modelId
stringlengths 4
112
| sha
stringlengths 40
40
| lastModified
stringlengths 24
24
| tags
list | pipeline_tag
stringclasses 29
values | private
bool 1
class | author
stringlengths 2
38
⌀ | config
null | id
stringlengths 4
112
| downloads
float64 0
36.8M
⌀ | likes
float64 0
712
⌀ | library_name
stringclasses 17
values | __index_level_0__
int64 0
38.5k
| readme
stringlengths 0
186k
|
---|---|---|---|---|---|---|---|---|---|---|---|---|---|
google/multiberts-seed_2-step_1800k | 65f204330ef345286cd6bb9c59b2167a920468da | 2021-11-06T02:06:17.000Z | [
"pytorch",
"tf",
"bert",
"pretraining",
"en",
"arxiv:2106.16163",
"arxiv:1908.08962",
"transformers",
"multiberts",
"multiberts-seed_2",
"multiberts-seed_2-step_1800k",
"license:apache-2.0"
]
| null | false | google | null | google/multiberts-seed_2-step_1800k | 2 | null | transformers | 24,000 | ---
language: en
tags:
- multiberts
- multiberts-seed_2
- multiberts-seed_2-step_1800k
license: apache-2.0
---
# MultiBERTs, Intermediate Checkpoint - Seed 2, Step 1800k
MultiBERTs is a collection of checkpoints and a statistical library to support
robust research on BERT. We provide 25 BERT-base models trained with
similar hyper-parameters as
[the original BERT model](https://github.com/google-research/bert) but
with different random seeds, which causes variations in the initial weights and order of
training instances. The aim is to distinguish findings that apply to a specific
artifact (i.e., a particular instance of the model) from those that apply to the
more general procedure.
We also provide 140 intermediate checkpoints captured
during the course of pre-training (we saved 28 checkpoints for the first 5 runs).
The models were originally released through
[http://goo.gle/multiberts](http://goo.gle/multiberts). We describe them in our
paper
[The MultiBERTs: BERT Reproductions for Robustness Analysis](https://arxiv.org/abs/2106.16163).
This is model #2, captured at step 1800k (max: 2000k, i.e., 2M steps).
## Model Description
This model was captured during a reproduction of
[BERT-base uncased](https://github.com/google-research/bert), for English: it
is a Transformers model pretrained on a large corpus of English data, using the
Masked Language Modelling (MLM) and the Next Sentence Prediction (NSP)
objectives.
The intended uses, limitations, training data and training procedure for the fully trained model are similar
to [BERT-base uncased](https://github.com/google-research/bert). Two major
differences with the original model:
* We pre-trained the MultiBERTs models for 2 million steps using sequence
length 512 (instead of 1 million steps using sequence length 128 then 512).
* We used an alternative version of Wikipedia and Books Corpus, initially
collected for [Turc et al., 2019](https://arxiv.org/abs/1908.08962).
This is a best-effort reproduction, and so it is probable that differences with
the original model have gone unnoticed. The performance of MultiBERTs on GLUE after full training is oftentimes comparable to that of original
BERT, but we found significant differences on the dev set of SQuAD (MultiBERTs outperforms original BERT).
See our [technical report](https://arxiv.org/abs/2106.16163) for more details.
### How to use
Using code from
[BERT-base uncased](https://huggingface.co/bert-base-uncased), here is an example based on
Tensorflow:
```
from transformers import BertTokenizer, TFBertModel
tokenizer = BertTokenizer.from_pretrained('google/multiberts-seed_2-step_1800k')
model = TFBertModel.from_pretrained("google/multiberts-seed_2-step_1800k")
text = "Replace me by any text you'd like."
encoded_input = tokenizer(text, return_tensors='tf')
output = model(encoded_input)
```
PyTorch version:
```
from transformers import BertTokenizer, BertModel
tokenizer = BertTokenizer.from_pretrained('google/multiberts-seed_2-step_1800k')
model = BertModel.from_pretrained("google/multiberts-seed_2-step_1800k")
text = "Replace me by any text you'd like."
encoded_input = tokenizer(text, return_tensors='pt')
output = model(**encoded_input)
```
## Citation info
```bibtex
@article{sellam2021multiberts,
title={The MultiBERTs: BERT Reproductions for Robustness Analysis},
author={Thibault Sellam and Steve Yadlowsky and Jason Wei and Naomi Saphra and Alexander D'Amour and Tal Linzen and Jasmijn Bastings and Iulia Turc and Jacob Eisenstein and Dipanjan Das and Ian Tenney and Ellie Pavlick},
journal={arXiv preprint arXiv:2106.16163},
year={2021}
}
```
|
google/multiberts-seed_2-step_1900k | 271eed868855d8e78e84d57f53b51d82c7800bc8 | 2021-11-06T02:08:03.000Z | [
"pytorch",
"tf",
"bert",
"pretraining",
"en",
"arxiv:2106.16163",
"arxiv:1908.08962",
"transformers",
"multiberts",
"multiberts-seed_2",
"multiberts-seed_2-step_1900k",
"license:apache-2.0"
]
| null | false | google | null | google/multiberts-seed_2-step_1900k | 2 | null | transformers | 24,001 | ---
language: en
tags:
- multiberts
- multiberts-seed_2
- multiberts-seed_2-step_1900k
license: apache-2.0
---
# MultiBERTs, Intermediate Checkpoint - Seed 2, Step 1900k
MultiBERTs is a collection of checkpoints and a statistical library to support
robust research on BERT. We provide 25 BERT-base models trained with
similar hyper-parameters as
[the original BERT model](https://github.com/google-research/bert) but
with different random seeds, which causes variations in the initial weights and order of
training instances. The aim is to distinguish findings that apply to a specific
artifact (i.e., a particular instance of the model) from those that apply to the
more general procedure.
We also provide 140 intermediate checkpoints captured
during the course of pre-training (we saved 28 checkpoints for the first 5 runs).
The models were originally released through
[http://goo.gle/multiberts](http://goo.gle/multiberts). We describe them in our
paper
[The MultiBERTs: BERT Reproductions for Robustness Analysis](https://arxiv.org/abs/2106.16163).
This is model #2, captured at step 1900k (max: 2000k, i.e., 2M steps).
## Model Description
This model was captured during a reproduction of
[BERT-base uncased](https://github.com/google-research/bert), for English: it
is a Transformers model pretrained on a large corpus of English data, using the
Masked Language Modelling (MLM) and the Next Sentence Prediction (NSP)
objectives.
The intended uses, limitations, training data and training procedure for the fully trained model are similar
to [BERT-base uncased](https://github.com/google-research/bert). Two major
differences with the original model:
* We pre-trained the MultiBERTs models for 2 million steps using sequence
length 512 (instead of 1 million steps using sequence length 128 then 512).
* We used an alternative version of Wikipedia and Books Corpus, initially
collected for [Turc et al., 2019](https://arxiv.org/abs/1908.08962).
This is a best-effort reproduction, and so it is probable that differences with
the original model have gone unnoticed. The performance of MultiBERTs on GLUE after full training is oftentimes comparable to that of original
BERT, but we found significant differences on the dev set of SQuAD (MultiBERTs outperforms original BERT).
See our [technical report](https://arxiv.org/abs/2106.16163) for more details.
### How to use
Using code from
[BERT-base uncased](https://huggingface.co/bert-base-uncased), here is an example based on
Tensorflow:
```
from transformers import BertTokenizer, TFBertModel
tokenizer = BertTokenizer.from_pretrained('google/multiberts-seed_2-step_1900k')
model = TFBertModel.from_pretrained("google/multiberts-seed_2-step_1900k")
text = "Replace me by any text you'd like."
encoded_input = tokenizer(text, return_tensors='tf')
output = model(encoded_input)
```
PyTorch version:
```
from transformers import BertTokenizer, BertModel
tokenizer = BertTokenizer.from_pretrained('google/multiberts-seed_2-step_1900k')
model = BertModel.from_pretrained("google/multiberts-seed_2-step_1900k")
text = "Replace me by any text you'd like."
encoded_input = tokenizer(text, return_tensors='pt')
output = model(**encoded_input)
```
## Citation info
```bibtex
@article{sellam2021multiberts,
title={The MultiBERTs: BERT Reproductions for Robustness Analysis},
author={Thibault Sellam and Steve Yadlowsky and Jason Wei and Naomi Saphra and Alexander D'Amour and Tal Linzen and Jasmijn Bastings and Iulia Turc and Jacob Eisenstein and Dipanjan Das and Ian Tenney and Ellie Pavlick},
journal={arXiv preprint arXiv:2106.16163},
year={2021}
}
```
|
google/multiberts-seed_2-step_500k | 5159428a0989623e6c705beb4d110d3e3bff6ffb | 2021-11-06T01:44:21.000Z | [
"pytorch",
"tf",
"bert",
"pretraining",
"en",
"arxiv:2106.16163",
"arxiv:1908.08962",
"transformers",
"multiberts",
"multiberts-seed_2",
"multiberts-seed_2-step_500k",
"license:apache-2.0"
]
| null | false | google | null | google/multiberts-seed_2-step_500k | 2 | null | transformers | 24,002 | ---
language: en
tags:
- multiberts
- multiberts-seed_2
- multiberts-seed_2-step_500k
license: apache-2.0
---
# MultiBERTs, Intermediate Checkpoint - Seed 2, Step 500k
MultiBERTs is a collection of checkpoints and a statistical library to support
robust research on BERT. We provide 25 BERT-base models trained with
similar hyper-parameters as
[the original BERT model](https://github.com/google-research/bert) but
with different random seeds, which causes variations in the initial weights and order of
training instances. The aim is to distinguish findings that apply to a specific
artifact (i.e., a particular instance of the model) from those that apply to the
more general procedure.
We also provide 140 intermediate checkpoints captured
during the course of pre-training (we saved 28 checkpoints for the first 5 runs).
The models were originally released through
[http://goo.gle/multiberts](http://goo.gle/multiberts). We describe them in our
paper
[The MultiBERTs: BERT Reproductions for Robustness Analysis](https://arxiv.org/abs/2106.16163).
This is model #2, captured at step 500k (max: 2000k, i.e., 2M steps).
## Model Description
This model was captured during a reproduction of
[BERT-base uncased](https://github.com/google-research/bert), for English: it
is a Transformers model pretrained on a large corpus of English data, using the
Masked Language Modelling (MLM) and the Next Sentence Prediction (NSP)
objectives.
The intended uses, limitations, training data and training procedure for the fully trained model are similar
to [BERT-base uncased](https://github.com/google-research/bert). Two major
differences with the original model:
* We pre-trained the MultiBERTs models for 2 million steps using sequence
length 512 (instead of 1 million steps using sequence length 128 then 512).
* We used an alternative version of Wikipedia and Books Corpus, initially
collected for [Turc et al., 2019](https://arxiv.org/abs/1908.08962).
This is a best-effort reproduction, and so it is probable that differences with
the original model have gone unnoticed. The performance of MultiBERTs on GLUE after full training is oftentimes comparable to that of original
BERT, but we found significant differences on the dev set of SQuAD (MultiBERTs outperforms original BERT).
See our [technical report](https://arxiv.org/abs/2106.16163) for more details.
### How to use
Using code from
[BERT-base uncased](https://huggingface.co/bert-base-uncased), here is an example based on
Tensorflow:
```
from transformers import BertTokenizer, TFBertModel
tokenizer = BertTokenizer.from_pretrained('google/multiberts-seed_2-step_500k')
model = TFBertModel.from_pretrained("google/multiberts-seed_2-step_500k")
text = "Replace me by any text you'd like."
encoded_input = tokenizer(text, return_tensors='tf')
output = model(encoded_input)
```
PyTorch version:
```
from transformers import BertTokenizer, BertModel
tokenizer = BertTokenizer.from_pretrained('google/multiberts-seed_2-step_500k')
model = BertModel.from_pretrained("google/multiberts-seed_2-step_500k")
text = "Replace me by any text you'd like."
encoded_input = tokenizer(text, return_tensors='pt')
output = model(**encoded_input)
```
## Citation info
```bibtex
@article{sellam2021multiberts,
title={The MultiBERTs: BERT Reproductions for Robustness Analysis},
author={Thibault Sellam and Steve Yadlowsky and Jason Wei and Naomi Saphra and Alexander D'Amour and Tal Linzen and Jasmijn Bastings and Iulia Turc and Jacob Eisenstein and Dipanjan Das and Ian Tenney and Ellie Pavlick},
journal={arXiv preprint arXiv:2106.16163},
year={2021}
}
```
|
google/multiberts-seed_2-step_600k | 04dfbd13247e1c0d6880deb708d360632819427a | 2021-11-06T01:45:59.000Z | [
"pytorch",
"tf",
"bert",
"pretraining",
"en",
"arxiv:2106.16163",
"arxiv:1908.08962",
"transformers",
"multiberts",
"multiberts-seed_2",
"multiberts-seed_2-step_600k",
"license:apache-2.0"
]
| null | false | google | null | google/multiberts-seed_2-step_600k | 2 | null | transformers | 24,003 | ---
language: en
tags:
- multiberts
- multiberts-seed_2
- multiberts-seed_2-step_600k
license: apache-2.0
---
# MultiBERTs, Intermediate Checkpoint - Seed 2, Step 600k
MultiBERTs is a collection of checkpoints and a statistical library to support
robust research on BERT. We provide 25 BERT-base models trained with
similar hyper-parameters as
[the original BERT model](https://github.com/google-research/bert) but
with different random seeds, which causes variations in the initial weights and order of
training instances. The aim is to distinguish findings that apply to a specific
artifact (i.e., a particular instance of the model) from those that apply to the
more general procedure.
We also provide 140 intermediate checkpoints captured
during the course of pre-training (we saved 28 checkpoints for the first 5 runs).
The models were originally released through
[http://goo.gle/multiberts](http://goo.gle/multiberts). We describe them in our
paper
[The MultiBERTs: BERT Reproductions for Robustness Analysis](https://arxiv.org/abs/2106.16163).
This is model #2, captured at step 600k (max: 2000k, i.e., 2M steps).
## Model Description
This model was captured during a reproduction of
[BERT-base uncased](https://github.com/google-research/bert), for English: it
is a Transformers model pretrained on a large corpus of English data, using the
Masked Language Modelling (MLM) and the Next Sentence Prediction (NSP)
objectives.
The intended uses, limitations, training data and training procedure for the fully trained model are similar
to [BERT-base uncased](https://github.com/google-research/bert). Two major
differences with the original model:
* We pre-trained the MultiBERTs models for 2 million steps using sequence
length 512 (instead of 1 million steps using sequence length 128 then 512).
* We used an alternative version of Wikipedia and Books Corpus, initially
collected for [Turc et al., 2019](https://arxiv.org/abs/1908.08962).
This is a best-effort reproduction, and so it is probable that differences with
the original model have gone unnoticed. The performance of MultiBERTs on GLUE after full training is oftentimes comparable to that of original
BERT, but we found significant differences on the dev set of SQuAD (MultiBERTs outperforms original BERT).
See our [technical report](https://arxiv.org/abs/2106.16163) for more details.
### How to use
Using code from
[BERT-base uncased](https://huggingface.co/bert-base-uncased), here is an example based on
Tensorflow:
```
from transformers import BertTokenizer, TFBertModel
tokenizer = BertTokenizer.from_pretrained('google/multiberts-seed_2-step_600k')
model = TFBertModel.from_pretrained("google/multiberts-seed_2-step_600k")
text = "Replace me by any text you'd like."
encoded_input = tokenizer(text, return_tensors='tf')
output = model(encoded_input)
```
PyTorch version:
```
from transformers import BertTokenizer, BertModel
tokenizer = BertTokenizer.from_pretrained('google/multiberts-seed_2-step_600k')
model = BertModel.from_pretrained("google/multiberts-seed_2-step_600k")
text = "Replace me by any text you'd like."
encoded_input = tokenizer(text, return_tensors='pt')
output = model(**encoded_input)
```
## Citation info
```bibtex
@article{sellam2021multiberts,
title={The MultiBERTs: BERT Reproductions for Robustness Analysis},
author={Thibault Sellam and Steve Yadlowsky and Jason Wei and Naomi Saphra and Alexander D'Amour and Tal Linzen and Jasmijn Bastings and Iulia Turc and Jacob Eisenstein and Dipanjan Das and Ian Tenney and Ellie Pavlick},
journal={arXiv preprint arXiv:2106.16163},
year={2021}
}
```
|
google/multiberts-seed_2-step_800k | 02178e8202d205e5773864b9cc348f5af23059b3 | 2021-11-06T01:49:23.000Z | [
"pytorch",
"tf",
"bert",
"pretraining",
"en",
"arxiv:2106.16163",
"arxiv:1908.08962",
"transformers",
"multiberts",
"multiberts-seed_2",
"multiberts-seed_2-step_800k",
"license:apache-2.0"
]
| null | false | google | null | google/multiberts-seed_2-step_800k | 2 | null | transformers | 24,004 | ---
language: en
tags:
- multiberts
- multiberts-seed_2
- multiberts-seed_2-step_800k
license: apache-2.0
---
# MultiBERTs, Intermediate Checkpoint - Seed 2, Step 800k
MultiBERTs is a collection of checkpoints and a statistical library to support
robust research on BERT. We provide 25 BERT-base models trained with
similar hyper-parameters as
[the original BERT model](https://github.com/google-research/bert) but
with different random seeds, which causes variations in the initial weights and order of
training instances. The aim is to distinguish findings that apply to a specific
artifact (i.e., a particular instance of the model) from those that apply to the
more general procedure.
We also provide 140 intermediate checkpoints captured
during the course of pre-training (we saved 28 checkpoints for the first 5 runs).
The models were originally released through
[http://goo.gle/multiberts](http://goo.gle/multiberts). We describe them in our
paper
[The MultiBERTs: BERT Reproductions for Robustness Analysis](https://arxiv.org/abs/2106.16163).
This is model #2, captured at step 800k (max: 2000k, i.e., 2M steps).
## Model Description
This model was captured during a reproduction of
[BERT-base uncased](https://github.com/google-research/bert), for English: it
is a Transformers model pretrained on a large corpus of English data, using the
Masked Language Modelling (MLM) and the Next Sentence Prediction (NSP)
objectives.
The intended uses, limitations, training data and training procedure for the fully trained model are similar
to [BERT-base uncased](https://github.com/google-research/bert). Two major
differences with the original model:
* We pre-trained the MultiBERTs models for 2 million steps using sequence
length 512 (instead of 1 million steps using sequence length 128 then 512).
* We used an alternative version of Wikipedia and Books Corpus, initially
collected for [Turc et al., 2019](https://arxiv.org/abs/1908.08962).
This is a best-effort reproduction, and so it is probable that differences with
the original model have gone unnoticed. The performance of MultiBERTs on GLUE after full training is oftentimes comparable to that of original
BERT, but we found significant differences on the dev set of SQuAD (MultiBERTs outperforms original BERT).
See our [technical report](https://arxiv.org/abs/2106.16163) for more details.
### How to use
Using code from
[BERT-base uncased](https://huggingface.co/bert-base-uncased), here is an example based on
Tensorflow:
```
from transformers import BertTokenizer, TFBertModel
tokenizer = BertTokenizer.from_pretrained('google/multiberts-seed_2-step_800k')
model = TFBertModel.from_pretrained("google/multiberts-seed_2-step_800k")
text = "Replace me by any text you'd like."
encoded_input = tokenizer(text, return_tensors='tf')
output = model(encoded_input)
```
PyTorch version:
```
from transformers import BertTokenizer, BertModel
tokenizer = BertTokenizer.from_pretrained('google/multiberts-seed_2-step_800k')
model = BertModel.from_pretrained("google/multiberts-seed_2-step_800k")
text = "Replace me by any text you'd like."
encoded_input = tokenizer(text, return_tensors='pt')
output = model(**encoded_input)
```
## Citation info
```bibtex
@article{sellam2021multiberts,
title={The MultiBERTs: BERT Reproductions for Robustness Analysis},
author={Thibault Sellam and Steve Yadlowsky and Jason Wei and Naomi Saphra and Alexander D'Amour and Tal Linzen and Jasmijn Bastings and Iulia Turc and Jacob Eisenstein and Dipanjan Das and Ian Tenney and Ellie Pavlick},
journal={arXiv preprint arXiv:2106.16163},
year={2021}
}
```
|
google/multiberts-seed_3-step_1900k | 2b2ab91a25b88566ecad948060c3ae8e38ea5e7b | 2021-11-06T02:58:09.000Z | [
"pytorch",
"tf",
"bert",
"pretraining",
"en",
"arxiv:2106.16163",
"arxiv:1908.08962",
"transformers",
"multiberts",
"multiberts-seed_3",
"multiberts-seed_3-step_1900k",
"license:apache-2.0"
]
| null | false | google | null | google/multiberts-seed_3-step_1900k | 2 | null | transformers | 24,005 | ---
language: en
tags:
- multiberts
- multiberts-seed_3
- multiberts-seed_3-step_1900k
license: apache-2.0
---
# MultiBERTs, Intermediate Checkpoint - Seed 3, Step 1900k
MultiBERTs is a collection of checkpoints and a statistical library to support
robust research on BERT. We provide 25 BERT-base models trained with
similar hyper-parameters as
[the original BERT model](https://github.com/google-research/bert) but
with different random seeds, which causes variations in the initial weights and order of
training instances. The aim is to distinguish findings that apply to a specific
artifact (i.e., a particular instance of the model) from those that apply to the
more general procedure.
We also provide 140 intermediate checkpoints captured
during the course of pre-training (we saved 28 checkpoints for the first 5 runs).
The models were originally released through
[http://goo.gle/multiberts](http://goo.gle/multiberts). We describe them in our
paper
[The MultiBERTs: BERT Reproductions for Robustness Analysis](https://arxiv.org/abs/2106.16163).
This is model #3, captured at step 1900k (max: 2000k, i.e., 2M steps).
## Model Description
This model was captured during a reproduction of
[BERT-base uncased](https://github.com/google-research/bert), for English: it
is a Transformers model pretrained on a large corpus of English data, using the
Masked Language Modelling (MLM) and the Next Sentence Prediction (NSP)
objectives.
The intended uses, limitations, training data and training procedure for the fully trained model are similar
to [BERT-base uncased](https://github.com/google-research/bert). Two major
differences with the original model:
* We pre-trained the MultiBERTs models for 2 million steps using sequence
length 512 (instead of 1 million steps using sequence length 128 then 512).
* We used an alternative version of Wikipedia and Books Corpus, initially
collected for [Turc et al., 2019](https://arxiv.org/abs/1908.08962).
This is a best-effort reproduction, and so it is probable that differences with
the original model have gone unnoticed. The performance of MultiBERTs on GLUE after full training is oftentimes comparable to that of original
BERT, but we found significant differences on the dev set of SQuAD (MultiBERTs outperforms original BERT).
See our [technical report](https://arxiv.org/abs/2106.16163) for more details.
### How to use
Using code from
[BERT-base uncased](https://huggingface.co/bert-base-uncased), here is an example based on
Tensorflow:
```
from transformers import BertTokenizer, TFBertModel
tokenizer = BertTokenizer.from_pretrained('google/multiberts-seed_3-step_1900k')
model = TFBertModel.from_pretrained("google/multiberts-seed_3-step_1900k")
text = "Replace me by any text you'd like."
encoded_input = tokenizer(text, return_tensors='tf')
output = model(encoded_input)
```
PyTorch version:
```
from transformers import BertTokenizer, BertModel
tokenizer = BertTokenizer.from_pretrained('google/multiberts-seed_3-step_1900k')
model = BertModel.from_pretrained("google/multiberts-seed_3-step_1900k")
text = "Replace me by any text you'd like."
encoded_input = tokenizer(text, return_tensors='pt')
output = model(**encoded_input)
```
## Citation info
```bibtex
@article{sellam2021multiberts,
title={The MultiBERTs: BERT Reproductions for Robustness Analysis},
author={Thibault Sellam and Steve Yadlowsky and Jason Wei and Naomi Saphra and Alexander D'Amour and Tal Linzen and Jasmijn Bastings and Iulia Turc and Jacob Eisenstein and Dipanjan Das and Ian Tenney and Ellie Pavlick},
journal={arXiv preprint arXiv:2106.16163},
year={2021}
}
```
|
google/multiberts-seed_3-step_200k | ae6080735c646999469bc91372aee1fb83a155a5 | 2021-11-06T02:28:36.000Z | [
"pytorch",
"tf",
"bert",
"pretraining",
"en",
"arxiv:2106.16163",
"arxiv:1908.08962",
"transformers",
"multiberts",
"multiberts-seed_3",
"multiberts-seed_3-step_200k",
"license:apache-2.0"
]
| null | false | google | null | google/multiberts-seed_3-step_200k | 2 | null | transformers | 24,006 | ---
language: en
tags:
- multiberts
- multiberts-seed_3
- multiberts-seed_3-step_200k
license: apache-2.0
---
# MultiBERTs, Intermediate Checkpoint - Seed 3, Step 200k
MultiBERTs is a collection of checkpoints and a statistical library to support
robust research on BERT. We provide 25 BERT-base models trained with
similar hyper-parameters as
[the original BERT model](https://github.com/google-research/bert) but
with different random seeds, which causes variations in the initial weights and order of
training instances. The aim is to distinguish findings that apply to a specific
artifact (i.e., a particular instance of the model) from those that apply to the
more general procedure.
We also provide 140 intermediate checkpoints captured
during the course of pre-training (we saved 28 checkpoints for the first 5 runs).
The models were originally released through
[http://goo.gle/multiberts](http://goo.gle/multiberts). We describe them in our
paper
[The MultiBERTs: BERT Reproductions for Robustness Analysis](https://arxiv.org/abs/2106.16163).
This is model #3, captured at step 200k (max: 2000k, i.e., 2M steps).
## Model Description
This model was captured during a reproduction of
[BERT-base uncased](https://github.com/google-research/bert), for English: it
is a Transformers model pretrained on a large corpus of English data, using the
Masked Language Modelling (MLM) and the Next Sentence Prediction (NSP)
objectives.
The intended uses, limitations, training data and training procedure for the fully trained model are similar
to [BERT-base uncased](https://github.com/google-research/bert). Two major
differences with the original model:
* We pre-trained the MultiBERTs models for 2 million steps using sequence
length 512 (instead of 1 million steps using sequence length 128 then 512).
* We used an alternative version of Wikipedia and Books Corpus, initially
collected for [Turc et al., 2019](https://arxiv.org/abs/1908.08962).
This is a best-effort reproduction, and so it is probable that differences with
the original model have gone unnoticed. The performance of MultiBERTs on GLUE after full training is oftentimes comparable to that of original
BERT, but we found significant differences on the dev set of SQuAD (MultiBERTs outperforms original BERT).
See our [technical report](https://arxiv.org/abs/2106.16163) for more details.
### How to use
Using code from
[BERT-base uncased](https://huggingface.co/bert-base-uncased), here is an example based on
Tensorflow:
```
from transformers import BertTokenizer, TFBertModel
tokenizer = BertTokenizer.from_pretrained('google/multiberts-seed_3-step_200k')
model = TFBertModel.from_pretrained("google/multiberts-seed_3-step_200k")
text = "Replace me by any text you'd like."
encoded_input = tokenizer(text, return_tensors='tf')
output = model(encoded_input)
```
PyTorch version:
```
from transformers import BertTokenizer, BertModel
tokenizer = BertTokenizer.from_pretrained('google/multiberts-seed_3-step_200k')
model = BertModel.from_pretrained("google/multiberts-seed_3-step_200k")
text = "Replace me by any text you'd like."
encoded_input = tokenizer(text, return_tensors='pt')
output = model(**encoded_input)
```
## Citation info
```bibtex
@article{sellam2021multiberts,
title={The MultiBERTs: BERT Reproductions for Robustness Analysis},
author={Thibault Sellam and Steve Yadlowsky and Jason Wei and Naomi Saphra and Alexander D'Amour and Tal Linzen and Jasmijn Bastings and Iulia Turc and Jacob Eisenstein and Dipanjan Das and Ian Tenney and Ellie Pavlick},
journal={arXiv preprint arXiv:2106.16163},
year={2021}
}
```
|
google/multiberts-seed_3-step_60k | 8668b7a48d2eb49f4339807afff8b3fa42909342 | 2021-11-06T02:16:32.000Z | [
"pytorch",
"tf",
"bert",
"pretraining",
"en",
"arxiv:2106.16163",
"arxiv:1908.08962",
"transformers",
"multiberts",
"multiberts-seed_3",
"multiberts-seed_3-step_60k",
"license:apache-2.0"
]
| null | false | google | null | google/multiberts-seed_3-step_60k | 2 | null | transformers | 24,007 | ---
language: en
tags:
- multiberts
- multiberts-seed_3
- multiberts-seed_3-step_60k
license: apache-2.0
---
# MultiBERTs, Intermediate Checkpoint - Seed 3, Step 60k
MultiBERTs is a collection of checkpoints and a statistical library to support
robust research on BERT. We provide 25 BERT-base models trained with
similar hyper-parameters as
[the original BERT model](https://github.com/google-research/bert) but
with different random seeds, which causes variations in the initial weights and order of
training instances. The aim is to distinguish findings that apply to a specific
artifact (i.e., a particular instance of the model) from those that apply to the
more general procedure.
We also provide 140 intermediate checkpoints captured
during the course of pre-training (we saved 28 checkpoints for the first 5 runs).
The models were originally released through
[http://goo.gle/multiberts](http://goo.gle/multiberts). We describe them in our
paper
[The MultiBERTs: BERT Reproductions for Robustness Analysis](https://arxiv.org/abs/2106.16163).
This is model #3, captured at step 60k (max: 2000k, i.e., 2M steps).
## Model Description
This model was captured during a reproduction of
[BERT-base uncased](https://github.com/google-research/bert), for English: it
is a Transformers model pretrained on a large corpus of English data, using the
Masked Language Modelling (MLM) and the Next Sentence Prediction (NSP)
objectives.
The intended uses, limitations, training data and training procedure for the fully trained model are similar
to [BERT-base uncased](https://github.com/google-research/bert). Two major
differences with the original model:
* We pre-trained the MultiBERTs models for 2 million steps using sequence
length 512 (instead of 1 million steps using sequence length 128 then 512).
* We used an alternative version of Wikipedia and Books Corpus, initially
collected for [Turc et al., 2019](https://arxiv.org/abs/1908.08962).
This is a best-effort reproduction, and so it is probable that differences with
the original model have gone unnoticed. The performance of MultiBERTs on GLUE after full training is oftentimes comparable to that of original
BERT, but we found significant differences on the dev set of SQuAD (MultiBERTs outperforms original BERT).
See our [technical report](https://arxiv.org/abs/2106.16163) for more details.
### How to use
Using code from
[BERT-base uncased](https://huggingface.co/bert-base-uncased), here is an example based on
Tensorflow:
```
from transformers import BertTokenizer, TFBertModel
tokenizer = BertTokenizer.from_pretrained('google/multiberts-seed_3-step_60k')
model = TFBertModel.from_pretrained("google/multiberts-seed_3-step_60k")
text = "Replace me by any text you'd like."
encoded_input = tokenizer(text, return_tensors='tf')
output = model(encoded_input)
```
PyTorch version:
```
from transformers import BertTokenizer, BertModel
tokenizer = BertTokenizer.from_pretrained('google/multiberts-seed_3-step_60k')
model = BertModel.from_pretrained("google/multiberts-seed_3-step_60k")
text = "Replace me by any text you'd like."
encoded_input = tokenizer(text, return_tensors='pt')
output = model(**encoded_input)
```
## Citation info
```bibtex
@article{sellam2021multiberts,
title={The MultiBERTs: BERT Reproductions for Robustness Analysis},
author={Thibault Sellam and Steve Yadlowsky and Jason Wei and Naomi Saphra and Alexander D'Amour and Tal Linzen and Jasmijn Bastings and Iulia Turc and Jacob Eisenstein and Dipanjan Das and Ian Tenney and Ellie Pavlick},
journal={arXiv preprint arXiv:2106.16163},
year={2021}
}
```
|
google/multiberts-seed_3-step_80k | 02402bb9ed0e0ace5c4725a926aa4398690fd981 | 2021-11-06T02:18:12.000Z | [
"pytorch",
"tf",
"bert",
"pretraining",
"en",
"arxiv:2106.16163",
"arxiv:1908.08962",
"transformers",
"multiberts",
"multiberts-seed_3",
"multiberts-seed_3-step_80k",
"license:apache-2.0"
]
| null | false | google | null | google/multiberts-seed_3-step_80k | 2 | null | transformers | 24,008 | ---
language: en
tags:
- multiberts
- multiberts-seed_3
- multiberts-seed_3-step_80k
license: apache-2.0
---
# MultiBERTs, Intermediate Checkpoint - Seed 3, Step 80k
MultiBERTs is a collection of checkpoints and a statistical library to support
robust research on BERT. We provide 25 BERT-base models trained with
similar hyper-parameters as
[the original BERT model](https://github.com/google-research/bert) but
with different random seeds, which causes variations in the initial weights and order of
training instances. The aim is to distinguish findings that apply to a specific
artifact (i.e., a particular instance of the model) from those that apply to the
more general procedure.
We also provide 140 intermediate checkpoints captured
during the course of pre-training (we saved 28 checkpoints for the first 5 runs).
The models were originally released through
[http://goo.gle/multiberts](http://goo.gle/multiberts). We describe them in our
paper
[The MultiBERTs: BERT Reproductions for Robustness Analysis](https://arxiv.org/abs/2106.16163).
This is model #3, captured at step 80k (max: 2000k, i.e., 2M steps).
## Model Description
This model was captured during a reproduction of
[BERT-base uncased](https://github.com/google-research/bert), for English: it
is a Transformers model pretrained on a large corpus of English data, using the
Masked Language Modelling (MLM) and the Next Sentence Prediction (NSP)
objectives.
The intended uses, limitations, training data and training procedure for the fully trained model are similar
to [BERT-base uncased](https://github.com/google-research/bert). Two major
differences with the original model:
* We pre-trained the MultiBERTs models for 2 million steps using sequence
length 512 (instead of 1 million steps using sequence length 128 then 512).
* We used an alternative version of Wikipedia and Books Corpus, initially
collected for [Turc et al., 2019](https://arxiv.org/abs/1908.08962).
This is a best-effort reproduction, and so it is probable that differences with
the original model have gone unnoticed. The performance of MultiBERTs on GLUE after full training is oftentimes comparable to that of original
BERT, but we found significant differences on the dev set of SQuAD (MultiBERTs outperforms original BERT).
See our [technical report](https://arxiv.org/abs/2106.16163) for more details.
### How to use
Using code from
[BERT-base uncased](https://huggingface.co/bert-base-uncased), here is an example based on
Tensorflow:
```
from transformers import BertTokenizer, TFBertModel
tokenizer = BertTokenizer.from_pretrained('google/multiberts-seed_3-step_80k')
model = TFBertModel.from_pretrained("google/multiberts-seed_3-step_80k")
text = "Replace me by any text you'd like."
encoded_input = tokenizer(text, return_tensors='tf')
output = model(encoded_input)
```
PyTorch version:
```
from transformers import BertTokenizer, BertModel
tokenizer = BertTokenizer.from_pretrained('google/multiberts-seed_3-step_80k')
model = BertModel.from_pretrained("google/multiberts-seed_3-step_80k")
text = "Replace me by any text you'd like."
encoded_input = tokenizer(text, return_tensors='pt')
output = model(**encoded_input)
```
## Citation info
```bibtex
@article{sellam2021multiberts,
title={The MultiBERTs: BERT Reproductions for Robustness Analysis},
author={Thibault Sellam and Steve Yadlowsky and Jason Wei and Naomi Saphra and Alexander D'Amour and Tal Linzen and Jasmijn Bastings and Iulia Turc and Jacob Eisenstein and Dipanjan Das and Ian Tenney and Ellie Pavlick},
journal={arXiv preprint arXiv:2106.16163},
year={2021}
}
```
|
google/multiberts-seed_4-step_1900k | d2b8ff6818d08bc028f21417c103d2d0be799817 | 2021-11-06T03:49:07.000Z | [
"pytorch",
"tf",
"bert",
"pretraining",
"en",
"arxiv:2106.16163",
"arxiv:1908.08962",
"transformers",
"multiberts",
"multiberts-seed_4",
"multiberts-seed_4-step_1900k",
"license:apache-2.0"
]
| null | false | google | null | google/multiberts-seed_4-step_1900k | 2 | null | transformers | 24,009 | ---
language: en
tags:
- multiberts
- multiberts-seed_4
- multiberts-seed_4-step_1900k
license: apache-2.0
---
# MultiBERTs, Intermediate Checkpoint - Seed 4, Step 1900k
MultiBERTs is a collection of checkpoints and a statistical library to support
robust research on BERT. We provide 25 BERT-base models trained with
similar hyper-parameters as
[the original BERT model](https://github.com/google-research/bert) but
with different random seeds, which causes variations in the initial weights and order of
training instances. The aim is to distinguish findings that apply to a specific
artifact (i.e., a particular instance of the model) from those that apply to the
more general procedure.
We also provide 140 intermediate checkpoints captured
during the course of pre-training (we saved 28 checkpoints for the first 5 runs).
The models were originally released through
[http://goo.gle/multiberts](http://goo.gle/multiberts). We describe them in our
paper
[The MultiBERTs: BERT Reproductions for Robustness Analysis](https://arxiv.org/abs/2106.16163).
This is model #4, captured at step 1900k (max: 2000k, i.e., 2M steps).
## Model Description
This model was captured during a reproduction of
[BERT-base uncased](https://github.com/google-research/bert), for English: it
is a Transformers model pretrained on a large corpus of English data, using the
Masked Language Modelling (MLM) and the Next Sentence Prediction (NSP)
objectives.
The intended uses, limitations, training data and training procedure for the fully trained model are similar
to [BERT-base uncased](https://github.com/google-research/bert). Two major
differences with the original model:
* We pre-trained the MultiBERTs models for 2 million steps using sequence
length 512 (instead of 1 million steps using sequence length 128 then 512).
* We used an alternative version of Wikipedia and Books Corpus, initially
collected for [Turc et al., 2019](https://arxiv.org/abs/1908.08962).
This is a best-effort reproduction, and so it is probable that differences with
the original model have gone unnoticed. The performance of MultiBERTs on GLUE after full training is oftentimes comparable to that of original
BERT, but we found significant differences on the dev set of SQuAD (MultiBERTs outperforms original BERT).
See our [technical report](https://arxiv.org/abs/2106.16163) for more details.
### How to use
Using code from
[BERT-base uncased](https://huggingface.co/bert-base-uncased), here is an example based on
Tensorflow:
```
from transformers import BertTokenizer, TFBertModel
tokenizer = BertTokenizer.from_pretrained('google/multiberts-seed_4-step_1900k')
model = TFBertModel.from_pretrained("google/multiberts-seed_4-step_1900k")
text = "Replace me by any text you'd like."
encoded_input = tokenizer(text, return_tensors='tf')
output = model(encoded_input)
```
PyTorch version:
```
from transformers import BertTokenizer, BertModel
tokenizer = BertTokenizer.from_pretrained('google/multiberts-seed_4-step_1900k')
model = BertModel.from_pretrained("google/multiberts-seed_4-step_1900k")
text = "Replace me by any text you'd like."
encoded_input = tokenizer(text, return_tensors='pt')
output = model(**encoded_input)
```
## Citation info
```bibtex
@article{sellam2021multiberts,
title={The MultiBERTs: BERT Reproductions for Robustness Analysis},
author={Thibault Sellam and Steve Yadlowsky and Jason Wei and Naomi Saphra and Alexander D'Amour and Tal Linzen and Jasmijn Bastings and Iulia Turc and Jacob Eisenstein and Dipanjan Das and Ian Tenney and Ellie Pavlick},
journal={arXiv preprint arXiv:2106.16163},
year={2021}
}
```
|
google/multiberts-seed_4-step_700k | 3d584d8805ba53a364d8bc2358e1ce64918ec093 | 2021-11-06T03:28:51.000Z | [
"pytorch",
"tf",
"bert",
"pretraining",
"en",
"arxiv:2106.16163",
"arxiv:1908.08962",
"transformers",
"multiberts",
"multiberts-seed_4",
"multiberts-seed_4-step_700k",
"license:apache-2.0"
]
| null | false | google | null | google/multiberts-seed_4-step_700k | 2 | null | transformers | 24,010 | ---
language: en
tags:
- multiberts
- multiberts-seed_4
- multiberts-seed_4-step_700k
license: apache-2.0
---
# MultiBERTs, Intermediate Checkpoint - Seed 4, Step 700k
MultiBERTs is a collection of checkpoints and a statistical library to support
robust research on BERT. We provide 25 BERT-base models trained with
similar hyper-parameters as
[the original BERT model](https://github.com/google-research/bert) but
with different random seeds, which causes variations in the initial weights and order of
training instances. The aim is to distinguish findings that apply to a specific
artifact (i.e., a particular instance of the model) from those that apply to the
more general procedure.
We also provide 140 intermediate checkpoints captured
during the course of pre-training (we saved 28 checkpoints for the first 5 runs).
The models were originally released through
[http://goo.gle/multiberts](http://goo.gle/multiberts). We describe them in our
paper
[The MultiBERTs: BERT Reproductions for Robustness Analysis](https://arxiv.org/abs/2106.16163).
This is model #4, captured at step 700k (max: 2000k, i.e., 2M steps).
## Model Description
This model was captured during a reproduction of
[BERT-base uncased](https://github.com/google-research/bert), for English: it
is a Transformers model pretrained on a large corpus of English data, using the
Masked Language Modelling (MLM) and the Next Sentence Prediction (NSP)
objectives.
The intended uses, limitations, training data and training procedure for the fully trained model are similar
to [BERT-base uncased](https://github.com/google-research/bert). Two major
differences with the original model:
* We pre-trained the MultiBERTs models for 2 million steps using sequence
length 512 (instead of 1 million steps using sequence length 128 then 512).
* We used an alternative version of Wikipedia and Books Corpus, initially
collected for [Turc et al., 2019](https://arxiv.org/abs/1908.08962).
This is a best-effort reproduction, and so it is probable that differences with
the original model have gone unnoticed. The performance of MultiBERTs on GLUE after full training is oftentimes comparable to that of original
BERT, but we found significant differences on the dev set of SQuAD (MultiBERTs outperforms original BERT).
See our [technical report](https://arxiv.org/abs/2106.16163) for more details.
### How to use
Using code from
[BERT-base uncased](https://huggingface.co/bert-base-uncased), here is an example based on
Tensorflow:
```
from transformers import BertTokenizer, TFBertModel
tokenizer = BertTokenizer.from_pretrained('google/multiberts-seed_4-step_700k')
model = TFBertModel.from_pretrained("google/multiberts-seed_4-step_700k")
text = "Replace me by any text you'd like."
encoded_input = tokenizer(text, return_tensors='tf')
output = model(encoded_input)
```
PyTorch version:
```
from transformers import BertTokenizer, BertModel
tokenizer = BertTokenizer.from_pretrained('google/multiberts-seed_4-step_700k')
model = BertModel.from_pretrained("google/multiberts-seed_4-step_700k")
text = "Replace me by any text you'd like."
encoded_input = tokenizer(text, return_tensors='pt')
output = model(**encoded_input)
```
## Citation info
```bibtex
@article{sellam2021multiberts,
title={The MultiBERTs: BERT Reproductions for Robustness Analysis},
author={Thibault Sellam and Steve Yadlowsky and Jason Wei and Naomi Saphra and Alexander D'Amour and Tal Linzen and Jasmijn Bastings and Iulia Turc and Jacob Eisenstein and Dipanjan Das and Ian Tenney and Ellie Pavlick},
journal={arXiv preprint arXiv:2106.16163},
year={2021}
}
```
|
google/t5-efficient-base-dl6 | 5b76c68a7670a8c6b7f684d451c3f8a70ef9bc9a | 2022-02-15T10:52:09.000Z | [
"pytorch",
"tf",
"jax",
"t5",
"text2text-generation",
"en",
"dataset:c4",
"arxiv:2109.10686",
"transformers",
"deep-narrow",
"license:apache-2.0",
"autotrain_compatible"
]
| text2text-generation | false | google | null | google/t5-efficient-base-dl6 | 2 | null | transformers | 24,011 | ---
language:
- en
datasets:
- c4
tags:
- deep-narrow
inference: false
license: apache-2.0
---
# T5-Efficient-BASE-DL6 (Deep-Narrow version)
T5-Efficient-BASE-DL6 is a variation of [Google's original T5](https://ai.googleblog.com/2020/02/exploring-transfer-learning-with-t5.html) following the [T5 model architecture](https://huggingface.co/docs/transformers/model_doc/t5).
It is a *pretrained-only* checkpoint and was released with the
paper **[Scale Efficiently: Insights from Pre-training and Fine-tuning Transformers](https://arxiv.org/abs/2109.10686)**
by *Yi Tay, Mostafa Dehghani, Jinfeng Rao, William Fedus, Samira Abnar, Hyung Won Chung, Sharan Narang, Dani Yogatama, Ashish Vaswani, Donald Metzler*.
In a nutshell, the paper indicates that a **Deep-Narrow** model architecture is favorable for **downstream** performance compared to other model architectures
of similar parameter count.
To quote the paper:
> We generally recommend a DeepNarrow strategy where the model’s depth is preferentially increased
> before considering any other forms of uniform scaling across other dimensions. This is largely due to
> how much depth influences the Pareto-frontier as shown in earlier sections of the paper. Specifically, a
> tall small (deep and narrow) model is generally more efficient compared to the base model. Likewise,
> a tall base model might also generally more efficient compared to a large model. We generally find
> that, regardless of size, even if absolute performance might increase as we continue to stack layers,
> the relative gain of Pareto-efficiency diminishes as we increase the layers, converging at 32 to 36
> layers. Finally, we note that our notion of efficiency here relates to any one compute dimension, i.e.,
> params, FLOPs or throughput (speed). We report all three key efficiency metrics (number of params,
> FLOPS and speed) and leave this decision to the practitioner to decide which compute dimension to
> consider.
To be more precise, *model depth* is defined as the number of transformer blocks that are stacked sequentially.
A sequence of word embeddings is therefore processed sequentially by each transformer block.
## Details model architecture
This model checkpoint - **t5-efficient-base-dl6** - is of model type **Base** with the following variations:
- **dl** is **6**
It has **166.29** million parameters and thus requires *ca.* **665.15 MB** of memory in full precision (*fp32*)
or **332.57 MB** of memory in half precision (*fp16* or *bf16*).
A summary of the *original* T5 model architectures can be seen here:
| Model | nl (el/dl) | ff | dm | kv | nh | #Params|
| ----| ---- | ---- | ---- | ---- | ---- | ----|
| Tiny | 4/4 | 1024 | 256 | 32 | 4 | 16M|
| Mini | 4/4 | 1536 | 384 | 32 | 8 | 31M|
| Small | 6/6 | 2048 | 512 | 32 | 8 | 60M|
| Base | 12/12 | 3072 | 768 | 64 | 12 | 220M|
| Large | 24/24 | 4096 | 1024 | 64 | 16 | 738M|
| Xl | 24/24 | 16384 | 1024 | 128 | 32 | 3B|
| XXl | 24/24 | 65536 | 1024 | 128 | 128 | 11B|
whereas the following abbreviations are used:
| Abbreviation | Definition |
| ----| ---- |
| nl | Number of transformer blocks (depth) |
| dm | Dimension of embedding vector (output vector of transformers block) |
| kv | Dimension of key/value projection matrix |
| nh | Number of attention heads |
| ff | Dimension of intermediate vector within transformer block (size of feed-forward projection matrix) |
| el | Number of transformer blocks in the encoder (encoder depth) |
| dl | Number of transformer blocks in the decoder (decoder depth) |
| sh | Signifies that attention heads are shared |
| skv | Signifies that key-values projection matrices are tied |
If a model checkpoint has no specific, *el* or *dl* than both the number of encoder- and decoder layers correspond to *nl*.
## Pre-Training
The checkpoint was pretrained on the [Colossal, Cleaned version of Common Crawl (C4)](https://huggingface.co/datasets/c4) for 524288 steps using
the span-based masked language modeling (MLM) objective.
## Fine-Tuning
**Note**: This model is a **pretrained** checkpoint and has to be fine-tuned for practical usage.
The checkpoint was pretrained in English and is therefore only useful for English NLP tasks.
You can follow on of the following examples on how to fine-tune the model:
*PyTorch*:
- [Summarization](https://github.com/huggingface/transformers/tree/master/examples/pytorch/summarization)
- [Question Answering](https://github.com/huggingface/transformers/blob/master/examples/pytorch/question-answering/run_seq2seq_qa.py)
- [Text Classification](https://github.com/huggingface/transformers/tree/master/examples/pytorch/text-classification) - *Note*: You will have to slightly adapt the training example here to make it work with an encoder-decoder model.
*Tensorflow*:
- [Summarization](https://github.com/huggingface/transformers/tree/master/examples/tensorflow/summarization)
- [Text Classification](https://github.com/huggingface/transformers/tree/master/examples/tensorflow/text-classification) - *Note*: You will have to slightly adapt the training example here to make it work with an encoder-decoder model.
*JAX/Flax*:
- [Summarization](https://github.com/huggingface/transformers/tree/master/examples/flax/summarization)
- [Text Classification](https://github.com/huggingface/transformers/tree/master/examples/flax/text-classification) - *Note*: You will have to slightly adapt the training example here to make it work with an encoder-decoder model.
## Downstream Performance
TODO: Add table if available
## Computational Complexity
TODO: Add table if available
## More information
We strongly recommend the reader to go carefully through the original paper **[Scale Efficiently: Insights from Pre-training and Fine-tuning Transformers](https://arxiv.org/abs/2109.10686)** to get a more nuanced understanding of this model checkpoint.
As explained in the following [issue](https://github.com/google-research/google-research/issues/986#issuecomment-1035051145), checkpoints including the *sh* or *skv*
model architecture variations have *not* been ported to Transformers as they are probably of limited practical usage and are lacking a more detailed description. Those checkpoints are kept [here](https://huggingface.co/NewT5SharedHeadsSharedKeyValues) as they might be ported potentially in the future. |
google/t5-efficient-base-ff2000 | 375aec73fb9734dd41a8c0cc1bf7fc389506a4f3 | 2022-02-15T10:52:46.000Z | [
"pytorch",
"tf",
"jax",
"t5",
"text2text-generation",
"en",
"dataset:c4",
"arxiv:2109.10686",
"transformers",
"deep-narrow",
"license:apache-2.0",
"autotrain_compatible"
]
| text2text-generation | false | google | null | google/t5-efficient-base-ff2000 | 2 | null | transformers | 24,012 | ---
language:
- en
datasets:
- c4
tags:
- deep-narrow
inference: false
license: apache-2.0
---
# T5-Efficient-BASE-FF2000 (Deep-Narrow version)
T5-Efficient-BASE-FF2000 is a variation of [Google's original T5](https://ai.googleblog.com/2020/02/exploring-transfer-learning-with-t5.html) following the [T5 model architecture](https://huggingface.co/docs/transformers/model_doc/t5).
It is a *pretrained-only* checkpoint and was released with the
paper **[Scale Efficiently: Insights from Pre-training and Fine-tuning Transformers](https://arxiv.org/abs/2109.10686)**
by *Yi Tay, Mostafa Dehghani, Jinfeng Rao, William Fedus, Samira Abnar, Hyung Won Chung, Sharan Narang, Dani Yogatama, Ashish Vaswani, Donald Metzler*.
In a nutshell, the paper indicates that a **Deep-Narrow** model architecture is favorable for **downstream** performance compared to other model architectures
of similar parameter count.
To quote the paper:
> We generally recommend a DeepNarrow strategy where the model’s depth is preferentially increased
> before considering any other forms of uniform scaling across other dimensions. This is largely due to
> how much depth influences the Pareto-frontier as shown in earlier sections of the paper. Specifically, a
> tall small (deep and narrow) model is generally more efficient compared to the base model. Likewise,
> a tall base model might also generally more efficient compared to a large model. We generally find
> that, regardless of size, even if absolute performance might increase as we continue to stack layers,
> the relative gain of Pareto-efficiency diminishes as we increase the layers, converging at 32 to 36
> layers. Finally, we note that our notion of efficiency here relates to any one compute dimension, i.e.,
> params, FLOPs or throughput (speed). We report all three key efficiency metrics (number of params,
> FLOPS and speed) and leave this decision to the practitioner to decide which compute dimension to
> consider.
To be more precise, *model depth* is defined as the number of transformer blocks that are stacked sequentially.
A sequence of word embeddings is therefore processed sequentially by each transformer block.
## Details model architecture
This model checkpoint - **t5-efficient-base-ff2000** - is of model type **Base** with the following variations:
- **ff** is **2000**
It has **185.18** million parameters and thus requires *ca.* **740.73 MB** of memory in full precision (*fp32*)
or **370.37 MB** of memory in half precision (*fp16* or *bf16*).
A summary of the *original* T5 model architectures can be seen here:
| Model | nl (el/dl) | ff | dm | kv | nh | #Params|
| ----| ---- | ---- | ---- | ---- | ---- | ----|
| Tiny | 4/4 | 1024 | 256 | 32 | 4 | 16M|
| Mini | 4/4 | 1536 | 384 | 32 | 8 | 31M|
| Small | 6/6 | 2048 | 512 | 32 | 8 | 60M|
| Base | 12/12 | 3072 | 768 | 64 | 12 | 220M|
| Large | 24/24 | 4096 | 1024 | 64 | 16 | 738M|
| Xl | 24/24 | 16384 | 1024 | 128 | 32 | 3B|
| XXl | 24/24 | 65536 | 1024 | 128 | 128 | 11B|
whereas the following abbreviations are used:
| Abbreviation | Definition |
| ----| ---- |
| nl | Number of transformer blocks (depth) |
| dm | Dimension of embedding vector (output vector of transformers block) |
| kv | Dimension of key/value projection matrix |
| nh | Number of attention heads |
| ff | Dimension of intermediate vector within transformer block (size of feed-forward projection matrix) |
| el | Number of transformer blocks in the encoder (encoder depth) |
| dl | Number of transformer blocks in the decoder (decoder depth) |
| sh | Signifies that attention heads are shared |
| skv | Signifies that key-values projection matrices are tied |
If a model checkpoint has no specific, *el* or *dl* than both the number of encoder- and decoder layers correspond to *nl*.
## Pre-Training
The checkpoint was pretrained on the [Colossal, Cleaned version of Common Crawl (C4)](https://huggingface.co/datasets/c4) for 524288 steps using
the span-based masked language modeling (MLM) objective.
## Fine-Tuning
**Note**: This model is a **pretrained** checkpoint and has to be fine-tuned for practical usage.
The checkpoint was pretrained in English and is therefore only useful for English NLP tasks.
You can follow on of the following examples on how to fine-tune the model:
*PyTorch*:
- [Summarization](https://github.com/huggingface/transformers/tree/master/examples/pytorch/summarization)
- [Question Answering](https://github.com/huggingface/transformers/blob/master/examples/pytorch/question-answering/run_seq2seq_qa.py)
- [Text Classification](https://github.com/huggingface/transformers/tree/master/examples/pytorch/text-classification) - *Note*: You will have to slightly adapt the training example here to make it work with an encoder-decoder model.
*Tensorflow*:
- [Summarization](https://github.com/huggingface/transformers/tree/master/examples/tensorflow/summarization)
- [Text Classification](https://github.com/huggingface/transformers/tree/master/examples/tensorflow/text-classification) - *Note*: You will have to slightly adapt the training example here to make it work with an encoder-decoder model.
*JAX/Flax*:
- [Summarization](https://github.com/huggingface/transformers/tree/master/examples/flax/summarization)
- [Text Classification](https://github.com/huggingface/transformers/tree/master/examples/flax/text-classification) - *Note*: You will have to slightly adapt the training example here to make it work with an encoder-decoder model.
## Downstream Performance
TODO: Add table if available
## Computational Complexity
TODO: Add table if available
## More information
We strongly recommend the reader to go carefully through the original paper **[Scale Efficiently: Insights from Pre-training and Fine-tuning Transformers](https://arxiv.org/abs/2109.10686)** to get a more nuanced understanding of this model checkpoint.
As explained in the following [issue](https://github.com/google-research/google-research/issues/986#issuecomment-1035051145), checkpoints including the *sh* or *skv*
model architecture variations have *not* been ported to Transformers as they are probably of limited practical usage and are lacking a more detailed description. Those checkpoints are kept [here](https://huggingface.co/NewT5SharedHeadsSharedKeyValues) as they might be ported potentially in the future. |
google/t5-efficient-large-dl12 | fc3c40afdfd60e9e5783582e3ec97860cd34788b | 2022-02-15T10:54:42.000Z | [
"pytorch",
"tf",
"jax",
"t5",
"text2text-generation",
"en",
"dataset:c4",
"arxiv:2109.10686",
"transformers",
"deep-narrow",
"license:apache-2.0",
"autotrain_compatible"
]
| text2text-generation | false | google | null | google/t5-efficient-large-dl12 | 2 | null | transformers | 24,013 | ---
language:
- en
datasets:
- c4
tags:
- deep-narrow
inference: false
license: apache-2.0
---
# T5-Efficient-LARGE-DL12 (Deep-Narrow version)
T5-Efficient-LARGE-DL12 is a variation of [Google's original T5](https://ai.googleblog.com/2020/02/exploring-transfer-learning-with-t5.html) following the [T5 model architecture](https://huggingface.co/docs/transformers/model_doc/t5).
It is a *pretrained-only* checkpoint and was released with the
paper **[Scale Efficiently: Insights from Pre-training and Fine-tuning Transformers](https://arxiv.org/abs/2109.10686)**
by *Yi Tay, Mostafa Dehghani, Jinfeng Rao, William Fedus, Samira Abnar, Hyung Won Chung, Sharan Narang, Dani Yogatama, Ashish Vaswani, Donald Metzler*.
In a nutshell, the paper indicates that a **Deep-Narrow** model architecture is favorable for **downstream** performance compared to other model architectures
of similar parameter count.
To quote the paper:
> We generally recommend a DeepNarrow strategy where the model’s depth is preferentially increased
> before considering any other forms of uniform scaling across other dimensions. This is largely due to
> how much depth influences the Pareto-frontier as shown in earlier sections of the paper. Specifically, a
> tall small (deep and narrow) model is generally more efficient compared to the base model. Likewise,
> a tall base model might also generally more efficient compared to a large model. We generally find
> that, regardless of size, even if absolute performance might increase as we continue to stack layers,
> the relative gain of Pareto-efficiency diminishes as we increase the layers, converging at 32 to 36
> layers. Finally, we note that our notion of efficiency here relates to any one compute dimension, i.e.,
> params, FLOPs or throughput (speed). We report all three key efficiency metrics (number of params,
> FLOPS and speed) and leave this decision to the practitioner to decide which compute dimension to
> consider.
To be more precise, *model depth* is defined as the number of transformer blocks that are stacked sequentially.
A sequence of word embeddings is therefore processed sequentially by each transformer block.
## Details model architecture
This model checkpoint - **t5-efficient-large-dl12** - is of model type **Large** with the following variations:
- **dl** is **12**
It has **536.34** million parameters and thus requires *ca.* **2145.37 MB** of memory in full precision (*fp32*)
or **1072.69 MB** of memory in half precision (*fp16* or *bf16*).
A summary of the *original* T5 model architectures can be seen here:
| Model | nl (el/dl) | ff | dm | kv | nh | #Params|
| ----| ---- | ---- | ---- | ---- | ---- | ----|
| Tiny | 4/4 | 1024 | 256 | 32 | 4 | 16M|
| Mini | 4/4 | 1536 | 384 | 32 | 8 | 31M|
| Small | 6/6 | 2048 | 512 | 32 | 8 | 60M|
| Base | 12/12 | 3072 | 768 | 64 | 12 | 220M|
| Large | 24/24 | 4096 | 1024 | 64 | 16 | 738M|
| Xl | 24/24 | 16384 | 1024 | 128 | 32 | 3B|
| XXl | 24/24 | 65536 | 1024 | 128 | 128 | 11B|
whereas the following abbreviations are used:
| Abbreviation | Definition |
| ----| ---- |
| nl | Number of transformer blocks (depth) |
| dm | Dimension of embedding vector (output vector of transformers block) |
| kv | Dimension of key/value projection matrix |
| nh | Number of attention heads |
| ff | Dimension of intermediate vector within transformer block (size of feed-forward projection matrix) |
| el | Number of transformer blocks in the encoder (encoder depth) |
| dl | Number of transformer blocks in the decoder (decoder depth) |
| sh | Signifies that attention heads are shared |
| skv | Signifies that key-values projection matrices are tied |
If a model checkpoint has no specific, *el* or *dl* than both the number of encoder- and decoder layers correspond to *nl*.
## Pre-Training
The checkpoint was pretrained on the [Colossal, Cleaned version of Common Crawl (C4)](https://huggingface.co/datasets/c4) for 524288 steps using
the span-based masked language modeling (MLM) objective.
## Fine-Tuning
**Note**: This model is a **pretrained** checkpoint and has to be fine-tuned for practical usage.
The checkpoint was pretrained in English and is therefore only useful for English NLP tasks.
You can follow on of the following examples on how to fine-tune the model:
*PyTorch*:
- [Summarization](https://github.com/huggingface/transformers/tree/master/examples/pytorch/summarization)
- [Question Answering](https://github.com/huggingface/transformers/blob/master/examples/pytorch/question-answering/run_seq2seq_qa.py)
- [Text Classification](https://github.com/huggingface/transformers/tree/master/examples/pytorch/text-classification) - *Note*: You will have to slightly adapt the training example here to make it work with an encoder-decoder model.
*Tensorflow*:
- [Summarization](https://github.com/huggingface/transformers/tree/master/examples/tensorflow/summarization)
- [Text Classification](https://github.com/huggingface/transformers/tree/master/examples/tensorflow/text-classification) - *Note*: You will have to slightly adapt the training example here to make it work with an encoder-decoder model.
*JAX/Flax*:
- [Summarization](https://github.com/huggingface/transformers/tree/master/examples/flax/summarization)
- [Text Classification](https://github.com/huggingface/transformers/tree/master/examples/flax/text-classification) - *Note*: You will have to slightly adapt the training example here to make it work with an encoder-decoder model.
## Downstream Performance
TODO: Add table if available
## Computational Complexity
TODO: Add table if available
## More information
We strongly recommend the reader to go carefully through the original paper **[Scale Efficiently: Insights from Pre-training and Fine-tuning Transformers](https://arxiv.org/abs/2109.10686)** to get a more nuanced understanding of this model checkpoint.
As explained in the following [issue](https://github.com/google-research/google-research/issues/986#issuecomment-1035051145), checkpoints including the *sh* or *skv*
model architecture variations have *not* been ported to Transformers as they are probably of limited practical usage and are lacking a more detailed description. Those checkpoints are kept [here](https://huggingface.co/NewT5SharedHeadsSharedKeyValues) as they might be ported potentially in the future. |
google/t5-efficient-small-dl8 | 11320d618027c8e2ddfcb41ddaae8e15d97b27a6 | 2022-02-15T10:56:37.000Z | [
"pytorch",
"tf",
"jax",
"t5",
"text2text-generation",
"en",
"dataset:c4",
"arxiv:2109.10686",
"transformers",
"deep-narrow",
"license:apache-2.0",
"autotrain_compatible"
]
| text2text-generation | false | google | null | google/t5-efficient-small-dl8 | 2 | null | transformers | 24,014 | ---
language:
- en
datasets:
- c4
tags:
- deep-narrow
inference: false
license: apache-2.0
---
# T5-Efficient-SMALL-DL8 (Deep-Narrow version)
T5-Efficient-SMALL-DL8 is a variation of [Google's original T5](https://ai.googleblog.com/2020/02/exploring-transfer-learning-with-t5.html) following the [T5 model architecture](https://huggingface.co/docs/transformers/model_doc/t5).
It is a *pretrained-only* checkpoint and was released with the
paper **[Scale Efficiently: Insights from Pre-training and Fine-tuning Transformers](https://arxiv.org/abs/2109.10686)**
by *Yi Tay, Mostafa Dehghani, Jinfeng Rao, William Fedus, Samira Abnar, Hyung Won Chung, Sharan Narang, Dani Yogatama, Ashish Vaswani, Donald Metzler*.
In a nutshell, the paper indicates that a **Deep-Narrow** model architecture is favorable for **downstream** performance compared to other model architectures
of similar parameter count.
To quote the paper:
> We generally recommend a DeepNarrow strategy where the model’s depth is preferentially increased
> before considering any other forms of uniform scaling across other dimensions. This is largely due to
> how much depth influences the Pareto-frontier as shown in earlier sections of the paper. Specifically, a
> tall small (deep and narrow) model is generally more efficient compared to the base model. Likewise,
> a tall base model might also generally more efficient compared to a large model. We generally find
> that, regardless of size, even if absolute performance might increase as we continue to stack layers,
> the relative gain of Pareto-efficiency diminishes as we increase the layers, converging at 32 to 36
> layers. Finally, we note that our notion of efficiency here relates to any one compute dimension, i.e.,
> params, FLOPs or throughput (speed). We report all three key efficiency metrics (number of params,
> FLOPS and speed) and leave this decision to the practitioner to decide which compute dimension to
> consider.
To be more precise, *model depth* is defined as the number of transformer blocks that are stacked sequentially.
A sequence of word embeddings is therefore processed sequentially by each transformer block.
## Details model architecture
This model checkpoint - **t5-efficient-small-dl8** - is of model type **Small** with the following variations:
- **dl** is **8**
It has **68.92** million parameters and thus requires *ca.* **275.66 MB** of memory in full precision (*fp32*)
or **137.83 MB** of memory in half precision (*fp16* or *bf16*).
A summary of the *original* T5 model architectures can be seen here:
| Model | nl (el/dl) | ff | dm | kv | nh | #Params|
| ----| ---- | ---- | ---- | ---- | ---- | ----|
| Tiny | 4/4 | 1024 | 256 | 32 | 4 | 16M|
| Mini | 4/4 | 1536 | 384 | 32 | 8 | 31M|
| Small | 6/6 | 2048 | 512 | 32 | 8 | 60M|
| Base | 12/12 | 3072 | 768 | 64 | 12 | 220M|
| Large | 24/24 | 4096 | 1024 | 64 | 16 | 738M|
| Xl | 24/24 | 16384 | 1024 | 128 | 32 | 3B|
| XXl | 24/24 | 65536 | 1024 | 128 | 128 | 11B|
whereas the following abbreviations are used:
| Abbreviation | Definition |
| ----| ---- |
| nl | Number of transformer blocks (depth) |
| dm | Dimension of embedding vector (output vector of transformers block) |
| kv | Dimension of key/value projection matrix |
| nh | Number of attention heads |
| ff | Dimension of intermediate vector within transformer block (size of feed-forward projection matrix) |
| el | Number of transformer blocks in the encoder (encoder depth) |
| dl | Number of transformer blocks in the decoder (decoder depth) |
| sh | Signifies that attention heads are shared |
| skv | Signifies that key-values projection matrices are tied |
If a model checkpoint has no specific, *el* or *dl* than both the number of encoder- and decoder layers correspond to *nl*.
## Pre-Training
The checkpoint was pretrained on the [Colossal, Cleaned version of Common Crawl (C4)](https://huggingface.co/datasets/c4) for 524288 steps using
the span-based masked language modeling (MLM) objective.
## Fine-Tuning
**Note**: This model is a **pretrained** checkpoint and has to be fine-tuned for practical usage.
The checkpoint was pretrained in English and is therefore only useful for English NLP tasks.
You can follow on of the following examples on how to fine-tune the model:
*PyTorch*:
- [Summarization](https://github.com/huggingface/transformers/tree/master/examples/pytorch/summarization)
- [Question Answering](https://github.com/huggingface/transformers/blob/master/examples/pytorch/question-answering/run_seq2seq_qa.py)
- [Text Classification](https://github.com/huggingface/transformers/tree/master/examples/pytorch/text-classification) - *Note*: You will have to slightly adapt the training example here to make it work with an encoder-decoder model.
*Tensorflow*:
- [Summarization](https://github.com/huggingface/transformers/tree/master/examples/tensorflow/summarization)
- [Text Classification](https://github.com/huggingface/transformers/tree/master/examples/tensorflow/text-classification) - *Note*: You will have to slightly adapt the training example here to make it work with an encoder-decoder model.
*JAX/Flax*:
- [Summarization](https://github.com/huggingface/transformers/tree/master/examples/flax/summarization)
- [Text Classification](https://github.com/huggingface/transformers/tree/master/examples/flax/text-classification) - *Note*: You will have to slightly adapt the training example here to make it work with an encoder-decoder model.
## Downstream Performance
TODO: Add table if available
## Computational Complexity
TODO: Add table if available
## More information
We strongly recommend the reader to go carefully through the original paper **[Scale Efficiently: Insights from Pre-training and Fine-tuning Transformers](https://arxiv.org/abs/2109.10686)** to get a more nuanced understanding of this model checkpoint.
As explained in the following [issue](https://github.com/google-research/google-research/issues/986#issuecomment-1035051145), checkpoints including the *sh* or *skv*
model architecture variations have *not* been ported to Transformers as they are probably of limited practical usage and are lacking a more detailed description. Those checkpoints are kept [here](https://huggingface.co/NewT5SharedHeadsSharedKeyValues) as they might be ported potentially in the future. |
google/t5-efficient-small-el16-dl2 | a17fe8750aa034eefa2983df496e47ed9aca1460 | 2022-02-15T10:56:56.000Z | [
"pytorch",
"tf",
"jax",
"t5",
"text2text-generation",
"en",
"dataset:c4",
"arxiv:2109.10686",
"transformers",
"deep-narrow",
"license:apache-2.0",
"autotrain_compatible"
]
| text2text-generation | false | google | null | google/t5-efficient-small-el16-dl2 | 2 | null | transformers | 24,015 | ---
language:
- en
datasets:
- c4
tags:
- deep-narrow
inference: false
license: apache-2.0
---
# T5-Efficient-SMALL-EL16-DL2 (Deep-Narrow version)
T5-Efficient-SMALL-EL16-DL2 is a variation of [Google's original T5](https://ai.googleblog.com/2020/02/exploring-transfer-learning-with-t5.html) following the [T5 model architecture](https://huggingface.co/docs/transformers/model_doc/t5).
It is a *pretrained-only* checkpoint and was released with the
paper **[Scale Efficiently: Insights from Pre-training and Fine-tuning Transformers](https://arxiv.org/abs/2109.10686)**
by *Yi Tay, Mostafa Dehghani, Jinfeng Rao, William Fedus, Samira Abnar, Hyung Won Chung, Sharan Narang, Dani Yogatama, Ashish Vaswani, Donald Metzler*.
In a nutshell, the paper indicates that a **Deep-Narrow** model architecture is favorable for **downstream** performance compared to other model architectures
of similar parameter count.
To quote the paper:
> We generally recommend a DeepNarrow strategy where the model’s depth is preferentially increased
> before considering any other forms of uniform scaling across other dimensions. This is largely due to
> how much depth influences the Pareto-frontier as shown in earlier sections of the paper. Specifically, a
> tall small (deep and narrow) model is generally more efficient compared to the base model. Likewise,
> a tall base model might also generally more efficient compared to a large model. We generally find
> that, regardless of size, even if absolute performance might increase as we continue to stack layers,
> the relative gain of Pareto-efficiency diminishes as we increase the layers, converging at 32 to 36
> layers. Finally, we note that our notion of efficiency here relates to any one compute dimension, i.e.,
> params, FLOPs or throughput (speed). We report all three key efficiency metrics (number of params,
> FLOPS and speed) and leave this decision to the practitioner to decide which compute dimension to
> consider.
To be more precise, *model depth* is defined as the number of transformer blocks that are stacked sequentially.
A sequence of word embeddings is therefore processed sequentially by each transformer block.
## Details model architecture
This model checkpoint - **t5-efficient-small-el16-dl2** - is of model type **Small** with the following variations:
- **el** is **16**
- **dl** is **2**
It has **75.21** million parameters and thus requires *ca.* **300.83 MB** of memory in full precision (*fp32*)
or **150.42 MB** of memory in half precision (*fp16* or *bf16*).
A summary of the *original* T5 model architectures can be seen here:
| Model | nl (el/dl) | ff | dm | kv | nh | #Params|
| ----| ---- | ---- | ---- | ---- | ---- | ----|
| Tiny | 4/4 | 1024 | 256 | 32 | 4 | 16M|
| Mini | 4/4 | 1536 | 384 | 32 | 8 | 31M|
| Small | 6/6 | 2048 | 512 | 32 | 8 | 60M|
| Base | 12/12 | 3072 | 768 | 64 | 12 | 220M|
| Large | 24/24 | 4096 | 1024 | 64 | 16 | 738M|
| Xl | 24/24 | 16384 | 1024 | 128 | 32 | 3B|
| XXl | 24/24 | 65536 | 1024 | 128 | 128 | 11B|
whereas the following abbreviations are used:
| Abbreviation | Definition |
| ----| ---- |
| nl | Number of transformer blocks (depth) |
| dm | Dimension of embedding vector (output vector of transformers block) |
| kv | Dimension of key/value projection matrix |
| nh | Number of attention heads |
| ff | Dimension of intermediate vector within transformer block (size of feed-forward projection matrix) |
| el | Number of transformer blocks in the encoder (encoder depth) |
| dl | Number of transformer blocks in the decoder (decoder depth) |
| sh | Signifies that attention heads are shared |
| skv | Signifies that key-values projection matrices are tied |
If a model checkpoint has no specific, *el* or *dl* than both the number of encoder- and decoder layers correspond to *nl*.
## Pre-Training
The checkpoint was pretrained on the [Colossal, Cleaned version of Common Crawl (C4)](https://huggingface.co/datasets/c4) for 524288 steps using
the span-based masked language modeling (MLM) objective.
## Fine-Tuning
**Note**: This model is a **pretrained** checkpoint and has to be fine-tuned for practical usage.
The checkpoint was pretrained in English and is therefore only useful for English NLP tasks.
You can follow on of the following examples on how to fine-tune the model:
*PyTorch*:
- [Summarization](https://github.com/huggingface/transformers/tree/master/examples/pytorch/summarization)
- [Question Answering](https://github.com/huggingface/transformers/blob/master/examples/pytorch/question-answering/run_seq2seq_qa.py)
- [Text Classification](https://github.com/huggingface/transformers/tree/master/examples/pytorch/text-classification) - *Note*: You will have to slightly adapt the training example here to make it work with an encoder-decoder model.
*Tensorflow*:
- [Summarization](https://github.com/huggingface/transformers/tree/master/examples/tensorflow/summarization)
- [Text Classification](https://github.com/huggingface/transformers/tree/master/examples/tensorflow/text-classification) - *Note*: You will have to slightly adapt the training example here to make it work with an encoder-decoder model.
*JAX/Flax*:
- [Summarization](https://github.com/huggingface/transformers/tree/master/examples/flax/summarization)
- [Text Classification](https://github.com/huggingface/transformers/tree/master/examples/flax/text-classification) - *Note*: You will have to slightly adapt the training example here to make it work with an encoder-decoder model.
## Downstream Performance
TODO: Add table if available
## Computational Complexity
TODO: Add table if available
## More information
We strongly recommend the reader to go carefully through the original paper **[Scale Efficiently: Insights from Pre-training and Fine-tuning Transformers](https://arxiv.org/abs/2109.10686)** to get a more nuanced understanding of this model checkpoint.
As explained in the following [issue](https://github.com/google-research/google-research/issues/986#issuecomment-1035051145), checkpoints including the *sh* or *skv*
model architecture variations have *not* been ported to Transformers as they are probably of limited practical usage and are lacking a more detailed description. Those checkpoints are kept [here](https://huggingface.co/NewT5SharedHeadsSharedKeyValues) as they might be ported potentially in the future. |
google/t5-efficient-small-el8-dl4 | d46c8d93c05a40fe910117029b86c05aa4a4dcf9 | 2022-02-15T10:50:08.000Z | [
"pytorch",
"tf",
"jax",
"t5",
"text2text-generation",
"en",
"dataset:c4",
"arxiv:2109.10686",
"transformers",
"deep-narrow",
"license:apache-2.0",
"autotrain_compatible"
]
| text2text-generation | false | google | null | google/t5-efficient-small-el8-dl4 | 2 | null | transformers | 24,016 | ---
language:
- en
datasets:
- c4
tags:
- deep-narrow
inference: false
license: apache-2.0
---
# T5-Efficient-SMALL-EL8-DL4 (Deep-Narrow version)
T5-Efficient-SMALL-EL8-DL4 is a variation of [Google's original T5](https://ai.googleblog.com/2020/02/exploring-transfer-learning-with-t5.html) following the [T5 model architecture](https://huggingface.co/docs/transformers/model_doc/t5).
It is a *pretrained-only* checkpoint and was released with the
paper **[Scale Efficiently: Insights from Pre-training and Fine-tuning Transformers](https://arxiv.org/abs/2109.10686)**
by *Yi Tay, Mostafa Dehghani, Jinfeng Rao, William Fedus, Samira Abnar, Hyung Won Chung, Sharan Narang, Dani Yogatama, Ashish Vaswani, Donald Metzler*.
In a nutshell, the paper indicates that a **Deep-Narrow** model architecture is favorable for **downstream** performance compared to other model architectures
of similar parameter count.
To quote the paper:
> We generally recommend a DeepNarrow strategy where the model’s depth is preferentially increased
> before considering any other forms of uniform scaling across other dimensions. This is largely due to
> how much depth influences the Pareto-frontier as shown in earlier sections of the paper. Specifically, a
> tall small (deep and narrow) model is generally more efficient compared to the base model. Likewise,
> a tall base model might also generally more efficient compared to a large model. We generally find
> that, regardless of size, even if absolute performance might increase as we continue to stack layers,
> the relative gain of Pareto-efficiency diminishes as we increase the layers, converging at 32 to 36
> layers. Finally, we note that our notion of efficiency here relates to any one compute dimension, i.e.,
> params, FLOPs or throughput (speed). We report all three key efficiency metrics (number of params,
> FLOPS and speed) and leave this decision to the practitioner to decide which compute dimension to
> consider.
To be more precise, *model depth* is defined as the number of transformer blocks that are stacked sequentially.
A sequence of word embeddings is therefore processed sequentially by each transformer block.
## Details model architecture
This model checkpoint - **t5-efficient-small-el8-dl4** - is of model type **Small** with the following variations:
- **el** is **8**
- **dl** is **4**
It has **58.42** million parameters and thus requires *ca.* **233.69 MB** of memory in full precision (*fp32*)
or **116.84 MB** of memory in half precision (*fp16* or *bf16*).
A summary of the *original* T5 model architectures can be seen here:
| Model | nl (el/dl) | ff | dm | kv | nh | #Params|
| ----| ---- | ---- | ---- | ---- | ---- | ----|
| Tiny | 4/4 | 1024 | 256 | 32 | 4 | 16M|
| Mini | 4/4 | 1536 | 384 | 32 | 8 | 31M|
| Small | 6/6 | 2048 | 512 | 32 | 8 | 60M|
| Base | 12/12 | 3072 | 768 | 64 | 12 | 220M|
| Large | 24/24 | 4096 | 1024 | 64 | 16 | 738M|
| Xl | 24/24 | 16384 | 1024 | 128 | 32 | 3B|
| XXl | 24/24 | 65536 | 1024 | 128 | 128 | 11B|
whereas the following abbreviations are used:
| Abbreviation | Definition |
| ----| ---- |
| nl | Number of transformer blocks (depth) |
| dm | Dimension of embedding vector (output vector of transformers block) |
| kv | Dimension of key/value projection matrix |
| nh | Number of attention heads |
| ff | Dimension of intermediate vector within transformer block (size of feed-forward projection matrix) |
| el | Number of transformer blocks in the encoder (encoder depth) |
| dl | Number of transformer blocks in the decoder (decoder depth) |
| sh | Signifies that attention heads are shared |
| skv | Signifies that key-values projection matrices are tied |
If a model checkpoint has no specific, *el* or *dl* than both the number of encoder- and decoder layers correspond to *nl*.
## Pre-Training
The checkpoint was pretrained on the [Colossal, Cleaned version of Common Crawl (C4)](https://huggingface.co/datasets/c4) for 524288 steps using
the span-based masked language modeling (MLM) objective.
## Fine-Tuning
**Note**: This model is a **pretrained** checkpoint and has to be fine-tuned for practical usage.
The checkpoint was pretrained in English and is therefore only useful for English NLP tasks.
You can follow on of the following examples on how to fine-tune the model:
*PyTorch*:
- [Summarization](https://github.com/huggingface/transformers/tree/master/examples/pytorch/summarization)
- [Question Answering](https://github.com/huggingface/transformers/blob/master/examples/pytorch/question-answering/run_seq2seq_qa.py)
- [Text Classification](https://github.com/huggingface/transformers/tree/master/examples/pytorch/text-classification) - *Note*: You will have to slightly adapt the training example here to make it work with an encoder-decoder model.
*Tensorflow*:
- [Summarization](https://github.com/huggingface/transformers/tree/master/examples/tensorflow/summarization)
- [Text Classification](https://github.com/huggingface/transformers/tree/master/examples/tensorflow/text-classification) - *Note*: You will have to slightly adapt the training example here to make it work with an encoder-decoder model.
*JAX/Flax*:
- [Summarization](https://github.com/huggingface/transformers/tree/master/examples/flax/summarization)
- [Text Classification](https://github.com/huggingface/transformers/tree/master/examples/flax/text-classification) - *Note*: You will have to slightly adapt the training example here to make it work with an encoder-decoder model.
## Downstream Performance
TODO: Add table if available
## Computational Complexity
TODO: Add table if available
## More information
We strongly recommend the reader to go carefully through the original paper **[Scale Efficiently: Insights from Pre-training and Fine-tuning Transformers](https://arxiv.org/abs/2109.10686)** to get a more nuanced understanding of this model checkpoint.
As explained in the following [issue](https://github.com/google-research/google-research/issues/986#issuecomment-1035051145), checkpoints including the *sh* or *skv*
model architecture variations have *not* been ported to Transformers as they are probably of limited practical usage and are lacking a more detailed description. Those checkpoints are kept [here](https://huggingface.co/NewT5SharedHeadsSharedKeyValues) as they might be ported potentially in the future. |
google/t5-efficient-tiny-dl6 | 61c31f15834a01a2105602f50f56a98bb1ee6b7f | 2022-02-15T10:54:21.000Z | [
"pytorch",
"tf",
"jax",
"t5",
"text2text-generation",
"en",
"dataset:c4",
"arxiv:2109.10686",
"transformers",
"deep-narrow",
"license:apache-2.0",
"autotrain_compatible"
]
| text2text-generation | false | google | null | google/t5-efficient-tiny-dl6 | 2 | null | transformers | 24,017 | ---
language:
- en
datasets:
- c4
tags:
- deep-narrow
inference: false
license: apache-2.0
---
# T5-Efficient-TINY-DL6 (Deep-Narrow version)
T5-Efficient-TINY-DL6 is a variation of [Google's original T5](https://ai.googleblog.com/2020/02/exploring-transfer-learning-with-t5.html) following the [T5 model architecture](https://huggingface.co/docs/transformers/model_doc/t5).
It is a *pretrained-only* checkpoint and was released with the
paper **[Scale Efficiently: Insights from Pre-training and Fine-tuning Transformers](https://arxiv.org/abs/2109.10686)**
by *Yi Tay, Mostafa Dehghani, Jinfeng Rao, William Fedus, Samira Abnar, Hyung Won Chung, Sharan Narang, Dani Yogatama, Ashish Vaswani, Donald Metzler*.
In a nutshell, the paper indicates that a **Deep-Narrow** model architecture is favorable for **downstream** performance compared to other model architectures
of similar parameter count.
To quote the paper:
> We generally recommend a DeepNarrow strategy where the model’s depth is preferentially increased
> before considering any other forms of uniform scaling across other dimensions. This is largely due to
> how much depth influences the Pareto-frontier as shown in earlier sections of the paper. Specifically, a
> tall small (deep and narrow) model is generally more efficient compared to the base model. Likewise,
> a tall base model might also generally more efficient compared to a large model. We generally find
> that, regardless of size, even if absolute performance might increase as we continue to stack layers,
> the relative gain of Pareto-efficiency diminishes as we increase the layers, converging at 32 to 36
> layers. Finally, we note that our notion of efficiency here relates to any one compute dimension, i.e.,
> params, FLOPs or throughput (speed). We report all three key efficiency metrics (number of params,
> FLOPS and speed) and leave this decision to the practitioner to decide which compute dimension to
> consider.
To be more precise, *model depth* is defined as the number of transformer blocks that are stacked sequentially.
A sequence of word embeddings is therefore processed sequentially by each transformer block.
## Details model architecture
This model checkpoint - **t5-efficient-tiny-dl6** - is of model type **Tiny** with the following variations:
- **dl** is **6**
It has **23.98** million parameters and thus requires *ca.* **95.94 MB** of memory in full precision (*fp32*)
or **47.97 MB** of memory in half precision (*fp16* or *bf16*).
A summary of the *original* T5 model architectures can be seen here:
| Model | nl (el/dl) | ff | dm | kv | nh | #Params|
| ----| ---- | ---- | ---- | ---- | ---- | ----|
| Tiny | 4/4 | 1024 | 256 | 32 | 4 | 16M|
| Mini | 4/4 | 1536 | 384 | 32 | 8 | 31M|
| Small | 6/6 | 2048 | 512 | 32 | 8 | 60M|
| Base | 12/12 | 3072 | 768 | 64 | 12 | 220M|
| Large | 24/24 | 4096 | 1024 | 64 | 16 | 738M|
| Xl | 24/24 | 16384 | 1024 | 128 | 32 | 3B|
| XXl | 24/24 | 65536 | 1024 | 128 | 128 | 11B|
whereas the following abbreviations are used:
| Abbreviation | Definition |
| ----| ---- |
| nl | Number of transformer blocks (depth) |
| dm | Dimension of embedding vector (output vector of transformers block) |
| kv | Dimension of key/value projection matrix |
| nh | Number of attention heads |
| ff | Dimension of intermediate vector within transformer block (size of feed-forward projection matrix) |
| el | Number of transformer blocks in the encoder (encoder depth) |
| dl | Number of transformer blocks in the decoder (decoder depth) |
| sh | Signifies that attention heads are shared |
| skv | Signifies that key-values projection matrices are tied |
If a model checkpoint has no specific, *el* or *dl* than both the number of encoder- and decoder layers correspond to *nl*.
## Pre-Training
The checkpoint was pretrained on the [Colossal, Cleaned version of Common Crawl (C4)](https://huggingface.co/datasets/c4) for 524288 steps using
the span-based masked language modeling (MLM) objective.
## Fine-Tuning
**Note**: This model is a **pretrained** checkpoint and has to be fine-tuned for practical usage.
The checkpoint was pretrained in English and is therefore only useful for English NLP tasks.
You can follow on of the following examples on how to fine-tune the model:
*PyTorch*:
- [Summarization](https://github.com/huggingface/transformers/tree/master/examples/pytorch/summarization)
- [Question Answering](https://github.com/huggingface/transformers/blob/master/examples/pytorch/question-answering/run_seq2seq_qa.py)
- [Text Classification](https://github.com/huggingface/transformers/tree/master/examples/pytorch/text-classification) - *Note*: You will have to slightly adapt the training example here to make it work with an encoder-decoder model.
*Tensorflow*:
- [Summarization](https://github.com/huggingface/transformers/tree/master/examples/tensorflow/summarization)
- [Text Classification](https://github.com/huggingface/transformers/tree/master/examples/tensorflow/text-classification) - *Note*: You will have to slightly adapt the training example here to make it work with an encoder-decoder model.
*JAX/Flax*:
- [Summarization](https://github.com/huggingface/transformers/tree/master/examples/flax/summarization)
- [Text Classification](https://github.com/huggingface/transformers/tree/master/examples/flax/text-classification) - *Note*: You will have to slightly adapt the training example here to make it work with an encoder-decoder model.
## Downstream Performance
TODO: Add table if available
## Computational Complexity
TODO: Add table if available
## More information
We strongly recommend the reader to go carefully through the original paper **[Scale Efficiently: Insights from Pre-training and Fine-tuning Transformers](https://arxiv.org/abs/2109.10686)** to get a more nuanced understanding of this model checkpoint.
As explained in the following [issue](https://github.com/google-research/google-research/issues/986#issuecomment-1035051145), checkpoints including the *sh* or *skv*
model architecture variations have *not* been ported to Transformers as they are probably of limited practical usage and are lacking a more detailed description. Those checkpoints are kept [here](https://huggingface.co/NewT5SharedHeadsSharedKeyValues) as they might be ported potentially in the future. |
google/t5-efficient-tiny-dl8 | e961034a5df01dbab4f3427b58f6aa4c9279d841 | 2022-02-15T10:54:24.000Z | [
"pytorch",
"tf",
"jax",
"t5",
"text2text-generation",
"en",
"dataset:c4",
"arxiv:2109.10686",
"transformers",
"deep-narrow",
"license:apache-2.0",
"autotrain_compatible"
]
| text2text-generation | false | google | null | google/t5-efficient-tiny-dl8 | 2 | null | transformers | 24,018 | ---
language:
- en
datasets:
- c4
tags:
- deep-narrow
inference: false
license: apache-2.0
---
# T5-Efficient-TINY-DL8 (Deep-Narrow version)
T5-Efficient-TINY-DL8 is a variation of [Google's original T5](https://ai.googleblog.com/2020/02/exploring-transfer-learning-with-t5.html) following the [T5 model architecture](https://huggingface.co/docs/transformers/model_doc/t5).
It is a *pretrained-only* checkpoint and was released with the
paper **[Scale Efficiently: Insights from Pre-training and Fine-tuning Transformers](https://arxiv.org/abs/2109.10686)**
by *Yi Tay, Mostafa Dehghani, Jinfeng Rao, William Fedus, Samira Abnar, Hyung Won Chung, Sharan Narang, Dani Yogatama, Ashish Vaswani, Donald Metzler*.
In a nutshell, the paper indicates that a **Deep-Narrow** model architecture is favorable for **downstream** performance compared to other model architectures
of similar parameter count.
To quote the paper:
> We generally recommend a DeepNarrow strategy where the model’s depth is preferentially increased
> before considering any other forms of uniform scaling across other dimensions. This is largely due to
> how much depth influences the Pareto-frontier as shown in earlier sections of the paper. Specifically, a
> tall small (deep and narrow) model is generally more efficient compared to the base model. Likewise,
> a tall base model might also generally more efficient compared to a large model. We generally find
> that, regardless of size, even if absolute performance might increase as we continue to stack layers,
> the relative gain of Pareto-efficiency diminishes as we increase the layers, converging at 32 to 36
> layers. Finally, we note that our notion of efficiency here relates to any one compute dimension, i.e.,
> params, FLOPs or throughput (speed). We report all three key efficiency metrics (number of params,
> FLOPS and speed) and leave this decision to the practitioner to decide which compute dimension to
> consider.
To be more precise, *model depth* is defined as the number of transformer blocks that are stacked sequentially.
A sequence of word embeddings is therefore processed sequentially by each transformer block.
## Details model architecture
This model checkpoint - **t5-efficient-tiny-dl8** - is of model type **Tiny** with the following variations:
- **dl** is **8**
It has **26.09** million parameters and thus requires *ca.* **104.34 MB** of memory in full precision (*fp32*)
or **52.17 MB** of memory in half precision (*fp16* or *bf16*).
A summary of the *original* T5 model architectures can be seen here:
| Model | nl (el/dl) | ff | dm | kv | nh | #Params|
| ----| ---- | ---- | ---- | ---- | ---- | ----|
| Tiny | 4/4 | 1024 | 256 | 32 | 4 | 16M|
| Mini | 4/4 | 1536 | 384 | 32 | 8 | 31M|
| Small | 6/6 | 2048 | 512 | 32 | 8 | 60M|
| Base | 12/12 | 3072 | 768 | 64 | 12 | 220M|
| Large | 24/24 | 4096 | 1024 | 64 | 16 | 738M|
| Xl | 24/24 | 16384 | 1024 | 128 | 32 | 3B|
| XXl | 24/24 | 65536 | 1024 | 128 | 128 | 11B|
whereas the following abbreviations are used:
| Abbreviation | Definition |
| ----| ---- |
| nl | Number of transformer blocks (depth) |
| dm | Dimension of embedding vector (output vector of transformers block) |
| kv | Dimension of key/value projection matrix |
| nh | Number of attention heads |
| ff | Dimension of intermediate vector within transformer block (size of feed-forward projection matrix) |
| el | Number of transformer blocks in the encoder (encoder depth) |
| dl | Number of transformer blocks in the decoder (decoder depth) |
| sh | Signifies that attention heads are shared |
| skv | Signifies that key-values projection matrices are tied |
If a model checkpoint has no specific, *el* or *dl* than both the number of encoder- and decoder layers correspond to *nl*.
## Pre-Training
The checkpoint was pretrained on the [Colossal, Cleaned version of Common Crawl (C4)](https://huggingface.co/datasets/c4) for 524288 steps using
the span-based masked language modeling (MLM) objective.
## Fine-Tuning
**Note**: This model is a **pretrained** checkpoint and has to be fine-tuned for practical usage.
The checkpoint was pretrained in English and is therefore only useful for English NLP tasks.
You can follow on of the following examples on how to fine-tune the model:
*PyTorch*:
- [Summarization](https://github.com/huggingface/transformers/tree/master/examples/pytorch/summarization)
- [Question Answering](https://github.com/huggingface/transformers/blob/master/examples/pytorch/question-answering/run_seq2seq_qa.py)
- [Text Classification](https://github.com/huggingface/transformers/tree/master/examples/pytorch/text-classification) - *Note*: You will have to slightly adapt the training example here to make it work with an encoder-decoder model.
*Tensorflow*:
- [Summarization](https://github.com/huggingface/transformers/tree/master/examples/tensorflow/summarization)
- [Text Classification](https://github.com/huggingface/transformers/tree/master/examples/tensorflow/text-classification) - *Note*: You will have to slightly adapt the training example here to make it work with an encoder-decoder model.
*JAX/Flax*:
- [Summarization](https://github.com/huggingface/transformers/tree/master/examples/flax/summarization)
- [Text Classification](https://github.com/huggingface/transformers/tree/master/examples/flax/text-classification) - *Note*: You will have to slightly adapt the training example here to make it work with an encoder-decoder model.
## Downstream Performance
TODO: Add table if available
## Computational Complexity
TODO: Add table if available
## More information
We strongly recommend the reader to go carefully through the original paper **[Scale Efficiently: Insights from Pre-training and Fine-tuning Transformers](https://arxiv.org/abs/2109.10686)** to get a more nuanced understanding of this model checkpoint.
As explained in the following [issue](https://github.com/google-research/google-research/issues/986#issuecomment-1035051145), checkpoints including the *sh* or *skv*
model architecture variations have *not* been ported to Transformers as they are probably of limited practical usage and are lacking a more detailed description. Those checkpoints are kept [here](https://huggingface.co/NewT5SharedHeadsSharedKeyValues) as they might be ported potentially in the future. |
google/t5-xxl-ssm-wqo | da3431783746255a5589fdce382b0b4a92132711 | 2020-12-07T08:47:04.000Z | [
"pytorch",
"tf",
"t5",
"text2text-generation",
"en",
"dataset:c4",
"dataset:wikipedia",
"dataset:web_questions",
"arxiv:2002.08909",
"arxiv:1910.10683",
"transformers",
"license:apache-2.0",
"autotrain_compatible"
]
| text2text-generation | false | google | null | google/t5-xxl-ssm-wqo | 2 | null | transformers | 24,019 | ---
language: en
datasets:
- c4
- wikipedia
- web_questions
license: apache-2.0
---
[Google's T5](https://ai.googleblog.com/2020/02/exploring-transfer-learning-with-t5.html) for **Closed Book Question Answering**.
The model was pre-trained using T5's denoising objective on [C4](https://huggingface.co/datasets/c4), subsequently additionally pre-trained using [REALM](https://arxiv.org/pdf/2002.08909.pdf)'s salient span masking objective on [Wikipedia](https://huggingface.co/datasets/wikipedia), and finally fine-tuned on [Web Questions (WQ)](https://huggingface.co/datasets/web_questions).
**Note**: The model was fine-tuned on 90% of the train splits of [Web Questions (WQ)](https://huggingface.co/datasets/web_questions) for 20k steps and validated on the held-out 10% of the train split.
Other community Checkpoints: [here](https://huggingface.co/models?search=ssm)
Paper: [How Much Knowledge Can You Pack
Into the Parameters of a Language Model?](https://arxiv.org/abs/1910.10683.pdf)
Authors: *Adam Roberts, Colin Raffel, Noam Shazeer*
## Results on Web Questions - Test Set
|Id | link | Exact Match |
|---|---|---|
|T5-11b|https://huggingface.co/google/t5-11b-ssm-wqo|40.8|
|**T5-xxl**|**https://huggingface.co/google/t5-xxl-ssm-wqo**|**42.8**|
## Usage
The model can be used as follows for **closed book question answering**:
```python
from transformers import AutoModelForSeq2SeqLM, AutoTokenizer
t5_qa_model = AutoModelForSeq2SeqLM.from_pretrained("google/t5-xxl-ssm-wqo")
t5_tok = AutoTokenizer.from_pretrained("google/t5-xxl-ssm-wqo")
input_ids = t5_tok("When was Franklin D. Roosevelt born?", return_tensors="pt").input_ids
gen_output = t5_qa_model.generate(input_ids)[0]
print(t5_tok.decode(gen_output, skip_special_tokens=True))
```
## Abstract
It has recently been observed that neural language models trained on unstructured text can implicitly store and retrieve knowledge using natural language queries. In this short paper, we measure the practical utility of this approach by fine-tuning pre-trained models to answer questions without access to any external context or knowledge. We show that this approach scales with model size and performs competitively with open-domain systems that explicitly retrieve answers from an external knowledge source when answering questions. To facilitate reproducibility and future work, we release our code and trained models at https://goo.gle/t5-cbqa.
 |
google/tapas-tiny-masklm | 4f6ed0e42165c2c8ff3766d08b516252b8e16bd6 | 2021-11-29T14:14:53.000Z | [
"pytorch",
"tf",
"tapas",
"fill-mask",
"transformers",
"autotrain_compatible"
]
| fill-mask | false | google | null | google/tapas-tiny-masklm | 2 | null | transformers | 24,020 | This model corresponds to **tapas_masklm_tiny_reset** of the [original repository](https://github.com/google-research/tapas).
Here's how you can use it:
```python
from transformers import TapasTokenizer, TapasForMaskedLM
import pandas as pd
import torch
tokenizer = TapasTokenizer.from_pretrained("google/tapas-tiny-masklm")
model = TapasForMaskedLM.from_pretrained("google/tapas-tiny-masklm")
data = {'Actors': ["Brad Pitt", "Leonardo Di Caprio", "George Clooney"],
'Age': ["56", "45", "59"],
'Number of movies': ["87", "53", "69"]
}
table = pd.DataFrame.from_dict(data)
query = "How many movies has Leonardo [MASK] Caprio played in?"
# prepare inputs
inputs = tokenizer(table=table, queries=query, padding="max_length", return_tensors="pt")
# forward pass
outputs = model(**inputs)
# return top 5 values and predictions
masked_index = torch.nonzero(inputs.input_ids.squeeze() == tokenizer.mask_token_id, as_tuple=False)
logits = outputs.logits[0, masked_index.item(), :]
probs = logits.softmax(dim=0)
values, predictions = probs.topk(5)
for value, pred in zip(values, predictions):
print(f"{tokenizer.decode([pred])} with confidence {value}")
``` |
groar/gpt-neo-1.3B-finetuned-escape3 | 0444b22f1fa2b0686e772dd095a579c2119cb042 | 2022-02-14T15:17:25.000Z | [
"pytorch",
"tensorboard",
"gpt_neo",
"text-generation",
"transformers",
"generated_from_trainer",
"license:apache-2.0",
"model-index"
]
| text-generation | false | groar | null | groar/gpt-neo-1.3B-finetuned-escape3 | 2 | null | transformers | 24,021 | ---
license: apache-2.0
tags:
- generated_from_trainer
model-index:
- name: gpt-neo-1.3B-finetuned-escape3
results: []
---
<!-- This model card has been generated automatically according to the information the Trainer had access to. You
should probably proofread and complete it, then remove this comment. -->
# gpt-neo-1.3B-finetuned-escape3
This model is a fine-tuned version of [EleutherAI/gpt-neo-1.3B](https://huggingface.co/EleutherAI/gpt-neo-1.3B) on the None dataset.
## Model description
More information needed
## Intended uses & limitations
More information needed
## Training and evaluation data
More information needed
## Training procedure
### Training hyperparameters
The following hyperparameters were used during training:
- learning_rate: 2e-05
- train_batch_size: 8
- eval_batch_size: 8
- seed: 42
- optimizer: Adam with betas=(0.9,0.999) and epsilon=1e-08
- lr_scheduler_type: linear
- num_epochs: 30
### Framework versions
- Transformers 4.16.2
- Pytorch 1.10.0+cu111
- Datasets 1.18.3
- Tokenizers 0.11.0
|
gusintheshell/DialoGPT-small-rickbot | e78c153437ae404b84edaec9a27feae14f2fa7b4 | 2021-08-29T00:42:21.000Z | [
"pytorch",
"gpt2",
"text-generation",
"transformers",
"conversational"
]
| conversational | false | gusintheshell | null | gusintheshell/DialoGPT-small-rickbot | 2 | null | transformers | 24,022 | ---
tags:
- conversational
---
# Rick bot |
gwangjogong/quora-insincere-electra-small | 33e68d584bc7afdf0f9aa171382ca8a9a176102a | 2021-06-17T05:11:09.000Z | [
"pytorch",
"electra",
"text-classification",
"transformers"
]
| text-classification | false | gwangjogong | null | gwangjogong/quora-insincere-electra-small | 2 | null | transformers | 24,023 | Entry not found |
haji2438/bertweet-base-SNS_BRANDS_50k | 4a908f42c664f512e530e55ae8900fc42eca3ebb | 2022-01-24T03:51:35.000Z | [
"pytorch",
"tensorboard",
"roberta",
"fill-mask",
"transformers",
"generated_from_trainer",
"model-index",
"autotrain_compatible"
]
| fill-mask | false | haji2438 | null | haji2438/bertweet-base-SNS_BRANDS_50k | 2 | null | transformers | 24,024 | ---
tags:
- generated_from_trainer
model-index:
- name: bertweet-base-SNS_BRANDS_50k
results: []
---
<!-- This model card has been generated automatically according to the information the Trainer had access to. You
should probably proofread and complete it, then remove this comment. -->
# bertweet-base-SNS_BRANDS_50k
This model is a fine-tuned version of [vinai/bertweet-base](https://huggingface.co/vinai/bertweet-base) on the None dataset.
It achieves the following results on the evaluation set:
- Loss: 0.0490
## Model description
More information needed
## Intended uses & limitations
More information needed
## Training and evaluation data
More information needed
## Training procedure
### Training hyperparameters
The following hyperparameters were used during training:
- learning_rate: 2e-05
- train_batch_size: 8
- eval_batch_size: 8
- seed: 42
- optimizer: Adam with betas=(0.9,0.999) and epsilon=1e-08
- lr_scheduler_type: linear
- lr_scheduler_warmup_steps: 500
- num_epochs: 4
### Training results
| Training Loss | Epoch | Step | Validation Loss |
|:-------------:|:-----:|:----:|:---------------:|
| 0.0787 | 1.0 | 1465 | 0.0751 |
| 0.0662 | 2.0 | 2930 | 0.0628 |
| 0.053 | 3.0 | 4395 | 0.0531 |
| 0.0452 | 4.0 | 5860 | 0.0490 |
### Framework versions
- Transformers 4.15.0
- Pytorch 1.10.0+cu111
- Datasets 1.18.0
- Tokenizers 0.10.3
|
haji2438/test_Com_bertweet_fine_tuned | 393839a912f51af2520af11cb60fda8fdc10e586 | 2022-01-10T18:26:35.000Z | [
"pytorch",
"roberta",
"text-classification",
"transformers"
]
| text-classification | false | haji2438 | null | haji2438/test_Com_bertweet_fine_tuned | 2 | null | transformers | 24,025 | Entry not found |
halimara/model_sentence_bert | 724f7b915e8c162c932f3af9eeb0ed57ab19ffa7 | 2021-10-01T13:40:15.000Z | [
"pytorch",
"bert",
"feature-extraction",
"transformers"
]
| feature-extraction | false | halimara | null | halimara/model_sentence_bert | 2 | null | transformers | 24,026 | |
ham19za/model | 2183b8ff1e25f178af8fa1553ef64b4f25a1c670 | 2021-09-03T14:09:34.000Z | [
"pytorch",
"gpt_neo",
"text-generation",
"transformers"
]
| text-generation | false | ham19za | null | ham19za/model | 2 | null | transformers | 24,027 | Entry not found |
hama/Doctor_Bot | ced1db33f321cbe2a3e769a5302a5d023ff9bc7d | 2021-09-11T18:05:05.000Z | [
"pytorch",
"gpt2",
"text-generation",
"transformers",
"conversational"
]
| conversational | false | hama | null | hama/Doctor_Bot | 2 | null | transformers | 24,028 | ---
tags:
- conversational
---
# DOC DialoGPT Model |
hama/me0.01 | 97a234007c2d681971377dd094f4aeabc49c42dd | 2021-11-06T19:48:16.000Z | [
"pytorch",
"gpt2",
"text-generation",
"transformers",
"conversational"
]
| conversational | false | hama | null | hama/me0.01 | 2 | null | transformers | 24,029 | ---
tags:
- conversational
---
# me 101 |
hangmu/9.4AIstudy | b0cf06ca8bc620707c16fa2914b1e639dbe5298b | 2021-09-05T11:42:00.000Z | [
"pytorch",
"bert",
"fill-mask",
"transformers",
"autotrain_compatible"
]
| fill-mask | false | hangmu | null | hangmu/9.4AIstudy | 2 | null | transformers | 24,030 | Entry not found |
hanseokhyeon/bert-badword-base | ba4de31ec337cc66567c446d58e87d5286daa3ca | 2021-05-19T18:00:19.000Z | [
"pytorch",
"jax",
"bert",
"text-classification",
"transformers"
]
| text-classification | false | hanseokhyeon | null | hanseokhyeon/bert-badword-base | 2 | null | transformers | 24,031 | Entry not found |
hanseokhyeon/bert-badword-puri-2400 | 0ffff51964b9712baa9aff8883a5354d4533fd3f | 2021-05-19T18:10:27.000Z | [
"pytorch",
"jax",
"bert",
"text-classification",
"transformers"
]
| text-classification | false | hanseokhyeon | null | hanseokhyeon/bert-badword-puri-2400 | 2 | null | transformers | 24,032 | Entry not found |
harish/AStitchInLanguageModels-Task2_EN_BERTTokenizedNoPreTrain | 060fcb8ac3a9ac72066887d79a85b2b3e7218dd1 | 2021-08-20T12:00:01.000Z | [
"pytorch",
"bert",
"fill-mask",
"transformers",
"autotrain_compatible"
]
| fill-mask | false | harish | null | harish/AStitchInLanguageModels-Task2_EN_BERTTokenizedNoPreTrain | 2 | null | transformers | 24,033 | Entry not found |
harish/AStitchInLanguageModels-Task2_PT_mBERTTokenizedSelectReplacePreTrain | 6fc4eb06fdc396ec727e10f00c13b48b4cc9f6f2 | 2021-08-20T12:25:17.000Z | [
"pytorch",
"bert",
"fill-mask",
"transformers",
"autotrain_compatible"
]
| fill-mask | false | harish | null | harish/AStitchInLanguageModels-Task2_PT_mBERTTokenizedSelectReplacePreTrain | 2 | null | transformers | 24,034 | Entry not found |
harish/BERT-Plus-CxG-100K | 86e9d73c5b5cc2b6981f005aa21028c17057ae02 | 2021-05-19T18:21:36.000Z | [
"pytorch",
"jax",
"bert",
"transformers"
]
| null | false | harish | null | harish/BERT-Plus-CxG-100K | 2 | null | transformers | 24,035 | Entry not found |
harish/BERT-Plus-CxG-20K | f88b8b555a123f18ae7ca1f27125b98ea77f4bcb | 2021-05-19T18:22:32.000Z | [
"pytorch",
"jax",
"bert",
"transformers"
]
| null | false | harish | null | harish/BERT-Plus-CxG-20K | 2 | null | transformers | 24,036 | Entry not found |
harish/EN-AStitchTask1A-BERTBaseCased-FalseFalse-0-3-BEST | 1fe324eae610d1d7073a21c03b610572c4789630 | 2021-09-05T00:05:50.000Z | [
"pytorch",
"bert",
"text-classification",
"transformers"
]
| text-classification | false | harish | null | harish/EN-AStitchTask1A-BERTBaseCased-FalseFalse-0-3-BEST | 2 | null | transformers | 24,037 | Entry not found |
harish/EN-AStitchTask1A-BERTBaseCased-TrueTrue-0-3-BEST | f0c98c90fbcd7ad2e4ae04eecebf7c11949dc5e1 | 2021-09-05T00:13:30.000Z | [
"pytorch",
"bert",
"text-classification",
"transformers"
]
| text-classification | false | harish | null | harish/EN-AStitchTask1A-BERTBaseCased-TrueTrue-0-3-BEST | 2 | null | transformers | 24,038 | Entry not found |
harish/PT-FalseFalse-0_2_BEST | 15549cb7ceccc13ba1e8133cba4ec2ce01ca4d6b | 2021-05-19T18:32:16.000Z | [
"pytorch",
"jax",
"bert",
"text-classification",
"transformers"
]
| text-classification | false | harish | null | harish/PT-FalseFalse-0_2_BEST | 2 | null | transformers | 24,039 | Entry not found |
harish/PT-UP-xlmR-FalseFalse-0_0_BEST | 2b0f74d7ce7b74f04371b84aa48984c83106c0d2 | 2021-06-28T14:36:22.000Z | [
"pytorch",
"xlm-roberta",
"text-classification",
"transformers"
]
| text-classification | false | harish | null | harish/PT-UP-xlmR-FalseFalse-0_0_BEST | 2 | null | transformers | 24,040 | Entry not found |
harish/PT-UP-xlmR-OneShot-FalseTrue-0_2_BEST | 9895698f8f2faba143e214cb7bf36120bc9fbafa | 2021-06-28T15:52:47.000Z | [
"pytorch",
"xlm-roberta",
"text-classification",
"transformers"
]
| text-classification | false | harish | null | harish/PT-UP-xlmR-OneShot-FalseTrue-0_2_BEST | 2 | null | transformers | 24,041 | Entry not found |
harish/PT-UP-xlmR-TrueTrue-0_4_BEST | 81e97e0226f8be2cd6823c76481cf7616d2d2305 | 2021-06-28T14:33:05.000Z | [
"pytorch",
"xlm-roberta",
"text-classification",
"transformers"
]
| text-classification | false | harish | null | harish/PT-UP-xlmR-TrueTrue-0_4_BEST | 2 | null | transformers | 24,042 | Entry not found |
harish/PT-XLM_R-FalseFalse-0_2_BEST | e93cf5ff336bf7366bf272aa1a8d12a9ce430060 | 2021-05-09T10:25:56.000Z | [
"pytorch",
"xlm-roberta",
"text-classification",
"transformers"
]
| text-classification | false | harish | null | harish/PT-XLM_R-FalseFalse-0_2_BEST | 2 | null | transformers | 24,043 | Entry not found |
harish/PT-XLM_R-FalseTrue-0_2_BEST | 519749c184df23243ca80cd537cb2023ea70d7e4 | 2021-05-09T00:56:11.000Z | [
"pytorch",
"xlm-roberta",
"text-classification",
"transformers"
]
| text-classification | false | harish | null | harish/PT-XLM_R-FalseTrue-0_2_BEST | 2 | null | transformers | 24,044 | Entry not found |
harish/PT-v3-dev-test-all-PreTrain-e6-all | cb57a32346a71544a5445a6a01e122094fdea423 | 2021-05-19T18:49:04.000Z | [
"pytorch",
"jax",
"bert",
"fill-mask",
"transformers",
"autotrain_compatible"
]
| fill-mask | false | harish | null | harish/PT-v3-dev-test-all-PreTrain-e6-all | 2 | null | transformers | 24,045 | Entry not found |
haritzpuerto/MetaQA | 4d9a6d913c833415e627bc174638befab2b2c9a0 | 2022-02-15T10:57:45.000Z | [
"pytorch",
"bert",
"transformers"
]
| null | false | haritzpuerto | null | haritzpuerto/MetaQA | 2 | null | transformers | 24,046 | Entry not found |
helmi0695/det5-base | 6ed35e141c201080775c1b3f39974d8569afda49 | 2021-10-18T15:05:13.000Z | [
"pytorch",
"t5",
"text2text-generation",
"transformers",
"autotrain_compatible"
]
| text2text-generation | false | helmi0695 | null | helmi0695/det5-base | 2 | null | transformers | 24,047 | Entry not found |
hendrixcosta/bertoso | 7ad47d03cc4f43d9bac48729adf66fd8a5f27bd0 | 2021-05-19T18:58:51.000Z | [
"pytorch",
"jax",
"bert",
"question-answering",
"transformers",
"autotrain_compatible"
]
| question-answering | false | hendrixcosta | null | hendrixcosta/bertoso | 2 | null | transformers | 24,048 | Entry not found |
hf-internal-testing/tiny-random-hubert | 8132718dad3c31c2dd3d5e13b988a94681b01408 | 2021-09-17T19:25:54.000Z | [
"pytorch",
"tf",
"hubert",
"transformers"
]
| null | false | hf-internal-testing | null | hf-internal-testing/tiny-random-hubert | 2 | null | transformers | 24,049 | Entry not found |
hf-internal-testing/tiny-random-ibert | e42afa46847fd616ea8ab641f6b7d1471d263649 | 2021-09-17T19:26:06.000Z | [
"pytorch",
"ibert",
"transformers"
]
| null | false | hf-internal-testing | null | hf-internal-testing/tiny-random-ibert | 2 | null | transformers | 24,050 | Entry not found |
hf-test/xls-r-ab-test | 3287e21137ac96500ff1d4d915d7a472462ac0f3 | 2022-01-09T00:36:20.000Z | [
"pytorch",
"tensorboard",
"wav2vec2",
"automatic-speech-recognition",
"ab",
"dataset:common_voice",
"transformers",
"mozilla-foundation/common_voice_7_0",
"generated_from_trainer",
"model-index"
]
| automatic-speech-recognition | false | hf-test | null | hf-test/xls-r-ab-test | 2 | null | transformers | 24,051 | ---
language:
- ab
tags:
- automatic-speech-recognition
- mozilla-foundation/common_voice_7_0
- generated_from_trainer
datasets:
- common_voice
model-index:
- name: ''
results: []
---
<!-- This model card has been generated automatically according to the information the Trainer had access to. You
should probably proofread and complete it, then remove this comment. -->
#
This model is a fine-tuned version of [hf-test/xls-r-dummy](https://huggingface.co/hf-test/xls-r-dummy) on the MOZILLA-FOUNDATION/COMMON_VOICE_7_0 - AB dataset.
It achieves the following results on the evaluation set:
- Loss: 156.8787
- Wer: 1.3460
## Model description
More information needed
## Intended uses & limitations
More information needed
## Training and evaluation data
More information needed
## Training procedure
### Training hyperparameters
The following hyperparameters were used during training:
- learning_rate: 0.0003
- train_batch_size: 2
- eval_batch_size: 8
- seed: 42
- optimizer: Adam with betas=(0.9,0.999) and epsilon=1e-08
- lr_scheduler_type: linear
- training_steps: 10
- mixed_precision_training: Native AMP
### Training results
### Framework versions
- Transformers 4.16.0.dev0
- Pytorch 1.10.0
- Datasets 1.16.1
- Tokenizers 0.10.3
|
hfl/chinese-legal-electra-large-discriminator | 0f99abbeb1c316db79055e591c8f5d0eeff48d18 | 2021-01-22T05:19:50.000Z | [
"pytorch",
"tf",
"electra",
"zh",
"arxiv:2004.13922",
"transformers",
"license:apache-2.0"
]
| null | false | hfl | null | hfl/chinese-legal-electra-large-discriminator | 2 | 1 | transformers | 24,052 | ---
language:
- zh
license: "apache-2.0"
---
# This model is specifically designed for legal domain.
## Chinese ELECTRA
Google and Stanford University released a new pre-trained model called ELECTRA, which has a much compact model size and relatively competitive performance compared to BERT and its variants.
For further accelerating the research of the Chinese pre-trained model, the Joint Laboratory of HIT and iFLYTEK Research (HFL) has released the Chinese ELECTRA models based on the official code of ELECTRA.
ELECTRA-small could reach similar or even higher scores on several NLP tasks with only 1/10 parameters compared to BERT and its variants.
This project is based on the official code of ELECTRA: [https://github.com/google-research/electra](https://github.com/google-research/electra)
You may also interested in,
- Chinese BERT series: https://github.com/ymcui/Chinese-BERT-wwm
- Chinese ELECTRA: https://github.com/ymcui/Chinese-ELECTRA
- Chinese XLNet: https://github.com/ymcui/Chinese-XLNet
- Knowledge Distillation Toolkit - TextBrewer: https://github.com/airaria/TextBrewer
More resources by HFL: https://github.com/ymcui/HFL-Anthology
## Citation
If you find our resource or paper is useful, please consider including the following citation in your paper.
- https://arxiv.org/abs/2004.13922
```
@inproceedings{cui-etal-2020-revisiting,
title = "Revisiting Pre-Trained Models for {C}hinese Natural Language Processing",
author = "Cui, Yiming and
Che, Wanxiang and
Liu, Ting and
Qin, Bing and
Wang, Shijin and
Hu, Guoping",
booktitle = "Proceedings of the 2020 Conference on Empirical Methods in Natural Language Processing: Findings",
month = nov,
year = "2020",
address = "Online",
publisher = "Association for Computational Linguistics",
url = "https://www.aclweb.org/anthology/2020.findings-emnlp.58",
pages = "657--668",
}
``` |
hgiyt/ar-monomodel-mberttok | 6d8274e4241e46e2afdbb273410714f6c3ac030b | 2021-05-19T19:28:13.000Z | [
"pytorch",
"jax",
"bert",
"fill-mask",
"transformers",
"autotrain_compatible"
]
| fill-mask | false | hgiyt | null | hgiyt/ar-monomodel-mberttok | 2 | null | transformers | 24,053 | Entry not found |
hgiyt/fi-mbertmodel-monotok-adapter | b061973ee057da70c54f3afbcd83e202536dd40e | 2021-05-19T19:32:58.000Z | [
"pytorch",
"jax",
"bert",
"fill-mask",
"transformers",
"autotrain_compatible"
]
| fill-mask | false | hgiyt | null | hgiyt/fi-mbertmodel-monotok-adapter | 2 | null | transformers | 24,054 | Entry not found |
hgiyt/id-mbertmodel-mberttok | 1846a3610d8b87628037c9fcadf37d4adc43c640 | 2021-05-19T19:39:54.000Z | [
"pytorch",
"jax",
"bert",
"fill-mask",
"transformers",
"autotrain_compatible"
]
| fill-mask | false | hgiyt | null | hgiyt/id-mbertmodel-mberttok | 2 | null | transformers | 24,055 | Entry not found |
hgiyt/id-monomodel-mberttok | 6d47929ad98d1919f1c242fcf2d27c83ef0e9c25 | 2021-05-19T19:43:23.000Z | [
"pytorch",
"jax",
"bert",
"fill-mask",
"transformers",
"autotrain_compatible"
]
| fill-mask | false | hgiyt | null | hgiyt/id-monomodel-mberttok | 2 | null | transformers | 24,056 | Entry not found |
hgiyt/tr-monomodel-mberttok | 3425aa866472fa7a9c4aa61b560618b68b178250 | 2021-05-19T19:56:10.000Z | [
"pytorch",
"jax",
"bert",
"fill-mask",
"transformers",
"autotrain_compatible"
]
| fill-mask | false | hgiyt | null | hgiyt/tr-monomodel-mberttok | 2 | null | transformers | 24,057 | Entry not found |
hgiyt/tr-monomodel-monotok | 8cf390dfa3b2abfe8ce631ad1f4b4609f43ac57f | 2021-05-19T19:58:31.000Z | [
"pytorch",
"jax",
"bert",
"fill-mask",
"transformers",
"autotrain_compatible"
]
| fill-mask | false | hgiyt | null | hgiyt/tr-monomodel-monotok | 2 | null | transformers | 24,058 | Entry not found |
hiiamsid/est5-base-qg | 2bdf024494b04f6bdf27ccb7b551d6813933fcc0 | 2021-10-07T09:26:49.000Z | [
"pytorch",
"t5",
"text2text-generation",
"es",
"transformers",
"spanish",
"question generation",
"qg",
"license:mit",
"autotrain_compatible"
]
| text2text-generation | false | hiiamsid | null | hiiamsid/est5-base-qg | 2 | null | transformers | 24,059 | ---
language: ["es"]
tags:
- spanish
- question generation
- qg
Datasets:
- SQUAD
license: mit
---
This is the finetuned model of hiiamsid/est5-base for Question Generation task.
* Here input is the context only and output is questions. No information regarding answers were given to model.
* Unfortunately, due to lack of sufficient resources it is fine tuned with batch_size=10 and num_seq_len=256. So, if too large context is given model may not get information about last portions.
```
from transformers import T5ForConditionalGeneration, T5Tokenizer
MODEL_NAME = 'hiiamsid/est5-base-qg'
model = T5ForConditionalGeneration.from_pretrained(MODEL_NAME)
tokenizer = T5Tokenizer.from_pretrained(MODEL_NAME)
model.cuda();
model.eval();
def generate_question(text, beams=10, grams=2, num_return_seq=10,max_size=256):
x = tokenizer(text, return_tensors='pt', padding=True).to(model.device)
out = model.generate(**x, no_repeat_ngram_size=grams, num_beams=beams, num_return_sequences=num_return_seq, max_length=max_size)
return tokenizer.decode(out[0], skip_special_tokens=True)
print(generate_question('Any context in spanish from which question is to be generated'))
```
## Citing & Authors
- Datasets : [squad_es](https://huggingface.co/datasets/squad_es)
- Model : [hiiamsid/est5-base](hiiamsid/est5-base) |
hiiii23/distilbert-base-uncased-finetuned-squad | 3e2cc6b3aa8a5a92b1b3cdec6f9f6462dc0db108 | 2021-10-10T13:02:48.000Z | [
"pytorch",
"tensorboard",
"distilbert",
"question-answering",
"dataset:squad",
"transformers",
"generated_from_trainer",
"license:apache-2.0",
"model-index",
"autotrain_compatible"
]
| question-answering | false | hiiii23 | null | hiiii23/distilbert-base-uncased-finetuned-squad | 2 | null | transformers | 24,060 | ---
license: apache-2.0
tags:
- generated_from_trainer
datasets:
- squad
model-index:
- name: distilbert-base-uncased-finetuned-squad
results: []
---
<!-- This model card has been generated automatically according to the information the Trainer had access to. You
should probably proofread and complete it, then remove this comment. -->
# distilbert-base-uncased-finetuned-squad
This model is a fine-tuned version of [distilbert-base-uncased](https://huggingface.co/distilbert-base-uncased) on the squad dataset.
## Model description
More information needed
## Intended uses & limitations
More information needed
## Training and evaluation data
More information needed
## Training procedure
### Training hyperparameters
The following hyperparameters were used during training:
- learning_rate: 2e-05
- train_batch_size: 16
- eval_batch_size: 16
- seed: 42
- optimizer: Adam with betas=(0.9,0.999) and epsilon=1e-08
- lr_scheduler_type: linear
- num_epochs: 3
### Framework versions
- Transformers 4.11.3
- Pytorch 1.9.0+cu111
- Datasets 1.12.1
- Tokenizers 0.10.3
|
hireddivas/DialoGPT-small-ray | 37a21aab8fda420112840bf785bf740a41334996 | 2021-11-03T05:56:23.000Z | [
"pytorch",
"gpt2",
"text-generation",
"transformers",
"conversational"
]
| conversational | false | hireddivas | null | hireddivas/DialoGPT-small-ray | 2 | null | transformers | 24,061 | ---
tags:
- conversational
---
GPT-2 chatbot - talk to Ray Smuckles |
hireddivas/dialoGPT-small-phil | 22d4bb31d2f37255fb4763c6930cdbb67de4afbc | 2021-11-01T05:27:59.000Z | [
"pytorch",
"gpt2",
"text-generation",
"transformers",
"conversational"
]
| conversational | false | hireddivas | null | hireddivas/dialoGPT-small-phil | 2 | null | transformers | 24,062 | ---
tags:
- conversational
---
GPT-2 model trained on Phil from Eastenders |
hkunlp/from_all_T5_large_prefix_sql2text2 | 3e8223b2c6bc17680eb331ff4a5a8f44259d0115 | 2022-01-11T18:18:48.000Z | [
"pytorch",
"t5",
"transformers"
]
| null | false | hkunlp | null | hkunlp/from_all_T5_large_prefix_sql2text2 | 2 | null | transformers | 24,063 | Entry not found |
hkunlp/from_all_T5_large_prefix_webqsp2 | 9837dc5f771988435c5074b173584d805ed5e8bd | 2022-01-11T16:27:43.000Z | [
"pytorch",
"t5",
"transformers"
]
| null | false | hkunlp | null | hkunlp/from_all_T5_large_prefix_webqsp2 | 2 | null | transformers | 24,064 | Entry not found |
hongjehong/hjh_kobart_summarizer | e1216c8976ada2b22070f0c66f3fed261a4eaf84 | 2021-10-11T16:14:54.000Z | [
"pytorch",
"bart",
"text2text-generation",
"transformers",
"autotrain_compatible"
]
| text2text-generation | false | hongjehong | null | hongjehong/hjh_kobart_summarizer | 2 | null | transformers | 24,065 | Entry not found |
howey/bert-base-uncased-mrpc | 23d4cf0be1560d96f26add1b1182d0e1ab4fb8cb | 2021-05-26T14:47:44.000Z | [
"pytorch",
"bert",
"text-classification",
"transformers"
]
| text-classification | false | howey | null | howey/bert-base-uncased-mrpc | 2 | null | transformers | 24,066 | Entry not found |
howey/electra-base-cola | 573ea54f9d4eb2e1f4b2aecf5d08539da4b7772c | 2021-05-24T08:40:52.000Z | [
"pytorch",
"electra",
"text-classification",
"transformers"
]
| text-classification | false | howey | null | howey/electra-base-cola | 2 | null | transformers | 24,067 | Entry not found |
howey/electra-base-qnli | bd0cb1cc9b114acf69cd514abaf5093ec3ec71c2 | 2021-05-18T15:10:19.000Z | [
"pytorch",
"electra",
"text-classification",
"transformers"
]
| text-classification | false | howey | null | howey/electra-base-qnli | 2 | null | transformers | 24,068 | Entry not found |
howey/electra-large-cola | 52a16bfbad0533fc40701b41a79290810c9c4f2f | 2021-06-04T06:25:15.000Z | [
"pytorch",
"electra",
"text-classification",
"transformers"
]
| text-classification | false | howey | null | howey/electra-large-cola | 2 | 1 | transformers | 24,069 | Entry not found |
howey/electra-large-mrpc | 88206cbd71e3a0f6d19ddcaadb156f51905e6be1 | 2021-06-04T06:14:31.000Z | [
"pytorch",
"electra",
"text-classification",
"transformers"
]
| text-classification | false | howey | null | howey/electra-large-mrpc | 2 | null | transformers | 24,070 | Entry not found |
howey/electra-large-qnli | 452b134794df5ccf2582cfbab173fe1de7202575 | 2021-06-04T06:31:46.000Z | [
"pytorch",
"electra",
"text-classification",
"transformers"
]
| text-classification | false | howey | null | howey/electra-large-qnli | 2 | null | transformers | 24,071 | Entry not found |
howey/electra-large-stsb | cf06fc0884d5f08e4e11c3c0a844c0634e1b50cf | 2021-06-04T06:28:12.000Z | [
"pytorch",
"electra",
"text-classification",
"transformers"
]
| text-classification | false | howey | null | howey/electra-large-stsb | 2 | null | transformers | 24,072 | Entry not found |
howey/roberta-large-rte | 6b154931035643eb223908224c5c5aa9cf56d829 | 2021-06-03T14:16:01.000Z | [
"pytorch",
"roberta",
"text-classification",
"transformers"
]
| text-classification | false | howey | null | howey/roberta-large-rte | 2 | null | transformers | 24,073 | Entry not found |
howey/roberta-large-stsb | 9bc1f6c22f899f46a8d7a9d835b0759148ff240e | 2021-06-03T11:42:49.000Z | [
"pytorch",
"roberta",
"text-classification",
"transformers"
]
| text-classification | false | howey | null | howey/roberta-large-stsb | 2 | null | transformers | 24,074 | Entry not found |
hrdipto/wav2vec2-xls-r-timit-tokenizer | 995ecf7fd736a934f449765fcebba000358e921c | 2021-12-21T11:49:30.000Z | [
"pytorch",
"tensorboard",
"wav2vec2",
"automatic-speech-recognition",
"transformers",
"generated_from_trainer",
"license:apache-2.0",
"model-index"
]
| automatic-speech-recognition | false | hrdipto | null | hrdipto/wav2vec2-xls-r-timit-tokenizer | 2 | null | transformers | 24,075 | ---
license: apache-2.0
tags:
- generated_from_trainer
model-index:
- name: wav2vec2-xls-r-timit-tokenizer
results: []
---
<!-- This model card has been generated automatically according to the information the Trainer had access to. You
should probably proofread and complete it, then remove this comment. -->
# wav2vec2-xls-r-timit-tokenizer
This model is a fine-tuned version of [facebook/wav2vec2-xls-r-300m](https://huggingface.co/facebook/wav2vec2-xls-r-300m) on the None dataset.
It achieves the following results on the evaluation set:
- Loss: 0.4285
- Wer: 0.3662
## Model description
More information needed
## Intended uses & limitations
More information needed
## Training and evaluation data
More information needed
## Training procedure
### Training hyperparameters
The following hyperparameters were used during training:
- learning_rate: 0.0003
- train_batch_size: 16
- eval_batch_size: 8
- seed: 42
- gradient_accumulation_steps: 2
- total_train_batch_size: 32
- optimizer: Adam with betas=(0.9,0.999) and epsilon=1e-08
- lr_scheduler_type: linear
- lr_scheduler_warmup_steps: 500
- num_epochs: 30
- mixed_precision_training: Native AMP
### Training results
| Training Loss | Epoch | Step | Validation Loss | Wer |
|:-------------:|:-----:|:----:|:---------------:|:------:|
| 2.1571 | 4.03 | 500 | 0.5235 | 0.5098 |
| 0.2001 | 8.06 | 1000 | 0.4172 | 0.4375 |
| 0.0968 | 12.1 | 1500 | 0.4562 | 0.4016 |
| 0.0607 | 16.13 | 2000 | 0.4640 | 0.4050 |
| 0.0409 | 20.16 | 2500 | 0.4688 | 0.3914 |
| 0.0273 | 24.19 | 3000 | 0.4414 | 0.3763 |
| 0.0181 | 28.22 | 3500 | 0.4285 | 0.3662 |
### Framework versions
- Transformers 4.11.3
- Pytorch 1.10.0+cu111
- Datasets 1.13.3
- Tokenizers 0.10.3
|
huggingartists/25-17 | 14ac1f8fa22e35a60f1f08610a36e75ce72f5904 | 2021-09-10T12:55:59.000Z | [
"pytorch",
"jax",
"gpt2",
"text-generation",
"en",
"dataset:huggingartists/25-17",
"transformers",
"huggingartists",
"lyrics",
"lm-head",
"causal-lm"
]
| text-generation | false | huggingartists | null | huggingartists/25-17 | 2 | null | transformers | 24,076 | ---
language: en
datasets:
- huggingartists/25-17
tags:
- huggingartists
- lyrics
- lm-head
- causal-lm
widget:
- text: "I am"
---
<div class="inline-flex flex-col" style="line-height: 1.5;">
<div class="flex">
<div
style="display:DISPLAY_1; margin-left: auto; margin-right: auto; width: 92px; height:92px; border-radius: 50%; background-size: cover; background-image: url('https://images.genius.com/4fedc5dd2830a874a5274bf1cac62002.1000x1000x1.jpg')">
</div>
</div>
<div style="text-align: center; margin-top: 3px; font-size: 16px; font-weight: 800">🤖 HuggingArtists Model 🤖</div>
<div style="text-align: center; font-size: 16px; font-weight: 800">25/17</div>
<a href="https://genius.com/artists/25-17">
<div style="text-align: center; font-size: 14px;">@25-17</div>
</a>
</div>
I was made with [huggingartists](https://github.com/AlekseyKorshuk/huggingartists).
Create your own bot based on your favorite artist with [the demo](https://colab.research.google.com/github/AlekseyKorshuk/huggingartists/blob/master/huggingartists-demo.ipynb)!
## How does it work?
To understand how the model was developed, check the [W&B report](https://wandb.ai/huggingartists/huggingartists/reportlist).
## Training data
The model was trained on lyrics from 25/17.
Dataset is available [here](https://huggingface.co/datasets/huggingartists/25-17).
And can be used with:
```python
from datasets import load_dataset
dataset = load_dataset("huggingartists/25-17")
```
[Explore the data](https://wandb.ai/huggingartists/huggingartists/runs/1iuytbjp/artifacts), which is tracked with [W&B artifacts](https://docs.wandb.com/artifacts) at every step of the pipeline.
## Training procedure
The model is based on a pre-trained [GPT-2](https://huggingface.co/gpt2) which is fine-tuned on 25/17's lyrics.
Hyperparameters and metrics are recorded in the [W&B training run](https://wandb.ai/huggingartists/huggingartists/runs/knv4l4gw) for full transparency and reproducibility.
At the end of training, [the final model](https://wandb.ai/huggingartists/huggingartists/runs/knv4l4gw/artifacts) is logged and versioned.
## How to use
You can use this model directly with a pipeline for text generation:
```python
from transformers import pipeline
generator = pipeline('text-generation',
model='huggingartists/25-17')
generator("I am", num_return_sequences=5)
```
Or with Transformers library:
```python
from transformers import AutoTokenizer, AutoModelWithLMHead
tokenizer = AutoTokenizer.from_pretrained("huggingartists/25-17")
model = AutoModelWithLMHead.from_pretrained("huggingartists/25-17")
```
## Limitations and bias
The model suffers from [the same limitations and bias as GPT-2](https://huggingface.co/gpt2#limitations-and-bias).
In addition, the data present in the user's tweets further affects the text generated by the model.
## About
*Built by Aleksey Korshuk*
[](https://github.com/AlekseyKorshuk)
[](https://twitter.com/intent/follow?screen_name=alekseykorshuk)
[](https://t.me/joinchat/_CQ04KjcJ-4yZTky)
For more details, visit the project repository.
[](https://github.com/AlekseyKorshuk/huggingartists)
|
huggingartists/5opka | 54d1722eacd367b5dc9bdedb2e0373e14b6acb9e | 2021-09-16T15:23:49.000Z | [
"pytorch",
"jax",
"gpt2",
"text-generation",
"en",
"dataset:huggingartists/5opka",
"transformers",
"huggingartists",
"lyrics",
"lm-head",
"causal-lm"
]
| text-generation | false | huggingartists | null | huggingartists/5opka | 2 | null | transformers | 24,077 | ---
language: en
datasets:
- huggingartists/5opka
tags:
- huggingartists
- lyrics
- lm-head
- causal-lm
widget:
- text: "I am"
---
<div class="inline-flex flex-col" style="line-height: 1.5;">
<div class="flex">
<div
style="display:DISPLAY_1; margin-left: auto; margin-right: auto; width: 92px; height:92px; border-radius: 50%; background-size: cover; background-image: url('https://images.genius.com/c56dce03a151e17a9626e55e6c295bb1.1000x1000x1.jpg')">
</div>
</div>
<div style="text-align: center; margin-top: 3px; font-size: 16px; font-weight: 800">🤖 HuggingArtists Model 🤖</div>
<div style="text-align: center; font-size: 16px; font-weight: 800">5opka</div>
<a href="https://genius.com/artists/5opka">
<div style="text-align: center; font-size: 14px;">@5opka</div>
</a>
</div>
I was made with [huggingartists](https://github.com/AlekseyKorshuk/huggingartists).
Create your own bot based on your favorite artist with [the demo](https://colab.research.google.com/github/AlekseyKorshuk/huggingartists/blob/master/huggingartists-demo.ipynb)!
## How does it work?
To understand how the model was developed, check the [W&B report](https://wandb.ai/huggingartists/huggingartists/reportlist).
## Training data
The model was trained on lyrics from 5opka.
Dataset is available [here](https://huggingface.co/datasets/huggingartists/5opka).
And can be used with:
```python
from datasets import load_dataset
dataset = load_dataset("huggingartists/5opka")
```
[Explore the data](https://wandb.ai/huggingartists/huggingartists/runs/1o2s4fw8/artifacts), which is tracked with [W&B artifacts](https://docs.wandb.com/artifacts) at every step of the pipeline.
## Training procedure
The model is based on a pre-trained [GPT-2](https://huggingface.co/gpt2) which is fine-tuned on 5opka's lyrics.
Hyperparameters and metrics are recorded in the [W&B training run](https://wandb.ai/huggingartists/huggingartists/runs/3vitposx) for full transparency and reproducibility.
At the end of training, [the final model](https://wandb.ai/huggingartists/huggingartists/runs/3vitposx/artifacts) is logged and versioned.
## How to use
You can use this model directly with a pipeline for text generation:
```python
from transformers import pipeline
generator = pipeline('text-generation',
model='huggingartists/5opka')
generator("I am", num_return_sequences=5)
```
Or with Transformers library:
```python
from transformers import AutoTokenizer, AutoModelWithLMHead
tokenizer = AutoTokenizer.from_pretrained("huggingartists/5opka")
model = AutoModelWithLMHead.from_pretrained("huggingartists/5opka")
```
## Limitations and bias
The model suffers from [the same limitations and bias as GPT-2](https://huggingface.co/gpt2#limitations-and-bias).
In addition, the data present in the user's tweets further affects the text generated by the model.
## About
*Built by Aleksey Korshuk*
[](https://github.com/AlekseyKorshuk)
[](https://twitter.com/intent/follow?screen_name=alekseykorshuk)
[](https://t.me/joinchat/_CQ04KjcJ-4yZTky)
For more details, visit the project repository.
[](https://github.com/AlekseyKorshuk/huggingartists)
|
huggingartists/aikko | 78249774ba43454f90ea19f1c50008a14db87e7f | 2021-09-12T14:10:49.000Z | [
"pytorch",
"jax",
"gpt2",
"text-generation",
"en",
"dataset:huggingartists/aikko",
"transformers",
"huggingartists",
"lyrics",
"lm-head",
"causal-lm"
]
| text-generation | false | huggingartists | null | huggingartists/aikko | 2 | null | transformers | 24,078 | ---
language: en
datasets:
- huggingartists/aikko
tags:
- huggingartists
- lyrics
- lm-head
- causal-lm
widget:
- text: "I am"
---
<div class="inline-flex flex-col" style="line-height: 1.5;">
<div class="flex">
<div
style="display:DISPLAY_1; margin-left: auto; margin-right: auto; width: 92px; height:92px; border-radius: 50%; background-size: cover; background-image: url('https://images.genius.com/a1a40316d1405fa83df2a21923d64168.1000x1000x1.jpg')">
</div>
</div>
<div style="text-align: center; margin-top: 3px; font-size: 16px; font-weight: 800">🤖 HuggingArtists Model 🤖</div>
<div style="text-align: center; font-size: 16px; font-weight: 800">aikko</div>
<a href="https://genius.com/artists/aikko">
<div style="text-align: center; font-size: 14px;">@aikko</div>
</a>
</div>
I was made with [huggingartists](https://github.com/AlekseyKorshuk/huggingartists).
Create your own bot based on your favorite artist with [the demo](https://colab.research.google.com/github/AlekseyKorshuk/huggingartists/blob/master/huggingartists-demo.ipynb)!
## How does it work?
To understand how the model was developed, check the [W&B report](https://wandb.ai/huggingartists/huggingartists/reportlist).
## Training data
The model was trained on lyrics from aikko.
Dataset is available [here](https://huggingface.co/datasets/huggingartists/aikko).
And can be used with:
```python
from datasets import load_dataset
dataset = load_dataset("huggingartists/aikko")
```
[Explore the data](https://wandb.ai/huggingartists/huggingartists/runs/1cfdpsrg/artifacts), which is tracked with [W&B artifacts](https://docs.wandb.com/artifacts) at every step of the pipeline.
## Training procedure
The model is based on a pre-trained [GPT-2](https://huggingface.co/gpt2) which is fine-tuned on aikko's lyrics.
Hyperparameters and metrics are recorded in the [W&B training run](https://wandb.ai/huggingartists/huggingartists/runs/oesyn53g) for full transparency and reproducibility.
At the end of training, [the final model](https://wandb.ai/huggingartists/huggingartists/runs/oesyn53g/artifacts) is logged and versioned.
## How to use
You can use this model directly with a pipeline for text generation:
```python
from transformers import pipeline
generator = pipeline('text-generation',
model='huggingartists/aikko')
generator("I am", num_return_sequences=5)
```
Or with Transformers library:
```python
from transformers import AutoTokenizer, AutoModelWithLMHead
tokenizer = AutoTokenizer.from_pretrained("huggingartists/aikko")
model = AutoModelWithLMHead.from_pretrained("huggingartists/aikko")
```
## Limitations and bias
The model suffers from [the same limitations and bias as GPT-2](https://huggingface.co/gpt2#limitations-and-bias).
In addition, the data present in the user's tweets further affects the text generated by the model.
## About
*Built by Aleksey Korshuk*
[](https://github.com/AlekseyKorshuk)
[](https://twitter.com/intent/follow?screen_name=alekseykorshuk)
[](https://t.me/joinchat/_CQ04KjcJ-4yZTky)
For more details, visit the project repository.
[](https://github.com/AlekseyKorshuk/huggingartists)
|
huggingartists/architects | 424167f586c0c06f3310d5cd0309f1bb1c04c132 | 2021-11-11T18:00:17.000Z | [
"pytorch",
"jax",
"gpt2",
"text-generation",
"en",
"dataset:huggingartists/architects",
"transformers",
"huggingartists",
"lyrics",
"lm-head",
"causal-lm"
]
| text-generation | false | huggingartists | null | huggingartists/architects | 2 | null | transformers | 24,079 | ---
language: en
datasets:
- huggingartists/architects
tags:
- huggingartists
- lyrics
- lm-head
- causal-lm
widget:
- text: "I am"
---
<div class="inline-flex flex-col" style="line-height: 1.5;">
<div class="flex">
<div
style="display:DISPLAY_1; margin-left: auto; margin-right: auto; width: 92px; height:92px; border-radius: 50%; background-size: cover; background-image: url('https://images.genius.com/d2cd8787bdf913fc1518987f971c6bd3.960x960x1.jpg')">
</div>
</div>
<div style="text-align: center; margin-top: 3px; font-size: 16px; font-weight: 800">🤖 HuggingArtists Model 🤖</div>
<div style="text-align: center; font-size: 16px; font-weight: 800">Architects</div>
<a href="https://genius.com/artists/architects">
<div style="text-align: center; font-size: 14px;">@architects</div>
</a>
</div>
I was made with [huggingartists](https://github.com/AlekseyKorshuk/huggingartists).
Create your own bot based on your favorite artist with [the demo](https://colab.research.google.com/github/AlekseyKorshuk/huggingartists/blob/master/huggingartists-demo.ipynb)!
## How does it work?
To understand how the model was developed, check the [W&B report](https://wandb.ai/huggingartists/huggingartists/reportlist).
## Training data
The model was trained on lyrics from Architects.
Dataset is available [here](https://huggingface.co/datasets/huggingartists/architects).
And can be used with:
```python
from datasets import load_dataset
dataset = load_dataset("huggingartists/architects")
```
[Explore the data](https://wandb.ai/huggingartists/huggingartists/runs/licizuue/artifacts), which is tracked with [W&B artifacts](https://docs.wandb.com/artifacts) at every step of the pipeline.
## Training procedure
The model is based on a pre-trained [GPT-2](https://huggingface.co/gpt2) which is fine-tuned on Architects's lyrics.
Hyperparameters and metrics are recorded in the [W&B training run](https://wandb.ai/huggingartists/huggingartists/runs/1a9mrzf8) for full transparency and reproducibility.
At the end of training, [the final model](https://wandb.ai/huggingartists/huggingartists/runs/1a9mrzf8/artifacts) is logged and versioned.
## How to use
You can use this model directly with a pipeline for text generation:
```python
from transformers import pipeline
generator = pipeline('text-generation',
model='huggingartists/architects')
generator("I am", num_return_sequences=5)
```
Or with Transformers library:
```python
from transformers import AutoTokenizer, AutoModelWithLMHead
tokenizer = AutoTokenizer.from_pretrained("huggingartists/architects")
model = AutoModelWithLMHead.from_pretrained("huggingartists/architects")
```
## Limitations and bias
The model suffers from [the same limitations and bias as GPT-2](https://huggingface.co/gpt2#limitations-and-bias).
In addition, the data present in the user's tweets further affects the text generated by the model.
## About
*Built by Aleksey Korshuk*
[](https://github.com/AlekseyKorshuk)
[](https://twitter.com/intent/follow?screen_name=alekseykorshuk)
[](https://t.me/joinchat/_CQ04KjcJ-4yZTky)
For more details, visit the project repository.
[](https://github.com/AlekseyKorshuk/huggingartists)
|
huggingartists/bones | 52c43eb599f2f704dc2cb344ecd3bdc3694a9297 | 2021-10-02T23:03:17.000Z | [
"pytorch",
"jax",
"gpt2",
"text-generation",
"en",
"dataset:huggingartists/bones",
"transformers",
"huggingartists",
"lyrics",
"lm-head",
"causal-lm"
]
| text-generation | false | huggingartists | null | huggingartists/bones | 2 | null | transformers | 24,080 | ---
language: en
datasets:
- huggingartists/bones
tags:
- huggingartists
- lyrics
- lm-head
- causal-lm
widget:
- text: "I am"
---
<div class="inline-flex flex-col" style="line-height: 1.5;">
<div class="flex">
<div
style="display:DISPLAY_1; margin-left: auto; margin-right: auto; width: 92px; height:92px; border-radius: 50%; background-size: cover; background-image: url('https://images.genius.com/564dc935d7c601860b155b359d8ddf9d.1000x1000x1.png')">
</div>
</div>
<div style="text-align: center; margin-top: 3px; font-size: 16px; font-weight: 800">🤖 HuggingArtists Model 🤖</div>
<div style="text-align: center; font-size: 16px; font-weight: 800">BONES</div>
<a href="https://genius.com/artists/bones">
<div style="text-align: center; font-size: 14px;">@bones</div>
</a>
</div>
I was made with [huggingartists](https://github.com/AlekseyKorshuk/huggingartists).
Create your own bot based on your favorite artist with [the demo](https://colab.research.google.com/github/AlekseyKorshuk/huggingartists/blob/master/huggingartists-demo.ipynb)!
## How does it work?
To understand how the model was developed, check the [W&B report](https://wandb.ai/huggingartists/huggingartists/reportlist).
## Training data
The model was trained on lyrics from BONES.
Dataset is available [here](https://huggingface.co/datasets/huggingartists/bones).
And can be used with:
```python
from datasets import load_dataset
dataset = load_dataset("huggingartists/bones")
```
[Explore the data](https://wandb.ai/huggingartists/huggingartists/runs/26h7sojw/artifacts), which is tracked with [W&B artifacts](https://docs.wandb.com/artifacts) at every step of the pipeline.
## Training procedure
The model is based on a pre-trained [GPT-2](https://huggingface.co/gpt2) which is fine-tuned on BONES's lyrics.
Hyperparameters and metrics are recorded in the [W&B training run](https://wandb.ai/huggingartists/huggingartists/runs/1yr1mvc2) for full transparency and reproducibility.
At the end of training, [the final model](https://wandb.ai/huggingartists/huggingartists/runs/1yr1mvc2/artifacts) is logged and versioned.
## How to use
You can use this model directly with a pipeline for text generation:
```python
from transformers import pipeline
generator = pipeline('text-generation',
model='huggingartists/bones')
generator("I am", num_return_sequences=5)
```
Or with Transformers library:
```python
from transformers import AutoTokenizer, AutoModelWithLMHead
tokenizer = AutoTokenizer.from_pretrained("huggingartists/bones")
model = AutoModelWithLMHead.from_pretrained("huggingartists/bones")
```
## Limitations and bias
The model suffers from [the same limitations and bias as GPT-2](https://huggingface.co/gpt2#limitations-and-bias).
In addition, the data present in the user's tweets further affects the text generated by the model.
## About
*Built by Aleksey Korshuk*
[](https://github.com/AlekseyKorshuk)
[](https://twitter.com/intent/follow?screen_name=alekseykorshuk)
[](https://t.me/joinchat/_CQ04KjcJ-4yZTky)
For more details, visit the project repository.
[](https://github.com/AlekseyKorshuk/huggingartists)
|
huggingartists/boris-grebenshikov | 9cb590c72d7a827a4842368d2caf8f2b33855028 | 2021-11-10T08:53:52.000Z | [
"pytorch",
"jax",
"gpt2",
"text-generation",
"en",
"dataset:huggingartists/boris-grebenshikov",
"transformers",
"huggingartists",
"lyrics",
"lm-head",
"causal-lm"
]
| text-generation | false | huggingartists | null | huggingartists/boris-grebenshikov | 2 | null | transformers | 24,081 | ---
language: en
datasets:
- huggingartists/boris-grebenshikov
tags:
- huggingartists
- lyrics
- lm-head
- causal-lm
widget:
- text: "I am"
---
<div class="inline-flex flex-col" style="line-height: 1.5;">
<div class="flex">
<div
style="display:DISPLAY_1; margin-left: auto; margin-right: auto; width: 92px; height:92px; border-radius: 50%; background-size: cover; background-image: url('https://images.genius.com/491c2f003f52c9837809b86faef7b764.1000x1000x1.jpg')">
</div>
</div>
<div style="text-align: center; margin-top: 3px; font-size: 16px; font-weight: 800">🤖 HuggingArtists Model 🤖</div>
<div style="text-align: center; font-size: 16px; font-weight: 800">Борис Гребенщиков (Boris Grebenshikov)</div>
<a href="https://genius.com/artists/boris-grebenshikov">
<div style="text-align: center; font-size: 14px;">@boris-grebenshikov</div>
</a>
</div>
I was made with [huggingartists](https://github.com/AlekseyKorshuk/huggingartists).
Create your own bot based on your favorite artist with [the demo](https://colab.research.google.com/github/AlekseyKorshuk/huggingartists/blob/master/huggingartists-demo.ipynb)!
## How does it work?
To understand how the model was developed, check the [W&B report](https://wandb.ai/huggingartists/huggingartists/reportlist).
## Training data
The model was trained on lyrics from Борис Гребенщиков (Boris Grebenshikov).
Dataset is available [here](https://huggingface.co/datasets/huggingartists/boris-grebenshikov).
And can be used with:
```python
from datasets import load_dataset
dataset = load_dataset("huggingartists/boris-grebenshikov")
```
[Explore the data](https://wandb.ai/huggingartists/huggingartists/runs/3nb43gls/artifacts), which is tracked with [W&B artifacts](https://docs.wandb.com/artifacts) at every step of the pipeline.
## Training procedure
The model is based on a pre-trained [GPT-2](https://huggingface.co/gpt2) which is fine-tuned on Борис Гребенщиков (Boris Grebenshikov)'s lyrics.
Hyperparameters and metrics are recorded in the [W&B training run](https://wandb.ai/huggingartists/huggingartists/runs/34p8ye7k) for full transparency and reproducibility.
At the end of training, [the final model](https://wandb.ai/huggingartists/huggingartists/runs/34p8ye7k/artifacts) is logged and versioned.
## How to use
You can use this model directly with a pipeline for text generation:
```python
from transformers import pipeline
generator = pipeline('text-generation',
model='huggingartists/boris-grebenshikov')
generator("I am", num_return_sequences=5)
```
Or with Transformers library:
```python
from transformers import AutoTokenizer, AutoModelWithLMHead
tokenizer = AutoTokenizer.from_pretrained("huggingartists/boris-grebenshikov")
model = AutoModelWithLMHead.from_pretrained("huggingartists/boris-grebenshikov")
```
## Limitations and bias
The model suffers from [the same limitations and bias as GPT-2](https://huggingface.co/gpt2#limitations-and-bias).
In addition, the data present in the user's tweets further affects the text generated by the model.
## About
*Built by Aleksey Korshuk*
[](https://github.com/AlekseyKorshuk)
[](https://twitter.com/intent/follow?screen_name=alekseykorshuk)
[](https://t.me/joinchat/_CQ04KjcJ-4yZTky)
For more details, visit the project repository.
[](https://github.com/AlekseyKorshuk/huggingartists)
|
huggingartists/duran-duran | 0a2c0e460c897379905e4643c8d81f876731af51 | 2021-08-10T12:53:45.000Z | [
"pytorch",
"jax",
"gpt2",
"text-generation",
"en",
"dataset:huggingartists/duran-duran",
"transformers",
"huggingartists",
"lyrics",
"lm-head",
"causal-lm"
]
| text-generation | false | huggingartists | null | huggingartists/duran-duran | 2 | null | transformers | 24,082 | ---
language: en
datasets:
- huggingartists/duran-duran
tags:
- huggingartists
- lyrics
- lm-head
- causal-lm
widget:
- text: "I am"
---
<div class="inline-flex flex-col" style="line-height: 1.5;">
<div class="flex">
<div
style="display:DISPLAY_1; margin-left: auto; margin-right: auto; width: 92px; height:92px; border-radius: 50%; background-size: cover; background-image: url('https://images.genius.com/95697394e4f58c9aa507e408f51008db.1000x1000x1.jpg')">
</div>
</div>
<div style="text-align: center; margin-top: 3px; font-size: 16px; font-weight: 800">🤖 HuggingArtists Model 🤖</div>
<div style="text-align: center; font-size: 16px; font-weight: 800">Duran Duran</div>
<a href="https://genius.com/artists/duran-duran">
<div style="text-align: center; font-size: 14px;">@duran-duran</div>
</a>
</div>
I was made with [huggingartists](https://github.com/AlekseyKorshuk/huggingartists).
Create your own bot based on your favorite artist with [the demo](https://colab.research.google.com/github/AlekseyKorshuk/huggingartists/blob/master/huggingartists-demo.ipynb)!
## How does it work?
To understand how the model was developed, check the [W&B report](https://wandb.ai/huggingartists/huggingartists/reportlist).
## Training data
The model was trained on lyrics from Duran Duran.
Dataset is available [here](https://huggingface.co/datasets/huggingartists/duran-duran).
And can be used with:
```python
from datasets import load_dataset
dataset = load_dataset("huggingartists/duran-duran")
```
[Explore the data](https://wandb.ai/huggingartists/huggingartists/runs/dy133fuf/artifacts), which is tracked with [W&B artifacts](https://docs.wandb.com/artifacts) at every step of the pipeline.
## Training procedure
The model is based on a pre-trained [GPT-2](https://huggingface.co/gpt2) which is fine-tuned on Duran Duran's lyrics.
Hyperparameters and metrics are recorded in the [W&B training run](https://wandb.ai/huggingartists/huggingartists/runs/386u7cc3) for full transparency and reproducibility.
At the end of training, [the final model](https://wandb.ai/huggingartists/huggingartists/runs/386u7cc3/artifacts) is logged and versioned.
## How to use
You can use this model directly with a pipeline for text generation:
```python
from transformers import pipeline
generator = pipeline('text-generation',
model='huggingartists/duran-duran')
generator("I am", num_return_sequences=5)
```
Or with Transformers library:
```python
from transformers import AutoTokenizer, AutoModelWithLMHead
tokenizer = AutoTokenizer.from_pretrained("huggingartists/duran-duran")
model = AutoModelWithLMHead.from_pretrained("huggingartists/duran-duran")
```
## Limitations and bias
The model suffers from [the same limitations and bias as GPT-2](https://huggingface.co/gpt2#limitations-and-bias).
In addition, the data present in the user's tweets further affects the text generated by the model.
## About
*Built by Aleksey Korshuk*
[](https://github.com/AlekseyKorshuk)
[](https://twitter.com/intent/follow?screen_name=alekseykorshuk)
[](https://t.me/joinchat/_CQ04KjcJ-4yZTky)
For more details, visit the project repository.
[](https://github.com/AlekseyKorshuk/huggingartists)
|
huggingartists/egor-letov | e355cf06d0bb232ad73e1f3d8b8c6a2ac01faef7 | 2022-02-13T20:16:48.000Z | [
"pytorch",
"jax",
"gpt2",
"text-generation",
"en",
"dataset:huggingartists/egor-letov",
"transformers",
"huggingartists",
"lyrics",
"lm-head",
"causal-lm"
]
| text-generation | false | huggingartists | null | huggingartists/egor-letov | 2 | null | transformers | 24,083 | ---
language: en
datasets:
- huggingartists/egor-letov
tags:
- huggingartists
- lyrics
- lm-head
- causal-lm
widget:
- text: "I am"
---
<div class="inline-flex flex-col" style="line-height: 1.5;">
<div class="flex">
<div
style="display:DISPLAY_1; margin-left: auto; margin-right: auto; width: 92px; height:92px; border-radius: 50%; background-size: cover; background-image: url('https://images.genius.com/faa3dae99bf1fe365927608fd55c745a.330x330x1.jpg')">
</div>
</div>
<div style="text-align: center; margin-top: 3px; font-size: 16px; font-weight: 800">🤖 HuggingArtists Model 🤖</div>
<div style="text-align: center; font-size: 16px; font-weight: 800">Егор Летов (Egor Letov)</div>
<a href="https://genius.com/artists/egor-letov">
<div style="text-align: center; font-size: 14px;">@egor-letov</div>
</a>
</div>
I was made with [huggingartists](https://github.com/AlekseyKorshuk/huggingartists).
Create your own bot based on your favorite artist with [the demo](https://colab.research.google.com/github/AlekseyKorshuk/huggingartists/blob/master/huggingartists-demo.ipynb)!
## How does it work?
To understand how the model was developed, check the [W&B report](https://wandb.ai/huggingartists/huggingartists/reportlist).
## Training data
The model was trained on lyrics from Егор Летов (Egor Letov).
Dataset is available [here](https://huggingface.co/datasets/huggingartists/egor-letov).
And can be used with:
```python
from datasets import load_dataset
dataset = load_dataset("huggingartists/egor-letov")
```
[Explore the data](https://wandb.ai/huggingartists/huggingartists/runs/1omrcegx/artifacts), which is tracked with [W&B artifacts](https://docs.wandb.com/artifacts) at every step of the pipeline.
## Training procedure
The model is based on a pre-trained [GPT-2](https://huggingface.co/gpt2) which is fine-tuned on Егор Летов (Egor Letov)'s lyrics.
Hyperparameters and metrics are recorded in the [W&B training run](https://wandb.ai/huggingartists/huggingartists/runs/3lk60u9h) for full transparency and reproducibility.
At the end of training, [the final model](https://wandb.ai/huggingartists/huggingartists/runs/3lk60u9h/artifacts) is logged and versioned.
## How to use
You can use this model directly with a pipeline for text generation:
```python
from transformers import pipeline
generator = pipeline('text-generation',
model='huggingartists/egor-letov')
generator("I am", num_return_sequences=5)
```
Or with Transformers library:
```python
from transformers import AutoTokenizer, AutoModelWithLMHead
tokenizer = AutoTokenizer.from_pretrained("huggingartists/egor-letov")
model = AutoModelWithLMHead.from_pretrained("huggingartists/egor-letov")
```
## Limitations and bias
The model suffers from [the same limitations and bias as GPT-2](https://huggingface.co/gpt2#limitations-and-bias).
In addition, the data present in the user's tweets further affects the text generated by the model.
## About
*Built by Aleksey Korshuk*
[](https://github.com/AlekseyKorshuk)
[](https://twitter.com/intent/follow?screen_name=alekseykorshuk)
[](https://t.me/joinchat/_CQ04KjcJ-4yZTky)
For more details, visit the project repository.
[](https://github.com/AlekseyKorshuk/huggingartists)
|
huggingartists/florence-the-machine | 909dd0ec2c77404c9cbb635f24d06e951dbda11f | 2021-08-10T09:03:06.000Z | [
"pytorch",
"jax",
"gpt2",
"text-generation",
"en",
"dataset:huggingartists/florence-the-machine",
"transformers",
"huggingartists",
"lyrics",
"lm-head",
"causal-lm"
]
| text-generation | false | huggingartists | null | huggingartists/florence-the-machine | 2 | null | transformers | 24,084 | ---
language: en
datasets:
- huggingartists/florence-the-machine
tags:
- huggingartists
- lyrics
- lm-head
- causal-lm
widget:
- text: "I am"
---
<div class="inline-flex flex-col" style="line-height: 1.5;">
<div class="flex">
<div
style="display:DISPLAY_1; margin-left: auto; margin-right: auto; width: 92px; height:92px; border-radius: 50%; background-size: cover; background-image: url('https://images.genius.com/99d09eb55276442d715ac14f06173a4e.1000x1000x1.jpg')">
</div>
</div>
<div style="text-align: center; margin-top: 3px; font-size: 16px; font-weight: 800">🤖 HuggingArtists Model 🤖</div>
<div style="text-align: center; font-size: 16px; font-weight: 800">Florence + The Machine</div>
<a href="https://genius.com/artists/florence-the-machine">
<div style="text-align: center; font-size: 14px;">@florence-the-machine</div>
</a>
</div>
I was made with [huggingartists](https://github.com/AlekseyKorshuk/huggingartists).
Create your own bot based on your favorite artist with [the demo](https://colab.research.google.com/github/AlekseyKorshuk/huggingartists/blob/master/huggingartists-demo.ipynb)!
## How does it work?
To understand how the model was developed, check the [W&B report](https://wandb.ai/huggingartists/huggingartists/reportlist).
## Training data
The model was trained on lyrics from Florence + The Machine.
Dataset is available [here](https://huggingface.co/datasets/huggingartists/florence-the-machine).
And can be used with:
```python
from datasets import load_dataset
dataset = load_dataset("huggingartists/florence-the-machine")
```
[Explore the data](https://wandb.ai/huggingartists/huggingartists/runs/icjt5evm/artifacts), which is tracked with [W&B artifacts](https://docs.wandb.com/artifacts) at every step of the pipeline.
## Training procedure
The model is based on a pre-trained [GPT-2](https://huggingface.co/gpt2) which is fine-tuned on Florence + The Machine's lyrics.
Hyperparameters and metrics are recorded in the [W&B training run](https://wandb.ai/huggingartists/huggingartists/runs/1zfb9y24) for full transparency and reproducibility.
At the end of training, [the final model](https://wandb.ai/huggingartists/huggingartists/runs/1zfb9y24/artifacts) is logged and versioned.
## How to use
You can use this model directly with a pipeline for text generation:
```python
from transformers import pipeline
generator = pipeline('text-generation',
model='huggingartists/florence-the-machine')
generator("I am", num_return_sequences=5)
```
Or with Transformers library:
```python
from transformers import AutoTokenizer, AutoModelWithLMHead
tokenizer = AutoTokenizer.from_pretrained("huggingartists/florence-the-machine")
model = AutoModelWithLMHead.from_pretrained("huggingartists/florence-the-machine")
```
## Limitations and bias
The model suffers from [the same limitations and bias as GPT-2](https://huggingface.co/gpt2#limitations-and-bias).
In addition, the data present in the user's tweets further affects the text generated by the model.
## About
*Built by Aleksey Korshuk*
[](https://github.com/AlekseyKorshuk)
[](https://twitter.com/intent/follow?screen_name=alekseykorshuk)
[](https://t.me/joinchat/_CQ04KjcJ-4yZTky)
For more details, visit the project repository.
[](https://github.com/AlekseyKorshuk/huggingartists)
|
huggingartists/john-lennon | 366cb36d88836cba9ef362c3f46ab5f81af07489 | 2021-09-10T10:37:44.000Z | [
"pytorch",
"jax",
"gpt2",
"text-generation",
"en",
"dataset:huggingartists/john-lennon",
"transformers",
"huggingartists",
"lyrics",
"lm-head",
"causal-lm"
]
| text-generation | false | huggingartists | null | huggingartists/john-lennon | 2 | null | transformers | 24,085 | ---
language: en
datasets:
- huggingartists/john-lennon
tags:
- huggingartists
- lyrics
- lm-head
- causal-lm
widget:
- text: "I am"
---
<div class="inline-flex flex-col" style="line-height: 1.5;">
<div class="flex">
<div
style="display:DISPLAY_1; margin-left: auto; margin-right: auto; width: 92px; height:92px; border-radius: 50%; background-size: cover; background-image: url('https://images.genius.com/de14b272004b51dea8071e7cba21cbac.1000x1000x1.jpg')">
</div>
</div>
<div style="text-align: center; margin-top: 3px; font-size: 16px; font-weight: 800">🤖 HuggingArtists Model 🤖</div>
<div style="text-align: center; font-size: 16px; font-weight: 800">John Lennon</div>
<a href="https://genius.com/artists/john-lennon">
<div style="text-align: center; font-size: 14px;">@john-lennon</div>
</a>
</div>
I was made with [huggingartists](https://github.com/AlekseyKorshuk/huggingartists).
Create your own bot based on your favorite artist with [the demo](https://colab.research.google.com/github/AlekseyKorshuk/huggingartists/blob/master/huggingartists-demo.ipynb)!
## How does it work?
To understand how the model was developed, check the [W&B report](https://wandb.ai/huggingartists/huggingartists/reportlist).
## Training data
The model was trained on lyrics from John Lennon.
Dataset is available [here](https://huggingface.co/datasets/huggingartists/john-lennon).
And can be used with:
```python
from datasets import load_dataset
dataset = load_dataset("huggingartists/john-lennon")
```
[Explore the data](https://wandb.ai/huggingartists/huggingartists/runs/f3d8fseh/artifacts), which is tracked with [W&B artifacts](https://docs.wandb.com/artifacts) at every step of the pipeline.
## Training procedure
The model is based on a pre-trained [GPT-2](https://huggingface.co/gpt2) which is fine-tuned on John Lennon's lyrics.
Hyperparameters and metrics are recorded in the [W&B training run](https://wandb.ai/huggingartists/huggingartists/runs/36mtogkg) for full transparency and reproducibility.
At the end of training, [the final model](https://wandb.ai/huggingartists/huggingartists/runs/36mtogkg/artifacts) is logged and versioned.
## How to use
You can use this model directly with a pipeline for text generation:
```python
from transformers import pipeline
generator = pipeline('text-generation',
model='huggingartists/john-lennon')
generator("I am", num_return_sequences=5)
```
Or with Transformers library:
```python
from transformers import AutoTokenizer, AutoModelWithLMHead
tokenizer = AutoTokenizer.from_pretrained("huggingartists/john-lennon")
model = AutoModelWithLMHead.from_pretrained("huggingartists/john-lennon")
```
## Limitations and bias
The model suffers from [the same limitations and bias as GPT-2](https://huggingface.co/gpt2#limitations-and-bias).
In addition, the data present in the user's tweets further affects the text generated by the model.
## About
*Built by Aleksey Korshuk*
[](https://github.com/AlekseyKorshuk)
[](https://twitter.com/intent/follow?screen_name=alekseykorshuk)
[](https://t.me/joinchat/_CQ04KjcJ-4yZTky)
For more details, visit the project repository.
[](https://github.com/AlekseyKorshuk/huggingartists)
|
huggingartists/lady-gaga | ca0671f5f5916768122e5b22521944b92bfb113e | 2022-03-08T20:28:19.000Z | [
"pytorch",
"jax",
"gpt2",
"text-generation",
"en",
"dataset:huggingartists/lady-gaga",
"transformers",
"huggingartists",
"lyrics",
"lm-head",
"causal-lm"
]
| text-generation | false | huggingartists | null | huggingartists/lady-gaga | 2 | null | transformers | 24,086 | ---
language: en
datasets:
- huggingartists/lady-gaga
tags:
- huggingartists
- lyrics
- lm-head
- causal-lm
widget:
- text: "I am"
---
<div class="inline-flex flex-col" style="line-height: 1.5;">
<div class="flex">
<div
style="display:DISPLAY_1; margin-left: auto; margin-right: auto; width: 92px; height:92px; border-radius: 50%; background-size: cover; background-image: url('https://images.genius.com/e7e76c378cb43b4b1ff03947d5c0481a.400x400x1.jpg')">
</div>
</div>
<div style="text-align: center; margin-top: 3px; font-size: 16px; font-weight: 800">🤖 HuggingArtists Model 🤖</div>
<div style="text-align: center; font-size: 16px; font-weight: 800">Lady Gaga</div>
<a href="https://genius.com/artists/lady-gaga">
<div style="text-align: center; font-size: 14px;">@lady-gaga</div>
</a>
</div>
I was made with [huggingartists](https://github.com/AlekseyKorshuk/huggingartists).
Create your own bot based on your favorite artist with [the demo](https://colab.research.google.com/github/AlekseyKorshuk/huggingartists/blob/master/huggingartists-demo.ipynb)!
## How does it work?
To understand how the model was developed, check the [W&B report](https://wandb.ai/huggingartists/huggingartists/reportlist).
## Training data
The model was trained on lyrics from Lady Gaga.
Dataset is available [here](https://huggingface.co/datasets/huggingartists/lady-gaga).
And can be used with:
```python
from datasets import load_dataset
dataset = load_dataset("huggingartists/lady-gaga")
```
[Explore the data](https://wandb.ai/huggingartists/huggingartists/runs/17c0d4ej/artifacts), which is tracked with [W&B artifacts](https://docs.wandb.com/artifacts) at every step of the pipeline.
## Training procedure
The model is based on a pre-trained [GPT-2](https://huggingface.co/gpt2) which is fine-tuned on Lady Gaga's lyrics.
Hyperparameters and metrics are recorded in the [W&B training run](https://wandb.ai/huggingartists/huggingartists/runs/2j7yp9qd) for full transparency and reproducibility.
At the end of training, [the final model](https://wandb.ai/huggingartists/huggingartists/runs/2j7yp9qd/artifacts) is logged and versioned.
## How to use
You can use this model directly with a pipeline for text generation:
```python
from transformers import pipeline
generator = pipeline('text-generation',
model='huggingartists/lady-gaga')
generator("I am", num_return_sequences=5)
```
Or with Transformers library:
```python
from transformers import AutoTokenizer, AutoModelWithLMHead
tokenizer = AutoTokenizer.from_pretrained("huggingartists/lady-gaga")
model = AutoModelWithLMHead.from_pretrained("huggingartists/lady-gaga")
```
## Limitations and bias
The model suffers from [the same limitations and bias as GPT-2](https://huggingface.co/gpt2#limitations-and-bias).
In addition, the data present in the user's tweets further affects the text generated by the model.
## About
*Built by Aleksey Korshuk*
[](https://github.com/AlekseyKorshuk)
[](https://twitter.com/intent/follow?screen_name=alekseykorshuk)
[](https://t.me/joinchat/_CQ04KjcJ-4yZTky)
For more details, visit the project repository.
[](https://github.com/AlekseyKorshuk/huggingartists)
|
huggingartists/led-zeppelin | a16dd5fa0e56e9257a89e38b6c2d33b8be0f6e06 | 2022-02-15T22:19:29.000Z | [
"pytorch",
"jax",
"gpt2",
"text-generation",
"en",
"dataset:huggingartists/led-zeppelin",
"transformers",
"huggingartists",
"lyrics",
"lm-head",
"causal-lm"
]
| text-generation | false | huggingartists | null | huggingartists/led-zeppelin | 2 | null | transformers | 24,087 | ---
language: en
datasets:
- huggingartists/led-zeppelin
tags:
- huggingartists
- lyrics
- lm-head
- causal-lm
widget:
- text: "I am"
---
<div class="inline-flex flex-col" style="line-height: 1.5;">
<div class="flex">
<div
style="display:DISPLAY_1; margin-left: auto; margin-right: auto; width: 92px; height:92px; border-radius: 50%; background-size: cover; background-image: url('https://images.genius.com/e4763bba12e6411077a3e573cd290da0.433x433x1.jpg')">
</div>
</div>
<div style="text-align: center; margin-top: 3px; font-size: 16px; font-weight: 800">🤖 HuggingArtists Model 🤖</div>
<div style="text-align: center; font-size: 16px; font-weight: 800">Led Zeppelin</div>
<a href="https://genius.com/artists/led-zeppelin">
<div style="text-align: center; font-size: 14px;">@led-zeppelin</div>
</a>
</div>
I was made with [huggingartists](https://github.com/AlekseyKorshuk/huggingartists).
Create your own bot based on your favorite artist with [the demo](https://colab.research.google.com/github/AlekseyKorshuk/huggingartists/blob/master/huggingartists-demo.ipynb)!
## How does it work?
To understand how the model was developed, check the [W&B report](https://wandb.ai/huggingartists/huggingartists/reportlist).
## Training data
The model was trained on lyrics from Led Zeppelin.
Dataset is available [here](https://huggingface.co/datasets/huggingartists/led-zeppelin).
And can be used with:
```python
from datasets import load_dataset
dataset = load_dataset("huggingartists/led-zeppelin")
```
[Explore the data](https://wandb.ai/huggingartists/huggingartists/runs/cpexpb1w/artifacts), which is tracked with [W&B artifacts](https://docs.wandb.com/artifacts) at every step of the pipeline.
## Training procedure
The model is based on a pre-trained [GPT-2](https://huggingface.co/gpt2) which is fine-tuned on Led Zeppelin's lyrics.
Hyperparameters and metrics are recorded in the [W&B training run](https://wandb.ai/huggingartists/huggingartists/runs/bna2epba) for full transparency and reproducibility.
At the end of training, [the final model](https://wandb.ai/huggingartists/huggingartists/runs/bna2epba/artifacts) is logged and versioned.
## How to use
You can use this model directly with a pipeline for text generation:
```python
from transformers import pipeline
generator = pipeline('text-generation',
model='huggingartists/led-zeppelin')
generator("I am", num_return_sequences=5)
```
Or with Transformers library:
```python
from transformers import AutoTokenizer, AutoModelWithLMHead
tokenizer = AutoTokenizer.from_pretrained("huggingartists/led-zeppelin")
model = AutoModelWithLMHead.from_pretrained("huggingartists/led-zeppelin")
```
## Limitations and bias
The model suffers from [the same limitations and bias as GPT-2](https://huggingface.co/gpt2#limitations-and-bias).
In addition, the data present in the user's tweets further affects the text generated by the model.
## About
*Built by Aleksey Korshuk*
[](https://github.com/AlekseyKorshuk)
[](https://twitter.com/intent/follow?screen_name=alekseykorshuk)
[](https://t.me/joinchat/_CQ04KjcJ-4yZTky)
For more details, visit the project repository.
[](https://github.com/AlekseyKorshuk/huggingartists)
|
huggingartists/madonna | d439efe98de06421da387560af3b81b51ba10037 | 2022-06-16T21:35:29.000Z | [
"pytorch",
"jax",
"gpt2",
"text-generation",
"en",
"dataset:huggingartists/madonna",
"transformers",
"huggingartists",
"lyrics",
"lm-head",
"causal-lm"
]
| text-generation | false | huggingartists | null | huggingartists/madonna | 2 | null | transformers | 24,088 | ---
language: en
datasets:
- huggingartists/madonna
tags:
- huggingartists
- lyrics
- lm-head
- causal-lm
widget:
- text: "I am"
---
<div class="inline-flex flex-col" style="line-height: 1.5;">
<div class="flex">
<div
style="display:DISPLAY_1; margin-left: auto; margin-right: auto; width: 92px; height:92px; border-radius: 50%; background-size: cover; background-image: url('https://images.genius.com/676c1c425eaa8e7600136c56af6dfada.1000x1000x1.jpg')">
</div>
</div>
<div style="text-align: center; margin-top: 3px; font-size: 16px; font-weight: 800">🤖 HuggingArtists Model 🤖</div>
<div style="text-align: center; font-size: 16px; font-weight: 800">Madonna</div>
<a href="https://genius.com/artists/madonna">
<div style="text-align: center; font-size: 14px;">@madonna</div>
</a>
</div>
I was made with [huggingartists](https://github.com/AlekseyKorshuk/huggingartists).
Create your own bot based on your favorite artist with [the demo](https://colab.research.google.com/github/AlekseyKorshuk/huggingartists/blob/master/huggingartists-demo.ipynb)!
## How does it work?
To understand how the model was developed, check the [W&B report](https://wandb.ai/huggingartists/huggingartists/reportlist).
## Training data
The model was trained on lyrics from Madonna.
Dataset is available [here](https://huggingface.co/datasets/huggingartists/madonna).
And can be used with:
```python
from datasets import load_dataset
dataset = load_dataset("huggingartists/madonna")
```
[Explore the data](https://wandb.ai/huggingartists/huggingartists/runs/2abhif57/artifacts), which is tracked with [W&B artifacts](https://docs.wandb.com/artifacts) at every step of the pipeline.
## Training procedure
The model is based on a pre-trained [GPT-2](https://huggingface.co/gpt2) which is fine-tuned on Madonna's lyrics.
Hyperparameters and metrics are recorded in the [W&B training run](https://wandb.ai/huggingartists/huggingartists/runs/2eok9fmu) for full transparency and reproducibility.
At the end of training, [the final model](https://wandb.ai/huggingartists/huggingartists/runs/2eok9fmu/artifacts) is logged and versioned.
## How to use
You can use this model directly with a pipeline for text generation:
```python
from transformers import pipeline
generator = pipeline('text-generation',
model='huggingartists/madonna')
generator("I am", num_return_sequences=5)
```
Or with Transformers library:
```python
from transformers import AutoTokenizer, AutoModelWithLMHead
tokenizer = AutoTokenizer.from_pretrained("huggingartists/madonna")
model = AutoModelWithLMHead.from_pretrained("huggingartists/madonna")
```
## Limitations and bias
The model suffers from [the same limitations and bias as GPT-2](https://huggingface.co/gpt2#limitations-and-bias).
In addition, the data present in the user's tweets further affects the text generated by the model.
## About
*Built by Aleksey Korshuk*
[](https://github.com/AlekseyKorshuk)
[](https://twitter.com/intent/follow?screen_name=alekseykorshuk)
[](https://t.me/joinchat/_CQ04KjcJ-4yZTky)
For more details, visit the project repository.
[](https://github.com/AlekseyKorshuk/huggingartists)
|
huggingartists/mayot | c0ecdce0f18fa2e97ec8c0ff42f7968ca055e7c7 | 2021-09-29T17:40:26.000Z | [
"pytorch",
"jax",
"gpt2",
"text-generation",
"en",
"dataset:huggingartists/mayot",
"transformers",
"huggingartists",
"lyrics",
"lm-head",
"causal-lm"
]
| text-generation | false | huggingartists | null | huggingartists/mayot | 2 | null | transformers | 24,089 | ---
language: en
datasets:
- huggingartists/mayot
tags:
- huggingartists
- lyrics
- lm-head
- causal-lm
widget:
- text: "I am"
---
<div class="inline-flex flex-col" style="line-height: 1.5;">
<div class="flex">
<div
style="display:DISPLAY_1; margin-left: auto; margin-right: auto; width: 92px; height:92px; border-radius: 50%; background-size: cover; background-image: url('https://images.genius.com/1d4b4adcdf1f58e1899ee5557375ef7c.1000x1000x1.jpg')">
</div>
</div>
<div style="text-align: center; margin-top: 3px; font-size: 16px; font-weight: 800">🤖 HuggingArtists Model 🤖</div>
<div style="text-align: center; font-size: 16px; font-weight: 800">MAYOT</div>
<a href="https://genius.com/artists/mayot">
<div style="text-align: center; font-size: 14px;">@mayot</div>
</a>
</div>
I was made with [huggingartists](https://github.com/AlekseyKorshuk/huggingartists).
Create your own bot based on your favorite artist with [the demo](https://colab.research.google.com/github/AlekseyKorshuk/huggingartists/blob/master/huggingartists-demo.ipynb)!
## How does it work?
To understand how the model was developed, check the [W&B report](https://wandb.ai/huggingartists/huggingartists/reportlist).
## Training data
The model was trained on lyrics from MAYOT.
Dataset is available [here](https://huggingface.co/datasets/huggingartists/mayot).
And can be used with:
```python
from datasets import load_dataset
dataset = load_dataset("huggingartists/mayot")
```
[Explore the data](https://wandb.ai/huggingartists/huggingartists/runs/lf4wcx85/artifacts), which is tracked with [W&B artifacts](https://docs.wandb.com/artifacts) at every step of the pipeline.
## Training procedure
The model is based on a pre-trained [GPT-2](https://huggingface.co/gpt2) which is fine-tuned on MAYOT's lyrics.
Hyperparameters and metrics are recorded in the [W&B training run](https://wandb.ai/huggingartists/huggingartists/runs/1uulibm2) for full transparency and reproducibility.
At the end of training, [the final model](https://wandb.ai/huggingartists/huggingartists/runs/1uulibm2/artifacts) is logged and versioned.
## How to use
You can use this model directly with a pipeline for text generation:
```python
from transformers import pipeline
generator = pipeline('text-generation',
model='huggingartists/mayot')
generator("I am", num_return_sequences=5)
```
Or with Transformers library:
```python
from transformers import AutoTokenizer, AutoModelWithLMHead
tokenizer = AutoTokenizer.from_pretrained("huggingartists/mayot")
model = AutoModelWithLMHead.from_pretrained("huggingartists/mayot")
```
## Limitations and bias
The model suffers from [the same limitations and bias as GPT-2](https://huggingface.co/gpt2#limitations-and-bias).
In addition, the data present in the user's tweets further affects the text generated by the model.
## About
*Built by Aleksey Korshuk*
[](https://github.com/AlekseyKorshuk)
[](https://twitter.com/intent/follow?screen_name=alekseykorshuk)
[](https://t.me/joinchat/_CQ04KjcJ-4yZTky)
For more details, visit the project repository.
[](https://github.com/AlekseyKorshuk/huggingartists)
|
huggingartists/rex-orange-county | 8a1569494d4a7c8944fc83cc01c0b231e1301e77 | 2021-09-12T20:31:29.000Z | [
"pytorch",
"jax",
"gpt2",
"text-generation",
"en",
"dataset:huggingartists/rex-orange-county",
"transformers",
"huggingartists",
"lyrics",
"lm-head",
"causal-lm"
]
| text-generation | false | huggingartists | null | huggingartists/rex-orange-county | 2 | null | transformers | 24,090 | ---
language: en
datasets:
- huggingartists/rex-orange-county
tags:
- huggingartists
- lyrics
- lm-head
- causal-lm
widget:
- text: "I am"
---
<div class="inline-flex flex-col" style="line-height: 1.5;">
<div class="flex">
<div
style="display:DISPLAY_1; margin-left: auto; margin-right: auto; width: 92px; height:92px; border-radius: 50%; background-size: cover; background-image: url('https://images.genius.com/348ad82a8d34eaff777b6743ca0f2d70.400x400x1.jpg')">
</div>
</div>
<div style="text-align: center; margin-top: 3px; font-size: 16px; font-weight: 800">🤖 HuggingArtists Model 🤖</div>
<div style="text-align: center; font-size: 16px; font-weight: 800">Rex Orange County</div>
<a href="https://genius.com/artists/rex-orange-county">
<div style="text-align: center; font-size: 14px;">@rex-orange-county</div>
</a>
</div>
I was made with [huggingartists](https://github.com/AlekseyKorshuk/huggingartists).
Create your own bot based on your favorite artist with [the demo](https://colab.research.google.com/github/AlekseyKorshuk/huggingartists/blob/master/huggingartists-demo.ipynb)!
## How does it work?
To understand how the model was developed, check the [W&B report](https://wandb.ai/huggingartists/huggingartists/reportlist).
## Training data
The model was trained on lyrics from Rex Orange County.
Dataset is available [here](https://huggingface.co/datasets/huggingartists/rex-orange-county).
And can be used with:
```python
from datasets import load_dataset
dataset = load_dataset("huggingartists/rex-orange-county")
```
[Explore the data](https://wandb.ai/huggingartists/huggingartists/runs/3by3xc64/artifacts), which is tracked with [W&B artifacts](https://docs.wandb.com/artifacts) at every step of the pipeline.
## Training procedure
The model is based on a pre-trained [GPT-2](https://huggingface.co/gpt2) which is fine-tuned on Rex Orange County's lyrics.
Hyperparameters and metrics are recorded in the [W&B training run](https://wandb.ai/huggingartists/huggingartists/runs/1bwctmad) for full transparency and reproducibility.
At the end of training, [the final model](https://wandb.ai/huggingartists/huggingartists/runs/1bwctmad/artifacts) is logged and versioned.
## How to use
You can use this model directly with a pipeline for text generation:
```python
from transformers import pipeline
generator = pipeline('text-generation',
model='huggingartists/rex-orange-county')
generator("I am", num_return_sequences=5)
```
Or with Transformers library:
```python
from transformers import AutoTokenizer, AutoModelWithLMHead
tokenizer = AutoTokenizer.from_pretrained("huggingartists/rex-orange-county")
model = AutoModelWithLMHead.from_pretrained("huggingartists/rex-orange-county")
```
## Limitations and bias
The model suffers from [the same limitations and bias as GPT-2](https://huggingface.co/gpt2#limitations-and-bias).
In addition, the data present in the user's tweets further affects the text generated by the model.
## About
*Built by Aleksey Korshuk*
[](https://github.com/AlekseyKorshuk)
[](https://twitter.com/intent/follow?screen_name=alekseykorshuk)
[](https://t.me/joinchat/_CQ04KjcJ-4yZTky)
For more details, visit the project repository.
[](https://github.com/AlekseyKorshuk/huggingartists)
|
huggingartists/shadowraze | 29b8eef4d680bcc471852e73b21acd64020f2488 | 2021-10-15T02:02:54.000Z | [
"pytorch",
"jax",
"gpt2",
"text-generation",
"en",
"dataset:huggingartists/shadowraze",
"transformers",
"huggingartists",
"lyrics",
"lm-head",
"causal-lm"
]
| text-generation | false | huggingartists | null | huggingartists/shadowraze | 2 | null | transformers | 24,091 | ---
language: en
datasets:
- huggingartists/shadowraze
tags:
- huggingartists
- lyrics
- lm-head
- causal-lm
widget:
- text: "I am"
---
<div class="inline-flex flex-col" style="line-height: 1.5;">
<div class="flex">
<div
style="display:DISPLAY_1; margin-left: auto; margin-right: auto; width: 92px; height:92px; border-radius: 50%; background-size: cover; background-image: url('https://images.genius.com/e2576b95c2049862de20cbd0f1a4e0d7.1000x1000x1.jpg')">
</div>
</div>
<div style="text-align: center; margin-top: 3px; font-size: 16px; font-weight: 800">🤖 HuggingArtists Model 🤖</div>
<div style="text-align: center; font-size: 16px; font-weight: 800">shadowraze</div>
<a href="https://genius.com/artists/shadowraze">
<div style="text-align: center; font-size: 14px;">@shadowraze</div>
</a>
</div>
I was made with [huggingartists](https://github.com/AlekseyKorshuk/huggingartists).
Create your own bot based on your favorite artist with [the demo](https://colab.research.google.com/github/AlekseyKorshuk/huggingartists/blob/master/huggingartists-demo.ipynb)!
## How does it work?
To understand how the model was developed, check the [W&B report](https://wandb.ai/huggingartists/huggingartists/reportlist).
## Training data
The model was trained on lyrics from shadowraze.
Dataset is available [here](https://huggingface.co/datasets/huggingartists/shadowraze).
And can be used with:
```python
from datasets import load_dataset
dataset = load_dataset("huggingartists/shadowraze")
```
[Explore the data](https://wandb.ai/huggingartists/huggingartists/runs/pkbkflsq/artifacts), which is tracked with [W&B artifacts](https://docs.wandb.com/artifacts) at every step of the pipeline.
## Training procedure
The model is based on a pre-trained [GPT-2](https://huggingface.co/gpt2) which is fine-tuned on shadowraze's lyrics.
Hyperparameters and metrics are recorded in the [W&B training run](https://wandb.ai/huggingartists/huggingartists/runs/tiu2mjo1) for full transparency and reproducibility.
At the end of training, [the final model](https://wandb.ai/huggingartists/huggingartists/runs/tiu2mjo1/artifacts) is logged and versioned.
## How to use
You can use this model directly with a pipeline for text generation:
```python
from transformers import pipeline
generator = pipeline('text-generation',
model='huggingartists/shadowraze')
generator("I am", num_return_sequences=5)
```
Or with Transformers library:
```python
from transformers import AutoTokenizer, AutoModelWithLMHead
tokenizer = AutoTokenizer.from_pretrained("huggingartists/shadowraze")
model = AutoModelWithLMHead.from_pretrained("huggingartists/shadowraze")
```
## Limitations and bias
The model suffers from [the same limitations and bias as GPT-2](https://huggingface.co/gpt2#limitations-and-bias).
In addition, the data present in the user's tweets further affects the text generated by the model.
## About
*Built by Aleksey Korshuk*
[](https://github.com/AlekseyKorshuk)
[](https://twitter.com/intent/follow?screen_name=alekseykorshuk)
[](https://t.me/joinchat/_CQ04KjcJ-4yZTky)
For more details, visit the project repository.
[](https://github.com/AlekseyKorshuk/huggingartists)
|
huggingartists/slava-marlow | 20c3f884844989fa4371d003c51d9eefd40f71ed | 2021-10-16T10:37:58.000Z | [
"pytorch",
"jax",
"gpt2",
"text-generation",
"en",
"dataset:huggingartists/slava-marlow",
"transformers",
"huggingartists",
"lyrics",
"lm-head",
"causal-lm"
]
| text-generation | false | huggingartists | null | huggingartists/slava-marlow | 2 | null | transformers | 24,092 | ---
language: en
datasets:
- huggingartists/slava-marlow
tags:
- huggingartists
- lyrics
- lm-head
- causal-lm
widget:
- text: "I am"
---
<div class="inline-flex flex-col" style="line-height: 1.5;">
<div class="flex">
<div
style="display:DISPLAY_1; margin-left: auto; margin-right: auto; width: 92px; height:92px; border-radius: 50%; background-size: cover; background-image: url('https://images.genius.com/e308b1bc9eeb159ecfa9d807d715f095.1000x1000x1.jpg')">
</div>
</div>
<div style="text-align: center; margin-top: 3px; font-size: 16px; font-weight: 800">🤖 HuggingArtists Model 🤖</div>
<div style="text-align: center; font-size: 16px; font-weight: 800">SLAVA MARLOW</div>
<a href="https://genius.com/artists/slava-marlow">
<div style="text-align: center; font-size: 14px;">@slava-marlow</div>
</a>
</div>
I was made with [huggingartists](https://github.com/AlekseyKorshuk/huggingartists).
Create your own bot based on your favorite artist with [the demo](https://colab.research.google.com/github/AlekseyKorshuk/huggingartists/blob/master/huggingartists-demo.ipynb)!
## How does it work?
To understand how the model was developed, check the [W&B report](https://wandb.ai/huggingartists/huggingartists/reportlist).
## Training data
The model was trained on lyrics from SLAVA MARLOW.
Dataset is available [here](https://huggingface.co/datasets/huggingartists/slava-marlow).
And can be used with:
```python
from datasets import load_dataset
dataset = load_dataset("huggingartists/slava-marlow")
```
[Explore the data](https://wandb.ai/huggingartists/huggingartists/runs/1fdcz1s5/artifacts), which is tracked with [W&B artifacts](https://docs.wandb.com/artifacts) at every step of the pipeline.
## Training procedure
The model is based on a pre-trained [GPT-2](https://huggingface.co/gpt2) which is fine-tuned on SLAVA MARLOW's lyrics.
Hyperparameters and metrics are recorded in the [W&B training run](https://wandb.ai/huggingartists/huggingartists/runs/ro4q353s) for full transparency and reproducibility.
At the end of training, [the final model](https://wandb.ai/huggingartists/huggingartists/runs/ro4q353s/artifacts) is logged and versioned.
## How to use
You can use this model directly with a pipeline for text generation:
```python
from transformers import pipeline
generator = pipeline('text-generation',
model='huggingartists/slava-marlow')
generator("I am", num_return_sequences=5)
```
Or with Transformers library:
```python
from transformers import AutoTokenizer, AutoModelWithLMHead
tokenizer = AutoTokenizer.from_pretrained("huggingartists/slava-marlow")
model = AutoModelWithLMHead.from_pretrained("huggingartists/slava-marlow")
```
## Limitations and bias
The model suffers from [the same limitations and bias as GPT-2](https://huggingface.co/gpt2#limitations-and-bias).
In addition, the data present in the user's tweets further affects the text generated by the model.
## About
*Built by Aleksey Korshuk*
[](https://github.com/AlekseyKorshuk)
[](https://twitter.com/intent/follow?screen_name=alekseykorshuk)
[](https://t.me/joinchat/_CQ04KjcJ-4yZTky)
For more details, visit the project repository.
[](https://github.com/AlekseyKorshuk/huggingartists)
|
huggingartists/system-of-a-down | 1d377cd8aa06534364f99932e353a5a049fcaa67 | 2021-09-12T12:08:09.000Z | [
"pytorch",
"jax",
"gpt2",
"text-generation",
"en",
"dataset:huggingartists/system-of-a-down",
"transformers",
"huggingartists",
"lyrics",
"lm-head",
"causal-lm"
]
| text-generation | false | huggingartists | null | huggingartists/system-of-a-down | 2 | null | transformers | 24,093 | ---
language: en
datasets:
- huggingartists/system-of-a-down
tags:
- huggingartists
- lyrics
- lm-head
- causal-lm
widget:
- text: "I am"
---
<div class="inline-flex flex-col" style="line-height: 1.5;">
<div class="flex">
<div
style="display:DISPLAY_1; margin-left: auto; margin-right: auto; width: 92px; height:92px; border-radius: 50%; background-size: cover; background-image: url('https://images.genius.com/5688d59e74bfc07b0531636114f56c1e.1000x1000x1.jpg')">
</div>
</div>
<div style="text-align: center; margin-top: 3px; font-size: 16px; font-weight: 800">🤖 HuggingArtists Model 🤖</div>
<div style="text-align: center; font-size: 16px; font-weight: 800">System of a Down</div>
<a href="https://genius.com/artists/system-of-a-down">
<div style="text-align: center; font-size: 14px;">@system-of-a-down</div>
</a>
</div>
I was made with [huggingartists](https://github.com/AlekseyKorshuk/huggingartists).
Create your own bot based on your favorite artist with [the demo](https://colab.research.google.com/github/AlekseyKorshuk/huggingartists/blob/master/huggingartists-demo.ipynb)!
## How does it work?
To understand how the model was developed, check the [W&B report](https://wandb.ai/huggingartists/huggingartists/reportlist).
## Training data
The model was trained on lyrics from System of a Down.
Dataset is available [here](https://huggingface.co/datasets/huggingartists/system-of-a-down).
And can be used with:
```python
from datasets import load_dataset
dataset = load_dataset("huggingartists/system-of-a-down")
```
[Explore the data](https://wandb.ai/huggingartists/huggingartists/runs/3m1sikv8/artifacts), which is tracked with [W&B artifacts](https://docs.wandb.com/artifacts) at every step of the pipeline.
## Training procedure
The model is based on a pre-trained [GPT-2](https://huggingface.co/gpt2) which is fine-tuned on System of a Down's lyrics.
Hyperparameters and metrics are recorded in the [W&B training run](https://wandb.ai/huggingartists/huggingartists/runs/wf3qe4yi) for full transparency and reproducibility.
At the end of training, [the final model](https://wandb.ai/huggingartists/huggingartists/runs/wf3qe4yi/artifacts) is logged and versioned.
## How to use
You can use this model directly with a pipeline for text generation:
```python
from transformers import pipeline
generator = pipeline('text-generation',
model='huggingartists/system-of-a-down')
generator("I am", num_return_sequences=5)
```
Or with Transformers library:
```python
from transformers import AutoTokenizer, AutoModelWithLMHead
tokenizer = AutoTokenizer.from_pretrained("huggingartists/system-of-a-down")
model = AutoModelWithLMHead.from_pretrained("huggingartists/system-of-a-down")
```
## Limitations and bias
The model suffers from [the same limitations and bias as GPT-2](https://huggingface.co/gpt2#limitations-and-bias).
In addition, the data present in the user's tweets further affects the text generated by the model.
## About
*Built by Aleksey Korshuk*
[](https://github.com/AlekseyKorshuk)
[](https://twitter.com/intent/follow?screen_name=alekseykorshuk)
[](https://t.me/joinchat/_CQ04KjcJ-4yZTky)
For more details, visit the project repository.
[](https://github.com/AlekseyKorshuk/huggingartists)
|
huggingartists/the-notorious-big | 999a3ba5104445275f065a938bdbb22d5876c619 | 2021-10-08T17:26:01.000Z | [
"pytorch",
"jax",
"gpt2",
"text-generation",
"en",
"dataset:huggingartists/the-notorious-big",
"transformers",
"huggingartists",
"lyrics",
"lm-head",
"causal-lm"
]
| text-generation | false | huggingartists | null | huggingartists/the-notorious-big | 2 | null | transformers | 24,094 | ---
language: en
datasets:
- huggingartists/the-notorious-big
tags:
- huggingartists
- lyrics
- lm-head
- causal-lm
widget:
- text: "I am"
---
<div class="inline-flex flex-col" style="line-height: 1.5;">
<div class="flex">
<div
style="display:DISPLAY_1; margin-left: auto; margin-right: auto; width: 92px; height:92px; border-radius: 50%; background-size: cover; background-image: url('https://images.genius.com/664976b54a605d6ac0df2415a8ccac16.564x564x1.jpg')">
</div>
</div>
<div style="text-align: center; margin-top: 3px; font-size: 16px; font-weight: 800">🤖 HuggingArtists Model 🤖</div>
<div style="text-align: center; font-size: 16px; font-weight: 800">The Notorious B.I.G.</div>
<a href="https://genius.com/artists/the-notorious-big">
<div style="text-align: center; font-size: 14px;">@the-notorious-big</div>
</a>
</div>
I was made with [huggingartists](https://github.com/AlekseyKorshuk/huggingartists).
Create your own bot based on your favorite artist with [the demo](https://colab.research.google.com/github/AlekseyKorshuk/huggingartists/blob/master/huggingartists-demo.ipynb)!
## How does it work?
To understand how the model was developed, check the [W&B report](https://wandb.ai/huggingartists/huggingartists/reportlist).
## Training data
The model was trained on lyrics from The Notorious B.I.G..
Dataset is available [here](https://huggingface.co/datasets/huggingartists/the-notorious-big).
And can be used with:
```python
from datasets import load_dataset
dataset = load_dataset("huggingartists/the-notorious-big")
```
[Explore the data](https://wandb.ai/huggingartists/huggingartists/runs/wkvasju4/artifacts), which is tracked with [W&B artifacts](https://docs.wandb.com/artifacts) at every step of the pipeline.
## Training procedure
The model is based on a pre-trained [GPT-2](https://huggingface.co/gpt2) which is fine-tuned on The Notorious B.I.G.'s lyrics.
Hyperparameters and metrics are recorded in the [W&B training run](https://wandb.ai/huggingartists/huggingartists/runs/1coezuy2) for full transparency and reproducibility.
At the end of training, [the final model](https://wandb.ai/huggingartists/huggingartists/runs/1coezuy2/artifacts) is logged and versioned.
## How to use
You can use this model directly with a pipeline for text generation:
```python
from transformers import pipeline
generator = pipeline('text-generation',
model='huggingartists/the-notorious-big')
generator("I am", num_return_sequences=5)
```
Or with Transformers library:
```python
from transformers import AutoTokenizer, AutoModelWithLMHead
tokenizer = AutoTokenizer.from_pretrained("huggingartists/the-notorious-big")
model = AutoModelWithLMHead.from_pretrained("huggingartists/the-notorious-big")
```
## Limitations and bias
The model suffers from [the same limitations and bias as GPT-2](https://huggingface.co/gpt2#limitations-and-bias).
In addition, the data present in the user's tweets further affects the text generated by the model.
## About
*Built by Aleksey Korshuk*
[](https://github.com/AlekseyKorshuk)
[](https://twitter.com/intent/follow?screen_name=alekseykorshuk)
[](https://t.me/joinchat/_CQ04KjcJ-4yZTky)
For more details, visit the project repository.
[](https://github.com/AlekseyKorshuk/huggingartists)
|
huggingartists/the-the-pigs | 97e49981e119e69bbe5616a3a0086252f578384e | 2021-09-11T06:35:36.000Z | [
"pytorch",
"jax",
"gpt2",
"text-generation",
"en",
"dataset:huggingartists/the-the-pigs",
"transformers",
"huggingartists",
"lyrics",
"lm-head",
"causal-lm"
]
| text-generation | false | huggingartists | null | huggingartists/the-the-pigs | 2 | null | transformers | 24,095 | ---
language: en
datasets:
- huggingartists/the-the-pigs
tags:
- huggingartists
- lyrics
- lm-head
- causal-lm
widget:
- text: "I am"
---
<div class="inline-flex flex-col" style="line-height: 1.5;">
<div class="flex">
<div
style="display:DISPLAY_1; margin-left: auto; margin-right: auto; width: 92px; height:92px; border-radius: 50%; background-size: cover; background-image: url('https://images.genius.com/2f1fd1b951237ad3387096f392d41fa5.720x720x1.jpg')">
</div>
</div>
<div style="text-align: center; margin-top: 3px; font-size: 16px; font-weight: 800">🤖 HuggingArtists Model 🤖</div>
<div style="text-align: center; font-size: 16px; font-weight: 800">The ‘’Вепри’’ (The Pigs)</div>
<a href="https://genius.com/artists/the-the-pigs">
<div style="text-align: center; font-size: 14px;">@the-the-pigs</div>
</a>
</div>
I was made with [huggingartists](https://github.com/AlekseyKorshuk/huggingartists).
Create your own bot based on your favorite artist with [the demo](https://colab.research.google.com/github/AlekseyKorshuk/huggingartists/blob/master/huggingartists-demo.ipynb)!
## How does it work?
To understand how the model was developed, check the [W&B report](https://wandb.ai/huggingartists/huggingartists/reportlist).
## Training data
The model was trained on lyrics from The ‘’Вепри’’ (The Pigs).
Dataset is available [here](https://huggingface.co/datasets/huggingartists/the-the-pigs).
And can be used with:
```python
from datasets import load_dataset
dataset = load_dataset("huggingartists/the-the-pigs")
```
[Explore the data](https://wandb.ai/huggingartists/huggingartists/runs/7yh65db9/artifacts), which is tracked with [W&B artifacts](https://docs.wandb.com/artifacts) at every step of the pipeline.
## Training procedure
The model is based on a pre-trained [GPT-2](https://huggingface.co/gpt2) which is fine-tuned on The ‘’Вепри’’ (The Pigs)'s lyrics.
Hyperparameters and metrics are recorded in the [W&B training run](https://wandb.ai/huggingartists/huggingartists/runs/65gj1lk1) for full transparency and reproducibility.
At the end of training, [the final model](https://wandb.ai/huggingartists/huggingartists/runs/65gj1lk1/artifacts) is logged and versioned.
## How to use
You can use this model directly with a pipeline for text generation:
```python
from transformers import pipeline
generator = pipeline('text-generation',
model='huggingartists/the-the-pigs')
generator("I am", num_return_sequences=5)
```
Or with Transformers library:
```python
from transformers import AutoTokenizer, AutoModelWithLMHead
tokenizer = AutoTokenizer.from_pretrained("huggingartists/the-the-pigs")
model = AutoModelWithLMHead.from_pretrained("huggingartists/the-the-pigs")
```
## Limitations and bias
The model suffers from [the same limitations and bias as GPT-2](https://huggingface.co/gpt2#limitations-and-bias).
In addition, the data present in the user's tweets further affects the text generated by the model.
## About
*Built by Aleksey Korshuk*
[](https://github.com/AlekseyKorshuk)
[](https://twitter.com/intent/follow?screen_name=alekseykorshuk)
[](https://t.me/joinchat/_CQ04KjcJ-4yZTky)
For more details, visit the project repository.
[](https://github.com/AlekseyKorshuk/huggingartists)
|
huggingartists/till-lindemann | 7a017ad13ad0356c6106df796df8d44cfac0a5f3 | 2021-09-15T11:46:53.000Z | [
"pytorch",
"jax",
"gpt2",
"text-generation",
"en",
"dataset:huggingartists/till-lindemann",
"transformers",
"huggingartists",
"lyrics",
"lm-head",
"causal-lm"
]
| text-generation | false | huggingartists | null | huggingartists/till-lindemann | 2 | null | transformers | 24,096 | ---
language: en
datasets:
- huggingartists/till-lindemann
tags:
- huggingartists
- lyrics
- lm-head
- causal-lm
widget:
- text: "I am"
---
<div class="inline-flex flex-col" style="line-height: 1.5;">
<div class="flex">
<div
style="display:DISPLAY_1; margin-left: auto; margin-right: auto; width: 92px; height:92px; border-radius: 50%; background-size: cover; background-image: url('https://images.genius.com/48d6ca7ca17a9dfc9ad3034e71533a89.1000x1000x1.jpg')">
</div>
</div>
<div style="text-align: center; margin-top: 3px; font-size: 16px; font-weight: 800">🤖 HuggingArtists Model 🤖</div>
<div style="text-align: center; font-size: 16px; font-weight: 800">Till Lindemann</div>
<a href="https://genius.com/artists/till-lindemann">
<div style="text-align: center; font-size: 14px;">@till-lindemann</div>
</a>
</div>
I was made with [huggingartists](https://github.com/AlekseyKorshuk/huggingartists).
Create your own bot based on your favorite artist with [the demo](https://colab.research.google.com/github/AlekseyKorshuk/huggingartists/blob/master/huggingartists-demo.ipynb)!
## How does it work?
To understand how the model was developed, check the [W&B report](https://wandb.ai/huggingartists/huggingartists/reportlist).
## Training data
The model was trained on lyrics from Till Lindemann.
Dataset is available [here](https://huggingface.co/datasets/huggingartists/till-lindemann).
And can be used with:
```python
from datasets import load_dataset
dataset = load_dataset("huggingartists/till-lindemann")
```
[Explore the data](https://wandb.ai/huggingartists/huggingartists/runs/2xh6fyqt/artifacts), which is tracked with [W&B artifacts](https://docs.wandb.com/artifacts) at every step of the pipeline.
## Training procedure
The model is based on a pre-trained [GPT-2](https://huggingface.co/gpt2) which is fine-tuned on Till Lindemann's lyrics.
Hyperparameters and metrics are recorded in the [W&B training run](https://wandb.ai/huggingartists/huggingartists/runs/32ohf092) for full transparency and reproducibility.
At the end of training, [the final model](https://wandb.ai/huggingartists/huggingartists/runs/32ohf092/artifacts) is logged and versioned.
## How to use
You can use this model directly with a pipeline for text generation:
```python
from transformers import pipeline
generator = pipeline('text-generation',
model='huggingartists/till-lindemann')
generator("I am", num_return_sequences=5)
```
Or with Transformers library:
```python
from transformers import AutoTokenizer, AutoModelWithLMHead
tokenizer = AutoTokenizer.from_pretrained("huggingartists/till-lindemann")
model = AutoModelWithLMHead.from_pretrained("huggingartists/till-lindemann")
```
## Limitations and bias
The model suffers from [the same limitations and bias as GPT-2](https://huggingface.co/gpt2#limitations-and-bias).
In addition, the data present in the user's tweets further affects the text generated by the model.
## About
*Built by Aleksey Korshuk*
[](https://github.com/AlekseyKorshuk)
[](https://twitter.com/intent/follow?screen_name=alekseykorshuk)
[](https://t.me/joinchat/_CQ04KjcJ-4yZTky)
For more details, visit the project repository.
[](https://github.com/AlekseyKorshuk/huggingartists)
|
huggingartists/twenty-one-pilots | 377bdcaecb61b592b2cf0d38705de1406b378727 | 2021-08-14T06:54:43.000Z | [
"pytorch",
"jax",
"gpt2",
"text-generation",
"en",
"dataset:huggingartists/twenty-one-pilots",
"transformers",
"huggingartists",
"lyrics",
"lm-head",
"causal-lm"
]
| text-generation | false | huggingartists | null | huggingartists/twenty-one-pilots | 2 | null | transformers | 24,097 | ---
language: en
datasets:
- huggingartists/twenty-one-pilots
tags:
- huggingartists
- lyrics
- lm-head
- causal-lm
widget:
- text: "I am"
---
<div class="inline-flex flex-col" style="line-height: 1.5;">
<div class="flex">
<div
style="display:DISPLAY_1; margin-left: auto; margin-right: auto; width: 92px; height:92px; border-radius: 50%; background-size: cover; background-image: url('https://images.genius.com/5ab9e38cf86aa170734fea1731610abc.1000x1000x1.jpg')">
</div>
</div>
<div style="text-align: center; margin-top: 3px; font-size: 16px; font-weight: 800">🤖 HuggingArtists Model 🤖</div>
<div style="text-align: center; font-size: 16px; font-weight: 800">twenty one pilots</div>
<a href="https://genius.com/artists/twenty-one-pilots">
<div style="text-align: center; font-size: 14px;">@twenty-one-pilots</div>
</a>
</div>
I was made with [huggingartists](https://github.com/AlekseyKorshuk/huggingartists).
Create your own bot based on your favorite artist with [the demo](https://colab.research.google.com/github/AlekseyKorshuk/huggingartists/blob/master/huggingartists-demo.ipynb)!
## How does it work?
To understand how the model was developed, check the [W&B report](https://wandb.ai/huggingartists/huggingartists/reportlist).
## Training data
The model was trained on lyrics from twenty one pilots.
Dataset is available [here](https://huggingface.co/datasets/huggingartists/twenty-one-pilots).
And can be used with:
```python
from datasets import load_dataset
dataset = load_dataset("huggingartists/twenty-one-pilots")
```
[Explore the data](https://wandb.ai/huggingartists/huggingartists/runs/2wr3j4nk/artifacts), which is tracked with [W&B artifacts](https://docs.wandb.com/artifacts) at every step of the pipeline.
## Training procedure
The model is based on a pre-trained [GPT-2](https://huggingface.co/gpt2) which is fine-tuned on twenty one pilots's lyrics.
Hyperparameters and metrics are recorded in the [W&B training run](https://wandb.ai/huggingartists/huggingartists/runs/3jhgvd5t) for full transparency and reproducibility.
At the end of training, [the final model](https://wandb.ai/huggingartists/huggingartists/runs/3jhgvd5t/artifacts) is logged and versioned.
## How to use
You can use this model directly with a pipeline for text generation:
```python
from transformers import pipeline
generator = pipeline('text-generation',
model='huggingartists/twenty-one-pilots')
generator("I am", num_return_sequences=5)
```
Or with Transformers library:
```python
from transformers import AutoTokenizer, AutoModelWithLMHead
tokenizer = AutoTokenizer.from_pretrained("huggingartists/twenty-one-pilots")
model = AutoModelWithLMHead.from_pretrained("huggingartists/twenty-one-pilots")
```
## Limitations and bias
The model suffers from [the same limitations and bias as GPT-2](https://huggingface.co/gpt2#limitations-and-bias).
In addition, the data present in the user's tweets further affects the text generated by the model.
## About
*Built by Aleksey Korshuk*
[](https://github.com/AlekseyKorshuk)
[](https://twitter.com/intent/follow?screen_name=alekseykorshuk)
[](https://t.me/joinchat/_CQ04KjcJ-4yZTky)
For more details, visit the project repository.
[](https://github.com/AlekseyKorshuk/huggingartists)
|
huggingtweets/aaroisosaari | 2b59c4db0980965ba374e00b2a1b59d497d85e46 | 2021-05-21T17:19:58.000Z | [
"pytorch",
"jax",
"gpt2",
"text-generation",
"en",
"transformers",
"huggingtweets"
]
| text-generation | false | huggingtweets | null | huggingtweets/aaroisosaari | 2 | null | transformers | 24,098 | ---
language: en
thumbnail: https://www.huggingtweets.com/aaroisosaari/1603799247165/predictions.png
tags:
- huggingtweets
widget:
- text: "My dream is"
---
<link rel="stylesheet" href="https://unpkg.com/@tailwindcss/[email protected]/dist/typography.min.css">
<style>
@media (prefers-color-scheme: dark) {
.prose { color: #E2E8F0 !important; }
.prose h2, .prose h3, .prose a, .prose thead { color: #F7FAFC !important; }
}
</style>
<section class='prose'>
<div>
<div style="width: 132px; height:132px; border-radius: 50%; background-size: cover; background-image: url('https://pbs.twimg.com/profile_images/1314155784771600384/07jkE1Oq_400x400.jpg')">
</div>
<div style="margin-top: 8px; font-size: 19px; font-weight: 800">Aaro Isosaari 🤖 AI Bot </div>
<div style="font-size: 15px; color: #657786">@aaroisosaari bot</div>
</div>
I was made with [huggingtweets](https://github.com/borisdayma/huggingtweets).
Create your own bot based on your favorite user with [the demo](https://colab.research.google.com/github/borisdayma/huggingtweets/blob/master/huggingtweets-demo.ipynb)!
## How does it work?
The model uses the following pipeline.
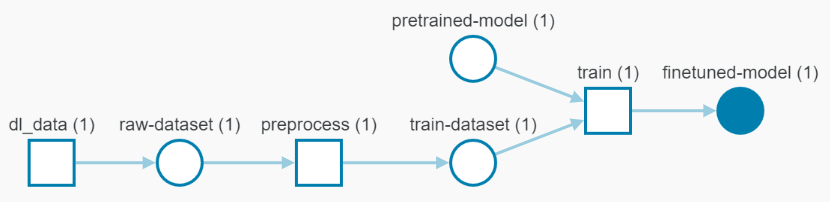
To understand how the model was developed, check the [W&B report](https://app.wandb.ai/wandb/huggingtweets/reports/HuggingTweets-Train-a-model-to-generate-tweets--VmlldzoxMTY5MjI).
## Training data
The model was trained on [@aaroisosaari's tweets](https://twitter.com/aaroisosaari).
<table style='border-width:0'>
<thead style='border-width:0'>
<tr style='border-width:0 0 1px 0; border-color: #CBD5E0'>
<th style='border-width:0'>Data</th>
<th style='border-width:0'>Quantity</th>
</tr>
</thead>
<tbody style='border-width:0'>
<tr style='border-width:0 0 1px 0; border-color: #E2E8F0'>
<td style='border-width:0'>Tweets downloaded</td>
<td style='border-width:0'>435</td>
</tr>
<tr style='border-width:0 0 1px 0; border-color: #E2E8F0'>
<td style='border-width:0'>Retweets</td>
<td style='border-width:0'>246</td>
</tr>
<tr style='border-width:0 0 1px 0; border-color: #E2E8F0'>
<td style='border-width:0'>Short tweets</td>
<td style='border-width:0'>28</td>
</tr>
<tr style='border-width:0'>
<td style='border-width:0'>Tweets kept</td>
<td style='border-width:0'>161</td>
</tr>
</tbody>
</table>
[Explore the data](https://app.wandb.ai/wandb/huggingtweets/runs/2j18yjsh/artifacts), which is tracked with [W&B artifacts](https://docs.wandb.com/artifacts) at every step of the pipeline.
## Training procedure
The model is based on a pre-trained [GPT-2](https://huggingface.co/gpt2) which is fine-tuned on @aaroisosaari's tweets.
Hyperparameters and metrics are recorded in the [W&B training run](https://app.wandb.ai/wandb/huggingtweets/runs/12mzkb8p) for full transparency and reproducibility.
At the end of training, [the final model](https://app.wandb.ai/wandb/huggingtweets/runs/12mzkb8p/artifacts) is logged and versioned.
## Intended uses & limitations
### How to use
You can use this model directly with a pipeline for text generation:
<pre><code><span style="color:#03A9F4">from</span> transformers <span style="color:#03A9F4">import</span> pipeline
generator = pipeline(<span style="color:#FF9800">'text-generation'</span>,
model=<span style="color:#FF9800">'huggingtweets/aaroisosaari'</span>)
generator(<span style="color:#FF9800">"My dream is"</span>, num_return_sequences=<span style="color:#8BC34A">5</span>)</code></pre>
### Limitations and bias
The model suffers from [the same limitations and bias as GPT-2](https://huggingface.co/gpt2#limitations-and-bias).
In addition, the data present in the user's tweets further affects the text generated by the model.
## About
*Built by Boris Dayma*
</section>
[](https://twitter.com/intent/follow?screen_name=borisdayma)
<section class='prose'>
For more details, visit the project repository.
</section>
[](https://github.com/borisdayma/huggingtweets)
<!--- random size file --> |
huggingtweets/adhitadselvaraj | a6a1c7840f1c0a04d6fd683e7336db43dfed1549 | 2021-05-21T17:39:36.000Z | [
"pytorch",
"jax",
"gpt2",
"text-generation",
"en",
"transformers",
"huggingtweets"
]
| text-generation | false | huggingtweets | null | huggingtweets/adhitadselvaraj | 2 | null | transformers | 24,099 | ---
language: en
thumbnail: https://github.com/borisdayma/huggingtweets/blob/master/img/logo_share.png?raw=true
tags:
- huggingtweets
widget:
- text: "My dream is"
---
<link rel="stylesheet" href="https://unpkg.com/@tailwindcss/[email protected]/dist/typography.min.css">
<style>
@media (prefers-color-scheme: dark) {
.prose { color: #E2E8F0 !important; }
.prose h2, .prose h3, .prose a, .prose thead { color: #F7FAFC !important; }
}
</style>
<section class='prose'>
<div>
<div style="width: 132px; height:132px; border-radius: 50%; background-size: cover; background-image: url('http://pbs.twimg.com/profile_images/1295249742801203206/F3Wl-EIy_400x400.jpg')">
</div>
<div style="margin-top: 8px; font-size: 19px; font-weight: 800">Adhita 😷 🤖 AI Bot </div>
<div style="font-size: 15px; color: #657786">@adhitadselvaraj bot</div>
</div>
I was made with [huggingtweets](https://github.com/borisdayma/huggingtweets).
Create your own bot based on your favorite user with [the demo](https://colab.research.google.com/github/borisdayma/huggingtweets/blob/master/huggingtweets-demo.ipynb)!
## How does it work?
The model uses the following pipeline.
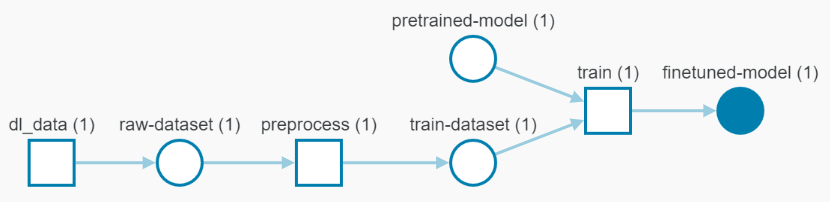
To understand how the model was developed, check the [W&B report](https://app.wandb.ai/wandb/huggingtweets/reports/HuggingTweets-Train-a-model-to-generate-tweets--VmlldzoxMTY5MjI).
## Training data
The model was trained on [@adhitadselvaraj's tweets](https://twitter.com/adhitadselvaraj).
<table style='border-width:0'>
<thead style='border-width:0'>
<tr style='border-width:0 0 1px 0; border-color: #CBD5E0'>
<th style='border-width:0'>Data</th>
<th style='border-width:0'>Quantity</th>
</tr>
</thead>
<tbody style='border-width:0'>
<tr style='border-width:0 0 1px 0; border-color: #E2E8F0'>
<td style='border-width:0'>Tweets downloaded</td>
<td style='border-width:0'>3209</td>
</tr>
<tr style='border-width:0 0 1px 0; border-color: #E2E8F0'>
<td style='border-width:0'>Retweets</td>
<td style='border-width:0'>649</td>
</tr>
<tr style='border-width:0 0 1px 0; border-color: #E2E8F0'>
<td style='border-width:0'>Short tweets</td>
<td style='border-width:0'>521</td>
</tr>
<tr style='border-width:0'>
<td style='border-width:0'>Tweets kept</td>
<td style='border-width:0'>2039</td>
</tr>
</tbody>
</table>
[Explore the data](https://app.wandb.ai/wandb/huggingtweets/runs/27pmox1m/artifacts), which is tracked with [W&B artifacts](https://docs.wandb.com/artifacts) at every step of the pipeline.
## Training procedure
The model is based on a pre-trained [GPT-2](https://huggingface.co/gpt2) which is fine-tuned on @adhitadselvaraj's tweets.
Hyperparameters and metrics are recorded in the [W&B training run](https://app.wandb.ai/wandb/huggingtweets/runs/3m21mvy6) for full transparency and reproducibility.
At the end of training, [the final model](https://app.wandb.ai/wandb/huggingtweets/runs/3m21mvy6/artifacts) is logged and versioned.
## Intended uses & limitations
### How to use
You can use this model directly with a pipeline for text generation:
<pre><code><span style="color:#03A9F4">from</span> transformers <span style="color:#03A9F4">import</span> pipeline
generator = pipeline(<span style="color:#FF9800">'text-generation'</span>,
model=<span style="color:#FF9800">'huggingtweets/adhitadselvaraj'</span>)
generator(<span style="color:#FF9800">"My dream is"</span>, num_return_sequences=<span style="color:#8BC34A">5</span>)</code></pre>
### Limitations and bias
The model suffers from [the same limitations and bias as GPT-2](https://huggingface.co/gpt2#limitations-and-bias).
In addition, the data present in the user's tweets further affects the text generated by the model.
## About
*Built by Boris Dayma*
</section>
[](https://twitter.com/borisdayma)
<section class='prose'>
For more details, visit the project repository.
</section>
[](https://github.com/borisdayma/huggingtweets)
|
Subsets and Splits
No community queries yet
The top public SQL queries from the community will appear here once available.