modelId
stringlengths 4
112
| sha
stringlengths 40
40
| lastModified
stringlengths 24
24
| tags
sequence | pipeline_tag
stringclasses 29
values | private
bool 1
class | author
stringlengths 2
38
⌀ | config
null | id
stringlengths 4
112
| downloads
float64 0
36.8M
⌀ | likes
float64 0
712
⌀ | library_name
stringclasses 17
values | __index_level_0__
int64 0
38.5k
| readme
stringlengths 0
186k
|
---|---|---|---|---|---|---|---|---|---|---|---|---|---|
GroNLP/wav2vec2-dutch-base | 67936cfc475473608f6763087a2a6006e3b7f5c7 | 2022-03-11T16:04:18.000Z | [
"pytorch",
"wav2vec2",
"pretraining",
"nl",
"transformers",
"speech"
] | null | false | GroNLP | null | GroNLP/wav2vec2-dutch-base | 1 | null | transformers | 30,800 | ---
language: nl
tags:
- speech
---
# Wav2Vec2-Dutch-Base
A Dutch Wav2Vec2 model. This model is created by further pre-training the original English [`facebook/wav2vec2-base`](https://huggingface.co/facebook/wav2vec2-base) model on Dutch speech from [Het Corpus Gesproken Nederlands](https://taalmaterialen.ivdnt.org/download/tstc-corpus-gesproken-nederlands/).
This model is one of two Dutch Wav2Vec2 models:
- [`GroNLP/wav2vec2-dutch-base`](https://huggingface.co/GroNLP/wav2vec2-dutch-base) (this model)
- [`GroNLP/wav2vec2-dutch-large`](https://huggingface.co/GroNLP/wav2vec2-dutch-large) |
clapika2010/beers_finetuned | 1d173e5332840df34eed9ab2269da4ef3e268026 | 2022-03-24T17:44:32.000Z | [
"pytorch",
"roberta",
"fill-mask",
"transformers",
"autotrain_compatible"
] | fill-mask | false | clapika2010 | null | clapika2010/beers_finetuned | 1 | null | transformers | 30,801 | Entry not found |
zhiweitong/bart-large-nq-qg | e20e9b726637dd2a94c1b235bcd8f9957f21b90e | 2022-03-15T08:14:50.000Z | [
"pytorch",
"bart",
"text2text-generation",
"transformers",
"autotrain_compatible"
] | text2text-generation | false | zhiweitong | null | zhiweitong/bart-large-nq-qg | 1 | null | transformers | 30,802 | Entry not found |
wooihen/xlm-roberta-base-finetuned-panx-de | ec8d6527200dd635755bafe85bfb98676e30684e | 2022-07-27T06:37:57.000Z | [
"pytorch",
"tensorboard",
"xlm-roberta",
"token-classification",
"dataset:xtreme",
"transformers",
"generated_from_trainer",
"license:mit",
"model-index",
"autotrain_compatible"
] | token-classification | false | wooihen | null | wooihen/xlm-roberta-base-finetuned-panx-de | 1 | null | transformers | 30,803 | ---
license: mit
tags:
- generated_from_trainer
datasets:
- xtreme
metrics:
- f1
model-index:
- name: xlm-roberta-base-finetuned-panx-de
results:
- task:
name: Token Classification
type: token-classification
dataset:
name: xtreme
type: xtreme
args: PAN-X.de
metrics:
- name: F1
type: f1
value: 0.8648740833380706
---
<!-- This model card has been generated automatically according to the information the Trainer had access to. You
should probably proofread and complete it, then remove this comment. -->
# xlm-roberta-base-finetuned-panx-de
This model is a fine-tuned version of [xlm-roberta-base](https://huggingface.co/xlm-roberta-base) on the xtreme dataset.
It achieves the following results on the evaluation set:
- Loss: 0.1365
- F1: 0.8649
## Model description
More information needed
## Intended uses & limitations
More information needed
## Training and evaluation data
More information needed
## Training procedure
### Training hyperparameters
The following hyperparameters were used during training:
- learning_rate: 5e-05
- train_batch_size: 24
- eval_batch_size: 24
- seed: 42
- optimizer: Adam with betas=(0.9,0.999) and epsilon=1e-08
- lr_scheduler_type: linear
- num_epochs: 3
### Training results
| Training Loss | Epoch | Step | Validation Loss | F1 |
|:-------------:|:-----:|:----:|:---------------:|:------:|
| 0.2553 | 1.0 | 525 | 0.1575 | 0.8279 |
| 0.1284 | 2.0 | 1050 | 0.1386 | 0.8463 |
| 0.0813 | 3.0 | 1575 | 0.1365 | 0.8649 |
### Framework versions
- Transformers 4.11.3
- Pytorch 1.12.0+cu113
- Datasets 1.16.1
- Tokenizers 0.10.3
|
benjaminbeilharz/bert2bert-empathetic-dialogues | 50c064bf9ca470ce53229953cac7effbda0c7a9e | 2022-03-12T08:01:42.000Z | [
"pytorch",
"encoder-decoder",
"text2text-generation",
"transformers",
"autotrain_compatible"
] | text2text-generation | false | benjaminbeilharz | null | benjaminbeilharz/bert2bert-empathetic-dialogues | 1 | null | transformers | 30,804 | Entry not found |
benjaminbeilharz/dialoGPT-small-conditioned2nextturn | 5175b611e49c72f6895f3dcc5768ee2ed1ae1b30 | 2022-03-12T08:22:17.000Z | [
"pytorch",
"gpt2",
"text-generation",
"transformers"
] | text-generation | false | benjaminbeilharz | null | benjaminbeilharz/dialoGPT-small-conditioned2nextturn | 1 | null | transformers | 30,805 | Entry not found |
lilitket/wav2vec2-large-xls-r-300m-hyAM_batch4 | ab24b00bd3d2e91f2a264c028f0db63f882c61f0 | 2022-03-12T14:42:05.000Z | [
"pytorch",
"wav2vec2",
"automatic-speech-recognition",
"transformers"
] | automatic-speech-recognition | false | lilitket | null | lilitket/wav2vec2-large-xls-r-300m-hyAM_batch4 | 1 | null | transformers | 30,806 | Entry not found |
lilitket/wav2vec2-large-xls-r-300m-hyAM_batch2_grad2 | b8d3f938cf8e3e3314e79ea086e1910fab39fb88 | 2022-03-12T14:47:26.000Z | [
"pytorch",
"wav2vec2",
"automatic-speech-recognition",
"transformers"
] | automatic-speech-recognition | false | lilitket | null | lilitket/wav2vec2-large-xls-r-300m-hyAM_batch2_grad2 | 1 | null | transformers | 30,807 | Entry not found |
cammy/bart-large-cnn-weaksup-original-100k | e0594a55d87cf2e4df166a4243267d1c671cbe93 | 2022-03-13T00:10:30.000Z | [
"pytorch",
"bart",
"text2text-generation",
"transformers",
"generated_from_trainer",
"license:mit",
"model-index",
"autotrain_compatible"
] | text2text-generation | false | cammy | null | cammy/bart-large-cnn-weaksup-original-100k | 1 | null | transformers | 30,808 | ---
license: mit
tags:
- generated_from_trainer
metrics:
- rouge
model-index:
- name: bart-large-cnn-weaksup-original-100k
results: []
---
<!-- This model card has been generated automatically according to the information the Trainer had access to. You
should probably proofread and complete it, then remove this comment. -->
# bart-large-cnn-weaksup-original-100k
This model is a fine-tuned version of [facebook/bart-large-cnn](https://huggingface.co/facebook/bart-large-cnn) on an unknown dataset.
It achieves the following results on the evaluation set:
- Loss: 1.5931
- Rouge1: 30.4429
- Rouge2: 15.6691
- Rougel: 24.1975
- Rougelsum: 27.4761
- Gen Len: 68.4568
## Model description
More information needed
## Intended uses & limitations
More information needed
## Training and evaluation data
More information needed
## Training procedure
### Training hyperparameters
The following hyperparameters were used during training:
- learning_rate: 2e-05
- train_batch_size: 1
- eval_batch_size: 1
- seed: 42
- optimizer: Adam with betas=(0.9,0.999) and epsilon=1e-08
- lr_scheduler_type: linear
- num_epochs: 1
- mixed_precision_training: Native AMP
### Training results
| Training Loss | Epoch | Step | Validation Loss | Rouge1 | Rouge2 | Rougel | Rougelsum | Gen Len |
|:-------------:|:-----:|:------:|:---------------:|:-------:|:-------:|:-------:|:---------:|:-------:|
| 1.261 | 1.0 | 100000 | 1.5931 | 30.4429 | 15.6691 | 24.1975 | 27.4761 | 68.4568 |
### Framework versions
- Transformers 4.16.2
- Pytorch 1.10.2
- Datasets 1.18.3
- Tokenizers 0.11.0
|
lijingxin/pegasus-samsum | 0fe1a97c62c599e534e24ebb0067d97cccda65a3 | 2022-03-12T15:33:54.000Z | [
"pytorch",
"tensorboard",
"pegasus",
"text2text-generation",
"dataset:samsum",
"transformers",
"generated_from_trainer",
"model-index",
"autotrain_compatible"
] | text2text-generation | false | lijingxin | null | lijingxin/pegasus-samsum | 1 | null | transformers | 30,809 | ---
tags:
- generated_from_trainer
datasets:
- samsum
model-index:
- name: pegasus-samsum
results: []
---
<!-- This model card has been generated automatically according to the information the Trainer had access to. You
should probably proofread and complete it, then remove this comment. -->
# pegasus-samsum
This model is a fine-tuned version of [google/pegasus-cnn_dailymail](https://huggingface.co/google/pegasus-cnn_dailymail) on the samsum dataset.
It achieves the following results on the evaluation set:
- Loss: 1.4874
## Model description
More information needed
## Intended uses & limitations
More information needed
## Training and evaluation data
More information needed
## Training procedure
### Training hyperparameters
The following hyperparameters were used during training:
- learning_rate: 5e-05
- train_batch_size: 1
- eval_batch_size: 1
- seed: 42
- gradient_accumulation_steps: 16
- total_train_batch_size: 16
- optimizer: Adam with betas=(0.9,0.999) and epsilon=1e-08
- lr_scheduler_type: linear
- lr_scheduler_warmup_steps: 500
- num_epochs: 1
### Training results
| Training Loss | Epoch | Step | Validation Loss |
|:-------------:|:-----:|:----:|:---------------:|
| 1.702 | 0.54 | 500 | 1.4874 |
### Framework versions
- Transformers 4.11.3
- Pytorch 1.9.1
- Datasets 1.16.1
- Tokenizers 0.10.3
|
lilitket/wav2vec2-large-xls-r-300m-hyAM_batch4_lr4 | 406552bbd87be87885e0127b5bc58af5c262eca2 | 2022-03-12T16:32:36.000Z | [
"pytorch",
"wav2vec2",
"automatic-speech-recognition",
"transformers"
] | automatic-speech-recognition | false | lilitket | null | lilitket/wav2vec2-large-xls-r-300m-hyAM_batch4_lr4 | 1 | null | transformers | 30,810 | Entry not found |
lilitket/wav2vec2-large-xls-r-300m-hyAM_batch4_lr16 | 769ae80f473bedf06b00bde4af0b9fe7a0f07cb6 | 2022-03-12T17:45:46.000Z | [
"pytorch",
"wav2vec2",
"automatic-speech-recognition",
"transformers"
] | automatic-speech-recognition | false | lilitket | null | lilitket/wav2vec2-large-xls-r-300m-hyAM_batch4_lr16 | 1 | null | transformers | 30,811 | Entry not found |
lilitket/xls-r-300m-hyAM_batch4_lr8e-05_warmup400 | f943b9be536811222bcd467437c616d76c85246b | 2022-03-12T21:09:24.000Z | [
"pytorch",
"wav2vec2",
"automatic-speech-recognition",
"transformers"
] | automatic-speech-recognition | false | lilitket | null | lilitket/xls-r-300m-hyAM_batch4_lr8e-05_warmup400 | 1 | null | transformers | 30,812 | Entry not found |
snoop2head/Deep-Shallow-Ko2En | cc0d7056b89f4c35f6efd287ff7cb7d74a75a6d1 | 2022-03-14T13:03:45.000Z | [
"pytorch",
"transformer",
"transformers"
] | null | false | snoop2head | null | snoop2head/Deep-Shallow-Ko2En | 1 | null | transformers | 30,813 | Entry not found |
beston91/gpt2_large_ft_mult_1k | b928295c1a4c7914959ec1938a358ec2ea8702e4 | 2022-03-13T00:56:23.000Z | [
"pytorch",
"gpt2",
"text-generation",
"transformers"
] | text-generation | false | beston91 | null | beston91/gpt2_large_ft_mult_1k | 1 | null | transformers | 30,814 | Entry not found |
cammy/bart-large-cnn-weaksup-100-NOpad-early | ab3d69b266687b3f1e64445eab65329ccf81f288 | 2022-03-13T05:24:09.000Z | [
"pytorch",
"bart",
"text2text-generation",
"transformers",
"generated_from_trainer",
"license:mit",
"model-index",
"autotrain_compatible"
] | text2text-generation | false | cammy | null | cammy/bart-large-cnn-weaksup-100-NOpad-early | 1 | null | transformers | 30,815 | ---
license: mit
tags:
- generated_from_trainer
metrics:
- rouge
model-index:
- name: bart-large-cnn-weaksup-100-NOpad-early
results: []
---
<!-- This model card has been generated automatically according to the information the Trainer had access to. You
should probably proofread and complete it, then remove this comment. -->
# bart-large-cnn-weaksup-100-NOpad-early
This model is a fine-tuned version of [facebook/bart-large-cnn](https://huggingface.co/facebook/bart-large-cnn) on an unknown dataset.
It achieves the following results on the evaluation set:
- Loss: 2.0768
- Rouge1: 28.7908
- Rouge2: 10.6989
- Rougel: 20.534
- Rougelsum: 24.1294
- Gen Len: 68.5
## Model description
More information needed
## Intended uses & limitations
More information needed
## Training and evaluation data
More information needed
## Training procedure
### Training hyperparameters
The following hyperparameters were used during training:
- learning_rate: 2e-05
- train_batch_size: 1
- eval_batch_size: 1
- seed: 42
- optimizer: Adam with betas=(0.9,0.999) and epsilon=1e-08
- lr_scheduler_type: linear
- num_epochs: 3
- mixed_precision_training: Native AMP
### Training results
| Training Loss | Epoch | Step | Validation Loss | Rouge1 | Rouge2 | Rougel | Rougelsum | Gen Len |
|:-------------:|:-----:|:----:|:---------------:|:-------:|:-------:|:-------:|:---------:|:-------:|
| No log | 1.0 | 100 | 1.8905 | 31.1534 | 13.7074 | 21.6489 | 27.0709 | 64.2 |
| No log | 2.0 | 200 | 2.0768 | 28.7908 | 10.6989 | 20.534 | 24.1294 | 68.5 |
### Framework versions
- Transformers 4.16.2
- Pytorch 1.10.2
- Datasets 1.18.3
- Tokenizers 0.11.0
|
cammy/bart-large-cnn-weaksup-1000-NOpad-early | acd98d85777e8c4df21407c0e15b803d41f353ce | 2022-03-13T05:51:27.000Z | [
"pytorch",
"bart",
"text2text-generation",
"transformers",
"generated_from_trainer",
"license:mit",
"model-index",
"autotrain_compatible"
] | text2text-generation | false | cammy | null | cammy/bart-large-cnn-weaksup-1000-NOpad-early | 1 | null | transformers | 30,816 | ---
license: mit
tags:
- generated_from_trainer
metrics:
- rouge
model-index:
- name: bart-large-cnn-weaksup-1000-NOpad-early
results: []
---
<!-- This model card has been generated automatically according to the information the Trainer had access to. You
should probably proofread and complete it, then remove this comment. -->
# bart-large-cnn-weaksup-1000-NOpad-early
This model is a fine-tuned version of [facebook/bart-large-cnn](https://huggingface.co/facebook/bart-large-cnn) on an unknown dataset.
It achieves the following results on the evaluation set:
- Loss: 1.9082
- Rouge1: 26.9663
- Rouge2: 11.3027
- Rougel: 20.7327
- Rougelsum: 23.5965
- Gen Len: 67.19
## Model description
More information needed
## Intended uses & limitations
More information needed
## Training and evaluation data
More information needed
## Training procedure
### Training hyperparameters
The following hyperparameters were used during training:
- learning_rate: 2e-05
- train_batch_size: 1
- eval_batch_size: 1
- seed: 42
- optimizer: Adam with betas=(0.9,0.999) and epsilon=1e-08
- lr_scheduler_type: linear
- num_epochs: 3
- mixed_precision_training: Native AMP
### Training results
| Training Loss | Epoch | Step | Validation Loss | Rouge1 | Rouge2 | Rougel | Rougelsum | Gen Len |
|:-------------:|:-----:|:----:|:---------------:|:-------:|:-------:|:-------:|:---------:|:-------:|
| 1.4775 | 1.0 | 1000 | 1.6796 | 27.208 | 12.01 | 20.8401 | 24.1333 | 66.06 |
| 0.6972 | 2.0 | 2000 | 1.9082 | 26.9663 | 11.3027 | 20.7327 | 23.5965 | 67.19 |
### Framework versions
- Transformers 4.16.2
- Pytorch 1.10.2
- Datasets 1.18.3
- Tokenizers 0.11.0
|
cammy/bart-large-cnn-weaksup-10k-NOpad-early | b46947cb5ff7cf947bae0a6b95cd07d6c2707a07 | 2022-03-13T08:16:48.000Z | [
"pytorch",
"bart",
"text2text-generation",
"transformers",
"generated_from_trainer",
"license:mit",
"model-index",
"autotrain_compatible"
] | text2text-generation | false | cammy | null | cammy/bart-large-cnn-weaksup-10k-NOpad-early | 1 | null | transformers | 30,817 | ---
license: mit
tags:
- generated_from_trainer
metrics:
- rouge
model-index:
- name: bart-large-cnn-weaksup-10k-NOpad-early
results: []
---
<!-- This model card has been generated automatically according to the information the Trainer had access to. You
should probably proofread and complete it, then remove this comment. -->
# bart-large-cnn-weaksup-10k-NOpad-early
This model is a fine-tuned version of [facebook/bart-large-cnn](https://huggingface.co/facebook/bart-large-cnn) on an unknown dataset.
It achieves the following results on the evaluation set:
- Loss: 1.7883
- Rouge1: 26.9755
- Rouge2: 12.4975
- Rougel: 21.0743
- Rougelsum: 23.9303
- Gen Len: 69.549
## Model description
More information needed
## Intended uses & limitations
More information needed
## Training and evaluation data
More information needed
## Training procedure
### Training hyperparameters
The following hyperparameters were used during training:
- learning_rate: 2e-05
- train_batch_size: 1
- eval_batch_size: 1
- seed: 42
- optimizer: Adam with betas=(0.9,0.999) and epsilon=1e-08
- lr_scheduler_type: linear
- num_epochs: 3
- mixed_precision_training: Native AMP
### Training results
| Training Loss | Epoch | Step | Validation Loss | Rouge1 | Rouge2 | Rougel | Rougelsum | Gen Len |
|:-------------:|:-----:|:-----:|:---------------:|:-------:|:-------:|:-------:|:---------:|:-------:|
| 1.4657 | 1.0 | 10000 | 1.7295 | 27.973 | 13.2818 | 21.8493 | 25.0101 | 67.831 |
| 0.8522 | 2.0 | 20000 | 1.7883 | 26.9755 | 12.4975 | 21.0743 | 23.9303 | 69.549 |
### Framework versions
- Transformers 4.16.2
- Pytorch 1.10.2
- Datasets 1.18.3
- Tokenizers 0.11.0
|
Splend1dchan/t5lephone-mnli | ad9db3c55a31888ac384f769b5f4d61ec99dff8c | 2022-03-13T06:42:43.000Z | [
"pytorch",
"t5",
"text2text-generation",
"transformers",
"autotrain_compatible"
] | text2text-generation | false | Splend1dchan | null | Splend1dchan/t5lephone-mnli | 1 | null | transformers | 30,818 | Entry not found |
cammy/bart-large-cnn-100-lit-evalMA-NOpad1 | e21ca352058c8572d6400913954b85b7e1e7b768 | 2022-03-13T09:49:45.000Z | [
"pytorch",
"bart",
"text2text-generation",
"transformers",
"autotrain_compatible"
] | text2text-generation | false | cammy | null | cammy/bart-large-cnn-100-lit-evalMA-NOpad1 | 1 | null | transformers | 30,819 | Entry not found |
cammy/bart-large-cnn-1000-lit-evalMA-NOpad | fcb3033ebfc4e366f475be6f814e46637a9ff8d4 | 2022-03-13T10:50:26.000Z | [
"pytorch",
"bart",
"text2text-generation",
"transformers",
"generated_from_trainer",
"license:mit",
"model-index",
"autotrain_compatible"
] | text2text-generation | false | cammy | null | cammy/bart-large-cnn-1000-lit-evalMA-NOpad | 1 | null | transformers | 30,820 | ---
license: mit
tags:
- generated_from_trainer
metrics:
- rouge
model-index:
- name: bart-large-cnn-1000-lit-evalMA-NOpad
results: []
---
<!-- This model card has been generated automatically according to the information the Trainer had access to. You
should probably proofread and complete it, then remove this comment. -->
# bart-large-cnn-1000-lit-evalMA-NOpad
This model is a fine-tuned version of [facebook/bart-large-cnn](https://huggingface.co/facebook/bart-large-cnn) on an unknown dataset.
It achieves the following results on the evaluation set:
- Loss: 1.9804
- Rouge1: 27.2698
- Rouge2: 11.8561
- Rougel: 20.5948
- Rougelsum: 23.5497
- Gen Len: 67.67
## Model description
More information needed
## Intended uses & limitations
More information needed
## Training and evaluation data
More information needed
## Training procedure
### Training hyperparameters
The following hyperparameters were used during training:
- learning_rate: 2e-05
- train_batch_size: 1
- eval_batch_size: 1
- seed: 42
- optimizer: Adam with betas=(0.9,0.999) and epsilon=1e-08
- lr_scheduler_type: linear
- num_epochs: 5
- mixed_precision_training: Native AMP
### Training results
| Training Loss | Epoch | Step | Validation Loss | Rouge1 | Rouge2 | Rougel | Rougelsum | Gen Len |
|:-------------:|:-----:|:----:|:---------------:|:-------:|:-------:|:-------:|:---------:|:-------:|
| 1.5372 | 1.0 | 1000 | 1.7499 | 27.7275 | 12.7894 | 21.1334 | 24.4929 | 66.31 |
| 0.7344 | 2.0 | 2000 | 1.9804 | 27.2698 | 11.8561 | 20.5948 | 23.5497 | 67.67 |
### Framework versions
- Transformers 4.16.2
- Pytorch 1.10.2
- Datasets 1.18.3
- Tokenizers 0.11.0
|
cammy/bart-large-cnn-100-lit-evalMA-NOpad2 | 0d38c437db512f15277026bb8f675577c8a2eb74 | 2022-03-13T11:11:08.000Z | [
"pytorch",
"bart",
"text2text-generation",
"transformers",
"generated_from_trainer",
"license:mit",
"model-index",
"autotrain_compatible"
] | text2text-generation | false | cammy | null | cammy/bart-large-cnn-100-lit-evalMA-NOpad2 | 1 | null | transformers | 30,821 | ---
license: mit
tags:
- generated_from_trainer
metrics:
- rouge
model-index:
- name: bart-large-cnn-100-lit-evalMA-NOpad2
results: []
---
<!-- This model card has been generated automatically according to the information the Trainer had access to. You
should probably proofread and complete it, then remove this comment. -->
# bart-large-cnn-100-lit-evalMA-NOpad2
This model is a fine-tuned version of [facebook/bart-large-cnn](https://huggingface.co/facebook/bart-large-cnn) on an unknown dataset.
It achieves the following results on the evaluation set:
- Loss: 1.2126
- Rouge1: 25.6196
- Rouge2: 7.2753
- Rougel: 18.0987
- Rougelsum: 20.8416
- Gen Len: 67.3
## Model description
More information needed
## Intended uses & limitations
More information needed
## Training and evaluation data
More information needed
## Training procedure
### Training hyperparameters
The following hyperparameters were used during training:
- learning_rate: 2e-05
- train_batch_size: 1
- eval_batch_size: 1
- seed: 42
- optimizer: Adam with betas=(0.9,0.999) and epsilon=1e-08
- lr_scheduler_type: linear
- num_epochs: 5
- mixed_precision_training: Native AMP
### Training results
| Training Loss | Epoch | Step | Validation Loss | Rouge1 | Rouge2 | Rougel | Rougelsum | Gen Len |
|:-------------:|:-----:|:----:|:---------------:|:-------:|:------:|:-------:|:---------:|:-------:|
| No log | 1.0 | 100 | 1.0890 | 23.5493 | 8.9875 | 17.1471 | 20.1643 | 67.8 |
| No log | 2.0 | 200 | 1.2126 | 25.6196 | 7.2753 | 18.0987 | 20.8416 | 67.3 |
### Framework versions
- Transformers 4.16.2
- Pytorch 1.10.2
- Datasets 1.18.3
- Tokenizers 0.11.0
|
Danik51002/finetuned | cc58daa628b40505718a1cc1632e38deeadd50e5 | 2022-03-27T08:26:55.000Z | [
"pytorch",
"tensorboard",
"gpt2",
"text-generation",
"transformers",
"generated_from_trainer",
"model-index"
] | text-generation | false | Danik51002 | null | Danik51002/finetuned | 1 | null | transformers | 30,822 | ---
tags:
- generated_from_trainer
model-index:
- name: finetuned
results: []
---
<!-- This model card has been generated automatically according to the information the Trainer had access to. You
should probably proofread and complete it, then remove this comment. -->
# finetuned
This model is a fine-tuned version of [sberbank-ai/rugpt3small_based_on_gpt2](https://huggingface.co/sberbank-ai/rugpt3small_based_on_gpt2) on an unknown dataset.
## Model description
More information needed
## Intended uses & limitations
More information needed
## Training and evaluation data
More information needed
## Training procedure
### Training hyperparameters
The following hyperparameters were used during training:
- learning_rate: 5e-05
- train_batch_size: 42
- eval_batch_size: 42
- seed: 42
- gradient_accumulation_steps: 20
- total_train_batch_size: 840
- optimizer: Adam with betas=(0.9,0.999) and epsilon=1e-08
- lr_scheduler_type: linear
- lr_scheduler_warmup_steps: 15
- num_epochs: 300
### Training results
### Framework versions
- Transformers 4.17.0
- Pytorch 1.10.0+cu111
- Tokenizers 0.11.6
|
Devendr/wav2vec2-large-xls-r-300m-hindi | fccc51f49bf5eab58613f089584df90e88aa0266 | 2022-03-13T14:44:09.000Z | [
"pytorch",
"tensorboard",
"wav2vec2",
"automatic-speech-recognition",
"dataset:common_voice",
"transformers",
"generated_from_trainer",
"license:apache-2.0",
"model-index"
] | automatic-speech-recognition | false | Devendr | null | Devendr/wav2vec2-large-xls-r-300m-hindi | 1 | null | transformers | 30,823 | ---
license: apache-2.0
tags:
- generated_from_trainer
datasets:
- common_voice
model-index:
- name: wav2vec2-large-xls-r-300m-hindi
results: []
---
<!-- This model card has been generated automatically according to the information the Trainer had access to. You
should probably proofread and complete it, then remove this comment. -->
# wav2vec2-large-xls-r-300m-hindi
This model is a fine-tuned version of [facebook/wav2vec2-xls-r-300m](https://huggingface.co/facebook/wav2vec2-xls-r-300m) on the common_voice dataset.
## Model description
More information needed
## Intended uses & limitations
More information needed
## Training and evaluation data
More information needed
## Training procedure
### Training hyperparameters
The following hyperparameters were used during training:
- learning_rate: 0.0003
- train_batch_size: 16
- eval_batch_size: 8
- seed: 42
- gradient_accumulation_steps: 2
- total_train_batch_size: 32
- optimizer: Adam with betas=(0.9,0.999) and epsilon=1e-08
- lr_scheduler_type: linear
- lr_scheduler_warmup_steps: 500
- num_epochs: 30
- mixed_precision_training: Native AMP
### Training results
### Framework versions
- Transformers 4.11.3
- Pytorch 1.10.0+cu111
- Datasets 1.18.3
- Tokenizers 0.10.3
|
Danik51002/NewModel | 92cb4a92eb05d4e441538c56809d84fbc9dbd30e | 2022-03-27T12:52:39.000Z | [
"pytorch",
"tensorboard",
"gpt2",
"text-generation",
"transformers",
"generated_from_trainer",
"model-index"
] | text-generation | false | Danik51002 | null | Danik51002/NewModel | 1 | null | transformers | 30,824 | ---
tags:
- generated_from_trainer
model-index:
- name: NewModel
results: []
---
<!-- This model card has been generated automatically according to the information the Trainer had access to. You
should probably proofread and complete it, then remove this comment. -->
# NewModel
This model is a fine-tuned version of [sberbank-ai/rugpt3small_based_on_gpt2](https://huggingface.co/sberbank-ai/rugpt3small_based_on_gpt2) on an unknown dataset.
## Model description
More information needed
## Intended uses & limitations
More information needed
## Training and evaluation data
More information needed
## Training procedure
### Training hyperparameters
The following hyperparameters were used during training:
- learning_rate: 5e-05
- train_batch_size: 42
- eval_batch_size: 42
- seed: 42
- gradient_accumulation_steps: 20
- total_train_batch_size: 840
- optimizer: Adam with betas=(0.9,0.999) and epsilon=1e-08
- lr_scheduler_type: linear
- lr_scheduler_warmup_steps: 15
- num_epochs: 200
### Training results
### Framework versions
- Transformers 4.17.0
- Pytorch 1.10.0+cu111
- Tokenizers 0.11.6
|
tau/test | a548349261d3c82c7821d7a5d3eb3bf591ccfad8 | 2022-03-13T17:20:05.000Z | [
"pytorch",
"t5",
"text2text-generation",
"transformers",
"autotrain_compatible"
] | text2text-generation | false | tau | null | tau/test | 1 | null | transformers | 30,825 | Entry not found |
qahq/CL-AraBERTv0.1-base | 1da981a391df548f060730678b806e0bd79010b5 | 2022-03-21T16:04:15.000Z | [
"pytorch",
"bert",
"fill-mask",
"transformers",
"license:apache-2.0",
"autotrain_compatible"
] | fill-mask | false | qahq | null | qahq/CL-AraBERTv0.1-base | 1 | null | transformers | 30,826 | ---
license: apache-2.0
---
|
lilitket/20220314-084927 | cc90e2f52342a2163e933a57df86be1cbf3e804a | 2022-03-14T13:26:06.000Z | [
"pytorch",
"wav2vec2",
"automatic-speech-recognition",
"transformers"
] | automatic-speech-recognition | false | lilitket | null | lilitket/20220314-084927 | 1 | null | transformers | 30,827 | Entry not found |
Kalaoke/embeddings_dense_model | 7038a8f1ad9d4073c1553d61f1727f95c43e6f61 | 2022-03-14T09:54:04.000Z | [
"pytorch",
"distilbert",
"feature-extraction",
"sentence-transformers",
"sentence-similarity"
] | sentence-similarity | false | Kalaoke | null | Kalaoke/embeddings_dense_model | 1 | null | sentence-transformers | 30,828 | ---
pipeline_tag: sentence-similarity
tags:
- sentence-transformers
- feature-extraction
- sentence-similarity
---
# Kalaoke/embeddings_dense_model
This is a [sentence-transformers](https://www.SBERT.net) model: It maps sentences & paragraphs to a 50 dimensional dense vector space and can be used for tasks like clustering or semantic search.
<!--- Describe your model here -->
## Usage (Sentence-Transformers)
Using this model becomes easy when you have [sentence-transformers](https://www.SBERT.net) installed:
```
pip install -U sentence-transformers
```
Then you can use the model like this:
```python
from sentence_transformers import SentenceTransformer
sentences = ["This is an example sentence", "Each sentence is converted"]
model = SentenceTransformer('Kalaoke/embeddings_dense_model')
embeddings = model.encode(sentences)
print(embeddings)
```
## Evaluation Results
<!--- Describe how your model was evaluated -->
For an automated evaluation of this model, see the *Sentence Embeddings Benchmark*: [https://seb.sbert.net](https://seb.sbert.net?model_name=Kalaoke/embeddings_dense_model)
## Training
The model was trained with the parameters:
**DataLoader**:
`torch.utils.data.dataloader.DataLoader` of length 1050 with parameters:
```
{'batch_size': 32, 'sampler': 'torch.utils.data.sampler.RandomSampler', 'batch_sampler': 'torch.utils.data.sampler.BatchSampler'}
```
**Loss**:
`sentence_transformers.losses.CosineSimilarityLoss.CosineSimilarityLoss`
Parameters of the fit()-Method:
```
{
"epochs": 3,
"evaluation_steps": 1000,
"evaluator": "sentence_transformers.evaluation.BinaryClassificationEvaluator.BinaryClassificationEvaluator",
"max_grad_norm": 1,
"optimizer_class": "<class 'transformers.optimization.AdamW'>",
"optimizer_params": {
"lr": 2e-05
},
"scheduler": "WarmupLinear",
"steps_per_epoch": null,
"warmup_steps": 315,
"weight_decay": 0.01
}
```
## Full Model Architecture
```
SentenceTransformer(
(0): Transformer({'max_seq_length': 512, 'do_lower_case': False}) with Transformer model: DistilBertModel
(1): Pooling({'word_embedding_dimension': 768, 'pooling_mode_cls_token': False, 'pooling_mode_mean_tokens': True, 'pooling_mode_max_tokens': False, 'pooling_mode_mean_sqrt_len_tokens': False})
(2): Asym(
(topic-0): Dense({'in_features': 768, 'out_features': 50, 'bias': False, 'activation_function': 'torch.nn.modules.activation.Tanh'})
(title-0): Dense({'in_features': 768, 'out_features': 50, 'bias': False, 'activation_function': 'torch.nn.modules.activation.Tanh'})
)
)
```
## Citing & Authors
<!--- Describe where people can find more information --> |
sanchit-gandhi/wav2vec2-2-bart-large-no-adapter | f09c487bf0af0c95eab6b1eceb28518a352a1d90 | 2022-03-14T21:45:57.000Z | [
"pytorch",
"tensorboard",
"speech-encoder-decoder",
"automatic-speech-recognition",
"dataset:librispeech_asr",
"transformers",
"generated_from_trainer",
"model-index"
] | automatic-speech-recognition | false | sanchit-gandhi | null | sanchit-gandhi/wav2vec2-2-bart-large-no-adapter | 1 | null | transformers | 30,829 | ---
tags:
- generated_from_trainer
datasets:
- librispeech_asr
model-index:
- name: ''
results: []
---
<!-- This model card has been generated automatically according to the information the Trainer had access to. You
should probably proofread and complete it, then remove this comment. -->
#
This model was trained from scratch on the librispeech_asr dataset.
It achieves the following results on the evaluation set:
- Loss: 5.6120
- Wer: 1.0267
## Model description
More information needed
## Intended uses & limitations
More information needed
## Training and evaluation data
More information needed
## Training procedure
### Training hyperparameters
The following hyperparameters were used during training:
- learning_rate: 3e-05
- train_batch_size: 8
- eval_batch_size: 8
- seed: 42
- gradient_accumulation_steps: 4
- total_train_batch_size: 32
- optimizer: Adam with betas=(0.9,0.999) and epsilon=1e-08
- lr_scheduler_type: linear
- lr_scheduler_warmup_steps: 500
- num_epochs: 5.0
- mixed_precision_training: Native AMP
### Training results
| Training Loss | Epoch | Step | Validation Loss | Wer |
|:-------------:|:-----:|:----:|:---------------:|:------:|
| 6.7189 | 0.56 | 500 | 6.9796 | 0.9350 |
| 6.5068 | 1.12 | 1000 | 6.4823 | 1.3923 |
| 6.4601 | 1.68 | 1500 | 6.1801 | 1.1578 |
| 6.1802 | 2.24 | 2000 | 6.0002 | 1.7750 |
| 6.0888 | 2.8 | 2500 | 5.8453 | 1.7581 |
| 6.0993 | 3.36 | 3000 | 5.7702 | 1.4096 |
| 6.0851 | 3.92 | 3500 | 5.6634 | 1.0944 |
| 5.9357 | 4.48 | 4000 | 5.6120 | 1.0267 |
### Framework versions
- Transformers 4.17.0.dev0
- Pytorch 1.10.2+cu113
- Datasets 1.18.3
- Tokenizers 0.11.0
|
vamsibanda/bert-large-cased-onnx | bd7d6f1b51e77e2b8e90e063db990e86e5545b0a | 2022-07-23T04:17:19.000Z | [
"pytorch",
"onnx",
"bert",
"feature-extraction",
"transformers"
] | feature-extraction | false | vamsibanda | null | vamsibanda/bert-large-cased-onnx | 1 | null | transformers | 30,830 | |
GPL/scifact-distilbert-tas-b-gpl-self_miner | fbf6d0feec31523a165aee0f06fb35fdac0ab262 | 2022-03-14T14:17:30.000Z | [
"pytorch",
"distilbert",
"feature-extraction",
"sentence-transformers",
"sentence-similarity",
"transformers"
] | sentence-similarity | false | GPL | null | GPL/scifact-distilbert-tas-b-gpl-self_miner | 1 | null | sentence-transformers | 30,831 | ---
pipeline_tag: sentence-similarity
tags:
- sentence-transformers
- feature-extraction
- sentence-similarity
- transformers
---
# {MODEL_NAME}
This is a [sentence-transformers](https://www.SBERT.net) model: It maps sentences & paragraphs to a 768 dimensional dense vector space and can be used for tasks like clustering or semantic search.
<!--- Describe your model here -->
## Usage (Sentence-Transformers)
Using this model becomes easy when you have [sentence-transformers](https://www.SBERT.net) installed:
```
pip install -U sentence-transformers
```
Then you can use the model like this:
```python
from sentence_transformers import SentenceTransformer
sentences = ["This is an example sentence", "Each sentence is converted"]
model = SentenceTransformer('{MODEL_NAME}')
embeddings = model.encode(sentences)
print(embeddings)
```
## Usage (HuggingFace Transformers)
Without [sentence-transformers](https://www.SBERT.net), you can use the model like this: First, you pass your input through the transformer model, then you have to apply the right pooling-operation on-top of the contextualized word embeddings.
```python
from transformers import AutoTokenizer, AutoModel
import torch
def cls_pooling(model_output, attention_mask):
return model_output[0][:,0]
# Sentences we want sentence embeddings for
sentences = ['This is an example sentence', 'Each sentence is converted']
# Load model from HuggingFace Hub
tokenizer = AutoTokenizer.from_pretrained('{MODEL_NAME}')
model = AutoModel.from_pretrained('{MODEL_NAME}')
# Tokenize sentences
encoded_input = tokenizer(sentences, padding=True, truncation=True, return_tensors='pt')
# Compute token embeddings
with torch.no_grad():
model_output = model(**encoded_input)
# Perform pooling. In this case, cls pooling.
sentence_embeddings = cls_pooling(model_output, encoded_input['attention_mask'])
print("Sentence embeddings:")
print(sentence_embeddings)
```
## Evaluation Results
<!--- Describe how your model was evaluated -->
For an automated evaluation of this model, see the *Sentence Embeddings Benchmark*: [https://seb.sbert.net](https://seb.sbert.net?model_name={MODEL_NAME})
## Training
The model was trained with the parameters:
**DataLoader**:
`torch.utils.data.dataloader.DataLoader` of length 140000 with parameters:
```
{'batch_size': 32, 'sampler': 'torch.utils.data.sampler.SequentialSampler', 'batch_sampler': 'torch.utils.data.sampler.BatchSampler'}
```
**Loss**:
`gpl.toolkit.loss.MarginDistillationLoss`
Parameters of the fit()-Method:
```
{
"epochs": 1,
"evaluation_steps": 0,
"evaluator": "NoneType",
"max_grad_norm": 1,
"optimizer_class": "<class 'transformers.optimization.AdamW'>",
"optimizer_params": {
"lr": 2e-05
},
"scheduler": "WarmupLinear",
"steps_per_epoch": 140000,
"warmup_steps": 1000,
"weight_decay": 0.01
}
```
## Full Model Architecture
```
SentenceTransformer(
(0): Transformer({'max_seq_length': 350, 'do_lower_case': False}) with Transformer model: DistilBertModel
(1): Pooling({'word_embedding_dimension': 768, 'pooling_mode_cls_token': True, 'pooling_mode_mean_tokens': False, 'pooling_mode_max_tokens': False, 'pooling_mode_mean_sqrt_len_tokens': False})
)
```
## Citing & Authors
<!--- Describe where people can find more information --> |
GPL/trec-covid-v2-distilbert-tas-b-gpl-self_miner | d080b40c4d945c5e1f22b342dc36dcfd410c64b3 | 2022-03-14T14:18:03.000Z | [
"pytorch",
"distilbert",
"feature-extraction",
"sentence-transformers",
"sentence-similarity",
"transformers"
] | sentence-similarity | false | GPL | null | GPL/trec-covid-v2-distilbert-tas-b-gpl-self_miner | 1 | null | sentence-transformers | 30,832 | ---
pipeline_tag: sentence-similarity
tags:
- sentence-transformers
- feature-extraction
- sentence-similarity
- transformers
---
# {MODEL_NAME}
This is a [sentence-transformers](https://www.SBERT.net) model: It maps sentences & paragraphs to a 768 dimensional dense vector space and can be used for tasks like clustering or semantic search.
<!--- Describe your model here -->
## Usage (Sentence-Transformers)
Using this model becomes easy when you have [sentence-transformers](https://www.SBERT.net) installed:
```
pip install -U sentence-transformers
```
Then you can use the model like this:
```python
from sentence_transformers import SentenceTransformer
sentences = ["This is an example sentence", "Each sentence is converted"]
model = SentenceTransformer('{MODEL_NAME}')
embeddings = model.encode(sentences)
print(embeddings)
```
## Usage (HuggingFace Transformers)
Without [sentence-transformers](https://www.SBERT.net), you can use the model like this: First, you pass your input through the transformer model, then you have to apply the right pooling-operation on-top of the contextualized word embeddings.
```python
from transformers import AutoTokenizer, AutoModel
import torch
def cls_pooling(model_output, attention_mask):
return model_output[0][:,0]
# Sentences we want sentence embeddings for
sentences = ['This is an example sentence', 'Each sentence is converted']
# Load model from HuggingFace Hub
tokenizer = AutoTokenizer.from_pretrained('{MODEL_NAME}')
model = AutoModel.from_pretrained('{MODEL_NAME}')
# Tokenize sentences
encoded_input = tokenizer(sentences, padding=True, truncation=True, return_tensors='pt')
# Compute token embeddings
with torch.no_grad():
model_output = model(**encoded_input)
# Perform pooling. In this case, cls pooling.
sentence_embeddings = cls_pooling(model_output, encoded_input['attention_mask'])
print("Sentence embeddings:")
print(sentence_embeddings)
```
## Evaluation Results
<!--- Describe how your model was evaluated -->
For an automated evaluation of this model, see the *Sentence Embeddings Benchmark*: [https://seb.sbert.net](https://seb.sbert.net?model_name={MODEL_NAME})
## Training
The model was trained with the parameters:
**DataLoader**:
`torch.utils.data.dataloader.DataLoader` of length 140000 with parameters:
```
{'batch_size': 32, 'sampler': 'torch.utils.data.sampler.SequentialSampler', 'batch_sampler': 'torch.utils.data.sampler.BatchSampler'}
```
**Loss**:
`gpl.toolkit.loss.MarginDistillationLoss`
Parameters of the fit()-Method:
```
{
"epochs": 1,
"evaluation_steps": 0,
"evaluator": "NoneType",
"max_grad_norm": 1,
"optimizer_class": "<class 'transformers.optimization.AdamW'>",
"optimizer_params": {
"lr": 2e-05
},
"scheduler": "WarmupLinear",
"steps_per_epoch": 140000,
"warmup_steps": 1000,
"weight_decay": 0.01
}
```
## Full Model Architecture
```
SentenceTransformer(
(0): Transformer({'max_seq_length': 350, 'do_lower_case': False}) with Transformer model: DistilBertModel
(1): Pooling({'word_embedding_dimension': 768, 'pooling_mode_cls_token': True, 'pooling_mode_mean_tokens': False, 'pooling_mode_max_tokens': False, 'pooling_mode_mean_sqrt_len_tokens': False})
)
```
## Citing & Authors
<!--- Describe where people can find more information --> |
GPL/cqadupstack-distilbert-tas-b-gpl-self_miner | 2b939ed344e8c50d24a8571e422fa9d46a787752 | 2022-03-14T14:18:20.000Z | [
"pytorch",
"distilbert",
"feature-extraction",
"sentence-transformers",
"sentence-similarity",
"transformers"
] | sentence-similarity | false | GPL | null | GPL/cqadupstack-distilbert-tas-b-gpl-self_miner | 1 | null | sentence-transformers | 30,833 | ---
pipeline_tag: sentence-similarity
tags:
- sentence-transformers
- feature-extraction
- sentence-similarity
- transformers
---
# {MODEL_NAME}
This is a [sentence-transformers](https://www.SBERT.net) model: It maps sentences & paragraphs to a 768 dimensional dense vector space and can be used for tasks like clustering or semantic search.
<!--- Describe your model here -->
## Usage (Sentence-Transformers)
Using this model becomes easy when you have [sentence-transformers](https://www.SBERT.net) installed:
```
pip install -U sentence-transformers
```
Then you can use the model like this:
```python
from sentence_transformers import SentenceTransformer
sentences = ["This is an example sentence", "Each sentence is converted"]
model = SentenceTransformer('{MODEL_NAME}')
embeddings = model.encode(sentences)
print(embeddings)
```
## Usage (HuggingFace Transformers)
Without [sentence-transformers](https://www.SBERT.net), you can use the model like this: First, you pass your input through the transformer model, then you have to apply the right pooling-operation on-top of the contextualized word embeddings.
```python
from transformers import AutoTokenizer, AutoModel
import torch
def cls_pooling(model_output, attention_mask):
return model_output[0][:,0]
# Sentences we want sentence embeddings for
sentences = ['This is an example sentence', 'Each sentence is converted']
# Load model from HuggingFace Hub
tokenizer = AutoTokenizer.from_pretrained('{MODEL_NAME}')
model = AutoModel.from_pretrained('{MODEL_NAME}')
# Tokenize sentences
encoded_input = tokenizer(sentences, padding=True, truncation=True, return_tensors='pt')
# Compute token embeddings
with torch.no_grad():
model_output = model(**encoded_input)
# Perform pooling. In this case, cls pooling.
sentence_embeddings = cls_pooling(model_output, encoded_input['attention_mask'])
print("Sentence embeddings:")
print(sentence_embeddings)
```
## Evaluation Results
<!--- Describe how your model was evaluated -->
For an automated evaluation of this model, see the *Sentence Embeddings Benchmark*: [https://seb.sbert.net](https://seb.sbert.net?model_name={MODEL_NAME})
## Training
The model was trained with the parameters:
**DataLoader**:
`torch.utils.data.dataloader.DataLoader` of length 140000 with parameters:
```
{'batch_size': 32, 'sampler': 'torch.utils.data.sampler.SequentialSampler', 'batch_sampler': 'torch.utils.data.sampler.BatchSampler'}
```
**Loss**:
`gpl.toolkit.loss.MarginDistillationLoss`
Parameters of the fit()-Method:
```
{
"epochs": 1,
"evaluation_steps": 0,
"evaluator": "NoneType",
"max_grad_norm": 1,
"optimizer_class": "<class 'transformers.optimization.AdamW'>",
"optimizer_params": {
"lr": 2e-05
},
"scheduler": "WarmupLinear",
"steps_per_epoch": 140000,
"warmup_steps": 1000,
"weight_decay": 0.01
}
```
## Full Model Architecture
```
SentenceTransformer(
(0): Transformer({'max_seq_length': 350, 'do_lower_case': False}) with Transformer model: DistilBertModel
(1): Pooling({'word_embedding_dimension': 768, 'pooling_mode_cls_token': True, 'pooling_mode_mean_tokens': False, 'pooling_mode_max_tokens': False, 'pooling_mode_mean_sqrt_len_tokens': False})
)
```
## Citing & Authors
<!--- Describe where people can find more information --> |
GPL/robust04-distilbert-tas-b-gpl-self_miner | 094a95ffc73f3e3c2fb45bd964a8bbc818f25f3b | 2022-03-14T14:18:37.000Z | [
"pytorch",
"distilbert",
"feature-extraction",
"sentence-transformers",
"sentence-similarity",
"transformers"
] | sentence-similarity | false | GPL | null | GPL/robust04-distilbert-tas-b-gpl-self_miner | 1 | null | sentence-transformers | 30,834 | ---
pipeline_tag: sentence-similarity
tags:
- sentence-transformers
- feature-extraction
- sentence-similarity
- transformers
---
# {MODEL_NAME}
This is a [sentence-transformers](https://www.SBERT.net) model: It maps sentences & paragraphs to a 768 dimensional dense vector space and can be used for tasks like clustering or semantic search.
<!--- Describe your model here -->
## Usage (Sentence-Transformers)
Using this model becomes easy when you have [sentence-transformers](https://www.SBERT.net) installed:
```
pip install -U sentence-transformers
```
Then you can use the model like this:
```python
from sentence_transformers import SentenceTransformer
sentences = ["This is an example sentence", "Each sentence is converted"]
model = SentenceTransformer('{MODEL_NAME}')
embeddings = model.encode(sentences)
print(embeddings)
```
## Usage (HuggingFace Transformers)
Without [sentence-transformers](https://www.SBERT.net), you can use the model like this: First, you pass your input through the transformer model, then you have to apply the right pooling-operation on-top of the contextualized word embeddings.
```python
from transformers import AutoTokenizer, AutoModel
import torch
def cls_pooling(model_output, attention_mask):
return model_output[0][:,0]
# Sentences we want sentence embeddings for
sentences = ['This is an example sentence', 'Each sentence is converted']
# Load model from HuggingFace Hub
tokenizer = AutoTokenizer.from_pretrained('{MODEL_NAME}')
model = AutoModel.from_pretrained('{MODEL_NAME}')
# Tokenize sentences
encoded_input = tokenizer(sentences, padding=True, truncation=True, return_tensors='pt')
# Compute token embeddings
with torch.no_grad():
model_output = model(**encoded_input)
# Perform pooling. In this case, cls pooling.
sentence_embeddings = cls_pooling(model_output, encoded_input['attention_mask'])
print("Sentence embeddings:")
print(sentence_embeddings)
```
## Evaluation Results
<!--- Describe how your model was evaluated -->
For an automated evaluation of this model, see the *Sentence Embeddings Benchmark*: [https://seb.sbert.net](https://seb.sbert.net?model_name={MODEL_NAME})
## Training
The model was trained with the parameters:
**DataLoader**:
`torch.utils.data.dataloader.DataLoader` of length 140000 with parameters:
```
{'batch_size': 32, 'sampler': 'torch.utils.data.sampler.SequentialSampler', 'batch_sampler': 'torch.utils.data.sampler.BatchSampler'}
```
**Loss**:
`gpl.toolkit.loss.MarginDistillationLoss`
Parameters of the fit()-Method:
```
{
"epochs": 1,
"evaluation_steps": 0,
"evaluator": "NoneType",
"max_grad_norm": 1,
"optimizer_class": "<class 'transformers.optimization.AdamW'>",
"optimizer_params": {
"lr": 2e-05
},
"scheduler": "WarmupLinear",
"steps_per_epoch": 140000,
"warmup_steps": 1000,
"weight_decay": 0.01
}
```
## Full Model Architecture
```
SentenceTransformer(
(0): Transformer({'max_seq_length': 350, 'do_lower_case': False}) with Transformer model: DistilBertModel
(1): Pooling({'word_embedding_dimension': 768, 'pooling_mode_cls_token': True, 'pooling_mode_mean_tokens': False, 'pooling_mode_max_tokens': False, 'pooling_mode_mean_sqrt_len_tokens': False})
)
```
## Citing & Authors
<!--- Describe where people can find more information --> |
GPL/trec-covid-distilbert-tas-b-gpl-self_miner | ffd171f8480dbcd9970aefae9f92f21b15df3a7e | 2022-03-14T14:22:13.000Z | [
"pytorch",
"distilbert",
"feature-extraction",
"sentence-transformers",
"sentence-similarity",
"transformers"
] | sentence-similarity | false | GPL | null | GPL/trec-covid-distilbert-tas-b-gpl-self_miner | 1 | null | sentence-transformers | 30,835 | ---
pipeline_tag: sentence-similarity
tags:
- sentence-transformers
- feature-extraction
- sentence-similarity
- transformers
---
# {MODEL_NAME}
This is a [sentence-transformers](https://www.SBERT.net) model: It maps sentences & paragraphs to a 768 dimensional dense vector space and can be used for tasks like clustering or semantic search.
<!--- Describe your model here -->
## Usage (Sentence-Transformers)
Using this model becomes easy when you have [sentence-transformers](https://www.SBERT.net) installed:
```
pip install -U sentence-transformers
```
Then you can use the model like this:
```python
from sentence_transformers import SentenceTransformer
sentences = ["This is an example sentence", "Each sentence is converted"]
model = SentenceTransformer('{MODEL_NAME}')
embeddings = model.encode(sentences)
print(embeddings)
```
## Usage (HuggingFace Transformers)
Without [sentence-transformers](https://www.SBERT.net), you can use the model like this: First, you pass your input through the transformer model, then you have to apply the right pooling-operation on-top of the contextualized word embeddings.
```python
from transformers import AutoTokenizer, AutoModel
import torch
def cls_pooling(model_output, attention_mask):
return model_output[0][:,0]
# Sentences we want sentence embeddings for
sentences = ['This is an example sentence', 'Each sentence is converted']
# Load model from HuggingFace Hub
tokenizer = AutoTokenizer.from_pretrained('{MODEL_NAME}')
model = AutoModel.from_pretrained('{MODEL_NAME}')
# Tokenize sentences
encoded_input = tokenizer(sentences, padding=True, truncation=True, return_tensors='pt')
# Compute token embeddings
with torch.no_grad():
model_output = model(**encoded_input)
# Perform pooling. In this case, cls pooling.
sentence_embeddings = cls_pooling(model_output, encoded_input['attention_mask'])
print("Sentence embeddings:")
print(sentence_embeddings)
```
## Evaluation Results
<!--- Describe how your model was evaluated -->
For an automated evaluation of this model, see the *Sentence Embeddings Benchmark*: [https://seb.sbert.net](https://seb.sbert.net?model_name={MODEL_NAME})
## Training
The model was trained with the parameters:
**DataLoader**:
`torch.utils.data.dataloader.DataLoader` of length 140000 with parameters:
```
{'batch_size': 32, 'sampler': 'torch.utils.data.sampler.SequentialSampler', 'batch_sampler': 'torch.utils.data.sampler.BatchSampler'}
```
**Loss**:
`gpl.toolkit.loss.MarginDistillationLoss`
Parameters of the fit()-Method:
```
{
"epochs": 1,
"evaluation_steps": 0,
"evaluator": "NoneType",
"max_grad_norm": 1,
"optimizer_class": "<class 'transformers.optimization.AdamW'>",
"optimizer_params": {
"lr": 2e-05
},
"scheduler": "WarmupLinear",
"steps_per_epoch": 140000,
"warmup_steps": 1000,
"weight_decay": 0.01
}
```
## Full Model Architecture
```
SentenceTransformer(
(0): Transformer({'max_seq_length': 350, 'do_lower_case': False}) with Transformer model: DistilBertModel
(1): Pooling({'word_embedding_dimension': 768, 'pooling_mode_cls_token': True, 'pooling_mode_mean_tokens': False, 'pooling_mode_max_tokens': False, 'pooling_mode_mean_sqrt_len_tokens': False})
)
```
## Citing & Authors
<!--- Describe where people can find more information --> |
GPL/arguana-distilbert-tas-b-gpl-self_miner | eaa663cdd4c130371aeb61ecb3573c911fdbb871 | 2022-03-14T14:22:47.000Z | [
"pytorch",
"distilbert",
"feature-extraction",
"sentence-transformers",
"sentence-similarity",
"transformers"
] | sentence-similarity | false | GPL | null | GPL/arguana-distilbert-tas-b-gpl-self_miner | 1 | null | sentence-transformers | 30,836 | ---
pipeline_tag: sentence-similarity
tags:
- sentence-transformers
- feature-extraction
- sentence-similarity
- transformers
---
# {MODEL_NAME}
This is a [sentence-transformers](https://www.SBERT.net) model: It maps sentences & paragraphs to a 768 dimensional dense vector space and can be used for tasks like clustering or semantic search.
<!--- Describe your model here -->
## Usage (Sentence-Transformers)
Using this model becomes easy when you have [sentence-transformers](https://www.SBERT.net) installed:
```
pip install -U sentence-transformers
```
Then you can use the model like this:
```python
from sentence_transformers import SentenceTransformer
sentences = ["This is an example sentence", "Each sentence is converted"]
model = SentenceTransformer('{MODEL_NAME}')
embeddings = model.encode(sentences)
print(embeddings)
```
## Usage (HuggingFace Transformers)
Without [sentence-transformers](https://www.SBERT.net), you can use the model like this: First, you pass your input through the transformer model, then you have to apply the right pooling-operation on-top of the contextualized word embeddings.
```python
from transformers import AutoTokenizer, AutoModel
import torch
def cls_pooling(model_output, attention_mask):
return model_output[0][:,0]
# Sentences we want sentence embeddings for
sentences = ['This is an example sentence', 'Each sentence is converted']
# Load model from HuggingFace Hub
tokenizer = AutoTokenizer.from_pretrained('{MODEL_NAME}')
model = AutoModel.from_pretrained('{MODEL_NAME}')
# Tokenize sentences
encoded_input = tokenizer(sentences, padding=True, truncation=True, return_tensors='pt')
# Compute token embeddings
with torch.no_grad():
model_output = model(**encoded_input)
# Perform pooling. In this case, cls pooling.
sentence_embeddings = cls_pooling(model_output, encoded_input['attention_mask'])
print("Sentence embeddings:")
print(sentence_embeddings)
```
## Evaluation Results
<!--- Describe how your model was evaluated -->
For an automated evaluation of this model, see the *Sentence Embeddings Benchmark*: [https://seb.sbert.net](https://seb.sbert.net?model_name={MODEL_NAME})
## Training
The model was trained with the parameters:
**DataLoader**:
`torch.utils.data.dataloader.DataLoader` of length 140000 with parameters:
```
{'batch_size': 32, 'sampler': 'torch.utils.data.sampler.SequentialSampler', 'batch_sampler': 'torch.utils.data.sampler.BatchSampler'}
```
**Loss**:
`gpl.toolkit.loss.MarginDistillationLoss`
Parameters of the fit()-Method:
```
{
"epochs": 1,
"evaluation_steps": 0,
"evaluator": "NoneType",
"max_grad_norm": 1,
"optimizer_class": "<class 'transformers.optimization.AdamW'>",
"optimizer_params": {
"lr": 2e-05
},
"scheduler": "WarmupLinear",
"steps_per_epoch": 140000,
"warmup_steps": 1000,
"weight_decay": 0.01
}
```
## Full Model Architecture
```
SentenceTransformer(
(0): Transformer({'max_seq_length': 350, 'do_lower_case': False}) with Transformer model: DistilBertModel
(1): Pooling({'word_embedding_dimension': 768, 'pooling_mode_cls_token': True, 'pooling_mode_mean_tokens': False, 'pooling_mode_max_tokens': False, 'pooling_mode_mean_sqrt_len_tokens': False})
)
```
## Citing & Authors
<!--- Describe where people can find more information --> |
GPL/climate-fever-distilbert-tas-b-gpl-self_miner | cc55804d1f95f8ac52505d69d896abd58db490d3 | 2022-03-14T14:23:05.000Z | [
"pytorch",
"distilbert",
"feature-extraction",
"sentence-transformers",
"sentence-similarity",
"transformers"
] | sentence-similarity | false | GPL | null | GPL/climate-fever-distilbert-tas-b-gpl-self_miner | 1 | null | sentence-transformers | 30,837 | ---
pipeline_tag: sentence-similarity
tags:
- sentence-transformers
- feature-extraction
- sentence-similarity
- transformers
---
# {MODEL_NAME}
This is a [sentence-transformers](https://www.SBERT.net) model: It maps sentences & paragraphs to a 768 dimensional dense vector space and can be used for tasks like clustering or semantic search.
<!--- Describe your model here -->
## Usage (Sentence-Transformers)
Using this model becomes easy when you have [sentence-transformers](https://www.SBERT.net) installed:
```
pip install -U sentence-transformers
```
Then you can use the model like this:
```python
from sentence_transformers import SentenceTransformer
sentences = ["This is an example sentence", "Each sentence is converted"]
model = SentenceTransformer('{MODEL_NAME}')
embeddings = model.encode(sentences)
print(embeddings)
```
## Usage (HuggingFace Transformers)
Without [sentence-transformers](https://www.SBERT.net), you can use the model like this: First, you pass your input through the transformer model, then you have to apply the right pooling-operation on-top of the contextualized word embeddings.
```python
from transformers import AutoTokenizer, AutoModel
import torch
def cls_pooling(model_output, attention_mask):
return model_output[0][:,0]
# Sentences we want sentence embeddings for
sentences = ['This is an example sentence', 'Each sentence is converted']
# Load model from HuggingFace Hub
tokenizer = AutoTokenizer.from_pretrained('{MODEL_NAME}')
model = AutoModel.from_pretrained('{MODEL_NAME}')
# Tokenize sentences
encoded_input = tokenizer(sentences, padding=True, truncation=True, return_tensors='pt')
# Compute token embeddings
with torch.no_grad():
model_output = model(**encoded_input)
# Perform pooling. In this case, cls pooling.
sentence_embeddings = cls_pooling(model_output, encoded_input['attention_mask'])
print("Sentence embeddings:")
print(sentence_embeddings)
```
## Evaluation Results
<!--- Describe how your model was evaluated -->
For an automated evaluation of this model, see the *Sentence Embeddings Benchmark*: [https://seb.sbert.net](https://seb.sbert.net?model_name={MODEL_NAME})
## Training
The model was trained with the parameters:
**DataLoader**:
`torch.utils.data.dataloader.DataLoader` of length 140000 with parameters:
```
{'batch_size': 32, 'sampler': 'torch.utils.data.sampler.SequentialSampler', 'batch_sampler': 'torch.utils.data.sampler.BatchSampler'}
```
**Loss**:
`gpl.toolkit.loss.MarginDistillationLoss`
Parameters of the fit()-Method:
```
{
"epochs": 1,
"evaluation_steps": 0,
"evaluator": "NoneType",
"max_grad_norm": 1,
"optimizer_class": "<class 'transformers.optimization.AdamW'>",
"optimizer_params": {
"lr": 2e-05
},
"scheduler": "WarmupLinear",
"steps_per_epoch": 140000,
"warmup_steps": 1000,
"weight_decay": 0.01
}
```
## Full Model Architecture
```
SentenceTransformer(
(0): Transformer({'max_seq_length': 350, 'do_lower_case': False}) with Transformer model: DistilBertModel
(1): Pooling({'word_embedding_dimension': 768, 'pooling_mode_cls_token': True, 'pooling_mode_mean_tokens': False, 'pooling_mode_max_tokens': False, 'pooling_mode_mean_sqrt_len_tokens': False})
)
```
## Citing & Authors
<!--- Describe where people can find more information --> |
GPL/hotpotqa-distilbert-tas-b-gpl-self_miner | a397243c5cabc9deb692ed0749d085621780646f | 2022-03-14T14:23:55.000Z | [
"pytorch",
"distilbert",
"feature-extraction",
"sentence-transformers",
"sentence-similarity",
"transformers"
] | sentence-similarity | false | GPL | null | GPL/hotpotqa-distilbert-tas-b-gpl-self_miner | 1 | null | sentence-transformers | 30,838 | ---
pipeline_tag: sentence-similarity
tags:
- sentence-transformers
- feature-extraction
- sentence-similarity
- transformers
---
# {MODEL_NAME}
This is a [sentence-transformers](https://www.SBERT.net) model: It maps sentences & paragraphs to a 768 dimensional dense vector space and can be used for tasks like clustering or semantic search.
<!--- Describe your model here -->
## Usage (Sentence-Transformers)
Using this model becomes easy when you have [sentence-transformers](https://www.SBERT.net) installed:
```
pip install -U sentence-transformers
```
Then you can use the model like this:
```python
from sentence_transformers import SentenceTransformer
sentences = ["This is an example sentence", "Each sentence is converted"]
model = SentenceTransformer('{MODEL_NAME}')
embeddings = model.encode(sentences)
print(embeddings)
```
## Usage (HuggingFace Transformers)
Without [sentence-transformers](https://www.SBERT.net), you can use the model like this: First, you pass your input through the transformer model, then you have to apply the right pooling-operation on-top of the contextualized word embeddings.
```python
from transformers import AutoTokenizer, AutoModel
import torch
def cls_pooling(model_output, attention_mask):
return model_output[0][:,0]
# Sentences we want sentence embeddings for
sentences = ['This is an example sentence', 'Each sentence is converted']
# Load model from HuggingFace Hub
tokenizer = AutoTokenizer.from_pretrained('{MODEL_NAME}')
model = AutoModel.from_pretrained('{MODEL_NAME}')
# Tokenize sentences
encoded_input = tokenizer(sentences, padding=True, truncation=True, return_tensors='pt')
# Compute token embeddings
with torch.no_grad():
model_output = model(**encoded_input)
# Perform pooling. In this case, cls pooling.
sentence_embeddings = cls_pooling(model_output, encoded_input['attention_mask'])
print("Sentence embeddings:")
print(sentence_embeddings)
```
## Evaluation Results
<!--- Describe how your model was evaluated -->
For an automated evaluation of this model, see the *Sentence Embeddings Benchmark*: [https://seb.sbert.net](https://seb.sbert.net?model_name={MODEL_NAME})
## Training
The model was trained with the parameters:
**DataLoader**:
`torch.utils.data.dataloader.DataLoader` of length 140000 with parameters:
```
{'batch_size': 32, 'sampler': 'torch.utils.data.sampler.SequentialSampler', 'batch_sampler': 'torch.utils.data.sampler.BatchSampler'}
```
**Loss**:
`gpl.toolkit.loss.MarginDistillationLoss`
Parameters of the fit()-Method:
```
{
"epochs": 1,
"evaluation_steps": 0,
"evaluator": "NoneType",
"max_grad_norm": 1,
"optimizer_class": "<class 'transformers.optimization.AdamW'>",
"optimizer_params": {
"lr": 2e-05
},
"scheduler": "WarmupLinear",
"steps_per_epoch": 140000,
"warmup_steps": 1000,
"weight_decay": 0.01
}
```
## Full Model Architecture
```
SentenceTransformer(
(0): Transformer({'max_seq_length': 350, 'do_lower_case': False}) with Transformer model: DistilBertModel
(1): Pooling({'word_embedding_dimension': 768, 'pooling_mode_cls_token': True, 'pooling_mode_mean_tokens': False, 'pooling_mode_max_tokens': False, 'pooling_mode_mean_sqrt_len_tokens': False})
)
```
## Citing & Authors
<!--- Describe where people can find more information --> |
GPL/nfcorpus-distilbert-tas-b-gpl-self_miner | 0317af0d0f20eca4beda75168394591cac07bd66 | 2022-03-14T14:24:13.000Z | [
"pytorch",
"distilbert",
"feature-extraction",
"sentence-transformers",
"sentence-similarity",
"transformers"
] | sentence-similarity | false | GPL | null | GPL/nfcorpus-distilbert-tas-b-gpl-self_miner | 1 | null | sentence-transformers | 30,839 | ---
pipeline_tag: sentence-similarity
tags:
- sentence-transformers
- feature-extraction
- sentence-similarity
- transformers
---
# {MODEL_NAME}
This is a [sentence-transformers](https://www.SBERT.net) model: It maps sentences & paragraphs to a 768 dimensional dense vector space and can be used for tasks like clustering or semantic search.
<!--- Describe your model here -->
## Usage (Sentence-Transformers)
Using this model becomes easy when you have [sentence-transformers](https://www.SBERT.net) installed:
```
pip install -U sentence-transformers
```
Then you can use the model like this:
```python
from sentence_transformers import SentenceTransformer
sentences = ["This is an example sentence", "Each sentence is converted"]
model = SentenceTransformer('{MODEL_NAME}')
embeddings = model.encode(sentences)
print(embeddings)
```
## Usage (HuggingFace Transformers)
Without [sentence-transformers](https://www.SBERT.net), you can use the model like this: First, you pass your input through the transformer model, then you have to apply the right pooling-operation on-top of the contextualized word embeddings.
```python
from transformers import AutoTokenizer, AutoModel
import torch
def cls_pooling(model_output, attention_mask):
return model_output[0][:,0]
# Sentences we want sentence embeddings for
sentences = ['This is an example sentence', 'Each sentence is converted']
# Load model from HuggingFace Hub
tokenizer = AutoTokenizer.from_pretrained('{MODEL_NAME}')
model = AutoModel.from_pretrained('{MODEL_NAME}')
# Tokenize sentences
encoded_input = tokenizer(sentences, padding=True, truncation=True, return_tensors='pt')
# Compute token embeddings
with torch.no_grad():
model_output = model(**encoded_input)
# Perform pooling. In this case, cls pooling.
sentence_embeddings = cls_pooling(model_output, encoded_input['attention_mask'])
print("Sentence embeddings:")
print(sentence_embeddings)
```
## Evaluation Results
<!--- Describe how your model was evaluated -->
For an automated evaluation of this model, see the *Sentence Embeddings Benchmark*: [https://seb.sbert.net](https://seb.sbert.net?model_name={MODEL_NAME})
## Training
The model was trained with the parameters:
**DataLoader**:
`torch.utils.data.dataloader.DataLoader` of length 140000 with parameters:
```
{'batch_size': 32, 'sampler': 'torch.utils.data.sampler.SequentialSampler', 'batch_sampler': 'torch.utils.data.sampler.BatchSampler'}
```
**Loss**:
`gpl.toolkit.loss.MarginDistillationLoss`
Parameters of the fit()-Method:
```
{
"epochs": 1,
"evaluation_steps": 0,
"evaluator": "NoneType",
"max_grad_norm": 1,
"optimizer_class": "<class 'transformers.optimization.AdamW'>",
"optimizer_params": {
"lr": 2e-05
},
"scheduler": "WarmupLinear",
"steps_per_epoch": 140000,
"warmup_steps": 1000,
"weight_decay": 0.01
}
```
## Full Model Architecture
```
SentenceTransformer(
(0): Transformer({'max_seq_length': 350, 'do_lower_case': False}) with Transformer model: DistilBertModel
(1): Pooling({'word_embedding_dimension': 768, 'pooling_mode_cls_token': True, 'pooling_mode_mean_tokens': False, 'pooling_mode_max_tokens': False, 'pooling_mode_mean_sqrt_len_tokens': False})
)
```
## Citing & Authors
<!--- Describe where people can find more information --> |
GPL/nq-distilbert-tas-b-gpl-self_miner | 8de5a0f14b51a9f0f54f6bdfa65d347cc35b3bcc | 2022-03-14T14:24:29.000Z | [
"pytorch",
"distilbert",
"feature-extraction",
"sentence-transformers",
"sentence-similarity",
"transformers"
] | sentence-similarity | false | GPL | null | GPL/nq-distilbert-tas-b-gpl-self_miner | 1 | null | sentence-transformers | 30,840 | ---
pipeline_tag: sentence-similarity
tags:
- sentence-transformers
- feature-extraction
- sentence-similarity
- transformers
---
# {MODEL_NAME}
This is a [sentence-transformers](https://www.SBERT.net) model: It maps sentences & paragraphs to a 768 dimensional dense vector space and can be used for tasks like clustering or semantic search.
<!--- Describe your model here -->
## Usage (Sentence-Transformers)
Using this model becomes easy when you have [sentence-transformers](https://www.SBERT.net) installed:
```
pip install -U sentence-transformers
```
Then you can use the model like this:
```python
from sentence_transformers import SentenceTransformer
sentences = ["This is an example sentence", "Each sentence is converted"]
model = SentenceTransformer('{MODEL_NAME}')
embeddings = model.encode(sentences)
print(embeddings)
```
## Usage (HuggingFace Transformers)
Without [sentence-transformers](https://www.SBERT.net), you can use the model like this: First, you pass your input through the transformer model, then you have to apply the right pooling-operation on-top of the contextualized word embeddings.
```python
from transformers import AutoTokenizer, AutoModel
import torch
def cls_pooling(model_output, attention_mask):
return model_output[0][:,0]
# Sentences we want sentence embeddings for
sentences = ['This is an example sentence', 'Each sentence is converted']
# Load model from HuggingFace Hub
tokenizer = AutoTokenizer.from_pretrained('{MODEL_NAME}')
model = AutoModel.from_pretrained('{MODEL_NAME}')
# Tokenize sentences
encoded_input = tokenizer(sentences, padding=True, truncation=True, return_tensors='pt')
# Compute token embeddings
with torch.no_grad():
model_output = model(**encoded_input)
# Perform pooling. In this case, cls pooling.
sentence_embeddings = cls_pooling(model_output, encoded_input['attention_mask'])
print("Sentence embeddings:")
print(sentence_embeddings)
```
## Evaluation Results
<!--- Describe how your model was evaluated -->
For an automated evaluation of this model, see the *Sentence Embeddings Benchmark*: [https://seb.sbert.net](https://seb.sbert.net?model_name={MODEL_NAME})
## Training
The model was trained with the parameters:
**DataLoader**:
`torch.utils.data.dataloader.DataLoader` of length 140000 with parameters:
```
{'batch_size': 32, 'sampler': 'torch.utils.data.sampler.SequentialSampler', 'batch_sampler': 'torch.utils.data.sampler.BatchSampler'}
```
**Loss**:
`gpl.toolkit.loss.MarginDistillationLoss`
Parameters of the fit()-Method:
```
{
"epochs": 1,
"evaluation_steps": 0,
"evaluator": "NoneType",
"max_grad_norm": 1,
"optimizer_class": "<class 'transformers.optimization.AdamW'>",
"optimizer_params": {
"lr": 2e-05
},
"scheduler": "WarmupLinear",
"steps_per_epoch": 140000,
"warmup_steps": 1000,
"weight_decay": 0.01
}
```
## Full Model Architecture
```
SentenceTransformer(
(0): Transformer({'max_seq_length': 350, 'do_lower_case': False}) with Transformer model: DistilBertModel
(1): Pooling({'word_embedding_dimension': 768, 'pooling_mode_cls_token': True, 'pooling_mode_mean_tokens': False, 'pooling_mode_max_tokens': False, 'pooling_mode_mean_sqrt_len_tokens': False})
)
```
## Citing & Authors
<!--- Describe where people can find more information --> |
GPL/trec-news-distilbert-tas-b-gpl-self_miner | 92aad421fc6cb4fa62d4c038c24cb7775678b01b | 2022-03-14T14:25:19.000Z | [
"pytorch",
"distilbert",
"feature-extraction",
"sentence-transformers",
"sentence-similarity",
"transformers"
] | sentence-similarity | false | GPL | null | GPL/trec-news-distilbert-tas-b-gpl-self_miner | 1 | null | sentence-transformers | 30,841 | ---
pipeline_tag: sentence-similarity
tags:
- sentence-transformers
- feature-extraction
- sentence-similarity
- transformers
---
# {MODEL_NAME}
This is a [sentence-transformers](https://www.SBERT.net) model: It maps sentences & paragraphs to a 768 dimensional dense vector space and can be used for tasks like clustering or semantic search.
<!--- Describe your model here -->
## Usage (Sentence-Transformers)
Using this model becomes easy when you have [sentence-transformers](https://www.SBERT.net) installed:
```
pip install -U sentence-transformers
```
Then you can use the model like this:
```python
from sentence_transformers import SentenceTransformer
sentences = ["This is an example sentence", "Each sentence is converted"]
model = SentenceTransformer('{MODEL_NAME}')
embeddings = model.encode(sentences)
print(embeddings)
```
## Usage (HuggingFace Transformers)
Without [sentence-transformers](https://www.SBERT.net), you can use the model like this: First, you pass your input through the transformer model, then you have to apply the right pooling-operation on-top of the contextualized word embeddings.
```python
from transformers import AutoTokenizer, AutoModel
import torch
def cls_pooling(model_output, attention_mask):
return model_output[0][:,0]
# Sentences we want sentence embeddings for
sentences = ['This is an example sentence', 'Each sentence is converted']
# Load model from HuggingFace Hub
tokenizer = AutoTokenizer.from_pretrained('{MODEL_NAME}')
model = AutoModel.from_pretrained('{MODEL_NAME}')
# Tokenize sentences
encoded_input = tokenizer(sentences, padding=True, truncation=True, return_tensors='pt')
# Compute token embeddings
with torch.no_grad():
model_output = model(**encoded_input)
# Perform pooling. In this case, cls pooling.
sentence_embeddings = cls_pooling(model_output, encoded_input['attention_mask'])
print("Sentence embeddings:")
print(sentence_embeddings)
```
## Evaluation Results
<!--- Describe how your model was evaluated -->
For an automated evaluation of this model, see the *Sentence Embeddings Benchmark*: [https://seb.sbert.net](https://seb.sbert.net?model_name={MODEL_NAME})
## Training
The model was trained with the parameters:
**DataLoader**:
`torch.utils.data.dataloader.DataLoader` of length 140000 with parameters:
```
{'batch_size': 32, 'sampler': 'torch.utils.data.sampler.SequentialSampler', 'batch_sampler': 'torch.utils.data.sampler.BatchSampler'}
```
**Loss**:
`gpl.toolkit.loss.MarginDistillationLoss`
Parameters of the fit()-Method:
```
{
"epochs": 1,
"evaluation_steps": 0,
"evaluator": "NoneType",
"max_grad_norm": 1,
"optimizer_class": "<class 'transformers.optimization.AdamW'>",
"optimizer_params": {
"lr": 2e-05
},
"scheduler": "WarmupLinear",
"steps_per_epoch": 140000,
"warmup_steps": 1000,
"weight_decay": 0.01
}
```
## Full Model Architecture
```
SentenceTransformer(
(0): Transformer({'max_seq_length': 350, 'do_lower_case': False}) with Transformer model: DistilBertModel
(1): Pooling({'word_embedding_dimension': 768, 'pooling_mode_cls_token': True, 'pooling_mode_mean_tokens': False, 'pooling_mode_max_tokens': False, 'pooling_mode_mean_sqrt_len_tokens': False})
)
```
## Citing & Authors
<!--- Describe where people can find more information --> |
GPL/webis-touche2020-distilbert-tas-b-gpl-self_miner | c4e87e105b5f934cd2b5b7743d7cca6615539e68 | 2022-03-14T14:25:36.000Z | [
"pytorch",
"distilbert",
"feature-extraction",
"sentence-transformers",
"sentence-similarity",
"transformers"
] | sentence-similarity | false | GPL | null | GPL/webis-touche2020-distilbert-tas-b-gpl-self_miner | 1 | null | sentence-transformers | 30,842 | ---
pipeline_tag: sentence-similarity
tags:
- sentence-transformers
- feature-extraction
- sentence-similarity
- transformers
---
# {MODEL_NAME}
This is a [sentence-transformers](https://www.SBERT.net) model: It maps sentences & paragraphs to a 768 dimensional dense vector space and can be used for tasks like clustering or semantic search.
<!--- Describe your model here -->
## Usage (Sentence-Transformers)
Using this model becomes easy when you have [sentence-transformers](https://www.SBERT.net) installed:
```
pip install -U sentence-transformers
```
Then you can use the model like this:
```python
from sentence_transformers import SentenceTransformer
sentences = ["This is an example sentence", "Each sentence is converted"]
model = SentenceTransformer('{MODEL_NAME}')
embeddings = model.encode(sentences)
print(embeddings)
```
## Usage (HuggingFace Transformers)
Without [sentence-transformers](https://www.SBERT.net), you can use the model like this: First, you pass your input through the transformer model, then you have to apply the right pooling-operation on-top of the contextualized word embeddings.
```python
from transformers import AutoTokenizer, AutoModel
import torch
def cls_pooling(model_output, attention_mask):
return model_output[0][:,0]
# Sentences we want sentence embeddings for
sentences = ['This is an example sentence', 'Each sentence is converted']
# Load model from HuggingFace Hub
tokenizer = AutoTokenizer.from_pretrained('{MODEL_NAME}')
model = AutoModel.from_pretrained('{MODEL_NAME}')
# Tokenize sentences
encoded_input = tokenizer(sentences, padding=True, truncation=True, return_tensors='pt')
# Compute token embeddings
with torch.no_grad():
model_output = model(**encoded_input)
# Perform pooling. In this case, cls pooling.
sentence_embeddings = cls_pooling(model_output, encoded_input['attention_mask'])
print("Sentence embeddings:")
print(sentence_embeddings)
```
## Evaluation Results
<!--- Describe how your model was evaluated -->
For an automated evaluation of this model, see the *Sentence Embeddings Benchmark*: [https://seb.sbert.net](https://seb.sbert.net?model_name={MODEL_NAME})
## Training
The model was trained with the parameters:
**DataLoader**:
`torch.utils.data.dataloader.DataLoader` of length 140000 with parameters:
```
{'batch_size': 32, 'sampler': 'torch.utils.data.sampler.SequentialSampler', 'batch_sampler': 'torch.utils.data.sampler.BatchSampler'}
```
**Loss**:
`gpl.toolkit.loss.MarginDistillationLoss`
Parameters of the fit()-Method:
```
{
"epochs": 1,
"evaluation_steps": 0,
"evaluator": "NoneType",
"max_grad_norm": 1,
"optimizer_class": "<class 'transformers.optimization.AdamW'>",
"optimizer_params": {
"lr": 2e-05
},
"scheduler": "WarmupLinear",
"steps_per_epoch": 140000,
"warmup_steps": 1000,
"weight_decay": 0.01
}
```
## Full Model Architecture
```
SentenceTransformer(
(0): Transformer({'max_seq_length': 350, 'do_lower_case': False}) with Transformer model: DistilBertModel
(1): Pooling({'word_embedding_dimension': 768, 'pooling_mode_cls_token': True, 'pooling_mode_mean_tokens': False, 'pooling_mode_max_tokens': False, 'pooling_mode_mean_sqrt_len_tokens': False})
)
```
## Citing & Authors
<!--- Describe where people can find more information --> |
GPL/scidocs-distilbert-tas-b-gpl-self_miner | 16e528b0d28189eacd46c7253088cf2e7f829ef2 | 2022-03-14T14:26:01.000Z | [
"pytorch",
"distilbert",
"feature-extraction",
"sentence-transformers",
"sentence-similarity",
"transformers"
] | sentence-similarity | false | GPL | null | GPL/scidocs-distilbert-tas-b-gpl-self_miner | 1 | null | sentence-transformers | 30,843 | ---
pipeline_tag: sentence-similarity
tags:
- sentence-transformers
- feature-extraction
- sentence-similarity
- transformers
---
# {MODEL_NAME}
This is a [sentence-transformers](https://www.SBERT.net) model: It maps sentences & paragraphs to a 768 dimensional dense vector space and can be used for tasks like clustering or semantic search.
<!--- Describe your model here -->
## Usage (Sentence-Transformers)
Using this model becomes easy when you have [sentence-transformers](https://www.SBERT.net) installed:
```
pip install -U sentence-transformers
```
Then you can use the model like this:
```python
from sentence_transformers import SentenceTransformer
sentences = ["This is an example sentence", "Each sentence is converted"]
model = SentenceTransformer('{MODEL_NAME}')
embeddings = model.encode(sentences)
print(embeddings)
```
## Usage (HuggingFace Transformers)
Without [sentence-transformers](https://www.SBERT.net), you can use the model like this: First, you pass your input through the transformer model, then you have to apply the right pooling-operation on-top of the contextualized word embeddings.
```python
from transformers import AutoTokenizer, AutoModel
import torch
def cls_pooling(model_output, attention_mask):
return model_output[0][:,0]
# Sentences we want sentence embeddings for
sentences = ['This is an example sentence', 'Each sentence is converted']
# Load model from HuggingFace Hub
tokenizer = AutoTokenizer.from_pretrained('{MODEL_NAME}')
model = AutoModel.from_pretrained('{MODEL_NAME}')
# Tokenize sentences
encoded_input = tokenizer(sentences, padding=True, truncation=True, return_tensors='pt')
# Compute token embeddings
with torch.no_grad():
model_output = model(**encoded_input)
# Perform pooling. In this case, cls pooling.
sentence_embeddings = cls_pooling(model_output, encoded_input['attention_mask'])
print("Sentence embeddings:")
print(sentence_embeddings)
```
## Evaluation Results
<!--- Describe how your model was evaluated -->
For an automated evaluation of this model, see the *Sentence Embeddings Benchmark*: [https://seb.sbert.net](https://seb.sbert.net?model_name={MODEL_NAME})
## Training
The model was trained with the parameters:
**DataLoader**:
`torch.utils.data.dataloader.DataLoader` of length 140000 with parameters:
```
{'batch_size': 32, 'sampler': 'torch.utils.data.sampler.SequentialSampler', 'batch_sampler': 'torch.utils.data.sampler.BatchSampler'}
```
**Loss**:
`gpl.toolkit.loss.MarginDistillationLoss`
Parameters of the fit()-Method:
```
{
"epochs": 1,
"evaluation_steps": 0,
"evaluator": "NoneType",
"max_grad_norm": 1,
"optimizer_class": "<class 'transformers.optimization.AdamW'>",
"optimizer_params": {
"lr": 2e-05
},
"scheduler": "WarmupLinear",
"steps_per_epoch": 140000,
"warmup_steps": 1000,
"weight_decay": 0.01
}
```
## Full Model Architecture
```
SentenceTransformer(
(0): Transformer({'max_seq_length': 350, 'do_lower_case': False}) with Transformer model: DistilBertModel
(1): Pooling({'word_embedding_dimension': 768, 'pooling_mode_cls_token': True, 'pooling_mode_mean_tokens': False, 'pooling_mode_max_tokens': False, 'pooling_mode_mean_sqrt_len_tokens': False})
)
```
## Citing & Authors
<!--- Describe where people can find more information --> |
Splend1dchan/byt5base-glue-mnli | 224d0023af8cbe3f0afeacd5987a8d9fdaad526f | 2022-03-14T17:09:50.000Z | [
"pytorch",
"t5",
"text2text-generation",
"transformers",
"autotrain_compatible"
] | text2text-generation | false | Splend1dchan | null | Splend1dchan/byt5base-glue-mnli | 1 | null | transformers | 30,844 | Entry not found |
anton-l/xls-r-300m-mbart-large-50 | 2665011af4960abd82e9532dc783a2a1461c0926 | 2022-03-14T21:19:20.000Z | [
"pytorch",
"speech-encoder-decoder",
"automatic-speech-recognition",
"transformers",
"license:apache-2.0"
] | automatic-speech-recognition | false | anton-l | null | anton-l/xls-r-300m-mbart-large-50 | 1 | null | transformers | 30,845 | ---
license: apache-2.0
---
A freshly initialized seq2seq model |
peterhsu/codeparrot-ds-accelerate | 30dbb932e78e8b4e58dd9e812dac0ee90a0d2090 | 2022-03-15T20:59:04.000Z | [
"pytorch",
"gpt2",
"text-generation",
"transformers"
] | text-generation | false | peterhsu | null | peterhsu/codeparrot-ds-accelerate | 1 | null | transformers | 30,846 | Entry not found |
tau/fewsion_1024_0.3_3150 | b78280012c63a6095332c959a734e3759f6bde4d | 2022-03-15T07:23:24.000Z | [
"pytorch",
"t5",
"text2text-generation",
"transformers",
"autotrain_compatible"
] | text2text-generation | false | tau | null | tau/fewsion_1024_0.3_3150 | 1 | null | transformers | 30,847 | Entry not found |
zuppif/resnetd-26 | a39caedee7d972ea8484be1cdb0e4b2d1ea1a9ea | 2022-03-17T09:09:16.000Z | [
"pytorch",
"resnetd",
"transformers"
] | null | false | zuppif | null | zuppif/resnetd-26 | 1 | null | transformers | 30,848 | Entry not found |
zuppif/resnetd-34 | 2554e1e8672ca97467d71f75e1d12bdaaf2cab58 | 2022-03-17T09:10:19.000Z | [
"pytorch",
"resnetd",
"transformers"
] | null | false | zuppif | null | zuppif/resnetd-34 | 1 | null | transformers | 30,849 | Entry not found |
zuppif/resnetd-50 | 252bd5a1c46413962046c276af8cb77ca52bd64c | 2022-03-17T09:11:34.000Z | [
"pytorch",
"resnetd",
"transformers"
] | null | false | zuppif | null | zuppif/resnetd-50 | 1 | null | transformers | 30,850 | Entry not found |
zuppif/resnetd-152 | ea1c0a8f5a465ba9daffa11403ebed27e90f947d | 2022-03-17T09:16:03.000Z | [
"pytorch",
"resnetd",
"transformers"
] | null | false | zuppif | null | zuppif/resnetd-152 | 1 | null | transformers | 30,851 | Entry not found |
Francesc/distilbert-base-uncased-finetuned-imdb-accelerate | a5e6157621b950577ee5852de2f980111eaa3d67 | 2022-03-15T18:44:43.000Z | [
"pytorch",
"distilbert",
"fill-mask",
"transformers",
"autotrain_compatible"
] | fill-mask | false | Francesc | null | Francesc/distilbert-base-uncased-finetuned-imdb-accelerate | 1 | null | transformers | 30,852 | Entry not found |
abinternet143/t5-small-finetuned-xsum | 91decf121b984de2925c975285305ec511957ab6 | 2022-03-16T20:53:29.000Z | [
"pytorch",
"tensorboard",
"t5",
"text2text-generation",
"dataset:xsum",
"transformers",
"generated_from_trainer",
"license:apache-2.0",
"model-index",
"autotrain_compatible"
] | text2text-generation | false | abinternet143 | null | abinternet143/t5-small-finetuned-xsum | 1 | null | transformers | 30,853 | ---
license: apache-2.0
tags:
- generated_from_trainer
datasets:
- xsum
model-index:
- name: t5-small-finetuned-xsum
results: []
---
<!-- This model card has been generated automatically according to the information the Trainer had access to. You
should probably proofread and complete it, then remove this comment. -->
# t5-small-finetuned-xsum
This model is a fine-tuned version of [t5-small](https://huggingface.co/t5-small) on the xsum dataset.
## Model description
More information needed
## Intended uses & limitations
More information needed
## Training and evaluation data
More information needed
## Training procedure
### Training hyperparameters
The following hyperparameters were used during training:
- learning_rate: 2e-05
- train_batch_size: 64
- eval_batch_size: 64
- seed: 42
- optimizer: Adam with betas=(0.9,0.999) and epsilon=1e-08
- lr_scheduler_type: linear
- num_epochs: 1
- mixed_precision_training: Native AMP
### Framework versions
- Transformers 4.17.0
- Pytorch 1.11.0a0+bfe5ad2
- Datasets 2.0.0
- Tokenizers 0.11.6
|
mfleck/wav2vec2-large-xls-r-300m-slowenian-with-lm | f0f07335ace860813a6db5dddf3283093f791fc5 | 2022-03-15T16:15:30.000Z | [
"pytorch",
"wav2vec2",
"automatic-speech-recognition",
"transformers",
"generated_from_trainer",
"license:apache-2.0",
"model-index"
] | automatic-speech-recognition | false | mfleck | null | mfleck/wav2vec2-large-xls-r-300m-slowenian-with-lm | 1 | null | transformers | 30,854 | ---
license: apache-2.0
tags:
- generated_from_trainer
model-index:
- name: wav2vec2-large-xls-r-300m-slowenian-with-lm
results: []
---
<!-- This model card has been generated automatically according to the information the Trainer had access to. You
should probably proofread and complete it, then remove this comment. -->
# wav2vec2-large-xls-r-300m-slowenian-with-lm
This model is a fine-tuned version of [facebook/wav2vec2-xls-r-300m](https://huggingface.co/facebook/wav2vec2-xls-r-300m) on the None dataset.
It achieves the following results on the evaluation set:
- Loss: 0.3935
- Wer: 0.3480
## Model description
More information needed
## Intended uses & limitations
More information needed
## Training and evaluation data
More information needed
## Training procedure
### Training hyperparameters
The following hyperparameters were used during training:
- learning_rate: 0.0001
- train_batch_size: 32
- eval_batch_size: 8
- seed: 42
- gradient_accumulation_steps: 2
- total_train_batch_size: 64
- optimizer: Adam with betas=(0.9,0.999) and epsilon=1e-08
- lr_scheduler_type: linear
- lr_scheduler_warmup_steps: 50
- num_epochs: 30
- mixed_precision_training: Native AMP
### Training results
| Training Loss | Epoch | Step | Validation Loss | Wer |
|:-------------:|:-----:|:----:|:---------------:|:------:|
| 7.9937 | 2.5 | 100 | 3.1565 | 1.0 |
| 3.0466 | 5.0 | 200 | 3.0009 | 0.9992 |
| 2.9708 | 7.5 | 300 | 2.9494 | 0.9992 |
| 2.0519 | 10.0 | 400 | 0.8874 | 0.7290 |
| 0.5773 | 12.5 | 500 | 0.5258 | 0.5037 |
| 0.3427 | 15.0 | 600 | 0.4767 | 0.4649 |
| 0.2612 | 17.5 | 700 | 0.4549 | 0.4209 |
| 0.212 | 20.0 | 800 | 0.4294 | 0.3860 |
| 0.1748 | 22.5 | 900 | 0.4085 | 0.3769 |
| 0.1587 | 25.0 | 1000 | 0.4017 | 0.3673 |
| 0.1435 | 27.5 | 1100 | 0.3927 | 0.3538 |
| 0.1314 | 30.0 | 1200 | 0.3935 | 0.3480 |
### Framework versions
- Transformers 4.17.0
- Pytorch 1.9.0+cu111
- Datasets 1.18.4
- Tokenizers 0.11.6
|
apkbala107/tamilroberto | 7e55c38b099d681b62244e6a5604b2752a840ef2 | 2022-03-15T15:13:41.000Z | [
"pytorch",
"tensorboard",
"roberta",
"fill-mask",
"transformers",
"license:cc",
"autotrain_compatible"
] | fill-mask | false | apkbala107 | null | apkbala107/tamilroberto | 1 | null | transformers | 30,855 | ---
license: cc
---
|
torbenal/MiniLMv2-L6-H384-RoBERTa-Large | 1834a50e5b15a672da345f2b8ff656186adf99f0 | 2022-03-15T15:30:53.000Z | [
"pytorch",
"roberta",
"fill-mask",
"transformers",
"autotrain_compatible"
] | fill-mask | false | torbenal | null | torbenal/MiniLMv2-L6-H384-RoBERTa-Large | 1 | null | transformers | 30,856 | # MiniLM v2
Microsoft's MiniLM v2 L6 H384 distilled from RoBERTa-Large \
Found [here](https://github.com/microsoft/unilm/tree/master/minilm) |
lijingxin/bert-base-uncased-issues-128 | 07073a0739292e699ebf1b4116282e72edd073e7 | 2022-03-16T03:19:04.000Z | [
"pytorch",
"bert",
"fill-mask",
"transformers",
"generated_from_trainer",
"license:apache-2.0",
"model-index",
"autotrain_compatible"
] | fill-mask | false | lijingxin | null | lijingxin/bert-base-uncased-issues-128 | 1 | null | transformers | 30,857 | ---
license: apache-2.0
tags:
- generated_from_trainer
model-index:
- name: bert-base-uncased-issues-128
results: []
---
<!-- This model card has been generated automatically according to the information the Trainer had access to. You
should probably proofread and complete it, then remove this comment. -->
# bert-base-uncased-issues-128
This model is a fine-tuned version of [bert-base-uncased](https://huggingface.co/bert-base-uncased) on the None dataset.
It achieves the following results on the evaluation set:
- Loss: 1.2540
## Model description
More information needed
## Intended uses & limitations
More information needed
## Training and evaluation data
More information needed
## Training procedure
### Training hyperparameters
The following hyperparameters were used during training:
- learning_rate: 5e-05
- train_batch_size: 32
- eval_batch_size: 8
- seed: 42
- optimizer: Adam with betas=(0.9,0.999) and epsilon=1e-08
- lr_scheduler_type: linear
- num_epochs: 16
### Training results
| Training Loss | Epoch | Step | Validation Loss |
|:-------------:|:-----:|:----:|:---------------:|
| 2.0981 | 1.0 | 291 | 1.6917 |
| 1.6493 | 2.0 | 582 | 1.4357 |
| 1.4831 | 3.0 | 873 | 1.3923 |
| 1.3957 | 4.0 | 1164 | 1.4056 |
| 1.3339 | 5.0 | 1455 | 1.1944 |
| 1.2936 | 6.0 | 1746 | 1.2888 |
| 1.2458 | 7.0 | 2037 | 1.2715 |
| 1.2004 | 8.0 | 2328 | 1.1992 |
| 1.1785 | 9.0 | 2619 | 1.1726 |
| 1.1389 | 10.0 | 2910 | 1.2157 |
| 1.1313 | 11.0 | 3201 | 1.1977 |
| 1.0935 | 12.0 | 3492 | 1.1794 |
| 1.0826 | 13.0 | 3783 | 1.2260 |
| 1.0729 | 14.0 | 4074 | 1.1549 |
| 1.0599 | 15.0 | 4365 | 1.1269 |
| 1.0538 | 16.0 | 4656 | 1.2540 |
### Framework versions
- Transformers 4.11.3
- Pytorch 1.10.2
- Datasets 1.16.1
- Tokenizers 0.10.3
|
newtonkwan/gpt2-xl-ft-with-non-challenging-1k | e517214a052bc530b3b2afba180aa41745216539 | 2022-03-15T16:14:22.000Z | [
"pytorch",
"gpt2",
"text-generation",
"transformers"
] | text-generation | false | newtonkwan | null | newtonkwan/gpt2-xl-ft-with-non-challenging-1k | 1 | null | transformers | 30,858 | Entry not found |
facebook/regnet-x-008 | 58a01db88b994f5ff97c8acff097bea5dc2bd776 | 2022-06-30T10:14:24.000Z | [
"pytorch",
"tf",
"regnet",
"image-classification",
"dataset:imagenet-1k",
"arxiv:2003.13678",
"transformers",
"vision",
"license:apache-2.0"
] | image-classification | false | facebook | null | facebook/regnet-x-008 | 1 | null | transformers | 30,859 | ---
license: apache-2.0
tags:
- vision
- image-classification
datasets:
- imagenet-1k
widget:
- src: https://huggingface.co/datasets/mishig/sample_images/resolve/main/tiger.jpg
example_title: Tiger
- src: https://huggingface.co/datasets/mishig/sample_images/resolve/main/teapot.jpg
example_title: Teapot
- src: https://huggingface.co/datasets/mishig/sample_images/resolve/main/palace.jpg
example_title: Palace
---
# RegNet
RegNet model trained on imagenet-1k. It was introduced in the paper [Designing Network Design Spaces](https://arxiv.org/abs/2003.13678) and first released in [this repository](https://github.com/facebookresearch/pycls).
Disclaimer: The team releasing RegNet did not write a model card for this model so this model card has been written by the Hugging Face team.
## Model description
The authors design search spaces to perform Neural Architecture Search (NAS). They first start from a high dimensional search space and iteratively reduce the search space by empirically applying constraints based on the best-performing models sampled by the current search space.
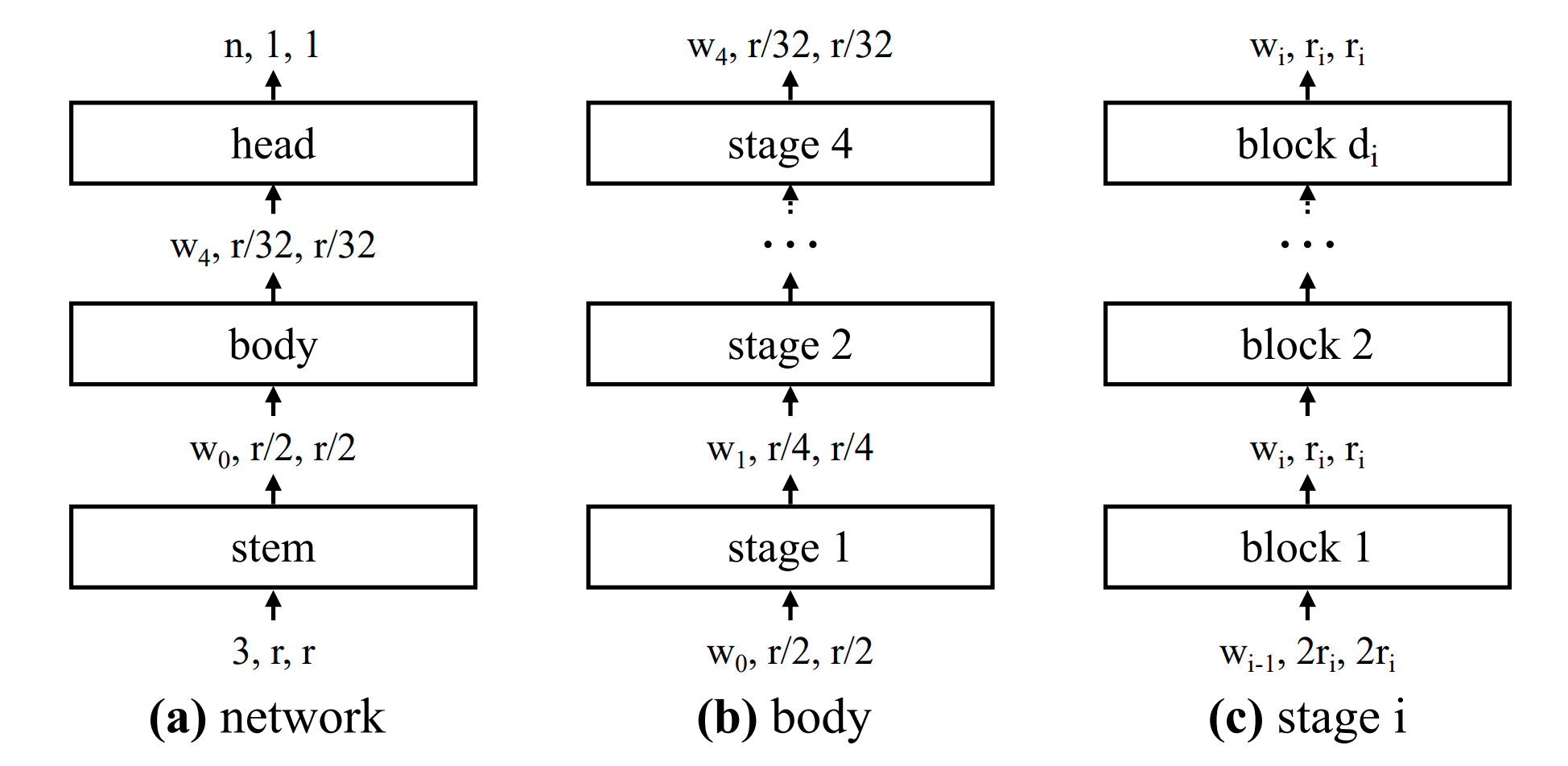
## Intended uses & limitations
You can use the raw model for image classification. See the [model hub](https://huggingface.co/models?search=regnet) to look for
fine-tuned versions on a task that interests you.
### How to use
Here is how to use this model:
```python
>>> from transformers import AutoFeatureExtractor, RegNetForImageClassification
>>> import torch
>>> from datasets import load_dataset
>>> dataset = load_dataset("huggingface/cats-image")
>>> image = dataset["test"]["image"][0]
>>> feature_extractor = AutoFeatureExtractor.from_pretrained("zuppif/regnet-y-040")
>>> model = RegNetForImageClassification.from_pretrained("zuppif/regnet-y-040")
>>> inputs = feature_extractor(image, return_tensors="pt")
>>> with torch.no_grad():
... logits = model(**inputs).logits
>>> # model predicts one of the 1000 ImageNet classes
>>> predicted_label = logits.argmax(-1).item()
>>> print(model.config.id2label[predicted_label])
'tabby, tabby cat'
```
For more code examples, we refer to the [documentation](https://huggingface.co/docs/transformers/master/en/model_doc/regnet). |
facebook/regnet-x-064 | ec98667114da4f67037d019a0eb9e99c51c589f6 | 2022-06-30T10:14:43.000Z | [
"pytorch",
"tf",
"regnet",
"image-classification",
"dataset:imagenet-1k",
"arxiv:2003.13678",
"transformers",
"vision",
"license:apache-2.0"
] | image-classification | false | facebook | null | facebook/regnet-x-064 | 1 | null | transformers | 30,860 | ---
license: apache-2.0
tags:
- vision
- image-classification
datasets:
- imagenet-1k
widget:
- src: https://huggingface.co/datasets/mishig/sample_images/resolve/main/tiger.jpg
example_title: Tiger
- src: https://huggingface.co/datasets/mishig/sample_images/resolve/main/teapot.jpg
example_title: Teapot
- src: https://huggingface.co/datasets/mishig/sample_images/resolve/main/palace.jpg
example_title: Palace
---
# RegNet
RegNet model trained on imagenet-1k. It was introduced in the paper [Designing Network Design Spaces](https://arxiv.org/abs/2003.13678) and first released in [this repository](https://github.com/facebookresearch/pycls).
Disclaimer: The team releasing RegNet did not write a model card for this model so this model card has been written by the Hugging Face team.
## Model description
The authors design search spaces to perform Neural Architecture Search (NAS). They first start from a high dimensional search space and iteratively reduce the search space by empirically applying constraints based on the best-performing models sampled by the current search space.
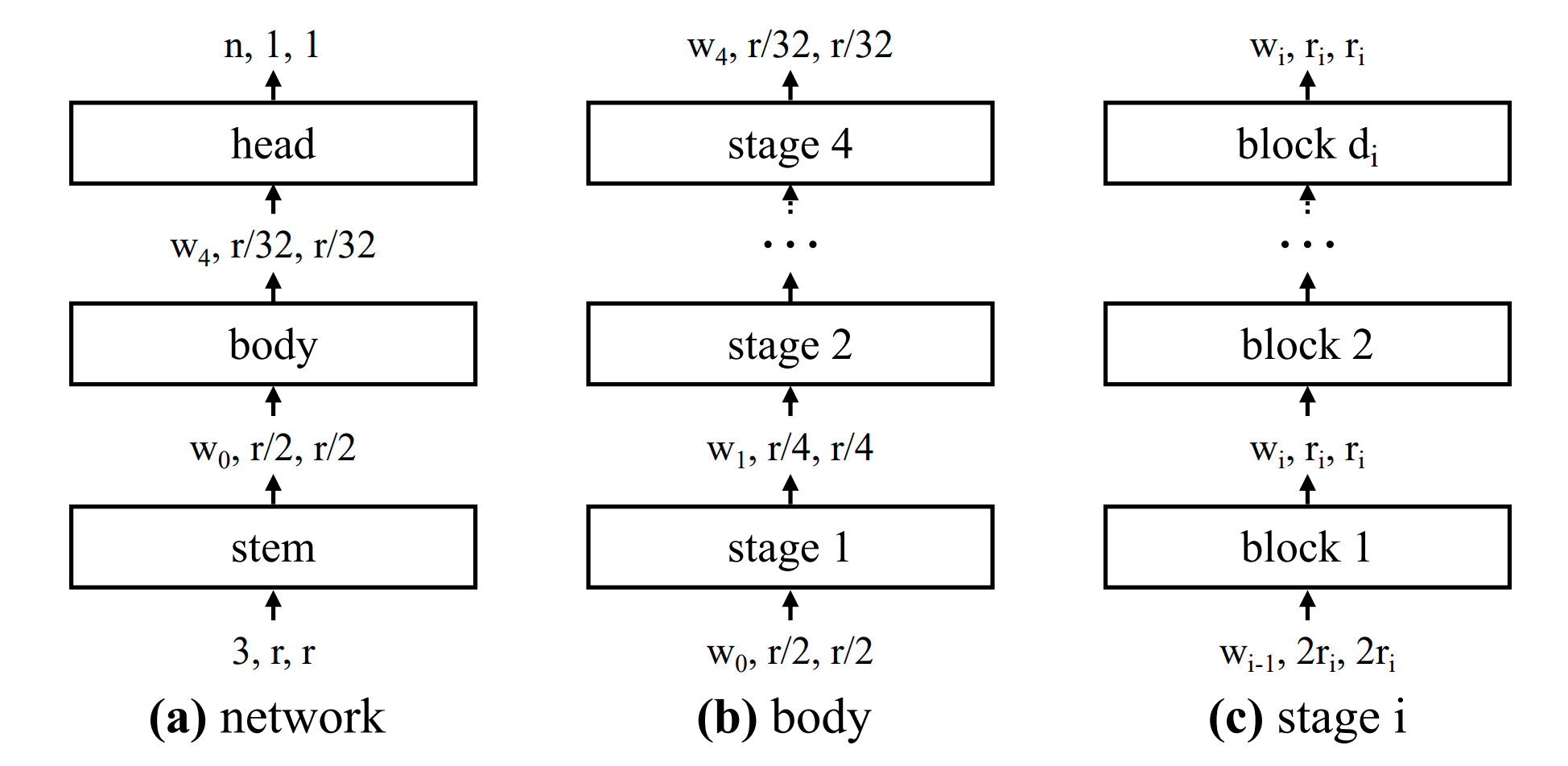
## Intended uses & limitations
You can use the raw model for image classification. See the [model hub](https://huggingface.co/models?search=regnet) to look for
fine-tuned versions on a task that interests you.
### How to use
Here is how to use this model:
```python
>>> from transformers import AutoFeatureExtractor, RegNetForImageClassification
>>> import torch
>>> from datasets import load_dataset
>>> dataset = load_dataset("huggingface/cats-image")
>>> image = dataset["test"]["image"][0]
>>> feature_extractor = AutoFeatureExtractor.from_pretrained("zuppif/regnet-y-040")
>>> model = RegNetForImageClassification.from_pretrained("zuppif/regnet-y-040")
>>> inputs = feature_extractor(image, return_tensors="pt")
>>> with torch.no_grad():
... logits = model(**inputs).logits
>>> # model predicts one of the 1000 ImageNet classes
>>> predicted_label = logits.argmax(-1).item()
>>> print(model.config.id2label[predicted_label])
'tabby, tabby cat'
```
For more code examples, we refer to the [documentation](https://huggingface.co/docs/transformers/master/en/model_doc/regnet). |
anton-l/xtreme_s_xlsr_300m_minds14_old_splits | 9d475abdf4ba91ca74c069270ce4938e0e0443ac | 2022-03-17T22:23:22.000Z | [
"pytorch",
"tensorboard",
"wav2vec2",
"audio-classification",
"transformers",
"automatic-speech-recognition",
"google/xtreme_s",
"generated_from_trainer",
"license:apache-2.0",
"model-index"
] | automatic-speech-recognition | false | anton-l | null | anton-l/xtreme_s_xlsr_300m_minds14_old_splits | 1 | 1 | transformers | 30,861 | ---
license: apache-2.0
tags:
- automatic-speech-recognition
- google/xtreme_s
- generated_from_trainer
metrics:
- f1
- accuracy
model-index:
- name: xtreme_s_xlsr_minds14
results: []
---
<!-- This model card has been generated automatically according to the information the Trainer had access to. You
should probably proofread and complete it, then remove this comment. -->
# xtreme_s_xlsr_minds14
This model is a fine-tuned version of [facebook/wav2vec2-xls-r-300m](https://huggingface.co/facebook/wav2vec2-xls-r-300m) on the GOOGLE/XTREME_S - MINDS14 dataset.
It achieves the following results on the evaluation set:
- Loss: 0.2890
- F1: 0.9474
- Accuracy: 0.9470
## Model description
More information needed
## Intended uses & limitations
More information needed
## Training and evaluation data
More information needed
## Training procedure
### Training hyperparameters
The following hyperparameters were used during training:
- learning_rate: 0.0003
- train_batch_size: 32
- eval_batch_size: 8
- seed: 42
- distributed_type: multi-GPU
- num_devices: 2
- total_train_batch_size: 64
- total_eval_batch_size: 16
- optimizer: Adam with betas=(0.9,0.999) and epsilon=1e-08
- lr_scheduler_type: linear
- lr_scheduler_warmup_steps: 1500
- num_epochs: 50.0
- mixed_precision_training: Native AMP
### Training results
| Training Loss | Epoch | Step | Validation Loss | F1 | Accuracy |
|:-------------:|:-----:|:----:|:---------------:|:------:|:--------:|
| 2.551 | 2.7 | 200 | 2.5855 | 0.0407 | 0.1201 |
| 1.6934 | 5.41 | 400 | 1.5072 | 0.5862 | 0.6085 |
| 0.5914 | 8.11 | 600 | 0.7274 | 0.8270 | 0.8232 |
| 0.3896 | 10.81 | 800 | 0.4402 | 0.8905 | 0.8890 |
| 0.5052 | 13.51 | 1000 | 0.4483 | 0.8837 | 0.8829 |
| 0.4806 | 16.22 | 1200 | 0.4981 | 0.8784 | 0.8787 |
| 0.2103 | 18.92 | 1400 | 0.4957 | 0.8810 | 0.8817 |
| 0.4198 | 21.62 | 1600 | 0.5161 | 0.8927 | 0.8921 |
| 0.11 | 24.32 | 1800 | 0.4456 | 0.8923 | 0.8902 |
| 0.1233 | 27.03 | 2000 | 0.3858 | 0.9016 | 0.9012 |
| 0.1827 | 29.73 | 2200 | 0.3765 | 0.9162 | 0.9159 |
| 0.1235 | 32.43 | 2400 | 0.3716 | 0.9134 | 0.9128 |
| 0.1873 | 35.14 | 2600 | 0.3080 | 0.9314 | 0.9311 |
| 0.017 | 37.84 | 2800 | 0.2629 | 0.9415 | 0.9409 |
| 0.0436 | 40.54 | 3000 | 0.3159 | 0.9397 | 0.9390 |
| 0.0455 | 43.24 | 3200 | 0.2963 | 0.9393 | 0.9390 |
| 0.046 | 45.95 | 3400 | 0.2914 | 0.9457 | 0.9451 |
| 0.0042 | 48.65 | 3600 | 0.2890 | 0.9474 | 0.9470 |
### Framework versions
- Transformers 4.18.0.dev0
- Pytorch 1.10.2+cu113
- Datasets 1.18.4.dev0
- Tokenizers 0.11.6
|
krinal214/bert-3lang | a00a250b59b9971d1cb7f3a819a7bee993a39dda | 2022-03-15T23:30:47.000Z | [
"pytorch",
"tensorboard",
"bert",
"question-answering",
"dataset:tydiqa",
"transformers",
"generated_from_trainer",
"license:apache-2.0",
"model-index",
"autotrain_compatible"
] | question-answering | false | krinal214 | null | krinal214/bert-3lang | 1 | null | transformers | 30,862 | ---
license: apache-2.0
tags:
- generated_from_trainer
datasets:
- tydiqa
model-index:
- name: bert-3lang
results: []
---
<!-- This model card has been generated automatically according to the information the Trainer had access to. You
should probably proofread and complete it, then remove this comment. -->
# bert-3lang
This model is a fine-tuned version of [bert-base-multilingual-cased](https://huggingface.co/bert-base-multilingual-cased) on the tydiqa dataset.
It achieves the following results on the evaluation set:
- Loss: 0.6422
## Model description
More information needed
## Intended uses & limitations
More information needed
## Training and evaluation data
More information needed
## Training procedure
### Training hyperparameters
The following hyperparameters were used during training:
- learning_rate: 2e-05
- train_batch_size: 16
- eval_batch_size: 16
- seed: 42
- optimizer: Adam with betas=(0.9,0.999) and epsilon=1e-08
- lr_scheduler_type: linear
- num_epochs: 1
### Training results
| Training Loss | Epoch | Step | Validation Loss |
|:-------------:|:-----:|:----:|:---------------:|
| 1.8161 | 1.0 | 905 | 0.6422 |
### Framework versions
- Transformers 4.15.0
- Pytorch 1.9.1
- Datasets 2.0.0
- Tokenizers 0.10.3
|
aytugkaya/xlm-roberta-base-finetuned-panx-de | 5668c34844a76963b04f317f11136ca270043012 | 2022-03-16T02:12:08.000Z | [
"pytorch",
"xlm-roberta",
"token-classification",
"dataset:xtreme",
"transformers",
"generated_from_trainer",
"license:mit",
"model-index",
"autotrain_compatible"
] | token-classification | false | aytugkaya | null | aytugkaya/xlm-roberta-base-finetuned-panx-de | 1 | null | transformers | 30,863 | ---
license: mit
tags:
- generated_from_trainer
datasets:
- xtreme
metrics:
- f1
model-index:
- name: xlm-roberta-base-finetuned-panx-de
results:
- task:
name: Token Classification
type: token-classification
dataset:
name: xtreme
type: xtreme
args: PAN-X.de
metrics:
- name: F1
type: f1
value: 0.8650707909251151
---
<!-- This model card has been generated automatically according to the information the Trainer had access to. You
should probably proofread and complete it, then remove this comment. -->
# xlm-roberta-base-finetuned-panx-de
This model is a fine-tuned version of [xlm-roberta-base](https://huggingface.co/xlm-roberta-base) on the xtreme dataset.
It achieves the following results on the evaluation set:
- Loss: 0.1474
- F1: 0.8651
## Model description
More information needed
## Intended uses & limitations
More information needed
## Training and evaluation data
More information needed
## Training procedure
### Training hyperparameters
The following hyperparameters were used during training:
- learning_rate: 5e-05
- train_batch_size: 12
- eval_batch_size: 12
- seed: 42
- optimizer: Adam with betas=(0.9,0.999) and epsilon=1e-08
- lr_scheduler_type: linear
- num_epochs: 3
### Training results
| Training Loss | Epoch | Step | Validation Loss | F1 |
|:-------------:|:-----:|:----:|:---------------:|:------:|
| 0.2498 | 1.0 | 1049 | 0.1835 | 0.8213 |
| 0.1293 | 2.0 | 2098 | 0.1448 | 0.8481 |
| 0.0788 | 3.0 | 3147 | 0.1474 | 0.8651 |
### Framework versions
- Transformers 4.17.0
- Pytorch 1.10.2+cu102
- Datasets 1.18.3
- Tokenizers 0.11.6
|
saghar/MiniLMv2-L6-H768-distilled-from-RoBERTa-Large-finetuned-wikitext103 | bbdd1bc288229d42452cf0220fb679d51c21ecae | 2022-03-18T19:10:05.000Z | [
"pytorch",
"roberta",
"fill-mask",
"dataset:wikitext",
"transformers",
"generated_from_trainer",
"model-index",
"autotrain_compatible"
] | fill-mask | false | saghar | null | saghar/MiniLMv2-L6-H768-distilled-from-RoBERTa-Large-finetuned-wikitext103 | 1 | null | transformers | 30,864 | ---
tags:
- generated_from_trainer
datasets:
- wikitext
model-index:
- name: MiniLMv2-L6-H768-distilled-from-RoBERTa-Large-finetuned-wikitext103
results: []
---
<!-- This model card has been generated automatically according to the information the Trainer had access to. You
should probably proofread and complete it, then remove this comment. -->
# MiniLMv2-L6-H768-distilled-from-RoBERTa-Large-finetuned-wikitext103
This model is a fine-tuned version of [nreimers/MiniLMv2-L6-H768-distilled-from-RoBERTa-Large](https://huggingface.co/nreimers/MiniLMv2-L6-H768-distilled-from-RoBERTa-Large) on the wikitext dataset.
It achieves the following results on the evaluation set:
- Loss: 3.7556
## Model description
More information needed
## Intended uses & limitations
More information needed
## Training and evaluation data
More information needed
## Training procedure
### Training hyperparameters
The following hyperparameters were used during training:
- learning_rate: 2e-05
- train_batch_size: 32
- eval_batch_size: 32
- seed: 42
- optimizer: Adam with betas=(0.9,0.999) and epsilon=1e-08
- lr_scheduler_type: linear
- num_epochs: 3.0
### Training results
| Training Loss | Epoch | Step | Validation Loss |
|:-------------:|:-----:|:----:|:---------------:|
| 4.6806 | 1.0 | 3125 | 3.9691 |
| 4.0441 | 2.0 | 6250 | 3.7885 |
| 3.9509 | 3.0 | 9375 | 3.7556 |
### Framework versions
- Transformers 4.16.2
- Pytorch 1.8.1
- Datasets 1.11.0
- Tokenizers 0.10.3
|
Neulvo/distilbert-base-uncased-finetuned-imdb | 4f62b00524d5c447baacf8dd6bece3790865a447 | 2022-03-16T06:05:40.000Z | [
"pytorch",
"tensorboard",
"distilbert",
"fill-mask",
"dataset:imdb",
"transformers",
"generated_from_trainer",
"license:apache-2.0",
"model-index",
"autotrain_compatible"
] | fill-mask | false | Neulvo | null | Neulvo/distilbert-base-uncased-finetuned-imdb | 1 | null | transformers | 30,865 | ---
license: apache-2.0
tags:
- generated_from_trainer
datasets:
- imdb
model-index:
- name: distilbert-base-uncased-finetuned-imdb
results: []
---
<!-- This model card has been generated automatically according to the information the Trainer had access to. You
should probably proofread and complete it, then remove this comment. -->
# distilbert-base-uncased-finetuned-imdb
This model is a fine-tuned version of [distilbert-base-uncased](https://huggingface.co/distilbert-base-uncased) on the imdb dataset.
It achieves the following results on the evaluation set:
- Loss: 2.4717
## Model description
More information needed
## Intended uses & limitations
More information needed
## Training and evaluation data
More information needed
## Training procedure
### Training hyperparameters
The following hyperparameters were used during training:
- learning_rate: 2e-05
- train_batch_size: 64
- eval_batch_size: 64
- seed: 42
- optimizer: Adam with betas=(0.9,0.999) and epsilon=1e-08
- lr_scheduler_type: linear
- num_epochs: 3.0
- mixed_precision_training: Native AMP
### Training results
| Training Loss | Epoch | Step | Validation Loss |
|:-------------:|:-----:|:----:|:---------------:|
| 2.7071 | 1.0 | 157 | 2.4942 |
| 2.5754 | 2.0 | 314 | 2.4235 |
| 2.5426 | 3.0 | 471 | 2.4361 |
### Framework versions
- Transformers 4.16.2
- Pytorch 1.10.2
- Datasets 1.18.3
- Tokenizers 0.11.0
|
ScandinavianMrT/gpt2_prefinetune_SARC_1epoch_withcontext | e5751d38c0ca3d9969eb95c93255255cadcc36fb | 2022-03-16T07:23:51.000Z | [
"pytorch",
"gpt2",
"text-generation",
"transformers",
"generated_from_trainer",
"license:mit",
"model-index"
] | text-generation | false | ScandinavianMrT | null | ScandinavianMrT/gpt2_prefinetune_SARC_1epoch_withcontext | 1 | null | transformers | 30,866 | ---
license: mit
tags:
- generated_from_trainer
model-index:
- name: gpt2_prefinetune_SARC_1epoch_withcontext
results: []
---
<!-- This model card has been generated automatically according to the information the Trainer had access to. You
should probably proofread and complete it, then remove this comment. -->
# gpt2_prefinetune_SARC_1epoch_withcontext
This model is a fine-tuned version of [gpt2](https://huggingface.co/gpt2) on the None dataset.
It achieves the following results on the evaluation set:
- Loss: 3.7899
## Model description
More information needed
## Intended uses & limitations
More information needed
## Training and evaluation data
More information needed
## Training procedure
### Training hyperparameters
The following hyperparameters were used during training:
- learning_rate: 2e-05
- train_batch_size: 8
- eval_batch_size: 8
- seed: 42
- optimizer: Adam with betas=(0.9,0.999) and epsilon=1e-08
- lr_scheduler_type: linear
- num_epochs: 1
### Training results
| Training Loss | Epoch | Step | Validation Loss |
|:-------------:|:-----:|:-----:|:---------------:|
| 3.8788 | 1.0 | 14028 | 3.7899 |
### Framework versions
- Transformers 4.17.0
- Pytorch 1.10.0+cu111
- Datasets 2.0.0
- Tokenizers 0.11.6
|
triet1102/bert-base-cased-GoogleRE | 9a859b6d285d446a0eb6356c53aa74baa18fa2be | 2022-03-17T10:37:20.000Z | [
"pytorch",
"bert",
"fill-mask",
"transformers",
"autotrain_compatible"
] | fill-mask | false | triet1102 | null | triet1102/bert-base-cased-GoogleRE | 1 | null | transformers | 30,867 | Entry not found |
Nadav/MacSQuAD | 0ef052112814ad1b676f8aa1ccddbcc7a431dd16 | 2022-03-17T18:20:05.000Z | [
"pytorch",
"bert",
"question-answering",
"transformers",
"license:afl-3.0",
"autotrain_compatible"
] | question-answering | false | Nadav | null | Nadav/MacSQuAD | 1 | null | transformers | 30,868 | ---
license: afl-3.0
---
A MacBERTh model fine-tuned on SQuAD_v2. Hopefully, this will allow the model to perform well on QA tasks on historical texts.
Finetune parameters:
```
training_args = TrainingArguments(
output_dir="./results",
evaluation_strategy="epoch",
learning_rate=3e-5,
per_device_train_batch_size=64,
per_device_eval_batch_size=64,
num_train_epochs=2,
weight_decay=0.01,
lr_scheduler_type=SchedulerType.LINEAR,
warmup_ratio=0.2
)
```
Evaluation metrics on the validation set of SQuAD_v2:
```
{'exact': 49.49886296639434, 'f1': 53.9199170778635, 'total': 11873, 'HasAns_exact': 60.08771929824562, 'HasAns_f1': 68.94250598270429, 'HasAns_total': 5928, 'NoAns_exact': 38.940285954583686, 'NoAns_f1': 38.940285954583686, 'NoAns_total': 5945, 'best_exact': 50.5095595047587, 'best_exact_thresh': 0.0, 'best_f1': 51.75825524534494, 'best_f1_thresh': 0.0}
``` |
krinal214/zero_shot | 50576657396ff78582c637891740e8add571e69d | 2022-03-16T12:41:46.000Z | [
"pytorch",
"tensorboard",
"bert",
"question-answering",
"dataset:squad",
"transformers",
"generated_from_trainer",
"license:apache-2.0",
"model-index",
"autotrain_compatible"
] | question-answering | false | krinal214 | null | krinal214/zero_shot | 1 | null | transformers | 30,869 | ---
license: apache-2.0
tags:
- generated_from_trainer
datasets:
- squad
model-index:
- name: zero_last
results: []
---
<!-- This model card has been generated automatically according to the information the Trainer had access to. You
should probably proofread and complete it, then remove this comment. -->
# zero_last
This model is a fine-tuned version of [bert-base-multilingual-cased](https://huggingface.co/bert-base-multilingual-cased) on the squad dataset.
It achieves the following results on the evaluation set:
- Loss: 1.9190
## Model description
More information needed
## Intended uses & limitations
More information needed
## Training and evaluation data
More information needed
## Training procedure
### Training hyperparameters
The following hyperparameters were used during training:
- learning_rate: 2e-05
- train_batch_size: 16
- eval_batch_size: 16
- seed: 42
- optimizer: Adam with betas=(0.9,0.999) and epsilon=1e-08
- lr_scheduler_type: linear
- num_epochs: 1
### Training results
| Training Loss | Epoch | Step | Validation Loss |
|:-------------:|:-----:|:----:|:---------------:|
| 0.9816 | 1.0 | 5557 | 1.9190 |
### Framework versions
- Transformers 4.15.0
- Pytorch 1.9.1
- Datasets 2.0.0
- Tokenizers 0.10.3
|
osanseviero/distilbert-base-uncased-finetuned-squad-d5716d28 | f9bc73352dc9cfb4cc89f138aa27dc2ebb177580 | 2022-06-27T07:23:07.000Z | [
"pytorch",
"distilbert",
"fill-mask",
"en",
"dataset:squad",
"arxiv:1910.01108",
"transformers",
"question-answering",
"license:apache-2.0",
"model-index",
"autotrain_compatible"
] | question-answering | false | osanseviero | null | osanseviero/distilbert-base-uncased-finetuned-squad-d5716d28 | 1 | null | transformers | 30,870 | ---
language:
- en
thumbnail: https://github.com/karanchahal/distiller/blob/master/distiller.jpg
tags:
- question-answering
license: apache-2.0
datasets:
- squad
metrics:
- squad
model-index:
- name: osanseviero/distilbert-base-uncased-finetuned-squad-d5716d28
results:
- task:
type: question-answering
name: Question Answering
dataset:
name: adversarial_qa
type: adversarial_qa
config: adversarialQA
split: train
metrics:
- name: Loss
type: loss
value: 4.052208423614502
verified: true
---
# DistilBERT with a second step of distillation
## Model description
This model replicates the "DistilBERT (D)" model from Table 2 of the [DistilBERT paper](https://arxiv.org/pdf/1910.01108.pdf). In this approach, a DistilBERT student is fine-tuned on SQuAD v1.1, but with a BERT model (also fine-tuned on SQuAD v1.1) acting as a teacher for a second step of task-specific distillation.
In this version, the following pre-trained models were used:
* Student: `distilbert-base-uncased`
* Teacher: `lewtun/bert-base-uncased-finetuned-squad-v1`
## Training data
This model was trained on the SQuAD v1.1 dataset which can be obtained from the `datasets` library as follows:
```python
from datasets import load_dataset
squad = load_dataset('squad')
```
## Training procedure
## Eval results
| | Exact Match | F1 |
|------------------|-------------|------|
| DistilBERT paper | 79.1 | 86.9 |
| Ours | 78.4 | 86.5 |
The scores were calculated using the `squad` metric from `datasets`.
### BibTeX entry and citation info
```bibtex
@misc{sanh2020distilbert,
title={DistilBERT, a distilled version of BERT: smaller, faster, cheaper and lighter},
author={Victor Sanh and Lysandre Debut and Julien Chaumond and Thomas Wolf},
year={2020},
eprint={1910.01108},
archivePrefix={arXiv},
primaryClass={cs.CL}
}
``` |
Neulvo/marian-finetuned-kde4-en-to-fr-accelerate | 86a5043f267e822cf30f6f189742a54aa1348d91 | 2022-03-16T15:53:38.000Z | [
"pytorch",
"marian",
"text2text-generation",
"transformers",
"autotrain_compatible"
] | text2text-generation | false | Neulvo | null | Neulvo/marian-finetuned-kde4-en-to-fr-accelerate | 1 | null | transformers | 30,871 | Entry not found |
mondovero/distilgpt2_fine_tuned_coder_custom | 6aaa7a36d28cf91e609b152789beedd749770b37 | 2022-03-16T16:12:45.000Z | [
"pytorch",
"gpt2",
"text-generation",
"transformers"
] | text-generation | false | mondovero | null | mondovero/distilgpt2_fine_tuned_coder_custom | 1 | null | transformers | 30,872 | Entry not found |
microsoft/resnet-26 | 5ca407f2074c8fd2c4ffefe3b75fbb4323c0ddc1 | 2022-07-01T17:33:42.000Z | [
"pytorch",
"tf",
"resnet",
"image-classification",
"transformers"
] | image-classification | false | microsoft | null | microsoft/resnet-26 | 1 | null | transformers | 30,873 | Entry not found |
apkbala107/tamilroberta | bbf77438dd3eadfbbc2a62217dc3b0b6350b2d57 | 2022-03-16T16:06:23.000Z | [
"pytorch",
"tensorboard",
"roberta",
"fill-mask",
"transformers",
"license:cc",
"autotrain_compatible"
] | fill-mask | false | apkbala107 | null | apkbala107/tamilroberta | 1 | null | transformers | 30,874 | ---
license: cc
---
|
anton-l/xls-r-300m-bart-base | 51acc16047f2a5dc237e546e68b880553992b177 | 2022-03-16T17:27:16.000Z | [
"pytorch",
"speech-encoder-decoder",
"automatic-speech-recognition",
"transformers",
"license:apache-2.0"
] | automatic-speech-recognition | false | anton-l | null | anton-l/xls-r-300m-bart-base | 1 | null | transformers | 30,875 | ---
license: apache-2.0
---
|
horsbug98/Part_2_mBERT_Model_E2 | c5c3c3289f660b4459a4d431a6a7c59d1f916915 | 2022-03-16T17:25:02.000Z | [
"pytorch",
"bert",
"question-answering",
"dataset:tydiqa",
"transformers",
"generated_from_trainer",
"license:apache-2.0",
"model-index",
"autotrain_compatible"
] | question-answering | false | horsbug98 | null | horsbug98/Part_2_mBERT_Model_E2 | 1 | null | transformers | 30,876 | ---
license: apache-2.0
tags:
- generated_from_trainer
datasets:
- tydiqa
model-index:
- name: debug_mbert_task2_2
results: []
---
<!-- This model card has been generated automatically according to the information the Trainer had access to. You
should probably proofread and complete it, then remove this comment. -->
# debug_mbert_task2_2
This model is a fine-tuned version of [bert-base-multilingual-cased](https://huggingface.co/bert-base-multilingual-cased) on the tydiqa secondary_task dataset.
## Model description
More information needed
## Intended uses & limitations
More information needed
## Training and evaluation data
More information needed
## Training procedure
### Training hyperparameters
The following hyperparameters were used during training:
- learning_rate: 3e-05
- train_batch_size: 12
- eval_batch_size: 8
- seed: 42
- optimizer: Adam with betas=(0.9,0.999) and epsilon=1e-08
- lr_scheduler_type: linear
- num_epochs: 2.0
### Training results
### Framework versions
- Transformers 4.15.0
- Pytorch 1.9.1
- Datasets 2.0.0
- Tokenizers 0.10.3
|
horsbug98/Part_2_XLM_Model_E1 | dba7818f994199e15f35bf70bf98d5f70185f36e | 2022-03-30T18:29:46.000Z | [
"pytorch",
"xlm-roberta",
"question-answering",
"dataset:tydiqa",
"transformers",
"generated_from_trainer",
"license:mit",
"model-index",
"autotrain_compatible"
] | question-answering | false | horsbug98 | null | horsbug98/Part_2_XLM_Model_E1 | 1 | null | transformers | 30,877 | ---
license: mit
tags:
- generated_from_trainer
datasets:
- tydiqa
model-index:
- name: debug_xlm_task2_1
results: []
---
<!-- This model card has been generated automatically according to the information the Trainer had access to. You
should probably proofread and complete it, then remove this comment. -->
# debug_xlm_task2_1
This model is a fine-tuned version of [xlm-roberta-base](https://huggingface.co/xlm-roberta-base) on the tydiqa secondary_task dataset.
## Model description
More information needed
## Intended uses & limitations
More information needed
## Training and evaluation data
More information needed
## Training procedure
### Training hyperparameters
The following hyperparameters were used during training:
- learning_rate: 3e-05
- train_batch_size: 12
- eval_batch_size: 8
- seed: 42
- optimizer: Adam with betas=(0.9,0.999) and epsilon=1e-08
- lr_scheduler_type: linear
- num_epochs: 1.0
### Training results
### Framework versions
- Transformers 4.15.0
- Pytorch 1.9.1
- Datasets 2.0.0
- Tokenizers 0.10.3
|
horsbug98/Part_2_BERT_Multilingual_Dutch_Model_E1 | 65a4a825acc3cae701be64a2eefd152f9f239151 | 2022-03-16T18:09:32.000Z | [
"pytorch",
"bert",
"question-answering",
"dataset:tydiqa",
"transformers",
"generated_from_trainer",
"model-index",
"autotrain_compatible"
] | question-answering | false | horsbug98 | null | horsbug98/Part_2_BERT_Multilingual_Dutch_Model_E1 | 1 | null | transformers | 30,878 | ---
tags:
- generated_from_trainer
datasets:
- tydiqa
model-index:
- name: debug_bert_finetuned_dutch_task2_1
results: []
---
<!-- This model card has been generated automatically according to the information the Trainer had access to. You
should probably proofread and complete it, then remove this comment. -->
# debug_bert_finetuned_dutch_task2_1
This model is a fine-tuned version of [henryk/bert-base-multilingual-cased-finetuned-dutch-squad2](https://huggingface.co/henryk/bert-base-multilingual-cased-finetuned-dutch-squad2) on the tydiqa secondary_task dataset.
## Model description
More information needed
## Intended uses & limitations
More information needed
## Training and evaluation data
More information needed
## Training procedure
### Training hyperparameters
The following hyperparameters were used during training:
- learning_rate: 3e-05
- train_batch_size: 12
- eval_batch_size: 8
- seed: 42
- optimizer: Adam with betas=(0.9,0.999) and epsilon=1e-08
- lr_scheduler_type: linear
- num_epochs: 1.0
### Training results
### Framework versions
- Transformers 4.15.0
- Pytorch 1.9.1
- Datasets 2.0.0
- Tokenizers 0.10.3
|
DrishtiSharma/poem-gen-gpt2-small-spanish | 2852d4354c52396f999d825297f98180ff6f1814 | 2022-03-16T18:46:26.000Z | [
"pytorch",
"tensorboard",
"gpt2",
"text-generation",
"transformers",
"generated_from_trainer",
"license:apache-2.0",
"model-index"
] | text-generation | false | DrishtiSharma | null | DrishtiSharma/poem-gen-gpt2-small-spanish | 1 | null | transformers | 30,879 | ---
license: apache-2.0
tags:
- generated_from_trainer
model-index:
- name: poem-gen-gpt2-small-spanish
results: []
---
<!-- This model card has been generated automatically according to the information the Trainer had access to. You
should probably proofread and complete it, then remove this comment. -->
# poem-gen-gpt2-small-spanish
This model is a fine-tuned version of [datificate/gpt2-small-spanish](https://huggingface.co/datificate/gpt2-small-spanish) on an unknown dataset.
It achieves the following results on the evaluation set:
- Loss: 3.9229
## Model description
More information needed
## Intended uses & limitations
More information needed
## Training and evaluation data
More information needed
## Training procedure
### Training hyperparameters
The following hyperparameters were used during training:
- learning_rate: 2e-05
- train_batch_size: 4
- eval_batch_size: 4
- seed: 42
- optimizer: Adam with betas=(0.9,0.999) and epsilon=1e-08
- lr_scheduler_type: linear
- num_epochs: 3.0
### Training results
| Training Loss | Epoch | Step | Validation Loss |
|:-------------:|:-----:|:----:|:---------------:|
| 4.2121 | 1.0 | 2569 | 3.9954 |
| 4.0612 | 2.0 | 5138 | 3.9375 |
| 3.9988 | 3.0 | 7707 | 3.9229 |
### Framework versions
- Transformers 4.17.0
- Pytorch 1.10.0+cu111
- Datasets 2.0.0
- Tokenizers 0.11.6
|
horsbug98/Part_1_mBERT_Model_E1 | 7a924df2247d833b3b4ac4fe4ad9bfb23f87f0b9 | 2022-03-16T18:48:12.000Z | [
"pytorch",
"bert",
"question-answering",
"dataset:tydiqa",
"transformers",
"generated_from_trainer",
"model-index",
"autotrain_compatible"
] | question-answering | false | horsbug98 | null | horsbug98/Part_1_mBERT_Model_E1 | 1 | null | transformers | 30,880 | ---
tags:
- generated_from_trainer
datasets:
- tydiqa
model-index:
- name: debug_bert_finetuned_dutch_task2_1
results: []
---
<!-- This model card has been generated automatically according to the information the Trainer had access to. You
should probably proofread and complete it, then remove this comment. -->
# debug_bert_finetuned_dutch_task2_1
This model is a fine-tuned version of [henryk/bert-base-multilingual-cased-finetuned-dutch-squad2](https://huggingface.co/henryk/bert-base-multilingual-cased-finetuned-dutch-squad2) on the tydiqa secondary_task dataset.
## Model description
More information needed
## Intended uses & limitations
More information needed
## Training and evaluation data
More information needed
## Training procedure
### Training hyperparameters
The following hyperparameters were used during training:
- learning_rate: 3e-05
- train_batch_size: 12
- eval_batch_size: 8
- seed: 42
- optimizer: Adam with betas=(0.9,0.999) and epsilon=1e-08
- lr_scheduler_type: linear
- num_epochs: 1.0
### Training results
### Framework versions
- Transformers 4.15.0
- Pytorch 1.9.1
- Datasets 2.0.0
- Tokenizers 0.10.3
|
negfir/Distill_4L_2ep | 3edc3b88a77e5d6faed210fbc742f3686889cf0a | 2022-03-16T19:14:10.000Z | [
"pytorch",
"distilbert",
"fill-mask",
"transformers",
"autotrain_compatible"
] | fill-mask | false | negfir | null | negfir/Distill_4L_2ep | 1 | null | transformers | 30,881 | Entry not found |
newtonkwan/gpt2-xl-ft-2 | 24a8ed66fdf945468c1599c44b6270fb5ad69e66 | 2022-03-16T21:04:23.000Z | [
"pytorch",
"tensorboard",
"gpt2",
"text-generation",
"transformers",
"generated_from_trainer",
"model-index"
] | text-generation | false | newtonkwan | null | newtonkwan/gpt2-xl-ft-2 | 1 | null | transformers | 30,882 | ---
tags:
- generated_from_trainer
model-index:
- name: gpt2-xl-ft-2
results: []
---
<!-- This model card has been generated automatically according to the information the Trainer had access to. You
should probably proofread and complete it, then remove this comment. -->
# gpt2-xl-ft-2
This model is a fine-tuned version of [gpt2-xl](https://huggingface.co/gpt2-xl) on an unknown dataset.
It achieves the following results on the evaluation set:
- Loss: 1.6371
## Model description
More information needed
## Intended uses & limitations
More information needed
## Training and evaluation data
More information needed
## Training procedure
### Training hyperparameters
The following hyperparameters were used during training:
- learning_rate: 0.0005
- train_batch_size: 4
- eval_batch_size: 4
- seed: 2022
- gradient_accumulation_steps: 32
- total_train_batch_size: 128
- optimizer: Adam with betas=(0.9,0.999) and epsilon=1e-08
- lr_scheduler_type: linear
- lr_scheduler_warmup_steps: 100.0
- num_epochs: 4
### Training results
| Training Loss | Epoch | Step | Validation Loss |
|:-------------:|:-----:|:----:|:---------------:|
| No log | 0.99 | 62 | 1.5080 |
| No log | 1.99 | 124 | 1.5119 |
| No log | 2.99 | 186 | 1.5765 |
| No log | 3.99 | 248 | 1.6371 |
### Framework versions
- Transformers 4.17.0
- Pytorch 1.10.0+cu111
- Datasets 2.0.0
- Tokenizers 0.11.6
### Perplexity
Score: 27.79615592956543
### Dataset Size
Size: 10000 |
anton-l/xtreme_s_xlsr_covost2_fr_en | b184a9ee527f7fc489bf7b0f7bf81ee55ea1a704 | 2022-03-17T11:58:49.000Z | [
"pytorch",
"tensorboard",
"speech-encoder-decoder",
"automatic-speech-recognition",
"transformers"
] | automatic-speech-recognition | false | anton-l | null | anton-l/xtreme_s_xlsr_covost2_fr_en | 1 | null | transformers | 30,883 | Entry not found |
saghar/TinyBERT_General_6L_768D-finetuned-wikitext103 | 29151bbd0c201654aef0dbc11abc08751e27b075 | 2022-03-17T06:14:16.000Z | [
"pytorch",
"bert",
"fill-mask",
"dataset:wikitext",
"transformers",
"generated_from_trainer",
"model-index",
"autotrain_compatible"
] | fill-mask | false | saghar | null | saghar/TinyBERT_General_6L_768D-finetuned-wikitext103 | 1 | null | transformers | 30,884 | ---
tags:
- generated_from_trainer
datasets:
- wikitext
model-index:
- name: TinyBERT_General_6L_768D-finetuned-wikitext103
results: []
---
<!-- This model card has been generated automatically according to the information the Trainer had access to. You
should probably proofread and complete it, then remove this comment. -->
# TinyBERT_General_6L_768D-finetuned-wikitext103
This model is a fine-tuned version of [huawei-noah/TinyBERT_General_6L_768D](https://huggingface.co/huawei-noah/TinyBERT_General_6L_768D) on the wikitext dataset.
It achieves the following results on the evaluation set:
- Loss: 3.3768
## Model description
More information needed
## Intended uses & limitations
More information needed
## Training and evaluation data
More information needed
## Training procedure
### Training hyperparameters
The following hyperparameters were used during training:
- learning_rate: 2e-05
- train_batch_size: 32
- eval_batch_size: 32
- seed: 42
- optimizer: Adam with betas=(0.9,0.999) and epsilon=1e-08
- lr_scheduler_type: linear
- num_epochs: 3.0
### Training results
| Training Loss | Epoch | Step | Validation Loss |
|:-------------:|:-----:|:----:|:---------------:|
| 4.1792 | 1.0 | 3125 | 3.5465 |
| 3.6726 | 2.0 | 6250 | 3.4226 |
| 3.6065 | 3.0 | 9375 | 3.3768 |
### Framework versions
- Transformers 4.16.2
- Pytorch 1.8.1
- Datasets 1.11.0
- Tokenizers 0.10.3
|
wypoon/bert-base-uncased-mlm | d6ee550be7b80d7022b88783c5ba165498f4d8cc | 2022-03-16T23:55:53.000Z | [
"pytorch",
"bert",
"fill-mask",
"transformers",
"generated_from_trainer",
"license:apache-2.0",
"model-index",
"autotrain_compatible"
] | fill-mask | false | wypoon | null | wypoon/bert-base-uncased-mlm | 1 | null | transformers | 30,885 | ---
license: apache-2.0
tags:
- generated_from_trainer
model-index:
- name: bert-base-uncased-mlm
results: []
---
<!-- This model card has been generated automatically according to the information the Trainer had access to. You
should probably proofread and complete it, then remove this comment. -->
# bert-base-uncased-mlm
This model is a fine-tuned version of [bert-base-uncased](https://huggingface.co/bert-base-uncased) on an unknown dataset.
It achieves the following results on the evaluation set:
- Loss: 1.7425
## Model description
More information needed
## Intended uses & limitations
More information needed
## Training and evaluation data
More information needed
## Training procedure
### Training hyperparameters
The following hyperparameters were used during training:
- learning_rate: 5e-05
- train_batch_size: 32
- eval_batch_size: 8
- seed: 42
- optimizer: Adam with betas=(0.9,0.999) and epsilon=1e-08
- lr_scheduler_type: linear
- num_epochs: 16
### Training results
| Training Loss | Epoch | Step | Validation Loss |
|:-------------:|:-----:|:----:|:---------------:|
| 2.2248 | 1.0 | 38 | 1.9818 |
| 1.9124 | 2.0 | 76 | 1.8334 |
| 1.8166 | 3.0 | 114 | 1.7863 |
| 1.7414 | 4.0 | 152 | 1.9024 |
| 1.6727 | 5.0 | 190 | 1.7832 |
| 1.5969 | 6.0 | 228 | 1.8033 |
| 1.5023 | 7.0 | 266 | 1.5792 |
| 1.4593 | 8.0 | 304 | 1.7809 |
| 1.4825 | 9.0 | 342 | 1.6362 |
| 1.3928 | 10.0 | 380 | 1.6409 |
| 1.386 | 11.0 | 418 | 1.6855 |
| 1.3579 | 12.0 | 456 | 1.7348 |
| 1.2951 | 13.0 | 494 | 1.6956 |
| 1.3187 | 14.0 | 532 | 1.8408 |
| 1.3065 | 15.0 | 570 | 1.6207 |
| 1.3496 | 16.0 | 608 | 1.7425 |
### Framework versions
- Transformers 4.17.0
- Pytorch 1.10.0+cu111
- Datasets 2.0.0
- Tokenizers 0.11.6
|
newtonkwan/gpt2-xl-ft-3 | 149e2ccd93d42fbd32cc82ec15073e212e57023f | 2022-03-17T10:47:43.000Z | [
"pytorch",
"tensorboard",
"gpt2",
"text-generation",
"transformers",
"generated_from_trainer",
"model-index"
] | text-generation | false | newtonkwan | null | newtonkwan/gpt2-xl-ft-3 | 1 | null | transformers | 30,886 | ---
tags:
- generated_from_trainer
model-index:
- name: gpt2-xl-ft-3
results: []
---
<!-- This model card has been generated automatically according to the information the Trainer had access to. You
should probably proofread and complete it, then remove this comment. -->
# gpt2-xl-ft-3
This model is a fine-tuned version of [gpt2-xl](https://huggingface.co/gpt2-xl) on an unknown dataset.
It achieves the following results on the evaluation set:
- Loss: 1.4315
## Model description
More information needed
## Intended uses & limitations
More information needed
## Training and evaluation data
More information needed
## Training procedure
### Training hyperparameters
The following hyperparameters were used during training:
- learning_rate: 0.0005
- train_batch_size: 4
- eval_batch_size: 4
- seed: 2022
- gradient_accumulation_steps: 32
- total_train_batch_size: 128
- optimizer: Adam with betas=(0.9,0.999) and epsilon=1e-08
- lr_scheduler_type: linear
- lr_scheduler_warmup_steps: 100.0
- num_epochs: 4
### Training results
| Training Loss | Epoch | Step | Validation Loss |
|:-------------:|:-----:|:----:|:---------------:|
| No log | 1.0 | 156 | 1.3062 |
| No log | 2.0 | 312 | 1.3141 |
| No log | 3.0 | 468 | 1.3810 |
| 1.1725 | 4.0 | 624 | 1.4315 |
### Framework versions
- Transformers 4.17.0
- Pytorch 1.10.0+cu111
- Datasets 2.0.0
- Tokenizers 0.11.6
### Perplexity
Score: 138.43353271484375
### Dataset Size
Size: 25000
|
MolePatrol/Olbot | 588257f152f9295996d78d9512aa674e4eb3ff0f | 2022-03-23T21:14:51.000Z | [
"pytorch",
"gpt2",
"text-generation",
"transformers",
"conversational"
] | conversational | false | MolePatrol | null | MolePatrol/Olbot | 1 | null | transformers | 30,887 | ---
tags:
- conversational
---
# My Awesome Model |
cammy/bart-large-cnn-100-lit-evalMA-ga | e0e591a5433886403c3bab497a357c08641ca6c5 | 2022-03-17T02:46:15.000Z | [
"pytorch",
"tensorboard",
"bart",
"text2text-generation",
"transformers",
"autotrain_compatible"
] | text2text-generation | false | cammy | null | cammy/bart-large-cnn-100-lit-evalMA-ga | 1 | null | transformers | 30,888 | Entry not found |
libalabala/mt5-small-finetuned-amazon-en-es | 942027a143164fd3068962c2e4e8c4e24f0f39df | 2022-03-24T07:00:11.000Z | [
"pytorch",
"tensorboard",
"mt5",
"text2text-generation",
"transformers",
"summarization",
"generated_from_trainer",
"license:apache-2.0",
"model-index",
"autotrain_compatible"
] | summarization | false | libalabala | null | libalabala/mt5-small-finetuned-amazon-en-es | 1 | null | transformers | 30,889 | ---
license: apache-2.0
tags:
- summarization
- generated_from_trainer
metrics:
- rouge
model-index:
- name: mt5-small-finetuned-amazon-en-es
results: []
---
<!-- This model card has been generated automatically according to the information the Trainer had access to. You
should probably proofread and complete it, then remove this comment. -->
# mt5-small-finetuned-amazon-en-es
This model is a fine-tuned version of [google/mt5-small](https://huggingface.co/google/mt5-small) on the None dataset.
It achieves the following results on the evaluation set:
- Loss: 3.1997
- Rouge1: 16.7312
- Rouge2: 8.6607
- Rougel: 16.1846
- Rougelsum: 16.2411
## Model description
More information needed
## Intended uses & limitations
More information needed
## Training and evaluation data
More information needed
## Training procedure
### Training hyperparameters
The following hyperparameters were used during training:
- learning_rate: 5.6e-05
- train_batch_size: 8
- eval_batch_size: 8
- seed: 42
- optimizer: Adam with betas=(0.9,0.999) and epsilon=1e-08
- lr_scheduler_type: linear
- num_epochs: 3
### Training results
| Training Loss | Epoch | Step | Validation Loss | Rouge1 | Rouge2 | Rougel | Rougelsum |
|:-------------:|:-----:|:----:|:---------------:|:-------:|:------:|:-------:|:---------:|
| 7.0772 | 1.0 | 1209 | 3.3307 | 12.4644 | 4.0353 | 12.0167 | 12.0722 |
| 4.0223 | 2.0 | 2418 | 3.2257 | 15.338 | 7.0168 | 14.7769 | 14.8391 |
| 3.8018 | 3.0 | 3627 | 3.1997 | 16.7312 | 8.6607 | 16.1846 | 16.2411 |
### Framework versions
- Transformers 4.17.0
- Pytorch 1.10.0+cu111
- Datasets 2.0.0
- Tokenizers 0.11.6
|
Jungwonchang/wav2vec2-large-xls-r-300m-vietnamese-colab | 53ace321d93a09b0bdba6114f678aa4b37eff471 | 2022-03-17T11:55:20.000Z | [
"pytorch",
"tensorboard",
"wav2vec2",
"automatic-speech-recognition",
"dataset:common_voice",
"transformers",
"generated_from_trainer",
"license:apache-2.0",
"model-index"
] | automatic-speech-recognition | false | Jungwonchang | null | Jungwonchang/wav2vec2-large-xls-r-300m-vietnamese-colab | 1 | null | transformers | 30,890 | ---
license: apache-2.0
tags:
- generated_from_trainer
datasets:
- common_voice
model-index:
- name: wav2vec2-large-xls-r-300m-vietnamese-colab
results: []
---
<!-- This model card has been generated automatically according to the information the Trainer had access to. You
should probably proofread and complete it, then remove this comment. -->
# wav2vec2-large-xls-r-300m-vietnamese-colab
This model is a fine-tuned version of [facebook/wav2vec2-xls-r-300m](https://huggingface.co/facebook/wav2vec2-xls-r-300m) on the common_voice dataset.
## Model description
More information needed
## Intended uses & limitations
More information needed
## Training and evaluation data
More information needed
## Training procedure
### Training hyperparameters
The following hyperparameters were used during training:
- learning_rate: 0.0003
- train_batch_size: 16
- eval_batch_size: 8
- seed: 42
- gradient_accumulation_steps: 2
- total_train_batch_size: 32
- optimizer: Adam with betas=(0.9,0.999) and epsilon=1e-08
- lr_scheduler_type: linear
- lr_scheduler_warmup_steps: 500
- num_epochs: 30
- mixed_precision_training: Native AMP
### Training results
### Framework versions
- Transformers 4.11.3
- Pytorch 1.10.0+cu111
- Datasets 1.18.3
- Tokenizers 0.10.3
|
mideind/IceBERT-ic3 | afb51e2a601dafcb5a21c90645bc393bb0802852 | 2022-03-17T14:03:37.000Z | [
"pytorch",
"roberta",
"fill-mask",
"is",
"arxiv:2201.05601",
"transformers",
"icelandic",
"masked-lm",
"license:agpl-3.0",
"autotrain_compatible"
] | fill-mask | false | mideind | null | mideind/IceBERT-ic3 | 1 | null | transformers | 30,891 | ---
language: is
widget:
- text: Má bjóða þér <mask> í kvöld?
- text: Forseti <mask> er ágæt.
- text: Súpan var <mask> á bragðið.
tags:
- roberta
- icelandic
- masked-lm
- pytorch
license: agpl-3.0
---
# IceBERT-ic3
This model was trained with fairseq using the RoBERTa-base architecture. It is one of many models we have trained for Icelandic, see the paper referenced below for further details. The training data used is shown in the table below.
| Dataset | Size | Tokens |
|------------------------------------------------------|---------|--------|
| Icelandic Common Crawl Corpus (IC3) | 4.9 GB | 824M |
## Citation
The model is described in this paper [https://arxiv.org/abs/2201.05601](https://arxiv.org/abs/2201.05601). Please cite the paper if you make use of the model.
```
@article{DBLP:journals/corr/abs-2201-05601,
author = {V{\'{e}}steinn Sn{\ae}bjarnarson and
Haukur Barri S{\'{\i}}monarson and
P{\'{e}}tur Orri Ragnarsson and
Svanhv{\'{\i}}t Lilja Ing{\'{o}}lfsd{\'{o}}ttir and
Haukur P{\'{a}}ll J{\'{o}}nsson and
Vilhj{\'{a}}lmur {\TH}orsteinsson and
Hafsteinn Einarsson},
title = {A Warm Start and a Clean Crawled Corpus - {A} Recipe for Good Language
Models},
journal = {CoRR},
volume = {abs/2201.05601},
year = {2022},
url = {https://arxiv.org/abs/2201.05601},
eprinttype = {arXiv},
eprint = {2201.05601},
timestamp = {Thu, 20 Jan 2022 14:21:35 +0100},
biburl = {https://dblp.org/rec/journals/corr/abs-2201-05601.bib},
bibsource = {dblp computer science bibliography, https://dblp.org}
}
```
|
davidlopez/distilbert-base-uncased-go-emotion-EnkelMode-cyberblue | c56d5fa0cac4f052a92412cde1018562fe2f7080 | 2022-03-17T14:32:17.000Z | [
"pytorch",
"distilbert",
"transformers"
] | null | false | davidlopez | null | davidlopez/distilbert-base-uncased-go-emotion-EnkelMode-cyberblue | 1 | null | transformers | 30,892 | Entry not found |
negfir/BERT_6L | 4f88b96f1a78524941d33a04412338eff7989bd9 | 2022-03-17T14:47:31.000Z | [
"pytorch",
"bert",
"fill-mask",
"transformers",
"autotrain_compatible"
] | fill-mask | false | negfir | null | negfir/BERT_6L | 1 | null | transformers | 30,893 | Entry not found |
newtonkwan/gpt2-xl-ft-4 | e3ea0458f25f8f63edd3da2e482423b7a1ae87db | 2022-03-17T16:38:08.000Z | [
"pytorch",
"tensorboard",
"gpt2",
"text-generation",
"transformers",
"generated_from_trainer",
"model-index"
] | text-generation | false | newtonkwan | null | newtonkwan/gpt2-xl-ft-4 | 1 | null | transformers | 30,894 | ---
tags:
- generated_from_trainer
model-index:
- name: gpt2-xl-ft-4
results: []
---
<!-- This model card has been generated automatically according to the information the Trainer had access to. You
should probably proofread and complete it, then remove this comment. -->
# gpt2-xl-ft-4
This model is a fine-tuned version of [gpt2-xl](https://huggingface.co/gpt2-xl) on an unknown dataset.
It achieves the following results on the evaluation set:
- Loss: 1.2823
## Model description
More information needed
## Intended uses & limitations
More information needed
## Training and evaluation data
More information needed
## Training procedure
### Training hyperparameters
The following hyperparameters were used during training:
- learning_rate: 0.0005
- train_batch_size: 8
- eval_batch_size: 8
- seed: 2022
- gradient_accumulation_steps: 32
- total_train_batch_size: 256
- optimizer: Adam with betas=(0.9,0.999) and epsilon=1e-08
- lr_scheduler_type: linear
- lr_scheduler_warmup_steps: 100.0
- num_epochs: 4
### Training results
| Training Loss | Epoch | Step | Validation Loss |
|:-------------:|:-----:|:----:|:---------------:|
| No log | 0.96 | 15 | 3.5549 |
| No log | 1.96 | 30 | 1.4216 |
| No log | 2.96 | 45 | 1.2969 |
| No log | 3.96 | 60 | 1.2823 |
### Framework versions
- Transformers 4.17.0
- Pytorch 1.10.0+cu111
- Datasets 2.0.0
- Tokenizers 0.11.6
### Perplexity
Score: 35.67070770263672
### Dataset Size
Size: 5000 |
Graphcore/bert-base-uncased-squad | 3eb3ca0534cd06e4524883c53eabfa9ef00d5f23 | 2022-05-25T18:30:44.000Z | [
"pytorch",
"bert",
"question-answering",
"dataset:squad",
"transformers",
"generated_from_trainer",
"license:apache-2.0",
"model-index",
"autotrain_compatible"
] | question-answering | false | Graphcore | null | Graphcore/bert-base-uncased-squad | 1 | 1 | transformers | 30,895 | ---
license: apache-2.0
tags:
- generated_from_trainer
datasets:
- squad
model-index:
- name: Graphcore/bert-base-uncased-squad
results: []
---
# Graphcore/bert-base-uncased-squad
Optimum Graphcore is a new open-source library and toolkit that enables developers to access IPU-optimized models certified by Hugging Face. It is an extension of Transformers, providing a set of performance optimization tools enabling maximum efficiency to train and run models on Graphcore’s IPUs - a completely new kind of massively parallel processor to accelerate machine intelligence. Learn more about how to take train Transformer models faster with IPUs at [hf.co/hardware/graphcore](https://huggingface.co/hardware/graphcore).
Through HuggingFace Optimum, Graphcore released ready-to-use IPU-trained model checkpoints and IPU configuration files to make it easy to train models with maximum efficiency in the IPU. Optimum shortens the development lifecycle of your AI models by letting you plug-and-play any public dataset and allows a seamless integration to our State-of-the-art hardware giving you a quicker time-to-value for your AI project.
## Model description
BERT (Bidirectional Encoder Representations from Transformers) is a transformers model which is designed to pretrain bidirectional representations from unlabelled texts. It enables easy and fast fine-tuning for different downstream tasks such as Sequence Classification, Named Entity Recognition, Question Answering, Multiple Choice and MaskedLM.
It was trained with two objectives in pretraining : Masked language modelling (MLM) and Next sentence prediction(NSP). First, MLM is different from traditional LM which sees the words one after another while BERT allows the model to learn a bidirectional representation. In addition to MLM, NSP is used for jointly pertaining text-pair representations.
It reduces the need of many engineering efforts for building task specific architectures through pre-trained representation. And achieves state-of-the-art performance on a large suite of sentence-level and token-level tasks.
## Intended uses & limitations
This model is a fine-tuned version of [Graphcore/bert-base-uncased](https://huggingface.co/Graphcore/bert-base-uncased) on the squad dataset.
## Training and evaluation data
Trained on squad dataset:
- [HuggingFace/squad](https://huggingface.co/datasets/squad)
## Training procedure
Model was trained on 16 Graphcore Mk2 IPUs using the [optimum-graphcore](https://github.com/huggingface/optimum-graphcore) library.
Command line:
```
python examples/question-answering/run_qa.py \
--model_name_or_path Graphcore/bert-base-uncased \
--ipu_config_name Graphcore/bert-base-ipu \
--dataset_name squad \
--do_train \
--do_eval \
--num_train_epochs 3 \
--per_device_train_batch_size 2 \
--per_device_eval_batch_size 2 \
--gradient_accumulation_steps 16 \
--pod_type pod16 \
--learning_rate 9e-5 \
--max_seq_length 384 \
--doc_stride 128 \
--seed 42\
--lr_scheduler_type linear \
--loss_scaling 64 \
--weight_decay 0.01 \
--warmup_ratio 0.2 \
--logging_steps 1 \
--save_steps 50 \
--dataloader_num_workers 64 \
--ipu_config_overrides "embedding_serialization_factor=2" \
--output_dir squad_v2_bert_base \
--overwrite_output_dir
```
### Training hyperparameters
The following hyperparameters were used during training:
- learning_rate: 6e-05
- train_batch_size: 2
- eval_batch_size: 2
- seed: 42
- distributed_type: IPU
- gradient_accumulation_steps: 16
- total_train_batch_size: 256
- total_eval_batch_size: 32
- optimizer: Adam with betas=(0.9,0.999) and epsilon=1e-08
- lr_scheduler_type: linear
- lr_scheduler_warmup_ratio: 0.1
- num_epochs: 3.0
- training precision: Mixed Precision
### Training results
```
{
"epoch": 3.0,
"eval_exact_match": 81.79754020813624,
"eval_f1": 88.84840994541061,
"eval_samples": 10784
}
```
### Framework versions
- Transformers 4.18.0.dev0
- Pytorch 1.10.0+cpu
- Datasets 1.18.4
- Tokenizers 0.11.6
|
sileod/genqa | f3c2d228e77c55a8c7e8c979df520909505e0f32 | 2022-03-25T09:39:20.000Z | [
"pytorch",
"bart",
"text2text-generation",
"transformers",
"autotrain_compatible"
] | text2text-generation | false | sileod | null | sileod/genqa | 1 | null | transformers | 30,896 | Entry not found |
beston91/gpt2-xl-ft-logits-5k | be5bba696495b3c74ad358f296b60f3ebd6fcd43 | 2022-03-18T02:54:46.000Z | [
"pytorch",
"tensorboard",
"gpt2",
"text-generation",
"transformers",
"generated_from_trainer",
"model-index"
] | text-generation | false | beston91 | null | beston91/gpt2-xl-ft-logits-5k | 1 | null | transformers | 30,897 | ---
tags:
- generated_from_trainer
model-index:
- name: gpt2-xl-vanilla-debiased-5000
results: []
---
<!-- This model card has been generated automatically according to the information the Trainer had access to. You
should probably proofread and complete it, then remove this comment. -->
# gpt2-xl-vanilla-debiased-5000
This model is a fine-tuned version of [gpt2-xl](https://huggingface.co/gpt2-xl) on an unknown dataset.
It achieves the following results on the evaluation set:
- Loss: 7.0371
## Model description
More information needed
## Intended uses & limitations
More information needed
## Training and evaluation data
More information needed
## Training procedure
### Training hyperparameters
The following hyperparameters were used during training:
- learning_rate: 5e-06
- train_batch_size: 4
- eval_batch_size: 4
- seed: 42
- gradient_accumulation_steps: 32
- total_train_batch_size: 128
- optimizer: Adam with betas=(0.9,0.999) and epsilon=1e-08
- lr_scheduler_type: linear
- lr_scheduler_warmup_steps: 100.0
- num_epochs: 4
- mixed_precision_training: Native AMP
### Training results
| Training Loss | Epoch | Step | Validation Loss |
|:-------------:|:-----:|:----:|:---------------:|
| No log | 0.99 | 27 | 6.1985 |
| No log | 1.99 | 54 | 6.4583 |
| No log | 2.99 | 81 | 6.7709 |
| No log | 3.99 | 108 | 7.0371 |
### Framework versions
- Transformers 4.17.0
- Pytorch 1.10.0+cu111
- Datasets 2.0.0
- Tokenizers 0.11.6
|
willcai/wav2vec2_common_voice_accents_4 | 8fd5ad5dbb834bdf733081bd8cc31f3f0d163be0 | 2022-03-18T11:11:03.000Z | [
"pytorch",
"wav2vec2",
"automatic-speech-recognition",
"dataset:common_voice",
"transformers",
"generated_from_trainer",
"license:apache-2.0",
"model-index"
] | automatic-speech-recognition | false | willcai | null | willcai/wav2vec2_common_voice_accents_4 | 1 | null | transformers | 30,898 | ---
license: apache-2.0
tags:
- generated_from_trainer
datasets:
- common_voice
model-index:
- name: wav2vec2_common_voice_accents_4
results: []
---
<!-- This model card has been generated automatically according to the information the Trainer had access to. You
should probably proofread and complete it, then remove this comment. -->
# wav2vec2_common_voice_accents_4
This model is a fine-tuned version of [facebook/wav2vec2-xls-r-300m](https://huggingface.co/facebook/wav2vec2-xls-r-300m) on the common_voice dataset.
It achieves the following results on the evaluation set:
- Loss: 0.0047
## Model description
More information needed
## Intended uses & limitations
More information needed
## Training and evaluation data
More information needed
## Training procedure
### Training hyperparameters
The following hyperparameters were used during training:
- learning_rate: 0.0003
- train_batch_size: 48
- eval_batch_size: 4
- seed: 42
- distributed_type: multi-GPU
- num_devices: 8
- total_train_batch_size: 384
- total_eval_batch_size: 32
- optimizer: Adam with betas=(0.9,0.999) and epsilon=1e-08
- lr_scheduler_type: linear
- lr_scheduler_warmup_steps: 500
- num_epochs: 30
- mixed_precision_training: Native AMP
### Training results
| Training Loss | Epoch | Step | Validation Loss |
|:-------------:|:-----:|:----:|:---------------:|
| 4.615 | 1.28 | 400 | 0.8202 |
| 0.3778 | 2.56 | 800 | 0.1587 |
| 0.2229 | 3.85 | 1200 | 0.1027 |
| 0.1799 | 5.13 | 1600 | 0.0879 |
| 0.1617 | 6.41 | 2000 | 0.0772 |
| 0.1474 | 7.69 | 2400 | 0.0625 |
| 0.134 | 8.97 | 2800 | 0.0498 |
| 0.1213 | 10.26 | 3200 | 0.0429 |
| 0.1186 | 11.54 | 3600 | 0.0434 |
| 0.1118 | 12.82 | 4000 | 0.0312 |
| 0.1026 | 14.1 | 4400 | 0.0365 |
| 0.0951 | 15.38 | 4800 | 0.0321 |
| 0.0902 | 16.67 | 5200 | 0.0262 |
| 0.0843 | 17.95 | 5600 | 0.0208 |
| 0.0744 | 19.23 | 6000 | 0.0140 |
| 0.0718 | 20.51 | 6400 | 0.0204 |
| 0.0694 | 21.79 | 6800 | 0.0133 |
| 0.0636 | 23.08 | 7200 | 0.0104 |
| 0.0609 | 24.36 | 7600 | 0.0084 |
| 0.0559 | 25.64 | 8000 | 0.0050 |
| 0.0527 | 26.92 | 8400 | 0.0089 |
| 0.0495 | 28.21 | 8800 | 0.0058 |
| 0.0471 | 29.49 | 9200 | 0.0047 |
### Framework versions
- Transformers 4.17.0
- Pytorch 1.10.2+cu102
- Datasets 1.18.4
- Tokenizers 0.11.6
|
calebcsjm/reverse_text_flipped_tokens_HarryPotter | 427641ec385ae2c80be9d49e794964a615ee2de4 | 2022-03-18T03:31:13.000Z | [
"pytorch",
"tensorboard",
"gpt2",
"text-generation",
"transformers"
] | text-generation | false | calebcsjm | null | calebcsjm/reverse_text_flipped_tokens_HarryPotter | 1 | null | transformers | 30,899 | Entry not found |
Subsets and Splits
No saved queries yet
Save your SQL queries to embed, download, and access them later. Queries will appear here once saved.