modelId
stringlengths 4
112
| sha
stringlengths 40
40
| lastModified
stringlengths 24
24
| tags
sequence | pipeline_tag
stringclasses 29
values | private
bool 1
class | author
stringlengths 2
38
⌀ | config
null | id
stringlengths 4
112
| downloads
float64 0
36.8M
⌀ | likes
float64 0
712
⌀ | library_name
stringclasses 17
values | __index_level_0__
int64 0
38.5k
| readme
stringlengths 0
186k
|
---|---|---|---|---|---|---|---|---|---|---|---|---|---|
abdoutony207/m2m100_418M-evaluated-en-to-ar-2000instancesUNMULTI-leaningRate2e-05-batchSize8 | 2d53ae4972896b4c8a6f5e905ddbb70789dd7246 | 2022-06-12T17:06:11.000Z | [
"pytorch",
"tensorboard",
"m2m_100",
"text2text-generation",
"transformers",
"autotrain_compatible"
] | text2text-generation | false | abdoutony207 | null | abdoutony207/m2m100_418M-evaluated-en-to-ar-2000instancesUNMULTI-leaningRate2e-05-batchSize8 | 2 | null | transformers | 26,300 | Entry not found |
erickfm/t5-base-finetuned-bias-sweep-9ac77936 | 01494b3d896168a91d3f45313542b7abc9ea0c37 | 2022-06-12T16:55:12.000Z | [
"pytorch",
"t5",
"text2text-generation",
"transformers",
"autotrain_compatible"
] | text2text-generation | false | erickfm | null | erickfm/t5-base-finetuned-bias-sweep-9ac77936 | 2 | null | transformers | 26,301 | Entry not found |
nlokam99/ada_sample_2 | 251bd11fb4954fdb36ba60b5af265c0dcb2a4569 | 2022-06-12T17:40:42.000Z | [
"pytorch",
"gpt2",
"text-generation",
"transformers",
"conversational",
"license:mit"
] | conversational | false | nlokam99 | null | nlokam99/ada_sample_2 | 2 | null | transformers | 26,302 | ---
thumbnail: https://huggingface.co/front/thumbnails/dialogpt.png
tags:
- conversational
license: mit
--- |
abdoutony207/m2m100_418M-evaluated-en-to-ar-2000instancesopus-leaningRate2e-05-batchSize8 | a5fe12d82308e5f7ec51cd951edd6e94676ec4fc | 2022-06-12T18:54:40.000Z | [
"pytorch",
"tensorboard",
"m2m_100",
"text2text-generation",
"transformers",
"autotrain_compatible"
] | text2text-generation | false | abdoutony207 | null | abdoutony207/m2m100_418M-evaluated-en-to-ar-2000instancesopus-leaningRate2e-05-batchSize8 | 2 | null | transformers | 26,303 | Entry not found |
robbespo/opus-mt-en-ro-finetuned-en-to-ro | f53d4e9d1eadd2464e21f96f1394080ccd3aeafc | 2022-06-13T21:43:26.000Z | [
"pytorch",
"tensorboard",
"marian",
"text2text-generation",
"dataset:wmt16",
"transformers",
"generated_from_trainer",
"license:apache-2.0",
"model-index",
"autotrain_compatible"
] | text2text-generation | false | robbespo | null | robbespo/opus-mt-en-ro-finetuned-en-to-ro | 2 | null | transformers | 26,304 | ---
license: apache-2.0
tags:
- generated_from_trainer
datasets:
- wmt16
metrics:
- bleu
model-index:
- name: opus-mt-en-ro-finetuned-en-to-ro
results:
- task:
name: Sequence-to-sequence Language Modeling
type: text2text-generation
dataset:
name: wmt16
type: wmt16
args: ro-en
metrics:
- name: Bleu
type: bleu
value: 28.1507
---
<!-- This model card has been generated automatically according to the information the Trainer had access to. You
should probably proofread and complete it, then remove this comment. -->
# opus-mt-en-ro-finetuned-en-to-ro
This model is a fine-tuned version of [Helsinki-NLP/opus-mt-en-ro](https://huggingface.co/Helsinki-NLP/opus-mt-en-ro) on the wmt16 dataset.
It achieves the following results on the evaluation set:
- Loss: 1.2886
- Bleu: 28.1507
- Gen Len: 34.1136
## Model description
More information needed
## Intended uses & limitations
More information needed
## Training and evaluation data
More information needed
## Training procedure
### Training hyperparameters
The following hyperparameters were used during training:
- learning_rate: 2e-05
- train_batch_size: 16
- eval_batch_size: 16
- seed: 42
- optimizer: Adam with betas=(0.9,0.999) and epsilon=1e-08
- lr_scheduler_type: linear
- num_epochs: 1
- mixed_precision_training: Native AMP
### Training results
| Training Loss | Epoch | Step | Validation Loss | Bleu | Gen Len |
|:-------------:|:-----:|:-----:|:---------------:|:-------:|:-------:|
| 0.7437 | 1.0 | 38145 | 1.2886 | 28.1507 | 34.1136 |
### Framework versions
- Transformers 4.19.4
- Pytorch 1.11.0+cu113
- Datasets 2.2.2
- Tokenizers 0.12.1
|
Deborah/custom-model | 0a6de78a23962022fa4e59d16aa160462c56efc7 | 2022-06-12T21:12:54.000Z | [
"pytorch",
"bert",
"token-classification",
"transformers",
"autotrain_compatible"
] | token-classification | false | Deborah | null | Deborah/custom-model | 2 | null | transformers | 26,305 | Entry not found |
rohitsroch/hybrid_utt-clusterrank_bart-base_dialogsum_sum | 999588a01f8072e3888e04111526f6a76787bdd4 | 2022-06-12T23:19:41.000Z | [
"pytorch",
"bart",
"text2text-generation",
"en",
"dataset:yulongchen/DialogSum",
"transformers",
"dialogue-summarization",
"license:apache-2.0",
"autotrain_compatible"
] | text2text-generation | false | rohitsroch | null | rohitsroch/hybrid_utt-clusterrank_bart-base_dialogsum_sum | 2 | null | transformers | 26,306 | ---
language:
- en
license: apache-2.0
tags:
- dialogue-summarization
model_index:
- name: hybrid_utt-clusterrank_bart-base_dialogsum_sum
results:
- task:
name: Summarization
type: summarization
datasets:
- yulongchen/DialogSum
---
## Paper
## [Domain Adapted Abstractive Summarization of Dialogue using Transfer Learning](https://dl.acm.org/doi/10.1145/3508546.3508640)
Authors: *Rohit Sroch*
## Abstract
Recently, the abstractive dialogue summarization task has been gaining a lot of attention from researchers. Also, unlike news articles and documents with well-structured text, dialogue differs in the sense that it often comes from two or more interlocutors, exchanging information with each other and having an inherent hierarchical structure based on the sequence of utterances by different speakers. This paper proposes a simple but effective hybrid approach that consists of two modules and uses transfer learning by leveraging pretrained language models (PLMs) to generate an abstractive summary. The first module highlights important utterances, capturing the utterance level relationship by adapting an auto-encoding model like BERT based on the unsupervised or supervised method. And then, the second module generates a concise abstractive summary by adapting encoder-decoder models like T5, BART, and PEGASUS. Experiment results on benchmark datasets show that our approach achieves a state-of-the-art performance by adapting to dialogue scenarios and can also be helpful in low-resource settings for domain adaptation.
*Rohit Sroch. 2021. Domain Adapted Abstractive Summarization of Dialogue using Transfer Learning. In 2021 4th International Conference on Algorithms, Computing and Artificial Intelligence (ACAI'21). Association for Computing Machinery, New York, NY, USA, Article 94, 1–6. https://doi.org/10.1145/3508546.3508640*
# hybrid_utt-clusterrank_bart-base_dialogsum_sum
This model is a fine-tuned version of [facebook/bart-base](https://huggingface.co/facebook/bart-base) on DialogSum dataset for dialogue summarization task.
## Model description
More information needed
### Training hyperparameters
The following hyperparameters were used during training:
- learning_rate: 5e-5
- train_batch_size: 8
- eval_batch_size: 8
- seed: 42
- optimizer: Adam with betas=(0.9,0.999) and epsilon=1e-08
- lr_scheduler_type: linear
- lr_scheduler_warmup_ratio: 0.1
- num_epochs: 10.0
- label_smoothing_factor: 0.1
### Results on Test Set
- predict_gen_len = 32.37
- predict_rouge1 = **43.3999**
- predict_rouge2 = **17.3447**
- predict_rougeL = **35.1421**
- predict_rougeLsum = **38.1883**
- predict_samples = 500
- predict_samples_per_second = 9.506
- predict_steps_per_second = 1.198
### Framework versions
- Transformers>=4.8.0
- Pytorch>=1.6.0
- Datasets>=1.10.2
- Tokenizers>=0.10.3
If you use this model, please cite the following paper:
```
@inproceedings{10.1145/3508546.3508640,
author = {Sroch, Rohit},
title = {Domain Adapted Abstractive Summarization of Dialogue Using Transfer Learning},
year = {2021},
isbn = {9781450385053},
publisher = {Association for Computing Machinery},
address = {New York, NY, USA},
url = {https://doi.org/10.1145/3508546.3508640},
doi = {10.1145/3508546.3508640},
articleno = {94},
numpages = {6},
keywords = {encoder-decoder, T5, abstractive summary, PEGASUS, BART, dialogue summarization, PLMs, BERT},
location = {Sanya, China},
series = {ACAI'21}
}
``` |
BigSalmon/GPTNeo350MInformalToFormalLincoln8 | cb9d7a7399f015a33927a95fc56b2275ce80f160 | 2022-07-26T01:39:08.000Z | [
"pytorch",
"gpt_neo",
"text-generation",
"transformers"
] | text-generation | false | BigSalmon | null | BigSalmon/GPTNeo350MInformalToFormalLincoln8 | 2 | null | transformers | 26,307 | Trained on this model: https://huggingface.co/xhyi/PT_GPTNEO350_ATG/tree/main
```
from transformers import AutoTokenizer, AutoModelForCausalLM
tokenizer = AutoTokenizer.from_pretrained("BigSalmon/GPTNeo350MInformalToFormalLincoln8")
model = AutoModelForCausalLM.from_pretrained("BigSalmon/GPTNeo350MInformalToFormalLincoln8")
```
```
How To Make Prompt:
informal english: i am very ready to do that just that.
Translated into the Style of Abraham Lincoln: you can assure yourself of my readiness to work toward this end.
Translated into the Style of Abraham Lincoln: please be assured that i am most ready to undertake this laborious task.
***
informal english: space is huge and needs to be explored.
Translated into the Style of Abraham Lincoln: space awaits traversal, a new world whose boundaries are endless.
Translated into the Style of Abraham Lincoln: space is a ( limitless / boundless ) expanse, a vast virgin domain awaiting exploration.
***
informal english: corn fields are all across illinois, visible once you leave chicago.
Translated into the Style of Abraham Lincoln: corn fields ( permeate illinois / span the state of illinois / ( occupy / persist in ) all corners of illinois / line the horizon of illinois / envelop the landscape of illinois ), manifesting themselves visibly as one ventures beyond chicago.
informal english:
```
```
infill: chrome extensions [MASK] accomplish everyday tasks.
Translated into the Style of Abraham Lincoln: chrome extensions ( expedite the ability to / unlock the means to more readily ) accomplish everyday tasks.
infill: at a time when nintendo has become inflexible, [MASK] consoles that are tethered to a fixed iteration, sega diligently curates its legacy of classic video games on handheld devices.
Translated into the Style of Abraham Lincoln: at a time when nintendo has become inflexible, ( stubbornly [MASK] on / firmly set on / unyielding in its insistence on ) consoles that are tethered to a fixed iteration, sega diligently curates its legacy of classic video games on handheld devices.
infill:
```
```
Essay Intro (Warriors vs. Rockets in Game 7):
text: eagerly anticipated by fans, game 7's are the highlight of the post-season.
text: ever-building in suspense, game 7's have the crowd captivated.
***
Essay Intro (South Korean TV Is Becoming Popular):
text: maturing into a bona fide paragon of programming, south korean television ( has much to offer / entertains without fail / never disappoints ).
text: increasingly held in critical esteem, south korean television continues to impress.
text: at the forefront of quality content, south korea is quickly achieving celebrity status.
***
Essay Intro (
```
```
Search: What is the definition of Checks and Balances?
https://en.wikipedia.org/wiki/Checks_and_balances
Checks and Balances is the idea of having a system where each and every action in government should be subject to one or more checks that would not allow one branch or the other to overly dominate.
https://www.harvard.edu/glossary/Checks_and_Balances
Checks and Balances is a system that allows each branch of government to limit the powers of the other branches in order to prevent abuse of power
https://www.law.cornell.edu/library/constitution/Checks_and_Balances
Checks and Balances is a system of separation through which branches of government can control the other, thus preventing excess power.
***
Search: What is the definition of Separation of Powers?
https://en.wikipedia.org/wiki/Separation_of_powers
The separation of powers is a principle in government, whereby governmental powers are separated into different branches, each with their own set of powers, that are prevent one branch from aggregating too much power.
https://www.yale.edu/tcf/Separation_of_Powers.html
Separation of Powers is the division of governmental functions between the executive, legislative and judicial branches, clearly demarcating each branch's authority, in the interest of ensuring that individual liberty or security is not undermined.
***
Search: What is the definition of Connection of Powers?
https://en.wikipedia.org/wiki/Connection_of_powers
Connection of Powers is a feature of some parliamentary forms of government where different branches of government are intermingled, typically the executive and legislative branches.
https://simple.wikipedia.org/wiki/Connection_of_powers
The term Connection of Powers describes a system of government in which there is overlap between different parts of the government.
***
Search: What is the definition of
```
```
Search: What are phrase synonyms for "second-guess"?
https://www.powerthesaurus.org/second-guess/synonyms
Shortest to Longest:
- feel dubious about
- raise an eyebrow at
- wrinkle their noses at
- cast a jaundiced eye at
- teeter on the fence about
***
Search: What are phrase synonyms for "mean to newbies"?
https://www.powerthesaurus.org/mean_to_newbies/synonyms
Shortest to Longest:
- readiness to balk at rookies
- absence of tolerance for novices
- hostile attitude toward newcomers
***
Search: What are phrase synonyms for "make use of"?
https://www.powerthesaurus.org/make_use_of/synonyms
Shortest to Longest:
- call upon
- glean value from
- reap benefits from
- derive utility from
- seize on the merits of
- draw on the strength of
- tap into the potential of
***
Search: What are phrase synonyms for "hurting itself"?
https://www.powerthesaurus.org/hurting_itself/synonyms
Shortest to Longest:
- erring
- slighting itself
- forfeiting its integrity
- doing itself a disservice
- evincing a lack of backbone
***
Search: What are phrase synonyms for "
```
```
- nebraska
- unicamerical legislature
- different from federal house and senate
text: featuring a unicameral legislature, nebraska's political system stands in stark contrast to the federal model, comprised of a house and senate.
***
- penny has practically no value
- should be taken out of circulation
- just as other coins have been in us history
- lost use
- value not enough
- to make environmental consequences worthy
text: all but valueless, the penny should be retired. as with other coins in american history, it has become defunct. too minute to warrant the environmental consequences of its production, it has outlived its usefulness.
***
-
```
```
original: sports teams are profitable for owners. [MASK], their valuations experience a dramatic uptick.
infill: sports teams are profitable for owners. ( accumulating vast sums / stockpiling treasure / realizing benefits / cashing in / registering robust financials / scoring on balance sheets ), their valuations experience a dramatic uptick.
***
original:
```
```
wordy: classical music is becoming less popular more and more.
Translate into Concise Text: interest in classic music is fading.
***
wordy:
```
```
sweet: savvy voters ousted him.
longer: voters who were informed delivered his defeat.
***
sweet:
```
```
1: commercial space company spacex plans to launch a whopping 52 flights in 2022.
2: spacex, a commercial space company, intends to undertake a total of 52 flights in 2022.
3: in 2022, commercial space company spacex has its sights set on undertaking 52 flights.
4: 52 flights are in the pipeline for 2022, according to spacex, a commercial space company.
5: a commercial space company, spacex aims to conduct 52 flights in 2022.
***
1:
```
Keywords to sentences or sentence.
```
ngos are characterized by:
□ voluntary citizens' group that is organized on a local, national or international level
□ encourage political participation
□ often serve humanitarian functions
□ work for social, economic, or environmental change
***
what are the drawbacks of living near an airbnb?
□ noise
□ parking
□ traffic
□ security
□ strangers
***
```
```
original: musicals generally use spoken dialogue as well as songs to convey the story. operas are usually fully sung.
adapted: musicals generally use spoken dialogue as well as songs to convey the story. ( in a stark departure / on the other hand / in contrast / by comparison / at odds with this practice / far from being alike / in defiance of this standard / running counter to this convention ), operas are usually fully sung.
***
original: akoya and tahitian are types of pearls. akoya pearls are mostly white, and tahitian pearls are naturally dark.
adapted: akoya and tahitian are types of pearls. ( a far cry from being indistinguishable / easily distinguished / on closer inspection / setting them apart / not to be mistaken for one another / hardly an instance of mere synonymy / differentiating the two ), akoya pearls are mostly white, and tahitian pearls are naturally dark.
***
original:
```
```
original: had trouble deciding.
translated into journalism speak: wrestled with the question, agonized over the matter, furrowed their brows in contemplation.
***
original:
```
```
input: not loyal
1800s english: ( two-faced / inimical / perfidious / duplicitous / mendacious / double-dealing / shifty ).
***
input:
``` |
enoriega/kw_pubmed_vanilla_sentence_10000_0.0003 | 1cb5bd8bb555ac0798b06fae67f7a92e16df2021 | 2022-06-13T13:27:59.000Z | [
"pytorch",
"tensorboard",
"bert",
"fill-mask",
"transformers",
"autotrain_compatible"
] | fill-mask | false | enoriega | null | enoriega/kw_pubmed_vanilla_sentence_10000_0.0003 | 2 | null | transformers | 26,308 | Entry not found |
enoriega/kw_pubmed_vanilla_document_10000_0.0003 | d38124b35eb4ff00fbabaf5c7e8e90b9745670a8 | 2022-06-13T13:29:52.000Z | [
"pytorch",
"tensorboard",
"bert",
"fill-mask",
"transformers",
"autotrain_compatible"
] | fill-mask | false | enoriega | null | enoriega/kw_pubmed_vanilla_document_10000_0.0003 | 2 | null | transformers | 26,309 | Entry not found |
samba/samba-sentiments-fine-tuned | 83804a37b4f564283174f8ac4fb0f4bb27741bda | 2022-06-13T02:26:52.000Z | [
"pytorch",
"roberta",
"text-classification",
"transformers",
"license:apache-2.0"
] | text-classification | false | samba | null | samba/samba-sentiments-fine-tuned | 2 | null | transformers | 26,310 | ---
license: apache-2.0
---
|
Priya9/wav2vec2-large-xls-r-300m-turkish-colab | 87f5772ae326bd17885cdbc29090dcd3cafaa70f | 2022-06-13T11:18:47.000Z | [
"pytorch",
"tensorboard",
"wav2vec2",
"automatic-speech-recognition",
"dataset:common_voice",
"transformers",
"generated_from_trainer",
"license:apache-2.0",
"model-index"
] | automatic-speech-recognition | false | Priya9 | null | Priya9/wav2vec2-large-xls-r-300m-turkish-colab | 2 | null | transformers | 26,311 | ---
license: apache-2.0
tags:
- generated_from_trainer
datasets:
- common_voice
model-index:
- name: wav2vec2-large-xls-r-300m-turkish-colab
results: []
---
<!-- This model card has been generated automatically according to the information the Trainer had access to. You
should probably proofread and complete it, then remove this comment. -->
# wav2vec2-large-xls-r-300m-turkish-colab
This model is a fine-tuned version of [facebook/wav2vec2-xls-r-300m](https://huggingface.co/facebook/wav2vec2-xls-r-300m) on the common_voice dataset.
It achieves the following results on the evaluation set:
- Loss: 0.3859
- Wer: 0.4680
## Model description
More information needed
## Intended uses & limitations
More information needed
## Training and evaluation data
More information needed
## Training procedure
### Training hyperparameters
The following hyperparameters were used during training:
- learning_rate: 0.0003
- train_batch_size: 16
- eval_batch_size: 8
- seed: 42
- gradient_accumulation_steps: 2
- total_train_batch_size: 32
- optimizer: Adam with betas=(0.9,0.999) and epsilon=1e-08
- lr_scheduler_type: linear
- lr_scheduler_warmup_steps: 500
- num_epochs: 10
- mixed_precision_training: Native AMP
### Training results
| Training Loss | Epoch | Step | Validation Loss | Wer |
|:-------------:|:-----:|:----:|:---------------:|:------:|
| 3.8707 | 3.67 | 400 | 0.6588 | 0.7110 |
| 0.3955 | 7.34 | 800 | 0.3859 | 0.4680 |
### Framework versions
- Transformers 4.11.3
- Pytorch 1.10.0+cu113
- Datasets 1.18.3
- Tokenizers 0.10.3
|
PSW/samsum_reverse_train_distilbart_xsum_9-6_min10max2000_topp0.6_topk50_epoch3 | 9a0c73f83bb8a2c009e2982e06d2a701b7f87a5f | 2022-06-13T05:06:07.000Z | [
"pytorch",
"bart",
"text2text-generation",
"transformers",
"autotrain_compatible"
] | text2text-generation | false | PSW | null | PSW/samsum_reverse_train_distilbart_xsum_9-6_min10max2000_topp0.6_topk50_epoch3 | 2 | null | transformers | 26,312 | Entry not found |
PSW/samsum_reverse_train_distilbart_xsum_9-6_min10max2000_topp0.6_topk40_epoch3 | cd1b6cb31af462684dbb7ed6ab30428c915f1406 | 2022-06-13T06:36:10.000Z | [
"pytorch",
"bart",
"text2text-generation",
"transformers",
"autotrain_compatible"
] | text2text-generation | false | PSW | null | PSW/samsum_reverse_train_distilbart_xsum_9-6_min10max2000_topp0.6_topk40_epoch3 | 2 | null | transformers | 26,313 | Entry not found |
sasuke/bert-base-uncased-finetuned-sst2-finetuned-sst2 | a7d20bceee614abc735e0a64163e76c3634ad1f7 | 2022-06-13T09:25:58.000Z | [
"pytorch",
"tensorboard",
"bert",
"text-classification",
"transformers"
] | text-classification | false | sasuke | null | sasuke/bert-base-uncased-finetuned-sst2-finetuned-sst2 | 2 | null | transformers | 26,314 | Entry not found |
Marscen/distilbert-base-uncased-finetuned-squad | 5751e3bbe747333aed01b904a3b24ffa1184f7d5 | 2022-06-14T09:21:08.000Z | [
"pytorch",
"tensorboard",
"distilbert",
"question-answering",
"dataset:squad_v2",
"transformers",
"generated_from_trainer",
"license:apache-2.0",
"model-index",
"autotrain_compatible"
] | question-answering | false | Marscen | null | Marscen/distilbert-base-uncased-finetuned-squad | 2 | null | transformers | 26,315 | ---
license: apache-2.0
tags:
- generated_from_trainer
datasets:
- squad_v2
model-index:
- name: distilbert-base-uncased-finetuned-squad
results: []
---
<!-- This model card has been generated automatically according to the information the Trainer had access to. You
should probably proofread and complete it, then remove this comment. -->
# distilbert-base-uncased-finetuned-squad
This model is a fine-tuned version of [distilbert-base-uncased](https://huggingface.co/distilbert-base-uncased) on the squad_v2 dataset.
It achieves the following results on the evaluation set:
- Loss: 1.4052
## Model description
More information needed
## Intended uses & limitations
More information needed
## Training and evaluation data
More information needed
## Training procedure
### Training hyperparameters
The following hyperparameters were used during training:
- learning_rate: 2e-05
- train_batch_size: 16
- eval_batch_size: 16
- seed: 42
- optimizer: Adam with betas=(0.9,0.999) and epsilon=1e-08
- lr_scheduler_type: linear
- num_epochs: 3
### Training results
| Training Loss | Epoch | Step | Validation Loss |
|:-------------:|:-----:|:-----:|:---------------:|
| 1.2178 | 1.0 | 8235 | 1.1827 |
| 0.9355 | 2.0 | 16470 | 1.3283 |
| 0.761 | 3.0 | 24705 | 1.4052 |
### Framework versions
- Transformers 4.19.4
- Pytorch 1.8.1+cu111
- Datasets 2.2.2
- Tokenizers 0.12.1
|
tayyaba/autotrain-pan-977432399 | 824cbbbd8f315848ebcbb0a75deb83e5e66c61ce | 2022-06-13T10:13:31.000Z | [
"pytorch",
"roberta",
"text-classification",
"en",
"dataset:tayyaba/autotrain-data-pan",
"transformers",
"autotrain",
"co2_eq_emissions"
] | text-classification | false | tayyaba | null | tayyaba/autotrain-pan-977432399 | 2 | null | transformers | 26,316 | ---
tags: autotrain
language: en
widget:
- text: "I love AutoTrain 🤗"
datasets:
- tayyaba/autotrain-data-pan
co2_eq_emissions: 27.081173251466467
---
# Model Trained Using AutoTrain
- Problem type: Binary Classification
- Model ID: 977432399
- CO2 Emissions (in grams): 27.081173251466467
## Validation Metrics
- Loss: 0.277687668800354
- Accuracy: 0.8841666666666667
- Precision: 0.9185918591859186
- Recall: 0.9277777777777778
- AUC: 0.9422805555555556
- F1: 0.9231619679380874
## Usage
You can use cURL to access this model:
```
$ curl -X POST -H "Authorization: Bearer YOUR_API_KEY" -H "Content-Type: application/json" -d '{"inputs": "I love AutoTrain"}' https://api-inference.huggingface.co/models/tayyaba/autotrain-pan-977432399
```
Or Python API:
```
from transformers import AutoModelForSequenceClassification, AutoTokenizer
model = AutoModelForSequenceClassification.from_pretrained("tayyaba/autotrain-pan-977432399", use_auth_token=True)
tokenizer = AutoTokenizer.from_pretrained("tayyaba/autotrain-pan-977432399", use_auth_token=True)
inputs = tokenizer("I love AutoTrain", return_tensors="pt")
outputs = model(**inputs)
``` |
84rry/84rry-xls-r-300M-AR-improved | 2f264c17e9f2fe306a5d2a60973487f67ad78959 | 2022-06-14T00:12:14.000Z | [
"pytorch",
"wav2vec2",
"automatic-speech-recognition",
"transformers"
] | automatic-speech-recognition | false | 84rry | null | 84rry/84rry-xls-r-300M-AR-improved | 2 | null | transformers | 26,317 | Entry not found |
LDD/bert_wwm_new_ext | 5b49ed05da4242422f013f8e2692e76676fdab49 | 2022-06-14T05:30:44.000Z | [
"pytorch",
"bert",
"feature-extraction",
"transformers"
] | feature-extraction | false | LDD | null | LDD/bert_wwm_new_ext | 2 | null | transformers | 26,318 | 在LDD/wwm的基础上进行新闻语料库的增量预训练 |
kazed/AraBART-finetuned-xlsum-arabic | f529516b9e01f06d13d133953ca2c30a13426fbb | 2022-06-14T09:35:58.000Z | [
"pytorch",
"tensorboard",
"mbart",
"text2text-generation",
"transformers",
"autotrain_compatible"
] | text2text-generation | false | kazed | null | kazed/AraBART-finetuned-xlsum-arabic | 2 | null | transformers | 26,319 | Entry not found |
jcrbsa/pt-gpt2vit | 2e54776a1fffbea523c735a230a726ed8e0eea17 | 2022-06-17T08:36:13.000Z | [
"pytorch",
"jax",
"vision-encoder-decoder",
"pt",
"transformers"
] | null | false | jcrbsa | null | jcrbsa/pt-gpt2vit | 2 | 1 | transformers | 26,320 | ---
language:
- pt
---
Image Captioning in Portuguese trained with ViT and GPT2
[DEMO](https://huggingface.co/spaces/adalbertojunior/image_captioning_portuguese)
Research supported with Cloud TPUs from Google's TPU Research Cloud (TRC) |
zdreiosis/ff_analysis_4 | 4da009843d31deb0dadeb2bc1dabb72aa98c66bf | 2022-06-14T09:44:05.000Z | [
"pytorch",
"tensorboard",
"bert",
"text-classification",
"transformers",
"gen_ffa",
"generated_from_trainer",
"license:apache-2.0",
"model-index"
] | text-classification | false | zdreiosis | null | zdreiosis/ff_analysis_4 | 2 | null | transformers | 26,321 | ---
license: apache-2.0
tags:
- gen_ffa
- generated_from_trainer
metrics:
- f1
- accuracy
model-index:
- name: ff_analysis_4
results: []
---
<!-- This model card has been generated automatically according to the information the Trainer had access to. You
should probably proofread and complete it, then remove this comment. -->
# ff_analysis_4
This model is a fine-tuned version of [zdreiosis/ff_analysis_4](https://huggingface.co/zdreiosis/ff_analysis_4) on the None dataset.
It achieves the following results on the evaluation set:
- Loss: 0.0022
- F1: 1.0
- Roc Auc: 1.0
- Accuracy: 1.0
## Model description
More information needed
## Intended uses & limitations
More information needed
## Training and evaluation data
More information needed
## Training procedure
### Training hyperparameters
The following hyperparameters were used during training:
- learning_rate: 2e-05
- train_batch_size: 32
- eval_batch_size: 32
- seed: 42
- optimizer: Adam with betas=(0.9,0.999) and epsilon=1e-08
- lr_scheduler_type: linear
- num_epochs: 30
### Training results
| Training Loss | Epoch | Step | Validation Loss | F1 | Roc Auc | Accuracy |
|:-------------:|:-----:|:----:|:---------------:|:---:|:-------:|:--------:|
| No log | 1.47 | 50 | 0.0055 | 1.0 | 1.0 | 1.0 |
| No log | 2.94 | 100 | 0.0052 | 1.0 | 1.0 | 1.0 |
| No log | 4.41 | 150 | 0.0044 | 1.0 | 1.0 | 1.0 |
| No log | 5.88 | 200 | 0.0037 | 1.0 | 1.0 | 1.0 |
| No log | 7.35 | 250 | 0.0030 | 1.0 | 1.0 | 1.0 |
| No log | 8.82 | 300 | 0.0030 | 1.0 | 1.0 | 1.0 |
| No log | 10.29 | 350 | 0.0028 | 1.0 | 1.0 | 1.0 |
| No log | 11.76 | 400 | 0.0027 | 1.0 | 1.0 | 1.0 |
| No log | 13.24 | 450 | 0.0025 | 1.0 | 1.0 | 1.0 |
| 0.0078 | 14.71 | 500 | 0.0022 | 1.0 | 1.0 | 1.0 |
| 0.0078 | 16.18 | 550 | 0.0025 | 1.0 | 1.0 | 1.0 |
| 0.0078 | 17.65 | 600 | 0.0023 | 1.0 | 1.0 | 1.0 |
| 0.0078 | 19.12 | 650 | 0.0022 | 1.0 | 1.0 | 1.0 |
| 0.0078 | 20.59 | 700 | 0.0022 | 1.0 | 1.0 | 1.0 |
| 0.0078 | 22.06 | 750 | 0.0021 | 1.0 | 1.0 | 1.0 |
| 0.0078 | 23.53 | 800 | 0.0020 | 1.0 | 1.0 | 1.0 |
| 0.0078 | 25.0 | 850 | 0.0020 | 1.0 | 1.0 | 1.0 |
| 0.0078 | 26.47 | 900 | 0.0019 | 1.0 | 1.0 | 1.0 |
| 0.0078 | 27.94 | 950 | 0.0019 | 1.0 | 1.0 | 1.0 |
| 0.0025 | 29.41 | 1000 | 0.0019 | 1.0 | 1.0 | 1.0 |
### Framework versions
- Transformers 4.15.0
- Pytorch 1.11.0+cu113
- Datasets 2.2.2
- Tokenizers 0.10.3
|
jkhan447/sarcasm-detection-RoBerta-base-POS | f418c5fe78ccda8a991e22c6e9afbaabada0f148 | 2022-06-14T09:55:14.000Z | [
"pytorch",
"tensorboard",
"roberta",
"text-classification",
"transformers",
"generated_from_trainer",
"license:mit",
"model-index"
] | text-classification | false | jkhan447 | null | jkhan447/sarcasm-detection-RoBerta-base-POS | 2 | null | transformers | 26,322 | ---
license: mit
tags:
- generated_from_trainer
metrics:
- accuracy
model-index:
- name: sarcasm-detection-RoBerta-base-POS
results: []
---
<!-- This model card has been generated automatically according to the information the Trainer had access to. You
should probably proofread and complete it, then remove this comment. -->
# sarcasm-detection-RoBerta-base-POS
This model is a fine-tuned version of [roberta-base](https://huggingface.co/roberta-base) on the None dataset.
It achieves the following results on the evaluation set:
- Loss: 0.6651
- Accuracy: 0.607
## Model description
More information needed
## Intended uses & limitations
More information needed
## Training and evaluation data
More information needed
## Training procedure
### Training hyperparameters
The following hyperparameters were used during training:
- learning_rate: 2e-05
- train_batch_size: 16
- eval_batch_size: 16
- seed: 42
- optimizer: Adam with betas=(0.9,0.999) and epsilon=1e-08
- lr_scheduler_type: linear
- num_epochs: 50
### Training results
### Framework versions
- Transformers 4.19.4
- Pytorch 1.11.0+cu113
- Datasets 2.2.2
- Tokenizers 0.12.1
|
Priya9/wav2vec2-large-xls-r-300m-tamil-colab | ff21f3e46e680fe3370414acbbd07819ada83cc6 | 2022-06-14T16:51:10.000Z | [
"pytorch",
"tensorboard",
"wav2vec2",
"automatic-speech-recognition",
"dataset:common_voice",
"transformers",
"generated_from_trainer",
"license:apache-2.0",
"model-index"
] | automatic-speech-recognition | false | Priya9 | null | Priya9/wav2vec2-large-xls-r-300m-tamil-colab | 2 | null | transformers | 26,323 | ---
license: apache-2.0
tags:
- generated_from_trainer
datasets:
- common_voice
model-index:
- name: wav2vec2-large-xls-r-300m-tamil-colab
results: []
---
<!-- This model card has been generated automatically according to the information the Trainer had access to. You
should probably proofread and complete it, then remove this comment. -->
# wav2vec2-large-xls-r-300m-tamil-colab
This model is a fine-tuned version of [facebook/wav2vec2-xls-r-300m](https://huggingface.co/facebook/wav2vec2-xls-r-300m) on the common_voice dataset.
It achieves the following results on the evaluation set:
- Loss: 0.5869
- Wer: 0.7266
## Model description
More information needed
## Intended uses & limitations
More information needed
## Training and evaluation data
More information needed
## Training procedure
### Training hyperparameters
The following hyperparameters were used during training:
- learning_rate: 0.0003
- train_batch_size: 16
- eval_batch_size: 8
- seed: 42
- gradient_accumulation_steps: 2
- total_train_batch_size: 32
- optimizer: Adam with betas=(0.9,0.999) and epsilon=1e-08
- lr_scheduler_type: linear
- lr_scheduler_warmup_steps: 500
- num_epochs: 10
- mixed_precision_training: Native AMP
### Training results
| Training Loss | Epoch | Step | Validation Loss | Wer |
|:-------------:|:-----:|:----:|:---------------:|:------:|
| 5.2913 | 3.39 | 400 | 1.0961 | 0.9474 |
| 0.5857 | 6.78 | 800 | 0.5869 | 0.7266 |
### Framework versions
- Transformers 4.11.3
- Pytorch 1.10.0+cu113
- Datasets 1.18.3
- Tokenizers 0.10.3
|
miemBertProject/miem-scibert-linguistic | bc79b5407baaa62f854306edb9e8700f95934109 | 2022-06-14T13:53:15.000Z | [
"pytorch",
"bert",
"fill-mask",
"transformers",
"autotrain_compatible"
] | fill-mask | false | miemBertProject | null | miemBertProject/miem-scibert-linguistic | 2 | null | transformers | 26,324 | Entry not found |
emergix/distilbert-base-uncased-finetuned-imdb | 800a3662e7c435fd26150a7c49c36b39d4fbfdf3 | 2022-06-15T09:27:01.000Z | [
"pytorch",
"tensorboard",
"distilbert",
"fill-mask",
"dataset:imdb",
"transformers",
"generated_from_trainer",
"license:apache-2.0",
"model-index",
"autotrain_compatible"
] | fill-mask | false | emergix | null | emergix/distilbert-base-uncased-finetuned-imdb | 2 | null | transformers | 26,325 | ---
license: apache-2.0
tags:
- generated_from_trainer
datasets:
- imdb
model-index:
- name: distilbert-base-uncased-finetuned-imdb
results: []
---
<!-- This model card has been generated automatically according to the information the Trainer had access to. You
should probably proofread and complete it, then remove this comment. -->
# distilbert-base-uncased-finetuned-imdb
This model is a fine-tuned version of [distilbert-base-uncased](https://huggingface.co/distilbert-base-uncased) on the imdb dataset.
It achieves the following results on the evaluation set:
- Loss: 2.4626
## Model description
More information needed
## Intended uses & limitations
More information needed
## Training and evaluation data
More information needed
## Training procedure
### Training hyperparameters
The following hyperparameters were used during training:
- learning_rate: 2e-05
- train_batch_size: 64
- eval_batch_size: 64
- seed: 42
- optimizer: Adam with betas=(0.9,0.999) and epsilon=1e-08
- lr_scheduler_type: linear
- num_epochs: 3.0
### Training results
| Training Loss | Epoch | Step | Validation Loss |
|:-------------:|:-----:|:----:|:---------------:|
| 2.6963 | 1.0 | 157 | 2.5091 |
| 2.5737 | 2.0 | 314 | 2.4515 |
| 2.5496 | 3.0 | 471 | 2.3946 |
### Framework versions
- Transformers 4.19.4
- Pytorch 1.13.0.dev20220609
- Datasets 2.2.1
- Tokenizers 0.11.6
|
Sebabrata/lmv2-g-aadhaar-236doc-06-14 | a56329876a97d6c03ad59c82fcf40e12d6188e15 | 2022-06-14T15:12:43.000Z | [
"pytorch",
"tensorboard",
"layoutlmv2",
"token-classification",
"transformers",
"generated_from_trainer",
"license:cc-by-nc-sa-4.0",
"model-index",
"autotrain_compatible"
] | token-classification | false | Sebabrata | null | Sebabrata/lmv2-g-aadhaar-236doc-06-14 | 2 | null | transformers | 26,326 | ---
license: cc-by-nc-sa-4.0
tags:
- generated_from_trainer
model-index:
- name: lmv2-g-aadhaar-236doc-06-14
results: []
---
<!-- This model card has been generated automatically according to the information the Trainer had access to. You
should probably proofread and complete it, then remove this comment. -->
# lmv2-g-aadhaar-236doc-06-14
This model is a fine-tuned version of [microsoft/layoutlmv2-base-uncased](https://huggingface.co/microsoft/layoutlmv2-base-uncased) on the None dataset.
It achieves the following results on the evaluation set:
- Loss: 0.0427
- Aadhaar Precision: 0.9783
- Aadhaar Recall: 1.0
- Aadhaar F1: 0.9890
- Aadhaar Number: 45
- Dob Precision: 0.9787
- Dob Recall: 1.0
- Dob F1: 0.9892
- Dob Number: 46
- Gender Precision: 1.0
- Gender Recall: 0.9787
- Gender F1: 0.9892
- Gender Number: 47
- Name Precision: 0.9574
- Name Recall: 0.9375
- Name F1: 0.9474
- Name Number: 48
- Overall Precision: 0.9785
- Overall Recall: 0.9785
- Overall F1: 0.9785
- Overall Accuracy: 0.9939
## Model description
More information needed
## Intended uses & limitations
More information needed
## Training and evaluation data
More information needed
## Training procedure
### Training hyperparameters
The following hyperparameters were used during training:
- learning_rate: 4e-05
- train_batch_size: 1
- eval_batch_size: 1
- seed: 42
- optimizer: Adam with betas=(0.9,0.999) and epsilon=1e-08
- lr_scheduler_type: constant
- num_epochs: 30
### Training results
| Training Loss | Epoch | Step | Validation Loss | Aadhaar Precision | Aadhaar Recall | Aadhaar F1 | Aadhaar Number | Dob Precision | Dob Recall | Dob F1 | Dob Number | Gender Precision | Gender Recall | Gender F1 | Gender Number | Name Precision | Name Recall | Name F1 | Name Number | Overall Precision | Overall Recall | Overall F1 | Overall Accuracy |
|:-------------:|:-----:|:----:|:---------------:|:-----------------:|:--------------:|:----------:|:--------------:|:-------------:|:----------:|:------:|:----------:|:----------------:|:-------------:|:---------:|:-------------:|:--------------:|:-----------:|:-------:|:-----------:|:-----------------:|:--------------:|:----------:|:----------------:|
| 1.0024 | 1.0 | 188 | 0.5819 | 0.9348 | 0.9556 | 0.9451 | 45 | 1.0 | 1.0 | 1.0 | 46 | 1.0 | 0.9574 | 0.9783 | 47 | 0.5172 | 0.625 | 0.5660 | 48 | 0.8410 | 0.8817 | 0.8609 | 0.9744 |
| 0.4484 | 2.0 | 376 | 0.3263 | 0.8980 | 0.9778 | 0.9362 | 45 | 1.0 | 1.0 | 1.0 | 46 | 1.0 | 0.9787 | 0.9892 | 47 | 0.6842 | 0.8125 | 0.7429 | 48 | 0.8838 | 0.9409 | 0.9115 | 0.9733 |
| 0.2508 | 3.0 | 564 | 0.2230 | 0.9318 | 0.9111 | 0.9213 | 45 | 1.0 | 1.0 | 1.0 | 46 | 1.0 | 0.9787 | 0.9892 | 47 | 0.8913 | 0.8542 | 0.8723 | 48 | 0.9560 | 0.9355 | 0.9457 | 0.9811 |
| 0.165 | 4.0 | 752 | 0.1728 | 0.9362 | 0.9778 | 0.9565 | 45 | 1.0 | 1.0 | 1.0 | 46 | 1.0 | 0.9787 | 0.9892 | 47 | 0.8444 | 0.7917 | 0.8172 | 48 | 0.9457 | 0.9355 | 0.9405 | 0.9844 |
| 0.1081 | 5.0 | 940 | 0.0987 | 0.8958 | 0.9556 | 0.9247 | 45 | 1.0 | 1.0 | 1.0 | 46 | 1.0 | 0.9787 | 0.9892 | 47 | 1.0 | 0.9167 | 0.9565 | 48 | 0.9728 | 0.9624 | 0.9676 | 0.9928 |
| 0.0834 | 6.0 | 1128 | 0.0984 | 0.8980 | 0.9778 | 0.9362 | 45 | 1.0 | 1.0 | 1.0 | 46 | 1.0 | 0.9574 | 0.9783 | 47 | 0.8148 | 0.9167 | 0.8627 | 48 | 0.9227 | 0.9624 | 0.9421 | 0.9833 |
| 0.0676 | 7.0 | 1316 | 0.0773 | 0.9362 | 0.9778 | 0.9565 | 45 | 1.0 | 1.0 | 1.0 | 46 | 1.0 | 0.9787 | 0.9892 | 47 | 0.9111 | 0.8542 | 0.8817 | 48 | 0.9620 | 0.9516 | 0.9568 | 0.9894 |
| 0.0572 | 8.0 | 1504 | 0.0786 | 0.8235 | 0.9333 | 0.8750 | 45 | 1.0 | 1.0 | 1.0 | 46 | 1.0 | 0.9787 | 0.9892 | 47 | 0.8936 | 0.875 | 0.8842 | 48 | 0.9263 | 0.9462 | 0.9362 | 0.9872 |
| 0.0481 | 9.0 | 1692 | 0.0576 | 0.9375 | 1.0 | 0.9677 | 45 | 1.0 | 1.0 | 1.0 | 46 | 1.0 | 0.9787 | 0.9892 | 47 | 0.9362 | 0.9167 | 0.9263 | 48 | 0.9679 | 0.9731 | 0.9705 | 0.99 |
| 0.0349 | 10.0 | 1880 | 0.0610 | 0.9574 | 1.0 | 0.9783 | 45 | 1.0 | 1.0 | 1.0 | 46 | 1.0 | 0.9787 | 0.9892 | 47 | 0.8958 | 0.8958 | 0.8958 | 48 | 0.9626 | 0.9677 | 0.9651 | 0.9894 |
| 0.0287 | 11.0 | 2068 | 0.0978 | 0.9091 | 0.8889 | 0.8989 | 45 | 1.0 | 1.0 | 1.0 | 46 | 1.0 | 0.9787 | 0.9892 | 47 | 0.9348 | 0.8958 | 0.9149 | 48 | 0.9615 | 0.9409 | 0.9511 | 0.985 |
| 0.0297 | 12.0 | 2256 | 0.0993 | 0.9375 | 1.0 | 0.9677 | 45 | 1.0 | 1.0 | 1.0 | 46 | 1.0 | 0.9787 | 0.9892 | 47 | 0.7959 | 0.8125 | 0.8041 | 48 | 0.9312 | 0.9462 | 0.9387 | 0.9833 |
| 0.0395 | 13.0 | 2444 | 0.0824 | 0.9362 | 0.9778 | 0.9565 | 45 | 1.0 | 1.0 | 1.0 | 46 | 1.0 | 0.9787 | 0.9892 | 47 | 0.875 | 0.875 | 0.875 | 48 | 0.9519 | 0.9570 | 0.9544 | 0.9872 |
| 0.0333 | 14.0 | 2632 | 0.0788 | 0.8913 | 0.9111 | 0.9011 | 45 | 1.0 | 1.0 | 1.0 | 46 | 1.0 | 0.9787 | 0.9892 | 47 | 0.9556 | 0.8958 | 0.9247 | 48 | 0.9617 | 0.9462 | 0.9539 | 0.9867 |
| 0.0356 | 15.0 | 2820 | 0.0808 | 0.84 | 0.9333 | 0.8842 | 45 | 1.0 | 1.0 | 1.0 | 46 | 1.0 | 0.9787 | 0.9892 | 47 | 0.9565 | 0.9167 | 0.9362 | 48 | 0.9468 | 0.9570 | 0.9519 | 0.9867 |
| 0.0192 | 16.0 | 3008 | 0.0955 | 0.8462 | 0.9778 | 0.9072 | 45 | 0.9787 | 1.0 | 0.9892 | 46 | 0.9583 | 0.9787 | 0.9684 | 47 | 0.9070 | 0.8125 | 0.8571 | 48 | 0.9211 | 0.9409 | 0.9309 | 0.9822 |
| 0.016 | 17.0 | 3196 | 0.0936 | 0.9130 | 0.9333 | 0.9231 | 45 | 1.0 | 1.0 | 1.0 | 46 | 1.0 | 0.9787 | 0.9892 | 47 | 0.9318 | 0.8542 | 0.8913 | 48 | 0.9615 | 0.9409 | 0.9511 | 0.9867 |
| 0.0218 | 18.0 | 3384 | 0.1009 | 0.9545 | 0.9333 | 0.9438 | 45 | 1.0 | 1.0 | 1.0 | 46 | 1.0 | 0.9787 | 0.9892 | 47 | 0.8571 | 0.875 | 0.8660 | 48 | 0.9514 | 0.9462 | 0.9488 | 0.9844 |
| 0.0165 | 19.0 | 3572 | 0.0517 | 0.9574 | 1.0 | 0.9783 | 45 | 1.0 | 1.0 | 1.0 | 46 | 1.0 | 0.9787 | 0.9892 | 47 | 0.9333 | 0.875 | 0.9032 | 48 | 0.9728 | 0.9624 | 0.9676 | 0.9906 |
| 0.0198 | 20.0 | 3760 | 0.0890 | 0.9167 | 0.9778 | 0.9462 | 45 | 1.0 | 1.0 | 1.0 | 46 | 1.0 | 0.9787 | 0.9892 | 47 | 0.9149 | 0.8958 | 0.9053 | 48 | 0.9572 | 0.9624 | 0.9598 | 0.9867 |
| 0.0077 | 21.0 | 3948 | 0.0835 | 0.9574 | 1.0 | 0.9783 | 45 | 1.0 | 1.0 | 1.0 | 46 | 1.0 | 0.9787 | 0.9892 | 47 | 0.88 | 0.9167 | 0.8980 | 48 | 0.9577 | 0.9731 | 0.9653 | 0.9872 |
| 0.0088 | 22.0 | 4136 | 0.0427 | 0.9783 | 1.0 | 0.9890 | 45 | 0.9787 | 1.0 | 0.9892 | 46 | 1.0 | 0.9787 | 0.9892 | 47 | 0.9574 | 0.9375 | 0.9474 | 48 | 0.9785 | 0.9785 | 0.9785 | 0.9939 |
| 0.0078 | 23.0 | 4324 | 0.0597 | 0.9574 | 1.0 | 0.9783 | 45 | 1.0 | 1.0 | 1.0 | 46 | 1.0 | 0.9787 | 0.9892 | 47 | 0.8654 | 0.9375 | 0.9 | 48 | 0.9529 | 0.9785 | 0.9655 | 0.9889 |
| 0.0178 | 24.0 | 4512 | 0.0524 | 0.9574 | 1.0 | 0.9783 | 45 | 1.0 | 1.0 | 1.0 | 46 | 1.0 | 0.9787 | 0.9892 | 47 | 1.0 | 0.875 | 0.9333 | 48 | 0.9890 | 0.9624 | 0.9755 | 0.9922 |
| 0.012 | 25.0 | 4700 | 0.0637 | 0.9375 | 1.0 | 0.9677 | 45 | 1.0 | 1.0 | 1.0 | 46 | 1.0 | 0.9787 | 0.9892 | 47 | 0.8491 | 0.9375 | 0.8911 | 48 | 0.9430 | 0.9785 | 0.9604 | 0.9867 |
| 0.0135 | 26.0 | 4888 | 0.0668 | 0.9184 | 1.0 | 0.9574 | 45 | 1.0 | 1.0 | 1.0 | 46 | 1.0 | 0.9787 | 0.9892 | 47 | 0.86 | 0.8958 | 0.8776 | 48 | 0.9424 | 0.9677 | 0.9549 | 0.9867 |
| 0.0123 | 27.0 | 5076 | 0.0713 | 0.9565 | 0.9778 | 0.9670 | 45 | 1.0 | 1.0 | 1.0 | 46 | 1.0 | 0.9787 | 0.9892 | 47 | 0.9375 | 0.9375 | 0.9375 | 48 | 0.9731 | 0.9731 | 0.9731 | 0.9911 |
| 0.0074 | 28.0 | 5264 | 0.0675 | 0.9362 | 0.9778 | 0.9565 | 45 | 1.0 | 1.0 | 1.0 | 46 | 1.0 | 0.9787 | 0.9892 | 47 | 0.9 | 0.9375 | 0.9184 | 48 | 0.9577 | 0.9731 | 0.9653 | 0.99 |
| 0.0051 | 29.0 | 5452 | 0.0713 | 0.9362 | 0.9778 | 0.9565 | 45 | 1.0 | 1.0 | 1.0 | 46 | 1.0 | 0.9787 | 0.9892 | 47 | 0.9167 | 0.9167 | 0.9167 | 48 | 0.9626 | 0.9677 | 0.9651 | 0.9906 |
| 0.0027 | 30.0 | 5640 | 0.0725 | 0.9362 | 0.9778 | 0.9565 | 45 | 1.0 | 1.0 | 1.0 | 46 | 1.0 | 0.9787 | 0.9892 | 47 | 0.9167 | 0.9167 | 0.9167 | 48 | 0.9626 | 0.9677 | 0.9651 | 0.9906 |
### Framework versions
- Transformers 4.20.0.dev0
- Pytorch 1.11.0+cu113
- Datasets 2.2.2
- Tokenizers 0.12.1
|
LDD/bert_from_scratch_wwm_new | 51f1b8ef0e2c7e0ea88b0fb78b48abf957c61fd8 | 2022-06-14T14:32:41.000Z | [
"pytorch",
"bert",
"feature-extraction",
"transformers"
] | feature-extraction | false | LDD | null | LDD/bert_from_scratch_wwm_new | 2 | null | transformers | 26,327 | 用新闻数据集从头开始进行全词mask预训练bert |
plncmm/bert-clinical-scratch-wl-es | e9314ad921431b0c0b69c84149ce3dfe5810b324 | 2022-06-20T13:44:24.000Z | [
"pytorch",
"bert",
"fill-mask",
"transformers",
"generated_from_trainer",
"model-index",
"autotrain_compatible"
] | fill-mask | false | plncmm | null | plncmm/bert-clinical-scratch-wl-es | 2 | null | transformers | 26,328 | ---
tags:
- generated_from_trainer
model-index:
- name: bert-clincal-scratch-wl-es
results: []
---
<!-- This model card has been generated automatically according to the information the Trainer had access to. You
should probably proofread and complete it, then remove this comment. -->
# bert-clincal-scratch-wl-es
This model is a fine-tuned version of [dccuchile/bert-base-spanish-wwm-uncased](https://huggingface.co/dccuchile/bert-base-spanish-wwm-uncased) on an unknown dataset.
## Model description
More information needed
## Intended uses & limitations
More information needed
## Training and evaluation data
More information needed
## Training procedure
### Training hyperparameters
The following hyperparameters were used during training:
- learning_rate: 5e-05
- train_batch_size: 32
- eval_batch_size: 16
- seed: 42
- optimizer: Adam with betas=(0.9,0.999) and epsilon=1e-08
- lr_scheduler_type: linear
- num_epochs: 10.0
### Training results
### Framework versions
- Transformers 4.20.0.dev0
- Pytorch 1.11.0+cu113
- Datasets 2.2.1
- Tokenizers 0.12.1
|
Marscen/roberta-base-squad2-finetuned-squad2 | 58731f568177b37ca24fb27d5dbec4f5104652d4 | 2022-06-15T16:15:26.000Z | [
"pytorch",
"tensorboard",
"roberta",
"question-answering",
"dataset:squad_v2",
"transformers",
"generated_from_trainer",
"license:cc-by-4.0",
"model-index",
"autotrain_compatible"
] | question-answering | false | Marscen | null | Marscen/roberta-base-squad2-finetuned-squad2 | 2 | null | transformers | 26,329 | ---
license: cc-by-4.0
tags:
- generated_from_trainer
datasets:
- squad_v2
model-index:
- name: roberta-base-squad2-finetuned-squad2
results: []
---
<!-- This model card has been generated automatically according to the information the Trainer had access to. You
should probably proofread and complete it, then remove this comment. -->
# roberta-base-squad2-finetuned-squad2
This model is a fine-tuned version of [deepset/roberta-base-squad2](https://huggingface.co/deepset/roberta-base-squad2) on the squad_v2 dataset.
It achieves the following results on the evaluation set:
- Loss: 0.8815
## Model description
More information needed
## Intended uses & limitations
More information needed
## Training and evaluation data
More information needed
## Training procedure
### Training hyperparameters
The following hyperparameters were used during training:
- learning_rate: 3e-05
- train_batch_size: 8
- eval_batch_size: 8
- seed: 42
- optimizer: Adam with betas=(0.9,0.999) and epsilon=1e-08
- lr_scheduler_type: linear
- num_epochs: 1
### Training results
| Training Loss | Epoch | Step | Validation Loss |
|:-------------:|:-----:|:-----:|:---------------:|
| 0.6979 | 1.0 | 16478 | 0.8815 |
### Framework versions
- Transformers 4.19.4
- Pytorch 1.8.1+cu111
- Datasets 2.2.2
- Tokenizers 0.12.1
|
emergix/distilbert-base-uncased-finetuned-imdb-accelerate | 11b4f92d0b243c510597395f7722c36767b22d7f | 2022-06-15T10:00:48.000Z | [
"pytorch",
"distilbert",
"fill-mask",
"transformers",
"autotrain_compatible"
] | fill-mask | false | emergix | null | emergix/distilbert-base-uncased-finetuned-imdb-accelerate | 2 | null | transformers | 26,330 | Entry not found |
kravchenko/uk-mt5-small-gec-tokenized | 41d25f62dd1c1c3ad2f8ad355bf7b554245c50c6 | 2022-06-14T20:14:26.000Z | [
"pytorch",
"mt5",
"text2text-generation",
"transformers",
"autotrain_compatible"
] | text2text-generation | false | kravchenko | null | kravchenko/uk-mt5-small-gec-tokenized | 2 | null | transformers | 26,331 | Entry not found |
lmqg/t5-base-squadshifts-reddit | f9a4166f40a15c47ad356efcbdbaeb9e64b3f200 | 2022-06-15T00:06:10.000Z | [
"pytorch",
"t5",
"text2text-generation",
"transformers",
"autotrain_compatible"
] | text2text-generation | false | lmqg | null | lmqg/t5-base-squadshifts-reddit | 2 | null | transformers | 26,332 | Entry not found |
shoubhik/TrOCR_finetune | 70bc939d4bc958bfc9d28fb2de64188409ea2e99 | 2022-06-16T11:14:26.000Z | [
"pytorch",
"vision-encoder-decoder",
"transformers"
] | null | false | shoubhik | null | shoubhik/TrOCR_finetune | 2 | null | transformers | 26,333 | Entry not found |
jkhan447/sarcasm-detection-Bert-base-uncased-POS | 2672864c80e36172ca74fb430f398b815e610236 | 2022-06-15T07:17:36.000Z | [
"pytorch",
"tensorboard",
"bert",
"text-classification",
"transformers",
"generated_from_trainer",
"license:apache-2.0",
"model-index"
] | text-classification | false | jkhan447 | null | jkhan447/sarcasm-detection-Bert-base-uncased-POS | 2 | null | transformers | 26,334 | ---
license: apache-2.0
tags:
- generated_from_trainer
metrics:
- accuracy
model-index:
- name: sarcasm-detection-Bert-base-uncased-POS
results: []
---
<!-- This model card has been generated automatically according to the information the Trainer had access to. You
should probably proofread and complete it, then remove this comment. -->
# sarcasm-detection-Bert-base-uncased-POS
This model is a fine-tuned version of [bert-base-uncased](https://huggingface.co/bert-base-uncased) on the None dataset.
It achieves the following results on the evaluation set:
- Loss: 3.1904
- Accuracy: 0.591
## Model description
More information needed
## Intended uses & limitations
More information needed
## Training and evaluation data
More information needed
## Training procedure
### Training hyperparameters
The following hyperparameters were used during training:
- learning_rate: 2e-05
- train_batch_size: 16
- eval_batch_size: 16
- seed: 42
- optimizer: Adam with betas=(0.9,0.999) and epsilon=1e-08
- lr_scheduler_type: linear
- num_epochs: 50
### Training results
### Framework versions
- Transformers 4.19.4
- Pytorch 1.11.0+cu113
- Datasets 2.3.0
- Tokenizers 0.12.1
|
totoro4007/cryptoroberta-base-all-finetuned | 5ab7e685b3d10d1bfee75a3114efba593f58cba5 | 2022-06-15T04:39:56.000Z | [
"pytorch",
"xlm-roberta",
"text-classification",
"transformers"
] | text-classification | false | totoro4007 | null | totoro4007/cryptoroberta-base-all-finetuned | 2 | null | transformers | 26,335 | Entry not found |
ApoTro/slovak-t5-small | 2dbcfc7b8b4d960193ff83a08004dfea9bce4bb7 | 2022-06-16T17:49:45.000Z | [
"pytorch",
"jax",
"t5",
"text2text-generation",
"sk",
"dataset:oscar",
"transformers",
"license:mit",
"autotrain_compatible"
] | text2text-generation | false | ApoTro | null | ApoTro/slovak-t5-small | 2 | null | transformers | 26,336 | ---
language: sk
license: mit
datasets:
- oscar
---
# SlovakT5-small
This model was trained on slightly adapted code from [run_t5_mlm_flax.py](https://github.com/huggingface/transformers/tree/main/examples/flax/language-modeling).
If you want to know about training details or evaluation results, see [SlovakT5_report.pdf](https://huggingface.co/ApoTro/slovak-t5-small/resolve/main/SlovakT5_report.pdf). For evaluation, you can also run [SlovakT5_eval.ipynb](https://colab.research.google.com/github/richardcepka/notebooks/blob/main/SlovakT5_eval.ipynb).
### How to use
SlovakT5-small can be fine-tuned for a lot of different downstream tasks. For example, NER:
```python
from transformers import AutoTokenizer, T5ForConditionalGeneration
tokenizer = AutoTokenizer.from_pretrained("ApoTro/slovak-t5-small")
model = T5ForConditionalGeneration.from_pretrained("ApoTro/slovak-t5-small")
input_ids = tokenizer("ner veta: Do druhého kola postúpili Robert Fico a Andrej Kiska s rozdielom 4,0%.", return_tensors="pt").input_ids
labels = tokenizer("per: Robert Fico | per: Andrej Kiska", return_tensors="pt").input_ids
# the forward function automatically creates the correct decoder_input_ids
loss = model(input_ids=input_ids, labels=labels).loss
loss.item()
``` |
erickfm/kind-sweep-3 | 2d51e3d31a6941d20795a67a5fad138089eb4c03 | 2022-06-15T06:27:07.000Z | [
"pytorch",
"t5",
"text2text-generation",
"transformers",
"autotrain_compatible"
] | text2text-generation | false | erickfm | null | erickfm/kind-sweep-3 | 2 | null | transformers | 26,337 | Entry not found |
erickfm/fast-sweep-2 | 12d431978bee6ea2e2557eeff55b653cd7a63a44 | 2022-06-15T06:28:02.000Z | [
"pytorch",
"t5",
"text2text-generation",
"transformers",
"autotrain_compatible"
] | text2text-generation | false | erickfm | null | erickfm/fast-sweep-2 | 2 | null | transformers | 26,338 | Entry not found |
chandrasutrisnotjhong/dummy-model | 9cd64d4145e2309e83f483cef0097979c042b6ac | 2022-06-15T06:34:47.000Z | [
"pytorch",
"camembert",
"fill-mask",
"transformers",
"autotrain_compatible"
] | fill-mask | false | chandrasutrisnotjhong | null | chandrasutrisnotjhong/dummy-model | 2 | null | transformers | 26,339 | Entry not found |
soyul/wav2vec2-base-finetuned-ks | f0068bf76ce3dec3027afce00720b4da2486472c | 2022-06-15T06:56:18.000Z | [
"pytorch",
"wav2vec2",
"audio-classification",
"transformers"
] | audio-classification | false | soyul | null | soyul/wav2vec2-base-finetuned-ks | 2 | null | transformers | 26,340 | Entry not found |
Vanmas/dummy-model | 2198bdee976a416f10a814005c0a45624f30cbad | 2022-06-15T07:25:27.000Z | [
"pytorch",
"camembert",
"fill-mask",
"transformers",
"autotrain_compatible"
] | fill-mask | false | Vanmas | null | Vanmas/dummy-model | 2 | null | transformers | 26,341 | Entry not found |
amartyobanerjee/marian-finetuned-kde4-en-to-fr | 9cd4a41e89c69e73c8536c29981da914a3e577e8 | 2022-06-15T11:45:17.000Z | [
"pytorch",
"tensorboard",
"marian",
"text2text-generation",
"dataset:kde4",
"transformers",
"translation",
"generated_from_trainer",
"license:apache-2.0",
"model-index",
"autotrain_compatible"
] | translation | false | amartyobanerjee | null | amartyobanerjee/marian-finetuned-kde4-en-to-fr | 2 | null | transformers | 26,342 | ---
license: apache-2.0
tags:
- translation
- generated_from_trainer
datasets:
- kde4
metrics:
- bleu
model-index:
- name: marian-finetuned-kde4-en-to-fr
results:
- task:
name: Sequence-to-sequence Language Modeling
type: text2text-generation
dataset:
name: kde4
type: kde4
args: en-fr
metrics:
- name: Bleu
type: bleu
value: 52.83242564204547
---
<!-- This model card has been generated automatically according to the information the Trainer had access to. You
should probably proofread and complete it, then remove this comment. -->
# marian-finetuned-kde4-en-to-fr
This model is a fine-tuned version of [Helsinki-NLP/opus-mt-en-fr](https://huggingface.co/Helsinki-NLP/opus-mt-en-fr) on the kde4 dataset.
It achieves the following results on the evaluation set:
- Loss: 0.8560
- Bleu: 52.8324
## Model description
More information needed
## Intended uses & limitations
More information needed
## Training and evaluation data
More information needed
## Training procedure
### Training hyperparameters
The following hyperparameters were used during training:
- learning_rate: 2e-05
- train_batch_size: 32
- eval_batch_size: 64
- seed: 42
- optimizer: Adam with betas=(0.9,0.999) and epsilon=1e-08
- lr_scheduler_type: linear
- num_epochs: 3
- mixed_precision_training: Native AMP
### Training results
### Framework versions
- Transformers 4.19.4
- Pytorch 1.11.0+cu113
- Datasets 2.3.0
- Tokenizers 0.12.1
|
tuni/xlm-roberta-large-xnli-finetuned-mnli | 73a99f47202dc12ab7ae5fe93ca8cddf31773d1a | 2022-06-15T21:46:28.000Z | [
"pytorch",
"tensorboard",
"xlm-roberta",
"text-classification",
"dataset:glue",
"transformers",
"generated_from_trainer",
"license:mit",
"model-index"
] | text-classification | false | tuni | null | tuni/xlm-roberta-large-xnli-finetuned-mnli | 2 | null | transformers | 26,343 | ---
license: mit
tags:
- generated_from_trainer
datasets:
- glue
metrics:
- accuracy
model-index:
- name: xlm-roberta-large-xnli-finetuned-mnli
results:
- task:
name: Text Classification
type: text-classification
dataset:
name: glue
type: glue
args: mnli
metrics:
- name: Accuracy
type: accuracy
value: 0.8548888888888889
---
<!-- This model card has been generated automatically according to the information the Trainer had access to. You
should probably proofread and complete it, then remove this comment. -->
# xlm-roberta-large-xnli-finetuned-mnli
This model is a fine-tuned version of [joeddav/xlm-roberta-large-xnli](https://huggingface.co/joeddav/xlm-roberta-large-xnli) on the glue dataset.
It achieves the following results on the evaluation set:
- Loss: 1.2542
- Accuracy: 0.8549
## Model description
More information needed
## Intended uses & limitations
More information needed
## Training and evaluation data
More information needed
## Training procedure
### Training hyperparameters
The following hyperparameters were used during training:
- learning_rate: 2e-05
- train_batch_size: 4
- eval_batch_size: 4
- seed: 42
- optimizer: Adam with betas=(0.9,0.999) and epsilon=1e-08
- lr_scheduler_type: linear
- num_epochs: 5
### Training results
| Training Loss | Epoch | Step | Validation Loss | Accuracy |
|:-------------:|:-----:|:-----:|:---------------:|:--------:|
| 0.7468 | 1.0 | 2250 | 0.8551 | 0.8348 |
| 0.567 | 2.0 | 4500 | 0.8935 | 0.8377 |
| 0.318 | 3.0 | 6750 | 0.9892 | 0.8492 |
| 0.1146 | 4.0 | 9000 | 1.2373 | 0.8446 |
| 0.0383 | 5.0 | 11250 | 1.2542 | 0.8549 |
### Framework versions
- Transformers 4.19.4
- Pytorch 1.11.0+cu113
- Datasets 2.3.0
- Tokenizers 0.12.1
|
big-kek/medium-korzh | 497099b935313612eae8ceb93817690def9ced93 | 2022-07-15T07:05:03.000Z | [
"pytorch",
"gpt2",
"text-generation",
"transformers"
] | text-generation | false | big-kek | null | big-kek/medium-korzh | 2 | null | transformers | 26,344 | Entry not found |
huggingtweets/asadabukhalil | e417f0e3673138760bed0e375a096b7f6ffcaab6 | 2022-06-15T14:51:26.000Z | [
"pytorch",
"gpt2",
"text-generation",
"en",
"transformers",
"huggingtweets"
] | text-generation | false | huggingtweets | null | huggingtweets/asadabukhalil | 2 | null | transformers | 26,345 | ---
language: en
thumbnail: http://www.huggingtweets.com/asadabukhalil/1655304601394/predictions.png
tags:
- huggingtweets
widget:
- text: "My dream is"
---
<div class="inline-flex flex-col" style="line-height: 1.5;">
<div class="flex">
<div
style="display:inherit; margin-left: 4px; margin-right: 4px; width: 92px; height:92px; border-radius: 50%; background-size: cover; background-image: url('https://pbs.twimg.com/profile_images/1057819958573297665/748muZdj_400x400.jpg')">
</div>
<div
style="display:none; margin-left: 4px; margin-right: 4px; width: 92px; height:92px; border-radius: 50%; background-size: cover; background-image: url('')">
</div>
<div
style="display:none; margin-left: 4px; margin-right: 4px; width: 92px; height:92px; border-radius: 50%; background-size: cover; background-image: url('')">
</div>
</div>
<div style="text-align: center; margin-top: 3px; font-size: 16px; font-weight: 800">🤖 AI BOT 🤖</div>
<div style="text-align: center; font-size: 16px; font-weight: 800">asad abukhalil أسعد أبو خليل</div>
<div style="text-align: center; font-size: 14px;">@asadabukhalil</div>
</div>
I was made with [huggingtweets](https://github.com/borisdayma/huggingtweets).
Create your own bot based on your favorite user with [the demo](https://colab.research.google.com/github/borisdayma/huggingtweets/blob/master/huggingtweets-demo.ipynb)!
## How does it work?
The model uses the following pipeline.
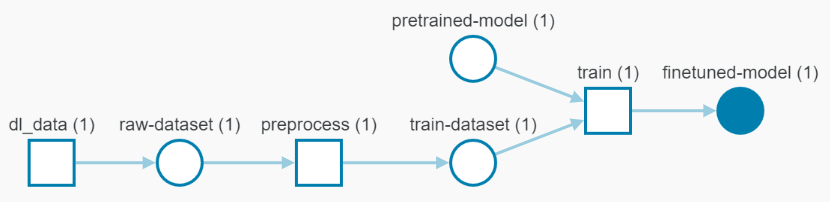
To understand how the model was developed, check the [W&B report](https://wandb.ai/wandb/huggingtweets/reports/HuggingTweets-Train-a-Model-to-Generate-Tweets--VmlldzoxMTY5MjI).
## Training data
The model was trained on tweets from asad abukhalil أسعد أبو خليل.
| Data | asad abukhalil أسعد أبو خليل |
| --- | --- |
| Tweets downloaded | 3231 |
| Retweets | 741 |
| Short tweets | 336 |
| Tweets kept | 2154 |
[Explore the data](https://wandb.ai/wandb/huggingtweets/runs/3py8vdcj/artifacts), which is tracked with [W&B artifacts](https://docs.wandb.com/artifacts) at every step of the pipeline.
## Training procedure
The model is based on a pre-trained [GPT-2](https://huggingface.co/gpt2) which is fine-tuned on @asadabukhalil's tweets.
Hyperparameters and metrics are recorded in the [W&B training run](https://wandb.ai/wandb/huggingtweets/runs/34eyno08) for full transparency and reproducibility.
At the end of training, [the final model](https://wandb.ai/wandb/huggingtweets/runs/34eyno08/artifacts) is logged and versioned.
## How to use
You can use this model directly with a pipeline for text generation:
```python
from transformers import pipeline
generator = pipeline('text-generation',
model='huggingtweets/asadabukhalil')
generator("My dream is", num_return_sequences=5)
```
## Limitations and bias
The model suffers from [the same limitations and bias as GPT-2](https://huggingface.co/gpt2#limitations-and-bias).
In addition, the data present in the user's tweets further affects the text generated by the model.
## About
*Built by Boris Dayma*
[](https://twitter.com/intent/follow?screen_name=borisdayma)
For more details, visit the project repository.
[](https://github.com/borisdayma/huggingtweets)
|
kcarnold/inquisitive1 | b9586946bd783e89f0b25b9b6e81a0c77f5a768d | 2022-06-15T14:54:36.000Z | [
"pytorch",
"bart",
"text2text-generation",
"transformers",
"autotrain_compatible"
] | text2text-generation | false | kcarnold | null | kcarnold/inquisitive1 | 2 | null | transformers | 26,346 | Entry not found |
erickfm/peach-sweep-6 | a902972e7a68beb711c1402fb8223a5fce58685d | 2022-06-15T16:37:29.000Z | [
"pytorch",
"t5",
"text2text-generation",
"transformers",
"autotrain_compatible"
] | text2text-generation | false | erickfm | null | erickfm/peach-sweep-6 | 2 | null | transformers | 26,347 | Entry not found |
erickfm/bumbling-sweep-4 | 44acce6d2c336af87039b24c1546e4f73fabf3a5 | 2022-06-15T17:45:23.000Z | [
"pytorch",
"t5",
"text2text-generation",
"transformers",
"autotrain_compatible"
] | text2text-generation | false | erickfm | null | erickfm/bumbling-sweep-4 | 2 | null | transformers | 26,348 | Entry not found |
erickfm/logical-sweep-8 | ca2c072f4da140fb3ccf1da7bd92a19f96b63e5f | 2022-06-15T19:43:47.000Z | [
"pytorch",
"t5",
"text2text-generation",
"transformers",
"autotrain_compatible"
] | text2text-generation | false | erickfm | null | erickfm/logical-sweep-8 | 2 | null | transformers | 26,349 | Entry not found |
lmqg/t5-large-squadshifts-reddit | 044342d2c8b3d59af24396aaf4376d20e254383a | 2022-06-16T19:17:03.000Z | [
"pytorch",
"t5",
"text2text-generation",
"transformers",
"autotrain_compatible"
] | text2text-generation | false | lmqg | null | lmqg/t5-large-squadshifts-reddit | 2 | null | transformers | 26,350 | Entry not found |
Nadav/roberta-base-squad-finetuned-on-runaways-en | af8ea2acb805de2f69f93a579bf53849d94cb54e | 2022-06-19T13:33:37.000Z | [
"pytorch",
"roberta",
"question-answering",
"transformers",
"autotrain_compatible"
] | question-answering | false | Nadav | null | Nadav/roberta-base-squad-finetuned-on-runaways-en | 2 | null | transformers | 26,351 | Entry not found |
income/jpq-gpl-webis-touche2020-document_encoder-base-msmarco-distilbert-tas-b | 5e4836b48eb6b068861bd47870349dc490b29084 | 2022-06-15T21:55:19.000Z | [
"pytorch",
"distilbert",
"transformers",
"license:apache-2.0"
] | null | false | income | null | income/jpq-gpl-webis-touche2020-document_encoder-base-msmarco-distilbert-tas-b | 2 | null | transformers | 26,352 | ---
license: apache-2.0
---
|
income/jpq-genq-hotpotqa-document_encoder-base-msmarco-distilbert-tas-b | e9994f27514db30df53cece294f2f67fe21b618a | 2022-06-15T22:10:37.000Z | [
"pytorch",
"distilbert",
"transformers",
"license:apache-2.0"
] | null | false | income | null | income/jpq-genq-hotpotqa-document_encoder-base-msmarco-distilbert-tas-b | 2 | null | transformers | 26,353 | ---
license: apache-2.0
---
|
webshop/il-choice-bert-image_1 | 7051b8bd6e7aca03dac467e2cef998f104034d83 | 2022-06-16T01:15:57.000Z | [
"pytorch",
"bert",
"transformers"
] | null | false | webshop | null | webshop/il-choice-bert-image_1 | 2 | null | transformers | 26,354 | Entry not found |
YYSH/Test-demo-colab | f4515e5a51d649a2a6d45d2d9e1ef33e1d395a72 | 2022-06-16T04:40:10.000Z | [
"pytorch",
"tensorboard",
"wav2vec2",
"automatic-speech-recognition",
"transformers",
"generated_from_trainer",
"model-index"
] | automatic-speech-recognition | false | YYSH | null | YYSH/Test-demo-colab | 2 | null | transformers | 26,355 | ---
tags:
- generated_from_trainer
model-index:
- name: Test-demo-colab
results: []
---
<!-- This model card has been generated automatically according to the information the Trainer had access to. You
should probably proofread and complete it, then remove this comment. -->
# Test-demo-colab
This model was trained from scratch on the None dataset.
It achieves the following results on the evaluation set:
- Loss: 0.9479
- Wer: 0.6856
## Model description
More information needed
## Intended uses & limitations
More information needed
## Training and evaluation data
More information needed
## Training procedure
### Training hyperparameters
The following hyperparameters were used during training:
- learning_rate: 0.0001
- train_batch_size: 8
- eval_batch_size: 8
- seed: 42
- optimizer: Adam with betas=(0.9,0.999) and epsilon=1e-08
- lr_scheduler_type: linear
- lr_scheduler_warmup_steps: 1000
- num_epochs: 30
- mixed_precision_training: Native AMP
### Training results
| Training Loss | Epoch | Step | Validation Loss | Wer |
|:-------------:|:-----:|:-----:|:---------------:|:------:|
| 4.2676 | 1.0 | 500 | 2.2725 | 1.0013 |
| 2.0086 | 2.01 | 1000 | 1.2788 | 0.8053 |
| 1.6389 | 3.01 | 1500 | 1.1333 | 0.7458 |
| 1.4908 | 4.02 | 2000 | 1.0369 | 0.7356 |
| 1.4137 | 5.02 | 2500 | 0.9894 | 0.7111 |
| 1.3507 | 6.02 | 3000 | 0.9394 | 0.7098 |
| 1.3101 | 7.03 | 3500 | 0.9531 | 0.6966 |
| 1.2682 | 8.03 | 4000 | 0.9255 | 0.6892 |
| 1.239 | 9.04 | 4500 | 0.9222 | 0.6818 |
| 1.2161 | 10.04 | 5000 | 0.9079 | 0.6911 |
| 1.1871 | 11.04 | 5500 | 0.9100 | 0.7033 |
| 1.1688 | 12.05 | 6000 | 0.9080 | 0.6924 |
| 1.1383 | 13.05 | 6500 | 0.9097 | 0.6910 |
| 1.1304 | 14.06 | 7000 | 0.9052 | 0.6810 |
| 1.1181 | 15.06 | 7500 | 0.9025 | 0.6847 |
| 1.0905 | 16.06 | 8000 | 0.9296 | 0.6832 |
| 1.0744 | 17.07 | 8500 | 0.9120 | 0.6912 |
| 1.0675 | 18.07 | 9000 | 0.9039 | 0.6864 |
| 1.0511 | 19.08 | 9500 | 0.9157 | 0.7004 |
| 1.0401 | 20.08 | 10000 | 0.9259 | 0.6792 |
| 1.0319 | 21.08 | 10500 | 0.9478 | 0.6976 |
| 1.0194 | 22.09 | 11000 | 0.9438 | 0.6820 |
| 1.0117 | 23.09 | 11500 | 0.9577 | 0.6891 |
| 1.0038 | 24.1 | 12000 | 0.9670 | 0.6918 |
| 0.9882 | 25.1 | 12500 | 0.9579 | 0.6884 |
| 0.9979 | 26.1 | 13000 | 0.9502 | 0.6869 |
| 0.9767 | 27.11 | 13500 | 0.9537 | 0.6833 |
| 0.964 | 28.11 | 14000 | 0.9525 | 0.6880 |
| 0.9867 | 29.12 | 14500 | 0.9479 | 0.6856 |
### Framework versions
- Transformers 4.17.0
- Pytorch 1.11.0+cu113
- Datasets 1.18.3
- Tokenizers 0.12.1
|
jhliu/ClinicalAdaptation-PubMedBERT-base-uncased-MIMIC-segment | 0fc5dd15aa8c755a009ff3db11d5be61fd9020fc | 2022-06-16T06:29:11.000Z | [
"pytorch",
"bert",
"fill-mask",
"transformers",
"autotrain_compatible"
] | fill-mask | false | jhliu | null | jhliu/ClinicalAdaptation-PubMedBERT-base-uncased-MIMIC-segment | 2 | null | transformers | 26,356 | Entry not found |
jhliu/ClinicalAdaptation-PubMedBERT-base-uncased-MIMIC-note | d2d3faba9a0c235a5f435d17c14ccdf4a235f2f0 | 2022-06-16T06:30:33.000Z | [
"pytorch",
"bert",
"fill-mask",
"transformers",
"autotrain_compatible"
] | fill-mask | false | jhliu | null | jhliu/ClinicalAdaptation-PubMedBERT-base-uncased-MIMIC-note | 2 | null | transformers | 26,357 | Entry not found |
waboucay/camembert-base-finetuned-repnum_wl-rua_wl_3_classes | 18bfdfedfbac6262662139fe8e9dd31f509711cc | 2022-06-16T07:44:53.000Z | [
"pytorch",
"camembert",
"text-classification",
"fr",
"transformers",
"nli"
] | text-classification | false | waboucay | null | waboucay/camembert-base-finetuned-repnum_wl-rua_wl_3_classes | 2 | null | transformers | 26,358 | ---
language:
- fr
tags:
- nli
metrics:
- f1
---
## Eval results
We obtain the following results on ```validation``` and ```test``` sets:
| Set | F1<sub>micro</sub> | F1<sub>macro</sub> |
|------------|--------------------|--------------------|
| validation | 75.6 | 75.3 |
| test | 76.1 | 75.8 |
|
waboucay/camembert-base-finetuned-rua_wl_3_classes | c66534100002c1987d06d5ba143c7d003af6bbd1 | 2022-06-16T07:39:30.000Z | [
"pytorch",
"camembert",
"text-classification",
"fr",
"transformers",
"nli"
] | text-classification | false | waboucay | null | waboucay/camembert-base-finetuned-rua_wl_3_classes | 2 | null | transformers | 26,359 | ---
language:
- fr
tags:
- nli
metrics:
- f1
---
## Eval results
We obtain the following results on ```validation``` and ```test``` sets:
| Set | F1<sub>micro</sub> | F1<sub>macro</sub> |
|------------|--------------------|--------------------|
| validation | 73.5 | 73.3 |
| test | 73.8 | 73.6 |
|
waboucay/camembert-large-finetuned-repnum_wl | 9fbec8ce3bff220f5798e99979e555013a9ea90d | 2022-06-16T09:46:51.000Z | [
"pytorch",
"camembert",
"text-classification",
"fr",
"transformers",
"nli"
] | text-classification | false | waboucay | null | waboucay/camembert-large-finetuned-repnum_wl | 2 | null | transformers | 26,360 | ---
language:
- fr
tags:
- nli
metrics:
- f1
---
## Eval results
We obtain the following results on ```validation``` and ```test``` sets:
| Set | F1<sub>micro</sub> | F1<sub>macro</sub> |
|------------|--------------------|--------------------|
| validation | 80.4 | 80.4 |
| test | 80.6 | 80.6 | |
erickfm/playful-sweep-1 | eeb396d9f1b6dfa424294074491959d3736875eb | 2022-06-16T10:06:14.000Z | [
"pytorch",
"t5",
"text2text-generation",
"transformers",
"autotrain_compatible"
] | text2text-generation | false | erickfm | null | erickfm/playful-sweep-1 | 2 | null | transformers | 26,361 | Entry not found |
eleldar/rubert-base-cased-sentence | d967214810056c323500989024fbfec8cd06984d | 2022-06-16T11:16:20.000Z | [
"pytorch",
"jax",
"bert",
"feature-extraction",
"transformers"
] | feature-extraction | false | eleldar | null | eleldar/rubert-base-cased-sentence | 2 | null | transformers | 26,362 | Model for API: https://github.com/eleldar/Punctuation |
waboucay/camembert-large-finetuned-rua_wl | 35eb8efce217c966f92c6a4e84784091168a1239 | 2022-06-16T12:02:25.000Z | [
"pytorch",
"camembert",
"text-classification",
"fr",
"transformers",
"nli"
] | text-classification | false | waboucay | null | waboucay/camembert-large-finetuned-rua_wl | 2 | null | transformers | 26,363 | ---
language:
- fr
tags:
- nli
metrics:
- f1
---
## Eval results
We obtain the following results on ```validation``` and ```test``` sets:
| Set | F1<sub>micro</sub> | F1<sub>macro</sub> |
|------------|--------------------|--------------------|
| validation | 74.8 | 74.5 |
| test | 74.8 | 74.6 | |
income/bpr-gpl-scifact-base-msmarco-distilbert-tas-b | 09cbc73e04b182603c561cc341582b8372f08c2b | 2022-06-16T18:05:25.000Z | [
"pytorch",
"distilbert",
"feature-extraction",
"sentence-transformers",
"sentence-similarity",
"transformers"
] | sentence-similarity | false | income | null | income/bpr-gpl-scifact-base-msmarco-distilbert-tas-b | 2 | null | sentence-transformers | 26,364 | ---
pipeline_tag: sentence-similarity
tags:
- sentence-transformers
- feature-extraction
- sentence-similarity
- transformers
---
# {MODEL_NAME}
This is a [sentence-transformers](https://www.SBERT.net) model: It maps sentences & paragraphs to a 768 dimensional dense vector space and can be used for tasks like clustering or semantic search.
<!--- Describe your model here -->
## Usage (Sentence-Transformers)
Using this model becomes easy when you have [sentence-transformers](https://www.SBERT.net) installed:
```
pip install -U sentence-transformers
```
Then you can use the model like this:
```python
from sentence_transformers import SentenceTransformer
sentences = ["This is an example sentence", "Each sentence is converted"]
model = SentenceTransformer('{MODEL_NAME}')
embeddings = model.encode(sentences)
print(embeddings)
```
## Usage (HuggingFace Transformers)
Without [sentence-transformers](https://www.SBERT.net), you can use the model like this: First, you pass your input through the transformer model, then you have to apply the right pooling-operation on-top of the contextualized word embeddings.
```python
from transformers import AutoTokenizer, AutoModel
import torch
#Mean Pooling - Take attention mask into account for correct averaging
def mean_pooling(model_output, attention_mask):
token_embeddings = model_output[0] #First element of model_output contains all token embeddings
input_mask_expanded = attention_mask.unsqueeze(-1).expand(token_embeddings.size()).float()
return torch.sum(token_embeddings * input_mask_expanded, 1) / torch.clamp(input_mask_expanded.sum(1), min=1e-9)
# Sentences we want sentence embeddings for
sentences = ['This is an example sentence', 'Each sentence is converted']
# Load model from HuggingFace Hub
tokenizer = AutoTokenizer.from_pretrained('{MODEL_NAME}')
model = AutoModel.from_pretrained('{MODEL_NAME}')
# Tokenize sentences
encoded_input = tokenizer(sentences, padding=True, truncation=True, return_tensors='pt')
# Compute token embeddings
with torch.no_grad():
model_output = model(**encoded_input)
# Perform pooling. In this case, max pooling.
sentence_embeddings = mean_pooling(model_output, encoded_input['attention_mask'])
print("Sentence embeddings:")
print(sentence_embeddings)
```
## Evaluation Results
<!--- Describe how your model was evaluated -->
For an automated evaluation of this model, see the *Sentence Embeddings Benchmark*: [https://seb.sbert.net](https://seb.sbert.net?model_name={MODEL_NAME})
## Training
The model was trained with the parameters:
**DataLoader**:
`torch.utils.data.dataloader.DataLoader` of length 481 with parameters:
```
{'batch_size': 32, 'sampler': 'torch.utils.data.sampler.RandomSampler', 'batch_sampler': 'torch.utils.data.sampler.BatchSampler'}
```
**Loss**:
`gpl.toolkit.loss.MarginDistillationLoss`
Parameters of the fit()-Method:
```
{
"callback": null,
"epochs": 10,
"evaluation_steps": 0,
"evaluator": "NoneType",
"max_grad_norm": 1,
"optimizer_class": "<class 'transformers.optimization.AdamW'>",
"optimizer_params": {
"lr": 2e-05
},
"scheduler": "WarmupLinear",
"steps_per_epoch": null,
"warmup_steps": 1000,
"weight_decay": 0.01
}
```
## Full Model Architecture
```
SentenceTransformer(
(0): Transformer({'max_seq_length': 350, 'do_lower_case': False}) with Transformer model: DistilBertModel
(1): Pooling({'word_embedding_dimension': 768, 'pooling_mode_cls_token': False, 'pooling_mode_mean_tokens': True, 'pooling_mode_max_tokens': False, 'pooling_mode_mean_sqrt_len_tokens': False})
)
```
## Citing & Authors
<!--- Describe where people can find more information --> |
huggingtweets/netflixinator | a7f1ac3685b91501741926d52e6c533a2c3bccec | 2022-06-16T18:12:59.000Z | [
"pytorch",
"gpt2",
"text-generation",
"en",
"transformers",
"huggingtweets"
] | text-generation | false | huggingtweets | null | huggingtweets/netflixinator | 2 | null | transformers | 26,365 | ---
language: en
thumbnail: http://www.huggingtweets.com/netflixinator/1655403174774/predictions.png
tags:
- huggingtweets
widget:
- text: "My dream is"
---
<div class="inline-flex flex-col" style="line-height: 1.5;">
<div class="flex">
<div
style="display:inherit; margin-left: 4px; margin-right: 4px; width: 92px; height:92px; border-radius: 50%; background-size: cover; background-image: url('https://pbs.twimg.com/profile_images/1417287754434727936/38RRdVlp_400x400.jpg')">
</div>
<div
style="display:none; margin-left: 4px; margin-right: 4px; width: 92px; height:92px; border-radius: 50%; background-size: cover; background-image: url('')">
</div>
<div
style="display:none; margin-left: 4px; margin-right: 4px; width: 92px; height:92px; border-radius: 50%; background-size: cover; background-image: url('')">
</div>
</div>
<div style="text-align: center; margin-top: 3px; font-size: 16px; font-weight: 800">🤖 AI BOT 🤖</div>
<div style="text-align: center; font-size: 16px; font-weight: 800">Fourtoffee #RussiavsUkraine #NewDeal4Animation</div>
<div style="text-align: center; font-size: 14px;">@netflixinator</div>
</div>
I was made with [huggingtweets](https://github.com/borisdayma/huggingtweets).
Create your own bot based on your favorite user with [the demo](https://colab.research.google.com/github/borisdayma/huggingtweets/blob/master/huggingtweets-demo.ipynb)!
## How does it work?
The model uses the following pipeline.
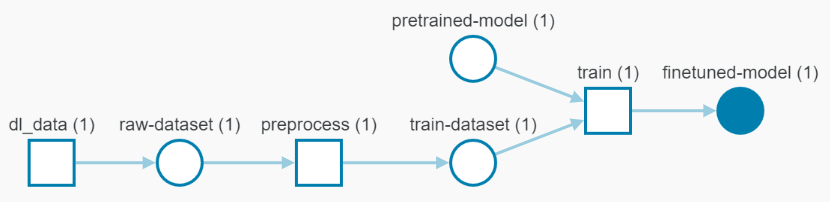
To understand how the model was developed, check the [W&B report](https://wandb.ai/wandb/huggingtweets/reports/HuggingTweets-Train-a-Model-to-Generate-Tweets--VmlldzoxMTY5MjI).
## Training data
The model was trained on tweets from Fourtoffee #RussiavsUkraine #NewDeal4Animation.
| Data | Fourtoffee #RussiavsUkraine #NewDeal4Animation |
| --- | --- |
| Tweets downloaded | 797 |
| Retweets | 7 |
| Short tweets | 59 |
| Tweets kept | 731 |
[Explore the data](https://wandb.ai/wandb/huggingtweets/runs/lgk6jsh5/artifacts), which is tracked with [W&B artifacts](https://docs.wandb.com/artifacts) at every step of the pipeline.
## Training procedure
The model is based on a pre-trained [GPT-2](https://huggingface.co/gpt2) which is fine-tuned on @netflixinator's tweets.
Hyperparameters and metrics are recorded in the [W&B training run](https://wandb.ai/wandb/huggingtweets/runs/3vysavz9) for full transparency and reproducibility.
At the end of training, [the final model](https://wandb.ai/wandb/huggingtweets/runs/3vysavz9/artifacts) is logged and versioned.
## How to use
You can use this model directly with a pipeline for text generation:
```python
from transformers import pipeline
generator = pipeline('text-generation',
model='huggingtweets/netflixinator')
generator("My dream is", num_return_sequences=5)
```
## Limitations and bias
The model suffers from [the same limitations and bias as GPT-2](https://huggingface.co/gpt2#limitations-and-bias).
In addition, the data present in the user's tweets further affects the text generated by the model.
## About
*Built by Boris Dayma*
[](https://twitter.com/intent/follow?screen_name=borisdayma)
For more details, visit the project repository.
[](https://github.com/borisdayma/huggingtweets)
|
philmunz/poc_finetuned | 8ce2ce55fa564041605e9c67905682210f02ed14 | 2022-06-16T19:10:11.000Z | [
"pytorch",
"bert",
"text-classification",
"transformers"
] | text-classification | false | philmunz | null | philmunz/poc_finetuned | 2 | null | transformers | 26,366 | Entry not found |
S2312dal/M2_MLM | 798abd5c7ada831de3d6d647fdc3dd0866247225 | 2022-06-16T19:42:48.000Z | [
"pytorch",
"tensorboard",
"roberta",
"fill-mask",
"transformers",
"generated_from_trainer",
"license:mit",
"model-index",
"autotrain_compatible"
] | fill-mask | false | S2312dal | null | S2312dal/M2_MLM | 2 | null | transformers | 26,367 | ---
license: mit
tags:
- generated_from_trainer
model-index:
- name: M2_MLM
results: []
---
<!-- This model card has been generated automatically according to the information the Trainer had access to. You
should probably proofread and complete it, then remove this comment. -->
# M2_MLM
This model is a fine-tuned version of [roberta-base](https://huggingface.co/roberta-base) on an unknown dataset.
It achieves the following results on the evaluation set:
- Loss: 1.3686
## Model description
More information needed
## Intended uses & limitations
More information needed
## Training and evaluation data
More information needed
## Training procedure
### Training hyperparameters
The following hyperparameters were used during training:
- learning_rate: 2e-05
- train_batch_size: 16
- eval_batch_size: 16
- seed: 42
- optimizer: Adam with betas=(0.9,0.999) and epsilon=1e-08
- lr_scheduler_type: linear
- num_epochs: 3.0
- mixed_precision_training: Native AMP
### Training results
| Training Loss | Epoch | Step | Validation Loss |
|:-------------:|:-----:|:----:|:---------------:|
| 1.5955 | 1.0 | 25 | 1.4376 |
| 1.4736 | 2.0 | 50 | 1.2969 |
| 1.3925 | 3.0 | 75 | 1.3163 |
### Framework versions
- Transformers 4.20.0
- Pytorch 1.11.0+cu113
- Datasets 2.3.2
- Tokenizers 0.12.1
|
chlab/efficientnet_47_planet_detection | 011d96ca1c1718a3c234fda2826f8147ccb8bb4f | 2022-06-17T14:24:09.000Z | [
"pytorch",
"Python 3.7+",
"dataset:imagenet",
"dataset:imagenet-21k",
"transformers",
"vision",
"image-classification",
"license:apache-2.0"
] | image-classification | false | chlab | null | chlab/efficientnet_47_planet_detection | 2 | null | transformers | 26,368 | ---
language:
- Python 3.7+
license: apache-2.0
tags:
- vision
- image-classification
datasets:
- imagenet
- imagenet-21k
widget:
- src: https://huggingface.co/datasets/mishig/sample_images/resolve/main/tiger.jpg
example_title: Tiger
- src: https://huggingface.co/datasets/mishig/sample_images/resolve/main/teapot.jpg
example_title: Teapot
- src: https://huggingface.co/datasets/mishig/sample_images/resolve/main/palace.jpg
example_title: Palace
---
# Efficientnetv2 (47 channels) |
ZipperXYZ/DialoGPT-medium-TheWorldMachine | 1efe8e2fe02b99cf35a0706bc0af0d00d9b68291 | 2022-06-16T22:22:29.000Z | [
"pytorch",
"gpt2",
"text-generation",
"transformers",
"conversational"
] | conversational | false | ZipperXYZ | null | ZipperXYZ/DialoGPT-medium-TheWorldMachine | 2 | null | transformers | 26,369 | ---
tags:
- conversational
---
# The world machine DialoGPT model |
AlyxTheKitten/DialoGPT-medium-AgedBlaine-2 | 86e3452e72408f35f9a24547ebb42377c326a6d4 | 2022-06-17T01:15:54.000Z | [
"pytorch",
"gpt2",
"text-generation",
"transformers",
"conversational"
] | conversational | false | AlyxTheKitten | null | AlyxTheKitten/DialoGPT-medium-AgedBlaine-2 | 2 | null | transformers | 26,370 | ---
tags:
- conversational
---
# AgedBlaine DialoGPT Model 2 |
Danastos/triviaqa_bert_el_4 | 101fa9984d0a331141929afc45e7a6a28c8e432b | 2022-06-19T12:06:32.000Z | [
"pytorch",
"bert",
"question-answering",
"transformers",
"autotrain_compatible"
] | question-answering | false | Danastos | null | Danastos/triviaqa_bert_el_4 | 2 | null | transformers | 26,371 | Entry not found |
eslamxm/mbart-finetuned-fa | 59d62b2a86a40f4da3ffc1c2fe1ed39ead6c5936 | 2022-06-18T13:40:54.000Z | [
"pytorch",
"tensorboard",
"mbart",
"text2text-generation",
"dataset:pn_summary",
"transformers",
"summarization",
"fa",
"Abstractive Summarization",
"generated_from_trainer",
"model-index",
"autotrain_compatible"
] | summarization | false | eslamxm | null | eslamxm/mbart-finetuned-fa | 2 | null | transformers | 26,372 | ---
tags:
- summarization
- fa
- mbart
- Abstractive Summarization
- generated_from_trainer
datasets:
- pn_summary
model-index:
- name: mbart-finetuned-fa
results: []
---
<!-- This model card has been generated automatically according to the information the Trainer had access to. You
should probably proofread and complete it, then remove this comment. -->
# mbart-finetuned-fa
This model is a fine-tuned version of [facebook/mbart-large-50](https://huggingface.co/facebook/mbart-large-50) on the pn_summary dataset.
It achieves the following results on the evaluation set:
- Loss: 3.2877
- Rouge-1: 44.07
- Rouge-2: 25.81
- Rouge-l: 38.96
- Gen Len: 41.7
- Bertscore: 78.95
## Model description
More information needed
## Intended uses & limitations
More information needed
## Training and evaluation data
More information needed
## Training procedure
### Training hyperparameters
The following hyperparameters were used during training:
- learning_rate: 0.0005
- train_batch_size: 4
- eval_batch_size: 4
- seed: 42
- gradient_accumulation_steps: 8
- total_train_batch_size: 32
- optimizer: Adam with betas=(0.9,0.999) and epsilon=1e-08
- lr_scheduler_type: linear
- lr_scheduler_warmup_steps: 250
- num_epochs: 5
- label_smoothing_factor: 0.1
### Training results
### Framework versions
- Transformers 4.20.0
- Pytorch 1.11.0+cu113
- Datasets 2.3.2
- Tokenizers 0.12.1
|
hjds0923/distilbert-base-uncased-finetuned-squad | 3cc40aaaabf4c2ab703d1f51ae116b3145093eb5 | 2022-06-17T10:08:57.000Z | [
"pytorch",
"tensorboard",
"distilbert",
"question-answering",
"dataset:squad",
"transformers",
"generated_from_trainer",
"license:apache-2.0",
"model-index",
"autotrain_compatible"
] | question-answering | false | hjds0923 | null | hjds0923/distilbert-base-uncased-finetuned-squad | 2 | null | transformers | 26,373 | ---
license: apache-2.0
tags:
- generated_from_trainer
datasets:
- squad
model-index:
- name: distilbert-base-uncased-finetuned-squad
results: []
---
<!-- This model card has been generated automatically according to the information the Trainer had access to. You
should probably proofread and complete it, then remove this comment. -->
# distilbert-base-uncased-finetuned-squad
This model is a fine-tuned version of [distilbert-base-uncased](https://huggingface.co/distilbert-base-uncased) on the squad dataset.
It achieves the following results on the evaluation set:
- eval_loss: 1.1675
- eval_runtime: 146.876
- eval_samples_per_second: 73.422
- eval_steps_per_second: 4.589
- epoch: 1.0
- step: 5533
## Model description
More information needed
## Intended uses & limitations
More information needed
## Training and evaluation data
More information needed
## Training procedure
### Training hyperparameters
The following hyperparameters were used during training:
- learning_rate: 2e-05
- train_batch_size: 16
- eval_batch_size: 16
- seed: 42
- optimizer: Adam with betas=(0.9,0.999) and epsilon=1e-08
- lr_scheduler_type: linear
- num_epochs: 3
### Framework versions
- Transformers 4.20.0
- Pytorch 1.11.0+cu113
- Datasets 2.3.2
- Tokenizers 0.12.1
|
bugdaryan/distilbert-base-uncased-finetuned-squad | 8e785c02d47dc06982946eaa31d6841bc96bdedc | 2022-06-17T14:18:48.000Z | [
"pytorch",
"tensorboard",
"distilbert",
"question-answering",
"dataset:squad_v2",
"transformers",
"generated_from_trainer",
"license:apache-2.0",
"model-index",
"autotrain_compatible"
] | question-answering | false | bugdaryan | null | bugdaryan/distilbert-base-uncased-finetuned-squad | 2 | null | transformers | 26,374 | ---
license: apache-2.0
tags:
- generated_from_trainer
datasets:
- squad_v2
model-index:
- name: distilbert-base-uncased-finetuned-squad
results: []
---
<!-- This model card has been generated automatically according to the information the Trainer had access to. You
should probably proofread and complete it, then remove this comment. -->
# distilbert-base-uncased-finetuned-squad
This model is a fine-tuned version of [distilbert-base-uncased](https://huggingface.co/distilbert-base-uncased) on the squad_v2 dataset.
## Model description
More information needed
## Intended uses & limitations
More information needed
## Training and evaluation data
More information needed
## Training procedure
### Training hyperparameters
The following hyperparameters were used during training:
- learning_rate: 2e-05
- train_batch_size: 32
- eval_batch_size: 32
- seed: 42
- optimizer: Adam with betas=(0.9,0.999) and epsilon=1e-08
- lr_scheduler_type: linear
- num_epochs: 3
### Framework versions
- Transformers 4.20.0
- Pytorch 1.11.0+cu113
- Datasets 2.3.2
- Tokenizers 0.12.1
|
waboucay/camembert-large-finetuned-xnli_fr | 23304c83e5562b0065a220b7629c1de7ec8edc47 | 2022-06-17T11:59:21.000Z | [
"pytorch",
"camembert",
"text-classification",
"fr",
"transformers",
"nli"
] | text-classification | false | waboucay | null | waboucay/camembert-large-finetuned-xnli_fr | 2 | null | transformers | 26,375 | ---
language:
- fr
tags:
- nli
metrics:
- f1
---
## Eval results
We obtain the following results on ```validation``` and ```test``` sets:
| Set | F1<sub>micro</sub> | F1<sub>macro</sub> |
|------------|--------------------|--------------------|
| validation | 92.9 | 92.1 |
| test | 91.7 | 90.7 | |
philmunz/poc_finetuned_ud | 829a2dd2938241ece83f50e05237c6daf737fd39 | 2022-06-17T16:12:51.000Z | [
"pytorch",
"bert",
"text-classification",
"transformers"
] | text-classification | false | philmunz | null | philmunz/poc_finetuned_ud | 2 | null | transformers | 26,376 | Entry not found |
Ryna/wav2vec2-large-xlsr-53-Enlgish-FT-ASCEND-colab | 206b5bce3308164017a1ef7c29ce3cd78d6317a0 | 2022-06-17T20:08:51.000Z | [
"pytorch",
"tensorboard",
"wav2vec2",
"automatic-speech-recognition",
"dataset:ascend",
"transformers",
"generated_from_trainer",
"license:apache-2.0",
"model-index"
] | automatic-speech-recognition | false | Ryna | null | Ryna/wav2vec2-large-xlsr-53-Enlgish-FT-ASCEND-colab | 2 | null | transformers | 26,377 | ---
license: apache-2.0
tags:
- generated_from_trainer
datasets:
- ascend
model-index:
- name: wav2vec2-large-xlsr-53-Enlgish-FT-ASCEND-colab
results: []
---
<!-- This model card has been generated automatically according to the information the Trainer had access to. You
should probably proofread and complete it, then remove this comment. -->
# wav2vec2-large-xlsr-53-Enlgish-FT-ASCEND-colab
This model is a fine-tuned version of [jonatasgrosman/wav2vec2-large-xlsr-53-english](https://huggingface.co/jonatasgrosman/wav2vec2-large-xlsr-53-english) on the ascend dataset.
## Model description
More information needed
## Intended uses & limitations
More information needed
## Training and evaluation data
More information needed
## Training procedure
### Training hyperparameters
The following hyperparameters were used during training:
- learning_rate: 0.0003
- train_batch_size: 16
- eval_batch_size: 8
- seed: 42
- gradient_accumulation_steps: 10000
- total_train_batch_size: 160000
- optimizer: Adam with betas=(0.9,0.999) and epsilon=1e-08
- lr_scheduler_type: linear
- lr_scheduler_warmup_steps: 500
- num_epochs: 30
- mixed_precision_training: Native AMP
### Training results
### Framework versions
- Transformers 4.20.0
- Pytorch 1.11.0+cu113
- Datasets 2.3.2
- Tokenizers 0.12.1
|
wiselinjayajos/dummy-model | 82c7de83f72abecee175d236b656e9d30ce17557 | 2022-06-17T16:44:17.000Z | [
"pytorch",
"camembert",
"fill-mask",
"transformers",
"autotrain_compatible"
] | fill-mask | false | wiselinjayajos | null | wiselinjayajos/dummy-model | 2 | null | transformers | 26,378 | Entry not found |
gemasphi/laprador | 9f976f83e6e63b18a47e9a84a407fd115d3b3c5c | 2022-06-17T19:42:04.000Z | [
"pytorch",
"bert",
"feature-extraction",
"sentence-transformers",
"sentence-similarity",
"transformers"
] | sentence-similarity | false | gemasphi | null | gemasphi/laprador | 2 | null | sentence-transformers | 26,379 | ---
pipeline_tag: sentence-similarity
tags:
- sentence-transformers
- feature-extraction
- sentence-similarity
- transformers
---
# gemasphi/laprador
This is a [sentence-transformers](https://www.SBERT.net) model: It maps sentences & paragraphs to a 768 dimensional dense vector space and can be used for tasks like clustering or semantic search.
<!--- Describe your model here -->
## Usage (Sentence-Transformers)
Using this model becomes easy when you have [sentence-transformers](https://www.SBERT.net) installed:
```
pip install -U sentence-transformers
```
Then you can use the model like this:
```python
from sentence_transformers import SentenceTransformer
sentences = ["This is an example sentence", "Each sentence is converted"]
model = SentenceTransformer('gemasphi/laprador')
embeddings = model.encode(sentences)
print(embeddings)
```
## Usage (HuggingFace Transformers)
Without [sentence-transformers](https://www.SBERT.net), you can use the model like this: First, you pass your input through the transformer model, then you have to apply the right pooling-operation on-top of the contextualized word embeddings.
```python
from transformers import AutoTokenizer, AutoModel
import torch
#Mean Pooling - Take attention mask into account for correct averaging
def mean_pooling(model_output, attention_mask):
token_embeddings = model_output[0] #First element of model_output contains all token embeddings
input_mask_expanded = attention_mask.unsqueeze(-1).expand(token_embeddings.size()).float()
return torch.sum(token_embeddings * input_mask_expanded, 1) / torch.clamp(input_mask_expanded.sum(1), min=1e-9)
# Sentences we want sentence embeddings for
sentences = ['This is an example sentence', 'Each sentence is converted']
# Load model from HuggingFace Hub
tokenizer = AutoTokenizer.from_pretrained('gemasphi/laprador')
model = AutoModel.from_pretrained('gemasphi/laprador')
# Tokenize sentences
encoded_input = tokenizer(sentences, padding=True, truncation=True, return_tensors='pt')
# Compute token embeddings
with torch.no_grad():
model_output = model(**encoded_input)
# Perform pooling. In this case, mean pooling.
sentence_embeddings = mean_pooling(model_output, encoded_input['attention_mask'])
print("Sentence embeddings:")
print(sentence_embeddings)
```
## Evaluation Results
<!--- Describe how your model was evaluated -->
For an automated evaluation of this model, see the *Sentence Embeddings Benchmark*: [https://seb.sbert.net](https://seb.sbert.net?model_name=gemasphi/laprador)
## Full Model Architecture
```
SentenceTransformer(
(0): Transformer({'max_seq_length': 350, 'do_lower_case': False}) with Transformer model: BertModel
(1): Pooling({'word_embedding_dimension': 768, 'pooling_mode_cls_token': False, 'pooling_mode_mean_tokens': True, 'pooling_mode_max_tokens': False, 'pooling_mode_mean_sqrt_len_tokens': False})
)
```
## Citing & Authors
<!--- Describe where people can find more information --> |
nmcahill/TJ-classifier | ce051bb1f76acf3d48bc43ac970d3a64f1aae9e3 | 2022-06-17T21:22:10.000Z | [
"pytorch",
"distilbert",
"text-classification",
"transformers"
] | text-classification | false | nmcahill | null | nmcahill/TJ-classifier | 2 | null | transformers | 26,380 | Entry not found |
aminnaghavi/wav2vec2-base-dataset_asr-demo-colab | 9935df7d3b8209a82ece9bffd9bf9abfb5e56e5e | 2022-06-20T13:23:04.000Z | [
"pytorch",
"tensorboard",
"hubert",
"automatic-speech-recognition",
"dataset:superb",
"transformers",
"generated_from_trainer",
"license:apache-2.0",
"model-index"
] | automatic-speech-recognition | false | aminnaghavi | null | aminnaghavi/wav2vec2-base-dataset_asr-demo-colab | 2 | null | transformers | 26,381 | ---
license: apache-2.0
tags:
- generated_from_trainer
datasets:
- superb
model-index:
- name: wav2vec2-base-dataset_asr-demo-colab
results: []
---
<!-- This model card has been generated automatically according to the information the Trainer had access to. You
should probably proofread and complete it, then remove this comment. -->
# wav2vec2-base-dataset_asr-demo-colab
This model is a fine-tuned version of [ntu-spml/distilhubert](https://huggingface.co/ntu-spml/distilhubert) on the superb dataset.
It achieves the following results on the evaluation set:
- Loss: 295.0834
- Wer: 0.8282
## Model description
More information needed
## Intended uses & limitations
More information needed
## Training and evaluation data
More information needed
## Training procedure
### Training hyperparameters
The following hyperparameters were used during training:
- learning_rate: 0.001
- train_batch_size: 32
- eval_batch_size: 8
- seed: 42
- optimizer: Adam with betas=(0.9,0.999) and epsilon=1e-08
- lr_scheduler_type: linear
- lr_scheduler_warmup_steps: 250
- num_epochs: 5
- mixed_precision_training: Native AMP
### Training results
| Training Loss | Epoch | Step | Validation Loss | Wer |
|:-------------:|:-----:|:----:|:---------------:|:------:|
| 5638.536 | 1.6 | 500 | 409.4785 | 0.8556 |
| 2258.6455 | 3.19 | 1000 | 326.0520 | 0.8369 |
| 1389.4919 | 4.79 | 1500 | 295.0834 | 0.8282 |
### Framework versions
- Transformers 4.20.0
- Pytorch 1.11.0+cu113
- Datasets 2.3.2
- Tokenizers 0.12.1
|
janeel/muppet-roberta-base-finetuned-squad | 07ff0b3ac21b3cb9b6339591014c41e15c0d86b1 | 2022-06-18T07:57:35.000Z | [
"pytorch",
"tensorboard",
"roberta",
"question-answering",
"dataset:squad_v2",
"transformers",
"generated_from_trainer",
"license:mit",
"model-index",
"autotrain_compatible"
] | question-answering | false | janeel | null | janeel/muppet-roberta-base-finetuned-squad | 2 | null | transformers | 26,382 | ---
license: mit
tags:
- generated_from_trainer
datasets:
- squad_v2
model-index:
- name: muppet-roberta-base-finetuned-squad
results: []
---
<!-- This model card has been generated automatically according to the information the Trainer had access to. You
should probably proofread and complete it, then remove this comment. -->
# muppet-roberta-base-finetuned-squad
This model is a fine-tuned version of [facebook/muppet-roberta-base](https://huggingface.co/facebook/muppet-roberta-base) on the squad_v2 dataset.
It achieves the following results on the evaluation set:
- Loss: 0.9017
## Model description
More information needed
## Intended uses & limitations
More information needed
## Training and evaluation data
More information needed
## Training procedure
### Training hyperparameters
The following hyperparameters were used during training:
- learning_rate: 2e-05
- train_batch_size: 16
- eval_batch_size: 16
- seed: 42
- optimizer: Adam with betas=(0.9,0.999) and epsilon=1e-08
- lr_scheduler_type: linear
- num_epochs: 2
### Training results
| Training Loss | Epoch | Step | Validation Loss |
|:-------------:|:-----:|:-----:|:---------------:|
| 0.7007 | 1.0 | 8239 | 0.7905 |
| 0.4719 | 2.0 | 16478 | 0.9017 |
### Framework versions
- Transformers 4.20.0
- Pytorch 1.11.0+cu113
- Datasets 2.3.2
- Tokenizers 0.12.1
|
Nadav/robbert-base-squad-finetuned-on-runaways-nl | 5590413a3d18ead9f8665a59ece01dda93aa055a | 2022-06-18T08:59:19.000Z | [
"pytorch",
"roberta",
"question-answering",
"transformers",
"autotrain_compatible"
] | question-answering | false | Nadav | null | Nadav/robbert-base-squad-finetuned-on-runaways-nl | 2 | null | transformers | 26,383 | Entry not found |
eugenetanjc/wav2vec2-base-timit-demo-google-colab | 20809d10719d820d9a17f4631ad6b32f33756179 | 2022-06-24T02:12:22.000Z | [
"pytorch",
"tensorboard",
"wav2vec2",
"automatic-speech-recognition",
"transformers",
"generated_from_trainer",
"license:apache-2.0",
"model-index"
] | automatic-speech-recognition | false | eugenetanjc | null | eugenetanjc/wav2vec2-base-timit-demo-google-colab | 2 | null | transformers | 26,384 | ---
license: apache-2.0
tags:
- generated_from_trainer
model-index:
- name: wav2vec2-base-timit-demo-google-colab
results: []
---
<!-- This model card has been generated automatically according to the information the Trainer had access to. You
should probably proofread and complete it, then remove this comment. -->
# wav2vec2-base-timit-demo-google-colab
This model is a fine-tuned version of [facebook/wav2vec2-base-960h](https://huggingface.co/facebook/wav2vec2-base-960h) on the None dataset.
## Model description
More information needed
## Intended uses & limitations
More information needed
## Training and evaluation data
More information needed
## Training procedure
### Training hyperparameters
The following hyperparameters were used during training:
- learning_rate: 0.0001
- train_batch_size: 8
- eval_batch_size: 8
- seed: 42
- optimizer: Adam with betas=(0.9,0.999) and epsilon=1e-08
- lr_scheduler_type: linear
- lr_scheduler_warmup_steps: 1000
- num_epochs: 30
- mixed_precision_training: Native AMP
### Training results
### Framework versions
- Transformers 4.17.0
- Pytorch 1.11.0+cu113
- Datasets 1.18.3
- Tokenizers 0.12.1
|
tuni/xlm-roberta-large-xnli-finetuned-mnli-SJP-v2 | 596b4a1a8259d1b476c104375653bd9dcf41833c | 2022-06-18T13:48:56.000Z | [
"pytorch",
"tensorboard",
"xlm-roberta",
"text-classification",
"dataset:swiss_judgment_prediction",
"transformers",
"generated_from_trainer",
"license:mit",
"model-index"
] | text-classification | false | tuni | null | tuni/xlm-roberta-large-xnli-finetuned-mnli-SJP-v2 | 2 | null | transformers | 26,385 | ---
license: mit
tags:
- generated_from_trainer
datasets:
- swiss_judgment_prediction
metrics:
- accuracy
model-index:
- name: xlm-roberta-large-xnli-finetuned-mnli-SJP-v2
results:
- task:
name: Text Classification
type: text-classification
dataset:
name: swiss_judgment_prediction
type: swiss_judgment_prediction
args: all_languages
metrics:
- name: Accuracy
type: accuracy
value: 0.5954285714285714
---
<!-- This model card has been generated automatically according to the information the Trainer had access to. You
should probably proofread and complete it, then remove this comment. -->
# xlm-roberta-large-xnli-finetuned-mnli-SJP-v2
This model is a fine-tuned version of [joeddav/xlm-roberta-large-xnli](https://huggingface.co/joeddav/xlm-roberta-large-xnli) on the swiss_judgment_prediction dataset.
It achieves the following results on the evaluation set:
- Loss: 0.8093
- Accuracy: 0.5954
## Model description
More information needed
## Intended uses & limitations
More information needed
## Training and evaluation data
More information needed
## Training procedure
### Training hyperparameters
The following hyperparameters were used during training:
- learning_rate: 2e-05
- train_batch_size: 2
- eval_batch_size: 2
- seed: 42
- optimizer: Adam with betas=(0.9,0.999) and epsilon=1e-08
- lr_scheduler_type: linear
- num_epochs: 5
### Training results
| Training Loss | Epoch | Step | Validation Loss | Accuracy |
|:-------------:|:-----:|:----:|:---------------:|:--------:|
| No log | 1.0 | 5 | 0.8879 | 0.5191 |
| No log | 2.0 | 10 | 0.8093 | 0.5954 |
| No log | 3.0 | 15 | 2.4452 | 0.3176 |
| No log | 4.0 | 20 | 3.6636 | 0.3084 |
| No log | 5.0 | 25 | 3.7687 | 0.3393 |
### Framework versions
- Transformers 4.20.0
- Pytorch 1.11.0+cu113
- Datasets 2.3.2
- Tokenizers 0.12.1
|
theojolliffe/bart-cnn-science-v4-e6-manual | 185ad5b677d5881893da808357d7232835cf6822 | 2022-06-18T14:42:13.000Z | [
"pytorch",
"tensorboard",
"bart",
"text2text-generation",
"transformers",
"generated_from_trainer",
"license:mit",
"model-index",
"autotrain_compatible"
] | text2text-generation | false | theojolliffe | null | theojolliffe/bart-cnn-science-v4-e6-manual | 2 | null | transformers | 26,386 | ---
license: mit
tags:
- generated_from_trainer
metrics:
- rouge
model-index:
- name: bart-cnn-science-v4-e6-manual
results: []
---
<!-- This model card has been generated automatically according to the information the Trainer had access to. You
should probably proofread and complete it, then remove this comment. -->
# bart-cnn-science-v4-e6-manual
This model is a fine-tuned version of [theojolliffe/bart-cnn-science](https://huggingface.co/theojolliffe/bart-cnn-science) on an unknown dataset.
It achieves the following results on the evaluation set:
- Loss: 2.0752
- Rouge1: 49.2922
- Rouge2: 27.0916
- Rougel: 29.2754
- Rougelsum: 46.4762
- Gen Len: 140.8
## Model description
More information needed
## Intended uses & limitations
More information needed
## Training and evaluation data
More information needed
## Training procedure
### Training hyperparameters
The following hyperparameters were used during training:
- learning_rate: 2e-05
- train_batch_size: 2
- eval_batch_size: 2
- seed: 42
- optimizer: Adam with betas=(0.9,0.999) and epsilon=1e-08
- lr_scheduler_type: linear
- num_epochs: 6
- mixed_precision_training: Native AMP
### Training results
| Training Loss | Epoch | Step | Validation Loss | Rouge1 | Rouge2 | Rougel | Rougelsum | Gen Len |
|:-------------:|:-----:|:----:|:---------------:|:-------:|:-------:|:-------:|:---------:|:-------:|
| No log | 1.0 | 42 | 1.5703 | 45.3807 | 24.4571 | 27.901 | 42.7866 | 142.0 |
| No log | 2.0 | 84 | 1.5729 | 46.8902 | 24.2952 | 27.8304 | 43.9581 | 142.0 |
| No log | 3.0 | 126 | 1.7025 | 48.836 | 26.917 | 30.1325 | 45.7887 | 142.0 |
| No log | 4.0 | 168 | 1.8526 | 48.906 | 26.8641 | 30.4677 | 46.1825 | 139.2 |
| No log | 5.0 | 210 | 1.9818 | 51.145 | 28.834 | 30.1862 | 48.6876 | 141.8 |
| No log | 6.0 | 252 | 2.0752 | 49.2922 | 27.0916 | 29.2754 | 46.4762 | 140.8 |
### Framework versions
- Transformers 4.20.0
- Pytorch 1.11.0+cu113
- Datasets 2.3.2
- Tokenizers 0.12.1
|
theojolliffe/bart-cnn-science-v3-e2-v4-e4-manual | 61f0fbb3f8c99f7733db6b7f83f8deed502ccd2b | 2022-06-18T16:45:26.000Z | [
"pytorch",
"tensorboard",
"bart",
"text2text-generation",
"transformers",
"generated_from_trainer",
"license:mit",
"model-index",
"autotrain_compatible"
] | text2text-generation | false | theojolliffe | null | theojolliffe/bart-cnn-science-v3-e2-v4-e4-manual | 2 | null | transformers | 26,387 | ---
license: mit
tags:
- generated_from_trainer
metrics:
- rouge
model-index:
- name: bart-cnn-science-v3-e2-v4-e4-manual
results: []
---
<!-- This model card has been generated automatically according to the information the Trainer had access to. You
should probably proofread and complete it, then remove this comment. -->
# bart-cnn-science-v3-e2-v4-e4-manual
This model is a fine-tuned version of [theojolliffe/bart-cnn-science-v3-e2](https://huggingface.co/theojolliffe/bart-cnn-science-v3-e2) on an unknown dataset.
It achieves the following results on the evaluation set:
- Loss: 1.1223
- Rouge1: 50.8519
- Rouge2: 30.3314
- Rougel: 31.5149
- Rougelsum: 48.4389
- Gen Len: 142.0
## Model description
More information needed
## Intended uses & limitations
More information needed
## Training and evaluation data
More information needed
## Training procedure
### Training hyperparameters
The following hyperparameters were used during training:
- learning_rate: 2e-05
- train_batch_size: 2
- eval_batch_size: 2
- seed: 42
- optimizer: Adam with betas=(0.9,0.999) and epsilon=1e-08
- lr_scheduler_type: linear
- num_epochs: 4
- mixed_precision_training: Native AMP
### Training results
| Training Loss | Epoch | Step | Validation Loss | Rouge1 | Rouge2 | Rougel | Rougelsum | Gen Len |
|:-------------:|:-----:|:----:|:---------------:|:-------:|:-------:|:-------:|:---------:|:-------:|
| No log | 1.0 | 42 | 0.9420 | 53.5234 | 33.6131 | 35.8383 | 51.1499 | 142.0 |
| No log | 2.0 | 84 | 0.9439 | 52.388 | 32.1451 | 35.2339 | 49.6554 | 142.0 |
| No log | 3.0 | 126 | 1.0321 | 56.2765 | 37.671 | 39.2693 | 53.5596 | 142.0 |
| No log | 4.0 | 168 | 1.1223 | 50.8519 | 30.3314 | 31.5149 | 48.4389 | 142.0 |
### Framework versions
- Transformers 4.20.0
- Pytorch 1.11.0+cu113
- Datasets 2.3.2
- Tokenizers 0.12.1
|
tuni/xlm-roberta-large-xnli-finetuned-mnli-SJP-v3 | 9557eb8cba0c8e7c813173f457493478e8681eb1 | 2022-06-20T00:40:09.000Z | [
"pytorch",
"tensorboard",
"xlm-roberta",
"text-classification",
"dataset:swiss_judgment_prediction",
"transformers",
"generated_from_trainer",
"license:mit",
"model-index"
] | text-classification | false | tuni | null | tuni/xlm-roberta-large-xnli-finetuned-mnli-SJP-v3 | 2 | null | transformers | 26,388 | ---
license: mit
tags:
- generated_from_trainer
datasets:
- swiss_judgment_prediction
model-index:
- name: xlm-roberta-large-xnli-finetuned-mnli-SJP-v3
results: []
---
<!-- This model card has been generated automatically according to the information the Trainer had access to. You
should probably proofread and complete it, then remove this comment. -->
# xlm-roberta-large-xnli-finetuned-mnli-SJP-v3
This model is a fine-tuned version of [joeddav/xlm-roberta-large-xnli](https://huggingface.co/joeddav/xlm-roberta-large-xnli) on the swiss_judgment_prediction dataset.
It achieves the following results on the evaluation set:
- eval_loss: 5.4348
- eval_accuracy: 0.3352
- eval_runtime: 588.81
- eval_samples_per_second: 8.492
- eval_steps_per_second: 4.246
- epoch: 14.0
- step: 70
## Model description
More information needed
## Intended uses & limitations
More information needed
## Training and evaluation data
More information needed
## Training procedure
### Training hyperparameters
The following hyperparameters were used during training:
- learning_rate: 2e-05
- train_batch_size: 2
- eval_batch_size: 2
- seed: 42
- optimizer: Adam with betas=(0.9,0.999) and epsilon=1e-08
- lr_scheduler_type: linear
- num_epochs: 20
### Framework versions
- Transformers 4.20.0
- Pytorch 1.11.0+cu113
- Datasets 2.3.2
- Tokenizers 0.12.1
|
huggingtweets/svelounsegreto | 196eb366ef2b37eb5685ce8604b4fa6bc0f580ef | 2022-06-18T18:31:10.000Z | [
"pytorch",
"gpt2",
"text-generation",
"en",
"transformers",
"huggingtweets"
] | text-generation | false | huggingtweets | null | huggingtweets/svelounsegreto | 2 | null | transformers | 26,389 | ---
language: en
thumbnail: http://www.huggingtweets.com/svelounsegreto/1655577065862/predictions.png
tags:
- huggingtweets
widget:
- text: "My dream is"
---
<div class="inline-flex flex-col" style="line-height: 1.5;">
<div class="flex">
<div
style="display:inherit; margin-left: 4px; margin-right: 4px; width: 92px; height:92px; border-radius: 50%; background-size: cover; background-image: url('https://pbs.twimg.com/profile_images/1532495934944432147/fnWmG59I_400x400.jpg')">
</div>
<div
style="display:none; margin-left: 4px; margin-right: 4px; width: 92px; height:92px; border-radius: 50%; background-size: cover; background-image: url('')">
</div>
<div
style="display:none; margin-left: 4px; margin-right: 4px; width: 92px; height:92px; border-radius: 50%; background-size: cover; background-image: url('')">
</div>
</div>
<div style="text-align: center; margin-top: 3px; font-size: 16px; font-weight: 800">🤖 AI BOT 🤖</div>
<div style="text-align: center; font-size: 16px; font-weight: 800">TiSveloUnSegreto</div>
<div style="text-align: center; font-size: 14px;">@svelounsegreto</div>
</div>
I was made with [huggingtweets](https://github.com/borisdayma/huggingtweets).
Create your own bot based on your favorite user with [the demo](https://colab.research.google.com/github/borisdayma/huggingtweets/blob/master/huggingtweets-demo.ipynb)!
## How does it work?
The model uses the following pipeline.
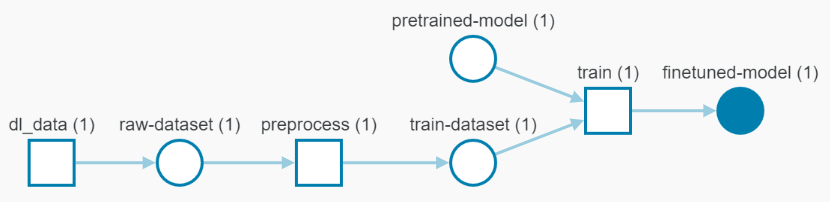
To understand how the model was developed, check the [W&B report](https://wandb.ai/wandb/huggingtweets/reports/HuggingTweets-Train-a-Model-to-Generate-Tweets--VmlldzoxMTY5MjI).
## Training data
The model was trained on tweets from TiSveloUnSegreto.
| Data | TiSveloUnSegreto |
| --- | --- |
| Tweets downloaded | 233 |
| Retweets | 0 |
| Short tweets | 0 |
| Tweets kept | 233 |
[Explore the data](https://wandb.ai/wandb/huggingtweets/runs/2dufvfue/artifacts), which is tracked with [W&B artifacts](https://docs.wandb.com/artifacts) at every step of the pipeline.
## Training procedure
The model is based on a pre-trained [GPT-2](https://huggingface.co/gpt2) which is fine-tuned on @svelounsegreto's tweets.
Hyperparameters and metrics are recorded in the [W&B training run](https://wandb.ai/wandb/huggingtweets/runs/16tsvbvd) for full transparency and reproducibility.
At the end of training, [the final model](https://wandb.ai/wandb/huggingtweets/runs/16tsvbvd/artifacts) is logged and versioned.
## How to use
You can use this model directly with a pipeline for text generation:
```python
from transformers import pipeline
generator = pipeline('text-generation',
model='huggingtweets/svelounsegreto')
generator("My dream is", num_return_sequences=5)
```
## Limitations and bias
The model suffers from [the same limitations and bias as GPT-2](https://huggingface.co/gpt2#limitations-and-bias).
In addition, the data present in the user's tweets further affects the text generated by the model.
## About
*Built by Boris Dayma*
[](https://twitter.com/intent/follow?screen_name=borisdayma)
For more details, visit the project repository.
[](https://github.com/borisdayma/huggingtweets)
|
jhliu/ClinicalNoteBERT-base-uncased-NTP-MIMIC-note | aacfcbe68c190eab234a4df69e8939169af4666f | 2022-06-20T01:12:19.000Z | [
"pytorch",
"bert",
"transformers"
] | null | false | jhliu | null | jhliu/ClinicalNoteBERT-base-uncased-NTP-MIMIC-note | 2 | null | transformers | 26,390 | Entry not found |
Lvxue/distilled_mt5-base_20ep | b3835b5e7c626b0a78d3631b8ffa5950d69ec1c1 | 2022-06-19T09:41:17.000Z | [
"pytorch",
"mt5",
"text2text-generation",
"transformers",
"autotrain_compatible"
] | text2text-generation | false | Lvxue | null | Lvxue/distilled_mt5-base_20ep | 2 | null | transformers | 26,391 | Entry not found |
pparkji/new_ipa_wav2vec2_timit | d3426555ce30b387f11500b4703336798079432f | 2022-06-19T06:50:21.000Z | [
"pytorch",
"wav2vec2",
"automatic-speech-recognition",
"transformers"
] | automatic-speech-recognition | false | pparkji | null | pparkji/new_ipa_wav2vec2_timit | 2 | null | transformers | 26,392 | Entry not found |
sanskar/ReviewSystem | 2bab41a5de60350bf472e13d112f953df878ea13 | 2022-06-20T10:52:03.000Z | [
"pytorch",
"distilbert",
"text-classification",
"transformers",
"license:apache-2.0"
] | text-classification | false | sanskar | null | sanskar/ReviewSystem | 2 | null | transformers | 26,393 | |
kjunelee/distilbert-base-uncased-finetuned-clinc | e46496c7923ceca5d78801266bfb9a7d3f124349 | 2022-06-19T20:23:59.000Z | [
"pytorch",
"tensorboard",
"distilbert",
"text-classification",
"transformers"
] | text-classification | false | kjunelee | null | kjunelee/distilbert-base-uncased-finetuned-clinc | 2 | null | transformers | 26,394 | Entry not found |
vjosap/finetuning-sentiment-model-3000-samples | 1b801b1f882065c1320344a6e466e13a4618088a | 2022-06-19T10:48:45.000Z | [
"pytorch",
"tensorboard",
"distilbert",
"text-classification",
"transformers",
"generated_from_trainer",
"license:apache-2.0",
"model-index"
] | text-classification | false | vjosap | null | vjosap/finetuning-sentiment-model-3000-samples | 2 | null | transformers | 26,395 | ---
license: apache-2.0
tags:
- generated_from_trainer
metrics:
- accuracy
- f1
model-index:
- name: finetuning-sentiment-model-3000-samples
results: []
---
<!-- This model card has been generated automatically according to the information the Trainer had access to. You
should probably proofread and complete it, then remove this comment. -->
# finetuning-sentiment-model-3000-samples
This model is a fine-tuned version of [distilbert-base-uncased](https://huggingface.co/distilbert-base-uncased) on an unknown dataset.
It achieves the following results on the evaluation set:
- Loss: 0.2903
- Accuracy: 0.88
- F1: 0.8808
## Model description
More information needed
## Intended uses & limitations
More information needed
## Training and evaluation data
More information needed
## Training procedure
### Training hyperparameters
The following hyperparameters were used during training:
- learning_rate: 2e-05
- train_batch_size: 16
- eval_batch_size: 16
- seed: 42
- optimizer: Adam with betas=(0.9,0.999) and epsilon=1e-08
- lr_scheduler_type: linear
- num_epochs: 2
### Training results
### Framework versions
- Transformers 4.20.0
- Pytorch 1.11.0+cu113
- Tokenizers 0.12.1
|
wiselinjayajos/bert-finetuned-squad | 8ecce2ac107ccc21bd635174192bc87c379f4b90 | 2022-06-20T23:35:59.000Z | [
"pytorch",
"tensorboard",
"bert",
"question-answering",
"dataset:squad",
"transformers",
"generated_from_trainer",
"license:apache-2.0",
"model-index",
"autotrain_compatible"
] | question-answering | false | wiselinjayajos | null | wiselinjayajos/bert-finetuned-squad | 2 | null | transformers | 26,396 | ---
license: apache-2.0
tags:
- generated_from_trainer
datasets:
- squad
model-index:
- name: bert-finetuned-squad
results: []
---
<!-- This model card has been generated automatically according to the information the Trainer had access to. You
should probably proofread and complete it, then remove this comment. -->
# bert-finetuned-squad
This model is a fine-tuned version of [bert-base-cased](https://huggingface.co/bert-base-cased) on the squad dataset.
## Model description
More information needed
## Intended uses & limitations
More information needed
## Training and evaluation data
More information needed
## Training procedure
### Training hyperparameters
The following hyperparameters were used during training:
- learning_rate: 2e-05
- train_batch_size: 8
- eval_batch_size: 8
- seed: 42
- optimizer: Adam with betas=(0.9,0.999) and epsilon=1e-08
- lr_scheduler_type: linear
- num_epochs: 3
### Training results
### Framework versions
- Transformers 4.20.0
- Pytorch 1.11.0+cu113
- Datasets 2.3.2
- Tokenizers 0.12.1
|
Sealgair/DialoGPT-medium-Eyden | 0ab4fa0efc742ec9d09c4012569f8eb91be2839d | 2022-06-19T16:16:41.000Z | [
"pytorch",
"gpt2",
"text-generation",
"transformers",
"conversational"
] | conversational | false | Sealgair | null | Sealgair/DialoGPT-medium-Eyden | 2 | null | transformers | 26,397 | ---
tags:
- conversational
---
# DialoGPT Model based on my sms history |
anas-awadalla/prophetnet-large-squad | e2bbc2ca2336e76cc3d3eb26a5c8387573ccb05f | 2022-06-19T19:16:54.000Z | [
"pytorch",
"tensorboard",
"prophetnet",
"text2text-generation",
"dataset:squad",
"transformers",
"generated_from_trainer",
"model-index",
"autotrain_compatible"
] | text2text-generation | false | anas-awadalla | null | anas-awadalla/prophetnet-large-squad | 2 | null | transformers | 26,398 | ---
tags:
- generated_from_trainer
datasets:
- squad
model-index:
- name: prophetnet-large-squad
results: []
---
<!-- This model card has been generated automatically according to the information the Trainer had access to. You
should probably proofread and complete it, then remove this comment. -->
# prophetnet-large-squad
This model is a fine-tuned version of [microsoft/prophetnet-large-uncased](https://huggingface.co/microsoft/prophetnet-large-uncased) on the squad dataset.
## Model description
More information needed
## Intended uses & limitations
More information needed
## Training and evaluation data
More information needed
## Training procedure
### Training hyperparameters
The following hyperparameters were used during training:
- learning_rate: 3e-05
- train_batch_size: 128
- eval_batch_size: 8
- seed: 42
- distributed_type: multi-GPU
- num_devices: 2
- total_train_batch_size: 256
- total_eval_batch_size: 16
- optimizer: Adam with betas=(0.9,0.999) and epsilon=1e-08
- lr_scheduler_type: linear
- num_epochs: 2.0
### Training results
### Framework versions
- Transformers 4.20.0.dev0
- Pytorch 1.11.0+cu113
- Datasets 2.0.0
- Tokenizers 0.11.6
|
anas-awadalla/prompt-tuned-t5-base-num-tokens-100-squad | 5c69b23ac2d0330168e6957971e83bd7f7b5e360 | 2022-06-20T15:56:39.000Z | [
"pytorch",
"tensorboard",
"dataset:squad",
"transformers",
"generated_from_trainer",
"license:apache-2.0",
"model-index"
] | null | false | anas-awadalla | null | anas-awadalla/prompt-tuned-t5-base-num-tokens-100-squad | 2 | null | transformers | 26,399 | ---
license: apache-2.0
tags:
- generated_from_trainer
datasets:
- squad
model-index:
- name: prompt-tuned-t5-base-num-tokens-100-squad
results: []
---
<!-- This model card has been generated automatically according to the information the Trainer had access to. You
should probably proofread and complete it, then remove this comment. -->
# prompt-tuned-t5-base-num-tokens-100-squad
This model is a fine-tuned version of [google/t5-base-lm-adapt](https://huggingface.co/google/t5-base-lm-adapt) on the squad dataset.
## Model description
More information needed
## Intended uses & limitations
More information needed
## Training and evaluation data
More information needed
## Training procedure
### Training hyperparameters
The following hyperparameters were used during training:
- learning_rate: 0.3
- train_batch_size: 8
- eval_batch_size: 8
- seed: 42
- distributed_type: multi-GPU
- num_devices: 2
- gradient_accumulation_steps: 2
- total_train_batch_size: 32
- total_eval_batch_size: 16
- optimizer: Adam with betas=(0.9,0.999) and epsilon=1e-08
- lr_scheduler_type: linear
- training_steps: 30000
### Training results
### Framework versions
- Transformers 4.20.0.dev0
- Pytorch 1.11.0+cu113
- Datasets 2.0.0
- Tokenizers 0.11.6
|
Subsets and Splits
No community queries yet
The top public SQL queries from the community will appear here once available.