Spaces:
Running
on
CPU Upgrade
Running
on
CPU Upgrade
## Use Cases | |
### Style transfer | |
One of the most popular use cases of image-to-image is style transfer. Style transfer models can convert a normal photography into a painting in the style of a famous painter. | |
## Task Variants | |
### Image inpainting | |
Image inpainting is widely used during photography editing to remove unwanted objects, such as poles, wires, or sensor | |
dust. | |
### Image colorization | |
Old or black and white images can be brought up to life using an image colorization model. | |
### Super Resolution | |
Super-resolution models increase the resolution of an image, allowing for higher-quality viewing and printing. | |
## Inference | |
You can use pipelines for image-to-image in 🧨diffusers library to easily use image-to-image models. See an example for `StableDiffusionImg2ImgPipeline` below. | |
```python | |
from PIL import Image | |
from diffusers import StableDiffusionImg2ImgPipeline | |
model_id_or_path = "runwayml/stable-diffusion-v1-5" | |
pipe = StableDiffusionImg2ImgPipeline.from_pretrained(model_id_or_path, torch_dtype=torch.float16) | |
pipe = pipe.to(cuda) | |
init_image = Image.open("mountains_image.jpeg").convert("RGB").resize((768, 512)) | |
prompt = "A fantasy landscape, trending on artstation" | |
images = pipe(prompt=prompt, image=init_image, strength=0.75, guidance_scale=7.5).images | |
images[0].save("fantasy_landscape.png") | |
``` | |
You can use [huggingface.js](https://github.com/huggingface/huggingface.js) to infer image-to-image models on Hugging Face Hub. | |
```javascript | |
import { HfInference } from "@huggingface/inference"; | |
const inference = new HfInference(HF_ACCESS_TOKEN); | |
await inference.imageToImage({ | |
data: await (await fetch("image")).blob(), | |
model: "timbrooks/instruct-pix2pix", | |
parameters: { | |
prompt: "Deblur this image", | |
}, | |
}); | |
``` | |
## ControlNet | |
Controlling the outputs of diffusion models only with a text prompt is a challenging problem. ControlNet is a neural network model that provides image-based control to diffusion models. Control images can be edges or other landmarks extracted from a source image. | |
Many ControlNet models were trained in our community event, JAX Diffusers sprint. You can see the full list of the ControlNet models available [here](https://huggingface.co/spaces/jax-diffusers-event/leaderboard). | |
## Most Used Model for the Task | |
Pix2Pix is a popular model used for image-to-image translation tasks. It is based on a conditional-GAN (generative adversarial network) where instead of a noise vector a 2D image is given as input. More information about Pix2Pix can be retrieved from this [link](https://phillipi.github.io/pix2pix/) where the associated paper and the GitHub repository can be found. | |
The images below show some examples extracted from the Pix2Pix paper. This model can be applied to various use cases. It is capable of relatively simpler things, e.g., converting a grayscale image to its colored version. But more importantly, it can generate realistic pictures from rough sketches (can be seen in the purse example) or from painting-like images (can be seen in the street and facade examples below). | |
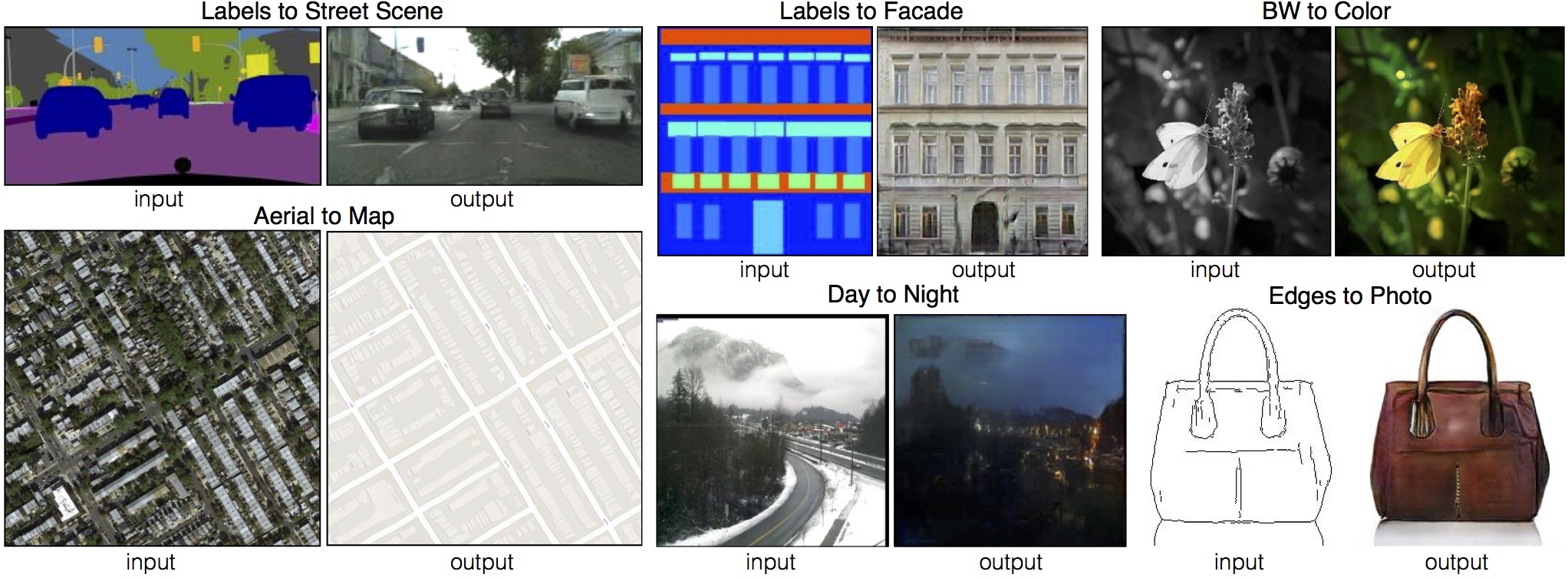 | |
## Useful Resources | |
- [Image-to-image guide with diffusers](https://huggingface.co/docs/diffusers/using-diffusers/img2img) | |
- [Train your ControlNet with diffusers 🧨](https://huggingface.co/blog/train-your-controlnet) | |
- [Ultra fast ControlNet with 🧨 Diffusers](https://huggingface.co/blog/controlnet) | |
## References | |
[1] P. Isola, J. -Y. Zhu, T. Zhou and A. A. Efros, "Image-to-Image Translation with Conditional Adversarial Networks," 2017 IEEE Conference on Computer Vision and Pattern Recognition (CVPR), 2017, pp. 5967-5976, doi: 10.1109/CVPR.2017.632. | |
This page was made possible thanks to the efforts of [Paul Gafton](https://github.com/Paul92) and [Osman Alenbey](https://huggingface.co/osman93). | |