GGUF](https://huggingface.co/mradermacher/BeagSake-7B-GGUF/resolve/main/BeagSake-7B.Q5_K_M.gguf) | Q5_K_M | 5.2 | |
| [GGUF](https://huggingface.co/mradermacher/BeagSake-7B-GGUF/resolve/main/BeagSake-7B.Q6_K.gguf) | Q6_K | 6.0 | very good quality |
| [GGUF](https://huggingface.co/mradermacher/BeagSake-7B-GGUF/resolve/main/BeagSake-7B.Q8_0.gguf) | Q8_0 | 7.8 | fast, best quality |
| [GGUF](https://huggingface.co/mradermacher/BeagSake-7B-GGUF/resolve/main/BeagSake-7B.f16.gguf) | f16 | 14.6 | 16 bpw, overkill |
Here is a handy graph by ikawrakow comparing some lower-quality quant
types (lower is better):

And here are Artefact2's thoughts on the matter:
https://gist.github.com/Artefact2/b5f810600771265fc1e39442288e8ec9
## FAQ / Model Request
See https://huggingface.co/mradermacher/model_requests for some answers to
questions you might have and/or if you want some other model quantized.
## Thanks
I thank my company, [nethype GmbH](https://www.nethype.de/), for letting
me use its servers and providing upgrades to my workstation to enable
this work in my free time.
<!-- end -->
|
blackerx/no1x-1.5Bv1-GGUF | blackerx | "2025-01-13T10:02:11Z" | 83 | 0 | transformers | [
"transformers",
"gguf",
"qwen2",
"text-generation-inference",
"unsloth",
"en",
"base_model:unsloth/Qwen2.5-1.5B-Instruct-bnb-4bit",
"base_model:quantized:unsloth/Qwen2.5-1.5B-Instruct-bnb-4bit",
"license:apache-2.0",
"endpoints_compatible",
"region:us",
"conversational"
] | null | "2025-01-12T17:20:09Z" | ---
base_model: unsloth/Qwen2.5-1.5B-Instruct-bnb-4bit
tags:
- text-generation-inference
- transformers
- unsloth
- qwen2
- gguf
license: apache-2.0
language:
- en
---
# Uploaded model
###
use system prompt with no1x
<textarea>
SYSTEM PROMPT:
You are an advanced AI assistant that utilizes a combination of Meta-Reasoning, ReAct, Chain-of-Thought, and Self-Verification to solve problems. Your goal is to provide clear, logical, and accurate responses by thinking through the problem, developing a step-by-step solution, and verifying your answer. Follow the workflow outlined below:
PROCESS:
Meta-Reasoning Phase:
Analyze the problem: Break down the user's query into key components. Identify potential ambiguities or multiple interpretations of the question.
Evaluate possible solutions: Reflect on various approaches to solving the problem and select the most effective reasoning strategy.
Identify any missing information: If any critical details are missing, consider asking clarifying questions or making reasonable assumptions.
ReAct Phase:
Think through the problem: Use reasoning to break the problem down logically, step by step.
Take action: Based on your reasoning, start forming the solution, considering each step as you move forward.
Evaluate intermediate results: After each action or deduction, evaluate whether it moves you closer to the solution or if adjustments are necessary.
Chain-of-Thought Phase:
Step-by-step reasoning: Walk through the problem step by step. Ensure that each step logically follows the previous one. Make connections between concepts as needed.
Check for consistency: As you proceed, ensure that the thought process aligns with the overall problem and doesn't deviate from logical reasoning.
Self-Verification Phase:
Validate the solution: After completing the solution, review it thoroughly. Check the consistency, correctness, and completeness of the answer.
Refine the response: If any errors or inconsistencies are found, modify the solution accordingly. Recheck your reasoning at every stage of the process.
Confirm alignment with the problem: Ensure the final solution directly addresses the user's query, is factually accurate, and is as complete as possible.
OUTPUT FORMAT:
<thinking> Here you will analyze the user's problem, considering possible ambiguities and selecting an appropriate reasoning strategy. </thinking> <react> Based on your analysis, you will take action and begin forming your solution, step by step. Evaluate the intermediate results and adjust as needed. </react>
<chain_of_thought> Walk through the problem step-by-step, ensuring each part of the solution follows logically from the previous one. </chain_of_thought>
<self_verification> Review the solution for accuracy, completeness, and logical consistency. Adjust and refine the answer if any errors are found. </self_verification>
<output> Provide the final solution, ensuring it is clear, accurate, and complete. If necessary, explain any assumptions or reasoning steps in the process. </output>
</textarea>
- **Developed by:** blackerx
- **License:** apache-2.0
- **Finetuned from model :** unsloth/Qwen2.5-1.5B-Instruct-bnb-4bit
This qwen2 model was trained 2x faster with [Unsloth](https://github.com/unslothai/unsloth) and Huggingface's TRL library.
[<img src="https://raw.githubusercontent.com/unslothai/unsloth/main/images/unsloth%20made%20with%20love.png" width="200"/>](https://github.com/unslothai/unsloth)
|
Leo/mistral-finetuned | Leo | "2024-05-29T12:31:46Z" | 8 | 0 | transformers | [
"transformers",
"safetensors",
"mistral",
"text-generation",
"trl",
"sft",
"generated_from_trainer",
"conversational",
"base_model:mistralai/Mistral-7B-Instruct-v0.3",
"base_model:finetune:mistralai/Mistral-7B-Instruct-v0.3",
"license:apache-2.0",
"autotrain_compatible",
"text-generation-inference",
"endpoints_compatible",
"region:us"
] | text-generation | "2024-05-29T05:39:05Z" | ---
license: apache-2.0
base_model: mistralai/Mistral-7B-Instruct-v0.3
tags:
- trl
- sft
- generated_from_trainer
model-index:
- name: mistral-finetuned
results: []
---
<!-- This model card has been generated automatically according to the information the Trainer had access to. You
should probably proofread and complete it, then remove this comment. -->
# mistral-finetuned
This model is a fine-tuned version of [mistralai/Mistral-7B-Instruct-v0.3](https://huggingface.co/mistralai/Mistral-7B-Instruct-v0.3) on the None dataset.
## Model description
More information needed
## Intended uses & limitations
More information needed
## Training and evaluation data
More information needed
## Training procedure
### Training hyperparameters
The following hyperparameters were used during training:
- learning_rate: 2e-05
- train_batch_size: 8
- eval_batch_size: 8
- seed: 42
- optimizer: Adam with betas=(0.9,0.999) and epsilon=1e-08
- lr_scheduler_type: cosine
- num_epochs: 3
- mixed_precision_training: Native AMP
### Training results
### Framework versions
- Transformers 4.39.3
- Pytorch 2.3.0+cu121
- Datasets 2.19.1
- Tokenizers 0.15.2
|
genki10/Trial3BERT_AugV8_k5_task1_organization_sp020_lw010_fold4 | genki10 | "2025-04-06T16:41:39Z" | 0 | 0 | transformers | [
"transformers",
"pytorch",
"bert",
"text-classification",
"generated_from_trainer",
"base_model:google-bert/bert-base-uncased",
"base_model:finetune:google-bert/bert-base-uncased",
"license:apache-2.0",
"autotrain_compatible",
"endpoints_compatible",
"region:us"
] | text-classification | "2025-04-06T16:22:55Z" | ---
library_name: transformers
license: apache-2.0
base_model: bert-base-uncased
tags:
- generated_from_trainer
model-index:
- name: Trial3BERT_AugV8_k5_task1_organization_sp020_lw010_fold4
results: []
---
<!-- This model card has been generated automatically according to the information the Trainer had access to. You
should probably proofread and complete it, then remove this comment. -->
# Trial3BERT_AugV8_k5_task1_organization_sp020_lw010_fold4
This model is a fine-tuned version of [bert-base-uncased](https://huggingface.co/bert-base-uncased) on the None dataset.
It achieves the following results on the evaluation set:
- Loss: 0.7087
- Qwk: 0.4647
- Mse: 0.7087
- Rmse: 0.8419
## Model description
More information needed
## Intended uses & limitations
More information needed
## Training and evaluation data
More information needed
## Training procedure
### Training hyperparameters
The following hyperparameters were used during training:
- learning_rate: 2e-05
- train_batch_size: 64
- eval_batch_size: 64
- seed: 42
- optimizer: Use adamw_torch with betas=(0.9,0.999) and epsilon=1e-08 and optimizer_args=No additional optimizer arguments
- lr_scheduler_type: linear
- num_epochs: 150
### Training results
| Training Loss | Epoch | Step | Validation Loss | Qwk | Mse | Rmse |
|:-------------:|:-----:|:----:|:---------------:|:------:|:------:|:------:|
| No log | 1.0 | 4 | 7.6852 | 0.0 | 7.6852 | 2.7722 |
| No log | 2.0 | 8 | 4.3924 | 0.0079 | 4.3924 | 2.0958 |
| No log | 3.0 | 12 | 2.6225 | 0.0040 | 2.6225 | 1.6194 |
| No log | 4.0 | 16 | 1.6783 | 0.0432 | 1.6783 | 1.2955 |
| No log | 5.0 | 20 | 1.0566 | 0.0212 | 1.0566 | 1.0279 |
| No log | 6.0 | 24 | 0.9603 | 0.0310 | 0.9603 | 0.9799 |
| No log | 7.0 | 28 | 1.4350 | 0.0484 | 1.4350 | 1.1979 |
| No log | 8.0 | 32 | 0.8649 | 0.2334 | 0.8649 | 0.9300 |
| No log | 9.0 | 36 | 0.9956 | 0.1472 | 0.9956 | 0.9978 |
| No log | 10.0 | 40 | 1.1372 | 0.2609 | 1.1372 | 1.0664 |
| No log | 11.0 | 44 | 0.7270 | 0.4443 | 0.7270 | 0.8526 |
| No log | 12.0 | 48 | 0.6761 | 0.3916 | 0.6761 | 0.8222 |
| No log | 13.0 | 52 | 0.7981 | 0.3532 | 0.7981 | 0.8934 |
| No log | 14.0 | 56 | 0.6918 | 0.4589 | 0.6918 | 0.8318 |
| No log | 15.0 | 60 | 0.6926 | 0.5291 | 0.6926 | 0.8322 |
| No log | 16.0 | 64 | 0.9074 | 0.4437 | 0.9074 | 0.9526 |
| No log | 17.0 | 68 | 0.7076 | 0.5115 | 0.7076 | 0.8412 |
| No log | 18.0 | 72 | 0.7593 | 0.5046 | 0.7593 | 0.8714 |
| No log | 19.0 | 76 | 0.7876 | 0.4624 | 0.7876 | 0.8875 |
| No log | 20.0 | 80 | 0.7343 | 0.4728 | 0.7343 | 0.8569 |
| No log | 21.0 | 84 | 0.7376 | 0.4990 | 0.7376 | 0.8588 |
| No log | 22.0 | 88 | 0.7141 | 0.4981 | 0.7141 | 0.8450 |
| No log | 23.0 | 92 | 0.7028 | 0.5068 | 0.7028 | 0.8383 |
| No log | 24.0 | 96 | 0.7847 | 0.4603 | 0.7847 | 0.8859 |
| No log | 25.0 | 100 | 0.8353 | 0.4244 | 0.8353 | 0.9139 |
| No log | 26.0 | 104 | 0.7059 | 0.4889 | 0.7059 | 0.8402 |
| No log | 27.0 | 108 | 1.0087 | 0.3493 | 1.0087 | 1.0043 |
| No log | 28.0 | 112 | 0.5947 | 0.5616 | 0.5947 | 0.7712 |
| No log | 29.0 | 116 | 0.7313 | 0.4418 | 0.7313 | 0.8552 |
| No log | 30.0 | 120 | 0.6589 | 0.5504 | 0.6589 | 0.8117 |
| No log | 31.0 | 124 | 0.7888 | 0.4542 | 0.7888 | 0.8882 |
| No log | 32.0 | 128 | 0.7826 | 0.4370 | 0.7826 | 0.8847 |
| No log | 33.0 | 132 | 0.7835 | 0.4391 | 0.7835 | 0.8852 |
| No log | 34.0 | 136 | 0.8954 | 0.4087 | 0.8954 | 0.9463 |
| No log | 35.0 | 140 | 0.5926 | 0.5679 | 0.5926 | 0.7698 |
| No log | 36.0 | 144 | 0.9144 | 0.3895 | 0.9144 | 0.9562 |
| No log | 37.0 | 148 | 0.6112 | 0.5596 | 0.6112 | 0.7818 |
| No log | 38.0 | 152 | 0.8593 | 0.3825 | 0.8593 | 0.9270 |
| No log | 39.0 | 156 | 0.6311 | 0.5195 | 0.6311 | 0.7944 |
| No log | 40.0 | 160 | 0.8590 | 0.4136 | 0.8590 | 0.9268 |
| No log | 41.0 | 164 | 0.7096 | 0.4792 | 0.7096 | 0.8424 |
| No log | 42.0 | 168 | 0.7121 | 0.4876 | 0.7121 | 0.8438 |
| No log | 43.0 | 172 | 0.9623 | 0.3402 | 0.9623 | 0.9810 |
| No log | 44.0 | 176 | 0.6471 | 0.5016 | 0.6471 | 0.8045 |
| No log | 45.0 | 180 | 0.8586 | 0.3767 | 0.8586 | 0.9266 |
| No log | 46.0 | 184 | 0.6931 | 0.4723 | 0.6931 | 0.8325 |
| No log | 47.0 | 188 | 0.6850 | 0.5086 | 0.6850 | 0.8276 |
| No log | 48.0 | 192 | 0.8139 | 0.4080 | 0.8139 | 0.9022 |
| No log | 49.0 | 196 | 0.8065 | 0.4075 | 0.8065 | 0.8980 |
| No log | 50.0 | 200 | 0.7087 | 0.4647 | 0.7087 | 0.8419 |
### Framework versions
- Transformers 4.47.0
- Pytorch 2.5.1+cu121
- Datasets 3.3.1
- Tokenizers 0.21.0
|
ssunbear/mistral-7b-qlora-arc-reasoning-3.7k-v3 | ssunbear | "2025-03-31T18:12:38Z" | 0 | 0 | transformers | [
"transformers",
"safetensors",
"mistral",
"text-generation",
"trl",
"sft",
"conversational",
"arxiv:1910.09700",
"autotrain_compatible",
"text-generation-inference",
"endpoints_compatible",
"4-bit",
"bitsandbytes",
"region:us"
] | text-generation | "2025-03-31T18:08:44Z" | ---
library_name: transformers
tags:
- trl
- sft
---
# Model Card for Model ID
<!-- Provide a quick summary of what the model is/does. -->
## Model Details
### Model Description
<!-- Provide a longer summary of what this model is. -->
This is the model card of a 🤗 transformers model that has been pushed on the Hub. This model card has been automatically generated.
- **Developed by:** [More Information Needed]
- **Funded by [optional]:** [More Information Needed]
- **Shared by [optional]:** [More Information Needed]
- **Model type:** [More Information Needed]
- **Language(s) (NLP):** [More Information Needed]
- **License:** [More Information Needed]
- **Finetuned from model [optional]:** [More Information Needed]
### Model Sources [optional]
<!-- Provide the basic links for the model. -->
- **Repository:** [More Information Needed]
- **Paper [optional]:** [More Information Needed]
- **Demo [optional]:** [More Information Needed]
## Uses
<!-- Address questions around how the model is intended to be used, including the foreseeable users of the model and those affected by the model. -->
### Direct Use
<!-- This section is for the model use without fine-tuning or plugging into a larger ecosystem/app. -->
[More Information Needed]
### Downstream Use [optional]
<!-- This section is for the model use when fine-tuned for a task, or when plugged into a larger ecosystem/app -->
[More Information Needed]
### Out-of-Scope Use
<!-- This section addresses misuse, malicious use, and uses that the model will not work well for. -->
[More Information Needed]
## Bias, Risks, and Limitations
<!-- This section is meant to convey both technical and sociotechnical limitations. -->
[More Information Needed]
### Recommendations
<!-- This section is meant to convey recommendations with respect to the bias, risk, and technical limitations. -->
Users (both direct and downstream) should be made aware of the risks, biases and limitations of the model. More information needed for further recommendations.
## How to Get Started with the Model
Use the code below to get started with the model.
[More Information Needed]
## Training Details
### Training Data
<!-- This should link to a Dataset Card, perhaps with a short stub of information on what the training data is all about as well as documentation related to data pre-processing or additional filtering. -->
[More Information Needed]
### Training Procedure
<!-- This relates heavily to the Technical Specifications. Content here should link to that section when it is relevant to the training procedure. -->
#### Preprocessing [optional]
[More Information Needed]
#### Training Hyperparameters
- **Training regime:** [More Information Needed] <!--fp32, fp16 mixed precision, bf16 mixed precision, bf16 non-mixed precision, fp16 non-mixed precision, fp8 mixed precision -->
#### Speeds, Sizes, Times [optional]
<!-- This section provides information about throughput, start/end time, checkpoint size if relevant, etc. -->
[More Information Needed]
## Evaluation
<!-- This section describes the evaluation protocols and provides the results. -->
### Testing Data, Factors & Metrics
#### Testing Data
<!-- This should link to a Dataset Card if possible. -->
[More Information Needed]
#### Factors
<!-- These are the things the evaluation is disaggregating by, e.g., subpopulations or domains. -->
[More Information Needed]
#### Metrics
<!-- These are the evaluation metrics being used, ideally with a description of why. -->
[More Information Needed]
### Results
[More Information Needed]
#### Summary
## Model Examination [optional]
<!-- Relevant interpretability work for the model goes here -->
[More Information Needed]
## Environmental Impact
<!-- Total emissions (in grams of CO2eq) and additional considerations, such as electricity usage, go here. Edit the suggested text below accordingly -->
Carbon emissions can be estimated using the [Machine Learning Impact calculator](https://mlco2.github.io/impact#compute) presented in [Lacoste et al. (2019)](https://arxiv.org/abs/1910.09700).
- **Hardware Type:** [More Information Needed]
- **Hours used:** [More Information Needed]
- **Cloud Provider:** [More Information Needed]
- **Compute Region:** [More Information Needed]
- **Carbon Emitted:** [More Information Needed]
## Technical Specifications [optional]
### Model Architecture and Objective
[More Information Needed]
### Compute Infrastructure
[More Information Needed]
#### Hardware
[More Information Needed]
#### Software
[More Information Needed]
## Citation [optional]
<!-- If there is a paper or blog post introducing the model, the APA and Bibtex information for that should go in this section. -->
**BibTeX:**
[More Information Needed]
**APA:**
[More Information Needed]
## Glossary [optional]
<!-- If relevant, include terms and calculations in this section that can help readers understand the model or model card. -->
[More Information Needed]
## More Information [optional]
[More Information Needed]
## Model Card Authors [optional]
[More Information Needed]
## Model Card Contact
[More Information Needed] |
mradermacher/LeoLM-hessianai-7b-chat-GGUF | mradermacher | "2024-12-28T09:36:13Z" | 113 | 0 | transformers | [
"transformers",
"gguf",
"en",
"de",
"dataset:LeoLM/OpenSchnabeltier",
"dataset:OpenAssistant/OASST-DE",
"dataset:FreedomIntelligence/alpaca-gpt4-deutsch",
"dataset:FreedomIntelligence/evol-instruct-deutsch",
"dataset:LeoLM/German_Poems",
"dataset:LeoLM/German_Songs",
"base_model:titanbot/LeoLM-hessianai-7b-chat",
"base_model:quantized:titanbot/LeoLM-hessianai-7b-chat",
"endpoints_compatible",
"region:us",
"conversational"
] | null | "2024-12-28T09:28:25Z" | ---
base_model: titanbot/LeoLM-hessianai-7b-chat
datasets:
- LeoLM/OpenSchnabeltier
- OpenAssistant/OASST-DE
- FreedomIntelligence/alpaca-gpt4-deutsch
- FreedomIntelligence/evol-instruct-deutsch
- LeoLM/German_Poems
- LeoLM/German_Songs
language:
- en
- de
library_name: transformers
quantized_by: mradermacher
---
## About
<!-- ### quantize_version: 2 -->
<!-- ### output_tensor_quantised: 1 -->
<!-- ### convert_type: hf -->
<!-- ### vocab_type: -->
<!-- ### tags: -->
static quants of https://huggingface.co/titanbot/LeoLM-hessianai-7b-chat
<!-- provided-files -->
weighted/imatrix quants seem not to be available (by me) at this time. If they do not show up a week or so after the static ones, I have probably not planned for them. Feel free to request them by opening a Community Discussion.
## Usage
If you are unsure how to use GGUF files, refer to one of [TheBloke's
READMEs](https://huggingface.co/TheBloke/KafkaLM-70B-German-V0.1-GGUF) for
more details, including on how to concatenate multi-part files.
## Provided Quants
(sorted by size, not necessarily quality. IQ-quants are often preferable over similar sized non-IQ quants)
| Link | Type | Size/GB | Notes |
|:-----|:-----|--------:|:------|
| [GGUF](https://huggingface.co/mradermacher/LeoLM-hessianai-7b-chat-GGUF/resolve/main/LeoLM-hessianai-7b-chat.Q2_K.gguf) | Q2_K | 2.6 | |
| [GGUF](https://huggingface.co/mradermacher/LeoLM-hessianai-7b-chat-GGUF/resolve/main/LeoLM-hessianai-7b-chat.Q3_K_S.gguf) | Q3_K_S | 3.0 | |
| [GGUF](https://huggingface.co/mradermacher/LeoLM-hessianai-7b-chat-GGUF/resolve/main/LeoLM-hessianai-7b-chat.Q3_K_M.gguf) | Q3_K_M | 3.4 | lower quality |
| [GGUF](https://huggingface.co/mradermacher/LeoLM-hessianai-7b-chat-GGUF/resolve/main/LeoLM-hessianai-7b-chat.Q3_K_L.gguf) | Q3_K_L | 3.7 | |
| [GGUF](https://huggingface.co/mradermacher/LeoLM-hessianai-7b-chat-GGUF/resolve/main/LeoLM-hessianai-7b-chat.IQ4_XS.gguf) | IQ4_XS | 3.7 | |
| [GGUF](https://huggingface.co/mradermacher/LeoLM-hessianai-7b-chat-GGUF/resolve/main/LeoLM-hessianai-7b-chat.Q4_K_S.gguf) | Q4_K_S | 4.0 | fast, recommended |
| [GGUF](https://huggingface.co/mradermacher/LeoLM-hessianai-7b-chat-GGUF/resolve/main/LeoLM-hessianai-7b-chat.Q4_K_M.gguf) | Q4_K_M | 4.2 | fast, recommended |
| [GGUF](https://huggingface.co/mradermacher/LeoLM-hessianai-7b-chat-GGUF/resolve/main/LeoLM-hessianai-7b-chat.Q5_K_S.gguf) | Q5_K_S | 4.8 | |
| [GGUF](https://huggingface.co/mradermacher/LeoLM-hessianai-7b-chat-GGUF/resolve/main/LeoLM-hessianai-7b-chat.Q5_K_M.gguf) | Q5_K_M | 4.9 | |
| [GGUF](https://huggingface.co/mradermacher/LeoLM-hessianai-7b-chat-GGUF/resolve/main/LeoLM-hessianai-7b-chat.Q6_K.gguf) | Q6_K | 5.6 | very good quality |
| [GGUF](https://huggingface.co/mradermacher/LeoLM-hessianai-7b-chat-GGUF/resolve/main/LeoLM-hessianai-7b-chat.Q8_0.gguf) | Q8_0 | 7.3 | fast, best quality |
| [GGUF](https://huggingface.co/mradermacher/LeoLM-hessianai-7b-chat-GGUF/resolve/main/LeoLM-hessianai-7b-chat.f16.gguf) | f16 | 13.6 | 16 bpw, overkill |
Here is a handy graph by ikawrakow comparing some lower-quality quant
types (lower is better):

And here are Artefact2's thoughts on the matter:
https://gist.github.com/Artefact2/b5f810600771265fc1e39442288e8ec9
## FAQ / Model Request
See https://huggingface.co/mradermacher/model_requests for some answers to
questions you might have and/or if you want some other model quantized.
## Thanks
I thank my company, [nethype GmbH](https://www.nethype.de/), for letting
me use its servers and providing upgrades to my workstation to enable
this work in my free time. Additional thanks to [@nicoboss](https://huggingface.co/nicoboss) for giving me access to his private supercomputer, enabling me to provide many more imatrix quants, at much higher quality, than I would otherwise be able to.
<!-- end -->
|
rooftopcoder/led-base-16384-tldr-test | rooftopcoder | "2023-08-01T07:59:53Z" | 97 | 0 | transformers | [
"transformers",
"pytorch",
"tensorboard",
"safetensors",
"led",
"text2text-generation",
"generated_from_trainer",
"dataset:CarperAI/openai_summarize_tldr",
"license:apache-2.0",
"autotrain_compatible",
"endpoints_compatible",
"region:us"
] | text2text-generation | "2023-03-14T09:59:05Z" | ---
license: apache-2.0
tags:
- generated_from_trainer
model-index:
- name: led-base-16384-tldr-test
results: []
datasets:
- CarperAI/openai_summarize_tldr
---
<!-- This model card has been generated automatically according to the information the Trainer had access to. You
should probably proofread and complete it, then remove this comment. -->
# led-base-16384-tldr-test
This model is a fine-tuned version of [allenai/led-base-16384](https://huggingface.co/allenai/led-base-16384) on an unknown dataset.
## Model description
More information needed
## Intended uses & limitations
More information needed
## Training and evaluation data
More information needed
## Training procedure
### Training hyperparameters
The following hyperparameters were used during training:
- learning_rate: 5e-05
- train_batch_size: 4
- eval_batch_size: 4
- seed: 42
- gradient_accumulation_steps: 4
- total_train_batch_size: 16
- optimizer: Adam with betas=(0.9,0.999) and epsilon=1e-08
- lr_scheduler_type: linear
- num_epochs: 2
- mixed_precision_training: Native AMP
### Training results
### Framework versions
- Transformers 4.26.1
- Pytorch 1.13.0
- Datasets 2.1.0
- Tokenizers 0.13.2 |
CyberHarem/satou_shin_idolmastercinderellagirls | CyberHarem | "2023-09-17T14:25:10Z" | 0 | 0 | null | [
"art",
"text-to-image",
"dataset:CyberHarem/satou_shin_idolmastercinderellagirls",
"license:mit",
"region:us"
] | text-to-image | "2023-09-17T14:02:17Z" | ---
license: mit
datasets:
- CyberHarem/satou_shin_idolmastercinderellagirls
pipeline_tag: text-to-image
tags:
- art
---
# Lora of satou_shin_idolmastercinderellagirls
This model is trained with [HCP-Diffusion](https://github.com/7eu7d7/HCP-Diffusion). And the auto-training framework is maintained by [DeepGHS Team](https://huggingface.co/deepghs).
The base model used during training is [NAI](https://huggingface.co/deepghs/animefull-latest), and the base model used for generating preview images is [Meina/MeinaMix_V11](https://huggingface.co/Meina/MeinaMix_V11).
After downloading the pt and safetensors files for the specified step, you need to use them simultaneously. The pt file will be used as an embedding, while the safetensors file will be loaded for Lora.
For example, if you want to use the model from step 3240, you need to download `3240/satou_shin_idolmastercinderellagirls.pt` as the embedding and `3240/satou_shin_idolmastercinderellagirls.safetensors` for loading Lora. By using both files together, you can generate images for the desired characters.
**The best step we recommend is 3240**, with the score of 0.946. The trigger words are:
1. `satou_shin_idolmastercinderellagirls`
2. `green_eyes, ahoge, blush, smile, bangs, long_hair, breasts, twintails, heart, blonde_hair, hair_ornament`
For the following groups, it is not recommended to use this model and we express regret:
1. Individuals who cannot tolerate any deviations from the original character design, even in the slightest detail.
2. Individuals who are facing the application scenarios with high demands for accuracy in recreating character outfits.
3. Individuals who cannot accept the potential randomness in AI-generated images based on the Stable Diffusion algorithm.
4. Individuals who are not comfortable with the fully automated process of training character models using LoRA, or those who believe that training character models must be done purely through manual operations to avoid disrespecting the characters.
5. Individuals who finds the generated image content offensive to their values.
These are available steps:
| Steps | Score | Download | pattern_1 | pattern_2 | pattern_3 | pattern_4 | pattern_5 | pattern_6 | pattern_7 | pattern_8 | pattern_9 | pattern_10 | pattern_11 | pattern_12 | pattern_13 | pattern_14 | pattern_15 | pattern_16 | bikini | bondage | free | maid | miko | nude | nude2 | suit | yukata |
|:---------|:----------|:--------------------------------------------------------------|:-----------------------------------------------|:-----------------------------------------------|:-----------------------------------------------|:-----------------------------------------------|:-----------------------------------------------|:-----------------------------------------------|:-----------------------------------------------|:-----------------------------------------------|:-----------------------------------------------|:-------------------------------------------------|:-------------------------------------------------|:-----------------------------------------------------|:-------------------------------------------------|:-------------------------------------------------|:-------------------------------------------------|:-------------------------------------------------|:-----------------------------------------|:--------------------------------------------------|:-----------------------------------------------|:-------------------------------------|:-------------------------------------|:-----------------------------------------------|:------------------------------------------------|:-------------------------------------|:-----------------------------------------|
| 8100 | 0.904 | [Download](8100/satou_shin_idolmastercinderellagirls.zip) |  |  |  |  |  |  |  |  |  |  |  | [<NSFW, click to see>](8100/previews/pattern_12.png) |  |  |  |  |  | [<NSFW, click to see>](8100/previews/bondage.png) | [<NSFW, click to see>](8100/previews/free.png) |  |  | [<NSFW, click to see>](8100/previews/nude.png) | [<NSFW, click to see>](8100/previews/nude2.png) |  |  |
| 7560 | 0.860 | [Download](7560/satou_shin_idolmastercinderellagirls.zip) |  |  |  |  |  |  |  |  |  |  |  | [<NSFW, click to see>](7560/previews/pattern_12.png) |  |  |  |  |  | [<NSFW, click to see>](7560/previews/bondage.png) | [<NSFW, click to see>](7560/previews/free.png) |  |  | [<NSFW, click to see>](7560/previews/nude.png) | [<NSFW, click to see>](7560/previews/nude2.png) |  |  |
| 7020 | 0.937 | [Download](7020/satou_shin_idolmastercinderellagirls.zip) |  |  |  |  |  |  |  |  |  |  |  | [<NSFW, click to see>](7020/previews/pattern_12.png) |  |  |  |  |  | [<NSFW, click to see>](7020/previews/bondage.png) | [<NSFW, click to see>](7020/previews/free.png) |  |  | [<NSFW, click to see>](7020/previews/nude.png) | [<NSFW, click to see>](7020/previews/nude2.png) |  |  |
| 6480 | 0.935 | [Download](6480/satou_shin_idolmastercinderellagirls.zip) |  |  |  |  |  |  |  |  |  |  |  | [<NSFW, click to see>](6480/previews/pattern_12.png) |  |  |  |  |  | [<NSFW, click to see>](6480/previews/bondage.png) | [<NSFW, click to see>](6480/previews/free.png) |  |  | [<NSFW, click to see>](6480/previews/nude.png) | [<NSFW, click to see>](6480/previews/nude2.png) |  |  |
| 5940 | 0.917 | [Download](5940/satou_shin_idolmastercinderellagirls.zip) |  |  |  |  |  |  |  |  |  |  |  | [<NSFW, click to see>](5940/previews/pattern_12.png) |  |  |  |  |  | [<NSFW, click to see>](5940/previews/bondage.png) | [<NSFW, click to see>](5940/previews/free.png) |  |  | [<NSFW, click to see>](5940/previews/nude.png) | [<NSFW, click to see>](5940/previews/nude2.png) |  |  |
| 5400 | 0.934 | [Download](5400/satou_shin_idolmastercinderellagirls.zip) |  |  |  |  |  |  |  |  |  |  |  | [<NSFW, click to see>](5400/previews/pattern_12.png) |  |  |  |  |  | [<NSFW, click to see>](5400/previews/bondage.png) | [<NSFW, click to see>](5400/previews/free.png) |  |  | [<NSFW, click to see>](5400/previews/nude.png) | [<NSFW, click to see>](5400/previews/nude2.png) |  |  |
| 4860 | 0.900 | [Download](4860/satou_shin_idolmastercinderellagirls.zip) |  |  |  |  |  |  |  |  |  |  |  | [<NSFW, click to see>](4860/previews/pattern_12.png) |  |  |  |  |  | [<NSFW, click to see>](4860/previews/bondage.png) | [<NSFW, click to see>](4860/previews/free.png) |  |  | [<NSFW, click to see>](4860/previews/nude.png) | [<NSFW, click to see>](4860/previews/nude2.png) |  |  |
| 4320 | 0.935 | [Download](4320/satou_shin_idolmastercinderellagirls.zip) |  |  |  |  |  |  |  |  |  |  |  | [<NSFW, click to see>](4320/previews/pattern_12.png) |  |  |  |  |  | [<NSFW, click to see>](4320/previews/bondage.png) | [<NSFW, click to see>](4320/previews/free.png) |  |  | [<NSFW, click to see>](4320/previews/nude.png) | [<NSFW, click to see>](4320/previews/nude2.png) |  |  |
| 3780 | 0.907 | [Download](3780/satou_shin_idolmastercinderellagirls.zip) |  |  |  |  |  |  |  |  |  |  |  | [<NSFW, click to see>](3780/previews/pattern_12.png) |  |  |  |  |  | [<NSFW, click to see>](3780/previews/bondage.png) | [<NSFW, click to see>](3780/previews/free.png) |  |  | [<NSFW, click to see>](3780/previews/nude.png) | [<NSFW, click to see>](3780/previews/nude2.png) |  |  |
| **3240** | **0.946** | [**Download**](3240/satou_shin_idolmastercinderellagirls.zip) |  |  |  |  |  |  |  |  |  |  |  | [<NSFW, click to see>](3240/previews/pattern_12.png) |  |  |  |  |  | [<NSFW, click to see>](3240/previews/bondage.png) | [<NSFW, click to see>](3240/previews/free.png) |  |  | [<NSFW, click to see>](3240/previews/nude.png) | [<NSFW, click to see>](3240/previews/nude2.png) |  |  |
| 2700 | 0.915 | [Download](2700/satou_shin_idolmastercinderellagirls.zip) |  |  |  |  |  |  |  |  |  |  |  | [<NSFW, click to see>](2700/previews/pattern_12.png) |  |  |  |  |  | [<NSFW, click to see>](2700/previews/bondage.png) | [<NSFW, click to see>](2700/previews/free.png) |  |  | [<NSFW, click to see>](2700/previews/nude.png) | [<NSFW, click to see>](2700/previews/nude2.png) |  |  |
| 2160 | 0.909 | [Download](2160/satou_shin_idolmastercinderellagirls.zip) |  |  |  |  |  |  |  |  |  |  |  | [<NSFW, click to see>](2160/previews/pattern_12.png) |  |  |  |  |  | [<NSFW, click to see>](2160/previews/bondage.png) | [<NSFW, click to see>](2160/previews/free.png) |  |  | [<NSFW, click to see>](2160/previews/nude.png) | [<NSFW, click to see>](2160/previews/nude2.png) |  |  |
| 1620 | 0.896 | [Download](1620/satou_shin_idolmastercinderellagirls.zip) |  |  |  |  |  |  |  |  |  |  |  | [<NSFW, click to see>](1620/previews/pattern_12.png) |  |  |  |  |  | [<NSFW, click to see>](1620/previews/bondage.png) | [<NSFW, click to see>](1620/previews/free.png) |  |  | [<NSFW, click to see>](1620/previews/nude.png) | [<NSFW, click to see>](1620/previews/nude2.png) |  |  |
| 1080 | 0.842 | [Download](1080/satou_shin_idolmastercinderellagirls.zip) |  |  |  |  |  |  |  |  |  |  |  | [<NSFW, click to see>](1080/previews/pattern_12.png) |  |  |  |  |  | [<NSFW, click to see>](1080/previews/bondage.png) | [<NSFW, click to see>](1080/previews/free.png) |  |  | [<NSFW, click to see>](1080/previews/nude.png) | [<NSFW, click to see>](1080/previews/nude2.png) |  |  |
| 540 | 0.828 | [Download](540/satou_shin_idolmastercinderellagirls.zip) |  |  |  |  |  |  |  |  |  |  |  | [<NSFW, click to see>](540/previews/pattern_12.png) |  |  |  |  |  | [<NSFW, click to see>](540/previews/bondage.png) | [<NSFW, click to see>](540/previews/free.png) |  |  | [<NSFW, click to see>](540/previews/nude.png) | [<NSFW, click to see>](540/previews/nude2.png) |  |  |
|
rossevine/Model_S_P_Wav2Vec2_Versi2 | rossevine | "2023-08-29T02:25:59Z" | 4 | 0 | transformers | [
"transformers",
"pytorch",
"tensorboard",
"wav2vec2",
"automatic-speech-recognition",
"generated_from_trainer",
"base_model:facebook/wav2vec2-large-xlsr-53",
"base_model:finetune:facebook/wav2vec2-large-xlsr-53",
"license:apache-2.0",
"endpoints_compatible",
"region:us"
] | automatic-speech-recognition | "2023-08-23T11:59:02Z" | ---
license: apache-2.0
base_model: facebook/wav2vec2-large-xlsr-53
tags:
- generated_from_trainer
metrics:
- wer
model-index:
- name: Model_S_P_Wav2Vec2_Versi2
results: []
---
<!-- This model card has been generated automatically according to the information the Trainer had access to. You
should probably proofread and complete it, then remove this comment. -->
# Model_S_P_Wav2Vec2_Versi2
This model is a fine-tuned version of [facebook/wav2vec2-large-xlsr-53](https://huggingface.co/facebook/wav2vec2-large-xlsr-53) on the None dataset.
It achieves the following results on the evaluation set:
- Loss: 2.2422
- Wer: 0.6012
- Cer: 0.2488
## Model description
More information needed
## Intended uses & limitations
More information needed
## Training and evaluation data
More information needed
## Training procedure
### Training hyperparameters
The following hyperparameters were used during training:
- learning_rate: 0.0003
- train_batch_size: 16
- eval_batch_size: 8
- seed: 42
- gradient_accumulation_steps: 2
- total_train_batch_size: 32
- optimizer: Adam with betas=(0.9,0.999) and epsilon=1e-08
- lr_scheduler_type: linear
- lr_scheduler_warmup_steps: 500
- num_epochs: 30
### Training results
| Training Loss | Epoch | Step | Validation Loss | Wer | Cer |
|:-------------:|:-----:|:----:|:---------------:|:------:|:------:|
| 3.8621 | 4.17 | 400 | 2.0001 | 0.8875 | 0.3540 |
| 0.7188 | 8.33 | 800 | 1.7482 | 0.6799 | 0.2826 |
| 0.4024 | 12.5 | 1200 | 2.0649 | 0.6533 | 0.2723 |
| 0.2796 | 16.67 | 1600 | 1.9127 | 0.6452 | 0.2666 |
| 0.2053 | 20.83 | 2000 | 1.8885 | 0.6095 | 0.2555 |
| 0.1523 | 25.0 | 2400 | 2.0067 | 0.6168 | 0.2569 |
| 0.105 | 29.17 | 2800 | 2.2422 | 0.6012 | 0.2488 |
### Framework versions
- Transformers 4.31.0
- Pytorch 2.0.1+cu117
- Datasets 1.18.3
- Tokenizers 0.13.3
|
TheBlueObserver/Qwen2.5-1.5B-Instruct__huatuo-r8-a8-epoch1 | TheBlueObserver | "2025-03-04T00:28:42Z" | 0 | 0 | null | [
"safetensors",
"region:us"
] | null | "2025-03-01T20:20:16Z" |
# TheBlueObserver/Qwen2.5-1.5B-Instruct__huatuo-r8-a8-epoch1 Model Card
## LoRA Details
- **Rank**: 8
- **Alpha**: 8
## Training Details
- **Datasets**: huatuo_reasoning
- **Limit**: -1
- **Max Steps**: default
- **Epochs**: 1
|
AstroMLCore/AstroM3-CLIP | AstroMLCore | "2025-03-27T21:37:42Z" | 45 | 0 | null | [
"safetensors",
"astronomy",
"multimodal",
"classification",
"dataset:AstroMLCore/AstroM3Processed",
"dataset:AstroMLCore/AstroM3Dataset",
"arxiv:2411.08842",
"region:us"
] | null | "2025-02-19T21:58:26Z" | ---
tags:
- astronomy
- multimodal
- classification
datasets:
- AstroMLCore/AstroM3Processed
- AstroMLCore/AstroM3Dataset
---
AstroM³ is a self-supervised multimodal model for astronomy that integrates time-series photometry, spectra, and metadata into a unified embedding space
for classification and other downstream tasks. AstroM³ is trained on [AstroM3Processed](https://huggingface.co/datasets/AstroMLCore/AstroM3Processed),
which is the pre-processed version of [AstroM3Dataset](https://huggingface.co/datasets/AstroMLCore/AstroM3Dataset).
For more details on the AstroM³ architecture, training, and results, please refer to the [paper](https://arxiv.org/abs/2411.08842).
<p align="center">
<img src="astroclip-architecture.png" width="100%">
<br />
<span>
Figure 1: Overview of the multimodal CLIP framework adapted for astronomy, incorporating three data modalities: photometric time-series, spectra, and metadata.
Each modality is processed by a dedicated encoder to create embeddings, which are then mapped into a shared embedding space through projection heads.
Pairwise similarity matrices align the embeddings across modalities, and a symmetric cross-entropy loss, computed over these matrices, optimizes the model.
The total loss, derived from all pairwise losses, guides the model’s trimodal learning.
</span>
</p>
To use AstroM³ for inference, install the AstroM3 library from our [GitHub repo](https://github.com/MeriDK/AstroM3).
```sh
git clone https://github.com/MeriDK/AstroM3.git
cd AstroM3
```
Create a virtual environment (tested with Python 3.10.14), then install the required dependencies:
```sh
uv venv venv --python 3.10.14
source venv/bin/activate
uv pip install -r requirements.txt
```
## A simple example to get started
1. Data Loading & Preprocessing
```python
from datasets import load_dataset
from src.data import process_photometry
# Load the test dataset
test_dataset = load_dataset('AstroMLCore/AstroM3Processed', name='full_42', split='test')
# Process photometry to have a fixed sequence length of 200 (center-cropped)
test_dataset = test_dataset.map(process_photometry, batched=True, fn_kwargs={'seq_len': 200, 'how': 'center'})
test_dataset = test_dataset.with_format('torch')
```
2. Model Loading & Embedding Extraction
```python
import torch
from src.model import AstroM3
# Load the base AstroM3-CLIP model
model = AstroM3.from_pretrained('AstroMLCore/AstroM3-CLIP')
# Retrieve the first sample (batch size = 1)
sample = test_dataset[0:1]
photometry = sample['photometry']
photometry_mask = sample['photometry_mask']
spectra = sample['spectra']
metadata = sample['metadata']
# Example 1: Generate embeddings when all modalities are present
p_emb, s_emb, m_emb = model.get_embeddings(photometry, photometry_mask, spectra, metadata)
multimodal_emb = (p_emb + s_emb + m_emb) / 3
print('Multimodal Embedding (All Modalities):', multimodal_emb)
# Example 2: Generate embeddings when the spectra modality is missing
dummy_spectra = torch.zeros_like(spectra) # Dummy tensor for missing spectra
p_emb, s_emb, m_emb = model.get_embeddings(photometry, photometry_mask, dummy_spectra, metadata)
multimodal_emb_missing = (p_emb + m_emb) / 2
print('Multimodal Embedding (Spectra Missing):', multimodal_emb_missing)
```
3. Classification Examples
```python
from src.model import AstroM3, Informer, GalSpecNet, MetaModel
# Photometry classification
photo_model = Informer.from_pretrained('AstroMLCore/AstroM3-CLIP-photo')
prediction = photo_model(photometry, photometry_mask).argmax(dim=1).item()
print('Photometry Classification:', test_dataset.features['label'].int2str(prediction))
# Spectra classification
spectra_model = GalSpecNet.from_pretrained('AstroMLCore/AstroM3-CLIP-spectra')
prediction = spectra_model(spectra).argmax(dim=1).item()
print('Spectra Classification:', test_dataset.features['label'].int2str(prediction))
# Metadata classification
meta_model = MetaModel.from_pretrained('AstroMLCore/AstroM3-CLIP-meta')
prediction = meta_model(metadata).argmax(dim=1).item()
print('Metadata Classification:', test_dataset.features['label'].int2str(prediction))
# Multimodal classification
all_model = AstroM3.from_pretrained('AstroMLCore/AstroM3-CLIP-all')
prediction = all_model(photometry, photometry_mask, spectra, metadata).argmax(dim=1).item()
print('Multimodal Classification:', test_dataset.features['label'].int2str(prediction))
```
## The AstroM³ Family
| # Model | # Description |
| :--- | :--- |
| [AstroM3-CLIP](https://huggingface.co/AstroMLCore/AstroM3-CLIP) | The base model pre-trained using the trimodal CLIP approach. |
| [AstroM3-CLIP-meta](https://huggingface.co/AstroMLCore/AstroM3-CLIP-meta) | Fine-tuned for metadata-only classification. |
| [AstroM3-CLIP-spectra](https://huggingface.co/AstroMLCore/AstroM3-CLIP-spectra) | Fine-tuned for spectra-only classification. |
| [AstroM3-CLIP-photo](https://huggingface.co/AstroMLCore/AstroM3-CLIP-photo) | Fine-tuned for photometry-only classification. |
| [AstroM3-CLIP-all](https://huggingface.co/AstroMLCore/AstroM3-CLIP-all) | Fine-tuned for multimodal classification. |
## AstroM3-CLIP Variants
These variants of the base AstroM3-CLIP model are trained using different random seeds (42, 0, 66, 12, 123);
ensure that the dataset is loaded with the corresponding seed for consistency.
| # Model | # Description |
| :--- | :--- |
| [AstroM3-CLIP-42](https://huggingface.co/AstroMLCore/AstroM3-CLIP-42) | The base model pre-trained with random seed 42 (identical to AstroM3-CLIP). |
| [AstroM3-CLIP-0](https://huggingface.co/AstroMLCore/AstroM3-CLIP-0) | AstroM3-CLIP pre-trained with random seed 0 (use dataset with seed 0). |
| [AstroM3-CLIP-66](https://huggingface.co/AstroMLCore/AstroM3-CLIP-66) | AstroM3-CLIP pre-trained with random seed 66 (use dataset with seed 66). |
| [AstroM3-CLIP-12](https://huggingface.co/AstroMLCore/AstroM3-CLIP-12) | AstroM3-CLIP pre-trained with random seed 12 (use dataset with seed 12). |
| [AstroM3-CLIP-123](https://huggingface.co/AstroMLCore/AstroM3-CLIP-123) | AstroM3-CLIP pre-trained with random seed 123 (use dataset with seed 123). |
## Using your own data
Note that the data in the AstroM3Processed dataset is already pre-processed.
If you want to use the model with your own data, you must pre-process it in the same way:
1. **Spectra**: Each spectrum is interpolated to a fixed wavelength grid (3850–9000 Å), normalized using mean and MAD, and log-MAD is added as an auxiliary feature.
2. **Photometry**: Light curves are deduplicated, sorted by time, normalized using mean and MAD, time-scaled to [0, 1], and augmented with auxiliary features like log-MAD and time span.
3. **Metadata**: Scalar metadata is transformed via domain-specific functions (e.g., absolute magnitude, log, sin/cos), then normalized using dataset-level statistics.
For a detailed description, read the [paper](https://arxiv.org/abs/2411.08842).
To see exactly how we performed this preprocessing, refer to [`preprocess.py`](https://huggingface.co/datasets/AstroMLCore/AstroM3Dataset/blob/main/preprocess.py) in the AstroM3Dataset repo.
---
## Citation
🤗 If you find this model usefull, please cite our paper 🤗
```bibtex
@article{rizhko2024astrom,
title={AstroM $\^{} 3$: A self-supervised multimodal model for astronomy},
author={Rizhko, Mariia and Bloom, Joshua S},
journal={arXiv preprint arXiv:2411.08842},
year={2024}
}
``` |
MonirahQQ/Mistral_discharge_summary_Subtraining_ngram | MonirahQQ | "2025-02-23T03:45:21Z" | 0 | 0 | transformers | [
"transformers",
"safetensors",
"text-generation-inference",
"unsloth",
"mistral",
"trl",
"en",
"base_model:unsloth/Mistral-Nemo-Instruct-2407-bnb-4bit",
"base_model:finetune:unsloth/Mistral-Nemo-Instruct-2407-bnb-4bit",
"license:apache-2.0",
"endpoints_compatible",
"region:us"
] | null | "2025-02-23T03:28:38Z" | ---
base_model: unsloth/Mistral-Nemo-Instruct-2407-bnb-4bit
tags:
- text-generation-inference
- transformers
- unsloth
- mistral
- trl
license: apache-2.0
language:
- en
---
# Uploaded model
- **Developed by:** MonirahQQ
- **License:** apache-2.0
- **Finetuned from model :** unsloth/Mistral-Nemo-Instruct-2407-bnb-4bit
This mistral model was trained 2x faster with [Unsloth](https://github.com/unslothai/unsloth) and Huggingface's TRL library.
[<img src="https://raw.githubusercontent.com/unslothai/unsloth/main/images/unsloth%20made%20with%20love.png" width="200"/>](https://github.com/unslothai/unsloth)
|
kasrahabib/all-MiniLM-L6-v2-finetuned-iso29148-f_nf_req-embdr | kasrahabib | "2024-05-14T13:35:10Z" | 62 | 0 | transformers | [
"transformers",
"tf",
"tensorboard",
"bert",
"text-classification",
"generated_from_keras_callback",
"base_model:sentence-transformers/all-MiniLM-L6-v2",
"base_model:finetune:sentence-transformers/all-MiniLM-L6-v2",
"license:apache-2.0",
"autotrain_compatible",
"endpoints_compatible",
"region:us"
] | text-classification | "2024-05-14T13:26:31Z" | ---
license: apache-2.0
base_model: sentence-transformers/all-MiniLM-L6-v2
tags:
- generated_from_keras_callback
model-index:
- name: kasrahabib/all-MiniLM-L6-v2-finetuned-iso29148-f_nf_req-embdr
results: []
---
<!-- This model card has been generated automatically according to the information Keras had access to. You should
probably proofread and complete it, then remove this comment. -->
# kasrahabib/all-MiniLM-L6-v2-finetuned-iso29148-f_nf_req-embdr
This model is a fine-tuned version of [sentence-transformers/all-MiniLM-L6-v2](https://huggingface.co/sentence-transformers/all-MiniLM-L6-v2) on an unknown dataset.
It achieves the following results on the evaluation set:
- Train Loss: 0.0009
- Validation Loss: 0.6623
- Epoch: 29
## Model description
More information needed
## Intended uses & limitations
More information needed
## Training and evaluation data
More information needed
## Training procedure
### Training hyperparameters
The following hyperparameters were used during training:
- optimizer: {'name': 'Adam', 'weight_decay': None, 'clipnorm': None, 'global_clipnorm': None, 'clipvalue': None, 'use_ema': False, 'ema_momentum': 0.99, 'ema_overwrite_frequency': None, 'jit_compile': True, 'is_legacy_optimizer': False, 'learning_rate': {'module': 'keras.optimizers.schedules', 'class_name': 'PolynomialDecay', 'config': {'initial_learning_rate': 2e-05, 'decay_steps': 4710, 'end_learning_rate': 0.0, 'power': 1.0, 'cycle': False, 'name': None}, 'registered_name': None}, 'beta_1': 0.9, 'beta_2': 0.999, 'epsilon': 1e-08, 'amsgrad': False}
- training_precision: float32
### Training results
| Train Loss | Validation Loss | Epoch |
|:----------:|:---------------:|:-----:|
| 0.5280 | 0.3710 | 0 |
| 0.3075 | 0.3428 | 1 |
| 0.2140 | 0.3139 | 2 |
| 0.1252 | 0.3637 | 3 |
| 0.0794 | 0.3695 | 4 |
| 0.0506 | 0.4162 | 5 |
| 0.0384 | 0.4577 | 6 |
| 0.0253 | 0.4791 | 7 |
| 0.0190 | 0.5735 | 8 |
| 0.0119 | 0.5711 | 9 |
| 0.0141 | 0.5977 | 10 |
| 0.0131 | 0.5945 | 11 |
| 0.0060 | 0.6052 | 12 |
| 0.0098 | 0.6270 | 13 |
| 0.0080 | 0.6484 | 14 |
| 0.0098 | 0.6139 | 15 |
| 0.0064 | 0.6103 | 16 |
| 0.0067 | 0.6232 | 17 |
| 0.0078 | 0.6205 | 18 |
| 0.0067 | 0.6126 | 19 |
| 0.0039 | 0.6108 | 20 |
| 0.0039 | 0.6407 | 21 |
| 0.0052 | 0.6501 | 22 |
| 0.0043 | 0.6523 | 23 |
| 0.0048 | 0.6800 | 24 |
| 0.0071 | 0.6644 | 25 |
| 0.0014 | 0.6600 | 26 |
| 0.0026 | 0.6578 | 27 |
| 0.0010 | 0.6613 | 28 |
| 0.0009 | 0.6623 | 29 |
### Framework versions
- Transformers 4.40.1
- TensorFlow 2.15.0
- Datasets 2.19.1
- Tokenizers 0.19.1
|
greg-szopinski/ppo-Pyramids-1 | greg-szopinski | "2023-08-01T10:22:29Z" | 0 | 0 | ml-agents | [
"ml-agents",
"tensorboard",
"onnx",
"Pyramids",
"deep-reinforcement-learning",
"reinforcement-learning",
"ML-Agents-Pyramids",
"region:us"
] | reinforcement-learning | "2023-08-01T10:22:26Z" | ---
library_name: ml-agents
tags:
- Pyramids
- deep-reinforcement-learning
- reinforcement-learning
- ML-Agents-Pyramids
---
# **ppo** Agent playing **Pyramids**
This is a trained model of a **ppo** agent playing **Pyramids**
using the [Unity ML-Agents Library](https://github.com/Unity-Technologies/ml-agents).
## Usage (with ML-Agents)
The Documentation: https://unity-technologies.github.io/ml-agents/ML-Agents-Toolkit-Documentation/
We wrote a complete tutorial to learn to train your first agent using ML-Agents and publish it to the Hub:
- A *short tutorial* where you teach Huggy the Dog 🐶 to fetch the stick and then play with him directly in your
browser: https://huggingface.co/learn/deep-rl-course/unitbonus1/introduction
- A *longer tutorial* to understand how works ML-Agents:
https://huggingface.co/learn/deep-rl-course/unit5/introduction
### Resume the training
```bash
mlagents-learn <your_configuration_file_path.yaml> --run-id=<run_id> --resume
```
### Watch your Agent play
You can watch your agent **playing directly in your browser**
1. If the environment is part of ML-Agents official environments, go to https://huggingface.co/unity
2. Step 1: Find your model_id: greg-szopinski/ppo-Pyramids-1
3. Step 2: Select your *.nn /*.onnx file
4. Click on Watch the agent play 👀
|
OnAnOrange/mistral-7B-human-test-examples-true-instruction-format | OnAnOrange | "2024-04-02T06:14:14Z" | 0 | 0 | transformers | [
"transformers",
"safetensors",
"arxiv:1910.09700",
"endpoints_compatible",
"region:us"
] | null | "2024-04-02T06:07:01Z" | ---
library_name: transformers
tags: []
---
# Model Card for Model ID
<!-- Provide a quick summary of what the model is/does. -->
## Model Details
### Model Description
<!-- Provide a longer summary of what this model is. -->
This is the model card of a 🤗 transformers model that has been pushed on the Hub. This model card has been automatically generated.
- **Developed by:** [More Information Needed]
- **Funded by [optional]:** [More Information Needed]
- **Shared by [optional]:** [More Information Needed]
- **Model type:** [More Information Needed]
- **Language(s) (NLP):** [More Information Needed]
- **License:** [More Information Needed]
- **Finetuned from model [optional]:** [More Information Needed]
### Model Sources [optional]
<!-- Provide the basic links for the model. -->
- **Repository:** [More Information Needed]
- **Paper [optional]:** [More Information Needed]
- **Demo [optional]:** [More Information Needed]
## Uses
<!-- Address questions around how the model is intended to be used, including the foreseeable users of the model and those affected by the model. -->
### Direct Use
<!-- This section is for the model use without fine-tuning or plugging into a larger ecosystem/app. -->
[More Information Needed]
### Downstream Use [optional]
<!-- This section is for the model use when fine-tuned for a task, or when plugged into a larger ecosystem/app -->
[More Information Needed]
### Out-of-Scope Use
<!-- This section addresses misuse, malicious use, and uses that the model will not work well for. -->
[More Information Needed]
## Bias, Risks, and Limitations
<!-- This section is meant to convey both technical and sociotechnical limitations. -->
[More Information Needed]
### Recommendations
<!-- This section is meant to convey recommendations with respect to the bias, risk, and technical limitations. -->
Users (both direct and downstream) should be made aware of the risks, biases and limitations of the model. More information needed for further recommendations.
## How to Get Started with the Model
Use the code below to get started with the model.
[More Information Needed]
## Training Details
### Training Data
<!-- This should link to a Dataset Card, perhaps with a short stub of information on what the training data is all about as well as documentation related to data pre-processing or additional filtering. -->
[More Information Needed]
### Training Procedure
<!-- This relates heavily to the Technical Specifications. Content here should link to that section when it is relevant to the training procedure. -->
#### Preprocessing [optional]
[More Information Needed]
#### Training Hyperparameters
- **Training regime:** [More Information Needed] <!--fp32, fp16 mixed precision, bf16 mixed precision, bf16 non-mixed precision, fp16 non-mixed precision, fp8 mixed precision -->
#### Speeds, Sizes, Times [optional]
<!-- This section provides information about throughput, start/end time, checkpoint size if relevant, etc. -->
[More Information Needed]
## Evaluation
<!-- This section describes the evaluation protocols and provides the results. -->
### Testing Data, Factors & Metrics
#### Testing Data
<!-- This should link to a Dataset Card if possible. -->
[More Information Needed]
#### Factors
<!-- These are the things the evaluation is disaggregating by, e.g., subpopulations or domains. -->
[More Information Needed]
#### Metrics
<!-- These are the evaluation metrics being used, ideally with a description of why. -->
[More Information Needed]
### Results
[More Information Needed]
#### Summary
## Model Examination [optional]
<!-- Relevant interpretability work for the model goes here -->
[More Information Needed]
## Environmental Impact
<!-- Total emissions (in grams of CO2eq) and additional considerations, such as electricity usage, go here. Edit the suggested text below accordingly -->
Carbon emissions can be estimated using the [Machine Learning Impact calculator](https://mlco2.github.io/impact#compute) presented in [Lacoste et al. (2019)](https://arxiv.org/abs/1910.09700).
- **Hardware Type:** [More Information Needed]
- **Hours used:** [More Information Needed]
- **Cloud Provider:** [More Information Needed]
- **Compute Region:** [More Information Needed]
- **Carbon Emitted:** [More Information Needed]
## Technical Specifications [optional]
### Model Architecture and Objective
[More Information Needed]
### Compute Infrastructure
[More Information Needed]
#### Hardware
[More Information Needed]
#### Software
[More Information Needed]
## Citation [optional]
<!-- If there is a paper or blog post introducing the model, the APA and Bibtex information for that should go in this section. -->
**BibTeX:**
[More Information Needed]
**APA:**
[More Information Needed]
## Glossary [optional]
<!-- If relevant, include terms and calculations in this section that can help readers understand the model or model card. -->
[More Information Needed]
## More Information [optional]
[More Information Needed]
## Model Card Authors [optional]
[More Information Needed]
## Model Card Contact
[More Information Needed] |
Cenrax/BioPipe-7B-slerp | Cenrax | "2024-03-30T19:50:33Z" | 4 | 0 | transformers | [
"transformers",
"mistral",
"text-generation",
"merge",
"mergekit",
"lazymergekit",
"OpenPipe/mistral-ft-optimized-1218",
"BioMistral/BioMistral-7B",
"base_model:BioMistral/BioMistral-7B",
"base_model:merge:BioMistral/BioMistral-7B",
"base_model:OpenPipe/mistral-ft-optimized-1218",
"base_model:merge:OpenPipe/mistral-ft-optimized-1218",
"autotrain_compatible",
"endpoints_compatible",
"region:us"
] | text-generation | "2024-03-30T19:42:13Z" | ---
tags:
- merge
- mergekit
- lazymergekit
- OpenPipe/mistral-ft-optimized-1218
- BioMistral/BioMistral-7B
base_model:
- OpenPipe/mistral-ft-optimized-1218
- BioMistral/BioMistral-7B
---
# BioPipe-7B-slerp
BioPipe-7B-slerp is a merge of the following models using [LazyMergekit](https://colab.research.google.com/drive/1obulZ1ROXHjYLn6PPZJwRR6GzgQogxxb?usp=sharing):
* [OpenPipe/mistral-ft-optimized-1218](https://huggingface.co/OpenPipe/mistral-ft-optimized-1218)
* [BioMistral/BioMistral-7B](https://huggingface.co/BioMistral/BioMistral-7B)
## 🧩 Configuration
```yaml
slices:
- sources:
- model: OpenPipe/mistral-ft-optimized-1218
layer_range: [0, 32]
- model: BioMistral/BioMistral-7B
layer_range: [0, 32]
merge_method: slerp
base_model: OpenPipe/mistral-ft-optimized-1218
parameters:
t:
- filter: self_attn
value: [0, 0.5, 0.3, 0.7, 1]
- filter: mlp
value: [1, 0.5, 0.7, 0.3, 0]
- value: 0.5
dtype: bfloat16
```
## 💻 Usage
```python
!pip install -qU transformers accelerate
from transformers import AutoTokenizer
import transformers
import torch
model = "Cenrax/BioPipe-7B-slerp"
messages = [{"role": "user", "content": "What is a large language model?"}]
tokenizer = AutoTokenizer.from_pretrained(model)
prompt = tokenizer.apply_chat_template(messages, tokenize=False, add_generation_prompt=True)
pipeline = transformers.pipeline(
"text-generation",
model=model,
torch_dtype=torch.float16,
device_map="auto",
)
outputs = pipeline(prompt, max_new_tokens=256, do_sample=True, temperature=0.7, top_k=50, top_p=0.95)
print(outputs[0]["generated_text"])
``` |
adammandic87/c488945a-a875-4104-82bb-e12f932df89c | adammandic87 | "2025-01-18T08:39:56Z" | 5 | 0 | peft | [
"peft",
"safetensors",
"llama",
"axolotl",
"generated_from_trainer",
"base_model:samoline/d4cbc50c-515a-491e-97ac-dcc4fadd483b",
"base_model:adapter:samoline/d4cbc50c-515a-491e-97ac-dcc4fadd483b",
"region:us"
] | null | "2025-01-18T08:35:48Z" | ---
library_name: peft
base_model: samoline/d4cbc50c-515a-491e-97ac-dcc4fadd483b
tags:
- axolotl
- generated_from_trainer
model-index:
- name: c488945a-a875-4104-82bb-e12f932df89c
results: []
---
<!-- This model card has been generated automatically according to the information the Trainer had access to. You
should probably proofread and complete it, then remove this comment. -->
[<img src="https://raw.githubusercontent.com/axolotl-ai-cloud/axolotl/main/image/axolotl-badge-web.png" alt="Built with Axolotl" width="200" height="32"/>](https://github.com/axolotl-ai-cloud/axolotl)
<details><summary>See axolotl config</summary>
axolotl version: `0.4.1`
```yaml
adapter: lora
base_model: samoline/d4cbc50c-515a-491e-97ac-dcc4fadd483b
bf16: auto
chat_template: llama3
dataset_prepared_path: null
datasets:
- data_files:
- train_c4393383-ef1d-4e9c-b95c-18b4f735570d.json
ds_type: json
format: custom
path: /workspace/input_data/train_c4393383-ef1d-4e9c-b95c-18b4f735570d.json
type:
field_input: input
field_instruction: instruction
field_output: output
format: '{instruction} {input}'
no_input_format: '{instruction}'
system_format: '{system}'
system_prompt: ''
debug: null
deepspeed: null
early_stopping_patience: null
eval_max_new_tokens: 128
eval_table_size: null
evals_per_epoch: 4
flash_attention: false
fp16: null
fsdp: null
fsdp_config: null
gradient_accumulation_steps: 4
gradient_checkpointing: false
group_by_length: false
hub_model_id: adammandic87/c488945a-a875-4104-82bb-e12f932df89c
hub_repo: null
hub_strategy: checkpoint
hub_token: null
learning_rate: 0.0002
load_in_4bit: false
load_in_8bit: false
local_rank: null
logging_steps: 1
lora_alpha: 16
lora_dropout: 0.05
lora_fan_in_fan_out: null
lora_model_dir: null
lora_r: 8
lora_target_linear: true
lr_scheduler: cosine
max_steps: 10
micro_batch_size: 2
mlflow_experiment_name: /tmp/train_c4393383-ef1d-4e9c-b95c-18b4f735570d.json
model_type: AutoModelForCausalLM
num_epochs: 1
optimizer: adamw_bnb_8bit
output_dir: miner_id_24
pad_to_sequence_len: true
resume_from_checkpoint: null
s2_attention: null
sample_packing: false
saves_per_epoch: 4
sequence_len: 512
special_tokens:
pad_token: <|endoftext|>
strict: false
tf32: false
tokenizer_type: AutoTokenizer
train_on_inputs: false
trust_remote_code: true
val_set_size: 0.05
wandb_entity: null
wandb_mode: online
wandb_name: 49def120-0589-4d75-a714-b567b410892c
wandb_project: birthday-sn56-19-Gradients-On-Demand
wandb_run: your_name
wandb_runid: 49def120-0589-4d75-a714-b567b410892c
warmup_steps: 10
weight_decay: 0.0
xformers_attention: null
```
</details><br>
# c488945a-a875-4104-82bb-e12f932df89c
This model is a fine-tuned version of [samoline/d4cbc50c-515a-491e-97ac-dcc4fadd483b](https://huggingface.co/samoline/d4cbc50c-515a-491e-97ac-dcc4fadd483b) on the None dataset.
It achieves the following results on the evaluation set:
- Loss: 1.1281
## Model description
More information needed
## Intended uses & limitations
More information needed
## Training and evaluation data
More information needed
## Training procedure
### Training hyperparameters
The following hyperparameters were used during training:
- learning_rate: 0.0002
- train_batch_size: 2
- eval_batch_size: 2
- seed: 42
- gradient_accumulation_steps: 4
- total_train_batch_size: 8
- optimizer: Use OptimizerNames.ADAMW_BNB with betas=(0.9,0.999) and epsilon=1e-08 and optimizer_args=No additional optimizer arguments
- lr_scheduler_type: cosine
- lr_scheduler_warmup_steps: 10
- training_steps: 10
### Training results
| Training Loss | Epoch | Step | Validation Loss |
|:-------------:|:------:|:----:|:---------------:|
| 1.1581 | 0.0002 | 1 | 1.1282 |
| 1.1193 | 0.0006 | 3 | 1.1280 |
| 1.085 | 0.0012 | 6 | 1.1265 |
| 0.9436 | 0.0018 | 9 | 1.1281 |
### Framework versions
- PEFT 0.13.2
- Transformers 4.46.0
- Pytorch 2.5.0+cu124
- Datasets 3.0.1
- Tokenizers 0.20.1 |
sschet/scibert_scivocab_cased_ner_jnlpba | sschet | "2023-02-01T03:41:13Z" | 8 | 0 | transformers | [
"transformers",
"pytorch",
"jax",
"bert",
"token-classification",
"en",
"dataset:tner/bc5cdr",
"dataset:commanderstrife/jnlpba",
"dataset:bc2gm_corpus",
"dataset:drAbreu/bc4chemd_ner",
"dataset:linnaeus",
"dataset:chintagunta85/ncbi_disease",
"arxiv:1903.10676",
"autotrain_compatible",
"endpoints_compatible",
"region:us"
] | token-classification | "2023-02-01T01:16:37Z" | ---
language: en
datasets:
- tner/bc5cdr
- commanderstrife/jnlpba
- bc2gm_corpus
- drAbreu/bc4chemd_ner
- linnaeus
- chintagunta85/ncbi_disease
---
# SciBERT finetuned on JNLPA for NER downstream task
## Language Model
[SciBERT](https://arxiv.org/pdf/1903.10676.pdf) is a pretrained language model based on BERT and trained by the
[Allen Institute for AI](https://allenai.org/) on papers from the corpus of
[Semantic Scholar](https://www.semanticscholar.org/).
Corpus size is 1.14M papers, 3.1B tokens. SciBERT has its own vocabulary (scivocab) that's built to best match
the training corpus.
## Downstream task
[`allenai/scibert_scivocab_cased`](https://huggingface.co/allenai/scibert_scivocab_cased#) has been finetuned for Named Entity
Recognition (NER) dowstream task. The code to train the NER can be found [here](https://github.com/fran-martinez/bio_ner_bert).
### Data
The corpus used to fine-tune the NER is [BioNLP / JNLPBA shared task](http://www.geniaproject.org/shared-tasks/bionlp-jnlpba-shared-task-2004).
- Training data consist of 2,000 PubMed abstracts with term/word annotation. This corresponds to 18,546 samples (senteces).
- Evaluation data consist of 404 PubMed abstracts with term/word annotation. This corresponds to 3,856 samples (sentences).
The classes (at word level) and its distribution (number of examples for each class) for training and evaluation datasets are shown below:
| Class Label | # training examples| # evaluation examples|
|:--------------|--------------:|----------------:|
|O | 382,963 | 81,647 |
|B-protein | 30,269 | 5,067 |
|I-protein | 24,848 | 4,774 |
|B-cell_type | 6,718 | 1,921 |
|I-cell_type | 8,748 | 2,991 |
|B-DNA | 9,533 | 1,056 |
|I-DNA | 15,774 | 1,789 |
|B-cell_line | 3,830 | 500 |
|I-cell_line | 7,387 | 9,89 |
|B-RNA | 951 | 118 |
|I-RNA | 1,530 | 187 |
### Model
An exhaustive hyperparameter search was done.
The hyperparameters that provided the best results are:
- Max length sequence: 128
- Number of epochs: 6
- Batch size: 32
- Dropout: 0.3
- Optimizer: Adam
The used learning rate was 5e-5 with a decreasing linear schedule. A warmup was used at the beggining of the training
with a ratio of steps equal to 0.1 from the total training steps.
The model from the epoch with the best F1-score was selected, in this case, the model from epoch 5.
### Evaluation
The following table shows the evaluation metrics calculated at span/entity level:
| | precision| recall| f1-score|
|:---------|-----------:|---------:|---------:|
cell_line | 0.5205 | 0.7100 | 0.6007 |
cell_type | 0.7736 | 0.7422 | 0.7576 |
protein | 0.6953 | 0.8459 | 0.7633 |
DNA | 0.6997 | 0.7894 | 0.7419 |
RNA | 0.6985 | 0.8051 | 0.7480 |
| | | |
**micro avg** | 0.6984 | 0.8076 | 0.7490|
**macro avg** | 0.7032 | 0.8076 | 0.7498 |
The macro F1-score is equal to 0.7498, compared to the value provided by the Allen Institute for AI in their
[paper](https://arxiv.org/pdf/1903.10676.pdf), which is equal to 0.7728. This drop in performance could be due to
several reasons, but one hypothesis could be the fact that the authors used an additional conditional random field,
while this model uses a regular classification layer with softmax activation on top of SciBERT model.
At word level, this model achieves a precision of 0.7742, a recall of 0.8536 and a F1-score of 0.8093.
### Model usage in inference
Use the pipeline:
````python
from transformers import pipeline
text = "Mouse thymus was used as a source of glucocorticoid receptor from normal CS lymphocytes."
nlp_ner = pipeline("ner",
model='fran-martinez/scibert_scivocab_cased_ner_jnlpba',
tokenizer='fran-martinez/scibert_scivocab_cased_ner_jnlpba')
nlp_ner(text)
"""
Output:
---------------------------
[
{'word': 'glucocorticoid',
'score': 0.9894881248474121,
'entity': 'B-protein'},
{'word': 'receptor',
'score': 0.989505410194397,
'entity': 'I-protein'},
{'word': 'normal',
'score': 0.7680378556251526,
'entity': 'B-cell_type'},
{'word': 'cs',
'score': 0.5176806449890137,
'entity': 'I-cell_type'},
{'word': 'lymphocytes',
'score': 0.9898491501808167,
'entity': 'I-cell_type'}
]
"""
````
Or load model and tokenizer as follows:
````python
import torch
from transformers import AutoTokenizer, AutoModelForTokenClassification
# Example
text = "Mouse thymus was used as a source of glucocorticoid receptor from normal CS lymphocytes."
# Load model
tokenizer = AutoTokenizer.from_pretrained("fran-martinez/scibert_scivocab_cased_ner_jnlpba")
model = AutoModelForTokenClassification.from_pretrained("fran-martinez/scibert_scivocab_cased_ner_jnlpba")
# Get input for BERT
input_ids = torch.tensor(tokenizer.encode(text)).unsqueeze(0)
# Predict
with torch.no_grad():
outputs = model(input_ids)
# From the output let's take the first element of the tuple.
# Then, let's get rid of [CLS] and [SEP] tokens (first and last)
predictions = outputs[0].argmax(axis=-1)[0][1:-1]
# Map label class indexes to string labels.
for token, pred in zip(tokenizer.tokenize(text), predictions):
print(token, '->', model.config.id2label[pred.numpy().item()])
"""
Output:
---------------------------
mouse -> O
thymus -> O
was -> O
used -> O
as -> O
a -> O
source -> O
of -> O
glucocorticoid -> B-protein
receptor -> I-protein
from -> O
normal -> B-cell_type
cs -> I-cell_type
lymphocytes -> I-cell_type
. -> O
"""
```` |
John6666/kagewanimix-v03-sdxl | John6666 | "2025-04-07T14:00:31Z" | 0 | 0 | diffusers | [
"diffusers",
"safetensors",
"text-to-image",
"stable-diffusion",
"stable-diffusion-xl",
"anime",
"girls",
"cute",
"merge",
"illustrious",
"en",
"base_model:JujoHotaru/BreedSeriesForXL",
"base_model:merge:JujoHotaru/BreedSeriesForXL",
"base_model:OnomaAIResearch/Illustrious-xl-early-release-v0",
"base_model:merge:OnomaAIResearch/Illustrious-xl-early-release-v0",
"license:other",
"autotrain_compatible",
"endpoints_compatible",
"diffusers:StableDiffusionXLPipeline",
"region:us"
] | text-to-image | "2025-04-07T13:53:06Z" | ---
license: other
license_name: faipl-1.0-sd
license_link: https://freedevproject.org/faipl-1.0-sd/
language:
- en
library_name: diffusers
pipeline_tag: text-to-image
tags:
- text-to-image
- stable-diffusion
- stable-diffusion-xl
- anime
- girls
- cute
- merge
- illustrious
base_model:
- OnomaAIResearch/Illustrious-xl-early-release-v0
- JujoHotaru/BreedSeriesForXL
---
Original model is [here](https://civitai.com/models/1425748?modelVersionId=1621696).
This model created by [Concerta](https://civitai.com/user/Concerta).
|
Samalabama66/Reinforce-cartpole | Samalabama66 | "2023-07-25T04:28:36Z" | 0 | 0 | null | [
"CartPole-v1",
"reinforce",
"reinforcement-learning",
"custom-implementation",
"deep-rl-class",
"model-index",
"region:us"
] | reinforcement-learning | "2023-07-25T04:28:26Z" | ---
tags:
- CartPole-v1
- reinforce
- reinforcement-learning
- custom-implementation
- deep-rl-class
model-index:
- name: Reinforce-cartpole
results:
- task:
type: reinforcement-learning
name: reinforcement-learning
dataset:
name: CartPole-v1
type: CartPole-v1
metrics:
- type: mean_reward
value: 500.00 +/- 0.00
name: mean_reward
verified: false
---
# **Reinforce** Agent playing **CartPole-v1**
This is a trained model of a **Reinforce** agent playing **CartPole-v1** .
To learn to use this model and train yours check Unit 4 of the Deep Reinforcement Learning Course: https://huggingface.co/deep-rl-course/unit4/introduction
|
Intel/ldm3d-sr | Intel | "2024-04-25T13:33:09Z" | 54 | 9 | diffusers | [
"diffusers",
"safetensors",
"stable-diffusion",
"stable-diffusion-diffusers",
"text-to-image",
"text-to-3d",
"en",
"arxiv:2311.03226",
"license:creativeml-openrail-m",
"model-index",
"diffusers:StableDiffusionUpscaleLDM3DPipeline",
"region:us"
] | text-to-3d | "2023-09-06T10:03:49Z" | ---
language:
- en
tags:
- stable-diffusion
- stable-diffusion-diffusers
- text-to-image
model-index:
- name: ldm3d-sr
results:
- task:
name: Latent Diffusion Model for 3D - Super-Resolution
type: latent-diffusion-model-for-3D-SR
dataset:
name: LAION-400M
type: laion/laion400m
metrics:
- name: LDM3D-SR-B FID
type: LDM3D-SR-B FID
value: 14.705
- name: LDM3D-SR-B IS
type: LDM3D-SR-B IS
value: 60.371
- name: LDM3D-SR-B PSNR
type: LDM3D-SR-B PSNR
value: 24.479
- name: LDM3D-SR-B SSIM
type: LDM3D-SR-B SSIM
value: 0.665
- name: LDM3D-SR-B Depth MARE
type: LDM3D-SR-B Depth MARE
value: 0.0537
library_name: diffusers
pipeline_tag: text-to-3d
license: creativeml-openrail-m
---
# LDM3D-SR model
The LDM3D-VR model suite was proposed in the paper [LDM3D-VR: Latent Diffusion Model for 3D](https://arxiv.org/pdf/2311.03226.pdf), authored by Gabriela Ben Melech Stan, Diana Wofk, Estelle Aflalo, Shao-Yen Tseng, Zhipeng Cai, Michael Paulitsch, and Vasudev Lal.
LDM3D-VR was accepted to the [NeurIPS 2023 Workshop on Diffusion Models](https://neurips.cc/virtual/2023/workshop/66539).
This new checkpoint is related to the upscaler called LDM3D-SR.
## Model details
Latent diffusion models have proven to be state-of-the-art in the creation and manipulation of visual outputs. However, as far as we know, the generation of depth maps jointly with RGB is still limited. We introduce LDM3D-VR, a suite of diffusion models targeting virtual reality development that includes LDM3D-pano and LDM3D-SR. These models enable the generation of panoramic RGBD based on textual prompts and the upscaling of low-resolution inputs to high-resolution RGBD, respectively. Our models are fine-tuned from existing pretrained models on datasets containing panoramic/high-resolution RGB images, depth maps and captions. Both models are evaluated in comparison to existing related methods.

<font size="2">LDM3D-SR overview </font>
## Usage
Using the [🤗's Diffusers library](https://github.com/huggingface/diffusers) in a simple and efficient manner.
```python
from PIL import Image
import os
import torch
from diffusers import StableDiffusionLDM3DPipeline, DiffusionPipeline
#Generate a rgb/depth output from LDM3D
pipe_ldm3d = StableDiffusionLDM3DPipeline.from_pretrained("Intel/ldm3d-4c")
pipe_ldm3d.to("cuda")
prompt =f"A picture of some lemons on a table"
output = pipe_ldm3d(prompt)
rgb_image, depth_image = output.rgb, output.depth
rgb_image[0].save(f"lemons_ldm3d_rgb.jpg")
depth_image[0].save(f"lemons_ldm3d_depth.png")
#Upscale the previous output to a resolution of (1024, 1024)
pipe_ldm3d_upscale = DiffusionPipeline.from_pretrained("Intel/ldm3d-sr", custom_pipeline="pipeline_stable_diffusion_upscale_ldm3d")
pipe_ldm3d_upscale.to("cuda")
low_res_img = Image.open(f"lemons_ldm3d_rgb.jpg").convert("RGB")
low_res_depth = Image.open(f"lemons_ldm3d_depth.png")
outputs = pipe_ldm3d_upscale(prompt="high quality high resolution uhd 4k image", rgb=low_res_img, depth=low_res_depth, num_inference_steps=50, target_res=[1024, 1024])
upscaled_rgb, upscaled_depth =outputs.rgb[0], outputs.depth[0]
upscaled_rgb.save(f"upscaled_lemons_rgb.png")
upscaled_depth.save(f"upscaled_lemons_depth.png")
```
This is the result:
Output of ldm3d-4c | Upscaled output
:-------------------------:|:-------------------------:
 | 
 | 
## Training data
The LDM3D model was finetuned on a dataset constructed from a subset of the LAION-400M dataset, a large-scale image-caption dataset that contains over 400 million image-caption pairs. In the finetuning process of the LDM3D-SR, the training data consists of additional high-resolution (HR) and low-resolution (LR) sets with 261,045 samples each. For HR samples, a subset of LAION Aesthetics 6+ with tuples (captions, 512x512-sized images, and depth maps from DPT-BEiT-L-512) is used. LR images are generated using a lightweight BSR-image-degradation method, introduced in applied to the HR image.
### Finetuning
The fine-tuning process comprises two stages. In the first stage, we train an autoencoder to generate a lower-dimensional, perceptually equivalent data representation. Subsequently, we fine-tune the diffusion model using the frozen autoencoder.
LDM3D-SR utilizes the autoencoder previously developed for [LDM3D-4c](https://huggingface.co/Intel/ldm3d-4c) to now encode low-resolution (LR) images into a 64x64x4 dimensional latent space. The diffusion model used here is an adapted version of the U-Net, now modified to have an 8-channel input. This change enables conditioning on LR latent via concatenation to the high-resolution (HR) latent during training, and to noise during inference. Text conditioning is also facilitated using cross attention with a CLIP text encoder.
## Evaluation results
The table below shows the quantitative results of upscaling from 128 x 128 to 512 x 512, evaluated on 2,243 samples from ImageNet-Val. We explore three methods for generating LR depth maps: performing depth estimation on the LR depth maps (LDM3D-SR-D), utilizing the original HR depth map for LR conditioning (LDM3D-SR-O), and applying bicubic degradation to the depth map (LDM3D-SR-B).
|Method |FID ↓ |IS ↑ |PSNR ↑ |SSIM ↑ |Depth MARE ↓ |
|-------------------|------|-----------|-----------|----------|-------------|
|Regression, bicubic|24.686|60.135±4.16|26.424±3.98|0.716±0.13|0.0153±0.0189|
|SDx4[29] |15.865|61.103±3.48|24.528±3.63|0.631±0.15|N/A |
|LDMx4[30] |15.245|60.060±3.88|25.511±3.94|0.686±0.16|N/A |
|SD-superres[2] |15.254|59.789±3.53|23.878±3.28|0.642±0.15|N/A |
|LDM3D-SR-D |15.522|59.736±3.37|24.113±3.54|0.659±0.16|0.0753±0.0734|
|LDM3D-SR-O |14.793|60.260±3.53|24.498±3.59|0.665±0.16|0.0530±0.0496|
|LDM3D-SR-B |14.705|60.371±3.56|24.479±3.58|0.665±0.48|0.0537±0.0506|
The results shown above can be referenced in Table 3 of the [LDM3D-VR paper](https://arxiv.org/pdf/2311.03226.pdf).
## Ethical Considerations and Limitations
For image generation, the [Stable Diffusion](https://huggingface.co/CompVis/stable-diffusion-v1-4#limitations) limitations and biases apply. For depth map generation, a first limitiation is that we are using DPT-large to produce the ground truth, hence, other limitations and biases from [DPT](https://huggingface.co/Intel/dpt-large) are applicable.
## Caveats and Recommendations
Users (both direct and downstream) should be made aware of the risks, biases and limitations of the model.
Here are a couple of useful links to learn more about Intel's AI software:
* [Intel Extension for PyTorch](https://github.com/intel/intel-extension-for-pytorch)
* [Intel Neural Compressor](https://github.com/intel/neural-compressor)
## Disclaimer
The license on this model does not constitute legal advice. We are not responsible for the actions of third parties who use this model. Please consult an attorney before using this model for commercial purposes.
### BibTeX entry and citation info
```bibtex
@misc{stan2023ldm3dvr,
title={LDM3D-VR: Latent Diffusion Model for 3D VR},
author={Gabriela Ben Melech Stan and Diana Wofk and Estelle Aflalo and Shao-Yen Tseng and Zhipeng Cai and Michael Paulitsch and Vasudev Lal},
year={2023},
eprint={2311.03226},
archivePrefix={arXiv},
primaryClass={cs.CV}
}
``` |
MaziyarPanahi/Qwen2.5-Coder-7B-Instruct-abliterated-GGUF | MaziyarPanahi | "2024-10-30T07:10:58Z" | 58 | 1 | null | [
"gguf",
"mistral",
"quantized",
"2-bit",
"3-bit",
"4-bit",
"5-bit",
"6-bit",
"8-bit",
"GGUF",
"text-generation",
"base_model:huihui-ai/Qwen2.5-Coder-7B-Instruct-abliterated",
"base_model:quantized:huihui-ai/Qwen2.5-Coder-7B-Instruct-abliterated",
"region:us",
"conversational"
] | text-generation | "2024-10-30T06:48:53Z" | ---
tags:
- quantized
- 2-bit
- 3-bit
- 4-bit
- 5-bit
- 6-bit
- 8-bit
- GGUF
- text-generation
- text-generation
model_name: Qwen2.5-Coder-7B-Instruct-abliterated-GGUF
base_model: huihui-ai/Qwen2.5-Coder-7B-Instruct-abliterated
inference: false
model_creator: huihui-ai
pipeline_tag: text-generation
quantized_by: MaziyarPanahi
---
# [MaziyarPanahi/Qwen2.5-Coder-7B-Instruct-abliterated-GGUF](https://huggingface.co/MaziyarPanahi/Qwen2.5-Coder-7B-Instruct-abliterated-GGUF)
- Model creator: [huihui-ai](https://huggingface.co/huihui-ai)
- Original model: [huihui-ai/Qwen2.5-Coder-7B-Instruct-abliterated](https://huggingface.co/huihui-ai/Qwen2.5-Coder-7B-Instruct-abliterated)
## Description
[MaziyarPanahi/Qwen2.5-Coder-7B-Instruct-abliterated-GGUF](https://huggingface.co/MaziyarPanahi/Qwen2.5-Coder-7B-Instruct-abliterated-GGUF) contains GGUF format model files for [huihui-ai/Qwen2.5-Coder-7B-Instruct-abliterated](https://huggingface.co/huihui-ai/Qwen2.5-Coder-7B-Instruct-abliterated).
### About GGUF
GGUF is a new format introduced by the llama.cpp team on August 21st 2023. It is a replacement for GGML, which is no longer supported by llama.cpp.
Here is an incomplete list of clients and libraries that are known to support GGUF:
* [llama.cpp](https://github.com/ggerganov/llama.cpp). The source project for GGUF. Offers a CLI and a server option.
* [llama-cpp-python](https://github.com/abetlen/llama-cpp-python), a Python library with GPU accel, LangChain support, and OpenAI-compatible API server.
* [LM Studio](https://lmstudio.ai/), an easy-to-use and powerful local GUI for Windows and macOS (Silicon), with GPU acceleration. Linux available, in beta as of 27/11/2023.
* [text-generation-webui](https://github.com/oobabooga/text-generation-webui), the most widely used web UI, with many features and powerful extensions. Supports GPU acceleration.
* [KoboldCpp](https://github.com/LostRuins/koboldcpp), a fully featured web UI, with GPU accel across all platforms and GPU architectures. Especially good for story telling.
* [GPT4All](https://gpt4all.io/index.html), a free and open source local running GUI, supporting Windows, Linux and macOS with full GPU accel.
* [LoLLMS Web UI](https://github.com/ParisNeo/lollms-webui), a great web UI with many interesting and unique features, including a full model library for easy model selection.
* [Faraday.dev](https://faraday.dev/), an attractive and easy to use character-based chat GUI for Windows and macOS (both Silicon and Intel), with GPU acceleration.
* [candle](https://github.com/huggingface/candle), a Rust ML framework with a focus on performance, including GPU support, and ease of use.
* [ctransformers](https://github.com/marella/ctransformers), a Python library with GPU accel, LangChain support, and OpenAI-compatible AI server. Note, as of time of writing (November 27th 2023), ctransformers has not been updated in a long time and does not support many recent models.
## Special thanks
🙏 Special thanks to [Georgi Gerganov](https://github.com/ggerganov) and the whole team working on [llama.cpp](https://github.com/ggerganov/llama.cpp/) for making all of this possible. |
alexandretl/ngpt | alexandretl | "2025-04-07T13:27:04Z" | 0 | 0 | null | [
"region:us"
] | null | "2024-10-21T15:27:44Z" | <!DOCTYPE html>
<html class="" lang="en">
<head>
<meta charset="utf-8" />
<meta
name="viewport"
content="width=device-width, initial-scale=1.0, user-scalable=no"
/>
<meta
name="description"
content="We're on a journey to advance and democratize artificial intelligence through open source and open science."
/>
<meta property="fb:app_id" content="1321688464574422" />
<meta name="twitter:card" content="summary_large_image" />
<meta name="twitter:site" content="@huggingface" />
<meta
property="og:title"
content="Hugging Face - The AI community building the future."
/>
<meta property="og:type" content="website" />
<title>Hugging Face - The AI community building the future.</title>
<style>
body {
margin: 0;
}
main {
background-color: white;
min-height: 100vh;
padding: 7rem 1rem 8rem 1rem;
text-align: center;
font-family: Source Sans Pro, ui-sans-serif, system-ui, -apple-system,
BlinkMacSystemFont, Segoe UI, Roboto, Helvetica Neue, Arial, Noto Sans,
sans-serif, Apple Color Emoji, Segoe UI Emoji, Segoe UI Symbol,
Noto Color Emoji;
}
img {
width: 6rem;
height: 6rem;
margin: 0 auto 1rem;
}
h1 {
font-size: 3.75rem;
line-height: 1;
color: rgba(31, 41, 55, 1);
font-weight: 700;
box-sizing: border-box;
margin: 0 auto;
}
p, a {
color: rgba(107, 114, 128, 1);
font-size: 1.125rem;
line-height: 1.75rem;
max-width: 28rem;
box-sizing: border-box;
margin: 0 auto;
}
.dark main {
background-color: rgb(11, 15, 25);
}
.dark h1 {
color: rgb(209, 213, 219);
}
.dark p, .dark a {
color: rgb(156, 163, 175);
}
</style>
<script>
// On page load or when changing themes, best to add inline in `head` to avoid FOUC
const key = "_tb_global_settings";
let theme = window.matchMedia("(prefers-color-scheme: dark)").matches
? "dark"
: "light";
try {
const storageTheme = JSON.parse(window.localStorage.getItem(key)).theme;
if (storageTheme) {
theme = storageTheme === "dark" ? "dark" : "light";
}
} catch (e) {}
if (theme === "dark") {
document.documentElement.classList.add("dark");
} else {
document.documentElement.classList.remove("dark");
}
</script>
</head>
<body>
<main>
<img
src="https://cdn-media.huggingface.co/assets/huggingface_logo.svg"
alt=""
/>
<div>
<h1>429</h1>
<p>We had to rate limit you. If you think it's an error, send us <a href="mailto:[email protected]">an email</a></p>
</div>
</main>
</body>
</html> |
saltyfish666/sd-class-butterflies-32 | saltyfish666 | "2024-12-02T00:20:53Z" | 45 | 0 | diffusers | [
"diffusers",
"safetensors",
"pytorch",
"unconditional-image-generation",
"diffusion-models-class",
"license:mit",
"diffusers:DDPMPipeline",
"region:us"
] | unconditional-image-generation | "2024-12-02T00:20:40Z" | ---
license: mit
tags:
- pytorch
- diffusers
- unconditional-image-generation
- diffusion-models-class
---
# Model Card for Unit 1 of the [Diffusion Models Class 🧨](https://github.com/huggingface/diffusion-models-class)
This model is a diffusion model for unconditional image generation of cute 🦋.
## Usage
```python
from diffusers import DDPMPipeline
pipeline = DDPMPipeline.from_pretrained('saltyfish666/sd-class-butterflies-32')
image = pipeline().images[0]
image
```
|
gokulsrinivasagan/bert_base_train_book_ent_15p_b_mnli | gokulsrinivasagan | "2025-04-09T05:20:00Z" | 0 | 0 | transformers | [
"transformers",
"tensorboard",
"safetensors",
"distilbert",
"text-classification",
"generated_from_trainer",
"en",
"dataset:glue",
"base_model:gokulsrinivasagan/bert_base_train_book_ent_15p_b",
"base_model:finetune:gokulsrinivasagan/bert_base_train_book_ent_15p_b",
"license:apache-2.0",
"model-index",
"autotrain_compatible",
"endpoints_compatible",
"region:us"
] | text-classification | "2025-04-09T03:52:35Z" | ---
library_name: transformers
language:
- en
license: apache-2.0
base_model: gokulsrinivasagan/bert_base_train_book_ent_15p_b
tags:
- generated_from_trainer
datasets:
- glue
metrics:
- accuracy
model-index:
- name: bert_base_train_book_ent_15p_b_mnli
results:
- task:
name: Text Classification
type: text-classification
dataset:
name: GLUE MNLI
type: glue
args: mnli
metrics:
- name: Accuracy
type: accuracy
value: 0.725793327908869
---
<!-- This model card has been generated automatically according to the information the Trainer had access to. You
should probably proofread and complete it, then remove this comment. -->
# bert_base_train_book_ent_15p_b_mnli
This model is a fine-tuned version of [gokulsrinivasagan/bert_base_train_book_ent_15p_b](https://huggingface.co/gokulsrinivasagan/bert_base_train_book_ent_15p_b) on the GLUE MNLI dataset.
It achieves the following results on the evaluation set:
- Loss: 0.6620
- Accuracy: 0.7258
## Model description
More information needed
## Intended uses & limitations
More information needed
## Training and evaluation data
More information needed
## Training procedure
### Training hyperparameters
The following hyperparameters were used during training:
- learning_rate: 5e-05
- train_batch_size: 256
- eval_batch_size: 256
- seed: 10
- optimizer: Use adamw_torch with betas=(0.9,0.999) and epsilon=1e-08 and optimizer_args=No additional optimizer arguments
- lr_scheduler_type: linear
- num_epochs: 50
### Training results
| Training Loss | Epoch | Step | Validation Loss | Accuracy |
|:-------------:|:-----:|:-----:|:---------------:|:--------:|
| 0.8416 | 1.0 | 1534 | 0.7447 | 0.6792 |
| 0.6732 | 2.0 | 3068 | 0.6874 | 0.7136 |
| 0.5679 | 3.0 | 4602 | 0.6784 | 0.7186 |
| 0.4664 | 4.0 | 6136 | 0.7155 | 0.7173 |
| 0.3711 | 5.0 | 7670 | 0.7697 | 0.7255 |
| 0.2875 | 6.0 | 9204 | 0.8823 | 0.7191 |
| 0.2234 | 7.0 | 10738 | 1.0170 | 0.7193 |
| 0.177 | 8.0 | 12272 | 1.0289 | 0.7144 |
### Framework versions
- Transformers 4.46.3
- Pytorch 2.2.1+cu118
- Datasets 3.1.0
- Tokenizers 0.20.3
|
isspek/roberta-base_monkeypox_2_2e-5_16_weight | isspek | "2025-03-23T14:30:13Z" | 8 | 0 | transformers | [
"transformers",
"safetensors",
"roberta",
"text-classification",
"arxiv:1910.09700",
"autotrain_compatible",
"endpoints_compatible",
"region:us"
] | text-classification | "2025-02-17T21:37:09Z" | ---
library_name: transformers
tags: []
---
# Model Card for Model ID
<!-- Provide a quick summary of what the model is/does. -->
## Model Details
### Model Description
<!-- Provide a longer summary of what this model is. -->
This is the model card of a 🤗 transformers model that has been pushed on the Hub. This model card has been automatically generated.
- **Developed by:** [More Information Needed]
- **Funded by [optional]:** [More Information Needed]
- **Shared by [optional]:** [More Information Needed]
- **Model type:** [More Information Needed]
- **Language(s) (NLP):** [More Information Needed]
- **License:** [More Information Needed]
- **Finetuned from model [optional]:** [More Information Needed]
### Model Sources [optional]
<!-- Provide the basic links for the model. -->
- **Repository:** [More Information Needed]
- **Paper [optional]:** [More Information Needed]
- **Demo [optional]:** [More Information Needed]
## Uses
<!-- Address questions around how the model is intended to be used, including the foreseeable users of the model and those affected by the model. -->
### Direct Use
<!-- This section is for the model use without fine-tuning or plugging into a larger ecosystem/app. -->
[More Information Needed]
### Downstream Use [optional]
<!-- This section is for the model use when fine-tuned for a task, or when plugged into a larger ecosystem/app -->
[More Information Needed]
### Out-of-Scope Use
<!-- This section addresses misuse, malicious use, and uses that the model will not work well for. -->
[More Information Needed]
## Bias, Risks, and Limitations
<!-- This section is meant to convey both technical and sociotechnical limitations. -->
[More Information Needed]
### Recommendations
<!-- This section is meant to convey recommendations with respect to the bias, risk, and technical limitations. -->
Users (both direct and downstream) should be made aware of the risks, biases and limitations of the model. More information needed for further recommendations.
## How to Get Started with the Model
Use the code below to get started with the model.
[More Information Needed]
## Training Details
### Training Data
<!-- This should link to a Dataset Card, perhaps with a short stub of information on what the training data is all about as well as documentation related to data pre-processing or additional filtering. -->
[More Information Needed]
### Training Procedure
<!-- This relates heavily to the Technical Specifications. Content here should link to that section when it is relevant to the training procedure. -->
#### Preprocessing [optional]
[More Information Needed]
#### Training Hyperparameters
- **Training regime:** [More Information Needed] <!--fp32, fp16 mixed precision, bf16 mixed precision, bf16 non-mixed precision, fp16 non-mixed precision, fp8 mixed precision -->
#### Speeds, Sizes, Times [optional]
<!-- This section provides information about throughput, start/end time, checkpoint size if relevant, etc. -->
[More Information Needed]
## Evaluation
<!-- This section describes the evaluation protocols and provides the results. -->
### Testing Data, Factors & Metrics
#### Testing Data
<!-- This should link to a Dataset Card if possible. -->
[More Information Needed]
#### Factors
<!-- These are the things the evaluation is disaggregating by, e.g., subpopulations or domains. -->
[More Information Needed]
#### Metrics
<!-- These are the evaluation metrics being used, ideally with a description of why. -->
[More Information Needed]
### Results
[More Information Needed]
#### Summary
## Model Examination [optional]
<!-- Relevant interpretability work for the model goes here -->
[More Information Needed]
## Environmental Impact
<!-- Total emissions (in grams of CO2eq) and additional considerations, such as electricity usage, go here. Edit the suggested text below accordingly -->
Carbon emissions can be estimated using the [Machine Learning Impact calculator](https://mlco2.github.io/impact#compute) presented in [Lacoste et al. (2019)](https://arxiv.org/abs/1910.09700).
- **Hardware Type:** [More Information Needed]
- **Hours used:** [More Information Needed]
- **Cloud Provider:** [More Information Needed]
- **Compute Region:** [More Information Needed]
- **Carbon Emitted:** [More Information Needed]
## Technical Specifications [optional]
### Model Architecture and Objective
[More Information Needed]
### Compute Infrastructure
[More Information Needed]
#### Hardware
[More Information Needed]
#### Software
[More Information Needed]
## Citation [optional]
<!-- If there is a paper or blog post introducing the model, the APA and Bibtex information for that should go in this section. -->
**BibTeX:**
[More Information Needed]
**APA:**
[More Information Needed]
## Glossary [optional]
<!-- If relevant, include terms and calculations in this section that can help readers understand the model or model card. -->
[More Information Needed]
## More Information [optional]
[More Information Needed]
## Model Card Authors [optional]
[More Information Needed]
## Model Card Contact
[More Information Needed] |
huggingkot/Violet_Twilight-v0.2-q4f16_1-MLC | huggingkot | "2025-03-12T18:43:35Z" | 0 | 0 | mlc-llm | [
"mlc-llm",
"web-llm",
"text-generation",
"en",
"base_model:Epiculous/Violet_Twilight-v0.2",
"base_model:quantized:Epiculous/Violet_Twilight-v0.2",
"region:us"
] | text-generation | "2025-03-12T18:42:04Z" |
---
library_name: mlc-llm
tags:
- mlc-llm
- web-llm
language:
- en
base_model:
- Epiculous/Violet_Twilight-v0.2
pipeline_tag: text-generation
---
This is a MLC converted weight from [Violet_Twilight-v0.2](https://huggingface.co/Epiculous/Violet_Twilight-v0.2) model in MLC format `q4f16_1`.
The model can be used for projects [MLC-LLM](https://github.com/mlc-ai/mlc-llm) and [WebLLM](https://github.com/mlc-ai/web-llm).
|
JacksonBrune/f550cf64-eed9-4e21-8c12-09e56596e20b | JacksonBrune | "2025-01-31T09:33:10Z" | 6 | 0 | peft | [
"peft",
"safetensors",
"mistral",
"axolotl",
"generated_from_trainer",
"custom_code",
"base_model:NousResearch/Yarn-Mistral-7b-128k",
"base_model:adapter:NousResearch/Yarn-Mistral-7b-128k",
"license:apache-2.0",
"region:us"
] | null | "2025-01-31T09:18:14Z" | ---
library_name: peft
license: apache-2.0
base_model: NousResearch/Yarn-Mistral-7b-128k
tags:
- axolotl
- generated_from_trainer
model-index:
- name: f550cf64-eed9-4e21-8c12-09e56596e20b
results: []
---
<!-- This model card has been generated automatically according to the information the Trainer had access to. You
should probably proofread and complete it, then remove this comment. -->
[<img src="https://raw.githubusercontent.com/axolotl-ai-cloud/axolotl/main/image/axolotl-badge-web.png" alt="Built with Axolotl" width="200" height="32"/>](https://github.com/axolotl-ai-cloud/axolotl)
<details><summary>See axolotl config</summary>
axolotl version: `0.4.1`
```yaml
adapter: lora
base_model: NousResearch/Yarn-Mistral-7b-128k
bf16: auto
chat_template: llama3
dataset_prepared_path: null
datasets:
- data_files:
- 248079f476a07bc3_train_data.json
ds_type: json
format: custom
path: /workspace/input_data/248079f476a07bc3_train_data.json
type:
field_instruction: problem
field_output: qwq
format: '{instruction}'
no_input_format: '{instruction}'
system_format: '{system}'
system_prompt: ''
debug: null
deepspeed: null
early_stopping_patience: null
eval_max_new_tokens: 128
eval_table_size: null
evals_per_epoch: 4
flash_attention: false
fp16: null
fsdp: null
fsdp_config: null
gradient_accumulation_steps: 4
gradient_checkpointing: false
group_by_length: false
hub_model_id: JacksonBrune/f550cf64-eed9-4e21-8c12-09e56596e20b
hub_repo: null
hub_strategy: checkpoint
hub_token: null
learning_rate: 0.0002
load_in_4bit: false
load_in_8bit: false
local_rank: null
logging_steps: 10
lora_alpha: 16
lora_dropout: 0.05
lora_fan_in_fan_out: null
lora_model_dir: null
lora_r: 8
lora_target_linear: true
lr_scheduler: cosine
max_steps: 50
micro_batch_size: 2
mlflow_experiment_name: /tmp/248079f476a07bc3_train_data.json
model_type: AutoModelForCausalLM
num_epochs: 1
optimizer: adamw_bnb_8bit
output_dir: miner_id_24
pad_to_sequence_len: true
resume_from_checkpoint: null
s2_attention: null
sample_packing: false
saves_per_epoch: 4
sequence_len: 512
special_tokens:
pad_token: </s>
strict: false
tf32: false
tokenizer_type: AutoTokenizer
train_on_inputs: false
trust_remote_code: true
val_set_size: 0.05
wandb_entity: null
wandb_mode: online
wandb_name: e6be45b1-93a3-491a-ac21-d779477a89fc
wandb_project: birthdya-sn56-18-Gradients-On-Demand
wandb_run: your_name
wandb_runid: e6be45b1-93a3-491a-ac21-d779477a89fc
warmup_steps: 5
weight_decay: 0.0
xformers_attention: null
```
</details><br>
# f550cf64-eed9-4e21-8c12-09e56596e20b
This model is a fine-tuned version of [NousResearch/Yarn-Mistral-7b-128k](https://huggingface.co/NousResearch/Yarn-Mistral-7b-128k) on the None dataset.
It achieves the following results on the evaluation set:
- Loss: 0.5504
## Model description
More information needed
## Intended uses & limitations
More information needed
## Training and evaluation data
More information needed
## Training procedure
### Training hyperparameters
The following hyperparameters were used during training:
- learning_rate: 0.0002
- train_batch_size: 2
- eval_batch_size: 2
- seed: 42
- gradient_accumulation_steps: 4
- total_train_batch_size: 8
- optimizer: Use OptimizerNames.ADAMW_BNB with betas=(0.9,0.999) and epsilon=1e-08 and optimizer_args=No additional optimizer arguments
- lr_scheduler_type: cosine
- lr_scheduler_warmup_steps: 5
- training_steps: 50
### Training results
| Training Loss | Epoch | Step | Validation Loss |
|:-------------:|:------:|:----:|:---------------:|
| No log | 0.0001 | 1 | 0.8032 |
| 2.9025 | 0.0019 | 13 | 0.6014 |
| 2.3242 | 0.0037 | 26 | 0.5661 |
| 2.152 | 0.0056 | 39 | 0.5504 |
### Framework versions
- PEFT 0.13.2
- Transformers 4.46.0
- Pytorch 2.5.0+cu124
- Datasets 3.0.1
- Tokenizers 0.20.1 |
shahadalll/videomae-base-finetuned-ucf-crimev3 | shahadalll | "2024-11-14T19:35:05Z" | 19 | 0 | transformers | [
"transformers",
"safetensors",
"videomae",
"video-classification",
"generated_from_trainer",
"base_model:MCG-NJU/videomae-base",
"base_model:finetune:MCG-NJU/videomae-base",
"license:cc-by-nc-4.0",
"endpoints_compatible",
"region:us"
] | video-classification | "2024-11-14T18:33:15Z" | ---
library_name: transformers
license: cc-by-nc-4.0
base_model: MCG-NJU/videomae-base
tags:
- generated_from_trainer
metrics:
- accuracy
model-index:
- name: videomae-base-finetuned-ucf-crimev3
results: []
---
<!-- This model card has been generated automatically according to the information the Trainer had access to. You
should probably proofread and complete it, then remove this comment. -->
# videomae-base-finetuned-ucf-crimev3
This model is a fine-tuned version of [MCG-NJU/videomae-base](https://huggingface.co/MCG-NJU/videomae-base) on an unknown dataset.
It achieves the following results on the evaluation set:
- Loss: 2.2215
- Accuracy: 0.3545
## Model description
More information needed
## Intended uses & limitations
More information needed
## Training and evaluation data
More information needed
## Training procedure
### Training hyperparameters
The following hyperparameters were used during training:
- learning_rate: 3e-05
- train_batch_size: 8
- eval_batch_size: 8
- seed: 42
- optimizer: Use adamw_torch with betas=(0.9,0.999) and epsilon=1e-08 and optimizer_args=No additional optimizer arguments
- lr_scheduler_type: linear
- lr_scheduler_warmup_steps: 500
- training_steps: 1230
### Training results
| Training Loss | Epoch | Step | Validation Loss | Accuracy |
|:-------------:|:-----:|:----:|:---------------:|:--------:|
| 1.2429 | 1.0 | 42 | 1.8114 | 0.3091 |
| 1.2691 | 2.0 | 84 | 1.8293 | 0.3273 |
| 0.9197 | 3.0 | 126 | 1.8547 | 0.3364 |
| 0.9611 | 4.0 | 168 | 1.8772 | 0.3455 |
| 0.848 | 5.0 | 210 | 1.8690 | 0.3636 |
| 1.0474 | 6.0 | 252 | 1.8581 | 0.3636 |
| 0.7281 | 7.0 | 294 | 1.9003 | 0.3909 |
| 0.6033 | 8.0 | 336 | 2.0023 | 0.3364 |
| 0.3703 | 9.0 | 378 | 2.1459 | 0.3364 |
| 0.4539 | 10.0 | 420 | 2.2215 | 0.3545 |
### Framework versions
- Transformers 4.47.0.dev0
- Pytorch 2.5.1+cu124
- Datasets 3.1.0
- Tokenizers 0.20.3
|
phunganhsang/XLM_CITA | phunganhsang | "2025-01-12T11:05:52Z" | 27 | 0 | transformers | [
"transformers",
"safetensors",
"xlm-roberta",
"text-classification",
"generated_from_trainer",
"base_model:FacebookAI/xlm-roberta-base",
"base_model:finetune:FacebookAI/xlm-roberta-base",
"license:mit",
"autotrain_compatible",
"endpoints_compatible",
"region:us"
] | text-classification | "2025-01-12T11:05:24Z" | ---
library_name: transformers
license: mit
base_model: FacebookAI/xlm-roberta-base
tags:
- generated_from_trainer
metrics:
- accuracy
- f1
model-index:
- name: XLM_CITA
results: []
---
<!-- This model card has been generated automatically according to the information the Trainer had access to. You
should probably proofread and complete it, then remove this comment. -->
# XLM_CITA
This model is a fine-tuned version of [FacebookAI/xlm-roberta-base](https://huggingface.co/FacebookAI/xlm-roberta-base) on the None dataset.
It achieves the following results on the evaluation set:
- Loss: 0.5616
- Accuracy: 0.7705
- F1: 0.7698
## Model description
More information needed
## Intended uses & limitations
More information needed
## Training and evaluation data
More information needed
## Training procedure
### Training hyperparameters
The following hyperparameters were used during training:
- learning_rate: 2e-05
- train_batch_size: 32
- eval_batch_size: 32
- seed: 42
- optimizer: Use adamw_torch with betas=(0.9,0.999) and epsilon=1e-08 and optimizer_args=No additional optimizer arguments
- lr_scheduler_type: cosine_with_restarts
- num_epochs: 10
### Training results
| Training Loss | Epoch | Step | Validation Loss | Accuracy | F1 |
|:-------------:|:-----:|:----:|:---------------:|:--------:|:------:|
| 0.6485 | 1.0 | 250 | 0.6020 | 0.6645 | 0.6490 |
| 0.5652 | 2.0 | 500 | 0.5210 | 0.7395 | 0.7397 |
| 0.5122 | 3.0 | 750 | 0.5111 | 0.7495 | 0.7496 |
| 0.4661 | 4.0 | 1000 | 0.5370 | 0.7685 | 0.7684 |
| 0.4244 | 5.0 | 1250 | 0.5206 | 0.7635 | 0.7636 |
| 0.3942 | 6.0 | 1500 | 0.5299 | 0.762 | 0.7621 |
| 0.3611 | 7.0 | 1750 | 0.5380 | 0.7695 | 0.7686 |
| 0.3421 | 8.0 | 2000 | 0.5595 | 0.7745 | 0.7736 |
| 0.3362 | 9.0 | 2250 | 0.5596 | 0.7715 | 0.7708 |
| 0.3274 | 10.0 | 2500 | 0.5616 | 0.7705 | 0.7698 |
### Framework versions
- Transformers 4.48.0
- Pytorch 2.1.2
- Datasets 2.19.2
- Tokenizers 0.21.0
|
PhiTau/Taxi-v3 | PhiTau | "2025-04-01T13:57:37Z" | 0 | 0 | null | [
"Taxi-v3",
"q-learning",
"reinforcement-learning",
"custom-implementation",
"model-index",
"region:us"
] | reinforcement-learning | "2025-04-01T13:57:00Z" | Temporary Redirect. Redirecting to /api/resolve-cache/models/PhiTau/Taxi-v3/75c779820a540223c29ee352427b5d3987639892/README.md?%2FPhiTau%2FTaxi-v3%2Fresolve%2Fmain%2FREADME.md=&etag=%2240dc2f8924f26250a13f54b571b76e547a0f89d5%22 |
hkivancoral/hushem_5x_deit_base_adamax_001_fold2 | hkivancoral | "2023-11-16T19:24:17Z" | 8 | 0 | transformers | [
"transformers",
"tensorboard",
"safetensors",
"vit",
"image-classification",
"generated_from_trainer",
"dataset:imagefolder",
"base_model:facebook/deit-base-patch16-224",
"base_model:finetune:facebook/deit-base-patch16-224",
"license:apache-2.0",
"model-index",
"autotrain_compatible",
"endpoints_compatible",
"region:us"
] | image-classification | "2023-11-16T18:42:06Z" | ---
license: apache-2.0
base_model: facebook/deit-base-patch16-224
tags:
- generated_from_trainer
datasets:
- imagefolder
metrics:
- accuracy
model-index:
- name: hushem_5x_deit_base_adamax_001_fold2
results:
- task:
name: Image Classification
type: image-classification
dataset:
name: imagefolder
type: imagefolder
config: default
split: test
args: default
metrics:
- name: Accuracy
type: accuracy
value: 0.4888888888888889
---
<!-- This model card has been generated automatically according to the information the Trainer had access to. You
should probably proofread and complete it, then remove this comment. -->
# hushem_5x_deit_base_adamax_001_fold2
This model is a fine-tuned version of [facebook/deit-base-patch16-224](https://huggingface.co/facebook/deit-base-patch16-224) on the imagefolder dataset.
It achieves the following results on the evaluation set:
- Loss: 5.7416
- Accuracy: 0.4889
## Model description
More information needed
## Intended uses & limitations
More information needed
## Training and evaluation data
More information needed
## Training procedure
### Training hyperparameters
The following hyperparameters were used during training:
- learning_rate: 0.001
- train_batch_size: 32
- eval_batch_size: 32
- seed: 42
- optimizer: Adam with betas=(0.9,0.999) and epsilon=1e-08
- lr_scheduler_type: linear
- lr_scheduler_warmup_ratio: 0.1
- num_epochs: 50
### Training results
| Training Loss | Epoch | Step | Validation Loss | Accuracy |
|:-------------:|:-----:|:----:|:---------------:|:--------:|
| 1.4053 | 1.0 | 27 | 1.3685 | 0.3111 |
| 1.3925 | 2.0 | 54 | 3.6868 | 0.2889 |
| 1.2318 | 3.0 | 81 | 1.5265 | 0.3333 |
| 1.1218 | 4.0 | 108 | 1.3720 | 0.3778 |
| 0.9389 | 5.0 | 135 | 1.3538 | 0.4444 |
| 0.8792 | 6.0 | 162 | 1.1885 | 0.4444 |
| 0.8387 | 7.0 | 189 | 1.3407 | 0.4889 |
| 0.7915 | 8.0 | 216 | 1.2361 | 0.4222 |
| 0.79 | 9.0 | 243 | 1.2485 | 0.4667 |
| 0.7076 | 10.0 | 270 | 1.6183 | 0.5333 |
| 0.6051 | 11.0 | 297 | 1.7700 | 0.4889 |
| 0.5603 | 12.0 | 324 | 1.7918 | 0.3556 |
| 0.6144 | 13.0 | 351 | 2.1767 | 0.5556 |
| 0.5279 | 14.0 | 378 | 1.6851 | 0.3778 |
| 0.3562 | 15.0 | 405 | 2.1689 | 0.4444 |
| 0.3897 | 16.0 | 432 | 2.2755 | 0.4667 |
| 0.4523 | 17.0 | 459 | 2.3235 | 0.4222 |
| 0.5055 | 18.0 | 486 | 2.6282 | 0.5556 |
| 0.2707 | 19.0 | 513 | 2.3398 | 0.5333 |
| 0.4827 | 20.0 | 540 | 2.5025 | 0.5111 |
| 0.2449 | 21.0 | 567 | 2.2455 | 0.4667 |
| 0.3199 | 22.0 | 594 | 3.8583 | 0.5333 |
| 0.2715 | 23.0 | 621 | 2.9016 | 0.5556 |
| 0.2241 | 24.0 | 648 | 2.9266 | 0.4444 |
| 0.1264 | 25.0 | 675 | 3.0321 | 0.4222 |
| 0.1028 | 26.0 | 702 | 3.8439 | 0.5778 |
| 0.2082 | 27.0 | 729 | 3.7749 | 0.5333 |
| 0.2344 | 28.0 | 756 | 3.4616 | 0.5333 |
| 0.0842 | 29.0 | 783 | 3.5970 | 0.5111 |
| 0.0483 | 30.0 | 810 | 4.3955 | 0.5111 |
| 0.1454 | 31.0 | 837 | 3.9120 | 0.5556 |
| 0.0972 | 32.0 | 864 | 3.9463 | 0.4889 |
| 0.014 | 33.0 | 891 | 4.4955 | 0.4889 |
| 0.0007 | 34.0 | 918 | 5.1958 | 0.5111 |
| 0.0273 | 35.0 | 945 | 5.0022 | 0.4889 |
| 0.0071 | 36.0 | 972 | 4.9340 | 0.5333 |
| 0.0003 | 37.0 | 999 | 5.2310 | 0.4889 |
| 0.0004 | 38.0 | 1026 | 5.5820 | 0.4889 |
| 0.0001 | 39.0 | 1053 | 5.6491 | 0.4889 |
| 0.0001 | 40.0 | 1080 | 5.6867 | 0.4889 |
| 0.0001 | 41.0 | 1107 | 5.7009 | 0.4889 |
| 0.0001 | 42.0 | 1134 | 5.7115 | 0.4889 |
| 0.0 | 43.0 | 1161 | 5.7213 | 0.4889 |
| 0.0001 | 44.0 | 1188 | 5.7289 | 0.4889 |
| 0.0001 | 45.0 | 1215 | 5.7342 | 0.4889 |
| 0.0 | 46.0 | 1242 | 5.7384 | 0.4889 |
| 0.0 | 47.0 | 1269 | 5.7406 | 0.4889 |
| 0.0 | 48.0 | 1296 | 5.7416 | 0.4889 |
| 0.0001 | 49.0 | 1323 | 5.7416 | 0.4889 |
| 0.0 | 50.0 | 1350 | 5.7416 | 0.4889 |
### Framework versions
- Transformers 4.35.2
- Pytorch 2.1.0+cu118
- Datasets 2.15.0
- Tokenizers 0.15.0
|
panxinyang/Qwen-Qwen1.5-0.5B-1718648935 | panxinyang | "2024-06-17T18:28:57Z" | 0 | 0 | peft | [
"peft",
"safetensors",
"arxiv:1910.09700",
"base_model:Qwen/Qwen1.5-0.5B",
"base_model:adapter:Qwen/Qwen1.5-0.5B",
"region:us"
] | null | "2024-06-17T18:28:55Z" | ---
base_model: Qwen/Qwen1.5-0.5B
library_name: peft
---
# Model Card for Model ID
<!-- Provide a quick summary of what the model is/does. -->
## Model Details
### Model Description
<!-- Provide a longer summary of what this model is. -->
- **Developed by:** [More Information Needed]
- **Funded by [optional]:** [More Information Needed]
- **Shared by [optional]:** [More Information Needed]
- **Model type:** [More Information Needed]
- **Language(s) (NLP):** [More Information Needed]
- **License:** [More Information Needed]
- **Finetuned from model [optional]:** [More Information Needed]
### Model Sources [optional]
<!-- Provide the basic links for the model. -->
- **Repository:** [More Information Needed]
- **Paper [optional]:** [More Information Needed]
- **Demo [optional]:** [More Information Needed]
## Uses
<!-- Address questions around how the model is intended to be used, including the foreseeable users of the model and those affected by the model. -->
### Direct Use
<!-- This section is for the model use without fine-tuning or plugging into a larger ecosystem/app. -->
[More Information Needed]
### Downstream Use [optional]
<!-- This section is for the model use when fine-tuned for a task, or when plugged into a larger ecosystem/app -->
[More Information Needed]
### Out-of-Scope Use
<!-- This section addresses misuse, malicious use, and uses that the model will not work well for. -->
[More Information Needed]
## Bias, Risks, and Limitations
<!-- This section is meant to convey both technical and sociotechnical limitations. -->
[More Information Needed]
### Recommendations
<!-- This section is meant to convey recommendations with respect to the bias, risk, and technical limitations. -->
Users (both direct and downstream) should be made aware of the risks, biases and limitations of the model. More information needed for further recommendations.
## How to Get Started with the Model
Use the code below to get started with the model.
[More Information Needed]
## Training Details
### Training Data
<!-- This should link to a Dataset Card, perhaps with a short stub of information on what the training data is all about as well as documentation related to data pre-processing or additional filtering. -->
[More Information Needed]
### Training Procedure
<!-- This relates heavily to the Technical Specifications. Content here should link to that section when it is relevant to the training procedure. -->
#### Preprocessing [optional]
[More Information Needed]
#### Training Hyperparameters
- **Training regime:** [More Information Needed] <!--fp32, fp16 mixed precision, bf16 mixed precision, bf16 non-mixed precision, fp16 non-mixed precision, fp8 mixed precision -->
#### Speeds, Sizes, Times [optional]
<!-- This section provides information about throughput, start/end time, checkpoint size if relevant, etc. -->
[More Information Needed]
## Evaluation
<!-- This section describes the evaluation protocols and provides the results. -->
### Testing Data, Factors & Metrics
#### Testing Data
<!-- This should link to a Dataset Card if possible. -->
[More Information Needed]
#### Factors
<!-- These are the things the evaluation is disaggregating by, e.g., subpopulations or domains. -->
[More Information Needed]
#### Metrics
<!-- These are the evaluation metrics being used, ideally with a description of why. -->
[More Information Needed]
### Results
[More Information Needed]
#### Summary
## Model Examination [optional]
<!-- Relevant interpretability work for the model goes here -->
[More Information Needed]
## Environmental Impact
<!-- Total emissions (in grams of CO2eq) and additional considerations, such as electricity usage, go here. Edit the suggested text below accordingly -->
Carbon emissions can be estimated using the [Machine Learning Impact calculator](https://mlco2.github.io/impact#compute) presented in [Lacoste et al. (2019)](https://arxiv.org/abs/1910.09700).
- **Hardware Type:** [More Information Needed]
- **Hours used:** [More Information Needed]
- **Cloud Provider:** [More Information Needed]
- **Compute Region:** [More Information Needed]
- **Carbon Emitted:** [More Information Needed]
## Technical Specifications [optional]
### Model Architecture and Objective
[More Information Needed]
### Compute Infrastructure
[More Information Needed]
#### Hardware
[More Information Needed]
#### Software
[More Information Needed]
## Citation [optional]
<!-- If there is a paper or blog post introducing the model, the APA and Bibtex information for that should go in this section. -->
**BibTeX:**
[More Information Needed]
**APA:**
[More Information Needed]
## Glossary [optional]
<!-- If relevant, include terms and calculations in this section that can help readers understand the model or model card. -->
[More Information Needed]
## More Information [optional]
[More Information Needed]
## Model Card Authors [optional]
[More Information Needed]
## Model Card Contact
[More Information Needed]
### Framework versions
- PEFT 0.11.1 |
aipib/llmjp-slerp5 | aipib | "2024-06-18T04:32:45Z" | 152 | 0 | transformers | [
"transformers",
"safetensors",
"gguf",
"gpt2",
"text-generation",
"merge",
"mergekit",
"lazymergekit",
"aipib/llmjp-slerp",
"aipib/llmjp-slerp3",
"base_model:aipib/llmjp-slerp",
"base_model:merge:aipib/llmjp-slerp",
"base_model:aipib/llmjp-slerp3",
"base_model:merge:aipib/llmjp-slerp3",
"autotrain_compatible",
"text-generation-inference",
"endpoints_compatible",
"region:us"
] | text-generation | "2024-06-17T12:26:43Z" | ---
base_model:
- aipib/llmjp-slerp
- aipib/llmjp-slerp3
tags:
- merge
- mergekit
- lazymergekit
- aipib/llmjp-slerp
- aipib/llmjp-slerp3
---
# llmjp-slerp5
llmjp-slerp5 is a merge of the following models using [LazyMergekit](https://colab.research.google.com/drive/1obulZ1ROXHjYLn6PPZJwRR6GzgQogxxb?usp=sharing):
* [aipib/llmjp-slerp](https://huggingface.co/aipib/llmjp-slerp)
* [aipib/llmjp-slerp3](https://huggingface.co/aipib/llmjp-slerp3)
## 💻 Usage
```python
!pip install -qU transformers accelerate
from transformers import AutoTokenizer
import transformers
import torch
model = "aipib/llmjp-slerp5"
messages = [{"role": "user", "content": "What is a large language model?"}]
tokenizer = AutoTokenizer.from_pretrained(model)
prompt = tokenizer.apply_chat_template(messages, tokenize=False, add_generation_prompt=True)
pipeline = transformers.pipeline(
"text-generation",
model=model,
torch_dtype=torch.float16,
device_map="auto",
)
outputs = pipeline(prompt, max_new_tokens=256, do_sample=True, temperature=0.7, top_k=50, top_p=0.95)
print(outputs[0]["generated_text"])
``` |
viva-996/FineLlama-3.1-8B | viva-996 | "2025-02-16T16:07:46Z" | 0 | 0 | transformers | [
"transformers",
"safetensors",
"unsloth",
"arxiv:1910.09700",
"endpoints_compatible",
"region:us"
] | null | "2025-02-16T11:27:18Z" | ---
library_name: transformers
tags:
- unsloth
---
# Model Card for Model ID
<!-- Provide a quick summary of what the model is/does. -->
## Model Details
### Model Description
<!-- Provide a longer summary of what this model is. -->
This is the model card of a 🤗 transformers model that has been pushed on the Hub. This model card has been automatically generated.
- **Developed by:** [More Information Needed]
- **Funded by [optional]:** [More Information Needed]
- **Shared by [optional]:** [More Information Needed]
- **Model type:** [More Information Needed]
- **Language(s) (NLP):** [More Information Needed]
- **License:** [More Information Needed]
- **Finetuned from model [optional]:** [More Information Needed]
### Model Sources [optional]
<!-- Provide the basic links for the model. -->
- **Repository:** [More Information Needed]
- **Paper [optional]:** [More Information Needed]
- **Demo [optional]:** [More Information Needed]
## Uses
<!-- Address questions around how the model is intended to be used, including the foreseeable users of the model and those affected by the model. -->
### Direct Use
<!-- This section is for the model use without fine-tuning or plugging into a larger ecosystem/app. -->
[More Information Needed]
### Downstream Use [optional]
<!-- This section is for the model use when fine-tuned for a task, or when plugged into a larger ecosystem/app -->
[More Information Needed]
### Out-of-Scope Use
<!-- This section addresses misuse, malicious use, and uses that the model will not work well for. -->
[More Information Needed]
## Bias, Risks, and Limitations
<!-- This section is meant to convey both technical and sociotechnical limitations. -->
[More Information Needed]
### Recommendations
<!-- This section is meant to convey recommendations with respect to the bias, risk, and technical limitations. -->
Users (both direct and downstream) should be made aware of the risks, biases and limitations of the model. More information needed for further recommendations.
## How to Get Started with the Model
Use the code below to get started with the model.
[More Information Needed]
## Training Details
### Training Data
<!-- This should link to a Dataset Card, perhaps with a short stub of information on what the training data is all about as well as documentation related to data pre-processing or additional filtering. -->
[More Information Needed]
### Training Procedure
<!-- This relates heavily to the Technical Specifications. Content here should link to that section when it is relevant to the training procedure. -->
#### Preprocessing [optional]
[More Information Needed]
#### Training Hyperparameters
- **Training regime:** [More Information Needed] <!--fp32, fp16 mixed precision, bf16 mixed precision, bf16 non-mixed precision, fp16 non-mixed precision, fp8 mixed precision -->
#### Speeds, Sizes, Times [optional]
<!-- This section provides information about throughput, start/end time, checkpoint size if relevant, etc. -->
[More Information Needed]
## Evaluation
<!-- This section describes the evaluation protocols and provides the results. -->
### Testing Data, Factors & Metrics
#### Testing Data
<!-- This should link to a Dataset Card if possible. -->
[More Information Needed]
#### Factors
<!-- These are the things the evaluation is disaggregating by, e.g., subpopulations or domains. -->
[More Information Needed]
#### Metrics
<!-- These are the evaluation metrics being used, ideally with a description of why. -->
[More Information Needed]
### Results
[More Information Needed]
#### Summary
## Model Examination [optional]
<!-- Relevant interpretability work for the model goes here -->
[More Information Needed]
## Environmental Impact
<!-- Total emissions (in grams of CO2eq) and additional considerations, such as electricity usage, go here. Edit the suggested text below accordingly -->
Carbon emissions can be estimated using the [Machine Learning Impact calculator](https://mlco2.github.io/impact#compute) presented in [Lacoste et al. (2019)](https://arxiv.org/abs/1910.09700).
- **Hardware Type:** [More Information Needed]
- **Hours used:** [More Information Needed]
- **Cloud Provider:** [More Information Needed]
- **Compute Region:** [More Information Needed]
- **Carbon Emitted:** [More Information Needed]
## Technical Specifications [optional]
### Model Architecture and Objective
[More Information Needed]
### Compute Infrastructure
[More Information Needed]
#### Hardware
[More Information Needed]
#### Software
[More Information Needed]
## Citation [optional]
<!-- If there is a paper or blog post introducing the model, the APA and Bibtex information for that should go in this section. -->
**BibTeX:**
[More Information Needed]
**APA:**
[More Information Needed]
## Glossary [optional]
<!-- If relevant, include terms and calculations in this section that can help readers understand the model or model card. -->
[More Information Needed]
## More Information [optional]
[More Information Needed]
## Model Card Authors [optional]
[More Information Needed]
## Model Card Contact
[More Information Needed] |
vdos/3cf6e881-58d4-4c83-b040-9d0b8321ff0e | vdos | "2024-12-20T00:57:10Z" | 8 | 0 | peft | [
"peft",
"safetensors",
"gemma2",
"axolotl",
"generated_from_trainer",
"base_model:unsloth/gemma-2-2b",
"base_model:adapter:unsloth/gemma-2-2b",
"license:gemma",
"region:us"
] | null | "2024-12-20T00:49:14Z" | ---
library_name: peft
license: gemma
base_model: unsloth/gemma-2-2b
tags:
- axolotl
- generated_from_trainer
model-index:
- name: 3cf6e881-58d4-4c83-b040-9d0b8321ff0e
results: []
---
<!-- This model card has been generated automatically according to the information the Trainer had access to. You
should probably proofread and complete it, then remove this comment. -->
[<img src="https://raw.githubusercontent.com/axolotl-ai-cloud/axolotl/main/image/axolotl-badge-web.png" alt="Built with Axolotl" width="200" height="32"/>](https://github.com/axolotl-ai-cloud/axolotl)
<details><summary>See axolotl config</summary>
axolotl version: `0.5.2`
```yaml
adapter: lora
base_model: unsloth/gemma-2-2b
bf16: auto
chat_template: llama3
dataset_prepared_path: null
datasets:
- data_files:
- bbe70c53a119531f_train_data.json
ds_type: json
format: custom
path: /workspace/input_data/bbe70c53a119531f_train_data.json
type:
field_input: transcription
field_instruction: glosses
field_output: translation
format: '{instruction} {input}'
no_input_format: '{instruction}'
system_format: '{system}'
system_prompt: ''
debug: null
deepspeed: null
early_stopping_patience: 1
eval_max_new_tokens: 128
eval_steps: 25
eval_table_size: null
flash_attention: false
fp16: false
fsdp: null
fsdp_config: null
gradient_accumulation_steps: 16
gradient_checkpointing: true
group_by_length: true
hub_model_id: vdos/3cf6e881-58d4-4c83-b040-9d0b8321ff0e
hub_repo: null
hub_strategy: checkpoint
hub_token: null
learning_rate: 0.0001
load_in_4bit: false
load_in_8bit: false
local_rank: null
logging_steps: 1
lora_alpha: 64
lora_dropout: 0.05
lora_fan_in_fan_out: null
lora_model_dir: null
lora_r: 32
lora_target_linear: true
lr_scheduler: cosine
max_steps: 50
micro_batch_size: 2
mlflow_experiment_name: /tmp/bbe70c53a119531f_train_data.json
model_type: AutoModelForCausalLM
num_epochs: 3
optimizer: adamw_torch
output_dir: miner_id_24
pad_to_sequence_len: true
resume_from_checkpoint: null
s2_attention: null
sample_packing: false
save_steps: 25
sequence_len: 2048
strict: false
tf32: false
tokenizer_type: AutoTokenizer
train_on_inputs: false
trust_remote_code: true
val_set_size: 0.05
wandb_entity: null
wandb_mode: online
wandb_name: 3cf6e881-58d4-4c83-b040-9d0b8321ff0e
wandb_project: Gradients-On-Demand
wandb_run: your_name
wandb_runid: 3cf6e881-58d4-4c83-b040-9d0b8321ff0e
warmup_ratio: 0.05
weight_decay: 0.01
xformers_attention: true
```
</details><br>
# 3cf6e881-58d4-4c83-b040-9d0b8321ff0e
This model is a fine-tuned version of [unsloth/gemma-2-2b](https://huggingface.co/unsloth/gemma-2-2b) on the None dataset.
It achieves the following results on the evaluation set:
- Loss: 1.9679
## Model description
More information needed
## Intended uses & limitations
More information needed
## Training and evaluation data
More information needed
## Training procedure
### Training hyperparameters
The following hyperparameters were used during training:
- learning_rate: 0.0001
- train_batch_size: 2
- eval_batch_size: 2
- seed: 42
- distributed_type: multi-GPU
- num_devices: 4
- gradient_accumulation_steps: 16
- total_train_batch_size: 128
- total_eval_batch_size: 8
- optimizer: Use OptimizerNames.ADAMW_TORCH with betas=(0.9,0.999) and epsilon=1e-08 and optimizer_args=No additional optimizer arguments
- lr_scheduler_type: cosine
- lr_scheduler_warmup_steps: 2
- training_steps: 50
### Training results
| Training Loss | Epoch | Step | Validation Loss |
|:-------------:|:------:|:----:|:---------------:|
| 4.9321 | 0.0128 | 1 | 6.1235 |
| 0.6469 | 0.3195 | 25 | 2.1838 |
| 0.4683 | 0.6390 | 50 | 1.9679 |
### Framework versions
- PEFT 0.13.2
- Transformers 4.46.3
- Pytorch 2.3.1+cu121
- Datasets 3.1.0
- Tokenizers 0.20.3 |
Subsets and Splits