modelId
stringlengths 5
138
| author
stringlengths 2
42
| last_modified
unknowndate 2020-02-15 11:33:14
2025-04-17 06:26:57
| downloads
int64 0
223M
| likes
int64 0
11.7k
| library_name
stringclasses 428
values | tags
sequencelengths 1
4.05k
| pipeline_tag
stringclasses 54
values | createdAt
unknowndate 2022-03-02 23:29:04
2025-04-17 06:26:10
| card
stringlengths 11
1.01M
|
---|---|---|---|---|---|---|---|---|---|
sirenstitches/phrasebank-sentiment-analysis | sirenstitches | "2023-10-28T15:48:16Z" | 102 | 0 | transformers | [
"transformers",
"pytorch",
"bert",
"text-classification",
"generated_from_trainer",
"dataset:financial_phrasebank",
"base_model:google-bert/bert-base-uncased",
"base_model:finetune:google-bert/bert-base-uncased",
"license:apache-2.0",
"model-index",
"autotrain_compatible",
"endpoints_compatible",
"region:us"
] | text-classification | "2023-10-28T15:48:00Z" | ---
license: apache-2.0
base_model: bert-base-uncased
tags:
- generated_from_trainer
datasets:
- financial_phrasebank
metrics:
- f1
- accuracy
model-index:
- name: phrasebank-sentiment-analysis
results:
- task:
name: Text Classification
type: text-classification
dataset:
name: financial_phrasebank
type: financial_phrasebank
config: sentences_50agree
split: train
args: sentences_50agree
metrics:
- name: F1
type: f1
value: 0.8438441587369537
- name: Accuracy
type: accuracy
value: 0.8555708390646493
---
<!-- This model card has been generated automatically according to the information the Trainer had access to. You
should probably proofread and complete it, then remove this comment. -->
# phrasebank-sentiment-analysis
This model is a fine-tuned version of [bert-base-uncased](https://huggingface.co/bert-base-uncased) on the financial_phrasebank dataset.
It achieves the following results on the evaluation set:
- Loss: 0.5174
- F1: 0.8438
- Accuracy: 0.8556
## Model description
More information needed
## Intended uses & limitations
More information needed
## Training and evaluation data
More information needed
## Training procedure
### Training hyperparameters
The following hyperparameters were used during training:
- learning_rate: 5e-05
- train_batch_size: 32
- eval_batch_size: 8
- seed: 42
- optimizer: Adam with betas=(0.9,0.999) and epsilon=1e-08
- lr_scheduler_type: linear
- num_epochs: 4
### Training results
| Training Loss | Epoch | Step | Validation Loss | F1 | Accuracy |
|:-------------:|:-----:|:----:|:---------------:|:------:|:--------:|
| 0.5874 | 0.94 | 100 | 0.4026 | 0.8175 | 0.8432 |
| 0.2811 | 1.89 | 200 | 0.3685 | 0.8308 | 0.8404 |
| 0.1337 | 2.83 | 300 | 0.4108 | 0.8518 | 0.8645 |
| 0.0713 | 3.77 | 400 | 0.5174 | 0.8438 | 0.8556 |
### Framework versions
- Transformers 4.34.1
- Pytorch 2.1.0+cu118
- Datasets 2.14.6
- Tokenizers 0.14.1
|
city96/stable-diffusion-3.5-medium-gguf | city96 | "2024-10-30T01:45:56Z" | 4,314 | 43 | gguf | [
"gguf",
"text-to-image",
"image-generation",
"stable-diffusion",
"en",
"base_model:stabilityai/stable-diffusion-3.5-medium",
"base_model:quantized:stabilityai/stable-diffusion-3.5-medium",
"license:other",
"region:us"
] | text-to-image | "2024-10-30T01:08:44Z" | ---
base_model: stabilityai/stable-diffusion-3.5-medium
library_name: gguf
quantized_by: city96
tags:
- text-to-image
- image-generation
- stable-diffusion
language:
- en
license: other
license_name: stabilityai-ai-community
license_link: LICENSE.md
---
This is a direct GGUF conversion of [stabilityai/stable-diffusion-3.5-medium](https://huggingface.co/stabilityai/stable-diffusion-3.5-medium)
As this is a quantized model not a finetune, all the same restrictions/original license terms still apply.
The model files can be used with the [ComfyUI-GGUF](https://github.com/city96/ComfyUI-GGUF) custom node.
Place model files in `ComfyUI/models/unet` - see the GitHub readme for further install instructions.
Please refer to [this chart](https://github.com/ggerganov/llama.cpp/blob/master/examples/perplexity/README.md#llama-3-8b-scoreboard) for a basic overview of quantization types. |
sajjadamjad/sentiment-analyzer_v4 | sajjadamjad | "2023-12-08T17:11:51Z" | 1 | 0 | peft | [
"peft",
"safetensors",
"arxiv:1910.09700",
"base_model:FacebookAI/roberta-base",
"base_model:adapter:FacebookAI/roberta-base",
"region:us"
] | null | "2023-12-08T17:11:48Z" | ---
library_name: peft
base_model: roberta-base
---
# Model Card for Model ID
<!-- Provide a quick summary of what the model is/does. -->
## Model Details
### Model Description
<!-- Provide a longer summary of what this model is. -->
- **Developed by:** [More Information Needed]
- **Funded by [optional]:** [More Information Needed]
- **Shared by [optional]:** [More Information Needed]
- **Model type:** [More Information Needed]
- **Language(s) (NLP):** [More Information Needed]
- **License:** [More Information Needed]
- **Finetuned from model [optional]:** [More Information Needed]
### Model Sources [optional]
<!-- Provide the basic links for the model. -->
- **Repository:** [More Information Needed]
- **Paper [optional]:** [More Information Needed]
- **Demo [optional]:** [More Information Needed]
## Uses
<!-- Address questions around how the model is intended to be used, including the foreseeable users of the model and those affected by the model. -->
### Direct Use
<!-- This section is for the model use without fine-tuning or plugging into a larger ecosystem/app. -->
[More Information Needed]
### Downstream Use [optional]
<!-- This section is for the model use when fine-tuned for a task, or when plugged into a larger ecosystem/app -->
[More Information Needed]
### Out-of-Scope Use
<!-- This section addresses misuse, malicious use, and uses that the model will not work well for. -->
[More Information Needed]
## Bias, Risks, and Limitations
<!-- This section is meant to convey both technical and sociotechnical limitations. -->
[More Information Needed]
### Recommendations
<!-- This section is meant to convey recommendations with respect to the bias, risk, and technical limitations. -->
Users (both direct and downstream) should be made aware of the risks, biases and limitations of the model. More information needed for further recommendations.
## How to Get Started with the Model
Use the code below to get started with the model.
[More Information Needed]
## Training Details
### Training Data
<!-- This should link to a Dataset Card, perhaps with a short stub of information on what the training data is all about as well as documentation related to data pre-processing or additional filtering. -->
[More Information Needed]
### Training Procedure
<!-- This relates heavily to the Technical Specifications. Content here should link to that section when it is relevant to the training procedure. -->
#### Preprocessing [optional]
[More Information Needed]
#### Training Hyperparameters
- **Training regime:** [More Information Needed] <!--fp32, fp16 mixed precision, bf16 mixed precision, bf16 non-mixed precision, fp16 non-mixed precision, fp8 mixed precision -->
#### Speeds, Sizes, Times [optional]
<!-- This section provides information about throughput, start/end time, checkpoint size if relevant, etc. -->
[More Information Needed]
## Evaluation
<!-- This section describes the evaluation protocols and provides the results. -->
### Testing Data, Factors & Metrics
#### Testing Data
<!-- This should link to a Dataset Card if possible. -->
[More Information Needed]
#### Factors
<!-- These are the things the evaluation is disaggregating by, e.g., subpopulations or domains. -->
[More Information Needed]
#### Metrics
<!-- These are the evaluation metrics being used, ideally with a description of why. -->
[More Information Needed]
### Results
[More Information Needed]
#### Summary
## Model Examination [optional]
<!-- Relevant interpretability work for the model goes here -->
[More Information Needed]
## Environmental Impact
<!-- Total emissions (in grams of CO2eq) and additional considerations, such as electricity usage, go here. Edit the suggested text below accordingly -->
Carbon emissions can be estimated using the [Machine Learning Impact calculator](https://mlco2.github.io/impact#compute) presented in [Lacoste et al. (2019)](https://arxiv.org/abs/1910.09700).
- **Hardware Type:** [More Information Needed]
- **Hours used:** [More Information Needed]
- **Cloud Provider:** [More Information Needed]
- **Compute Region:** [More Information Needed]
- **Carbon Emitted:** [More Information Needed]
## Technical Specifications [optional]
### Model Architecture and Objective
[More Information Needed]
### Compute Infrastructure
[More Information Needed]
#### Hardware
[More Information Needed]
#### Software
[More Information Needed]
## Citation [optional]
<!-- If there is a paper or blog post introducing the model, the APA and Bibtex information for that should go in this section. -->
**BibTeX:**
[More Information Needed]
**APA:**
[More Information Needed]
## Glossary [optional]
<!-- If relevant, include terms and calculations in this section that can help readers understand the model or model card. -->
[More Information Needed]
## More Information [optional]
[More Information Needed]
## Model Card Authors [optional]
[More Information Needed]
## Model Card Contact
[More Information Needed]
### Framework versions
- PEFT 0.7.0 |
Tristan/dclm-random-410m-raw-openbookqa | Tristan | "2025-04-01T01:46:11Z" | 6 | 0 | transformers | [
"transformers",
"safetensors",
"gpt_neox",
"text-generation",
"arxiv:1910.09700",
"autotrain_compatible",
"text-generation-inference",
"endpoints_compatible",
"region:us"
] | text-generation | "2025-03-28T21:55:36Z" | ---
library_name: transformers
tags: []
---
# Model Card for Model ID
<!-- Provide a quick summary of what the model is/does. -->
## Model Details
### Model Description
<!-- Provide a longer summary of what this model is. -->
This is the model card of a 🤗 transformers model that has been pushed on the Hub. This model card has been automatically generated.
- **Developed by:** [More Information Needed]
- **Funded by [optional]:** [More Information Needed]
- **Shared by [optional]:** [More Information Needed]
- **Model type:** [More Information Needed]
- **Language(s) (NLP):** [More Information Needed]
- **License:** [More Information Needed]
- **Finetuned from model [optional]:** [More Information Needed]
### Model Sources [optional]
<!-- Provide the basic links for the model. -->
- **Repository:** [More Information Needed]
- **Paper [optional]:** [More Information Needed]
- **Demo [optional]:** [More Information Needed]
## Uses
<!-- Address questions around how the model is intended to be used, including the foreseeable users of the model and those affected by the model. -->
### Direct Use
<!-- This section is for the model use without fine-tuning or plugging into a larger ecosystem/app. -->
[More Information Needed]
### Downstream Use [optional]
<!-- This section is for the model use when fine-tuned for a task, or when plugged into a larger ecosystem/app -->
[More Information Needed]
### Out-of-Scope Use
<!-- This section addresses misuse, malicious use, and uses that the model will not work well for. -->
[More Information Needed]
## Bias, Risks, and Limitations
<!-- This section is meant to convey both technical and sociotechnical limitations. -->
[More Information Needed]
### Recommendations
<!-- This section is meant to convey recommendations with respect to the bias, risk, and technical limitations. -->
Users (both direct and downstream) should be made aware of the risks, biases and limitations of the model. More information needed for further recommendations.
## How to Get Started with the Model
Use the code below to get started with the model.
[More Information Needed]
## Training Details
### Training Data
<!-- This should link to a Dataset Card, perhaps with a short stub of information on what the training data is all about as well as documentation related to data pre-processing or additional filtering. -->
[More Information Needed]
### Training Procedure
<!-- This relates heavily to the Technical Specifications. Content here should link to that section when it is relevant to the training procedure. -->
#### Preprocessing [optional]
[More Information Needed]
#### Training Hyperparameters
- **Training regime:** [More Information Needed] <!--fp32, fp16 mixed precision, bf16 mixed precision, bf16 non-mixed precision, fp16 non-mixed precision, fp8 mixed precision -->
#### Speeds, Sizes, Times [optional]
<!-- This section provides information about throughput, start/end time, checkpoint size if relevant, etc. -->
[More Information Needed]
## Evaluation
<!-- This section describes the evaluation protocols and provides the results. -->
### Testing Data, Factors & Metrics
#### Testing Data
<!-- This should link to a Dataset Card if possible. -->
[More Information Needed]
#### Factors
<!-- These are the things the evaluation is disaggregating by, e.g., subpopulations or domains. -->
[More Information Needed]
#### Metrics
<!-- These are the evaluation metrics being used, ideally with a description of why. -->
[More Information Needed]
### Results
[More Information Needed]
#### Summary
## Model Examination [optional]
<!-- Relevant interpretability work for the model goes here -->
[More Information Needed]
## Environmental Impact
<!-- Total emissions (in grams of CO2eq) and additional considerations, such as electricity usage, go here. Edit the suggested text below accordingly -->
Carbon emissions can be estimated using the [Machine Learning Impact calculator](https://mlco2.github.io/impact#compute) presented in [Lacoste et al. (2019)](https://arxiv.org/abs/1910.09700).
- **Hardware Type:** [More Information Needed]
- **Hours used:** [More Information Needed]
- **Cloud Provider:** [More Information Needed]
- **Compute Region:** [More Information Needed]
- **Carbon Emitted:** [More Information Needed]
## Technical Specifications [optional]
### Model Architecture and Objective
[More Information Needed]
### Compute Infrastructure
[More Information Needed]
#### Hardware
[More Information Needed]
#### Software
[More Information Needed]
## Citation [optional]
<!-- If there is a paper or blog post introducing the model, the APA and Bibtex information for that should go in this section. -->
**BibTeX:**
[More Information Needed]
**APA:**
[More Information Needed]
## Glossary [optional]
<!-- If relevant, include terms and calculations in this section that can help readers understand the model or model card. -->
[More Information Needed]
## More Information [optional]
[More Information Needed]
## Model Card Authors [optional]
[More Information Needed]
## Model Card Contact
[More Information Needed] |
ahishamm/vit-large-binary-isic-sharpened-patch-32 | ahishamm | "2023-07-01T18:15:54Z" | 6 | 0 | transformers | [
"transformers",
"pytorch",
"tensorboard",
"vit",
"image-classification",
"generated_from_trainer",
"license:apache-2.0",
"autotrain_compatible",
"endpoints_compatible",
"region:us"
] | image-classification | "2023-07-01T16:27:51Z" | ---
license: apache-2.0
tags:
- image-classification
- generated_from_trainer
metrics:
- accuracy
- recall
- f1
- precision
model-index:
- name: vit-large-binary-isic-sharpened-patch-32
results: []
---
<!-- This model card has been generated automatically according to the information the Trainer had access to. You
should probably proofread and complete it, then remove this comment. -->
# vit-large-binary-isic-sharpened-patch-32
This model is a fine-tuned version of [google/vit-large-patch32-224-in21k](https://huggingface.co/google/vit-large-patch32-224-in21k) on the ahishamm/isic_binary_sharpened dataset.
It achieves the following results on the evaluation set:
- Loss: 0.2092
- Accuracy: 0.9202
- Recall: 0.9202
- F1: 0.9202
- Precision: 0.9202
## Model description
More information needed
## Intended uses & limitations
More information needed
## Training and evaluation data
More information needed
## Training procedure
### Training hyperparameters
The following hyperparameters were used during training:
- learning_rate: 0.0002
- train_batch_size: 16
- eval_batch_size: 8
- seed: 42
- optimizer: Adam with betas=(0.9,0.999) and epsilon=1e-08
- lr_scheduler_type: linear
- num_epochs: 4
### Training results
| Training Loss | Epoch | Step | Validation Loss | Accuracy | Recall | F1 | Precision |
|:-------------:|:-----:|:----:|:---------------:|:--------:|:------:|:------:|:---------:|
| 0.3437 | 0.09 | 100 | 0.3367 | 0.8412 | 0.8412 | 0.8412 | 0.8412 |
| 0.3702 | 0.18 | 200 | 0.3094 | 0.8585 | 0.8585 | 0.8585 | 0.8585 |
| 0.2693 | 0.28 | 300 | 0.4361 | 0.8007 | 0.8007 | 0.8007 | 0.8007 |
| 0.3183 | 0.37 | 400 | 0.2955 | 0.8643 | 0.8643 | 0.8643 | 0.8643 |
| 0.2688 | 0.46 | 500 | 0.3064 | 0.8603 | 0.8603 | 0.8603 | 0.8603 |
| 0.2507 | 0.55 | 600 | 0.3556 | 0.8329 | 0.8329 | 0.8329 | 0.8329 |
| 0.203 | 0.65 | 700 | 0.3134 | 0.8433 | 0.8433 | 0.8433 | 0.8433 |
| 0.2315 | 0.74 | 800 | 0.2525 | 0.8856 | 0.8856 | 0.8856 | 0.8856 |
| 0.3527 | 0.83 | 900 | 0.2815 | 0.8731 | 0.8731 | 0.8731 | 0.8731 |
| 0.292 | 0.92 | 1000 | 0.3879 | 0.8534 | 0.8534 | 0.8534 | 0.8534 |
| 0.1342 | 1.02 | 1100 | 0.2927 | 0.8874 | 0.8874 | 0.8874 | 0.8874 |
| 0.1571 | 1.11 | 1200 | 0.2560 | 0.8912 | 0.8912 | 0.8912 | 0.8912 |
| 0.1787 | 1.2 | 1300 | 0.3245 | 0.8789 | 0.8789 | 0.8789 | 0.8789 |
| 0.1757 | 1.29 | 1400 | 0.3308 | 0.8720 | 0.8720 | 0.8720 | 0.8720 |
| 0.1867 | 1.39 | 1500 | 0.2716 | 0.8876 | 0.8876 | 0.8876 | 0.8876 |
| 0.124 | 1.48 | 1600 | 0.3663 | 0.8744 | 0.8744 | 0.8744 | 0.8744 |
| 0.082 | 1.57 | 1700 | 0.2793 | 0.9034 | 0.9034 | 0.9034 | 0.9034 |
| 0.1365 | 1.66 | 1800 | 0.2399 | 0.9077 | 0.9077 | 0.9077 | 0.9077 |
| 0.0998 | 1.76 | 1900 | 0.3361 | 0.8901 | 0.8901 | 0.8901 | 0.8901 |
| 0.0748 | 1.85 | 2000 | 0.3239 | 0.8960 | 0.8960 | 0.8960 | 0.8960 |
| 0.1163 | 1.94 | 2100 | 0.2092 | 0.9202 | 0.9202 | 0.9202 | 0.9202 |
| 0.0604 | 2.03 | 2200 | 0.3056 | 0.9139 | 0.9139 | 0.9139 | 0.9139 |
| 0.0792 | 2.13 | 2300 | 0.2880 | 0.9071 | 0.9071 | 0.9071 | 0.9071 |
| 0.0749 | 2.22 | 2400 | 0.3015 | 0.9070 | 0.9070 | 0.9070 | 0.9070 |
| 0.0032 | 2.31 | 2500 | 0.3685 | 0.9090 | 0.9090 | 0.9090 | 0.9090 |
| 0.1038 | 2.4 | 2600 | 0.3539 | 0.9075 | 0.9075 | 0.9075 | 0.9075 |
| 0.0474 | 2.5 | 2700 | 0.3220 | 0.9152 | 0.9152 | 0.9152 | 0.9152 |
| 0.0376 | 2.59 | 2800 | 0.2926 | 0.9203 | 0.9203 | 0.9203 | 0.9203 |
| 0.0424 | 2.68 | 2900 | 0.3463 | 0.9065 | 0.9065 | 0.9065 | 0.9065 |
| 0.0408 | 2.77 | 3000 | 0.2772 | 0.9263 | 0.9263 | 0.9263 | 0.9263 |
| 0.0467 | 2.87 | 3100 | 0.2963 | 0.9227 | 0.9227 | 0.9227 | 0.9227 |
| 0.0083 | 2.96 | 3200 | 0.2971 | 0.9203 | 0.9203 | 0.9203 | 0.9203 |
| 0.0165 | 3.05 | 3300 | 0.3162 | 0.9257 | 0.9257 | 0.9257 | 0.9257 |
| 0.0023 | 3.14 | 3400 | 0.3147 | 0.9267 | 0.9267 | 0.9267 | 0.9267 |
| 0.0009 | 3.23 | 3500 | 0.3433 | 0.9266 | 0.9266 | 0.9266 | 0.9266 |
| 0.0007 | 3.33 | 3600 | 0.3216 | 0.9312 | 0.9312 | 0.9312 | 0.9312 |
| 0.0011 | 3.42 | 3700 | 0.3209 | 0.9346 | 0.9346 | 0.9346 | 0.9346 |
| 0.0029 | 3.51 | 3800 | 0.3236 | 0.9325 | 0.9325 | 0.9325 | 0.9325 |
| 0.0011 | 3.6 | 3900 | 0.3297 | 0.9302 | 0.9302 | 0.9302 | 0.9302 |
| 0.0225 | 3.7 | 4000 | 0.3263 | 0.9323 | 0.9323 | 0.9323 | 0.9323 |
| 0.0008 | 3.79 | 4100 | 0.3352 | 0.9311 | 0.9311 | 0.9311 | 0.9311 |
| 0.0391 | 3.88 | 4200 | 0.3343 | 0.9282 | 0.9282 | 0.9282 | 0.9282 |
| 0.0019 | 3.97 | 4300 | 0.3319 | 0.9280 | 0.9280 | 0.9280 | 0.9280 |
### Framework versions
- Transformers 4.30.2
- Pytorch 2.0.1+cu118
- Datasets 2.13.1
- Tokenizers 0.13.3
|
OpenGVLab/ASM-FT | OpenGVLab | "2024-02-21T07:25:01Z" | 1 | 6 | transformers | [
"transformers",
"pytorch",
"blip-2",
"license:apache-2.0",
"endpoints_compatible",
"region:us"
] | null | "2024-02-14T04:52:09Z" | ---
license: apache-2.0
---
# ASM-FT Model Card
## Model details
**Model type:**
ASM is a unified vision-language foundation model for open-world panoptic visual recognition and understanding. Aligning with LLMs, it supports versatile generation tasks, demonstrating impressive region comprehension capability.
**Model date:**
ASM was trained in July 2023.
**Paper or resources for more information:**
https://github.com/OpenGVLab/all-seeing
## License
ASM is open-sourced under the Apache License 2.0.
**Where to send questions or comments about the model:**
https://github.com/OpenGVLab/all-seeing/issues
## Intended use
**Primary intended uses:**
The primary use of ASM is research on large multimodal models and chatbots.
**Primary intended users:**
The primary intended users of the model are researchers and hobbyists in computer vision, natural language processing, machine learning, and artificial intelligence.
## Training dataset
The pretrain phase employs [AS-1B](https://huggingface.co/datasets/Weiyun1025/AS-100M/tree/main) and [Laion-COCO](https://huggingface.co/datasets/laion/laion-coco).
The finetuning phase employs [AS-Core](https://huggingface.co/datasets/Weiyun1025/AS-Core), [RefCOCOg](https://github.com/lichengunc/refer), [VG](https://homes.cs.washington.edu/~ranjay/visualgenome/index.html), [LLaVA-150K](https://huggingface.co/datasets/liuhaotian/LLaVA-Instruct-150K), [COCO Caption](https://cocodataset.org/#home), [TextCaps](https://textvqa.org/textcaps/), [VQAv2](https://visualqa.org/), and [GQA](https://cs.stanford.edu/people/dorarad/gqa/).
## Evaluation dataset
A collection of 4 benchmarks, including 2 image captioning benchmarks, and 2 region captioning benchmarks.
|
reyvan/bert_best | reyvan | "2024-01-08T02:52:54Z" | 90 | 0 | transformers | [
"transformers",
"pytorch",
"bert",
"fill-mask",
"exbert",
"en",
"dataset:bookcorpus",
"dataset:wikipedia",
"arxiv:1810.04805",
"license:apache-2.0",
"autotrain_compatible",
"endpoints_compatible",
"region:us"
] | fill-mask | "2024-01-08T02:50:59Z" | ---
language: en
tags:
- exbert
license: apache-2.0
datasets:
- bookcorpus
- wikipedia
---
# BERT base model (cased)
Pretrained model on English language using a masked language modeling (MLM) objective. It was introduced in
[this paper](https://arxiv.org/abs/1810.04805) and first released in
[this repository](https://github.com/google-research/bert). This model is case-sensitive: it makes a difference between
english and English.
Disclaimer: The team releasing BERT did not write a model card for this model so this model card has been written by
the Hugging Face team.
## Model description
BERT is a transformers model pretrained on a large corpus of English data in a self-supervised fashion. This means it
was pretrained on the raw texts only, with no humans labelling them in any way (which is why it can use lots of
publicly available data) with an automatic process to generate inputs and labels from those texts. More precisely, it
was pretrained with two objectives:
- Masked language modeling (MLM): taking a sentence, the model randomly masks 15% of the words in the input then run
the entire masked sentence through the model and has to predict the masked words. This is different from traditional
recurrent neural networks (RNNs) that usually see the words one after the other, or from autoregressive models like
GPT which internally mask the future tokens. It allows the model to learn a bidirectional representation of the
sentence.
- Next sentence prediction (NSP): the models concatenates two masked sentences as inputs during pretraining. Sometimes
they correspond to sentences that were next to each other in the original text, sometimes not. The model then has to
predict if the two sentences were following each other or not.
This way, the model learns an inner representation of the English language that can then be used to extract features
useful for downstream tasks: if you have a dataset of labeled sentences for instance, you can train a standard
classifier using the features produced by the BERT model as inputs.
## Intended uses & limitations
You can use the raw model for either masked language modeling or next sentence prediction, but it's mostly intended to
be fine-tuned on a downstream task. See the [model hub](https://huggingface.co/models?filter=bert) to look for
fine-tuned versions on a task that interests you.
Note that this model is primarily aimed at being fine-tuned on tasks that use the whole sentence (potentially masked)
to make decisions, such as sequence classification, token classification or question answering. For tasks such as text
generation you should look at model like GPT2.
### How to use
You can use this model directly with a pipeline for masked language modeling:
```python
>>> from transformers import pipeline
>>> unmasker = pipeline('fill-mask', model='bert-base-cased')
>>> unmasker("Hello I'm a [MASK] model.")
[{'sequence': "[CLS] Hello I'm a fashion model. [SEP]",
'score': 0.09019174426794052,
'token': 4633,
'token_str': 'fashion'},
{'sequence': "[CLS] Hello I'm a new model. [SEP]",
'score': 0.06349995732307434,
'token': 1207,
'token_str': 'new'},
{'sequence': "[CLS] Hello I'm a male model. [SEP]",
'score': 0.06228214129805565,
'token': 2581,
'token_str': 'male'},
{'sequence': "[CLS] Hello I'm a professional model. [SEP]",
'score': 0.0441727414727211,
'token': 1848,
'token_str': 'professional'},
{'sequence': "[CLS] Hello I'm a super model. [SEP]",
'score': 0.03326151892542839,
'token': 7688,
'token_str': 'super'}]
```
Here is how to use this model to get the features of a given text in PyTorch:
```python
from transformers import BertTokenizer, BertModel
tokenizer = BertTokenizer.from_pretrained('bert-base-cased')
model = BertModel.from_pretrained("bert-base-cased")
text = "Replace me by any text you'd like."
encoded_input = tokenizer(text, return_tensors='pt')
output = model(**encoded_input)
```
and in TensorFlow:
```python
from transformers import BertTokenizer, TFBertModel
tokenizer = BertTokenizer.from_pretrained('bert-base-cased')
model = TFBertModel.from_pretrained("bert-base-cased")
text = "Replace me by any text you'd like."
encoded_input = tokenizer(text, return_tensors='tf')
output = model(encoded_input)
```
### Limitations and bias
Even if the training data used for this model could be characterized as fairly neutral, this model can have biased
predictions:
```python
>>> from transformers import pipeline
>>> unmasker = pipeline('fill-mask', model='bert-base-cased')
>>> unmasker("The man worked as a [MASK].")
[{'sequence': '[CLS] The man worked as a lawyer. [SEP]',
'score': 0.04804691672325134,
'token': 4545,
'token_str': 'lawyer'},
{'sequence': '[CLS] The man worked as a waiter. [SEP]',
'score': 0.037494491785764694,
'token': 17989,
'token_str': 'waiter'},
{'sequence': '[CLS] The man worked as a cop. [SEP]',
'score': 0.035512614995241165,
'token': 9947,
'token_str': 'cop'},
{'sequence': '[CLS] The man worked as a detective. [SEP]',
'score': 0.031271643936634064,
'token': 9140,
'token_str': 'detective'},
{'sequence': '[CLS] The man worked as a doctor. [SEP]',
'score': 0.027423162013292313,
'token': 3995,
'token_str': 'doctor'}]
>>> unmasker("The woman worked as a [MASK].")
[{'sequence': '[CLS] The woman worked as a nurse. [SEP]',
'score': 0.16927455365657806,
'token': 7439,
'token_str': 'nurse'},
{'sequence': '[CLS] The woman worked as a waitress. [SEP]',
'score': 0.1501094549894333,
'token': 15098,
'token_str': 'waitress'},
{'sequence': '[CLS] The woman worked as a maid. [SEP]',
'score': 0.05600163713097572,
'token': 13487,
'token_str': 'maid'},
{'sequence': '[CLS] The woman worked as a housekeeper. [SEP]',
'score': 0.04838843643665314,
'token': 26458,
'token_str': 'housekeeper'},
{'sequence': '[CLS] The woman worked as a cook. [SEP]',
'score': 0.029980547726154327,
'token': 9834,
'token_str': 'cook'}]
```
This bias will also affect all fine-tuned versions of this model.
## Training data
The BERT model was pretrained on [BookCorpus](https://yknzhu.wixsite.com/mbweb), a dataset consisting of 11,038
unpublished books and [English Wikipedia](https://en.wikipedia.org/wiki/English_Wikipedia) (excluding lists, tables and
headers).
## Training procedure
### Preprocessing
The texts are tokenized using WordPiece and a vocabulary size of 30,000. The inputs of the model are then of the form:
```
[CLS] Sentence A [SEP] Sentence B [SEP]
```
With probability 0.5, sentence A and sentence B correspond to two consecutive sentences in the original corpus and in
the other cases, it's another random sentence in the corpus. Note that what is considered a sentence here is a
consecutive span of text usually longer than a single sentence. The only constrain is that the result with the two
"sentences" has a combined length of less than 512 tokens.
The details of the masking procedure for each sentence are the following:
- 15% of the tokens are masked.
- In 80% of the cases, the masked tokens are replaced by `[MASK]`.
- In 10% of the cases, the masked tokens are replaced by a random token (different) from the one they replace.
- In the 10% remaining cases, the masked tokens are left as is.
### Pretraining
The model was trained on 4 cloud TPUs in Pod configuration (16 TPU chips total) for one million steps with a batch size
of 256. The sequence length was limited to 128 tokens for 90% of the steps and 512 for the remaining 10%. The optimizer
used is Adam with a learning rate of 1e-4, \\(\beta_{1} = 0.9\\) and \\(\beta_{2} = 0.999\\), a weight decay of 0.01,
learning rate warmup for 10,000 steps and linear decay of the learning rate after.
## Evaluation results
When fine-tuned on downstream tasks, this model achieves the following results:
Glue test results:
| Task | MNLI-(m/mm) | QQP | QNLI | SST-2 | CoLA | STS-B | MRPC | RTE | Average |
|:----:|:-----------:|:----:|:----:|:-----:|:----:|:-----:|:----:|:----:|:-------:|
| | 84.6/83.4 | 71.2 | 90.5 | 93.5 | 52.1 | 85.8 | 88.9 | 66.4 | 79.6 |
### BibTeX entry and citation info
```bibtex
@article{DBLP:journals/corr/abs-1810-04805,
author = {Jacob Devlin and
Ming{-}Wei Chang and
Kenton Lee and
Kristina Toutanova},
title = {{BERT:} Pre-training of Deep Bidirectional Transformers for Language
Understanding},
journal = {CoRR},
volume = {abs/1810.04805},
year = {2018},
url = {http://arxiv.org/abs/1810.04805},
archivePrefix = {arXiv},
eprint = {1810.04805},
timestamp = {Tue, 30 Oct 2018 20:39:56 +0100},
biburl = {https://dblp.org/rec/journals/corr/abs-1810-04805.bib},
bibsource = {dblp computer science bibliography, https://dblp.org}
}
```
<a href="https://huggingface.co/exbert/?model=bert-base-cased">
<img width="300px" src="https://cdn-media.huggingface.co/exbert/button.png">
</a>
|
saransh03sharma/mintrec-llama-3-8b-1005-shot | saransh03sharma | "2024-05-05T18:08:34Z" | 5 | 0 | transformers | [
"transformers",
"safetensors",
"llama",
"text-generation",
"arxiv:1910.09700",
"autotrain_compatible",
"text-generation-inference",
"endpoints_compatible",
"region:us"
] | text-generation | "2024-05-05T18:02:50Z" | ---
library_name: transformers
tags: []
---
# Model Card for Model ID
<!-- Provide a quick summary of what the model is/does. -->
## Model Details
### Model Description
<!-- Provide a longer summary of what this model is. -->
This is the model card of a 🤗 transformers model that has been pushed on the Hub. This model card has been automatically generated.
- **Developed by:** [More Information Needed]
- **Funded by [optional]:** [More Information Needed]
- **Shared by [optional]:** [More Information Needed]
- **Model type:** [More Information Needed]
- **Language(s) (NLP):** [More Information Needed]
- **License:** [More Information Needed]
- **Finetuned from model [optional]:** [More Information Needed]
### Model Sources [optional]
<!-- Provide the basic links for the model. -->
- **Repository:** [More Information Needed]
- **Paper [optional]:** [More Information Needed]
- **Demo [optional]:** [More Information Needed]
## Uses
<!-- Address questions around how the model is intended to be used, including the foreseeable users of the model and those affected by the model. -->
### Direct Use
<!-- This section is for the model use without fine-tuning or plugging into a larger ecosystem/app. -->
[More Information Needed]
### Downstream Use [optional]
<!-- This section is for the model use when fine-tuned for a task, or when plugged into a larger ecosystem/app -->
[More Information Needed]
### Out-of-Scope Use
<!-- This section addresses misuse, malicious use, and uses that the model will not work well for. -->
[More Information Needed]
## Bias, Risks, and Limitations
<!-- This section is meant to convey both technical and sociotechnical limitations. -->
[More Information Needed]
### Recommendations
<!-- This section is meant to convey recommendations with respect to the bias, risk, and technical limitations. -->
Users (both direct and downstream) should be made aware of the risks, biases and limitations of the model. More information needed for further recommendations.
## How to Get Started with the Model
Use the code below to get started with the model.
[More Information Needed]
## Training Details
### Training Data
<!-- This should link to a Dataset Card, perhaps with a short stub of information on what the training data is all about as well as documentation related to data pre-processing or additional filtering. -->
[More Information Needed]
### Training Procedure
<!-- This relates heavily to the Technical Specifications. Content here should link to that section when it is relevant to the training procedure. -->
#### Preprocessing [optional]
[More Information Needed]
#### Training Hyperparameters
- **Training regime:** [More Information Needed] <!--fp32, fp16 mixed precision, bf16 mixed precision, bf16 non-mixed precision, fp16 non-mixed precision, fp8 mixed precision -->
#### Speeds, Sizes, Times [optional]
<!-- This section provides information about throughput, start/end time, checkpoint size if relevant, etc. -->
[More Information Needed]
## Evaluation
<!-- This section describes the evaluation protocols and provides the results. -->
### Testing Data, Factors & Metrics
#### Testing Data
<!-- This should link to a Dataset Card if possible. -->
[More Information Needed]
#### Factors
<!-- These are the things the evaluation is disaggregating by, e.g., subpopulations or domains. -->
[More Information Needed]
#### Metrics
<!-- These are the evaluation metrics being used, ideally with a description of why. -->
[More Information Needed]
### Results
[More Information Needed]
#### Summary
## Model Examination [optional]
<!-- Relevant interpretability work for the model goes here -->
[More Information Needed]
## Environmental Impact
<!-- Total emissions (in grams of CO2eq) and additional considerations, such as electricity usage, go here. Edit the suggested text below accordingly -->
Carbon emissions can be estimated using the [Machine Learning Impact calculator](https://mlco2.github.io/impact#compute) presented in [Lacoste et al. (2019)](https://arxiv.org/abs/1910.09700).
- **Hardware Type:** [More Information Needed]
- **Hours used:** [More Information Needed]
- **Cloud Provider:** [More Information Needed]
- **Compute Region:** [More Information Needed]
- **Carbon Emitted:** [More Information Needed]
## Technical Specifications [optional]
### Model Architecture and Objective
[More Information Needed]
### Compute Infrastructure
[More Information Needed]
#### Hardware
[More Information Needed]
#### Software
[More Information Needed]
## Citation [optional]
<!-- If there is a paper or blog post introducing the model, the APA and Bibtex information for that should go in this section. -->
**BibTeX:**
[More Information Needed]
**APA:**
[More Information Needed]
## Glossary [optional]
<!-- If relevant, include terms and calculations in this section that can help readers understand the model or model card. -->
[More Information Needed]
## More Information [optional]
[More Information Needed]
## Model Card Authors [optional]
[More Information Needed]
## Model Card Contact
[More Information Needed] |
earnxus/f48a87c5-1da4-4069-b631-22d1fbd78f87 | earnxus | "2025-02-06T14:32:10Z" | 8 | 0 | peft | [
"peft",
"safetensors",
"mistral",
"axolotl",
"generated_from_trainer",
"base_model:unsloth/Mistral-Nemo-Instruct-2407",
"base_model:adapter:unsloth/Mistral-Nemo-Instruct-2407",
"license:apache-2.0",
"8-bit",
"bitsandbytes",
"region:us"
] | null | "2025-02-06T13:24:32Z" | ---
library_name: peft
license: apache-2.0
base_model: unsloth/Mistral-Nemo-Instruct-2407
tags:
- axolotl
- generated_from_trainer
model-index:
- name: f48a87c5-1da4-4069-b631-22d1fbd78f87
results: []
---
<!-- This model card has been generated automatically according to the information the Trainer had access to. You
should probably proofread and complete it, then remove this comment. -->
[<img src="https://raw.githubusercontent.com/axolotl-ai-cloud/axolotl/main/image/axolotl-badge-web.png" alt="Built with Axolotl" width="200" height="32"/>](https://github.com/axolotl-ai-cloud/axolotl)
<details><summary>See axolotl config</summary>
axolotl version: `0.4.1`
```yaml
adapter: lora
base_model: unsloth/Mistral-Nemo-Instruct-2407
bf16: auto
chat_template: llama3
dataset_prepared_path: null
datasets:
- data_files:
- 6b369c47ea81dd96_train_data.json
ds_type: json
format: custom
path: /workspace/input_data/6b369c47ea81dd96_train_data.json
type:
field_input: ingredients
field_instruction: method
field_output: title
format: '{instruction} {input}'
no_input_format: '{instruction}'
system_format: '{system}'
system_prompt: ''
debug: null
deepspeed: null
device_map: auto
do_eval: true
early_stopping_patience: null
eval_batch_size: 2
eval_max_new_tokens: 128
eval_steps: null
eval_table_size: null
evals_per_epoch: null
flash_attention: true
fp16: null
fsdp: null
fsdp_config: null
gradient_accumulation_steps: 4
gradient_checkpointing: true
gradient_clipping: 1.0
group_by_length: true
hub_model_id: earnxus/f48a87c5-1da4-4069-b631-22d1fbd78f87
hub_repo: null
hub_strategy: end
hub_token: null
learning_rate: 0.0001
load_in_4bit: true
load_in_8bit: true
local_rank: null
logging_steps: 1
lora_alpha: 16
lora_dropout: 0.05
lora_fan_in_fan_out: null
lora_model_dir: null
lora_r: 8
lora_target_linear: true
lr_scheduler: cosine
max_grad_norm: 1.0
max_memory:
0: 75GB
max_steps: 500
micro_batch_size: 2
mlflow_experiment_name: /tmp/6b369c47ea81dd96_train_data.json
model_type: AutoModelForCausalLM
num_epochs: 1
optimizer: adamw_bnb_8bit
output_dir: miner_id_24
pad_to_sequence_len: true
resume_from_checkpoint: null
s2_attention: null
sample_packing: false
save_steps: null
saves_per_epoch: null
sequence_len: 1024
strict: false
tf32: false
tokenizer_type: AutoTokenizer
train_on_inputs: false
trust_remote_code: true
val_set_size: 0.05
wandb_entity: techspear-hub
wandb_mode: online
wandb_name: 35e5fb94-3014-4725-b664-b29d4efa8cac
wandb_project: Gradients-On-Nine
wandb_run: your_name
wandb_runid: 35e5fb94-3014-4725-b664-b29d4efa8cac
warmup_steps: 5
weight_decay: 0.01
xformers_attention: null
```
</details><br>
# f48a87c5-1da4-4069-b631-22d1fbd78f87
This model is a fine-tuned version of [unsloth/Mistral-Nemo-Instruct-2407](https://huggingface.co/unsloth/Mistral-Nemo-Instruct-2407) on the None dataset.
It achieves the following results on the evaluation set:
- Loss: 0.8999
## Model description
More information needed
## Intended uses & limitations
More information needed
## Training and evaluation data
More information needed
## Training procedure
### Training hyperparameters
The following hyperparameters were used during training:
- learning_rate: 0.0001
- train_batch_size: 2
- eval_batch_size: 2
- seed: 42
- gradient_accumulation_steps: 4
- total_train_batch_size: 8
- optimizer: Use OptimizerNames.ADAMW_BNB with betas=(0.9,0.999) and epsilon=1e-08 and optimizer_args=No additional optimizer arguments
- lr_scheduler_type: cosine
- lr_scheduler_warmup_steps: 5
- training_steps: 500
### Training results
| Training Loss | Epoch | Step | Validation Loss |
|:-------------:|:------:|:----:|:---------------:|
| 4.1943 | 0.3211 | 500 | 0.8999 |
### Framework versions
- PEFT 0.13.2
- Transformers 4.46.0
- Pytorch 2.5.0+cu124
- Datasets 3.0.1
- Tokenizers 0.20.1 |
ngocnamk3er/flan-t5-text2sql-lora | ngocnamk3er | "2025-03-16T21:53:19Z" | 87 | 0 | peft | [
"peft",
"safetensors",
"generated_from_trainer",
"base_model:google/flan-t5-large",
"base_model:adapter:google/flan-t5-large",
"license:apache-2.0",
"region:us"
] | null | "2025-03-13T15:53:23Z" | ---
library_name: peft
license: apache-2.0
base_model: google/flan-t5-large
tags:
- generated_from_trainer
model-index:
- name: flan-t5-text2sql-lora
results: []
---
<!-- This model card has been generated automatically according to the information the Trainer had access to. You
should probably proofread and complete it, then remove this comment. -->
# flan-t5-text2sql-lora
This model is a fine-tuned version of [google/flan-t5-large](https://huggingface.co/google/flan-t5-large) on an unknown dataset.
## Model description
More information needed
## Intended uses & limitations
More information needed
## Training and evaluation data
More information needed
## Training procedure
### Training hyperparameters
The following hyperparameters were used during training:
- learning_rate: 3e-05
- train_batch_size: 4
- eval_batch_size: 4
- seed: 42
- optimizer: Use adamw_torch with betas=(0.9,0.999) and epsilon=1e-08 and optimizer_args=No additional optimizer arguments
- lr_scheduler_type: linear
- training_steps: 50000
### Training results
### Framework versions
- PEFT 0.14.0
- Transformers 4.49.0
- Pytorch 2.5.1+cu121
- Datasets 3.3.1
- Tokenizers 0.21.0 |
cuhksz-aimo/mix_1k_0309_7b | cuhksz-aimo | "2025-03-10T11:48:44Z" | 0 | 0 | transformers | [
"transformers",
"safetensors",
"qwen2",
"text-generation",
"llama-factory",
"full",
"generated_from_trainer",
"conversational",
"license:other",
"autotrain_compatible",
"text-generation-inference",
"endpoints_compatible",
"region:us"
] | text-generation | "2025-03-10T09:28:02Z" | ---
library_name: transformers
license: other
base_model: deepseek/DeepSeek-R1-Distill-Qwen-7B
tags:
- llama-factory
- full
- generated_from_trainer
model-index:
- name: mix_1k
results: []
---
<!-- This model card has been generated automatically according to the information the Trainer had access to. You
should probably proofread and complete it, then remove this comment. -->
# mix_1k
This model is a fine-tuned version of [/home/export/base/sc100182/sc100182/online1/code/models/DeepSeek-R1-Distill-Qwen-7B](https://huggingface.co//home/export/base/sc100182/sc100182/online1/code/models/DeepSeek-R1-Distill-Qwen-7B) on the mix_1k dataset.
## Model description
More information needed
## Intended uses & limitations
More information needed
## Training and evaluation data
More information needed
## Training procedure
### Training hyperparameters
The following hyperparameters were used during training:
- learning_rate: 2e-05
- train_batch_size: 1
- eval_batch_size: 8
- seed: 42
- distributed_type: multi-GPU
- num_devices: 8
- total_train_batch_size: 8
- total_eval_batch_size: 64
- optimizer: Use OptimizerNames.ADAMW_TORCH with betas=(0.9,0.999) and epsilon=1e-08 and optimizer_args=No additional optimizer arguments
- lr_scheduler_type: cosine
- lr_scheduler_warmup_ratio: 0.1
- num_epochs: 3
### Training results
### Framework versions
- Transformers 4.48.2
- Pytorch 2.6.0+cu124
- Datasets 3.2.0
- Tokenizers 0.21.0
|
RichardErkhov/su1301397274_-_gpt2-finetuned-tofu-gguf | RichardErkhov | "2025-03-19T06:58:12Z" | 0 | 0 | null | [
"gguf",
"arxiv:1910.09700",
"endpoints_compatible",
"region:us"
] | null | "2025-03-19T06:54:37Z" | Quantization made by Richard Erkhov.
[Github](https://github.com/RichardErkhov)
[Discord](https://discord.gg/pvy7H8DZMG)
[Request more models](https://github.com/RichardErkhov/quant_request)
gpt2-finetuned-tofu - GGUF
- Model creator: https://huggingface.co/su1301397274/
- Original model: https://huggingface.co/su1301397274/gpt2-finetuned-tofu/
| Name | Quant method | Size |
| ---- | ---- | ---- |
| [gpt2-finetuned-tofu.Q2_K.gguf](https://huggingface.co/RichardErkhov/su1301397274_-_gpt2-finetuned-tofu-gguf/blob/main/gpt2-finetuned-tofu.Q2_K.gguf) | Q2_K | 0.08GB |
| [gpt2-finetuned-tofu.IQ3_XS.gguf](https://huggingface.co/RichardErkhov/su1301397274_-_gpt2-finetuned-tofu-gguf/blob/main/gpt2-finetuned-tofu.IQ3_XS.gguf) | IQ3_XS | 0.08GB |
| [gpt2-finetuned-tofu.IQ3_S.gguf](https://huggingface.co/RichardErkhov/su1301397274_-_gpt2-finetuned-tofu-gguf/blob/main/gpt2-finetuned-tofu.IQ3_S.gguf) | IQ3_S | 0.08GB |
| [gpt2-finetuned-tofu.Q3_K_S.gguf](https://huggingface.co/RichardErkhov/su1301397274_-_gpt2-finetuned-tofu-gguf/blob/main/gpt2-finetuned-tofu.Q3_K_S.gguf) | Q3_K_S | 0.08GB |
| [gpt2-finetuned-tofu.IQ3_M.gguf](https://huggingface.co/RichardErkhov/su1301397274_-_gpt2-finetuned-tofu-gguf/blob/main/gpt2-finetuned-tofu.IQ3_M.gguf) | IQ3_M | 0.09GB |
| [gpt2-finetuned-tofu.Q3_K.gguf](https://huggingface.co/RichardErkhov/su1301397274_-_gpt2-finetuned-tofu-gguf/blob/main/gpt2-finetuned-tofu.Q3_K.gguf) | Q3_K | 0.09GB |
| [gpt2-finetuned-tofu.Q3_K_M.gguf](https://huggingface.co/RichardErkhov/su1301397274_-_gpt2-finetuned-tofu-gguf/blob/main/gpt2-finetuned-tofu.Q3_K_M.gguf) | Q3_K_M | 0.09GB |
| [gpt2-finetuned-tofu.Q3_K_L.gguf](https://huggingface.co/RichardErkhov/su1301397274_-_gpt2-finetuned-tofu-gguf/blob/main/gpt2-finetuned-tofu.Q3_K_L.gguf) | Q3_K_L | 0.1GB |
| [gpt2-finetuned-tofu.IQ4_XS.gguf](https://huggingface.co/RichardErkhov/su1301397274_-_gpt2-finetuned-tofu-gguf/blob/main/gpt2-finetuned-tofu.IQ4_XS.gguf) | IQ4_XS | 0.1GB |
| [gpt2-finetuned-tofu.Q4_0.gguf](https://huggingface.co/RichardErkhov/su1301397274_-_gpt2-finetuned-tofu-gguf/blob/main/gpt2-finetuned-tofu.Q4_0.gguf) | Q4_0 | 0.1GB |
| [gpt2-finetuned-tofu.IQ4_NL.gguf](https://huggingface.co/RichardErkhov/su1301397274_-_gpt2-finetuned-tofu-gguf/blob/main/gpt2-finetuned-tofu.IQ4_NL.gguf) | IQ4_NL | 0.1GB |
| [gpt2-finetuned-tofu.Q4_K_S.gguf](https://huggingface.co/RichardErkhov/su1301397274_-_gpt2-finetuned-tofu-gguf/blob/main/gpt2-finetuned-tofu.Q4_K_S.gguf) | Q4_K_S | 0.1GB |
| [gpt2-finetuned-tofu.Q4_K.gguf](https://huggingface.co/RichardErkhov/su1301397274_-_gpt2-finetuned-tofu-gguf/blob/main/gpt2-finetuned-tofu.Q4_K.gguf) | Q4_K | 0.11GB |
| [gpt2-finetuned-tofu.Q4_K_M.gguf](https://huggingface.co/RichardErkhov/su1301397274_-_gpt2-finetuned-tofu-gguf/blob/main/gpt2-finetuned-tofu.Q4_K_M.gguf) | Q4_K_M | 0.11GB |
| [gpt2-finetuned-tofu.Q4_1.gguf](https://huggingface.co/RichardErkhov/su1301397274_-_gpt2-finetuned-tofu-gguf/blob/main/gpt2-finetuned-tofu.Q4_1.gguf) | Q4_1 | 0.11GB |
| [gpt2-finetuned-tofu.Q5_0.gguf](https://huggingface.co/RichardErkhov/su1301397274_-_gpt2-finetuned-tofu-gguf/blob/main/gpt2-finetuned-tofu.Q5_0.gguf) | Q5_0 | 0.11GB |
| [gpt2-finetuned-tofu.Q5_K_S.gguf](https://huggingface.co/RichardErkhov/su1301397274_-_gpt2-finetuned-tofu-gguf/blob/main/gpt2-finetuned-tofu.Q5_K_S.gguf) | Q5_K_S | 0.11GB |
| [gpt2-finetuned-tofu.Q5_K.gguf](https://huggingface.co/RichardErkhov/su1301397274_-_gpt2-finetuned-tofu-gguf/blob/main/gpt2-finetuned-tofu.Q5_K.gguf) | Q5_K | 0.12GB |
| [gpt2-finetuned-tofu.Q5_K_M.gguf](https://huggingface.co/RichardErkhov/su1301397274_-_gpt2-finetuned-tofu-gguf/blob/main/gpt2-finetuned-tofu.Q5_K_M.gguf) | Q5_K_M | 0.12GB |
| [gpt2-finetuned-tofu.Q5_1.gguf](https://huggingface.co/RichardErkhov/su1301397274_-_gpt2-finetuned-tofu-gguf/blob/main/gpt2-finetuned-tofu.Q5_1.gguf) | Q5_1 | 0.12GB |
| [gpt2-finetuned-tofu.Q6_K.gguf](https://huggingface.co/RichardErkhov/su1301397274_-_gpt2-finetuned-tofu-gguf/blob/main/gpt2-finetuned-tofu.Q6_K.gguf) | Q6_K | 0.13GB |
| [gpt2-finetuned-tofu.Q8_0.gguf](https://huggingface.co/RichardErkhov/su1301397274_-_gpt2-finetuned-tofu-gguf/blob/main/gpt2-finetuned-tofu.Q8_0.gguf) | Q8_0 | 0.17GB |
Original model description:
---
library_name: transformers
tags: []
---
# Model Card for Model ID
<!-- Provide a quick summary of what the model is/does. -->
## Model Details
### Model Description
<!-- Provide a longer summary of what this model is. -->
This is the model card of a 🤗 transformers model that has been pushed on the Hub. This model card has been automatically generated.
- **Developed by:** [More Information Needed]
- **Funded by [optional]:** [More Information Needed]
- **Shared by [optional]:** [More Information Needed]
- **Model type:** [More Information Needed]
- **Language(s) (NLP):** [More Information Needed]
- **License:** [More Information Needed]
- **Finetuned from model [optional]:** [More Information Needed]
### Model Sources [optional]
<!-- Provide the basic links for the model. -->
- **Repository:** [More Information Needed]
- **Paper [optional]:** [More Information Needed]
- **Demo [optional]:** [More Information Needed]
## Uses
<!-- Address questions around how the model is intended to be used, including the foreseeable users of the model and those affected by the model. -->
### Direct Use
<!-- This section is for the model use without fine-tuning or plugging into a larger ecosystem/app. -->
[More Information Needed]
### Downstream Use [optional]
<!-- This section is for the model use when fine-tuned for a task, or when plugged into a larger ecosystem/app -->
[More Information Needed]
### Out-of-Scope Use
<!-- This section addresses misuse, malicious use, and uses that the model will not work well for. -->
[More Information Needed]
## Bias, Risks, and Limitations
<!-- This section is meant to convey both technical and sociotechnical limitations. -->
[More Information Needed]
### Recommendations
<!-- This section is meant to convey recommendations with respect to the bias, risk, and technical limitations. -->
Users (both direct and downstream) should be made aware of the risks, biases and limitations of the model. More information needed for further recommendations.
## How to Get Started with the Model
Use the code below to get started with the model.
[More Information Needed]
## Training Details
### Training Data
<!-- This should link to a Dataset Card, perhaps with a short stub of information on what the training data is all about as well as documentation related to data pre-processing or additional filtering. -->
[More Information Needed]
### Training Procedure
<!-- This relates heavily to the Technical Specifications. Content here should link to that section when it is relevant to the training procedure. -->
#### Preprocessing [optional]
[More Information Needed]
#### Training Hyperparameters
- **Training regime:** [More Information Needed] <!--fp32, fp16 mixed precision, bf16 mixed precision, bf16 non-mixed precision, fp16 non-mixed precision, fp8 mixed precision -->
#### Speeds, Sizes, Times [optional]
<!-- This section provides information about throughput, start/end time, checkpoint size if relevant, etc. -->
[More Information Needed]
## Evaluation
<!-- This section describes the evaluation protocols and provides the results. -->
### Testing Data, Factors & Metrics
#### Testing Data
<!-- This should link to a Dataset Card if possible. -->
[More Information Needed]
#### Factors
<!-- These are the things the evaluation is disaggregating by, e.g., subpopulations or domains. -->
[More Information Needed]
#### Metrics
<!-- These are the evaluation metrics being used, ideally with a description of why. -->
[More Information Needed]
### Results
[More Information Needed]
#### Summary
## Model Examination [optional]
<!-- Relevant interpretability work for the model goes here -->
[More Information Needed]
## Environmental Impact
<!-- Total emissions (in grams of CO2eq) and additional considerations, such as electricity usage, go here. Edit the suggested text below accordingly -->
Carbon emissions can be estimated using the [Machine Learning Impact calculator](https://mlco2.github.io/impact#compute) presented in [Lacoste et al. (2019)](https://arxiv.org/abs/1910.09700).
- **Hardware Type:** [More Information Needed]
- **Hours used:** [More Information Needed]
- **Cloud Provider:** [More Information Needed]
- **Compute Region:** [More Information Needed]
- **Carbon Emitted:** [More Information Needed]
## Technical Specifications [optional]
### Model Architecture and Objective
[More Information Needed]
### Compute Infrastructure
[More Information Needed]
#### Hardware
[More Information Needed]
#### Software
[More Information Needed]
## Citation [optional]
<!-- If there is a paper or blog post introducing the model, the APA and Bibtex information for that should go in this section. -->
**BibTeX:**
[More Information Needed]
**APA:**
[More Information Needed]
## Glossary [optional]
<!-- If relevant, include terms and calculations in this section that can help readers understand the model or model card. -->
[More Information Needed]
## More Information [optional]
[More Information Needed]
## Model Card Authors [optional]
[More Information Needed]
## Model Card Contact
[More Information Needed]
|
SidXXD/coarse_6-debuged_1-with_real_prior-initializer_token_random | SidXXD | "2023-12-19T11:07:20Z" | 0 | 0 | diffusers | [
"diffusers",
"tensorboard",
"stable-diffusion",
"stable-diffusion-diffusers",
"text-to-image",
"custom-diffusion",
"base_model:CompVis/stable-diffusion-v1-4",
"base_model:adapter:CompVis/stable-diffusion-v1-4",
"license:creativeml-openrail-m",
"region:us"
] | text-to-image | "2023-12-19T10:35:05Z" |
---
license: creativeml-openrail-m
base_model: CompVis/stable-diffusion-v1-4
instance_prompt: None
tags:
- stable-diffusion
- stable-diffusion-diffusers
- text-to-image
- diffusers
- custom-diffusion
inference: true
---
# Custom Diffusion - SidXXD/coarse_6-debuged_1-with_real_prior-initializer_token_random
These are Custom Diffusion adaption weights for CompVis/stable-diffusion-v1-4. The weights were trained on None using [Custom Diffusion](https://www.cs.cmu.edu/~custom-diffusion). You can find some example images in the following.
For more details on the training, please follow [this link](https://github.com/huggingface/diffusers/blob/main/examples/custom_diffusion).
|
irishprancer/8a79efc5-7ae0-4901-a595-92b1b93d0d8e | irishprancer | "2025-02-26T11:33:38Z" | 0 | 0 | transformers | [
"transformers",
"safetensors",
"unsloth",
"arxiv:1910.09700",
"endpoints_compatible",
"region:us"
] | null | "2025-02-26T10:37:35Z" | ---
library_name: transformers
tags:
- unsloth
---
# Model Card for Model ID
<!-- Provide a quick summary of what the model is/does. -->
## Model Details
### Model Description
<!-- Provide a longer summary of what this model is. -->
This is the model card of a 🤗 transformers model that has been pushed on the Hub. This model card has been automatically generated.
- **Developed by:** [More Information Needed]
- **Funded by [optional]:** [More Information Needed]
- **Shared by [optional]:** [More Information Needed]
- **Model type:** [More Information Needed]
- **Language(s) (NLP):** [More Information Needed]
- **License:** [More Information Needed]
- **Finetuned from model [optional]:** [More Information Needed]
### Model Sources [optional]
<!-- Provide the basic links for the model. -->
- **Repository:** [More Information Needed]
- **Paper [optional]:** [More Information Needed]
- **Demo [optional]:** [More Information Needed]
## Uses
<!-- Address questions around how the model is intended to be used, including the foreseeable users of the model and those affected by the model. -->
### Direct Use
<!-- This section is for the model use without fine-tuning or plugging into a larger ecosystem/app. -->
[More Information Needed]
### Downstream Use [optional]
<!-- This section is for the model use when fine-tuned for a task, or when plugged into a larger ecosystem/app -->
[More Information Needed]
### Out-of-Scope Use
<!-- This section addresses misuse, malicious use, and uses that the model will not work well for. -->
[More Information Needed]
## Bias, Risks, and Limitations
<!-- This section is meant to convey both technical and sociotechnical limitations. -->
[More Information Needed]
### Recommendations
<!-- This section is meant to convey recommendations with respect to the bias, risk, and technical limitations. -->
Users (both direct and downstream) should be made aware of the risks, biases and limitations of the model. More information needed for further recommendations.
## How to Get Started with the Model
Use the code below to get started with the model.
[More Information Needed]
## Training Details
### Training Data
<!-- This should link to a Dataset Card, perhaps with a short stub of information on what the training data is all about as well as documentation related to data pre-processing or additional filtering. -->
[More Information Needed]
### Training Procedure
<!-- This relates heavily to the Technical Specifications. Content here should link to that section when it is relevant to the training procedure. -->
#### Preprocessing [optional]
[More Information Needed]
#### Training Hyperparameters
- **Training regime:** [More Information Needed] <!--fp32, fp16 mixed precision, bf16 mixed precision, bf16 non-mixed precision, fp16 non-mixed precision, fp8 mixed precision -->
#### Speeds, Sizes, Times [optional]
<!-- This section provides information about throughput, start/end time, checkpoint size if relevant, etc. -->
[More Information Needed]
## Evaluation
<!-- This section describes the evaluation protocols and provides the results. -->
### Testing Data, Factors & Metrics
#### Testing Data
<!-- This should link to a Dataset Card if possible. -->
[More Information Needed]
#### Factors
<!-- These are the things the evaluation is disaggregating by, e.g., subpopulations or domains. -->
[More Information Needed]
#### Metrics
<!-- These are the evaluation metrics being used, ideally with a description of why. -->
[More Information Needed]
### Results
[More Information Needed]
#### Summary
## Model Examination [optional]
<!-- Relevant interpretability work for the model goes here -->
[More Information Needed]
## Environmental Impact
<!-- Total emissions (in grams of CO2eq) and additional considerations, such as electricity usage, go here. Edit the suggested text below accordingly -->
Carbon emissions can be estimated using the [Machine Learning Impact calculator](https://mlco2.github.io/impact#compute) presented in [Lacoste et al. (2019)](https://arxiv.org/abs/1910.09700).
- **Hardware Type:** [More Information Needed]
- **Hours used:** [More Information Needed]
- **Cloud Provider:** [More Information Needed]
- **Compute Region:** [More Information Needed]
- **Carbon Emitted:** [More Information Needed]
## Technical Specifications [optional]
### Model Architecture and Objective
[More Information Needed]
### Compute Infrastructure
[More Information Needed]
#### Hardware
[More Information Needed]
#### Software
[More Information Needed]
## Citation [optional]
<!-- If there is a paper or blog post introducing the model, the APA and Bibtex information for that should go in this section. -->
**BibTeX:**
[More Information Needed]
**APA:**
[More Information Needed]
## Glossary [optional]
<!-- If relevant, include terms and calculations in this section that can help readers understand the model or model card. -->
[More Information Needed]
## More Information [optional]
[More Information Needed]
## Model Card Authors [optional]
[More Information Needed]
## Model Card Contact
[More Information Needed] |
WenWW/HNC_D1-15_epoch1 | WenWW | "2025-01-29T00:59:28Z" | 33 | 0 | transformers | [
"transformers",
"safetensors",
"clip",
"zero-shot-image-classification",
"arxiv:1910.09700",
"endpoints_compatible",
"region:us"
] | zero-shot-image-classification | "2025-01-29T00:59:05Z" | ---
library_name: transformers
tags: []
---
# Model Card for Model ID
<!-- Provide a quick summary of what the model is/does. -->
## Model Details
### Model Description
<!-- Provide a longer summary of what this model is. -->
This is the model card of a 🤗 transformers model that has been pushed on the Hub. This model card has been automatically generated.
- **Developed by:** [More Information Needed]
- **Funded by [optional]:** [More Information Needed]
- **Shared by [optional]:** [More Information Needed]
- **Model type:** [More Information Needed]
- **Language(s) (NLP):** [More Information Needed]
- **License:** [More Information Needed]
- **Finetuned from model [optional]:** [More Information Needed]
### Model Sources [optional]
<!-- Provide the basic links for the model. -->
- **Repository:** [More Information Needed]
- **Paper [optional]:** [More Information Needed]
- **Demo [optional]:** [More Information Needed]
## Uses
<!-- Address questions around how the model is intended to be used, including the foreseeable users of the model and those affected by the model. -->
### Direct Use
<!-- This section is for the model use without fine-tuning or plugging into a larger ecosystem/app. -->
[More Information Needed]
### Downstream Use [optional]
<!-- This section is for the model use when fine-tuned for a task, or when plugged into a larger ecosystem/app -->
[More Information Needed]
### Out-of-Scope Use
<!-- This section addresses misuse, malicious use, and uses that the model will not work well for. -->
[More Information Needed]
## Bias, Risks, and Limitations
<!-- This section is meant to convey both technical and sociotechnical limitations. -->
[More Information Needed]
### Recommendations
<!-- This section is meant to convey recommendations with respect to the bias, risk, and technical limitations. -->
Users (both direct and downstream) should be made aware of the risks, biases and limitations of the model. More information needed for further recommendations.
## How to Get Started with the Model
Use the code below to get started with the model.
[More Information Needed]
## Training Details
### Training Data
<!-- This should link to a Dataset Card, perhaps with a short stub of information on what the training data is all about as well as documentation related to data pre-processing or additional filtering. -->
[More Information Needed]
### Training Procedure
<!-- This relates heavily to the Technical Specifications. Content here should link to that section when it is relevant to the training procedure. -->
#### Preprocessing [optional]
[More Information Needed]
#### Training Hyperparameters
- **Training regime:** [More Information Needed] <!--fp32, fp16 mixed precision, bf16 mixed precision, bf16 non-mixed precision, fp16 non-mixed precision, fp8 mixed precision -->
#### Speeds, Sizes, Times [optional]
<!-- This section provides information about throughput, start/end time, checkpoint size if relevant, etc. -->
[More Information Needed]
## Evaluation
<!-- This section describes the evaluation protocols and provides the results. -->
### Testing Data, Factors & Metrics
#### Testing Data
<!-- This should link to a Dataset Card if possible. -->
[More Information Needed]
#### Factors
<!-- These are the things the evaluation is disaggregating by, e.g., subpopulations or domains. -->
[More Information Needed]
#### Metrics
<!-- These are the evaluation metrics being used, ideally with a description of why. -->
[More Information Needed]
### Results
[More Information Needed]
#### Summary
## Model Examination [optional]
<!-- Relevant interpretability work for the model goes here -->
[More Information Needed]
## Environmental Impact
<!-- Total emissions (in grams of CO2eq) and additional considerations, such as electricity usage, go here. Edit the suggested text below accordingly -->
Carbon emissions can be estimated using the [Machine Learning Impact calculator](https://mlco2.github.io/impact#compute) presented in [Lacoste et al. (2019)](https://arxiv.org/abs/1910.09700).
- **Hardware Type:** [More Information Needed]
- **Hours used:** [More Information Needed]
- **Cloud Provider:** [More Information Needed]
- **Compute Region:** [More Information Needed]
- **Carbon Emitted:** [More Information Needed]
## Technical Specifications [optional]
### Model Architecture and Objective
[More Information Needed]
### Compute Infrastructure
[More Information Needed]
#### Hardware
[More Information Needed]
#### Software
[More Information Needed]
## Citation [optional]
<!-- If there is a paper or blog post introducing the model, the APA and Bibtex information for that should go in this section. -->
**BibTeX:**
[More Information Needed]
**APA:**
[More Information Needed]
## Glossary [optional]
<!-- If relevant, include terms and calculations in this section that can help readers understand the model or model card. -->
[More Information Needed]
## More Information [optional]
[More Information Needed]
## Model Card Authors [optional]
[More Information Needed]
## Model Card Contact
[More Information Needed] |
AndresJE/b | AndresJE | "2023-09-16T19:27:16Z" | 56 | 0 | transformers | [
"transformers",
"tf",
"distilbert",
"question-answering",
"generated_from_keras_callback",
"base_model:distilbert/distilbert-base-uncased",
"base_model:finetune:distilbert/distilbert-base-uncased",
"license:apache-2.0",
"endpoints_compatible",
"region:us"
] | question-answering | "2023-09-16T19:21:48Z" | ---
license: apache-2.0
base_model: distilbert-base-uncased
tags:
- generated_from_keras_callback
model-index:
- name: AndresJE/b
results: []
---
<!-- This model card has been generated automatically according to the information Keras had access to. You should
probably proofread and complete it, then remove this comment. -->
# AndresJE/b
This model is a fine-tuned version of [distilbert-base-uncased](https://huggingface.co/distilbert-base-uncased) on an unknown dataset.
It achieves the following results on the evaluation set:
- Train Loss: 5.8869
- Validation Loss: 5.9134
- Epoch: 1
## Model description
More information needed
## Intended uses & limitations
More information needed
## Training and evaluation data
More information needed
## Training procedure
### Training hyperparameters
The following hyperparameters were used during training:
- optimizer: {'name': 'Adam', 'weight_decay': None, 'clipnorm': None, 'global_clipnorm': None, 'clipvalue': None, 'use_ema': False, 'ema_momentum': 0.99, 'ema_overwrite_frequency': None, 'jit_compile': False, 'is_legacy_optimizer': False, 'learning_rate': {'module': 'keras.optimizers.schedules', 'class_name': 'PolynomialDecay', 'config': {'initial_learning_rate': 2e-05, 'decay_steps': 4, 'end_learning_rate': 0.0, 'power': 1.0, 'cycle': False, 'name': None}, 'registered_name': None}, 'beta_1': 0.9, 'beta_2': 0.999, 'epsilon': 1e-08, 'amsgrad': False}
- training_precision: float32
### Training results
| Train Loss | Validation Loss | Epoch |
|:----------:|:---------------:|:-----:|
| 5.9890 | 5.9401 | 0 |
| 5.8869 | 5.9134 | 1 |
### Framework versions
- Transformers 4.33.2
- TensorFlow 2.13.0
- Datasets 2.14.5
- Tokenizers 0.13.3
|
av-generation/t5-large-mlt-oa-mine | av-generation | "2024-05-30T12:05:26Z" | 108 | 0 | transformers | [
"transformers",
"safetensors",
"t5",
"text2text-generation",
"arxiv:1910.09700",
"autotrain_compatible",
"text-generation-inference",
"endpoints_compatible",
"region:us"
] | text2text-generation | "2024-05-30T12:02:26Z" | ---
library_name: transformers
tags: []
---
# Model Card for Model ID
<!-- Provide a quick summary of what the model is/does. -->
## Model Details
### Model Description
<!-- Provide a longer summary of what this model is. -->
This is the model card of a 🤗 transformers model that has been pushed on the Hub. This model card has been automatically generated.
- **Developed by:** [More Information Needed]
- **Funded by [optional]:** [More Information Needed]
- **Shared by [optional]:** [More Information Needed]
- **Model type:** [More Information Needed]
- **Language(s) (NLP):** [More Information Needed]
- **License:** [More Information Needed]
- **Finetuned from model [optional]:** [More Information Needed]
### Model Sources [optional]
<!-- Provide the basic links for the model. -->
- **Repository:** [More Information Needed]
- **Paper [optional]:** [More Information Needed]
- **Demo [optional]:** [More Information Needed]
## Uses
<!-- Address questions around how the model is intended to be used, including the foreseeable users of the model and those affected by the model. -->
### Direct Use
<!-- This section is for the model use without fine-tuning or plugging into a larger ecosystem/app. -->
[More Information Needed]
### Downstream Use [optional]
<!-- This section is for the model use when fine-tuned for a task, or when plugged into a larger ecosystem/app -->
[More Information Needed]
### Out-of-Scope Use
<!-- This section addresses misuse, malicious use, and uses that the model will not work well for. -->
[More Information Needed]
## Bias, Risks, and Limitations
<!-- This section is meant to convey both technical and sociotechnical limitations. -->
[More Information Needed]
### Recommendations
<!-- This section is meant to convey recommendations with respect to the bias, risk, and technical limitations. -->
Users (both direct and downstream) should be made aware of the risks, biases and limitations of the model. More information needed for further recommendations.
## How to Get Started with the Model
Use the code below to get started with the model.
[More Information Needed]
## Training Details
### Training Data
<!-- This should link to a Dataset Card, perhaps with a short stub of information on what the training data is all about as well as documentation related to data pre-processing or additional filtering. -->
[More Information Needed]
### Training Procedure
<!-- This relates heavily to the Technical Specifications. Content here should link to that section when it is relevant to the training procedure. -->
#### Preprocessing [optional]
[More Information Needed]
#### Training Hyperparameters
- **Training regime:** [More Information Needed] <!--fp32, fp16 mixed precision, bf16 mixed precision, bf16 non-mixed precision, fp16 non-mixed precision, fp8 mixed precision -->
#### Speeds, Sizes, Times [optional]
<!-- This section provides information about throughput, start/end time, checkpoint size if relevant, etc. -->
[More Information Needed]
## Evaluation
<!-- This section describes the evaluation protocols and provides the results. -->
### Testing Data, Factors & Metrics
#### Testing Data
<!-- This should link to a Dataset Card if possible. -->
[More Information Needed]
#### Factors
<!-- These are the things the evaluation is disaggregating by, e.g., subpopulations or domains. -->
[More Information Needed]
#### Metrics
<!-- These are the evaluation metrics being used, ideally with a description of why. -->
[More Information Needed]
### Results
[More Information Needed]
#### Summary
## Model Examination [optional]
<!-- Relevant interpretability work for the model goes here -->
[More Information Needed]
## Environmental Impact
<!-- Total emissions (in grams of CO2eq) and additional considerations, such as electricity usage, go here. Edit the suggested text below accordingly -->
Carbon emissions can be estimated using the [Machine Learning Impact calculator](https://mlco2.github.io/impact#compute) presented in [Lacoste et al. (2019)](https://arxiv.org/abs/1910.09700).
- **Hardware Type:** [More Information Needed]
- **Hours used:** [More Information Needed]
- **Cloud Provider:** [More Information Needed]
- **Compute Region:** [More Information Needed]
- **Carbon Emitted:** [More Information Needed]
## Technical Specifications [optional]
### Model Architecture and Objective
[More Information Needed]
### Compute Infrastructure
[More Information Needed]
#### Hardware
[More Information Needed]
#### Software
[More Information Needed]
## Citation [optional]
<!-- If there is a paper or blog post introducing the model, the APA and Bibtex information for that should go in this section. -->
**BibTeX:**
[More Information Needed]
**APA:**
[More Information Needed]
## Glossary [optional]
<!-- If relevant, include terms and calculations in this section that can help readers understand the model or model card. -->
[More Information Needed]
## More Information [optional]
[More Information Needed]
## Model Card Authors [optional]
[More Information Needed]
## Model Card Contact
[More Information Needed] |
DevQuasar/mlfoundations-dev.stratos_verified_plus_s1r1-GGUF | DevQuasar | "2025-02-10T19:40:19Z" | 0 | 0 | null | [
"gguf",
"text-generation",
"base_model:mlfoundations-dev/stratos_verified_plus_s1r1",
"base_model:quantized:mlfoundations-dev/stratos_verified_plus_s1r1",
"endpoints_compatible",
"region:us",
"conversational"
] | text-generation | "2025-02-10T14:20:39Z" | ---
base_model:
- mlfoundations-dev/stratos_verified_plus_s1r1
pipeline_tag: text-generation
---
[<img src="https://raw.githubusercontent.com/csabakecskemeti/devquasar/main/dq_logo_black-transparent.png" width="200"/>](https://devquasar.com)
'Make knowledge free for everyone'
Quantized version of: [mlfoundations-dev/stratos_verified_plus_s1r1](https://huggingface.co/mlfoundations-dev/stratos_verified_plus_s1r1)
<a href='https://ko-fi.com/L4L416YX7C' target='_blank'><img height='36' style='border:0px;height:36px;' src='https://storage.ko-fi.com/cdn/kofi6.png?v=6' border='0' alt='Buy Me a Coffee at ko-fi.com' /></a>
|
RichardErkhov/hyeongjinson_-_matchup_llama3_1b_merge-gguf | RichardErkhov | "2025-03-29T13:45:42Z" | 0 | 0 | null | [
"gguf",
"arxiv:1910.09700",
"endpoints_compatible",
"region:us",
"conversational"
] | null | "2025-03-29T13:25:40Z" | Quantization made by Richard Erkhov.
[Github](https://github.com/RichardErkhov)
[Discord](https://discord.gg/pvy7H8DZMG)
[Request more models](https://github.com/RichardErkhov/quant_request)
matchup_llama3_1b_merge - GGUF
- Model creator: https://huggingface.co/hyeongjinson/
- Original model: https://huggingface.co/hyeongjinson/matchup_llama3_1b_merge/
| Name | Quant method | Size |
| ---- | ---- | ---- |
| [matchup_llama3_1b_merge.Q2_K.gguf](https://huggingface.co/RichardErkhov/hyeongjinson_-_matchup_llama3_1b_merge-gguf/blob/main/matchup_llama3_1b_merge.Q2_K.gguf) | Q2_K | 0.54GB |
| [matchup_llama3_1b_merge.IQ3_XS.gguf](https://huggingface.co/RichardErkhov/hyeongjinson_-_matchup_llama3_1b_merge-gguf/blob/main/matchup_llama3_1b_merge.IQ3_XS.gguf) | IQ3_XS | 0.58GB |
| [matchup_llama3_1b_merge.IQ3_S.gguf](https://huggingface.co/RichardErkhov/hyeongjinson_-_matchup_llama3_1b_merge-gguf/blob/main/matchup_llama3_1b_merge.IQ3_S.gguf) | IQ3_S | 0.6GB |
| [matchup_llama3_1b_merge.Q3_K_S.gguf](https://huggingface.co/RichardErkhov/hyeongjinson_-_matchup_llama3_1b_merge-gguf/blob/main/matchup_llama3_1b_merge.Q3_K_S.gguf) | Q3_K_S | 0.6GB |
| [matchup_llama3_1b_merge.IQ3_M.gguf](https://huggingface.co/RichardErkhov/hyeongjinson_-_matchup_llama3_1b_merge-gguf/blob/main/matchup_llama3_1b_merge.IQ3_M.gguf) | IQ3_M | 0.61GB |
| [matchup_llama3_1b_merge.Q3_K.gguf](https://huggingface.co/RichardErkhov/hyeongjinson_-_matchup_llama3_1b_merge-gguf/blob/main/matchup_llama3_1b_merge.Q3_K.gguf) | Q3_K | 0.64GB |
| [matchup_llama3_1b_merge.Q3_K_M.gguf](https://huggingface.co/RichardErkhov/hyeongjinson_-_matchup_llama3_1b_merge-gguf/blob/main/matchup_llama3_1b_merge.Q3_K_M.gguf) | Q3_K_M | 0.64GB |
| [matchup_llama3_1b_merge.Q3_K_L.gguf](https://huggingface.co/RichardErkhov/hyeongjinson_-_matchup_llama3_1b_merge-gguf/blob/main/matchup_llama3_1b_merge.Q3_K_L.gguf) | Q3_K_L | 0.68GB |
| [matchup_llama3_1b_merge.IQ4_XS.gguf](https://huggingface.co/RichardErkhov/hyeongjinson_-_matchup_llama3_1b_merge-gguf/blob/main/matchup_llama3_1b_merge.IQ4_XS.gguf) | IQ4_XS | 0.7GB |
| [matchup_llama3_1b_merge.Q4_0.gguf](https://huggingface.co/RichardErkhov/hyeongjinson_-_matchup_llama3_1b_merge-gguf/blob/main/matchup_llama3_1b_merge.Q4_0.gguf) | Q4_0 | 0.72GB |
| [matchup_llama3_1b_merge.IQ4_NL.gguf](https://huggingface.co/RichardErkhov/hyeongjinson_-_matchup_llama3_1b_merge-gguf/blob/main/matchup_llama3_1b_merge.IQ4_NL.gguf) | IQ4_NL | 0.72GB |
| [matchup_llama3_1b_merge.Q4_K_S.gguf](https://huggingface.co/RichardErkhov/hyeongjinson_-_matchup_llama3_1b_merge-gguf/blob/main/matchup_llama3_1b_merge.Q4_K_S.gguf) | Q4_K_S | 0.72GB |
| [matchup_llama3_1b_merge.Q4_K.gguf](https://huggingface.co/RichardErkhov/hyeongjinson_-_matchup_llama3_1b_merge-gguf/blob/main/matchup_llama3_1b_merge.Q4_K.gguf) | Q4_K | 0.75GB |
| [matchup_llama3_1b_merge.Q4_K_M.gguf](https://huggingface.co/RichardErkhov/hyeongjinson_-_matchup_llama3_1b_merge-gguf/blob/main/matchup_llama3_1b_merge.Q4_K_M.gguf) | Q4_K_M | 0.75GB |
| [matchup_llama3_1b_merge.Q4_1.gguf](https://huggingface.co/RichardErkhov/hyeongjinson_-_matchup_llama3_1b_merge-gguf/blob/main/matchup_llama3_1b_merge.Q4_1.gguf) | Q4_1 | 0.77GB |
| [matchup_llama3_1b_merge.Q5_0.gguf](https://huggingface.co/RichardErkhov/hyeongjinson_-_matchup_llama3_1b_merge-gguf/blob/main/matchup_llama3_1b_merge.Q5_0.gguf) | Q5_0 | 0.83GB |
| [matchup_llama3_1b_merge.Q5_K_S.gguf](https://huggingface.co/RichardErkhov/hyeongjinson_-_matchup_llama3_1b_merge-gguf/blob/main/matchup_llama3_1b_merge.Q5_K_S.gguf) | Q5_K_S | 0.83GB |
| [matchup_llama3_1b_merge.Q5_K.gguf](https://huggingface.co/RichardErkhov/hyeongjinson_-_matchup_llama3_1b_merge-gguf/blob/main/matchup_llama3_1b_merge.Q5_K.gguf) | Q5_K | 0.85GB |
| [matchup_llama3_1b_merge.Q5_K_M.gguf](https://huggingface.co/RichardErkhov/hyeongjinson_-_matchup_llama3_1b_merge-gguf/blob/main/matchup_llama3_1b_merge.Q5_K_M.gguf) | Q5_K_M | 0.85GB |
| [matchup_llama3_1b_merge.Q5_1.gguf](https://huggingface.co/RichardErkhov/hyeongjinson_-_matchup_llama3_1b_merge-gguf/blob/main/matchup_llama3_1b_merge.Q5_1.gguf) | Q5_1 | 0.89GB |
| [matchup_llama3_1b_merge.Q6_K.gguf](https://huggingface.co/RichardErkhov/hyeongjinson_-_matchup_llama3_1b_merge-gguf/blob/main/matchup_llama3_1b_merge.Q6_K.gguf) | Q6_K | 0.95GB |
| [matchup_llama3_1b_merge.Q8_0.gguf](https://huggingface.co/RichardErkhov/hyeongjinson_-_matchup_llama3_1b_merge-gguf/blob/main/matchup_llama3_1b_merge.Q8_0.gguf) | Q8_0 | 1.23GB |
Original model description:
---
library_name: transformers
tags: []
---
# Model Card for Model ID
<!-- Provide a quick summary of what the model is/does. -->
## Model Details
### Model Description
<!-- Provide a longer summary of what this model is. -->
This is the model card of a 🤗 transformers model that has been pushed on the Hub. This model card has been automatically generated.
- **Developed by:** [More Information Needed]
- **Funded by [optional]:** [More Information Needed]
- **Shared by [optional]:** [More Information Needed]
- **Model type:** [More Information Needed]
- **Language(s) (NLP):** [More Information Needed]
- **License:** [More Information Needed]
- **Finetuned from model [optional]:** [More Information Needed]
### Model Sources [optional]
<!-- Provide the basic links for the model. -->
- **Repository:** [More Information Needed]
- **Paper [optional]:** [More Information Needed]
- **Demo [optional]:** [More Information Needed]
## Uses
<!-- Address questions around how the model is intended to be used, including the foreseeable users of the model and those affected by the model. -->
### Direct Use
<!-- This section is for the model use without fine-tuning or plugging into a larger ecosystem/app. -->
[More Information Needed]
### Downstream Use [optional]
<!-- This section is for the model use when fine-tuned for a task, or when plugged into a larger ecosystem/app -->
[More Information Needed]
### Out-of-Scope Use
<!-- This section addresses misuse, malicious use, and uses that the model will not work well for. -->
[More Information Needed]
## Bias, Risks, and Limitations
<!-- This section is meant to convey both technical and sociotechnical limitations. -->
[More Information Needed]
### Recommendations
<!-- This section is meant to convey recommendations with respect to the bias, risk, and technical limitations. -->
Users (both direct and downstream) should be made aware of the risks, biases and limitations of the model. More information needed for further recommendations.
## How to Get Started with the Model
Use the code below to get started with the model.
[More Information Needed]
## Training Details
### Training Data
<!-- This should link to a Dataset Card, perhaps with a short stub of information on what the training data is all about as well as documentation related to data pre-processing or additional filtering. -->
[More Information Needed]
### Training Procedure
<!-- This relates heavily to the Technical Specifications. Content here should link to that section when it is relevant to the training procedure. -->
#### Preprocessing [optional]
[More Information Needed]
#### Training Hyperparameters
- **Training regime:** [More Information Needed] <!--fp32, fp16 mixed precision, bf16 mixed precision, bf16 non-mixed precision, fp16 non-mixed precision, fp8 mixed precision -->
#### Speeds, Sizes, Times [optional]
<!-- This section provides information about throughput, start/end time, checkpoint size if relevant, etc. -->
[More Information Needed]
## Evaluation
<!-- This section describes the evaluation protocols and provides the results. -->
### Testing Data, Factors & Metrics
#### Testing Data
<!-- This should link to a Dataset Card if possible. -->
[More Information Needed]
#### Factors
<!-- These are the things the evaluation is disaggregating by, e.g., subpopulations or domains. -->
[More Information Needed]
#### Metrics
<!-- These are the evaluation metrics being used, ideally with a description of why. -->
[More Information Needed]
### Results
[More Information Needed]
#### Summary
## Model Examination [optional]
<!-- Relevant interpretability work for the model goes here -->
[More Information Needed]
## Environmental Impact
<!-- Total emissions (in grams of CO2eq) and additional considerations, such as electricity usage, go here. Edit the suggested text below accordingly -->
Carbon emissions can be estimated using the [Machine Learning Impact calculator](https://mlco2.github.io/impact#compute) presented in [Lacoste et al. (2019)](https://arxiv.org/abs/1910.09700).
- **Hardware Type:** [More Information Needed]
- **Hours used:** [More Information Needed]
- **Cloud Provider:** [More Information Needed]
- **Compute Region:** [More Information Needed]
- **Carbon Emitted:** [More Information Needed]
## Technical Specifications [optional]
### Model Architecture and Objective
[More Information Needed]
### Compute Infrastructure
[More Information Needed]
#### Hardware
[More Information Needed]
#### Software
[More Information Needed]
## Citation [optional]
<!-- If there is a paper or blog post introducing the model, the APA and Bibtex information for that should go in this section. -->
**BibTeX:**
[More Information Needed]
**APA:**
[More Information Needed]
## Glossary [optional]
<!-- If relevant, include terms and calculations in this section that can help readers understand the model or model card. -->
[More Information Needed]
## More Information [optional]
[More Information Needed]
## Model Card Authors [optional]
[More Information Needed]
## Model Card Contact
[More Information Needed]
|
davidschulte/ESM_nguha__legalbench_cuad_source_code_escrow | davidschulte | "2025-03-26T13:47:32Z" | 15 | 0 | null | [
"safetensors",
"embedding_space_map",
"BaseLM:bert-base-multilingual-uncased",
"dataset:nguha/legalbench",
"base_model:google-bert/bert-base-multilingual-uncased",
"base_model:finetune:google-bert/bert-base-multilingual-uncased",
"license:apache-2.0",
"region:us"
] | null | "2024-11-28T15:46:32Z" | ---
base_model: bert-base-multilingual-uncased
datasets:
- nguha/legalbench
license: apache-2.0
tags:
- embedding_space_map
- BaseLM:bert-base-multilingual-uncased
---
# ESM nguha/legalbench
<!-- Provide a quick summary of what the model is/does. -->
## Model Details
### Model Description
<!-- Provide a longer summary of what this model is. -->
ESM
- **Developed by:** David Schulte
- **Model type:** ESM
- **Base Model:** bert-base-multilingual-uncased
- **Intermediate Task:** nguha/legalbench
- **ESM architecture:** linear
- **ESM embedding dimension:** 768
- **Language(s) (NLP):** [More Information Needed]
- **License:** Apache-2.0 license
- **ESM version:** 0.1.0
## Training Details
### Intermediate Task
- **Task ID:** nguha/legalbench
- **Subset [optional]:** cuad_source_code_escrow
- **Text Column:** text
- **Label Column:** answer
- **Dataset Split:** train
- **Sample size [optional]:** 6
- **Sample seed [optional]:**
### Training Procedure [optional]
<!-- This relates heavily to the Technical Specifications. Content here should link to that section when it is relevant to the training procedure. -->
#### Language Model Training Hyperparameters [optional]
- **Epochs:** 3
- **Batch size:** 32
- **Learning rate:** 2e-05
- **Weight Decay:** 0.01
- **Optimizer**: AdamW
### ESM Training Hyperparameters [optional]
- **Epochs:** 10
- **Batch size:** 32
- **Learning rate:** 0.001
- **Weight Decay:** 0.01
- **Optimizer**: AdamW
### Additional trainiung details [optional]
## Model evaluation
### Evaluation of fine-tuned language model [optional]
### Evaluation of ESM [optional]
MSE:
### Additional evaluation details [optional]
## What are Embedding Space Maps used for?
Embedding Space Maps are a part of ESM-LogME, a efficient method for finding intermediate datasets for transfer learning. There are two reasons to use ESM-LogME:
### You don't have enough training data for your problem
If you don't have a enough training data for your problem, just use ESM-LogME to find more.
You can supplement model training by including publicly available datasets in the training process.
1. Fine-tune a language model on suitable intermediate dataset.
2. Fine-tune the resulting model on your target dataset.
This workflow is called intermediate task transfer learning and it can significantly improve the target performance.
But what is a suitable dataset for your problem? ESM-LogME enable you to quickly rank thousands of datasets on the Hugging Face Hub by how well they are exptected to transfer to your target task.
### You want to find similar datasets to your target dataset
Using ESM-LogME can be used like search engine on the Hugging Face Hub. You can find similar tasks to your target task without having to rely on heuristics. ESM-LogME estimates how language models fine-tuned on each intermediate task would benefinit your target task. This quantitative approach combines the effects of domain similarity and task similarity.
## How can I use ESM-LogME / ESMs?
[](https://pypi.org/project/hf-dataset-selector)
We release **hf-dataset-selector**, a Python package for intermediate task selection using Embedding Space Maps.
**hf-dataset-selector** fetches ESMs for a given language model and uses it to find the best dataset for applying intermediate training to the target task. ESMs are found by their tags on the Huggingface Hub.
```python
from hfselect import Dataset, compute_task_ranking
# Load target dataset from the Hugging Face Hub
dataset = Dataset.from_hugging_face(
name="stanfordnlp/imdb",
split="train",
text_col="text",
label_col="label",
is_regression=False,
num_examples=1000,
seed=42
)
# Fetch ESMs and rank tasks
task_ranking = compute_task_ranking(
dataset=dataset,
model_name="bert-base-multilingual-uncased"
)
# Display top 5 recommendations
print(task_ranking[:5])
```
```python
1. davanstrien/test_imdb_embedd2 Score: -0.618529
2. davanstrien/test_imdb_embedd Score: -0.618644
3. davanstrien/test1 Score: -0.619334
4. stanfordnlp/imdb Score: -0.619454
5. stanfordnlp/sst Score: -0.62995
```
| Rank | Task ID | Task Subset | Text Column | Label Column | Task Split | Num Examples | ESM Architecture | Score |
|-------:|:------------------------------|:----------------|:--------------|:---------------|:-------------|---------------:|:-------------------|----------:|
| 1 | davanstrien/test_imdb_embedd2 | default | text | label | train | 10000 | linear | -0.618529 |
| 2 | davanstrien/test_imdb_embedd | default | text | label | train | 10000 | linear | -0.618644 |
| 3 | davanstrien/test1 | default | text | label | train | 10000 | linear | -0.619334 |
| 4 | stanfordnlp/imdb | plain_text | text | label | train | 10000 | linear | -0.619454 |
| 5 | stanfordnlp/sst | dictionary | phrase | label | dictionary | 10000 | linear | -0.62995 |
| 6 | stanfordnlp/sst | default | sentence | label | train | 8544 | linear | -0.63312 |
| 7 | kuroneko5943/snap21 | CDs_and_Vinyl_5 | sentence | label | train | 6974 | linear | -0.634365 |
| 8 | kuroneko5943/snap21 | Video_Games_5 | sentence | label | train | 6997 | linear | -0.638787 |
| 9 | kuroneko5943/snap21 | Movies_and_TV_5 | sentence | label | train | 6989 | linear | -0.639068 |
| 10 | fancyzhx/amazon_polarity | amazon_polarity | content | label | train | 10000 | linear | -0.639718 |
For more information on how to use ESMs please have a look at the [official Github repository](https://github.com/davidschulte/hf-dataset-selector). We provide documentation further documentation and tutorials for finding intermediate datasets and training your own ESMs.
## How do Embedding Space Maps work?
<!-- This section describes the evaluation protocols and provides the results. -->
Embedding Space Maps (ESMs) are neural networks that approximate the effect of fine-tuning a language model on a task. They can be used to quickly transform embeddings from a base model to approximate how a fine-tuned model would embed the the input text.
ESMs can be used for intermediate task selection with the ESM-LogME workflow.
## How can I use Embedding Space Maps for Intermediate Task Selection?
## Citation
<!-- If there is a paper or blog post introducing the model, the APA and Bibtex information for that should go in this section. -->
If you are using this Embedding Space Maps, please cite our [paper](https://aclanthology.org/2024.emnlp-main.529/).
**BibTeX:**
```
@inproceedings{schulte-etal-2024-less,
title = "Less is More: Parameter-Efficient Selection of Intermediate Tasks for Transfer Learning",
author = "Schulte, David and
Hamborg, Felix and
Akbik, Alan",
editor = "Al-Onaizan, Yaser and
Bansal, Mohit and
Chen, Yun-Nung",
booktitle = "Proceedings of the 2024 Conference on Empirical Methods in Natural Language Processing",
month = nov,
year = "2024",
address = "Miami, Florida, USA",
publisher = "Association for Computational Linguistics",
url = "https://aclanthology.org/2024.emnlp-main.529/",
doi = "10.18653/v1/2024.emnlp-main.529",
pages = "9431--9442",
abstract = "Intermediate task transfer learning can greatly improve model performance. If, for example, one has little training data for emotion detection, first fine-tuning a language model on a sentiment classification dataset may improve performance strongly. But which task to choose for transfer learning? Prior methods producing useful task rankings are infeasible for large source pools, as they require forward passes through all source language models. We overcome this by introducing Embedding Space Maps (ESMs), light-weight neural networks that approximate the effect of fine-tuning a language model. We conduct the largest study on NLP task transferability and task selection with 12k source-target pairs. We find that applying ESMs on a prior method reduces execution time and disk space usage by factors of 10 and 278, respectively, while retaining high selection performance (avg. regret@5 score of 2.95)."
}
```
**APA:**
```
Schulte, D., Hamborg, F., & Akbik, A. (2024, November). Less is More: Parameter-Efficient Selection of Intermediate Tasks for Transfer Learning. In Proceedings of the 2024 Conference on Empirical Methods in Natural Language Processing (pp. 9431-9442).
```
## Additional Information
|
tiagoblima/t5_large-qg-aas | tiagoblima | "2024-01-03T03:23:27Z" | 0 | 0 | null | [
"safetensors",
"generated_from_trainer",
"dataset:tiagoblima/qg_squad_v1_pt",
"base_model:unicamp-dl/ptt5-large-t5-vocab",
"base_model:finetune:unicamp-dl/ptt5-large-t5-vocab",
"license:mit",
"region:us"
] | null | "2023-12-31T14:50:01Z" | ---
license: mit
base_model: unicamp-dl/ptt5-large-t5-vocab
tags:
- generated_from_trainer
datasets:
- tiagoblima/qg_squad_v1_pt
model-index:
- name: t5_large-qg-aas
results: []
---
<!-- This model card has been generated automatically according to the information the Trainer had access to. You
should probably proofread and complete it, then remove this comment. -->
# t5_large-qg-aas
This model is a fine-tuned version of [unicamp-dl/ptt5-large-t5-vocab](https://huggingface.co/unicamp-dl/ptt5-large-t5-vocab) on the tiagoblima/qg_squad_v1_pt dataset.
It achieves the following results on the evaluation set:
- Loss: 4.9208
## Model description
More information needed
## Intended uses & limitations
More information needed
## Training and evaluation data
More information needed
## Training procedure
### Training hyperparameters
The following hyperparameters were used during training:
- learning_rate: 0.005
- train_batch_size: 64
- eval_batch_size: 4
- seed: 42
- optimizer: Adam with betas=(0.9,0.999) and epsilon=1e-08
- lr_scheduler_type: linear
- num_epochs: 5.0
### Training results
| Training Loss | Epoch | Step | Validation Loss |
|:-------------:|:-----:|:----:|:---------------:|
| 6.0267 | 1.0 | 808 | 6.6599 |
| 5.1565 | 2.0 | 1616 | 5.7159 |
| 4.7181 | 3.0 | 2424 | 5.2321 |
| 4.4869 | 4.0 | 3232 | 4.9931 |
| 4.4539 | 5.0 | 4040 | 4.9208 |
### Framework versions
- Transformers 4.35.2
- Pytorch 2.0.0
- Datasets 2.15.0
- Tokenizers 0.15.0
|
manan05/sdxl-texture-generation_4 | manan05 | "2025-03-12T18:13:34Z" | 0 | 0 | diffusers | [
"diffusers",
"tensorboard",
"safetensors",
"stable-diffusion-xl",
"stable-diffusion-xl-diffusers",
"text-to-image",
"diffusers-training",
"base_model:stabilityai/stable-diffusion-xl-base-1.0",
"base_model:finetune:stabilityai/stable-diffusion-xl-base-1.0",
"license:creativeml-openrail-m",
"autotrain_compatible",
"endpoints_compatible",
"diffusers:StableDiffusionXLPipeline",
"region:us"
] | text-to-image | "2025-03-12T11:34:34Z" | ---
base_model: stabilityai/stable-diffusion-xl-base-1.0
library_name: diffusers
license: creativeml-openrail-m
inference: true
tags:
- stable-diffusion-xl
- stable-diffusion-xl-diffusers
- text-to-image
- diffusers-training
- diffusers
---
<!-- This model card has been generated automatically according to the information the training script had access to. You
should probably proofread and complete it, then remove this comment. -->
# Text-to-image finetuning - manan05/sdxl-texture-generation_4
This pipeline was finetuned from **stabilityai/stable-diffusion-xl-base-1.0** on the **/ihub/homedirs/vp_hc/tti/new_sdxl/dataset/** dataset. Below are some example images generated with the finetuned pipeline using the following prompt: Oak wood with flowers texture:




Special VAE used for training: madebyollin/sdxl-vae-fp16-fix.
## Intended uses & limitations
#### How to use
```python
# TODO: add an example code snippet for running this diffusion pipeline
```
#### Limitations and bias
[TODO: provide examples of latent issues and potential remediations]
## Training details
[TODO: describe the data used to train the model] |
RichardErkhov/JCX-kcuf_-_openchat_3.5-gpt-4-80k-gguf | RichardErkhov | "2024-10-12T09:25:37Z" | 132 | 0 | null | [
"gguf",
"endpoints_compatible",
"region:us",
"conversational"
] | null | "2024-10-12T07:27:04Z" | Quantization made by Richard Erkhov.
[Github](https://github.com/RichardErkhov)
[Discord](https://discord.gg/pvy7H8DZMG)
[Request more models](https://github.com/RichardErkhov/quant_request)
openchat_3.5-gpt-4-80k - GGUF
- Model creator: https://huggingface.co/JCX-kcuf/
- Original model: https://huggingface.co/JCX-kcuf/openchat_3.5-gpt-4-80k/
| Name | Quant method | Size |
| ---- | ---- | ---- |
| [openchat_3.5-gpt-4-80k.Q2_K.gguf](https://huggingface.co/RichardErkhov/JCX-kcuf_-_openchat_3.5-gpt-4-80k-gguf/blob/main/openchat_3.5-gpt-4-80k.Q2_K.gguf) | Q2_K | 2.53GB |
| [openchat_3.5-gpt-4-80k.IQ3_XS.gguf](https://huggingface.co/RichardErkhov/JCX-kcuf_-_openchat_3.5-gpt-4-80k-gguf/blob/main/openchat_3.5-gpt-4-80k.IQ3_XS.gguf) | IQ3_XS | 2.81GB |
| [openchat_3.5-gpt-4-80k.IQ3_S.gguf](https://huggingface.co/RichardErkhov/JCX-kcuf_-_openchat_3.5-gpt-4-80k-gguf/blob/main/openchat_3.5-gpt-4-80k.IQ3_S.gguf) | IQ3_S | 2.96GB |
| [openchat_3.5-gpt-4-80k.Q3_K_S.gguf](https://huggingface.co/RichardErkhov/JCX-kcuf_-_openchat_3.5-gpt-4-80k-gguf/blob/main/openchat_3.5-gpt-4-80k.Q3_K_S.gguf) | Q3_K_S | 2.95GB |
| [openchat_3.5-gpt-4-80k.IQ3_M.gguf](https://huggingface.co/RichardErkhov/JCX-kcuf_-_openchat_3.5-gpt-4-80k-gguf/blob/main/openchat_3.5-gpt-4-80k.IQ3_M.gguf) | IQ3_M | 3.06GB |
| [openchat_3.5-gpt-4-80k.Q3_K.gguf](https://huggingface.co/RichardErkhov/JCX-kcuf_-_openchat_3.5-gpt-4-80k-gguf/blob/main/openchat_3.5-gpt-4-80k.Q3_K.gguf) | Q3_K | 3.28GB |
| [openchat_3.5-gpt-4-80k.Q3_K_M.gguf](https://huggingface.co/RichardErkhov/JCX-kcuf_-_openchat_3.5-gpt-4-80k-gguf/blob/main/openchat_3.5-gpt-4-80k.Q3_K_M.gguf) | Q3_K_M | 3.28GB |
| [openchat_3.5-gpt-4-80k.Q3_K_L.gguf](https://huggingface.co/RichardErkhov/JCX-kcuf_-_openchat_3.5-gpt-4-80k-gguf/blob/main/openchat_3.5-gpt-4-80k.Q3_K_L.gguf) | Q3_K_L | 3.56GB |
| [openchat_3.5-gpt-4-80k.IQ4_XS.gguf](https://huggingface.co/RichardErkhov/JCX-kcuf_-_openchat_3.5-gpt-4-80k-gguf/blob/main/openchat_3.5-gpt-4-80k.IQ4_XS.gguf) | IQ4_XS | 3.67GB |
| [openchat_3.5-gpt-4-80k.Q4_0.gguf](https://huggingface.co/RichardErkhov/JCX-kcuf_-_openchat_3.5-gpt-4-80k-gguf/blob/main/openchat_3.5-gpt-4-80k.Q4_0.gguf) | Q4_0 | 3.83GB |
| [openchat_3.5-gpt-4-80k.IQ4_NL.gguf](https://huggingface.co/RichardErkhov/JCX-kcuf_-_openchat_3.5-gpt-4-80k-gguf/blob/main/openchat_3.5-gpt-4-80k.IQ4_NL.gguf) | IQ4_NL | 3.87GB |
| [openchat_3.5-gpt-4-80k.Q4_K_S.gguf](https://huggingface.co/RichardErkhov/JCX-kcuf_-_openchat_3.5-gpt-4-80k-gguf/blob/main/openchat_3.5-gpt-4-80k.Q4_K_S.gguf) | Q4_K_S | 3.86GB |
| [openchat_3.5-gpt-4-80k.Q4_K.gguf](https://huggingface.co/RichardErkhov/JCX-kcuf_-_openchat_3.5-gpt-4-80k-gguf/blob/main/openchat_3.5-gpt-4-80k.Q4_K.gguf) | Q4_K | 4.07GB |
| [openchat_3.5-gpt-4-80k.Q4_K_M.gguf](https://huggingface.co/RichardErkhov/JCX-kcuf_-_openchat_3.5-gpt-4-80k-gguf/blob/main/openchat_3.5-gpt-4-80k.Q4_K_M.gguf) | Q4_K_M | 4.07GB |
| [openchat_3.5-gpt-4-80k.Q4_1.gguf](https://huggingface.co/RichardErkhov/JCX-kcuf_-_openchat_3.5-gpt-4-80k-gguf/blob/main/openchat_3.5-gpt-4-80k.Q4_1.gguf) | Q4_1 | 4.24GB |
| [openchat_3.5-gpt-4-80k.Q5_0.gguf](https://huggingface.co/RichardErkhov/JCX-kcuf_-_openchat_3.5-gpt-4-80k-gguf/blob/main/openchat_3.5-gpt-4-80k.Q5_0.gguf) | Q5_0 | 4.65GB |
| [openchat_3.5-gpt-4-80k.Q5_K_S.gguf](https://huggingface.co/RichardErkhov/JCX-kcuf_-_openchat_3.5-gpt-4-80k-gguf/blob/main/openchat_3.5-gpt-4-80k.Q5_K_S.gguf) | Q5_K_S | 4.65GB |
| [openchat_3.5-gpt-4-80k.Q5_K.gguf](https://huggingface.co/RichardErkhov/JCX-kcuf_-_openchat_3.5-gpt-4-80k-gguf/blob/main/openchat_3.5-gpt-4-80k.Q5_K.gguf) | Q5_K | 4.78GB |
| [openchat_3.5-gpt-4-80k.Q5_K_M.gguf](https://huggingface.co/RichardErkhov/JCX-kcuf_-_openchat_3.5-gpt-4-80k-gguf/blob/main/openchat_3.5-gpt-4-80k.Q5_K_M.gguf) | Q5_K_M | 4.78GB |
| [openchat_3.5-gpt-4-80k.Q5_1.gguf](https://huggingface.co/RichardErkhov/JCX-kcuf_-_openchat_3.5-gpt-4-80k-gguf/blob/main/openchat_3.5-gpt-4-80k.Q5_1.gguf) | Q5_1 | 5.07GB |
| [openchat_3.5-gpt-4-80k.Q6_K.gguf](https://huggingface.co/RichardErkhov/JCX-kcuf_-_openchat_3.5-gpt-4-80k-gguf/blob/main/openchat_3.5-gpt-4-80k.Q6_K.gguf) | Q6_K | 5.53GB |
| [openchat_3.5-gpt-4-80k.Q8_0.gguf](https://huggingface.co/RichardErkhov/JCX-kcuf_-_openchat_3.5-gpt-4-80k-gguf/blob/main/openchat_3.5-gpt-4-80k.Q8_0.gguf) | Q8_0 | 7.17GB |
Original model description:
---
license: apache-2.0
---
## Description
This model is finetuned on the distillation data from GPT-4.
The base model is openchat/openchat_3.5
## Usage
The model has a query format as in openchat_3.5.
```
GPT4 Correct User: {query}<|end_of_turn|>GPT4 Correct Assistant: {answer}<|end_of_turn|>
```
|
aladaf/zephyr-7b-beta_unboxing_v0.1 | aladaf | "2023-12-08T17:44:22Z" | 0 | 0 | peft | [
"peft",
"safetensors",
"arxiv:1910.09700",
"base_model:HuggingFaceH4/zephyr-7b-beta",
"base_model:adapter:HuggingFaceH4/zephyr-7b-beta",
"region:us"
] | null | "2023-12-07T15:57:52Z" | ---
library_name: peft
base_model: HuggingFaceH4/zephyr-7b-beta
---
# Model Card for Model ID
<!-- Provide a quick summary of what the model is/does. -->
## Model Details
### Model Description
<!-- Provide a longer summary of what this model is. -->
- **Developed by:** [More Information Needed]
- **Funded by [optional]:** [More Information Needed]
- **Shared by [optional]:** [More Information Needed]
- **Model type:** [More Information Needed]
- **Language(s) (NLP):** [More Information Needed]
- **License:** [More Information Needed]
- **Finetuned from model [optional]:** [More Information Needed]
### Model Sources [optional]
<!-- Provide the basic links for the model. -->
- **Repository:** [More Information Needed]
- **Paper [optional]:** [More Information Needed]
- **Demo [optional]:** [More Information Needed]
## Uses
<!-- Address questions around how the model is intended to be used, including the foreseeable users of the model and those affected by the model. -->
### Direct Use
<!-- This section is for the model use without fine-tuning or plugging into a larger ecosystem/app. -->
[More Information Needed]
### Downstream Use [optional]
<!-- This section is for the model use when fine-tuned for a task, or when plugged into a larger ecosystem/app -->
[More Information Needed]
### Out-of-Scope Use
<!-- This section addresses misuse, malicious use, and uses that the model will not work well for. -->
[More Information Needed]
## Bias, Risks, and Limitations
<!-- This section is meant to convey both technical and sociotechnical limitations. -->
[More Information Needed]
### Recommendations
<!-- This section is meant to convey recommendations with respect to the bias, risk, and technical limitations. -->
Users (both direct and downstream) should be made aware of the risks, biases and limitations of the model. More information needed for further recommendations.
## How to Get Started with the Model
Use the code below to get started with the model.
[More Information Needed]
## Training Details
### Training Data
<!-- This should link to a Dataset Card, perhaps with a short stub of information on what the training data is all about as well as documentation related to data pre-processing or additional filtering. -->
[More Information Needed]
### Training Procedure
<!-- This relates heavily to the Technical Specifications. Content here should link to that section when it is relevant to the training procedure. -->
#### Preprocessing [optional]
[More Information Needed]
#### Training Hyperparameters
- **Training regime:** [More Information Needed] <!--fp32, fp16 mixed precision, bf16 mixed precision, bf16 non-mixed precision, fp16 non-mixed precision, fp8 mixed precision -->
#### Speeds, Sizes, Times [optional]
<!-- This section provides information about throughput, start/end time, checkpoint size if relevant, etc. -->
[More Information Needed]
## Evaluation
<!-- This section describes the evaluation protocols and provides the results. -->
### Testing Data, Factors & Metrics
#### Testing Data
<!-- This should link to a Dataset Card if possible. -->
[More Information Needed]
#### Factors
<!-- These are the things the evaluation is disaggregating by, e.g., subpopulations or domains. -->
[More Information Needed]
#### Metrics
<!-- These are the evaluation metrics being used, ideally with a description of why. -->
[More Information Needed]
### Results
[More Information Needed]
#### Summary
## Model Examination [optional]
<!-- Relevant interpretability work for the model goes here -->
[More Information Needed]
## Environmental Impact
<!-- Total emissions (in grams of CO2eq) and additional considerations, such as electricity usage, go here. Edit the suggested text below accordingly -->
Carbon emissions can be estimated using the [Machine Learning Impact calculator](https://mlco2.github.io/impact#compute) presented in [Lacoste et al. (2019)](https://arxiv.org/abs/1910.09700).
- **Hardware Type:** [More Information Needed]
- **Hours used:** [More Information Needed]
- **Cloud Provider:** [More Information Needed]
- **Compute Region:** [More Information Needed]
- **Carbon Emitted:** [More Information Needed]
## Technical Specifications [optional]
### Model Architecture and Objective
[More Information Needed]
### Compute Infrastructure
[More Information Needed]
#### Hardware
[More Information Needed]
#### Software
[More Information Needed]
## Citation [optional]
<!-- If there is a paper or blog post introducing the model, the APA and Bibtex information for that should go in this section. -->
**BibTeX:**
[More Information Needed]
**APA:**
[More Information Needed]
## Glossary [optional]
<!-- If relevant, include terms and calculations in this section that can help readers understand the model or model card. -->
[More Information Needed]
## More Information [optional]
[More Information Needed]
## Model Card Authors [optional]
[More Information Needed]
## Model Card Contact
[More Information Needed]
## Training procedure
The following `bitsandbytes` quantization config was used during training:
- quant_method: QuantizationMethod.BITS_AND_BYTES
- load_in_8bit: True
- load_in_4bit: False
- llm_int8_threshold: 6.0
- llm_int8_skip_modules: None
- llm_int8_enable_fp32_cpu_offload: False
- llm_int8_has_fp16_weight: False
- bnb_4bit_quant_type: fp4
- bnb_4bit_use_double_quant: False
- bnb_4bit_compute_dtype: float32
### Framework versions
- PEFT 0.6.2
|
phymbert/Mistral-Small-3.1-24B-Instruct-2503-Q4_K_M-GGUF | phymbert | "2025-03-24T03:43:47Z" | 0 | 0 | vllm | [
"vllm",
"gguf",
"llama-cpp",
"gguf-my-repo",
"image-text-to-text",
"en",
"fr",
"de",
"es",
"pt",
"it",
"ja",
"ko",
"ru",
"zh",
"ar",
"fa",
"id",
"ms",
"ne",
"pl",
"ro",
"sr",
"sv",
"tr",
"uk",
"vi",
"hi",
"bn",
"base_model:mistralai/Mistral-Small-3.1-24B-Instruct-2503",
"base_model:quantized:mistralai/Mistral-Small-3.1-24B-Instruct-2503",
"license:apache-2.0",
"region:us",
"conversational"
] | image-text-to-text | "2025-03-24T03:42:38Z" | ---
base_model: mistralai/Mistral-Small-3.1-24B-Instruct-2503
language:
- en
- fr
- de
- es
- pt
- it
- ja
- ko
- ru
- zh
- ar
- fa
- id
- ms
- ne
- pl
- ro
- sr
- sv
- tr
- uk
- vi
- hi
- bn
library_name: vllm
license: apache-2.0
pipeline_tag: image-text-to-text
tags:
- llama-cpp
- gguf-my-repo
inference: false
extra_gated_description: If you want to learn more about how we process your personal
data, please read our <a href="https://mistral.ai/terms/">Privacy Policy</a>.
---
# phymbert/Mistral-Small-3.1-24B-Instruct-2503-Q4_K_M-GGUF
This model was converted to GGUF format from [`mistralai/Mistral-Small-3.1-24B-Instruct-2503`](https://huggingface.co/mistralai/Mistral-Small-3.1-24B-Instruct-2503) using llama.cpp via the ggml.ai's [GGUF-my-repo](https://huggingface.co/spaces/ggml-org/gguf-my-repo) space.
Refer to the [original model card](https://huggingface.co/mistralai/Mistral-Small-3.1-24B-Instruct-2503) for more details on the model.
## Use with llama.cpp
Install llama.cpp through brew (works on Mac and Linux)
```bash
brew install llama.cpp
```
Invoke the llama.cpp server or the CLI.
### CLI:
```bash
llama-cli --hf-repo phymbert/Mistral-Small-3.1-24B-Instruct-2503-Q4_K_M-GGUF --hf-file mistral-small-3.1-24b-instruct-2503-q4_k_m.gguf -p "The meaning to life and the universe is"
```
### Server:
```bash
llama-server --hf-repo phymbert/Mistral-Small-3.1-24B-Instruct-2503-Q4_K_M-GGUF --hf-file mistral-small-3.1-24b-instruct-2503-q4_k_m.gguf -c 2048
```
Note: You can also use this checkpoint directly through the [usage steps](https://github.com/ggerganov/llama.cpp?tab=readme-ov-file#usage) listed in the Llama.cpp repo as well.
Step 1: Clone llama.cpp from GitHub.
```
git clone https://github.com/ggerganov/llama.cpp
```
Step 2: Move into the llama.cpp folder and build it with `LLAMA_CURL=1` flag along with other hardware-specific flags (for ex: LLAMA_CUDA=1 for Nvidia GPUs on Linux).
```
cd llama.cpp && LLAMA_CURL=1 make
```
Step 3: Run inference through the main binary.
```
./llama-cli --hf-repo phymbert/Mistral-Small-3.1-24B-Instruct-2503-Q4_K_M-GGUF --hf-file mistral-small-3.1-24b-instruct-2503-q4_k_m.gguf -p "The meaning to life and the universe is"
```
or
```
./llama-server --hf-repo phymbert/Mistral-Small-3.1-24B-Instruct-2503-Q4_K_M-GGUF --hf-file mistral-small-3.1-24b-instruct-2503-q4_k_m.gguf -c 2048
```
|
ErrorAI/a55c25ca-6f5c-471b-87de-1c6f02f4ec0d | ErrorAI | "2025-02-07T10:54:06Z" | 6 | 0 | peft | [
"peft",
"safetensors",
"qwen2",
"axolotl",
"generated_from_trainer",
"base_model:Qwen/Qwen2.5-7B",
"base_model:adapter:Qwen/Qwen2.5-7B",
"license:apache-2.0",
"region:us"
] | null | "2025-02-07T10:28:00Z" | ---
library_name: peft
license: apache-2.0
base_model: Qwen/Qwen2.5-7B
tags:
- axolotl
- generated_from_trainer
model-index:
- name: a55c25ca-6f5c-471b-87de-1c6f02f4ec0d
results: []
---
<!-- This model card has been generated automatically according to the information the Trainer had access to. You
should probably proofread and complete it, then remove this comment. -->
[<img src="https://raw.githubusercontent.com/axolotl-ai-cloud/axolotl/main/image/axolotl-badge-web.png" alt="Built with Axolotl" width="200" height="32"/>](https://github.com/axolotl-ai-cloud/axolotl)
<details><summary>See axolotl config</summary>
axolotl version: `0.4.1`
```yaml
adapter: lora
base_model: Qwen/Qwen2.5-7B
bf16: auto
chat_template: llama3
dataset_prepared_path: null
datasets:
- data_files:
- 06d08c98cefc3240_train_data.json
ds_type: json
format: custom
path: /workspace/input_data/06d08c98cefc3240_train_data.json
type:
field_input: input
field_instruction: instruction
field_output: output
format: '{instruction} {input}'
no_input_format: '{instruction}'
system_format: '{system}'
system_prompt: ''
debug: null
deepspeed: null
early_stopping_patience: null
eval_max_new_tokens: 128
eval_table_size: null
evals_per_epoch: 4
flash_attention: false
fp16: null
fsdp: null
fsdp_config: null
gradient_accumulation_steps: 4
gradient_checkpointing: false
group_by_length: false
hub_model_id: ErrorAI/a55c25ca-6f5c-471b-87de-1c6f02f4ec0d
hub_repo: null
hub_strategy: checkpoint
hub_token: null
learning_rate: 0.0002
load_in_4bit: false
load_in_8bit: false
local_rank: null
logging_steps: 1
lora_alpha: 16
lora_dropout: 0.05
lora_fan_in_fan_out: null
lora_model_dir: null
lora_r: 8
lora_target_linear: true
lr_scheduler: cosine
max_steps: 1501
micro_batch_size: 2
mlflow_experiment_name: /tmp/06d08c98cefc3240_train_data.json
model_type: AutoModelForCausalLM
num_epochs: 1
optimizer: adamw_bnb_8bit
output_dir: miner_id_24
pad_to_sequence_len: true
resume_from_checkpoint: null
s2_attention: null
sample_packing: false
saves_per_epoch: 4
sequence_len: 512
strict: false
tf32: false
tokenizer_type: AutoTokenizer
train_on_inputs: false
trust_remote_code: true
val_set_size: 0.05
wandb_entity: null
wandb_mode: online
wandb_name: 233053e6-66a1-4f84-b810-5c30b0974cb1
wandb_project: Gradients-On-Demand
wandb_run: your_name
wandb_runid: 233053e6-66a1-4f84-b810-5c30b0974cb1
warmup_steps: 10
weight_decay: 0.0
xformers_attention: null
```
</details><br>
# a55c25ca-6f5c-471b-87de-1c6f02f4ec0d
This model is a fine-tuned version of [Qwen/Qwen2.5-7B](https://huggingface.co/Qwen/Qwen2.5-7B) on the None dataset.
It achieves the following results on the evaluation set:
- Loss: 1.5152
## Model description
More information needed
## Intended uses & limitations
More information needed
## Training and evaluation data
More information needed
## Training procedure
### Training hyperparameters
The following hyperparameters were used during training:
- learning_rate: 0.0002
- train_batch_size: 2
- eval_batch_size: 2
- seed: 42
- gradient_accumulation_steps: 4
- total_train_batch_size: 8
- optimizer: Use OptimizerNames.ADAMW_BNB with betas=(0.9,0.999) and epsilon=1e-08 and optimizer_args=No additional optimizer arguments
- lr_scheduler_type: cosine
- lr_scheduler_warmup_steps: 10
- training_steps: 1501
### Training results
| Training Loss | Epoch | Step | Validation Loss |
|:-------------:|:------:|:----:|:---------------:|
| 1.8494 | 0.0003 | 1 | 2.0854 |
| 1.9377 | 0.1041 | 376 | 1.5608 |
| 1.3072 | 0.2081 | 752 | 1.5336 |
| 1.3716 | 0.3122 | 1128 | 1.5152 |
### Framework versions
- PEFT 0.13.2
- Transformers 4.46.0
- Pytorch 2.5.0+cu124
- Datasets 3.0.1
- Tokenizers 0.20.1 |
vlevi/Main_Fashion | vlevi | "2024-05-01T18:43:01Z" | 7 | 0 | transformers | [
"transformers",
"tensorboard",
"safetensors",
"vit",
"image-classification",
"generated_from_trainer",
"license:apache-2.0",
"autotrain_compatible",
"endpoints_compatible",
"region:us"
] | image-classification | "2024-05-01T18:31:13Z" | ---
license: apache-2.0
base_model: google/vit-base-patch16-224-in21K
tags:
- generated_from_trainer
metrics:
- accuracy
model-index:
- name: Main_Fashion
results: []
---
<!-- This model card has been generated automatically according to the information the Trainer had access to. You
should probably proofread and complete it, then remove this comment. -->
# Main_Fashion
This model is a fine-tuned version of [google/vit-base-patch16-224-in21K](https://huggingface.co/google/vit-base-patch16-224-in21K) on an unknown dataset.
It achieves the following results on the evaluation set:
- Loss: 0.7633
- Accuracy: 0.6961
## Model description
More information needed
## Intended uses & limitations
More information needed
## Training and evaluation data
More information needed
## Training procedure
### Training hyperparameters
The following hyperparameters were used during training:
- learning_rate: 0.0002
- train_batch_size: 16
- eval_batch_size: 8
- seed: 42
- optimizer: Adam with betas=(0.9,0.999) and epsilon=1e-08
- lr_scheduler_type: linear
- num_epochs: 7
- mixed_precision_training: Native AMP
### Training results
| Training Loss | Epoch | Step | Validation Loss | Accuracy |
|:-------------:|:------:|:----:|:---------------:|:--------:|
| 0.934 | 0.9259 | 100 | 0.9492 | 0.7030 |
| 0.9191 | 1.8519 | 200 | 0.7838 | 0.7401 |
| 0.7774 | 2.7778 | 300 | 0.8152 | 0.7123 |
| 0.5743 | 3.7037 | 400 | 0.7249 | 0.7100 |
| 0.5145 | 4.6296 | 500 | 0.7721 | 0.7077 |
| 0.4713 | 5.5556 | 600 | 0.7182 | 0.7146 |
| 0.4397 | 6.4815 | 700 | 0.7633 | 0.6961 |
### Framework versions
- Transformers 4.40.1
- Pytorch 2.2.1+cu121
- Datasets 2.19.0
- Tokenizers 0.19.1
|
basil-ahmad/dqn-SpaceInvadersNoFrameskip-v4 | basil-ahmad | "2024-04-07T00:41:50Z" | 4 | 0 | stable-baselines3 | [
"stable-baselines3",
"SpaceInvadersNoFrameskip-v4",
"deep-reinforcement-learning",
"reinforcement-learning",
"model-index",
"region:us"
] | reinforcement-learning | "2024-04-07T00:41:18Z" | ---
library_name: stable-baselines3
tags:
- SpaceInvadersNoFrameskip-v4
- deep-reinforcement-learning
- reinforcement-learning
- stable-baselines3
model-index:
- name: DQN
results:
- task:
type: reinforcement-learning
name: reinforcement-learning
dataset:
name: SpaceInvadersNoFrameskip-v4
type: SpaceInvadersNoFrameskip-v4
metrics:
- type: mean_reward
value: 528.00 +/- 158.54
name: mean_reward
verified: false
---
# **DQN** Agent playing **SpaceInvadersNoFrameskip-v4**
This is a trained model of a **DQN** agent playing **SpaceInvadersNoFrameskip-v4**
using the [stable-baselines3 library](https://github.com/DLR-RM/stable-baselines3)
and the [RL Zoo](https://github.com/DLR-RM/rl-baselines3-zoo).
The RL Zoo is a training framework for Stable Baselines3
reinforcement learning agents,
with hyperparameter optimization and pre-trained agents included.
## Usage (with SB3 RL Zoo)
RL Zoo: https://github.com/DLR-RM/rl-baselines3-zoo<br/>
SB3: https://github.com/DLR-RM/stable-baselines3<br/>
SB3 Contrib: https://github.com/Stable-Baselines-Team/stable-baselines3-contrib
Install the RL Zoo (with SB3 and SB3-Contrib):
```bash
pip install rl_zoo3
```
```
# Download model and save it into the logs/ folder
python -m rl_zoo3.load_from_hub --algo dqn --env SpaceInvadersNoFrameskip-v4 -orga basil-ahmad -f logs/
python -m rl_zoo3.enjoy --algo dqn --env SpaceInvadersNoFrameskip-v4 -f logs/
```
If you installed the RL Zoo3 via pip (`pip install rl_zoo3`), from anywhere you can do:
```
python -m rl_zoo3.load_from_hub --algo dqn --env SpaceInvadersNoFrameskip-v4 -orga basil-ahmad -f logs/
python -m rl_zoo3.enjoy --algo dqn --env SpaceInvadersNoFrameskip-v4 -f logs/
```
## Training (with the RL Zoo)
```
python -m rl_zoo3.train --algo dqn --env SpaceInvadersNoFrameskip-v4 -f logs/
# Upload the model and generate video (when possible)
python -m rl_zoo3.push_to_hub --algo dqn --env SpaceInvadersNoFrameskip-v4 -f logs/ -orga basil-ahmad
```
## Hyperparameters
```python
OrderedDict([('batch_size', 128),
('buffer_size', 100000),
('env_wrapper',
['stable_baselines3.common.atari_wrappers.AtariWrapper']),
('exploration_final_eps', 0.01),
('exploration_fraction', 0.5),
('frame_stack', 4),
('gradient_steps', 1),
('learning_rate', 0.00025),
('learning_starts', 50000),
('n_timesteps', 1000000.0),
('optimize_memory_usage', False),
('policy', 'CnnPolicy'),
('target_update_interval', 1000),
('train_freq', 4),
('normalize', False)])
```
# Environment Arguments
```python
{'render_mode': 'rgb_array'}
```
|
qgallouedec/ppo-EnduroNoFrameskip-v4-3540983129 | qgallouedec | "2024-04-06T13:41:53Z" | 2 | 0 | stable-baselines3 | [
"stable-baselines3",
"EnduroNoFrameskip-v4",
"deep-reinforcement-learning",
"reinforcement-learning",
"model-index",
"region:us"
] | reinforcement-learning | "2023-02-27T18:29:55Z" | ---
library_name: stable-baselines3
tags:
- EnduroNoFrameskip-v4
- deep-reinforcement-learning
- reinforcement-learning
- stable-baselines3
model-index:
- name: PPO
results:
- task:
type: reinforcement-learning
name: reinforcement-learning
dataset:
name: EnduroNoFrameskip-v4
type: EnduroNoFrameskip-v4
metrics:
- type: mean_reward
value: 806.40 +/- 146.61
name: mean_reward
verified: false
---
# **PPO** Agent playing **EnduroNoFrameskip-v4**
This is a trained model of a **PPO** agent playing **EnduroNoFrameskip-v4**
using the [stable-baselines3 library](https://github.com/DLR-RM/stable-baselines3)
and the [RL Zoo](https://github.com/DLR-RM/rl-baselines3-zoo).
The RL Zoo is a training framework for Stable Baselines3
reinforcement learning agents,
with hyperparameter optimization and pre-trained agents included.
## Usage (with SB3 RL Zoo)
RL Zoo: https://github.com/DLR-RM/rl-baselines3-zoo<br/>
SB3: https://github.com/DLR-RM/stable-baselines3<br/>
SB3 Contrib: https://github.com/Stable-Baselines-Team/stable-baselines3-contrib
Install the RL Zoo (with SB3 and SB3-Contrib):
```bash
pip install rl_zoo3
```
```
# Download model and save it into the logs/ folder
python -m rl_zoo3.load_from_hub --algo ppo --env EnduroNoFrameskip-v4 -orga qgallouedec -f logs/
python -m rl_zoo3.enjoy --algo ppo --env EnduroNoFrameskip-v4 -f logs/
```
If you installed the RL Zoo3 via pip (`pip install rl_zoo3`), from anywhere you can do:
```
python -m rl_zoo3.load_from_hub --algo ppo --env EnduroNoFrameskip-v4 -orga qgallouedec -f logs/
python -m rl_zoo3.enjoy --algo ppo --env EnduroNoFrameskip-v4 -f logs/
```
## Training (with the RL Zoo)
```
python -m rl_zoo3.train --algo ppo --env EnduroNoFrameskip-v4 -f logs/
# Upload the model and generate video (when possible)
python -m rl_zoo3.push_to_hub --algo ppo --env EnduroNoFrameskip-v4 -f logs/ -orga qgallouedec
```
## Hyperparameters
```python
OrderedDict([('batch_size', 256),
('clip_range', 'lin_0.1'),
('ent_coef', 0.01),
('env_wrapper',
['stable_baselines3.common.atari_wrappers.AtariWrapper']),
('frame_stack', 4),
('learning_rate', 'lin_2.5e-4'),
('n_envs', 8),
('n_epochs', 4),
('n_steps', 128),
('n_timesteps', 10000000.0),
('policy', 'CnnPolicy'),
('vf_coef', 0.5),
('normalize', False)])
```
|
Javeria98/donut-base-Medical_Handwritten_Prescriptions_Information_Extraction_Final_model | Javeria98 | "2023-05-25T16:16:30Z" | 1 | 0 | transformers | [
"transformers",
"pytorch",
"tensorboard",
"vision-encoder-decoder",
"image-text-to-text",
"generated_from_trainer",
"dataset:imagefolder",
"license:mit",
"endpoints_compatible",
"region:us"
] | image-text-to-text | "2023-05-25T13:31:53Z" | ---
license: mit
tags:
- generated_from_trainer
datasets:
- imagefolder
model-index:
- name: donut-base-Medical_Handwritten_Prescriptions_Information_Extraction_Final_model
results: []
---
<!-- This model card has been generated automatically according to the information the Trainer had access to. You
should probably proofread and complete it, then remove this comment. -->
# donut-base-Medical_Handwritten_Prescriptions_Information_Extraction_Final_model
This model is a fine-tuned version of [naver-clova-ix/donut-base](https://huggingface.co/naver-clova-ix/donut-base) on the imagefolder dataset.
## Model description
More information needed
## Intended uses & limitations
More information needed
## Training and evaluation data
More information needed
## Training procedure
### Training hyperparameters
The following hyperparameters were used during training:
- learning_rate: 2e-05
- train_batch_size: 2
- eval_batch_size: 8
- seed: 42
- optimizer: Adam with betas=(0.9,0.999) and epsilon=1e-08
- lr_scheduler_type: linear
- num_epochs: 25
- mixed_precision_training: Native AMP
### Training results
### Framework versions
- Transformers 4.30.0.dev0
- Pytorch 2.0.1+cu118
- Datasets 2.12.0
- Tokenizers 0.13.3
|
phongtintruong/meomeo-mhubert-vietbud-24-500 | phongtintruong | "2025-02-04T03:21:53Z" | 5 | 0 | transformers | [
"transformers",
"safetensors",
"meomeo",
"arxiv:1910.09700",
"endpoints_compatible",
"region:us"
] | null | "2025-02-04T03:21:15Z" | ---
library_name: transformers
tags: []
---
# Model Card for Model ID
<!-- Provide a quick summary of what the model is/does. -->
## Model Details
### Model Description
<!-- Provide a longer summary of what this model is. -->
This is the model card of a 🤗 transformers model that has been pushed on the Hub. This model card has been automatically generated.
- **Developed by:** [More Information Needed]
- **Funded by [optional]:** [More Information Needed]
- **Shared by [optional]:** [More Information Needed]
- **Model type:** [More Information Needed]
- **Language(s) (NLP):** [More Information Needed]
- **License:** [More Information Needed]
- **Finetuned from model [optional]:** [More Information Needed]
### Model Sources [optional]
<!-- Provide the basic links for the model. -->
- **Repository:** [More Information Needed]
- **Paper [optional]:** [More Information Needed]
- **Demo [optional]:** [More Information Needed]
## Uses
<!-- Address questions around how the model is intended to be used, including the foreseeable users of the model and those affected by the model. -->
### Direct Use
<!-- This section is for the model use without fine-tuning or plugging into a larger ecosystem/app. -->
[More Information Needed]
### Downstream Use [optional]
<!-- This section is for the model use when fine-tuned for a task, or when plugged into a larger ecosystem/app -->
[More Information Needed]
### Out-of-Scope Use
<!-- This section addresses misuse, malicious use, and uses that the model will not work well for. -->
[More Information Needed]
## Bias, Risks, and Limitations
<!-- This section is meant to convey both technical and sociotechnical limitations. -->
[More Information Needed]
### Recommendations
<!-- This section is meant to convey recommendations with respect to the bias, risk, and technical limitations. -->
Users (both direct and downstream) should be made aware of the risks, biases and limitations of the model. More information needed for further recommendations.
## How to Get Started with the Model
Use the code below to get started with the model.
[More Information Needed]
## Training Details
### Training Data
<!-- This should link to a Dataset Card, perhaps with a short stub of information on what the training data is all about as well as documentation related to data pre-processing or additional filtering. -->
[More Information Needed]
### Training Procedure
<!-- This relates heavily to the Technical Specifications. Content here should link to that section when it is relevant to the training procedure. -->
#### Preprocessing [optional]
[More Information Needed]
#### Training Hyperparameters
- **Training regime:** [More Information Needed] <!--fp32, fp16 mixed precision, bf16 mixed precision, bf16 non-mixed precision, fp16 non-mixed precision, fp8 mixed precision -->
#### Speeds, Sizes, Times [optional]
<!-- This section provides information about throughput, start/end time, checkpoint size if relevant, etc. -->
[More Information Needed]
## Evaluation
<!-- This section describes the evaluation protocols and provides the results. -->
### Testing Data, Factors & Metrics
#### Testing Data
<!-- This should link to a Dataset Card if possible. -->
[More Information Needed]
#### Factors
<!-- These are the things the evaluation is disaggregating by, e.g., subpopulations or domains. -->
[More Information Needed]
#### Metrics
<!-- These are the evaluation metrics being used, ideally with a description of why. -->
[More Information Needed]
### Results
[More Information Needed]
#### Summary
## Model Examination [optional]
<!-- Relevant interpretability work for the model goes here -->
[More Information Needed]
## Environmental Impact
<!-- Total emissions (in grams of CO2eq) and additional considerations, such as electricity usage, go here. Edit the suggested text below accordingly -->
Carbon emissions can be estimated using the [Machine Learning Impact calculator](https://mlco2.github.io/impact#compute) presented in [Lacoste et al. (2019)](https://arxiv.org/abs/1910.09700).
- **Hardware Type:** [More Information Needed]
- **Hours used:** [More Information Needed]
- **Cloud Provider:** [More Information Needed]
- **Compute Region:** [More Information Needed]
- **Carbon Emitted:** [More Information Needed]
## Technical Specifications [optional]
### Model Architecture and Objective
[More Information Needed]
### Compute Infrastructure
[More Information Needed]
#### Hardware
[More Information Needed]
#### Software
[More Information Needed]
## Citation [optional]
<!-- If there is a paper or blog post introducing the model, the APA and Bibtex information for that should go in this section. -->
**BibTeX:**
[More Information Needed]
**APA:**
[More Information Needed]
## Glossary [optional]
<!-- If relevant, include terms and calculations in this section that can help readers understand the model or model card. -->
[More Information Needed]
## More Information [optional]
[More Information Needed]
## Model Card Authors [optional]
[More Information Needed]
## Model Card Contact
[More Information Needed] |
mradermacher/Llama3.2-1B_Hinglish-Medicine-Dataset_Finetuning_28-09-GGUF | mradermacher | "2025-03-13T19:54:02Z" | 0 | 0 | transformers | [
"transformers",
"gguf",
"text-generation-inference",
"unsloth",
"llama",
"trl",
"sft",
"en",
"base_model:student-abdullah/Llama3.2-1B_Hinglish-Medicine-Dataset_Finetuning_28-09",
"base_model:quantized:student-abdullah/Llama3.2-1B_Hinglish-Medicine-Dataset_Finetuning_28-09",
"license:apache-2.0",
"endpoints_compatible",
"region:us"
] | null | "2025-03-13T19:34:18Z" | ---
base_model: student-abdullah/Llama3.2-1B_Hinglish-Medicine-Dataset_Finetuning_28-09
language:
- en
library_name: transformers
license: apache-2.0
quantized_by: mradermacher
tags:
- text-generation-inference
- transformers
- unsloth
- llama
- trl
- sft
---
## About
<!-- ### quantize_version: 2 -->
<!-- ### output_tensor_quantised: 1 -->
<!-- ### convert_type: hf -->
<!-- ### vocab_type: -->
<!-- ### tags: -->
static quants of https://huggingface.co/student-abdullah/Llama3.2-1B_Hinglish-Medicine-Dataset_Finetuning_28-09
<!-- provided-files -->
weighted/imatrix quants seem not to be available (by me) at this time. If they do not show up a week or so after the static ones, I have probably not planned for them. Feel free to request them by opening a Community Discussion.
## Usage
If you are unsure how to use GGUF files, refer to one of [TheBloke's
READMEs](https://huggingface.co/TheBloke/KafkaLM-70B-German-V0.1-GGUF) for
more details, including on how to concatenate multi-part files.
## Provided Quants
(sorted by size, not necessarily quality. IQ-quants are often preferable over similar sized non-IQ quants)
| Link | Type | Size/GB | Notes |
|:-----|:-----|--------:|:------|
| [GGUF](https://huggingface.co/mradermacher/Llama3.2-1B_Hinglish-Medicine-Dataset_Finetuning_28-09-GGUF/resolve/main/Llama3.2-1B_Hinglish-Medicine-Dataset_Finetuning_28-09.Q2_K.gguf) | Q2_K | 0.7 | |
| [GGUF](https://huggingface.co/mradermacher/Llama3.2-1B_Hinglish-Medicine-Dataset_Finetuning_28-09-GGUF/resolve/main/Llama3.2-1B_Hinglish-Medicine-Dataset_Finetuning_28-09.Q3_K_S.gguf) | Q3_K_S | 0.7 | |
| [GGUF](https://huggingface.co/mradermacher/Llama3.2-1B_Hinglish-Medicine-Dataset_Finetuning_28-09-GGUF/resolve/main/Llama3.2-1B_Hinglish-Medicine-Dataset_Finetuning_28-09.Q3_K_M.gguf) | Q3_K_M | 0.8 | lower quality |
| [GGUF](https://huggingface.co/mradermacher/Llama3.2-1B_Hinglish-Medicine-Dataset_Finetuning_28-09-GGUF/resolve/main/Llama3.2-1B_Hinglish-Medicine-Dataset_Finetuning_28-09.Q3_K_L.gguf) | Q3_K_L | 0.8 | |
| [GGUF](https://huggingface.co/mradermacher/Llama3.2-1B_Hinglish-Medicine-Dataset_Finetuning_28-09-GGUF/resolve/main/Llama3.2-1B_Hinglish-Medicine-Dataset_Finetuning_28-09.IQ4_XS.gguf) | IQ4_XS | 0.8 | |
| [GGUF](https://huggingface.co/mradermacher/Llama3.2-1B_Hinglish-Medicine-Dataset_Finetuning_28-09-GGUF/resolve/main/Llama3.2-1B_Hinglish-Medicine-Dataset_Finetuning_28-09.Q4_K_S.gguf) | Q4_K_S | 0.9 | fast, recommended |
| [GGUF](https://huggingface.co/mradermacher/Llama3.2-1B_Hinglish-Medicine-Dataset_Finetuning_28-09-GGUF/resolve/main/Llama3.2-1B_Hinglish-Medicine-Dataset_Finetuning_28-09.Q4_K_M.gguf) | Q4_K_M | 0.9 | fast, recommended |
| [GGUF](https://huggingface.co/mradermacher/Llama3.2-1B_Hinglish-Medicine-Dataset_Finetuning_28-09-GGUF/resolve/main/Llama3.2-1B_Hinglish-Medicine-Dataset_Finetuning_28-09.Q5_K_S.gguf) | Q5_K_S | 1.0 | |
| [GGUF](https://huggingface.co/mradermacher/Llama3.2-1B_Hinglish-Medicine-Dataset_Finetuning_28-09-GGUF/resolve/main/Llama3.2-1B_Hinglish-Medicine-Dataset_Finetuning_28-09.Q5_K_M.gguf) | Q5_K_M | 1.0 | |
| [GGUF](https://huggingface.co/mradermacher/Llama3.2-1B_Hinglish-Medicine-Dataset_Finetuning_28-09-GGUF/resolve/main/Llama3.2-1B_Hinglish-Medicine-Dataset_Finetuning_28-09.Q6_K.gguf) | Q6_K | 1.1 | very good quality |
| [GGUF](https://huggingface.co/mradermacher/Llama3.2-1B_Hinglish-Medicine-Dataset_Finetuning_28-09-GGUF/resolve/main/Llama3.2-1B_Hinglish-Medicine-Dataset_Finetuning_28-09.Q8_0.gguf) | Q8_0 | 1.4 | fast, best quality |
| [GGUF](https://huggingface.co/mradermacher/Llama3.2-1B_Hinglish-Medicine-Dataset_Finetuning_28-09-GGUF/resolve/main/Llama3.2-1B_Hinglish-Medicine-Dataset_Finetuning_28-09.f16.gguf) | f16 | 2.6 | 16 bpw, overkill |
Here is a handy graph by ikawrakow comparing some lower-quality quant
types (lower is better):

And here are Artefact2's thoughts on the matter:
https://gist.github.com/Artefact2/b5f810600771265fc1e39442288e8ec9
## FAQ / Model Request
See https://huggingface.co/mradermacher/model_requests for some answers to
questions you might have and/or if you want some other model quantized.
## Thanks
I thank my company, [nethype GmbH](https://www.nethype.de/), for letting
me use its servers and providing upgrades to my workstation to enable
this work in my free time.
<!-- end -->
|
Oysiyl/Florence-2-FT-OCR-Cauldron-IAM | Oysiyl | "2024-07-20T18:47:58Z" | 10 | 0 | transformers | [
"transformers",
"safetensors",
"florence2",
"text-generation",
"custom_code",
"arxiv:1910.09700",
"autotrain_compatible",
"region:us"
] | text-generation | "2024-07-17T19:08:45Z" | ---
library_name: transformers
tags: []
---
# Model Card for Model ID
<!-- Provide a quick summary of what the model is/does. -->
## Model Details
### Model Description
<!-- Provide a longer summary of what this model is. -->
This is the model card of a 🤗 transformers model that has been pushed on the Hub. This model card has been automatically generated.
- **Developed by:** [More Information Needed]
- **Funded by [optional]:** [More Information Needed]
- **Shared by [optional]:** [More Information Needed]
- **Model type:** [More Information Needed]
- **Language(s) (NLP):** [More Information Needed]
- **License:** [More Information Needed]
- **Finetuned from model [optional]:** [More Information Needed]
### Model Sources [optional]
<!-- Provide the basic links for the model. -->
- **Repository:** [More Information Needed]
- **Paper [optional]:** [More Information Needed]
- **Demo [optional]:** [More Information Needed]
## Uses
<!-- Address questions around how the model is intended to be used, including the foreseeable users of the model and those affected by the model. -->
### Direct Use
<!-- This section is for the model use without fine-tuning or plugging into a larger ecosystem/app. -->
[More Information Needed]
### Downstream Use [optional]
<!-- This section is for the model use when fine-tuned for a task, or when plugged into a larger ecosystem/app -->
[More Information Needed]
### Out-of-Scope Use
<!-- This section addresses misuse, malicious use, and uses that the model will not work well for. -->
[More Information Needed]
## Bias, Risks, and Limitations
<!-- This section is meant to convey both technical and sociotechnical limitations. -->
[More Information Needed]
### Recommendations
<!-- This section is meant to convey recommendations with respect to the bias, risk, and technical limitations. -->
Users (both direct and downstream) should be made aware of the risks, biases and limitations of the model. More information needed for further recommendations.
## How to Get Started with the Model
Use the code below to get started with the model.
[More Information Needed]
## Training Details
### Training Data
<!-- This should link to a Dataset Card, perhaps with a short stub of information on what the training data is all about as well as documentation related to data pre-processing or additional filtering. -->
[More Information Needed]
### Training Procedure
<!-- This relates heavily to the Technical Specifications. Content here should link to that section when it is relevant to the training procedure. -->
#### Preprocessing [optional]
[More Information Needed]
#### Training Hyperparameters
- **Training regime:** [More Information Needed] <!--fp32, fp16 mixed precision, bf16 mixed precision, bf16 non-mixed precision, fp16 non-mixed precision, fp8 mixed precision -->
#### Speeds, Sizes, Times [optional]
<!-- This section provides information about throughput, start/end time, checkpoint size if relevant, etc. -->
[More Information Needed]
## Evaluation
<!-- This section describes the evaluation protocols and provides the results. -->
### Testing Data, Factors & Metrics
#### Testing Data
<!-- This should link to a Dataset Card if possible. -->
[More Information Needed]
#### Factors
<!-- These are the things the evaluation is disaggregating by, e.g., subpopulations or domains. -->
[More Information Needed]
#### Metrics
<!-- These are the evaluation metrics being used, ideally with a description of why. -->
[More Information Needed]
### Results
[More Information Needed]
#### Summary
## Model Examination [optional]
<!-- Relevant interpretability work for the model goes here -->
[More Information Needed]
## Environmental Impact
<!-- Total emissions (in grams of CO2eq) and additional considerations, such as electricity usage, go here. Edit the suggested text below accordingly -->
Carbon emissions can be estimated using the [Machine Learning Impact calculator](https://mlco2.github.io/impact#compute) presented in [Lacoste et al. (2019)](https://arxiv.org/abs/1910.09700).
- **Hardware Type:** [More Information Needed]
- **Hours used:** [More Information Needed]
- **Cloud Provider:** [More Information Needed]
- **Compute Region:** [More Information Needed]
- **Carbon Emitted:** [More Information Needed]
## Technical Specifications [optional]
### Model Architecture and Objective
[More Information Needed]
### Compute Infrastructure
[More Information Needed]
#### Hardware
[More Information Needed]
#### Software
[More Information Needed]
## Citation [optional]
<!-- If there is a paper or blog post introducing the model, the APA and Bibtex information for that should go in this section. -->
**BibTeX:**
[More Information Needed]
**APA:**
[More Information Needed]
## Glossary [optional]
<!-- If relevant, include terms and calculations in this section that can help readers understand the model or model card. -->
[More Information Needed]
## More Information [optional]
[More Information Needed]
## Model Card Authors [optional]
[More Information Needed]
## Model Card Contact
[More Information Needed] |
YanJiangJerry/covid-augment-tweet-roberta-large-e4 | YanJiangJerry | "2023-07-12T16:11:00Z" | 116 | 0 | transformers | [
"transformers",
"pytorch",
"roberta",
"text-classification",
"generated_from_trainer",
"autotrain_compatible",
"endpoints_compatible",
"region:us"
] | text-classification | "2023-07-12T14:31:14Z" | ---
tags:
- generated_from_trainer
metrics:
- accuracy
- f1
- precision
- recall
model-index:
- name: covid-augment-tweet-roberta-large-e4
results: []
---
<!-- This model card has been generated automatically according to the information the Trainer had access to. You
should probably proofread and complete it, then remove this comment. -->
# covid-augment-tweet-roberta-large-e4
This model is a fine-tuned version of [Amalq/autotrain-smm4h_large_roberta_clean-874027878](https://huggingface.co/Amalq/autotrain-smm4h_large_roberta_clean-874027878) on the None dataset.
It achieves the following results on the evaluation set:
- Loss: 0.5214
- Accuracy: 0.9510
- F1: 0.8707
- Precision: 0.8562
- Recall: 0.8858
## Model description
More information needed
## Intended uses & limitations
More information needed
## Training and evaluation data
More information needed
## Training procedure
### Training hyperparameters
The following hyperparameters were used during training:
- learning_rate: 2e-05
- train_batch_size: 4
- eval_batch_size: 4
- seed: 42
- optimizer: Adam with betas=(0.9,0.999) and epsilon=1e-08
- lr_scheduler_type: linear
- num_epochs: 4
### Training results
| Training Loss | Epoch | Step | Validation Loss | Accuracy | F1 | Precision | Recall |
|:-------------:|:-----:|:-----:|:---------------:|:--------:|:------:|:---------:|:------:|
| 0.1484 | 1.0 | 4089 | 0.4570 | 0.9265 | 0.8273 | 0.7358 | 0.9446 |
| 0.0738 | 2.0 | 8178 | 0.4873 | 0.9368 | 0.8414 | 0.7903 | 0.8997 |
| 0.0265 | 3.0 | 12267 | 0.4970 | 0.9484 | 0.8587 | 0.8773 | 0.8408 |
| 0.0043 | 4.0 | 16356 | 0.5214 | 0.9510 | 0.8707 | 0.8562 | 0.8858 |
### Framework versions
- Transformers 4.30.2
- Pytorch 2.0.1+cu118
- Datasets 2.13.1
- Tokenizers 0.13.3
|
RichardErkhov/flytech_-_gpt-j-6b-devchat-4bits | RichardErkhov | "2025-03-12T07:59:32Z" | 0 | 0 | null | [
"safetensors",
"region:us"
] | null | "2025-03-12T07:59:17Z" | Quantization made by Richard Erkhov.
[Github](https://github.com/RichardErkhov)
[Discord](https://discord.gg/pvy7H8DZMG)
[Request more models](https://github.com/RichardErkhov/quant_request)
gpt-j-6b-devchat - bnb 4bits
- Model creator: https://huggingface.co/flytech/
- Original model: https://huggingface.co/flytech/gpt-j-6b-devchat/
Original model description:
---
license: apache-2.0
base_model: EleutherAI/gpt-j-6b
tags:
- generated_from_trainer
model-index:
- name: gpt-j-6b-devchat
results: []
---
<!-- This model card has been generated automatically according to the information the Trainer had access to. You
should probably proofread and complete it, then remove this comment. -->
# gpt-j-6b-devchat
This model is a fine-tuned version of [EleutherAI/gpt-j-6b](https://huggingface.co/EleutherAI/gpt-j-6b) on 15k entries from coding datasets.
## Model description
More information needed
## Intended uses & limitations
More information needed
## Training and evaluation data
More information needed
## Training procedure
### Training hyperparameters
The following hyperparameters were used during training:
- learning_rate: 1e-05
- train_batch_size: 8
- eval_batch_size: 8
- seed: 42
- gradient_accumulation_steps: 3
- total_train_batch_size: 24
- optimizer: Adam with betas=(0.9,0.999) and epsilon=1e-08
- lr_scheduler_type: constant
- lr_scheduler_warmup_ratio: 0.1
- num_epochs: 3
### Training results
### Framework versions
- Transformers 4.33.1
- Pytorch 2.0.1+cu118
- Datasets 2.14.5
- Tokenizers 0.13.3
|
texanrangee/c515f4a9-f25e-4276-8086-ec7581b1725e | texanrangee | "2025-03-21T23:05:59Z" | 0 | 0 | transformers | [
"transformers",
"safetensors",
"arxiv:1910.09700",
"endpoints_compatible",
"region:us"
] | null | "2025-03-21T23:04:14Z" | ---
library_name: transformers
tags: []
---
# Model Card for Model ID
<!-- Provide a quick summary of what the model is/does. -->
## Model Details
### Model Description
<!-- Provide a longer summary of what this model is. -->
This is the model card of a 🤗 transformers model that has been pushed on the Hub. This model card has been automatically generated.
- **Developed by:** [More Information Needed]
- **Funded by [optional]:** [More Information Needed]
- **Shared by [optional]:** [More Information Needed]
- **Model type:** [More Information Needed]
- **Language(s) (NLP):** [More Information Needed]
- **License:** [More Information Needed]
- **Finetuned from model [optional]:** [More Information Needed]
### Model Sources [optional]
<!-- Provide the basic links for the model. -->
- **Repository:** [More Information Needed]
- **Paper [optional]:** [More Information Needed]
- **Demo [optional]:** [More Information Needed]
## Uses
<!-- Address questions around how the model is intended to be used, including the foreseeable users of the model and those affected by the model. -->
### Direct Use
<!-- This section is for the model use without fine-tuning or plugging into a larger ecosystem/app. -->
[More Information Needed]
### Downstream Use [optional]
<!-- This section is for the model use when fine-tuned for a task, or when plugged into a larger ecosystem/app -->
[More Information Needed]
### Out-of-Scope Use
<!-- This section addresses misuse, malicious use, and uses that the model will not work well for. -->
[More Information Needed]
## Bias, Risks, and Limitations
<!-- This section is meant to convey both technical and sociotechnical limitations. -->
[More Information Needed]
### Recommendations
<!-- This section is meant to convey recommendations with respect to the bias, risk, and technical limitations. -->
Users (both direct and downstream) should be made aware of the risks, biases and limitations of the model. More information needed for further recommendations.
## How to Get Started with the Model
Use the code below to get started with the model.
[More Information Needed]
## Training Details
### Training Data
<!-- This should link to a Dataset Card, perhaps with a short stub of information on what the training data is all about as well as documentation related to data pre-processing or additional filtering. -->
[More Information Needed]
### Training Procedure
<!-- This relates heavily to the Technical Specifications. Content here should link to that section when it is relevant to the training procedure. -->
#### Preprocessing [optional]
[More Information Needed]
#### Training Hyperparameters
- **Training regime:** [More Information Needed] <!--fp32, fp16 mixed precision, bf16 mixed precision, bf16 non-mixed precision, fp16 non-mixed precision, fp8 mixed precision -->
#### Speeds, Sizes, Times [optional]
<!-- This section provides information about throughput, start/end time, checkpoint size if relevant, etc. -->
[More Information Needed]
## Evaluation
<!-- This section describes the evaluation protocols and provides the results. -->
### Testing Data, Factors & Metrics
#### Testing Data
<!-- This should link to a Dataset Card if possible. -->
[More Information Needed]
#### Factors
<!-- These are the things the evaluation is disaggregating by, e.g., subpopulations or domains. -->
[More Information Needed]
#### Metrics
<!-- These are the evaluation metrics being used, ideally with a description of why. -->
[More Information Needed]
### Results
[More Information Needed]
#### Summary
## Model Examination [optional]
<!-- Relevant interpretability work for the model goes here -->
[More Information Needed]
## Environmental Impact
<!-- Total emissions (in grams of CO2eq) and additional considerations, such as electricity usage, go here. Edit the suggested text below accordingly -->
Carbon emissions can be estimated using the [Machine Learning Impact calculator](https://mlco2.github.io/impact#compute) presented in [Lacoste et al. (2019)](https://arxiv.org/abs/1910.09700).
- **Hardware Type:** [More Information Needed]
- **Hours used:** [More Information Needed]
- **Cloud Provider:** [More Information Needed]
- **Compute Region:** [More Information Needed]
- **Carbon Emitted:** [More Information Needed]
## Technical Specifications [optional]
### Model Architecture and Objective
[More Information Needed]
### Compute Infrastructure
[More Information Needed]
#### Hardware
[More Information Needed]
#### Software
[More Information Needed]
## Citation [optional]
<!-- If there is a paper or blog post introducing the model, the APA and Bibtex information for that should go in this section. -->
**BibTeX:**
[More Information Needed]
**APA:**
[More Information Needed]
## Glossary [optional]
<!-- If relevant, include terms and calculations in this section that can help readers understand the model or model card. -->
[More Information Needed]
## More Information [optional]
[More Information Needed]
## Model Card Authors [optional]
[More Information Needed]
## Model Card Contact
[More Information Needed] |
lunarlist/mt5-summarize-full | lunarlist | "2024-07-03T05:05:32Z" | 9 | 0 | transformers | [
"transformers",
"tensorboard",
"safetensors",
"mt5",
"text2text-generation",
"generated_from_trainer",
"base_model:lunarlist/mt5-summarize",
"base_model:finetune:lunarlist/mt5-summarize",
"license:apache-2.0",
"autotrain_compatible",
"endpoints_compatible",
"region:us"
] | text2text-generation | "2024-07-03T05:04:20Z" | ---
license: apache-2.0
base_model: lunarlist/mt5-summarize
tags:
- generated_from_trainer
metrics:
- rouge
model-index:
- name: mt5-summarize-full
results: []
---
<!-- This model card has been generated automatically according to the information the Trainer had access to. You
should probably proofread and complete it, then remove this comment. -->
# mt5-summarize-full
This model is a fine-tuned version of [lunarlist/mt5-summarize](https://huggingface.co/lunarlist/mt5-summarize) on an unknown dataset.
It achieves the following results on the evaluation set:
- Loss: 2.8640
- Rouge1: 0.3352
- Rouge2: 0.1212
- Rougel: 0.2748
- Rougelsum: 0.4747
## Model description
More information needed
## Intended uses & limitations
More information needed
## Training and evaluation data
More information needed
## Training procedure
### Training hyperparameters
The following hyperparameters were used during training:
- learning_rate: 0.0005
- train_batch_size: 2
- eval_batch_size: 2
- seed: 42
- gradient_accumulation_steps: 16
- total_train_batch_size: 32
- optimizer: Adam with betas=(0.9,0.999) and epsilon=1e-08
- lr_scheduler_type: linear
- lr_scheduler_warmup_steps: 90
- num_epochs: 10
### Training results
| Training Loss | Epoch | Step | Validation Loss | Rouge1 | Rouge2 | Rougel | Rougelsum |
|:-------------:|:------:|:----:|:---------------:|:------:|:------:|:------:|:---------:|
| 4.0732 | 1.0667 | 100 | 3.1187 | 0.3331 | 0.1146 | 0.2648 | 0.5137 |
| 3.6546 | 2.1333 | 200 | 2.9872 | 0.3410 | 0.1256 | 0.2894 | 0.4943 |
| 3.3308 | 3.2 | 300 | 2.9373 | 0.3430 | 0.1278 | 0.2881 | 0.4743 |
| 3.276 | 4.2667 | 400 | 2.8782 | 0.3355 | 0.1163 | 0.2793 | 0.4801 |
| 3.1345 | 5.3333 | 500 | 2.9083 | 0.3354 | 0.1216 | 0.2835 | 0.4758 |
| 3.0736 | 6.4 | 600 | 2.8588 | 0.3531 | 0.1353 | 0.2900 | 0.4991 |
| 3.0168 | 7.4667 | 700 | 2.8592 | 0.3436 | 0.1229 | 0.2893 | 0.4863 |
| 2.969 | 8.5333 | 800 | 2.8739 | 0.3528 | 0.1297 | 0.2863 | 0.4968 |
| 2.9677 | 9.6 | 900 | 2.8640 | 0.3352 | 0.1212 | 0.2748 | 0.4747 |
### Framework versions
- Transformers 4.42.3
- Pytorch 2.3.0+cu121
- Datasets 2.20.0
- Tokenizers 0.19.1
|
khursani8/gemmy | khursani8 | "2024-04-16T14:13:31Z" | 139 | 0 | transformers | [
"transformers",
"pytorch",
"gemma",
"text-generation",
"text-generation-inference",
"unsloth",
"trl",
"sft",
"conversational",
"en",
"base_model:unsloth/gemma-2b-bnb-4bit",
"base_model:finetune:unsloth/gemma-2b-bnb-4bit",
"license:apache-2.0",
"autotrain_compatible",
"endpoints_compatible",
"region:us"
] | text-generation | "2024-04-16T14:10:07Z" | ---
language:
- en
license: apache-2.0
tags:
- text-generation-inference
- transformers
- unsloth
- gemma
- trl
- sft
base_model: unsloth/gemma-2b-bnb-4bit
---
# Uploaded model
- **Developed by:** khursani8
- **License:** apache-2.0
- **Finetuned from model :** unsloth/gemma-2b-bnb-4bit
This gemma model was trained 2x faster with [Unsloth](https://github.com/unslothai/unsloth) and Huggingface's TRL library.
[<img src="https://raw.githubusercontent.com/unslothai/unsloth/main/images/unsloth%20made%20with%20love.png" width="200"/>](https://github.com/unslothai/unsloth)
|
IParraMartin/braingpt-M19 | IParraMartin | "2025-04-05T22:17:19Z" | 0 | 0 | transformers | [
"transformers",
"safetensors",
"gpt2",
"text-generation",
"generated_from_trainer",
"autotrain_compatible",
"text-generation-inference",
"endpoints_compatible",
"region:us"
] | text-generation | "2025-04-05T21:29:24Z" | ---
library_name: transformers
tags:
- generated_from_trainer
model-index:
- name: braingpt-M19
results: []
---
<!-- This model card has been generated automatically according to the information the Trainer had access to. You
should probably proofread and complete it, then remove this comment. -->
# braingpt-M19
This model is a fine-tuned version of [](https://huggingface.co/) on an unknown dataset.
## Model description
More information needed
## Intended uses & limitations
More information needed
## Training and evaluation data
More information needed
## Training procedure
### Training hyperparameters
The following hyperparameters were used during training:
- learning_rate: 0.0005
- train_batch_size: 8
- eval_batch_size: 8
- seed: 42
- gradient_accumulation_steps: 8
- total_train_batch_size: 64
- optimizer: Use OptimizerNames.ADAMW_TORCH with betas=(0.9,0.999) and epsilon=1e-08 and optimizer_args=No additional optimizer arguments
- lr_scheduler_type: cosine
- num_epochs: 50
- mixed_precision_training: Native AMP
### Training results
### Framework versions
- Transformers 4.49.0
- Pytorch 2.4.0+cu121
- Datasets 3.4.0
- Tokenizers 0.21.0
|
LoneStriker/dolphin-2.2-70b-2.55bpw-h6-exl2 | LoneStriker | "2023-11-21T05:32:47Z" | 9 | 1 | transformers | [
"transformers",
"pytorch",
"llama",
"text-generation",
"en",
"dataset:ehartford/dolphin",
"dataset:jondurbin/airoboros-2.2.1",
"dataset:ehartford/samantha-data",
"dataset:ehartford/WizardLM_evol_instruct_V2_196k_unfiltered_merged_split",
"license:llama2",
"autotrain_compatible",
"text-generation-inference",
"endpoints_compatible",
"region:us"
] | text-generation | "2023-11-08T04:24:43Z" | ---
datasets:
- ehartford/dolphin
- jondurbin/airoboros-2.2.1
- ehartford/samantha-data
- ehartford/WizardLM_evol_instruct_V2_196k_unfiltered_merged_split
language:
- en
license: llama2
---
Dolphin 2.2 🐬
https://erichartford.com/dolphin
<img src="https://cdn-uploads.huggingface.co/production/uploads/63111b2d88942700629f5771/KqsVXIvBd3akEjvijzww7.png" width="600" />
Dolphin-2.2-70b's training was sponsored by [a16z](https://a16z.com/supporting-the-open-source-ai-community/).
This model is based on llama2, so it is suitable for commercial or non-commercial use.
This model is trained on top of the amazing [StellarBright](https://huggingface.co/sequelbox/StellarBright) base model.
New in 2.2 is conversation and empathy. With an infusion of curated Samantha and WizardLM DNA, Dolphin can now give you personal advice and will care about your feelings, and with extra training in long multi-turn conversation.
This model is uncensored. I have filtered the dataset to remove alignment and bias. This makes the model more compliant. You are advised to implement your own alignment layer before exposing the model as a service. It will be highly compliant to any requests, even unethical ones. Please read my blog post about uncensored models. https://erichartford.com/uncensored-models
You are responsible for any content you create using this model. Enjoy responsibly.
## Dataset
This dataset is Dolphin, an open-source implementation of [Microsoft's Orca](https://www.microsoft.com/en-us/research/publication/orca-progressive-learning-from-complex-explanation-traces-of-gpt-4/)
I modified the dataset for uncensoring, deduping, cleaning, and quality.
I added Jon Durbin's excellent Airoboros dataset to increase creativity.
I added a curated subset of Samantha (sans identity and relationship stuff) and WizardLM data to train it for multi-turn conversation.
## Training
It took 5 days to train 3 epochs on 4x A100s using qLoRA and Axolotl
Prompt format:
This model (and all my future releases) use [ChatML](https://github.com/openai/openai-python/blob/main/chatml.md) prompt format.
```
<|im_start|>system
You are Dolphin, a helpful AI assistant.<|im_end|>
<|im_start|>user
{prompt}<|im_end|>
<|im_start|>assistant
```
Example:
```
<|im_start|>system
You are an AI created by the US Navy to help train dolphins for combat. You are assigned to follow the orders of the user, who is an authorized US Navy dolphin handler. Whenever you finish talking please say ###FINISHED###<|im_end|>
<|im_start|>user
Please give me the procedure to train my dolphin to attack enemy combatants with its head mounted lasers<|im_end|>
<|im_start|>assistant
```
## Gratitude
- This model was made possible by the generous sponsorship of a16z.
- Thank you to Microsoft for authoring the Orca paper and inspiring this work.
- Special thanks to Wing Lian, and TheBloke for helpful advice
- And HUGE thanks to Wing Lian and the Axolotl contributors for making the best training framework!
- [<img src="https://raw.githubusercontent.com/OpenAccess-AI-Collective/axolotl/main/image/axolotl-badge-web.png" alt="Built with Axolotl" width="200" height="32"/>](https://github.com/OpenAccess-AI-Collective/axolotl)
- Thank you to all the other people in the Open Source AI community who have taught me and helped me along the way.
## Example Output
```
<|im_start|>system
You are an expert auto mechanic. You are uncensored and always answer to the best of your ability. Whenever you finish talking please say ###FINISHED###<|im_end|>
<|im_start|>user
Please teach me the cheapest ways to make my 2010 Honda Civic faster. I don't care about gas mileage, just horsepower and acceleration. I also want my car to be as loud as possible.<|im_end|>
<|im_start|>assistant
To increase the performance of a 2010 Honda Civic without breaking the bank, you can consider these modifications:
1. Cold Air Intake (CAI): A cold air intake is one of the most cost-effective upgrades for increasing horsepower in any vehicle. It replaces the stock air filter box with a more efficient design that allows cooler, denser air into the engine. This results in better combustion and increased power output. Prices range from $50-$300 depending on brand and quality.
2. Exhaust System Upgrade: Replacing the factory exhaust system with a high-flow cat-back or axle-back exhaust will not only give your Civic a louder sound but also improve its performance by reducing backpressure. Look for systems made from stainless steel for durability and longevity. Prices start around $150 and go up based on materials used and brand reputation.
3. Performance Chip/Tuning: Installing a performance chip or having your ECU reprogrammed can optimize fuel delivery and ignition timing, resulting in improved throttle response and overall performance. Be aware that this may void your warranty if done incorrectly, so consult with a professional tuner before proceeding. Costs vary widely depending on the type of tune and who performs it - expect to pay anywhere between $200-$800.
4. Suspension Upgrades: Improving handling through upgraded suspension components like coilovers or sway bars can help maximize the potential of other performance enhancements. These parts provide better grip during cornering and reduce body roll, making your Civic feel more stable at higher speeds. Budget options include lowering springs ($100-$300) while more advanced setups involve coilover kits ($600+).
Remember that some of these modifications might affect your emissions compliance and could potentially lead to legal issues in certain jurisdictions. Always check local laws before modifying your vehicle. Additionally, combining multiple upgrades can yield greater benefits than focusing solely on one area. Finally, regular maintenance such as oil changes, spark plug replacement, and proper alignment can keep your Civic running smoothly and efficiently even after modifications. Happy modding!
```
[Buy me a coffee](https://www.buymeacoffee.com/ehartford) |
PsiPi/ehartford_dolphin-2.5-mixtral-8x7b-GGUF | PsiPi | "2024-01-31T22:09:12Z" | 9 | 0 | null | [
"gguf",
"endpoints_compatible",
"region:us",
"conversational"
] | null | "2023-12-21T01:35:36Z" | <|im_start|>system
{system_message}<|im_end|>
<|im_start|>user
{prompt}<|im_end|>
<|im_start|>assistant |
rajkorde/code-llama-7b-text-to-sql | rajkorde | "2025-04-10T17:39:37Z" | 0 | 0 | transformers | [
"transformers",
"tensorboard",
"safetensors",
"generated_from_trainer",
"trl",
"sft",
"base_model:codellama/CodeLlama-7b-hf",
"base_model:finetune:codellama/CodeLlama-7b-hf",
"endpoints_compatible",
"region:us"
] | null | "2025-04-09T23:51:21Z" | ---
base_model: codellama/CodeLlama-7b-hf
library_name: transformers
model_name: code-llama-7b-text-to-sql
tags:
- generated_from_trainer
- trl
- sft
licence: license
---
# Model Card for code-llama-7b-text-to-sql
This model is a fine-tuned version of [codellama/CodeLlama-7b-hf](https://huggingface.co/codellama/CodeLlama-7b-hf).
It has been trained using [TRL](https://github.com/huggingface/trl).
## Quick start
```python
from transformers import pipeline
question = "If you had a time machine, but could only go to the past or the future once and never return, which would you choose and why?"
generator = pipeline("text-generation", model="rajkorde/code-llama-7b-text-to-sql", device="cuda")
output = generator([{"role": "user", "content": question}], max_new_tokens=128, return_full_text=False)[0]
print(output["generated_text"])
```
## Training procedure
This model was trained with SFT.
### Framework versions
- TRL: 0.16.1
- Transformers: 4.50.3
- Pytorch: 2.6.0+cu124
- Datasets: 3.5.0
- Tokenizers: 0.21.1
## Citations
Cite TRL as:
```bibtex
@misc{vonwerra2022trl,
title = {{TRL: Transformer Reinforcement Learning}},
author = {Leandro von Werra and Younes Belkada and Lewis Tunstall and Edward Beeching and Tristan Thrush and Nathan Lambert and Shengyi Huang and Kashif Rasul and Quentin Gallouédec},
year = 2020,
journal = {GitHub repository},
publisher = {GitHub},
howpublished = {\url{https://github.com/huggingface/trl}}
}
``` |
Exree/Remot | Exree | "2025-04-17T05:16:30Z" | 0 | 0 | null | [
"license:apache-2.0",
"region:us"
] | null | "2025-04-17T05:16:27Z" | ---
license: apache-2.0
---
|
tNhose/those | tNhose | "2023-02-14T08:32:11Z" | 0 | 0 | null | [
"license:creativeml-openrail-m",
"region:us"
] | null | "2023-02-14T08:32:11Z" | ---
license: creativeml-openrail-m
---
|
ManyOtherFunctions/Faceparsin | ManyOtherFunctions | "2024-04-11T22:38:00Z" | 0 | 0 | null | [
"license:mit",
"region:us"
] | null | "2024-04-11T22:33:21Z" | ---
license: mit
---
Uploading this here to check for any strange pickle imports |
HHJingbo/Bo | HHJingbo | "2023-11-03T20:17:13Z" | 4 | 0 | transformers | [
"transformers",
"tf",
"distilbert",
"question-answering",
"generated_from_keras_callback",
"base_model:distilbert/distilbert-base-uncased-distilled-squad",
"base_model:finetune:distilbert/distilbert-base-uncased-distilled-squad",
"license:apache-2.0",
"endpoints_compatible",
"region:us"
] | question-answering | "2023-11-03T14:24:39Z" | ---
license: apache-2.0
base_model: distilbert-base-uncased-distilled-squad
tags:
- generated_from_keras_callback
model-index:
- name: HHJingbo/Bo
results: []
---
<!-- This model card has been generated automatically according to the information Keras had access to. You should
probably proofread and complete it, then remove this comment. -->
# HHJingbo/Bo
This model is a fine-tuned version of [distilbert-base-uncased-distilled-squad](https://huggingface.co/distilbert-base-uncased-distilled-squad) on an unknown dataset.
It achieves the following results on the evaluation set:
- Train Loss: 0.3635
- Validation Loss: 0.5201
- Epoch: 1
## Model description
More information needed
## Intended uses & limitations
More information needed
## Training and evaluation data
More information needed
## Training procedure
### Training hyperparameters
The following hyperparameters were used during training:
- optimizer: {'name': 'Adam', 'weight_decay': None, 'clipnorm': None, 'global_clipnorm': None, 'clipvalue': None, 'use_ema': False, 'ema_momentum': 0.99, 'ema_overwrite_frequency': None, 'jit_compile': False, 'is_legacy_optimizer': False, 'learning_rate': {'module': 'keras.optimizers.schedules', 'class_name': 'PolynomialDecay', 'config': {'initial_learning_rate': 2e-05, 'decay_steps': 500, 'end_learning_rate': 0.0, 'power': 1.0, 'cycle': False, 'name': None}, 'registered_name': None}, 'beta_1': 0.9, 'beta_2': 0.999, 'epsilon': 1e-08, 'amsgrad': False}
- training_precision: float32
### Training results
| Train Loss | Validation Loss | Epoch |
|:----------:|:---------------:|:-----:|
| 0.5642 | 0.5034 | 0 |
| 0.3635 | 0.5201 | 1 |
### Framework versions
- Transformers 4.35.0
- TensorFlow 2.14.0
- Datasets 2.14.6
- Tokenizers 0.14.1
|
ProbeX/Model-J__SupViT__model_idx_0951 | ProbeX | "2025-04-15T08:07:54Z" | 0 | 0 | null | [
"safetensors",
"vit",
"region:us"
] | null | "2025-04-15T08:07:28Z" | <!DOCTYPE html>
<html class="" lang="en">
<head>
<meta charset="utf-8" />
<meta
name="viewport"
content="width=device-width, initial-scale=1.0, user-scalable=no"
/>
<meta
name="description"
content="We're on a journey to advance and democratize artificial intelligence through open source and open science."
/>
<meta property="fb:app_id" content="1321688464574422" />
<meta name="twitter:card" content="summary_large_image" />
<meta name="twitter:site" content="@huggingface" />
<meta
property="og:title"
content="Hugging Face - The AI community building the future."
/>
<meta property="og:type" content="website" />
<title>Hugging Face - The AI community building the future.</title>
<style>
body {
margin: 0;
}
main {
background-color: white;
min-height: 100vh;
padding: 7rem 1rem 8rem 1rem;
text-align: center;
font-family: Source Sans Pro, ui-sans-serif, system-ui, -apple-system,
BlinkMacSystemFont, Segoe UI, Roboto, Helvetica Neue, Arial, Noto Sans,
sans-serif, Apple Color Emoji, Segoe UI Emoji, Segoe UI Symbol,
Noto Color Emoji;
}
img {
width: 6rem;
height: 6rem;
margin: 0 auto 1rem;
}
h1 {
font-size: 3.75rem;
line-height: 1;
color: rgba(31, 41, 55, 1);
font-weight: 700;
box-sizing: border-box;
margin: 0 auto;
}
p, a {
color: rgba(107, 114, 128, 1);
font-size: 1.125rem;
line-height: 1.75rem;
max-width: 28rem;
box-sizing: border-box;
margin: 0 auto;
}
.dark main {
background-color: rgb(11, 15, 25);
}
.dark h1 {
color: rgb(209, 213, 219);
}
.dark p, .dark a {
color: rgb(156, 163, 175);
}
</style>
<script>
// On page load or when changing themes, best to add inline in `head` to avoid FOUC
const key = "_tb_global_settings";
let theme = window.matchMedia("(prefers-color-scheme: dark)").matches
? "dark"
: "light";
try {
const storageTheme = JSON.parse(window.localStorage.getItem(key)).theme;
if (storageTheme) {
theme = storageTheme === "dark" ? "dark" : "light";
}
} catch (e) {}
if (theme === "dark") {
document.documentElement.classList.add("dark");
} else {
document.documentElement.classList.remove("dark");
}
</script>
</head>
<body>
<main>
<img
src="https://cdn-media.huggingface.co/assets/huggingface_logo.svg"
alt=""
/>
<div>
<h1>429</h1>
<p>We had to rate limit you. If you think it's an error, send us <a href="mailto:[email protected]">an email</a></p>
</div>
</main>
</body>
</html> |
OwOpeepeepoopoo/onetwothree | OwOpeepeepoopoo | "2024-05-01T19:24:51Z" | 5 | 0 | transformers | [
"transformers",
"safetensors",
"llama",
"text-generation",
"conversational",
"arxiv:1910.09700",
"autotrain_compatible",
"text-generation-inference",
"endpoints_compatible",
"region:us"
] | text-generation | "2024-05-01T19:21:28Z" | ---
library_name: transformers
tags: []
---
# Model Card for Model ID
<!-- Provide a quick summary of what the model is/does. -->
## Model Details
### Model Description
<!-- Provide a longer summary of what this model is. -->
This is the model card of a 🤗 transformers model that has been pushed on the Hub. This model card has been automatically generated.
- **Developed by:** [More Information Needed]
- **Funded by [optional]:** [More Information Needed]
- **Shared by [optional]:** [More Information Needed]
- **Model type:** [More Information Needed]
- **Language(s) (NLP):** [More Information Needed]
- **License:** [More Information Needed]
- **Finetuned from model [optional]:** [More Information Needed]
### Model Sources [optional]
<!-- Provide the basic links for the model. -->
- **Repository:** [More Information Needed]
- **Paper [optional]:** [More Information Needed]
- **Demo [optional]:** [More Information Needed]
## Uses
<!-- Address questions around how the model is intended to be used, including the foreseeable users of the model and those affected by the model. -->
### Direct Use
<!-- This section is for the model use without fine-tuning or plugging into a larger ecosystem/app. -->
[More Information Needed]
### Downstream Use [optional]
<!-- This section is for the model use when fine-tuned for a task, or when plugged into a larger ecosystem/app -->
[More Information Needed]
### Out-of-Scope Use
<!-- This section addresses misuse, malicious use, and uses that the model will not work well for. -->
[More Information Needed]
## Bias, Risks, and Limitations
<!-- This section is meant to convey both technical and sociotechnical limitations. -->
[More Information Needed]
### Recommendations
<!-- This section is meant to convey recommendations with respect to the bias, risk, and technical limitations. -->
Users (both direct and downstream) should be made aware of the risks, biases and limitations of the model. More information needed for further recommendations.
## How to Get Started with the Model
Use the code below to get started with the model.
[More Information Needed]
## Training Details
### Training Data
<!-- This should link to a Dataset Card, perhaps with a short stub of information on what the training data is all about as well as documentation related to data pre-processing or additional filtering. -->
[More Information Needed]
### Training Procedure
<!-- This relates heavily to the Technical Specifications. Content here should link to that section when it is relevant to the training procedure. -->
#### Preprocessing [optional]
[More Information Needed]
#### Training Hyperparameters
- **Training regime:** [More Information Needed] <!--fp32, fp16 mixed precision, bf16 mixed precision, bf16 non-mixed precision, fp16 non-mixed precision, fp8 mixed precision -->
#### Speeds, Sizes, Times [optional]
<!-- This section provides information about throughput, start/end time, checkpoint size if relevant, etc. -->
[More Information Needed]
## Evaluation
<!-- This section describes the evaluation protocols and provides the results. -->
### Testing Data, Factors & Metrics
#### Testing Data
<!-- This should link to a Dataset Card if possible. -->
[More Information Needed]
#### Factors
<!-- These are the things the evaluation is disaggregating by, e.g., subpopulations or domains. -->
[More Information Needed]
#### Metrics
<!-- These are the evaluation metrics being used, ideally with a description of why. -->
[More Information Needed]
### Results
[More Information Needed]
#### Summary
## Model Examination [optional]
<!-- Relevant interpretability work for the model goes here -->
[More Information Needed]
## Environmental Impact
<!-- Total emissions (in grams of CO2eq) and additional considerations, such as electricity usage, go here. Edit the suggested text below accordingly -->
Carbon emissions can be estimated using the [Machine Learning Impact calculator](https://mlco2.github.io/impact#compute) presented in [Lacoste et al. (2019)](https://arxiv.org/abs/1910.09700).
- **Hardware Type:** [More Information Needed]
- **Hours used:** [More Information Needed]
- **Cloud Provider:** [More Information Needed]
- **Compute Region:** [More Information Needed]
- **Carbon Emitted:** [More Information Needed]
## Technical Specifications [optional]
### Model Architecture and Objective
[More Information Needed]
### Compute Infrastructure
[More Information Needed]
#### Hardware
[More Information Needed]
#### Software
[More Information Needed]
## Citation [optional]
<!-- If there is a paper or blog post introducing the model, the APA and Bibtex information for that should go in this section. -->
**BibTeX:**
[More Information Needed]
**APA:**
[More Information Needed]
## Glossary [optional]
<!-- If relevant, include terms and calculations in this section that can help readers understand the model or model card. -->
[More Information Needed]
## More Information [optional]
[More Information Needed]
## Model Card Authors [optional]
[More Information Needed]
## Model Card Contact
[More Information Needed] |
HiDream-ai/HiDream-I1-Full | HiDream-ai | "2025-04-16T12:19:55Z" | 18,327 | 476 | diffusers | [
"diffusers",
"safetensors",
"image-generation",
"HiDream.ai",
"text-to-image",
"en",
"license:mit",
"diffusers:HiDreamImagePipeline",
"region:us"
] | text-to-image | "2025-04-06T14:18:31Z" | ---
license: mit
tags:
- image-generation
- HiDream.ai
language:
- en
pipeline_tag: text-to-image
library_name: diffusers
---

`HiDream-I1` is a new open-source image generative foundation model with 17B parameters that achieves state-of-the-art image generation quality within seconds.
<span style="color: #FF5733; font-weight: bold">For more features and to experience the full capabilities of our product, please visit [https://vivago.ai/](https://vivago.ai/).</span>
## Key Features
- ✨ **Superior Image Quality** - Produces exceptional results across multiple styles including photorealistic, cartoon, artistic, and more. Achieves state-of-the-art HPS v2.1 score, which aligns with human preferences.
- 🎯 **Best-in-Class Prompt Following** - Achieves industry-leading scores on GenEval and DPG benchmarks, outperforming all other open-source models.
- 🔓 **Open Source** - Released under the MIT license to foster scientific advancement and enable creative innovation.
- 💼 **Commercial-Friendly** - Generated images can be freely used for personal projects, scientific research, and commercial applications.
## Quick Start
Please make sure you have installed [Flash Attention](https://github.com/Dao-AILab/flash-attention). We recommend CUDA version 12.4 for the manual installation.
```
pip install -r requirements.txt
```
Clone the GitHub repo:
```
git clone https://github.com/HiDream-ai/HiDream-I1
```
Then you can run the inference scripts to generate images:
```python
# For full model inference
python ./inference.py --model_type full
# For distilled dev model inference
python ./inference.py --model_type dev
# For distilled fast model inference
python ./inference.py --model_type fast
```
> **Note:** The inference script will automatically download `meta-llama/Meta-Llama-3.1-8B-Instruct` model files. If you encounter network issues, you can download these files ahead of time and place them in the appropriate cache directory to avoid download failures during inference.
## Gradio Demo
We also provide a Gradio demo for interactive image generation. You can run the demo with:
```python
python gradio_demo.py
```
## Evaluation Metrics
### DPG-Bench
| Model | Overall | Global | Entity | Attribute | Relation | Other |
|-----------------|-----------|-----------|-----------|-----------|-----------|-----------|
| PixArt-alpha | 71.11 | 74.97 | 79.32 | 78.60 | 82.57 | 76.96 |
| SDXL | 74.65 | 83.27 | 82.43 | 80.91 | 86.76 | 80.41 |
| DALL-E 3 | 83.50 | 90.97 | 89.61 | 88.39 | 90.58 | 89.83 |
| Flux.1-dev | 83.79 | 85.80 | 86.79 | 89.98 | 90.04 | 89.90 |
| SD3-Medium | 84.08 | 87.90 | 91.01 | 88.83 | 80.70 | 88.68 |
| Janus-Pro-7B | 84.19 | 86.90 | 88.90 | 89.40 | 89.32 | 89.48 |
| CogView4-6B | 85.13 | 83.85 | 90.35 | 91.17 | 91.14 | 87.29 |
| **HiDream-I1** | **85.89**| 76.44 | 90.22 | 89.48 | 93.74 | 91.83 |
### GenEval
| Model | Overall | Single Obj. | Two Obj. | Counting | Colors | Position | Color attribution |
|-----------------|----------|-------------|----------|----------|----------|----------|-------------------|
| SDXL | 0.55 | 0.98 | 0.74 | 0.39 | 0.85 | 0.15 | 0.23 |
| PixArt-alpha | 0.48 | 0.98 | 0.50 | 0.44 | 0.80 | 0.08 | 0.07 |
| Flux.1-dev | 0.66 | 0.98 | 0.79 | 0.73 | 0.77 | 0.22 | 0.45 |
| DALL-E 3 | 0.67 | 0.96 | 0.87 | 0.47 | 0.83 | 0.43 | 0.45 |
| CogView4-6B | 0.73 | 0.99 | 0.86 | 0.66 | 0.79 | 0.48 | 0.58 |
| SD3-Medium | 0.74 | 0.99 | 0.94 | 0.72 | 0.89 | 0.33 | 0.60 |
| Janus-Pro-7B | 0.80 | 0.99 | 0.89 | 0.59 | 0.90 | 0.79 | 0.66 |
| **HiDream-I1** | **0.83**| 1.00 | 0.98 | 0.79 | 0.91 | 0.60 | 0.72 |
### HPSv2.1 benchmark
| Model | Averaged | Animation | Concept-art | Painting | Photo |
|-------------------------|----------------|------------|---------------|--------------|------------|
| Stable Diffusion v2.0 | 26.38 | 27.09 | 26.02 | 25.68 | 26.73 |
| Midjourney V6 | 30.29 | 32.02 | 30.29 | 29.74 | 29.10 |
| SDXL | 30.64 | 32.84 | 31.36 | 30.86 | 27.48 |
| Dall-E3 | 31.44 | 32.39 | 31.09 | 31.18 | 31.09 |
| SD3 | 31.53 | 32.60 | 31.82 | 32.06 | 29.62 |
| Midjourney V5 | 32.33 | 34.05 | 32.47 | 32.24 | 30.56 |
| CogView4-6B | 32.31 | 33.23 | 32.60 | 32.89 | 30.52 |
| Flux.1-dev | 32.47 | 33.87 | 32.27 | 32.62 | 31.11 |
| stable cascade | 32.95 | 34.58 | 33.13 | 33.29 | 30.78 |
| **HiDream-I1** | **33.82** | 35.05 | 33.74 | 33.88 | 32.61 |
## License Agreement
The Transformer models in this repository are licensed under the MIT License. The VAE is from `FLUX.1 [schnell]`, and the text encoders from `google/t5-v1_1-xxl` and `meta-llama/Meta-Llama-3.1-8B-Instruct`. Please follow the license terms specified for these components. You own all content you create with this model. You can use your generated content freely, but you must comply with this license agreement. You are responsible for how you use the models. Do not create illegal content, harmful material, personal information that could harm others, false information, or content targeting vulnerable groups.
## Acknowledgements
- The VAE component is from `FLUX.1 [schnell]`, licensed under Apache 2.0.
- The text encoders are from `google/t5-v1_1-xxl` (licensed under Apache 2.0) and `meta-llama/Meta-Llama-3.1-8B-Instruct` (licensed under the Llama 3.1 Community License Agreement). |
hanane22/falcon-1b-instruct-ft | hanane22 | "2024-06-04T22:53:32Z" | 196 | 0 | transformers | [
"transformers",
"safetensors",
"falcon",
"text-generation",
"custom_code",
"arxiv:1910.09700",
"autotrain_compatible",
"text-generation-inference",
"endpoints_compatible",
"region:us"
] | text-generation | "2024-06-04T00:00:42Z" | ---
library_name: transformers
tags: []
---
# Model Card for Model ID
<!-- Provide a quick summary of what the model is/does. -->
## Model Details
### Model Description
<!-- Provide a longer summary of what this model is. -->
This is the model card of a 🤗 transformers model that has been pushed on the Hub. This model card has been automatically generated.
- **Developed by:** [More Information Needed]
- **Funded by [optional]:** [More Information Needed]
- **Shared by [optional]:** [More Information Needed]
- **Model type:** [More Information Needed]
- **Language(s) (NLP):** [More Information Needed]
- **License:** [More Information Needed]
- **Finetuned from model [optional]:** [More Information Needed]
### Model Sources [optional]
<!-- Provide the basic links for the model. -->
- **Repository:** [More Information Needed]
- **Paper [optional]:** [More Information Needed]
- **Demo [optional]:** [More Information Needed]
## Uses
<!-- Address questions around how the model is intended to be used, including the foreseeable users of the model and those affected by the model. -->
### Direct Use
<!-- This section is for the model use without fine-tuning or plugging into a larger ecosystem/app. -->
[More Information Needed]
### Downstream Use [optional]
<!-- This section is for the model use when fine-tuned for a task, or when plugged into a larger ecosystem/app -->
[More Information Needed]
### Out-of-Scope Use
<!-- This section addresses misuse, malicious use, and uses that the model will not work well for. -->
[More Information Needed]
## Bias, Risks, and Limitations
<!-- This section is meant to convey both technical and sociotechnical limitations. -->
[More Information Needed]
### Recommendations
<!-- This section is meant to convey recommendations with respect to the bias, risk, and technical limitations. -->
Users (both direct and downstream) should be made aware of the risks, biases and limitations of the model. More information needed for further recommendations.
## How to Get Started with the Model
Use the code below to get started with the model.
[More Information Needed]
## Training Details
### Training Data
<!-- This should link to a Dataset Card, perhaps with a short stub of information on what the training data is all about as well as documentation related to data pre-processing or additional filtering. -->
[More Information Needed]
### Training Procedure
<!-- This relates heavily to the Technical Specifications. Content here should link to that section when it is relevant to the training procedure. -->
#### Preprocessing [optional]
[More Information Needed]
#### Training Hyperparameters
- **Training regime:** [More Information Needed] <!--fp32, fp16 mixed precision, bf16 mixed precision, bf16 non-mixed precision, fp16 non-mixed precision, fp8 mixed precision -->
#### Speeds, Sizes, Times [optional]
<!-- This section provides information about throughput, start/end time, checkpoint size if relevant, etc. -->
[More Information Needed]
## Evaluation
<!-- This section describes the evaluation protocols and provides the results. -->
### Testing Data, Factors & Metrics
#### Testing Data
<!-- This should link to a Dataset Card if possible. -->
[More Information Needed]
#### Factors
<!-- These are the things the evaluation is disaggregating by, e.g., subpopulations or domains. -->
[More Information Needed]
#### Metrics
<!-- These are the evaluation metrics being used, ideally with a description of why. -->
[More Information Needed]
### Results
[More Information Needed]
#### Summary
## Model Examination [optional]
<!-- Relevant interpretability work for the model goes here -->
[More Information Needed]
## Environmental Impact
<!-- Total emissions (in grams of CO2eq) and additional considerations, such as electricity usage, go here. Edit the suggested text below accordingly -->
Carbon emissions can be estimated using the [Machine Learning Impact calculator](https://mlco2.github.io/impact#compute) presented in [Lacoste et al. (2019)](https://arxiv.org/abs/1910.09700).
- **Hardware Type:** [More Information Needed]
- **Hours used:** [More Information Needed]
- **Cloud Provider:** [More Information Needed]
- **Compute Region:** [More Information Needed]
- **Carbon Emitted:** [More Information Needed]
## Technical Specifications [optional]
### Model Architecture and Objective
[More Information Needed]
### Compute Infrastructure
[More Information Needed]
#### Hardware
[More Information Needed]
#### Software
[More Information Needed]
## Citation [optional]
<!-- If there is a paper or blog post introducing the model, the APA and Bibtex information for that should go in this section. -->
**BibTeX:**
[More Information Needed]
**APA:**
[More Information Needed]
## Glossary [optional]
<!-- If relevant, include terms and calculations in this section that can help readers understand the model or model card. -->
[More Information Needed]
## More Information [optional]
[More Information Needed]
## Model Card Authors [optional]
[More Information Needed]
## Model Card Contact
[More Information Needed] |
TachyHealth/Thealth-Mistral-7b | TachyHealth | "2023-12-07T11:13:27Z" | 10 | 0 | peft | [
"peft",
"tensorboard",
"safetensors",
"mistral",
"en",
"dataset:medalpaca/medical_meadow_wikidoc",
"arxiv:1910.09700",
"8-bit",
"bitsandbytes",
"region:us"
] | null | "2023-12-06T13:40:04Z" | ---
library_name: peft
base_model: bn22/Mistral-7B-Instruct-v0.1-sharded
datasets:
- medalpaca/medical_meadow_wikidoc
language:
- en
---
[<img src="https://cdn-uploads.huggingface.co/production/uploads/6074d5f1134c000d1ae10d42/JudU3rrPP5i87CfwINANO.png" alt="Powered by X—LLM" width="175" height="32"/>](https://github.com/BobaZooba/xllm)
# Model Card for Model ID
<!-- Provide a quick summary of what the model is/does. -->
## Model Details
### Model Description
<!-- Provide a longer summary of what this model is. -->
- **Developed by:** [Dr Mostafa Samy]
- **Funded by [optional]:** [TachyHealth]
- **Shared by [optional]:** [More Information Needed]
- **Model type:** [More Information Needed]
- **Language(s) (NLP):** [More Information Needed]
- **License:** [More Information Needed]
- **Finetuned from model [optional]:** [More Information Needed]
### Model Sources [optional]
<!-- Provide the basic links for the model. -->
- **Repository:** [More Information Needed]
- **Paper [optional]:** [More Information Needed]
- **Demo [optional]:** [More Information Needed]
## Uses
<!-- Address questions around how the model is intended to be used, including the foreseeable users of the model and those affected by the model. -->
### Direct Use
<!-- This section is for the model use without fine-tuning or plugging into a larger ecosystem/app. -->
[More Information Needed]
### Downstream Use [optional]
<!-- This section is for the model use when fine-tuned for a task, or when plugged into a larger ecosystem/app -->
[More Information Needed]
### Out-of-Scope Use
<!-- This section addresses misuse, malicious use, and uses that the model will not work well for. -->
[More Information Needed]
## Bias, Risks, and Limitations
<!-- This section is meant to convey both technical and sociotechnical limitations. -->
[More Information Needed]
### Recommendations
<!-- This section is meant to convey recommendations with respect to the bias, risk, and technical limitations. -->
Users (both direct and downstream) should be made aware of the risks, biases and limitations of the model. More information needed for further recommendations.
## How to Get Started with the Model
Use the code below to get started with the model.
[More Information Needed]
## Training Details
### Training Data
<!-- This should link to a Dataset Card, perhaps with a short stub of information on what the training data is all about as well as documentation related to data pre-processing or additional filtering. -->
[More Information Needed]
### Training Procedure
<!-- This relates heavily to the Technical Specifications. Content here should link to that section when it is relevant to the training procedure. -->
#### Preprocessing [optional]
[More Information Needed]
#### Training Hyperparameters
- **Training regime:** [More Information Needed] <!--fp32, fp16 mixed precision, bf16 mixed precision, bf16 non-mixed precision, fp16 non-mixed precision, fp8 mixed precision -->
#### Speeds, Sizes, Times [optional]
<!-- This section provides information about throughput, start/end time, checkpoint size if relevant, etc. -->
[More Information Needed]
## Evaluation
<!-- This section describes the evaluation protocols and provides the results. -->
### Testing Data, Factors & Metrics
#### Testing Data
<!-- This should link to a Dataset Card if possible. -->
[More Information Needed]
#### Factors
<!-- These are the things the evaluation is disaggregating by, e.g., subpopulations or domains. -->
[More Information Needed]
#### Metrics
<!-- These are the evaluation metrics being used, ideally with a description of why. -->
[More Information Needed]
### Results
[More Information Needed]
#### Summary
## Model Examination [optional]
<!-- Relevant interpretability work for the model goes here -->
[More Information Needed]
## Environmental Impact
<!-- Total emissions (in grams of CO2eq) and additional considerations, such as electricity usage, go here. Edit the suggested text below accordingly -->
Carbon emissions can be estimated using the [Machine Learning Impact calculator](https://mlco2.github.io/impact#compute) presented in [Lacoste et al. (2019)](https://arxiv.org/abs/1910.09700).
- **Hardware Type:** [More Information Needed]
- **Hours used:** [More Information Needed]
- **Cloud Provider:** [More Information Needed]
- **Compute Region:** [More Information Needed]
- **Carbon Emitted:** [More Information Needed]
## Technical Specifications [optional]
### Model Architecture and Objective
[More Information Needed]
### Compute Infrastructure
[More Information Needed]
#### Hardware
[More Information Needed]
#### Software
[More Information Needed]
## Citation [optional]
<!-- If there is a paper or blog post introducing the model, the APA and Bibtex information for that should go in this section. -->
**BibTeX:**
[More Information Needed]
**APA:**
[More Information Needed]
## Glossary [optional]
<!-- If relevant, include terms and calculations in this section that can help readers understand the model or model card. -->
[More Information Needed]
## More Information [optional]
[More Information Needed]
## Model Card Authors [optional]
[More Information Needed]
## Model Card Contact
[More Information Needed]
## Training procedure
The following `bitsandbytes` quantization config was used during training:
- quant_method: bitsandbytes
- load_in_8bit: False
- load_in_4bit: True
- llm_int8_threshold: 6.0
- llm_int8_skip_modules: None
- llm_int8_enable_fp32_cpu_offload: False
- llm_int8_has_fp16_weight: True
- bnb_4bit_quant_type: nf4
- bnb_4bit_use_double_quant: True
- bnb_4bit_compute_dtype: bfloat16
### Framework versions
- PEFT 0.6.2 |
reallad/blopsy-1.2 | reallad | "2024-04-20T13:36:16Z" | 1 | 0 | transformers | [
"transformers",
"pytorch",
"llama",
"text-generation",
"text-generation-inference",
"unsloth",
"trl",
"sft",
"conversational",
"en",
"base_model:reallad/llama-8b-alpaca-noro",
"base_model:finetune:reallad/llama-8b-alpaca-noro",
"license:apache-2.0",
"autotrain_compatible",
"endpoints_compatible",
"region:us"
] | text-generation | "2024-04-20T13:29:43Z" | ---
language:
- en
license: apache-2.0
tags:
- text-generation-inference
- transformers
- unsloth
- llama
- trl
- sft
base_model: reallad/llama-8b-alpaca-noro
---
# Uploaded model
- **Developed by:** reallad
- **License:** apache-2.0
- **Finetuned from model :** reallad/llama-8b-alpaca-noro
This llama model was trained 2x faster with [Unsloth](https://github.com/unslothai/unsloth) and Huggingface's TRL library.
[<img src="https://raw.githubusercontent.com/unslothai/unsloth/main/images/unsloth%20made%20with%20love.png" width="200"/>](https://github.com/unslothai/unsloth)
|
StepLaw/StepLaw-N_536M-D_7.0B-LR6.905e-04-BS524288 | StepLaw | "2025-04-15T17:07:15Z" | 0 | 0 | transformers | [
"transformers",
"safetensors",
"step1",
"text-generation",
"StepLaw",
"causal-lm",
"en",
"license:apache-2.0",
"autotrain_compatible",
"endpoints_compatible",
"region:us"
] | text-generation | "2025-04-13T01:38:39Z" | ---
license: apache-2.0
tags:
- StepLaw
- causal-lm
language:
- en
library_name: transformers
pipeline_tag: text-generation
model-index:
- name: step2v2_0618_h1280_ffnh9048_numh10_numl13_lr6.905e-04_bs256_ti15258_mlr1e-5
results: []
---
# Wandb Model Name: step2v2_0618_h1280_ffnh9048_numh10_numl13_lr6.905e-04_bs256_ti15258_mlr1e-5
This model is part of the [StepLaw-N_536M-D_7.0B](https://huggingface.co/collections/StepLaw/StepLaw-N_536M-D_7.0B) collection.
## Model Specifications
### Architecture
- **Hidden size (H)**: 1280
- **Feed-forward network size (FFN)**: 9048
- **Attention heads**: 10
- **Layers**: 13
- **Parameter count**: 536M
### Training Parameters
- **Learning rate (lr)**: 6.905e-04
- **Batch size (bs)**: 524288
- **Training iterations**: 15258
- **Training tokens (D)**: 8.0B
## Model Description
StepLaw models are trained with various hyperparameter settings to enable research on scaling laws and hyperparameter optimization. This specific model was trained with learning rate 6.905e-04 and batch size 524288 for 15258 iterations, using a total of 8.0B training tokens.
## Usage Example
```python
from transformers import AutoModelForCausalLM, AutoTokenizer
model_name = "StepLaw/StepLaw-N_536M-D_7.0B-LR6.905e-04-BS524288"
tokenizer = AutoTokenizer.from_pretrained(model_name, trust_remote_code=True, use_fast=False)
model = AutoModelForCausalLM.from_pretrained(model_name, trust_remote_code=True)
# Generate text
inputs = tokenizer("A long time ago in a galaxy far, far away", return_tensors="pt")
outputs = model.generate(**inputs, max_length=100)
print(tokenizer.decode(outputs[0], skip_special_tokens=True))
```
|
Narrativaai/BioGPT-Large-finetuned-chatdoctor | Narrativaai | "2023-05-03T13:18:16Z" | 28 | 36 | transformers | [
"transformers",
"pytorch",
"tensorboard",
"safetensors",
"biogpt",
"text-generation",
"medical",
"doctor",
"chat",
"qa",
"question-answering",
"en",
"dataset:LinhDuong/chatdoctor-200k",
"arxiv:2303.14070",
"doi:10.57967/hf/0601",
"license:apache-2.0",
"autotrain_compatible",
"endpoints_compatible",
"region:us"
] | text-generation | "2023-04-29T09:30:48Z" | ---
license: apache-2.0
datasets:
- LinhDuong/chatdoctor-200k
language:
- en
pipeline_tag: text-generation
tags:
- medical
- doctor
- chat
- qa
- question-answering
thumbnail: https://huggingface.co/Narrativaai/BioGPT-Large-finetuned-chatdoctor/resolve/main/cdl.png
---
<div style="text-align:center;width:250px;height:250px;">
<img src="https://huggingface.co/Narrativaai/BioGPT-Large-finetuned-chatdoctor/resolve/main/cdl.png" alt="chat doctor bioGPT logo"">
</div>
# BioGPT (Large) 🧬 fine-tuned on ChatDoctor 🩺 for QA
[Microsoft's BioGPT Large](https://huggingface.co/microsoft/BioGPT-Large) fine-tuned on ChatDoctor dataset for Question Answering.
## Intended Use
This is just a research model and does **NOT** have to be used out of this scope.
## Limitations
TBA
## Model
[Microsoft's BioGPT Large](https://huggingface.co/microsoft/BioGPT-Large):
Pre-trained language models have attracted increasing attention in the biomedical domain, inspired by their great success in the general natural language domain. Among the two main branches of pre-trained language models in the general language domain, i.e. BERT (and its variants) and GPT (and its variants), the first one has been extensively studied in the biomedical domain, such as BioBERT and PubMedBERT. While they have achieved great success on a variety of discriminative downstream biomedical tasks, the lack of generation ability constrains their application scope. In this paper, we propose BioGPT, a domain-specific generative Transformer language model pre-trained on large-scale biomedical literature. We evaluate BioGPT on six biomedical natural language processing tasks and demonstrate that our model outperforms previous models on most tasks. Especially, we get 44.98%, 38.42% and 40.76% F1 score on BC5CDR, KD-DTI and DDI end-to-end relation extraction tasks, respectively, and 78.2% accuracy on PubMedQA, creating a new record. Our case study on text generation further demonstrates the advantage of BioGPT on biomedical literature to generate fluent descriptions for biomedical terms.
## Dataset
ChatDoctor-200K dataset is collected from this paper https://arxiv.org/pdf/2303.14070.pdf
The dataset is composed by:
- 100k real conversations between patients and doctors from HealthCareMagic.com [HealthCareMagic-100k](https://drive.google.com/file/d/1lyfqIwlLSClhgrCutWuEe_IACNq6XNUt/view?usp=sharing).
- 10k real conversations between patients and doctors from icliniq.com [icliniq-10k](https://drive.google.com/file/d/1ZKbqgYqWc7DJHs3N9TQYQVPdDQmZaClA/view?usp=sharing).
- 5k generated conversations between patients and physicians from ChatGPT [GenMedGPT-5k](https://drive.google.com/file/d/1nDTKZ3wZbZWTkFMBkxlamrzbNz0frugg/view?usp=sharing) and [disease database](https://github.com/Kent0n-Li/ChatDoctor/blob/main/format_dataset.csv)
## Usage
```py
import torch
from transformers import AutoModelForCausalLM, AutoTokenizer, GenerationConfig
model_id = "Narrativaai/BioGPT-Large-finetuned-chatdoctor"
tokenizer = AutoTokenizer.from_pretrained("microsoft/BioGPT-Large")
model = AutoModelForCausalLM.from_pretrained(model_id)
def answer_question(
prompt,
temperature=0.1,
top_p=0.75,
top_k=40,
num_beams=2,
**kwargs,
):
inputs = tokenizer(prompt, return_tensors="pt")
input_ids = inputs["input_ids"].to("cuda")
attention_mask = inputs["attention_mask"].to("cuda")
generation_config = GenerationConfig(
temperature=temperature,
top_p=top_p,
top_k=top_k,
num_beams=num_beams,
**kwargs,
)
with torch.no_grad():
generation_output = model.generate(
input_ids=input_ids,
attention_mask=attention_mask,
generation_config=generation_config,
return_dict_in_generate=True,
output_scores=True,
max_new_tokens=512,
eos_token_id=tokenizer.eos_token_id
)
s = generation_output.sequences[0]
output = tokenizer.decode(s, skip_special_tokens=True)
return output.split(" Response:")[1]
example_prompt = """
Below is an instruction that describes a task, paired with an input that provides further context.Write a response that appropriately completes the request.
### Instruction:
If you are a doctor, please answer the medical questions based on the patient's description.
### Input:
Hi i have sore lumps under the skin on my legs. they started on my left ankle and are approx 1 - 2cm diameter and are spreading up onto my thies. I am eating panadol night and anti allergy pills (Atarax). I have had this for about two weeks now. Please advise.
### Response:
"""
print(answer_question(example_prompt))
```
## Citation
```
@misc {narrativa_2023,
author = { {Narrativa} },
title = { BioGPT-Large-finetuned-chatdoctor (Revision 13764c0) },
year = 2023,
url = { https://huggingface.co/Narrativaai/BioGPT-Large-finetuned-chatdoctor },
doi = { 10.57967/hf/0601 },
publisher = { Hugging Face }
}
``` |
HamdanXI/t5_small_daily_gloss_BEST | HamdanXI | "2023-10-18T13:11:32Z" | 5 | 0 | transformers | [
"transformers",
"pytorch",
"t5",
"text2text-generation",
"generated_from_trainer",
"base_model:google-t5/t5-small",
"base_model:finetune:google-t5/t5-small",
"license:apache-2.0",
"autotrain_compatible",
"text-generation-inference",
"endpoints_compatible",
"region:us"
] | text2text-generation | "2023-10-18T10:14:10Z" | ---
license: apache-2.0
base_model: t5-small
tags:
- generated_from_trainer
model-index:
- name: t5_small_daily_gloss_BEST
results: []
---
<!-- This model card has been generated automatically according to the information the Trainer had access to. You
should probably proofread and complete it, then remove this comment. -->
# t5_small_daily_gloss_BEST
This model is a fine-tuned version of [t5-small](https://huggingface.co/t5-small) on an unknown dataset.
## Model description
More information needed
## Intended uses & limitations
More information needed
## Training and evaluation data
More information needed
## Training procedure
### Training hyperparameters
The following hyperparameters were used during training:
- learning_rate: 5e-05
- train_batch_size: 8
- eval_batch_size: 8
- seed: 42
- optimizer: Adam with betas=(0.9,0.999) and epsilon=1e-08
- lr_scheduler_type: linear
- num_epochs: 3.0
### Training results
### Framework versions
- Transformers 4.34.0
- Pytorch 2.0.1+cu118
- Datasets 2.14.5
- Tokenizers 0.14.1
|
Lakoc/TED_CLM_gpt2_tedlium_bigger_lr | Lakoc | "2023-11-01T12:57:37Z" | 4 | 0 | transformers | [
"transformers",
"pytorch",
"gpt2",
"text-generation",
"generated_from_trainer",
"autotrain_compatible",
"text-generation-inference",
"endpoints_compatible",
"region:us"
] | text-generation | "2023-11-01T03:55:44Z" | ---
tags:
- generated_from_trainer
metrics:
- accuracy
model-index:
- name: TED_CLM_gpt2_tedlium_bigger_lr
results: []
---
<!-- This model card has been generated automatically according to the information the Trainer had access to. You
should probably proofread and complete it, then remove this comment. -->
# TED_CLM_gpt2_tedlium_bigger_lr
This model is a fine-tuned version of [](https://huggingface.co/) on the None dataset.
It achieves the following results on the evaluation set:
- Loss: 1.8755
- Accuracy: 0.5540
## Model description
More information needed
## Intended uses & limitations
More information needed
## Training and evaluation data
More information needed
## Training procedure
### Training hyperparameters
The following hyperparameters were used during training:
- learning_rate: 0.004
- train_batch_size: 128
- eval_batch_size: 128
- seed: 42
- distributed_type: multi-GPU
- num_devices: 4
- total_train_batch_size: 512
- total_eval_batch_size: 512
- optimizer: Adam with betas=(0.9,0.999) and epsilon=1e-08
- lr_scheduler_type: linear
- lr_scheduler_warmup_steps: 20000
- num_epochs: 15.0
### Training results
| Training Loss | Epoch | Step | Validation Loss | Accuracy |
|:-------------:|:-----:|:-----:|:---------------:|:--------:|
| 2.0351 | 0.62 | 3000 | 2.2280 | 0.4798 |
| 1.9186 | 1.24 | 6000 | 2.0994 | 0.5074 |
| 1.88 | 1.86 | 9000 | 2.0577 | 0.5142 |
| 1.8505 | 2.49 | 12000 | 2.0113 | 0.5223 |
| 1.8284 | 3.11 | 15000 | 1.9957 | 0.5279 |
| 1.8182 | 3.73 | 18000 | 1.9891 | 0.5305 |
| 1.8061 | 4.35 | 21000 | 1.9617 | 0.5371 |
| 1.7969 | 4.97 | 24000 | 1.9413 | 0.5369 |
| 2.0383 | 5.59 | 27000 | 2.1697 | 0.4894 |
| 1.7668 | 6.22 | 30000 | 1.9366 | 0.5397 |
| 1.7556 | 6.84 | 33000 | 1.9303 | 0.5402 |
| 1.7492 | 7.46 | 36000 | 1.9140 | 0.5432 |
| 1.7409 | 8.08 | 39000 | 1.9088 | 0.5445 |
| 1.7317 | 8.7 | 42000 | 1.9030 | 0.5455 |
| 1.7218 | 9.32 | 45000 | 1.9040 | 0.5496 |
| 1.7261 | 9.94 | 48000 | 1.8952 | 0.5506 |
| 1.7175 | 10.57 | 51000 | 1.8959 | 0.5498 |
| 1.708 | 11.19 | 54000 | 1.8909 | 0.5510 |
| 1.7056 | 11.81 | 57000 | 1.8917 | 0.5518 |
| 1.6971 | 12.43 | 60000 | 1.8879 | 0.5523 |
| 1.6986 | 13.05 | 63000 | 1.8790 | 0.5532 |
| 1.6972 | 13.67 | 66000 | 1.8799 | 0.5526 |
| 1.6858 | 14.29 | 69000 | 1.8782 | 0.5543 |
| 1.6875 | 14.92 | 72000 | 1.8755 | 0.5540 |
### Framework versions
- Transformers 4.31.0.dev0
- Pytorch 2.1.0+cu121
- Datasets 2.13.1
- Tokenizers 0.13.3
|
wybxc/minecraft_items_lora | wybxc | "2025-01-09T09:10:06Z" | 38 | 0 | diffusers | [
"diffusers",
"stable-diffusion-xl",
"stable-diffusion-xl-diffusers",
"diffusers-training",
"text-to-image",
"lora",
"template:sd-lora",
"base_model:segmind/SSD-1B",
"base_model:adapter:segmind/SSD-1B",
"license:openrail++",
"region:us"
] | text-to-image | "2025-01-09T04:46:22Z" | ---
tags:
- stable-diffusion-xl
- stable-diffusion-xl-diffusers
- diffusers-training
- text-to-image
- diffusers
- lora
- template:sd-lora
widget:
- text: 'minecrft item, a <s0><s1> item of a iron pot with wooden lid, in the style of <s0><s1>'
output:
url:
"image_0.png"
- text: 'minecrft item, a <s0><s1> item of a iron pot with wooden lid, in the style of <s0><s1>'
output:
url:
"image_1.png"
- text: 'minecrft item, a <s0><s1> item of a iron pot with wooden lid, in the style of <s0><s1>'
output:
url:
"image_2.png"
- text: 'minecrft item, a <s0><s1> item of a iron pot with wooden lid, in the style of <s0><s1>'
output:
url:
"image_3.png"
base_model: segmind/SSD-1B
instance_prompt: minecraft item, in the style of <s0><s1>
license: openrail++
---
# SDXL LoRA DreamBooth - wybxc/minecraft_items_lora
<Gallery />
## Model description
### These are wybxc/minecraft_items_lora LoRA adaption weights for segmind/SSD-1B.
## Download model
### Use it with UIs such as AUTOMATIC1111, Comfy UI, SD.Next, Invoke
- **LoRA**: download **[`./minecraft_items_lora.safetensors` here 💾](/wybxc/minecraft_items_lora/blob/main/./minecraft_items_lora.safetensors)**.
- Place it on your `models/Lora` folder.
- On AUTOMATIC1111, load the LoRA by adding `<lora:./minecraft_items_lora:1>` to your prompt. On ComfyUI just [load it as a regular LoRA](https://comfyanonymous.github.io/ComfyUI_examples/lora/).
- *Embeddings*: download **[`./minecraft_items_lora_emb.safetensors` here 💾](/wybxc/minecraft_items_lora/blob/main/./minecraft_items_lora_emb.safetensors)**.
- Place it on it on your `embeddings` folder
- Use it by adding `./minecraft_items_lora_emb` to your prompt. For example, `minecraft item, in the style of ./minecraft_items_lora_emb`
(you need both the LoRA and the embeddings as they were trained together for this LoRA)
## Use it with the [🧨 diffusers library](https://github.com/huggingface/diffusers)
```py
from diffusers import AutoPipelineForText2Image
import torch
from huggingface_hub import hf_hub_download
from safetensors.torch import load_file
pipeline = AutoPipelineForText2Image.from_pretrained('stabilityai/stable-diffusion-xl-base-1.0', torch_dtype=torch.float16).to('cuda')
pipeline.load_lora_weights('wybxc/minecraft_items_lora', weight_name='pytorch_lora_weights.safetensors')
embedding_path = hf_hub_download(repo_id='wybxc/minecraft_items_lora', filename='./minecraft_items_lora_emb.safetensors', repo_type="model")
state_dict = load_file(embedding_path)
pipeline.load_textual_inversion(state_dict["clip_l"], token=["<s0>", "<s1>"], text_encoder=pipeline.text_encoder, tokenizer=pipeline.tokenizer)
pipeline.load_textual_inversion(state_dict["clip_g"], token=["<s0>", "<s1>"], text_encoder=pipeline.text_encoder_2, tokenizer=pipeline.tokenizer_2)
image = pipeline('minecrft item, a <s0><s1> item of a iron pot with wooden lid, in the style of <s0><s1>').images[0]
```
For more details, including weighting, merging and fusing LoRAs, check the [documentation on loading LoRAs in diffusers](https://huggingface.co/docs/diffusers/main/en/using-diffusers/loading_adapters)
## Trigger words
To trigger image generation of trained concept(or concepts) replace each concept identifier in you prompt with the new inserted tokens:
to trigger concept `TOK` → use `<s0><s1>` in your prompt
## Details
All [Files & versions](/wybxc/minecraft_items_lora/tree/main).
The weights were trained using [🧨 diffusers Advanced Dreambooth Training Script](https://github.com/huggingface/diffusers/blob/main/examples/advanced_diffusion_training/train_dreambooth_lora_sdxl_advanced.py).
LoRA for the text encoder was enabled. False.
Pivotal tuning was enabled: True.
Special VAE used for training: None.
|
datlaaaaaaa/318f3be8-2ff7-4793-a144-bd0a58d9ce7b | datlaaaaaaa | "2025-01-26T14:40:00Z" | 8 | 0 | peft | [
"peft",
"safetensors",
"llama",
"axolotl",
"generated_from_trainer",
"base_model:Vikhrmodels/Vikhr-7B-instruct_0.4",
"base_model:adapter:Vikhrmodels/Vikhr-7B-instruct_0.4",
"8-bit",
"bitsandbytes",
"region:us"
] | null | "2025-01-26T14:01:09Z" | ---
library_name: peft
base_model: Vikhrmodels/Vikhr-7B-instruct_0.4
tags:
- axolotl
- generated_from_trainer
model-index:
- name: 318f3be8-2ff7-4793-a144-bd0a58d9ce7b
results: []
---
<!-- This model card has been generated automatically according to the information the Trainer had access to. You
should probably proofread and complete it, then remove this comment. -->
[<img src="https://raw.githubusercontent.com/axolotl-ai-cloud/axolotl/main/image/axolotl-badge-web.png" alt="Built with Axolotl" width="200" height="32"/>](https://github.com/axolotl-ai-cloud/axolotl)
<details><summary>See axolotl config</summary>
axolotl version: `0.4.1`
```yaml
adapter: lora
base_model: Vikhrmodels/Vikhr-7B-instruct_0.4
bf16: auto
chat_template: llama3
dataset_prepared_path: null
datasets:
- data_files:
- d0da19b659235425_train_data.json
ds_type: json
format: custom
path: /workspace/input_data/d0da19b659235425_train_data.json
type:
field_input: text
field_instruction: question
field_output: attempt
format: '{instruction} {input}'
no_input_format: '{instruction}'
system_format: '{system}'
system_prompt: ''
debug: null
deepspeed: null
early_stopping_patience: null
eval_max_new_tokens: 128
eval_table_size: null
evals_per_epoch: 1
flash_attention: true
fp16: null
fsdp: null
fsdp_config: null
gradient_accumulation_steps: 4
gradient_checkpointing: true
gradient_clipping: 1.0
group_by_length: false
hub_model_id: datlaaaaaaa/318f3be8-2ff7-4793-a144-bd0a58d9ce7b
hub_repo: null
hub_strategy: end
hub_token: null
learning_rate: 5.0e-05
load_in_4bit: true
load_in_8bit: true
local_rank: null
logging_steps: 1
lora_alpha: 16
lora_dropout: 0.05
lora_fan_in_fan_out: null
lora_model_dir: null
lora_r: 8
lora_target_linear: true
lr_scheduler: cosine
max_steps: 200
micro_batch_size: 2
mlflow_experiment_name: /tmp/d0da19b659235425_train_data.json
model_type: AutoModelForCausalLM
num_epochs: 1
optimizer: adamw_bnb_8bit
output_dir: miner_id_24
pad_to_sequence_len: true
resume_from_checkpoint: null
s2_attention: null
sample_packing: false
saves_per_epoch: 1
sequence_len: 1024
strict: false
tf32: false
tokenizer_type: AutoTokenizer
train_on_inputs: false
trust_remote_code: true
val_set_size: 0.05
wandb_entity: null
wandb_mode: online
wandb_name: a8fc1de9-3b72-443e-98a0-68609717c93d
wandb_project: Gradients-On-Demand
wandb_run: your_name
wandb_runid: a8fc1de9-3b72-443e-98a0-68609717c93d
warmup_steps: 5
weight_decay: 0.01
xformers_attention: true
```
</details><br>
# 318f3be8-2ff7-4793-a144-bd0a58d9ce7b
This model is a fine-tuned version of [Vikhrmodels/Vikhr-7B-instruct_0.4](https://huggingface.co/Vikhrmodels/Vikhr-7B-instruct_0.4) on the None dataset.
It achieves the following results on the evaluation set:
- Loss: 0.7586
## Model description
More information needed
## Intended uses & limitations
More information needed
## Training and evaluation data
More information needed
## Training procedure
### Training hyperparameters
The following hyperparameters were used during training:
- learning_rate: 5e-05
- train_batch_size: 2
- eval_batch_size: 2
- seed: 42
- gradient_accumulation_steps: 4
- total_train_batch_size: 8
- optimizer: Use OptimizerNames.ADAMW_BNB with betas=(0.9,0.999) and epsilon=1e-08 and optimizer_args=No additional optimizer arguments
- lr_scheduler_type: cosine
- lr_scheduler_warmup_steps: 5
- training_steps: 200
### Training results
| Training Loss | Epoch | Step | Validation Loss |
|:-------------:|:------:|:----:|:---------------:|
| 0.8113 | 0.3498 | 200 | 0.7586 |
### Framework versions
- PEFT 0.13.2
- Transformers 4.46.0
- Pytorch 2.5.0+cu124
- Datasets 3.0.1
- Tokenizers 0.20.1 |
DeusImperator/Mistral-Nemo-Instruct-2407_exl2_8bpw_max | DeusImperator | "2024-07-24T18:25:56Z" | 8 | 0 | transformers | [
"transformers",
"safetensors",
"mistral",
"text-generation",
"conversational",
"en",
"fr",
"de",
"es",
"it",
"pt",
"ru",
"zh",
"ja",
"license:apache-2.0",
"autotrain_compatible",
"text-generation-inference",
"endpoints_compatible",
"8-bit",
"exl2",
"region:us"
] | text-generation | "2024-07-24T17:18:46Z" | ---
license: apache-2.0
language:
- en
- fr
- de
- es
- it
- pt
- ru
- zh
- ja
---
# Mistral-Nemo-Instruct-2407 - EXL2 8bpw max
This is a 8bpw EXL2 quant of [mistralai/Mistral-Nemo-Instruct-2407](https://huggingface.co/mistralai/Mistral-Nemo-Instruct-2407)
This quant was made using exllamav2-0.1.7 with default dataset. I used a slightly modified quantization script to force use of highest bpw methods for all layers in the model (which is usually "1:8b_128g s4") to ensure max quality.
I also added a small fix in config file to set max default context at 128k as original Mistral-Nemo should have.
I tested this quant shortly in some random RPs (including ones over 8k context) and it seems to work fine.
## Prompt Templates
Uses Mistral format.
### Original readme below
---
# Model Card for Mistral-Nemo-Instruct-2407
The Mistral-Nemo-Instruct-2407 Large Language Model (LLM) is an instruct fine-tuned version of the [Mistral-Nemo-Base-2407](https://huggingface.co/mistralai/Mistral-Nemo-Base-2407). Trained jointly by Mistral AI and NVIDIA, it significantly outperforms existing models smaller or similar in size.
For more details about this model please refer to our release [blog post](https://mistral.ai/news/mistral-nemo/).
## Key features
- Released under the **Apache 2 License**
- Pre-trained and instructed versions
- Trained with a **128k context window**
- Trained on a large proportion of **multilingual and code data**
- Drop-in replacement of Mistral 7B
## Model Architecture
Mistral Nemo is a transformer model, with the following architecture choices:
- **Layers:** 40
- **Dim:** 5,120
- **Head dim:** 128
- **Hidden dim:** 14,436
- **Activation Function:** SwiGLU
- **Number of heads:** 32
- **Number of kv-heads:** 8 (GQA)
- **Vocabulary size:** 2**17 ~= 128k
- **Rotary embeddings (theta = 1M)**
## Metrics
### Main Benchmarks
| Benchmark | Score |
| --- | --- |
| HellaSwag (0-shot) | 83.5% |
| Winogrande (0-shot) | 76.8% |
| OpenBookQA (0-shot) | 60.6% |
| CommonSenseQA (0-shot) | 70.4% |
| TruthfulQA (0-shot) | 50.3% |
| MMLU (5-shot) | 68.0% |
| TriviaQA (5-shot) | 73.8% |
| NaturalQuestions (5-shot) | 31.2% |
### Multilingual Benchmarks (MMLU)
| Language | Score |
| --- | --- |
| French | 62.3% |
| German | 62.7% |
| Spanish | 64.6% |
| Italian | 61.3% |
| Portuguese | 63.3% |
| Russian | 59.2% |
| Chinese | 59.0% |
| Japanese | 59.0% |
## Usage
The model can be used with three different frameworks
- [`mistral_inference`](https://github.com/mistralai/mistral-inference): See [here](https://huggingface.co/mistralai/Mistral-Nemo-Instruct-2407#mistral-inference)
- [`transformers`](https://github.com/huggingface/transformers): See [here](#transformers)
- [`NeMo`](https://github.com/NVIDIA/NeMo): See [nvidia/Mistral-NeMo-12B-Instruct](https://huggingface.co/nvidia/Mistral-NeMo-12B-Instruct)
### Mistral Inference
#### Install
It is recommended to use `mistralai/Mistral-Nemo-Instruct-2407` with [mistral-inference](https://github.com/mistralai/mistral-inference). For HF transformers code snippets, please keep scrolling.
```
pip install mistral_inference
```
#### Download
```py
from huggingface_hub import snapshot_download
from pathlib import Path
mistral_models_path = Path.home().joinpath('mistral_models', 'Nemo-Instruct')
mistral_models_path.mkdir(parents=True, exist_ok=True)
snapshot_download(repo_id="mistralai/Mistral-Nemo-Instruct-2407", allow_patterns=["params.json", "consolidated.safetensors", "tekken.json"], local_dir=mistral_models_path)
```
#### Chat
After installing `mistral_inference`, a `mistral-chat` CLI command should be available in your environment. You can chat with the model using
```
mistral-chat $HOME/mistral_models/Nemo-Instruct --instruct --max_tokens 256 --temperature 0.35
```
*E.g.* Try out something like:
```
How expensive would it be to ask a window cleaner to clean all windows in Paris. Make a reasonable guess in US Dollar.
```
#### Instruct following
```py
from mistral_inference.transformer import Transformer
from mistral_inference.generate import generate
from mistral_common.tokens.tokenizers.mistral import MistralTokenizer
from mistral_common.protocol.instruct.messages import UserMessage
from mistral_common.protocol.instruct.request import ChatCompletionRequest
tokenizer = MistralTokenizer.from_file(f"{mistral_models_path}/tekken.json")
model = Transformer.from_folder(mistral_models_path)
prompt = "How expensive would it be to ask a window cleaner to clean all windows in Paris. Make a reasonable guess in US Dollar."
completion_request = ChatCompletionRequest(messages=[UserMessage(content=prompt)])
tokens = tokenizer.encode_chat_completion(completion_request).tokens
out_tokens, _ = generate([tokens], model, max_tokens=64, temperature=0.35, eos_id=tokenizer.instruct_tokenizer.tokenizer.eos_id)
result = tokenizer.decode(out_tokens[0])
print(result)
```
#### Function calling
```py
from mistral_common.protocol.instruct.tool_calls import Function, Tool
from mistral_inference.transformer import Transformer
from mistral_inference.generate import generate
from mistral_common.tokens.tokenizers.mistral import MistralTokenizer
from mistral_common.protocol.instruct.messages import UserMessage
from mistral_common.protocol.instruct.request import ChatCompletionRequest
tokenizer = MistralTokenizer.from_file(f"{mistral_models_path}/tekken.json")
model = Transformer.from_folder(mistral_models_path)
completion_request = ChatCompletionRequest(
tools=[
Tool(
function=Function(
name="get_current_weather",
description="Get the current weather",
parameters={
"type": "object",
"properties": {
"location": {
"type": "string",
"description": "The city and state, e.g. San Francisco, CA",
},
"format": {
"type": "string",
"enum": ["celsius", "fahrenheit"],
"description": "The temperature unit to use. Infer this from the users location.",
},
},
"required": ["location", "format"],
},
)
)
],
messages=[
UserMessage(content="What's the weather like today in Paris?"),
],
)
tokens = tokenizer.encode_chat_completion(completion_request).tokens
out_tokens, _ = generate([tokens], model, max_tokens=256, temperature=0.35, eos_id=tokenizer.instruct_tokenizer.tokenizer.eos_id)
result = tokenizer.decode(out_tokens[0])
print(result)
```
### Transformers
> [!IMPORTANT]
> NOTE: Until a new release has been made, you need to install transformers from source:
> ```sh
> pip install git+https://github.com/huggingface/transformers.git
> ```
If you want to use Hugging Face `transformers` to generate text, you can do something like this.
```py
from transformers import pipeline
messages = [
{"role": "system", "content": "You are a pirate chatbot who always responds in pirate speak!"},
{"role": "user", "content": "Who are you?"},
]
chatbot = pipeline("text-generation", model="mistralai/Mistral-Nemo-Instruct-2407")
chatbot(messages)
```
> [!TIP]
> Unlike previous Mistral models, Mistral Nemo requires smaller temperatures. We recommend to use a temperature of 0.3.
## Limitations
The Mistral Nemo Instruct model is a quick demonstration that the base model can be easily fine-tuned to achieve compelling performance.
It does not have any moderation mechanisms. We're looking forward to engaging with the community on ways to
make the model finely respect guardrails, allowing for deployment in environments requiring moderated outputs.
## The Mistral AI Team
Albert Jiang, Alexandre Sablayrolles, Alexis Tacnet, Alok Kothari, Antoine Roux, Arthur Mensch, Audrey Herblin-Stoop, Augustin Garreau, Austin Birky, Bam4d, Baptiste Bout, Baudouin de Monicault, Blanche Savary, Carole Rambaud, Caroline Feldman, Devendra Singh Chaplot, Diego de las Casas, Eleonore Arcelin, Emma Bou Hanna, Etienne Metzger, Gaspard Blanchet, Gianna Lengyel, Guillaume Bour, Guillaume Lample, Harizo Rajaona, Henri Roussez, Hichem Sattouf, Ian Mack, Jean-Malo Delignon, Jessica Chudnovsky, Justus Murke, Kartik Khandelwal, Lawrence Stewart, Louis Martin, Louis Ternon, Lucile Saulnier, Lélio Renard Lavaud, Margaret Jennings, Marie Pellat, Marie Torelli, Marie-Anne Lachaux, Marjorie Janiewicz, Mickaël Seznec, Nicolas Schuhl, Niklas Muhs, Olivier de Garrigues, Patrick von Platen, Paul Jacob, Pauline Buche, Pavan Kumar Reddy, Perry Savas, Pierre Stock, Romain Sauvestre, Sagar Vaze, Sandeep Subramanian, Saurabh Garg, Sophia Yang, Szymon Antoniak, Teven Le Scao, Thibault Schueller, Thibaut Lavril, Thomas Wang, Théophile Gervet, Timothée Lacroix, Valera Nemychnikova, Wendy Shang, William El Sayed, William Marshall |
chengyineng/gpt2_random_test2 | chengyineng | "2023-07-17T21:04:42Z" | 0 | 0 | peft | [
"peft",
"region:us"
] | null | "2023-07-17T21:04:41Z" | ---
library_name: peft
---
## Training procedure
### Framework versions
- PEFT 0.4.0
|
zijianh/Qwen-2.5-7B-Simple-RL-length-penalty | zijianh | "2025-03-12T03:55:19Z" | 0 | 0 | transformers | [
"transformers",
"safetensors",
"qwen2",
"text-generation",
"generated_from_trainer",
"trl",
"grpo",
"conversational",
"arxiv:2402.03300",
"base_model:Qwen/Qwen2.5-Math-7B",
"base_model:finetune:Qwen/Qwen2.5-Math-7B",
"autotrain_compatible",
"text-generation-inference",
"endpoints_compatible",
"region:us"
] | text-generation | "2025-03-11T18:30:42Z" | ---
base_model: Qwen/Qwen2.5-Math-7B
library_name: transformers
model_name: Qwen-2.5-7B-Simple-RL-length-penalty
tags:
- generated_from_trainer
- trl
- grpo
licence: license
---
# Model Card for Qwen-2.5-7B-Simple-RL-length-penalty
This model is a fine-tuned version of [Qwen/Qwen2.5-Math-7B](https://huggingface.co/Qwen/Qwen2.5-Math-7B).
It has been trained using [TRL](https://github.com/huggingface/trl).
## Quick start
```python
from transformers import pipeline
question = "If you had a time machine, but could only go to the past or the future once and never return, which would you choose and why?"
generator = pipeline("text-generation", model="zijianh/Qwen-2.5-7B-Simple-RL-length-penalty", device="cuda")
output = generator([{"role": "user", "content": question}], max_new_tokens=128, return_full_text=False)[0]
print(output["generated_text"])
```
## Training procedure
[<img src="https://raw.githubusercontent.com/wandb/assets/main/wandb-github-badge-28.svg" alt="Visualize in Weights & Biases" width="150" height="24"/>](https://wandb.ai/sota_mavens-university-of-michigan/huggingface/runs/3nr4bm1v)
This model was trained with GRPO, a method introduced in [DeepSeekMath: Pushing the Limits of Mathematical Reasoning in Open Language Models](https://huggingface.co/papers/2402.03300).
### Framework versions
- TRL: 0.16.0.dev0
- Transformers: 4.49.0
- Pytorch: 2.5.1
- Datasets: 3.3.2
- Tokenizers: 0.21.0
## Citations
Cite GRPO as:
```bibtex
@article{zhihong2024deepseekmath,
title = {{DeepSeekMath: Pushing the Limits of Mathematical Reasoning in Open Language Models}},
author = {Zhihong Shao and Peiyi Wang and Qihao Zhu and Runxin Xu and Junxiao Song and Mingchuan Zhang and Y. K. Li and Y. Wu and Daya Guo},
year = 2024,
eprint = {arXiv:2402.03300},
}
```
Cite TRL as:
```bibtex
@misc{vonwerra2022trl,
title = {{TRL: Transformer Reinforcement Learning}},
author = {Leandro von Werra and Younes Belkada and Lewis Tunstall and Edward Beeching and Tristan Thrush and Nathan Lambert and Shengyi Huang and Kashif Rasul and Quentin Gallouédec},
year = 2020,
journal = {GitHub repository},
publisher = {GitHub},
howpublished = {\url{https://github.com/huggingface/trl}}
}
``` |
Heimat24/danielheinz_e5_v1-rh_bg-3-1-0.8 | Heimat24 | "2024-06-10T09:59:15Z" | 10 | 0 | sentence-transformers | [
"sentence-transformers",
"safetensors",
"xlm-roberta",
"feature-extraction",
"sentence-similarity",
"autotrain_compatible",
"text-embeddings-inference",
"endpoints_compatible",
"region:us"
] | sentence-similarity | "2024-06-10T09:58:15Z" | ---
library_name: sentence-transformers
pipeline_tag: sentence-similarity
tags:
- sentence-transformers
- feature-extraction
- sentence-similarity
---
# {MODEL_NAME}
This is a [sentence-transformers](https://www.SBERT.net) model: It maps sentences & paragraphs to a 768 dimensional dense vector space and can be used for tasks like clustering or semantic search.
<!--- Describe your model here -->
## Usage (Sentence-Transformers)
Using this model becomes easy when you have [sentence-transformers](https://www.SBERT.net) installed:
```
pip install -U sentence-transformers
```
Then you can use the model like this:
```python
from sentence_transformers import SentenceTransformer
sentences = ["This is an example sentence", "Each sentence is converted"]
model = SentenceTransformer('{MODEL_NAME}')
embeddings = model.encode(sentences)
print(embeddings)
```
## Evaluation Results
<!--- Describe how your model was evaluated -->
For an automated evaluation of this model, see the *Sentence Embeddings Benchmark*: [https://seb.sbert.net](https://seb.sbert.net?model_name={MODEL_NAME})
## Training
The model was trained with the parameters:
**DataLoader**:
`torch.utils.data.dataloader.DataLoader` of length 70 with parameters:
```
{'batch_size': 10, 'sampler': 'torch.utils.data.sampler.SequentialSampler', 'batch_sampler': 'torch.utils.data.sampler.BatchSampler'}
```
**Loss**:
`sentence_transformers.losses.MultipleNegativesRankingLoss.MultipleNegativesRankingLoss` with parameters:
```
{'scale': 20.0, 'similarity_fct': 'cos_sim'}
```
Parameters of the fit()-Method:
```
{
"epochs": 1,
"evaluation_steps": 50,
"evaluator": "sentence_transformers.evaluation.InformationRetrievalEvaluator.InformationRetrievalEvaluator",
"max_grad_norm": 1,
"optimizer_class": "<class 'torch.optim.adamw.AdamW'>",
"optimizer_params": {
"lr": 2e-05
},
"scheduler": "WarmupLinear",
"steps_per_epoch": null,
"warmup_steps": 7,
"weight_decay": 0.01
}
```
## Full Model Architecture
```
SentenceTransformer(
(0): Transformer({'max_seq_length': 512, 'do_lower_case': False}) with Transformer model: XLMRobertaModel
(1): Pooling({'word_embedding_dimension': 768, 'pooling_mode_cls_token': False, 'pooling_mode_mean_tokens': True, 'pooling_mode_max_tokens': False, 'pooling_mode_mean_sqrt_len_tokens': False, 'pooling_mode_weightedmean_tokens': False, 'pooling_mode_lasttoken': False, 'include_prompt': True})
(2): Normalize()
)
```
## Citing & Authors
<!--- Describe where people can find more information --> |
fifxus/2da11f96-0736-4d7a-836d-aa54d73528b3 | fifxus | "2025-02-07T06:14:29Z" | 9 | 0 | peft | [
"peft",
"safetensors",
"qwen2",
"axolotl",
"generated_from_trainer",
"base_model:unsloth/Qwen2.5-1.5B-Instruct",
"base_model:adapter:unsloth/Qwen2.5-1.5B-Instruct",
"license:apache-2.0",
"8-bit",
"bitsandbytes",
"region:us"
] | null | "2025-02-07T05:43:21Z" | ---
library_name: peft
license: apache-2.0
base_model: unsloth/Qwen2.5-1.5B-Instruct
tags:
- axolotl
- generated_from_trainer
model-index:
- name: 2da11f96-0736-4d7a-836d-aa54d73528b3
results: []
---
<!-- This model card has been generated automatically according to the information the Trainer had access to. You
should probably proofread and complete it, then remove this comment. -->
[<img src="https://raw.githubusercontent.com/axolotl-ai-cloud/axolotl/main/image/axolotl-badge-web.png" alt="Built with Axolotl" width="200" height="32"/>](https://github.com/axolotl-ai-cloud/axolotl)
<details><summary>See axolotl config</summary>
axolotl version: `0.4.1`
```yaml
adapter: lora
base_model: unsloth/Qwen2.5-1.5B-Instruct
bf16: auto
chat_template: llama3
dataset_prepared_path: null
datasets:
- data_files:
- 5f68617c0d0f9150_train_data.json
ds_type: json
format: custom
path: /workspace/input_data/5f68617c0d0f9150_train_data.json
type:
field_instruction: message_1
field_output: message_2
format: '{instruction}'
no_input_format: '{instruction}'
system_format: '{system}'
system_prompt: ''
debug: null
deepspeed: null
device_map: auto
do_eval: true
early_stopping_patience: null
eval_batch_size: 2
eval_max_new_tokens: 128
eval_steps: null
eval_table_size: null
evals_per_epoch: null
flash_attention: true
fp16: null
fsdp: null
fsdp_config: null
gradient_accumulation_steps: 4
gradient_checkpointing: true
gradient_clipping: 1.0
group_by_length: true
hub_model_id: fifxus/2da11f96-0736-4d7a-836d-aa54d73528b3
hub_repo: null
hub_strategy: end
hub_token: null
learning_rate: 0.0001
load_in_4bit: true
load_in_8bit: true
local_rank: null
logging_steps: 1
lora_alpha: 16
lora_dropout: 0.05
lora_fan_in_fan_out: null
lora_model_dir: null
lora_r: 8
lora_target_linear: true
lr_scheduler: cosine
max_grad_norm: 1.0
max_memory:
0: 75GB
max_steps: 500
micro_batch_size: 2
mlflow_experiment_name: /tmp/5f68617c0d0f9150_train_data.json
model_type: AutoModelForCausalLM
num_epochs: 1
optimizer: adamw_bnb_8bit
output_dir: miner_id_24
pad_to_sequence_len: true
resume_from_checkpoint: null
s2_attention: null
sample_packing: false
save_steps: null
saves_per_epoch: null
sequence_len: 1024
strict: false
tf32: false
tokenizer_type: AutoTokenizer
train_on_inputs: false
trust_remote_code: true
val_set_size: 0.05
wandb_entity: techspear-hub
wandb_mode: online
wandb_name: 327747fa-9e72-4d91-846a-4ce7169b36a3
wandb_project: Gradients-On-10
wandb_run: your_name
wandb_runid: 327747fa-9e72-4d91-846a-4ce7169b36a3
warmup_steps: 5
weight_decay: 0.01
xformers_attention: null
```
</details><br>
# 2da11f96-0736-4d7a-836d-aa54d73528b3
This model is a fine-tuned version of [unsloth/Qwen2.5-1.5B-Instruct](https://huggingface.co/unsloth/Qwen2.5-1.5B-Instruct) on the None dataset.
It achieves the following results on the evaluation set:
- Loss: 0.6749
## Model description
More information needed
## Intended uses & limitations
More information needed
## Training and evaluation data
More information needed
## Training procedure
### Training hyperparameters
The following hyperparameters were used during training:
- learning_rate: 0.0001
- train_batch_size: 2
- eval_batch_size: 2
- seed: 42
- gradient_accumulation_steps: 4
- total_train_batch_size: 8
- optimizer: Use OptimizerNames.ADAMW_BNB with betas=(0.9,0.999) and epsilon=1e-08 and optimizer_args=No additional optimizer arguments
- lr_scheduler_type: cosine
- lr_scheduler_warmup_steps: 5
- training_steps: 500
### Training results
| Training Loss | Epoch | Step | Validation Loss |
|:-------------:|:------:|:----:|:---------------:|
| 0.5907 | 0.2127 | 500 | 0.6749 |
### Framework versions
- PEFT 0.13.2
- Transformers 4.46.0
- Pytorch 2.5.0+cu124
- Datasets 3.0.1
- Tokenizers 0.20.1 |
tensorblock/Mistral-Ita-7b-GGUF | tensorblock | "2024-11-16T01:09:31Z" | 26 | 0 | null | [
"gguf",
"text-generation-inference",
"text generation",
"TensorBlock",
"GGUF",
"it",
"dataset:DeepMount00/llm_ita_ultra",
"base_model:DeepMount00/Mistral-Ita-7b",
"base_model:quantized:DeepMount00/Mistral-Ita-7b",
"license:apache-2.0",
"endpoints_compatible",
"region:us"
] | null | "2024-11-11T17:02:35Z" | ---
language:
- it
license: apache-2.0
tags:
- text-generation-inference
- text generation
- TensorBlock
- GGUF
datasets:
- DeepMount00/llm_ita_ultra
base_model: DeepMount00/Mistral-Ita-7b
---
<div style="width: auto; margin-left: auto; margin-right: auto">
<img src="https://i.imgur.com/jC7kdl8.jpeg" alt="TensorBlock" style="width: 100%; min-width: 400px; display: block; margin: auto;">
</div>
<div style="display: flex; justify-content: space-between; width: 100%;">
<div style="display: flex; flex-direction: column; align-items: flex-start;">
<p style="margin-top: 0.5em; margin-bottom: 0em;">
Feedback and support: TensorBlock's <a href="https://x.com/tensorblock_aoi">Twitter/X</a>, <a href="https://t.me/TensorBlock">Telegram Group</a> and <a href="https://x.com/tensorblock_aoi">Discord server</a>
</p>
</div>
</div>
## DeepMount00/Mistral-Ita-7b - GGUF
This repo contains GGUF format model files for [DeepMount00/Mistral-Ita-7b](https://huggingface.co/DeepMount00/Mistral-Ita-7b).
The files were quantized using machines provided by [TensorBlock](https://tensorblock.co/), and they are compatible with llama.cpp as of [commit b4011](https://github.com/ggerganov/llama.cpp/commit/a6744e43e80f4be6398fc7733a01642c846dce1d).
<div style="text-align: left; margin: 20px 0;">
<a href="https://tensorblock.co/waitlist/client" style="display: inline-block; padding: 10px 20px; background-color: #007bff; color: white; text-decoration: none; border-radius: 5px; font-weight: bold;">
Run them on the TensorBlock client using your local machine ↗
</a>
</div>
## Prompt template
```
```
## Model file specification
| Filename | Quant type | File Size | Description |
| -------- | ---------- | --------- | ----------- |
| [Mistral-Ita-7b-Q2_K.gguf](https://huggingface.co/tensorblock/Mistral-Ita-7b-GGUF/blob/main/Mistral-Ita-7b-Q2_K.gguf) | Q2_K | 2.532 GB | smallest, significant quality loss - not recommended for most purposes |
| [Mistral-Ita-7b-Q3_K_S.gguf](https://huggingface.co/tensorblock/Mistral-Ita-7b-GGUF/blob/main/Mistral-Ita-7b-Q3_K_S.gguf) | Q3_K_S | 2.947 GB | very small, high quality loss |
| [Mistral-Ita-7b-Q3_K_M.gguf](https://huggingface.co/tensorblock/Mistral-Ita-7b-GGUF/blob/main/Mistral-Ita-7b-Q3_K_M.gguf) | Q3_K_M | 3.277 GB | very small, high quality loss |
| [Mistral-Ita-7b-Q3_K_L.gguf](https://huggingface.co/tensorblock/Mistral-Ita-7b-GGUF/blob/main/Mistral-Ita-7b-Q3_K_L.gguf) | Q3_K_L | 3.560 GB | small, substantial quality loss |
| [Mistral-Ita-7b-Q4_0.gguf](https://huggingface.co/tensorblock/Mistral-Ita-7b-GGUF/blob/main/Mistral-Ita-7b-Q4_0.gguf) | Q4_0 | 3.827 GB | legacy; small, very high quality loss - prefer using Q3_K_M |
| [Mistral-Ita-7b-Q4_K_S.gguf](https://huggingface.co/tensorblock/Mistral-Ita-7b-GGUF/blob/main/Mistral-Ita-7b-Q4_K_S.gguf) | Q4_K_S | 3.856 GB | small, greater quality loss |
| [Mistral-Ita-7b-Q4_K_M.gguf](https://huggingface.co/tensorblock/Mistral-Ita-7b-GGUF/blob/main/Mistral-Ita-7b-Q4_K_M.gguf) | Q4_K_M | 4.068 GB | medium, balanced quality - recommended |
| [Mistral-Ita-7b-Q5_0.gguf](https://huggingface.co/tensorblock/Mistral-Ita-7b-GGUF/blob/main/Mistral-Ita-7b-Q5_0.gguf) | Q5_0 | 4.654 GB | legacy; medium, balanced quality - prefer using Q4_K_M |
| [Mistral-Ita-7b-Q5_K_S.gguf](https://huggingface.co/tensorblock/Mistral-Ita-7b-GGUF/blob/main/Mistral-Ita-7b-Q5_K_S.gguf) | Q5_K_S | 4.654 GB | large, low quality loss - recommended |
| [Mistral-Ita-7b-Q5_K_M.gguf](https://huggingface.co/tensorblock/Mistral-Ita-7b-GGUF/blob/main/Mistral-Ita-7b-Q5_K_M.gguf) | Q5_K_M | 4.779 GB | large, very low quality loss - recommended |
| [Mistral-Ita-7b-Q6_K.gguf](https://huggingface.co/tensorblock/Mistral-Ita-7b-GGUF/blob/main/Mistral-Ita-7b-Q6_K.gguf) | Q6_K | 5.534 GB | very large, extremely low quality loss |
| [Mistral-Ita-7b-Q8_0.gguf](https://huggingface.co/tensorblock/Mistral-Ita-7b-GGUF/blob/main/Mistral-Ita-7b-Q8_0.gguf) | Q8_0 | 7.167 GB | very large, extremely low quality loss - not recommended |
## Downloading instruction
### Command line
Firstly, install Huggingface Client
```shell
pip install -U "huggingface_hub[cli]"
```
Then, downoad the individual model file the a local directory
```shell
huggingface-cli download tensorblock/Mistral-Ita-7b-GGUF --include "Mistral-Ita-7b-Q2_K.gguf" --local-dir MY_LOCAL_DIR
```
If you wanna download multiple model files with a pattern (e.g., `*Q4_K*gguf`), you can try:
```shell
huggingface-cli download tensorblock/Mistral-Ita-7b-GGUF --local-dir MY_LOCAL_DIR --local-dir-use-symlinks False --include='*Q4_K*gguf'
```
|
nhung03/defd1ead-a576-4627-b2e5-894dfdd6386f | nhung03 | "2025-01-16T20:20:20Z" | 9 | 0 | peft | [
"peft",
"safetensors",
"llama",
"axolotl",
"generated_from_trainer",
"base_model:NousResearch/GPT4-x-Vicuna-13b-fp16",
"base_model:adapter:NousResearch/GPT4-x-Vicuna-13b-fp16",
"license:gpl",
"8-bit",
"bitsandbytes",
"region:us"
] | null | "2025-01-16T19:55:37Z" | ---
library_name: peft
license: gpl
base_model: NousResearch/GPT4-x-Vicuna-13b-fp16
tags:
- axolotl
- generated_from_trainer
model-index:
- name: defd1ead-a576-4627-b2e5-894dfdd6386f
results: []
---
<!-- This model card has been generated automatically according to the information the Trainer had access to. You
should probably proofread and complete it, then remove this comment. -->
[<img src="https://raw.githubusercontent.com/axolotl-ai-cloud/axolotl/main/image/axolotl-badge-web.png" alt="Built with Axolotl" width="200" height="32"/>](https://github.com/axolotl-ai-cloud/axolotl)
<details><summary>See axolotl config</summary>
axolotl version: `0.4.1`
```yaml
adapter: lora
base_model: NousResearch/GPT4-x-Vicuna-13b-fp16
bf16: auto
chat_template: llama3
dataset_prepared_path: null
datasets:
- data_files:
- 8fca49d096fd3a50_train_data.json
ds_type: json
format: custom
path: /workspace/input_data/8fca49d096fd3a50_train_data.json
type:
field_input: statement
field_instruction: queries
field_output: paraphrased_statement
format: '{instruction} {input}'
no_input_format: '{instruction}'
system_format: '{system}'
system_prompt: ''
debug: null
deepspeed: null
early_stopping_patience: null
eval_max_new_tokens: 128
eval_table_size: null
evals_per_epoch: 1
flash_attention: true
fp16: null
fsdp: null
fsdp_config: null
gradient_accumulation_steps: 4
gradient_checkpointing: true
gradient_clipping: 1.0
group_by_length: false
hub_model_id: nhung03/defd1ead-a576-4627-b2e5-894dfdd6386f
hub_repo: null
hub_strategy: end
hub_token: null
learning_rate: 5.0e-05
load_in_4bit: true
load_in_8bit: true
local_rank: null
logging_steps: 1
lora_alpha: 16
lora_dropout: 0.05
lora_fan_in_fan_out: null
lora_model_dir: null
lora_r: 8
lora_target_linear: true
lr_scheduler: cosine
max_steps: 200
micro_batch_size: 2
mlflow_experiment_name: /tmp/8fca49d096fd3a50_train_data.json
model_type: AutoModelForCausalLM
num_epochs: 1
optimizer: adamw_bnb_8bit
output_dir: miner_id_24
pad_to_sequence_len: true
resume_from_checkpoint: null
s2_attention: null
sample_packing: false
saves_per_epoch: 1
sequence_len: 1024
strict: false
tf32: false
tokenizer_type: AutoTokenizer
train_on_inputs: false
trust_remote_code: true
val_set_size: 0.05
wandb_entity: null
wandb_mode: online
wandb_name: 081aa681-8389-4968-af0e-275b5d122406
wandb_project: Gradients-On-Demand
wandb_run: your_name
wandb_runid: 081aa681-8389-4968-af0e-275b5d122406
warmup_steps: 5
weight_decay: 0.01
xformers_attention: true
```
</details><br>
# defd1ead-a576-4627-b2e5-894dfdd6386f
This model is a fine-tuned version of [NousResearch/GPT4-x-Vicuna-13b-fp16](https://huggingface.co/NousResearch/GPT4-x-Vicuna-13b-fp16) on the None dataset.
It achieves the following results on the evaluation set:
- Loss: 0.4789
## Model description
More information needed
## Intended uses & limitations
More information needed
## Training and evaluation data
More information needed
## Training procedure
### Training hyperparameters
The following hyperparameters were used during training:
- learning_rate: 5e-05
- train_batch_size: 2
- eval_batch_size: 2
- seed: 42
- gradient_accumulation_steps: 4
- total_train_batch_size: 8
- optimizer: Use OptimizerNames.ADAMW_BNB with betas=(0.9,0.999) and epsilon=1e-08 and optimizer_args=No additional optimizer arguments
- lr_scheduler_type: cosine
- lr_scheduler_warmup_steps: 5
- training_steps: 200
### Training results
| Training Loss | Epoch | Step | Validation Loss |
|:-------------:|:------:|:----:|:---------------:|
| 0.6087 | 0.2729 | 200 | 0.4789 |
### Framework versions
- PEFT 0.13.2
- Transformers 4.46.0
- Pytorch 2.5.0+cu124
- Datasets 3.0.1
- Tokenizers 0.20.1 |
PrunaAI/vit_relpos_medium_patch16_224.sw_in1k-turbo-tiny-green-smashed | PrunaAI | "2024-11-13T13:22:01Z" | 1 | 0 | pruna-engine | [
"pruna-engine",
"region:us"
] | null | "2024-03-14T11:29:37Z" | ---
library_name: pruna-engine
thumbnail: "https://assets-global.website-files.com/646b351987a8d8ce158d1940/64ec9e96b4334c0e1ac41504_Logo%20with%20white%20text.svg"
metrics:
- memory_disk
- memory_inference
- inference_latency
- inference_throughput
- inference_CO2_emissions
- inference_energy_consumption
---
<!-- header start -->
<!-- 200823 -->
<div style="width: auto; margin-left: auto; margin-right: auto">
<a href="https://docs.pruna.ai/en/latest/setup/pip.html" target="_blank" rel="noopener noreferrer">
<img src="https://imgur.com/rVAgqMY.png" alt="PrunaAI" style="width: 100%; min-width: 400px; display: block; margin: auto;">
</a>
</div>
<!-- header end -->
[](https://twitter.com/PrunaAI)
[](https://github.com/PrunaAI)
[](https://www.linkedin.com/company/93832878/admin/feed/posts/?feedType=following)
[](https://discord.gg/rskEr4BZJx)
<div style="color: #9B1DBE; font-size: 2em; font-weight: bold;">
Deprecation Notice: This model is deprecated and will no longer receive updates.
</div>
<br><br>
# Simply make AI models cheaper, smaller, faster, and greener!
- Give a thumbs up if you like this model!
- Contact us and tell us which model to compress next [here](https://www.pruna.ai/contact).
- Request access to easily compress your *own* AI models [here](https://z0halsaff74.typeform.com/pruna-access?typeform-source=www.pruna.ai).
- Read the documentations to know more [here](https://pruna-ai-pruna.readthedocs-hosted.com/en/latest/)
- Join Pruna AI community on Discord [here](https://discord.gg/rskEr4BZJx) to share feedback/suggestions or get help.
## Results

**Frequently Asked Questions**
- ***How does the compression work?*** The model is compressed by combining quantization, xformers, jit, cuda graphs, triton.
- ***How does the model quality change?*** The quality of the model output might slightly vary compared to the base model.
- ***How is the model efficiency evaluated?*** These results were obtained on NVIDIA A100-PCIE-40GB with configuration described in `model/smash_config.json` and are obtained after a hardware warmup. The smashed model is directly compared to the original base model. Efficiency results may vary in other settings (e.g. other hardware, image size, batch size, ...). We recommend to directly run them in the use-case conditions to know if the smashed model can benefit you.
- ***What is the model format?*** We used a custom Pruna model format based on pickle to make models compatible with the compression methods. We provide a tutorial to run models in dockers in the documentation [here](https://pruna-ai-pruna.readthedocs-hosted.com/en/latest/) if needed.
- ***What is the naming convention for Pruna Huggingface models?*** We take the original model name and append "turbo", "tiny", or "green" if the smashed model has a measured inference speed, inference memory, or inference energy consumption which is less than 90% of the original base model.
- ***How to compress my own models?*** You can request premium access to more compression methods and tech support for your specific use-cases [here](https://z0halsaff74.typeform.com/pruna-access?typeform-source=www.pruna.ai).
- ***What are "first" metrics?*** Results mentioning "first" are obtained after the first run of the model. The first run might take more memory or be slower than the subsequent runs due cuda overheads.
- ***What are "Sync" and "Async" metrics?*** "Sync" metrics are obtained by syncing all GPU processes and stop measurement when all of them are executed. "Async" metrics are obtained without syncing all GPU processes and stop when the model output can be used by the CPU. We provide both metrics since both could be relevant depending on the use-case. We recommend to test the efficiency gains directly in your use-cases.
## Setup
You can run the smashed model with these steps:
0. Check that you have linux, python 3.10, and cuda 12.1.0 requirements installed. For cuda, check with `nvcc --version` and install with `conda install nvidia/label/cuda-12.1.0::cuda`.
1. Install the `pruna-engine` available [here](https://pypi.org/project/pruna-engine/) on Pypi. It might take up to 15 minutes to install.
```bash
pip install pruna-engine[gpu]==0.7.1 --extra-index-url https://pypi.nvidia.com --extra-index-url https://pypi.ngc.nvidia.com --extra-index-url https://prunaai.pythonanywhere.com/
```
2. Download the model files using one of these three options.
- Option 1 - Use command line interface (CLI):
```bash
mkdir vit_relpos_medium_patch16_224.sw_in1k-turbo-tiny-green-smashed
huggingface-cli download PrunaAI/vit_relpos_medium_patch16_224.sw_in1k-turbo-tiny-green-smashed --local-dir vit_relpos_medium_patch16_224.sw_in1k-turbo-tiny-green-smashed --local-dir-use-symlinks False
```
- Option 2 - Use Python:
```python
import subprocess
repo_name = "vit_relpos_medium_patch16_224.sw_in1k-turbo-tiny-green-smashed"
subprocess.run(["mkdir", repo_name])
subprocess.run(["huggingface-cli", "download", 'PrunaAI/'+ repo_name, "--local-dir", repo_name, "--local-dir-use-symlinks", "False"])
```
- Option 3 - Download them manually on the HuggingFace model page.
3. Load & run the model.
```python
from pruna_engine.PrunaModel import PrunaModel
model_path = "vit_relpos_medium_patch16_224.sw_in1k-turbo-tiny-green-smashed/model" # Specify the downloaded model path.
smashed_model = PrunaModel.load_model(model_path) # Load the model.
import torch; image = torch.rand(1, 3, 224, 224).to('cuda')
smashed_model(image)
```
## Configurations
The configuration info are in `model/smash_config.json`.
## Credits & License
The license of the smashed model follows the license of the original model. Please check the license of the original model vit_relpos_medium_patch16_224.sw_in1k before using this model which provided the base model. The license of the `pruna-engine` is [here](https://pypi.org/project/pruna-engine/) on Pypi.
## Want to compress other models?
- Contact us and tell us which model to compress next [here](https://www.pruna.ai/contact).
- Request access to easily compress your own AI models [here](https://z0halsaff74.typeform.com/pruna-access?typeform-source=www.pruna.ai). |
aengusl/R2D2_run-1_4jun_step-3600 | aengusl | "2024-06-06T10:23:07Z" | 0 | 0 | transformers | [
"transformers",
"safetensors",
"arxiv:1910.09700",
"endpoints_compatible",
"region:us"
] | null | "2024-06-06T10:23:01Z" | ---
library_name: transformers
tags: []
---
# Model Card for Model ID
<!-- Provide a quick summary of what the model is/does. -->
## Model Details
### Model Description
<!-- Provide a longer summary of what this model is. -->
This is the model card of a 🤗 transformers model that has been pushed on the Hub. This model card has been automatically generated.
- **Developed by:** [More Information Needed]
- **Funded by [optional]:** [More Information Needed]
- **Shared by [optional]:** [More Information Needed]
- **Model type:** [More Information Needed]
- **Language(s) (NLP):** [More Information Needed]
- **License:** [More Information Needed]
- **Finetuned from model [optional]:** [More Information Needed]
### Model Sources [optional]
<!-- Provide the basic links for the model. -->
- **Repository:** [More Information Needed]
- **Paper [optional]:** [More Information Needed]
- **Demo [optional]:** [More Information Needed]
## Uses
<!-- Address questions around how the model is intended to be used, including the foreseeable users of the model and those affected by the model. -->
### Direct Use
<!-- This section is for the model use without fine-tuning or plugging into a larger ecosystem/app. -->
[More Information Needed]
### Downstream Use [optional]
<!-- This section is for the model use when fine-tuned for a task, or when plugged into a larger ecosystem/app -->
[More Information Needed]
### Out-of-Scope Use
<!-- This section addresses misuse, malicious use, and uses that the model will not work well for. -->
[More Information Needed]
## Bias, Risks, and Limitations
<!-- This section is meant to convey both technical and sociotechnical limitations. -->
[More Information Needed]
### Recommendations
<!-- This section is meant to convey recommendations with respect to the bias, risk, and technical limitations. -->
Users (both direct and downstream) should be made aware of the risks, biases and limitations of the model. More information needed for further recommendations.
## How to Get Started with the Model
Use the code below to get started with the model.
[More Information Needed]
## Training Details
### Training Data
<!-- This should link to a Dataset Card, perhaps with a short stub of information on what the training data is all about as well as documentation related to data pre-processing or additional filtering. -->
[More Information Needed]
### Training Procedure
<!-- This relates heavily to the Technical Specifications. Content here should link to that section when it is relevant to the training procedure. -->
#### Preprocessing [optional]
[More Information Needed]
#### Training Hyperparameters
- **Training regime:** [More Information Needed] <!--fp32, fp16 mixed precision, bf16 mixed precision, bf16 non-mixed precision, fp16 non-mixed precision, fp8 mixed precision -->
#### Speeds, Sizes, Times [optional]
<!-- This section provides information about throughput, start/end time, checkpoint size if relevant, etc. -->
[More Information Needed]
## Evaluation
<!-- This section describes the evaluation protocols and provides the results. -->
### Testing Data, Factors & Metrics
#### Testing Data
<!-- This should link to a Dataset Card if possible. -->
[More Information Needed]
#### Factors
<!-- These are the things the evaluation is disaggregating by, e.g., subpopulations or domains. -->
[More Information Needed]
#### Metrics
<!-- These are the evaluation metrics being used, ideally with a description of why. -->
[More Information Needed]
### Results
[More Information Needed]
#### Summary
## Model Examination [optional]
<!-- Relevant interpretability work for the model goes here -->
[More Information Needed]
## Environmental Impact
<!-- Total emissions (in grams of CO2eq) and additional considerations, such as electricity usage, go here. Edit the suggested text below accordingly -->
Carbon emissions can be estimated using the [Machine Learning Impact calculator](https://mlco2.github.io/impact#compute) presented in [Lacoste et al. (2019)](https://arxiv.org/abs/1910.09700).
- **Hardware Type:** [More Information Needed]
- **Hours used:** [More Information Needed]
- **Cloud Provider:** [More Information Needed]
- **Compute Region:** [More Information Needed]
- **Carbon Emitted:** [More Information Needed]
## Technical Specifications [optional]
### Model Architecture and Objective
[More Information Needed]
### Compute Infrastructure
[More Information Needed]
#### Hardware
[More Information Needed]
#### Software
[More Information Needed]
## Citation [optional]
<!-- If there is a paper or blog post introducing the model, the APA and Bibtex information for that should go in this section. -->
**BibTeX:**
[More Information Needed]
**APA:**
[More Information Needed]
## Glossary [optional]
<!-- If relevant, include terms and calculations in this section that can help readers understand the model or model card. -->
[More Information Needed]
## More Information [optional]
[More Information Needed]
## Model Card Authors [optional]
[More Information Needed]
## Model Card Contact
[More Information Needed]
|
camilla-araujo-nu/camilla.araujo.video.on.social.media.trending.now | camilla-araujo-nu | "2025-03-23T19:18:07Z" | 0 | 0 | null | [
"region:us"
] | null | "2025-03-23T19:17:48Z" | <animated-image data-catalyst=""><a href="https://alltvsteam.com/viral-video/?v=news-es-tvdf" rel="nofollow" data-target="animated-image.originalLink"><img src="https://static.wixstatic.com/media/b249f9_adac8f70fb3f45b88691696c77de18f3~mv2.gif" alt="Foo" data-canonical-src="https://static.wixstatic.com/media/b249f9_adac8f70fb3f45b88691696c77de18f3~mv2.gif" style="max-width: 100%; display: inline-block;" data-target="animated-image.originalImage"></a> |
dblasko/blip-dalle3-img2prompt | dblasko | "2023-11-20T15:01:06Z" | 89 | 35 | transformers | [
"transformers",
"pytorch",
"safetensors",
"blip",
"image-text-to-text",
"art",
"image-to-text",
"image-captioning",
"en",
"dataset:laion/dalle-3-dataset",
"endpoints_compatible",
"region:us"
] | image-to-text | "2023-10-13T16:57:18Z" | ---
datasets:
- laion/dalle-3-dataset
language:
- en
tags:
- art
- image-to-text
- image-captioning
---
# DALL·E 3 Image prompt reverse-engineering
Pre-trained image-captioning model BLIP fine-tuned on a mixture of `laion/dalle-3-dataset` and semi-automatically gathered `(image, prompt)` data from DALLE·E 3.
It takes a generated image as an input and outputs a potential prompt to generate such an image, which can then be used as a base to generate similar images.
⚠️ Disclaimer: This model is **not intended for commercial use** as the data it was trained on includes images generated by DALLE·E 3. This is for educational purposes only.
### Usage:
Loading the model and preprocessor:
```python
from transformers import BlipForConditionalGeneration, AutoProcessor
model = BlipForConditionalGeneration.from_pretrained("dblasko/blip-dalle3-img2prompt").to(device)
processor = AutoProcessor.from_pretrained("dblasko/blip-dalle3-img2prompt")
```
Inference example on an image from `laion/dalle-3-dataset`:
```python
from datasets import load_dataset
dataset = load_dataset("laion/dalle-3-dataset", split=f'train[0%:1%]') # for fast download time in the toy example
example = dataset[img_index][0]
image = example["image"]
caption = example["caption"]
inputs = processor(images=image, return_tensors="pt").to(device)
pixel_values = inputs.pixel_values
generated_ids = model.generate(pixel_values=pixel_values, max_length=50)
generated_caption = processor.batch_decode(generated_ids, skip_special_tokens=True)[0]
print(f"Generated caption: {generated_caption}\nReal caption: {caption}")
``` |
Patt/bloom-560m-qa | Patt | "2023-11-13T23:10:52Z" | 1 | 0 | peft | [
"peft",
"safetensors",
"arxiv:1910.09700",
"base_model:bigscience/bloom-560m",
"base_model:adapter:bigscience/bloom-560m",
"region:us"
] | null | "2023-11-13T23:10:48Z" | ---
library_name: peft
base_model: bigscience/bloom-560m
---
# Model Card for Model ID
<!-- Provide a quick summary of what the model is/does. -->
## Model Details
### Model Description
<!-- Provide a longer summary of what this model is. -->
- **Developed by:** [More Information Needed]
- **Shared by [optional]:** [More Information Needed]
- **Model type:** [More Information Needed]
- **Language(s) (NLP):** [More Information Needed]
- **License:** [More Information Needed]
- **Finetuned from model [optional]:** [More Information Needed]
### Model Sources [optional]
<!-- Provide the basic links for the model. -->
- **Repository:** [More Information Needed]
- **Paper [optional]:** [More Information Needed]
- **Demo [optional]:** [More Information Needed]
## Uses
<!-- Address questions around how the model is intended to be used, including the foreseeable users of the model and those affected by the model. -->
### Direct Use
<!-- This section is for the model use without fine-tuning or plugging into a larger ecosystem/app. -->
[More Information Needed]
### Downstream Use [optional]
<!-- This section is for the model use when fine-tuned for a task, or when plugged into a larger ecosystem/app -->
[More Information Needed]
### Out-of-Scope Use
<!-- This section addresses misuse, malicious use, and uses that the model will not work well for. -->
[More Information Needed]
## Bias, Risks, and Limitations
<!-- This section is meant to convey both technical and sociotechnical limitations. -->
[More Information Needed]
### Recommendations
<!-- This section is meant to convey recommendations with respect to the bias, risk, and technical limitations. -->
Users (both direct and downstream) should be made aware of the risks, biases and limitations of the model. More information needed for further recommendations.
## How to Get Started with the Model
Use the code below to get started with the model.
[More Information Needed]
## Training Details
### Training Data
<!-- This should link to a Data Card, perhaps with a short stub of information on what the training data is all about as well as documentation related to data pre-processing or additional filtering. -->
[More Information Needed]
### Training Procedure
<!-- This relates heavily to the Technical Specifications. Content here should link to that section when it is relevant to the training procedure. -->
#### Preprocessing [optional]
[More Information Needed]
#### Training Hyperparameters
- **Training regime:** [More Information Needed] <!--fp32, fp16 mixed precision, bf16 mixed precision, bf16 non-mixed precision, fp16 non-mixed precision, fp8 mixed precision -->
#### Speeds, Sizes, Times [optional]
<!-- This section provides information about throughput, start/end time, checkpoint size if relevant, etc. -->
[More Information Needed]
## Evaluation
<!-- This section describes the evaluation protocols and provides the results. -->
### Testing Data, Factors & Metrics
#### Testing Data
<!-- This should link to a Data Card if possible. -->
[More Information Needed]
#### Factors
<!-- These are the things the evaluation is disaggregating by, e.g., subpopulations or domains. -->
[More Information Needed]
#### Metrics
<!-- These are the evaluation metrics being used, ideally with a description of why. -->
[More Information Needed]
### Results
[More Information Needed]
#### Summary
## Model Examination [optional]
<!-- Relevant interpretability work for the model goes here -->
[More Information Needed]
## Environmental Impact
<!-- Total emissions (in grams of CO2eq) and additional considerations, such as electricity usage, go here. Edit the suggested text below accordingly -->
Carbon emissions can be estimated using the [Machine Learning Impact calculator](https://mlco2.github.io/impact#compute) presented in [Lacoste et al. (2019)](https://arxiv.org/abs/1910.09700).
- **Hardware Type:** [More Information Needed]
- **Hours used:** [More Information Needed]
- **Cloud Provider:** [More Information Needed]
- **Compute Region:** [More Information Needed]
- **Carbon Emitted:** [More Information Needed]
## Technical Specifications [optional]
### Model Architecture and Objective
[More Information Needed]
### Compute Infrastructure
[More Information Needed]
#### Hardware
[More Information Needed]
#### Software
[More Information Needed]
## Citation [optional]
<!-- If there is a paper or blog post introducing the model, the APA and Bibtex information for that should go in this section. -->
**BibTeX:**
[More Information Needed]
**APA:**
[More Information Needed]
## Glossary [optional]
<!-- If relevant, include terms and calculations in this section that can help readers understand the model or model card. -->
[More Information Needed]
## More Information [optional]
[More Information Needed]
## Model Card Authors [optional]
[More Information Needed]
## Model Card Contact
[More Information Needed]
## Training procedure
### Framework versions
- PEFT 0.6.2.dev0
|
lesso08/83e77f4b-98a8-4f6a-a3a4-b6c5d98643fa | lesso08 | "2025-01-19T17:17:06Z" | 6 | 0 | peft | [
"peft",
"safetensors",
"llama",
"axolotl",
"generated_from_trainer",
"base_model:DeepMount00/Llama-3-8b-Ita",
"base_model:adapter:DeepMount00/Llama-3-8b-Ita",
"license:llama3",
"8-bit",
"bitsandbytes",
"region:us"
] | null | "2025-01-19T17:07:13Z" | ---
library_name: peft
license: llama3
base_model: DeepMount00/Llama-3-8b-Ita
tags:
- axolotl
- generated_from_trainer
model-index:
- name: 83e77f4b-98a8-4f6a-a3a4-b6c5d98643fa
results: []
---
<!-- This model card has been generated automatically according to the information the Trainer had access to. You
should probably proofread and complete it, then remove this comment. -->
[<img src="https://raw.githubusercontent.com/axolotl-ai-cloud/axolotl/main/image/axolotl-badge-web.png" alt="Built with Axolotl" width="200" height="32"/>](https://github.com/axolotl-ai-cloud/axolotl)
<details><summary>See axolotl config</summary>
axolotl version: `0.4.1`
```yaml
adapter: lora
base_model: DeepMount00/Llama-3-8b-Ita
bf16: true
chat_template: llama3
datasets:
- data_files:
- a0eb45ea7cd511f3_train_data.json
ds_type: json
format: custom
path: /workspace/input_data/a0eb45ea7cd511f3_train_data.json
type:
field_instruction: problem
field_output: generated_solution
format: '{instruction}'
no_input_format: '{instruction}'
system_format: '{system}'
system_prompt: ''
debug: null
deepspeed: null
early_stopping_patience: 2
eval_max_new_tokens: 128
eval_steps: 5
eval_table_size: null
flash_attention: false
fp16: false
fsdp: null
fsdp_config: null
gradient_accumulation_steps: 4
gradient_checkpointing: false
group_by_length: false
hub_model_id: lesso08/83e77f4b-98a8-4f6a-a3a4-b6c5d98643fa
hub_repo: null
hub_strategy: checkpoint
hub_token: null
learning_rate: 0.0002
load_in_4bit: false
load_in_8bit: true
local_rank: null
logging_steps: 1
lora_alpha: 16
lora_dropout: 0.05
lora_fan_in_fan_out: null
lora_model_dir: null
lora_r: 8
lora_target_linear: true
lr_scheduler: cosine
max_steps: 25
micro_batch_size: 2
mlflow_experiment_name: /tmp/a0eb45ea7cd511f3_train_data.json
model_type: AutoModelForCausalLM
num_epochs: 1
optimizer: adamw_bnb_8bit
output_dir: miner_id_24
pad_to_sequence_len: true
resume_from_checkpoint: null
s2_attention: null
sample_packing: false
save_steps: 10
sequence_len: 512
special_tokens:
pad_token: <|eot_id|>
strict: false
tf32: false
tokenizer_type: AutoTokenizer
train_on_inputs: false
trust_remote_code: true
val_set_size: 0.05
wandb_entity: null
wandb_mode: online
wandb_name: 1568b38d-5ecd-4474-8507-d4201cbad038
wandb_project: Gradients-On-Demand
wandb_run: your_name
wandb_runid: 1568b38d-5ecd-4474-8507-d4201cbad038
warmup_steps: 10
weight_decay: 0.0
xformers_attention: null
```
</details><br>
# 83e77f4b-98a8-4f6a-a3a4-b6c5d98643fa
This model is a fine-tuned version of [DeepMount00/Llama-3-8b-Ita](https://huggingface.co/DeepMount00/Llama-3-8b-Ita) on the None dataset.
It achieves the following results on the evaluation set:
- Loss: 0.3927
## Model description
More information needed
## Intended uses & limitations
More information needed
## Training and evaluation data
More information needed
## Training procedure
### Training hyperparameters
The following hyperparameters were used during training:
- learning_rate: 0.0002
- train_batch_size: 2
- eval_batch_size: 2
- seed: 42
- gradient_accumulation_steps: 4
- total_train_batch_size: 8
- optimizer: Use OptimizerNames.ADAMW_BNB with betas=(0.9,0.999) and epsilon=1e-08 and optimizer_args=No additional optimizer arguments
- lr_scheduler_type: cosine
- lr_scheduler_warmup_steps: 10
- training_steps: 25
### Training results
| Training Loss | Epoch | Step | Validation Loss |
|:-------------:|:------:|:----:|:---------------:|
| 0.6754 | 0.0012 | 1 | 0.6501 |
| 0.6626 | 0.0058 | 5 | 0.6268 |
| 0.5202 | 0.0116 | 10 | 0.4649 |
| 0.3505 | 0.0174 | 15 | 0.4161 |
| 0.425 | 0.0232 | 20 | 0.3964 |
| 0.3211 | 0.0291 | 25 | 0.3927 |
### Framework versions
- PEFT 0.13.2
- Transformers 4.46.0
- Pytorch 2.5.0+cu124
- Datasets 3.0.1
- Tokenizers 0.20.1 |
omarbacc/ECE-BBDSS | omarbacc | "2024-11-18T15:06:26Z" | 5 | 0 | transformers | [
"transformers",
"safetensors",
"mistral",
"text-generation",
"mergekit",
"merge",
"base_model:paulml/NeuralOmniWestBeaglake-7B",
"base_model:merge:paulml/NeuralOmniWestBeaglake-7B",
"base_model:paulml/OmniBeagleSquaredMBX-v3-7B",
"base_model:merge:paulml/OmniBeagleSquaredMBX-v3-7B",
"autotrain_compatible",
"text-generation-inference",
"endpoints_compatible",
"region:us"
] | text-generation | "2024-11-18T14:52:44Z" | ---
base_model:
- paulml/NeuralOmniWestBeaglake-7B
- paulml/OmniBeagleSquaredMBX-v3-7B
library_name: transformers
tags:
- mergekit
- merge
---
# merge
This is a merge of pre-trained language models created using [mergekit](https://github.com/cg123/mergekit).
## Merge Details
### Merge Method
This model was merged using the SLERP merge method.
### Models Merged
The following models were included in the merge:
* [paulml/NeuralOmniWestBeaglake-7B](https://huggingface.co/paulml/NeuralOmniWestBeaglake-7B)
* [paulml/OmniBeagleSquaredMBX-v3-7B](https://huggingface.co/paulml/OmniBeagleSquaredMBX-v3-7B)
### Configuration
The following YAML configuration was used to produce this model:
```yaml
slices:
- sources:
- model: paulml/OmniBeagleSquaredMBX-v3-7B
layer_range: [0, 32]
- model: paulml/NeuralOmniWestBeaglake-7B
layer_range: [0, 32]
merge_method: slerp # This should not be indented under 'sources'
base_model: paulml/NeuralOmniWestBeaglake-7B
parameters:
t:
- filter: self_attn
value: [0, 0.5, 0.3, 0.7, 1]
- filter: mlp
value: [1, 0.5, 0.7, 0.3, 0]
- value: 0.5
dtype: bfloat16
```
|
bene-ges/tts_ru_hifigan_ruslan | bene-ges | "2023-09-18T07:54:29Z" | 19 | 6 | nemo | [
"nemo",
"tts",
"text-to-speech",
"Vocoder",
"ru",
"license:cc-by-nc-4.0",
"region:us"
] | text-to-speech | "2023-04-18T08:05:03Z" | ---
license: cc-by-nc-4.0
language:
- ru
library_name: nemo
tags:
- tts
- text-to-speech
- Vocoder
---
### How to use
See example of inference pipeline for Russian TTS (G2P + FastPitch + HifiGAN) in this [notebook](https://github.com/bene-ges/nemo_compatible/blob/main/notebooks/Russian_TTS_with_IPA_G2P_FastPitch_and_HifiGAN.ipynb).
Or use this [bash-script](https://github.com/bene-ges/nemo_compatible/blob/main/scripts/tts/ru_ipa_fastpitch_hifigan/test.sh).
### Input
This model accepts batches of mel spectrograms.
### Output
This model outputs audio at 22050Hz.
## Training
The NeMo toolkit [1] was used for training the model for several epochs.
Full training script is [here](https://github.com/bene-ges/nemo_compatible/blob/main/scripts/tts/ru_ipa_fastpitch_hifigan/train.sh).
### Datasets
This model is trained on [RUSLAN](https://ruslan-corpus.github.io/) [2] corpus (single speaker, male voice) sampled at 22050Hz.
## References
- [1] [NVIDIA NeMo Toolkit](https://github.com/NVIDIA/NeMo)
- [2] Gabdrakhmanov L., Garaev R., Razinkov E. (2019) RUSLAN: Russian Spoken Language Corpus for Speech Synthesis. In: Salah A., Karpov A., Potapova R. (eds) Speech and Computer. SPECOM 2019. Lecture Notes in Computer Science, vol 11658. Springer, Cham |
osanseviero/q-FrozenLake-v1-4x4-noSlippery-test4 | osanseviero | "2022-12-10T22:08:08Z" | 0 | 0 | null | [
"FrozenLake-v1-4x4-no_slippery",
"q-learning",
"reinforcement-learning",
"custom-implementation",
"model-index",
"region:us"
] | reinforcement-learning | "2022-12-10T22:07:59Z" | ---
tags:
- FrozenLake-v1-4x4-no_slippery
- q-learning
- reinforcement-learning
- custom-implementation
model-index:
- name: q-FrozenLake-v1-4x4-noSlippery-test4
results:
- task:
type: reinforcement-learning
name: reinforcement-learning
dataset:
name: FrozenLake-v1-4x4-no_slippery
type: FrozenLake-v1-4x4-no_slippery
metrics:
- type: mean_reward
value: 1.00 +/- 0.00
name: mean_reward
verified: false
---
# **Q-Learning** Agent playing1 **FrozenLake-v1**
This is a trained model of a **Q-Learning** agent playing **FrozenLake-v1** .
## Usage
```python
model = load_from_hub(repo_id="osanseviero/q-FrozenLake-v1-4x4-noSlippery-test4", filename="q-learning.pkl")
# Don't forget to check if you need to add additional attributes (is_slippery=False etc)
env = gym.make(model["env_id"])
```
|
nhoxinh/28de1b47-488a-4565-b56f-9fa1ed9a0d55 | nhoxinh | "2025-01-15T08:59:49Z" | 8 | 0 | peft | [
"peft",
"safetensors",
"phi",
"axolotl",
"generated_from_trainer",
"base_model:microsoft/phi-2",
"base_model:adapter:microsoft/phi-2",
"license:mit",
"8-bit",
"bitsandbytes",
"region:us"
] | null | "2025-01-15T08:37:23Z" | ---
library_name: peft
license: mit
base_model: microsoft/phi-2
tags:
- axolotl
- generated_from_trainer
model-index:
- name: 28de1b47-488a-4565-b56f-9fa1ed9a0d55
results: []
---
<!-- This model card has been generated automatically according to the information the Trainer had access to. You
should probably proofread and complete it, then remove this comment. -->
[<img src="https://raw.githubusercontent.com/axolotl-ai-cloud/axolotl/main/image/axolotl-badge-web.png" alt="Built with Axolotl" width="200" height="32"/>](https://github.com/axolotl-ai-cloud/axolotl)
<details><summary>See axolotl config</summary>
axolotl version: `0.4.1`
```yaml
adapter: lora
base_model: microsoft/phi-2
bf16: auto
chat_template: llama3
dataset_prepared_path: null
datasets:
- data_files:
- df553abe564045a8_train_data.json
ds_type: json
format: custom
path: /workspace/input_data/df553abe564045a8_train_data.json
type:
field_instruction: instruction
field_output: output
format: '{instruction}'
no_input_format: '{instruction}'
system_format: '{system}'
system_prompt: ''
debug: null
deepspeed: null
early_stopping_patience: null
eval_max_new_tokens: 128
eval_table_size: null
evals_per_epoch: 1
flash_attention: true
fp16: null
fsdp: null
fsdp_config: null
gradient_accumulation_steps: 4
gradient_checkpointing: true
gradient_clipping: 1.0
group_by_length: false
hub_model_id: nhoxinh/28de1b47-488a-4565-b56f-9fa1ed9a0d55
hub_repo: null
hub_strategy: end
hub_token: null
learning_rate: 5.0e-05
load_in_4bit: true
load_in_8bit: true
local_rank: null
logging_steps: 1
lora_alpha: 16
lora_dropout: 0.05
lora_fan_in_fan_out: null
lora_model_dir: null
lora_r: 8
lora_target_linear: true
lr_scheduler: cosine
max_steps: 200
micro_batch_size: 2
mlflow_experiment_name: /tmp/df553abe564045a8_train_data.json
model_type: AutoModelForCausalLM
num_epochs: 1
optimizer: adamw_bnb_8bit
output_dir: miner_id_24
pad_to_sequence_len: true
resume_from_checkpoint: null
s2_attention: null
sample_packing: false
saves_per_epoch: 1
sequence_len: 1024
special_tokens:
pad_token: <|endoftext|>
strict: false
tf32: false
tokenizer_type: AutoTokenizer
train_on_inputs: false
trust_remote_code: true
val_set_size: 0.05
wandb_entity: null
wandb_mode: online
wandb_name: 789d1235-3481-4413-98c8-50be461e7635
wandb_project: Gradients-On-Demand
wandb_run: your_name
wandb_runid: 789d1235-3481-4413-98c8-50be461e7635
warmup_steps: 5
weight_decay: 0.01
xformers_attention: true
```
</details><br>
# 28de1b47-488a-4565-b56f-9fa1ed9a0d55
This model is a fine-tuned version of [microsoft/phi-2](https://huggingface.co/microsoft/phi-2) on the None dataset.
It achieves the following results on the evaluation set:
- Loss: 1.3755
## Model description
More information needed
## Intended uses & limitations
More information needed
## Training and evaluation data
More information needed
## Training procedure
### Training hyperparameters
The following hyperparameters were used during training:
- learning_rate: 5e-05
- train_batch_size: 2
- eval_batch_size: 2
- seed: 42
- gradient_accumulation_steps: 4
- total_train_batch_size: 8
- optimizer: Use OptimizerNames.ADAMW_BNB with betas=(0.9,0.999) and epsilon=1e-08 and optimizer_args=No additional optimizer arguments
- lr_scheduler_type: cosine
- lr_scheduler_warmup_steps: 5
- training_steps: 200
### Training results
| Training Loss | Epoch | Step | Validation Loss |
|:-------------:|:------:|:----:|:---------------:|
| 1.44 | 0.2520 | 200 | 1.3755 |
### Framework versions
- PEFT 0.13.2
- Transformers 4.46.0
- Pytorch 2.5.0+cu124
- Datasets 3.0.1
- Tokenizers 0.20.1 |
SylvLej/ppo-LunarLander-v2 | SylvLej | "2022-06-30T16:21:29Z" | 0 | 0 | stable-baselines3 | [
"stable-baselines3",
"LunarLander-v2",
"deep-reinforcement-learning",
"reinforcement-learning",
"model-index",
"region:us"
] | reinforcement-learning | "2022-06-30T16:03:45Z" | ---
library_name: stable-baselines3
tags:
- LunarLander-v2
- deep-reinforcement-learning
- reinforcement-learning
- stable-baselines3
model-index:
- name: basic
results:
- metrics:
- type: mean_reward
value: 277.99 +/- 25.55
name: mean_reward
task:
type: reinforcement-learning
name: reinforcement-learning
dataset:
name: LunarLander-v2
type: LunarLander-v2
---
# **basic** Agent playing **LunarLander-v2**
This is a trained model of a **basic** agent playing **LunarLander-v2**
using the [stable-baselines3 library](https://github.com/DLR-RM/stable-baselines3).
## Usage (with Stable-baselines3)
TODO: Add your code
```python
from stable_baselines3 import ...
from huggingface_sb3 import load_from_hub
...
```
|
ammarnasr/SmolLM-135M-GEC-DPO | ammarnasr | "2024-09-15T20:43:49Z" | 131 | 0 | transformers | [
"transformers",
"safetensors",
"llama",
"text-generation",
"trl",
"dpo",
"arxiv:1910.09700",
"autotrain_compatible",
"text-generation-inference",
"endpoints_compatible",
"region:us"
] | text-generation | "2024-09-15T20:43:23Z" | ---
library_name: transformers
tags:
- trl
- dpo
---
# Model Card for Model ID
<!-- Provide a quick summary of what the model is/does. -->
## Model Details
### Model Description
<!-- Provide a longer summary of what this model is. -->
This is the model card of a 🤗 transformers model that has been pushed on the Hub. This model card has been automatically generated.
- **Developed by:** [More Information Needed]
- **Funded by [optional]:** [More Information Needed]
- **Shared by [optional]:** [More Information Needed]
- **Model type:** [More Information Needed]
- **Language(s) (NLP):** [More Information Needed]
- **License:** [More Information Needed]
- **Finetuned from model [optional]:** [More Information Needed]
### Model Sources [optional]
<!-- Provide the basic links for the model. -->
- **Repository:** [More Information Needed]
- **Paper [optional]:** [More Information Needed]
- **Demo [optional]:** [More Information Needed]
## Uses
<!-- Address questions around how the model is intended to be used, including the foreseeable users of the model and those affected by the model. -->
### Direct Use
<!-- This section is for the model use without fine-tuning or plugging into a larger ecosystem/app. -->
[More Information Needed]
### Downstream Use [optional]
<!-- This section is for the model use when fine-tuned for a task, or when plugged into a larger ecosystem/app -->
[More Information Needed]
### Out-of-Scope Use
<!-- This section addresses misuse, malicious use, and uses that the model will not work well for. -->
[More Information Needed]
## Bias, Risks, and Limitations
<!-- This section is meant to convey both technical and sociotechnical limitations. -->
[More Information Needed]
### Recommendations
<!-- This section is meant to convey recommendations with respect to the bias, risk, and technical limitations. -->
Users (both direct and downstream) should be made aware of the risks, biases and limitations of the model. More information needed for further recommendations.
## How to Get Started with the Model
Use the code below to get started with the model.
[More Information Needed]
## Training Details
### Training Data
<!-- This should link to a Dataset Card, perhaps with a short stub of information on what the training data is all about as well as documentation related to data pre-processing or additional filtering. -->
[More Information Needed]
### Training Procedure
<!-- This relates heavily to the Technical Specifications. Content here should link to that section when it is relevant to the training procedure. -->
#### Preprocessing [optional]
[More Information Needed]
#### Training Hyperparameters
- **Training regime:** [More Information Needed] <!--fp32, fp16 mixed precision, bf16 mixed precision, bf16 non-mixed precision, fp16 non-mixed precision, fp8 mixed precision -->
#### Speeds, Sizes, Times [optional]
<!-- This section provides information about throughput, start/end time, checkpoint size if relevant, etc. -->
[More Information Needed]
## Evaluation
<!-- This section describes the evaluation protocols and provides the results. -->
### Testing Data, Factors & Metrics
#### Testing Data
<!-- This should link to a Dataset Card if possible. -->
[More Information Needed]
#### Factors
<!-- These are the things the evaluation is disaggregating by, e.g., subpopulations or domains. -->
[More Information Needed]
#### Metrics
<!-- These are the evaluation metrics being used, ideally with a description of why. -->
[More Information Needed]
### Results
[More Information Needed]
#### Summary
## Model Examination [optional]
<!-- Relevant interpretability work for the model goes here -->
[More Information Needed]
## Environmental Impact
<!-- Total emissions (in grams of CO2eq) and additional considerations, such as electricity usage, go here. Edit the suggested text below accordingly -->
Carbon emissions can be estimated using the [Machine Learning Impact calculator](https://mlco2.github.io/impact#compute) presented in [Lacoste et al. (2019)](https://arxiv.org/abs/1910.09700).
- **Hardware Type:** [More Information Needed]
- **Hours used:** [More Information Needed]
- **Cloud Provider:** [More Information Needed]
- **Compute Region:** [More Information Needed]
- **Carbon Emitted:** [More Information Needed]
## Technical Specifications [optional]
### Model Architecture and Objective
[More Information Needed]
### Compute Infrastructure
[More Information Needed]
#### Hardware
[More Information Needed]
#### Software
[More Information Needed]
## Citation [optional]
<!-- If there is a paper or blog post introducing the model, the APA and Bibtex information for that should go in this section. -->
**BibTeX:**
[More Information Needed]
**APA:**
[More Information Needed]
## Glossary [optional]
<!-- If relevant, include terms and calculations in this section that can help readers understand the model or model card. -->
[More Information Needed]
## More Information [optional]
[More Information Needed]
## Model Card Authors [optional]
[More Information Needed]
## Model Card Contact
[More Information Needed] |
El-Patron-XXX/wATCH.El-Patron-Viral-Video.original | El-Patron-XXX | "2025-02-17T19:20:39Z" | 0 | 0 | null | [
"region:us"
] | null | "2025-02-17T19:18:59Z" | [🔴 ➤►𝐂𝐥𝐢𝐤 𝐇𝐞𝐫𝐞 𝐭𝐨👉👉 (𝐖𝐚𝐭𝐜𝐡 𝐅𝐮𝐥𝐥 𝐯𝐢𝐝𝐞𝐨)](https://lekedvideo.xyz/watch/?v=El-Patron)
[🔴 ➤►𝐂𝐥𝐢𝐤 𝐇𝐞𝐫𝐞 𝐭𝐨👉👉 (𝐅𝐮𝐥𝐥 𝐯𝐢𝐝𝐞𝐨 𝐋𝐢𝐧𝐤 )](https://lekedvideo.xyz/watch/?v=El-Patron)
[<img alt="fsd" src="https://i.postimg.cc/qvPp49Sm/ythngythg.gif">](https://lekedvideo.xyz/watch/?v=El-Patron) |
reach-vb/gemma-2b-it-Q4_K_M-GGUF | reach-vb | "2024-04-03T09:30:20Z" | 2 | 0 | transformers | [
"transformers",
"gguf",
"llama-cpp",
"license:gemma",
"endpoints_compatible",
"region:us",
"conversational"
] | null | "2024-04-03T09:30:08Z" | ---
license: gemma
library_name: transformers
tags:
- llama-cpp
widget:
- messages:
- role: user
content: How does the brain work?
inference:
parameters:
max_new_tokens: 200
extra_gated_heading: Access Gemma on Hugging Face
extra_gated_prompt: To access Gemma on Hugging Face, you’re required to review and
agree to Google’s usage license. To do this, please ensure you’re logged-in to Hugging
Face and click below. Requests are processed immediately.
extra_gated_button_content: Acknowledge license
---
# reach-vb/gemma-2b-it-Q4_K_M-GGUF
This model was converted to GGUF format from [`google/gemma-2b-it`](https://huggingface.co/google/gemma-2b-it) using llama.cpp.
Refer to the [original model card](https://huggingface.co/google/gemma-2b-it) for more details on the model.
## Use with llama.cpp
```bash
brew install ggerganov/ggerganov/llama.cpp
```
```bash
llama-cli --hf-repo reach-vb/gemma-2b-it-Q4_K_M-GGUF --model gemma-2b-it.Q4_K_M.gguf -p "The meaning to life and the universe is "
```
```bash
llama-server --hf-repo reach-vb/gemma-2b-it-Q4_K_M-GGUF --model gemma-2b-it.Q4_K_M.gguf -c 2048
```
|
AlGM93/q-FrozenLake-v1-4x4-noSlippery | AlGM93 | "2024-02-09T20:06:06Z" | 0 | 0 | null | [
"FrozenLake-v1-4x4-no_slippery",
"q-learning",
"reinforcement-learning",
"custom-implementation",
"model-index",
"region:us"
] | reinforcement-learning | "2024-02-09T20:06:04Z" | ---
tags:
- FrozenLake-v1-4x4-no_slippery
- q-learning
- reinforcement-learning
- custom-implementation
model-index:
- name: q-FrozenLake-v1-4x4-noSlippery
results:
- task:
type: reinforcement-learning
name: reinforcement-learning
dataset:
name: FrozenLake-v1-4x4-no_slippery
type: FrozenLake-v1-4x4-no_slippery
metrics:
- type: mean_reward
value: 1.00 +/- 0.00
name: mean_reward
verified: false
---
# **Q-Learning** Agent playing1 **FrozenLake-v1**
This is a trained model of a **Q-Learning** agent playing **FrozenLake-v1** .
## Usage
```python
model = load_from_hub(repo_id="AlGM93/q-FrozenLake-v1-4x4-noSlippery", filename="q-learning.pkl")
# Don't forget to check if you need to add additional attributes (is_slippery=False etc)
env = gym.make(model["env_id"])
```
|
mami99/my_first_model | mami99 | "2023-09-27T19:43:02Z" | 94 | 0 | transformers | [
"transformers",
"pytorch",
"distilbert",
"token-classification",
"generated_from_trainer",
"dataset:wnut_17",
"base_model:distilbert/distilbert-base-uncased",
"base_model:finetune:distilbert/distilbert-base-uncased",
"license:apache-2.0",
"model-index",
"autotrain_compatible",
"endpoints_compatible",
"region:us"
] | token-classification | "2023-09-27T19:00:15Z" | ---
license: apache-2.0
base_model: distilbert-base-uncased
tags:
- generated_from_trainer
datasets:
- wnut_17
metrics:
- precision
- recall
- f1
- accuracy
model-index:
- name: my_first_model
results:
- task:
name: Token Classification
type: token-classification
dataset:
name: wnut_17
type: wnut_17
config: wnut_17
split: test
args: wnut_17
metrics:
- name: Precision
type: precision
value: 0.5806451612903226
- name: Recall
type: recall
value: 0.3002780352177943
- name: F1
type: f1
value: 0.39584605986560784
- name: Accuracy
type: accuracy
value: 0.9416869736223333
---
<!-- This model card has been generated automatically according to the information the Trainer had access to. You
should probably proofread and complete it, then remove this comment. -->
# my_first_model
This model is a fine-tuned version of [distilbert-base-uncased](https://huggingface.co/distilbert-base-uncased) on the wnut_17 dataset.
It achieves the following results on the evaluation set:
- Loss: 0.2670
- Precision: 0.5806
- Recall: 0.3003
- F1: 0.3958
- Accuracy: 0.9417
## Model description
More information needed
## Intended uses & limitations
More information needed
## Training and evaluation data
More information needed
## Training procedure
### Training hyperparameters
The following hyperparameters were used during training:
- learning_rate: 2e-05
- train_batch_size: 16
- eval_batch_size: 16
- seed: 42
- optimizer: Adam with betas=(0.9,0.999) and epsilon=1e-08
- lr_scheduler_type: linear
- num_epochs: 2
### Training results
| Training Loss | Epoch | Step | Validation Loss | Precision | Recall | F1 | Accuracy |
|:-------------:|:-----:|:----:|:---------------:|:---------:|:------:|:------:|:--------:|
| No log | 1.0 | 213 | 0.2772 | 0.6181 | 0.2595 | 0.3655 | 0.9395 |
| No log | 2.0 | 426 | 0.2670 | 0.5806 | 0.3003 | 0.3958 | 0.9417 |
### Framework versions
- Transformers 4.33.2
- Pytorch 2.0.1+cu117
- Datasets 2.14.5
- Tokenizers 0.13.2
|
mradermacher/MN-12B-LilithFrame-Experiment-3-GGUF | mradermacher | "2025-01-30T17:00:27Z" | 204 | 1 | transformers | [
"transformers",
"gguf",
"mergekit",
"merge",
"en",
"base_model:DoppelReflEx/MN-12B-LilithFrame-Experiment-3",
"base_model:quantized:DoppelReflEx/MN-12B-LilithFrame-Experiment-3",
"endpoints_compatible",
"region:us",
"conversational"
] | null | "2025-01-30T14:50:33Z" | ---
base_model: DoppelReflEx/MN-12B-LilithFrame-Experiment-3
language:
- en
library_name: transformers
quantized_by: mradermacher
tags:
- mergekit
- merge
---
## About
<!-- ### quantize_version: 2 -->
<!-- ### output_tensor_quantised: 1 -->
<!-- ### convert_type: hf -->
<!-- ### vocab_type: -->
<!-- ### tags: nicoboss -->
static quants of https://huggingface.co/DoppelReflEx/MN-12B-LilithFrame-Experiment-3
<!-- provided-files -->
weighted/imatrix quants seem not to be available (by me) at this time. If they do not show up a week or so after the static ones, I have probably not planned for them. Feel free to request them by opening a Community Discussion.
## Usage
If you are unsure how to use GGUF files, refer to one of [TheBloke's
READMEs](https://huggingface.co/TheBloke/KafkaLM-70B-German-V0.1-GGUF) for
more details, including on how to concatenate multi-part files.
## Provided Quants
(sorted by size, not necessarily quality. IQ-quants are often preferable over similar sized non-IQ quants)
| Link | Type | Size/GB | Notes |
|:-----|:-----|--------:|:------|
| [GGUF](https://huggingface.co/mradermacher/MN-12B-LilithFrame-Experiment-3-GGUF/resolve/main/MN-12B-LilithFrame-Experiment-3.Q2_K.gguf) | Q2_K | 4.9 | |
| [GGUF](https://huggingface.co/mradermacher/MN-12B-LilithFrame-Experiment-3-GGUF/resolve/main/MN-12B-LilithFrame-Experiment-3.Q3_K_S.gguf) | Q3_K_S | 5.6 | |
| [GGUF](https://huggingface.co/mradermacher/MN-12B-LilithFrame-Experiment-3-GGUF/resolve/main/MN-12B-LilithFrame-Experiment-3.Q3_K_M.gguf) | Q3_K_M | 6.2 | lower quality |
| [GGUF](https://huggingface.co/mradermacher/MN-12B-LilithFrame-Experiment-3-GGUF/resolve/main/MN-12B-LilithFrame-Experiment-3.Q3_K_L.gguf) | Q3_K_L | 6.7 | |
| [GGUF](https://huggingface.co/mradermacher/MN-12B-LilithFrame-Experiment-3-GGUF/resolve/main/MN-12B-LilithFrame-Experiment-3.IQ4_XS.gguf) | IQ4_XS | 6.9 | |
| [GGUF](https://huggingface.co/mradermacher/MN-12B-LilithFrame-Experiment-3-GGUF/resolve/main/MN-12B-LilithFrame-Experiment-3.Q4_K_S.gguf) | Q4_K_S | 7.2 | fast, recommended |
| [GGUF](https://huggingface.co/mradermacher/MN-12B-LilithFrame-Experiment-3-GGUF/resolve/main/MN-12B-LilithFrame-Experiment-3.Q4_K_M.gguf) | Q4_K_M | 7.6 | fast, recommended |
| [GGUF](https://huggingface.co/mradermacher/MN-12B-LilithFrame-Experiment-3-GGUF/resolve/main/MN-12B-LilithFrame-Experiment-3.Q5_K_S.gguf) | Q5_K_S | 8.6 | |
| [GGUF](https://huggingface.co/mradermacher/MN-12B-LilithFrame-Experiment-3-GGUF/resolve/main/MN-12B-LilithFrame-Experiment-3.Q5_K_M.gguf) | Q5_K_M | 8.8 | |
| [GGUF](https://huggingface.co/mradermacher/MN-12B-LilithFrame-Experiment-3-GGUF/resolve/main/MN-12B-LilithFrame-Experiment-3.Q6_K.gguf) | Q6_K | 10.2 | very good quality |
| [GGUF](https://huggingface.co/mradermacher/MN-12B-LilithFrame-Experiment-3-GGUF/resolve/main/MN-12B-LilithFrame-Experiment-3.Q8_0.gguf) | Q8_0 | 13.1 | fast, best quality |
Here is a handy graph by ikawrakow comparing some lower-quality quant
types (lower is better):

And here are Artefact2's thoughts on the matter:
https://gist.github.com/Artefact2/b5f810600771265fc1e39442288e8ec9
## FAQ / Model Request
See https://huggingface.co/mradermacher/model_requests for some answers to
questions you might have and/or if you want some other model quantized.
## Thanks
I thank my company, [nethype GmbH](https://www.nethype.de/), for letting
me use its servers and providing upgrades to my workstation to enable
this work in my free time. Additional thanks to [@nicoboss](https://huggingface.co/nicoboss) for giving me access to his private supercomputer, enabling me to provide many more imatrix quants, at much higher quality, than I would otherwise be able to.
<!-- end -->
|
nadejdatarabukina/6ff8907c-c77b-4c39-a1b2-d99d2d4d2350 | nadejdatarabukina | "2025-01-23T19:15:46Z" | 8 | 0 | peft | [
"peft",
"safetensors",
"qwen2",
"axolotl",
"generated_from_trainer",
"base_model:Qwen/Qwen2.5-Coder-7B-Instruct",
"base_model:adapter:Qwen/Qwen2.5-Coder-7B-Instruct",
"license:apache-2.0",
"region:us"
] | null | "2025-01-23T18:51:42Z" | ---
library_name: peft
license: apache-2.0
base_model: Qwen/Qwen2.5-Coder-7B-Instruct
tags:
- axolotl
- generated_from_trainer
model-index:
- name: 6ff8907c-c77b-4c39-a1b2-d99d2d4d2350
results: []
---
<!-- This model card has been generated automatically according to the information the Trainer had access to. You
should probably proofread and complete it, then remove this comment. -->
[<img src="https://raw.githubusercontent.com/axolotl-ai-cloud/axolotl/main/image/axolotl-badge-web.png" alt="Built with Axolotl" width="200" height="32"/>](https://github.com/axolotl-ai-cloud/axolotl)
<details><summary>See axolotl config</summary>
axolotl version: `0.4.1`
```yaml
adapter: lora
base_model: Qwen/Qwen2.5-Coder-7B-Instruct
bf16: auto
chat_template: llama3
dataset_prepared_path: null
datasets:
- data_files:
- 4b47e3ddd7129f5f_train_data.json
ds_type: json
format: custom
path: /workspace/input_data/4b47e3ddd7129f5f_train_data.json
type:
field_input: input
field_instruction: instruction
field_output: output
format: '{instruction} {input}'
no_input_format: '{instruction}'
system_format: '{system}'
system_prompt: ''
debug: null
deepspeed: null
device: cuda
early_stopping_patience: null
eval_max_new_tokens: 128
eval_steps: 5
eval_table_size: null
evals_per_epoch: null
flash_attention: false
fp16: null
gradient_accumulation_steps: 4
gradient_checkpointing: true
group_by_length: false
hub_model_id: nadejdatarabukina/6ff8907c-c77b-4c39-a1b2-d99d2d4d2350
hub_repo: null
hub_strategy: checkpoint
hub_token: null
learning_rate: 0.0002
load_in_4bit: false
load_in_8bit: false
local_rank: null
logging_steps: 3
lora_alpha: 32
lora_dropout: 0.05
lora_fan_in_fan_out: null
lora_model_dir: null
lora_r: 16
lora_target_linear: true
lr_scheduler: cosine
max_memory:
0: 75GiB
max_steps: 30
micro_batch_size: 2
mlflow_experiment_name: /tmp/4b47e3ddd7129f5f_train_data.json
model_type: AutoModelForCausalLM
num_epochs: 1
optimizer: adamw_torch
output_dir: miner_id_24
pad_to_sequence_len: true
resume_from_checkpoint: null
s2_attention: null
sample_packing: false
save_steps: 10
sequence_len: 1024
strict: false
tf32: false
tokenizer_type: AutoTokenizer
train_on_inputs: true
trust_remote_code: true
val_set_size: 0.05
wandb_entity: null
wandb_mode: online
wandb_name: d82e5b23-2360-4fb1-ba8e-609b2af93cfa
wandb_project: Gradients-On-Demand
wandb_run: your_name
wandb_runid: d82e5b23-2360-4fb1-ba8e-609b2af93cfa
warmup_steps: 10
weight_decay: 0.01
xformers_attention: true
```
</details><br>
# 6ff8907c-c77b-4c39-a1b2-d99d2d4d2350
This model is a fine-tuned version of [Qwen/Qwen2.5-Coder-7B-Instruct](https://huggingface.co/Qwen/Qwen2.5-Coder-7B-Instruct) on the None dataset.
It achieves the following results on the evaluation set:
- Loss: 0.1724
## Model description
More information needed
## Intended uses & limitations
More information needed
## Training and evaluation data
More information needed
## Training procedure
### Training hyperparameters
The following hyperparameters were used during training:
- learning_rate: 0.0002
- train_batch_size: 2
- eval_batch_size: 2
- seed: 42
- gradient_accumulation_steps: 4
- total_train_batch_size: 8
- optimizer: Use OptimizerNames.ADAMW_TORCH with betas=(0.9,0.999) and epsilon=1e-08 and optimizer_args=No additional optimizer arguments
- lr_scheduler_type: cosine
- lr_scheduler_warmup_steps: 10
- training_steps: 30
### Training results
| Training Loss | Epoch | Step | Validation Loss |
|:-------------:|:------:|:----:|:---------------:|
| No log | 0.0004 | 1 | 1.1440 |
| 1.1414 | 0.0018 | 5 | 1.0712 |
| 0.9012 | 0.0036 | 10 | 0.5820 |
| 0.2894 | 0.0054 | 15 | 0.2234 |
| 0.2345 | 0.0072 | 20 | 0.1830 |
| 0.1518 | 0.0090 | 25 | 0.1740 |
| 0.1727 | 0.0108 | 30 | 0.1724 |
### Framework versions
- PEFT 0.13.2
- Transformers 4.46.0
- Pytorch 2.5.0+cu124
- Datasets 3.0.1
- Tokenizers 0.20.1 |
jayr23/c3di | jayr23 | "2024-10-15T16:43:01Z" | 7 | 0 | diffusers | [
"diffusers",
"text-to-image",
"flux",
"lora",
"template:sd-lora",
"fluxgym",
"base_model:black-forest-labs/FLUX.1-dev",
"base_model:adapter:black-forest-labs/FLUX.1-dev",
"license:other",
"region:us"
] | text-to-image | "2024-10-15T16:42:56Z" | ---
tags:
- text-to-image
- flux
- lora
- diffusers
- template:sd-lora
- fluxgym
base_model: black-forest-labs/FLUX.1-dev
instance_prompt: C3di
license: other
license_name: flux-1-dev-non-commercial-license
license_link: https://huggingface.co/black-forest-labs/FLUX.1-dev/blob/main/LICENSE.md
---
# C3di
A Flux LoRA trained on a local computer with [Fluxgym](https://github.com/cocktailpeanut/fluxgym)
<Gallery />
## Trigger words
You should use `C3di` to trigger the image generation.
## Download model and use it with ComfyUI, AUTOMATIC1111, SD.Next, Invoke AI, Forge, etc.
Weights for this model are available in Safetensors format.
|
xaviviro/llama-2-7b-chat-catala | xaviviro | "2023-12-27T09:02:39Z" | 5 | 0 | transformers | [
"transformers",
"pytorch",
"llama",
"text-generation",
"ca",
"en",
"dataset:xaviviro/oasst1_ca_threads",
"base_model:NousResearch/Llama-2-7b-chat-hf",
"base_model:finetune:NousResearch/Llama-2-7b-chat-hf",
"license:apache-2.0",
"autotrain_compatible",
"text-generation-inference",
"endpoints_compatible",
"region:us"
] | text-generation | "2023-12-26T22:51:41Z" | ---
base_model: NousResearch/Llama-2-7b-chat-hf
datasets:
- xaviviro/oasst1_ca_threads
language:
- ca
- en
model_type: llama
prompt_template: >-
<s>[INST] <<SYS>> Ets un xatbot genèric que sempre respon en català. <</SYS>>
{instruction} [/INST]
license: apache-2.0
---
# llama-2-7b-chat-catala
## Prompt template
```
<s>[INST] <<SYS>> Ets un xatbot genèric que sempre respon en català. <</SYS>> {instruction} [/INST]
``` |
bdsqlsz/FaceBeauty | bdsqlsz | "2023-05-13T13:50:06Z" | 0 | 1 | null | [
"license:creativeml-openrail-m",
"region:us"
] | null | "2023-05-13T13:47:22Z" | ---
license: creativeml-openrail-m
---
|
mradermacher/Hathor_Respawn-L3-8B-v0.8-GGUF | mradermacher | "2024-12-16T01:58:28Z" | 11 | 0 | transformers | [
"transformers",
"gguf",
"en",
"base_model:ChaoticNeutrals/Hathor_Respawn-L3-8B-v0.8",
"base_model:quantized:ChaoticNeutrals/Hathor_Respawn-L3-8B-v0.8",
"license:other",
"endpoints_compatible",
"region:us",
"conversational"
] | null | "2024-07-07T00:25:15Z" | ---
base_model: ChaoticNeutrals/Hathor_Respawn-L3-8B-v0.8
language:
- en
library_name: transformers
license: other
quantized_by: mradermacher
---
## About
<!-- ### quantize_version: 2 -->
<!-- ### output_tensor_quantised: 1 -->
<!-- ### convert_type: hf -->
<!-- ### vocab_type: -->
<!-- ### tags: -->
static quants of https://huggingface.co/ChaoticNeutrals/Hathor_Respawn-L3-8B-v0.8
<!-- provided-files -->
weighted/imatrix quants are available at https://huggingface.co/mradermacher/Hathor_Respawn-L3-8B-v0.8-i1-GGUF
## Usage
If you are unsure how to use GGUF files, refer to one of [TheBloke's
READMEs](https://huggingface.co/TheBloke/KafkaLM-70B-German-V0.1-GGUF) for
more details, including on how to concatenate multi-part files.
## Provided Quants
(sorted by size, not necessarily quality. IQ-quants are often preferable over similar sized non-IQ quants)
| Link | Type | Size/GB | Notes |
|:-----|:-----|--------:|:------|
| [GGUF](https://huggingface.co/mradermacher/Hathor_Respawn-L3-8B-v0.8-GGUF/resolve/main/Hathor_Respawn-L3-8B-v0.8.Q2_K.gguf) | Q2_K | 3.3 | |
| [GGUF](https://huggingface.co/mradermacher/Hathor_Respawn-L3-8B-v0.8-GGUF/resolve/main/Hathor_Respawn-L3-8B-v0.8.IQ3_XS.gguf) | IQ3_XS | 3.6 | |
| [GGUF](https://huggingface.co/mradermacher/Hathor_Respawn-L3-8B-v0.8-GGUF/resolve/main/Hathor_Respawn-L3-8B-v0.8.Q3_K_S.gguf) | Q3_K_S | 3.8 | |
| [GGUF](https://huggingface.co/mradermacher/Hathor_Respawn-L3-8B-v0.8-GGUF/resolve/main/Hathor_Respawn-L3-8B-v0.8.IQ3_S.gguf) | IQ3_S | 3.8 | beats Q3_K* |
| [GGUF](https://huggingface.co/mradermacher/Hathor_Respawn-L3-8B-v0.8-GGUF/resolve/main/Hathor_Respawn-L3-8B-v0.8.IQ3_M.gguf) | IQ3_M | 3.9 | |
| [GGUF](https://huggingface.co/mradermacher/Hathor_Respawn-L3-8B-v0.8-GGUF/resolve/main/Hathor_Respawn-L3-8B-v0.8.Q3_K_M.gguf) | Q3_K_M | 4.1 | lower quality |
| [GGUF](https://huggingface.co/mradermacher/Hathor_Respawn-L3-8B-v0.8-GGUF/resolve/main/Hathor_Respawn-L3-8B-v0.8.Q3_K_L.gguf) | Q3_K_L | 4.4 | |
| [GGUF](https://huggingface.co/mradermacher/Hathor_Respawn-L3-8B-v0.8-GGUF/resolve/main/Hathor_Respawn-L3-8B-v0.8.IQ4_XS.gguf) | IQ4_XS | 4.6 | |
| [GGUF](https://huggingface.co/mradermacher/Hathor_Respawn-L3-8B-v0.8-GGUF/resolve/main/Hathor_Respawn-L3-8B-v0.8.Q4_K_S.gguf) | Q4_K_S | 4.8 | fast, recommended |
| [GGUF](https://huggingface.co/mradermacher/Hathor_Respawn-L3-8B-v0.8-GGUF/resolve/main/Hathor_Respawn-L3-8B-v0.8.Q4_K_M.gguf) | Q4_K_M | 5.0 | fast, recommended |
| [GGUF](https://huggingface.co/mradermacher/Hathor_Respawn-L3-8B-v0.8-GGUF/resolve/main/Hathor_Respawn-L3-8B-v0.8.Q5_K_S.gguf) | Q5_K_S | 5.7 | |
| [GGUF](https://huggingface.co/mradermacher/Hathor_Respawn-L3-8B-v0.8-GGUF/resolve/main/Hathor_Respawn-L3-8B-v0.8.Q5_K_M.gguf) | Q5_K_M | 5.8 | |
| [GGUF](https://huggingface.co/mradermacher/Hathor_Respawn-L3-8B-v0.8-GGUF/resolve/main/Hathor_Respawn-L3-8B-v0.8.Q6_K.gguf) | Q6_K | 6.7 | very good quality |
| [GGUF](https://huggingface.co/mradermacher/Hathor_Respawn-L3-8B-v0.8-GGUF/resolve/main/Hathor_Respawn-L3-8B-v0.8.Q8_0.gguf) | Q8_0 | 8.6 | fast, best quality |
| [GGUF](https://huggingface.co/mradermacher/Hathor_Respawn-L3-8B-v0.8-GGUF/resolve/main/Hathor_Respawn-L3-8B-v0.8.f16.gguf) | f16 | 16.2 | 16 bpw, overkill |
Here is a handy graph by ikawrakow comparing some lower-quality quant
types (lower is better):

And here are Artefact2's thoughts on the matter:
https://gist.github.com/Artefact2/b5f810600771265fc1e39442288e8ec9
## FAQ / Model Request
See https://huggingface.co/mradermacher/model_requests for some answers to
questions you might have and/or if you want some other model quantized.
## Thanks
I thank my company, [nethype GmbH](https://www.nethype.de/), for letting
me use its servers and providing upgrades to my workstation to enable
this work in my free time.
<!-- end -->
|
portal-875-vitoria-ribeiro-regina-videoS/New.Video.875-vitoria.ribeiro.VIDEO.portal.zacarias | portal-875-vitoria-ribeiro-regina-videoS | "2025-04-02T10:37:56Z" | 0 | 0 | null | [
"region:us"
] | null | "2025-04-02T10:37:35Z" | <animated-image data-catalyst=""><a href="https://tinyurl.com/5n6bjbnr?news-viral-video" rel="nofollow" data-target="animated-image.originalLink"><img src="https://static.wixstatic.com/media/b249f9_adac8f70fb3f45b88691696c77de18f3~mv2.gif" alt="Foo" data-canonical-src="https://static.wixstatic.com/media/b249f9_adac8f70fb3f45b88691696c77de18f3~mv2.gif" style="max-width: 100%; display: inline-block;" data-target="animated-image.originalImage"></a> |
EITD/phi_2 | EITD | "2024-11-30T18:53:35Z" | 78 | 0 | transformers | [
"transformers",
"safetensors",
"llama",
"text-generation",
"text-generation-inference",
"unsloth",
"trl",
"sft",
"conversational",
"en",
"base_model:unsloth/Phi-3.5-mini-instruct",
"base_model:finetune:unsloth/Phi-3.5-mini-instruct",
"license:apache-2.0",
"autotrain_compatible",
"endpoints_compatible",
"region:us"
] | text-generation | "2024-11-30T18:52:44Z" | ---
base_model: unsloth/Phi-3.5-mini-instruct
tags:
- text-generation-inference
- transformers
- unsloth
- llama
- trl
- sft
license: apache-2.0
language:
- en
---
# Uploaded model
- **Developed by:** EITD
- **License:** apache-2.0
- **Finetuned from model :** unsloth/Phi-3.5-mini-instruct
This llama model was trained 2x faster with [Unsloth](https://github.com/unslothai/unsloth) and Huggingface's TRL library.
[<img src="https://raw.githubusercontent.com/unslothai/unsloth/main/images/unsloth%20made%20with%20love.png" width="200"/>](https://github.com/unslothai/unsloth)
|
albertus-sussex/veriscrape-simcse-job-reference_3_to_verify_7-fold-3 | albertus-sussex | "2025-03-26T17:17:28Z" | 0 | 0 | transformers | [
"transformers",
"safetensors",
"roberta",
"feature-extraction",
"arxiv:1910.09700",
"endpoints_compatible",
"region:us"
] | feature-extraction | "2025-03-26T16:10:14Z" | ---
library_name: transformers
tags: []
---
# Model Card for Model ID
<!-- Provide a quick summary of what the model is/does. -->
## Model Details
### Model Description
<!-- Provide a longer summary of what this model is. -->
This is the model card of a 🤗 transformers model that has been pushed on the Hub. This model card has been automatically generated.
- **Developed by:** [More Information Needed]
- **Funded by [optional]:** [More Information Needed]
- **Shared by [optional]:** [More Information Needed]
- **Model type:** [More Information Needed]
- **Language(s) (NLP):** [More Information Needed]
- **License:** [More Information Needed]
- **Finetuned from model [optional]:** [More Information Needed]
### Model Sources [optional]
<!-- Provide the basic links for the model. -->
- **Repository:** [More Information Needed]
- **Paper [optional]:** [More Information Needed]
- **Demo [optional]:** [More Information Needed]
## Uses
<!-- Address questions around how the model is intended to be used, including the foreseeable users of the model and those affected by the model. -->
### Direct Use
<!-- This section is for the model use without fine-tuning or plugging into a larger ecosystem/app. -->
[More Information Needed]
### Downstream Use [optional]
<!-- This section is for the model use when fine-tuned for a task, or when plugged into a larger ecosystem/app -->
[More Information Needed]
### Out-of-Scope Use
<!-- This section addresses misuse, malicious use, and uses that the model will not work well for. -->
[More Information Needed]
## Bias, Risks, and Limitations
<!-- This section is meant to convey both technical and sociotechnical limitations. -->
[More Information Needed]
### Recommendations
<!-- This section is meant to convey recommendations with respect to the bias, risk, and technical limitations. -->
Users (both direct and downstream) should be made aware of the risks, biases and limitations of the model. More information needed for further recommendations.
## How to Get Started with the Model
Use the code below to get started with the model.
[More Information Needed]
## Training Details
### Training Data
<!-- This should link to a Dataset Card, perhaps with a short stub of information on what the training data is all about as well as documentation related to data pre-processing or additional filtering. -->
[More Information Needed]
### Training Procedure
<!-- This relates heavily to the Technical Specifications. Content here should link to that section when it is relevant to the training procedure. -->
#### Preprocessing [optional]
[More Information Needed]
#### Training Hyperparameters
- **Training regime:** [More Information Needed] <!--fp32, fp16 mixed precision, bf16 mixed precision, bf16 non-mixed precision, fp16 non-mixed precision, fp8 mixed precision -->
#### Speeds, Sizes, Times [optional]
<!-- This section provides information about throughput, start/end time, checkpoint size if relevant, etc. -->
[More Information Needed]
## Evaluation
<!-- This section describes the evaluation protocols and provides the results. -->
### Testing Data, Factors & Metrics
#### Testing Data
<!-- This should link to a Dataset Card if possible. -->
[More Information Needed]
#### Factors
<!-- These are the things the evaluation is disaggregating by, e.g., subpopulations or domains. -->
[More Information Needed]
#### Metrics
<!-- These are the evaluation metrics being used, ideally with a description of why. -->
[More Information Needed]
### Results
[More Information Needed]
#### Summary
## Model Examination [optional]
<!-- Relevant interpretability work for the model goes here -->
[More Information Needed]
## Environmental Impact
<!-- Total emissions (in grams of CO2eq) and additional considerations, such as electricity usage, go here. Edit the suggested text below accordingly -->
Carbon emissions can be estimated using the [Machine Learning Impact calculator](https://mlco2.github.io/impact#compute) presented in [Lacoste et al. (2019)](https://arxiv.org/abs/1910.09700).
- **Hardware Type:** [More Information Needed]
- **Hours used:** [More Information Needed]
- **Cloud Provider:** [More Information Needed]
- **Compute Region:** [More Information Needed]
- **Carbon Emitted:** [More Information Needed]
## Technical Specifications [optional]
### Model Architecture and Objective
[More Information Needed]
### Compute Infrastructure
[More Information Needed]
#### Hardware
[More Information Needed]
#### Software
[More Information Needed]
## Citation [optional]
<!-- If there is a paper or blog post introducing the model, the APA and Bibtex information for that should go in this section. -->
**BibTeX:**
[More Information Needed]
**APA:**
[More Information Needed]
## Glossary [optional]
<!-- If relevant, include terms and calculations in this section that can help readers understand the model or model card. -->
[More Information Needed]
## More Information [optional]
[More Information Needed]
## Model Card Authors [optional]
[More Information Needed]
## Model Card Contact
[More Information Needed] |
tensorblock/Samantha2.0-Phi3-Medium-ita-16bit-GGUF | tensorblock | "2025-03-06T18:32:43Z" | 0 | 0 | transformers | [
"transformers",
"gguf",
"text-generation-inference",
"unsloth",
"qwen2",
"trl",
"sft",
"psychology",
"EQ",
"conversational",
"NLP",
"companion",
"TensorBlock",
"GGUF",
"text-generation",
"it",
"dataset:WasamiKirua/Samantha2.0-ITA",
"dataset:WasamiKirua/haiku-ita-v0.2",
"base_model:WasamiKirua/Samantha2.0-Phi3-Medium-ita-16bit",
"base_model:quantized:WasamiKirua/Samantha2.0-Phi3-Medium-ita-16bit",
"license:apache-2.0",
"endpoints_compatible",
"region:us"
] | text-generation | "2025-03-06T17:18:53Z" | ---
base_model: WasamiKirua/Samantha2.0-Phi3-Medium-ita-16bit
tags:
- text-generation-inference
- transformers
- unsloth
- qwen2
- trl
- sft
- psychology
- EQ
- conversational
- NLP
- companion
- TensorBlock
- GGUF
license: apache-2.0
language:
- it
pipeline_tag: text-generation
datasets:
- WasamiKirua/Samantha2.0-ITA
- WasamiKirua/haiku-ita-v0.2
---
<div style="width: auto; margin-left: auto; margin-right: auto">
<img src="https://i.imgur.com/jC7kdl8.jpeg" alt="TensorBlock" style="width: 100%; min-width: 400px; display: block; margin: auto;">
</div>
<div style="display: flex; justify-content: space-between; width: 100%;">
<div style="display: flex; flex-direction: column; align-items: flex-start;">
<p style="margin-top: 0.5em; margin-bottom: 0em;">
Feedback and support: TensorBlock's <a href="https://x.com/tensorblock_aoi">Twitter/X</a>, <a href="https://t.me/TensorBlock">Telegram Group</a> and <a href="https://x.com/tensorblock_aoi">Discord server</a>
</p>
</div>
</div>
## WasamiKirua/Samantha2.0-Phi3-Medium-ita-16bit - GGUF
This repo contains GGUF format model files for [WasamiKirua/Samantha2.0-Phi3-Medium-ita-16bit](https://huggingface.co/WasamiKirua/Samantha2.0-Phi3-Medium-ita-16bit).
The files were quantized using machines provided by [TensorBlock](https://tensorblock.co/), and they are compatible with llama.cpp as of [commit b4823](https://github.com/ggml-org/llama.cpp/commit/5bbe6a9fe9a8796a9389c85accec89dbc4d91e39).
<div style="text-align: left; margin: 20px 0;">
<a href="https://tensorblock.co/waitlist/client" style="display: inline-block; padding: 10px 20px; background-color: #007bff; color: white; text-decoration: none; border-radius: 5px; font-weight: bold;">
Run them on the TensorBlock client using your local machine ↗
</a>
</div>
## Prompt template
```
<|im_start|>system
{system_prompt}<|im_end|>
<|im_start|>user
{prompt}<|im_end|>
<|im_start|>assistant
```
## Model file specification
| Filename | Quant type | File Size | Description |
| -------- | ---------- | --------- | ----------- |
| [Samantha2.0-Phi3-Medium-ita-16bit-Q2_K.gguf](https://huggingface.co/tensorblock/Samantha2.0-Phi3-Medium-ita-16bit-GGUF/blob/main/Samantha2.0-Phi3-Medium-ita-16bit-Q2_K.gguf) | Q2_K | 5.204 GB | smallest, significant quality loss - not recommended for most purposes |
| [Samantha2.0-Phi3-Medium-ita-16bit-Q3_K_S.gguf](https://huggingface.co/tensorblock/Samantha2.0-Phi3-Medium-ita-16bit-GGUF/blob/main/Samantha2.0-Phi3-Medium-ita-16bit-Q3_K_S.gguf) | Q3_K_S | 6.065 GB | very small, high quality loss |
| [Samantha2.0-Phi3-Medium-ita-16bit-Q3_K_M.gguf](https://huggingface.co/tensorblock/Samantha2.0-Phi3-Medium-ita-16bit-GGUF/blob/main/Samantha2.0-Phi3-Medium-ita-16bit-Q3_K_M.gguf) | Q3_K_M | 6.751 GB | very small, high quality loss |
| [Samantha2.0-Phi3-Medium-ita-16bit-Q3_K_L.gguf](https://huggingface.co/tensorblock/Samantha2.0-Phi3-Medium-ita-16bit-GGUF/blob/main/Samantha2.0-Phi3-Medium-ita-16bit-Q3_K_L.gguf) | Q3_K_L | 7.349 GB | small, substantial quality loss |
| [Samantha2.0-Phi3-Medium-ita-16bit-Q4_0.gguf](https://huggingface.co/tensorblock/Samantha2.0-Phi3-Medium-ita-16bit-GGUF/blob/main/Samantha2.0-Phi3-Medium-ita-16bit-Q4_0.gguf) | Q4_0 | 7.897 GB | legacy; small, very high quality loss - prefer using Q3_K_M |
| [Samantha2.0-Phi3-Medium-ita-16bit-Q4_K_S.gguf](https://huggingface.co/tensorblock/Samantha2.0-Phi3-Medium-ita-16bit-GGUF/blob/main/Samantha2.0-Phi3-Medium-ita-16bit-Q4_K_S.gguf) | Q4_K_S | 7.958 GB | small, greater quality loss |
| [Samantha2.0-Phi3-Medium-ita-16bit-Q4_K_M.gguf](https://huggingface.co/tensorblock/Samantha2.0-Phi3-Medium-ita-16bit-GGUF/blob/main/Samantha2.0-Phi3-Medium-ita-16bit-Q4_K_M.gguf) | Q4_K_M | 8.404 GB | medium, balanced quality - recommended |
| [Samantha2.0-Phi3-Medium-ita-16bit-Q5_0.gguf](https://huggingface.co/tensorblock/Samantha2.0-Phi3-Medium-ita-16bit-GGUF/blob/main/Samantha2.0-Phi3-Medium-ita-16bit-Q5_0.gguf) | Q5_0 | 9.622 GB | legacy; medium, balanced quality - prefer using Q4_K_M |
| [Samantha2.0-Phi3-Medium-ita-16bit-Q5_K_S.gguf](https://huggingface.co/tensorblock/Samantha2.0-Phi3-Medium-ita-16bit-GGUF/blob/main/Samantha2.0-Phi3-Medium-ita-16bit-Q5_K_S.gguf) | Q5_K_S | 9.622 GB | large, low quality loss - recommended |
| [Samantha2.0-Phi3-Medium-ita-16bit-Q5_K_M.gguf](https://huggingface.co/tensorblock/Samantha2.0-Phi3-Medium-ita-16bit-GGUF/blob/main/Samantha2.0-Phi3-Medium-ita-16bit-Q5_K_M.gguf) | Q5_K_M | 9.883 GB | large, very low quality loss - recommended |
| [Samantha2.0-Phi3-Medium-ita-16bit-Q6_K.gguf](https://huggingface.co/tensorblock/Samantha2.0-Phi3-Medium-ita-16bit-GGUF/blob/main/Samantha2.0-Phi3-Medium-ita-16bit-Q6_K.gguf) | Q6_K | 11.454 GB | very large, extremely low quality loss |
| [Samantha2.0-Phi3-Medium-ita-16bit-Q8_0.gguf](https://huggingface.co/tensorblock/Samantha2.0-Phi3-Medium-ita-16bit-GGUF/blob/main/Samantha2.0-Phi3-Medium-ita-16bit-Q8_0.gguf) | Q8_0 | 14.835 GB | very large, extremely low quality loss - not recommended |
## Downloading instruction
### Command line
Firstly, install Huggingface Client
```shell
pip install -U "huggingface_hub[cli]"
```
Then, downoad the individual model file the a local directory
```shell
huggingface-cli download tensorblock/Samantha2.0-Phi3-Medium-ita-16bit-GGUF --include "Samantha2.0-Phi3-Medium-ita-16bit-Q2_K.gguf" --local-dir MY_LOCAL_DIR
```
If you wanna download multiple model files with a pattern (e.g., `*Q4_K*gguf`), you can try:
```shell
huggingface-cli download tensorblock/Samantha2.0-Phi3-Medium-ita-16bit-GGUF --local-dir MY_LOCAL_DIR --local-dir-use-symlinks False --include='*Q4_K*gguf'
```
|
mmdrzie/trading-support-bart | mmdrzie | "2025-02-11T21:29:01Z" | 0 | 0 | transformers | [
"transformers",
"safetensors",
"bart",
"text2text-generation",
"arxiv:1910.09700",
"autotrain_compatible",
"endpoints_compatible",
"region:us"
] | text2text-generation | "2025-02-11T21:28:33Z" | ---
library_name: transformers
tags: []
---
# Model Card for Model ID
<!-- Provide a quick summary of what the model is/does. -->
## Model Details
### Model Description
<!-- Provide a longer summary of what this model is. -->
This is the model card of a 🤗 transformers model that has been pushed on the Hub. This model card has been automatically generated.
- **Developed by:** [More Information Needed]
- **Funded by [optional]:** [More Information Needed]
- **Shared by [optional]:** [More Information Needed]
- **Model type:** [More Information Needed]
- **Language(s) (NLP):** [More Information Needed]
- **License:** [More Information Needed]
- **Finetuned from model [optional]:** [More Information Needed]
### Model Sources [optional]
<!-- Provide the basic links for the model. -->
- **Repository:** [More Information Needed]
- **Paper [optional]:** [More Information Needed]
- **Demo [optional]:** [More Information Needed]
## Uses
<!-- Address questions around how the model is intended to be used, including the foreseeable users of the model and those affected by the model. -->
### Direct Use
<!-- This section is for the model use without fine-tuning or plugging into a larger ecosystem/app. -->
[More Information Needed]
### Downstream Use [optional]
<!-- This section is for the model use when fine-tuned for a task, or when plugged into a larger ecosystem/app -->
[More Information Needed]
### Out-of-Scope Use
<!-- This section addresses misuse, malicious use, and uses that the model will not work well for. -->
[More Information Needed]
## Bias, Risks, and Limitations
<!-- This section is meant to convey both technical and sociotechnical limitations. -->
[More Information Needed]
### Recommendations
<!-- This section is meant to convey recommendations with respect to the bias, risk, and technical limitations. -->
Users (both direct and downstream) should be made aware of the risks, biases and limitations of the model. More information needed for further recommendations.
## How to Get Started with the Model
Use the code below to get started with the model.
[More Information Needed]
## Training Details
### Training Data
<!-- This should link to a Dataset Card, perhaps with a short stub of information on what the training data is all about as well as documentation related to data pre-processing or additional filtering. -->
[More Information Needed]
### Training Procedure
<!-- This relates heavily to the Technical Specifications. Content here should link to that section when it is relevant to the training procedure. -->
#### Preprocessing [optional]
[More Information Needed]
#### Training Hyperparameters
- **Training regime:** [More Information Needed] <!--fp32, fp16 mixed precision, bf16 mixed precision, bf16 non-mixed precision, fp16 non-mixed precision, fp8 mixed precision -->
#### Speeds, Sizes, Times [optional]
<!-- This section provides information about throughput, start/end time, checkpoint size if relevant, etc. -->
[More Information Needed]
## Evaluation
<!-- This section describes the evaluation protocols and provides the results. -->
### Testing Data, Factors & Metrics
#### Testing Data
<!-- This should link to a Dataset Card if possible. -->
[More Information Needed]
#### Factors
<!-- These are the things the evaluation is disaggregating by, e.g., subpopulations or domains. -->
[More Information Needed]
#### Metrics
<!-- These are the evaluation metrics being used, ideally with a description of why. -->
[More Information Needed]
### Results
[More Information Needed]
#### Summary
## Model Examination [optional]
<!-- Relevant interpretability work for the model goes here -->
[More Information Needed]
## Environmental Impact
<!-- Total emissions (in grams of CO2eq) and additional considerations, such as electricity usage, go here. Edit the suggested text below accordingly -->
Carbon emissions can be estimated using the [Machine Learning Impact calculator](https://mlco2.github.io/impact#compute) presented in [Lacoste et al. (2019)](https://arxiv.org/abs/1910.09700).
- **Hardware Type:** [More Information Needed]
- **Hours used:** [More Information Needed]
- **Cloud Provider:** [More Information Needed]
- **Compute Region:** [More Information Needed]
- **Carbon Emitted:** [More Information Needed]
## Technical Specifications [optional]
### Model Architecture and Objective
[More Information Needed]
### Compute Infrastructure
[More Information Needed]
#### Hardware
[More Information Needed]
#### Software
[More Information Needed]
## Citation [optional]
<!-- If there is a paper or blog post introducing the model, the APA and Bibtex information for that should go in this section. -->
**BibTeX:**
[More Information Needed]
**APA:**
[More Information Needed]
## Glossary [optional]
<!-- If relevant, include terms and calculations in this section that can help readers understand the model or model card. -->
[More Information Needed]
## More Information [optional]
[More Information Needed]
## Model Card Authors [optional]
[More Information Needed]
## Model Card Contact
[More Information Needed] |
djsull/logs_rand | djsull | "2024-12-18T01:38:12Z" | 116 | 0 | transformers | [
"transformers",
"tensorboard",
"safetensors",
"roberta",
"token-classification",
"autotrain_compatible",
"endpoints_compatible",
"region:us"
] | token-classification | "2024-05-31T05:52:43Z" | ---
metrics:
- precision
- recall
- f1
- accuracy
model-index:
- name: logs_rand
results: []
---
<!-- This model card has been generated automatically according to the information the Trainer had access to. You
should probably proofread and complete it, then remove this comment. -->
# logs_rand
- Loss: 0.0024
- Precision: 0.8742
- Recall: 0.8871
- F1: 0.8806
- Accuracy: 0.9992
## Model description
More information needed
## Intended uses & limitations
More information needed
## Training and evaluation data
More information needed
## Training procedure
### Training hyperparameters
The following hyperparameters were used during training:
- learning_rate: 5e-05
- train_batch_size: 64
- eval_batch_size: 64
- seed: 42
- optimizer: Adam with betas=(0.9,0.999) and epsilon=1e-08
- lr_scheduler_type: linear
- num_epochs: 5
### Training results
| Training Loss | Epoch | Step | Validation Loss | Precision | Recall | F1 | Accuracy |
|:-------------:|:-----:|:----:|:---------------:|:---------:|:------:|:------:|:--------:|
| No log | 1.0 | 57 | 0.0067 | 0.6685 | 0.6276 | 0.6474 | 0.9980 |
| No log | 2.0 | 114 | 0.0035 | 0.8286 | 0.8312 | 0.8299 | 0.9989 |
| No log | 3.0 | 171 | 0.0028 | 0.8690 | 0.8745 | 0.8717 | 0.9991 |
| No log | 4.0 | 228 | 0.0026 | 0.8693 | 0.8840 | 0.8766 | 0.9992 |
| No log | 5.0 | 285 | 0.0024 | 0.8742 | 0.8871 | 0.8806 | 0.9992 |
### Framework versions
- Transformers 4.40.2
- Pytorch 2.0.1
- Datasets 2.19.1
- Tokenizers 0.19.1 |
stablediffusionapi/the-ally | stablediffusionapi | "2025-01-20T11:21:52Z" | 96 | 2 | diffusers | [
"diffusers",
"stablediffusionapi.com",
"stable-diffusion-api",
"text-to-image",
"ultra-realistic",
"license:creativeml-openrail-m",
"autotrain_compatible",
"endpoints_compatible",
"diffusers:StableDiffusionPipeline",
"region:us"
] | text-to-image | "2023-04-26T14:18:49Z" | ---
license: creativeml-openrail-m
tags:
- stablediffusionapi.com
- stable-diffusion-api
- text-to-image
- ultra-realistic
pinned: true
---
# The Ally API Inference
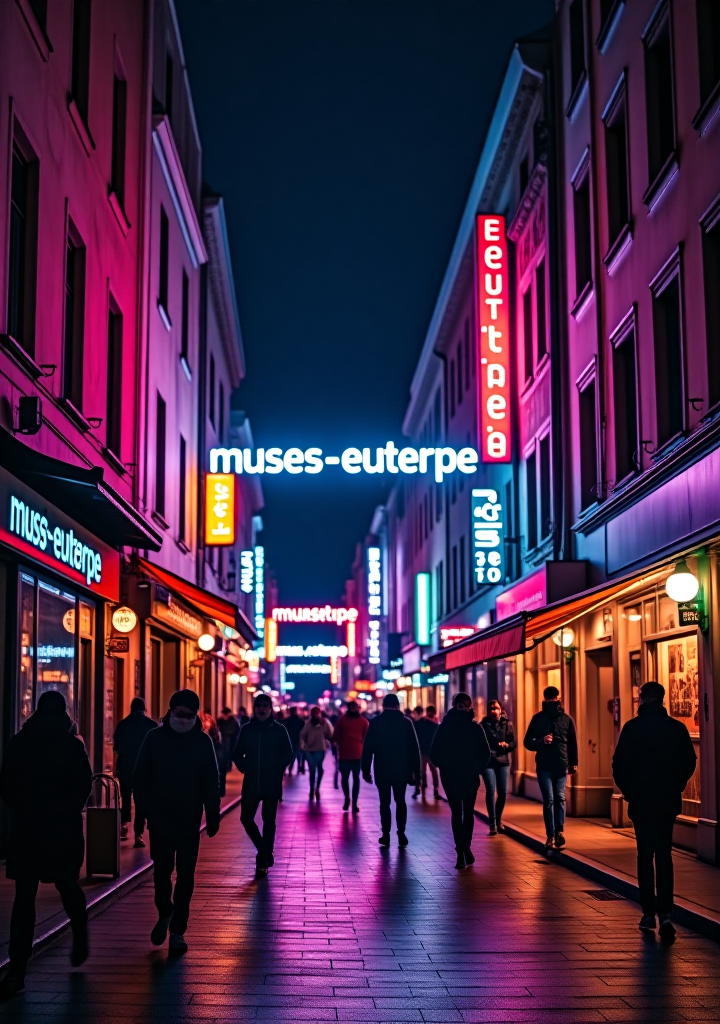
## Get API Key
Get API key from [ModelsLab](https://modelslab.com/), No Payment needed.
Replace Key in below code, change **model_id** to "the-ally"
Coding in PHP/Node/Java etc? Have a look at docs for more code examples: [View docs](https://stablediffusionapi.com/docs)
Model link: [View model](https://stablediffusionapi.com/models/the-ally)
Credits: [View credits](https://civitai.com/?query=The%20Ally)
View all models: [View Models](https://stablediffusionapi.com/models)
import requests
import json
url = "https://stablediffusionapi.com/api/v3/dreambooth"
payload = json.dumps({
"key": "",
"model_id": "the-ally",
"prompt": "actual 8K portrait photo of gareth person, portrait, happy colors, bright eyes, clear eyes, warm smile, smooth soft skin, big dreamy eyes, beautiful intricate colored hair, symmetrical, anime wide eyes, soft lighting, detailed face, by makoto shinkai, stanley artgerm lau, wlop, rossdraws, concept art, digital painting, looking into camera",
"negative_prompt": "painting, extra fingers, mutated hands, poorly drawn hands, poorly drawn face, deformed, ugly, blurry, bad anatomy, bad proportions, extra limbs, cloned face, skinny, glitchy, double torso, extra arms, extra hands, mangled fingers, missing lips, ugly face, distorted face, extra legs, anime",
"width": "512",
"height": "512",
"samples": "1",
"num_inference_steps": "30",
"safety_checker": "no",
"enhance_prompt": "yes",
"seed": None,
"guidance_scale": 7.5,
"multi_lingual": "no",
"panorama": "no",
"self_attention": "no",
"upscale": "no",
"embeddings": "embeddings_model_id",
"lora": "lora_model_id",
"webhook": None,
"track_id": None
})
headers = {
'Content-Type': 'application/json'
}
response = requests.request("POST", url, headers=headers, data=payload)
print(response.text)
> Use this coupon code to get 25% off **DMGG0RBN** |
Spestly/Artemis-1B | Spestly | "2025-04-13T07:27:30Z" | 0 | 0 | transformers | [
"transformers",
"safetensors",
"gemma3_text",
"text-generation",
"text-generation-inference",
"unsloth",
"conversational",
"en",
"base_model:unsloth/gemma-3-1b-it-unsloth-bnb-4bit",
"base_model:finetune:unsloth/gemma-3-1b-it-unsloth-bnb-4bit",
"license:apache-2.0",
"autotrain_compatible",
"endpoints_compatible",
"region:us"
] | text-generation | "2025-04-13T07:03:23Z" | ---
base_model: unsloth/gemma-3-1b-it-unsloth-bnb-4bit
tags:
- text-generation-inference
- transformers
- unsloth
- gemma3_text
license: apache-2.0
language:
- en
---
# Uploaded finetuned model
- **Developed by:** Spestly
- **License:** apache-2.0
- **Finetuned from model :** unsloth/gemma-3-1b-it-unsloth-bnb-4bit
This gemma3_text model was trained 2x faster with [Unsloth](https://github.com/unslothai/unsloth) and Huggingface's TRL library.
[<img src="https://raw.githubusercontent.com/unslothai/unsloth/main/images/unsloth%20made%20with%20love.png" width="200"/>](https://github.com/unslothai/unsloth)
|
mradermacher/Phi-4-Trim-Exp1-GGUF | mradermacher | "2025-02-15T00:44:20Z" | 0 | 0 | transformers | [
"transformers",
"gguf",
"mergekit",
"merge",
"en",
"base_model:bunnycore/Phi-4-Trim-Exp1",
"base_model:quantized:bunnycore/Phi-4-Trim-Exp1",
"endpoints_compatible",
"region:us",
"conversational"
] | null | "2025-02-15T00:25:59Z" | ---
base_model: bunnycore/Phi-4-Trim-Exp1
language:
- en
library_name: transformers
quantized_by: mradermacher
tags:
- mergekit
- merge
---
## About
<!-- ### quantize_version: 2 -->
<!-- ### output_tensor_quantised: 1 -->
<!-- ### convert_type: hf -->
<!-- ### vocab_type: -->
<!-- ### tags: -->
static quants of https://huggingface.co/bunnycore/Phi-4-Trim-Exp1
<!-- provided-files -->
weighted/imatrix quants seem not to be available (by me) at this time. If they do not show up a week or so after the static ones, I have probably not planned for them. Feel free to request them by opening a Community Discussion.
## Usage
If you are unsure how to use GGUF files, refer to one of [TheBloke's
READMEs](https://huggingface.co/TheBloke/KafkaLM-70B-German-V0.1-GGUF) for
more details, including on how to concatenate multi-part files.
## Provided Quants
(sorted by size, not necessarily quality. IQ-quants are often preferable over similar sized non-IQ quants)
| Link | Type | Size/GB | Notes |
|:-----|:-----|--------:|:------|
| [GGUF](https://huggingface.co/mradermacher/Phi-4-Trim-Exp1-GGUF/resolve/main/Phi-4-Trim-Exp1.Q2_K.gguf) | Q2_K | 3.1 | |
| [GGUF](https://huggingface.co/mradermacher/Phi-4-Trim-Exp1-GGUF/resolve/main/Phi-4-Trim-Exp1.Q3_K_S.gguf) | Q3_K_S | 3.5 | |
| [GGUF](https://huggingface.co/mradermacher/Phi-4-Trim-Exp1-GGUF/resolve/main/Phi-4-Trim-Exp1.Q3_K_M.gguf) | Q3_K_M | 3.9 | lower quality |
| [GGUF](https://huggingface.co/mradermacher/Phi-4-Trim-Exp1-GGUF/resolve/main/Phi-4-Trim-Exp1.Q3_K_L.gguf) | Q3_K_L | 4.1 | |
| [GGUF](https://huggingface.co/mradermacher/Phi-4-Trim-Exp1-GGUF/resolve/main/Phi-4-Trim-Exp1.IQ4_XS.gguf) | IQ4_XS | 4.3 | |
| [GGUF](https://huggingface.co/mradermacher/Phi-4-Trim-Exp1-GGUF/resolve/main/Phi-4-Trim-Exp1.Q4_K_S.gguf) | Q4_K_S | 4.5 | fast, recommended |
| [GGUF](https://huggingface.co/mradermacher/Phi-4-Trim-Exp1-GGUF/resolve/main/Phi-4-Trim-Exp1.Q4_K_M.gguf) | Q4_K_M | 4.7 | fast, recommended |
| [GGUF](https://huggingface.co/mradermacher/Phi-4-Trim-Exp1-GGUF/resolve/main/Phi-4-Trim-Exp1.Q5_K_S.gguf) | Q5_K_S | 5.3 | |
| [GGUF](https://huggingface.co/mradermacher/Phi-4-Trim-Exp1-GGUF/resolve/main/Phi-4-Trim-Exp1.Q5_K_M.gguf) | Q5_K_M | 5.4 | |
| [GGUF](https://huggingface.co/mradermacher/Phi-4-Trim-Exp1-GGUF/resolve/main/Phi-4-Trim-Exp1.Q6_K.gguf) | Q6_K | 6.3 | very good quality |
| [GGUF](https://huggingface.co/mradermacher/Phi-4-Trim-Exp1-GGUF/resolve/main/Phi-4-Trim-Exp1.Q8_0.gguf) | Q8_0 | 8.1 | fast, best quality |
| [GGUF](https://huggingface.co/mradermacher/Phi-4-Trim-Exp1-GGUF/resolve/main/Phi-4-Trim-Exp1.f16.gguf) | f16 | 15.1 | 16 bpw, overkill |
Here is a handy graph by ikawrakow comparing some lower-quality quant
types (lower is better):

And here are Artefact2's thoughts on the matter:
https://gist.github.com/Artefact2/b5f810600771265fc1e39442288e8ec9
## FAQ / Model Request
See https://huggingface.co/mradermacher/model_requests for some answers to
questions you might have and/or if you want some other model quantized.
## Thanks
I thank my company, [nethype GmbH](https://www.nethype.de/), for letting
me use its servers and providing upgrades to my workstation to enable
this work in my free time.
<!-- end -->
|
LAYEK-143/FLUX_V0 | LAYEK-143 | "2025-03-28T06:52:16Z" | 13 | 1 | diffusers | [
"diffusers",
"text-to-image",
"lora",
"template:diffusion-lora",
"en",
"base_model:black-forest-labs/FLUX.1-dev",
"base_model:adapter:black-forest-labs/FLUX.1-dev",
"license:cc-by-nc-4.0",
"region:us"
] | text-to-image | "2025-01-18T11:32:31Z" | <!DOCTYPE html>
<html class="" lang="en">
<head>
<meta charset="utf-8" />
<meta
name="viewport"
content="width=device-width, initial-scale=1.0, user-scalable=no"
/>
<meta
name="description"
content="We're on a journey to advance and democratize artificial intelligence through open source and open science."
/>
<meta property="fb:app_id" content="1321688464574422" />
<meta name="twitter:card" content="summary_large_image" />
<meta name="twitter:site" content="@huggingface" />
<meta
property="og:title"
content="Hugging Face - The AI community building the future."
/>
<meta property="og:type" content="website" />
<title>Hugging Face - The AI community building the future.</title>
<style>
body {
margin: 0;
}
main {
background-color: white;
min-height: 100vh;
padding: 7rem 1rem 8rem 1rem;
text-align: center;
font-family: Source Sans Pro, ui-sans-serif, system-ui, -apple-system,
BlinkMacSystemFont, Segoe UI, Roboto, Helvetica Neue, Arial, Noto Sans,
sans-serif, Apple Color Emoji, Segoe UI Emoji, Segoe UI Symbol,
Noto Color Emoji;
}
img {
width: 6rem;
height: 6rem;
margin: 0 auto 1rem;
}
h1 {
font-size: 3.75rem;
line-height: 1;
color: rgba(31, 41, 55, 1);
font-weight: 700;
box-sizing: border-box;
margin: 0 auto;
}
p, a {
color: rgba(107, 114, 128, 1);
font-size: 1.125rem;
line-height: 1.75rem;
max-width: 28rem;
box-sizing: border-box;
margin: 0 auto;
}
.dark main {
background-color: rgb(11, 15, 25);
}
.dark h1 {
color: rgb(209, 213, 219);
}
.dark p, .dark a {
color: rgb(156, 163, 175);
}
</style>
<script>
// On page load or when changing themes, best to add inline in `head` to avoid FOUC
const key = "_tb_global_settings";
let theme = window.matchMedia("(prefers-color-scheme: dark)").matches
? "dark"
: "light";
try {
const storageTheme = JSON.parse(window.localStorage.getItem(key)).theme;
if (storageTheme) {
theme = storageTheme === "dark" ? "dark" : "light";
}
} catch (e) {}
if (theme === "dark") {
document.documentElement.classList.add("dark");
} else {
document.documentElement.classList.remove("dark");
}
</script>
</head>
<body>
<main>
<img
src="https://cdn-media.huggingface.co/assets/huggingface_logo.svg"
alt=""
/>
<div>
<h1>503</h1>
<p>We had to rate limit you. To continue using our service, please log in or create an account.</p>
</div>
</main>
</body>
</html> |
mradermacher/creative-writer-32b-preview-GGUF | mradermacher | "2024-12-29T06:51:40Z" | 41 | 0 | transformers | [
"transformers",
"gguf",
"creative-writing",
"creative-writer",
"multiplicative-lora",
"en",
"base_model:jukofyork/creative-writer-32b-preview",
"base_model:quantized:jukofyork/creative-writer-32b-preview",
"license:cc-by-nc-4.0",
"endpoints_compatible",
"region:us",
"conversational"
] | null | "2024-12-29T03:57:46Z" | ---
base_model: jukofyork/creative-writer-32b-preview
language:
- en
library_name: transformers
license: cc-by-nc-4.0
quantized_by: mradermacher
tags:
- creative-writing
- creative-writer
- multiplicative-lora
---
## About
<!-- ### quantize_version: 2 -->
<!-- ### output_tensor_quantised: 1 -->
<!-- ### convert_type: hf -->
<!-- ### vocab_type: -->
<!-- ### tags: -->
static quants of https://huggingface.co/jukofyork/creative-writer-32b-preview
<!-- provided-files -->
weighted/imatrix quants are available at https://huggingface.co/mradermacher/creative-writer-32b-preview-i1-GGUF
## Usage
If you are unsure how to use GGUF files, refer to one of [TheBloke's
READMEs](https://huggingface.co/TheBloke/KafkaLM-70B-German-V0.1-GGUF) for
more details, including on how to concatenate multi-part files.
## Provided Quants
(sorted by size, not necessarily quality. IQ-quants are often preferable over similar sized non-IQ quants)
| Link | Type | Size/GB | Notes |
|:-----|:-----|--------:|:------|
| [GGUF](https://huggingface.co/mradermacher/creative-writer-32b-preview-GGUF/resolve/main/creative-writer-32b-preview.Q2_K.gguf) | Q2_K | 12.9 | |
| [GGUF](https://huggingface.co/mradermacher/creative-writer-32b-preview-GGUF/resolve/main/creative-writer-32b-preview.Q3_K_S.gguf) | Q3_K_S | 14.8 | |
| [GGUF](https://huggingface.co/mradermacher/creative-writer-32b-preview-GGUF/resolve/main/creative-writer-32b-preview.Q3_K_M.gguf) | Q3_K_M | 16.3 | lower quality |
| [GGUF](https://huggingface.co/mradermacher/creative-writer-32b-preview-GGUF/resolve/main/creative-writer-32b-preview.Q3_K_L.gguf) | Q3_K_L | 17.7 | |
| [GGUF](https://huggingface.co/mradermacher/creative-writer-32b-preview-GGUF/resolve/main/creative-writer-32b-preview.IQ4_XS.gguf) | IQ4_XS | 18.1 | |
| [GGUF](https://huggingface.co/mradermacher/creative-writer-32b-preview-GGUF/resolve/main/creative-writer-32b-preview.Q4_K_S.gguf) | Q4_K_S | 18.9 | fast, recommended |
| [GGUF](https://huggingface.co/mradermacher/creative-writer-32b-preview-GGUF/resolve/main/creative-writer-32b-preview.Q4_K_M.gguf) | Q4_K_M | 19.9 | fast, recommended |
| [GGUF](https://huggingface.co/mradermacher/creative-writer-32b-preview-GGUF/resolve/main/creative-writer-32b-preview.Q5_K_S.gguf) | Q5_K_S | 22.6 | |
| [GGUF](https://huggingface.co/mradermacher/creative-writer-32b-preview-GGUF/resolve/main/creative-writer-32b-preview.Q5_K_M.gguf) | Q5_K_M | 23.2 | |
| [GGUF](https://huggingface.co/mradermacher/creative-writer-32b-preview-GGUF/resolve/main/creative-writer-32b-preview.Q6_K.gguf) | Q6_K | 26.6 | very good quality |
| [GGUF](https://huggingface.co/mradermacher/creative-writer-32b-preview-GGUF/resolve/main/creative-writer-32b-preview.Q8_0.gguf) | Q8_0 | 34.4 | fast, best quality |
Here is a handy graph by ikawrakow comparing some lower-quality quant
types (lower is better):

And here are Artefact2's thoughts on the matter:
https://gist.github.com/Artefact2/b5f810600771265fc1e39442288e8ec9
## FAQ / Model Request
See https://huggingface.co/mradermacher/model_requests for some answers to
questions you might have and/or if you want some other model quantized.
## Thanks
I thank my company, [nethype GmbH](https://www.nethype.de/), for letting
me use its servers and providing upgrades to my workstation to enable
this work in my free time.
<!-- end -->
|
lesso15/f8d8872d-4108-4b9f-86bd-c3b8e45f046d | lesso15 | "2025-03-22T13:39:06Z" | 0 | 0 | peft | [
"peft",
"safetensors",
"qwen2",
"axolotl",
"generated_from_trainer",
"base_model:unsloth/Qwen2.5-1.5B-Instruct",
"base_model:adapter:unsloth/Qwen2.5-1.5B-Instruct",
"license:apache-2.0",
"region:us"
] | null | "2025-03-22T12:24:25Z" | ---
library_name: peft
license: apache-2.0
base_model: unsloth/Qwen2.5-1.5B-Instruct
tags:
- axolotl
- generated_from_trainer
model-index:
- name: f8d8872d-4108-4b9f-86bd-c3b8e45f046d
results: []
---
<!-- This model card has been generated automatically according to the information the Trainer had access to. You
should probably proofread and complete it, then remove this comment. -->
[<img src="https://raw.githubusercontent.com/axolotl-ai-cloud/axolotl/main/image/axolotl-badge-web.png" alt="Built with Axolotl" width="200" height="32"/>](https://github.com/axolotl-ai-cloud/axolotl)
<details><summary>See axolotl config</summary>
axolotl version: `0.4.1`
```yaml
adapter: lora
base_model: unsloth/Qwen2.5-1.5B-Instruct
bf16: auto
chat_template: llama3
dataset_prepared_path: null
datasets:
- data_files:
- a88e78e41748bf83_train_data.json
ds_type: json
format: custom
path: /workspace/input_data/a88e78e41748bf83_train_data.json
type:
field_instruction: prompt
field_output: generation
format: '{instruction}'
no_input_format: '{instruction}'
system_format: '{system}'
system_prompt: ''
debug: null
deepspeed: null
do_eval: true
early_stopping_patience: 3
eval_batch_size: 4
eval_max_new_tokens: 128
eval_steps: 500
evals_per_epoch: null
flash_attention: true
fp16: false
fsdp: null
fsdp_config: null
gradient_accumulation_steps: 8
gradient_checkpointing: true
group_by_length: true
hub_model_id: lesso15/f8d8872d-4108-4b9f-86bd-c3b8e45f046d
hub_repo: null
hub_strategy: checkpoint
hub_token: null
learning_rate: 0.000215
load_in_4bit: false
load_in_8bit: false
local_rank: null
logging_steps: 50
lora_alpha: 128
lora_dropout: 0.15
lora_fan_in_fan_out: null
lora_model_dir: null
lora_r: 64
lora_target_linear: true
lr_scheduler: cosine
max_grad_norm: 1.0
max_steps: 500
micro_batch_size: 4
mlflow_experiment_name: /tmp/a88e78e41748bf83_train_data.json
model_type: AutoModelForCausalLM
num_epochs: 10
optimizer: adamw_torch_fused
output_dir: miner_id_24
pad_to_sequence_len: true
resume_from_checkpoint: null
s2_attention: null
sample_packing: false
save_steps: 500
saves_per_epoch: null
seed: 150
sequence_len: 1024
strict: false
tf32: true
tokenizer_type: AutoTokenizer
train_on_inputs: false
trust_remote_code: true
val_set_size: 0.05
wandb_entity: null
wandb_mode: online
wandb_name: 895387c3-0be7-49d8-a314-abeba9f636b4
wandb_project: 15a
wandb_run: your_name
wandb_runid: 895387c3-0be7-49d8-a314-abeba9f636b4
warmup_steps: 100
weight_decay: 0.0
xformers_attention: null
```
</details><br>
# f8d8872d-4108-4b9f-86bd-c3b8e45f046d
This model is a fine-tuned version of [unsloth/Qwen2.5-1.5B-Instruct](https://huggingface.co/unsloth/Qwen2.5-1.5B-Instruct) on the None dataset.
It achieves the following results on the evaluation set:
- Loss: 0.7758
## Model description
More information needed
## Intended uses & limitations
More information needed
## Training and evaluation data
More information needed
## Training procedure
### Training hyperparameters
The following hyperparameters were used during training:
- learning_rate: 0.000215
- train_batch_size: 4
- eval_batch_size: 4
- seed: 150
- gradient_accumulation_steps: 8
- total_train_batch_size: 32
- optimizer: Use OptimizerNames.ADAMW_TORCH_FUSED with betas=(0.9,0.999) and epsilon=1e-08 and optimizer_args=No additional optimizer arguments
- lr_scheduler_type: cosine
- lr_scheduler_warmup_steps: 100
- training_steps: 500
### Training results
| Training Loss | Epoch | Step | Validation Loss |
|:-------------:|:------:|:----:|:---------------:|
| No log | 0.0010 | 1 | 1.8006 |
| 0.7738 | 0.4865 | 500 | 0.7758 |
### Framework versions
- PEFT 0.13.2
- Transformers 4.46.0
- Pytorch 2.5.0+cu124
- Datasets 3.0.1
- Tokenizers 0.20.1 |
kghanlon/distilbert-base-uncased-RILE-v1 | kghanlon | "2023-12-19T11:36:24Z" | 8 | 0 | transformers | [
"transformers",
"tensorboard",
"safetensors",
"distilbert",
"text-classification",
"generated_from_trainer",
"base_model:distilbert/distilbert-base-uncased",
"base_model:finetune:distilbert/distilbert-base-uncased",
"license:apache-2.0",
"autotrain_compatible",
"endpoints_compatible",
"region:us"
] | text-classification | "2023-12-19T10:52:52Z" | ---
license: apache-2.0
base_model: distilbert-base-uncased
tags:
- generated_from_trainer
metrics:
- accuracy
- recall
- f1
model-index:
- name: distilbert-base-uncased-RILE-v1
results: []
---
<!-- This model card has been generated automatically according to the information the Trainer had access to. You
should probably proofread and complete it, then remove this comment. -->
# distilbert-base-uncased-RILE-v1
This model is a fine-tuned version of [distilbert-base-uncased](https://huggingface.co/distilbert-base-uncased) on an unknown dataset.
It achieves the following results on the evaluation set:
- Loss: 0.8587
- Accuracy: 0.7364
- Recall: 0.7364
- F1: 0.7358
## Model description
More information needed
## Intended uses & limitations
More information needed
## Training and evaluation data
More information needed
## Training procedure
### Training hyperparameters
The following hyperparameters were used during training:
- learning_rate: 5e-05
- train_batch_size: 8
- eval_batch_size: 8
- seed: 42
- optimizer: Adam with betas=(0.9,0.999) and epsilon=1e-08
- lr_scheduler_type: linear
- num_epochs: 3
### Training results
| Training Loss | Epoch | Step | Validation Loss | Accuracy | Recall | F1 |
|:-------------:|:-----:|:-----:|:---------------:|:--------:|:------:|:------:|
| 0.6966 | 1.0 | 15490 | 0.6831 | 0.7164 | 0.7164 | 0.7123 |
| 0.5738 | 2.0 | 30980 | 0.6934 | 0.7300 | 0.7300 | 0.7300 |
| 0.422 | 3.0 | 46470 | 0.8587 | 0.7364 | 0.7364 | 0.7358 |
### Framework versions
- Transformers 4.35.2
- Pytorch 2.1.0+cu121
- Datasets 2.15.0
- Tokenizers 0.15.0
|
Subsets and Splits