html_url
stringlengths 48
51
| title
stringlengths 1
290
| comments
sequencelengths 0
30
| body
stringlengths 0
228k
⌀ | number
int64 2
7.08k
|
---|---|---|---|---|
https://github.com/huggingface/datasets/issues/5677 | Dataset.map() crashes when any column contains more than 1000 empty dictionaries | [] | ### Describe the bug
`Dataset.map()` crashes any time any column contains more than `writer_batch_size` (default 1000) empty dictionaries, regardless of whether the column is being operated on. The error does not occur if the dictionaries are non-empty.
### Steps to reproduce the bug
Example:
```
import datasets
def add_one(example):
example["col2"] += 1
return example
n = 1001 # crashes
# n = 999 # works
ds = datasets.Dataset.from_dict({"col1": [{}] * n, "col2": [1] * n})
ds = ds.map(add_one, writer_batch_size=1000)
```
### Expected behavior
Above code should not crash
### Environment info
- `datasets` version: 2.10.1
- Platform: Linux-5.4.0-120-generic-x86_64-with-glibc2.10
- Python version: 3.8.15
- PyArrow version: 9.0.0
- Pandas version: 1.5.3 | 5,677 |
https://github.com/huggingface/datasets/issues/5675 | Filter datasets by language code | [
"The dataset still can be found, if instead of using the search form you just enter the language code in the url, like https://huggingface.co/datasets?language=language:myv. \r\n\r\nBut of course having a more complete list of languages in the search form (or just a fallback to the language codes, if they are missing from the code=>language mapping) would be much more convenient!",
"Hi! I've opened a PR to make these languages searchable on the Hub.",
"Thanks @mariosasko!\r\nDo you think it is possible to turn this into a more scalable pipeline? Such as:\r\n1. Looping through all the datasets on the hub and collecting the set of all their language codes;\r\n2. Selecting the codes not covered yet in `Language.ts`\r\n3. Looking up their codes at https://iso639-3.sil.org/code_tables/639/data\r\n4. Adding all the newly found language codes to `Language.ts`",
"@avidale This has been discussed in https://github.com/huggingface/datasets/issues/4881, so also feel free to share your opinion there."
] | Hi! I use the language search field on https://huggingface.co/datasets
However, some of the datasets tagged by ISO language code are not accessible by this search form.
For example, [myv_ru_2022](https://huggingface.co/datasets/slone/myv_ru_2022) is has `myv` language tag but it is not included in Languages search form.
I've also noticed the same problem with `mhr` (see https://huggingface.co/datasets/AigizK/mari-russian-parallel-corpora) | 5,675 |
https://github.com/huggingface/datasets/issues/5674 | Stored XSS | [
"Hi! You can contact `[email protected]` to report this vulnerability."
] | x | 5,674 |
https://github.com/huggingface/datasets/issues/5672 | Pushing dataset to hub crash | [
"Hi ! It's been fixed by https://github.com/huggingface/datasets/pull/5598. We're doing a new release tomorrow with the fix and you'll be able to push your 100k images ;)\r\n\r\nBasically `push_to_hub` used to fail if the remote repository already exists and has a README.md without dataset_info in the YAML tags.\r\n\r\nIn the meantime you can install datasets from source",
"Hi @lhoestq ,\r\n\r\nWhat version of datasets library fix this case? I am using the last `v2.10.1` and I get the same error.",
"We just released 2.11 which includes a fix :)"
] | ### Describe the bug
Uploading a dataset with `push_to_hub()` fails without error description.
### Steps to reproduce the bug
Hey there,
I've built a image dataset of 100k images + text pair as described here https://huggingface.co/docs/datasets/image_dataset#imagefolder
Now I'm trying to push it to the hub but I'm running into issues. First, I tried doing it via git directly, I added all the files in git lfs and pushed but I got hit with an error saying huggingface only accept up to 10k files in a folder.
So I'm now trying with the `push_to_hub()` func as follow:
```python
from datasets import load_dataset
import os
dataset = load_dataset("imagefolder", data_dir="./data", split="train")
dataset.push_to_hub("tzvc/organization-logos", token=os.environ.get('HF_TOKEN'))
```
But again, this produces an error:
```
Resolving data files: 100%|███████████████████████████████████████████████████████████████████████████████████████████████████████| 100212/100212 [00:00<00:00, 439108.61it/s]
Downloading and preparing dataset imagefolder/default to /home/contact_theochampion/.cache/huggingface/datasets/imagefolder/default-20567ffc703aa314/0.0.0/37fbb85cc714a338bea574ac6c7d0b5be5aff46c1862c1989b20e0771199e93f...
Downloading data files: 100%|█████████████████████████████████████████████████████████████████████████████████████████████████████| 100211/100211 [00:00<00:00, 149323.73it/s]
Downloading data files: 100%|████████████████████████████████████████████████████████████████████████████████████████████████████████████████| 1/1 [00:00<00:00, 15947.92it/s]
Extracting data files: 100%|██████████████████████████████████████████████████████████████████████████████████████████████████████████████████| 1/1 [00:00<00:00, 2245.34it/s]
Dataset imagefolder downloaded and prepared to /home/contact_theochampion/.cache/huggingface/datasets/imagefolder/default-20567ffc703aa314/0.0.0/37fbb85cc714a338bea574ac6c7d0b5be5aff46c1862c1989b20e0771199e93f. Subsequent calls will reuse this data.
Resuming upload of the dataset shards.
Pushing dataset shards to the dataset hub: 100%|██████████████████████████████████████████████████████████████████████████████████████████████| 14/14 [00:31<00:00, 2.24s/it]
Downloading metadata: 100%|███████████████████████████████████████████████████████████████████████████████████████████████████████████████████| 118/118 [00:00<00:00, 225kB/s]
Traceback (most recent call last):
File "/home/contact_theochampion/organization-logos/push_to_hub.py", line 5, in <module>
dataset.push_to_hub("tzvc/organization-logos", token=os.environ.get('HF_TOKEN'))
File "/home/contact_theochampion/.local/lib/python3.9/site-packages/datasets/arrow_dataset.py", line 5245, in push_to_hub
repo_info = dataset_infos[next(iter(dataset_infos))]
StopIteration
```
What could be happening here ?
### Expected behavior
The dataset is pushed to the hub
### Environment info
- `datasets` version: 2.10.1
- Platform: Linux-5.10.0-21-cloud-amd64-x86_64-with-glibc2.31
- Python version: 3.9.2
- PyArrow version: 11.0.0
- Pandas version: 1.5.3 | 5,672 |
https://github.com/huggingface/datasets/issues/5671 | How to use `load_dataset('glue', 'cola')` | [
"Sounds like an issue with incompatible `transformers` dependencies versions.\r\n\r\nCan you try to update `transformers` ?\r\n\r\nEDIT: I checked the `transformers` dependencies and it seems like you need `tokenizers>=0.10.1,<0.11` with `transformers==4.5.1`\r\n\r\nEDIT2: this old version of `datasets` seems to import `transformers` but it's no longer the case, so you could also simply update `datasets` and `transformers` won't be imported",
"Thank you for advising me to update these libraries versions.\r\n\r\nI can implement codes using `datasets==2.10.1` and `transformers==4.27.3`"
] | ### Describe the bug
I'm new to use HuggingFace datasets but I cannot use `load_dataset('glue', 'cola')`.
- I was stacked by the following problem:
```python
from datasets import load_dataset
cola_dataset = load_dataset('glue', 'cola')
---------------------------------------------------------------------------
InvalidVersion Traceback (most recent call last)
File <timed exec>:1
(Omit because of long error message)
File /usr/local/lib/python3.8/site-packages/packaging/version.py:197, in Version.__init__(self, version)
195 match = self._regex.search(version)
196 if not match:
--> 197 raise InvalidVersion(f"Invalid version: '{version}'")
199 # Store the parsed out pieces of the version
200 self._version = _Version(
201 epoch=int(match.group("epoch")) if match.group("epoch") else 0,
202 release=tuple(int(i) for i in match.group("release").split(".")),
(...)
208 local=_parse_local_version(match.group("local")),
209 )
InvalidVersion: Invalid version: '0.10.1,<0.11'
```
- You can check this full error message in my repository: [MLOps-Basics/week_0_project_setup/experimental_notebooks/data_exploration.ipynb](https://github.com/makinzm/MLOps-Basics/blob/eabab4b837880607d9968d3fa687c70177b2affd/week_0_project_setup/experimental_notebooks/data_exploration.ipynb)
### Steps to reproduce the bug
- This is my repository to reproduce: [MLOps-Basics/week_0_project_setup](https://github.com/makinzm/MLOps-Basics/tree/eabab4b837880607d9968d3fa687c70177b2affd/week_0_project_setup)
1. cd `/DockerImage` and command `docker build . -t week0`
2. cd `/` and command `docker-compose up`
3. Run `experimental_notebooks/data_exploration.ipynb`
----
Just to be sure, I wrote down Dockerfile and requirements.txt
- Dockerfile
```Dockerfile
FROM python:3.8
WORKDIR /root/working
RUN apt-get update && \
apt-get install -y python3-dev python3-pip python3-venv && \
apt-get clean && \
rm -rf /var/lib/apt/lists/*
COPY requirements.txt .
RUN pip3 install --no-cache-dir jupyter notebook && pip install --no-cache-dir -r requirements.txt
CMD ["bash"]
```
- requirements.txt
```txt
pytorch-lightning==1.2.10
datasets==1.6.2
transformers==4.5.1
scikit-learn==0.24.2
```
### Expected behavior
There is no bug to implement `load_dataset('glue', 'cola')`
### Environment info
I already wrote it. | 5,671 |
https://github.com/huggingface/datasets/issues/5670 | Unable to load multi class classification datasets | [
"Hi ! This sounds related to https://github.com/huggingface/datasets/issues/5406\r\n\r\nUpdating `datasets` fixes the issue ;)",
"Thanks @lhoestq!\r\n\r\nI'll close this issue now."
] | ### Describe the bug
I've been playing around with huggingface library, mostly with `datasets` and wanted to download the multi class classification datasets to fine tune BERT on this task. ([link](https://huggingface.co/docs/transformers/training#train-with-pytorch-trainer)).
While loading the dataset, I'm getting the following error snippet.
```
---------------------------------------------------------------------------
TypeError Traceback (most recent call last)
Cell In[44], line 3
1 from datasets import load_dataset
----> 3 imdb_dataset = load_dataset("yelp_review_full")
4 imdb_dataset
File /work/pi_adrozdov_umass_edu/syerawar_umass_edu/envs/vadops/lib/python3.10/site-packages/datasets/load.py:1719, in load_dataset(path, name, data_dir, data_files, split, cache_dir, features, download_config, download_mode, ignore_verifications, keep_in_memory, save_infos, revision, use_auth_token, task, streaming, **config_kwargs)
1716 ignore_verifications = ignore_verifications or save_infos
1718 # Create a dataset builder
-> 1719 builder_instance = load_dataset_builder(
1720 path=path,
1721 name=name,
1722 data_dir=data_dir,
1723 data_files=data_files,
1724 cache_dir=cache_dir,
1725 features=features,
1726 download_config=download_config,
1727 download_mode=download_mode,
1728 revision=revision,
1729 use_auth_token=use_auth_token,
1730 **config_kwargs,
1731 )
1733 # Return iterable dataset in case of streaming
1734 if streaming:
File /work/pi_adrozdov_umass_edu/syerawar_umass_edu/envs/vadops/lib/python3.10/site-packages/datasets/load.py:1523, in load_dataset_builder(path, name, data_dir, data_files, cache_dir, features, download_config, download_mode, revision, use_auth_token, **config_kwargs)
1520 raise ValueError(error_msg)
1522 # Instantiate the dataset builder
-> 1523 builder_instance: DatasetBuilder = builder_cls(
1524 cache_dir=cache_dir,
1525 config_name=config_name,
1526 data_dir=data_dir,
1527 data_files=data_files,
1528 hash=hash,
1529 features=features,
1530 use_auth_token=use_auth_token,
1531 **builder_kwargs,
1532 **config_kwargs,
1533 )
1535 return builder_instance
File /work/pi_adrozdov_umass_edu/syerawar_umass_edu/envs/vadops/lib/python3.10/site-packages/datasets/builder.py:1292, in GeneratorBasedBuilder.__init__(self, writer_batch_size, *args, **kwargs)
1291 def __init__(self, *args, writer_batch_size=None, **kwargs):
-> 1292 super().__init__(*args, **kwargs)
1293 # Batch size used by the ArrowWriter
1294 # It defines the number of samples that are kept in memory before writing them
1295 # and also the length of the arrow chunks
1296 # None means that the ArrowWriter will use its default value
1297 self._writer_batch_size = writer_batch_size or self.DEFAULT_WRITER_BATCH_SIZE
File /work/pi_adrozdov_umass_edu/syerawar_umass_edu/envs/vadops/lib/python3.10/site-packages/datasets/builder.py:312, in DatasetBuilder.__init__(self, cache_dir, config_name, hash, base_path, info, features, use_auth_token, repo_id, data_files, data_dir, name, **config_kwargs)
309 # prepare info: DatasetInfo are a standardized dataclass across all datasets
310 # Prefill datasetinfo
311 if info is None:
--> 312 info = self.get_exported_dataset_info()
313 info.update(self._info())
314 info.builder_name = self.name
File /work/pi_adrozdov_umass_edu/syerawar_umass_edu/envs/vadops/lib/python3.10/site-packages/datasets/builder.py:412, in DatasetBuilder.get_exported_dataset_info(self)
400 def get_exported_dataset_info(self) -> DatasetInfo:
401 """Empty DatasetInfo if doesn't exist
402
403 Example:
(...)
410 ```
411 """
--> 412 return self.get_all_exported_dataset_infos().get(self.config.name, DatasetInfo())
File /work/pi_adrozdov_umass_edu/syerawar_umass_edu/envs/vadops/lib/python3.10/site-packages/datasets/builder.py:398, in DatasetBuilder.get_all_exported_dataset_infos(cls)
385 @classmethod
386 def get_all_exported_dataset_infos(cls) -> DatasetInfosDict:
387 """Empty dict if doesn't exist
388
389 Example:
(...)
396 ```
397 """
--> 398 return DatasetInfosDict.from_directory(cls.get_imported_module_dir())
File /work/pi_adrozdov_umass_edu/syerawar_umass_edu/envs/vadops/lib/python3.10/site-packages/datasets/info.py:370, in DatasetInfosDict.from_directory(cls, dataset_infos_dir)
368 dataset_metadata = DatasetMetadata.from_readme(Path(dataset_infos_dir) / "README.md")
369 if "dataset_info" in dataset_metadata:
--> 370 return cls.from_metadata(dataset_metadata)
371 if os.path.exists(os.path.join(dataset_infos_dir, config.DATASETDICT_INFOS_FILENAME)):
372 # this is just to have backward compatibility with dataset_infos.json files
373 with open(os.path.join(dataset_infos_dir, config.DATASETDICT_INFOS_FILENAME), encoding="utf-8") as f:
File /work/pi_adrozdov_umass_edu/syerawar_umass_edu/envs/vadops/lib/python3.10/site-packages/datasets/info.py:396, in DatasetInfosDict.from_metadata(cls, dataset_metadata)
387 return cls(
388 {
389 dataset_info_yaml_dict.get("config_name", "default"): DatasetInfo._from_yaml_dict(
(...)
393 }
394 )
395 else:
--> 396 dataset_info = DatasetInfo._from_yaml_dict(dataset_metadata["dataset_info"])
397 dataset_info.config_name = dataset_metadata["dataset_info"].get("config_name", "default")
398 return cls({dataset_info.config_name: dataset_info})
File /work/pi_adrozdov_umass_edu/syerawar_umass_edu/envs/vadops/lib/python3.10/site-packages/datasets/info.py:332, in DatasetInfo._from_yaml_dict(cls, yaml_data)
330 yaml_data = copy.deepcopy(yaml_data)
331 if yaml_data.get("features") is not None:
--> 332 yaml_data["features"] = Features._from_yaml_list(yaml_data["features"])
333 if yaml_data.get("splits") is not None:
334 yaml_data["splits"] = SplitDict._from_yaml_list(yaml_data["splits"])
File /work/pi_adrozdov_umass_edu/syerawar_umass_edu/envs/vadops/lib/python3.10/site-packages/datasets/features/features.py:1745, in Features._from_yaml_list(cls, yaml_data)
1742 else:
1743 raise TypeError(f"Expected a dict or a list but got {type(obj)}: {obj}")
-> 1745 return cls.from_dict(from_yaml_inner(yaml_data))
File /work/pi_adrozdov_umass_edu/syerawar_umass_edu/envs/vadops/lib/python3.10/site-packages/datasets/features/features.py:1741, in Features._from_yaml_list.<locals>.from_yaml_inner(obj)
1739 elif isinstance(obj, list):
1740 names = [_feature.pop("name") for _feature in obj]
-> 1741 return {name: from_yaml_inner(_feature) for name, _feature in zip(names, obj)}
1742 else:
1743 raise TypeError(f"Expected a dict or a list but got {type(obj)}: {obj}")
File /work/pi_adrozdov_umass_edu/syerawar_umass_edu/envs/vadops/lib/python3.10/site-packages/datasets/features/features.py:1741, in <dictcomp>(.0)
1739 elif isinstance(obj, list):
1740 names = [_feature.pop("name") for _feature in obj]
-> 1741 return {name: from_yaml_inner(_feature) for name, _feature in zip(names, obj)}
1742 else:
1743 raise TypeError(f"Expected a dict or a list but got {type(obj)}: {obj}")
File /work/pi_adrozdov_umass_edu/syerawar_umass_edu/envs/vadops/lib/python3.10/site-packages/datasets/features/features.py:1736, in Features._from_yaml_list.<locals>.from_yaml_inner(obj)
1734 return {"_type": snakecase_to_camelcase(obj["dtype"])}
1735 else:
-> 1736 return from_yaml_inner(obj["dtype"])
1737 else:
1738 return {"_type": snakecase_to_camelcase(_type), **unsimplify(obj)[_type]}
File /work/pi_adrozdov_umass_edu/syerawar_umass_edu/envs/vadops/lib/python3.10/site-packages/datasets/features/features.py:1738, in Features._from_yaml_list.<locals>.from_yaml_inner(obj)
1736 return from_yaml_inner(obj["dtype"])
1737 else:
-> 1738 return {"_type": snakecase_to_camelcase(_type), **unsimplify(obj)[_type]}
1739 elif isinstance(obj, list):
1740 names = [_feature.pop("name") for _feature in obj]
File /work/pi_adrozdov_umass_edu/syerawar_umass_edu/envs/vadops/lib/python3.10/site-packages/datasets/features/features.py:1706, in Features._from_yaml_list.<locals>.unsimplify(feature)
1704 if isinstance(feature.get("class_label"), dict) and isinstance(feature["class_label"].get("names"), dict):
1705 label_ids = sorted(feature["class_label"]["names"])
-> 1706 if label_ids and label_ids != list(range(label_ids[-1] + 1)):
1707 raise ValueError(
1708 f"ClassLabel expected a value for all label ids [0:{label_ids[-1] + 1}] but some ids are missing."
1709 )
1710 feature["class_label"]["names"] = [feature["class_label"]["names"][label_id] for label_id in label_ids]
TypeError: can only concatenate str (not "int") to str
```
The same issue happens when I try to load `go-emotions` multi class classification dataset. Could somebody guide me on how to fix this issue?
### Steps to reproduce the bug
Run the following code snippet in a python script/ notebook cell:
```
from datasets import load_dataset
yelp_dataset = load_dataset("yelp_review_full")
yelp_dataset
```
### Expected behavior
The dataset should be loaded perfectly, which showing the train, test and unsupervised splits with the basic data statistics
### Environment info
- `datasets` version: 2.6.1
- Platform: Linux-5.4.0-124-generic-x86_64-with-glibc2.31
- Python version: 3.10.9
- PyArrow version: 8.0.0
- Pandas version: 1.5.3 | 5,670 |
https://github.com/huggingface/datasets/issues/5669 | Almost identical datasets, huge performance difference | [
"Do I miss something here?",
"Hi! \r\n\r\nThe first dataset stores images as bytes (the \"image\" column type is `datasets.Image()`) and decodes them as `PIL.Image` objects and the second dataset stores them as variable-length lists (the \"image\" column type is `datasets.Sequence(...)`)), so I guess going from `arrow bytes -> NumPy -> decoding as PIL.Image -> PyTorch` is faster than going from `arrow list -> NumPy -> PyTorch`. \r\n\r\nTo store image bytes in the second example, you can do the following:\r\n\r\n```python\r\ndef transform(example):\r\n example[\"image2\"] = cv2.imread(example[\"image_file_path\"])\r\n return example\r\n\r\nfeatures = dataset.features.copy()\r\ndel features[\"image\"]\r\nfeatures[\"image2\"] = datasets.Image()\r\ndataset2 = dataset.map(transform, remove_columns=[\"image\"], features=features)\r\n\r\nfor x in DataLoader(dataset2.with_format(\"torch\"), batch_size=16, shuffle=True, num_workers=8):\r\n pass\r\n```",
"Thanks, @mariosasko. I could not understand why a (decoded) sequence should be MUCH slower than an encoded image (that must be decoded every time). At any rate, I tried you suggestion. It made the `map` step to run extremely slow (consumes all the 16GB of memory and starts swapping)\r\n\r\nI tried also the easiest (as I see it) scenario, where images are kept as bytes, but it made things even worse: not only it was extremely slow, but also crashes\r\n\r\n```python\r\n\r\ndef transform(example):\r\n example[\"image2\"] = cv2.imread(example[\"image_file_path\"]).tobytes()\r\n return example\r\n\r\ndataset2 = dataset.map(transform, remove_columns=[\"image\"])\r\n\r\nfor x in DataLoader(dataset2.with_format(\"torch\"), batch_size=16, shuffle=True, num_workers=8):\r\n pass\r\n\r\n\r\nResource temporarily unavailable (src/thread.cpp:269)\r\nOutput exceeds the size limit. Open the full output data in a text editor\r\n---------------------------------------------------------------------------\r\nRuntimeError Traceback (most recent call last)\r\nFile ~/virtenvs/py310/lib/python3.10/site-packages/torch/utils/data/dataloader.py:1133, in _MultiProcessingDataLoaderIter._try_get_data(self, timeout)\r\n 1132 try:\r\n-> 1133 data = self._data_queue.get(timeout=timeout)\r\n 1134 return (True, data)\r\n\r\nFile ~/virtenvs/py310/lib/python3.10/multiprocessing/queues.py:113, in Queue.get(self, block, timeout)\r\n 112 timeout = deadline - time.monotonic()\r\n--> 113 if not self._poll(timeout):\r\n 114 raise Empty\r\n\r\nFile ~/virtenvs/py310/lib/python3.10/multiprocessing/connection.py:257, in _ConnectionBase.poll(self, timeout)\r\n 256 self._check_readable()\r\n--> 257 return self._poll(timeout)\r\n\r\nFile ~/virtenvs/py310/lib/python3.10/multiprocessing/connection.py:424, in Connection._poll(self, timeout)\r\n 423 def _poll(self, timeout):\r\n--> 424 r = wait([self], timeout)\r\n 425 return bool(r)\r\n\r\nFile ~/virtenvs/py310/lib/python3.10/multiprocessing/connection.py:931, in wait(object_list, timeout)\r\n 930 while True:\r\n--> 931 ready = selector.select(timeout)\r\n 932 if ready:\r\n...\r\n-> 1146 raise RuntimeError('DataLoader worker (pid(s) {}) exited unexpectedly'.format(pids_str)) from e\r\n 1147 if isinstance(e, queue.Empty):\r\n 1148 return (False, None)\r\n\r\nRuntimeError: DataLoader worker (pid(s) 195393) exited unexpectedly\r\nResource temporarily unavailable (src/thread.cpp:269)\r\nResource temporarily unavailable (src/thread.cpp:269)\r\nResource temporarily unavailable (src/thread.cpp:269)\r\nResource temporarily unavailable (src/thread.cpp:269)\r\nResource temporarily unavailable (src/thread.cpp:269)\r\n```\r\n",
"Correction: the `beans` dataset stores the image file paths, not the bytes.\r\n\r\nFor your use case, I think it makes more sense to use `with_tranform` than `map` and lazily decode images with `cv2.imread` when indexing an example/batch:\r\n```python\r\nimport cv2\r\n\r\ndef transform(batch):\r\n batch[\"image2\"] = np.stack([cv2.imread(image_file_path) for image_file_path in batch[\"image_file_path\"]])\r\n return batch\r\n\r\ndataset = dataset.with_transform(transform)\r\n```\r\n",
"This is incorrect.\n\nDid you try to run it? dataset[0] returns a tensor of numbers. dataset2[0]\nreturns the same tensor, but after a few long seconds. Looping over a\nthousand of images cannot take 15 minutes.\n\nOn Fri, 24 Mar 2023 at 19:28 Mario Šaško ***@***.***> wrote:\n\n> Correction: the beans dataset stores the image file paths, not the bytes.\n>\n> For your use case, I think it makes more sense to use with_tranform than\n> map and lazily decode images with cv2.imread when accessing an\n> example/batch:\n>\n> import cv2\n> def transform(batch):\n> batch[\"image2\"] = np.stack([cv2.imread(image_file_path) for image_file_path in batch[\"image_file_path\"]])\n> return batch\n> dataset = dataset.with_transform(transform)\n>\n> —\n> Reply to this email directly, view it on GitHub\n> <https://github.com/huggingface/datasets/issues/5669#issuecomment-1483084347>,\n> or unsubscribe\n> <https://github.com/notifications/unsubscribe-auth/AASS73SHRWXIQX6SCYCJ7ITW5XDUDANCNFSM6AAAAAAWFSHWEM>\n> .\n> You are receiving this because you authored the thread.Message ID:\n> ***@***.***>\n>\n",
"I updated the transform with the NumPy -> PyTorch conversion.\r\n\r\nI'm sharing the entire code:\r\n```python\r\nimport cv2\r\nimport numpy as np\r\nimport datasets\r\nimport torch\r\nfrom datasets import load_dataset\r\nfrom torch.utils.data import DataLoader\r\n\r\ndataset = load_dataset(\"beans\", split=\"train\")\r\n\r\ndef transform(batch):\r\n # # Pillow decodes as RGB\r\n # batch[\"image\"] = torch.stack([torch.from_numpy(cv2.cvtColor(cv2.imread(image_file_path), cv2.COLOR_BGR2RGB)) for image_file_path in batch[\"image_file_path\"]])\r\n batch[\"image\"] = torch.stack([torch.from_numpy(cv2.imread(image_file_path)) for image_file_path in batch[\"image_file_path\"]])\r\n batch[\"labels\"] = torch.tensor(batch[\"labels\"])\r\n return batch\r\n\r\ndataset2 = dataset.cast_column(\"image\", datasets.Image(decode=False)).with_transform(transform)\r\n\r\nfor x in DataLoader(dataset2, batch_size=16, shuffle=True, num_workers=8):\r\n pass\r\n```\r\n\r\nThis code is ≈ 10% faster on my machine than the default decoding with Pillow and `.with_format(\"torch\")`.",
"Thanks, @mariosasko \r\nMy question remain unanswered though. Why is the `map`ed dataset so slow? My understanding is that a dataset of numpy arrays should be must faster than a dataset that has to decode images into numpy arrays every time one accesses an item. "
] | ### Describe the bug
I am struggling to understand (huge) performance difference between two datasets that are almost identical.
### Steps to reproduce the bug
# Fast (normal) dataset speed:
```python
import cv2
from datasets import load_dataset
from torch.utils.data import DataLoader
dataset = load_dataset("beans", split="train")
for x in DataLoader(dataset.with_format("torch"), batch_size=16, shuffle=True, num_workers=8):
pass
```
The above pass over the dataset takes about 1.5 seconds on my computer.
However, if I re-create (almost) the same dataset, the sweep takes HUGE amount of time: 15 minutes. Steps to reproduce:
```python
def transform(example):
example["image2"] = cv2.imread(example["image_file_path"])
return example
dataset2 = dataset.map(transform, remove_columns=["image"])
for x in DataLoader(dataset2.with_format("torch"), batch_size=16, shuffle=True, num_workers=8):
pass
```
### Expected behavior
Same timings
### Environment info
python==3.10.9
datasets==2.10.1 | 5,669 |
https://github.com/huggingface/datasets/issues/5666 | Support tensorflow 2.12.0 in CI | [] | Once we find out the root cause of:
- #5663
we should revert the temporary pin on tensorflow introduced by:
- #5664 | 5,666 |
https://github.com/huggingface/datasets/issues/5665 | Feature request: IterableDataset.push_to_hub | [
"+1",
"+1"
] | ### Feature request
It'd be great to have a lazy push to hub, similar to the lazy loading we have with `IterableDataset`.
Suppose you'd like to filter [LAION](https://huggingface.co/datasets/laion/laion400m) based on certain conditions, but as LAION doesn't fit into your disk, you'd like to leverage streaming:
```
from datasets import load_dataset
dataset = load_dataset("laion/laion400m", streaming=True, split="train")
```
Then you could filter the dataset based on certain conditions:
```
filtered_dataset = dataset.filter(lambda example: example['HEIGHT'] > 400)
```
In order to persist this dataset and push it back to the hub, one currently needs to first load the entire filtered dataset on disk and then push:
```
from datasets import Dataset
Dataset.from_generator(filtered_dataset.__iter__).push_to_hub(...)
```
It would be great if we can instead lazy push to the data to the hub (basically stream the data to the hub), not being limited by our disk size:
```
filtered_dataset.push_to_hub("my-filtered-dataset")
```
### Motivation
This feature would be very useful for people that want to filter huge datasets without having to load the entire dataset or a filtered version thereof on their local disk.
### Your contribution
Happy to test out a PR :) | 5,665 |
https://github.com/huggingface/datasets/issues/5663 | CI is broken: ModuleNotFoundError: jax requires jaxlib to be installed | [] | CI test_py310 is broken: see https://github.com/huggingface/datasets/actions/runs/4498945505/jobs/7916194236?pr=5662
```
FAILED tests/test_arrow_dataset.py::BaseDatasetTest::test_map_jax_in_memory - ModuleNotFoundError: jax requires jaxlib to be installed. See https://github.com/google/jax#installation for installation instructions.
FAILED tests/test_arrow_dataset.py::BaseDatasetTest::test_map_jax_on_disk - ModuleNotFoundError: jax requires jaxlib to be installed. See https://github.com/google/jax#installation for installation instructions.
FAILED tests/test_formatting.py::FormatterTest::test_jax_formatter - ModuleNotFoundError: jax requires jaxlib to be installed. See https://github.com/google/jax#installation for installation instructions.
FAILED tests/test_formatting.py::FormatterTest::test_jax_formatter_audio - ModuleNotFoundError: jax requires jaxlib to be installed. See https://github.com/google/jax#installation for installation instructions.
FAILED tests/test_formatting.py::FormatterTest::test_jax_formatter_device - ModuleNotFoundError: jax requires jaxlib to be installed. See https://github.com/google/jax#installation for installation instructions.
FAILED tests/test_formatting.py::FormatterTest::test_jax_formatter_image - ModuleNotFoundError: jax requires jaxlib to be installed. See https://github.com/google/jax#installation for installation instructions.
FAILED tests/test_formatting.py::FormatterTest::test_jax_formatter_jnp_array_kwargs - ModuleNotFoundError: jax requires jaxlib to be installed. See https://github.com/google/jax#installation for installation instructions.
FAILED tests/features/test_features.py::CastToPythonObjectsTest::test_cast_to_python_objects_jax - ModuleNotFoundError: jax requires jaxlib to be installed. See https://github.com/google/jax#installation for installation instructions.
===== 8 failed, 2147 passed, 10 skipped, 37 warnings in 228.69s (0:03:48) ======
``` | 5,663 |
https://github.com/huggingface/datasets/issues/5661 | CI is broken: Unnecessary `dict` comprehension | [] | CI check_code_quality is broken:
```
src/datasets/arrow_dataset.py:3267:35: C416 [*] Unnecessary `dict` comprehension (rewrite using `dict()`)
Found 1 error.
``` | 5,661 |
https://github.com/huggingface/datasets/issues/5660 | integration with imbalanced-learn | [
"You can convert any dataset to pandas to be used with imbalanced-learn using `.to_pandas()`\r\n\r\nOtherwise if you want to keep a `Dataset` object and still use e.g. [make_imbalance](https://imbalanced-learn.org/stable/references/generated/imblearn.datasets.make_imbalance.html#imblearn.datasets.make_imbalance), you just need to pass the list of rows ids and labels:\r\n\r\n```python\r\nrow_indices = list(range(len(dataset)))\r\nresampled_row_indices, _ = make_imbalance(\r\n row_indices,\r\n dataset[\"label\"],\r\n sampling_strategy={0: 25, 1: 50, 2: 50},\r\n random_state=RANDOM_STATE,\r\n)\r\n\r\nresampled_dataset = dataset.select(resampled_row_indices)\r\n```"
] | ### Feature request
Wouldn't it be great if the various class balancing operations from imbalanced-learn were available as part of datasets?
### Motivation
I'm trying to use imbalanced-learn to balance a dataset, but it's not clear how to get the two to interoperate - what would be great would be some examples. I've looked online, asked gpt-4, but so far not making much progress.
### Your contribution
If I can get this working myself I can submit a PR with example code to go in the docs | 5,660 |
https://github.com/huggingface/datasets/issues/5659 | [Audio] Soundfile/libsndfile requirements too stringent for decoding mp3 files | [
"cc @polinaeterna @lhoestq ",
"@sanchit-gandhi can you please also post the logs of `pip install soundfile==0.12.1`? To check what wheel is being installed or if it's being built from source (I think it's the latter case). \r\nRequired `libsndfile` binary **should** be bundeled with `soundfile` wheel but I assume it **might not** be the case for some non standard Linux distributions. \r\nThe only solution for using `soundfile` here is to build [`libsndfile`](https://github.com/libsndfile/libsndfile) from source:\r\n\r\n```bash\r\ngit clone https://github.com/libsndfile/libsndfile.git\r\ncd libsndfile/\r\nautoreconf -vif\r\n./configure --enable-werror \r\nmake\r\nmake install\r\n```\r\nfor this, some building libraries should be installed, for Debian/Ubuntu it's like:\r\n```bash\r\napt install autoconf autogen automake build-essential libasound2-dev \\\r\n libflac-dev libogg-dev libtool libvorbis-dev libopus-dev libmp3lame-dev \\\r\n libmpg123-dev pkg-config python\r\n```\r\nbut for other Linux distributions it might be different.\r\n\r\nWhen the binary is compiled, it should be put into location where `soundfile` would search for it (the directory is named `_soundfile_data`), it depends on where`libsdfile` (from the previous step) and `soundfile` were installed, might be something like this:\r\n\r\n```bash\r\ncp /usr/local/lib/libsndfile.so /usr/local/lib/python3.7/dist-packages/_soundfile_data/\r\ncp /usr/local/lib/libsndfile.la /usr/local/lib/python3.7/dist-packages/_soundfile_data/\r\n```\r\n\r\nAnother solution is to not use `soundfile` and apply custom processing function with `torchaudio` while setting `decode=False` in `Audio` feature and passing custom function to `.map`. ",
"Not sure if it may help, but you could also try updating `pip` before installing soundfile",
"@lhoestq @sanchit-gandhi. I encountered the same error (also on the TPU v4) when trying to run `datasets` from source.\r\n\r\nDowngrading soundfile with `pip install soundfile==0.12.0` seems to fix the issue for me.",
"Maybe let's open an issue at https://github.com/bastibe/python-soundfile/issues in case they might know why you get `OSError: cannot load library 'libsndfile.so'` ?",
"> @sanchit-gandhi can you please also post the logs of `pip install soundfile==0.12.1`? To check what wheel is being installed or if it's being built from source (I think it's the latter case). Required `libsndfile` binary **should** be bundeled with `soundfile` wheel but I assume it **might not** be the case for some non standard Linux distributions. The only solution for using `soundfile` here is to build [`libsndfile`](https://github.com/libsndfile/libsndfile) from source:\r\n> \r\n> ```shell\r\n> git clone https://github.com/libsndfile/libsndfile.git\r\n> cd libsndfile/\r\n> autoreconf -vif\r\n> ./configure --enable-werror \r\n> make\r\n> make install\r\n> ```\r\n\r\nThis fixed the issue for me. After installing libsndfile as described above, I had to uninstall soundfile and re-install it with this command. `pip install \"soundfile>=0.12.1\"`",
"Thank you so much for the comprehensive instructions @polinaeterna! Also confirming that they worked for me 🤗 In my case, I had to run several of these commands under \"sudo\" for privileges, but otherwise this workaround gave a successful `libsndfile` install:\r\n\r\n1. Grab source code:\r\n```\r\ngit clone https://github.com/libsndfile/libsndfile.git\r\n```\r\n\r\n2. Set up a build environment:\r\n```\r\nsudo apt install autoconf autogen automake build-essential libasound2-dev \\\r\n libflac-dev libogg-dev libtool libvorbis-dev libopus-dev libmp3lame-dev \\\r\n libmpg123-dev pkg-config python\r\n```\r\n\r\n3. Build and test `libsndfile`:\r\n\r\n```\r\nautoreconf -vif\r\n./configure --enable-werror\r\nsudo make\r\nsudo make check\r\n```\r\n\r\n4. Create `_soundfile_data` submodule (if it does not exist already):\r\n```\r\nsudo mkdir /usr/local/lib/python3.8/dist-packages/_soundfile_data/\r\n```\r\n\r\n5. Copy `libsndfile` files into submodule:\r\n```\r\nsudo cp /usr/local/lib/libsndfile.* /usr/local/lib/python3.8/dist-packages/_soundfile_data/\r\n```",
"On a different machine, I also tried separately by first upgrading pip, then installing soundfile. This worked too! Thanks @lhoestq 🙌",
"> @sanchit-gandhi can you please also post the logs of `pip install soundfile==0.12.1`? To check what wheel is being installed or if it's being built from source (I think it's the latter case). Required `libsndfile` binary **should** be bundeled with `soundfile` wheel but I assume it **might not** be the case for some non standard Linux distributions. The only solution for using `soundfile` here is to build [`libsndfile`](https://github.com/libsndfile/libsndfile) from source:\r\n> \r\n> ```shell\r\n> git clone https://github.com/libsndfile/libsndfile.git\r\n> cd libsndfile/\r\n> autoreconf -vif\r\n> ./configure --enable-werror \r\n> make\r\n> make install\r\n> ```\r\n> \r\n> for this, some building libraries should be installed, for Debian/Ubuntu it's like:\r\n> \r\n> ```shell\r\n> apt install autoconf autogen automake build-essential libasound2-dev \\\r\n> libflac-dev libogg-dev libtool libvorbis-dev libopus-dev libmp3lame-dev \\\r\n> libmpg123-dev pkg-config python\r\n> ```\r\n> \r\n> but for other Linux distributions it might be different.\r\n> \r\n> When the binary is compiled, it should be put into location where `soundfile` would search for it (the directory is named `_soundfile_data`), it depends on where`libsdfile` (from the previous step) and `soundfile` were installed, might be something like this:\r\n> \r\n> ```shell\r\n> cp /usr/local/lib/libsndfile.so /usr/local/lib/python3.7/dist-packages/_soundfile_data/\r\n> cp /usr/local/lib/libsndfile.la /usr/local/lib/python3.7/dist-packages/_soundfile_data/\r\n> ```\r\n> \r\n> Another solution is to not use `soundfile` and apply custom processing function with `torchaudio` while setting `decode=False` in `Audio` feature and passing custom function to `.map`.\r\n\r\nThanks, the solution solved my problem. \r\n\r\n1. Purge uninstall libsndfile, uninstall python-soundfile.\r\n2. Build libsndfile from source code and install.\r\n3. Build python-soundfile from source code and install\r\n4. Well done.",
"> Thank you so much for the comprehensive instructions @polinaeterna! Also confirming that they worked for me 🤗 In my case, I had to run several of these commands under \"sudo\" for privileges, but otherwise this workaround gave a successful `libsndfile` install:\r\n> \r\n> 1. Grab source code:\r\n> \r\n> ```\r\n> git clone https://github.com/libsndfile/libsndfile.git\r\n> ```\r\n> \r\n> 2. Set up a build environment:\r\n> \r\n> ```\r\n> sudo apt install autoconf autogen automake build-essential libasound2-dev \\\r\n> libflac-dev libogg-dev libtool libvorbis-dev libopus-dev libmp3lame-dev \\\r\n> libmpg123-dev pkg-config python\r\n> ```\r\n> \r\n> 3. Build and test `libsndfile`:\r\n> \r\n> ```\r\n> autoreconf -vif\r\n> ./configure --enable-werror\r\n> sudo make\r\n> sudo make check\r\n> ```\r\n> \r\n> 4. Create `_soundfile_data` submodule (if it does not exist already):\r\n> \r\n> ```\r\n> sudo mkdir /usr/local/lib/python3.8/dist-packages/_soundfile_data/\r\n> ```\r\n> \r\n> 5. Copy `libsndfile` files into submodule:\r\n> \r\n> ```\r\n> sudo cp /usr/local/lib/libsndfile.* /usr/local/lib/python3.8/dist-packages/_soundfile_data/\r\n> ```\r\n\r\nI had to run 'make install' or the `/usr/local/lib/libsndfile.*` files didn't exist.\r\n\r\nIt's working though!",
"I had the same issue but it is working now! Thanks for all of your comments!",
"I had the same issue on SageMaker but not on Colab;\r\nThe `soundfile` versioning was fine.\r\n\r\n my approach to solve it was to match {\"numpy\", \"numba\"} exact versions\r\n\r\n```\r\n! pip install \"numpy==1.23.5\"\r\n! pip install \"numpy==0.58.1\"\r\n\r\n```\r\nthe numbers are from Colab where successfully I could do the job.\r\n\r\n",
"> Thank you so much for the comprehensive instructions @polinaeterna! Also confirming that they worked for me 🤗 In my case, I had to run several of these commands under \"sudo\" for privileges, but otherwise this workaround gave a successful `libsndfile` install:\r\n> \r\n> 1. Grab source code:\r\n> \r\n> ```\r\n> git clone https://github.com/libsndfile/libsndfile.git\r\n> ```\r\n> \r\n> 2. Set up a build environment:\r\n> \r\n> ```\r\n> sudo apt install autoconf autogen automake build-essential libasound2-dev \\\r\n> libflac-dev libogg-dev libtool libvorbis-dev libopus-dev libmp3lame-dev \\\r\n> libmpg123-dev pkg-config python\r\n> ```\r\n> \r\n> 3. Build and test `libsndfile`:\r\n> \r\n> ```\r\n> autoreconf -vif\r\n> ./configure --enable-werror\r\n> sudo make\r\n> sudo make check\r\n> ```\r\n> \r\n> 4. Create `_soundfile_data` submodule (if it does not exist already):\r\n> \r\n> ```\r\n> sudo mkdir /usr/local/lib/python3.8/dist-packages/_soundfile_data/\r\n> ```\r\n> \r\n> 5. Copy `libsndfile` files into submodule:\r\n> \r\n> ```\r\n> sudo cp /usr/local/lib/libsndfile.* /usr/local/lib/python3.8/dist-packages/_soundfile_data/\r\n> ```\r\n\r\nIt works and don't forget to \"apt uninstall libsndfile1\" after installing it from source code."
] | ### Describe the bug
I'm encountering several issues trying to load mp3 audio files using `datasets` on a TPU v4.
The PR https://github.com/huggingface/datasets/pull/5573 updated the audio loading logic to rely solely on the `soundfile`/`libsndfile` libraries for loading audio samples, regardless of their file type.
The installation guide suggests that `libsndfile` is bundled in when `soundfile` is pip installed:
https://github.com/huggingface/datasets/blob/e1af108015e43f9df8734a1faeeaeb9eafce3971/docs/source/installation.md?plain=1#L70-L71
However, just pip installing `soundfile==0.12.1` throws an error that `libsndfile` is missing:
```
pip install soundfile==0.12.1
```
Then:
```python
>>> soundfile
>>> soundfile.__libsndfile_version__
```
<details>
<summary> Traceback (most recent call last): </summary>
```
File "/home/sanchitgandhi/hf/lib/python3.8/site-packages/soundfile.py", line 161, in <module>
import _soundfile_data # ImportError if this doesn't exist
ModuleNotFoundError: No module named '_soundfile_data'
During handling of the above exception, another exception occurred:
Traceback (most recent call last):
File "/home/sanchitgandhi/hf/lib/python3.8/site-packages/soundfile.py", line 170, in <module>
raise OSError('sndfile library not found using ctypes.util.find_library')
OSError: sndfile library not found using ctypes.util.find_library
During handling of the above exception, another exception occurred:
Traceback (most recent call last):
File "<string>", line 1, in <module>
File "/home/sanchitgandhi/hf/lib/python3.8/site-packages/soundfile.py", line 192, in <module>
_snd = _ffi.dlopen(_explicit_libname)
OSError: cannot load library 'libsndfile.so': libsndfile.so: cannot open shared object file: No such file or directory
```
</details>
Thus, I've followed the official instructions for installing the `soundfile` package from https://github.com/bastibe/python-soundfile#installation, which states that `libsndfile` needs to be installed separately as:
```
pip install --upgrade soundfile
sudo apt install libsndfile1
```
We can now import `soundfile`:
```python
>>> import soundfile
>>> soundfile.__version__
'0.12.1'
>>> soundfile.__libsndfile_version__
'1.0.28'
```
We see that we have `soundfile==0.12.1`, which matches the `datasets[audio]` package constraints:
https://github.com/huggingface/datasets/blob/e1af108015e43f9df8734a1faeeaeb9eafce3971/setup.py#L144-L147
But we have `libsndfile==1.0.28`, which is too low for decoding mp3 files:
https://github.com/huggingface/datasets/blob/e1af108015e43f9df8734a1faeeaeb9eafce3971/src/datasets/config.py#L136-L138
Updating/upgrading the `libsndfile` doesn't change this:
```
sudo apt-get update
sudo apt-get upgrade
```
Is there any other suggestion for how to get a compatible `libsndfile` version? Currently, the version bundled with Ubuntu `apt-get` is too low for decoding mp3 files.
Maybe we could add this under `setup.py` such that we install the correct `libsndfile` version when we do `pip install datasets[audio]`? IMO this would help circumvent such version issues.
### Steps to reproduce the bug
Environment described above. Loading mp3 files:
```python
from datasets import load_dataset
common_voice_es = load_dataset("common_voice", "es", split="validation", streaming=True)
print(next(iter(common_voice_es)))
```
```python
---------------------------------------------------------------------------
RuntimeError Traceback (most recent call last)
Cell In[4], line 2
1 common_voice_es = load_dataset("common_voice", "es", split="validation", streaming=True)
----> 2 print(next(iter(common_voice_es)))
File ~/datasets/src/datasets/iterable_dataset.py:941, in IterableDataset.__iter__(self)
937 for key, example in ex_iterable:
938 if self.features:
939 # `IterableDataset` automatically fills missing columns with None.
940 # This is done with `_apply_feature_types_on_example`.
--> 941 yield _apply_feature_types_on_example(
942 example, self.features, token_per_repo_id=self._token_per_repo_id
943 )
944 else:
945 yield example
File ~/datasets/src/datasets/iterable_dataset.py:700, in _apply_feature_types_on_example(example, features, token_per_repo_id)
698 encoded_example = features.encode_example(example)
699 # Decode example for Audio feature, e.g.
--> 700 decoded_example = features.decode_example(encoded_example, token_per_repo_id=token_per_repo_id)
701 return decoded_example
File ~/datasets/src/datasets/features/features.py:1864, in Features.decode_example(self, example, token_per_repo_id)
1850 def decode_example(self, example: dict, token_per_repo_id: Optional[Dict[str, Union[str, bool, None]]] = None):
1851 """Decode example with custom feature decoding.
1852
1853 Args:
(...)
1861 `dict[str, Any]`
1862 """
-> 1864 return {
1865 column_name: decode_nested_example(feature, value, token_per_repo_id=token_per_repo_id)
1866 if self._column_requires_decoding[column_name]
1867 else value
1868 for column_name, (feature, value) in zip_dict(
1869 {key: value for key, value in self.items() if key in example}, example
1870 )
1871 }
File ~/datasets/src/datasets/features/features.py:1865, in <dictcomp>(.0)
1850 def decode_example(self, example: dict, token_per_repo_id: Optional[Dict[str, Union[str, bool, None]]] = None):
1851 """Decode example with custom feature decoding.
1852
1853 Args:
(...)
1861 `dict[str, Any]`
1862 """
1864 return {
-> 1865 column_name: decode_nested_example(feature, value, token_per_repo_id=token_per_repo_id)
1866 if self._column_requires_decoding[column_name]
1867 else value
1868 for column_name, (feature, value) in zip_dict(
1869 {key: value for key, value in self.items() if key in example}, example
1870 )
1871 }
File ~/datasets/src/datasets/features/features.py:1308, in decode_nested_example(schema, obj, token_per_repo_id)
1305 elif isinstance(schema, (Audio, Image)):
1306 # we pass the token to read and decode files from private repositories in streaming mode
1307 if obj is not None and schema.decode:
-> 1308 return schema.decode_example(obj, token_per_repo_id=token_per_repo_id)
1309 return obj
File ~/datasets/src/datasets/features/audio.py:167, in Audio.decode_example(self, value, token_per_repo_id)
162 raise RuntimeError(
163 "Decoding 'opus' files requires system library 'libsndfile'>=1.0.31, "
164 'You can try to update `soundfile` python library: `pip install "soundfile>=0.12.1"`. '
165 )
166 elif not config.IS_MP3_SUPPORTED and audio_format == "mp3":
--> 167 raise RuntimeError(
168 "Decoding 'mp3' files requires system library 'libsndfile'>=1.1.0, "
169 'You can try to update `soundfile` python library: `pip install "soundfile>=0.12.1"`. '
170 )
172 if file is None:
173 token_per_repo_id = token_per_repo_id or {}
RuntimeError: Decoding 'mp3' files requires system library 'libsndfile'>=1.1.0, You can try to update `soundfile` python library: `pip install "soundfile>=0.12.1"`.
```
### Expected behavior
Load mp3 files!
### Environment info
- `datasets` version: 2.10.2.dev0
- Platform: Linux-5.13.0-1023-gcp-x86_64-with-glibc2.29
- Python version: 3.8.10
- Huggingface_hub version: 0.13.1
- PyArrow version: 11.0.0
- Pandas version: 1.5.3
- Soundfile version: 0.12.1
- Libsndfile version: 1.0.28 | 5,659 |
https://github.com/huggingface/datasets/issues/5654 | Offset overflow when executing Dataset.map | [
"Upd. the above code works if we replace `25` with `1`, but the result value at key \"hr\" is not a tensor but a list of lists of lists of uint8.\r\n\r\nAdding `train_data.set_format(\"torch\")` after map fixes this, but the original issue remains\r\n\r\n",
"As a workaround, one can replace\r\n`return {\"hr\": torch.stack([crop_transf(tensor) for _ in range(25)])}`\r\nwith\r\n`return {f\"hr_crop_{i}\": crop_transf(tensor) for i in range(25)}`\r\nand then choose appropriate crop randomly in further processing, but I still don't understand why the original approach doesn't work(\r\n"
] | ### Describe the bug
Hi, I'm trying to use `.map` method to cache multiple random crops from the image to speed up data processing during training, as the image size is too big.
The map function executes all iterations, and then returns the following error:
```bash
Traceback (most recent call last):
File "/home/ubuntu/miniconda3/envs/enhancement/lib/python3.8/site-packages/datasets/arrow_dataset.py", line 3353, in _map_single
writer.finalize() # close_stream=bool(buf_writer is None)) # We only close if we are writing in a file
File "/home/ubuntu/miniconda3/envs/enhancement/lib/python3.8/site-packages/datasets/arrow_writer.py", line 582, in finalize
self.write_examples_on_file()
File "/home/ubuntu/miniconda3/envs/enhancement/lib/python3.8/site-packages/datasets/arrow_writer.py", line 446, in write_examples_on_file
self.write_batch(batch_examples=batch_examples)
File "/home/ubuntu/miniconda3/envs/enhancement/lib/python3.8/site-packages/datasets/arrow_writer.py", line 555, in write_batch
self.write_table(pa_table, writer_batch_size)
File "/home/ubuntu/miniconda3/envs/enhancement/lib/python3.8/site-packages/datasets/arrow_writer.py", line 567, in write_table
pa_table = pa_table.combine_chunks()
File "pyarrow/table.pxi", line 3315, in pyarrow.lib.Table.combine_chunks
File "pyarrow/error.pxi", line 144, in pyarrow.lib.pyarrow_internal_check_status
File "pyarrow/error.pxi", line 100, in pyarrow.lib.check_status
pyarrow.lib.ArrowInvalid: offset overflow while concatenating arrays
```
Here is the minimal code (`/home/datasets/DIV2K_train_HR` is just a folder of images that can be replaced by any appropriate):
### Steps to reproduce the bug
```python
from glob import glob
import torch
from datasets import Dataset, Image
from torchvision.transforms import PILToTensor, RandomCrop
file_paths = glob("/home/datasets/DIV2K_train_HR/*")
to_tensor = PILToTensor()
crop_transf = RandomCrop(size=256)
def prepare_data(example):
tensor = to_tensor(example["image"].convert("RGB"))
return {"hr": torch.stack([crop_transf(tensor) for _ in range(25)])}
train_data = Dataset.from_dict({"image": file_paths}).cast_column("image", Image())
train_data = train_data.map(
prepare_data,
cache_file_name="/home/datasets/DIV2K_train_HR_crops.tmp",
desc="Caching multiple random crops of image",
remove_columns="image",
)
print(train_data[0].keys(), train_data[0]["hr"].shape)
```
### Expected behavior
Cached file is stored at `"/home/datasets/DIV2K_train_HR_crops.tmp"`, output is `dict_keys(['hr']) torch.Size([25, 3, 256, 256])`
### Environment info
- `datasets` version: 2.10.1
- Platform: Linux-5.15.0-67-generic-x86_64-with-glibc2.10
- Python version: 3.8.16
- PyArrow version: 11.0.0
- Pandas version: 1.5.3
- Pytorch version: 2.0.0+cu117
- torchvision version: 0.15.1+cu117 | 5,654 |
https://github.com/huggingface/datasets/issues/5653 | Doc: save_to_disk, `num_proc` will affect `num_shards`, but it's not documented | [
"I agree this should be documented"
] | ### Describe the bug
[`num_proc`](https://huggingface.co/docs/datasets/main/en/package_reference/main_classes#datasets.DatasetDict.save_to_disk.num_proc) will affect `num_shards`, but it's not documented
### Steps to reproduce the bug
Nothing to reproduce
### Expected behavior
[document of `num_shards`](https://huggingface.co/docs/datasets/main/en/package_reference/main_classes#datasets.DatasetDict.save_to_disk.num_shards) explicitly says that it depends on `max_shard_size`, it should also mention `num_proc`.
### Environment info
datasets main document | 5,653 |
https://github.com/huggingface/datasets/issues/5651 | expanduser in save_to_disk | [
"`save_to_disk` should indeed expand `~`. Marking it as a \"good first issue\".",
"#self-assign\r\n\r\nFile path to code: \r\n\r\nhttps://github.com/huggingface/datasets/blob/2.13.0/src/datasets/arrow_dataset.py#L1364\r\n\r\n@RmZeta2718 I created a pull request for this issue. ",
"Hello, \r\nIt says `save_to_disk` is deprecated in 2.8.0, so the alternative to this will be `storage_options`? \r\n\r\nhttps://huggingface.co/docs/datasets/package_reference/main_classes#datasets.Dataset.save_to_disk",
"@ashikshafi08 I think you misunderstood the warning. The method `save_to_disk` is not deprecated only the optional parameter `fs`.\r\nAlso @benjaminbrown038 as I cannot find your PR I would like to work on this if you don't mind.",
"@mariosasko It's been several months and the PR is not reviewed. Could you please take a look? I assume this is not complicated and could be merged fairly soon."
] | ### Describe the bug
save_to_disk() does not expand `~`
1. `dataset = load_datasets("any dataset")`
2. `dataset.save_to_disk("~/data")`
3. a folder named "~" created in current folder
4. FileNotFoundError is raised, because the expanded path does not exist (`/home/<user>/data`)
related issue https://github.com/huggingface/transformers/issues/10628
### Steps to reproduce the bug
As described above.
### Expected behavior
expanduser correctly
### Environment info
- datasets 2.10.1
- python 3.10 | 5,651 |
https://github.com/huggingface/datasets/issues/5650 | load_dataset can't work correct with my image data | [
"Can you post a reproducible code snippet of what you tried to do?\r\n\r\n",
"> Can you post a reproducible code snippet of what you tried to do?\n> \n> \n\n```python\nfrom datasets import load_dataset\n\ndataset = load_dataset(\"my_folder_name\", split=\"train\")\n```",
"hi @WiNE-iNEFF ! can you please also tell a bit more about how your data is structured (directory structure and filenames patterns)?",
"> hi @WiNE-iNEFF ! can you please also tell a bit more about how your data is structured (directory structure and filenames patterns)?\n\nAll file have format .png converted in RGBA. \nIn main folder \"MyData\" contain 4 folder with images. In function load_dataset i use folder \"MyData\"",
"@WiNE-iNEFF I'm sorry there is still not enough information to answer your question :( For now I can only assume that your [filenames contain split names](https://huggingface.co/docs/datasets/repository_structure#splits-and-file-names) which are somehow incorrectly parsed. \r\nWhat would be the output if you omit `split` while loading? Like just\r\n```python\r\nds = load_dataset(\"MyData\")\r\nprint(ds)\r\n```\r\n\r\n",
"> @WiNE-iNEFF I'm sorry there is still not enough information to answer your question :( For now I can only assume that your [filenames contain split names](https://huggingface.co/docs/datasets/repository_structure#splits-and-file-names) which are somehow incorrectly parsed. \n> What would be the output if you omit `split` while loading? Like just\n> ```python\n> ds = load_dataset(\"MyData\")\n> print(ds)\n> ```\n> \n> \n\n```python\nDataset({\n features: ['image', 'label'],\n num_rows: 4\n})\n```",
"@WiNE-iNEFF My only guess is that 4 images in your data have `\"train\"` string in their names (something like `\"train_image_0.png\"`) and others do not and the loader ignores all the files that do not contain split name in filename. If it's true, please try to remove \"train\" from filenames. Or maybe they are inside a directory named \"train\", then the directory should be renamed (unless you want to put only these 4 specific images to the train but apparently you do not).\r\n\r\nIf there is a bug I cannot investigate it unfortunately because I cannot reproduce your case without some data samples. ",
"> @WiNE-iNEFF My only guess is that 4 images in your data have `\"train\"` string in their names (something like `\"train_image_0.png\"`) and others do not and the loader ignores all the files that do not contain split name in filename. If it's true, please try to remove \"train\" from filenames. Or maybe they are inside a directory named \"train\", then the directory should be renamed (unless you want to put only these 4 specific images to the train but apparently you do not).\n> \n> If there is a bug I cannot investigate it unfortunately because I cannot reproduce your case without some data samples. \n\nI checked my files and some of them do have the words train, valid and test in their names, but the number of such images is more than 500, not 4.",
"@WiNE-iNEFF Probably they are named inconsistently so that the correct pattern for which files should correspond to which split cannot be inferred. You can make it clearer to the loader by removing split names from filenames and putting files in separate folder for each split (you can take a look at the [documentation for imagefolder](https://huggingface.co/docs/datasets/image_dataset#imagefolder)):\r\n```\r\n Fuaimeanna2/\r\n├─ test\r\n│ ├─ label_0\r\n│ │ ├── filename_0.jpg\r\n│ │ └── filename_1.jpg\r\n│ │ └── ...\r\n│ ├─ label_1\r\n│ │ └── ...\r\n│ ├─ label_2\r\n│ │ └── ...\r\n│ └─ label_3\r\n│ └── ...\r\n├─ train\r\n│ ├─ label_0\r\n│ │ └── ...\r\n│ ├─ label_1\r\n│ │ └── ...\r\n│ ├─ label_2\r\n│ │ └── ...\r\n│ └─ label_3\r\n│ └── ...\r\n└── validation\r\n ├─ label_0\r\n │ └── ...\r\n ├─ label_1\r\n │ └── ...\r\n ├─ label_2\r\n │ └── ...\r\n └─ label_3\r\n └── ...\r\n```",
"> @WiNE-iNEFF Probably they are named inconsistently so that the correct pattern for which files should correspond to which split cannot be inferred. You can make it clearer to the loader by removing split names from filenames and putting files in separate folder for each split (you can take a look at the [documentation for imagefolder](https://huggingface.co/docs/datasets/image_dataset#imagefolder)):\n> ```\n> Fuaimeanna2/\n> ├─ test\n> │ ├─ label_0\n> │ │ ├── filename_0.jpg\n> │ │ └── filename_1.jpg\n> │ │ └── ...\n> │ ├─ label_1\n> │ │ └── ...\n> │ ├─ label_2\n> │ │ └── ...\n> │ └─ label_3\n> │ └── ...\n> ├─ train\n> │ ├─ label_0\n> │ │ └── ...\n> │ ├─ label_1\n> │ │ └── ...\n> │ ├─ label_2\n> │ │ └── ...\n> │ └─ label_3\n> │ └── ...\n> └── validation\n> ├─ label_0\n> │ └── ...\n> ├─ label_1\n> │ └── ...\n> ├─ label_2\n> │ └── ...\n> └─ label_3\n> └── ...\n> ```\n\nI have read this documentation more than once. It just wasn't a problem before.",
"Hi,\r\n\r\nYou need to use:\r\n```\r\nfrom datasets import load_dataset\r\n\r\ndataset = load_dataset(\"imagefolder\", split=\"train\", data_dir=\"path_to_your_folder\")\r\n```\r\ninstead of \r\n```\r\nfrom datasets import load_dataset\r\n\r\ndataset = load_dataset(\"my_folder_name\", split=\"train\")\r\n```\r\nTo create an image dataset from your local folders.",
"> Hi,\r\n> \r\n> You need to use:\r\n> \r\n> ```\r\n> from datasets import load_dataset\r\n> \r\n> dataset = load_dataset(\"imagefolder\", split=\"train\", data_dir=\"path_to_your_folder\")\r\n> ```\r\n> \r\n> instead of\r\n> \r\n> ```\r\n> from datasets import load_dataset\r\n> \r\n> dataset = load_dataset(\"my_folder_name\", split=\"train\")\r\n> ```\r\n> \r\n> To create an image dataset from your local folders.\r\n\r\nThank you, but even using the method that you wrote above absolutely nothing changes, especially without using data_dir on my other data everything works fine",
"@WiNE-iNEFF have you tried the suggestion I posted above? with removing split names from filenames and structuring files in folders? \r\n\r\n\r\n> even using the method that you wrote above absolutely nothing changes\r\n\r\nfyi - nothing changed because these two approaches are basically the same. it's just that when you pass your data directory as a dataset name (`load_dataset(\"my_folder_name\"`), not as `data_dir` (`load_dataset(\"imagefolder\", data_dir=\"my_folder_name\"`), `datasets` infers what module to use (`imagefolder` in your case) automatically, by file extensions.",
"Oh I didn't know that! OK but in any case, not sure why the image builder isn't working for @WiNE-iNEFF. But it's hard for us to help if we can't reproduce. I'd just check the structure of the folders, see if the splits are correctly set up, etc.",
"> @WiNE-iNEFF have you tried the suggestion I posted above? with removing split names from filenames and structuring files in folders? \n> \n> \n> > even using the method that you wrote above absolutely nothing changes\n> \n> fyi - nothing changed because these two approaches are basically the same. it's just that when you pass your data directory as a dataset name (`load_dataset(\"my_folder_name\"`), not as `data_dir` (`load_dataset(\"imagefolder\", data_dir=\"my_folder_name\"`), `datasets` infers what module to use (`imagefolder` in your case) automatically, by file extensions.\n\nI'll try to try your method over the next few days, then I'll write it turned out ",
"> @WiNE-iNEFF have you tried the suggestion I posted above? with removing split names from filenames and structuring files in folders? \n> \n> \n> > even using the method that you wrote above absolutely nothing changes\n> \n> fyi - nothing changed because these two approaches are basically the same. it's just that when you pass your data directory as a dataset name (`load_dataset(\"my_folder_name\"`), not as `data_dir` (`load_dataset(\"imagefolder\", data_dir=\"my_folder_name\"`), `datasets` infers what module to use (`imagefolder` in your case) automatically, by file extensions.\n\nI tried creating a `train` folder and put my image folders in it. As a result, all 18,000 images were loaded. ",
"@WiNE-iNEFF great! So to explain what happened according to my assumptions:\r\n\r\nWhen you use a standard packaged loader (like `imagefolder`, `csv`, `jsonl`, and so on) and load your data like `load_dataset(\"my_folder_name\")` or `load_dataset(\"imagefolder\", data_dir=\"my_folder_name\"`, the library searches for patterns to divide files into splits. This is described a bit in [this doc](https://huggingface.co/docs/datasets/v2.10.0/en/repository_structure#splits-and-file-names). And the order to search for patterns is the following:\r\n1. first it checks for [pattern like `data/<split_name>-xxxxx-of-xxxxx`](https://huggingface.co/docs/datasets/v2.10.0/en/repository_structure#custom-split-names) (which allows to pass custom split names)\r\n2. then for directories named as splits (if you have directories named `train`, `test` etc.)\r\n3. then for [splits in filenames](https://huggingface.co/docs/datasets/v2.10.0/en/repository_structure#splits-and-file-names) (like if you have files named `train-image.jpg`, `test_0.jpg`, ...)\r\n4. then if no pattern was found, it treats all files as belonging to a single `train` split\r\n\r\nThe code is [here](https://github.com/huggingface/datasets/blob/main/src/datasets/data_files.py#L215).\r\nSo I assume that in your case, since you didn't have directories for splits (pattern 2), some files that included split keywords (pattern 3) were included and others were ignored as not matching the pattern. And when you added `train` directory, the pattern for directories (pattern 2) was triggered first and everything worked as expected. Everything worked in your previous cases probably because you didn't have split names keywords in filenames, so all the files ended up being a part of a single train split (pattern 4).\r\n\r\nAnother way to mitigate this apart from structuring your data according to the patterns is to explicitly state with files belong to which splits by passing them with `data_files` parameter:\r\n```python\r\nload_dataset(\"my_folder_name\", data_files={\"train\": \"**\"}) # to tell that all files should be included \r\n```\r\n\r\nNow I see that this order should be explained in documentation and also referenced in sections for packaged modules like `imagefolder`, thank you for pointing this out. \r\n\r\n \r\n",
"@NielsRogge @polinaeterna I have a similar problem when reading my dataset. I want to use DETR for object detection, but my data is in YOLO format. With a dataset of 10k images, yolo format involves having 10k labels. As far as I read regarding [COCO format](https://auto.gluon.ai/stable/tutorials/multimodal/object_detection/data_preparation/convert_data_to_coco_format.html), there must be one JSON per split. However, as I post in the [Hugging Face forum](https://discuss.huggingface.co/t/prepare-dataset-from-yolo-format-to-coco-for-detr/34894), when it is read, the number of rows is 1, which does not make sense. \r\nThe instruction to read the train-val-test splits are: \r\n```python\r\nfrom datasets import load_dataset\r\ndata_files = {\r\n\t\"train\": './train_labels.json',\r\n\t\"validation\": './val_labels.json',\r\n\t\"test\": './test_labels.json'\r\n}\r\ndataset = load_dataset(\"json\", data_files=data_files)\r\n```\r\nAn example of the short version of the json file I read, to reproduce my error, is the following: \r\n\r\n``` json\r\n{\r\n \"info\": {},\r\n \"licenses\": [],\r\n \"images\": [\r\n {\r\n \"id\": 1,\r\n \"file_name\": \"aceca_100.mp4frame21.png\",\r\n \"width\": 1280,\r\n \"height\": 720,\r\n \"pixel_values\": null,\r\n \"pixel_mask\": null\r\n },\r\n {\r\n \"id\": 2,\r\n \"file_name\": \"aceca_100.mp4frame24.png\",\r\n \"width\": 1280,\r\n \"height\": 720,\r\n \"pixel_values\": null,\r\n \"pixel_mask\": null\r\n },\r\n {\r\n \"id\": 3,\r\n \"file_name\": \"aceca_100.mp4frame25.png\",\r\n \"width\": 1280,\r\n \"height\": 720,\r\n \"pixel_values\": null,\r\n \"pixel_mask\": null}],\r\n \"annotations\": [\r\n {\r\n \"id\": 1,\r\n \"image_id\": 1,\r\n \"category_id\": 0,\r\n \"bbox\": [0.0, 278.21896388398557, 86.94096523844935, 156.0293445072134],\r\n \"area\": 13565.341816979679,\r\n \"iscrowd\": 0\r\n },\r\n {\r\n \"id\": 2,\r\n \"image_id\": 2,\r\n \"category_id\": 0,\r\n \"bbox\": [149.28851295721816, 297.6359759754418, 34.76802347007475, 98.03908698442889],\r\n \"area\": 3408.625277259324,\r\n \"iscrowd\": 0\r\n },\r\n {\r\n \"id\": 3,\r\n \"image_id\": 3,\r\n \"category_id\": 0,\r\n \"bbox\": [153.3817197549372, 300.168969412891, 31.787555842913775, 89.69583163436312],\r\n \"area\": 2851.2112569539095,\r\n \"iscrowd\": 0\r\n }\r\n ],\r\n \"categories\": [\r\n {\r\n \"id\": 0, \"name\": \"person\"\r\n }\r\n ]\r\n }\r\n```\r\nIf full files required, my email is [email protected]",
"Hi @Alberto1404, to load an object detection dataset it's recommended to make use of the metadata feature as explained [here](https://huggingface.co/docs/datasets/image_dataset#object-detection). ",
"Thank you @NielsRogge! It works!!!",
"You can now refer to https://huggingface.co/docs/datasets/repository_structure to learn about the `datasets`' data files inference, so I'm closing this issue."
] | I have about 20000 images in my folder which divided into 4 folders with class names.
When i use load_dataset("my_folder_name", split="train") this function create dataset in which there are only 4 images, the remaining 19000 images were not added there. What is the problem and did not understand. Tried converting images and the like but absolutely nothing worked | 5,650 |
https://github.com/huggingface/datasets/issues/5649 | The index column created with .to_sql() is dependent on the batch_size when writing | [
"Thanks for reporting, @lsb. \r\n\r\nWe are investigating it.\r\n\r\nOn the other hand, please note that in the next `datasets` release, the index will not be created by default (see #5583). If you would like to have it, you will need to explicitly pass `index=True`. ",
"I think this is low enough priority for me to close this as Won't Fix. If I need any primary keys I can generate them beforehand. Feel free to reopen."
] | ### Describe the bug
It seems like the "index" column is designed to be unique? The values are only unique per batch. The SQL index is not a unique index.
This can be a problem, for instance, when building a faiss index on a dataset and then trying to match up ids with a sql export.
### Steps to reproduce the bug
```
from datasets import Dataset
import sqlite3
db = sqlite3.connect(":memory:")
nice_numbers = Dataset.from_dict({"nice_number": range(101,106)})
nice_numbers.to_sql("nice1", db, batch_size=1)
nice_numbers.to_sql("nice2", db, batch_size=2)
print(db.execute("select * from nice1").fetchall()) # [(0, 101), (0, 102), (0, 103), (0, 104), (0, 105)]
print(db.execute("select * from nice2").fetchall()) # [(0, 101), (1, 102), (0, 103), (1, 104), (0, 105)]
```
### Expected behavior
I expected the "index" column to be unique
### Environment info
```
% datasets-cli env
Copy-and-paste the text below in your GitHub issue.
- `datasets` version: 2.10.1
- Platform: macOS-13.2.1-arm64-arm-64bit
- Python version: 3.9.6
- PyArrow version: 7.0.0
- Pandas version: 1.5.2
zsh: segmentation fault datasets-cli env
``` | 5,649 |
https://github.com/huggingface/datasets/issues/5648 | flatten_indices doesn't work with pandas format | [
"Thanks for reporting! This can be fixed by setting the format to `arrow` in `flatten_indices` and restoring the original format after the flattening. I'm working on a PR that reduces the number of the `flatten_indices` calls in our codebase and makes `flatten_indices` a no-op when a dataset does not have an indices mapping, so I'll incorporate the fix in that PR."
] | ### Describe the bug
Hi,
I noticed that `flatten_indices` throws an error when the batch format is `pandas`. This is probably due to the fact that flatten_indices uses map internally which doesn't accept dataframes as the transformation function output
### Steps to reproduce the bug
tabular_data = pd.DataFrame(np.random.randn(10,10))
tabular_data = datasets.arrow_dataset.Dataset.from_pandas(tabular_data)
tabular_data.with_format("pandas").select([0,1,2,3]).flatten_indices()
### Expected behavior
No error thrown
### Environment info
- `datasets` version: 2.10.1
- Python version: 3.9.5
- PyArrow version: 11.0.0
- Pandas version: 1.4.1 | 5,648 |
https://github.com/huggingface/datasets/issues/5647 | Make all print statements optional | [
"related to #5444 ",
"We now log these messages instead of printing them (addressed in #6019), so I'm closing this issue."
] | ### Feature request
Make all print statements optional to speed up the development
### Motivation
Im loading multiple tiny datasets and all the print statements make the loading slower
### Your contribution
I can help contribute | 5,647 |
https://github.com/huggingface/datasets/issues/5645 | Datasets map and select(range()) is giving dill error | [
"It looks like an error that we observed once in https://github.com/huggingface/datasets/pull/5166\r\n\r\nCan you try to update `datasets` ?\r\n\r\n```\r\npip install -U datasets\r\n```\r\n\r\nif it doesn't work, can you make sure you don't have packages installed that may modify `dill`'s behavior, such as `apache-beam` ?",
"@lhoestq That fixed the problem, Thanks :)"
] | ### Describe the bug
I'm using Huggingface Datasets library to load the dataset in google colab
When I do,
> data = train_dataset.select(range(10))
or
> train_datasets = train_dataset.map(
> process_data_to_model_inputs,
> batched=True,
> batch_size=batch_size,
> remove_columns=["article", "abstract"],
> )
I get following error: `module 'dill._dill' has no attribute 'log'`
I've tried downgrading the dill version from latest to 0.2.8, but no luck.
Stack trace:
> ---------------------------------------------------------------------------
> ModuleNotFoundError Traceback (most recent call last)
> /usr/local/lib/python3.9/dist-packages/datasets/utils/py_utils.py in _no_cache_fields(obj)
> 367 try:
> --> 368 import transformers as tr
> 369
>
> ModuleNotFoundError: No module named 'transformers'
>
> During handling of the above exception, another exception occurred:
>
> AttributeError Traceback (most recent call last)
> 17 frames
> <ipython-input-13-dd14813880a6> in <module>
> ----> 1 test = train_dataset.select(range(10))
>
> /usr/local/lib/python3.9/dist-packages/datasets/arrow_dataset.py in wrapper(*args, **kwargs)
> 155 }
> 156 # apply actual function
> --> 157 out: Union["Dataset", "DatasetDict"] = func(self, *args, **kwargs)
> 158 datasets: List["Dataset"] = list(out.values()) if isinstance(out, dict) else [out]
> 159 # re-apply format to the output
>
> /usr/local/lib/python3.9/dist-packages/datasets/fingerprint.py in wrapper(*args, **kwargs)
> 155 if kwargs.get(fingerprint_name) is None:
> 156 kwargs_for_fingerprint["fingerprint_name"] = fingerprint_name
> --> 157 kwargs[fingerprint_name] = update_fingerprint(
> 158 self._fingerprint, transform, kwargs_for_fingerprint
> 159 )
>
> /usr/local/lib/python3.9/dist-packages/datasets/fingerprint.py in update_fingerprint(fingerprint, transform, transform_args)
> 103 for key in sorted(transform_args):
> 104 hasher.update(key)
> --> 105 hasher.update(transform_args[key])
> 106 return hasher.hexdigest()
> 107
>
> /usr/local/lib/python3.9/dist-packages/datasets/fingerprint.py in update(self, value)
> 55 def update(self, value):
> 56 self.m.update(f"=={type(value)}==".encode("utf8"))
> ---> 57 self.m.update(self.hash(value).encode("utf-8"))
> 58
> 59 def hexdigest(self):
>
> /usr/local/lib/python3.9/dist-packages/datasets/fingerprint.py in hash(cls, value)
> 51 return cls.dispatch[type(value)](cls, value)
> 52 else:
> ---> 53 return cls.hash_default(value)
> 54
> 55 def update(self, value):
>
> /usr/local/lib/python3.9/dist-packages/datasets/fingerprint.py in hash_default(cls, value)
> 44 @classmethod
> 45 def hash_default(cls, value):
> ---> 46 return cls.hash_bytes(dumps(value))
> 47
> 48 @classmethod
>
> /usr/local/lib/python3.9/dist-packages/datasets/utils/py_utils.py in dumps(obj)
> 387 file = StringIO()
> 388 with _no_cache_fields(obj):
> --> 389 dump(obj, file)
> 390 return file.getvalue()
> 391
>
> /usr/local/lib/python3.9/dist-packages/datasets/utils/py_utils.py in dump(obj, file)
> 359 def dump(obj, file):
> 360 """pickle an object to a file"""
> --> 361 Pickler(file, recurse=True).dump(obj)
> 362 return
> 363
>
> /usr/local/lib/python3.9/dist-packages/dill/_dill.py in dump(self, obj)
> 392 return
> 393
> --> 394 def load_session(filename='/tmp/session.pkl', main=None):
> 395 """update the __main__ module with the state from the session file"""
> 396 if main is None: main = _main_module
>
> /usr/lib/python3.9/pickle.py in dump(self, obj)
> 485 if self.proto >= 4:
> 486 self.framer.start_framing()
> --> 487 self.save(obj)
> 488 self.write(STOP)
> 489 self.framer.end_framing()
>
> /usr/local/lib/python3.9/dist-packages/dill/_dill.py in save(self, obj, save_persistent_id)
> 386 pickler._byref = False # disable pickling by name reference
> 387 pickler._recurse = False # disable pickling recursion for globals
> --> 388 pickler._session = True # is best indicator of when pickling a session
> 389 pickler.dump(main)
> 390 finally:
>
> /usr/lib/python3.9/pickle.py in save(self, obj, save_persistent_id)
> 558 f = self.dispatch.get(t)
> 559 if f is not None:
> --> 560 f(self, obj) # Call unbound method with explicit self
> 561 return
> 562
>
> /usr/local/lib/python3.9/dist-packages/dill/_dill.py in save_singleton(pickler, obj)
>
> /usr/lib/python3.9/pickle.py in save_reduce(self, func, args, state, listitems, dictitems, state_setter, obj)
> 689 write(NEWOBJ)
> 690 else:
> --> 691 save(func)
> 692 save(args)
> 693 write(REDUCE)
>
> /usr/local/lib/python3.9/dist-packages/dill/_dill.py in save(self, obj, save_persistent_id)
> 386 pickler._byref = False # disable pickling by name reference
> 387 pickler._recurse = False # disable pickling recursion for globals
> --> 388 pickler._session = True # is best indicator of when pickling a session
> 389 pickler.dump(main)
> 390 finally:
>
> /usr/lib/python3.9/pickle.py in save(self, obj, save_persistent_id)
> 558 f = self.dispatch.get(t)
> 559 if f is not None:
> --> 560 f(self, obj) # Call unbound method with explicit self
> 561 return
> 562
>
> /usr/local/lib/python3.9/dist-packages/datasets/utils/py_utils.py in save_function(pickler, obj)
> 583 dill._dill.log.info("# F1")
> 584 else:
> --> 585 dill._dill.log.info("F2: %s" % obj)
> 586 name = getattr(obj, "__qualname__", getattr(obj, "__name__", None))
> 587 dill._dill.StockPickler.save_global(pickler, obj, name=name)
>
> AttributeError: module 'dill._dill' has no attribute 'log'
### Steps to reproduce the bug
After loading the dataset(eg: https://huggingface.co/datasets/scientific_papers) in google colab
do either
> data = train_dataset.select(range(10))
or
> train_datasets = train_dataset.map(
> process_data_to_model_inputs,
> batched=True,
> batch_size=batch_size,
> remove_columns=["article", "abstract"],
> )
### Expected behavior
The map and select function should work
### Environment info
dataset: https://huggingface.co/datasets/scientific_papers
dill = 0.3.6
python= 3.9.16
transformer = 4.2.0 | 5,645 |
https://github.com/huggingface/datasets/issues/5641 | Features cannot be named "self" | [] | ### Describe the bug
Hi,
I noticed that we cannot create a HuggingFace dataset from Pandas DataFrame with a column named `self`.
The error seems to be coming from arguments validation in the `Features.from_dict` function.
### Steps to reproduce the bug
```python
import datasets
dummy_pandas = pd.DataFrame([0,1,2,3], columns = ["self"])
datasets.arrow_dataset.Dataset.from_pandas(dummy_pandas)
```
### Expected behavior
No error thrown
### Environment info
- `datasets` version: 2.8.0
- Python version: 3.9.5
- PyArrow version: 6.0.1
- Pandas version: 1.4.1 | 5,641 |
https://github.com/huggingface/datasets/issues/5639 | Parquet file wrongly recognized as zip prevents loading a dataset | [] | ### Describe the bug
When trying to `load_dataset_builder` for `HuggingFaceGECLM/StackExchange_Mar2023`, extraction fails, because parquet file [devops-00000-of-00001-22fe902fd8702892.parquet](https://huggingface.co/datasets/HuggingFaceGECLM/StackExchange_Mar2023/resolve/1f8c9a2ab6f7d0f9ae904b8b922e4384592ae1a5/data/devops-00000-of-00001-22fe902fd8702892.parquet) is wrongly identified by python as being a zip not a parquet.
(Full thread on [Slack](https://huggingface.slack.com/archives/C02V51Q3800/p1678890880803599))
### Steps to reproduce the bug
```python
from datasets import load_dataset_builder
ds = load_dataset_builder("HuggingFaceGECLM/StackExchange_Mar2023")
```
### Expected behavior
Loading the file normally.
### Environment info
- `datasets` version: 2.3.2
- Platform: Linux-5.14.0-1058-oem-x86_64-with-glibc2.29
- Python version: 3.8.10
- PyArrow version: 8.0.0
- Pandas version: 1.4.3 | 5,639 |
https://github.com/huggingface/datasets/issues/5638 | xPath to implement all operations for Path | [
" I think https://github.com/fsspec/universal_pathlib is the project you are looking for.\r\n\r\n`xPath` has the methods often used in dataset scripts, and `mkdir` is not one of them (`dl_manager`'s role is to \"interact\" with the file system, so using `mkdir` is discouraged).",
"Right is there a difference between UPath and xPath? Typically is xPath less well implemented compared to Upath, ie missing some implementations of some methods? Or are there methods in xPath that are not implemented with UPath?",
"`xPath` is an internal component (it doesn't have a leading underscore in the name, but it should) not meant to be used outside of `datasets`, and it's only tested on HTTP URLs, not S3.\r\n\r\n",
"Okay I understand that xPath won't support my usecase. What I was perhaps getting to is why not use UPath in `datasets` instead of `xPath` if UPath seems to have strictly more robust implementations.",
"It seems like `universal_pathlib` does not support `fsspec` URL chaining (`::` is the chaining symbol) and \"compression\" filesystems (e.g., `zip`), but this is what we need to access and stream files from within an archive (e.g., we want to stream URLs such as this one: `zip://data.parquet::https://www.dummyurl.com/archive.zip`)"
] | ### Feature request
Current xPath implementation is a great extension of Path in order to work with remote objects. However some methods such as `mkdir` are not implemented correctly. It should instead rely on `fsspec` methods, instead of defaulting do `Path` methods which only work locally.
### Motivation
I'm using xPath to interact with remote objects.
### Your contribution
I could try to make a PR. I'm a bit unfamiliar with chaining right now. | 5,638 |
https://github.com/huggingface/datasets/issues/5637 | IterableDataset with_format does not support 'device' keyword for jax | [
"Hi! Yes, only `torch` is currently supported. Unlike `Dataset`, `IterableDataset` is not PyArrow-backed, so we cannot simply call `to_numpy` on the underlying subtables to format them numerically. Instead, we must manually convert examples to (numeric) arrays while preserving consistency with `Dataset`, which is not trivial, so this is still a to-do.",
"Any plans to support it in the future? Or would streaming dataset be left without support for jax and tensorflow?"
] | ### Describe the bug
As seen here: https://huggingface.co/docs/datasets/use_with_jax dataset.with_format() supports the keyword 'device', to put data on a specific device when loaded as jax. However, when called on an IterableDataset, I got the error `TypeError: with_format() got an unexpected keyword argument 'device'`
Looking over the code, it seems IterableDataset support only pytorch and no support for jax device keyword?
https://github.com/huggingface/datasets/blob/fc5c84f36684343bff3e424cb0fd1ac5ecdd66da/src/datasets/iterable_dataset.py#L1029
### Steps to reproduce the bug
1. Load an IterableDataset (tested in streaming mode)
2. Call with_format('jax',device=device)
### Expected behavior
I expect to call `with_format('jax', device=device)` as per [documentation](https://huggingface.co/docs/datasets/use_with_jax) without error
### Environment info
Tested with installing newest (dev) and also pip release (2.10.1).
- `datasets` version: 2.10.2.dev0
- Platform: Linux-5.15.89+-x86_64-with-debian-bullseye-sid
- Python version: 3.7.12
- Huggingface_hub version: 0.12.1
- PyArrow version: 11.0.0
- Pandas version: 1.3.5
| 5,637 |
https://github.com/huggingface/datasets/issues/5634 | Not all progress bars are showing up when they should for downloading dataset | [
"Hi! \r\n\r\nBy default, tqdm has `leave=True` to \"keep all traces of the progress bar upon the termination of iteration\". However, we use `leave=False` in some places (as of recently), which removes the bar once the iteration is over.\r\n\r\nI feel like our TQDM bars are noisy, so I think we should always set `leave=False` and also use the `delay` parameter to display progress bars only for tasks that take time (e.g., more than 3s). What do you think about this? Do you find these bars useful (after the dataset generation is over)?\r\n",
"Hi sorry for the late update. I think the problem still exists despite the `leave` flag\r\n\r\n<img width=\"1105\" alt=\"image\" src=\"https://user-images.githubusercontent.com/110427462/226501615-5b02fb02-fd5f-4eda-b1f7-a7ed6570892d.png\">\r\n\r\n\r\n```\r\nPackage Version\r\n------------------------ ---------\r\naiofiles 22.1.0\r\naiohttp 3.8.4\r\naiosignal 1.3.1\r\naiosqlite 0.18.0\r\nanyio 3.6.2\r\nappnope 0.1.3\r\nargon2-cffi 21.3.0\r\nargon2-cffi-bindings 21.2.0\r\narrow 1.2.3\r\nasttokens 2.2.1\r\nasync-generator 1.10\r\nasync-timeout 4.0.2\r\nattrs 22.2.0\r\nBabel 2.12.1\r\nbackcall 0.2.0\r\nbeautifulsoup4 4.11.2\r\nbleach 6.0.0\r\nbrotlipy 0.7.0\r\ncertifi 2022.12.7\r\ncffi 1.15.1\r\ncfgv 3.3.1\r\ncharset-normalizer 2.1.1\r\ncomm 0.1.2\r\nconda 22.9.0\r\nconda-package-handling 2.0.2\r\nconda_package_streaming 0.7.0\r\ncoverage 7.2.1\r\ncryptography 38.0.4\r\ndatasets 2.8.0\r\ndebugpy 1.6.6\r\ndecorator 5.1.1\r\ndefusedxml 0.7.1\r\ndill 0.3.6\r\ndistlib 0.3.6\r\ndistro 1.4.0\r\nentrypoints 0.4\r\nexceptiongroup 1.1.0\r\nexecuting 1.2.0\r\nfastjsonschema 2.16.3\r\nfilelock 3.9.0\r\nflaky 3.7.0\r\nfqdn 1.5.1\r\nfrozenlist 1.3.3\r\nfsspec 2023.3.0\r\nhuggingface-hub 0.10.1\r\nidentify 2.5.18\r\nidna 3.4\r\niniconfig 2.0.0\r\nipykernel 6.12.1\r\nipyparallel 8.4.1\r\nipython 7.32.0\r\nipython-genutils 0.2.0\r\nipywidgets 8.0.4\r\nisoduration 20.11.0\r\njedi 0.18.2\r\nJinja2 3.1.2\r\njson5 0.9.11\r\njsonpointer 2.3\r\njsonschema 4.17.3\r\njupyter_client 8.0.3\r\njupyter_core 5.2.0\r\njupyter-events 0.6.3\r\njupyter_server 2.4.0\r\njupyter_server_fileid 0.8.0\r\njupyter_server_terminals 0.4.4\r\njupyter_server_ydoc 0.6.1\r\njupyter-ydoc 0.2.2\r\njupyterlab 3.6.1\r\njupyterlab-pygments 0.2.2\r\njupyterlab_server 2.20.0\r\njupyterlab-widgets 3.0.5\r\nlibmambapy 1.1.0\r\nmamba 1.1.0\r\nMarkupSafe 2.1.2\r\nmatplotlib-inline 0.1.6\r\nmistune 2.0.5\r\nmultidict 6.0.4\r\nmultiprocess 0.70.14\r\nnbclassic 0.5.3\r\nnbclient 0.7.2\r\nnbconvert 7.2.9\r\nnbformat 5.7.3\r\nnest-asyncio 1.5.6\r\nnodeenv 1.7.0\r\nnotebook 6.5.3\r\nnotebook_shim 0.2.2\r\nnumpy 1.24.2\r\noutcome 1.2.0\r\npackaging 23.0\r\npandas 1.5.3\r\npandocfilters 1.5.0\r\nparso 0.8.3\r\npexpect 4.8.0\r\npickleshare 0.7.5\r\npip 22.3.1\r\nplatformdirs 3.0.0\r\nplotly 5.13.1\r\npluggy 1.0.0\r\npre-commit 3.1.0\r\nprometheus-client 0.16.0\r\nprompt-toolkit 3.0.38\r\npsutil 5.9.4\r\nptyprocess 0.7.0\r\npure-eval 0.2.2\r\npyarrow 11.0.0\r\npycosat 0.6.4\r\npycparser 2.21\r\nPygments 2.14.0\r\npyOpenSSL 22.1.0\r\npyrsistent 0.19.3\r\nPySocks 1.7.1\r\npytest 7.2.1\r\npytest-asyncio 0.20.3\r\npytest-cov 4.0.0\r\npytest-timeout 2.1.0\r\npython-dateutil 2.8.2\r\npython-json-logger 2.0.7\r\npytz 2022.7.1\r\nPyYAML 6.0\r\npyzmq 25.0.0\r\nrequests 2.28.1\r\nresponses 0.18.0\r\nrfc3339-validator 0.1.4\r\nrfc3986-validator 0.1.1\r\nruamel-yaml-conda 0.15.80\r\nSend2Trash 1.8.0\r\nsetuptools 65.6.3\r\nsimplegeneric 0.8.1\r\nsix 1.16.0\r\nsniffio 1.3.0\r\nsortedcontainers 2.4.0\r\nsoupsieve 2.4\r\nstack-data 0.6.2\r\ntenacity 8.2.2\r\nterminado 0.17.1\r\ntinycss2 1.2.1\r\ntomli 2.0.1\r\ntoolz 0.12.0\r\ntornado 6.2\r\ntqdm 4.65.0\r\ntraitlets 5.8.1\r\ntrio 0.22.0\r\ntyping_extensions 4.5.0\r\nuri-template 1.2.0\r\nurllib3 1.26.13\r\nvirtualenv 20.19.0\r\nwcwidth 0.2.6\r\nwebcolors 1.12\r\nwebencodings 0.5.1\r\nwebsocket-client 1.5.1\r\nwheel 0.38.4\r\nwidgetsnbextension 4.0.5\r\nxxhash 3.2.0\r\ny-py 0.5.9\r\nyarl 1.8.2\r\nypy-websocket 0.8.2\r\nzstandard 0.19.0\r\n```\r\n\r\nAny idea why this is happening? I debugged this to know the tqdm.pbar value is not being updated properly and its not the kernel not sending the comm messages to the IProgress bar"
] | ### Describe the bug
During downloading the rotten tomatoes dataset, not all progress bars are displayed properly. This might be related to [this ticket](https://github.com/huggingface/datasets/issues/5117) as it raised the same concern but its not clear if the fix solves this issue too.
ipywidgets
<img width="1243" alt="image" src="https://user-images.githubusercontent.com/110427462/224851138-13fee5b7-ab51-4883-b96f-1b9808782e3b.png">
tqdm
<img width="1251" alt="Screen Shot 2023-03-13 at 3 58 59 PM" src="https://user-images.githubusercontent.com/110427462/224851180-5feb7825-9250-4b1e-ad0c-f3172ac1eb78.png">
### Steps to reproduce the bug
1. Run this line
```
from datasets import load_dataset
rotten_tomatoes = load_dataset("rotten_tomatoes", split="train")
```
### Expected behavior
all progress bars for builder script, metadata, readme, training, validation, and test set
### Environment info
requirements.txt
```
aiofiles==22.1.0
aiohttp==3.8.4
aiosignal==1.3.1
aiosqlite==0.18.0
anyio==3.6.2
appnope==0.1.3
argon2-cffi==21.3.0
argon2-cffi-bindings==21.2.0
arrow==1.2.3
asttokens==2.2.1
async-generator==1.10
async-timeout==4.0.2
attrs==22.2.0
Babel==2.12.1
backcall==0.2.0
beautifulsoup4==4.11.2
bleach==6.0.0
brotlipy @ file:///Users/runner/miniforge3/conda-bld/brotlipy_1666764961872/work
certifi==2022.12.7
cffi @ file:///Users/runner/miniforge3/conda-bld/cffi_1671179414629/work
cfgv==3.3.1
charset-normalizer @ file:///home/conda/feedstock_root/build_artifacts/charset-normalizer_1661170624537/work
comm==0.1.2
conda==22.9.0
conda-package-handling @ file:///home/conda/feedstock_root/build_artifacts/conda-package-handling_1669907009957/work
conda_package_streaming @ file:///home/conda/feedstock_root/build_artifacts/conda-package-streaming_1669733752472/work
coverage==7.2.1
cryptography @ file:///Users/runner/miniforge3/conda-bld/cryptography_1669592251328/work
datasets==2.1.0
debugpy==1.6.6
decorator==5.1.1
defusedxml==0.7.1
dill==0.3.6
distlib==0.3.6
distro==1.4.0
entrypoints==0.4
exceptiongroup==1.1.0
executing==1.2.0
fastjsonschema==2.16.3
filelock==3.9.0
flaky==3.7.0
fqdn==1.5.1
frozenlist==1.3.3
fsspec==2023.3.0
huggingface-hub==0.10.1
identify==2.5.18
idna @ file:///home/conda/feedstock_root/build_artifacts/idna_1663625384323/work
iniconfig==2.0.0
ipykernel==6.12.1
ipyparallel==8.4.1
ipython==7.32.0
ipython-genutils==0.2.0
ipywidgets==8.0.4
isoduration==20.11.0
jedi==0.18.2
Jinja2==3.1.2
json5==0.9.11
jsonpointer==2.3
jsonschema==4.17.3
jupyter-events==0.6.3
jupyter-ydoc==0.2.2
jupyter_client==8.0.3
jupyter_core==5.2.0
jupyter_server==2.4.0
jupyter_server_fileid==0.8.0
jupyter_server_terminals==0.4.4
jupyter_server_ydoc==0.6.1
jupyterlab==3.6.1
jupyterlab-pygments==0.2.2
jupyterlab-widgets==3.0.5
jupyterlab_server==2.20.0
libmambapy @ file:///Users/runner/miniforge3/conda-bld/mamba-split_1671598370072/work/libmambapy
mamba @ file:///Users/runner/miniforge3/conda-bld/mamba-split_1671598370072/work/mamba
MarkupSafe==2.1.2
matplotlib-inline==0.1.6
mistune==2.0.5
multidict==6.0.4
multiprocess==0.70.14
nbclassic==0.5.3
nbclient==0.7.2
nbconvert==7.2.9
nbformat==5.7.3
nest-asyncio==1.5.6
nodeenv==1.7.0
notebook==6.5.3
notebook_shim==0.2.2
numpy==1.24.2
outcome==1.2.0
packaging==23.0
pandas==1.5.3
pandocfilters==1.5.0
parso==0.8.3
pexpect==4.8.0
pickleshare==0.7.5
platformdirs==3.0.0
plotly==5.13.1
pluggy==1.0.0
pre-commit==3.1.0
prometheus-client==0.16.0
prompt-toolkit==3.0.38
psutil==5.9.4
ptyprocess==0.7.0
pure-eval==0.2.2
pyarrow==11.0.0
pycosat @ file:///Users/runner/miniforge3/conda-bld/pycosat_1666836580084/work
pycparser @ file:///home/conda/feedstock_root/build_artifacts/pycparser_1636257122734/work
Pygments==2.14.0
pyOpenSSL @ file:///home/conda/feedstock_root/build_artifacts/pyopenssl_1665350324128/work
pyrsistent==0.19.3
PySocks @ file:///home/conda/feedstock_root/build_artifacts/pysocks_1661604839144/work
pytest==7.2.1
pytest-asyncio==0.20.3
pytest-cov==4.0.0
pytest-timeout==2.1.0
python-dateutil==2.8.2
python-json-logger==2.0.7
pytz==2022.7.1
PyYAML==6.0
pyzmq==25.0.0
requests @ file:///home/conda/feedstock_root/build_artifacts/requests_1661872987712/work
responses==0.18.0
rfc3339-validator==0.1.4
rfc3986-validator==0.1.1
ruamel-yaml-conda @ file:///Users/runner/miniforge3/conda-bld/ruamel_yaml_1666819760545/work
Send2Trash==1.8.0
simplegeneric==0.8.1
six==1.16.0
sniffio==1.3.0
sortedcontainers==2.4.0
soupsieve==2.4
stack-data==0.6.2
tenacity==8.2.2
terminado==0.17.1
tinycss2==1.2.1
tomli==2.0.1
toolz @ file:///home/conda/feedstock_root/build_artifacts/toolz_1657485559105/work
tornado==6.2
tqdm==4.64.1
traitlets==5.8.1
trio==0.22.0
typing_extensions==4.5.0
uri-template==1.2.0
urllib3 @ file:///home/conda/feedstock_root/build_artifacts/urllib3_1669259737463/work
virtualenv==20.19.0
wcwidth==0.2.6
webcolors==1.12
webencodings==0.5.1
websocket-client==1.5.1
widgetsnbextension==4.0.5
xxhash==3.2.0
y-py==0.5.9
yarl==1.8.2
ypy-websocket==0.8.2
zstandard==0.19.0
``` | 5,634 |
https://github.com/huggingface/datasets/issues/5633 | Cannot import datasets | [
"Okay, the issue was likely caused by mixing `conda` and `pip` usage - I forgot that I have already used `pip` in this environment previously and that it was 'spoiled' because of it. Creating another environment and installing `datasets` by pip with other packages from the `requirements.txt` file solved the problem."
] | ### Describe the bug
Hi,
I cannot even import the library :( I installed it by running:
```
$ conda install datasets
```
Then I realized I should maybe use the huggingface channel, because I encountered the error below, so I ran:
```
$ conda remove datasets
$ conda install -c huggingface datasets
```
Please see 'steps to reproduce the bug' for the specific error, as steps to reproduce is just importing the library
### Steps to reproduce the bug
```
$ python3
Python 3.8.15 (default, Nov 24 2022, 15:19:38)
[GCC 11.2.0] :: Anaconda, Inc. on linux
Type "help", "copyright", "credits" or "license" for more information.
>>> import datasets
Traceback (most recent call last):
File "<stdin>", line 1, in <module>
File "/home/jack/.conda/envs/jack_zpp/lib/python3.8/site-packages/datasets/__init__.py", line 33, in <module>
from .arrow_dataset import Dataset, concatenate_datasets
File "/home/jack/.conda/envs/jack_zpp/lib/python3.8/site-packages/datasets/arrow_dataset.py", line 59, in <module>
from .arrow_reader import ArrowReader
File "/home/jack/.conda/envs/jack_zpp/lib/python3.8/site-packages/datasets/arrow_reader.py", line 27, in <module>
import pyarrow.parquet as pq
File "/home/jack/.conda/envs/jack_zpp/lib/python3.8/site-packages/pyarrow/parquet/__init__.py", line 20, in <module>
from .core import *
File "/home/jack/.conda/envs/jack_zpp/lib/python3.8/site-packages/pyarrow/parquet/core.py", line 37, in <module>
from pyarrow._parquet import (ParquetReader, Statistics, # noqa
ImportError: cannot import name 'FileEncryptionProperties' from 'pyarrow._parquet' (/home/jack/.conda/envs/jack_zpp/lib/python3.8/site-packages/pyarrow/_parquet.cpython-38-x86_64-linux-gnu.so)
```
### Expected behavior
I would expect for the statement `import datasets` to cause no error
### Environment info
Output of `conda list`:
```
# packages in environment at /home/jack/.conda/envs/pbalawender_zpp:
#
# Name Version Build Channel
_libgcc_mutex 0.1 main
_openmp_mutex 5.1 1_gnu
abseil-cpp 20210324.2 h2531618_0
advertools 0.13.2 pypi_0 pypi
aiofiles 0.8.0 pypi_0 pypi
aiohttp 3.8.3 py38h5eee18b_0
aiosignal 1.2.0 pyhd3eb1b0_0
aiosqlite 0.17.0 pypi_0 pypi
anyio 3.6.2 pypi_0 pypi
aquirdturtle-collapsible-headings 3.1.0 pypi_0 pypi
argon2-cffi 21.3.0 pypi_0 pypi
argon2-cffi-bindings 21.2.0 pypi_0 pypi
arrow 1.2.3 pypi_0 pypi
arrow-cpp 3.0.0 py38h6b21186_4
asttokens 2.2.0 pypi_0 pypi
async-timeout 4.0.2 py38h06a4308_0
attrs 22.1.0 py38h06a4308_0
automat 22.10.0 pypi_0 pypi
aws-c-common 0.4.57 he6710b0_1
aws-c-event-stream 0.1.6 h2531618_5
aws-checksums 0.1.9 he6710b0_0
aws-sdk-cpp 1.8.185 hce553d0_0
babel 2.11.0 pypi_0 pypi
backcall 0.2.0 pyhd3eb1b0_0
beautifulsoup4 4.11.1 pypi_0 pypi
blas 1.0 mkl
bleach 5.0.1 pypi_0 pypi
boost-cpp 1.73.0 h27cfd23_11
bottleneck 1.3.5 py38h7deecbd_0
brotli 1.0.9 h5eee18b_7
brotli-bin 1.0.9 h5eee18b_7
brotlipy 0.7.0 py38h27cfd23_1003
bzip2 1.0.8 h7b6447c_0
c-ares 1.18.1 h7f8727e_0
ca-certificates 2023.01.10 h06a4308_0
certifi 2022.9.24 pypi_0 pypi
cffi 1.15.1 py38h5eee18b_3
charset-normalizer 2.1.1 pypi_0 pypi
click 8.1.3 pypi_0 pypi
constantly 15.1.0 pypi_0 pypi
contourpy 1.0.6 pypi_0 pypi
cryptography 38.0.4 pypi_0 pypi
cssselect 1.2.0 pypi_0 pypi
cudatoolkit 10.1.243 h8cb64d8_10 conda-forge
cycler 0.11.0 pypi_0 pypi
dacite 1.6.0 pypi_0 pypi
dataclasses 0.8 pyh6d0b6a4_7
datasets 1.18.4 py_0 huggingface
datetime 4.7 pypi_0 pypi
debugpy 1.6.4 pypi_0 pypi
decorator 5.1.1 pyhd3eb1b0_0
defusedxml 0.7.1 pypi_0 pypi
dill 0.3.6 py38h06a4308_0
docker-pycreds 0.4.0 pypi_0 pypi
double-conversion 3.1.5 he6710b0_1
entrypoints 0.4 py38h06a4308_0
executing 0.8.3 pyhd3eb1b0_0
filelock 3.8.0 pypi_0 pypi
flake8 6.0.0 pypi_0 pypi
flask 2.1.3 py38h06a4308_0
flit-core 3.6.0 pyhd3eb1b0_0
fonttools 4.38.0 pypi_0 pypi
fqdn 1.5.1 pypi_0 pypi
freetype 2.12.1 h4a9f257_0
frozenlist 1.3.3 py38h5eee18b_0
fsspec 2022.11.0 py38h06a4308_0
gensim 4.2.0 pypi_0 pypi
gflags 2.2.2 he6710b0_0
giflib 5.2.1 h5eee18b_3
gitdb 4.0.10 pypi_0 pypi
gitpython 3.1.30 pypi_0 pypi
glog 0.5.0 h2531618_0
grpc-cpp 1.39.0 hae934f6_5
huggingface-hub 0.11.1 pypi_0 pypi
huggingface_hub 0.13.1 py_0 huggingface
hyperlink 21.0.0 pypi_0 pypi
icu 58.2 he6710b0_3
idna 3.4 py38h06a4308_0
importlib-metadata 5.1.0 pypi_0 pypi
importlib_metadata 4.11.3 hd3eb1b0_0
importlib_resources 5.2.0 pyhd3eb1b0_1
incremental 22.10.0 pypi_0 pypi
intel-openmp 2021.4.0 h06a4308_3561
ipykernel 6.17.1 pyh210e3f2_0 conda-forge
ipython 8.7.0 pypi_0 pypi
ipython-genutils 0.2.0 pypi_0 pypi
ipywidgets 8.0.2 pyhd8ed1ab_1 conda-forge
isoduration 20.11.0 pypi_0 pypi
itemadapter 0.7.0 pypi_0 pypi
itemloaders 1.0.6 pypi_0 pypi
itsdangerous 2.0.1 pyhd3eb1b0_0
jedi 0.18.2 pypi_0 pypi
jinja2 3.1.2 py38h06a4308_0
jmespath 1.0.1 pypi_0 pypi
joblib 1.2.0 pypi_0 pypi
jpeg 9b h024ee3a_2
json5 0.9.10 pypi_0 pypi
jsonpickle 3.0.0 pypi_0 pypi
jsonpointer 2.3 pypi_0 pypi
jsonschema 4.17.3 py38h06a4308_0
jupyter-core 5.1.0 pypi_0 pypi
jupyter-events 0.5.0 pypi_0 pypi
jupyter-server 1.23.3 pypi_0 pypi
jupyter-server-fileid 0.6.0 pypi_0 pypi
jupyter-server-ydoc 0.4.0 pypi_0 pypi
jupyter-ydoc 0.2.2 pypi_0 pypi
jupyter_client 7.4.9 py38h06a4308_0
jupyter_core 5.2.0 py38h06a4308_0
jupyterlab 3.6.0a4 pypi_0 pypi
jupyterlab-pygments 0.2.2 pypi_0 pypi
jupyterlab-server 2.16.3 pypi_0 pypi
jupyterlab_widgets 3.0.3 pyhd8ed1ab_0 conda-forge
kiwisolver 1.4.4 pypi_0 pypi
krb5 1.19.4 h568e23c_0
lcms2 2.12 h3be6417_0
ld_impl_linux-64 2.38 h1181459_1
libboost 1.73.0 h3ff78a5_11
libbrotlicommon 1.0.9 h5eee18b_7
libbrotlidec 1.0.9 h5eee18b_7
libbrotlienc 1.0.9 h5eee18b_7
libcurl 7.88.1 h91b91d3_0
libedit 3.1.20221030 h5eee18b_0
libev 4.33 h7f8727e_1
libevent 2.1.12 h8f2d780_0
libffi 3.4.2 h6a678d5_6
libgcc-ng 11.2.0 h1234567_1
libgomp 11.2.0 h1234567_1
libnghttp2 1.46.0 hce63b2e_0
libpng 1.6.39 h5eee18b_0
libprotobuf 3.17.2 h4ff587b_1
libsodium 1.0.18 h7b6447c_0
libssh2 1.10.0 h8f2d780_0
libstdcxx-ng 11.2.0 h1234567_1
libthrift 0.14.2 hcc01f38_0
libtiff 4.1.0 h2733197_1
libuv 1.44.2 h5eee18b_0
libwebp 1.2.0 h89dd481_0
lz4-c 1.9.4 h6a678d5_0
markupsafe 2.1.1 py38h7f8727e_0
matplotlib 3.6.2 pypi_0 pypi
matplotlib-inline 0.1.6 py38h06a4308_0
mccabe 0.7.0 pypi_0 pypi
mistune 2.0.4 pypi_0 pypi
mkl 2021.4.0 h06a4308_640
mkl-service 2.4.0 py38h7f8727e_0
mkl_fft 1.3.1 py38hd3c417c_0
mkl_random 1.2.2 py38h51133e4_0
morfeusz2 1.99.6 pypi_0 pypi
multidict 6.0.2 py38h5eee18b_0
multiprocess 0.70.14 py38h06a4308_0
nbclassic 0.4.8 pypi_0 pypi
nbclient 0.7.2 pypi_0 pypi
nbconvert 7.2.5 pypi_0 pypi
nbformat 5.7.0 py38h06a4308_0
ncurses 6.4 h6a678d5_0
nest-asyncio 1.5.6 py38h06a4308_0
ninja 1.10.2 h06a4308_5
ninja-base 1.10.2 hd09550d_5
notebook 6.5.2 pypi_0 pypi
notebook-shim 0.2.2 pypi_0 pypi
numexpr 2.8.4 py38he184ba9_0
numpy 1.23.5 py38h14f4228_0
numpy-base 1.23.5 py38h31eccc5_0
oauthlib 3.2.2 pypi_0 pypi
opencv-python 4.6.0.66 pypi_0 pypi
openssl 1.1.1t h7f8727e_0
orc 1.6.9 ha97a36c_3
packaging 22.0 py38h06a4308_0
pandas 1.5.2 pypi_0 pypi
pandocfilters 1.5.0 pypi_0 pypi
parsel 1.7.0 pypi_0 pypi
parso 0.8.3 pyhd3eb1b0_0
pathlib 1.0.1 pypi_0 pypi
pathtools 0.1.2 pypi_0 pypi
pexpect 4.8.0 pyhd3eb1b0_3
pickleshare 0.7.5 pyhd3eb1b0_1003
pillow 9.3.0 pypi_0 pypi
pip 22.2.2 py38h06a4308_0
pkgutil-resolve-name 1.3.10 py38h06a4308_0
platformdirs 2.5.4 pypi_0 pypi
prometheus-client 0.15.0 pypi_0 pypi
promise 2.3 pypi_0 pypi
prompt-toolkit 3.0.33 pypi_0 pypi
protego 0.2.1 pypi_0 pypi
protobuf 4.21.12 pypi_0 pypi
psutil 5.9.0 py38h5eee18b_0
ptyprocess 0.7.0 pyhd3eb1b0_2
pure_eval 0.2.2 pyhd3eb1b0_0
pyarrow 10.0.1 pypi_0 pypi
pyasn1 0.4.8 pypi_0 pypi
pyasn1-modules 0.2.8 pypi_0 pypi
pycodestyle 2.10.0 pypi_0 pypi
pycparser 2.21 pyhd3eb1b0_0
pydispatcher 2.0.6 pypi_0 pypi
pyflakes 3.0.1 pypi_0 pypi
pygments 2.11.2 pyhd3eb1b0_0
pyopenssl 22.1.0 pypi_0 pypi
pyrsistent 0.18.0 py38heee7806_0
pysocks 1.7.1 py38h06a4308_0
python 3.8.15 h7a1cb2a_2
python-dateutil 2.8.2 pyhd3eb1b0_0
python-dotenv 0.21.0 pypi_0 pypi
python-fastjsonschema 2.16.2 py38h06a4308_0
python-json-logger 2.0.4 pypi_0 pypi
python-xxhash 2.0.2 py38h5eee18b_1
pytorch 1.7.1 py3.8_cuda10.1.243_cudnn7.6.3_0 pytorch
pytz 2022.6 pypi_0 pypi
pyyaml 6.0 py38h5eee18b_1
pyzmq 23.2.0 py38h6a678d5_0
queuelib 1.6.2 pypi_0 pypi
re2 2022.04.01 h295c915_0
readline 8.2 h5eee18b_0
regex 2022.10.31 pypi_0 pypi
requests 2.28.1 py38h06a4308_0
requests-file 1.5.1 pypi_0 pypi
requests-oauthlib 1.3.1 pypi_0 pypi
rfc3339-validator 0.1.4 pypi_0 pypi
rfc3986-validator 0.1.1 pypi_0 pypi
scikit-learn 1.1.3 pypi_0 pypi
scipy 1.9.3 pypi_0 pypi
scrapy 2.7.1 pypi_0 pypi
seaborn 0.12.1 pypi_0 pypi
send2trash 1.8.0 pypi_0 pypi
sentry-sdk 1.12.1 pypi_0 pypi
service-identity 21.1.0 pypi_0 pypi
setproctitle 1.3.2 pypi_0 pypi
setuptools 65.6.3 pypi_0 pypi
shortuuid 1.0.11 pypi_0 pypi
six 1.16.0 pyhd3eb1b0_1
smart-open 6.2.0 pypi_0 pypi
smmap 5.0.0 pypi_0 pypi
snappy 1.1.9 h295c915_0
sniffio 1.3.0 pypi_0 pypi
soupsieve 2.3.2.post1 pypi_0 pypi
sqlite 3.40.1 h5082296_0
stack-data 0.6.2 pypi_0 pypi
stack_data 0.2.0 pyhd3eb1b0_0
terminado 0.17.0 pypi_0 pypi
threadpoolctl 3.1.0 pypi_0 pypi
tinycss2 1.2.1 pypi_0 pypi
tk 8.6.12 h1ccaba5_0
tldextract 3.4.0 pypi_0 pypi
tokenizers 0.13.2 pypi_0 pypi
tomli 2.0.1 pypi_0 pypi
torchvision 0.8.2 py38_cu101 pytorch
tornado 6.2 py38h5eee18b_0
tqdm 4.64.1 py38h06a4308_0
traitlets 5.6.0 pypi_0 pypi
transformers 4.25.1 pypi_0 pypi
tweepy 4.12.1 pypi_0 pypi
twisted 22.10.0 pypi_0 pypi
twython 3.9.1 pypi_0 pypi
typing-extensions 4.4.0 py38h06a4308_0
typing_extensions 4.4.0 py38h06a4308_0
uri-template 1.2.0 pypi_0 pypi
uriparser 0.9.3 he6710b0_1
urllib3 1.26.13 pypi_0 pypi
utf8proc 2.6.1 h27cfd23_0
w3lib 2.1.0 pypi_0 pypi
wandb 0.13.7 pypi_0 pypi
wcwidth 0.2.5 pyhd3eb1b0_0
webcolors 1.12 pypi_0 pypi
webencodings 0.5.1 pypi_0 pypi
websocket-client 1.4.2 pypi_0 pypi
werkzeug 2.2.2 py38h06a4308_0
wheel 0.38.4 py38h06a4308_0
widgetsnbextension 4.0.3 py38h06a4308_0
xxhash 0.8.0 h7f8727e_3
xz 5.2.10 h5eee18b_1
y-py 0.5.4 pypi_0 pypi
yaml 0.2.5 h7b6447c_0
yarl 1.8.1 py38h5eee18b_0
ypy-websocket 0.5.0 pypi_0 pypi
zeromq 4.3.4 h2531618_0
zipp 3.11.0 py38h06a4308_0
zlib 1.2.13 h5eee18b_0
zope-interface 5.5.2 pypi_0 pypi
zstd 1.4.9 haebb681_0
```
| 5,633 |
https://github.com/huggingface/datasets/issues/5632 | Dataset cannot convert too large dictionnary | [
"Answered on the forum:\r\n\r\n> To fix the overflow error, we need to merge [support LargeListArray in pyarrow by xwwwwww · Pull Request #4800 · huggingface/datasets · GitHub](https://github.com/huggingface/datasets/pull/4800), which adds support for the large lists. However, before merging it, we need to come up with a cleaner API for large lists. I hope to find some time to address this before Datasets 3.0."
] | ### Describe the bug
Hello everyone!
I tried to build a new dataset with the command "dict_valid = datasets.Dataset.from_dict({'input_values': values_array})".
However, I have a very large dataset (~400Go) and it seems that dataset cannot handle this.
Indeed, I can create the dataset until a certain size of my dictionnary, and then I have the error "OverflowError: Python int too large to convert to C long".
Do you know how to solve this problem?
Unfortunately I cannot give a reproductible code because I cannot share a so large file, but you can find the code below (it's a test on only a part of the validation data ~10Go, but it's already the case).
Thank you!
### Steps to reproduce the bug
SAVE_DIR = './data/'
features = h5py.File(SAVE_DIR+'features.hdf5','r')
valid_data = features["validation"]["data/features"]
v_array_values = [np.float32(item[()]) for item in valid_data.values()]
for i in range(len(v_array_values)):
v_array_values[i] = v_array_values[i].round(decimals=5)
dict_valid = datasets.Dataset.from_dict({'input_values': v_array_values})
### Expected behavior
The code is expected to give me a Huggingface dataset.
### Environment info
python: 3.8.15
numpy: 1.22.3
datasets: 2.3.2
pyarrow: 8.0.0 | 5,632 |
https://github.com/huggingface/datasets/issues/5631 | Custom split names | [
"Hi!\r\n\r\nYou can also use names other than \"train\", \"validation\" and \"test\". As an example, check the [script](https://huggingface.co/datasets/mozilla-foundation/common_voice_11_0/blob/e095840f23f3dffc1056c078c2f9320dad9ca74d/common_voice_11_0.py#L139) of the Common Voice 11 dataset. "
] | ### Feature request
Hi,
I participated in multiple NLP tasks where there are more than just train, test, validation splits, there could be multiple validation sets or test sets. But it seems currently only those mentioned three splits supported. It would be nice to have the support for more splits on the hub. (currently i can have more splits when I am loading datasets from urls, but not hub)
### Motivation
Easier access to more splits
### Your contribution
No | 5,631 |
https://github.com/huggingface/datasets/issues/5629 | load_dataset gives "403" error when using Financial phrasebank | [
"Hi! You seem to be using an outdated version of `datasets` that downloads the older script version. To avoid the error, you can either pass `revision=\"main\"` to `load_dataset` (this can fail if a script uses newer features of the lib) or update your installation with `pip install -U datasets` (better solution)."
] | When I try to load this dataset, I receive the following error:
ConnectionError: Couldn't reach https://www.researchgate.net/profile/Pekka_Malo/publication/251231364_FinancialPhraseBank-v10/data/0c96051eee4fb1d56e000000/FinancialPhraseBank-v10.zip (error 403)
Has this been seen before? Thanks. The website loads when I try to access it manually. | 5,629 |
https://github.com/huggingface/datasets/issues/5627 | Unable to load AutoTrain-generated dataset from the hub | [
"The AutoTrain format is not supported right now. I think it would require a dedicated dataset builder",
"Okay, good to know. Thanks for the reply. For now I will just have to\nmanage the split manually before training, because I can’t find any way of\npulling out file indices or file names from the autogenerated split. The\nfile names field of the image dataset (loaded directly from arrow file) is\nmissing, just fyi (for anyone else this might be relevant too).\n\nOn Fri, Mar 10, 2023 at 7:02 PM Quentin Lhoest ***@***.***>\nwrote:\n\n> The AutoTrain format is not supported right now. I think it would require\n> a dedicated dataset builder\n>\n> —\n> Reply to this email directly, view it on GitHub\n> <https://github.com/huggingface/datasets/issues/5627#issuecomment-1464734308>,\n> or unsubscribe\n> <https://github.com/notifications/unsubscribe-auth/ACBJ4F5A353MCZ76OGRJ6CTW3PFI7ANCNFSM6AAAAAAVWXNUTE>\n> .\n> You are receiving this because you authored the thread.Message ID:\n> ***@***.***>\n>\n"
] | ### Describe the bug
DatasetGenerationError: An error occurred while generating the dataset -> ValueError: Couldn't cast ... because column names don't match
```
ValueError: Couldn't cast
_data_files: list<item: struct<filename: string>>
child 0, item: struct<filename: string>
child 0, filename: string
_fingerprint: string
_format_columns: list<item: string>
child 0, item: string
_format_kwargs: struct<>
_format_type: null
_indexes: struct<>
_output_all_columns: bool
_split: null
to
{'citation': Value(dtype='string', id=None), 'description': Value(dtype='string', id=None), 'features': {'image': {'_type': Value(dtype='string', id=None)}, 'target': {'names': Sequence(feature=Value(dtype='string', id=None), length=-1, id=None), '_type': Value(dtype='string', id=None)}}, 'homepage': Value(dtype='string', id=None), 'license': Value(dtype='string', id=None), 'splits': {'train': {'name': Value(dtype='string', id=None), 'num_bytes': Value(dtype='int64', id=None), 'num_examples': Value(dtype='int64', id=None), 'dataset_name': Value(dtype='null', id=None)}}}
because column names don't match
```
### Steps to reproduce the bug
Steps to reproduce:
1. `pip install datasets==2.10.1`
2. Attempt to load (private dataset). Note that I'm authenticated via ` huggingface-cli login`
```
from datasets import load_dataset
# load dataset
dataset = "ijmiller2/autotrain-data-betterbin-vision-10000"
dataset = load_dataset(dataset)
```
Here's the full traceback:
```Downloading and preparing dataset json/ijmiller2--autotrain-data-betterbin-vision-10000 to /Users/ian/.cache/huggingface/datasets/ijmiller2___json/ijmiller2--autotrain-data-betterbin-vision-10000-2eae034a9ff8a1a9/0.0.0/0f7e3662623656454fcd2b650f34e886a7db4b9104504885bd462096cc7a9f51...
Downloading data files: 100%|███████████████████████████████████████████████████████████████████████| 2/2 [00:00<00:00, 2383.80it/s]
Extracting data files: 100%|█████████████████████████████████████████████████████████████████████████| 2/2 [00:00<00:00, 505.95it/s]
---------------------------------------------------------------------------
ValueError Traceback (most recent call last)
File ~/anaconda3/envs/betterbin/lib/python3.8/site-packages/datasets/builder.py:1874, in ArrowBasedBuilder._prepare_split_single(self, gen_kwargs, fpath, file_format, max_shard_size, job_id)
1868 writer = writer_class(
1869 features=writer._features,
1870 path=fpath.replace("SSSSS", f"{shard_id:05d}").replace("JJJJJ", f"{job_id:05d}"),
1871 storage_options=self._fs.storage_options,
1872 embed_local_files=embed_local_files,
1873 )
-> 1874 writer.write_table(table)
1875 num_examples_progress_update += len(table)
File ~/anaconda3/envs/betterbin/lib/python3.8/site-packages/datasets/arrow_writer.py:568, in ArrowWriter.write_table(self, pa_table, writer_batch_size)
567 pa_table = pa_table.combine_chunks()
--> 568 pa_table = table_cast(pa_table, self._schema)
569 if self.embed_local_files:
File ~/anaconda3/envs/betterbin/lib/python3.8/site-packages/datasets/table.py:2312, in table_cast(table, schema)
2311 if table.schema != schema:
-> 2312 return cast_table_to_schema(table, schema)
2313 elif table.schema.metadata != schema.metadata:
File ~/anaconda3/envs/betterbin/lib/python3.8/site-packages/datasets/table.py:2270, in cast_table_to_schema(table, schema)
2269 if sorted(table.column_names) != sorted(features):
-> 2270 raise ValueError(f"Couldn't cast\n{table.schema}\nto\n{features}\nbecause column names don't match")
2271 arrays = [cast_array_to_feature(table[name], feature) for name, feature in features.items()]
ValueError: Couldn't cast
_data_files: list<item: struct<filename: string>>
child 0, item: struct<filename: string>
child 0, filename: string
_fingerprint: string
_format_columns: list<item: string>
child 0, item: string
_format_kwargs: struct<>
_format_type: null
_indexes: struct<>
_output_all_columns: bool
_split: null
to
{'citation': Value(dtype='string', id=None), 'description': Value(dtype='string', id=None), 'features': {'image': {'_type': Value(dtype='string', id=None)}, 'target': {'names': Sequence(feature=Value(dtype='string', id=None), length=-1, id=None), '_type': Value(dtype='string', id=None)}}, 'homepage': Value(dtype='string', id=None), 'license': Value(dtype='string', id=None), 'splits': {'train': {'name': Value(dtype='string', id=None), 'num_bytes': Value(dtype='int64', id=None), 'num_examples': Value(dtype='int64', id=None), 'dataset_name': Value(dtype='null', id=None)}}}
because column names don't match
The above exception was the direct cause of the following exception:
DatasetGenerationError Traceback (most recent call last)
Input In [8], in <cell line: 6>()
4 # load dataset
5 dataset = "ijmiller2/autotrain-data-betterbin-vision-10000"
----> 6 dataset = load_dataset(dataset)
File ~/anaconda3/envs/betterbin/lib/python3.8/site-packages/datasets/load.py:1782, in load_dataset(path, name, data_dir, data_files, split, cache_dir, features, download_config, download_mode, verification_mode, ignore_verifications, keep_in_memory, save_infos, revision, use_auth_token, task, streaming, num_proc, **config_kwargs)
1779 try_from_hf_gcs = path not in _PACKAGED_DATASETS_MODULES
1781 # Download and prepare data
-> 1782 builder_instance.download_and_prepare(
1783 download_config=download_config,
1784 download_mode=download_mode,
1785 verification_mode=verification_mode,
1786 try_from_hf_gcs=try_from_hf_gcs,
1787 num_proc=num_proc,
1788 )
1790 # Build dataset for splits
1791 keep_in_memory = (
1792 keep_in_memory if keep_in_memory is not None else is_small_dataset(builder_instance.info.dataset_size)
1793 )
File ~/anaconda3/envs/betterbin/lib/python3.8/site-packages/datasets/builder.py:872, in DatasetBuilder.download_and_prepare(self, output_dir, download_config, download_mode, verification_mode, ignore_verifications, try_from_hf_gcs, dl_manager, base_path, use_auth_token, file_format, max_shard_size, num_proc, storage_options, **download_and_prepare_kwargs)
870 if num_proc is not None:
871 prepare_split_kwargs["num_proc"] = num_proc
--> 872 self._download_and_prepare(
873 dl_manager=dl_manager,
874 verification_mode=verification_mode,
875 **prepare_split_kwargs,
876 **download_and_prepare_kwargs,
877 )
878 # Sync info
879 self.info.dataset_size = sum(split.num_bytes for split in self.info.splits.values())
File ~/anaconda3/envs/betterbin/lib/python3.8/site-packages/datasets/builder.py:967, in DatasetBuilder._download_and_prepare(self, dl_manager, verification_mode, **prepare_split_kwargs)
963 split_dict.add(split_generator.split_info)
965 try:
966 # Prepare split will record examples associated to the split
--> 967 self._prepare_split(split_generator, **prepare_split_kwargs)
968 except OSError as e:
969 raise OSError(
970 "Cannot find data file. "
971 + (self.manual_download_instructions or "")
972 + "\nOriginal error:\n"
973 + str(e)
974 ) from None
File ~/anaconda3/envs/betterbin/lib/python3.8/site-packages/datasets/builder.py:1749, in ArrowBasedBuilder._prepare_split(self, split_generator, file_format, num_proc, max_shard_size)
1747 job_id = 0
1748 with pbar:
-> 1749 for job_id, done, content in self._prepare_split_single(
1750 gen_kwargs=gen_kwargs, job_id=job_id, **_prepare_split_args
1751 ):
1752 if done:
1753 result = content
File ~/anaconda3/envs/betterbin/lib/python3.8/site-packages/datasets/builder.py:1892, in ArrowBasedBuilder._prepare_split_single(self, gen_kwargs, fpath, file_format, max_shard_size, job_id)
1890 if isinstance(e, SchemaInferenceError) and e.__context__ is not None:
1891 e = e.__context__
-> 1892 raise DatasetGenerationError("An error occurred while generating the dataset") from e
1894 yield job_id, True, (total_num_examples, total_num_bytes, writer._features, num_shards, shard_lengths)
DatasetGenerationError: An error occurred while generating the dataset
```
### Expected behavior
I'm ultimately trying to generate my own performance metrics on validation data (before putting an endpoint into production) and so was hoping to load all or at least the validation subset from the hub.
I'm expecting the `load_dataset()` function to work as shown in the documentation [here](https://huggingface.co/docs/datasets/loading#hugging-face-hub):
```python
dataset = load_dataset(
"lhoestq/custom_squad",
revision="main" # tag name, or branch name, or commit hash
)
```
### Environment info
- `datasets` version: 2.10.1
- Platform: macOS-13.2.1-arm64-arm-64bit
- Python version: 3.8.13
- PyArrow version: 9.0.0
- Pandas version: 1.4.4 | 5,627 |
https://github.com/huggingface/datasets/issues/5625 | Allow "jsonl" data type signifier | [
"You can use \"json\" instead. It doesn't work by extension names, but rather by dataset builder names, e.g. \"text\", \"imagefolder\", etc. I don't think the example in `transformers` is correct because of that",
"Yes, I understand the reasoning but this issue is to propose that the example in transformers (while incorrect) \"makes sense\" in terms of user expectation. So the question is whether it would be possible to add \"aliases\" for common types (like \"json\" and \"text\") based on common extensions (like jsonl and txt)?"
] | ### Feature request
`load_dataset` currently does not accept `jsonl` as type but only `json`.
### Motivation
I was working with one of the `run_translation` scripts and used my own datasets (`.jsonl`) as train_dataset. But the default code did not work because
```
FileNotFoundError: Couldn't find a dataset script at jsonl\jsonl.py or any data file in the same directory. Couldn't find 'jsonl' on the Hugging Face Hub either: FileNotFoundError: Dataset 'jsonl' doesn't exist on the Hub. If the repo is private or gated, make sure to log in with `huggingface-cli login`.
```
The reason is because the script has these lines to extract the data type by its extension. Therefore, the derived type is `jsonl` which is not recognized by datasets as the error above shows.
https://github.com/huggingface/transformers/blob/ade26bf9912f69e2110137443e4406d7dbe253e7/examples/pytorch/translation/run_translation.py#L342-L356
I suppose you could argue that this is the script's fault (in which case I'll do a PR over at `transformers`) but it makes sense to me to add `jsonl` as an alias to `json` in `datasets`.
### Your contribution
At the moment I cannot work on this. I think it can be as "easy" as having an alias for json, namely jsonl. | 5,625 |
https://github.com/huggingface/datasets/issues/5624 | glue datasets returning -1 for test split | [
"Hi @lithafnium, thanks for reporting.\r\n\r\nPlease note that you can use the \"Community\" tab in the corresponding dataset page to start any discussion: https://huggingface.co/datasets/glue/discussions\r\n\r\nIndeed this issue was already raised there (https://huggingface.co/datasets/glue/discussions/5) and answered: https://huggingface.co/datasets/glue/discussions/5#63907885937867f0cb3cde31\r\n> The test labels are not public.\r\n>\r\n> Note this dataset belongs to a benchmark: people send their predictions for the test split to GLUE (https://gluebenchmark.com/) and then they get a score in their leaderboard...\r\n"
] | ### Describe the bug
Downloading any dataset from GLUE has -1 as class labels for test split. Train and validation have regular 0/1 class labels. This is also present in the dataset card online.
### Steps to reproduce the bug
```
dataset = load_dataset("glue", "sst2")
for d in dataset:
# prints out -1
print(d["label"]
```
### Expected behavior
Expected behavior should be 0/1 instead of -1.
### Environment info
- `datasets` version: 2.4.0
- Platform: Linux-5.15.0-46-generic-x86_64-with-glibc2.17
- Python version: 3.8.16
- PyArrow version: 8.0.0
- Pandas version: 1.5.3
| 5,624 |
https://github.com/huggingface/datasets/issues/5618 | Unpin fsspec < 2023.3.0 once issue fixed | [] | Unpin `fsspec` upper version once root cause of our CI break is fixed.
See:
- #5614 | 5,618 |
https://github.com/huggingface/datasets/issues/5616 | CI is broken after fsspec-2023.3.0 release | [] | As reported by @lhoestq, our CI is broken after `fsspec` 2023.3.0 release:
```
FAILED tests/test_filesystem.py::test_compression_filesystems[Bz2FileSystem] - AssertionError: assert [{'created': ...: False, ...}] == ['file.txt']
At index 0 diff: {'name': 'file.txt', 'size': 70, 'type': 'file', 'created': 1678175677.1887748, 'islink': False, 'mode': 33188, 'uid': 1001, 'gid': 123, 'mtime': 1678175677.1887748, 'ino': 286957, 'nlink': 1} != 'file.txt'
Full diff:
[
- 'file.txt',
+ {'created': 1678175677.1887748,
+ 'gid': 123,
+ 'ino': 286957,
+ 'islink': False,
+ 'mode': 33188,
+ 'mtime': 1678175677.1887748,
+ 'name': 'file.txt',
+ 'nlink': 1,
+ 'size': 70,
+ 'type': 'file',
+ 'uid': 1001},
]
```
Also:
```
FAILED tests/test_filesystem.py::test_compression_filesystems[GzipFileSystem] - AssertionError: assert [{'created': ...: False, ...}] == ['file.txt']
FAILED tests/test_filesystem.py::test_compression_filesystems[Lz4FileSystem] - AssertionError: assert [{'created': ...: False, ...}] == ['file.txt']
FAILED tests/test_filesystem.py::test_compression_filesystems[XzFileSystem] - AssertionError: assert [{'created': ...: False, ...}] == ['file.txt']
FAILED tests/test_filesystem.py::test_compression_filesystems[ZstdFileSystem] - AssertionError: assert [{'created': ...: False, ...}] == ['file.txt']
===== 5 failed, 2134 passed, 18 skipped, 38 warnings in 157.21s (0:02:37) ======
```
See:
- fsspec/filesystem_spec#1205 | 5,616 |
https://github.com/huggingface/datasets/issues/5615 | IterableDataset.add_column is unable to accept another IterableDataset as a parameter. | [
"Hi! You can use `concatenate_datasets([ids1, ids2], axis=1)` to do this."
] | ### Describe the bug
`IterableDataset.add_column` occurs an exception when passing another `IterableDataset` as a parameter.
The method seems to accept only eager evaluated values.
https://github.com/huggingface/datasets/blob/35b789e8f6826b6b5a6b48fcc2416c890a1f326a/src/datasets/iterable_dataset.py#L1388-L1391
I wrote codes below to make it.
```py
def add_column(dataset: IterableDataset, name: str, add_dataset: IterableDataset, key: str) -> IterableDataset:
iter_add_dataset = iter(add_dataset)
def add_column_fn(example):
if name in example:
raise ValueError(f"Error when adding {name}: column {name} is already in the dataset.")
return {name: next(iter_add_dataset)[key]}
return dataset.map(add_column_fn)
```
Is there other way to do it? Or is it intended?
### Steps to reproduce the bug
Thie codes below occurs `NotImplementedError`
```py
from datasets import IterableDataset
def gen(num):
yield {f"col{num}": 1}
yield {f"col{num}": 2}
yield {f"col{num}": 3}
ids1 = IterableDataset.from_generator(gen, gen_kwargs={"num": 1})
ids2 = IterableDataset.from_generator(gen, gen_kwargs={"num": 2})
new_ids = ids1.add_column("new_col", ids1)
for row in new_ids:
print(row)
```
### Expected behavior
`IterableDataset.add_column` is able to task `IterableDataset` and lazy evaluated values as a parameter since IterableDataset is lazy evalued.
### Environment info
- `datasets` version: 2.8.0
- Platform: Linux-3.10.0-1160.36.2.el7.x86_64-x86_64-with-glibc2.17
- Python version: 3.9.7
- PyArrow version: 11.0.0
- Pandas version: 1.5.3
| 5,615 |
https://github.com/huggingface/datasets/issues/5613 | Version mismatch with multiprocess and dill on Python 3.10 | [
"Sorry, I just found https://github.com/apache/beam/issues/24458. It seems this issue is being worked on. ",
"Reopening, since I think the docs should inform the user of this problem. For example, [this page](https://huggingface.co/docs/datasets/installation) says \r\n> Datasets is tested on Python 3.7+.\r\n\r\nbut it should probably say that Beam Datasets do not work with Python 3.10 (or link to a known issues page). ",
"Same problem on Colab using a vanilla setup running :\r\nPython 3.10.11 \r\napache-beam 2.47.0\r\ndatasets 2.12.0",
"Same problem, \r\npy 3.10.11\r\napache-beam==2.47.0\r\ndatasets==2.12.0",
"I have made a workaround by forcing an install of the version of `multiprocess` version `0.70.15` (after installing `datasets` and `apache-beam`). I can confirm that (on Python 3.10 in [this colab notebook](https://colab.research.google.com/drive/1PTeGlshamFcJZix_GiS3vMXX_YzAhGv0?usp=sharing)) `datasets` can download pre-processed Wikipedia dumps and can download non-pre-processed dumps using `beam_runner=\"DirectRunner\"`. I don't know if/how other `beam_runner`s can be made compatible.",
"Same problem.\r\n\r\n```\r\npython = \"^3.10\"\r\napache-beam = { extras = [\"gcp\"], version = \"2.54.0\" }\r\ndatasets = \"^2.18.0\"\r\n```"
] | ### Describe the bug
Grabbing the latest version of `datasets` and `apache-beam` with `poetry` using Python 3.10 gives a crash at runtime. The crash is
```
File "/Users/adpauls/sc/git/DSI-transformers/data/NQ/create_NQ_train_vali.py", line 1, in <module>
import datasets
File "/Users/adpauls/Library/Caches/pypoetry/virtualenvs/yyy-oPbZ7mKM-py3.10/lib/python3.10/site-packages/datasets/__init__.py", line 43, in <module>
from .arrow_dataset import Dataset
File "/Users/adpauls/Library/Caches/pypoetry/virtualenvs/yyy-oPbZ7mKM-py3.10/lib/python3.10/site-packages/datasets/arrow_dataset.py", line 65, in <module>
from .arrow_reader import ArrowReader
File "/Users/adpauls/Library/Caches/pypoetry/virtualenvs/yyy-oPbZ7mKM-py3.10/lib/python3.10/site-packages/datasets/arrow_reader.py", line 30, in <module>
from .download.download_config import DownloadConfig
File "/Users/adpauls/Library/Caches/pypoetry/virtualenvs/yyy-oPbZ7mKM-py3.10/lib/python3.10/site-packages/datasets/download/__init__.py", line 9, in <module>
from .download_manager import DownloadManager, DownloadMode
File "/Users/adpauls/Library/Caches/pypoetry/virtualenvs/yyy-oPbZ7mKM-py3.10/lib/python3.10/site-packages/datasets/download/download_manager.py", line 35, in <module>
from ..utils.py_utils import NestedDataStructure, map_nested, size_str
File "/Users/adpauls/Library/Caches/pypoetry/virtualenvs/yyy-oPbZ7mKM-py3.10/lib/python3.10/site-packages/datasets/utils/py_utils.py", line 40, in <module>
import multiprocess.pool
File "/Users/adpauls/Library/Caches/pypoetry/virtualenvs/yyy-oPbZ7mKM-py3.10/lib/python3.10/site-packages/multiprocess/pool.py", line 609, in <module>
class ThreadPool(Pool):
File "/Users/adpauls/Library/Caches/pypoetry/virtualenvs/yyy-oPbZ7mKM-py3.10/lib/python3.10/site-packages/multiprocess/pool.py", line 611, in ThreadPool
from .dummy import Process
File "/Users/adpauls/Library/Caches/pypoetry/virtualenvs/yyy-oPbZ7mKM-py3.10/lib/python3.10/site-packages/multiprocess/dummy/__init__.py", line 87, in <module>
class Condition(threading._Condition):
AttributeError: module 'threading' has no attribute '_Condition'. Did you mean: 'Condition'?
```
I think this is a bad interaction of versions from `dill`, `multiprocess`, `apache-beam`, and `threading` from the Python (3.10) standard lib. Upgrading `multiprocess` to a version that does not crash like this is not possible because `apache-beam` pins `dill` to and old version:
```
Because multiprocess (0.70.10) depends on dill (>=0.3.2)
and apache-beam (2.45.0) depends on dill (>=0.3.1.1,<0.3.2), multiprocess (0.70.10) is incompatible with apache-beam (2.45.0).
And because no versions of apache-beam match >2.45.0,<3.0.0, multiprocess (0.70.10) is incompatible with apache-beam (>=2.45.0,<3.0.0).
So, because yyy depends on both apache-beam (^2.45.0) and multiprocess (0.70.10), version solving failed.
```
Perhaps it is not right to file a bug here, but I'm not totally sure whose fault it is. And in any case, this is an immediate blocker to using `datasets` out of the box.
Possibly related to https://github.com/huggingface/datasets/issues/5232.
### Steps to reproduce the bug
Steps to reproduce:
1. Make a poetry project with this configuration
```
[tool.poetry]
name = "yyy"
version = "0.1.0"
description = ""
authors = ["Adam Pauls <[email protected]>"]
readme = "README.md"
packages = [{ include = "xxx" }]
[tool.poetry.dependencies]
python = ">=3.10,<3.11"
datasets = "^2.10.1"
apache-beam = "^2.45.0"
[build-system]
requires = ["poetry-core"]
build-backend = "poetry.core.masonry.api"
```
2. `poetry install`.
3. `poetry run python -c "import datasets"`.
### Expected behavior
Script runs.
### Environment info
Python 3.10. Here are the versions installed by `poetry`:
```
•• Installing frozenlist (1.3.3)
• Installing idna (3.4)
• Installing multidict (6.0.4)
• Installing aiosignal (1.3.1)
• Installing async-timeout (4.0.2)
• Installing attrs (22.2.0)
• Installing certifi (2022.12.7)
• Installing charset-normalizer (3.1.0)
• Installing six (1.16.0)
• Installing urllib3 (1.26.14)
• Installing yarl (1.8.2)
• Installing aiohttp (3.8.4)
• Installing dill (0.3.1.1)
• Installing docopt (0.6.2)
• Installing filelock (3.9.0)
• Installing numpy (1.22.4)
• Installing pyparsing (3.0.9)
• Installing protobuf (3.19.4)
• Installing packaging (23.0)
• Installing python-dateutil (2.8.2)
• Installing pytz (2022.7.1)
• Installing pyyaml (6.0)
• Installing requests (2.28.2)
• Installing tqdm (4.65.0)
• Installing typing-extensions (4.5.0)
• Installing cloudpickle (2.2.1)
• Installing crcmod (1.7)
• Installing fastavro (1.7.2)
• Installing fasteners (0.18)
• Installing fsspec (2023.3.0)
• Installing grpcio (1.51.3)
• Installing hdfs (2.7.0)
• Installing httplib2 (0.20.4)
• Installing huggingface-hub (0.12.1)
• Installing multiprocess (0.70.9)
• Installing objsize (0.6.1)
• Installing orjson (3.8.7)
• Installing pandas (1.5.3)
• Installing proto-plus (1.22.2)
• Installing pyarrow (9.0.0)
• Installing pydot (1.4.2)
• Installing pymongo (3.13.0)
• Installing regex (2022.10.31)
• Installing responses (0.18.0)
• Installing xxhash (3.2.0)
• Installing zstandard (0.20.0)
• Installing apache-beam (2.45.0)
• Installing datasets (2.10.1)
``` | 5,613 |
https://github.com/huggingface/datasets/issues/5612 | Arrow map type in parquet files unsupported | [
"I'm attaching a minimal reproducible example:\r\n```python\r\nfrom datasets import load_dataset\r\nimport pyarrow as pa\r\nimport pyarrow.parquet as pq\r\n\r\ntable_with_map = pa.Table.from_pydict(\r\n {\"a\": [1, 2], \"b\": [[(\"a\", 2)], [(\"b\", 4)]]},\r\n schema=pa.schema({\"a\": pa.int32(), \"b\": pa.map_(pa.string(), pa.int32())})\r\n)\r\npq.write_table(table_with_map, \"parquet_with_map.parquet\")\r\ndset = load_dataset(\"parquet\", data_files=\"parquet_with_map.parquet\", split=\"train\") # error unless streaming=True\r\n``` \r\n\r\nFor a dataset generated with the packaged loaders (CSV, JSON, Parquet), `streaming=True` sets the dataset's features to `None` (unless explicitly provided in `load_dataset`), hence no error will be thrown as long as the features stay \"unresolved\" (resolving the features with `_resolve_features` will lead to an error).",
"I've also been wondering about datasets support for Arrow Map datatypes. I had a situation where I had a pandas series of dict[str, float] with hundreds of different possible key values (ie. not bounded), and this got converted to a sequence of structs where every single struct had the entire set of keys.\r\n\r\nI worked around it, by explicitly creating a sequence of [str, float], but given that pyarrow has an explicit Map datatype, it would be good to be able to explicitly cast/force this data type combination.",
"(feel free to ignore) polars will not support this type: https://github.com/pola-rs/polars/issues/3942#issuecomment-1202331210\r\n\r\n> Polars will not add the map dtype. It's benefit do not outweigh the extra complexity. Maybe we can investigate conversion of maps to struct. But I will have to explore that.",
"Looks like they chose to convert every instance with https://github.com/pola-rs/polars/pull/4226"
] | ### Describe the bug
When I try to load parquet files that were processed with Spark, I get the following issue:
`ValueError: Arrow type map<string, string ('warc_headers')> does not have a datasets dtype equivalent.`
Strangely, loading the dataset with `streaming=True` solves the issue.
### Steps to reproduce the bug
The dataset is private, but this can be reproduced with any dataset that has Arrow maps.
### Expected behavior
Loading the dataset no matter whether streaming is True or not.
### Environment info
- `datasets` version: 2.10.1
- Platform: Linux-5.15.0-1029-gcp-x86_64-with-glibc2.31
- Python version: 3.10.7
- PyArrow version: 8.0.0
- Pandas version: 1.4.2 | 5,612 |
https://github.com/huggingface/datasets/issues/5610 | use datasets streaming mode in trainer ddp mode cause memory leak | [
"Same problem, \r\ntransformers 4.28.1\r\ndatasets 2.12.0\r\n\r\nleak around 100Mb per 10 seconds when use dataloader_num_werker > 0 in training argumennts for transformer train, possile bug in transformers repo, but still not found solution :(\r\n",
"found an article described a problem, may be helpful for somebody:\r\nhttps://ppwwyyxx.com/blog/2022/Demystify-RAM-Usage-in-Multiprocess-DataLoader/\r\nI confirm, it`s not memory leak, after some time memory growing has stopped",
"\"After some time\" - from your description, it sounds like memory growth can happen for 12 hours+, even days, before it stops? That seems very scary."
] | ### Describe the bug
use datasets streaming mode in trainer ddp mode cause memory leak
### Steps to reproduce the bug
import os
import time
import datetime
import sys
import numpy as np
import random
import torch
from torch.utils.data import Dataset, DataLoader, random_split, RandomSampler, SequentialSampler,DistributedSampler,BatchSampler
torch.manual_seed(42)
from transformers import GPT2LMHeadModel, GPT2Tokenizer, GPT2Config, GPT2Model,DataCollatorForLanguageModeling,AutoModelForCausalLM
from transformers import AdamW, get_linear_schedule_with_warmup
hf_model_path ='./Wenzhong-GPT2-110M'
tokenizer = GPT2Tokenizer.from_pretrained(hf_model_path)
tokenizer.add_special_tokens({'pad_token': '<|pad|>'})
from datasets import load_dataset
gpus=8
max_len = 576
batch_size_node = 17
save_step = 5000
gradient_accumulation = 2
dataloader_num = 4
max_step = 351000*1000//batch_size_node//gradient_accumulation//gpus
#max_step = -1
print("total_step:%d"%(max_step))
import datasets
datasets.version
dataset = load_dataset("text", data_files="./gpt_data_v1/*",split='train',cache_dir='./dataset_cache',streaming=True)
print('load over')
shuffled_dataset = dataset.shuffle(seed=42)
print('shuffle over')
def dataset_tokener(example,max_lenth=max_len):
example['text'] = list(map(lambda x : x.strip()+'<|endoftext|>',example['text'] ))
return tokenizer(example['text'], truncation=True, max_length=max_lenth, padding="longest")
new_new_dataset = shuffled_dataset.map(dataset_tokener, batched=True, remove_columns=["text"])
print('map over')
configuration = GPT2Config.from_pretrained(hf_model_path, output_hidden_states=False)
model = AutoModelForCausalLM.from_pretrained(hf_model_path)
model.resize_token_embeddings(len(tokenizer))
seed_val = 42
random.seed(seed_val)
np.random.seed(seed_val)
torch.manual_seed(seed_val)
torch.cuda.manual_seed_all(seed_val)
from transformers import Trainer,TrainingArguments
import os
print("strat train")
training_args = TrainingArguments(output_dir="./test_trainer",
num_train_epochs=1.0,
report_to="none",
do_train=True,
dataloader_num_workers=dataloader_num,
local_rank=int(os.environ.get('LOCAL_RANK', -1)),
overwrite_output_dir=True,
logging_strategy='steps',
logging_first_step=True,
logging_dir="./logs",
log_on_each_node=False,
per_device_train_batch_size=batch_size_node,
warmup_ratio=0.03,
save_steps=save_step,
save_total_limit=5,
gradient_accumulation_steps=gradient_accumulation,
max_steps=max_step,
disable_tqdm=False,
data_seed=42
)
trainer = Trainer(
model=model,
args=training_args,
train_dataset=new_new_dataset,
eval_dataset=None,
tokenizer=tokenizer,
data_collator=DataCollatorForLanguageModeling(tokenizer,mlm=False),
#compute_metrics=compute_metrics if training_args.do_eval and not is_torch_tpu_available() else None,
#preprocess_logits_for_metrics=preprocess_logits_for_metrics
#if training_args.do_eval and not is_torch_tpu_available()
#else None,
)
trainer.train(resume_from_checkpoint=True)
### Expected behavior
use the train code uppper
my dataset ./gpt_data_v1 have 1000 files, each file size is 120mb
start cmd is : python -m torch.distributed.launch --nproc_per_node=8 my_train.py
here is result:
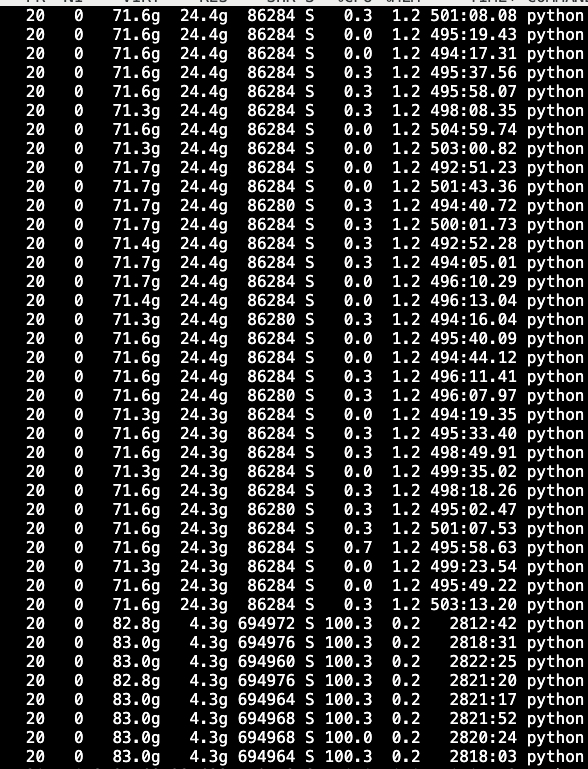
here is memory usage monitor in 12 hours

every dataloader work allocate over 24gb cpu memory
according to memory usage monitor in 12 hours,sometime small memory releases, but total memory usage is increase.
i think datasets streaming mode should not used so much memery,so maybe somewhere has memory leak.
### Environment info
pytorch 1.11.0
py 3.8
cuda 11.3
transformers 4.26.1
datasets 2.9.0
| 5,610 |
https://github.com/huggingface/datasets/issues/5609 | `load_from_disk` vs `load_dataset` performance. | [
"Hi! We've recently made some improvements to `save_to_disk`/`list_to_disk` (100x faster in some scenarios), so it would help if you could install `datasets` directly from `main` (`pip install git+https://github.com/huggingface/datasets.git`) and re-run the \"benchmark\".",
"Great to hear! I'll give it a try when I've got a moment.",
"@mariosasko is that fix released to pip in the meantime? Asking cause im facing still the same issue (regarding loading images from local paths):\r\n```\r\ndataset = load_dataset(\"csv\", cache_dir=\"cache\", data_files=[\"/STORAGE/DATA/mijam/vit/code/list_filtered.csv\"], num_proc=16, split=\"train\").cast_column(\"image\", Image())\r\ndataset = dataset.class_encode_column(\"label\")\r\n```\r\nquite fast. \r\n\r\nThen I do `save_to_disk()` and some time later:\r\n```\r\ndataset = load_from_disk('/STORAGE/DATA/mijam/accel/saved_arrow_big')\r\n```\r\nreally slow. In theory it should be quicked since it only loads arrow files, no conversions and so on.\r\n",
"@mjamroz I assume your CSV file stores image file paths. This means `save_to_disk` needs to embed the image bytes resulting in a much bigger Arrow file (than the initial one). Maybe specifying `num_shards` to make the Arrow files smaller can help (large Arrow files on some systems take a long time to load)."
] | ### Describe the bug
I have downloaded `openwebtext` (~12GB) and filtered out a small amount of junk (it's still huge). Now, I would like to use this filtered version for future work. It seems I have two choices:
1. Use `load_dataset` each time, relying on the cache mechanism, and re-run my filtering.
2. `save_to_disk` and then use `load_from_disk` to load the filtered version.
The performance of these two approaches is wildly different:
* Using `load_dataset` takes about 20 seconds to load the dataset, and a few seconds to re-filter (thanks to the brilliant filter/map caching)
* Using `load_from_disk` takes 14 minutes! And the second time I tried, the session just crashed (on a machine with 32GB of RAM)
I don't know if you'd call this a bug, but it seems like there shouldn't need to be two methods to load from disk, or that they should not take such wildly different amounts of time, or that one should not crash. Or maybe that the docs could offer some guidance about when to pick which method and why two methods exist, or just how do most people do it?
Something I couldn't work out from reading the docs was this: can I modify a dataset from the hub, save it (locally) and use `load_dataset` to load it? This [post seemed to suggest that the answer is no](https://discuss.huggingface.co/t/save-and-load-datasets/9260).
### Steps to reproduce the bug
See above
### Expected behavior
Load times should be about the same.
### Environment info
- `datasets` version: 2.9.0
- Platform: Linux-5.10.102.1-microsoft-standard-WSL2-x86_64-with-glibc2.31
- Python version: 3.10.8
- PyArrow version: 11.0.0
- Pandas version: 1.5.3 | 5,609 |
https://github.com/huggingface/datasets/issues/5608 | audiofolder only creates dataset of 13 rows (files) when the data folder it's reading from has 20,000 mp3 files. | [
"Hi!\r\n\r\n> naming convention of mp3 files\r\n\r\nYes, this could be the problem. MP3 files should end with `.mp3`/`.MP3` to be recognized as audio files.\r\n\r\nIf the file names are not the culprit, can you paste the audio folder's directory structure to help us reproduce the error (e.g., by running the `tree \"x\"` command)?",
"Hi! I'm sorry, I don't want to reveal my entire dataset, but here's a snippet (all of the mp3 files below are some of the ones not being recognized by audiofolder. Also, for another dataset, audiofolder loaded zero mp3 files because \"train\" was in the name of one of the mp3 files. \r\nmy_dataset\r\n├── data\r\n│ ├── VHA_Innovation_Stories_-_Day_2-123.mp3\r\n│ ├── VHA_Innovation_Stories_-_Day_2-124.mp3\r\n│ ├── ASSOCIATION_OF_GENERAL_PRACTITIONERS_OF_JAMAICA_NEPHROLOGY_CONFERENCE_-_JULY_3,_2022-93.mp3\r\n│ ├── ASSOCIATION_OF_GENERAL_PRACTITIONERS_OF_JAMAICA_NEPHROLOGY_CONFERENCE_-_JULY_3,_2022-94.mp3\r\n│ ├── ASSOCIATION_OF_GENERAL_PRACTITIONERS_OF_JAMAICA_NEPHROLOGY_CONFERENCE_-_JULY_3,_2022-95.mp3\r\n│ ├── Your_Impact\\357\\274\\232_Neurosurgery_equipment-5.mp3\r\n│ └── Your_Impact\\357\\274\\232_Neurosurgery_equipment-6.mp3\r\n└── metadata.csv\r\n\r\nHere's a few of the 13 files recognized by the dataset:\r\nBritish_Heart_Foundation_-_Your_guide_to_a_Coronary_Angiogram,_a_test_for_heart_disease-1.mp3\r\nBritish_Heart_Foundation_-_Your_guide_to_a_Coronary_Angiogram,_a_test_for_heart_disease-2.mp3\r\nBritish_Heart_Foundation_-_Your_guide_to_a_Coronary_Angiogram,_a_test_for_heart_disease-3.mp3\r\nIVP_⧸_IVU_test_Procedure_for_Kidneys_intravenous_pyelogram_-_medical_radiology_X-ray_ivp-1.mp3\r\nIVP_⧸_IVU_test_Procedure_for_Kidneys_intravenous_pyelogram_-_medical_radiology_X-ray_ivp-2.mp3"
] | ### Describe the bug
x = load_dataset("audiofolder", data_dir="x")
When running this, x is a dataset of 13 rows (files) when it should be 20,000 rows (files) as the data_dir "x" has 20,000 mp3 files. Does anyone know what could possibly cause this (naming convention of mp3 files, etc.)
### Steps to reproduce the bug
x = load_dataset("audiofolder", data_dir="x")
### Expected behavior
x = load_dataset("audiofolder", data_dir="x") should create a dataset of 20,000 rows (files).
### Environment info
- `datasets` version: 2.9.0
- Platform: Linux-3.10.0-1160.80.1.el7.x86_64-x86_64-with-glibc2.17
- Python version: 3.9.16
- PyArrow version: 11.0.0
- Pandas version: 1.5.3 | 5,608 |
https://github.com/huggingface/datasets/issues/5606 | Add `Dataset.to_list` to the API | [
"Hello, I have an interest in this issue.\r\nIs the `Dataset.to_dict` you are describing correct in the code here?\r\n\r\nhttps://github.com/huggingface/datasets/blob/35b789e8f6826b6b5a6b48fcc2416c890a1f326a/src/datasets/arrow_dataset.py#L4633-L4667",
"Yes, this is where `Dataset.to_dict` is defined.",
"#self-assign"
] | Since there is `Dataset.from_list` in the API, we should also add `Dataset.to_list` to be consistent.
Regarding the implementation, we can re-use `Dataset.to_dict`'s code and replace the `to_pydict` calls with `to_pylist`. | 5,606 |
https://github.com/huggingface/datasets/issues/5604 | Problems with downloading The Pile | [
"Hi! \r\n\r\n\r\nYou can specify `download_config=DownloadConfig(resume_download=True))` in `load_dataset` to resume the download when re-running the code after the timeout error:\r\n```python\r\nfrom datasets import load_dataset, DownloadConfig\r\ndataset = load_dataset('the_pile', split='train', cache_dir='F:\\datasets', download_config=DownloadConfig(resume_download=True))\r\n```\r\n\r\n",
"@mariosasko , I used your suggestion but its not saving anything , just stops and runs from the same point .\r\nbelow is the script to download and save on disk .\r\n\r\n```\r\nfrom datasets import load_dataset, DownloadConfig\r\n\r\n\r\n#load the Pile dataset from Hugging Face Datasets\r\n#dataset = load_dataset('the_pile')\r\ndataset = load_dataset('the_pile', split='train', cache_dir='datasets', download_config=DownloadConfig(resume_download=True))\r\n\r\n\r\n# save each file in the dataset to disk\r\nfor i, example in enumerate(dataset['train']):\r\n filename = f'pile_file_{i}.json'\r\n with open(filename, 'w') as f:\r\n f.write(str(example))\r\n\r\nprint(\"Finished saving Pile dataset files to disk.\")\r\n```\r\n",
"@mariosasko , it shows nothing in dataset folder\r\n\r\n```\r\n du -sh /mnt/nlp/hugging_face/*\r\n20K /mnt/nlp/hugging_face/datasets\r\n4.0K /mnt/nlp/hugging_face/download_pile.py\r\n```\r\n",
"@mariosasko \r\n\r\n```\r\nroot@d20f0ab8f4f8:/mnt/hugging_face# python3 download_pile.py\r\nNo config specified, defaulting to: the_pile/all\r\nDownloading and preparing dataset the_pile/all to /mnt/hugging_face/datasets/the_pile/all/0.0.0/6fadc480ecb32470826cbf5900a9558b791ce55d5e9a0fdc8ad653e7b64bb349...\r\nDownloading data files: 0%| | 0/3 [00:00<?, ?it/s]\r\n\r\n\r\n\r\n\r\n\r\nDownloading data: 70%|████████████████████████████████████████████████████████████████████▊ | 10.7G/15.2G [12:09<11:53, 6.36MB/s]\r\nDownloading data: 100%|██████████████████████████████████████████████████████████████████████████████████████████████████| 15.2G/15.2G [22:15<00:00, 7.25MB/s]\r\nDownloading data: 100%|██████████████████████████████████████████████████████████████████████████████████████████████████| 15.2G/15.2G [46:17<00:00, 5.48MB/s]\r\nDownloading data: 40%|██████████████████████████████████████▏ | 6.07G/15.3G [50:49<1:17:02, 1.99MB/s]\r\nTraceback (most recent call last):██████████████████████████▊ | 6.07G/15.3G [50:49<25:35:23, 99.9kB/s]\r\n File \"/usr/local/lib/python3.8/dist-packages/urllib3/response.py\", line 444, in _error_catcher\r\n yield\r\n File \"/usr/local/lib/python3.8/dist-packages/urllib3/response.py\", line 567, in read\r\n data = self._fp_read(amt) if not fp_closed else b\"\"\r\n File \"/usr/local/lib/python3.8/dist-packages/urllib3/response.py\", line 525, in _fp_read\r\n data = self._fp.read(chunk_amt)\r\n File \"/usr/lib/python3.8/http/client.py\", line 459, in read\r\n n = self.readinto(b)\r\n File \"/usr/lib/python3.8/http/client.py\", line 503, in readinto\r\n n = self.fp.readinto(b)\r\n File \"/usr/lib/python3.8/socket.py\", line 669, in readinto\r\n return self._sock.recv_into(b)\r\n File \"/usr/lib/python3.8/ssl.py\", line 1241, in recv_into\r\n return self.read(nbytes, buffer)\r\n File \"/usr/lib/python3.8/ssl.py\", line 1099, in read\r\n return self._sslobj.read(len, buffer)\r\nConnectionResetError: [Errno 104] Connection reset by peer\r\n\r\nDuring handling of the above exception, another exception occurred:\r\n\r\nTraceback (most recent call last):\r\n File \"/usr/local/lib/python3.8/dist-packages/requests/models.py\", line 816, in generate\r\n yield from self.raw.stream(chunk_size, decode_content=True)\r\n File \"/usr/local/lib/python3.8/dist-packages/urllib3/response.py\", line 628, in stream\r\n data = self.read(amt=amt, decode_content=decode_content)\r\n File \"/usr/local/lib/python3.8/dist-packages/urllib3/response.py\", line 593, in read\r\n raise IncompleteRead(self._fp_bytes_read, self.length_remaining)\r\n File \"/usr/lib/python3.8/contextlib.py\", line 131, in __exit__\r\n self.gen.throw(type, value, traceback)\r\n File \"/usr/local/lib/python3.8/dist-packages/urllib3/response.py\", line 461, in _error_catcher\r\n raise ProtocolError(\"Connection broken: %r\" % e, e)\r\nurllib3.exceptions.ProtocolError: (\"Connection broken: ConnectionResetError(104, 'Connection reset by peer')\", ConnectionResetError(104, 'Connection reset by peer'))\r\n\r\nDuring handling of the above exception, another exception occurred:\r\n\r\nTraceback (most recent call last):\r\n File \"download_pile.py\", line 6, in <module>\r\n dataset = load_dataset('the_pile', split='train', cache_dir='datasets', download_config=DownloadConfig(resume_download=True))\r\n File \"/usr/local/lib/python3.8/dist-packages/datasets/load.py\", line 1782, in load_dataset\r\n builder_instance.download_and_prepare(\r\n File \"/usr/local/lib/python3.8/dist-packages/datasets/builder.py\", line 872, in download_and_prepare\r\n self._download_and_prepare(\r\n File \"/usr/local/lib/python3.8/dist-packages/datasets/builder.py\", line 1649, in _download_and_prepare\r\n super()._download_and_prepare(\r\n File \"/usr/local/lib/python3.8/dist-packages/datasets/builder.py\", line 945, in _download_and_prepare\r\n split_generators = self._split_generators(dl_manager, **split_generators_kwargs)\r\n File \"/root/.cache/huggingface/modules/datasets_modules/datasets/the_pile/6fadc480ecb32470826cbf5900a9558b791ce55d5e9a0fdc8ad653e7b64bb349/the_pile.py\", line 192, in _split_generators\r\n data_dir = dl_manager.download(_DATA_URLS[self.config.name])\r\n File \"/usr/local/lib/python3.8/dist-packages/datasets/download/download_manager.py\", line 427, in download\r\n downloaded_path_or_paths = map_nested(\r\n File \"/usr/local/lib/python3.8/dist-packages/datasets/utils/py_utils.py\", line 443, in map_nested\r\n mapped = [\r\n File \"/usr/local/lib/python3.8/dist-packages/datasets/utils/py_utils.py\", line 444, in <listcomp>\r\n _single_map_nested((function, obj, types, None, True, None))\r\n File \"/usr/local/lib/python3.8/dist-packages/datasets/utils/py_utils.py\", line 363, in _single_map_nested\r\n mapped = [_single_map_nested((function, v, types, None, True, None)) for v in pbar]\r\n File \"/usr/local/lib/python3.8/dist-packages/datasets/utils/py_utils.py\", line 363, in <listcomp>\r\n mapped = [_single_map_nested((function, v, types, None, True, None)) for v in pbar]\r\n File \"/usr/local/lib/python3.8/dist-packages/datasets/utils/py_utils.py\", line 346, in _single_map_nested\r\n return function(data_struct)\r\n File \"/usr/local/lib/python3.8/dist-packages/datasets/download/download_manager.py\", line 453, in _download\r\n return cached_path(url_or_filename, download_config=download_config)\r\n File \"/usr/local/lib/python3.8/dist-packages/datasets/utils/file_utils.py\", line 182, in cached_path\r\n output_path = get_from_cache(\r\n File \"/usr/local/lib/python3.8/dist-packages/datasets/utils/file_utils.py\", line 575, in get_from_cache\r\n http_get(\r\n File \"/usr/local/lib/python3.8/dist-packages/datasets/utils/file_utils.py\", line 379, in http_get\r\n for chunk in response.iter_content(chunk_size=1024):\r\n File \"/usr/local/lib/python3.8/dist-packages/requests/models.py\", line 818, in generate\r\n raise ChunkedEncodingError(e)\r\nrequests.exceptions.ChunkedEncodingError: (\"Connection broken: ConnectionResetError(104, 'Connection reset by peer')\", ConnectionResetError(104, 'Connection reset by peer'))\r\n```\r\n",
"Users with slow internet speed are doomed (4MB/s). The dataset downloads fine at minimum speed 10MB/s.\n\nAlso, when the train splits were generated and then I removed the downloads folder to save up disk space, it started redownloading the whole dataset. Is there any way to use the already generated splits instead?",
"@sentialx @mariosasko , anytime on my above script , am I downloading and saving dataset correctly . Please suggest :)",
"@sentialx probably worth noting that `resume_download=True` doesn't directly save the dataset to disk, but instead just helps in resuming the dataset resume on interruption as @mariosasko mentions. resolving resumptions after a crash is [an open issue](https://github.com/huggingface/datasets/issues/5380) at the moment."
] | ### Describe the bug
The downloads in the screenshot seem to be interrupted after some time and the last download throws a "Read timed out" error.
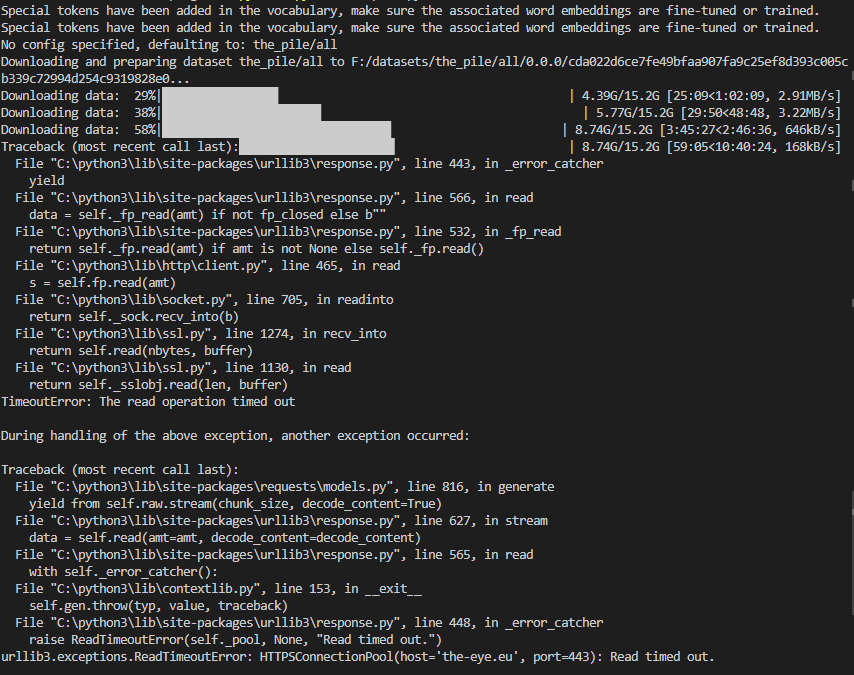
Here are the downloaded files:
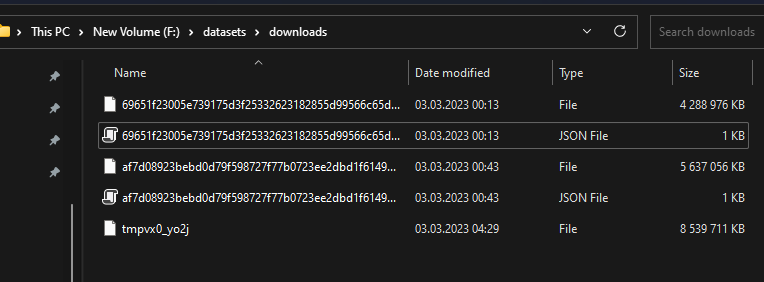
They should be all 14GB like here (https://the-eye.eu/public/AI/pile/train/).
Alternatively, can I somehow download the files by myself and use the datasets preparing script?
### Steps to reproduce the bug
dataset = load_dataset('the_pile', split='train', cache_dir='F:\datasets')
### Expected behavior
The files should be downloaded correctly.
### Environment info
- `datasets` version: 2.10.1
- Platform: Windows-10-10.0.22623-SP0
- Python version: 3.10.5
- PyArrow version: 9.0.0
- Pandas version: 1.4.2 | 5,604 |
https://github.com/huggingface/datasets/issues/5601 | Authorization error | [
"Hi! \r\n\r\nIt's better to report this kind of issue in the `huggingface_hub` repo, so if you still haven't resolved it, I suggest you open an issue there.",
"Yeah, I solved it. Problem was in osxkeychain. When I do `hugginface-cli login` it's add token with default account (username)`hg_user` but my repo contain other username. When I changed username in keychain - it works now."
] | ### Describe the bug
Get `Authorization error` when try to push data into hugginface datasets hub.
### Steps to reproduce the bug
I did all steps in the [tutorial](https://huggingface.co/docs/datasets/share),
1. `huggingface-cli login` with WRITE token
2. `git lfs install`
3. `git clone https://huggingface.co/datasets/namespace/your_dataset_name`
4.
```
cp /somewhere/data/*.json .
git lfs track *.json
git add .gitattributes
git add *.json
git commit -m "add json files"
```
but when I execute `git push` I got the error:
```
Uploading LFS objects: 0% (0/1), 0 B | 0 B/s, done.
batch response: Authorization error.
error: failed to push some refs to 'https://huggingface.co/datasets/zeusfsx/ukrainian-news'
```
Size of data ~100Gb. I have five json files - different parts.
### Expected behavior
All my data pushed into hub
### Environment info
- `datasets` version: 2.10.1
- Platform: macOS-13.2.1-arm64-arm-64bit
- Python version: 3.10.10
- PyArrow version: 11.0.0
- Pandas version: 1.5.3 | 5,601 |
https://github.com/huggingface/datasets/issues/5600 | Dataloader getitem not working for DreamboothDatasets | [
"Hi! \r\n\r\n> (see example of DreamboothDatasets)\r\n\r\n\r\nCould you please provide a link to it? If you are referring to the example in the `diffusers` repo, your issue is unrelated to `datasets` as that example uses `Dataset` from PyTorch to load data."
] | ### Describe the bug
Dataloader getitem is not working as before (see example of [DreamboothDatasets](https://github.com/huggingface/peft/blob/main/examples/lora_dreambooth/train_dreambooth.py#L451C14-L529))
moving Datasets to 2.8.0 solved the issue.
### Steps to reproduce the bug
1- using DreamBoothDataset to load some images
2- error after loading when trying to visualise the images
### Expected behavior
I was expecting a numpy array of the image
### Environment info
- Platform: Linux-5.10.147+-x86_64-with-glibc2.29
- Python version: 3.8.10
- PyArrow version: 9.0.0
- Pandas version: 1.3.5 | 5,600 |
https://github.com/huggingface/datasets/issues/5597 | in-place dataset update | [
"We won't support in-place modifications since `datasets` is based on the Apache Arrow format which doesn't support in-place modifications.\r\n\r\nIn your case the old dataset is garbage collected pretty quickly so you won't have memory issues.\r\n\r\nNote that datasets loaded from disk (memory mapped) are not loaded in memory, and therefore the new dataset actually use the same buffers as the old one.",
"Thank you for your detailed reply.\r\n\r\n> In your case the old dataset is garbage collected pretty quickly so you won't have memory issues.\r\n\r\nI understand this, but it still copies the old dataset to create the new one, is this correct? So maybe it is not memory-consuming, but time-consuming?",
"Indeed, and because of that it is more efficient to add multiple rows at once instead of one by one, using `concatenate_datasets` for example."
] | ### Motivation
For the circumstance that I creat an empty `Dataset` and keep appending new rows into it, I found that it leads to creating a new dataset at each call. It looks quite memory-consuming. I just wonder if there is any more efficient way to do this.
```python
from datasets import Dataset
ds = Dataset.from_list([])
ds.add_item({'a': [1, 2, 3], 'b': 4})
print(ds)
>>> Dataset({
>>> features: [],
>>> num_rows: 0
>>> })
ds = ds.add_item({'a': [1, 2, 3], 'b': 4})
print(ds)
>>> Dataset({
>>> features: ['a', 'b'],
>>> num_rows: 1
>>> })
```
### Feature request
Call for in-place dataset update functions, that update the existing `Dataset` in place without creating a new copy. The interface is supposed to keep the same style as PyTorch, such as the in-place version of a `function` is named `function_`. For example, the in-pace version of `add_item`, i.e., `add_item_`, immediately updates the `Dataset`.
```python
from datasets import Dataset
ds = Dataset.from_list([])
ds.add_item({'a': [1, 2, 3], 'b': 4})
print(ds)
>>> Dataset({
>>> features: [],
>>> num_rows: 0
>>> })
ds.add_item_({'a': [1, 2, 3], 'b': 4})
print(ds)
>>> Dataset({
>>> features: ['a', 'b'],
>>> num_rows: 1
>>> })
```
### Related Functions
* `.map`
* `.filter`
* `.add_item` | 5,597 |
https://github.com/huggingface/datasets/issues/5596 | [TypeError: Couldn't cast array of type] Can only load a subset of the dataset | [
"Apparently some JSON objects have a `\"labels\"` field. Since this field is not present in every object, you must specify all the fields types in the README.md\r\n\r\nEDIT: actually specifying the feature types doesn’t solve the issue, it raises an error because “labels” is missing in the data",
"We've updated the dataset to remove the extra `labels` field from some files, closing this issue. Thanks!",
"A similar error occurs in the Pile dataset (EleutherAI/the_pile)\r\n\r\nLoading the dataset produces the following error.\r\n\r\n```\r\nTypeError: Couldn't cast array of type\r\nstruct<file: string, id: string>\r\nto\r\n{'id': Value(dtype='string', id=None)}\r\n```\r\n",
"I think this was fixed in https://huggingface.co/datasets/EleutherAI/the_pile/discussions/11",
"i have the same problem ,how to solve :\r\n raise TypeError(f\"Couldn't cast array of type\\n{array.type}\\nto\\n{feature}\")\r\nTypeError: Couldn't cast array of type\r\nlist<item: string>\r\nto\r\n{'content': Value(dtype='string', id=None), 'role': Value(dtype='string', id=None)}"
] | ### Describe the bug
I'm trying to load this [dataset](https://huggingface.co/datasets/bigcode-data/the-stack-gh-issues) which consists of jsonl files and I get the following error:
```
casted_values = _c(array.values, feature[0])
File "/opt/conda/lib/python3.7/site-packages/datasets/table.py", line 1839, in wrapper
return func(array, *args, **kwargs)
File "/opt/conda/lib/python3.7/site-packages/datasets/table.py", line 2132, in cast_array_to_feature
raise TypeError(f"Couldn't cast array of type\n{array.type}\nto\n{feature}")
TypeError: Couldn't cast array of type
struct<type: string, action: string, datetime: timestamp[s], author: string, title: string, description: string, comment_id: int64, comment: string, labels: list<item: string>>
to
{'type': Value(dtype='string', id=None), 'action': Value(dtype='string', id=None), 'datetime': Value(dtype='timestamp[s]', id=None), 'author': Value(dtype='string', id=None), 'title': Value(dtype='string', id=None), 'description': Value(dtype='string', id=None), 'comment_id': Value(dtype='int64', id=None), 'comment': Value(dtype='string', id=None)}
```
But I can succesfully load a subset of the dataset, for example this works:
```python
ds = load_dataset('bigcode-data/the-stack-gh-issues', split="train", data_files=[f"data/data-{x}.jsonl" for x in range(10)])
```
and `ds.features` returns:
```
{'repo': Value(dtype='string', id=None),
'org': Value(dtype='string', id=None),
'issue_id': Value(dtype='int64', id=None),
'issue_number': Value(dtype='int64', id=None),
'pull_request': {'user_login': Value(dtype='string', id=None),
'repo': Value(dtype='string', id=None),
'number': Value(dtype='int64', id=None)},
'events': [{'type': Value(dtype='string', id=None),
'action': Value(dtype='string', id=None),
'datetime': Value(dtype='timestamp[s]', id=None),
'author': Value(dtype='string', id=None),
'title': Value(dtype='string', id=None),
'description': Value(dtype='string', id=None),
'comment_id': Value(dtype='int64', id=None),
'comment': Value(dtype='string', id=None)}]}
```
So I'm not sure if there's an issue with just some of the files. Grateful if you have any suggestions to fix the issue.
Side note:
I saw this related [issue](https://github.com/huggingface/datasets/issues/3637) and tried to write a loading script to have `events` as a `Sequence` and not `list` [here](https://huggingface.co/datasets/bigcode-data/the-stack-gh-issues/blob/main/loading.py) (the script was renamed). It worked with a subset locally but doesn't for the remote dataset it can't find https://huggingface.co/datasets/bigcode-data/the-stack-gh-issues/resolve/main/data.
### Steps to reproduce the bug
```python
from datasets import load_dataset
ds = load_dataset('bigcode-data/the-stack-gh-issues', split="train")
```
### Expected behavior
Load the entire dataset succesfully.
### Environment info
- `datasets` version: 2.10.1
- Platform: Linux-4.19.0-23-cloud-amd64-x86_64-with-debian-10.13
- Python version: 3.7.12
- PyArrow version: 9.0.0
- Pandas version: 1.3.4 | 5,596 |
https://github.com/huggingface/datasets/issues/5594 | Error while downloading the xtreme udpos dataset | [
"Hi! I cannot reproduce this error on my machine.\r\n\r\nThe raised error could mean that one of the downloaded files is corrupted. To verify this is not the case, you can run `load_dataset` as follows:\r\n```python\r\ntrain_dataset = load_dataset('xtreme', 'udpos.English', split=\"train\", cache_dir=args.cache_dir, download_mode=\"force_redownload\", verification_mode=\"all_checks\")\r\n```",
"Hi! Apologies for the delayed response! I tried the above and it doesn't solve the issue. Actually, the dataset gets downloaded most times, but sometimes this error occurs (at random afaik). Is it possible that there is a server issue for this particular dataset? I am able to download other datasets using the same code on the same machine with no issues :( I get this error now : \r\n```\r\nDownloading data: 16%|███████████████▌ | 55.9M/355M [04:45<25:25, 196kB/s]\r\nTraceback (most recent call last):\r\n File \"/home/skhanuja/Optimal-Resource-Allocation-for-Multilingual-Finetuning/src/train_al.py\", line 1107, in <module>\r\n main()\r\n File \"/home/skhanuja/Optimal-Resource-Allocation-for-Multilingual-Finetuning/src/train_al.py\", line 439, in main\r\n en_dataset = load_dataset(\"xtreme\", \"udpos.English\", split=\"train\", download_mode=\"force_redownload\", verification_mode=\"all_checks\")\r\n File \"/home/skhanuja/miniconda3/envs/multilingual_ft/lib/python3.10/site-packages/datasets/load.py\", line 1782, in load_dataset\r\n builder_instance.download_and_prepare(\r\n File \"/home/skhanuja/miniconda3/envs/multilingual_ft/lib/python3.10/site-packages/datasets/builder.py\", line 872, in download_and_prepare\r\n self._download_and_prepare(\r\n File \"/home/skhanuja/miniconda3/envs/multilingual_ft/lib/python3.10/site-packages/datasets/builder.py\", line 1649, in _download_and_prepare\r\n super()._download_and_prepare(\r\n File \"/home/skhanuja/miniconda3/envs/multilingual_ft/lib/python3.10/site-packages/datasets/builder.py\", line 949, in _download_and_prepare\r\n verify_checksums(\r\n File \"/home/skhanuja/miniconda3/envs/multilingual_ft/lib/python3.10/site-packages/datasets/utils/info_utils.py\", line 62, in verify_checksums\r\n raise NonMatchingChecksumError(\r\ndatasets.utils.info_utils.NonMatchingChecksumError: Checksums didn't match for dataset source files:\r\n['https://lindat.mff.cuni.cz/repository/xmlui/bitstream/handle/11234/1-3105/ud-treebanks-v2.5.tgz']\r\nSet `verification_mode='no_checks'` to skip checksums verification and ignore this error\r\n```",
"If this happens randomly, then this means the data file from the error message is not always downloaded correctly. \r\n\r\nThe only solution in this scenario is to download the dataset again by passing `download_mode=\"force_redownload\"` to the `load_dataset` call.",
"Wow. I effectively have to redownload a dataset of 1TB because of this now?\r\nBecause 3% of its parts are broken?\r\n\r\nWhy is this downloader library so sh*t and badly documented also? I found almost nothing on the net, at least finally this issue about the problem here.\r\nNo words to express how disappointed I am by that dataset tool provided by Huggingface here, which I sadly have to use because HF is the only place where the Dataset I plan to work with is hosted....\r\n\r\nI mean... checksum check after download... or hitting timeout of a part... and redownload if not matching... that's content of every junior developer training session.\r\n\r\nI added `verification_mode=\"all_checks\"`. And it really calculated checksums for 4096 parts of ~350 MB... But then did nothing and tried to extract still, hitting the error again. \r\n\r\nEDIT: Apparently it is able to fix it by getting a little help: Just delete the broken parts and associated files from `~/.cache/huggingface/datasets/downloads`",
"I'm getting it too, although just retrying fixed it. Nevertheless, the dataset is too large to have re-downloaded the whole thing, for it's probably just one file with an issue. It would be good to know if there's a way people could manually examine the files (first for sizes, then possibly checksums)... going to the web or elsewhere to compare and correct it by hand, if ever needed.",
"Okay, no, it got further but it is repeatedly giving me:\r\n```/home/jaggz/.cache/huggingface/modules/datasets_modules/datasets/mozilla-foundation--common_voice_11_0/3f27acf10f303eac5b6fbbbe02495aeddb46ecffdb0a2fe3507fcfbf89094631/common_voice_11_0.py\", line 195, in _generate_examples\r\nresult[\"audio\"] = {\"path\": path, \"bytes\": file.read()}\r\n^^^^^^^^^^^\r\nFile \"/usr/lib/python3.11/tarfile.py\", line 687, in read\r\nraise ReadError(\"unexpected end of data\")\r\ntarfile.ReadError: unexpected end of data\r\n\r\nThe above exception was the direct cause of the following exception:\r\n\r\nTraceback (most recent call last):\r\nFile \"/home/jaggz/src/transformers/examples/pytorch/speech-recognition/run_speech_recognition_seq2seq.py\", line 625, in <module>\r\nmain()\r\nFile \"/home/jaggz/src/transformers/examples/pytorch/speech-recognition/run_speech_recognition_seq2seq.py\", line 360, in main\r\nraw_datasets[\"train\"] = load_dataset(\r\n^^^^^^^^^^^^^\r\nFile \"/home/jaggz/venvs/pynow/lib/python3.11/site-packages/datasets/load.py\", line 2153, in load_dataset\r\nbuilder_instance.download_and_prepare(\r\nFile \"/home/jaggz/venvs/pynow/lib/python3.11/site-packages/datasets/builder.py\", line 954, in download_and_prepare\r\nself._download_and_prepare(\r\nFile \"/home/jaggz/venvs/pynow/lib/python3.11/site-packages/datasets/builder.py\", line 1717, in _download_and_prepare\r\nsuper()._download_and_prepare(\r\nFile \"/home/jaggz/venvs/pynow/lib/python3.11/site-packages/datasets/builder.py\", line 1049, in _download_and_prepare\r\nself._prepare_split(split_generator, **prepare_split_kwargs)\r\nFile \"/home/jaggz/venvs/pynow/lib/python3.11/site-packages/datasets/builder.py\", line 1555, in _prepare_split\r\nfor job_id, done, content in self._prepare_split_single(\r\nFile \"/home/jaggz/venvs/pynow/lib/python3.11/site-packages/datasets/builder.py\", line 1712, in _prepare_split_single\r\nraise DatasetGenerationError(\"An error occurred while generating the dataset\") from e\r\ndatasets.builder.DatasetGenerationError: An error occurred while generating the datase\r\n",
"@RuntimeRacer \r\n> EDIT: Apparently it is able to fix it by getting a little help: Just delete the broken parts and associated files from `~/.cache/huggingface/datasets/downloads`\r\n\r\nHow do you know the broken parts?\r\nMine's consistently erroring and.. yeah, really this thing should be able to check the files (but where's that even done)...\r\n\r\n2023-11-02 00:14:09.846055: I tensorflow/core/platform/cpu_feature_guard.cc:182] This TensorFlow binary is optimized to use available CPU instructions in performance-critical operations.\r\nTo enable the following instructions: AVX2 FMA, in other operations, rebuild TensorFlow with the appropriate compiler flags.\r\n/home/j/src/transformers/examples/pytorch/speech-recognition/run_speech_recognition_seq2seq.py:299: FutureWarning: The `use_auth_token` argument is deprecated and will be removed in v4.34. Please use `token` instead.\r\n warnings.warn(\r\n11/02/2023 00:14:37 - WARNING - __main__ - Process rank: 0, device: cuda:0, n_gpu: 1, distributed training: False, 16-bits training: True\r\n11/02/2023 00:14:37 - INFO - __main__ - Training/evaluation parameters Seq2SeqTrainingArguments(\r\n_n_gpu=1,\r\nadafactor=False,\r\nadam_beta1=0.9,\r\nadam_beta2=0.999,\r\n...\r\nlogging_dir=./whisper-tiny-en/runs/Nov02_00-14-28_jsys,\r\n...\r\nrun_name=./whisper-tiny-en,\r\n...\r\nweight_decay=0.0,\r\n)\r\n11/02/2023 00:14:37 - INFO - __main__ - Training/evaluation parameters Seq2SeqTrainingArguments(\r\n_n_gpu=1,\r\nadafactor=False,\r\n...\r\nlogging_dir=./whisper-tiny-en/runs/Nov02_00-14-28_jsys,\r\n...\r\nweight_decay=0.0,\r\n)\r\n\r\nDownloading data files: 0%| | 0/5 [00:00<?, ?it/s]\r\nDownloading data files: 100%|██████████| 5/5 [00:00<00:00, 2426.42it/s]\r\n\r\nExtracting data files: 0%| | 0/5 [00:00<?, ?it/s]\r\nExtracting data files: 100%|██████████| 5/5 [00:00<00:00, 421.16it/s]\r\n\r\nDownloading data files: 0%| | 0/5 [00:00<?, ?it/s]\r\nDownloading data files: 100%|██████████| 5/5 [00:00<00:00, 18707.87it/s]\r\n\r\nExtracting data files: 0%| | 0/5 [00:00<?, ?it/s]\r\nExtracting data files: 100%|██████████| 5/5 [00:00<00:00, 3754.97it/s]\r\n\r\nGenerating train split: 0 examples [00:00, ? examples/s]\r\n\r\nReading metadata...: 0it [00:00, ?it/s]\u001b[A\r\n...\r\nReading metadata...: 948736it [00:23, 40632.92it/s] \r\n\r\nGenerating train split: 1 examples [00:23, 23.37s/ examples]\r\n...\r\nGenerating train split: 948736 examples [08:28, 1866.15 examples/s]\r\n\r\nGenerating validation split: 0 examples [00:00, ? examples/s]\r\n\r\nReading metadata...: 0it [00:00, ?it/s]\u001b[A\r\n\r\nReading metadata...: 16089it [00:00, 157411.88it/s]\u001b[A\r\nReading metadata...: 16354it [00:00, 158233.27it/s]\r\n\r\nGenerating validation split: 1 examples [00:00, 7.60 examples/s]\r\nGenerating validation split: 16354 examples [00:14, 1154.77 examples/s]\r\n\r\nGenerating test split: 0 examples [00:00, ? examples/s]\r\n\r\nReading metadata...: 0it [00:00, ?it/s]\u001b[A\r\nReading metadata...: 16354it [00:00, 194855.03it/s]\r\n\r\nGenerating test split: 1 examples [00:00, 4.53 examples/s]\r\nGenerating test split: 16354 examples [00:07, 2105.43 examples/s]\r\n\r\nGenerating other split: 0 examples [00:00, ? examples/s]\r\n\r\nReading metadata...: 0it [00:00, ?it/s]\u001b[A\r\nReading metadata...: 290846it [00:01, 235823.90it/s]\r\n\r\nGenerating other split: 1 examples [00:01, 1.27s/ examples]\r\n...\r\nGenerating other split: 290846 examples [02:12, 2196.96 examples/s]\r\nGenerating invalidated split: 0 examples [00:00, ? examples/s]\r\nReading metadata...: 252599it [00:01, 241965.85it/s]\r\n\r\nGenerating invalidated split: 1 examples [00:01, 1.08s/ examples]\r\n...\r\nGenerating invalidated split: 60130 examples [00:34, 1764.14 examples/s]\r\nTraceback (most recent call last):\r\n File \"/home/j/venvs/pycur/lib/python3.11/site-packages/datasets/builder.py\", line 1676, in _prepare_split_single\r\n for key, record in generator:\r\n File \"/home/j/.cache/huggingface/modules/datasets_modules/datasets/mozilla-foundation--common_voice_11_0/3f27acf10f303eac5b6fbbbe02495aeddb46ecffdb0a2fe3507fcfbf89094631/common_voice_11_0.py\", line 195, in _generate_examples\r\n result[\"audio\"] = {\"path\": path, \"bytes\": file.read()}\r\n ^^^^^^^^^^^\r\n File \"/usr/lib/python3.11/tarfile.py\", line 687, in read\r\n raise ReadError(\"unexpected end of data\")\r\ntarfile.ReadError: unexpected end of data\r\n\r\nThe above exception was the direct cause of the following exception:\r\n\r\nTraceback (most recent call last):\r\n File \"/home/j/src/transformers/examples/pytorch/speech-recognition/run_speech_recognition_seq2seq.py\", line 625, in <module>\r\n main()\r\n File \"/home/j/src/transformers/examples/pytorch/speech-recognition/run_speech_recognition_seq2seq.py\", line 360, in main\r\n raw_datasets[\"train\"] = load_dataset(\r\n ^^^^^^^^^^^^^\r\n File \"/home/j/venvs/pycur/lib/python3.11/site-packages/datasets/load.py\", line 2153, in load_dataset\r\n builder_instance.download_and_prepare(\r\n File \"/home/j/venvs/pycur/lib/python3.11/site-packages/datasets/builder.py\", line 954, in download_and_prepare\r\n self._download_and_prepare(\r\n File \"/home/j/venvs/pycur/lib/python3.11/site-packages/datasets/builder.py\", line 1717, in _download_and_prepare\r\n super()._download_and_prepare(\r\n File \"/home/j/venvs/pycur/lib/python3.11/site-packages/datasets/builder.py\", line 1049, in _download_and_prepare\r\n self._prepare_split(split_generator, **prepare_split_kwargs)\r\n File \"/home/j/venvs/pycur/lib/python3.11/site-packages/datasets/builder.py\", line 1555, in _prepare_split\r\n for job_id, done, content in self._prepare_split_single(\r\n File \"/home/j/venvs/pycur/lib/python3.11/site-packages/datasets/builder.py\", line 1712, in _prepare_split_single\r\n raise DatasetGenerationError(\"An error occurred while generating the dataset\") from e\r\ndatasets.builder.DatasetGenerationError: An error occurred while generating the dataset\r\n",
"@jaggzh Hi, I actually came around with a fix for this, wasn't that easy to solve since there were a lot of hidden pitfalls in the code, and it's quite hacky, but I was able to download the full dataset.\r\n\r\nI just didn't create a PR for it yet since I was too lazy to create a fork and change my local repo's origin. 😅 \r\nLet me try to do this tonight, I'll give you a ping once it's up.\r\n\r\nEDIT: And no, what I wrote above about adding a param to the download config does NOT solve it apparently. A code fix is required here.",
"@jaggzh PR is up: https://github.com/huggingface/datasets/pull/6380\r\n\r\n🤞 on approval for merge to the main repo.",
"@mariosasko Can you re-open this? We really need some better diagnostics output, at the least, to locate which files are contributing, some checksum output, etc. I can't even tell if this is a mozilla...py issue or huggingface datasets or ....",
"@RuntimeRacer \r\nBeautiful, thank you so much. I patched with your PR and am re-running now.\r\n(I'm running this script: https://github.com/huggingface/transformers/blob/main/examples/pytorch/speech-recognition/run_speech_recognition_seq2seq.py)\r\nOkay, actually it failed; so now I'm running with verification_mode='all_checks' added to the load_data() call and it's re-running now. Wish me luck.\r\n(Note: It's generating checksums; I don't see an option that handles anything between basic_checks and all_checks -- Something checking dl'ed files' lengths would be a good common fix I'd think; corruption is more rare nowadays than a short file (although maybe your patch helps prevent that in the first place.) :}",
"@RuntimeRacer \r\nNo luck. Sigh.\r\n[Edit: My tmux copy didn't get some data. That was weird. I'm adding in the initial part of the output:]\r\n```\r\nDownloading data files: 100%|██████████| 5/5 [00:00<00:00, 2190.69it/s]\r\nComputing checksums: 100%|██████████| 41/41 [11:39<00:00, 17.05s/it] Extracting data files: 100%|██████████| 5/5 [00:00<00:00, 12.37it/s]\r\nDownloading data files: 100%|██████████| 5/5 [00:00<00:00, 107.64it/s]\r\nExtracting data files: 100%|██████████| 5/5 [00:00<00:00, 3149.82it/s]\r\nReading metadata...: 948736it [00:03, 243227.36it/s]s/s]\r\n...\r\n```\r\n```\r\n...\r\nReading metadata...: 252599it [00:01, 249267.71it/s]xamples/s]\r\nGenerating invalidated split: 60130 examples [00:31, 1916.33 examples/s]\r\nTraceback (most recent call last):\r\nFile \"/home/j/src/py/datasets/src/datasets/builder.py\", line 1676, in _prepare_split_single\r\nfor key, record in generator:\r\nFile \"/home/j/.cache/huggingface/modules/datasets_modules/datasets/mozilla-foundation--common_voice_11_0/3f27acf10f303eac5b6fbbbe02495aeddb46ecffdb0a2fe3507fcfbf89094631/common_voice_11_0.py\", line 195, in _generate_examples\r\nresult[\"audio\"] = {\"path\": path, \"bytes\": file.read()}\r\n^^^^^^^^^^^\r\nFile \"/usr/lib/python3.11/tarfile.py\", line 687, in read\r\nraise ReadError(\"unexpected end of data\")\r\ntarfile.ReadError: unexpected end of data\r\n\r\nThe above exception was the direct cause of the following exception:\r\n\r\nTraceback (most recent call last):\r\nFile \"/home/j/src/transformers/examples/pytorch/speech-recognition/run_speech_recognition_seq2seq.py\", line 627, in <module>\r\nmain()\r\nFile \"/home/j/src/transformers/examples/pytorch/speech-recognition/run_speech_recognition_seq2seq.py\", line 360, in main\r\nraw_datasets[\"train\"] = load_dataset(\r\n^^^^^^^^^^^^^\r\nFile \"/home/j/src/py/datasets/src/datasets/load.py\", line 2153, in load_dataset\r\nbuilder_instance.download_and_prepare(\r\nFile \"/home/j/src/py/datasets/src/datasets/builder.py\", line 954, in download_and_prepare\r\nself._download_and_prepare(\r\nFile \"/home/j/src/py/datasets/src/datasets/builder.py\", line 1717, in _download_and_prepare\r\nsuper()._download_and_prepare(\r\nFile \"/home/j/src/py/datasets/src/datasets/builder.py\", line 1049, in _download_and_prepare\r\nself._prepare_split(split_generator, **prepare_split_kwargs)\r\nFile \"/home/j/src/py/datasets/src/datasets/builder.py\", line 1555, in _prepare_split\r\nfor job_id, done, content in self._prepare_split_single(\r\nFile \"/home/j/src/py/datasets/src/datasets/builder.py\", line 1712\r\n```",
"I'm unable to reproduce this error. Based on https://github.com/psf/requests/issues/4956, newer releases of `urllib3` check the returned content length by default, so perhaps updating `requests` and `urllib3` to the latest versions (`pip install -U requests urllib3`) and loading the dataset with `datasets.load_dataset(\"xtreme\", \"udpos.English\", download_config=datasets.DownloadConfig(resume_download=True))` (re-run when it fails to resume the download) can fix the issue.",
"@jaggzh I think you will need to re-download the whole dataset with my patched code. Files which have already been downloaded and marked as complete by the broken downloader won't be detected even on re-run (I described that in the PR).\r\nI also had to download reazonspeech, which is over 1TB, twice. 🙈 \r\nFor re-download, you need to manually delete the dataset files from your local machine's huggingface download cache.\r\n\r\n@mariosasko Not sure how you tested it, but it's not an issue in `requests` or `urllib`. The problem is the huggingface downloader, which generates a nested download thread for the actual download I think.\r\nThe issue I had with the reazonspeech dataset (https://huggingface.co/datasets/reazon-research/reazonspeech/tree/main) basically was, that it started downloading a part, but sometimes the connection would 'starve' and only continue with a few kilobytes, and eventually stop receiving any data at all.\r\nSometimes it would even recover during the download and finish properly.\r\nHowever, if it did not recover, the request would hit the really generous default timeout (which is 100 seconds I think), however the exception thrown by the failure inside `urllib`, isn't captured or handled by the upper level downloader code of the `datasets` library.\r\n`datasets` even has a retry mechanism, which would continue interrupted downloads if they have the `.incomplete` suffix, which isn't cleared if, for example, a manual `CTRL+C` is sent by the user to the python process.\r\nBut: If it runs into that edge case I described above (TL;DR: connection starves after minutes + timeout exception which isn't captured), the cache downloader will consider the download as successful and remove the `.incomplete` suffix nevertheless, leaving the archive file in a corrupted state.\r\n\r\nHonestly, I spent hours on trying to figure out what was even going on and why the retry mechanics of the cache downloader didn't work at all.\r\nBut it is indeed an issue caused by the download process itself not receiving any info about actual content size and filesize size on disk of the archive to be downloaded, thus, having no direct control in case something fails on the request level.\r\n\r\nIMHO, this requires a major refactor of the way this part of the downloader works.\r\nYet I was able to quick-fix it by adding some synthetic Exception handling and explicit retry-handling in the code, als done in my PR.",
"@RuntimeRacer \r\nUgh. It took a day. I'm seeing if I can get some debug code in here to examine the files myself. (I'm not sure why checksum tests would fail, so, yeah, I think you're right -- this stuff needs some work. Going through ipdb right now to try to get some idea of what's going on in the code).",
"@RuntimeRacer Data can only be appended to the `.incomplete` files if `load_dataset` is called with `download_config=DownloadConfig(resume_download=True)`. \r\n\r\nWhere exactly does this exception happen (in the code)? The error stack trace would help a lot.",
"@mariosasko I do not have a trace of this exception nor do I know which type it is. I am honestly not even sure if an exception is thrown, or the process just aborts without error.\r\n\r\n> @RuntimeRacer Data can only be appended to the .incomplete files if load_dataset is called with download_config=DownloadConfig(resume_download=True).\r\n\r\nWell, I think I did a very clear explaination of the issue in the PR I shared, and the description above, but maybe I wasn't precise enough. Let me try to explain once more:\r\n\r\nWhat you mention here is the \"normal\" case, if the process is aborted. In this case, there will be files with `.incomplete` suffix, which the cache downloader can continue to download. That is correct.\r\n\r\nBUT: What I am talking about all the time is an edge case: if the download step crashes / timeouts internally, the cache downloader will NOT be aware of this, and REMOVES the `.incomplete` suffix.\r\nIt does NOT know that the file is incomplete when the `http_get` function returns and will remove the `.incomplete` suffix in any case once `http_get` returns.\r\nBut the problem is that `http_get` returns without failure, even if the download failed.\r\nAnd this is still a problem even with latest `urllib` and `requests` library.\r\n",
"@RuntimeRacer Updating `urllib3` and `requests` to the latest versions fixes the issue explained in this [blog](https://blog.petrzemek.net/2018/04/22/on-incomplete-http-reads-and-the-requests-library-in-python/) post. \r\n\r\nHowever, the issue explained above seems more similar to [this](https://stackoverflow.com/questions/52731196/python-3-6-5-requests-with-streaming-getting-stuck-in-iter-content-even-if-chun) one. To address it, we can reduce the default timeout to 10 seconds (btw, this was the initial value, but it was causing problems for some users) and expose a config variable so that users can easily control it. Additionally, we can re-run `http_get` similarly to https://github.com/huggingface/huggingface_hub/pull/1766 when the connection/timeout error happens to make the logic even more robust. Would this work for you? The last part is what you did in the PR, right?\r\n\r\n@jaggzh From all the datasets mentioned in this issue, `xtreme` is the only one that stores the data file checksums in the metadata. So, the checksum check has no effect when enabled for the rest of the datasets.",
"(I don't have any .incomplete files, just the extraction errors.)\r\nI was going through the code to try to relate filenames to the hex/hash files, but realized I might not need to.\r\nSo instead I coded up a script in bash to examine the tar files for validity (had an issue with bash subshells not adding to my array so I had cgpt recode it in perl).\r\n\r\n```perl\r\n#!/usr/bin/perl\r\nuse strict;\r\nuse warnings;\r\n\r\n# Initialize the array to store tar files\r\nmy @tars;\r\n\r\n# Open the current directory\r\nopendir(my $dh, '.') or die \"Cannot open directory: $!\";\r\n\r\n# Read files in the current directory\r\nwhile (my $f = readdir($dh)) {\r\n # Skip files ending with lock, json, or py\r\n next if $f =~ /\\.(lock|json|py)$/;\r\n\r\n # Use the `file` command to determine the type of file\r\n my $ft = `file \"$f\"`;\r\n\r\n # If it's a tar archive, add it to the list\r\n if ($ft =~ /tar archive/) {\r\n push @tars, $f;\r\n }\r\n}\r\n\r\nclosedir($dh);\r\n\r\nprint \"Final Tars count: \" . scalar(@tars) . \"\\n\";\r\n\r\n# Iterate over the tar files and check them\r\nforeach my $i (0 .. $#tars) {\r\n my $f = $tars[$i];\r\n printf '%d/%d ', $i+1, scalar(@tars);\r\n \r\n # Use `ls -lgG` to list the files, similar to the original bash script\r\n system(\"ls -lgG '$f'\");\r\n\r\n # Check the integrity of the tar file\r\n my $errfn = \"/tmp/$f.tarerr\";\r\n if (system(\"tar tf '$f' > /dev/null 2> '$errfn'\") != 0) {\r\n print \" BAD $f\\n\";\r\n print \" ERR: \";\r\n system(\"cat '$errfn'\");\r\n }\r\n\r\n # Remove the error file if it exists\r\n unlink $errfn if -e $errfn;\r\n}\r\n```\r\n\r\nThis found one hash file that errored in the tar extraction, and one small tmp* file that also was supposedly a tar and was erroring. I removed those two and re-data loaded.. it grabbed just what it needed and I'm on my way. Yay!\r\n\r\nSo... is there a way for the datasets api to get file sizes? That would be a very easy and fast test, leaving checksum slowdowns for extra-messed-up situations.\r\n\r\n",
"> @RuntimeRacer Updating `urllib3` and `requests` to the latest versions fixes the issue explained in this [blog](https://blog.petrzemek.net/2018/04/22/on-incomplete-http-reads-and-the-requests-library-in-python/) post.\r\n> \r\n> However, the issue explained above seems more similar to [this](https://stackoverflow.com/questions/52731196/python-3-6-5-requests-with-streaming-getting-stuck-in-iter-content-even-if-chun) one. To address it, we can reduce the default timeout to 10 seconds (btw, this was the initial value, but it was causing problems for some users) and expose a config variable so that users can easily control it. Additionally, we can re-run `http_get` similarly to [huggingface/huggingface_hub#1766](https://github.com/huggingface/huggingface_hub/pull/1766) when the connection/timeout error happens to make the logic even more robust. Would this work for you? The last part is what you did in the PR, right?\r\n> \r\n> @jaggzh From all the datasets mentioned in this issue, `xtreme` is the only one that stores the data file checksums in the metadata. So, the checksum check has no effect when enabled for the rest of the datasets.\r\n\r\n@mariosasko Well if you look at my commit date, you will see that I run into this problem still in October. The blog post you mention and the update in the pull request for `urllib` was from July: https://github.com/psf/requests/issues/4956#issuecomment-1648632935\r\n\r\nBut yeah the [issue on StackOverflow](https://stackoverflow.com/questions/52731196/python-3-6-5-requests-with-streaming-getting-stuck-in-iter-content-even-if-chun) you mentioned seems like that's the source issue I was running into there.\r\nI experimented with timeouts, but changing them didn't help to resolve the issue of the starving connection unfortunately.\r\nHowever, https://github.com/huggingface/huggingface_hub/pull/1766 seems like that could be working; it's very similar to my change. So yeah I think this would fix it probably.\r\n\r\nAlso I can confirm the checksum option did not work for [reazonspeech](https://huggingface.co/datasets/reazon-research/reazonspeech/tree/main) as well. So maybe it's a double edge case that only occurs for some datasets. 🤷♂️ ",
"Also, the hf urls to files -- while I can't see a way of getting a listing from the hf site side -- do include the file size in the http header response. So we do have a quick way of just verifying lengths for resume. (This message may not be interesting to you all).\r\n\r\nFirst, a json clip (mozilla-foundation___common_voice_11_0/en/11.0.0/3f27acf10f303eac5b6fbbbe02495aeddb46ecffdb0a2fe3507fcfbf89094631/dataset_info.json):\r\n\r\n* I don't know how specific this .json is to mozilla common voice\r\n* Note that *dataset_size* is not the dataset size :) DatasetInfo class docs indicate it might be their \"combined size in bytes of the Arrow tables for all splits.\"\r\n* *num_bytes*: does match the individual file size though, and matches the http header (further down)\r\n```\r\n{\r\n \"builder_name\" : \"common_voice_11_0\",\r\n...\r\n \"config_name\" : \"en\",\r\n \"dataset_name\" : \"common_voice_11_0\",\r\n \"dataset_size\" : 1680793952,\r\n...\r\n \"download_checksums\" : {\r\n...\r\n \"https://huggingface.co/datasets/mozilla-foundation/common_voice_11_0/resolve/main/audio/en/invalidated/en_invalidated_3.tar\" : {\r\n \"checksum\" : null,\r\n \"num_bytes\" : 2110853120\r\n },\r\n...\r\n```\r\n\r\n```bash\r\n~/.cache/huggingface/datasets/downloads$ ls -lgG b45f82cb87bab2c35361857fcd46042ab658b42c37dc9a455248c2866c9b8f40* | cut -c 14-\r\n```\r\n```\r\n2110853120 Nov 1 16:28 b45f82cb87bab2c35361857fcd46042ab658b42c37dc9a455248c2866c9b8f40\r\n148 Nov 1 16:28 b45f82cb87bab2c35361857fcd46042ab658b42c37dc9a455248c2866c9b8f40.json\r\n0 Nov 1 16:07 b45f82cb87bab2c35361857fcd46042ab658b42c37dc9a455248c2866c9b8f40.lock\r\n```\r\n\r\n* Note the -L to follow redirects. Two headers are below:\r\n\r\n```bash\r\n$ curl -I -L https://huggingface.co/datasets/mozilla-foundation/common_voice_11_0/resolve/main/audio/en/invalidated/en_invalidated_3.tar\r\n```\r\n```\r\nHTTP/2 302 \r\ncontent-type: text/plain; charset=utf-8\r\ncontent-length: 1215\r\nlocation: https://cdn-lfs.huggingface.co/repos/00/ce/00ce867b4ae70bd23a10b60c32a8626d87b2666fc088ad03f86b94788faff554/984086fc250badece2992e8be4d7c4430f7c1208fb8bf37dc7c4aecdc803b220?response-content-disposition=attachment%3B+filename*%3DUTF-8%27%27en_invalidated_3.tar%3B+filename%3D%22en_invalidated_3.tar%22%3B&response-content-type=application%2Fx-tar&Expires=1699389040&Policy=eyJTdGF0ZW1lbnQiOlt7IkNvbmRpdGlvbiI6eyJEYXRlTGVzc1RoYW4iOnsiQVdTOkVwb2NoVGltZSI6MTY5OTM4OTA0MH19LCJSZXNvdXJjZSI6Imh0dHBzOi8vY2RuLWxmcy5odWdnaW5nZmFjZS5jby9yZXBvcy8wMC9jZS8wMGNlODY3YjRhZTcwYmQyM2ExMGI2MGMzMmE4NjI2ZDg3YjI2NjZmYzA4OGFkMDNmODZiOTQ3ODhmYWZmNTU0Lzk4NDA4NmZjMjUwYmFkZWNlMjk5MmU4YmU0ZDdjNDQzMGY3YzEyMDhmYjhiZjM3ZGM3YzRhZWNkYzgwM2IyMjA%7EcmVzcG9uc2UtY29udGVudC1kaXNwb3NpdGlvbj0qJnJlc3BvbnNlLWNvbnRlbnQtdHlwZT0qIn1dfQ__&Signature=WYc32e75PqbKSAv3KTpG86ooFT6oOyDDQpCt1i2B8gVS10J3qvpZlDmxaBgnGlCCl7SRiAvhIQctgwooNtWbUeDqK3T4bAo0-OOrGCuVi-%7EKWUBcoHce7nHWpl%7Ex9ubHS%7EFoYcGB2SCEqh5fIgGjNV-VKRX6TSXkRto5bclQq4VCJKHufDsJ114A1V4Qu%7EYiRIWKG4Gi93Xv4OFhyWY0uqykvP5c0x02F%7ELX0m3WbW-eXBk6Fw2xnV1XLrEkdR-9Ax2vHqMYIIw6yV0wWEc1hxE393P9mMG1TNDj%7EXDuCoOaA7LbrwBCxai%7Ew2MopdPamTXyOia5-FnSqEdsV29v4Q__&Key-Pair-Id=KVTP0A1DKRTAX\r\ndate: Sat, 04 Nov 2023 20:30:40 GMT\r\nx-powered-by: huggingface-moon\r\nx-request-id: Root=1-6546a9f0-5e7f729d09bdb38e35649a7e\r\naccess-control-allow-origin: https://huggingface.co\r\nvary: Origin, Accept\r\naccess-control-expose-headers: X-Repo-Commit,X-Request-Id,X-Error-Code,X-Error-Message,ETag,Link,Accept-Ranges,Content-Range\r\nx-repo-commit: 23b4059922516c140711b91831aa3393a22e9b80\r\naccept-ranges: bytes\r\nx-linked-size: 2110853120\r\nx-linked-etag: \"984086fc250badece2992e8be4d7c4430f7c1208fb8bf37dc7c4aecdc803b220\"\r\nx-cache: Miss from cloudfront\r\nvia: 1.1 f31a6426ebd75ce4393909b12f5cbdcc.cloudfront.net (CloudFront)\r\nx-amz-cf-pop: LAX53-P4\r\nx-amz-cf-id: BcYMFcHVcxPome2IjAvx0ZU90G41QlNI_HEHDGDqCQaEPvrOsnsGXw==\r\n\r\nHTTP/2 200 \r\ncontent-type: application/x-tar\r\ncontent-length: 2110853120\r\ndate: Sat, 04 Nov 2023 20:19:35 GMT\r\nlast-modified: Fri, 18 Nov 2022 15:08:22 GMT\r\netag: \"acac28988e2f7e73b68e865179fbd008\"\r\nx-amz-storage-class: INTELLIGENT_TIERING\r\nx-amz-version-id: LgTuOcd9FGN4JnAXp26O.1v2VW42GPtF\r\ncontent-disposition: attachment; filename*=UTF-8''en_invalidated_3.tar; filename=\"en_invalidated_3.tar\";\r\naccept-ranges: bytes\r\nserver: AmazonS3\r\nx-cache: Hit from cloudfront\r\nvia: 1.1 d07c8167eda81d307ca96358727f505e.cloudfront.net (CloudFront)\r\nx-amz-cf-pop: LAX50-P5\r\nx-amz-cf-id: 6oNZg_V8U1M_JXsMHQAPuRmDfxbY2BnMUWcVH0nz3VnfEZCzF5lgkQ==\r\nage: 666\r\ncache-control: public, max-age=604800, immutable, s-maxage=604800\r\nvary: Origin\r\n\r\n```\r\n"
] | ### Describe the bug
Hi,
I am facing an error while downloading the xtreme udpos dataset using load_dataset. I have datasets 2.10.1 installed
```Downloading and preparing dataset xtreme/udpos.Arabic to /compute/tir-1-18/skhanuja/multilingual_ft/cache/data/xtreme/udpos.Arabic/1.0.0/29f5d57a48779f37ccb75cb8708d1095448aad0713b425bdc1ff9a4a128a56e4...
Downloading data: 16%|██████████████▏ | 56.9M/355M [03:11<16:43, 297kB/s]
Generating train split: 0%| | 0/6075 [00:00<?, ? examples/s]Traceback (most recent call last):
File "/home/skhanuja/miniconda3/envs/multilingual_ft/lib/python3.10/site-packages/datasets/builder.py", line 1608, in _prepare_split_single
for key, record in generator:
File "/home/skhanuja/.cache/huggingface/modules/datasets_modules/datasets/xtreme/29f5d57a48779f37ccb75cb8708d1095448aad0713b425bdc1ff9a4a128a56e4/xtreme.py", line 732, in _generate_examples
yield from UdposParser.generate_examples(config=self.config, filepath=filepath, **kwargs)
File "/home/skhanuja/.cache/huggingface/modules/datasets_modules/datasets/xtreme/29f5d57a48779f37ccb75cb8708d1095448aad0713b425bdc1ff9a4a128a56e4/xtreme.py", line 921, in generate_examples
for path, file in filepath:
File "/home/skhanuja/miniconda3/envs/multilingual_ft/lib/python3.10/site-packages/datasets/download/download_manager.py", line 158, in __iter__
yield from self.generator(*self.args, **self.kwargs)
File "/home/skhanuja/miniconda3/envs/multilingual_ft/lib/python3.10/site-packages/datasets/download/download_manager.py", line 211, in _iter_from_path
yield from cls._iter_tar(f)
File "/home/skhanuja/miniconda3/envs/multilingual_ft/lib/python3.10/site-packages/datasets/download/download_manager.py", line 167, in _iter_tar
for tarinfo in stream:
File "/home/skhanuja/miniconda3/envs/multilingual_ft/lib/python3.10/tarfile.py", line 2475, in __iter__
tarinfo = self.next()
File "/home/skhanuja/miniconda3/envs/multilingual_ft/lib/python3.10/tarfile.py", line 2344, in next
raise ReadError("unexpected end of data")
tarfile.ReadError: unexpected end of data
The above exception was the direct cause of the following exception:
Traceback (most recent call last):
File "/home/skhanuja/Optimal-Resource-Allocation-for-Multilingual-Finetuning/src/train_al.py", line 855, in <module>
main()
File "/home/skhanuja/Optimal-Resource-Allocation-for-Multilingual-Finetuning/src/train_al.py", line 487, in main
train_dataset = load_dataset(dataset_name, source_language, split="train", cache_dir=args.cache_dir, download_mode="force_redownload")
File "/home/skhanuja/miniconda3/envs/multilingual_ft/lib/python3.10/site-packages/datasets/load.py", line 1782, in load_dataset
builder_instance.download_and_prepare(
File "/home/skhanuja/miniconda3/envs/multilingual_ft/lib/python3.10/site-packages/datasets/builder.py", line 872, in download_and_prepare
self._download_and_prepare(
File "/home/skhanuja/miniconda3/envs/multilingual_ft/lib/python3.10/site-packages/datasets/builder.py", line 1649, in _download_and_prepare
super()._download_and_prepare(
File "/home/skhanuja/miniconda3/envs/multilingual_ft/lib/python3.10/site-packages/datasets/builder.py", line 967, in _download_and_prepare
self._prepare_split(split_generator, **prepare_split_kwargs)
File "/home/skhanuja/miniconda3/envs/multilingual_ft/lib/python3.10/site-packages/datasets/builder.py", line 1488, in _prepare_split
for job_id, done, content in self._prepare_split_single(
File "/home/skhanuja/miniconda3/envs/multilingual_ft/lib/python3.10/site-packages/datasets/builder.py", line 1644, in _prepare_split_single
raise DatasetGenerationError("An error occurred while generating the dataset") from e
datasets.builder.DatasetGenerationError: An error occurred while generating the dataset
```
### Steps to reproduce the bug
```
train_dataset = load_dataset('xtreme', 'udpos.English', split="train", cache_dir=args.cache_dir, download_mode="force_redownload")
```
### Expected behavior
Download the udpos dataset
### Environment info
- `datasets` version: 2.10.1
- Platform: Linux-3.10.0-957.1.3.el7.x86_64-x86_64-with-glibc2.17
- Python version: 3.10.8
- PyArrow version: 10.0.1
- Pandas version: 1.5.2 | 5,594 |
https://github.com/huggingface/datasets/issues/5586 | .sort() is broken when used after .filter(), only in 2.10.0 | [
"Thanks for reporting and thanks @mariosasko for fixing ! We just did a patch release `2.10.1` with the fix"
] | ### Describe the bug
Hi, thank you for your support!
It seems like the addition of multiple key sort (#5502) in 2.10.0 broke the `.sort()` method.
After filtering a dataset with `.filter()`, the `.sort()` seems to refer to the query_table index of the previous unfiltered dataset, resulting in an IndexError.
This only happens with the 2.10.0 release.
### Steps to reproduce the bug
```Python
from datasets import load_dataset
# dataset with length of 1104
ds = load_dataset('glue', 'ax')['test']
ds = ds.filter(lambda x: x['idx'] > 1100)
ds.sort('premise')
print('Done')
```
File "/home/dongkeun/datasets_test/test.py", line 5, in <module>
ds.sort('premise')
File "/home/dongkeun/miniconda3/envs/datasets_test/lib/python3.9/site-packages/datasets/arrow_dataset.py", line 528, in wrapper
out: Union["Dataset", "DatasetDict"] = func(self, *args, **kwargs)
File "/home/dongkeun/miniconda3/envs/datasets_test/lib/python3.9/site-packages/datasets/fingerprint.py", line 511, in wrapper
out = func(dataset, *args, **kwargs)
File "/home/dongkeun/miniconda3/envs/datasets_test/lib/python3.9/site-packages/datasets/arrow_dataset.py", line 3959, in sort
sort_table = query_table(
File "/home/dongkeun/miniconda3/envs/datasets_test/lib/python3.9/site-packages/datasets/formatting/formatting.py", line 588, in query_table
_check_valid_index_key(key, size)
File "/home/dongkeun/miniconda3/envs/datasets_test/lib/python3.9/site-packages/datasets/formatting/formatting.py", line 537, in _check_valid_index_key
_check_valid_index_key(max(key), size=size)
File "/home/dongkeun/miniconda3/envs/datasets_test/lib/python3.9/site-packages/datasets/formatting/formatting.py", line 531, in _check_valid_index_key
raise IndexError(f"Invalid key: {key} is out of bounds for size {size}")
IndexError: Invalid key: 1103 is out of bounds for size 3
### Expected behavior
It should sort the dataset and print "Done". Which it does on 2.9.0.
### Environment info
- `datasets` version: 2.10.0
- Platform: Linux-5.15.0-41-generic-x86_64-with-glibc2.31
- Python version: 3.9.16
- PyArrow version: 11.0.0
- Pandas version: 1.5.3 | 5,586 |
https://github.com/huggingface/datasets/issues/5585 | Cache is not transportable | [
"Hi ! No the cache is not transportable in general. It will work on a shared filesystem if you use the same python environment, but not across machines/os/environments.\r\n\r\nIn particular, reloading cached datasets does work, but reloading cached processed datasets (e.g. from `map`) may not work. This is because some hashes used by caching are based on pickle dumps of the function you pass to `map`.\r\n\r\nFinally you may copy the cache to another machine, but all the `cached-*.arrow` files are unlikely to be reloaded.",
"OK good to know. Thanks @lhoestq !"
] | ### Describe the bug
I would like to share cache between two machines (a Windows host machine and a WSL instance).
I run most my code in WSL. I have just run out of space in the virtual drive. Rather than expand the drive size, I plan to move to cache to the host Windows machine, thereby sharing the downloads.
I'm hoping that I can just copy/paste the cache files, but I notice that a lot of the file names start with the path name, e.g. `_home_davidg_.cache_huggingface_datasets_conll2003_default-451...98.lock` where `home/davidg` is where the cache is in WSL.
This seems to suggest that the cache is not portable/cannot be centralised or shared. Is this the case, or are the files that start with path names not integral to the caching mechanism? Because copying the cache files _seems_ to work, but I'm not filled with confidence that something isn't going to break.
A related issue, when trying to load a dataset that should come from cache (running in WSL, pointing to cache on the Windows host) it seemed to work fine, but it still uses a WSL directory for `.cache\huggingface\modules\datasets_modules`. I see nothing in the docs about this, or how to point it to a different place.
I have asked a related question on the forum: https://discuss.huggingface.co/t/is-datasets-cache-operating-system-agnostic/32656
### Steps to reproduce the bug
View the cache directory in WSL/Windows.
### Expected behavior
Cache can be shared between (virtual) machines and be transportable.
It would be nice to have a simple way to say "Dear Hugging Face packages, please put ALL your cache in `blah/de/blah`" and have all the Hugging Face packages respect that single location.
### Environment info
```
- `datasets` version: 2.9.0
- Platform: Linux-5.10.102.1-microsoft-standard-WSL2-x86_64-with-glibc2.31
- Python version: 3.10.8
- PyArrow version: 11.0.0
- Pandas version: 1.5.3
- ``` | 5,585 |
https://github.com/huggingface/datasets/issues/5584 | Unable to load coyo700M dataset | [
"Hi @manuaero \r\n\r\nThank you for your interest in the COYO dataset.\r\n\r\nOur dataset provides the img-url and alt-text in the form of a parquet, so to utilize the coyo dataset you will need to download it directly.\r\n\r\nWe provide a [guide](https://github.com/kakaobrain/coyo-dataset/blob/main/download/README.md) to download, so check it out.\r\n\r\nThank you."
] | ### Describe the bug
Seeing this error when downloading https://huggingface.co/datasets/kakaobrain/coyo-700m:
```ArrowInvalid: Parquet magic bytes not found in footer. Either the file is corrupted or this is not a parquet file.```
Full stack trace
```Downloading and preparing dataset parquet/kakaobrain--coyo-700m to /root/.cache/huggingface/datasets/kakaobrain___parquet/kakaobrain--coyo-700m-ae729692ae3e0073/0.0.0/2a3b91fbd88a2c90d1dbbb32b460cf621d31bd5b05b934492fdef7d8d6f236ec...
Downloading data files: 100%
1/1 [00:00<00:00, 63.35it/s]
Extracting data files: 100%
1/1 [00:00<00:00, 5.00it/s]
---------------------------------------------------------------------------
ArrowInvalid Traceback (most recent call last)
[/usr/local/lib/python3.8/dist-packages/datasets/builder.py](https://localhost:8080/#) in _prepare_split_single(self, gen_kwargs, fpath, file_format, max_shard_size, job_id)
1859 _time = time.time()
-> 1860 for _, table in generator:
1861 if max_shard_size is not None and writer._num_bytes > max_shard_size:
9 frames
ArrowInvalid: Parquet magic bytes not found in footer. Either the file is corrupted or this is not a parquet file.
The above exception was the direct cause of the following exception:
DatasetGenerationError Traceback (most recent call last)
[/usr/local/lib/python3.8/dist-packages/datasets/builder.py](https://localhost:8080/#) in _prepare_split_single(self, gen_kwargs, fpath, file_format, max_shard_size, job_id)
1890 if isinstance(e, SchemaInferenceError) and e.__context__ is not None:
1891 e = e.__context__
-> 1892 raise DatasetGenerationError("An error occurred while generating the dataset") from e
1893
1894 yield job_id, True, (total_num_examples, total_num_bytes, writer._features, num_shards, shard_lengths)
DatasetGenerationError: An error occurred while generating the dataset```
### Steps to reproduce the bug
```
from datasets import load_dataset
hf_dataset = load_dataset("kakaobrain/coyo-700m")
```
### Expected behavior
The above commands load the dataset successfully. Or handles exception and continue loading the remainder.
### Environment info
colab. any | 5,584 |
https://github.com/huggingface/datasets/issues/5581 | [DOC] Mistaken docs on set_format | [
"Thanks for reporting!"
] | ### Describe the bug
https://huggingface.co/docs/datasets/v2.10.0/en/package_reference/main_classes#datasets.Dataset.set_format
<img width="700" alt="image" src="https://user-images.githubusercontent.com/36224762/221506973-ae2e3991-60a7-4d4e-99f8-965c6eb61e59.png">
While actually running it will result in:
<img width="1094" alt="image" src="https://user-images.githubusercontent.com/36224762/221507032-007dab82-8781-4319-b21a-e6e4d40d97b3.png">
### Steps to reproduce the bug
_
### Expected behavior
_
### Environment info
- `datasets` version: 2.10.0
- Platform: Linux-5.10.147+-x86_64-with-glibc2.29
- Python version: 3.8.10
- PyArrow version: 9.0.0
- Pandas version: 1.3.5 | 5,581 |
https://github.com/huggingface/datasets/issues/5577 | Cannot load `the_pile_openwebtext2` | [
"Hi! I've merged a PR to use `int32` instead of `int8` for `reddit_scores`, so it should work now.\r\n\r\n"
] | ### Describe the bug
I met the same bug mentioned in #3053 which is never fixed. Because several `reddit_scores` are larger than `int8` even `int16`. https://huggingface.co/datasets/the_pile_openwebtext2/blob/main/the_pile_openwebtext2.py#L62
### Steps to reproduce the bug
```python3
from datasets import load_dataset
dataset = load_dataset("the_pile_openwebtext2")
```
### Expected behavior
load as normal.
### Environment info
- `datasets` version: 2.10.0
- Platform: Linux-5.4.143.bsk.7-amd64-x86_64-with-glibc2.31
- Python version: 3.9.2
- PyArrow version: 11.0.0
- Pandas version: 1.5.3 | 5,577 |
https://github.com/huggingface/datasets/issues/5576 | I was getting a similar error `pyarrow.lib.ArrowInvalid: Integer value 528 not in range: -128 to 127` - AFAICT, this is because the type specified for `reddit_scores` is `datasets.Sequence(datasets.Value("int8"))`, but the actual values can be well outside the max range for 8-bit integers. | [
"Duplicated issue."
] | I was getting a similar error `pyarrow.lib.ArrowInvalid: Integer value 528 not in range: -128 to 127` - AFAICT, this is because the type specified for `reddit_scores` is `datasets.Sequence(datasets.Value("int8"))`, but the actual values can be well outside the max range for 8-bit integers.
I worked around this by downloading the `the_pile_openwebtext2.py` and editing it to use local files and drop reddit scores as a column (not needed for my purposes).
_Originally posted by @tc-wolf in https://github.com/huggingface/datasets/issues/3053#issuecomment-1281392422_
| 5,576 |
https://github.com/huggingface/datasets/issues/5575 | Metadata for each column | [
"Hi! Indeed it would be useful to support this. PyArrow natively supports schema-level and column-level metadata, so implementing this should be straightforward. The API I have in mind would work as follows:\r\n```python\r\ncol_feature = Value(\"string\", metadata=\"Some column-level metadata\")\r\n\r\nfeatures = Features({\"col\": col_feature}, metadata=\"Some schema-level metadata\")\r\n```\r\n\r\nWDYT?",
"Sorry for the late reply, \r\nYes, I think this is the most straight-forward approach with the things that we already have.\r\n\r\n",
"@mariosasko Let me know how I can help.",
"Hi, is this feature to be implemented in the near future? It would be really nice if that would be the case! ",
"Hi, I also need this feature for tell my customer if any of the feature is encrypted with a certain key. "
] | ### Feature request
Being able to put some metadata for each column as a string or any other type.
### Motivation
I will bring the motivation by an example, lets say we are experimenting with embedding produced by some image encoder network, and we want to iterate through a couple of preprocessing and see which one works better in our downstream task, here as workaround right now what I do is the compute the hash of the preprocessing that the images went through as part of the new columns name, it would be nice to attach some kinda meta data in these scenarios to the each columns. metadata
### Your contribution
Maybe we could map another relational like database as the metadata? | 5,575 |
https://github.com/huggingface/datasets/issues/5574 | c4 dataset streaming fails with `FileNotFoundError` | [
"Also encountering this issue for every dataset I try to stream! Installed datasets from main:\r\n```\r\n- `datasets` version: 2.10.1.dev0\r\n- Platform: macOS-13.1-arm64-arm-64bit\r\n- Python version: 3.9.13\r\n- PyArrow version: 10.0.1\r\n- Pandas version: 1.5.2\r\n```\r\n\r\nRepro:\r\n```python\r\nfrom datasets import load_dataset\r\n\r\nspigi = load_dataset(\"kensho/spgispeech\", \"dev\", split=\"validation\", streaming=True, use_auth_token=True)\r\nsample = next(iter(spigi))\r\n```\r\n\r\n<details>\r\n<summary> Traceback </summary>\r\n\r\n```python\r\n---------------------------------------------------------------------------\r\nClientResponseError Traceback (most recent call last)\r\nFile ~/venv/lib/python3.9/site-packages/fsspec/implementations/http.py:407, in HTTPFileSystem._info(self, url, **kwargs)\r\n 405 try:\r\n 406 info.update(\r\n--> 407 await _file_info(\r\n 408 self.encode_url(url),\r\n 409 size_policy=policy,\r\n 410 session=session,\r\n 411 **self.kwargs,\r\n 412 **kwargs,\r\n 413 )\r\n 414 )\r\n 415 if info.get(\"size\") is not None:\r\n\r\nFile ~/venv/lib/python3.9/site-packages/fsspec/implementations/http.py:792, in _file_info(url, session, size_policy, **kwargs)\r\n 791 async with r:\r\n--> 792 r.raise_for_status()\r\n 794 # TODO:\r\n 795 # recognise lack of 'Accept-Ranges',\r\n 796 # or 'Accept-Ranges': 'none' (not 'bytes')\r\n 797 # to mean streaming only, no random access => return None\r\n\r\nFile ~/venv/lib/python3.9/site-packages/aiohttp/client_reqrep.py:1005, in ClientResponse.raise_for_status(self)\r\n 1004 self.release()\r\n-> 1005 raise ClientResponseError(\r\n 1006 self.request_info,\r\n 1007 self.history,\r\n 1008 status=self.status,\r\n 1009 message=self.reason,\r\n 1010 headers=self.headers,\r\n 1011 )\r\n\r\nClientResponseError: 403, message='Forbidden', url=URL('[https://cdn-lfs.huggingface.co/repos/e2/89/e28905247d6f48bb4edad5baf9b1bb4158e897a13fdf18bf3b8ee89ff8387ab8/46eca7431a7b6bad344bf451800e5b10cea1dd168f26d1027a6d9eb374b7fac3?response-content-disposition=attachment%3B+filename*%3DUTF-8''dev.csv%3B+filename%3D%22dev.csv%22%3B&response-content-type=text/csv&Expires=1677494732&Policy=eyJTdGF0ZW1lbnQiOlt7IlJlc291cmNlIjoiaHR0cHM6Ly9jZG4tbGZzLmh1Z2dpbmdmYWNlLmNvL3JlcG9zL2UyLzg5L2UyODkwNTI0N2Q2ZjQ4YmI0ZWRhZDViYWY5YjFiYjQxNThlODk3YTEzZmRmMThiZjNiOGVlODlmZjgzODdhYjgvNDZlY2E3NDMxYTdiNmJhZDM0NGJmNDUxODAwZTViMTBjZWExZGQxNjhmMjZkMTAyN2E2ZDllYjM3NGI3ZmFjMz9yZXNwb25zZS1jb250ZW50LWRpc3Bvc2l0aW9uPSomcmVzcG9uc2UtY29udGVudC10eXBlPXRleHQlMkZjc3YiLCJDb25kaXRpb24iOnsiRGF0ZUxlc3NUaGFuIjp7IkFXUzpFcG9jaFRpbWUiOjE2Nzc0OTQ3MzJ9fX1dfQ__&Signature=EzQB9f7xPckvqfFB6LzcyR-wzTnQCqtPDdWtQUzZ3QJ-gY-IHG5mxQITJgMr1nVTbJZrPmGAaDngMcPFUfSQa8RmCqYH~dZl-UGE8CO4neKNUT1DvA2WEvLDS4WaAJ3SN-9rX0uFb03~c1QS78cIgIRboYvf6ugKiJz86Bd7Vs~tcp201JFR0A6jIMseqApOnkb9d8dHMP3Ny~F6gO3Qf2QpEWM-QsDIyw2Kz2QV55nq8TsDpRYZCZo50~WwD~73Hej0PoDhEA1K37d19pa0CQhkaN-gjCrbT9xLabbvhJWa~ZkWcMdD0teCgjYqv1wKyvFXDAxukxLGEc7OBXVbYw__&Key-Pair-Id=KVTP0A1DKRTAX](https://cdn-lfs.huggingface.co/repos/e2/89/e28905247d6f48bb4edad5baf9b1bb4158e897a13fdf18bf3b8ee89ff8387ab8/46eca7431a7b6bad344bf451800e5b10cea1dd168f26d1027a6d9eb374b7fac3?response-content-disposition=attachment%3B+filename*%3DUTF-8%27%27dev.csv%3B+filename%3D%22dev.csv%22%3B&response-content-type=text/csv&Expires=1677494732&Policy=eyJTdGF0ZW1lbnQiOlt7IlJlc291cmNlIjoiaHR0cHM6Ly9jZG4tbGZzLmh1Z2dpbmdmYWNlLmNvL3JlcG9zL2UyLzg5L2UyODkwNTI0N2Q2ZjQ4YmI0ZWRhZDViYWY5YjFiYjQxNThlODk3YTEzZmRmMThiZjNiOGVlODlmZjgzODdhYjgvNDZlY2E3NDMxYTdiNmJhZDM0NGJmNDUxODAwZTViMTBjZWExZGQxNjhmMjZkMTAyN2E2ZDllYjM3NGI3ZmFjMz9yZXNwb25zZS1jb250ZW50LWRpc3Bvc2l0aW9uPSomcmVzcG9uc2UtY29udGVudC10eXBlPXRleHQlMkZjc3YiLCJDb25kaXRpb24iOnsiRGF0ZUxlc3NUaGFuIjp7IkFXUzpFcG9jaFRpbWUiOjE2Nzc0OTQ3MzJ9fX1dfQ__&Signature=EzQB9f7xPckvqfFB6LzcyR-wzTnQCqtPDdWtQUzZ3QJ-gY-IHG5mxQITJgMr1nVTbJZrPmGAaDngMcPFUfSQa8RmCqYH~dZl-UGE8CO4neKNUT1DvA2WEvLDS4WaAJ3SN-9rX0uFb03~c1QS78cIgIRboYvf6ugKiJz86Bd7Vs~tcp201JFR0A6jIMseqApOnkb9d8dHMP3Ny~F6gO3Qf2QpEWM-QsDIyw2Kz2QV55nq8TsDpRYZCZo50~WwD~73Hej0PoDhEA1K37d19pa0CQhkaN-gjCrbT9xLabbvhJWa~ZkWcMdD0teCgjYqv1wKyvFXDAxukxLGEc7OBXVbYw__&Key-Pair-Id=KVTP0A1DKRTAX)')\r\n\r\nThe above exception was the direct cause of the following exception:\r\n\r\nFileNotFoundError Traceback (most recent call last)\r\nCell In[5], line 4\r\n 1 from datasets import load_dataset\r\n 3 spigi = load_dataset(\"kensho/spgispeech\", \"dev\", split=\"validation\", streaming=True)\r\n----> 4 sample = next(iter(spigi))\r\n\r\nFile ~/datasets/src/datasets/iterable_dataset.py:937, in IterableDataset.__iter__(self)\r\n 934 yield from self._iter_pytorch(ex_iterable)\r\n 935 return\r\n--> 937 for key, example in ex_iterable:\r\n 938 if self.features:\r\n 939 # `IterableDataset` automatically fills missing columns with None.\r\n 940 # This is done with `_apply_feature_types_on_example`.\r\n 941 yield _apply_feature_types_on_example(\r\n 942 example, self.features, token_per_repo_id=self._token_per_repo_id\r\n 943 )\r\n\r\nFile ~/datasets/src/datasets/iterable_dataset.py:113, in ExamplesIterable.__iter__(self)\r\n 112 def __iter__(self):\r\n--> 113 yield from self.generate_examples_fn(**self.kwargs)\r\n\r\nFile ~/.cache/huggingface/modules/datasets_modules/datasets/kensho--spgispeech/5fbf75dd9ef795a9b5a673457d2cbaf0b8fa0de8fb62acbd1da338d83a41e2f0/spgispeech.py:186, in Spgispeech._generate_examples(self, local_extracted_archive_paths, archives, meta_path)\r\n 183 dict_keys = [\"wav_filename\", \"wav_filesize\", \"transcript\"]\r\n 185 logging.info(\"Reading metadata...\")\r\n--> 186 with open(meta_path, encoding=\"utf-8\") as f:\r\n 187 csvreader = csv.DictReader(f, delimiter=\"|\")\r\n 188 metadata = {x[\"wav_filename\"]: dict((k, x[k]) for k in dict_keys) for x in csvreader}\r\n\r\nFile ~/datasets/src/datasets/streaming.py:70, in extend_module_for_streaming.<locals>.wrap_auth.<locals>.wrapper(*args, **kwargs)\r\n 68 @wraps(function)\r\n 69 def wrapper(*args, **kwargs):\r\n---> 70 return function(*args, use_auth_token=use_auth_token, **kwargs)\r\n\r\nFile ~/datasets/src/datasets/download/streaming_download_manager.py:495, in xopen(file, mode, use_auth_token, *args, **kwargs)\r\n 493 kwargs = {**kwargs, **new_kwargs}\r\n 494 try:\r\n--> 495 file_obj = fsspec.open(file, mode=mode, *args, **kwargs).open()\r\n 496 except ValueError as e:\r\n 497 if str(e) == \"Cannot seek streaming HTTP file\":\r\n\r\nFile ~/venv/lib/python3.9/site-packages/fsspec/core.py:135, in OpenFile.open(self)\r\n 128 def open(self):\r\n 129 \"\"\"Materialise this as a real open file without context\r\n 130 \r\n 131 The OpenFile object should be explicitly closed to avoid enclosed file\r\n 132 instances persisting. You must, therefore, keep a reference to the OpenFile\r\n 133 during the life of the file-like it generates.\r\n 134 \"\"\"\r\n--> 135 return self.__enter__()\r\n\r\nFile ~/venv/lib/python3.9/site-packages/fsspec/core.py:103, in OpenFile.__enter__(self)\r\n 100 def __enter__(self):\r\n 101 mode = self.mode.replace(\"t\", \"\").replace(\"b\", \"\") + \"b\"\r\n--> 103 f = self.fs.open(self.path, mode=mode)\r\n 105 self.fobjects = [f]\r\n 107 if self.compression is not None:\r\n\r\nFile ~/venv/lib/python3.9/site-packages/fsspec/spec.py:1106, in AbstractFileSystem.open(self, path, mode, block_size, cache_options, compression, **kwargs)\r\n 1104 else:\r\n 1105 ac = kwargs.pop(\"autocommit\", not self._intrans)\r\n-> 1106 f = self._open(\r\n 1107 path,\r\n 1108 mode=mode,\r\n 1109 block_size=block_size,\r\n 1110 autocommit=ac,\r\n 1111 cache_options=cache_options,\r\n 1112 **kwargs,\r\n 1113 )\r\n 1114 if compression is not None:\r\n 1115 from fsspec.compression import compr\r\n\r\nFile ~/venv/lib/python3.9/site-packages/fsspec/implementations/http.py:346, in HTTPFileSystem._open(self, path, mode, block_size, autocommit, cache_type, cache_options, size, **kwargs)\r\n 344 kw[\"asynchronous\"] = self.asynchronous\r\n 345 kw.update(kwargs)\r\n--> 346 size = size or self.info(path, **kwargs)[\"size\"]\r\n 347 session = sync(self.loop, self.set_session)\r\n 348 if block_size and size:\r\n\r\nFile ~/venv/lib/python3.9/site-packages/fsspec/asyn.py:113, in sync_wrapper.<locals>.wrapper(*args, **kwargs)\r\n 110 @functools.wraps(func)\r\n 111 def wrapper(*args, **kwargs):\r\n 112 self = obj or args[0]\r\n--> 113 return sync(self.loop, func, *args, **kwargs)\r\n\r\nFile ~/venv/lib/python3.9/site-packages/fsspec/asyn.py:98, in sync(loop, func, timeout, *args, **kwargs)\r\n 96 raise FSTimeoutError from return_result\r\n 97 elif isinstance(return_result, BaseException):\r\n---> 98 raise return_result\r\n 99 else:\r\n 100 return return_result\r\n\r\nFile ~/venv/lib/python3.9/site-packages/fsspec/asyn.py:53, in _runner(event, coro, result, timeout)\r\n 51 coro = asyncio.wait_for(coro, timeout=timeout)\r\n 52 try:\r\n---> 53 result[0] = await coro\r\n 54 except Exception as ex:\r\n 55 result[0] = ex\r\n\r\nFile ~/venv/lib/python3.9/site-packages/fsspec/implementations/http.py:420, in HTTPFileSystem._info(self, url, **kwargs)\r\n 417 except Exception as exc:\r\n 418 if policy == \"get\":\r\n 419 # If get failed, then raise a FileNotFoundError\r\n--> 420 raise FileNotFoundError(url) from exc\r\n 421 logger.debug(str(exc))\r\n 423 return {\"name\": url, \"size\": None, **info, \"type\": \"file\"}\r\n\r\nFileNotFoundError: https://huggingface.co/datasets/kensho/spgispeech/resolve/main/data/meta/dev.csv\r\n```\r\n</details>",
"Hi ! We're investigating this issue, sorry for the inconvenience",
"This has been resolved ! Thanks for reporting",
"Wow, thanks for the very quick fix!",
"This problem now appears again, this time with an underlying HTTP 502 status code:\r\n\r\n```\r\naiohttp.client_exceptions.ClientResponseError: 502, message='Bad Gateway', url=URL('https://huggingface.co/datasets/allenai/c4/resolve/1ddc917116b730e1859edef32896ec5c16be51d0/en/c4-validation.00002-of-00008.json.gz')\r\n```",
"Re-executing a minute later, the underlying cause is an HTTP 403 status code, as reported yesterday:\r\n\r\n```\r\naiohttp.client_exceptions.ClientResponseError: 403, message='Forbidden', url=URL('https://cdn-lfs.huggingface.co/datasets/allenai/c4/4bf6b248b0f910dcde2cdf2118d6369d8208c8f9515ec29ab73e531f380b18e2?response-content-disposition=attachment%3B+filename*%3DUTF-8''c4-validation.00002-of-00008.json.gz%3B+filename%3D%22c4-validation.00002-of-00008.json.gz%22%3B&response-content-type=application/gzip&Expires=1677571273&Policy=eyJTdGF0ZW1lbnQiOlt7IlJlc291cmNlIjoiaHR0cHM6Ly9jZG4tbGZzLmh1Z2dpbmdmYWNlLmNvL2RhdGFzZXRzL2FsbGVuYWkvYzQvNGJmNmIyNDhiMGY5MTBkY2RlMmNkZjIxMThkNjM2OWQ4MjA4YzhmOTUxNWVjMjlhYjczZTUzMWYzODBiMThlMj9yZXNwb25zZS1jb250ZW50LWRpc3Bvc2l0aW9uPSomcmVzcG9uc2UtY29udGVudC10eXBlPWFwcGxpY2F0aW9uJTJGZ3ppcCIsIkNvbmRpdGlvbiI6eyJEYXRlTGVzc1RoYW4iOnsiQVdTOkVwb2NoVGltZSI6MTY3NzU3MTI3M319fV19&Signature=WW42NOKkLuX~xVB1QfbkqzdvGo2AOXpgbF3PjTXy6iKd~ffilr1N9ScPXfvTXqy5yvdhJg1G0xJy1zYtUjGAL8GEx3Av-0vIhpWMGYTM8XKEU5gYA9qt30oVtNph6TkTYSABrsYTaj-hzQL9WCgyapmjvG69ETMh4wj44r2rcbk4T3j0l6l4u76Gh~lyRSll3aK4qycdUwcyL7FECDu~0W1mJIJwKkCrWHhSpHJSshb-0ElwG71pq4eyQ5g2uxHdK6JbRF7loxUpRQQJ1vlk0EHXdw0wTMaQ9tqHy6xcrQd8Ep0Yvx3tUD8MR0vWOcbQKnL6LwPQByc8tkChlpjnig__&Key-Pair-Id=KVTP0A1DKRTAX')\r\n```",
"I'm facing the same problem. Interestingly using `wget` I can download the file. ",
"It's been resolved again ;)",
"> It's been resolved again ;)\r\n\r\nI'm experiencing the same issue when trying to load this dataset, `FileNotFoundError: https://huggingface.co/datasets/allenai/c4/resolve/1ddc917116b730e1859edef32896ec5c16be51d0/realnewslike/c4-train.00000-of-00512.json.gz`",
"Experiencing the same issues as above : `FileNotFoundError: https://huggingface.co/datasets/allenai/c4/resolve/1ddc917116b730e1859edef32896ec5c16be51d0/en/c4-train.00000-of-01024.json.gz\r\nIf the repo is private or gated, make sure to log in with `huggingface-cli login`.`\r\n\r\nHave made sure to login as well, issue persists.",
"> Experiencing the same issues as above : `FileNotFoundError: https://huggingface.co/datasets/allenai/c4/resolve/1ddc917116b730e1859edef32896ec5c16be51d0/en/c4-train.00000-of-01024.json.gz If the repo is private or gated, make sure to log in with `huggingface-cli login`.`\r\n> \r\n> Have made sure to login as well, issue persists.\r\n\r\nI meet the same issue",
"I meet the same issue"
] | ### Describe the bug
Loading the `c4` dataset in streaming mode with `load_dataset("c4", "en", split="validation", streaming=True)` and then using it fails with a `FileNotFoundException`.
### Steps to reproduce the bug
```python
from datasets import load_dataset
dataset = load_dataset("c4", "en", split="train", streaming=True)
next(iter(dataset))
```
causes a
```
FileNotFoundError: https://huggingface.co/datasets/allenai/c4/resolve/1ddc917116b730e1859edef32896ec5c16be51d0/en/c4-train.00000-of-01024.json.gz
```
I can download this file manually though e.g. by entering this URL in a browser.
There is an underlying HTTP 403 status code:
```
aiohttp.client_exceptions.ClientResponseError: 403, message='Forbidden', url=URL('https://cdn-lfs.huggingface.co/datasets/allenai/c4/8ef8d75b0e045dec4aa5123a671b4564466b0707086a7ed1ba8721626dfffbc9?response-content-disposition=attachment%3B+filename*%3DUTF-8''c4-train.00000-of-01024.json.gz%3B+filename%3D%22c4-train.00000-of-01024.json.gz%22%3B&response-content-type=application/gzip&Expires=1677483770&Policy=eyJTdGF0ZW1lbnQiOlt7IlJlc291cmNlIjoiaHR0cHM6Ly9jZG4tbGZzLmh1Z2dpbmdmYWNlLmNvL2RhdGFzZXRzL2FsbGVuYWkvYzQvOGVmOGQ3NWIwZTA0NWRlYzRhYTUxMjNhNjcxYjQ1NjQ0NjZiMDcwNzA4NmE3ZWQxYmE4NzIxNjI2ZGZmZmJjOT9yZXNwb25zZS1jb250ZW50LWRpc3Bvc2l0aW9uPSomcmVzcG9uc2UtY29udGVudC10eXBlPWFwcGxpY2F0aW9uJTJGZ3ppcCIsIkNvbmRpdGlvbiI6eyJEYXRlTGVzc1RoYW4iOnsiQVdTOkVwb2NoVGltZSI6MTY3NzQ4Mzc3MH19fV19&Signature=yjL3UeY72cf2xpnvPvD68eAYOEe2qtaUJV55sB-jnPskBJEMwpMJcBZvg2~GqXZdM3O-GWV-Z3CI~d4u5VCb4YZ-HlmOjr3VBYkvox2EKiXnBIhjMecf2UVUPtxhTa9kBVlWjqu4qKzB9gKXZF2Cwpp5ctLzapEaT2nnqF84RAL-rsqMA3I~M8vWWfivQsbBK63hMfgZqqKMgdWM0iKMaItveDl0ufQ29azMFmsR7qd8V7sU2Z-F1fAeohS8HpN9OOnClW34yi~YJ2AbgZJJBXA~qsylfVA0Qp7Q~yX~q4P8JF1vmJ2BjkiSbGrj3bAXOGugpOVU5msI52DT88yMdA__&Key-Pair-Id=KVTP0A1DKRTAX')
```
### Expected behavior
This should retrieve the first example from the C4 validation set. This worked a few days ago but stopped working now.
### Environment info
- `datasets` version: 2.9.0
- Platform: Linux-5.15.0-60-generic-x86_64-with-glibc2.31
- Python version: 3.9.16
- PyArrow version: 11.0.0
- Pandas version: 1.5.3
| 5,574 |
https://github.com/huggingface/datasets/issues/5572 | Datasets 2.10.0 does not reuse the dataset cache | [] | ### Describe the bug
download_mode="reuse_dataset_if_exists" will always consider that a dataset doesn't exist.
Specifically, upon losing an internet connection trying to load a dataset for a second time in ten seconds, a connection error results, showing a breakpoint of:
```
File ~/jupyterlab/.direnv/python-3.9.6/lib/python3.9/site-packages/datasets/load.py:1174, in dataset_module_factory(path, revision, download_config, download_mode, dynamic_modules_path, data_dir, data_files, **download_kwargs)
1165 except Exception as e: # noqa: catch any exception of hf_hub and consider that the dataset doesn't exist
1166 if isinstance(
1167 e,
1168 (
(...)
1172 ),
1173 ):
-> 1174 raise ConnectionError(f"Couldn't reach '{path}' on the Hub ({type(e).__name__})")
1175 elif "404" in str(e):
1176 msg = f"Dataset '{path}' doesn't exist on the Hub"
ConnectionError: Couldn't reach 'lsb/tenk' on the Hub (ConnectionError)
```
This has been around since at least v2.0.
### Steps to reproduce the bug
```
from datasets import load_dataset
import numpy as np
tenk = load_dataset("lsb/tenk") # ten thousand integers
print(np.average(tenk['train']['a'])) # prints 4999.5
### now disconnect your internet
tenk_too = load_dataset("lsb/tenk", download_mode="reuse_dataset_if_exists")
# Raises ConnectionError: Couldn't reach 'lsb/tenk' on the Hub (ConnectionError)
```
### Expected behavior
I expected that I would be able to reuse the dataset I just downloaded.
### Environment info
- `datasets` version: 2.10.0
- Platform: macOS-13.1-arm64-arm-64bit
- Python version: 3.9.6
- PyArrow version: 7.0.0
- Pandas version: 1.5.2 | 5,572 |
https://github.com/huggingface/datasets/issues/5571 | load_dataset fails for JSON in windows | [
"Hi! \r\n\r\nYou need to pass an input json file explicitly as `data_files` to `load_dataset` to avoid this error:\r\n```python\r\n ds = load_dataset(\"json\", data_files=args.input_json)\r\n```\r\n\r\n",
"Thanks it worked!"
] | ### Describe the bug
Steps:
1. Created a dataset in a Linux VM and created a small sample using dataset.to_json() method.
2. Downloaded the JSON file to my local Windows machine for working and saved in say - r"C:\Users\name\file.json"
3. I am reading the file in my local PyCharm - the location of python file is different than the location of the JSON.
4. When I read using load_dataset("json",args.input_json), it throws and error from builder.py.
raise InvalidConfigName(
f"Bad characters from black list '{invalid_windows_characters}' found in '{self.name}'. "
f"They could create issues when creating a directory for this config on Windows filesystem."
6. When I bring the data to the current directory, it works fine.
### Steps to reproduce the bug
Steps:
1. Created a dataset in a Linux VM and created a small sample using dataset.to_json() method.
2. Downloaded the JSON file to my local Windows machine for working and saved in say - r"C:\Users\name\file.json"
3. I am reading the file in my local PyCharm - the location of python file is different than the location of the JSON.
4. When I read using load_dataset("json",args.input_json), it throws and error from builder.py.
raise InvalidConfigName(
f"Bad characters from black list '{invalid_windows_characters}' found in '{self.name}'. "
f"They could create issues when creating a directory for this config on Windows filesystem."
6. When I bring the data to the current directory, it works fine.
### Expected behavior
Should be able to read from a path different than current directory in Windows machine.
### Environment info
datasets version: 2.3.1
python version: 3.8
Windows OS | 5,571 |
https://github.com/huggingface/datasets/issues/5570 | load_dataset gives FileNotFoundError on imagenet-1k if license is not accepted on the hub | [
"Hi, thanks for the feedback! Would it help to add a tip or note saying the dataset is gated and you need to accept the license before downloading it?",
"The error is now more informative:\r\n```\r\nFileNotFoundError: Couldn't find a dataset script at /content/imagenet-1k/imagenet-1k.py or any data file in the same directory. Couldn't find 'imagenet-1k' on the Hugging Face Hub either: FileNotFoundError: Dataset 'imagenet-1k' doesn't exist on the Hub. If the repo is private or gated, make sure to log in with `huggingface-cli login`.\r\n```\r\n\r\n"
] | ### Describe the bug
When calling ```load_dataset('imagenet-1k')``` FileNotFoundError is raised, if not logged in and if logged in with huggingface-cli but not having accepted the licence on the hub. There is no error once accepting.
### Steps to reproduce the bug
```
from datasets import load_dataset
imagenet = load_dataset("imagenet-1k", split="train", streaming=True)
FileNotFoundError: Couldn't find a dataset script at /content/imagenet-1k/imagenet-1k.py or any data file in the same directory. Couldn't find 'imagenet-1k' on the Hugging Face Hub either: FileNotFoundError: Dataset 'imagenet-1k' doesn't exist on the Hub
```
tested on a colab notebook.
### Expected behavior
I would expect a specific error indicating that I have to login then accept the dataset licence.
I find this bug very relevant as this code is on a guide on the [Huggingface documentation for Datasets](https://huggingface.co/docs/datasets/about_mapstyle_vs_iterable)
### Environment info
google colab cpu-only instance | 5,570 |
https://github.com/huggingface/datasets/issues/5568 | dataset.to_iterable_dataset() loses useful info like dataset features | [
"Hi ! Oh good catch. I think the features should be passed to `IterableDataset.from_generator()` in `to_iterable_dataset()` indeed.\r\n\r\nSetting this as a good first issue if someone would like to contribute, otherwise we can take care of it :)",
"#self-assign",
"seems like the feature parameter is missing from `return IterableDataset.from_generator(Dataset._iter_shards, gen_kwargs={\"shards\": shards})` hence it defaults to None."
] | ### Describe the bug
Hello,
I like the new `to_iterable_dataset` feature but I noticed something that seems to be missing.
When using `to_iterable_dataset` to transform your map style dataset into iterable dataset, you lose valuable metadata like the features.
These metadata are useful if you want to interleave iterable datasets, cast columns etc.
### Steps to reproduce the bug
```python
dataset = load_dataset("lhoestq/demo1")["train"]
print(dataset.features)
# {'id': Value(dtype='string', id=None), 'package_name': Value(dtype='string', id=None), 'review': Value(dtype='string', id=None), 'date': Value(dtype='string', id=None), 'star': Value(dtype='int64', id=None), 'version_id': Value(dtype='int64', id=None)}
dataset = dataset.to_iterable_dataset()
print(dataset.features)
# None
```
### Expected behavior
Keep the relevant information
### Environment info
datasets==2.10.0 | 5,568 |
https://github.com/huggingface/datasets/issues/5566 | Directly reading parquet files in a s3 bucket from the load_dataset method | [
"Hi ! I think is in the scope of this other issue: to https://github.com/huggingface/datasets/issues/5281 "
] | ### Feature request
Right now, we have to read the get the parquet file to the local storage. So having ability to read given the bucket directly address would be benificial
### Motivation
In a production set up, this feature can help us a lot. So we do not need move training datafiles in between storage.
### Your contribution
I am willing to help if there's anyway. | 5,566 |
https://github.com/huggingface/datasets/issues/5555 | `.shuffle` throwing error `ValueError: Protocol not known: parent` | [
"Hi ! The indices mapping is written in the same cachedirectory as your dataset.\r\n\r\nCan you run this to show your current cache directory ?\r\n```python\r\nprint(train_dataset.cache_files)\r\n```",
"```\r\n[{'filename': '.../train/dataset.arrow'}, {'filename': '.../train/dataset.arrow'}]\r\n```\r\n\r\nThese are the actual paths where `.hf` files are stored. ",
"I'm not aware of any `.hf` file ? What are you referring to ?\r\n\r\nAlso the error says \"Protocol unknown: parent\". Is there a chance you may have ended up with a path that contains this string `parent://` ?",
"I figured out why the issue was occuring but don't know the long-term fix.\r\nThe dataset I was trying to shuffle was loaded from a saved file which had `::` delimiter in filename. When I try with the exact same file without `::` in filename, it works as expected.\r\nQuick fix is to not use colons in filename. But if this is expected behaviour, this should be clearly stated in the documentation.\r\nThanks for help @lhoestq "
] | ### Describe the bug
```
---------------------------------------------------------------------------
ValueError Traceback (most recent call last)
Cell In [16], line 1
----> 1 train_dataset = train_dataset.shuffle()
File /opt/conda/envs/pytorch/lib/python3.9/site-packages/datasets/arrow_dataset.py:551, in transmit_format.<locals>.wrapper(*args, **kwargs)
544 self_format = {
545 "type": self._format_type,
546 "format_kwargs": self._format_kwargs,
547 "columns": self._format_columns,
548 "output_all_columns": self._output_all_columns,
549 }
550 # apply actual function
--> 551 out: Union["Dataset", "DatasetDict"] = func(self, *args, **kwargs)
552 datasets: List["Dataset"] = list(out.values()) if isinstance(out, dict) else [out]
553 # re-apply format to the output
File /opt/conda/envs/pytorch/lib/python3.9/site-packages/datasets/fingerprint.py:480, in fingerprint_transform.<locals>._fingerprint.<locals>.wrapper(*args, **kwargs)
476 validate_fingerprint(kwargs[fingerprint_name])
478 # Call actual function
--> 480 out = func(self, *args, **kwargs)
482 # Update fingerprint of in-place transforms + update in-place history of transforms
484 if inplace: # update after calling func so that the fingerprint doesn't change if the function fails
File /opt/conda/envs/pytorch/lib/python3.9/site-packages/datasets/arrow_dataset.py:3616, in Dataset.shuffle(self, seed, generator, keep_in_memory, load_from_cache_file, indices_cache_file_name, writer_batch_size, new_fingerprint)
3610 return self._new_dataset_with_indices(
3611 fingerprint=new_fingerprint, indices_cache_file_name=indices_cache_file_name
3612 )
3614 permutation = generator.permutation(len(self))
-> 3616 return self.select(
3617 indices=permutation,
3618 keep_in_memory=keep_in_memory,
3619 indices_cache_file_name=indices_cache_file_name if not keep_in_memory else None,
3620 writer_batch_size=writer_batch_size,
3621 new_fingerprint=new_fingerprint,
3622 )
File /opt/conda/envs/pytorch/lib/python3.9/site-packages/datasets/arrow_dataset.py:551, in transmit_format.<locals>.wrapper(*args, **kwargs)
544 self_format = {
545 "type": self._format_type,
546 "format_kwargs": self._format_kwargs,
547 "columns": self._format_columns,
548 "output_all_columns": self._output_all_columns,
549 }
550 # apply actual function
--> 551 out: Union["Dataset", "DatasetDict"] = func(self, *args, **kwargs)
552 datasets: List["Dataset"] = list(out.values()) if isinstance(out, dict) else [out]
553 # re-apply format to the output
File /opt/conda/envs/pytorch/lib/python3.9/site-packages/datasets/fingerprint.py:480, in fingerprint_transform.<locals>._fingerprint.<locals>.wrapper(*args, **kwargs)
476 validate_fingerprint(kwargs[fingerprint_name])
478 # Call actual function
--> 480 out = func(self, *args, **kwargs)
482 # Update fingerprint of in-place transforms + update in-place history of transforms
484 if inplace: # update after calling func so that the fingerprint doesn't change if the function fails
File /opt/conda/envs/pytorch/lib/python3.9/site-packages/datasets/arrow_dataset.py:3266, in Dataset.select(self, indices, keep_in_memory, indices_cache_file_name, writer_batch_size, new_fingerprint)
3263 return self._select_contiguous(start, length, new_fingerprint=new_fingerprint)
3265 # If not contiguous, we need to create a new indices mapping
-> 3266 return self._select_with_indices_mapping(
3267 indices,
3268 keep_in_memory=keep_in_memory,
3269 indices_cache_file_name=indices_cache_file_name,
3270 writer_batch_size=writer_batch_size,
3271 new_fingerprint=new_fingerprint,
3272 )
File /opt/conda/envs/pytorch/lib/python3.9/site-packages/datasets/arrow_dataset.py:551, in transmit_format.<locals>.wrapper(*args, **kwargs)
544 self_format = {
545 "type": self._format_type,
546 "format_kwargs": self._format_kwargs,
547 "columns": self._format_columns,
548 "output_all_columns": self._output_all_columns,
549 }
550 # apply actual function
--> 551 out: Union["Dataset", "DatasetDict"] = func(self, *args, **kwargs)
552 datasets: List["Dataset"] = list(out.values()) if isinstance(out, dict) else [out]
553 # re-apply format to the output
File /opt/conda/envs/pytorch/lib/python3.9/site-packages/datasets/fingerprint.py:480, in fingerprint_transform.<locals>._fingerprint.<locals>.wrapper(*args, **kwargs)
476 validate_fingerprint(kwargs[fingerprint_name])
478 # Call actual function
--> 480 out = func(self, *args, **kwargs)
482 # Update fingerprint of in-place transforms + update in-place history of transforms
484 if inplace: # update after calling func so that the fingerprint doesn't change if the function fails
File /opt/conda/envs/pytorch/lib/python3.9/site-packages/datasets/arrow_dataset.py:3389, in Dataset._select_with_indices_mapping(self, indices, keep_in_memory, indices_cache_file_name, writer_batch_size, new_fingerprint)
3387 logger.info(f"Caching indices mapping at {indices_cache_file_name}")
3388 tmp_file = tempfile.NamedTemporaryFile("wb", dir=os.path.dirname(indices_cache_file_name), delete=False)
-> 3389 writer = ArrowWriter(
3390 path=tmp_file.name, writer_batch_size=writer_batch_size, fingerprint=new_fingerprint, unit="indices"
3391 )
3393 indices = indices if isinstance(indices, list) else list(indices)
3395 size = len(self)
File /opt/conda/envs/pytorch/lib/python3.9/site-packages/datasets/arrow_writer.py:315, in ArrowWriter.__init__(self, schema, features, path, stream, fingerprint, writer_batch_size, hash_salt, check_duplicates, disable_nullable, update_features, with_metadata, unit, embed_local_files, storage_options)
312 self._disable_nullable = disable_nullable
314 if stream is None:
--> 315 fs_token_paths = fsspec.get_fs_token_paths(path, storage_options=storage_options)
316 self._fs: fsspec.AbstractFileSystem = fs_token_paths[0]
317 self._path = (
318 fs_token_paths[2][0]
319 if not is_remote_filesystem(self._fs)
320 else self._fs.unstrip_protocol(fs_token_paths[2][0])
321 )
File /opt/conda/envs/pytorch/lib/python3.9/site-packages/fsspec/core.py:593, in get_fs_token_paths(urlpath, mode, num, name_function, storage_options, protocol, expand)
591 else:
592 urlpath = stringify_path(urlpath)
--> 593 chain = _un_chain(urlpath, storage_options or {})
594 if len(chain) > 1:
595 inkwargs = {}
File /opt/conda/envs/pytorch/lib/python3.9/site-packages/fsspec/core.py:330, in _un_chain(path, kwargs)
328 for bit in reversed(bits):
329 protocol = split_protocol(bit)[0] or "file"
--> 330 cls = get_filesystem_class(protocol)
331 extra_kwargs = cls._get_kwargs_from_urls(bit)
332 kws = kwargs.get(protocol, {})
File /opt/conda/envs/pytorch/lib/python3.9/site-packages/fsspec/registry.py:240, in get_filesystem_class(protocol)
238 if protocol not in registry:
239 if protocol not in known_implementations:
--> 240 raise ValueError("Protocol not known: %s" % protocol)
241 bit = known_implementations[protocol]
242 try:
ValueError: Protocol not known: parent
```
This is what the `train_dataset` object looks like
```
Dataset({
features: ['label', 'input_ids', 'attention_mask'],
num_rows: 364166
})
```
### Steps to reproduce the bug
The `train_dataset` obj is created by concatenating two datasets
And then shuffle is called, but it throws the mentioned error.
### Expected behavior
Should shuffle the dataset properly.
### Environment info
- `datasets` version: 2.6.1
- Platform: Linux-5.15.0-1022-aws-x86_64-with-glibc2.31
- Python version: 3.9.13
- PyArrow version: 10.0.0
- Pandas version: 1.4.4
| 5,555 |
https://github.com/huggingface/datasets/issues/5548 | Apply flake8-comprehensions to codebase | [] | ### Feature request
Apply ruff flake8 comprehension checks to codebase.
### Motivation
This should strictly improve the performance / readability of the codebase by removing unnecessary iteration, function calls, etc. This should generate better Python bytecode which should strictly improve performance.
I already applied this fixes to PyTorch and Sympy with little issue and have opened PRs to diffusers and transformers todo this as well.
### Your contribution
Making a PR. | 5,548 |
https://github.com/huggingface/datasets/issues/5546 | Downloaded datasets do not cache at $HF_HOME | [
"Hi ! Can you make sure you set `HF_HOME` before importing `datasets` ?\r\n\r\nThen you can print\r\n```python\r\nprint(datasets.config.HF_CACHE_HOME)\r\nprint(datasets.config.HF_DATASETS_CACHE)\r\n```"
] | ### Describe the bug
In the huggingface course (https://huggingface.co/course/chapter3/2?fw=pt) it said that if we set HF_HOME, downloaded datasets would be cached at specified address but it does not. downloaded models from checkpoint names are downloaded and cached at HF_HOME but this is not the case for datasets, they are still cached at ~/.cache/huggingface/datasets.
### Steps to reproduce the bug
Run the following code
```
from datasets import load_dataset
raw_datasets = load_dataset("glue", "mrpc")
raw_datasets
```
it downloads and store dataset at ~/.cache/huggingface/datasets
### Expected behavior
to cache dataset at HF_HOME.
### Environment info
python 3.10.6
Kubuntu 22.04
HF_HOME located on a separate partition | 5,546 |
https://github.com/huggingface/datasets/issues/5543 | the pile datasets url seems to change back | [
"Thanks for reporting, @wjfwzzc.\r\n\r\nI am transferring this issue to the corresponding dataset on the Hub: https://huggingface.co/datasets/bookcorpusopen/discussions/1",
"Thank you. All fixes are done:\r\n- [x] https://huggingface.co/datasets/bookcorpusopen/discussions/2\r\n- [x] https://huggingface.co/datasets/the_pile/discussions/1\r\n- [x] https://huggingface.co/datasets/the_pile_books3/discussions/1\r\n- [x] https://huggingface.co/datasets/the_pile_openwebtext2/discussions/2\r\n- [x] https://huggingface.co/datasets/the_pile_stack_exchange/discussions/2"
] | ### Describe the bug
in #3627, the host url of the pile dataset became `https://mystic.the-eye.eu`. Now the new url is broken, but `https://the-eye.eu` seems to work again.
### Steps to reproduce the bug
```python3
from datasets import load_dataset
dataset = load_dataset("bookcorpusopen")
```
shows
```python3
ConnectionError: Couldn't reach https://mystic.the-eye.eu/public/AI/pile_preliminary_components/books1.tar.gz (ProxyError(MaxRetryError("HTTPSConnectionPool(host='mystic.the-eye.eu', port=443): Max retries exceeded with url: /public/AI/pile_pr
eliminary_components/books1.tar.gz (Caused by ProxyError('Cannot connect to proxy.', OSError('Tunnel connection failed: 504 Gateway Timeout')))")))
```
### Expected behavior
Downloading as normal.
### Environment info
- `datasets` version: 2.9.0
- Platform: Linux-5.4.143.bsk.7-amd64-x86_64-with-glibc2.31
- Python version: 3.9.2
- PyArrow version: 6.0.1
- Pandas version: 1.5.3 | 5,543 |
https://github.com/huggingface/datasets/issues/5541 | Flattening indices in selected datasets is extremely inefficient | [
"Running the script above on the branch https://github.com/huggingface/datasets/pull/5542 results in the expected behaviour:\r\n```\r\nNum chunks for original ds: 1\r\nOriginal ds save/load\r\nsave_to_disk -- RAM memory used: 0.671875 MB -- Total time: 0.255265 s\r\nload_from_disk -- RAM memory used: 42.796875 MB -- Total time: 0.014899 s\r\nNum chunks for original ds after reloading: 5000\r\n\r\nNum chunks for selected ds: 1\r\nflatten_indices -- RAM memory used: 42.546875 MB -- Total time: 23.735089 s\r\nNum chunks for selected ds after flattening: 5000\r\n\r\nSelected ds save/load\r\nsave_to_disk -- RAM memory used: 0.0 MB -- Total time: 0.287112 s\r\nload_from_disk -- RAM memory used: 38.84375 MB -- Total time: 0.014772 s\r\nNum chunks for selected ds after reloading: 5000\r\n```",
"Wouahouh super cool @marioga thanks a lot!",
"We just released `datasets==2.10.0` with this big improvement, thanks again @marioga "
] | ### Describe the bug
If we perform a `select` (or `shuffle`, `train_test_split`, etc.) operation on a dataset , we end up with a dataset with an `indices_table`. Currently, flattening such dataset consumes a lot of memory and the resulting flat dataset contains ChunkedArrays with as many chunks as there are rows. This is extremely inefficient and slows down the operations on the flat dataset, e.g., saving/loading the dataset to disk becomes really slow.
Perhaps more importantly, loading the dataset back from disk basically loads the whole table into RAM, as it cannot take advantage of memory mapping.
### Steps to reproduce the bug
The following script reproduces the issue:
```python
import gc
import os
import psutil
import tempfile
import time
from datasets import Dataset
DATASET_SIZE = 5000000
def profile(func):
def wrapper(*args, **kwargs):
mem_before = psutil.Process(os.getpid()).memory_info().rss / (1024 * 1024)
start = time.time()
# Run function here
out = func(*args, **kwargs)
end = time.time()
mem_after = psutil.Process(os.getpid()).memory_info().rss / (1024 * 1024)
print(f"{func.__name__} -- RAM memory used: {mem_after - mem_before} MB -- Total time: {end - start:.6f} s")
return out
return wrapper
def main():
ds = Dataset.from_list([{'col': i} for i in range(DATASET_SIZE)])
print(f"Num chunks for original ds: {ds.data['col'].num_chunks}")
with tempfile.TemporaryDirectory() as tmpdir:
path1 = os.path.join(tmpdir, 'ds1')
print("Original ds save/load")
profile(ds.save_to_disk)(path1)
ds_loaded = profile(Dataset.load_from_disk)(path1)
print(f"Num chunks for original ds after reloading: {ds_loaded.data['col'].num_chunks}")
print("")
ds_select = ds.select(reversed(range(len(ds))))
print(f"Num chunks for selected ds: {ds_select.data['col'].num_chunks}")
del ds
del ds_loaded
gc.collect()
# This would happen anyway when we call save_to_disk
ds_select = profile(ds_select.flatten_indices)()
print(f"Num chunks for selected ds after flattening: {ds_select.data['col'].num_chunks}")
print("")
path2 = os.path.join(tmpdir, 'ds2')
print("Selected ds save/load")
profile(ds_select.save_to_disk)(path2)
del ds_select
gc.collect()
ds_select_loaded = profile(Dataset.load_from_disk)(path2)
print(f"Num chunks for selected ds after reloading: {ds_select_loaded.data['col'].num_chunks}")
if __name__ == '__main__':
main()
```
Sample result:
```
Num chunks for original ds: 1
Original ds save/load
save_to_disk -- RAM memory used: 0.515625 MB -- Total time: 0.253888 s
load_from_disk -- RAM memory used: 42.765625 MB -- Total time: 0.015176 s
Num chunks for original ds after reloading: 5000
Num chunks for selected ds: 1
flatten_indices -- RAM memory used: 4852.609375 MB -- Total time: 46.116774 s
Num chunks for selected ds after flattening: 5000000
Selected ds save/load
save_to_disk -- RAM memory used: 1326.65625 MB -- Total time: 42.309825 s
load_from_disk -- RAM memory used: 2085.953125 MB -- Total time: 11.659137 s
Num chunks for selected ds after reloading: 5000000
```
### Expected behavior
Saving/loading the dataset should be much faster and consume almost no extra memory thanks to pyarrow memory mapping.
### Environment info
- `datasets` version: 2.9.1.dev0
- Platform: macOS-13.1-arm64-arm-64bit
- Python version: 3.10.8
- PyArrow version: 11.0.0
- Pandas version: 1.5.3 | 5,541 |
https://github.com/huggingface/datasets/issues/5539 | IndexError: invalid index of a 0-dim tensor. Use `tensor.item()` in Python or `tensor.item<T>()` in C++ to convert a 0-dim tensor to a number | [
"Hi! The `set_transform` does not apply a custom formatting transform on a single example but the entire batch, so the fixed version of your transform would look as follows:\r\n```python\r\nfrom datasets import load_dataset\r\nimport torch\r\n\r\ndataset = load_dataset(\"lambdalabs/pokemon-blip-captions\", split='train')\r\ndef t(batch):\r\n return {\"test\": torch.tensor([1] * len(batch[next(iter(batch))]))}\r\n \r\ndataset.set_transform(t)\r\nd_0 = dataset[0]\r\n```\r\n\r\nStill, the formatter's error message should mention that a dict of **sequences** is expected as the returned value (not just a dict) to make debugging easier.",
"I can take this",
"Fixed in #5553 ",
"> Hi! The `set_transform` does not apply a custom formatting transform on a single example but the entire batch, so the fixed version of your transform would look as follows:\r\n> \r\n> ```python\r\n> from datasets import load_dataset\r\n> import torch\r\n> \r\n> dataset = load_dataset(\"lambdalabs/pokemon-blip-captions\", split='train')\r\n> def t(batch):\r\n> return {\"test\": torch.tensor([1] * len(batch[next(iter(batch))]))}\r\n> \r\n> dataset.set_transform(t)\r\n> d_0 = dataset[0]\r\n> ```\r\n> \r\n> Still, the formatter's error message should mention that a dict of **sequences** is expected as the returned value (not just a dict) to make debugging easier.\r\n\r\nok, will change it according to suggestion. Thanks for the reply!"
] | ### Describe the bug
When dataset contains a 0-dim tensor, formatting.py raises a following error and fails.
```bash
Traceback (most recent call last):
File "<path>/lib/python3.8/site-packages/datasets/formatting/formatting.py", line 501, in format_row
return _unnest(formatted_batch)
File "<path>/lib/python3.8/site-packages/datasets/formatting/formatting.py", line 137, in _unnest
return {key: array[0] for key, array in py_dict.items()}
File "<path>/lib/python3.8/site-packages/datasets/formatting/formatting.py", line 137, in <dictcomp>
return {key: array[0] for key, array in py_dict.items()}
IndexError: invalid index of a 0-dim tensor. Use `tensor.item()` in Python or `tensor.item<T>()` in C++ to convert a 0-dim tensor to a number
```
### Steps to reproduce the bug
Load whichever dataset and add transform method to add 0-dim tensor. Or create/find a dataset containing 0-dim tensor. E.g.
```python
from datasets import load_dataset
import torch
dataset = load_dataset("lambdalabs/pokemon-blip-captions", split='train')
def t(batch):
return {"test": torch.tensor(1)}
dataset.set_transform(t)
d_0 = dataset[0]
```
### Expected behavior
Extractor will correctly get a row from the dataset, even if it contains 0-dim tensor.
### Environment info
`datasets==2.8.0`, but it looks like it is also applicable to main branch version (as of 16th February) | 5,539 |
https://github.com/huggingface/datasets/issues/5538 | load_dataset in seaborn is not working for me. getting this error. | [
"Hi! `seaborn`'s `load_dataset` pulls datasets from [here](https://github.com/mwaskom/seaborn-data) and not from our Hub, so this issue is not related to our library in any way and should be reported in their repo instead."
] | TimeoutError Traceback (most recent call last)
~\anaconda3\lib\urllib\request.py in do_open(self, http_class, req, **http_conn_args)
1345 try:
-> 1346 h.request(req.get_method(), req.selector, req.data, headers,
1347 encode_chunked=req.has_header('Transfer-encoding'))
~\anaconda3\lib\http\client.py in request(self, method, url, body, headers, encode_chunked)
1278 """Send a complete request to the server."""
-> 1279 self._send_request(method, url, body, headers, encode_chunked)
1280
~\anaconda3\lib\http\client.py in _send_request(self, method, url, body, headers, encode_chunked)
1324 body = _encode(body, 'body')
-> 1325 self.endheaders(body, encode_chunked=encode_chunked)
1326
~\anaconda3\lib\http\client.py in endheaders(self, message_body, encode_chunked)
1273 raise CannotSendHeader()
-> 1274 self._send_output(message_body, encode_chunked=encode_chunked)
1275
~\anaconda3\lib\http\client.py in _send_output(self, message_body, encode_chunked)
1033 del self._buffer[:]
-> 1034 self.send(msg)
1035
~\anaconda3\lib\http\client.py in send(self, data)
973 if self.auto_open:
--> 974 self.connect()
975 else:
~\anaconda3\lib\http\client.py in connect(self)
1440
-> 1441 super().connect()
1442
~\anaconda3\lib\http\client.py in connect(self)
944 """Connect to the host and port specified in __init__."""
--> 945 self.sock = self._create_connection(
946 (self.host,self.port), self.timeout, self.source_address)
~\anaconda3\lib\socket.py in create_connection(address, timeout, source_address)
843 try:
--> 844 raise err
845 finally:
~\anaconda3\lib\socket.py in create_connection(address, timeout, source_address)
831 sock.bind(source_address)
--> 832 sock.connect(sa)
833 # Break explicitly a reference cycle
TimeoutError: [WinError 10060] A connection attempt failed because the connected party did not properly respond after a period of time, or established connection failed because connected host has failed to respond
During handling of the above exception, another exception occurred:
URLError Traceback (most recent call last)
~\AppData\Local\Temp/ipykernel_12220/2927704185.py in <module>
1 import seaborn as sn
----> 2 iris = sn.load_dataset('iris')
~\anaconda3\lib\site-packages\seaborn\utils.py in load_dataset(name, cache, data_home, **kws)
594 if name not in get_dataset_names():
595 raise ValueError(f"'{name}' is not one of the example datasets.")
--> 596 urlretrieve(url, cache_path)
597 full_path = cache_path
598 else:
~\anaconda3\lib\urllib\request.py in urlretrieve(url, filename, reporthook, data)
237 url_type, path = _splittype(url)
238
--> 239 with contextlib.closing(urlopen(url, data)) as fp:
240 headers = fp.info()
241
~\anaconda3\lib\urllib\request.py in urlopen(url, data, timeout, cafile, capath, cadefault, context)
212 else:
213 opener = _opener
--> 214 return opener.open(url, data, timeout)
215
216 def install_opener(opener):
~\anaconda3\lib\urllib\request.py in open(self, fullurl, data, timeout)
515
516 sys.audit('urllib.Request', req.full_url, req.data, req.headers, req.get_method())
--> 517 response = self._open(req, data)
518
519 # post-process response
~\anaconda3\lib\urllib\request.py in _open(self, req, data)
532
533 protocol = req.type
--> 534 result = self._call_chain(self.handle_open, protocol, protocol +
535 '_open', req)
536 if result:
~\anaconda3\lib\urllib\request.py in _call_chain(self, chain, kind, meth_name, *args)
492 for handler in handlers:
493 func = getattr(handler, meth_name)
--> 494 result = func(*args)
495 if result is not None:
496 return result
~\anaconda3\lib\urllib\request.py in https_open(self, req)
1387
1388 def https_open(self, req):
-> 1389 return self.do_open(http.client.HTTPSConnection, req,
1390 context=self._context, check_hostname=self._check_hostname)
1391
~\anaconda3\lib\urllib\request.py in do_open(self, http_class, req, **http_conn_args)
1347 encode_chunked=req.has_header('Transfer-encoding'))
1348 except OSError as err: # timeout error
-> 1349 raise URLError(err)
1350 r = h.getresponse()
1351 except:
URLError: <urlopen error [WinError 10060] A connection attempt failed because the connected party did not properly respond after a period of time, or established connection failed because connected host has failed to respond>
| 5,538 |
https://github.com/huggingface/datasets/issues/5537 | Increase speed of data files resolution | [
"#self-assign",
"You were right, if `self.dir_cache` is not None in glob, it is exactly the same as what is returned by find, at least for all the tests we have, and some extended evaluation I did across a random sample of about 1000 datasets. \r\n\r\nThanks for the nice hints, and let me know if this is not exactly what we want here!\r\n\r\nsee PR: https://github.com/huggingface/datasets/pull/5704\r\n\r\n",
"I think we can make the data files resolution (significantly) faster in 2 steps:\r\n\r\n1. `glob` calls `find` (which in turn calls `ls`), so we need `find` to be fast, and this can be achieved by fetching all the entries in a single API call and avoiding calls to `ls`. Implementing this for `HfFileSystem.find` (the one in `huggingface_hub`) is on my TO-DO list.\r\n2. caching the repeated `find` calls in `_get_data_files_patterns` when the `data_files` patterns are not provided in `load_dataset`. To address this, we can introduce a `_resolve_single_pattern` function that would accept a filesystem object and a list of regex patterns to resolve. Then we can wrap this filesystem object in `_get_data_files_patterns` with an object that would cache the find calls before resolving the patterns with `_resolve_single_pattern`. (Feel free to suggest a cleaner implementation)\r\n\r\nWDYT?",
"Good idea :) \r\n\r\nFor 2:\r\n\r\nThat would work ! It's also possible to have a FileSystem with a cache on `.find` and use it inside the resolver passed to `_get_data_files_patterns`. Right now they're pretty simple:\r\n\r\n```python\r\n# for remote repositories\r\nresolver = partial(_resolve_single_pattern_in_dataset_repository, dataset_info, base_path=base_path)\r\n# for local\r\nresolver = partial(_resolve_single_pattern_locally, base_path)\r\n```",
"something like this maybe (with Quentin's reimplementation of `HfFilesystem.find`)?\r\n\r\n ```\r\n @lru_cache(max_size=None)\r\n def _find(self, path, maxdepth=None, withdirs=False, detail=False, **kwargs):\r\n```\r\n\r\nIn any case please let me know if I can help in any way!"
] | Certain datasets like `bigcode/the-stack-dedup` have so many files that loading them takes forever right from the data files resolution step.
`datasets` uses file patterns to check the structure of the repository but it takes too much time to iterate over and over again on all the data files.
This comes from `resolve_patterns_in_dataset_repository` which calls `_resolve_single_pattern_in_dataset_repository`, which iterates on all the files at
```python
glob_iter = [PurePath(filepath) for filepath in fs.glob(PurePath(pattern).as_posix()) if fs.isfile(filepath)]
```
but calling `glob` on such a dataset is too expensive. Indeed it calls `ls()` in `hffilesystem.py` too many times.
Maybe `glob` can be more optimized in `hffilesystem.py`, or the data files resolution can directly be implemented in the filesystem by checking its `dir_cache` ? | 5,537 |
https://github.com/huggingface/datasets/issues/5536 | Failure to hash function when using .map() | [
"Hi ! `enc` is not hashable:\r\n```python\r\nimport tiktoken\r\nfrom datasets.fingerprint import Hasher\r\n\r\nenc = tiktoken.get_encoding(\"gpt2\")\r\nHasher.hash(enc)\r\n# raises TypeError: cannot pickle 'builtins.CoreBPE' object\r\n```\r\nIt happens because it's not picklable, and because of that it's not possible to cache the result of `map`, hence the warning message.\r\n\r\nYou can find more details about caching here: https://huggingface.co/docs/datasets/about_cache\r\n\r\nYou can also provide your own unique hash in `map` if you want, with the `new_fingerprint` argument.\r\nOr disable caching using\r\n```python\r\nimport datasets\r\ndatasets.disable_caching()\r\n```",
"@lhoestq Thank you for the explanation and advice. Will relay all of this to the repo where this (non)issue arose. \r\n\r\nGreat job with huggingface! ",
"We made tiktoken tokenizers hashable in #5552, which is included in today's release `datasets==2.10.0`",
"Just a heads up that when I'm trying to use TikToken along with the a given Dataset `.map()` method, I am still met with the following error :\r\n\r\n```\r\n File \"/opt/conda/lib/python3.8/site-packages/dill/_dill.py\", line 388, in save\r\n StockPickler.save(self, obj, save_persistent_id)\r\n File \"/opt/conda/lib/python3.8/pickle.py\", line 578, in save\r\n rv = reduce(self.proto)\r\nTypeError: cannot pickle 'builtins.CoreBPE' object\r\n```\r\n\r\nMy current environment is running datasets v2.10.0.",
"cc @mariosasko ",
"@lhoestq @edhenry I am also seeing this, do you have any suggested solution?",
"With which `datasets` version ? Can you try to udpate ?",
"@lhoestq @edhenry I am on datasets version `'2.12.0'. I see the same `TypeError: cannot pickle 'builtins.CoreBPE' object` that others are seeing.",
"I am able to reproduce this on datasets 2.14.2. The `datasets.disable_caching()` doesn't work around it.\r\n\r\n@lhoestq - you might want to reopen this issue. Because of this issue folks won't be able run Karpathy's NanoGPT :(.",
"update: temporarily solved the problem by setting\r\n```\r\n--preprocess_num_workers 1\r\n```\r\n\r\n-------------\r\nI have met the same problem, here is my env:\r\n```\r\ndatasets 2.14.4\r\ntransformers 4.31.0\r\ntiktoken 0.4.0\r\ntorch 1.13.1\r\n```",
"@mengban I cannot reproduce the issue even with these versions installed. It would help if you could provide info about your system and the `pip list` output.",
"@mariosasko Please take a look at this\r\n```python\r\nfrom typing import Any\r\nfrom datasets import Dataset\r\nimport tiktoken\r\n\r\ndataset = Dataset.from_list([{\"n\": str(i)} for i in range(20)])\r\nenc = tiktoken.get_encoding(\"gpt2\")\r\n\r\n\r\nclass A:\r\n tokenizer = enc #tiktoken.get_encoding(\"gpt2\")\r\n\r\n def __call__(self, example) -> Any:\r\n ids = self.tokenizer.encode(example[\"n\"])\r\n example[\"len\"] = len(ids)\r\n return example\r\n\r\na = A()\r\n\r\ndef process(example):\r\n ids = a.tokenizer.encode(example[\"n\"])\r\n example[\"len\"] = len(ids)\r\n return example\r\n\r\n# success\r\ntokenized = dataset.map(process, desc=\"tiktoken\", num_proc=2)\r\n\r\n# raise TypeError: cannot pickle 'builtins.CoreBPE' object\r\ntokenized = dataset.map(a, desc=\"tiktoken\", num_proc=2)\r\n```\r\n\r\npip list\r\n```\r\ndatasets 2.14.4\r\ntiktoken 0.4.0\r\n```",
"Thanks @maxwellzh! Our `Hasher` works with this snippet, but the problem is running multiprocessing with a non-serializable `tiktoken.Encoding` object.\r\n\r\nInserting the following code before the `map` should fix this:\r\n```python\r\nimport copyreg\r\n\r\ndef pickle_Encoding(enc):\r\n return (functools.partial(tiktoken.core.Encoding, enc.name, pat_str=enc._pat_str, mergeable_ranks=enc._mergeable_ranks, special_tokens=enc._special_tokens), ())\r\n\r\ncopyreg.pickle(tiktoken.core.Encoding, pickle_Encoding)\r\n```\r\n\r\nBut the best fix would be implementing `__reduce__` for `tiktoken.Encoding` or `tiktoken.CoreBPE`. If I find time, I'll try to fix this in the `tiktoken` repo.",
"I think the right way to fix this would be to have new tokenizer instance for each process. This applies to many other tokenizers that don't support multi-process or have bugs. To do this, first define tokenizer factory class like this:\r\n\r\n```\r\n class TikTokenFactory:\r\n def __init__(self):\r\n self._enc = None\r\n self.eot_token = None\r\n\r\n def encode_ordinary(self, text):\r\n if self._enc is None:\r\n self._enc = tiktoken.get_encoding(\"gpt2\")\r\n self.eot_token = self._enc.eot_token\r\n return self._enc.encode_ordinary(text)\r\n```\r\n\r\nNow use this in `.map()` like this:\r\n\r\n```\r\n # tokenize the dataset\r\n tokenized = dataset.map(\r\n partial(process, TikTokenFactory()),\r\n remove_columns=['text'],\r\n desc=\"tokenizing the splits\",\r\n num_proc=max(1, cpu_count()//2),\r\n )\r\n```\r\n\r\nA full working example is here: https://github.com/sytelus/nanoGPT/blob/refactor/nanogpt_common/hf_data_prepare.py"
] | ### Describe the bug
_Parameter 'function'=<function process at 0x7f1ec4388af0> of the transform datasets.arrow_dataset.Dataset.\_map_single couldn't be hashed properly, a random hash was used instead. Make sure your transforms and parameters are serializable with pickle or dill for the dataset fingerprinting and caching to work. If you reuse this transform, the caching mechanism will consider it to be different from the previous calls and recompute everything. This warning is only showed once. Subsequent hashing failures won't be showed._
This issue with `.map()` happens for me consistently, as also described in closed issue #4506
Dataset indices can be individually serialized using dill and pickle without any errors. I'm using tiktoken to encode in the function passed to map(). Similarly, indices can be individually encoded without error.
### Steps to reproduce the bug
```py
from datasets import load_dataset
import tiktoken
dataset = load_dataset("stas/openwebtext-10k")
enc = tiktoken.get_encoding("gpt2")
tokenized = dataset.map(
process,
remove_columns=['text'],
desc="tokenizing the OWT splits",
)
def process(example):
ids = enc.encode(example['text'])
ids.append(enc.eot_token)
out = {'ids': ids, 'len': len(ids)}
return out
```
### Expected behavior
Should encode simple text objects.
### Environment info
Python versions tried: both 3.8 and 3.10.10
`PYTHONUTF8=1` as env variable
Datasets tried:
- stas/openwebtext-10k
- rotten_tomatoes
- local text file
OS: Ubuntu Linux 20.04
Package versions:
- torch 1.13.1
- dill 0.3.4 (if using 0.3.6 - same issue)
- datasets 2.9.0
- tiktoken 0.2.0
| 5,536 |
https://github.com/huggingface/datasets/issues/5534 | map() breaks at certain dataset size when using Array3D | [
"Hi! This code works for me locally or in Colab. What's the output of `python -c \"import pyarrow as pa; print(pa.__version__)\"` when you run it inside your environment?",
"Thanks for looking into this!\r\nThe output of `python -c \"import pyarrow as pa; print(pa.__version__)\"` is:\r\n```\r\n11.0.0\r\n```\r\n\r\nI did the following to setup the environment:\r\n```\r\nconda create -n datasets_debug python=3.9\r\nconda activate datasets_debug\r\npip install datasets==2.9.0\r\n```\r\n\r\nI just tested this on another machine (Ubuntu 18.04.6 LTS) with the same result as mentioned in the issue description.\r\n"
] | ### Describe the bug
`map()` magically breaks when using a `Array3D` feature and mapping it. I created a very simple dummy dataset (see below). When filtering it down to 95 elements I can apply map, but it breaks when filtering it down to just 96 entries with the following exception:
```
Traceback (most recent call last):
File "/home/arbi01/miniconda3/envs/tmp9/lib/python3.9/site-packages/datasets/arrow_dataset.py", line 3255, in _map_single
writer.finalize() # close_stream=bool(buf_writer is None)) # We only close if we are writing in a file
File "/home/arbi01/miniconda3/envs/tmp9/lib/python3.9/site-packages/datasets/arrow_writer.py", line 581, in finalize
self.write_examples_on_file()
File "/home/arbi01/miniconda3/envs/tmp9/lib/python3.9/site-packages/datasets/arrow_writer.py", line 440, in write_examples_on_file
batch_examples[col] = array_concat(arrays)
File "/home/arbi01/miniconda3/envs/tmp9/lib/python3.9/site-packages/datasets/table.py", line 1931, in array_concat
return _concat_arrays(arrays)
File "/home/arbi01/miniconda3/envs/tmp9/lib/python3.9/site-packages/datasets/table.py", line 1901, in _concat_arrays
return array_type.wrap_array(_concat_arrays([array.storage for array in arrays]))
File "/home/arbi01/miniconda3/envs/tmp9/lib/python3.9/site-packages/datasets/table.py", line 1922, in _concat_arrays
_concat_arrays([array.values for array in arrays]),
File "/home/arbi01/miniconda3/envs/tmp9/lib/python3.9/site-packages/datasets/table.py", line 1922, in _concat_arrays
_concat_arrays([array.values for array in arrays]),
File "/home/arbi01/miniconda3/envs/tmp9/lib/python3.9/site-packages/datasets/table.py", line 1920, in _concat_arrays
return pa.ListArray.from_arrays(
File "pyarrow/array.pxi", line 1997, in pyarrow.lib.ListArray.from_arrays
File "pyarrow/array.pxi", line 1527, in pyarrow.lib.Array.validate
File "pyarrow/error.pxi", line 100, in pyarrow.lib.check_status
pyarrow.lib.ArrowInvalid: Negative offsets in list array
During handling of the above exception, another exception occurred:
Traceback (most recent call last):
File "<stdin>", line 1, in <module>
File "/home/arbi01/miniconda3/envs/tmp9/lib/python3.9/site-packages/datasets/arrow_dataset.py", line 2815, in map
return self._map_single(
File "/home/arbi01/miniconda3/envs/tmp9/lib/python3.9/site-packages/datasets/arrow_dataset.py", line 546, in wrapper
out: Union["Dataset", "DatasetDict"] = func(self, *args, **kwargs)
File "/home/arbi01/miniconda3/envs/tmp9/lib/python3.9/site-packages/datasets/arrow_dataset.py", line 513, in wrapper
out: Union["Dataset", "DatasetDict"] = func(self, *args, **kwargs)
File "/home/arbi01/miniconda3/envs/tmp9/lib/python3.9/site-packages/datasets/fingerprint.py", line 480, in wrapper
out = func(self, *args, **kwargs)
File "/home/arbi01/miniconda3/envs/tmp9/lib/python3.9/site-packages/datasets/arrow_dataset.py", line 3259, in _map_single
writer.finalize()
File "/home/arbi01/miniconda3/envs/tmp9/lib/python3.9/site-packages/datasets/arrow_writer.py", line 581, in finalize
self.write_examples_on_file()
File "/home/arbi01/miniconda3/envs/tmp9/lib/python3.9/site-packages/datasets/arrow_writer.py", line 440, in write_examples_on_file
batch_examples[col] = array_concat(arrays)
File "/home/arbi01/miniconda3/envs/tmp9/lib/python3.9/site-packages/datasets/table.py", line 1931, in array_concat
return _concat_arrays(arrays)
File "/home/arbi01/miniconda3/envs/tmp9/lib/python3.9/site-packages/datasets/table.py", line 1901, in _concat_arrays
return array_type.wrap_array(_concat_arrays([array.storage for array in arrays]))
File "/home/arbi01/miniconda3/envs/tmp9/lib/python3.9/site-packages/datasets/table.py", line 1922, in _concat_arrays
_concat_arrays([array.values for array in arrays]),
File "/home/arbi01/miniconda3/envs/tmp9/lib/python3.9/site-packages/datasets/table.py", line 1922, in _concat_arrays
_concat_arrays([array.values for array in arrays]),
File "/home/arbi01/miniconda3/envs/tmp9/lib/python3.9/site-packages/datasets/table.py", line 1920, in _concat_arrays
return pa.ListArray.from_arrays(
File "pyarrow/array.pxi", line 1997, in pyarrow.lib.ListArray.from_arrays
File "pyarrow/array.pxi", line 1527, in pyarrow.lib.Array.validate
File "pyarrow/error.pxi", line 100, in pyarrow.lib.check_status
pyarrow.lib.ArrowInvalid: Negative offsets in list array
```
### Steps to reproduce the bug
1. put following dataset loading script into: debug/debug.py
```python
import datasets
import numpy as np
class DEBUG(datasets.GeneratorBasedBuilder):
"""DEBUG dataset."""
def _info(self):
return datasets.DatasetInfo(
features=datasets.Features(
{
"id": datasets.Value("uint8"),
"img_data": datasets.Array3D(shape=(3, 224, 224), dtype="uint8"),
},
),
supervised_keys=None,
)
def _split_generators(self, dl_manager):
return [datasets.SplitGenerator(name=datasets.Split.TRAIN)]
def _generate_examples(self):
for i in range(149):
image_np = np.zeros(shape=(3, 224, 224), dtype=np.int8).tolist()
yield f"id_{i}", {"id": i, "img_data": image_np}
```
2. try the following code:
```python
import datasets
def add_dummy_col(ex):
ex["dummy"] = "test"
return ex
ds = datasets.load_dataset(path="debug", split="train")
# works
ds_filtered_works = ds.filter(lambda example: example["id"] < 95)
print(f"filtered result size: {len(ds_filtered_works)}")
# output:
# filtered result size: 95
ds_mapped_works = ds_filtered_works.map(add_dummy_col)
# fails
ds_filtered_error = ds.filter(lambda example: example["id"] < 96)
print(f"filtered result size: {len(ds_filtered_error)}")
# output:
# filtered result size: 96
ds_mapped_error = ds_filtered_error.map(add_dummy_col)
```
### Expected behavior
The example code does not fail.
### Environment info
Python 3.9.16 (main, Jan 11 2023, 16:05:54); [GCC 11.2.0] :: Anaconda, Inc. on linux
datasets 2.9.0 | 5,534 |
https://github.com/huggingface/datasets/issues/5532 | train_test_split in arrow_dataset does not ensure to keep single classes in test set | [
"Hi! You can get this behavior by specifying `stratify_by_column=\"label\"` in `train_test_split`.\r\n\r\nThis is the full example:\r\n```python\r\nimport numpy as np\r\nfrom datasets import Dataset, ClassLabel\r\n\r\ndata = [\r\n {'label': 0, 'text': \"example1\"},\r\n {'label': 1, 'text': \"example2\"},\r\n {'label': 1, 'text': \"example3\"},\r\n {'label': 1, 'text': \"example4\"},\r\n {'label': 0, 'text': \"example5\"},\r\n {'label': 1, 'text': \"example6\"},\r\n {'label': 2, 'text': \"example7\"},\r\n {'label': 2, 'text': \"example8\"}\r\n]\r\n\r\nfor _ in range(10):\r\n data_set = Dataset.from_list(data)\r\n data_set = data_set.cast_column(\"label\", ClassLabel(num_classes=3))\r\n data_set = data_set.train_test_split(test_size=0.5, stratify_by_column=\"label\")\r\n unique_labels_train = np.unique(data_set[\"train\"][:][\"label\"])\r\n unique_labels_test = np.unique(data_set[\"test\"][:][\"label\"])\r\n assert len(unique_labels_train) >= len(unique_labels_test) \r\n```\r\n"
] | ### Describe the bug
When I have a dataset with very few (e.g. 1) examples per class and I call the train_test_split function on it, sometimes the single class will be in the test set. thus will never be considered for training.
### Steps to reproduce the bug
```
import numpy as np
from datasets import Dataset
data = [
{'label': 0, 'text': "example1"},
{'label': 1, 'text': "example2"},
{'label': 1, 'text': "example3"},
{'label': 1, 'text': "example4"},
{'label': 0, 'text': "example5"},
{'label': 1, 'text': "example6"},
{'label': 2, 'text': "example7"},
{'label': 2, 'text': "example8"}
]
for _ in range(10):
data_set = Dataset.from_list(data)
data_set = data_set.train_test_split(test_size=0.5)
data_set["train"]
unique_labels_train = np.unique(data_set["train"][:]["label"])
unique_labels_test = np.unique(data_set["test"][:]["label"])
assert len(unique_labels_train) >= len(unique_labels_test)
```
### Expected behavior
I expect to have every available class at least once in my training set.
### Environment info
- `datasets` version: 2.9.0
- Platform: Linux-5.15.65+-x86_64-with-debian-bullseye-sid
- Python version: 3.7.12
- PyArrow version: 11.0.0
- Pandas version: 1.3.5
| 5,532 |
https://github.com/huggingface/datasets/issues/5531 | Invalid Arrow data from JSONL | [] | This code fails:
```python
from datasets import Dataset
ds = Dataset.from_json(path_to_file)
ds.data.validate()
```
raises
```python
ArrowInvalid: Column 2: In chunk 1: Invalid: Struct child array #3 invalid: Invalid: Length spanned by list offsets (4064) larger than values array (length 4063)
```
This causes many issues for @TevenLeScao:
- `map` fails because it fails to rewrite invalid arrow arrays
```python
~/Desktop/hf/datasets/src/datasets/arrow_writer.py in write_examples_on_file(self)
438 if all(isinstance(row[0][col], (pa.Array, pa.ChunkedArray)) for row in self.current_examples):
439 arrays = [row[0][col] for row in self.current_examples]
--> 440 batch_examples[col] = array_concat(arrays)
441 else:
442 batch_examples[col] = [
~/Desktop/hf/datasets/src/datasets/table.py in array_concat(arrays)
1885
1886 if not _is_extension_type(array_type):
-> 1887 return pa.concat_arrays(arrays)
1888
1889 def _offsets_concat(offsets):
~/.virtualenvs/hf-datasets/lib/python3.7/site-packages/pyarrow/array.pxi in pyarrow.lib.concat_arrays()
~/.virtualenvs/hf-datasets/lib/python3.7/site-packages/pyarrow/error.pxi in pyarrow.lib.pyarrow_internal_check_status()
~/.virtualenvs/hf-datasets/lib/python3.7/site-packages/pyarrow/error.pxi in pyarrow.lib.check_status()
ArrowIndexError: array slice would exceed array length
```
- `to_dict()` **segfaults** ⚠️
```python
/Users/runner/work/crossbow/crossbow/arrow/cpp/src/arrow/array/data.cc:99: Check failed: (off) <= (length) Slice offset greater
than array length
```
To reproduce: unzip the archive and run the above code using `sanity_oscar_en.jsonl`
[sanity_oscar_en.jsonl.zip](https://github.com/huggingface/datasets/files/10734124/sanity_oscar_en.jsonl.zip)
PS: reading using pandas and converting to Arrow works though (note that the dataset lives in RAM in this case):
```python
ds = Dataset.from_pandas(pd.read_json(path_to_file, lines=True))
ds.data.validate()
```
| 5,531 |
https://github.com/huggingface/datasets/issues/5525 | TypeError: Couldn't cast array of type string to null | [
"Thanks for reporting, @TJ-Solergibert.\r\n\r\nWe cannot access your Colab notebook: `There was an error loading this notebook. Ensure that the file is accessible and try again.`\r\nCould you please make it publicly accessible?\r\n",
"I swear it's public, I've checked the settings and I've been able to open it in incognito mode.\r\n\r\nNotebook: https://colab.research.google.com/drive/1JCrS7FlGfu_kFqChMrwKZ_bpabnIMqbP?usp=sharing\r\n\r\nAnyway, this is the code to reproduce the error:\r\n\r\n```python3\r\nfrom datasets import ClassLabel\r\nfrom datasets import load_dataset\r\n\r\neuroparl_ds = load_dataset(\"tj-solergibert/Europarl-ST\")\r\n\r\nsource_lang = \"nl\"\r\nlanguages = list(europarl_ds[\"train\"][0][\"transcriptions\"].keys())\r\nClassLabels = ClassLabel(num_classes = len(languages), names = languages)\r\n\r\ndef map_label2id(example):\r\n example['dest_lang'] = ClassLabels.str2int(example['dest_lang'])\r\n return example\r\n\r\ndef unfold_transcriptions(example):\r\n for lang in languages:\r\n example[lang] = example[\"transcriptions\"][lang]\r\n return example\r\n\r\ndef unroll(batch, src_lang, dest_langs):\r\n source_t, dest_t, dest_l = [], [], []\r\n for lang in dest_langs: \r\n source_t += batch[src_lang]\r\n dest_t += batch[lang]\r\n dest_l += [lang]\r\n return_dict = {\"source_text\": source_t, \"dest_text\": dest_t, \"dest_lang\": dest_l}\r\n return return_dict\r\n\r\ndef preprocess_split(ds_split, src_lang):\r\n dest_langs = [x for x in languages if x != src_lang]\r\n\r\n ds_split = ds_split.map(unroll, fn_kwargs= {\"src_lang\": src_lang, \"dest_langs\": dest_langs}, batched = True, batch_size = 1, remove_columns= list(languages))\r\n ds_split = ds_split.filter(lambda x: x[\"source_text\"] != None and x[\"dest_text\"] != None) # Remove incomplete translations\r\n ds_split = ds_split.filter(lambda x: x[\"source_text\"] != \"None\" and x[\"dest_text\"] != \"None\")\r\n ds_split = ds_split.map(map_label2id) \r\n ds_split = ds_split.cast_column(\"dest_lang\", ClassLabels)\r\n return ds_split\r\n\r\ndef reset_cortas(example):\r\n for lang in languages:\r\n if isinstance(example[lang], str):\r\n if example[lang].isnumeric () or len(example[lang]) <= 5:\r\n example[lang] = \"None\"\r\n return example\r\n\r\ndef clean_dataset(dataset):\r\n # Remove columns\r\n dataset = dataset.remove_columns([\"original_speech\", \"original_language\", \"audio_path\", \"segment_start\", \"segment_end\"])\r\n # Unfold\r\n dataset = dataset.map(unfold_transcriptions, remove_columns = [\"transcriptions\"])\r\n dataset = dataset.map(reset_cortas)\r\n return dataset\r\n\r\nprocessed_europarl = clean_dataset(europarl_ds[\"test\"])\r\nnew_train_ds = preprocess_split(processed_europarl, 'nl')\r\n```",
"Thanks, @TJ-Solergibert. I can access your notebook now. Maybe it was just a temporary issue.\r\n\r\nAt first sight, it seems something related to your data: maybe some of the examples do not have all the transcriptions for all the languages. Then, some of them are null when unrolled. And when trying to concatenate with the other rows containing strings, the cast issue is raised (the arrays to be concatenated have different types).\r\n\r\nDo you think this could be the case?",
"See, in this example, \"nl\" and \"ro\" transcripts are null:\r\n```python\r\n>>> europarl_ds[\"test\"][:1]\r\n{'original_speech': ['− Señor Presidente, en primer lugar, quisiera felicitar al señor Seeber por el trabajo realizado, porque en su informe se recogen muchas de las preocupaciones manifestadas en esta'],\r\n 'original_language': ['es'],\r\n 'audio_path': ['es/audios/en.20081008.24.3-238.m4a'],\r\n 'segment_start': [0.6200000047683716],\r\n 'segment_end': [11.319999694824219],\r\n 'transcriptions': [{'de': '− Herr Präsident! Zunächst möchte ich Richard Seeber zu der von ihm geleisteten Arbeit gratulieren, denn sein Bericht greift viele der in diesem Haus zum Ausdruck gebrachten Anliegen',\r\n 'en': '− Mr President, firstly I would like to congratulate Mr Seeber on the work he has done, because his report picks up many of the concerns expressed in this',\r\n 'es': '− Señor Presidente, en primer lugar, quisiera felicitar al señor Seeber por el trabajo realizado, porque en su informe se recogen muchas de las preocupaciones manifestadas en esta',\r\n 'fr': '− Monsieur le Président, je voudrais tout d ’ abord féliciter M. Seeber pour le travail qu ’ il a effectué, parce que son rapport reprend beaucoup des inquiétudes exprimées au sein de cette',\r\n 'it': \"− Signor Presidente, mi congratulo innanzi tutto con l'onorevole Seeber per il lavoro svolto, perché la sua relazione accoglie molti dei timori espressi da quest'Aula\",\r\n 'nl': None,\r\n 'pl': '− Panie przewodniczący! Po pierwsze chciałabym pogratulować panu posłowi Seeberowi wykonanej pracy, ponieważ jego sprawozdanie podejmuje szereg podnoszonych w tej Izbie',\r\n 'pt': '− Senhor Presidente, começo por felicitar o senhor deputado Seeber pelo trabalho que desenvolveu em torno deste relatório, que retoma muitas das preocupações expressas nesta',\r\n 'ro': None}]}\r\n```\r\n```python\r\n>>> processed_europarl[0]\r\n{'de': '− Herr Präsident! Zunächst möchte ich Richard Seeber zu der von ihm geleisteten Arbeit gratulieren, denn sein Bericht greift viele der in diesem Haus zum Ausdruck gebrachten Anliegen',\r\n 'en': '− Mr President, firstly I would like to congratulate Mr Seeber on the work he has done, because his report picks up many of the concerns expressed in this',\r\n 'es': '− Señor Presidente, en primer lugar, quisiera felicitar al señor Seeber por el trabajo realizado, porque en su informe se recogen muchas de las preocupaciones manifestadas en esta',\r\n 'fr': '− Monsieur le Président, je voudrais tout d ’ abord féliciter M. Seeber pour le travail qu ’ il a effectué, parce que son rapport reprend beaucoup des inquiétudes exprimées au sein de cette',\r\n 'it': \"− Signor Presidente, mi congratulo innanzi tutto con l'onorevole Seeber per il lavoro svolto, perché la sua relazione accoglie molti dei timori espressi da quest'Aula\",\r\n 'nl': None,\r\n 'pl': '− Panie przewodniczący! Po pierwsze chciałabym pogratulować panu posłowi Seeberowi wykonanej pracy, ponieważ jego sprawozdanie podejmuje szereg podnoszonych w tej Izbie',\r\n 'pt': '− Senhor Presidente, começo por felicitar o senhor deputado Seeber pelo trabalho que desenvolveu em torno deste relatório, que retoma muitas das preocupações expressas nesta',\r\n 'ro': None}\r\n```",
"You can fix this issue by forcing the cast of None to str by hand:\r\n- If you replace this line:\r\n```python\r\nsource_t += batch[src_lang]\r\n```\r\n- With this line (because the batch size is 1):\r\n```python\r\nsource_t += [str(batch[src_lang][0])]\r\n```\r\n- Or with this line (if the batch size were larger than 1):\r\n```python\r\nsource_t += [str(text) for text in batch[src_lang]]\r\n```",
"Problem solved! Thanks @albertvillanova, now I have even increased the batch size and it's crazy fast :rocket: !"
] | ### Describe the bug
Processing a dataset I alredy uploaded to the Hub (https://huggingface.co/datasets/tj-solergibert/Europarl-ST) I found that for some splits and some languages (test split, source_lang = "nl") after applying a map function I get the mentioned error.
I alredy tried reseting the shorter strings (reset_cortas function). It only happends with NL, PL, RO and PT. It does not make sense since when processing the other languages I also use the corpus of those that fail and it does not cause any errors.
I suspect that the error may be in this direction:
We use cast_array_to_feature to support casting to custom types like Audio and Image # Also, when trying type "string", we don't want to convert integers or floats to "string". # We only do it if trying_type is False - since this is what the user asks for.
### Steps to reproduce the bug
Here I link a colab notebook to reproduce the error:
https://colab.research.google.com/drive/1JCrS7FlGfu_kFqChMrwKZ_bpabnIMqbP?authuser=1#scrollTo=FBAvlhMxIzpA
### Expected behavior
Data processing does not fail. A correct example can be seen here: https://huggingface.co/datasets/tj-solergibert/Europarl-ST-processed-mt-en
### Environment info
- `datasets` version: 2.9.0
- Platform: Linux-5.10.147+-x86_64-with-glibc2.29
- Python version: 3.8.10
- PyArrow version: 9.0.0
- Pandas version: 1.3.5 | 5,525 |
https://github.com/huggingface/datasets/issues/5523 | Checking that split name is correct happens only after the data is downloaded | [] | ### Describe the bug
Verification of split names (=indexing data by split) happens after downloading the data. So when the split name is incorrect, users learn about that only after the data is fully downloaded, for large datasets it might take a lot of time.
### Steps to reproduce the bug
Load any dataset with random split name, for example:
```python
from datasets import load_dataset
load_dataset("mozilla-foundation/common_voice_11_0", "en", split="blabla")
```
and the download will start smoothly, despite there is no split named "blabla".
### Expected behavior
Raise error when split name is incorrect.
### Environment info
`datasets==2.9.1.dev0` | 5,523 |
https://github.com/huggingface/datasets/issues/5520 | ClassLabel.cast_storage raises TypeError when called on an empty IntegerArray | [] | ### Describe the bug
`ClassLabel.cast_storage` raises `TypeError` when called on an empty `IntegerArray`.
### Steps to reproduce the bug
Minimal steps:
```python
import pyarrow as pa
from datasets import ClassLabel
ClassLabel(names=['foo', 'bar']).cast_storage(pa.array([], pa.int64()))
```
In practice, this bug arises in situations like the one below:
```python
from datasets import ClassLabel, Dataset, Features, Sequence
dataset = Dataset.from_dict({'labels': [[], []]}, features=Features({'labels': Sequence(ClassLabel(names=['foo', 'bar']))}))
# this raises TypeError
dataset.map(batched=True, batch_size=1)
```
### Expected behavior
`ClassLabel.cast_storage` should return an empty Int64Array.
### Environment info
- `datasets` version: 2.9.1.dev0
- Platform: Linux-4.15.0-1032-aws-x86_64-with-glibc2.27
- Python version: 3.10.6
- PyArrow version: 11.0.0
- Pandas version: 1.5.3 | 5,520 |
https://github.com/huggingface/datasets/issues/5517 | `with_format("numpy")` silently downcasts float64 to float32 features | [
"Hi! This behavior stems from these lines:\r\n\r\nhttps://github.com/huggingface/datasets/blob/b065547654efa0ec633cf373ac1512884c68b2e1/src/datasets/formatting/np_formatter.py#L45-L46\r\n\r\nI agree we should preserve the original type whenever possible and downcast explicitly with a warning.\r\n\r\n@lhoestq Do you remember why we need this \"default dtype\" logic in our formatters?",
"I was also wondering why the default type logic is needed. Me just deleting it is probably too naive of a solution.",
"Hmm I think the idea was to end up with the usual default precision for deep learning models - no matter how the data was stored or where it comes from.\r\n\r\nFor example in NLP we store tokens using an optimized low precision to save disk space, but when we set the format to `torch` we actually need to get `int64`. Although the need for a default for integers also comes from numpy not returning the same integer precision depending on your machine. Finally I guess we added a default for floats as well for consistency.\r\n\r\nI'm a bit embarrassed by this though, as a user I'd have expected to get the same precision indeed as well and get a zero copy view.",
"Will you fix this or should I open a PR?",
"Unfortunately removing it for integers is a breaking change for most `transformers` + `datasets` users for NLP (which is a common case). Removing it for floats is a breaking change for `transformers` + `datasets` for ASR as well. And it also is a breaking change for the other users relying on this behavior.\r\n\r\nTherefore I think that the only short term solution is for the user to provide `dtype=` manually and document better this behavior. We could also extend `dtype` to accept a value that means \"return the same dtype as the underlying storage\" and make it easier to do zero copy.",
"@lhoestq It should be fine to remove this conversion in Datasets 3.0, no? For now, we can warn the user (with a log message) about the future change when the default type is changed.",
"Let's see with the transformers team if it sounds reasonable ? We'd have to fix multiple example scripts though.\r\n\r\nIf it's not ok we can also explore keeping this behavior only for tokens and audio data.",
"IMO being coupled with Transformers can lead to unexpected behavior when one tries to use our lib without pairing it with Transformers, so I think it's still important to \"fix\" this, even if it means we will need to update Transformers' example scripts afterward.\r\n",
"Ideally let's update the `transformers` example scripts before the change :P",
"For others that run into the same issue: A temporary workaround for me is this:\r\n```python\r\ndef numpy_transform(batch):\r\n return {key: np.asarray(val) for key, val in batch.items()}\r\n\r\ndataset = dataset.with_transform(numpy_transform)\r\n```",
"This behavior (silent upcast from `int32` to `int64`) is also unexpected for the user in https://discuss.huggingface.co/t/standard-getitem-returns-wrong-data-type-for-arrays/62470/2",
"Hi, I stumbled on a variation that upcasts uint8 to int64. I would expect the dtype to be the same as it was when I generated the dataset.\r\n\r\n```\r\nimport numpy as np\r\nimport datasets as ds\r\n\r\nfoo = np.random.randint(0, 256, size=(5, 10, 10), dtype=np.uint8)\r\n\r\nfeatures = ds.Features({\"foo\": ds.Array2D((10, 10), \"uint8\")})\r\ndataset = ds.Dataset.from_dict({\"foo\": foo}, features=features)\r\ndataset.set_format(\"torch\")\r\nprint(\"feature dtype:\", dataset.features[\"foo\"].dtype)\r\nprint(\"array dtype:\", dataset[\"foo\"].dtype)\r\n\r\n# feature dtype: uint8\r\n# array dtype: torch.int64\r\n```\r\n",
"workaround to remove torch upcasting\r\n\r\n```\r\nimport datasets as ds\r\nimport torch\r\n\r\nclass FixedTorchFormatter(ds.formatting.TorchFormatter):\r\n def _tensorize(self, value):\r\n return torch.from_numpy(value)\r\n\r\n\r\nds.formatting._register_formatter(FixedTorchFormatter, \"torch\")\r\n```"
] | ### Describe the bug
When I create a dataset with a `float64` feature, then apply numpy formatting the returned numpy arrays are silently downcasted to `float32`.
### Steps to reproduce the bug
```python
import datasets
dataset = datasets.Dataset.from_dict({'a': [1.0, 2.0, 3.0]}).with_format("numpy")
print("feature dtype:", dataset.features['a'].dtype)
print("array dtype:", dataset['a'].dtype)
```
output:
```
feature dtype: float64
array dtype: float32
```
### Expected behavior
```
feature dtype: float64
array dtype: float64
```
### Environment info
- `datasets` version: 2.8.0
- Platform: Linux-5.4.0-135-generic-x86_64-with-glibc2.29
- Python version: 3.8.10
- PyArrow version: 10.0.1
- Pandas version: 1.4.4
### Suggested Fix
Changing [the `_tensorize` function of the numpy formatter](https://github.com/huggingface/datasets/blob/b065547654efa0ec633cf373ac1512884c68b2e1/src/datasets/formatting/np_formatter.py#L32) to
```python
def _tensorize(self, value):
if isinstance(value, (str, bytes, type(None))):
return value
elif isinstance(value, (np.character, np.ndarray)) and np.issubdtype(value.dtype, np.character):
return value
elif isinstance(value, np.number):
return value
return np.asarray(value, **self.np_array_kwargs)
```
fixes this particular issue for me. Not sure if this would break other tests. This should also avoid unnecessary copying of the array.
| 5,517 |
https://github.com/huggingface/datasets/issues/5514 | Improve inconsistency of `Dataset.map` interface for `load_from_cache_file` | [
"Hi, thanks for noticing this! We can't just remove the cache control as this allows us to control where the arrow files generated by the ops are written (cached on disk if enabled or a temporary directory if disabled). The right way to address this inconsistency would be by having `load_from_cache_file=None` by default everywhere.",
"Hi! Yes, this seems more plausible. I can implement that. One last thing is the type annotation `load_from_cache_file: bool = None`. Which I then would change to `load_from_cache_file: Optional[bool] = None`.",
"PR #5515 ",
"Yes, `Optional[bool]` is the correct type annotation and thanks for the PR."
] | ### Feature request
1. Replace the `load_from_cache_file` default value to `True`.
2. Remove or alter checks from `is_caching_enabled` logic.
### Motivation
I stumbled over an inconsistency in the `Dataset.map` interface. The documentation (and source) states for the parameter `load_from_cache_file`:
```
load_from_cache_file (`bool`, defaults to `True` if caching is enabled):
If a cache file storing the current computation from `function`
can be identified, use it instead of recomputing.
```
1. `load_from_cache_file` default value is `None`, while being annotated as `bool`
2. It is inconsistent with other method signatures like `filter`, that have the default value `True`
3. The logic is inconsistent, as the `map` method checks if caching is enabled through `is_caching_enabled`. This logic is not used for other similar methods.
### Your contribution
I am not fully aware of the logic behind caching checks. If this is just a inconsistency that historically grew, I would suggest to remove the `is_caching_enabled` logic as the "default" logic. Maybe someone can give insights, if environment variables have a higher priority than local variables or vice versa.
If this is clarified, I could adjust the source according to the "Feature request" section of this issue. | 5,514 |
https://github.com/huggingface/datasets/issues/5513 | Some functions use a param named `type` shouldn't that be avoided since it's a Python reserved name? | [
"Hi! Let's not do this - renaming it would be a breaking change, and going through the deprecation cycle is only worth it if it improves user experience.",
"Hi @mariosasko, ok it makes sense. Anyway, don't you think it's worth it at some point to start a deprecation cycle e.g. `fs` in `load_from_disk`? It doesn't affect user experience but it's for sure a bad practice IMO, but's up to you 😄 Feel free to close this issue otherwise!",
"I don't think deprecating a param name in this particular instance is worth the hassle, so I'm closing the issue 🙂.",
"Sure, makes sense @mariosasko thanks!"
] | Hi @mariosasko, @lhoestq, or whoever reads this! :)
After going through `ArrowDataset.set_format` I found out that the `type` param is actually named `type` which is a Python reserved name as you may already know, shouldn't that be renamed to `format_type` before the 3.0.0 is released?
Just wanted to get your input, and if applicable, tackle this issue myself! Thanks 🤗 | 5,513 |
https://github.com/huggingface/datasets/issues/5511 | Creating a dummy dataset from a bigger one | [
"Update `datasets` or downgrade `huggingface-hub` ;)\r\n\r\nThe `huggingface-hub` lib did a breaking change a few months ago, and you're using an old version of `datasets` that does't support it",
"Awesome thanks a lot! Everything works just fine with `datasets==2.9.0` :-) ",
"Getting same error with latest versions.\r\n\r\n\r\n```shell\r\n---------------------------------------------------------------------------\r\nTypeError Traceback (most recent call last)\r\nCell In[99], line 1\r\n----> 1 dataset.push_to_hub(\"mirfan899/kids_phoneme_asr\")\r\n\r\nFile /opt/conda/lib/python3.10/site-packages/datasets/arrow_dataset.py:3538, in Dataset.push_to_hub(self, repo_id, split, private, token, branch, shard_size, embed_external_files)\r\n 3493 def push_to_hub(\r\n 3494 self,\r\n 3495 repo_id: str,\r\n (...)\r\n 3501 embed_external_files: bool = True,\r\n 3502 ):\r\n 3503 \"\"\"Pushes the dataset to the hub.\r\n 3504 The dataset is pushed using HTTP requests and does not need to have neither git or git-lfs installed.\r\n 3505 \r\n (...)\r\n 3536 ```\r\n 3537 \"\"\"\r\n-> 3538 repo_id, split, uploaded_size, dataset_nbytes = self._push_parquet_shards_to_hub(\r\n 3539 repo_id=repo_id,\r\n 3540 split=split,\r\n 3541 private=private,\r\n 3542 token=token,\r\n 3543 branch=branch,\r\n 3544 shard_size=shard_size,\r\n 3545 embed_external_files=embed_external_files,\r\n 3546 )\r\n 3547 organization, dataset_name = repo_id.split(\"/\")\r\n 3548 info_to_dump = self.info.copy()\r\n\r\nFile /opt/conda/lib/python3.10/site-packages/datasets/arrow_dataset.py:3474, in Dataset._push_parquet_shards_to_hub(self, repo_id, split, private, token, branch, shard_size, embed_external_files)\r\n 3472 shard.to_parquet(buffer)\r\n 3473 uploaded_size += buffer.tell()\r\n-> 3474 _retry(\r\n 3475 api.upload_file,\r\n 3476 func_kwargs=dict(\r\n 3477 path_or_fileobj=buffer.getvalue(),\r\n 3478 path_in_repo=path_in_repo(index),\r\n 3479 repo_id=repo_id,\r\n 3480 token=token,\r\n 3481 repo_type=\"dataset\",\r\n 3482 revision=branch,\r\n 3483 identical_ok=True,\r\n 3484 ),\r\n 3485 exceptions=HTTPError,\r\n 3486 status_codes=[504],\r\n 3487 base_wait_time=2.0,\r\n 3488 max_retries=5,\r\n 3489 max_wait_time=20.0,\r\n 3490 )\r\n 3491 return repo_id, split, uploaded_size, dataset_nbytes\r\n\r\nFile /opt/conda/lib/python3.10/site-packages/datasets/utils/file_utils.py:330, in _retry(func, func_args, func_kwargs, exceptions, status_codes, max_retries, base_wait_time, max_wait_time)\r\n 328 while True:\r\n 329 try:\r\n--> 330 return func(*func_args, **func_kwargs)\r\n 331 except exceptions as err:\r\n 332 if retry >= max_retries or (status_codes and err.response.status_code not in status_codes):\r\n\r\nFile /opt/conda/lib/python3.10/site-packages/huggingface_hub/utils/_validators.py:120, in validate_hf_hub_args.<locals>._inner_fn(*args, **kwargs)\r\n 117 if check_use_auth_token:\r\n 118 kwargs = smoothly_deprecate_use_auth_token(fn_name=fn.__name__, has_token=has_token, kwargs=kwargs)\r\n--> 120 return fn(*args, **kwargs)\r\n\r\nTypeError: HfApi.upload_file() got an unexpected keyword argument 'identical_ok'\r\n```",
"Feel free to update `datasets` and `huggingface-hub`, it should fix it :)",
"I went ahead and upgraded both datasets and hub and still getting the same error\r\n",
"Which version do you have ? It's been a while since it has been fixed",
"huggingface 0.0.1\r\nhuggingface-hub 0.17.1\r\ndatasets 2.14.5\r\n\r\nstill has the issue!!",
"I face the same issue even after upgrading :/"
] | ### Describe the bug
I often want to create a dummy dataset from a bigger dataset for fast iteration when training. However, I'm having a hard time doing this especially when trying to upload the dataset to the Hub.
### Steps to reproduce the bug
```python
from datasets import load_dataset
dataset = load_dataset("lambdalabs/pokemon-blip-captions")
dataset["train"] = dataset["train"].select(range(20))
dataset.push_to_hub("patrickvonplaten/dummy_image_data")
```
gives:
```
~/python_bin/datasets/arrow_dataset.py in _push_parquet_shards_to_hub(self, repo_id, split, private, token, branch, max_shard_size, embed_external_files)
4003 base_wait_time=2.0,
4004 max_retries=5,
-> 4005 max_wait_time=20.0,
4006 )
4007 return repo_id, split, uploaded_size, dataset_nbytes
~/python_bin/datasets/utils/file_utils.py in _retry(func, func_args, func_kwargs, exceptions, status_codes, max_retries, base_wait_time, max_wait_time)
328 while True:
329 try:
--> 330 return func(*func_args, **func_kwargs)
331 except exceptions as err:
332 if retry >= max_retries or (status_codes and err.response.status_code not in status_codes):
~/hf/lib/python3.7/site-packages/huggingface_hub/utils/_validators.py in _inner_fn(*args, **kwargs)
122 )
123
--> 124 return fn(*args, **kwargs)
125
126 return _inner_fn # type: ignore
TypeError: upload_file() got an unexpected keyword argument 'identical_ok'
In [2]:
```
### Expected behavior
I would have expected this to work. It's for me the most intuitive way of creating a dummy dataset.
### Environment info
```
- `datasets` version: 2.1.1.dev0
- Platform: Linux-4.19.0-22-cloud-amd64-x86_64-with-debian-10.13
- Python version: 3.7.3
- PyArrow version: 11.0.0
- Pandas version: 1.3.5
``` | 5,511 |
https://github.com/huggingface/datasets/issues/5508 | Saving a dataset after setting format to torch doesn't work, but only if filtering | [
"Hey, I'm a research engineer working on language modelling wanting to contribute to open source. I was wondering if I could give it a shot?",
"Hi! This issue was fixed in https://github.com/huggingface/datasets/pull/4972, so please install `datasets>=2.5.0` to avoid it."
] | ### Describe the bug
Saving a dataset after setting format to torch doesn't work, but only if filtering
### Steps to reproduce the bug
```
a = Dataset.from_dict({"b": [1, 2]})
a.set_format('torch')
a.save_to_disk("test_save") # saves successfully
a.filter(None).save_to_disk("test_save_filter") # does not
>> [...] TypeError: Provided `function` which is applied to all elements of table returns a `dict` of types [<class 'torch.Tensor'>]. When using `batched=True`, make sure provided `function` returns a `dict` of types like `(<class 'list'>, <class 'numpy.ndarray'>)`.
# note: skipping the format change to torch lets this work.
### Expected behavior
Saving to work
### Environment info
- `datasets` version: 2.4.0
- Platform: Linux-6.1.9-arch1-1-x86_64-with-glibc2.36
- Python version: 3.10.9
- PyArrow version: 9.0.0
- Pandas version: 1.4.4 | 5,508 |
https://github.com/huggingface/datasets/issues/5507 | Optimise behaviour in respect to indices mapping | [] | _Originally [posted](https://huggingface.slack.com/archives/C02V51Q3800/p1675443873878489?thread_ts=1675418893.373479&cid=C02V51Q3800) on Slack_
Considering all this, perhaps for Datasets 3.0, we can do the following:
* [ ] have `continuous=True` by default in `.shard` (requested in the survey and makes more sense for us since it doesn't create an indices mapping)
* [x] allow calling `save_to_disk` on "unflattened" datasets
* [ ] remove "hidden" expensive calls in `save_to_disk`, `unique`, `concatenate_datasets`, etc. For instance, instead of silently calling `flatten_indices` where it's needed, it's probably better to be explicit (considering how expensive these ops can be) and raise an error instead | 5,507 |
https://github.com/huggingface/datasets/issues/5506 | IterableDataset and Dataset return different batch sizes when using Trainer with multiple GPUs | [
"Hi ! `datasets` doesn't do batching - the PyTorch DataLoader does and is created by the `Trainer`. Do you pass other arguments to training_args with respect to data loading ?\r\n\r\nAlso we recently released `.to_iterable_dataset` that does pretty much what you implemented, but using contiguous shards to get a better speed:\r\n```python\r\nif use_iterable_dataset:\r\n num_shards = 100\r\n dataset = dataset.to_iterable_dataset(num_shards=num_shards)\r\n```",
"This is the full set of training args passed. No training args were changed when switching dataset types.\r\n\r\n```python\r\ntraining_args = TrainingArguments(\r\n output_dir=\"./checkpoints\",\r\n overwrite_output_dir=True,\r\n num_train_epochs=1,\r\n per_device_train_batch_size=256,\r\n save_steps=2000,\r\n save_total_limit=4,\r\n prediction_loss_only=True,\r\n report_to='none',\r\n gradient_accumulation_steps=6,\r\n fp16=True,\r\n max_steps=60000,\r\n lr_scheduler_type='linear',\r\n warmup_ratio=0.1,\r\n logging_steps=100,\r\n weight_decay=0.01,\r\n adam_beta1=0.9,\r\n adam_beta2=0.98,\r\n adam_epsilon=1e-6,\r\n learning_rate=1e-4\r\n)\r\n```",
"I think the issue comes from `transformers`: https://github.com/huggingface/transformers/issues/21444",
"Makes sense. Given that it's a `transformers` issue and already being tracked, I'll close this out."
] | ### Describe the bug
I am training a Roberta model using 2 GPUs and the `Trainer` API with a batch size of 256.
Initially I used a standard `Dataset`, but had issues with slow data loading. After reading [this issue](https://github.com/huggingface/datasets/issues/2252), I swapped to loading my dataset as contiguous shards and passing those to an `IterableDataset`. I observed an unexpected drop in GPU memory utilization, and found the batch size returned from the model had been cut in half.
When using `Trainer` with 2 GPUs and a batch size of 256, `Dataset` returns a batch of size 512 (256 per GPU), while `IterableDataset` returns a batch size of 256 (256 total). My guess is `IterableDataset` isn't accounting for multiple cards.
### Steps to reproduce the bug
```python
import datasets
from datasets import IterableDataset
from transformers import RobertaConfig
from transformers import RobertaTokenizerFast
from transformers import RobertaForMaskedLM
from transformers import DataCollatorForLanguageModeling
from transformers import Trainer, TrainingArguments
use_iterable_dataset = True
def gen_from_shards(shards):
for shard in shards:
for example in shard:
yield example
dataset = datasets.load_from_disk('my_dataset.hf')
if use_iterable_dataset:
n_shards = 100
shards = [dataset.shard(num_shards=n_shards, index=i) for i in range(n_shards)]
dataset = IterableDataset.from_generator(gen_from_shards, gen_kwargs={"shards": shards})
tokenizer = RobertaTokenizerFast.from_pretrained("./my_tokenizer", max_len=160, use_fast=True)
config = RobertaConfig(
vocab_size=8248,
max_position_embeddings=256,
num_attention_heads=8,
num_hidden_layers=6,
type_vocab_size=1)
model = RobertaForMaskedLM(config=config)
data_collator = DataCollatorForLanguageModeling(tokenizer=tokenizer, mlm=True, mlm_probability=0.15)
training_args = TrainingArguments(
per_device_train_batch_size=256
# other args removed for brevity
)
trainer = Trainer(
model=model,
args=training_args,
data_collator=data_collator,
train_dataset=dataset,
)
trainer.train()
```
### Expected behavior
Expected `Dataset` and `IterableDataset` to have the same batch size behavior. If the current behavior is intentional, the batch size printout at the start of training should be updated. Currently, both dataset classes result in `Trainer` printing the same total batch size, even though the batch size sent to the GPUs are different.
### Environment info
datasets 2.7.1
transformers 4.25.1 | 5,506 |
https://github.com/huggingface/datasets/issues/5505 | PyTorch BatchSampler still loads from Dataset one-by-one | [
"This change seems to come from a few months ago in the PyTorch side. That's good news and it means we may not need to pass a batch_sampler as soon as we add `Dataset.__getitems__` to get the optimal speed :)\r\n\r\nThanks for reporting ! Would you like to open a PR to add `__getitems__` and remove this outdated documentation ?",
"Yeah I figured this was the sort of thing that probably once worked. I can confirm that you no longer need the batch sampler, just `batch_size=n` in the `DataLoader`.\r\n\r\nI'll pass on the PR, I'm flat out right now, sorry."
] | ### Describe the bug
In [the docs here](https://huggingface.co/docs/datasets/use_with_pytorch#use-a-batchsampler), it mentions the issue of the Dataset being read one-by-one, then states that using a BatchSampler resolves the issue.
I'm not sure if this is a mistake in the docs or the code, but it seems that the only way for a Dataset to be passed a list of indexes by PyTorch (instead of one index at a time) is to define a `__getitems__` method (note the plural) on the Dataset object, and since the HF Dataset doesn't have this, PyTorch executes [this line of code](https://github.com/pytorch/pytorch/blob/master/torch/utils/data/_utils/fetch.py#L58), reverting to fetching one-by-one.
### Steps to reproduce the bug
You can put a breakpoint in `Dataset.__getitem__()` or just print the args from there and see that it's called multiple times for a single `next(iter(dataloader))`, even when using the code from the docs:
```py
from torch.utils.data.sampler import BatchSampler, RandomSampler
batch_sampler = BatchSampler(RandomSampler(ds), batch_size=32, drop_last=False)
dataloader = DataLoader(ds, batch_sampler=batch_sampler)
```
### Expected behavior
The expected behaviour would be for it to fetch batches from the dataset, rather than one-by-one.
To demonstrate that there is room for improvement: once I have a HF dataset `ds`, if I just add this line:
```py
ds.__getitems__ = ds.__getitem__
```
...then the time taken to loop over the dataset improves considerably (for wikitext-103, from one minute to 13 seconds with batch size 32). Probably not a big deal in the grand scheme of things, but seems like an easy win.
### Environment info
- `datasets` version: 2.9.0
- Platform: Linux-5.10.102.1-microsoft-standard-WSL2-x86_64-with-glibc2.31
- Python version: 3.10.8
- PyArrow version: 10.0.1
- Pandas version: 1.5.3 | 5,505 |
https://github.com/huggingface/datasets/issues/5500 | WMT19 custom download checksum error | [
"I update the `datatsets` version and it works."
] | ### Describe the bug
I use the following scripts to download data from WMT19:
```python
import datasets
from datasets import inspect_dataset, load_dataset_builder
from wmt19.wmt_utils import _TRAIN_SUBSETS,_DEV_SUBSETS
## this is a must due to: https://discuss.huggingface.co/t/load-dataset-hangs-with-local-files/28034/3
if __name__ == '__main__':
dev_subsets,train_subsets = [],[]
for subset in _TRAIN_SUBSETS:
if subset.target=='en' and 'de' in subset.sources:
train_subsets.append(subset.name)
for subset in _DEV_SUBSETS:
if subset.target=='en' and 'de' in subset.sources:
dev_subsets.append(subset.name)
inspect_dataset("wmt19", "./wmt19")
builder = load_dataset_builder(
"./wmt19/wmt_utils.py",
language_pair=("de", "en"),
subsets={
datasets.Split.TRAIN: train_subsets,
datasets.Split.VALIDATION: dev_subsets,
},
)
builder.download_and_prepare()
ds = builder.as_dataset()
ds.to_json("../data/wmt19/ende/data.json")
```
And I got the following error:
```
Traceback (most recent call last): | 0/2 [00:00<?, ?obj/s]
File "draft.py", line 26, in <module>
builder.download_and_prepare() | 0/1 [00:00<?, ?obj/s]
File "/Users/hannibal046/anaconda3/lib/python3.8/site-packages/datasets/builder.py", line 605, in download_and_prepare
self._download_and_prepare(%| | 0/1 [00:00<?, ?obj/s]
File "/Users/hannibal046/anaconda3/lib/python3.8/site-packages/datasets/builder.py", line 1104, in _download_and_prepare
super()._download_and_prepare(dl_manager, verify_infos, check_duplicate_keys=verify_infos) | 0/1 [00:00<?, ?obj/s]
File "/Users/hannibal046/anaconda3/lib/python3.8/site-packages/datasets/builder.py", line 676, in _download_and_prepare
verify_checksums(s #13: 0%| | 0/1 [00:00<?, ?obj/s]
File "/Users/hannibal046/anaconda3/lib/python3.8/site-packages/datasets/utils/info_utils.py", line 35, in verify_checksums
raise UnexpectedDownloadedFile(str(set(recorded_checksums) - set(expected_checksums))) | 0/1 [00:00<?, ?obj/s]
datasets.utils.info_utils.UnexpectedDownloadedFile: {'https://s3.amazonaws.com/web-language-models/paracrawl/release1/paracrawl-release1.en-de.zipporah0-dedup-clean.tgz', 'https://huggingface.co/datasets/wmt/wmt13/resolve/main-zip/training-parallel-europarl-v7.zip', 'https://huggingface.co/datasets/wmt/wmt18/resolve/main-zip/translation-task/rapid2016.zip', 'https://huggingface.co/datasets/wmt/wmt18/resolve/main-zip/translation-task/training-parallel-nc-v13.zip', 'https://huggingface.co/datasets/wmt/wmt17/resolve/main-zip/translation-task/training-parallel-nc-v12.zip', 'https://huggingface.co/datasets/wmt/wmt14/resolve/main-zip/training-parallel-nc-v9.zip', 'https://huggingface.co/datasets/wmt/wmt15/resolve/main-zip/training-parallel-nc-v10.zip', 'https://huggingface.co/datasets/wmt/wmt16/resolve/main-zip/translation-task/training-parallel-nc-v11.zip'}
```
### Steps to reproduce the bug
see above
### Expected behavior
download data successfully
### Environment info
datasets==2.1.0
python==3.8
| 5,500 |
https://github.com/huggingface/datasets/issues/5499 | `load_dataset` has ~4 seconds of overhead for cached data | [
"Hi ! To skip the verification step that checks if newer data exist, you can enable offline mode with `HF_DATASETS_OFFLINE=1`.\r\n\r\nAlthough I agree this step should be much faster for datasets hosted on the HF Hub - we could just compare the commit hash from the local data and the remote git repository. We're not been leveraging the git commit hashes, since the library was built before we even had git repositories for each dataset on HF.",
"Thanks @lhoestq, for memory when I recorded those times I had `HF_DATASETS_OFFLINE` set."
] | ### Feature request
When loading a dataset that has been cached locally, the `load_dataset` function takes a lot longer than it should take to fetch the dataset from disk (or memory).
This is particularly noticeable for smaller datasets. For example, wikitext-2, comparing `load_data` (once cached) and `load_from_disk`, the `load_dataset` method takes 40 times longer.
⏱ 4.84s ⮜ load_dataset
⏱ 119ms ⮜ load_from_disk
### Motivation
I assume this is doing something like checking for a newer version.
If so, that's an age old problem: do you make the user wait _every single time they load from cache_ or do you do something like load from cache always, _then_ check for a newer version and alert if they have stale data. The decision usually revolves around what percentage of the time the data will have been updated, and how dangerous old data is.
For most datasets it's extremely unlikely that there will be a newer version on any given run, so 99% of the time this is just wasted time.
Maybe you don't want to make that decision for all users, but at least having the _option_ to not wait for checks would be an improvement.
### Your contribution
. | 5,499 |
https://github.com/huggingface/datasets/issues/5498 | TypeError: 'bool' object is not iterable when filtering a datasets.arrow_dataset.Dataset | [
"Hi! Instead of a single boolean, your filter function should return an iterable (of booleans) in the batched mode like so:\r\n```python\r\ntrain_dataset = train_dataset.filter(\r\n function=lambda batch: [image is not None for image in batch[\"image\"]], \r\n batched=True,\r\n batch_size=10)\r\n```\r\n\r\nPS: You can make this operation much faster by operating directly on the arrow data to skip the decoding part:\r\n```python\r\ntrain_dataset = train_dataset.with_format(\"arrow\")\r\ntrain_dataset = train_dataset.filter(\r\n function=lambda table: table[\"image\"].is_valid().to_pylist(), \r\n batched=True,\r\n batch_size=100)\r\ntrain_dataset = train_dataset.with_format(None)\r\n```",
"Thank a lot!",
"I hit the same issue and the error message isn't really clear on what's going wrong. It might be helpful to update the docs with a batched example."
] | ### Describe the bug
Hi,
Thanks for the amazing work on the library!
**Describe the bug**
I think I might have noticed a small bug in the filter method.
Having loaded a dataset using `load_dataset`, when I try to filter out empty entries with `batched=True`, I get a TypeError.
### Steps to reproduce the bug
```
train_dataset = train_dataset.filter(
function=lambda example: example["image"] is not None,
batched=True,
batch_size=10)
```
Error message:
```
File .../lib/python3.9/site-packages/datasets/fingerprint.py:480, in fingerprint_transform.<locals>._fingerprint.<locals>.wrapper(*args, **kwargs)
476 validate_fingerprint(kwargs[fingerprint_name])
478 # Call actual function
--> 480 out = func(self, *args, **kwargs)
...
-> 5666 indices_array = [i for i, to_keep in zip(indices, mask) if to_keep]
5667 if indices_mapping is not None:
5668 indices_array = pa.array(indices_array, type=pa.uint64())
TypeError: 'bool' object is not iterable
```
**Removing batched=True allows to bypass the issue.**
### Expected behavior
According to the doc, "[batch_size corresponds to the] number of examples per batch provided to function if batched = True", so we shouldn't need to remove the batchd=True arg?
source: https://huggingface.co/docs/datasets/v2.9.0/en/package_reference/main_classes#datasets.Dataset.filter
### Environment info
- `datasets` version: 2.9.0
- Platform: Linux-5.4.0-122-generic-x86_64-with-glibc2.31
- Python version: 3.9.10
- PyArrow version: 10.0.1
- Pandas version: 1.5.3 | 5,498 |
https://github.com/huggingface/datasets/issues/5496 | Add a `reduce` method | [
"Hi! Sure, feel free to open a PR, so we can see the API you have in mind.",
"I would like to give it a go! #self-assign",
"Closing as `Dataset.map` can be used instead (see https://github.com/huggingface/datasets/pull/5533#issuecomment-1440571658 and https://github.com/huggingface/datasets/pull/5533#issuecomment-1446403263)"
] | ### Feature request
Right now the `Dataset` class implements `map()` and `filter()`, but leaves out the third functional idiom popular among Python users: `reduce`.
### Motivation
A `reduce` method is often useful when calculating dataset statistics, for example, the occurrence of a particular n-gram or the average line length of a code dataset.
### Your contribution
I haven't contributed to `datasets` before, but I don't expect this will be too difficult, since the implementation will closely follow that of `map` and `filter`. I could have a crack over the weekend. | 5,496 |
https://github.com/huggingface/datasets/issues/5495 | to_tf_dataset fails with datetime UTC columns even if not included in columns argument | [
"Hi! This is indeed a bug in our zero-copy logic.\r\n\r\nTo fix it, instead of the line:\r\nhttps://github.com/huggingface/datasets/blob/7cfac43b980ab9e4a69c2328f085770996323005/src/datasets/features/features.py#L702\r\n\r\nwe should have:\r\n```python\r\nreturn pa.types.is_primitive(pa_type) and not (pa.types.is_boolean(pa_type) or pa.types.is_temporal(pa_type))\r\n```",
"@mariosasko submitted a small PR [here](https://github.com/huggingface/datasets/pull/5504)"
] | ### Describe the bug
There appears to be some eager behavior in `to_tf_dataset` that runs against every column in a dataset even if they aren't included in the columns argument. This is problematic with datetime UTC columns due to them not working with zero copy. If I don't have UTC information in my datetime column, then everything works as expected.
### Steps to reproduce the bug
```python
import numpy as np
import pandas as pd
from datasets import Dataset
df = pd.DataFrame(np.random.rand(2, 1), columns=["x"])
# df["dt"] = pd.to_datetime(["2023-01-01", "2023-01-01"]) # works fine
df["dt"] = pd.to_datetime(["2023-01-01 00:00:00.00000+00:00", "2023-01-01 00:00:00.00000+00:00"])
df.to_parquet("test.pq")
ds = Dataset.from_parquet("test.pq")
tf_ds = ds.to_tf_dataset(columns=["x"], batch_size=2, shuffle=True)
```
```
ArrowInvalid Traceback (most recent call last)
Cell In[1], line 12
8 df.to_parquet("test.pq")
11 ds = Dataset.from_parquet("test.pq")
---> 12 tf_ds = ds.to_tf_dataset(columns=["r"], batch_size=2, shuffle=True)
File ~/venv/lib/python3.8/site-packages/datasets/arrow_dataset.py:411, in TensorflowDatasetMixin.to_tf_dataset(self, batch_size, columns, shuffle, collate_fn, drop_remainder, collate_fn_args, label_cols, prefetch, num_workers)
407 dataset = self
409 # TODO(Matt, QL): deprecate the retention of label_ids and label
--> 411 output_signature, columns_to_np_types = dataset._get_output_signature(
412 dataset,
413 collate_fn=collate_fn,
414 collate_fn_args=collate_fn_args,
415 cols_to_retain=cols_to_retain,
416 batch_size=batch_size if drop_remainder else None,
417 )
419 if "labels" in output_signature:
420 if ("label_ids" in columns or "label" in columns) and "labels" not in columns:
File ~/venv/lib/python3.8/site-packages/datasets/arrow_dataset.py:254, in TensorflowDatasetMixin._get_output_signature(dataset, collate_fn, collate_fn_args, cols_to_retain, batch_size, num_test_batches)
252 for _ in range(num_test_batches):
253 indices = sample(range(len(dataset)), test_batch_size)
--> 254 test_batch = dataset[indices]
255 if cols_to_retain is not None:
256 test_batch = {key: value for key, value in test_batch.items() if key in cols_to_retain}
File ~/venv/lib/python3.8/site-packages/datasets/arrow_dataset.py:2590, in Dataset.__getitem__(self, key)
2588 def __getitem__(self, key): # noqa: F811
2589 """Can be used to index columns (by string names) or rows (by integer index or iterable of indices or bools)."""
-> 2590 return self._getitem(
2591 key,
2592 )
File ~/venv/lib/python3.8/site-packages/datasets/arrow_dataset.py:2575, in Dataset._getitem(self, key, **kwargs)
2573 formatter = get_formatter(format_type, features=self.features, **format_kwargs)
2574 pa_subtable = query_table(self._data, key, indices=self._indices if self._indices is not None else None)
-> 2575 formatted_output = format_table(
2576 pa_subtable, key, formatter=formatter, format_columns=format_columns, output_all_columns=output_all_columns
2577 )
2578 return formatted_output
File ~/venv/lib/python3.8/site-packages/datasets/formatting/formatting.py:634, in format_table(table, key, formatter, format_columns, output_all_columns)
632 python_formatter = PythonFormatter(features=None)
633 if format_columns is None:
--> 634 return formatter(pa_table, query_type=query_type)
635 elif query_type == "column":
636 if key in format_columns:
File ~/venv/lib/python3.8/site-packages/datasets/formatting/formatting.py:410, in Formatter.__call__(self, pa_table, query_type)
408 return self.format_column(pa_table)
409 elif query_type == "batch":
--> 410 return self.format_batch(pa_table)
File ~/venv/lib/python3.8/site-packages/datasets/formatting/np_formatter.py:78, in NumpyFormatter.format_batch(self, pa_table)
77 def format_batch(self, pa_table: pa.Table) -> Mapping:
---> 78 batch = self.numpy_arrow_extractor().extract_batch(pa_table)
79 batch = self.python_features_decoder.decode_batch(batch)
80 batch = self.recursive_tensorize(batch)
File ~/venv/lib/python3.8/site-packages/datasets/formatting/formatting.py:164, in NumpyArrowExtractor.extract_batch(self, pa_table)
163 def extract_batch(self, pa_table: pa.Table) -> dict:
--> 164 return {col: self._arrow_array_to_numpy(pa_table[col]) for col in pa_table.column_names}
File ~/venv/lib/python3.8/site-packages/datasets/formatting/formatting.py:164, in <dictcomp>(.0)
163 def extract_batch(self, pa_table: pa.Table) -> dict:
--> 164 return {col: self._arrow_array_to_numpy(pa_table[col]) for col in pa_table.column_names}
File ~/venv/lib/python3.8/site-packages/datasets/formatting/formatting.py:185, in NumpyArrowExtractor._arrow_array_to_numpy(self, pa_array)
181 else:
182 zero_copy_only = _is_zero_copy_only(pa_array.type) and all(
183 not _is_array_with_nulls(chunk) for chunk in pa_array.chunks
184 )
--> 185 array: List = [
186 row for chunk in pa_array.chunks for row in chunk.to_numpy(zero_copy_only=zero_copy_only)
187 ]
188 else:
189 if isinstance(pa_array.type, _ArrayXDExtensionType):
190 # don't call to_pylist() to preserve dtype of the fixed-size array
File ~/venv/lib/python3.8/site-packages/datasets/formatting/formatting.py:186, in <listcomp>(.0)
181 else:
182 zero_copy_only = _is_zero_copy_only(pa_array.type) and all(
183 not _is_array_with_nulls(chunk) for chunk in pa_array.chunks
184 )
185 array: List = [
--> 186 row for chunk in pa_array.chunks for row in chunk.to_numpy(zero_copy_only=zero_copy_only)
187 ]
188 else:
189 if isinstance(pa_array.type, _ArrayXDExtensionType):
190 # don't call to_pylist() to preserve dtype of the fixed-size array
File ~/venv/lib/python3.8/site-packages/pyarrow/array.pxi:1475, in pyarrow.lib.Array.to_numpy()
File ~/venv/lib/python3.8/site-packages/pyarrow/error.pxi:100, in pyarrow.lib.check_status()
ArrowInvalid: Needed to copy 1 chunks with 0 nulls, but zero_copy_only was True
```
### Expected behavior
I think there are two potential issues/fixes
1. Proper handling of datetime UTC columns (perhaps there is something incorrect with zero copy handling here)
2. Not eagerly running against every column in a dataset when the columns argument of `to_tf_dataset` specifies a subset of columns (although I'm not sure if this is unavoidable)
### Environment info
- `datasets` version: 2.9.0
- Platform: macOS-13.2-x86_64-i386-64bit
- Python version: 3.8.12
- PyArrow version: 11.0.0
- Pandas version: 1.5.3
| 5,495 |
https://github.com/huggingface/datasets/issues/5494 | Update audio installation doc page | [
"Totally agree, the docs should be in sync with our code.\r\n\r\nIndeed to avoid confusing users, I think we should have updated the docs at the same time as this PR:\r\n- #5167",
"@albertvillanova yeah sure I should have, but I forgot back then, sorry for that 😶",
"No, @polinaeterna, nothing to be sorry about.\r\n\r\nMy comment was for all of us datasets team, as a reminder: when making a PR, but also when reviewing some other's PR, we should not forget to update the corresponding docstring and doc pages. It is something we can improve if we help each other in reminding about it... :hugs: ",
"@polinaeterna I think we can close this issue now as we no longer use `torchaudio` for decoding."
] | Our [installation documentation page](https://huggingface.co/docs/datasets/installation#audio) says that one can use Datasets for mp3 only with `torchaudio<0.12`. `torchaudio>0.12` is actually supported too but requires a specific version of ffmpeg which is not easily installed on all linux versions but there is a custom ubuntu repo for it, we have insctructions in the code: https://github.com/huggingface/datasets/blob/main/src/datasets/features/audio.py#L327
So we should update the doc page. But first investigate [this issue](5488). | 5,494 |
https://github.com/huggingface/datasets/issues/5492 | Push_to_hub in a pull request | [
"Assigned to myself and will get to it in the next week, but if someone finds this issue annoying and wants to submit a PR before I do, just ping me here and I'll reassign :). ",
"I would like to be assigned to this issue, @nateraw . #self-assign"
] | Right now `ds.push_to_hub()` can push a dataset on `main` or on a new branch with `branch=`, but there is no way to open a pull request. Even passing `branch=refs/pr/x` doesn't seem to work: it tries to create a branch with that name
cc @nateraw
It should be possible to tweak the use of `huggingface_hub` in `push_to_hub` to make it open a PR or push to an existing PR | 5,492 |
https://github.com/huggingface/datasets/issues/5488 | Error loading MP3 files from CommonVoice | [
"Hi @kradonneoh, thanks for reporting.\r\n\r\nPlease note that to work with audio datasets (and specifically with MP3 files) we have detailed installation instructions in our docs: https://huggingface.co/docs/datasets/installation#audio\r\n- one of the requirements is torchaudio<0.12.0\r\n\r\nLet us know if the problem persists after having followed them.",
"I saw that and have followed it (hence the Expected Behavior section of the bug report). \r\n\r\nIs there no intention of updating to the latest version? It does limit the version of `torch` I can use, which isn’t ideal.",
"@kradonneoh hey! actually with `ffmpeg4` loading of mp3 files should work, so this is a not expected behavior and we need to investigate it. It works on my side with `torchaudio==0.13` and `ffmpeg==4.2.7`. Which `torchaudio` version do you use?\r\n\r\n`datasets` should support decoding of mp3 files with `torchaudio` when its version is `>0.12` but as you noted it requires `ffmpeg>4`, we need to fix this in the documentation, thank you for pointing to this! \r\n\r\nBut according to your traceback it seems that it tries to use [`libsndfile`](https://github.com/libsndfile/libsndfile) backend for mp3 decoding. And `libsndfile` library supports mp3 decoding starting from version 1.1.0 which on Linux has to be compiled from source for now afaik. \r\n\r\nfyi - we are aiming at getting rid of `torchaudio` dependency at all by the next major library release in favor of `libsndfile` too.",
"We now decode MP3 with `soundfile`, so I'm closing this issue"
] | ### Describe the bug
When loading a CommonVoice dataset with `datasets==2.9.0` and `torchaudio>=0.12.0`, I get an error reading the audio arrays:
```python
---------------------------------------------------------------------------
LibsndfileError Traceback (most recent call last)
~/.local/lib/python3.8/site-packages/datasets/features/audio.py in _decode_mp3(self, path_or_file)
310 try: # try torchaudio anyway because sometimes it works (depending on the os and os packages installed)
--> 311 array, sampling_rate = self._decode_mp3_torchaudio(path_or_file)
312 except RuntimeError:
~/.local/lib/python3.8/site-packages/datasets/features/audio.py in _decode_mp3_torchaudio(self, path_or_file)
351
--> 352 array, sampling_rate = torchaudio.load(path_or_file, format="mp3")
353 if self.sampling_rate and self.sampling_rate != sampling_rate:
~/.local/lib/python3.8/site-packages/torchaudio/backend/soundfile_backend.py in load(filepath, frame_offset, num_frames, normalize, channels_first, format)
204 """
--> 205 with soundfile.SoundFile(filepath, "r") as file_:
206 if file_.format != "WAV" or normalize:
~/.local/lib/python3.8/site-packages/soundfile.py in __init__(self, file, mode, samplerate, channels, subtype, endian, format, closefd)
654 format, subtype, endian)
--> 655 self._file = self._open(file, mode_int, closefd)
656 if set(mode).issuperset('r+') and self.seekable():
~/.local/lib/python3.8/site-packages/soundfile.py in _open(self, file, mode_int, closefd)
1212 err = _snd.sf_error(file_ptr)
-> 1213 raise LibsndfileError(err, prefix="Error opening {0!r}: ".format(self.name))
1214 if mode_int == _snd.SFM_WRITE:
LibsndfileError: Error opening <_io.BytesIO object at 0x7fa539462090>: File contains data in an unknown format.
```
I assume this is because there's some issue with the mp3 decoding process. I've verified that I have `ffmpeg>=4` (on a Linux distro), which appears to be the fallback backend for `torchaudio,` (at least according to #4889).
### Steps to reproduce the bug
```python
dataset = load_dataset("mozilla-foundation/common_voice_11_0", "be", split="train")
dataset[0]
```
### Expected behavior
Similar behavior to `torchaudio<0.12.0`, which doesn't result in a `LibsndfileError`
### Environment info
- `datasets` version: 2.9.0
- Platform: Linux-5.15.0-52-generic-x86_64-with-glibc2.29
- Python version: 3.8.10
- PyArrow version: 10.0.1
- Pandas version: 1.5.1 | 5,488 |
https://github.com/huggingface/datasets/issues/5487 | Incorrect filepath for dill module | [
"Hi! The correct path is still `dill._dill.XXXX` in the latest release. What do you get when you run `python -c \"import dill; print(dill.__version__)\"` in your environment?",
"`0.3.6` I feel like that's bad news, because it's probably not the issue.\r\n\r\nMy mistake, about the wrong path guess. I think I didn't notice that the first `dill` in the path isn't supposed to be included in the path specification in python.\r\n<img width=\"146\" alt=\"Screen Shot 2023-01-31 at 12 58 32 PM\" src=\"https://user-images.githubusercontent.com/35349273/215844209-74af6a8f-9bff-4c75-9495-44c658c8e9f7.png\">\r\n",
"Hi, @avivbrokman, this issue you report appeared only with old versions of dill. See:\r\n- #288\r\n\r\nAre you sure you are in the right Python environment?\r\n- Please note that Jupyter (where I guess you get the error) may have multiple execution backends (IPython kernels) that might be different from the Python environment your are using to get the dill version\r\n - Have you run `import dill; print(dill.__version__)` in the same Jupyter/IPython that you were using when you got the error while executing `import datasets`?",
"I'm using spyder, and I am still getting `0.3.6` for `dill`, so unfortunately #288 isn't applicable, I think. However, I found something odd that I believe is a clue: \r\n\r\n```\r\nimport inspect\r\nimport dill\r\n\r\ninspect.getfile(dill)\r\n>>> '/Users/avivbrokman/opt/anaconda3/lib/python3.9/site-packages/dill/__init__.py'\r\n```\r\n\r\nI checked out the directory, and there is no `dill` subdirectory within '/Users/avivbrokman/opt/anaconda3/lib/python3.9/site-packages/dill`, as there should be. Rather, `_dill.py` is in '/Users/avivbrokman/opt/anaconda3/lib/python3.9/site-packages/dill` itself. \r\n\r\n If I run `pip install dill` or `pip install --upgrade dill`, I get the message `Requirement already satisfied: dill in ./opt/anaconda3/lib/python3.9/site-packages (0.3.6)`. If I run `conda upgrade dill`, I get the message `Solving environment: failed with repodata from current_repodata.json, will retry with next repodata source.` a couple of times, followed by\r\n\r\n```\r\nSolving environment: failed\r\nSolving environment: / \r\nFound conflicts! Looking for incompatible packages.\r\n```\r\n\r\nAnd then terminal proceeds to list conflicts between different packages I have.\r\n\r\nThis is all very strange to me because I recently uninstalled and reinstalled `anaconda`.\r\n",
"As I said above, I guess this is not a problem with `datasets`. I think you have different Python environments: one with the new dill version (the one you get while using pip) and other with the old dill version (the one where you get the AttributeError).\r\n\r\nYou should update `dill` in the Python environment you are using within spyder.\r\n\r\nPlease note that the `_dill` module is present in the `dill` package since their 2.8.0 version."
] | ### Describe the bug
I installed the `datasets` package and when I try to `import` it, I get the following error:
```
Traceback (most recent call last):
File "/var/folders/jt/zw5g74ln6tqfdzsl8tx378j00000gn/T/ipykernel_3805/3458380017.py", line 1, in <module>
import datasets
File "/Users/avivbrokman/opt/anaconda3/lib/python3.9/site-packages/datasets/__init__.py", line 43, in <module>
from .arrow_dataset import Dataset
File "/Users/avivbrokman/opt/anaconda3/lib/python3.9/site-packages/datasets/arrow_dataset.py", line 66, in <module>
from .arrow_writer import ArrowWriter, OptimizedTypedSequence
File "/Users/avivbrokman/opt/anaconda3/lib/python3.9/site-packages/datasets/arrow_writer.py", line 27, in <module>
from .features import Features, Image, Value
File "/Users/avivbrokman/opt/anaconda3/lib/python3.9/site-packages/datasets/features/__init__.py", line 17, in <module>
from .audio import Audio
File "/Users/avivbrokman/opt/anaconda3/lib/python3.9/site-packages/datasets/features/audio.py", line 12, in <module>
from ..download.streaming_download_manager import xopen
File "/Users/avivbrokman/opt/anaconda3/lib/python3.9/site-packages/datasets/download/__init__.py", line 9, in <module>
from .download_manager import DownloadManager, DownloadMode
File "/Users/avivbrokman/opt/anaconda3/lib/python3.9/site-packages/datasets/download/download_manager.py", line 36, in <module>
from ..utils.py_utils import NestedDataStructure, map_nested, size_str
File "/Users/avivbrokman/opt/anaconda3/lib/python3.9/site-packages/datasets/utils/py_utils.py", line 602, in <module>
class Pickler(dill.Pickler):
File "/Users/avivbrokman/opt/anaconda3/lib/python3.9/site-packages/datasets/utils/py_utils.py", line 605, in Pickler
dispatch = dill._dill.MetaCatchingDict(dill.Pickler.dispatch.copy())
AttributeError: module 'dill' has no attribute '_dill'
```
Looking at the github source code for dill, it appears that `datasets` has a bug or is not compatible with the latest `dill`. Specifically, rather than `dill._dill.XXXX` it should be `dill.dill._dill.XXXX`. But given the popularity of `datasets` I feel confused about me being the first person to have this issue, so it makes me wonder if I'm misdiagnosing the issue.
### Steps to reproduce the bug
Install `dill` and `datasets` packages and then `import datasets`
### Expected behavior
I expect `datasets` to import.
### Environment info
- `datasets` version: 2.9.0
- Platform: macOS-10.16-x86_64-i386-64bit
- Python version: 3.9.13
- PyArrow version: 11.0.0
- Pandas version: 1.4.4 | 5,487 |
https://github.com/huggingface/datasets/issues/5486 | Adding `sep` to TextConfig | [
"Hi @omar-araboghli, thanks for your proposal.\r\n\r\nHave you tried to use \"csv\" loader instead of \"text\"? That already has a `sep` argument.",
"Hi @albertvillanova, thanks for the quick response!\r\n\r\nIndeed, I have been trying to use `csv` instead of `text`. However I am still not able to define range of rows as one sequence, that is achievable with passing `sample_by='paragraph'` to the `TextConfig`\r\n\r\nFor instance, the below code\r\n\r\n```python\r\nimport datasets\r\n\r\ndataset = datasets.load_dataset(\r\n path='csv',\r\n data_files={'train': TRAINING_SET_PATH},\r\n sep='\\t',\r\n header=None,\r\n column_names=['tokens', 'pos_tags', 'chunk_tags', 'ner_tags']\r\n)\r\n```\r\n\r\nleads to \r\n\r\n```python\r\ndataset\r\n>>> DatasetDict({\r\n train: Dataset({\r\n features: ['tokens', 'pos_tags', 'chunk_tags', 'ner_tags'],\r\n num_rows: 62543\r\n })\r\n})\r\n\r\ndataset['train'][0]\r\n>>> {'tokens': 'Distribution',\r\n 'pos_tags': 'NN',\r\n 'chunk_tags': 'O',\r\n 'ner_tags': 'O'\r\n}\r\n```\r\nIs there a way to deal with multiple csv rows as one dataset instance, where each column is a sequence of those rows ?"
] | I have a local a `.txt` file that follows the `CONLL2003` format which I need to load using `load_script`. However, by using `sample_by='line'`, one can only split the dataset into lines without splitting each line into columns. Would it be reasonable to add a `sep` argument in combination with `sample_by='paragraph'` to parse a paragraph into an array for each column ? If so, I am happy to contribute!
## Environment
* `python 3.8.10`
* `datasets 2.9.0`
## Snippet of `train.txt`
```txt
Distribution NN O O
and NN O O
dynamics NN O O
of NN O O
electron NN O B-RP
complexes NN O I-RP
in NN O O
cyanobacterial NN O B-R
membranes NN O I-R
The NN O O
occurrence NN O O
of NN O O
prostaglandin NN O B-R
F2α NN O I-R
in NN O O
Pharbitis NN O B-R
seedlings NN O I-R
grown NN O O
under NN O O
short NN O B-P
days NN O I-P
or NN O I-P
days NN O I-P
```
## Current Behaviour
```python
# defining 4 features ['tokens', 'pos_tags', 'chunk_tags', 'ner_tags'] here would fail with `ValueError: Length of names (4) does not match length of arrays (1)`
dataset = datasets.load_dataset(path='text', features=features, data_files={'train': 'train.txt'}, sample_by='line')
dataset['train']['tokens'][0]
>>> 'Distribution\tNN\tO\tO'
```
## Expected Behaviour / Suggestion
```python
# suppose we defined 4 features ['tokens', 'pos_tags', 'chunk_tags', 'ner_tags']
dataset = datasets.load_dataset(path='text', features=features, data_files={'train': 'train.txt'}, sample_by='paragraph', sep='\t')
dataset['train']['tokens'][0]
>>> ['Distribution', 'and', 'dynamics', ... ]
dataset['train']['ner_tags'][0]
>>> ['O', 'O', 'O', ... ]
``` | 5,486 |
https://github.com/huggingface/datasets/issues/5483 | Unable to upload dataset | [
"Seems to work now, perhaps it was something internal with our university's network."
] | ### Describe the bug
Uploading a simple dataset ends with an exception
### Steps to reproduce the bug
I created a new conda env with python 3.10, pip installed datasets and:
```python
>>> from datasets import load_dataset, load_from_disk, Dataset
>>> d = Dataset.from_dict({"text": ["hello"] * 2})
>>> d.push_to_hub("ttt111")
/home/olab/kirstain/anaconda3/envs/datasets/lib/python3.10/site-packages/huggingface_hub/utils/_hf_folder.py:92: UserWarning: A token has been found in `/a/home/cc/students/cs/kirstain/.huggingface/token`. This is the old path where tokens were stored. The new location is `/home/olab/kirstain/.cache/huggingface/token` which is configurable using `HF_HOME` environment variable. Your token has been copied to this new location. You can now safely delete the old token file manually or use `huggingface-cli logout`.
warnings.warn(
Creating parquet from Arrow format: 100%|████████████████████████████████████████████████████████████████████████████████████████████████████████████████████████████████████████████████████████████████████| 1/1 [00:00<00:00, 279.94ba/s]
Upload 1 LFS files: 0%| | 0/1 [00:02<?, ?it/s]
Pushing dataset shards to the dataset hub: 0%| | 0/1 [00:04<?, ?it/s]
Traceback (most recent call last):
File "/home/olab/kirstain/anaconda3/envs/datasets/lib/python3.10/site-packages/huggingface_hub/utils/_errors.py", line 264, in hf_raise_for_status
response.raise_for_status()
File "/home/olab/kirstain/anaconda3/envs/datasets/lib/python3.10/site-packages/requests/models.py", line 1021, in raise_for_status
raise HTTPError(http_error_msg, response=self)
requests.exceptions.HTTPError: 403 Client Error: Forbidden for url: https://s3.us-east-1.amazonaws.com/lfs.huggingface.co/repos/cf/0c/cf0c5ab8a3f729e5f57a8b79a36ecea64a31126f13218591c27ed9a1c7bd9b41/ece885a4bb6bbc8c1bb51b45542b805283d74590f72cd4c45d3ba76628570386?X-Amz-Algorithm=AWS4-HMAC-SHA256&X-Amz-Content-Sha256=UNSIGNED-PAYLOAD&X-Amz-Credential=AKIA4N7VTDGO27GPWFUO%2F20230128%2Fus-east-1%2Fs3%2Faws4_request&X-Amz-Date=20230128T151640Z&X-Amz-Expires=900&X-Amz-Signature=89e78e9a9d70add7ed93d453334f4f93c6f29d889d46750a1f2da04af73978db&X-Amz-SignedHeaders=host&x-amz-storage-class=INTELLIGENT_TIERING&x-id=PutObject
The above exception was the direct cause of the following exception:
Traceback (most recent call last):
File "/home/olab/kirstain/anaconda3/envs/datasets/lib/python3.10/site-packages/huggingface_hub/_commit_api.py", line 334, in _inner_upload_lfs_object
return _upload_lfs_object(
File "/home/olab/kirstain/anaconda3/envs/datasets/lib/python3.10/site-packages/huggingface_hub/_commit_api.py", line 391, in _upload_lfs_object
lfs_upload(
File "/home/olab/kirstain/anaconda3/envs/datasets/lib/python3.10/site-packages/huggingface_hub/lfs.py", line 273, in lfs_upload
_upload_single_part(
File "/home/olab/kirstain/anaconda3/envs/datasets/lib/python3.10/site-packages/huggingface_hub/lfs.py", line 305, in _upload_single_part
hf_raise_for_status(upload_res)
File "/home/olab/kirstain/anaconda3/envs/datasets/lib/python3.10/site-packages/huggingface_hub/utils/_errors.py", line 318, in hf_raise_for_status
raise HfHubHTTPError(str(e), response=response) from e
huggingface_hub.utils._errors.HfHubHTTPError: 403 Client Error: Forbidden for url: https://s3.us-east-1.amazonaws.com/lfs.huggingface.co/repos/cf/0c/cf0c5ab8a3f729e5f57a8b79a36ecea64a31126f13218591c27ed9a1c7bd9b41/ece885a4bb6bbc8c1bb51b45542b805283d74590f72cd4c45d3ba76628570386?X-Amz-Algorithm=AWS4-HMAC-SHA256&X-Amz-Content-Sha256=UNSIGNED-PAYLOAD&X-Amz-Credential=AKIA4N7VTDGO27GPWFUO%2F20230128%2Fus-east-1%2Fs3%2Faws4_request&X-Amz-Date=20230128T151640Z&X-Amz-Expires=900&X-Amz-Signature=89e78e9a9d70add7ed93d453334f4f93c6f29d889d46750a1f2da04af73978db&X-Amz-SignedHeaders=host&x-amz-storage-class=INTELLIGENT_TIERING&x-id=PutObject
The above exception was the direct cause of the following exception:
Traceback (most recent call last):
File "<stdin>", line 1, in <module>
File "/home/olab/kirstain/anaconda3/envs/datasets/lib/python3.10/site-packages/datasets/arrow_dataset.py", line 4909, in push_to_hub
repo_id, split, uploaded_size, dataset_nbytes, repo_files, deleted_size = self._push_parquet_shards_to_hub(
File "/home/olab/kirstain/anaconda3/envs/datasets/lib/python3.10/site-packages/datasets/arrow_dataset.py", line 4804, in _push_parquet_shards_to_hub
_retry(
File "/home/olab/kirstain/anaconda3/envs/datasets/lib/python3.10/site-packages/datasets/utils/file_utils.py", line 281, in _retry
return func(*func_args, **func_kwargs)
File "/home/olab/kirstain/anaconda3/envs/datasets/lib/python3.10/site-packages/huggingface_hub/utils/_validators.py", line 124, in _inner_fn
return fn(*args, **kwargs)
File "/home/olab/kirstain/anaconda3/envs/datasets/lib/python3.10/site-packages/huggingface_hub/hf_api.py", line 2537, in upload_file
commit_info = self.create_commit(
File "/home/olab/kirstain/anaconda3/envs/datasets/lib/python3.10/site-packages/huggingface_hub/utils/_validators.py", line 124, in _inner_fn
return fn(*args, **kwargs)
File "/home/olab/kirstain/anaconda3/envs/datasets/lib/python3.10/site-packages/huggingface_hub/hf_api.py", line 2346, in create_commit
upload_lfs_files(
File "/home/olab/kirstain/anaconda3/envs/datasets/lib/python3.10/site-packages/huggingface_hub/utils/_validators.py", line 124, in _inner_fn
return fn(*args, **kwargs)
File "/home/olab/kirstain/anaconda3/envs/datasets/lib/python3.10/site-packages/huggingface_hub/_commit_api.py", line 346, in upload_lfs_files
thread_map(
File "/home/olab/kirstain/anaconda3/envs/datasets/lib/python3.10/site-packages/tqdm/contrib/concurrent.py", line 94, in thread_map
return _executor_map(ThreadPoolExecutor, fn, *iterables, **tqdm_kwargs)
File "/home/olab/kirstain/anaconda3/envs/datasets/lib/python3.10/site-packages/tqdm/contrib/concurrent.py", line 76, in _executor_map
return list(tqdm_class(ex.map(fn, *iterables, **map_args), **kwargs))
File "/home/olab/kirstain/anaconda3/envs/datasets/lib/python3.10/site-packages/tqdm/std.py", line 1195, in __iter__
for obj in iterable:
File "/home/olab/kirstain/anaconda3/envs/datasets/lib/python3.10/concurrent/futures/_base.py", line 621, in result_iterator
yield _result_or_cancel(fs.pop())
File "/home/olab/kirstain/anaconda3/envs/datasets/lib/python3.10/concurrent/futures/_base.py", line 319, in _result_or_cancel
return fut.result(timeout)
File "/home/olab/kirstain/anaconda3/envs/datasets/lib/python3.10/concurrent/futures/_base.py", line 458, in result
return self.__get_result()
File "/home/olab/kirstain/anaconda3/envs/datasets/lib/python3.10/concurrent/futures/_base.py", line 403, in __get_result
raise self._exception
File "/home/olab/kirstain/anaconda3/envs/datasets/lib/python3.10/concurrent/futures/thread.py", line 58, in run
result = self.fn(*self.args, **self.kwargs)
File "/home/olab/kirstain/anaconda3/envs/datasets/lib/python3.10/site-packages/huggingface_hub/_commit_api.py", line 338, in _inner_upload_lfs_object
raise RuntimeError(
RuntimeError: Error while uploading 'data/train-00000-of-00001-6df93048e66df326.parquet' to the Hub.
```
### Expected behavior
The dataset should be uploaded without any exceptions
### Environment info
- `datasets` version: 2.9.0
- Platform: Linux-4.15.0-65-generic-x86_64-with-glibc2.27
- Python version: 3.10.9
- PyArrow version: 11.0.0
- Pandas version: 1.5.3
| 5,483 |
https://github.com/huggingface/datasets/issues/5482 | Reload features from Parquet metadata | [
"I'd be happy to have a look, if nobody else has started working on this yet @lhoestq. \r\n\r\nIt seems to me that for the `arrow` format features are currently attached as metadata [in `datasets.arrow_writer`](https://github.com/huggingface/datasets/blob/5f810b7011a8a4ab077a1847c024d2d9e267b065/src/datasets/arrow_writer.py#L412) and retrieved from the metadata at `load_dataset` time using [`datasets.features.features.from_arrow_schema`](https://github.com/huggingface/datasets/blob/5f810b7011a8a4ab077a1847c024d2d9e267b065/src/datasets/features/features.py#L1602). \r\n\r\nThis will need to be replicated for `parquet` via calls to [this api](https://arrow.apache.org/docs/python/generated/pyarrow.parquet.write_metadata.html) from `io.parquet.ParquetWriter` and `io.parquet.ParquetReader` [respectively](https://github.com/huggingface/datasets/blob/5f810b7011a8a4ab077a1847c024d2d9e267b065/src/datasets/io/parquet.py#L104).\r\n\r\nAny other important considerations?\r\n",
"Thanks @MFreidank ! That's correct :)\r\n\r\nReading the metadata to infer the features can be ideally done in the `parquet.py` file in `packaged_builder` when a parquet file is read. You can cast the arrow table to the schema you get from the features.arrow_schema",
"#self-assign"
] | The idea would be to allow this :
```python
ds.to_parquet("my_dataset/ds.parquet")
reloaded = load_dataset("my_dataset")
assert ds.features == reloaded.features
```
And it should also work with Image and Audio types (right now they're reloaded as a dict type)
This can be implemented by storing and reading the feature types in the parquet metadata, as we do for arrow files. | 5,482 |
https://github.com/huggingface/datasets/issues/5481 | Load a cached dataset as iterable | [
"Can I work on this issue? I am pretty new to this.",
"Hi ! Sure :) you can comment `#self-assign` to assign yourself to this issue.\r\n\r\nI can give you some pointers to get started:\r\n\r\n`load_dataset` works roughly this way:\r\n1. it instantiate a dataset builder using `load_dataset_builder()`\r\n2. the builder download and prepare the dataset as Arrow files in the cache using `download_and_prepare()`\r\n3. the builder returns a Dataset object with `as_dataset()`\r\n\r\nOne way to approach this would be to implement `as_iterable_dataset()` in `builder.py`.\r\n\r\nAnd similarly to `as_dataset()`, you can use the `ArrowReader`. It has a `get_file_instructions()` method that can be helpful. It gives you the files to read as list of dictionaries with those keys: `filename`, `skip` and `take`.\r\n\r\nThe `skip` and `take` arguments are used in case the user wants to load a subset of the dataset, e.g.\r\n```python\r\nload_dataset(..., split=\"train[:10]\")\r\n```\r\n\r\nLet me know if you have questions or if I can help :)",
"This use-case is a bit specific, and `load_dataset` already has enough parameters (plus, `streaming=True` also returns an iterable dataset, so we would have to explain the difference), so I think it would be better to add `IterableDataset.from_file` to the API (more flexible and aligned with the goal from https://github.com/huggingface/datasets/issues/3444) instead.",
"> This use-case is a bit specific\r\n\r\nThis allows to use `datasets` for large scale training where map-style datasets are too slow and use too much memory in PyTorch. So I would still consider adding it.\r\n\r\nAlternatively we could add this feature one level bellow:\r\n```python\r\nbuilder = load_dataset_builder(...)\r\nbuilder.download_and_prepare()\r\nids = builder.as_iterable_dataset()\r\n```",
"Yes, I see how this can be useful. Still, I think `Dataset.to_iterable` + `IterableDataset.from_file` would be much cleaner in terms of the API design (and more flexible since `load_dataset` can only access the \"initial\" (unprocessed) version of a dataset).\r\n\r\nAnd since it can be tricky to manually find the \"initial\" version of a dataset in the cache, maybe `load_dataset` could return an iterable dataset streamed from the cache if `streaming=True` and the cache is up-to-date. ",
"> This allows to use datasets for large scale training where map-style datasets are too slow and use too much memory in PyTorch.\r\n\r\nI second that. e.g. In my last experiment Oscar-en uses 16GB RSS RAM per process and when using multiple processes the host quickly runs out cpu memory. ",
">And since it can be tricky to manually find the \"initial\" version of a dataset in the cache, maybe load_dataset could return an iterable dataset streamed from the cache if streaming=True and the cache is up-to-date.\r\n\r\nThis is exactly the need on JeanZay (HPC) - I have the dataset cache ready, but the compute node is offline, so making streaming work off a local cache would address that need.\r\n\r\nIf you will have a working POC I can be the tester. ",
"> Yes, I see how this can be useful. Still, I think Dataset.to_iterable + IterableDataset.from_file would be much cleaner in terms of the API design (and more flexible since load_dataset can only access the \"initial\" (unprocessed) version of a dataset).\r\n\r\nI like `IterableDataset.from_file` as well. On the other hand `Dataset.to_iterable` first requires to load a Dataset object, which can take time depending on your hardware and your dataset size (sometimes 1h+).\r\n\r\n> And since it can be tricky to manually find the \"initial\" version of a dataset in the cache, maybe load_dataset could return an iterable dataset streamed from the cache if streaming=True and the cache is up-to-date.\r\n\r\nThat would definitely do the job. I was suggesting a different parameter just to make explicit the difference between\r\n- streaming from the raw data\r\n- streaming from the local cache\r\n\r\nBut I'd be fine with streaming from cache is the cache is up-to-date since it's always faster. We could log a message as usual to make it explicit that the cache is used",
"> I was suggesting a different parameter just to make explicit the difference between\r\n\r\nMosaicML's `streaming` library does the same (tries to stream from the local cache if possible), so logging a message should be explicit enough :).",
"Ok ! Sounds good then :)",
"Hi Both! It has been a while since my first issue so I am gonna go for this one ! #self-assign",
"#self-assign",
"I like idea of `IterableDataset.from_file`. ",
"https://github.com/huggingface/datasets/pull/5821 should be helpful to implement `IterableDataset.from_file`, since it defines a new ArrowExamplesIterable that takes an Arrow tables generator function (e.g. from a file) and can be used in an IterableDataset",
"@lhoestq I have just started working on this issue. ",
"@lhoestq Thank you for taking over.",
"So what's recommanded usage of `IterableDataset.from_file` and `load_dataset`? How about I have multiple arrow files and `load_dataset` is often convenient to handle that.",
"If you have multiple Arrow files you can load them using\r\n\r\n```python\r\nfrom datasets import load_dataset\r\n\r\ndata_files = {\"train\": [\"path/to/0.arrow\", \"path/to/1.arrow\", ..., \"path/to/n.arrow\"]}\r\n\r\nds = load_dataset(\"arrow\", data_files=data_files, streaming=True)\r\n```\r\n\r\nThis is equivalent to calling `IterableDataset.from_file` and `concatenate_datasets`."
] | The idea would be to allow something like
```python
ds = load_dataset("c4", "en", as_iterable=True)
```
To be used to train models. It would load an IterableDataset from the cached Arrow files.
Cc @stas00
Edit : from the discussions we may load from cache when streaming=True | 5,481 |
https://github.com/huggingface/datasets/issues/5479 | audiofolder works on local env, but creates empty dataset in a remote one, what dependencies could I be missing/outdated | [] | ### Describe the bug
I'm using a custom audio dataset (400+ audio files) in the correct format for audiofolder. Although loading the dataset with audiofolder works in one local setup, it doesn't in a remote one (it just creates an empty dataset). I have both ffmpeg and libndfile installed on both computers, what could be missing/need to be updated in the one that doesn't work? On the remote env, libsndfile is 1.0.28 and ffmpeg is 4.2.1.
from datasets import load_dataset
ds = load_dataset("audiofolder", data_dir="...")
Here is the output (should be generating 400+ rows):
Downloading and preparing dataset audiofolder/default to ...
Downloading data files: 0%| | 0/2 [00:00<?, ?it/s]
Downloading data files: 0it [00:00, ?it/s]
Extracting data files: 0it [00:00, ?it/s]
Generating train split: 0 examples [00:00, ? examples/s]
Dataset audiofolder downloaded and prepared to ... Subsequent calls will reuse this data.
0%| | 0/1 [00:00<?, ?it/s]
DatasetDict({
train: Dataset({
features: ['audio', 'transcription'],
num_rows: 1
})
})
Here is my pip environment in the one that doesn't work (uses torch 1.11.a0 from shared env):
Package Version
------------------- -------------------
aiofiles 22.1.0
aiohttp 3.8.3
aiosignal 1.3.1
altair 4.2.1
anyio 3.6.2
appdirs 1.4.4
argcomplete 2.0.0
argon2-cffi 20.1.0
astunparse 1.6.3
async-timeout 4.0.2
attrs 21.2.0
audioread 3.0.0
backcall 0.2.0
bleach 4.0.0
certifi 2021.10.8
cffi 1.14.6
charset-normalizer 2.0.12
click 8.1.3
contourpy 1.0.7
cycler 0.11.0
datasets 2.9.0
debugpy 1.4.1
decorator 5.0.9
defusedxml 0.7.1
dill 0.3.6
distlib 0.3.4
entrypoints 0.3
evaluate 0.4.0
expecttest 0.1.3
fastapi 0.89.1
ffmpy 0.3.0
filelock 3.6.0
fonttools 4.38.0
frozenlist 1.3.3
fsspec 2023.1.0
future 0.18.2
gradio 3.16.2
h11 0.14.0
httpcore 0.16.3
httpx 0.23.3
huggingface-hub 0.12.0
idna 3.3
ipykernel 6.2.0
ipython 7.26.0
ipython-genutils 0.2.0
ipywidgets 7.6.3
jedi 0.18.0
Jinja2 3.0.1
jiwer 2.5.1
joblib 1.2.0
jsonschema 3.2.0
jupyter 1.0.0
jupyter-client 6.1.12
jupyter-console 6.4.0
jupyter-core 4.7.1
jupyterlab-pygments 0.1.2
jupyterlab-widgets 1.0.0
kiwisolver 1.4.4
Levenshtein 0.20.2
librosa 0.9.2
linkify-it-py 1.0.3
llvmlite 0.39.1
markdown-it-py 2.1.0
MarkupSafe 2.0.1
matplotlib 3.6.3
matplotlib-inline 0.1.2
mdit-py-plugins 0.3.3
mdurl 0.1.2
mistune 0.8.4
multidict 6.0.4
multiprocess 0.70.14
nbclient 0.5.4
nbconvert 6.1.0
nbformat 5.1.3
nest-asyncio 1.5.1
notebook 6.4.3
numba 0.56.4
numpy 1.20.3
orjson 3.8.5
packaging 21.0
pandas 1.5.3
pandocfilters 1.4.3
parso 0.8.2
pexpect 4.8.0
pickleshare 0.7.5
Pillow 9.4.0
pip 22.3.1
pipx 1.1.0
platformdirs 2.5.2
pooch 1.6.0
prometheus-client 0.11.0
prompt-toolkit 3.0.19
psutil 5.9.0
ptyprocess 0.7.0
pyarrow 10.0.1
pycparser 2.20
pycryptodome 3.16.0
pydantic 1.10.4
pydub 0.25.1
Pygments 2.10.0
pyparsing 2.4.7
pyrsistent 0.18.0
python-dateutil 2.8.2
python-multipart 0.0.5
pytz 2022.7.1
PyYAML 6.0
pyzmq 22.2.1
qtconsole 5.1.1
QtPy 1.10.0
rapidfuzz 2.13.7
regex 2022.10.31
requests 2.27.1
resampy 0.4.2
responses 0.18.0
rfc3986 1.5.0
scikit-learn 1.2.1
scipy 1.6.3
Send2Trash 1.8.0
setuptools 65.5.1
shiboken6 6.3.1
shiboken6-generator 6.3.1
six 1.16.0
sniffio 1.3.0
soundfile 0.11.0
starlette 0.22.0
terminado 0.11.0
testpath 0.5.0
threadpoolctl 3.1.0
tokenizers 0.13.2
toolz 0.12.0
torch 1.11.0a0+gitunknown
tornado 6.1
tqdm 4.64.1
traitlets 5.0.5
transformers 4.27.0.dev0
types-dataclasses 0.6.4
typing_extensions 4.1.1
uc-micro-py 1.0.1
urllib3 1.26.9
userpath 1.8.0
uvicorn 0.20.0
virtualenv 20.14.1
wcwidth 0.2.5
webencodings 0.5.1
websockets 10.4
wheel 0.37.1
widgetsnbextension 3.5.1
xxhash 3.2.0
yarl 1.8.2
### Steps to reproduce the bug
Create a pip environment with the packages listed above (make sure ffmpeg and libsndfile is installed with same versions listed above).
Create a custom audio dataset and load it in with load_dataset("audiofolder", ...)
### Expected behavior
load_dataset should create a dataset with 400+ rows.
### Environment info
- `datasets` version: 2.9.0
- Platform: Linux-3.10.0-1160.80.1.el7.x86_64-x86_64-with-glibc2.17
- Python version: 3.9.0
- PyArrow version: 10.0.1
- Pandas version: 1.5.3 | 5,479 |
https://github.com/huggingface/datasets/issues/5477 | Unpin sqlalchemy once issue is fixed | [
"@albertvillanova It looks like that issue has been fixed so I made a PR to unpin sqlalchemy! ",
"The source issue:\r\n- https://github.com/pandas-dev/pandas/issues/40686\r\n\r\nhas been fixed:\r\n- https://github.com/pandas-dev/pandas/pull/48576\r\n\r\nThe fix was released yesterday (2023-04-03) only in `pandas-2.0.0`:\r\n- https://github.com/pandas-dev/pandas/releases/tag/v2.0.0\r\n\r\nbut it will not be back-ported to `pandas-1`:\r\n- https://github.com/pandas-dev/pandas/pull/48576#issuecomment-1466467159\r\n\r\nAlso note that `pandas-2.0.0` dropped support for Python 3.7:\r\n- https://github.com/pandas-dev/pandas/issues/41678\r\n- https://github.com/pandas-dev/pandas/pull/41989\r\n\r\nTherefore, we cannot unpin `sqlalchemy` until we drop support for Python 3.7 (these Python users cannot use `pandas-2`)."
] | Once the source issue is fixed:
- pandas-dev/pandas#51015
we should revert the pin introduced in:
- #5476 | 5,477 |
https://github.com/huggingface/datasets/issues/5475 | Dataset scan time is much slower than using native arrow | [
"Hi ! In your code you only iterate on the Arrow buffers - you don't actually load the data as python objects. For a fair comparison, you can modify your code using:\r\n```diff\r\n- for _ in range(0, len(table), bsz):\r\n- _ = {k:table[k][_ : _ + bsz] for k in cols}\r\n+ for _ in range(0, len(table), bsz):\r\n+ _ = {k:table[k][_ : _ + bsz].to_pylist() for k in cols}\r\n```\r\n\r\nI re-ran your code and got a speed ratio of 1.00x and 1.02x",
"Ah I see, datasets is implicitly making this conversion. Thanks for pointing that out!\r\n\r\nIf it's not too much, I would also suggest updating some of your docs with the same `.to_pylist()` conversion in the code snippet that follows [here](https://huggingface.co/course/chapter5/4?fw=pt#:~:text=let%E2%80%99s%20run%20a%20little%20speed%20test%20by%20iterating%20over%20all%20the%20elements%20in%20the%20PubMed%20Abstracts%20dataset%3A).",
"This code snippet shows `datasets` code that reads the Arrow data as python objects already, there is no need to add to_pylist. Or were you thinking about something else ?"
] | ### Describe the bug
I'm basically running the same scanning experiment from the tutorials https://huggingface.co/course/chapter5/4?fw=pt except now I'm comparing to a native pyarrow version.
I'm finding that the native pyarrow approach is much faster (2 orders of magnitude). Is there something I'm missing that explains this phenomenon?
### Steps to reproduce the bug
https://colab.research.google.com/drive/11EtHDaGAf1DKCpvYnAPJUW-LFfAcDzHY?usp=sharing
### Expected behavior
I expect scan times to be on par with using pyarrow directly.
### Environment info
standard colab environment | 5,475 |
Subsets and Splits
No community queries yet
The top public SQL queries from the community will appear here once available.