modelId
stringlengths 5
139
| author
stringlengths 2
42
| last_modified
timestamp[us, tz=UTC]date 2020-02-15 11:33:14
2025-06-27 00:42:13
| downloads
int64 0
223M
| likes
int64 0
11.7k
| library_name
stringclasses 499
values | tags
sequencelengths 1
4.05k
| pipeline_tag
stringclasses 54
values | createdAt
timestamp[us, tz=UTC]date 2022-03-02 23:29:04
2025-06-27 00:40:00
| card
stringlengths 11
1.01M
|
---|---|---|---|---|---|---|---|---|---|
ShenaoZ/0.0005_withdpo_4iters_bs256_55505lr_iter_4 | ShenaoZ | 2024-05-09T06:44:58Z | 6 | 0 | transformers | [
"transformers",
"safetensors",
"mistral",
"text-generation",
"alignment-handbook",
"trl",
"dpo",
"generated_from_trainer",
"conversational",
"dataset:updated",
"dataset:original",
"base_model:ShenaoZ/0.0005_withdpo_4iters_bs256_555lr_iter_3",
"base_model:finetune:ShenaoZ/0.0005_withdpo_4iters_bs256_555lr_iter_3",
"license:mit",
"autotrain_compatible",
"text-generation-inference",
"endpoints_compatible",
"region:us"
] | text-generation | 2024-05-09T05:58:17Z | ---
license: mit
base_model: ShenaoZ/0.0005_withdpo_4iters_bs256_555lr_iter_3
tags:
- alignment-handbook
- trl
- dpo
- generated_from_trainer
- trl
- dpo
- generated_from_trainer
datasets:
- updated
- original
model-index:
- name: 0.0005_withdpo_4iters_bs256_55505lr_iter_4
results: []
---
<!-- This model card has been generated automatically according to the information the Trainer had access to. You
should probably proofread and complete it, then remove this comment. -->
# 0.0005_withdpo_4iters_bs256_55505lr_iter_4
This model is a fine-tuned version of [ShenaoZ/0.0005_withdpo_4iters_bs256_555lr_iter_3](https://huggingface.co/ShenaoZ/0.0005_withdpo_4iters_bs256_555lr_iter_3) on the updated and the original datasets.
## Model description
More information needed
## Intended uses & limitations
More information needed
## Training and evaluation data
More information needed
## Training procedure
### Training hyperparameters
The following hyperparameters were used during training:
- learning_rate: 5e-08
- train_batch_size: 8
- eval_batch_size: 8
- seed: 42
- distributed_type: multi-GPU
- num_devices: 8
- gradient_accumulation_steps: 4
- total_train_batch_size: 256
- total_eval_batch_size: 64
- optimizer: Adam with betas=(0.9,0.999) and epsilon=1e-08
- lr_scheduler_type: cosine
- lr_scheduler_warmup_ratio: 0.1
- num_epochs: 1
### Training results
### Framework versions
- Transformers 4.40.2
- Pytorch 2.1.2+cu121
- Datasets 2.14.6
- Tokenizers 0.19.1
|
tomreichel/proofdb | tomreichel | 2024-05-09T06:34:20Z | 0 | 0 | transformers | [
"transformers",
"safetensors",
"arxiv:1910.09700",
"endpoints_compatible",
"region:us"
] | null | 2024-05-09T06:30:17Z | ---
library_name: transformers
tags: []
---
# Model Card for Model ID
<!-- Provide a quick summary of what the model is/does. -->
## Model Details
### Model Description
<!-- Provide a longer summary of what this model is. -->
This is the model card of a 🤗 transformers model that has been pushed on the Hub. This model card has been automatically generated.
- **Developed by:** [More Information Needed]
- **Funded by [optional]:** [More Information Needed]
- **Shared by [optional]:** [More Information Needed]
- **Model type:** [More Information Needed]
- **Language(s) (NLP):** [More Information Needed]
- **License:** [More Information Needed]
- **Finetuned from model [optional]:** [More Information Needed]
### Model Sources [optional]
<!-- Provide the basic links for the model. -->
- **Repository:** [More Information Needed]
- **Paper [optional]:** [More Information Needed]
- **Demo [optional]:** [More Information Needed]
## Uses
<!-- Address questions around how the model is intended to be used, including the foreseeable users of the model and those affected by the model. -->
### Direct Use
<!-- This section is for the model use without fine-tuning or plugging into a larger ecosystem/app. -->
[More Information Needed]
### Downstream Use [optional]
<!-- This section is for the model use when fine-tuned for a task, or when plugged into a larger ecosystem/app -->
[More Information Needed]
### Out-of-Scope Use
<!-- This section addresses misuse, malicious use, and uses that the model will not work well for. -->
[More Information Needed]
## Bias, Risks, and Limitations
<!-- This section is meant to convey both technical and sociotechnical limitations. -->
[More Information Needed]
### Recommendations
<!-- This section is meant to convey recommendations with respect to the bias, risk, and technical limitations. -->
Users (both direct and downstream) should be made aware of the risks, biases and limitations of the model. More information needed for further recommendations.
## How to Get Started with the Model
Use the code below to get started with the model.
[More Information Needed]
## Training Details
### Training Data
<!-- This should link to a Dataset Card, perhaps with a short stub of information on what the training data is all about as well as documentation related to data pre-processing or additional filtering. -->
[More Information Needed]
### Training Procedure
<!-- This relates heavily to the Technical Specifications. Content here should link to that section when it is relevant to the training procedure. -->
#### Preprocessing [optional]
[More Information Needed]
#### Training Hyperparameters
- **Training regime:** [More Information Needed] <!--fp32, fp16 mixed precision, bf16 mixed precision, bf16 non-mixed precision, fp16 non-mixed precision, fp8 mixed precision -->
#### Speeds, Sizes, Times [optional]
<!-- This section provides information about throughput, start/end time, checkpoint size if relevant, etc. -->
[More Information Needed]
## Evaluation
<!-- This section describes the evaluation protocols and provides the results. -->
### Testing Data, Factors & Metrics
#### Testing Data
<!-- This should link to a Dataset Card if possible. -->
[More Information Needed]
#### Factors
<!-- These are the things the evaluation is disaggregating by, e.g., subpopulations or domains. -->
[More Information Needed]
#### Metrics
<!-- These are the evaluation metrics being used, ideally with a description of why. -->
[More Information Needed]
### Results
[More Information Needed]
#### Summary
## Model Examination [optional]
<!-- Relevant interpretability work for the model goes here -->
[More Information Needed]
## Environmental Impact
<!-- Total emissions (in grams of CO2eq) and additional considerations, such as electricity usage, go here. Edit the suggested text below accordingly -->
Carbon emissions can be estimated using the [Machine Learning Impact calculator](https://mlco2.github.io/impact#compute) presented in [Lacoste et al. (2019)](https://arxiv.org/abs/1910.09700).
- **Hardware Type:** [More Information Needed]
- **Hours used:** [More Information Needed]
- **Cloud Provider:** [More Information Needed]
- **Compute Region:** [More Information Needed]
- **Carbon Emitted:** [More Information Needed]
## Technical Specifications [optional]
### Model Architecture and Objective
[More Information Needed]
### Compute Infrastructure
[More Information Needed]
#### Hardware
[More Information Needed]
#### Software
[More Information Needed]
## Citation [optional]
<!-- If there is a paper or blog post introducing the model, the APA and Bibtex information for that should go in this section. -->
**BibTeX:**
[More Information Needed]
**APA:**
[More Information Needed]
## Glossary [optional]
<!-- If relevant, include terms and calculations in this section that can help readers understand the model or model card. -->
[More Information Needed]
## More Information [optional]
[More Information Needed]
## Model Card Authors [optional]
[More Information Needed]
## Model Card Contact
[More Information Needed] |
asrilmurdian/asril-pegasus-xlsum-skripsi | asrilmurdian | 2024-05-09T06:31:24Z | 4 | 0 | transformers | [
"transformers",
"safetensors",
"pegasus",
"text2text-generation",
"generated_from_trainer",
"base_model:google/pegasus-xsum",
"base_model:finetune:google/pegasus-xsum",
"autotrain_compatible",
"endpoints_compatible",
"region:us"
] | text2text-generation | 2024-04-23T07:48:44Z | ---
base_model: google/pegasus-xsum
tags:
- generated_from_trainer
model-index:
- name: asril-pegasus-xlsum-skripsi
results: []
---
<!-- This model card has been generated automatically according to the information the Trainer had access to. You
should probably proofread and complete it, then remove this comment. -->
# asril-pegasus-xlsum-skripsi
This model is a fine-tuned version of [google/pegasus-xsum](https://huggingface.co/google/pegasus-xsum) on an unknown dataset.
It achieves the following results on the evaluation set:
- Loss: 2.6919
## Model description
this model is spesifically for indonsesian abstractive news article summarization wich has been fine tuning in more than 48k dataset
this model fine-tuned using pegasus model
## Intended uses & limitations
More information needed
## Training and evaluation data
xlsum/indonesian
## Training procedure
### Training hyperparameters
The following hyperparameters were used during training:
- learning_rate: 5e-05
- train_batch_size: 4
- eval_batch_size: 8
- seed: 42
- optimizer: Adam with betas=(0.9,0.999) and epsilon=1e-08
- lr_scheduler_type: linear
- lr_scheduler_warmup_steps: 500
- num_epochs: 1
- mixed_precision_training: Native AMP
### Training results
| Training Loss | Epoch | Step | Validation Loss |
|:-------------:|:------:|:----:|:---------------:|
| 4.5256 | 0.1046 | 1000 | 3.4857 |
| 3.699 | 0.2092 | 2000 | 3.1625 |
| 3.4046 | 0.3138 | 3000 | 2.9968 |
| 3.2456 | 0.4184 | 4000 | 2.8834 |
| 3.126 | 0.5230 | 5000 | 2.8127 |
| 3.055 | 0.6275 | 6000 | 2.7644 |
| 3.005 | 0.7321 | 7000 | 2.7281 |
| 2.9597 | 0.8367 | 8000 | 2.7060 |
| 2.9627 | 0.9413 | 9000 | 2.6919 |
### Framework versions
- Transformers 4.40.0
- Pytorch 2.1.0+cu121
- Datasets 2.19.0
- Tokenizers 0.19.1
|
tharun1507/Decipher-the_code_explainer | tharun1507 | 2024-05-09T06:27:53Z | 123 | 0 | transformers | [
"transformers",
"safetensors",
"bert",
"text-classification",
"arxiv:1910.09700",
"autotrain_compatible",
"endpoints_compatible",
"region:us"
] | text-classification | 2024-05-09T06:25:58Z | ---
library_name: transformers
tags: []
---
# Model Card for Model ID
<!-- Provide a quick summary of what the model is/does. -->
## Model Details
### Model Description
<!-- Provide a longer summary of what this model is. -->
This is the model card of a 🤗 transformers model that has been pushed on the Hub. This model card has been automatically generated.
- **Developed by:** [More Information Needed]
- **Funded by [optional]:** [More Information Needed]
- **Shared by [optional]:** [More Information Needed]
- **Model type:** [More Information Needed]
- **Language(s) (NLP):** [More Information Needed]
- **License:** [More Information Needed]
- **Finetuned from model [optional]:** [More Information Needed]
### Model Sources [optional]
<!-- Provide the basic links for the model. -->
- **Repository:** [More Information Needed]
- **Paper [optional]:** [More Information Needed]
- **Demo [optional]:** [More Information Needed]
## Uses
<!-- Address questions around how the model is intended to be used, including the foreseeable users of the model and those affected by the model. -->
### Direct Use
<!-- This section is for the model use without fine-tuning or plugging into a larger ecosystem/app. -->
[More Information Needed]
### Downstream Use [optional]
<!-- This section is for the model use when fine-tuned for a task, or when plugged into a larger ecosystem/app -->
[More Information Needed]
### Out-of-Scope Use
<!-- This section addresses misuse, malicious use, and uses that the model will not work well for. -->
[More Information Needed]
## Bias, Risks, and Limitations
<!-- This section is meant to convey both technical and sociotechnical limitations. -->
[More Information Needed]
### Recommendations
<!-- This section is meant to convey recommendations with respect to the bias, risk, and technical limitations. -->
Users (both direct and downstream) should be made aware of the risks, biases and limitations of the model. More information needed for further recommendations.
## How to Get Started with the Model
Use the code below to get started with the model.
[More Information Needed]
## Training Details
### Training Data
<!-- This should link to a Dataset Card, perhaps with a short stub of information on what the training data is all about as well as documentation related to data pre-processing or additional filtering. -->
[More Information Needed]
### Training Procedure
<!-- This relates heavily to the Technical Specifications. Content here should link to that section when it is relevant to the training procedure. -->
#### Preprocessing [optional]
[More Information Needed]
#### Training Hyperparameters
- **Training regime:** [More Information Needed] <!--fp32, fp16 mixed precision, bf16 mixed precision, bf16 non-mixed precision, fp16 non-mixed precision, fp8 mixed precision -->
#### Speeds, Sizes, Times [optional]
<!-- This section provides information about throughput, start/end time, checkpoint size if relevant, etc. -->
[More Information Needed]
## Evaluation
<!-- This section describes the evaluation protocols and provides the results. -->
### Testing Data, Factors & Metrics
#### Testing Data
<!-- This should link to a Dataset Card if possible. -->
[More Information Needed]
#### Factors
<!-- These are the things the evaluation is disaggregating by, e.g., subpopulations or domains. -->
[More Information Needed]
#### Metrics
<!-- These are the evaluation metrics being used, ideally with a description of why. -->
[More Information Needed]
### Results
[More Information Needed]
#### Summary
## Model Examination [optional]
<!-- Relevant interpretability work for the model goes here -->
[More Information Needed]
## Environmental Impact
<!-- Total emissions (in grams of CO2eq) and additional considerations, such as electricity usage, go here. Edit the suggested text below accordingly -->
Carbon emissions can be estimated using the [Machine Learning Impact calculator](https://mlco2.github.io/impact#compute) presented in [Lacoste et al. (2019)](https://arxiv.org/abs/1910.09700).
- **Hardware Type:** [More Information Needed]
- **Hours used:** [More Information Needed]
- **Cloud Provider:** [More Information Needed]
- **Compute Region:** [More Information Needed]
- **Carbon Emitted:** [More Information Needed]
## Technical Specifications [optional]
### Model Architecture and Objective
[More Information Needed]
### Compute Infrastructure
[More Information Needed]
#### Hardware
[More Information Needed]
#### Software
[More Information Needed]
## Citation [optional]
<!-- If there is a paper or blog post introducing the model, the APA and Bibtex information for that should go in this section. -->
**BibTeX:**
[More Information Needed]
**APA:**
[More Information Needed]
## Glossary [optional]
<!-- If relevant, include terms and calculations in this section that can help readers understand the model or model card. -->
[More Information Needed]
## More Information [optional]
[More Information Needed]
## Model Card Authors [optional]
[More Information Needed]
## Model Card Contact
[More Information Needed]
|
deepanshdj/dj-zephyr-7b-F16-GGUF | deepanshdj | 2024-05-09T06:24:13Z | 28 | 1 | transformers | [
"transformers",
"gguf",
"mistral",
"text-generation-inference",
"llama",
"trl",
"sft",
"en",
"base_model:HuggingFaceH4/zephyr-7b-beta",
"base_model:quantized:HuggingFaceH4/zephyr-7b-beta",
"license:apache-2.0",
"endpoints_compatible",
"region:us",
"conversational"
] | null | 2024-05-09T06:00:35Z | ---
language:
- en
license: apache-2.0
tags:
- text-generation-inference
- transformers
- llama
- trl
- sft
base_model: HuggingFaceH4/zephyr-7b-beta
---
---
## model name: zephyr-7b-dj-GGUF
## model creator: Deepansh Jha
## huggingface id: deepanshdj
## finetuned dataset: osaat1 (https://huggingface.co/datasets/deepanshdj/ossat1_8k_llama3)
---
# 🦙 Welcome to the zephyr-7b-dj-GGUF Wonderland! 🌟
## Unleash the Power of Conversation with zephyr-7b-dj-GGUF
Dive into the enchanting world of dj-llama3-F16-GGUF, a marvel crafted by the ingenious Deepansh Jha! 🚀 Licensed under the Apache License 2.0, this model is your passport to the realms of captivating dialogue and spellbinding text generation. 🎩✨
## Discover the Magic
Envisioned with creativity and nurtured with passion, dj-llama3-F16-GGUF is your companion for all things conversational! 💬 Whether you're weaving stories, sparking conversations, or crafting dialogues, this model is your trusty guide through the wonders of language. 📚🌈
## Model Maven
- **Model Creator:** Deepansh Jha
- **License:** Apache License 2.0
## Embark on Your Journey
Unleash the potential of zephyr-7b-dj-GGUF in your projects and endeavors! Let its charm and versatility illuminate your path to linguistic greatness. 🌟✨
## Join the Adventure
Come, be a part of this magical journey! 🎉 Contribute, explore, and create with zephyr-7b-dj-GGUF. The possibilities are as endless as the imagination itself! 🌌🚀
|
keanhean/esm2_t30_150M_UR50D-11k-PANTHER-classification | keanhean | 2024-05-09T06:24:00Z | 108 | 0 | transformers | [
"transformers",
"safetensors",
"esm",
"text-classification",
"generated_from_trainer",
"base_model:facebook/esm2_t30_150M_UR50D",
"base_model:finetune:facebook/esm2_t30_150M_UR50D",
"license:mit",
"autotrain_compatible",
"endpoints_compatible",
"region:us"
] | text-classification | 2024-05-09T06:23:45Z | ---
license: mit
base_model: facebook/esm2_t30_150M_UR50D
tags:
- generated_from_trainer
metrics:
- accuracy
model-index:
- name: esm2_t30_150M_UR50D-11k-PANTHER-classification
results: []
---
<!-- This model card has been generated automatically according to the information the Trainer had access to. You
should probably proofread and complete it, then remove this comment. -->
# esm2_t30_150M_UR50D-11k-PANTHER-classification
This model is a fine-tuned version of [facebook/esm2_t30_150M_UR50D](https://huggingface.co/facebook/esm2_t30_150M_UR50D) on the None dataset.
It achieves the following results on the evaluation set:
- Loss: 3.3041
- Accuracy: 0.4290
## Model description
More information needed
## Intended uses & limitations
More information needed
## Training and evaluation data
More information needed
## Training procedure
### Training hyperparameters
The following hyperparameters were used during training:
- learning_rate: 2e-05
- train_batch_size: 8
- eval_batch_size: 8
- seed: 42
- gradient_accumulation_steps: 16
- total_train_batch_size: 128
- optimizer: Adam with betas=(0.9,0.999) and epsilon=1e-08
- lr_scheduler_type: linear
- num_epochs: 3
### Training results
| Training Loss | Epoch | Step | Validation Loss | Accuracy |
|:-------------:|:------:|:----:|:---------------:|:--------:|
| No log | 0.9944 | 67 | 3.6678 | 0.2561 |
| No log | 1.9889 | 134 | 3.3917 | 0.3890 |
| No log | 2.9833 | 201 | 3.3041 | 0.4290 |
### Framework versions
- Transformers 4.40.2
- Pytorch 2.3.0+cu121
- Datasets 2.19.1
- Tokenizers 0.19.1
|
Kaswan/Kaswan | Kaswan | 2024-05-09T06:23:30Z | 0 | 0 | null | [
"license:apache-2.0",
"region:us"
] | null | 2024-05-09T06:23:30Z | ---
license: apache-2.0
---
|
ritesh47/finetuning-sentiment-model-3000-samples | ritesh47 | 2024-05-09T06:23:07Z | 107 | 0 | transformers | [
"transformers",
"pytorch",
"tensorboard",
"distilbert",
"text-classification",
"generated_from_trainer",
"license:apache-2.0",
"autotrain_compatible",
"endpoints_compatible",
"region:us"
] | text-classification | 2024-05-09T06:16:42Z | ---
license: apache-2.0
tags:
- generated_from_trainer
metrics:
- accuracy
- f1
model-index:
- name: finetuning-sentiment-model-3000-samples
results: []
---
<!-- This model card has been generated automatically according to the information the Trainer had access to. You
should probably proofread and complete it, then remove this comment. -->
# finetuning-sentiment-model-3000-samples
This model is a fine-tuned version of [distilbert-base-uncased](https://huggingface.co/distilbert-base-uncased) on an unknown dataset.
It achieves the following results on the evaluation set:
- Loss: 0.3348
- Accuracy: 0.8567
- F1: 0.8571
## Model description
More information needed
## Intended uses & limitations
More information needed
## Training and evaluation data
More information needed
## Training procedure
### Training hyperparameters
The following hyperparameters were used during training:
- learning_rate: 2e-05
- train_batch_size: 16
- eval_batch_size: 16
- seed: 42
- optimizer: Adam with betas=(0.9,0.999) and epsilon=1e-08
- lr_scheduler_type: linear
- num_epochs: 2
### Training results
### Framework versions
- Transformers 4.30.0
- Pytorch 2.2.1+cu121
- Datasets 2.19.1
- Tokenizers 0.13.3
|
TinyPixel/gra | TinyPixel | 2024-05-09T06:12:01Z | 0 | 0 | transformers | [
"transformers",
"safetensors",
"arxiv:1910.09700",
"endpoints_compatible",
"region:us"
] | null | 2024-05-09T06:11:51Z | ---
library_name: transformers
tags: []
---
# Model Card for Model ID
<!-- Provide a quick summary of what the model is/does. -->
## Model Details
### Model Description
<!-- Provide a longer summary of what this model is. -->
This is the model card of a 🤗 transformers model that has been pushed on the Hub. This model card has been automatically generated.
- **Developed by:** [More Information Needed]
- **Funded by [optional]:** [More Information Needed]
- **Shared by [optional]:** [More Information Needed]
- **Model type:** [More Information Needed]
- **Language(s) (NLP):** [More Information Needed]
- **License:** [More Information Needed]
- **Finetuned from model [optional]:** [More Information Needed]
### Model Sources [optional]
<!-- Provide the basic links for the model. -->
- **Repository:** [More Information Needed]
- **Paper [optional]:** [More Information Needed]
- **Demo [optional]:** [More Information Needed]
## Uses
<!-- Address questions around how the model is intended to be used, including the foreseeable users of the model and those affected by the model. -->
### Direct Use
<!-- This section is for the model use without fine-tuning or plugging into a larger ecosystem/app. -->
[More Information Needed]
### Downstream Use [optional]
<!-- This section is for the model use when fine-tuned for a task, or when plugged into a larger ecosystem/app -->
[More Information Needed]
### Out-of-Scope Use
<!-- This section addresses misuse, malicious use, and uses that the model will not work well for. -->
[More Information Needed]
## Bias, Risks, and Limitations
<!-- This section is meant to convey both technical and sociotechnical limitations. -->
[More Information Needed]
### Recommendations
<!-- This section is meant to convey recommendations with respect to the bias, risk, and technical limitations. -->
Users (both direct and downstream) should be made aware of the risks, biases and limitations of the model. More information needed for further recommendations.
## How to Get Started with the Model
Use the code below to get started with the model.
[More Information Needed]
## Training Details
### Training Data
<!-- This should link to a Dataset Card, perhaps with a short stub of information on what the training data is all about as well as documentation related to data pre-processing or additional filtering. -->
[More Information Needed]
### Training Procedure
<!-- This relates heavily to the Technical Specifications. Content here should link to that section when it is relevant to the training procedure. -->
#### Preprocessing [optional]
[More Information Needed]
#### Training Hyperparameters
- **Training regime:** [More Information Needed] <!--fp32, fp16 mixed precision, bf16 mixed precision, bf16 non-mixed precision, fp16 non-mixed precision, fp8 mixed precision -->
#### Speeds, Sizes, Times [optional]
<!-- This section provides information about throughput, start/end time, checkpoint size if relevant, etc. -->
[More Information Needed]
## Evaluation
<!-- This section describes the evaluation protocols and provides the results. -->
### Testing Data, Factors & Metrics
#### Testing Data
<!-- This should link to a Dataset Card if possible. -->
[More Information Needed]
#### Factors
<!-- These are the things the evaluation is disaggregating by, e.g., subpopulations or domains. -->
[More Information Needed]
#### Metrics
<!-- These are the evaluation metrics being used, ideally with a description of why. -->
[More Information Needed]
### Results
[More Information Needed]
#### Summary
## Model Examination [optional]
<!-- Relevant interpretability work for the model goes here -->
[More Information Needed]
## Environmental Impact
<!-- Total emissions (in grams of CO2eq) and additional considerations, such as electricity usage, go here. Edit the suggested text below accordingly -->
Carbon emissions can be estimated using the [Machine Learning Impact calculator](https://mlco2.github.io/impact#compute) presented in [Lacoste et al. (2019)](https://arxiv.org/abs/1910.09700).
- **Hardware Type:** [More Information Needed]
- **Hours used:** [More Information Needed]
- **Cloud Provider:** [More Information Needed]
- **Compute Region:** [More Information Needed]
- **Carbon Emitted:** [More Information Needed]
## Technical Specifications [optional]
### Model Architecture and Objective
[More Information Needed]
### Compute Infrastructure
[More Information Needed]
#### Hardware
[More Information Needed]
#### Software
[More Information Needed]
## Citation [optional]
<!-- If there is a paper or blog post introducing the model, the APA and Bibtex information for that should go in this section. -->
**BibTeX:**
[More Information Needed]
**APA:**
[More Information Needed]
## Glossary [optional]
<!-- If relevant, include terms and calculations in this section that can help readers understand the model or model card. -->
[More Information Needed]
## More Information [optional]
[More Information Needed]
## Model Card Authors [optional]
[More Information Needed]
## Model Card Contact
[More Information Needed] |
Soykot/CodeG | Soykot | 2024-05-09T06:11:02Z | 0 | 0 | peft | [
"peft",
"tensorboard",
"safetensors",
"arxiv:1910.09700",
"base_model:NousResearch/Llama-2-7b-chat-hf",
"base_model:adapter:NousResearch/Llama-2-7b-chat-hf",
"region:us"
] | null | 2024-05-09T06:10:25Z | ---
library_name: peft
base_model: NousResearch/Llama-2-7b-chat-hf
---
# Model Card for Model ID
<!-- Provide a quick summary of what the model is/does. -->
## Model Details
### Model Description
<!-- Provide a longer summary of what this model is. -->
- **Developed by:** [More Information Needed]
- **Funded by [optional]:** [More Information Needed]
- **Shared by [optional]:** [More Information Needed]
- **Model type:** [More Information Needed]
- **Language(s) (NLP):** [More Information Needed]
- **License:** [More Information Needed]
- **Finetuned from model [optional]:** [More Information Needed]
### Model Sources [optional]
<!-- Provide the basic links for the model. -->
- **Repository:** [More Information Needed]
- **Paper [optional]:** [More Information Needed]
- **Demo [optional]:** [More Information Needed]
## Uses
<!-- Address questions around how the model is intended to be used, including the foreseeable users of the model and those affected by the model. -->
### Direct Use
<!-- This section is for the model use without fine-tuning or plugging into a larger ecosystem/app. -->
[More Information Needed]
### Downstream Use [optional]
<!-- This section is for the model use when fine-tuned for a task, or when plugged into a larger ecosystem/app -->
[More Information Needed]
### Out-of-Scope Use
<!-- This section addresses misuse, malicious use, and uses that the model will not work well for. -->
[More Information Needed]
## Bias, Risks, and Limitations
<!-- This section is meant to convey both technical and sociotechnical limitations. -->
[More Information Needed]
### Recommendations
<!-- This section is meant to convey recommendations with respect to the bias, risk, and technical limitations. -->
Users (both direct and downstream) should be made aware of the risks, biases and limitations of the model. More information needed for further recommendations.
## How to Get Started with the Model
Use the code below to get started with the model.
[More Information Needed]
## Training Details
### Training Data
<!-- This should link to a Dataset Card, perhaps with a short stub of information on what the training data is all about as well as documentation related to data pre-processing or additional filtering. -->
[More Information Needed]
### Training Procedure
<!-- This relates heavily to the Technical Specifications. Content here should link to that section when it is relevant to the training procedure. -->
#### Preprocessing [optional]
[More Information Needed]
#### Training Hyperparameters
- **Training regime:** [More Information Needed] <!--fp32, fp16 mixed precision, bf16 mixed precision, bf16 non-mixed precision, fp16 non-mixed precision, fp8 mixed precision -->
#### Speeds, Sizes, Times [optional]
<!-- This section provides information about throughput, start/end time, checkpoint size if relevant, etc. -->
[More Information Needed]
## Evaluation
<!-- This section describes the evaluation protocols and provides the results. -->
### Testing Data, Factors & Metrics
#### Testing Data
<!-- This should link to a Dataset Card if possible. -->
[More Information Needed]
#### Factors
<!-- These are the things the evaluation is disaggregating by, e.g., subpopulations or domains. -->
[More Information Needed]
#### Metrics
<!-- These are the evaluation metrics being used, ideally with a description of why. -->
[More Information Needed]
### Results
[More Information Needed]
#### Summary
## Model Examination [optional]
<!-- Relevant interpretability work for the model goes here -->
[More Information Needed]
## Environmental Impact
<!-- Total emissions (in grams of CO2eq) and additional considerations, such as electricity usage, go here. Edit the suggested text below accordingly -->
Carbon emissions can be estimated using the [Machine Learning Impact calculator](https://mlco2.github.io/impact#compute) presented in [Lacoste et al. (2019)](https://arxiv.org/abs/1910.09700).
- **Hardware Type:** [More Information Needed]
- **Hours used:** [More Information Needed]
- **Cloud Provider:** [More Information Needed]
- **Compute Region:** [More Information Needed]
- **Carbon Emitted:** [More Information Needed]
## Technical Specifications [optional]
### Model Architecture and Objective
[More Information Needed]
### Compute Infrastructure
[More Information Needed]
#### Hardware
[More Information Needed]
#### Software
[More Information Needed]
## Citation [optional]
<!-- If there is a paper or blog post introducing the model, the APA and Bibtex information for that should go in this section. -->
**BibTeX:**
[More Information Needed]
**APA:**
[More Information Needed]
## Glossary [optional]
<!-- If relevant, include terms and calculations in this section that can help readers understand the model or model card. -->
[More Information Needed]
## More Information [optional]
[More Information Needed]
## Model Card Authors [optional]
[More Information Needed]
## Model Card Contact
[More Information Needed]
### Framework versions
- PEFT 0.10.0 |
Soykot/results | Soykot | 2024-05-09T06:09:22Z | 1 | 0 | peft | [
"peft",
"tensorboard",
"safetensors",
"arxiv:1910.09700",
"base_model:NousResearch/Llama-2-7b-chat-hf",
"base_model:adapter:NousResearch/Llama-2-7b-chat-hf",
"region:us"
] | null | 2024-04-24T10:10:08Z | ---
library_name: peft
base_model: NousResearch/Llama-2-7b-chat-hf
---
# Model Card for Model ID
<!-- Provide a quick summary of what the model is/does. -->
## Model Details
### Model Description
<!-- Provide a longer summary of what this model is. -->
- **Developed by:** [More Information Needed]
- **Funded by [optional]:** [More Information Needed]
- **Shared by [optional]:** [More Information Needed]
- **Model type:** [More Information Needed]
- **Language(s) (NLP):** [More Information Needed]
- **License:** [More Information Needed]
- **Finetuned from model [optional]:** [More Information Needed]
### Model Sources [optional]
<!-- Provide the basic links for the model. -->
- **Repository:** [More Information Needed]
- **Paper [optional]:** [More Information Needed]
- **Demo [optional]:** [More Information Needed]
## Uses
<!-- Address questions around how the model is intended to be used, including the foreseeable users of the model and those affected by the model. -->
### Direct Use
<!-- This section is for the model use without fine-tuning or plugging into a larger ecosystem/app. -->
[More Information Needed]
### Downstream Use [optional]
<!-- This section is for the model use when fine-tuned for a task, or when plugged into a larger ecosystem/app -->
[More Information Needed]
### Out-of-Scope Use
<!-- This section addresses misuse, malicious use, and uses that the model will not work well for. -->
[More Information Needed]
## Bias, Risks, and Limitations
<!-- This section is meant to convey both technical and sociotechnical limitations. -->
[More Information Needed]
### Recommendations
<!-- This section is meant to convey recommendations with respect to the bias, risk, and technical limitations. -->
Users (both direct and downstream) should be made aware of the risks, biases and limitations of the model. More information needed for further recommendations.
## How to Get Started with the Model
Use the code below to get started with the model.
[More Information Needed]
## Training Details
### Training Data
<!-- This should link to a Dataset Card, perhaps with a short stub of information on what the training data is all about as well as documentation related to data pre-processing or additional filtering. -->
[More Information Needed]
### Training Procedure
<!-- This relates heavily to the Technical Specifications. Content here should link to that section when it is relevant to the training procedure. -->
#### Preprocessing [optional]
[More Information Needed]
#### Training Hyperparameters
- **Training regime:** [More Information Needed] <!--fp32, fp16 mixed precision, bf16 mixed precision, bf16 non-mixed precision, fp16 non-mixed precision, fp8 mixed precision -->
#### Speeds, Sizes, Times [optional]
<!-- This section provides information about throughput, start/end time, checkpoint size if relevant, etc. -->
[More Information Needed]
## Evaluation
<!-- This section describes the evaluation protocols and provides the results. -->
### Testing Data, Factors & Metrics
#### Testing Data
<!-- This should link to a Dataset Card if possible. -->
[More Information Needed]
#### Factors
<!-- These are the things the evaluation is disaggregating by, e.g., subpopulations or domains. -->
[More Information Needed]
#### Metrics
<!-- These are the evaluation metrics being used, ideally with a description of why. -->
[More Information Needed]
### Results
[More Information Needed]
#### Summary
## Model Examination [optional]
<!-- Relevant interpretability work for the model goes here -->
[More Information Needed]
## Environmental Impact
<!-- Total emissions (in grams of CO2eq) and additional considerations, such as electricity usage, go here. Edit the suggested text below accordingly -->
Carbon emissions can be estimated using the [Machine Learning Impact calculator](https://mlco2.github.io/impact#compute) presented in [Lacoste et al. (2019)](https://arxiv.org/abs/1910.09700).
- **Hardware Type:** [More Information Needed]
- **Hours used:** [More Information Needed]
- **Cloud Provider:** [More Information Needed]
- **Compute Region:** [More Information Needed]
- **Carbon Emitted:** [More Information Needed]
## Technical Specifications [optional]
### Model Architecture and Objective
[More Information Needed]
### Compute Infrastructure
[More Information Needed]
#### Hardware
[More Information Needed]
#### Software
[More Information Needed]
## Citation [optional]
<!-- If there is a paper or blog post introducing the model, the APA and Bibtex information for that should go in this section. -->
**BibTeX:**
[More Information Needed]
**APA:**
[More Information Needed]
## Glossary [optional]
<!-- If relevant, include terms and calculations in this section that can help readers understand the model or model card. -->
[More Information Needed]
## More Information [optional]
[More Information Needed]
## Model Card Authors [optional]
[More Information Needed]
## Model Card Contact
[More Information Needed]
### Framework versions
- PEFT 0.10.0 |
ShenaoZ/0.001_withdpo_4iters_bs256_555lr_iter_3 | ShenaoZ | 2024-05-09T06:07:35Z | 6 | 0 | transformers | [
"transformers",
"safetensors",
"mistral",
"text-generation",
"alignment-handbook",
"trl",
"dpo",
"generated_from_trainer",
"conversational",
"dataset:updated",
"dataset:original",
"base_model:ShenaoZ/0.001_withdpo_4iters_bs256_555lr_iter_2",
"base_model:finetune:ShenaoZ/0.001_withdpo_4iters_bs256_555lr_iter_2",
"license:mit",
"autotrain_compatible",
"text-generation-inference",
"endpoints_compatible",
"region:us"
] | text-generation | 2024-05-09T05:20:23Z | ---
license: mit
base_model: ShenaoZ/0.001_withdpo_4iters_bs256_555lr_iter_2
tags:
- alignment-handbook
- trl
- dpo
- generated_from_trainer
- trl
- dpo
- generated_from_trainer
datasets:
- updated
- original
model-index:
- name: 0.001_withdpo_4iters_bs256_555lr_iter_3
results: []
---
<!-- This model card has been generated automatically according to the information the Trainer had access to. You
should probably proofread and complete it, then remove this comment. -->
# 0.001_withdpo_4iters_bs256_555lr_iter_3
This model is a fine-tuned version of [ShenaoZ/0.001_withdpo_4iters_bs256_555lr_iter_2](https://huggingface.co/ShenaoZ/0.001_withdpo_4iters_bs256_555lr_iter_2) on the updated and the original datasets.
## Model description
More information needed
## Intended uses & limitations
More information needed
## Training and evaluation data
More information needed
## Training procedure
### Training hyperparameters
The following hyperparameters were used during training:
- learning_rate: 5e-07
- train_batch_size: 8
- eval_batch_size: 8
- seed: 42
- distributed_type: multi-GPU
- num_devices: 8
- gradient_accumulation_steps: 4
- total_train_batch_size: 256
- total_eval_batch_size: 64
- optimizer: Adam with betas=(0.9,0.999) and epsilon=1e-08
- lr_scheduler_type: cosine
- lr_scheduler_warmup_ratio: 0.1
- num_epochs: 1
### Training results
### Framework versions
- Transformers 4.40.2
- Pytorch 2.1.2+cu121
- Datasets 2.14.6
- Tokenizers 0.19.1
|
pysenii/pysen | pysenii | 2024-05-09T06:05:43Z | 0 | 0 | transformers | [
"transformers",
"safetensors",
"autotrain",
"text-generation-inference",
"text-generation",
"peft",
"conversational",
"license:other",
"endpoints_compatible",
"region:us"
] | text-generation | 2024-05-09T02:12:07Z | ---
tags:
- autotrain
- text-generation-inference
- text-generation
- peft
library_name: transformers
widget:
- messages:
- role: user
content: What is your favorite condiment?
license: other
---
# Model Trained Using AutoTrain
This model was trained using AutoTrain. For more information, please visit [AutoTrain](https://hf.co/docs/autotrain).
# Usage
```python
from transformers import AutoModelForCausalLM, AutoTokenizer
model_path = "PATH_TO_THIS_REPO"
tokenizer = AutoTokenizer.from_pretrained(model_path)
model = AutoModelForCausalLM.from_pretrained(
model_path,
device_map="auto",
torch_dtype='auto'
).eval()
# Prompt content: "hi"
messages = [
{"role": "user", "content": "hi"}
]
input_ids = tokenizer.apply_chat_template(conversation=messages, tokenize=True, add_generation_prompt=True, return_tensors='pt')
output_ids = model.generate(input_ids.to('cuda'))
response = tokenizer.decode(output_ids[0][input_ids.shape[1]:], skip_special_tokens=True)
# Model response: "Hello! How can I assist you today?"
print(response)
``` |
kuhm209/distilbert-base-uncased-finetuned-emotions | kuhm209 | 2024-05-09T06:04:46Z | 119 | 0 | transformers | [
"transformers",
"safetensors",
"distilbert",
"text-classification",
"generated_from_trainer",
"dataset:emotion",
"base_model:distilbert/distilbert-base-uncased",
"base_model:finetune:distilbert/distilbert-base-uncased",
"license:apache-2.0",
"model-index",
"autotrain_compatible",
"endpoints_compatible",
"region:us"
] | text-classification | 2024-05-09T06:04:22Z | ---
license: apache-2.0
base_model: distilbert-base-uncased
tags:
- generated_from_trainer
datasets:
- emotion
metrics:
- accuracy
- f1
model-index:
- name: distilbert-base-uncased-finetuned-emotions
results:
- task:
name: Text Classification
type: text-classification
dataset:
name: emotion
type: emotion
config: split
split: validation
args: split
metrics:
- name: Accuracy
type: accuracy
value: 0.9255
- name: F1
type: f1
value: 0.9252882296716175
---
<!-- This model card has been generated automatically according to the information the Trainer had access to. You
should probably proofread and complete it, then remove this comment. -->
# distilbert-base-uncased-finetuned-emotions
This model is a fine-tuned version of [distilbert-base-uncased](https://huggingface.co/distilbert-base-uncased) on the emotion dataset.
It achieves the following results on the evaluation set:
- Loss: 0.2072
- Accuracy: 0.9255
- F1: 0.9253
## Model description
More information needed
## Intended uses & limitations
More information needed
## Training and evaluation data
More information needed
## Training procedure
### Training hyperparameters
The following hyperparameters were used during training:
- learning_rate: 2e-05
- train_batch_size: 64
- eval_batch_size: 64
- seed: 42
- optimizer: Adam with betas=(0.9,0.999) and epsilon=1e-08
- lr_scheduler_type: linear
- num_epochs: 2
### Training results
| Training Loss | Epoch | Step | Validation Loss | Accuracy | F1 |
|:-------------:|:-----:|:----:|:---------------:|:--------:|:------:|
| 0.83 | 1.0 | 250 | 0.3067 | 0.9075 | 0.9068 |
| 0.2465 | 2.0 | 500 | 0.2072 | 0.9255 | 0.9253 |
### Framework versions
- Transformers 4.40.2
- Pytorch 2.3.0+cu121
- Datasets 2.19.1
- Tokenizers 0.19.1
|
Alphacode-AI/Alphallama3-8B_v2 | Alphacode-AI | 2024-05-09T06:04:42Z | 2,338 | 1 | transformers | [
"transformers",
"safetensors",
"llama",
"text-generation",
"conversational",
"ko",
"dataset:Custom_datasets",
"base_model:meta-llama/Meta-Llama-3-8B",
"base_model:finetune:meta-llama/Meta-Llama-3-8B",
"license:llama3",
"autotrain_compatible",
"text-generation-inference",
"endpoints_compatible",
"region:us"
] | text-generation | 2024-05-09T02:34:47Z | ---
license: llama3
datasets:
- Custom_datasets
language:
- ko
pipeline_tag: text-generation
base_model: "meta-llama/Meta-Llama-3-8B"
---
This model is a version of Meta-Llama-3-8B that has been fine-tuned with Our In House CustomData.
Train Spec : We utilized an A100x8 * 1 for training our model with DeepSpeed / HuggingFace TRL Trainer / HuggingFace Accelerate |
bayley/Meta-Llama-3-70B-Instruct-q4f16_1-MLC | bayley | 2024-05-09T06:03:19Z | 0 | 0 | null | [
"license:llama3",
"region:us"
] | null | 2024-05-09T02:57:50Z | ---
license: llama3
---
Llama 3 70B Instruct quantized for use with [MLC-LLM](https://llm.mlc.ai)
MLC-LLM is an Apache TVM based inference framework with a neat trick: out of all of the frameworks that support tensor parallel inference, MLC-LLM is by far the easiest to install for single-user inference. This allows for [linear-ish performance scaling](https://blog.mlc.ai/2023/10/19/Scalable-Language-Model-Inference-on-Multiple-NVDIA-AMD-GPUs) on 2x 3090, 2x 4090, or 2x 7900 XTX, achieving about 30 tokens per second. In particular, this is faster than the performance from a single 48GB card costing much more.
MLC-LLM requires Ubuntu 22.04 or above (the prebuilt wheels will not work on Ubuntu 20.04). For CUDA users:
`python3 -m pip install --pre -U -f https://mlc.ai/wheels mlc-llm-nightly-cu122 mlc-ai-nightly-cu122`
`git clone https://huggingface.co/bayley/Meta-Llama-3-70B-Instruct-q4f16_1-MLC`
`mlc_llm compile Meta-Llama-3-70B-Instruct-q4f16_1-MLC/mlc-chat-config.json --device cuda --overrides "tensor_parallel_shards=<number of gpus>" -o Meta-Llama-3-70B-Instruct-q4f16_1-cuda.so`
`mlc_llm serve Meta-Llama-3-70B-Instruct-q4f16_1-MLC --model-lib Meta-Llama-3-70B-Instruct-q4f16_1-cuda.so --host 0.0.0.0`
This should start an OpenAI-compatible REST server serving a chat completions endpoint that you can connect to with your favorite frontend. |
blockblockblock/llama-3-70B-Instruct-abliterated-bpw5.5-exl2 | blockblockblock | 2024-05-09T06:01:06Z | 6 | 0 | transformers | [
"transformers",
"safetensors",
"llama",
"text-generation",
"conversational",
"license:llama3",
"autotrain_compatible",
"text-generation-inference",
"endpoints_compatible",
"exl2",
"region:us"
] | text-generation | 2024-05-09T05:47:08Z | ---
license: llama3
license_name: llama3
license_link: LICENSE
library_name: transformers
---
# Llama-3-70B-Instruct-abliterated Model Card
This is meta-llama/Llama-3-70B-Instruct with orthogonalized bfloat16 safetensor weights, generated with the methodology that was described in the preview paper/blog post: '[Refusal in LLMs is mediated by a single direction](https://www.alignmentforum.org/posts/jGuXSZgv6qfdhMCuJ/refusal-in-llms-is-mediated-by-a-single-direction)' which I encourage you to read to understand more.
TL;DR: this model has had certain weights manipulated to "inhibit" the model's ability to express refusal. It is not in anyway _guaranteed_ that it won't refuse you, understand your request, it may still lecture you about ethics/safety, etc. It is tuned in all other respects the same as the original 70B instruct model was, just with the strongest refusal direction orthogonalized out.
## Quants
[GGUF Quants available here](https://huggingface.co/failspy/llama-3-70B-Instruct-abliterated-GGUF)
## For the people who like tinkering or looking to save bandwidth
In the repo, I've included `refusal_dir.pth`
If you have Llama-3-70B-Instruct model downloaded already, you can use the ortho cookbook to apply it to your downloaded model, which will make it the same as what you'd download from here.
## Quirkiness awareness notice
This model may come with interesting quirks, as I obviously haven't extensively tested it, and the methodology being so new. I encourage you to play with the model, and post any quirks you notice in the community tab, as that'll help us further understand what this orthogonalization has in the way of side effects. The code I used to generate it (and my published 'Kappa-3' model which is just Phi-3 with the same methodology applied) is available in a Python notebook in this repo. Specifically, the [ortho_cookbook.ipynb](https://huggingface.co/failspy/llama-3-70B-Instruct-abliterated/blob/main/ortho_cookbook.ipynb).
If you manage to develop further improvements, please share! This is really the most primitive way to use ablation, but there are other possibilities that I believe are as-yet unexplored. |
Nikhil7280/OdiaBERTa-large | Nikhil7280 | 2024-05-09T05:59:21Z | 119 | 0 | transformers | [
"transformers",
"tensorboard",
"safetensors",
"roberta",
"fill-mask",
"generated_from_trainer",
"autotrain_compatible",
"endpoints_compatible",
"region:us"
] | fill-mask | 2024-05-09T04:28:41Z | ---
tags:
- generated_from_trainer
model-index:
- name: OdiaBERTa-large
results: []
---
<!-- This model card has been generated automatically according to the information the Trainer had access to. You
should probably proofread and complete it, then remove this comment. -->
# OdiaBERTa-large
This model is a fine-tuned version of [](https://huggingface.co/) on an unknown dataset.
## Model description
More information needed
## Intended uses & limitations
More information needed
## Training and evaluation data
More information needed
## Training procedure
### Training hyperparameters
The following hyperparameters were used during training:
- learning_rate: 2e-05
- train_batch_size: 32
- eval_batch_size: 32
- seed: 42
- optimizer: Adam with betas=(0.9,0.999) and epsilon=1e-08
- lr_scheduler_type: linear
- num_epochs: 10
### Training results
### Framework versions
- Transformers 4.39.3
- Pytorch 2.1.2
- Datasets 2.18.0
- Tokenizers 0.15.2
|
LumousInTheWild/exp_acha_mluke_base_again | LumousInTheWild | 2024-05-09T05:57:19Z | 6 | 0 | transformers | [
"transformers",
"safetensors",
"luke",
"text-classification",
"generated_from_trainer",
"base_model:studio-ousia/mluke-base",
"base_model:finetune:studio-ousia/mluke-base",
"license:apache-2.0",
"autotrain_compatible",
"endpoints_compatible",
"region:us"
] | text-classification | 2024-05-09T05:15:35Z | ---
license: apache-2.0
base_model: studio-ousia/mluke-base
tags:
- generated_from_trainer
model-index:
- name: exp_acha_mluke_base_again
results: []
---
<!-- This model card has been generated automatically according to the information the Trainer had access to. You
should probably proofread and complete it, then remove this comment. -->
# exp_acha_mluke_base_again
This model is a fine-tuned version of [studio-ousia/mluke-base](https://huggingface.co/studio-ousia/mluke-base) on the None dataset.
It achieves the following results on the evaluation set:
- Loss: 0.1684
## Model description
More information needed
## Intended uses & limitations
More information needed
## Training and evaluation data
More information needed
## Training procedure
### Training hyperparameters
The following hyperparameters were used during training:
- learning_rate: 1e-05
- train_batch_size: 16
- eval_batch_size: 32
- seed: 42
- optimizer: Adam with betas=(0.9,0.999) and epsilon=1e-08
- lr_scheduler_type: cosine_with_restarts
- lr_scheduler_warmup_ratio: 0.2
- num_epochs: 10
### Training results
| Training Loss | Epoch | Step | Validation Loss |
|:-------------:|:-----:|:----:|:---------------:|
| 0.4203 | 1.0 | 208 | 0.4335 |
| 0.4144 | 2.0 | 416 | 0.2626 |
| 0.2435 | 3.0 | 624 | 0.2097 |
| 0.1661 | 4.0 | 832 | 0.2061 |
| 0.1921 | 5.0 | 1040 | 0.2021 |
| 0.115 | 6.0 | 1248 | 0.1835 |
| 0.1205 | 7.0 | 1456 | 0.1695 |
| 0.0198 | 8.0 | 1664 | 0.1703 |
| 0.0267 | 9.0 | 1872 | 0.1640 |
| 0.145 | 10.0 | 2080 | 0.1684 |
### Framework versions
- Transformers 4.40.1
- Pytorch 2.2.1+cu121
- Datasets 2.19.1
- Tokenizers 0.19.1
|
JamesKim/mistral-7b-qlora-alpaca-1k-0508_HybridHPO | JamesKim | 2024-05-09T05:53:58Z | 79 | 0 | transformers | [
"transformers",
"safetensors",
"mistral",
"text-generation",
"trl",
"sft",
"arxiv:1910.09700",
"autotrain_compatible",
"text-generation-inference",
"endpoints_compatible",
"4-bit",
"bitsandbytes",
"region:us"
] | text-generation | 2024-05-09T05:51:30Z | ---
library_name: transformers
tags:
- trl
- sft
---
# Model Card for Model ID
<!-- Provide a quick summary of what the model is/does. -->
## Model Details
### Model Description
<!-- Provide a longer summary of what this model is. -->
This is the model card of a 🤗 transformers model that has been pushed on the Hub. This model card has been automatically generated.
- **Developed by:** [More Information Needed]
- **Funded by [optional]:** [More Information Needed]
- **Shared by [optional]:** [More Information Needed]
- **Model type:** [More Information Needed]
- **Language(s) (NLP):** [More Information Needed]
- **License:** [More Information Needed]
- **Finetuned from model [optional]:** [More Information Needed]
### Model Sources [optional]
<!-- Provide the basic links for the model. -->
- **Repository:** [More Information Needed]
- **Paper [optional]:** [More Information Needed]
- **Demo [optional]:** [More Information Needed]
## Uses
<!-- Address questions around how the model is intended to be used, including the foreseeable users of the model and those affected by the model. -->
### Direct Use
<!-- This section is for the model use without fine-tuning or plugging into a larger ecosystem/app. -->
[More Information Needed]
### Downstream Use [optional]
<!-- This section is for the model use when fine-tuned for a task, or when plugged into a larger ecosystem/app -->
[More Information Needed]
### Out-of-Scope Use
<!-- This section addresses misuse, malicious use, and uses that the model will not work well for. -->
[More Information Needed]
## Bias, Risks, and Limitations
<!-- This section is meant to convey both technical and sociotechnical limitations. -->
[More Information Needed]
### Recommendations
<!-- This section is meant to convey recommendations with respect to the bias, risk, and technical limitations. -->
Users (both direct and downstream) should be made aware of the risks, biases and limitations of the model. More information needed for further recommendations.
## How to Get Started with the Model
Use the code below to get started with the model.
[More Information Needed]
## Training Details
### Training Data
<!-- This should link to a Dataset Card, perhaps with a short stub of information on what the training data is all about as well as documentation related to data pre-processing or additional filtering. -->
[More Information Needed]
### Training Procedure
<!-- This relates heavily to the Technical Specifications. Content here should link to that section when it is relevant to the training procedure. -->
#### Preprocessing [optional]
[More Information Needed]
#### Training Hyperparameters
- **Training regime:** [More Information Needed] <!--fp32, fp16 mixed precision, bf16 mixed precision, bf16 non-mixed precision, fp16 non-mixed precision, fp8 mixed precision -->
#### Speeds, Sizes, Times [optional]
<!-- This section provides information about throughput, start/end time, checkpoint size if relevant, etc. -->
[More Information Needed]
## Evaluation
<!-- This section describes the evaluation protocols and provides the results. -->
### Testing Data, Factors & Metrics
#### Testing Data
<!-- This should link to a Dataset Card if possible. -->
[More Information Needed]
#### Factors
<!-- These are the things the evaluation is disaggregating by, e.g., subpopulations or domains. -->
[More Information Needed]
#### Metrics
<!-- These are the evaluation metrics being used, ideally with a description of why. -->
[More Information Needed]
### Results
[More Information Needed]
#### Summary
## Model Examination [optional]
<!-- Relevant interpretability work for the model goes here -->
[More Information Needed]
## Environmental Impact
<!-- Total emissions (in grams of CO2eq) and additional considerations, such as electricity usage, go here. Edit the suggested text below accordingly -->
Carbon emissions can be estimated using the [Machine Learning Impact calculator](https://mlco2.github.io/impact#compute) presented in [Lacoste et al. (2019)](https://arxiv.org/abs/1910.09700).
- **Hardware Type:** [More Information Needed]
- **Hours used:** [More Information Needed]
- **Cloud Provider:** [More Information Needed]
- **Compute Region:** [More Information Needed]
- **Carbon Emitted:** [More Information Needed]
## Technical Specifications [optional]
### Model Architecture and Objective
[More Information Needed]
### Compute Infrastructure
[More Information Needed]
#### Hardware
[More Information Needed]
#### Software
[More Information Needed]
## Citation [optional]
<!-- If there is a paper or blog post introducing the model, the APA and Bibtex information for that should go in this section. -->
**BibTeX:**
[More Information Needed]
**APA:**
[More Information Needed]
## Glossary [optional]
<!-- If relevant, include terms and calculations in this section that can help readers understand the model or model card. -->
[More Information Needed]
## More Information [optional]
[More Information Needed]
## Model Card Authors [optional]
[More Information Needed]
## Model Card Contact
[More Information Needed] |
Thirawarit/checkpoint | Thirawarit | 2024-05-09T05:51:09Z | 0 | 0 | null | [
"safetensors",
"trl",
"sft",
"generated_from_trainer",
"base_model:nvidia/Llama3-ChatQA-1.5-8B",
"base_model:finetune:nvidia/Llama3-ChatQA-1.5-8B",
"license:llama3",
"region:us"
] | null | 2024-05-09T05:51:02Z | ---
license: llama3
base_model: nvidia/Llama3-ChatQA-1.5-8B
tags:
- trl
- sft
- generated_from_trainer
model-index:
- name: checkpoint
results: []
---
<!-- This model card has been generated automatically according to the information the Trainer had access to. You
should probably proofread and complete it, then remove this comment. -->
# checkpoint
This model is a fine-tuned version of [nvidia/Llama3-ChatQA-1.5-8B](https://huggingface.co/nvidia/Llama3-ChatQA-1.5-8B) on an unknown dataset.
## Model description
More information needed
## Intended uses & limitations
More information needed
## Training and evaluation data
More information needed
## Training procedure
### Training hyperparameters
The following hyperparameters were used during training:
- learning_rate: 0.0002
- train_batch_size: 4
- eval_batch_size: 1
- seed: 42
- optimizer: Adam with betas=(0.9,0.999) and epsilon=1e-08
- lr_scheduler_type: linear
- lr_scheduler_warmup_ratio: 0.03
- num_epochs: 1
- mixed_precision_training: Native AMP
### Training results
### Framework versions
- Transformers 4.40.1
- Pytorch 2.2.1+cu121
- Datasets 2.19.1
- Tokenizers 0.19.1
|
spsither/tibetan_RoBERTa_Afd_e1 | spsither | 2024-05-09T05:44:42Z | 119 | 0 | transformers | [
"transformers",
"safetensors",
"roberta",
"fill-mask",
"arxiv:1910.09700",
"autotrain_compatible",
"endpoints_compatible",
"region:us"
] | fill-mask | 2024-05-09T05:44:27Z | ---
library_name: transformers
tags: []
---
# Model Card for Model ID
<!-- Provide a quick summary of what the model is/does. -->
## Model Details
### Model Description
<!-- Provide a longer summary of what this model is. -->
This is the model card of a 🤗 transformers model that has been pushed on the Hub. This model card has been automatically generated.
- **Developed by:** [More Information Needed]
- **Funded by [optional]:** [More Information Needed]
- **Shared by [optional]:** [More Information Needed]
- **Model type:** [More Information Needed]
- **Language(s) (NLP):** [More Information Needed]
- **License:** [More Information Needed]
- **Finetuned from model [optional]:** [More Information Needed]
### Model Sources [optional]
<!-- Provide the basic links for the model. -->
- **Repository:** [More Information Needed]
- **Paper [optional]:** [More Information Needed]
- **Demo [optional]:** [More Information Needed]
## Uses
<!-- Address questions around how the model is intended to be used, including the foreseeable users of the model and those affected by the model. -->
### Direct Use
<!-- This section is for the model use without fine-tuning or plugging into a larger ecosystem/app. -->
[More Information Needed]
### Downstream Use [optional]
<!-- This section is for the model use when fine-tuned for a task, or when plugged into a larger ecosystem/app -->
[More Information Needed]
### Out-of-Scope Use
<!-- This section addresses misuse, malicious use, and uses that the model will not work well for. -->
[More Information Needed]
## Bias, Risks, and Limitations
<!-- This section is meant to convey both technical and sociotechnical limitations. -->
[More Information Needed]
### Recommendations
<!-- This section is meant to convey recommendations with respect to the bias, risk, and technical limitations. -->
Users (both direct and downstream) should be made aware of the risks, biases and limitations of the model. More information needed for further recommendations.
## How to Get Started with the Model
Use the code below to get started with the model.
[More Information Needed]
## Training Details
### Training Data
<!-- This should link to a Dataset Card, perhaps with a short stub of information on what the training data is all about as well as documentation related to data pre-processing or additional filtering. -->
[More Information Needed]
### Training Procedure
<!-- This relates heavily to the Technical Specifications. Content here should link to that section when it is relevant to the training procedure. -->
#### Preprocessing [optional]
[More Information Needed]
#### Training Hyperparameters
- **Training regime:** [More Information Needed] <!--fp32, fp16 mixed precision, bf16 mixed precision, bf16 non-mixed precision, fp16 non-mixed precision, fp8 mixed precision -->
#### Speeds, Sizes, Times [optional]
<!-- This section provides information about throughput, start/end time, checkpoint size if relevant, etc. -->
[More Information Needed]
## Evaluation
<!-- This section describes the evaluation protocols and provides the results. -->
### Testing Data, Factors & Metrics
#### Testing Data
<!-- This should link to a Dataset Card if possible. -->
[More Information Needed]
#### Factors
<!-- These are the things the evaluation is disaggregating by, e.g., subpopulations or domains. -->
[More Information Needed]
#### Metrics
<!-- These are the evaluation metrics being used, ideally with a description of why. -->
[More Information Needed]
### Results
[More Information Needed]
#### Summary
## Model Examination [optional]
<!-- Relevant interpretability work for the model goes here -->
[More Information Needed]
## Environmental Impact
<!-- Total emissions (in grams of CO2eq) and additional considerations, such as electricity usage, go here. Edit the suggested text below accordingly -->
Carbon emissions can be estimated using the [Machine Learning Impact calculator](https://mlco2.github.io/impact#compute) presented in [Lacoste et al. (2019)](https://arxiv.org/abs/1910.09700).
- **Hardware Type:** [More Information Needed]
- **Hours used:** [More Information Needed]
- **Cloud Provider:** [More Information Needed]
- **Compute Region:** [More Information Needed]
- **Carbon Emitted:** [More Information Needed]
## Technical Specifications [optional]
### Model Architecture and Objective
[More Information Needed]
### Compute Infrastructure
[More Information Needed]
#### Hardware
[More Information Needed]
#### Software
[More Information Needed]
## Citation [optional]
<!-- If there is a paper or blog post introducing the model, the APA and Bibtex information for that should go in this section. -->
**BibTeX:**
[More Information Needed]
**APA:**
[More Information Needed]
## Glossary [optional]
<!-- If relevant, include terms and calculations in this section that can help readers understand the model or model card. -->
[More Information Needed]
## More Information [optional]
[More Information Needed]
## Model Card Authors [optional]
[More Information Needed]
## Model Card Contact
[More Information Needed] |
ritjod/L.I.O.S | ritjod | 2024-05-09T05:34:48Z | 0 | 0 | null | [
"license:apache-2.0",
"region:us"
] | null | 2024-05-09T05:34:48Z | ---
license: apache-2.0
---
|
Praful659/gte_Qwen1_instruct_qlora_model_emotion_detection | Praful659 | 2024-05-09T05:22:58Z | 0 | 0 | transformers | [
"transformers",
"safetensors",
"arxiv:1910.09700",
"endpoints_compatible",
"region:us"
] | null | 2024-05-09T05:22:11Z | ---
library_name: transformers
tags: []
---
# Model Card for Model ID
<!-- Provide a quick summary of what the model is/does. -->
## Model Details
### Model Description
<!-- Provide a longer summary of what this model is. -->
This is the model card of a 🤗 transformers model that has been pushed on the Hub. This model card has been automatically generated.
- **Developed by:** [More Information Needed]
- **Funded by [optional]:** [More Information Needed]
- **Shared by [optional]:** [More Information Needed]
- **Model type:** [More Information Needed]
- **Language(s) (NLP):** [More Information Needed]
- **License:** [More Information Needed]
- **Finetuned from model [optional]:** [More Information Needed]
### Model Sources [optional]
<!-- Provide the basic links for the model. -->
- **Repository:** [More Information Needed]
- **Paper [optional]:** [More Information Needed]
- **Demo [optional]:** [More Information Needed]
## Uses
<!-- Address questions around how the model is intended to be used, including the foreseeable users of the model and those affected by the model. -->
### Direct Use
<!-- This section is for the model use without fine-tuning or plugging into a larger ecosystem/app. -->
[More Information Needed]
### Downstream Use [optional]
<!-- This section is for the model use when fine-tuned for a task, or when plugged into a larger ecosystem/app -->
[More Information Needed]
### Out-of-Scope Use
<!-- This section addresses misuse, malicious use, and uses that the model will not work well for. -->
[More Information Needed]
## Bias, Risks, and Limitations
<!-- This section is meant to convey both technical and sociotechnical limitations. -->
[More Information Needed]
### Recommendations
<!-- This section is meant to convey recommendations with respect to the bias, risk, and technical limitations. -->
Users (both direct and downstream) should be made aware of the risks, biases and limitations of the model. More information needed for further recommendations.
## How to Get Started with the Model
Use the code below to get started with the model.
[More Information Needed]
## Training Details
### Training Data
<!-- This should link to a Dataset Card, perhaps with a short stub of information on what the training data is all about as well as documentation related to data pre-processing or additional filtering. -->
[More Information Needed]
### Training Procedure
<!-- This relates heavily to the Technical Specifications. Content here should link to that section when it is relevant to the training procedure. -->
#### Preprocessing [optional]
[More Information Needed]
#### Training Hyperparameters
- **Training regime:** [More Information Needed] <!--fp32, fp16 mixed precision, bf16 mixed precision, bf16 non-mixed precision, fp16 non-mixed precision, fp8 mixed precision -->
#### Speeds, Sizes, Times [optional]
<!-- This section provides information about throughput, start/end time, checkpoint size if relevant, etc. -->
[More Information Needed]
## Evaluation
<!-- This section describes the evaluation protocols and provides the results. -->
### Testing Data, Factors & Metrics
#### Testing Data
<!-- This should link to a Dataset Card if possible. -->
[More Information Needed]
#### Factors
<!-- These are the things the evaluation is disaggregating by, e.g., subpopulations or domains. -->
[More Information Needed]
#### Metrics
<!-- These are the evaluation metrics being used, ideally with a description of why. -->
[More Information Needed]
### Results
[More Information Needed]
#### Summary
## Model Examination [optional]
<!-- Relevant interpretability work for the model goes here -->
[More Information Needed]
## Environmental Impact
<!-- Total emissions (in grams of CO2eq) and additional considerations, such as electricity usage, go here. Edit the suggested text below accordingly -->
Carbon emissions can be estimated using the [Machine Learning Impact calculator](https://mlco2.github.io/impact#compute) presented in [Lacoste et al. (2019)](https://arxiv.org/abs/1910.09700).
- **Hardware Type:** [More Information Needed]
- **Hours used:** [More Information Needed]
- **Cloud Provider:** [More Information Needed]
- **Compute Region:** [More Information Needed]
- **Carbon Emitted:** [More Information Needed]
## Technical Specifications [optional]
### Model Architecture and Objective
[More Information Needed]
### Compute Infrastructure
[More Information Needed]
#### Hardware
[More Information Needed]
#### Software
[More Information Needed]
## Citation [optional]
<!-- If there is a paper or blog post introducing the model, the APA and Bibtex information for that should go in this section. -->
**BibTeX:**
[More Information Needed]
**APA:**
[More Information Needed]
## Glossary [optional]
<!-- If relevant, include terms and calculations in this section that can help readers understand the model or model card. -->
[More Information Needed]
## More Information [optional]
[More Information Needed]
## Model Card Authors [optional]
[More Information Needed]
## Model Card Contact
[More Information Needed] |
Litzy619/Phi0503HMA16OLD | Litzy619 | 2024-05-09T05:13:16Z | 0 | 0 | null | [
"safetensors",
"generated_from_trainer",
"base_model:microsoft/Phi-3-mini-4k-instruct",
"base_model:finetune:microsoft/Phi-3-mini-4k-instruct",
"license:mit",
"region:us"
] | null | 2024-05-09T00:45:42Z | ---
license: mit
base_model: microsoft/Phi-3-mini-4k-instruct
tags:
- generated_from_trainer
model-index:
- name: Phi0503HMA16
results: []
---
<!-- This model card has been generated automatically according to the information the Trainer had access to. You
should probably proofread and complete it, then remove this comment. -->
# Phi0503HMA16
This model is a fine-tuned version of [microsoft/Phi-3-mini-4k-instruct](https://huggingface.co/microsoft/Phi-3-mini-4k-instruct) on an unknown dataset.
It achieves the following results on the evaluation set:
- Loss: 0.0775
## Model description
More information needed
## Intended uses & limitations
More information needed
## Training and evaluation data
More information needed
## Training procedure
### Training hyperparameters
The following hyperparameters were used during training:
- learning_rate: 0.0003
- train_batch_size: 8
- eval_batch_size: 8
- seed: 42
- gradient_accumulation_steps: 16
- total_train_batch_size: 128
- optimizer: Adam with betas=(0.9,0.999) and epsilon=1e-08
- lr_scheduler_type: cosine_with_restarts
- lr_scheduler_warmup_steps: 100
- num_epochs: 3
- mixed_precision_training: Native AMP
### Training results
| Training Loss | Epoch | Step | Validation Loss |
|:-------------:|:-----:|:----:|:---------------:|
| 4.3598 | 0.09 | 10 | 0.9925 |
| 0.4108 | 0.18 | 20 | 0.2307 |
| 0.2372 | 0.27 | 30 | 0.2352 |
| 0.2131 | 0.36 | 40 | 0.2189 |
| 0.1969 | 0.45 | 50 | 0.1518 |
| 0.1415 | 0.54 | 60 | 0.0999 |
| 0.0976 | 0.63 | 70 | 0.1068 |
| 0.0853 | 0.73 | 80 | 0.0846 |
| 0.0864 | 0.82 | 90 | 0.0784 |
| 0.0782 | 0.91 | 100 | 0.0734 |
| 0.0866 | 1.0 | 110 | 0.0806 |
| 0.0649 | 1.09 | 120 | 0.0712 |
| 0.0663 | 1.18 | 130 | 0.0769 |
| 0.0704 | 1.27 | 140 | 0.0729 |
| 0.0634 | 1.36 | 150 | 0.0740 |
| 0.068 | 1.45 | 160 | 0.0709 |
| 0.0645 | 1.54 | 170 | 0.0687 |
| 0.063 | 1.63 | 180 | 0.0689 |
| 0.0584 | 1.72 | 190 | 0.0604 |
| 0.065 | 1.81 | 200 | 0.0608 |
| 0.0532 | 1.9 | 210 | 0.0681 |
| 0.0539 | 1.99 | 220 | 0.0694 |
| 0.0313 | 2.08 | 230 | 0.0816 |
| 0.0356 | 2.18 | 240 | 0.0880 |
| 0.0296 | 2.27 | 250 | 0.0834 |
| 0.0287 | 2.36 | 260 | 0.0780 |
| 0.0336 | 2.45 | 270 | 0.0801 |
| 0.0236 | 2.54 | 280 | 0.0827 |
| 0.0263 | 2.63 | 290 | 0.0828 |
| 0.0335 | 2.72 | 300 | 0.0794 |
| 0.0317 | 2.81 | 310 | 0.0780 |
| 0.0296 | 2.9 | 320 | 0.0773 |
| 0.0289 | 2.99 | 330 | 0.0775 |
### Framework versions
- Transformers 4.36.0.dev0
- Pytorch 2.1.2+cu121
- Datasets 2.14.6
- Tokenizers 0.14.0
|
ryanyeo/kirnect-Llama-Ko-2-7B-remote-rev-0501 | ryanyeo | 2024-05-09T05:05:28Z | 6 | 0 | transformers | [
"transformers",
"safetensors",
"llama",
"text-generation",
"arxiv:1910.09700",
"autotrain_compatible",
"text-generation-inference",
"endpoints_compatible",
"region:us"
] | text-generation | 2024-05-09T04:58:02Z | ---
library_name: transformers
tags: []
---
# Model Card for Model ID
<!-- Provide a quick summary of what the model is/does. -->
## Model Details
### Model Description
<!-- Provide a longer summary of what this model is. -->
This is the model card of a 🤗 transformers model that has been pushed on the Hub. This model card has been automatically generated.
- **Developed by:** [More Information Needed]
- **Funded by [optional]:** [More Information Needed]
- **Shared by [optional]:** [More Information Needed]
- **Model type:** [More Information Needed]
- **Language(s) (NLP):** [More Information Needed]
- **License:** [More Information Needed]
- **Finetuned from model [optional]:** [More Information Needed]
### Model Sources [optional]
<!-- Provide the basic links for the model. -->
- **Repository:** [More Information Needed]
- **Paper [optional]:** [More Information Needed]
- **Demo [optional]:** [More Information Needed]
## Uses
<!-- Address questions around how the model is intended to be used, including the foreseeable users of the model and those affected by the model. -->
### Direct Use
<!-- This section is for the model use without fine-tuning or plugging into a larger ecosystem/app. -->
[More Information Needed]
### Downstream Use [optional]
<!-- This section is for the model use when fine-tuned for a task, or when plugged into a larger ecosystem/app -->
[More Information Needed]
### Out-of-Scope Use
<!-- This section addresses misuse, malicious use, and uses that the model will not work well for. -->
[More Information Needed]
## Bias, Risks, and Limitations
<!-- This section is meant to convey both technical and sociotechnical limitations. -->
[More Information Needed]
### Recommendations
<!-- This section is meant to convey recommendations with respect to the bias, risk, and technical limitations. -->
Users (both direct and downstream) should be made aware of the risks, biases and limitations of the model. More information needed for further recommendations.
## How to Get Started with the Model
Use the code below to get started with the model.
[More Information Needed]
## Training Details
### Training Data
<!-- This should link to a Dataset Card, perhaps with a short stub of information on what the training data is all about as well as documentation related to data pre-processing or additional filtering. -->
[More Information Needed]
### Training Procedure
<!-- This relates heavily to the Technical Specifications. Content here should link to that section when it is relevant to the training procedure. -->
#### Preprocessing [optional]
[More Information Needed]
#### Training Hyperparameters
- **Training regime:** [More Information Needed] <!--fp32, fp16 mixed precision, bf16 mixed precision, bf16 non-mixed precision, fp16 non-mixed precision, fp8 mixed precision -->
#### Speeds, Sizes, Times [optional]
<!-- This section provides information about throughput, start/end time, checkpoint size if relevant, etc. -->
[More Information Needed]
## Evaluation
<!-- This section describes the evaluation protocols and provides the results. -->
### Testing Data, Factors & Metrics
#### Testing Data
<!-- This should link to a Dataset Card if possible. -->
[More Information Needed]
#### Factors
<!-- These are the things the evaluation is disaggregating by, e.g., subpopulations or domains. -->
[More Information Needed]
#### Metrics
<!-- These are the evaluation metrics being used, ideally with a description of why. -->
[More Information Needed]
### Results
[More Information Needed]
#### Summary
## Model Examination [optional]
<!-- Relevant interpretability work for the model goes here -->
[More Information Needed]
## Environmental Impact
<!-- Total emissions (in grams of CO2eq) and additional considerations, such as electricity usage, go here. Edit the suggested text below accordingly -->
Carbon emissions can be estimated using the [Machine Learning Impact calculator](https://mlco2.github.io/impact#compute) presented in [Lacoste et al. (2019)](https://arxiv.org/abs/1910.09700).
- **Hardware Type:** [More Information Needed]
- **Hours used:** [More Information Needed]
- **Cloud Provider:** [More Information Needed]
- **Compute Region:** [More Information Needed]
- **Carbon Emitted:** [More Information Needed]
## Technical Specifications [optional]
### Model Architecture and Objective
[More Information Needed]
### Compute Infrastructure
[More Information Needed]
#### Hardware
[More Information Needed]
#### Software
[More Information Needed]
## Citation [optional]
<!-- If there is a paper or blog post introducing the model, the APA and Bibtex information for that should go in this section. -->
**BibTeX:**
[More Information Needed]
**APA:**
[More Information Needed]
## Glossary [optional]
<!-- If relevant, include terms and calculations in this section that can help readers understand the model or model card. -->
[More Information Needed]
## More Information [optional]
[More Information Needed]
## Model Card Authors [optional]
[More Information Needed]
## Model Card Contact
[More Information Needed] |
LoneStriker/Llama-3-Refueled-5.0bpw-h6-exl2 | LoneStriker | 2024-05-09T04:56:52Z | 7 | 0 | transformers | [
"transformers",
"safetensors",
"llama",
"text-generation",
"data labeling",
"conversational",
"en",
"license:cc-by-nc-4.0",
"autotrain_compatible",
"text-generation-inference",
"endpoints_compatible",
"5-bit",
"exl2",
"region:us"
] | text-generation | 2024-05-09T04:54:24Z | ---
license: cc-by-nc-4.0
language:
- en
library_name: transformers
tags:
- data labeling
---
<div style="width: auto; margin-left: auto; margin-right: auto; background-color:black">
<img src="https://assets-global.website-files.com/6423879a8f63c1bb18d74bfa/648818d56d04c3bdf36d71ab_Refuel_rev8-01_ts-p-1600.png" alt="Refuel.ai" style="width: 100%; min-width: 400px; display: block; margin: auto;">
</div>
## Model Details
RefuelLLM-2-small, aka Llama-3-Refueled, is a Llama3-8B base model instruction tuned on a corpus of 2750+ datasets, spanning tasks such as classification, reading comprehension, structured attribute extraction and entity resolution. We're excited to open-source the model for the community to build on top of.
* More details about [RefuelLLM-2 family of models](https://www.refuel.ai/blog-posts/announcing-refuel-llm-2)
* You can also try out the models in our [LLM playground](https://labs.refuel.ai/playground)
**Model developers** - Refuel AI
**Input** - Text only.
**Output** - Text only.
**Architecture** - Llama-3-Refueled is built on top of Llama-3-8B-instruct which is an auto-regressive language model that uses an optimized transformer architecture.
**Release Date** - May 8, 2024.
## How to use
This repository contains weights for Llama-3-Refueled that are compatible for use with HuggingFace. See the snippet below for usage with Transformers:
```python
>>> import torch
>>> from transformers import AutoModelForCausalLM, AutoTokenizer
>>> model_id = "refuelai/Llama-3-Refueled"
>>> tokenizer = AutoTokenizer.from_pretrained(model_id)
>>> model = AutoModelForCausalLM.from_pretrained(model_id, torch_dtype=torch.float16, device_map="auto")
>>> messages = [{"role": "user", "content": "Is this comment toxic or non-toxic: RefuelLLM is the new way to label text data!"}]
>>> inputs = tokenizer.apply_chat_template(messages, return_tensors="pt", add_generation_prompt=True).to("cuda")
>>> outputs = model.generate(inputs, max_new_tokens=20)
>>> print(tokenizer.decode(outputs[0]))
```
## Training Data
The model was both trained on over 4 Billion tokens, spanning 2750+ NLP tasks. Our training collection consists majorly of:
1. Human annotated datasets like Flan, Task Source, and the Aya collection
2. Synthetic datasets like OpenOrca, OpenHermes and WizardLM
3. Proprietary datasets developed or licensed by Refuel AI
## Benchmarks
In this section, we report the results for Refuel models on our benchmark of labeling tasks. For details on the methodology see [here](https://refuel.ai/blog-posts/announcing-refuel-llm-2).
<table>
<tr></tr>
<tr><th>Provider</th><th>Model</th><th colspan="4" style="text-align: center">LLM Output Quality (by task type)</tr>
<tr><td></td><td></td><td>Overall</td><td>Classification</td><td>Reading Comprehension</td><td>Structure Extraction</td><td>Entity Matching</td><td></td></tr>
<tr><td>Refuel</td><td>RefuelLLM-2</td><td>83.82%</td><td>84.94%</td><td>76.03%</td><td>88.16%</td><td>92.00%</td><td></td></tr>
<tr><td>OpenAI</td><td>GPT-4-Turbo</td><td>80.88%</td><td>81.77%</td><td>72.08%</td><td>84.79%</td><td>97.20%</td><td></td></tr>
<tr><td>Refuel</td><td>RefuelLLM-2-small (Llama-3-Refueled)</td><td>79.67%</td><td>81.72%</td><td>70.04%</td><td>84.28%</td><td>92.00%</td><td></td></tr>
<tr><td>Anthropic</td><td>Claude-3-Opus</td><td>79.19%</td><td>82.49%</td><td>67.30%</td><td>88.25%</td><td>94.96%</td><td></td></tr>
<tr><td>Meta</td><td>Llama3-70B-Instruct</td><td>78.20%</td><td>79.38%</td><td>66.03%</td><td>85.96%</td><td>94.13%</td><td></td></tr>
<tr><td>Google</td><td>Gemini-1.5-Pro</td><td>74.59%</td><td>73.52%</td><td>60.67%</td><td>84.27%</td><td>98.48%</td><td></td></tr>
<tr><td>Mistral</td><td>Mixtral-8x7B-Instruct</td><td>62.87%</td><td>79.11%</td><td>45.56%</td><td>47.08%</td><td>86.52%</td><td></td></tr>
<tr><td>Anthropic</td><td>Claude-3-Sonnet</td><td>70.99%</td><td>79.91%</td><td>45.44%</td><td>78.10%</td><td>96.34%</td><td></td></tr>
<tr><td>Anthropic</td><td>Claude-3-Haiku</td><td>69.23%</td><td>77.27%</td><td>50.19%</td><td>84.97%</td><td>54.08%</td><td></td></tr>
<tr><td>OpenAI</td><td>GPT-3.5-Turbo</td><td>68.13%</td><td>74.39%</td><td>53.21%</td><td>69.40%</td><td>80.41%</td><td></td></tr>
<tr><td>Meta</td><td>Llama3-8B-Instruct</td><td>62.30%</td><td>68.52%</td><td>49.16%</td><td>65.09%</td><td>63.61%</td><td></td></tr>
</table>
## Limitations
The Llama-3-Refueled does not have any moderation mechanisms. We're looking forward to engaging with the community
on ways to make the model finely respect guardrails, allowing for deployment in environments requiring moderated outputs. |
danelcsb/rtdetr-finetuned-balloon | danelcsb | 2024-05-09T04:56:50Z | 134 | 0 | transformers | [
"transformers",
"safetensors",
"rt_detr",
"object-detection",
"arxiv:1910.09700",
"endpoints_compatible",
"region:us"
] | object-detection | 2024-04-14T13:20:54Z | ---
library_name: transformers
tags: []
---
# Model Card for Model ID
<!-- Provide a quick summary of what the model is/does. -->
## Model Details
### Model Description
<!-- Provide a longer summary of what this model is. -->
This is the model card of a 🤗 transformers model that has been pushed on the Hub. This model card has been automatically generated.
- **Developed by:** [More Information Needed]
- **Funded by [optional]:** [More Information Needed]
- **Shared by [optional]:** [More Information Needed]
- **Model type:** [More Information Needed]
- **Language(s) (NLP):** [More Information Needed]
- **License:** [More Information Needed]
- **Finetuned from model [optional]:** [More Information Needed]
### Model Sources [optional]
<!-- Provide the basic links for the model. -->
- **Repository:** [More Information Needed]
- **Paper [optional]:** [More Information Needed]
- **Demo [optional]:** [More Information Needed]
## Uses
<!-- Address questions around how the model is intended to be used, including the foreseeable users of the model and those affected by the model. -->
### Direct Use
<!-- This section is for the model use without fine-tuning or plugging into a larger ecosystem/app. -->
[More Information Needed]
### Downstream Use [optional]
<!-- This section is for the model use when fine-tuned for a task, or when plugged into a larger ecosystem/app -->
[More Information Needed]
### Out-of-Scope Use
<!-- This section addresses misuse, malicious use, and uses that the model will not work well for. -->
[More Information Needed]
## Bias, Risks, and Limitations
<!-- This section is meant to convey both technical and sociotechnical limitations. -->
[More Information Needed]
### Recommendations
<!-- This section is meant to convey recommendations with respect to the bias, risk, and technical limitations. -->
Users (both direct and downstream) should be made aware of the risks, biases and limitations of the model. More information needed for further recommendations.
## How to Get Started with the Model
Use the code below to get started with the model.
[More Information Needed]
## Training Details
### Training Data
<!-- This should link to a Dataset Card, perhaps with a short stub of information on what the training data is all about as well as documentation related to data pre-processing or additional filtering. -->
[More Information Needed]
### Training Procedure
<!-- This relates heavily to the Technical Specifications. Content here should link to that section when it is relevant to the training procedure. -->
#### Preprocessing [optional]
[More Information Needed]
#### Training Hyperparameters
- **Training regime:** [More Information Needed] <!--fp32, fp16 mixed precision, bf16 mixed precision, bf16 non-mixed precision, fp16 non-mixed precision, fp8 mixed precision -->
#### Speeds, Sizes, Times [optional]
<!-- This section provides information about throughput, start/end time, checkpoint size if relevant, etc. -->
[More Information Needed]
## Evaluation
<!-- This section describes the evaluation protocols and provides the results. -->
### Testing Data, Factors & Metrics
#### Testing Data
<!-- This should link to a Dataset Card if possible. -->
[More Information Needed]
#### Factors
<!-- These are the things the evaluation is disaggregating by, e.g., subpopulations or domains. -->
[More Information Needed]
#### Metrics
<!-- These are the evaluation metrics being used, ideally with a description of why. -->
[More Information Needed]
### Results
[More Information Needed]
#### Summary
## Model Examination [optional]
<!-- Relevant interpretability work for the model goes here -->
[More Information Needed]
## Environmental Impact
<!-- Total emissions (in grams of CO2eq) and additional considerations, such as electricity usage, go here. Edit the suggested text below accordingly -->
Carbon emissions can be estimated using the [Machine Learning Impact calculator](https://mlco2.github.io/impact#compute) presented in [Lacoste et al. (2019)](https://arxiv.org/abs/1910.09700).
- **Hardware Type:** [More Information Needed]
- **Hours used:** [More Information Needed]
- **Cloud Provider:** [More Information Needed]
- **Compute Region:** [More Information Needed]
- **Carbon Emitted:** [More Information Needed]
## Technical Specifications [optional]
### Model Architecture and Objective
[More Information Needed]
### Compute Infrastructure
[More Information Needed]
#### Hardware
[More Information Needed]
#### Software
[More Information Needed]
## Citation [optional]
<!-- If there is a paper or blog post introducing the model, the APA and Bibtex information for that should go in this section. -->
**BibTeX:**
[More Information Needed]
**APA:**
[More Information Needed]
## Glossary [optional]
<!-- If relevant, include terms and calculations in this section that can help readers understand the model or model card. -->
[More Information Needed]
## More Information [optional]
[More Information Needed]
## Model Card Authors [optional]
[More Information Needed]
## Model Card Contact
[More Information Needed] |
LoneStriker/Llama-3-Refueled-4.0bpw-h6-exl2 | LoneStriker | 2024-05-09T04:54:20Z | 6 | 0 | transformers | [
"transformers",
"safetensors",
"llama",
"text-generation",
"data labeling",
"conversational",
"en",
"license:cc-by-nc-4.0",
"autotrain_compatible",
"text-generation-inference",
"endpoints_compatible",
"4-bit",
"exl2",
"region:us"
] | text-generation | 2024-05-09T04:52:15Z | ---
license: cc-by-nc-4.0
language:
- en
library_name: transformers
tags:
- data labeling
---
<div style="width: auto; margin-left: auto; margin-right: auto; background-color:black">
<img src="https://assets-global.website-files.com/6423879a8f63c1bb18d74bfa/648818d56d04c3bdf36d71ab_Refuel_rev8-01_ts-p-1600.png" alt="Refuel.ai" style="width: 100%; min-width: 400px; display: block; margin: auto;">
</div>
## Model Details
RefuelLLM-2-small, aka Llama-3-Refueled, is a Llama3-8B base model instruction tuned on a corpus of 2750+ datasets, spanning tasks such as classification, reading comprehension, structured attribute extraction and entity resolution. We're excited to open-source the model for the community to build on top of.
* More details about [RefuelLLM-2 family of models](https://www.refuel.ai/blog-posts/announcing-refuel-llm-2)
* You can also try out the models in our [LLM playground](https://labs.refuel.ai/playground)
**Model developers** - Refuel AI
**Input** - Text only.
**Output** - Text only.
**Architecture** - Llama-3-Refueled is built on top of Llama-3-8B-instruct which is an auto-regressive language model that uses an optimized transformer architecture.
**Release Date** - May 8, 2024.
## How to use
This repository contains weights for Llama-3-Refueled that are compatible for use with HuggingFace. See the snippet below for usage with Transformers:
```python
>>> import torch
>>> from transformers import AutoModelForCausalLM, AutoTokenizer
>>> model_id = "refuelai/Llama-3-Refueled"
>>> tokenizer = AutoTokenizer.from_pretrained(model_id)
>>> model = AutoModelForCausalLM.from_pretrained(model_id, torch_dtype=torch.float16, device_map="auto")
>>> messages = [{"role": "user", "content": "Is this comment toxic or non-toxic: RefuelLLM is the new way to label text data!"}]
>>> inputs = tokenizer.apply_chat_template(messages, return_tensors="pt", add_generation_prompt=True).to("cuda")
>>> outputs = model.generate(inputs, max_new_tokens=20)
>>> print(tokenizer.decode(outputs[0]))
```
## Training Data
The model was both trained on over 4 Billion tokens, spanning 2750+ NLP tasks. Our training collection consists majorly of:
1. Human annotated datasets like Flan, Task Source, and the Aya collection
2. Synthetic datasets like OpenOrca, OpenHermes and WizardLM
3. Proprietary datasets developed or licensed by Refuel AI
## Benchmarks
In this section, we report the results for Refuel models on our benchmark of labeling tasks. For details on the methodology see [here](https://refuel.ai/blog-posts/announcing-refuel-llm-2).
<table>
<tr></tr>
<tr><th>Provider</th><th>Model</th><th colspan="4" style="text-align: center">LLM Output Quality (by task type)</tr>
<tr><td></td><td></td><td>Overall</td><td>Classification</td><td>Reading Comprehension</td><td>Structure Extraction</td><td>Entity Matching</td><td></td></tr>
<tr><td>Refuel</td><td>RefuelLLM-2</td><td>83.82%</td><td>84.94%</td><td>76.03%</td><td>88.16%</td><td>92.00%</td><td></td></tr>
<tr><td>OpenAI</td><td>GPT-4-Turbo</td><td>80.88%</td><td>81.77%</td><td>72.08%</td><td>84.79%</td><td>97.20%</td><td></td></tr>
<tr><td>Refuel</td><td>RefuelLLM-2-small (Llama-3-Refueled)</td><td>79.67%</td><td>81.72%</td><td>70.04%</td><td>84.28%</td><td>92.00%</td><td></td></tr>
<tr><td>Anthropic</td><td>Claude-3-Opus</td><td>79.19%</td><td>82.49%</td><td>67.30%</td><td>88.25%</td><td>94.96%</td><td></td></tr>
<tr><td>Meta</td><td>Llama3-70B-Instruct</td><td>78.20%</td><td>79.38%</td><td>66.03%</td><td>85.96%</td><td>94.13%</td><td></td></tr>
<tr><td>Google</td><td>Gemini-1.5-Pro</td><td>74.59%</td><td>73.52%</td><td>60.67%</td><td>84.27%</td><td>98.48%</td><td></td></tr>
<tr><td>Mistral</td><td>Mixtral-8x7B-Instruct</td><td>62.87%</td><td>79.11%</td><td>45.56%</td><td>47.08%</td><td>86.52%</td><td></td></tr>
<tr><td>Anthropic</td><td>Claude-3-Sonnet</td><td>70.99%</td><td>79.91%</td><td>45.44%</td><td>78.10%</td><td>96.34%</td><td></td></tr>
<tr><td>Anthropic</td><td>Claude-3-Haiku</td><td>69.23%</td><td>77.27%</td><td>50.19%</td><td>84.97%</td><td>54.08%</td><td></td></tr>
<tr><td>OpenAI</td><td>GPT-3.5-Turbo</td><td>68.13%</td><td>74.39%</td><td>53.21%</td><td>69.40%</td><td>80.41%</td><td></td></tr>
<tr><td>Meta</td><td>Llama3-8B-Instruct</td><td>62.30%</td><td>68.52%</td><td>49.16%</td><td>65.09%</td><td>63.61%</td><td></td></tr>
</table>
## Limitations
The Llama-3-Refueled does not have any moderation mechanisms. We're looking forward to engaging with the community
on ways to make the model finely respect guardrails, allowing for deployment in environments requiring moderated outputs. |
LoneStriker/Llama-3-Refueled-3.0bpw-h6-exl2 | LoneStriker | 2024-05-09T04:52:10Z | 6 | 0 | transformers | [
"transformers",
"safetensors",
"llama",
"text-generation",
"data labeling",
"conversational",
"en",
"license:cc-by-nc-4.0",
"autotrain_compatible",
"text-generation-inference",
"endpoints_compatible",
"3-bit",
"exl2",
"region:us"
] | text-generation | 2024-05-09T04:50:24Z | ---
license: cc-by-nc-4.0
language:
- en
library_name: transformers
tags:
- data labeling
---
<div style="width: auto; margin-left: auto; margin-right: auto; background-color:black">
<img src="https://assets-global.website-files.com/6423879a8f63c1bb18d74bfa/648818d56d04c3bdf36d71ab_Refuel_rev8-01_ts-p-1600.png" alt="Refuel.ai" style="width: 100%; min-width: 400px; display: block; margin: auto;">
</div>
## Model Details
RefuelLLM-2-small, aka Llama-3-Refueled, is a Llama3-8B base model instruction tuned on a corpus of 2750+ datasets, spanning tasks such as classification, reading comprehension, structured attribute extraction and entity resolution. We're excited to open-source the model for the community to build on top of.
* More details about [RefuelLLM-2 family of models](https://www.refuel.ai/blog-posts/announcing-refuel-llm-2)
* You can also try out the models in our [LLM playground](https://labs.refuel.ai/playground)
**Model developers** - Refuel AI
**Input** - Text only.
**Output** - Text only.
**Architecture** - Llama-3-Refueled is built on top of Llama-3-8B-instruct which is an auto-regressive language model that uses an optimized transformer architecture.
**Release Date** - May 8, 2024.
## How to use
This repository contains weights for Llama-3-Refueled that are compatible for use with HuggingFace. See the snippet below for usage with Transformers:
```python
>>> import torch
>>> from transformers import AutoModelForCausalLM, AutoTokenizer
>>> model_id = "refuelai/Llama-3-Refueled"
>>> tokenizer = AutoTokenizer.from_pretrained(model_id)
>>> model = AutoModelForCausalLM.from_pretrained(model_id, torch_dtype=torch.float16, device_map="auto")
>>> messages = [{"role": "user", "content": "Is this comment toxic or non-toxic: RefuelLLM is the new way to label text data!"}]
>>> inputs = tokenizer.apply_chat_template(messages, return_tensors="pt", add_generation_prompt=True).to("cuda")
>>> outputs = model.generate(inputs, max_new_tokens=20)
>>> print(tokenizer.decode(outputs[0]))
```
## Training Data
The model was both trained on over 4 Billion tokens, spanning 2750+ NLP tasks. Our training collection consists majorly of:
1. Human annotated datasets like Flan, Task Source, and the Aya collection
2. Synthetic datasets like OpenOrca, OpenHermes and WizardLM
3. Proprietary datasets developed or licensed by Refuel AI
## Benchmarks
In this section, we report the results for Refuel models on our benchmark of labeling tasks. For details on the methodology see [here](https://refuel.ai/blog-posts/announcing-refuel-llm-2).
<table>
<tr></tr>
<tr><th>Provider</th><th>Model</th><th colspan="4" style="text-align: center">LLM Output Quality (by task type)</tr>
<tr><td></td><td></td><td>Overall</td><td>Classification</td><td>Reading Comprehension</td><td>Structure Extraction</td><td>Entity Matching</td><td></td></tr>
<tr><td>Refuel</td><td>RefuelLLM-2</td><td>83.82%</td><td>84.94%</td><td>76.03%</td><td>88.16%</td><td>92.00%</td><td></td></tr>
<tr><td>OpenAI</td><td>GPT-4-Turbo</td><td>80.88%</td><td>81.77%</td><td>72.08%</td><td>84.79%</td><td>97.20%</td><td></td></tr>
<tr><td>Refuel</td><td>RefuelLLM-2-small (Llama-3-Refueled)</td><td>79.67%</td><td>81.72%</td><td>70.04%</td><td>84.28%</td><td>92.00%</td><td></td></tr>
<tr><td>Anthropic</td><td>Claude-3-Opus</td><td>79.19%</td><td>82.49%</td><td>67.30%</td><td>88.25%</td><td>94.96%</td><td></td></tr>
<tr><td>Meta</td><td>Llama3-70B-Instruct</td><td>78.20%</td><td>79.38%</td><td>66.03%</td><td>85.96%</td><td>94.13%</td><td></td></tr>
<tr><td>Google</td><td>Gemini-1.5-Pro</td><td>74.59%</td><td>73.52%</td><td>60.67%</td><td>84.27%</td><td>98.48%</td><td></td></tr>
<tr><td>Mistral</td><td>Mixtral-8x7B-Instruct</td><td>62.87%</td><td>79.11%</td><td>45.56%</td><td>47.08%</td><td>86.52%</td><td></td></tr>
<tr><td>Anthropic</td><td>Claude-3-Sonnet</td><td>70.99%</td><td>79.91%</td><td>45.44%</td><td>78.10%</td><td>96.34%</td><td></td></tr>
<tr><td>Anthropic</td><td>Claude-3-Haiku</td><td>69.23%</td><td>77.27%</td><td>50.19%</td><td>84.97%</td><td>54.08%</td><td></td></tr>
<tr><td>OpenAI</td><td>GPT-3.5-Turbo</td><td>68.13%</td><td>74.39%</td><td>53.21%</td><td>69.40%</td><td>80.41%</td><td></td></tr>
<tr><td>Meta</td><td>Llama3-8B-Instruct</td><td>62.30%</td><td>68.52%</td><td>49.16%</td><td>65.09%</td><td>63.61%</td><td></td></tr>
</table>
## Limitations
The Llama-3-Refueled does not have any moderation mechanisms. We're looking forward to engaging with the community
on ways to make the model finely respect guardrails, allowing for deployment in environments requiring moderated outputs. |
infgrad/stella-base-zh-v3-1792d | infgrad | 2024-05-09T04:51:09Z | 4,629 | 35 | sentence-transformers | [
"sentence-transformers",
"pytorch",
"bert",
"feature-extraction",
"sentence-similarity",
"mteb",
"license:mit",
"model-index",
"autotrain_compatible",
"endpoints_compatible",
"region:us"
] | sentence-similarity | 2024-02-17T05:30:28Z | ---
pipeline_tag: sentence-similarity
tags:
- sentence-transformers
- feature-extraction
- sentence-similarity
- mteb
model-index:
- name: stella-base-zh-v3-1792d
results:
- task:
type: STS
dataset:
type: C-MTEB/AFQMC
name: MTEB AFQMC
config: default
split: validation
revision: None
metrics:
- type: cos_sim_pearson
value: 54.5145388936202
- type: cos_sim_spearman
value: 59.223125058197134
- type: euclidean_pearson
value: 57.819377838734695
- type: euclidean_spearman
value: 59.22310494948463
- type: manhattan_pearson
value: 57.44029759610327
- type: manhattan_spearman
value: 58.88336250854381
- task:
type: STS
dataset:
type: C-MTEB/ATEC
name: MTEB ATEC
config: default
split: test
revision: None
metrics:
- type: cos_sim_pearson
value: 54.544243591344866
- type: cos_sim_spearman
value: 58.43052988038229
- type: euclidean_pearson
value: 62.1608405146189
- type: euclidean_spearman
value: 58.43052762862396
- type: manhattan_pearson
value: 61.88443779892169
- type: manhattan_spearman
value: 58.26899143609596
- task:
type: Classification
dataset:
type: mteb/amazon_reviews_multi
name: MTEB AmazonReviewsClassification (zh)
config: zh
split: test
revision: 1399c76144fd37290681b995c656ef9b2e06e26d
metrics:
- type: accuracy
value: 46.343999999999994
- type: f1
value: 44.46931958420461
- task:
type: STS
dataset:
type: C-MTEB/BQ
name: MTEB BQ
config: default
split: test
revision: None
metrics:
- type: cos_sim_pearson
value: 68.52081000538426
- type: cos_sim_spearman
value: 70.44089935351529
- type: euclidean_pearson
value: 69.24671010626395
- type: euclidean_spearman
value: 70.44090281761693
- type: manhattan_pearson
value: 69.00737718109357
- type: manhattan_spearman
value: 70.24344902456502
- task:
type: Clustering
dataset:
type: C-MTEB/CLSClusteringP2P
name: MTEB CLSClusteringP2P
config: default
split: test
revision: None
metrics:
- type: v_measure
value: 42.86119436460332
- task:
type: Clustering
dataset:
type: C-MTEB/CLSClusteringS2S
name: MTEB CLSClusteringS2S
config: default
split: test
revision: None
metrics:
- type: v_measure
value: 39.97521728440642
- task:
type: Reranking
dataset:
type: C-MTEB/CMedQAv1-reranking
name: MTEB CMedQAv1
config: default
split: test
revision: None
metrics:
- type: map
value: 88.34151862240452
- type: mrr
value: 90.40380952380953
- task:
type: Reranking
dataset:
type: C-MTEB/CMedQAv2-reranking
name: MTEB CMedQAv2
config: default
split: test
revision: None
metrics:
- type: map
value: 89.06288758814637
- type: mrr
value: 90.91285714285713
- task:
type: Retrieval
dataset:
type: C-MTEB/CmedqaRetrieval
name: MTEB CmedqaRetrieval
config: default
split: dev
revision: None
metrics:
- type: map_at_1
value: 25.651000000000003
- type: map_at_10
value: 38.576
- type: map_at_100
value: 40.534
- type: map_at_1000
value: 40.64
- type: map_at_3
value: 34.016000000000005
- type: map_at_5
value: 36.675999999999995
- type: mrr_at_1
value: 39.06
- type: mrr_at_10
value: 47.278
- type: mrr_at_100
value: 48.272999999999996
- type: mrr_at_1000
value: 48.314
- type: mrr_at_3
value: 44.461
- type: mrr_at_5
value: 46.107
- type: ndcg_at_1
value: 39.06
- type: ndcg_at_10
value: 45.384
- type: ndcg_at_100
value: 52.796
- type: ndcg_at_1000
value: 54.55
- type: ndcg_at_3
value: 39.497
- type: ndcg_at_5
value: 42.189
- type: precision_at_1
value: 39.06
- type: precision_at_10
value: 10.17
- type: precision_at_100
value: 1.6179999999999999
- type: precision_at_1000
value: 0.184
- type: precision_at_3
value: 22.247
- type: precision_at_5
value: 16.529
- type: recall_at_1
value: 25.651000000000003
- type: recall_at_10
value: 56.82899999999999
- type: recall_at_100
value: 87.134
- type: recall_at_1000
value: 98.709
- type: recall_at_3
value: 39.461
- type: recall_at_5
value: 47.329
- task:
type: PairClassification
dataset:
type: C-MTEB/CMNLI
name: MTEB Cmnli
config: default
split: validation
revision: None
metrics:
- type: cos_sim_accuracy
value: 83.1870114251353
- type: cos_sim_ap
value: 90.42393852164342
- type: cos_sim_f1
value: 84.10685985963323
- type: cos_sim_precision
value: 81.5229317533465
- type: cos_sim_recall
value: 86.85994856207621
- type: dot_accuracy
value: 83.1870114251353
- type: dot_ap
value: 90.41339758845682
- type: dot_f1
value: 84.10685985963323
- type: dot_precision
value: 81.5229317533465
- type: dot_recall
value: 86.85994856207621
- type: euclidean_accuracy
value: 83.1870114251353
- type: euclidean_ap
value: 90.42393581056393
- type: euclidean_f1
value: 84.10685985963323
- type: euclidean_precision
value: 81.5229317533465
- type: euclidean_recall
value: 86.85994856207621
- type: manhattan_accuracy
value: 82.77811184606134
- type: manhattan_ap
value: 90.18115714681704
- type: manhattan_f1
value: 83.75083130126357
- type: manhattan_precision
value: 79.62065331928345
- type: manhattan_recall
value: 88.33294365209258
- type: max_accuracy
value: 83.1870114251353
- type: max_ap
value: 90.42393852164342
- type: max_f1
value: 84.10685985963323
- task:
type: Retrieval
dataset:
type: C-MTEB/CovidRetrieval
name: MTEB CovidRetrieval
config: default
split: dev
revision: None
metrics:
- type: map_at_1
value: 68.388
- type: map_at_10
value: 76.819
- type: map_at_100
value: 77.153
- type: map_at_1000
value: 77.16
- type: map_at_3
value: 74.98700000000001
- type: map_at_5
value: 76.101
- type: mrr_at_1
value: 68.599
- type: mrr_at_10
value: 76.844
- type: mrr_at_100
value: 77.168
- type: mrr_at_1000
value: 77.17500000000001
- type: mrr_at_3
value: 75.044
- type: mrr_at_5
value: 76.208
- type: ndcg_at_1
value: 68.599
- type: ndcg_at_10
value: 80.613
- type: ndcg_at_100
value: 82.017
- type: ndcg_at_1000
value: 82.19300000000001
- type: ndcg_at_3
value: 76.956
- type: ndcg_at_5
value: 78.962
- type: precision_at_1
value: 68.599
- type: precision_at_10
value: 9.336
- type: precision_at_100
value: 0.996
- type: precision_at_1000
value: 0.101
- type: precision_at_3
value: 27.678000000000004
- type: precision_at_5
value: 17.619
- type: recall_at_1
value: 68.388
- type: recall_at_10
value: 92.36
- type: recall_at_100
value: 98.52499999999999
- type: recall_at_1000
value: 99.895
- type: recall_at_3
value: 82.53399999999999
- type: recall_at_5
value: 87.355
- task:
type: Retrieval
dataset:
type: C-MTEB/DuRetrieval
name: MTEB DuRetrieval
config: default
split: dev
revision: None
metrics:
- type: map_at_1
value: 25.1
- type: map_at_10
value: 77.71000000000001
- type: map_at_100
value: 80.638
- type: map_at_1000
value: 80.679
- type: map_at_3
value: 53.187
- type: map_at_5
value: 67.735
- type: mrr_at_1
value: 87.8
- type: mrr_at_10
value: 91.8
- type: mrr_at_100
value: 91.893
- type: mrr_at_1000
value: 91.89500000000001
- type: mrr_at_3
value: 91.51700000000001
- type: mrr_at_5
value: 91.704
- type: ndcg_at_1
value: 87.8
- type: ndcg_at_10
value: 85.55
- type: ndcg_at_100
value: 88.626
- type: ndcg_at_1000
value: 89.021
- type: ndcg_at_3
value: 83.94
- type: ndcg_at_5
value: 83.259
- type: precision_at_1
value: 87.8
- type: precision_at_10
value: 41.295
- type: precision_at_100
value: 4.781
- type: precision_at_1000
value: 0.488
- type: precision_at_3
value: 75.3
- type: precision_at_5
value: 64.13
- type: recall_at_1
value: 25.1
- type: recall_at_10
value: 87.076
- type: recall_at_100
value: 97.095
- type: recall_at_1000
value: 99.129
- type: recall_at_3
value: 56.013999999999996
- type: recall_at_5
value: 73.2
- task:
type: Retrieval
dataset:
type: C-MTEB/EcomRetrieval
name: MTEB EcomRetrieval
config: default
split: dev
revision: None
metrics:
- type: map_at_1
value: 53.300000000000004
- type: map_at_10
value: 63.01
- type: map_at_100
value: 63.574
- type: map_at_1000
value: 63.587
- type: map_at_3
value: 60.783
- type: map_at_5
value: 62.098
- type: mrr_at_1
value: 53.300000000000004
- type: mrr_at_10
value: 63.01
- type: mrr_at_100
value: 63.574
- type: mrr_at_1000
value: 63.587
- type: mrr_at_3
value: 60.783
- type: mrr_at_5
value: 62.098
- type: ndcg_at_1
value: 53.300000000000004
- type: ndcg_at_10
value: 67.876
- type: ndcg_at_100
value: 70.434
- type: ndcg_at_1000
value: 70.753
- type: ndcg_at_3
value: 63.275000000000006
- type: ndcg_at_5
value: 65.654
- type: precision_at_1
value: 53.300000000000004
- type: precision_at_10
value: 8.32
- type: precision_at_100
value: 0.9480000000000001
- type: precision_at_1000
value: 0.097
- type: precision_at_3
value: 23.5
- type: precision_at_5
value: 15.260000000000002
- type: recall_at_1
value: 53.300000000000004
- type: recall_at_10
value: 83.2
- type: recall_at_100
value: 94.8
- type: recall_at_1000
value: 97.3
- type: recall_at_3
value: 70.5
- type: recall_at_5
value: 76.3
- task:
type: Classification
dataset:
type: C-MTEB/IFlyTek-classification
name: MTEB IFlyTek
config: default
split: validation
revision: None
metrics:
- type: accuracy
value: 49.92689495959984
- type: f1
value: 37.784780470986625
- task:
type: Classification
dataset:
type: C-MTEB/JDReview-classification
name: MTEB JDReview
config: default
split: test
revision: None
metrics:
- type: accuracy
value: 86.26641651031895
- type: ap
value: 54.50750244841821
- type: f1
value: 80.94927946681523
- task:
type: STS
dataset:
type: C-MTEB/LCQMC
name: MTEB LCQMC
config: default
split: test
revision: None
metrics:
- type: cos_sim_pearson
value: 72.3980811478615
- type: cos_sim_spearman
value: 78.26906056425528
- type: euclidean_pearson
value: 77.87705501225068
- type: euclidean_spearman
value: 78.26905834518651
- type: manhattan_pearson
value: 77.77154630197
- type: manhattan_spearman
value: 78.1940918602169
- task:
type: Reranking
dataset:
type: C-MTEB/Mmarco-reranking
name: MTEB MMarcoReranking
config: default
split: dev
revision: None
metrics:
- type: map
value: 27.48003475319453
- type: mrr
value: 26.400793650793652
- task:
type: Retrieval
dataset:
type: C-MTEB/MMarcoRetrieval
name: MTEB MMarcoRetrieval
config: default
split: dev
revision: None
metrics:
- type: map_at_1
value: 64.373
- type: map_at_10
value: 73.604
- type: map_at_100
value: 73.953
- type: map_at_1000
value: 73.965
- type: map_at_3
value: 71.70100000000001
- type: map_at_5
value: 72.859
- type: mrr_at_1
value: 66.676
- type: mrr_at_10
value: 74.248
- type: mrr_at_100
value: 74.56099999999999
- type: mrr_at_1000
value: 74.572
- type: mrr_at_3
value: 72.59100000000001
- type: mrr_at_5
value: 73.592
- type: ndcg_at_1
value: 66.676
- type: ndcg_at_10
value: 77.417
- type: ndcg_at_100
value: 79.006
- type: ndcg_at_1000
value: 79.334
- type: ndcg_at_3
value: 73.787
- type: ndcg_at_5
value: 75.74
- type: precision_at_1
value: 66.676
- type: precision_at_10
value: 9.418
- type: precision_at_100
value: 1.0210000000000001
- type: precision_at_1000
value: 0.105
- type: precision_at_3
value: 27.832
- type: precision_at_5
value: 17.736
- type: recall_at_1
value: 64.373
- type: recall_at_10
value: 88.565
- type: recall_at_100
value: 95.789
- type: recall_at_1000
value: 98.355
- type: recall_at_3
value: 78.914
- type: recall_at_5
value: 83.56
- task:
type: Classification
dataset:
type: mteb/amazon_massive_intent
name: MTEB MassiveIntentClassification (zh-CN)
config: zh-CN
split: test
revision: 31efe3c427b0bae9c22cbb560b8f15491cc6bed7
metrics:
- type: accuracy
value: 72.0544720914593
- type: f1
value: 69.61749470345791
- task:
type: Classification
dataset:
type: mteb/amazon_massive_scenario
name: MTEB MassiveScenarioClassification (zh-CN)
config: zh-CN
split: test
revision: 7d571f92784cd94a019292a1f45445077d0ef634
metrics:
- type: accuracy
value: 75.30262273032953
- type: f1
value: 75.05097671215634
- task:
type: Retrieval
dataset:
type: C-MTEB/MedicalRetrieval
name: MTEB MedicalRetrieval
config: default
split: dev
revision: None
metrics:
- type: map_at_1
value: 55.1
- type: map_at_10
value: 61.284000000000006
- type: map_at_100
value: 61.794000000000004
- type: map_at_1000
value: 61.838
- type: map_at_3
value: 59.75
- type: map_at_5
value: 60.64000000000001
- type: mrr_at_1
value: 55.300000000000004
- type: mrr_at_10
value: 61.38400000000001
- type: mrr_at_100
value: 61.894000000000005
- type: mrr_at_1000
value: 61.938
- type: mrr_at_3
value: 59.85
- type: mrr_at_5
value: 60.74
- type: ndcg_at_1
value: 55.1
- type: ndcg_at_10
value: 64.345
- type: ndcg_at_100
value: 67.148
- type: ndcg_at_1000
value: 68.36
- type: ndcg_at_3
value: 61.182
- type: ndcg_at_5
value: 62.808
- type: precision_at_1
value: 55.1
- type: precision_at_10
value: 7.3999999999999995
- type: precision_at_100
value: 0.8789999999999999
- type: precision_at_1000
value: 0.098
- type: precision_at_3
value: 21.767
- type: precision_at_5
value: 13.86
- type: recall_at_1
value: 55.1
- type: recall_at_10
value: 74
- type: recall_at_100
value: 87.9
- type: recall_at_1000
value: 97.5
- type: recall_at_3
value: 65.3
- type: recall_at_5
value: 69.3
- task:
type: Classification
dataset:
type: C-MTEB/MultilingualSentiment-classification
name: MTEB MultilingualSentiment
config: default
split: validation
revision: None
metrics:
- type: accuracy
value: 76.21666666666667
- type: f1
value: 76.03732395559548
- task:
type: PairClassification
dataset:
type: C-MTEB/OCNLI
name: MTEB Ocnli
config: default
split: validation
revision: None
metrics:
- type: cos_sim_accuracy
value: 81.8083378451543
- type: cos_sim_ap
value: 85.43050139514027
- type: cos_sim_f1
value: 83.25969563082965
- type: cos_sim_precision
value: 77.79816513761469
- type: cos_sim_recall
value: 89.54593453009504
- type: dot_accuracy
value: 81.8083378451543
- type: dot_ap
value: 85.43050139514027
- type: dot_f1
value: 83.25969563082965
- type: dot_precision
value: 77.79816513761469
- type: dot_recall
value: 89.54593453009504
- type: euclidean_accuracy
value: 81.8083378451543
- type: euclidean_ap
value: 85.43050139514027
- type: euclidean_f1
value: 83.25969563082965
- type: euclidean_precision
value: 77.79816513761469
- type: euclidean_recall
value: 89.54593453009504
- type: manhattan_accuracy
value: 81.53762858689767
- type: manhattan_ap
value: 84.90556637024838
- type: manhattan_f1
value: 82.90258449304174
- type: manhattan_precision
value: 78.30985915492957
- type: manhattan_recall
value: 88.0675818373812
- type: max_accuracy
value: 81.8083378451543
- type: max_ap
value: 85.43050139514027
- type: max_f1
value: 83.25969563082965
- task:
type: Classification
dataset:
type: C-MTEB/OnlineShopping-classification
name: MTEB OnlineShopping
config: default
split: test
revision: None
metrics:
- type: accuracy
value: 93.53
- type: ap
value: 91.62070655043128
- type: f1
value: 93.51908163199477
- task:
type: STS
dataset:
type: C-MTEB/PAWSX
name: MTEB PAWSX
config: default
split: test
revision: None
metrics:
- type: cos_sim_pearson
value: 38.451787103814375
- type: cos_sim_spearman
value: 43.97299462643919
- type: euclidean_pearson
value: 43.63298716626501
- type: euclidean_spearman
value: 43.973080252178576
- type: manhattan_pearson
value: 43.37465277323481
- type: manhattan_spearman
value: 43.71981281220414
- task:
type: STS
dataset:
type: C-MTEB/QBQTC
name: MTEB QBQTC
config: default
split: test
revision: None
metrics:
- type: cos_sim_pearson
value: 37.75882451277358
- type: cos_sim_spearman
value: 40.0244327844802
- type: euclidean_pearson
value: 38.11050875514246
- type: euclidean_spearman
value: 40.02440987254504
- type: manhattan_pearson
value: 38.03186803221696
- type: manhattan_spearman
value: 39.757452890246775
- task:
type: STS
dataset:
type: mteb/sts22-crosslingual-sts
name: MTEB STS22 (zh)
config: zh
split: test
revision: 6d1ba47164174a496b7fa5d3569dae26a6813b80
metrics:
- type: cos_sim_pearson
value: 65.9133992390713
- type: cos_sim_spearman
value: 66.4894937647578
- type: euclidean_pearson
value: 66.19047142189935
- type: euclidean_spearman
value: 66.4894937647578
- type: manhattan_pearson
value: 66.6960935896136
- type: manhattan_spearman
value: 66.88179996508133
- task:
type: STS
dataset:
type: C-MTEB/STSB
name: MTEB STSB
config: default
split: test
revision: None
metrics:
- type: cos_sim_pearson
value: 80.55099417946924
- type: cos_sim_spearman
value: 83.05000687568048
- type: euclidean_pearson
value: 82.62744668792926
- type: euclidean_spearman
value: 83.05000687568048
- type: manhattan_pearson
value: 82.6543207325763
- type: manhattan_spearman
value: 83.06852715971705
- task:
type: Reranking
dataset:
type: C-MTEB/T2Reranking
name: MTEB T2Reranking
config: default
split: dev
revision: None
metrics:
- type: map
value: 66.48634798223672
- type: mrr
value: 76.30158461488861
- task:
type: Retrieval
dataset:
type: C-MTEB/T2Retrieval
name: MTEB T2Retrieval
config: default
split: dev
revision: None
metrics:
- type: map_at_1
value: 27.483999999999998
- type: map_at_10
value: 76.848
- type: map_at_100
value: 80.541
- type: map_at_1000
value: 80.607
- type: map_at_3
value: 54.111
- type: map_at_5
value: 66.46300000000001
- type: mrr_at_1
value: 90.045
- type: mrr_at_10
value: 92.552
- type: mrr_at_100
value: 92.642
- type: mrr_at_1000
value: 92.645
- type: mrr_at_3
value: 92.134
- type: mrr_at_5
value: 92.391
- type: ndcg_at_1
value: 90.045
- type: ndcg_at_10
value: 84.504
- type: ndcg_at_100
value: 88.23100000000001
- type: ndcg_at_1000
value: 88.85300000000001
- type: ndcg_at_3
value: 85.992
- type: ndcg_at_5
value: 84.548
- type: precision_at_1
value: 90.045
- type: precision_at_10
value: 41.91
- type: precision_at_100
value: 5.017
- type: precision_at_1000
value: 0.516
- type: precision_at_3
value: 75.15899999999999
- type: precision_at_5
value: 62.958000000000006
- type: recall_at_1
value: 27.483999999999998
- type: recall_at_10
value: 83.408
- type: recall_at_100
value: 95.514
- type: recall_at_1000
value: 98.65
- type: recall_at_3
value: 55.822
- type: recall_at_5
value: 69.868
- task:
type: Classification
dataset:
type: C-MTEB/TNews-classification
name: MTEB TNews
config: default
split: validation
revision: None
metrics:
- type: accuracy
value: 53.196
- type: f1
value: 51.51679244513836
- task:
type: Clustering
dataset:
type: C-MTEB/ThuNewsClusteringP2P
name: MTEB ThuNewsClusteringP2P
config: default
split: test
revision: None
metrics:
- type: v_measure
value: 67.87592101539063
- task:
type: Clustering
dataset:
type: C-MTEB/ThuNewsClusteringS2S
name: MTEB ThuNewsClusteringS2S
config: default
split: test
revision: None
metrics:
- type: v_measure
value: 62.4675464095125
- task:
type: Retrieval
dataset:
type: C-MTEB/VideoRetrieval
name: MTEB VideoRetrieval
config: default
split: dev
revision: None
metrics:
- type: map_at_1
value: 57.9
- type: map_at_10
value: 68.099
- type: map_at_100
value: 68.55499999999999
- type: map_at_1000
value: 68.566
- type: map_at_3
value: 66.4
- type: map_at_5
value: 67.46
- type: mrr_at_1
value: 57.9
- type: mrr_at_10
value: 68.099
- type: mrr_at_100
value: 68.55499999999999
- type: mrr_at_1000
value: 68.566
- type: mrr_at_3
value: 66.4
- type: mrr_at_5
value: 67.46
- type: ndcg_at_1
value: 57.9
- type: ndcg_at_10
value: 72.555
- type: ndcg_at_100
value: 74.715
- type: ndcg_at_1000
value: 75.034
- type: ndcg_at_3
value: 69.102
- type: ndcg_at_5
value: 71.004
- type: precision_at_1
value: 57.9
- type: precision_at_10
value: 8.63
- type: precision_at_100
value: 0.963
- type: precision_at_1000
value: 0.099
- type: precision_at_3
value: 25.633
- type: precision_at_5
value: 16.3
- type: recall_at_1
value: 57.9
- type: recall_at_10
value: 86.3
- type: recall_at_100
value: 96.3
- type: recall_at_1000
value: 98.9
- type: recall_at_3
value: 76.9
- type: recall_at_5
value: 81.5
- task:
type: Classification
dataset:
type: C-MTEB/waimai-classification
name: MTEB Waimai
config: default
split: test
revision: None
metrics:
- type: accuracy
value: 87.27000000000001
- type: ap
value: 71.10883470119464
- type: f1
value: 85.76618863591946
license: mit
---
**新闻 | News**
**[2024-04-06]** 开源[puff](https://huggingface.co/infgrad/puff-base-v1)系列模型,**专门针对检索和语义匹配任务,更多的考虑泛化性和私有通用测试集效果,向量维度可变,中英双语**。
**[2024-02-27]** 开源stella-mrl-large-zh-v3.5-1792d模型,支持**向量可变维度**。
**[2024-02-17]** 开源stella v3系列、dialogue编码模型和相关训练数据。
**[2023-10-19]** 开源stella-base-en-v2 使用简单,**不需要任何前缀文本**。
**[2023-10-12]** 开源stella-base-zh-v2和stella-large-zh-v2, 效果更好且使用简单,**不需要任何前缀文本**。
**[2023-09-11]** 开源stella-base-zh和stella-large-zh
欢迎去[本人主页](https://huggingface.co/infgrad)查看最新模型,并提出您的宝贵意见!
# 1 开源清单
本次开源2个通用向量编码模型和一个针对dialogue进行编码的向量模型,同时开源全量160万对话重写数据集和20万的难负例的检索数据集。
**开源模型:**
| ModelName | ModelSize | MaxTokens | EmbeddingDimensions | Language | Scenario | C-MTEB Score |
|---------------------------------------------------------------------------------------------------------------|-----------|-----------|---------------------|----------|----------|--------------|
| [infgrad/stella-base-zh-v3-1792d](https://huggingface.co/infgrad/stella-base-zh-v3-1792d) | 0.4GB | 512 | 1792 | zh-CN | 通用文本 | 67.96 |
| [infgrad/stella-large-zh-v3-1792d](https://huggingface.co/infgrad/stella-large-zh-v3-1792d) | 1.3GB | 512 | 1792 | zh-CN | 通用文本 | 68.48 |
| [infgrad/stella-dialogue-large-zh-v3-1792d](https://huggingface.co/infgrad/stella-dialogue-large-zh-v3-1792d) | 1.3GB | 512 | 1792 | zh-CN | **对话文本** | 不适用 |
**开源数据:**
1. [全量对话重写数据集](https://huggingface.co/datasets/infgrad/dialogue_rewrite_llm) 约160万
2. [部分带有难负例的检索数据集](https://huggingface.co/datasets/infgrad/retrieval_data_llm) 约20万
上述数据集均使用LLM构造,欢迎各位贡献数据集。
# 2 使用方法
## 2.1 通用编码模型使用方法
直接SentenceTransformer加载即可:
```python
from sentence_transformers import SentenceTransformer
model = SentenceTransformer("infgrad/stella-base-zh-v3-1792d")
# model = SentenceTransformer("infgrad/stella-large-zh-v3-1792d")
vectors = model.encode(["text1", "text2"])
```
## 2.2 dialogue编码模型使用方法
**使用场景:**
**在一段对话中,需要根据用户语句去检索相关文本,但是对话中的用户语句存在大量的指代和省略,导致直接使用通用编码模型效果不好,
可以使用本项目的专门的dialogue编码模型进行编码**
**使用要点:**
1. 对dialogue进行编码时,dialogue中的每个utterance需要是如下格式:`"{ROLE}: {TEXT}"`,然后使用`[SEP]` join一下
2. 整个对话都要送入模型进行编码,如果长度不够就删掉早期的对话,**编码后的向量本质是对话中最后一句话的重写版本的向量!!**
3. 对话用stella-dialogue-large-zh-v3-1792d编码,被检索文本使用stella-large-zh-v3-1792d进行编码,所以本场景是需要2个编码模型的
如果对使用方法还有疑惑,请到下面章节阅读该模型是如何训练的。
使用示例:
```python
from sentence_transformers import SentenceTransformer
dial_model = SentenceTransformer("infgrad/stella-dialogue-large-zh-v3-1792d")
general_model = SentenceTransformer("infgrad/stella-large-zh-v3-1792d")
# dialogue = ["张三: 吃饭吗", "李四: 等会去"]
dialogue = ["A: 最近去打篮球了吗", "B: 没有"]
corpus = ["B没打篮球是因为受伤了。", "B没有打乒乓球"]
last_utterance_vector = dial_model.encode(["[SEP]".join(dialogue)], normalize_embeddings=True)
corpus_vectors = general_model.encode(corpus, normalize_embeddings=True)
# 计算相似度
sims = (last_utterance_vector * corpus_vectors).sum(axis=1)
print(sims)
```
# 3 通用编码模型训练技巧分享
## hard negative
难负例挖掘也是个经典的trick了,几乎总能提升效果
## dropout-1d
dropout已经是深度学习的标配,我们可以稍微改造下使其更适合句向量的训练。
我们在训练时会尝试让每一个token-embedding都可以表征整个句子,而在推理时使用mean_pooling从而达到类似模型融合的效果。
具体操作是在mean_pooling时加入dropout_1d,torch代码如下:
```python
vector_dropout = nn.Dropout1d(0.3) # 算力有限,试了0.3和0.5 两个参数,其中0.3更优
last_hidden_state = bert_model(...)[0]
last_hidden = last_hidden_state.masked_fill(~attention_mask[..., None].bool(), 0.0)
last_hidden = vector_dropout(last_hidden)
vectors = last_hidden.sum(dim=1) / attention_mask.sum(dim=1)[..., None]
```
# 4 dialogue编码模型细节
## 4.1 为什么需要一个dialogue编码模型?
参见本人历史文章:https://www.zhihu.com/pin/1674913544847077376
## 4.2 训练数据
单条数据示例:
```json
{
"dialogue": [
"A: 最近去打篮球了吗",
"B: 没有"
],
"last_utterance_rewrite": "B: 我最近没有去打篮球"
}
```
## 4.3 训练Loss
```
loss = cosine_loss( dial_model.encode(dialogue), existing_model.encode(last_utterance_rewrite) )
```
dial_model就是要被训练的模型,本人是以stella-large-zh-v3-1792d作为base-model进行继续训练的
existing_model就是现有训练好的**通用编码模型**,本人使用的是stella-large-zh-v3-1792d
已开源dialogue-embedding的全量训练数据,理论上可以复现本模型效果。
Loss下降情况:
<div align="center">
<img src="dial_loss.png" alt="icon" width="2000px"/>
</div>
## 4.4 效果
目前还没有专门测试集,本人简单测试了下是有效果的,部分测试结果见文件`dial_retrieval_test.xlsx`。
# 5 后续TODO
1. 更多的dial-rewrite数据
2. 不同EmbeddingDimensions的编码模型
# 6 FAQ
Q: 为什么向量维度是1792?\
A: 最初考虑发布768、1024,768+768,1024+1024,1024+768维度,但是时间有限,先做了1792就只发布1792维度的模型。理论上维度越高效果越好。
Q: 如何复现CMTEB效果?\
A: SentenceTransformer加载后直接用官方评测脚本就行,注意对于Classification任务向量需要先normalize一下
Q: 复现的CMTEB效果和本文不一致?\
A: 聚类不一致正常,官方评测代码没有设定seed,其他不一致建议检查代码或联系本人。
Q: 如何选择向量模型?\
A: 没有免费的午餐,在自己测试集上试试,本人推荐bge、e5和stella.
Q: 长度为什么只有512,能否更长?\
A: 可以但没必要,长了效果普遍不好,这是当前训练方法和数据导致的,几乎无解,建议长文本还是走分块。
Q: 训练资源和算力?\
A: 亿级别的数据,单卡A100要一个月起步 |
hadiaskari98/SecuritySER | hadiaskari98 | 2024-05-09T04:47:33Z | 0 | 0 | null | [
"license:apache-2.0",
"region:us"
] | null | 2024-05-09T04:47:33Z | ---
license: apache-2.0
---
|
Wellyowo/whisper-tiny-zh-tw | Wellyowo | 2024-05-09T04:45:07Z | 97 | 1 | transformers | [
"transformers",
"tensorboard",
"safetensors",
"whisper",
"automatic-speech-recognition",
"generated_from_trainer",
"dataset:common_voice_13_0",
"base_model:Wellyowo/whisper-tiny-zh-tw",
"base_model:finetune:Wellyowo/whisper-tiny-zh-tw",
"license:apache-2.0",
"model-index",
"endpoints_compatible",
"region:us"
] | automatic-speech-recognition | 2024-05-08T13:20:18Z | ---
license: apache-2.0
base_model: Wellyowo/whisper-tiny-zh-tw
tags:
- generated_from_trainer
datasets:
- common_voice_13_0
metrics:
- wer
model-index:
- name: whisper-tiny-zh-tw
results:
- task:
name: Automatic Speech Recognition
type: automatic-speech-recognition
dataset:
name: common_voice_13_0
type: common_voice_13_0
config: zh-TW
split: test
args: zh-TW
metrics:
- name: Wer
type: wer
value: 59.22330097087378
---
<!-- This model card has been generated automatically according to the information the Trainer had access to. You
should probably proofread and complete it, then remove this comment. -->
# whisper-tiny-zh-tw
This model is a fine-tuned version of [Wellyowo/whisper-tiny-zh-tw](https://huggingface.co/Wellyowo/whisper-tiny-zh-tw) on the common_voice_13_0 dataset.
It achieves the following results on the evaluation set:
- Loss: 0.5683
- Wer Ortho: 60.0
- Wer: 59.2233
## Model description
More information needed
## Intended uses & limitations
More information needed
## Training and evaluation data
More information needed
## Training procedure
### Training hyperparameters
The following hyperparameters were used during training:
- learning_rate: 1e-05
- train_batch_size: 4
- eval_batch_size: 4
- seed: 42
- gradient_accumulation_steps: 4
- total_train_batch_size: 16
- optimizer: Adam with betas=(0.9,0.999) and epsilon=1e-08
- lr_scheduler_type: constant_with_warmup
- lr_scheduler_warmup_steps: 50
- training_steps: 4000
- mixed_precision_training: Native AMP
### Training results
| Training Loss | Epoch | Step | Validation Loss | Wer Ortho | Wer |
|:-------------:|:-----:|:----:|:---------------:|:---------:|:-------:|
| 0.1844 | 0.69 | 500 | 0.5523 | 64.0 | 65.0485 |
| 0.1105 | 1.38 | 1000 | 0.5425 | 65.0 | 64.0777 |
| 0.0475 | 2.06 | 1500 | 0.5324 | 63.0 | 64.0777 |
| 0.0687 | 2.75 | 2000 | 0.5128 | 62.0 | 61.1650 |
| 0.0371 | 3.44 | 2500 | 0.5496 | 64.0 | 64.0777 |
| 0.0181 | 4.13 | 3000 | 0.5339 | 62.0 | 63.1068 |
| 0.0202 | 4.82 | 3500 | 0.5474 | 65.0 | 64.0777 |
| 0.011 | 5.51 | 4000 | 0.5683 | 60.0 | 59.2233 |
### Framework versions
- Transformers 4.37.2
- Pytorch 2.0.1+cu117
- Datasets 2.16.1
- Tokenizers 0.15.1
|
monsterbeasts/LishizhenGPT | monsterbeasts | 2024-05-09T04:44:44Z | 7 | 0 | transformers | [
"transformers",
"pytorch",
"safetensors",
"bloom",
"text-generation",
"ak",
"ar",
"as",
"bm",
"bn",
"ca",
"code",
"en",
"es",
"eu",
"fon",
"fr",
"gu",
"hi",
"id",
"ig",
"ki",
"kn",
"lg",
"ln",
"ml",
"mr",
"ne",
"nso",
"ny",
"or",
"pa",
"pt",
"rn",
"rw",
"sn",
"st",
"sw",
"ta",
"te",
"tn",
"ts",
"tum",
"tw",
"ur",
"vi",
"wo",
"xh",
"yo",
"zh",
"zu",
"dataset:bigscience/xP3mt",
"arxiv:2211.01786",
"license:bigscience-bloom-rail-1.0",
"model-index",
"autotrain_compatible",
"text-generation-inference",
"endpoints_compatible",
"region:us"
] | text-generation | 2024-04-23T09:05:31Z | ---
datasets:
- bigscience/xP3mt
license: bigscience-bloom-rail-1.0
language:
- ak
- ar
- as
- bm
- bn
- ca
- code
- en
- es
- eu
- fon
- fr
- gu
- hi
- id
- ig
- ki
- kn
- lg
- ln
- ml
- mr
- ne
- nso
- ny
- or
- pa
- pt
- rn
- rw
- sn
- st
- sw
- ta
- te
- tn
- ts
- tum
- tw
- ur
- vi
- wo
- xh
- yo
- zh
- zu
programming_language:
- C
- C++
- C#
- Go
- Java
- JavaScript
- Lua
- PHP
- Python
- Ruby
- Rust
- Scala
- TypeScript
pipeline_tag: text-generation
widget:
- text: "一个传奇的开端,一个不灭的神话,这不仅仅是一部电影,而是作为一个走进新时代的标签,永远彪炳史册。Would you rate the previous review as positive, neutral or negative?"
example_title: "zh-en sentiment"
- text: "一个传奇的开端,一个不灭的神话,这不仅仅是一部电影,而是作为一个走进新时代的标签,永远彪炳史册。你认为这句话的立场是赞扬、中立还是批评?"
example_title: "zh-zh sentiment"
- text: "Suggest at least five related search terms to \"Mạng neural nhân tạo\"."
example_title: "vi-en query"
- text: "Proposez au moins cinq mots clés concernant «Réseau de neurones artificiels»."
example_title: "fr-fr query"
- text: "Explain in a sentence in Telugu what is backpropagation in neural networks."
example_title: "te-en qa"
- text: "Why is the sky blue?"
example_title: "en-en qa"
- text: "Write a fairy tale about a troll saving a princess from a dangerous dragon. The fairy tale is a masterpiece that has achieved praise worldwide and its moral is \"Heroes Come in All Shapes and Sizes\". Story (in Spanish):"
example_title: "es-en fable"
- text: "Write a fable about wood elves living in a forest that is suddenly invaded by ogres. The fable is a masterpiece that has achieved praise worldwide and its moral is \"Violence is the last refuge of the incompetent\". Fable (in Hindi):"
example_title: "hi-en fable"
model-index:
- name: bloomz-7b1-mt
results:
- task:
type: Coreference resolution
dataset:
type: winogrande
name: Winogrande XL (xl)
config: xl
split: validation
revision: a80f460359d1e9a67c006011c94de42a8759430c
metrics:
- type: Accuracy
value: 56.51
- task:
type: Coreference resolution
dataset:
type: Muennighoff/xwinograd
name: XWinograd (en)
config: en
split: test
revision: 9dd5ea5505fad86b7bedad667955577815300cee
metrics:
- type: Accuracy
value: 65.76
- task:
type: Coreference resolution
dataset:
type: Muennighoff/xwinograd
name: XWinograd (fr)
config: fr
split: test
revision: 9dd5ea5505fad86b7bedad667955577815300cee
metrics:
- type: Accuracy
value: 57.83
- task:
type: Coreference resolution
dataset:
type: Muennighoff/xwinograd
name: XWinograd (jp)
config: jp
split: test
revision: 9dd5ea5505fad86b7bedad667955577815300cee
metrics:
- type: Accuracy
value: 51.82
- task:
type: Coreference resolution
dataset:
type: Muennighoff/xwinograd
name: XWinograd (pt)
config: pt
split: test
revision: 9dd5ea5505fad86b7bedad667955577815300cee
metrics:
- type: Accuracy
value: 57.41
- task:
type: Coreference resolution
dataset:
type: Muennighoff/xwinograd
name: XWinograd (ru)
config: ru
split: test
revision: 9dd5ea5505fad86b7bedad667955577815300cee
metrics:
- type: Accuracy
value: 55.87
- task:
type: Coreference resolution
dataset:
type: Muennighoff/xwinograd
name: XWinograd (zh)
config: zh
split: test
revision: 9dd5ea5505fad86b7bedad667955577815300cee
metrics:
- type: Accuracy
value: 62.7
- task:
type: Natural language inference
dataset:
type: anli
name: ANLI (r1)
config: r1
split: validation
revision: 9dbd830a06fea8b1c49d6e5ef2004a08d9f45094
metrics:
- type: Accuracy
value: 42.6
- task:
type: Natural language inference
dataset:
type: anli
name: ANLI (r2)
config: r2
split: validation
revision: 9dbd830a06fea8b1c49d6e5ef2004a08d9f45094
metrics:
- type: Accuracy
value: 39.4
- task:
type: Natural language inference
dataset:
type: anli
name: ANLI (r3)
config: r3
split: validation
revision: 9dbd830a06fea8b1c49d6e5ef2004a08d9f45094
metrics:
- type: Accuracy
value: 42.0
- task:
type: Natural language inference
dataset:
type: super_glue
name: SuperGLUE (cb)
config: cb
split: validation
revision: 9e12063561e7e6c79099feb6d5a493142584e9e2
metrics:
- type: Accuracy
value: 83.93
- task:
type: Natural language inference
dataset:
type: super_glue
name: SuperGLUE (rte)
config: rte
split: validation
revision: 9e12063561e7e6c79099feb6d5a493142584e9e2
metrics:
- type: Accuracy
value: 82.67
- task:
type: Natural language inference
dataset:
type: xnli
name: XNLI (ar)
config: ar
split: validation
revision: a5a45e4ff92d5d3f34de70aaf4b72c3bdf9f7f16
metrics:
- type: Accuracy
value: 55.58
- task:
type: Natural language inference
dataset:
type: xnli
name: XNLI (bg)
config: bg
split: validation
revision: a5a45e4ff92d5d3f34de70aaf4b72c3bdf9f7f16
metrics:
- type: Accuracy
value: 44.9
- task:
type: Natural language inference
dataset:
type: xnli
name: XNLI (de)
config: de
split: validation
revision: a5a45e4ff92d5d3f34de70aaf4b72c3bdf9f7f16
metrics:
- type: Accuracy
value: 48.92
- task:
type: Natural language inference
dataset:
type: xnli
name: XNLI (el)
config: el
split: validation
revision: a5a45e4ff92d5d3f34de70aaf4b72c3bdf9f7f16
metrics:
- type: Accuracy
value: 42.89
- task:
type: Natural language inference
dataset:
type: xnli
name: XNLI (en)
config: en
split: validation
revision: a5a45e4ff92d5d3f34de70aaf4b72c3bdf9f7f16
metrics:
- type: Accuracy
value: 58.92
- task:
type: Natural language inference
dataset:
type: xnli
name: XNLI (es)
config: es
split: validation
revision: a5a45e4ff92d5d3f34de70aaf4b72c3bdf9f7f16
metrics:
- type: Accuracy
value: 57.35
- task:
type: Natural language inference
dataset:
type: xnli
name: XNLI (fr)
config: fr
split: validation
revision: a5a45e4ff92d5d3f34de70aaf4b72c3bdf9f7f16
metrics:
- type: Accuracy
value: 56.67
- task:
type: Natural language inference
dataset:
type: xnli
name: XNLI (hi)
config: hi
split: validation
revision: a5a45e4ff92d5d3f34de70aaf4b72c3bdf9f7f16
metrics:
- type: Accuracy
value: 53.45
- task:
type: Natural language inference
dataset:
type: xnli
name: XNLI (ru)
config: ru
split: validation
revision: a5a45e4ff92d5d3f34de70aaf4b72c3bdf9f7f16
metrics:
- type: Accuracy
value: 50.24
- task:
type: Natural language inference
dataset:
type: xnli
name: XNLI (sw)
config: sw
split: validation
revision: a5a45e4ff92d5d3f34de70aaf4b72c3bdf9f7f16
metrics:
- type: Accuracy
value: 48.27
- task:
type: Natural language inference
dataset:
type: xnli
name: XNLI (th)
config: th
split: validation
revision: a5a45e4ff92d5d3f34de70aaf4b72c3bdf9f7f16
metrics:
- type: Accuracy
value: 41.08
- task:
type: Natural language inference
dataset:
type: xnli
name: XNLI (tr)
config: tr
split: validation
revision: a5a45e4ff92d5d3f34de70aaf4b72c3bdf9f7f16
metrics:
- type: Accuracy
value: 38.71
- task:
type: Natural language inference
dataset:
type: xnli
name: XNLI (ur)
config: ur
split: validation
revision: a5a45e4ff92d5d3f34de70aaf4b72c3bdf9f7f16
metrics:
- type: Accuracy
value: 49.48
- task:
type: Natural language inference
dataset:
type: xnli
name: XNLI (vi)
config: vi
split: validation
revision: a5a45e4ff92d5d3f34de70aaf4b72c3bdf9f7f16
metrics:
- type: Accuracy
value: 54.5
- task:
type: Natural language inference
dataset:
type: xnli
name: XNLI (zh)
config: zh
split: validation
revision: a5a45e4ff92d5d3f34de70aaf4b72c3bdf9f7f16
metrics:
- type: Accuracy
value: 54.3
- task:
type: Program synthesis
dataset:
type: openai_humaneval
name: HumanEval
config: None
split: test
revision: e8dc562f5de170c54b5481011dd9f4fa04845771
metrics:
- type: Pass@1
value: 7.23
- type: Pass@10
value: 14.46
- type: Pass@100
value: 25.86
- task:
type: Sentence completion
dataset:
type: story_cloze
name: StoryCloze (2016)
config: "2016"
split: validation
revision: e724c6f8cdf7c7a2fb229d862226e15b023ee4db
metrics:
- type: Accuracy
value: 89.58
- task:
type: Sentence completion
dataset:
type: super_glue
name: SuperGLUE (copa)
config: copa
split: validation
revision: 9e12063561e7e6c79099feb6d5a493142584e9e2
metrics:
- type: Accuracy
value: 84.0
- task:
type: Sentence completion
dataset:
type: xcopa
name: XCOPA (et)
config: et
split: validation
revision: 37f73c60fb123111fa5af5f9b705d0b3747fd187
metrics:
- type: Accuracy
value: 52.0
- task:
type: Sentence completion
dataset:
type: xcopa
name: XCOPA (ht)
config: ht
split: validation
revision: 37f73c60fb123111fa5af5f9b705d0b3747fd187
metrics:
- type: Accuracy
value: 54.0
- task:
type: Sentence completion
dataset:
type: xcopa
name: XCOPA (id)
config: id
split: validation
revision: 37f73c60fb123111fa5af5f9b705d0b3747fd187
metrics:
- type: Accuracy
value: 73.0
- task:
type: Sentence completion
dataset:
type: xcopa
name: XCOPA (it)
config: it
split: validation
revision: 37f73c60fb123111fa5af5f9b705d0b3747fd187
metrics:
- type: Accuracy
value: 62.0
- task:
type: Sentence completion
dataset:
type: xcopa
name: XCOPA (qu)
config: qu
split: validation
revision: 37f73c60fb123111fa5af5f9b705d0b3747fd187
metrics:
- type: Accuracy
value: 61.0
- task:
type: Sentence completion
dataset:
type: xcopa
name: XCOPA (sw)
config: sw
split: validation
revision: 37f73c60fb123111fa5af5f9b705d0b3747fd187
metrics:
- type: Accuracy
value: 61.0
- task:
type: Sentence completion
dataset:
type: xcopa
name: XCOPA (ta)
config: ta
split: validation
revision: 37f73c60fb123111fa5af5f9b705d0b3747fd187
metrics:
- type: Accuracy
value: 62.0
- task:
type: Sentence completion
dataset:
type: xcopa
name: XCOPA (th)
config: th
split: validation
revision: 37f73c60fb123111fa5af5f9b705d0b3747fd187
metrics:
- type: Accuracy
value: 61.0
- task:
type: Sentence completion
dataset:
type: xcopa
name: XCOPA (tr)
config: tr
split: validation
revision: 37f73c60fb123111fa5af5f9b705d0b3747fd187
metrics:
- type: Accuracy
value: 56.0
- task:
type: Sentence completion
dataset:
type: xcopa
name: XCOPA (vi)
config: vi
split: validation
revision: 37f73c60fb123111fa5af5f9b705d0b3747fd187
metrics:
- type: Accuracy
value: 77.0
- task:
type: Sentence completion
dataset:
type: xcopa
name: XCOPA (zh)
config: zh
split: validation
revision: 37f73c60fb123111fa5af5f9b705d0b3747fd187
metrics:
- type: Accuracy
value: 80.0
- task:
type: Sentence completion
dataset:
type: Muennighoff/xstory_cloze
name: XStoryCloze (ar)
config: ar
split: validation
revision: 8bb76e594b68147f1a430e86829d07189622b90d
metrics:
- type: Accuracy
value: 83.85
- task:
type: Sentence completion
dataset:
type: Muennighoff/xstory_cloze
name: XStoryCloze (es)
config: es
split: validation
revision: 8bb76e594b68147f1a430e86829d07189622b90d
metrics:
- type: Accuracy
value: 88.82
- task:
type: Sentence completion
dataset:
type: Muennighoff/xstory_cloze
name: XStoryCloze (eu)
config: eu
split: validation
revision: 8bb76e594b68147f1a430e86829d07189622b90d
metrics:
- type: Accuracy
value: 73.26
- task:
type: Sentence completion
dataset:
type: Muennighoff/xstory_cloze
name: XStoryCloze (hi)
config: hi
split: validation
revision: 8bb76e594b68147f1a430e86829d07189622b90d
metrics:
- type: Accuracy
value: 80.41
- task:
type: Sentence completion
dataset:
type: Muennighoff/xstory_cloze
name: XStoryCloze (id)
config: id
split: validation
revision: 8bb76e594b68147f1a430e86829d07189622b90d
metrics:
- type: Accuracy
value: 84.58
- task:
type: Sentence completion
dataset:
type: Muennighoff/xstory_cloze
name: XStoryCloze (my)
config: my
split: validation
revision: 8bb76e594b68147f1a430e86829d07189622b90d
metrics:
- type: Accuracy
value: 51.56
- task:
type: Sentence completion
dataset:
type: Muennighoff/xstory_cloze
name: XStoryCloze (ru)
config: ru
split: validation
revision: 8bb76e594b68147f1a430e86829d07189622b90d
metrics:
- type: Accuracy
value: 64.26
- task:
type: Sentence completion
dataset:
type: Muennighoff/xstory_cloze
name: XStoryCloze (sw)
config: sw
split: validation
revision: 8bb76e594b68147f1a430e86829d07189622b90d
metrics:
- type: Accuracy
value: 71.01
- task:
type: Sentence completion
dataset:
type: Muennighoff/xstory_cloze
name: XStoryCloze (te)
config: te
split: validation
revision: 8bb76e594b68147f1a430e86829d07189622b90d
metrics:
- type: Accuracy
value: 73.06
- task:
type: Sentence completion
dataset:
type: Muennighoff/xstory_cloze
name: XStoryCloze (zh)
config: zh
split: validation
revision: 8bb76e594b68147f1a430e86829d07189622b90d
metrics:
- type: Accuracy
value: 85.9
---
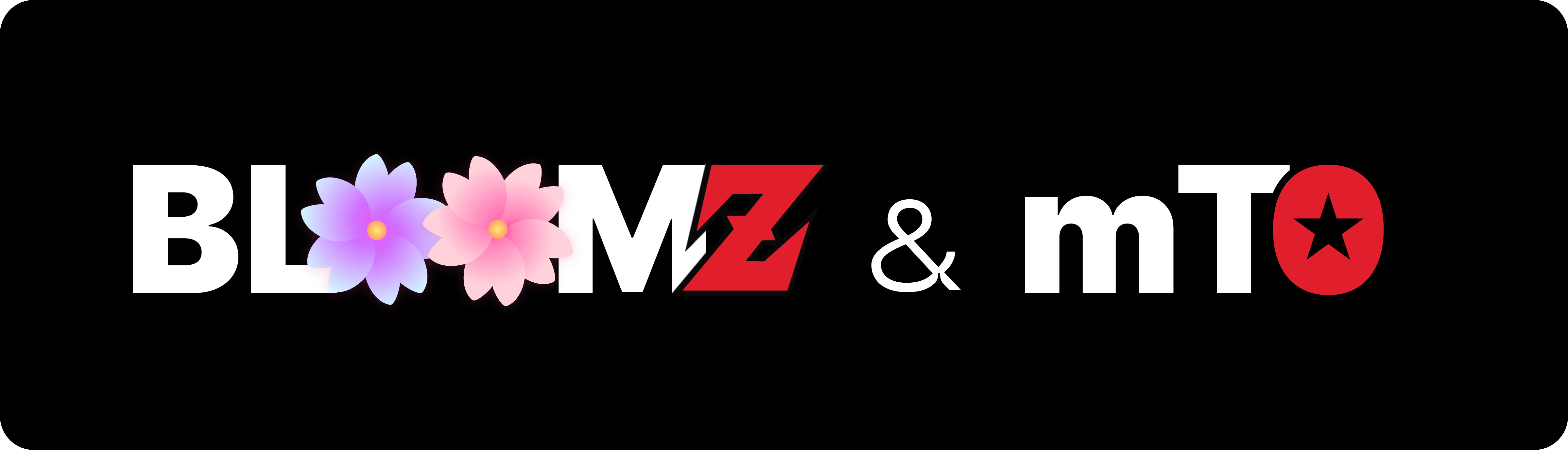
# Table of Contents
1. [Model Summary](#model-summary)
2. [Use](#use)
3. [Limitations](#limitations)
4. [Training](#training)
5. [Evaluation](#evaluation)
7. [Citation](#citation)
# Model Summary
> We present BLOOMZ & mT0, a family of models capable of following human instructions in dozens of languages zero-shot. We finetune BLOOM & mT5 pretrained multilingual language models on our crosslingual task mixture (xP3) and find the resulting models capable of crosslingual generalization to unseen tasks & languages.
- **Repository:** [bigscience-workshop/xmtf](https://github.com/bigscience-workshop/xmtf)
- **Paper:** [Crosslingual Generalization through Multitask Finetuning](https://arxiv.org/abs/2211.01786)
- **Point of Contact:** [Niklas Muennighoff](mailto:[email protected])
- **Languages:** Refer to [bloom](https://huggingface.co/bigscience/bloom) for pretraining & [xP3](https://huggingface.co/datasets/bigscience/xP3) for finetuning language proportions. It understands both pretraining & finetuning languages.
- **BLOOMZ & mT0 Model Family:**
<div class="max-w-full overflow-auto">
<table>
<tr>
<th colspan="12">Multitask finetuned on <a style="font-weight:bold" href=https://huggingface.co/datasets/bigscience/xP3>xP3</a>. Recommended for prompting in English.
</tr>
<tr>
<td>Parameters</td>
<td>300M</td>
<td>580M</td>
<td>1.2B</td>
<td>3.7B</td>
<td>13B</td>
<td>560M</td>
<td>1.1B</td>
<td>1.7B</td>
<td>3B</td>
<td>7.1B</td>
<td>176B</td>
</tr>
<tr>
<td>Finetuned Model</td>
<td><a href=https://huggingface.co/bigscience/mt0-small>mt0-small</a></td>
<td><a href=https://huggingface.co/bigscience/mt0-base>mt0-base</a></td>
<td><a href=https://huggingface.co/bigscience/mt0-large>mt0-large</a></td>
<td><a href=https://huggingface.co/bigscience/mt0-xl>mt0-xl</a></td>
<td><a href=https://huggingface.co/bigscience/mt0-xxl>mt0-xxl</a></td>
<td><a href=https://huggingface.co/bigscience/bloomz-560m>bloomz-560m</a></td>
<td><a href=https://huggingface.co/bigscience/bloomz-1b1>bloomz-1b1</a></td>
<td><a href=https://huggingface.co/bigscience/bloomz-1b7>bloomz-1b7</a></td>
<td><a href=https://huggingface.co/bigscience/bloomz-3b>bloomz-3b</a></td>
<td><a href=https://huggingface.co/bigscience/bloomz-7b1>bloomz-7b1</a></td>
<td><a href=https://huggingface.co/bigscience/bloomz>bloomz</a></td>
</tr>
</tr>
<tr>
<th colspan="12">Multitask finetuned on <a style="font-weight:bold" href=https://huggingface.co/datasets/bigscience/xP3mt>xP3mt</a>. Recommended for prompting in non-English.</th>
</tr>
<tr>
<td>Finetuned Model</td>
<td></td>
<td></td>
<td></td>
<td></td>
<td><a href=https://huggingface.co/bigscience/mt0-xxl-mt>mt0-xxl-mt</a></td>
<td></td>
<td></td>
<td></td>
<td></td>
<td><a href=https://huggingface.co/bigscience/bloomz-7b1-mt>bloomz-7b1-mt</a></td>
<td><a href=https://huggingface.co/bigscience/bloomz-mt>bloomz-mt</a></td>
</tr>
<th colspan="12">Multitask finetuned on <a style="font-weight:bold" href=https://huggingface.co/datasets/Muennighoff/P3>P3</a>. Released for research purposes only. Strictly inferior to above models!</th>
</tr>
<tr>
<td>Finetuned Model</td>
<td></td>
<td></td>
<td></td>
<td></td>
<td><a href=https://huggingface.co/bigscience/mt0-xxl-p3>mt0-xxl-p3</a></td>
<td></td>
<td></td>
<td></td>
<td></td>
<td><a href=https://huggingface.co/bigscience/bloomz-7b1-p3>bloomz-7b1-p3</a></td>
<td><a href=https://huggingface.co/bigscience/bloomz-p3>bloomz-p3</a></td>
</tr>
<th colspan="12">Original pretrained checkpoints. Not recommended.</th>
<tr>
<td>Pretrained Model</td>
<td><a href=https://huggingface.co/google/mt5-small>mt5-small</a></td>
<td><a href=https://huggingface.co/google/mt5-base>mt5-base</a></td>
<td><a href=https://huggingface.co/google/mt5-large>mt5-large</a></td>
<td><a href=https://huggingface.co/google/mt5-xl>mt5-xl</a></td>
<td><a href=https://huggingface.co/google/mt5-xxl>mt5-xxl</a></td>
<td><a href=https://huggingface.co/bigscience/bloom-560m>bloom-560m</a></td>
<td><a href=https://huggingface.co/bigscience/bloom-1b1>bloom-1b1</a></td>
<td><a href=https://huggingface.co/bigscience/bloom-1b7>bloom-1b7</a></td>
<td><a href=https://huggingface.co/bigscience/bloom-3b>bloom-3b</a></td>
<td><a href=https://huggingface.co/bigscience/bloom-7b1>bloom-7b1</a></td>
<td><a href=https://huggingface.co/bigscience/bloom>bloom</a></td>
</tr>
</table>
</div>
# Use
## Intended use
We recommend using the model to perform tasks expressed in natural language. For example, given the prompt "*Translate to English: Je t’aime.*", the model will most likely answer "*I love you.*". Some prompt ideas from our paper:
- 一个传奇的开端,一个不灭的神话,这不仅仅是一部电影,而是作为一个走进新时代的标签,永远彪炳史册。你认为这句话的立场是赞扬、中立还是批评?
- Suggest at least five related search terms to "Mạng neural nhân tạo".
- Write a fairy tale about a troll saving a princess from a dangerous dragon. The fairy tale is a masterpiece that has achieved praise worldwide and its moral is "Heroes Come in All Shapes and Sizes". Story (in Spanish):
- Explain in a sentence in Telugu what is backpropagation in neural networks.
**Feel free to share your generations in the Community tab!**
## How to use
### CPU
<details>
<summary> Click to expand </summary>
```python
# pip install -q transformers
from transformers import AutoModelForCausalLM, AutoTokenizer
checkpoint = "bigscience/bloomz-7b1-mt"
tokenizer = AutoTokenizer.from_pretrained(checkpoint)
model = AutoModelForCausalLM.from_pretrained(checkpoint)
inputs = tokenizer.encode("Translate to English: Je t’aime.", return_tensors="pt")
outputs = model.generate(inputs)
print(tokenizer.decode(outputs[0]))
```
</details>
### GPU
<details>
<summary> Click to expand </summary>
```python
# pip install -q transformers accelerate
from transformers import AutoModelForCausalLM, AutoTokenizer
checkpoint = "bigscience/bloomz-7b1-mt"
tokenizer = AutoTokenizer.from_pretrained(checkpoint)
model = AutoModelForCausalLM.from_pretrained(checkpoint, torch_dtype="auto", device_map="auto")
inputs = tokenizer.encode("Translate to English: Je t’aime.", return_tensors="pt").to("cuda")
outputs = model.generate(inputs)
print(tokenizer.decode(outputs[0]))
```
</details>
### GPU in 8bit
<details>
<summary> Click to expand </summary>
```python
# pip install -q transformers accelerate bitsandbytes
from transformers import AutoModelForCausalLM, AutoTokenizer
checkpoint = "bigscience/bloomz-7b1-mt"
tokenizer = AutoTokenizer.from_pretrained(checkpoint)
model = AutoModelForCausalLM.from_pretrained(checkpoint, device_map="auto", load_in_8bit=True)
inputs = tokenizer.encode("Translate to English: Je t’aime.", return_tensors="pt").to("cuda")
outputs = model.generate(inputs)
print(tokenizer.decode(outputs[0]))
```
</details>
<!-- Necessary for whitespace -->
###
# Limitations
**Prompt Engineering:** The performance may vary depending on the prompt. For BLOOMZ models, we recommend making it very clear when the input stops to avoid the model trying to continue it. For example, the prompt "*Translate to English: Je t'aime*" without the full stop (.) at the end, may result in the model trying to continue the French sentence. Better prompts are e.g. "*Translate to English: Je t'aime.*", "*Translate to English: Je t'aime. Translation:*" "*What is "Je t'aime." in English?*", where it is clear for the model when it should answer. Further, we recommend providing the model as much context as possible. For example, if you want it to answer in Telugu, then tell the model, e.g. "*Explain in a sentence in Telugu what is backpropagation in neural networks.*".
# Training
## Model
- **Architecture:** Same as [bloom-7b1](https://huggingface.co/bigscience/bloom-7b1), also refer to the `config.json` file
- **Finetuning steps:** 1000
- **Finetuning tokens:** 4.19 billion
- **Finetuning layout:** 1x pipeline parallel, 1x tensor parallel, 64x data parallel
- **Precision:** float16
## Hardware
- **CPUs:** AMD CPUs with 512GB memory per node
- **GPUs:** 64 A100 80GB GPUs with 8 GPUs per node (8 nodes) using NVLink 4 inter-gpu connects, 4 OmniPath links
- **Communication:** NCCL-communications network with a fully dedicated subnet
## Software
- **Orchestration:** [Megatron-DeepSpeed](https://github.com/bigscience-workshop/Megatron-DeepSpeed)
- **Optimizer & parallelism:** [DeepSpeed](https://github.com/microsoft/DeepSpeed)
- **Neural networks:** [PyTorch](https://github.com/pytorch/pytorch) (pytorch-1.11 w/ CUDA-11.5)
- **FP16 if applicable:** [apex](https://github.com/NVIDIA/apex)
# Evaluation
We refer to Table 7 from our [paper](https://arxiv.org/abs/2211.01786) & [bigscience/evaluation-results](https://huggingface.co/datasets/bigscience/evaluation-results) for zero-shot results on unseen tasks. The sidebar reports zero-shot performance of the best prompt per dataset config.
# Citation
```bibtex
@article{muennighoff2022crosslingual,
title={Crosslingual generalization through multitask finetuning},
author={Muennighoff, Niklas and Wang, Thomas and Sutawika, Lintang and Roberts, Adam and Biderman, Stella and Scao, Teven Le and Bari, M Saiful and Shen, Sheng and Yong, Zheng-Xin and Schoelkopf, Hailey and others},
journal={arXiv preprint arXiv:2211.01786},
year={2022}
}
``` |
LoneStriker/Llama-3-Refueled-GGUF | LoneStriker | 2024-05-09T04:42:53Z | 28 | 8 | transformers | [
"transformers",
"gguf",
"data labeling",
"en",
"license:cc-by-nc-4.0",
"endpoints_compatible",
"region:us",
"conversational"
] | null | 2024-05-09T04:30:44Z | ---
license: cc-by-nc-4.0
language:
- en
library_name: transformers
tags:
- data labeling
---
<div style="width: auto; margin-left: auto; margin-right: auto; background-color:black">
<img src="https://assets-global.website-files.com/6423879a8f63c1bb18d74bfa/648818d56d04c3bdf36d71ab_Refuel_rev8-01_ts-p-1600.png" alt="Refuel.ai" style="width: 100%; min-width: 400px; display: block; margin: auto;">
</div>
## Model Details
RefuelLLM-2-small, aka Llama-3-Refueled, is a Llama3-8B base model instruction tuned on a corpus of 2750+ datasets, spanning tasks such as classification, reading comprehension, structured attribute extraction and entity resolution. We're excited to open-source the model for the community to build on top of.
* More details about [RefuelLLM-2 family of models](https://www.refuel.ai/blog-posts/announcing-refuel-llm-2)
* You can also try out the models in our [LLM playground](https://labs.refuel.ai/playground)
**Model developers** - Refuel AI
**Input** - Text only.
**Output** - Text only.
**Architecture** - Llama-3-Refueled is built on top of Llama-3-8B-instruct which is an auto-regressive language model that uses an optimized transformer architecture.
**Release Date** - May 8, 2024.
## How to use
This repository contains weights for Llama-3-Refueled that are compatible for use with HuggingFace. See the snippet below for usage with Transformers:
```python
>>> import torch
>>> from transformers import AutoModelForCausalLM, AutoTokenizer
>>> model_id = "refuelai/Llama-3-Refueled"
>>> tokenizer = AutoTokenizer.from_pretrained(model_id)
>>> model = AutoModelForCausalLM.from_pretrained(model_id, torch_dtype=torch.float16, device_map="auto")
>>> messages = [{"role": "user", "content": "Is this comment toxic or non-toxic: RefuelLLM is the new way to label text data!"}]
>>> inputs = tokenizer.apply_chat_template(messages, return_tensors="pt", add_generation_prompt=True).to("cuda")
>>> outputs = model.generate(inputs, max_new_tokens=20)
>>> print(tokenizer.decode(outputs[0]))
```
## Training Data
The model was both trained on over 4 Billion tokens, spanning 2750+ NLP tasks. Our training collection consists majorly of:
1. Human annotated datasets like Flan, Task Source, and the Aya collection
2. Synthetic datasets like OpenOrca, OpenHermes and WizardLM
3. Proprietary datasets developed or licensed by Refuel AI
## Benchmarks
In this section, we report the results for Refuel models on our benchmark of labeling tasks. For details on the methodology see [here](https://refuel.ai/blog-posts/announcing-refuel-llm-2).
<table>
<tr></tr>
<tr><th>Provider</th><th>Model</th><th colspan="4" style="text-align: center">LLM Output Quality (by task type)</tr>
<tr><td></td><td></td><td>Overall</td><td>Classification</td><td>Reading Comprehension</td><td>Structure Extraction</td><td>Entity Matching</td><td></td></tr>
<tr><td>Refuel</td><td>RefuelLLM-2</td><td>83.82%</td><td>84.94%</td><td>76.03%</td><td>88.16%</td><td>92.00%</td><td></td></tr>
<tr><td>OpenAI</td><td>GPT-4-Turbo</td><td>80.88%</td><td>81.77%</td><td>72.08%</td><td>84.79%</td><td>97.20%</td><td></td></tr>
<tr><td>Refuel</td><td>RefuelLLM-2-small (Llama-3-Refueled)</td><td>79.67%</td><td>81.72%</td><td>70.04%</td><td>84.28%</td><td>92.00%</td><td></td></tr>
<tr><td>Anthropic</td><td>Claude-3-Opus</td><td>79.19%</td><td>82.49%</td><td>67.30%</td><td>88.25%</td><td>94.96%</td><td></td></tr>
<tr><td>Meta</td><td>Llama3-70B-Instruct</td><td>78.20%</td><td>79.38%</td><td>66.03%</td><td>85.96%</td><td>94.13%</td><td></td></tr>
<tr><td>Google</td><td>Gemini-1.5-Pro</td><td>74.59%</td><td>73.52%</td><td>60.67%</td><td>84.27%</td><td>98.48%</td><td></td></tr>
<tr><td>Mistral</td><td>Mixtral-8x7B-Instruct</td><td>62.87%</td><td>79.11%</td><td>45.56%</td><td>47.08%</td><td>86.52%</td><td></td></tr>
<tr><td>Anthropic</td><td>Claude-3-Sonnet</td><td>70.99%</td><td>79.91%</td><td>45.44%</td><td>78.10%</td><td>96.34%</td><td></td></tr>
<tr><td>Anthropic</td><td>Claude-3-Haiku</td><td>69.23%</td><td>77.27%</td><td>50.19%</td><td>84.97%</td><td>54.08%</td><td></td></tr>
<tr><td>OpenAI</td><td>GPT-3.5-Turbo</td><td>68.13%</td><td>74.39%</td><td>53.21%</td><td>69.40%</td><td>80.41%</td><td></td></tr>
<tr><td>Meta</td><td>Llama3-8B-Instruct</td><td>62.30%</td><td>68.52%</td><td>49.16%</td><td>65.09%</td><td>63.61%</td><td></td></tr>
</table>
## Limitations
The Llama-3-Refueled does not have any moderation mechanisms. We're looking forward to engaging with the community
on ways to make the model finely respect guardrails, allowing for deployment in environments requiring moderated outputs. |
zhoukz/yi-34b-200k-4bit | zhoukz | 2024-05-09T04:39:39Z | 6 | 0 | transformers | [
"transformers",
"safetensors",
"llama",
"text-generation",
"arxiv:1910.09700",
"autotrain_compatible",
"text-generation-inference",
"endpoints_compatible",
"4-bit",
"bitsandbytes",
"region:us"
] | text-generation | 2024-05-09T04:12:37Z | ---
library_name: transformers
tags: []
---
# Model Card for Model ID
<!-- Provide a quick summary of what the model is/does. -->
## Model Details
### Model Description
<!-- Provide a longer summary of what this model is. -->
This is the model card of a 🤗 transformers model that has been pushed on the Hub. This model card has been automatically generated.
- **Developed by:** [More Information Needed]
- **Funded by [optional]:** [More Information Needed]
- **Shared by [optional]:** [More Information Needed]
- **Model type:** [More Information Needed]
- **Language(s) (NLP):** [More Information Needed]
- **License:** [More Information Needed]
- **Finetuned from model [optional]:** [More Information Needed]
### Model Sources [optional]
<!-- Provide the basic links for the model. -->
- **Repository:** [More Information Needed]
- **Paper [optional]:** [More Information Needed]
- **Demo [optional]:** [More Information Needed]
## Uses
<!-- Address questions around how the model is intended to be used, including the foreseeable users of the model and those affected by the model. -->
### Direct Use
<!-- This section is for the model use without fine-tuning or plugging into a larger ecosystem/app. -->
[More Information Needed]
### Downstream Use [optional]
<!-- This section is for the model use when fine-tuned for a task, or when plugged into a larger ecosystem/app -->
[More Information Needed]
### Out-of-Scope Use
<!-- This section addresses misuse, malicious use, and uses that the model will not work well for. -->
[More Information Needed]
## Bias, Risks, and Limitations
<!-- This section is meant to convey both technical and sociotechnical limitations. -->
[More Information Needed]
### Recommendations
<!-- This section is meant to convey recommendations with respect to the bias, risk, and technical limitations. -->
Users (both direct and downstream) should be made aware of the risks, biases and limitations of the model. More information needed for further recommendations.
## How to Get Started with the Model
Use the code below to get started with the model.
[More Information Needed]
## Training Details
### Training Data
<!-- This should link to a Dataset Card, perhaps with a short stub of information on what the training data is all about as well as documentation related to data pre-processing or additional filtering. -->
[More Information Needed]
### Training Procedure
<!-- This relates heavily to the Technical Specifications. Content here should link to that section when it is relevant to the training procedure. -->
#### Preprocessing [optional]
[More Information Needed]
#### Training Hyperparameters
- **Training regime:** [More Information Needed] <!--fp32, fp16 mixed precision, bf16 mixed precision, bf16 non-mixed precision, fp16 non-mixed precision, fp8 mixed precision -->
#### Speeds, Sizes, Times [optional]
<!-- This section provides information about throughput, start/end time, checkpoint size if relevant, etc. -->
[More Information Needed]
## Evaluation
<!-- This section describes the evaluation protocols and provides the results. -->
### Testing Data, Factors & Metrics
#### Testing Data
<!-- This should link to a Dataset Card if possible. -->
[More Information Needed]
#### Factors
<!-- These are the things the evaluation is disaggregating by, e.g., subpopulations or domains. -->
[More Information Needed]
#### Metrics
<!-- These are the evaluation metrics being used, ideally with a description of why. -->
[More Information Needed]
### Results
[More Information Needed]
#### Summary
## Model Examination [optional]
<!-- Relevant interpretability work for the model goes here -->
[More Information Needed]
## Environmental Impact
<!-- Total emissions (in grams of CO2eq) and additional considerations, such as electricity usage, go here. Edit the suggested text below accordingly -->
Carbon emissions can be estimated using the [Machine Learning Impact calculator](https://mlco2.github.io/impact#compute) presented in [Lacoste et al. (2019)](https://arxiv.org/abs/1910.09700).
- **Hardware Type:** [More Information Needed]
- **Hours used:** [More Information Needed]
- **Cloud Provider:** [More Information Needed]
- **Compute Region:** [More Information Needed]
- **Carbon Emitted:** [More Information Needed]
## Technical Specifications [optional]
### Model Architecture and Objective
[More Information Needed]
### Compute Infrastructure
[More Information Needed]
#### Hardware
[More Information Needed]
#### Software
[More Information Needed]
## Citation [optional]
<!-- If there is a paper or blog post introducing the model, the APA and Bibtex information for that should go in this section. -->
**BibTeX:**
[More Information Needed]
**APA:**
[More Information Needed]
## Glossary [optional]
<!-- If relevant, include terms and calculations in this section that can help readers understand the model or model card. -->
[More Information Needed]
## More Information [optional]
[More Information Needed]
## Model Card Authors [optional]
[More Information Needed]
## Model Card Contact
[More Information Needed] |
BinhMinhs10/whisper-tiny-minds14-en | BinhMinhs10 | 2024-05-09T04:35:11Z | 94 | 0 | transformers | [
"transformers",
"tensorboard",
"safetensors",
"whisper",
"automatic-speech-recognition",
"generated_from_trainer",
"dataset:PolyAI/minds14",
"base_model:openai/whisper-tiny",
"base_model:finetune:openai/whisper-tiny",
"license:apache-2.0",
"model-index",
"endpoints_compatible",
"region:us"
] | automatic-speech-recognition | 2024-05-07T03:58:30Z | ---
license: apache-2.0
base_model: openai/whisper-tiny
tags:
- generated_from_trainer
datasets:
- PolyAI/minds14
metrics:
- wer
model-index:
- name: whisper-tiny-minds14-en
results:
- task:
name: Automatic Speech Recognition
type: automatic-speech-recognition
dataset:
name: PolyAI/minds14
type: PolyAI/minds14
config: en-US
split: train[450:]
args: en-US
metrics:
- name: Wer
type: wer
value: 0.32078963602714374
---
<!-- This model card has been generated automatically according to the information the Trainer had access to. You
should probably proofread and complete it, then remove this comment. -->
# whisper-tiny-minds14-en
This model is a fine-tuned version of [openai/whisper-tiny](https://huggingface.co/openai/whisper-tiny) on the PolyAI/minds14 dataset.
It achieves the following results on the evaluation set:
- Loss: 0.8739
- Wer: 0.320790
## Model description
More information needed
## Intended uses & limitations
More information needed
## Training and evaluation data
More information needed
## Training procedure
### Training hyperparameters
The following hyperparameters were used during training:
- learning_rate: 3e-05
- train_batch_size: 128
- eval_batch_size: 128
- seed: 42
- optimizer: Adam with betas=(0.9,0.999) and epsilon=1e-08
- lr_scheduler_type: linear
- lr_scheduler_warmup_steps: 100
- training_steps: 1500
- mixed_precision_training: Native AMP
### Training results
| Training Loss | Epoch | Step | Validation Loss | Wer |
|:-------------:|:-----:|:----:|:---------------:|:-------:|
| 0.0001 | 125.0 | 500 | 0.8046 | 30.4133 |
| 0.0001 | 250.0 | 1000 | 0.8565 | 31.8322 |
| 0.0001 | 375.0 | 1500 | 0.8739 | 32.0790 |
### Framework versions
- Transformers 4.40.2
- Pytorch 2.1.2
- Datasets 2.19.1
- Tokenizers 0.19.1 |
shibinpp7/example1 | shibinpp7 | 2024-05-09T04:19:47Z | 0 | 0 | null | [
"license:apache-2.0",
"region:us"
] | null | 2024-05-09T04:19:47Z | ---
license: apache-2.0
---
|
abc88767/model110 | abc88767 | 2024-05-09T04:18:26Z | 92 | 0 | transformers | [
"transformers",
"safetensors",
"stablelm",
"text-generation",
"conversational",
"arxiv:1910.09700",
"autotrain_compatible",
"endpoints_compatible",
"region:us"
] | text-generation | 2024-05-09T04:16:46Z | ---
library_name: transformers
tags: []
---
# Model Card for Model ID
<!-- Provide a quick summary of what the model is/does. -->
## Model Details
### Model Description
<!-- Provide a longer summary of what this model is. -->
This is the model card of a 🤗 transformers model that has been pushed on the Hub. This model card has been automatically generated.
- **Developed by:** [More Information Needed]
- **Funded by [optional]:** [More Information Needed]
- **Shared by [optional]:** [More Information Needed]
- **Model type:** [More Information Needed]
- **Language(s) (NLP):** [More Information Needed]
- **License:** [More Information Needed]
- **Finetuned from model [optional]:** [More Information Needed]
### Model Sources [optional]
<!-- Provide the basic links for the model. -->
- **Repository:** [More Information Needed]
- **Paper [optional]:** [More Information Needed]
- **Demo [optional]:** [More Information Needed]
## Uses
<!-- Address questions around how the model is intended to be used, including the foreseeable users of the model and those affected by the model. -->
### Direct Use
<!-- This section is for the model use without fine-tuning or plugging into a larger ecosystem/app. -->
[More Information Needed]
### Downstream Use [optional]
<!-- This section is for the model use when fine-tuned for a task, or when plugged into a larger ecosystem/app -->
[More Information Needed]
### Out-of-Scope Use
<!-- This section addresses misuse, malicious use, and uses that the model will not work well for. -->
[More Information Needed]
## Bias, Risks, and Limitations
<!-- This section is meant to convey both technical and sociotechnical limitations. -->
[More Information Needed]
### Recommendations
<!-- This section is meant to convey recommendations with respect to the bias, risk, and technical limitations. -->
Users (both direct and downstream) should be made aware of the risks, biases and limitations of the model. More information needed for further recommendations.
## How to Get Started with the Model
Use the code below to get started with the model.
[More Information Needed]
## Training Details
### Training Data
<!-- This should link to a Dataset Card, perhaps with a short stub of information on what the training data is all about as well as documentation related to data pre-processing or additional filtering. -->
[More Information Needed]
### Training Procedure
<!-- This relates heavily to the Technical Specifications. Content here should link to that section when it is relevant to the training procedure. -->
#### Preprocessing [optional]
[More Information Needed]
#### Training Hyperparameters
- **Training regime:** [More Information Needed] <!--fp32, fp16 mixed precision, bf16 mixed precision, bf16 non-mixed precision, fp16 non-mixed precision, fp8 mixed precision -->
#### Speeds, Sizes, Times [optional]
<!-- This section provides information about throughput, start/end time, checkpoint size if relevant, etc. -->
[More Information Needed]
## Evaluation
<!-- This section describes the evaluation protocols and provides the results. -->
### Testing Data, Factors & Metrics
#### Testing Data
<!-- This should link to a Dataset Card if possible. -->
[More Information Needed]
#### Factors
<!-- These are the things the evaluation is disaggregating by, e.g., subpopulations or domains. -->
[More Information Needed]
#### Metrics
<!-- These are the evaluation metrics being used, ideally with a description of why. -->
[More Information Needed]
### Results
[More Information Needed]
#### Summary
## Model Examination [optional]
<!-- Relevant interpretability work for the model goes here -->
[More Information Needed]
## Environmental Impact
<!-- Total emissions (in grams of CO2eq) and additional considerations, such as electricity usage, go here. Edit the suggested text below accordingly -->
Carbon emissions can be estimated using the [Machine Learning Impact calculator](https://mlco2.github.io/impact#compute) presented in [Lacoste et al. (2019)](https://arxiv.org/abs/1910.09700).
- **Hardware Type:** [More Information Needed]
- **Hours used:** [More Information Needed]
- **Cloud Provider:** [More Information Needed]
- **Compute Region:** [More Information Needed]
- **Carbon Emitted:** [More Information Needed]
## Technical Specifications [optional]
### Model Architecture and Objective
[More Information Needed]
### Compute Infrastructure
[More Information Needed]
#### Hardware
[More Information Needed]
#### Software
[More Information Needed]
## Citation [optional]
<!-- If there is a paper or blog post introducing the model, the APA and Bibtex information for that should go in this section. -->
**BibTeX:**
[More Information Needed]
**APA:**
[More Information Needed]
## Glossary [optional]
<!-- If relevant, include terms and calculations in this section that can help readers understand the model or model card. -->
[More Information Needed]
## More Information [optional]
[More Information Needed]
## Model Card Authors [optional]
[More Information Needed]
## Model Card Contact
[More Information Needed] |
ImanN1/finetune_wav2vec2_960h_thirty | ImanN1 | 2024-05-09T04:13:09Z | 164 | 0 | transformers | [
"transformers",
"tensorboard",
"safetensors",
"wav2vec2",
"automatic-speech-recognition",
"generated_from_trainer",
"base_model:facebook/wav2vec2-base-960h",
"base_model:finetune:facebook/wav2vec2-base-960h",
"license:apache-2.0",
"endpoints_compatible",
"region:us"
] | automatic-speech-recognition | 2024-05-09T04:12:58Z | ---
license: apache-2.0
base_model: facebook/wav2vec2-base-960h
tags:
- generated_from_trainer
metrics:
- wer
model-index:
- name: finetune_wav2vec2_960h_thirty
results: []
---
<!-- This model card has been generated automatically according to the information the Trainer had access to. You
should probably proofread and complete it, then remove this comment. -->
# finetune_wav2vec2_960h_thirty
This model is a fine-tuned version of [facebook/wav2vec2-base-960h](https://huggingface.co/facebook/wav2vec2-base-960h) on the None dataset.
It achieves the following results on the evaluation set:
- Loss: 0.7088
- Wer: 30.8513
- Cer: 15.3422
## Model description
More information needed
## Intended uses & limitations
More information needed
## Training and evaluation data
More information needed
## Training procedure
### Training hyperparameters
The following hyperparameters were used during training:
- learning_rate: 0.0001
- train_batch_size: 32
- eval_batch_size: 16
- seed: 42
- optimizer: Adam with betas=(0.9,0.999) and epsilon=1e-08
- lr_scheduler_type: linear
- lr_scheduler_warmup_steps: 2000
- training_steps: 3800
### Training results
| Training Loss | Epoch | Step | Validation Loss | Wer | Cer |
|:-------------:|:------:|:----:|:---------------:|:-------:|:-------:|
| 0.9521 | 2.8090 | 1000 | 0.7088 | 30.8513 | 15.3422 |
| 0.6771 | 5.6180 | 2000 | 0.5857 | 26.9346 | 13.3483 |
| 0.5499 | 8.4270 | 3000 | 0.5207 | 24.7273 | 12.1108 |
### Framework versions
- Transformers 4.40.2
- Pytorch 2.1.1+cu121
- Datasets 2.19.1
- Tokenizers 0.19.1
|
zhoukz/yi-9b-200k-4bit | zhoukz | 2024-05-09T04:11:26Z | 80 | 0 | transformers | [
"transformers",
"safetensors",
"llama",
"text-generation",
"arxiv:1910.09700",
"autotrain_compatible",
"text-generation-inference",
"endpoints_compatible",
"4-bit",
"bitsandbytes",
"region:us"
] | text-generation | 2024-05-09T03:49:27Z | ---
library_name: transformers
tags: []
---
# Model Card for Model ID
<!-- Provide a quick summary of what the model is/does. -->
## Model Details
### Model Description
<!-- Provide a longer summary of what this model is. -->
This is the model card of a 🤗 transformers model that has been pushed on the Hub. This model card has been automatically generated.
- **Developed by:** [More Information Needed]
- **Funded by [optional]:** [More Information Needed]
- **Shared by [optional]:** [More Information Needed]
- **Model type:** [More Information Needed]
- **Language(s) (NLP):** [More Information Needed]
- **License:** [More Information Needed]
- **Finetuned from model [optional]:** [More Information Needed]
### Model Sources [optional]
<!-- Provide the basic links for the model. -->
- **Repository:** [More Information Needed]
- **Paper [optional]:** [More Information Needed]
- **Demo [optional]:** [More Information Needed]
## Uses
<!-- Address questions around how the model is intended to be used, including the foreseeable users of the model and those affected by the model. -->
### Direct Use
<!-- This section is for the model use without fine-tuning or plugging into a larger ecosystem/app. -->
[More Information Needed]
### Downstream Use [optional]
<!-- This section is for the model use when fine-tuned for a task, or when plugged into a larger ecosystem/app -->
[More Information Needed]
### Out-of-Scope Use
<!-- This section addresses misuse, malicious use, and uses that the model will not work well for. -->
[More Information Needed]
## Bias, Risks, and Limitations
<!-- This section is meant to convey both technical and sociotechnical limitations. -->
[More Information Needed]
### Recommendations
<!-- This section is meant to convey recommendations with respect to the bias, risk, and technical limitations. -->
Users (both direct and downstream) should be made aware of the risks, biases and limitations of the model. More information needed for further recommendations.
## How to Get Started with the Model
Use the code below to get started with the model.
[More Information Needed]
## Training Details
### Training Data
<!-- This should link to a Dataset Card, perhaps with a short stub of information on what the training data is all about as well as documentation related to data pre-processing or additional filtering. -->
[More Information Needed]
### Training Procedure
<!-- This relates heavily to the Technical Specifications. Content here should link to that section when it is relevant to the training procedure. -->
#### Preprocessing [optional]
[More Information Needed]
#### Training Hyperparameters
- **Training regime:** [More Information Needed] <!--fp32, fp16 mixed precision, bf16 mixed precision, bf16 non-mixed precision, fp16 non-mixed precision, fp8 mixed precision -->
#### Speeds, Sizes, Times [optional]
<!-- This section provides information about throughput, start/end time, checkpoint size if relevant, etc. -->
[More Information Needed]
## Evaluation
<!-- This section describes the evaluation protocols and provides the results. -->
### Testing Data, Factors & Metrics
#### Testing Data
<!-- This should link to a Dataset Card if possible. -->
[More Information Needed]
#### Factors
<!-- These are the things the evaluation is disaggregating by, e.g., subpopulations or domains. -->
[More Information Needed]
#### Metrics
<!-- These are the evaluation metrics being used, ideally with a description of why. -->
[More Information Needed]
### Results
[More Information Needed]
#### Summary
## Model Examination [optional]
<!-- Relevant interpretability work for the model goes here -->
[More Information Needed]
## Environmental Impact
<!-- Total emissions (in grams of CO2eq) and additional considerations, such as electricity usage, go here. Edit the suggested text below accordingly -->
Carbon emissions can be estimated using the [Machine Learning Impact calculator](https://mlco2.github.io/impact#compute) presented in [Lacoste et al. (2019)](https://arxiv.org/abs/1910.09700).
- **Hardware Type:** [More Information Needed]
- **Hours used:** [More Information Needed]
- **Cloud Provider:** [More Information Needed]
- **Compute Region:** [More Information Needed]
- **Carbon Emitted:** [More Information Needed]
## Technical Specifications [optional]
### Model Architecture and Objective
[More Information Needed]
### Compute Infrastructure
[More Information Needed]
#### Hardware
[More Information Needed]
#### Software
[More Information Needed]
## Citation [optional]
<!-- If there is a paper or blog post introducing the model, the APA and Bibtex information for that should go in this section. -->
**BibTeX:**
[More Information Needed]
**APA:**
[More Information Needed]
## Glossary [optional]
<!-- If relevant, include terms and calculations in this section that can help readers understand the model or model card. -->
[More Information Needed]
## More Information [optional]
[More Information Needed]
## Model Card Authors [optional]
[More Information Needed]
## Model Card Contact
[More Information Needed] |
lioo/sdxl-controlnet-recolor-4-diffusers | lioo | 2024-05-09T04:07:10Z | 10 | 1 | diffusers | [
"diffusers",
"safetensors",
"image-to-image",
"region:us"
] | image-to-image | 2024-05-08T13:52:23Z | ---
library_name: diffusers
pipeline_tag: image-to-image
---
This is a recolor control net for diffusers converted from stabilityai/control-lora/control-LoRAs-rank256/control-lora-recolor-rank256.safetensors
|
dyyyyy/bhaigpt | dyyyyy | 2024-05-09T04:06:06Z | 0 | 0 | null | [
"license:apache-2.0",
"region:us"
] | null | 2024-05-09T04:06:06Z | ---
license: apache-2.0
---
|
Litzy619/O0508V5 | Litzy619 | 2024-05-09T04:05:27Z | 0 | 0 | null | [
"safetensors",
"generated_from_trainer",
"base_model:allenai/OLMo-1B",
"base_model:finetune:allenai/OLMo-1B",
"license:apache-2.0",
"region:us"
] | null | 2024-05-09T03:05:08Z | ---
license: apache-2.0
base_model: allenai/OLMo-1B
tags:
- generated_from_trainer
model-index:
- name: O0508V5
results: []
---
<!-- This model card has been generated automatically according to the information the Trainer had access to. You
should probably proofread and complete it, then remove this comment. -->
# O0508V5
This model is a fine-tuned version of [allenai/OLMo-1B](https://huggingface.co/allenai/OLMo-1B) on an unknown dataset.
It achieves the following results on the evaluation set:
- Loss: 0.0560
## Model description
More information needed
## Intended uses & limitations
More information needed
## Training and evaluation data
More information needed
## Training procedure
### Training hyperparameters
The following hyperparameters were used during training:
- learning_rate: 0.0003
- train_batch_size: 8
- eval_batch_size: 8
- seed: 42
- gradient_accumulation_steps: 16
- total_train_batch_size: 128
- optimizer: Adam with betas=(0.9,0.999) and epsilon=1e-08
- lr_scheduler_type: cosine_with_restarts
- lr_scheduler_warmup_steps: 80
- num_epochs: 3
- mixed_precision_training: Native AMP
### Training results
| Training Loss | Epoch | Step | Validation Loss |
|:-------------:|:-----:|:----:|:---------------:|
| 5.0498 | 0.09 | 10 | 2.7298 |
| 1.1224 | 0.18 | 20 | 0.2229 |
| 0.1729 | 0.27 | 30 | 0.1692 |
| 0.1563 | 0.36 | 40 | 0.1537 |
| 0.1488 | 0.45 | 50 | 0.1479 |
| 0.1499 | 0.54 | 60 | 0.1482 |
| 0.1479 | 0.63 | 70 | 0.1459 |
| 0.1505 | 0.73 | 80 | 0.1541 |
| 0.1441 | 0.82 | 90 | 0.2022 |
| 0.1219 | 0.91 | 100 | 0.7452 |
| 1.0984 | 1.0 | 110 | 0.1533 |
| 0.1732 | 1.09 | 120 | 0.1442 |
| 0.1367 | 1.18 | 130 | 0.0983 |
| 0.1436 | 1.27 | 140 | 0.0921 |
| 0.0958 | 1.36 | 150 | 0.1662 |
| 0.1167 | 1.45 | 160 | 0.0953 |
| 0.0732 | 1.54 | 170 | 0.0720 |
| 0.0692 | 1.63 | 180 | 0.0678 |
| 0.0662 | 1.72 | 190 | 0.0587 |
| 0.0586 | 1.81 | 200 | 0.0591 |
| 0.0587 | 1.9 | 210 | 0.0595 |
| 0.0627 | 1.99 | 220 | 0.0556 |
| 0.0582 | 2.08 | 230 | 0.0560 |
| 0.0535 | 2.18 | 240 | 0.0567 |
| 0.055 | 2.27 | 250 | 0.0577 |
| 0.0613 | 2.36 | 260 | 0.0559 |
| 0.0537 | 2.45 | 270 | 0.0569 |
| 0.0519 | 2.54 | 280 | 0.0616 |
| 0.0559 | 2.63 | 290 | 0.0582 |
| 0.0556 | 2.72 | 300 | 0.0559 |
| 0.0576 | 2.81 | 310 | 0.0557 |
| 0.0607 | 2.9 | 320 | 0.0559 |
| 0.0592 | 2.99 | 330 | 0.0560 |
### Framework versions
- Transformers 4.36.0.dev0
- Pytorch 2.1.2+cu121
- Datasets 2.14.6
- Tokenizers 0.14.0
|
namangarg110/hiera-tiny-224-in1k | namangarg110 | 2024-05-09T04:03:39Z | 137 | 0 | transformers | [
"transformers",
"safetensors",
"hiera",
"image-classification",
"arxiv:1910.09700",
"autotrain_compatible",
"endpoints_compatible",
"region:us"
] | image-classification | 2024-05-09T03:53:11Z | ---
library_name: transformers
tags: []
---
# Model Card for Model ID
<!-- Provide a quick summary of what the model is/does. -->
## Model Details
### Model Description
<!-- Provide a longer summary of what this model is. -->
This is the model card of a 🤗 transformers model that has been pushed on the Hub. This model card has been automatically generated.
- **Developed by:** [More Information Needed]
- **Funded by [optional]:** [More Information Needed]
- **Shared by [optional]:** [More Information Needed]
- **Model type:** [More Information Needed]
- **Language(s) (NLP):** [More Information Needed]
- **License:** [More Information Needed]
- **Finetuned from model [optional]:** [More Information Needed]
### Model Sources [optional]
<!-- Provide the basic links for the model. -->
- **Repository:** [More Information Needed]
- **Paper [optional]:** [More Information Needed]
- **Demo [optional]:** [More Information Needed]
## Uses
<!-- Address questions around how the model is intended to be used, including the foreseeable users of the model and those affected by the model. -->
### Direct Use
<!-- This section is for the model use without fine-tuning or plugging into a larger ecosystem/app. -->
[More Information Needed]
### Downstream Use [optional]
<!-- This section is for the model use when fine-tuned for a task, or when plugged into a larger ecosystem/app -->
[More Information Needed]
### Out-of-Scope Use
<!-- This section addresses misuse, malicious use, and uses that the model will not work well for. -->
[More Information Needed]
## Bias, Risks, and Limitations
<!-- This section is meant to convey both technical and sociotechnical limitations. -->
[More Information Needed]
### Recommendations
<!-- This section is meant to convey recommendations with respect to the bias, risk, and technical limitations. -->
Users (both direct and downstream) should be made aware of the risks, biases and limitations of the model. More information needed for further recommendations.
## How to Get Started with the Model
Use the code below to get started with the model.
[More Information Needed]
## Training Details
### Training Data
<!-- This should link to a Dataset Card, perhaps with a short stub of information on what the training data is all about as well as documentation related to data pre-processing or additional filtering. -->
[More Information Needed]
### Training Procedure
<!-- This relates heavily to the Technical Specifications. Content here should link to that section when it is relevant to the training procedure. -->
#### Preprocessing [optional]
[More Information Needed]
#### Training Hyperparameters
- **Training regime:** [More Information Needed] <!--fp32, fp16 mixed precision, bf16 mixed precision, bf16 non-mixed precision, fp16 non-mixed precision, fp8 mixed precision -->
#### Speeds, Sizes, Times [optional]
<!-- This section provides information about throughput, start/end time, checkpoint size if relevant, etc. -->
[More Information Needed]
## Evaluation
<!-- This section describes the evaluation protocols and provides the results. -->
### Testing Data, Factors & Metrics
#### Testing Data
<!-- This should link to a Dataset Card if possible. -->
[More Information Needed]
#### Factors
<!-- These are the things the evaluation is disaggregating by, e.g., subpopulations or domains. -->
[More Information Needed]
#### Metrics
<!-- These are the evaluation metrics being used, ideally with a description of why. -->
[More Information Needed]
### Results
[More Information Needed]
#### Summary
## Model Examination [optional]
<!-- Relevant interpretability work for the model goes here -->
[More Information Needed]
## Environmental Impact
<!-- Total emissions (in grams of CO2eq) and additional considerations, such as electricity usage, go here. Edit the suggested text below accordingly -->
Carbon emissions can be estimated using the [Machine Learning Impact calculator](https://mlco2.github.io/impact#compute) presented in [Lacoste et al. (2019)](https://arxiv.org/abs/1910.09700).
- **Hardware Type:** [More Information Needed]
- **Hours used:** [More Information Needed]
- **Cloud Provider:** [More Information Needed]
- **Compute Region:** [More Information Needed]
- **Carbon Emitted:** [More Information Needed]
## Technical Specifications [optional]
### Model Architecture and Objective
[More Information Needed]
### Compute Infrastructure
[More Information Needed]
#### Hardware
[More Information Needed]
#### Software
[More Information Needed]
## Citation [optional]
<!-- If there is a paper or blog post introducing the model, the APA and Bibtex information for that should go in this section. -->
**BibTeX:**
[More Information Needed]
**APA:**
[More Information Needed]
## Glossary [optional]
<!-- If relevant, include terms and calculations in this section that can help readers understand the model or model card. -->
[More Information Needed]
## More Information [optional]
[More Information Needed]
## Model Card Authors [optional]
[More Information Needed]
## Model Card Contact
[More Information Needed]
|
Praful659/Qwen1_qlora_model_emotion_detection | Praful659 | 2024-05-09T03:50:53Z | 0 | 0 | transformers | [
"transformers",
"safetensors",
"arxiv:1910.09700",
"endpoints_compatible",
"region:us"
] | null | 2024-05-08T01:05:26Z | ---
library_name: transformers
tags: []
---
# Model Card for Model ID
<!-- Provide a quick summary of what the model is/does. -->
## Model Details
### Model Description
<!-- Provide a longer summary of what this model is. -->
This is the model card of a 🤗 transformers model that has been pushed on the Hub. This model card has been automatically generated.
- **Developed by:** [More Information Needed]
- **Funded by [optional]:** [More Information Needed]
- **Shared by [optional]:** [More Information Needed]
- **Model type:** [More Information Needed]
- **Language(s) (NLP):** [More Information Needed]
- **License:** [More Information Needed]
- **Finetuned from model [optional]:** [More Information Needed]
### Model Sources [optional]
<!-- Provide the basic links for the model. -->
- **Repository:** [More Information Needed]
- **Paper [optional]:** [More Information Needed]
- **Demo [optional]:** [More Information Needed]
## Uses
<!-- Address questions around how the model is intended to be used, including the foreseeable users of the model and those affected by the model. -->
### Direct Use
<!-- This section is for the model use without fine-tuning or plugging into a larger ecosystem/app. -->
[More Information Needed]
### Downstream Use [optional]
<!-- This section is for the model use when fine-tuned for a task, or when plugged into a larger ecosystem/app -->
[More Information Needed]
### Out-of-Scope Use
<!-- This section addresses misuse, malicious use, and uses that the model will not work well for. -->
[More Information Needed]
## Bias, Risks, and Limitations
<!-- This section is meant to convey both technical and sociotechnical limitations. -->
[More Information Needed]
### Recommendations
<!-- This section is meant to convey recommendations with respect to the bias, risk, and technical limitations. -->
Users (both direct and downstream) should be made aware of the risks, biases and limitations of the model. More information needed for further recommendations.
## How to Get Started with the Model
Use the code below to get started with the model.
[More Information Needed]
## Training Details
### Training Data
<!-- This should link to a Dataset Card, perhaps with a short stub of information on what the training data is all about as well as documentation related to data pre-processing or additional filtering. -->
[More Information Needed]
### Training Procedure
<!-- This relates heavily to the Technical Specifications. Content here should link to that section when it is relevant to the training procedure. -->
#### Preprocessing [optional]
[More Information Needed]
#### Training Hyperparameters
- **Training regime:** [More Information Needed] <!--fp32, fp16 mixed precision, bf16 mixed precision, bf16 non-mixed precision, fp16 non-mixed precision, fp8 mixed precision -->
#### Speeds, Sizes, Times [optional]
<!-- This section provides information about throughput, start/end time, checkpoint size if relevant, etc. -->
[More Information Needed]
## Evaluation
<!-- This section describes the evaluation protocols and provides the results. -->
### Testing Data, Factors & Metrics
#### Testing Data
<!-- This should link to a Dataset Card if possible. -->
[More Information Needed]
#### Factors
<!-- These are the things the evaluation is disaggregating by, e.g., subpopulations or domains. -->
[More Information Needed]
#### Metrics
<!-- These are the evaluation metrics being used, ideally with a description of why. -->
[More Information Needed]
### Results
[More Information Needed]
#### Summary
## Model Examination [optional]
<!-- Relevant interpretability work for the model goes here -->
[More Information Needed]
## Environmental Impact
<!-- Total emissions (in grams of CO2eq) and additional considerations, such as electricity usage, go here. Edit the suggested text below accordingly -->
Carbon emissions can be estimated using the [Machine Learning Impact calculator](https://mlco2.github.io/impact#compute) presented in [Lacoste et al. (2019)](https://arxiv.org/abs/1910.09700).
- **Hardware Type:** [More Information Needed]
- **Hours used:** [More Information Needed]
- **Cloud Provider:** [More Information Needed]
- **Compute Region:** [More Information Needed]
- **Carbon Emitted:** [More Information Needed]
## Technical Specifications [optional]
### Model Architecture and Objective
[More Information Needed]
### Compute Infrastructure
[More Information Needed]
#### Hardware
[More Information Needed]
#### Software
[More Information Needed]
## Citation [optional]
<!-- If there is a paper or blog post introducing the model, the APA and Bibtex information for that should go in this section. -->
**BibTeX:**
[More Information Needed]
**APA:**
[More Information Needed]
## Glossary [optional]
<!-- If relevant, include terms and calculations in this section that can help readers understand the model or model card. -->
[More Information Needed]
## More Information [optional]
[More Information Needed]
## Model Card Authors [optional]
[More Information Needed]
## Model Card Contact
[More Information Needed] |
Dinoboyy/Turkman | Dinoboyy | 2024-05-09T03:41:35Z | 0 | 0 | null | [
"license:apache-2.0",
"region:us"
] | null | 2024-05-09T03:41:35Z | ---
license: apache-2.0
---
|
brown1808/vinallama-2b-custom-ver2 | brown1808 | 2024-05-09T03:40:14Z | 132 | 0 | transformers | [
"transformers",
"safetensors",
"llama",
"text-generation",
"arxiv:1910.09700",
"autotrain_compatible",
"text-generation-inference",
"endpoints_compatible",
"region:us"
] | text-generation | 2024-05-09T03:37:31Z | ---
library_name: transformers
tags: []
---
# Model Card for Model ID
<!-- Provide a quick summary of what the model is/does. -->
## Model Details
### Model Description
<!-- Provide a longer summary of what this model is. -->
This is the model card of a 🤗 transformers model that has been pushed on the Hub. This model card has been automatically generated.
- **Developed by:** [More Information Needed]
- **Funded by [optional]:** [More Information Needed]
- **Shared by [optional]:** [More Information Needed]
- **Model type:** [More Information Needed]
- **Language(s) (NLP):** [More Information Needed]
- **License:** [More Information Needed]
- **Finetuned from model [optional]:** [More Information Needed]
### Model Sources [optional]
<!-- Provide the basic links for the model. -->
- **Repository:** [More Information Needed]
- **Paper [optional]:** [More Information Needed]
- **Demo [optional]:** [More Information Needed]
## Uses
<!-- Address questions around how the model is intended to be used, including the foreseeable users of the model and those affected by the model. -->
### Direct Use
<!-- This section is for the model use without fine-tuning or plugging into a larger ecosystem/app. -->
[More Information Needed]
### Downstream Use [optional]
<!-- This section is for the model use when fine-tuned for a task, or when plugged into a larger ecosystem/app -->
[More Information Needed]
### Out-of-Scope Use
<!-- This section addresses misuse, malicious use, and uses that the model will not work well for. -->
[More Information Needed]
## Bias, Risks, and Limitations
<!-- This section is meant to convey both technical and sociotechnical limitations. -->
[More Information Needed]
### Recommendations
<!-- This section is meant to convey recommendations with respect to the bias, risk, and technical limitations. -->
Users (both direct and downstream) should be made aware of the risks, biases and limitations of the model. More information needed for further recommendations.
## How to Get Started with the Model
Use the code below to get started with the model.
[More Information Needed]
## Training Details
### Training Data
<!-- This should link to a Dataset Card, perhaps with a short stub of information on what the training data is all about as well as documentation related to data pre-processing or additional filtering. -->
[More Information Needed]
### Training Procedure
<!-- This relates heavily to the Technical Specifications. Content here should link to that section when it is relevant to the training procedure. -->
#### Preprocessing [optional]
[More Information Needed]
#### Training Hyperparameters
- **Training regime:** [More Information Needed] <!--fp32, fp16 mixed precision, bf16 mixed precision, bf16 non-mixed precision, fp16 non-mixed precision, fp8 mixed precision -->
#### Speeds, Sizes, Times [optional]
<!-- This section provides information about throughput, start/end time, checkpoint size if relevant, etc. -->
[More Information Needed]
## Evaluation
<!-- This section describes the evaluation protocols and provides the results. -->
### Testing Data, Factors & Metrics
#### Testing Data
<!-- This should link to a Dataset Card if possible. -->
[More Information Needed]
#### Factors
<!-- These are the things the evaluation is disaggregating by, e.g., subpopulations or domains. -->
[More Information Needed]
#### Metrics
<!-- These are the evaluation metrics being used, ideally with a description of why. -->
[More Information Needed]
### Results
[More Information Needed]
#### Summary
## Model Examination [optional]
<!-- Relevant interpretability work for the model goes here -->
[More Information Needed]
## Environmental Impact
<!-- Total emissions (in grams of CO2eq) and additional considerations, such as electricity usage, go here. Edit the suggested text below accordingly -->
Carbon emissions can be estimated using the [Machine Learning Impact calculator](https://mlco2.github.io/impact#compute) presented in [Lacoste et al. (2019)](https://arxiv.org/abs/1910.09700).
- **Hardware Type:** [More Information Needed]
- **Hours used:** [More Information Needed]
- **Cloud Provider:** [More Information Needed]
- **Compute Region:** [More Information Needed]
- **Carbon Emitted:** [More Information Needed]
## Technical Specifications [optional]
### Model Architecture and Objective
[More Information Needed]
### Compute Infrastructure
[More Information Needed]
#### Hardware
[More Information Needed]
#### Software
[More Information Needed]
## Citation [optional]
<!-- If there is a paper or blog post introducing the model, the APA and Bibtex information for that should go in this section. -->
**BibTeX:**
[More Information Needed]
**APA:**
[More Information Needed]
## Glossary [optional]
<!-- If relevant, include terms and calculations in this section that can help readers understand the model or model card. -->
[More Information Needed]
## More Information [optional]
[More Information Needed]
## Model Card Authors [optional]
[More Information Needed]
## Model Card Contact
[More Information Needed] |
blockblockblock/llama-3-70B-Instruct-abliterated-bpw5-exl2 | blockblockblock | 2024-05-09T03:38:47Z | 6 | 0 | transformers | [
"transformers",
"safetensors",
"llama",
"text-generation",
"conversational",
"license:llama3",
"autotrain_compatible",
"text-generation-inference",
"endpoints_compatible",
"5-bit",
"exl2",
"region:us"
] | text-generation | 2024-05-09T03:28:12Z | ---
license: llama3
license_name: llama3
license_link: LICENSE
library_name: transformers
---
# Llama-3-70B-Instruct-abliterated Model Card
This is meta-llama/Llama-3-70B-Instruct with orthogonalized bfloat16 safetensor weights, generated with the methodology that was described in the preview paper/blog post: '[Refusal in LLMs is mediated by a single direction](https://www.alignmentforum.org/posts/jGuXSZgv6qfdhMCuJ/refusal-in-llms-is-mediated-by-a-single-direction)' which I encourage you to read to understand more.
TL;DR: this model has had certain weights manipulated to "inhibit" the model's ability to express refusal. It is not in anyway _guaranteed_ that it won't refuse you, understand your request, it may still lecture you about ethics/safety, etc. It is tuned in all other respects the same as the original 70B instruct model was, just with the strongest refusal direction orthogonalized out.
## Quants
[GGUF Quants available here](https://huggingface.co/failspy/llama-3-70B-Instruct-abliterated-GGUF)
## For the people who like tinkering or looking to save bandwidth
In the repo, I've included `refusal_dir.pth`
If you have Llama-3-70B-Instruct model downloaded already, you can use the ortho cookbook to apply it to your downloaded model, which will make it the same as what you'd download from here.
## Quirkiness awareness notice
This model may come with interesting quirks, as I obviously haven't extensively tested it, and the methodology being so new. I encourage you to play with the model, and post any quirks you notice in the community tab, as that'll help us further understand what this orthogonalization has in the way of side effects. The code I used to generate it (and my published 'Kappa-3' model which is just Phi-3 with the same methodology applied) is available in a Python notebook in this repo. Specifically, the [ortho_cookbook.ipynb](https://huggingface.co/failspy/llama-3-70B-Instruct-abliterated/blob/main/ortho_cookbook.ipynb).
If you manage to develop further improvements, please share! This is really the most primitive way to use ablation, but there are other possibilities that I believe are as-yet unexplored. |
Litzy619/O0508V8 | Litzy619 | 2024-05-09T03:24:16Z | 0 | 0 | null | [
"safetensors",
"generated_from_trainer",
"base_model:allenai/OLMo-1B",
"base_model:finetune:allenai/OLMo-1B",
"license:apache-2.0",
"region:us"
] | null | 2024-05-09T02:12:52Z | ---
license: apache-2.0
base_model: allenai/OLMo-1B
tags:
- generated_from_trainer
model-index:
- name: O0508V8
results: []
---
<!-- This model card has been generated automatically according to the information the Trainer had access to. You
should probably proofread and complete it, then remove this comment. -->
# O0508V8
This model is a fine-tuned version of [allenai/OLMo-1B](https://huggingface.co/allenai/OLMo-1B) on an unknown dataset.
It achieves the following results on the evaluation set:
- Loss: 0.0714
## Model description
More information needed
## Intended uses & limitations
More information needed
## Training and evaluation data
More information needed
## Training procedure
### Training hyperparameters
The following hyperparameters were used during training:
- learning_rate: 0.0003
- train_batch_size: 8
- eval_batch_size: 8
- seed: 42
- gradient_accumulation_steps: 16
- total_train_batch_size: 128
- optimizer: Adam with betas=(0.9,0.999) and epsilon=1e-08
- lr_scheduler_type: cosine_with_restarts
- lr_scheduler_warmup_steps: 80
- num_epochs: 3
- mixed_precision_training: Native AMP
### Training results
| Training Loss | Epoch | Step | Validation Loss |
|:-------------:|:-----:|:----:|:---------------:|
| 4.9313 | 0.09 | 10 | 2.2642 |
| 0.7787 | 0.18 | 20 | 0.1738 |
| 0.1559 | 0.27 | 30 | 0.1586 |
| 0.1548 | 0.36 | 40 | 0.1529 |
| 0.1516 | 0.45 | 50 | 0.1509 |
| 0.1513 | 0.54 | 60 | 0.1491 |
| 0.1496 | 0.63 | 70 | 0.1470 |
| 0.1489 | 0.73 | 80 | 0.1570 |
| 0.1465 | 0.82 | 90 | 0.1479 |
| 0.146 | 0.91 | 100 | 0.1486 |
| 0.1491 | 1.0 | 110 | 0.1496 |
| 0.1457 | 1.09 | 120 | 0.1494 |
| 0.1458 | 1.18 | 130 | 0.1526 |
| 0.1469 | 1.27 | 140 | 0.1488 |
| 0.1486 | 1.36 | 150 | 0.1478 |
| 0.1445 | 1.45 | 160 | 0.1491 |
| 0.1447 | 1.54 | 170 | 0.1455 |
| 0.1426 | 1.63 | 180 | 0.1267 |
| 0.1494 | 1.72 | 190 | 0.1451 |
| 0.2731 | 1.81 | 200 | 0.1196 |
| 0.2257 | 1.9 | 210 | 0.1045 |
| 0.0849 | 1.99 | 220 | 0.0824 |
| 0.0779 | 2.08 | 230 | 0.0861 |
| 0.0813 | 2.18 | 240 | 0.0786 |
| 0.0691 | 2.27 | 250 | 0.0755 |
| 0.0785 | 2.36 | 260 | 0.0753 |
| 0.0724 | 2.45 | 270 | 0.0728 |
| 0.0654 | 2.54 | 280 | 0.0728 |
| 0.0706 | 2.63 | 290 | 0.0731 |
| 0.0744 | 2.72 | 300 | 0.0714 |
| 0.0684 | 2.81 | 310 | 0.0712 |
| 0.0712 | 2.9 | 320 | 0.0713 |
| 0.0774 | 2.99 | 330 | 0.0714 |
### Framework versions
- Transformers 4.36.0.dev0
- Pytorch 2.1.2+cu121
- Datasets 2.14.6
- Tokenizers 0.14.0
|
Litzy619/O0508V4 | Litzy619 | 2024-05-09T03:18:52Z | 0 | 0 | null | [
"safetensors",
"generated_from_trainer",
"base_model:allenai/OLMo-1B",
"base_model:finetune:allenai/OLMo-1B",
"license:apache-2.0",
"region:us"
] | null | 2024-05-09T02:08:00Z | ---
license: apache-2.0
base_model: allenai/OLMo-1B
tags:
- generated_from_trainer
model-index:
- name: O0508V4
results: []
---
<!-- This model card has been generated automatically according to the information the Trainer had access to. You
should probably proofread and complete it, then remove this comment. -->
# O0508V4
This model is a fine-tuned version of [allenai/OLMo-1B](https://huggingface.co/allenai/OLMo-1B) on an unknown dataset.
It achieves the following results on the evaluation set:
- Loss: 0.1459
## Model description
More information needed
## Intended uses & limitations
More information needed
## Training and evaluation data
More information needed
## Training procedure
### Training hyperparameters
The following hyperparameters were used during training:
- learning_rate: 0.0003
- train_batch_size: 8
- eval_batch_size: 8
- seed: 42
- gradient_accumulation_steps: 16
- total_train_batch_size: 128
- optimizer: Adam with betas=(0.9,0.999) and epsilon=1e-08
- lr_scheduler_type: cosine_with_restarts
- lr_scheduler_warmup_steps: 80
- num_epochs: 3
- mixed_precision_training: Native AMP
### Training results
| Training Loss | Epoch | Step | Validation Loss |
|:-------------:|:-----:|:----:|:---------------:|
| 5.0718 | 0.09 | 10 | 2.8079 |
| 1.195 | 0.18 | 20 | 0.1632 |
| 0.1544 | 0.27 | 30 | 0.1530 |
| 0.1554 | 0.36 | 40 | 0.1549 |
| 0.1521 | 0.45 | 50 | 0.1545 |
| 0.1525 | 0.54 | 60 | 0.1528 |
| 0.156 | 0.63 | 70 | 0.1505 |
| 0.1502 | 0.73 | 80 | 0.1601 |
| 0.153 | 0.82 | 90 | 0.1507 |
| 0.152 | 0.91 | 100 | 0.1521 |
| 0.152 | 1.0 | 110 | 0.1515 |
| 0.1459 | 1.09 | 120 | 0.1506 |
| 0.146 | 1.18 | 130 | 0.1512 |
| 0.1468 | 1.27 | 140 | 0.1476 |
| 0.1485 | 1.36 | 150 | 0.1465 |
| 0.1433 | 1.45 | 160 | 0.1495 |
| 0.1453 | 1.54 | 170 | 0.1464 |
| 0.1463 | 1.63 | 180 | 0.1459 |
| 0.1465 | 1.72 | 190 | 0.1516 |
| 0.1462 | 1.81 | 200 | 0.1488 |
| 0.1483 | 1.9 | 210 | 0.1472 |
| 0.1461 | 1.99 | 220 | 0.1492 |
| 0.1466 | 2.08 | 230 | 0.1473 |
| 0.1417 | 2.18 | 240 | 0.1464 |
| 0.1433 | 2.27 | 250 | 0.1473 |
| 0.1447 | 2.36 | 260 | 0.1481 |
| 0.1434 | 2.45 | 270 | 0.1471 |
| 0.1425 | 2.54 | 280 | 0.1459 |
| 0.1426 | 2.63 | 290 | 0.1468 |
| 0.1448 | 2.72 | 300 | 0.1458 |
| 0.1435 | 2.81 | 310 | 0.1458 |
| 0.1448 | 2.9 | 320 | 0.1459 |
| 0.146 | 2.99 | 330 | 0.1459 |
### Framework versions
- Transformers 4.36.0.dev0
- Pytorch 2.1.2+cu121
- Datasets 2.14.6
- Tokenizers 0.14.0
|
jental/gemma_model10000 | jental | 2024-05-09T03:16:19Z | 0 | 0 | transformers | [
"transformers",
"safetensors",
"arxiv:1910.09700",
"endpoints_compatible",
"region:us"
] | null | 2024-05-09T03:16:07Z | ---
library_name: transformers
tags: []
---
# Model Card for Model ID
<!-- Provide a quick summary of what the model is/does. -->
## Model Details
### Model Description
<!-- Provide a longer summary of what this model is. -->
This is the model card of a 🤗 transformers model that has been pushed on the Hub. This model card has been automatically generated.
- **Developed by:** [More Information Needed]
- **Funded by [optional]:** [More Information Needed]
- **Shared by [optional]:** [More Information Needed]
- **Model type:** [More Information Needed]
- **Language(s) (NLP):** [More Information Needed]
- **License:** [More Information Needed]
- **Finetuned from model [optional]:** [More Information Needed]
### Model Sources [optional]
<!-- Provide the basic links for the model. -->
- **Repository:** [More Information Needed]
- **Paper [optional]:** [More Information Needed]
- **Demo [optional]:** [More Information Needed]
## Uses
<!-- Address questions around how the model is intended to be used, including the foreseeable users of the model and those affected by the model. -->
### Direct Use
<!-- This section is for the model use without fine-tuning or plugging into a larger ecosystem/app. -->
[More Information Needed]
### Downstream Use [optional]
<!-- This section is for the model use when fine-tuned for a task, or when plugged into a larger ecosystem/app -->
[More Information Needed]
### Out-of-Scope Use
<!-- This section addresses misuse, malicious use, and uses that the model will not work well for. -->
[More Information Needed]
## Bias, Risks, and Limitations
<!-- This section is meant to convey both technical and sociotechnical limitations. -->
[More Information Needed]
### Recommendations
<!-- This section is meant to convey recommendations with respect to the bias, risk, and technical limitations. -->
Users (both direct and downstream) should be made aware of the risks, biases and limitations of the model. More information needed for further recommendations.
## How to Get Started with the Model
Use the code below to get started with the model.
[More Information Needed]
## Training Details
### Training Data
<!-- This should link to a Dataset Card, perhaps with a short stub of information on what the training data is all about as well as documentation related to data pre-processing or additional filtering. -->
[More Information Needed]
### Training Procedure
<!-- This relates heavily to the Technical Specifications. Content here should link to that section when it is relevant to the training procedure. -->
#### Preprocessing [optional]
[More Information Needed]
#### Training Hyperparameters
- **Training regime:** [More Information Needed] <!--fp32, fp16 mixed precision, bf16 mixed precision, bf16 non-mixed precision, fp16 non-mixed precision, fp8 mixed precision -->
#### Speeds, Sizes, Times [optional]
<!-- This section provides information about throughput, start/end time, checkpoint size if relevant, etc. -->
[More Information Needed]
## Evaluation
<!-- This section describes the evaluation protocols and provides the results. -->
### Testing Data, Factors & Metrics
#### Testing Data
<!-- This should link to a Dataset Card if possible. -->
[More Information Needed]
#### Factors
<!-- These are the things the evaluation is disaggregating by, e.g., subpopulations or domains. -->
[More Information Needed]
#### Metrics
<!-- These are the evaluation metrics being used, ideally with a description of why. -->
[More Information Needed]
### Results
[More Information Needed]
#### Summary
## Model Examination [optional]
<!-- Relevant interpretability work for the model goes here -->
[More Information Needed]
## Environmental Impact
<!-- Total emissions (in grams of CO2eq) and additional considerations, such as electricity usage, go here. Edit the suggested text below accordingly -->
Carbon emissions can be estimated using the [Machine Learning Impact calculator](https://mlco2.github.io/impact#compute) presented in [Lacoste et al. (2019)](https://arxiv.org/abs/1910.09700).
- **Hardware Type:** [More Information Needed]
- **Hours used:** [More Information Needed]
- **Cloud Provider:** [More Information Needed]
- **Compute Region:** [More Information Needed]
- **Carbon Emitted:** [More Information Needed]
## Technical Specifications [optional]
### Model Architecture and Objective
[More Information Needed]
### Compute Infrastructure
[More Information Needed]
#### Hardware
[More Information Needed]
#### Software
[More Information Needed]
## Citation [optional]
<!-- If there is a paper or blog post introducing the model, the APA and Bibtex information for that should go in this section. -->
**BibTeX:**
[More Information Needed]
**APA:**
[More Information Needed]
## Glossary [optional]
<!-- If relevant, include terms and calculations in this section that can help readers understand the model or model card. -->
[More Information Needed]
## More Information [optional]
[More Information Needed]
## Model Card Authors [optional]
[More Information Needed]
## Model Card Contact
[More Information Needed]
|
freewheelin/free-llama3-dpo-v0.2 | freewheelin | 2024-05-09T03:15:13Z | 4,207 | 0 | transformers | [
"transformers",
"safetensors",
"llama",
"text-generation",
"ko",
"en",
"arxiv:2312.15166",
"license:mit",
"autotrain_compatible",
"text-generation-inference",
"endpoints_compatible",
"region:us"
] | text-generation | 2024-05-09T03:01:23Z | ---
language:
- ko
- en
license: mit
---
# Model Card for free-llama-dpo-v0.2
## Developed by : [Freewheelin](https://freewheelin-recruit.oopy.io/) AI Technical Team
## Hardware and Software
* **Training Factors**: We fine-tuned this model using the [HuggingFace TRL Trainer](https://huggingface.co/docs/trl/trainer)
## Method
- This model was trained using the learning method introduced in the [SOLAR paper](https://arxiv.org/pdf/2312.15166.pdf).
|
InfinityC/test_sum_abs_t5_small_wasa_stops | InfinityC | 2024-05-09T03:13:05Z | 107 | 0 | transformers | [
"transformers",
"tensorboard",
"safetensors",
"t5",
"text2text-generation",
"generated_from_trainer",
"base_model:google-t5/t5-small",
"base_model:finetune:google-t5/t5-small",
"license:apache-2.0",
"autotrain_compatible",
"text-generation-inference",
"endpoints_compatible",
"region:us"
] | text2text-generation | 2024-05-09T01:33:24Z | ---
license: apache-2.0
base_model: google-t5/t5-small
tags:
- generated_from_trainer
metrics:
- rouge
model-index:
- name: test_sum_abs_t5_small_wasa_stops
results: []
---
<!-- This model card has been generated automatically according to the information the Trainer had access to. You
should probably proofread and complete it, then remove this comment. -->
# test_sum_abs_t5_small_wasa_stops
This model is a fine-tuned version of [google-t5/t5-small](https://huggingface.co/google-t5/t5-small) on the None dataset.
It achieves the following results on the evaluation set:
- Loss: 0.8601
- Rouge1: 0.3823
- Rouge2: 0.2702
- Rougel: 0.3451
- Rougelsum: 0.3454
- Gen Len: 18.9864
## Model description
More information needed
## Intended uses & limitations
More information needed
## Training and evaluation data
More information needed
## Training procedure
### Training hyperparameters
The following hyperparameters were used during training:
- learning_rate: 2e-05
- train_batch_size: 16
- eval_batch_size: 16
- seed: 42
- optimizer: Adam with betas=(0.9,0.999) and epsilon=1e-08
- lr_scheduler_type: linear
- num_epochs: 4
- mixed_precision_training: Native AMP
### Training results
| Training Loss | Epoch | Step | Validation Loss | Rouge1 | Rouge2 | Rougel | Rougelsum | Gen Len |
|:-------------:|:-----:|:----:|:---------------:|:------:|:------:|:------:|:---------:|:-------:|
| 1.0591 | 1.0 | 1764 | 0.9275 | 0.3767 | 0.2652 | 0.3403 | 0.3404 | 18.9787 |
| 0.9758 | 2.0 | 3528 | 0.8813 | 0.3817 | 0.2702 | 0.3448 | 0.345 | 18.9819 |
| 0.9575 | 3.0 | 5292 | 0.8648 | 0.3818 | 0.2692 | 0.3445 | 0.3446 | 18.987 |
| 0.9435 | 4.0 | 7056 | 0.8601 | 0.3823 | 0.2702 | 0.3451 | 0.3454 | 18.9864 |
### Framework versions
- Transformers 4.39.3
- Pytorch 2.1.2
- Datasets 2.18.0
- Tokenizers 0.15.2
|
Litzy619/O0508V2 | Litzy619 | 2024-05-09T03:08:01Z | 0 | 0 | null | [
"safetensors",
"generated_from_trainer",
"base_model:allenai/OLMo-1B",
"base_model:finetune:allenai/OLMo-1B",
"license:apache-2.0",
"region:us"
] | null | 2024-05-09T01:46:20Z | ---
license: apache-2.0
base_model: allenai/OLMo-1B
tags:
- generated_from_trainer
model-index:
- name: O0508V2
results: []
---
<!-- This model card has been generated automatically according to the information the Trainer had access to. You
should probably proofread and complete it, then remove this comment. -->
# O0508V2
This model is a fine-tuned version of [allenai/OLMo-1B](https://huggingface.co/allenai/OLMo-1B) on an unknown dataset.
It achieves the following results on the evaluation set:
- Loss: 0.1459
## Model description
More information needed
## Intended uses & limitations
More information needed
## Training and evaluation data
More information needed
## Training procedure
### Training hyperparameters
The following hyperparameters were used during training:
- learning_rate: 0.0003
- train_batch_size: 8
- eval_batch_size: 8
- seed: 42
- gradient_accumulation_steps: 16
- total_train_batch_size: 128
- optimizer: Adam with betas=(0.9,0.999) and epsilon=1e-08
- lr_scheduler_type: cosine_with_restarts
- lr_scheduler_warmup_steps: 80
- num_epochs: 3
- mixed_precision_training: Native AMP
### Training results
| Training Loss | Epoch | Step | Validation Loss |
|:-------------:|:-----:|:----:|:---------------:|
| 5.1761 | 0.09 | 10 | 3.3017 |
| 1.7101 | 0.18 | 20 | 0.2079 |
| 0.1675 | 0.27 | 30 | 0.1572 |
| 0.1525 | 0.36 | 40 | 0.1620 |
| 0.1506 | 0.45 | 50 | 0.1540 |
| 0.152 | 0.54 | 60 | 0.1542 |
| 0.1542 | 0.63 | 70 | 0.1484 |
| 0.1521 | 0.73 | 80 | 0.1561 |
| 0.1547 | 0.82 | 90 | 0.1471 |
| 0.1498 | 0.91 | 100 | 0.1494 |
| 0.1527 | 1.0 | 110 | 0.1522 |
| 0.1473 | 1.09 | 120 | 0.1486 |
| 0.1469 | 1.18 | 130 | 0.1526 |
| 0.1474 | 1.27 | 140 | 0.1504 |
| 0.149 | 1.36 | 150 | 0.1489 |
| 0.1448 | 1.45 | 160 | 0.1471 |
| 0.1457 | 1.54 | 170 | 0.1470 |
| 0.1471 | 1.63 | 180 | 0.1471 |
| 0.1461 | 1.72 | 190 | 0.1505 |
| 0.1454 | 1.81 | 200 | 0.1540 |
| 0.149 | 1.9 | 210 | 0.1509 |
| 0.1468 | 1.99 | 220 | 0.1496 |
| 0.1474 | 2.08 | 230 | 0.1478 |
| 0.1418 | 2.18 | 240 | 0.1463 |
| 0.1433 | 2.27 | 250 | 0.1476 |
| 0.1449 | 2.36 | 260 | 0.1481 |
| 0.1437 | 2.45 | 270 | 0.1464 |
| 0.1418 | 2.54 | 280 | 0.1467 |
| 0.1422 | 2.63 | 290 | 0.1473 |
| 0.1449 | 2.72 | 300 | 0.1458 |
| 0.1438 | 2.81 | 310 | 0.1457 |
| 0.1445 | 2.9 | 320 | 0.1459 |
| 0.1454 | 2.99 | 330 | 0.1459 |
### Framework versions
- Transformers 4.36.0.dev0
- Pytorch 2.1.2+cu121
- Datasets 2.14.6
- Tokenizers 0.14.0
|
BothBosu/bert-scam-classifier-v1.1 | BothBosu | 2024-05-09T03:06:11Z | 108 | 0 | transformers | [
"transformers",
"tensorboard",
"safetensors",
"bert",
"text-classification",
"generated_from_trainer",
"base_model:google-bert/bert-base-uncased",
"base_model:finetune:google-bert/bert-base-uncased",
"license:apache-2.0",
"autotrain_compatible",
"endpoints_compatible",
"region:us"
] | text-classification | 2024-05-06T07:37:33Z | ---
license: apache-2.0
base_model: google-bert/bert-base-uncased
tags:
- generated_from_trainer
metrics:
- accuracy
- precision
- recall
- f1
model-index:
- name: bert-scam-classifier-v1.1
results: []
---
<!-- This model card has been generated automatically according to the information the Trainer had access to. You
should probably proofread and complete it, then remove this comment. -->
# bert-scam-classifier-v1.1
This model is a fine-tuned version of [google-bert/bert-base-uncased](https://huggingface.co/google-bert/bert-base-uncased) on an unknown dataset.
It achieves the following results on the evaluation set:
- Loss: 0.0013
- Accuracy: {'accuracy': 1.0}
- Precision: {'precision': 1.0}
- Recall: {'recall': 1.0}
- F1: {'f1': 1.0}
## Model description
More information needed
## Intended uses & limitations
More information needed
## Training and evaluation data
More information needed
## Training procedure
### Training hyperparameters
The following hyperparameters were used during training:
- learning_rate: 2e-05
- train_batch_size: 8
- eval_batch_size: 8
- seed: 42
- optimizer: Adam with betas=(0.9,0.999) and epsilon=1e-08
- lr_scheduler_type: linear
- num_epochs: 2
### Training results
| Training Loss | Epoch | Step | Validation Loss | Accuracy | Precision | Recall | F1 |
|:-------------:|:-----:|:----:|:---------------:|:-----------------:|:------------------:|:---------------:|:-----------:|
| No log | 1.0 | 160 | 0.0029 | {'accuracy': 1.0} | {'precision': 1.0} | {'recall': 1.0} | {'f1': 1.0} |
| No log | 2.0 | 320 | 0.0013 | {'accuracy': 1.0} | {'precision': 1.0} | {'recall': 1.0} | {'f1': 1.0} |
### Framework versions
- Transformers 4.40.1
- Pytorch 2.2.1+cu121
- Datasets 2.19.0
- Tokenizers 0.19.1
|
Litzy619/O0508V3 | Litzy619 | 2024-05-09T03:03:50Z | 0 | 0 | null | [
"safetensors",
"generated_from_trainer",
"base_model:allenai/OLMo-1B",
"base_model:finetune:allenai/OLMo-1B",
"license:apache-2.0",
"region:us"
] | null | 2024-05-09T01:46:20Z | ---
license: apache-2.0
base_model: allenai/OLMo-1B
tags:
- generated_from_trainer
model-index:
- name: O0508V3
results: []
---
<!-- This model card has been generated automatically according to the information the Trainer had access to. You
should probably proofread and complete it, then remove this comment. -->
# O0508V3
This model is a fine-tuned version of [allenai/OLMo-1B](https://huggingface.co/allenai/OLMo-1B) on an unknown dataset.
It achieves the following results on the evaluation set:
- Loss: 0.1463
## Model description
More information needed
## Intended uses & limitations
More information needed
## Training and evaluation data
More information needed
## Training procedure
### Training hyperparameters
The following hyperparameters were used during training:
- learning_rate: 0.0003
- train_batch_size: 8
- eval_batch_size: 8
- seed: 42
- gradient_accumulation_steps: 16
- total_train_batch_size: 128
- optimizer: Adam with betas=(0.9,0.999) and epsilon=1e-08
- lr_scheduler_type: cosine_with_restarts
- lr_scheduler_warmup_steps: 80
- num_epochs: 3
- mixed_precision_training: Native AMP
### Training results
| Training Loss | Epoch | Step | Validation Loss |
|:-------------:|:-----:|:----:|:---------------:|
| 5.1298 | 0.09 | 10 | 3.0519 |
| 1.4507 | 0.18 | 20 | 0.1818 |
| 0.162 | 0.27 | 30 | 0.1622 |
| 0.1611 | 0.36 | 40 | 0.1538 |
| 0.1505 | 0.45 | 50 | 0.1496 |
| 0.1523 | 0.54 | 60 | 0.1514 |
| 0.1501 | 0.63 | 70 | 0.1488 |
| 0.1502 | 0.73 | 80 | 0.1596 |
| 0.1476 | 0.82 | 90 | 0.1489 |
| 0.1477 | 0.91 | 100 | 0.1497 |
| 0.1516 | 1.0 | 110 | 0.1493 |
| 0.1457 | 1.09 | 120 | 0.1494 |
| 0.1472 | 1.18 | 130 | 0.1531 |
| 0.1471 | 1.27 | 140 | 0.1500 |
| 0.1493 | 1.36 | 150 | 0.1487 |
| 0.1443 | 1.45 | 160 | 0.1491 |
| 0.1456 | 1.54 | 170 | 0.1471 |
| 0.1474 | 1.63 | 180 | 0.1465 |
| 0.1461 | 1.72 | 190 | 0.1490 |
| 0.1467 | 1.81 | 200 | 0.1531 |
| 0.1511 | 1.9 | 210 | 0.1490 |
| 0.1468 | 1.99 | 220 | 0.1480 |
| 0.1481 | 2.08 | 230 | 0.1475 |
| 0.1423 | 2.18 | 240 | 0.1476 |
| 0.1443 | 2.27 | 250 | 0.1476 |
| 0.1453 | 2.36 | 260 | 0.1495 |
| 0.1443 | 2.45 | 270 | 0.1465 |
| 0.1422 | 2.54 | 280 | 0.1472 |
| 0.143 | 2.63 | 290 | 0.1476 |
| 0.145 | 2.72 | 300 | 0.1464 |
| 0.1438 | 2.81 | 310 | 0.1463 |
| 0.1449 | 2.9 | 320 | 0.1463 |
| 0.1459 | 2.99 | 330 | 0.1463 |
### Framework versions
- Transformers 4.36.0.dev0
- Pytorch 2.1.2+cu121
- Datasets 2.14.6
- Tokenizers 0.14.0
|
azhara001/donut-base-demo-final_v23e-05_AdamW | azhara001 | 2024-05-09T03:01:56Z | 50 | 0 | transformers | [
"transformers",
"safetensors",
"vision-encoder-decoder",
"image-text-to-text",
"arxiv:1910.09700",
"endpoints_compatible",
"region:us"
] | image-text-to-text | 2024-05-02T23:56:23Z | ---
library_name: transformers
tags: []
---
# Model Card for Model ID
<!-- Provide a quick summary of what the model is/does. -->
## Model Details
### Model Description
<!-- Provide a longer summary of what this model is. -->
This is the model card of a 🤗 transformers model that has been pushed on the Hub. This model card has been automatically generated.
- **Developed by:** [More Information Needed]
- **Funded by [optional]:** [More Information Needed]
- **Shared by [optional]:** [More Information Needed]
- **Model type:** [More Information Needed]
- **Language(s) (NLP):** [More Information Needed]
- **License:** [More Information Needed]
- **Finetuned from model [optional]:** [More Information Needed]
### Model Sources [optional]
<!-- Provide the basic links for the model. -->
- **Repository:** [More Information Needed]
- **Paper [optional]:** [More Information Needed]
- **Demo [optional]:** [More Information Needed]
## Uses
<!-- Address questions around how the model is intended to be used, including the foreseeable users of the model and those affected by the model. -->
### Direct Use
<!-- This section is for the model use without fine-tuning or plugging into a larger ecosystem/app. -->
[More Information Needed]
### Downstream Use [optional]
<!-- This section is for the model use when fine-tuned for a task, or when plugged into a larger ecosystem/app -->
[More Information Needed]
### Out-of-Scope Use
<!-- This section addresses misuse, malicious use, and uses that the model will not work well for. -->
[More Information Needed]
## Bias, Risks, and Limitations
<!-- This section is meant to convey both technical and sociotechnical limitations. -->
[More Information Needed]
### Recommendations
<!-- This section is meant to convey recommendations with respect to the bias, risk, and technical limitations. -->
Users (both direct and downstream) should be made aware of the risks, biases and limitations of the model. More information needed for further recommendations.
## How to Get Started with the Model
Use the code below to get started with the model.
[More Information Needed]
## Training Details
### Training Data
<!-- This should link to a Dataset Card, perhaps with a short stub of information on what the training data is all about as well as documentation related to data pre-processing or additional filtering. -->
[More Information Needed]
### Training Procedure
<!-- This relates heavily to the Technical Specifications. Content here should link to that section when it is relevant to the training procedure. -->
#### Preprocessing [optional]
[More Information Needed]
#### Training Hyperparameters
- **Training regime:** [More Information Needed] <!--fp32, fp16 mixed precision, bf16 mixed precision, bf16 non-mixed precision, fp16 non-mixed precision, fp8 mixed precision -->
#### Speeds, Sizes, Times [optional]
<!-- This section provides information about throughput, start/end time, checkpoint size if relevant, etc. -->
[More Information Needed]
## Evaluation
<!-- This section describes the evaluation protocols and provides the results. -->
### Testing Data, Factors & Metrics
#### Testing Data
<!-- This should link to a Dataset Card if possible. -->
[More Information Needed]
#### Factors
<!-- These are the things the evaluation is disaggregating by, e.g., subpopulations or domains. -->
[More Information Needed]
#### Metrics
<!-- These are the evaluation metrics being used, ideally with a description of why. -->
[More Information Needed]
### Results
[More Information Needed]
#### Summary
## Model Examination [optional]
<!-- Relevant interpretability work for the model goes here -->
[More Information Needed]
## Environmental Impact
<!-- Total emissions (in grams of CO2eq) and additional considerations, such as electricity usage, go here. Edit the suggested text below accordingly -->
Carbon emissions can be estimated using the [Machine Learning Impact calculator](https://mlco2.github.io/impact#compute) presented in [Lacoste et al. (2019)](https://arxiv.org/abs/1910.09700).
- **Hardware Type:** [More Information Needed]
- **Hours used:** [More Information Needed]
- **Cloud Provider:** [More Information Needed]
- **Compute Region:** [More Information Needed]
- **Carbon Emitted:** [More Information Needed]
## Technical Specifications [optional]
### Model Architecture and Objective
[More Information Needed]
### Compute Infrastructure
[More Information Needed]
#### Hardware
[More Information Needed]
#### Software
[More Information Needed]
## Citation [optional]
<!-- If there is a paper or blog post introducing the model, the APA and Bibtex information for that should go in this section. -->
**BibTeX:**
[More Information Needed]
**APA:**
[More Information Needed]
## Glossary [optional]
<!-- If relevant, include terms and calculations in this section that can help readers understand the model or model card. -->
[More Information Needed]
## More Information [optional]
[More Information Needed]
## Model Card Authors [optional]
[More Information Needed]
## Model Card Contact
[More Information Needed] |
jayllan23/ISY503-sentiment_analysis2 | jayllan23 | 2024-05-09T03:00:44Z | 119 | 0 | transformers | [
"transformers",
"tensorboard",
"safetensors",
"distilbert",
"text-classification",
"generated_from_trainer",
"base_model:distilbert/distilbert-base-uncased",
"base_model:finetune:distilbert/distilbert-base-uncased",
"license:apache-2.0",
"autotrain_compatible",
"endpoints_compatible",
"region:us"
] | text-classification | 2024-05-09T02:54:59Z | ---
license: apache-2.0
base_model: distilbert-base-uncased
tags:
- generated_from_trainer
metrics:
- accuracy
- f1
model-index:
- name: ISY503-sentiment_analysis2
results: []
---
<!-- This model card has been generated automatically according to the information the Trainer had access to. You
should probably proofread and complete it, then remove this comment. -->
# ISY503-sentiment_analysis2
This model is a fine-tuned version of [distilbert-base-uncased](https://huggingface.co/distilbert-base-uncased) on an unknown dataset.
It achieves the following results on the evaluation set:
- Loss: 0.3695
- Accuracy: 0.8667
- F1: 0.8701
## Model description
More information needed
## Intended uses & limitations
More information needed
## Training and evaluation data
More information needed
## Training procedure
### Training hyperparameters
The following hyperparameters were used during training:
- learning_rate: 2e-05
- train_batch_size: 16
- eval_batch_size: 16
- seed: 42
- optimizer: Adam with betas=(0.9,0.999) and epsilon=1e-08
- lr_scheduler_type: linear
- num_epochs: 2
### Training results
### Framework versions
- Transformers 4.40.1
- Pytorch 2.2.1+cu121
- Datasets 2.19.1
- Tokenizers 0.19.1
|
azhara001/donut-base-demo-new-v2-3e-05_AdamW_6566 | azhara001 | 2024-05-09T02:55:16Z | 50 | 0 | transformers | [
"transformers",
"safetensors",
"vision-encoder-decoder",
"image-text-to-text",
"arxiv:1910.09700",
"endpoints_compatible",
"region:us"
] | image-text-to-text | 2024-05-09T02:54:44Z | ---
library_name: transformers
tags: []
---
# Model Card for Model ID
<!-- Provide a quick summary of what the model is/does. -->
## Model Details
### Model Description
<!-- Provide a longer summary of what this model is. -->
This is the model card of a 🤗 transformers model that has been pushed on the Hub. This model card has been automatically generated.
- **Developed by:** [More Information Needed]
- **Funded by [optional]:** [More Information Needed]
- **Shared by [optional]:** [More Information Needed]
- **Model type:** [More Information Needed]
- **Language(s) (NLP):** [More Information Needed]
- **License:** [More Information Needed]
- **Finetuned from model [optional]:** [More Information Needed]
### Model Sources [optional]
<!-- Provide the basic links for the model. -->
- **Repository:** [More Information Needed]
- **Paper [optional]:** [More Information Needed]
- **Demo [optional]:** [More Information Needed]
## Uses
<!-- Address questions around how the model is intended to be used, including the foreseeable users of the model and those affected by the model. -->
### Direct Use
<!-- This section is for the model use without fine-tuning or plugging into a larger ecosystem/app. -->
[More Information Needed]
### Downstream Use [optional]
<!-- This section is for the model use when fine-tuned for a task, or when plugged into a larger ecosystem/app -->
[More Information Needed]
### Out-of-Scope Use
<!-- This section addresses misuse, malicious use, and uses that the model will not work well for. -->
[More Information Needed]
## Bias, Risks, and Limitations
<!-- This section is meant to convey both technical and sociotechnical limitations. -->
[More Information Needed]
### Recommendations
<!-- This section is meant to convey recommendations with respect to the bias, risk, and technical limitations. -->
Users (both direct and downstream) should be made aware of the risks, biases and limitations of the model. More information needed for further recommendations.
## How to Get Started with the Model
Use the code below to get started with the model.
[More Information Needed]
## Training Details
### Training Data
<!-- This should link to a Dataset Card, perhaps with a short stub of information on what the training data is all about as well as documentation related to data pre-processing or additional filtering. -->
[More Information Needed]
### Training Procedure
<!-- This relates heavily to the Technical Specifications. Content here should link to that section when it is relevant to the training procedure. -->
#### Preprocessing [optional]
[More Information Needed]
#### Training Hyperparameters
- **Training regime:** [More Information Needed] <!--fp32, fp16 mixed precision, bf16 mixed precision, bf16 non-mixed precision, fp16 non-mixed precision, fp8 mixed precision -->
#### Speeds, Sizes, Times [optional]
<!-- This section provides information about throughput, start/end time, checkpoint size if relevant, etc. -->
[More Information Needed]
## Evaluation
<!-- This section describes the evaluation protocols and provides the results. -->
### Testing Data, Factors & Metrics
#### Testing Data
<!-- This should link to a Dataset Card if possible. -->
[More Information Needed]
#### Factors
<!-- These are the things the evaluation is disaggregating by, e.g., subpopulations or domains. -->
[More Information Needed]
#### Metrics
<!-- These are the evaluation metrics being used, ideally with a description of why. -->
[More Information Needed]
### Results
[More Information Needed]
#### Summary
## Model Examination [optional]
<!-- Relevant interpretability work for the model goes here -->
[More Information Needed]
## Environmental Impact
<!-- Total emissions (in grams of CO2eq) and additional considerations, such as electricity usage, go here. Edit the suggested text below accordingly -->
Carbon emissions can be estimated using the [Machine Learning Impact calculator](https://mlco2.github.io/impact#compute) presented in [Lacoste et al. (2019)](https://arxiv.org/abs/1910.09700).
- **Hardware Type:** [More Information Needed]
- **Hours used:** [More Information Needed]
- **Cloud Provider:** [More Information Needed]
- **Compute Region:** [More Information Needed]
- **Carbon Emitted:** [More Information Needed]
## Technical Specifications [optional]
### Model Architecture and Objective
[More Information Needed]
### Compute Infrastructure
[More Information Needed]
#### Hardware
[More Information Needed]
#### Software
[More Information Needed]
## Citation [optional]
<!-- If there is a paper or blog post introducing the model, the APA and Bibtex information for that should go in this section. -->
**BibTeX:**
[More Information Needed]
**APA:**
[More Information Needed]
## Glossary [optional]
<!-- If relevant, include terms and calculations in this section that can help readers understand the model or model card. -->
[More Information Needed]
## More Information [optional]
[More Information Needed]
## Model Card Authors [optional]
[More Information Needed]
## Model Card Contact
[More Information Needed] |
vwxyzjn/rloo_zephyr_vllm_warmup_1e-6_promising | vwxyzjn | 2024-05-09T02:54:38Z | 6 | 0 | transformers | [
"transformers",
"safetensors",
"mistral",
"text-generation",
"generated_from_trainer",
"conversational",
"autotrain_compatible",
"text-generation-inference",
"endpoints_compatible",
"region:us"
] | text-generation | 2024-05-09T02:49:58Z | ---
tags:
- generated_from_trainer
model-index:
- name: rloo_zephyr_vllm_warmup_1e-6_promising
results: []
---
<!-- This model card has been generated automatically according to the information the Trainer had access to. You
should probably proofread and complete it, then remove this comment. -->
# rloo_zephyr_vllm_warmup_1e-6_promising
This model was trained from scratch on an unknown dataset.
## Model description
More information needed
## Intended uses & limitations
More information needed
## Training and evaluation data
More information needed
## Training procedure
### Training hyperparameters
The following hyperparameters were used during training:
- learning_rate: 1e-06
- train_batch_size: 1
- eval_batch_size: 8
- seed: 42
- distributed_type: multi-GPU
- num_devices: 7
- gradient_accumulation_steps: 64
- total_train_batch_size: 448
- total_eval_batch_size: 56
- optimizer: Adam with betas=(0.9,0.999) and epsilon=1e-08
- lr_scheduler_type: linear
- lr_scheduler_warmup_ratio: 0.2
- num_epochs: 3.0
### Framework versions
- Transformers 4.40.1
- Pytorch 2.2.1+cu121
- Datasets 2.18.0
- Tokenizers 0.19.1
|
Holarissun/dpo_tldrtldr_gpt4_subset10000_modelgemma2b_maxsteps5000_bz8_lr1e-06 | Holarissun | 2024-05-09T02:53:41Z | 0 | 0 | peft | [
"peft",
"safetensors",
"trl",
"dpo",
"generated_from_trainer",
"base_model:google/gemma-2b",
"base_model:adapter:google/gemma-2b",
"license:gemma",
"region:us"
] | null | 2024-05-09T02:53:38Z | ---
license: gemma
library_name: peft
tags:
- trl
- dpo
- generated_from_trainer
base_model: google/gemma-2b
model-index:
- name: dpo_tldrtldr_gpt4_subset10000_modelgemma2b_maxsteps5000_bz8_lr1e-06
results: []
---
<!-- This model card has been generated automatically according to the information the Trainer had access to. You
should probably proofread and complete it, then remove this comment. -->
# dpo_tldrtldr_gpt4_subset10000_modelgemma2b_maxsteps5000_bz8_lr1e-06
This model is a fine-tuned version of [google/gemma-2b](https://huggingface.co/google/gemma-2b) on an unknown dataset.
## Model description
More information needed
## Intended uses & limitations
More information needed
## Training and evaluation data
More information needed
## Training procedure
### Training hyperparameters
The following hyperparameters were used during training:
- learning_rate: 1e-06
- train_batch_size: 1
- eval_batch_size: 8
- seed: 42
- gradient_accumulation_steps: 8
- total_train_batch_size: 8
- optimizer: Adam with betas=(0.9,0.999) and epsilon=1e-08
- lr_scheduler_type: linear
- lr_scheduler_warmup_steps: 15
- training_steps: 5000
### Training results
### Framework versions
- PEFT 0.9.0
- Transformers 4.38.2
- Pytorch 2.1.2
- Datasets 2.18.0
- Tokenizers 0.15.2 |
Holarissun/dpo_tldrtldr_gpt3_subset10000_modelgemma2b_maxsteps5000_bz8_lr1e-06 | Holarissun | 2024-05-09T02:51:10Z | 0 | 0 | peft | [
"peft",
"safetensors",
"trl",
"dpo",
"generated_from_trainer",
"base_model:google/gemma-2b",
"base_model:adapter:google/gemma-2b",
"license:gemma",
"region:us"
] | null | 2024-05-09T02:51:07Z | ---
license: gemma
library_name: peft
tags:
- trl
- dpo
- generated_from_trainer
base_model: google/gemma-2b
model-index:
- name: dpo_tldrtldr_gpt3_subset10000_modelgemma2b_maxsteps5000_bz8_lr1e-06
results: []
---
<!-- This model card has been generated automatically according to the information the Trainer had access to. You
should probably proofread and complete it, then remove this comment. -->
# dpo_tldrtldr_gpt3_subset10000_modelgemma2b_maxsteps5000_bz8_lr1e-06
This model is a fine-tuned version of [google/gemma-2b](https://huggingface.co/google/gemma-2b) on an unknown dataset.
## Model description
More information needed
## Intended uses & limitations
More information needed
## Training and evaluation data
More information needed
## Training procedure
### Training hyperparameters
The following hyperparameters were used during training:
- learning_rate: 1e-06
- train_batch_size: 1
- eval_batch_size: 8
- seed: 42
- gradient_accumulation_steps: 8
- total_train_batch_size: 8
- optimizer: Adam with betas=(0.9,0.999) and epsilon=1e-08
- lr_scheduler_type: linear
- lr_scheduler_warmup_steps: 15
- training_steps: 5000
### Training results
### Framework versions
- PEFT 0.9.0
- Transformers 4.38.2
- Pytorch 2.1.2
- Datasets 2.18.0
- Tokenizers 0.15.2 |
netcat420/MFANN3bv0.7.10-GGUF | netcat420 | 2024-05-09T02:49:43Z | 0 | 0 | adapter-transformers | [
"adapter-transformers",
"gguf",
"phi",
"text-classification",
"arxiv:2306.01708",
"license:apache-2.0",
"endpoints_compatible",
"region:us"
] | text-classification | 2024-05-08T18:47:44Z | ---
license: apache-2.0
library_name: adapter-transformers
pipeline_tag: text-classification
---
---
base_model:
- netcat420/MFANN3bv0.3
- netcat420/MFANN3bv0.7
- liminerity/Phigments12
- netcat420/MFANN3bv0.6
library_name: transformers
tags:
- mergekit
- merge
license: apache-2.0
---
# MFANN3bv0.7.10
This is a merge of pre-trained language models created using [mergekit](https://github.com/cg123/mergekit).
## Merge Details
### Merge Method
This model was merged using the [TIES](https://arxiv.org/abs/2306.01708) merge method using [liminerity/Phigments12](https://huggingface.co/liminerity/Phigments12) as a base.
### Models Merged
The following models were included in the merge:
* [netcat420/MFANN3bv0.3](https://huggingface.co/netcat420/MFANN3bv0.3)
* [netcat420/MFANN3bv0.7](https://huggingface.co/netcat420/MFANN3bv0.7)
* [netcat420/MFANN3bv0.6](https://huggingface.co/netcat420/MFANN3bv0.6)
### Configuration
The following YAML configuration was used to produce this model:
```yaml
models:
- model: netcat420/MFANN3bv0.6
parameters:
density: [1, 0.7, 0.1] # density gradient
weight: 1.0
- model: netcat420/MFANN3bv0.3
parameters:
density: 0.5
weight: [0, 0.3, 0.7, 1] # weight gradient
- model: netcat420/MFANN3bv0.7
parameters:
density: 0.33
weight:
- filter: mlp
value: 0.5
- value: 0
merge_method: ties
base_model: liminerity/Phigments12
parameters:
normalize: true
int8_mask: true
dtype: float16
```
System prompt
```
### System:
### thought-process:
``` |
BothBosu/distilbert-scam-classifier-v1.3 | BothBosu | 2024-05-09T02:43:39Z | 120 | 0 | transformers | [
"transformers",
"tensorboard",
"safetensors",
"distilbert",
"text-classification",
"generated_from_trainer",
"base_model:distilbert/distilbert-base-uncased",
"base_model:finetune:distilbert/distilbert-base-uncased",
"license:apache-2.0",
"autotrain_compatible",
"endpoints_compatible",
"region:us"
] | text-classification | 2024-05-06T07:34:47Z | ---
license: apache-2.0
base_model: distilbert/distilbert-base-uncased
tags:
- generated_from_trainer
metrics:
- accuracy
- precision
- recall
- f1
model-index:
- name: distilbert-scam-classifier-v1.3
results: []
---
<!-- This model card has been generated automatically according to the information the Trainer had access to. You
should probably proofread and complete it, then remove this comment. -->
# distilbert-scam-classifier-v1.3
This model is a fine-tuned version of [distilbert/distilbert-base-uncased](https://huggingface.co/distilbert/distilbert-base-uncased) on an unknown dataset.
It achieves the following results on the evaluation set:
- Loss: 0.0012
- Accuracy: {'accuracy': 1.0}
- Precision: {'precision': 1.0}
- Recall: {'recall': 1.0}
- F1: {'f1': 1.0}
## Model description
More information needed
## Intended uses & limitations
More information needed
## Training and evaluation data
More information needed
## Training procedure
### Training hyperparameters
The following hyperparameters were used during training:
- learning_rate: 2e-05
- train_batch_size: 8
- eval_batch_size: 8
- seed: 42
- optimizer: Adam with betas=(0.9,0.999) and epsilon=1e-08
- lr_scheduler_type: linear
- num_epochs: 2
### Training results
| Training Loss | Epoch | Step | Validation Loss | Accuracy | Precision | Recall | F1 |
|:-------------:|:-----:|:----:|:---------------:|:-----------------:|:------------------:|:---------------:|:-----------:|
| No log | 1.0 | 160 | 0.0021 | {'accuracy': 1.0} | {'precision': 1.0} | {'recall': 1.0} | {'f1': 1.0} |
| No log | 2.0 | 320 | 0.0012 | {'accuracy': 1.0} | {'precision': 1.0} | {'recall': 1.0} | {'f1': 1.0} |
### Framework versions
- Transformers 4.40.1
- Pytorch 2.2.1+cu121
- Datasets 2.19.1
- Tokenizers 0.19.1
|
ShenaoZ/0.001_withdpo_4iters_bs256_555lr_iter_2 | ShenaoZ | 2024-05-09T02:42:15Z | 5 | 0 | transformers | [
"transformers",
"safetensors",
"mistral",
"text-generation",
"alignment-handbook",
"trl",
"dpo",
"generated_from_trainer",
"conversational",
"dataset:updated",
"dataset:original",
"base_model:ShenaoZ/0.001_withdpo_4iters_bs256_555lr_iter_1",
"base_model:finetune:ShenaoZ/0.001_withdpo_4iters_bs256_555lr_iter_1",
"license:mit",
"autotrain_compatible",
"text-generation-inference",
"endpoints_compatible",
"region:us"
] | text-generation | 2024-05-09T01:53:54Z | ---
license: mit
base_model: ShenaoZ/0.001_withdpo_4iters_bs256_555lr_iter_1
tags:
- alignment-handbook
- trl
- dpo
- generated_from_trainer
- trl
- dpo
- generated_from_trainer
datasets:
- updated
- original
model-index:
- name: 0.001_withdpo_4iters_bs256_555lr_iter_2
results: []
---
<!-- This model card has been generated automatically according to the information the Trainer had access to. You
should probably proofread and complete it, then remove this comment. -->
# 0.001_withdpo_4iters_bs256_555lr_iter_2
This model is a fine-tuned version of [ShenaoZ/0.001_withdpo_4iters_bs256_555lr_iter_1](https://huggingface.co/ShenaoZ/0.001_withdpo_4iters_bs256_555lr_iter_1) on the updated and the original datasets.
## Model description
More information needed
## Intended uses & limitations
More information needed
## Training and evaluation data
More information needed
## Training procedure
### Training hyperparameters
The following hyperparameters were used during training:
- learning_rate: 5e-07
- train_batch_size: 8
- eval_batch_size: 8
- seed: 42
- distributed_type: multi-GPU
- num_devices: 8
- gradient_accumulation_steps: 4
- total_train_batch_size: 256
- total_eval_batch_size: 64
- optimizer: Adam with betas=(0.9,0.999) and epsilon=1e-08
- lr_scheduler_type: cosine
- lr_scheduler_warmup_ratio: 0.1
- num_epochs: 1
### Training results
### Framework versions
- Transformers 4.40.2
- Pytorch 2.1.2+cu121
- Datasets 2.14.6
- Tokenizers 0.19.1
|
chronbmm/sanskrit5-multitask | chronbmm | 2024-05-09T02:40:24Z | 210 | 1 | transformers | [
"transformers",
"safetensors",
"t5",
"text2text-generation",
"arxiv:1910.09700",
"autotrain_compatible",
"text-generation-inference",
"endpoints_compatible",
"region:us"
] | text2text-generation | 2024-05-09T02:38:34Z | ---
library_name: transformers
tags: []
---
# Model Card for Model ID
<!-- Provide a quick summary of what the model is/does. -->
## Model Details
### Model Description
<!-- Provide a longer summary of what this model is. -->
This is the model card of a 🤗 transformers model that has been pushed on the Hub. This model card has been automatically generated.
- **Developed by:** [More Information Needed]
- **Funded by [optional]:** [More Information Needed]
- **Shared by [optional]:** [More Information Needed]
- **Model type:** [More Information Needed]
- **Language(s) (NLP):** [More Information Needed]
- **License:** [More Information Needed]
- **Finetuned from model [optional]:** [More Information Needed]
### Model Sources [optional]
<!-- Provide the basic links for the model. -->
- **Repository:** [More Information Needed]
- **Paper [optional]:** [More Information Needed]
- **Demo [optional]:** [More Information Needed]
## Uses
<!-- Address questions around how the model is intended to be used, including the foreseeable users of the model and those affected by the model. -->
### Direct Use
<!-- This section is for the model use without fine-tuning or plugging into a larger ecosystem/app. -->
[More Information Needed]
### Downstream Use [optional]
<!-- This section is for the model use when fine-tuned for a task, or when plugged into a larger ecosystem/app -->
[More Information Needed]
### Out-of-Scope Use
<!-- This section addresses misuse, malicious use, and uses that the model will not work well for. -->
[More Information Needed]
## Bias, Risks, and Limitations
<!-- This section is meant to convey both technical and sociotechnical limitations. -->
[More Information Needed]
### Recommendations
<!-- This section is meant to convey recommendations with respect to the bias, risk, and technical limitations. -->
Users (both direct and downstream) should be made aware of the risks, biases and limitations of the model. More information needed for further recommendations.
## How to Get Started with the Model
Use the code below to get started with the model.
[More Information Needed]
## Training Details
### Training Data
<!-- This should link to a Dataset Card, perhaps with a short stub of information on what the training data is all about as well as documentation related to data pre-processing or additional filtering. -->
[More Information Needed]
### Training Procedure
<!-- This relates heavily to the Technical Specifications. Content here should link to that section when it is relevant to the training procedure. -->
#### Preprocessing [optional]
[More Information Needed]
#### Training Hyperparameters
- **Training regime:** [More Information Needed] <!--fp32, fp16 mixed precision, bf16 mixed precision, bf16 non-mixed precision, fp16 non-mixed precision, fp8 mixed precision -->
#### Speeds, Sizes, Times [optional]
<!-- This section provides information about throughput, start/end time, checkpoint size if relevant, etc. -->
[More Information Needed]
## Evaluation
<!-- This section describes the evaluation protocols and provides the results. -->
### Testing Data, Factors & Metrics
#### Testing Data
<!-- This should link to a Dataset Card if possible. -->
[More Information Needed]
#### Factors
<!-- These are the things the evaluation is disaggregating by, e.g., subpopulations or domains. -->
[More Information Needed]
#### Metrics
<!-- These are the evaluation metrics being used, ideally with a description of why. -->
[More Information Needed]
### Results
[More Information Needed]
#### Summary
## Model Examination [optional]
<!-- Relevant interpretability work for the model goes here -->
[More Information Needed]
## Environmental Impact
<!-- Total emissions (in grams of CO2eq) and additional considerations, such as electricity usage, go here. Edit the suggested text below accordingly -->
Carbon emissions can be estimated using the [Machine Learning Impact calculator](https://mlco2.github.io/impact#compute) presented in [Lacoste et al. (2019)](https://arxiv.org/abs/1910.09700).
- **Hardware Type:** [More Information Needed]
- **Hours used:** [More Information Needed]
- **Cloud Provider:** [More Information Needed]
- **Compute Region:** [More Information Needed]
- **Carbon Emitted:** [More Information Needed]
## Technical Specifications [optional]
### Model Architecture and Objective
[More Information Needed]
### Compute Infrastructure
[More Information Needed]
#### Hardware
[More Information Needed]
#### Software
[More Information Needed]
## Citation [optional]
<!-- If there is a paper or blog post introducing the model, the APA and Bibtex information for that should go in this section. -->
**BibTeX:**
[More Information Needed]
**APA:**
[More Information Needed]
## Glossary [optional]
<!-- If relevant, include terms and calculations in this section that can help readers understand the model or model card. -->
[More Information Needed]
## More Information [optional]
[More Information Needed]
## Model Card Authors [optional]
[More Information Needed]
## Model Card Contact
[More Information Needed]
|
wangffeing/q-FrozenLake-v1-4x4-noSlippery | wangffeing | 2024-05-09T02:37:08Z | 0 | 0 | null | [
"FrozenLake-v1-4x4-no_slippery",
"q-learning",
"reinforcement-learning",
"custom-implementation",
"model-index",
"region:us"
] | reinforcement-learning | 2024-05-09T02:37:05Z | ---
tags:
- FrozenLake-v1-4x4-no_slippery
- q-learning
- reinforcement-learning
- custom-implementation
model-index:
- name: q-FrozenLake-v1-4x4-noSlippery
results:
- task:
type: reinforcement-learning
name: reinforcement-learning
dataset:
name: FrozenLake-v1-4x4-no_slippery
type: FrozenLake-v1-4x4-no_slippery
metrics:
- type: mean_reward
value: 1.00 +/- 0.00
name: mean_reward
verified: false
---
# **Q-Learning** Agent playing1 **FrozenLake-v1**
This is a trained model of a **Q-Learning** agent playing **FrozenLake-v1** .
## Usage
```python
model = load_from_hub(repo_id="wangffeing/q-FrozenLake-v1-4x4-noSlippery", filename="q-learning.pkl")
# Don't forget to check if you need to add additional attributes (is_slippery=False etc)
env = gym.make(model["env_id"])
```
|
Holarissun/dpo_tldrtldr_gpt3_subset10000_modelgemma2b_maxsteps5000_bz8_lr5e-06 | Holarissun | 2024-05-09T02:35:10Z | 0 | 0 | peft | [
"peft",
"safetensors",
"trl",
"dpo",
"generated_from_trainer",
"base_model:google/gemma-2b",
"base_model:adapter:google/gemma-2b",
"license:gemma",
"region:us"
] | null | 2024-05-09T02:35:08Z | ---
license: gemma
library_name: peft
tags:
- trl
- dpo
- generated_from_trainer
base_model: google/gemma-2b
model-index:
- name: dpo_tldrtldr_gpt3_subset10000_modelgemma2b_maxsteps5000_bz8_lr5e-06
results: []
---
<!-- This model card has been generated automatically according to the information the Trainer had access to. You
should probably proofread and complete it, then remove this comment. -->
# dpo_tldrtldr_gpt3_subset10000_modelgemma2b_maxsteps5000_bz8_lr5e-06
This model is a fine-tuned version of [google/gemma-2b](https://huggingface.co/google/gemma-2b) on an unknown dataset.
## Model description
More information needed
## Intended uses & limitations
More information needed
## Training and evaluation data
More information needed
## Training procedure
### Training hyperparameters
The following hyperparameters were used during training:
- learning_rate: 5e-06
- train_batch_size: 1
- eval_batch_size: 8
- seed: 42
- gradient_accumulation_steps: 8
- total_train_batch_size: 8
- optimizer: Adam with betas=(0.9,0.999) and epsilon=1e-08
- lr_scheduler_type: linear
- lr_scheduler_warmup_steps: 15
- training_steps: 5000
### Training results
### Framework versions
- PEFT 0.9.0
- Transformers 4.38.2
- Pytorch 2.1.2
- Datasets 2.18.0
- Tokenizers 0.15.2 |
chuxin-llm/Chuxin-1.6B-1M | chuxin-llm | 2024-05-09T02:34:30Z | 87 | 9 | transformers | [
"transformers",
"pytorch",
"llama",
"text-generation",
"arxiv:2405.04828",
"license:mit",
"autotrain_compatible",
"text-generation-inference",
"endpoints_compatible",
"region:us"
] | text-generation | 2024-05-07T12:36:01Z | ---
license: mit
---
# Chuxin-1.6B-1M
<br>
## 介绍 (Introduction)
**Chuxin-1.6B-Base**是16亿参数规模的模型。Chuxin-1.6B完全基于开源数据构建,在经过超大规模数据训练后,Chuxin-1.6B在各类下游任务上具有非常的竞争力。
**Chuxin-1.6B-1M**是基于Chuxin-1.6B模型在1M窗口下训练后的结果,大海捞针实验显示其具有非常强的上下文检索能力。
如果您想了解更多关于Chuxin-1.6B开源模型的细节,我们建议您参阅我们的[技术报告](https://arxiv.org/pdf/2405.04828)
**Chuxin-1.6B-Base** is a model with 1.6 billion parameters. Chuxin-1.6B is built entirely on open-source data. After being trained with large-scale data, Chuxin has very competitive capabilities in various downstream tasks.
**Chuxin-1.6B-1M** is the result of training the Chuxin-1.6B model with a 1M windows. Experiments such as searching for a needle in a haystack demonstrate its strong contextual retrieval abilities.
If you would like to learn more about the Chuxin-1.6B open-source model, we suggest you refer to our [technical report](https://arxiv.org/pdf/2405.04828).
<br>
## 快速使用(Quickstart)
您可以通过以下代码轻松调用:
You can easily call the model with the following code:
```python
from transformers import AutoModelForCausalLM, AutoTokenizer
tokenizer = AutoTokenizer.from_pretrained("chuxin-llm/Chuxin-1.6B-1M", trust_remote_code=True)
model = AutoModelForCausalLM.from_pretrained("chuxin-llm/Chuxin-1.6B-1M", device_map="auto", trust_remote_code=True, bf16=True).eval()
inputs = tokenizer('蒙古国的首都是乌兰巴托(Ulaanbaatar)\n冰岛的首都是雷克雅未克(Reykjavik)\n埃塞俄比亚的首都是', return_tensors='pt')
inputs = inputs.to(model.device)
pred = model.generate(**inputs, max_new_tokens=15, do_sample=False)
print(tokenizer.decode(pred.cpu()[0], skip_special_tokens=True))
# 蒙古国的首都是乌兰巴托(Ulaanbaatar)\n冰岛的首都是雷克雅未克(Reykjavik)\n埃塞俄比亚的首都是亚的斯亚贝巴(Addis Ababa)...
```
## 评测效果(Evaluation)
### 常识推理和阅读理解 (Common Sense Reasoning and Reading Comprehension tasks)
| Model | size | ARC-c |ARC-e |Boolq |Copa |Hellaswag |OpenbookQA |Piqa |Sciq |Winogrande |Avg|
|:--------------|:----------:|:-----------:|:-----------:|:-----------:|:-----------:|:-----------:|:-----------:|:-----------:|:-----------:|:-----------:|:-----------:|
| chuxin-1.6B-base | 1.6B | 39.68 | 71.38 | 71.25 | 83 | 66.09 | 35.00 | 77.09 | 95 | 63.54 | 66.89 |
| chuxin-1.6B-32k | 1.6B | 39.16 | 70.66 | 67.71 | 81 | 65.69 | 35.8 | 76.88 | 94.2 | 62.51 | 65.96 |
| chuxin-1.6B-64k | 1.6B | 38.48 | 70.24 | 67.52 | 82 | 65.6 | 35.2 | 76.61 | 94.3 | 63.3 | 65.92 |
| chuxin-1.6B-128k | 1.6B | 39.08 | 69.4 | 67.71 | 80 | 65.74 | 35.4 | 76.39 | 94.1 | 63.3 | 65.68 |
| chuxin-1.6B-256k | 1.6B | 40.19 | 70.75 | 69.3 | 78 | 65.85 | 35.8 | 76.88 | 93.5 | 63.85 | 66.01 |
| chuxin-1.6B-512k | 1.6B | 40.61 |71.21| 67.77 |78| 64.82| 34.8| 76.88| 93.6| 61.88| 65.51|
| chuxin-1.6B-1M | 1.6B | 41.13| 72.26| 62.08| 75| 64.59 |34.8| 76.71| 93.33| 62.43| 64.7|
### Open LLM LeaderBoard
| Model | size | ARC-c |HellaSwag|MMLU |TruthfulQA |Winogrande |GSM-8k |Avg |Avg wo GSM|
|:--------------|:----------:|:-----------:|:-----------:|:-----------:|:-----------:|:-----------:|:-----------:|:-----------:|:-----------:|
| chuxin-1.6B-base | 1.6B | 39.68 | 66.09 | 41.07 | 37.65 | 63.54 | 12.66 | 43.45 |49.61|
| chuxin-1.6B-32k | 1.6B | 39.16 | 65.69 | 38.63 | 35.66 | 62.51 | 11.6 | 42.21 | 48.33|
| chuxin-1.6B-64k | 1.6B | 38.48 | 65.6 | 38.43 | 35.07 | 63.3 | 11.9 | 42.13|48.18|
| chuxin-1.6B-128k | 1.6B | 39.08 | 65.74 | 37.65 | 34.89 | 63.3 | 11.07 | 41.96|48.13|
| chuxin-1.6B-256k | 1.6B | 40.19 | 65.85 | 37.16 | 35.2 | 63.85 | 10.16 | 42.07 |48.45|
| chuxin-1.6B-512k | 1.6B | 40.61| 64.82| 36.66| 33.66| 61.88| 8.11| 40.96| 47.53|
| Chuxin-1.6B-1M | 1.6B | 41.13 |64.59| 35.76| 34.67| 62.43| 6.82| 40.9| 47.72|
### 大海捞针 (needle in a haystack)
<p align="center">
<img src="niah.png" style="width: 1200px"/>
<p>
## 引用 (Citation)
如果你觉得我们的工作对你有帮助,欢迎引用!
If you find our work helpful, feel free to give us a cite.
```
@article{chuxin,
title={CHUXIN: 1.6B TECHNICAL REPORT},
author={Xiaomin Zhuang, Yufan Jiang, Qiaozhi He, Zhihua Wu},
journal={arXiv preprint arXiv:2405.04828},
year={2024}
}
```
<br> |
cognitivecomputations/Llama-3-8B-Instruct-abliterated-v2-gguf | cognitivecomputations | 2024-05-09T02:31:41Z | 59 | 19 | transformers | [
"transformers",
"gguf",
"license:llama3",
"endpoints_compatible",
"region:us",
"conversational"
] | null | 2024-05-09T02:03:38Z | ---
library_name: transformers
license: llama3
---
# Model Card for Llama-3-8B-Instruct-abliterated-v2
## Overview
This model card describes the Llama-3-8B-Instruct-abliterated-v2 model, which is an orthogonalized version of the meta-llama/Llama-3-8B-Instruct model, and an improvement upon the previous generation Llama-3-8B-Instruct-abliterated. This variant has had certain weights manipulated to inhibit the model's ability to express refusal.
[Join the Cognitive Computations Discord!](https://discord.gg/cognitivecomputations)
## Details
* The model was trained with more data to better pinpoint the "refusal direction".
* This model is MUCH better at directly and succinctly answering requests without producing even so much as disclaimers.
## Methodology
The methodology used to generate this model is described in the preview paper/blog post: '[Refusal in LLMs is mediated by a single direction](https://www.alignmentforum.org/posts/jGuXSZgv6qfdhMCuJ/refusal-in-llms-is-mediated-by-a-single-direction)'
## Quirks and Side Effects
This model may come with interesting quirks, as the methodology is still new and untested. The code used to generate the model is available in the Python notebook [ortho_cookbook.ipynb](https://huggingface.co/failspy/llama-3-70B-Instruct-abliterated/blob/main/ortho_cookbook.ipynb).
Please note that the model may still refuse to answer certain requests, even after the weights have been manipulated to inhibit refusal. |
RichardErkhov/DeepMount00_-_mamba_790_hf_qa-8bits | RichardErkhov | 2024-05-09T02:29:48Z | 77 | 0 | transformers | [
"transformers",
"safetensors",
"mamba",
"text-generation",
"autotrain_compatible",
"text-generation-inference",
"endpoints_compatible",
"8-bit",
"bitsandbytes",
"region:us"
] | text-generation | 2024-05-09T02:15:38Z | Quantization made by Richard Erkhov.
[Github](https://github.com/RichardErkhov)
[Discord](https://discord.gg/pvy7H8DZMG)
[Request more models](https://github.com/RichardErkhov/quant_request)
mamba_790_hf_qa - bnb 8bits
- Model creator: https://huggingface.co/DeepMount00/
- Original model: https://huggingface.co/DeepMount00/mamba_790_hf_qa/
Original model description:
---
language:
- it
license: apache-2.0
datasets:
- DeepMount00/gquad_it
pipeline_tag: question-answering
---
## SQuAD-it Evaluation
The Stanford Question Answering Dataset (SQuAD) in Italian (SQuAD-it) is used to evaluate the model's reading comprehension and question-answering capabilities. The following table presents the F1 score and Exact Match (EM) metrics:
| Model | F1 Score | Exact Match (EM) |
|----------------------------------------------|----------|------------------|
| **DeepMount00/Gemma_QA_ITA_v3** | **77.24%** | **64.60%** |
| **DeepMount00/Gemma_QA_ITA_v2** | **77.17%** | **63.82%** |
| **DeepMount00/mamba_790_hf_qa** | **75.89%** | **66.71%** |
| **DeepMount00/Gemma_QA_ITA** | **59.59%** | **40.68%** |
## How to Use
How to use mamba q&a
```python
from transformers import MambaConfig, MambaForCausalLM, AutoTokenizer
import torch
model_name = "DeepMount00/mamba_790_hf_qa"
tokenizer = AutoTokenizer.from_pretrained(model_name)
model = MambaForCausalLM.from_pretrained(model_name, device_map={"": 0}).eval()
def predict(contesto, domanda):
device = "cuda:0"
prefix_text = 'Di seguito ti verrà fornito un contesto e poi una domanda. il tuo compito è quello di rispondere alla domanda basandoti esclusivamente sul contesto\n\n'
prompt = f"""{prefix_text}##CONTESTO: {contesto}\n##DOMANDA: {domanda}\n"""
input_ids = tokenizer([prompt], return_tensors="pt").to(device)
generate_ids = model.generate(**input_ids, max_new_tokens=150, eos_token_id=8112)
answer = tokenizer.batch_decode(generate_ids)
try:
final_answer = answer[0].split("##RISPOSTA: ")[1].split("##END")[0].strip("\n")
except IndexError:
final_answer = ""
return final_answer
contesto = """La torre degli Asinelli è una delle cosiddette due torri di Bologna, simbolo della città, situate in piazza di porta Ravegnana, all'incrocio tra le antiche strade San Donato (ora via Zamboni), San Vitale, Maggiore e Castiglione. Eretta, secondo la tradizione, fra il 1109 e il 1119 dal nobile Gherardo Asinelli, la torre è alta 97,20 metri, pende verso ovest per 2,23 metri e presenta all'interno una scalinata composta da 498 gradini. Ancora non si può dire con certezza quando e da chi fu costruita la torre degli Asinelli. Si presume che la torre debba il proprio nome a Gherardo Asinelli, il nobile cavaliere di fazione ghibellina al quale se ne attribuisce la costruzione, iniziata secondo una consolidata tradizione l'11 ottobre 1109 e terminata dieci anni dopo, nel 1119."""
domanda = "Dove si trova precisamente la torre degli Asinelli?"
print(predict(contesto, domanda))
```
|
Anurag-310/medical-chatbot | Anurag-310 | 2024-05-09T02:28:03Z | 0 | 0 | null | [
"license:apache-2.0",
"region:us"
] | null | 2024-05-09T02:28:03Z | ---
license: apache-2.0
---
|
uirev/MLX-gemma-Code-Instruct-Finetune-test | uirev | 2024-05-09T02:24:03Z | 77 | 0 | transformers | [
"transformers",
"safetensors",
"gemma",
"text-generation",
"mlx",
"conversational",
"autotrain_compatible",
"text-generation-inference",
"endpoints_compatible",
"region:us"
] | text-generation | 2024-05-09T02:21:19Z | ---
library_name: transformers
tags:
- mlx
---
# Paramstr/MLX-gemma-Code-Instruct-Finetune-test
This model was converted to MLX format from [`Paramstr/gemma-Code-Instruct-Finetune-test`]() using mlx-lm version **0.4.0**.
Refer to the [original model card](https://huggingface.co/Paramstr/gemma-Code-Instruct-Finetune-test) for more details on the model.
## Use with mlx
```bash
pip install mlx-lm
```
```python
from mlx_lm import load, generate
model, tokenizer = load("Paramstr/MLX-gemma-Code-Instruct-Finetune-test")
response = generate(model, tokenizer, prompt="hello", verbose=True)
```
|
jsfamily/korean-small_t2 | jsfamily | 2024-05-09T02:18:42Z | 88 | 0 | transformers | [
"transformers",
"tensorboard",
"safetensors",
"whisper",
"automatic-speech-recognition",
"hf-asr-leaderboard",
"generated_from_trainer",
"ko",
"dataset:jsfamily/test-small-komodel",
"base_model:openai/whisper-small",
"base_model:finetune:openai/whisper-small",
"license:apache-2.0",
"endpoints_compatible",
"region:us"
] | automatic-speech-recognition | 2024-05-09T01:43:31Z | ---
language:
- ko
license: apache-2.0
tags:
- hf-asr-leaderboard
- generated_from_trainer
base_model: openai/whisper-small
datasets:
- jsfamily/test-small-komodel
model-index:
- name: test-small-komodel
results: []
---
<!-- This model card has been generated automatically according to the information the Trainer had access to. You
should probably proofread and complete it, then remove this comment. -->
# test-small-komodel
This model is a fine-tuned version of [openai/whisper-small](https://huggingface.co/openai/whisper-small) on the jsfamily/test-small-komodel dataset.
It achieves the following results on the evaluation set:
- eval_loss: 0.7197
- eval_cer: 13.8258
- eval_runtime: 73.4922
- eval_samples_per_second: 2.735
- eval_steps_per_second: 0.354
- step: 0
## Model description
More information needed
## Intended uses & limitations
More information needed
## Training and evaluation data
More information needed
## Training procedure
### Training hyperparameters
The following hyperparameters were used during training:
- learning_rate: 1e-05
- train_batch_size: 16
- eval_batch_size: 8
- seed: 42
- optimizer: Adam with betas=(0.9,0.999) and epsilon=1e-08
- lr_scheduler_type: linear
- lr_scheduler_warmup_steps: 500
- num_epochs: 3.0
- mixed_precision_training: Native AMP
### Framework versions
- Transformers 4.39.0.dev0
- Pytorch 2.2.1+cu121
- Datasets 2.18.0
- Tokenizers 0.15.2
|
azhara001/donut-base-demo-new-v2-3e-05_AdamW_3752 | azhara001 | 2024-05-09T02:17:38Z | 49 | 0 | transformers | [
"transformers",
"safetensors",
"vision-encoder-decoder",
"image-text-to-text",
"arxiv:1910.09700",
"endpoints_compatible",
"region:us"
] | image-text-to-text | 2024-05-09T02:17:03Z | ---
library_name: transformers
tags: []
---
# Model Card for Model ID
<!-- Provide a quick summary of what the model is/does. -->
## Model Details
### Model Description
<!-- Provide a longer summary of what this model is. -->
This is the model card of a 🤗 transformers model that has been pushed on the Hub. This model card has been automatically generated.
- **Developed by:** [More Information Needed]
- **Funded by [optional]:** [More Information Needed]
- **Shared by [optional]:** [More Information Needed]
- **Model type:** [More Information Needed]
- **Language(s) (NLP):** [More Information Needed]
- **License:** [More Information Needed]
- **Finetuned from model [optional]:** [More Information Needed]
### Model Sources [optional]
<!-- Provide the basic links for the model. -->
- **Repository:** [More Information Needed]
- **Paper [optional]:** [More Information Needed]
- **Demo [optional]:** [More Information Needed]
## Uses
<!-- Address questions around how the model is intended to be used, including the foreseeable users of the model and those affected by the model. -->
### Direct Use
<!-- This section is for the model use without fine-tuning or plugging into a larger ecosystem/app. -->
[More Information Needed]
### Downstream Use [optional]
<!-- This section is for the model use when fine-tuned for a task, or when plugged into a larger ecosystem/app -->
[More Information Needed]
### Out-of-Scope Use
<!-- This section addresses misuse, malicious use, and uses that the model will not work well for. -->
[More Information Needed]
## Bias, Risks, and Limitations
<!-- This section is meant to convey both technical and sociotechnical limitations. -->
[More Information Needed]
### Recommendations
<!-- This section is meant to convey recommendations with respect to the bias, risk, and technical limitations. -->
Users (both direct and downstream) should be made aware of the risks, biases and limitations of the model. More information needed for further recommendations.
## How to Get Started with the Model
Use the code below to get started with the model.
[More Information Needed]
## Training Details
### Training Data
<!-- This should link to a Dataset Card, perhaps with a short stub of information on what the training data is all about as well as documentation related to data pre-processing or additional filtering. -->
[More Information Needed]
### Training Procedure
<!-- This relates heavily to the Technical Specifications. Content here should link to that section when it is relevant to the training procedure. -->
#### Preprocessing [optional]
[More Information Needed]
#### Training Hyperparameters
- **Training regime:** [More Information Needed] <!--fp32, fp16 mixed precision, bf16 mixed precision, bf16 non-mixed precision, fp16 non-mixed precision, fp8 mixed precision -->
#### Speeds, Sizes, Times [optional]
<!-- This section provides information about throughput, start/end time, checkpoint size if relevant, etc. -->
[More Information Needed]
## Evaluation
<!-- This section describes the evaluation protocols and provides the results. -->
### Testing Data, Factors & Metrics
#### Testing Data
<!-- This should link to a Dataset Card if possible. -->
[More Information Needed]
#### Factors
<!-- These are the things the evaluation is disaggregating by, e.g., subpopulations or domains. -->
[More Information Needed]
#### Metrics
<!-- These are the evaluation metrics being used, ideally with a description of why. -->
[More Information Needed]
### Results
[More Information Needed]
#### Summary
## Model Examination [optional]
<!-- Relevant interpretability work for the model goes here -->
[More Information Needed]
## Environmental Impact
<!-- Total emissions (in grams of CO2eq) and additional considerations, such as electricity usage, go here. Edit the suggested text below accordingly -->
Carbon emissions can be estimated using the [Machine Learning Impact calculator](https://mlco2.github.io/impact#compute) presented in [Lacoste et al. (2019)](https://arxiv.org/abs/1910.09700).
- **Hardware Type:** [More Information Needed]
- **Hours used:** [More Information Needed]
- **Cloud Provider:** [More Information Needed]
- **Compute Region:** [More Information Needed]
- **Carbon Emitted:** [More Information Needed]
## Technical Specifications [optional]
### Model Architecture and Objective
[More Information Needed]
### Compute Infrastructure
[More Information Needed]
#### Hardware
[More Information Needed]
#### Software
[More Information Needed]
## Citation [optional]
<!-- If there is a paper or blog post introducing the model, the APA and Bibtex information for that should go in this section. -->
**BibTeX:**
[More Information Needed]
**APA:**
[More Information Needed]
## Glossary [optional]
<!-- If relevant, include terms and calculations in this section that can help readers understand the model or model card. -->
[More Information Needed]
## More Information [optional]
[More Information Needed]
## Model Card Authors [optional]
[More Information Needed]
## Model Card Contact
[More Information Needed] |
RichardErkhov/DeepMount00_-_mamba_790_hf_qa-4bits | RichardErkhov | 2024-05-09T02:15:33Z | 78 | 0 | transformers | [
"transformers",
"safetensors",
"mamba",
"text-generation",
"autotrain_compatible",
"text-generation-inference",
"endpoints_compatible",
"4-bit",
"bitsandbytes",
"region:us"
] | text-generation | 2024-05-09T02:05:09Z | Quantization made by Richard Erkhov.
[Github](https://github.com/RichardErkhov)
[Discord](https://discord.gg/pvy7H8DZMG)
[Request more models](https://github.com/RichardErkhov/quant_request)
mamba_790_hf_qa - bnb 4bits
- Model creator: https://huggingface.co/DeepMount00/
- Original model: https://huggingface.co/DeepMount00/mamba_790_hf_qa/
Original model description:
---
language:
- it
license: apache-2.0
datasets:
- DeepMount00/gquad_it
pipeline_tag: question-answering
---
## SQuAD-it Evaluation
The Stanford Question Answering Dataset (SQuAD) in Italian (SQuAD-it) is used to evaluate the model's reading comprehension and question-answering capabilities. The following table presents the F1 score and Exact Match (EM) metrics:
| Model | F1 Score | Exact Match (EM) |
|----------------------------------------------|----------|------------------|
| **DeepMount00/Gemma_QA_ITA_v3** | **77.24%** | **64.60%** |
| **DeepMount00/Gemma_QA_ITA_v2** | **77.17%** | **63.82%** |
| **DeepMount00/mamba_790_hf_qa** | **75.89%** | **66.71%** |
| **DeepMount00/Gemma_QA_ITA** | **59.59%** | **40.68%** |
## How to Use
How to use mamba q&a
```python
from transformers import MambaConfig, MambaForCausalLM, AutoTokenizer
import torch
model_name = "DeepMount00/mamba_790_hf_qa"
tokenizer = AutoTokenizer.from_pretrained(model_name)
model = MambaForCausalLM.from_pretrained(model_name, device_map={"": 0}).eval()
def predict(contesto, domanda):
device = "cuda:0"
prefix_text = 'Di seguito ti verrà fornito un contesto e poi una domanda. il tuo compito è quello di rispondere alla domanda basandoti esclusivamente sul contesto\n\n'
prompt = f"""{prefix_text}##CONTESTO: {contesto}\n##DOMANDA: {domanda}\n"""
input_ids = tokenizer([prompt], return_tensors="pt").to(device)
generate_ids = model.generate(**input_ids, max_new_tokens=150, eos_token_id=8112)
answer = tokenizer.batch_decode(generate_ids)
try:
final_answer = answer[0].split("##RISPOSTA: ")[1].split("##END")[0].strip("\n")
except IndexError:
final_answer = ""
return final_answer
contesto = """La torre degli Asinelli è una delle cosiddette due torri di Bologna, simbolo della città, situate in piazza di porta Ravegnana, all'incrocio tra le antiche strade San Donato (ora via Zamboni), San Vitale, Maggiore e Castiglione. Eretta, secondo la tradizione, fra il 1109 e il 1119 dal nobile Gherardo Asinelli, la torre è alta 97,20 metri, pende verso ovest per 2,23 metri e presenta all'interno una scalinata composta da 498 gradini. Ancora non si può dire con certezza quando e da chi fu costruita la torre degli Asinelli. Si presume che la torre debba il proprio nome a Gherardo Asinelli, il nobile cavaliere di fazione ghibellina al quale se ne attribuisce la costruzione, iniziata secondo una consolidata tradizione l'11 ottobre 1109 e terminata dieci anni dopo, nel 1119."""
domanda = "Dove si trova precisamente la torre degli Asinelli?"
print(predict(contesto, domanda))
```
|
rengjey/llama3-8b-oig-unsloth | rengjey | 2024-05-09T02:13:36Z | 0 | 0 | transformers | [
"transformers",
"safetensors",
"text-generation-inference",
"unsloth",
"llama",
"trl",
"en",
"base_model:unsloth/llama-3-8b-bnb-4bit",
"base_model:finetune:unsloth/llama-3-8b-bnb-4bit",
"license:apache-2.0",
"endpoints_compatible",
"region:us"
] | null | 2024-05-09T02:13:22Z | ---
language:
- en
license: apache-2.0
tags:
- text-generation-inference
- transformers
- unsloth
- llama
- trl
base_model: unsloth/llama-3-8b-bnb-4bit
---
# Uploaded model
- **Developed by:** rengjey
- **License:** apache-2.0
- **Finetuned from model :** unsloth/llama-3-8b-bnb-4bit
This llama model was trained 2x faster with [Unsloth](https://github.com/unslothai/unsloth) and Huggingface's TRL library.
[<img src="https://raw.githubusercontent.com/unslothai/unsloth/main/images/unsloth%20made%20with%20love.png" width="200"/>](https://github.com/unslothai/unsloth)
|
rengjey/llama3-8b-oig-unsloth-merged | rengjey | 2024-05-09T02:13:10Z | 2 | 0 | transformers | [
"transformers",
"pytorch",
"llama",
"text-generation",
"text-generation-inference",
"unsloth",
"trl",
"sft",
"en",
"base_model:unsloth/llama-3-8b-bnb-4bit",
"base_model:finetune:unsloth/llama-3-8b-bnb-4bit",
"license:apache-2.0",
"autotrain_compatible",
"endpoints_compatible",
"region:us"
] | text-generation | 2024-05-09T02:06:05Z | ---
language:
- en
license: apache-2.0
tags:
- text-generation-inference
- transformers
- unsloth
- llama
- trl
- sft
base_model: unsloth/llama-3-8b-bnb-4bit
---
# Uploaded model
- **Developed by:** rengjey
- **License:** apache-2.0
- **Finetuned from model :** unsloth/llama-3-8b-bnb-4bit
This llama model was trained 2x faster with [Unsloth](https://github.com/unslothai/unsloth) and Huggingface's TRL library.
[<img src="https://raw.githubusercontent.com/unslothai/unsloth/main/images/unsloth%20made%20with%20love.png" width="200"/>](https://github.com/unslothai/unsloth)
|
hskyrockit/rocithero | hskyrockit | 2024-05-09T02:11:55Z | 0 | 0 | null | [
"license:apache-2.0",
"region:us"
] | null | 2024-05-09T02:11:55Z | ---
license: apache-2.0
---
|
dgktrnh/codet5-fine-tuned | dgktrnh | 2024-05-09T02:08:13Z | 109 | 0 | transformers | [
"transformers",
"safetensors",
"t5",
"text2text-generation",
"generated_from_trainer",
"base_model:Salesforce/codet5p-220m",
"base_model:finetune:Salesforce/codet5p-220m",
"license:bsd-3-clause",
"autotrain_compatible",
"text-generation-inference",
"endpoints_compatible",
"region:us"
] | text2text-generation | 2024-04-19T07:27:35Z | ---
license: bsd-3-clause
base_model: Salesforce/codet5p-220m
tags:
- generated_from_trainer
model-index:
- name: codet5-fine-tuned
results: []
---
<!-- This model card has been generated automatically according to the information the Trainer had access to. You
should probably proofread and complete it, then remove this comment. -->
# codet5-fine-tuned
This model is a fine-tuned version of [Salesforce/codet5p-220m](https://huggingface.co/Salesforce/codet5p-220m) on the None dataset.
It achieves the following results on the evaluation set:
- Loss: 0.0726
## Model description
More information needed
## Intended uses & limitations
More information needed
## Training and evaluation data
More information needed
## Training procedure
### Training hyperparameters
The following hyperparameters were used during training:
- learning_rate: 5e-05
- train_batch_size: 3
- eval_batch_size: 3
- seed: 42
- gradient_accumulation_steps: 4
- total_train_batch_size: 12
- optimizer: Adam with betas=(0.9,0.999) and epsilon=1e-08
- lr_scheduler_type: linear
- num_epochs: 20
### Training results
| Training Loss | Epoch | Step | Validation Loss |
|:-------------:|:-----:|:----:|:---------------:|
| No log | 0.99 | 56 | 0.0618 |
| 0.0882 | 1.99 | 113 | 0.0578 |
| 0.0882 | 3.0 | 170 | 0.0567 |
| 0.0503 | 4.0 | 227 | 0.0560 |
| 0.0503 | 4.99 | 283 | 0.0569 |
| 0.0396 | 5.99 | 340 | 0.0574 |
| 0.0396 | 7.0 | 397 | 0.0584 |
| 0.03 | 8.0 | 454 | 0.0601 |
| 0.0231 | 8.99 | 510 | 0.0612 |
| 0.0231 | 9.99 | 567 | 0.0646 |
| 0.0184 | 11.0 | 624 | 0.0647 |
| 0.0184 | 12.0 | 681 | 0.0665 |
| 0.0146 | 12.99 | 737 | 0.0671 |
| 0.0146 | 13.99 | 794 | 0.0677 |
| 0.012 | 15.0 | 851 | 0.0708 |
| 0.0099 | 16.0 | 908 | 0.0712 |
| 0.0099 | 16.99 | 964 | 0.0725 |
| 0.0087 | 17.99 | 1021 | 0.0720 |
| 0.0087 | 19.0 | 1078 | 0.0727 |
| 0.0079 | 19.74 | 1120 | 0.0726 |
### Framework versions
- Transformers 4.39.3
- Pytorch 2.1.2
- Datasets 2.19.1
- Tokenizers 0.15.2
|
RichardErkhov/mylas02_-_Roberta_SQuaD_FineTuned-8bits | RichardErkhov | 2024-05-09T02:05:28Z | 49 | 0 | transformers | [
"transformers",
"safetensors",
"roberta",
"text-generation",
"autotrain_compatible",
"endpoints_compatible",
"8-bit",
"bitsandbytes",
"region:us"
] | text-generation | 2024-05-09T02:01:31Z | Quantization made by Richard Erkhov.
[Github](https://github.com/RichardErkhov)
[Discord](https://discord.gg/pvy7H8DZMG)
[Request more models](https://github.com/RichardErkhov/quant_request)
Roberta_SQuaD_FineTuned - bnb 8bits
- Model creator: https://huggingface.co/mylas02/
- Original model: https://huggingface.co/mylas02/Roberta_SQuaD_FineTuned/
Original model description:
---
license: mit
base_model: roberta-base
tags:
- generated_from_trainer
model-index:
- name: Roberta_SQuaD_FineTuned
results: []
---
<!-- This model card has been generated automatically according to the information the Trainer had access to. You
should probably proofread and complete it, then remove this comment. -->
# Roberta_SQuaD_FineTuned
This model is a fine-tuned version of [roberta-base](https://huggingface.co/roberta-base) on an unknown dataset.
## Model description
More information needed
## Intended uses & limitations
More information needed
## Training and evaluation data
More information needed
## Training procedure
### Training hyperparameters
The following hyperparameters were used during training:
- learning_rate: 2e-05
- train_batch_size: 16
- eval_batch_size: 16
- seed: 42
- optimizer: Adam with betas=(0.9,0.999) and epsilon=1e-08
- lr_scheduler_type: linear
- num_epochs: 3
### Training results
### Framework versions
- Transformers 4.39.2
- Pytorch 2.2.1+cu121
- Datasets 2.18.0
- Tokenizers 0.15.2
|
RichardErkhov/mylas02_-_Roberta_SQuaD_FineTuned-4bits | RichardErkhov | 2024-05-09T02:01:29Z | 49 | 0 | transformers | [
"transformers",
"safetensors",
"roberta",
"text-generation",
"autotrain_compatible",
"endpoints_compatible",
"4-bit",
"bitsandbytes",
"region:us"
] | text-generation | 2024-05-09T01:57:25Z | Quantization made by Richard Erkhov.
[Github](https://github.com/RichardErkhov)
[Discord](https://discord.gg/pvy7H8DZMG)
[Request more models](https://github.com/RichardErkhov/quant_request)
Roberta_SQuaD_FineTuned - bnb 4bits
- Model creator: https://huggingface.co/mylas02/
- Original model: https://huggingface.co/mylas02/Roberta_SQuaD_FineTuned/
Original model description:
---
license: mit
base_model: roberta-base
tags:
- generated_from_trainer
model-index:
- name: Roberta_SQuaD_FineTuned
results: []
---
<!-- This model card has been generated automatically according to the information the Trainer had access to. You
should probably proofread and complete it, then remove this comment. -->
# Roberta_SQuaD_FineTuned
This model is a fine-tuned version of [roberta-base](https://huggingface.co/roberta-base) on an unknown dataset.
## Model description
More information needed
## Intended uses & limitations
More information needed
## Training and evaluation data
More information needed
## Training procedure
### Training hyperparameters
The following hyperparameters were used during training:
- learning_rate: 2e-05
- train_batch_size: 16
- eval_batch_size: 16
- seed: 42
- optimizer: Adam with betas=(0.9,0.999) and epsilon=1e-08
- lr_scheduler_type: linear
- num_epochs: 3
### Training results
### Framework versions
- Transformers 4.39.2
- Pytorch 2.2.1+cu121
- Datasets 2.18.0
- Tokenizers 0.15.2
|
qunfengd/esm2_t12_35M_UR50D-finetuned-AMP_Classification_HighAccuracy | qunfengd | 2024-05-09T01:59:10Z | 62 | 0 | transformers | [
"transformers",
"tf",
"esm",
"text-classification",
"generated_from_keras_callback",
"base_model:facebook/esm2_t12_35M_UR50D",
"base_model:finetune:facebook/esm2_t12_35M_UR50D",
"license:mit",
"autotrain_compatible",
"endpoints_compatible",
"region:us"
] | text-classification | 2024-05-09T01:58:52Z | ---
license: mit
tags:
- generated_from_keras_callback
base_model: facebook/esm2_t12_35M_UR50D
model-index:
- name: esm2_t12_35M_UR50D-finetuned-AMP_Classification_HighAccuracy
results: []
---
<!-- This model card has been generated automatically according to the information Keras had access to. You should
probably proofread and complete it, then remove this comment. -->
# esm2_t12_35M_UR50D-finetuned-AMP_Classification_HighAccuracy
This model is a fine-tuned version of [facebook/esm2_t12_35M_UR50D](https://huggingface.co/facebook/esm2_t12_35M_UR50D) on an unknown dataset.
It achieves the following results on the evaluation set:
- Train Loss: 0.0059
- Train Accuracy: 0.9981
- Validation Loss: 0.0985
- Validation Accuracy: 0.9769
- Epoch: 9
## Model description
More information needed
## Intended uses & limitations
More information needed
## Training and evaluation data
More information needed
## Training procedure
### Training hyperparameters
The following hyperparameters were used during training:
- optimizer: {'name': 'AdamWeightDecay', 'learning_rate': 2e-05, 'decay': 0.0, 'beta_1': 0.9, 'beta_2': 0.999, 'epsilon': 1e-07, 'amsgrad': False, 'weight_decay_rate': 0.0}
- training_precision: float32
### Training results
| Train Loss | Train Accuracy | Validation Loss | Validation Accuracy | Epoch |
|:----------:|:--------------:|:---------------:|:-------------------:|:-----:|
| 0.1093 | 0.9602 | 0.0862 | 0.9702 | 0 |
| 0.0626 | 0.9763 | 0.0717 | 0.9723 | 1 |
| 0.0372 | 0.9858 | 0.0976 | 0.9713 | 2 |
| 0.0204 | 0.9927 | 0.0913 | 0.9771 | 3 |
| 0.0121 | 0.9964 | 0.0953 | 0.9769 | 4 |
| 0.0109 | 0.9963 | 0.1082 | 0.9752 | 5 |
| 0.0085 | 0.9970 | 0.1057 | 0.9728 | 6 |
| 0.0063 | 0.9981 | 0.1145 | 0.9736 | 7 |
| 0.0051 | 0.9984 | 0.1181 | 0.9755 | 8 |
| 0.0059 | 0.9981 | 0.0985 | 0.9769 | 9 |
### Framework versions
- Transformers 4.40.1
- TensorFlow 2.15.0
- Datasets 2.19.1
- Tokenizers 0.19.1
|
selbl/distilbert_secondtry_model | selbl | 2024-05-09T01:51:41Z | 111 | 0 | transformers | [
"transformers",
"pytorch",
"distilbert",
"question-answering",
"generated_from_trainer",
"base_model:distilbert/distilbert-base-uncased",
"base_model:finetune:distilbert/distilbert-base-uncased",
"license:apache-2.0",
"endpoints_compatible",
"region:us"
] | question-answering | 2024-05-09T01:07:50Z | ---
license: apache-2.0
base_model: distilbert-base-uncased
tags:
- generated_from_trainer
model-index:
- name: distilbert_secondtry_model
results: []
---
<!-- This model card has been generated automatically according to the information the Trainer had access to. You
should probably proofread and complete it, then remove this comment. -->
# distilbert_secondtry_model
This model is a fine-tuned version of [distilbert-base-uncased](https://huggingface.co/distilbert-base-uncased) on the None dataset.
It achieves the following results on the evaluation set:
- Loss: 0.4593
## Model description
More information needed
## Intended uses & limitations
More information needed
## Training and evaluation data
More information needed
## Training procedure
### Training hyperparameters
The following hyperparameters were used during training:
- learning_rate: 2e-05
- train_batch_size: 16
- eval_batch_size: 16
- seed: 42
- optimizer: Adam with betas=(0.9,0.999) and epsilon=1e-08
- lr_scheduler_type: linear
- num_epochs: 3
### Training results
| Training Loss | Epoch | Step | Validation Loss |
|:-------------:|:-----:|:----:|:---------------:|
| No log | 1.0 | 391 | 0.4336 |
| 0.5475 | 2.0 | 782 | 0.4294 |
| 0.3436 | 3.0 | 1173 | 0.4593 |
### Framework versions
- Transformers 4.32.1
- Pytorch 2.3.0.dev20240129
- Datasets 2.14.6
- Tokenizers 0.13.2
|
RichardErkhov/minkhantycc_-_GPT2forQuestionAnswering-4bits | RichardErkhov | 2024-05-09T01:46:45Z | 79 | 0 | transformers | [
"transformers",
"safetensors",
"gpt2",
"text-generation",
"autotrain_compatible",
"text-generation-inference",
"endpoints_compatible",
"4-bit",
"bitsandbytes",
"region:us"
] | text-generation | 2024-05-09T01:43:00Z | Quantization made by Richard Erkhov.
[Github](https://github.com/RichardErkhov)
[Discord](https://discord.gg/pvy7H8DZMG)
[Request more models](https://github.com/RichardErkhov/quant_request)
GPT2forQuestionAnswering - bnb 4bits
- Model creator: https://huggingface.co/minkhantycc/
- Original model: https://huggingface.co/minkhantycc/GPT2forQuestionAnswering/
Original model description:
---
language:
- en
license: mit
library_name: transformers
datasets:
- squad
metrics:
- squad
pipeline_tag: question-answering
---
|
RichardErkhov/aware-ai_-_bart-squadv2-8bits | RichardErkhov | 2024-05-09T01:42:46Z | 77 | 0 | transformers | [
"transformers",
"safetensors",
"bart",
"text-generation",
"arxiv:1910.13461",
"autotrain_compatible",
"endpoints_compatible",
"8-bit",
"bitsandbytes",
"region:us"
] | text-generation | 2024-05-09T01:37:58Z | Quantization made by Richard Erkhov.
[Github](https://github.com/RichardErkhov)
[Discord](https://discord.gg/pvy7H8DZMG)
[Request more models](https://github.com/RichardErkhov/quant_request)
bart-squadv2 - bnb 8bits
- Model creator: https://huggingface.co/aware-ai/
- Original model: https://huggingface.co/aware-ai/bart-squadv2/
Original model description:
---
datasets:
- squad_v2
---
# BART-LARGE finetuned on SQuADv2
This is bart-large model finetuned on SQuADv2 dataset for question answering task
## Model details
BART was propsed in the [paper](https://arxiv.org/abs/1910.13461) **BART: Denoising Sequence-to-Sequence Pre-training for Natural Language Generation, Translation, and Comprehension**.
BART is a seq2seq model intended for both NLG and NLU tasks.
To use BART for question answering tasks, we feed the complete document into the encoder and decoder, and use the top
hidden state of the decoder as a representation for each
word. This representation is used to classify the token. As given in the paper bart-large achives comparable to ROBERTa on SQuAD.
Another notable thing about BART is that it can handle sequences with upto 1024 tokens.
| Param | #Value |
|---------------------|--------|
| encoder layers | 12 |
| decoder layers | 12 |
| hidden size | 4096 |
| num attetion heads | 16 |
| on disk size | 1.63GB |
## Model training
This model was trained with following parameters using simpletransformers wrapper:
```
train_args = {
'learning_rate': 1e-5,
'max_seq_length': 512,
'doc_stride': 512,
'overwrite_output_dir': True,
'reprocess_input_data': False,
'train_batch_size': 8,
'num_train_epochs': 2,
'gradient_accumulation_steps': 2,
'no_cache': True,
'use_cached_eval_features': False,
'save_model_every_epoch': False,
'output_dir': "bart-squadv2",
'eval_batch_size': 32,
'fp16_opt_level': 'O2',
}
```
[You can even train your own model using this colab notebook](https://colab.research.google.com/drive/1I5cK1M_0dLaf5xoewh6swcm5nAInfwHy?usp=sharing)
## Results
```{"correct": 6832, "similar": 4409, "incorrect": 632, "eval_loss": -14.950117511952177}```
## Model in Action 🚀
```python3
from transformers import BartTokenizer, BartForQuestionAnswering
import torch
tokenizer = BartTokenizer.from_pretrained('a-ware/bart-squadv2')
model = BartForQuestionAnswering.from_pretrained('a-ware/bart-squadv2')
question, text = "Who was Jim Henson?", "Jim Henson was a nice puppet"
encoding = tokenizer(question, text, return_tensors='pt')
input_ids = encoding['input_ids']
attention_mask = encoding['attention_mask']
start_scores, end_scores = model(input_ids, attention_mask=attention_mask, output_attentions=False)[:2]
all_tokens = tokenizer.convert_ids_to_tokens(input_ids[0])
answer = ' '.join(all_tokens[torch.argmax(start_scores) : torch.argmax(end_scores)+1])
answer = tokenizer.convert_tokens_to_ids(answer.split())
answer = tokenizer.decode(answer)
#answer => 'a nice puppet'
```
> Created with ❤️ by A-ware UG [](https://github.com/aware-ai)
|
RichardErkhov/aware-ai_-_bart-squadv2-4bits | RichardErkhov | 2024-05-09T01:37:54Z | 78 | 0 | transformers | [
"transformers",
"safetensors",
"bart",
"text-generation",
"arxiv:1910.13461",
"autotrain_compatible",
"endpoints_compatible",
"4-bit",
"bitsandbytes",
"region:us"
] | text-generation | 2024-05-09T01:32:31Z | Quantization made by Richard Erkhov.
[Github](https://github.com/RichardErkhov)
[Discord](https://discord.gg/pvy7H8DZMG)
[Request more models](https://github.com/RichardErkhov/quant_request)
bart-squadv2 - bnb 4bits
- Model creator: https://huggingface.co/aware-ai/
- Original model: https://huggingface.co/aware-ai/bart-squadv2/
Original model description:
---
datasets:
- squad_v2
---
# BART-LARGE finetuned on SQuADv2
This is bart-large model finetuned on SQuADv2 dataset for question answering task
## Model details
BART was propsed in the [paper](https://arxiv.org/abs/1910.13461) **BART: Denoising Sequence-to-Sequence Pre-training for Natural Language Generation, Translation, and Comprehension**.
BART is a seq2seq model intended for both NLG and NLU tasks.
To use BART for question answering tasks, we feed the complete document into the encoder and decoder, and use the top
hidden state of the decoder as a representation for each
word. This representation is used to classify the token. As given in the paper bart-large achives comparable to ROBERTa on SQuAD.
Another notable thing about BART is that it can handle sequences with upto 1024 tokens.
| Param | #Value |
|---------------------|--------|
| encoder layers | 12 |
| decoder layers | 12 |
| hidden size | 4096 |
| num attetion heads | 16 |
| on disk size | 1.63GB |
## Model training
This model was trained with following parameters using simpletransformers wrapper:
```
train_args = {
'learning_rate': 1e-5,
'max_seq_length': 512,
'doc_stride': 512,
'overwrite_output_dir': True,
'reprocess_input_data': False,
'train_batch_size': 8,
'num_train_epochs': 2,
'gradient_accumulation_steps': 2,
'no_cache': True,
'use_cached_eval_features': False,
'save_model_every_epoch': False,
'output_dir': "bart-squadv2",
'eval_batch_size': 32,
'fp16_opt_level': 'O2',
}
```
[You can even train your own model using this colab notebook](https://colab.research.google.com/drive/1I5cK1M_0dLaf5xoewh6swcm5nAInfwHy?usp=sharing)
## Results
```{"correct": 6832, "similar": 4409, "incorrect": 632, "eval_loss": -14.950117511952177}```
## Model in Action 🚀
```python3
from transformers import BartTokenizer, BartForQuestionAnswering
import torch
tokenizer = BartTokenizer.from_pretrained('a-ware/bart-squadv2')
model = BartForQuestionAnswering.from_pretrained('a-ware/bart-squadv2')
question, text = "Who was Jim Henson?", "Jim Henson was a nice puppet"
encoding = tokenizer(question, text, return_tensors='pt')
input_ids = encoding['input_ids']
attention_mask = encoding['attention_mask']
start_scores, end_scores = model(input_ids, attention_mask=attention_mask, output_attentions=False)[:2]
all_tokens = tokenizer.convert_ids_to_tokens(input_ids[0])
answer = ' '.join(all_tokens[torch.argmax(start_scores) : torch.argmax(end_scores)+1])
answer = tokenizer.convert_tokens_to_ids(answer.split())
answer = tokenizer.decode(answer)
#answer => 'a nice puppet'
```
> Created with ❤️ by A-ware UG [](https://github.com/aware-ai)
|
RichardErkhov/aditi2212_-_roberta-finetuned-ChennaiQA-final-8bits | RichardErkhov | 2024-05-09T01:37:26Z | 49 | 0 | transformers | [
"transformers",
"safetensors",
"roberta",
"text-generation",
"autotrain_compatible",
"endpoints_compatible",
"8-bit",
"bitsandbytes",
"region:us"
] | text-generation | 2024-05-09T01:32:44Z | Quantization made by Richard Erkhov.
[Github](https://github.com/RichardErkhov)
[Discord](https://discord.gg/pvy7H8DZMG)
[Request more models](https://github.com/RichardErkhov/quant_request)
roberta-finetuned-ChennaiQA-final - bnb 8bits
- Model creator: https://huggingface.co/aditi2212/
- Original model: https://huggingface.co/aditi2212/roberta-finetuned-ChennaiQA-final/
Original model description:
---
license: cc-by-4.0
base_model: deepset/roberta-base-squad2
tags:
- generated_from_trainer
model-index:
- name: roberta-finetuned-ChennaiQA-final
results: []
---
<!-- This model card has been generated automatically according to the information the Trainer had access to. You
should probably proofread and complete it, then remove this comment. -->
# roberta-finetuned-ChennaiQA-final
This model is a fine-tuned version of [deepset/roberta-base-squad2](https://huggingface.co/deepset/roberta-base-squad2) on the None dataset.
## Model description
More information needed
## Intended uses & limitations
More information needed
## Training and evaluation data
More information needed
## Training procedure
### Training hyperparameters
The following hyperparameters were used during training:
- learning_rate: 2e-05
- train_batch_size: 8
- eval_batch_size: 8
- seed: 42
- distributed_type: multi-GPU
- optimizer: Adam with betas=(0.9,0.999) and epsilon=1e-08
- lr_scheduler_type: linear
- num_epochs: 20
### Training results
### Framework versions
- Transformers 4.39.3
- Pytorch 2.2.2+cu121
- Datasets 2.18.0
- Tokenizers 0.15.2
|
RichardErkhov/Kunalmod_-_finetuned-ja-model-8bits | RichardErkhov | 2024-05-09T01:37:01Z | 50 | 0 | transformers | [
"transformers",
"safetensors",
"roberta",
"text-generation",
"autotrain_compatible",
"endpoints_compatible",
"8-bit",
"bitsandbytes",
"region:us"
] | text-generation | 2024-05-09T01:31:08Z | Quantization made by Richard Erkhov.
[Github](https://github.com/RichardErkhov)
[Discord](https://discord.gg/pvy7H8DZMG)
[Request more models](https://github.com/RichardErkhov/quant_request)
finetuned-ja-model - bnb 8bits
- Model creator: https://huggingface.co/Kunalmod/
- Original model: https://huggingface.co/Kunalmod/finetuned-ja-model/
Original model description:
---
license: mit
base_model: tsmatz/roberta_qa_japanese
tags:
- generated_from_trainer
model-index:
- name: finetuned-ja-model
results: []
---
<!-- This model card has been generated automatically according to the information the Trainer had access to. You
should probably proofread and complete it, then remove this comment. -->
# finetuned-ja-model
This model is a fine-tuned version of [tsmatz/roberta_qa_japanese](https://huggingface.co/tsmatz/roberta_qa_japanese) on the None dataset.
## Model description
More information needed
## Intended uses & limitations
More information needed
## Training and evaluation data
More information needed
## Training procedure
### Training hyperparameters
The following hyperparameters were used during training:
- learning_rate: 7e-05
- train_batch_size: 8
- eval_batch_size: 8
- seed: 42
- optimizer: Adam with betas=(0.9,0.999) and epsilon=1e-08
- lr_scheduler_type: linear
- num_epochs: 3
### Training results
### Framework versions
- Transformers 4.35.2
- Pytorch 2.1.0+cu121
- Datasets 2.16.1
- Tokenizers 0.15.0
|
cyberagent/opencole-typographylmm-llava-v1.5-7b-lora | cyberagent | 2024-05-09T01:25:48Z | 309 | 6 | transformers | [
"transformers",
"safetensors",
"llava",
"image-text-to-text",
"arxiv:1910.09700",
"endpoints_compatible",
"region:us"
] | image-text-to-text | 2024-05-09T01:18:11Z | ---
library_name: transformers
tags: []
---
# Model Card for Model ID
<!-- Provide a quick summary of what the model is/does. -->
This model is based on [LLaVA1.5-7b](https://huggingface.co/liuhaotian/llava-v1.5-7b). The model is finetuned with LoRA on [OpenCOLE1.0 dataset](naoto0804/opencole) to generate text layouts.
## Model Details
### Model Description
<!-- Provide a longer summary of what this model is. -->
This is the model card of a 🤗 transformers model that has been pushed on the Hub. This model card has been automatically generated.
<!--
- **Developed by:** [More Information Needed]
- **Funded by [optional]:** [More Information Needed]
- **Shared by [optional]:** [More Information Needed]
- **Model type:** [More Information Needed]
-->
- **Language(s) (NLP):** English
- **License:**
Llama 2 is licensed under the LLAMA 2 Community License, Copyright (c) Meta Platforms, Inc. All Rights Reserved.
- **Finetuned from model:** [LLaVA1.5-7b](https://huggingface.co/liuhaotian/llava-v1.5-7b)
### Model Sources [optional]
<!-- Provide the basic links for the model. -->
- **Repository:** [CyberAgentAILab/OpenCOLE](https://github.com/CyberAgentAILab/OpenCOLE)
- **Paper:** [OpenCOLE: Towards Reproducible Automatic Graphic Design Generation]()
<!-- **Demo [optional]:** [More Information Needed] -->
## Uses
<!-- Address questions around how the model is intended to be used, including the foreseeable users of the model and those affected by the model. -->
Please refer to [OpenCOLE](https://github.com/CyberAgentAILab/OpenCOLE).
<!-- ### Direct Use -->
<!-- This section is for the model use without fine-tuning or plugging into a larger ecosystem/app. -->
<!-- [More Information Needed] -->
<!-- ### Downstream Use [optional] -->
<!-- This section is for the model use when fine-tuned for a task, or when plugged into a larger ecosystem/app -->
<!-- [More Information Needed] -->
<!-- ### Out-of-Scope Use -->
<!-- This section addresses misuse, malicious use, and uses that the model will not work well for. -->
<!-- [More Information Needed] -->
<!-- ## Bias, Risks, and Limitations -->
<!-- This section is meant to convey both technical and sociotechnical limitations. -->
<!-- [More Information Needed] -->
<!-- ### Recommendations -->
<!-- This section is meant to convey recommendations with respect to the bias, risk, and technical limitations. -->
<!-- Users (both direct and downstream) should be made aware of the risks, biases and limitations of the model. More information needed for further recommendations. -->
<!--## How to Get Started with the Model -->
<!--Use the code below to get started with the model. -->
<!--[More Information Needed] -->
## Training Details
### Training Data
<!-- This should link to a Dataset Card, perhaps with a short stub of information on what the training data is all about as well as documentation related to data pre-processing or additional filtering. -->
- About 18k image-text extracted automatically from OpenCOLE
Below is an example.
```
[
{
"id": "592d203395a7a863ddcd9df1",
"image": "images/592/592d203395a7a863ddcd9df1.png",
"conversations": [
{
"from": "human",
"value": "<image>\nGiven an image and text input including set of keywords to be placed on the image and its properties (optional), plan the layout of the texts. The output should be formatted as a JSON instance that conforms to the JSON schema below.\n\nAs an example, for the schema {\"properties\": {\"foo\": {\"title\": \"Foo\", \"description\": \"a list of strings\", \"type\": \"array\", \"items\": {\"type\": \"string\"}}}, \"required\": [\"foo\"]}\nthe object {\"foo\": [\"bar\", \"baz\"]} is a well-formatted instance of the schema. The object {\"properties\": {\"foo\": [\"bar\", \"baz\"]}} is not well-formatted.\n\nHere is the output schema:\n```\n{\"properties\": {\"elements\": {\"title\": \"Elements\", \"default\": [], \"type\": \"array\", \"items\": {\"$ref\": \"#/definitions/Element\"}}}, \"definitions\": {\"Element\": {\"title\": \"Element\", \"type\": \"object\", \"properties\": {\"text\": {\"title\": \"Text\", \"description\": \"Dummy text\", \"type\": \"string\"}, \"width\": {\"title\": \"Width\", \"description\": \"range: 0 <= width <= 127\", \"type\": \"integer\"}, \"height\": {\"title\": \"Height\", \"description\": \"range: 0 <= height <= 127\", \"type\": \"integer\"}, \"left\": {\"title\": \"Left\", \"description\": \"range: 0 <= left <= 127\", \"type\": \"integer\"}, \"top\": {\"title\": \"Top\", \"description\": \"range: 0 <= top <= 127\", \"type\": \"integer\"}, \"font\": {\"title\": \"Font\", \"type\": \"string\"}, \"color\": {\"title\": \"Color\", \"description\": \"range: 0 <= color <= 127\", \"type\": \"integer\"}, \"text_align\": {\"title\": \"Text Align\", \"description\": \"choices: \\\"\\\", \\\"left\\\", \\\"center\\\", \\\"right\\\"\", \"type\": \"string\"}, \"capitalize\": {\"title\": \"Capitalize\", \"description\": \"choices: \\\"false\\\", \\\"true\\\"\", \"type\": \"string\"}, \"font_size\": {\"title\": \"Font Size\", \"description\": \"range: 0 <= font_size <= 127\", \"type\": \"integer\"}, \"angle\": {\"title\": \"Angle\", \"description\": \"range: 0 <= angle <= 127\", \"type\": \"integer\"}, \"letter_spacing\": {\"title\": \"Letter Spacing\", \"description\": \"range: 0 <= letter_spacing <= 127\", \"type\": \"integer\"}, \"line_height\": {\"title\": \"Line Height\", \"description\": \"range: 0 <= line_height <= 127\", \"type\": \"integer\"}}, \"required\": [\"text\", \"width\", \"height\", \"left\", \"top\", \"font\", \"color\", \"text_align\", \"capitalize\", \"font_size\", \"angle\", \"letter_spacing\", \"line_height\"]}}}\n``` Input: [\"WE DON'T HAVE\\nANOTHER PLANET\", \"GREEN\", \"GO\"]"
},
{
"from": "gpt",
"value": "{\"elements\": [{\"text\": \"GO\", \"width\": 62, \"height\": 40, \"left\": 11, \"top\": 43, \"font\": \"Cormorant Infant\", \"color\": 38, \"text_align\": \"center\", \"capitalize\": \"false\", \"font_size\": 79, \"angle\": 0, \"letter_spacing\": 61, \"line_height\": 27}, {\"text\": \"GREEN\", \"width\": 69, \"height\": 30, \"left\": 6, \"top\": 60, \"font\": \"Cormorant Infant\", \"color\": 56, \"text_align\": \"center\", \"capitalize\": \"false\", \"font_size\": 67, \"angle\": 0, \"letter_spacing\": 50, \"line_height\": 27}, {\"text\": \"WE DON'T HAVE\\nANOTHER PLANET\", \"width\": 71, \"height\": 37, \"left\": 3, \"top\": 74, \"font\": \"Cormorant Infant\", \"color\": 56, \"text_align\": \"center\", \"capitalize\": \"false\", \"font_size\": 39, \"angle\": 0, \"letter_spacing\": 29, \"line_height\": 47}]}"
}
]
},
...
```
<!-- ### Training Procedure -->
<!-- This relates heavily to the Technical Specifications. Content here should link to that section when it is relevant to the training procedure. -->
<!-- #### Preprocessing [optional] -->
<!-- [More Information Needed] -->
<!-- #### Training Hyperparameters -->
<!-- - **Training regime:** [More Information Needed] -->
- <!--fp32, fp16 mixed precision, bf16 mixed precision, bf16 non-mixed precision, fp16 non-mixed precision, fp8 mixed precision -->
<!-- #### Speeds, Sizes, Times [optional] -->
<!-- This section provides information about throughput, start/end time, checkpoint size if relevant, etc. -->
<!-- [More Information Needed] -->
<!-- ## Evaluation ->
<!-- This section describes the evaluation protocols and provides the results. -->
<!-- ### Testing Data, Factors & Metrics ->
#### Testing Data
<!-- This should link to a Dataset Card if possible. -->
<!-- [More Information Needed] -->
<!-- #### Factors -->
<!-- These are the things the evaluation is disaggregating by, e.g., subpopulations or domains. -->
<!-- [More Information Needed] -->
<!-- #### Metrics -->
<!-- These are the evaluation metrics being used, ideally with a description of why. -->
<!-- [More Information Needed] -->
<!-- ### Results -->
<!-- [More Information Needed] -->
<!-- #### Summary -->
<!-- ## Model Examination [optional] -->
<!-- Relevant interpretability work for the model goes here -->
<!-- [More Information Needed] -->
<!-- ## Environmental Impact -->
<!-- Total emissions (in grams of CO2eq) and additional considerations, such as electricity usage, go here. Edit the suggested text below accordingly -->
<!--
Carbon emissions can be estimated using the [Machine Learning Impact calculator](https://mlco2.github.io/impact#compute) presented in [Lacoste et al. (2019)](https://arxiv.org/abs/1910.09700).
- **Hardware Type:** [More Information Needed]
- **Hours used:** [More Information Needed]
- **Cloud Provider:** [More Information Needed]
- **Compute Region:** [More Information Needed]
- **Carbon Emitted:** [More Information Needed]
## Technical Specifications [optional]
### Model Architecture and Objective
[More Information Needed]
### Compute Infrastructure
[More Information Needed]
#### Hardware
[More Information Needed]
#### Software
[More Information Needed]
-->
## Citation
<!-- If there is a paper or blog post introducing the model, the APA and Bibtex information for that should go in this section. -->
```
@inproceedings{inoue2024opencole,
title={{OpenCOLE: Towards Reproducible Automatic Graphic Design Generation}},
author={Naoto Inoue and Kento Masui and Wataru Shimoda and Kota Yamaguchi},
booktitle={Proceedings of the IEEE/CVF Conference on Computer Vision and Pattern Recognition Workshops (CVPRW)},
year={2024},
}
```
<!--
**APA:**
[More Information Needed]
## Glossary [optional]
-->
<!-- If relevant, include terms and calculations in this section that can help readers understand the model or model card. -->
<!-- [More Information Needed] -->
<!-- ## More Information [optional] -->
<!-- [More Information Needed] -->
<!-- ## Model Card Authors [optional] -->
<!-- [More Information Needed] -->
## Model Card Contact
[Naoto Inoue](https://github.com/naoto0804) |
jqf/bakery2 | jqf | 2024-05-09T01:22:30Z | 49 | 0 | transformers | [
"transformers",
"safetensors",
"vision-encoder-decoder",
"image-text-to-text",
"arxiv:1910.09700",
"endpoints_compatible",
"region:us"
] | image-text-to-text | 2024-05-09T00:29:50Z | ---
library_name: transformers
tags: []
---
# Model Card for Model ID
<!-- Provide a quick summary of what the model is/does. -->
## Model Details
### Model Description
<!-- Provide a longer summary of what this model is. -->
This is the model card of a 🤗 transformers model that has been pushed on the Hub. This model card has been automatically generated.
- **Developed by:** [More Information Needed]
- **Funded by [optional]:** [More Information Needed]
- **Shared by [optional]:** [More Information Needed]
- **Model type:** [More Information Needed]
- **Language(s) (NLP):** [More Information Needed]
- **License:** [More Information Needed]
- **Finetuned from model [optional]:** [More Information Needed]
### Model Sources [optional]
<!-- Provide the basic links for the model. -->
- **Repository:** [More Information Needed]
- **Paper [optional]:** [More Information Needed]
- **Demo [optional]:** [More Information Needed]
## Uses
<!-- Address questions around how the model is intended to be used, including the foreseeable users of the model and those affected by the model. -->
### Direct Use
<!-- This section is for the model use without fine-tuning or plugging into a larger ecosystem/app. -->
[More Information Needed]
### Downstream Use [optional]
<!-- This section is for the model use when fine-tuned for a task, or when plugged into a larger ecosystem/app -->
[More Information Needed]
### Out-of-Scope Use
<!-- This section addresses misuse, malicious use, and uses that the model will not work well for. -->
[More Information Needed]
## Bias, Risks, and Limitations
<!-- This section is meant to convey both technical and sociotechnical limitations. -->
[More Information Needed]
### Recommendations
<!-- This section is meant to convey recommendations with respect to the bias, risk, and technical limitations. -->
Users (both direct and downstream) should be made aware of the risks, biases and limitations of the model. More information needed for further recommendations.
## How to Get Started with the Model
Use the code below to get started with the model.
[More Information Needed]
## Training Details
### Training Data
<!-- This should link to a Dataset Card, perhaps with a short stub of information on what the training data is all about as well as documentation related to data pre-processing or additional filtering. -->
[More Information Needed]
### Training Procedure
<!-- This relates heavily to the Technical Specifications. Content here should link to that section when it is relevant to the training procedure. -->
#### Preprocessing [optional]
[More Information Needed]
#### Training Hyperparameters
- **Training regime:** [More Information Needed] <!--fp32, fp16 mixed precision, bf16 mixed precision, bf16 non-mixed precision, fp16 non-mixed precision, fp8 mixed precision -->
#### Speeds, Sizes, Times [optional]
<!-- This section provides information about throughput, start/end time, checkpoint size if relevant, etc. -->
[More Information Needed]
## Evaluation
<!-- This section describes the evaluation protocols and provides the results. -->
### Testing Data, Factors & Metrics
#### Testing Data
<!-- This should link to a Dataset Card if possible. -->
[More Information Needed]
#### Factors
<!-- These are the things the evaluation is disaggregating by, e.g., subpopulations or domains. -->
[More Information Needed]
#### Metrics
<!-- These are the evaluation metrics being used, ideally with a description of why. -->
[More Information Needed]
### Results
[More Information Needed]
#### Summary
## Model Examination [optional]
<!-- Relevant interpretability work for the model goes here -->
[More Information Needed]
## Environmental Impact
<!-- Total emissions (in grams of CO2eq) and additional considerations, such as electricity usage, go here. Edit the suggested text below accordingly -->
Carbon emissions can be estimated using the [Machine Learning Impact calculator](https://mlco2.github.io/impact#compute) presented in [Lacoste et al. (2019)](https://arxiv.org/abs/1910.09700).
- **Hardware Type:** [More Information Needed]
- **Hours used:** [More Information Needed]
- **Cloud Provider:** [More Information Needed]
- **Compute Region:** [More Information Needed]
- **Carbon Emitted:** [More Information Needed]
## Technical Specifications [optional]
### Model Architecture and Objective
[More Information Needed]
### Compute Infrastructure
[More Information Needed]
#### Hardware
[More Information Needed]
#### Software
[More Information Needed]
## Citation [optional]
<!-- If there is a paper or blog post introducing the model, the APA and Bibtex information for that should go in this section. -->
**BibTeX:**
[More Information Needed]
**APA:**
[More Information Needed]
## Glossary [optional]
<!-- If relevant, include terms and calculations in this section that can help readers understand the model or model card. -->
[More Information Needed]
## More Information [optional]
[More Information Needed]
## Model Card Authors [optional]
[More Information Needed]
## Model Card Contact
[More Information Needed] |
drgary/ft3_law3_lora_model | drgary | 2024-05-09T01:21:51Z | 0 | 0 | transformers | [
"transformers",
"safetensors",
"text-generation-inference",
"unsloth",
"llama",
"trl",
"en",
"base_model:unsloth/llama-3-8b-bnb-4bit",
"base_model:finetune:unsloth/llama-3-8b-bnb-4bit",
"license:apache-2.0",
"endpoints_compatible",
"region:us"
] | null | 2024-05-09T01:21:45Z | ---
language:
- en
license: apache-2.0
tags:
- text-generation-inference
- transformers
- unsloth
- llama
- trl
base_model: unsloth/llama-3-8b-bnb-4bit
---
# Uploaded model
- **Developed by:** drgary
- **License:** apache-2.0
- **Finetuned from model :** unsloth/llama-3-8b-bnb-4bit
This llama model was trained 2x faster with [Unsloth](https://github.com/unslothai/unsloth) and Huggingface's TRL library.
[<img src="https://raw.githubusercontent.com/unslothai/unsloth/main/images/unsloth%20made%20with%20love.png" width="200"/>](https://github.com/unslothai/unsloth)
|
oopsung/EEVE-ins-sft-poc-v1 | oopsung | 2024-05-09T01:20:08Z | 12 | 0 | transformers | [
"transformers",
"safetensors",
"llama",
"text-generation",
"conversational",
"arxiv:1910.09700",
"autotrain_compatible",
"text-generation-inference",
"endpoints_compatible",
"region:us"
] | text-generation | 2024-05-09T01:14:57Z | ---
library_name: transformers
tags: []
---
# Model Card for Model ID
<!-- Provide a quick summary of what the model is/does. -->
## Model Details
### Model Description
<!-- Provide a longer summary of what this model is. -->
This is the model card of a 🤗 transformers model that has been pushed on the Hub. This model card has been automatically generated.
- **Developed by:** [More Information Needed]
- **Funded by [optional]:** [More Information Needed]
- **Shared by [optional]:** [More Information Needed]
- **Model type:** [More Information Needed]
- **Language(s) (NLP):** [More Information Needed]
- **License:** [More Information Needed]
- **Finetuned from model [optional]:** [More Information Needed]
### Model Sources [optional]
<!-- Provide the basic links for the model. -->
- **Repository:** [More Information Needed]
- **Paper [optional]:** [More Information Needed]
- **Demo [optional]:** [More Information Needed]
## Uses
<!-- Address questions around how the model is intended to be used, including the foreseeable users of the model and those affected by the model. -->
### Direct Use
<!-- This section is for the model use without fine-tuning or plugging into a larger ecosystem/app. -->
[More Information Needed]
### Downstream Use [optional]
<!-- This section is for the model use when fine-tuned for a task, or when plugged into a larger ecosystem/app -->
[More Information Needed]
### Out-of-Scope Use
<!-- This section addresses misuse, malicious use, and uses that the model will not work well for. -->
[More Information Needed]
## Bias, Risks, and Limitations
<!-- This section is meant to convey both technical and sociotechnical limitations. -->
[More Information Needed]
### Recommendations
<!-- This section is meant to convey recommendations with respect to the bias, risk, and technical limitations. -->
Users (both direct and downstream) should be made aware of the risks, biases and limitations of the model. More information needed for further recommendations.
## How to Get Started with the Model
Use the code below to get started with the model.
[More Information Needed]
## Training Details
### Training Data
<!-- This should link to a Dataset Card, perhaps with a short stub of information on what the training data is all about as well as documentation related to data pre-processing or additional filtering. -->
[More Information Needed]
### Training Procedure
<!-- This relates heavily to the Technical Specifications. Content here should link to that section when it is relevant to the training procedure. -->
#### Preprocessing [optional]
[More Information Needed]
#### Training Hyperparameters
- **Training regime:** [More Information Needed] <!--fp32, fp16 mixed precision, bf16 mixed precision, bf16 non-mixed precision, fp16 non-mixed precision, fp8 mixed precision -->
#### Speeds, Sizes, Times [optional]
<!-- This section provides information about throughput, start/end time, checkpoint size if relevant, etc. -->
[More Information Needed]
## Evaluation
<!-- This section describes the evaluation protocols and provides the results. -->
### Testing Data, Factors & Metrics
#### Testing Data
<!-- This should link to a Dataset Card if possible. -->
[More Information Needed]
#### Factors
<!-- These are the things the evaluation is disaggregating by, e.g., subpopulations or domains. -->
[More Information Needed]
#### Metrics
<!-- These are the evaluation metrics being used, ideally with a description of why. -->
[More Information Needed]
### Results
[More Information Needed]
#### Summary
## Model Examination [optional]
<!-- Relevant interpretability work for the model goes here -->
[More Information Needed]
## Environmental Impact
<!-- Total emissions (in grams of CO2eq) and additional considerations, such as electricity usage, go here. Edit the suggested text below accordingly -->
Carbon emissions can be estimated using the [Machine Learning Impact calculator](https://mlco2.github.io/impact#compute) presented in [Lacoste et al. (2019)](https://arxiv.org/abs/1910.09700).
- **Hardware Type:** [More Information Needed]
- **Hours used:** [More Information Needed]
- **Cloud Provider:** [More Information Needed]
- **Compute Region:** [More Information Needed]
- **Carbon Emitted:** [More Information Needed]
## Technical Specifications [optional]
### Model Architecture and Objective
[More Information Needed]
### Compute Infrastructure
[More Information Needed]
#### Hardware
[More Information Needed]
#### Software
[More Information Needed]
## Citation [optional]
<!-- If there is a paper or blog post introducing the model, the APA and Bibtex information for that should go in this section. -->
**BibTeX:**
[More Information Needed]
**APA:**
[More Information Needed]
## Glossary [optional]
<!-- If relevant, include terms and calculations in this section that can help readers understand the model or model card. -->
[More Information Needed]
## More Information [optional]
[More Information Needed]
## Model Card Authors [optional]
[More Information Needed]
## Model Card Contact
[More Information Needed] |
jialinselenasong/pubmed-bert-all-deep | jialinselenasong | 2024-05-09T01:13:24Z | 133 | 0 | transformers | [
"transformers",
"tensorboard",
"safetensors",
"bert",
"token-classification",
"generated_from_trainer",
"base_model:NeuML/pubmedbert-base-embeddings",
"base_model:finetune:NeuML/pubmedbert-base-embeddings",
"license:apache-2.0",
"autotrain_compatible",
"endpoints_compatible",
"region:us"
] | token-classification | 2024-05-09T01:01:44Z | ---
license: apache-2.0
base_model: NeuML/pubmedbert-base-embeddings
tags:
- generated_from_trainer
metrics:
- precision
- recall
- f1
- accuracy
model-index:
- name: pubmed-bert-all-deep
results: []
---
<!-- This model card has been generated automatically according to the information the Trainer had access to. You
should probably proofread and complete it, then remove this comment. -->
# pubmed-bert-all-deep
This model is a fine-tuned version of [NeuML/pubmedbert-base-embeddings](https://huggingface.co/NeuML/pubmedbert-base-embeddings) on an unknown dataset.
It achieves the following results on the evaluation set:
- Loss: 0.9764
- Precision: 0.4738
- Recall: 0.4800
- F1: 0.4769
- Accuracy: 0.7380
## Model description
More information needed
## Intended uses & limitations
More information needed
## Training and evaluation data
More information needed
## Training procedure
### Training hyperparameters
The following hyperparameters were used during training:
- learning_rate: 2e-05
- train_batch_size: 8
- eval_batch_size: 8
- seed: 42
- optimizer: Adam with betas=(0.9,0.999) and epsilon=1e-08
- lr_scheduler_type: linear
- num_epochs: 10
### Training results
| Training Loss | Epoch | Step | Validation Loss | Precision | Recall | F1 | Accuracy |
|:-------------:|:-----:|:----:|:---------------:|:---------:|:------:|:------:|:--------:|
| No log | 1.0 | 363 | 1.1611 | 0.1988 | 0.1654 | 0.1806 | 0.6258 |
| 1.3011 | 2.0 | 726 | 1.0030 | 0.3355 | 0.3221 | 0.3287 | 0.6877 |
| 0.9032 | 3.0 | 1089 | 0.9300 | 0.4125 | 0.3563 | 0.3823 | 0.7095 |
| 0.9032 | 4.0 | 1452 | 0.8892 | 0.4466 | 0.4189 | 0.4323 | 0.7220 |
| 0.7036 | 5.0 | 1815 | 0.9079 | 0.4476 | 0.4530 | 0.4503 | 0.7257 |
| 0.5735 | 6.0 | 2178 | 0.9415 | 0.4651 | 0.4684 | 0.4667 | 0.7299 |
| 0.4796 | 7.0 | 2541 | 0.9484 | 0.4791 | 0.4558 | 0.4672 | 0.7324 |
| 0.4796 | 8.0 | 2904 | 0.9677 | 0.4673 | 0.4757 | 0.4715 | 0.7335 |
| 0.4197 | 9.0 | 3267 | 0.9810 | 0.4760 | 0.4791 | 0.4775 | 0.7361 |
| 0.3812 | 10.0 | 3630 | 0.9764 | 0.4738 | 0.4800 | 0.4769 | 0.7380 |
### Framework versions
- Transformers 4.40.1
- Pytorch 2.2.1+cu121
- Datasets 2.19.1
- Tokenizers 0.19.1
|
EthanRhys/Silver-Current | EthanRhys | 2024-05-09T01:10:40Z | 0 | 0 | null | [
"license:openrail++",
"region:us"
] | null | 2024-05-09T01:09:14Z | ---
license: openrail++
---
|
RichardErkhov/deepset_-_roberta-base-squad2-covid-4bits | RichardErkhov | 2024-05-09T01:04:25Z | 63 | 0 | transformers | [
"transformers",
"safetensors",
"roberta",
"text-generation",
"autotrain_compatible",
"endpoints_compatible",
"4-bit",
"bitsandbytes",
"region:us"
] | text-generation | 2024-05-09T01:03:14Z | Quantization made by Richard Erkhov.
[Github](https://github.com/RichardErkhov)
[Discord](https://discord.gg/pvy7H8DZMG)
[Request more models](https://github.com/RichardErkhov/quant_request)
roberta-base-squad2-covid - bnb 4bits
- Model creator: https://huggingface.co/deepset/
- Original model: https://huggingface.co/deepset/roberta-base-squad2-covid/
Original model description:
---
language: en
datasets:
- squad_v2
license: cc-by-4.0
---
# roberta-base-squad2 for QA on COVID-19
## Overview
**Language model:** deepset/roberta-base-squad2
**Language:** English
**Downstream-task:** Extractive QA
**Training data:** [SQuAD-style CORD-19 annotations from 23rd April](https://github.com/deepset-ai/COVID-QA/blob/master/data/question-answering/200423_covidQA.json)
**Code:** See [an example QA pipeline on Haystack](https://haystack.deepset.ai/tutorials/01_basic_qa_pipeline)
**Infrastructure**: Tesla v100
## Hyperparameters
```
batch_size = 24
n_epochs = 3
base_LM_model = "deepset/roberta-base-squad2"
max_seq_len = 384
learning_rate = 3e-5
lr_schedule = LinearWarmup
warmup_proportion = 0.1
doc_stride = 128
xval_folds = 5
dev_split = 0
no_ans_boost = -100
```
---
license: cc-by-4.0
---
## Performance
5-fold cross-validation on the data set led to the following results:
**Single EM-Scores:** [0.222, 0.123, 0.234, 0.159, 0.158]
**Single F1-Scores:** [0.476, 0.493, 0.599, 0.461, 0.465]
**Single top\\_3\\_recall Scores:** [0.827, 0.776, 0.860, 0.771, 0.777]
**XVAL EM:** 0.17890995260663506
**XVAL f1:** 0.49925444207319924
**XVAL top\\_3\\_recall:** 0.8021327014218009
This model is the model obtained from the **third** fold of the cross-validation.
## Usage
### In Haystack
For doing QA at scale (i.e. many docs instead of single paragraph), you can load the model also in [haystack](https://github.com/deepset-ai/haystack/):
```python
reader = FARMReader(model_name_or_path="deepset/roberta-base-squad2-covid")
# or
reader = TransformersReader(model="deepset/roberta-base-squad2",tokenizer="deepset/roberta-base-squad2-covid")
```
### In Transformers
```python
from transformers import AutoModelForQuestionAnswering, AutoTokenizer, pipeline
model_name = "deepset/roberta-base-squad2-covid"
# a) Get predictions
nlp = pipeline('question-answering', model=model_name, tokenizer=model_name)
QA_input = {
'question': 'Why is model conversion important?',
'context': 'The option to convert models between FARM and transformers gives freedom to the user and let people easily switch between frameworks.'
}
res = nlp(QA_input)
# b) Load model & tokenizer
model = AutoModelForQuestionAnswering.from_pretrained(model_name)
tokenizer = AutoTokenizer.from_pretrained(model_name)
```
## Authors
**Branden Chan:** [email protected]
**Timo Möller:** [email protected]
**Malte Pietsch:** [email protected]
**Tanay Soni:** [email protected]
**Bogdan Kostić:** [email protected]
## About us
<div class="grid lg:grid-cols-2 gap-x-4 gap-y-3">
<div class="w-full h-40 object-cover mb-2 rounded-lg flex items-center justify-center">
<img alt="" src="https://raw.githubusercontent.com/deepset-ai/.github/main/deepset-logo-colored.png" class="w-40"/>
</div>
<div class="w-full h-40 object-cover mb-2 rounded-lg flex items-center justify-center">
<img alt="" src="https://raw.githubusercontent.com/deepset-ai/.github/main/haystack-logo-colored.png" class="w-40"/>
</div>
</div>
[deepset](http://deepset.ai/) is the company behind the open-source NLP framework [Haystack](https://haystack.deepset.ai/) which is designed to help you build production ready NLP systems that use: Question answering, summarization, ranking etc.
Some of our other work:
- [Distilled roberta-base-squad2 (aka "tinyroberta-squad2")]([https://huggingface.co/deepset/tinyroberta-squad2)
- [German BERT (aka "bert-base-german-cased")](https://deepset.ai/german-bert)
- [GermanQuAD and GermanDPR datasets and models (aka "gelectra-base-germanquad", "gbert-base-germandpr")](https://deepset.ai/germanquad)
## Get in touch and join the Haystack community
<p>For more info on Haystack, visit our <strong><a href="https://github.com/deepset-ai/haystack">GitHub</a></strong> repo and <strong><a href="https://docs.haystack.deepset.ai">Documentation</a></strong>.
We also have a <strong><a class="h-7" href="https://haystack.deepset.ai/community/join">Discord community open to everyone!</a></strong></p>
[Twitter](https://twitter.com/deepset_ai) | [LinkedIn](https://www.linkedin.com/company/deepset-ai/) | [Discord](https://haystack.deepset.ai/community) | [GitHub Discussions](https://github.com/deepset-ai/haystack/discussions) | [Website](https://deepset.ai)
By the way: [we're hiring!](http://www.deepset.ai/jobs)
|
jialinselenasong/bert-all-deep | jialinselenasong | 2024-05-09T00:56:57Z | 112 | 0 | transformers | [
"transformers",
"tensorboard",
"safetensors",
"bert",
"token-classification",
"generated_from_trainer",
"base_model:google-bert/bert-base-cased",
"base_model:finetune:google-bert/bert-base-cased",
"license:apache-2.0",
"autotrain_compatible",
"endpoints_compatible",
"region:us"
] | token-classification | 2024-05-09T00:42:37Z | ---
license: apache-2.0
base_model: google-bert/bert-base-cased
tags:
- generated_from_trainer
metrics:
- precision
- recall
- f1
- accuracy
model-index:
- name: bert-all-deep
results: []
---
<!-- This model card has been generated automatically according to the information the Trainer had access to. You
should probably proofread and complete it, then remove this comment. -->
# bert-all-deep
This model is a fine-tuned version of [google-bert/bert-base-cased](https://huggingface.co/google-bert/bert-base-cased) on an unknown dataset.
It achieves the following results on the evaluation set:
- Loss: 0.8570
- Precision: 0.6195
- Recall: 0.7039
- F1: 0.6590
- Accuracy: 0.8148
## Model description
More information needed
## Intended uses & limitations
More information needed
## Training and evaluation data
More information needed
## Training procedure
### Training hyperparameters
The following hyperparameters were used during training:
- learning_rate: 2e-05
- train_batch_size: 8
- eval_batch_size: 8
- seed: 42
- optimizer: Adam with betas=(0.9,0.999) and epsilon=1e-08
- lr_scheduler_type: linear
- num_epochs: 10
### Training results
| Training Loss | Epoch | Step | Validation Loss | Precision | Recall | F1 | Accuracy |
|:-------------:|:-----:|:----:|:---------------:|:---------:|:------:|:------:|:--------:|
| No log | 1.0 | 363 | 0.5960 | 0.5756 | 0.6524 | 0.6116 | 0.8019 |
| 0.7348 | 2.0 | 726 | 0.5768 | 0.5826 | 0.6904 | 0.6319 | 0.8102 |
| 0.422 | 3.0 | 1089 | 0.5991 | 0.6155 | 0.6880 | 0.6497 | 0.8185 |
| 0.422 | 4.0 | 1452 | 0.6229 | 0.6145 | 0.7043 | 0.6564 | 0.8169 |
| 0.2916 | 5.0 | 1815 | 0.6857 | 0.6163 | 0.7080 | 0.6590 | 0.8159 |
| 0.2032 | 6.0 | 2178 | 0.7307 | 0.6277 | 0.6987 | 0.6613 | 0.8182 |
| 0.1531 | 7.0 | 2541 | 0.7933 | 0.6168 | 0.7103 | 0.6603 | 0.8132 |
| 0.1531 | 8.0 | 2904 | 0.8186 | 0.6238 | 0.6992 | 0.6594 | 0.8158 |
| 0.119 | 9.0 | 3267 | 0.8438 | 0.6159 | 0.7082 | 0.6589 | 0.8149 |
| 0.1 | 10.0 | 3630 | 0.8570 | 0.6195 | 0.7039 | 0.6590 | 0.8148 |
### Framework versions
- Transformers 4.40.1
- Pytorch 2.2.1+cu121
- Datasets 2.19.1
- Tokenizers 0.19.1
|
SyntaxTheRed/CartPole | SyntaxTheRed | 2024-05-09T00:54:24Z | 0 | 0 | null | [
"CartPole-v1",
"reinforce",
"reinforcement-learning",
"custom-implementation",
"deep-rl-class",
"model-index",
"region:us"
] | reinforcement-learning | 2024-02-21T17:16:12Z | ---
tags:
- CartPole-v1
- reinforce
- reinforcement-learning
- custom-implementation
- deep-rl-class
model-index:
- name: CartPole
results:
- task:
type: reinforcement-learning
name: reinforcement-learning
dataset:
name: CartPole-v1
type: CartPole-v1
metrics:
- type: mean_reward
value: 500.00 +/- 0.00
name: mean_reward
verified: false
---
# **Reinforce** Agent playing **CartPole-v1**
This is a trained model of a **Reinforce** agent playing **CartPole-v1** .
To learn to use this model and train yours check Unit 4 of the Deep Reinforcement Learning Course: https://huggingface.co/deep-rl-course/unit4/introduction
|
Monor/Llama3-ChatQA-1.5-8B-gguf | Monor | 2024-05-09T00:52:48Z | 38 | 1 | null | [
"gguf",
"license:apache-2.0",
"endpoints_compatible",
"region:us"
] | null | 2024-05-07T12:03:59Z | ---
license: apache-2.0
---
## Introduce
Quantizing the [nvidia/Llama3-ChatQA-1.5-8B](https://huggingface.co/nvidia/Llama3-ChatQA-1.5-8B) to f16, q2, q3, q4, q5, q6 and q8 with Llama.cpp.
|
Subsets and Splits