modelId
stringlengths 4
112
| sha
stringlengths 40
40
| lastModified
stringlengths 24
24
| tags
sequence | pipeline_tag
stringclasses 29
values | private
bool 1
class | author
stringlengths 2
38
⌀ | config
null | id
stringlengths 4
112
| downloads
float64 0
36.8M
⌀ | likes
float64 0
712
⌀ | library_name
stringclasses 17
values | __index_level_0__
int64 0
38.5k
| readme
stringlengths 0
186k
|
---|---|---|---|---|---|---|---|---|---|---|---|---|---|
uclanlp/plbart-multi_task-static | bb0218751170a541477bc83531916a8be2db651b | 2022-03-02T07:40:03.000Z | [
"pytorch",
"plbart",
"text2text-generation",
"transformers",
"autotrain_compatible"
] | text2text-generation | false | uclanlp | null | uclanlp/plbart-multi_task-static | 0 | null | transformers | 36,200 | Entry not found |
uclanlp/plbart-refine-java-small | 3806d817c9b07550770b91b260ced92979b8313d | 2021-11-09T17:09:52.000Z | [
"pytorch",
"plbart",
"text2text-generation",
"transformers",
"autotrain_compatible"
] | text2text-generation | false | uclanlp | null | uclanlp/plbart-refine-java-small | 0 | null | transformers | 36,201 | Entry not found |
uclanlp/plbart-single_task-en_java | fc01f16a888897fed6d7d82f670133d60c62f9b7 | 2022-03-02T07:05:00.000Z | [
"pytorch",
"plbart",
"text2text-generation",
"transformers",
"autotrain_compatible"
] | text2text-generation | false | uclanlp | null | uclanlp/plbart-single_task-en_java | 0 | null | transformers | 36,202 | Entry not found |
uclanlp/plbart-single_task-en_ruby | 8907b197998a22b4b07f0edd1eb094baba140fa6 | 2022-03-02T07:06:19.000Z | [
"pytorch",
"plbart",
"text2text-generation",
"transformers",
"autotrain_compatible"
] | text2text-generation | false | uclanlp | null | uclanlp/plbart-single_task-en_ruby | 0 | null | transformers | 36,203 | Entry not found |
uclanlp/plbart-single_task-interpreted-generation | 286e091ef6f6873e510b57d95bd2399dc81c26be | 2022-03-02T07:19:36.000Z | [
"pytorch",
"plbart",
"text2text-generation",
"transformers",
"autotrain_compatible"
] | text2text-generation | false | uclanlp | null | uclanlp/plbart-single_task-interpreted-generation | 0 | null | transformers | 36,204 | Entry not found |
uclanlp/plbart-single_task-js_en | e756d919dff5bb87746bf2078f69b107a3cda9fd | 2022-03-02T07:02:26.000Z | [
"pytorch",
"plbart",
"text2text-generation",
"transformers",
"autotrain_compatible"
] | text2text-generation | false | uclanlp | null | uclanlp/plbart-single_task-js_en | 0 | null | transformers | 36,205 | Entry not found |
uclanlp/visualbert-nlvr2-pre | 09a7ebd71066465e9d909de1e1c6c0aecdbf7645 | 2021-05-31T11:12:02.000Z | [
"pytorch",
"visual_bert",
"pretraining",
"transformers"
] | null | false | uclanlp | null | uclanlp/visualbert-nlvr2-pre | 0 | null | transformers | 36,206 | Entry not found |
uclanlp/visualbert-vcr-coco-pre | d83463c00c8ae5d7b1b4c8ffdccf1698172f1390 | 2021-05-31T11:27:41.000Z | [
"pytorch",
"visual_bert",
"pretraining",
"transformers"
] | null | false | uclanlp | null | uclanlp/visualbert-vcr-coco-pre | 0 | null | transformers | 36,207 | Entry not found |
ueb1/IceBERT-finetuned-ner | 23ec9afdcab6a37b42b0e1a0b1b315a321b7eac3 | 2021-10-05T21:28:47.000Z | [
"pytorch",
"tensorboard",
"roberta",
"token-classification",
"dataset:mim_gold_ner",
"transformers",
"generated_from_trainer",
"license:gpl-3.0",
"model-index",
"autotrain_compatible"
] | token-classification | false | ueb1 | null | ueb1/IceBERT-finetuned-ner | 0 | null | transformers | 36,208 | ---
license: gpl-3.0
tags:
- generated_from_trainer
datasets:
- mim_gold_ner
metrics:
- precision
- recall
- f1
- accuracy
model-index:
- name: IceBERT-finetuned-ner
results:
- task:
name: Token Classification
type: token-classification
dataset:
name: mim_gold_ner
type: mim_gold_ner
args: mim-gold-ner
metrics:
- name: Precision
type: precision
value: 0.8926985693142575
- name: Recall
type: recall
value: 0.8648584060222249
- name: F1
type: f1
value: 0.8785579899253504
- name: Accuracy
type: accuracy
value: 0.985303647287535
---
<!-- This model card has been generated automatically according to the information the Trainer had access to. You
should probably proofread and complete it, then remove this comment. -->
# IceBERT-finetuned-ner
This model is a fine-tuned version of [vesteinn/IceBERT](https://huggingface.co/vesteinn/IceBERT) on the mim_gold_ner dataset.
It achieves the following results on the evaluation set:
- Loss: 0.0799
- Precision: 0.8927
- Recall: 0.8649
- F1: 0.8786
- Accuracy: 0.9853
## Model description
More information needed
## Intended uses & limitations
More information needed
## Training and evaluation data
More information needed
## Training procedure
### Training hyperparameters
The following hyperparameters were used during training:
- learning_rate: 2e-05
- train_batch_size: 16
- eval_batch_size: 16
- seed: 42
- optimizer: Adam with betas=(0.9,0.999) and epsilon=1e-08
- lr_scheduler_type: linear
- num_epochs: 3
### Training results
| Training Loss | Epoch | Step | Validation Loss | Precision | Recall | F1 | Accuracy |
|:-------------:|:-----:|:----:|:---------------:|:---------:|:------:|:------:|:--------:|
| 0.0528 | 1.0 | 2904 | 0.0774 | 0.8784 | 0.8529 | 0.8655 | 0.9829 |
| 0.0258 | 2.0 | 5808 | 0.0742 | 0.8769 | 0.8705 | 0.8737 | 0.9843 |
| 0.0166 | 3.0 | 8712 | 0.0799 | 0.8927 | 0.8649 | 0.8786 | 0.9853 |
### Framework versions
- Transformers 4.11.2
- Pytorch 1.9.0+cu102
- Datasets 1.12.1
- Tokenizers 0.10.3
|
ueb1/XLMR-ENIS-finetuned-ner | 266212dd5b06e3cbb0da4da23898632db2fff7a5 | 2021-10-05T23:19:15.000Z | [
"pytorch",
"tensorboard",
"xlm-roberta",
"token-classification",
"dataset:mim_gold_ner",
"transformers",
"generated_from_trainer",
"license:agpl-3.0",
"model-index",
"autotrain_compatible"
] | token-classification | false | ueb1 | null | ueb1/XLMR-ENIS-finetuned-ner | 0 | null | transformers | 36,209 | ---
license: agpl-3.0
tags:
- generated_from_trainer
datasets:
- mim_gold_ner
metrics:
- precision
- recall
- f1
- accuracy
model-index:
- name: XLMR-ENIS-finetuned-ner
results:
- task:
name: Token Classification
type: token-classification
dataset:
name: mim_gold_ner
type: mim_gold_ner
args: mim-gold-ner
metrics:
- name: Precision
type: precision
value: 0.8685291700903862
- name: Recall
type: recall
value: 0.841273450824332
- name: F1
type: f1
value: 0.8546840706942359
- name: Accuracy
type: accuracy
value: 0.9824748714976435
---
<!-- This model card has been generated automatically according to the information the Trainer had access to. You
should probably proofread and complete it, then remove this comment. -->
# XLMR-ENIS-finetuned-ner
This model is a fine-tuned version of [vesteinn/XLMR-ENIS](https://huggingface.co/vesteinn/XLMR-ENIS) on the mim_gold_ner dataset.
It achieves the following results on the evaluation set:
- Loss: 0.0940
- Precision: 0.8685
- Recall: 0.8413
- F1: 0.8547
- Accuracy: 0.9825
## Model description
More information needed
## Intended uses & limitations
More information needed
## Training and evaluation data
More information needed
## Training procedure
### Training hyperparameters
The following hyperparameters were used during training:
- learning_rate: 2e-05
- train_batch_size: 16
- eval_batch_size: 16
- seed: 42
- optimizer: Adam with betas=(0.9,0.999) and epsilon=1e-08
- lr_scheduler_type: linear
- num_epochs: 3
### Training results
| Training Loss | Epoch | Step | Validation Loss | Precision | Recall | F1 | Accuracy |
|:-------------:|:-----:|:----:|:---------------:|:---------:|:------:|:------:|:--------:|
| 0.0564 | 1.0 | 2904 | 0.0943 | 0.8505 | 0.8118 | 0.8307 | 0.9798 |
| 0.0321 | 2.0 | 5808 | 0.0907 | 0.8610 | 0.8235 | 0.8419 | 0.9814 |
| 0.0198 | 3.0 | 8712 | 0.0940 | 0.8685 | 0.8413 | 0.8547 | 0.9825 |
### Framework versions
- Transformers 4.11.2
- Pytorch 1.9.0+cu102
- Datasets 1.12.1
- Tokenizers 0.10.3
|
ufal/byt5-small-multilexnorm2021-da | 84554f95d050988a09516c0aaf76e50d85ca7d32 | 2021-10-15T20:41:10.000Z | [
"pytorch",
"t5",
"text2text-generation",
"da",
"dataset:mc4",
"dataset:wikipedia",
"dataset:multilexnorm",
"arxiv:2105.13626",
"arxiv:1907.06292",
"transformers",
"lexical normalization",
"license:apache-2.0",
"autotrain_compatible"
] | text2text-generation | false | ufal | null | ufal/byt5-small-multilexnorm2021-da | 0 | 1 | transformers | 36,210 | ---
language: da
datasets:
- mc4
- wikipedia
- multilexnorm
tags:
- lexical normalization
license: apache-2.0
---
# Fine-tuned ByT5-small for MultiLexNorm (Danish version)

This is the official release of the fine-tuned models for **the winning entry** to the [*W-NUT 2021: Multilingual Lexical Normalization (MultiLexNorm)* shared task](https://noisy-text.github.io/2021/multi-lexnorm.html), which evaluates lexical-normalization systems on 12 social media datasets in 11 languages.
Our system is based on [ByT5](https://arxiv.org/abs/2105.13626), which we first pre-train on synthetic data and then fine-tune on authentic normalization data. It achieves the best performance by a wide margin in intrinsic evaluation, and also the best performance in extrinsic evaluation through dependency parsing. In addition to these fine-tuned models, we also release the source files on [GitHub](https://github.com/ufal/multilexnorm2021) and an interactive demo on [Google Colab](https://colab.research.google.com/drive/1rxpI8IlKk-D2crFqi2hdzbTBIezqgsCg?usp=sharing).
## How to use
The model was *not* fine-tuned in a standard sentence-to-sentence setting – instead, it was tailored to the token-to-token definition of MultiLexNorm data. Please refer to [**the interactive demo on Colab notebook**](https://colab.research.google.com/drive/1rxpI8IlKk-D2crFqi2hdzbTBIezqgsCg?usp=sharing) to learn how to use these models.
## How to cite
```bibtex
@inproceedings{wnut-ufal,
title= "{ÚFAL} at {MultiLexNorm} 2021: Improving Multilingual Lexical Normalization by Fine-tuning {ByT5}",
author = "Samuel, David and Straka, Milan",
booktitle = "Proceedings of the 7th Workshop on Noisy User-generated Text (W-NUT 2021)",
year = "2021",
publisher = "Association for Computational Linguistics",
address = "Punta Cana, Dominican Republic"
}
```
## ByT5 - Small
ByT5 is a tokenizer-free version of [Google's T5](https://ai.googleblog.com/2020/02/exploring-transfer-learning-with-t5.html) and generally follows the architecture of [MT5](https://huggingface.co/google/mt5-small).
ByT5 was only pre-trained on [mC4](https://www.tensorflow.org/datasets/catalog/c4#c4multilingual) excluding any supervised training with an average span-mask of 20 UTF-8 characters. Therefore, this model has to be fine-tuned before it is useable on a downstream task.
ByT5 works especially well on noisy text data,*e.g.*, `google/byt5-small` significantly outperforms [mt5-small](https://huggingface.co/google/mt5-small) on [TweetQA](https://arxiv.org/abs/1907.06292).
Paper: [ByT5: Towards a token-free future with pre-trained byte-to-byte models](https://arxiv.org/abs/2105.13626)
Authors: *Linting Xue, Aditya Barua, Noah Constant, Rami Al-Rfou, Sharan Narang, Mihir Kale, Adam Roberts, Colin Raffel*
|
ufal/byt5-small-multilexnorm2021-iden | bba2b1a6439cd3fbfd85a581100685500012d5f4 | 2021-10-20T12:31:18.000Z | [
"pytorch",
"t5",
"text2text-generation",
"id",
"en",
"dataset:mc4",
"dataset:wikipedia",
"dataset:multilexnorm",
"arxiv:2105.13626",
"arxiv:1907.06292",
"transformers",
"lexical normalization",
"license:apache-2.0",
"autotrain_compatible"
] | text2text-generation | false | ufal | null | ufal/byt5-small-multilexnorm2021-iden | 0 | null | transformers | 36,211 | ---
language:
- id
- en
datasets:
- mc4
- wikipedia
- multilexnorm
tags:
- lexical normalization
license: apache-2.0
---
# Fine-tuned ByT5-small for MultiLexNorm (Indonesian-English version)

This is the official release of the fine-tuned models for **the winning entry** to the [*W-NUT 2021: Multilingual Lexical Normalization (MultiLexNorm)* shared task](https://noisy-text.github.io/2021/multi-lexnorm.html), which evaluates lexical-normalization systems on 12 social media datasets in 11 languages.
Our system is based on [ByT5](https://arxiv.org/abs/2105.13626), which we first pre-train on synthetic data and then fine-tune on authentic normalization data. It achieves the best performance by a wide margin in intrinsic evaluation, and also the best performance in extrinsic evaluation through dependency parsing. In addition to these fine-tuned models, we also release the source files on [GitHub](https://github.com/ufal/multilexnorm2021) and an interactive demo on [Google Colab](https://colab.research.google.com/drive/1rxpI8IlKk-D2crFqi2hdzbTBIezqgsCg?usp=sharing).
## How to use
The model was *not* fine-tuned in a standard sentence-to-sentence setting – instead, it was tailored to the token-to-token definition of MultiLexNorm data. Please refer to [**the interactive demo on Colab notebook**](https://colab.research.google.com/drive/1rxpI8IlKk-D2crFqi2hdzbTBIezqgsCg?usp=sharing) to learn how to use these models.
## How to cite
```bibtex
@inproceedings{wnut-ufal,
title= "{ÚFAL} at {MultiLexNorm} 2021: Improving Multilingual Lexical Normalization by Fine-tuning {ByT5}",
author = "Samuel, David and Straka, Milan",
booktitle = "Proceedings of the 7th Workshop on Noisy User-generated Text (W-NUT 2021)",
year = "2021",
publisher = "Association for Computational Linguistics",
address = "Punta Cana, Dominican Republic"
}
```
## ByT5 - Small
ByT5 is a tokenizer-free version of [Google's T5](https://ai.googleblog.com/2020/02/exploring-transfer-learning-with-t5.html) and generally follows the architecture of [MT5](https://huggingface.co/google/mt5-small).
ByT5 was only pre-trained on [mC4](https://www.tensorflow.org/datasets/catalog/c4#c4multilingual) excluding any supervised training with an average span-mask of 20 UTF-8 characters. Therefore, this model has to be fine-tuned before it is useable on a downstream task.
ByT5 works especially well on noisy text data,*e.g.*, `google/byt5-small` significantly outperforms [mt5-small](https://huggingface.co/google/mt5-small) on [TweetQA](https://arxiv.org/abs/1907.06292).
Paper: [ByT5: Towards a token-free future with pre-trained byte-to-byte models](https://arxiv.org/abs/2105.13626)
Authors: *Linting Xue, Aditya Barua, Noah Constant, Rami Al-Rfou, Sharan Narang, Mihir Kale, Adam Roberts, Colin Raffel*
|
ufal/byt5-small-multilexnorm2021-it | 0383500e1e59ce6cea155d59de8225228cbc6ef6 | 2021-10-20T12:38:22.000Z | [
"pytorch",
"t5",
"text2text-generation",
"it",
"dataset:mc4",
"dataset:wikipedia",
"dataset:multilexnorm",
"arxiv:2105.13626",
"arxiv:1907.06292",
"transformers",
"lexical normalization",
"license:apache-2.0",
"autotrain_compatible"
] | text2text-generation | false | ufal | null | ufal/byt5-small-multilexnorm2021-it | 0 | null | transformers | 36,212 | ---
language: it
datasets:
- mc4
- wikipedia
- multilexnorm
tags:
- lexical normalization
license: apache-2.0
---
# Fine-tuned ByT5-small for MultiLexNorm (Italian version)

This is the official release of the fine-tuned models for **the winning entry** to the [*W-NUT 2021: Multilingual Lexical Normalization (MultiLexNorm)* shared task](https://noisy-text.github.io/2021/multi-lexnorm.html), which evaluates lexical-normalization systems on 12 social media datasets in 11 languages.
Our system is based on [ByT5](https://arxiv.org/abs/2105.13626), which we first pre-train on synthetic data and then fine-tune on authentic normalization data. It achieves the best performance by a wide margin in intrinsic evaluation, and also the best performance in extrinsic evaluation through dependency parsing. In addition to these fine-tuned models, we also release the source files on [GitHub](https://github.com/ufal/multilexnorm2021) and an interactive demo on [Google Colab](https://colab.research.google.com/drive/1rxpI8IlKk-D2crFqi2hdzbTBIezqgsCg?usp=sharing).
## How to use
The model was *not* fine-tuned in a standard sentence-to-sentence setting – instead, it was tailored to the token-to-token definition of MultiLexNorm data. Please refer to [**the interactive demo on Colab notebook**](https://colab.research.google.com/drive/1rxpI8IlKk-D2crFqi2hdzbTBIezqgsCg?usp=sharing) to learn how to use these models.
## How to cite
```bibtex
@inproceedings{wnut-ufal,
title= "{ÚFAL} at {MultiLexNorm} 2021: Improving Multilingual Lexical Normalization by Fine-tuning {ByT5}",
author = "Samuel, David and Straka, Milan",
booktitle = "Proceedings of the 7th Workshop on Noisy User-generated Text (W-NUT 2021)",
year = "2021",
publisher = "Association for Computational Linguistics",
address = "Punta Cana, Dominican Republic"
}
```
## ByT5 - Small
ByT5 is a tokenizer-free version of [Google's T5](https://ai.googleblog.com/2020/02/exploring-transfer-learning-with-t5.html) and generally follows the architecture of [MT5](https://huggingface.co/google/mt5-small).
ByT5 was only pre-trained on [mC4](https://www.tensorflow.org/datasets/catalog/c4#c4multilingual) excluding any supervised training with an average span-mask of 20 UTF-8 characters. Therefore, this model has to be fine-tuned before it is useable on a downstream task.
ByT5 works especially well on noisy text data,*e.g.*, `google/byt5-small` significantly outperforms [mt5-small](https://huggingface.co/google/mt5-small) on [TweetQA](https://arxiv.org/abs/1907.06292).
Paper: [ByT5: Towards a token-free future with pre-trained byte-to-byte models](https://arxiv.org/abs/2105.13626)
Authors: *Linting Xue, Aditya Barua, Noah Constant, Rami Al-Rfou, Sharan Narang, Mihir Kale, Adam Roberts, Colin Raffel*
|
ufal/byt5-small-multilexnorm2021-nl | 8dbe3a718cad034e3463c8cdee483ab88382aa9f | 2021-10-20T12:42:44.000Z | [
"pytorch",
"t5",
"text2text-generation",
"nl",
"dataset:mc4",
"dataset:wikipedia",
"dataset:multilexnorm",
"arxiv:2105.13626",
"arxiv:1907.06292",
"transformers",
"lexical normalization",
"license:apache-2.0",
"autotrain_compatible"
] | text2text-generation | false | ufal | null | ufal/byt5-small-multilexnorm2021-nl | 0 | null | transformers | 36,213 | ---
language: nl
datasets:
- mc4
- wikipedia
- multilexnorm
tags:
- lexical normalization
license: apache-2.0
---
# Fine-tuned ByT5-small for MultiLexNorm (Dutch version)

This is the official release of the fine-tuned models for **the winning entry** to the [*W-NUT 2021: Multilingual Lexical Normalization (MultiLexNorm)* shared task](https://noisy-text.github.io/2021/multi-lexnorm.html), which evaluates lexical-normalization systems on 12 social media datasets in 11 languages.
Our system is based on [ByT5](https://arxiv.org/abs/2105.13626), which we first pre-train on synthetic data and then fine-tune on authentic normalization data. It achieves the best performance by a wide margin in intrinsic evaluation, and also the best performance in extrinsic evaluation through dependency parsing. In addition to these fine-tuned models, we also release the source files on [GitHub](https://github.com/ufal/multilexnorm2021) and an interactive demo on [Google Colab](https://colab.research.google.com/drive/1rxpI8IlKk-D2crFqi2hdzbTBIezqgsCg?usp=sharing).
## How to use
The model was *not* fine-tuned in a standard sentence-to-sentence setting – instead, it was tailored to the token-to-token definition of MultiLexNorm data. Please refer to [**the interactive demo on Colab notebook**](https://colab.research.google.com/drive/1rxpI8IlKk-D2crFqi2hdzbTBIezqgsCg?usp=sharing) to learn how to use these models.
## How to cite
```bibtex
@inproceedings{wnut-ufal,
title= "{ÚFAL} at {MultiLexNorm} 2021: Improving Multilingual Lexical Normalization by Fine-tuning {ByT5}",
author = "Samuel, David and Straka, Milan",
booktitle = "Proceedings of the 7th Workshop on Noisy User-generated Text (W-NUT 2021)",
year = "2021",
publisher = "Association for Computational Linguistics",
address = "Punta Cana, Dominican Republic"
}
```
## ByT5 - Small
ByT5 is a tokenizer-free version of [Google's T5](https://ai.googleblog.com/2020/02/exploring-transfer-learning-with-t5.html) and generally follows the architecture of [MT5](https://huggingface.co/google/mt5-small).
ByT5 was only pre-trained on [mC4](https://www.tensorflow.org/datasets/catalog/c4#c4multilingual) excluding any supervised training with an average span-mask of 20 UTF-8 characters. Therefore, this model has to be fine-tuned before it is useable on a downstream task.
ByT5 works especially well on noisy text data,*e.g.*, `google/byt5-small` significantly outperforms [mt5-small](https://huggingface.co/google/mt5-small) on [TweetQA](https://arxiv.org/abs/1907.06292).
Paper: [ByT5: Towards a token-free future with pre-trained byte-to-byte models](https://arxiv.org/abs/2105.13626)
Authors: *Linting Xue, Aditya Barua, Noah Constant, Rami Al-Rfou, Sharan Narang, Mihir Kale, Adam Roberts, Colin Raffel*
|
ufal/byt5-small-multilexnorm2021-tr | 0ff1dc175218b32053eb226923126779415229a9 | 2021-10-20T12:56:48.000Z | [
"pytorch",
"t5",
"text2text-generation",
"tr",
"dataset:mc4",
"dataset:wikipedia",
"dataset:multilexnorm",
"arxiv:2105.13626",
"arxiv:1907.06292",
"transformers",
"lexical normalization",
"license:apache-2.0",
"autotrain_compatible"
] | text2text-generation | false | ufal | null | ufal/byt5-small-multilexnorm2021-tr | 0 | null | transformers | 36,214 | ---
language: tr
datasets:
- mc4
- wikipedia
- multilexnorm
tags:
- lexical normalization
license: apache-2.0
---
# Fine-tuned ByT5-small for MultiLexNorm (Turkish version)

This is the official release of the fine-tuned models for **the winning entry** to the [*W-NUT 2021: Multilingual Lexical Normalization (MultiLexNorm)* shared task](https://noisy-text.github.io/2021/multi-lexnorm.html), which evaluates lexical-normalization systems on 12 social media datasets in 11 languages.
Our system is based on [ByT5](https://arxiv.org/abs/2105.13626), which we first pre-train on synthetic data and then fine-tune on authentic normalization data. It achieves the best performance by a wide margin in intrinsic evaluation, and also the best performance in extrinsic evaluation through dependency parsing. In addition to these fine-tuned models, we also release the source files on [GitHub](https://github.com/ufal/multilexnorm2021) and an interactive demo on [Google Colab](https://colab.research.google.com/drive/1rxpI8IlKk-D2crFqi2hdzbTBIezqgsCg?usp=sharing).
## How to use
The model was *not* fine-tuned in a standard sentence-to-sentence setting – instead, it was tailored to the token-to-token definition of MultiLexNorm data. Please refer to [**the interactive demo on Colab notebook**](https://colab.research.google.com/drive/1rxpI8IlKk-D2crFqi2hdzbTBIezqgsCg?usp=sharing) to learn how to use these models.
## How to cite
```bibtex
@inproceedings{wnut-ufal,
title= "{ÚFAL} at {MultiLexNorm} 2021: Improving Multilingual Lexical Normalization by Fine-tuning {ByT5}",
author = "Samuel, David and Straka, Milan",
booktitle = "Proceedings of the 7th Workshop on Noisy User-generated Text (W-NUT 2021)",
year = "2021",
publisher = "Association for Computational Linguistics",
address = "Punta Cana, Dominican Republic"
}
```
## ByT5 - Small
ByT5 is a tokenizer-free version of [Google's T5](https://ai.googleblog.com/2020/02/exploring-transfer-learning-with-t5.html) and generally follows the architecture of [MT5](https://huggingface.co/google/mt5-small).
ByT5 was only pre-trained on [mC4](https://www.tensorflow.org/datasets/catalog/c4#c4multilingual) excluding any supervised training with an average span-mask of 20 UTF-8 characters. Therefore, this model has to be fine-tuned before it is useable on a downstream task.
ByT5 works especially well on noisy text data,*e.g.*, `google/byt5-small` significantly outperforms [mt5-small](https://huggingface.co/google/mt5-small) on [TweetQA](https://arxiv.org/abs/1907.06292).
Paper: [ByT5: Towards a token-free future with pre-trained byte-to-byte models](https://arxiv.org/abs/2105.13626)
Authors: *Linting Xue, Aditya Barua, Noah Constant, Rami Al-Rfou, Sharan Narang, Mihir Kale, Adam Roberts, Colin Raffel*
|
unicamp-dl/mt5-base-mmarco-v1 | 0dc8b8dd0eb9e3fa45389a6dc34c872b07292654 | 2022-01-05T21:30:24.000Z | [
"pytorch",
"mt5",
"text2text-generation",
"pt",
"dataset:msmarco",
"arxiv:2108.13897",
"transformers",
"msmarco",
"t5",
"tensorflow",
"pt-br",
"license:mit",
"autotrain_compatible"
] | text2text-generation | false | unicamp-dl | null | unicamp-dl/mt5-base-mmarco-v1 | 0 | null | transformers | 36,215 | ---
language: pt
license: mit
tags:
- msmarco
- t5
- pytorch
- tensorflow
- pt
- pt-br
datasets:
- msmarco
widget:
- text: "Texto de exemplo em português"
inference: false
---
# mt5-base Reranker finetuned on mMARCO
## Introduction
mt5-base-mmarco-v1 is a mT5-based model fine-tuned on a multilingual translated version of MS MARCO passage dataset. This dataset, named Multi MS MARCO, is formed by 9 complete MS MARCO passages collection in 9 different languages. In the version v1, the datasets were translated using [Helsinki](https://huggingface.co/Helsinki-NLP) NMT models.
Further information about the dataset or the translation method can be found on our paper [**mMARCO: A Multilingual Version of MS MARCO Passage Ranking Dataset**](https://arxiv.org/abs/2108.13897) and [mMARCO](https://github.com/unicamp-dl/mMARCO) repository.
## Usage
```python
from transformers import T5Tokenizer, MT5ForConditionalGeneration
model_name = 'unicamp-dl/mt5-base-mmarco-v1'
tokenizer = T5Tokenizer.from_pretrained(model_name)
model = MT5ForConditionalGeneration.from_pretrained(model_name)
```
# Citation
If you use mt5-base-mmarco-v1, please cite:
@misc{bonifacio2021mmarco,
title={mMARCO: A Multilingual Version of MS MARCO Passage Ranking Dataset},
author={Luiz Henrique Bonifacio and Vitor Jeronymo and Hugo Queiroz Abonizio and Israel Campiotti and Marzieh Fadaee and and Roberto Lotufo and Rodrigo Nogueira},
year={2021},
eprint={2108.13897},
archivePrefix={arXiv},
primaryClass={cs.CL}
} |
unknownTransformer/wav2vec2-large-xlsr-german | 937e7487d86c7fc963d05fbe42efd0e31e23ae47 | 2021-05-11T08:43:09.000Z | [
"pytorch",
"wav2vec2",
"automatic-speech-recognition",
"transformers"
] | automatic-speech-recognition | false | unknownTransformer | null | unknownTransformer/wav2vec2-large-xlsr-german | 0 | null | transformers | 36,216 | Bad Modell for Research Purposes! |
upskyy/kobart-summarization-v2 | 79ff33e1a4d8c5bc35684f8ff031194fb68eee82 | 2021-10-03T14:46:43.000Z | [
"pytorch",
"bart",
"text2text-generation",
"transformers",
"autotrain_compatible"
] | text2text-generation | false | upskyy | null | upskyy/kobart-summarization-v2 | 0 | 1 | transformers | 36,217 | Entry not found |
usami/t5-small-finetuned-xsum | d94209374b8c500723fdf48da5a67b43729af779 | 2022-01-31T11:28:06.000Z | [
"pytorch",
"t5",
"text2text-generation",
"transformers",
"autotrain_compatible"
] | text2text-generation | false | usami | null | usami/t5-small-finetuned-xsum | 0 | null | transformers | 36,218 | Entry not found |
uva-irlab/quretec | 1ea91bdff782f22e94439d736677d44f3d8153ff | 2021-08-26T14:06:47.000Z | [
"pytorch",
"bert",
"en",
"dataset:uva-irlab/canard_quretec",
"arxiv:2005.11723",
"transformers",
"conversational-search",
"model-index"
] | null | false | uva-irlab | null | uva-irlab/quretec | 0 | null | transformers | 36,219 | ---
language:
- en
tags:
- conversational-search # Example: audio
metrics:
- f1
datasets:
- uva-irlab/canard_quretec
model-index:
- name: QuReTec
results:
- task:
name: Conversational search # Example: Speech Recognition
type: conversational # Example: automatic-speech-recognition
dataset:
name: CANARD # Example: Common Voice zh-CN
type: canard # Example: common_voice
metrics:
- name: Micro F1 # Example: Test WER
type: f1 # Example: wer
value: 68.7 # Example: 20.90
- name: Micro Recall
type: recall
value: 66.1
- name: Micro Precision
type: precision
value: 71.5
---
# QuReTec: query resolution model
QuReTeC is a query resolution model. It finds the relevant terms in a question history.
It is based on **bert-large-uncased** with a max sequence length of 300.
# Config details
Training and evaluation was done using the following BertConfig:
```json
BertConfig {
"_name_or_path": "uva-irlab/quretec",
"architectures": ["BertForMaskedLM"],
"attention_probs_dropout_prob": 0.1,
"finetuning_task": "ner",
"gradient_checkpointing": false,
"hidden_act": "gelu",
"hidden_dropout_prob": 0.4,
"hidden_size": 1024,
"id2label": {
"0": "[PAD]",
"1": "O",
"2": "REL",
"3": "[CLS]",
"4": "[SEP]"
},
"initializer_range": 0.02,
"intermediate_size": 4096,
"label2id": {
"O": 1,
"REL": 2,
"[CLS]": 3,
"[PAD]": 0,
"[SEP]": 4
},
"layer_norm_eps": 1e-12,
"max_position_embeddings": 512,
"model_type": "bert",
"num_attention_heads": 16,
"num_hidden_layers": 24,
"pad_token_id": 0,
"position_embedding_type": "absolute",
"transformers_version": "4.6.1",
"type_vocab_size": 2,
"use_cache": true,
"vocab_size": 30522
}
```
# Original authors
QuReTeC model from the published SIGIR 2020 paper: Query Resolution for Conversational Search with Limited Supervision by N. Voskarides, D. Li, P. Ren, E. Kanoulas and M. de Rijke. [[pdf]](https://arxiv.org/abs/2005.11723).
# Contributions
Uploaded by G. Scheuer ([website](https://giguruscheuer.com)) |
uyeongjae/distilgpt2-finetuned-wikitext2 | 88f0ba1a40ec5f90080628b22d249b3c0348351f | 2021-09-17T05:34:07.000Z | [
"pytorch",
"tensorboard",
"gpt2",
"text-generation",
"transformers",
"generated_from_trainer",
"license:apache-2.0",
"model-index"
] | text-generation | false | uyeongjae | null | uyeongjae/distilgpt2-finetuned-wikitext2 | 0 | null | transformers | 36,220 | ---
license: apache-2.0
tags:
- generated_from_trainer
datasets:
- null
model-index:
- name: distilgpt2-finetuned-wikitext2
results:
- task:
name: Causal Language Modeling
type: text-generation
---
<!-- This model card has been generated automatically according to the information the Trainer had access to. You
should probably proofread and complete it, then remove this comment. -->
# distilgpt2-finetuned-wikitext2
This model is a fine-tuned version of [distilgpt2](https://huggingface.co/distilgpt2) on the None dataset.
It achieves the following results on the evaluation set:
- Loss: 3.6426
## Model description
More information needed
## Intended uses & limitations
More information needed
## Training and evaluation data
More information needed
## Training procedure
### Training hyperparameters
The following hyperparameters were used during training:
- learning_rate: 2e-05
- train_batch_size: 8
- eval_batch_size: 8
- seed: 42
- optimizer: Adam with betas=(0.9,0.999) and epsilon=1e-08
- lr_scheduler_type: linear
- num_epochs: 3.0
### Training results
| Training Loss | Epoch | Step | Validation Loss |
|:-------------:|:-----:|:----:|:---------------:|
| 3.5974 | 1.0 | 2334 | 3.6426 |
| 3.5891 | 2.0 | 4668 | 3.6426 |
| 3.572 | 3.0 | 7002 | 3.6426 |
### Framework versions
- Transformers 4.10.2
- Pytorch 1.7.1
- Datasets 1.11.0
- Tokenizers 0.10.3
|
vachevkd/qna-t5sm-squad-v01 | 9e10cfa8524fb1a811b16059f70e208eacd1119a | 2021-12-19T15:56:04.000Z | [
"pytorch",
"t5",
"text2text-generation",
"transformers",
"autotrain_compatible"
] | text2text-generation | false | vachevkd | null | vachevkd/qna-t5sm-squad-v01 | 0 | null | transformers | 36,221 | Entry not found |
vachonni/wav2vec2-large-xls-r-300m-da-colab | 72000fcd3dd1135626794fb8393b1df6b8ce3181 | 2022-01-14T12:14:53.000Z | [
"pytorch",
"tensorboard",
"wav2vec2",
"automatic-speech-recognition",
"transformers",
"generated_from_trainer",
"license:apache-2.0",
"model-index"
] | automatic-speech-recognition | false | vachonni | null | vachonni/wav2vec2-large-xls-r-300m-da-colab | 0 | null | transformers | 36,222 | ---
license: apache-2.0
tags:
- generated_from_trainer
model-index:
- name: wav2vec2-large-xls-r-300m-da-colab
results: []
---
<!-- This model card has been generated automatically according to the information the Trainer had access to. You
should probably proofread and complete it, then remove this comment. -->
# wav2vec2-large-xls-r-300m-da-colab
This model is a fine-tuned version of [Alvenir/wav2vec2-base-da](https://huggingface.co/Alvenir/wav2vec2-base-da) on the None dataset.
## Model description
More information needed
## Intended uses & limitations
More information needed
## Training and evaluation data
More information needed
## Training procedure
### Training hyperparameters
The following hyperparameters were used during training:
- learning_rate: 0.0003
- train_batch_size: 16
- eval_batch_size: 8
- seed: 42
- gradient_accumulation_steps: 2
- total_train_batch_size: 32
- optimizer: Adam with betas=(0.9,0.999) and epsilon=1e-08
- lr_scheduler_type: linear
- lr_scheduler_warmup_steps: 500
- num_epochs: 30
- mixed_precision_training: Native AMP
### Training results
### Framework versions
- Transformers 4.11.3
- Pytorch 1.10.0+cu111
- Datasets 1.13.3
- Tokenizers 0.10.3
|
valarikv/DialoGPT-small-bateman | b63220afe67ac1d60122612fa7fd0a3c14a4c23c | 2022-01-02T17:39:58.000Z | [
"pytorch",
"gpt2",
"text-generation",
"transformers",
"conversational"
] | conversational | false | valarikv | null | valarikv/DialoGPT-small-bateman | 0 | null | transformers | 36,223 | ---
tags:
- conversational
---
# Patrick Bateman DialoGPT Model
|
valurank/paraphrase-mpnet-base-v2-offensive | bf4e92804d69c64d880b1341616ceac053100185 | 2022-06-08T20:33:14.000Z | [
"pytorch",
"mpnet",
"feature-extraction",
"sentence-transformers",
"sentence-similarity",
"transformers",
"license:other"
] | sentence-similarity | false | valurank | null | valurank/paraphrase-mpnet-base-v2-offensive | 0 | null | sentence-transformers | 36,224 | ---
pipeline_tag: sentence-similarity
license: other
tags:
- sentence-transformers
- feature-extraction
- sentence-similarity
- transformers
---
# valurank/paraphrase-mpnet-base-v2-offensive
This is a [sentence-transformers](https://www.SBERT.net) model: It maps sentences & paragraphs to a 768 dimensional dense vector space and can be used for tasks like clustering or semantic search.
<!--- Describe your model here -->
## Usage (Sentence-Transformers)
Using this model becomes easy when you have [sentence-transformers](https://www.SBERT.net) installed:
```
pip install -U sentence-transformers
```
Then you can use the model like this:
```python
from sentence_transformers import SentenceTransformer
sentences = ["This is an example sentence", "Each sentence is converted"]
model = SentenceTransformer('valurank/paraphrase-mpnet-base-v2-offensive')
embeddings = model.encode(sentences)
print(embeddings)
```
## Usage (HuggingFace Transformers)
Without [sentence-transformers](https://www.SBERT.net), you can use the model like this: First, you pass your input through the transformer model, then you have to apply the right pooling-operation on-top of the contextualized word embeddings.
```python
from transformers import AutoTokenizer, AutoModel
import torch
#Mean Pooling - Take attention mask into account for correct averaging
def mean_pooling(model_output, attention_mask):
token_embeddings = model_output[0] #First element of model_output contains all token embeddings
input_mask_expanded = attention_mask.unsqueeze(-1).expand(token_embeddings.size()).float()
return torch.sum(token_embeddings * input_mask_expanded, 1) / torch.clamp(input_mask_expanded.sum(1), min=1e-9)
# Sentences we want sentence embeddings for
sentences = ['This is an example sentence', 'Each sentence is converted']
# Load model from HuggingFace Hub
tokenizer = AutoTokenizer.from_pretrained('valurank/paraphrase-mpnet-base-v2-offensive')
model = AutoModel.from_pretrained('valurank/paraphrase-mpnet-base-v2-offensive')
# Tokenize sentences
encoded_input = tokenizer(sentences, padding=True, truncation=True, return_tensors='pt')
# Compute token embeddings
with torch.no_grad():
model_output = model(**encoded_input)
# Perform pooling. In this case, mean pooling.
sentence_embeddings = mean_pooling(model_output, encoded_input['attention_mask'])
print("Sentence embeddings:")
print(sentence_embeddings)
```
## Evaluation Results
<!--- Describe how your model was evaluated -->
For an automated evaluation of this model, see the *Sentence Embeddings Benchmark*: [https://seb.sbert.net](https://seb.sbert.net?model_name=valurank/paraphrase-mpnet-base-v2-offensive)
## Training
The model was trained with the parameters:
**DataLoader**:
`torch.utils.data.dataloader.DataLoader` of length 1280 with parameters:
```
{'batch_size': 16, 'sampler': 'torch.utils.data.sampler.RandomSampler', 'batch_sampler': 'torch.utils.data.sampler.BatchSampler'}
```
**Loss**:
`sentence_transformers.losses.CosineSimilarityLoss.CosineSimilarityLoss`
Parameters of the fit()-Method:
```
{
"epochs": 1,
"evaluation_steps": 0,
"evaluator": "NoneType",
"max_grad_norm": 1,
"optimizer_class": "<class 'transformers.optimization.AdamW'>",
"optimizer_params": {
"lr": 2e-05
},
"scheduler": "WarmupLinear",
"steps_per_epoch": null,
"warmup_steps": 10,
"weight_decay": 0.01
}
```
## Full Model Architecture
```
SentenceTransformer(
(0): Transformer({'max_seq_length': 512, 'do_lower_case': False}) with Transformer model: MPNetModel
(1): Pooling({'word_embedding_dimension': 768, 'pooling_mode_cls_token': False, 'pooling_mode_mean_tokens': True, 'pooling_mode_max_tokens': False, 'pooling_mode_mean_sqrt_len_tokens': False})
)
```
## Citing & Authors
<!--- Describe where people can find more information --> |
vanadhi/bert-base-uncased-fiqa-flm-sq-flit | 4198977ffd736e54399f7e331c7560b0a8c02333 | 2021-12-25T18:44:16.000Z | [
"pytorch",
"bert",
"question-answering",
"transformers",
"generated_from_trainer",
"model-index",
"autotrain_compatible"
] | question-answering | false | vanadhi | null | vanadhi/bert-base-uncased-fiqa-flm-sq-flit | 0 | null | transformers | 36,225 | ---
tags:
- generated_from_trainer
model-index:
- name: bert-base-uncased-fiqa-flm-sq-flit
results: []
---
<!-- This model card has been generated automatically according to the information the Trainer had access to. You
should probably proofread and complete it, then remove this comment. -->
# bert-base-uncased-fiqa-flm-sq-flit
This model is a fine-tuned version of bert-base-uncased on a custom dataset created for question answering in
financial domain.
## Model description
BERT is a transformers model pretrained on a large corpus of English data in a self-supervised fashion.
The model was further processed as below for the specific downstream QA task.
1. Pretrained for domain adaptation with Masked language modeling (MLM) objective with
the FIQA challenge Opinion-based QA task is available here - https://drive.google.com/file/d/1BlWaV-qVPfpGyJoWQJU9bXQgWCATgxEP/view
2. Pretrained with MLM objective with custom generated dataset for Banking and Finance.
3. Fine Tuned with SQuAD V2 dataset for QA task adaptation.
4. Fine Tuned with custom labeled dataset in SQuAD format for domain and task adaptation.
## Intended uses & limitations
The model is intended to be used for a custom Questions Answering system in the BFSI domain.
## Training and evaluation data
More information needed
## Training procedure
### Training hyperparameters
The following hyperparameters were used during training:
- learning_rate: 2e-05
- train_batch_size: 8
- eval_batch_size: 8
- seed: 42
- optimizer: Adam with betas=(0.9,0.999) and epsilon=1e-08
- lr_scheduler_type: linear
- lr_scheduler_warmup_ratio: 0.2
- num_epochs: 2.0
### Training results
### Framework versions
- Transformers 4.15.0.dev0
- Pytorch 1.10.0+cu111
- Datasets 1.16.1
- Tokenizers 0.10.3
|
vanessahahn/bert-fr-de-en-ar-twitter | d69b1c2b7ab774664dc454acf8a8a92eaa521e3c | 2021-06-08T19:17:23.000Z | [
"pytorch"
] | null | false | vanessahahn | null | vanessahahn/bert-fr-de-en-ar-twitter | 0 | null | null | 36,226 | Entry not found |
vasilis/wav2vec2-large-xlsr-53-estonian | 6009cf85eefc3b2d44cff706b15542f481c3d10a | 2021-04-15T09:21:31.000Z | [
"pytorch",
"wav2vec2",
"et",
"dataset:common_voice",
"dataset:NST Estonian ASR Database",
"transformers",
"audio",
"automatic-speech-recognition",
"speech",
"xlsr-fine-tuning-week",
"license:apache-2.0",
"model-index"
] | automatic-speech-recognition | false | vasilis | null | vasilis/wav2vec2-large-xlsr-53-estonian | 0 | null | transformers | 36,227 | ---
language: et
datasets:
- common_voice
- NST Estonian ASR Database
metrics:
- wer
- cer
tags:
- audio
- automatic-speech-recognition
- speech
- xlsr-fine-tuning-week
license: apache-2.0
model-index:
- name: XLSR Wav2Vec2 Large 53 - Estonian by Vasilis
results:
- task:
name: Speech Recognition
type: automatic-speech-recognition
dataset:
name: Common Voice et
type: common_voice
args: et
metrics:
- name: Test WER
type: wer
value: 30.658320
- name: Test CER
type: cer
value: 5.261490
---
# Wav2Vec2-Large-XLSR-53-Estonian
Fine-tuned [facebook/wav2vec2-large-xlsr-53](https://huggingface.co/facebook/wav2vec2-large-xlsr-53) on Estonian using the [Common Voice](https://huggingface.co/datasets/common_voice).
When using this model, make sure that your speech input is sampled at 16kHz.
## Usage
The model can be used directly (without a language model) as follows:
```python
import torch
import torchaudio
from datasets import load_dataset
from transformers import Wav2Vec2ForCTC, Wav2Vec2Processor
test_dataset = load_dataset("common_voice", "et", split="test[:2%]") #TODO: replace {lang_id} in your language code here. Make sure the code is one of the *ISO codes* of [this](https://huggingface.co/languages) site.
processor = Wav2Vec2Processor.from_pretrained("vasilis/wav2vec2-large-xlsr-53-Estonian") #TODO: replace {model_id} with your model id. The model id consists of {your_username}/{your_modelname}, *e.g.* `elgeish/wav2vec2-large-xlsr-53-arabic`
model = Wav2Vec2ForCTC.from_pretrained("vasilis/wav2vec2-large-xlsr-53-Estonian") #TODO: replace {model_id} with your model id. The model id consists of {your_username}/{your_modelname}, *e.g.* `elgeish/wav2vec2-large-xlsr-53-arabic`
resampler = torchaudio.transforms.Resample(48_000, 16_000)
# Preprocessing the datasets.
# We need to read the aduio files as arrays
def speech_file_to_array_fn(batch):
speech_array, sampling_rate = torchaudio.load(batch["path"])
batch["speech"] = resampler(speech_array).squeeze().numpy()
return batch
test_dataset = test_dataset.map(speech_file_to_array_fn)
inputs = processor(test_dataset["speech"][:2], sampling_rate=16_000, return_tensors="pt", padding=True)
with torch.no_grad():
logits = model(inputs.input_values, attention_mask=inputs.attention_mask).logits
predicted_ids = torch.argmax(logits, dim=-1)
print("Prediction:", processor.batch_decode(predicted_ids))
print("Reference:", test_dataset["sentence"][:2])
```
## Evaluation
The model can be evaluated as follows on the Estonian test data of Common Voice.
```python
import torch
import torchaudio
from datasets import load_dataset, load_metric
from transformers import Wav2Vec2ForCTC, Wav2Vec2Processor
import re
test_dataset = load_dataset("common_voice", "et", split="test")
wer = load_metric("wer")
processor = Wav2Vec2Processor.from_pretrained("vasilis/wav2vec2-large-xlsr-53-Estonian")
model = Wav2Vec2ForCTC.from_pretrained("vasilis/wav2vec2-large-xlsr-53-Estonian")
model.to("cuda")
chars_to_ignore_regex = "[\,\?\.\!\-\;\:\"\“\%\‘\”\�\']" # TODO: adapt this list to include all special characters you removed from the data
resampler = {
48_000: torchaudio.transforms.Resample(48_000, 16_000),
44100: torchaudio.transforms.Resample(44100, 16_000),
32000: torchaudio.transforms.Resample(32000, 16_000)
}
# Preprocessing the datasets.
# We need to read the aduio files as arrays
def speech_file_to_array_fn(batch):
batch["sentence"] = re.sub(chars_to_ignore_regex, '', batch["sentence"]).lower()
speech_array, sampling_rate = torchaudio.load(batch["path"])
batch["speech"] = resampler[sampling_rate](speech_array).squeeze().numpy()
return batch
test_dataset = test_dataset.map(speech_file_to_array_fn)
# Preprocessing the datasets.
# We need to read the aduio files as arrays
def evaluate(batch):
inputs = processor(batch["speech"], sampling_rate=16_000, return_tensors="pt", padding=True)
with torch.no_grad():
logits = model(inputs.input_values.to("cuda"), attention_mask=inputs.attention_mask.to("cuda")).logits
pred_ids = torch.argmax(logits, dim=-1)
batch["pred_strings"] = processor.batch_decode(pred_ids)
return batch
result = test_dataset.map(evaluate, batched=True, batch_size=8)
print("WER: {:2f}".format(100 * wer.compute(predictions=result["pred_strings"], references=result["sentence"])))
print("CER: {:2f}".format(100 * wer.compute(predictions=[" ".join(list(entry)) for entry in result["pred_strings"]], references=[" ".join(list(entry)) for entry in result["sentence"]])))
```
**Test Result**: 30.658320 %
## Training
Common voice `train` and `validation` sets were used for finetuning
for 20000 steps (approx. 116 epochs). Both the `feature extractor` (`Wav2Vec2FeatureExtractor`) and
`feature projection` (`Wav2Vec2FeatureProjection`) layer were frozen. Only the `encoder` layer (`Wav2Vec2EncoderStableLayerNorm`) was finetuned.
|
vasilis/wav2vec2-large-xlsr-53-swedish | d73c028f85b72430cb191016b519af9fa3a7f8ca | 2021-04-09T12:23:23.000Z | [
"pytorch",
"wav2vec2",
"sv-SE",
"dataset:common_voice",
"dataset:NST Swedish ASR Database",
"transformers",
"audio",
"automatic-speech-recognition",
"speech",
"xlsr-fine-tuning-week",
"license:apache-2.0",
"model-index"
] | automatic-speech-recognition | false | vasilis | null | vasilis/wav2vec2-large-xlsr-53-swedish | 0 | 1 | transformers | 36,228 | ---
language: sv-SE
datasets:
- common_voice
- NST Swedish ASR Database
metrics:
- wer
- cer
tags:
- audio
- automatic-speech-recognition
- speech
- xlsr-fine-tuning-week
license: apache-2.0
model-index:
- name: V XLSR Wav2Vec2 Large 53 - Swedish
results:
- task:
name: Speech Recognition
type: automatic-speech-recognition
dataset:
name: Common Voice sv-SE
type: common_voice
args: sv-SE
metrics:
- name: Test WER
type: wer
value: 14.695793
- name: Test CER
type: cer
value: 5.264666
---
# Wav2Vec2-Large-XLSR-53-Swedish
Fine-tuned [facebook/wav2vec2-large-xlsr-53](https://huggingface.co/facebook/wav2vec2-large-xlsr-53) on Swedish using the [Common Voice](https://huggingface.co/datasets/common_voice) and parts for the [NST Swedish ASR Database](https://www.nb.no/sprakbanken/en/resource-catalogue/oai-nb-no-sbr-16/).
When using this model, make sure that your speech input is sampled at 16kHz.
## Usage
The model can be used directly (without a language model) as follows:
```python
import torch
import torchaudio
from datasets import load_dataset
from transformers import Wav2Vec2ForCTC, Wav2Vec2Processor
test_dataset = load_dataset("common_voice", "sv-SE", split="test[:2%]") #TODO: replace {lang_id} in your language code here. Make sure the code is one of the *ISO codes* of [this](https://huggingface.co/languages) site.
processor = Wav2Vec2Processor.from_pretrained("vasilis/wav2vec2-large-xlsr-53-swedish") #TODO: replace {model_id} with your model id. The model id consists of {your_username}/{your_modelname}, *e.g.* `elgeish/wav2vec2-large-xlsr-53-arabic`
model = Wav2Vec2ForCTC.from_pretrained("vasilis/wav2vec2-large-xlsr-53-swedish") #TODO: replace {model_id} with your model id. The model id consists of {your_username}/{your_modelname}, *e.g.* `elgeish/wav2vec2-large-xlsr-53-arabic`
resampler = torchaudio.transforms.Resample(48_000, 16_000)
# Preprocessing the datasets.
# We need to read the aduio files as arrays
def speech_file_to_array_fn(batch):
speech_array, sampling_rate = torchaudio.load(batch["path"])
batch["speech"] = resampler(speech_array).squeeze().numpy()
return batch
test_dataset = test_dataset.map(speech_file_to_array_fn)
inputs = processor(test_dataset["speech"][:2], sampling_rate=16_000, return_tensors="pt", padding=True)
with torch.no_grad():
logits = model(inputs.input_values, attention_mask=inputs.attention_mask).logits
predicted_ids = torch.argmax(logits, dim=-1)
print("Prediction:", processor.batch_decode(predicted_ids))
print("Reference:", test_dataset["sentence"][:2])
```
## Evaluation
The model can be evaluated as follows on the Swedish test data of Common Voice.
```python
import torch
import torchaudio
from datasets import load_dataset, load_metric
from transformers import Wav2Vec2ForCTC, Wav2Vec2Processor
import re
test_dataset = load_dataset("common_voice", "sv-SE", split="test")
wer = load_metric("wer")
processor = Wav2Vec2Processor.from_pretrained("vasilis/wav2vec2-large-xlsr-53-swedish")
model = Wav2Vec2ForCTC.from_pretrained("vasilis/wav2vec2-large-xlsr-53-swedish")
model.to("cuda")
chars_to_ignore_regex = "[\,\?\.\!\-\;\:\"\“\%\‘\”\�\']" # TODO: adapt this list to include all special characters you removed from the data
resampler = {
48_000: torchaudio.transforms.Resample(48_000, 16_000),
44100: torchaudio.transforms.Resample(44100, 16_000),
32000: torchaudio.transforms.Resample(32000, 16_000)
}
# Preprocessing the datasets.
# We need to read the aduio files as arrays
def speech_file_to_array_fn(batch):
batch["sentence"] = re.sub(chars_to_ignore_regex, '', batch["sentence"]).lower()
speech_array, sampling_rate = torchaudio.load(batch["path"])
batch["speech"] = resampler[sampling_rate](speech_array).squeeze().numpy()
return batch
test_dataset = test_dataset.map(speech_file_to_array_fn)
# Preprocessing the datasets.
# We need to read the aduio files as arrays
def evaluate(batch):
inputs = processor(batch["speech"], sampling_rate=16_000, return_tensors="pt", padding=True)
with torch.no_grad():
logits = model(inputs.input_values.to("cuda"), attention_mask=inputs.attention_mask.to("cuda")).logits
pred_ids = torch.argmax(logits, dim=-1)
batch["pred_strings"] = processor.batch_decode(pred_ids)
return batch
result = test_dataset.map(evaluate, batched=True, batch_size=8)
print("WER: {:2f}".format(100 * wer.compute(predictions=result["pred_strings"], references=result["sentence"])))
print("CER: {:2f}".format(100 * wer.compute(predictions=[" ".join(list(entry)) for entry in result["pred_strings"]], references=[" ".join(list(entry)) for entry in result["sentence"]])))
```
**Test Result**: 14.695793 %
## Training
As first step used Common Voice train dataset and parts from NST
as can be found [here](https://github.com/se-asr/nst/tree/master).
Part of NST where removed using this mask
```python
mask = [(5 < len(x.split()) < 20) and np.average([len(entry) for entry in x.split()]) > 5 for x in dataset['transcript'].tolist()]
```
After training like this for 20000 steps the model was finetuned on all of nst data using the mask
```python
mask = [(1 < len(x.split()) < 25) and np.average([len(entry) for entry in x.split()]) > 3 for x in dataset['transcript'].tolist()]
```
and all of common voice for 100000 more steps approximately 16 epochs.
|
vasilis/xls-r-et-V-3 | c9ef0cfd07761dd95511799fbd88916d4689ae97 | 2022-03-24T11:54:23.000Z | [
"pytorch",
"tensorboard",
"wav2vec2",
"automatic-speech-recognition",
"et",
"dataset:mozilla-foundation/common_voice_8_0",
"transformers",
"mozilla-foundation/common_voice_8_0",
"robust-speech-event",
"generated_from_trainer",
"hf-asr-leaderboard",
"license:apache-2.0",
"model-index"
] | automatic-speech-recognition | false | vasilis | null | vasilis/xls-r-et-V-3 | 0 | null | transformers | 36,229 | ---
language:
- et
license: apache-2.0
tags:
- automatic-speech-recognition
- mozilla-foundation/common_voice_8_0
- et
- robust-speech-event
- generated_from_trainer
- hf-asr-leaderboard
datasets:
- mozilla-foundation/common_voice_8_0
model-index:
- name: XLS-R-1B - Estonian
results:
- task:
name: Automatic Speech Recognition
type: automatic-speech-recognition
dataset:
name: Common Voice 8
type: mozilla-foundation/common_voice_8_0
args: et
metrics:
- name: Test WER
type: wer
value: 52.47
- name: Test CER
type: cer
value: 12.59
- task:
name: Automatic Speech Recognition
type: automatic-speech-recognition
dataset:
name: Robust Speech Event - Dev Data
type: speech-recognition-community-v2/dev_data
args: sv
metrics:
- name: Test WER
type: wer
value: 61.02
- name: Test CER
type: cer
value: 21.08
- task:
name: Automatic Speech Recognition
type: automatic-speech-recognition
dataset:
name: Robust Speech Event - Dev Data
type: speech-recognition-community-v2/dev_data
args: et
metrics:
- name: Test WER
type: wer
value: 59.23
- task:
name: Automatic Speech Recognition
type: automatic-speech-recognition
dataset:
name: Robust Speech Event - Test Data
type: speech-recognition-community-v2/eval_data
args: et
metrics:
- name: Test WER
type: wer
value: 69.08
---
<!-- This model card has been generated automatically according to the information the Trainer had access to. You
should probably proofread and complete it, then remove this comment. -->
#
This model is a fine-tuned version of [facebook/wav2vec2-xls-r-1b](https://huggingface.co/facebook/wav2vec2-xls-r-1b) on the MOZILLA-FOUNDATION/COMMON_VOICE_8_0 - ET dataset.
It achieves the following results on the evaluation set:
- Loss: 0.8824
- Wer: 0.5246
## Model description
More information needed
## Intended uses & limitations
More information needed
## Training and evaluation data
More information needed
## Training procedure
### Training hyperparameters
The following hyperparameters were used during training:
- learning_rate: 7e-05
- train_batch_size: 32
- eval_batch_size: 32
- seed: 42
- optimizer: Adam with betas=(0.9,0.999) and epsilon=1e-08
- lr_scheduler_type: linear
- lr_scheduler_warmup_steps: 500
- training_steps: 25000
- mixed_precision_training: Native AMP
### Training results
| Training Loss | Epoch | Step | Validation Loss | Wer |
|:-------------:|:------:|:-----:|:---------------:|:------:|
| 1.0296 | 2.79 | 500 | 0.8106 | 0.8029 |
| 0.9339 | 5.59 | 1000 | 0.7419 | 0.7932 |
| 0.8925 | 8.38 | 1500 | 0.7137 | 0.7706 |
| 0.8484 | 11.17 | 2000 | 0.7020 | 0.7677 |
| 0.7521 | 13.97 | 2500 | 0.7043 | 0.7375 |
| 0.719 | 16.76 | 3000 | 0.6617 | 0.7428 |
| 0.656 | 19.55 | 3500 | 0.6388 | 0.7202 |
| 0.6085 | 22.35 | 4000 | 0.6211 | 0.6960 |
| 0.5598 | 25.14 | 4500 | 0.6132 | 0.6644 |
| 0.4969 | 27.93 | 5000 | 0.6065 | 0.6521 |
| 0.4638 | 30.73 | 5500 | 0.6978 | 0.6577 |
| 0.4385 | 33.52 | 6000 | 0.5994 | 0.6565 |
| 0.396 | 36.31 | 6500 | 0.6170 | 0.6258 |
| 0.3861 | 39.11 | 7000 | 0.6486 | 0.6217 |
| 0.3602 | 41.9 | 7500 | 0.6508 | 0.6115 |
| 0.3251 | 44.69 | 8000 | 0.7022 | 0.6253 |
| 0.3197 | 47.49 | 8500 | 0.7706 | 0.6215 |
| 0.3013 | 50.28 | 9000 | 0.6419 | 0.5999 |
| 0.2813 | 53.07 | 9500 | 0.6908 | 0.5959 |
| 0.286 | 55.87 | 10000 | 0.7151 | 0.5916 |
| 0.2645 | 58.66 | 10500 | 0.7181 | 0.5860 |
| 0.2535 | 61.45 | 11000 | 0.7877 | 0.5979 |
| 0.247 | 64.25 | 11500 | 0.8199 | 0.6129 |
| 0.2412 | 67.04 | 12000 | 0.7679 | 0.5884 |
| 0.2404 | 69.83 | 12500 | 0.7266 | 0.5816 |
| 0.2293 | 72.63 | 13000 | 0.7928 | 0.5795 |
| 0.2176 | 75.42 | 13500 | 0.7916 | 0.5846 |
| 0.2143 | 78.21 | 14000 | 0.7954 | 0.5765 |
| 0.2185 | 81.01 | 14500 | 0.8317 | 0.5907 |
| 0.2057 | 83.8 | 15000 | 0.8016 | 0.5851 |
| 0.1895 | 86.59 | 15500 | 0.8080 | 0.5679 |
| 0.1883 | 89.39 | 16000 | 0.8103 | 0.5712 |
| 0.1802 | 92.18 | 16500 | 0.8383 | 0.5644 |
| 0.1826 | 94.97 | 17000 | 0.8799 | 0.5657 |
| 0.1717 | 97.77 | 17500 | 0.8620 | 0.5709 |
| 0.1701 | 100.56 | 18000 | 0.8717 | 0.5662 |
| 0.1623 | 103.35 | 18500 | 0.8534 | 0.5594 |
| 0.158 | 106.15 | 19000 | 0.8595 | 0.5546 |
| 0.1508 | 108.94 | 19500 | 0.8574 | 0.5545 |
| 0.142 | 111.73 | 20000 | 0.8671 | 0.5537 |
| 0.1395 | 114.53 | 20500 | 0.8436 | 0.5525 |
| 0.1373 | 117.32 | 21000 | 0.8808 | 0.5482 |
| 0.1338 | 120.11 | 21500 | 0.9024 | 0.5418 |
| 0.1278 | 122.91 | 22000 | 0.9143 | 0.5409 |
| 0.1207 | 125.7 | 22500 | 0.8917 | 0.5358 |
| 0.1203 | 128.49 | 23000 | 0.9041 | 0.5341 |
| 0.1083 | 131.28 | 23500 | 0.8884 | 0.5341 |
| 0.1147 | 134.08 | 24000 | 0.8910 | 0.5255 |
| 0.1129 | 136.87 | 24500 | 0.8826 | 0.5241 |
| 0.1029 | 139.66 | 25000 | 0.8824 | 0.5246 |
### Framework versions
- Transformers 4.16.0.dev0
- Pytorch 1.10.1+cu102
- Datasets 1.17.1.dev0
- Tokenizers 0.11.0
|
vasudevgupta/abnet-iwslt14-de-en | 1e179e7b78c093e9df7fdedc2cc5185d8be2495b | 2021-02-03T07:18:19.000Z | [
"pytorch",
"transformers"
] | null | false | vasudevgupta | null | vasudevgupta/abnet-iwslt14-de-en | 0 | null | transformers | 36,230 | Entry not found |
verissimomanoel/RobertaTwitterBR | acd7f90b385f70226df04e13ff12eb8838cd9316 | 2021-05-20T22:53:32.000Z | [
"pytorch",
"jax",
"roberta",
"fill-mask",
"transformers",
"autotrain_compatible"
] | fill-mask | false | verissimomanoel | null | verissimomanoel/RobertaTwitterBR | 0 | null | transformers | 36,231 | ### Twitter RoBERTa BR
This is a RoBERTa Twitter in Portuguese model trained on ~7M tweets.
The results will be posted in the future.
### Example of using
```
tokenizer = AutoTokenizer.from_pretrained("verissimomanoel/RobertaTwitterBR")
model = AutoModel.from_pretrained("verissimomanoel/RobertaTwitterBR")
```
|
vesteinn/IceBERT-finetuned-ner | 2ce6b46533b4fc7b526ea1bf5220ef08a714a502 | 2021-09-29T16:17:30.000Z | [
"pytorch",
"tensorboard",
"roberta",
"token-classification",
"dataset:mim_gold_ner",
"transformers",
"generated_from_trainer",
"license:gpl-3.0",
"model-index",
"autotrain_compatible"
] | token-classification | false | vesteinn | null | vesteinn/IceBERT-finetuned-ner | 0 | null | transformers | 36,232 | ---
license: gpl-3.0
tags:
- generated_from_trainer
datasets:
- mim_gold_ner
metrics:
- precision
- recall
- f1
- accuracy
model-index:
- name: IceBERT-finetuned-ner
results:
- task:
name: Token Classification
type: token-classification
dataset:
name: mim_gold_ner
type: mim_gold_ner
args: mim-gold-ner
metrics:
- name: Precision
type: precision
value: 0.8870349771350884
- name: Recall
type: recall
value: 0.8575696021029992
- name: F1
type: f1
value: 0.8720534629404617
- name: Accuracy
type: accuracy
value: 0.9848236357672584
---
<!-- This model card has been generated automatically according to the information the Trainer had access to. You
should probably proofread and complete it, then remove this comment. -->
# IceBERT-finetuned-ner
This model is a fine-tuned version of [vesteinn/IceBERT](https://huggingface.co/vesteinn/IceBERT) on the mim_gold_ner dataset.
It achieves the following results on the evaluation set:
- Loss: 0.0815
- Precision: 0.8870
- Recall: 0.8576
- F1: 0.8721
- Accuracy: 0.9848
## Model description
More information needed
## Intended uses & limitations
More information needed
## Training and evaluation data
More information needed
## Training procedure
### Training hyperparameters
The following hyperparameters were used during training:
- learning_rate: 2e-05
- train_batch_size: 16
- eval_batch_size: 16
- seed: 42
- optimizer: Adam with betas=(0.9,0.999) and epsilon=1e-08
- lr_scheduler_type: linear
- num_epochs: 3
### Training results
| Training Loss | Epoch | Step | Validation Loss | Precision | Recall | F1 | Accuracy |
|:-------------:|:-----:|:----:|:---------------:|:---------:|:------:|:------:|:--------:|
| 0.0536 | 1.0 | 2904 | 0.0749 | 0.8749 | 0.8426 | 0.8585 | 0.9831 |
| 0.0269 | 2.0 | 5808 | 0.0754 | 0.8734 | 0.8471 | 0.8600 | 0.9840 |
| 0.0173 | 3.0 | 8712 | 0.0815 | 0.8870 | 0.8576 | 0.8721 | 0.9848 |
### Framework versions
- Transformers 4.11.0
- Pytorch 1.9.0+cu102
- Datasets 1.12.1
- Tokenizers 0.10.3
|
vesteinn/IceBERT-ner | 2e2adcc9c0ce8a16ad2a675206962396140fdda2 | 2021-09-29T09:35:31.000Z | [
"pytorch",
"tensorboard",
"roberta",
"token-classification",
"dataset:mim_gold_ner",
"transformers",
"generated_from_trainer",
"license:gpl-3.0",
"model-index",
"autotrain_compatible"
] | token-classification | false | vesteinn | null | vesteinn/IceBERT-ner | 0 | null | transformers | 36,233 | ---
license: gpl-3.0
tags:
- generated_from_trainer
datasets:
- mim_gold_ner
metrics:
- precision
- recall
- f1
- accuracy
widget:
- text: Systurnar Guðrún og Monique átu einar á McDonalds og horfðu á Stöð 2, þar glitti í Bruce Willis leika í Die Hard 2.
model-index:
- name: IceBERT-finetuned-ner
results:
- task:
name: Token Classification
type: token-classification
dataset:
name: mim_gold_ner
type: mim_gold_ner
args: mim-gold-ner
metrics:
- name: Precision
type: precision
value: 0.9351994710160899
- name: Recall
type: recall
value: 0.9440427188786294
- name: F1
type: f1
value: 0.9396002878813043
- name: Accuracy
type: accuracy
value: 0.9920330921021648
---
<!-- This model card has been generated automatically according to the information the Trainer had access to. You
should probably proofread and complete it, then remove this comment. -->
# IceBERT-finetuned-ner
This model is a fine-tuned version of [vesteinn/IceBERT](https://huggingface.co/vesteinn/IceBERT) on the mim_gold_ner dataset.
It achieves the following results on the evaluation set:
- Loss: 0.0347
- Precision: 0.9352
- Recall: 0.9440
- F1: 0.9396
- Accuracy: 0.9920
## Model description
More information needed
## Intended uses & limitations
More information needed
## Training and evaluation data
More information needed
## Training procedure
### Training hyperparameters
The following hyperparameters were used during training:
- learning_rate: 2e-05
- train_batch_size: 16
- eval_batch_size: 16
- seed: 42
- optimizer: Adam with betas=(0.9,0.999) and epsilon=1e-08
- lr_scheduler_type: linear
- num_epochs: 3
### Training results
| Training Loss | Epoch | Step | Validation Loss | Precision | Recall | F1 | Accuracy |
|:-------------:|:-----:|:----:|:---------------:|:---------:|:------:|:------:|:--------:|
| 0.0568 | 1.0 | 2929 | 0.0386 | 0.9114 | 0.9162 | 0.9138 | 0.9897 |
| 0.0325 | 2.0 | 5858 | 0.0325 | 0.9300 | 0.9363 | 0.9331 | 0.9912 |
| 0.0184 | 3.0 | 8787 | 0.0347 | 0.9352 | 0.9440 | 0.9396 | 0.9920 |
### Framework versions
- Transformers 4.11.0
- Pytorch 1.9.0+cu102
- Datasets 1.12.1
- Tokenizers 0.10.3
|
victen/xlm-roberta-base-finetuned-panx-de | e648ee12e05661e9a38a8886504d3758d9f3e7a5 | 2022-02-09T10:49:12.000Z | [
"pytorch",
"tensorboard",
"xlm-roberta",
"token-classification",
"dataset:xtreme",
"transformers",
"generated_from_trainer",
"license:mit",
"model-index",
"autotrain_compatible"
] | token-classification | false | victen | null | victen/xlm-roberta-base-finetuned-panx-de | 0 | null | transformers | 36,234 | ---
license: mit
tags:
- generated_from_trainer
datasets:
- xtreme
metrics:
- f1
model-index:
- name: xlm-roberta-base-finetuned-panx-de
results:
- task:
name: Token Classification
type: token-classification
dataset:
name: xtreme
type: xtreme
args: PAN-X.de
metrics:
- name: F1
type: f1
value: 0.8591260810195721
---
<!-- This model card has been generated automatically according to the information the Trainer had access to. You
should probably proofread and complete it, then remove this comment. -->
# xlm-roberta-base-finetuned-panx-de
This model is a fine-tuned version of [xlm-roberta-base](https://huggingface.co/xlm-roberta-base) on the xtreme dataset.
It achieves the following results on the evaluation set:
- Loss: 0.1352
- F1: 0.8591
## Model description
More information needed
## Intended uses & limitations
More information needed
## Training and evaluation data
More information needed
## Training procedure
### Training hyperparameters
The following hyperparameters were used during training:
- learning_rate: 5e-05
- train_batch_size: 24
- eval_batch_size: 24
- seed: 42
- optimizer: Adam with betas=(0.9,0.999) and epsilon=1e-08
- lr_scheduler_type: linear
- num_epochs: 3
### Training results
| Training Loss | Epoch | Step | Validation Loss | F1 |
|:-------------:|:-----:|:----:|:---------------:|:------:|
| 0.257 | 1.0 | 525 | 0.1512 | 0.8302 |
| 0.1305 | 2.0 | 1050 | 0.1401 | 0.8447 |
| 0.0817 | 3.0 | 1575 | 0.1352 | 0.8591 |
### Framework versions
- Transformers 4.11.3
- Pytorch 1.10.0+cu111
- Datasets 1.16.1
- Tokenizers 0.10.3
|
vijote/DialoGPT-small-Morty | 368986d7b4fcedfa61349367c4d0bd68985ae3e5 | 2022-01-09T15:09:05.000Z | [
"pytorch",
"conversational"
] | conversational | false | vijote | null | vijote/DialoGPT-small-Morty | 0 | null | null | 36,235 | ---
tags:
- conversational
---
# Morty DialoGPT Model test |
vincentlu073/legal-zh-multi-span-bio | 9fd22d974cf7f68e2d89b013102cea28ae8658a1 | 2021-05-20T09:00:04.000Z | [
"pytorch",
"bert",
"transformers"
] | null | false | vincentlu073 | null | vincentlu073/legal-zh-multi-span-bio | 0 | null | transformers | 36,236 | Entry not found |
visualjoyce/transformers4vl-vilbert-mt | abbc7f5c86c7f54be82af98af7a1eb178b568b0e | 2021-06-22T13:08:27.000Z | [
"pytorch",
"vilbert",
"transformers"
] | null | false | visualjoyce | null | visualjoyce/transformers4vl-vilbert-mt | 0 | 2 | transformers | 36,237 | Entry not found |
visualjoyce/transformers4vl-vilbert | 8be5d1a7bfed1c31736ca767c5acda9b53979c25 | 2021-06-22T12:56:49.000Z | [
"pytorch",
"vilbert",
"transformers"
] | null | false | visualjoyce | null | visualjoyce/transformers4vl-vilbert | 0 | 1 | transformers | 36,238 | Entry not found |
vitusya/distilbert-base-uncased-finetuned-squad | 4853079eae9296e857898071ce98d077f1cdb7b9 | 2021-11-23T21:15:03.000Z | [
"pytorch",
"distilbert",
"question-answering",
"dataset:squad",
"transformers",
"generated_from_trainer",
"license:apache-2.0",
"model-index",
"autotrain_compatible"
] | question-answering | false | vitusya | null | vitusya/distilbert-base-uncased-finetuned-squad | 0 | null | transformers | 36,239 | ---
license: apache-2.0
tags:
- generated_from_trainer
datasets:
- squad
model-index:
- name: distilbert-base-uncased-finetuned-squad
results: []
---
<!-- This model card has been generated automatically according to the information the Trainer had access to. You
should probably proofread and complete it, then remove this comment. -->
# distilbert-base-uncased-finetuned-squad
This model is a fine-tuned version of [distilbert-base-uncased](https://huggingface.co/distilbert-base-uncased) on the squad dataset.
It achieves the following results on the evaluation set:
- Loss: 1.1610
## Model description
More information needed
## Intended uses & limitations
More information needed
## Training and evaluation data
More information needed
## Training procedure
### Training hyperparameters
The following hyperparameters were used during training:
- learning_rate: 2e-05
- train_batch_size: 16
- eval_batch_size: 16
- seed: 42
- optimizer: Adam with betas=(0.9,0.999) and epsilon=1e-08
- lr_scheduler_type: linear
- num_epochs: 3
### Training results
| Training Loss | Epoch | Step | Validation Loss |
|:-------------:|:-----:|:-----:|:---------------:|
| 1.2137 | 1.0 | 5533 | 1.1625 |
| 0.9496 | 2.0 | 11066 | 1.1263 |
| 0.7591 | 3.0 | 16599 | 1.1610 |
### Framework versions
- Transformers 4.13.0.dev0
- Pytorch 1.10.0+cu102
- Datasets 1.15.1
- Tokenizers 0.10.3
|
vivek-g-2009/DialoGPT-medium-harrypotter | bea55e2fd901385b1bb7794767e593ca924e2981 | 2021-08-27T08:16:15.000Z | [
"pytorch",
"gpt2",
"text-generation",
"transformers",
"conversational"
] | conversational | false | vivek-g-2009 | null | vivek-g-2009/DialoGPT-medium-harrypotter | 0 | null | transformers | 36,240 | ---
tags:
- conversational
---
# Harry Potter DialoGPT Model |
vkorennoy/gpt2_first | 62dcd17adf4b96129fa85cdd57383ee0bc699cda | 2021-11-21T20:38:32.000Z | [
"pytorch",
"gpt2",
"text-generation",
"transformers"
] | text-generation | false | vkorennoy | null | vkorennoy/gpt2_first | 0 | null | transformers | 36,241 | Entry not found |
vkrishnamoorthy/distilbert-base-uncased-finetuned-squad | 9ce50e66c3a3ed067e99de500fbde2e76b6a6449 | 2022-02-28T19:27:07.000Z | [
"pytorch",
"distilbert",
"question-answering",
"transformers",
"autotrain_compatible"
] | question-answering | false | vkrishnamoorthy | null | vkrishnamoorthy/distilbert-base-uncased-finetuned-squad | 0 | null | transformers | 36,242 | Entry not found |
vlco-o/NLboto_o-aki-dialogpt | a72a8d1dc8abf973c6720a9b0aa54a26254dfdf7 | 2021-12-14T17:08:19.000Z | [
"pytorch",
"gpt2",
"text-generation",
"transformers",
"conversational"
] | conversational | false | vlco-o | null | vlco-o/NLboto_o-aki-dialogpt | 0 | null | transformers | 36,243 | ---
tags:
- conversational
---
# NLboto_o aki |
vlco-o/NLboto_o-small-dialogpt | 6f28022ec0f456334edf06cd17d824685e9bfd89 | 2021-12-10T23:29:23.000Z | [
"pytorch",
"gpt2",
"text-generation",
"transformers",
"conversational"
] | conversational | false | vlco-o | null | vlco-o/NLboto_o-small-dialogpt | 0 | null | transformers | 36,244 | ---
tags:
- conversational
---
# NLboto_o model |
vneralla/xlrs-53-finnish | cdac12fbcbee86ac8a447793db62db9016818e53 | 2022-03-08T08:59:34.000Z | [
"pytorch",
"jax",
"wav2vec2",
"pretraining",
"multilingual",
"dataset:common_voice",
"arxiv:2006.13979",
"transformers",
"speech",
"automatic-speech-recognition",
"license:apache-2.0"
] | automatic-speech-recognition | false | vneralla | null | vneralla/xlrs-53-finnish | 0 | null | transformers | 36,245 | ---
language: multilingual
datasets:
- common_voice
tags:
- speech
- automatic-speech-recognition
license: apache-2.0
---
# Wav2Vec2-XLSR-53
[Facebook's XLSR-Wav2Vec2](https://ai.facebook.com/blog/wav2vec-20-learning-the-structure-of-speech-from-raw-audio/)
The base model pretrained on 16kHz sampled speech audio. When using the model make sure that your speech input is also sampled at 16Khz. Note that this model should be fine-tuned on a downstream task, like Automatic Speech Recognition. Check out [this blog](https://huggingface.co/blog/fine-tune-wav2vec2-english) for more information.
[Paper](https://arxiv.org/abs/2006.13979)
Authors: Alexis Conneau, Alexei Baevski, Ronan Collobert, Abdelrahman Mohamed, Michael Auli
**Abstract**
This paper presents XLSR which learns cross-lingual speech representations by pretraining a single model from the raw waveform of speech in multiple languages. We build on wav2vec 2.0 which is trained by solving a contrastive task over masked latent speech representations and jointly learns a quantization of the latents shared across languages. The resulting model is fine-tuned on labeled data and experiments show that cross-lingual pretraining significantly outperforms monolingual pretraining. On the CommonVoice benchmark, XLSR shows a relative phoneme error rate reduction of 72% compared to the best known results. On BABEL, our approach improves word error rate by 16% relative compared to a comparable system. Our approach enables a single multilingual speech recognition model which is competitive to strong individual models. Analysis shows that the latent discrete speech representations are shared across languages with increased sharing for related languages. We hope to catalyze research in low-resource speech understanding by releasing XLSR-53, a large model pretrained in 53 languages.
The original model can be found under https://github.com/pytorch/fairseq/tree/master/examples/wav2vec#wav2vec-20.
# Usage
See [this notebook](https://colab.research.google.com/github/patrickvonplaten/notebooks/blob/master/Fine_Tune_XLSR_Wav2Vec2_on_Turkish_ASR_with_%F0%9F%A4%97_Transformers.ipynb) for more information on how to fine-tune the model.
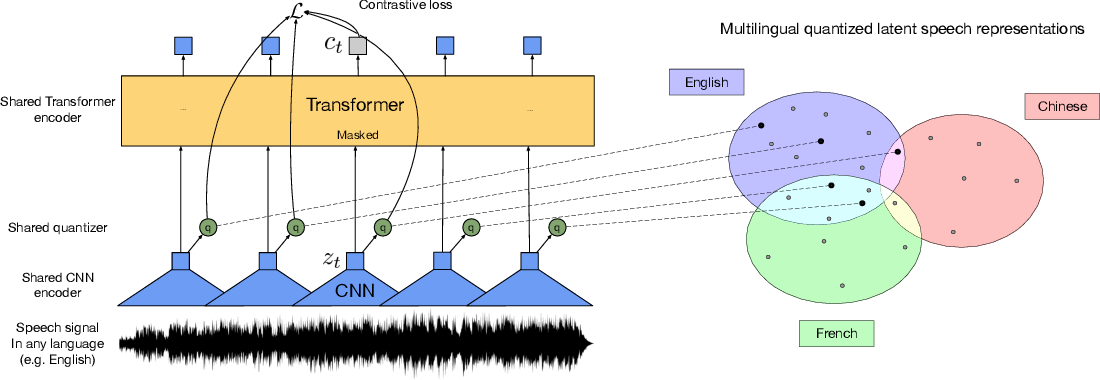
|
vocab-transformers/dense_encoder-msmarco-bert-base-word2vec256k_emb_updated | 1474220b3b21ae781efeb3398e74455eb4409446 | 2022-02-21T20:13:25.000Z | [
"pytorch",
"bert",
"feature-extraction",
"sentence-transformers",
"sentence-similarity",
"transformers"
] | sentence-similarity | false | vocab-transformers | null | vocab-transformers/dense_encoder-msmarco-bert-base-word2vec256k_emb_updated | 0 | null | sentence-transformers | 36,246 | ---
pipeline_tag: sentence-similarity
tags:
- sentence-transformers
- feature-extraction
- sentence-similarity
- transformers
---
# dense_encoder-msmarco-bert-base-word2vec256k
**Note: Token embeddings where updated!**
This model is based on [msmarco-word2vec256000-bert-base-uncased](https://huggingface.co/nicoladecao/msmarco-word2vec256000-bert-base-uncased) with a 256k sized vocabulary initialized with word2vec.
It has been trained on MS MARCO using [MarginMSELoss](https://github.com/UKPLab/sentence-transformers/blob/master/examples/training/ms_marco/train_bi-encoder_margin-mse.py). See the train_script.py in this repository.
Performance:
- MS MARCO dev: (evaluating) (MRR@10)
- TREC-DL 2019: 67.56 (nDCG@10)
- TREC-DL 2020: 71.26 (nDCG@10)
## Usage (Sentence-Transformers)
This is a [sentence-transformers](https://www.SBERT.net) model: It maps sentences & paragraphs to a 768 dimensional dense vector space and can be used for tasks like clustering or semantic search.
<!--- Describe your model here -->
Using this model becomes easy when you have [sentence-transformers](https://www.SBERT.net) installed:
```
pip install -U sentence-transformers
```
Then you can use the model like this:
```python
from sentence_transformers import SentenceTransformer
sentences = ["This is an example sentence", "Each sentence is converted"]
model = SentenceTransformer('{MODEL_NAME}')
embeddings = model.encode(sentences)
print(embeddings)
```
## Usage (HuggingFace Transformers)
Without [sentence-transformers](https://www.SBERT.net), you can use the model like this: First, you pass your input through the transformer model, then you have to apply the right pooling-operation on-top of the contextualized word embeddings.
```python
from transformers import AutoTokenizer, AutoModel
import torch
#Mean Pooling - Take attention mask into account for correct averaging
def mean_pooling(model_output, attention_mask):
token_embeddings = model_output[0] #First element of model_output contains all token embeddings
input_mask_expanded = attention_mask.unsqueeze(-1).expand(token_embeddings.size()).float()
return torch.sum(token_embeddings * input_mask_expanded, 1) / torch.clamp(input_mask_expanded.sum(1), min=1e-9)
# Sentences we want sentence embeddings for
sentences = ['This is an example sentence', 'Each sentence is converted']
# Load model from HuggingFace Hub
tokenizer = AutoTokenizer.from_pretrained('{MODEL_NAME}')
model = AutoModel.from_pretrained('{MODEL_NAME}')
# Tokenize sentences
encoded_input = tokenizer(sentences, padding=True, truncation=True, return_tensors='pt')
# Compute token embeddings
with torch.no_grad():
model_output = model(**encoded_input)
# Perform pooling. In this case, mean pooling.
sentence_embeddings = mean_pooling(model_output, encoded_input['attention_mask'])
print("Sentence embeddings:")
print(sentence_embeddings)
```
## Evaluation Results
<!--- Describe how your model was evaluated -->
For an automated evaluation of this model, see the *Sentence Embeddings Benchmark*: [https://seb.sbert.net](https://seb.sbert.net?model_name={MODEL_NAME})
## Training
The model was trained with the parameters:
**DataLoader**:
`torch.utils.data.dataloader.DataLoader` of length 15716 with parameters:
```
{'batch_size': 32, 'sampler': 'torch.utils.data.sampler.RandomSampler', 'batch_sampler': 'torch.utils.data.sampler.BatchSampler'}
```
**Loss**:
`sentence_transformers.losses.MarginMSELoss.MarginMSELoss`
Parameters of the fit()-Method:
```
{
"epochs": 30,
"evaluation_steps": 0,
"evaluator": "NoneType",
"max_grad_norm": 1,
"optimizer_class": "<class 'transformers.optimization.AdamW'>",
"optimizer_params": {
"lr": 2e-05
},
"scheduler": "WarmupLinear",
"steps_per_epoch": null,
"warmup_steps": 1000,
"weight_decay": 0.01
}
```
## Full Model Architecture
```
SentenceTransformer(
(0): Transformer({'max_seq_length': 250, 'do_lower_case': False}) with Transformer model: BertModel
(1): Pooling({'word_embedding_dimension': 768, 'pooling_mode_cls_token': False, 'pooling_mode_mean_tokens': True, 'pooling_mode_max_tokens': False, 'pooling_mode_mean_sqrt_len_tokens': False})
)
```
## Citing & Authors
<!--- Describe where people can find more information --> |
vocab-transformers/msmarco-distilbert-word2vec256k-MLM_230k | 77b49f5e2fd91fdd9f4e849a8823260dcda4c9fc | 2022-02-22T08:25:00.000Z | [
"pytorch",
"distilbert",
"fill-mask",
"transformers",
"autotrain_compatible"
] | fill-mask | false | vocab-transformers | null | vocab-transformers/msmarco-distilbert-word2vec256k-MLM_230k | 0 | null | transformers | 36,247 | # Model
This model is based on [nicoladecao/msmarco-word2vec256000-distilbert-base-uncased](https://huggingface.co/nicoladecao/msmarco-word2vec256000-distilbert-base-uncased) with a 256k sized vocabulary initialized with word2vec.
This model has been trained with MLM on the MS MARCO corpus collection for 230k steps. See train_mlm.py for the train script. It was run on 2x V100 GPUs. The word embedding matrix was frozen.
|
vocab-transformers/msmarco-distilbert-word2vec256k-MLM_785k_emb_updated | 9b4adde48c4980358bd462ffc7e51597b5c7095f | 2022-02-21T20:12:43.000Z | [
"pytorch",
"distilbert",
"fill-mask",
"transformers",
"autotrain_compatible"
] | fill-mask | false | vocab-transformers | null | vocab-transformers/msmarco-distilbert-word2vec256k-MLM_785k_emb_updated | 0 | null | transformers | 36,248 | # Model
This model is based on [nicoladecao/msmarco-word2vec256000-distilbert-base-uncased](https://huggingface.co/nicoladecao/msmarco-word2vec256000-distilbert-base-uncased) with a 256k sized vocabulary initialized with word2vec.
This model has been trained with MLM on the MS MARCO corpus collection for 785k steps. See train_mlm.py for the train script. It was run on 2x V100 GPUs.
**Note: Token embeddings where updated!**
|
voidful/part-10000 | 3ff430ce7244991b0ab9696273d9efe5caa68a0d | 2022-01-22T16:59:54.000Z | [
"pytorch",
"bart",
"text2text-generation",
"transformers",
"autotrain_compatible"
] | text2text-generation | false | voidful | null | voidful/part-10000 | 0 | null | transformers | 36,249 | Entry not found |
voidful/part-1100000 | 0019b95784fc1d048db441b1d3d5982336cd91f3 | 2022-03-04T15:59:29.000Z | [
"pytorch",
"bart",
"feature-extraction",
"transformers",
"license:afl-3.0"
] | feature-extraction | false | voidful | null | voidful/part-1100000 | 0 | null | transformers | 36,250 | ---
license: afl-3.0
---
|
vppvgit/Finetuned | 39be53d68647763f27403bada83e9443f8553350 | 2021-11-18T15:17:35.000Z | [
"pytorch",
"bert",
"fill-mask",
"transformers",
"generated_from_trainer",
"model-index",
"autotrain_compatible"
] | fill-mask | false | vppvgit | null | vppvgit/Finetuned | 0 | null | transformers | 36,251 | ---
tags:
- generated_from_trainer
datasets:
- null
model-index:
- name: BibliBERT
results:
- task:
name: Masked Language Modeling
type: fill-mask
---
<!-- This model card has been generated automatically according to the information the Trainer had access to. You
should probably proofread and complete it, then remove this comment. -->
# BibliBERT
This model is a fine-tuned version of [dbmdz/bert-base-italian-xxl-cased](https://huggingface.co/dbmdz/bert-base-italian-xxl-cased) on the None dataset.
It achieves the following results on the evaluation set:
- Loss: 0.7784
## Model description
More information needed
## Intended uses & limitations
More information needed
## Training and evaluation data
More information needed
## Training procedure
### Training hyperparameters
The following hyperparameters were used during training:
- learning_rate: 2e-05
- train_batch_size: 8
- eval_batch_size: 8
- seed: 0
- optimizer: Adam with betas=(0.9,0.999) and epsilon=1e-08
- lr_scheduler_type: linear
- num_epochs: 50
### Training results
| Training Loss | Epoch | Step | Validation Loss |
|:-------------:|:-----:|:------:|:---------------:|
| 1.5764 | 1.0 | 16528 | 1.5214 |
| 1.4572 | 2.0 | 33056 | 1.4201 |
| 1.3787 | 3.0 | 49584 | 1.3728 |
| 1.3451 | 4.0 | 66112 | 1.3245 |
| 1.3066 | 5.0 | 82640 | 1.2614 |
| 1.2447 | 6.0 | 99168 | 1.2333 |
| 1.2172 | 7.0 | 115696 | 1.2149 |
| 1.2079 | 8.0 | 132224 | 1.1853 |
| 1.2167 | 9.0 | 148752 | 1.1586 |
| 1.2056 | 10.0 | 165280 | 1.1503 |
| 1.1307 | 11.0 | 181808 | 1.1224 |
| 1.1689 | 12.0 | 198336 | 1.1074 |
| 1.1007 | 13.0 | 214864 | 1.0924 |
| 1.0901 | 14.0 | 231392 | 1.0659 |
| 1.0667 | 15.0 | 247920 | 1.0650 |
| 1.0434 | 16.0 | 264448 | 1.0362 |
| 1.0333 | 17.0 | 280976 | 1.0250 |
| 1.0342 | 18.0 | 297504 | 1.0198 |
| 1.0059 | 19.0 | 314032 | 0.9950 |
| 0.9719 | 20.0 | 330560 | 0.9836 |
| 0.9863 | 21.0 | 347088 | 0.9873 |
| 0.9781 | 22.0 | 363616 | 0.9724 |
| 0.9369 | 23.0 | 380144 | 0.9599 |
| 0.9578 | 24.0 | 396672 | 0.9557 |
| 0.9253 | 25.0 | 413200 | 0.9400 |
| 0.9441 | 26.0 | 429728 | 0.9222 |
| 0.9138 | 27.0 | 446256 | 0.9140 |
| 0.882 | 28.0 | 462784 | 0.9045 |
| 0.864 | 29.0 | 479312 | 0.8880 |
| 0.8632 | 30.0 | 495840 | 0.9023 |
| 0.8342 | 32.0 | 528896 | 0.8740 |
| 0.8037 | 34.0 | 561952 | 0.8647 |
| 0.8119 | 37.0 | 611536 | 0.8358 |
| 0.8011 | 38.0 | 628064 | 0.8252 |
| 0.786 | 39.0 | 644592 | 0.8228 |
| 0.7697 | 41.0 | 677648 | 0.8138 |
| 0.7485 | 42.0 | 694176 | 0.8104 |
| 0.7689 | 43.0 | 710704 | 0.8018 |
| 0.7401 | 45.0 | 743760 | 0.7957 |
| 0.7031 | 47.0 | 776816 | 0.7726 |
| 0.7578 | 48.0 | 793344 | 0.7864 |
| 0.7298 | 49.0 | 809872 | 0.7775 |
| 0.707 | 50.0 | 826400 | 0.7784 |
### Framework versions
- Transformers 4.10.3
- Pytorch 1.9.0+cu102
- Datasets 1.12.1
- Tokenizers 0.10.3
|
vr25/fin_RoBERTa-v1 | 4218aaa689c120e8f20bfaaa0f818d84f4e3a751 | 2021-05-20T23:06:21.000Z | [
"pytorch",
"jax",
"roberta",
"fill-mask",
"transformers",
"autotrain_compatible"
] | fill-mask | false | vr25 | null | vr25/fin_RoBERTa-v1 | 0 | null | transformers | 36,252 | Entry not found |
vuiseng9/bert-base-squadv1-block-pruning-hybrid-filled-lt-nncf-57.92sparse-lt | 41d4286f142fef9dc5d5ffad1711ea963e22b525 | 2022-01-18T17:45:15.000Z | [
"pytorch",
"onnx",
"bert",
"transformers"
] | null | false | vuiseng9 | null | vuiseng9/bert-base-squadv1-block-pruning-hybrid-filled-lt-nncf-57.92sparse-lt | 0 | null | transformers | 36,253 | This model is a downstream optimization of [```vuiseng9/bert-base-squadv1-block-pruning-hybrid-filled-lt```](https://huggingface.co/vuiseng9/bert-base-squadv1-block-pruning-hybrid-filled-lt) using [OpenVINO/NNCF](https://github.com/openvinotoolkit/nncf). Applied optimization includes:
1. magnitude sparsification at 57.92% upon initialization so that sparsity over all linear layers of bert-base is at 90%. Parameters are ranked globally via thier absolute norm. Only linear layers of self-attention and ffnn are targeted.
2. Custom distillation with large model ```bert-large-uncased-whole-word-masking-finetuned-squad```
```
eval_exact_match = 80.4447
eval_f1 = 87.7678
eval_samples = 10784
```
# Setup
```bash
# OpenVINO/NNCF
git clone https://github.com/vuiseng9/nncf && cd nncf
git checkout tld-poc
git reset --hard 1dec7afe7a4b567c059fcf287ea2c234980fded2
python setup.py develop
pip install -r examples/torch/requirements.txt
# Huggingface nn_pruning
git clone https://github.com/vuiseng9/nn_pruning && cd nn_pruning
git checkout reproduce-evaluation
git reset --hard 2d4e196d694c465e43e5fbce6c3836d0a60e1446
pip install -e ".[dev]"
# Huggingface Transformers
git clone https://github.com/vuiseng9/transformers && cd transformers
git checkout tld-poc
git reset --hard 10a1e29d84484e48fd106f58957d9ffc89dc43c5
pip install -e .
head -n 1 examples/pytorch/question-answering/requirements.txt | xargs -i pip install {}
# Additional dependencies
pip install onnx
```
# Train
```bash
git clone https://huggingface.co/vuiseng9/bert-base-squadv1-block-pruning-hybrid-filled-lt
BASE_MODEL=/path/to/cloned_repo_above #to-revise
wget https://huggingface.co/vuiseng9/bert-base-squadv1-block-pruning-hybrid-filled-lt-nncf-57.92sparse-lt/raw/main/nncf_bert_squad_sparsity.json
NNCF_CFG=/path/to/downloaded_nncf_cfg_above #to-revise
OUTROOT=/path/to/train_output_root #to-revise
WORKDIR=transformers/examples/pytorch/question-answering #to-revise
RUNID=bert-base-squadv1-block-pruning-hybrid-filled-lt-nncf-57.92sparse-lt
cd $WORKDIR
OUTDIR=$OUTROOT/$RUNID
mkdir -p $OUTDIR
export CUDA_VISIBLE_DEVICES=0
NEPOCH=5
python run_qa.py \
--model_name_or_path vuiseng9/bert-base-squadv1-block-pruning-hybrid \
--optimize_model_before_eval \
--optimized_checkpoint $BASE_MODEL \
--dataset_name squad \
--do_eval \
--do_train \
--evaluation_strategy steps \
--eval_steps 250 \
--learning_rate 3e-5 \
--lr_scheduler_type cosine_with_restarts \
--warmup_ratio 0.25 \
--cosine_cycles 1 \
--teacher bert-large-uncased-whole-word-masking-finetuned-squad \
--teacher_ratio 0.9 \
--num_train_epochs $NEPOCH \
--per_device_eval_batch_size 128 \
--per_device_train_batch_size 16 \
--max_seq_length 384 \
--doc_stride 128 \
--save_steps 250 \
--nncf_config $NNCF_CFG \
--logging_steps 1 \
--overwrite_output_dir \
--run_name $RUNID \
--output_dir $OUTDIR
```
# Eval
This repo must be cloned locally.
```bash
git clone https://huggingface.co/vuiseng9/bert-base-squadv1-block-pruning-hybrid-filled-lt-nncf-57.92sparse-lt
MODELROOT=/path/to/cloned_repo_above #to-revise
export CUDA_VISIBLE_DEVICES=0
OUTDIR=eval-bert-base-squadv1-block-pruning-hybrid-filled-lt-nncf-57.92sparse-lt
WORKDIR=transformers/examples/pytorch/question-answering #to-revise
cd $WORKDIR
mkdir $OUTDIR
nohup python run_qa.py \
--model_name_or_path vuiseng9/bert-base-squadv1-block-pruning-hybrid \
--dataset_name squad \
--optimize_model_before_eval \
--qat_checkpoint $MODELROOT/checkpoint-20000 \
--nncf_config $MODELROOT/nncf_bert_squad_sparsity.json \
--to_onnx $OUTDIR/bert-base-squadv1-block-pruning-hybrid-filled-lt-nncf-57.92sparse-lt.onnx \
--do_eval \
--per_device_eval_batch_size 128 \
--max_seq_length 384 \
--doc_stride 128 \
--overwrite_output_dir \
--output_dir $OUTDIR 2>&1 | tee $OUTDIR/run.log &
```
|
vuiseng9/bert-base-squadv1-block-pruning-hybrid-filled-lt-qat-lt | c29e0005529372bdd374205eeff551dbf01956c9 | 2022-02-08T22:58:30.000Z | [
"pytorch",
"onnx",
"bert",
"transformers"
] | null | false | vuiseng9 | null | vuiseng9/bert-base-squadv1-block-pruning-hybrid-filled-lt-qat-lt | 0 | null | transformers | 36,254 | This model is a downstream optimization of [```vuiseng9/bert-base-squadv1-block-pruning-hybrid-filled-lt```](https://huggingface.co/vuiseng9/bert-base-squadv1-block-pruning-hybrid-filled-lt) using [OpenVINO/NNCF](https://github.com/openvinotoolkit/nncf). Applied optimization includes:
1. NNCF Quantize-Aware Training - Symmetric 8-bit for both weight and activation on all learnable layers.
2. Custom distillation with large model ```bert-large-uncased-whole-word-masking-finetuned-squad```
```
eval_exact_match = 80.7001
eval_f1 = 87.9777
eval_samples = 10784
```
# Setup
```bash
# OpenVINO/NNCF
git clone https://github.com/vuiseng9/nncf && cd nncf
git checkout tld-poc
git reset --hard 1dec7afe7a4b567c059fcf287ea2c234980fded2
python setup.py develop
pip install -r examples/torch/requirements.txt
# Huggingface nn_pruning
git clone https://github.com/vuiseng9/nn_pruning && cd nn_pruning
git checkout reproduce-evaluation
git reset --hard 2d4e196d694c465e43e5fbce6c3836d0a60e1446
pip install -e ".[dev]"
# Huggingface Transformers
git clone https://github.com/vuiseng9/transformers && cd transformers
git checkout tld-poc
git reset --hard 10a1e29d84484e48fd106f58957d9ffc89dc43c5
pip install -e .
head -n 1 examples/pytorch/question-answering/requirements.txt | xargs -i pip install {}
# Additional dependencies
pip install onnx
```
# Train
```bash
git clone https://huggingface.co/vuiseng9/bert-base-squadv1-block-pruning-hybrid-filled-lt
BASE_MODEL=/path/to/cloned_repo_above #to-revise
wget https://huggingface.co/vuiseng9/bert-base-squadv1-block-pruning-hybrid-filled-lt-qat-lt/raw/main/nncf_bert_squad_qat.json
NNCF_CFG=/path/to/downloaded_nncf_cfg_above #to-revise
OUTROOT=/path/to/train_output_root #to-revise
WORKDIR=transformers/examples/pytorch/question-answering #to-revise
RUNID=bert-base-squadv1-block-pruning-hybrid-filled-lt-qat-lt
cd $WORKDIR
OUTDIR=$OUTROOT/$RUNID
mkdir -p $OUTDIR
export CUDA_VISIBLE_DEVICES=0
NEPOCH=5
python run_qa.py \
--model_name_or_path vuiseng9/bert-base-squadv1-block-pruning-hybrid \
--optimize_model_before_eval \
--optimized_checkpoint $BASE_MODEL \
--dataset_name squad \
--do_eval \
--do_train \
--evaluation_strategy steps \
--eval_steps 250 \
--learning_rate 3e-5 \
--lr_scheduler_type cosine_with_restarts \
--warmup_ratio 0.25 \
--cosine_cycles 1 \
--teacher bert-large-uncased-whole-word-masking-finetuned-squad \
--teacher_ratio 0.9 \
--num_train_epochs $NEPOCH \
--per_device_eval_batch_size 128 \
--per_device_train_batch_size 16 \
--max_seq_length 384 \
--doc_stride 128 \
--save_steps 250 \
--nncf_config $NNCF_CFG \
--logging_steps 1 \
--overwrite_output_dir \
--run_name $RUNID \
--output_dir $OUTDIR
```
# Eval
This repo must be cloned locally.
```bash
git clone https://huggingface.co/vuiseng9/bert-base-squadv1-block-pruning-hybrid-filled-lt-qat-lt
MODELROOT=/path/to/cloned_repo_above #to-revise
export CUDA_VISIBLE_DEVICES=0
OUTDIR=eval-bert-base-squadv1-block-pruning-hybrid-filled-lt-qat-lt
WORKDIR=transformers/examples/pytorch/question-answering #to-revise
cd $WORKDIR
mkdir $OUTDIR
nohup python run_qa.py \
--model_name_or_path vuiseng9/bert-base-squadv1-block-pruning-hybrid \
--dataset_name squad \
--optimize_model_before_eval \
--qat_checkpoint $MODELROOT/checkpoint-26750 \
--nncf_config $MODELROOT/nncf_bert_squad_qat.json \
--to_onnx $OUTDIR/bert-base-squadv1-block-pruning-hybrid-filled-lt-qat-lt.onnx \
--do_eval \
--per_device_eval_batch_size 128 \
--max_seq_length 384 \
--doc_stride 128 \
--overwrite_output_dir \
--output_dir $OUTDIR 2>&1 | tee $OUTDIR/run.log &
```
### tile-alignment
to evaluate tile-alignment checkpoint, add ```--tile_alignment``` and point ```--qat_checkpoint``` to checkpoint with 'tilealigned' postfix. Use branch ```tld-poc``` with commit id ```c525c52cq``` |
vuiseng9/bert-base-squadv1-block-pruning-hybrid-filled-lt | 37ca5f0ad8a7c8000512aa5e7e3776b68803debd | 2022-01-09T03:11:21.000Z | [
"pytorch",
"bert",
"question-answering",
"arxiv:2109.04838",
"transformers",
"autotrain_compatible"
] | question-answering | false | vuiseng9 | null | vuiseng9/bert-base-squadv1-block-pruning-hybrid-filled-lt | 0 | null | transformers | 36,255 | This model is a downstream fine-tuning of [```vuiseng9/bert-base-squadv1-block-pruning-hybrid```](https://huggingface.co/vuiseng9/bert-base-squadv1-block-pruning-hybrid). "filled" means unstructured fine-grained sparsified parameters are allowed to learn during fine-tuning. "lt" means distillation of larger model as teacher, i.e. ```bert-large-uncased-whole-word-masking-finetuned-squad```
```
eval_exact_match = 80.3311
eval_f1 = 87.69
eval_samples = 10784
```
This model is a replication of [block pruning paper](https://arxiv.org/abs/2109.04838) with its open-sourced codebase (forked and modified).
To reproduce this model, pls follow [documentation here](https://github.com/vuiseng9/nn_pruning/blob/reproduce-evaluation/reproduce-eval/readme.md) until step 3.
# Eval
The model cannot be evaluated with HF QA example out-of-the-box as the final dimension of the model architecture has been realized. Follow the custom setup below.
```bash
# OpenVINO/NNCF
git clone https://github.com/vuiseng9/nncf && cd nncf
git checkout tld-poc
git reset --hard 1dec7afe7a4b567c059fcf287ea2c234980fded2
python setup.py develop
pip install -r examples/torch/requirements.txt
# Huggingface nn_pruning
git clone https://github.com/vuiseng9/nn_pruning && cd nn_pruning
git checkout reproduce-evaluation
git reset --hard 2d4e196d694c465e43e5fbce6c3836d0a60e1446
pip install -e ".[dev]"
# Huggingface Transformers
git clone https://github.com/vuiseng9/transformers && cd transformers
git checkout tld-poc
git reset --hard 10a1e29d84484e48fd106f58957d9ffc89dc43c5
pip install -e .
head -n 1 examples/pytorch/question-answering/requirements.txt | xargs -i pip install {}
```
This repo must be cloned locally.
```bash
git clone https://huggingface.co/vuiseng9/bert-base-squadv1-block-pruning-hybrid-filled-lt
```
Add ```--optimize_model_before_eval``` and ```--optimized_checkpoint /path/to/clone``` during evaluation.
```bash
export CUDA_VISIBLE_DEVICES=0
OUTDIR=eval-bert-base-squadv1-block-pruning-hybrid-filled-lt-cropped
WORKDIR=transformers/examples/pytorch/question-answering
cd $WORKDIR
mkdir $OUTDIR
nohup python run_qa.py \
--model_name_or_path vuiseng9/bert-base-squadv1-block-pruning-hybrid \
--dataset_name squad \
--optimize_model_before_eval \
--optimized_checkpoint /path/to/clone/bert-base-squadv1-block-pruning-hybrid-filled-lt \
--do_eval \
--per_device_eval_batch_size 128 \
--max_seq_length 384 \
--doc_stride 128 \
--overwrite_output_dir \
--output_dir $OUTDIR 2>&1 | tee $OUTDIR/run.log &
```
|
vuiseng9/bert-base-squadv1-block-pruning-hybrid | 346f622a12af43f4b8ad86a56ef209fc9e4788c4 | 2022-01-09T03:12:11.000Z | [
"pytorch",
"bert",
"question-answering",
"arxiv:2109.04838",
"transformers",
"autotrain_compatible"
] | question-answering | false | vuiseng9 | null | vuiseng9/bert-base-squadv1-block-pruning-hybrid | 0 | null | transformers | 36,256 | BERT-base tuned for Squadv1.1 is pruned with movement pruning algorithm in hybrid fashion, i.e. 32x32 block for self-attention layers, per-dimension grain size for ffn layers.
```
eval_exact_match = 78.5241
eval_f1 = 86.4138
eval_samples = 10784
```
This model is a replication of [block pruning paper](https://arxiv.org/abs/2109.04838) with its open-sourced codebase (forked and modified).
To reproduce this model, pls follow [documentation here](https://github.com/vuiseng9/nn_pruning/blob/reproduce-evaluation/reproduce-eval/readme.md) until step 2.
# Eval
The model can be evaluated out-of-the-box with HF QA example. Note that only pruned self-attention heads are discarded where pruned ffn dimension are sparsified instead of removal. Verified in v4.13.0, v4.9.1.
```bash
export CUDA_VISIBLE_DEVICES=0
OUTDIR=eval-bert-base-squadv1-block-pruning-hybrid
WORKDIR=transformers/examples/pytorch/question-answering
cd $WORKDIR
mkdir $OUTDIR
nohup python run_qa.py \
--model_name_or_path vuiseng9/bert-base-squadv1-block-pruning-hybrid \
--dataset_name squad \
--do_eval \
--per_device_eval_batch_size 16 \
--max_seq_length 384 \
--doc_stride 128 \
--overwrite_output_dir \
--output_dir $OUTDIR 2>&1 | tee $OUTDIR/run.log &
```
If the intent is to observe inference acceleration, the pruned structure in the model must be "cropped"/discarded. Follow the custom setup below.
```bash
# OpenVINO/NNCF
git clone https://github.com/vuiseng9/nncf && cd nncf
git checkout tld-poc
git reset --hard 1dec7afe7a4b567c059fcf287ea2c234980fded2
python setup.py develop
pip install -r examples/torch/requirements.txt
# Huggingface nn_pruning
git clone https://github.com/vuiseng9/nn_pruning && cd nn_pruning
git checkout reproduce-evaluation
git reset --hard 2d4e196d694c465e43e5fbce6c3836d0a60e1446
pip install -e ".[dev]"
# Huggingface Transformers
git clone https://github.com/vuiseng9/transformers && cd transformers
git checkout tld-poc
git reset --hard 10a1e29d84484e48fd106f58957d9ffc89dc43c5
pip install -e .
head -n 1 examples/pytorch/question-answering/requirements.txt | xargs -i pip install {}
```
Add ```--optimize_model_before_eval``` during evaluation.
```bash
export CUDA_VISIBLE_DEVICES=0
OUTDIR=eval-bert-base-squadv1-block-pruning-hybrid-cropped
WORKDIR=transformers/examples/pytorch/question-answering
cd $WORKDIR
mkdir $OUTDIR
nohup python run_qa.py \
--model_name_or_path vuiseng9/bert-base-squadv1-block-pruning-hybrid \
--dataset_name squad \
--optimize_model_before_eval \
--do_eval \
--per_device_eval_batch_size 128 \
--max_seq_length 384 \
--doc_stride 128 \
--overwrite_output_dir \
--output_dir $OUTDIR 2>&1 | tee $OUTDIR/run.log &
```
|
vuiseng9/bert-base-uncased-squadv1-85.4-sparse | 6b75846ad406107255044e5b4ed78290d215c506 | 2021-11-11T18:13:01.000Z | [
"pytorch",
"tf",
"bert",
"transformers"
] | null | false | vuiseng9 | null | vuiseng9/bert-base-uncased-squadv1-85.4-sparse | 0 | null | transformers | 36,257 | * A set of unstructured sparse bert-base-uncased models fine-tuned for SQuADv1.
* Tensorflow models are created using ```TFAutoModelForQuestionAnswering.from_pretrained(..., from_pt=True)``` and ```model.save_pretrained(tf_pth)```.
* Observed issue - loss in model translation, discrepancy observed in evaluation between pytorch and tensorflow models.
* Table below is evaluated in HF's transformers v4.9.2. Sparsity is normalized to dense layers in attention heads and FFNN.
* Evaluation cli:
```bash
python run_qa.py \
--model_name_or_path <model identifier> \
--dataset_name squad \
--do_eval \
--per_device_eval_batch_size 384 \
--max_seq_length 68 \
--doc_stride 26 \
--output_dir /tmp/eval-squad
```
| | HF Model Hub Identifier | sparsity | em (pytorch) | em (tf) | f1 (pytorch) | f1 (tf) |
|---:|:------------------------------------------------------------------------------------------------------------------------|-----------:|---------------:|----------:|---------------:|----------:|
| 0 | [vuiseng9/bert-base-uncased-squadv1-85.4-sparse](https://huggingface.co/vuiseng9/bert-base-uncased-squadv1-85.4-sparse) | 85.4 | 69.9338 | 14.2573 | 77.6861 | 23.4917 |
| 1 | [vuiseng9/bert-base-uncased-squadv1-72.9-sparse](https://huggingface.co/vuiseng9/bert-base-uncased-squadv1-72.9-sparse) | 72.9 | 74.6358 | 31.0596 | 82.2555 | 39.8446 |
| 2 | [vuiseng9/bert-base-uncased-squadv1-65.1-sparse](https://huggingface.co/vuiseng9/bert-base-uncased-squadv1-65.1-sparse) | 65.1 | 76.1306 | 43.0274 | 83.4117 | 51.4300 |
| 3 | [vuiseng9/bert-base-uncased-squadv1-59.6-sparse](https://huggingface.co/vuiseng9/bert-base-uncased-squadv1-59.6-sparse) | 59.6 | 76.8590 | 50.4920 | 84.1267 | 59.0881 |
| 4 | [vuiseng9/bert-base-uncased-squadv1-52.0-sparse](https://huggingface.co/vuiseng9/bert-base-uncased-squadv1-52.0-sparse) | 52.0 | 78.0038 | 54.2857 | 85.2000 | 62.2914 | |
vvn/en-to-it-marianmt | 7eca316f445ea1fd14ab7a5bdc05c20b97a6a68c | 2021-07-27T16:50:28.000Z | [
"pytorch",
"marian",
"text2text-generation",
"transformers",
"autotrain_compatible"
] | text2text-generation | false | vvn | null | vvn/en-to-it-marianmt | 0 | null | transformers | 36,258 | Fine-Tuned MarianMT translation model for translating text from English to Italian.
Checkpoint of pre-trained model = Helsinki-NLP/opus-mt-en-it.
Trained using custom training loop with PyTorch on Colab for 2 epochs.
Link to the GitHub repo containing Google Colab notebook: https://github.com/vanadnarayane26/Maverick_2.0_Translation_layer/blob/main/En_to_it_marianmt.ipynb |
w11wo/lao-roberta-base-pos-tagger | 65f510328cf3faf09034dc417ad5257492ba03c4 | 2021-12-07T05:14:57.000Z | [
"pytorch",
"roberta",
"token-classification",
"lo",
"arxiv:1907.11692",
"transformers",
"lao-roberta-base-pos-tagger",
"license:mit",
"autotrain_compatible"
] | token-classification | false | w11wo | null | w11wo/lao-roberta-base-pos-tagger | 0 | null | transformers | 36,259 | ---
language: lo
tags:
- lao-roberta-base-pos-tagger
license: mit
widget:
- text: "ຮ້ອງ ມ່ວນ ແທ້ ສຽງດີ ອິຫຼີ"
---
## Lao RoBERTa Base POS Tagger
Lao RoBERTa Base POS Tagger is a part-of-speech token-classification model based on the [RoBERTa](https://arxiv.org/abs/1907.11692) model. The model was originally the pre-trained [Lao RoBERTa Base](https://huggingface.co/w11wo/lao-roberta-base) model, which is then fine-tuned on the [`Yunshan Cup 2020`](https://github.com/GKLMIP/Yunshan-Cup-2020) dataset consisting of tag-labelled Lao corpus.
After training, the model achieved an evaluation accuracy of 83.14%. On the benchmark test set, the model achieved an accuracy of 83.30%.
Hugging Face's `Trainer` class from the [Transformers](https://huggingface.co/transformers) library was used to train the model. PyTorch was used as the backend framework during training, but the model remains compatible with other frameworks nonetheless.
## Model
| Model | #params | Arch. | Training/Validation data (text) |
| ----------------------------- | ------- | ------------ | ------------------------------- |
| `lao-roberta-base-pos-tagger` | 124M | RoBERTa Base | `Yunshan Cup 2020` |
## Evaluation Results
The model was trained for 15 epochs, with a batch size of 8, a learning rate of 5e-5, with cosine annealing to 0. The best model was loaded at the end.
| Epoch | Training Loss | Validation Loss | Accuracy |
| ----- | ------------- | --------------- | -------- |
| 1 | 1.026100 | 0.733780 | 0.746021 |
| 2 | 0.646900 | 0.659625 | 0.775688 |
| 3 | 0.500400 | 0.576214 | 0.798523 |
| 4 | 0.385400 | 0.606503 | 0.805269 |
| 5 | 0.288000 | 0.652493 | 0.809092 |
| 6 | 0.204600 | 0.671678 | 0.815216 |
| 7 | 0.145200 | 0.704693 | 0.818209 |
| 8 | 0.098700 | 0.830561 | 0.816998 |
| 9 | 0.066100 | 0.883329 | 0.825232 |
| 10 | 0.043900 | 0.933347 | 0.825664 |
| 11 | 0.027200 | 0.992055 | 0.828449 |
| 12 | 0.017300 | 1.054874 | 0.830819 |
| 13 | 0.011500 | 1.081638 | 0.830940 |
| 14 | 0.008500 | 1.094252 | 0.831304 |
| 15 | 0.007400 | 1.097428 | 0.831442 |
## How to Use
### As Token Classifier
```python
from transformers import pipeline
pretrained_name = "w11wo/lao-roberta-base-pos-tagger"
nlp = pipeline(
"token-classification",
model=pretrained_name,
tokenizer=pretrained_name
)
nlp("ຮ້ອງ ມ່ວນ ແທ້ ສຽງດີ ອິຫຼີ")
```
## Disclaimer
Do consider the biases which come from both the pre-trained RoBERTa model and the `Yunshan Cup 2020` dataset that may be carried over into the results of this model.
## Author
Lao RoBERTa Base POS Tagger was trained and evaluated by [Wilson Wongso](https://w11wo.github.io/). All computation and development are done on Google Colaboratory using their free GPU access.
|
wadeed/DialogGPT-small-chandlerbingg | b5182c72f0273c99e1f7b20fb71420c4d5d548bc | 2021-12-10T12:25:54.000Z | [
"pytorch",
"gpt2",
"text-generation",
"transformers",
"conversational"
] | conversational | false | wadeed | null | wadeed/DialogGPT-small-chandlerbingg | 0 | null | transformers | 36,260 | ---
tags:
- conversational
---
#Chandler Bing DialoGPT Model |
wbmitcast/mymode03 | aaf3ad3feabb8c2a5681d045ba4b8b7879853760 | 2021-10-06T09:04:11.000Z | [
"pytorch",
"bert",
"fill-mask",
"transformers",
"autotrain_compatible"
] | fill-mask | false | wbmitcast | null | wbmitcast/mymode03 | 0 | null | transformers | 36,261 | Entry not found |
wbmitcast/mymodel04 | 598d680943405e4b28625b74fb921a8fb05ca91a | 2021-10-06T11:24:48.000Z | [
"pytorch",
"bert",
"fill-mask",
"transformers",
"autotrain_compatible"
] | fill-mask | false | wbmitcast | null | wbmitcast/mymodel04 | 0 | null | transformers | 36,262 | Entry not found |
we-are-groot/narrative_gen | f217239cf6bae7e38159d1d2f0fe089e57e5b8cb | 2022-02-16T16:18:11.000Z | [
"pytorch",
"t5",
"text2text-generation",
"transformers",
"autotrain_compatible"
] | text2text-generation | false | we-are-groot | null | we-are-groot/narrative_gen | 0 | null | transformers | 36,263 | Entry not found |
webshell/wav2vec2-base-fine-tune-timit | 10c2d7c65c293fb248f34bb8db0ce5b1f84ee8d2 | 2021-12-10T09:58:10.000Z | [
"pytorch",
"tensorboard",
"wav2vec2",
"automatic-speech-recognition",
"transformers",
"generated_from_trainer",
"license:apache-2.0",
"model-index"
] | automatic-speech-recognition | false | webshell | null | webshell/wav2vec2-base-fine-tune-timit | 0 | null | transformers | 36,264 | ---
license: apache-2.0
tags:
- generated_from_trainer
model-index:
- name: wav2vec2-base-fine-tune-timit
results: []
---
<!-- This model card has been generated automatically according to the information the Trainer had access to. You
should probably proofread and complete it, then remove this comment. -->
# wav2vec2-base-fine-tune-timit
This model is a fine-tuned version of [facebook/wav2vec2-base](https://huggingface.co/facebook/wav2vec2-base) on the None dataset.
It achieves the following results on the evaluation set:
- Loss: 0.4451
- Wer: 0.3422
## Model description
More information needed
## Intended uses & limitations
More information needed
## Training and evaluation data
More information needed
## Training procedure
### Training hyperparameters
The following hyperparameters were used during training:
- learning_rate: 0.0001
- train_batch_size: 32
- eval_batch_size: 8
- seed: 42
- optimizer: Adam with betas=(0.9,0.999) and epsilon=1e-08
- lr_scheduler_type: linear
- lr_scheduler_warmup_steps: 1000
- num_epochs: 30
- mixed_precision_training: Native AMP
### Training results
| Training Loss | Epoch | Step | Validation Loss | Wer |
|:-------------:|:-----:|:----:|:---------------:|:------:|
| 3.6487 | 4.0 | 500 | 1.9065 | 1.0411 |
| 0.8742 | 8.0 | 1000 | 0.4658 | 0.4720 |
| 0.3084 | 12.0 | 1500 | 0.4367 | 0.4010 |
| 0.1825 | 16.0 | 2000 | 0.4403 | 0.3817 |
| 0.1334 | 20.0 | 2500 | 0.4577 | 0.3625 |
| 0.1114 | 24.0 | 3000 | 0.4456 | 0.3537 |
| 0.0835 | 28.0 | 3500 | 0.4451 | 0.3422 |
### Framework versions
- Transformers 4.11.3
- Pytorch 1.10.0+cu111
- Datasets 1.13.3
- Tokenizers 0.10.3
|
wenrenbutong/model_name1 | cd0b3a11795e665b87a28fbabc8bb4d9bbee7e08 | 2021-07-18T09:41:24.000Z | [
"pytorch",
"bert",
"fill-mask",
"transformers",
"autotrain_compatible"
] | fill-mask | false | wenrenbutong | null | wenrenbutong/model_name1 | 0 | null | transformers | 36,265 | Entry not found |
wesam266/wav2vec2-large-xlsr-53_english | 738cc7d6c99790623a74148847ebbc1c7ca1482c | 2022-01-23T02:40:28.000Z | [
"pytorch",
"wav2vec2",
"automatic-speech-recognition",
"transformers",
"generated_from_trainer",
"license:apache-2.0",
"model-index"
] | automatic-speech-recognition | false | wesam266 | null | wesam266/wav2vec2-large-xlsr-53_english | 0 | null | transformers | 36,266 | ---
license: apache-2.0
tags:
- generated_from_trainer
model-index:
- name: wav2vec2-large-xlsr-53_english
results: []
---
<!-- This model card has been generated automatically according to the information the Trainer had access to. You
should probably proofread and complete it, then remove this comment. -->
# wav2vec2-large-xlsr-53_english
This model is a fine-tuned version of [facebook/wav2vec2-large-xlsr-53](https://huggingface.co/facebook/wav2vec2-large-xlsr-53) on the None dataset.
It achieves the following results on the evaluation set:
- Loss: 0.2620
- Wer: 0.1916
## Model description
More information needed
## Intended uses & limitations
More information needed
## Training and evaluation data
More information needed
## Training procedure
### Training hyperparameters
The following hyperparameters were used during training:
- learning_rate: 0.0005
- train_batch_size: 32
- eval_batch_size: 8
- seed: 42
- gradient_accumulation_steps: 2
- total_train_batch_size: 64
- optimizer: Adam with betas=(0.9,0.999) and epsilon=1e-08
- lr_scheduler_type: linear
- lr_scheduler_warmup_steps: 500
- num_epochs: 5
- mixed_precision_training: Native AMP
### Training results
| Training Loss | Epoch | Step | Validation Loss | Wer |
|:-------------:|:-----:|:-----:|:---------------:|:------:|
| 3.0506 | 0.12 | 250 | 3.0206 | 0.9999 |
| 1.4381 | 0.25 | 500 | 1.0267 | 0.6323 |
| 1.0903 | 0.37 | 750 | 0.5841 | 0.3704 |
| 1.0384 | 0.5 | 1000 | 0.5156 | 0.3348 |
| 0.9658 | 0.62 | 1250 | 0.4721 | 0.3221 |
| 0.9184 | 0.74 | 1500 | 0.4301 | 0.3213 |
| 0.8939 | 0.87 | 1750 | 0.4188 | 0.2884 |
| 0.9051 | 0.99 | 2000 | 0.3852 | 0.2807 |
| 0.563 | 1.12 | 2250 | 0.3752 | 0.2804 |
| 0.6122 | 1.24 | 2500 | 0.3745 | 0.2732 |
| 0.6213 | 1.36 | 2750 | 0.3671 | 0.2575 |
| 0.5839 | 1.49 | 3000 | 0.3560 | 0.2578 |
| 0.615 | 1.61 | 3250 | 0.3555 | 0.2536 |
| 0.5557 | 1.74 | 3500 | 0.3511 | 0.2485 |
| 0.5497 | 1.86 | 3750 | 0.3364 | 0.2425 |
| 0.5412 | 1.98 | 4000 | 0.3253 | 0.2418 |
| 0.2834 | 2.11 | 4250 | 0.3293 | 0.2322 |
| 0.2723 | 2.23 | 4500 | 0.3157 | 0.2322 |
| 0.2713 | 2.35 | 4750 | 0.3148 | 0.2304 |
| 0.2878 | 2.48 | 5000 | 0.3143 | 0.2286 |
| 0.2776 | 2.6 | 5250 | 0.3122 | 0.2250 |
| 0.2553 | 2.73 | 5500 | 0.3003 | 0.2234 |
| 0.278 | 2.85 | 5750 | 0.2973 | 0.2198 |
| 0.2445 | 2.97 | 6000 | 0.2938 | 0.2180 |
| 0.4361 | 3.1 | 6250 | 0.2914 | 0.2132 |
| 0.3979 | 3.22 | 6500 | 0.2916 | 0.2125 |
| 0.4221 | 3.35 | 6750 | 0.2879 | 0.2113 |
| 0.4051 | 3.47 | 7000 | 0.2819 | 0.2100 |
| 0.4218 | 3.59 | 7250 | 0.2812 | 0.2072 |
| 0.4201 | 3.72 | 7500 | 0.2772 | 0.2055 |
| 0.3515 | 3.84 | 7750 | 0.2747 | 0.2031 |
| 0.4021 | 3.97 | 8000 | 0.2702 | 0.2018 |
| 0.4304 | 4.09 | 8250 | 0.2721 | 0.2007 |
| 0.3923 | 4.21 | 8500 | 0.2689 | 0.1991 |
| 0.3824 | 4.34 | 8750 | 0.2692 | 0.1980 |
| 0.3743 | 4.46 | 9000 | 0.2718 | 0.1950 |
| 0.3771 | 4.59 | 9250 | 0.2653 | 0.1950 |
| 0.4048 | 4.71 | 9500 | 0.2649 | 0.1934 |
| 0.3539 | 4.83 | 9750 | 0.2638 | 0.1919 |
| 0.3498 | 4.96 | 10000 | 0.2620 | 0.1916 |
### Framework versions
- Transformers 4.11.3
- Pytorch 1.10.1+cu113
- Datasets 1.17.0
- Tokenizers 0.10.3
|
widyanto/IndoT5-small-qg-hl | 60f5639a9f45b70fb350c45e3210a70f5803be7a | 2021-08-23T13:11:34.000Z | [
"pytorch",
"t5",
"feature-extraction",
"transformers"
] | feature-extraction | false | widyanto | null | widyanto/IndoT5-small-qg-hl | 0 | null | transformers | 36,267 | Entry not found |
wiktor7245/finetuning_m2m_de_pl | ac8fed1f13f8cf04d617154764124bc93d388779 | 2021-10-03T15:15:24.000Z | [
"pytorch",
"m2m_100",
"text2text-generation",
"transformers",
"autotrain_compatible"
] | text2text-generation | false | wiktor7245 | null | wiktor7245/finetuning_m2m_de_pl | 0 | null | transformers | 36,268 | Entry not found |
willemjan/indo1 | 90d31d516e910064681b47a5b8739efbe9f36fc5 | 2022-02-07T09:14:26.000Z | [
"pytorch",
"bert",
"fill-mask",
"transformers",
"license:cc-by-nc-3.0",
"autotrain_compatible"
] | fill-mask | false | willemjan | null | willemjan/indo1 | 0 | null | transformers | 36,269 | ---
license: cc-by-nc-3.0
---
|
willemjan/indo2 | 8ed69bbbdeee9c29de3cbac0c3671c84cd5ee90d | 2022-02-07T09:17:20.000Z | [
"pytorch",
"bert",
"fill-mask",
"transformers",
"license:cc-by-nc-3.0",
"autotrain_compatible"
] | fill-mask | false | willemjan | null | willemjan/indo2 | 0 | null | transformers | 36,270 | ---
license: cc-by-nc-3.0
---
|
willemjan/nl1 | 42a023aa1153bcfca58eea52da16348d65337e2b | 2022-02-07T08:44:23.000Z | [
"pytorch",
"bert",
"fill-mask",
"transformers",
"license:cc-by-nc-3.0",
"autotrain_compatible"
] | fill-mask | false | willemjan | null | willemjan/nl1 | 0 | null | transformers | 36,271 | ---
license: cc-by-nc-3.0
---
|
willemjan/spa | 6707db380d441eb99d1911f99316515f406a0167 | 2022-02-07T09:21:31.000Z | [
"pytorch",
"bert",
"fill-mask",
"transformers",
"license:cc-by-nc-sa-3.0",
"autotrain_compatible"
] | fill-mask | false | willemjan | null | willemjan/spa | 0 | null | transformers | 36,272 | ---
license: cc-by-nc-sa-3.0
---
|
wjc123/qa_finetuned | 19f64440ea49491e85416d203c371fed6bc346d0 | 2021-12-12T08:21:12.000Z | [
"pytorch",
"roberta",
"question-answering",
"transformers",
"autotrain_compatible"
] | question-answering | false | wjc123 | null | wjc123/qa_finetuned | 0 | null | transformers | 36,273 | Entry not found |
wjching/DialoGPT-small-ricksanchez | 5bae126e91ee1688d0f702c94acba7ec64978103 | 2021-08-28T07:41:39.000Z | [
"pytorch",
"gpt2",
"text-generation",
"transformers",
"conversational"
] | conversational | false | wjching | null | wjching/DialoGPT-small-ricksanchez | 0 | null | transformers | 36,274 | ---
tags:
- conversational
---
# Rick Sanchez DialoGPT Model |
wolfrage89/annual_report_translation_id_en | a059bd9165b64b2cbaf050d73d17817021f0c17c | 2022-01-27T13:01:46.000Z | [
"pytorch",
"marian",
"text2text-generation",
"transformers",
"autotrain_compatible"
] | text2text-generation | false | wolfrage89 | null | wolfrage89/annual_report_translation_id_en | 0 | 3 | transformers | 36,275 | ### Finetuned on annual report sentence pair
This marianMT has been further finetuned on annual report sentence pairs
## Test out at huggingface spaces!
https://huggingface.co/spaces/wolfrage89/finance_domain_translation_marianMT
## Sample colab notebook
https://colab.research.google.com/drive/1H57vwiah7n1JXvXYMqJ8dklrIuU6Cljb?usp=sharing
## How to use
```python
!pip install transformers
!pip install sentencepiece
from transformers import MarianMTModel, MarianTokenizer
tokenizer = MarianTokenizer.from_pretrained("wolfrage89/annual_report_translation_id_en")
model = MarianMTModel.from_pretrained("wolfrage89/annual_report_translation_id_en")
#tokenizing bahasa sentence
bahasa_sentence = "Interpretasi ini merupakan interpretasi atas PSAK 46: Pajak Penghasilan yang bertujuan untuk mengklarifikasi dan memberikan panduan dalam merefleksikan ketidakpastian perlakuan pajak penghasilan dalam laporan keuangan."
tokenized_bahasa_sentence = tokenizer([bahasa_sentence], return_tensors='pt', max_length=104, truncation=True)
#feeding tokenized sentence into model, the max_legnth have been set to 104 as the model was trained mostly on sentences with this length
translated_tokens = model.generate(**tokenized_bahasa_sentence, max_length=104)[0]
## decoding the tokens to get english sentence
english_sentence = tokenizer.decode(translated_tokens, skip_special_tokens=True)
print(english_sentence)
# This interpretation is an interpretation of PSAK 46: Income Tax that aims to clarify and provide guidance in reflecting the uncertainty of income tax treatments in the financial statements.
```
### opus-mt-id-en (original model)
* source languages: id
* target languages: en
* OPUS readme: [id-en](https://github.com/Helsinki-NLP/OPUS-MT-train/blob/master/models/id-en/README.md)
|
wudi7758521521/bert_cn | e6a081099ccf15f3e18b21462db8bda9c4ef4937 | 2021-07-30T05:21:13.000Z | [
"pytorch",
"bert",
"fill-mask",
"transformers",
"autotrain_compatible"
] | fill-mask | false | wudi7758521521 | null | wudi7758521521/bert_cn | 0 | null | transformers | 36,276 | Entry not found |
wudi7758521521/model_name | 96ded66eec7cc695ecaa61225906806abde397a4 | 2021-07-18T08:50:09.000Z | [
"pytorch",
"bert",
"fill-mask",
"transformers",
"autotrain_compatible"
] | fill-mask | false | wudi7758521521 | null | wudi7758521521/model_name | 0 | null | transformers | 36,277 | Entry not found |
xhyi/distilLED1_08_31_2021_v1 | e16a98892e18fdde855271d2c6e12cd52d215fc6 | 2021-08-31T09:05:37.000Z | [
"pytorch",
"led",
"text2text-generation",
"transformers",
"autotrain_compatible"
] | text2text-generation | false | xhyi | null | xhyi/distilLED1_08_31_2021_v1 | 0 | null | transformers | 36,278 | Entry not found |
xhyi/distilLED2_08_31_2021_v4 | 1acdcb6ed0062a119d1165e30cc7d38b1c60d06e | 2021-09-01T01:36:13.000Z | [
"pytorch",
"led",
"text2text-generation",
"transformers",
"autotrain_compatible"
] | text2text-generation | false | xhyi | null | xhyi/distilLED2_08_31_2021_v4 | 0 | null | transformers | 36,279 | Entry not found |
xhyi/distilLED4_09_01_2021_v6_2 | 04a969ebc405e0d9be14ffc84427e41c92731185 | 2021-09-02T06:28:25.000Z | [
"pytorch",
"led",
"text2text-generation",
"transformers",
"autotrain_compatible"
] | text2text-generation | false | xhyi | null | xhyi/distilLED4_09_01_2021_v6_2 | 0 | null | transformers | 36,280 | Step Training Loss Validation Loss Rouge2 Precision Rouge2 Recall Rouge2 Fmeasure
100 3.049500 2.605496 0.172300 0.186900 0.151200
200 3.019400 2.567277 0.165100 0.189400 0.145000
300 3.014400 2.538830 0.157000 0.179200 0.134200
400 2.867200 2.490068 0.163600 0.177100 0.136200
500 2.723700 2.465870 0.168400 0.195700 0.152300
600 2.925400 2.452575 0.169500 0.210100 0.159400
700 2.878900 2.440204 0.173400 0.198000 0.155800
800 3.156500 2.423908 0.172900 0.196300 0.152800
+ 440 steps before
total = 1240 steps |
xiaoheiqaq/DialoGPT-smallharrypotter | 5e7e95b6e0a7a20e71bf51a8c294fe90a5510aee | 2021-09-23T02:23:39.000Z | [
"pytorch",
"gpt2",
"text-generation",
"transformers",
"conversational"
] | conversational | false | xiaoheiqaq | null | xiaoheiqaq/DialoGPT-smallharrypotter | 0 | null | transformers | 36,281 | ---
tags:
- conversational
---
# Harry Potter DialoGPT Model |
xinyang47/ai12 | 12f1adc77867a921be4458919a5d00ad7e3dfb24 | 2022-02-11T08:30:25.000Z | [
"pytorch",
"bert",
"fill-mask",
"transformers",
"autotrain_compatible"
] | fill-mask | false | xinyang47 | null | xinyang47/ai12 | 0 | null | transformers | 36,282 | Entry not found |
xinyang47/ai12_cn | 5cf7b28f80e31361408244bd4468ead54188c821 | 2022-02-11T09:44:04.000Z | [
"pytorch",
"bert",
"fill-mask",
"transformers",
"autotrain_compatible"
] | fill-mask | false | xinyang47 | null | xinyang47/ai12_cn | 0 | null | transformers | 36,283 | Entry not found |
xkang/distilbert-base-uncased-finetuned-imdb-accelerate | 5e3a80349786a144d8d039614a79bed94a885ac3 | 2021-12-27T07:41:02.000Z | [
"pytorch",
"distilbert",
"fill-mask",
"transformers",
"autotrain_compatible"
] | fill-mask | false | xkang | null | xkang/distilbert-base-uncased-finetuned-imdb-accelerate | 0 | null | transformers | 36,284 | Entry not found |
xsway/wav2vec2-large-xlsr-georgian | b29b81945f5fa2e35fbb05da5256815d8cc71e20 | 2021-03-29T21:07:53.000Z | [
"pytorch",
"wav2vec2",
"automatic-speech-recognition",
"ka",
"dataset:common_voice",
"transformers",
"audio",
"speech",
"xlsr-fine-tuning-week",
"license:apache-2.0",
"model-index"
] | automatic-speech-recognition | false | xsway | null | xsway/wav2vec2-large-xlsr-georgian | 0 | null | transformers | 36,285 | ---
language: ka
datasets:
- common_voice
metrics:
- wer
tags:
- audio
- automatic-speech-recognition
- speech
- xlsr-fine-tuning-week
license: apache-2.0
model-index:
- name: XLSR Wav2Vec finetuned for Georgian
results:
- task:
name: Speech Recognition
type: automatic-speech-recognition
dataset:
name: Common Voice ka
type: common_voice
args: ka
metrics:
- name: Test WER
type: wer
value: 45.28
---
# Wav2Vec2-Large-XLSR-53-Georgian
Fine-tuned [facebook/wav2vec2-large-xlsr-53](https://huggingface.co/facebook/wav2vec2-large-xlsr-53) on Georgian using the [Common Voice](https://huggingface.co/datasets/common_voice).
When using this model, make sure that your speech input is sampled at 16kHz.
## Usage
The model can be used directly (without a language model) as follows:
```python
import librosa
import torch
import torchaudio
from datasets import load_dataset
from transformers import Wav2Vec2ForCTC, Wav2Vec2Processor
test_dataset = load_dataset("common_voice", "ka", split="test[:2%]")
processor = Wav2Vec2Processor.from_pretrained("xsway/wav2vec2-large-xlsr-georgian")
model = Wav2Vec2ForCTC.from_pretrained("xsway/wav2vec2-large-xlsr-georgian")
resampler = lambda sampling_rate, y: librosa.resample(y.numpy().squeeze(), sampling_rate, 16_000)
# Preprocessing the datasets.
# We need to read the audio files as arrays
def speech_file_to_array_fn(batch):
\\\\tspeech_array, sampling_rate = torchaudio.load(batch["path"])
\\\\tbatch["speech"] = resampler(sampling_rate, speech_array).squeeze()
\\\\treturn batch
test_dataset = test_dataset.map(speech_file_to_array_fn)
inputs = processor(test_dataset["speech"][:2], sampling_rate=16_000, return_tensors="pt", padding=True)
with torch.no_grad():
\\\\tlogits = model(inputs.input_values, attention_mask=inputs.attention_mask).logits
predicted_ids = torch.argmax(logits, dim=-1)
print("Prediction:", processor.batch_decode(predicted_ids))
print("Reference:", test_dataset["sentence"][:2])
```
## Evaluation
The model can be evaluated as follows on the Georgian test data of Common Voice.
```python
import torch
import torchaudio
from datasets import load_dataset, load_metric
from transformers import Wav2Vec2ForCTC, Wav2Vec2Processor
import re
import librosa
test_dataset = load_dataset("common_voice", "ka", split="test")
wer = load_metric("wer")
processor = Wav2Vec2Processor.from_pretrained("xsway/wav2vec2-large-xlsr-georgian")
model = Wav2Vec2ForCTC.from_pretrained("xsway/wav2vec2-large-xlsr-georgian")
model.to("cuda")
chars_to_ignore_regex = '[\\\\\\\\,\\\\\\\\?\\\\\\\\.\\\\\\\\!\\\\\\\\-\\\\\\\\;\\\\\\\\:\\\\\\\\"\\\\\\\\“]'
resampler = lambda sampling_rate, y: librosa.resample(y.numpy().squeeze(), sampling_rate, 16_000)
# Preprocessing the datasets.
# We need to read the audio files as arrays
def speech_file_to_array_fn(batch):
batch["sentence"] = re.sub(chars_to_ignore_regex, '', batch["sentence"]).lower()
speech_array, sampling_rate = torchaudio.load(batch["path"])
batch["speech"] = resampler(sampling_rate, speech_array).squeeze()
return batch
test_dataset = test_dataset.map(speech_file_to_array_fn)
# Preprocessing the datasets.
# We need to read the audio files as arrays
def evaluate(batch):
inputs = processor(batch["speech"], sampling_rate=16_000, return_tensors="pt", padding=True)
with torch.no_grad():
logits = model(inputs.input_values.to("cuda"), attention_mask=inputs.attention_mask.to("cuda")).logits
pred_ids = torch.argmax(logits, dim=-1)
batch["pred_strings"] = processor.batch_decode(pred_ids)
return batch
result = test_dataset.map(evaluate, batched=True, batch_size=8)
print("WER: {:2f}".format(100 * wer.compute(predictions=result["pred_strings"], references=result["sentence"])))
```
**Test Result**: 45.28 %
## Training
The Common Voice `train`, `validation` datasets were used for training.
The script used for training can be found [here](...)
|
xujiacheng127/anchi-bert | 791927047feb2b8c2d9cca15f669f4514f094a8b | 2022-02-15T12:01:06.000Z | [
"pytorch"
] | null | false | xujiacheng127 | null | xujiacheng127/anchi-bert | 0 | null | null | 36,286 | import json
import requests
headers = {"Authorization": f"Bearer {API_TOKEN}"}
API_URL = "https://api-inference.huggingface.co/models/bert-base-uncased"
def query(payload):
data = json.dumps(payload)
response = requests.request("POST", API_URL, headers=headers, data=data)
return json.loads(response.content.decode("utf-8"))
data = query({"inputs": "The answer to the universe is [MASK]."}) |
yahya1994/DialoGPT-small-DN-L | 9e3cc45576ef0ef381926a8909e3ef65df642e9b | 2021-09-11T02:02:21.000Z | [
"pytorch",
"gpt2",
"text-generation",
"transformers",
"conversational"
] | conversational | false | yahya1994 | null | yahya1994/DialoGPT-small-DN-L | 0 | null | transformers | 36,287 | ---
tags:
- conversational
---
# L dialog |
yahya1994/DialoGPT-small-DN-Light | 6a1adccef77241b31d69e57993b7577b3c6cafd7 | 2021-09-09T20:59:27.000Z | [
"pytorch",
"gpt2",
"text-generation",
"transformers",
"conversational"
] | conversational | false | yahya1994 | null | yahya1994/DialoGPT-small-DN-Light | 0 | null | transformers | 36,288 | ---
tags:
- conversational
---
# Light dialog |
yahya1994/DialoGPT-small-DN-Ryuk | 0279b7e3b1fec99bc1042721e093f652e001d705 | 2021-09-07T18:20:29.000Z | [
"pytorch",
"gpt2",
"text-generation",
"transformers",
"conversational"
] | conversational | false | yahya1994 | null | yahya1994/DialoGPT-small-DN-Ryuk | 0 | null | transformers | 36,289 | ---
tags:
- conversational
---
# Ryuk dialog |
yahya1994/DialoGPT-small-ReZero-Subaru | 177f49117acb3e3ff25a89fa2ff4874b6a5bd5e3 | 2021-09-17T23:04:49.000Z | [
"pytorch",
"gpt2",
"text-generation",
"transformers",
"conversational"
] | conversational | false | yahya1994 | null | yahya1994/DialoGPT-small-ReZero-Subaru | 0 | null | transformers | 36,290 | ---
tags:
- conversational
---
# Subaru dialog |
yair/HeadlineGeneration-sagemaker | aaaec00599b2fe7f830a9c9a2ba890ec2814443d | 2021-05-17T05:39:29.000Z | [
"pytorch",
"bart",
"text2text-generation",
"transformers",
"autotrain_compatible"
] | text2text-generation | false | yair | null | yair/HeadlineGeneration-sagemaker | 0 | null | transformers | 36,291 |
---
language: en
tags:
- sagemaker
- bart
- summarization
license: apache-2.0
|
yair/HeadlineGeneration-sagemaker2 | 219eb6b792a3096c20b39ee2ddce15b1af34825e | 2021-05-18T08:45:49.000Z | [
"pytorch",
"bart",
"text2text-generation",
"transformers",
"autotrain_compatible"
] | text2text-generation | false | yair | null | yair/HeadlineGeneration-sagemaker2 | 0 | null | transformers | 36,292 |
---
language: en
tags:
- sagemaker
- bart
- summarization
license: apache-2.0
- Training 3000 examples
|
yamako/dummy-model | 5544bb242919cd4ddf14bc4f66003b644d25e54b | 2021-09-07T11:32:36.000Z | [
"pytorch",
"camembert",
"fill-mask",
"transformers",
"autotrain_compatible"
] | fill-mask | false | yamako | null | yamako/dummy-model | 0 | null | transformers | 36,293 | Entry not found |
yancong/distilbert-base-uncased-finetuned-existence | e8526ad32154696a132c0bf6b3f740e0a3af132e | 2022-02-22T20:56:03.000Z | [
"pytorch",
"tensorboard",
"distilbert",
"fill-mask",
"transformers",
"generated_from_trainer",
"license:apache-2.0",
"model-index",
"autotrain_compatible"
] | fill-mask | false | yancong | null | yancong/distilbert-base-uncased-finetuned-existence | 0 | null | transformers | 36,294 | ---
license: apache-2.0
tags:
- generated_from_trainer
model-index:
- name: distilbert-base-uncased-finetuned-existence
results: []
---
<!-- This model card has been generated automatically according to the information the Trainer had access to. You
should probably proofread and complete it, then remove this comment. -->
# distilbert-base-uncased-finetuned-existence
This model is a fine-tuned version of [distilbert-base-uncased](https://huggingface.co/distilbert-base-uncased) on an unknown dataset.
It achieves the following results on the evaluation set:
- Loss: 1.7925
## Model description
More information needed
## Intended uses & limitations
More information needed
## Training and evaluation data
More information needed
## Training procedure
### Training hyperparameters
The following hyperparameters were used during training:
- learning_rate: 2e-05
- train_batch_size: 64
- eval_batch_size: 64
- seed: 42
- optimizer: Adam with betas=(0.9,0.999) and epsilon=1e-08
- lr_scheduler_type: linear
- num_epochs: 3.0
### Training results
| Training Loss | Epoch | Step | Validation Loss |
|:-------------:|:-----:|:----:|:---------------:|
| 2.9532 | 1.0 | 221 | 2.1697 |
| 2.0959 | 2.0 | 442 | 1.9725 |
| 1.9277 | 3.0 | 663 | 1.7944 |
### Framework versions
- Transformers 4.16.2
- Pytorch 1.10.1
- Datasets 1.18.3
- Tokenizers 0.11.0
|
yancong/distilbert-base-uncased-finetuned-mi | e9520ca46d230e064c871fb1c9348b5648a0d740 | 2022-02-22T21:47:23.000Z | [
"pytorch",
"tensorboard",
"distilbert",
"fill-mask",
"transformers",
"generated_from_trainer",
"license:apache-2.0",
"model-index",
"autotrain_compatible"
] | fill-mask | false | yancong | null | yancong/distilbert-base-uncased-finetuned-mi | 0 | null | transformers | 36,295 | ---
license: apache-2.0
tags:
- generated_from_trainer
model-index:
- name: distilbert-base-uncased-finetuned-mi
results: []
---
<!-- This model card has been generated automatically according to the information the Trainer had access to. You
should probably proofread and complete it, then remove this comment. -->
# distilbert-base-uncased-finetuned-mi
This model is a fine-tuned version of [distilbert-base-uncased](https://huggingface.co/distilbert-base-uncased) on an unknown dataset.
It achieves the following results on the evaluation set:
- Loss: 1.8606
## Model description
More information needed
## Intended uses & limitations
More information needed
## Training and evaluation data
More information needed
## Training procedure
### Training hyperparameters
The following hyperparameters were used during training:
- learning_rate: 2e-05
- train_batch_size: 64
- eval_batch_size: 64
- seed: 42
- optimizer: Adam with betas=(0.9,0.999) and epsilon=1e-08
- lr_scheduler_type: linear
- num_epochs: 3.0
### Training results
| Training Loss | Epoch | Step | Validation Loss |
|:-------------:|:-----:|:----:|:---------------:|
| 3.1069 | 1.0 | 97 | 2.3524 |
| 2.1677 | 2.0 | 194 | 1.9426 |
| 1.9197 | 3.0 | 291 | 2.0536 |
### Framework versions
- Transformers 4.16.2
- Pytorch 1.10.1
- Datasets 1.18.3
- Tokenizers 0.11.0
|
yancong/distilbert-base-uncased-finetuned-quantifier | de950ec0b19429f07f74fd7952b8445b8a9f42d2 | 2022-02-22T02:57:28.000Z | [
"pytorch",
"tensorboard",
"distilbert",
"fill-mask",
"transformers",
"generated_from_trainer",
"license:apache-2.0",
"model-index",
"autotrain_compatible"
] | fill-mask | false | yancong | null | yancong/distilbert-base-uncased-finetuned-quantifier | 0 | null | transformers | 36,296 | ---
license: apache-2.0
tags:
- generated_from_trainer
model-index:
- name: distilbert-base-uncased-finetuned-quantifier
results: []
---
<!-- This model card has been generated automatically according to the information the Trainer had access to. You
should probably proofread and complete it, then remove this comment. -->
# distilbert-base-uncased-finetuned-quantifier
This model is a fine-tuned version of [distilbert-base-uncased](https://huggingface.co/distilbert-base-uncased) on an unknown dataset.
It achieves the following results on the evaluation set:
- Loss: 1.7478
## Model description
More information needed
## Intended uses & limitations
More information needed
## Training and evaluation data
More information needed
## Training procedure
### Training hyperparameters
The following hyperparameters were used during training:
- learning_rate: 2e-05
- train_batch_size: 64
- eval_batch_size: 64
- seed: 42
- optimizer: Adam with betas=(0.9,0.999) and epsilon=1e-08
- lr_scheduler_type: linear
- num_epochs: 3.0
### Training results
| Training Loss | Epoch | Step | Validation Loss |
|:-------------:|:-----:|:----:|:---------------:|
| 3.2007 | 1.0 | 94 | 2.3496 |
| 2.2332 | 2.0 | 188 | 1.8656 |
| 2.0141 | 3.0 | 282 | 1.8479 |
### Framework versions
- Transformers 4.16.2
- Pytorch 1.10.1
- Datasets 1.18.3
- Tokenizers 0.11.0
|
yarik921/Teflon_0.1 | 44d5c39ec9f1a15d0007693284ee35324ff2fed2 | 2021-12-14T09:19:49.000Z | [
"pytorch",
"wav2vec2",
"automatic-speech-recognition",
"transformers"
] | automatic-speech-recognition | false | yarik921 | null | yarik921/Teflon_0.1 | 0 | null | transformers | 36,297 | Entry not found |
yazdipour/text-to-sparql-t5-small-2021-10-17_18-47 | f0309db6e13def837a3d09766ef290707a6cc43a | 2021-10-17T19:48:35.000Z | [
"pytorch",
"tensorboard",
"t5",
"text2text-generation",
"transformers",
"generated_from_trainer",
"license:apache-2.0",
"model-index",
"autotrain_compatible"
] | text2text-generation | false | yazdipour | null | yazdipour/text-to-sparql-t5-small-2021-10-17_18-47 | 0 | null | transformers | 36,298 | ---
license: apache-2.0
tags:
- generated_from_trainer
datasets:
- null
metrics:
- f1
model-index:
- name: text-to-sparql-t5-small-2021-10-17_18-47
results:
- task:
name: Sequence-to-sequence Language Modeling
type: text2text-generation
metrics:
- name: F1
type: f1
value: 0.2345714420080185
---
<!-- This model card has been generated automatically according to the information the Trainer had access to. You
should probably proofread and complete it, then remove this comment. -->
# text-to-sparql-t5-small-2021-10-17_18-47
This model is a fine-tuned version of [t5-small](https://huggingface.co/t5-small) on the None dataset.
It achieves the following results on the evaluation set:
- Loss: 0.5258
- Gen Len: 19.0
- P: 0.4582
- R: 0.0278
- F1: 0.2346
- Score: 3.5848
- Bleu-precisions: [82.57739877107295, 62.13358857503344, 48.43062944877681, 41.90172321318059]
- Bleu-bp: 0.0631
## Model description
More information needed
## Intended uses & limitations
More information needed
## Training and evaluation data
More information needed
## Training procedure
### Training hyperparameters
The following hyperparameters were used during training:
- learning_rate: 2e-05
- train_batch_size: 8
- eval_batch_size: 8
- seed: 42
- optimizer: Adam with betas=(0.9,0.999) and epsilon=1e-08
- lr_scheduler_type: linear
- num_epochs: 1
- mixed_precision_training: Native AMP
### Training results
| Training Loss | Epoch | Step | Validation Loss | Gen Len | P | R | F1 | Score | Bleu-precisions | Bleu-bp |
|:-------------:|:-----:|:----:|:---------------:|:-------:|:------:|:------:|:------:|:------:|:----------------------------------------------------------------------------:|:-------:|
| 0.7575 | 1.0 | 4807 | 0.5258 | 19.0 | 0.4582 | 0.0278 | 0.2346 | 3.5848 | [82.57739877107295, 62.13358857503344, 48.43062944877681, 41.90172321318059] | 0.0631 |
### Framework versions
- Transformers 4.10.0
- Pytorch 1.9.0+cu111
- Datasets 1.13.3
- Tokenizers 0.10.3
|
yazdipour/text-to-sparql-t5-small-2021-10-18_09-32 | 5b97314b671c8a525c081e77785ab8374f874e52 | 2021-10-18T10:33:05.000Z | [
"pytorch",
"tensorboard",
"t5",
"text2text-generation",
"transformers",
"generated_from_trainer",
"license:apache-2.0",
"model-index",
"autotrain_compatible"
] | text2text-generation | false | yazdipour | null | yazdipour/text-to-sparql-t5-small-2021-10-18_09-32 | 0 | null | transformers | 36,299 | ---
license: apache-2.0
tags:
- generated_from_trainer
datasets:
- null
metrics:
- f1
model-index:
- name: text-to-sparql-t5-small-2021-10-18_09-32
results:
- task:
name: Sequence-to-sequence Language Modeling
type: text2text-generation
metrics:
- name: F1
type: f1
value: 0.26458749175071716
---
<!-- This model card has been generated automatically according to the information the Trainer had access to. You
should probably proofread and complete it, then remove this comment. -->
# text-to-sparql-t5-small-2021-10-18_09-32
This model is a fine-tuned version of [t5-small](https://huggingface.co/t5-small) on the None dataset.
It achieves the following results on the evaluation set:
- Loss: 0.5119
- Gen Len: 19.0
- P: 0.4884
- R: 0.0583
- F1: 0.2646
- Score: 3.5425
- Bleu-precisions: [82.80295919500207, 62.695879280325016, 50.2215675749897, 44.03052700138759]
- Bleu-bp: 0.0609
## Model description
More information needed
## Intended uses & limitations
More information needed
## Training and evaluation data
More information needed
## Training procedure
### Training hyperparameters
The following hyperparameters were used during training:
- learning_rate: 2e-05
- train_batch_size: 8
- eval_batch_size: 8
- seed: 42
- optimizer: Adam with betas=(0.9,0.999) and epsilon=1e-08
- lr_scheduler_type: linear
- num_epochs: 1
- mixed_precision_training: Native AMP
### Training results
| Training Loss | Epoch | Step | Validation Loss | Gen Len | P | R | F1 | Score | Bleu-precisions | Bleu-bp |
|:-------------:|:-----:|:----:|:---------------:|:-------:|:------:|:------:|:------:|:------:|:----------------------------------------------------------------------------:|:-------:|
| 0.7088 | 1.0 | 4772 | 0.5119 | 19.0 | 0.4884 | 0.0583 | 0.2646 | 3.5425 | [82.80295919500207, 62.695879280325016, 50.2215675749897, 44.03052700138759] | 0.0609 |
### Framework versions
- Transformers 4.10.0
- Pytorch 1.9.0+cu111
- Datasets 1.13.3
- Tokenizers 0.10.3
|
Subsets and Splits
No community queries yet
The top public SQL queries from the community will appear here once available.