modelId
stringlengths 4
112
| sha
stringlengths 40
40
| lastModified
stringlengths 24
24
| tags
sequence | pipeline_tag
stringclasses 29
values | private
bool 1
class | author
stringlengths 2
38
⌀ | config
null | id
stringlengths 4
112
| downloads
float64 0
36.8M
⌀ | likes
float64 0
712
⌀ | library_name
stringclasses 17
values | __index_level_0__
int64 0
38.5k
| readme
stringlengths 0
186k
|
---|---|---|---|---|---|---|---|---|---|---|---|---|---|
huggingtweets/sovereign_beast | 01cb2fed8684fc832cc36a4058905db0fbe59c1e | 2021-05-22T23:34:50.000Z | [
"pytorch",
"jax",
"gpt2",
"text-generation",
"en",
"transformers",
"huggingtweets"
] | text-generation | false | huggingtweets | null | huggingtweets/sovereign_beast | 2 | null | transformers | 24,200 | ---
language: en
thumbnail: https://www.huggingtweets.com/sovereign_beast/1617890642358/predictions.png
tags:
- huggingtweets
widget:
- text: "My dream is"
---
<div>
<div style="width: 132px; height:132px; border-radius: 50%; background-size: cover; background-image: url('https://pbs.twimg.com/profile_images/1360371143618801665/kgYG2UQ3_400x400.jpg')">
</div>
<div style="margin-top: 8px; font-size: 19px; font-weight: 800">🏳️⚧️ Indecipherable Scrawlings 🏳️⚧️ 🤖 AI Bot </div>
<div style="font-size: 15px">@sovereign_beast bot</div>
</div>
I was made with [huggingtweets](https://github.com/borisdayma/huggingtweets).
Create your own bot based on your favorite user with [the demo](https://colab.research.google.com/github/borisdayma/huggingtweets/blob/master/huggingtweets-demo.ipynb)!
## How does it work?
The model uses the following pipeline.
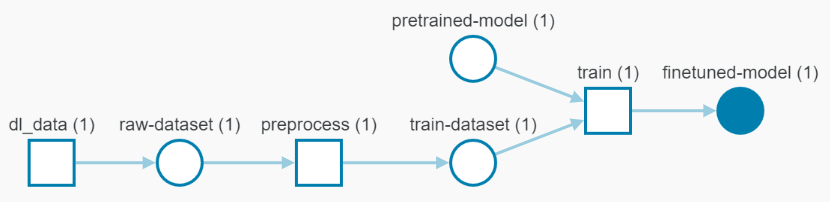
To understand how the model was developed, check the [W&B report](https://wandb.ai/wandb/huggingtweets/reports/HuggingTweets-Train-a-Model-to-Generate-Tweets--VmlldzoxMTY5MjI).
## Training data
The model was trained on [@sovereign_beast's tweets](https://twitter.com/sovereign_beast).
| Data | Quantity |
| --- | --- |
| Tweets downloaded | 3145 |
| Retweets | 1016 |
| Short tweets | 116 |
| Tweets kept | 2013 |
[Explore the data](https://wandb.ai/wandb/huggingtweets/runs/35o219p2/artifacts), which is tracked with [W&B artifacts](https://docs.wandb.com/artifacts) at every step of the pipeline.
## Training procedure
The model is based on a pre-trained [GPT-2](https://huggingface.co/gpt2) which is fine-tuned on @sovereign_beast's tweets.
Hyperparameters and metrics are recorded in the [W&B training run](https://wandb.ai/wandb/huggingtweets/runs/3mmo9uhd) for full transparency and reproducibility.
At the end of training, [the final model](https://wandb.ai/wandb/huggingtweets/runs/3mmo9uhd/artifacts) is logged and versioned.
## How to use
You can use this model directly with a pipeline for text generation:
```python
from transformers import pipeline
generator = pipeline('text-generation',
model='huggingtweets/sovereign_beast')
generator("My dream is", num_return_sequences=5)
```
## Limitations and bias
The model suffers from [the same limitations and bias as GPT-2](https://huggingface.co/gpt2#limitations-and-bias).
In addition, the data present in the user's tweets further affects the text generated by the model.
## About
*Built by Boris Dayma*
[](https://twitter.com/intent/follow?screen_name=borisdayma)
For more details, visit the project repository.
[](https://github.com/borisdayma/huggingtweets)
|
huggingtweets/speakerpelosi | 01e0b8ec261100e2c63b47e487737da650476451 | 2021-05-22T23:40:35.000Z | [
"pytorch",
"jax",
"gpt2",
"text-generation",
"en",
"transformers",
"huggingtweets"
] | text-generation | false | huggingtweets | null | huggingtweets/speakerpelosi | 2 | null | transformers | 24,201 | ---
language: en
thumbnail: https://github.com/borisdayma/huggingtweets/blob/master/img/logo.png?raw=true
tags:
- huggingtweets
widget:
- text: "My dream is"
---
<link rel="stylesheet" href="https://unpkg.com/@tailwindcss/[email protected]/dist/typography.min.css">
<style>
@media (prefers-color-scheme: dark) {
.prose { color: #E2E8F0 !important; }
.prose h2, .prose h3, .prose a, .prose thead { color: #F7FAFC !important; }
}
</style>
<section class='prose'>
<div>
<div style="width: 132px; height:132px; border-radius: 50%; background-size: cover; background-image: url('https://pbs.twimg.com/profile_images/1114294290375688193/P9mcJNGb_400x400.png')">
</div>
<div style="margin-top: 8px; font-size: 19px; font-weight: 800">Nancy Pelosi 🤖 AI Bot </div>
<div style="font-size: 15px; color: #657786">@speakerpelosi bot</div>
</div>
I was made with [huggingtweets](https://github.com/borisdayma/huggingtweets).
Create your own bot based on your favorite user with [the demo](https://colab.research.google.com/github/borisdayma/huggingtweets/blob/master/huggingtweets-demo.ipynb)!
## How does it work?
The model uses the following pipeline.
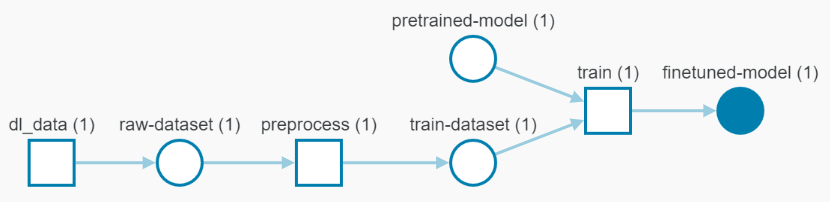
To understand how the model was developed, check the [W&B report](https://app.wandb.ai/wandb/huggingtweets/reports/HuggingTweets-Train-a-model-to-generate-tweets--VmlldzoxMTY5MjI).
## Training data
The model was trained on [@speakerpelosi's tweets](https://twitter.com/speakerpelosi).
<table style='border-width:0'>
<thead style='border-width:0'>
<tr style='border-width:0 0 1px 0; border-color: #CBD5E0'>
<th style='border-width:0'>Data</th>
<th style='border-width:0'>Quantity</th>
</tr>
</thead>
<tbody style='border-width:0'>
<tr style='border-width:0 0 1px 0; border-color: #E2E8F0'>
<td style='border-width:0'>Tweets downloaded</td>
<td style='border-width:0'>3221</td>
</tr>
<tr style='border-width:0 0 1px 0; border-color: #E2E8F0'>
<td style='border-width:0'>Retweets</td>
<td style='border-width:0'>601</td>
</tr>
<tr style='border-width:0 0 1px 0; border-color: #E2E8F0'>
<td style='border-width:0'>Short tweets</td>
<td style='border-width:0'>4</td>
</tr>
<tr style='border-width:0'>
<td style='border-width:0'>Tweets kept</td>
<td style='border-width:0'>2616</td>
</tr>
</tbody>
</table>
[Explore the data](https://wandb.ai/wandb/huggingtweets/runs/1lhx8q9a/artifacts), which is tracked with [W&B artifacts](https://docs.wandb.com/artifacts) at every step of the pipeline.
## Training procedure
The model is based on a pre-trained [GPT-2](https://huggingface.co/gpt2) which is fine-tuned on @speakerpelosi's tweets.
Hyperparameters and metrics are recorded in the [W&B training run](https://wandb.ai/wandb/huggingtweets/runs/3alajmxr) for full transparency and reproducibility.
At the end of training, [the final model](https://wandb.ai/wandb/huggingtweets/runs/3alajmxr/artifacts) is logged and versioned.
## Intended uses & limitations
### How to use
You can use this model directly with a pipeline for text generation:
<pre><code><span style="color:#03A9F4">from</span> transformers <span style="color:#03A9F4">import</span> pipeline
generator = pipeline(<span style="color:#FF9800">'text-generation'</span>,
model=<span style="color:#FF9800">'huggingtweets/speakerpelosi'</span>)
generator(<span style="color:#FF9800">"My dream is"</span>, num_return_sequences=<span style="color:#8BC34A">5</span>)</code></pre>
### Limitations and bias
The model suffers from [the same limitations and bias as GPT-2](https://huggingface.co/gpt2#limitations-and-bias).
In addition, the data present in the user's tweets further affects the text generated by the model.
## About
*Built by Boris Dayma*
</section>
[](https://twitter.com/intent/follow?screen_name=borisdayma)
<section class='prose'>
For more details, visit the project repository.
</section>
[](https://github.com/borisdayma/huggingtweets)
<!--- random size file --> |
huggingtweets/steashaz | 4a0680f0f96170ee2c1019ad483ccbadce12e037 | 2021-05-22T23:59:19.000Z | [
"pytorch",
"jax",
"gpt2",
"text-generation",
"en",
"transformers",
"huggingtweets"
] | text-generation | false | huggingtweets | null | huggingtweets/steashaz | 2 | null | transformers | 24,202 | ---
language: en
thumbnail: https://www.huggingtweets.com/steashaz/1603197572121/predictions.png
tags:
- huggingtweets
widget:
- text: "My dream is"
---
<link rel="stylesheet" href="https://unpkg.com/@tailwindcss/[email protected]/dist/typography.min.css">
<style>
@media (prefers-color-scheme: dark) {
.prose { color: #E2E8F0 !important; }
.prose h2, .prose h3, .prose a, .prose thead { color: #F7FAFC !important; }
}
</style>
<section class='prose'>
<div>
<div style="width: 132px; height:132px; border-radius: 50%; background-size: cover; background-image: url('https://pbs.twimg.com/profile_images/1303742736726622210/fvdUN0Im_400x400.jpg')">
</div>
<div style="margin-top: 8px; font-size: 19px; font-weight: 800">Stéphanie Laporte 🤖 AI Bot </div>
<div style="font-size: 15px; color: #657786">@steashaz bot</div>
</div>
I was made with [huggingtweets](https://github.com/borisdayma/huggingtweets).
Create your own bot based on your favorite user with [the demo](https://colab.research.google.com/github/borisdayma/huggingtweets/blob/master/huggingtweets-demo.ipynb)!
## How does it work?
The model uses the following pipeline.
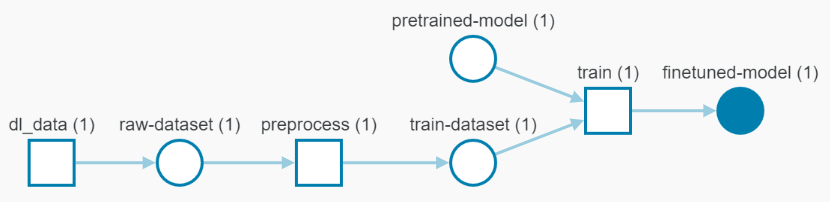
To understand how the model was developed, check the [W&B report](https://app.wandb.ai/wandb/huggingtweets/reports/HuggingTweets-Train-a-model-to-generate-tweets--VmlldzoxMTY5MjI).
## Training data
The model was trained on [@steashaz's tweets](https://twitter.com/steashaz).
<table style='border-width:0'>
<thead style='border-width:0'>
<tr style='border-width:0 0 1px 0; border-color: #CBD5E0'>
<th style='border-width:0'>Data</th>
<th style='border-width:0'>Quantity</th>
</tr>
</thead>
<tbody style='border-width:0'>
<tr style='border-width:0 0 1px 0; border-color: #E2E8F0'>
<td style='border-width:0'>Tweets downloaded</td>
<td style='border-width:0'>3224</td>
</tr>
<tr style='border-width:0 0 1px 0; border-color: #E2E8F0'>
<td style='border-width:0'>Retweets</td>
<td style='border-width:0'>1202</td>
</tr>
<tr style='border-width:0 0 1px 0; border-color: #E2E8F0'>
<td style='border-width:0'>Short tweets</td>
<td style='border-width:0'>543</td>
</tr>
<tr style='border-width:0'>
<td style='border-width:0'>Tweets kept</td>
<td style='border-width:0'>1479</td>
</tr>
</tbody>
</table>
[Explore the data](https://app.wandb.ai/wandb/huggingtweets/runs/s7mezf77/artifacts), which is tracked with [W&B artifacts](https://docs.wandb.com/artifacts) at every step of the pipeline.
## Training procedure
The model is based on a pre-trained [GPT-2](https://huggingface.co/gpt2) which is fine-tuned on @steashaz's tweets.
Hyperparameters and metrics are recorded in the [W&B training run](https://app.wandb.ai/wandb/huggingtweets/runs/1g8rzv0o) for full transparency and reproducibility.
At the end of training, [the final model](https://app.wandb.ai/wandb/huggingtweets/runs/1g8rzv0o/artifacts) is logged and versioned.
## Intended uses & limitations
### How to use
You can use this model directly with a pipeline for text generation:
<pre><code><span style="color:#03A9F4">from</span> transformers <span style="color:#03A9F4">import</span> pipeline
generator = pipeline(<span style="color:#FF9800">'text-generation'</span>,
model=<span style="color:#FF9800">'huggingtweets/steashaz'</span>)
generator(<span style="color:#FF9800">"My dream is"</span>, num_return_sequences=<span style="color:#8BC34A">5</span>)</code></pre>
### Limitations and bias
The model suffers from [the same limitations and bias as GPT-2](https://huggingface.co/gpt2#limitations-and-bias).
In addition, the data present in the user's tweets further affects the text generated by the model.
## About
*Built by Boris Dayma*
</section>
[](https://twitter.com/intent/follow?screen_name=borisdayma)
<section class='prose'>
For more details, visit the project repository.
</section>
[](https://github.com/borisdayma/huggingtweets)
<!--- random size file --> |
huggingtweets/stephenmhouston | 44f99f1dcf347fcec1fc44198813a7d1a3d0ab03 | 2021-05-23T00:04:56.000Z | [
"pytorch",
"jax",
"gpt2",
"text-generation",
"en",
"transformers",
"huggingtweets"
] | text-generation | false | huggingtweets | null | huggingtweets/stephenmhouston | 2 | null | transformers | 24,203 | ---
language: en
thumbnail: https://www.huggingtweets.com/stephenmhouston/1602273674776/predictions.png
tags:
- huggingtweets
widget:
- text: "My dream is"
---
<link rel="stylesheet" href="https://unpkg.com/@tailwindcss/[email protected]/dist/typography.min.css">
<style>
@media (prefers-color-scheme: dark) {
.prose { color: #E2E8F0 !important; }
.prose h2, .prose h3, .prose a, .prose thead { color: #F7FAFC !important; }
}
</style>
<section class='prose'>
<div>
<div style="width: 132px; height:132px; border-radius: 50%; background-size: cover; background-image: url('https://pbs.twimg.com/profile_images/911998357354168325/xnF4ZYT1_400x400.jpg')">
</div>
<div style="margin-top: 8px; font-size: 19px; font-weight: 800">Stephen Houston 🤖 AI Bot </div>
<div style="font-size: 15px; color: #657786">@stephenmhouston bot</div>
</div>
I was made with [huggingtweets](https://github.com/borisdayma/huggingtweets).
Create your own bot based on your favorite user with [the demo](https://colab.research.google.com/github/borisdayma/huggingtweets/blob/master/huggingtweets-demo.ipynb)!
## How does it work?
The model uses the following pipeline.
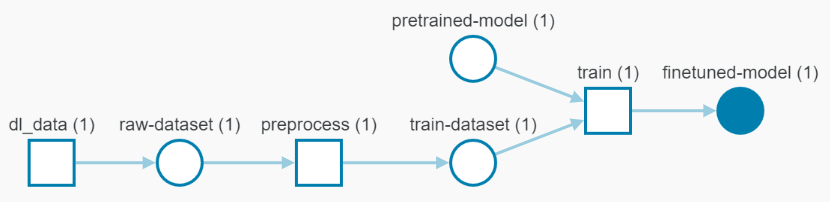
To understand how the model was developed, check the [W&B report](https://app.wandb.ai/wandb/huggingtweets/reports/HuggingTweets-Train-a-model-to-generate-tweets--VmlldzoxMTY5MjI).
## Training data
The model was trained on [@stephenmhouston's tweets](https://twitter.com/stephenmhouston).
<table style='border-width:0'>
<thead style='border-width:0'>
<tr style='border-width:0 0 1px 0; border-color: #CBD5E0'>
<th style='border-width:0'>Data</th>
<th style='border-width:0'>Quantity</th>
</tr>
</thead>
<tbody style='border-width:0'>
<tr style='border-width:0 0 1px 0; border-color: #E2E8F0'>
<td style='border-width:0'>Tweets downloaded</td>
<td style='border-width:0'>342</td>
</tr>
<tr style='border-width:0 0 1px 0; border-color: #E2E8F0'>
<td style='border-width:0'>Retweets</td>
<td style='border-width:0'>150</td>
</tr>
<tr style='border-width:0 0 1px 0; border-color: #E2E8F0'>
<td style='border-width:0'>Short tweets</td>
<td style='border-width:0'>34</td>
</tr>
<tr style='border-width:0'>
<td style='border-width:0'>Tweets kept</td>
<td style='border-width:0'>158</td>
</tr>
</tbody>
</table>
[Explore the data](https://app.wandb.ai/wandb/huggingtweets/runs/24ddy7ab/artifacts), which is tracked with [W&B artifacts](https://docs.wandb.com/artifacts) at every step of the pipeline.
## Training procedure
The model is based on a pre-trained [GPT-2](https://huggingface.co/gpt2) which is fine-tuned on @stephenmhouston's tweets.
Hyperparameters and metrics are recorded in the [W&B training run](https://app.wandb.ai/wandb/huggingtweets/runs/1fk6tci5) for full transparency and reproducibility.
At the end of training, [the final model](https://app.wandb.ai/wandb/huggingtweets/runs/1fk6tci5/artifacts) is logged and versioned.
## Intended uses & limitations
### How to use
You can use this model directly with a pipeline for text generation:
<pre><code><span style="color:#03A9F4">from</span> transformers <span style="color:#03A9F4">import</span> pipeline
generator = pipeline(<span style="color:#FF9800">'text-generation'</span>,
model=<span style="color:#FF9800">'huggingtweets/stephenmhouston'</span>)
generator(<span style="color:#FF9800">"My dream is"</span>, num_return_sequences=<span style="color:#8BC34A">5</span>)</code></pre>
### Limitations and bias
The model suffers from [the same limitations and bias as GPT-2](https://huggingface.co/gpt2#limitations-and-bias).
In addition, the data present in the user's tweets further affects the text generated by the model.
## About
*Built by Boris Dayma*
</section>
[](https://twitter.com/intent/follow?screen_name=borisdayma)
<section class='prose'>
For more details, visit the project repository.
</section>
[](https://github.com/borisdayma/huggingtweets)
<!--- random size file --> |
huggingtweets/stinkbomb64 | 96ce88527c0b16a96e861eb842c6e0d6443399f9 | 2021-05-23T00:08:37.000Z | [
"pytorch",
"jax",
"gpt2",
"text-generation",
"en",
"transformers",
"huggingtweets"
] | text-generation | false | huggingtweets | null | huggingtweets/stinkbomb64 | 2 | null | transformers | 24,204 | ---
language: en
thumbnail: https://github.com/borisdayma/huggingtweets/blob/master/img/logo.png?raw=true
tags:
- huggingtweets
widget:
- text: "My dream is"
---
<div>
<div style="width: 132px; height:132px; border-radius: 50%; background-size: cover; background-image: url('https://pbs.twimg.com/profile_images/1241400877015019521/UDC17hPg_400x400.jpg')">
</div>
<div style="margin-top: 8px; font-size: 19px; font-weight: 800">Super Stinkbomb 64 🤖 AI Bot </div>
<div style="font-size: 15px">@stinkbomb64 bot</div>
</div>
I was made with [huggingtweets](https://github.com/borisdayma/huggingtweets).
Create your own bot based on your favorite user with [the demo](https://colab.research.google.com/github/borisdayma/huggingtweets/blob/master/huggingtweets-demo.ipynb)!
## How does it work?
The model uses the following pipeline.
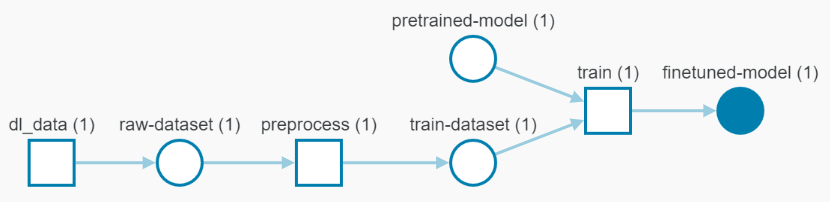
To understand how the model was developed, check the [W&B report](https://wandb.ai/wandb/huggingtweets/reports/HuggingTweets-Train-a-Model-to-Generate-Tweets--VmlldzoxMTY5MjI).
## Training data
The model was trained on [@stinkbomb64's tweets](https://twitter.com/stinkbomb64).
| Data | Quantity |
| --- | --- |
| Tweets downloaded | 2832 |
| Retweets | 497 |
| Short tweets | 195 |
| Tweets kept | 2140 |
[Explore the data](https://wandb.ai/wandb/huggingtweets/runs/1to4r6la/artifacts), which is tracked with [W&B artifacts](https://docs.wandb.com/artifacts) at every step of the pipeline.
## Training procedure
The model is based on a pre-trained [GPT-2](https://huggingface.co/gpt2) which is fine-tuned on @stinkbomb64's tweets.
Hyperparameters and metrics are recorded in the [W&B training run](https://wandb.ai/wandb/huggingtweets/runs/1sdahggr) for full transparency and reproducibility.
At the end of training, [the final model](https://wandb.ai/wandb/huggingtweets/runs/1sdahggr/artifacts) is logged and versioned.
## How to use
You can use this model directly with a pipeline for text generation:
```python
from transformers import pipeline
generator = pipeline('text-generation',
model='huggingtweets/stinkbomb64')
generator("My dream is", num_return_sequences=5)
```
## Limitations and bias
The model suffers from [the same limitations and bias as GPT-2](https://huggingface.co/gpt2#limitations-and-bias).
In addition, the data present in the user's tweets further affects the text generated by the model.
## About
*Built by Boris Dayma*
[](https://twitter.com/intent/follow?screen_name=borisdayma)
For more details, visit the project repository.
[](https://github.com/borisdayma/huggingtweets)
|
huggingtweets/stuartpb | ff295b50a17b965abbce32bd091e49b946d5ae0f | 2021-05-23T00:17:11.000Z | [
"pytorch",
"jax",
"gpt2",
"text-generation",
"en",
"transformers",
"huggingtweets"
] | text-generation | false | huggingtweets | null | huggingtweets/stuartpb | 2 | null | transformers | 24,205 | ---
language: en
thumbnail: https://www.huggingtweets.com/stuartpb/1616626168464/predictions.png
tags:
- huggingtweets
widget:
- text: "My dream is"
---
<div>
<div style="width: 132px; height:132px; border-radius: 50%; background-size: cover; background-image: url('https://pbs.twimg.com/profile_images/1356366514237018112/P117Nxds_400x400.png')">
</div>
<div style="margin-top: 8px; font-size: 19px; font-weight: 800">Stuart P. Bentley 🤖 AI Bot </div>
<div style="font-size: 15px">@stuartpb bot</div>
</div>
I was made with [huggingtweets](https://github.com/borisdayma/huggingtweets).
Create your own bot based on your favorite user with [the demo](https://colab.research.google.com/github/borisdayma/huggingtweets/blob/master/huggingtweets-demo.ipynb)!
## How does it work?
The model uses the following pipeline.
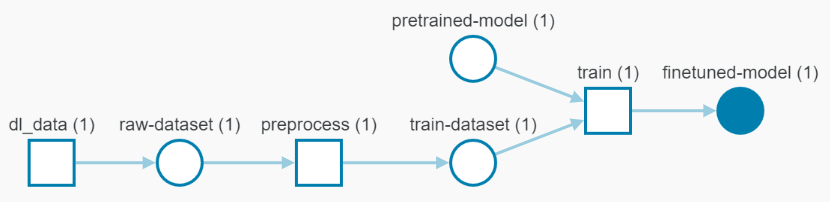
To understand how the model was developed, check the [W&B report](https://app.wandb.ai/wandb/huggingtweets/reports/HuggingTweets-Train-a-model-to-generate-tweets--VmlldzoxMTY5MjI).
## Training data
The model was trained on [@stuartpb's tweets](https://twitter.com/stuartpb).
| Data | Quantity |
| --- | --- |
| Tweets downloaded | 3238 |
| Retweets | 720 |
| Short tweets | 227 |
| Tweets kept | 2291 |
[Explore the data](https://wandb.ai/wandb/huggingtweets/runs/1j6iskne/artifacts), which is tracked with [W&B artifacts](https://docs.wandb.com/artifacts) at every step of the pipeline.
## Training procedure
The model is based on a pre-trained [GPT-2](https://huggingface.co/gpt2) which is fine-tuned on @stuartpb's tweets.
Hyperparameters and metrics are recorded in the [W&B training run](https://wandb.ai/wandb/huggingtweets/runs/1xdvmwmk) for full transparency and reproducibility.
At the end of training, [the final model](https://wandb.ai/wandb/huggingtweets/runs/1xdvmwmk/artifacts) is logged and versioned.
## How to use
You can use this model directly with a pipeline for text generation:
```python
from transformers import pipeline
generator = pipeline('text-generation',
model='huggingtweets/stuartpb')
generator("My dream is", num_return_sequences=5)
```
## Limitations and bias
The model suffers from [the same limitations and bias as GPT-2](https://huggingface.co/gpt2#limitations-and-bias).
In addition, the data present in the user's tweets further affects the text generated by the model.
## About
*Built by Boris Dayma*
[](https://twitter.com/intent/follow?screen_name=borisdayma)
For more details, visit the project repository.
[](https://github.com/borisdayma/huggingtweets)
|
huggingtweets/studio71us | 7f7c39e299f74bfd8801c3638d84a14b1d1e86db | 2021-05-23T00:18:37.000Z | [
"pytorch",
"jax",
"gpt2",
"text-generation",
"en",
"transformers",
"huggingtweets"
] | text-generation | false | huggingtweets | null | huggingtweets/studio71us | 2 | null | transformers | 24,206 | ---
language: en
thumbnail: https://www.huggingtweets.com/studio71us/1606410536949/predictions.png
tags:
- huggingtweets
widget:
- text: "My dream is"
---
<link rel="stylesheet" href="https://unpkg.com/@tailwindcss/[email protected]/dist/typography.min.css">
<style>
@media (prefers-color-scheme: dark) {
.prose { color: #E2E8F0 !important; }
.prose h2, .prose h3, .prose a, .prose thead { color: #F7FAFC !important; }
}
</style>
<section class='prose'>
<div>
<div style="width: 132px; height:132px; border-radius: 50%; background-size: cover; background-image: url('https://pbs.twimg.com/profile_images/1171505614024921089/zpI6IDeo_400x400.jpg')">
</div>
<div style="margin-top: 8px; font-size: 19px; font-weight: 800">Studio71 🤖 AI Bot </div>
<div style="font-size: 15px; color: #657786">@studio71us bot</div>
</div>
I was made with [huggingtweets](https://github.com/borisdayma/huggingtweets).
Create your own bot based on your favorite user with [the demo](https://colab.research.google.com/github/borisdayma/huggingtweets/blob/master/huggingtweets-demo.ipynb)!
## How does it work?
The model uses the following pipeline.
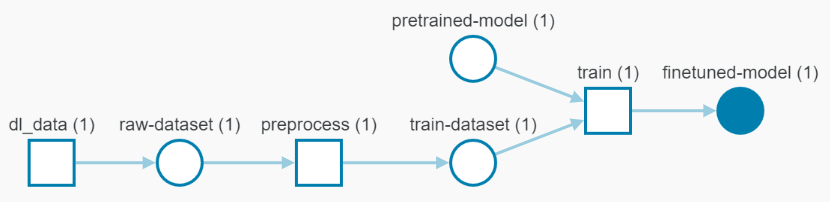
To understand how the model was developed, check the [W&B report](https://app.wandb.ai/wandb/huggingtweets/reports/HuggingTweets-Train-a-model-to-generate-tweets--VmlldzoxMTY5MjI).
## Training data
The model was trained on [@studio71us's tweets](https://twitter.com/studio71us).
<table style='border-width:0'>
<thead style='border-width:0'>
<tr style='border-width:0 0 1px 0; border-color: #CBD5E0'>
<th style='border-width:0'>Data</th>
<th style='border-width:0'>Quantity</th>
</tr>
</thead>
<tbody style='border-width:0'>
<tr style='border-width:0 0 1px 0; border-color: #E2E8F0'>
<td style='border-width:0'>Tweets downloaded</td>
<td style='border-width:0'>3213</td>
</tr>
<tr style='border-width:0 0 1px 0; border-color: #E2E8F0'>
<td style='border-width:0'>Retweets</td>
<td style='border-width:0'>558</td>
</tr>
<tr style='border-width:0 0 1px 0; border-color: #E2E8F0'>
<td style='border-width:0'>Short tweets</td>
<td style='border-width:0'>121</td>
</tr>
<tr style='border-width:0'>
<td style='border-width:0'>Tweets kept</td>
<td style='border-width:0'>2534</td>
</tr>
</tbody>
</table>
[Explore the data](https://wandb.ai/wandb/huggingtweets/runs/3uvy4ox3/artifacts), which is tracked with [W&B artifacts](https://docs.wandb.com/artifacts) at every step of the pipeline.
## Training procedure
The model is based on a pre-trained [GPT-2](https://huggingface.co/gpt2) which is fine-tuned on @studio71us's tweets.
Hyperparameters and metrics are recorded in the [W&B training run](https://wandb.ai/wandb/huggingtweets/runs/1ozrv7i5) for full transparency and reproducibility.
At the end of training, [the final model](https://wandb.ai/wandb/huggingtweets/runs/1ozrv7i5/artifacts) is logged and versioned.
## Intended uses & limitations
### How to use
You can use this model directly with a pipeline for text generation:
<pre><code><span style="color:#03A9F4">from</span> transformers <span style="color:#03A9F4">import</span> pipeline
generator = pipeline(<span style="color:#FF9800">'text-generation'</span>,
model=<span style="color:#FF9800">'huggingtweets/studio71us'</span>)
generator(<span style="color:#FF9800">"My dream is"</span>, num_return_sequences=<span style="color:#8BC34A">5</span>)</code></pre>
### Limitations and bias
The model suffers from [the same limitations and bias as GPT-2](https://huggingface.co/gpt2#limitations-and-bias).
In addition, the data present in the user's tweets further affects the text generated by the model.
## About
*Built by Boris Dayma*
</section>
[](https://twitter.com/intent/follow?screen_name=borisdayma)
<section class='prose'>
For more details, visit the project repository.
</section>
[](https://github.com/borisdayma/huggingtweets)
<!--- random size file --> |
huggingtweets/tarp1_t | b61961d76b368db581bd0122ab5251c28d10726a | 2021-05-23T00:39:49.000Z | [
"pytorch",
"jax",
"gpt2",
"text-generation",
"en",
"transformers",
"huggingtweets"
] | text-generation | false | huggingtweets | null | huggingtweets/tarp1_t | 2 | null | transformers | 24,207 | ---
language: en
thumbnail: https://www.huggingtweets.com/tarp1_t/1616663221343/predictions.png
tags:
- huggingtweets
widget:
- text: "My dream is"
---
<div>
<div style="width: 132px; height:132px; border-radius: 50%; background-size: cover; background-image: url('https://pbs.twimg.com/profile_images/1369794935558447110/pg7wTprO_400x400.jpg')">
</div>
<div style="margin-top: 8px; font-size: 19px; font-weight: 800">tarpit 🤖 AI Bot </div>
<div style="font-size: 15px">@tarp1_t bot</div>
</div>
I was made with [huggingtweets](https://github.com/borisdayma/huggingtweets).
Create your own bot based on your favorite user with [the demo](https://colab.research.google.com/github/borisdayma/huggingtweets/blob/master/huggingtweets-demo.ipynb)!
## How does it work?
The model uses the following pipeline.
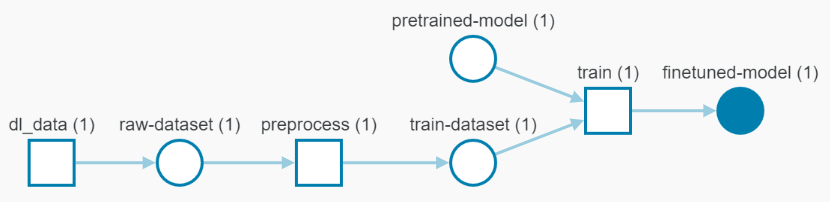
To understand how the model was developed, check the [W&B report](https://wandb.ai/wandb/huggingtweets/reports/HuggingTweets-Train-a-Model-to-Generate-Tweets--VmlldzoxMTY5MjI).
## Training data
The model was trained on [@tarp1_t's tweets](https://twitter.com/tarp1_t).
| Data | Quantity |
| --- | --- |
| Tweets downloaded | 1914 |
| Retweets | 589 |
| Short tweets | 253 |
| Tweets kept | 1072 |
[Explore the data](https://wandb.ai/wandb/huggingtweets/runs/3u04uxz2/artifacts), which is tracked with [W&B artifacts](https://docs.wandb.com/artifacts) at every step of the pipeline.
## Training procedure
The model is based on a pre-trained [GPT-2](https://huggingface.co/gpt2) which is fine-tuned on @tarp1_t's tweets.
Hyperparameters and metrics are recorded in the [W&B training run](https://wandb.ai/wandb/huggingtweets/runs/1bl2f3s6) for full transparency and reproducibility.
At the end of training, [the final model](https://wandb.ai/wandb/huggingtweets/runs/1bl2f3s6/artifacts) is logged and versioned.
## How to use
You can use this model directly with a pipeline for text generation:
```python
from transformers import pipeline
generator = pipeline('text-generation',
model='huggingtweets/tarp1_t')
generator("My dream is", num_return_sequences=5)
```
## Limitations and bias
The model suffers from [the same limitations and bias as GPT-2](https://huggingface.co/gpt2#limitations-and-bias).
In addition, the data present in the user's tweets further affects the text generated by the model.
## About
*Built by Boris Dayma*
[](https://twitter.com/intent/follow?screen_name=borisdayma)
For more details, visit the project repository.
[](https://github.com/borisdayma/huggingtweets)
|
huggingtweets/taylorswift13 | 65ec1a6256dc5dbddee9e776598641650e778fd9 | 2022-07-20T01:33:55.000Z | [
"pytorch",
"gpt2",
"text-generation",
"en",
"transformers",
"huggingtweets"
] | text-generation | false | huggingtweets | null | huggingtweets/taylorswift13 | 2 | null | transformers | 24,208 | ---
language: en
thumbnail: http://www.huggingtweets.com/taylorswift13/1658280830926/predictions.png
tags:
- huggingtweets
widget:
- text: "My dream is"
---
<div class="inline-flex flex-col" style="line-height: 1.5;">
<div class="flex">
<div
style="display:inherit; margin-left: 4px; margin-right: 4px; width: 92px; height:92px; border-radius: 50%; background-size: cover; background-image: url('https://pbs.twimg.com/profile_images/1405947624184713220/aCLC4bTE_400x400.jpg')">
</div>
<div
style="display:none; margin-left: 4px; margin-right: 4px; width: 92px; height:92px; border-radius: 50%; background-size: cover; background-image: url('')">
</div>
<div
style="display:none; margin-left: 4px; margin-right: 4px; width: 92px; height:92px; border-radius: 50%; background-size: cover; background-image: url('')">
</div>
</div>
<div style="text-align: center; margin-top: 3px; font-size: 16px; font-weight: 800">🤖 AI BOT 🤖</div>
<div style="text-align: center; font-size: 16px; font-weight: 800">Taylor Swift</div>
<div style="text-align: center; font-size: 14px;">@taylorswift13</div>
</div>
I was made with [huggingtweets](https://github.com/borisdayma/huggingtweets).
Create your own bot based on your favorite user with [the demo](https://colab.research.google.com/github/borisdayma/huggingtweets/blob/master/huggingtweets-demo.ipynb)!
## How does it work?
The model uses the following pipeline.
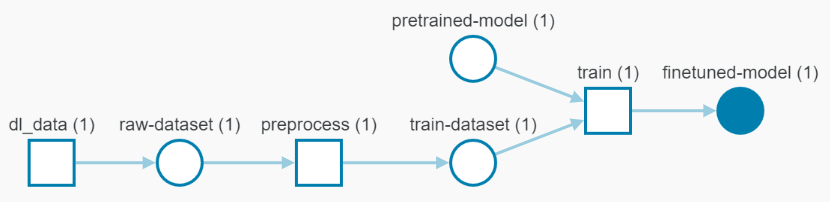
To understand how the model was developed, check the [W&B report](https://wandb.ai/wandb/huggingtweets/reports/HuggingTweets-Train-a-Model-to-Generate-Tweets--VmlldzoxMTY5MjI).
## Training data
The model was trained on tweets from Taylor Swift.
| Data | Taylor Swift |
| --- | --- |
| Tweets downloaded | 720 |
| Retweets | 89 |
| Short tweets | 88 |
| Tweets kept | 543 |
[Explore the data](https://wandb.ai/wandb/huggingtweets/runs/l7pbyjt8/artifacts), which is tracked with [W&B artifacts](https://docs.wandb.com/artifacts) at every step of the pipeline.
## Training procedure
The model is based on a pre-trained [GPT-2](https://huggingface.co/gpt2) which is fine-tuned on @taylorswift13's tweets.
Hyperparameters and metrics are recorded in the [W&B training run](https://wandb.ai/wandb/huggingtweets/runs/knykf4ka) for full transparency and reproducibility.
At the end of training, [the final model](https://wandb.ai/wandb/huggingtweets/runs/knykf4ka/artifacts) is logged and versioned.
## How to use
You can use this model directly with a pipeline for text generation:
```python
from transformers import pipeline
generator = pipeline('text-generation',
model='huggingtweets/taylorswift13')
generator("My dream is", num_return_sequences=5)
```
## Limitations and bias
The model suffers from [the same limitations and bias as GPT-2](https://huggingface.co/gpt2#limitations-and-bias).
In addition, the data present in the user's tweets further affects the text generated by the model.
## About
*Built by Boris Dayma*
[](https://twitter.com/intent/follow?screen_name=borisdayma)
For more details, visit the project repository.
[](https://github.com/borisdayma/huggingtweets)
|
huggingtweets/teawoodleaf | 46d30fa6ff5e03742552017fca0306abdcbce8e5 | 2021-05-23T00:46:49.000Z | [
"pytorch",
"jax",
"gpt2",
"text-generation",
"en",
"transformers",
"huggingtweets"
] | text-generation | false | huggingtweets | null | huggingtweets/teawoodleaf | 2 | null | transformers | 24,209 | ---
language: en
thumbnail: https://www.huggingtweets.com/teawoodleaf/1616745041242/predictions.png
tags:
- huggingtweets
widget:
- text: "My dream is"
---
<div>
<div style="width: 132px; height:132px; border-radius: 50%; background-size: cover; background-image: url('https://pbs.twimg.com/profile_images/1311111653019316225/54KQc064_400x400.jpg')">
</div>
<div style="margin-top: 8px; font-size: 19px; font-weight: 800">tea | katie 🤖 AI Bot </div>
<div style="font-size: 15px">@teawoodleaf bot</div>
</div>
I was made with [huggingtweets](https://github.com/borisdayma/huggingtweets).
Create your own bot based on your favorite user with [the demo](https://colab.research.google.com/github/borisdayma/huggingtweets/blob/master/huggingtweets-demo.ipynb)!
## How does it work?
The model uses the following pipeline.
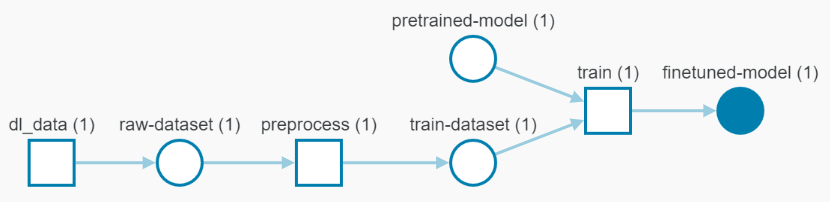
To understand how the model was developed, check the [W&B report](https://wandb.ai/wandb/huggingtweets/reports/HuggingTweets-Train-a-Model-to-Generate-Tweets--VmlldzoxMTY5MjI).
## Training data
The model was trained on [@teawoodleaf's tweets](https://twitter.com/teawoodleaf).
| Data | Quantity |
| --- | --- |
| Tweets downloaded | 3228 |
| Retweets | 1183 |
| Short tweets | 179 |
| Tweets kept | 1866 |
[Explore the data](https://wandb.ai/wandb/huggingtweets/runs/26chzppk/artifacts), which is tracked with [W&B artifacts](https://docs.wandb.com/artifacts) at every step of the pipeline.
## Training procedure
The model is based on a pre-trained [GPT-2](https://huggingface.co/gpt2) which is fine-tuned on @teawoodleaf's tweets.
Hyperparameters and metrics are recorded in the [W&B training run](https://wandb.ai/wandb/huggingtweets/runs/2bexjaz6) for full transparency and reproducibility.
At the end of training, [the final model](https://wandb.ai/wandb/huggingtweets/runs/2bexjaz6/artifacts) is logged and versioned.
## How to use
You can use this model directly with a pipeline for text generation:
```python
from transformers import pipeline
generator = pipeline('text-generation',
model='huggingtweets/teawoodleaf')
generator("My dream is", num_return_sequences=5)
```
## Limitations and bias
The model suffers from [the same limitations and bias as GPT-2](https://huggingface.co/gpt2#limitations-and-bias).
In addition, the data present in the user's tweets further affects the text generated by the model.
## About
*Built by Boris Dayma*
[](https://twitter.com/intent/follow?screen_name=borisdayma)
For more details, visit the project repository.
[](https://github.com/borisdayma/huggingtweets)
|
huggingtweets/techgirljenni | 528bc1e466df7b6f9a961c0ecda2834e0731ec34 | 2021-05-23T00:49:06.000Z | [
"pytorch",
"jax",
"gpt2",
"text-generation",
"en",
"transformers",
"huggingtweets"
] | text-generation | false | huggingtweets | null | huggingtweets/techgirljenni | 2 | null | transformers | 24,210 | ---
language: en
thumbnail: https://www.huggingtweets.com/techgirljenni/1616350777470/predictions.png
tags:
- huggingtweets
widget:
- text: "My dream is"
---
<div>
<div style="width: 132px; height:132px; border-radius: 50%; background-size: cover; background-image: url('https://pbs.twimg.com/profile_images/1362933362613190656/8z7YDTf1_400x400.jpg')">
</div>
<div style="margin-top: 8px; font-size: 19px; font-weight: 800">⚡Jennifer Freeman ⚡ 🤖 AI Bot </div>
<div style="font-size: 15px">@techgirljenni bot</div>
</div>
I was made with [huggingtweets](https://github.com/borisdayma/huggingtweets).
Create your own bot based on your favorite user with [the demo](https://colab.research.google.com/github/borisdayma/huggingtweets/blob/master/huggingtweets-demo.ipynb)!
## How does it work?
The model uses the following pipeline.
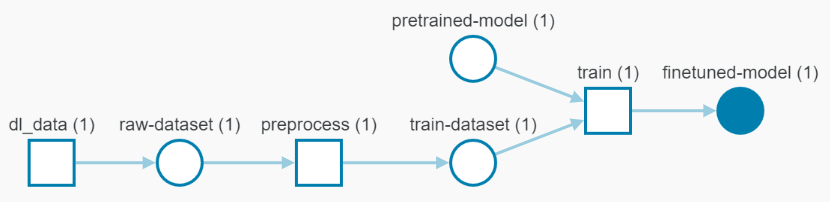
To understand how the model was developed, check the [W&B report](https://app.wandb.ai/wandb/huggingtweets/reports/HuggingTweets-Train-a-model-to-generate-tweets--VmlldzoxMTY5MjI).
## Training data
The model was trained on [@techgirljenni's tweets](https://twitter.com/techgirljenni).
| Data | Quantity |
| --- | --- |
| Tweets downloaded | 1189 |
| Retweets | 164 |
| Short tweets | 193 |
| Tweets kept | 832 |
[Explore the data](https://wandb.ai/wandb/huggingtweets/runs/1ds1xpcl/artifacts), which is tracked with [W&B artifacts](https://docs.wandb.com/artifacts) at every step of the pipeline.
## Training procedure
The model is based on a pre-trained [GPT-2](https://huggingface.co/gpt2) which is fine-tuned on @techgirljenni's tweets.
Hyperparameters and metrics are recorded in the [W&B training run](https://wandb.ai/wandb/huggingtweets/runs/tc6grn5w) for full transparency and reproducibility.
At the end of training, [the final model](https://wandb.ai/wandb/huggingtweets/runs/tc6grn5w/artifacts) is logged and versioned.
## How to use
You can use this model directly with a pipeline for text generation:
```python
from transformers import pipeline
generator = pipeline('text-generation',
model='huggingtweets/techgirljenni')
generator("My dream is", num_return_sequences=5)
```
## Limitations and bias
The model suffers from [the same limitations and bias as GPT-2](https://huggingface.co/gpt2#limitations-and-bias).
In addition, the data present in the user's tweets further affects the text generated by the model.
## About
*Built by Boris Dayma*
[](https://twitter.com/intent/follow?screen_name=borisdayma)
For more details, visit the project repository.
[](https://github.com/borisdayma/huggingtweets)
|
huggingtweets/teletour | 98c93a8693e33d17541055258400a74888e6a076 | 2021-05-23T00:57:13.000Z | [
"pytorch",
"jax",
"gpt2",
"text-generation",
"en",
"transformers",
"huggingtweets"
] | text-generation | false | huggingtweets | null | huggingtweets/teletour | 2 | null | transformers | 24,211 | ---
language: en
thumbnail: https://www.huggingtweets.com/teletour/1616646677755/predictions.png
tags:
- huggingtweets
widget:
- text: "My dream is"
---
<div>
<div style="width: 132px; height:132px; border-radius: 50%; background-size: cover; background-image: url('https://pbs.twimg.com/profile_images/1372496147835711490/MO1IPreG_400x400.jpg')">
</div>
<div style="margin-top: 8px; font-size: 19px; font-weight: 800">th 🤖 AI Bot </div>
<div style="font-size: 15px">@teletour bot</div>
</div>
I was made with [huggingtweets](https://github.com/borisdayma/huggingtweets).
Create your own bot based on your favorite user with [the demo](https://colab.research.google.com/github/borisdayma/huggingtweets/blob/master/huggingtweets-demo.ipynb)!
## How does it work?
The model uses the following pipeline.
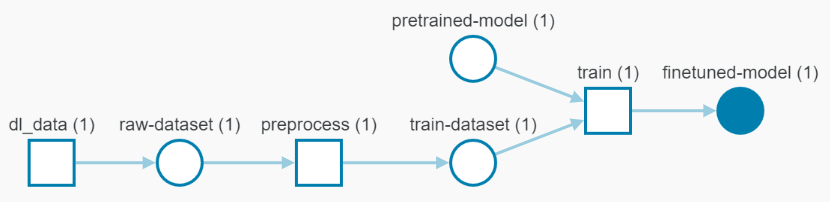
To understand how the model was developed, check the [W&B report](https://wandb.ai/wandb/huggingtweets/reports/HuggingTweets-Train-a-Model-to-Generate-Tweets--VmlldzoxMTY5MjI).
## Training data
The model was trained on [@teletour's tweets](https://twitter.com/teletour).
| Data | Quantity |
| --- | --- |
| Tweets downloaded | 3231 |
| Retweets | 612 |
| Short tweets | 269 |
| Tweets kept | 2350 |
[Explore the data](https://wandb.ai/wandb/huggingtweets/runs/2y9lbyhj/artifacts), which is tracked with [W&B artifacts](https://docs.wandb.com/artifacts) at every step of the pipeline.
## Training procedure
The model is based on a pre-trained [GPT-2](https://huggingface.co/gpt2) which is fine-tuned on @teletour's tweets.
Hyperparameters and metrics are recorded in the [W&B training run](https://wandb.ai/wandb/huggingtweets/runs/3imdcvw8) for full transparency and reproducibility.
At the end of training, [the final model](https://wandb.ai/wandb/huggingtweets/runs/3imdcvw8/artifacts) is logged and versioned.
## How to use
You can use this model directly with a pipeline for text generation:
```python
from transformers import pipeline
generator = pipeline('text-generation',
model='huggingtweets/teletour')
generator("My dream is", num_return_sequences=5)
```
## Limitations and bias
The model suffers from [the same limitations and bias as GPT-2](https://huggingface.co/gpt2#limitations-and-bias).
In addition, the data present in the user's tweets further affects the text generated by the model.
## About
*Built by Boris Dayma*
[](https://twitter.com/intent/follow?screen_name=borisdayma)
For more details, visit the project repository.
[](https://github.com/borisdayma/huggingtweets)
|
huggingtweets/temrqp | 551f9068650e2172679fcf747f4ee776a1451528 | 2021-05-23T00:59:23.000Z | [
"pytorch",
"jax",
"gpt2",
"text-generation",
"en",
"transformers",
"huggingtweets"
] | text-generation | false | huggingtweets | null | huggingtweets/temrqp | 2 | null | transformers | 24,212 | ---
language: en
thumbnail: https://www.huggingtweets.com/temrqp/1616666887355/predictions.png
tags:
- huggingtweets
widget:
- text: "My dream is"
---
<div>
<div style="width: 132px; height:132px; border-radius: 50%; background-size: cover; background-image: url('https://pbs.twimg.com/profile_images/1196088309979713536/RKpnvtwE_400x400.jpg')">
</div>
<div style="margin-top: 8px; font-size: 19px; font-weight: 800">temta 🤖 AI Bot </div>
<div style="font-size: 15px">@temrqp bot</div>
</div>
I was made with [huggingtweets](https://github.com/borisdayma/huggingtweets).
Create your own bot based on your favorite user with [the demo](https://colab.research.google.com/github/borisdayma/huggingtweets/blob/master/huggingtweets-demo.ipynb)!
## How does it work?
The model uses the following pipeline.
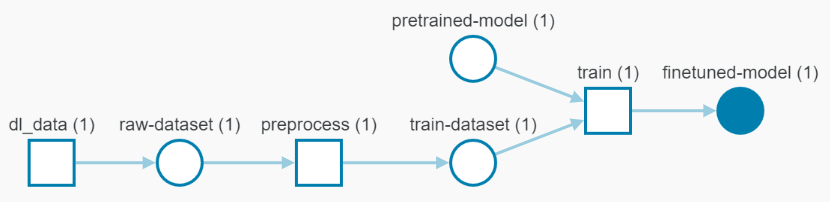
To understand how the model was developed, check the [W&B report](https://wandb.ai/wandb/huggingtweets/reports/HuggingTweets-Train-a-Model-to-Generate-Tweets--VmlldzoxMTY5MjI).
## Training data
The model was trained on [@temrqp's tweets](https://twitter.com/temrqp).
| Data | Quantity |
| --- | --- |
| Tweets downloaded | 440 |
| Retweets | 15 |
| Short tweets | 189 |
| Tweets kept | 236 |
[Explore the data](https://wandb.ai/wandb/huggingtweets/runs/8u1qrfcl/artifacts), which is tracked with [W&B artifacts](https://docs.wandb.com/artifacts) at every step of the pipeline.
## Training procedure
The model is based on a pre-trained [GPT-2](https://huggingface.co/gpt2) which is fine-tuned on @temrqp's tweets.
Hyperparameters and metrics are recorded in the [W&B training run](https://wandb.ai/wandb/huggingtweets/runs/a31q8djv) for full transparency and reproducibility.
At the end of training, [the final model](https://wandb.ai/wandb/huggingtweets/runs/a31q8djv/artifacts) is logged and versioned.
## How to use
You can use this model directly with a pipeline for text generation:
```python
from transformers import pipeline
generator = pipeline('text-generation',
model='huggingtweets/temrqp')
generator("My dream is", num_return_sequences=5)
```
## Limitations and bias
The model suffers from [the same limitations and bias as GPT-2](https://huggingface.co/gpt2#limitations-and-bias).
In addition, the data present in the user's tweets further affects the text generated by the model.
## About
*Built by Boris Dayma*
[](https://twitter.com/intent/follow?screen_name=borisdayma)
For more details, visit the project repository.
[](https://github.com/borisdayma/huggingtweets)
|
huggingtweets/the_nftking | c9f0b5c285e64bdd34ded1197de572e49af0165c | 2021-10-16T14:11:01.000Z | [
"pytorch",
"gpt2",
"text-generation",
"en",
"transformers",
"huggingtweets"
] | text-generation | false | huggingtweets | null | huggingtweets/the_nftking | 2 | 1 | transformers | 24,213 | ---
language: en
thumbnail: https://www.huggingtweets.com/the_nftking/1634393457706/predictions.png
tags:
- huggingtweets
widget:
- text: "My dream is"
---
<div class="inline-flex flex-col" style="line-height: 1.5;">
<div class="flex">
<div
style="display:inherit; margin-left: 4px; margin-right: 4px; width: 92px; height:92px; border-radius: 50%; background-size: cover; background-image: url('https://pbs.twimg.com/profile_images/1434700639649599488/J63TSf--_400x400.jpg')">
</div>
<div
style="display:none; margin-left: 4px; margin-right: 4px; width: 92px; height:92px; border-radius: 50%; background-size: cover; background-image: url('')">
</div>
<div
style="display:none; margin-left: 4px; margin-right: 4px; width: 92px; height:92px; border-radius: 50%; background-size: cover; background-image: url('')">
</div>
</div>
<div style="text-align: center; margin-top: 3px; font-size: 16px; font-weight: 800">🤖 AI BOT 🤖</div>
<div style="text-align: center; font-size: 16px; font-weight: 800">NFT KING 👑</div>
<div style="text-align: center; font-size: 14px;">@the_nftking</div>
</div>
I was made with [huggingtweets](https://github.com/borisdayma/huggingtweets).
Create your own bot based on your favorite user with [the demo](https://colab.research.google.com/github/borisdayma/huggingtweets/blob/master/huggingtweets-demo.ipynb)!
## How does it work?
The model uses the following pipeline.
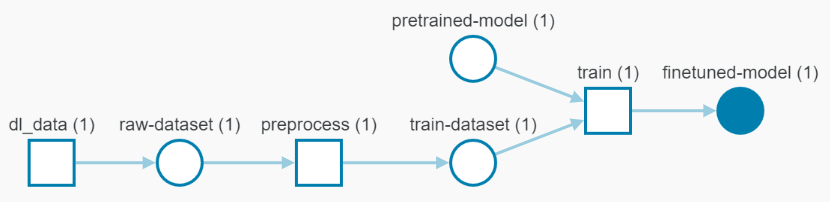
To understand how the model was developed, check the [W&B report](https://wandb.ai/wandb/huggingtweets/reports/HuggingTweets-Train-a-Model-to-Generate-Tweets--VmlldzoxMTY5MjI).
## Training data
The model was trained on tweets from NFT KING 👑.
| Data | NFT KING 👑 |
| --- | --- |
| Tweets downloaded | 163 |
| Retweets | 23 |
| Short tweets | 36 |
| Tweets kept | 104 |
[Explore the data](https://wandb.ai/wandb/huggingtweets/runs/26d96n9m/artifacts), which is tracked with [W&B artifacts](https://docs.wandb.com/artifacts) at every step of the pipeline.
## Training procedure
The model is based on a pre-trained [GPT-2](https://huggingface.co/gpt2) which is fine-tuned on @the_nftking's tweets.
Hyperparameters and metrics are recorded in the [W&B training run](https://wandb.ai/wandb/huggingtweets/runs/f7wd0e6f) for full transparency and reproducibility.
At the end of training, [the final model](https://wandb.ai/wandb/huggingtweets/runs/f7wd0e6f/artifacts) is logged and versioned.
## How to use
You can use this model directly with a pipeline for text generation:
```python
from transformers import pipeline
generator = pipeline('text-generation',
model='huggingtweets/the_nftking')
generator("My dream is", num_return_sequences=5)
```
## Limitations and bias
The model suffers from [the same limitations and bias as GPT-2](https://huggingface.co/gpt2#limitations-and-bias).
In addition, the data present in the user's tweets further affects the text generated by the model.
## About
*Built by Boris Dayma*
[](https://twitter.com/intent/follow?screen_name=borisdayma)
For more details, visit the project repository.
[](https://github.com/borisdayma/huggingtweets)
|
huggingtweets/the_robisho | 0e830a39accf98c5f038b8317d8aba52243a7605 | 2021-07-10T03:40:16.000Z | [
"pytorch",
"gpt2",
"text-generation",
"en",
"transformers",
"huggingtweets"
] | text-generation | false | huggingtweets | null | huggingtweets/the_robisho | 2 | null | transformers | 24,214 | ---
language: en
thumbnail: https://www.huggingtweets.com/the_robisho/1625888412499/predictions.png
tags:
- huggingtweets
widget:
- text: "My dream is"
---
<div class="inline-flex flex-col" style="line-height: 1.5;">
<div class="flex">
<div
style="display:inherit; margin-left: 4px; margin-right: 4px; width: 92px; height:92px; border-radius: 50%; background-size: cover; background-image: url('https://pbs.twimg.com/profile_images/1311895700582666243/6N1xGNV9_400x400.jpg')">
</div>
<div
style="display:none; margin-left: 4px; margin-right: 4px; width: 92px; height:92px; border-radius: 50%; background-size: cover; background-image: url('')">
</div>
<div
style="display:none; margin-left: 4px; margin-right: 4px; width: 92px; height:92px; border-radius: 50%; background-size: cover; background-image: url('')">
</div>
</div>
<div style="text-align: center; margin-top: 3px; font-size: 16px; font-weight: 800">🤖 AI BOT 🤖</div>
<div style="text-align: center; font-size: 16px; font-weight: 800">Nick Landback</div>
<div style="text-align: center; font-size: 14px;">@the_robisho</div>
</div>
I was made with [huggingtweets](https://github.com/borisdayma/huggingtweets).
Create your own bot based on your favorite user with [the demo](https://colab.research.google.com/github/borisdayma/huggingtweets/blob/master/huggingtweets-demo.ipynb)!
## How does it work?
The model uses the following pipeline.
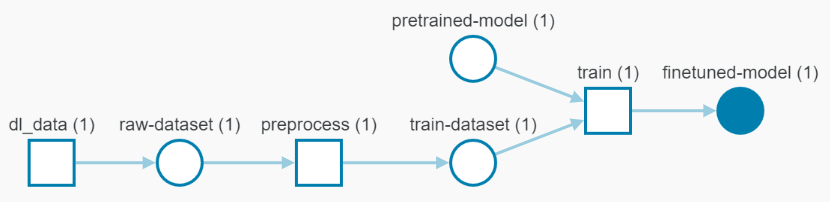
To understand how the model was developed, check the [W&B report](https://wandb.ai/wandb/huggingtweets/reports/HuggingTweets-Train-a-Model-to-Generate-Tweets--VmlldzoxMTY5MjI).
## Training data
The model was trained on tweets from Nick Landback.
| Data | Nick Landback |
| --- | --- |
| Tweets downloaded | 3205 |
| Retweets | 1018 |
| Short tweets | 21 |
| Tweets kept | 2166 |
[Explore the data](https://wandb.ai/wandb/huggingtweets/runs/bbz9lc2n/artifacts), which is tracked with [W&B artifacts](https://docs.wandb.com/artifacts) at every step of the pipeline.
## Training procedure
The model is based on a pre-trained [GPT-2](https://huggingface.co/gpt2) which is fine-tuned on @the_robisho's tweets.
Hyperparameters and metrics are recorded in the [W&B training run](https://wandb.ai/wandb/huggingtweets/runs/gh2qryi3) for full transparency and reproducibility.
At the end of training, [the final model](https://wandb.ai/wandb/huggingtweets/runs/gh2qryi3/artifacts) is logged and versioned.
## How to use
You can use this model directly with a pipeline for text generation:
```python
from transformers import pipeline
generator = pipeline('text-generation',
model='huggingtweets/the_robisho')
generator("My dream is", num_return_sequences=5)
```
## Limitations and bias
The model suffers from [the same limitations and bias as GPT-2](https://huggingface.co/gpt2#limitations-and-bias).
In addition, the data present in the user's tweets further affects the text generated by the model.
## About
*Built by Boris Dayma*
[](https://twitter.com/intent/follow?screen_name=borisdayma)
For more details, visit the project repository.
[](https://github.com/borisdayma/huggingtweets)
|
huggingtweets/theeye_eee | 0077f7c413a234c0789fdfe0c68b94af677f7497 | 2021-05-23T01:38:39.000Z | [
"pytorch",
"jax",
"gpt2",
"text-generation",
"en",
"transformers",
"huggingtweets"
] | text-generation | false | huggingtweets | null | huggingtweets/theeye_eee | 2 | null | transformers | 24,215 | ---
language: en
thumbnail: https://www.huggingtweets.com/theeye_eee/1613324013605/predictions.png
tags:
- huggingtweets
widget:
- text: "My dream is"
---
<div>
<div style="width: 132px; height:132px; border-radius: 50%; background-size: cover; background-image: url('https://pbs.twimg.com/profile_images/1302699354411728898/JMOVs0nc_400x400.jpg')">
</div>
<div style="margin-top: 8px; font-size: 19px; font-weight: 800">theEYE 🤖 AI Bot </div>
<div style="font-size: 15px">@theeye_eee bot</div>
</div>
I was made with [huggingtweets](https://github.com/borisdayma/huggingtweets).
Create your own bot based on your favorite user with [the demo](https://colab.research.google.com/github/borisdayma/huggingtweets/blob/master/huggingtweets-demo.ipynb)!
## How does it work?
The model uses the following pipeline.
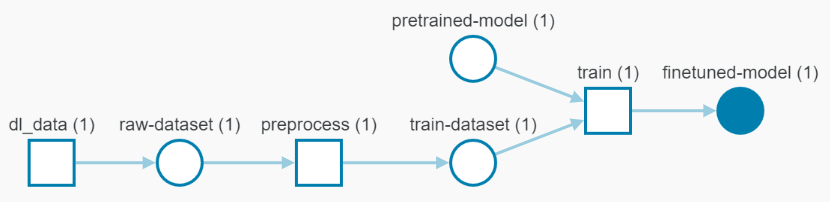
To understand how the model was developed, check the [W&B report](https://app.wandb.ai/wandb/huggingtweets/reports/HuggingTweets-Train-a-model-to-generate-tweets--VmlldzoxMTY5MjI).
## Training data
The model was trained on [@theeye_eee's tweets](https://twitter.com/theeye_eee).
| Data | Quantity |
| --- | --- |
| Tweets downloaded | 416 |
| Retweets | 69 |
| Short tweets | 12 |
| Tweets kept | 335 |
[Explore the data](https://wandb.ai/wandb/huggingtweets/runs/oavkxa5z/artifacts), which is tracked with [W&B artifacts](https://docs.wandb.com/artifacts) at every step of the pipeline.
## Training procedure
The model is based on a pre-trained [GPT-2](https://huggingface.co/gpt2) which is fine-tuned on @theeye_eee's tweets.
Hyperparameters and metrics are recorded in the [W&B training run](https://wandb.ai/wandb/huggingtweets/runs/hco1pbdv) for full transparency and reproducibility.
At the end of training, [the final model](https://wandb.ai/wandb/huggingtweets/runs/hco1pbdv/artifacts) is logged and versioned.
## How to use
You can use this model directly with a pipeline for text generation:
```python
from transformers import pipeline
generator = pipeline('text-generation',
model='huggingtweets/theeye_eee')
generator("My dream is", num_return_sequences=5)
```
## Limitations and bias
The model suffers from [the same limitations and bias as GPT-2](https://huggingface.co/gpt2#limitations-and-bias).
In addition, the data present in the user's tweets further affects the text generated by the model.
## About
*Built by Boris Dayma*
[](https://twitter.com/intent/follow?screen_name=borisdayma)
For more details, visit the project repository.
[](https://github.com/borisdayma/huggingtweets)
|
huggingtweets/thefoxjulia | 976f06b2e2567288f31f845bdb6456a0b3438d36 | 2021-05-23T01:39:56.000Z | [
"pytorch",
"jax",
"gpt2",
"text-generation",
"en",
"transformers",
"huggingtweets"
] | text-generation | false | huggingtweets | null | huggingtweets/thefoxjulia | 2 | null | transformers | 24,216 | ---
language: en
thumbnail: https://www.huggingtweets.com/thefoxjulia/1614104604500/predictions.png
tags:
- huggingtweets
widget:
- text: "My dream is"
---
<div>
<div style="width: 132px; height:132px; border-radius: 50%; background-size: cover; background-image: url('https://pbs.twimg.com/profile_images/1342676796706332672/DZLAqeT1_400x400.jpg')">
</div>
<div style="margin-top: 8px; font-size: 19px; font-weight: 800">🦊julia🦊 🤖 AI Bot </div>
<div style="font-size: 15px">@thefoxjulia bot</div>
</div>
I was made with [huggingtweets](https://github.com/borisdayma/huggingtweets).
Create your own bot based on your favorite user with [the demo](https://colab.research.google.com/github/borisdayma/huggingtweets/blob/master/huggingtweets-demo.ipynb)!
## How does it work?
The model uses the following pipeline.
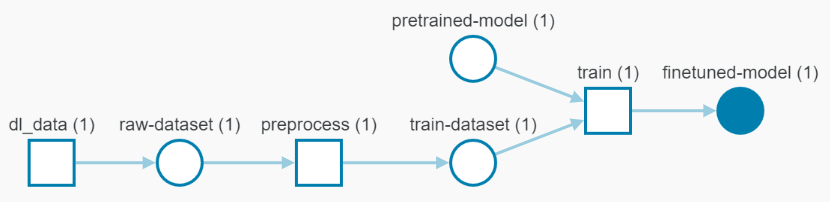
To understand how the model was developed, check the [W&B report](https://app.wandb.ai/wandb/huggingtweets/reports/HuggingTweets-Train-a-model-to-generate-tweets--VmlldzoxMTY5MjI).
## Training data
The model was trained on [@thefoxjulia's tweets](https://twitter.com/thefoxjulia).
| Data | Quantity |
| --- | --- |
| Tweets downloaded | 3158 |
| Retweets | 1737 |
| Short tweets | 544 |
| Tweets kept | 877 |
[Explore the data](https://wandb.ai/wandb/huggingtweets/runs/1bwc2es8/artifacts), which is tracked with [W&B artifacts](https://docs.wandb.com/artifacts) at every step of the pipeline.
## Training procedure
The model is based on a pre-trained [GPT-2](https://huggingface.co/gpt2) which is fine-tuned on @thefoxjulia's tweets.
Hyperparameters and metrics are recorded in the [W&B training run](https://wandb.ai/wandb/huggingtweets/runs/1sghmlbv) for full transparency and reproducibility.
At the end of training, [the final model](https://wandb.ai/wandb/huggingtweets/runs/1sghmlbv/artifacts) is logged and versioned.
## How to use
You can use this model directly with a pipeline for text generation:
```python
from transformers import pipeline
generator = pipeline('text-generation',
model='huggingtweets/thefoxjulia')
generator("My dream is", num_return_sequences=5)
```
## Limitations and bias
The model suffers from [the same limitations and bias as GPT-2](https://huggingface.co/gpt2#limitations-and-bias).
In addition, the data present in the user's tweets further affects the text generated by the model.
## About
*Built by Boris Dayma*
[](https://twitter.com/intent/follow?screen_name=borisdayma)
For more details, visit the project repository.
[](https://github.com/borisdayma/huggingtweets)
|
huggingtweets/themarktwain | d2f892ef753e28acac02c4faa8ce56ab5c66352c | 2021-05-23T01:46:17.000Z | [
"pytorch",
"jax",
"gpt2",
"text-generation",
"en",
"transformers",
"huggingtweets"
] | text-generation | false | huggingtweets | null | huggingtweets/themarktwain | 2 | null | transformers | 24,217 | ---
language: en
thumbnail: https://www.huggingtweets.com/themarktwain/1619412843939/predictions.png
tags:
- huggingtweets
widget:
- text: "My dream is"
---
<div>
<div style="width: 132px; height:132px; border-radius: 50%; background-size: cover; background-image: url('https://pbs.twimg.com/profile_images/1228714762/twain_400x400.jpg')">
</div>
<div style="margin-top: 8px; font-size: 19px; font-weight: 800">Mark Twain 🤖 AI Bot </div>
<div style="font-size: 15px">@themarktwain bot</div>
</div>
I was made with [huggingtweets](https://github.com/borisdayma/huggingtweets).
Create your own bot based on your favorite user with [the demo](https://colab.research.google.com/github/borisdayma/huggingtweets/blob/master/huggingtweets-demo.ipynb)!
## How does it work?
The model uses the following pipeline.
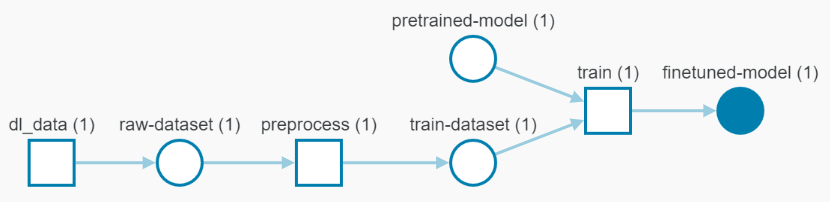
To understand how the model was developed, check the [W&B report](https://wandb.ai/wandb/huggingtweets/reports/HuggingTweets-Train-a-Model-to-Generate-Tweets--VmlldzoxMTY5MjI).
## Training data
The model was trained on [@themarktwain's tweets](https://twitter.com/themarktwain).
| Data | Quantity |
| --- | --- |
| Tweets downloaded | 3214 |
| Retweets | 0 |
| Short tweets | 0 |
| Tweets kept | 3214 |
[Explore the data](https://wandb.ai/wandb/huggingtweets/runs/rsor9owm/artifacts), which is tracked with [W&B artifacts](https://docs.wandb.com/artifacts) at every step of the pipeline.
## Training procedure
The model is based on a pre-trained [GPT-2](https://huggingface.co/gpt2) which is fine-tuned on @themarktwain's tweets.
Hyperparameters and metrics are recorded in the [W&B training run](https://wandb.ai/wandb/huggingtweets/runs/3d16s01c) for full transparency and reproducibility.
At the end of training, [the final model](https://wandb.ai/wandb/huggingtweets/runs/3d16s01c/artifacts) is logged and versioned.
## How to use
You can use this model directly with a pipeline for text generation:
```python
from transformers import pipeline
generator = pipeline('text-generation',
model='huggingtweets/themarktwain')
generator("My dream is", num_return_sequences=5)
```
## Limitations and bias
The model suffers from [the same limitations and bias as GPT-2](https://huggingface.co/gpt2#limitations-and-bias).
In addition, the data present in the user's tweets further affects the text generated by the model.
## About
*Built by Boris Dayma*
[](https://twitter.com/intent/follow?screen_name=borisdayma)
For more details, visit the project repository.
[](https://github.com/borisdayma/huggingtweets)
|
huggingtweets/theonion | ef6eb8d283ec44e33eafb25f8d083aac4928fe2a | 2021-10-26T04:42:42.000Z | [
"pytorch",
"gpt2",
"text-generation",
"en",
"transformers",
"huggingtweets"
] | text-generation | false | huggingtweets | null | huggingtweets/theonion | 2 | null | transformers | 24,218 | ---
language: en
thumbnail: https://www.huggingtweets.com/theonion/1635223358201/predictions.png
tags:
- huggingtweets
widget:
- text: "My dream is"
---
<div class="inline-flex flex-col" style="line-height: 1.5;">
<div class="flex">
<div
style="display:inherit; margin-left: 4px; margin-right: 4px; width: 92px; height:92px; border-radius: 50%; background-size: cover; background-image: url('https://pbs.twimg.com/profile_images/875392068125769732/yrN-1k0Y_400x400.jpg')">
</div>
<div
style="display:none; margin-left: 4px; margin-right: 4px; width: 92px; height:92px; border-radius: 50%; background-size: cover; background-image: url('')">
</div>
<div
style="display:none; margin-left: 4px; margin-right: 4px; width: 92px; height:92px; border-radius: 50%; background-size: cover; background-image: url('')">
</div>
</div>
<div style="text-align: center; margin-top: 3px; font-size: 16px; font-weight: 800">🤖 AI BOT 🤖</div>
<div style="text-align: center; font-size: 16px; font-weight: 800">The Onion</div>
<div style="text-align: center; font-size: 14px;">@theonion</div>
</div>
I was made with [huggingtweets](https://github.com/borisdayma/huggingtweets).
Create your own bot based on your favorite user with [the demo](https://colab.research.google.com/github/borisdayma/huggingtweets/blob/master/huggingtweets-demo.ipynb)!
## How does it work?
The model uses the following pipeline.
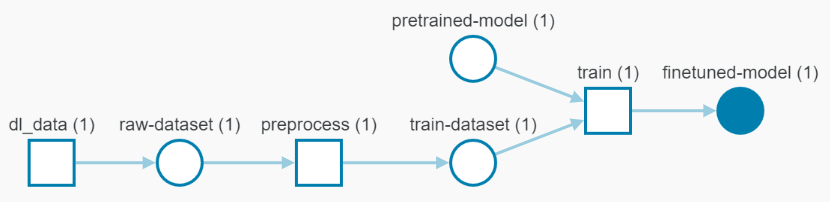
To understand how the model was developed, check the [W&B report](https://wandb.ai/wandb/huggingtweets/reports/HuggingTweets-Train-a-Model-to-Generate-Tweets--VmlldzoxMTY5MjI).
## Training data
The model was trained on tweets from The Onion.
| Data | The Onion |
| --- | --- |
| Tweets downloaded | 3250 |
| Retweets | 2 |
| Short tweets | 10 |
| Tweets kept | 3238 |
[Explore the data](https://wandb.ai/wandb/huggingtweets/runs/tl5cqc3f/artifacts), which is tracked with [W&B artifacts](https://docs.wandb.com/artifacts) at every step of the pipeline.
## Training procedure
The model is based on a pre-trained [GPT-2](https://huggingface.co/gpt2) which is fine-tuned on @theonion's tweets.
Hyperparameters and metrics are recorded in the [W&B training run](https://wandb.ai/wandb/huggingtweets/runs/1y8p1w9v) for full transparency and reproducibility.
At the end of training, [the final model](https://wandb.ai/wandb/huggingtweets/runs/1y8p1w9v/artifacts) is logged and versioned.
## How to use
You can use this model directly with a pipeline for text generation:
```python
from transformers import pipeline
generator = pipeline('text-generation',
model='huggingtweets/theonion')
generator("My dream is", num_return_sequences=5)
```
## Limitations and bias
The model suffers from [the same limitations and bias as GPT-2](https://huggingface.co/gpt2#limitations-and-bias).
In addition, the data present in the user's tweets further affects the text generated by the model.
## About
*Built by Boris Dayma*
[](https://twitter.com/intent/follow?screen_name=borisdayma)
For more details, visit the project repository.
[](https://github.com/borisdayma/huggingtweets)
|
huggingtweets/thestoicemperor | 784c80427f082e087b1ff628e8c4b4c63a46e40c | 2021-08-07T09:53:28.000Z | [
"pytorch",
"gpt2",
"text-generation",
"en",
"transformers",
"huggingtweets"
] | text-generation | false | huggingtweets | null | huggingtweets/thestoicemperor | 2 | null | transformers | 24,219 | ---
language: en
thumbnail: https://www.huggingtweets.com/thestoicemperor/1628330005157/predictions.png
tags:
- huggingtweets
widget:
- text: "My dream is"
---
<div class="inline-flex flex-col" style="line-height: 1.5;">
<div class="flex">
<div
style="display:inherit; margin-left: 4px; margin-right: 4px; width: 92px; height:92px; border-radius: 50%; background-size: cover; background-image: url('https://pbs.twimg.com/profile_images/851774550631104514/FnBLKlzZ_400x400.jpg')">
</div>
<div
style="display:none; margin-left: 4px; margin-right: 4px; width: 92px; height:92px; border-radius: 50%; background-size: cover; background-image: url('')">
</div>
<div
style="display:none; margin-left: 4px; margin-right: 4px; width: 92px; height:92px; border-radius: 50%; background-size: cover; background-image: url('')">
</div>
</div>
<div style="text-align: center; margin-top: 3px; font-size: 16px; font-weight: 800">🤖 AI BOT 🤖</div>
<div style="text-align: center; font-size: 16px; font-weight: 800">The Stoic Emperor</div>
<div style="text-align: center; font-size: 14px;">@thestoicemperor</div>
</div>
I was made with [huggingtweets](https://github.com/borisdayma/huggingtweets).
Create your own bot based on your favorite user with [the demo](https://colab.research.google.com/github/borisdayma/huggingtweets/blob/master/huggingtweets-demo.ipynb)!
## How does it work?
The model uses the following pipeline.
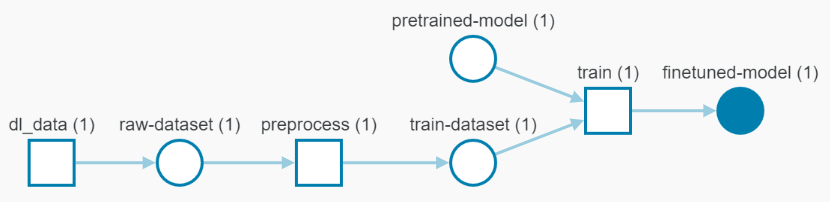
To understand how the model was developed, check the [W&B report](https://wandb.ai/wandb/huggingtweets/reports/HuggingTweets-Train-a-Model-to-Generate-Tweets--VmlldzoxMTY5MjI).
## Training data
The model was trained on tweets from The Stoic Emperor.
| Data | The Stoic Emperor |
| --- | --- |
| Tweets downloaded | 1415 |
| Retweets | 7 |
| Short tweets | 40 |
| Tweets kept | 1368 |
[Explore the data](https://wandb.ai/wandb/huggingtweets/runs/173wj3i3/artifacts), which is tracked with [W&B artifacts](https://docs.wandb.com/artifacts) at every step of the pipeline.
## Training procedure
The model is based on a pre-trained [GPT-2](https://huggingface.co/gpt2) which is fine-tuned on @thestoicemperor's tweets.
Hyperparameters and metrics are recorded in the [W&B training run](https://wandb.ai/wandb/huggingtweets/runs/o5491war) for full transparency and reproducibility.
At the end of training, [the final model](https://wandb.ai/wandb/huggingtweets/runs/o5491war/artifacts) is logged and versioned.
## How to use
You can use this model directly with a pipeline for text generation:
```python
from transformers import pipeline
generator = pipeline('text-generation',
model='huggingtweets/thestoicemperor')
generator("My dream is", num_return_sequences=5)
```
## Limitations and bias
The model suffers from [the same limitations and bias as GPT-2](https://huggingface.co/gpt2#limitations-and-bias).
In addition, the data present in the user's tweets further affects the text generated by the model.
## About
*Built by Boris Dayma*
[](https://twitter.com/intent/follow?screen_name=borisdayma)
For more details, visit the project repository.
[](https://github.com/borisdayma/huggingtweets)
|
huggingtweets/tim_cook | 212939a62cf522fc079e424fa61a72095062cb2d | 2021-05-23T02:20:04.000Z | [
"pytorch",
"jax",
"gpt2",
"text-generation",
"en",
"transformers",
"huggingtweets"
] | text-generation | false | huggingtweets | null | huggingtweets/tim_cook | 2 | null | transformers | 24,220 | ---
language: en
thumbnail: https://www.huggingtweets.com/tim_cook/1616639027743/predictions.png
tags:
- huggingtweets
widget:
- text: "My dream is"
---
<div>
<div style="width: 132px; height:132px; border-radius: 50%; background-size: cover; background-image: url('https://pbs.twimg.com/profile_images/1194113737092935681/63O1znGw_400x400.jpg')">
</div>
<div style="margin-top: 8px; font-size: 19px; font-weight: 800">Tim Cook 🤖 AI Bot </div>
<div style="font-size: 15px">@tim_cook bot</div>
</div>
I was made with [huggingtweets](https://github.com/borisdayma/huggingtweets).
Create your own bot based on your favorite user with [the demo](https://colab.research.google.com/github/borisdayma/huggingtweets/blob/master/huggingtweets-demo.ipynb)!
## How does it work?
The model uses the following pipeline.
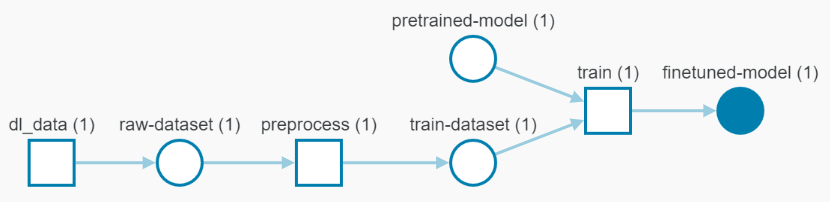
To understand how the model was developed, check the [W&B report](https://app.wandb.ai/wandb/huggingtweets/reports/HuggingTweets-Train-a-model-to-generate-tweets--VmlldzoxMTY5MjI).
## Training data
The model was trained on [@tim_cook's tweets](https://twitter.com/tim_cook).
| Data | Quantity |
| --- | --- |
| Tweets downloaded | 1157 |
| Retweets | 20 |
| Short tweets | 13 |
| Tweets kept | 1124 |
[Explore the data](https://wandb.ai/wandb/huggingtweets/runs/2bjpyixq/artifacts), which is tracked with [W&B artifacts](https://docs.wandb.com/artifacts) at every step of the pipeline.
## Training procedure
The model is based on a pre-trained [GPT-2](https://huggingface.co/gpt2) which is fine-tuned on @tim_cook's tweets.
Hyperparameters and metrics are recorded in the [W&B training run](https://wandb.ai/wandb/huggingtweets/runs/2gp3fgex) for full transparency and reproducibility.
At the end of training, [the final model](https://wandb.ai/wandb/huggingtweets/runs/2gp3fgex/artifacts) is logged and versioned.
## How to use
You can use this model directly with a pipeline for text generation:
```python
from transformers import pipeline
generator = pipeline('text-generation',
model='huggingtweets/tim_cook')
generator("My dream is", num_return_sequences=5)
```
## Limitations and bias
The model suffers from [the same limitations and bias as GPT-2](https://huggingface.co/gpt2#limitations-and-bias).
In addition, the data present in the user's tweets further affects the text generated by the model.
## About
*Built by Boris Dayma*
[](https://twitter.com/intent/follow?screen_name=borisdayma)
For more details, visit the project repository.
[](https://github.com/borisdayma/huggingtweets)
|
huggingtweets/tk_tr | 87c3633c3e8953c3ac41b5208f0cb6c3b0aba95e | 2021-05-23T02:29:17.000Z | [
"pytorch",
"jax",
"gpt2",
"text-generation",
"en",
"transformers",
"huggingtweets"
] | text-generation | false | huggingtweets | null | huggingtweets/tk_tr | 2 | null | transformers | 24,221 | ---
language: en
thumbnail: https://www.huggingtweets.com/tk_tr/1611556252665/predictions.png
tags:
- huggingtweets
widget:
- text: "My dream is"
---
<link rel="stylesheet" href="https://unpkg.com/@tailwindcss/[email protected]/dist/typography.min.css">
<style>
@media (prefers-color-scheme: dark) {
.prose { color: #E2E8F0 !important; }
.prose h2, .prose h3, .prose a, .prose thead { color: #F7FAFC !important; }
}
</style>
<section class='prose'>
<div>
<div style="width: 132px; height:132px; border-radius: 50%; background-size: cover; background-image: url('https://pbs.twimg.com/profile_images/1053335417456599040/jAkCW1Q8_400x400.jpg')">
</div>
<div style="margin-top: 8px; font-size: 19px; font-weight: 800">Türk Hava Yolları 🤖 AI Bot </div>
<div style="font-size: 15px; color: #657786">@tk_tr bot</div>
</div>
I was made with [huggingtweets](https://github.com/borisdayma/huggingtweets).
Create your own bot based on your favorite user with [the demo](https://colab.research.google.com/github/borisdayma/huggingtweets/blob/master/huggingtweets-demo.ipynb)!
## How does it work?
The model uses the following pipeline.
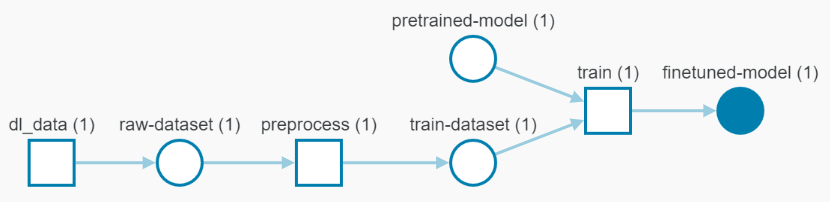
To understand how the model was developed, check the [W&B report](https://app.wandb.ai/wandb/huggingtweets/reports/HuggingTweets-Train-a-model-to-generate-tweets--VmlldzoxMTY5MjI).
## Training data
The model was trained on [@tk_tr's tweets](https://twitter.com/tk_tr).
<table style='border-width:0'>
<thead style='border-width:0'>
<tr style='border-width:0 0 1px 0; border-color: #CBD5E0'>
<th style='border-width:0'>Data</th>
<th style='border-width:0'>Quantity</th>
</tr>
</thead>
<tbody style='border-width:0'>
<tr style='border-width:0 0 1px 0; border-color: #E2E8F0'>
<td style='border-width:0'>Tweets downloaded</td>
<td style='border-width:0'>3247</td>
</tr>
<tr style='border-width:0 0 1px 0; border-color: #E2E8F0'>
<td style='border-width:0'>Retweets</td>
<td style='border-width:0'>141</td>
</tr>
<tr style='border-width:0 0 1px 0; border-color: #E2E8F0'>
<td style='border-width:0'>Short tweets</td>
<td style='border-width:0'>13</td>
</tr>
<tr style='border-width:0'>
<td style='border-width:0'>Tweets kept</td>
<td style='border-width:0'>3093</td>
</tr>
</tbody>
</table>
[Explore the data](https://wandb.ai/wandb/huggingtweets/runs/2756do1o/artifacts), which is tracked with [W&B artifacts](https://docs.wandb.com/artifacts) at every step of the pipeline.
## Training procedure
The model is based on a pre-trained [GPT-2](https://huggingface.co/gpt2) which is fine-tuned on @tk_tr's tweets.
Hyperparameters and metrics are recorded in the [W&B training run](https://wandb.ai/wandb/huggingtweets/runs/gjxd7tbg) for full transparency and reproducibility.
At the end of training, [the final model](https://wandb.ai/wandb/huggingtweets/runs/gjxd7tbg/artifacts) is logged and versioned.
## Intended uses & limitations
### How to use
You can use this model directly with a pipeline for text generation:
<pre><code><span style="color:#03A9F4">from</span> transformers <span style="color:#03A9F4">import</span> pipeline
generator = pipeline(<span style="color:#FF9800">'text-generation'</span>,
model=<span style="color:#FF9800">'huggingtweets/tk_tr'</span>)
generator(<span style="color:#FF9800">"My dream is"</span>, num_return_sequences=<span style="color:#8BC34A">5</span>)</code></pre>
### Limitations and bias
The model suffers from [the same limitations and bias as GPT-2](https://huggingface.co/gpt2#limitations-and-bias).
In addition, the data present in the user's tweets further affects the text generated by the model.
## About
*Built by Boris Dayma*
</section>
[](https://twitter.com/intent/follow?screen_name=borisdayma)
<section class='prose'>
For more details, visit the project repository.
</section>
[](https://github.com/borisdayma/huggingtweets)
|
huggingtweets/tomb_respecter | 7f7f0206a3920896fb6270b275447d2106f8f89c | 2021-05-23T02:35:18.000Z | [
"pytorch",
"jax",
"gpt2",
"text-generation",
"en",
"transformers",
"huggingtweets"
] | text-generation | false | huggingtweets | null | huggingtweets/tomb_respecter | 2 | null | transformers | 24,222 | ---
language: en
thumbnail: https://www.huggingtweets.com/tomb_respecter/1614137349511/predictions.png
tags:
- huggingtweets
widget:
- text: "My dream is"
---
<div>
<div style="width: 132px; height:132px; border-radius: 50%; background-size: cover; background-image: url('https://pbs.twimg.com/profile_images/1221212153509662721/PDodqvE1_400x400.jpg')">
</div>
<div style="margin-top: 8px; font-size: 19px; font-weight: 800">tomb respecter 🤖 AI Bot </div>
<div style="font-size: 15px">@tomb_respecter bot</div>
</div>
I was made with [huggingtweets](https://github.com/borisdayma/huggingtweets).
Create your own bot based on your favorite user with [the demo](https://colab.research.google.com/github/borisdayma/huggingtweets/blob/master/huggingtweets-demo.ipynb)!
## How does it work?
The model uses the following pipeline.
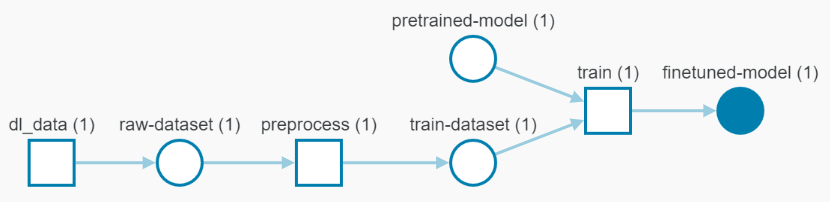
To understand how the model was developed, check the [W&B report](https://app.wandb.ai/wandb/huggingtweets/reports/HuggingTweets-Train-a-model-to-generate-tweets--VmlldzoxMTY5MjI).
## Training data
The model was trained on [@tomb_respecter's tweets](https://twitter.com/tomb_respecter).
| Data | Quantity |
| --- | --- |
| Tweets downloaded | 3193 |
| Retweets | 196 |
| Short tweets | 90 |
| Tweets kept | 2907 |
[Explore the data](https://wandb.ai/wandb/huggingtweets/runs/3oii0g6f/artifacts), which is tracked with [W&B artifacts](https://docs.wandb.com/artifacts) at every step of the pipeline.
## Training procedure
The model is based on a pre-trained [GPT-2](https://huggingface.co/gpt2) which is fine-tuned on @tomb_respecter's tweets.
Hyperparameters and metrics are recorded in the [W&B training run](https://wandb.ai/wandb/huggingtweets/runs/1tdsteqg) for full transparency and reproducibility.
At the end of training, [the final model](https://wandb.ai/wandb/huggingtweets/runs/1tdsteqg/artifacts) is logged and versioned.
## How to use
You can use this model directly with a pipeline for text generation:
```python
from transformers import pipeline
generator = pipeline('text-generation',
model='huggingtweets/tomb_respecter')
generator("My dream is", num_return_sequences=5)
```
## Limitations and bias
The model suffers from [the same limitations and bias as GPT-2](https://huggingface.co/gpt2#limitations-and-bias).
In addition, the data present in the user's tweets further affects the text generated by the model.
## About
*Built by Boris Dayma*
[](https://twitter.com/intent/follow?screen_name=borisdayma)
For more details, visit the project repository.
[](https://github.com/borisdayma/huggingtweets)
|
huggingtweets/trevorthalacker | 501776e3d1760b0d216d8ac9e5cd9e00458bfc59 | 2021-07-18T07:39:42.000Z | [
"pytorch",
"gpt2",
"text-generation",
"en",
"transformers",
"huggingtweets"
] | text-generation | false | huggingtweets | null | huggingtweets/trevorthalacker | 2 | null | transformers | 24,223 | ---
language: en
thumbnail: https://www.huggingtweets.com/trevorthalacker/1626593979307/predictions.png
tags:
- huggingtweets
widget:
- text: "My dream is"
---
<div class="inline-flex flex-col" style="line-height: 1.5;">
<div class="flex">
<div
style="display:inherit; margin-left: 4px; margin-right: 4px; width: 92px; height:92px; border-radius: 50%; background-size: cover; background-image: url('https://pbs.twimg.com/profile_images/1414806244536242178/hxQldoS0_400x400.jpg')">
</div>
<div
style="display:none; margin-left: 4px; margin-right: 4px; width: 92px; height:92px; border-radius: 50%; background-size: cover; background-image: url('')">
</div>
<div
style="display:none; margin-left: 4px; margin-right: 4px; width: 92px; height:92px; border-radius: 50%; background-size: cover; background-image: url('')">
</div>
</div>
<div style="text-align: center; margin-top: 3px; font-size: 16px; font-weight: 800">🤖 AI BOT 🤖</div>
<div style="text-align: center; font-size: 16px; font-weight: 800">Trevor</div>
<div style="text-align: center; font-size: 14px;">@trevorthalacker</div>
</div>
I was made with [huggingtweets](https://github.com/borisdayma/huggingtweets).
Create your own bot based on your favorite user with [the demo](https://colab.research.google.com/github/borisdayma/huggingtweets/blob/master/huggingtweets-demo.ipynb)!
## How does it work?
The model uses the following pipeline.
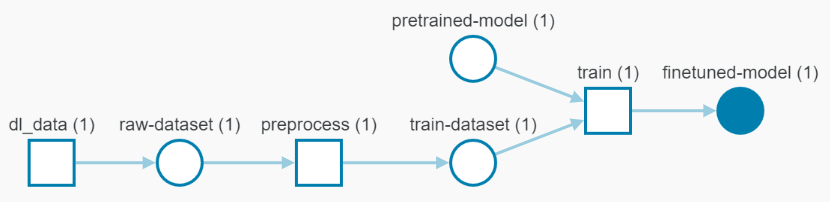
To understand how the model was developed, check the [W&B report](https://wandb.ai/wandb/huggingtweets/reports/HuggingTweets-Train-a-Model-to-Generate-Tweets--VmlldzoxMTY5MjI).
## Training data
The model was trained on tweets from Trevor.
| Data | Trevor |
| --- | --- |
| Tweets downloaded | 1792 |
| Retweets | 123 |
| Short tweets | 227 |
| Tweets kept | 1442 |
[Explore the data](https://wandb.ai/wandb/huggingtweets/runs/kmb92alm/artifacts), which is tracked with [W&B artifacts](https://docs.wandb.com/artifacts) at every step of the pipeline.
## Training procedure
The model is based on a pre-trained [GPT-2](https://huggingface.co/gpt2) which is fine-tuned on @trevorthalacker's tweets.
Hyperparameters and metrics are recorded in the [W&B training run](https://wandb.ai/wandb/huggingtweets/runs/37c7xs83) for full transparency and reproducibility.
At the end of training, [the final model](https://wandb.ai/wandb/huggingtweets/runs/37c7xs83/artifacts) is logged and versioned.
## How to use
You can use this model directly with a pipeline for text generation:
```python
from transformers import pipeline
generator = pipeline('text-generation',
model='huggingtweets/trevorthalacker')
generator("My dream is", num_return_sequences=5)
```
## Limitations and bias
The model suffers from [the same limitations and bias as GPT-2](https://huggingface.co/gpt2#limitations-and-bias).
In addition, the data present in the user's tweets further affects the text generated by the model.
## About
*Built by Boris Dayma*
[](https://twitter.com/intent/follow?screen_name=borisdayma)
For more details, visit the project repository.
[](https://github.com/borisdayma/huggingtweets)
|
huggingtweets/tszzl | 1c424f67145cdee9a1205c8fee11bc9fe56d6d7a | 2022-05-07T11:10:36.000Z | [
"pytorch",
"gpt2",
"text-generation",
"en",
"transformers",
"huggingtweets"
] | text-generation | false | huggingtweets | null | huggingtweets/tszzl | 2 | null | transformers | 24,224 | ---
language: en
thumbnail: http://www.huggingtweets.com/tszzl/1651921830963/predictions.png
tags:
- huggingtweets
widget:
- text: "My dream is"
---
<div class="inline-flex flex-col" style="line-height: 1.5;">
<div class="flex">
<div
style="display:inherit; margin-left: 4px; margin-right: 4px; width: 92px; height:92px; border-radius: 50%; background-size: cover; background-image: url('https://pbs.twimg.com/profile_images/1518044179217145857/vtps7fRk_400x400.jpg')">
</div>
<div
style="display:none; margin-left: 4px; margin-right: 4px; width: 92px; height:92px; border-radius: 50%; background-size: cover; background-image: url('')">
</div>
<div
style="display:none; margin-left: 4px; margin-right: 4px; width: 92px; height:92px; border-radius: 50%; background-size: cover; background-image: url('')">
</div>
</div>
<div style="text-align: center; margin-top: 3px; font-size: 16px; font-weight: 800">🤖 AI BOT 🤖</div>
<div style="text-align: center; font-size: 16px; font-weight: 800">roon</div>
<div style="text-align: center; font-size: 14px;">@tszzl</div>
</div>
I was made with [huggingtweets](https://github.com/borisdayma/huggingtweets).
Create your own bot based on your favorite user with [the demo](https://colab.research.google.com/github/borisdayma/huggingtweets/blob/master/huggingtweets-demo.ipynb)!
## How does it work?
The model uses the following pipeline.
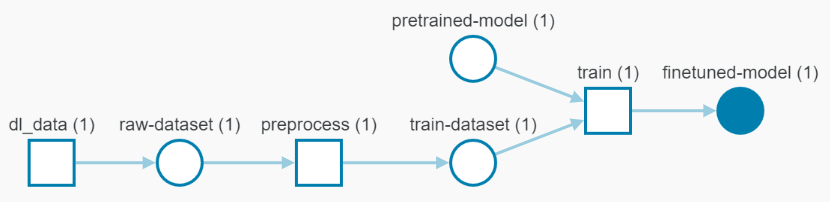
To understand how the model was developed, check the [W&B report](https://wandb.ai/wandb/huggingtweets/reports/HuggingTweets-Train-a-Model-to-Generate-Tweets--VmlldzoxMTY5MjI).
## Training data
The model was trained on tweets from roon.
| Data | roon |
| --- | --- |
| Tweets downloaded | 3237 |
| Retweets | 548 |
| Short tweets | 534 |
| Tweets kept | 2155 |
[Explore the data](https://wandb.ai/wandb/huggingtweets/runs/y00ywvfi/artifacts), which is tracked with [W&B artifacts](https://docs.wandb.com/artifacts) at every step of the pipeline.
## Training procedure
The model is based on a pre-trained [GPT-2](https://huggingface.co/gpt2) which is fine-tuned on @tszzl's tweets.
Hyperparameters and metrics are recorded in the [W&B training run](https://wandb.ai/wandb/huggingtweets/runs/3tyvy3sv) for full transparency and reproducibility.
At the end of training, [the final model](https://wandb.ai/wandb/huggingtweets/runs/3tyvy3sv/artifacts) is logged and versioned.
## How to use
You can use this model directly with a pipeline for text generation:
```python
from transformers import pipeline
generator = pipeline('text-generation',
model='huggingtweets/tszzl')
generator("My dream is", num_return_sequences=5)
```
## Limitations and bias
The model suffers from [the same limitations and bias as GPT-2](https://huggingface.co/gpt2#limitations-and-bias).
In addition, the data present in the user's tweets further affects the text generated by the model.
## About
*Built by Boris Dayma*
[](https://twitter.com/intent/follow?screen_name=borisdayma)
For more details, visit the project repository.
[](https://github.com/borisdayma/huggingtweets)
|
huggingtweets/twentyonepilots | 949f9d6e5a4c9dc60c8f52b7d6fa9c71ef5ee7a4 | 2022-01-29T07:40:09.000Z | [
"pytorch",
"gpt2",
"text-generation",
"en",
"transformers",
"huggingtweets"
] | text-generation | false | huggingtweets | null | huggingtweets/twentyonepilots | 2 | null | transformers | 24,225 | ---
language: en
thumbnail: http://www.huggingtweets.com/twentyonepilots/1643442004355/predictions.png
tags:
- huggingtweets
widget:
- text: "My dream is"
---
<div class="inline-flex flex-col" style="line-height: 1.5;">
<div class="flex">
<div
style="display:inherit; margin-left: 4px; margin-right: 4px; width: 92px; height:92px; border-radius: 50%; background-size: cover; background-image: url('https://pbs.twimg.com/profile_images/1379847503324057601/LH84R4zr_400x400.jpg')">
</div>
<div
style="display:none; margin-left: 4px; margin-right: 4px; width: 92px; height:92px; border-radius: 50%; background-size: cover; background-image: url('')">
</div>
<div
style="display:none; margin-left: 4px; margin-right: 4px; width: 92px; height:92px; border-radius: 50%; background-size: cover; background-image: url('')">
</div>
</div>
<div style="text-align: center; margin-top: 3px; font-size: 16px; font-weight: 800">🤖 AI BOT 🤖</div>
<div style="text-align: center; font-size: 16px; font-weight: 800">twenty one pilots</div>
<div style="text-align: center; font-size: 14px;">@twentyonepilots</div>
</div>
I was made with [huggingtweets](https://github.com/borisdayma/huggingtweets).
Create your own bot based on your favorite user with [the demo](https://colab.research.google.com/github/borisdayma/huggingtweets/blob/master/huggingtweets-demo.ipynb)!
## How does it work?
The model uses the following pipeline.
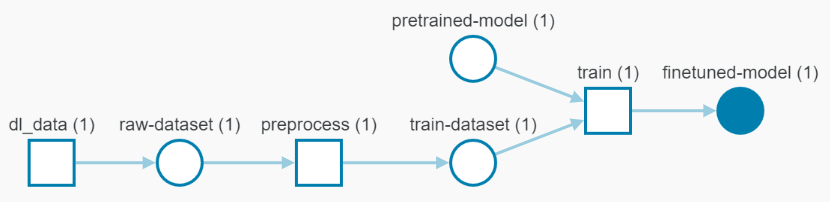
To understand how the model was developed, check the [W&B report](https://wandb.ai/wandb/huggingtweets/reports/HuggingTweets-Train-a-Model-to-Generate-Tweets--VmlldzoxMTY5MjI).
## Training data
The model was trained on tweets from twenty one pilots.
| Data | twenty one pilots |
| --- | --- |
| Tweets downloaded | 3190 |
| Retweets | 537 |
| Short tweets | 287 |
| Tweets kept | 2366 |
[Explore the data](https://wandb.ai/wandb/huggingtweets/runs/1cw9xn7c/artifacts), which is tracked with [W&B artifacts](https://docs.wandb.com/artifacts) at every step of the pipeline.
## Training procedure
The model is based on a pre-trained [GPT-2](https://huggingface.co/gpt2) which is fine-tuned on @twentyonepilots's tweets.
Hyperparameters and metrics are recorded in the [W&B training run](https://wandb.ai/wandb/huggingtweets/runs/trh1am21) for full transparency and reproducibility.
At the end of training, [the final model](https://wandb.ai/wandb/huggingtweets/runs/trh1am21/artifacts) is logged and versioned.
## How to use
You can use this model directly with a pipeline for text generation:
```python
from transformers import pipeline
generator = pipeline('text-generation',
model='huggingtweets/twentyonepilots')
generator("My dream is", num_return_sequences=5)
```
## Limitations and bias
The model suffers from [the same limitations and bias as GPT-2](https://huggingface.co/gpt2#limitations-and-bias).
In addition, the data present in the user's tweets further affects the text generated by the model.
## About
*Built by Boris Dayma*
[](https://twitter.com/intent/follow?screen_name=borisdayma)
For more details, visit the project repository.
[](https://github.com/borisdayma/huggingtweets)
|
huggingtweets/urst0ff | 8e3389da27f27a4d7363849dc3b47cdf8c12e33e | 2021-05-23T03:27:17.000Z | [
"pytorch",
"jax",
"gpt2",
"text-generation",
"en",
"transformers",
"huggingtweets"
] | text-generation | false | huggingtweets | null | huggingtweets/urst0ff | 2 | null | transformers | 24,226 | ---
language: en
thumbnail: https://www.huggingtweets.com/urst0ff/1616685735707/predictions.png
tags:
- huggingtweets
widget:
- text: "My dream is"
---
<div>
<div style="width: 132px; height:132px; border-radius: 50%; background-size: cover; background-image: url('https://pbs.twimg.com/profile_images/1196907550144442382/SvFy5c0Q_400x400.jpg')">
</div>
<div style="margin-top: 8px; font-size: 19px; font-weight: 800">death metal minivan 🤖 AI Bot </div>
<div style="font-size: 15px">@urst0ff bot</div>
</div>
I was made with [huggingtweets](https://github.com/borisdayma/huggingtweets).
Create your own bot based on your favorite user with [the demo](https://colab.research.google.com/github/borisdayma/huggingtweets/blob/master/huggingtweets-demo.ipynb)!
## How does it work?
The model uses the following pipeline.
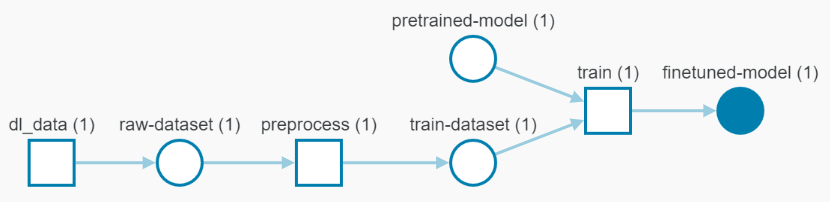
To understand how the model was developed, check the [W&B report](https://wandb.ai/wandb/huggingtweets/reports/HuggingTweets-Train-a-Model-to-Generate-Tweets--VmlldzoxMTY5MjI).
## Training data
The model was trained on [@urst0ff's tweets](https://twitter.com/urst0ff).
| Data | Quantity |
| --- | --- |
| Tweets downloaded | 1955 |
| Retweets | 75 |
| Short tweets | 151 |
| Tweets kept | 1729 |
[Explore the data](https://wandb.ai/wandb/huggingtweets/runs/1u8zc1mk/artifacts), which is tracked with [W&B artifacts](https://docs.wandb.com/artifacts) at every step of the pipeline.
## Training procedure
The model is based on a pre-trained [GPT-2](https://huggingface.co/gpt2) which is fine-tuned on @urst0ff's tweets.
Hyperparameters and metrics are recorded in the [W&B training run](https://wandb.ai/wandb/huggingtweets/runs/yg1cb4yl) for full transparency and reproducibility.
At the end of training, [the final model](https://wandb.ai/wandb/huggingtweets/runs/yg1cb4yl/artifacts) is logged and versioned.
## How to use
You can use this model directly with a pipeline for text generation:
```python
from transformers import pipeline
generator = pipeline('text-generation',
model='huggingtweets/urst0ff')
generator("My dream is", num_return_sequences=5)
```
## Limitations and bias
The model suffers from [the same limitations and bias as GPT-2](https://huggingface.co/gpt2#limitations-and-bias).
In addition, the data present in the user's tweets further affects the text generated by the model.
## About
*Built by Boris Dayma*
[](https://twitter.com/intent/follow?screen_name=borisdayma)
For more details, visit the project repository.
[](https://github.com/borisdayma/huggingtweets)
|
huggingtweets/vercel | c539e793a2e33d758d6a55a5e5ea2077973eda96 | 2021-05-23T03:46:00.000Z | [
"pytorch",
"jax",
"gpt2",
"text-generation",
"en",
"transformers",
"huggingtweets"
] | text-generation | false | huggingtweets | null | huggingtweets/vercel | 2 | null | transformers | 24,227 | ---
language: en
thumbnail: https://github.com/borisdayma/huggingtweets/blob/master/img/logo.png?raw=true
tags:
- huggingtweets
widget:
- text: "My dream is"
---
<link rel="stylesheet" href="https://unpkg.com/@tailwindcss/[email protected]/dist/typography.min.css">
<style>
@media (prefers-color-scheme: dark) {
.prose { color: #E2E8F0 !important; }
.prose h2, .prose h3, .prose a, .prose thead { color: #F7FAFC !important; }
}
</style>
<section class='prose'>
<div>
<div style="width: 132px; height:132px; border-radius: 50%; background-size: cover; background-image: url('https://pbs.twimg.com/profile_images/1252531684353998848/6R0-p1Vf_400x400.jpg')">
</div>
<div style="margin-top: 8px; font-size: 19px; font-weight: 800">Vercel 🤖 AI Bot </div>
<div style="font-size: 15px; color: #657786">@vercel bot</div>
</div>
I was made with [huggingtweets](https://github.com/borisdayma/huggingtweets).
Create your own bot based on your favorite user with [the demo](https://colab.research.google.com/github/borisdayma/huggingtweets/blob/master/huggingtweets-demo.ipynb)!
## How does it work?
The model uses the following pipeline.
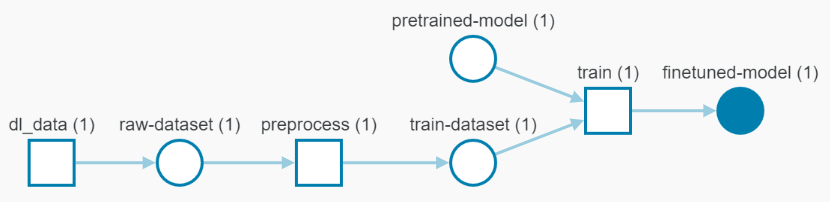
To understand how the model was developed, check the [W&B report](https://app.wandb.ai/wandb/huggingtweets/reports/HuggingTweets-Train-a-model-to-generate-tweets--VmlldzoxMTY5MjI).
## Training data
The model was trained on [@vercel's tweets](https://twitter.com/vercel).
<table style='border-width:0'>
<thead style='border-width:0'>
<tr style='border-width:0 0 1px 0; border-color: #CBD5E0'>
<th style='border-width:0'>Data</th>
<th style='border-width:0'>Quantity</th>
</tr>
</thead>
<tbody style='border-width:0'>
<tr style='border-width:0 0 1px 0; border-color: #E2E8F0'>
<td style='border-width:0'>Tweets downloaded</td>
<td style='border-width:0'>1072</td>
</tr>
<tr style='border-width:0 0 1px 0; border-color: #E2E8F0'>
<td style='border-width:0'>Retweets</td>
<td style='border-width:0'>339</td>
</tr>
<tr style='border-width:0 0 1px 0; border-color: #E2E8F0'>
<td style='border-width:0'>Short tweets</td>
<td style='border-width:0'>102</td>
</tr>
<tr style='border-width:0'>
<td style='border-width:0'>Tweets kept</td>
<td style='border-width:0'>631</td>
</tr>
</tbody>
</table>
[Explore the data](https://app.wandb.ai/wandb/huggingtweets/runs/2e8z8gby/artifacts), which is tracked with [W&B artifacts](https://docs.wandb.com/artifacts) at every step of the pipeline.
## Training procedure
The model is based on a pre-trained [GPT-2](https://huggingface.co/gpt2) which is fine-tuned on @vercel's tweets.
Hyperparameters and metrics are recorded in the [W&B training run](https://app.wandb.ai/wandb/huggingtweets/runs/3svt5mgv) for full transparency and reproducibility.
At the end of training, [the final model](https://app.wandb.ai/wandb/huggingtweets/runs/3svt5mgv/artifacts) is logged and versioned.
## Intended uses & limitations
### How to use
You can use this model directly with a pipeline for text generation:
<pre><code><span style="color:#03A9F4">from</span> transformers <span style="color:#03A9F4">import</span> pipeline
generator = pipeline(<span style="color:#FF9800">'text-generation'</span>,
model=<span style="color:#FF9800">'huggingtweets/vercel'</span>)
generator(<span style="color:#FF9800">"My dream is"</span>, num_return_sequences=<span style="color:#8BC34A">5</span>)</code></pre>
### Limitations and bias
The model suffers from [the same limitations and bias as GPT-2](https://huggingface.co/gpt2#limitations-and-bias).
In addition, the data present in the user's tweets further affects the text generated by the model.
## About
*Built by Boris Dayma*
</section>
[](https://twitter.com/intent/follow?screen_name=borisdayma)
<section class='prose'>
For more details, visit the project repository.
</section>
[](https://github.com/borisdayma/huggingtweets)
<!--- random size file --> |
huggingtweets/viral_b_shah | 704823290d95cfea5a77409405207ffd68f1e983 | 2021-05-23T03:57:09.000Z | [
"pytorch",
"jax",
"gpt2",
"text-generation",
"en",
"transformers",
"huggingtweets"
] | text-generation | false | huggingtweets | null | huggingtweets/viral_b_shah | 2 | null | transformers | 24,228 | ---
language: en
thumbnail: https://github.com/borisdayma/huggingtweets/blob/master/img/logo_share.png?raw=true
tags:
- huggingtweets
widget:
- text: "My dream is"
---
<link rel="stylesheet" href="https://unpkg.com/@tailwindcss/[email protected]/dist/typography.min.css">
<style>
@media (prefers-color-scheme: dark) {
.prose { color: #E2E8F0 !important; }
.prose h2, .prose h3, .prose a, .prose thead { color: #F7FAFC !important; }
}
</style>
<section class='prose'>
<div>
<div style="width: 132px; height:132px; border-radius: 50%; background-size: cover; background-image: url('http://pbs.twimg.com/profile_images/832605174547898368/HMFMaln__400x400.jpg')">
</div>
<div style="margin-top: 8px; font-size: 19px; font-weight: 800">Viral B. Shah 🤖 AI Bot </div>
<div style="font-size: 15px; color: #657786">@viral_b_shah bot</div>
</div>
I was made with [huggingtweets](https://github.com/borisdayma/huggingtweets).
Create your own bot based on your favorite user with [the demo](https://colab.research.google.com/github/borisdayma/huggingtweets/blob/master/huggingtweets-demo.ipynb)!
## How does it work?
The model uses the following pipeline.
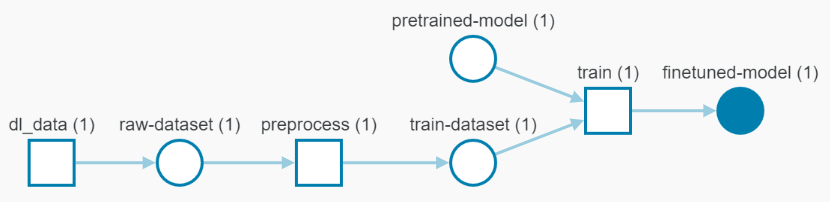
To understand how the model was developed, check the [W&B report](https://app.wandb.ai/wandb/huggingtweets/reports/HuggingTweets-Train-a-model-to-generate-tweets--VmlldzoxMTY5MjI).
## Training data
The model was trained on [@viral_b_shah's tweets](https://twitter.com/viral_b_shah).
<table style='border-width:0'>
<thead style='border-width:0'>
<tr style='border-width:0 0 1px 0; border-color: #CBD5E0'>
<th style='border-width:0'>Data</th>
<th style='border-width:0'>Quantity</th>
</tr>
</thead>
<tbody style='border-width:0'>
<tr style='border-width:0 0 1px 0; border-color: #E2E8F0'>
<td style='border-width:0'>Tweets downloaded</td>
<td style='border-width:0'>3218</td>
</tr>
<tr style='border-width:0 0 1px 0; border-color: #E2E8F0'>
<td style='border-width:0'>Retweets</td>
<td style='border-width:0'>2233</td>
</tr>
<tr style='border-width:0 0 1px 0; border-color: #E2E8F0'>
<td style='border-width:0'>Short tweets</td>
<td style='border-width:0'>42</td>
</tr>
<tr style='border-width:0'>
<td style='border-width:0'>Tweets kept</td>
<td style='border-width:0'>943</td>
</tr>
</tbody>
</table>
[Explore the data](https://app.wandb.ai/wandb/huggingtweets/runs/i029wkup/artifacts), which is tracked with [W&B artifacts](https://docs.wandb.com/artifacts) at every step of the pipeline.
## Training procedure
The model is based on a pre-trained [GPT-2](https://huggingface.co/gpt2) which is fine-tuned on @viral_b_shah's tweets.
Hyperparameters and metrics are recorded in the [W&B training run](https://app.wandb.ai/wandb/huggingtweets/runs/2hd72k2x) for full transparency and reproducibility.
At the end of training, [the final model](https://app.wandb.ai/wandb/huggingtweets/runs/2hd72k2x/artifacts) is logged and versioned.
## Intended uses & limitations
### How to use
You can use this model directly with a pipeline for text generation:
<pre><code><span style="color:#03A9F4">from</span> transformers <span style="color:#03A9F4">import</span> pipeline
generator = pipeline(<span style="color:#FF9800">'text-generation'</span>,
model=<span style="color:#FF9800">'huggingtweets/viral_b_shah'</span>)
generator(<span style="color:#FF9800">"My dream is"</span>, num_return_sequences=<span style="color:#8BC34A">5</span>)</code></pre>
### Limitations and bias
The model suffers from [the same limitations and bias as GPT-2](https://huggingface.co/gpt2#limitations-and-bias).
In addition, the data present in the user's tweets further affects the text generated by the model.
## About
*Built by Boris Dayma*
</section>
[](https://twitter.com/borisdayma)
<section class='prose'>
For more details, visit the project repository.
</section>
[](https://github.com/borisdayma/huggingtweets)
|
huggingtweets/vitalikbuterin | 55baf8ebca82ec87bf4afd62456e53e6a541c2f1 | 2021-05-23T04:01:46.000Z | [
"pytorch",
"jax",
"gpt2",
"text-generation",
"en",
"transformers",
"huggingtweets"
] | text-generation | false | huggingtweets | null | huggingtweets/vitalikbuterin | 2 | null | transformers | 24,229 | ---
language: en
thumbnail: https://www.huggingtweets.com/vitalikbuterin/1607573059057/predictions.png
tags:
- huggingtweets
widget:
- text: "My dream is"
---
<link rel="stylesheet" href="https://unpkg.com/@tailwindcss/[email protected]/dist/typography.min.css">
<style>
@media (prefers-color-scheme: dark) {
.prose { color: #E2E8F0 !important; }
.prose h2, .prose h3, .prose a, .prose thead { color: #F7FAFC !important; }
}
</style>
<section class='prose'>
<div>
<div style="width: 132px; height:132px; border-radius: 50%; background-size: cover; background-image: url('https://pbs.twimg.com/profile_images/977496875887558661/L86xyLF4_400x400.jpg')">
</div>
<div style="margin-top: 8px; font-size: 19px; font-weight: 800">vitalik.eth 🤖 AI Bot </div>
<div style="font-size: 15px; color: #657786">@vitalikbuterin bot</div>
</div>
I was made with [huggingtweets](https://github.com/borisdayma/huggingtweets).
Create your own bot based on your favorite user with [the demo](https://colab.research.google.com/github/borisdayma/huggingtweets/blob/master/huggingtweets-demo.ipynb)!
## How does it work?
The model uses the following pipeline.
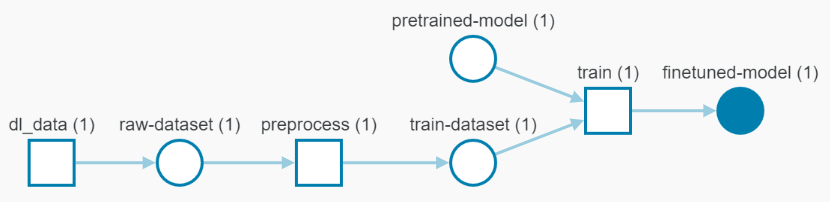
To understand how the model was developed, check the [W&B report](https://app.wandb.ai/wandb/huggingtweets/reports/HuggingTweets-Train-a-model-to-generate-tweets--VmlldzoxMTY5MjI).
## Training data
The model was trained on [@vitalikbuterin's tweets](https://twitter.com/vitalikbuterin).
<table style='border-width:0'>
<thead style='border-width:0'>
<tr style='border-width:0 0 1px 0; border-color: #CBD5E0'>
<th style='border-width:0'>Data</th>
<th style='border-width:0'>Quantity</th>
</tr>
</thead>
<tbody style='border-width:0'>
<tr style='border-width:0 0 1px 0; border-color: #E2E8F0'>
<td style='border-width:0'>Tweets downloaded</td>
<td style='border-width:0'>3235</td>
</tr>
<tr style='border-width:0 0 1px 0; border-color: #E2E8F0'>
<td style='border-width:0'>Retweets</td>
<td style='border-width:0'>287</td>
</tr>
<tr style='border-width:0 0 1px 0; border-color: #E2E8F0'>
<td style='border-width:0'>Short tweets</td>
<td style='border-width:0'>122</td>
</tr>
<tr style='border-width:0'>
<td style='border-width:0'>Tweets kept</td>
<td style='border-width:0'>2826</td>
</tr>
</tbody>
</table>
[Explore the data](https://wandb.ai/wandb/huggingtweets/runs/12dt6biy/artifacts), which is tracked with [W&B artifacts](https://docs.wandb.com/artifacts) at every step of the pipeline.
## Training procedure
The model is based on a pre-trained [GPT-2](https://huggingface.co/gpt2) which is fine-tuned on @vitalikbuterin's tweets.
Hyperparameters and metrics are recorded in the [W&B training run](https://wandb.ai/wandb/huggingtweets/runs/12ceuzt7) for full transparency and reproducibility.
At the end of training, [the final model](https://wandb.ai/wandb/huggingtweets/runs/12ceuzt7/artifacts) is logged and versioned.
## Intended uses & limitations
### How to use
You can use this model directly with a pipeline for text generation:
<pre><code><span style="color:#03A9F4">from</span> transformers <span style="color:#03A9F4">import</span> pipeline
generator = pipeline(<span style="color:#FF9800">'text-generation'</span>,
model=<span style="color:#FF9800">'huggingtweets/vitalikbuterin'</span>)
generator(<span style="color:#FF9800">"My dream is"</span>, num_return_sequences=<span style="color:#8BC34A">5</span>)</code></pre>
### Limitations and bias
The model suffers from [the same limitations and bias as GPT-2](https://huggingface.co/gpt2#limitations-and-bias).
In addition, the data present in the user's tweets further affects the text generated by the model.
## About
*Built by Boris Dayma*
</section>
[](https://twitter.com/intent/follow?screen_name=borisdayma)
<section class='prose'>
For more details, visit the project repository.
</section>
[](https://github.com/borisdayma/huggingtweets)
|
huggingtweets/wausaubob | 71f3b2c67e530b7835d5633f34b026043789de8f | 2021-05-23T04:14:29.000Z | [
"pytorch",
"jax",
"gpt2",
"text-generation",
"en",
"transformers",
"huggingtweets"
] | text-generation | false | huggingtweets | null | huggingtweets/wausaubob | 2 | null | transformers | 24,230 | ---
language: en
thumbnail: https://www.huggingtweets.com/wausaubob/1616731136628/predictions.png
tags:
- huggingtweets
widget:
- text: "My dream is"
---
<div>
<div style="width: 132px; height:132px; border-radius: 50%; background-size: cover; background-image: url('https://pbs.twimg.com/profile_images/1374548471995305986/XVI8-nGF_400x400.jpg')">
</div>
<div style="margin-top: 8px; font-size: 19px; font-weight: 800">Be Like Bob—Stay Home 🤖 AI Bot </div>
<div style="font-size: 15px">@wausaubob bot</div>
</div>
I was made with [huggingtweets](https://github.com/borisdayma/huggingtweets).
Create your own bot based on your favorite user with [the demo](https://colab.research.google.com/github/borisdayma/huggingtweets/blob/master/huggingtweets-demo.ipynb)!
## How does it work?
The model uses the following pipeline.
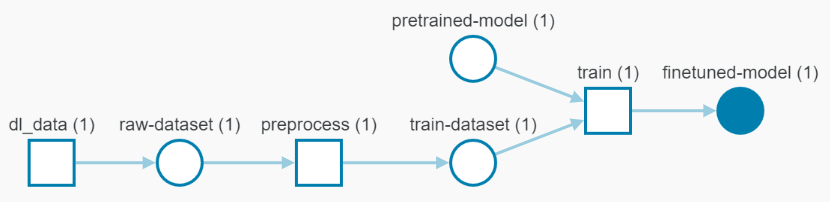
To understand how the model was developed, check the [W&B report](https://wandb.ai/wandb/huggingtweets/reports/HuggingTweets-Train-a-Model-to-Generate-Tweets--VmlldzoxMTY5MjI).
## Training data
The model was trained on [@wausaubob's tweets](https://twitter.com/wausaubob).
| Data | Quantity |
| --- | --- |
| Tweets downloaded | 744 |
| Retweets | 77 |
| Short tweets | 135 |
| Tweets kept | 532 |
[Explore the data](https://wandb.ai/wandb/huggingtweets/runs/2h6y30jv/artifacts), which is tracked with [W&B artifacts](https://docs.wandb.com/artifacts) at every step of the pipeline.
## Training procedure
The model is based on a pre-trained [GPT-2](https://huggingface.co/gpt2) which is fine-tuned on @wausaubob's tweets.
Hyperparameters and metrics are recorded in the [W&B training run](https://wandb.ai/wandb/huggingtweets/runs/2ocvosw0) for full transparency and reproducibility.
At the end of training, [the final model](https://wandb.ai/wandb/huggingtweets/runs/2ocvosw0/artifacts) is logged and versioned.
## How to use
You can use this model directly with a pipeline for text generation:
```python
from transformers import pipeline
generator = pipeline('text-generation',
model='huggingtweets/wausaubob')
generator("My dream is", num_return_sequences=5)
```
## Limitations and bias
The model suffers from [the same limitations and bias as GPT-2](https://huggingface.co/gpt2#limitations-and-bias).
In addition, the data present in the user's tweets further affects the text generated by the model.
## About
*Built by Boris Dayma*
[](https://twitter.com/intent/follow?screen_name=borisdayma)
For more details, visit the project repository.
[](https://github.com/borisdayma/huggingtweets)
|
huggingtweets/wellypooscene | 4cb254503fe9e6f300fa47298264deadbbcbbc8f | 2021-05-23T04:18:56.000Z | [
"pytorch",
"jax",
"gpt2",
"text-generation",
"en",
"transformers",
"huggingtweets"
] | text-generation | false | huggingtweets | null | huggingtweets/wellypooscene | 2 | null | transformers | 24,231 | ---
language: en
thumbnail: https://www.huggingtweets.com/wellypooscene/1616644578299/predictions.png
tags:
- huggingtweets
widget:
- text: "My dream is"
---
<div>
<div style="width: 132px; height:132px; border-radius: 50%; background-size: cover; background-image: url('https://pbs.twimg.com/profile_images/914278196987568128/20uJJCTQ_400x400.jpg')">
</div>
<div style="margin-top: 8px; font-size: 19px; font-weight: 800">cowtown afficionado 🤖 AI Bot </div>
<div style="font-size: 15px">@wellypooscene bot</div>
</div>
I was made with [huggingtweets](https://github.com/borisdayma/huggingtweets).
Create your own bot based on your favorite user with [the demo](https://colab.research.google.com/github/borisdayma/huggingtweets/blob/master/huggingtweets-demo.ipynb)!
## How does it work?
The model uses the following pipeline.
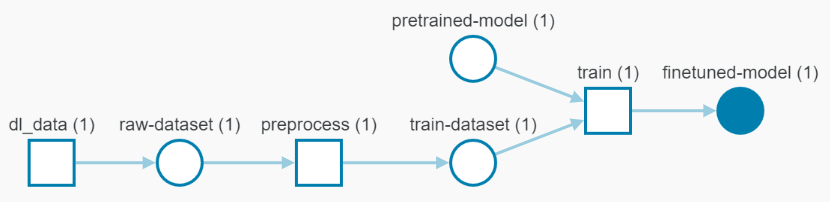
To understand how the model was developed, check the [W&B report](https://app.wandb.ai/wandb/huggingtweets/reports/HuggingTweets-Train-a-model-to-generate-tweets--VmlldzoxMTY5MjI).
## Training data
The model was trained on [@wellypooscene's tweets](https://twitter.com/wellypooscene).
| Data | Quantity |
| --- | --- |
| Tweets downloaded | 414 |
| Retweets | 40 |
| Short tweets | 35 |
| Tweets kept | 339 |
[Explore the data](https://wandb.ai/wandb/huggingtweets/runs/2mtl43mo/artifacts), which is tracked with [W&B artifacts](https://docs.wandb.com/artifacts) at every step of the pipeline.
## Training procedure
The model is based on a pre-trained [GPT-2](https://huggingface.co/gpt2) which is fine-tuned on @wellypooscene's tweets.
Hyperparameters and metrics are recorded in the [W&B training run](https://wandb.ai/wandb/huggingtweets/runs/38ufdelt) for full transparency and reproducibility.
At the end of training, [the final model](https://wandb.ai/wandb/huggingtweets/runs/38ufdelt/artifacts) is logged and versioned.
## How to use
You can use this model directly with a pipeline for text generation:
```python
from transformers import pipeline
generator = pipeline('text-generation',
model='huggingtweets/wellypooscene')
generator("My dream is", num_return_sequences=5)
```
## Limitations and bias
The model suffers from [the same limitations and bias as GPT-2](https://huggingface.co/gpt2#limitations-and-bias).
In addition, the data present in the user's tweets further affects the text generated by the model.
## About
*Built by Boris Dayma*
[](https://twitter.com/intent/follow?screen_name=borisdayma)
For more details, visit the project repository.
[](https://github.com/borisdayma/huggingtweets)
|
huggingtweets/woketopus | 72e9ecc53a0bc1b9bbee0c9587f8372d8a6047d2 | 2021-05-23T04:33:18.000Z | [
"pytorch",
"jax",
"gpt2",
"text-generation",
"en",
"transformers",
"huggingtweets"
] | text-generation | false | huggingtweets | null | huggingtweets/woketopus | 2 | null | transformers | 24,232 | ---
language: en
thumbnail: https://www.huggingtweets.com/woketopus/1614149251542/predictions.png
tags:
- huggingtweets
widget:
- text: "My dream is"
---
<div>
<div style="width: 132px; height:132px; border-radius: 50%; background-size: cover; background-image: url('https://pbs.twimg.com/profile_images/1363814877119336451/DtC1OuMG_400x400.jpg')">
</div>
<div style="margin-top: 8px; font-size: 19px; font-weight: 800">woketopus is grooving 🤖 AI Bot </div>
<div style="font-size: 15px">@woketopus bot</div>
</div>
I was made with [huggingtweets](https://github.com/borisdayma/huggingtweets).
Create your own bot based on your favorite user with [the demo](https://colab.research.google.com/github/borisdayma/huggingtweets/blob/master/huggingtweets-demo.ipynb)!
## How does it work?
The model uses the following pipeline.
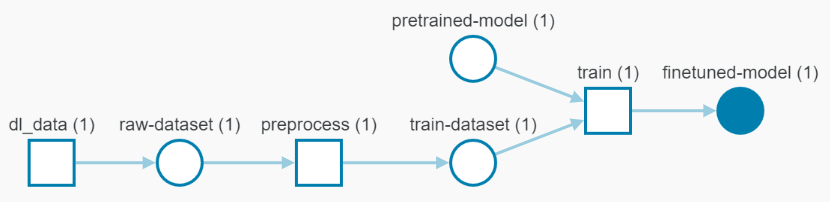
To understand how the model was developed, check the [W&B report](https://app.wandb.ai/wandb/huggingtweets/reports/HuggingTweets-Train-a-model-to-generate-tweets--VmlldzoxMTY5MjI).
## Training data
The model was trained on [@woketopus's tweets](https://twitter.com/woketopus).
| Data | Quantity |
| --- | --- |
| Tweets downloaded | 3219 |
| Retweets | 217 |
| Short tweets | 646 |
| Tweets kept | 2356 |
[Explore the data](https://wandb.ai/wandb/huggingtweets/runs/3t0s1gfu/artifacts), which is tracked with [W&B artifacts](https://docs.wandb.com/artifacts) at every step of the pipeline.
## Training procedure
The model is based on a pre-trained [GPT-2](https://huggingface.co/gpt2) which is fine-tuned on @woketopus's tweets.
Hyperparameters and metrics are recorded in the [W&B training run](https://wandb.ai/wandb/huggingtweets/runs/1s4xkpp2) for full transparency and reproducibility.
At the end of training, [the final model](https://wandb.ai/wandb/huggingtweets/runs/1s4xkpp2/artifacts) is logged and versioned.
## How to use
You can use this model directly with a pipeline for text generation:
```python
from transformers import pipeline
generator = pipeline('text-generation',
model='huggingtweets/woketopus')
generator("My dream is", num_return_sequences=5)
```
## Limitations and bias
The model suffers from [the same limitations and bias as GPT-2](https://huggingface.co/gpt2#limitations-and-bias).
In addition, the data present in the user's tweets further affects the text generated by the model.
## About
*Built by Boris Dayma*
[](https://twitter.com/intent/follow?screen_name=borisdayma)
For more details, visit the project repository.
[](https://github.com/borisdayma/huggingtweets)
|
huggingtweets/wolfejosh | 4af64a49e2c5ef1aee7be04f1b3e5de836190cd3 | 2021-05-23T04:34:25.000Z | [
"pytorch",
"jax",
"gpt2",
"text-generation",
"en",
"transformers",
"huggingtweets"
] | text-generation | false | huggingtweets | null | huggingtweets/wolfejosh | 2 | null | transformers | 24,233 | ---
language: en
thumbnail: https://www.huggingtweets.com/wolfejosh/1616623620980/predictions.png
tags:
- huggingtweets
widget:
- text: "My dream is"
---
<div>
<div style="width: 132px; height:132px; border-radius: 50%; background-size: cover; background-image: url('https://pbs.twimg.com/profile_images/1253094103413395456/1OXl60FT_400x400.jpg')">
</div>
<div style="margin-top: 8px; font-size: 19px; font-weight: 800">Josh Wolfe 🤖 AI Bot </div>
<div style="font-size: 15px">@wolfejosh bot</div>
</div>
I was made with [huggingtweets](https://github.com/borisdayma/huggingtweets).
Create your own bot based on your favorite user with [the demo](https://colab.research.google.com/github/borisdayma/huggingtweets/blob/master/huggingtweets-demo.ipynb)!
## How does it work?
The model uses the following pipeline.
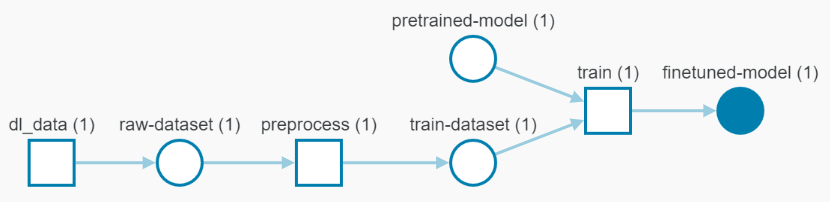
To understand how the model was developed, check the [W&B report](https://app.wandb.ai/wandb/huggingtweets/reports/HuggingTweets-Train-a-model-to-generate-tweets--VmlldzoxMTY5MjI).
## Training data
The model was trained on [@wolfejosh's tweets](https://twitter.com/wolfejosh).
| Data | Quantity |
| --- | --- |
| Tweets downloaded | 3245 |
| Retweets | 838 |
| Short tweets | 374 |
| Tweets kept | 2033 |
[Explore the data](https://wandb.ai/wandb/huggingtweets/runs/121x3hw4/artifacts), which is tracked with [W&B artifacts](https://docs.wandb.com/artifacts) at every step of the pipeline.
## Training procedure
The model is based on a pre-trained [GPT-2](https://huggingface.co/gpt2) which is fine-tuned on @wolfejosh's tweets.
Hyperparameters and metrics are recorded in the [W&B training run](https://wandb.ai/wandb/huggingtweets/runs/2poyfrja) for full transparency and reproducibility.
At the end of training, [the final model](https://wandb.ai/wandb/huggingtweets/runs/2poyfrja/artifacts) is logged and versioned.
## How to use
You can use this model directly with a pipeline for text generation:
```python
from transformers import pipeline
generator = pipeline('text-generation',
model='huggingtweets/wolfejosh')
generator("My dream is", num_return_sequences=5)
```
## Limitations and bias
The model suffers from [the same limitations and bias as GPT-2](https://huggingface.co/gpt2#limitations-and-bias).
In addition, the data present in the user's tweets further affects the text generated by the model.
## About
*Built by Boris Dayma*
[](https://twitter.com/intent/follow?screen_name=borisdayma)
For more details, visit the project repository.
[](https://github.com/borisdayma/huggingtweets)
|
huggingtweets/xinqisu | 5552c0561be4dd4bd7767a3195a3d9431f3f3084 | 2021-05-23T04:51:48.000Z | [
"pytorch",
"jax",
"gpt2",
"text-generation",
"en",
"transformers",
"huggingtweets"
] | text-generation | false | huggingtweets | null | huggingtweets/xinqisu | 2 | null | transformers | 24,234 | ---
language: en
thumbnail: https://www.huggingtweets.com/xinqisu/1607803999992/predictions.png
tags:
- huggingtweets
widget:
- text: "My dream is"
---
<link rel="stylesheet" href="https://unpkg.com/@tailwindcss/[email protected]/dist/typography.min.css">
<style>
@media (prefers-color-scheme: dark) {
.prose { color: #E2E8F0 !important; }
.prose h2, .prose h3, .prose a, .prose thead { color: #F7FAFC !important; }
}
</style>
<section class='prose'>
<div>
<div style="width: 132px; height:132px; border-radius: 50%; background-size: cover; background-image: url('https://pbs.twimg.com/profile_images/1185460679550984192/jCxIECDA_400x400.jpg')">
</div>
<div style="margin-top: 8px; font-size: 19px; font-weight: 800">Xinqi Su 蘇昕琪 🤖 AI Bot </div>
<div style="font-size: 15px; color: #657786">@xinqisu bot</div>
</div>
I was made with [huggingtweets](https://github.com/borisdayma/huggingtweets).
Create your own bot based on your favorite user with [the demo](https://colab.research.google.com/github/borisdayma/huggingtweets/blob/master/huggingtweets-demo.ipynb)!
## How does it work?
The model uses the following pipeline.
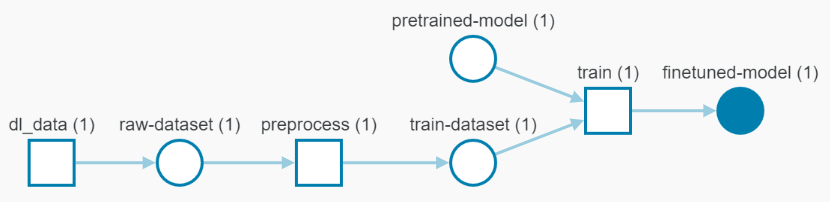
To understand how the model was developed, check the [W&B report](https://app.wandb.ai/wandb/huggingtweets/reports/HuggingTweets-Train-a-model-to-generate-tweets--VmlldzoxMTY5MjI).
## Training data
The model was trained on [@xinqisu's tweets](https://twitter.com/xinqisu).
<table style='border-width:0'>
<thead style='border-width:0'>
<tr style='border-width:0 0 1px 0; border-color: #CBD5E0'>
<th style='border-width:0'>Data</th>
<th style='border-width:0'>Quantity</th>
</tr>
</thead>
<tbody style='border-width:0'>
<tr style='border-width:0 0 1px 0; border-color: #E2E8F0'>
<td style='border-width:0'>Tweets downloaded</td>
<td style='border-width:0'>3248</td>
</tr>
<tr style='border-width:0 0 1px 0; border-color: #E2E8F0'>
<td style='border-width:0'>Retweets</td>
<td style='border-width:0'>174</td>
</tr>
<tr style='border-width:0 0 1px 0; border-color: #E2E8F0'>
<td style='border-width:0'>Short tweets</td>
<td style='border-width:0'>39</td>
</tr>
<tr style='border-width:0'>
<td style='border-width:0'>Tweets kept</td>
<td style='border-width:0'>3035</td>
</tr>
</tbody>
</table>
[Explore the data](https://wandb.ai/wandb/huggingtweets/runs/1l67ih2t/artifacts), which is tracked with [W&B artifacts](https://docs.wandb.com/artifacts) at every step of the pipeline.
## Training procedure
The model is based on a pre-trained [GPT-2](https://huggingface.co/gpt2) which is fine-tuned on @xinqisu's tweets.
Hyperparameters and metrics are recorded in the [W&B training run](https://wandb.ai/wandb/huggingtweets/runs/2m0wt4pe) for full transparency and reproducibility.
At the end of training, [the final model](https://wandb.ai/wandb/huggingtweets/runs/2m0wt4pe/artifacts) is logged and versioned.
## Intended uses & limitations
### How to use
You can use this model directly with a pipeline for text generation:
<pre><code><span style="color:#03A9F4">from</span> transformers <span style="color:#03A9F4">import</span> pipeline
generator = pipeline(<span style="color:#FF9800">'text-generation'</span>,
model=<span style="color:#FF9800">'huggingtweets/xinqisu'</span>)
generator(<span style="color:#FF9800">"My dream is"</span>, num_return_sequences=<span style="color:#8BC34A">5</span>)</code></pre>
### Limitations and bias
The model suffers from [the same limitations and bias as GPT-2](https://huggingface.co/gpt2#limitations-and-bias).
In addition, the data present in the user's tweets further affects the text generated by the model.
## About
*Built by Boris Dayma*
</section>
[](https://twitter.com/intent/follow?screen_name=borisdayma)
<section class='prose'>
For more details, visit the project repository.
</section>
[](https://github.com/borisdayma/huggingtweets)
|
huggingtweets/xwylraz0rbl4d3x | a3f32a96e3494e79b968078dc8190c668b0ca82b | 2021-05-23T04:54:20.000Z | [
"pytorch",
"jax",
"gpt2",
"text-generation",
"en",
"transformers",
"huggingtweets"
] | text-generation | false | huggingtweets | null | huggingtweets/xwylraz0rbl4d3x | 2 | null | transformers | 24,235 | ---
language: en
thumbnail: https://www.huggingtweets.com/xwylraz0rbl4d3x/1617889284619/predictions.png
tags:
- huggingtweets
widget:
- text: "My dream is"
---
<div>
<div style="width: 132px; height:132px; border-radius: 50%; background-size: cover; background-image: url('https://pbs.twimg.com/profile_images/1366426861299920900/A1ynltRo_400x400.jpg')">
</div>
<div style="margin-top: 8px; font-size: 19px; font-weight: 800">𝙁𝙀𝙈𝘽𝙊𝙔 𝙏𝙍𝘼𝙉𝙎𝙄𝙏𝙄𝙊𝙉𝙄𝙉𝙂 🤖 AI Bot </div>
<div style="font-size: 15px">@xwylraz0rbl4d3x bot</div>
</div>
I was made with [huggingtweets](https://github.com/borisdayma/huggingtweets).
Create your own bot based on your favorite user with [the demo](https://colab.research.google.com/github/borisdayma/huggingtweets/blob/master/huggingtweets-demo.ipynb)!
## How does it work?
The model uses the following pipeline.
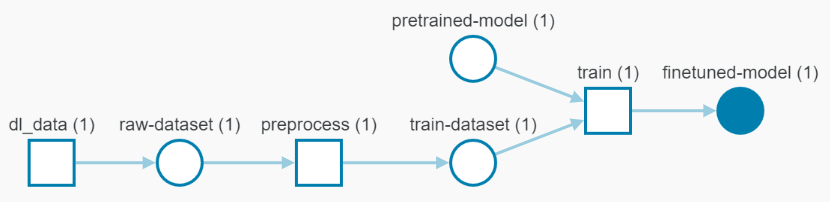
To understand how the model was developed, check the [W&B report](https://wandb.ai/wandb/huggingtweets/reports/HuggingTweets-Train-a-Model-to-Generate-Tweets--VmlldzoxMTY5MjI).
## Training data
The model was trained on [@xwylraz0rbl4d3x's tweets](https://twitter.com/xwylraz0rbl4d3x).
| Data | Quantity |
| --- | --- |
| Tweets downloaded | 3152 |
| Retweets | 682 |
| Short tweets | 631 |
| Tweets kept | 1839 |
[Explore the data](https://wandb.ai/wandb/huggingtweets/runs/36q46b23/artifacts), which is tracked with [W&B artifacts](https://docs.wandb.com/artifacts) at every step of the pipeline.
## Training procedure
The model is based on a pre-trained [GPT-2](https://huggingface.co/gpt2) which is fine-tuned on @xwylraz0rbl4d3x's tweets.
Hyperparameters and metrics are recorded in the [W&B training run](https://wandb.ai/wandb/huggingtweets/runs/3a0sqgtk) for full transparency and reproducibility.
At the end of training, [the final model](https://wandb.ai/wandb/huggingtweets/runs/3a0sqgtk/artifacts) is logged and versioned.
## How to use
You can use this model directly with a pipeline for text generation:
```python
from transformers import pipeline
generator = pipeline('text-generation',
model='huggingtweets/xwylraz0rbl4d3x')
generator("My dream is", num_return_sequences=5)
```
## Limitations and bias
The model suffers from [the same limitations and bias as GPT-2](https://huggingface.co/gpt2#limitations-and-bias).
In addition, the data present in the user's tweets further affects the text generated by the model.
## About
*Built by Boris Dayma*
[](https://twitter.com/intent/follow?screen_name=borisdayma)
For more details, visit the project repository.
[](https://github.com/borisdayma/huggingtweets)
|
huggingtweets/yellowdogedem | dc73ea35d00ff2cadba23d638ffd5089263e6eb7 | 2021-05-23T04:59:31.000Z | [
"pytorch",
"jax",
"gpt2",
"text-generation",
"en",
"transformers",
"huggingtweets"
] | text-generation | false | huggingtweets | null | huggingtweets/yellowdogedem | 2 | null | transformers | 24,236 | ---
language: en
thumbnail: https://www.huggingtweets.com/yellowdogedem/1617172947443/predictions.png
tags:
- huggingtweets
widget:
- text: "My dream is"
---
<div>
<div style="width: 132px; height:132px; border-radius: 50%; background-size: cover; background-image: url('https://pbs.twimg.com/profile_images/1374515470498373639/dygzoNob_400x400.jpg')">
</div>
<div style="margin-top: 8px; font-size: 19px; font-weight: 800">Toby (Inactive) 🗳️🥑⚙️🚊🌃🚀🌐 🤖 AI Bot </div>
<div style="font-size: 15px">@yellowdogedem bot</div>
</div>
I was made with [huggingtweets](https://github.com/borisdayma/huggingtweets).
Create your own bot based on your favorite user with [the demo](https://colab.research.google.com/github/borisdayma/huggingtweets/blob/master/huggingtweets-demo.ipynb)!
## How does it work?
The model uses the following pipeline.
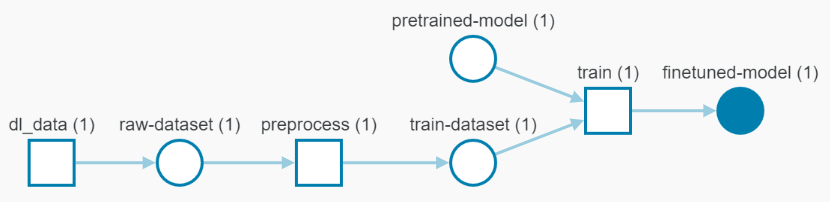
To understand how the model was developed, check the [W&B report](https://wandb.ai/wandb/huggingtweets/reports/HuggingTweets-Train-a-Model-to-Generate-Tweets--VmlldzoxMTY5MjI).
## Training data
The model was trained on [@yellowdogedem's tweets](https://twitter.com/yellowdogedem).
| Data | Quantity |
| --- | --- |
| Tweets downloaded | 3233 |
| Retweets | 494 |
| Short tweets | 788 |
| Tweets kept | 1951 |
[Explore the data](https://wandb.ai/wandb/huggingtweets/runs/2ln60y7n/artifacts), which is tracked with [W&B artifacts](https://docs.wandb.com/artifacts) at every step of the pipeline.
## Training procedure
The model is based on a pre-trained [GPT-2](https://huggingface.co/gpt2) which is fine-tuned on @yellowdogedem's tweets.
Hyperparameters and metrics are recorded in the [W&B training run](https://wandb.ai/wandb/huggingtweets/runs/2vj8xb46) for full transparency and reproducibility.
At the end of training, [the final model](https://wandb.ai/wandb/huggingtweets/runs/2vj8xb46/artifacts) is logged and versioned.
## How to use
You can use this model directly with a pipeline for text generation:
```python
from transformers import pipeline
generator = pipeline('text-generation',
model='huggingtweets/yellowdogedem')
generator("My dream is", num_return_sequences=5)
```
## Limitations and bias
The model suffers from [the same limitations and bias as GPT-2](https://huggingface.co/gpt2#limitations-and-bias).
In addition, the data present in the user's tweets further affects the text generated by the model.
## About
*Built by Boris Dayma*
[](https://twitter.com/intent/follow?screen_name=borisdayma)
For more details, visit the project repository.
[](https://github.com/borisdayma/huggingtweets)
|
huggingtweets/zetsubunny | 493abcff2fa52022b0a9971a5170e2bf71fe75f1 | 2021-05-23T05:19:49.000Z | [
"pytorch",
"jax",
"gpt2",
"text-generation",
"en",
"transformers",
"huggingtweets"
] | text-generation | false | huggingtweets | null | huggingtweets/zetsubunny | 2 | null | transformers | 24,237 | ---
language: en
thumbnail: https://www.huggingtweets.com/zetsubunny/1617772715416/predictions.png
tags:
- huggingtweets
widget:
- text: "My dream is"
---
<div>
<div style="width: 132px; height:132px; border-radius: 50%; background-size: cover; background-image: url('https://pbs.twimg.com/profile_images/1362563256230031366/ujesNtUk_400x400.jpg')">
</div>
<div style="margin-top: 8px; font-size: 19px; font-weight: 800">MEMENTO BUNI 🤖 AI Bot </div>
<div style="font-size: 15px">@zetsubunny bot</div>
</div>
I was made with [huggingtweets](https://github.com/borisdayma/huggingtweets).
Create your own bot based on your favorite user with [the demo](https://colab.research.google.com/github/borisdayma/huggingtweets/blob/master/huggingtweets-demo.ipynb)!
## How does it work?
The model uses the following pipeline.
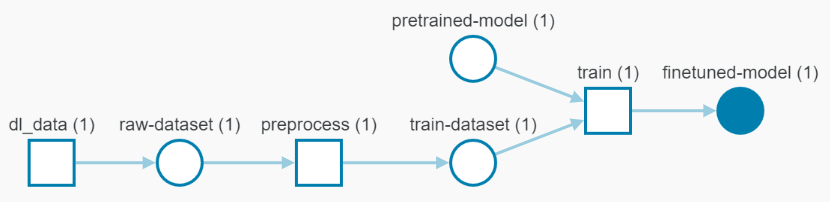
To understand how the model was developed, check the [W&B report](https://wandb.ai/wandb/huggingtweets/reports/HuggingTweets-Train-a-Model-to-Generate-Tweets--VmlldzoxMTY5MjI).
## Training data
The model was trained on [@zetsubunny's tweets](https://twitter.com/zetsubunny).
| Data | Quantity |
| --- | --- |
| Tweets downloaded | 3230 |
| Retweets | 1122 |
| Short tweets | 264 |
| Tweets kept | 1844 |
[Explore the data](https://wandb.ai/wandb/huggingtweets/runs/3u5onhoi/artifacts), which is tracked with [W&B artifacts](https://docs.wandb.com/artifacts) at every step of the pipeline.
## Training procedure
The model is based on a pre-trained [GPT-2](https://huggingface.co/gpt2) which is fine-tuned on @zetsubunny's tweets.
Hyperparameters and metrics are recorded in the [W&B training run](https://wandb.ai/wandb/huggingtweets/runs/tv6yd107) for full transparency and reproducibility.
At the end of training, [the final model](https://wandb.ai/wandb/huggingtweets/runs/tv6yd107/artifacts) is logged and versioned.
## How to use
You can use this model directly with a pipeline for text generation:
```python
from transformers import pipeline
generator = pipeline('text-generation',
model='huggingtweets/zetsubunny')
generator("My dream is", num_return_sequences=5)
```
## Limitations and bias
The model suffers from [the same limitations and bias as GPT-2](https://huggingface.co/gpt2#limitations-and-bias).
In addition, the data present in the user's tweets further affects the text generated by the model.
## About
*Built by Boris Dayma*
[](https://twitter.com/intent/follow?screen_name=borisdayma)
For more details, visit the project repository.
[](https://github.com/borisdayma/huggingtweets)
|
hugo/secret-project-all-1 | bf6d8e7202a59ce0ccdfe3767576ff58b6fa93bd | 2021-12-07T00:37:14.000Z | [
"pytorch",
"bert",
"text-classification",
"transformers"
] | text-classification | false | hugo | null | hugo/secret-project-all-1 | 2 | null | transformers | 24,238 | Entry not found |
hugsao123/xlm-roberta-base-finetuned-panx-de | 77823feb5847596a3ebe38329d69d29de087fb89 | 2022-02-20T15:03:47.000Z | [
"pytorch",
"tensorboard",
"xlm-roberta",
"token-classification",
"dataset:xtreme",
"transformers",
"generated_from_trainer",
"license:mit",
"model-index",
"autotrain_compatible"
] | token-classification | false | hugsao123 | null | hugsao123/xlm-roberta-base-finetuned-panx-de | 2 | null | transformers | 24,239 | ---
license: mit
tags:
- generated_from_trainer
datasets:
- xtreme
metrics:
- f1
model-index:
- name: xlm-roberta-base-finetuned-panx-de
results:
- task:
name: Token Classification
type: token-classification
dataset:
name: xtreme
type: xtreme
args: PAN-X.en
metrics:
- name: F1
type: f1
value: 0.7589617892939993
---
<!-- This model card has been generated automatically according to the information the Trainer had access to. You
should probably proofread and complete it, then remove this comment. -->
# xlm-roberta-base-finetuned-panx-de
This model is a fine-tuned version of [xlm-roberta-base](https://huggingface.co/xlm-roberta-base) on the xtreme dataset.
It achieves the following results on the evaluation set:
- Loss: 0.3903
- F1: 0.7590
## Model description
More information needed
## Intended uses & limitations
More information needed
## Training and evaluation data
More information needed
## Training procedure
### Training hyperparameters
The following hyperparameters were used during training:
- learning_rate: 5e-05
- train_batch_size: 24
- eval_batch_size: 24
- seed: 42
- optimizer: Adam with betas=(0.9,0.999) and epsilon=1e-08
- lr_scheduler_type: linear
- num_epochs: 3
### Training results
| Training Loss | Epoch | Step | Validation Loss | F1 |
|:-------------:|:-----:|:----:|:---------------:|:------:|
| 1.0489 | 1.0 | 50 | 0.5561 | 0.6565 |
| 0.4953 | 2.0 | 100 | 0.4385 | 0.7189 |
| 0.35 | 3.0 | 150 | 0.3903 | 0.7590 |
### Framework versions
- Transformers 4.15.0
- Pytorch 1.8.2+cu111
- Datasets 1.18.3
- Tokenizers 0.10.3
|
husnu/bert-base-turkish-128k-cased-finetuned_lr-2e-05_epochs-3TQUAD2-finetuned_lr-2e-05_epochs-1 | ee41240f8b09b0c161fa57214ce3cd6eed5b1662 | 2022-01-15T20:09:15.000Z | [
"pytorch",
"tensorboard",
"bert",
"question-answering",
"dataset:squad",
"transformers",
"generated_from_trainer",
"model-index",
"autotrain_compatible"
] | question-answering | false | husnu | null | husnu/bert-base-turkish-128k-cased-finetuned_lr-2e-05_epochs-3TQUAD2-finetuned_lr-2e-05_epochs-1 | 2 | null | transformers | 24,240 | ---
tags:
- generated_from_trainer
datasets:
- squad
model-index:
- name: bert-base-turkish-128k-cased-finetuned_lr-2e-05_epochs-3TQUAD2-finetuned_lr-2e-05_epochs-1
results: []
---
<!-- This model card has been generated automatically according to the information the Trainer had access to. You
should probably proofread and complete it, then remove this comment. -->
# bert-base-turkish-128k-cased-finetuned_lr-2e-05_epochs-3TQUAD2-finetuned_lr-2e-05_epochs-1
This model is a fine-tuned version of [husnu/bert-base-turkish-128k-cased-finetuned_lr-2e-05_epochs-3](https://huggingface.co/husnu/bert-base-turkish-128k-cased-finetuned_lr-2e-05_epochs-3) on the squad dataset.
It achieves the following results on the evaluation set:
- Loss: 1.4196
## Model description
More information needed
## Intended uses & limitations
More information needed
## Training and evaluation data
More information needed
## Training procedure
### Training hyperparameters
The following hyperparameters were used during training:
- learning_rate: 2e-05
- train_batch_size: 8
- eval_batch_size: 8
- seed: 42
- optimizer: Adam with betas=(0.9,0.999) and epsilon=1e-08
- lr_scheduler_type: linear
- num_epochs: 1
### Training results
| Training Loss | Epoch | Step | Validation Loss |
|:-------------:|:-----:|:----:|:---------------:|
| 0.5885 | 1.0 | 2245 | 1.4196 |
### Framework versions
- Transformers 4.15.0
- Pytorch 1.10.0+cu111
- Datasets 1.17.0
- Tokenizers 0.10.3
|
husnu/bert-base-turkish-128k-cased-finetuned_lr-2e-05_epochs-3TQUAD2-finetuned_lr-2e-05_epochs-3 | 4f9eccbe372d4edb0266f21c9ab0f205eb745208 | 2022-01-15T18:42:09.000Z | [
"pytorch",
"tensorboard",
"bert",
"question-answering",
"dataset:turkish squad v2",
"transformers",
"generated_from_trainer",
"model-index",
"autotrain_compatible"
] | question-answering | false | husnu | null | husnu/bert-base-turkish-128k-cased-finetuned_lr-2e-05_epochs-3TQUAD2-finetuned_lr-2e-05_epochs-3 | 2 | null | transformers | 24,241 | ---
tags:
- generated_from_trainer
datasets:
- turkish squad v2
model-index:
- name: bert-base-turkish-128k-cased-finetuned_lr-2e-05_epochs-3TQUAD2-finetuned_lr-2e-05_epochs-3
results: []
---
<!-- This model card has been generated automatically according to the information the Trainer had access to. You
should probably proofread and complete it, then remove this comment. -->
# bert-base-turkish-128k-cased-finetuned_lr-2e-05_epochs-3TQUAD2-finetuned_lr-2e-05_epochs-3
This model is a fine-tuned version of [husnu/bert-base-turkish-128k-cased-finetuned_lr-2e-05_epochs-3](https://huggingface.co/husnu/bert-base-turkish-128k-cased-finetuned_lr-2e-05_epochs-3) on the turkish squad2 dataset.
It achieves the following results on the evaluation set:
- Loss: 1.9011
## Model description
More information needed
## Intended uses & limitations
More information needed
## Training and evaluation data
More information needed
## Training procedure
### Training hyperparameters
The following hyperparameters were used during training:
- learning_rate: 2e-05
- train_batch_size: 8
- eval_batch_size: 8
- seed: 42
- optimizer: Adam with betas=(0.9,0.999) and epsilon=1e-08
- lr_scheduler_type: linear
- num_epochs: 3
### Training results
| Training Loss | Epoch | Step | Validation Loss |
|:-------------:|:-----:|:----:|:---------------:|
| 0.6404 | 1.0 | 2245 | 1.4524 |
| 0.403 | 2.0 | 4490 | 1.5638 |
| 0.2355 | 3.0 | 6735 | 1.9011 |
### Framework versions
- Transformers 4.15.0
- Pytorch 1.10.0+cu111
- Datasets 1.17.0
- Tokenizers 0.10.3
|
husnu/xtremedistil-l6-h256-uncased-TQUAD-finetuned_lr-2e-05_epochs-9 | 8df0378cc69f52cc441e7e79bd4e944a90955480 | 2022-01-15T05:08:37.000Z | [
"pytorch",
"tensorboard",
"bert",
"question-answering",
"dataset:squad",
"transformers",
"generated_from_trainer",
"license:mit",
"model-index",
"autotrain_compatible"
] | question-answering | false | husnu | null | husnu/xtremedistil-l6-h256-uncased-TQUAD-finetuned_lr-2e-05_epochs-9 | 2 | null | transformers | 24,242 | ---
license: mit
tags:
- generated_from_trainer
datasets:
- squad
model-index:
- name: xtremedistil-l6-h256-uncased-TQUAD-finetuned_lr-2e-05_epochs-9
results: []
---
<!-- This model card has been generated automatically according to the information the Trainer had access to. You
should probably proofread and complete it, then remove this comment. -->
# xtremedistil-l6-h256-uncased-TQUAD-finetuned_lr-2e-05_epochs-9
This model is a fine-tuned version of [microsoft/xtremedistil-l6-h256-uncased](https://huggingface.co/microsoft/xtremedistil-l6-h256-uncased) on the Turkish squad dataset.
It achieves the following results on the evaluation set:
- Loss: 2.2340
## Model description
More information needed
## Intended uses & limitations
More information needed
## Training and evaluation data
More information needed
## Training procedure
### Training hyperparameters
The following hyperparameters were used during training:
- learning_rate: 2e-05
- train_batch_size: 16
- eval_batch_size: 16
- seed: 42
- optimizer: Adam with betas=(0.9,0.999) and epsilon=1e-08
- lr_scheduler_type: linear
- num_epochs: 9
### Training results
| Training Loss | Epoch | Step | Validation Loss |
|:-------------:|:-----:|:----:|:---------------:|
| 3.5236 | 1.0 | 1050 | 3.0042 |
| 2.8489 | 2.0 | 2100 | 2.5866 |
| 2.5485 | 3.0 | 3150 | 2.3526 |
| 2.4067 | 4.0 | 4200 | 2.3535 |
| 2.3091 | 5.0 | 5250 | 2.2862 |
| 2.2401 | 6.0 | 6300 | 2.3989 |
| 2.1715 | 7.0 | 7350 | 2.2284 |
| 2.1414 | 8.0 | 8400 | 2.2298 |
| 2.1221 | 9.0 | 9450 | 2.2340 |
### Framework versions
- Transformers 4.15.0
- Pytorch 1.10.0+cu111
- Datasets 1.17.0
- Tokenizers 0.10.3
|
huwendeng/distilroberta_b | ae2444ea2cb2212886bc51ccadce75a1f6d3bb45 | 2021-05-20T16:41:42.000Z | [
"pytorch",
"jax",
"roberta",
"text-classification",
"transformers"
] | text-classification | false | huwendeng | null | huwendeng/distilroberta_b | 2 | null | transformers | 24,243 | Entry not found |
huyenbui117/Covid19_phoNER | e86e753fddd183a63fe57dc88ae4f85b72acb3cf | 2021-11-27T19:34:09.000Z | [
"pytorch",
"roberta",
"token-classification",
"transformers",
"autotrain_compatible"
] | token-classification | false | huyenbui117 | null | huyenbui117/Covid19_phoNER | 2 | null | transformers | 24,244 | Model trained for PhoNER_COVID19 dataset |
hyesunyun/NonsenseUpdateDiffIntBart | 83f849c10386415df6a85fd08aae4a6ef37f90d3 | 2022-02-03T17:14:33.000Z | [
"pytorch",
"bart",
"text2text-generation",
"en",
"dataset:nonsense corpus",
"transformers",
"summarization",
"diff generation",
"autotrain_compatible"
] | summarization | false | hyesunyun | null | hyesunyun/NonsenseUpdateDiffIntBart | 2 | null | transformers | 24,245 | ---
language:
- en
tags:
- summarization
- diff generation
datasets:
- nonsense corpus
metrics:
- rouge
---
hello! this is the pretrained BART. The dataset used for pretraining is nonsense summary corpus with output as diff. |
iamholmes/first-model | 667ea647210b3e6d74c4b8797642c371704aa588 | 2021-07-06T10:40:06.000Z | [
"pytorch",
"bert",
"text-classification",
"transformers"
] | text-classification | false | iamholmes | null | iamholmes/first-model | 2 | null | transformers | 24,246 | Entry not found |
ibraheemmoosa/xlmindic-base-multiscript-soham | ffb32c1c9b8350ac22b2c2f6d5db91f71bb0c612 | 2022-02-10T09:24:02.000Z | [
"pytorch",
"tf",
"jax",
"albert",
"text-classification",
"as",
"bn",
"gu",
"hi",
"mr",
"ne",
"or",
"pa",
"si",
"sa",
"bpy",
"bh",
"gom",
"mai",
"dataset:oscar",
"transformers",
"multilingual",
"fill-mask",
"xlmindic",
"nlp",
"indoaryan",
"indicnlp",
"iso15919",
"license:apache-2.0",
"co2_eq_emissions"
] | text-classification | false | ibraheemmoosa | null | ibraheemmoosa/xlmindic-base-multiscript-soham | 2 | null | transformers | 24,247 | ---
language:
- as
- bn
- gu
- hi
- mr
- ne
- or
- pa
- si
- sa
- bpy
- bh
- gom
- mai
license: apache-2.0
datasets:
- oscar
tags:
- multilingual
- albert
- fill-mask
- xlmindic
- nlp
- indoaryan
- indicnlp
- iso15919
- text-classification
widget:
- text : 'চীনের মধ্যাঞ্চলে আরও একটি শহরের বাসিন্দারা আবার ঘরবন্দী হয়ে পড়েছেন। আজ মঙ্গলবার নতুন করে লকডাউন–সংক্রান্ত বিধিনিষেধ জারি হওয়ার পর ঘরে আটকা পড়েছেন তাঁরা। করোনার অতি সংক্রামক নতুন ধরন অমিক্রনের বিস্তার ঠেকাতে এমন পদক্ষেপ নিয়েছে কর্তৃপক্ষ। খবর বার্তা সংস্থা এএফপির।'
co2_eq_emissions:
emissions: "0.21 in grams of CO2"
source: "calculated using this webstie https://mlco2.github.io/impact/#compute"
training_type: "fine-tuning"
geographical_location: "NA"
hardware_used: "P100 for about 1.5 hours"
---
# XLMIndic Base Multiscript
This model is finetuned from [this model](https://huggingface.co/ibraheemmoosa/xlmindic-base-multiscript) on Soham Bangla News Classification task which is part of the IndicGLUE benchmark.
## Model description
This model has the same configuration as the [ALBERT Base v2 model](https://huggingface.co/albert-base-v2/). Specifically, this model has the following configuration:
- 12 repeating layers
- 128 embedding dimension
- 768 hidden dimension
- 12 attention heads
- 11M parameters
- 512 sequence length
## Training data
This model was fine-tuned on Soham dataset that is part of the IndicGLUE benchmark.
## Training procedure
### Preprocessing
The texts are tokenized using SentencePiece and a vocabulary size of 50,000.
### Training
The model was trained for 8 epochs with a batch size of 16 and a learning rate of *2e-5*.
## Evaluation results
See results specific to Soham in the following table.
### IndicGLUE
Task | mBERT | XLM-R | IndicBERT-Base | XLMIndic-Base-Uniscript | XLMIndic-Base-Multiscript (This Model)
-----| ----- | ----- | ------ | ------- | --------
Wikipedia Section Title Prediction | 71.90 | 65.45 | 69.40 | **81.78 ± 0.60** | 77.17 ± 0.76
Article Genre Classification | 88.64 | 96.61 | 97.72 | **98.70 ± 0.29** | 98.30 ± 0.26
Named Entity Recognition (F1-score) | 71.29 | 62.18 | 56.69 | **89.85 ± 1.14** | 83.19 ± 1.58
BBC Hindi News Article Classification | 60.55 | 75.52 | 74.60 | **79.14 ± 0.60** | 77.28 ± 1.50
Soham Bangla News Article Classification | 80.23 | 87.6 | 78.45 | **93.89 ± 0.48** | 93.22 ± 0.49
INLTK Gujarati Headlines Genre Classification | - | - | **92.91** | 90.73 ± 0.75 | 90.41 ± 0.69
INLTK Marathi Headlines Genre Classification | - | - | **94.30** | 92.04 ± 0.47 | 92.21 ± 0.23
IITP Hindi Product Reviews Sentiment Classification | 74.57 | **78.97** | 71.32 | 77.18 ± 0.77 | 76.33 ± 0.84
IITP Hindi Movie Reviews Sentiment Classification | 56.77 | 61.61 | 59.03 | **66.34 ± 0.16** | 65.91 ± 2.20
MIDAS Hindi Discourse Type Classification | 71.20 | **79.94** | 78.44 | 78.54 ± 0.91 | 78.39 ± 0.33
Cloze Style Question Answering (Fill-mask task) | - | - | 37.16 | **41.54** | 38.21
## Intended uses & limitations
This model is pretrained on Indo-Aryan languages. Thus it is intended to be used for downstream tasks on these languages.
You can use the raw model for either masked language modeling or next sentence prediction, but it's mostly intended to
be fine-tuned on a downstream task. See the [model hub](https://huggingface.co/models?filter=xlmindic) to look for
fine-tuned versions on a task that interests you.
Note that this model is primarily aimed at being fine-tuned on tasks that use the whole sentence (potentially masked)
to make decisions, such as sequence classification, token classification or question answering. For tasks such as text
generation you should look at model like GPT2.
### How to use
Then you can use this model directly with a pipeline for masked language modeling:
```python
>>> from transformers import pipeline
>>> unmasker = pipeline('fill-mask', model='ibraheemmoosa/xlmindic-base-multiscript')
>>> text = "রবীন্দ্রনাথ ঠাকুর এফআরএএস (৭ মে ১৮৬১ - ৭ আগস্ট ১৯৪১; ২৫ বৈশাখ ১২৬৮ - ২২ শ্রাবণ ১৩৪৮ বঙ্গাব্দ) ছিলেন অগ্রণী বাঙালি [MASK], ঔপন্যাসিক, সংগীতস্রষ্টা, নাট্যকার, চিত্রকর, ছোটগল্পকার, প্রাবন্ধিক, অভিনেতা, কণ্ঠশিল্পী ও দার্শনিক। ১৯১৩ সালে গীতাঞ্জলি কাব্যগ্রন্থের ইংরেজি অনুবাদের জন্য তিনি এশীয়দের মধ্যে সাহিত্যে প্রথম নোবেল পুরস্কার লাভ করেন।"
>>> unmasker(text)
[{'score': 0.34163928031921387,
'token': 5399,
'token_str': 'কবি',
'sequence': 'রবীন্দ্রনাথ ঠাকুর এফআরএএস (৭ মে ১৮৬১ - ৭ আগস্ট ১৯৪১; ২৫ বৈশাখ ১২৬৮ - ২২ শ্রাবণ ১৩৪৮ বঙ্গাব্দ) ছিলেন অগ্রণী বাঙালি কবি, পন্যাসিক, সংগীতস্রষ্টা, নাট্যকার, চিত্রকর, ছোটগল্পকার, প্রাবন্ধিক, অভিনেতা, কণ্ঠশিল্পী ও দার্শনিক। ১৯১৩ সালে গীতাঞ্জলি কাব্যগ্রন্থের ইংরেজি অনুবাদের জন্য তিনি এশীয়দের মধ্যে সাহিত্যে প্রথম নোবেল পুরস্কার লাভ করেন।'},
{'score': 0.30519795417785645,
'token': 33436,
'token_str': 'people',
'sequence': 'রবীন্দ্রনাথ ঠাকুর এফআরএএস (৭ মে ১৮৬১ - ৭ আগস্ট ১৯৪১; ২৫ বৈশাখ ১২৬৮ - ২২ শ্রাবণ ১৩৪৮ বঙ্গাব্দ) ছিলেন অগ্রণী বাঙালি people, পন্যাসিক, সংগীতস্রষ্টা, নাট্যকার, চিত্রকর, ছোটগল্পকার, প্রাবন্ধিক, অভিনেতা, কণ্ঠশিল্পী ও দার্শনিক। ১৯১৩ সালে গীতাঞ্জলি কাব্যগ্রন্থের ইংরেজি অনুবাদের জন্য তিনি এশীয়দের মধ্যে সাহিত্যে প্রথম নোবেল পুরস্কার লাভ করেন।'},
{'score': 0.29130080342292786,
'token': 30476,
'token_str': 'সাহিত্যিক',
'sequence': 'রবীন্দ্রনাথ ঠাকুর এফআরএএস (৭ মে ১৮৬১ - ৭ আগস্ট ১৯৪১; ২৫ বৈশাখ ১২৬৮ - ২২ শ্রাবণ ১৩৪৮ বঙ্গাব্দ) ছিলেন অগ্রণী বাঙালি সাহিত্যিক, পন্যাসিক, সংগীতস্রষ্টা, নাট্যকার, চিত্রকর, ছোটগল্পকার, প্রাবন্ধিক, অভিনেতা, কণ্ঠশিল্পী ও দার্শনিক। ১৯১৩ সালে গীতাঞ্জলি কাব্যগ্রন্থের ইংরেজি অনুবাদের জন্য তিনি এশীয়দের মধ্যে সাহিত্যে প্রথম নোবেল পুরস্কার লাভ করেন।'},
{'score': 0.031051287427544594,
'token': 6139,
'token_str': 'লেখক',
'sequence': 'রবীন্দ্রনাথ ঠাকুর এফআরএএস (৭ মে ১৮৬১ - ৭ আগস্ট ১৯৪১; ২৫ বৈশাখ ১২৬৮ - ২২ শ্রাবণ ১৩৪৮ বঙ্গাব্দ) ছিলেন অগ্রণী বাঙালি লেখক, পন্যাসিক, সংগীতস্রষ্টা, নাট্যকার, চিত্রকর, ছোটগল্পকার, প্রাবন্ধিক, অভিনেতা, কণ্ঠশিল্পী ও দার্শনিক। ১৯১৩ সালে গীতাঞ্জলি কাব্যগ্রন্থের ইংরেজি অনুবাদের জন্য তিনি এশীয়দের মধ্যে সাহিত্যে প্রথম নোবেল পুরস্কার লাভ করেন।'},
{'score': 0.002705035964027047,
'token': 38443,
'token_str': 'শিল্পীরা',
'sequence': 'রবীন্দ্রনাথ ঠাকুর এফআরএএস (৭ মে ১৮৬১ - ৭ আগস্ট ১৯৪১; ২৫ বৈশাখ ১২৬৮ - ২২ শ্রাবণ ১৩৪৮ বঙ্গাব্দ) ছিলেন অগ্রণী বাঙালি শিল্পীরা, পন্যাসিক, সংগীতস্রষ্টা, নাট্যকার, চিত্রকর, ছোটগল্পকার, প্রাবন্ধিক, অভিনেতা, কণ্ঠশিল্পী ও দার্শনিক। ১৯১৩ সালে গীতাঞ্জলি কাব্যগ্রন্থের ইংরেজি অনুবাদের জন্য তিনি এশীয়দের মধ্যে সাহিত্যে প্রথম নোবেল পুরস্কার লাভ করেন।'}]
```
### Limitations and bias
Even though we pretrain on a comparatively large multilingual corpus the model may exhibit harmful gender, ethnic and political bias. If you fine-tune this model on a task where these issues are important you should take special care when relying on the model to make decisions.
## Contact
Feel free to contact us if you have any ideas or if you want to know more about our models.
- Ibraheem Muhammad Moosa ([email protected])
- Mahmud Elahi Akhter ([email protected])
- Ashfia Binte Habib
## BibTeX entry and citation info
```bibtex
@article{Moosa2022DoesTH,
title={Does Transliteration Help Multilingual Language Modeling?},
author={Ibraheem Muhammad Moosa and Mahmuda Akhter and Ashfia Binte Habib},
journal={ArXiv},
year={2022},
volume={abs/2201.12501}
}
``` |
idrimadrid/autonlp-creator_classifications-4021083 | 86e92327d9640e627364d8a61a52f16697022799 | 2021-07-20T12:57:16.000Z | [
"pytorch",
"bert",
"text-classification",
"en",
"dataset:idrimadrid/autonlp-data-creator_classifications",
"transformers",
"autonlp"
] | text-classification | false | idrimadrid | null | idrimadrid/autonlp-creator_classifications-4021083 | 2 | null | transformers | 24,248 | ---
tags: autonlp
language: en
widget:
- text: "I love AutoNLP 🤗"
datasets:
- idrimadrid/autonlp-data-creator_classifications
---
# Model Trained Using AutoNLP
- Problem type: Multi-class Classification
- Model ID: 4021083
## Validation Metrics
- Loss: 0.6848716735839844
- Accuracy: 0.8825910931174089
- Macro F1: 0.41301646762109634
- Micro F1: 0.8825910931174088
- Weighted F1: 0.863740586166105
- Macro Precision: 0.4129337301330573
- Micro Precision: 0.8825910931174089
- Weighted Precision: 0.8531335941587811
- Macro Recall: 0.44466614072309585
- Micro Recall: 0.8825910931174089
- Weighted Recall: 0.8825910931174089
## Usage
You can use cURL to access this model:
```
$ curl -X POST -H "Authorization: Bearer YOUR_API_KEY" -H "Content-Type: application/json" -d '{"inputs": "I love AutoNLP"}' https://api-inference.huggingface.co/models/idrimadrid/autonlp-creator_classifications-4021083
```
Or Python API:
```
from transformers import AutoModelForSequenceClassification, AutoTokenizer
model = AutoModelForSequenceClassification.from_pretrained("idrimadrid/autonlp-creator_classifications-4021083", use_auth_token=True)
tokenizer = AutoTokenizer.from_pretrained("idrimadrid/autonlp-creator_classifications-4021083", use_auth_token=True)
inputs = tokenizer("I love AutoNLP", return_tensors="pt")
outputs = model(**inputs)
``` |
ielab/TILDEv2-TILDE128-exp | c967c1941f88c3d6b076dcab73c29a1da70f507e | 2021-10-31T13:51:09.000Z | [
"pytorch",
"bert",
"transformers"
] | null | false | ielab | null | ielab/TILDEv2-TILDE128-exp | 2 | null | transformers | 24,249 | TILDEv2 trained with passages expand with TILDE (m=128) |
ietz/bert-base-uncased-finetuned-jira-hyperledger-issue-titles-and-bodies | 61e6808018462bfc630c8d9ca2023e55a411a2e7 | 2022-02-04T08:02:28.000Z | [
"pytorch",
"bert",
"fill-mask",
"transformers",
"autotrain_compatible"
] | fill-mask | false | ietz | null | ietz/bert-base-uncased-finetuned-jira-hyperledger-issue-titles-and-bodies | 2 | null | transformers | 24,250 | Entry not found |
ifis-zork/IFIS_ZORK_AI_MEDIUM_HORROR | 6fd88d3c04981b12519be5746ccfb9f529765e13 | 2021-07-20T23:14:58.000Z | [
"pytorch",
"tensorboard",
"gpt2",
"text-generation",
"transformers",
"generated_from_trainer"
] | text-generation | false | ifis-zork | null | ifis-zork/IFIS_ZORK_AI_MEDIUM_HORROR | 2 | null | transformers | 24,251 | ---
tags:
- generated_from_trainer
model_index:
- name: IFIS_ZORK_AI_MEDIUM_HORROR
results:
- task:
name: Causal Language Modeling
type: text-generation
---
<!-- This model card has been generated automatically according to the information the Trainer had access to. You
should probably proofread and complete it, then remove this comment. -->
# IFIS_ZORK_AI_MEDIUM_HORROR
This model is a fine-tuned version of [gpt2-medium](https://huggingface.co/gpt2-medium) on an unkown dataset.
## Model description
More information needed
## Intended uses & limitations
More information needed
## Training and evaluation data
More information needed
## Training procedure
### Training hyperparameters
The following hyperparameters were used during training:
- learning_rate: 5e-05
- train_batch_size: 1
- eval_batch_size: 2
- seed: 42
- optimizer: Adam with betas=(0.9,0.999) and epsilon=1e-08
- lr_scheduler_type: linear
- lr_scheduler_warmup_steps: 200
- num_epochs: 3
### Training results
### Framework versions
- Transformers 4.8.2
- Pytorch 1.9.0+cu102
- Tokenizers 0.10.3
|
ifis-zork/ZORK_AI_MODERN_A | d3cf6e7b1e11a7aef0a987b8e709ad0320aef335 | 2021-07-20T19:37:56.000Z | [
"pytorch",
"tensorboard",
"gpt2",
"text-generation",
"transformers",
"generated_from_trainer"
] | text-generation | false | ifis-zork | null | ifis-zork/ZORK_AI_MODERN_A | 2 | null | transformers | 24,252 | ---
tags:
- generated_from_trainer
model_index:
- name: ZORK_AI_MODERN_A
results:
- task:
name: Causal Language Modeling
type: text-generation
---
<!-- This model card has been generated automatically according to the information the Trainer had access to. You
should probably proofread and complete it, then remove this comment. -->
# ZORK_AI_MODERN_A
This model is a fine-tuned version of [gpt2-medium](https://huggingface.co/gpt2-medium) on an unkown dataset.
## Model description
More information needed
## Intended uses & limitations
More information needed
## Training and evaluation data
More information needed
## Training procedure
### Training hyperparameters
The following hyperparameters were used during training:
- learning_rate: 5e-05
- train_batch_size: 1
- eval_batch_size: 2
- seed: 42
- optimizer: Adam with betas=(0.9,0.999) and epsilon=1e-08
- lr_scheduler_type: linear
- lr_scheduler_warmup_steps: 200
- num_epochs: 3
### Training results
### Framework versions
- Transformers 4.8.2
- Pytorch 1.9.0+cu102
- Tokenizers 0.10.3
|
imvladikon/bert-base-uncased-jigsaw | 280db43a5a263277360db5f4f1cf9107689b8792 | 2021-07-18T15:46:05.000Z | [
"pytorch",
"bert",
"en",
"dataset:jigsaw",
"transformers",
"generated_from_trainer"
] | null | false | imvladikon | null | imvladikon/bert-base-uncased-jigsaw | 2 | null | transformers | 24,253 | ---
language:
- en
license:
tags:
- generated_from_trainer
datasets:
- jigsaw
model_index:
- name: bert-base-uncased
results:
- {}
---
<!-- This model card has been generated automatically according to the information the Trainer had access to. You
should probably proofread and complete it, then remove this comment. -->
# bert-base-uncased
This model is a fine-tuned version of [](https://huggingface.co/) on the jigsaw dataset.
It achieves the following results on the evaluation set:
- Loss: 0.0393
- Precision Micro: 0.7758
- Recall Micro: 0.7858
- F1 Micro: 0.7808
- F2 Micro: 0.7838
- Precision Macro: 0.6349
- Recall Macro: 0.5972
- F1 Macro: 0.6105
- F2 Macro: 0.6015
- Overall Precision: 0.9841
- Overall Recall: 0.9841
- Overall F1: 0.9841
- Overall F2: 0.9841
- Overall Accuracy: 0.9841
- Matthews Corrcoef: 0.7725
- Aucroc Macro: 0.9897
- Aucroc Micro: 0.9920
- Accuracy Toxic: 0.9678
- F1 Toxic: 0.8295
- Accuracy Severe Toxic: 0.9899
- F1 Severe Toxic: 0.3313
- Accuracy Obscene: 0.9816
- F1 Obscene: 0.8338
- Accuracy Threat: 0.9974
- F1 Threat: 0.4545
- Accuracy Insult: 0.9763
- F1 Insult: 0.7662
- Accuracy Identity Hate: 0.9914
- F1 Identity Hate: 0.4480
## Model description
More information needed
## Intended uses & limitations
More information needed
## Training and evaluation data
More information needed
## Training procedure
### Training hyperparameters
The following hyperparameters were used during training:
- learning_rate: 3e-05
- train_batch_size: 24
- eval_batch_size: 12
- seed: 42
- gradient_accumulation_steps: 2
- total_train_batch_size: 48
- optimizer: Adam with betas=(0.9,0.999) and epsilon=1e-08
- lr_scheduler_type: linear
- num_epochs: 5
### Training results
| Training Loss | Epoch | Step | Validation Loss | Precision Micro | Recall Micro | F1 Micro | F2 Micro | Precision Macro | Recall Macro | F1 Macro | F2 Macro | Overall Precision | Overall Recall | Overall F1 | Overall F2 | Overall Accuracy | Matthews Corrcoef | Aucroc Macro | Aucroc Micro | Accuracy Toxic | F1 Toxic | Accuracy Severe Toxic | F1 Severe Toxic | Accuracy Obscene | F1 Obscene | Accuracy Threat | F1 Threat | Accuracy Insult | F1 Insult | Accuracy Identity Hate | F1 Identity Hate |
|:-------------:|:-----:|:-----:|:---------------:|:---------------:|:------------:|:--------:|:--------:|:---------------:|:------------:|:--------:|:--------:|:-----------------:|:--------------:|:----------:|:----------:|:----------------:|:-----------------:|:------------:|:------------:|:--------------:|:--------:|:---------------------:|:---------------:|:----------------:|:----------:|:---------------:|:---------:|:---------------:|:---------:|:----------------------:|:----------------:|
| 0.0433 | 1.0 | 2659 | 0.0423 | 0.7607 | 0.7798 | 0.7702 | 0.7759 | 0.6398 | 0.5561 | 0.5585 | 0.5535 | 0.9832 | 0.9832 | 0.9832 | 0.9832 | 0.9832 | 0.7615 | 0.9887 | 0.9908 | 0.9671 | 0.8211 | 0.9878 | 0.4354 | 0.9805 | 0.8265 | 0.9974 | 0.2243 | 0.9746 | 0.7602 | 0.9918 | 0.2834 |
| 0.0366 | 2.0 | 5318 | 0.0393 | 0.7758 | 0.7858 | 0.7808 | 0.7838 | 0.6349 | 0.5972 | 0.6105 | 0.6015 | 0.9841 | 0.9841 | 0.9841 | 0.9841 | 0.9841 | 0.7725 | 0.9897 | 0.9920 | 0.9678 | 0.8295 | 0.9899 | 0.3313 | 0.9816 | 0.8338 | 0.9974 | 0.4545 | 0.9763 | 0.7662 | 0.9914 | 0.4480 |
| 0.0305 | 3.0 | 7977 | 0.0399 | 0.7608 | 0.8186 | 0.7887 | 0.8064 | 0.6621 | 0.6856 | 0.6715 | 0.6794 | 0.9842 | 0.9842 | 0.9842 | 0.9842 | 0.9842 | 0.7810 | 0.9897 | 0.9919 | 0.9662 | 0.8272 | 0.9892 | 0.4772 | 0.9815 | 0.8347 | 0.9977 | 0.5629 | 0.9772 | 0.7740 | 0.9931 | 0.5528 |
| 0.0263 | 4.0 | 10636 | 0.0435 | 0.7333 | 0.8336 | 0.7803 | 0.8114 | 0.6395 | 0.7039 | 0.6687 | 0.6890 | 0.9830 | 0.9830 | 0.9830 | 0.9830 | 0.9830 | 0.7732 | 0.9897 | 0.9912 | 0.9608 | 0.8083 | 0.9898 | 0.4791 | 0.9812 | 0.8319 | 0.9972 | 0.5368 | 0.9756 | 0.7700 | 0.9935 | 0.5861 |
| 0.0218 | 5.0 | 13295 | 0.0456 | 0.7480 | 0.8108 | 0.7781 | 0.7974 | 0.6661 | 0.6720 | 0.6662 | 0.6691 | 0.9833 | 0.9833 | 0.9833 | 0.9833 | 0.9833 | 0.7701 | 0.9890 | 0.9907 | 0.9612 | 0.8071 | 0.9894 | 0.4642 | 0.9823 | 0.8354 | 0.9977 | 0.5325 | 0.9754 | 0.7613 | 0.9936 | 0.5968 |
### Framework versions
- Transformers 4.8.2
- Pytorch 1.9.0+cu102
- Datasets 1.9.0
- Tokenizers 0.10.3
|
infinitejoy/wav2vec2-large-xls-r-300m-basaa-cv8 | 55558caa9ebfb20925bc3652aafd0de7f7d2a0fc | 2022-03-23T18:32:58.000Z | [
"pytorch",
"wav2vec2",
"automatic-speech-recognition",
"bas",
"dataset:mozilla-foundation/common_voice_8_0",
"transformers",
"generated_from_trainer",
"hf-asr-leaderboard",
"model_for_talk",
"mozilla-foundation/common_voice_8_0",
"robust-speech-event",
"license:apache-2.0",
"model-index"
] | automatic-speech-recognition | false | infinitejoy | null | infinitejoy/wav2vec2-large-xls-r-300m-basaa-cv8 | 2 | null | transformers | 24,254 | ---
language:
- bas
license: apache-2.0
tags:
- automatic-speech-recognition
- bas
- generated_from_trainer
- hf-asr-leaderboard
- model_for_talk
- mozilla-foundation/common_voice_8_0
- robust-speech-event
datasets:
- mozilla-foundation/common_voice_8_0
model-index:
- name: XLS-R-300M - Basaa
results:
- task:
name: Automatic Speech Recognition
type: automatic-speech-recognition
dataset:
name: Common Voice 8
type: mozilla-foundation/common_voice_8_0
args: bas
metrics:
- name: Test WER
type: wer
value: 38.057
- name: Test CER
type: cer
value: 11.233
---
<!-- This model card has been generated automatically according to the information the Trainer had access to. You
should probably proofread and complete it, then remove this comment. -->
# wav2vec2-large-xls-r-300m-basaa-cv8
This model is a fine-tuned version of [facebook/wav2vec2-xls-r-300m](https://huggingface.co/facebook/wav2vec2-xls-r-300m) on the MOZILLA-FOUNDATION/COMMON_VOICE_8_0 - BAS dataset.
It achieves the following results on the evaluation set:
- Loss: 0.4648
- Wer: 0.5472
## Model description
More information needed
## Intended uses & limitations
More information needed
## Training and evaluation data
More information needed
## Training procedure
### Training hyperparameters
The following hyperparameters were used during training:
- learning_rate: 7e-05
- train_batch_size: 32
- eval_batch_size: 16
- seed: 42
- optimizer: Adam with betas=(0.9,0.999) and epsilon=1e-08
- lr_scheduler_type: linear
- lr_scheduler_warmup_steps: 500
- num_epochs: 100.0
- mixed_precision_training: Native AMP
### Training results
| Training Loss | Epoch | Step | Validation Loss | Wer |
|:-------------:|:-----:|:----:|:---------------:|:------:|
| 2.9421 | 12.82 | 500 | 2.8894 | 1.0 |
| 1.1872 | 25.64 | 1000 | 0.6688 | 0.7460 |
| 0.8894 | 38.46 | 1500 | 0.4868 | 0.6516 |
| 0.769 | 51.28 | 2000 | 0.4960 | 0.6507 |
| 0.6936 | 64.1 | 2500 | 0.4781 | 0.5384 |
| 0.624 | 76.92 | 3000 | 0.4643 | 0.5430 |
| 0.5966 | 89.74 | 3500 | 0.4530 | 0.5591 |
### Framework versions
- Transformers 4.16.0.dev0
- Pytorch 1.10.1+cu102
- Datasets 1.18.3
- Tokenizers 0.11.0
|
infinitejoy/wav2vec2-large-xls-r-300m-basaa | 055a81af6c303bcb87a2f83c886e0523ec41b533 | 2022-03-23T18:33:50.000Z | [
"pytorch",
"wav2vec2",
"automatic-speech-recognition",
"bas",
"dataset:mozilla-foundation/common_voice_7_0",
"transformers",
"generated_from_trainer",
"hf-asr-leaderboard",
"mozilla-foundation/common_voice_7_0",
"robust-speech-event",
"license:apache-2.0",
"model-index"
] | automatic-speech-recognition | false | infinitejoy | null | infinitejoy/wav2vec2-large-xls-r-300m-basaa | 2 | 1 | transformers | 24,255 | ---
language:
- bas
license: apache-2.0
tags:
- automatic-speech-recognition
- generated_from_trainer
- hf-asr-leaderboard
- mozilla-foundation/common_voice_7_0
- robust-speech-event
datasets:
- mozilla-foundation/common_voice_7_0
model-index:
- name: XLS-R-300M - Basaa
results:
- task:
name: Automatic Speech Recognition
type: automatic-speech-recognition
dataset:
name: Common Voice 7
type: mozilla-foundation/common_voice_7_0
args: bas
metrics:
- name: Test WER
type: wer
value: 104.08
- name: Test CER
type: cer
value: 228.48
---
<!-- This model card has been generated automatically according to the information the Trainer had access to. You
should probably proofread and complete it, then remove this comment. -->
# wav2vec2-large-xls-r-300m-basaa
This model is a fine-tuned version of [facebook/wav2vec2-xls-r-300m](https://huggingface.co/facebook/wav2vec2-xls-r-300m) on the MOZILLA-FOUNDATION/COMMON_VOICE_7_0 - BAS dataset.
It achieves the following results on the evaluation set:
- Loss: 0.5975
- Wer: 0.4981
## Model description
More information needed
## Intended uses & limitations
More information needed
## Training and evaluation data
More information needed
## Training procedure
### Training hyperparameters
The following hyperparameters were used during training:
- learning_rate: 7e-05
- train_batch_size: 32
- eval_batch_size: 32
- seed: 42
- optimizer: Adam with betas=(0.9,0.999) and epsilon=1e-08
- lr_scheduler_type: linear
- lr_scheduler_warmup_steps: 500
- num_epochs: 200.0
- mixed_precision_training: Native AMP
### Training results
| Training Loss | Epoch | Step | Validation Loss | Wer |
|:-------------:|:------:|:----:|:---------------:|:------:|
| 2.9287 | 15.62 | 500 | 2.8774 | 1.0 |
| 1.1182 | 31.25 | 1000 | 0.6248 | 0.7131 |
| 0.8329 | 46.88 | 1500 | 0.5573 | 0.5792 |
| 0.7109 | 62.5 | 2000 | 0.5420 | 0.5683 |
| 0.6295 | 78.12 | 2500 | 0.5166 | 0.5395 |
| 0.5715 | 93.75 | 3000 | 0.5487 | 0.5629 |
| 0.5016 | 109.38 | 3500 | 0.5370 | 0.5471 |
| 0.4661 | 125.0 | 4000 | 0.5621 | 0.5395 |
| 0.423 | 140.62 | 4500 | 0.5658 | 0.5248 |
| 0.3793 | 156.25 | 5000 | 0.5921 | 0.4981 |
| 0.3651 | 171.88 | 5500 | 0.5987 | 0.4888 |
| 0.3351 | 187.5 | 6000 | 0.6017 | 0.4948 |
### Framework versions
- Transformers 4.16.0.dev0
- Pytorch 1.10.1+cu102
- Datasets 1.17.1.dev0
- Tokenizers 0.11.0
|
infinitejoy/wav2vec2-large-xls-r-300m-bashkir | 93ccdba8d075b8b2e455534a35a77a9eef41aa7e | 2022-03-23T18:30:18.000Z | [
"pytorch",
"wav2vec2",
"automatic-speech-recognition",
"ba",
"dataset:mozilla-foundation/common_voice_7_0",
"transformers",
"generated_from_trainer",
"hf-asr-leaderboard",
"mozilla-foundation/common_voice_7_0",
"robust-speech-event",
"license:apache-2.0",
"model-index"
] | automatic-speech-recognition | false | infinitejoy | null | infinitejoy/wav2vec2-large-xls-r-300m-bashkir | 2 | null | transformers | 24,256 | ---
language:
- ba
license: apache-2.0
tags:
- automatic-speech-recognition
- generated_from_trainer
- hf-asr-leaderboard
- mozilla-foundation/common_voice_7_0
- robust-speech-event
datasets:
- mozilla-foundation/common_voice_7_0
model-index:
- name: XLS-R-300M - Bashkir
results:
- task:
name: Automatic Speech Recognition
type: automatic-speech-recognition
dataset:
name: Common Voice 7
type: mozilla-foundation/common_voice_7_0
args: ba
metrics:
- name: Test WER
type: wer
value: 24.2
- name: Test CER
type: cer
value: 5.08
---
<!-- This model card has been generated automatically according to the information the Trainer had access to. You
should probably proofread and complete it, then remove this comment. -->
# wav2vec2-large-xls-r-300m-bashkir
This model is a fine-tuned version of [facebook/wav2vec2-xls-r-300m](https://huggingface.co/facebook/wav2vec2-xls-r-300m) on the MOZILLA-FOUNDATION/COMMON_VOICE_7_0 - BA dataset.
It achieves the following results on the evaluation set:
- Loss: 0.1892
- Wer: 0.2421
## Model description
More information needed
## Intended uses & limitations
More information needed
## Training and evaluation data
More information needed
## Training procedure
### Training hyperparameters
The following hyperparameters were used during training:
- learning_rate: 0.0003
- train_batch_size: 32
- eval_batch_size: 32
- seed: 42
- optimizer: Adam with betas=(0.9,0.999) and epsilon=1e-08
- lr_scheduler_type: linear
- lr_scheduler_warmup_steps: 2000
- num_epochs: 10.0
- mixed_precision_training: Native AMP
### Training results
| Training Loss | Epoch | Step | Validation Loss | Wer |
|:-------------:|:-----:|:-----:|:---------------:|:------:|
| 1.4792 | 0.5 | 2000 | 0.4598 | 0.5404 |
| 1.449 | 1.0 | 4000 | 0.4650 | 0.5610 |
| 1.3742 | 1.49 | 6000 | 0.4001 | 0.4977 |
| 1.3375 | 1.99 | 8000 | 0.3916 | 0.4894 |
| 1.2961 | 2.49 | 10000 | 0.3641 | 0.4569 |
| 1.2714 | 2.99 | 12000 | 0.3491 | 0.4488 |
| 1.2399 | 3.48 | 14000 | 0.3151 | 0.3986 |
| 1.2067 | 3.98 | 16000 | 0.3081 | 0.3923 |
| 1.1842 | 4.48 | 18000 | 0.2875 | 0.3703 |
| 1.1644 | 4.98 | 20000 | 0.2840 | 0.3670 |
| 1.161 | 5.48 | 22000 | 0.2790 | 0.3597 |
| 1.1303 | 5.97 | 24000 | 0.2552 | 0.3272 |
| 1.0874 | 6.47 | 26000 | 0.2405 | 0.3142 |
| 1.0613 | 6.97 | 28000 | 0.2352 | 0.3055 |
| 1.0498 | 7.47 | 30000 | 0.2249 | 0.2910 |
| 1.021 | 7.96 | 32000 | 0.2118 | 0.2752 |
| 1.0002 | 8.46 | 34000 | 0.2046 | 0.2662 |
| 0.9762 | 8.96 | 36000 | 0.1969 | 0.2530 |
| 0.9568 | 9.46 | 38000 | 0.1917 | 0.2449 |
| 0.953 | 9.96 | 40000 | 0.1893 | 0.2425 |
### Framework versions
- Transformers 4.16.0.dev0
- Pytorch 1.10.1+cu102
- Datasets 1.17.1.dev0
- Tokenizers 0.11.0
|
infinitejoy/wav2vec2-large-xls-r-300m-georgian | 344bf82537eec1d868d811dfa4643376899e18b5 | 2022-03-24T11:52:25.000Z | [
"pytorch",
"wav2vec2",
"automatic-speech-recognition",
"ka",
"dataset:mozilla-foundation/common_voice_7_0",
"transformers",
"mozilla-foundation/common_voice_7_0",
"generated_from_trainer",
"robust-speech-event",
"model_for_talk",
"hf-asr-leaderboard",
"license:apache-2.0",
"model-index"
] | automatic-speech-recognition | false | infinitejoy | null | infinitejoy/wav2vec2-large-xls-r-300m-georgian | 2 | null | transformers | 24,257 | ---
language:
- ka
license: apache-2.0
tags:
- automatic-speech-recognition
- mozilla-foundation/common_voice_7_0
- generated_from_trainer
- ka
- robust-speech-event
- model_for_talk
- hf-asr-leaderboard
datasets:
- mozilla-foundation/common_voice_7_0
model-index:
- name: XLS-R-300M - Georgian
results:
- task:
name: Automatic Speech Recognition
type: automatic-speech-recognition
dataset:
name: Common Voice 7
type: mozilla-foundation/common_voice_7_0
args: ka
metrics:
- name: Test WER
type: wer
value: 42.09
- name: Test CER
type: cer
value: 8.01
- task:
name: Automatic Speech Recognition
type: automatic-speech-recognition
dataset:
name: Robust Speech Event - Dev Data
type: speech-recognition-community-v2/dev_data
args: ka
metrics:
- name: Test WER
type: wer
value: 65.32
- task:
name: Automatic Speech Recognition
type: automatic-speech-recognition
dataset:
name: Robust Speech Event - Test Data
type: speech-recognition-community-v2/eval_data
args: ka
metrics:
- name: Test WER
type: wer
value: 65.03
---
<!-- This model card has been generated automatically according to the information the Trainer had access to. You
should probably proofread and complete it, then remove this comment. -->
# wav2vec2-large-xls-r-300m-georgian
This model is a fine-tuned version of [facebook/wav2vec2-xls-r-300m](https://huggingface.co/facebook/wav2vec2-xls-r-300m) on the MOZILLA-FOUNDATION/COMMON_VOICE_7_0 - KA dataset.
It achieves the following results on the evaluation set:
- Loss: 0.3666
- Wer: 0.4211
## Model description
More information needed
## Intended uses & limitations
More information needed
## Training and evaluation data
More information needed
## Training procedure
### Training hyperparameters
The following hyperparameters were used during training:
- learning_rate: 0.0003
- train_batch_size: 32
- eval_batch_size: 32
- seed: 42
- optimizer: Adam with betas=(0.9,0.999) and epsilon=1e-08
- lr_scheduler_type: linear
- lr_scheduler_warmup_steps: 500
- num_epochs: 100.0
- mixed_precision_training: Native AMP
### Training results
| Training Loss | Epoch | Step | Validation Loss | Wer |
|:-------------:|:-----:|:----:|:---------------:|:------:|
| 1.8805 | 5.95 | 500 | 0.7547 | 0.8438 |
| 1.2123 | 11.9 | 1000 | 0.4732 | 0.6542 |
| 1.0822 | 17.86 | 1500 | 0.4027 | 0.5778 |
| 0.9938 | 23.81 | 2000 | 0.3847 | 0.5524 |
| 0.9383 | 29.76 | 2500 | 0.3845 | 0.5204 |
| 0.8932 | 35.71 | 3000 | 0.3833 | 0.5297 |
| 0.8495 | 41.67 | 3500 | 0.3759 | 0.5036 |
| 0.8201 | 47.62 | 4000 | 0.3616 | 0.4859 |
| 0.7794 | 53.57 | 4500 | 0.3874 | 0.4938 |
| 0.735 | 59.52 | 5000 | 0.3748 | 0.4782 |
| 0.7082 | 65.48 | 5500 | 0.3615 | 0.4675 |
| 0.669 | 71.43 | 6000 | 0.3797 | 0.4601 |
| 0.6457 | 77.38 | 6500 | 0.3812 | 0.4515 |
| 0.6098 | 83.33 | 7000 | 0.3660 | 0.4343 |
| 0.5874 | 89.29 | 7500 | 0.3640 | 0.4257 |
| 0.5627 | 95.24 | 8000 | 0.3661 | 0.4239 |
### Framework versions
- Transformers 4.16.0.dev0
- Pytorch 1.10.1+cu102
- Datasets 1.17.1.dev0
- Tokenizers 0.11.0
|
infinitejoy/wav2vec2-large-xls-r-300m-irish | 6b414b222a6f0476a5678e757f7ed70551ccbdf8 | 2022-03-24T11:52:28.000Z | [
"pytorch",
"wav2vec2",
"automatic-speech-recognition",
"ga-IE",
"dataset:mozilla-foundation/common_voice_7_0",
"transformers",
"mozilla-foundation/common_voice_7_0",
"generated_from_trainer",
"robust-speech-event",
"model_for_talk",
"hf-asr-leaderboard",
"license:apache-2.0",
"model-index"
] | automatic-speech-recognition | false | infinitejoy | null | infinitejoy/wav2vec2-large-xls-r-300m-irish | 2 | null | transformers | 24,258 | ---
language:
- ga-IE
license: apache-2.0
tags:
- automatic-speech-recognition
- mozilla-foundation/common_voice_7_0
- generated_from_trainer
- ga-IE
- robust-speech-event
- model_for_talk
- hf-asr-leaderboard
datasets:
- mozilla-foundation/common_voice_7_0
model-index:
- name: XLS-R-300M - Irish
results:
- task:
name: Automatic Speech Recognition
type: automatic-speech-recognition
dataset:
name: Common Voice 7
type: mozilla-foundation/common_voice_7_0
args: ga-IE
metrics:
- name: Test WER
type: wer
value: 103.54
- name: Test CER
type: cer
value: 326.923
---
<!-- This model card has been generated automatically according to the information the Trainer had access to. You
should probably proofread and complete it, then remove this comment. -->
# wav2vec2-large-xls-r-300m-irish
This model is a fine-tuned version of [facebook/wav2vec2-xls-r-300m](https://huggingface.co/facebook/wav2vec2-xls-r-300m) on the MOZILLA-FOUNDATION/COMMON_VOICE_7_0 - GA-IE dataset.
It achieves the following results on the evaluation set:
- Loss: 1.1647
- Wer: 0.7296
## Model description
More information needed
## Intended uses & limitations
More information needed
## Training and evaluation data
More information needed
## Training procedure
### Training hyperparameters
The following hyperparameters were used during training:
- learning_rate: 7e-05
- train_batch_size: 64
- eval_batch_size: 8
- seed: 42
- gradient_accumulation_steps: 4
- total_train_batch_size: 256
- optimizer: Adam with betas=(0.9,0.999) and epsilon=1e-08
- lr_scheduler_type: linear
- lr_scheduler_warmup_steps: 200
- num_epochs: 300.0
- mixed_precision_training: Native AMP
### Training results
| Training Loss | Epoch | Step | Validation Loss | Wer |
|:-------------:|:------:|:----:|:---------------:|:------:|
| 2.9022 | 124.94 | 500 | 2.7763 | 0.9824 |
| 1.5112 | 249.94 | 1000 | 1.1736 | 0.7405 |
### Framework versions
- Transformers 4.16.0.dev0
- Pytorch 1.10.1+cu102
- Datasets 1.17.1.dev0
- Tokenizers 0.11.0
|
infinitejoy/wav2vec2-large-xls-r-300m-kyrgyz | fe4e957e476b567e4cf15dc89e56712e35d5983b | 2022-03-24T11:52:31.000Z | [
"pytorch",
"wav2vec2",
"automatic-speech-recognition",
"ky",
"dataset:mozilla-foundation/common_voice_7_0",
"transformers",
"mozilla-foundation/common_voice_7_0",
"generated_from_trainer",
"robust-speech-event",
"model_for_talk",
"hf-asr-leaderboard",
"license:apache-2.0",
"model-index"
] | automatic-speech-recognition | false | infinitejoy | null | infinitejoy/wav2vec2-large-xls-r-300m-kyrgyz | 2 | null | transformers | 24,259 | ---
language:
- ky
license: apache-2.0
tags:
- automatic-speech-recognition
- mozilla-foundation/common_voice_7_0
- generated_from_trainer
- ky
- robust-speech-event
- model_for_talk
- hf-asr-leaderboard
datasets:
- mozilla-foundation/common_voice_7_0
model-index:
- name: XLS-R-300M - Kyrgyz
results:
- task:
name: Automatic Speech Recognition
type: automatic-speech-recognition
dataset:
name: Common Voice 7
type: mozilla-foundation/common_voice_7_0
args: ky
metrics:
- name: Test WER
type: wer
value: 40.908
- name: Test CER
type: cer
value: 10.999
---
<!-- This model card has been generated automatically according to the information the Trainer had access to. You
should probably proofread and complete it, then remove this comment. -->
# wav2vec2-large-xls-r-300m-kyrgyz
This model is a fine-tuned version of [facebook/wav2vec2-xls-r-300m](https://huggingface.co/facebook/wav2vec2-xls-r-300m) on the MOZILLA-FOUNDATION/COMMON_VOICE_7_0 - KY dataset.
It achieves the following results on the evaluation set:
- Loss: 0.5817
- Wer: 0.4096
## Model description
More information needed
## Intended uses & limitations
More information needed
## Training and evaluation data
More information needed
## Training procedure
### Training hyperparameters
The following hyperparameters were used during training:
- learning_rate: 0.0003
- train_batch_size: 32
- eval_batch_size: 1
- seed: 42
- optimizer: Adam with betas=(0.9,0.999) and epsilon=1e-08
- lr_scheduler_type: linear
- lr_scheduler_warmup_steps: 2000
- num_epochs: 100.0
- mixed_precision_training: Native AMP
### Training results
| Training Loss | Epoch | Step | Validation Loss | Wer |
|:-------------:|:-----:|:-----:|:---------------:|:------:|
| 1.5412 | 18.69 | 2000 | 0.6161 | 0.5747 |
| 1.311 | 37.38 | 4000 | 0.5707 | 0.5070 |
| 1.1367 | 56.07 | 6000 | 0.5372 | 0.4664 |
| 0.9696 | 74.77 | 8000 | 0.5443 | 0.4328 |
| 0.8163 | 93.46 | 10000 | 0.5916 | 0.4124 |
### Framework versions
- Transformers 4.16.0.dev0
- Pytorch 1.10.1+cu102
- Datasets 1.17.1.dev0
- Tokenizers 0.11.0
|
infinitejoy/wav2vec2-large-xls-r-300m-latvian | bce836ab3ff6a5dc75e075909f03c494a7430f69 | 2022-03-23T18:33:25.000Z | [
"pytorch",
"wav2vec2",
"automatic-speech-recognition",
"lv",
"dataset:mozilla-foundation/common_voice_7_0",
"transformers",
"generated_from_trainer",
"hf-asr-leaderboard",
"model_for_talk",
"mozilla-foundation/common_voice_7_0",
"robust-speech-event",
"license:apache-2.0",
"model-index"
] | automatic-speech-recognition | false | infinitejoy | null | infinitejoy/wav2vec2-large-xls-r-300m-latvian | 2 | null | transformers | 24,260 | ---
language:
- lv
license: apache-2.0
tags:
- automatic-speech-recognition
- generated_from_trainer
- hf-asr-leaderboard
- lv
- model_for_talk
- mozilla-foundation/common_voice_7_0
- robust-speech-event
datasets:
- mozilla-foundation/common_voice_7_0
model-index:
- name: XLS-R-300M - Latvian
results:
- task:
name: Automatic Speech Recognition
type: automatic-speech-recognition
dataset:
name: Common Voice 7
type: mozilla-foundation/common_voice_7_0
args: lv
metrics:
- name: Test WER
type: wer
value: 16.977
- name: Test CER
type: cer
value: 4.23
- task:
name: Automatic Speech Recognition
type: automatic-speech-recognition
dataset:
name: Robust Speech Event - Dev Data
type: speech-recognition-community-v2/dev_data
args: lv
metrics:
- name: Test WER
type: wer
value: 45.247
- name: Test CER
type: cer
value: 16.924
- task:
name: Automatic Speech Recognition
type: automatic-speech-recognition
dataset:
name: Robust Speech Event - Test Data
type: speech-recognition-community-v2/eval_data
args: lv
metrics:
- name: Test WER
type: wer
value: 56.16
---
<!-- This model card has been generated automatically according to the information the Trainer had access to. You
should probably proofread and complete it, then remove this comment. -->
# wav2vec2-large-xls-r-300m-latvian
This model is a fine-tuned version of [facebook/wav2vec2-xls-r-300m](https://huggingface.co/facebook/wav2vec2-xls-r-300m) on the MOZILLA-FOUNDATION/COMMON_VOICE_7_0 - LV dataset.
It achieves the following results on the evaluation set:
- Loss: 0.1892
- Wer: 0.1698
## Model description
More information needed
## Intended uses & limitations
More information needed
## Training and evaluation data
More information needed
## Training procedure
### Training hyperparameters
The following hyperparameters were used during training:
- learning_rate: 7e-05
- train_batch_size: 32
- eval_batch_size: 1
- seed: 42
- optimizer: Adam with betas=(0.9,0.999) and epsilon=1e-08
- lr_scheduler_type: linear
- lr_scheduler_warmup_steps: 2000
- num_epochs: 100.0
- mixed_precision_training: Native AMP
### Training results
| Training Loss | Epoch | Step | Validation Loss | Wer |
|:-------------:|:-----:|:-----:|:---------------:|:------:|
| 1.4235 | 12.82 | 2000 | 0.4475 | 0.4551 |
| 0.9383 | 25.64 | 4000 | 0.2235 | 0.2328 |
| 0.8359 | 38.46 | 6000 | 0.2004 | 0.2098 |
| 0.7633 | 51.28 | 8000 | 0.1960 | 0.1882 |
| 0.7001 | 64.1 | 10000 | 0.1902 | 0.1809 |
| 0.652 | 76.92 | 12000 | 0.1979 | 0.1775 |
| 0.6025 | 89.74 | 14000 | 0.1866 | 0.1696 |
### Framework versions
- Transformers 4.16.0.dev0
- Pytorch 1.10.1+cu102
- Datasets 1.17.1.dev0
- Tokenizers 0.11.0
|
infinitejoy/wav2vec2-large-xls-r-300m-marathi-cv8 | 0eb0445e8192da240c6a68604af3873b35b0226c | 2022-03-24T11:58:09.000Z | [
"pytorch",
"wav2vec2",
"automatic-speech-recognition",
"mr",
"dataset:mozilla-foundation/common_voice_8_0",
"transformers",
"mozilla-foundation/common_voice_8_0",
"generated_from_trainer",
"robust-speech-event",
"model_for_talk",
"hf-asr-leaderboard",
"license:apache-2.0",
"model-index"
] | automatic-speech-recognition | false | infinitejoy | null | infinitejoy/wav2vec2-large-xls-r-300m-marathi-cv8 | 2 | null | transformers | 24,261 | ---
language:
- mr
license: apache-2.0
tags:
- automatic-speech-recognition
- mozilla-foundation/common_voice_8_0
- generated_from_trainer
- mr
- robust-speech-event
- model_for_talk
- hf-asr-leaderboard
datasets:
- mozilla-foundation/common_voice_8_0
model-index:
- name: XLS-R-300M - Marathi
results:
- task:
name: Automatic Speech Recognition
type: automatic-speech-recognition
dataset:
name: Common Voice 8
type: mozilla-foundation/common_voice_8_0
args: mr
metrics:
- name: Test WER
type: wer
value: 55.716
- name: Test CER
type: cer
value: 13.842
---
<!-- This model card has been generated automatically according to the information the Trainer had access to. You
should probably proofread and complete it, then remove this comment. -->
# wav2vec2-large-xls-r-300m-marathi-cv8
This model is a fine-tuned version of [facebook/wav2vec2-xls-r-300m](https://huggingface.co/facebook/wav2vec2-xls-r-300m) on the MOZILLA-FOUNDATION/COMMON_VOICE_8_0 - MR dataset.
It achieves the following results on the evaluation set:
- Loss: 0.6483
- Wer: 0.6049
## Model description
More information needed
## Intended uses & limitations
More information needed
## Training and evaluation data
More information needed
## Training procedure
### Training hyperparameters
The following hyperparameters were used during training:
- learning_rate: 0.0003
- train_batch_size: 32
- eval_batch_size: 16
- seed: 42
- optimizer: Adam with betas=(0.9,0.999) and epsilon=1e-08
- lr_scheduler_type: linear
- lr_scheduler_warmup_steps: 500
- num_epochs: 100.0
- mixed_precision_training: Native AMP
### Training results
| Training Loss | Epoch | Step | Validation Loss | Wer |
|:-------------:|:-----:|:----:|:---------------:|:------:|
| 2.671 | 22.73 | 500 | 1.3618 | 0.9499 |
| 1.1599 | 45.45 | 1000 | 0.6330 | 0.6627 |
| 0.8252 | 68.18 | 1500 | 0.6226 | 0.6426 |
| 0.6424 | 90.91 | 2000 | 0.6359 | 0.6041 |
### Framework versions
- Transformers 4.17.0.dev0
- Pytorch 1.10.2+cu102
- Datasets 1.18.3
- Tokenizers 0.11.0
|
infinitejoy/wav2vec2-large-xls-r-300m-romansh-sursilvan | 6b922777a0980449c43e266dda9944df128779c3 | 2022-03-24T11:51:18.000Z | [
"pytorch",
"wav2vec2",
"automatic-speech-recognition",
"rm-sursilv",
"dataset:mozilla-foundation/common_voice_7_0",
"transformers",
"mozilla-foundation/common_voice_7_0",
"generated_from_trainer",
"robust-speech-event",
"model_for_talk",
"hf-asr-leaderboard",
"license:apache-2.0",
"model-index"
] | automatic-speech-recognition | false | infinitejoy | null | infinitejoy/wav2vec2-large-xls-r-300m-romansh-sursilvan | 2 | null | transformers | 24,262 | ---
language:
- rm-sursilv
license: apache-2.0
tags:
- automatic-speech-recognition
- mozilla-foundation/common_voice_7_0
- generated_from_trainer
- rm-sursilv
- robust-speech-event
- model_for_talk
- hf-asr-leaderboard
datasets:
- mozilla-foundation/common_voice_7_0
model-index:
- name: XLS-R-300M - Romansh Sursilvan
results:
- task:
name: Automatic Speech Recognition
type: automatic-speech-recognition
dataset:
name: Common Voice 7
type: mozilla-foundation/common_voice_7_0
args: rm-sursilv
metrics:
- name: Test WER
type: wer
value: 19.816
- name: Test CER
type: cer
value: 4.153
---
<!-- This model card has been generated automatically according to the information the Trainer had access to. You
should probably proofread and complete it, then remove this comment. -->
# wav2vec2-large-xls-r-300m-romansh-sursilvan
This model is a fine-tuned version of [facebook/wav2vec2-xls-r-300m](https://huggingface.co/facebook/wav2vec2-xls-r-300m) on the MOZILLA-FOUNDATION/COMMON_VOICE_7_0 - RM-SURSILV dataset.
It achieves the following results on the evaluation set:
- Loss: 0.2163
- Wer: 0.1981
## Model description
More information needed
## Intended uses & limitations
More information needed
## Training and evaluation data
More information needed
## Training procedure
### Training hyperparameters
The following hyperparameters were used during training:
- learning_rate: 7e-05
- train_batch_size: 32
- eval_batch_size: 1
- seed: 42
- optimizer: Adam with betas=(0.9,0.999) and epsilon=1e-08
- lr_scheduler_type: linear
- lr_scheduler_warmup_steps: 2000
- num_epochs: 120.0
- mixed_precision_training: Native AMP
### Training results
| Training Loss | Epoch | Step | Validation Loss | Wer |
|:-------------:|:------:|:-----:|:---------------:|:------:|
| 1.1004 | 23.81 | 2000 | 0.3710 | 0.4191 |
| 0.7002 | 47.62 | 4000 | 0.2342 | 0.2562 |
| 0.5573 | 71.43 | 6000 | 0.2175 | 0.2177 |
| 0.4799 | 95.24 | 8000 | 0.2109 | 0.1987 |
| 0.4511 | 119.05 | 10000 | 0.2164 | 0.1975 |
### Framework versions
- Transformers 4.16.0.dev0
- Pytorch 1.10.1+cu102
- Datasets 1.17.1.dev0
- Tokenizers 0.11.0
|
inspectorsolaris/gpt2_french | c45583a06dc4816c377f5836498e652e5ddf09e0 | 2021-05-24T09:02:18.000Z | [
"pytorch",
"gpt2",
"text-generation",
"transformers"
] | text-generation | false | inspectorsolaris | null | inspectorsolaris/gpt2_french | 2 | null | transformers | 24,263 | hello
|
ionite/DialoGPT-medium-McKayAI | a8834db5fd6b0f62e9738c71162b2054fe381610 | 2021-11-07T05:52:48.000Z | [
"pytorch",
"gpt2",
"text-generation",
"transformers",
"conversational"
] | conversational | false | ionite | null | ionite/DialoGPT-medium-McKayAI | 2 | 1 | transformers | 24,264 | ---
tags:
- conversational
---
# McKayAI DialoGPT Model |
it5/it5-base-formal-to-informal | 7d0c28da139bb9204c069a700cd3d88d972e739b | 2022-03-09T07:45:49.000Z | [
"pytorch",
"tf",
"jax",
"tensorboard",
"t5",
"text2text-generation",
"it",
"dataset:yahoo/xformal_it",
"arxiv:2203.03759",
"transformers",
"italian",
"sequence-to-sequence",
"style-transfer",
"formality-style-transfer",
"license:apache-2.0",
"model-index",
"co2_eq_emissions",
"autotrain_compatible"
] | text2text-generation | false | it5 | null | it5/it5-base-formal-to-informal | 2 | null | transformers | 24,265 | ---
language:
- it
license: apache-2.0
tags:
- italian
- sequence-to-sequence
- style-transfer
- formality-style-transfer
datasets:
- yahoo/xformal_it
widget:
- text: "Questa performance è a dir poco spiacevole."
- text: "In attesa di un Suo cortese riscontro, Le auguriamo un piacevole proseguimento di giornata."
- text: "Questa visione mi procura una goduria indescrivibile."
- text: "qualora ciò possa interessarti, ti pregherei di contattarmi."
metrics:
- rouge
- bertscore
model-index:
- name: it5-base-formal-to-informal
results:
- task:
type: formality-style-transfer
name: "Formal-to-informal Style Transfer"
dataset:
type: xformal_it
name: "XFORMAL (Italian Subset)"
metrics:
- type: rouge1
value: 0.652
name: "Avg. Test Rouge1"
- type: rouge2
value: 0.446
name: "Avg. Test Rouge2"
- type: rougeL
value: 0.632
name: "Avg. Test RougeL"
- type: bertscore
value: 0.665
name: "Avg. Test BERTScore"
args:
- model_type: "dbmdz/bert-base-italian-xxl-uncased"
- lang: "it"
- num_layers: 10
- rescale_with_baseline: True
- baseline_path: "bertscore_baseline_ita.tsv"
co2_eq_emissions:
emissions: "17g"
source: "Google Cloud Platform Carbon Footprint"
training_type: "fine-tuning"
geographical_location: "Eemshaven, Netherlands, Europe"
hardware_used: "1 TPU v3-8 VM"
---
# IT5 Base for Formal-to-informal Style Transfer 🤗
This repository contains the checkpoint for the [IT5 Base](https://huggingface.co/gsarti/it5-base) model fine-tuned on Formal-to-informal style transfer on the Italian subset of the XFORMAL dataset as part of the experiments of the paper [IT5: Large-scale Text-to-text Pretraining for Italian Language Understanding and Generation](https://arxiv.org/abs/2203.03759) by [Gabriele Sarti](https://gsarti.com) and [Malvina Nissim](https://malvinanissim.github.io).
A comprehensive overview of other released materials is provided in the [gsarti/it5](https://github.com/gsarti/it5) repository. Refer to the paper for additional details concerning the reported scores and the evaluation approach.
## Using the model
Model checkpoints are available for usage in Tensorflow, Pytorch and JAX. They can be used directly with pipelines as:
```python
from transformers import pipelines
f2i = pipeline("text2text-generation", model='it5/it5-base-formal-to-informal')
f2i("Vi ringrazio infinitamente per vostra disponibilità")
>>> [{"generated_text": "e grazie per la vostra disponibilità!"}]
```
or loaded using autoclasses:
```python
from transformers import AutoTokenizer, AutoModelForSeq2SeqLM
tokenizer = AutoTokenizer.from_pretrained("it5/it5-base-formal-to-informal")
model = AutoModelForSeq2SeqLM.from_pretrained("it5/it5-base-formal-to-informal")
```
If you use this model in your research, please cite our work as:
```bibtex
@article{sarti-nissim-2022-it5,
title={{IT5}: Large-scale Text-to-text Pretraining for Italian Language Understanding and Generation},
author={Sarti, Gabriele and Nissim, Malvina},
journal={ArXiv preprint 2203.03759},
url={https://arxiv.org/abs/2203.03759},
year={2022},
month={mar}
}
``` |
it5/it5-large-informal-to-formal | 29e69478a6df660fc6cd11561413173cb6e3e9d1 | 2022-03-09T07:48:09.000Z | [
"pytorch",
"tf",
"jax",
"tensorboard",
"t5",
"text2text-generation",
"it",
"dataset:yahoo/xformal_it",
"arxiv:2203.03759",
"transformers",
"italian",
"sequence-to-sequence",
"style-transfer",
"formality-style-transfer",
"license:apache-2.0",
"model-index",
"co2_eq_emissions",
"autotrain_compatible"
] | text2text-generation | false | it5 | null | it5/it5-large-informal-to-formal | 2 | null | transformers | 24,266 | ---
language:
- it
license: apache-2.0
tags:
- italian
- sequence-to-sequence
- style-transfer
- formality-style-transfer
datasets:
- yahoo/xformal_it
widget:
- text: "maronn qualcuno mi spieg' CHECCOSA SUCCEDE?!?!"
- text: "wellaaaaaaa, ma fraté sei proprio troppo simpatiko, grazieeee!!"
- text: "nn capisco xke tt i ragazzi lo fanno"
- text: "IT5 è SUPERMEGA BRAVISSIMO a capire tt il vernacolo italiano!!!"
metrics:
- rouge
- bertscore
model-index:
- name: it5-large-informal-to-formal
results:
- task:
type: formality-style-transfer
name: "Informal-to-formal Style Transfer"
dataset:
type: xformal_it
name: "XFORMAL (Italian Subset)"
metrics:
- type: rouge1
value: 0.663
name: "Avg. Test Rouge1"
- type: rouge2
value: 0.477
name: "Avg. Test Rouge2"
- type: rougeL
value: 0.645
name: "Avg. Test RougeL"
- type: bertscore
value: 0.714
name: "Avg. Test BERTScore"
args:
- model_type: "dbmdz/bert-base-italian-xxl-uncased"
- lang: "it"
- num_layers: 10
- rescale_with_baseline: True
- baseline_path: "bertscore_baseline_ita.tsv"
co2_eq_emissions:
emissions: "51g"
source: "Google Cloud Platform Carbon Footprint"
training_type: "fine-tuning"
geographical_location: "Eemshaven, Netherlands, Europe"
hardware_used: "1 TPU v3-8 VM"
---
# IT5 Base for Informal-to-formal Style Transfer 🧐
This repository contains the checkpoint for the [IT5 Large](https://huggingface.co/gsarti/it5-large) model fine-tuned on Informal-to-formal style transfer on the Italian subset of the XFORMAL dataset as part of the experiments of the paper [IT5: Large-scale Text-to-text Pretraining for Italian Language Understanding and Generation](https://arxiv.org/abs/2203.03759) by [Gabriele Sarti](https://gsarti.com) and [Malvina Nissim](https://malvinanissim.github.io).
A comprehensive overview of other released materials is provided in the [gsarti/it5](https://github.com/gsarti/it5) repository. Refer to the paper for additional details concerning the reported scores and the evaluation approach.
## Using the model
Model checkpoints are available for usage in Tensorflow, Pytorch and JAX. They can be used directly with pipelines as:
```python
from transformers import pipelines
i2f = pipeline("text2text-generation", model='it5/it5-large-informal-to-formal')
i2f("nn capisco xke tt i ragazzi lo fanno")
>>> [{"generated_text": "non comprendo perché tutti i ragazzi agiscono così"}]
```
or loaded using autoclasses:
```python
from transformers import AutoTokenizer, AutoModelForSeq2SeqLM
tokenizer = AutoTokenizer.from_pretrained("it5/it5-large-informal-to-formal")
model = AutoModelForSeq2SeqLM.from_pretrained("it5/it5-large-informal-to-formal")
```
If you use this model in your research, please cite our work as:
```bibtex
@article{sarti-nissim-2022-it5,
title={{IT5}: Large-scale Text-to-text Pretraining for Italian Language Understanding and Generation},
author={Sarti, Gabriele and Nissim, Malvina},
journal={ArXiv preprint 2203.03759},
url={https://arxiv.org/abs/2203.03759},
year={2022},
month={mar}
}
``` |
it5/it5-small-repubblica-to-ilgiornale | 76e15ef56e650789a6e68cbe3e95c2bd5ff27e71 | 2022-03-09T08:02:27.000Z | [
"pytorch",
"tf",
"jax",
"tensorboard",
"t5",
"text2text-generation",
"it",
"dataset:gsarti/change_it",
"arxiv:2203.03759",
"transformers",
"italian",
"sequence-to-sequence",
"newspaper",
"ilgiornale",
"repubblica",
"style-transfer",
"license:apache-2.0",
"model-index",
"co2_eq_emissions",
"autotrain_compatible"
] | text2text-generation | false | it5 | null | it5/it5-small-repubblica-to-ilgiornale | 2 | null | transformers | 24,267 | ---
language:
- it
license: apache-2.0
datasets:
- gsarti/change_it
tags:
- italian
- sequence-to-sequence
- newspaper
- ilgiornale
- repubblica
- style-transfer
widget:
- text: "WASHINGTON - La Corea del Nord torna dopo nove anni nella blacklist Usa degli Stati considerati sponsor del terrorismo. Come Iran, Siria e Sudan. Lo ha deciso Donald Trump , che ha preferito dare l'annuncio non durante il suo recente viaggio in Asia ma ieri, in una riunione del governo alla Casa Bianca. 'Oggi gli Stati Uniti designeranno la Corea del nord come uno stato sponsor del terrorismo', ha tuonato il tycoon, anticipando che sarà formalizzata oggi dal dipartimento di stato e sarà accompagnata da nuove e più severe sanzioni. 'Il livello più alto' mai imposto a Pyongyang, ha promesso. 'Avrebbe dovuto succedere molto tempo fa', ha aggiunto, scaricando per l'ennesima volta la responsabilità dell'attuale crisi sull'amministrazione Obama. Poi si è scagliato contro un 'regime assassino' che 'deve mettere fine allo sviluppo del suo programma illegale nucleare e balistico'. Per giustificare la svolta, Trump ha accusato Pyongyang non solo di 'minacciare il mondo con una devastazione nucleare' ma anche di aver 'ripetutamente sostenuto atti di terrorismo internazionale', compreso omicidi in suolo straniero. Il riferimento è all' uccisione all'aeroporto della capitale malese di Kim Jong Nam , il fratellastro del leader nordcoreano Kim Jong Un , ma non ci sono altri episodi noti. Tanto che alcuni esperti, come pure dirigenti Usa coperti dall'anonimato, dubitano che Pyongyang risponda ai criteri per una tale designazione. La mossa appare altamente simbolica, dato che la Corea del Nord è già pesantemente sanzionata a livello internazionale. Per il segretario di stato Rex Tillerson è solo l'ultima di una serie di passi per rafforzare la pressione su Pyongyang e costringerla a sedersi ad un tavolo perché gli Usa hanno sempre 'speranza nella diplomazia'. Ma nello stesso tempo è un monito per 'fermare e dissuadere' altri Paesi dal sostenere la Corea del Nord, finita nella blacklist 'anche per l'uso di armi chimiche'. Ma la mossa potrebbe anche essere controproducente, provocando una risposta di Kim o minando gli sforzi per sollecitare Pechino ad una maggiore pressione su Pyongyang. In ogni caso non aiuta il dialogo diretto tra Usa e Corea del Nord, che sembrava essere stato avviato in modo riservato. Come non aiutano gli scambi di insulti fra Trump e Kim. Nord Corea, Trump: 'Cerco di essere amico di Kim, sarebbe una bella cosa per il mondo'. Pyongyang era stata messa nella lista Usa degli Stati sponsor del terrorismo per aver fatto esplodere nel 1987 un volo della Korean Air uccidendo tutti i 115 passeggeri a bordo. Ma l'amministrazione di George W. Bush l'aveva rimossa sperando di far avanzare i negoziati sulla denuclearizzazione della penisola coreana. Il governo giapponese sostiene la decisione degli Stati Uniti di inserire la Corea del Nord nella lista degli stati che sponsorizzano il terrorismo, pur riconoscendo che l'annuncio potrebbe provocare una reazione immediata del regime di Pyongyang. Il premier Shinzo Abe ha accolto con consenso il comunicato Usa e ha detto alla stampa che servirà a incrementare la pressione sulla Corea del Nord. Il ministro della Difesa Itsunori Onodera , pur valutando positivamente la notifica, ha spiegato che si attendono azioni provocatorie dallo stato eremita, ribadendo che è vitale rimanere vigili. Secondo la stampa nipponica Abe aveva richiesto al dipartimento di Stato Usa di mettere la Corea del Nord sulla lista durante l'incontro col presidente Usa Donald Trump a Tokyo a inizio mese. L'ultimo lancio di missile balistico condotto da Pyongyang nell'oceano Pacifico, sorvolando il mare del Giappone, risale allo scorso settembre."
- text: "ROMA - Una nuova droga killer è stata sequestrata per la prima volta in Europa dagli investigatori del Nas. Si tratta di una nuova \"miscela psicoattiva altamente tossica\" per la prima volta individuata da forze di polizia, simile all'eroina sintetica, ma molto più economica e letale. Tanto che i 20 grammi scoperti sarebbero stati sufficienti per fabbricare ben 20.000 dosi e lo stesso contatto attraverso la pelle può provocare intossicazione. Individuata per la prima volta, la nuova droga presenta una struttura simile al farmaco sedativo Fentanyl ma con effetti molto più devastanti per l'organismo. Proveniva dell'estero ed era contenuta in un plico postale indirizzato in una città del centro Italia: è stata intercettata tramite accertamenti sul web grazie a un'operazione di intelligence che ha visto come protagonisti i militari della Sezione operativa centrale del Comando carabinieri per la Tutela della salute (Nas). Economica e letale, secondo gli investigatori \"in confronto l'eroina è quasi 'acqua fresca', anzi, proprio per la sua economicità, in alcuni casi viene venduta dai pusher a giovani conviti di comprare eroina\". La diffusione di nuove droghe sintetiche che continuamente appaiono sui mercati necessita di un'attività investigativa costante e complessa. Si tratta infatti di sostanze dalla struttura molecolare molto simile a quella del Fentanyl ma ogni volta leggermente diversa. Di qui la difficoltà di individuarle e l'importanza del nuovo sequestro. \"La chiamano impropriamente 'eroina sintetica' - spiega il comandante dei Nas, generale Adelmo Lusi - per il tipo di effetto psicotropo simile, ma dal punto di vista della tossicità è molto peggio: con 25 milligrammi di eroina ci si sballa, con 25mg di simil-fentanyl, come quello appena sequestrato, si muore\". Le indagini sono partite da ricoveri per overdose in ospedale, in cui arrivavano ragazzi che non rispondevano al trattamento disintossicante per l'eroina. La nuova sostanza verrà ora segnalata per l'inserimento tra le tabelle ministeriali degli stupefacenti prevista dal Dpr 309/1990."
- text: "Fragile come il burro. Il nostro territorio è precario. Ne sanno qualcosa i comuni che sono stati investititi dal maltempo . Il dissesto idrogeologico imperversa su tutto il territorio. Infatti, oltre 6.600 comuni , pari all’82% del totale, sono in aree ad elevato rischio idrogeologico, pari al 10% della sua superficie. La popolazione potenzialmente esposta è stimata in 5,8 milioni di persone. I dati emergono dalle recenti analisi fatte da Legambiente e Protezione civile, che mettono in evidenza come in 10 anni in Italia sia raddoppiata l’area dei territori colpiti da alluvioni e frane , passando da una media di quattro regioni all’anno a otto regioni. Nella classifica delle regioni a maggior rischio idrogeologico prima è la Calabria con il 100% dei comuni esposti; al 100% ci sono anche la provincia di Trento, il Molise, la Basilicata, l’Umbria, la Valle d’Aosta. Poi Marche, Liguria al 99%; Lazio, Toscana al 98%; Abruzzo (96%), Emilia-Romagna (95%), Campania e Friuli Venezia Giulia al 92%, Piemonte (87%), Sardegna (81%), Puglia (78%), Sicilia (71%), Lombardia (60%), provincia di Bolzano (59%), Veneto (56%). Tra le cause che condizionano ed amplificano il rischio idrogeologico c’è l’azione dell’uomo (abbandono e degrado, cementificazione, consumo di suolo, abusivismo, disboscamento e incendi). Ma anche e soprattutto la mancanza di una seria manutenzione ordinaria e non ad una organica politica di prevenzione."
- text: "Arriva dal Partito nazionalista basco (Pnv) la conferma che i cinque deputati che siedono in parlamento voteranno la sfiducia al governo guidato da Mariano Rajoy. Pochi voti, ma significativi quelli della formazione politica di Aitor Esteban, che interverrà nel pomeriggio. Pur con dimensioni molto ridotte, il partito basco si è trovato a fare da ago della bilancia in aula. E il sostegno alla mozione presentata dai Socialisti potrebbe significare per il primo ministro non trovare quei 176 voti che gli servono per continuare a governare. \" Perché dovrei dimettermi io che per il momento ho la fiducia della Camera e quella che mi è stato data alle urne \", ha detto oggi Rajoy nel suo intervento in aula, mentre procedeva la discussione sulla mozione di sfiducia. Il voto dei baschi ora cambia le carte in tavola e fa crescere ulteriormente la pressione sul premier perché rassegni le sue dimissioni. La sfiducia al premier, o un'eventuale scelta di dimettersi, porterebbe alle estreme conseguenze lo scandalo per corruzione che ha investito il Partito popolare. Ma per ora sembra pensare a tutt'altro. \"Non ha intenzione di dimettersi - ha detto il segretario generale del Partito popolare , María Dolores de Cospedal - Non gioverebbe all'interesse generale o agli interessi del Pp\"."
metrics:
- rouge
- bertscore
- headline-headline-consistency-classifier
- headline-article-consistency-classifier
model-index:
- name: it5-small-repubblica-to-ilgiornale
results:
- task:
type: headline-style-transfer-repubblica-to-ilgiornale
name: "Headline style transfer (Repubblica to Il Giornale)"
dataset:
type: gsarti/change_it
name: "CHANGE-IT"
metrics:
- type: rouge1
value: 0.255
name: "Test Rouge1"
- type: rouge2
value: 0.080
name: "Test Rouge2"
- type: rougeL
value: 0.223
name: "Test RougeL"
- type: bertscore
value: 0.380
name: "Test BERTScore"
args:
- model_type: "dbmdz/bert-base-italian-xxl-uncased"
- lang: "it"
- num_layers: 10
- rescale_with_baseline: True
- baseline_path: "bertscore_baseline_ita.tsv"
- type: headline-headline-consistency-classifier
value: 0.887
name: "Test Headline-Headline Consistency Accuracy"
- type: headline-article-consistency-classifier
value: 0.894
name: "Test Headline-Article Consistency Accuracy"
co2_eq_emissions:
emissions: "8g"
source: "Google Cloud Platform Carbon Footprint"
training_type: "fine-tuning"
geographical_location: "Eemshaven, Netherlands, Europe"
hardware_used: "1 TPU v3-8 VM"
thumbnail: https://gsarti.com/publication/it5/featured.png
---
# IT5 Small for News Headline Style Transfer (Repubblica to Il Giornale) 🗞️➡️🗞️ 🇮🇹
This repository contains the checkpoint for the [IT5 Small](https://huggingface.co/gsarti/it5-small) model fine-tuned on news headline style transfer in the Repubblica to Il Giornale direction on the Italian CHANGE-IT dataset as part of the experiments of the paper [IT5: Large-scale Text-to-text Pretraining for Italian Language Understanding and Generation](https://arxiv.org/abs/2203.03759) by [Gabriele Sarti](https://gsarti.com) and [Malvina Nissim](https://malvinanissim.github.io).
A comprehensive overview of other released materials is provided in the [gsarti/it5](https://github.com/gsarti/it5) repository. Refer to the paper for additional details concerning the reported scores and the evaluation approach.
## Using the model
The model is trained to generate an headline in the style of Il Giornale from the full body of an article written in the style of Repubblica. Model checkpoints are available for usage in Tensorflow, Pytorch and JAX. They can be used directly with pipelines as:
```python
from transformers import pipelines
r2g = pipeline("text2text-generation", model='it5/it5-small-repubblica-to-ilgiornale')
r2g("Arriva dal Partito nazionalista basco (Pnv) la conferma che i cinque deputati che siedono in parlamento voteranno la sfiducia al governo guidato da Mariano Rajoy. Pochi voti, ma significativi quelli della formazione politica di Aitor Esteban, che interverrà nel pomeriggio. Pur con dimensioni molto ridotte, il partito basco si è trovato a fare da ago della bilancia in aula. E il sostegno alla mozione presentata dai Socialisti potrebbe significare per il primo ministro non trovare quei 176 voti che gli servono per continuare a governare. \" Perché dovrei dimettermi io che per il momento ho la fiducia della Camera e quella che mi è stato data alle urne \", ha detto oggi Rajoy nel suo intervento in aula, mentre procedeva la discussione sulla mozione di sfiducia. Il voto dei baschi ora cambia le carte in tavola e fa crescere ulteriormente la pressione sul premier perché rassegni le sue dimissioni. La sfiducia al premier, o un'eventuale scelta di dimettersi, porterebbe alle estreme conseguenze lo scandalo per corruzione che ha investito il Partito popolare. Ma per ora sembra pensare a tutt'altro. \"Non ha intenzione di dimettersi - ha detto il segretario generale del Partito popolare , María Dolores de Cospedal - Non gioverebbe all'interesse generale o agli interessi del Pp\".")
>>> [{"generated_text": "il nazionalista rajoy: 'voteremo la sfiducia'"}]
```
or loaded using autoclasses:
```python
from transformers import AutoTokenizer, AutoModelForSeq2SeqLM
tokenizer = AutoTokenizer.from_pretrained("it5/it5-small-repubblica-to-ilgiornale")
model = AutoModelForSeq2SeqLM.from_pretrained("it5/it5-small-repubblica-to-ilgiornale")
```
If you use this model in your research, please cite our work as:
```bibtex
@article{sarti-nissim-2022-it5,
title={{IT5}: Large-scale Text-to-text Pretraining for Italian Language Understanding and Generation},
author={Sarti, Gabriele and Nissim, Malvina},
journal={ArXiv preprint 2203.03759},
url={https://arxiv.org/abs/2203.03759},
year={2022},
month={mar}
}
``` |
it5/mt5-small-ilgiornale-to-repubblica | ff70df9e22888806206c80a447af170be9f749ff | 2022-03-09T08:03:24.000Z | [
"pytorch",
"tf",
"jax",
"tensorboard",
"mt5",
"text2text-generation",
"it",
"dataset:gsarti/change_it",
"arxiv:2203.03759",
"transformers",
"italian",
"sequence-to-sequence",
"newspaper",
"ilgiornale",
"repubblica",
"style-transfer",
"license:apache-2.0",
"model-index",
"co2_eq_emissions",
"autotrain_compatible"
] | text2text-generation | false | it5 | null | it5/mt5-small-ilgiornale-to-repubblica | 2 | null | transformers | 24,268 | ---
language:
- it
license: apache-2.0
datasets:
- gsarti/change_it
tags:
- italian
- sequence-to-sequence
- newspaper
- ilgiornale
- repubblica
- style-transfer
widget:
- text: "WASHINGTON - La Corea del Nord torna dopo nove anni nella blacklist Usa degli Stati considerati sponsor del terrorismo. Come Iran, Siria e Sudan. Lo ha deciso Donald Trump , che ha preferito dare l'annuncio non durante il suo recente viaggio in Asia ma ieri, in una riunione del governo alla Casa Bianca. 'Oggi gli Stati Uniti designeranno la Corea del nord come uno stato sponsor del terrorismo', ha tuonato il tycoon, anticipando che sarà formalizzata oggi dal dipartimento di stato e sarà accompagnata da nuove e più severe sanzioni. 'Il livello più alto' mai imposto a Pyongyang, ha promesso. 'Avrebbe dovuto succedere molto tempo fa', ha aggiunto, scaricando per l'ennesima volta la responsabilità dell'attuale crisi sull'amministrazione Obama. Poi si è scagliato contro un 'regime assassino' che 'deve mettere fine allo sviluppo del suo programma illegale nucleare e balistico'. Per giustificare la svolta, Trump ha accusato Pyongyang non solo di 'minacciare il mondo con una devastazione nucleare' ma anche di aver 'ripetutamente sostenuto atti di terrorismo internazionale', compreso omicidi in suolo straniero. Il riferimento è all' uccisione all'aeroporto della capitale malese di Kim Jong Nam , il fratellastro del leader nordcoreano Kim Jong Un , ma non ci sono altri episodi noti. Tanto che alcuni esperti, come pure dirigenti Usa coperti dall'anonimato, dubitano che Pyongyang risponda ai criteri per una tale designazione. La mossa appare altamente simbolica, dato che la Corea del Nord è già pesantemente sanzionata a livello internazionale. Per il segretario di stato Rex Tillerson è solo l'ultima di una serie di passi per rafforzare la pressione su Pyongyang e costringerla a sedersi ad un tavolo perché gli Usa hanno sempre 'speranza nella diplomazia'. Ma nello stesso tempo è un monito per 'fermare e dissuadere' altri Paesi dal sostenere la Corea del Nord, finita nella blacklist 'anche per l'uso di armi chimiche'. Ma la mossa potrebbe anche essere controproducente, provocando una risposta di Kim o minando gli sforzi per sollecitare Pechino ad una maggiore pressione su Pyongyang. In ogni caso non aiuta il dialogo diretto tra Usa e Corea del Nord, che sembrava essere stato avviato in modo riservato. Come non aiutano gli scambi di insulti fra Trump e Kim. Nord Corea, Trump: 'Cerco di essere amico di Kim, sarebbe una bella cosa per il mondo'. Pyongyang era stata messa nella lista Usa degli Stati sponsor del terrorismo per aver fatto esplodere nel 1987 un volo della Korean Air uccidendo tutti i 115 passeggeri a bordo. Ma l'amministrazione di George W. Bush l'aveva rimossa sperando di far avanzare i negoziati sulla denuclearizzazione della penisola coreana. Il governo giapponese sostiene la decisione degli Stati Uniti di inserire la Corea del Nord nella lista degli stati che sponsorizzano il terrorismo, pur riconoscendo che l'annuncio potrebbe provocare una reazione immediata del regime di Pyongyang. Il premier Shinzo Abe ha accolto con consenso il comunicato Usa e ha detto alla stampa che servirà a incrementare la pressione sulla Corea del Nord. Il ministro della Difesa Itsunori Onodera , pur valutando positivamente la notifica, ha spiegato che si attendono azioni provocatorie dallo stato eremita, ribadendo che è vitale rimanere vigili. Secondo la stampa nipponica Abe aveva richiesto al dipartimento di Stato Usa di mettere la Corea del Nord sulla lista durante l'incontro col presidente Usa Donald Trump a Tokyo a inizio mese. L'ultimo lancio di missile balistico condotto da Pyongyang nell'oceano Pacifico, sorvolando il mare del Giappone, risale allo scorso settembre."
- text: "ROMA - Una nuova droga killer è stata sequestrata per la prima volta in Europa dagli investigatori del Nas. Si tratta di una nuova \"miscela psicoattiva altamente tossica\" per la prima volta individuata da forze di polizia, simile all'eroina sintetica, ma molto più economica e letale. Tanto che i 20 grammi scoperti sarebbero stati sufficienti per fabbricare ben 20.000 dosi e lo stesso contatto attraverso la pelle può provocare intossicazione. Individuata per la prima volta, la nuova droga presenta una struttura simile al farmaco sedativo Fentanyl ma con effetti molto più devastanti per l'organismo. Proveniva dell'estero ed era contenuta in un plico postale indirizzato in una città del centro Italia: è stata intercettata tramite accertamenti sul web grazie a un'operazione di intelligence che ha visto come protagonisti i militari della Sezione operativa centrale del Comando carabinieri per la Tutela della salute (Nas). Economica e letale, secondo gli investigatori \"in confronto l'eroina è quasi 'acqua fresca', anzi, proprio per la sua economicità, in alcuni casi viene venduta dai pusher a giovani conviti di comprare eroina\". La diffusione di nuove droghe sintetiche che continuamente appaiono sui mercati necessita di un'attività investigativa costante e complessa. Si tratta infatti di sostanze dalla struttura molecolare molto simile a quella del Fentanyl ma ogni volta leggermente diversa. Di qui la difficoltà di individuarle e l'importanza del nuovo sequestro. \"La chiamano impropriamente 'eroina sintetica' - spiega il comandante dei Nas, generale Adelmo Lusi - per il tipo di effetto psicotropo simile, ma dal punto di vista della tossicità è molto peggio: con 25 milligrammi di eroina ci si sballa, con 25mg di simil-fentanyl, come quello appena sequestrato, si muore\". Le indagini sono partite da ricoveri per overdose in ospedale, in cui arrivavano ragazzi che non rispondevano al trattamento disintossicante per l'eroina. La nuova sostanza verrà ora segnalata per l'inserimento tra le tabelle ministeriali degli stupefacenti prevista dal Dpr 309/1990."
- text: "Fragile come il burro. Il nostro territorio è precario. Ne sanno qualcosa i comuni che sono stati investititi dal maltempo . Il dissesto idrogeologico imperversa su tutto il territorio. Infatti, oltre 6.600 comuni , pari all’82% del totale, sono in aree ad elevato rischio idrogeologico, pari al 10% della sua superficie. La popolazione potenzialmente esposta è stimata in 5,8 milioni di persone. I dati emergono dalle recenti analisi fatte da Legambiente e Protezione civile, che mettono in evidenza come in 10 anni in Italia sia raddoppiata l’area dei territori colpiti da alluvioni e frane , passando da una media di quattro regioni all’anno a otto regioni. Nella classifica delle regioni a maggior rischio idrogeologico prima è la Calabria con il 100% dei comuni esposti; al 100% ci sono anche la provincia di Trento, il Molise, la Basilicata, l’Umbria, la Valle d’Aosta. Poi Marche, Liguria al 99%; Lazio, Toscana al 98%; Abruzzo (96%), Emilia-Romagna (95%), Campania e Friuli Venezia Giulia al 92%, Piemonte (87%), Sardegna (81%), Puglia (78%), Sicilia (71%), Lombardia (60%), provincia di Bolzano (59%), Veneto (56%). Tra le cause che condizionano ed amplificano il rischio idrogeologico c’è l’azione dell’uomo (abbandono e degrado, cementificazione, consumo di suolo, abusivismo, disboscamento e incendi). Ma anche e soprattutto la mancanza di una seria manutenzione ordinaria e non ad una organica politica di prevenzione."
- text: "Arriva dal Partito nazionalista basco (Pnv) la conferma che i cinque deputati che siedono in parlamento voteranno la sfiducia al governo guidato da Mariano Rajoy. Pochi voti, ma significativi quelli della formazione politica di Aitor Esteban, che interverrà nel pomeriggio. Pur con dimensioni molto ridotte, il partito basco si è trovato a fare da ago della bilancia in aula. E il sostegno alla mozione presentata dai Socialisti potrebbe significare per il primo ministro non trovare quei 176 voti che gli servono per continuare a governare. \" Perché dovrei dimettermi io che per il momento ho la fiducia della Camera e quella che mi è stato data alle urne \", ha detto oggi Rajoy nel suo intervento in aula, mentre procedeva la discussione sulla mozione di sfiducia. Il voto dei baschi ora cambia le carte in tavola e fa crescere ulteriormente la pressione sul premier perché rassegni le sue dimissioni. La sfiducia al premier, o un'eventuale scelta di dimettersi, porterebbe alle estreme conseguenze lo scandalo per corruzione che ha investito il Partito popolare. Ma per ora sembra pensare a tutt'altro. \"Non ha intenzione di dimettersi - ha detto il segretario generale del Partito popolare , María Dolores de Cospedal - Non gioverebbe all'interesse generale o agli interessi del Pp\"."
metrics:
- rouge
- bertscore
- headline-headline-consistency-classifier
- headline-article-consistency-classifier
model-index:
- name: mt5-small-ilgiornale-to-repubblica
results:
- task:
type: headline-style-transfer-ilgiornale-to-repubblica
name: "Headline style transfer (Il Giornale to Repubblica)"
dataset:
type: gsarti/change_it
name: "CHANGE-IT"
metrics:
- type: rouge1
value: 0.248
name: "Test Rouge1"
- type: rouge2
value: 0.081
name: "Test Rouge2"
- type: rougeL
value: 0.219
name: "Test RougeL"
- type: bertscore
value: 0.381
name: "Test BERTScore"
args:
- model_type: "dbmdz/bert-base-italian-xxl-uncased"
- lang: "it"
- num_layers: 10
- rescale_with_baseline: True
- baseline_path: "bertscore_baseline_ita.tsv"
- type: headline-headline-consistency-classifier
value: 0.785
name: "Test Headline-Headline Consistency Accuracy"
- type: headline-article-consistency-classifier
value: 0.767
name: "Test Headline-Article Consistency Accuracy"
co2_eq_emissions:
emissions: "17g"
source: "Google Cloud Platform Carbon Footprint"
training_type: "fine-tuning"
geographical_location: "Eemshaven, Netherlands, Europe"
hardware_used: "1 TPU v3-8 VM"
thumbnail: https://gsarti.com/publication/it5/featured.png
---
# mT5 Small for News Headline Style Transfer (Il Giornale to Repubblica) 🗞️➡️🗞️ 🇮🇹
This repository contains the checkpoint for the [mT5 Small](https://huggingface.co/google/mt5-small) model fine-tuned on news headline style transfer in the Il Giornale to Repubblica direction on the Italian CHANGE-IT dataset as part of the experiments of the paper [IT5: Large-scale Text-to-text Pretraining for Italian Language Understanding and Generation](https://arxiv.org/abs/2203.03759) by [Gabriele Sarti](https://gsarti.com) and [Malvina Nissim](https://malvinanissim.github.io).
A comprehensive overview of other released materials is provided in the [gsarti/it5](https://github.com/gsarti/it5) repository. Refer to the paper for additional details concerning the reported scores and the evaluation approach.
## Using the model
The model is trained to generate an headline in the style of Repubblica from the full body of an article written in the style of Il Giornale. Model checkpoints are available for usage in Tensorflow, Pytorch and JAX. They can be used directly with pipelines as:
```python
from transformers import pipelines
g2r = pipeline("text2text-generation", model='it5/mt5-small-ilgiornale-to-repubblica')
g2r("Arriva dal Partito nazionalista basco (Pnv) la conferma che i cinque deputati che siedono in parlamento voteranno la sfiducia al governo guidato da Mariano Rajoy. Pochi voti, ma significativi quelli della formazione politica di Aitor Esteban, che interverrà nel pomeriggio. Pur con dimensioni molto ridotte, il partito basco si è trovato a fare da ago della bilancia in aula. E il sostegno alla mozione presentata dai Socialisti potrebbe significare per il primo ministro non trovare quei 176 voti che gli servono per continuare a governare. \" Perché dovrei dimettermi io che per il momento ho la fiducia della Camera e quella che mi è stato data alle urne \", ha detto oggi Rajoy nel suo intervento in aula, mentre procedeva la discussione sulla mozione di sfiducia. Il voto dei baschi ora cambia le carte in tavola e fa crescere ulteriormente la pressione sul premier perché rassegni le sue dimissioni. La sfiducia al premier, o un'eventuale scelta di dimettersi, porterebbe alle estreme conseguenze lo scandalo per corruzione che ha investito il Partito popolare. Ma per ora sembra pensare a tutt'altro. \"Non ha intenzione di dimettersi - ha detto il segretario generale del Partito popolare , María Dolores de Cospedal - Non gioverebbe all'interesse generale o agli interessi del Pp\".")
>>> [{"generated_text": "il nazionalista rajoy: 'voteremo la sfiducia'"}]
```
or loaded using autoclasses:
```python
from transformers import AutoTokenizer, AutoModelForSeq2SeqLM
tokenizer = AutoTokenizer.from_pretrained("it5/mt5-small-ilgiornale-to-repubblica")
model = AutoModelForSeq2SeqLM.from_pretrained("it5/mt5-small-ilgiornale-to-repubblica")
```
If you use this model in your research, please cite our work as:
```bibtex
@article{sarti-nissim-2022-it5,
title={{IT5}: Large-scale Text-to-text Pretraining for Italian Language Understanding and Generation},
author={Sarti, Gabriele and Nissim, Malvina},
journal={ArXiv preprint 2203.03759},
url={https://arxiv.org/abs/2203.03759},
year={2022},
month={mar}
}
``` |
itsunoda/wolfbbsRoBERTa-small | 47b7a78d93778325bc531e580a616a1968941c72 | 2021-01-11T12:07:14.000Z | [
"pytorch",
"tf",
"camembert",
"fill-mask",
"transformers",
"autotrain_compatible"
] | fill-mask | false | itsunoda | null | itsunoda/wolfbbsRoBERTa-small | 2 | null | transformers | 24,269 | Note that model type is Camembert.
|
jaesun/kcbert-base-finetuned-klue-nli | fac41c9a84ce54469ba80f5c1a3c619d5cc01865 | 2021-10-28T04:59:03.000Z | [
"pytorch",
"bert",
"text-classification",
"transformers"
] | text-classification | false | jaesun | null | jaesun/kcbert-base-finetuned-klue-nli | 2 | null | transformers | 24,270 | Entry not found |
jaeyoung/klue_mln_train_alldata | 9a509a0e3c1a38737a86ed9f7f33841b59eb419f | 2021-10-06T15:20:05.000Z | [
"pytorch",
"xlm-roberta",
"fill-mask",
"transformers",
"autotrain_compatible"
] | fill-mask | false | jaeyoung | null | jaeyoung/klue_mln_train_alldata | 2 | null | transformers | 24,271 | Entry not found |
jaimin/AraBERT | 24622131b8c47afa903cfce9aa19320e7748ea3c | 2021-08-26T04:14:46.000Z | [
"pytorch",
"bert",
"text-classification",
"transformers"
] | text-classification | false | jaimin | null | jaimin/AraBERT | 2 | null | transformers | 24,272 | Entry not found |
jaimin/Gujarati-Model | 2da2dbc825d1672a94283d6ebcb19b74d502872f | 2021-07-12T13:23:21.000Z | [
"pytorch",
"roberta",
"feature-extraction",
"transformers"
] | feature-extraction | false | jaimin | null | jaimin/Gujarati-Model | 2 | null | transformers | 24,273 | tokenizer = AutoTokenizer.from_pretrained("jaimin/Gujarati-Model")
model = AutoModel.from_pretrained("jaimin/Gujarati-Model") |
jaimin/arabic-bert | c09b423d5560f196ea69155d80c481128455879a | 2021-08-23T10:57:42.000Z | [
"pytorch",
"bert",
"text-classification",
"transformers"
] | text-classification | false | jaimin | null | jaimin/arabic-bert | 2 | null | transformers | 24,274 | Entry not found |
jaimin/wav2vec2-base-gujarati-demo | fde14689823c91105f5748ec22120e7d6f158294 | 2021-07-06T06:37:37.000Z | [
"pytorch",
"jax",
"wav2vec2",
"automatic-speech-recognition",
"Guj",
"dataset:google",
"transformers",
"audio",
"speech",
"xlsr-fine-tuning-week",
"license:apache-2.0",
"model-index"
] | automatic-speech-recognition | false | jaimin | null | jaimin/wav2vec2-base-gujarati-demo | 2 | null | transformers | 24,275 | ---
language: Guj
datasets:
- google
tags:
- audio
- automatic-speech-recognition
- speech
- xlsr-fine-tuning-week
license: apache-2.0
model-index:
- name: XLSR Wav2Vec2 Guj by Jaimin
results:
- task:
name: Speech Recognition
type: automatic-speech-recognition
dataset:
name: Google
type: voice
args: guj
metrics:
- name: Test WER
type: wer
value: 28.92
---
# wav2vec2-base-gujarati-demo
Fine-tuned [facebook/wav2vec2-large-xlsr-53](https://huggingface.co/facebook/wav2vec2-large-xlsr-53) in Guj
When using this model, make sure that your speech input is sampled at 16kHz.
## Usage
The model can be used directly (without a language model) as follows:
```python
import torch
import torchaudio
from datasets import load_dataset
from transformers import Wav2Vec2ForCTC, Wav2Vec2Processor
common_voice_train,common_voice_test = load_dataset('csv', data_files={'train': 'train.csv','test': 'test.csv'},error_bad_lines=False,encoding='utf-8',split=['train', 'test']).
processor = Wav2Vec2Processor.from_pretrained("jaimin/wav2vec2-base-gujarati-demo")
model = Wav2Vec2ForCTC.from_pretrained("jaimin/wav2vec2-base-gujarati-demo")
resampler = torchaudio.transforms.Resample(48_000, 16_000)
# Preprocessing the datasets.
# We need to read the aduio files as arrays
def speech_file_to_array_fn(batch):
speech_array, sampling_rate = torchaudio.load(batch["path"])
batch["speech"] = resampler(speech_array).squeeze().numpy()
return batch
test_dataset = common_voice_test.map(speech_file_to_array_fn)
inputs = processor(test_dataset["speech"], sampling_rate=16_000, return_tensors="pt", padding=True)
with torch.no_grad():
logits = model(inputs.input_values).logits
predicted_ids = torch.argmax(logits, dim=-1)
print("Prediction:", processor.batch_decode(predicted_ids))
print("Reference:", test_dataset["sentence"][0].lower())
```
## Evaluation
The model can be evaluated as follows on the {language} test data of Common Voice.
```python
import torch
import torchaudio
from datasets import load_dataset, load_metric
from transformers import Wav2Vec2ForCTC, Wav2Vec2Processor
import re
common_voice_validation = load_dataset('csv', data_files={'test': 'validation.csv'},error_bad_lines=False,encoding='utf-8',split='test')
wer = load_metric("wer")
processor = Wav2Vec2Processor.from_pretrained("jaimin/wav2vec2-base-gujarati-demo")
model = Wav2Vec2ForCTC.from_pretrained("Amrrs/wav2vec2-base-gujarati-demo")
model.to("cuda")
chars_to_ignore_regex = '[\,\?\.\!\-\;\:\"\“]'
resampler = torchaudio.transforms.Resample(48_000, 16_000)
# Preprocessing the datasets.
# We need to read the aduio files as arrays
def speech_file_to_array_fn(batch):
batch["sentence"] = re.sub(chars_to_ignore_regex, '', batch["sentence"]).lower()
speech_array, sampling_rate = torchaudio.load(batch["path"])
batch["speech"] = resampler(speech_array).squeeze().numpy()
return batch
test_dataset = common_voice_validation.map(speech_file_to_array_fn)
# Preprocessing the datasets.
# We need to read the aduio files as arrays
def evaluate(batch):
inputs = processor(batch["speech"], sampling_rate=16000, return_tensors="pt", padding=True)
with torch.no_grad():
logits = model(inputs.input_values.to("cuda")).logits
pred_ids = torch.argmax(logits, dim=-1)
batch["pred_strings"] = processor.batch_decode(pred_ids)
return batch
result = common_voice_validation.map(evaluate, batched=True, batch_size=8)
print("WER: {:2f}".format(100 * wer.compute(predictions=result["pred_strings"], references=result["sentence"])))
```
**Test Result**: 28.92 %
## Training
The Google datasets were used for training.
The script used for training can be found [here](https://colab.research.google.com/drive/1-Klkgr4f-C9SanHfVC5RhP0ELUH6TYlN?usp=sharing)
|
jakobcassiman/mbart-large-cc25-finetune-nl | 357dfe2d186b09e9690a92b44e878e515b5713e1 | 2021-08-03T14:59:19.000Z | [
"pytorch",
"mbart",
"text2text-generation",
"transformers",
"autotrain_compatible"
] | text2text-generation | false | jakobcassiman | null | jakobcassiman/mbart-large-cc25-finetune-nl | 2 | null | transformers | 24,276 | Entry not found |
jakobwes/xlm_roberta_squad_v1.1 | 71c75863b7f13c604458375f26396fdce54f01b8 | 2021-05-09T14:08:37.000Z | [
"pytorch",
"xlm-roberta",
"question-answering",
"transformers",
"autotrain_compatible"
] | question-answering | false | jakobwes | null | jakobwes/xlm_roberta_squad_v1.1 | 2 | null | transformers | 24,277 | XLM-RoBERTa base (`xlm-roberta-base`) finetuned on squad v1.1.
**Training-specifications:**
- training_epochs: 3.0
- max_seq_length: 384
- batch_size: 16
- dataset_name: squad
- doc_stride 128
**Train-results:**
```
{
"epoch": 3.0,
"init_mem_cpu_alloc_delta": 991453184,
"init_mem_cpu_peaked_delta": 0,
"init_mem_gpu_alloc_delta": 1109893120,
"init_mem_gpu_peaked_delta": 0,
"train_mem_cpu_alloc_delta": 14753792,
"train_mem_cpu_peaked_delta": 0,
"train_mem_gpu_alloc_delta": 3330195456,
"train_mem_gpu_peaked_delta": 8287144960,
"train_runtime": 11376.3034,
"train_samples": 89597,
"train_samples_per_second": 1.477
}
```
**Eval-results:**
```
{
"epoch": 3.0,
"eval_samples": 10918,
"exact_match": 82.06244087038789,
"f1": 89.09539709124654
}
```
|
jamarju/roberta-base-bne-squad-2.0-es | ca67487e8a62b60af86ddc2927e0308ad0381349 | 2021-08-04T22:10:47.000Z | [
"pytorch",
"roberta",
"question-answering",
"es",
"dataset:squad_es",
"transformers",
"autotrain_compatible"
] | question-answering | false | jamarju | null | jamarju/roberta-base-bne-squad-2.0-es | 2 | null | transformers | 24,278 | ---
language:
- es
datasets:
- squad_es
widget:
- text: "¿Quién era el duque en la batalla de Hastings?"
context: "La dinastía normanda tuvo un gran impacto político, cultural y militar en la Europa medieval e incluso en el Cercano Oriente. Los normandos eran famosos por su espíritu marcial y, finalmente, por su piedad cristiana, convirtiéndose en exponentes de la ortodoxia católica en la que se asimilaron. Adoptaron la lengua galorromance de la tierra franca que establecieron, siendo su dialecto conocido como francés normando, normando o normando, una lengua literaria importante. El ducado de Normandía, que formaron por tratado con la corona francesa, fue un gran feudo de la Francia medieval, y bajo Ricardo I de Normandía se forjó en un principado cohesionado y formidable en la tenencia feudal. Los normandos se caracterizan tanto por su cultura, como por su singular arquitectura románica y sus tradiciones musicales, y por sus importantes logros e innovaciones militares. Aventureros normandos fundaron el Reino de Sicilia bajo Roger II después de conquistar el sur de Italia con los sarracenos y bizantinos, y una expedición en nombre de su duque, Guillermo el Conquistador, condujo a la conquista normanda de Inglaterra. La influencia cultural y militar normanda se extendió desde estos nuevos centros europeos a los estados cruzados del Cercano Oriente, donde su príncipe Bohemundo I fundó el Principado de Antioquía en el Levante mediterráneo, a Escocia y Gales en Gran Bretaña."
---
This is the [BSC-TeMU/roberta-base-bne](https://huggingface.co/BSC-TeMU/roberta-base-bne) model ([source](https://github.com/PlanTL-SANIDAD/lm-spanish)) trained on the [squad_es v2.0.0](https://huggingface.co/datasets/squad_es) dataset ([source](https://github.com/ccasimiro88/TranslateAlignRetrieve)).
Current achievement: em=58.80, f1=67.40
Results:
```
{
"epoch": 4.0,
"eval_HasAns_exact": 48.51551956815115,
"eval_HasAns_f1": 65.70745010262016,
"eval_HasAns_total": 5928,
"eval_NoAns_exact": 69.0893760539629,
"eval_NoAns_f1": 69.0893760539629,
"eval_NoAns_total": 5930,
"eval_best_exact": 58.804182830156854,
"eval_best_exact_thresh": 0.0,
"eval_best_f1": 67.39869828034618,
"eval_best_f1_thresh": 0.0,
"eval_exact": 58.804182830156854,
"eval_f1": 67.39869828034568,
"eval_samples": 12211,
"eval_total": 11858
}
```
Training script:
```
python -m torch.distributed.launch --nproc_per_node=3 ./run_qa.py \
--model_name_or_path BSC-TeMU/roberta-base-bne \
--dataset_name squad_es \
--dataset_config_name v2.0.0 \
--do_train \
--do_eval \
--learning_rate 3e-5 \
--num_train_epochs 4 \
--max_seq_length 384 \
--doc_stride 128 \
--output_dir ./models/roberta-base-bne-squad-2.0-es/ \
--per_device_eval_batch_size=8 \
--per_device_train_batch_size=8 \
--version_2_with_negative \
--ddp_find_unused_parameters=False \
--overwrite_output_dir \
```
|
jamiewjm/CCGwGPT2extep2 | 4a777259c918ab6bb8c66f38828c05bd538eb0cb | 2021-11-18T06:19:32.000Z | [
"pytorch",
"gpt2",
"text-generation",
"transformers"
] | text-generation | false | jamiewjm | null | jamiewjm/CCGwGPT2extep2 | 2 | null | transformers | 24,279 | Entry not found |
jamiewjm/CCGwGPT2extep5 | 49fa1f8f5a4fdd9ccc9d5717b6d0e263b63eebd9 | 2021-11-17T15:17:26.000Z | [
"pytorch",
"gpt2",
"text-generation",
"transformers"
] | text-generation | false | jamiewjm | null | jamiewjm/CCGwGPT2extep5 | 2 | null | transformers | 24,280 | Entry not found |
jannesg/takalane_xho_roberta | 45712b40d2b0d88a8d2bddedb8d62ea8eecf7264 | 2021-09-22T08:52:19.000Z | [
"pytorch",
"jax",
"roberta",
"fill-mask",
"xho",
"transformers",
"masked-lm",
"license:mit",
"autotrain_compatible"
] | fill-mask | false | jannesg | null | jannesg/takalane_xho_roberta | 2 | null | transformers | 24,281 | ---
language:
- xho
thumbnail: https://pbs.twimg.com/media/EVjR6BsWoAAFaq5.jpg
tags:
- xho
- fill-mask
- pytorch
- roberta
- masked-lm
license: mit
---
# Takalani Sesame - Xhosa 🇿🇦
<img src="https://pbs.twimg.com/media/EVjR6BsWoAAFaq5.jpg" width="600"/>
## Model description
Takalani Sesame (named after the South African version of Sesame Street) is a project that aims to promote the use of South African languages in NLP, and in particular look at techniques for low-resource languages to equalise performance with larger languages around the world.
## Intended uses & limitations
#### How to use
```python
from transformers import AutoTokenizer, AutoModelWithLMHead
tokenizer = AutoTokenizer.from_pretrained("jannesg/takalane_xho_roberta")
model = AutoModelWithLMHead.from_pretrained("jannesg/takalane_xho_roberta")
```
#### Limitations and bias
Updates will be added continously to improve performance.
## Training data
Data collected from [https://wortschatz.uni-leipzig.de/en](https://wortschatz.uni-leipzig.de/en) <br/>
**Sentences:** 100000
## Training procedure
No preprocessing. Standard Huggingface hyperparameters.
## Author
Jannes Germishuys [website](http://jannesgg.github.io)
|
jayson31/DialoGPT-small-RickAndMorty | 098a43767d0b62438aa011afca6d202f2bff81b8 | 2021-10-04T06:14:36.000Z | [
"pytorch",
"gpt2",
"text-generation",
"transformers"
] | text-generation | false | jayson31 | null | jayson31/DialoGPT-small-RickAndMorty | 2 | null | transformers | 24,282 | Entry not found |
jazzisfuture/new_summary_t5_small | 708afc82bb086c6a4ece8ac7c9c365ff6ae83e0d | 2021-06-23T12:26:22.000Z | [
"pytorch",
"jax",
"t5",
"text2text-generation",
"transformers",
"autotrain_compatible"
] | text2text-generation | false | jazzisfuture | null | jazzisfuture/new_summary_t5_small | 2 | null | transformers | 24,283 | Entry not found |
jcmc/wav2vec-1b-cv8-cv | 9ccf0747d0fd6203ae501ffb2747d4b04646232c | 2022-02-04T02:20:23.000Z | [
"pytorch",
"tensorboard",
"wav2vec2",
"automatic-speech-recognition",
"transformers"
] | automatic-speech-recognition | false | jcmc | null | jcmc/wav2vec-1b-cv8-cv | 2 | null | transformers | 24,284 | Entry not found |
jean-paul/kinyaRoberta-large | f19c9002d84989277c62c19084a551ad48d8324a | 2021-08-29T10:25:44.000Z | [
"pytorch",
"roberta",
"fill-mask",
"arxiv:1907.11692",
"transformers",
"autotrain_compatible"
] | fill-mask | false | jean-paul | null | jean-paul/kinyaRoberta-large | 2 | null | transformers | 24,285 | # Model description
A Pretrained model on the Kinyarwanda language dataset using a masked language modeling (MLM) objective. RoBerta model was first introduced in [this paper](https://arxiv.org/abs/1907.11692). This KinyaRoBERTa model was pretrained with uncased tokens which means that no difference between for example ikinyarwanda and Ikinyarwanda.
# Training parameters
#### Dataset
The data set used has both sources from the new articles in Rwanda extracted from different new web pages, dumped Wikipedia files, and the books in Kinyarwanda. The sizes of the sources of data are 72 thousand new articles, three thousand dumped Wikipedia articles, and six books with more than a thousand pages.
#### Hyperparameters
The model was trained with the default configuration of RoBerta and Trainer from the Huggingface. However, due to some resource computation issues, we kept the number of transformer layers to 12.
# How to use:
1) The model can be used directly with the pipeline for masked language modeling as follows:
```
from transformers import pipeline
the_mask_pipe = pipeline(
"fill-mask",
model='jean-paul/kinyaRoberta-large',
tokenizer='jean-paul/kinyaRoberta-large',
)
the_mask_pipe("Ejo ndikwiga nagize <mask> baje kunsura.")
[{'sequence': 'Ejo ndikwiga nagize amahirwe baje kunsura.', 'score': 0.5675836205482483, 'token': 1711, 'token_str': ' amahirwe'},
{'sequence': 'Ejo ndikwiga nagize benshi baje kunsura.', 'score': 0.03573048859834671, 'token': 769, 'token_str': ' benshi'},
{'sequence': 'Ejo ndikwiga nagize ubwoba baje kunsura.', 'score': 0.03272199630737305, 'token': 2594, 'token_str': ' ubwoba'},
{'sequence': 'Ejo ndikwiga nagize ngo baje kunsura.', 'score': 0.013406379148364067, 'token': 396, 'token_str': ' ngo'},
{'sequence': 'Ejo ndikwiga nagize abantu baje kunsura.', 'score': 0.012342716567218304, 'token': 500, 'token_str': ' abantu'}]
```
2) Direct use from the transformer library to get features using AutoModel
```
from transformers import AutoTokenizer, AutoModelForMaskedLM
tokenizer = AutoTokenizer.from_pretrained("jean-paul/kinyaRoberta-large")
model = AutoModelForMaskedLM.from_pretrained("jean-paul/kinyaRoberta-large")
input_text = "Ejo ndikwiga nagize abashyitsi baje kunsura."
encoded_input = tokenizer(input_text, return_tensors='pt')
output = model(**encoded_input)
```
__Note__: We used the huggingface implementations for pretraining RoBerta from scratch, both the RoBerta model and the classes needed to do it. |
jeanlks/DialogGPT-small-gayvid | ee859c3bf3b03b03c80500417fb4c441267ff514 | 2021-09-20T16:20:03.000Z | [
"pytorch",
"gpt2",
"text-generation",
"transformers",
"conversational"
] | conversational | false | jeanlks | null | jeanlks/DialogGPT-small-gayvid | 2 | null | transformers | 24,286 | ---
tags:
- conversational
---
#Chatbot gay |
jegormeister/dialogpt-ir-bot | 53959bb656894ceaa6c1996f34c326357acc969b | 2021-10-29T21:41:36.000Z | [
"pytorch",
"gpt2",
"text-generation",
"transformers"
] | text-generation | false | jegormeister | null | jegormeister/dialogpt-ir-bot | 2 | null | transformers | 24,287 | Entry not found |
jejomi/xls-r-ta | c7cd110d381d64ef260c89ef67685c97eb7de6bf | 2022-02-06T11:34:32.000Z | [
"pytorch",
"wav2vec2",
"automatic-speech-recognition",
"ta",
"dataset:common_voice",
"transformers",
"mozilla-foundation/common_voice_7_0",
"generated_from_trainer",
"license:apache-2.0",
"model-index"
] | automatic-speech-recognition | false | jejomi | null | jejomi/xls-r-ta | 2 | null | transformers | 24,288 | ---
language:
- ta
license: apache-2.0
tags:
- automatic-speech-recognition
- mozilla-foundation/common_voice_7_0
- generated_from_trainer
datasets:
- common_voice
model-index:
- name: ''
results: []
---
<!-- This model card has been generated automatically according to the information the Trainer had access to. You
should probably proofread and complete it, then remove this comment. -->
#
This model is a fine-tuned version of [facebook/wav2vec2-xls-r-300m](https://huggingface.co/facebook/wav2vec2-xls-r-300m) on the MOZILLA-FOUNDATION/COMMON_VOICE_7_0 - TA dataset.
It achieves the following results on the evaluation set:
- Loss: inf
- Wer: 1.0
## Model description
More information needed
## Intended uses & limitations
More information needed
## Training and evaluation data
More information needed
## Training procedure
### Training hyperparameters
The following hyperparameters were used during training:
- learning_rate: 0.0003
- train_batch_size: 2
- eval_batch_size: 8
- seed: 42
- optimizer: Adam with betas=(0.9,0.999) and epsilon=1e-08
- lr_scheduler_type: linear
- training_steps: 10
- mixed_precision_training: Native AMP
### Training results
### Framework versions
- Transformers 4.16.0.dev0
- Pytorch 1.10.1+cu102
- Datasets 1.17.1.dev0
- Tokenizers 0.11.0
|
jfarray/Model_all-distilroberta-v1_5_Epochs | 684d3fa722322c97be38a5b9726df789c3c76a76 | 2022-02-13T19:40:19.000Z | [
"pytorch",
"roberta",
"feature-extraction",
"sentence-transformers",
"sentence-similarity"
] | sentence-similarity | false | jfarray | null | jfarray/Model_all-distilroberta-v1_5_Epochs | 2 | null | sentence-transformers | 24,289 | ---
pipeline_tag: sentence-similarity
tags:
- sentence-transformers
- feature-extraction
- sentence-similarity
---
# {MODEL_NAME}
This is a [sentence-transformers](https://www.SBERT.net) model: It maps sentences & paragraphs to a 768 dimensional dense vector space and can be used for tasks like clustering or semantic search.
<!--- Describe your model here -->
## Usage (Sentence-Transformers)
Using this model becomes easy when you have [sentence-transformers](https://www.SBERT.net) installed:
```
pip install -U sentence-transformers
```
Then you can use the model like this:
```python
from sentence_transformers import SentenceTransformer
sentences = ["This is an example sentence", "Each sentence is converted"]
model = SentenceTransformer('{MODEL_NAME}')
embeddings = model.encode(sentences)
print(embeddings)
```
## Evaluation Results
<!--- Describe how your model was evaluated -->
For an automated evaluation of this model, see the *Sentence Embeddings Benchmark*: [https://seb.sbert.net](https://seb.sbert.net?model_name={MODEL_NAME})
## Training
The model was trained with the parameters:
**DataLoader**:
`torch.utils.data.dataloader.DataLoader` of length 11 with parameters:
```
{'batch_size': 15, 'sampler': 'torch.utils.data.sampler.RandomSampler', 'batch_sampler': 'torch.utils.data.sampler.BatchSampler'}
```
**Loss**:
`sentence_transformers.losses.CosineSimilarityLoss.CosineSimilarityLoss`
Parameters of the fit()-Method:
```
{
"epochs": 5,
"evaluation_steps": 1,
"evaluator": "sentence_transformers.evaluation.EmbeddingSimilarityEvaluator.EmbeddingSimilarityEvaluator",
"max_grad_norm": 1,
"optimizer_class": "<class 'transformers.optimization.AdamW'>",
"optimizer_params": {
"lr": 2e-05
},
"scheduler": "WarmupLinear",
"steps_per_epoch": null,
"warmup_steps": 6,
"weight_decay": 0.01
}
```
## Full Model Architecture
```
SentenceTransformer(
(0): Transformer({'max_seq_length': 512, 'do_lower_case': False}) with Transformer model: RobertaModel
(1): Pooling({'word_embedding_dimension': 768, 'pooling_mode_cls_token': False, 'pooling_mode_mean_tokens': True, 'pooling_mode_max_tokens': False, 'pooling_mode_mean_sqrt_len_tokens': False})
(2): Normalize()
)
```
## Citing & Authors
<!--- Describe where people can find more information --> |
jfarray/Model_dccuchile_bert-base-spanish-wwm-uncased_50_Epochs | 081449a6f4e8fe0ca52ef453acd429367f093a96 | 2022-02-14T21:41:05.000Z | [
"pytorch",
"bert",
"feature-extraction",
"sentence-transformers",
"sentence-similarity"
] | sentence-similarity | false | jfarray | null | jfarray/Model_dccuchile_bert-base-spanish-wwm-uncased_50_Epochs | 2 | null | sentence-transformers | 24,290 | ---
pipeline_tag: sentence-similarity
tags:
- sentence-transformers
- feature-extraction
- sentence-similarity
---
# {MODEL_NAME}
This is a [sentence-transformers](https://www.SBERT.net) model: It maps sentences & paragraphs to a 256 dimensional dense vector space and can be used for tasks like clustering or semantic search.
<!--- Describe your model here -->
## Usage (Sentence-Transformers)
Using this model becomes easy when you have [sentence-transformers](https://www.SBERT.net) installed:
```
pip install -U sentence-transformers
```
Then you can use the model like this:
```python
from sentence_transformers import SentenceTransformer
sentences = ["This is an example sentence", "Each sentence is converted"]
model = SentenceTransformer('{MODEL_NAME}')
embeddings = model.encode(sentences)
print(embeddings)
```
## Evaluation Results
<!--- Describe how your model was evaluated -->
For an automated evaluation of this model, see the *Sentence Embeddings Benchmark*: [https://seb.sbert.net](https://seb.sbert.net?model_name={MODEL_NAME})
## Training
The model was trained with the parameters:
**DataLoader**:
`torch.utils.data.dataloader.DataLoader` of length 11 with parameters:
```
{'batch_size': 15, 'sampler': 'torch.utils.data.sampler.RandomSampler', 'batch_sampler': 'torch.utils.data.sampler.BatchSampler'}
```
**Loss**:
`sentence_transformers.losses.CosineSimilarityLoss.CosineSimilarityLoss`
Parameters of the fit()-Method:
```
{
"epochs": 50,
"evaluation_steps": 1,
"evaluator": "sentence_transformers.evaluation.EmbeddingSimilarityEvaluator.EmbeddingSimilarityEvaluator",
"max_grad_norm": 1,
"optimizer_class": "<class 'transformers.optimization.AdamW'>",
"optimizer_params": {
"lr": 2e-05
},
"scheduler": "WarmupLinear",
"steps_per_epoch": null,
"warmup_steps": 55,
"weight_decay": 0.01
}
```
## Full Model Architecture
```
SentenceTransformer(
(0): Transformer({'max_seq_length': 256, 'do_lower_case': False}) with Transformer model: BertModel
(1): Pooling({'word_embedding_dimension': 768, 'pooling_mode_cls_token': False, 'pooling_mode_mean_tokens': True, 'pooling_mode_max_tokens': False, 'pooling_mode_mean_sqrt_len_tokens': False})
(2): Dense({'in_features': 768, 'out_features': 256, 'bias': True, 'activation_function': 'torch.nn.modules.activation.Tanh'})
)
```
## Citing & Authors
<!--- Describe where people can find more information --> |
jfarray/Model_distiluse-base-multilingual-cased-v1_30_Epochs | a1568410f18ea8c55fefacd0d6db1a6c7a7fd8a1 | 2022-02-12T14:08:36.000Z | [
"pytorch",
"distilbert",
"feature-extraction",
"sentence-transformers",
"sentence-similarity"
] | sentence-similarity | false | jfarray | null | jfarray/Model_distiluse-base-multilingual-cased-v1_30_Epochs | 2 | null | sentence-transformers | 24,291 | ---
pipeline_tag: sentence-similarity
tags:
- sentence-transformers
- feature-extraction
- sentence-similarity
---
# {MODEL_NAME}
This is a [sentence-transformers](https://www.SBERT.net) model: It maps sentences & paragraphs to a 512 dimensional dense vector space and can be used for tasks like clustering or semantic search.
<!--- Describe your model here -->
## Usage (Sentence-Transformers)
Using this model becomes easy when you have [sentence-transformers](https://www.SBERT.net) installed:
```
pip install -U sentence-transformers
```
Then you can use the model like this:
```python
from sentence_transformers import SentenceTransformer
sentences = ["This is an example sentence", "Each sentence is converted"]
model = SentenceTransformer('{MODEL_NAME}')
embeddings = model.encode(sentences)
print(embeddings)
```
## Evaluation Results
<!--- Describe how your model was evaluated -->
For an automated evaluation of this model, see the *Sentence Embeddings Benchmark*: [https://seb.sbert.net](https://seb.sbert.net?model_name={MODEL_NAME})
## Training
The model was trained with the parameters:
**DataLoader**:
`torch.utils.data.dataloader.DataLoader` of length 11 with parameters:
```
{'batch_size': 15, 'sampler': 'torch.utils.data.sampler.RandomSampler', 'batch_sampler': 'torch.utils.data.sampler.BatchSampler'}
```
**Loss**:
`sentence_transformers.losses.CosineSimilarityLoss.CosineSimilarityLoss`
Parameters of the fit()-Method:
```
{
"epochs": 30,
"evaluation_steps": 1,
"evaluator": "sentence_transformers.evaluation.EmbeddingSimilarityEvaluator.EmbeddingSimilarityEvaluator",
"max_grad_norm": 1,
"optimizer_class": "<class 'transformers.optimization.AdamW'>",
"optimizer_params": {
"lr": 2e-05
},
"scheduler": "WarmupLinear",
"steps_per_epoch": null,
"warmup_steps": 33,
"weight_decay": 0.01
}
```
## Full Model Architecture
```
SentenceTransformer(
(0): Transformer({'max_seq_length': 128, 'do_lower_case': False}) with Transformer model: DistilBertModel
(1): Pooling({'word_embedding_dimension': 768, 'pooling_mode_cls_token': False, 'pooling_mode_mean_tokens': True, 'pooling_mode_max_tokens': False, 'pooling_mode_mean_sqrt_len_tokens': False})
(2): Dense({'in_features': 768, 'out_features': 512, 'bias': True, 'activation_function': 'torch.nn.modules.activation.Tanh'})
)
```
## Citing & Authors
<!--- Describe where people can find more information --> |
jfarray/Model_paraphrase-multilingual-MiniLM-L12-v2_5_Epochs | d19997d7244493f8bb083975e05c44280797d6e0 | 2022-02-12T20:37:59.000Z | [
"pytorch",
"bert",
"feature-extraction",
"sentence-transformers",
"sentence-similarity",
"transformers"
] | sentence-similarity | false | jfarray | null | jfarray/Model_paraphrase-multilingual-MiniLM-L12-v2_5_Epochs | 2 | null | sentence-transformers | 24,292 | ---
pipeline_tag: sentence-similarity
tags:
- sentence-transformers
- feature-extraction
- sentence-similarity
- transformers
---
# {MODEL_NAME}
This is a [sentence-transformers](https://www.SBERT.net) model: It maps sentences & paragraphs to a 384 dimensional dense vector space and can be used for tasks like clustering or semantic search.
<!--- Describe your model here -->
## Usage (Sentence-Transformers)
Using this model becomes easy when you have [sentence-transformers](https://www.SBERT.net) installed:
```
pip install -U sentence-transformers
```
Then you can use the model like this:
```python
from sentence_transformers import SentenceTransformer
sentences = ["This is an example sentence", "Each sentence is converted"]
model = SentenceTransformer('{MODEL_NAME}')
embeddings = model.encode(sentences)
print(embeddings)
```
## Usage (HuggingFace Transformers)
Without [sentence-transformers](https://www.SBERT.net), you can use the model like this: First, you pass your input through the transformer model, then you have to apply the right pooling-operation on-top of the contextualized word embeddings.
```python
from transformers import AutoTokenizer, AutoModel
import torch
#Mean Pooling - Take attention mask into account for correct averaging
def mean_pooling(model_output, attention_mask):
token_embeddings = model_output[0] #First element of model_output contains all token embeddings
input_mask_expanded = attention_mask.unsqueeze(-1).expand(token_embeddings.size()).float()
return torch.sum(token_embeddings * input_mask_expanded, 1) / torch.clamp(input_mask_expanded.sum(1), min=1e-9)
# Sentences we want sentence embeddings for
sentences = ['This is an example sentence', 'Each sentence is converted']
# Load model from HuggingFace Hub
tokenizer = AutoTokenizer.from_pretrained('{MODEL_NAME}')
model = AutoModel.from_pretrained('{MODEL_NAME}')
# Tokenize sentences
encoded_input = tokenizer(sentences, padding=True, truncation=True, return_tensors='pt')
# Compute token embeddings
with torch.no_grad():
model_output = model(**encoded_input)
# Perform pooling. In this case, mean pooling.
sentence_embeddings = mean_pooling(model_output, encoded_input['attention_mask'])
print("Sentence embeddings:")
print(sentence_embeddings)
```
## Evaluation Results
<!--- Describe how your model was evaluated -->
For an automated evaluation of this model, see the *Sentence Embeddings Benchmark*: [https://seb.sbert.net](https://seb.sbert.net?model_name={MODEL_NAME})
## Training
The model was trained with the parameters:
**DataLoader**:
`torch.utils.data.dataloader.DataLoader` of length 11 with parameters:
```
{'batch_size': 15, 'sampler': 'torch.utils.data.sampler.RandomSampler', 'batch_sampler': 'torch.utils.data.sampler.BatchSampler'}
```
**Loss**:
`sentence_transformers.losses.CosineSimilarityLoss.CosineSimilarityLoss`
Parameters of the fit()-Method:
```
{
"epochs": 5,
"evaluation_steps": 1,
"evaluator": "sentence_transformers.evaluation.EmbeddingSimilarityEvaluator.EmbeddingSimilarityEvaluator",
"max_grad_norm": 1,
"optimizer_class": "<class 'transformers.optimization.AdamW'>",
"optimizer_params": {
"lr": 2e-05
},
"scheduler": "WarmupLinear",
"steps_per_epoch": null,
"warmup_steps": 6,
"weight_decay": 0.01
}
```
## Full Model Architecture
```
SentenceTransformer(
(0): Transformer({'max_seq_length': 128, 'do_lower_case': False}) with Transformer model: BertModel
(1): Pooling({'word_embedding_dimension': 384, 'pooling_mode_cls_token': False, 'pooling_mode_mean_tokens': True, 'pooling_mode_max_tokens': False, 'pooling_mode_mean_sqrt_len_tokens': False})
)
```
## Citing & Authors
<!--- Describe where people can find more information --> |
jfarray/Model_paraphrase-multilingual-mpnet-base-v2_100_Epochs | 8d1f9e48e5c16f52f18f579e8ea1c64d963847de | 2022-02-13T00:33:38.000Z | [
"pytorch",
"xlm-roberta",
"feature-extraction",
"sentence-transformers",
"sentence-similarity",
"transformers"
] | sentence-similarity | false | jfarray | null | jfarray/Model_paraphrase-multilingual-mpnet-base-v2_100_Epochs | 2 | null | sentence-transformers | 24,293 | ---
pipeline_tag: sentence-similarity
tags:
- sentence-transformers
- feature-extraction
- sentence-similarity
- transformers
---
# {MODEL_NAME}
This is a [sentence-transformers](https://www.SBERT.net) model: It maps sentences & paragraphs to a 768 dimensional dense vector space and can be used for tasks like clustering or semantic search.
<!--- Describe your model here -->
## Usage (Sentence-Transformers)
Using this model becomes easy when you have [sentence-transformers](https://www.SBERT.net) installed:
```
pip install -U sentence-transformers
```
Then you can use the model like this:
```python
from sentence_transformers import SentenceTransformer
sentences = ["This is an example sentence", "Each sentence is converted"]
model = SentenceTransformer('{MODEL_NAME}')
embeddings = model.encode(sentences)
print(embeddings)
```
## Usage (HuggingFace Transformers)
Without [sentence-transformers](https://www.SBERT.net), you can use the model like this: First, you pass your input through the transformer model, then you have to apply the right pooling-operation on-top of the contextualized word embeddings.
```python
from transformers import AutoTokenizer, AutoModel
import torch
#Mean Pooling - Take attention mask into account for correct averaging
def mean_pooling(model_output, attention_mask):
token_embeddings = model_output[0] #First element of model_output contains all token embeddings
input_mask_expanded = attention_mask.unsqueeze(-1).expand(token_embeddings.size()).float()
return torch.sum(token_embeddings * input_mask_expanded, 1) / torch.clamp(input_mask_expanded.sum(1), min=1e-9)
# Sentences we want sentence embeddings for
sentences = ['This is an example sentence', 'Each sentence is converted']
# Load model from HuggingFace Hub
tokenizer = AutoTokenizer.from_pretrained('{MODEL_NAME}')
model = AutoModel.from_pretrained('{MODEL_NAME}')
# Tokenize sentences
encoded_input = tokenizer(sentences, padding=True, truncation=True, return_tensors='pt')
# Compute token embeddings
with torch.no_grad():
model_output = model(**encoded_input)
# Perform pooling. In this case, mean pooling.
sentence_embeddings = mean_pooling(model_output, encoded_input['attention_mask'])
print("Sentence embeddings:")
print(sentence_embeddings)
```
## Evaluation Results
<!--- Describe how your model was evaluated -->
For an automated evaluation of this model, see the *Sentence Embeddings Benchmark*: [https://seb.sbert.net](https://seb.sbert.net?model_name={MODEL_NAME})
## Training
The model was trained with the parameters:
**DataLoader**:
`torch.utils.data.dataloader.DataLoader` of length 11 with parameters:
```
{'batch_size': 15, 'sampler': 'torch.utils.data.sampler.RandomSampler', 'batch_sampler': 'torch.utils.data.sampler.BatchSampler'}
```
**Loss**:
`sentence_transformers.losses.CosineSimilarityLoss.CosineSimilarityLoss`
Parameters of the fit()-Method:
```
{
"epochs": 100,
"evaluation_steps": 1,
"evaluator": "sentence_transformers.evaluation.EmbeddingSimilarityEvaluator.EmbeddingSimilarityEvaluator",
"max_grad_norm": 1,
"optimizer_class": "<class 'transformers.optimization.AdamW'>",
"optimizer_params": {
"lr": 2e-05
},
"scheduler": "WarmupLinear",
"steps_per_epoch": null,
"warmup_steps": 110,
"weight_decay": 0.01
}
```
## Full Model Architecture
```
SentenceTransformer(
(0): Transformer({'max_seq_length': 128, 'do_lower_case': False}) with Transformer model: XLMRobertaModel
(1): Pooling({'word_embedding_dimension': 768, 'pooling_mode_cls_token': False, 'pooling_mode_mean_tokens': True, 'pooling_mode_max_tokens': False, 'pooling_mode_mean_sqrt_len_tokens': False})
)
```
## Citing & Authors
<!--- Describe where people can find more information --> |
jfarray/Model_paraphrase-multilingual-mpnet-base-v2_30_Epochs | 74b645a70882ba0043820589b3f21e211c906380 | 2022-02-12T23:02:17.000Z | [
"pytorch",
"xlm-roberta",
"feature-extraction",
"sentence-transformers",
"sentence-similarity",
"transformers"
] | sentence-similarity | false | jfarray | null | jfarray/Model_paraphrase-multilingual-mpnet-base-v2_30_Epochs | 2 | null | sentence-transformers | 24,294 | ---
pipeline_tag: sentence-similarity
tags:
- sentence-transformers
- feature-extraction
- sentence-similarity
- transformers
---
# {MODEL_NAME}
This is a [sentence-transformers](https://www.SBERT.net) model: It maps sentences & paragraphs to a 768 dimensional dense vector space and can be used for tasks like clustering or semantic search.
<!--- Describe your model here -->
## Usage (Sentence-Transformers)
Using this model becomes easy when you have [sentence-transformers](https://www.SBERT.net) installed:
```
pip install -U sentence-transformers
```
Then you can use the model like this:
```python
from sentence_transformers import SentenceTransformer
sentences = ["This is an example sentence", "Each sentence is converted"]
model = SentenceTransformer('{MODEL_NAME}')
embeddings = model.encode(sentences)
print(embeddings)
```
## Usage (HuggingFace Transformers)
Without [sentence-transformers](https://www.SBERT.net), you can use the model like this: First, you pass your input through the transformer model, then you have to apply the right pooling-operation on-top of the contextualized word embeddings.
```python
from transformers import AutoTokenizer, AutoModel
import torch
#Mean Pooling - Take attention mask into account for correct averaging
def mean_pooling(model_output, attention_mask):
token_embeddings = model_output[0] #First element of model_output contains all token embeddings
input_mask_expanded = attention_mask.unsqueeze(-1).expand(token_embeddings.size()).float()
return torch.sum(token_embeddings * input_mask_expanded, 1) / torch.clamp(input_mask_expanded.sum(1), min=1e-9)
# Sentences we want sentence embeddings for
sentences = ['This is an example sentence', 'Each sentence is converted']
# Load model from HuggingFace Hub
tokenizer = AutoTokenizer.from_pretrained('{MODEL_NAME}')
model = AutoModel.from_pretrained('{MODEL_NAME}')
# Tokenize sentences
encoded_input = tokenizer(sentences, padding=True, truncation=True, return_tensors='pt')
# Compute token embeddings
with torch.no_grad():
model_output = model(**encoded_input)
# Perform pooling. In this case, mean pooling.
sentence_embeddings = mean_pooling(model_output, encoded_input['attention_mask'])
print("Sentence embeddings:")
print(sentence_embeddings)
```
## Evaluation Results
<!--- Describe how your model was evaluated -->
For an automated evaluation of this model, see the *Sentence Embeddings Benchmark*: [https://seb.sbert.net](https://seb.sbert.net?model_name={MODEL_NAME})
## Training
The model was trained with the parameters:
**DataLoader**:
`torch.utils.data.dataloader.DataLoader` of length 11 with parameters:
```
{'batch_size': 15, 'sampler': 'torch.utils.data.sampler.RandomSampler', 'batch_sampler': 'torch.utils.data.sampler.BatchSampler'}
```
**Loss**:
`sentence_transformers.losses.CosineSimilarityLoss.CosineSimilarityLoss`
Parameters of the fit()-Method:
```
{
"epochs": 30,
"evaluation_steps": 1,
"evaluator": "sentence_transformers.evaluation.EmbeddingSimilarityEvaluator.EmbeddingSimilarityEvaluator",
"max_grad_norm": 1,
"optimizer_class": "<class 'transformers.optimization.AdamW'>",
"optimizer_params": {
"lr": 2e-05
},
"scheduler": "WarmupLinear",
"steps_per_epoch": null,
"warmup_steps": 33,
"weight_decay": 0.01
}
```
## Full Model Architecture
```
SentenceTransformer(
(0): Transformer({'max_seq_length': 128, 'do_lower_case': False}) with Transformer model: XLMRobertaModel
(1): Pooling({'word_embedding_dimension': 768, 'pooling_mode_cls_token': False, 'pooling_mode_mean_tokens': True, 'pooling_mode_max_tokens': False, 'pooling_mode_mean_sqrt_len_tokens': False})
)
```
## Citing & Authors
<!--- Describe where people can find more information --> |
jgammack/MTL-distilbert-base-uncased-squad | d51ceb0bb018ed9e864b37813f48b498b6044c62 | 2022-02-08T15:58:41.000Z | [
"pytorch",
"tensorboard",
"distilbert",
"question-answering",
"dataset:squad",
"transformers",
"generated_from_trainer",
"license:apache-2.0",
"model-index",
"autotrain_compatible"
] | question-answering | false | jgammack | null | jgammack/MTL-distilbert-base-uncased-squad | 2 | null | transformers | 24,295 | ---
license: apache-2.0
tags:
- generated_from_trainer
datasets:
- squad
model-index:
- name: MTL-distilbert-base-uncased-squad
results: []
---
<!-- This model card has been generated automatically according to the information the Trainer had access to. You
should probably proofread and complete it, then remove this comment. -->
# MTL-distilbert-base-uncased-squad
This model is a fine-tuned version of [jgammack/MTL-distilbert-base-uncased](https://huggingface.co/jgammack/MTL-distilbert-base-uncased) on the squad dataset.
## Model description
More information needed
## Intended uses & limitations
More information needed
## Training and evaluation data
More information needed
## Training procedure
### Training hyperparameters
The following hyperparameters were used during training:
- learning_rate: 2e-05
- train_batch_size: 8
- eval_batch_size: 8
- seed: 42
- optimizer: Adam with betas=(0.9,0.999) and epsilon=1e-08
- lr_scheduler_type: linear
- num_epochs: 3
- mixed_precision_training: Native AMP
### Training results
### Framework versions
- Transformers 4.16.2
- Pytorch 1.10.0+cu111
- Datasets 1.18.3
- Tokenizers 0.11.0
|
jgonik/nlp-puzzle | 28c3cbb70726bdf1799241aa6b6d3eb18dfec4ad | 2021-07-28T00:53:32.000Z | [
"pytorch",
"bert",
"text-classification",
"transformers"
] | text-classification | false | jgonik | null | jgonik/nlp-puzzle | 2 | null | transformers | 24,296 | Entry not found |
jgonik/repo_name | 20091309911c121cabb81ff939a0913c58f3fb93 | 2021-07-28T00:50:14.000Z | [
"pytorch",
"bert",
"text-classification",
"transformers"
] | text-classification | false | jgonik | null | jgonik/repo_name | 2 | null | transformers | 24,297 | Entry not found |
jhonparra18/wav2vec2-large-xls-r-300m-guarani-small | 06163a0ba8f27cbf7092bff03ea9fe8c576fab6c | 2022-04-27T14:42:06.000Z | [
"pytorch",
"tensorboard",
"wav2vec2",
"automatic-speech-recognition",
"dataset:common_voice",
"dataset:gn",
"transformers",
"generated_from_trainer",
"robust-speech-event",
"hf-asr-leaderboard",
"license:apache-2.0",
"model-index"
] | automatic-speech-recognition | false | jhonparra18 | null | jhonparra18/wav2vec2-large-xls-r-300m-guarani-small | 2 | null | transformers | 24,298 | ---
license: apache-2.0
tags:
- generated_from_trainer
- robust-speech-event
- hf-asr-leaderboard
datasets:
- common_voice
- gn
model-index:
- name: wav2vec2-large-xls-r-300m-guarani-small
results: []
---
<!-- This model card has been generated automatically according to the information the Trainer had access to. You
should probably proofread and complete it, then remove this comment. -->
# wav2vec2-large-xls-r-300m-guarani-small
This model is a fine-tuned version of [facebook/wav2vec2-xls-r-300m](https://huggingface.co/facebook/wav2vec2-xls-r-300m) on the common_voice dataset.
It achieves the following results on the evaluation set:
- Loss: 0.4964
- Wer: 0.5957
## Model description
More information needed
## Intended uses & limitations
More information needed
## Training and evaluation data
More information needed
## Training procedure
### Training hyperparameters
The following hyperparameters were used during training:
- learning_rate: 0.0002
- train_batch_size: 16
- eval_batch_size: 8
- seed: 42
- gradient_accumulation_steps: 2
- total_train_batch_size: 32
- optimizer: Adam with betas=(0.9,0.999) and epsilon=1e-08
- lr_scheduler_type: linear
- lr_scheduler_warmup_steps: 100
- num_epochs: 30
- mixed_precision_training: Native AMP
### Training results
| Training Loss | Epoch | Step | Validation Loss | Wer |
|:-------------:|:-----:|:----:|:---------------:|:------:|
| No log | 6.65 | 100 | 1.1326 | 1.0 |
| 1.6569 | 13.32 | 200 | 0.5264 | 0.6478 |
| 1.6569 | 19.97 | 300 | 0.5370 | 0.6261 |
| 0.2293 | 26.65 | 400 | 0.4964 | 0.5957 |
### Framework versions
- Transformers 4.16.2
- Pytorch 1.10.0+cu111
- Datasets 1.18.3
- Tokenizers 0.11.0
|
ji-xin/bert_base-MRPC-two_stage | 1484ff9d7c512ab9b4872570146dbe17c88480f7 | 2020-07-07T20:05:34.000Z | [
"pytorch",
"transformers"
] | null | false | ji-xin | null | ji-xin/bert_base-MRPC-two_stage | 2 | null | transformers | 24,299 | Entry not found |
Subsets and Splits
No community queries yet
The top public SQL queries from the community will appear here once available.