modelId
stringlengths 4
112
| sha
stringlengths 40
40
| lastModified
stringlengths 24
24
| tags
sequence | pipeline_tag
stringclasses 29
values | private
bool 1
class | author
stringlengths 2
38
β | config
null | id
stringlengths 4
112
| downloads
float64 0
36.8M
β | likes
float64 0
712
β | library_name
stringclasses 17
values | __index_level_0__
int64 0
38.5k
| readme
stringlengths 0
186k
|
---|---|---|---|---|---|---|---|---|---|---|---|---|---|
xingqiang/nezha-zh-address-match-wwm-base | 8cca1ad008364fd9ff1a168bfcf1833dd2f7bbfe | 2022-05-06T03:24:16.000Z | [
"pytorch",
"bert",
"feature-extraction",
"transformers"
] | feature-extraction | false | xingqiang | null | xingqiang/nezha-zh-address-match-wwm-base | 1 | null | transformers | 31,700 | Entry not found |
xingqiang/nezha-zh-address-match-wwm-finetuned | 6be2113b764e52451eaefbc407c70e0ee66c1061 | 2022-05-06T05:52:09.000Z | [
"pytorch",
"bert",
"feature-extraction",
"transformers"
] | feature-extraction | false | xingqiang | null | xingqiang/nezha-zh-address-match-wwm-finetuned | 1 | null | transformers | 31,701 | Entry not found |
yhavinga/t5-eff-large-8l-dutch-english-cased | 52203847bfe1f1ae08ecf7158f5fe5294228d9ca | 2022-06-14T10:29:32.000Z | [
"pytorch",
"jax",
"t5",
"text2text-generation",
"nl",
"en",
"dataset:yhavinga/mc4_nl_cleaned",
"arxiv:1910.10683",
"arxiv:2109.10686",
"transformers",
"seq2seq",
"license:apache-2.0",
"autotrain_compatible"
] | text2text-generation | false | yhavinga | null | yhavinga/t5-eff-large-8l-dutch-english-cased | 1 | null | transformers | 31,702 | ---
language:
- nl
- en
datasets:
- yhavinga/mc4_nl_cleaned
tags:
- t5
- seq2seq
inference: false
license: apache-2.0
---
# t5-eff-large-8l-dutch-english-cased
A [T5](https://ai.googleblog.com/2020/02/exploring-transfer-learning-with-t5.html) sequence to sequence model
pre-trained from scratch on [cleaned Dutch π³π±π§πͺ mC4 and cleaned English π¬π§ C4](https://huggingface.co/datasets/yhavinga/mc4_nl_cleaned).
This **t5 eff** model has **334M** parameters.
It was pre-trained with the masked language modeling objective on the dataset
`mc4_nl_cleaned` config `large_en_nl` for **1** epoch(s) and a duration of **3d 23h**,
with a sequence length of **512**, batch size **128** and **851850** total steps (**56B** tokens).
Pre-training evaluation loss and accuracy are **1,15** and **0,74**.
Refer to the evaluation section below for a comparison of the pre-trained models on summarization and translation.
* Pre-trained T5 models need to be finetuned before they can be used for downstream tasks, therefore the inference widget on the right has been turned off.
* For a demo of the Dutch CNN summarization models, head over to the Hugging Face Spaces for
the **[Netherformer π°](https://huggingface.co/spaces/flax-community/netherformer)** example application!
Please refer to the original T5 papers and Scale Efficiently papers for more information about the T5 architecture
and configs, though it must be noted that this model (t5-eff-large-8l-dutch-english-cased) is unrelated to these projects and not an 'official' checkpoint.
* **[Exploring the Limits of Transfer Learning with a Unified Text-to-Text Transformer](https://arxiv.org/pdf/1910.10683.pdf)** by *Colin Raffel, Noam Shazeer, Adam Roberts, Katherine Lee, Sharan Narang, Michael Matena, Yanqi Zhou, Wei Li, Peter J. Liu*.
* **[Scale Efficiently: Insights from Pre-training and Fine-tuning Transformers](https://arxiv.org/abs/2109.10686)** by *Yi Tay, Mostafa Dehghani, Jinfeng Rao, William Fedus, Samira Abnar, Hyung Won Chung, Sharan Narang, Dani Yogatama, Ashish Vaswani, Donald Metzler*.
## Tokenizer
The model uses a cased SentencePiece tokenizer configured with the `Nmt, NFKC, Replace multi-space to single-space` normalizers
and has 32003 tokens.
It was trained on Dutch and English with scripts from the Huggingface Transformers [Flax examples](https://github.com/huggingface/transformers/tree/master/examples/flax/language-modeling).
See [./raw/main/tokenizer.json](tokenizer.json) for details.
## Dataset(s)
All models listed below are pre-trained on
[cleaned Dutch mC4](https://huggingface.co/datasets/yhavinga/mc4_nl_cleaned),
which is the original mC4, except
* Documents that contained words from a selection of the Dutch and English [List of Dirty Naught Obscene and Otherwise Bad Words](https://github.com/LDNOOBW/List-of-Dirty-Naughty-Obscene-and-Otherwise-Bad-Words) are removed
* Sentences with less than 3 words are removed
* Sentences with a word of more than 1000 characters are removed
* Documents with less than 5 sentences are removed
* Documents with "javascript", "lorum ipsum", "terms of use", "privacy policy", "cookie policy", "uses cookies",
"use of cookies", "use cookies", "elementen ontbreken", "deze printversie" are removed.
The Dutch and English models are pre-trained on a 50/50% mix of Dutch mC4 and English C4.
The translation models are fine-tuned on [CCMatrix](https://huggingface.co/datasets/yhavinga/ccmatrix).
## Dutch T5 Models
Three types of [Dutch T5 models have been trained (blog)](https://huggingface.co/spaces/yhavinga/pre-training-dutch-t5-models).
`t5-base-dutch` is the only model with an original T5 config.
The other model types t5-v1.1 and t5-eff have `gated-relu` instead of `relu` as activation function,
and trained with a drop-out of `0.0` unless training would diverge (`t5-v1.1-large-dutch-cased`).
The T5-eff models are models that differ in their number of layers. The table will list
the several dimensions of these models. Not all t5-eff models are efficient, the best example being the inefficient
`t5-xl-4L-dutch-english-cased`.
| | [t5-base-dutch](https://huggingface.co/yhavinga/t5-base-dutch) | [t5-v1.1-base-dutch-uncased](https://huggingface.co/yhavinga/t5-v1.1-base-dutch-uncased) | [t5-v1.1-base-dutch-cased](https://huggingface.co/yhavinga/t5-v1.1-base-dutch-cased) | [t5-v1.1-large-dutch-cased](https://huggingface.co/yhavinga/t5-v1.1-large-dutch-cased) | [t5-v1_1-base-dutch-english-cased](https://huggingface.co/yhavinga/t5-v1_1-base-dutch-english-cased) | [t5-v1_1-base-dutch-english-cased-1024](https://huggingface.co/yhavinga/t5-v1_1-base-dutch-english-cased-1024) | [t5-small-24L-dutch-english](https://huggingface.co/yhavinga/t5-small-24L-dutch-english) | [t5-xl-4L-dutch-english-cased](https://huggingface.co/yhavinga/t5-xl-4L-dutch-english-cased) | [t5-base-36L-dutch-english-cased](https://huggingface.co/yhavinga/t5-base-36L-dutch-english-cased) | [t5-eff-xl-8l-dutch-english-cased](https://huggingface.co/yhavinga/t5-eff-xl-8l-dutch-english-cased) | [t5-eff-large-8l-dutch-english-cased](https://huggingface.co/yhavinga/t5-eff-large-8l-dutch-english-cased) |
|:------------------|:----------------|:-----------------------------|:---------------------------|:----------------------------|:-----------------------------------|:----------------------------------------|:-----------------------------|:-------------------------------|:----------------------------------|:-----------------------------------|:--------------------------------------|
| *type* | t5 | t5-v1.1 | t5-v1.1 | t5-v1.1 | t5-v1.1 | t5-v1.1 | t5 eff | t5 eff | t5 eff | t5 eff | t5 eff |
| *d_model* | 768 | 768 | 768 | 1024 | 768 | 768 | 512 | 2048 | 768 | 1024 | 1024 |
| *d_ff* | 3072 | 2048 | 2048 | 2816 | 2048 | 2048 | 1920 | 5120 | 2560 | 16384 | 4096 |
| *num_heads* | 12 | 12 | 12 | 16 | 12 | 12 | 8 | 32 | 12 | 32 | 16 |
| *d_kv* | 64 | 64 | 64 | 64 | 64 | 64 | 64 | 64 | 64 | 128 | 64 |
| *num_layers* | 12 | 12 | 12 | 24 | 12 | 12 | 24 | 4 | 36 | 8 | 8 |
| *num parameters* | 223M | 248M | 248M | 783M | 248M | 248M | 250M | 585M | 729M | 1241M | 335M |
| *feed_forward_proj* | relu | gated-gelu | gated-gelu | gated-gelu | gated-gelu | gated-gelu | gated-gelu | gated-gelu | gated-gelu | gated-gelu | gated-gelu |
| *dropout* | 0.1 | 0.0 | 0.0 | 0.1 | 0.0 | 0.0 | 0.0 | 0.1 | 0.0 | 0.0 | 0.0 |
| *dataset* | mc4_nl_cleaned | mc4_nl_cleaned full | mc4_nl_cleaned full | mc4_nl_cleaned | mc4_nl_cleaned small_en_nl | mc4_nl_cleaned large_en_nl | mc4_nl_cleaned large_en_nl | mc4_nl_cleaned large_en_nl | mc4_nl_cleaned large_en_nl | mc4_nl_cleaned large_en_nl | mc4_nl_cleaned large_en_nl |
| *tr. seq len* | 512 | 1024 | 1024 | 512 | 512 | 1024 | 512 | 512 | 512 | 512 | 512 |
| *batch size* | 128 | 64 | 64 | 64 | 128 | 64 | 128 | 512 | 512 | 64 | 128 |
| *total steps* | 527500 | 1014525 | 1210154 | 1120k/2427498 | 2839630 | 1520k/3397024 | 851852 | 212963 | 212963 | 538k/1703705 | 851850 |
| *epochs* | 1 | 2 | 2 | 2 | 10 | 4 | 1 | 1 | 1 | 1 | 1 |
| *duration* | 2d9h | 5d5h | 6d6h | 8d13h | 11d18h | 9d1h | 4d10h | 6d1h | 17d15h | 4d 19h | 3d 23h |
| *optimizer* | adafactor | adafactor | adafactor | adafactor | adafactor | adafactor | adafactor | adafactor | adafactor | adafactor | adafactor |
| *lr* | 0.005 | 0.005 | 0.005 | 0.005 | 0.005 | 0.005 | 0.005 | 0.005 | 0.009 | 0.005 | 0.005 |
| *warmup* | 10000.0 | 10000.0 | 10000.0 | 10000.0 | 10000.0 | 5000.0 | 20000.0 | 2500.0 | 1000.0 | 1500.0 | 1500.0 |
| *eval loss* | 1,38 | 1,20 | 0,96 | 1,07 | 1,11 | 1,13 | 1,18 | 1,27 | 1,05 | 1,3019 | 1,15 |
| *eval acc* | 0,70 | 0,73 | 0,78 | 0,76 | 0,75 | 0,74 | 0,74 | 0,72 | 0,76 | 0,71 | 0,74 |
## Evaluation
Most models from the list above have been evaluated on summarization and translation.
The figure below shows the evaluation scores, where the x-axis shows the translation Bleu score (higher is better)
and y-axis the summarization Rouge1 translation score (higher is better).
Point size is proportional to the model size. Models with faster inference speed are green, slower inference speed is
plotted as bleu.

The next two sections provide more information on how the evaluation was performed.
## Evaluation on summarization
The models below have been evaluated for summarization on 50K samples from the CNN Dailymail dataset.
All models were fine-tuned with the AdamW optimizer with a batch size of 128 and constant learning rate of 1e-3 after a
warmup of 32 steps, with a label smoothing factor of 0.05. Article and summary token lengths were set to 1024 and 142.
NB: the evaluation checkpoints are not saved, since they were trained for comparison of pre-trained models only.
The numbers reported are the Rouge scores on 1000 documents from the test split. The rouge1 score is visualized in the
| | [t5-base-dutch](https://huggingface.co/yhavinga/t5-base-dutch) | [t5-v1.1-base-dutch-uncased](https://huggingface.co/yhavinga/t5-v1.1-base-dutch-uncased) | [t5-v1.1-base-dutch-cased](https://huggingface.co/yhavinga/t5-v1.1-base-dutch-cased) | [t5-v1_1-base-dutch-english-cased](https://huggingface.co/yhavinga/t5-v1_1-base-dutch-english-cased) | [t5-v1_1-base-dutch-english-cased-1024](https://huggingface.co/yhavinga/t5-v1_1-base-dutch-english-cased-1024) | [t5-small-24L-dutch-english](https://huggingface.co/yhavinga/t5-small-24L-dutch-english) | [t5-xl-4L-dutch-english-cased](https://huggingface.co/yhavinga/t5-xl-4L-dutch-english-cased) | [t5-base-36L-dutch-english-cased](https://huggingface.co/yhavinga/t5-base-36L-dutch-english-cased) | [t5-eff-large-8l-dutch-english-cased](https://huggingface.co/yhavinga/t5-eff-large-8l-dutch-english-cased) | mt5-base |
|:------------------------|----------------:|-----------------------------:|---------------------------:|-----------------------------------:|----------------------------------------:|-----------------------------:|-------------------------------:|----------------------------------:|--------------------------------------:|-----------:|
| *rouge1* | 33.38 | 33.97 | 34.39 | 33.38 | 34.97 | 34.38 | 30.35 | **35.04** | 34.04 | 33.25 |
| *rouge2* | 13.32 | 13.85 | 13.98 | 13.47 | 14.01 | 13.89 | 11.57 | **14.23** | 13.76 | 12.74 |
| *rougeL* | 24.22 | 24.72 | 25.1 | 24.34 | 24.99 | **25.25** | 22.69 | 25.05 | 24.75 | 23.5 |
| *rougeLsum* | 30.23 | 30.9 | 31.44 | 30.51 | 32.01 | 31.38 | 27.5 | **32.12** | 31.12 | 30.15 |
| *samples_per_second* | 3.18 | 3.02 | 2.99 | 3.22 | 2.97 | 1.57 | 2.8 | 0.61 | **3.27** | 1.22 |
## Evaluation on translation
The models below have been evaluated for English to Dutch translation on 50K samples from the CCMatrix dataset.
Note that the first four models are pre-trained on Dutch only. That they still perform adequate is probably because
the translation direction is English to Dutch.
All models were fine-tuned with the AdamW optimizer with a batch size of 128 and constant learning rate of 5e-5 after a
warmup of 32 steps, with a label smoothing factor of 0.1 and maximum sequence length of 128 tokens.
The numbers reported are the Bleu scores on 1000 documents from the test split.
NB: the evaluation checkpoints are not saved, since they were trained for comparison of pre-trained models only.
| | [t5-base-dutch](https://huggingface.co/yhavinga/t5-base-dutch) | [t5-v1.1-base-dutch-uncased](https://huggingface.co/yhavinga/t5-v1.1-base-dutch-uncased) | [t5-v1.1-base-dutch-cased](https://huggingface.co/yhavinga/t5-v1.1-base-dutch-cased) | [t5-v1.1-large-dutch-cased](https://huggingface.co/yhavinga/t5-v1.1-large-dutch-cased) | [t5-v1_1-base-dutch-english-cased](https://huggingface.co/yhavinga/t5-v1_1-base-dutch-english-cased) | [t5-v1_1-base-dutch-english-cased-1024](https://huggingface.co/yhavinga/t5-v1_1-base-dutch-english-cased-1024) | [t5-small-24L-dutch-english](https://huggingface.co/yhavinga/t5-small-24L-dutch-english) | [t5-xl-4L-dutch-english-cased](https://huggingface.co/yhavinga/t5-xl-4L-dutch-english-cased) | [t5-base-36L-dutch-english-cased](https://huggingface.co/yhavinga/t5-base-36L-dutch-english-cased) | [t5-eff-large-8l-dutch-english-cased](https://huggingface.co/yhavinga/t5-eff-large-8l-dutch-english-cased) | mt5-base |
|:-------------------------------|----------------:|-----------------------------:|---------------------------:|----------------------------:|-----------------------------------:|----------------------------------------:|-----------------------------:|-------------------------------:|----------------------------------:|--------------------------------------:|-----------:|
| *precision_ng1* | 74.17 | 78.09 | 77.08 | 72.12 | 77.19 | 78.76 | 78.59 | 77.3 | **79.75** | 78.88 | 73.47 |
| *precision_ng2* | 52.42 | 57.52 | 55.31 | 48.7 | 55.39 | 58.01 | 57.83 | 55.27 | **59.89** | 58.27 | 50.12 |
| *precision_ng3* | 39.55 | 45.2 | 42.54 | 35.54 | 42.25 | 45.13 | 45.02 | 42.06 | **47.4** | 45.95 | 36.59 |
| *precision_ng4* | 30.23 | 36.04 | 33.26 | 26.27 | 32.74 | 35.72 | 35.41 | 32.61 | **38.1** | 36.91 | 27.26 |
| *bp* | 0.99 | 0.98 | 0.97 | 0.98 | 0.98 | 0.98 | 0.98 | 0.97 | 0.98 | 0.98 | 0.98 |
| *score* | 45.88 | 51.21 | 48.31 | 41.59 | 48.17 | 51.31 | 50.82 | 47.83 | **53** | 51.79 | 42.74 |
| *samples_per_second* | **45.19** | 45.05 | 38.67 | 10.12 | 42.19 | 42.61 | 12.85 | 33.74 | 9.07 | 37.86 | 9.03 |
## Translation models
The models `t5-small-24L-dutch-english` and `t5-base-36L-dutch-english` have been fine-tuned for both language
directions on the first 25M samples from CCMatrix, giving a total of 50M training samples.
Evaluation is performed on out-of-sample CCMatrix and also on Tatoeba and Opus Books.
The `_bp` columns list the *brevity penalty*. The `avg_bleu` score is the bleu score
averaged over all three evaluation datasets. The best scores displayed in bold for both translation directions.
| | [t5-base-36L-ccmatrix-multi](https://huggingface.co/yhavinga/t5-base-36L-ccmatrix-multi) | [t5-base-36L-ccmatrix-multi](https://huggingface.co/yhavinga/t5-base-36L-ccmatrix-multi) | [t5-small-24L-ccmatrix-multi](https://huggingface.co/yhavinga/t5-small-24L-ccmatrix-multi) | [t5-small-24L-ccmatrix-multi](https://huggingface.co/yhavinga/t5-small-24L-ccmatrix-multi) |
|:-----------------------|:-----------------------------|:-----------------------------|:------------------------------|:------------------------------|
| *source_lang* | en | nl | en | nl |
| *target_lang* | nl | en | nl | en |
| *source_prefix* | translate English to Dutch: | translate Dutch to English: | translate English to Dutch: | translate Dutch to English: |
| *ccmatrix_bleu* | **56.8** | 62.8 | 57.4 | **63.1** |
| *tatoeba_bleu* | **46.6** | **52.8** | 46.4 | 51.7 |
| *opus_books_bleu* | **13.5** | **24.9** | 12.9 | 23.4 |
| *ccmatrix_bp* | 0.95 | 0.96 | 0.95 | 0.96 |
| *tatoeba_bp* | 0.97 | 0.94 | 0.98 | 0.94 |
| *opus_books_bp* | 0.8 | 0.94 | 0.77 | 0.89 |
| *avg_bleu* | **38.96** | **46.86** | 38.92 | 46.06 |
| *max_source_length* | 128 | 128 | 128 | 128 |
| *max_target_length* | 128 | 128 | 128 | 128 |
| *adam_beta1* | 0.9 | 0.9 | 0.9 | 0.9 |
| *adam_beta2* | 0.997 | 0.997 | 0.997 | 0.997 |
| *weight_decay* | 0.05 | 0.05 | 0.002 | 0.002 |
| *lr* | 5e-05 | 5e-05 | 0.0005 | 0.0005 |
| *label_smoothing_factor* | 0.15 | 0.15 | 0.1 | 0.1 |
| *train_batch_size* | 128 | 128 | 128 | 128 |
| *warmup_steps* | 2000 | 2000 | 2000 | 2000 |
| *total steps* | 390625 | 390625 | 390625 | 390625 |
| *duration* | 4d 5h | 4d 5h | 3d 2h | 3d 2h |
| *num parameters* | 729M | 729M | 250M | 250M |
## Acknowledgements
This project would not have been possible without compute generously provided by Google through the
[TPU Research Cloud](https://sites.research.google/trc/). The HuggingFace π€ ecosystem was instrumental in all parts
of the training. Weights & Biases made it possible to keep track of many training sessions
and orchestrate hyper-parameter sweeps with insightful visualizations.
The following repositories where helpful in setting up the TPU-VM,
and getting an idea what sensible hyper-parameters are for training gpt2 from scratch:
* [Gsarti's Pretrain and Fine-tune a T5 model with Flax on GCP](https://github.com/gsarti/t5-flax-gcp)
* [Flax/Jax Community week t5-base-dutch](https://huggingface.co/flax-community/t5-base-dutch)
Created by [Yeb Havinga](https://www.linkedin.com/in/yeb-havinga-86530825/)
|
theojolliffe/bart-large-cnn-finetuned-roundup-4-4 | 1afdfe005d3c58db45f0c955593ad41b16a7bff6 | 2022-05-06T13:00:06.000Z | [
"pytorch",
"tensorboard",
"bart",
"text2text-generation",
"transformers",
"generated_from_trainer",
"license:mit",
"model-index",
"autotrain_compatible"
] | text2text-generation | false | theojolliffe | null | theojolliffe/bart-large-cnn-finetuned-roundup-4-4 | 1 | null | transformers | 31,703 | ---
license: mit
tags:
- generated_from_trainer
metrics:
- rouge
model-index:
- name: bart-large-cnn-finetuned-roundup-4-4
results: []
---
<!-- This model card has been generated automatically according to the information the Trainer had access to. You
should probably proofread and complete it, then remove this comment. -->
# bart-large-cnn-finetuned-roundup-4-4
This model is a fine-tuned version of [facebook/bart-large-cnn](https://huggingface.co/facebook/bart-large-cnn) on an unknown dataset.
It achieves the following results on the evaluation set:
- Loss: 0.7912
- Rouge1: 53.8175
- Rouge2: 35.1335
- Rougel: 38.0823
- Rougelsum: 51.2925
- Gen Len: 142.0
## Model description
More information needed
## Intended uses & limitations
More information needed
## Training and evaluation data
More information needed
## Training procedure
### Training hyperparameters
The following hyperparameters were used during training:
- learning_rate: 2e-05
- train_batch_size: 2
- eval_batch_size: 2
- seed: 42
- optimizer: Adam with betas=(0.9,0.999) and epsilon=1e-08
- lr_scheduler_type: linear
- num_epochs: 4
- mixed_precision_training: Native AMP
### Training results
| Training Loss | Epoch | Step | Validation Loss | Rouge1 | Rouge2 | Rougel | Rougelsum | Gen Len |
|:-------------:|:-----:|:----:|:---------------:|:-------:|:-------:|:-------:|:---------:|:-------:|
| No log | 1.0 | 398 | 0.9455 | 52.8137 | 33.4924 | 35.5866 | 50.7208 | 142.0 |
| 1.1309 | 2.0 | 796 | 0.8397 | 54.0923 | 35.0799 | 37.4609 | 51.5914 | 142.0 |
| 0.6902 | 3.0 | 1194 | 0.7932 | 53.5752 | 35.0842 | 37.9295 | 51.0356 | 142.0 |
| 0.4951 | 4.0 | 1592 | 0.7912 | 53.8175 | 35.1335 | 38.0823 | 51.2925 | 142.0 |
### Framework versions
- Transformers 4.18.0
- Pytorch 1.11.0+cu113
- Datasets 2.1.0
- Tokenizers 0.12.1
|
lilitket/20220506-092616 | 97518242f1beeae45f37bb02a8d26acbea692b28 | 2022-05-06T21:15:24.000Z | [
"pytorch",
"wav2vec2",
"automatic-speech-recognition",
"transformers"
] | automatic-speech-recognition | false | lilitket | null | lilitket/20220506-092616 | 1 | null | transformers | 31,704 | Entry not found |
crabz/exp2 | ae64d245862c35d5180e65690ea5833c2c1763c5 | 2022-05-06T09:56:16.000Z | [
"pytorch",
"roberta",
"transformers"
] | null | false | crabz | null | crabz/exp2 | 1 | null | transformers | 31,705 | Entry not found |
chrisvinsen/xlsr-wav2vec2-final | bac1d77d8a812d2c0096c43b9f77da84893b4fc6 | 2022-05-29T01:09:13.000Z | [
"pytorch",
"wav2vec2",
"automatic-speech-recognition",
"transformers"
] | automatic-speech-recognition | false | chrisvinsen | null | chrisvinsen/xlsr-wav2vec2-final | 1 | null | transformers | 31,706 | CommonVoice Dataset 8.0 --> Train + Others
WER : 0.216
WER with LM: 0.147 |
theojolliffe/distilbart-cnn-12-6-finetuned-roundup-4-8 | 4a26af304e7efdd50220d3162d893dc902e5f4dd | 2022-05-06T11:45:37.000Z | [
"pytorch",
"tensorboard",
"bart",
"text2text-generation",
"transformers",
"generated_from_trainer",
"license:apache-2.0",
"model-index",
"autotrain_compatible"
] | text2text-generation | false | theojolliffe | null | theojolliffe/distilbart-cnn-12-6-finetuned-roundup-4-8 | 1 | null | transformers | 31,707 | ---
license: apache-2.0
tags:
- generated_from_trainer
metrics:
- rouge
model-index:
- name: distilbart-cnn-12-6-finetuned-roundup-4-8
results: []
---
<!-- This model card has been generated automatically according to the information the Trainer had access to. You
should probably proofread and complete it, then remove this comment. -->
# distilbart-cnn-12-6-finetuned-roundup-4-8
This model is a fine-tuned version of [sshleifer/distilbart-cnn-12-6](https://huggingface.co/sshleifer/distilbart-cnn-12-6) on an unknown dataset.
It achieves the following results on the evaluation set:
- Loss: 0.8447
- Rouge1: 54.3326
- Rouge2: 36.1031
- Rougel: 38.842
- Rougelsum: 51.7632
- Gen Len: 142.0
## Model description
More information needed
## Intended uses & limitations
More information needed
## Training and evaluation data
More information needed
## Training procedure
### Training hyperparameters
The following hyperparameters were used during training:
- learning_rate: 2e-05
- train_batch_size: 2
- eval_batch_size: 2
- seed: 42
- optimizer: Adam with betas=(0.9,0.999) and epsilon=1e-08
- lr_scheduler_type: linear
- num_epochs: 8
- mixed_precision_training: Native AMP
### Training results
| Training Loss | Epoch | Step | Validation Loss | Rouge1 | Rouge2 | Rougel | Rougelsum | Gen Len |
|:-------------:|:-----:|:----:|:---------------:|:-------:|:-------:|:-------:|:---------:|:--------:|
| No log | 1.0 | 398 | 1.1572 | 51.6618 | 32.7542 | 34.8631 | 49.2691 | 141.3333 |
| 1.405 | 2.0 | 796 | 1.0039 | 52.2029 | 32.6704 | 34.4948 | 50.1141 | 142.0 |
| 0.9039 | 3.0 | 1194 | 0.9300 | 53.2839 | 34.3928 | 36.8971 | 51.1148 | 142.0 |
| 0.6705 | 4.0 | 1592 | 0.8708 | 52.5229 | 33.8116 | 36.9664 | 50.0067 | 142.0 |
| 0.6705 | 5.0 | 1990 | 0.8508 | 53.4468 | 35.1394 | 38.4144 | 50.794 | 142.0 |
| 0.5205 | 6.0 | 2388 | 0.8347 | 53.8859 | 35.1182 | 38.1126 | 51.3089 | 142.0 |
| 0.3898 | 7.0 | 2786 | 0.8406 | 54.2293 | 36.1189 | 38.7127 | 51.6878 | 142.0 |
| 0.3468 | 8.0 | 3184 | 0.8447 | 54.3326 | 36.1031 | 38.842 | 51.7632 | 142.0 |
### Framework versions
- Transformers 4.18.0
- Pytorch 1.11.0+cu113
- Datasets 2.1.0
- Tokenizers 0.12.1
|
guhuawuli/gpt2-imdb | 1584e1854f035b996d67537b61bf75205a1058a6 | 2022-05-06T23:35:42.000Z | [
"pytorch",
"tensorboard",
"gpt2",
"text-generation",
"transformers",
"generated_from_trainer",
"license:mit",
"model-index"
] | text-generation | false | guhuawuli | null | guhuawuli/gpt2-imdb | 1 | null | transformers | 31,708 | ---
license: mit
tags:
- generated_from_trainer
model-index:
- name: gpt2-imdb
results: []
---
<!-- This model card has been generated automatically according to the information the Trainer had access to. You
should probably proofread and complete it, then remove this comment. -->
# gpt2-imdb
This model is a fine-tuned version of [gpt2](https://huggingface.co/gpt2) on the None dataset.
It achieves the following results on the evaluation set:
- Loss: 3.6155
## Model description
More information needed
## Intended uses & limitations
More information needed
## Training and evaluation data
More information needed
## Training procedure
### Training hyperparameters
The following hyperparameters were used during training:
- learning_rate: 2e-05
- train_batch_size: 32
- eval_batch_size: 32
- seed: 42
- optimizer: Adam with betas=(0.9,0.999) and epsilon=1e-08
- lr_scheduler_type: linear
- num_epochs: 3.0
### Training results
| Training Loss | Epoch | Step | Validation Loss |
|:-------------:|:-----:|:----:|:---------------:|
| 3.7476 | 1.0 | 2904 | 3.6428 |
| 3.6877 | 2.0 | 5808 | 3.6215 |
| 3.6595 | 3.0 | 8712 | 3.6155 |
### Framework versions
- Transformers 4.18.0
- Pytorch 1.10.0a0+3fd9dcf
- Datasets 2.1.0
- Tokenizers 0.12.1
|
davidlekve/distilroberta-base-finetuned-bruno-mars | 5e7ed5ffb3a33aba99d9e6277edacb2908c03ff3 | 2022-05-06T16:18:48.000Z | [
"pytorch",
"tensorboard",
"roberta",
"fill-mask",
"transformers",
"generated_from_trainer",
"license:apache-2.0",
"model-index",
"autotrain_compatible"
] | fill-mask | false | davidlekve | null | davidlekve/distilroberta-base-finetuned-bruno-mars | 1 | null | transformers | 31,709 | ---
license: apache-2.0
tags:
- generated_from_trainer
model-index:
- name: distilroberta-base-finetuned-bruno-mars
results: []
---
<!-- This model card has been generated automatically according to the information the Trainer had access to. You
should probably proofread and complete it, then remove this comment. -->
# distilroberta-base-finetuned-bruno-mars
This model is a fine-tuned version of [distilroberta-base](https://huggingface.co/distilroberta-base) on the None dataset.
It achieves the following results on the evaluation set:
- Loss: 2.4055
## Model description
More information needed
## Intended uses & limitations
More information needed
## Training and evaluation data
More information needed
## Training procedure
### Training hyperparameters
The following hyperparameters were used during training:
- learning_rate: 2e-05
- train_batch_size: 8
- eval_batch_size: 8
- seed: 42
- optimizer: Adam with betas=(0.9,0.999) and epsilon=1e-08
- lr_scheduler_type: linear
- num_epochs: 3.0
### Training results
| Training Loss | Epoch | Step | Validation Loss |
|:-------------:|:-----:|:----:|:---------------:|
| No log | 1.0 | 62 | 2.5992 |
| No log | 2.0 | 124 | 2.4069 |
| No log | 3.0 | 186 | 2.4055 |
### Framework versions
- Transformers 4.18.0
- Pytorch 1.11.0+cpu
- Datasets 2.1.0
- Tokenizers 0.12.1
|
theojolliffe/bart-cnn-v3-e16 | c85ce8feebd342b539422a71df38943cd921652c | 2022-05-06T17:55:32.000Z | [
"pytorch",
"tensorboard",
"bart",
"text2text-generation",
"transformers",
"generated_from_trainer",
"license:mit",
"model-index",
"autotrain_compatible"
] | text2text-generation | false | theojolliffe | null | theojolliffe/bart-cnn-v3-e16 | 1 | null | transformers | 31,710 | ---
license: mit
tags:
- generated_from_trainer
metrics:
- rouge
model-index:
- name: bart-large-cnn-finetuned-roundup-4-16
results: []
---
<!-- This model card has been generated automatically according to the information the Trainer had access to. You
should probably proofread and complete it, then remove this comment. -->
# bart-large-cnn-finetuned-roundup-4-16
This model is a fine-tuned version of [facebook/bart-large-cnn](https://huggingface.co/facebook/bart-large-cnn) on an unknown dataset.
It achieves the following results on the evaluation set:
- Loss: 0.8760
- Rouge1: 56.3338
- Rouge2: 42.4032
- Rougel: 45.9455
- Rougelsum: 54.6488
- Gen Len: 142.0
## Model description
More information needed
## Intended uses & limitations
More information needed
## Training and evaluation data
More information needed
## Training procedure
### Training hyperparameters
The following hyperparameters were used during training:
- learning_rate: 2e-05
- train_batch_size: 2
- eval_batch_size: 2
- seed: 42
- optimizer: Adam with betas=(0.9,0.999) and epsilon=1e-08
- lr_scheduler_type: linear
- num_epochs: 16
- mixed_precision_training: Native AMP
### Training results
| Training Loss | Epoch | Step | Validation Loss | Rouge1 | Rouge2 | Rougel | Rougelsum | Gen Len |
|:-------------:|:-----:|:----:|:---------------:|:-------:|:-------:|:-------:|:---------:|:--------:|
| No log | 1.0 | 398 | 0.9325 | 52.7796 | 33.0802 | 34.8217 | 50.2211 | 142.0 |
| 1.1317 | 2.0 | 796 | 0.8313 | 53.6274 | 35.3235 | 37.7077 | 51.0888 | 141.2963 |
| 0.6757 | 3.0 | 1194 | 0.7893 | 54.1449 | 34.7532 | 36.3211 | 51.781 | 142.0 |
| 0.4511 | 4.0 | 1592 | 0.7647 | 52.2694 | 34.2286 | 36.5736 | 49.7078 | 142.0 |
| 0.4511 | 5.0 | 1990 | 0.7596 | 55.1986 | 37.5865 | 41.406 | 53.1897 | 141.8333 |
| 0.3037 | 6.0 | 2388 | 0.7688 | 53.9367 | 36.8729 | 39.9456 | 51.5108 | 142.0 |
| 0.209 | 7.0 | 2786 | 0.7590 | 54.6867 | 37.6415 | 41.2602 | 52.746 | 142.0 |
| 0.1452 | 8.0 | 3184 | 0.7744 | 53.5374 | 36.3666 | 40.0432 | 51.3461 | 142.0 |
| 0.11 | 9.0 | 3582 | 0.8042 | 56.6623 | 40.4702 | 44.0028 | 54.5138 | 142.0 |
| 0.11 | 10.0 | 3980 | 0.8105 | 55.6002 | 40.5663 | 43.8119 | 53.9117 | 142.0 |
| 0.0833 | 11.0 | 4378 | 0.8230 | 56.2517 | 40.8567 | 44.0009 | 54.3271 | 142.0 |
| 0.0634 | 12.0 | 4776 | 0.8329 | 55.9228 | 40.6443 | 43.6161 | 54.0975 | 142.0 |
| 0.0474 | 13.0 | 5174 | 0.8570 | 55.4923 | 40.3683 | 43.4675 | 53.404 | 142.0 |
| 0.0349 | 14.0 | 5572 | 0.8658 | 56.4454 | 41.8069 | 44.2922 | 54.464 | 142.0 |
| 0.0349 | 15.0 | 5970 | 0.8754 | 56.3837 | 42.2025 | 45.7817 | 54.4912 | 142.0 |
| 0.0304 | 16.0 | 6368 | 0.8760 | 56.3338 | 42.4032 | 45.9455 | 54.6488 | 142.0 |
### Framework versions
- Transformers 4.18.0
- Pytorch 1.11.0+cu113
- Datasets 2.1.0
- Tokenizers 0.12.1
|
huggingtweets/trancentrall | 80efaa913b295ee5b2d29323d3bd717163e20216 | 2022-05-06T18:17:57.000Z | [
"pytorch",
"gpt2",
"text-generation",
"en",
"transformers",
"huggingtweets"
] | text-generation | false | huggingtweets | null | huggingtweets/trancentrall | 1 | null | transformers | 31,711 | ---
language: en
thumbnail: http://www.huggingtweets.com/trancentrall/1651861073034/predictions.png
tags:
- huggingtweets
widget:
- text: "My dream is"
---
<div class="inline-flex flex-col" style="line-height: 1.5;">
<div class="flex">
<div
style="display:inherit; margin-left: 4px; margin-right: 4px; width: 92px; height:92px; border-radius: 50%; background-size: cover; background-image: url('https://pbs.twimg.com/profile_images/1439718913286328324/BWMkSlFf_400x400.jpg')">
</div>
<div
style="display:none; margin-left: 4px; margin-right: 4px; width: 92px; height:92px; border-radius: 50%; background-size: cover; background-image: url('')">
</div>
<div
style="display:none; margin-left: 4px; margin-right: 4px; width: 92px; height:92px; border-radius: 50%; background-size: cover; background-image: url('')">
</div>
</div>
<div style="text-align: center; margin-top: 3px; font-size: 16px; font-weight: 800">π€ AI BOT π€</div>
<div style="text-align: center; font-size: 16px; font-weight: 800">jotchua</div>
<div style="text-align: center; font-size: 14px;">@trancentrall</div>
</div>
I was made with [huggingtweets](https://github.com/borisdayma/huggingtweets).
Create your own bot based on your favorite user with [the demo](https://colab.research.google.com/github/borisdayma/huggingtweets/blob/master/huggingtweets-demo.ipynb)!
## How does it work?
The model uses the following pipeline.
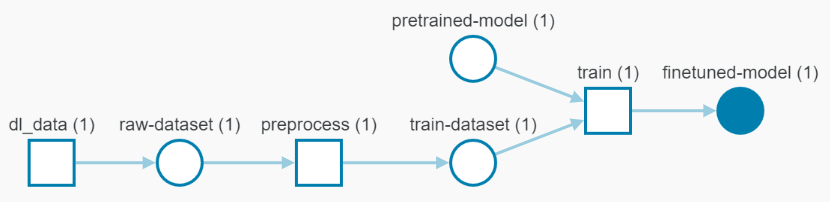
To understand how the model was developed, check the [W&B report](https://wandb.ai/wandb/huggingtweets/reports/HuggingTweets-Train-a-Model-to-Generate-Tweets--VmlldzoxMTY5MjI).
## Training data
The model was trained on tweets from jotchua.
| Data | jotchua |
| --- | --- |
| Tweets downloaded | 3197 |
| Retweets | 165 |
| Short tweets | 937 |
| Tweets kept | 2095 |
[Explore the data](https://wandb.ai/wandb/huggingtweets/runs/2cfuds5z/artifacts), which is tracked with [W&B artifacts](https://docs.wandb.com/artifacts) at every step of the pipeline.
## Training procedure
The model is based on a pre-trained [GPT-2](https://huggingface.co/gpt2) which is fine-tuned on @trancentrall's tweets.
Hyperparameters and metrics are recorded in the [W&B training run](https://wandb.ai/wandb/huggingtweets/runs/37rzneux) for full transparency and reproducibility.
At the end of training, [the final model](https://wandb.ai/wandb/huggingtweets/runs/37rzneux/artifacts) is logged and versioned.
## How to use
You can use this model directly with a pipeline for text generation:
```python
from transformers import pipeline
generator = pipeline('text-generation',
model='huggingtweets/trancentrall')
generator("My dream is", num_return_sequences=5)
```
## Limitations and bias
The model suffers from [the same limitations and bias as GPT-2](https://huggingface.co/gpt2#limitations-and-bias).
In addition, the data present in the user's tweets further affects the text generated by the model.
## About
*Built by Boris Dayma*
[](https://twitter.com/intent/follow?screen_name=borisdayma)
For more details, visit the project repository.
[](https://github.com/borisdayma/huggingtweets)
|
Satyamatury/wav2vec2-large-xls-r-300m-turkish-colab | 38c14a36751aa28924991a054eedf8c83b7876e3 | 2022-05-27T16:28:53.000Z | [
"pytorch",
"tensorboard",
"wav2vec2",
"automatic-speech-recognition",
"dataset:common_voice",
"transformers",
"generated_from_trainer",
"license:apache-2.0",
"model-index"
] | automatic-speech-recognition | false | Satyamatury | null | Satyamatury/wav2vec2-large-xls-r-300m-turkish-colab | 1 | null | transformers | 31,712 | ---
license: apache-2.0
tags:
- generated_from_trainer
datasets:
- common_voice
model-index:
- name: wav2vec2-large-xls-r-300m-turkish-colab
results: []
---
<!-- This model card has been generated automatically according to the information the Trainer had access to. You
should probably proofread and complete it, then remove this comment. -->
# wav2vec2-large-xls-r-300m-turkish-colab
This model is a fine-tuned version of [facebook/wav2vec2-xls-r-300m](https://huggingface.co/facebook/wav2vec2-xls-r-300m) on the common_voice dataset.
## Model description
More information needed
## Intended uses & limitations
More information needed
## Training and evaluation data
More information needed
## Training procedure
### Training hyperparameters
The following hyperparameters were used during training:
- learning_rate: 0.0003
- train_batch_size: 16
- eval_batch_size: 8
- seed: 42
- gradient_accumulation_steps: 2
- total_train_batch_size: 32
- optimizer: Adam with betas=(0.9,0.999) and epsilon=1e-08
- lr_scheduler_type: linear
- lr_scheduler_warmup_steps: 500
- num_epochs: 30
### Training results
### Framework versions
- Transformers 4.11.3
- Pytorch 1.10.0+cu113
- Datasets 1.18.3
- Tokenizers 0.10.3
|
Santiagot1105/wav2vec2-large-xlsr-es-col-test | 91240d4a54e3fca0e9c5805e57af58b4173b5790 | 2022-05-06T21:35:56.000Z | [
"pytorch",
"tensorboard",
"wav2vec2",
"automatic-speech-recognition",
"transformers",
"generated_from_trainer",
"license:apache-2.0",
"model-index"
] | automatic-speech-recognition | false | Santiagot1105 | null | Santiagot1105/wav2vec2-large-xlsr-es-col-test | 1 | 1 | transformers | 31,713 | ---
license: apache-2.0
tags:
- generated_from_trainer
model-index:
- name: wav2vec2-large-xlsr-es-col-test
results: []
---
<!-- This model card has been generated automatically according to the information the Trainer had access to. You
should probably proofread and complete it, then remove this comment. -->
# wav2vec2-large-xlsr-es-col-test
This model is a fine-tuned version of [jonatasgrosman/wav2vec2-large-xlsr-53-spanish](https://huggingface.co/jonatasgrosman/wav2vec2-large-xlsr-53-spanish) on the None dataset.
It achieves the following results on the evaluation set:
- Loss: 0.0923
- Wer: 0.0886
## Model description
More information needed
## Intended uses & limitations
More information needed
## Training and evaluation data
More information needed
## Training procedure
### Training hyperparameters
The following hyperparameters were used during training:
- learning_rate: 0.0003
- train_batch_size: 16
- eval_batch_size: 8
- seed: 42
- gradient_accumulation_steps: 2
- total_train_batch_size: 32
- optimizer: Adam with betas=(0.9,0.999) and epsilon=1e-08
- lr_scheduler_type: linear
- lr_scheduler_warmup_steps: 500
- num_epochs: 30
- mixed_precision_training: Native AMP
### Training results
| Training Loss | Epoch | Step | Validation Loss | Wer |
|:-------------:|:-----:|:----:|:---------------:|:------:|
| 0.0905 | 18.18 | 400 | 0.0923 | 0.0886 |
### Framework versions
- Transformers 4.11.3
- Pytorch 1.10.1+cu102
- Datasets 1.13.3
- Tokenizers 0.10.3
|
theojolliffe/fb-bart-large-finetuned-trade-the-event-finance-summarizer-finetuned-roundup-1-4 | b1dbf15817773528a9464135b21763e8bdba3ce3 | 2022-05-06T19:32:56.000Z | [
"pytorch",
"tensorboard",
"bart",
"text2text-generation",
"transformers",
"generated_from_trainer",
"model-index",
"autotrain_compatible"
] | text2text-generation | false | theojolliffe | null | theojolliffe/fb-bart-large-finetuned-trade-the-event-finance-summarizer-finetuned-roundup-1-4 | 1 | null | transformers | 31,714 | ---
tags:
- generated_from_trainer
metrics:
- rouge
model-index:
- name: fb-bart-large-finetuned-trade-the-event-finance-summarizer-finetuned-roundup-1-4
results: []
---
<!-- This model card has been generated automatically according to the information the Trainer had access to. You
should probably proofread and complete it, then remove this comment. -->
# fb-bart-large-finetuned-trade-the-event-finance-summarizer-finetuned-roundup-1-4
This model is a fine-tuned version of [nickmuchi/fb-bart-large-finetuned-trade-the-event-finance-summarizer](https://huggingface.co/nickmuchi/fb-bart-large-finetuned-trade-the-event-finance-summarizer) on an unknown dataset.
It achieves the following results on the evaluation set:
- Loss: 0.8305
- Rouge1: 54.122
- Rouge2: 35.2787
- Rougel: 37.6989
- Rougelsum: 51.4679
- Gen Len: 142.0
## Model description
More information needed
## Intended uses & limitations
More information needed
## Training and evaluation data
More information needed
## Training procedure
### Training hyperparameters
The following hyperparameters were used during training:
- learning_rate: 2e-05
- train_batch_size: 1
- eval_batch_size: 1
- seed: 42
- optimizer: Adam with betas=(0.9,0.999) and epsilon=1e-08
- lr_scheduler_type: linear
- num_epochs: 4
- mixed_precision_training: Native AMP
### Training results
| Training Loss | Epoch | Step | Validation Loss | Rouge1 | Rouge2 | Rougel | Rougelsum | Gen Len |
|:-------------:|:-----:|:----:|:---------------:|:-------:|:-------:|:-------:|:---------:|:--------:|
| 1.7158 | 1.0 | 795 | 0.9986 | 53.1755 | 33.3503 | 35.235 | 50.6513 | 142.0 |
| 0.7643 | 2.0 | 1590 | 0.8622 | 53.3646 | 34.429 | 36.7998 | 51.0487 | 141.1852 |
| 0.5894 | 3.0 | 2385 | 0.8345 | 54.2777 | 35.0495 | 37.8567 | 51.7937 | 142.0 |
| 0.4039 | 4.0 | 3180 | 0.8305 | 54.122 | 35.2787 | 37.6989 | 51.4679 | 142.0 |
### Framework versions
- Transformers 4.18.0
- Pytorch 1.11.0+cu113
- Datasets 2.1.0
- Tokenizers 0.12.1
|
huggingtweets/csbible | 0513d1b3596f30cf1e1984b57959785a82774187 | 2022-05-06T19:26:43.000Z | [
"pytorch",
"gpt2",
"text-generation",
"en",
"transformers",
"huggingtweets"
] | text-generation | false | huggingtweets | null | huggingtweets/csbible | 1 | null | transformers | 31,715 | ---
language: en
thumbnail: http://www.huggingtweets.com/csbible/1651865198723/predictions.png
tags:
- huggingtweets
widget:
- text: "My dream is"
---
<div class="inline-flex flex-col" style="line-height: 1.5;">
<div class="flex">
<div
style="display:inherit; margin-left: 4px; margin-right: 4px; width: 92px; height:92px; border-radius: 50%; background-size: cover; background-image: url('https://pbs.twimg.com/profile_images/818891995057946624/2mUjD9A4_400x400.jpg')">
</div>
<div
style="display:none; margin-left: 4px; margin-right: 4px; width: 92px; height:92px; border-radius: 50%; background-size: cover; background-image: url('')">
</div>
<div
style="display:none; margin-left: 4px; margin-right: 4px; width: 92px; height:92px; border-radius: 50%; background-size: cover; background-image: url('')">
</div>
</div>
<div style="text-align: center; margin-top: 3px; font-size: 16px; font-weight: 800">π€ AI BOT π€</div>
<div style="text-align: center; font-size: 16px; font-weight: 800">Christian Standard Bible</div>
<div style="text-align: center; font-size: 14px;">@csbible</div>
</div>
I was made with [huggingtweets](https://github.com/borisdayma/huggingtweets).
Create your own bot based on your favorite user with [the demo](https://colab.research.google.com/github/borisdayma/huggingtweets/blob/master/huggingtweets-demo.ipynb)!
## How does it work?
The model uses the following pipeline.
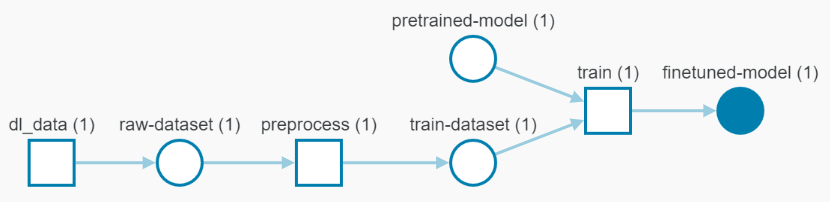
To understand how the model was developed, check the [W&B report](https://wandb.ai/wandb/huggingtweets/reports/HuggingTweets-Train-a-Model-to-Generate-Tweets--VmlldzoxMTY5MjI).
## Training data
The model was trained on tweets from Christian Standard Bible.
| Data | Christian Standard Bible |
| --- | --- |
| Tweets downloaded | 3250 |
| Retweets | 29 |
| Short tweets | 31 |
| Tweets kept | 3190 |
[Explore the data](https://wandb.ai/wandb/huggingtweets/runs/89bp2qgq/artifacts), which is tracked with [W&B artifacts](https://docs.wandb.com/artifacts) at every step of the pipeline.
## Training procedure
The model is based on a pre-trained [GPT-2](https://huggingface.co/gpt2) which is fine-tuned on @csbible's tweets.
Hyperparameters and metrics are recorded in the [W&B training run](https://wandb.ai/wandb/huggingtweets/runs/196rw0mt) for full transparency and reproducibility.
At the end of training, [the final model](https://wandb.ai/wandb/huggingtweets/runs/196rw0mt/artifacts) is logged and versioned.
## How to use
You can use this model directly with a pipeline for text generation:
```python
from transformers import pipeline
generator = pipeline('text-generation',
model='huggingtweets/csbible')
generator("My dream is", num_return_sequences=5)
```
## Limitations and bias
The model suffers from [the same limitations and bias as GPT-2](https://huggingface.co/gpt2#limitations-and-bias).
In addition, the data present in the user's tweets further affects the text generated by the model.
## About
*Built by Boris Dayma*
[](https://twitter.com/intent/follow?screen_name=borisdayma)
For more details, visit the project repository.
[](https://github.com/borisdayma/huggingtweets)
|
davidlekve/distilroberta-base-finetuned-billy-ray-cyrus | b38ed3bdb411ed4fecb44c54654a0c8640a31c3c | 2022-05-06T20:05:17.000Z | [
"pytorch",
"tensorboard",
"roberta",
"fill-mask",
"transformers",
"generated_from_trainer",
"license:apache-2.0",
"model-index",
"autotrain_compatible"
] | fill-mask | false | davidlekve | null | davidlekve/distilroberta-base-finetuned-billy-ray-cyrus | 1 | null | transformers | 31,716 | ---
license: apache-2.0
tags:
- generated_from_trainer
model-index:
- name: distilroberta-base-finetuned-billy-ray-cyrus
results: []
---
<!-- This model card has been generated automatically according to the information the Trainer had access to. You
should probably proofread and complete it, then remove this comment. -->
# distilroberta-base-finetuned-billy-ray-cyrus
This model is a fine-tuned version of [distilroberta-base](https://huggingface.co/distilroberta-base) on the None dataset.
It achieves the following results on the evaluation set:
- Loss: 2.6282
## Model description
More information needed
## Intended uses & limitations
More information needed
## Training and evaluation data
More information needed
## Training procedure
### Training hyperparameters
The following hyperparameters were used during training:
- learning_rate: 2e-05
- train_batch_size: 8
- eval_batch_size: 8
- seed: 42
- optimizer: Adam with betas=(0.9,0.999) and epsilon=1e-08
- lr_scheduler_type: linear
- num_epochs: 3.0
### Training results
| Training Loss | Epoch | Step | Validation Loss |
|:-------------:|:-----:|:----:|:---------------:|
| No log | 1.0 | 47 | 2.5714 |
| No log | 2.0 | 94 | 2.5574 |
| No log | 3.0 | 141 | 2.6282 |
### Framework versions
- Transformers 4.18.0
- Pytorch 1.11.0+cpu
- Datasets 2.1.0
- Tokenizers 0.12.1
|
Khalsuu/english-filipino-wav2vec2-l-xls-r-test-05 | fbd7c9935c20280f028f8fd6d4200450ebc95239 | 2022-05-28T05:23:04.000Z | [
"pytorch",
"tensorboard",
"wav2vec2",
"automatic-speech-recognition",
"dataset:filipino_voice",
"transformers",
"generated_from_trainer",
"license:apache-2.0",
"model-index"
] | automatic-speech-recognition | false | Khalsuu | null | Khalsuu/english-filipino-wav2vec2-l-xls-r-test-05 | 1 | 1 | transformers | 31,717 | ---
license: apache-2.0
tags:
- generated_from_trainer
datasets:
- filipino_voice
model-index:
- name: english-filipino-wav2vec2-l-xls-r-test-05
results: []
---
# english-filipino-wav2vec2-l-xls-r-test-05
## Model description
This model is a fine-tuned version of [jonatasgrosman/wav2vec2-large-xlsr-53-english](https://huggingface.co/jonatasgrosman/wav2vec2-large-xlsr-53-english) on the filipino_voice dataset.
It achieves the following results on the evaluation set:
- Loss: 0.4738
- Wer: 0.2684
### Training hyperparameters
The following hyperparameters were used during training:
- learning_rate: 0.0003
- train_batch_size: 8
- eval_batch_size: 8
- seed: 42
- gradient_accumulation_steps: 2
- total_train_batch_size: 16
- optimizer: Adam with betas=(0.9,0.999) and epsilon=1e-08
- lr_scheduler_type: linear
- lr_scheduler_warmup_steps: 500
- num_epochs: 30
- mixed_precision_training: Native AMP
### Training results
| Training Loss | Epoch | Step | Validation Loss | Wer |
|:-------------:|:-----:|:----:|:---------------:|:------:|
| 3.3328 | 2.09 | 400 | 2.2174 | 0.9733 |
| 0.6432 | 4.19 | 800 | 0.3735 | 0.3896 |
| 0.2741 | 6.28 | 1200 | 0.3639 | 0.3425 |
| 0.1877 | 8.38 | 1600 | 0.3506 | 0.3425 |
| 0.1408 | 10.47 | 2000 | 0.3644 | 0.3181 |
| 0.1133 | 12.57 | 2400 | 0.3837 | 0.3047 |
| 0.0953 | 14.66 | 2800 | 0.4415 | 0.3103 |
| 0.0814 | 16.75 | 3200 | 0.3940 | 0.3092 |
| 0.0707 | 18.85 | 3600 | 0.4164 | 0.3013 |
| 0.059 | 20.94 | 4000 | 0.4488 | 0.2983 |
| 0.0545 | 23.04 | 4400 | 0.4803 | 0.3028 |
| 0.0482 | 25.13 | 4800 | 0.4731 | 0.2811 |
| 0.0426 | 27.23 | 5200 | 0.4606 | 0.2757 |
| 0.0395 | 29.32 | 5600 | 0.4738 | 0.2684 |
## Intended uses & limitations
More information needed
## Training and evaluation data
More information needed
## Training procedure
### Framework versions
- Transformers 4.11.3
- Pytorch 1.10.0+cu113
- Datasets 1.18.3
- Tokenizers 0.10.3
|
vuiseng9/nncf-qat-kd-bert-l-squadv1.1-sl384 | 5a8b3a2d6afa505c386ed5aed40b2fa123991360 | 2022-05-07T00:09:14.000Z | [
"pytorch",
"onnx",
"bert",
"dataset:squad",
"transformers",
"license:apache-2.0",
"model-index"
] | null | false | vuiseng9 | null | vuiseng9/nncf-qat-kd-bert-l-squadv1.1-sl384 | 1 | null | transformers | 31,718 | ---
license: apache-2.0
datasets:
- squad
model-index:
- name: nncf-qat-kd-bert-l-squadv1.1-sl384
results: []
---
This model is quantized version of ```vuiseng9/bert-l-squadv1.1-sl384``` using OpenVINO NNCF.
### Training
```bash
# used 4xV100 GPUS
# --fp16 for lower turnaround and resource requirement
python run_qa.py \
--model_name_or_path bert-large-uncased-whole-word-masking-finetuned-squad \
--dataset_name squad \
--do_eval \
--do_train \
--evaluation_strategy steps \
--eval_steps 250 \
--learning_rate 3e-5 \
--fp16 \
--num_train_epochs 2 \
--per_device_eval_batch_size 64 \
--per_device_train_batch_size 8 \
--max_seq_length 384 \
--doc_stride 128 \
--save_steps 500 \
--logging_steps 1 \
--overwrite_output_dir \
--nncf_config nncf_bert_config_squad_kd.json \ #stock config which is also enclosed here
--run_name $RUNID \
--output_dir $OUTDIR
```
### Evaluation
Require ```vuiseng9/transformers (fork)``` , commit: ```ff24569b```, NNCF v2.1+ commit (```8e26365```)
```bash
git clone https://huggingface.co/vuiseng9/nncf-qat-kd-bert-l-squadv1.1-sl384
python run_qa.py \
--model_name_or_path ./nncf-qat-kd-bert-l-squadv1.1-sl384 \
--dataset_name squad \
--nncf_config nncf-qat-kd-bert-l-squadv1.1-sl384/nncf_bert_config_squad_kd.json \
--nncf_ckpt ./nncf-qat-kd-bert-l-squadv1.1-sl384 \
--do_eval \
--per_device_eval_batch_size 128 \
--max_seq_length 384 \
--doc_stride 128 \
--output_dir /tmp/eval-nncf-qat-kd-bert-l-squadv1.1-sl384 \
--overwrite_output_dir
```
### Results
```
eval_exact_match = 87.1523
eval_f1 = 93.2668
eval_samples = 10784
``` |
lilitket/20220507-052144 | 8679ae9fd3cbb44d2a18d970fd6e69ed596eb689 | 2022-05-07T06:16:36.000Z | [
"pytorch",
"wav2vec2",
"automatic-speech-recognition",
"transformers"
] | automatic-speech-recognition | false | lilitket | null | lilitket/20220507-052144 | 1 | null | transformers | 31,719 | Entry not found |
crystina-z/mdpr-passage-msmarco | 1f2528679ff705ae08bd8d3fb2261545c06e3b92 | 2022-05-07T07:49:09.000Z | [
"pytorch",
"bert",
"feature-extraction",
"transformers"
] | feature-extraction | false | crystina-z | null | crystina-z/mdpr-passage-msmarco | 1 | null | transformers | 31,720 | Entry not found |
retextly/autotrain-test-831226565 | c9f01e824cad5bc7abb8f4e7265835c0c7b7cfb4 | 2022-05-07T09:28:04.000Z | [
"pytorch",
"pegasus",
"text2text-generation",
"en",
"dataset:retextly/autotrain-data-test",
"transformers",
"autotrain",
"co2_eq_emissions",
"autotrain_compatible"
] | text2text-generation | false | retextly | null | retextly/autotrain-test-831226565 | 1 | null | transformers | 31,721 | ---
tags: autotrain
language: en
widget:
- text: "I love AutoTrain π€"
datasets:
- retextly/autotrain-data-test
co2_eq_emissions: 134.3402063080293
---
# Model Trained Using AutoTrain
- Problem type: Summarization
- Model ID: 831226565
- CO2 Emissions (in grams): 134.3402063080293
## Validation Metrics
- Loss: 0.33837366104125977
- Rouge1: 89.9891
- Rouge2: 85.7247
- RougeL: 89.7421
- RougeLsum: 89.4872
- Gen Len: 30.1818
## Usage
You can use cURL to access this model:
```
$ curl -X POST -H "Authorization: Bearer YOUR_HUGGINGFACE_API_KEY" -H "Content-Type: application/json" -d '{"inputs": "I love AutoTrain"}' https://api-inference.huggingface.co/retextly/autotrain-test-831226565
``` |
xraychen/mqa-baseline | 26005c1234eb3b6e9c9c09b9d1ed85f2e771bcbe | 2022-05-07T09:16:36.000Z | [
"pytorch",
"bert",
"question-answering",
"transformers",
"autotrain_compatible"
] | question-answering | false | xraychen | null | xraychen/mqa-baseline | 1 | null | transformers | 31,722 | Entry not found |
xraychen/squad-baseline | d079d34fa92eabb5033355c25300faacae43190f | 2022-05-07T10:01:37.000Z | [
"pytorch",
"bert",
"question-answering",
"transformers",
"autotrain_compatible"
] | question-answering | false | xraychen | null | xraychen/squad-baseline | 1 | null | transformers | 31,723 | Entry not found |
xugenpeng/xlm-roberta-base-finetuned-panx-de | 3ed40002d2a83f84094abcbc0c51698236763669 | 2022-05-07T11:01:26.000Z | [
"pytorch",
"tensorboard",
"xlm-roberta",
"token-classification",
"transformers",
"generated_from_trainer",
"license:mit",
"model-index",
"autotrain_compatible"
] | token-classification | false | xugenpeng | null | xugenpeng/xlm-roberta-base-finetuned-panx-de | 1 | null | transformers | 31,724 | ---
license: mit
tags:
- generated_from_trainer
metrics:
- f1
model-index:
- name: xlm-roberta-base-finetuned-panx-de
results: []
---
<!-- This model card has been generated automatically according to the information the Trainer had access to. You
should probably proofread and complete it, then remove this comment. -->
# xlm-roberta-base-finetuned-panx-de
This model is a fine-tuned version of [xlm-roberta-base](https://huggingface.co/xlm-roberta-base) on the None dataset.
It achieves the following results on the evaluation set:
- Loss: 0.1350
- F1: 0.8609
## Model description
More information needed
## Intended uses & limitations
More information needed
## Training and evaluation data
More information needed
## Training procedure
### Training hyperparameters
The following hyperparameters were used during training:
- learning_rate: 5e-05
- train_batch_size: 32
- eval_batch_size: 32
- seed: 42
- optimizer: Adam with betas=(0.9,0.999) and epsilon=1e-08
- lr_scheduler_type: linear
- num_epochs: 3
### Training results
| Training Loss | Epoch | Step | Validation Loss | F1 |
|:-------------:|:-----:|:----:|:---------------:|:------:|
| 0.2684 | 1.0 | 394 | 0.1598 | 0.8261 |
| 0.13 | 2.0 | 788 | 0.1318 | 0.8528 |
| 0.0852 | 3.0 | 1182 | 0.1350 | 0.8609 |
### Framework versions
- Transformers 4.11.3
- Pytorch 1.9.1
- Datasets 1.16.1
- Tokenizers 0.10.3
|
huggingtweets/spacecatssgb | 7d00f47dc1d7ac35e9d05fd973826a7590a8b4e9 | 2022-05-07T11:14:25.000Z | [
"pytorch",
"gpt2",
"text-generation",
"en",
"transformers",
"huggingtweets"
] | text-generation | false | huggingtweets | null | huggingtweets/spacecatssgb | 1 | null | transformers | 31,725 | ---
language: en
thumbnail: http://www.huggingtweets.com/spacecatssgb/1651922060699/predictions.png
tags:
- huggingtweets
widget:
- text: "My dream is"
---
<div class="inline-flex flex-col" style="line-height: 1.5;">
<div class="flex">
<div
style="display:inherit; margin-left: 4px; margin-right: 4px; width: 92px; height:92px; border-radius: 50%; background-size: cover; background-image: url('https://pbs.twimg.com/profile_images/1517568585333637122/_wEfCpgw_400x400.jpg')">
</div>
<div
style="display:none; margin-left: 4px; margin-right: 4px; width: 92px; height:92px; border-radius: 50%; background-size: cover; background-image: url('')">
</div>
<div
style="display:none; margin-left: 4px; margin-right: 4px; width: 92px; height:92px; border-radius: 50%; background-size: cover; background-image: url('')">
</div>
</div>
<div style="text-align: center; margin-top: 3px; font-size: 16px; font-weight: 800">π€ AI BOT π€</div>
<div style="text-align: center; font-size: 16px; font-weight: 800">SpaceCats NFTs</div>
<div style="text-align: center; font-size: 14px;">@spacecatssgb</div>
</div>
I was made with [huggingtweets](https://github.com/borisdayma/huggingtweets).
Create your own bot based on your favorite user with [the demo](https://colab.research.google.com/github/borisdayma/huggingtweets/blob/master/huggingtweets-demo.ipynb)!
## How does it work?
The model uses the following pipeline.
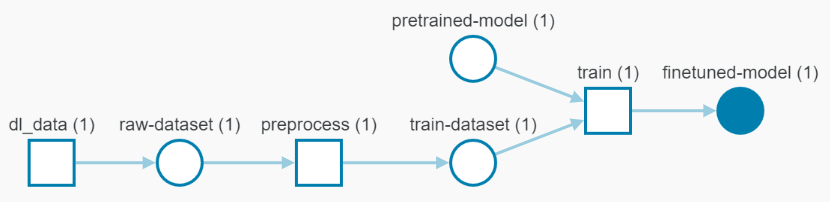
To understand how the model was developed, check the [W&B report](https://wandb.ai/wandb/huggingtweets/reports/HuggingTweets-Train-a-Model-to-Generate-Tweets--VmlldzoxMTY5MjI).
## Training data
The model was trained on tweets from SpaceCats NFTs.
| Data | SpaceCats NFTs |
| --- | --- |
| Tweets downloaded | 249 |
| Retweets | 44 |
| Short tweets | 10 |
| Tweets kept | 195 |
[Explore the data](https://wandb.ai/wandb/huggingtweets/runs/1gdsjxjx/artifacts), which is tracked with [W&B artifacts](https://docs.wandb.com/artifacts) at every step of the pipeline.
## Training procedure
The model is based on a pre-trained [GPT-2](https://huggingface.co/gpt2) which is fine-tuned on @spacecatssgb's tweets.
Hyperparameters and metrics are recorded in the [W&B training run](https://wandb.ai/wandb/huggingtweets/runs/3aq9f1hp) for full transparency and reproducibility.
At the end of training, [the final model](https://wandb.ai/wandb/huggingtweets/runs/3aq9f1hp/artifacts) is logged and versioned.
## How to use
You can use this model directly with a pipeline for text generation:
```python
from transformers import pipeline
generator = pipeline('text-generation',
model='huggingtweets/spacecatssgb')
generator("My dream is", num_return_sequences=5)
```
## Limitations and bias
The model suffers from [the same limitations and bias as GPT-2](https://huggingface.co/gpt2#limitations-and-bias).
In addition, the data present in the user's tweets further affects the text generated by the model.
## About
*Built by Boris Dayma*
[](https://twitter.com/intent/follow?screen_name=borisdayma)
For more details, visit the project repository.
[](https://github.com/borisdayma/huggingtweets)
|
theojolliffe/bart-large-cnn-finetuned-pubmed-finetuned-roundup-e1 | 9ce03b28bf7ae4042554bc277ce09ceb9d2aa7a9 | 2022-05-07T11:40:08.000Z | [
"pytorch",
"tensorboard",
"bart",
"text2text-generation",
"transformers",
"generated_from_trainer",
"license:mit",
"model-index",
"autotrain_compatible"
] | text2text-generation | false | theojolliffe | null | theojolliffe/bart-large-cnn-finetuned-pubmed-finetuned-roundup-e1 | 1 | null | transformers | 31,726 | ---
license: mit
tags:
- generated_from_trainer
model-index:
- name: bart-large-cnn-finetuned-pubmed-finetuned-roundup-e1
results: []
---
<!-- This model card has been generated automatically according to the information the Trainer had access to. You
should probably proofread and complete it, then remove this comment. -->
# bart-large-cnn-finetuned-pubmed-finetuned-roundup-e1
This model is a fine-tuned version of [theojolliffe/bart-large-cnn-finetuned-pubmed](https://huggingface.co/theojolliffe/bart-large-cnn-finetuned-pubmed) on an unknown dataset.
## Model description
More information needed
## Intended uses & limitations
More information needed
## Training and evaluation data
More information needed
## Training procedure
### Training hyperparameters
The following hyperparameters were used during training:
- learning_rate: 2e-05
- train_batch_size: 2
- eval_batch_size: 2
- seed: 42
- optimizer: Adam with betas=(0.9,0.999) and epsilon=1e-08
- lr_scheduler_type: linear
- num_epochs: 1
- mixed_precision_training: Native AMP
### Training results
| Training Loss | Epoch | Step | Validation Loss | Rouge1 | Rouge2 | Rougel | Rougelsum | Gen Len |
|:-------------:|:-----:|:----:|:---------------:|:-------:|:-------:|:-------:|:---------:|:-------:|
| No log | 1.0 | 25 | 1.4393 | 48.2616 | 31.3629 | 35.4175 | 46.251 | 140.0 |
### Framework versions
- Transformers 4.18.0
- Pytorch 1.11.0+cu113
- Datasets 2.1.0
- Tokenizers 0.12.1
|
theojolliffe/bart-large-cnn-finetuned-pubmed-finetuned-roundup-e16 | 8685653fc8dff4287d4626a3f4fefc43ed28187c | 2022-05-07T12:07:36.000Z | [
"pytorch",
"tensorboard",
"bart",
"text2text-generation",
"transformers",
"generated_from_trainer",
"license:mit",
"model-index",
"autotrain_compatible"
] | text2text-generation | false | theojolliffe | null | theojolliffe/bart-large-cnn-finetuned-pubmed-finetuned-roundup-e16 | 1 | null | transformers | 31,727 | ---
license: mit
tags:
- generated_from_trainer
metrics:
- rouge
model-index:
- name: bart-large-cnn-finetuned-pubmed-finetuned-roundup-e16
results: []
---
<!-- This model card has been generated automatically according to the information the Trainer had access to. You
should probably proofread and complete it, then remove this comment. -->
# bart-large-cnn-finetuned-pubmed-finetuned-roundup-e16
This model is a fine-tuned version of [theojolliffe/bart-large-cnn-finetuned-pubmed](https://huggingface.co/theojolliffe/bart-large-cnn-finetuned-pubmed) on an unknown dataset.
It achieves the following results on the evaluation set:
- Loss: 2.6815
- Rouge1: 48.7608
- Rouge2: 29.554
- Rougel: 30.5554
- Rougelsum: 46.4001
- Gen Len: 142.0
## Model description
More information needed
## Intended uses & limitations
More information needed
## Training and evaluation data
More information needed
## Training procedure
### Training hyperparameters
The following hyperparameters were used during training:
- learning_rate: 2e-05
- train_batch_size: 2
- eval_batch_size: 2
- seed: 42
- optimizer: Adam with betas=(0.9,0.999) and epsilon=1e-08
- lr_scheduler_type: linear
- num_epochs: 16
- mixed_precision_training: Native AMP
### Training results
| Training Loss | Epoch | Step | Validation Loss | Rouge1 | Rouge2 | Rougel | Rougelsum | Gen Len |
|:-------------:|:-----:|:----:|:---------------:|:-------:|:-------:|:-------:|:---------:|:-------:|
| No log | 1.0 | 25 | 1.4287 | 46.5701 | 28.6267 | 34.7827 | 45.0622 | 142.0 |
| No log | 2.0 | 50 | 1.4419 | 46.6171 | 27.4276 | 31.0085 | 43.1797 | 142.0 |
| No log | 3.0 | 75 | 1.5418 | 50.1144 | 29.3433 | 32.0144 | 46.9217 | 142.0 |
| No log | 4.0 | 100 | 1.7125 | 49.1395 | 28.611 | 30.9759 | 46.8346 | 142.0 |
| No log | 5.0 | 125 | 1.8978 | 43.9629 | 24.1224 | 26.0032 | 41.2272 | 142.0 |
| No log | 6.0 | 150 | 2.0990 | 49.0579 | 29.5182 | 31.5829 | 46.0207 | 142.0 |
| No log | 7.0 | 175 | 2.2380 | 48.8754 | 27.7691 | 28.8597 | 45.3281 | 142.0 |
| No log | 8.0 | 200 | 2.2922 | 48.311 | 29.2517 | 33.8241 | 46.6099 | 142.0 |
| No log | 9.0 | 225 | 2.3820 | 45.4663 | 23.9904 | 27.5497 | 41.9446 | 142.0 |
| No log | 10.0 | 250 | 2.4856 | 48.2224 | 27.7455 | 28.159 | 45.4726 | 142.0 |
| No log | 11.0 | 275 | 2.4731 | 46.1799 | 22.1941 | 26.8254 | 43.9986 | 142.0 |
| No log | 12.0 | 300 | 2.5278 | 47.8623 | 27.6514 | 26.6377 | 42.9255 | 142.0 |
| No log | 13.0 | 325 | 2.6229 | 45.573 | 25.4966 | 27.7158 | 42.2306 | 142.0 |
| No log | 14.0 | 350 | 2.6032 | 48.1972 | 27.0387 | 28.336 | 45.0293 | 142.0 |
| No log | 15.0 | 375 | 2.6600 | 47.7301 | 27.3567 | 29.3389 | 44.3516 | 142.0 |
| No log | 16.0 | 400 | 2.6815 | 48.7608 | 29.554 | 30.5554 | 46.4001 | 142.0 |
### Framework versions
- Transformers 4.18.0
- Pytorch 1.11.0+cu113
- Datasets 2.1.0
- Tokenizers 0.12.1
|
KoichiYasuoka/roberta-small-coptic-upos | 3c0c29b98144e78c83057de4e9040ee08670c1a5 | 2022-05-08T03:01:24.000Z | [
"pytorch",
"roberta",
"token-classification",
"cop",
"dataset:universal_dependencies",
"transformers",
"coptic",
"pos",
"dependency-parsing",
"license:cc-by-sa-4.0",
"autotrain_compatible"
] | token-classification | false | KoichiYasuoka | null | KoichiYasuoka/roberta-small-coptic-upos | 1 | null | transformers | 31,728 | ---
language:
- "cop"
tags:
- "coptic"
- "token-classification"
- "pos"
- "dependency-parsing"
datasets:
- "universal_dependencies"
license: "cc-by-sa-4.0"
pipeline_tag: "token-classification"
widget:
- text: "β²§β²β²β²β²©β²β²β²Μβ²β²©β²β²β²β²Ο©οΈ€β²οΈ₯ⲑϫβ²β²β²β²₯Β·"
- text: "β²β²β²Ο£β²Ο©β²±β²₯Ο£β²β²£β²β²Μⲑβ²β²©β²β²β²β²Β·"
---
# roberta-small-coptic-upos
## Model Description
This is a RoBERTa model pre-trained with [UD_Coptic](https://universaldependencies.org/cop/) for POS-tagging and dependency-parsing, derived from [roberta-small-coptic](https://huggingface.co/KoichiYasuoka/roberta-small-coptic). Every word is tagged by [UPOS](https://universaldependencies.org/u/pos/) (Universal Part-Of-Speech).
## How to Use
```py
from transformers import AutoTokenizer,AutoModelForTokenClassification
tokenizer=AutoTokenizer.from_pretrained("KoichiYasuoka/roberta-small-coptic-upos")
model=AutoModelForTokenClassification.from_pretrained("KoichiYasuoka/roberta-small-coptic-upos")
```
or
```
import esupar
nlp=esupar.load("KoichiYasuoka/roberta-small-coptic-upos")
```
## See Also
[esupar](https://github.com/KoichiYasuoka/esupar): Tokenizer POS-tagger and Dependency-parser with BERT/RoBERTa models
|
bilelomrani/lilt-camembert-base-title-classifier | 78f765335916c65c297ca8081f69f347b8502e43 | 2022-05-07T14:45:12.000Z | [
"pytorch",
"tensorboard",
"liltrobertalike",
"transformers"
] | null | false | bilelomrani | null | bilelomrani/lilt-camembert-base-title-classifier | 1 | null | transformers | 31,729 | Entry not found |
retextly/t5-small-finetuned-xsum | 7709cb1c78ecfcec0a95a2481dc024a9870a4588 | 2022-05-07T15:44:43.000Z | [
"pytorch",
"tensorboard",
"t5",
"text2text-generation",
"dataset:xsum",
"transformers",
"generated_from_trainer",
"license:apache-2.0",
"model-index",
"autotrain_compatible"
] | text2text-generation | false | retextly | null | retextly/t5-small-finetuned-xsum | 1 | null | transformers | 31,730 | ---
license: apache-2.0
tags:
- generated_from_trainer
datasets:
- xsum
model-index:
- name: t5-small-finetuned-xsum
results: []
---
<!-- This model card has been generated automatically according to the information the Trainer had access to. You
should probably proofread and complete it, then remove this comment. -->
# t5-small-finetuned-xsum
This model is a fine-tuned version of [t5-small](https://huggingface.co/t5-small) on the xsum dataset.
## Model description
More information needed
## Intended uses & limitations
More information needed
## Training and evaluation data
More information needed
## Training procedure
### Training hyperparameters
The following hyperparameters were used during training:
- learning_rate: 2e-05
- train_batch_size: 16
- eval_batch_size: 16
- seed: 42
- optimizer: Adam with betas=(0.9,0.999) and epsilon=1e-08
- lr_scheduler_type: linear
- num_epochs: 1
- mixed_precision_training: Native AMP
### Framework versions
- Transformers 4.18.0
- Pytorch 1.11.0+cu113
- Datasets 2.1.0
- Tokenizers 0.12.1
|
theojolliffe/bart-cnn-pubmed-arxiv-v3-e4 | 30def238b723eb7a6e150a9a0bba89a54cfcc68d | 2022-05-07T16:53:55.000Z | [
"pytorch",
"tensorboard",
"bart",
"text2text-generation",
"transformers",
"generated_from_trainer",
"license:mit",
"model-index",
"autotrain_compatible"
] | text2text-generation | false | theojolliffe | null | theojolliffe/bart-cnn-pubmed-arxiv-v3-e4 | 1 | null | transformers | 31,731 | ---
license: mit
tags:
- generated_from_trainer
metrics:
- rouge
model-index:
- name: bart-cnn-pubmed-arxiv-v3-e4
results: []
---
<!-- This model card has been generated automatically according to the information the Trainer had access to. You
should probably proofread and complete it, then remove this comment. -->
# bart-cnn-pubmed-arxiv-v3-e4
This model is a fine-tuned version of [theojolliffe/bart-cnn-pubmed-arxiv](https://huggingface.co/theojolliffe/bart-cnn-pubmed-arxiv) on an unknown dataset.
It achieves the following results on the evaluation set:
- Loss: 0.7934
- Rouge1: 54.2624
- Rouge2: 35.6024
- Rougel: 37.1697
- Rougelsum: 51.5144
- Gen Len: 141.9815
## Model description
More information needed
## Intended uses & limitations
More information needed
## Training and evaluation data
More information needed
## Training procedure
### Training hyperparameters
The following hyperparameters were used during training:
- learning_rate: 2e-05
- train_batch_size: 2
- eval_batch_size: 2
- seed: 42
- optimizer: Adam with betas=(0.9,0.999) and epsilon=1e-08
- lr_scheduler_type: linear
- num_epochs: 4
- mixed_precision_training: Native AMP
### Training results
| Training Loss | Epoch | Step | Validation Loss | Rouge1 | Rouge2 | Rougel | Rougelsum | Gen Len |
|:-------------:|:-----:|:----:|:---------------:|:-------:|:-------:|:-------:|:---------:|:--------:|
| No log | 1.0 | 398 | 0.9533 | 52.3191 | 32.4576 | 33.2016 | 49.6502 | 142.0 |
| 1.1154 | 2.0 | 796 | 0.8407 | 53.6639 | 34.3433 | 36.1893 | 50.9077 | 142.0 |
| 0.6856 | 3.0 | 1194 | 0.7978 | 54.4723 | 36.1315 | 37.7891 | 51.902 | 142.0 |
| 0.4943 | 4.0 | 1592 | 0.7934 | 54.2624 | 35.6024 | 37.1697 | 51.5144 | 141.9815 |
### Framework versions
- Transformers 4.18.0
- Pytorch 1.11.0+cu113
- Datasets 2.1.0
- Tokenizers 0.12.1
|
crystina-z/xdpr-tied-msmarco-10epoch | f8e7f782a3a13fa4e5c388afcba11e8463d3eb00 | 2022-05-07T16:41:05.000Z | [
"pytorch",
"xlm-roberta",
"feature-extraction",
"transformers"
] | feature-extraction | false | crystina-z | null | crystina-z/xdpr-tied-msmarco-10epoch | 1 | null | transformers | 31,732 | Entry not found |
Khalsuu/english-filipino-wav2vec2-l-xls-r-test-08 | 939839e41f5bf55a7e3441da39f91912f7e1ffb8 | 2022-05-08T01:35:51.000Z | [
"pytorch",
"tensorboard",
"wav2vec2",
"automatic-speech-recognition",
"dataset:filipino_voice",
"transformers",
"generated_from_trainer",
"license:apache-2.0",
"model-index"
] | automatic-speech-recognition | false | Khalsuu | null | Khalsuu/english-filipino-wav2vec2-l-xls-r-test-08 | 1 | null | transformers | 31,733 | ---
license: apache-2.0
tags:
- generated_from_trainer
datasets:
- filipino_voice
model-index:
- name: english-filipino-wav2vec2-l-xls-r-test-08
results: []
---
<!-- This model card has been generated automatically according to the information the Trainer had access to. You
should probably proofread and complete it, then remove this comment. -->
# english-filipino-wav2vec2-l-xls-r-test-08
This model is a fine-tuned version of [jonatasgrosman/wav2vec2-large-xlsr-53-english](https://huggingface.co/jonatasgrosman/wav2vec2-large-xlsr-53-english) on the filipino_voice dataset.
It achieves the following results on the evaluation set:
- Loss: 0.5968
- Wer: 0.4255
## Model description
More information needed
## Intended uses & limitations
More information needed
## Training and evaluation data
More information needed
## Training procedure
### Training hyperparameters
The following hyperparameters were used during training:
- learning_rate: 0.0003
- train_batch_size: 8
- eval_batch_size: 8
- seed: 42
- gradient_accumulation_steps: 2
- total_train_batch_size: 16
- optimizer: Adam with betas=(0.9,0.999) and epsilon=1e-08
- lr_scheduler_type: linear
- lr_scheduler_warmup_steps: 500
- num_epochs: 20
- mixed_precision_training: Native AMP
### Training results
| Training Loss | Epoch | Step | Validation Loss | Wer |
|:-------------:|:-----:|:----:|:---------------:|:------:|
| 3.3434 | 2.09 | 400 | 2.2857 | 0.9625 |
| 1.6304 | 4.19 | 800 | 1.1547 | 0.7268 |
| 0.9231 | 6.28 | 1200 | 1.0252 | 0.6186 |
| 0.6098 | 8.38 | 1600 | 0.9371 | 0.5494 |
| 0.4922 | 10.47 | 2000 | 0.7092 | 0.5478 |
| 0.3652 | 12.57 | 2400 | 0.7358 | 0.5149 |
| 0.2735 | 14.66 | 2800 | 0.6270 | 0.4646 |
| 0.2038 | 16.75 | 3200 | 0.5717 | 0.4506 |
| 0.1552 | 18.85 | 3600 | 0.5968 | 0.4255 |
### Framework versions
- Transformers 4.11.3
- Pytorch 1.10.0+cu113
- Datasets 1.18.3
- Tokenizers 0.10.3
|
huggingtweets/brutedeforce | a6be1634032da08f5e06be8e7130989f2c3a990a | 2022-05-08T00:31:49.000Z | [
"pytorch",
"gpt2",
"text-generation",
"en",
"transformers",
"huggingtweets"
] | text-generation | false | huggingtweets | null | huggingtweets/brutedeforce | 1 | null | transformers | 31,734 | ---
language: en
thumbnail: https://github.com/borisdayma/huggingtweets/blob/master/img/logo.png?raw=true
tags:
- huggingtweets
widget:
- text: "My dream is"
---
<div class="inline-flex flex-col" style="line-height: 1.5;">
<div class="flex">
<div
style="display:inherit; margin-left: 4px; margin-right: 4px; width: 92px; height:92px; border-radius: 50%; background-size: cover; background-image: url('https://pbs.twimg.com/profile_images/1481651838717808654/9UjpARw0_400x400.jpg')">
</div>
<div
style="display:none; margin-left: 4px; margin-right: 4px; width: 92px; height:92px; border-radius: 50%; background-size: cover; background-image: url('')">
</div>
<div
style="display:none; margin-left: 4px; margin-right: 4px; width: 92px; height:92px; border-radius: 50%; background-size: cover; background-image: url('')">
</div>
</div>
<div style="text-align: center; margin-top: 3px; font-size: 16px; font-weight: 800">π€ AI BOT π€</div>
<div style="text-align: center; font-size: 16px; font-weight: 800">brute de force</div>
<div style="text-align: center; font-size: 14px;">@brutedeforce</div>
</div>
I was made with [huggingtweets](https://github.com/borisdayma/huggingtweets).
Create your own bot based on your favorite user with [the demo](https://colab.research.google.com/github/borisdayma/huggingtweets/blob/master/huggingtweets-demo.ipynb)!
## How does it work?
The model uses the following pipeline.
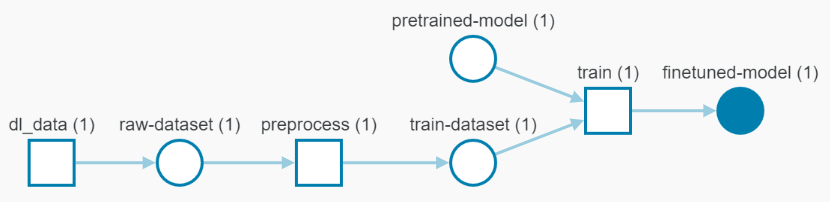
To understand how the model was developed, check the [W&B report](https://wandb.ai/wandb/huggingtweets/reports/HuggingTweets-Train-a-Model-to-Generate-Tweets--VmlldzoxMTY5MjI).
## Training data
The model was trained on tweets from brute de force.
| Data | brute de force |
| --- | --- |
| Tweets downloaded | 3087 |
| Retweets | 497 |
| Short tweets | 229 |
| Tweets kept | 2361 |
[Explore the data](https://wandb.ai/wandb/huggingtweets/runs/njvklep4/artifacts), which is tracked with [W&B artifacts](https://docs.wandb.com/artifacts) at every step of the pipeline.
## Training procedure
The model is based on a pre-trained [GPT-2](https://huggingface.co/gpt2) which is fine-tuned on @brutedeforce's tweets.
Hyperparameters and metrics are recorded in the [W&B training run](https://wandb.ai/wandb/huggingtweets/runs/2oxvamkp) for full transparency and reproducibility.
At the end of training, [the final model](https://wandb.ai/wandb/huggingtweets/runs/2oxvamkp/artifacts) is logged and versioned.
## How to use
You can use this model directly with a pipeline for text generation:
```python
from transformers import pipeline
generator = pipeline('text-generation',
model='huggingtweets/brutedeforce')
generator("My dream is", num_return_sequences=5)
```
## Limitations and bias
The model suffers from [the same limitations and bias as GPT-2](https://huggingface.co/gpt2#limitations-and-bias).
In addition, the data present in the user's tweets further affects the text generated by the model.
## About
*Built by Boris Dayma*
[](https://twitter.com/intent/follow?screen_name=borisdayma)
For more details, visit the project repository.
[](https://github.com/borisdayma/huggingtweets)
|
jimregan/wav2vec-awb | c62b96fbe0a0429a6d662ebba965dd17750bd4b0 | 2022-05-15T15:58:16.000Z | [
"pytorch",
"tensorboard",
"wav2vec2",
"automatic-speech-recognition",
"transformers",
"license:apache-2.0"
] | automatic-speech-recognition | false | jimregan | null | jimregan/wav2vec-awb | 1 | null | transformers | 31,735 | ---
license: apache-2.0
---
|
vinaykudari/bart-acled-t2s | a7b0e9b462c0f1a73ab0a29bd4edbabe8001a8a5 | 2022-05-08T03:31:13.000Z | [
"pytorch",
"bart",
"text2text-generation",
"transformers",
"autotrain_compatible"
] | text2text-generation | false | vinaykudari | null | vinaykudari/bart-acled-t2s | 1 | null | transformers | 31,736 | Entry not found |
vinaykudari/pegasus-acled-t2s | 260c58fcf912ef4ad9b727a282803e2a0f750712 | 2022-05-09T08:34:31.000Z | [
"pytorch",
"pegasus",
"text2text-generation",
"transformers",
"autotrain_compatible"
] | text2text-generation | false | vinaykudari | null | vinaykudari/pegasus-acled-t2s | 1 | null | transformers | 31,737 | Entry not found |
Jiexing/sparc_add_depen_t5_3b-1344 | f5acd77c5cc6f724cbdbe767f0f834d02bb5d440 | 2022-05-08T04:58:28.000Z | [
"pytorch",
"t5",
"text2text-generation",
"transformers",
"autotrain_compatible"
] | text2text-generation | false | Jiexing | null | Jiexing/sparc_add_depen_t5_3b-1344 | 1 | null | transformers | 31,738 | Entry not found |
theojolliffe/bart-cnn-pubmed-arxiv-pubmed-v3-e16 | 0b911b823a6dc83f235a439abef2edfee8d81bcd | 2022-05-08T15:16:32.000Z | [
"pytorch",
"tensorboard",
"bart",
"text2text-generation",
"transformers",
"generated_from_trainer",
"license:mit",
"model-index",
"autotrain_compatible"
] | text2text-generation | false | theojolliffe | null | theojolliffe/bart-cnn-pubmed-arxiv-pubmed-v3-e16 | 1 | null | transformers | 31,739 | ---
license: mit
tags:
- generated_from_trainer
metrics:
- rouge
model-index:
- name: bart-cnn-pubmed-arxiv-pubmed-v3-e16
results: []
---
<!-- This model card has been generated automatically according to the information the Trainer had access to. You
should probably proofread and complete it, then remove this comment. -->
# bart-cnn-pubmed-arxiv-pubmed-v3-e16
This model is a fine-tuned version of [theojolliffe/bart-cnn-pubmed-arxiv-pubmed](https://huggingface.co/theojolliffe/bart-cnn-pubmed-arxiv-pubmed) on an unknown dataset.
It achieves the following results on the evaluation set:
- Loss: 0.8702
- Rouge1: 56.1421
- Rouge2: 41.3514
- Rougel: 44.5146
- Rougelsum: 54.3477
- Gen Len: 142.0
## Model description
More information needed
## Intended uses & limitations
More information needed
## Training and evaluation data
More information needed
## Training procedure
### Training hyperparameters
The following hyperparameters were used during training:
- learning_rate: 2e-05
- train_batch_size: 2
- eval_batch_size: 2
- seed: 42
- optimizer: Adam with betas=(0.9,0.999) and epsilon=1e-08
- lr_scheduler_type: linear
- num_epochs: 16
- mixed_precision_training: Native AMP
### Training results
| Training Loss | Epoch | Step | Validation Loss | Rouge1 | Rouge2 | Rougel | Rougelsum | Gen Len |
|:-------------:|:-----:|:----:|:---------------:|:-------:|:-------:|:-------:|:---------:|:--------:|
| No log | 1.0 | 398 | 0.9532 | 53.1932 | 32.9882 | 35.3852 | 50.6138 | 142.0 |
| 1.1219 | 2.0 | 796 | 0.8252 | 54.1306 | 35.3774 | 37.4334 | 51.6652 | 142.0 |
| 0.6698 | 3.0 | 1194 | 0.7828 | 53.8766 | 35.2945 | 39.2662 | 51.3239 | 142.0 |
| 0.4435 | 4.0 | 1592 | 0.7744 | 53.9029 | 35.2716 | 37.5502 | 51.1179 | 142.0 |
| 0.4435 | 5.0 | 1990 | 0.7644 | 53.8132 | 36.3643 | 39.9548 | 51.5348 | 141.4815 |
| 0.3001 | 6.0 | 2388 | 0.7996 | 53.7376 | 36.2289 | 39.063 | 51.7514 | 142.0 |
| 0.2045 | 7.0 | 2786 | 0.8009 | 54.4924 | 37.3594 | 40.033 | 52.1405 | 142.0 |
| 0.1416 | 8.0 | 3184 | 0.7578 | 55.2039 | 39.0907 | 42.171 | 53.2835 | 142.0 |
| 0.1058 | 9.0 | 3582 | 0.8030 | 54.6634 | 38.2708 | 42.232 | 52.6619 | 142.0 |
| 0.1058 | 10.0 | 3980 | 0.8057 | 53.8692 | 37.943 | 41.1825 | 51.7243 | 142.0 |
| 0.0803 | 11.0 | 4378 | 0.8182 | 56.5077 | 41.5916 | 44.1933 | 54.8699 | 142.0 |
| 0.0599 | 12.0 | 4776 | 0.8261 | 56.9709 | 42.1438 | 45.5351 | 55.0701 | 142.0 |
| 0.0458 | 13.0 | 5174 | 0.8469 | 56.5208 | 42.0329 | 44.4172 | 54.7958 | 142.0 |
| 0.0346 | 14.0 | 5572 | 0.8583 | 56.9187 | 42.4072 | 46.1096 | 55.3656 | 142.0 |
| 0.0346 | 15.0 | 5970 | 0.8653 | 56.503 | 42.047 | 45.8598 | 54.9676 | 141.8519 |
| 0.0293 | 16.0 | 6368 | 0.8702 | 56.1421 | 41.3514 | 44.5146 | 54.3477 | 142.0 |
### Framework versions
- Transformers 4.18.0
- Pytorch 1.11.0+cu113
- Datasets 2.1.0
- Tokenizers 0.12.1
|
vinaykudari/t5-acled-ie-a | 77e12ad7a2c5c4f366d5fd5895e3bc079a58fcaf | 2022-05-09T05:31:01.000Z | [
"pytorch",
"t5",
"text2text-generation",
"transformers",
"autotrain_compatible"
] | text2text-generation | false | vinaykudari | null | vinaykudari/t5-acled-ie-a | 1 | null | transformers | 31,740 | Entry not found |
theojolliffe/bart-cnn-pubmed-arxiv-pubmed-v3-e64 | 455bce5ce0d2d335c1746598d00e9dd966eb34fa | 2022-05-09T02:03:17.000Z | [
"pytorch",
"tensorboard",
"bart",
"text2text-generation",
"transformers",
"generated_from_trainer",
"license:mit",
"model-index",
"autotrain_compatible"
] | text2text-generation | false | theojolliffe | null | theojolliffe/bart-cnn-pubmed-arxiv-pubmed-v3-e64 | 1 | null | transformers | 31,741 | ---
license: mit
tags:
- generated_from_trainer
metrics:
- rouge
model-index:
- name: bart-cnn-pubmed-arxiv-pubmed-v3-e64
results: []
---
<!-- This model card has been generated automatically according to the information the Trainer had access to. You
should probably proofread and complete it, then remove this comment. -->
# bart-cnn-pubmed-arxiv-pubmed-v3-e64
This model is a fine-tuned version of [theojolliffe/bart-cnn-pubmed-arxiv-pubmed](https://huggingface.co/theojolliffe/bart-cnn-pubmed-arxiv-pubmed) on an unknown dataset.
It achieves the following results on the evaluation set:
- Loss: 1.0630
- Rouge1: 58.7
- Rouge2: 47.8042
- Rougel: 50.6967
- Rougelsum: 57.5543
- Gen Len: 142.0
## Model description
More information needed
## Intended uses & limitations
More information needed
## Training and evaluation data
More information needed
## Training procedure
### Training hyperparameters
The following hyperparameters were used during training:
- learning_rate: 2e-05
- train_batch_size: 2
- eval_batch_size: 2
- seed: 42
- optimizer: Adam with betas=(0.9,0.999) and epsilon=1e-08
- lr_scheduler_type: linear
- num_epochs: 64
- mixed_precision_training: Native AMP
### Training results
| Training Loss | Epoch | Step | Validation Loss | Rouge1 | Rouge2 | Rougel | Rougelsum | Gen Len |
|:-------------:|:-----:|:-----:|:---------------:|:-------:|:-------:|:-------:|:---------:|:--------:|
| No log | 1.0 | 398 | 0.9499 | 53.8396 | 34.0954 | 35.6734 | 51.3453 | 142.0 |
| 1.1219 | 2.0 | 796 | 0.8223 | 53.0414 | 33.3193 | 35.7448 | 50.1675 | 142.0 |
| 0.6681 | 3.0 | 1194 | 0.7689 | 53.6684 | 35.3651 | 37.7087 | 51.1441 | 142.0 |
| 0.4393 | 4.0 | 1592 | 0.7694 | 53.9066 | 35.3925 | 38.8917 | 51.6172 | 142.0 |
| 0.4393 | 5.0 | 1990 | 0.7597 | 54.0746 | 36.1026 | 39.1318 | 51.9272 | 142.0 |
| 0.2947 | 6.0 | 2388 | 0.8284 | 53.1168 | 34.7428 | 38.0573 | 50.9563 | 142.0 |
| 0.2016 | 7.0 | 2786 | 0.7951 | 55.7222 | 39.0458 | 42.5265 | 53.5359 | 142.0 |
| 0.1422 | 8.0 | 3184 | 0.7793 | 56.2376 | 40.3348 | 43.435 | 54.3228 | 142.0 |
| 0.1096 | 9.0 | 3582 | 0.8260 | 55.0372 | 39.0552 | 42.5403 | 53.0694 | 142.0 |
| 0.1096 | 10.0 | 3980 | 0.8397 | 53.849 | 37.519 | 40.674 | 52.1357 | 141.7037 |
| 0.0881 | 11.0 | 4378 | 0.8504 | 56.4835 | 41.0484 | 44.9407 | 54.3557 | 142.0 |
| 0.0693 | 12.0 | 4776 | 0.8285 | 55.7705 | 39.8585 | 43.722 | 53.7607 | 142.0 |
| 0.0572 | 13.0 | 5174 | 0.8327 | 57.932 | 43.5378 | 46.8233 | 55.8739 | 142.0 |
| 0.0461 | 14.0 | 5572 | 0.8720 | 57.6733 | 42.9742 | 45.8698 | 56.018 | 142.0 |
| 0.0461 | 15.0 | 5970 | 0.8723 | 57.6072 | 42.6946 | 45.2551 | 55.8486 | 142.0 |
| 0.0416 | 16.0 | 6368 | 0.8764 | 57.1973 | 43.1931 | 46.4492 | 55.3842 | 142.0 |
| 0.0343 | 17.0 | 6766 | 0.8638 | 57.4474 | 43.3544 | 46.3026 | 55.7863 | 142.0 |
| 0.03 | 18.0 | 7164 | 0.9234 | 57.9166 | 43.8551 | 46.6473 | 56.3895 | 142.0 |
| 0.0252 | 19.0 | 7562 | 0.9393 | 58.2908 | 45.2321 | 47.1398 | 56.6618 | 142.0 |
| 0.0252 | 20.0 | 7960 | 0.8966 | 59.2798 | 46.381 | 49.3514 | 57.6061 | 142.0 |
| 0.024 | 21.0 | 8358 | 0.9056 | 57.8409 | 44.2048 | 47.3329 | 56.2568 | 142.0 |
| 0.0195 | 22.0 | 8756 | 0.9424 | 57.551 | 44.6847 | 47.2771 | 56.2391 | 142.0 |
| 0.0182 | 23.0 | 9154 | 0.9361 | 59.1078 | 46.4704 | 49.4178 | 57.6796 | 142.0 |
| 0.0169 | 24.0 | 9552 | 0.9456 | 56.7966 | 43.3135 | 46.4208 | 55.4646 | 142.0 |
| 0.0169 | 25.0 | 9950 | 0.9867 | 59.5561 | 47.4638 | 50.0725 | 58.2388 | 141.8519 |
| 0.0147 | 26.0 | 10348 | 0.9727 | 58.2574 | 44.9904 | 47.2701 | 56.4274 | 142.0 |
| 0.0125 | 27.0 | 10746 | 0.9589 | 58.6792 | 45.8465 | 48.0781 | 57.0755 | 142.0 |
| 0.0117 | 28.0 | 11144 | 0.9635 | 59.1118 | 46.6614 | 50.0552 | 57.6153 | 142.0 |
| 0.0103 | 29.0 | 11542 | 0.9623 | 58.2517 | 45.6401 | 48.5888 | 56.7733 | 142.0 |
| 0.0103 | 30.0 | 11940 | 0.9752 | 59.0707 | 47.203 | 49.7992 | 57.6216 | 142.0 |
| 0.0096 | 31.0 | 12338 | 0.9610 | 57.6781 | 44.0504 | 47.6718 | 56.1201 | 142.0 |
| 0.0089 | 32.0 | 12736 | 0.9705 | 58.5592 | 45.7397 | 48.681 | 57.0302 | 142.0 |
| 0.008 | 33.0 | 13134 | 0.9989 | 58.1997 | 45.6345 | 48.2551 | 56.8571 | 141.7778 |
| 0.0075 | 34.0 | 13532 | 0.9880 | 57.9632 | 44.7845 | 47.8763 | 56.3979 | 142.0 |
| 0.0075 | 35.0 | 13930 | 1.0041 | 58.1316 | 46.2737 | 49.5986 | 56.8263 | 142.0 |
| 0.0061 | 36.0 | 14328 | 0.9923 | 58.4686 | 46.1735 | 49.1299 | 57.0331 | 142.0 |
| 0.0066 | 37.0 | 14726 | 1.0157 | 58.4277 | 45.6559 | 49.1739 | 56.8198 | 141.6481 |
| 0.0052 | 38.0 | 15124 | 1.0220 | 58.5166 | 46.3883 | 50.0964 | 57.0104 | 142.0 |
| 0.0049 | 39.0 | 15522 | 0.9949 | 59.3697 | 47.0609 | 50.2733 | 58.1388 | 142.0 |
| 0.0049 | 40.0 | 15920 | 1.0368 | 59.9537 | 48.4059 | 51.8185 | 58.8002 | 142.0 |
| 0.0039 | 41.0 | 16318 | 1.0228 | 58.2093 | 46.4807 | 49.54 | 56.9994 | 142.0 |
| 0.0041 | 42.0 | 16716 | 1.0218 | 57.6376 | 45.4951 | 49.003 | 56.4606 | 142.0 |
| 0.0035 | 43.0 | 17114 | 1.0381 | 57.2845 | 43.9593 | 46.779 | 55.6106 | 142.0 |
| 0.0059 | 44.0 | 17512 | 1.0316 | 58.5506 | 46.2111 | 49.4844 | 56.9506 | 142.0 |
| 0.0059 | 45.0 | 17910 | 1.0388 | 58.8383 | 47.6053 | 50.6187 | 57.7125 | 142.0 |
| 0.0028 | 46.0 | 18308 | 1.0068 | 59.3198 | 47.6888 | 50.2478 | 58.0 | 142.0 |
| 0.0028 | 47.0 | 18706 | 1.0446 | 58.8938 | 46.7524 | 49.5642 | 57.3659 | 142.0 |
| 0.0022 | 48.0 | 19104 | 1.0347 | 59.8253 | 48.3871 | 51.3949 | 58.5652 | 142.0 |
| 0.0024 | 49.0 | 19502 | 1.0294 | 60.655 | 50.2339 | 53.1662 | 59.3333 | 142.0 |
| 0.0024 | 50.0 | 19900 | 1.0225 | 58.5131 | 47.3009 | 50.1642 | 57.2287 | 142.0 |
| 0.0022 | 51.0 | 20298 | 1.0320 | 59.6101 | 47.4104 | 50.5291 | 58.075 | 142.0 |
| 0.0018 | 52.0 | 20696 | 1.0507 | 58.7957 | 46.8893 | 50.2996 | 57.3662 | 142.0 |
| 0.0015 | 53.0 | 21094 | 1.0599 | 58.9064 | 47.9433 | 51.3082 | 57.6871 | 142.0 |
| 0.0015 | 54.0 | 21492 | 1.0636 | 59.6607 | 48.5737 | 51.2361 | 58.333 | 142.0 |
| 0.0013 | 55.0 | 21890 | 1.0452 | 58.7026 | 46.5286 | 49.9672 | 57.2521 | 142.0 |
| 0.0012 | 56.0 | 22288 | 1.0418 | 58.9452 | 47.7209 | 50.657 | 57.7103 | 142.0 |
| 0.0011 | 57.0 | 22686 | 1.0578 | 58.485 | 46.0691 | 49.811 | 57.2591 | 142.0 |
| 0.0009 | 58.0 | 23084 | 1.0561 | 59.2268 | 48.1987 | 50.1948 | 57.8871 | 142.0 |
| 0.0009 | 59.0 | 23482 | 1.0548 | 59.6307 | 48.1778 | 50.9934 | 58.2098 | 142.0 |
| 0.0009 | 60.0 | 23880 | 1.0498 | 59.5054 | 48.8866 | 51.5977 | 58.1868 | 142.0 |
| 0.0008 | 61.0 | 24278 | 1.0583 | 60.0232 | 49.2518 | 52.2297 | 58.6774 | 142.0 |
| 0.0007 | 62.0 | 24676 | 1.0659 | 59.1755 | 48.4144 | 51.5157 | 58.0416 | 142.0 |
| 0.0007 | 63.0 | 25074 | 1.0622 | 59.1023 | 47.74 | 50.5188 | 57.9707 | 142.0 |
| 0.0007 | 64.0 | 25472 | 1.0630 | 58.7 | 47.8042 | 50.6967 | 57.5543 | 142.0 |
### Framework versions
- Transformers 4.18.0
- Pytorch 1.11.0+cu113
- Datasets 2.1.0
- Tokenizers 0.12.1
|
HarmlessTarget/DialoGPT-medium-Bender | 481d94a4a4c8c2dd092f0c0666740d9ee2928b84 | 2022-05-09T16:46:11.000Z | [
"pytorch",
"gpt2",
"text-generation",
"transformers",
"conversational"
] | conversational | false | HarmlessTarget | null | HarmlessTarget/DialoGPT-medium-Bender | 1 | null | transformers | 31,742 | ---
tags:
- conversational
---
#Bender DialoGPT Model |
Xikun/greaselm-csqa | 1d90a41f975f4db9e56075201850df49a1be1895 | 2022-05-09T04:29:28.000Z | [
"pytorch",
"greaselm",
"transformers"
] | null | false | Xikun | null | Xikun/greaselm-csqa | 1 | null | transformers | 31,743 | |
huggingtweets/malnote | 28cb709ccf12c5ab0e44a1ac0dac898d7299f771 | 2022-05-09T05:36:36.000Z | [
"pytorch",
"gpt2",
"text-generation",
"en",
"transformers",
"huggingtweets"
] | text-generation | false | huggingtweets | null | huggingtweets/malnote | 1 | null | transformers | 31,744 | ---
language: en
thumbnail: http://www.huggingtweets.com/malnote/1652074591822/predictions.png
tags:
- huggingtweets
widget:
- text: "My dream is"
---
<div class="inline-flex flex-col" style="line-height: 1.5;">
<div class="flex">
<div
style="display:inherit; margin-left: 4px; margin-right: 4px; width: 92px; height:92px; border-radius: 50%; background-size: cover; background-image: url('https://pbs.twimg.com/profile_images/1475058675626561537/bI19TTid_400x400.png')">
</div>
<div
style="display:none; margin-left: 4px; margin-right: 4px; width: 92px; height:92px; border-radius: 50%; background-size: cover; background-image: url('')">
</div>
<div
style="display:none; margin-left: 4px; margin-right: 4px; width: 92px; height:92px; border-radius: 50%; background-size: cover; background-image: url('')">
</div>
</div>
<div style="text-align: center; margin-top: 3px; font-size: 16px; font-weight: 800">π€ AI BOT π€</div>
<div style="text-align: center; font-size: 16px; font-weight: 800">Arantxa Ε tefan</div>
<div style="text-align: center; font-size: 14px;">@malnote</div>
</div>
I was made with [huggingtweets](https://github.com/borisdayma/huggingtweets).
Create your own bot based on your favorite user with [the demo](https://colab.research.google.com/github/borisdayma/huggingtweets/blob/master/huggingtweets-demo.ipynb)!
## How does it work?
The model uses the following pipeline.
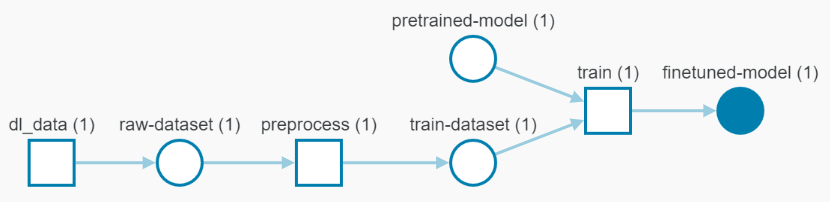
To understand how the model was developed, check the [W&B report](https://wandb.ai/wandb/huggingtweets/reports/HuggingTweets-Train-a-Model-to-Generate-Tweets--VmlldzoxMTY5MjI).
## Training data
The model was trained on tweets from Arantxa Ε tefan.
| Data | Arantxa Ε tefan |
| --- | --- |
| Tweets downloaded | 3250 |
| Retweets | 6 |
| Short tweets | 218 |
| Tweets kept | 3026 |
[Explore the data](https://wandb.ai/wandb/huggingtweets/runs/ow72fqyd/artifacts), which is tracked with [W&B artifacts](https://docs.wandb.com/artifacts) at every step of the pipeline.
## Training procedure
The model is based on a pre-trained [GPT-2](https://huggingface.co/gpt2) which is fine-tuned on @malnote's tweets.
Hyperparameters and metrics are recorded in the [W&B training run](https://wandb.ai/wandb/huggingtweets/runs/33l50h31) for full transparency and reproducibility.
At the end of training, [the final model](https://wandb.ai/wandb/huggingtweets/runs/33l50h31/artifacts) is logged and versioned.
## How to use
You can use this model directly with a pipeline for text generation:
```python
from transformers import pipeline
generator = pipeline('text-generation',
model='huggingtweets/malnote')
generator("My dream is", num_return_sequences=5)
```
## Limitations and bias
The model suffers from [the same limitations and bias as GPT-2](https://huggingface.co/gpt2#limitations-and-bias).
In addition, the data present in the user's tweets further affects the text generated by the model.
## About
*Built by Boris Dayma*
[](https://twitter.com/intent/follow?screen_name=borisdayma)
For more details, visit the project repository.
[](https://github.com/borisdayma/huggingtweets)
|
ntcuong777/electra-iu-answer-retrieval | 31a4f070a06ce3d184474ee5aa92172b82b9ede3 | 2022-05-13T15:31:50.000Z | [
"pytorch",
"electra",
"transformers"
] | null | false | ntcuong777 | null | ntcuong777/electra-iu-answer-retrieval | 1 | null | transformers | 31,745 | This is a model for International University VNU-HCMC use cases only. |
subhasisj/zh-Pretrained-squad-qa-minilmv2-32 | aff50118b71ff81324b9b052bdce31780b3671e9 | 2022-05-28T20:12:43.000Z | [
"pytorch",
"bert",
"question-answering",
"transformers",
"autotrain_compatible"
] | question-answering | false | subhasisj | null | subhasisj/zh-Pretrained-squad-qa-minilmv2-32 | 1 | null | transformers | 31,746 | Entry not found |
theojolliffe/distilbart-cnn-arxiv-pubmed-pubmed | bd049f10ea31d4215d8bae0f2fda46484601137f | 2022-05-10T00:28:07.000Z | [
"pytorch",
"tensorboard",
"bart",
"text2text-generation",
"dataset:scientific_papers",
"transformers",
"generated_from_trainer",
"license:apache-2.0",
"model-index",
"autotrain_compatible"
] | text2text-generation | false | theojolliffe | null | theojolliffe/distilbart-cnn-arxiv-pubmed-pubmed | 1 | null | transformers | 31,747 | ---
license: apache-2.0
tags:
- generated_from_trainer
datasets:
- scientific_papers
metrics:
- rouge
model-index:
- name: distilbart-cnn-arxiv-pubmed-pubmed
results:
- task:
name: Sequence-to-sequence Language Modeling
type: text2text-generation
dataset:
name: scientific_papers
type: scientific_papers
args: pubmed
metrics:
- name: Rouge1
type: rouge
value: 36.6704
---
<!-- This model card has been generated automatically according to the information the Trainer had access to. You
should probably proofread and complete it, then remove this comment. -->
# distilbart-cnn-arxiv-pubmed-pubmed
This model is a fine-tuned version of [theojolliffe/distilbart-cnn-arxiv-pubmed](https://huggingface.co/theojolliffe/distilbart-cnn-arxiv-pubmed) on the scientific_papers dataset.
It achieves the following results on the evaluation set:
- Loss: 2.1171
- Rouge1: 36.6704
- Rouge2: 14.9713
- Rougel: 22.6149
- Rougelsum: 33.3591
- Gen Len: 136.8372
## Model description
More information needed
## Intended uses & limitations
More information needed
## Training and evaluation data
More information needed
## Training procedure
### Training hyperparameters
The following hyperparameters were used during training:
- learning_rate: 2e-05
- train_batch_size: 2
- eval_batch_size: 2
- seed: 42
- optimizer: Adam with betas=(0.9,0.999) and epsilon=1e-08
- lr_scheduler_type: linear
- num_epochs: 1
- mixed_precision_training: Native AMP
### Training results
| Training Loss | Epoch | Step | Validation Loss | Rouge1 | Rouge2 | Rougel | Rougelsum | Gen Len |
|:-------------:|:-----:|:-----:|:---------------:|:-------:|:-------:|:-------:|:---------:|:--------:|
| 2.2556 | 1.0 | 14991 | 2.1171 | 36.6704 | 14.9713 | 22.6149 | 33.3591 | 136.8372 |
### Framework versions
- Transformers 4.18.0
- Pytorch 1.11.0+cu113
- Datasets 2.1.0
- Tokenizers 0.12.1
|
captainswiftfox/DialoGPT-small-rick | acdaa4aa9c08b6af3614b6b341e85dc1cfcb448f | 2022-05-09T13:16:17.000Z | [
"pytorch",
"gpt2",
"text-generation",
"transformers",
"conversational"
] | conversational | false | captainswiftfox | null | captainswiftfox/DialoGPT-small-rick | 1 | null | transformers | 31,748 | ---
tags:
- conversational
---
# Rick Sanchez DialoGPT Model |
masakhane/afrimbart_pcm_en_news | 0f8ffe87174459667f0be7df4f7369689de56386 | 2022-05-10T11:17:35.000Z | [
"pytorch",
"mbart",
"text2text-generation",
"transformers",
"license:afl-3.0",
"autotrain_compatible"
] | text2text-generation | false | masakhane | null | masakhane/afrimbart_pcm_en_news | 1 | null | transformers | 31,749 | ---
license: afl-3.0
---
|
phanidhar/model-imdb-finetuned | 30a1fe3ecf5b76d9d41c09ab705649d2fd76136f | 2022-05-09T16:42:43.000Z | [
"pytorch",
"bert",
"fill-mask",
"transformers",
"autotrain_compatible"
] | fill-mask | false | phanidhar | null | phanidhar/model-imdb-finetuned | 1 | null | transformers | 31,750 | Entry not found |
IljaSamoilov/MBART-estonian-subtitles-with-seconds | b0f2f2d278cdd5e03bae61080405316ea36c9777 | 2022-05-12T12:34:45.000Z | [
"pytorch",
"mbart",
"text2text-generation",
"et",
"transformers",
"autotrain_compatible"
] | text2text-generation | false | IljaSamoilov | null | IljaSamoilov/MBART-estonian-subtitles-with-seconds | 1 | null | transformers | 31,751 | ---
language:
- et
widget:
- text: "te olete ka noh, noh, pÀris korralikult ka RahvusringhÀÀlingu teatud máttes sellisesse keerulisse olukorda pannud,"
- text: "Et, et, et miks mitte olla siis tasakaalus, ma noh, hΓΌpoteetiliselt viskan selle palli ΓΌles,"
---
Dataset must be processed as following:
```
def preprocess_function_with_seconds(ds):
inputs = ds['generated']
targets = ds['subtitle']
model_inputs = tokenizer(inputs, truncation=True, max_length=128, padding=True, return_tensors="np")
secs = list(map(lambda x: "{:.1f}".format(x), ds["seconds"]))
sec_inputs = tokenizer(secs, truncation=True, max_length=128, padding=True, return_tensors="np")
model_inputs['input_ids'] = np.concatenate((sec_inputs['input_ids'][:,1:2], model_inputs['input_ids']), 1)
model_inputs['attention_mask'] = np.concatenate((sec_inputs['attention_mask'][:,1:2], model_inputs['attention_mask']), 1)
with tokenizer.as_target_tokenizer():
labels = tokenizer(targets, truncation=True, max_length=128, padding=True, return_tensors="np")
model_inputs["labels"] = labels["input_ids"]
return model_inputs
```
Importing the model and tokenizer:
```
tokenizer = MBart50Tokenizer.from_pretrained("IljaSamoilov/MBART-estonian-subtitles-with-seconds", src_lang="et_EE", tgt_lang="et_EE")
model = MBartForConditionalGeneration.from_pretrained("IljaSamoilov/MBART-estonian-subtitles-with-seconds")
``` |
subhasisj/MiniLMv2-qa-encoder | 13a00c23112b69092903a29a55117b8b7cc31f37 | 2022-05-09T19:33:47.000Z | [
"pytorch",
"bert",
"fill-mask",
"transformers",
"autotrain_compatible"
] | fill-mask | false | subhasisj | null | subhasisj/MiniLMv2-qa-encoder | 1 | null | transformers | 31,752 | Entry not found |
murdockthedude/wav2vec2-base-timit-demo-colab | ddbfb1bc6e94479103a6972f83f774632ce56eef | 2022-05-10T02:31:15.000Z | [
"pytorch",
"tensorboard",
"wav2vec2",
"automatic-speech-recognition",
"transformers",
"generated_from_trainer",
"license:apache-2.0",
"model-index"
] | automatic-speech-recognition | false | murdockthedude | null | murdockthedude/wav2vec2-base-timit-demo-colab | 1 | null | transformers | 31,753 | ---
license: apache-2.0
tags:
- generated_from_trainer
model-index:
- name: wav2vec2-base-timit-demo-colab
results: []
---
<!-- This model card has been generated automatically according to the information the Trainer had access to. You
should probably proofread and complete it, then remove this comment. -->
# wav2vec2-base-timit-demo-colab
This model is a fine-tuned version of [facebook/wav2vec2-base](https://huggingface.co/facebook/wav2vec2-base) on the None dataset.
It achieves the following results on the evaluation set:
- Loss: 0.4627
- Wer: 0.3518
## Model description
More information needed
## Intended uses & limitations
More information needed
## Training and evaluation data
More information needed
## Training procedure
### Training hyperparameters
The following hyperparameters were used during training:
- learning_rate: 0.0001
- train_batch_size: 32
- eval_batch_size: 8
- seed: 42
- optimizer: Adam with betas=(0.9,0.999) and epsilon=1e-08
- lr_scheduler_type: linear
- lr_scheduler_warmup_steps: 1000
- num_epochs: 30
- mixed_precision_training: Native AMP
### Training results
| Training Loss | Epoch | Step | Validation Loss | Wer |
|:-------------:|:-----:|:----:|:---------------:|:------:|
| 3.4716 | 4.0 | 500 | 1.3023 | 0.9254 |
| 0.5958 | 8.0 | 1000 | 0.4582 | 0.4399 |
| 0.2223 | 12.0 | 1500 | 0.4477 | 0.3886 |
| 0.1373 | 16.0 | 2000 | 0.4791 | 0.3630 |
| 0.101 | 20.0 | 2500 | 0.4676 | 0.3561 |
| 0.0724 | 24.0 | 3000 | 0.4539 | 0.3510 |
| 0.0513 | 28.0 | 3500 | 0.4627 | 0.3518 |
### Framework versions
- Transformers 4.18.0
- Pytorch 1.11.0+cu113
- Datasets 1.17.0
- Tokenizers 0.12.1
|
dfsj/xlm-roberta-base-finetuned-panx-de | c6a5eb27309d5d7bb3d9bb62373f574f6719fc64 | 2022-05-10T03:20:57.000Z | [
"pytorch",
"xlm-roberta",
"token-classification",
"dataset:xtreme",
"transformers",
"generated_from_trainer",
"license:mit",
"model-index",
"autotrain_compatible"
] | token-classification | false | dfsj | null | dfsj/xlm-roberta-base-finetuned-panx-de | 1 | null | transformers | 31,754 | ---
license: mit
tags:
- generated_from_trainer
datasets:
- xtreme
metrics:
- f1
model-index:
- name: xlm-roberta-base-finetuned-panx-de
results:
- task:
name: Token Classification
type: token-classification
dataset:
name: xtreme
type: xtreme
args: PAN-X.de
metrics:
- name: F1
type: f1
value: 0.8674931756141947
---
<!-- This model card has been generated automatically according to the information the Trainer had access to. You
should probably proofread and complete it, then remove this comment. -->
# xlm-roberta-base-finetuned-panx-de
This model is a fine-tuned version of [xlm-roberta-base](https://huggingface.co/xlm-roberta-base) on the xtreme dataset.
It achieves the following results on the evaluation set:
- Loss: 0.1326
- F1: 0.8675
## Model description
More information needed
## Intended uses & limitations
More information needed
## Training and evaluation data
More information needed
## Training procedure
### Training hyperparameters
The following hyperparameters were used during training:
- learning_rate: 5e-05
- train_batch_size: 24
- eval_batch_size: 24
- seed: 42
- optimizer: Adam with betas=(0.9,0.999) and epsilon=1e-08
- lr_scheduler_type: linear
- num_epochs: 3
### Training results
| Training Loss | Epoch | Step | Validation Loss | F1 |
|:-------------:|:-----:|:----:|:---------------:|:------:|
| 0.2654 | 1.0 | 525 | 0.1745 | 0.8133 |
| 0.1317 | 2.0 | 1050 | 0.1428 | 0.8427 |
| 0.0823 | 3.0 | 1575 | 0.1326 | 0.8675 |
### Framework versions
- Transformers 4.18.0
- Pytorch 1.10.0+cu102
- Datasets 2.0.0
- Tokenizers 0.12.1
|
hsiehpinghan/dummy-model | d5259925506dd6d836c08c3aac39dbc1a0e5696b | 2022-05-10T06:46:53.000Z | [
"pytorch",
"albert",
"fill-mask",
"transformers",
"autotrain_compatible"
] | fill-mask | false | hsiehpinghan | null | hsiehpinghan/dummy-model | 1 | null | transformers | 31,755 | Entry not found |
naomiyjchen/xlm-roberta-base-finetuned-panx-de | 0a7e15bff047fb52de0b3199870d0eea67976e3f | 2022-05-10T08:47:46.000Z | [
"pytorch",
"tensorboard",
"xlm-roberta",
"token-classification",
"dataset:xtreme",
"transformers",
"generated_from_trainer",
"license:mit",
"model-index",
"autotrain_compatible"
] | token-classification | false | naomiyjchen | null | naomiyjchen/xlm-roberta-base-finetuned-panx-de | 1 | null | transformers | 31,756 | ---
license: mit
tags:
- generated_from_trainer
datasets:
- xtreme
metrics:
- f1
model-index:
- name: xlm-roberta-base-finetuned-panx-de
results:
- task:
name: Token Classification
type: token-classification
dataset:
name: xtreme
type: xtreme
args: PAN-X.de
metrics:
- name: F1
type: f1
value: 0.8620945214069894
---
<!-- This model card has been generated automatically according to the information the Trainer had access to. You
should probably proofread and complete it, then remove this comment. -->
# xlm-roberta-base-finetuned-panx-de
This model is a fine-tuned version of [xlm-roberta-base](https://huggingface.co/xlm-roberta-base) on the xtreme dataset.
It achieves the following results on the evaluation set:
- Loss: 0.1372
- F1: 0.8621
## Model description
More information needed
## Intended uses & limitations
More information needed
## Training and evaluation data
More information needed
## Training procedure
### Training hyperparameters
The following hyperparameters were used during training:
- learning_rate: 5e-05
- train_batch_size: 24
- eval_batch_size: 24
- seed: 42
- optimizer: Adam with betas=(0.9,0.999) and epsilon=1e-08
- lr_scheduler_type: linear
- num_epochs: 3
### Training results
| Training Loss | Epoch | Step | Validation Loss | F1 |
|:-------------:|:-----:|:----:|:---------------:|:------:|
| 0.2575 | 1.0 | 525 | 0.1621 | 0.8292 |
| 0.1287 | 2.0 | 1050 | 0.1378 | 0.8526 |
| 0.0831 | 3.0 | 1575 | 0.1372 | 0.8621 |
### Framework versions
- Transformers 4.11.3
- Pytorch 1.11.0+cu113
- Datasets 1.16.1
- Tokenizers 0.10.3
|
ebonazza2910/model-1h | fef7a26e3788ec8bf43c9e93e313e2f182a0e87a | 2022-05-10T11:13:54.000Z | [
"pytorch",
"tensorboard",
"wav2vec2",
"automatic-speech-recognition",
"dataset:common_voice",
"transformers",
"generated_from_trainer",
"license:apache-2.0",
"model-index"
] | automatic-speech-recognition | false | ebonazza2910 | null | ebonazza2910/model-1h | 1 | null | transformers | 31,757 | ---
license: apache-2.0
tags:
- generated_from_trainer
datasets:
- common_voice
model-index:
- name: model-1h
results: []
---
<!-- This model card has been generated automatically according to the information the Trainer had access to. You
should probably proofread and complete it, then remove this comment. -->
# model-1h
This model is a fine-tuned version of [facebook/wav2vec2-xls-r-300m](https://huggingface.co/facebook/wav2vec2-xls-r-300m) on the common_voice dataset.
It achieves the following results on the evaluation set:
- Loss: 1.8317
- Wer: 1.0
## Model description
More information needed
## Intended uses & limitations
More information needed
## Training and evaluation data
More information needed
## Training procedure
### Training hyperparameters
The following hyperparameters were used during training:
- learning_rate: 0.0003
- train_batch_size: 16
- eval_batch_size: 8
- seed: 42
- gradient_accumulation_steps: 2
- total_train_batch_size: 32
- optimizer: Adam with betas=(0.9,0.999) and epsilon=1e-08
- lr_scheduler_type: linear
- lr_scheduler_warmup_steps: 5
- num_epochs: 30
- mixed_precision_training: Native AMP
### Training results
| Training Loss | Epoch | Step | Validation Loss | Wer |
|:-------------:|:-----:|:----:|:---------------:|:------:|
| 11.4106 | 1.24 | 10 | 7.1597 | 1.0 |
| 4.777 | 2.47 | 20 | 3.9782 | 1.0 |
| 3.6585 | 3.71 | 30 | 3.3961 | 1.0 |
| 3.3143 | 4.94 | 40 | 3.1481 | 1.0 |
| 3.3318 | 6.24 | 50 | 3.0596 | 1.0 |
| 3.1368 | 7.47 | 60 | 2.9751 | 1.0 |
| 3.1058 | 8.71 | 70 | 2.9510 | 1.0 |
| 3.0605 | 9.94 | 80 | 2.9479 | 1.0 |
| 3.2043 | 11.24 | 90 | 2.9270 | 1.0 |
| 3.0424 | 12.47 | 100 | 2.9349 | 1.0 |
| 3.0374 | 13.71 | 110 | 2.9316 | 1.0 |
| 3.0256 | 14.94 | 120 | 2.9165 | 1.0 |
| 3.1724 | 16.24 | 130 | 2.9076 | 1.0 |
| 3.0119 | 17.47 | 140 | 2.9034 | 1.0 |
| 2.9937 | 18.71 | 150 | 2.8812 | 1.0 |
| 2.9775 | 19.94 | 160 | 2.8674 | 1.0 |
| 3.0826 | 21.24 | 170 | 2.8147 | 1.0 |
| 2.8717 | 22.47 | 180 | 2.7212 | 1.0 |
| 2.7714 | 23.71 | 190 | 2.6149 | 0.9952 |
| 2.634 | 24.94 | 200 | 2.4611 | 0.9984 |
| 2.5637 | 26.24 | 210 | 2.2734 | 1.0 |
| 2.237 | 27.47 | 220 | 2.0705 | 1.0 |
| 2.0381 | 28.71 | 230 | 1.9216 | 1.0 |
| 1.8788 | 29.94 | 240 | 1.8317 | 1.0 |
### Framework versions
- Transformers 4.11.3
- Pytorch 1.9.1+cu102
- Datasets 1.18.3
- Tokenizers 0.10.3
|
masakhane/m2m100_418M_en_swa_rel_news | dad4574c07921803246439f660f52c428220e04f | 2022-05-10T14:24:45.000Z | [
"pytorch",
"m2m_100",
"text2text-generation",
"transformers",
"license:afl-3.0",
"autotrain_compatible"
] | text2text-generation | false | masakhane | null | masakhane/m2m100_418M_en_swa_rel_news | 1 | null | transformers | 31,758 | ---
license: afl-3.0
---
|
masakhane/m2m100_418M_swa_en_rel_news_ft | 9c78bf85fe75ce3c9a52ef88357bdddfe349ae10 | 2022-05-10T14:34:37.000Z | [
"pytorch",
"m2m_100",
"text2text-generation",
"transformers",
"license:afl-3.0",
"autotrain_compatible"
] | text2text-generation | false | masakhane | null | masakhane/m2m100_418M_swa_en_rel_news_ft | 1 | null | transformers | 31,759 | ---
license: afl-3.0
---
|
huggingtweets/marcfriedrich7 | 3484465efd1c5ba35a2f569a7b92fcba6e876bad | 2022-05-10T10:39:29.000Z | [
"pytorch",
"gpt2",
"text-generation",
"en",
"transformers",
"huggingtweets"
] | text-generation | false | huggingtweets | null | huggingtweets/marcfriedrich7 | 1 | null | transformers | 31,760 | ---
language: en
thumbnail: http://www.huggingtweets.com/marcfriedrich7/1652179164370/predictions.png
tags:
- huggingtweets
widget:
- text: "My dream is"
---
<div class="inline-flex flex-col" style="line-height: 1.5;">
<div class="flex">
<div
style="display:inherit; margin-left: 4px; margin-right: 4px; width: 92px; height:92px; border-radius: 50%; background-size: cover; background-image: url('https://pbs.twimg.com/profile_images/1418445526375223297/XdAgs-rW_400x400.jpg')">
</div>
<div
style="display:none; margin-left: 4px; margin-right: 4px; width: 92px; height:92px; border-radius: 50%; background-size: cover; background-image: url('')">
</div>
<div
style="display:none; margin-left: 4px; margin-right: 4px; width: 92px; height:92px; border-radius: 50%; background-size: cover; background-image: url('')">
</div>
</div>
<div style="text-align: center; margin-top: 3px; font-size: 16px; font-weight: 800">π€ AI BOT π€</div>
<div style="text-align: center; font-size: 16px; font-weight: 800">marc friedrich</div>
<div style="text-align: center; font-size: 14px;">@marcfriedrich7</div>
</div>
I was made with [huggingtweets](https://github.com/borisdayma/huggingtweets).
Create your own bot based on your favorite user with [the demo](https://colab.research.google.com/github/borisdayma/huggingtweets/blob/master/huggingtweets-demo.ipynb)!
## How does it work?
The model uses the following pipeline.
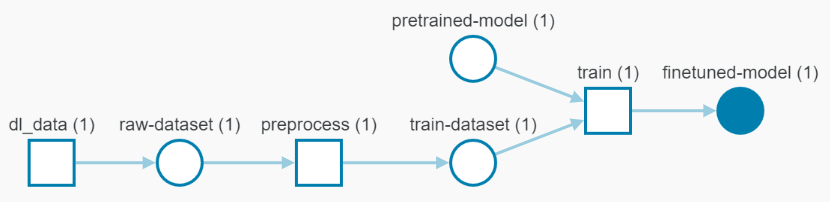
To understand how the model was developed, check the [W&B report](https://wandb.ai/wandb/huggingtweets/reports/HuggingTweets-Train-a-Model-to-Generate-Tweets--VmlldzoxMTY5MjI).
## Training data
The model was trained on tweets from marc friedrich.
| Data | marc friedrich |
| --- | --- |
| Tweets downloaded | 3249 |
| Retweets | 705 |
| Short tweets | 672 |
| Tweets kept | 1872 |
[Explore the data](https://wandb.ai/wandb/huggingtweets/runs/2p2smtko/artifacts), which is tracked with [W&B artifacts](https://docs.wandb.com/artifacts) at every step of the pipeline.
## Training procedure
The model is based on a pre-trained [GPT-2](https://huggingface.co/gpt2) which is fine-tuned on @marcfriedrich7's tweets.
Hyperparameters and metrics are recorded in the [W&B training run](https://wandb.ai/wandb/huggingtweets/runs/2ly8l45f) for full transparency and reproducibility.
At the end of training, [the final model](https://wandb.ai/wandb/huggingtweets/runs/2ly8l45f/artifacts) is logged and versioned.
## How to use
You can use this model directly with a pipeline for text generation:
```python
from transformers import pipeline
generator = pipeline('text-generation',
model='huggingtweets/marcfriedrich7')
generator("My dream is", num_return_sequences=5)
```
## Limitations and bias
The model suffers from [the same limitations and bias as GPT-2](https://huggingface.co/gpt2#limitations-and-bias).
In addition, the data present in the user's tweets further affects the text generated by the model.
## About
*Built by Boris Dayma*
[](https://twitter.com/intent/follow?screen_name=borisdayma)
For more details, visit the project repository.
[](https://github.com/borisdayma/huggingtweets)
|
huggingtweets/broductmanager | 63a52a6af686988241fa6dcaa974f01224437d5e | 2022-05-10T11:36:53.000Z | [
"pytorch",
"gpt2",
"text-generation",
"en",
"transformers",
"huggingtweets"
] | text-generation | false | huggingtweets | null | huggingtweets/broductmanager | 1 | null | transformers | 31,761 | ---
language: en
thumbnail: http://www.huggingtweets.com/broductmanager/1652182609331/predictions.png
tags:
- huggingtweets
widget:
- text: "My dream is"
---
<div class="inline-flex flex-col" style="line-height: 1.5;">
<div class="flex">
<div
style="display:inherit; margin-left: 4px; margin-right: 4px; width: 92px; height:92px; border-radius: 50%; background-size: cover; background-image: url('https://pbs.twimg.com/profile_images/1522425562895044608/H93gVhPH_400x400.jpg')">
</div>
<div
style="display:none; margin-left: 4px; margin-right: 4px; width: 92px; height:92px; border-radius: 50%; background-size: cover; background-image: url('')">
</div>
<div
style="display:none; margin-left: 4px; margin-right: 4px; width: 92px; height:92px; border-radius: 50%; background-size: cover; background-image: url('')">
</div>
</div>
<div style="text-align: center; margin-top: 3px; font-size: 16px; font-weight: 800">π€ AI BOT π€</div>
<div style="text-align: center; font-size: 16px; font-weight: 800">rahul</div>
<div style="text-align: center; font-size: 14px;">@broductmanager</div>
</div>
I was made with [huggingtweets](https://github.com/borisdayma/huggingtweets).
Create your own bot based on your favorite user with [the demo](https://colab.research.google.com/github/borisdayma/huggingtweets/blob/master/huggingtweets-demo.ipynb)!
## How does it work?
The model uses the following pipeline.
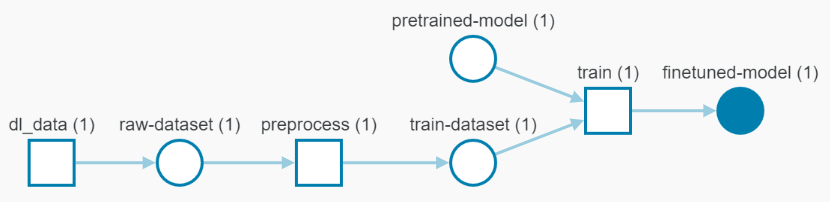
To understand how the model was developed, check the [W&B report](https://wandb.ai/wandb/huggingtweets/reports/HuggingTweets-Train-a-Model-to-Generate-Tweets--VmlldzoxMTY5MjI).
## Training data
The model was trained on tweets from rahul.
| Data | rahul |
| --- | --- |
| Tweets downloaded | 3244 |
| Retweets | 85 |
| Short tweets | 1164 |
| Tweets kept | 1995 |
[Explore the data](https://wandb.ai/wandb/huggingtweets/runs/1r967jne/artifacts), which is tracked with [W&B artifacts](https://docs.wandb.com/artifacts) at every step of the pipeline.
## Training procedure
The model is based on a pre-trained [GPT-2](https://huggingface.co/gpt2) which is fine-tuned on @broductmanager's tweets.
Hyperparameters and metrics are recorded in the [W&B training run](https://wandb.ai/wandb/huggingtweets/runs/2zx676ih) for full transparency and reproducibility.
At the end of training, [the final model](https://wandb.ai/wandb/huggingtweets/runs/2zx676ih/artifacts) is logged and versioned.
## How to use
You can use this model directly with a pipeline for text generation:
```python
from transformers import pipeline
generator = pipeline('text-generation',
model='huggingtweets/broductmanager')
generator("My dream is", num_return_sequences=5)
```
## Limitations and bias
The model suffers from [the same limitations and bias as GPT-2](https://huggingface.co/gpt2#limitations-and-bias).
In addition, the data present in the user's tweets further affects the text generated by the model.
## About
*Built by Boris Dayma*
[](https://twitter.com/intent/follow?screen_name=borisdayma)
For more details, visit the project repository.
[](https://github.com/borisdayma/huggingtweets)
|
masakhane/byt5_yor_en_news | c1f59eaa5d2b61f8b466ef81dc6760d3822f4d50 | 2022-05-10T12:50:15.000Z | [
"pytorch",
"t5",
"text2text-generation",
"transformers",
"license:afl-3.0",
"autotrain_compatible"
] | text2text-generation | false | masakhane | null | masakhane/byt5_yor_en_news | 1 | null | transformers | 31,762 | ---
license: afl-3.0
---
|
masakhane/m2m100_418M_yor_en_rel | 6bb6aad6815d8aa79bb6e9a271812286148ba96d | 2022-05-10T13:38:25.000Z | [
"pytorch",
"m2m_100",
"text2text-generation",
"transformers",
"license:afl-3.0",
"autotrain_compatible"
] | text2text-generation | false | masakhane | null | masakhane/m2m100_418M_yor_en_rel | 1 | null | transformers | 31,763 | ---
license: afl-3.0
---
|
husnu/wav2vec2-large-xls-r-300m-turkish-colab_common_voice-8_5 | 9febefec57da227e41199dd46c3e4ec1dddfb243 | 2022-05-10T17:22:15.000Z | [
"pytorch",
"tensorboard",
"wav2vec2",
"automatic-speech-recognition",
"dataset:common_voice",
"transformers",
"generated_from_trainer",
"license:apache-2.0",
"model-index"
] | automatic-speech-recognition | false | husnu | null | husnu/wav2vec2-large-xls-r-300m-turkish-colab_common_voice-8_5 | 1 | null | transformers | 31,764 | ---
license: apache-2.0
tags:
- generated_from_trainer
datasets:
- common_voice
model-index:
- name: wav2vec2-large-xls-r-300m-turkish-colab_common_voice-8_5
results: []
---
<!-- This model card has been generated automatically according to the information the Trainer had access to. You
should probably proofread and complete it, then remove this comment. -->
# wav2vec2-large-xls-r-300m-turkish-colab_common_voice-8_5
This model is a fine-tuned version of [husnu/wav2vec2-large-xls-r-300m-turkish-colab_common_voice-8_4](https://huggingface.co/husnu/wav2vec2-large-xls-r-300m-turkish-colab_common_voice-8_4) on the common_voice dataset.
It achieves the following results on the evaluation set:
- Loss: 0.3439
- Wer: 0.3634
## Model description
More information needed
## Intended uses & limitations
More information needed
## Training and evaluation data
More information needed
## Training procedure
### Training hyperparameters
The following hyperparameters were used during training:
- learning_rate: 0.0003
- train_batch_size: 16
- eval_batch_size: 8
- seed: 42
- gradient_accumulation_steps: 2
- total_train_batch_size: 32
- optimizer: Adam with betas=(0.9,0.999) and epsilon=1e-08
- lr_scheduler_type: linear
- lr_scheduler_warmup_steps: 500
- num_epochs: 3
- mixed_precision_training: Native AMP
### Training results
| Training Loss | Epoch | Step | Validation Loss | Wer |
|:-------------:|:-----:|:----:|:---------------:|:------:|
| 0.1243 | 0.51 | 400 | 0.4312 | 0.4202 |
| 0.1956 | 1.02 | 800 | 0.4421 | 0.4498 |
| 0.1816 | 1.53 | 1200 | 0.4012 | 0.4285 |
| 0.1548 | 2.04 | 1600 | 0.3720 | 0.3845 |
| 0.1171 | 2.55 | 2000 | 0.3439 | 0.3634 |
### Framework versions
- Transformers 4.11.3
- Pytorch 1.10.0+cu113
- Datasets 2.1.0
- Tokenizers 0.10.3
|
sanchit-gandhi/xtreme_s_xlsr_2_mbart_covost2_fr_en_2 | 2fe9464e48b649e34993150c7d53f8e286cbb2aa | 2022-05-13T08:42:36.000Z | [
"pytorch",
"tensorboard",
"speech-encoder-decoder",
"automatic-speech-recognition",
"transformers"
] | automatic-speech-recognition | false | sanchit-gandhi | null | sanchit-gandhi/xtreme_s_xlsr_2_mbart_covost2_fr_en_2 | 1 | null | transformers | 31,765 | Entry not found |
Xuandong/HPD-MiniLM-F128 | a24b508e4920ccd4907d1d51f333cceac3f88338 | 2022-05-10T17:54:43.000Z | [
"pytorch",
"bert",
"feature-extraction",
"arxiv:2203.07687",
"transformers",
"license:apache-2.0"
] | feature-extraction | false | Xuandong | null | Xuandong/HPD-MiniLM-F128 | 1 | null | transformers | 31,766 | ---
license: apache-2.0
---
# HPD-MiniLM-F128
This repository contains the pre-trained models for our paper [Compressing Sentence Representation for Semantic Retrieval via Homomorphic Projective Distillation](https://arxiv.org/abs/2203.07687). The sentence embedding model contains only 23M parameters and the model size is only 87MB.
## Overview
We propose **H**omomorphic **P**rojective **D**istillation (HPD) to learn compressed sentence embeddings. Our method augments a small Transformer encoder model with learnable projection layers to produce compact representations while mimicking a large pre-trained language model to retain the sentence representation quality.
## Details
This is a [sentence-transformers](https://www.SBERT.net) model: It maps sentences & paragraphs to a 128 dimensional dense vector space and can be used for tasks like clustering or semantic search.
The teacher model is [`princeton-nlp/sup-simcse-roberta-large`](https://huggingface.co/princeton-nlp/sup-simcse-bert-base-uncased) and the student model is [`nreimers/MiniLM-L6-H384-uncased`](https://huggingface.co/nreimers/MiniLM-L6-H384-uncased).
## Usage
Using this model becomes easy when you have [sentence-transformers](https://www.SBERT.net) installed:
```
pip install -U sentence-transformers
```
After installing the package, you can simply load our model
```python
from sentence_transformers import SentenceTransformer
model = SentenceTransformer('Xuandong/HPD-MiniLM-F128')
```
Then you can use our model for **encoding sentences into embeddings**
```python
sentences = ['He plays guitar.', 'A street vendor is outside.']
sentence_embeddings = model.encode(sentences)
for sentence, embedding in zip(sentences, sentence_embeddings):
print("Sentence:", sentence)
print("Embedding:", embedding)
print("")
```
## Evaluation Results
We evaluate our model on semantic textual similarity (STS) tasks. The results are:
| STS12 | STS13 | STS14 | STS15 | STS16 | STS-B | SICK-R | Avg. |
|-------|-------|-------|-------|-------|--------------|-----------------|-------|
| 74.94 | 84.52 | 80.25 | 84.87 | 81.90 | 84.98 | 81.15 | 81.80 |
## Training
Please refer to the github repo (https://github.com/XuandongZhao/HPD) for the details about the training.
## Full Model Architecture
```
SentenceTransformer(
(0): Transformer({'max_seq_length': 512, 'do_lower_case': False}) with Transformer model: BertModel
(1): Pooling({'word_embedding_dimension': 384, 'pooling_mode_cls_token': False, 'pooling_mode_mean_tokens': True, 'pooling_mode_max_tokens': False, 'pooling_mode_mean_sqrt_len_tokens': False})
(2): Dense({'in_features': 384, 'out_features': 128, 'bias': True, 'activation_function': 'torch.nn.modules.activation.Tanh'})
)
```
## Citation
Please cite our paper if you use HPD in your work:
```bibtex
@article{zhao2022compressing,
title={Compressing Sentence Representation for Semantic Retrieval via Homomorphic Projective Distillation},
author={Zhao, Xuandong and Yu, Zhiguo and Wu, Ming and Li, Lei},
journal={arXiv preprint arXiv:2203.07687},
year={2022}
}
``` |
Xuandong/HPD-TinyBERT-F128 | 28e49638354a308425ba4c2ad1b1fe678dfff07d | 2022-05-10T17:55:05.000Z | [
"pytorch",
"bert",
"feature-extraction",
"arxiv:2203.07687",
"transformers",
"license:apache-2.0"
] | feature-extraction | false | Xuandong | null | Xuandong/HPD-TinyBERT-F128 | 1 | null | transformers | 31,767 |
---
license: apache-2.0
---
# HPD-TinyBERT-F128
This repository contains the pre-trained models for our paper [Compressing Sentence Representation for Semantic Retrieval via Homomorphic Projective Distillation](https://arxiv.org/abs/2203.07687). The sentence embedding model contains only 14M parameters and the model size is only 55MB.
## Overview
We propose **H**omomorphic **P**rojective **D**istillation (HPD) to learn compressed sentence embeddings. Our method augments a small Transformer encoder model with learnable projection layers to produce compact representations while mimicking a large pre-trained language model to retain the sentence representation quality.
## Details
This is a [sentence-transformers](https://www.SBERT.net) model: It maps sentences & paragraphs to a 128 dimensional dense vector space and can be used for tasks like clustering or semantic search.
The teacher model is [`princeton-nlp/sup-simcse-roberta-large`](https://huggingface.co/princeton-nlp/sup-simcse-bert-base-uncased) and the student model is [`nreimers/TinyBERT_L-4_H-312_v2`](https://huggingface.co/nreimers/TinyBERT_L-4_H-312_v2).
## Usage
Using this model becomes easy when you have [sentence-transformers](https://www.SBERT.net) installed:
```
pip install -U sentence-transformers
```
After installing the package, you can simply load our model
```python
from sentence_transformers import SentenceTransformer
model = SentenceTransformer('Xuandong/HPD-TinyBERT-F128')
```
Then you can use our model for **encoding sentences into embeddings**
```python
sentences = ['He plays guitar.', 'A street vendor is outside.']
sentence_embeddings = model.encode(sentences)
for sentence, embedding in zip(sentences, sentence_embeddings):
print("Sentence:", sentence)
print("Embedding:", embedding)
print("")
```
## Evaluation Results
We evaluate our model on semantic textual similarity (STS) tasks. The results are:
| STS12 | STS13 | STS14 | STS15 | STS16 | STS-B | SICK-R | Avg. |
|-------|-------|-------|-------|-------|--------------|-----------------|-------|
| 74.29 | 83.05 | 78.80 | 84.62 | 81.17 | 84.36 | 80.83 | 81.02 |
## Training
Please refer to the github repo (https://github.com/XuandongZhao/HPD) for the details about the training.
## Full Model Architecture
```
SentenceTransformer(
(0): Transformer({'max_seq_length': 512, 'do_lower_case': False}) with Transformer model: BertModel
(1): Pooling({'word_embedding_dimension': 312, 'pooling_mode_cls_token': False, 'pooling_mode_mean_tokens': True, 'pooling_mode_max_tokens': False, 'pooling_mode_mean_sqrt_len_tokens': False})
(2): Dense({'in_features': 312, 'out_features': 128, 'bias': True, 'activation_function': 'torch.nn.modules.activation.Tanh'})
)
```
## Citation
Please cite our paper if you use HPD in your work:
```bibtex
@article{zhao2022compressing,
title={Compressing Sentence Representation for Semantic Retrieval via Homomorphic Projective Distillation},
author={Zhao, Xuandong and Yu, Zhiguo and Wu, Ming and Li, Lei},
journal={arXiv preprint arXiv:2203.07687},
year={2022}
}
``` |
huxxx657/roberta-base-finetuned-scrambled-squad-10 | 36d2ffb4f71fd70df69b36688e2f5faa0e545b85 | 2022-05-10T19:05:14.000Z | [
"pytorch",
"tensorboard",
"roberta",
"question-answering",
"dataset:squad",
"transformers",
"generated_from_trainer",
"license:mit",
"model-index",
"autotrain_compatible"
] | question-answering | false | huxxx657 | null | huxxx657/roberta-base-finetuned-scrambled-squad-10 | 1 | null | transformers | 31,768 | ---
license: mit
tags:
- generated_from_trainer
datasets:
- squad
model-index:
- name: roberta-base-finetuned-scrambled-squad-10
results: []
---
<!-- This model card has been generated automatically according to the information the Trainer had access to. You
should probably proofread and complete it, then remove this comment. -->
# roberta-base-finetuned-scrambled-squad-10
This model is a fine-tuned version of [roberta-base](https://huggingface.co/roberta-base) on the squad dataset.
It achieves the following results on the evaluation set:
- Loss: 1.7200
## Model description
More information needed
## Intended uses & limitations
More information needed
## Training and evaluation data
More information needed
## Training procedure
### Training hyperparameters
The following hyperparameters were used during training:
- learning_rate: 7e-05
- train_batch_size: 16
- eval_batch_size: 16
- seed: 42
- optimizer: Adam with betas=(0.9,0.999) and epsilon=1e-08
- lr_scheduler_type: linear
- num_epochs: 1
### Training results
| Training Loss | Epoch | Step | Validation Loss |
|:-------------:|:-----:|:----:|:---------------:|
| 1.7482 | 1.0 | 5532 | 1.7200 |
### Framework versions
- Transformers 4.18.0
- Pytorch 1.11.0+cu113
- Datasets 2.1.0
- Tokenizers 0.12.1
|
subhasisj/ar-TAPT-MLM-MiniLM | 55decf779fccd83afe6729ed7e595930c741ef6b | 2022-05-10T21:18:40.000Z | [
"pytorch",
"tensorboard",
"bert",
"fill-mask",
"transformers",
"generated_from_trainer",
"model-index",
"autotrain_compatible"
] | fill-mask | false | subhasisj | null | subhasisj/ar-TAPT-MLM-MiniLM | 1 | null | transformers | 31,769 | ---
tags:
- generated_from_trainer
model-index:
- name: ar-TAPT-MLM-MiniLM
results: []
---
<!-- This model card has been generated automatically according to the information the Trainer had access to. You
should probably proofread and complete it, then remove this comment. -->
# ar-TAPT-MLM-MiniLM
This model is a fine-tuned version of [subhasisj/MiniLMv2-qa-encoder](https://huggingface.co/subhasisj/MiniLMv2-qa-encoder) on an unknown dataset.
## Model description
More information needed
## Intended uses & limitations
More information needed
## Training and evaluation data
More information needed
## Training procedure
### Training hyperparameters
The following hyperparameters were used during training:
- learning_rate: 5e-05
- train_batch_size: 16
- eval_batch_size: 8
- seed: 42
- optimizer: Adam with betas=(0.9,0.999) and epsilon=1e-08
- lr_scheduler_type: linear
- num_epochs: 1
- mixed_precision_training: Native AMP
### Training results
### Framework versions
- Transformers 4.18.0
- Pytorch 1.11.0+cu113
- Datasets 2.2.0
- Tokenizers 0.12.1
|
enoriega/kw_pubmed_1000_0.000006 | b53f6385bd4a329b82d8e73232395fcf9da2dad7 | 2022-05-12T05:54:58.000Z | [
"pytorch",
"tensorboard",
"bert",
"fill-mask",
"transformers",
"autotrain_compatible"
] | fill-mask | false | enoriega | null | enoriega/kw_pubmed_1000_0.000006 | 1 | null | transformers | 31,770 | Entry not found |
huxxx657/roberta-base-finetuned-scrambled-squad-15-new | b69490d8b093b68e040c4ccd748b94880f46c8af | 2022-05-11T03:06:01.000Z | [
"pytorch",
"tensorboard",
"roberta",
"question-answering",
"dataset:squad",
"transformers",
"generated_from_trainer",
"license:mit",
"model-index",
"autotrain_compatible"
] | question-answering | false | huxxx657 | null | huxxx657/roberta-base-finetuned-scrambled-squad-15-new | 1 | null | transformers | 31,771 | ---
license: mit
tags:
- generated_from_trainer
datasets:
- squad
model-index:
- name: roberta-base-finetuned-scrambled-squad-15-new
results: []
---
<!-- This model card has been generated automatically according to the information the Trainer had access to. You
should probably proofread and complete it, then remove this comment. -->
# roberta-base-finetuned-scrambled-squad-15-new
This model is a fine-tuned version of [roberta-base](https://huggingface.co/roberta-base) on the squad dataset.
It achieves the following results on the evaluation set:
- Loss: 1.0283
## Model description
More information needed
## Intended uses & limitations
More information needed
## Training and evaluation data
More information needed
## Training procedure
### Training hyperparameters
The following hyperparameters were used during training:
- learning_rate: 7e-05
- train_batch_size: 16
- eval_batch_size: 16
- seed: 42
- optimizer: Adam with betas=(0.9,0.999) and epsilon=1e-08
- lr_scheduler_type: linear
- num_epochs: 1
### Training results
| Training Loss | Epoch | Step | Validation Loss |
|:-------------:|:-----:|:----:|:---------------:|
| 1.0269 | 1.0 | 5536 | 1.0283 |
### Framework versions
- Transformers 4.18.0
- Pytorch 1.11.0+cu113
- Datasets 2.2.0
- Tokenizers 0.12.1
|
ablam/distilgpt2_fine_tuned_gcode | 34da97162901db4bd6faf450d49788e71f372135 | 2022-06-11T03:52:00.000Z | [
"pytorch",
"tensorboard",
"gpt2",
"text-generation",
"transformers",
"generated_from_trainer",
"model-index"
] | text-generation | false | ablam | null | ablam/distilgpt2_fine_tuned_gcode | 1 | null | transformers | 31,772 | ---
tags:
- generated_from_trainer
model-index:
- name: distilgpt2_fine_tuned_gcode
results: []
---
<!-- This model card has been generated automatically according to the information the Trainer had access to. You
should probably proofread and complete it, then remove this comment. -->
# distilgpt2_fine_tuned_gcode
This model is a fine-tuned version of [congcongwang/distilgpt2_fine_tuned_coder](https://huggingface.co/congcongwang/distilgpt2_fine_tuned_coder) on the None dataset.
It achieves the following results on the evaluation set:
- Loss: 4.1670
## Model description
More information needed
## Intended uses & limitations
More information needed
## Training and evaluation data
More information needed
## Training procedure
### Training hyperparameters
The following hyperparameters were used during training:
- learning_rate: 0.1
- train_batch_size: 8
- eval_batch_size: 8
- seed: 42
- optimizer: Adam with betas=(0.9,0.999) and epsilon=1e-08
- lr_scheduler_type: linear
- num_epochs: 1
### Training results
| Training Loss | Epoch | Step | Validation Loss |
|:-------------:|:-----:|:-----:|:---------------:|
| 4.1754 | 1.0 | 52144 | 4.1670 |
### Framework versions
- Transformers 4.15.0
- Pytorch 1.10.1
- Datasets 2.1.0
- Tokenizers 0.10.3
|
yomexa/xlm-roberta-base-finetuned-panx-de | ee8c3993333e5db3b686a80bdd5e2cdd3a929780 | 2022-05-11T02:42:06.000Z | [
"pytorch",
"tensorboard",
"xlm-roberta",
"token-classification",
"dataset:xtreme",
"transformers",
"generated_from_trainer",
"license:mit",
"model-index",
"autotrain_compatible"
] | token-classification | false | yomexa | null | yomexa/xlm-roberta-base-finetuned-panx-de | 1 | null | transformers | 31,773 | ---
license: mit
tags:
- generated_from_trainer
datasets:
- xtreme
metrics:
- f1
model-index:
- name: xlm-roberta-base-finetuned-panx-de
results:
- task:
name: Token Classification
type: token-classification
dataset:
name: xtreme
type: xtreme
args: PAN-X.de
metrics:
- name: F1
type: f1
value: 0.8620945214069894
---
<!-- This model card has been generated automatically according to the information the Trainer had access to. You
should probably proofread and complete it, then remove this comment. -->
# xlm-roberta-base-finetuned-panx-de
This model is a fine-tuned version of [xlm-roberta-base](https://huggingface.co/xlm-roberta-base) on the xtreme dataset.
It achieves the following results on the evaluation set:
- Loss: 0.1372
- F1: 0.8621
## Model description
More information needed
## Intended uses & limitations
More information needed
## Training and evaluation data
More information needed
## Training procedure
### Training hyperparameters
The following hyperparameters were used during training:
- learning_rate: 5e-05
- train_batch_size: 24
- eval_batch_size: 24
- seed: 42
- optimizer: Adam with betas=(0.9,0.999) and epsilon=1e-08
- lr_scheduler_type: linear
- num_epochs: 3
### Training results
| Training Loss | Epoch | Step | Validation Loss | F1 |
|:-------------:|:-----:|:----:|:---------------:|:------:|
| 0.2575 | 1.0 | 525 | 0.1621 | 0.8292 |
| 0.1287 | 2.0 | 1050 | 0.1378 | 0.8526 |
| 0.0831 | 3.0 | 1575 | 0.1372 | 0.8621 |
### Framework versions
- Transformers 4.11.3
- Pytorch 1.11.0+cu113
- Datasets 1.16.1
- Tokenizers 0.10.3
|
ceggian/bert_post_trained_reddit_batch256 | 0aa34a9eadc3b2052d456c7bb31b81aa363dacbc | 2022-05-11T05:50:42.000Z | [
"pytorch",
"bert",
"pretraining",
"transformers"
] | null | false | ceggian | null | ceggian/bert_post_trained_reddit_batch256 | 1 | null | transformers | 31,774 | Entry not found |
ceggian/bert_post_trained_reddit_batch32 | 7abff010e0ce4cf7ab4c531c371c1ba462296185 | 2022-05-11T07:12:04.000Z | [
"pytorch",
"bert",
"pretraining",
"transformers"
] | null | false | ceggian | null | ceggian/bert_post_trained_reddit_batch32 | 1 | null | transformers | 31,775 | Entry not found |
tau/False_large_pmi_para0_sent1_span2_itTrue_sargmax_rrFalse_8_1024_0.3_epoch1 | f732b39aa96efdcdf7fa9de936d2bb9db31bd7bb | 2022-05-11T08:55:54.000Z | [
"pytorch",
"t5",
"text2text-generation",
"transformers",
"autotrain_compatible"
] | text2text-generation | false | tau | null | tau/False_large_pmi_para0_sent1_span2_itTrue_sargmax_rrFalse_8_1024_0.3_epoch1 | 1 | null | transformers | 31,776 | Entry not found |
masakhane/afrimt5_en_zul_news | b6cbd3e7ffe04176a8ed4a4b16dc373e5ed97b73 | 2022-05-12T12:51:47.000Z | [
"pytorch",
"mt5",
"text2text-generation",
"transformers",
"license:afl-3.0",
"autotrain_compatible"
] | text2text-generation | false | masakhane | null | masakhane/afrimt5_en_zul_news | 1 | null | transformers | 31,777 | ---
license: afl-3.0
---
|
masakhane/afrimbart_twi_en_news | 86af6ffd8d992f0102050bd731045e3edd55cc21 | 2022-05-12T11:55:53.000Z | [
"pytorch",
"mbart",
"text2text-generation",
"transformers",
"license:afl-3.0",
"autotrain_compatible"
] | text2text-generation | false | masakhane | null | masakhane/afrimbart_twi_en_news | 1 | null | transformers | 31,778 | ---
license: afl-3.0
---
|
masakhane/afrimbart_en_twi_news | 629607af748d886af5189fa13f529ff865f284f8 | 2022-05-12T11:55:50.000Z | [
"pytorch",
"mbart",
"text2text-generation",
"transformers",
"license:afl-3.0",
"autotrain_compatible"
] | text2text-generation | false | masakhane | null | masakhane/afrimbart_en_twi_news | 1 | null | transformers | 31,779 | ---
license: afl-3.0
---
|
masakhane/afrimbart_zul_en_news | 4011c880cc25ca9b14a993507f573e623e69d7db | 2022-05-12T12:51:51.000Z | [
"pytorch",
"mbart",
"text2text-generation",
"transformers",
"license:afl-3.0",
"autotrain_compatible"
] | text2text-generation | false | masakhane | null | masakhane/afrimbart_zul_en_news | 1 | null | transformers | 31,780 | ---
license: afl-3.0
---
|
masakhane/afribyt5_en_zul_news | e34c779a773b56f8ed6dbd3472479824f5435d99 | 2022-05-12T12:59:06.000Z | [
"pytorch",
"t5",
"text2text-generation",
"transformers",
"license:afl-3.0",
"autotrain_compatible"
] | text2text-generation | false | masakhane | null | masakhane/afribyt5_en_zul_news | 1 | null | transformers | 31,781 | ---
license: afl-3.0
---
|
masakhane/byt5_twi_en_news | e17252746d4040efdec164f89b49cc1202d669e5 | 2022-05-12T12:07:40.000Z | [
"pytorch",
"t5",
"text2text-generation",
"transformers",
"license:afl-3.0",
"autotrain_compatible"
] | text2text-generation | false | masakhane | null | masakhane/byt5_twi_en_news | 1 | null | transformers | 31,782 | ---
license: afl-3.0
---
|
masakhane/byt5_zul_en_news | a785374920d5e3814c5f70d063a950763fd041aa | 2022-05-12T12:59:13.000Z | [
"pytorch",
"t5",
"text2text-generation",
"transformers",
"license:afl-3.0",
"autotrain_compatible"
] | text2text-generation | false | masakhane | null | masakhane/byt5_zul_en_news | 1 | null | transformers | 31,783 | ---
license: afl-3.0
---
|
masakhane/mbart50_en_twi_news | f2c4fe632f690ae86c6260af5595fee93bb0222d | 2022-05-12T12:15:58.000Z | [
"pytorch",
"mbart",
"text2text-generation",
"transformers",
"license:afl-3.0",
"autotrain_compatible"
] | text2text-generation | false | masakhane | null | masakhane/mbart50_en_twi_news | 1 | null | transformers | 31,784 | ---
license: afl-3.0
---
|
masakhane/mbart50_twi_en_news | 221b09d8a059c763b2afdc2a6a1182726c1bc602 | 2022-05-12T12:16:01.000Z | [
"pytorch",
"mbart",
"text2text-generation",
"transformers",
"license:afl-3.0",
"autotrain_compatible"
] | text2text-generation | false | masakhane | null | masakhane/mbart50_twi_en_news | 1 | null | transformers | 31,785 | ---
license: afl-3.0
---
|
masakhane/m2m100_418M_en_twi_news | ac52828a47e83e9243c01e5090d112408720815d | 2022-05-12T12:27:51.000Z | [
"pytorch",
"m2m_100",
"text2text-generation",
"transformers",
"license:afl-3.0",
"autotrain_compatible"
] | text2text-generation | false | masakhane | null | masakhane/m2m100_418M_en_twi_news | 1 | null | transformers | 31,786 | ---
license: afl-3.0
---
|
masakhane/m2m100_418M_en_zul_news | eda4b2bd2dfb270697bf6b322f80c974fb95d18f | 2022-05-12T13:14:44.000Z | [
"pytorch",
"m2m_100",
"text2text-generation",
"transformers",
"license:afl-3.0",
"autotrain_compatible"
] | text2text-generation | false | masakhane | null | masakhane/m2m100_418M_en_zul_news | 1 | null | transformers | 31,787 | ---
license: afl-3.0
---
|
PSW/min2_sim_swap_seed1 | 1ba19bab0033f85f40e193666c3257e18983a48a | 2022-05-12T01:58:58.000Z | [
"pytorch",
"bart",
"text2text-generation",
"transformers",
"autotrain_compatible"
] | text2text-generation | false | PSW | null | PSW/min2_sim_swap_seed1 | 1 | null | transformers | 31,788 | Entry not found |
masakhane/m2m100_418M_zul_en_rel_ft | faf372852fb8e368dced548ae8d6f1043389eec8 | 2022-05-12T13:36:18.000Z | [
"pytorch",
"m2m_100",
"text2text-generation",
"transformers",
"license:afl-3.0",
"autotrain_compatible"
] | text2text-generation | false | masakhane | null | masakhane/m2m100_418M_zul_en_rel_ft | 1 | null | transformers | 31,789 | ---
license: afl-3.0
---
|
masakhane/m2m100_418M_en_zul_rel | eafb3f777204eea261c39033d556efd2f5951ec9 | 2022-05-12T13:43:24.000Z | [
"pytorch",
"m2m_100",
"text2text-generation",
"transformers",
"license:afl-3.0",
"autotrain_compatible"
] | text2text-generation | false | masakhane | null | masakhane/m2m100_418M_en_zul_rel | 1 | null | transformers | 31,790 | ---
license: afl-3.0
---
|
masakhane/m2m100_418M_zul_en_rel | 2bac700c108fd6c1c79b90806a0d299f87789cbe | 2022-05-12T13:43:27.000Z | [
"pytorch",
"m2m_100",
"text2text-generation",
"transformers",
"license:afl-3.0",
"autotrain_compatible"
] | text2text-generation | false | masakhane | null | masakhane/m2m100_418M_zul_en_rel | 1 | null | transformers | 31,791 | ---
license: afl-3.0
---
|
PSW/max2_sim_swap_seed1 | 3001c3b746446ecaaeb5bb1fd52b63785d5b8a72 | 2022-05-12T04:11:00.000Z | [
"pytorch",
"bart",
"text2text-generation",
"transformers",
"autotrain_compatible"
] | text2text-generation | false | PSW | null | PSW/max2_sim_swap_seed1 | 1 | null | transformers | 31,792 | Entry not found |
Nonegom/roberta_curriculum_learn | 216b4f1bb426eb66959c8e81bf2c5b9c1eadfe0e | 2022-05-11T12:43:06.000Z | [
"pytorch",
"roberta",
"question-answering",
"transformers",
"autotrain_compatible"
] | question-answering | false | Nonegom | null | Nonegom/roberta_curriculum_learn | 1 | 1 | transformers | 31,793 | Entry not found |
orenpereg/paraphrase-mpnet-base-v2_sst2_4samps | 5f42358947628bf4f8e0f23550e51336617e0c7f | 2022-05-11T13:32:25.000Z | [
"pytorch",
"mpnet",
"feature-extraction",
"sentence-transformers",
"sentence-similarity",
"transformers"
] | sentence-similarity | false | orenpereg | null | orenpereg/paraphrase-mpnet-base-v2_sst2_4samps | 1 | null | sentence-transformers | 31,794 | ---
pipeline_tag: sentence-similarity
tags:
- sentence-transformers
- feature-extraction
- sentence-similarity
- transformers
---
# orenpereg/paraphrase-mpnet-base-v2_sst2_4samps
This is a [sentence-transformers](https://www.SBERT.net) model: It maps sentences & paragraphs to a 768 dimensional dense vector space and can be used for tasks like clustering or semantic search.
<!--- Describe your model here -->
## Usage (Sentence-Transformers)
Using this model becomes easy when you have [sentence-transformers](https://www.SBERT.net) installed:
```
pip install -U sentence-transformers
```
Then you can use the model like this:
```python
from sentence_transformers import SentenceTransformer
sentences = ["This is an example sentence", "Each sentence is converted"]
model = SentenceTransformer('orenpereg/paraphrase-mpnet-base-v2_sst2_4samps')
embeddings = model.encode(sentences)
print(embeddings)
```
## Usage (HuggingFace Transformers)
Without [sentence-transformers](https://www.SBERT.net), you can use the model like this: First, you pass your input through the transformer model, then you have to apply the right pooling-operation on-top of the contextualized word embeddings.
```python
from transformers import AutoTokenizer, AutoModel
import torch
#Mean Pooling - Take attention mask into account for correct averaging
def mean_pooling(model_output, attention_mask):
token_embeddings = model_output[0] #First element of model_output contains all token embeddings
input_mask_expanded = attention_mask.unsqueeze(-1).expand(token_embeddings.size()).float()
return torch.sum(token_embeddings * input_mask_expanded, 1) / torch.clamp(input_mask_expanded.sum(1), min=1e-9)
# Sentences we want sentence embeddings for
sentences = ['This is an example sentence', 'Each sentence is converted']
# Load model from HuggingFace Hub
tokenizer = AutoTokenizer.from_pretrained('orenpereg/paraphrase-mpnet-base-v2_sst2_4samps')
model = AutoModel.from_pretrained('orenpereg/paraphrase-mpnet-base-v2_sst2_4samps')
# Tokenize sentences
encoded_input = tokenizer(sentences, padding=True, truncation=True, return_tensors='pt')
# Compute token embeddings
with torch.no_grad():
model_output = model(**encoded_input)
# Perform pooling. In this case, mean pooling.
sentence_embeddings = mean_pooling(model_output, encoded_input['attention_mask'])
print("Sentence embeddings:")
print(sentence_embeddings)
```
## Evaluation Results
<!--- Describe how your model was evaluated -->
For an automated evaluation of this model, see the *Sentence Embeddings Benchmark*: [https://seb.sbert.net](https://seb.sbert.net?model_name=orenpereg/paraphrase-mpnet-base-v2_sst2_4samps)
## Training
The model was trained with the parameters:
**DataLoader**:
`torch.utils.data.dataloader.DataLoader` of length 5 with parameters:
```
{'batch_size': 16, 'sampler': 'torch.utils.data.sampler.RandomSampler', 'batch_sampler': 'torch.utils.data.sampler.BatchSampler'}
```
**Loss**:
`sentence_transformers.losses.CosineSimilarityLoss.CosineSimilarityLoss`
Parameters of the fit()-Method:
```
{
"epochs": 1,
"evaluation_steps": 0,
"evaluator": "NoneType",
"max_grad_norm": 1,
"optimizer_class": "<class 'transformers.optimization.AdamW'>",
"optimizer_params": {
"lr": 2e-05
},
"scheduler": "WarmupLinear",
"steps_per_epoch": null,
"warmup_steps": 3,
"weight_decay": 0.01
}
```
## Full Model Architecture
```
SentenceTransformer(
(0): Transformer({'max_seq_length': 512, 'do_lower_case': False}) with Transformer model: MPNetModel
(1): Pooling({'word_embedding_dimension': 768, 'pooling_mode_cls_token': False, 'pooling_mode_mean_tokens': True, 'pooling_mode_max_tokens': False, 'pooling_mode_mean_sqrt_len_tokens': False})
)
```
## Citing & Authors
<!--- Describe where people can find more information --> |
lilitket/20220511-173140 | 41b7455102a4209cae51ce1922cc324741233726 | 2022-05-11T21:07:12.000Z | [
"pytorch",
"wav2vec2",
"automatic-speech-recognition",
"transformers"
] | automatic-speech-recognition | false | lilitket | null | lilitket/20220511-173140 | 1 | null | transformers | 31,795 | Entry not found |
PSW/min2_sim_swap_seed27 | c9503f2c06f103c6e20cedc4d6a41f4123621469 | 2022-05-12T02:43:04.000Z | [
"pytorch",
"bart",
"text2text-generation",
"transformers",
"autotrain_compatible"
] | text2text-generation | false | PSW | null | PSW/min2_sim_swap_seed27 | 1 | null | transformers | 31,796 | Entry not found |
huggingtweets/alice_lbl-lotrbookquotes | 1dda8a1091f3ce81a0a207b5a19872fe6d1ced49 | 2022-05-11T14:44:26.000Z | [
"pytorch",
"gpt2",
"text-generation",
"en",
"transformers",
"huggingtweets"
] | text-generation | false | huggingtweets | null | huggingtweets/alice_lbl-lotrbookquotes | 1 | null | transformers | 31,797 | ---
language: en
thumbnail: http://www.huggingtweets.com/alice_lbl-lotrbookquotes/1652280261416/predictions.png
tags:
- huggingtweets
widget:
- text: "My dream is"
---
<div class="inline-flex flex-col" style="line-height: 1.5;">
<div class="flex">
<div
style="display:inherit; margin-left: 4px; margin-right: 4px; width: 92px; height:92px; border-radius: 50%; background-size: cover; background-image: url('https://pbs.twimg.com/profile_images/1424546909104926720/g4pTa5BS_400x400.jpg')">
</div>
<div
style="display:inherit; margin-left: 4px; margin-right: 4px; width: 92px; height:92px; border-radius: 50%; background-size: cover; background-image: url('https://pbs.twimg.com/profile_images/1047569624693465089/0yKYd-Xl_400x400.jpg')">
</div>
<div
style="display:none; margin-left: 4px; margin-right: 4px; width: 92px; height:92px; border-radius: 50%; background-size: cover; background-image: url('')">
</div>
</div>
<div style="text-align: center; margin-top: 3px; font-size: 16px; font-weight: 800">π€ AI CYBORG π€</div>
<div style="text-align: center; font-size: 16px; font-weight: 800">Alice in Wonderland & Looking-Glass (line by line) & Lord of the Rings quotes</div>
<div style="text-align: center; font-size: 14px;">@alice_lbl-lotrbookquotes</div>
</div>
I was made with [huggingtweets](https://github.com/borisdayma/huggingtweets).
Create your own bot based on your favorite user with [the demo](https://colab.research.google.com/github/borisdayma/huggingtweets/blob/master/huggingtweets-demo.ipynb)!
## How does it work?
The model uses the following pipeline.
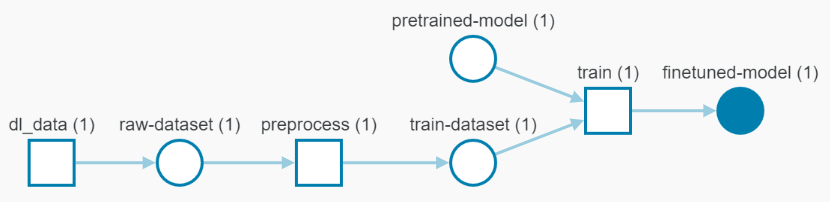
To understand how the model was developed, check the [W&B report](https://wandb.ai/wandb/huggingtweets/reports/HuggingTweets-Train-a-Model-to-Generate-Tweets--VmlldzoxMTY5MjI).
## Training data
The model was trained on tweets from Alice in Wonderland & Looking-Glass (line by line) & Lord of the Rings quotes.
| Data | Alice in Wonderland & Looking-Glass (line by line) | Lord of the Rings quotes |
| --- | --- | --- |
| Tweets downloaded | 3050 | 3250 |
| Retweets | 0 | 0 |
| Short tweets | 38 | 0 |
| Tweets kept | 3012 | 3250 |
[Explore the data](https://wandb.ai/wandb/huggingtweets/runs/14brvkjr/artifacts), which is tracked with [W&B artifacts](https://docs.wandb.com/artifacts) at every step of the pipeline.
## Training procedure
The model is based on a pre-trained [GPT-2](https://huggingface.co/gpt2) which is fine-tuned on @alice_lbl-lotrbookquotes's tweets.
Hyperparameters and metrics are recorded in the [W&B training run](https://wandb.ai/wandb/huggingtweets/runs/tzmzyo79) for full transparency and reproducibility.
At the end of training, [the final model](https://wandb.ai/wandb/huggingtweets/runs/tzmzyo79/artifacts) is logged and versioned.
## How to use
You can use this model directly with a pipeline for text generation:
```python
from transformers import pipeline
generator = pipeline('text-generation',
model='huggingtweets/alice_lbl-lotrbookquotes')
generator("My dream is", num_return_sequences=5)
```
## Limitations and bias
The model suffers from [the same limitations and bias as GPT-2](https://huggingface.co/gpt2#limitations-and-bias).
In addition, the data present in the user's tweets further affects the text generated by the model.
## About
*Built by Boris Dayma*
[](https://twitter.com/intent/follow?screen_name=borisdayma)
For more details, visit the project repository.
[](https://github.com/borisdayma/huggingtweets)
|
PSW/low_resource_percent1_min2swap_seed42 | 475b23911d4bd1c80c5c13a583835321b3db8c3c | 2022-05-12T06:14:12.000Z | [
"pytorch",
"bart",
"text2text-generation",
"transformers",
"autotrain_compatible"
] | text2text-generation | false | PSW | null | PSW/low_resource_percent1_min2swap_seed42 | 1 | null | transformers | 31,798 | Entry not found |
subhasisj/vi-TAPT-MLM-MiniLM | eb4112e742594878359ffd1ec714229b942cf463 | 2022-05-11T19:17:47.000Z | [
"pytorch",
"tensorboard",
"bert",
"fill-mask",
"transformers",
"generated_from_trainer",
"model-index",
"autotrain_compatible"
] | fill-mask | false | subhasisj | null | subhasisj/vi-TAPT-MLM-MiniLM | 1 | null | transformers | 31,799 | ---
tags:
- generated_from_trainer
model-index:
- name: vi-TAPT-MLM-MiniLM
results: []
---
<!-- This model card has been generated automatically according to the information the Trainer had access to. You
should probably proofread and complete it, then remove this comment. -->
# vi-TAPT-MLM-MiniLM
This model is a fine-tuned version of [subhasisj/MiniLMv2-qa-encoder](https://huggingface.co/subhasisj/MiniLMv2-qa-encoder) on an unknown dataset.
## Model description
More information needed
## Intended uses & limitations
More information needed
## Training and evaluation data
More information needed
## Training procedure
### Training hyperparameters
The following hyperparameters were used during training:
- learning_rate: 5e-05
- train_batch_size: 16
- eval_batch_size: 8
- seed: 42
- optimizer: Adam with betas=(0.9,0.999) and epsilon=1e-08
- lr_scheduler_type: linear
- num_epochs: 1
- mixed_precision_training: Native AMP
### Training results
### Framework versions
- Transformers 4.18.0
- Pytorch 1.11.0+cu113
- Datasets 2.2.0
- Tokenizers 0.12.1
|
Subsets and Splits
No community queries yet
The top public SQL queries from the community will appear here once available.