html_url
stringlengths 48
51
| title
stringlengths 1
290
| comments
sequencelengths 0
30
| body
stringlengths 0
228k
⌀ | number
int64 2
7.08k
|
---|---|---|---|---|
https://github.com/huggingface/datasets/issues/5474 | Column project operation on `datasets.Dataset` | [
"Hi ! This would be a nice addition indeed :) This sounds like a duplicate of https://github.com/huggingface/datasets/issues/5468\r\n\r\n> Not sure. Some of my PRs are still open and some do not have any discussions.\r\n\r\nSorry to hear that, feel free to ping me on those PRs"
] | ### Feature request
There is no operation to select a subset of columns of original dataset. Expected API follows.
```python
a = Dataset.from_dict({
'int': [0, 1, 2]
'char': ['a', 'b', 'c'],
'none': [None] * 3,
})
b = a.project('int', 'char') # usually, .select()
print(a.column_names) # stdout: ['int', 'char', 'none']
print(b.column_names) # stdout: ['int', 'char']
```
Method project can easily accept not only column names (as a `str)` but univariant function applied to corresponding column as an example. Or keyword arguments can be used in order to rename columns in advance (see `pandas`, `pyspark`, `pyarrow`, and SQL)..
### Motivation
Projection is a typical operation in every data processing library. And it is a basic block of a well-known data manipulation language like SQL. Without this operation `datasets.Dataset` interface is not complete.
### Your contribution
Not sure. Some of my PRs are still open and some do not have any discussions. | 5,474 |
https://github.com/huggingface/datasets/issues/5468 | Allow opposite of remove_columns on Dataset and DatasetDict | [
"Hi! I agree it would be nice to have a method like that. Instead of `keep_columns`, we can name it `select_columns` to be more aligned with PyArrow's naming convention (`pa.Table.select`).",
"Hi, I am a newbie to open source and would like to contribute. @mariosasko can I take up this issue ?",
"Hey, I also want to work on this issue I am a newbie to open source. ",
"This sounds related to https://github.com/huggingface/datasets/issues/5474\r\n\r\nI'm fine with `select_columns`, or we could also override `select` to also accept a list of columns maybe ?",
"@lhoestq, I am planning to add a member function to the dataset class to perform the selection operation. Do you think its the right way to proceed? or there is a better option ?",
"Unless @mariosasko thinks otherwise, I think it can go in `Dataset.select()` :)\r\nThough some parameters like keep_in_memory, indices_cache_file_name or writer_batch_size wouldn't when selecting columns, so we would need to update the docstring as well",
"If someone wants to give it a shot, feel free to comment `#self-assign` and it will assign the issue to you.\r\n\r\nFeel free to ping us here if you have questions or if we can help :)",
"I would rather have this functionality as a separate method. IMO it's always better to be explicit than to have an API where a single method can do different/uncorrelated things (somewhat reminds me of Pandas, and there is probably a good reason why PyArrow is more rigid in this aspect).",
"In the end I also think it would be nice to have it as a separate method, this way we can also have it for `IterableDataset` (which can't have `select` for indices)"
] | ### Feature request
In this blog post https://huggingface.co/blog/audio-datasets, I noticed the following code:
```python
COLUMNS_TO_KEEP = ["text", "audio"]
all_columns = gigaspeech["train"].column_names
columns_to_remove = set(all_columns) - set(COLUMNS_TO_KEEP)
gigaspeech = gigaspeech.remove_columns(columns_to_remove)
```
This kind of thing happens a lot when you don't need to keep all columns from the dataset. It would be more convenient (and less error prone) if you could just write:
```python
gigaspeech = gigaspeech.keep_columns(["text", "audio"])
```
Internally, `keep_columns` could still call `remove_columns`, but it expresses more clearly what the user's intent is.
### Motivation
Less code to write for the user of the dataset.
### Your contribution
- | 5,468 |
https://github.com/huggingface/datasets/issues/5465 | audiofolder creates empty dataset even though the dataset passed in follows the correct structure | [] | ### Describe the bug
The structure of my dataset folder called "my_dataset" is : data metadata.csv
The data folder consists of all mp3 files and metadata.csv consist of file locations like 'data/...mp3 and transcriptions. There's 400+ mp3 files and corresponding transcriptions for my dataset.
When I run the following:
ds = load_dataset("audiofolder", data_dir="my_dataset")
I get:
Using custom data configuration default-...
Downloading and preparing dataset audiofolder/default to /...
Downloading data files: 0%| | 0/2 [00:00<?, ?it/s]
Downloading data files: 0it [00:00, ?it/s]
Extracting data files: 0it [00:00, ?it/s]
Generating train split: 0 examples [00:00, ? examples/s]
Dataset audiofolder downloaded and prepared to /.... Subsequent calls will reuse this data.
0%| | 0/1 [00:00<?, ?it/s]
DatasetDict({
train: Dataset({
features: ['audio', 'transcription'],
num_rows: 1
})
})
### Steps to reproduce the bug
Create a dataset folder called 'my_dataset' with a subfolder called 'data' that has mp3 files. Also, create metadata.csv that has file locations like 'data/...mp3' and their corresponding transcription.
Run:
ds = load_dataset("audiofolder", data_dir="my_dataset")
### Expected behavior
It should generate a dataset with numerous rows.
### Environment info
Run on Jupyter notebook | 5,465 |
https://github.com/huggingface/datasets/issues/5464 | NonMatchingChecksumError for hendrycks_test | [
"Thanks for reporting, @sarahwie.\r\n\r\nPlease note this issue was already fixed in `datasets` 2.6.0 version:\r\n- #5040\r\n\r\nIf you update your `datasets` version, you will be able to load the dataset:\r\n```\r\npip install -U datasets\r\n```",
"Oops, missed that I needed to upgrade. Thanks!"
] | ### Describe the bug
The checksum of the file has likely changed on the remote host.
### Steps to reproduce the bug
`dataset = nlp.load_dataset("hendrycks_test", "anatomy")`
### Expected behavior
no error thrown
### Environment info
- `datasets` version: 2.2.1
- Platform: macOS-13.1-arm64-arm-64bit
- Python version: 3.9.13
- PyArrow version: 9.0.0
- Pandas version: 1.5.1 | 5,464 |
https://github.com/huggingface/datasets/issues/5461 | Discrepancy in `nyu_depth_v2` dataset | [
"Ccing @dwofk (the author of `fast-depth`). \r\n\r\nThanks, @awsaf49 for reporting this. I believe this is because the NYU Depth V2 shipped from `fast-depth` is already preprocessed. \r\n\r\nIf you think it might be better to have the NYU Depth V2 dataset from BTS [here](https://huggingface.co/datasets/sayakpaul/nyu_depth_v2) feel free to open a PR, I am happy to provide guidance :) ",
"Good catch ! Ideally it would be nice to have the datasets in the raw form, this way users can choose whatever processing they want to apply",
"> Ccing @dwofk (the author of `fast-depth`).\r\n> \r\n> Thanks, @awsaf49 for reporting this. I believe this is because the NYU Depth V2 shipped from `fast-depth` is already preprocessed.\r\n> \r\n> If you think it might be better to have the NYU Depth V2 dataset from BTS [here](https://huggingface.co/datasets/sayakpaul/nyu_depth_v2) feel free to open a PR, I am happy to provide guidance :)\r\n\r\n@sayakpaul I would love to create a PR on this. As this will be my first PR here, some guidance would be helpful.\r\n\r\nNeed a bit of advice on the dataset, there are three publicly available datasets. Which one should I consider for PR?\r\n1. [BTS](https://github.com/cleinc/bts): Containst train/test: 36K/654 data, dtype = `uint16` hence more precise\r\n2. [DenseDepth](https://github.com/ialhashim/DenseDepth) It contains train/test: 50K/654 data, dtype = `uint8` hence less precise\r\n3. [Official](https://cs.nyu.edu/~silberman/datasets/nyu_depth_v2.html#raw_parts): Size is big 400GB+, requires **MatLab** code for fixing **projection** and **sync**, DataType: `pgm` and `dump` hence can't be used directly.\r\n\r\ncc: @lhoestq\r\n\r\n",
"I think BTS. Repositories like https://github.com/vinvino02/GLPDepth usually use BTS. Also, just for clarity, the PR will be to https://huggingface.co/datasets/sayakpaul/nyu_depth_v2. Once we have worked it out, we can update the following things:\r\n\r\n* https://github.com/huggingface/blog/pull/718\r\n* https://huggingface.co/docs/datasets/main/en/depth_estimation\r\n\r\nDon't worry about it if it seems overwhelming. We will work it out together :) \r\n\r\n@lhoestq what do you think? ",
"@sayakpaul If I get this right I have to,\r\n1. Create a PR on https://huggingface.co/datasets/sayakpaul/nyu_depth_v2\r\n2. Create a PR on https://github.com/huggingface/blog\r\n3. Create a PR on https://github.com/huggingface/datasets to update https://github.com/huggingface/datasets/blob/main/docs/source/depth_estimation.mdx",
"The last two are low-hanging fruits. Don't worry about them. ",
"Yup opening a PR to use BTS on https://huggingface.co/datasets/sayakpaul/nyu_depth_v2 sounds good :) Thanks for the help !",
"Finally, I have found the origin of the **discretized depth map**. When I first loaded the datasets from HF I noticed it was 30GB but in DenseDepth data is only 4GB with dtype=uint8. This means data from fast-depth (before loading to HF) must have high precision. So when I tried to dig deeper by directly loading depth_map from `h5py`, I found depth_map from `h5py` came with `float32`. But when the data is processed in HF with `datasets.Image()` it was directly converted to `uint8` from `float32` hence the **discretized** depth map.\r\nhttps://github.com/huggingface/datasets/blob/c78559cacbb0ca6e0bc8bfc313cc0359f8c23ead/src/datasets/features/image.py#L91-L93\r\n\r\n## Solutions:\r\n\r\n#### 1. Array2D\r\nUse `Array2D` feature with `float32` for depth_map \r\n\r\n* Code:\r\n```py\r\nFeatures({'depth_map': Array2D(shape=(480, 640), dtype='float32')})\r\n```\r\n* Pros:\r\nNo precision loss.\r\n\r\n* Cons:\r\nAs depth_map is saved as Array I think it can't be visuzlied in [hf.co/dataset](https://huggingface.co/datasets/sayakpaul/nyu_depth_v2) page like segmentation mask.\r\n\r\n#### 2. Uint16\r\nUse `uint16` as dtype for Image in `_h5_loader` for saving depth maps and accept `uint16` dtype in `datasets.Image()` feature.\r\n\r\n* Code\r\n```py\r\ndepth = np.array(h5f[\"depth\"])\r\ndepth /= 10.0 # [0, max_depth] -> [0, 1]\r\ndepth *= (2**16 -1) # transform from [0, 1] -> [0, 2^16 - 1]\r\ndepth = depth.astype('uint16')\r\n```\r\n* Pros:\r\n * We can visualize depth map in hf.co/datasets page like segmentation mask.\r\n * No need for post-processing.\r\n\r\n* Cons:\r\n * We need to make two change\r\n * Modify `_h5_loader` in https://huggingface.co/datasets/sayakpaul/nyu_depth_v2 to convert depth_map from `float32` to `uint16`.\r\n * Make sure `datasets.Image()` converts `np.ndarray` to `uint16` checking max value\r\n * Precision loss due to `float32` to `uint16`\r\n * Post-processing required for depth_map to transform from `[0, 2^16 - 1]` to `[0, max_depth]` before feeding them to model.",
"Thanks so much for digging into this. \r\n\r\nSince the second solution entails changes to core datatypes in `datasets`, I think it's better to go with the first solution. \r\n\r\n@lhoestq WDYT?",
"@sayakpaul Yes, Solution 1 requires minimal change and provides no precision loss. But I think support for `uint16` image would be a great addition as many datasets come with `uint16` image. For example [UW-Madison GI Tract Image Segmentation](https://www.kaggle.com/competitions/uw-madison-gi-tract-image-segmentation) dataset, here the image itself comes with `uint16` dtype rather than mask. So, saving `uint16` image with `uint8` will result in precision loss.\r\n\r\nPerhaps we can adapt solution 1 for this issue and Add support for `uint16` image separately?",
"Using Array2D makes it not practical to use to train a model - in `transformers` we expect an image type.\r\n\r\nThere is a pull request to support more precision than uint8 in Image() here: https://github.com/huggingface/datasets/pull/5365/files\r\n\r\nwe can probably merge it today and do a release right away",
"Fantastic, @lhoestq! \r\n\r\n@awsaf49 then let's wait for the PR to get merged and then take the next steps? ",
"Sure",
"The PR adds support for uint16 which is ok for BTS if I understand correctly, would it be ok for you ?",
"If the main issue with the current version of NYU we have on the Hub is related to the precision loss stemming from `Image()`, I'd prefer if `Image()` supported float32 as well. ",
"I also prefer `float32` as it offers more precision. But I'm not sure if we'll be able to visualize image with `float32` precision.",
"We could have a separate loading for the float32 one using Array2D, but I feel like it's less convenient to use due to the amount of disk space and because it's not an Image() type. That's why I think uint16 is a better solution for users",
"A bit confused here, If https://github.com/huggingface/datasets/pull/5365 gets merged won't this issue will be resolved automatically?",
"Yes in theory :)",
"actually float32 also seems to work in this PR (it just doesn't work for multi-channel)",
"In that case, a new PR isn't necessary, right?",
"Yep. I just tested from the PR and it works:\r\n```python\r\n>>> train_dataset = load_dataset(\"sayakpaul/nyu_depth_v2\", split=\"train\", streaming=True) \r\nDownloading readme: 100%|██████████████████| 8.71k/8.71k [00:00<00:00, 3.60MB/s]\r\n>>> next(iter(train_dataset))\r\n{'image': <PIL.PngImagePlugin.PngImageFile image mode=RGB size=640x480 at 0x1382ED7F0>,\r\n 'depth_map': <PIL.TiffImagePlugin.TiffImageFile image mode=F size=640x480 at 0x1382EDF28>}\r\n>>> x = next(iter(train_dataset))\r\n>>> np.asarray(x[\"depth_map\"]) \r\narray([[0. , 0. , 0. , ..., 0. , 0. ,\r\n 0. ],\r\n [0. , 0. , 0. , ..., 0. , 0. ,\r\n 0. ],\r\n [0. , 0. , 0. , ..., 0. , 0. ,\r\n 0. ],\r\n ...,\r\n [0. , 2.2861192, 2.2861192, ..., 2.234162 , 2.234162 ,\r\n 0. ],\r\n [0. , 2.2861192, 2.2861192, ..., 2.234162 , 2.234162 ,\r\n 0. ],\r\n [0. , 2.2861192, 2.2861192, ..., 2.234162 , 2.234162 ,\r\n 0. ]], dtype=float32)\r\n```",
"Great! the case is closed! This issue has been solved and I have to say, it was quite the thrill ride. I felt like Sherlock Holmes, solving a mystery and finding the bug🕵️♂️. But in all seriousness, it was a pleasure working on this issue and I'm glad we could get to the bottom of it.\r\n\r\nOn another note, should I consider closing the issue? I think we still need to make updates on https://github.com/huggingface/blog and https://github.com/huggingface/datasets/blob/main/docs/source/depth_estimation.mdx",
"Haha thanks Mr Holmes :p\r\n\r\nmaybe let's close this issue when we're done updating the blog post and the documentation",
"@awsaf49 thank you for your hard work! \r\n\r\nI am a little unsure why the other links need to be updated, though. They all rely on datasets internally. ",
"I think depth_map still shows discretized version. It would be nice to have corrected one.\r\n<img src=\"https://huggingface.co/datasets/huggingface/documentation-images/resolve/main/datasets/depth_est_target_viz.png\" width = 300>",
"Also, I think we need to make some changes in the code to visualize depth_map as it is `float32` . `plot.imshow()` supports either [0, 1] + float32 or [0. 255] + uint8",
"Oh yes! Do you want to start with the fixes? Please feel free to say no but I wanted to make sure your contributions are reflected properly in our doc and the blog :)",
"Yes I think that would be nice :)",
"I'll make the changes tomorrow. I hope it's okay..."
] | ### Describe the bug
I think there is a discrepancy between depth map of `nyu_depth_v2` dataset [here](https://huggingface.co/docs/datasets/main/en/depth_estimation) and actual depth map. Depth values somehow got **discretized/clipped** resulting in depth maps that are different from actual ones. Here is a side-by-side comparison,
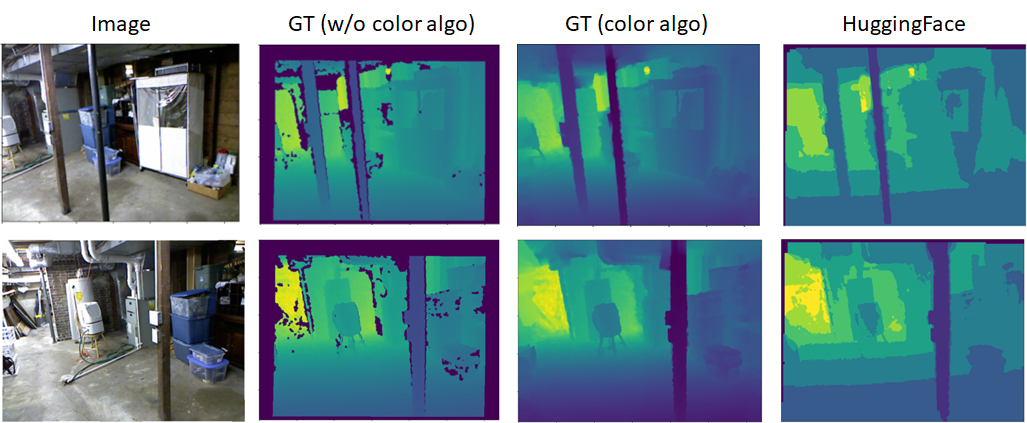
I tried to find the origin of this issue but sadly as I mentioned in tensorflow/datasets/issues/4674, the download link from `fast-depth` doesn't work anymore hence couldn't verify if the error originated there or during porting data from there to HF.
Hi @sayakpaul, as you worked on huggingface/datasets/issues/5255, if you still have access to that data could you please share the data or perhaps checkout this issue?
### Steps to reproduce the bug
This [notebook](https://colab.research.google.com/drive/1K3ZU8XUPRDOYD38MQS9nreQXJYitlKSW?usp=sharing#scrollTo=UEW7QSh0jf0i) from @sayakpaul could be used to generate depth maps and actual ground truths could be checked from this [dataset](https://www.kaggle.com/datasets/awsaf49/nyuv2-bts-dataset) from BTS repo.
> Note: BTS dataset has only 36K data compared to the train-test 50K. They sampled the data as adjacent frames look quite the same
### Expected behavior
Expected depth maps should be smooth rather than discrete/clipped.
### Environment info
- `datasets` version: 2.8.1.dev0
- Platform: Linux-5.10.147+-x86_64-with-glibc2.29
- Python version: 3.8.10
- PyArrow version: 9.0.0
- Pandas version: 1.3.5 | 5,461 |
https://github.com/huggingface/datasets/issues/5458 | slice split while streaming | [
"Hi! Yes, that's correct. When `streaming` is `True`, only split names can be specified as `split`, and for slicing, you have to use `.skip`/`.take` instead.\r\n\r\nE.g. \r\n`load_dataset(\"lhoestq/demo1\",revision=None, streaming=True, split=\"train[:3]\")`\r\n\r\nrewritten with `.skip`/`.take`:\r\n`load_dataset(\"lhoestq/demo1\",revision=None, streaming=True, split=\"train\").take(3)`\r\n\r\n\r\n",
"Thank you for your quick response!"
] | ### Describe the bug
When using the `load_dataset` function with streaming set to True, slicing splits is apparently not supported.
Did I miss this in the documentation?
### Steps to reproduce the bug
`load_dataset("lhoestq/demo1",revision=None, streaming=True, split="train[:3]")`
causes ValueError: Bad split: train[:3]. Available splits: ['train', 'test'] in builder.py, line 1213, in as_streaming_dataset
### Expected behavior
The first 3 entries of the dataset as a stream
### Environment info
- `datasets` version: 2.8.0
- Platform: Windows-10-10.0.19045-SP0
- Python version: 3.10.9
- PyArrow version: 10.0.1
- Pandas version: 1.5.2
| 5,458 |
https://github.com/huggingface/datasets/issues/5457 | prebuilt dataset relies on `downloads/extracted` | [
"Hi! \r\n\r\nThis issue is due to our audio/image datasets not being self-contained. This allows us to save disk space (files are written only once) but also leads to the issues like this one. We plan to make all our datasets self-contained in Datasets 3.0.\r\n\r\nIn the meantime, you can run the following map to ensure your dataset is self-contained:\r\n```python\r\nfrom datasets.table import embed_table_storage\r\n# load_dataset ...\r\ndset = dset.with_format(\"arrow\")\r\ndset.map(embed_table_storage, batched=True)\r\ndset = dset.with_format(\"python\")\r\n```\r\n",
"Understood. Thank you, Mario.\r\n\r\nPerhaps the solution could be very simple - move the extracted files into the directory of the cached dataset? Which would make it self-contained already and won't require waiting for a new major release. Unless I'm missing some back-compat nuance.\r\n\r\nBut regardless if X relies on Y - it could check if Y is still there when loading X. so not checking full consistency but just the top-level directory it relies on."
] | ### Describe the bug
I pre-built the dataset:
```
python -c 'import sys; from datasets import load_dataset; ds=load_dataset(sys.argv[1])' HuggingFaceM4/general-pmd-synthetic-testing
```
and it can be used just fine.
now I wipe out `downloads/extracted` and it no longer works.
```
rm -r ~/.cache/huggingface/datasets/downloads
```
That is I can still load it:
```
python -c 'import sys; from datasets import load_dataset; ds=load_dataset(sys.argv[1])' HuggingFaceM4/general-pmd-synthetic-testing
No config specified, defaulting to: general-pmd-synthetic-testing/100.unique
Found cached dataset general-pmd-synthetic-testing (/home/stas/.cache/huggingface/datasets/HuggingFaceM4___general-pmd-synthetic-testing/100.unique/1.1.1/86bc445e3e48cb5ef79de109eb4e54ff85b318cd55c3835c4ee8f86eae33d9d2)
```
but if I try to use it:
```
E stderr: Traceback (most recent call last):
E stderr: File "/mnt/nvme0/code/huggingface/m4-master-6/m4/training/main.py", line 116, in <module>
E stderr: train_loader, val_loader = get_dataloaders(
E stderr: File "/mnt/nvme0/code/huggingface/m4-master-6/m4/training/dataset.py", line 170, in get_dataloaders
E stderr: train_loader = get_dataloader_from_config(
E stderr: File "/mnt/nvme0/code/huggingface/m4-master-6/m4/training/dataset.py", line 443, in get_dataloader_from_config
E stderr: dataloader = get_dataloader(
E stderr: File "/mnt/nvme0/code/huggingface/m4-master-6/m4/training/dataset.py", line 264, in get_dataloader
E stderr: is_pmd = "meta" in hf_dataset[0] and "source" in hf_dataset[0]
E stderr: File "/mnt/nvme0/code/huggingface/datasets-master/src/datasets/arrow_dataset.py", line 2601, in __getitem__
E stderr: return self._getitem(
E stderr: File "/mnt/nvme0/code/huggingface/datasets-master/src/datasets/arrow_dataset.py", line 2586, in _getitem
E stderr: formatted_output = format_table(
E stderr: File "/mnt/nvme0/code/huggingface/datasets-master/src/datasets/formatting/formatting.py", line 634, in format_table
E stderr: return formatter(pa_table, query_type=query_type)
E stderr: File "/mnt/nvme0/code/huggingface/datasets-master/src/datasets/formatting/formatting.py", line 406, in __call__
E stderr: return self.format_row(pa_table)
E stderr: File "/mnt/nvme0/code/huggingface/datasets-master/src/datasets/formatting/formatting.py", line 442, in format_row
E stderr: row = self.python_features_decoder.decode_row(row)
E stderr: File "/mnt/nvme0/code/huggingface/datasets-master/src/datasets/formatting/formatting.py", line 225, in decode_row
E stderr: return self.features.decode_example(row) if self.features else row
E stderr: File "/mnt/nvme0/code/huggingface/datasets-master/src/datasets/features/features.py", line 1846, in decode_example
E stderr: return {
E stderr: File "/mnt/nvme0/code/huggingface/datasets-master/src/datasets/features/features.py", line 1847, in <dictcomp>
E stderr: column_name: decode_nested_example(feature, value, token_per_repo_id=token_per_repo_id)
E stderr: File "/mnt/nvme0/code/huggingface/datasets-master/src/datasets/features/features.py", line 1304, in decode_nested_example
E stderr: return decode_nested_example([schema.feature], obj)
E stderr: File "/mnt/nvme0/code/huggingface/datasets-master/src/datasets/features/features.py", line 1296, in decode_nested_example
E stderr: if decode_nested_example(sub_schema, first_elmt) != first_elmt:
E stderr: File "/mnt/nvme0/code/huggingface/datasets-master/src/datasets/features/features.py", line 1309, in decode_nested_example
E stderr: return schema.decode_example(obj, token_per_repo_id=token_per_repo_id)
E stderr: File "/mnt/nvme0/code/huggingface/datasets-master/src/datasets/features/image.py", line 144, in decode_example
E stderr: image = PIL.Image.open(path)
E stderr: File "/home/stas/anaconda3/envs/py38-pt113/lib/python3.8/site-packages/PIL/Image.py", line 3092, in open
E stderr: fp = builtins.open(filename, "rb")
E stderr: FileNotFoundError: [Errno 2] No such file or directory: '/mnt/nvme0/code/data/cache/huggingface/datasets/downloads/extracted/134227b9b94c4eccf19b205bf3021d4492d0227b9be6c2ddb6bf517d8d55a8cb/data/101/images_01.jpg'
```
Only if I wipe out the cached dir and rebuild then it starts working as `download/extracted` is back again with extracted files.
```
rm -r ~/.cache/huggingface/datasets/HuggingFaceM4___general-pmd-synthetic-testing
python -c 'import sys; from datasets import load_dataset; ds=load_dataset(sys.argv[1])' HuggingFaceM4/general-pmd-synthetic-testing
```
I think there are 2 issues here:
1. why does it still rely on extracted files after `arrow` files were printed - did I do something incorrectly when creating this dataset?
2. why doesn't the dataset know that it has been gutted and loads just fine? If it has a dependency on `download/extracted` then `load_dataset` should check if it's there and fail or force rebuilding. I am sure this could be a very expensive operation, so probably really solving #1 will not require this check. and this second item is probably an overkill. Other than perhaps if it had an optional `check_consistency` flag to do that.
### Environment info
datasets@main | 5,457 |
https://github.com/huggingface/datasets/issues/5454 | Save and resume the state of a DataLoader | [
"Something that'd be nice to have is \"manual update of state\". One of the learning from training LLMs is the ability to skip some batches whenever we notice huge spike might be handy.",
"Your outline spec is very sound and clear, @lhoestq - thank you!\r\n\r\n@thomasw21, indeed that would be a wonderful extra feature. In Megatron-Deepspeed we manually drained the dataloader for the range we wanted. I wasn't very satisfied with the way we did it, since its behavior would change if you were to do multiple range skips. I think it should remember all the ranges it skipped and not just skip the last range - since otherwise the data is inconsistent (but we probably should discuss this in a separate issue not to derail this much bigger one).",
"Hi there! I think this is a critical issue and have an urgent need for it, in my attempt to train on a super large-scale dataset using `datasets`. It is impossible to resume a time-consuming (like one month) experiment by iterating all seen data again, which could possibly cost several days.\r\n\r\n@stas00 @thomasw21 @lhoestq Any updates on this problem after 1 year passed?",
"any update?",
"No update so far, I wonder if someone implemented a resumable pytorch Sampler somwhere.\r\n\r\nThen regarding resuming a streaming dataset, we'd first like to have an efficient way to skip shards automatically but this is not implemented yet",
"I opened a draft here for IterableDataset: https://github.com/huggingface/datasets/pull/6658\r\n\r\n\r\n\r\n```python\r\n\"\"\"Requires https://github.com/huggingface/datasets/pull/6658 (WIP)\"\"\"\r\nfrom datasets import load_dataset\r\nfrom torch.utils.data import DataLoader\r\n\r\nds = load_dataset(..., streaming=True)\r\n# ds = ds.map(tokenize)\r\n# ds = ds.shuffle(seed=42, buffer_size=1000)\r\n\r\n# Init the dataset state_dict, or load it from a checkpoint\r\ndataset_state_dict = ds.state_dict()\r\n\r\n# Resumable training loop\r\nds.load_state_dict(dataset_state_dict)\r\ndataloader = DataLoader(ds, batch_size=batch_size)\r\nfor step, batch in enumerate(dataloader):\r\n ...\r\n if step % save_steps == 0:\r\n dataset_state_dict = ds.state_dict()\r\n```",
"Hi @lhoestq - can you provide more information and how to implement on saving and restoring vanilla DataLoader states with map-style datasets?\r\n\r\n",
"For now the easiest is probably to use the vanilla DataLoader only for batching and multiprocessing, and implement the resuming logic using a `Dataset` (it has `.select()` to skip examples) and a `dataset_state_dict`:\r\n\r\n\r\n```python\r\nfrom datasets import load_dataset\r\nfrom torch.utils.data import DataLoader\r\n\r\nds = load_dataset(...)\r\n# ds = ds.map(tokenize)\r\n# ds = ds.shuffle(seed=42)\r\n\r\n# Init the dataset state_dict, or load it from a checkpoint\r\ndataset_state_dict = {\"step\": 0} \r\n\r\n# Resumable training loop\r\nstart_step = dataset_state_dict[\"step\"]\r\ndataloader = DataLoader(ds.select(range(start_step * batch_size, len(ds))), batch_size=batch_size)\r\nfor step, batch in enumerate(dataloader, start=start_step):\r\n ...\r\n if step % save_steps == 0:\r\n dataset_state_dict = {\"step\": step}\r\n```",
"Hello, I found a similar implementation online that seems to solve your problem. https://github.com/facebookresearch/vissl/blob/main/vissl/data/data_helper.py#L93\r\nit looks like we can set_start_iter in StatefulDistributedSampler to implement the stateful resume requirement we want.\r\n\r\n",
"Hi y'all, @lhoestq I wanted to flag that we currently have a StatefulDataLoader in `pytorch/data/torchdata` that has state_dict/load_state_dict methods, which will call a dataset's state_dict/load_state_dict methods but also handle multiprocessing under the hood. Any chance we can collaborate on this and try to get them to work well together? Please have a look here for some basic examples: https://github.com/pytorch/data/tree/main/torchdata/stateful_dataloader#saving-and-loading-state ",
"Fantastic ! This will help pushing our IterableDataset state_dict implementation at https://github.com/huggingface/datasets/pull/6658 :) I'll check if there is anything missing to maker them work together, and add tests and some docs referring to the StatefulDataLoader :)",
"Ah I just saw this disclaimer in the torchdata README and it feels like people should not rely on it. Should the StatefulDataLoader live elsewhere @andrewkho ?\r\n\r\n> ⚠️ As of July 2023, we have paused active development on TorchData and have paused new releases. We have learnt a lot from building it and hearing from users, but also believe we need to re-evaluate the technical design and approach given how much the industry has changed since we began the project. During the rest of 2023 we will be re-evaluating our plans in this space. Please reach out if you suggestions or comments (please use https://github.com/pytorch/data/issues/1196 for feedback).",
"@lhoestq Good find, we are in the midst of updating this disclaimer as we're re-starting development and regular releases, though our approach will be to iterate on DL V1 (ie StatefulDataLoader) instead of continuing development on datapipes+DLV2. Let's discuss on a call at some point to figure out the best path forward! ",
"As a heads up, `IterableDataset` state_dict has been added in https://github.com/huggingface/datasets/pull/6658\r\n\r\n...and it works out of the box with the `torchdata` `StatefulDataLoader` :)\r\n\r\nSee the docs at https://huggingface.co/docs/datasets/main/en/use_with_pytorch#checkpoint-and-resume",
"amazing! Thank you, @lhoestq \r\n\r\ndoes it work with non-iterable dataset as well? the docs only mention iterable dataset",
"It's for iterable dataset only. For regular dataset I believe the sampler should implement state_dict, but maybe @andrewkho might know best how to resume a regular dataset with torchdata",
"@stas00 stateful dataloader will save and resume samplers for map style datasets. If no state_dict/load_state_dict is provided by the sampler, it will naively skip samples to fast forward. See here for more details https://github.com/pytorch/data/blob/main/torchdata/stateful_dataloader/README.md \n\nHope this helps! ",
"Thank you very much for clarifying that, Andrew.\r\n\r\n"
] | It would be nice when using `datasets` with a PyTorch DataLoader to be able to resume a training from a DataLoader state (e.g. to resume a training that crashed)
What I have in mind (but lmk if you have other ideas or comments):
For map-style datasets, this requires to have a PyTorch Sampler state that can be saved and reloaded per node and worker.
For iterable datasets, this requires to save the state of the dataset iterator, which includes:
- the current shard idx and row position in the current shard
- the epoch number
- the rng state
- the shuffle buffer
Right now you can already resume the data loading of an iterable dataset by using `IterableDataset.skip` but it takes a lot of time because it re-iterates on all the past data until it reaches the resuming point.
cc @stas00 @sgugger | 5,454 |
https://github.com/huggingface/datasets/issues/5451 | ImageFolder BadZipFile: Bad offset for central directory | [
"Hi ! Could you share the full stack trace ? Which dataset did you try to load ?\r\n\r\nit may be related to https://github.com/huggingface/datasets/pull/5640",
"The `BadZipFile` error means the ZIP file is corrupted, so I'm closing this issue as it's not directly related to `datasets`.",
"For others that find this issue following a `BadZipFile` error, I had the same problem because I had a file in a folder dataset `my-image.target` and the datasets library was incorrectly determining that the (PNG) file was a zip archive. When it tried to extract the file, this error occurred. \r\n\r\nUpdating to `datasets==2.12.0` fixed the problem for me."
] | ### Describe the bug
I'm getting the following exception:
```
lib/python3.10/zipfile.py:1353 in _RealGetContents │
│ │
│ 1350 │ │ # self.start_dir: Position of start of central directory │
│ 1351 │ │ self.start_dir = offset_cd + concat │
│ 1352 │ │ if self.start_dir < 0: │
│ ❱ 1353 │ │ │ raise BadZipFile("Bad offset for central directory") │
│ 1354 │ │ fp.seek(self.start_dir, 0) │
│ 1355 │ │ data = fp.read(size_cd) │
│ 1356 │ │ fp = io.BytesIO(data) │
╰──────────────────────────────────────────────────────────────────────────────────────────────────╯
BadZipFile: Bad offset for central directory
Extracting data files: 35%|█████████████████▊ | 38572/110812 [00:10<00:20, 3576.26it/s]
```
### Steps to reproduce the bug
```
load_dataset(
args.dataset_name,
args.dataset_config_name,
cache_dir=args.cache_dir,
),
```
### Expected behavior
loads the dataset
### Environment info
datasets==2.8.0
Python 3.10.8
Linux 129-146-3-202 5.15.0-52-generic #58~20.04.1-Ubuntu SMP Thu Oct 13 13:09:46 UTC 2022 x86_64 x86_64 x86_64 GNU/Linux | 5,451 |
https://github.com/huggingface/datasets/issues/5450 | to_tf_dataset with a TF collator causes bizarrely persistent slowdown | [
"wtf",
"Couldn't find what's causing this, this will need more investigation",
"A possible hint: The function it seems to be spending a lot of time in (when iterating over the original dataset) is `_get_mp` in the PIL JPEG decoder: \r\n\r\n",
"If \"mp\" is multiprocessing, this might suggest some kind of negative interaction between the JPEG decoder and TF's handling of processes/threads. Note that we haven't merged the parallel `to_tf_dataset` PR yet, so it's not caused by that PR!",
"Update: MP isn't multiprocessing at all, it's an internal PIL method for loading metadata from JPEG files. No idea why that would be a bottleneck, but I'll see if a Python profiler can't figure out where the time is actually being spent.",
"After further profiling, the slowdown is in the C methods for JPEG decoding that are included as part of PIL. Because Python profilers can't inspect inside that, I don't have any further information on which lines exactly are responsible for the slowdown or why.\r\n\r\nIn the meantime, I'm going to suggest switching from `return_tensors=\"tf\"` to `return_tensors=\"np\"` in most of our `transformers` code - this generally works better for pre-processing. Two relevant PRs are [here](https://github.com/huggingface/transformers/pull/21266) and [here](https://github.com/huggingface/notebooks/pull/308).",
"Closing this issue as we've done what we can with this one! "
] | ### Describe the bug
This will make more sense if you take a look at [a Colab notebook that reproduces this issue.](https://colab.research.google.com/drive/1rxyeciQFWJTI0WrZ5aojp4Ls1ut18fNH?usp=sharing)
Briefly, there are several datasets that, when you iterate over them with `to_tf_dataset` **and** a data collator that returns `tf` tensors, become very slow. We haven't been able to figure this one out - it can be intermittent, and we have no idea what could possibly cause it. The weirdest thing is that **the slowdown affects other attempts to access the underlying dataset**. If you try to iterate over the `tf.data.Dataset`, then interrupt execution, and then try to iterate over the original dataset, the original dataset is now also very slow! This is true even if the dataset format is not set to `tf` - the iteration is slow even though it's not calling TF at all!
There is a simple workaround for this - we can simply get our data collators to return `np` tensors. When we do this, the bug is never triggered and everything is fine. In general, `np` is preferred for this kind of preprocessing work anyway, when the preprocessing is not going to be compiled into a pure `tf.data` pipeline! However, the issue is fascinating, and the TF team were wondering if anyone in datasets (cc @lhoestq @mariosasko) might have an idea of what could cause this.
### Steps to reproduce the bug
Run the attached Colab.
### Expected behavior
The slowdown should go away, or at least not persist after we stop iterating over the `tf.data.Dataset`
### Environment info
The issue occurs on multiple versions of Python and TF, both on local machines and on Colab.
All testing was done using the latest versions of `transformers` and `datasets` from `main` | 5,450 |
https://github.com/huggingface/datasets/issues/5448 | Support fsspec 2023.1.0 in CI | [] | Once we find out the root cause of:
- #5445
we should revert the temporary pin on fsspec introduced by:
- #5447 | 5,448 |
https://github.com/huggingface/datasets/issues/5445 | CI tests are broken: AttributeError: 'mappingproxy' object has no attribute 'target' | [] | CI tests are broken, raising `AttributeError: 'mappingproxy' object has no attribute 'target'`. See: https://github.com/huggingface/datasets/actions/runs/3966497597/jobs/6797384185
```
...
ERROR tests/test_streaming_download_manager.py::TestxPath::test_xpath_rglob[mock://top_level-date=2019-10-0[1-4]/*-expected_paths4] - AttributeError: 'mappingproxy' object has no attribute 'target'
===== 2076 passed, 19 skipped, 15 warnings, 47 errors in 115.54s (0:01:55) =====
``` | 5,445 |
https://github.com/huggingface/datasets/issues/5444 | info messages logged as warnings | [
"Looks like a duplicate of https://github.com/huggingface/datasets/issues/1948. \r\n\r\nI also think these should be logged as INFO messages, but let's see what @lhoestq thinks.",
"It can be considered unexpected to see a `map` function return instantaneously. The warning is here to explain this case by mentioning that the cache was used. I don't expect first time users (only seeing warnings) to guess that the cache works this way",
"Oh, so it's intentional? Do all Hugging Face packages use `warning` when using cache?\r\nI guess feel free to close this issue then.",
"Yes it's intentional for `map`. For `load_dataset` it's also intentional but for a different reason: it shows where in the cache the dataset is located, in case the user wants to clear the cache.",
"OK I see. It's surprising to me that these are considered \"something unexpected happened\", the concept of cache is pretty common.\r\n\r\nHas a user every actually complained that they ran their code once, and it took a minute while the data downloaded, then ran their code again and it ran really fast (and completed successfully) but they were so baffled by the fact that it ran quickly, _and_ didn't set the log level to INFO, _and_ hadn't read the docs (or thought about it) to know that datasets are cached, that they logged an issue asking that this information be output as a warning every time they run their code?\r\n\r\nThat seems like a very niche scenario to cater for, given that the side effect is to flood the console with irrelevant warnings for every other user every other time they run a bit of `datasets` code. And the real world impact is that people TURN OFF warnings, which is a pretty bad habit to get into.\r\n\r\nAnyhoo, if there's no chance I'm going to change your mind, please close the issue :)",
"I see your point and I'm not closed to switching to INFO, but I think those logs are important to make the library less opaque. I also just checked `transformers` scripts and they default to INFO which is nice. However for colab users the default is still WARNING iirc, and it counts as one of the main env where `datasets` is used.\r\n\r\nWe also use progress bars a lot in `datasets`, that are shown if the logger is at the WARNING level. But we offer a function to disable the progress bars if necessary.",
"These kinds of messages are logged as INFO in Transformers, so we should probably be consistent with them"
] | ### Describe the bug
Code in `datasets` is using `logger.warning` when it should be using `logger.info`.
Some of these are probably a matter of opinion, but I think anything starting with `logger.warning(f"Loading chached` clearly falls into the info category.
Definitions from the Python docs for reference:
* INFO: Confirmation that things are working as expected.
* WARNING: An indication that something unexpected happened, or indicative of some problem in the near future (e.g. ‘disk space low’). The software is still working as expected.
In theory, a user should be able to resolve things such that there are no warnings.
### Steps to reproduce the bug
Load any dataset that's already cached.
### Expected behavior
No output when log level is at the default WARNING level.
### Environment info
- `datasets` version: 2.8.0
- Platform: Linux-5.10.102.1-microsoft-standard-WSL2-x86_64-with-glibc2.31
- Python version: 3.10.8
- PyArrow version: 9.0.0
- Pandas version: 1.5.2 | 5,444 |
https://github.com/huggingface/datasets/issues/5442 | OneDrive Integrations with HF Datasets | [
"Hi! \r\n\r\nWe use [`fsspec`](https://github.com/fsspec/filesystem_spec) to integrate with storage providers. You can find more info (and the usage examples) in [our docs](https://huggingface.co/docs/datasets/v2.8.0/filesystems#download-and-prepare-a-dataset-into-a-cloud-storage).\r\n\r\n[`gdrivefs`](https://github.com/fsspec/gdrivefs) makes it possible to use Google Drive as a storage service in Datasets, but this is not the case for OneDrive, since its[ Python SDK](https://github.com/OneDrive/onedrive-sdk-python) is not integrated with `fsspec`. Can you please request the integration with `fsspec` in their repo to address this limitation?",
"I'm closing this issue as implementing a fsspec-compliant OneDrive filesystem is not our responsibility."
] | ### Feature request
First of all , I would like to thank all community who are developed DataSet storage and make it free available
How to integrate our Onedrive account or any other possible storage clouds (like google drive,...) with the **HF** datasets section.
For example, if I have **50GB** on my **Onedrive** account and I want to move between drive and Hugging face repo or vis versa
### Motivation
make the dataset section more flexible with other possible storage
like the integration between Google Collab and Google drive the storage
### Your contribution
Can be done using Hugging face CLI | 5,442 |
https://github.com/huggingface/datasets/issues/5439 | [dataset request] Add Common Voice 12.0 | [
"@polinaeterna any tentative date on when the Common Voice 12.0 dataset will be added ?",
"This dataset is now hosted on the Hub here: https://huggingface.co/datasets/mozilla-foundation/common_voice_12_0"
] | ### Feature request
Please add the common voice 12_0 datasets. Apart from English, a significant amount of audio-data has been added to the other minor-language datasets.
### Motivation
The dataset link:
https://commonvoice.mozilla.org/en/datasets
| 5,439 |
https://github.com/huggingface/datasets/issues/5437 | Can't load png dataset with 4 channel (RGBA) | [
"Hi! Can you please share the directory structure of your image folder and the `load_dataset` call? We decode images with Pillow, and Pillow supports RGBA PNGs, so this shouldn't be a problem.\r\n\r\n",
"> Hi! Can you please share the directory structure of your image folder and the `load_dataset` call? We decode images with Pillow, and Pillow supports RGBA PNGs, so this shouldn't be a problem.\n> \n> \n\nI have only 1 folder that I use in the load_dataset function with the name \"IMGDATA\" and all my 9000 images are located in this folder.\n`\nfrom datasets import load_dataset\n\ndataset = load_dataset(\"IMGDATA\")\n`\nAt the same time, using another data set with images consisting of 3 RGB channels, everything works",
"Okay, I figured out what was wrong. When uploading my dataset via Google Drive, the images broke and Pillow couldn't open them. As a result, I solved the problem by downloading the ZIP archive"
] | I try to create dataset which contains about 9000 png images 64x64 in size, and they are all 4-channel (RGBA). When trying to use load_dataset() then a dataset is created from only 2 images. What exactly interferes I can not understand.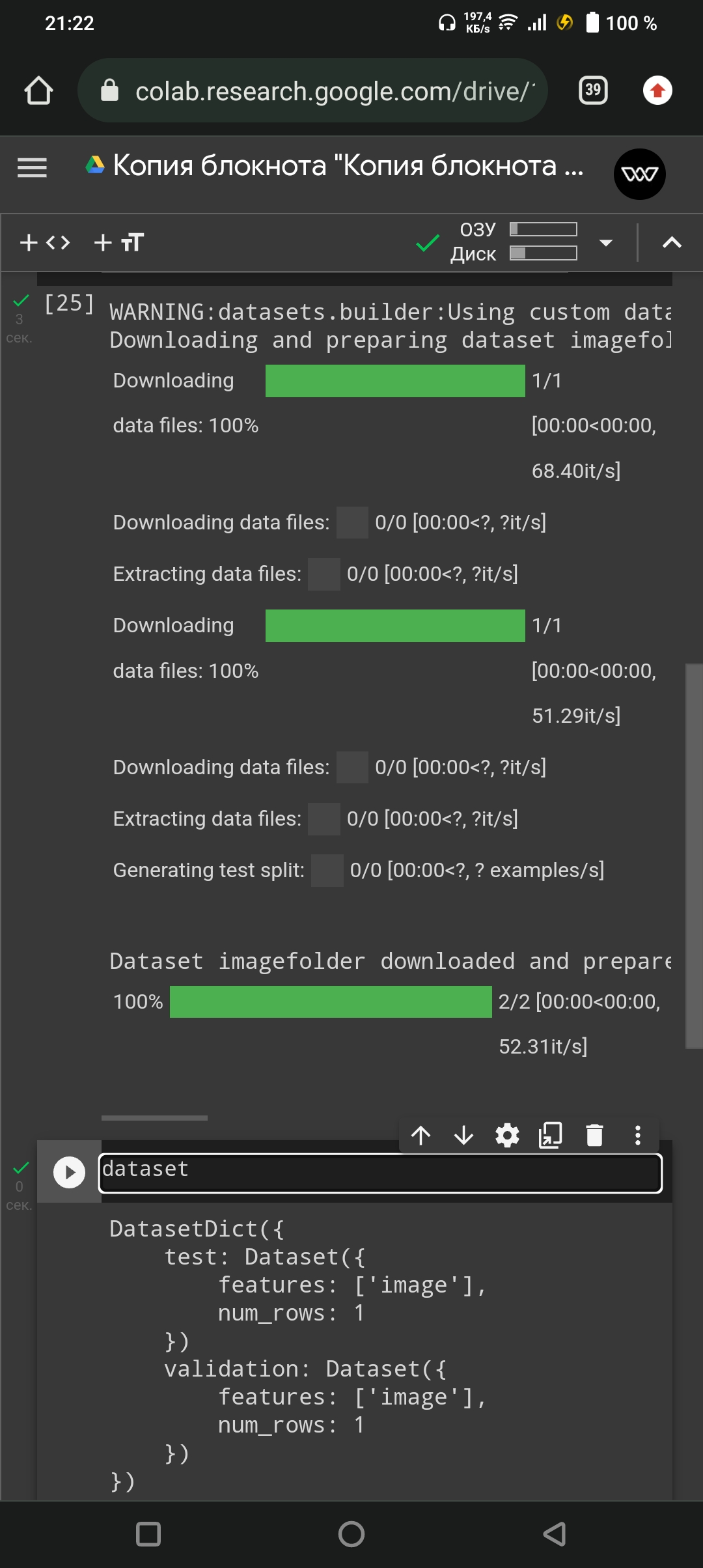 | 5,437 |
https://github.com/huggingface/datasets/issues/5435 | Wrong statement in "Load a Dataset in Streaming mode" leads to data leakage | [
"Just for your information, Tensorflow confirmed this issue [here.](https://github.com/tensorflow/tensorflow/issues/59279)",
"Thanks for reporting, @HaoyuYang59.\r\n\r\nPlease note that these are different \"dataset\" objects: our docs refer to Hugging Face `datasets.Dataset` and not to TensorFlow `tf.data.Dataset`.\r\n\r\nOur `datasets.Dataset.shuffle` method does not have a `reshuffle_each_iteration` argument. Therefore, I would say the statement in our docs is True because they refer to `datasets.Dataset.shuffle`, `datasets.Dataset.skip` and `datasets.Dataset.take`.\r\n\r\nI think this issue is restricted to TensorFlow dataset, and this would be addressed by them in the issue you opened in their repo: https://github.com/tensorflow/tensorflow/issues/59279",
"Also note that you are referring to an outdated documentation page: datasets 1.10.2 version\r\n\r\nCurrent datasets version is 2.8.0 and the corresponding documentation page is: https://huggingface.co/docs/datasets/stream#split-dataset",
"Hi @albertvillanova thanks for your reply and your explaination here. \r\n\r\nSorry for the confusion as I'm not actually a user of your repo and I just happen to find the thread by Google (and didn't read carefully).\r\n\r\nGreat to know that and you made everything very clear now.\r\n\r\nThanks for your time and sorry for the consusion.\r\n\r\nWishing you a wonderful time. \r\n\r\nRegards"
] | ### Describe the bug
In the [Split your dataset with take and skip](https://huggingface.co/docs/datasets/v1.10.2/dataset_streaming.html#split-your-dataset-with-take-and-skip), it states:
> Using take (or skip) prevents future calls to shuffle from shuffling the dataset shards order, otherwise the taken examples could come from other shards. In this case it only uses the shuffle buffer. Therefore it is advised to shuffle the dataset before splitting using take or skip. See more details in the [Shuffling the dataset: shuffle](https://huggingface.co/docs/datasets/v1.10.2/dataset_streaming.html#iterable-dataset-shuffling) section.`
>> \# You can also create splits from a shuffled dataset
>> train_dataset = shuffled_dataset.skip(1000)
>> eval_dataset = shuffled_dataset.take(1000)
Where the shuffled dataset comes from:
`shuffled_dataset = dataset.shuffle(buffer_size=10_000, seed=42)`
At least in Tensorflow 2.9/2.10/2.11, [docs](https://www.tensorflow.org/api_docs/python/tf/data/Dataset#shuffle) states the `reshuffle_each_iteration` argument is `True` by default. This means the dataset would be shuffled after each epoch, and as a result **the validation data would leak into training test**.
### Steps to reproduce the bug
N/A
### Expected behavior
The `reshuffle_each_iteration` argument should be set to `False`.
### Environment info
Tensorflow 2.9/2.10/2.11 | 5,435 |
https://github.com/huggingface/datasets/issues/5434 | sample_dataset module not found | [
"Hi! Can you describe what the actual error is?",
"working on the setfit example script\r\n\r\n from setfit import SetFitModel, SetFitTrainer, sample_dataset\r\n\r\nImportError: cannot import name 'sample_dataset' from 'setfit' (C:\\Python\\Python38\\lib\\site-packages\\setfit\\__init__.py)\r\n\r\n apart from that, I also had to hack these loads to import thses modules:\r\n from datasets.load import load_dataset \r\n from datasets.arrow_dataset import Dataset\r\n from datasets.dataset_dict import DatasetDict",
"Hi! This issue is related to the [SetFit](https://github.com/huggingface/setfit) project, so can you please open it there?"
] | null | 5,434 |
https://github.com/huggingface/datasets/issues/5433 | Support latest Docker image in CI benchmarks | [
"Sorry, it was us:[^1] https://github.com/iterative/cml/pull/1317 & https://github.com/iterative/cml/issues/1319#issuecomment-1385599559; should be fixed with [v0.18.17](https://github.com/iterative/cml/releases/tag/v0.18.17).\r\n\r\n[^1]: More or less, see https://github.com/yargs/yargs/issues/873.",
"Opened https://github.com/huggingface/datasets/pull/5436 unpinning again the container image.",
"Hi @0x2b3bfa0, thanks a lot for the investigation, the context about the the root cause and for fixing it!!\r\n\r\nWe are reviewing your PR to unpin the container image."
] | Once we find out the root cause of:
- #5431
we should revert the temporary pin on the Docker image version introduced by:
- #5432 | 5,433 |
https://github.com/huggingface/datasets/issues/5431 | CI benchmarks are broken: Unknown arguments: runnerPath, path | [] | Our CI benchmarks are broken, raising `Unknown arguments` error: https://github.com/huggingface/datasets/actions/runs/3932397079/jobs/6724905161
```
Unknown arguments: runnerPath, path
```
Stack trace:
```
100%|██████████| 500/500 [00:01<00:00, 338.98ba/s]
Updating lock file 'dvc.lock'
To track the changes with git, run:
git add dvc.lock
To enable auto staging, run:
dvc config core.autostage true
Use `dvc push` to send your updates to remote storage.
cml send-comment <markdown file>
Global Options:
--log Logging verbosity
[string] [choices: "error", "warn", "info", "debug"] [default: "info"]
--driver Git provider where the repository is hosted
[string] [choices: "github", "gitlab", "bitbucket"] [default: infer from the
environment]
--repo Repository URL or slug
[string] [default: infer from the environment]
--driver-token, --token CI driver personal/project access token (PAT)
[string] [default: infer from the environment]
--help Show help [boolean]
Options:
--target Comment type (`commit`, `pr`, `commit/f00bar`,
`pr/42`, `issue/1337`),default is automatic (`pr`
but fallback to `commit`). [string]
--watch Watch for changes and automatically update the
comment [boolean]
--publish Upload any local images found in the Markdown
report [boolean] [default: true]
--publish-url Self-hosted image server URL
[string] [default: "https://asset.cml.dev/"]
--publish-native, --native Uses driver's native capabilities to upload assets
instead of CML's storage; not available on GitHub
[boolean]
--watermark-title Hidden comment marker (used for targeting in
subsequent `cml comment update`); "{workflow}" &
"{run}" are auto-replaced [string] [default: ""]
Unknown arguments: runnerPath, path
Error: Process completed with exit code 1.
```
Issue reported to iterative/cml:
- iterative/cml#1319 | 5,431 |
https://github.com/huggingface/datasets/issues/5430 | Support Apache Beam >= 2.44.0 | [
"Some of the shard files now have 0 number of rows.\r\n\r\nWe have opened an issue in the Apache Beam repo:\r\n- https://github.com/apache/beam/issues/25041"
] | Once we find out the root cause of:
- #5426
we should revert the temporary pin on apache-beam introduced by:
- #5429 | 5,430 |
https://github.com/huggingface/datasets/issues/5428 | Load/Save FAISS index using fsspec | [
"Hi! Sure, feel free to submit a PR. Maybe if we want to be consistent with the existing API, it would be cleaner to directly add support for `fsspec` paths in `Dataset.load_faiss_index`/`Dataset.save_faiss_index` in the same manner as it was done in `Dataset.load_from_disk`/`Dataset.save_to_disk`.",
"That's a great idea! I'll do that instead. "
] | ### Feature request
From what I understand `faiss` already support this [link](https://github.com/facebookresearch/faiss/wiki/Index-IO,-cloning-and-hyper-parameter-tuning#generic-io-support)
I would like to use a stream as input to `Dataset.load_faiss_index` and `Dataset.save_faiss_index`.
### Motivation
In my case, I'm saving faiss index in cloud storage and use `fsspec` to load them. It would be ideal if I could send the stream directly instead of copying the file locally (or mounting the bucket) and then load the index.
### Your contribution
I can submit the PR | 5,428 |
https://github.com/huggingface/datasets/issues/5427 | Unable to download dataset id_clickbait | [
"Thanks for reporting, @ilos-vigil.\r\n\r\nWe have transferred this issue to the corresponding dataset on the Hugging Face Hub: https://huggingface.co/datasets/id_clickbait/discussions/1 "
] | ### Describe the bug
I tried to download dataset `id_clickbait`, but receive this error message.
```
FileNotFoundError: Couldn't find file at https://md-datasets-cache-zipfiles-prod.s3.eu-west-1.amazonaws.com/k42j7x2kpn-1.zip
```
When i open the link using browser, i got this XML data.
```xml
<?xml version="1.0" encoding="UTF-8"?>
<Error><Code>NoSuchBucket</Code><Message>The specified bucket does not exist</Message><BucketName>md-datasets-cache-zipfiles-prod</BucketName><RequestId>NVRM6VEEQD69SD00</RequestId><HostId>W/SPDxLGvlCGi0OD6d7mSDvfOAUqLAfvs9nTX50BkJrjMny+X9Jnqp/Li2lG9eTUuT4MUkAA2jjTfCrCiUmu7A==</HostId></Error>
```
### Steps to reproduce the bug
Code snippet:
```
from datasets import load_dataset
load_dataset('id_clickbait', 'annotated')
load_dataset('id_clickbait', 'raw')
```
Link to Kaggle notebook: https://www.kaggle.com/code/ilosvigil/bug-check-on-id-clickbait-dataset
### Expected behavior
Successfully download and load `id_newspaper` dataset.
### Environment info
- `datasets` version: 2.8.0
- Platform: Linux-5.15.65+-x86_64-with-debian-bullseye-sid
- Python version: 3.7.12
- PyArrow version: 8.0.0
- Pandas version: 1.3.5 | 5,427 |
https://github.com/huggingface/datasets/issues/5426 | CI tests are broken: SchemaInferenceError | [] | CI test (unit, ubuntu-latest, deps-minimum) is broken, raising a `SchemaInferenceError`: see https://github.com/huggingface/datasets/actions/runs/3930901593/jobs/6721492004
```
FAILED tests/test_beam.py::BeamBuilderTest::test_download_and_prepare_sharded - datasets.arrow_writer.SchemaInferenceError: Please pass `features` or at least one example when writing data
```
Stack trace:
```
______________ BeamBuilderTest.test_download_and_prepare_sharded _______________
[gw1] linux -- Python 3.7.15 /opt/hostedtoolcache/Python/3.7.15/x64/bin/python
self = <tests.test_beam.BeamBuilderTest testMethod=test_download_and_prepare_sharded>
@require_beam
def test_download_and_prepare_sharded(self):
import apache_beam as beam
original_write_parquet = beam.io.parquetio.WriteToParquet
expected_num_examples = len(get_test_dummy_examples())
with tempfile.TemporaryDirectory() as tmp_cache_dir:
builder = DummyBeamDataset(cache_dir=tmp_cache_dir, beam_runner="DirectRunner")
with patch("apache_beam.io.parquetio.WriteToParquet") as write_parquet_mock:
write_parquet_mock.side_effect = partial(original_write_parquet, num_shards=2)
> builder.download_and_prepare()
tests/test_beam.py:97:
_ _ _ _ _ _ _ _ _ _ _ _ _ _ _ _ _ _ _ _ _ _ _ _ _ _ _ _ _ _ _ _ _ _ _ _ _ _ _ _
/opt/hostedtoolcache/Python/3.7.15/x64/lib/python3.7/site-packages/datasets/builder.py:864: in download_and_prepare
**download_and_prepare_kwargs,
/opt/hostedtoolcache/Python/3.7.15/x64/lib/python3.7/site-packages/datasets/builder.py:1976: in _download_and_prepare
num_examples, num_bytes = beam_writer.finalize(metrics.query(m_filter))
/opt/hostedtoolcache/Python/3.7.15/x64/lib/python3.7/site-packages/datasets/arrow_writer.py:694: in finalize
shard_num_bytes, _ = parquet_to_arrow(source, destination)
/opt/hostedtoolcache/Python/3.7.15/x64/lib/python3.7/site-packages/datasets/arrow_writer.py:740: in parquet_to_arrow
num_bytes, num_examples = writer.finalize()
_ _ _ _ _ _ _ _ _ _ _ _ _ _ _ _ _ _ _ _ _ _ _ _ _ _ _ _ _ _ _ _ _ _ _ _ _ _ _ _
self = <datasets.arrow_writer.ArrowWriter object at 0x7f6dcbb3e810>
close_stream = True
def finalize(self, close_stream=True):
self.write_rows_on_file()
# In case current_examples < writer_batch_size, but user uses finalize()
if self._check_duplicates:
self.check_duplicate_keys()
# Re-intializing to empty list for next batch
self.hkey_record = []
self.write_examples_on_file()
# If schema is known, infer features even if no examples were written
if self.pa_writer is None and self.schema:
self._build_writer(self.schema)
if self.pa_writer is not None:
self.pa_writer.close()
self.pa_writer = None
if close_stream:
self.stream.close()
else:
if close_stream:
self.stream.close()
> raise SchemaInferenceError("Please pass `features` or at least one example when writing data")
E datasets.arrow_writer.SchemaInferenceError: Please pass `features` or at least one example when writing data
/opt/hostedtoolcache/Python/3.7.15/x64/lib/python3.7/site-packages/datasets/arrow_writer.py:593: SchemaInferenceError
``` | 5,426 |
https://github.com/huggingface/datasets/issues/5425 | Sort on multiple keys with datasets.Dataset.sort() | [
"Hi! \r\n\r\n`Dataset.sort` calls `df.sort_values` internally, and `df.sort_values` brings all the \"sort\" columns in memory, so sorting on multiple keys could be very expensive. This makes me think that maybe we can replace `df.sort_values` with `pyarrow.compute.sort_indices` - the latter can also sort on multiple keys and currently loads the data into memory; however, there is a plan to eventually implement \"memory-map\" friendly kernels for the Arrow compute ops (using the Acero execution engine). \r\n\r\nSo to address this issue, you should replace `df.sort_values` with `pyarrow.compute.sort_indices` in `Dataset.sort` and adjust the signature of this function (deprecate the `kind` parameter, etc.).\r\n\r\nPS: Feel free to ping us if you need some additional help/pointers",
"@mariosasko If I understand the code right, using `pyarrow.compute.sort_indices` would also require changes to the `select` method if it is meant to sort multiple keys. That's because `select` only accepts 1D input for `indices`, not an iterable or similar which would be required for multiple keys unless you want some looping over selects. Doesn't seem that straight-forward but I might be missing something here... ",
"@MichlF No, it doesn't require modifying select because sorting on multiple keys also returns a 1D array.\r\n\r\nIt's easier to understand with an example:\r\n```python\r\n>>> import pyarrow as pa\r\n>>> import pyarrow.compute as pc\r\n>>> table = pa.table({\r\n... \"name\": [\"John\", \"Eve\", \"Peter\", \"John\"],\r\n... \"surname\": [\"Johnson\", \"Smith\", \"Smith\", \"Doe\"],\r\n... \"age\": [20, 40, 30, 50],\r\n... })\r\n>>> indices = pc.sort_indices(table, sort_keys=[(\"name\", \"ascending\"), (\"surname\", \"ascending\")])\r\n>>> print(indices)\r\n[\r\n 1,\r\n 3,\r\n 0,\r\n 2\r\n]\r\n```\r\n\r\n",
"Thanks for clarifying.\r\nI can prepare a PR to address this issue. This would be my first PR here so I have a few maybe silly questions but:\r\n- What is the preferred input type of `sort_keys` for the sort method? A sequence with name, order tuples like pyarrow's `sort_indices` requires?\r\n- What about backwards compatability: is it supposed to also accept the old way of calling sort() or should both `column` and `kind` be deprecated?\r\n- If `sort_keys` is provided in the same format as for pyarrow's `sort_indices` - i.e. along with order for each column -, `reverse` doesn't make much sense either and should be deprecated as well I assume.",
"I think we can have the following signature:\r\n```python\r\ndef sort(\r\n self,\r\n column_names: Union[str, Sequence[str]],\r\n reverse: Union[bool, Sequence[bool]] = False,\r\n kind=\"deprecated\",\r\n null_placement: str = \"last\",\r\n keep_in_memory: bool = False,\r\n load_from_cache_file: bool = True,\r\n indices_cache_file_name: Optional[str] = None,\r\n writer_batch_size: Optional[int] = 1000,\r\n new_fingerprint: Optional[str] = None,\r\n ) -> \"Dataset\":\r\n``` \r\n\r\nSo we should:\r\n* rename`column` to `column_names`. `column` is a positional argument, so it's OK to rename it (not marked as positional-only with \"/\", but still should be fine)\r\n* deprecate `kind`\r\n* keep `reverse` instead of introducing `sort_keys`, but we should allow passing a list of booleans that defines the sort order of each column from `column_names` to it (`reverse = False` would be equal to `[False] * len(column_names)` and `reverse = True` to `[True] * len(column_names)`)",
"I am pretty much done with the PR. Just one clarification: `Sequence` in `arrow_dataset.py` is a custom dataclass from `features.py` instead of the `type.hinting` class `Sequence` from Python. Do you suggest using that custom `Sequence` class somehow ? Otherwise signature currently reads instead:\r\n```Python\r\n def sort(\r\n self,\r\n column_names: Union[str, List[str]],\r\n reverse: Union[bool, List[bool]] = False,\r\n kind = \"deprecated\",\r\n null_placement: str = \"last\",\r\n keep_in_memory: bool = False,\r\n load_from_cache_file: bool = True,\r\n indices_cache_file_name: Optional[str] = None,\r\n writer_batch_size: Optional[int] = 1000,\r\n new_fingerprint: Optional[str] = None,\r\n )\r\n```\r\n\r\nAlso, to maintain backwards compatibility, I added conditionals for `null_placement`, because pyarrow's `null_placement` only accepts `at_start` and `at_end`, and not `last` and `first`.\r\nIf that is all good, I think I can open the PR.",
"I meant `typing.Sequence` (`datasets.Sequence` is a feature type). \r\n\r\nRegarding `null_placement`, I think we can support both `at_start` and `at_end`, and `last` and `first` (for backward compatibility; convert internally to `at_end` and `at_start` respectively).",
"> I meant typing.Sequence (datasets.Sequence is a feature type).\r\n\r\nSorry, I actually meant `typing.Sequence` and not `type.hinting`. However, the issue is still that `dataset.Sequence` is imported in `arrow_dataset.py` so I cannot import and use `typing.Sequence` for the `sort`'s signature without overwriting the `dataset.Sequence` import. The latter is used in the `align_labels_with_mapping` method so it's a necessary import for `arrow_dataset.py`. \r\nTo import `typing.Sequence` as something else than `Sequence` to avoid overwriting may only be confusing and doesn't seem good practice!? The other solution is to keep `List` type hinting as in the signature I posted in my previous post but this excludes other Sequence types and may cause problems further down the line.\r\nPlease advise,\r\nThanks for all the clarifications!",
"You can avoid the name collision by renaming `typing.Sequence` to `Sequence_` when importing:\r\n```python\r\nfrom typing import Sequence as Sequence_\r\n```",
"Resolved via #5502 "
] | ### Feature request
From discussion on forum: https://discuss.huggingface.co/t/datasets-dataset-sort-does-not-preserve-ordering/29065/1
`sort()` does not preserve ordering, and it does not support sorting on multiple columns, nor a key function.
The suggested solution:
> ... having something similar to pandas and be able to specify multiple columns for sorting. We’re already using pandas under the hood to do the sorting in datasets.
The suggested workaround:
> convert your dataset to pandas and use `df.sort_values()`
### Motivation
Preserved ordering when sorting is very handy when one needs to sort on multiple columns, A and B, so that e.g. whenever A is equal for two or more rows, B is kept sorted.
Having a parameter to do this in 🤗datasets would be cleaner than going through pandas and back, and it wouldn't add much complexity to the library.
Alternatives:
- the possibility to specify multiple keys to sort by with decreasing priority (suggested solution),
- the ability to provide a key function for sorting, so that one can manually specify the sorting criteria.
### Your contribution
I'll be happy to contribute by submitting a PR. Will get documented on `CONTRIBUTING.MD`.
Would love to get thoughts on this, if anyone has anything to add. | 5,425 |
https://github.com/huggingface/datasets/issues/5424 | When applying `ReadInstruction` to custom load it's not DatasetDict but list of Dataset? | [
"Hi! You can get a `DatasetDict` if you pass a dictionary with read instructions as follows:\r\n```python\r\ninstructions = [\r\n ReadInstruction(split_name=\"train\", from_=0, to=10, unit='%', rounding='closest'),\r\n ReadInstruction(split_name=\"dev\", from_=0, to=10, unit='%', rounding='closest'),\r\n ReadInstruction(split_name=\"test\", from_=0, to=5, unit='%', rounding='closest')\r\n]\r\n\r\ndataset = load_dataset('csv', data_dir=\"data/\", data_files={\"train\":\"train.tsv\", \"dev\":\"dev.tsv\", \"test\":\"test.tsv\"}, delimiter=\"\\t\", split={inst.split_name: inst for inst in instructions})\r\n```\r\n"
] | ### Describe the bug
I am loading datasets from custom `tsv` files stored locally and applying split instructions for each split. Although the ReadInstruction is being applied correctly and I was expecting it to be `DatasetDict` but instead it is a list of `Dataset`.
### Steps to reproduce the bug
Steps to reproduce the behaviour:
1. Import
`from datasets import load_dataset, ReadInstruction`
2. Instruction to load the dataset
```
instructions = [
ReadInstruction(split_name="train", from_=0, to=10, unit='%', rounding='closest'),
ReadInstruction(split_name="dev", from_=0, to=10, unit='%', rounding='closest'),
ReadInstruction(split_name="test", from_=0, to=5, unit='%', rounding='closest')
]
```
3. Load
`dataset = load_dataset('csv', data_dir="data/", data_files={"train":"train.tsv", "dev":"dev.tsv", "test":"test.tsv"}, delimiter="\t", split=instructions)`
### Expected behavior
**Current behaviour**
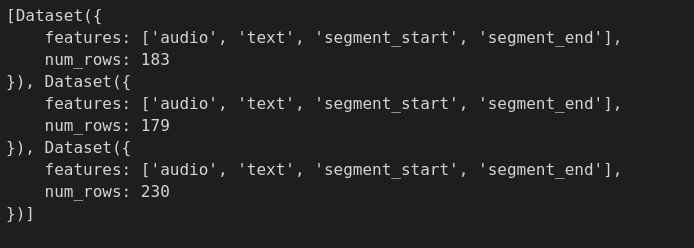
:
**Expected behaviour**
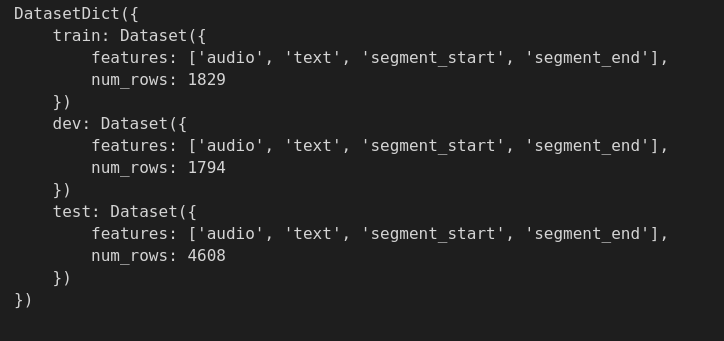
### Environment info
``datasets==2.8.0
``
`Python==3.8.5
`
`Platform - Ubuntu 20.04.4 LTS` | 5,424 |
https://github.com/huggingface/datasets/issues/5422 | Datasets load error for saved github issues | [
"I can confirm that the error exists!\r\nI'm trying to read 3 parquet files locally:\r\n```python\r\nfrom datasets import load_dataset, Features, Value, ClassLabel\r\n\r\nreview_dataset = load_dataset(\r\n \"parquet\",\r\n data_files={\r\n \"train\": os.path.join(sentiment_analysis_data_path, \"train.parquet\"),\r\n \"validation\": os.path.join(sentiment_analysis_data_path, \"validation.parquet\"),\r\n \"test\": os.path.join(sentiment_analysis_data_path, \"test.parquet\"),\r\n },\r\n)\r\n```\r\n\r\nBut you can fix it, by specifying `features` for `load_dataset()` function like this:\r\n```python\r\nfrom datasets import load_dataset, Features, Value, ClassLabel\r\n\r\nfeatures = Features(\r\n {\r\n \"label\": ClassLabel(\r\n num_classes=3,\r\n names=[\"negative\", \"neutral\", \"positive\"],\r\n ),\r\n \"text\": Value(dtype=\"string\"),\r\n }\r\n)\r\n\r\nreview_dataset = load_dataset(\r\n \"parquet\",\r\n data_files={\r\n \"train\": os.path.join(sentiment_analysis_data_path, \"train.parquet\"),\r\n \"validation\": os.path.join(sentiment_analysis_data_path, \"validation.parquet\"),\r\n \"test\": os.path.join(sentiment_analysis_data_path, \"test.parquet\"),\r\n },\r\n features=features,\r\n)\r\n\r\nprint(review_dataset)\r\n```",
"@Extremesarova I think this is a different issue, but understand using features could be a work-around.\r\nIt seems the field `closed_at` is `null` in many cases.\r\n\r\nI've not found a way to specify only a single feature without (succesfully) specifiying the full and quite detailed set of expected features. Using this features set gives an error the column names don't match.\r\n`features = Features({'closed_at': Value(dtype='timestamp[s]', id=None)})`\r\n\r\n",
"Found this when searching for the same error, looks like based on #3965 it's just an issue with the data. I found that changing `df = pd.DataFrame.from_records(all_issues)` to `df = pd.DataFrame.from_records(all_issues).dropna(axis=1, how='all').drop(['milestone'], axis=1)` from the fetch_issues function fixed the issue. \r\nThe \"milestone\" column seemed to be problematic (only ~50 non null rows) and dropped any columns that were all null as well just in case.",
"I have this same issue. I saved a dataset to disk and now I can't load it.",
"Ok the solution was to use load_from_disk instead of load_dataset.",
"Hi @folterj , I faced same issue while creating `issues_dataset` (https://huggingface.co/learn/nlp-course/chapter5/5?fw=pt). The fix which worked for me was loading the *.jsonl file as pd.read_json and then converting it into a Dataset using datasets API.\r\n```\r\nimport pandas as pd\r\ndf=pd.read_json(\"datasets-issues.jsonl\", lines=True)\r\ndf.head()\r\n\r\nfrom datasets import Dataset\r\nissues_dataset = Dataset.from_pandas(df)\r\nissues_dataset\r\nsample = issues_dataset.shuffle(seed=666).select(range(3))\r\nsample[0]\r\n```",
"I understand some work-around suggestions would be to not use load_dataset(), and instead using a different API function. Another alternative would be using the same function using streaming, as I had already suggested in my original post.\r\nHowever, the fact remains that there is an issue in this function as reported."
] | ### Describe the bug
Loading a previously downloaded & saved dataset as described in the HuggingFace course:
issues_dataset = load_dataset("json", data_files="issues/datasets-issues.jsonl", split="train")
Gives this error:
datasets.builder.DatasetGenerationError: An error occurred while generating the dataset
A work-around I found was to use streaming.
### Steps to reproduce the bug
Reproduce by executing the code provided:
https://huggingface.co/course/chapter5/5?fw=pt
From the heading:
'let’s create a function that can download all the issues from a GitHub repository'
### Expected behavior
No error
### Environment info
Datasets version 2.8.0. Note that version 2.6.1 gives the same error (related to null timestamp).
**[EDIT]**
This is the complete error trace confirming the issue is related to the timestamp (`Couldn't cast array of type timestamp[s] to null`)
```
Using custom data configuration default-950028611d2860c8
Downloading and preparing dataset json/default to [...]/.cache/huggingface/datasets/json/default-950028611d2860c8/0.0.0/0f7e3662623656454fcd2b650f34e886a7db4b9104504885bd462096cc7a9f51...
Downloading data files: 100%|██████████| 1/1 [00:00<?, ?it/s]
Extracting data files: 100%|██████████| 1/1 [00:00<00:00, 500.63it/s]
Generating train split: 2619 examples [00:00, 7155.72 examples/s]Traceback (most recent call last):
File "[...]\miniconda3\envs\HuggingFace\lib\site-packages\datasets\builder.py", line 1831, in _prepare_split_single
writer.write_table(table)
File "[...]\miniconda3\envs\HuggingFace\lib\site-packages\datasets\arrow_writer.py", line 567, in write_table
pa_table = table_cast(pa_table, self._schema)
File "[...]\miniconda3\envs\HuggingFace\lib\site-packages\datasets\table.py", line 2282, in table_cast
return cast_table_to_schema(table, schema)
File "[...]\miniconda3\envs\HuggingFace\lib\site-packages\datasets\table.py", line 2241, in cast_table_to_schema
arrays = [cast_array_to_feature(table[name], feature) for name, feature in features.items()]
File "[...]\miniconda3\envs\HuggingFace\lib\site-packages\datasets\table.py", line 2241, in <listcomp>
arrays = [cast_array_to_feature(table[name], feature) for name, feature in features.items()]
File "[...]\miniconda3\envs\HuggingFace\lib\site-packages\datasets\table.py", line 1807, in wrapper
return pa.chunked_array([func(chunk, *args, **kwargs) for chunk in array.chunks])
File "[...]\miniconda3\envs\HuggingFace\lib\site-packages\datasets\table.py", line 1807, in <listcomp>
return pa.chunked_array([func(chunk, *args, **kwargs) for chunk in array.chunks])
File "[...]\miniconda3\envs\HuggingFace\lib\site-packages\datasets\table.py", line 2035, in cast_array_to_feature
arrays = [_c(array.field(name), subfeature) for name, subfeature in feature.items()]
File "[...]\miniconda3\envs\HuggingFace\lib\site-packages\datasets\table.py", line 2035, in <listcomp>
arrays = [_c(array.field(name), subfeature) for name, subfeature in feature.items()]
File "[...]\miniconda3\envs\HuggingFace\lib\site-packages\datasets\table.py", line 1809, in wrapper
return func(array, *args, **kwargs)
File "[...]\miniconda3\envs\HuggingFace\lib\site-packages\datasets\table.py", line 2101, in cast_array_to_feature
return array_cast(array, feature(), allow_number_to_str=allow_number_to_str)
File "[...]\miniconda3\envs\HuggingFace\lib\site-packages\datasets\table.py", line 1809, in wrapper
return func(array, *args, **kwargs)
File "[...]\miniconda3\envs\HuggingFace\lib\site-packages\datasets\table.py", line 1990, in array_cast
raise TypeError(f"Couldn't cast array of type {array.type} to {pa_type}")
TypeError: Couldn't cast array of type timestamp[s] to null
The above exception was the direct cause of the following exception:
Traceback (most recent call last):
File "C:\Program Files\JetBrains\PyCharm 2022.1.3\plugins\python\helpers\pydev\pydevconsole.py", line 364, in runcode
coro = func()
File "<input>", line 1, in <module>
File "C:\Program Files\JetBrains\PyCharm 2022.1.3\plugins\python\helpers\pydev\_pydev_bundle\pydev_umd.py", line 198, in runfile
pydev_imports.execfile(filename, global_vars, local_vars) # execute the script
File "C:\Program Files\JetBrains\PyCharm 2022.1.3\plugins\python\helpers\pydev\_pydev_imps\_pydev_execfile.py", line 18, in execfile
exec(compile(contents+"\n", file, 'exec'), glob, loc)
File "[...]\PycharmProjects\TransformersTesting\dataset_issues.py", line 20, in <module>
issues_dataset = load_dataset("json", data_files="issues/datasets-issues.jsonl", split="train")
File "[...]\miniconda3\envs\HuggingFace\lib\site-packages\datasets\load.py", line 1757, in load_dataset
builder_instance.download_and_prepare(
File "[...]\miniconda3\envs\HuggingFace\lib\site-packages\datasets\builder.py", line 860, in download_and_prepare
self._download_and_prepare(
File "[...]\miniconda3\envs\HuggingFace\lib\site-packages\datasets\builder.py", line 953, in _download_and_prepare
self._prepare_split(split_generator, **prepare_split_kwargs)
File "[...]\miniconda3\envs\HuggingFace\lib\site-packages\datasets\builder.py", line 1706, in _prepare_split
for job_id, done, content in self._prepare_split_single(
File "[...]\miniconda3\envs\HuggingFace\lib\site-packages\datasets\builder.py", line 1849, in _prepare_split_single
raise DatasetGenerationError("An error occurred while generating the dataset") from e
datasets.builder.DatasetGenerationError: An error occurred while generating the dataset
Generating train split: 2619 examples [00:19, 7155.72 examples/s]
``` | 5,422 |
https://github.com/huggingface/datasets/issues/5421 | Support case-insensitive Hub dataset name in load_dataset | [
"Closing as case-insensitivity should be only for URL redirection on the Hub. In the APIs, we will only support the canonical name (https://github.com/huggingface/moon-landing/pull/2399#issuecomment-1382085611)"
] | ### Feature request
The dataset name on the Hub is case-insensitive (see https://github.com/huggingface/moon-landing/pull/2399, internal issue), i.e., https://huggingface.co/datasets/GLUE redirects to https://huggingface.co/datasets/glue.
Ideally, we could load the glue dataset using the following:
```
from datasets import load_dataset
load_dataset('GLUE', 'cola')
```
It breaks because the loading script `GLUE.py` does not exist (`glue.py` should be selected instead).
Minor additional comment: in other cases without a loading script, we can load the dataset, but the automatically generated config name depends on the casing:
- `load_dataset('severo/danish-wit')` generates the config name `severo--danish-wit-e6fda5b070deb133`, while
- `load_dataset('severo/danish-WIT')` generates the config name `severo--danish-WIT-e6fda5b070deb133`
### Motivation
To follow the same UX on the Hub and in the datasets library.
### Your contribution
... | 5,421 |
https://github.com/huggingface/datasets/issues/5419 | label_column='labels' in datasets.TextClassification and 'label' or 'label_ids' in transformers.DataColator | [
"Hi! Thanks for pointing out this inconsistency. Changing the default value at this point is probably not worth it, considering we've started discussing the state of the task API internally - we will most likely deprecate the current one and replace it with a more robust solution that relies on the `train_eval_index` field stored in the YAML section of the dataset cards.",
"The task templates API has been deprecated (will be removed in version 3.0), so I'm closing this issue."
] | ### Describe the bug
When preparing a dataset for a task using `datasets.TextClassification`, the output feature is named `labels`. When preparing the trainer using the `transformers.DataCollator` the default column name is `label` if binary or `label_ids` if multi-class problem.
It is required to rename the column accordingly to the expected name : `label` or `label_ids`
### Steps to reproduce the bug
```python
from datasets import TextClassification, AutoTokenized, DataCollatorWithPadding
ds_prepared = my_dataset.prepare_for_task(TextClassification(text_column='TEXT', label_column='MY_LABEL_COLUMN_1_OR_0'))
print(ds_prepared)
tokenizer = AutoTokenizer.from_pretrained("distilbert-base-uncased")
ds_tokenized = ds_prepared.map(lambda x: tokenizer(x['text'], truncation=True), batched=True)
print(ds_tokenized)
data_collator = DataCollatorWithPadding(tokenizer=tokenizer, return_tensors="tf")
tf_data = model.prepare_tf_dataset(ds_tokenized, shuffle=True, batch_size=16, collate_fn=data_collator)
print(tf_data)
```
### Expected behavior
Without renaming the the column, the target column is not in the final tf_data since it is not in the column name expected by the data_collator.
To correct this, we have to rename the column:
```python
ds_prepared = my_dataset.prepare_for_task(TextClassification(text_column='TEXT', label_column='MY_LABEL_COLUMN_1_OR_0')).rename_column('labels', 'label')
```
### Environment info
- `datasets` version: 2.8.0
- Platform: Linux-5.15.79.1-microsoft-standard-WSL2-x86_64-with-glibc2.35
- Python version: 3.10.6
- PyArrow version: 10.0.1
- Pandas version: 1.5.2
- `transformers` version: 4.26.0.dev0
- Platform: Linux-5.15.79.1-microsoft-standard-WSL2-x86_64-with-glibc2.35
- Python version: 3.10.6
- Huggingface_hub version: 0.11.1
- PyTorch version (GPU?): not installed (NA)
- Tensorflow version (GPU?): 2.11.0 (True)
- Flax version (CPU?/GPU?/TPU?): not installed (NA)
- Jax version: not installed
- JaxLib version: not installed
- Using GPU in script?: <fill in>
- Using distributed or parallel set-up in script?: <fill in> | 5,419 |
https://github.com/huggingface/datasets/issues/5418 | Add ProgressBar for `to_parquet` | [
"Thanks for your proposal, @zanussbaum. Yes, I agree that would definitely be a nice feature to have!",
"@albertvillanova I’m happy to make a quick PR for the feature! let me know ",
"That would be awesome ! You can comment `#self-assign` to assign you to this issue and open a PR :) Will be happy to review",
"Closing as this has been merged @lhoestq "
] | ### Feature request
Add a progress bar for `Dataset.to_parquet`, similar to how `to_json` works.
### Motivation
It's a bit frustrating to not know how long a dataset will take to write to file and if it's stuck or not without a progress bar
### Your contribution
Sure I can help if needed | 5,418 |
https://github.com/huggingface/datasets/issues/5415 | RuntimeError: Sharding is ambiguous for this dataset | [] | ### Describe the bug
When loading some datasets, a RuntimeError is raised.
For example, for "ami" dataset: https://huggingface.co/datasets/ami/discussions/3
```
.../huggingface/datasets/src/datasets/builder.py in _prepare_split(self, split_generator, check_duplicate_keys, file_format, num_proc, max_shard_size)
1415 fpath = path_join(self._output_dir, fname)
1416
-> 1417 num_input_shards = _number_of_shards_in_gen_kwargs(split_generator.gen_kwargs)
1418 if num_input_shards <= 1 and num_proc is not None:
1419 logger.warning(
.../huggingface/datasets/src/datasets/utils/sharding.py in _number_of_shards_in_gen_kwargs(gen_kwargs)
10 lists_lengths = {key: len(value) for key, value in gen_kwargs.items() if isinstance(value, list)}
11 if len(set(lists_lengths.values())) > 1:
---> 12 raise RuntimeError(
13 (
14 "Sharding is ambiguous for this dataset: "
RuntimeError: Sharding is ambiguous for this dataset: we found several data sources lists of different lengths, and we don't know over which list we should parallelize:
- key samples_paths has length 6
- key ids has length 7
- key verification_ids has length 6
To fix this, check the 'gen_kwargs' and make sure to use lists only for data sources, and use tuples otherwise. In the end there should only be one single list, or several lists with the same length.
```
This behavior was introduced when implementing multiprocessing by PR:
- #5107
### Steps to reproduce the bug
```python
ds = load_dataset("ami", "microphone-single", split="train", revision="2d7620bb7c3f1aab9f329615c3bdb598069d907a")
```
### Expected behavior
No error raised.
### Environment info
Since datasets 2.7.0 | 5,415 |
https://github.com/huggingface/datasets/issues/5414 | Sharding error with Multilingual LibriSpeech | [
"Thanks for reporting, @Nithin-Holla.\r\n\r\nThis is a known issue for multiple datasets and we are investigating it:\r\n- See e.g.: https://huggingface.co/datasets/ami/discussions/3",
"Main issue:\r\n- #5415",
"@albertvillanova Thanks! As a workaround for now, can I use the dataset in streaming mode?",
"Yes, @Nithin-Holla, in the meantime you can use this dataset in streaming mode."
] | ### Describe the bug
Loading the German Multilingual LibriSpeech dataset results in a RuntimeError regarding sharding with the following stacktrace:
```
Downloading and preparing dataset multilingual_librispeech/german to /home/nithin/datadrive/cache/huggingface/datasets/facebook___multilingual_librispeech/german/2.1.0/1904af50f57a5c370c9364cc337699cfe496d4e9edcae6648a96be23086362d0...
Downloading data files: 100%
3/3 [00:00<00:00, 107.23it/s]
Downloading data files: 100%
1/1 [00:00<00:00, 35.08it/s]
Downloading data files: 100%
6/6 [00:00<00:00, 303.36it/s]
Downloading data files: 100%
3/3 [00:00<00:00, 130.37it/s]
Downloading data files: 100%
1049/1049 [00:00<00:00, 4491.40it/s]
Downloading data files: 100%
37/37 [00:00<00:00, 1096.78it/s]
Downloading data files: 100%
40/40 [00:00<00:00, 1003.93it/s]
Extracting data files: 100%
3/3 [00:11<00:00, 2.62s/it]
Generating train split:
469942/0 [34:13<00:00, 273.21 examples/s]
Output exceeds the size limit. Open the full output data in a text editor
---------------------------------------------------------------------------
RuntimeError Traceback (most recent call last)
<ipython-input-14-74fa6d092bdc> in <module>
----> 1 mls = load_dataset(MLS_DATASET,
2 LANGUAGE,
3 cache_dir="~/datadrive/cache/huggingface/datasets",
4 ignore_verifications=True)
/anaconda/envs/py38_default/lib/python3.8/site-packages/datasets/load.py in load_dataset(path, name, data_dir, data_files, split, cache_dir, features, download_config, download_mode, ignore_verifications, keep_in_memory, save_infos, revision, use_auth_token, task, streaming, num_proc, **config_kwargs)
1755
1756 # Download and prepare data
-> 1757 builder_instance.download_and_prepare(
1758 download_config=download_config,
1759 download_mode=download_mode,
/anaconda/envs/py38_default/lib/python3.8/site-packages/datasets/builder.py in download_and_prepare(self, output_dir, download_config, download_mode, ignore_verifications, try_from_hf_gcs, dl_manager, base_path, use_auth_token, file_format, max_shard_size, num_proc, storage_options, **download_and_prepare_kwargs)
858 if num_proc is not None:
859 prepare_split_kwargs["num_proc"] = num_proc
--> 860 self._download_and_prepare(
861 dl_manager=dl_manager,
862 verify_infos=verify_infos,
/anaconda/envs/py38_default/lib/python3.8/site-packages/datasets/builder.py in _download_and_prepare(self, dl_manager, verify_infos, **prepare_splits_kwargs)
1609
1610 def _download_and_prepare(self, dl_manager, verify_infos, **prepare_splits_kwargs):
...
RuntimeError: Sharding is ambiguous for this dataset: we found several data sources lists of different lengths, and we don't know over which list we should parallelize:
- key audio_archives has length 1049
- key local_extracted_archive has length 1049
- key limited_ids_paths has length 1
To fix this, check the 'gen_kwargs' and make sure to use lists only for data sources, and use tuples otherwise. In the end there should only be one single list, or several lists with the same length.
```
### Steps to reproduce the bug
Here is the code to reproduce it:
```python
from datasets import load_dataset
MLS_DATASET = "facebook/multilingual_librispeech"
LANGUAGE = "german"
mls = load_dataset(MLS_DATASET,
LANGUAGE,
cache_dir="~/datadrive/cache/huggingface/datasets",
ignore_verifications=True)
```
### Expected behavior
The expected behaviour is that the dataset is successfully loaded.
### Environment info
- `datasets` version: 2.8.0
- Platform: Linux-5.4.0-1094-azure-x86_64-with-glibc2.10
- Python version: 3.8.8
- PyArrow version: 10.0.1
- Pandas version: 1.2.4 | 5,414 |
https://github.com/huggingface/datasets/issues/5413 | concatenate_datasets fails when two dataset with shards > 1 and unequal shard numbers | [
"Hi ! Thanks for reporting :)\r\n\r\nI managed to reproduce the hub using\r\n```python\r\n\r\nfrom datasets import concatenate_datasets, Dataset, load_from_disk\r\n\r\nDataset.from_dict({\"a\": range(9)}).save_to_disk(\"tmp/ds1\")\r\nds1 = load_from_disk(\"tmp/ds1\")\r\nds1 = concatenate_datasets([ds1, ds1])\r\n\r\nDataset.from_dict({\"b\": range(6)}).save_to_disk(\"tmp/ds2\")\r\nds2 = load_from_disk(\"tmp/ds2\")\r\nds2 = concatenate_datasets([ds2, ds2, ds2])\r\n\r\nconcatenate_datasets([ds1, ds2], axis=1)\r\n```\r\nand I get\r\n```python\r\nTraceback (most recent call last): \r\n File \"test.py\", line 98, in <module>\r\n dds = concatenate_datasets([ds1, ds2], axis=1)\r\n File \"/Users/.../datasets/combine.py\", line 182, in concatenate_datasets\r\n return _concatenate_map_style_datasets(dsets, info=info, split=split, axis=axis)\r\n File \"/Users/.../datasets/arrow_dataset.py\", line 5499, in _concatenate_map_style_datasets\r\n table = concat_tables([dset._data for dset in dsets], axis=axis)\r\n File \"/Users/.../datasets/table.py\", line 1778, in concat_tables\r\n return ConcatenationTable.from_tables(tables, axis=axis)\r\n File \"/Users/.../datasets/table.py\", line 1483, in from_tables\r\n blocks = _extend_blocks(blocks, table_blocks, axis=axis)\r\n File \"/Users/.../datasets/table.py\", line 1477, in _extend_blocks\r\n result[i].extend(row_blocks)\r\nIndexError: list index out of range\r\n```\r\n\r\nIt appears to happen when the two datasets have a number of shards that is not the same"
] | ### Describe the bug
When using `concatenate_datasets([dataset1, dataset2], axis = 1)` to concatenate two datasets with shards > 1, it fails:
```
File "/home/xzg/anaconda3/envs/tri-transfer/lib/python3.9/site-packages/datasets/combine.py", line 182, in concatenate_datasets
return _concatenate_map_style_datasets(dsets, info=info, split=split, axis=axis)
File "/home/xzg/anaconda3/envs/tri-transfer/lib/python3.9/site-packages/datasets/arrow_dataset.py", line 5499, in _concatenate_map_style_datasets
table = concat_tables([dset._data for dset in dsets], axis=axis)
File "/home/xzg/anaconda3/envs/tri-transfer/lib/python3.9/site-packages/datasets/table.py", line 1778, in concat_tables
return ConcatenationTable.from_tables(tables, axis=axis)
File "/home/xzg/anaconda3/envs/tri-transfer/lib/python3.9/site-packages/datasets/table.py", line 1483, in from_tables
blocks = _extend_blocks(blocks, table_blocks, axis=axis)
File "/home/xzg/anaconda3/envs/tri-transfer/lib/python3.9/site-packages/datasets/table.py", line 1477, in _extend_blocks
result[i].extend(row_blocks)
IndexError: list index out of range
```
### Steps to reproduce the bug
dataset = concatenate_datasets([dataset1, dataset2], axis = 1)
### Expected behavior
The datasets are correctly concatenated.
### Environment info
datasets==2.8.0 | 5,413 |
https://github.com/huggingface/datasets/issues/5412 | load_dataset() cannot find dataset_info.json with multiple training runs in parallel | [
"Hi ! It fails because the dataset is already being prepared by your first run. I'd encourage you to prepare your dataset before using it for multiple trainings.\r\n\r\nYou can also specify another cache directory by passing `cache_dir=` to `load_dataset()`.",
"Thank you! What do you mean by prepare it beforehand? I am unclear how to conduct dataset preparation outside of using the `load_dataset` function.",
"You can have a separate script that does load_dataset + map + save_to_disk to save your prepared dataset somewhere. Then in your training script you can reload the dataset with load_from_disk",
"Thank you! I believe I was running additional map steps after loading, resulting in the cache conflict. "
] | ### Describe the bug
I have a custom local dataset in JSON form. I am trying to do multiple training runs in parallel. The first training run runs with no issue. However, when I start another run on another GPU, the following code throws this error.
If there is a workaround to ignore the cache I think that would solve my problem too.
I am using datasets version 2.8.0.
### Steps to reproduce the bug
1. Start training run of GPU 0 loading dataset from
```
load_dataset(
"json",
data_files=tr_dataset_path,
split=f"train",
download_mode="force_redownload",
)
```
2. While GPU 0 is training, start an identical run on GPU 1. GPU 1 will produce the following error:
```
Traceback (most recent call last):
File "/local-scratch1/data/mt/code/qq/train.py", line 198, in <module>
main()
File "/home/username/.local/lib/python3.8/site-packages/click/core.py", line 1130, in __call__
return self.main(*args, **kwargs)
File "/home/username/.local/lib/python3.8/site-packages/click/core.py", line 1055, in main
rv = self.invoke(ctx)
File "/home/username/.local/lib/python3.8/site-packages/click/core.py", line 1404, in invoke
return ctx.invoke(self.callback, **ctx.params)
File "/home/username/.local/lib/python3.8/site-packages/click/core.py", line 760, in invoke
return __callback(*args, **kwargs)
File "/local-scratch1/data/mt/code/qq/train.py", line 113, in main
load_dataset(
File "/home/username/miniconda3/envs/qq3/lib/python3.8/site-packages/datasets/load.py", line 1734, in load_dataset
builder_instance = load_dataset_builder(
File "/home/username/miniconda3/envs/qq3/lib/python3.8/site-packages/datasets/load.py", line 1518, in load_dataset_builder
builder_instance: DatasetBuilder = builder_cls(
File "/home/username/miniconda3/envs/qq3/lib/python3.8/site-packages/datasets/builder.py", line 366, in __init__
self.info = DatasetInfo.from_directory(self._cache_dir)
File "/home/username/miniconda3/envs/qq3/lib/python3.8/site-packages/datasets/info.py", line 313, in from_directory
with fs.open(path_join(dataset_info_dir, config.DATASET_INFO_FILENAME), "r", encoding="utf-8") as f:
File "/home/username/miniconda3/envs/qq3/lib/python3.8/site-packages/fsspec/spec.py", line 1094, in open
self.open(
File "/home/username/miniconda3/envs/qq3/lib/python3.8/site-packages/fsspec/spec.py", line 1106, in open
f = self._open(
File "/home/username/miniconda3/envs/qq3/lib/python3.8/site-packages/fsspec/implementations/local.py", line 175, in _open
return LocalFileOpener(path, mode, fs=self, **kwargs)
File "/home/username/miniconda3/envs/qq3/lib/python3.8/site-packages/fsspec/implementations/local.py", line 273, in __init__
self._open()
File "/home/username/miniconda3/envs/qq3/lib/python3.8/site-packages/fsspec/implementations/local.py", line 278, in _open
self.f = open(self.path, mode=self.mode)
FileNotFoundError: [Errno 2] No such file or directory: '/home/username/.cache/huggingface/datasets/json/default-43d06a4aedb25e6d/0.0.0/0f7e3662623656454fcd2b650f34e886a7db4b9104504885bd462096cc7a9f51/dataset_info.json'
```
### Expected behavior
Expected behavior: 2nd GPU training run should run the same as 1st GPU training run.
### Environment info
Copy-and-paste the text below in your GitHub issue.
- `datasets` version: 2.8.0
- Platform: Linux-5.4.0-120-generic-x86_64-with-glibc2.10
- Python version: 3.8.15
- PyArrow version: 9.0.0
- Pandas version: 1.5.2 | 5,412 |
https://github.com/huggingface/datasets/issues/5408 | dataset map function could not be hash properly | [
"Hi ! On macos I tried with\r\n- py 3.9.11\r\n- datasets 2.8.0\r\n- transformers 4.25.1\r\n- dill 0.3.4\r\n\r\nand I was able to hash `prepare_dataset` correctly:\r\n```python\r\nfrom datasets.fingerprint import Hasher\r\nHasher.hash(prepare_dataset)\r\n```\r\n\r\nWhat version of transformers do you have ? Can you try to call `Hasher.hash` on the the tokenizer and the feature extractor to see which one can't be hashed ?",
"Thanks for your prompt reply.\r\n\r\nI update datasets version to 2.8.0 and the warning is gong."
] | ### Describe the bug
I follow the [blog post](https://huggingface.co/blog/fine-tune-whisper#building-a-demo) to finetune a Cantonese transcribe model.
When using map function to prepare dataset, following warning pop out:
`common_voice = common_voice.map(prepare_dataset,
remove_columns=common_voice.column_names["train"], num_proc=1)`
> Parameter 'function'=<function prepare_dataset at 0x000001D1D9D79A60> of the transform datasets.arrow_dataset.Dataset._map_single couldn't be hashed properly, a random hash was used instead. Make sure your transforms and parameters are serializable with pickle or dill for the dataset fingerprinting and caching to work. If you reuse this transform, the caching mechanism will consider it to be different from the previous calls and recompute everything. This warning is only showed once. Subsequent hashing failures won't be showed.
I read https://github.com/huggingface/datasets/issues/4521 and https://github.com/huggingface/datasets/issues/3178 but cannot solve the issue.
### Steps to reproduce the bug
```python
from datasets import load_dataset, DatasetDict
common_voice = DatasetDict()
common_voice["train"] = load_dataset("mozilla-foundation/common_voice_11_0", "zh-HK",
split="train+validation")
common_voice["test"] = load_dataset("mozilla-foundation/common_voice_11_0", "zh-HK",
split="test")
common_voice = common_voice.remove_columns(["accent", "age", "client_id", "down_votes", "gender", "locale", "path", "segment", "up_votes"])
from transformers import WhisperFeatureExtractor, WhisperTokenizer, WhisperProcessor
feature_extractor = WhisperFeatureExtractor.from_pretrained("openai/whisper-small")
tokenizer = WhisperTokenizer.from_pretrained("openai/whisper-small", language="chinese", task="transcribe")
processor = WhisperProcessor.from_pretrained("openai/whisper-small", language="chinese", task="transcribe")
from datasets import Audio
common_voice = common_voice.cast_column("audio", Audio(sampling_rate=16000))
def prepare_dataset(batch):
# load and resample audio data from 48 to 16kHz
audio = batch["audio"]
# compute log-Mel input features from input audio array
batch["input_features"] = feature_extractor(audio["array"],
sampling_rate=audio["sampling_rate"]).input_features[0]
# encode target text to label ids
batch["labels"] = tokenizer(batch["sentence"]).input_ids
return batch
common_voice = common_voice.map(prepare_dataset,
remove_columns=common_voice.column_names["train"], num_proc=1)
```
### Expected behavior
Should be no warning shown.
### Environment info
- `datasets` version: 2.7.0
- Platform: Windows-10-10.0.19045-SP0
- Python version: 3.9.12
- PyArrow version: 8.0.0
- Pandas version: 1.3.5
- dill version: 0.3.4
- multiprocess version: 0.70.12.2 | 5,408 |
https://github.com/huggingface/datasets/issues/5407 | Datasets.from_sql() generates deprecation warning | [
"Thanks for reporting @msummerfield. We are fixing it."
] | ### Describe the bug
Calling `Datasets.from_sql()` generates a warning:
`.../site-packages/datasets/builder.py:712: FutureWarning: 'use_auth_token' was deprecated in version 2.7.1 and will be removed in 3.0.0. Pass 'use_auth_token' to the initializer/'load_dataset_builder' instead.`
### Steps to reproduce the bug
Any valid call to `Datasets.from_sql()` will produce the deprecation warning.
### Expected behavior
No warning.
The fix should be simply to remove the parameter `use_auth_token` from the call to `builder.download_and_prepare()` at line 43 of `io/sql.py` (it is set to `None` anyway, and is not needed).
### Environment info
- `datasets` version: 2.8.0
- Platform: Linux-4.15.0-169-generic-x86_64-with-glibc2.27
- Python version: 3.9.15
- PyArrow version: 10.0.1
- Pandas version: 1.5.2
| 5,407 |
https://github.com/huggingface/datasets/issues/5406 | [2.6.1][2.7.0] Upgrade `datasets` to fix `TypeError: can only concatenate str (not "int") to str` | [
"I still get this error on 2.9.0\r\n<img width=\"1925\" alt=\"image\" src=\"https://user-images.githubusercontent.com/7208470/215597359-2f253c76-c472-4612-8099-d3a74d16eb29.png\">\r\n",
"Hi ! I just tested locally and or colab and it works fine for 2.9 on `sst2`.\r\n\r\nAlso the code that is shown in your stack trace is not present in the 2.9 source code - so I'm wondering how you installed `datasets` that could cause this ? (you can check by searching for `[0:{label_ids[-1] + 1}]` in the [2.9 codebase](https://github.dev/huggingface/datasets/tree/b5672a956d5de864e6f5550e493527d962d6ae55) - it doesn't find anything)\r\n\r\nAnyway you can try uninstalling `datasets` and install it again",
"For what it's worth, I've also gotten this error on 2.9.0, and I've tried uninstalling an reinstalling\r\n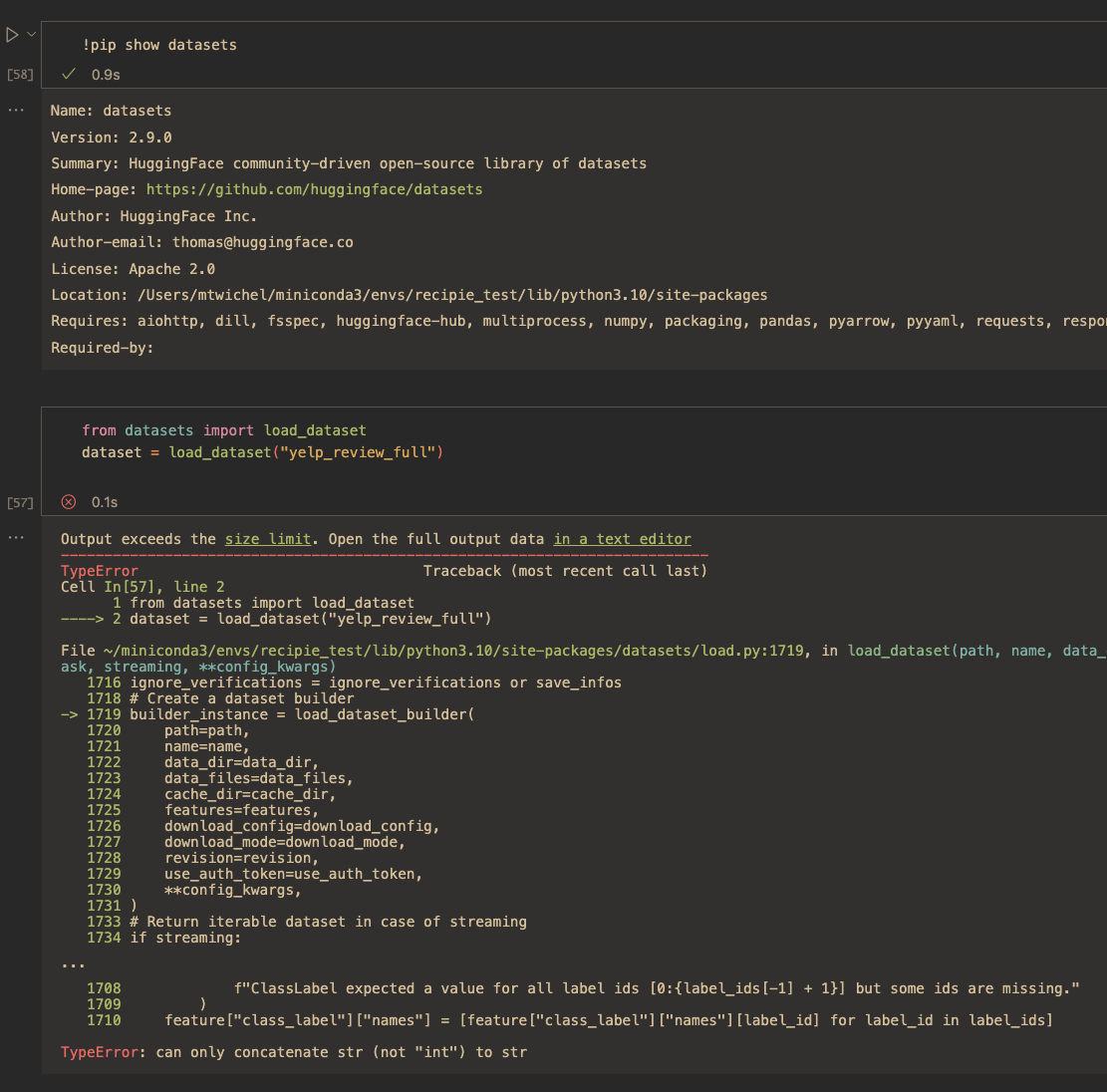\r\n\r\nI'm very new to this package (I was following this tutorial: https://huggingface.co/docs/transformers/training), so there's a good chance I was doing something wrong 😅 but thought I'd pass along the feedback",
"@ntrpnr @mtwichel Did you install `datasets` with conda ?\r\n\r\nI suspect that `datasets` 2.9 on conda still have this issue for some reason. When I install `datasets` with `pip` I don't have this error.",
"> @ntrpnr @mtwichel Did you install datasets with conda ?\r\n\r\nI did yeah, I wonder if that's the issue",
"I just checked on conda at https://anaconda.org/HuggingFace/datasets/files\r\n\r\nand everything looks fine, I got\r\n```python\r\n\r\nf\"ClassLabel expected a value for all label ids [0:{int(label_ids[-1]) + 1}] but some ids are missing.\"\r\n```\r\nas expected in features.py line 1760 (notice the \"int()\") to not have the TypeError.\r\n\r\nFrom where on conda did you install `datasets` ? You should use the `HuggingFace` official channel\r\n\r\nedit: the conda-forge one [here](https://anaconda.org/conda-forge/datasets/files) seems ok as well",
"Could you also try this in your notebook ? In case your python kernel doesn't match the `pip` environment in your shell\r\n```python\r\nimport datasets; datasets.__version__\r\n```\r\nand\r\n```\r\n!which python\r\n```\r\n```python\r\nimport sys; sys.executable\r\n```",
"Mmmm, just a potential clue:\r\n\r\nWhere are you running your Python code? Is it the Spyder IDE?\r\n\r\nI have recently seen some users reporting conflicting Python environments while using Spyder...\r\n\r\nMaybe related:\r\n- #5487",
"Other potential clue:\r\n- Had you already imported `datasets` before pip-updating it? You should first update datasets, before importing it. Otherwise, you need to restart the kernel after updating it.",
"I installed `datasets` with Conda using `conda install datasets` and got this issue.\r\n\r\nThen I tried to reinstall using\r\n`\r\nconda install -c huggingface -c conda-forge datasets\r\n`\r\nThe issue is now fixed.",
"I'm still getting this error on 2.13.0"
] | `datasets` 2.6.1 and 2.7.0 started to stop supporting datasets like IMDB, ConLL or MNIST datasets.
When loading a dataset using 2.6.1 or 2.7.0, you may this error when loading certain datasets:
```python
TypeError: can only concatenate str (not "int") to str
```
This is because we started to update the metadata of those datasets to a format that is not supported in 2.6.1 and 2.7.0
This change is required or those datasets won't be supported by the Hugging Face Hub.
Therefore if you encounter this error or if you're using `datasets` 2.6.1 or 2.7.0, we encourage you to update to a newer version.
For example, versions 2.6.2 and 2.7.1 patch this issue.
```python
pip install -U datasets
```
All the datasets affected are the ones with a ClassLabel feature type and YAML "dataset_info" metadata. More info [here](https://github.com/huggingface/datasets/issues/5275).
We apologize for the inconvenience. | 5,406 |
https://github.com/huggingface/datasets/issues/5405 | size_in_bytes the same for all splits | [
"Hi @Breakend,\r\n\r\nIndeed, the attribute `size_in_bytes` refers to the size of the entire dataset configuration, for all splits (size of downloaded files + Arrow files), not the specific split.\r\nThis is also the case for `download_size` (downloaded files) and `dataset_size` (Arrow files).\r\n\r\nThe size of the Arrow files for a specific split can be accessed: e.g. size of the \"test\" split only\r\n```python\r\nds[\"train\"].info.splits[\"test\"].num_bytes\r\n```\r\n\r\nI agree this is confusing and maybe we should improve it."
] | ### Describe the bug
Hi, it looks like whenever you pull a dataset and get size_in_bytes, it returns the same size for all splits (and that size is the combined size of all splits). It seems like this shouldn't be the intended behavior since it is misleading. Here's an example:
```
>>> from datasets import load_dataset
>>> x = load_dataset("glue", "wnli")
Found cached dataset glue (/Users/breakend/.cache/huggingface/datasets/glue/wnli/1.0.0/dacbe3125aa31d7f70367a07a8a9e72a5a0bfeb5fc42e75c9db75b96da6053ad)
100%|██████████████████████████████████████████████████████████████████████████████████████████████████████████████████████████████████████████████████████████| 3/3 [00:00<00:00, 1097.70it/s]
>>> x["train"].size_in_bytes
186159
>>> x["validation"].size_in_bytes
186159
>>> x["test"].size_in_bytes
186159
>>>
```
### Steps to reproduce the bug
```
>>> from datasets import load_dataset
>>> x = load_dataset("glue", "wnli")
>>> x["train"].size_in_bytes
186159
>>> x["validation"].size_in_bytes
186159
>>> x["test"].size_in_bytes
186159
```
### Expected behavior
The expected behavior is that it should return the separate sizes for all splits.
### Environment info
- `datasets` version: 2.7.1
- Platform: macOS-12.5-arm64-arm-64bit
- Python version: 3.10.8
- PyArrow version: 10.0.1
- Pandas version: 1.5.2 | 5,405 |
https://github.com/huggingface/datasets/issues/5404 | Better integration of BIG-bench | [
"Hi, I made my version : https://huggingface.co/datasets/tasksource/bigbench"
] | ### Feature request
Ideally, it would be nice to have a maintained PyPI package for `bigbench`.
### Motivation
We'd like to allow anyone to access, explore and use any task.
### Your contribution
@lhoestq has opened an issue in their repo:
- https://github.com/google/BIG-bench/issues/906 | 5,404 |
https://github.com/huggingface/datasets/issues/5402 | Missing state.json when creating a cloud dataset using a dataset_builder | [
"`load_from_disk` must be used on datasets saved using `save_to_disk`: they correspond to fully serialized datasets including their state.\r\n\r\nOn the other hand, `download_and_prepare` just downloads the raw data and convert them to arrow (or parquet if you want). We are working on allowing you to reload a dataset saved on S3 with `download_and_prepare` using `load_dataset` in #5281 \r\n\r\nFor now I'd encourage you to keep using `save_to_disk`",
"Thanks, I'll follow that issue. \r\n\r\nI was following the [cloud storage](https://huggingface.co/docs/datasets/filesystems) docs section and perhaps I'm missing some part of the flow; start with `load_dataset_builder` + `download_and_prepare`. You say I need an explicit `save_to_disk` but what object needs to be saved? the builder? is that related to the other issue?",
"Right now `load_dataset_builder` + `download_and_prepare` is to be used with tools like dask or spark, but `load_dataset` will support private cloud storage soon as well so you'll be able to reload the dataset with `datasets`.\r\n\r\nRight now the only function that can load a dataset from a cloud storage is `load_from_disk`, that must be used with a dataset serialized with `save_to_disk`."
] | ### Describe the bug
Using `load_dataset_builder` to create a builder, run `download_and_prepare` do upload it to S3. However when trying to load it, there are missing `state.json` files. Complete example:
```python
from aiobotocore.session import AioSession as Session
from datasets import load_from_disk, load_datase, load_dataset_builder
import s3fs
storage_options = {"session": Session()}
fs = s3fs.S3FileSystem(**storage_options)
output_dir = "s3://bucket/imdb"
builder = load_dataset_builder("imdb")
builder.download_and_prepare(output_dir, storage_options=storage_options)
load_from_disk(output_dir, fs=fs) # ERROR
# [Errno 2] No such file or directory: '/tmp/tmpy22yys8o/bucket/imdb/state.json'
```
As a comparison, if you use the non lazy `load_dataset`, it works and the S3 folder has different structure + state.json files. Example:
```python
from aiobotocore.session import AioSession as Session
from datasets import load_from_disk, load_dataset, load_dataset_builder
import s3fs
storage_options = {"session": Session()}
fs = s3fs.S3FileSystem(**storage_options)
output_dir = "s3://bucket/imdb"
dataset = load_dataset("imdb",)
dataset.save_to_disk(output_dir, fs=fs)
load_from_disk(output_dir, fs=fs) # WORKS
```
You still want the 1st option for the laziness and the parquet conversion. Thanks!
### Steps to reproduce the bug
```python
from aiobotocore.session import AioSession as Session
from datasets import load_from_disk, load_datase, load_dataset_builder
import s3fs
storage_options = {"session": Session()}
fs = s3fs.S3FileSystem(**storage_options)
output_dir = "s3://bucket/imdb"
builder = load_dataset_builder("imdb")
builder.download_and_prepare(output_dir, storage_options=storage_options)
load_from_disk(output_dir, fs=fs) # ERROR
# [Errno 2] No such file or directory: '/tmp/tmpy22yys8o/bucket/imdb/state.json'
```
BTW, you need the AioSession as s3fs is now based on aiobotocore, see https://github.com/fsspec/s3fs/issues/385.
### Expected behavior
Expected to be able to load the dataset from S3.
### Environment info
```
s3fs 2022.11.0
s3transfer 0.6.0
datasets 2.8.0
aiobotocore 2.4.2
boto3 1.24.59
botocore 1.27.59
```
python 3.7.15. | 5,402 |
https://github.com/huggingface/datasets/issues/5399 | Got disconnected from remote data host. Retrying in 5sec [2/20] | [] | ### Describe the bug
While trying to upload my image dataset of a CSV file type to huggingface by running the below code. The dataset consists of a little over 100k of image-caption pairs
### Steps to reproduce the bug
```
df = pd.read_csv('x.csv', encoding='utf-8-sig')
features = Features({
'link': Image(decode=True),
'caption': Value(dtype='string'),
})
#make sure u r logged in to HF
ds = Dataset.from_pandas(df, features=features)
ds.features
ds.push_to_hub("x/x")
```
I got the below error and It always stops at the same progress
```
100%|██████████| 4/4 [23:53<00:00, 358.48s/ba]
100%|██████████| 4/4 [24:37<00:00, 369.47s/ba]%|▍ | 1/22 [00:06<02:09, 6.16s/it]
100%|██████████| 4/4 [25:00<00:00, 375.15s/ba]%|▉ | 2/22 [25:54<2:36:15, 468.80s/it]
100%|██████████| 4/4 [24:53<00:00, 373.29s/ba]%|█▎ | 3/22 [51:01<4:07:07, 780.39s/it]
100%|██████████| 4/4 [24:01<00:00, 360.34s/ba]%|█▊ | 4/22 [1:17:00<5:04:07, 1013.74s/it]
100%|██████████| 4/4 [23:59<00:00, 359.91s/ba]%|██▎ | 5/22 [1:41:07<5:24:06, 1143.90s/it]
100%|██████████| 4/4 [24:16<00:00, 364.06s/ba]%|██▋ | 6/22 [2:05:14<5:29:15, 1234.74s/it]
100%|██████████| 4/4 [25:24<00:00, 381.10s/ba]%|███▏ | 7/22 [2:29:38<5:25:52, 1303.52s/it]
100%|██████████| 4/4 [25:24<00:00, 381.24s/ba]%|███▋ | 8/22 [2:56:02<5:23:46, 1387.58s/it]
100%|██████████| 4/4 [25:08<00:00, 377.23s/ba]%|████ | 9/22 [3:22:24<5:13:17, 1445.97s/it]
100%|██████████| 4/4 [24:11<00:00, 362.87s/ba]%|████▌ | 10/22 [3:48:24<4:56:02, 1480.19s/it]
100%|██████████| 4/4 [24:44<00:00, 371.11s/ba]%|█████ | 11/22 [4:12:42<4:30:10, 1473.66s/it]
100%|██████████| 4/4 [24:35<00:00, 368.81s/ba]%|█████▍ | 12/22 [4:37:34<4:06:29, 1478.98s/it]
100%|██████████| 4/4 [24:02<00:00, 360.67s/ba]%|█████▉ | 13/22 [5:03:24<3:45:04, 1500.45s/it]
100%|██████████| 4/4 [24:07<00:00, 361.78s/ba]%|██████▎ | 14/22 [5:27:33<3:17:59, 1484.97s/it]
100%|██████████| 4/4 [23:39<00:00, 354.85s/ba]%|██████▊ | 15/22 [5:51:48<2:52:10, 1475.82s/it]
Pushing dataset shards to the dataset hub: 73%|███████▎ | 16/22 [6:16:58<2:28:37, 1486.31s/it]Got disconnected from remote data host. Retrying in 5sec [1/20]
Got disconnected from remote data host. Retrying in 5sec [2/20]
Got disconnected from remote data host. Retrying in 5sec [3/20]
Got disconnected from remote data host. Retrying in 5sec [4/20]
Got disconnected from remote data host. Retrying in 5sec [5/20]
Got disconnected from remote data host. Retrying in 5sec [6/20]
Got disconnected from remote data host. Retrying in 5sec [7/20]
Got disconnected from remote data host. Retrying in 5sec [8/20]
Got disconnected from remote data host. Retrying in 5sec [9/20]
...
Got disconnected from remote data host. Retrying in 5sec [19/20]
Got disconnected from remote data host. Retrying in 5sec [20/20]
75%|███████▌ | 3/4 [24:47<08:15, 495.86s/ba]
Pushing dataset shards to the dataset hub: 73%|███████▎ | 16/22 [6:41:46<2:30:39, 1506.65s/it]
Output exceeds the size limit. Open the full output data in a text editor
---------------------------------------------------------------------------
ConnectionError Traceback (most recent call last)
<ipython-input-1-dbf8530779e9> in <module>
16 ds.features
```
### Expected behavior
I was trying to upload an image dataset and expected it to be fully uploaded
### Environment info
- `datasets` version: 2.8.0
- Platform: Windows-10-10.0.19041-SP0
- Python version: 3.7.9
- PyArrow version: 10.0.1
- Pandas version: 1.3.5 | 5,399 |
https://github.com/huggingface/datasets/issues/5398 | Unpin pydantic | [] | Once `pydantic` fixes their issue in their 1.10.3 version, unpin it.
See issue:
- #5394
See temporary fix:
- #5395 | 5,398 |
https://github.com/huggingface/datasets/issues/5394 | CI error: TypeError: dataclass_transform() got an unexpected keyword argument 'field_specifiers' | [
"I still getting the same error :\r\n\r\n`python -m spacy download fr_core_news_lg\r\n`.\r\n`import spacy`",
"@MFatnassi, this issue and the corresponding fix only affect our Continuous Integration testing environment.\r\n\r\nNote that `datasets` does not depend on `spacy`."
] | ### Describe the bug
While installing the dependencies, the CI raises a TypeError:
```
Traceback (most recent call last):
File "/opt/hostedtoolcache/Python/3.7.15/x64/lib/python3.7/runpy.py", line 183, in _run_module_as_main
mod_name, mod_spec, code = _get_module_details(mod_name, _Error)
File "/opt/hostedtoolcache/Python/3.7.15/x64/lib/python3.7/runpy.py", line 142, in _get_module_details
return _get_module_details(pkg_main_name, error)
File "/opt/hostedtoolcache/Python/3.7.15/x64/lib/python3.7/runpy.py", line 109, in _get_module_details
__import__(pkg_name)
File "/opt/hostedtoolcache/Python/3.7.15/x64/lib/python3.7/site-packages/spacy/__init__.py", line 6, in <module>
from .errors import setup_default_warnings
File "/opt/hostedtoolcache/Python/3.7.15/x64/lib/python3.7/site-packages/spacy/errors.py", line 2, in <module>
from .compat import Literal
File "/opt/hostedtoolcache/Python/3.7.15/x64/lib/python3.7/site-packages/spacy/compat.py", line 3, in <module>
from thinc.util import copy_array
File "/opt/hostedtoolcache/Python/3.7.15/x64/lib/python3.7/site-packages/thinc/__init__.py", line 5, in <module>
from .config import registry
File "/opt/hostedtoolcache/Python/3.7.15/x64/lib/python3.7/site-packages/thinc/config.py", line 2, in <module>
import confection
File "/opt/hostedtoolcache/Python/3.7.15/x64/lib/python3.7/site-packages/confection/__init__.py", line 10, in <module>
from pydantic import BaseModel, create_model, ValidationError, Extra
File "pydantic/__init__.py", line 2, in init pydantic.__init__
File "pydantic/dataclasses.py", line 46, in init pydantic.dataclasses
# | None | Attribute is set to None. |
File "pydantic/main.py", line 121, in init pydantic.main
TypeError: dataclass_transform() got an unexpected keyword argument 'field_specifiers'
```
See: https://github.com/huggingface/datasets/actions/runs/3793736481/jobs/6466356565
### Steps to reproduce the bug
```shell
pip install .[tests,metrics-tests]
python -m spacy download en_core_web_sm
```
### Expected behavior
No error.
### Environment info
See: https://github.com/huggingface/datasets/actions/runs/3793736481/jobs/6466356565 | 5,394 |
https://github.com/huggingface/datasets/issues/5391 | Whisper Event - RuntimeError: The size of tensor a (504) must match the size of tensor b (448) at non-singleton dimension 1 100% 1000/1000 [2:52:21<00:00, 10.34s/it] | [
"Hey @catswithbats! Super sorry for the late reply! This is happening because there is data with label length (504) that exceeds the model's max length (448). \r\n\r\nThere are two options here:\r\n1. Increase the model's `max_length` parameter: \r\n```python\r\nmodel.config.max_length = 512\r\n```\r\n2. Filter data with labels longer than max length: https://discuss.huggingface.co/t/open-to-the-community-whisper-fine-tuning-event/26681/21?u=sanchit-gandhi\r\n\r\nNote that the datasets repo is reserved for issues directly related to the HF datasets library. Issues related to custom fine-tuning implementations are more applicable to the HF Forum: https://discuss.huggingface.co. You're more likely to get a response by posting your issue in the most applicable place and boost the chance of someone sharing a working solution!",
"@sanchit-gandhi Thank you for all your work on this topic.\r\n\r\nI'm finding that changing the `max_length` value does not make this error go away."
] | Done in a VM with a GPU (Ubuntu) following the [Whisper Event - PYTHON](https://github.com/huggingface/community-events/tree/main/whisper-fine-tuning-event#python-script) instructions.
Attempted using [RuntimeError: he size of tensor a (504) must match the size of tensor b (448) at non-singleton dimension 1 100% 1000/1000 - WEB](https://discuss.huggingface.co/t/trainer-runtimeerror-the-size-of-tensor-a-462-must-match-the-size-of-tensor-b-448-at-non-singleton-dimension-1/26010/10 ) - another person experiencing the same issue. But could not resolve the issue with the google/fleurs data. __Not clear what can be modified in the PY code to resolve the input data size mismatch, as the training data is already very small__.
Tried posting on Discord, @sanchit-gandhi and @vaibhavs10. Was hoping that the event is over and some input/help is now available. [Hugging Face - whisper-small-amet](https://huggingface.co/drmeeseeks/whisper-small-amet).
The paper [Robust Speech Recognition via Large-Scale Weak Supervision](https://arxiv.org/abs/2212.04356) am_et is a low resource language (Table E), with the WER results ranging from 120-229, based on model size. (Whisper small WER=120.2).
# ---> Initial Training Output
/usr/local/lib/python3.8/dist-packages/transformers/optimization.py:306: FutureWarning: This implementation of AdamW is deprecated and will be removed in a future version. Use the PyTorch implementation torch.optim.AdamW instead, or set `no_deprecation_warning=True` to disable this warning
warnings.warn(
[INFO|trainer.py:1641] 2022-12-18 05:23:28,799 >> ***** Running training *****
[INFO|trainer.py:1642] 2022-12-18 05:23:28,799 >> Num examples = 446
[INFO|trainer.py:1643] 2022-12-18 05:23:28,799 >> Num Epochs = 72
[INFO|trainer.py:1644] 2022-12-18 05:23:28,799 >> Instantaneous batch size per device = 16
[INFO|trainer.py:1645] 2022-12-18 05:23:28,799 >> Total train batch size (w. parallel, distributed & accumulation) = 32
[INFO|trainer.py:1646] 2022-12-18 05:23:28,799 >> Gradient Accumulation steps = 2
[INFO|trainer.py:1647] 2022-12-18 05:23:28,800 >> Total optimization steps = 1000
[INFO|trainer.py:1648] 2022-12-18 05:23:28,801 >> Number of trainable parameters = 241734912
# ---> Error
14% 9/65 [07:07<48:34, 52.04s/it][INFO|configuration_utils.py:523] 2022-12-18 05:03:07,941 >> Generate config GenerationConfig {
"begin_suppress_tokens": [
220,
50257
],
"bos_token_id": 50257,
"decoder_start_token_id": 50258,
"eos_token_id": 50257,
"max_length": 448,
"pad_token_id": 50257,
"transformers_version": "4.26.0.dev0",
"use_cache": false
}
Traceback (most recent call last):
File "run_speech_recognition_seq2seq_streaming.py", line 629, in <module>
main()
File "run_speech_recognition_seq2seq_streaming.py", line 578, in main
train_result = trainer.train(resume_from_checkpoint=checkpoint)
File "/usr/local/lib/python3.8/dist-packages/transformers/trainer.py", line 1534, in train
return inner_training_loop(
File "/usr/local/lib/python3.8/dist-packages/transformers/trainer.py", line 1859, in _inner_training_loop
self._maybe_log_save_evaluate(tr_loss, model, trial, epoch, ignore_keys_for_eval)
File "/usr/local/lib/python3.8/dist-packages/transformers/trainer.py", line 2122, in _maybe_log_save_evaluate
metrics = self.evaluate(ignore_keys=ignore_keys_for_eval)
File "/usr/local/lib/python3.8/dist-packages/transformers/trainer_seq2seq.py", line 78, in evaluate
return super().evaluate(eval_dataset, ignore_keys=ignore_keys, metric_key_prefix=metric_key_prefix)
File "/usr/local/lib/python3.8/dist-packages/transformers/trainer.py", line 2818, in evaluate
output = eval_loop(
File "/usr/local/lib/python3.8/dist-packages/transformers/trainer.py", line 3000, in evaluation_loop
loss, logits, labels = self.prediction_step(model, inputs, prediction_loss_only, ignore_keys=ignore_keys)
File "/usr/local/lib/python3.8/dist-packages/transformers/trainer_seq2seq.py", line 213, in prediction_step
outputs = model(**inputs)
File "/usr/local/lib/python3.8/dist-packages/torch/nn/modules/module.py", line 1190, in _call_impl
return forward_call(*input, **kwargs)
File "/usr/local/lib/python3.8/dist-packages/transformers/models/whisper/modeling_whisper.py", line 1197, in forward
outputs = self.model(
File "/usr/local/lib/python3.8/dist-packages/torch/nn/modules/module.py", line 1190, in _call_impl
return forward_call(*input, **kwargs)
File "/usr/local/lib/python3.8/dist-packages/transformers/models/whisper/modeling_whisper.py", line 1066, in forward
decoder_outputs = self.decoder(
File "/usr/local/lib/python3.8/dist-packages/torch/nn/modules/module.py", line 1190, in _call_impl
return forward_call(*input, **kwargs)
File "/usr/local/lib/python3.8/dist-packages/transformers/models/whisper/modeling_whisper.py", line 873, in forward
hidden_states = inputs_embeds + positions
RuntimeError: The size of tensor a (504) must match the size of tensor b (448) at non-singleton dimension 1
100% 1000/1000 [2:52:21<00:00, 10.34s/it]
| 5,391 |
https://github.com/huggingface/datasets/issues/5390 | Error when pushing to the CI hub | [
"Hmmm, git bisect tells me that the behavior is the same since https://github.com/huggingface/datasets/commit/67e65c90e9490810b89ee140da11fdd13c356c9c (3 Oct), i.e. https://github.com/huggingface/datasets/pull/4926",
"Maybe related to the discussions in https://github.com/huggingface/datasets/pull/5196",
"Maybe the current version of moonlanding in Hub CI is the issue.\r\n\r\nI relaunched tests that were working two days ago: now they are failing. https://github.com/huggingface/datasets-server/commit/746414449cae4b311733f8a76e5b3b4ca73b38a9 for example\r\n\r\ncc @huggingface/moon-landing ",
"Hi! I don't think this has anything to do with `datasets`. Hub CI seems to be the culprit - the identical failure can be found in [this](https://github.com/huggingface/datasets/pull/5389) PR (with unrelated changes) opened today.",
"OK! Thanks for looking at it. Closing then."
] | ### Describe the bug
Note that it's a special case where the Hub URL is "https://hub-ci.huggingface.co", which does not appear if we do the same on the Hub (https://huggingface.co).
The call to `dataset.push_to_hub(` fails:
```
Pushing dataset shards to the dataset hub: 100%|██████████████████████████████████████████████████████████████████████████████████████████████████████████████████████████████████████████████████████████████████████████████████████████████████| 1/1 [00:01<00:00, 1.93s/it]
Traceback (most recent call last):
File "reproduce_hubci.py", line 16, in <module>
dataset.push_to_hub(repo_id=repo_id, private=False, token=USER_TOKEN, embed_external_files=True)
File "/home/slesage/hf/datasets/src/datasets/arrow_dataset.py", line 5025, in push_to_hub
HfApi(endpoint=config.HF_ENDPOINT).upload_file(
File "/home/slesage/.pyenv/versions/datasets/lib/python3.8/site-packages/huggingface_hub/hf_api.py", line 1346, in upload_file
raise err
File "/home/slesage/.pyenv/versions/datasets/lib/python3.8/site-packages/huggingface_hub/hf_api.py", line 1337, in upload_file
r.raise_for_status()
File "/home/slesage/.pyenv/versions/datasets/lib/python3.8/site-packages/requests/models.py", line 953, in raise_for_status
raise HTTPError(http_error_msg, response=self)
requests.exceptions.HTTPError: 400 Client Error: Bad Request for url: https://hub-ci.huggingface.co/api/datasets/__DUMMY_DATASETS_SERVER_USER__/bug-16718047265472/upload/main/README.md
```
### Steps to reproduce the bug
```python
# reproduce.py
from datasets import Dataset
import time
USER = "__DUMMY_DATASETS_SERVER_USER__"
USER_TOKEN = "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"
dataset = Dataset.from_dict({"a": [1, 2, 3]})
repo_id = f"{USER}/bug-{int(time.time() * 10e3)}"
dataset.push_to_hub(repo_id=repo_id, private=False, token=USER_TOKEN, embed_external_files=True)
```
```bash
$ HF_ENDPOINT="https://hub-ci.huggingface.co" python reproduce.py
```
### Expected behavior
No error and the dataset should be uploaded to the Hub with the README file (which generates the error).
### Environment info
- `datasets` version: 2.8.0
- Platform: Linux-5.15.0-1026-aws-x86_64-with-glibc2.35
- Python version: 3.9.15
- PyArrow version: 7.0.0
- Pandas version: 1.5.2
| 5,390 |
https://github.com/huggingface/datasets/issues/5388 | Getting Value Error while loading a dataset.. | [
"Hi! I can't reproduce this error locally (Mac) or in Colab. What version of `datasets` are you using?",
"Hi [mariosasko](https://github.com/mariosasko), the datasets version is '2.8.0'.",
"@valmetisrinivas you get that error because you imported `datasets` (and thus `fsspec`) before installing `zstandard`.\r\n\r\nPlease, restart your Colab runtime and execute the install commands before importing `datasets`:\r\n```python\r\n!pip install datasets\r\n!pip install zstandard\r\n\r\nfrom datasets import load_dataset\r\n\r\nds = load_dataset(\r\n \"json\",\r\n data_files=\"https://the-eye.eu/public/AI/pile_preliminary_components/FreeLaw_Opinions.jsonl.zst\",\r\n split=\"train\",\r\n streaming=True,\r\n)\r\nnext(iter(ds))\r\n```",
"> @valmetisrinivas you get that error because you imported `datasets` (and thus `fsspec`) before installing `zstandard`.\r\n> \r\n> Please, restart your Colab runtime and execute the install commands before importing `datasets`:\r\n> \r\n> ```python\r\n> !pip install datasets\r\n> !pip install zstandard\r\n> \r\n> from datasets import load_dataset\r\n> \r\n> ds = load_dataset(\r\n> \"json\",\r\n> data_files=\"https://the-eye.eu/public/AI/pile_preliminary_components/FreeLaw_Opinions.jsonl.zst\",\r\n> split=\"train\",\r\n> streaming=True,\r\n> )\r\n> next(iter(ds))\r\n> ```\r\n\r\nI guess that was the problem, importing datasets before the installation of zstandard. Thank you for the feedback. "
] | ### Describe the bug
I am trying to load a dataset using Hugging Face Datasets load_dataset method. I am getting the value error as show below. Can someone help with this? I am using Windows laptop and Google Colab notebook.
```
WARNING:datasets.builder:Using custom data configuration default-a1d9e8eaedd958cd
---------------------------------------------------------------------------
ValueError Traceback (most recent call last)
[<ipython-input-12-5b4fdcb8e6d5>](https://localhost:8080/#) in <module>
6 )
7
----> 8 next(iter(law_dataset_streamed))
17 frames
[/usr/local/lib/python3.8/dist-packages/fsspec/core.py](https://localhost:8080/#) in get_compression(urlpath, compression)
485 compression = infer_compression(urlpath)
486 if compression is not None and compression not in compr:
--> 487 raise ValueError("Compression type %s not supported" % compression)
488 return compression
489
ValueError: Compression type zstd not supported
```
### Steps to reproduce the bug
```
!pip install zstandard
from datasets import load_dataset
lds = load_dataset(
"json",
data_files="https://the-eye.eu/public/AI/pile_preliminary_components/FreeLaw_Opinions.jsonl.zst",
split="train",
streaming=True,
)
```
### Expected behavior
I expect an iterable object as the output 'lds' to be created.
### Environment info
Windows laptop with Google Colab notebook | 5,388 |
https://github.com/huggingface/datasets/issues/5387 | Missing documentation page : improve-performance | [
"Hi! Our documentation builder does not support links to sections, hence the bug. This is the link it should point to https://huggingface.co/docs/datasets/v2.8.0/en/cache#improve-performance."
] | ### Describe the bug
Trying to access https://huggingface.co/docs/datasets/v2.8.0/en/package_reference/cache#improve-performance, the page is missing.
The link is in here : https://huggingface.co/docs/datasets/v2.8.0/en/package_reference/loading_methods#datasets.load_dataset.keep_in_memory
### Steps to reproduce the bug
Access the page and see it's missing.
### Expected behavior
Not missing page
### Environment info
Doesn't matter | 5,387 |
https://github.com/huggingface/datasets/issues/5386 | `max_shard_size` in `datasets.push_to_hub()` breaks with large files | [
"Hi! \r\n\r\nThis behavior stems from the fact that we don't always embed image bytes in the underlying arrow table, which can lead to bad size estimation (we use the first 1000 table rows to [estimate](https://github.com/huggingface/datasets/blob/9a7272cd4222383a5b932b0083a4cc173fda44e8/src/datasets/arrow_dataset.py#L4627) the external file size). We plan to address this in the next major release by always embedding external bytes. In the meantime, you can either shuffle the dataset with `.shuffle().flatten_indices()` to make the estimation more precise or embed the bytes in the table like so:\r\n```python\r\nfrom datasets.table import embed_table_storage\r\nformat = ds.format\r\nds = ds.with_format(\"arrow\")\r\nds = ds.map(embed_table_storage, batched=True)\r\nds = ds.with_format(**format)\r\n...\r\nds.push_to_hub(...)\r\n```",
"Embedding the bytes worked like charm. Thanks @mariosasko!"
] | ### Describe the bug
`max_shard_size` parameter for `datasets.push_to_hub()` works unreliably with large files, generating shard files that are way past the specified limit.
In my private dataset, which contains unprocessed images of all sizes (up to `~100MB` per file), I've encountered cases where `max_shard_size='100MB'` results in shard files that are `>2GB` in size. Setting `max_shard_size` to another value, such as `1GB` or `500MB` does not fix this problem.
**The real problem is this:** When the shard file size grows too big, the entire dataset breaks because of #4721 and ultimately https://issues.apache.org/jira/browse/ARROW-5030. Since `max_shard_size` does not let one accurately control the size of the shard files, it becomes very easy to build a large dataset without any warnings that it will be broken -- even when you think you are mitigating this problem by setting `max_shard_size`.
```
File " /path/to/sd-test-suite-v1/venv/lib/site-packages/datasets/builder.py", line 1763, in _prepare_split_single
for _, table in generator:
File " /path/to/sd-test-suite-v1/venv/lib/site-packages/datasets/packaged_modules/parquet/parquet.py", line 69, in _generate_tables
for batch_idx, record_batch in enumerate(
File "pyarrow/_parquet.pyx", line 1323, in iter_batches
File "pyarrow/error.pxi", line 121, in pyarrow.lib.check_status
pyarrow.lib.ArrowNotImplementedError: Nested data conversions not implemented for chunked array outputs
```
### Steps to reproduce the bug
1. Clone [example repo](https://github.com/salieri/hf-dataset-shard-size-bug)
2. Follow steps in [README.md](https://github.com/salieri/hf-dataset-shard-size-bug/blob/main/README.md)
3. After uploading the dataset, you will see that the shard file size varies between `30MB` and `200MB` -- way beyond the `max_shard_size='75MB'` limit (example: `train-00003-of-00131...` is `155MB` in [here](https://huggingface.co/datasets/slri/shard-size-test/tree/main/data))
(Note that this example repo does not generate shard files that are so large that they would trigger #4721)
### Expected behavior
The shard file size should remain below or equal to `max_shard_size`.
### Environment info
- `datasets` version: 2.8.0
- Platform: Linux-5.10.157-139.675.amzn2.aarch64-aarch64-with-glibc2.17
- Python version: 3.7.15
- PyArrow version: 10.0.1
- Pandas version: 1.3.5 | 5,386 |
https://github.com/huggingface/datasets/issues/5385 | Is `fs=` deprecated in `load_from_disk()` as well? | [
"Hi! Yes, we should deprecate the `fs` param here. Would you be interested in submitting a PR? ",
"> Hi! Yes, we should deprecate the `fs` param here. Would you be interested in submitting a PR?\r\n\r\nYeah I can do that sometime next week. Should the storage_options be a new arg here? I’ll look around for anywhere else where fs is an arg.",
"Closed by #5393."
] | ### Describe the bug
The `fs=` argument was deprecated from `Dataset.save_to_disk` and `Dataset.load_from_disk` in favor of automagically figuring it out via fsspec:
https://github.com/huggingface/datasets/blob/9a7272cd4222383a5b932b0083a4cc173fda44e8/src/datasets/arrow_dataset.py#L1339-L1340
Is there a reason the same thing shouldn't also apply to `datasets.load.load_from_disk()` as well ?
https://github.com/huggingface/datasets/blob/9a7272cd4222383a5b932b0083a4cc173fda44e8/src/datasets/load.py#L1779
### Steps to reproduce the bug
n/a
### Expected behavior
n/a
### Environment info
n/a | 5,385 |
https://github.com/huggingface/datasets/issues/5383 | IterableDataset missing column_names, differs from Dataset interface | [
"Another example is that `IterableDataset.map` does not have `fn_kwargs`, among other arguments. It makes it harder to convert code from Dataset to IterableDataset.",
"Hi! `fn_kwargs` was added to `IterableDataset.map` in `datasets 2.5.0`, so please update your installation (`pip install -U datasets`) to use it.\r\n\r\nRegarding `column_names`, I agree we should add this property to `IterableDataset`. In the meantime, you can use `list(dataset.features.keys())` instead.",
"Thanks! That's great news.\n\nOn Thu, Dec 22, 2022, 07:48 Mario Šaško ***@***.***> wrote:\n\n> Hi! fn_kwargs was added to IterableDataset.map in datasets 2.5.0, so\n> please update your installation (pip install -U datasets) to use it.\n>\n> Regarding column_names, I agree we should add this property to\n> IterableDataset. In the meantime, you can use\n> list(dataset.features.keys()) instead.\n>\n> —\n> Reply to this email directly, view it on GitHub\n> <https://github.com/huggingface/datasets/issues/5383#issuecomment-1362993633>,\n> or unsubscribe\n> <https://github.com/notifications/unsubscribe-auth/AAHD6N2EQUFEOUFDW3VHSILWORZ45ANCNFSM6AAAAAATGKWVGM>\n> .\n> You are receiving this because you authored the thread.Message ID:\n> ***@***.***>\n>\n",
"I'm marking this issue as a \"good first issue\", as it makes sense to have `IterableDataset.column_names` in the API. Besides the case when `features` are `None` (e.g., `features` are `None` after `map`), in which we can also return `column_names` as `None`, adding this property should be straightforward,",
"Hi @mariosasko, I can work on this if that's ok?",
"Yes! I've assigned you the issue."
] | ### Describe the bug
The documentation on [Stream](https://huggingface.co/docs/datasets/v1.18.2/stream.html) seems to imply that IterableDataset behaves just like a Dataset. However, examples like
```
dataset.map(augment_data, batched=True, remove_columns=dataset.column_names, ...)
```
will not work because `.column_names` does not exist on IterableDataset. I cannot find any clear explanation on why this is not available, is it an oversight? We do have `iterable_ds.features` available.
### Steps to reproduce the bug
See above
### Expected behavior
Dataset and IterableDataset would be expected to have the same interface, with any differences noted in the documentation.
### Environment info
n/a | 5,383 |
https://github.com/huggingface/datasets/issues/5381 | Wrong URL for the_pile dataset | [
"Hi! This error can happen if there is a local file/folder with the same name as the requested dataset. And to avoid it, rename the local file/folder.\r\n\r\nSoon, it will be possible to explicitly request a Hub dataset as follows:https://github.com/huggingface/datasets/issues/5228#issuecomment-1313494020"
] | ### Describe the bug
When trying to load `the_pile` dataset from the library, I get a `FileNotFound` error.
### Steps to reproduce the bug
Steps to reproduce:
Run:
```
from datasets import load_dataset
dataset = load_dataset("the_pile")
```
I get the output:
"name": "FileNotFoundError",
"message": "Unable to resolve any data file that matches '['**']' at /storage/store/work/lgrinszt/memorization/the_pile with any supported extension ['csv', 'tsv', 'json', 'jsonl', 'parquet', 'txt', 'blp', 'bmp', 'dib', 'bufr', 'cur', 'pcx', 'dcx', 'dds', 'ps', 'eps', 'fit', 'fits', 'fli', 'flc', 'ftc', 'ftu', 'gbr', 'gif', 'grib', 'h5', 'hdf', 'png', 'apng', 'jp2', 'j2k', 'jpc', 'jpf', 'jpx', 'j2c', 'icns', 'ico', 'im', 'iim', 'tif', 'tiff', 'jfif', 'jpe', 'jpg', 'jpeg', 'mpg', 'mpeg', 'msp', 'pcd', 'pxr', 'pbm', 'pgm', 'ppm', 'pnm', 'psd', 'bw', 'rgb', 'rgba', 'sgi', 'ras', 'tga', 'icb', 'vda', 'vst', 'webp', 'wmf', 'emf', 'xbm', 'xpm', 'BLP', 'BMP', 'DIB', 'BUFR', 'CUR', 'PCX', 'DCX', 'DDS', 'PS', 'EPS', 'FIT', 'FITS', 'FLI', 'FLC', 'FTC', 'FTU', 'GBR', 'GIF', 'GRIB', 'H5', 'HDF', 'PNG', 'APNG', 'JP2', 'J2K', 'JPC', 'JPF', 'JPX', 'J2C', 'ICNS', 'ICO', 'IM', 'IIM', 'TIF', 'TIFF', 'JFIF', 'JPE', 'JPG', 'JPEG', 'MPG', 'MPEG', 'MSP', 'PCD', 'PXR', 'PBM', 'PGM', 'PPM', 'PNM', 'PSD', 'BW', 'RGB', 'RGBA', 'SGI', 'RAS', 'TGA', 'ICB', 'VDA', 'VST', 'WEBP', 'WMF', 'EMF', 'XBM', 'XPM', 'aiff', 'au', 'avr', 'caf', 'flac', 'htk', 'svx', 'mat4', 'mat5', 'mpc2k', 'ogg', 'paf', 'pvf', 'raw', 'rf64', 'sd2', 'sds', 'ircam', 'voc', 'w64', 'wav', 'nist', 'wavex', 'wve', 'xi', 'mp3', 'opus', 'AIFF', 'AU', 'AVR', 'CAF', 'FLAC', 'HTK', 'SVX', 'MAT4', 'MAT5', 'MPC2K', 'OGG', 'PAF', 'PVF', 'RAW', 'RF64', 'SD2', 'SDS', 'IRCAM', 'VOC', 'W64', 'WAV', 'NIST', 'WAVEX', 'WVE', 'XI', 'MP3', 'OPUS', 'zip']"
### Expected behavior
`the_pile` dataset should be dowloaded.
### Environment info
- `datasets` version: 2.7.1
- Platform: Linux-4.15.0-112-generic-x86_64-with-glibc2.27
- Python version: 3.10.8
- PyArrow version: 10.0.1
- Pandas version: 1.5.2 | 5,381 |
https://github.com/huggingface/datasets/issues/5380 | Improve dataset `.skip()` speed in streaming mode | [
"Hi! I agree `skip` can be inefficient to use in the current state.\r\n\r\nTo make it fast, we could use \"statistics\" stored in Parquet metadata and read only the chunks needed to form a dataset. \r\n\r\nAnd thanks to the \"datasets-server\" project, which aims to store the Parquet versions of the Hub datasets (only the smaller datasets are covered currently), this solution can also be applied to datasets stored in formats other than Parquet. (cc @severo)",
"@mariosasko do the current parquet files created by the datasets-server already have the required \"statistics\"? If not, please open an issue on https://github.com/huggingface/datasets-server with some details to make sure we implement it.",
"Yes, nothing has to be changed on the datasets-server side. What I mean by \"statistics\" is that we can use the \"row_group\" metadata embedded in a Parquet file (by default) to fetch the requested rows more efficiently.",
"Glad to see the feature could be of interest. \r\n\r\nI'm sure there are many possible ways to implement this feature. I don't know enough about the datasets-server, but I guess that it is not instantaneous, in the sense that user-owned private datasets might need hours or days until they are ported to the datasets-server (if at all), which could be cumbersome. Having optionally that information in the `dataset_infos.json` file would make it easier for users to control the skip process a bit.",
"re: statistics:\r\n\r\n- https://arrow.apache.org/docs/python/generated/pyarrow.parquet.FileMetaData.html\r\n- https://arrow.apache.org/docs/python/generated/pyarrow.parquet.RowGroupMetaData.html\r\n\r\n```python\r\n>>> import pyarrow.parquet as pq\r\n>>> import hffs\r\n>>> fs = hffs.HfFileSystem(\"glue\", repo_type=\"dataset\", revision=\"refs/convert/parquet\")\r\n>>> metadata = pq.read_metadata(\"ax/glue-test.parquet\", filesystem=fs)\r\n>>> metadata\r\n<pyarrow._parquet.FileMetaData object at 0x7f4537cec400>\r\n created_by: parquet-cpp-arrow version 7.0.0\r\n num_columns: 4\r\n num_rows: 1104\r\n num_row_groups: 2\r\n format_version: 1.0\r\n serialized_size: 2902\r\n>>> metadata.row_group(0)\r\n<pyarrow._parquet.RowGroupMetaData object at 0x7f45564bcbd0>\r\n num_columns: 4\r\n num_rows: 1000\r\n total_byte_size: 164474\r\n>>> metadata.row_group(1)\r\n<pyarrow._parquet.RowGroupMetaData object at 0x7f455005c400>\r\n num_columns: 4\r\n num_rows: 104\r\n total_byte_size: 13064\r\n```",
"> user-owned private datasets might need hours or days until they are ported to the datasets-server (if at all)\r\n\r\nprivate datasets are not supported yet (https://github.com/huggingface/datasets-server/issues/39)",
"@versae `Dataset.push_to_hub` writes shards in Parquet, so this solution would also work for such datasets (immediately after the push). ",
"@mariosasko that is right. However, there are still a good amount of datasets for which the shards are created manually. In our very specific case, we create medium-sized datasets (rarely over 100-200GB) of both text and audio, we prepare the shards by hand and then upload then. It would be great to have immediate access to this download skipping feature for them too.",
"From looking at Arrow's source, it seems Parquet stores metadata at the end, which means one needs to iterate over a Parquet file's data before accessing its metadata. We could mimic Dask to address this \"limitation\" and write metadata in a `_metadata`/`_common_metadata` file in `to_parquet`/`push_to_hub`, which we could then use to optimize reads (if present). Plus, it's handy that PyArrow can also parse these metadata files.",
"So if Parquet metadata needs to be in its own file anyway, why not implement this skipping feature by storing the example counts per shard in `dataset_infos.json`? That would allow:\r\n- Support both private and public datasets\r\n- Immediate access to the feature upon uploading of shards\r\n- Use any dataset, not only those uploaded using `.push_to_hub()`\r\n\r\nA proper Parquet metadata file could still be created and \"overwrite\" the `dataset_infos.json` info in the datasets-server."
] | ### Feature request
Add extra information to the `dataset_infos.json` file to include the number of samples/examples in each shard, for example in a new field `num_examples` alongside `num_bytes`. The `.skip()` function could use this information to ignore the download of a shard when in streaming mode, which AFAICT it should speed up the skipping process.
### Motivation
When resuming from a checkpoint after a crashed run, using `dataset.skip()` is very convenient to recover the exact state of the data and to not train again over the same examples (assuming same seed, no shuffling). However, I have noticed that for audio datasets in streaming mode this is very costly in terms of time, as shards need to be downloaded every time before skipping the right number of examples.
### Your contribution
I took a look already at the code, but it seems a change like this is way deeper than I am able to manage, as it touches the library in several parts. I could give it a try but might need some guidance on the internals. | 5,380 |
https://github.com/huggingface/datasets/issues/5378 | The dataset "the_pile", subset "enron_emails" , load_dataset() failure | [
"Thanks for reporting @shaoyuta. We are investigating it.\r\n\r\nWe are transferring the issue to \"the_pile\" Community tab on the Hub: https://huggingface.co/datasets/the_pile/discussions/4"
] | ### Describe the bug
When run
"datasets.load_dataset("the_pile","enron_emails")" failure

### Steps to reproduce the bug
Run below code in python cli:
>>> import datasets
>>> datasets.load_dataset("the_pile","enron_emails")
### Expected behavior
Load dataset "the_pile", "enron_emails" successfully.
### Environment info
Copy-and-paste the text below in your GitHub issue.
- `datasets` version: 2.7.1
- Platform: Linux-5.15.0-53-generic-x86_64-with-glibc2.35
- Python version: 3.10.6
- PyArrow version: 10.0.0
- Pandas version: 1.4.3
| 5,378 |
https://github.com/huggingface/datasets/issues/5374 | Using too many threads results in: Got disconnected from remote data host. Retrying in 5sec | [
"The data files are hosted on HF at https://huggingface.co/datasets/allenai/c4/tree/main\r\n\r\nYou have 200 runs streaming the same files in parallel. So this is probably a Hub limitation. Maybe rate limiting ? cc @julien-c \r\n\r\nMaybe you can also try to reduce the number of HTTP requests by increasing the block size of each request. This can be done by increasing `DEFAULT_BLOCK_SIZE` in `fsspec.implementations.http`. Default is `5 * 2**20` (5MiB)\r\n\r\nAnyway maybe it's just better to save the dataset locally in that case ?",
"you don't get an HTTP error code or something in your stack trace? Kinda hard to debug with this info",
"You could try to re-run using this `datasets` branch: [raise-err-when-disconnect](https://github.com/huggingface/datasets/compare/raise-err-when-disconnect?expand=1)\r\nIt should raise the fsspec error",
"The weird thing is that I already have it saved locally & it seems to indeed be using the cached one 🧐 ; I'm also using offline mode, so I don't think it has something to do with the Hub.\r\n```\r\nWARNING:datasets.load:Using the latest cached version of the module from /users/muennighoff/.cache/huggingface/modules/datasets_modules/datasets/c4/df532b158939272d032cc63ef19cd5b83e9b4d00c922b833e4cb18b2e9869b01 (last modified on Mon Dec 12 10:45:02 2022) since it couldn't be found locally at c4.\r\n```\r\n\r\n",
"No, you passed `streaming=True` so it streams the data from the Hub.\r\nThis message just shows that you use the cached version of the `c4` **module**, aka the python script that is run to generate the examples from the raw data files.\r\n\r\nMaybe the offline mode should also disable `fsspec`/`aiohttp` HTTP calls in `datasets` and not just the `requests` ones.",
"> This message just shows that you use the cached version of the c4 module\r\n\r\nAh my bad you're right about the module, but it's also using the downloaded & cached c4 dataset. There's no internet during the runs so it wouldn't work otherwise",
"You don't have internet, therefore you get an error while trying to stream ;)"
] | ### Describe the bug
`streaming_download_manager` seems to disconnect if too many runs access the same underlying dataset 🧐
The code works fine for me if I have ~100 runs in parallel, but disconnects once scaling to 200.
Possibly related:
- https://github.com/huggingface/datasets/pull/3100
- https://github.com/huggingface/datasets/pull/3050
### Steps to reproduce the bug
Running
```python
c4 = datasets.load_dataset("c4", "en", split="train", streaming=True).skip(args.start).take(args.end-args.start)
df = pd.DataFrame(c4, index=None)
```
with different start & end arguments on 200 CPUs in parallel yields:
```
WARNING:datasets.load:Using the latest cached version of the module from /users/muennighoff/.cache/huggingface/modules/datasets_modules/datasets/c4/df532b158939272d032cc63ef19cd5b83e9b4d00c922b833e4cb18b2e9869b01 (last modified on Mon Dec 12 10:45:02 2022) since it couldn't be found locally at c4.
WARNING:datasets.download.streaming_download_manager:Got disconnected from remote data host. Retrying in 5sec [1/20]
WARNING:datasets.download.streaming_download_manager:Got disconnected from remote data host. Retrying in 5sec [2/20]
WARNING:datasets.download.streaming_download_manager:Got disconnected from remote data host. Retrying in 5sec [3/20]
WARNING:datasets.download.streaming_download_manager:Got disconnected from remote data host. Retrying in 5sec [4/20]
WARNING:datasets.download.streaming_download_manager:Got disconnected from remote data host. Retrying in 5sec [5/20]
WARNING:datasets.download.streaming_download_manager:Got disconnected from remote data host. Retrying in 5sec [6/20]
WARNING:datasets.download.streaming_download_manager:Got disconnected from remote data host. Retrying in 5sec [7/20]
WARNING:datasets.download.streaming_download_manager:Got disconnected from remote data host. Retrying in 5sec [8/20]
WARNING:datasets.download.streaming_download_manager:Got disconnected from remote data host. Retrying in 5sec [9/20]
WARNING:datasets.download.streaming_download_manager:Got disconnected from remote data host. Retrying in 5sec [10/20]
WARNING:datasets.download.streaming_download_manager:Got disconnected from remote data host. Retrying in 5sec [11/20]
WARNING:datasets.download.streaming_download_manager:Got disconnected from remote data host. Retrying in 5sec [12/20]
WARNING:datasets.download.streaming_download_manager:Got disconnected from remote data host. Retrying in 5sec [13/20]
WARNING:datasets.download.streaming_download_manager:Got disconnected from remote data host. Retrying in 5sec [14/20]
WARNING:datasets.download.streaming_download_manager:Got disconnected from remote data host. Retrying in 5sec [15/20]
WARNING:datasets.download.streaming_download_manager:Got disconnected from remote data host. Retrying in 5sec [16/20]
WARNING:datasets.download.streaming_download_manager:Got disconnected from remote data host. Retrying in 5sec [17/20]
WARNING:datasets.download.streaming_download_manager:Got disconnected from remote data host. Retrying in 5sec [18/20]
WARNING:datasets.download.streaming_download_manager:Got disconnected from remote data host. Retrying in 5sec [19/20]
WARNING:datasets.download.streaming_download_manager:Got disconnected from remote data host. Retrying in 5sec [20/20]
╭───────────────────── Traceback (most recent call last) ──────────────────────╮
│ /pfs/lustrep4/scratch/project_462000119/muennighoff/dec-2022-tasky/inference │
│ _c4.py:68 in <module> │
│ │
│ 65 │ model.eval() │
│ 66 │ │
│ 67 │ c4 = datasets.load_dataset("c4", "en", split="train", streaming=Tru │
│ ❱ 68 │ df = pd.DataFrame(c4, index=None) │
│ 69 │ texts = df["text"].to_list() │
│ 70 │ preds = batch_inference(texts, batch_size=args.batch_size) │
│ 71 │
│ │
│ /opt/cray/pe/python/3.9.12.1/lib/python3.9/site-packages/pandas/core/frame.p │
│ y:684 in __init__ │
│ │
│ 681 │ │ # For data is list-like, or Iterable (will consume into list │
│ 682 │ │ elif is_list_like(data): │
│ 683 │ │ │ if not isinstance(data, (abc.Sequence, ExtensionArray)): │
│ ❱ 684 │ │ │ │ data = list(data) │
│ 685 │ │ │ if len(data) > 0: │
│ 686 │ │ │ │ if is_dataclass(data[0]): │
│ 687 │ │ │ │ │ data = dataclasses_to_dicts(data) │
│ │
│ /pfs/lustrep4/scratch/project_462000119/muennighoff/nov-2022-bettercom/venv/ │
│ lib/python3.9/site-packages/datasets/iterable_dataset.py:751 in __iter__ │
│ │
│ 748 │ │ yield from ex_iterable.shard_data_sources(shard_idx) │
│ 749 │ │
│ 750 │ def __iter__(self): │
│ ❱ 751 │ │ for key, example in self._iter(): │
│ 752 │ │ │ if self.features: │
│ 753 │ │ │ │ # `IterableDataset` automatically fills missing colum │
│ 754 │ │ │ │ # This is done with `_apply_feature_types`. │
│ │
│ /pfs/lustrep4/scratch/project_462000119/muennighoff/nov-2022-bettercom/venv/ │
│ lib/python3.9/site-packages/datasets/iterable_dataset.py:741 in _iter │
│ │
│ 738 │ │ │ ex_iterable = self._ex_iterable.shuffle_data_sources(self │
│ 739 │ │ else: │
│ 740 │ │ │ ex_iterable = self._ex_iterable │
│ ❱ 741 │ │ yield from ex_iterable │
│ 742 │ │
│ 743 │ def _iter_shard(self, shard_idx: int): │
│ 744 │ │ if self._shuffling: │
│ │
│ /pfs/lustrep4/scratch/project_462000119/muennighoff/nov-2022-bettercom/venv/ │
│ lib/python3.9/site-packages/datasets/iterable_dataset.py:617 in __iter__ │
│ │
│ 614 │ │ self.n = n │
│ 615 │ │
│ 616 │ def __iter__(self): │
│ ❱ 617 │ │ yield from islice(self.ex_iterable, self.n) │
│ 618 │ │
│ 619 │ def shuffle_data_sources(self, generator: np.random.Generator) -> │
│ 620 │ │ """Doesn't shuffle the wrapped examples iterable since it wou │
│ │
│ /pfs/lustrep4/scratch/project_462000119/muennighoff/nov-2022-bettercom/venv/ │
│ lib/python3.9/site-packages/datasets/iterable_dataset.py:594 in __iter__ │
│ │
│ 591 │ │
│ 592 │ def __iter__(self): │
│ 593 │ │ #ex_iterator = iter(self.ex_iterable) │
│ ❱ 594 │ │ yield from islice(self.ex_iterable, self.n, None) │
│ 595 │ │ #for _ in range(self.n): │
│ 596 │ │ # next(ex_iterator) │
│ 597 │ │ #yield from islice(ex_iterator, self.n, None) │
│ │
│ /pfs/lustrep4/scratch/project_462000119/muennighoff/nov-2022-bettercom/venv/ │
│ lib/python3.9/site-packages/datasets/iterable_dataset.py:106 in __iter__ │
│ │
│ 103 │ │ self.kwargs = kwargs │
│ 104 │ │
│ 105 │ def __iter__(self): │
│ ❱ 106 │ │ yield from self.generate_examples_fn(**self.kwargs) │
│ 107 │ │
│ 108 │ def shuffle_data_sources(self, generator: np.random.Generator) -> │
│ 109 │ │ return ShardShuffledExamplesIterable(self.generate_examples_f │
│ │
│ /users/muennighoff/.cache/huggingface/modules/datasets_modules/datasets/c4/d │
│ f532b158939272d032cc63ef19cd5b83e9b4d00c922b833e4cb18b2e9869b01/c4.py:89 in │
│ _generate_examples │
│ │
│ 86 │ │ for filepath in filepaths: │
│ 87 │ │ │ logger.info("generating examples from = %s", filepath) │
│ 88 │ │ │ with gzip.open(open(filepath, "rb"), "rt", encoding="utf-8" │
│ ❱ 89 │ │ │ │ for line in f: │
│ 90 │ │ │ │ │ if line: │
│ 91 │ │ │ │ │ │ example = json.loads(line) │
│ 92 │ │ │ │ │ │ yield id_, example │
│ │
│ /opt/cray/pe/python/3.9.12.1/lib/python3.9/gzip.py:313 in read1 │
│ │
│ 310 │ │ │
│ 311 │ │ if size < 0: │
│ 312 │ │ │ size = io.DEFAULT_BUFFER_SIZE │
│ ❱ 313 │ │ return self._buffer.read1(size) │
│ 314 │ │
│ 315 │ def peek(self, n): │
│ 316 │ │ self._check_not_closed() │
│ │
│ /opt/cray/pe/python/3.9.12.1/lib/python3.9/_compression.py:68 in readinto │
│ │
│ 65 │ │
│ 66 │ def readinto(self, b): │
│ 67 │ │ with memoryview(b) as view, view.cast("B") as byte_view: │
│ ❱ 68 │ │ │ data = self.read(len(byte_view)) │
│ 69 │ │ │ byte_view[:len(data)] = data │
│ 70 │ │ return len(data) │
│ 71 │
│ │
│ /opt/cray/pe/python/3.9.12.1/lib/python3.9/gzip.py:493 in read │
│ │
│ 490 │ │ │ │ self._new_member = False │
│ 491 │ │ │ │
│ 492 │ │ │ # Read a chunk of data from the file │
│ ❱ 493 │ │ │ buf = self._fp.read(io.DEFAULT_BUFFER_SIZE) │
│ 494 │ │ │ │
│ 495 │ │ │ uncompress = self._decompressor.decompress(buf, size) │
│ 496 │ │ │ if self._decompressor.unconsumed_tail != b"": │
│ │
│ /opt/cray/pe/python/3.9.12.1/lib/python3.9/gzip.py:96 in read │
│ │
│ 93 │ │ │ read = self._read │
│ 94 │ │ │ self._read = None │
│ 95 │ │ │ return self._buffer[read:] + \ │
│ ❱ 96 │ │ │ │ self.file.read(size-self._length+read) │
│ 97 │ │
│ 98 │ def prepend(self, prepend=b''): │
│ 99 │ │ if self._read is None: │
│ │
│ /pfs/lustrep4/scratch/project_462000119/muennighoff/nov-2022-bettercom/venv/ │
│ lib/python3.9/site-packages/datasets/download/streaming_download_manager.py: │
│ 365 in read_with_retries │
│ │
│ 362 │ │ │ │ ) │
│ 363 │ │ │ │ time.sleep(config.STREAMING_READ_RETRY_INTERVAL) │
│ 364 │ │ else: │
│ ❱ 365 │ │ │ raise ConnectionError("Server Disconnected") │
│ 366 │ │ return out │
│ 367 │ │
│ 368 │ file_obj.read = read_with_retries │
╰──────────────────────────────────────────────────────────────────────────────╯
ConnectionError: Server Disconnected
```
### Expected behavior
There should be no disconnect I think.
### Environment info
```
datasets=2.7.0
Python 3.9.12
``` | 5,374 |
https://github.com/huggingface/datasets/issues/5371 | Add a robustness benchmark dataset for vision | [
"Ccing @nazneenrajani @lvwerra @osanseviero "
] | ### Name
ImageNet-C
### Paper
Benchmarking Neural Network Robustness to Common Corruptions and Perturbations
### Data
https://github.com/hendrycks/robustness
### Motivation
It's a known fact that vision models are brittle when they meet with slightly corrupted and perturbed data. This is also correlated to the robustness aspects of vision models.
Researchers use different benchmark datasets to evaluate the robustness aspects of vision models. ImageNet-C is one of them.
Having this dataset in 🤗 Datasets would allow researchers to evaluate and study the robustness aspects of vision models. Since the metric associated with these evaluations is top-1 accuracy, researchers should be able to easily take advantage of the evaluation benchmarks on the Hub and perform comprehensive reporting.
ImageNet-C is a large dataset. Once it's in, it can act as a reference and we can also reach out to the authors of the other robustness benchmark datasets in vision, such as ObjectNet, WILDS, Metashift, etc. These datasets cater to different aspects. For example, ObjectNet is related to assessing how well a model performs under sub-population shifts.
Related thread: https://huggingface.slack.com/archives/C036H4A5U8Z/p1669994598060499 | 5,371 |
https://github.com/huggingface/datasets/issues/5363 | Dataset.from_generator() crashes on simple example | [] | null | 5,363 |
https://github.com/huggingface/datasets/issues/5362 | Run 'GPT-J' failure due to download dataset fail (' ConnectionError: Couldn't reach http://eaidata.bmk.sh/data/enron_emails.jsonl.zst ' ) | [
"Thanks for reporting, @shaoyuta.\r\n\r\nWe have checked and yes, apparently there is an issue with the server hosting the data of the \"enron_emails\" subset of \"the_pile\" dataset: http://eaidata.bmk.sh/data/enron_emails.jsonl.zst\r\nIt seems to be down: The connection has timed out.\r\n\r\nPlease note that at the Hugging Face Hub, we are not hosting their data for this dataset, but only a script that downloads the data from their servers. We are updating the data URL to one in another server.\r\n\r\nIn the meantime, please note that you can train your model in the entire \"the_pile\" dataset, by passing the \"all\" config (instead of the \"enron_emails\" one).",
"We have transferred this issue to the corresponding dataset Community tab: https://huggingface.co/datasets/the_pile/discussions/2\r\n\r\nPlease, follow the updates there."
] | ### Describe the bug
Run model "GPT-J" with dataset "the_pile" fail.
The fail out is as below:
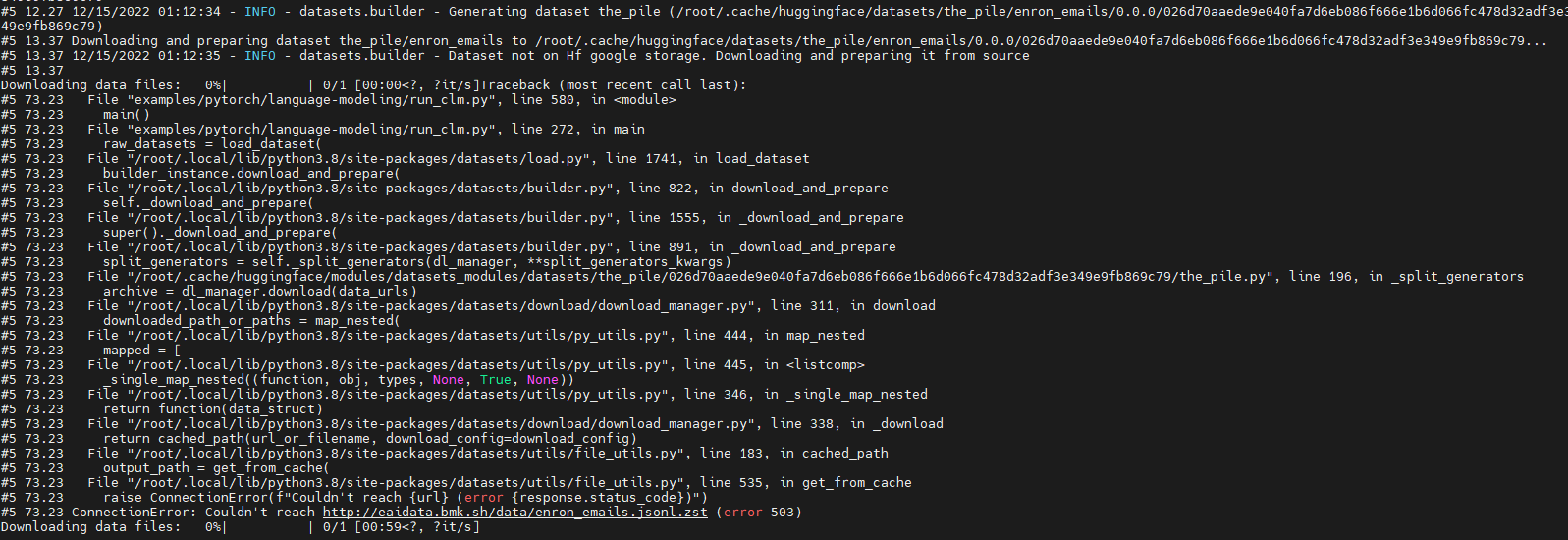
Looks like which is due to "http://eaidata.bmk.sh/data/enron_emails.jsonl.zst" unreachable .
### Steps to reproduce the bug
Steps to reproduce this issue:
git clone https://github.com/huggingface/transformers
cd transformers
python examples/pytorch/language-modeling/run_clm.py --model_name_or_path EleutherAI/gpt-j-6B --dataset_name the_pile --dataset_config_name enron_emails --do_eval --output_dir /tmp/output --overwrite_output_dir
### Expected behavior
This issue looks like due to "http://eaidata.bmk.sh/data/enron_emails.jsonl.zst " couldn't be reached.
Is there another way to download the dataset "the_pile" ?
Is there another way to cache the dataset "the_pile" but not let the hg to download it when runtime ?
### Environment info
huggingface_hub version: 0.11.1
Platform: Linux-5.15.0-52-generic-x86_64-with-glibc2.35
Python version: 3.9.12
Running in iPython ?: No
Running in notebook ?: No
Running in Google Colab ?: No
Token path ?: /home/taosy/.huggingface/token
Has saved token ?: False
Configured git credential helpers:
FastAI: N/A
Tensorflow: N/A
Torch: N/A
Jinja2: N/A
Graphviz: N/A
Pydot: N/A | 5,362 |
https://github.com/huggingface/datasets/issues/5361 | How concatenate `Audio` elements using batch mapping | [
"You can try something like this ?\r\n```python\r\ndef mapper_function(batch):\r\n return {\"concatenated_audio\": [np.concatenate([audio[\"array\"] for audio in batch[\"audio\"]])]}\r\n\r\ndataset = dataset.map(\r\n mapper_function,\r\n batched=True,\r\n batch_size=3,\r\n remove_columns=list(dataset.features),\r\n)\r\n```",
"Thanks for the snippet!\r\n\r\nOne more question. I wonder why those two mappers are working so different that one taking 4 sec while other taking over 1 min :\r\n\r\n```python\r\n%%time\r\ndef mapper_function1(batch):\r\n # list_audio\r\n return {\r\n \"audio\": [\r\n {\r\n \"array\": np.concatenate([audio[\"array\"] for audio in batch[\"audio\"]]),\r\n \"sampling_rate\": 16_000,\r\n }\r\n ]\r\n }\r\n\r\ndataset.map(\r\n mapper_function1,\r\n batched=True,\r\n batch_size=3,\r\n remove_columns=list(dataset.features),\r\n)\r\n\r\n# 100%\r\n# 135/135 [01:13<00:00, 1.93ba/s]\r\n# CPU times: user 1min 10s, sys: 3.21 s, total: 1min 13s\r\n# Wall time: 1min 13s\r\n# Dataset({\r\n# features: ['audio'],\r\n# num_rows: 135\r\n# })\r\n\r\n# --------------------------------\r\n%%time\r\ndef mapper_function2(batch):\r\n # list_audio\r\n return {\"audio\": [np.concatenate([audio[\"array\"] for audio in batch[\"audio\"]])]}\r\n\r\ndataset.map(\r\n mapper_function2,\r\n batched=True,\r\n batch_size=3,\r\n remove_columns=list(dataset.features),\r\n)\r\n\r\n# 100%\r\n# 135/135 [00:03<00:00, 40.69ba/s]\r\n# CPU times: user 1.88 s, sys: 1.48 s, total: 3.36 s\r\n# Wall time: 4.8 s\r\n# Dataset({\r\n# features: ['audio'],\r\n# num_rows: 135\r\n# })\r\n```\r\n",
"In the first one you get a dataset with an Audio type, and in the second one you get a dataset with a sequence of floats type.\r\n\r\nThe Audio type encodes the data as WAV to save disk space, so it takes more time to create.\r\nThe Audio type is automatically inferred because you modify the column \"audio\" which was already an Audio type. If you name it to something else, type inference will use a type struct with array and sampling rate fields."
] | ### Describe the bug
I am trying to do concatenate audios in a dataset e.g. `google/fleurs`.
```python
print(dataset)
# Dataset({
# features: ['path', 'audio'],
# num_rows: 24
# })
def mapper_function(batch):
# to merge every 3 audio
# np.concatnate(audios[i: i+3]) for i in range(i, len(batch), 3)
dataset = dataset.map(mapper_function, batch=True, batch_size=24)
print(dataset)
# Expected output:
# Dataset({
# features: ['path', 'audio'],
# num_rows: 8
# })
```
I tried to construct `result={}` dictionary inside the mapper function, I just found it will not work because it needs `byte` also needed :((
I'd appreciate if your share any use cases similar to my problem or any solutions really. Thanks!
cc: @lhoestq
### Steps to reproduce the bug
1. load audio dataset
2. try to merge every k audios and return as one
### Expected behavior
Merged dataset with a fewer rows. If we merge every 3 rows, then `n // 3` number of examples.
### Environment info
- `datasets` version: 2.1.0
- Platform: Linux-5.15.65+-x86_64-with-debian-bullseye-sid
- Python version: 3.7.12
- PyArrow version: 8.0.0
- Pandas version: 1.3.5 | 5,361 |
https://github.com/huggingface/datasets/issues/5360 | IterableDataset returns duplicated data using PyTorch DDP | [
"If you use huggingface trainer, you will find the trainer has wrapped a `IterableDatasetShard` to avoid duplication.\r\nSee:\r\nhttps://github.com/huggingface/transformers/blob/dfd818420dcbad68e05a502495cf666d338b2bfb/src/transformers/trainer.py#L835\r\n",
"If you want to support it by datasets natively, maybe we also need to change the code in `transformers` ?",
"Opened https://github.com/huggingface/transformers/issues/20770 to discuss this :)",
"Maybe something like this then ?\r\n```python\r\nfrom datasets.distributed import split_dataset_by_node\r\nds = split_dataset_by_node(ds, rank=rank, world_size=world_size)\r\n```\r\n\r\nFor map-style datasets the implementation is trivial (it can simply use `.shard()`).\r\n\r\nFor iterable datasets we would need to implement a new ExamplesIterable that would only iterate on a subset of the (possibly shuffled and re-shuffled after each epoch) list of shards, based on the rank and world size.",
"My plan is to skip examples by default to not end up with duplicates.\r\n\r\nAnd if a dataset has a number of shards that is a factor of the world size, then I'd make it more optimized by distributing the shards evenly across nodes instead.",
"Opened a PR here: https://github.com/huggingface/datasets/pull/5369\r\n\r\nfeel free to play with it and share your feedbacks :)",
"@lhoestq I add shuffle after split_dataset_by_node, duplicated data still exist. \r\nFor example, we have a directory named `mock_pretraining_data`, which has three files, `part-00000`, `part-00002`,`part-00002`. \r\nText in `part-00000` is like this: \r\n{\"id\": 0}\r\n{\"id\": 1}\r\n{\"id\": 2}\r\n{\"id\": 3}\r\n{\"id\": 4}\r\n{\"id\": 5}\r\n{\"id\": 6}\r\n{\"id\": 7}\r\n{\"id\": 8}\r\n{\"id\": 9}\r\n\r\nand `part-00001`\r\n{\"id\": 10}\r\n{\"id\": 11}\r\n{\"id\": 12}\r\n{\"id\": 13}\r\n{\"id\": 14}\r\n{\"id\": 15}\r\n{\"id\": 16}\r\n{\"id\": 17}\r\n{\"id\": 18}\r\n{\"id\": 19}\r\n\r\nand `part-00002`\r\n{\"id\": 20}\r\n{\"id\": 21}\r\n{\"id\": 22}\r\n{\"id\": 23}\r\n{\"id\": 24}\r\n{\"id\": 25}\r\n{\"id\": 26}\r\n{\"id\": 27}\r\n{\"id\": 28}\r\n{\"id\": 29}\r\n\r\nAnd code in `test_dist.py` like this,\r\n```python\r\nimport torch\r\nfrom torch.utils.data import Dataset, DataLoader\r\nfrom datasets import load_dataset\r\nimport os\r\nfrom transformers import AutoTokenizer, NezhaForPreTraining\r\nfrom transformers import AdamW, get_linear_schedule_with_warmup\r\nimport torch.nn.functional as F\r\nimport torch.nn as nn\r\nimport torch.distributed as dist\r\nfrom datasets.distributed import split_dataset_by_node\r\nfrom torch.nn.parallel import DistributedDataParallel as DDP\r\n\r\nos.environ[\"CUDA_VISIBLE_DEVICES\"] = '5,6,7'\r\n\r\ndist.init_process_group(\"nccl\")\r\nlocal_rank = int(os.environ['LOCAL_RANK'])\r\nworld_size = torch.distributed.get_world_size()\r\ndevice = torch.device('cuda', local_rank)\r\ndata_dir = './'\r\n\r\ndef load_trainset(train_path):\r\n dataset = load_dataset('json', data_dir=os.path.join(data_dir, train_path), split='train', streaming=True)\r\n return dataset\r\n\r\ndef collate_fn(examples):\r\n input_ids = []\r\n for example in examples:\r\n input_ids.append(example['id'])\r\n return torch.LongTensor(input_ids).to(device)\r\n\r\n\r\ndataset = load_trainset('mock_pretraining_data')\r\ndataset = split_dataset_by_node(dataset, rank=local_rank, world_size=world_size).shuffle(buffer_size=512)\r\n# train_sampler = torch.utils.data.distributed.DistributedSampler(dataset)\r\nbatch_size = 3\r\nprint('batch_size: {}'.format(batch_size))\r\ntrain_dataloader = DataLoader(dataset, batch_size=batch_size, collate_fn=collate_fn)\r\n\r\nfor x in train_dataloader:\r\n print({'rank': local_rank, 'id': x})\r\n```\r\nrun `python -m torch.distributed.launch --nproc_per_node=3 test_dist.py`\r\nThe output is\r\n```\r\n{'rank': 1, 'id': tensor([12, 15, 14], device='cuda:1')}\r\n{'rank': 1, 'id': tensor([16, 10, 18], device='cuda:1')}\r\n{'rank': 1, 'id': tensor([17, 13, 19], device='cuda:1')}\r\n{'rank': 1, 'id': tensor([11], device='cuda:1')}\r\n{'rank': 0, 'id': tensor([0, 2, 9], device='cuda:0')}\r\n{'rank': 0, 'id': tensor([4, 8, 1], device='cuda:0')}\r\n{'rank': 0, 'id': tensor([5, 3, 6], device='cuda:0')}\r\n{'rank': 0, 'id': tensor([7], device='cuda:0')}\r\n{'rank': 2, 'id': tensor([13, 15, 14], device='cuda:2')}\r\n{'rank': 2, 'id': tensor([19, 17, 18], device='cuda:2')}\r\n{'rank': 2, 'id': tensor([12, 16, 11], device='cuda:2')}\r\n{'rank': 2, 'id': tensor([10], device='cuda:2')}\r\n```\r\n`part-00001` is loaded twice, `part-00002` isn't loaded.\r\n\r\nIf I run `python -m torch.distributed.launch --nproc_per_node=2 test_dist.py`\r\nThe output is weirder,many numbers appear twice\r\n```\r\n{'rank': 1, 'id': tensor([26, 8, 13], device='cuda:1')}\r\n{'rank': 1, 'id': tensor([22, 19, 20], device='cuda:1')}\r\n{'rank': 1, 'id': tensor([12, 28, 11], device='cuda:1')}\r\n{'rank': 1, 'id': tensor([24, 2, 14], device='cuda:1')}\r\n{'rank': 1, 'id': tensor([ 6, 27, 3], device='cuda:1')}\r\n{'rank': 0, 'id': tensor([ 8, 25, 1], device='cuda:0')}\r\n{'rank': 0, 'id': tensor([20, 4, 12], device='cuda:0')}\r\n{'rank': 0, 'id': tensor([14, 29, 5], device='cuda:0')}\r\n{'rank': 0, 'id': tensor([ 7, 18, 23], device='cuda:0')}\r\n{'rank': 0, 'id': tensor([19, 17, 11], device='cuda:0')}\r\n``` ",
"Hi ! Thanks for reporting, you need to pass `seed=` to `shuffle()` or the processes won't use the same seed to shuffle the shards order before assigning each shard to a node.\r\n\r\nThe issue is that the workers are not using the same seed to shuffle the shards before splitting the shards list by node.",
"Opened https://github.com/huggingface/datasets/issues/5696",
"I have the same issue\r\n```\r\nds['train'] = load_dataset(streaming=True)\r\nds['train'] = split_dataset_by_node(ds['train'], rank=int(os.environ[\"RANK\"]), world_size=int(os.environ[\"WORLD_SIZE\"]))\r\nvectorized_datasets = ds.map(\r\n prepare_dataset,\r\n remove_columns=raw_datasets_features,\r\n).with_format(\"torch\")\r\n\r\nvectorized_datasets[\"train\"] = vectorized_datasets[\"train\"].shuffle(\r\n buffer_size=500,\r\n seed=42,\r\n)\r\n\r\ndef prepare_dataset(batch):\r\n ....\r\n print(f\"sentence: {batch['sentence']}, target_text: {batch['target_text']}\")\r\n return batch\r\n```\r\nWhen using split_dataset_by_node(), the data being read is indeed different for each GPU ID.\r\n\r\n```\r\ntrainer = Trainer(\r\n model=model,\r\n data_collator=data_collator,\r\n args=training_args,\r\n compute_metrics=compute_metrics,\r\n train_dataset=vectorized_datasets[\"train\"] if training_args.do_train else None,\r\n eval_dataset=vectorized_datasets[\"eval\"] if training_args.do_eval else None,\r\n tokenizer=processor,\r\n callbacks=[ShuffleCallback()],\r\n )\r\n...\r\ntrain_result = trainer.train(resume_from_checkpoint=checkpoint)\r\n```\r\nHowever, when I execute trainer.train(), the data being read is different from what I expected.\r\nBecause I print the batch value in prepare_dataset() , I observe that the data is the same for each GPU ID.\r\n\r\nHow should I handle this issue?\r\n\r\n\r\n",
"There are two ways an iterable dataset can be split by node:\r\n1. if the number of shards is a factor of number of GPUs: in that case the shards are evenly distributed per GPU\r\n2. otherwise, each GPU iterate on the data and at the end keeps 1 sample out of n(GPUs) - skipping the others.\r\n\r\nIn case 2. it's therefore possible to have the same examples passed to `prepare_dataset` for each GPU.\r\n\r\nThis doesn't sound optimized though, because it runs the preprocessing on samples that won't be used in the end.\r\n\r\nCould you open a new issue so that we can discuss about this and find a solution ?"
] | As mentioned in https://github.com/huggingface/datasets/issues/3423, when using PyTorch DDP the dataset ends up with duplicated data. We already check for the PyTorch `worker_info` for single node, but we should also check for `torch.distributed.get_world_size()` and `torch.distributed.get_rank()` | 5,360 |
https://github.com/huggingface/datasets/issues/5354 | Consider using "Sequence" instead of "List" | [
"Hi! Linking a comment to provide more info on the issue: https://stackoverflow.com/a/39458225. This means we should replace all (most of) the occurrences of `List` with `Sequence` in function signatures.\r\n\r\n@tranhd95 Would you be interested in submitting a PR?",
"Hi all! I tried to reproduce this issue and didn't work for me. Also in your example i noticed that the variables have different names: `list_of_filenames` and `list_of_files`, could this be related to that?\r\n```python\r\n#I found random data in parquet format:\r\n!wget \"https://github.com/Teradata/kylo/raw/master/samples/sample-data/parquet/userdata1.parquet\"\r\n!wget \"https://github.com/Teradata/kylo/raw/master/samples/sample-data/parquet/userdata2.parquet\"\r\n\r\n#Then i try reproduce\r\nlist_of_files = [\"userdata1.parquet\", \"userdata2.parquet\"]\r\nds = Dataset.from_parquet(list_of_files)\r\n```\r\n**My output:**\r\n```python\r\nWARNING:datasets.builder:Using custom data configuration default-e287d097dc54e046\r\nDownloading and preparing dataset parquet/default to /root/.cache/huggingface/datasets/parquet/default-e287d097dc54e046/0.0.0/2a3b91fbd88a2c90d1dbbb32b460cf621d31bd5b05b934492fdef7d8d6f236ec...\r\nDownloading data files: 100%\r\n1/1 [00:00<00:00, 40.38it/s]\r\nExtracting data files: 100%\r\n1/1 [00:00<00:00, 23.43it/s]\r\nDataset parquet downloaded and prepared to /root/.cache/huggingface/datasets/parquet/default-e287d097dc54e046/0.0.0/2a3b91fbd88a2c90d1dbbb32b460cf621d31bd5b05b934492fdef7d8d6f236ec. Subsequent calls will reuse this data.\r\n```\r\nP.S. This is my first experience with open source. So do not judge strictly if I do not understand something)",
"@dantema There is indeed a typo in variable names. Nevertheless, I'm sorry if I was not clear but the output is from `mypy` type checker. You can run the code snippet without issues. The problem is with the type checking.",
"However, I found out that the type annotation is actually misleading. The [`from_parquet`](https://github.com/huggingface/datasets/blob/5ef1ab1cc06c2b7a574bf2df454cd9fcb071ccb2/src/datasets/arrow_dataset.py#L1039) method should also accept list of [`PathLike`](https://github.com/huggingface/datasets/blob/main/src/datasets/utils/typing.py#L8) objects which includes [`os.PathLike`](https://docs.python.org/3/library/os.html#os.PathLike). But if I would ran the code snippet below, an exception is thrown.\r\n\r\n**Code**\r\n```py\r\nfrom pathlib import Path\r\n\r\nlist_of_filenames = [Path(\"foo.parquet\"), Path(\"bar.parquet\")]\r\nds = Dataset.from_parquet(list_of_filenames)\r\n```\r\n**Output**\r\n```py\r\n[/usr/local/lib/python3.8/dist-packages/datasets/arrow_dataset.py](https://localhost:8080/#) in from_parquet(path_or_paths, split, features, cache_dir, keep_in_memory, columns, **kwargs)\r\n 1071 from .io.parquet import ParquetDatasetReader\r\n 1072 \r\n-> 1073 return ParquetDatasetReader(\r\n 1074 path_or_paths,\r\n 1075 split=split,\r\n\r\n[/usr/local/lib/python3.8/dist-packages/datasets/io/parquet.py](https://localhost:8080/#) in __init__(self, path_or_paths, split, features, cache_dir, keep_in_memory, streaming, **kwargs)\r\n 35 path_or_paths = path_or_paths if isinstance(path_or_paths, dict) else {self.split: path_or_paths}\r\n 36 hash = _PACKAGED_DATASETS_MODULES[\"parquet\"][1]\r\n---> 37 self.builder = Parquet(\r\n 38 cache_dir=cache_dir,\r\n 39 data_files=path_or_paths,\r\n\r\n[/usr/local/lib/python3.8/dist-packages/datasets/builder.py](https://localhost:8080/#) in __init__(self, cache_dir, config_name, hash, base_path, info, features, use_auth_token, repo_id, data_files, data_dir, name, **config_kwargs)\r\n 298 \r\n 299 if data_files is not None and not isinstance(data_files, DataFilesDict):\r\n--> 300 data_files = DataFilesDict.from_local_or_remote(\r\n 301 sanitize_patterns(data_files), base_path=base_path, use_auth_token=use_auth_token\r\n 302 )\r\n\r\n[/usr/local/lib/python3.8/dist-packages/datasets/data_files.py](https://localhost:8080/#) in from_local_or_remote(cls, patterns, base_path, allowed_extensions, use_auth_token)\r\n 794 for key, patterns_for_key in patterns.items():\r\n 795 out[key] = (\r\n--> 796 DataFilesList.from_local_or_remote(\r\n 797 patterns_for_key,\r\n 798 base_path=base_path,\r\n\r\n[/usr/local/lib/python3.8/dist-packages/datasets/data_files.py](https://localhost:8080/#) in from_local_or_remote(cls, patterns, base_path, allowed_extensions, use_auth_token)\r\n 762 ) -> \"DataFilesList\":\r\n 763 base_path = base_path if base_path is not None else str(Path().resolve())\r\n--> 764 data_files = resolve_patterns_locally_or_by_urls(base_path, patterns, allowed_extensions)\r\n 765 origin_metadata = _get_origin_metadata_locally_or_by_urls(data_files, use_auth_token=use_auth_token)\r\n 766 return cls(data_files, origin_metadata)\r\n\r\n[/usr/local/lib/python3.8/dist-packages/datasets/data_files.py](https://localhost:8080/#) in resolve_patterns_locally_or_by_urls(base_path, patterns, allowed_extensions)\r\n 357 data_files = []\r\n 358 for pattern in patterns:\r\n--> 359 if is_remote_url(pattern):\r\n 360 data_files.append(Url(pattern))\r\n 361 else:\r\n\r\n[/usr/local/lib/python3.8/dist-packages/datasets/utils/file_utils.py](https://localhost:8080/#) in is_remote_url(url_or_filename)\r\n 62 \r\n 63 def is_remote_url(url_or_filename: str) -> bool:\r\n---> 64 parsed = urlparse(url_or_filename)\r\n 65 return parsed.scheme in (\"http\", \"https\", \"s3\", \"gs\", \"hdfs\", \"ftp\")\r\n 66 \r\n\r\n[/usr/lib/python3.8/urllib/parse.py](https://localhost:8080/#) in urlparse(url, scheme, allow_fragments)\r\n 373 Note that we don't break the components up in smaller bits\r\n 374 (e.g. netloc is a single string) and we don't expand % escapes.\"\"\"\r\n--> 375 url, scheme, _coerce_result = _coerce_args(url, scheme)\r\n 376 splitresult = urlsplit(url, scheme, allow_fragments)\r\n 377 scheme, netloc, url, query, fragment = splitresult\r\n\r\n[/usr/lib/python3.8/urllib/parse.py](https://localhost:8080/#) in _coerce_args(*args)\r\n 125 if str_input:\r\n 126 return args + (_noop,)\r\n--> 127 return _decode_args(args) + (_encode_result,)\r\n 128 \r\n 129 # Result objects are more helpful than simple tuples\r\n\r\n[/usr/lib/python3.8/urllib/parse.py](https://localhost:8080/#) in _decode_args(args, encoding, errors)\r\n 109 def _decode_args(args, encoding=_implicit_encoding,\r\n 110 errors=_implicit_errors):\r\n--> 111 return tuple(x.decode(encoding, errors) if x else '' for x in args)\r\n 112 \r\n 113 def _coerce_args(*args):\r\n\r\n[/usr/lib/python3.8/urllib/parse.py](https://localhost:8080/#) in <genexpr>(.0)\r\n 109 def _decode_args(args, encoding=_implicit_encoding,\r\n 110 errors=_implicit_errors):\r\n--> 111 return tuple(x.decode(encoding, errors) if x else '' for x in args)\r\n 112 \r\n 113 def _coerce_args(*args):\r\n\r\nAttributeError: 'PosixPath' object has no attribute 'decode'\r\n```\r\n\r\n@mariosasko Should I create a new issue? ",
"@mariosasko I would like to take this issue up. ",
"@avinashsai Hi, I've assigned you the issue.\r\n\r\n@tranhd95 Yes, feel free to report this in a new issue.",
"@avinashsai Are you still working on this? If not I would like to give it a try.",
"@mariosasko I would like to take this issue up!",
"Hi @tranhd95 @mariosasko ,I hope you all are doing well.\r\n\r\nI am interested in this issue, is this still open and unresolved ?\r\n\r\nThanks and Regards"
] | ### Feature request
Hi, please consider using `Sequence` type annotation instead of `List` in function arguments such as in [`Dataset.from_parquet()`](https://github.com/huggingface/datasets/blob/main/src/datasets/arrow_dataset.py#L1088). It leads to type checking errors, see below.
**How to reproduce**
```py
list_of_filenames = ["foo.parquet", "bar.parquet"]
ds = Dataset.from_parquet(list_of_filenames)
```
**Expected mypy output:**
```
Success: no issues found
```
**Actual mypy output:**
```py
test.py:19: error: Argument 1 to "from_parquet" of "Dataset" has incompatible type "List[str]"; expected "Union[Union[str, bytes, PathLike[Any]], List[Union[str, bytes, PathLike[Any]]]]" [arg-type]
test.py:19: note: "List" is invariant -- see https://mypy.readthedocs.io/en/stable/common_issues.html#variance
test.py:19: note: Consider using "Sequence" instead, which is covariant
```
**Env:** mypy 0.991, Python 3.10.0, datasets 2.7.1 | 5,354 |
https://github.com/huggingface/datasets/issues/5353 | Support remote file systems for `Audio` | [
"Just seen https://github.com/huggingface/datasets/issues/5281"
] | ### Feature request
Hi there!
It would be super cool if `Audio()`, and potentially other features, could read files from a remote file system.
### Motivation
Large amounts of data is often stored in buckets. `load_from_disk` is able to retrieve data from cloud storage but to my knowledge actually copies the datasets across first, so if you're working off a system with smaller disk specs (like a VM), you can run out of space very quickly.
### Your contribution
Something like this (for Google Cloud Platform in this instance):
```python
from datasets import Dataset, Audio
import gcsfs
fs = gcsfs.GCSFileSystem()
list_of_audio_fp = {'audio': ['1', '2', '3']}
ds = Dataset.from_dict(list_of_audio_fp)
ds = ds.cast_column("audio", Audio(sampling_rate=16000, fs=fs))
```
Under the hood:
```python
import librosa
from io import BytesIO
def load_audio(fp, sampling_rate=None, fs=None):
if fs is not None:
with fs.open(fp, 'rb') as f:
arr, sr = librosa.load(BytesIO(f), sr=sampling_rate)
else:
# Perform existing io operations
```
Written from memory so some things could be wrong. | 5,353 |
https://github.com/huggingface/datasets/issues/5352 | __init__() got an unexpected keyword argument 'input_size' | [
"Hi @J-shel, thanks for reporting.\r\n\r\nI think the issue comes from your call to `load_dataset`. As first argument, you should pass:\r\n- either the name of your dataset (\"mrf\") if this is already published on the Hub\r\n- or the path to the loading script of your dataset (\"path/to/your/local/mrf.py\").",
"Hi, following your suggestion, I changed my call to load_dataset. Below is the latest:\r\nreader = load_dataset('data/mrf.py',\"default\", input_size=1024, split=split, streaming=True, keep_in_memory=None)\r\nHowever, I still got the same error.\r\nI have one question that is if I only define input_size=2048 in BUILDER_CONFIGS, may I specify input_size=1024 when loading the dataset? Cause I found that I could only specify name=\"default\" since I only define name=\"default\" in BUILDER_CONFIGS."
] | ### Describe the bug
I try to define a custom configuration with a input_size attribute following the instructions by "Specifying several dataset configurations" in https://huggingface.co/docs/datasets/v1.2.1/add_dataset.html
But when I load the dataset, I got an error "__init__() got an unexpected keyword argument 'input_size'"
### Steps to reproduce the bug
Following is the code to define the dataset:
class CsvConfig(datasets.BuilderConfig):
"""BuilderConfig for CSV."""
input_size: int = 2048
class MRF(datasets.ArrowBasedBuilder):
"""Archival MRF data"""
BUILDER_CONFIG_CLASS = CsvConfig
VERSION = datasets.Version("1.0.0")
BUILDER_CONFIGS = [
CsvConfig(name="default", version=VERSION, description="MRF data", input_size=2048),
]
...
def _generate_examples(self):
input_size = self.config.input_size
if input_size > 1000:
numin = 10000
else:
numin = 15000
Below is the code to load the dataset:
reader = load_dataset("default", input_size=1024)
### Expected behavior
I hope to pass the "input_size" parameter to MRF datasets, and change "input_size" to any value when loading the datasets.
### Environment info
- `datasets` version: 2.5.1
- Platform: Linux-4.18.0-305.3.1.el8.x86_64-x86_64-with-glibc2.31
- Python version: 3.9.12
- PyArrow version: 9.0.0
- Pandas version: 1.5.0 | 5,352 |
https://github.com/huggingface/datasets/issues/5351 | Do we need to implement `_prepare_split`? | [
"Hi! `DatasetBuilder` is a parent class for concrete builders: `GeneratorBasedBuilder`, `ArrowBasedBuilder` and `BeamBasedBuilder`. When writing a builder script, these classes are the ones you should inherit from. And since all of them implement `_prepare_split`, you only have to implement the three methods mentioned above.",
"Thanks so much @mariosasko for the fast response! I've been referencing [this page in the docs](https://huggingface.co/docs/datasets/v2.4.0/en/about_dataset_load) because it it pretty comprehensive in terms of what we have to do and I figured since we subclass the `BuilderConfig` the same pattern would hold, but I've also seen the page with those sub-classed builders as well, so that fills in a knowledge gap for me.",
"cc @stevhliu who may have some ideas on how to improve this part of the docs.",
"one more question for my understanding @mariosasko. the requirement of a loading script has always seemed counterintuitive to me. if i have to provide a script with every dataset, what is the point of using `datasets` if we're doing all the work of loading it, I can just do that in my code and skip the datasets integration (this of course discounts other potential benefits around metadata management, etc., my example is just simplest use case though for the sake of discussion).\r\n\r\nso i figured I would implement my own `BuilderConfig` and `DatasetBuilder` to handle that portion of it and not have to make a script. i _thought_ this would result in `datasets` (via `download_and_prepare`) then making me something that I could load using `load_dataset` moving forward.\r\n\r\nConcretely, i envisioned this pattern being possible:\r\n\r\n ```\r\nclass MyBuilderConfig(BuilderConfig):\r\n def __init__(self, name=\"my_named_dataset\", ...):\r\n super().__init__(name, ...)\r\n\r\nclass MyDatasetBuilder(GeneratorBasedBuilder):\r\n BUILDER_CONFIG_CLASS = MyBuilderConfig\r\n ....\r\n\r\nmy_builder = MyDatasetBuilder(...)\r\n\r\n# this doesn't exactly work like I thought; I don't get a dataset back, but NoneType instead\r\n# though I can see it loading the files and it generates the cache, etc.\r\nmy_dataset = my_builder.download_and_prepare()\r\n\r\n# load the dataset in the future by referencing it by name and loading from the cached arrow version\r\nnew_instance_of_my_dataset = load_dataset(\"my_named_dataset\")\r\n```\r\n\r\nI've seen references to the `save_to_disk` method which might be the next step I need in order to load it by name, in which case, that makes sense, then i just need to debug why `download_and_prepare` isn't returning me a dataset, but I feel like I still have a larger conceptual knowledge gap on how to use the library correctly.\r\n\r\nThanks again in advance!",
"> the requirement of a loading script has always seemed counterintuitive to me\r\n\r\nThis is a requirement only for datasets not stored in standard formats such as CSV, JSON, SQL, Parquet, ImageFolder, etc. \r\n\r\n> if i have to provide a script with every dataset, what is the point of using datasets if we're doing all the work of loading it, I can just do that in my code and skip the datasets integration (this of course discounts other potential benefits around metadata management, etc., my example is just simplest use case though for the sake of discussion)\r\n\r\nOur README/documentation lists the main features... \r\n\r\nOne of the main ones is that our library makes it easy to work with datasets larger than RAM (thanks to Arrow and the caching mechanism), and this is not trivial to implement.\r\n\r\nRegarding the step-by-step builder, this is the pattern:\r\n```python\r\nfrom datasets import load_dataset_builder\r\nbuilder = load_dataset_builder(\"path/to/script\") # or direct instantiation with MyDatasetBuilder(...)\r\nbuilder.download_and_prepare()\r\ndset = builder.as_dataset()\r\n```",
"ok, that makes sense. thank you @mariosasko. I realized i'd never looked on the hub at any of the files associated with any datasets. just did that now and it appears that i'll need to have a script regardless _but_ that will just contain my custom config and builder classes, so without realizing it I was already making my script, I just need to wrap that in a file that sits alongside my data (I looked at Glue and realized I was already doing what I thought didn't make sense to have to do, lol).\r\n\r\n`download_and_prepare` isn't returning me a dataset though, but I'll look into that and open another issue if I can't figure it out.",
"`download_and_prepare` downloads and prepares the arrow files. You need to call `as_dataset` on the builder to get the dataset.",
"ok, I think I was assigning the output of `builder.download_and_prepare` but it's an inplace op, so that explains the `NoneType` i was getting back. Now I'm getting:\r\n\r\n```\r\nArrowInvalid Traceback (most recent call last)\r\n<ipython-input-7-3ed50fb87c70> in <module>\r\n----> 1 ds = dataset_builder.as_dataset()\r\n\r\n/databricks/python/lib/python3.8/site-packages/datasets/builder.py in as_dataset(self, split, run_post_process, ignore_verifications, in_memory)\r\n 1020 \r\n 1021 # Create a dataset for each of the given splits\r\n-> 1022 datasets = map_nested(\r\n 1023 partial(\r\n 1024 self._build_single_dataset,\r\n\r\n/databricks/python/lib/python3.8/site-packages/datasets/utils/py_utils.py in map_nested(function, data_struct, dict_only, map_list, map_tuple, map_numpy, num_proc, parallel_min_length, types, disable_tqdm, desc)\r\n 442 num_proc = 1\r\n 443 if num_proc <= 1 or len(iterable) < parallel_min_length:\r\n--> 444 mapped = [\r\n 445 _single_map_nested((function, obj, types, None, True, None))\r\n 446 for obj in logging.tqdm(iterable, disable=disable_tqdm, desc=desc)\r\n\r\n/databricks/python/lib/python3.8/site-packages/datasets/utils/py_utils.py in <listcomp>(.0)\r\n 443 if num_proc <= 1 or len(iterable) < parallel_min_length:\r\n 444 mapped = [\r\n--> 445 _single_map_nested((function, obj, types, None, True, None))\r\n 446 for obj in logging.tqdm(iterable, disable=disable_tqdm, desc=desc)\r\n 447 ]\r\n\r\n/databricks/python/lib/python3.8/site-packages/datasets/utils/py_utils.py in _single_map_nested(args)\r\n 344 # Singleton first to spare some computation\r\n 345 if not isinstance(data_struct, dict) and not isinstance(data_struct, types):\r\n--> 346 return function(data_struct)\r\n 347 \r\n 348 # Reduce logging to keep things readable in multiprocessing with tqdm\r\n\r\n/databricks/python/lib/python3.8/site-packages/datasets/builder.py in _build_single_dataset(self, split, run_post_process, ignore_verifications, in_memory)\r\n 1051 \r\n 1052 # Build base dataset\r\n-> 1053 ds = self._as_dataset(\r\n 1054 split=split,\r\n 1055 in_memory=in_memory,\r\n\r\n/databricks/python/lib/python3.8/site-packages/datasets/builder.py in _as_dataset(self, split, in_memory)\r\n 1120 \"\"\"\r\n 1121 cache_dir = self._fs._strip_protocol(self._output_dir)\r\n-> 1122 dataset_kwargs = ArrowReader(cache_dir, self.info).read(\r\n 1123 name=self.name,\r\n 1124 instructions=split,\r\n\r\n/databricks/python/lib/python3.8/site-packages/datasets/arrow_reader.py in read(self, name, instructions, split_infos, in_memory)\r\n 236 msg = f'Instruction \"{instructions}\" corresponds to no data!'\r\n 237 raise ValueError(msg)\r\n--> 238 return self.read_files(files=files, original_instructions=instructions, in_memory=in_memory)\r\n 239 \r\n 240 def read_files(\r\n\r\n/databricks/python/lib/python3.8/site-packages/datasets/arrow_reader.py in read_files(self, files, original_instructions, in_memory)\r\n 257 \"\"\"\r\n 258 # Prepend path to filename\r\n--> 259 pa_table = self._read_files(files, in_memory=in_memory)\r\n 260 # If original_instructions is not None, convert it to a human-readable NamedSplit\r\n 261 if original_instructions is not None:\r\n\r\n/databricks/python/lib/python3.8/site-packages/datasets/arrow_reader.py in _read_files(self, files, in_memory)\r\n 192 f[\"filename\"] = os.path.join(self._path, f[\"filename\"])\r\n 193 for f_dict in files:\r\n--> 194 pa_table: Table = self._get_table_from_filename(f_dict, in_memory=in_memory)\r\n 195 pa_tables.append(pa_table)\r\n 196 pa_tables = [t for t in pa_tables if len(t) > 0]\r\n\r\n/databricks/python/lib/python3.8/site-packages/datasets/arrow_reader.py in _get_table_from_filename(self, filename_skip_take, in_memory)\r\n 327 filename_skip_take[\"take\"] if \"take\" in filename_skip_take else None,\r\n 328 )\r\n--> 329 table = ArrowReader.read_table(filename, in_memory=in_memory)\r\n 330 if take == -1:\r\n 331 take = len(table) - skip\r\n\r\n/databricks/python/lib/python3.8/site-packages/datasets/arrow_reader.py in read_table(filename, in_memory)\r\n 348 \"\"\"\r\n 349 table_cls = InMemoryTable if in_memory else MemoryMappedTable\r\n--> 350 return table_cls.from_file(filename)\r\n 351 \r\n 352 \r\n\r\n/databricks/python/lib/python3.8/site-packages/datasets/table.py in from_file(cls, filename, replays)\r\n 1034 @classmethod\r\n 1035 def from_file(cls, filename: str, replays=None):\r\n-> 1036 table = _memory_mapped_arrow_table_from_file(filename)\r\n 1037 table = cls._apply_replays(table, replays)\r\n 1038 return cls(table, filename, replays)\r\n\r\n/databricks/python/lib/python3.8/site-packages/datasets/table.py in _memory_mapped_arrow_table_from_file(filename)\r\n 48 def _memory_mapped_arrow_table_from_file(filename: str) -> pa.Table:\r\n 49 memory_mapped_stream = pa.memory_map(filename)\r\n---> 50 opened_stream = pa.ipc.open_stream(memory_mapped_stream)\r\n 51 pa_table = opened_stream.read_all()\r\n 52 return pa_table\r\n\r\n/databricks/python/lib/python3.8/site-packages/pyarrow/ipc.py in open_stream(source)\r\n 152 reader : RecordBatchStreamReader\r\n 153 \"\"\"\r\n--> 154 return RecordBatchStreamReader(source)\r\n 155 \r\n 156 \r\n\r\n/databricks/python/lib/python3.8/site-packages/pyarrow/ipc.py in __init__(self, source)\r\n 43 \r\n 44 def __init__(self, source):\r\n---> 45 self._open(source)\r\n 46 \r\n 47 \r\n\r\n/databricks/python/lib/python3.8/site-packages/pyarrow/ipc.pxi in pyarrow.lib._RecordBatchStreamReader._open()\r\n\r\n/databricks/python/lib/python3.8/site-packages/pyarrow/error.pxi in pyarrow.lib.pyarrow_internal_check_status()\r\n\r\n/databricks/python/lib/python3.8/site-packages/pyarrow/error.pxi in pyarrow.lib.check_status()\r\n\r\nArrowInvalid: Tried reading schema message, was null or length 0\r\n```\r\n\r\n",
"looks like my arrow files are all empty @mariosasko \r\n\r\n\r\n\r\n\r\ni also see the `incomplete_info.lock` file a level up too. seems like the data isn't being persisted to disk when I call `download_and_prepare`. is there something else i need to do before then, perhaps?",
"quick update @mariosasko. i got it working! i had to downgrade to `datasets==2.4.0`. testing other versions now and will let you know the results.",
"I've tested with every version of `datasets>2.4.0` and i get the same error with all of them."
] | ### Describe the bug
I'm not sure this is a bug or if it's just missing in the documentation, or i'm not doing something correctly, but I'm subclassing `DatasetBuilder` and getting the following error because on the `DatasetBuilder` class the `_prepare_split` method is abstract (as are the others we are required to implement, hence the genesis of my question):
```
Traceback (most recent call last):
File "/home/jason/source/python/prism_machine_learning/examples/create_hf_datasets.py", line 28, in <module>
dataset_builder.download_and_prepare()
File "/home/jason/.virtualenvs/pml/lib/python3.8/site-packages/datasets/builder.py", line 704, in download_and_prepare
self._download_and_prepare(
File "/home/jason/.virtualenvs/pml/lib/python3.8/site-packages/datasets/builder.py", line 793, in _download_and_prepare
self._prepare_split(split_generator, **prepare_split_kwargs)
File "/home/jason/.virtualenvs/pml/lib/python3.8/site-packages/datasets/builder.py", line 1124, in _prepare_split
raise NotImplementedError()
NotImplementedError
```
### Steps to reproduce the bug
I will share implementation if it turns out that everything should be working (i.e. we only need to implement those 3 methods the docs mention), but I don't want to distract from the original question.
### Expected behavior
I just need to know if there are additional methods we need to implement when subclassing `DatasetBuilder` besides what the documentation specifies -> `_info`, `_split_generators` and `_generate_examples`
### Environment info
- `datasets` version: 2.4.0
- Platform: Linux-5.4.0-135-generic-x86_64-with-glibc2.2.5
- Python version: 3.8.12
- PyArrow version: 7.0.0
- Pandas version: 1.4.1
| 5,351 |
https://github.com/huggingface/datasets/issues/5348 | The data downloaded in the download folder of the cache does not respect `umask` | [
"note, that `datasets` already did some of that umask fixing in the past and also at the hub - the recent work on the hub about the same: https://github.com/huggingface/huggingface_hub/pull/1220\r\n\r\nAlso I noticed that each file has a .json counterpart and the latter always has the correct perms:\r\n\r\n```\r\n-rw------- 1 uue59kq cnw 173M Dec 9 01:37 537596e64721e2ae3d98785b91d30fda0360c196a8224e29658ad629e7303a4d\r\n-rw-rw---- 1 uue59kq cnw 101 Dec 9 01:37 537596e64721e2ae3d98785b91d30fda0360c196a8224e29658ad629e7303a4d.json\r\n```\r\n\r\nso perhaps cheating is possible and syncing the perms between the 2 will do the trick."
] | ### Describe the bug
For a project on a cluster we are several users to share the same cache for the datasets library. And we have a problem with the permissions on the data downloaded in the cache.
Indeed, it seems that the data is downloaded by giving read and write permissions only to the user launching the command (and no permissions to the group). In our case, those permissions don't respect the `umask` of this user, which was `0007`.
Traceback:
```
Using custom data configuration default
Downloading and preparing dataset text_caps/default to /gpfswork/rech/cnw/commun/datasets/HuggingFaceM4___text_caps/default/1.0.0/2b9ad220cd90fcf2bfb454645bc54364711b83d6d39401ffdaf8cc40882e9141...
Downloading data files: 100%|████████████████████| 3/3 [00:00<00:00, 921.62it/s]
---------------------------------------------------------------------------
PermissionError Traceback (most recent call last)
Cell In [3], line 1
----> 1 ds = load_dataset(dataset_name)
File /gpfswork/rech/cnw/commun/conda/lucile-m4_3/lib/python3.8/site-packages/datasets/load.py:1746, in load_dataset(path, name, data_dir, data_files, split, cache_dir, features, download_config, download_mode, ignore_verifications, keep_in_memory, save_infos, revision, use_auth_token, task, streaming, **config_kwargs)
1743 try_from_hf_gcs = path not in _PACKAGED_DATASETS_MODULES
1745 # Download and prepare data
-> 1746 builder_instance.download_and_prepare(
1747 download_config=download_config,
1748 download_mode=download_mode,
1749 ignore_verifications=ignore_verifications,
1750 try_from_hf_gcs=try_from_hf_gcs,
1751 use_auth_token=use_auth_token,
1752 )
1754 # Build dataset for splits
1755 keep_in_memory = (
1756 keep_in_memory if keep_in_memory is not None else is_small_dataset(builder_instance.info.dataset_size)
1757 )
File /gpfswork/rech/cnw/commun/conda/lucile-m4_3/lib/python3.8/site-packages/datasets/builder.py:704, in DatasetBuilder.download_and_prepare(self, download_config, download_mode, ignore_verifications, try_from_hf_gcs, dl_manager, base_path, use_auth_token, **download_and_prepare_kwargs)
702 logger.warning("HF google storage unreachable. Downloading and preparing it from source")
703 if not downloaded_from_gcs:
--> 704 self._download_and_prepare(
705 dl_manager=dl_manager, verify_infos=verify_infos, **download_and_prepare_kwargs
706 )
707 # Sync info
708 self.info.dataset_size = sum(split.num_bytes for split in self.info.splits.values())
File /gpfswork/rech/cnw/commun/conda/lucile-m4_3/lib/python3.8/site-packages/datasets/builder.py:1227, in GeneratorBasedBuilder._download_and_prepare(self, dl_manager, verify_infos)
1226 def _download_and_prepare(self, dl_manager, verify_infos):
-> 1227 super()._download_and_prepare(dl_manager, verify_infos, check_duplicate_keys=verify_infos)
File /gpfswork/rech/cnw/commun/conda/lucile-m4_3/lib/python3.8/site-packages/datasets/builder.py:771, in DatasetBuilder._download_and_prepare(self, dl_manager, verify_infos, **prepare_split_kwargs)
769 split_dict = SplitDict(dataset_name=self.name)
770 split_generators_kwargs = self._make_split_generators_kwargs(prepare_split_kwargs)
--> 771 split_generators = self._split_generators(dl_manager, **split_generators_kwargs)
773 # Checksums verification
774 if verify_infos and dl_manager.record_checksums:
File /gpfswork/rech/cnw/commun/modules/datasets_modules/datasets/HuggingFaceM4--TextCaps/2b9ad220cd90fcf2bfb454645bc54364711b83d6d39401ffdaf8cc40882e9141/TextCaps.py:125, in TextCapsDataset._split_generators(self, dl_manager)
123 def _split_generators(self, dl_manager):
124 # urls = _URLS[self.config.name] # TODO later
--> 125 data_dir = dl_manager.download_and_extract(_URLS)
126 gen_kwargs = {
127 split_name: {
128 f"{dir_name}_path": Path(data_dir[dir_name][split_name])
(...)
133 for split_name in ["train", "val", "test"]
134 }
136 for split_name in ["train", "val", "test"]:
File /gpfswork/rech/cnw/commun/conda/lucile-m4_3/lib/python3.8/site-packages/datasets/download/download_manager.py:431, in DownloadManager.download_and_extract(self, url_or_urls)
415 def download_and_extract(self, url_or_urls):
416 """Download and extract given url_or_urls.
417
418 Is roughly equivalent to:
(...)
429 extracted_path(s): `str`, extracted paths of given URL(s).
430 """
--> 431 return self.extract(self.download(url_or_urls))
File /gpfswork/rech/cnw/commun/conda/lucile-m4_3/lib/python3.8/site-packages/datasets/download/download_manager.py:324, in DownloadManager.download(self, url_or_urls)
321 self.downloaded_paths.update(dict(zip(url_or_urls.flatten(), downloaded_path_or_paths.flatten())))
323 start_time = datetime.now()
--> 324 self._record_sizes_checksums(url_or_urls, downloaded_path_or_paths)
325 duration = datetime.now() - start_time
326 logger.info(f"Checksum Computation took {duration.total_seconds() // 60} min")
File /gpfswork/rech/cnw/commun/conda/lucile-m4_3/lib/python3.8/site-packages/datasets/download/download_manager.py:229, in DownloadManager._record_sizes_checksums(self, url_or_urls, downloaded_path_or_paths)
226 """Record size/checksum of downloaded files."""
227 for url, path in zip(url_or_urls.flatten(), downloaded_path_or_paths.flatten()):
228 # call str to support PathLike objects
--> 229 self._recorded_sizes_checksums[str(url)] = get_size_checksum_dict(
230 path, record_checksum=self.record_checksums
231 )
File /gpfswork/rech/cnw/commun/conda/lucile-m4_3/lib/python3.8/site-packages/datasets/utils/info_utils.py:82, in get_size_checksum_dict(path, record_checksum)
80 if record_checksum:
81 m = sha256()
---> 82 with open(path, "rb") as f:
83 for chunk in iter(lambda: f.read(1 << 20), b""):
84 m.update(chunk)
PermissionError: [Errno 13] Permission denied: '/gpfswork/rech/cnw/commun/datasets/downloads/1e6aa6d23190c30885194fabb193dce3874d902d7636b66315ee8aaa584e80d6'
```
### Steps to reproduce the bug
I think the following will reproduce the bug.
Given 2 users belonging to the same group with `umask` set to `0007`
- first run with User 1:
```python
from datasets import load_dataset
ds_name = "HuggingFaceM4/VQAv2"
ds = load_dataset(ds_name)
```
- then run with User 2:
```python
from datasets import load_dataset
ds_name = "HuggingFaceM4/TextCaps"
ds = load_dataset(ds_name)
```
### Expected behavior
No `PermissionError`
### Environment info
- `datasets` version: 2.4.0
- Platform: Linux-4.18.0-305.65.1.el8_4.x86_64-x86_64-with-glibc2.17
- Python version: 3.8.13
- PyArrow version: 7.0.0
- Pandas version: 1.4.2
| 5,348 |
https://github.com/huggingface/datasets/issues/5346 | [Quick poll] Give your opinion on the future of the Hugging Face Open Source ecosystem! | [
"As the survey is finished, can we close this issue, @LysandreJik ?",
"Yes! I'll post a public summary on the forums shortly.",
"Is the summary available? I would be interested in reading your findings."
] | Thanks to all of you, Datasets is just about to pass 15k stars!
Since the last survey, a lot has happened: the [diffusers](https://github.com/huggingface/diffusers), [evaluate](https://github.com/huggingface/evaluate) and [skops](https://github.com/skops-dev/skops) libraries were born. `timm` joined the Hugging Face ecosystem. There were 25 new releases of `transformers`, 21 new releases of `datasets`, 13 new releases of `accelerate`.
If you have a couple of minutes and want to participate in shaping the future of the ecosystem, please share your thoughts:
[**hf.co/oss-survey**](https://docs.google.com/forms/d/e/1FAIpQLSf4xFQKtpjr6I_l7OfNofqiR8s-WG6tcNbkchDJJf5gYD72zQ/viewform?usp=sf_link)
(please reply in the above feedback form rather than to this thread)
Thank you all on behalf of the HuggingFace team! 🤗 | 5,346 |
https://github.com/huggingface/datasets/issues/5345 | Wrong dtype for array in audio features | [
"After some more investigation, this is due to [this line of code](https://github.com/huggingface/datasets/blob/main/src/datasets/features/audio.py#L279). The function `sf.read(file)` should be updated to `sf.read(file, dtype=\"float32\")`\r\n\r\nIndeed, the default value in soundfile is `float64` ([see here](https://pysoundfile.readthedocs.io/en/latest/#soundfile.read)). \r\n",
"@qmeeus I agree, decoding of different audio formats should return the same dtypes indeed!\r\n\r\nBut note that here you are concatenating datasets with different sampling rates: 48000 for CommonVoice and 16000 for Voxpopuli. So you should cast them to the same sampling rate value before interleaving, for example:\r\n```\r\ncv = cv.cast_column(\"audio\", Audio(sampling_rate=16000))\r\n```\r\notherwise you would get the same error because features of the same column (\"audio\") are not the same.\r\n\r\nAlso, the error you get is unexpected. Could you please confirm that you use the latest main version of the `datasets`? We had an issue that could lead to an error like this after using `rename_column` method, but it was fixed in https://github.com/huggingface/datasets/pull/5287 ",
"Hi Polina,\r\nSorry for the late answer\r\nIt is possible that the issue was due to a bug that is now fixed. I installed an editable version of datasets from github, but I don't recall whether I had updated it at the time of the issue. My research led me to other directions so I did not follow through on the interleave datasets.\r\n"
] | ### Describe the bug
When concatenating/interleaving different datasets, I stumble into an error because the features can't be aligned. After some investigation, I understood that the audio arrays had different dtypes, namely `float32` and `float64`. Consequently, the datasets cannot be merged.
### Steps to reproduce the bug
For example, for `facebook/voxpopuli` and `mozilla-foundation/common_voice_11_0`:
```
from datasets import load_dataset, interleave_datasets
covost = load_dataset("mozilla-foundation/common_voice_11_0", "en", split="train", streaming=True)
voxpopuli = datasets.load_dataset("facebook/voxpopuli", "nl", split="train", streaming=True)
sample_cv, = covost.take(1)
sample_vp, = voxpopuli.take(1)
assert sample_cv["audio"]["array"].dtype == sample_vp["audio"]["array"].dtype
# Fails
dataset = interleave_datasets([covost, voxpopuli])
# ValueError: The features can't be aligned because the key audio of features {'audio_id': Value(dtype='string', id=None), 'language': Value(dtype='int64', id=None), 'audio': {'array': Sequence(feature=Value(dtype='float64', id=None), length=-1, id=None), 'path': Value(dtype='string', id=None), 'sampling_rate': Value(dtype='int64', id=None)}, 'normalized_text': Value(dtype='string', id=None), 'gender': Value(dtype='string', id=None), 'speaker_id': Value(dtype='string', id=None), 'is_gold_transcript': Value(dtype='bool', id=None), 'accent': Value(dtype='string', id=None), 'sentence': Value(dtype='string', id=None)} has unexpected type - {'array': Sequence(feature=Value(dtype='float64', id=None), length=-1, id=None), 'path': Value(dtype='string', id=None), 'sampling_rate': Value(dtype='int64', id=None)} (expected either Audio(sampling_rate=16000, mono=True, decode=True, id=None) or Value("null").
```
### Expected behavior
The audio should be loaded to arrays with a unique dtype (I guess `float32`)
### Environment info
```
- `datasets` version: 2.7.1.dev0
- Platform: Linux-4.18.0-425.3.1.el8.x86_64-x86_64-with-glibc2.28
- Python version: 3.9.15
- PyArrow version: 10.0.1
- Pandas version: 1.5.2
``` | 5,345 |
https://github.com/huggingface/datasets/issues/5343 | T5 for Q&A produces truncated sentence | [] | Dear all, I am fine-tuning T5 for Q&A task using the MedQuAD ([GitHub - abachaa/MedQuAD: Medical Question Answering Dataset of 47,457 QA pairs created from 12 NIH websites](https://github.com/abachaa/MedQuAD)) dataset. In the dataset, there are many long answers with thousands of words. I have used pytorch_lightning to train the T5-large model. I have two questions.
For example, I set both the max_length, max_input_length, max_output_length to 128.
How to deal with those long answers? I just left them as is and the T5Tokenizer can automatically handle. I would assume the tokenizer just truncates an answer at the position of 128th word (or 127th). Is it possible that I manually split an answer into different parts, each part has 128 words; and then all these sub-answers serve as a separate answer to the same question?
Another question is that I get incomplete (truncated) answers when using the fine-tuned model in inference, even though the predicted answer is shorter than 128 words. I found a message posted 2 years ago saying that one should add at the end of texts when fine-tuning T5. I followed that but then got a warning message that duplicated were found. I am assuming that this is because the tokenizer truncates an answer text, thus is missing in the truncated answer, such that the end token is not produced in predicted answer. However, I am not sure. Can anybody point out how to address this issue?
Any suggestions are highly appreciated.
Below is some code snippet.
`
import pytorch_lightning as pl
from torch.utils.data import DataLoader
import torch
import numpy as np
import time
from pathlib import Path
from transformers import (
Adafactor,
T5ForConditionalGeneration,
T5Tokenizer,
get_linear_schedule_with_warmup
)
from torch.utils.data import RandomSampler
from question_answering.utils import *
class T5FineTuner(pl.LightningModule):
def __init__(self, hyparams):
super(T5FineTuner, self).__init__()
self.hyparams = hyparams
self.model = T5ForConditionalGeneration.from_pretrained(hyparams.model_name_or_path)
self.tokenizer = T5Tokenizer.from_pretrained(hyparams.tokenizer_name_or_path)
if self.hyparams.freeze_embeds:
self.freeze_embeds()
if self.hyparams.freeze_encoder:
self.freeze_params(self.model.get_encoder())
# assert_all_frozen()
self.step_count = 0
self.output_dir = Path(self.hyparams.output_dir)
n_observations_per_split = {
'train': self.hyparams.n_train,
'validation': self.hyparams.n_val,
'test': self.hyparams.n_test
}
self.n_obs = {k: v if v >= 0 else None for k, v in n_observations_per_split.items()}
self.em_score_list = []
self.subset_score_list = []
data_folder = r'C:\Datasets\MedQuAD-master'
self.train_data, self.val_data, self.test_data = load_medqa_data(data_folder)
def freeze_params(self, model):
for param in model.parameters():
param.requires_grad = False
def freeze_embeds(self):
try:
self.freeze_params(self.model.model.shared)
for d in [self.model.model.encoder, self.model.model.decoder]:
self.freeze_params(d.embed_positions)
self.freeze_params(d.embed_tokens)
except AttributeError:
self.freeze_params(self.model.shared)
for d in [self.model.encoder, self.model.decoder]:
self.freeze_params(d.embed_tokens)
def lmap(self, f, x):
return list(map(f, x))
def is_logger(self):
return self.trainer.proc_rank <= 0
def forward(self, input_ids, attention_mask=None, decoder_input_ids=None, decoder_attention_mask=None, labels=None):
return self.model(
input_ids,
attention_mask=attention_mask,
decoder_input_ids=decoder_input_ids,
decoder_attention_mask=decoder_attention_mask,
labels=labels
)
def _step(self, batch):
labels = batch['target_ids']
labels[labels[:, :] == self.tokenizer.pad_token_id] = -100
outputs = self(
input_ids = batch['source_ids'],
attention_mask=batch['source_mask'],
labels=labels,
decoder_attention_mask=batch['target_mask']
)
loss = outputs[0]
return loss
def ids_to_clean_text(self, generated_ids):
gen_text = self.tokenizer.batch_decode(generated_ids, skip_special_tokens=True, clean_up_tokenization_spaces=True)
return self.lmap(str.strip, gen_text)
def _generative_step(self, batch):
t0 = time.time()
generated_ids = self.model.generate(
batch["source_ids"],
attention_mask=batch["source_mask"],
use_cache=True,
decoder_attention_mask=batch['target_mask'],
max_length=128,
num_beams=2,
early_stopping=True
)
preds = self.ids_to_clean_text(generated_ids)
targets = self.ids_to_clean_text(batch["target_ids"])
gen_time = (time.time() - t0) / batch["source_ids"].shape[0]
loss = self._step(batch)
base_metrics = {'val_loss': loss}
summ_len = np.mean(self.lmap(len, generated_ids))
base_metrics.update(gen_time=gen_time, gen_len=summ_len, preds=preds, target=targets)
em_score, subset_match_score = calculate_scores(preds, targets)
self.em_score_list.append(em_score)
self.subset_score_list.append(subset_match_score)
em_score = torch.tensor(em_score, dtype=torch.float32)
subset_match_score = torch.tensor(subset_match_score, dtype=torch.float32)
base_metrics.update(em_score=em_score, subset_match_score=subset_match_score)
# rouge_results = self.rouge_metric.compute()
# rouge_dict = self.parse_score(rouge_results)
return base_metrics
def training_step(self, batch, batch_idx):
loss = self._step(batch)
tensorboard_logs = {'train_loss': loss}
return {'loss': loss, 'log': tensorboard_logs}
def training_epoch_end(self, outputs):
avg_train_loss = torch.stack([x['loss'] for x in outputs]).mean()
tensorboard_logs = {'avg_train_loss': avg_train_loss}
# return {'avg_train_loss': avg_train_loss, 'log': tensorboard_logs, 'progress_bar': tensorboard_logs}
def validation_step(self, batch, batch_idx):
return self._generative_step(batch)
def validation_epoch_end(self, outputs):
avg_loss = torch.stack([x['val_loss'] for x in outputs]).mean()
tensorboard_logs = {'val_loss': avg_loss}
if len(self.em_score_list) <= 2:
average_em_score = sum(self.em_score_list) / len(self.em_score_list)
average_subset_match_score = sum(self.subset_score_list) / len(self.subset_score_list)
else:
latest_em_score = self.em_score_list[:-2]
latest_subset_score = self.subset_score_list[:-2]
average_em_score = sum(latest_em_score) / len(latest_em_score)
average_subset_match_score = sum(latest_subset_score) / len(latest_subset_score)
average_em_score = torch.tensor(average_em_score, dtype=torch.float32)
average_subset_match_score = torch.tensor(average_subset_match_score, dtype=torch.float32)
tensorboard_logs.update(em_score=average_em_score, subset_match_score=average_subset_match_score)
self.target_gen = []
self.prediction_gen = []
return {
'avg_val_loss': avg_loss,
'em_score': average_em_score,
'subset_match_socre': average_subset_match_score,
'log': tensorboard_logs,
'progress_bar': tensorboard_logs
}
def configure_optimizers(self):
model = self.model
no_decay = ["bias", "LayerNorm.weight"]
optimizer_grouped_parameters = [
{
"params": [p for n, p in model.named_parameters() if not any(nd in n for nd in no_decay)],
"weight_decay": self.hyparams.weight_decay,
},
{
"params": [p for n, p in model.named_parameters() if any(nd in n for nd in no_decay)],
"weight_decay": 0.0,
},
]
optimizer = Adafactor(optimizer_grouped_parameters, lr=self.hyparams.learning_rate, scale_parameter=False,
relative_step=False)
self.opt = optimizer
return [optimizer]
def optimizer_step(self, epoch, batch_idx, optimizer, optimizer_idx, optimizer_closure=None,
on_tpu=False, using_native_amp=False, using_lbfgs=False):
optimizer.step(closure=optimizer_closure)
optimizer.zero_grad()
self.lr_scheduler.step()
def get_tqdm_dict(self):
tqdm_dict = {"loss": "{:.3f}".format(self.trainer.avg_loss), "lr": self.lr_scheduler.get_last_lr()[-1]}
return tqdm_dict
def train_dataloader(self):
n_samples = self.n_obs['train']
train_dataset = get_dataset(tokenizer=self.tokenizer, data=self.train_data, num_samples=n_samples,
args=self.hyparams)
sampler = RandomSampler(train_dataset)
dataloader = DataLoader(train_dataset, sampler=sampler, batch_size=self.hyparams.train_batch_size,
drop_last=True, num_workers=4)
# t_total = (
# (len(dataloader.dataset) // (self.hyparams.train_batch_size * max(1, self.hyparams.n_gpu)))
# // self.hyparams.gradient_accumulation_steps
# * float(self.hyparams.num_train_epochs)
# )
t_total = 100000
scheduler = get_linear_schedule_with_warmup(
self.opt, num_warmup_steps=self.hyparams.warmup_steps, num_training_steps=t_total
)
self.lr_scheduler = scheduler
return dataloader
def val_dataloader(self):
n_samples = self.n_obs['validation']
validation_dataset = get_dataset(tokenizer=self.tokenizer, data=self.val_data, num_samples=n_samples,
args=self.hyparams)
sampler = RandomSampler(validation_dataset)
return DataLoader(validation_dataset, shuffle=False, batch_size=self.hyparams.eval_batch_size, sampler=sampler, num_workers=4)
def test_dataloader(self):
n_samples = self.n_obs['test']
test_dataset = get_dataset(tokenizer=self.tokenizer, data=self.test_data, num_samples=n_samples, args=self.hyparams)
return DataLoader(test_dataset, batch_size=self.hyparams.eval_batch_size, num_workers=4)
def on_save_checkpoint(self, checkpoint):
save_path = self.output_dir.joinpath("best_tfmr")
self.model.config.save_step = self.step_count
self.model.save_pretrained(save_path)
self.tokenizer.save_pretrained(save_path)
import os
import argparse
import pytorch_lightning as pl
from question_answering.t5_closed_book import T5FineTuner
if __name__ == '__main__':
args_dict = dict(
output_dir="", # path to save the checkpoints
model_name_or_path='t5-large',
tokenizer_name_or_path='t5-large',
max_input_length=128,
max_output_length=128,
freeze_encoder=False,
freeze_embeds=False,
learning_rate=1e-5,
weight_decay=0.0,
adam_epsilon=1e-8,
warmup_steps=0,
train_batch_size=4,
eval_batch_size=4,
num_train_epochs=2,
gradient_accumulation_steps=10,
n_gpu=1,
resume_from_checkpoint=None,
val_check_interval=0.5,
n_val=4000,
n_train=-1,
n_test=-1,
early_stop_callback=False,
fp_16=False,
opt_level='O1',
max_grad_norm=1.0,
seed=101,
)
args_dict.update({'output_dir': 't5_large_MedQuAD_256', 'num_train_epochs': 100,
'train_batch_size': 16, 'eval_batch_size': 16, 'learning_rate': 1e-3})
args = argparse.Namespace(**args_dict)
checkpoint_callback = pl.callbacks.ModelCheckpoint(dirpath=args.output_dir, monitor="em_score", mode="max", save_top_k=1)
## If resuming from checkpoint, add an arg resume_from_checkpoint
train_params = dict(
accumulate_grad_batches=args.gradient_accumulation_steps,
gpus=args.n_gpu,
max_epochs=args.num_train_epochs,
# early_stop_callback=False,
precision=16 if args.fp_16 else 32,
# amp_level=args.opt_level,
# resume_from_checkpoint=args.resume_from_checkpoint,
gradient_clip_val=args.max_grad_norm,
checkpoint_callback=checkpoint_callback,
val_check_interval=args.val_check_interval,
# accelerator='dp'
# logger=wandb_logger,
# callbacks=[LoggingCallback()],
)
model = T5FineTuner(args)
trainer = pl.Trainer(**train_params)
trainer.fit(model)
` | 5,343 |
https://github.com/huggingface/datasets/issues/5342 | Emotion dataset cannot be downloaded | [
"Hi @cbarond there's already an open issue at https://github.com/dair-ai/emotion_dataset/issues/5, as the data seems to be missing now, so check that issue instead 👍🏻 ",
"Thanks @cbarond for reporting and @alvarobartt for pointing to the issue we opened in the author's repo.\r\n\r\nIndeed, this issue was first raised in the \"emotion\" dataset Community tab: https://huggingface.co/datasets/emotion/discussions/3\r\n\r\nI'm closing this issue and leave the issue above for the subsequent updates.\r\n\r\nDuplicate of: https://huggingface.co/datasets/emotion/discussions/3",
"try using \"SetFit/emotion\" instead",
"> try using \"SetFit/emotion\" instead\r\n\r\nI' replaced \"emotion\" with \"SetFit/Emotion\", but the code is getting stuck at\r\n\r\n`emotions = load_dataset(\"SetFit/emotion\")`\r\n\r\nI pause execution using the debugger, and it takes me to filelock.py:226\r\n\r\n`with self._thread_lock:`\r\n\r\nDo you know a way to get past this issue?",
"thanks @honeyimholm - worked for me",
"> try using \"SetFit/emotion\" instead\r\n\r\nIt really helps a lot, thank you!",
"The dataset loading script has been fixed: https://huggingface.co/datasets/emotion/discussions/4"
] | ### Describe the bug
The emotion dataset gives a FileNotFoundError. The full error is: `FileNotFoundError: Couldn't find file at https://www.dropbox.com/s/1pzkadrvffbqw6o/train.txt?dl=1`.
It was working yesterday (December 7, 2022), but stopped working today (December 8, 2022).
### Steps to reproduce the bug
```python
from datasets import load_dataset
dataset = load_dataset("emotion")
```
### Expected behavior
The dataset should load properly.
### Environment info
- `datasets` version: 2.7.1
- Platform: Windows-10-10.0.19045-SP0
- Python version: 3.9.13
- PyArrow version: 10.0.1
- Pandas version: 1.5.1 | 5,342 |
https://github.com/huggingface/datasets/issues/5338 | `map()` stops every 1000 steps | [
"Hi !\r\n\r\n> It starts using all the cores (I am not sure why because I did not pass num_proc)\r\n\r\nThe tokenizer uses Rust code that is multithreaded. And maybe the `feature_extractor` might run some things in parallel as well - but I'm not super familiar with its internals.\r\n\r\n> then progress bar stops at every 1k steps. (starts using a single core)\r\n\r\nEvery 1000 examples we flush the processed examples to disk. It is this way because Arrow is a columnar format: you must write data chunk by chunk. The processing in on hold while writing right now - maybe this can be improved in the future.",
"Hi @lhoestq \r\nThanks for the explanation! it was so helpful! Let me check why `feature_extractor` is running on multiple cpus."
] | ### Describe the bug
I am passing the following `prepare_dataset` function to `Dataset.map` (code is inspired from [here](https://github.com/huggingface/community-events/blob/main/whisper-fine-tuning-event/run_speech_recognition_seq2seq_streaming.py#L454))
```python3
def prepare_dataset(batch):
# load and resample audio data from 48 to 16kHz
audio = batch["audio"]
# compute log-Mel input features from input audio array
batch["input_features"] = feature_extractor(audio["array"], sampling_rate=audio["sampling_rate"]).input_features[0]
# encode target text to label ids
batch["labels"] = tokenizer(batch[text_column]).input_ids
return batch
...
train_ds = train_ds.map(prepare_dataset)
```
Here is the exact code I am running https://github.com/bayartsogt-ya/whisper-multiple-hf-datasets/blob/main/train.py#L70-L71
It starts using all the cores (I am not sure why because I did not pass `num_proc`)
then progress bar stops at every 1k steps. (starts using a single core)
then come back to using all the cores again.
link to [screen record](https://youtu.be/jPQpQQGp6Gc)
Can someone explain this process and maybe provide a way to improve this pipeline? cc: @lhoestq
### Steps to reproduce the bug
1. load the dataset
2. create a Whisper processor
3. create a `prepare_dataset` function
4. pass the function to `dataset.map(prepare_dataset)`
### Expected behavior
- Use a single core per a function
- not to stop at some point?
### Environment info
- `datasets` version: 2.7.1.dev0
- Platform: Linux-5.4.0-109-generic-x86_64-with-glibc2.27
- Python version: 3.8.10
- PyArrow version: 10.0.1
- Pandas version: 1.5.2 | 5,338 |
https://github.com/huggingface/datasets/issues/5337 | Support webdataset format | [
"I like the idea of having `webdataset` as an optional dependency to ensure our loader generates web datasets the same way as the main project.",
"Webdataset is the one of the most popular dataset formats for large scale computer vision tasks. Upvote for this issue. ",
"Any updates on this?",
"We haven't had the bandwidth to implement it so far, but if someone wants to give it a shot please don't hesitate ^^",
"Done in #6391 "
] | Webdataset is an efficient format for iterable datasets. It would be nice to support it in `datasets`, as discussed in https://github.com/rom1504/img2dataset/issues/234.
In particular it would be awesome to be able to load one using `load_dataset` in streaming mode (either from a local directory, or from a dataset on the Hugging Face Hub). Some datasets on the Hub are already in webdataset format.
It terms of implementation, we can have something similar to the Parquet loader.
I also think it's fine to have webdataset as an optional dependency. | 5,337 |
https://github.com/huggingface/datasets/issues/5332 | Passing numpy array to ClassLabel names causes ValueError | [
"Should `datasets` allow `ClassLabel` input parameter to be an `np.array` even though internally we need to cast it to a Python list? @lhoestq @mariosasko ",
"Hi! No, I don't think so. The `names` parameter is [annotated](https://github.com/huggingface/datasets/blob/582236640b9109988e5f7a16a8353696ffa09a16/src/datasets/features/features.py#L892) as `List[str]` (**NumPy arrays are not lists**), and considering that type checking is not a common practice in Python, I think we can leave the code as-is.",
"I appreciate it is the wrong type, and that type checking is not common, but I think there's a few circumstances that make it a good idea from a usability perspective.\r\n\r\nIt's quite a difficult error to debug because it comes from a utility function (so it's not immediately obvious which parameter caused it). What makes it even more difficult is the exception happens when the features instance is used to instantiate the dataset, **not** when when the wrong type is actually passed when the features is instantiated. When I was debugging the error, I didn't really consider it could be an issue with the features instance because it had instantiated fine. It's also not one of the more common exceptions caused by trying to use a non-list as a list.\r\n\r\nIt's also relatively easy to accidentally get a numpy array of class types (e.g. calling `unique()` on a pandas dataframe column). Additionally, passing in a `set` instead of the list (again, relatively easy because people may run `set(classes)` to generate uniques) causes an error when the features instance is used, albeit a slightly more obvious one.\r\n\r\nThe names list is already being processed and validated in the `__post_init__` method anyway, so it would not really be adding any complexity to check it is actually a list here too. I'm happy to contribute this change if you change your mind about whether it's worthwhile.",
"I agree that it's not easy to debug this issue, so perhaps we could add some basic type checking (e.g. `not isinstance(names, list)` -> error) to make debugging easier. Feel free to submit a PR.\r\n\r\n> Additionally, passing in a set instead of the list (again, relatively easy because people may run set(classes) to generate uniques) causes an error when the features instance is used, albeit a slightly more obvious one.\r\n\r\n`set` is an unordered structure (it's ordered in Python 3.6+, but this is CPython's implementation detail), and the order of ClassLabel `names` matters, so this doesn't require a fix.",
"What about checking for `Sequence` instead? I think users can pass a list or a tuple as well."
] | ### Describe the bug
If a numpy array is passed to the names argument of ClassLabel, creating a dataset with those features causes an error.
### Steps to reproduce the bug
https://colab.research.google.com/drive/1cV_es1PWZiEuus17n-2C-w0KEoEZ68IX
TLDR:
If I define my classes as:
```
my_classes = np.array(['one', 'two', 'three'])
```
Then this errors:
```py
features = Features({'value': Value('string'), 'label': ClassLabel(names=my_classes)})
dataset = Dataset.from_list(my_data, features=features)
```
```
ValueError Traceback (most recent call last)
[<ipython-input-8-a8a9d53ec82f>](https://localhost:8080/#) in <module>
----> 1 dataset = Dataset.from_list(my_data, features=features)
11 frames
[/usr/local/lib/python3.8/dist-packages/datasets/utils/py_utils.py](https://localhost:8080/#) in _asdict_inner(obj)
183 for f in fields(obj):
184 value = _asdict_inner(getattr(obj, f.name))
--> 185 if not f.init or value != f.default or f.metadata.get("include_in_asdict_even_if_is_default", False):
186 result[f.name] = value
187 return result
ValueError: The truth value of an array with more than one element is ambiguous. Use a.any() or a.all()
```
But this works:
```
features2 = Features({'value': Value('string'), 'label': ClassLabel(names=list(my_classes))})
dataset2 = Dataset.from_list(my_data, features=features2)
```
### Expected behavior
If I provide a numpy array of class names, I would expect either an error that the names list is the wrong type, or for it to be cast internally.
### Environment info
- `datasets` version: 2.7.1
- Platform: Linux-5.15.0-56-generic-x86_64-with-glibc2.10
- Python version: 3.8.15
- PyArrow version: 10.0.1
- Pandas version: 1.5.2
Additionally:
- Numpy version: 1.23.5
| 5,332 |
https://github.com/huggingface/datasets/issues/5326 | No documentation for main branch is built | [] | Since:
- #5250
- Commit: 703b84311f4ead83c7f79639f2dfa739295f0be6
the docs for main branch are no longer built.
The change introduced only triggers the docs building for releases. | 5,326 |
https://github.com/huggingface/datasets/issues/5325 | map(...batch_size=None) for IterableDataset | [
"Hi! I agree it makes sense for `IterableDataset.map` to support the `batch_size=None` case. This should be super easy to fix.",
"@mariosasko as this is something simple maybe I can include it as part of https://github.com/huggingface/datasets/pull/5311? Let me know :+1:",
"#self-assign",
"Feel free to close this @lhoestq as part of https://github.com/huggingface/datasets/pull/5336 :hugs:",
"Thanks again :)\r\n\r\n> For practical usages, an alternative to this would be to convert from an iterable dataset to a map-style dataset, but it is not obvious how to do this.\r\n\r\nThis is interesting as well, if anyone wants to explore"
] | ### Feature request
Dataset.map(...) allows batch_size to be None. It would be nice if IterableDataset did too.
### Motivation
Although it may seem a bit of a spurious request given that `IterableDataset` is meant for larger than memory datasets, but there are a couple of reasons why this might be nice.
One is that load_dataset(...) can return either IterableDataset or Dataset. mypy will then complain if batch_size=None even if we know it is Dataset. Of course we can do:
assert isinstance(d, datasets.DatasetDict)
But it is a mild inconvenience. What's more annoying is that whenever we use something like e.g. `combine_datasets(...)`, we end up with the union again, and so have to do the assert again.
Another is that we could actually end up with an IterableDataset small enough for memory in normal/correct usage, e.g. by filtering a massive IterableDataset.
For practical usages, an alternative to this would be to convert from an iterable dataset to a map-style dataset, but it is not obvious how to do this.
### Your contribution
Not this time. | 5,325 |
https://github.com/huggingface/datasets/issues/5324 | Fix docstrings and types in documentation that appears on the website | [
"I agree we have a mess with docstrings...",
"Ok, I believe we've cleaned up most of the old syntax we were using for the user-facing docs! There are still a couple of `:obj:`'s and `:class:` floating around in the docstrings we don't expose that I'll track down :)",
"Hi @polinaeterna @albertvillanova @stevhliu, I hope you all are doing well.\r\n\r\nIs this issue still unresolved as I am interested in it?",
"It should be mostly fixed for the user-facing APIs, but there may be some Sphinx syntax still lurking around in the non-public APIs. Feel free to open a PR to fix those if you catch any! 🤗 ",
"Thanks for your reply @stevhliu :)\r\nSure, I will try to find out the remaining and fix that.\r\n\r\n"
] | While I was working on https://github.com/huggingface/datasets/pull/5313 I've noticed that we have a mess in how we annotate types and format args and return values in the code. And some of it is displayed in the [Reference section](https://huggingface.co/docs/datasets/package_reference/builder_classes) of the documentation on the website.
Would be nice someday, maybe before releasing datasets 3.0.0, to unify it...... | 5,324 |
https://github.com/huggingface/datasets/issues/5323 | Duplicated Keys in Taskmaster-2 Dataset | [
"Thanks for reporting, @liaeh.\r\n\r\nWe are having a look at it. ",
"I have transferred the discussion to the Community tab of the dataset: https://huggingface.co/datasets/taskmaster2/discussions/1"
] | ### Describe the bug
Loading certain splits () of the taskmaster-2 dataset fails because of a DuplicatedKeysError. This occurs for the following domains: `'hotels', 'movies', 'music', 'sports'`. The domains `'flights', 'food-ordering', 'restaurant-search'` load fine.
Output:
### Steps to reproduce the bug
```
from datasets import load_dataset
dataset = load_dataset("taskmaster2", "music")
```
Output:
```
---------------------------------------------------------------------------
DuplicatedKeysError Traceback (most recent call last)
File ~/repos/tts-dataset/tts-dataset/venv/lib/python3.9/site-packages/datasets/builder.py:1532, in GeneratorBasedBuilder._prepare_split_single(self, arg)
[1531](file:///home/user/repos/tts-dataset/tts-dataset/venv/lib/python3.9/site-packages/datasets/builder.py?line=1530) example = self.info.features.encode_example(record) if self.info.features is not None else record
-> [1532](file:///home/user/repos/tts-dataset/tts-dataset/venv/lib/python3.9/site-packages/datasets/builder.py?line=1531) writer.write(example, key)
[1533](file:///home/user/repos/tts-dataset/tts-dataset/venv/lib/python3.9/site-packages/datasets/builder.py?line=1532) num_examples_progress_update += 1
File ~/repos/tts-dataset/tts-dataset/venv/lib/python3.9/site-packages/datasets/arrow_writer.py:475, in ArrowWriter.write(self, example, key, writer_batch_size)
[474](file:///home/user/repos/tts-dataset/tts-dataset/venv/lib/python3.9/site-packages/datasets/arrow_writer.py?line=473) if self._check_duplicates:
--> [475](file:///home/user/repos/tts-dataset/tts-dataset/venv/lib/python3.9/site-packages/datasets/arrow_writer.py?line=474) self.check_duplicate_keys()
[476](file:///home/user/repos/tts-dataset/tts-dataset/venv/lib/python3.9/site-packages/datasets/arrow_writer.py?line=475) # Re-intializing to empty list for next batch
File ~/repos/tts-dataset/tts-dataset/venv/lib/python3.9/site-packages/datasets/arrow_writer.py:492, in ArrowWriter.check_duplicate_keys(self)
[486](file:///home/user/repos/tts-dataset/tts-dataset/venv/lib/python3.9/site-packages/datasets/arrow_writer.py?line=485) duplicate_key_indices = [
[487](file:///home/user/repos/tts-dataset/tts-dataset/venv/lib/python3.9/site-packages/datasets/arrow_writer.py?line=486) str(self._num_examples + index)
[488](file:///home/user/repos/tts-dataset/tts-dataset/venv/lib/python3.9/site-packages/datasets/arrow_writer.py?line=487) for index, (duplicate_hash, _) in enumerate(self.hkey_record)
[489](file:///home/user/repos/tts-dataset/tts-dataset/venv/lib/python3.9/site-packages/datasets/arrow_writer.py?line=488) if duplicate_hash == hash
[490](file:///home/user/repos/tts-dataset/tts-dataset/venv/lib/python3.9/site-packages/datasets/arrow_writer.py?line=489) ]
--> [492](file:///home/user/repos/tts-dataset/tts-dataset/venv/lib/python3.9/site-packages/datasets/arrow_writer.py?line=491) raise DuplicatedKeysError(key, duplicate_key_indices)
[493](file:///home/user/repos/tts-dataset/tts-dataset/venv/lib/python3.9/site-packages/datasets/arrow_writer.py?line=492) else:
DuplicatedKeysError: Found multiple examples generated with the same key
The examples at index 858, 859 have the key dlg-89174425-d57a-4db7-a92b-165c3bff6735
During handling of the above exception, another exception occurred:
DuplicatedKeysError Traceback (most recent call last)
File ~/repos/tts-dataset/tts-dataset/venv/lib/python3.9/site-packages/datasets/builder.py:1541, in GeneratorBasedBuilder._prepare_split_single(self, arg)
[1540](file:///home/user/repos/tts-dataset/tts-dataset/venv/lib/python3.9/site-packages/datasets/builder.py?line=1539) num_shards = shard_id + 1
-> [1541](file:///home/user/repos/tts-dataset/tts-dataset/venv/lib/python3.9/site-packages/datasets/builder.py?line=1540) num_examples, num_bytes = writer.finalize()
[1542](file:///home/user/repos/tts-dataset/tts-dataset/venv/lib/python3.9/site-packages/datasets/builder.py?line=1541) writer.close()
File ~/repos/tts-dataset/tts-dataset/venv/lib/python3.9/site-packages/datasets/arrow_writer.py:563, in ArrowWriter.finalize(self, close_stream)
[562](file:///home/user/repos/tts-dataset/tts-dataset/venv/lib/python3.9/site-packages/datasets/arrow_writer.py?line=561) if self._check_duplicates:
--> [563](file:///home/user/repos/tts-dataset/tts-dataset/venv/lib/python3.9/site-packages/datasets/arrow_writer.py?line=562) self.check_duplicate_keys()
[564](file:///home/user/repos/tts-dataset/tts-dataset/venv/lib/python3.9/site-packages/datasets/arrow_writer.py?line=563) # Re-intializing to empty list for next batch
File ~/repos/tts-dataset/tts-dataset/venv/lib/python3.9/site-packages/datasets/arrow_writer.py:492, in ArrowWriter.check_duplicate_keys(self)
[486](file:///home/user/repos/tts-dataset/tts-dataset/venv/lib/python3.9/site-packages/datasets/arrow_writer.py?line=485) duplicate_key_indices = [
[487](file:///home/user/repos/tts-dataset/tts-dataset/venv/lib/python3.9/site-packages/datasets/arrow_writer.py?line=486) str(self._num_examples + index)
[488](file:///home/user/repos/tts-dataset/tts-dataset/venv/lib/python3.9/site-packages/datasets/arrow_writer.py?line=487) for index, (duplicate_hash, _) in enumerate(self.hkey_record)
[489](file:///home/user/repos/tts-dataset/tts-dataset/venv/lib/python3.9/site-packages/datasets/arrow_writer.py?line=488) if duplicate_hash == hash
[490](file:///home/user/repos/tts-dataset/tts-dataset/venv/lib/python3.9/site-packages/datasets/arrow_writer.py?line=489) ]
--> [492](file:///home/user/repos/tts-dataset/tts-dataset/venv/lib/python3.9/site-packages/datasets/arrow_writer.py?line=491) raise DuplicatedKeysError(key, duplicate_key_indices)
[493](file:///home/user/repos/tts-dataset/tts-dataset/venv/lib/python3.9/site-packages/datasets/arrow_writer.py?line=492) else:
DuplicatedKeysError: Found multiple examples generated with the same key
The examples at index 858, 859 have the key dlg-89174425-d57a-4db7-a92b-165c3bff6735
The above exception was the direct cause of the following exception:
DatasetGenerationError Traceback (most recent call last)
Cell In[23], line 1
----> 1 dataset = load_dataset("taskmaster2", "music")
File ~/repos/tts-dataset/tts-dataset/venv/lib/python3.9/site-packages/datasets/load.py:1741, in load_dataset(path, name, data_dir, data_files, split, cache_dir, features, download_config, download_mode, ignore_verifications, keep_in_memory, save_infos, revision, use_auth_token, task, streaming, num_proc, **config_kwargs)
[1738](file:///home/user/repos/tts-dataset/tts-dataset/venv/lib/python3.9/site-packages/datasets/load.py?line=1737) try_from_hf_gcs = path not in _PACKAGED_DATASETS_MODULES
[1740](file:///home/user/repos/tts-dataset/tts-dataset/venv/lib/python3.9/site-packages/datasets/load.py?line=1739) # Download and prepare data
-> [1741](file:///home/user/repos/tts-dataset/tts-dataset/venv/lib/python3.9/site-packages/datasets/load.py?line=1740) builder_instance.download_and_prepare(
[1742](file:///home/user/repos/tts-dataset/tts-dataset/venv/lib/python3.9/site-packages/datasets/load.py?line=1741) download_config=download_config,
[1743](file:///home/user/repos/tts-dataset/tts-dataset/venv/lib/python3.9/site-packages/datasets/load.py?line=1742) download_mode=download_mode,
[1744](file:///home/user/repos/tts-dataset/tts-dataset/venv/lib/python3.9/site-packages/datasets/load.py?line=1743) ignore_verifications=ignore_verifications,
[1745](file:///home/user/repos/tts-dataset/tts-dataset/venv/lib/python3.9/site-packages/datasets/load.py?line=1744) try_from_hf_gcs=try_from_hf_gcs,
[1746](file:///home/user/repos/tts-dataset/tts-dataset/venv/lib/python3.9/site-packages/datasets/load.py?line=1745) use_auth_token=use_auth_token,
[1747](file:///home/user/repos/tts-dataset/tts-dataset/venv/lib/python3.9/site-packages/datasets/load.py?line=1746) num_proc=num_proc,
[1748](file:///home/user/repos/tts-dataset/tts-dataset/venv/lib/python3.9/site-packages/datasets/load.py?line=1747) )
[1750](file:///home/user/repos/tts-dataset/tts-dataset/venv/lib/python3.9/site-packages/datasets/load.py?line=1749) # Build dataset for splits
[1751](file:///home/user/repos/tts-dataset/tts-dataset/venv/lib/python3.9/site-packages/datasets/load.py?line=1750) keep_in_memory = (
[1752](file:///home/user/repos/tts-dataset/tts-dataset/venv/lib/python3.9/site-packages/datasets/load.py?line=1751) keep_in_memory if keep_in_memory is not None else is_small_dataset(builder_instance.info.dataset_size)
[1753](file:///home/user/repos/tts-dataset/tts-dataset/venv/lib/python3.9/site-packages/datasets/load.py?line=1752) )
File ~/repos/tts-dataset/tts-dataset/venv/lib/python3.9/site-packages/datasets/builder.py:822, in DatasetBuilder.download_and_prepare(self, output_dir, download_config, download_mode, ignore_verifications, try_from_hf_gcs, dl_manager, base_path, use_auth_token, file_format, max_shard_size, num_proc, storage_options, **download_and_prepare_kwargs)
[820](file:///home/user/repos/tts-dataset/tts-dataset/venv/lib/python3.9/site-packages/datasets/builder.py?line=819) if num_proc is not None:
[821](file:///home/user/repos/tts-dataset/tts-dataset/venv/lib/python3.9/site-packages/datasets/builder.py?line=820) prepare_split_kwargs["num_proc"] = num_proc
--> [822](file:///home/user/repos/tts-dataset/tts-dataset/venv/lib/python3.9/site-packages/datasets/builder.py?line=821) self._download_and_prepare(
[823](file:///home/user/repos/tts-dataset/tts-dataset/venv/lib/python3.9/site-packages/datasets/builder.py?line=822) dl_manager=dl_manager,
[824](file:///home/user/repos/tts-dataset/tts-dataset/venv/lib/python3.9/site-packages/datasets/builder.py?line=823) verify_infos=verify_infos,
[825](file:///home/user/repos/tts-dataset/tts-dataset/venv/lib/python3.9/site-packages/datasets/builder.py?line=824) **prepare_split_kwargs,
[826](file:///home/user/repos/tts-dataset/tts-dataset/venv/lib/python3.9/site-packages/datasets/builder.py?line=825) **download_and_prepare_kwargs,
[827](file:///home/user/repos/tts-dataset/tts-dataset/venv/lib/python3.9/site-packages/datasets/builder.py?line=826) )
[828](file:///home/user/repos/tts-dataset/tts-dataset/venv/lib/python3.9/site-packages/datasets/builder.py?line=827) # Sync info
[829](file:///home/user/repos/tts-dataset/tts-dataset/venv/lib/python3.9/site-packages/datasets/builder.py?line=828) self.info.dataset_size = sum(split.num_bytes for split in self.info.splits.values())
File ~/repos/tts-dataset/tts-dataset/venv/lib/python3.9/site-packages/datasets/builder.py:1555, in GeneratorBasedBuilder._download_and_prepare(self, dl_manager, verify_infos, **prepare_splits_kwargs)
[1554](file:///home/user/repos/tts-dataset/tts-dataset/venv/lib/python3.9/site-packages/datasets/builder.py?line=1553) def _download_and_prepare(self, dl_manager, verify_infos, **prepare_splits_kwargs):
-> [1555](file:///home/user/repos/tts-dataset/tts-dataset/venv/lib/python3.9/site-packages/datasets/builder.py?line=1554) super()._download_and_prepare(
[1556](file:///home/user/repos/tts-dataset/tts-dataset/venv/lib/python3.9/site-packages/datasets/builder.py?line=1555) dl_manager, verify_infos, check_duplicate_keys=verify_infos, **prepare_splits_kwargs
[1557](file:///home/user/repos/tts-dataset/tts-dataset/venv/lib/python3.9/site-packages/datasets/builder.py?line=1556) )
File ~/repos/tts-dataset/tts-dataset/venv/lib/python3.9/site-packages/datasets/builder.py:913, in DatasetBuilder._download_and_prepare(self, dl_manager, verify_infos, **prepare_split_kwargs)
[909](file:///home/user/repos/tts-dataset/tts-dataset/venv/lib/python3.9/site-packages/datasets/builder.py?line=908) split_dict.add(split_generator.split_info)
[911](file:///home/user/repos/tts-dataset/tts-dataset/venv/lib/python3.9/site-packages/datasets/builder.py?line=910) try:
[912](file:///home/user/repos/tts-dataset/tts-dataset/venv/lib/python3.9/site-packages/datasets/builder.py?line=911) # Prepare split will record examples associated to the split
--> [913](file:///home/user/repos/tts-dataset/tts-dataset/venv/lib/python3.9/site-packages/datasets/builder.py?line=912) self._prepare_split(split_generator, **prepare_split_kwargs)
[914](file:///home/user/repos/tts-dataset/tts-dataset/venv/lib/python3.9/site-packages/datasets/builder.py?line=913) except OSError as e:
[915](file:///home/user/repos/tts-dataset/tts-dataset/venv/lib/python3.9/site-packages/datasets/builder.py?line=914) raise OSError(
[916](file:///home/user/repos/tts-dataset/tts-dataset/venv/lib/python3.9/site-packages/datasets/builder.py?line=915) "Cannot find data file. "
[917](file:///home/user/repos/tts-dataset/tts-dataset/venv/lib/python3.9/site-packages/datasets/builder.py?line=916) + (self.manual_download_instructions or "")
[918](file:///home/user/repos/tts-dataset/tts-dataset/venv/lib/python3.9/site-packages/datasets/builder.py?line=917) + "\nOriginal error:\n"
[919](file:///home/user/repos/tts-dataset/tts-dataset/venv/lib/python3.9/site-packages/datasets/builder.py?line=918) + str(e)
[920](file:///home/user/repos/tts-dataset/tts-dataset/venv/lib/python3.9/site-packages/datasets/builder.py?line=919) ) from None
File ~/repos/tts-dataset/tts-dataset/venv/lib/python3.9/site-packages/datasets/builder.py:1396, in GeneratorBasedBuilder._prepare_split(self, split_generator, check_duplicate_keys, file_format, num_proc, max_shard_size)
[1394](file:///home/user/repos/tts-dataset/tts-dataset/venv/lib/python3.9/site-packages/datasets/builder.py?line=1393) gen_kwargs = split_generator.gen_kwargs
[1395](file:///home/user/repos/tts-dataset/tts-dataset/venv/lib/python3.9/site-packages/datasets/builder.py?line=1394) job_id = 0
-> [1396](file:///home/user/repos/tts-dataset/tts-dataset/venv/lib/python3.9/site-packages/datasets/builder.py?line=1395) for job_id, done, content in self._prepare_split_single(
[1397](file:///home/user/repos/tts-dataset/tts-dataset/venv/lib/python3.9/site-packages/datasets/builder.py?line=1396) {"gen_kwargs": gen_kwargs, "job_id": job_id, **_prepare_split_args}
[1398](file:///home/user/repos/tts-dataset/tts-dataset/venv/lib/python3.9/site-packages/datasets/builder.py?line=1397) ):
[1399](file:///home/user/repos/tts-dataset/tts-dataset/venv/lib/python3.9/site-packages/datasets/builder.py?line=1398) if done:
[1400](file:///home/user/repos/tts-dataset/tts-dataset/venv/lib/python3.9/site-packages/datasets/builder.py?line=1399) result = content
File ~/repos/tts-dataset/tts-dataset/venv/lib/python3.9/site-packages/datasets/builder.py:1550, in GeneratorBasedBuilder._prepare_split_single(self, arg)
[1548](file:///home/user/repos/tts-dataset/tts-dataset/venv/lib/python3.9/site-packages/datasets/builder.py?line=1547) if isinstance(e, SchemaInferenceError) and e.__context__ is not None:
[1549](file:///home/user/repos/tts-dataset/tts-dataset/venv/lib/python3.9/site-packages/datasets/builder.py?line=1548) e = e.__context__
-> [1550](file:///home/user/repos/tts-dataset/tts-dataset/venv/lib/python3.9/site-packages/datasets/builder.py?line=1549) raise DatasetGenerationError("An error occurred while generating the dataset") from e
[1552](file:///home/user/repos/tts-dataset/tts-dataset/venv/lib/python3.9/site-packages/datasets/builder.py?line=1551) yield job_id, True, (total_num_examples, total_num_bytes, writer._features, num_shards, shard_lengths)
DatasetGenerationError: An error occurred while generating the dataset
```
### Expected behavior
Loads the dataset
### Environment info
- `datasets` version: 2.7.1
- Platform: Linux-5.13.0-40-generic-x86_64-with-glibc2.31
- Python version: 3.9.7
- PyArrow version: 10.0.1
- Pandas version: 1.5.2
| 5,323 |
https://github.com/huggingface/datasets/issues/5317 | `ImageFolder` performs poorly with large datasets | [
"Hi ! ImageFolder is made for small scale datasets indeed. For large scale image datasets you better group your images in TAR archives or Arrow/Parquet files. This is true not just for ImageFolder loading performance, but also because having millions of files is not ideal for your filesystem or when moving the data around.\r\n\r\nOption 1. use TAR archives\r\n\r\nI'd suggest you to take a look at how we load [Imagenet](https://huggingface.co/datasets/imagenet-1k/tree/main) for example. The dataset is sharded in multiple TAR archives and there is a [script](https://huggingface.co/datasets/imagenet-1k/blob/main/imagenet-1k.py) that iterates over the archives to load the images.\r\n\r\nOption 2. use Arrow/Parquet\r\n\r\nYou can load your images as an Arrow Dataset with\r\n```python\r\nfrom datasets import Dataset, Image, load_from_disk, load_dataset\r\n\r\nds = Dataset.from_dict({\"image\": list(glob.glob(\"path/to/dir/**/*.jpg\"))})\r\n\r\ndef add_metadata(example):\r\n ...\r\n\r\nds = ds.map(add_metadata, num_proc=16) # num_proc for multiprocessing\r\nds = ds.cast_column(\"image\", Image())\r\n\r\n# save as Arrow locally\r\nds.save_to_disk(\"output_dir\")\r\nreloaded = load_from_disk(\"output_dir\")\r\n\r\n# OR save as Parquet on the HF Hub\r\nds.push_to_hub(\"username/dataset_name\")\r\nreloaded = load_dataset(\"username/dataset_name\")\r\n# reloaded = load_dataset(\"username/dataset_name\", num_proc=16) # to use multiprocessing\r\n```\r\n\r\nPS: maybe we can actually have something similar to ImageFolder but for image archives at one point ?",
"@lhoestq Thanks!\r\n\r\nPerhaps it'd be worth adding a note on the documentation that `ImageFolder` is not intended for large datasets? This limitation is not intuitively obvious to someone who has not used it before, I think.",
"Thanks for the feedback @salieri! I opened #5329 to make it clear `ImageFolder` is not intended for large datasets. Please feel free to comment if you have any other feedback! 🙂 "
] | ### Describe the bug
While testing image dataset creation, I'm seeing significant performance bottlenecks with imagefolders when scanning a directory structure with large number of images.
## Setup
* Nested directories (5 levels deep)
* 3M+ images
* 1 `metadata.jsonl` file
## Performance Degradation Point 1
Degradation occurs because [`get_data_files_patterns`](https://github.com/huggingface/datasets/blob/main/src/datasets/data_files.py#L231-L243) runs the exact same scan for many different types of patterns, and there doesn't seem to be a way to easily limit this. It's controlled by the definition of [`ALL_DEFAULT_PATTERNS`](https://github.com/huggingface/datasets/blob/main/src/datasets/data_files.py#L82-L85).
One scan with 3M+ files takes about 10-15 minutes to complete on my setup, so having those extra scans really slows things down – from 10 minutes to 60+. Most of the scans return no matches, but they still take a significant amount of time to complete – hence the poor performance.
As a side effect, when this scan is run on 3M+ image files, Python also consumes up to 12 GB of RAM, which is not ideal.
## Performance Degradation Point 2
The second performance bottleneck is in [`PackagedDatasetModuleFactory.get_module`](https://github.com/huggingface/datasets/blob/d7dfbc83d68e87ba002c5eb2555f7a932e59038a/src/datasets/load.py#L707-L711), which calls `DataFilesDict.from_local_or_remote`.
It runs for a long time (60min+), consuming significant amounts of RAM – even more than the point 1 above. Based on `iostat -d 2`, it performs **zero** disk operations, which to me suggests that there is a code based bottleneck there that could be sorted out.
### Steps to reproduce the bug
```python
from datasets import load_dataset
import os
import huggingface_hub
dataset = load_dataset(
'imagefolder',
data_dir='/some/path',
# just to spell it out:
split=None,
drop_labels=True,
keep_in_memory=False
)
dataset.push_to_hub('account/dataset', private=True)
```
### Expected behavior
While it's certainly possible to write a custom loader to replace `ImageFolder` with, it'd be great if the off-the-shelf `ImageFolder` would by default have a setup that can scale to large datasets.
Or perhaps there could be a dedicated loader just for large datasets that trades off flexibility for performance? As in, maybe you have to define explicitly how you want it to work rather than it trying to guess your data structure like `_get_data_files_patterns()` does?
### Environment info
- `datasets` version: 2.7.1
- Platform: Linux-4.14.296-222.539.amzn2.x86_64-x86_64-with-glibc2.2.5
- Python version: 3.7.10
- PyArrow version: 10.0.1
- Pandas version: 1.3.5
| 5,317 |
https://github.com/huggingface/datasets/issues/5316 | Bug in sample_by="paragraph" | [
"Thanks for reporting, @adampauls.\r\n\r\nWe are having a look at it. "
] | ### Describe the bug
I think [this line](https://github.com/huggingface/datasets/blob/main/src/datasets/packaged_modules/text/text.py#L96) is wrong and should be `batch = f.read(self.config.chunksize)`. Otherwise it will never terminate because even when `f` is finished reading, `batch` will still be truthy from the last iteration.
### Steps to reproduce the bug
```
> cat test.txt
a b c
d e f
````
```python
>>> import datasets
>>> datasets.load_dataset("text", data_files={"train":"test.txt"}, sample_by="paragraph")
```
This will go on forever.
### Expected behavior
Terminates very quickly.
### Environment info
`version = "2.6.1"` but I think the bug is still there on main. | 5,316 |
https://github.com/huggingface/datasets/issues/5315 | Adding new splits to a dataset script with existing old splits info in metadata's `dataset_info` fails | [
"EDIT:\r\nI think in this case, the metadata files (either README or JSON) should not be read (i.e. `self.info.splits` should be None).\r\n\r\nOne idea: \r\n- I think ideally we should set this behavior when we pass `--save_info` to the CLI `test`\r\n- However, currently, the builder is unaware of this: `save_info` arg is not passed to it",
"> I think in this case\r\n\r\n@albertvillanova You mean in cases when the script was changed? \r\n\r\nI suggest that we:\r\n* add a check on the slice (like 'split_name[n%]) kind of format here: https://github.com/huggingface/datasets/blob/main/src/datasets/splits.py#L523 to catch things like this. \r\n* Error here happens before splits verification, but in `_prepare_split`, and `_prepare_split` doesn't perform any verification and don't know about it. so we can pass this parameter and take splits from `split_generator`, not from `split.info` in case when `verify_infos` is False\r\n* we can check if split **names** from split_generators and self.info.splits are the same **before** preparing splits (if `verify_info=True`) so that we don't spend time on generating unwanted data. \r\n* provide some user-friendly warnings about `ignore_verifications` parameter so that users know that if something is not matching they can ignore it\r\n\r\nI started it here: https://github.com/huggingface/datasets/pull/5327/files\r\n\r\nWhat do you think @albertvillanova ?",
"I edited my previous comment:\r\n- First I proposed setting `self.info.splits` to None when `ignore_verifications=True`\r\n - I thought it was the easiest implementation because `ignore_verifications` is passed to `DatasetBuilder.download_and_prepare`\r\n - However, afterwards, I realized this might not be a good idea for this use case:\r\n - A user wants to optimize the loading of the dataset, and passes `ignore_verifications=False` to avoid all the verifications\r\n - In this case, we want `self.info.splits` to be read from metadata file\r\n- Then, I thought that it might be better to set `self.info.splits` to None when we pass `--save_info` to the CLI test: if we are going to save the info to the metadata file, it makes no sense to read the info from the metadata file\r\n - This implementation is not so easy because the Builder knows nothing about `--save_info`\r\n\r\nI agree with you there are 2 things to be addressed here:\r\n- One is what I have just commented: `self.info.splits` should be None in this case\r\n- The other, a validation should be implemented when calling `make_file_instructions` and/or `SplitDict.__getitem__`, so that when passing \"training\" to it, we get a more descriptive error other than `TypeError: expected str, bytes or os.PathLike object, not NoneType` "
] | ### Describe the bug
If you first create a custom dataset with a specific set of splits, generate metadata with `datasets-cli test ... --save_info`, then change your script to include more splits, it fails.
That's what happened in https://huggingface.co/datasets/mrdbourke/food_vision_199_classes/discussions/2#6385fd1269634850f8ddff48.
### Steps to reproduce the bug
1. create a dataset with a custom split that returns, for example, only `"train"` split in `_splits_generators'`. specifically, if really want to reproduce, copy `https://huggingface.co/datasets/mrdbourke/food_vision_199_classes/blob/main/food_vision_199_classes.py
2. run `datasets-cli test dataset_script.py --save_info --all_configs` - this would generate metadata yaml in `README.md` that would contain info about splits, for example, like this:
```
splits:
- name: train
num_bytes: 2973286
num_examples: 19747
```
3. make changes to your script so that it returns another set of splits, for example, `"train"` and `"test"` (uncomment [these lines](https://huggingface.co/datasets/mrdbourke/food_vision_199_classes/blob/main/food_vision_199_classes.py#L271))
4. run `load_dataset` and get the following error:
```python
Traceback (most recent call last):
File "/home/daniel/code/pytorch/env/bin/datasets-cli", line 8, in <module>
sys.exit(main())
File "/home/daniel/code/pytorch/env/lib/python3.8/site-packages/datasets/commands/datasets_cli.py", line 39, in main
service.run()
File "/home/daniel/code/pytorch/env/lib/python3.8/site-packages/datasets/commands/test.py", line 141, in run
builder.download_and_prepare(
File "/home/daniel/code/pytorch/env/lib/python3.8/site-packages/datasets/builder.py", line 822, in download_and_prepare
self._download_and_prepare(
File "/home/daniel/code/pytorch/env/lib/python3.8/site-packages/datasets/builder.py", line 1555, in _download_and_prepare
super()._download_and_prepare(
File "/home/daniel/code/pytorch/env/lib/python3.8/site-packages/datasets/builder.py", line 913, in _download_and_prepare
self._prepare_split(split_generator, **prepare_split_kwargs)
File "/home/daniel/code/pytorch/env/lib/python3.8/site-packages/datasets/builder.py", line 1356, in _prepare_split
split_info = self.info.splits[split_generator.name]
File "/home/daniel/code/pytorch/env/lib/python3.8/site-packages/datasets/splits.py", line 525, in __getitem__
instructions = make_file_instructions(
File "/home/daniel/code/pytorch/env/lib/python3.8/site-packages/datasets/arrow_reader.py", line 111, in make_file_instructions
name2filenames = {
File "/home/daniel/code/pytorch/env/lib/python3.8/site-packages/datasets/arrow_reader.py", line 112, in <dictcomp>
info.name: filenames_for_dataset_split(
File "/home/daniel/code/pytorch/env/lib/python3.8/site-packages/datasets/naming.py", line 78, in filenames_for_dataset_split
prefix = filename_prefix_for_split(dataset_name, split)
File "/home/daniel/code/pytorch/env/lib/python3.8/site-packages/datasets/naming.py", line 57, in filename_prefix_for_split
if os.path.basename(name) != name:
File "/home/daniel/code/pytorch/env/lib/python3.8/posixpath.py", line 143, in basename
p = os.fspath(p)
TypeError: expected str, bytes or os.PathLike object, not NoneType
```
5. bonus: try to regenerate metadata in `README.md` with `datasets-cli` as in step 2 and get the same error.
This is because `dataset.info.splits` contains only `"train"` split so when we are doing `self.info.splits[split_generator.name]` it tries to infer smth like `info.splits['train[50%]']` and that's not the case and it fails.
### Expected behavior
to be discussed?
This can be solved by removing splits information from metadata file first. But I wonder if there is a better way.
### Environment info
- Datasets version: 2.7.1
- Python version: 3.8.13 | 5,315 |
https://github.com/huggingface/datasets/issues/5314 | Datasets: classification_report() got an unexpected keyword argument 'suffix' | [
"This seems similar to https://github.com/huggingface/datasets/issues/2512 Can you try to update seqeval ? ",
"@JonathanAlis also note that the metrics are deprecated in our `datasets` library.\r\n\r\nPlease, use the new library 🤗 Evaluate instead: https://huggingface.co/docs/evaluate"
] | https://github.com/huggingface/datasets/blob/main/metrics/seqeval/seqeval.py
> import datasets
predictions = [['O', 'O', 'B-MISC', 'I-MISC', 'I-MISC', 'I-MISC', 'O'], ['B-PER', 'I-PER', 'O']]
references = [['O', 'O', 'O', 'B-MISC', 'I-MISC', 'I-MISC', 'O'], ['B-PER', 'I-PER', 'O']]
seqeval = datasets.load_metric("seqeval")
results = seqeval.compute(predictions=predictions, references=references)
print(list(results.keys()))
print(results["overall_f1"])
print(results["PER"]["f1"])
It raises the error:
> TypeError: classification_report() got an unexpected keyword argument 'suffix'
For context, versions on my pip list -v
> datasets 1.12.1
seqeval 1.2.2 | 5,314 |
https://github.com/huggingface/datasets/issues/5306 | Can't use custom feature description when loading a dataset | [
"Forgot to actually convert the feature dict to a Feature object. Closing."
] | ### Describe the bug
I have created a feature dictionary to describe my datasets' column types, to use when loading the dataset, following [the doc](https://huggingface.co/docs/datasets/main/en/about_dataset_features). It crashes at dataset load.
### Steps to reproduce the bug
```python
# Creating features
task_list = [f"motif_G{i}" for i in range(19, 53)]
features = {t: Sequence(feature=Value(dtype="float64")) for t in task_list}
for col_name in ["class_label"]:
features[col_name] = Sequence(feature=Value(dtype="int64"))
for col_name in ["num_nodes"]:
features[col_name] = Value(dtype="int64")
for col_name in ["num_bridges", "num_cycles", "avg_shortest_path_len"]:
features[col_name] = Sequence(feature=Value(dtype="float64"))
for col_name in ["edge_attr", "node_feat", "edge_index"]:
features[col_name] = Sequence(feature=Sequence(feature=Value(dtype="int64")))
print(features)
dataset = load_dataset(path=f"graphs-datasets/unbalanced-motifs-500K", split="train", features=features)
```
Last line will crash and say 'TypeError: argument of type 'Sequence' is not iterable'.
Full stack:
```
Traceback (most recent call last):
File "pretrain_tokengt.py", line 131, in <module>
main(output_folder = "../workspace/pretraining",
File "pretrain_tokengt.py", line 52, in main
dataset = load_dataset(path=f"graphs-datasets/{dataset_name}", split="train", features=features)
File "huggingface_env/lib/python3.8/site-packages/datasets/load.py", line 1718, in load_dataset
builder_instance = load_dataset_builder(
File "huggingface_env/lib/python3.8/site-packages/datasets/load.py", line 1514, in load_dataset_builder
builder_instance: DatasetBuilder = builder_cls(
File "huggingface_env/lib/python3.8/site-packages/datasets/builder.py", line 321, in __init__
info.update(self._info())
File "huggingface_env/lib/python3.8/site-packages/datasets/packaged_modules/json/json.py", line 62, in _info
return datasets.DatasetInfo(features=self.config.features)
File "<string>", line 20, in __init__
File "huggingface_env/lib/python3.8/site-packages/datasets/info.py", line 155, in __post_init__
self.features = Features.from_dict(self.features)
File "huggingface_env/lib/python3.8/site-packages/datasets/features/features.py", line 1599, in from_dict
obj = generate_from_dict(dic)
File "huggingface_env/lib/python3.8/site-packages/datasets/features/features.py", line 1282, in generate_from_dict
return {key: generate_from_dict(value) for key, value in obj.items()}
File "huggingface_env/lib/python3.8/site-packages/datasets/features/features.py", line 1282, in <dictcomp>
return {key: generate_from_dict(value) for key, value in obj.items()}
File "huggingface_env/lib/python3.8/site-packages/datasets/features/features.py", line 1281, in generate_from_dict
if "_type" not in obj or isinstance(obj["_type"], dict):
TypeError: argument of type 'Sequence' is not iterable
```
### Expected behavior
For it not to crash.
### Environment info
- `datasets` version: 2.7.1
- Platform: Linux-5.14.0-1054-oem-x86_64-with-glibc2.29
- Python version: 3.8.10
- PyArrow version: 8.0.0
- Pandas version: 1.4.3 | 5,306 |
https://github.com/huggingface/datasets/issues/5305 | Dataset joelito/mc4_legal does not work with multiple files | [
"Thanks for reporting @JoelNiklaus.\r\n\r\nPlease note that since we moved all dataset loading scripts to the Hub, the issues and pull requests relative to specific datasets are directly handled on the Hub, in their Community tab. I'm transferring this issue there: https://huggingface.co/datasets/joelito/mc4_legal/discussions\r\n\r\nI am also having a look at the bug in your script.",
"Issue transferred to: https://huggingface.co/datasets/joelito/mc4_legal/discussions/1"
] | ### Describe the bug
The dataset https://huggingface.co/datasets/joelito/mc4_legal works for languages like bg with a single data file, but not for languages with multiple files like de. It shows zero rows for the de dataset.
joelniklaus@Joels-MacBook-Pro ~/N/P/C/L/p/m/mc4_legal (main) [1]> python test_mc4_legal.py (debug)
Found cached dataset mc4_legal (/Users/joelniklaus/.cache/huggingface/datasets/mc4_legal/de/0.0.0/fb6952a097180f8c936e2a7605525ff670354a344fc1a2c70107684d3f7cb02f)
Dataset({
features: ['index', 'url', 'timestamp', 'matches', 'text'],
num_rows: 0
})
joelniklaus@Joels-MacBook-Pro ~/N/P/C/L/p/m/mc4_legal (main)> python test_mc4_legal.py (debug)
Downloading and preparing dataset mc4_legal/bg to /Users/joelniklaus/.cache/huggingface/datasets/mc4_legal/bg/0.0.0/fb6952a097180f8c936e2a7605525ff670354a344fc1a2c70107684d3f7cb02f...
Downloading data files: 100%|███████████████████████████████████████████████████████████████████████████████████████████████████████████████████████████████████████████████████████████| 1/1 [00:00<00:00, 1240.55it/s]
Dataset mc4_legal downloaded and prepared to /Users/joelniklaus/.cache/huggingface/datasets/mc4_legal/bg/0.0.0/fb6952a097180f8c936e2a7605525ff670354a344fc1a2c70107684d3f7cb02f. Subsequent calls will reuse this data.
Dataset({
features: ['index', 'url', 'timestamp', 'matches', 'text'],
num_rows: 204
})
### Steps to reproduce the bug
import datasets
from datasets import load_dataset, get_dataset_config_names
language = "bg"
test = load_dataset("joelito/mc4_legal", language, split='train')
### Expected behavior
It should display the correct number of rows for the de dataset which should be a large number (thousands or more).
### Environment info
Package Version
------------------------ --------------
absl-py 1.3.0
aiohttp 3.8.1
aiosignal 1.2.0
astunparse 1.6.3
async-timeout 4.0.2
attrs 22.1.0
beautifulsoup4 4.11.1
blinker 1.4
blis 0.7.8
Bottleneck 1.3.4
brotlipy 0.7.0
cachetools 5.2.0
catalogue 2.0.7
certifi 2022.5.18.1
cffi 1.15.1
chardet 4.0.0
charset-normalizer 2.1.0
click 8.0.4
conllu 4.5.2
cryptography 38.0.1
cymem 2.0.6
datasets 2.6.1
dill 0.3.5.1
docker-pycreds 0.4.0
fasttext 0.9.2
fasttext-langdetect 1.0.3
filelock 3.0.12
flatbuffers 20210226132247
frozenlist 1.3.0
fsspec 2022.5.0
gast 0.4.0
gcloud 0.18.3
gitdb 4.0.9
GitPython 3.1.27
google-auth 2.9.0
google-auth-oauthlib 0.4.6
google-pasta 0.2.0
googleapis-common-protos 1.57.0
grpcio 1.47.0
h5py 3.7.0
httplib2 0.21.0
huggingface-hub 0.8.1
idna 3.4
importlib-metadata 4.12.0
Jinja2 3.1.2
joblib 1.0.1
keras 2.9.0
Keras-Preprocessing 1.1.2
langcodes 3.3.0
lxml 4.9.1
Markdown 3.3.7
MarkupSafe 2.1.1
mkl-fft 1.3.1
mkl-random 1.2.2
mkl-service 2.4.0
multidict 6.0.2
multiprocess 0.70.13
murmurhash 1.0.7
numexpr 2.8.1
numpy 1.22.3
oauth2client 4.1.3
oauthlib 3.2.1
opt-einsum 3.3.0
packaging 21.3
pandas 1.4.2
pathtools 0.1.2
pathy 0.6.1
pip 21.1.2
preshed 3.0.6
promise 2.3
protobuf 4.21.9
psutil 5.9.1
pyarrow 8.0.0
pyasn1 0.4.8
pyasn1-modules 0.2.8
pybind11 2.9.2
pycountry 22.3.5
pycparser 2.21
pydantic 1.8.2
PyJWT 2.4.0
pylzma 0.5.0
pyOpenSSL 22.0.0
pyparsing 3.0.4
PySocks 1.7.1
python-dateutil 2.8.2
pytz 2021.3
PyYAML 6.0
regex 2021.4.4
requests 2.28.1
requests-oauthlib 1.3.1
responses 0.18.0
rsa 4.8
sacremoses 0.0.45
scikit-learn 1.1.1
scipy 1.8.1
sentencepiece 0.1.96
sentry-sdk 1.6.0
setproctitle 1.2.3
setuptools 65.5.0
shortuuid 1.0.9
six 1.16.0
smart-open 5.2.1
smmap 5.0.0
soupsieve 2.3.2.post1
spacy 3.3.1
spacy-legacy 3.0.9
spacy-loggers 1.0.2
srsly 2.4.3
tabulate 0.8.9
tensorboard 2.9.1
tensorboard-data-server 0.6.1
tensorboard-plugin-wit 1.8.1
tensorflow 2.9.1
tensorflow-estimator 2.9.0
termcolor 2.1.0
thinc 8.0.17
threadpoolctl 3.1.0
tokenizers 0.12.1
torch 1.13.0
tqdm 4.64.0
transformers 4.20.1
typer 0.4.1
typing-extensions 4.3.0
Unidecode 1.3.6
urllib3 1.26.12
wandb 0.12.20
wasabi 0.9.1
web-anno-tsv 0.0.1
Werkzeug 2.1.2
wget 3.2
wheel 0.35.1
wrapt 1.14.1
xxhash 3.0.0
yarl 1.8.1
zipp 3.8.0
Python 3.8.10
| 5,305 |
https://github.com/huggingface/datasets/issues/5304 | timit_asr doesn't load the test split. | [
"The [timit_asr.py](https://huggingface.co/datasets/timit_asr/blob/main/timit_asr.py) script iterates over the WAV files per split directory using this:\r\n```python\r\nwav_paths = sorted(Path(data_dir).glob(f\"**/{split}/**/*.wav\"))\r\nwav_paths = wav_paths if wav_paths else sorted(Path(data_dir).glob(f\"**/{split.upper()}/**/*.WAV\"))\r\n```\r\n\r\nCan you check that there is a directory named \"test\" somewhere in your timit data directory ?"
] | ### Describe the bug
When I use the function ```timit = load_dataset('timit_asr', data_dir=data_dir)```, it only loads train split, not test split.
I tried to change the directory and filename to lower case to upper case for the test split, but it does not work at all.
```python
DatasetDict({
train: Dataset({
features: ['file', 'audio', 'text', 'phonetic_detail', 'word_detail', 'dialect_region', 'sentence_type', 'speaker_id', 'id'],
num_rows: 4620
})
test: Dataset({
features: ['file', 'audio', 'text', 'phonetic_detail', 'word_detail', 'dialect_region', 'sentence_type', 'speaker_id', 'id'],
num_rows: 0
})
})
```
The directory structure of both splits are same. (DIALECT_REGION / SPEAKER_CODE / DATA_FILES)
### Steps to reproduce the bug
1. just use ```timit = load_dataset('timit_asr', data_dir=data_dir)```
### Expected behavior
```python
DatasetDict({
train: Dataset({
features: ['file', 'audio', 'text', 'phonetic_detail', 'word_detail', 'dialect_region', 'sentence_type', 'speaker_id', 'id'],
num_rows: 4620
})
test: Dataset({
features: ['file', 'audio', 'text', 'phonetic_detail', 'word_detail', 'dialect_region', 'sentence_type', 'speaker_id', 'id'],
num_rows: 1680
})
})
```
### Environment info
- ubuntu 20.04
- python 3.9.13
- datasets 2.7.1 | 5,304 |
https://github.com/huggingface/datasets/issues/5298 | Bug in xopen with Windows pathnames | [] | Currently, `xopen` function has a bug with local Windows pathnames:
From its implementation:
```python
def xopen(file: str, mode="r", *args, **kwargs):
file = _as_posix(PurePath(file))
main_hop, *rest_hops = file.split("::")
if is_local_path(main_hop):
return open(file, mode, *args, **kwargs)
```
On a Windows machine, if we pass the argument:
```python
xopen("C:\\Users\\USERNAME\\filename.txt")
```
it returns
```python
open("C:/Users/USERNAME/filename.txt")
``` | 5,298 |
https://github.com/huggingface/datasets/issues/5296 | Bug in xjoin with Windows pathnames | [] | Currently, `xjoin` function has a bug with local Windows pathnames: instead of returning the OS-dependent join pathname, it always returns it in POSIX format.
```python
from datasets.download.streaming_download_manager import xjoin
path = xjoin("C:\\Users\\USERNAME", "filename.txt")
```
Join path should be:
```python
"C:\\Users\\USERNAME\\filename.txt"
```
However it is:
```python
"C:/Users/USERNAME/filename.txt"
``` | 5,296 |
https://github.com/huggingface/datasets/issues/5295 | Extractions failed when .zip file located on read-only path (e.g., SageMaker FastFile mode) | [
"Hi ! Thanks for reporting. Indeed the lock file should be placed in a directory with write permission (e.g. in the directory where the archive is extracted).",
"I opened https://github.com/huggingface/datasets/pull/5320 to fix this - it places the lock file in the cache directory instead of trying to put in next to the ZIP where it's read-only"
] | ### Describe the bug
Hi,
`load_dataset()` does not work .zip files located on a read-only directory. Looks like it's because Dataset creates a lock file in the [same directory](https://github.com/huggingface/datasets/blob/df4bdd365f2abb695f113cbf8856a925bc70901b/src/datasets/utils/extract.py) as the .zip file.
Encountered this when attempting `load_dataset()` on a datadir with SageMaker FastFile mode.
### Steps to reproduce the bug
```python
# Showing relevant lines only.
hyperparameters = {
"dataset_name": "ydshieh/coco_dataset_script",
"dataset_config_name": 2017,
"data_dir": "/opt/ml/input/data/coco",
"cache_dir": "/tmp/huggingface-cache", # Fix dataset complains out-of-space.
...
}
estimator = PyTorch(
base_job_name="clip",
source_dir="../src/sm-entrypoint",
entry_point="run_clip.py", # Transformers/src/examples/pytorch/contrastive-image-text/run_clip.py
framework_version="1.12",
py_version="py38",
hyperparameters=hyperparameters,
instance_count=1,
instance_type="ml.p3.16xlarge",
volume_size=100,
distribution={"smdistributed": {"dataparallel": {"enabled": True}}},
)
fast_file = lambda x: TrainingInput(x, input_mode='FastFile')
estimator.fit(
{
"pre-trained": fast_file("s3://vm-sagemakerr-us-east-1/clip/pre-trained-checkpoint/"),
"coco": fast_file("s3://vm-sagemakerr-us-east-1/clip/coco-zip-files/"),
}
)
```
Error message:
```text
ErrorMessage "OSError: [Errno 30] Read-only file system: '/opt/ml/input/data/coco/image_info_test2017.zip.lock'
"""
The above exception was the direct cause of the following exception
Traceback (most recent call last)
File "/opt/conda/lib/python3.8/runpy.py", line 194, in _run_module_as_main
return _run_code(code, main_globals, None,
File "/opt/conda/lib/python3.8/runpy.py", line 87, in _run_code
exec(code, run_globals)
File "/opt/conda/lib/python3.8/site-packages/mpi4py/__main__.py", line 7, in <module>
main()
File "/opt/conda/lib/python3.8/site-packages/mpi4py/run.py", line 198, in main
run_command_line(args)
File "/opt/conda/lib/python3.8/site-packages/mpi4py/run.py", line 47, in run_command_line
run_path(sys.argv[0], run_name='__main__')
File "/opt/conda/lib/python3.8/runpy.py", line 265, in run_path
return _run_module_code(code, init_globals, run_name,
File "/opt/conda/lib/python3.8/runpy.py", line 97, in _run_module_code
_run_code(code, mod_globals, init_globals,
File "run_clip_smddp.py", line 594, in <module>
File "run_clip_smddp.py", line 327, in main
dataset = load_dataset(
File "/opt/conda/lib/python3.8/site-packages/datasets/load.py", line 1741, in load_dataset
builder_instance.download_and_prepare(
File "/opt/conda/lib/python3.8/site-packages/datasets/builder.py", line 822, in download_and_prepare
self._download_and_prepare(
File "/opt/conda/lib/python3.8/site-packages/datasets/builder.py", line 1555, in _download_and_prepare
super()._download_and_prepare(
File "/opt/conda/lib/python3.8/site-packages/datasets/builder.py", line 891, in _download_and_prepare
split_generators = self._split_generators(dl_manager, **split_generators_kwargs)
File "/root/.cache/huggingface/modules/datasets_modules/datasets/ydshieh--coco_dataset_script/e033205c0266a54c10be132f9264f2a39dcf893e798f6756d224b1ff5078998f/coco_dataset_script.py", line 123, in _split_generators
archive_path = dl_manager.download_and_extract(_DL_URLS)
File "/opt/conda/lib/python3.8/site-packages/datasets/download/download_manager.py", line 447, in download_and_extract
return self.extract(self.download(url_or_urls))
File "/opt/conda/lib/python3.8/site-packages/datasets/download/download_manager.py", line 419, in extract
extracted_paths = map_nested(
File "/opt/conda/lib/python3.8/site-packages/datasets/utils/py_utils.py", line 472, in map_nested
mapped = pool.map(_single_map_nested, split_kwds)
File "/opt/conda/lib/python3.8/multiprocessing/pool.py", line 364, in map
return self._map_async(func, iterable, mapstar, chunksize).get()
File "/opt/conda/lib/python3.8/multiprocessing/pool.py", line 771, in get
raise self._value
OSError: [Errno 30] Read-only file system: '/opt/ml/input/data/coco/image_info_test2017.zip.lock'"
```
### Expected behavior
`load_dataset()` to succeed, just like when .zip file is passed in SageMaker File mode.
### Environment info
* datasets-2.7.1
* transformers-4.24.0
* python-3.8
* torch-1.12
* SageMaker PyTorch DLC | 5,295 |
https://github.com/huggingface/datasets/issues/5293 | Support streaming datasets with pathlib.Path.with_suffix | [] | Extend support for streaming datasets that use `pathlib.Path.with_suffix`.
This feature will be useful e.g. for datasets containing text files and annotated files with the same name but different extension. | 5,293 |
https://github.com/huggingface/datasets/issues/5292 | Missing documentation build for versions 2.7.1 and 2.6.2 | [
"- Build docs for 2.6.2:\r\n - Commit: a6a5a1cf4cdf1e0be65168aed5a327f543001fe8\r\n - Build docs GH Action: https://github.com/huggingface/datasets/actions/runs/3539470622/jobs/5941404044\r\n- Build docs for 2.7.1:\r\n - Commit: 5ef1ab1cc06c2b7a574bf2df454cd9fcb071ccb2\r\n - Build docs GH Action: https://github.com/huggingface/datasets/actions/runs/3539574442/jobs/5941636792"
] | After the patch releases [2.7.1](https://github.com/huggingface/datasets/releases/tag/2.7.1) and [2.6.2](https://github.com/huggingface/datasets/releases/tag/2.6.2), the online docs were not properly built (the build_documentation workflow was not triggered).
There was a fix by:
- #5291
However, both documentations were built from main branch, instead of their corresponding version branch.
We are rebuilding them. | 5,292 |
https://github.com/huggingface/datasets/issues/5288 | Lossy json serialization - deserialization of dataset info | [
"Hi ! JSON is a lossy format indeed. If you want to keep the feature types or other metadata I'd encourage you to store them as well. For example you can use `dataset.info.write_to_directory` and `DatasetInfo.from_directory` to store the feature types, split info, description, license etc."
] | ### Describe the bug
Saving a dataset to disk as json (using `to_json`) and then loading it again (using `load_dataset`) results in features whose labels are not type-cast correctly. In the code snippet below, `features.label` should have a label of type `ClassLabel` but has type `Value` instead.
### Steps to reproduce the bug
```
from datasets import load_dataset
def test_serdes_from_json(d):
dataset = load_dataset(d, split="train")
dataset.to_json('_test')
dataset_loaded = load_dataset("json", data_files='_test', split='train')
try:
assert dataset_loaded.info.features == dataset.info.features, "features unequal!"
except Exception as ex:
print(f'{ex}')
print(f'expected {dataset.info.features}, \nactual { dataset_loaded.info.features }')
test_serdes_from_json('rotten_tomatoes')
```
Output
```
features unequal!
expected {'text': Value(dtype='string', id=None), 'label': ClassLabel(names=['neg', 'pos'], id=None)},
actual {'text': Value(dtype='string', id=None), 'label': Value(dtype='int64', id=None)}
```
### Expected behavior
The deserialized `features.label` should have type `ClassLabel`.
### Environment info
- `datasets` version: 2.6.1
- Platform: Linux-5.10.144-127.601.amzn2.x86_64-x86_64-with-glibc2.17
- Python version: 3.7.13
- PyArrow version: 7.0.0
- Pandas version: 1.2.3 | 5,288 |
https://github.com/huggingface/datasets/issues/5286 | FileNotFoundError: Couldn't find file at https://dumps.wikimedia.org/enwiki/20220301/dumpstatus.json | [
"I found a solution \r\n\r\nIf you specifically install datasets==1.18 and then run\r\n\r\nimport datasets\r\nwiki = datasets.load_dataset('wikipedia', '20200501.en')\r\nthen this should work (it worked for me.)",
"I have the same problem here but installing datasets==1.18 wont work for me\r\n"
] | ### Describe the bug
I follow the steps provided on the website [https://huggingface.co/datasets/wikipedia](https://huggingface.co/datasets/wikipedia)
$ pip install apache_beam mwparserfromhell
>>> from datasets import load_dataset
>>> load_dataset("wikipedia", "20220301.en")
however this results in the following error:
raise MissingBeamOptions(
datasets.builder.MissingBeamOptions: Trying to generate a dataset using Apache Beam, yet no Beam Runner or PipelineOptions() has been provided in `load_dataset` or in the builder arguments. For big datasets it has to run on large-scale data processing tools like Dataflow, Spark, etc. More information about Apache Beam runners at https://beam.apache.org/documentation/runners/capability-matrix/
If you really want to run it locally because you feel like the Dataset is small enough, you can use the local beam runner called `DirectRunner` (you may run out of memory).
Example of usage:
`load_dataset('wikipedia', '20220301.en', beam_runner='DirectRunner')`
If I then prompt the system with:
>>> load_dataset('wikipedia', '20220301.en', beam_runner='DirectRunner')
the following error occurs:
raise FileNotFoundError(f"Couldn't find file at {url}")
FileNotFoundError: Couldn't find file at https://dumps.wikimedia.org/enwiki/20220301/dumpstatus.json
Here is the exact code:
Python 3.10.6 (main, Nov 2 2022, 18:53:38) [GCC 11.3.0] on linux
Type "help", "copyright", "credits" or "license" for more information.
>>> from datasets import load_dataset
>>> load_dataset('wikipedia', '20220301.en')
Downloading and preparing dataset wikipedia/20220301.en to /home/[EDITED]/.cache/huggingface/datasets/wikipedia/20220301.en/2.0.0/aa542ed919df55cc5d3347f42dd4521d05ca68751f50dbc32bae2a7f1e167559...
Downloading: 100%|████████████████████████████████████████████████████████████████████████████| 15.3k/15.3k [00:00<00:00, 22.2MB/s]
Traceback (most recent call last):
File "<stdin>", line 1, in <module>
File "/usr/local/lib/python3.10/dist-packages/datasets/load.py", line 1741, in load_dataset
builder_instance.download_and_prepare(
File "/usr/local/lib/python3.10/dist-packages/datasets/builder.py", line 822, in download_and_prepare
self._download_and_prepare(
File "/usr/local/lib/python3.10/dist-packages/datasets/builder.py", line 1879, in _download_and_prepare
raise MissingBeamOptions(
datasets.builder.MissingBeamOptions: Trying to generate a dataset using Apache Beam, yet no Beam Runner or PipelineOptions() has been provided in `load_dataset` or in the builder arguments. For big datasets it has to run on large-scale data processing tools like Dataflow, Spark, etc. More information about Apache Beam runners at https://beam.apache.org/documentation/runners/capability-matrix/
If you really want to run it locally because you feel like the Dataset is small enough, you can use the local beam runner called `DirectRunner` (you may run out of memory).
Example of usage:
`load_dataset('wikipedia', '20220301.en', beam_runner='DirectRunner')`
>>> load_dataset('wikipedia', '20220301.en', beam_runner='DirectRunner')
Downloading and preparing dataset wikipedia/20220301.en to /home/[EDITED]/.cache/huggingface/datasets/wikipedia/20220301.en/2.0.0/aa542ed919df55cc5d3347f42dd4521d05ca68751f50dbc32bae2a7f1e167559...
Downloading: 100%|████████████████████████████████████████████████████████████████████████████| 15.3k/15.3k [00:00<00:00, 18.8MB/s]
Downloading data files: 0%| | 0/1 [00:00<?, ?it/s]Traceback (most recent call last):
File "<stdin>", line 1, in <module>
File "/usr/local/lib/python3.10/dist-packages/datasets/load.py", line 1741, in load_dataset
builder_instance.download_and_prepare(
File "/usr/local/lib/python3.10/dist-packages/datasets/builder.py", line 822, in download_and_prepare
self._download_and_prepare(
File "/usr/local/lib/python3.10/dist-packages/datasets/builder.py", line 1909, in _download_and_prepare
super()._download_and_prepare(
File "/usr/local/lib/python3.10/dist-packages/datasets/builder.py", line 891, in _download_and_prepare
split_generators = self._split_generators(dl_manager, **split_generators_kwargs)
File "/home/rorytol/.cache/huggingface/modules/datasets_modules/datasets/wikipedia/aa542ed919df55cc5d3347f42dd4521d05ca68751f50dbc32bae2a7f1e167559/wikipedia.py", line 945, in _split_generators
downloaded_files = dl_manager.download_and_extract({"info": info_url})
File "/usr/local/lib/python3.10/dist-packages/datasets/download/download_manager.py", line 447, in download_and_extract
return self.extract(self.download(url_or_urls))
File "/usr/local/lib/python3.10/dist-packages/datasets/download/download_manager.py", line 311, in download
downloaded_path_or_paths = map_nested(
File "/usr/local/lib/python3.10/dist-packages/datasets/utils/py_utils.py", line 444, in map_nested
mapped = [
File "/usr/local/lib/python3.10/dist-packages/datasets/utils/py_utils.py", line 445, in <listcomp>
_single_map_nested((function, obj, types, None, True, None))
File "/usr/local/lib/python3.10/dist-packages/datasets/utils/py_utils.py", line 346, in _single_map_nested
return function(data_struct)
File "/usr/local/lib/python3.10/dist-packages/datasets/download/download_manager.py", line 338, in _download
return cached_path(url_or_filename, download_config=download_config)
File "/usr/local/lib/python3.10/dist-packages/datasets/utils/file_utils.py", line 183, in cached_path
output_path = get_from_cache(
File "/usr/local/lib/python3.10/dist-packages/datasets/utils/file_utils.py", line 530, in get_from_cache
raise FileNotFoundError(f"Couldn't find file at {url}")
FileNotFoundError: Couldn't find file at https://dumps.wikimedia.org/enwiki/20220301/dumpstatus.json
### Steps to reproduce the bug
$ pip install apache_beam mwparserfromhell
>>> from datasets import load_dataset
>>> load_dataset("wikipedia", "20220301.en")
>>> load_dataset('wikipedia', '20220301.en', beam_runner='DirectRunner')
### Expected behavior
Download the dataset
### Environment info
Running linux on a remote workstation operated through a macbook terminal
Python 3.10.6
| 5,286 |
https://github.com/huggingface/datasets/issues/5284 | Features of IterableDataset set to None by remove column | [
"Related to https://github.com/huggingface/datasets/issues/5245",
"#self-assign",
"Thanks @lhoestq and @alvarobartt!\r\n\r\nThis would be extremely helpful to have working for the Whisper fine-tuning event - we're **only** training using streaming mode, so it'll be quite important to have this feature working to make training as easy as possible!\r\n\r\n_c.f._ https://twitter.com/sanchitgandhi99/status/1592188332171493377",
"> Thanks @lhoestq and @alvarobartt!\n> \n> \n> \n> This would be extremely helpful to have working for the Whisper fine-tuning event - we're **only** training using streaming mode, so it'll be quite important to have this feature working to make training as easy as possible!\n> \n> \n> \n> _c.f._ https://twitter.com/sanchitgandhi99/status/1592188332171493377\n\nI'm almost done with at least a temporary fix to `rename_column`, `rename_columns`, and `remove_columns`, just trying to figure out how to extend it to the `map` function itself!\n\nI'll probably open the PR for review either tomorrow or Sunday hopefully! Glad I can help you and HuggingFace 🤗 ",
"Awesome - thank you so much for this PR @alvarobartt! Is much appreciated!",
"@sanchit-gandhi PR is ready and open for review at #5287, but there's still one issue I may need @lhoestq's input :hugs:",
"Let us know @sanchit-gandhi if you need a new release of `datasets` soon with this fix included :)",
"Thanks for the fix guys! We can direct people to install `datasets` from main if that's easier!",
"Hey guys, any update around this? I'm facing the same issue with a streamable dataset. ",
"Hi @asennoussi so this was already fixed and released as part of https://github.com/huggingface/datasets/releases/tag/2.8.0, so you should be able to install it as `pip install datasets==2.8.0` or just to use `pip install datasets --upgrade` to get the latest version, as of now, the https://github.com/huggingface/datasets/releases/tag/2.9.0 released last week! 🤗",
"Still facing the same issue though: \r\n```\r\nfrom datasets import IterableDatasetDict, load_dataset\r\n\r\nraw_datasets = vectorized_datasets = IterableDatasetDict()\r\n\r\n\r\nraw_datasets[\"train\"] = load_dataset(\"asennoussi/private\", split=\"train\", use_auth_token=True, streaming=True)\r\nraw_datasets[\"test\"] = load_dataset(\"asennoussi/private\", split=\"test\", use_auth_token=True, streaming=True)\r\n\r\nprint(\"Original features: \", raw_datasets['train'].features.keys())\r\n\r\n...\r\n\r\ndef prepare_dataset(batch):\r\n\r\n # load and (possibly) resample audio datato 16kHz\r\n audio = batch[\"audio\"]\r\n\r\n # compute log-Mel input features from input audio array \r\n batch[\"input_features\"] = processor.feature_extractor(audio[\"array\"], sampling_rate=audio[\"sampling_rate\"]).input_features[0]\r\n # compute input length of audio sample in seconds\r\n batch[\"input_length\"] = len(audio[\"array\"]) / audio[\"sampling_rate\"]\r\n \r\n # optional pre-processing steps\r\n transcription = batch[\"sentence\"]\r\n \r\n # encode target text to label ids\r\n batch[\"labels\"] = processor.tokenizer(transcription).input_ids\r\n batch[\"labels_length\"] = len(batch[\"labels\"])\r\n return batch\r\n...\r\nvectorized_datasets = vectorized_datasets.remove_columns(['input_length', 'labels_length']+list(next(iter(raw_datasets.values())).features))\r\nprint(\"Processed features: \", vectorized_datasets['train'].features)\r\nprint(\"First sample:\", next(iter(vectorized_datasets['train'])))\r\n\r\n```\r\n\r\nOutput: \r\n```\r\nOriginal features: dict_keys(['path', 'audio', 'sentence'])\r\nProcessed features: None\r\n```",
"Hmm weird, could you try to print\r\n\r\n```python\r\nprint(\"Processed features: \", vectorized_datasets['train'].features)\r\n```\r\n\r\nagain after iterating over the `vectorized_datasets`? In the code above, should be last line :)",
"Didn't seem to fix it: \r\n```\r\nOriginal features: dict_keys(['path', 'audio', 'sentence'])\r\nProcessed features: None\r\nProcessed features: None\r\n```",
"Actually the culprit looks to be this one: \r\n`vectorized_datasets = raw_datasets.map(prepare_dataset).with_format(\"torch\")`\r\nWhen I remove this line: `vectorized_datasets = vectorized_datasets.remove_columns(['input_length', 'labels_length']+list(next(iter(raw_datasets.values())).features))`\r\n\r\nI still get \r\n```\r\nProcessed features: None\r\n```",
"The culprit is definitely `.map` \r\nJust validated it. \r\nAny idea please? ",
"> The culprit is definitely `.map` Just validated it. Any idea please?\r\n\r\nYes, indeed `.map` losses the features, because AFAIK pre-fetching the data to infer the features is expensive and not ideal, that's part of this issue https://github.com/huggingface/datasets/issues/3888\r\n\r\nAnyway, now you can pass the `features` as a param to `.map` as follows:\r\n\r\n```python\r\nfrom datasets import Features\r\nvectorized_datasets = raw_datasets.map(\r\n prepare_dataset,\r\n features=Features(\r\n {\"path\": raw_datasets[\"train\"].info.features[\"path\"], \"audio\": raw_datasets[\"train\"].info.features[\"audio\"], \"sentence\": raw_datasets[\"train\"].info.features[\"sentence\"]}\r\n ),\r\n).with_format(\"torch\")\r\n```\r\n\r\nAlso, to let you know, when calling `.remove_columns` over an `IterableDataset`, the `features` are not lost, as well as `.rename_column` and `rename_columns` :)\r\n\r\nMore information about the latter at https://github.com/huggingface/datasets/pull/5287",
"@asennoussi alternatively you can just call `._resolve_features()` from your `IterableDataset` and it will pre-fetch the data to resolve the features, but note that feature-inference is not as accurate as if you manually specify which features and feature-types the `IterableDataset` has, as mentioned in the comment above, the alternative is to provide `features` param to `.map` :hugs:",
"Got it thanks a lot! "
] | ### Describe the bug
The `remove_column` method of the IterableDataset sets the dataset features to None.
### Steps to reproduce the bug
```python
from datasets import Audio, load_dataset
# load LS in streaming mode
dataset = load_dataset("librispeech_asr", "clean", split="validation", streaming=True)
# check original features
print("Original features: ", dataset.features.keys())
# define features to remove: we KEEP audio and text
COLUMNS_TO_REMOVE = ['chapter_id', 'speaker_id', 'file', 'id']
dataset = dataset.remove_columns(COLUMNS_TO_REMOVE)
# check processed features, uh-oh!
print("Processed features: ", dataset.features)
# streaming the first audio sample still works
print("First sample:", next(iter(ds)))
```
**Print Output:**
```
Original features: dict_keys(['file', 'audio', 'text', 'speaker_id', 'chapter_id', 'id'])
Processed features: None
First sample: {'audio': {'path': '2277-149896-0000.flac', 'array': array([ 0.00186157, 0.0005188 , 0.00024414, ..., -0.00097656,
-0.00109863, -0.00146484]), 'sampling_rate': 16000}, 'text': "HE WAS IN A FEVERED STATE OF MIND OWING TO THE BLIGHT HIS WIFE'S ACTION THREATENED TO CAST UPON HIS ENTIRE FUTURE"}
```
### Expected behavior
The features should be those **not** removed by the `remove_column` method, i.e. audio and text.
### Environment info
- `datasets` version: 2.7.1
- Platform: Linux-5.10.133+-x86_64-with-Ubuntu-18.04-bionic
- Python version: 3.7.15
- PyArrow version: 9.0.0
- Pandas version: 1.3.5
(Running on Google Colab for a blog post: https://colab.research.google.com/drive/1ySCQREPZEl4msLfxb79pYYOWjUZhkr9y#scrollTo=8pRDGiVmH2ml)
cc @polinaeterna @lhoestq | 5,284 |
https://github.com/huggingface/datasets/issues/5281 | Support cloud storage in load_dataset | [
"Or for example an archive on GitHub releases! Before I added support for JXL (locally only, PR still pending) I was considering hosting my files on GitHub instead...",
"+1 to this. I would like to use 'audiofolder' with a data_dir that's on S3, for example. I don't want to upload my dataset to the Hub, but I would find all the fingerprinting/caching features useful.",
"Adding to the conversation, Dask also uses `fsspec` for this feature.\r\n\r\n[Dask: How to connect to remote data](https://docs.dask.org/en/stable/how-to/connect-to-remote-data.html)\r\n\r\nHappy to help on this feature :D ",
"+1 to this feature request since I think it also tackles my use-case. I am collaborating with a team, working with a loading script which takes some time to generate the dataset artifacts. It would be very handy to use this as a cloud cache to avoid duplicating the effort. \r\n\r\nCurrently we could use `builder.download_and_prepare(path_to_cloud_storage, storage_options, ...)` to cache the artifacts to cloud storage, but then `builder.as_dataset()` yields `NotImplementedError: Loading a dataset cached in SomeCloudFileSystem is not supported`",
"Makes sense ! If you want to load locally a dataset that you download_and_prepared on a cloud storage, you would use `load_dataset(path_to_cloud_storage)` indeed. It would download the data from the cloud storage, cache them locally, and return a `Dataset`.",
"It seems currently the `cached_path` function handles all URLs by `get_from_cache` that only supports `ftp` and `http(s)` here:\r\nhttps://github.com/huggingface/datasets/blob/b5672a956d5de864e6f5550e493527d962d6ae55/src/datasets/utils/file_utils.py#L181\r\n\r\nI guess one can add another condition that handles `s3://` or `gs://` URLs via `fsspec` here.",
"I could use this functionality, so I put together a PR using @kyamagu's suggestion to use `fsspec` in `datasets.utils.file_utils`\r\n\r\nhttps://github.com/huggingface/datasets/pull/5580",
"Thanks @dwyatte for adding support for fsspec urls\r\n\r\nLet me just reopen this since the original issue is not resolved",
"I'm not yet understanding how to use https://github.com/huggingface/datasets/pull/5580 in order to use `load_dataset(data_files=\"s3://...\")`. Any help/example would be much appreciated :) thanks! ",
"It's still not officially supported x) But you can try to update `request_etag` in `file_utils.py` to use `fsspec_head` instead of `http_head`. It is responsible of getting the ETags of the remote files for caching. This change may do the trick for S3 urls",
"Thank you for your guys help on this and merging in #5580. I manually pulled the changes to my local datasets package (datasets.utils.file_utils.py) since it only seemed to be this file that was changed in the PR and I'm getting the error: \r\nInvalidSchema: No connection adapters were found for 's3://bucket/folder/'. I'm calling load_dataset using the S3 URI. When I use the S3 URL I get HTTPError: 403 Client Error. \r\nAm I not supposed to use the S3 URI? How do I pull in the changes from this merge? I'm running datasets 2.10.1. ",
"The current implementation depends on gcsfs/s3fs being able to authenticate through some other means e.g., environmental variables. For AWS, it looks like you can set `AWS_ACCESS_KEY_ID`, `AWS_SECRET_ACCESS_KEY`, and `AWS_SESSION_TOKEN`\r\n\r\nNote that while testing this just now, I did note a discrepancy between gcsfs and s3fs that we might want to address where gcsfs passes the timeout from `storage_options` [here](https://github.com/huggingface/datasets/blob/3e6269979fc80ae8939294d26298897f0db5b84d/src/datasets/utils/file_utils.py#L333) down into the `aiohttp.ClientSession.request`, but s3fs does not handle this (tries to pass to the `aiobotocore.session.AioSession` constructor raising `TypeError: __init__() got an unexpected keyword argument 'requests_timeout'`).\r\n\r\nIt seems like some work trying to unify kwargs across different fsspec implementations, so if the plan is to pass down `storage_options`, I wonder if we should just let users control the timeout (and other kwargs) using that and if not specified, use the default?",
"> Note that while testing this just now, I did note a discrepancy between gcsfs and s3fs that we might want to address where gcsfs passes the timeout from storage_options [here](https://github.com/huggingface/datasets/blob/3e6269979fc80ae8939294d26298897f0db5b84d/src/datasets/utils/file_utils.py#L333) down into the aiohttp.ClientSession.request, but s3fs does not handle this (tries to pass to the aiobotocore.session.AioSession constructor raising TypeError: __init__() got an unexpected keyword argument 'requests_timeout').\r\n\r\n> It seems like some work trying to unify kwargs across different fsspec implementations, so if the plan is to pass down storage_options, I wonder if we should just let users control the timeout (and other kwargs) and if not specified, use the default?\r\n\r\n@lhoestq here's a small PR for this: https://github.com/huggingface/datasets/pull/5673\r\n\r\n",
"@lhoestq sorry for being a little dense here but I am very keen to use fsspec / adlfs for for a larger image dataset I have for object detection. I have to keep it on Azure storage and would also like to avoid a full download or zipping (so use `load_dataset(..., streaming=True)`. So this development is godsend :) only... I am unable to make it work.\r\n\r\nWould you expect the setup to work for:\r\n- azure blob storage\r\n- image files (not the standard formats `json`, `parquet`....\r\n\r\n? I appreciate that you mostly focus on s3 but it seems that, similar to the [remaining cloud storage functionality](https://huggingface.co/docs/datasets/filesystems#cloud-storage), it should also work for Azure blob storage.\r\n\r\n\r\nI would imagine that something like (Streaming true or false):\r\n```python\r\nd = load_dataset(\"new_dataset.py\", storage_options=storage_options, split=\"train\")\r\n```\r\nwould work with \r\n```python\r\n# new_dataset.py\r\n....\r\n_URL=\"abfs://container/image_folder``` \r\n\r\narchive_path = dl_manager.download(_URL)\r\nsplit_metadata_paths = dl_manager.download(_METADATA_URLS)\r\nreturn [\r\n datasets.SplitGenerator(\r\n name=datasets.Split.TRAIN,\r\n gen_kwargs={\r\n \"annotation_file_path\": split_metadata_paths[\"train\"],\r\n \"files\": dl_manager.iter_files(archive_path)\r\n},\r\n ),\r\n...\r\n```\r\nbut I get \r\n```\r\nTraceback (most recent call last):\r\n... ^^^^^^^^^^^^^^^^^^^^^^^^^^^^^^^^^^^^^^^^^^^^^^^^^^^^^^^^^^^^^^^^^^^^^^^^^^^^^^^^^^^^^^^^^^^^^^^^^^^^^^^^^^^^^^^^^^^^^^^\r\n File \"~/miniconda3/envs/hf/lib/python3.11/site-packages/datasets/load.py\", line 1797, in load_dataset\r\n builder_instance.download_and_prepare(\r\n File \"~/miniconda3/envs/hf/lib/python3.11/site-packages/datasets/builder.py\", line 890, in download_and_prepare\r\n self._download_and_prepare(\r\n File \"~/miniconda3/envs/hf/lib/python3.11/site-packages/datasets/builder.py\", line 1649, in _download_and_prepare\r\n super()._download_and_prepare(\r\n File \"~/miniconda3/envs/hf/lib/python3.11/site-packages/datasets/builder.py\", line 963, in _download_and_prepare\r\n split_generators = self._split_generators(dl_manager, **split_generators_kwargs)\r\n ^^^^^^^^^^^^^^^^^^^^^^^^^^^^^^^^^^^^^^^^^^^^^^^^^^^^^^^^^^^^^\r\n File \"~/.cache/huggingface/modules/datasets_modules/datasets/new_dataset/dd26a081eab90074f41fa2c821b458424fde393cc73d3d8241aca956d1fb3aa0/new_dataset_script.py\", line 56, in _split_generators\r\n archive_path = dl_manager.download(_URL)\r\n ^^^^^^^^^^^^^^^^^^^^^^^^^\r\n File \"~/miniconda3/envs/hf/lib/python3.11/site-packages/datasets/download/download_manager.py\", line 427, in download\r\n downloaded_path_or_paths = map_nested(\r\n ^^^^^^^^^^^\r\n File \"~/miniconda3/envs/hf/lib/python3.11/site-packages/datasets/utils/py_utils.py\", line 435, in map_nested\r\n return function(data_struct)\r\n ^^^^^^^^^^^^^^^^^^^^^\r\n File \"~/miniconda3/envs/hf/lib/python3.11/site-packages/datasets/download/download_manager.py\", line 453, in _download\r\n return cached_path(url_or_filename, download_config=download_config)\r\n ^^^^^^^^^^^^^^^^^^^^^^^^^^^^^^^^^^^^^^^^^^^^^^^^^^^^^^^^^^^^^\r\n File \"~/miniconda3/envs/hf/lib/python3.11/site-packages/datasets/utils/file_utils.py\", line 206, in cached_path\r\n raise ValueError(f\"unable to parse {url_or_filename} as a URL or as a local path\")\r\nValueError: unable to parse abfs://container/image_folder as a URL or as a local path\r\n\r\n```\r\n",
"What version of `datasets` are you using ?",
"@lhoestq \r\nhello, i still have problem with loading json from S3:\r\n\r\n\r\nstorage_options = {\r\n \"key\": xxxx,\r\n \"secret\": xxx,\r\n \"endpoint_url\": xxxx\r\n}\r\npath = 's3://xxx/xxxxxxx.json'\r\ndataset = load_dataset(\"json\", data_files=path, storage_options=storage_options)\r\n\r\nand it throws an error:\r\nTypeError: AioSession.__init__() got an unexpected keyword argument 'hf'\r\nand I use the lastest 2.14.4_dev0 version",
"Hi @lhoestq, thanks for getting back to me :) you have been busy over the summer I see... I was on `2.12.0`. I have updated to `2.14.4`. \r\n\r\nNow `d = load_dataset(\"new_dataset.py\", storage_options=storage_options, split=\"train\", streaming=True)` works for Azure blob storage (with a local data loader script) when I explicitly list all blobs (I am struggling to make `fs.ls(<path>)` work in the script to make the list available to the download manager).\r\n\r\n Any chance that it could work out-of-the-box by supplying just the image folder, not the full list of image filenames? It seems that `dl_manager.download(_URL)` always wants one or more (possibly archived) files. In my situation, where I don't want to archive or download, it would be great to just supply the folder (seems reasonably doable with fsspec).\r\n\r\nLet me know if there is anything I can do to help.\r\n\r\nThanks,",
"> Any chance that it could work out-of-the-box by supplying just the image folder, not the full list of image filenames? It seems that dl_manager.download(_URL) always wants one or more (possibly archived) files. In my situation, where I don't want to archive or download, it would be great to just supply the folder (seems reasonably doable with fsspec).\r\n\r\n@mayorblock This is not supported right now, you have to use archives or implement a way to get the list by yourself\r\n\r\n> TypeError: AioSession.init() got an unexpected keyword argument 'hf'\r\n\r\n@hjq133 Can you update `fsspec` and try again ?\r\n\r\n```python\r\npip install -U fsspec\r\n```",
"thanks for your suggestion,it works now ! ",
"I'm seeing same problem as @hjq133 with following versions:\r\n\r\n```\r\ndatasets==2.15.0\r\n(venv) ➜ finetuning-llama2 git:(main) ✗ pip freeze | grep s3fs \r\ns3fs==2023.10.0\r\n(venv) ➜ finetuning-llama2 git:(main) ✗ pip freeze | grep fsspec\r\nfsspec==2023.10.0\r\n```",
"> @lhoestq hello, i still have problem with loading json from S3:\r\n> \r\n> storage_options = { \"key\": xxxx, \"secret\": xxx, \"endpoint_url\": xxxx } path = 's3://xxx/xxxxxxx.json' dataset = load_dataset(\"json\", data_files=path, storage_options=storage_options)\r\n> \r\n> and it throws an error: TypeError: AioSession.**init**() got an unexpected keyword argument 'hf' and I use the lastest 2.14.4_dev0 version\r\n\r\nI am trying to do the same thing, but the loading is just hanging, without any error. \r\n@lhoestq is there any documentation how to load from private s3 buckets?\r\n",
"Hi ! S3 support is still experimental. It seems like there is an extra `hf` field passed to the `s3fs` storage_options that causes this error. I just check the source code of `_prepare_single_hop_path_and_storage_options` and I think you can try passing explicitly your own `storage_options={\"s3\": {...}}`. Also note that it's generally better to load datasets from HF (we run extensive tests and benchmarks for speed and robustness)",
"That worked! Thanks\r\nIt seems thought that `data_dir=...` doesn't work on s3, only `data_files`.\r\n",
"@lhoestq Would this work either with an Azure Blob Storage Container or its respective Azure Machine Learning Datastore? If yes, what would that look like in code? I've tried a couple of combinations but no success so far, on the latest version of `datasets`. I need to migrate a dataset to the Azure cloud, `load_dataset(\"path_to_data\")` worked perfectly while the files were local only. Thank you!\r\n\r\n@mayorblock would you mind sharing how you got it to work? What did you pass as `storage_options`? Would it maybe work without a custom data loader script?",
"This ticket would be of so much help.",
"@lhoestq I've been using this feature for the last year on GCS without problem, but I think we need to fix an issue with S3 and then document the supported calling patterns to reduce confusion\r\n\r\nIt looks like `datasets` uses a default `DownloadConfig` which is where some potentially unintended storage options are getting passed to fsspec\r\n\r\n```\r\nDownloadConfig(\r\n\tcache_dir=None, \r\n\tforce_download=False, \r\n\tresume_download=False, \r\n\tlocal_files_only=False, \r\n\tproxies=None, \r\n\tuser_agent=None, \r\n\textract_compressed_file=False, \r\n\tforce_extract=False, \r\n\tdelete_extracted=False, \r\n\tuse_etag=True, \r\n\tnum_proc=None, \r\n\tmax_retries=1, \r\n\ttoken=None, \r\n\tignore_url_params=False, \r\n\tstorage_options={'hf': {'token': None, 'endpoint': 'https://huggingface.co'}}, \r\n\tdownload_desc=None\r\n)\r\n```\r\n(specifically the `storage_options={'hf': {'token': None, 'endpoint': 'https://huggingface.co'}}` part)\r\n\r\n`gcsfs` is robust to the extra key in storage options for whatever reason, but `s3fs` is not (haven't dug into why). I'm unable to test `adlfs` but it looks like people here got it working\r\n\r\nIs this an issue that needs fixed with s3fs? Or can we avoid passing these default storage options in some cases?\r\n\r\nUpdate: I think probably https://github.com/huggingface/datasets/pull/6127 is where these default storage options were introduced",
"Hmm not sure, maybe it has to do with `_prepare_single_hop_path_and_storage_options` returning the \"hf\" storage options when it shouldn't",
"Also running into this issue downloading a parquet dataset from S3 (Upload worked fine using [current main branch](https://github.com/huggingface/datasets/commit/90b896193a0a315789022580eb4c80305d168d4d)).\r\n`dataset = Dataset.from_parquet('s3://path-to-file')`\r\nraises \r\n`TypeError: AioSession.__init__() got an unexpected keyword argument 'hf'`\r\nFound that the issue is introduced in [#6028](https://github.com/huggingface/datasets/commit/14f6edd9222e577dccb962ed5338b79b73502fa5#diff-8a868b8c1127c5cbe7870bbeec30bd2cbec6696a378e65b66782a6935e3f412e)\r\n\r\nWhen commenting out the __post_init__ part to set 'hf', I am able to download the dataset."
] | Would be nice to be able to do
```python
data_files=["s3://..."] # or gs:// or any cloud storage path
storage_options = {...}
load_dataset(..., data_files=data_files, storage_options=storage_options)
```
The idea would be to use `fsspec` as in `download_and_prepare` and `save_to_disk`.
This has been requested several times already. Some users want to use their data from private cloud storage to train models
related:
https://github.com/huggingface/datasets/issues/3490
https://github.com/huggingface/datasets/issues/5244
[forum](https://discuss.huggingface.co/t/how-to-use-s3-path-with-load-dataset-with-streaming-true/25739/2) | 5,281 |
https://github.com/huggingface/datasets/issues/5280 | Import error | [
"Hi ! Can you \r\n```python\r\nimport platform\r\nprint(platform.python_version())\r\n```\r\nto see that it returns ?",
"Hi,\n\n3.8.13\n\nGet Outlook for Android<https://aka.ms/AAb9ysg>\n________________________________\nFrom: Quentin Lhoest ***@***.***>\nSent: Tuesday, November 22, 2022 2:37:02 PM\nTo: huggingface/datasets ***@***.***>\nCc: feketedavid1012 ***@***.***>; Author ***@***.***>\nSubject: Re: [huggingface/datasets] Import error (Issue #5280)\n\n\nHi ! Can you\n\nimport platform\nprint(platform.python_version())\n\nto see that it returns ?\n\n—\nReply to this email directly, view it on GitHub<https://github.com/huggingface/datasets/issues/5280#issuecomment-1323691385>, or unsubscribe<https://github.com/notifications/unsubscribe-auth/AJW7F5YGG32W6WABYC25NJTWJTD75ANCNFSM6AAAAAASHZJ2AU>.\nYou are receiving this because you authored the thread.Message ID: ***@***.***>\n",
"Then it should work as expected if you use the same python when using `datasets`\r\n\r\nPlease make sure you're running your code in the right environment",
"It's the right environment. But in if statement I have\n\"3.8.13\" < 3.7\nAnd in the error message is Python>=3.7 which is true in my case (3.8.13 is greater then 3.7), so I don't understand my python should be below the 3.7 which case the if statement is right, but the message is wrong, or above 3.7 which case if statement is wrong, error message is right.\n\nGet Outlook for Android<https://aka.ms/AAb9ysg>\n________________________________\nFrom: Quentin Lhoest ***@***.***>\nSent: Tuesday, November 22, 2022 2:41:43 PM\nTo: huggingface/datasets ***@***.***>\nCc: feketedavid1012 ***@***.***>; Author ***@***.***>\nSubject: Re: [huggingface/datasets] Import error (Issue #5280)\n\n\nThen it should work as expected if you use the same python when using datasets\n\nPlease make sure you're running your code in the right environment\n\n—\nReply to this email directly, view it on GitHub<https://github.com/huggingface/datasets/issues/5280#issuecomment-1323697094>, or unsubscribe<https://github.com/notifications/unsubscribe-auth/AJW7F54JURTAJJWWDO2QGI3WJTERPANCNFSM6AAAAAASHZJ2AU>.\nYou are receiving this because you authored the thread.Message ID: ***@***.***>\n",
"If you're having an error then you're not running your code in the right environment."
] | https://github.com/huggingface/datasets/blob/cd3d8e637cfab62d352a3f4e5e60e96597b5f0e9/src/datasets/__init__.py#L28
Hy,
I have error at the above line. I have python version 3.8.13, the message says I need python>=3.7, which is True, but I think the if statement not working properly (or the message wrong) | 5,280 |
https://github.com/huggingface/datasets/issues/5278 | load_dataset does not read jsonl metadata file properly | [
"Can you try to remove \"drop_labels=false\" ? It may force the loader to infer the labels instead of reading the metadata",
"Hi, thanks for responding. I tried that, but it does not change anything.",
"Can you try updating `datasets` ? Metadata support was added in `datasets` 2.4",
"Probably the issue, will report back asap!",
"Okay, now it seems to actually load the metadata and create the train_split, but it still says only returns \"image\" and \"label\", which is always 0 since all images are from same folder",
"> Can you try updating `datasets` ? Metadata support was added in `datasets` 2.4\r\n\r\nUpdate: This was the issue."
] | ### Describe the bug
Hi, I'm following [this page](https://huggingface.co/docs/datasets/image_dataset) to create a dataset of images and captions via an image folder and a metadata.json file, but I can't seem to get the dataloader to recognize the "text" column. It just spits out "image" and "label" as features.
Below is code to reproduce my exact example/problem.
### Steps to reproduce the bug
```ruby
dataset_link="19Unu89Ih_kP6zsE7f9Mkw8dy3NwHopRF"
id = dataset_link
output = 'Godardv01.zip'
gdown.download(id=id, output=output, quiet=False)
ds = load_dataset("imagefolder", data_dir="/kaggle/working/Volumes/TOSHIBA/Godard_imgs/Volumes/TOSHIBA/Godard_imgs/Full/train", split="train", drop_labels=False)
print(ds)
```
### Expected behavior
I would expect that it returned "image" and "text" columns from the code above.
### Environment info
- `datasets` version: 2.1.0
- Platform: Linux-5.15.65+-x86_64-with-debian-bullseye-sid
- Python version: 3.7.12
- PyArrow version: 5.0.0
- Pandas version: 1.3.5 | 5,278 |
https://github.com/huggingface/datasets/issues/5276 | Bug in downloading common_voice data and snall chunk of it to one's own hub | [
"Sounds like one of the file is not a valid one, can you make sure you uploaded valid mp3 files ?",
"Well I just sharded the original commonVoice dataset and pushed a small chunk of it in a private rep\n\nWhat did go wrong?\n\nHolen Sie sich Outlook für iOS<https://aka.ms/o0ukef>\n________________________________\nVon: Quentin Lhoest ***@***.***>\nGesendet: Tuesday, November 22, 2022 3:03:40 PM\nAn: huggingface/datasets ***@***.***>\nCc: capsabogdan ***@***.***>; Author ***@***.***>\nBetreff: Re: [huggingface/datasets] Bug in downloading common_voice data and snall chunk of it to one's own hub (Issue #5276)\n\n\nSounds like one of the file is not a valid one, can you make sure you uploaded valid mp3 files ?\n\n—\nReply to this email directly, view it on GitHub<https://github.com/huggingface/datasets/issues/5276#issuecomment-1323727434>, or unsubscribe<https://github.com/notifications/unsubscribe-auth/ALSIFOAPAL2V4TBJTSPMAULWJTHDZANCNFSM6AAAAAASHQJ63U>.\nYou are receiving this because you authored the thread.Message ID: ***@***.***>\n",
"It should be all good then !\r\nCould you share a link to your repository for me to investigate what went wrong ?",
"https://huggingface.co/datasets/DTU54DL/common-voice-test16k\n\nAm Di., 22. Nov. 2022 um 16:43 Uhr schrieb Quentin Lhoest <\n***@***.***>:\n\n> It should be all good then !\n> Could you share a link to your repository for me to investigate what went\n> wrong ?\n>\n> —\n> Reply to this email directly, view it on GitHub\n> <https://github.com/huggingface/datasets/issues/5276#issuecomment-1323876682>,\n> or unsubscribe\n> <https://github.com/notifications/unsubscribe-auth/ALSIFOEUJRZWXAM7DYA5VJDWJTS3NANCNFSM6AAAAAASHQJ63U>\n> .\n> You are receiving this because you authored the thread.Message ID:\n> ***@***.***>\n>\n",
"I see ! This is a bug with MP3 files.\r\n\r\nWhen we store audio data in parquet, we store the bytes and the file name. From the file name extension we know if it's a WAV, an MP3 or else. But here it looks like the paths are all None.\r\n\r\nIt looks like it comes from here:\r\n\r\nhttps://github.com/huggingface/datasets/blob/7feeb5648a63b6135a8259dedc3b1e19185ee4c7/src/datasets/features/audio.py#L212\r\n\r\nCc @polinaeterna maybe we should simply put the file name instead of None values ?",
"@lhoestq I remember we wanted to avoid storing redundant data but maybe it's not that crucial indeed to store one more string value. \r\nOr we can store paths only for mp3s, considering that for other formats we don't have such a problem with reading from bytes without format specified. ",
"It doesn't cost much to always store the file name IMO",
"thanks for the help!\n\ncan I do anything on my side? we are doing a DL project and we need the\ndata really quick.\n\nthanks\nbogdan\n\n> Message ID: ***@***.***>\n>\n",
"I opened a pull requests here: https://github.com/huggingface/datasets/pull/5285, we'll do a new release soon with this fix.\r\n\r\nOtherwise if you're really in a hurry you can install `datasets` from this PR",
"[image: image.png]\n\n> Message ID: ***@***.***>\n>\n",
"any idea on what's going wrong here?\n\nthanks\n\nAm So., 27. Nov. 2022 um 13:53 Uhr schrieb Bogdan Capsa <\n***@***.***>:\n\n> [image: image.png]\n>\n>> Message ID: ***@***.***>\n>>\n>\n",
"hi @capsabogdan! \r\ncould you please share more specifically what problem do you have now?",
"I have attached this screenshot above . can u pls help? So can not pip from pull request\r\n\r\n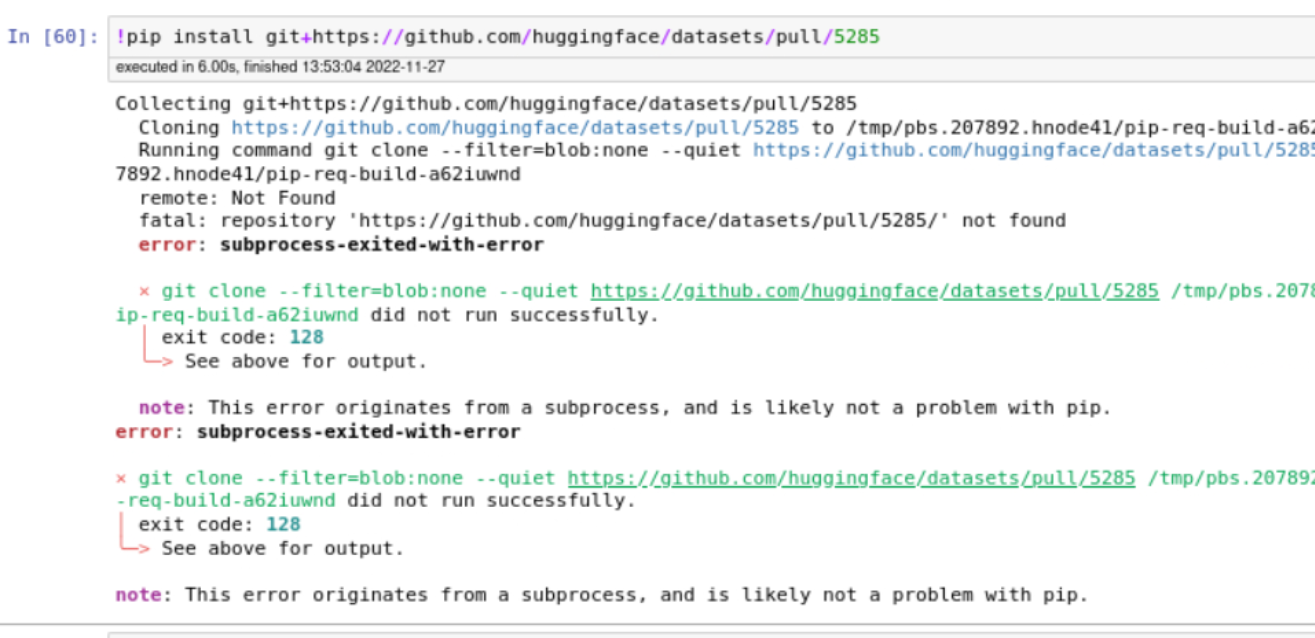\r\n",
"The pull request has been merged on `main`.\r\nYou can install `datasets` from `main` using\r\n```\r\npip install git+https://github.com/huggingface/datasets.git\r\n```",
"I've tried to load this dataset DTU54DL/common-voice-test16k, but am\ngetting the same error.\n\nSo the bug fix will fix only if I upload a new dataset, or also loading\npreviously uploaded datasets?\n\nthanks\n\nAm Mo., 28. Nov. 2022 um 19:51 Uhr schrieb Quentin Lhoest <\n***@***.***>:\n\n> The pull request has been merged on main.\n> You can install datasets from main using\n>\n> pip install git+https://github.com/huggingface/datasets.git\n>\n> —\n> Reply to this email directly, view it on GitHub\n> <https://github.com/huggingface/datasets/issues/5276#issuecomment-1329587334>,\n> or unsubscribe\n> <https://github.com/notifications/unsubscribe-auth/ALSIFOCNYYIGHM2EX3ZIO6DWKT5MXANCNFSM6AAAAAASHQJ63U>\n> .\n> You are receiving this because you were mentioned.Message ID:\n> ***@***.***>\n>\n",
"> So the bug fix will fix only if I upload a new dataset, or also loading\r\npreviously uploaded datasets?\r\n\r\nYou have to reupload the dataset, sorry for the inconvenience",
"thank you so much for the help! works like a charm!\n\nAm Di., 29. Nov. 2022 um 12:15 Uhr schrieb Quentin Lhoest <\n***@***.***>:\n\n> So the bug fix will fix only if I upload a new dataset, or also loading\n> previously uploaded datasets?\n>\n> You have to reupload the dataset, sorry for the inconvenience\n>\n> —\n> Reply to this email directly, view it on GitHub\n> <https://github.com/huggingface/datasets/issues/5276#issuecomment-1330468393>,\n> or unsubscribe\n> <https://github.com/notifications/unsubscribe-auth/ALSIFOBKEFZO57BAKY4IGW3WKXQUZANCNFSM6AAAAAASHQJ63U>\n> .\n> You are receiving this because you were mentioned.Message ID:\n> ***@***.***>\n>\n"
] | ### Describe the bug
I'm trying to load the common voice dataset. Currently there is no implementation to download just par tof the data, and I need just one part of it, without downloading the entire dataset
Help please?

### Steps to reproduce the bug
So here is what I have done:
1. Download common_voice data
2. Trim part of it and publish it to my own repo.
3. Download data from my own repo, but am getting this error.
### Expected behavior
There shouldn't be an error in downloading part of the data and publishing it to one's own repo
### Environment info
common_voice 11 | 5,276 |
https://github.com/huggingface/datasets/issues/5275 | YAML integer keys are not preserved Hub server-side | [
"@huggingface/datasets if you agree, I can make the bulk edit on the Hub to fix integer keys into strings.",
"Ok for me, and we can merge (internal) https://github.com/huggingface/moon-landing/pull/4609",
"FYI there are still 2k+ weekly users on `datasets` 2.6.1 which doesn't support the string label format for class labels. And among those, some are using datasets with class labels like imdb (60 users), conllpp (40), msra_ner (40), peoples_daily_enr (40), weibo_ner (30), conll2003 (20), etc. And renaming to string would break these users code.",
"but isn't `datasets 2.6.1` downloading files from the Hub with the corresponding tag? I thought we had something like this before",
"We're using `main` as models do. Some datasets need to be updated from time to time, e.g. when a link to download the data is dead.\r\n\r\nBut yea a year ago we had those tags, we just ended up not using them",
"I opened https://github.com/huggingface/datasets/issues/5406 to communicate on this. Let me know what you think, and if it sounds good to you I can pin this issue",
"So, is it OK to make the bulk edit on the Hub now or should we wait longer? If the latter, how long?",
"I think we can do it. If you want to be extra cautious you can do it for all datasets except imdb and conllpp for now which are actively used by 2.6.1 users. For those two we can keep the YAML like this for some more time, or alternatively use the old dataset_infos.json file",
"The bulk edit of canonical datasets (except imdb and conllpp) is running. \r\n\r\nSee e.g.: https://huggingface.co/datasets/acronym_identification/discussions/3\r\n\r\nEDITED: \r\nDone, except for \"universal_morphologies\", where I get\r\n```\r\nHTTPError: 413 Client Error: Payload Too Large for url: https://huggingface.co/api/validate-yaml\r\n```\r\n\r\nAlso not done for the datasets missing matadata \"dataset_info\":\r\n- mc4: https://huggingface.co/datasets/mc4/discussions/3\r\n- the_pile: https://huggingface.co/datasets/the_pile/discussions/6\r\n- timit_asr: https://huggingface.co/datasets/timit_asr/discussions/1",
"Thank you !",
"@lhoestq, there are 6 community datasets with YAML integer keys in their `dataset_info` `class_label`:\r\n- indonlp/indonlu\r\n- rcds/swiss_judgment_prediction\r\n- Jean-Baptiste/wikiner_fr\r\n- Bingsu/Cat_and_Dog\r\n- taskydata/tasky_or_not\r\n- RCC-MSU/collection3\r\n\r\nMaybe we could open a PR on them as well?",
"Let's do this then:\r\n\r\n- [x] [indonlp/indonlu](https://huggingface.co/datasets/indonlp/indonlu/discussions/3)\r\n- [x] rcds/swiss_judgment_prediction\r\n- [x] Jean-Baptiste/wikiner_fr\r\n- [x] Bingsu/Cat_and_Dog -> merged\r\n- [x] taskydata/tasky_or_not (was already using quotes)\r\n- [x] RCC-MSU/collection3\r\n\r\nEDIT: all done :)",
"@lhoestq I was not asking you to do it, but asking if you agree me to do it... :man_facepalming: \r\nAs I self-assigned this issue... :sweat_smile: "
] | After an internal discussion (https://github.com/huggingface/moon-landing/issues/4563):
- YAML integer keys are not preserved server-side: they are transformed to strings
- See for example this Hub PR: https://huggingface.co/datasets/acronym_identification/discussions/1/files
- Original:
```yaml
class_label:
names:
0: B-long
1: B-short
```
- Returned by the server:
```yaml
class_label:
names:
'0': B-long
'1': B-short
```
- They are planning to enforce only string keys
- Other projects already use interger-transformed-to string keys: e.g. `transformers` models `id2label`: https://huggingface.co/roberta-large-mnli/blob/main/config.json
```yaml
"id2label": {
"0": "CONTRADICTION",
"1": "NEUTRAL",
"2": "ENTAILMENT"
}
```
On the other hand, at `datasets` we are currently using YAML integer keys for `dataset_info` `class_label`.
Please note (thanks @lhoestq for pointing out) that previous versions (2.6 and 2.7) of `datasets` need being patched:
```python
In [18]: Features._from_yaml_list([{'dtype': {'class_label': {'names': {'0': 'neg', '1': 'pos'}}}, 'name': 'label'}])
---------------------------------------------------------------------------
TypeError Traceback (most recent call last)
<ipython-input-18-974f07eea526> in <module>
----> 1 Features._from_yaml_list(ry)
~/Desktop/hf/nlp/src/datasets/features/features.py in _from_yaml_list(cls, yaml_data)
1743 raise TypeError(f"Expected a dict or a list but got {type(obj)}: {obj}")
1744
-> 1745 return cls.from_dict(from_yaml_inner(yaml_data))
1746
1747 def encode_example(self, example):
~/Desktop/hf/nlp/src/datasets/features/features.py in from_yaml_inner(obj)
1739 elif isinstance(obj, list):
1740 names = [_feature.pop("name") for _feature in obj]
-> 1741 return {name: from_yaml_inner(_feature) for name, _feature in zip(names, obj)}
1742 else:
1743 raise TypeError(f"Expected a dict or a list but got {type(obj)}: {obj}")
~/Desktop/hf/nlp/src/datasets/features/features.py in <dictcomp>(.0)
1739 elif isinstance(obj, list):
1740 names = [_feature.pop("name") for _feature in obj]
-> 1741 return {name: from_yaml_inner(_feature) for name, _feature in zip(names, obj)}
1742 else:
1743 raise TypeError(f"Expected a dict or a list but got {type(obj)}: {obj}")
~/Desktop/hf/nlp/src/datasets/features/features.py in from_yaml_inner(obj)
1734 return {"_type": snakecase_to_camelcase(obj["dtype"])}
1735 else:
-> 1736 return from_yaml_inner(obj["dtype"])
1737 else:
1738 return {"_type": snakecase_to_camelcase(_type), **unsimplify(obj)[_type]}
~/Desktop/hf/nlp/src/datasets/features/features.py in from_yaml_inner(obj)
1736 return from_yaml_inner(obj["dtype"])
1737 else:
-> 1738 return {"_type": snakecase_to_camelcase(_type), **unsimplify(obj)[_type]}
1739 elif isinstance(obj, list):
1740 names = [_feature.pop("name") for _feature in obj]
~/Desktop/hf/nlp/src/datasets/features/features.py in unsimplify(feature)
1704 if isinstance(feature.get("class_label"), dict) and isinstance(feature["class_label"].get("names"), dict):
1705 label_ids = sorted(feature["class_label"]["names"])
-> 1706 if label_ids and label_ids != list(range(label_ids[-1] + 1)):
1707 raise ValueError(
1708 f"ClassLabel expected a value for all label ids [0:{label_ids[-1] + 1}] but some ids are missing."
TypeError: can only concatenate str (not "int") to str
```
TODO:
- [x] Remove YAML integer keys from `dataset_info` metadata
- [x] Make a patch release for affected `datasets` versions: 2.6 and 2.7
- [x] Communicate on the fix
- [x] Wait for adoption
- [x] Bulk edit the Hub to fix this in all canonical datasets | 5,275 |
https://github.com/huggingface/datasets/issues/5274 | load_dataset possibly broken for gated datasets? | [
"@BradleyHsu",
"Btw, thanks very much for finding the hub rollback temporary fix and bringing the issue to our attention @KhoomeiK!",
"I see the same issue when calling `load_dataset('poloclub/diffusiondb', 'large_random_1k')` with `datasets==2.7.1` and `huggingface-hub=0.11.0`. No issue with `datasets=2.6.1` and `huggingface_hub==0.10.1`.\r\n\r\nhttps://github.com/poloclub/diffusiondb/issues/7",
"I fixed my issue by specifying `repo_type` in `hf_hub_url()`. https://github.com/poloclub/diffusiondb/commit/9eb91c79aaca98b0515a0ce45778b8af65b84652\r\n\r\nI opened a PR on the Winoground's repo: https://huggingface.co/datasets/facebook/winoground/discussions/2",
"This is a bug in the script, indeed. The most robust fix is to use a relative path instead of `hf_hub_url`, which does not depend on `huggingface_hub`'s version 🙂. I've opened a PR here: https://huggingface.co/datasets/facebook/winoground/discussions/3.",
"Awesome, big thanks to both @xiaohk and @mariosasko!",
"so, if i reproduce the bug, what should i do ? with huggingface_hub0.13.3 dataset2.6.1",
"huggingface_hub.utils._validators.HFValidationError: Repo id must be in the form 'repo_name' or 'namespace/repo_name':\r\n\r\n tokenizer = AutoTokenizer.from_pretrained(ARGS.model_path, trust_remote_code=True)\r\n\r\nPlease handle automatically for local path and repo name inside, otherwise users always get confused about this",
"I think I'm also hitting this error, trying to load a model from a local path."
] | ### Describe the bug
When trying to download the [winoground dataset](https://huggingface.co/datasets/facebook/winoground), I get this error unless I roll back the version of huggingface-hub:
```
[/usr/local/lib/python3.7/dist-packages/huggingface_hub/utils/_validators.py](https://localhost:8080/#) in validate_repo_id(repo_id)
165 if repo_id.count("/") > 1:
166 raise HFValidationError(
--> 167 "Repo id must be in the form 'repo_name' or 'namespace/repo_name':"
168 f" '{repo_id}'. Use `repo_type` argument if needed."
169 )
HFValidationError: Repo id must be in the form 'repo_name' or 'namespace/repo_name': 'datasets/facebook/winoground'. Use `repo_type` argument if needed
```
### Steps to reproduce the bug
Install requirements:
```
pip install transformers
pip install datasets
# It works if you uncomment the following line, rolling back huggingface hub:
# pip install huggingface-hub==0.10.1
```
Then:
```
from datasets import load_dataset
auth_token = "" # Replace with an auth token, which you can get from your huggingface account: Profile -> Settings -> Access Tokens -> New Token
winoground = load_dataset("facebook/winoground", use_auth_token=auth_token)["test"]
```
### Expected behavior
Downloading of the datset
### Environment info
Just a google colab; see here: https://colab.research.google.com/drive/15wwOSte2CjTazdnCWYUm2VPlFbk2NGc0?usp=sharing | 5,274 |
https://github.com/huggingface/datasets/issues/5273 | download_mode="force_redownload" does not refresh cached dataset | [] | ### Describe the bug
`load_datasets` does not refresh dataset when features are imported from external file, even with `download_mode="force_redownload"`. The bug is not limited to nested fields, however it is more likely to occur with nested fields.
### Steps to reproduce the bug
To reproduce the bug 3 files are needed: `dataset.py` (contains dataset loading script), `schema.py` (contains features of dataset) and `main.py` (to run `load_datasets`)
`dataset.py`
```python
import datasets
from schema import features
class NewDataset(datasets.GeneratorBasedBuilder):
def _info(self):
return datasets.DatasetInfo(
features=features
)
def _split_generators(self, dl_manager):
return [
datasets.SplitGenerator(
name=datasets.Split.TRAIN
)
]
def _generate_examples(self):
data = [
{"id": 0, "nested": []},
{"id": 1, "nested": []}
]
for key, example in enumerate(data):
yield key, example
```
`schema.py`
```python
import datasets
features = datasets.Features(
{
"id": datasets.Value("int32"),
"nested": [
{"text": datasets.Value("string")}
]
}
)
```
`main.py`
```python
import datasets
a = datasets.load_dataset("dataset.py")
print(a["train"].info.features)
```
Now if `main.py` is run it prints the following correct output: `{'id': Value(dtype='int32', id=None), 'nested': [{'text': Value(dtype='string', id=None)}]}`. However, if f.e. the label of the feature "text" is changed to something else, f.e. to
`schema.py`
```python
import datasets
features = datasets.Features(
{
"id": datasets.Value("int32"),
"nested": [
{"textfoo": datasets.Value("string")}
]
}
)
```
`main.py` still prints `{'id': Value(dtype='int32', id=None), 'nested': [{'text': Value(dtype='string', id=None)}]}`, even if run with `download_mode="force_redownload"`. The only fix is to delete the folder in the cache.
### Expected behavior
The cached dataset is deleted and refreshed when using `load_datasets` with `download_mode="force_redownload"`.
### Environment info
- `datasets` version: 2.7.0
- Platform: Windows-10-10.0.19041-SP0
- Python version: 3.7.9
- PyArrow version: 10.0.0
- Pandas version: 1.3.5 | 5,273 |
https://github.com/huggingface/datasets/issues/5272 | Use pyarrow Tensor dtype | [
"Hi ! We're using the Arrow format for the datasets, and PyArrow tensors are not part of the Arrow format AFAIK:\r\n\r\n> There is no direct support in the arrow columnar format to store Tensors as column values.\r\n\r\nsource: https://github.com/apache/arrow/issues/4802#issuecomment-508494694",
"@wesm @rok its been around three years. any updates, regarding dataset arrow tensor support? 🙏 I know you must be very busy, would appreciate to learn what is the state of art. I saw the PR is still open [#8510](https://github.com/apache/arrow/pull/8510)",
"Hey @franz101 & @lhoestq!\r\nThere is a plan and a PR to create an [ExtensionArray of Tensors](https://github.com/apache/arrow/pull/8510) of equal sizes as well as a plan to do the same for Tensors of different sizes [ARROW-8714](https://issues.apache.org/jira/browse/ARROW-8714).",
"The work stalled a little because it was not clear where TensorArray would live. However Arrow community recently agreed to make a [well-known-extension-type document](https://lists.apache.org/thread/sxd5fhc42hb6svs79t3fd79gkqj83pfh) and I would like https://github.com/apache/arrow/pull/8510 to land there and add an implementation to C++/Python + another language. Is that something you would find beneficial to you?",
"that is a great update, thank you.\r\nit looks like this feature would benefit datasets implementation of [ArrayExtensionArray](https://github.com/huggingface/datasets/blob/9f2ff14673cac1f1ad56d80221a793f5938b68c7/src/datasets/features/features.py#L585-L641). Is that correct @eladsegal @lhoestq?\r\n\r\n",
"TensorArray sounds great ! Looking forward to it :)\r\n\r\nWe've had our own ExtensionArray for fixed shape tensors for a while now, hoping to see something more standardized by the arrow community.\r\n\r\nAlso super interested in the extension array for tensors of different sizes cc @mariosasko ",
"[FixedShapeTensor ExtensionType](https://github.com/apache/arrow/pull/8510) was merged and will be in Arrow 12.0.0 (release is planned mid April).\r\n",
"@rok Thanks for keeping us updated! I think it's best to introduce a new feature type that would use this extension type under the hood. I'll create an issue to discuss the design with the community in the coming days.\r\n\r\nAlso, is there a tentative time frame for the variable-shape Tensor extension type?",
"@mariosasko please tag me in the discussion, perhaps I can contribute.\r\n\r\nAs for the [variable shape tensor array](https://github.com/apache/arrow/issues/24868) - I'd be interested in working on it but didn't see much interest in community yet. Are you saying `huggingface/datasets` could use it?",
"pyarrow 12 is out 🎉, will have a look if I can work on it for the ExtensionArray",
"I think these two issues need to be fixed first on the Arrow side before adding the tensor feature type here: https://github.com/apache/arrow/issues/35573 and https://github.com/apache/arrow/issues/35599.\r\n\r\n@rok We've had a couple of requests for supporting variable-shape tensors on the forum/GH, but I did not manage to find the concrete issues using the search. TF/TFDS (and PyTorch with the `nested_tensor` API) support them, so it makes sense for us to do the same eventually (the Ray project has an [extension](https://github.com/ray-project/ray/blob/42a8d1489b37243f203120899a23d919dc85bf2a/python/ray/air/util/tensor_extensions/arrow.py#L634) type to support this case)",
"> @rok We've had a couple of requests for supporting variable-shape tensors on the forum/GH, but I did not manage to find the concrete issues using the search. TF/TFDS (and PyTorch with the `nested_tensor` API) support them, so it makes sense for us to do the same eventually (the Ray project has an [extension](https://github.com/ray-project/ray/blob/42a8d1489b37243f203120899a23d919dc85bf2a/python/ray/air/util/tensor_extensions/arrow.py#L634) type to support this case)\r\n\r\nThat does make sense indeed. We should probably also be careful about memory layout to enable zero-copy interface to TF/PyTorch.",
"So there is no way we can use [pyarrow.Tensor](https://arrow.apache.org/docs/python/generated/pyarrow.Tensor.html#pyarrow.Tensor) ?",
"Not with with the Arrow format, and therefore not in `datasets`. But they released a new [FixedShapeTensorArray](https://arrow.apache.org/docs/python/extending_types.html#fixed-size-tensor) to store tensors in Arrow format. We plan to support this in `datasets` at one point !",
"There is also an open issue to enable the conversion of `pyarrow.Tensor` to `pyarrow.FixedShapeTensorType`: https://github.com/apache/arrow/issues/35068. This way one could indirectly use `pyarrow.Tensor` in Arrow format.",
"We started a [mailing list discussion](https://lists.apache.org/thread/qc9qho0fg5ph1dns4hjq56hp4tj7rk1k) about potential `VariableShapeTensor` extension array, please check it out and give feedback. For more details here's also a PR https://github.com/apache/arrow/pull/37166."
] | ### Feature request
I was going the discussion of converting tensors to lists.
Is there a way to leverage pyarrow's Tensors for nested arrays / embeddings?
For example:
```python
import pyarrow as pa
import numpy as np
x = np.array([[2, 2, 4], [4, 5, 100]], np.int32)
pa.Tensor.from_numpy(x, dim_names=["dim1","dim2"])
```
[Apache docs](https://arrow.apache.org/docs/python/generated/pyarrow.Tensor.html)
Maybe this belongs into the pyarrow features / repo.
### Motivation
Working with big data, we need to make sure to use the best data structures and IO out there
### Your contribution
Can try to a PR if code changes necessary | 5,272 |
https://github.com/huggingface/datasets/issues/5270 | When len(_URLS) > 16, download will hang | [
"It can fix the bug temporarily.\r\n```python\r\nfrom datasets import DownloadConfig\r\nconfig = DownloadConfig(num_proc=8)\r\nIn [5]: dataset = load_dataset('Freed-Wu/kodak', split='test', download_config=config)\r\nDownloading and preparing dataset kodak/default to /home/wzy/.cache/huggingface/datasets/Freed-Wu___kodak/default/0.0.1/6cf51f2b3d686d24a33fe86945f9e16802def212325f9345cf3cbb1b9f5f4a57...\r\nDownloading data files #4: 100%|████████████████████████████████████████████████████████████████████████████████████████████████████████████████████████████████████████████████████████████| 3/3 [00:02<00:00, 1.39obj/s]\r\nDownloading data files #2: 100%|████████████████████████████████████████████████████████████████████████████████████████████████████████████████████████████████████████████████████████████| 3/3 [00:02<00:00, 1.38obj/s]\r\nDownloading data files #3: 100%|████████████████████████████████████████████████████████████████████████████████████████████████████████████████████████████████████████████████████████████| 3/3 [00:02<00:00, 1.13obj/s]\r\nDownloading data files #7: 100%|████████████████████████████████████████████████████████████████████████████████████████████████████████████████████████████████████████████████████████████| 3/3 [00:02<00:00, 1.09obj/s]\r\nDownloading data files #5: 100%|████████████████████████████████████████████████████████████████████████████████████████████████████████████████████████████████████████████████████████████| 3/3 [00:02<00:00, 1.08obj/s]\r\nDownloading data files #0: 100%|████████████████████████████████████████████████████████████████████████████████████████████████████████████████████████████████████████████████████████████| 3/3 [00:02<00:00, 1.08obj/s]\r\nDownloading data files #1: 100%|████████████████████████████████████████████████████████████████████████████████████████████████████████████████████████████████████████████████████████████| 3/3 [00:10<00:00, 3.36s/obj]\r\nDownloading data: 100%|██████████████████████████████████████████████████████████████████████████████████████████████████████████████████████████████████████████████████████████████████| 492k/492k [00:01<00:00, 253kB/s]\r\nDownloading data files #6: 100%|████████████████████████████████████████████████████████████████████████████████████████████████████████████████████████████████████████████████████████████| 3/3 [00:13<00:00, 4.63s/obj]\r\nExtracting data files #0: 100%|███████████████████████████████████████████████████████████████████████████████████████████████████████████████████████████████████████████████████████████| 3/3 [00:00<00:00, 1407.17obj/s]\r\nExtracting data files #1: 100%|███████████████████████████████████████████████████████████████████████████████████████████████████████████████████████████████████████████████████████████| 3/3 [00:00<00:00, 1325.91obj/s]\r\nExtracting data files #3: 100%|███████████████████████████████████████████████████████████████████████████████████████████████████████████████████████████████████████████████████████████| 3/3 [00:00<00:00, 1524.46obj/s]\r\nExtracting data files #2: 100%|███████████████████████████████████████████████████████████████████████████████████████████████████████████████████████████████████████████████████████████| 3/3 [00:00<00:00, 1404.66obj/s]\r\nExtracting data files #4: 100%|███████████████████████████████████████████████████████████████████████████████████████████████████████████████████████████████████████████████████████████| 3/3 [00:00<00:00, 1538.63obj/s]\r\nExtracting data files #6: 100%|███████████████████████████████████████████████████████████████████████████████████████████████████████████████████████████████████████████████████████████| 3/3 [00:00<00:00, 1711.73obj/s]\r\nExtracting data files #7: 100%|███████████████████████████████████████████████████████████████████████████████████████████████████████████████████████████████████████████████████████████| 3/3 [00:00<00:00, 2144.33obj/s]\r\nExtracting data files #5: 100%|███████████████████████████████████████████████████████████████████████████████████████████████████████████████████████████████████████████████████████████| 3/3 [00:00<00:00, 1964.85obj/s]\r\nDataset kodak downloaded and prepared to /home/wzy/.cache/huggingface/datasets/Freed-Wu___kodak/default/0.0.1/6cf51f2b3d686d24a33fe86945f9e16802def212325f9345cf3cbb1b9f5f4a57. Subsequent calls will reuse this data.\r\n```",
"Thanks for reporting ! This sounds like an issue with python multiprocessing. If we switch to multithreading for the downloads it should be much more robust - let me know if this is something you'd like to contribute, I'd be happy to help and give you some pointers",
"> an issue with python multiprocessing\r\n\r\nIf it is an issue with multiprocessing, should we report it to upstream?",
"Debugging this would require quite some work in my opinion, and I've often failed to make reproducible examples, since it's pretty correlated to one's environment + hardware. So I wouldn't spend too much time on this unless we manage to reproduce this on another machine consistently.\r\n\r\nInstead I'd encourage a more pragmatic fix that is: not create tons of processes (on regular machines it may slow things down anyway), and instead use multithreading by default.",
"I am not expert of python. I hear about python has GIL, which result in multi processing is worse than multi threading. So I am not sure if this change makes sense?\r\n\r\nAnd if this is a bug of multi processing, why not report to upstream and let them fix? And even if change it to multi threading, how can we make sure it can truly fix this problem?",
"Just my 2c. No offense.",
"> Just my 2c. No offense.\r\n\r\nsure np ^^\r\n\r\n> I hear about python has GIL, which result in multi processing is worse than multi threading. So I am not sure if this change makes sense?\r\n\r\nHere the bottleneck speed is the bandwidth used to download the files. When downloading, the GIL is released, so multithreading gives the same speed as multiprocessing.\r\n\r\n> And if this is a bug of multi processing, why not report to upstream and let them fix?\r\n\r\nUsually to fix a bug it's important to be able to reproduce it. This way you can share it, experiment with it, and then make sure it's fixed. Here I'm afraid it's not easy to reproduce. Though I think that spawning too many processes for your machine can lead to this kind of issues.\r\n\r\n> And even if change it to multi threading, how can we make sure it can truly fix this problem?\r\n\r\nMultithreading is more robust in python because IIRC there are less locks involved which are often the cause of code hanging for no reason."
] | ### Describe the bug
```python
In [9]: dataset = load_dataset('Freed-Wu/kodak', split='test')
Downloading: 100%|████████████████████████████████████████████████████████████████████████████████████████████████████████████████████████████████████████████████████████████████████| 2.53k/2.53k [00:00<00:00, 1.88MB/s]
[11/19/22 22:16:21] WARNING Using custom data configuration default builder.py:379
Downloading and preparing dataset kodak/default to /home/wzy/.cache/huggingface/datasets/Freed-Wu___kodak/default/0.0.1/bd1cc3434212e3e654f7e16ad618f8a1470b5982b086c91b1d6bc7187183c6e9...
Downloading: 100%|███████████████████████████████████████████████████████████████████████████████████████████████████████████████████████████████████████████████████████████████████████| 531k/531k [00:02<00:00, 239kB/s]
#10: 100%|██████████████████████████████████████████████████████████████████████████████████████████████████████████████████████████████████████████████████████████████████████████████████| 1/1 [00:04<00:00, 4.06s/obj]
Downloading: 100%|███████████████████████████████████████████████████████████████████████████████████████████████████████████████████████████████████████████████████████████████████████| 534k/534k [00:02<00:00, 193kB/s]
#14: 100%|██████████████████████████████████████████████████████████████████████████████████████████████████████████████████████████████████████████████████████████████████████████████████| 1/1 [00:04<00:00, 4.37s/obj]
Downloading: 100%|███████████████████████████████████████████████████████████████████████████████████████████████████████████████████████████████████████████████████████████████████████| 692k/692k [00:02<00:00, 269kB/s]
#12: 100%|██████████████████████████████████████████████████████████████████████████████████████████████████████████████████████████████████████████████████████████████████████████████████| 1/1 [00:04<00:00, 4.44s/obj]
Downloading: 100%|███████████████████████████████████████████████████████████████████████████████████████████████████████████████████████████████████████████████████████████████████████| 566k/566k [00:02<00:00, 210kB/s]
#5: 100%|███████████████████████████████████████████████████████████████████████████████████████████████████████████████████████████████████████████████████████████████████████████████████| 1/1 [00:04<00:00, 4.53s/obj]
Downloading: 100%|███████████████████████████████████████████████████████████████████████████████████████████████████████████████████████████████████████████████████████████████████████| 613k/613k [00:02<00:00, 235kB/s]
#13: 100%|██████████████████████████████████████████████████████████████████████████████████████████████████████████████████████████████████████████████████████████████████████████████████| 1/1 [00:04<00:00, 4.53s/obj]
Downloading: 100%|███████████████████████████████████████████████████████████████████████████████████████████████████████████████████████████████████████████████████████████████████████| 786k/786k [00:02<00:00, 342kB/s]
#3: 100%|███████████████████████████████████████████████████████████████████████████████████████████████████████████████████████████████████████████████████████████████████████████████████| 1/1 [00:04<00:00, 4.60s/obj]
Downloading: 100%|███████████████████████████████████████████████████████████████████████████████████████████████████████████████████████████████████████████████████████████████████████| 619k/619k [00:02<00:00, 254kB/s]
#4: 100%|███████████████████████████████████████████████████████████████████████████████████████████████████████████████████████████████████████████████████████████████████████████████████| 1/1 [00:04<00:00, 4.68s/obj]
Downloading: 100%|███████████████████████████████████████████████████████████████████████████████████████████████████████████████████████████████████████████████████████████████████████| 737k/737k [00:02<00:00, 271kB/s]
Downloading: 100%|███████████████████████████████████████████████████████████████████████████████████████████████████████████████████████████████████████████████████████████████████████| 788k/788k [00:02<00:00, 285kB/s]
#6: 100%|███████████████████████████████████████████████████████████████████████████████████████████████████████████████████████████████████████████████████████████████████████████████████| 1/1 [00:05<00:00, 5.04s/obj]
Downloading: 100%|███████████████████████████████████████████████████████████████████████████████████████████████████████████████████████████████████████████████████████████████████████| 618k/618k [00:04<00:00, 153kB/s]
#0: 100%|███████████████████████████████████████████████████████████████████████████████████████████████████████████████████████████████████████████████████████████████████████████████████| 2/2 [00:11<00:00, 5.69s/obj]
^CProcess ForkPoolWorker-47:
Process ForkPoolWorker-46:
Process ForkPoolWorker-36:
Process ForkPoolWorker-38:██████████████████████████████████████████████████████████████████████████████████████████████████████████████████████████████████████████████████████████████████| 1/1 [00:05<00:00, 5.04s/obj]
Process ForkPoolWorker-37:
Process ForkPoolWorker-45:
Process ForkPoolWorker-39:
Process ForkPoolWorker-43:
Process ForkPoolWorker-33:
Process ForkPoolWorker-18:
Traceback (most recent call last):
Traceback (most recent call last):
Traceback (most recent call last):
Traceback (most recent call last):
Traceback (most recent call last):
File "/usr/lib/python3.10/multiprocessing/process.py", line 314, in _bootstrap
self.run()
File "/usr/lib/python3.10/multiprocessing/process.py", line 314, in _bootstrap
self.run()
File "/usr/lib/python3.10/multiprocessing/process.py", line 314, in _bootstrap
self.run()
File "/usr/lib/python3.10/multiprocessing/process.py", line 108, in run
self._target(*self._args, **self._kwargs)
File "/usr/lib/python3.10/multiprocessing/process.py", line 314, in _bootstrap
self.run()
File "/usr/lib/python3.10/multiprocessing/pool.py", line 114, in worker
task = get()
File "/usr/lib/python3.10/multiprocessing/process.py", line 314, in _bootstrap
self.run()
File "/usr/lib/python3.10/multiprocessing/queues.py", line 364, in get
with self._rlock:
File "/usr/lib/python3.10/multiprocessing/process.py", line 108, in run
self._target(*self._args, **self._kwargs)
File "/usr/lib/python3.10/multiprocessing/synchronize.py", line 95, in __enter__
return self._semlock.__enter__()
File "/usr/lib/python3.10/multiprocessing/process.py", line 108, in run
self._target(*self._args, **self._kwargs)
File "/usr/lib/python3.10/multiprocessing/process.py", line 108, in run
self._target(*self._args, **self._kwargs)
File "/usr/lib/python3.10/multiprocessing/pool.py", line 114, in worker
task = get()
File "/usr/lib/python3.10/multiprocessing/pool.py", line 114, in worker
task = get()
File "/usr/lib/python3.10/multiprocessing/process.py", line 108, in run
self._target(*self._args, **self._kwargs)
File "/usr/lib/python3.10/multiprocessing/queues.py", line 364, in get
with self._rlock:
File "/usr/lib/python3.10/multiprocessing/pool.py", line 114, in worker
task = get()
File "/usr/lib/python3.10/multiprocessing/queues.py", line 364, in get
with self._rlock:
KeyboardInterrupt
File "/usr/lib/python3.10/multiprocessing/synchronize.py", line 95, in __enter__
return self._semlock.__enter__()
Traceback (most recent call last):
Traceback (most recent call last):
Traceback (most recent call last):
KeyboardInterrupt
File "/usr/lib/python3.10/multiprocessing/pool.py", line 114, in worker
task = get()
File "/usr/lib/python3.10/multiprocessing/queues.py", line 364, in get
with self._rlock:
File "/usr/lib/python3.10/multiprocessing/queues.py", line 364, in get
with self._rlock:
File "/usr/lib/python3.10/multiprocessing/synchronize.py", line 95, in __enter__
return self._semlock.__enter__()
File "/usr/lib/python3.10/multiprocessing/synchronize.py", line 95, in __enter__
return self._semlock.__enter__()
KeyboardInterrupt
File "/usr/lib/python3.10/multiprocessing/process.py", line 314, in _bootstrap
self.run()
File "/usr/lib/python3.10/multiprocessing/process.py", line 314, in _bootstrap
self.run()
KeyboardInterrupt
File "/usr/lib/python3.10/multiprocessing/process.py", line 314, in _bootstrap
self.run()
File "/usr/lib/python3.10/multiprocessing/process.py", line 108, in run
self._target(*self._args, **self._kwargs)
File "/usr/lib/python3.10/multiprocessing/process.py", line 108, in run
self._target(*self._args, **self._kwargs)
File "/usr/lib/python3.10/multiprocessing/pool.py", line 114, in worker
task = get()
File "/usr/lib/python3.10/multiprocessing/synchronize.py", line 95, in __enter__
return self._semlock.__enter__()
File "/usr/lib/python3.10/multiprocessing/process.py", line 108, in run
self._target(*self._args, **self._kwargs)
File "/usr/lib/python3.10/multiprocessing/pool.py", line 114, in worker
task = get()
File "/usr/lib/python3.10/multiprocessing/pool.py", line 114, in worker
task = get()
File "/usr/lib/python3.10/multiprocessing/queues.py", line 364, in get
with self._rlock:
File "/usr/lib/python3.10/multiprocessing/queues.py", line 365, in get
res = self._reader.recv_bytes()
File "/usr/lib/python3.10/multiprocessing/queues.py", line 364, in get
with self._rlock:
File "/usr/lib/python3.10/multiprocessing/synchronize.py", line 95, in __enter__
return self._semlock.__enter__()
KeyboardInterrupt
File "/usr/lib/python3.10/multiprocessing/synchronize.py", line 95, in __enter__
return self._semlock.__enter__()
File "/usr/lib/python3.10/multiprocessing/connection.py", line 221, in recv_bytes
buf = self._recv_bytes(maxlength)
KeyboardInterrupt
KeyboardInterrupt
File "/usr/lib/python3.10/multiprocessing/connection.py", line 419, in _recv_bytes
buf = self._recv(4)
File "/usr/lib/python3.10/multiprocessing/connection.py", line 384, in _recv
chunk = read(handle, remaining)
KeyboardInterrupt
Traceback (most recent call last):
File "/usr/lib/python3.10/multiprocessing/process.py", line 314, in _bootstrap
self.run()
File "/usr/lib/python3.10/multiprocessing/process.py", line 108, in run
self._target(*self._args, **self._kwargs)
File "/usr/lib/python3.10/multiprocessing/pool.py", line 114, in worker
task = get()
File "/usr/lib/python3.10/multiprocessing/queues.py", line 364, in get
with self._rlock:
File "/usr/lib/python3.10/multiprocessing/synchronize.py", line 95, in __enter__
return self._semlock.__enter__()
KeyboardInterrupt
Process ForkPoolWorker-20:
Process ForkPoolWorker-44:
Process ForkPoolWorker-22:
Traceback (most recent call last):
File "/usr/lib/python3.10/site-packages/urllib3/util/connection.py", line 85, in create_connection
sock.connect(sa)
ConnectionRefusedError: [Errno 111] Connection refused
During handling of the above exception, another exception occurred:
Traceback (most recent call last):
File "/usr/lib/python3.10/multiprocessing/process.py", line 314, in _bootstrap
self.run()
File "/usr/lib/python3.10/multiprocessing/process.py", line 108, in run
self._target(*self._args, **self._kwargs)
File "/usr/lib/python3.10/multiprocessing/pool.py", line 125, in worker
result = (True, func(*args, **kwds))
File "/usr/lib/python3.10/multiprocessing/pool.py", line 48, in mapstar
return list(map(*args))
File "/usr/lib/python3.10/site-packages/datasets/utils/py_utils.py", line 215, in _single_map_nested
mapped = [_single_map_nested((function, v, types, None, True)) for v in pbar]
File "/usr/lib/python3.10/site-packages/datasets/utils/py_utils.py", line 215, in <listcomp>
mapped = [_single_map_nested((function, v, types, None, True)) for v in pbar]
File "/usr/lib/python3.10/site-packages/datasets/utils/py_utils.py", line 197, in _single_map_nested
return function(data_struct)
File "/usr/lib/python3.10/site-packages/datasets/utils/download_manager.py", line 217, in _download
return cached_path(url_or_filename, download_config=download_config)
File "/usr/lib/python3.10/site-packages/datasets/utils/file_utils.py", line 298, in cached_path
output_path = get_from_cache(
File "/usr/lib/python3.10/site-packages/datasets/utils/file_utils.py", line 561, in get_from_cache
response = http_head(
File "/usr/lib/python3.10/site-packages/datasets/utils/file_utils.py", line 476, in http_head
response = _request_with_retry(
File "/usr/lib/python3.10/site-packages/datasets/utils/file_utils.py", line 405, in _request_with_retry
response = requests.request(method=method.upper(), url=url, timeout=timeout, **params)
File "/usr/lib/python3.10/site-packages/requests/api.py", line 59, in request
return session.request(method=method, url=url, **kwargs)
File "/usr/lib/python3.10/site-packages/requests/sessions.py", line 587, in request
resp = self.send(prep, **send_kwargs)
File "/usr/lib/python3.10/site-packages/requests/sessions.py", line 701, in send
r = adapter.send(request, **kwargs)
File "/usr/lib/python3.10/site-packages/requests/adapters.py", line 489, in send
resp = conn.urlopen(
File "/usr/lib/python3.10/site-packages/urllib3/connectionpool.py", line 703, in urlopen
httplib_response = self._make_request(
File "/usr/lib/python3.10/site-packages/urllib3/connectionpool.py", line 386, in _make_request
self._validate_conn(conn)
File "/usr/lib/python3.10/site-packages/urllib3/connectionpool.py", line 1042, in _validate_conn
conn.connect()
File "/usr/lib/python3.10/site-packages/urllib3/connection.py", line 358, in connect
self.sock = conn = self._new_conn()
File "/usr/lib/python3.10/site-packages/urllib3/connection.py", line 174, in _new_conn
conn = connection.create_connection(
File "/usr/lib/python3.10/site-packages/urllib3/util/connection.py", line 85, in create_connection
sock.connect(sa)
KeyboardInterrupt
#1: 0%| | 0/2 [03:00<?, ?obj/s]
Traceback (most recent call last):
Traceback (most recent call last):
File "/usr/lib/python3.10/multiprocessing/process.py", line 314, in _bootstrap
self.run()
File "/usr/lib/python3.10/multiprocessing/process.py", line 108, in run
self._target(*self._args, **self._kwargs)
File "/usr/lib/python3.10/multiprocessing/pool.py", line 125, in worker
result = (True, func(*args, **kwds))
File "/usr/lib/python3.10/multiprocessing/pool.py", line 48, in mapstar
return list(map(*args))
File "/usr/lib/python3.10/site-packages/datasets/utils/py_utils.py", line 215, in _single_map_nested
mapped = [_single_map_nested((function, v, types, None, True)) for v in pbar]
File "/usr/lib/python3.10/site-packages/datasets/utils/py_utils.py", line 215, in <listcomp>
mapped = [_single_map_nested((function, v, types, None, True)) for v in pbar]
File "/usr/lib/python3.10/site-packages/datasets/utils/py_utils.py", line 197, in _single_map_nested
return function(data_struct)
File "/usr/lib/python3.10/site-packages/datasets/utils/download_manager.py", line 217, in _download
return cached_path(url_or_filename, download_config=download_config)
File "/usr/lib/python3.10/site-packages/datasets/utils/file_utils.py", line 298, in cached_path
output_path = get_from_cache(
File "/usr/lib/python3.10/site-packages/datasets/utils/file_utils.py", line 659, in get_from_cache
http_get(
File "/usr/lib/python3.10/site-packages/datasets/utils/file_utils.py", line 442, in http_get
response = _request_with_retry(
File "/usr/lib/python3.10/site-packages/datasets/utils/file_utils.py", line 405, in _request_with_retry
response = requests.request(method=method.upper(), url=url, timeout=timeout, **params)
File "/usr/lib/python3.10/site-packages/requests/api.py", line 59, in request
return session.request(method=method, url=url, **kwargs)
File "/usr/lib/python3.10/site-packages/requests/sessions.py", line 587, in request
resp = self.send(prep, **send_kwargs)
File "/usr/lib/python3.10/site-packages/requests/sessions.py", line 701, in send
r = adapter.send(request, **kwargs)
File "/usr/lib/python3.10/site-packages/requests/adapters.py", line 489, in send
resp = conn.urlopen(
File "/usr/lib/python3.10/site-packages/urllib3/connectionpool.py", line 703, in urlopen
httplib_response = self._make_request(
File "/usr/lib/python3.10/site-packages/urllib3/connectionpool.py", line 386, in _make_request
self._validate_conn(conn)
File "/usr/lib/python3.10/multiprocessing/process.py", line 314, in _bootstrap
self.run()
File "/usr/lib/python3.10/site-packages/urllib3/connectionpool.py", line 1042, in _validate_conn
conn.connect()
File "/usr/lib/python3.10/multiprocessing/process.py", line 108, in run
self._target(*self._args, **self._kwargs)
File "/usr/lib/python3.10/site-packages/urllib3/connection.py", line 358, in connect
self.sock = conn = self._new_conn()
File "/usr/lib/python3.10/multiprocessing/pool.py", line 125, in worker
result = (True, func(*args, **kwds))
File "/usr/lib/python3.10/site-packages/urllib3/connection.py", line 174, in _new_conn
conn = connection.create_connection(
File "/usr/lib/python3.10/multiprocessing/pool.py", line 48, in mapstar
return list(map(*args))
File "/usr/lib/python3.10/site-packages/urllib3/util/connection.py", line 72, in create_connection
for res in socket.getaddrinfo(host, port, family, socket.SOCK_STREAM):
File "/usr/lib/python3.10/site-packages/datasets/utils/py_utils.py", line 215, in _single_map_nested
mapped = [_single_map_nested((function, v, types, None, True)) for v in pbar]
File "/usr/lib/python3.10/socket.py", line 955, in getaddrinfo
for res in _socket.getaddrinfo(host, port, family, type, proto, flags):
File "/usr/lib/python3.10/site-packages/datasets/utils/py_utils.py", line 215, in <listcomp>
mapped = [_single_map_nested((function, v, types, None, True)) for v in pbar]
File "/usr/lib/python3.10/site-packages/datasets/utils/py_utils.py", line 197, in _single_map_nested
return function(data_struct)
File "/usr/lib/python3.10/site-packages/datasets/utils/download_manager.py", line 217, in _download
return cached_path(url_or_filename, download_config=download_config)
KeyboardInterrupt
File "/usr/lib/python3.10/site-packages/datasets/utils/file_utils.py", line 298, in cached_path
output_path = get_from_cache(
File "/usr/lib/python3.10/site-packages/datasets/utils/file_utils.py", line 561, in get_from_cache
response = http_head(
File "/usr/lib/python3.10/site-packages/datasets/utils/file_utils.py", line 476, in http_head
response = _request_with_retry(
File "/usr/lib/python3.10/site-packages/datasets/utils/file_utils.py", line 405, in _request_with_retry
response = requests.request(method=method.upper(), url=url, timeout=timeout, **params)
File "/usr/lib/python3.10/site-packages/requests/api.py", line 59, in request
return session.request(method=method, url=url, **kwargs)
File "/usr/lib/python3.10/site-packages/requests/sessions.py", line 587, in request
resp = self.send(prep, **send_kwargs)
File "/usr/lib/python3.10/site-packages/requests/sessions.py", line 701, in send
r = adapter.send(request, **kwargs)
File "/usr/lib/python3.10/site-packages/requests/adapters.py", line 489, in send
resp = conn.urlopen(
File "/usr/lib/python3.10/site-packages/urllib3/connectionpool.py", line 703, in urlopen
httplib_response = self._make_request(
File "/usr/lib/python3.10/site-packages/urllib3/connectionpool.py", line 386, in _make_request
self._validate_conn(conn)
File "/usr/lib/python3.10/site-packages/urllib3/connectionpool.py", line 1042, in _validate_conn
conn.connect()
File "/usr/lib/python3.10/site-packages/urllib3/connection.py", line 358, in connect
self.sock = conn = self._new_conn()
File "/usr/lib/python3.10/site-packages/urllib3/connection.py", line 174, in _new_conn
conn = connection.create_connection(
File "/usr/lib/python3.10/site-packages/urllib3/util/connection.py", line 72, in create_connection
for res in socket.getaddrinfo(host, port, family, socket.SOCK_STREAM):
File "/usr/lib/python3.10/socket.py", line 955, in getaddrinfo
for res in _socket.getaddrinfo(host, port, family, type, proto, flags):
KeyboardInterrupt
#3: 0%| | 0/2 [03:00<?, ?obj/s]
#11: 0%| | 0/1 [00:49<?, ?obj/s]
Traceback (most recent call last):
File "/usr/lib/python3.10/site-packages/urllib3/util/connection.py", line 85, in create_connection
sock.connect(sa)
ConnectionRefusedError: [Errno 111] Connection refused
During handling of the above exception, another exception occurred:
Traceback (most recent call last):
File "/usr/lib/python3.10/multiprocessing/process.py", line 314, in _bootstrap
self.run()
File "/usr/lib/python3.10/multiprocessing/process.py", line 108, in run
self._target(*self._args, **self._kwargs)
File "/usr/lib/python3.10/multiprocessing/pool.py", line 125, in worker
result = (True, func(*args, **kwds))
File "/usr/lib/python3.10/multiprocessing/pool.py", line 48, in mapstar
return list(map(*args))
File "/usr/lib/python3.10/site-packages/datasets/utils/py_utils.py", line 215, in _single_map_nested
mapped = [_single_map_nested((function, v, types, None, True)) for v in pbar]
File "/usr/lib/python3.10/site-packages/datasets/utils/py_utils.py", line 215, in <listcomp>
mapped = [_single_map_nested((function, v, types, None, True)) for v in pbar]
File "/usr/lib/python3.10/site-packages/datasets/utils/py_utils.py", line 197, in _single_map_nested
return function(data_struct)
File "/usr/lib/python3.10/site-packages/datasets/utils/download_manager.py", line 217, in _download
return cached_path(url_or_filename, download_config=download_config)
File "/usr/lib/python3.10/site-packages/datasets/utils/file_utils.py", line 298, in cached_path
output_path = get_from_cache(
File "/usr/lib/python3.10/site-packages/datasets/utils/file_utils.py", line 561, in get_from_cache
response = http_head(
File "/usr/lib/python3.10/site-packages/datasets/utils/file_utils.py", line 476, in http_head
response = _request_with_retry(
File "/usr/lib/python3.10/site-packages/datasets/utils/file_utils.py", line 405, in _request_with_retry
response = requests.request(method=method.upper(), url=url, timeout=timeout, **params)
File "/usr/lib/python3.10/site-packages/requests/api.py", line 59, in request
return session.request(method=method, url=url, **kwargs)
File "/usr/lib/python3.10/site-packages/requests/sessions.py", line 587, in request
resp = self.send(prep, **send_kwargs)
File "/usr/lib/python3.10/site-packages/requests/sessions.py", line 723, in send
history = [resp for resp in gen]
File "/usr/lib/python3.10/site-packages/requests/sessions.py", line 723, in <listcomp>
history = [resp for resp in gen]
File "/usr/lib/python3.10/site-packages/requests/sessions.py", line 266, in resolve_redirects
resp = self.send(
File "/usr/lib/python3.10/site-packages/requests/sessions.py", line 701, in send
r = adapter.send(request, **kwargs)
File "/usr/lib/python3.10/site-packages/requests/adapters.py", line 489, in send
resp = conn.urlopen(
File "/usr/lib/python3.10/site-packages/urllib3/connectionpool.py", line 703, in urlopen
httplib_response = self._make_request(
File "/usr/lib/python3.10/site-packages/urllib3/connectionpool.py", line 386, in _make_request
self._validate_conn(conn)
File "/usr/lib/python3.10/site-packages/urllib3/connectionpool.py", line 1042, in _validate_conn
conn.connect()
File "/usr/lib/python3.10/site-packages/urllib3/connection.py", line 358, in connect
self.sock = conn = self._new_conn()
File "/usr/lib/python3.10/site-packages/urllib3/connection.py", line 174, in _new_conn
conn = connection.create_connection(
File "/usr/lib/python3.10/site-packages/urllib3/util/connection.py", line 85, in create_connection
sock.connect(sa)
KeyboardInterrupt
#5: 0%| | 0/1 [03:00<?, ?obj/s]
KeyboardInterrupt
Process ForkPoolWorker-42:
Traceback (most recent call last):
File "/usr/lib/python3.10/multiprocessing/process.py", line 314, in _bootstrap
self.run()
File "/usr/lib/python3.10/multiprocessing/process.py", line 108, in run
self._target(*self._args, **self._kwargs)
File "/usr/lib/python3.10/multiprocessing/pool.py", line 125, in worker
result = (True, func(*args, **kwds))
File "/usr/lib/python3.10/multiprocessing/pool.py", line 48, in mapstar
return list(map(*args))
File "/usr/lib/python3.10/site-packages/datasets/utils/py_utils.py", line 215, in _single_map_nested
mapped = [_single_map_nested((function, v, types, None, True)) for v in pbar]
File "/usr/lib/python3.10/site-packages/datasets/utils/py_utils.py", line 215, in <listcomp>
mapped = [_single_map_nested((function, v, types, None, True)) for v in pbar]
File "/usr/lib/python3.10/site-packages/datasets/utils/py_utils.py", line 197, in _single_map_nested
return function(data_struct)
File "/usr/lib/python3.10/site-packages/datasets/utils/download_manager.py", line 217, in _download
return cached_path(url_or_filename, download_config=download_config)
File "/usr/lib/python3.10/site-packages/datasets/utils/file_utils.py", line 298, in cached_path
output_path = get_from_cache(
File "/usr/lib/python3.10/site-packages/datasets/utils/file_utils.py", line 561, in get_from_cache
response = http_head(
File "/usr/lib/python3.10/site-packages/datasets/utils/file_utils.py", line 476, in http_head
response = _request_with_retry(
File "/usr/lib/python3.10/site-packages/datasets/utils/file_utils.py", line 405, in _request_with_retry
response = requests.request(method=method.upper(), url=url, timeout=timeout, **params)
File "/usr/lib/python3.10/site-packages/requests/api.py", line 59, in request
return session.request(method=method, url=url, **kwargs)
File "/usr/lib/python3.10/site-packages/requests/sessions.py", line 587, in request
resp = self.send(prep, **send_kwargs)
File "/usr/lib/python3.10/site-packages/requests/sessions.py", line 701, in send
r = adapter.send(request, **kwargs)
File "/usr/lib/python3.10/site-packages/requests/adapters.py", line 489, in send
resp = conn.urlopen(
File "/usr/lib/python3.10/site-packages/urllib3/connectionpool.py", line 703, in urlopen
httplib_response = self._make_request(
File "/usr/lib/python3.10/site-packages/urllib3/connectionpool.py", line 386, in _make_request
self._validate_conn(conn)
File "/usr/lib/python3.10/site-packages/urllib3/connectionpool.py", line 1042, in _validate_conn
conn.connect()
File "/usr/lib/python3.10/site-packages/urllib3/connection.py", line 358, in connect
self.sock = conn = self._new_conn()
File "/usr/lib/python3.10/site-packages/urllib3/connection.py", line 174, in _new_conn
conn = connection.create_connection(
File "/usr/lib/python3.10/site-packages/urllib3/util/connection.py", line 72, in create_connection
for res in socket.getaddrinfo(host, port, family, socket.SOCK_STREAM):
File "/usr/lib/python3.10/socket.py", line 955, in getaddrinfo
for res in _socket.getaddrinfo(host, port, family, type, proto, flags):
KeyboardInterrupt
#9: 0%| | 0/1 [00:51<?, ?obj/s]
```
### Steps to reproduce the bug
```python
"""Kodak.
Copyright 2020 The HuggingFace Datasets Authors and the current dataset script contributor.
Licensed under the Apache License, Version 2.0 (the "License");
you may not use this file except in compliance with the License.
You may obtain a copy of the License at
http://www.apache.org/licenses/LICENSE-2.0
Unless required by applicable law or agreed to in writing, software
distributed under the License is distributed on an "AS IS" BASIS,
WITHOUT WARRANTIES OR CONDITIONS OF ANY KIND, either express or implied.
See the License for the specific language governing permissions and
limitations under the License.
"""
import datasets
NUMBER = 17
_DESCRIPTION = """\
The pictures below link to lossless, true color (24 bits per pixel, aka "full
color") images. It is my understanding they have been released by the Eastman
Kodak Company for unrestricted usage. Many sites use them as a standard test
suite for compression testing, etc. Prior to this site, they were only
available in the Sun Raster format via ftp. This meant that the images could
not be previewed before downloading. Since their release, however, the lossless
PNG format has been incorporated into all the major browsers. Since PNG
supports 24-bit lossless color (which GIF and JPEG do not), it is now possible
to offer this browser-friendly access to the images.
"""
_HOMEPAGE = "https://r0k.us/graphics/kodak/"
_LICENSE = "GPLv3"
_URLS = [
f"https://github.com/MohamedBakrAli/Kodak-Lossless-True-Color-Image-Suite/raw/master/PhotoCD_PCD0992/{i}.png"
for i in range(1, 1 + NUMBER)
]
class Kodak(datasets.GeneratorBasedBuilder):
"""Kodak datasets."""
VERSION = datasets.Version("0.0.1")
def _info(self):
features = datasets.Features(
{
"image": datasets.Image(),
}
)
return datasets.DatasetInfo(
description=_DESCRIPTION,
features=features,
homepage=_HOMEPAGE,
license=_LICENSE,
)
def _split_generators(self, dl_manager):
"""Return SplitGenerators."""
file_paths = dl_manager.download_and_extract(_URLS)
return [
datasets.SplitGenerator(
name=datasets.Split.TEST,
gen_kwargs={
"file_paths": file_paths,
},
),
]
def _generate_examples(self, file_paths):
"""Yield examples."""
for file_path in file_paths:
yield file_path, {"image": file_path}
```
### Expected behavior
When `len(_URLS) < 16`, it works.
```python
In [3]: dataset = load_dataset('Freed-Wu/kodak', split='test')
Downloading: 100%|████████████████████████████████████████████████████████████████████████████████████████████████████████████████████████████████████████████████████████████████████| 2.53k/2.53k [00:00<00:00, 3.02MB/s]
[11/19/22 22:04:28] WARNING Using custom data configuration default builder.py:379
Downloading and preparing dataset kodak/default to /home/wzy/.cache/huggingface/datasets/Freed-Wu___kodak/default/0.0.1/d26017602a592b5bfa7e008127cdf9dec5af220c9068005f1b4eda036031f475...
Downloading: 100%|██████████████████████████████████████████████████████████████████████████████████████████████████████████████████████████████████████████████████████████████████████| 593k/593k [00:00<00:00, 2.88MB/s]
Downloading: 100%|███████████████████████████████████████████████████████████████████████████████████████████████████████████████████████████████████████████████████████████████████████| 621k/621k [00:03<00:00, 166kB/s]
Downloading: 100%|███████████████████████████████████████████████████████████████████████████████████████████████████████████████████████████████████████████████████████████████████████| 531k/531k [00:01<00:00, 366kB/s]
100%|██████████████████████████████████████████████████████████████████████████████████████████████████████████████████████████████████████████████████████████████████████████████████████| 16/16 [00:13<00:00, 1.18it/s]
100%|████████████████████████████████████████████████████████████████████████████████████████████████████████████████████████████████████████████████████████████████████████████████████| 16/16 [00:00<00:00, 3832.38it/s]
Dataset kodak downloaded and prepared to /home/wzy/.cache/huggingface/datasets/Freed-Wu___kodak/default/0.0.1/d26017602a592b5bfa7e008127cdf9dec5af220c9068005f1b4eda036031f475. Subsequent calls will reuse this data.
```
### Environment info
- `datasets` version: 2.7.0
- Platform: Linux-6.0.8-arch1-1-x86_64-with-glibc2.36
- Python version: 3.10.8
- PyArrow version: 9.0.0
- Pandas version: 1.4.4 | 5,270 |
https://github.com/huggingface/datasets/issues/5269 | Shell completions | [
"I don't think we need completion on the datasets-cli, since we're mainly developing huggingface-cli",
"I see."
] | ### Feature request
Like <https://github.com/huggingface/huggingface_hub/issues/1197>, datasets-cli maybe need it, too.
### Motivation
See above.
### Your contribution
Maybe. | 5,269 |
Subsets and Splits
No saved queries yet
Save your SQL queries to embed, download, and access them later. Queries will appear here once saved.