modelId
stringlengths 5
139
| author
stringlengths 2
42
| last_modified
timestamp[us, tz=UTC]date 2020-02-15 11:33:14
2025-07-12 18:27:22
| downloads
int64 0
223M
| likes
int64 0
11.7k
| library_name
stringclasses 518
values | tags
listlengths 1
4.05k
| pipeline_tag
stringclasses 55
values | createdAt
timestamp[us, tz=UTC]date 2022-03-02 23:29:04
2025-07-12 18:26:55
| card
stringlengths 11
1.01M
|
---|---|---|---|---|---|---|---|---|---|
huggingtweets/pink_rodent | huggingtweets | 2022-08-28T02:33:36Z | 107 | 0 | transformers | [
"transformers",
"pytorch",
"gpt2",
"text-generation",
"huggingtweets",
"en",
"autotrain_compatible",
"text-generation-inference",
"endpoints_compatible",
"region:us"
]
| text-generation | 2022-08-28T02:32:47Z | ---
language: en
thumbnail: http://www.huggingtweets.com/pink_rodent/1661654012124/predictions.png
tags:
- huggingtweets
widget:
- text: "My dream is"
---
<div class="inline-flex flex-col" style="line-height: 1.5;">
<div class="flex">
<div
style="display:inherit; margin-left: 4px; margin-right: 4px; width: 92px; height:92px; border-radius: 50%; background-size: cover; background-image: url('https://pbs.twimg.com/profile_images/1558011857838931968/JdtfxNhf_400x400.jpg')">
</div>
<div
style="display:none; margin-left: 4px; margin-right: 4px; width: 92px; height:92px; border-radius: 50%; background-size: cover; background-image: url('')">
</div>
<div
style="display:none; margin-left: 4px; margin-right: 4px; width: 92px; height:92px; border-radius: 50%; background-size: cover; background-image: url('')">
</div>
</div>
<div style="text-align: center; margin-top: 3px; font-size: 16px; font-weight: 800">π€ AI BOT π€</div>
<div style="text-align: center; font-size: 16px; font-weight: 800">mouse</div>
<div style="text-align: center; font-size: 14px;">@pink_rodent</div>
</div>
I was made with [huggingtweets](https://github.com/borisdayma/huggingtweets).
Create your own bot based on your favorite user with [the demo](https://colab.research.google.com/github/borisdayma/huggingtweets/blob/master/huggingtweets-demo.ipynb)!
## How does it work?
The model uses the following pipeline.
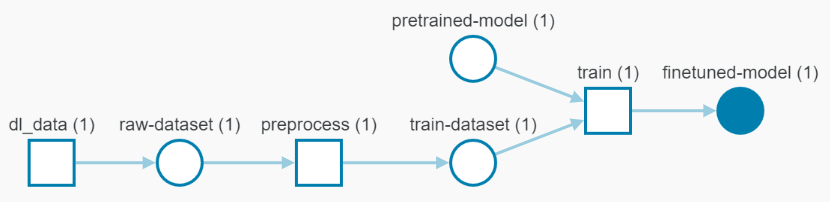
To understand how the model was developed, check the [W&B report](https://wandb.ai/wandb/huggingtweets/reports/HuggingTweets-Train-a-Model-to-Generate-Tweets--VmlldzoxMTY5MjI).
## Training data
The model was trained on tweets from mouse.
| Data | mouse |
| --- | --- |
| Tweets downloaded | 242 |
| Retweets | 48 |
| Short tweets | 55 |
| Tweets kept | 139 |
[Explore the data](https://wandb.ai/wandb/huggingtweets/runs/182s7hgh/artifacts), which is tracked with [W&B artifacts](https://docs.wandb.com/artifacts) at every step of the pipeline.
## Training procedure
The model is based on a pre-trained [GPT-2](https://huggingface.co/gpt2) which is fine-tuned on @pink_rodent's tweets.
Hyperparameters and metrics are recorded in the [W&B training run](https://wandb.ai/wandb/huggingtweets/runs/35lwy7go) for full transparency and reproducibility.
At the end of training, [the final model](https://wandb.ai/wandb/huggingtweets/runs/35lwy7go/artifacts) is logged and versioned.
## How to use
You can use this model directly with a pipeline for text generation:
```python
from transformers import pipeline
generator = pipeline('text-generation',
model='huggingtweets/pink_rodent')
generator("My dream is", num_return_sequences=5)
```
## Limitations and bias
The model suffers from [the same limitations and bias as GPT-2](https://huggingface.co/gpt2#limitations-and-bias).
In addition, the data present in the user's tweets further affects the text generated by the model.
## About
*Built by Boris Dayma*
[](https://twitter.com/intent/follow?screen_name=borisdayma)
For more details, visit the project repository.
[](https://github.com/borisdayma/huggingtweets)
|
paola-md/recipe-lr8e06-wd0.1-bs8 | paola-md | 2022-08-28T01:37:28Z | 164 | 0 | transformers | [
"transformers",
"pytorch",
"roberta",
"text-classification",
"generated_from_trainer",
"license:apache-2.0",
"autotrain_compatible",
"endpoints_compatible",
"region:us"
]
| text-classification | 2022-08-28T01:13:07Z | ---
license: apache-2.0
tags:
- generated_from_trainer
model-index:
- name: recipe-lr8e06-wd0.1-bs8
results: []
---
<!-- This model card has been generated automatically according to the information the Trainer had access to. You
should probably proofread and complete it, then remove this comment. -->
# recipe-lr8e06-wd0.1-bs8
This model is a fine-tuned version of [paola-md/recipe-distilroberta-Is](https://huggingface.co/paola-md/recipe-distilroberta-Is) on the None dataset.
It achieves the following results on the evaluation set:
- Loss: 0.2778
- Rmse: 0.5270
- Mse: 0.2778
- Mae: 0.4290
## Model description
More information needed
## Intended uses & limitations
More information needed
## Training and evaluation data
More information needed
## Training procedure
### Training hyperparameters
The following hyperparameters were used during training:
- learning_rate: 8e-06
- train_batch_size: 64
- eval_batch_size: 64
- seed: 42
- optimizer: Adam with betas=(0.9,0.999) and epsilon=1e-08
- lr_scheduler_type: linear
- num_epochs: 10
### Training results
| Training Loss | Epoch | Step | Validation Loss | Rmse | Mse | Mae |
|:-------------:|:-----:|:----:|:---------------:|:------:|:------:|:------:|
| 0.2766 | 1.0 | 2490 | 0.2741 | 0.5235 | 0.2741 | 0.4176 |
| 0.2739 | 2.0 | 4980 | 0.2773 | 0.5266 | 0.2773 | 0.4286 |
| 0.2726 | 3.0 | 7470 | 0.2778 | 0.5270 | 0.2778 | 0.4290 |
### Framework versions
- Transformers 4.19.0.dev0
- Pytorch 1.9.0+cu111
- Datasets 2.4.0
- Tokenizers 0.12.1
|
anas-awadalla/distilroberta-base-task-specific-distilation-on-squad | anas-awadalla | 2022-08-28T01:17:22Z | 10 | 0 | transformers | [
"transformers",
"pytorch",
"tensorboard",
"roberta",
"question-answering",
"generated_from_trainer",
"dataset:squad",
"license:apache-2.0",
"endpoints_compatible",
"region:us"
]
| question-answering | 2022-08-27T23:50:50Z | ---
license: apache-2.0
tags:
- generated_from_trainer
datasets:
- squad
model-index:
- name: distilroberta-base-task-specific-distilation-on-squad
results: []
---
<!-- This model card has been generated automatically according to the information the Trainer had access to. You
should probably proofread and complete it, then remove this comment. -->
# distilroberta-base-task-specific-distilation-on-squad
This model is a fine-tuned version of [distilroberta-base](https://huggingface.co/distilroberta-base) on the squad dataset.
## Model description
More information needed
## Intended uses & limitations
More information needed
## Training and evaluation data
More information needed
## Training procedure
### Training hyperparameters
The following hyperparameters were used during training:
- learning_rate: 3e-05
- train_batch_size: 32
- eval_batch_size: 8
- seed: 42
- optimizer: Adam with betas=(0.9,0.999) and epsilon=1e-08
- lr_scheduler_type: linear
- num_epochs: 2.0
### Training results
### Framework versions
- Transformers 4.20.0.dev0
- Pytorch 1.11.0+cu113
- Datasets 2.3.2
- Tokenizers 0.11.6
|
infiniteperplexity/xlm-roberta-base-finetuned-panx-de | infiniteperplexity | 2022-08-28T01:09:17Z | 105 | 0 | transformers | [
"transformers",
"pytorch",
"tensorboard",
"xlm-roberta",
"token-classification",
"generated_from_trainer",
"dataset:xtreme",
"license:mit",
"model-index",
"autotrain_compatible",
"endpoints_compatible",
"region:us"
]
| token-classification | 2022-08-28T00:45:38Z | ---
license: mit
tags:
- generated_from_trainer
datasets:
- xtreme
metrics:
- f1
model-index:
- name: xlm-roberta-base-finetuned-panx-de
results:
- task:
name: Token Classification
type: token-classification
dataset:
name: xtreme
type: xtreme
args: PAN-X.de
metrics:
- name: F1
type: f1
value: 0.8648740833380706
---
<!-- This model card has been generated automatically according to the information the Trainer had access to. You
should probably proofread and complete it, then remove this comment. -->
# xlm-roberta-base-finetuned-panx-de
This model is a fine-tuned version of [xlm-roberta-base](https://huggingface.co/xlm-roberta-base) on the xtreme dataset.
It achieves the following results on the evaluation set:
- Loss: 0.1365
- F1: 0.8649
## Model description
More information needed
## Intended uses & limitations
More information needed
## Training and evaluation data
More information needed
## Training procedure
### Training hyperparameters
The following hyperparameters were used during training:
- learning_rate: 5e-05
- train_batch_size: 24
- eval_batch_size: 24
- seed: 42
- optimizer: Adam with betas=(0.9,0.999) and epsilon=1e-08
- lr_scheduler_type: linear
- num_epochs: 3
### Training results
| Training Loss | Epoch | Step | Validation Loss | F1 |
|:-------------:|:-----:|:----:|:---------------:|:------:|
| 0.2553 | 1.0 | 525 | 0.1575 | 0.8279 |
| 0.1284 | 2.0 | 1050 | 0.1386 | 0.8463 |
| 0.0813 | 3.0 | 1575 | 0.1365 | 0.8649 |
### Framework versions
- Transformers 4.11.3
- Pytorch 1.12.1+cu113
- Datasets 1.16.1
- Tokenizers 0.10.3
|
paola-md/recipe-lr8e06-wd0.01-bs8 | paola-md | 2022-08-28T00:47:15Z | 163 | 0 | transformers | [
"transformers",
"pytorch",
"roberta",
"text-classification",
"generated_from_trainer",
"license:apache-2.0",
"autotrain_compatible",
"endpoints_compatible",
"region:us"
]
| text-classification | 2022-08-28T00:22:58Z | ---
license: apache-2.0
tags:
- generated_from_trainer
model-index:
- name: recipe-lr8e06-wd0.01-bs8
results: []
---
<!-- This model card has been generated automatically according to the information the Trainer had access to. You
should probably proofread and complete it, then remove this comment. -->
# recipe-lr8e06-wd0.01-bs8
This model is a fine-tuned version of [paola-md/recipe-distilroberta-Is](https://huggingface.co/paola-md/recipe-distilroberta-Is) on the None dataset.
It achieves the following results on the evaluation set:
- Loss: 0.2782
- Rmse: 0.5274
- Mse: 0.2782
- Mae: 0.4299
## Model description
More information needed
## Intended uses & limitations
More information needed
## Training and evaluation data
More information needed
## Training procedure
### Training hyperparameters
The following hyperparameters were used during training:
- learning_rate: 8e-06
- train_batch_size: 64
- eval_batch_size: 64
- seed: 42
- optimizer: Adam with betas=(0.9,0.999) and epsilon=1e-08
- lr_scheduler_type: linear
- num_epochs: 10
### Training results
| Training Loss | Epoch | Step | Validation Loss | Rmse | Mse | Mae |
|:-------------:|:-----:|:----:|:---------------:|:------:|:------:|:------:|
| 0.2766 | 1.0 | 2490 | 0.2739 | 0.5234 | 0.2739 | 0.4152 |
| 0.2739 | 2.0 | 4980 | 0.2769 | 0.5262 | 0.2769 | 0.4274 |
| 0.2725 | 3.0 | 7470 | 0.2782 | 0.5274 | 0.2782 | 0.4299 |
### Framework versions
- Transformers 4.19.0.dev0
- Pytorch 1.9.0+cu111
- Datasets 2.4.0
- Tokenizers 0.12.1
|
paola-md/recipe-lr2e05-wd0.02-bs8 | paola-md | 2022-08-28T00:22:11Z | 161 | 0 | transformers | [
"transformers",
"pytorch",
"roberta",
"text-classification",
"generated_from_trainer",
"license:apache-2.0",
"autotrain_compatible",
"endpoints_compatible",
"region:us"
]
| text-classification | 2022-08-27T23:57:54Z | ---
license: apache-2.0
tags:
- generated_from_trainer
model-index:
- name: recipe-lr2e05-wd0.02-bs8
results: []
---
<!-- This model card has been generated automatically according to the information the Trainer had access to. You
should probably proofread and complete it, then remove this comment. -->
# recipe-lr2e05-wd0.02-bs8
This model is a fine-tuned version of [paola-md/recipe-distilroberta-Is](https://huggingface.co/paola-md/recipe-distilroberta-Is) on the None dataset.
It achieves the following results on the evaluation set:
- Loss: 0.2767
- Rmse: 0.5260
- Mse: 0.2767
- Mae: 0.4245
## Model description
More information needed
## Intended uses & limitations
More information needed
## Training and evaluation data
More information needed
## Training procedure
### Training hyperparameters
The following hyperparameters were used during training:
- learning_rate: 2e-05
- train_batch_size: 64
- eval_batch_size: 64
- seed: 42
- optimizer: Adam with betas=(0.9,0.999) and epsilon=1e-08
- lr_scheduler_type: linear
- num_epochs: 10
### Training results
| Training Loss | Epoch | Step | Validation Loss | Rmse | Mse | Mae |
|:-------------:|:-----:|:----:|:---------------:|:------:|:------:|:------:|
| 0.2771 | 1.0 | 2490 | 0.2746 | 0.5240 | 0.2746 | 0.4201 |
| 0.2739 | 2.0 | 4980 | 0.2810 | 0.5301 | 0.2810 | 0.4329 |
| 0.2723 | 3.0 | 7470 | 0.2767 | 0.5260 | 0.2767 | 0.4245 |
### Framework versions
- Transformers 4.19.0.dev0
- Pytorch 1.9.0+cu111
- Datasets 2.4.0
- Tokenizers 0.12.1
|
paola-md/recipe-lr2e05-wd0.01-bs8 | paola-md | 2022-08-27T23:07:05Z | 163 | 0 | transformers | [
"transformers",
"pytorch",
"roberta",
"text-classification",
"generated_from_trainer",
"license:apache-2.0",
"autotrain_compatible",
"endpoints_compatible",
"region:us"
]
| text-classification | 2022-08-27T22:42:52Z | ---
license: apache-2.0
tags:
- generated_from_trainer
model-index:
- name: recipe-lr2e05-wd0.01-bs8
results: []
---
<!-- This model card has been generated automatically according to the information the Trainer had access to. You
should probably proofread and complete it, then remove this comment. -->
# recipe-lr2e05-wd0.01-bs8
This model is a fine-tuned version of [paola-md/recipe-distilroberta-Is](https://huggingface.co/paola-md/recipe-distilroberta-Is) on the None dataset.
It achieves the following results on the evaluation set:
- Loss: 0.2765
- Rmse: 0.5259
- Mse: 0.2765
- Mae: 0.4240
## Model description
More information needed
## Intended uses & limitations
More information needed
## Training and evaluation data
More information needed
## Training procedure
### Training hyperparameters
The following hyperparameters were used during training:
- learning_rate: 2e-05
- train_batch_size: 64
- eval_batch_size: 64
- seed: 42
- optimizer: Adam with betas=(0.9,0.999) and epsilon=1e-08
- lr_scheduler_type: linear
- num_epochs: 10
### Training results
| Training Loss | Epoch | Step | Validation Loss | Rmse | Mse | Mae |
|:-------------:|:-----:|:----:|:---------------:|:------:|:------:|:------:|
| 0.2771 | 1.0 | 2490 | 0.2743 | 0.5237 | 0.2743 | 0.4175 |
| 0.2739 | 2.0 | 4980 | 0.2801 | 0.5292 | 0.2801 | 0.4307 |
| 0.2723 | 3.0 | 7470 | 0.2765 | 0.5259 | 0.2765 | 0.4240 |
### Framework versions
- Transformers 4.19.0.dev0
- Pytorch 1.9.0+cu111
- Datasets 2.4.0
- Tokenizers 0.12.1
|
paola-md/recipe-lr1e05-wd0.02-bs16 | paola-md | 2022-08-27T22:42:16Z | 163 | 0 | transformers | [
"transformers",
"pytorch",
"roberta",
"text-classification",
"generated_from_trainer",
"license:apache-2.0",
"autotrain_compatible",
"endpoints_compatible",
"region:us"
]
| text-classification | 2022-08-27T22:25:06Z | ---
license: apache-2.0
tags:
- generated_from_trainer
model-index:
- name: recipe-lr1e05-wd0.02-bs16
results: []
---
<!-- This model card has been generated automatically according to the information the Trainer had access to. You
should probably proofread and complete it, then remove this comment. -->
# recipe-lr1e05-wd0.02-bs16
This model is a fine-tuned version of [paola-md/recipe-distilroberta-Is](https://huggingface.co/paola-md/recipe-distilroberta-Is) on the None dataset.
It achieves the following results on the evaluation set:
- Loss: 0.2793
- Rmse: 0.5285
- Mse: 0.2793
- Mae: 0.4342
## Model description
More information needed
## Intended uses & limitations
More information needed
## Training and evaluation data
More information needed
## Training procedure
### Training hyperparameters
The following hyperparameters were used during training:
- learning_rate: 1e-05
- train_batch_size: 128
- eval_batch_size: 128
- seed: 42
- optimizer: Adam with betas=(0.9,0.999) and epsilon=1e-08
- lr_scheduler_type: linear
- num_epochs: 10
### Training results
| Training Loss | Epoch | Step | Validation Loss | Rmse | Mse | Mae |
|:-------------:|:-----:|:----:|:---------------:|:------:|:------:|:------:|
| 0.2767 | 1.0 | 1245 | 0.2744 | 0.5239 | 0.2744 | 0.4125 |
| 0.2739 | 2.0 | 2490 | 0.2757 | 0.5250 | 0.2757 | 0.4212 |
| 0.2727 | 3.0 | 3735 | 0.2793 | 0.5285 | 0.2793 | 0.4342 |
### Framework versions
- Transformers 4.19.0.dev0
- Pytorch 1.9.0+cu111
- Datasets 2.4.0
- Tokenizers 0.12.1
|
paola-md/recipe-lr1e05-wd0.1-bs16 | paola-md | 2022-08-27T22:24:30Z | 163 | 0 | transformers | [
"transformers",
"pytorch",
"roberta",
"text-classification",
"generated_from_trainer",
"license:apache-2.0",
"autotrain_compatible",
"endpoints_compatible",
"region:us"
]
| text-classification | 2022-08-27T22:07:17Z | ---
license: apache-2.0
tags:
- generated_from_trainer
model-index:
- name: recipe-lr1e05-wd0.1-bs16
results: []
---
<!-- This model card has been generated automatically according to the information the Trainer had access to. You
should probably proofread and complete it, then remove this comment. -->
# recipe-lr1e05-wd0.1-bs16
This model is a fine-tuned version of [paola-md/recipe-distilroberta-Is](https://huggingface.co/paola-md/recipe-distilroberta-Is) on the None dataset.
It achieves the following results on the evaluation set:
- Loss: 0.2794
- Rmse: 0.5286
- Mse: 0.2794
- Mae: 0.4343
## Model description
More information needed
## Intended uses & limitations
More information needed
## Training and evaluation data
More information needed
## Training procedure
### Training hyperparameters
The following hyperparameters were used during training:
- learning_rate: 1e-05
- train_batch_size: 128
- eval_batch_size: 128
- seed: 42
- optimizer: Adam with betas=(0.9,0.999) and epsilon=1e-08
- lr_scheduler_type: linear
- num_epochs: 10
### Training results
| Training Loss | Epoch | Step | Validation Loss | Rmse | Mse | Mae |
|:-------------:|:-----:|:----:|:---------------:|:------:|:------:|:------:|
| 0.2767 | 1.0 | 1245 | 0.2744 | 0.5239 | 0.2744 | 0.4124 |
| 0.2739 | 2.0 | 2490 | 0.2757 | 0.5250 | 0.2757 | 0.4211 |
| 0.2727 | 3.0 | 3735 | 0.2794 | 0.5286 | 0.2794 | 0.4343 |
### Framework versions
- Transformers 4.19.0.dev0
- Pytorch 1.9.0+cu111
- Datasets 2.4.0
- Tokenizers 0.12.1
|
paola-md/recipe-lr1e05-wd0.01-bs16 | paola-md | 2022-08-27T21:48:54Z | 164 | 0 | transformers | [
"transformers",
"pytorch",
"roberta",
"text-classification",
"generated_from_trainer",
"license:apache-2.0",
"autotrain_compatible",
"endpoints_compatible",
"region:us"
]
| text-classification | 2022-08-27T21:31:43Z | ---
license: apache-2.0
tags:
- generated_from_trainer
model-index:
- name: recipe-lr1e05-wd0.01-bs16
results: []
---
<!-- This model card has been generated automatically according to the information the Trainer had access to. You
should probably proofread and complete it, then remove this comment. -->
# recipe-lr1e05-wd0.01-bs16
This model is a fine-tuned version of [paola-md/recipe-distilroberta-Is](https://huggingface.co/paola-md/recipe-distilroberta-Is) on the None dataset.
It achieves the following results on the evaluation set:
- Loss: 0.2793
- Rmse: 0.5285
- Mse: 0.2793
- Mae: 0.4342
## Model description
More information needed
## Intended uses & limitations
More information needed
## Training and evaluation data
More information needed
## Training procedure
### Training hyperparameters
The following hyperparameters were used during training:
- learning_rate: 1e-05
- train_batch_size: 128
- eval_batch_size: 128
- seed: 42
- optimizer: Adam with betas=(0.9,0.999) and epsilon=1e-08
- lr_scheduler_type: linear
- num_epochs: 10
### Training results
| Training Loss | Epoch | Step | Validation Loss | Rmse | Mse | Mae |
|:-------------:|:-----:|:----:|:---------------:|:------:|:------:|:------:|
| 0.2767 | 1.0 | 1245 | 0.2744 | 0.5239 | 0.2744 | 0.4124 |
| 0.2739 | 2.0 | 2490 | 0.2757 | 0.5251 | 0.2757 | 0.4212 |
| 0.2727 | 3.0 | 3735 | 0.2793 | 0.5285 | 0.2793 | 0.4342 |
### Framework versions
- Transformers 4.19.0.dev0
- Pytorch 1.9.0+cu111
- Datasets 2.4.0
- Tokenizers 0.12.1
|
jackoyoungblood/Reinforce-PongPolGrad | jackoyoungblood | 2022-08-27T21:43:41Z | 0 | 0 | null | [
"Pong-PLE-v0",
"reinforce",
"reinforcement-learning",
"custom-implementation",
"deep-rl-class",
"model-index",
"region:us"
]
| reinforcement-learning | 2022-08-27T21:41:20Z | ---
tags:
- Pong-PLE-v0
- reinforce
- reinforcement-learning
- custom-implementation
- deep-rl-class
model-index:
- name: Reinforce-PongPolGrad
results:
- task:
type: reinforcement-learning
name: reinforcement-learning
dataset:
name: Pong-PLE-v0
type: Pong-PLE-v0
metrics:
- type: mean_reward
value: -16.00 +/- 0.00
name: mean_reward
verified: false
---
# **Reinforce** Agent playing **Pong-PLE-v0**
This is a trained model of a **Reinforce** agent playing **Pong-PLE-v0** .
To learn to use this model and train yours check Unit 5 of the Deep Reinforcement Learning Class: https://github.com/huggingface/deep-rl-class/tree/main/unit5
|
paola-md/recipe-lr8e06-wd0.01-bs16 | paola-md | 2022-08-27T20:37:31Z | 163 | 0 | transformers | [
"transformers",
"pytorch",
"roberta",
"text-classification",
"generated_from_trainer",
"license:apache-2.0",
"autotrain_compatible",
"endpoints_compatible",
"region:us"
]
| text-classification | 2022-08-27T20:20:22Z | ---
license: apache-2.0
tags:
- generated_from_trainer
model-index:
- name: recipe-lr8e06-wd0.01-bs16
results: []
---
<!-- This model card has been generated automatically according to the information the Trainer had access to. You
should probably proofread and complete it, then remove this comment. -->
# recipe-lr8e06-wd0.01-bs16
This model is a fine-tuned version of [paola-md/recipe-distilroberta-Is](https://huggingface.co/paola-md/recipe-distilroberta-Is) on the None dataset.
It achieves the following results on the evaluation set:
- Loss: 0.2795
- Rmse: 0.5286
- Mse: 0.2795
- Mae: 0.4342
## Model description
More information needed
## Intended uses & limitations
More information needed
## Training and evaluation data
More information needed
## Training procedure
### Training hyperparameters
The following hyperparameters were used during training:
- learning_rate: 8e-06
- train_batch_size: 128
- eval_batch_size: 128
- seed: 42
- optimizer: Adam with betas=(0.9,0.999) and epsilon=1e-08
- lr_scheduler_type: linear
- num_epochs: 10
### Training results
| Training Loss | Epoch | Step | Validation Loss | Rmse | Mse | Mae |
|:-------------:|:-----:|:----:|:---------------:|:------:|:------:|:------:|
| 0.2767 | 1.0 | 1245 | 0.2745 | 0.5239 | 0.2745 | 0.4140 |
| 0.2741 | 2.0 | 2490 | 0.2760 | 0.5254 | 0.2760 | 0.4222 |
| 0.2729 | 3.0 | 3735 | 0.2795 | 0.5286 | 0.2795 | 0.4342 |
### Framework versions
- Transformers 4.19.0.dev0
- Pytorch 1.9.0+cu111
- Datasets 2.4.0
- Tokenizers 0.12.1
|
paola-md/recipe-lr2e05-wd0.02-bs16 | paola-md | 2022-08-27T20:19:45Z | 163 | 0 | transformers | [
"transformers",
"pytorch",
"roberta",
"text-classification",
"generated_from_trainer",
"license:apache-2.0",
"autotrain_compatible",
"endpoints_compatible",
"region:us"
]
| text-classification | 2022-08-27T20:02:35Z | ---
license: apache-2.0
tags:
- generated_from_trainer
model-index:
- name: recipe-lr2e05-wd0.02-bs16
results: []
---
<!-- This model card has been generated automatically according to the information the Trainer had access to. You
should probably proofread and complete it, then remove this comment. -->
# recipe-lr2e05-wd0.02-bs16
This model is a fine-tuned version of [paola-md/recipe-distilroberta-Is](https://huggingface.co/paola-md/recipe-distilroberta-Is) on the None dataset.
It achieves the following results on the evaluation set:
- Loss: 0.2780
- Rmse: 0.5272
- Mse: 0.2780
- Mae: 0.4313
## Model description
More information needed
## Intended uses & limitations
More information needed
## Training and evaluation data
More information needed
## Training procedure
### Training hyperparameters
The following hyperparameters were used during training:
- learning_rate: 2e-05
- train_batch_size: 128
- eval_batch_size: 128
- seed: 42
- optimizer: Adam with betas=(0.9,0.999) and epsilon=1e-08
- lr_scheduler_type: linear
- num_epochs: 10
### Training results
| Training Loss | Epoch | Step | Validation Loss | Rmse | Mse | Mae |
|:-------------:|:-----:|:----:|:---------------:|:------:|:------:|:------:|
| 0.277 | 1.0 | 1245 | 0.2743 | 0.5237 | 0.2743 | 0.4111 |
| 0.2738 | 2.0 | 2490 | 0.2814 | 0.5305 | 0.2814 | 0.4294 |
| 0.2725 | 3.0 | 3735 | 0.2780 | 0.5272 | 0.2780 | 0.4313 |
### Framework versions
- Transformers 4.19.0.dev0
- Pytorch 1.9.0+cu111
- Datasets 2.4.0
- Tokenizers 0.12.1
|
paola-md/recipe-lr2e05-wd0.1-bs16 | paola-md | 2022-08-27T20:01:59Z | 163 | 0 | transformers | [
"transformers",
"pytorch",
"roberta",
"text-classification",
"generated_from_trainer",
"license:apache-2.0",
"autotrain_compatible",
"endpoints_compatible",
"region:us"
]
| text-classification | 2022-08-27T19:44:53Z | ---
license: apache-2.0
tags:
- generated_from_trainer
model-index:
- name: recipe-lr2e05-wd0.1-bs16
results: []
---
<!-- This model card has been generated automatically according to the information the Trainer had access to. You
should probably proofread and complete it, then remove this comment. -->
# recipe-lr2e05-wd0.1-bs16
This model is a fine-tuned version of [paola-md/recipe-distilroberta-Is](https://huggingface.co/paola-md/recipe-distilroberta-Is) on the None dataset.
It achieves the following results on the evaluation set:
- Loss: 0.2783
- Rmse: 0.5275
- Mse: 0.2783
- Mae: 0.4319
## Model description
More information needed
## Intended uses & limitations
More information needed
## Training and evaluation data
More information needed
## Training procedure
### Training hyperparameters
The following hyperparameters were used during training:
- learning_rate: 2e-05
- train_batch_size: 128
- eval_batch_size: 128
- seed: 42
- optimizer: Adam with betas=(0.9,0.999) and epsilon=1e-08
- lr_scheduler_type: linear
- num_epochs: 10
### Training results
| Training Loss | Epoch | Step | Validation Loss | Rmse | Mse | Mae |
|:-------------:|:-----:|:----:|:---------------:|:------:|:------:|:------:|
| 0.2771 | 1.0 | 1245 | 0.2744 | 0.5238 | 0.2744 | 0.4105 |
| 0.2738 | 2.0 | 2490 | 0.2819 | 0.5309 | 0.2819 | 0.4298 |
| 0.2724 | 3.0 | 3735 | 0.2783 | 0.5275 | 0.2783 | 0.4319 |
### Framework versions
- Transformers 4.19.0.dev0
- Pytorch 1.9.0+cu111
- Datasets 2.4.0
- Tokenizers 0.12.1
|
theojolliffe/T5-model-1-feedback | theojolliffe | 2022-08-27T19:25:07Z | 7 | 0 | transformers | [
"transformers",
"pytorch",
"tensorboard",
"t5",
"text2text-generation",
"generated_from_trainer",
"license:apache-2.0",
"autotrain_compatible",
"text-generation-inference",
"endpoints_compatible",
"region:us"
]
| text2text-generation | 2022-08-26T21:31:43Z | ---
license: apache-2.0
tags:
- generated_from_trainer
model-index:
- name: T5-model-1-feedback
results: []
---
<!-- This model card has been generated automatically according to the information the Trainer had access to. You
should probably proofread and complete it, then remove this comment. -->
# T5-model-1-feedback
This model is a fine-tuned version of [theojolliffe/T5-model-1-d-4](https://huggingface.co/theojolliffe/T5-model-1-d-4) on the None dataset.
## Model description
More information needed
## Intended uses & limitations
More information needed
## Training and evaluation data
More information needed
## Training procedure
### Training hyperparameters
The following hyperparameters were used during training:
- learning_rate: 2e-05
- train_batch_size: 2
- eval_batch_size: 2
- seed: 42
- optimizer: Adam with betas=(0.9,0.999) and epsilon=1e-08
- lr_scheduler_type: linear
- num_epochs: 1
### Training results
| Training Loss | Epoch | Step | Validation Loss | Rouge1 | Rouge2 | Rougel | Rougelsum | Gen Len |
|:-------------:|:-----:|:----:|:---------------:|:-------:|:-------:|:-------:|:---------:|:-------:|
| No log | 1.0 | 130 | 0.4120 | 61.7277 | 46.2681 | 61.1325 | 61.2797 | 13.2632 |
### Framework versions
- Transformers 4.12.3
- Pytorch 1.9.0
- Datasets 1.18.0
- Tokenizers 0.10.3
|
curt-tigges/ppo-LunarLander-v2 | curt-tigges | 2022-08-27T19:12:38Z | 3 | 0 | stable-baselines3 | [
"stable-baselines3",
"LunarLander-v2",
"deep-reinforcement-learning",
"reinforcement-learning",
"model-index",
"region:us"
]
| reinforcement-learning | 2022-08-27T19:12:10Z | ---
library_name: stable-baselines3
tags:
- LunarLander-v2
- deep-reinforcement-learning
- reinforcement-learning
- stable-baselines3
model-index:
- name: PPO
results:
- metrics:
- type: mean_reward
value: 252.72 +/- 21.52
name: mean_reward
task:
type: reinforcement-learning
name: reinforcement-learning
dataset:
name: LunarLander-v2
type: LunarLander-v2
---
# **PPO** Agent playing **LunarLander-v2**
This is a trained model of a **PPO** agent playing **LunarLander-v2**
using the [stable-baselines3 library](https://github.com/DLR-RM/stable-baselines3).
## Usage (with Stable-baselines3)
TODO: Add your code
```python
from stable_baselines3 import ...
from huggingface_sb3 import load_from_hub
...
```
|
paola-md/recipe-gauss-wo-outliers | paola-md | 2022-08-27T17:24:48Z | 4 | 0 | transformers | [
"transformers",
"pytorch",
"roberta",
"text-classification",
"generated_from_trainer",
"license:apache-2.0",
"autotrain_compatible",
"endpoints_compatible",
"region:us"
]
| text-classification | 2022-08-27T16:33:27Z | ---
license: apache-2.0
tags:
- generated_from_trainer
model-index:
- name: recipe-gauss-wo-outliers
results: []
---
<!-- This model card has been generated automatically according to the information the Trainer had access to. You
should probably proofread and complete it, then remove this comment. -->
# recipe-gauss-wo-outliers
This model is a fine-tuned version of [paola-md/recipe-distilroberta-Is](https://huggingface.co/paola-md/recipe-distilroberta-Is) on the None dataset.
It achieves the following results on the evaluation set:
- Loss: 0.2885
- Rmse: 0.5371
- Mse: 0.2885
- Mae: 0.4213
## Model description
More information needed
## Intended uses & limitations
More information needed
## Training and evaluation data
More information needed
## Training procedure
### Training hyperparameters
The following hyperparameters were used during training:
- learning_rate: 2e-05
- train_batch_size: 128
- eval_batch_size: 128
- seed: 42
- optimizer: Adam with betas=(0.9,0.999) and epsilon=1e-08
- lr_scheduler_type: linear
- num_epochs: 10
### Training results
| Training Loss | Epoch | Step | Validation Loss | Rmse | Mse | Mae |
|:-------------:|:-----:|:-----:|:---------------:|:------:|:------:|:------:|
| 0.2768 | 1.0 | 1245 | 0.2747 | 0.5241 | 0.2747 | 0.4081 |
| 0.2737 | 2.0 | 2490 | 0.2793 | 0.5285 | 0.2793 | 0.4288 |
| 0.2722 | 3.0 | 3735 | 0.2792 | 0.5284 | 0.2792 | 0.4332 |
| 0.2703 | 4.0 | 4980 | 0.2770 | 0.5263 | 0.2770 | 0.4000 |
| 0.2682 | 5.0 | 6225 | 0.2758 | 0.5252 | 0.2758 | 0.4183 |
| 0.2658 | 6.0 | 7470 | 0.2792 | 0.5284 | 0.2792 | 0.4212 |
| 0.2631 | 7.0 | 8715 | 0.2769 | 0.5262 | 0.2769 | 0.4114 |
| 0.2599 | 8.0 | 9960 | 0.2802 | 0.5294 | 0.2802 | 0.4107 |
| 0.2572 | 9.0 | 11205 | 0.2885 | 0.5371 | 0.2885 | 0.4213 |
### Framework versions
- Transformers 4.19.0.dev0
- Pytorch 1.9.0+cu111
- Datasets 2.4.0
- Tokenizers 0.12.1
|
espnet/americasnlp22-asr-gvc | espnet | 2022-08-27T16:15:08Z | 1 | 0 | espnet | [
"espnet",
"audio",
"automatic-speech-recognition",
"gvc",
"dataset:americasnlp22",
"arxiv:1804.00015",
"license:cc-by-4.0",
"region:us"
]
| automatic-speech-recognition | 2022-06-06T19:07:35Z | ---
tags:
- espnet
- audio
- automatic-speech-recognition
language: gvc
datasets:
- americasnlp22
license: cc-by-4.0
---
## ESPnet2 ASR model
### `espnet/americasnlp22-asr-gvc`
This model was trained by Pavel Denisov using americasnlp22 recipe in [espnet](https://github.com/espnet/espnet/).
### Demo: How to use in ESPnet2
```bash
cd espnet
git checkout 66ca5df9f08b6084dbde4d9f312fa8ba0a47ecfc
pip install -e .
cd egs2/americasnlp22/asr1
./run.sh \
--skip_data_prep false \
--skip_train true \
--download_model espnet/americasnlp22-asr-gvc \
--lang gvc \
--local_data_opts "--lang gvc" \
--train_set train_gvc \
--valid_set dev_gvc \
--test_sets dev_gvc \
--gpu_inference false \
--inference_nj 8 \
--lm_train_text data/train_gvc/text \
--bpe_train_text data/train_gvc/text
```
<!-- Generated by scripts/utils/show_asr_result.sh -->
# RESULTS
## Environments
- date: `Sun Jun 5 03:29:33 CEST 2022`
- python version: `3.9.13 (main, May 18 2022, 00:00:00) [GCC 11.3.1 20220421 (Red Hat 11.3.1-2)]`
- espnet version: `espnet 202204`
- pytorch version: `pytorch 1.11.0+cu115`
- Git hash: `d55704daa36d3dd2ca24ae3162ac40d81957208c`
- Commit date: `Wed Jun 1 02:33:09 2022 +0200`
## asr_train_asr_transformer_raw_gvc_bpe100_sp
### WER
|dataset|Snt|Wrd|Corr|Sub|Del|Ins|Err|S.Err|
|---|---|---|---|---|---|---|---|---|
|decode_asr_asr_model_valid.cer_ctc.best/dev_gvc|253|2206|12.4|72.4|15.1|6.7|94.2|99.6|
### CER
|dataset|Snt|Wrd|Corr|Sub|Del|Ins|Err|S.Err|
|---|---|---|---|---|---|---|---|---|
|decode_asr_asr_model_valid.cer_ctc.best/dev_gvc|253|13453|64.7|15.5|19.9|10.2|45.6|99.6|
### TER
|dataset|Snt|Wrd|Corr|Sub|Del|Ins|Err|S.Err|
|---|---|---|---|---|---|---|---|---|
|decode_asr_asr_model_valid.cer_ctc.best/dev_gvc|253|10229|58.3|22.3|19.4|11.0|52.7|99.6|
## ASR config
<details><summary>expand</summary>
```
config: conf/train_asr_transformer.yaml
print_config: false
log_level: INFO
dry_run: false
iterator_type: sequence
output_dir: exp/asr_train_asr_transformer_raw_gvc_bpe100_sp
ngpu: 1
seed: 0
num_workers: 1
num_att_plot: 3
dist_backend: nccl
dist_init_method: env://
dist_world_size: null
dist_rank: null
local_rank: 0
dist_master_addr: null
dist_master_port: null
dist_launcher: null
multiprocessing_distributed: false
unused_parameters: false
sharded_ddp: false
cudnn_enabled: true
cudnn_benchmark: false
cudnn_deterministic: true
collect_stats: false
write_collected_feats: false
max_epoch: 15
patience: null
val_scheduler_criterion:
- valid
- loss
early_stopping_criterion:
- valid
- loss
- min
best_model_criterion:
- - valid
- cer_ctc
- min
keep_nbest_models: 1
nbest_averaging_interval: 0
grad_clip: 5.0
grad_clip_type: 2.0
grad_noise: false
accum_grad: 1
no_forward_run: false
resume: true
train_dtype: float32
use_amp: false
log_interval: null
use_matplotlib: true
use_tensorboard: true
use_wandb: false
wandb_project: null
wandb_id: null
wandb_entity: null
wandb_name: null
wandb_model_log_interval: -1
detect_anomaly: false
pretrain_path: null
init_param: []
ignore_init_mismatch: false
freeze_param:
- frontend.upstream.model.feature_extractor
- frontend.upstream.model.encoder.layers.0
- frontend.upstream.model.encoder.layers.1
- frontend.upstream.model.encoder.layers.2
- frontend.upstream.model.encoder.layers.3
- frontend.upstream.model.encoder.layers.4
- frontend.upstream.model.encoder.layers.5
- frontend.upstream.model.encoder.layers.6
- frontend.upstream.model.encoder.layers.7
- frontend.upstream.model.encoder.layers.8
- frontend.upstream.model.encoder.layers.9
- frontend.upstream.model.encoder.layers.10
- frontend.upstream.model.encoder.layers.11
- frontend.upstream.model.encoder.layers.12
- frontend.upstream.model.encoder.layers.13
- frontend.upstream.model.encoder.layers.14
- frontend.upstream.model.encoder.layers.15
- frontend.upstream.model.encoder.layers.16
- frontend.upstream.model.encoder.layers.17
- frontend.upstream.model.encoder.layers.18
- frontend.upstream.model.encoder.layers.19
- frontend.upstream.model.encoder.layers.20
- frontend.upstream.model.encoder.layers.21
num_iters_per_epoch: null
batch_size: 20
valid_batch_size: null
batch_bins: 200000
valid_batch_bins: null
train_shape_file:
- exp/asr_stats_raw_gvc_bpe100_sp/train/speech_shape
- exp/asr_stats_raw_gvc_bpe100_sp/train/text_shape.bpe
valid_shape_file:
- exp/asr_stats_raw_gvc_bpe100_sp/valid/speech_shape
- exp/asr_stats_raw_gvc_bpe100_sp/valid/text_shape.bpe
batch_type: numel
valid_batch_type: null
fold_length:
- 80000
- 150
sort_in_batch: descending
sort_batch: descending
multiple_iterator: false
chunk_length: 500
chunk_shift_ratio: 0.5
num_cache_chunks: 1024
train_data_path_and_name_and_type:
- - dump/raw/train_gvc_sp/wav.scp
- speech
- sound
- - dump/raw/train_gvc_sp/text
- text
- text
valid_data_path_and_name_and_type:
- - dump/raw/dev_gvc/wav.scp
- speech
- sound
- - dump/raw/dev_gvc/text
- text
- text
allow_variable_data_keys: false
max_cache_size: 0.0
max_cache_fd: 32
valid_max_cache_size: null
optim: adamw
optim_conf:
lr: 0.0001
scheduler: warmuplr
scheduler_conf:
warmup_steps: 300
token_list:
- <blank>
- <unk>
- β
- a
- ''''
- u
- i
- o
- h
- U
- .
- ro
- re
- ri
- ka
- s
- na
- p
- e
- βti
- t
- ':'
- d
- ha
- 'no'
- βhi
- m
- βni
- '~'
- Γ£
- ta
- βwa
- ti
- ','
- βto
- b
- n
- βkh
- ma
- r
- se
- w
- l
- k
- '"'
- Γ±
- Γ΅
- g
- (
- )
- v
- f
- '?'
- A
- K
- z
- Γ©
- T
- '!'
- D
- Γ³
- N
- Γ‘
- R
- P
- ΓΊ
- '0'
- Γ
- I
- '1'
- L
- '-'
- '8'
- E
- S
- Γ
- F
- '9'
- '6'
- G
- C
- x
- '3'
- '2'
- B
- W
- J
- H
- Y
- M
- j
- Γ§
- q
- c
- Γ
- '4'
- '7'
- O
- y
- <sos/eos>
init: null
input_size: null
ctc_conf:
dropout_rate: 0.0
ctc_type: builtin
reduce: true
ignore_nan_grad: true
joint_net_conf: null
use_preprocessor: true
token_type: bpe
bpemodel: data/gvc_token_list/bpe_unigram100/bpe.model
non_linguistic_symbols: null
cleaner: null
g2p: null
speech_volume_normalize: null
rir_scp: null
rir_apply_prob: 1.0
noise_scp: null
noise_apply_prob: 1.0
noise_db_range: '13_15'
frontend: s3prl
frontend_conf:
frontend_conf:
upstream: wav2vec2_url
upstream_ckpt: https://dl.fbaipublicfiles.com/fairseq/wav2vec/xlsr2_300m.pt
download_dir: ./hub
multilayer_feature: true
fs: 16k
specaug: null
specaug_conf: {}
normalize: utterance_mvn
normalize_conf: {}
model: espnet
model_conf:
ctc_weight: 1.0
lsm_weight: 0.0
length_normalized_loss: false
extract_feats_in_collect_stats: false
preencoder: linear
preencoder_conf:
input_size: 1024
output_size: 80
encoder: transformer
encoder_conf:
input_layer: conv2d2
num_blocks: 1
linear_units: 2048
dropout_rate: 0.2
output_size: 256
attention_heads: 8
attention_dropout_rate: 0.2
postencoder: null
postencoder_conf: {}
decoder: rnn
decoder_conf: {}
required:
- output_dir
- token_list
version: '202204'
distributed: false
```
</details>
### Citing ESPnet
```BibTex
@inproceedings{watanabe2018espnet,
author={Shinji Watanabe and Takaaki Hori and Shigeki Karita and Tomoki Hayashi and Jiro Nishitoba and Yuya Unno and Nelson Yalta and Jahn Heymann and Matthew Wiesner and Nanxin Chen and Adithya Renduchintala and Tsubasa Ochiai},
title={{ESPnet}: End-to-End Speech Processing Toolkit},
year={2018},
booktitle={Proceedings of Interspeech},
pages={2207--2211},
doi={10.21437/Interspeech.2018-1456},
url={http://dx.doi.org/10.21437/Interspeech.2018-1456}
}
```
or arXiv:
```bibtex
@misc{watanabe2018espnet,
title={ESPnet: End-to-End Speech Processing Toolkit},
author={Shinji Watanabe and Takaaki Hori and Shigeki Karita and Tomoki Hayashi and Jiro Nishitoba and Yuya Unno and Nelson Yalta and Jahn Heymann and Matthew Wiesner and Nanxin Chen and Adithya Renduchintala and Tsubasa Ochiai},
year={2018},
eprint={1804.00015},
archivePrefix={arXiv},
primaryClass={cs.CL}
}
```
|
espnet/americasnlp22-asr-gn | espnet | 2022-08-27T16:09:50Z | 1 | 0 | espnet | [
"espnet",
"audio",
"automatic-speech-recognition",
"gn",
"dataset:americasnlp22",
"arxiv:1804.00015",
"license:cc-by-4.0",
"region:us"
]
| automatic-speech-recognition | 2022-06-13T17:11:45Z | ---
tags:
- espnet
- audio
- automatic-speech-recognition
language: gn
datasets:
- americasnlp22
license: cc-by-4.0
---
## ESPnet2 ASR model
### `espnet/americasnlp22-asr-gn`
This model was trained by Pavel Denisov using americasnlp22 recipe in [espnet](https://github.com/espnet/espnet/).
### Demo: How to use in ESPnet2
Follow the [ESPnet installation instructions](https://espnet.github.io/espnet/installation.html)
if you haven't done that already.
```bash
cd espnet
git checkout fc62b1ce3e50c5ef8a2ac8cedb0d92ac41df54ca
pip install -e .
cd egs2/americasnlp22/asr1
./run.sh \
--skip_data_prep false \
--skip_train true \
--download_model espnet/americasnlp22-asr-gn \
--lang gn \
--local_data_opts "--lang gn" \
--train_set train_gn \
--valid_set dev_gn \
--test_sets dev_gn \
--gpu_inference false \
--inference_nj 8 \
--lm_train_text data/train_gn/text \
--bpe_train_text data/train_gn/text
```
<!-- Generated by scripts/utils/show_asr_result.sh -->
# RESULTS
## Environments
- date: `Sun Jun 5 12:17:58 CEST 2022`
- python version: `3.9.13 (main, May 18 2022, 00:00:00) [GCC 11.3.1 20220421 (Red Hat 11.3.1-2)]`
- espnet version: `espnet 202204`
- pytorch version: `pytorch 1.11.0+cu115`
- Git hash: `d55704daa36d3dd2ca24ae3162ac40d81957208c`
- Commit date: `Wed Jun 1 02:33:09 2022 +0200`
## asr_train_asr_transformer_raw_gn_bpe100_sp
### WER
|dataset|Snt|Wrd|Corr|Sub|Del|Ins|Err|S.Err|
|---|---|---|---|---|---|---|---|---|
|decode_asr_asr_model_valid.cer_ctc.best/dev_gn|93|391|11.5|73.7|14.8|12.5|101.0|100.0|
### CER
|dataset|Snt|Wrd|Corr|Sub|Del|Ins|Err|S.Err|
|---|---|---|---|---|---|---|---|---|
|decode_asr_asr_model_valid.cer_ctc.best/dev_gn|93|2946|83.4|7.9|8.7|8.7|25.3|100.0|
### TER
|dataset|Snt|Wrd|Corr|Sub|Del|Ins|Err|S.Err|
|---|---|---|---|---|---|---|---|---|
|decode_asr_asr_model_valid.cer_ctc.best/dev_gn|93|2439|76.6|13.5|9.9|8.7|32.1|100.0|
## ASR config
<details><summary>expand</summary>
```
config: conf/train_asr_transformer.yaml
print_config: false
log_level: INFO
dry_run: false
iterator_type: sequence
output_dir: exp/asr_train_asr_transformer_raw_gn_bpe100_sp
ngpu: 1
seed: 0
num_workers: 1
num_att_plot: 3
dist_backend: nccl
dist_init_method: env://
dist_world_size: null
dist_rank: null
local_rank: 0
dist_master_addr: null
dist_master_port: null
dist_launcher: null
multiprocessing_distributed: false
unused_parameters: false
sharded_ddp: false
cudnn_enabled: true
cudnn_benchmark: false
cudnn_deterministic: true
collect_stats: false
write_collected_feats: false
max_epoch: 15
patience: null
val_scheduler_criterion:
- valid
- loss
early_stopping_criterion:
- valid
- loss
- min
best_model_criterion:
- - valid
- cer_ctc
- min
keep_nbest_models: 1
nbest_averaging_interval: 0
grad_clip: 5.0
grad_clip_type: 2.0
grad_noise: false
accum_grad: 1
no_forward_run: false
resume: true
train_dtype: float32
use_amp: false
log_interval: null
use_matplotlib: true
use_tensorboard: true
use_wandb: false
wandb_project: null
wandb_id: null
wandb_entity: null
wandb_name: null
wandb_model_log_interval: -1
detect_anomaly: false
pretrain_path: null
init_param: []
ignore_init_mismatch: false
freeze_param:
- frontend.upstream.model.feature_extractor
- frontend.upstream.model.encoder.layers.0
- frontend.upstream.model.encoder.layers.1
- frontend.upstream.model.encoder.layers.2
- frontend.upstream.model.encoder.layers.3
- frontend.upstream.model.encoder.layers.4
- frontend.upstream.model.encoder.layers.5
- frontend.upstream.model.encoder.layers.6
- frontend.upstream.model.encoder.layers.7
- frontend.upstream.model.encoder.layers.8
- frontend.upstream.model.encoder.layers.9
- frontend.upstream.model.encoder.layers.10
- frontend.upstream.model.encoder.layers.11
- frontend.upstream.model.encoder.layers.12
- frontend.upstream.model.encoder.layers.13
- frontend.upstream.model.encoder.layers.14
- frontend.upstream.model.encoder.layers.15
- frontend.upstream.model.encoder.layers.16
- frontend.upstream.model.encoder.layers.17
- frontend.upstream.model.encoder.layers.18
- frontend.upstream.model.encoder.layers.19
- frontend.upstream.model.encoder.layers.20
- frontend.upstream.model.encoder.layers.21
num_iters_per_epoch: null
batch_size: 20
valid_batch_size: null
batch_bins: 200000
valid_batch_bins: null
train_shape_file:
- exp/asr_stats_raw_gn_bpe100_sp/train/speech_shape
- exp/asr_stats_raw_gn_bpe100_sp/train/text_shape.bpe
valid_shape_file:
- exp/asr_stats_raw_gn_bpe100_sp/valid/speech_shape
- exp/asr_stats_raw_gn_bpe100_sp/valid/text_shape.bpe
batch_type: numel
valid_batch_type: null
fold_length:
- 80000
- 150
sort_in_batch: descending
sort_batch: descending
multiple_iterator: false
chunk_length: 500
chunk_shift_ratio: 0.5
num_cache_chunks: 1024
train_data_path_and_name_and_type:
- - dump/raw/train_gn_sp/wav.scp
- speech
- sound
- - dump/raw/train_gn_sp/text
- text
- text
valid_data_path_and_name_and_type:
- - dump/raw/dev_gn/wav.scp
- speech
- sound
- - dump/raw/dev_gn/text
- text
- text
allow_variable_data_keys: false
max_cache_size: 0.0
max_cache_fd: 32
valid_max_cache_size: null
optim: adamw
optim_conf:
lr: 0.0001
scheduler: warmuplr
scheduler_conf:
warmup_steps: 300
token_list:
- <blank>
- <unk>
- β
- a
- i
- e
- o
- ''''
- .
- u
- '"'
- p
- r
- n
- y
- h
- β"
- βo
- Γ©
- re
- va
- pe
- s
- ra
- Γ‘
- he
- t
- mb
- g
- ka
- Γ£
- v
- ve
- je
- βha
- te
- k
- Γ±
- ha
- py
- ta
- ku
- αΊ½
- ja
- pa
- O
- mi
- Γ³
- mo
- j
- ko
- ΚΌ
- Γ±a
- me
- ma
- c
- M
- Γ
- H
- ΓΊ
- A
- Μ
- Γ΅
- Γ½
- m
- P
- U
- ','
- Ε©
- l
- α»Ή
- N
- Δ©
- E
- I
- J
- L
- Γ
- V
- S
- z
- '-'
- '?'
- Γ
- R
- G
- Y
- T
- K
- C
- d
- β
- B
- β
- β
- D
- b
- f
- q
- <sos/eos>
init: null
input_size: null
ctc_conf:
dropout_rate: 0.0
ctc_type: builtin
reduce: true
ignore_nan_grad: true
joint_net_conf: null
use_preprocessor: true
token_type: bpe
bpemodel: data/gn_token_list/bpe_unigram100/bpe.model
non_linguistic_symbols: null
cleaner: null
g2p: null
speech_volume_normalize: null
rir_scp: null
rir_apply_prob: 1.0
noise_scp: null
noise_apply_prob: 1.0
noise_db_range: '13_15'
frontend: s3prl
frontend_conf:
frontend_conf:
upstream: wav2vec2_url
upstream_ckpt: https://dl.fbaipublicfiles.com/fairseq/wav2vec/xlsr2_300m.pt
download_dir: ./hub
multilayer_feature: true
fs: 16k
specaug: null
specaug_conf: {}
normalize: utterance_mvn
normalize_conf: {}
model: espnet
model_conf:
ctc_weight: 1.0
lsm_weight: 0.0
length_normalized_loss: false
extract_feats_in_collect_stats: false
preencoder: linear
preencoder_conf:
input_size: 1024
output_size: 80
encoder: transformer
encoder_conf:
input_layer: conv2d2
num_blocks: 1
linear_units: 2048
dropout_rate: 0.2
output_size: 256
attention_heads: 8
attention_dropout_rate: 0.2
postencoder: null
postencoder_conf: {}
decoder: rnn
decoder_conf: {}
required:
- output_dir
- token_list
version: '202204'
distributed: false
```
</details>
### Citing ESPnet
```BibTex
@inproceedings{watanabe2018espnet,
author={Shinji Watanabe and Takaaki Hori and Shigeki Karita and Tomoki Hayashi and Jiro Nishitoba and Yuya Unno and Nelson Yalta and Jahn Heymann and Matthew Wiesner and Nanxin Chen and Adithya Renduchintala and Tsubasa Ochiai},
title={{ESPnet}: End-to-End Speech Processing Toolkit},
year={2018},
booktitle={Proceedings of Interspeech},
pages={2207--2211},
doi={10.21437/Interspeech.2018-1456},
url={http://dx.doi.org/10.21437/Interspeech.2018-1456}
}
```
or arXiv:
```bibtex
@misc{watanabe2018espnet,
title={ESPnet: End-to-End Speech Processing Toolkit},
author={Shinji Watanabe and Takaaki Hori and Shigeki Karita and Tomoki Hayashi and Jiro Nishitoba and Yuya Unno and Nelson Yalta and Jahn Heymann and Matthew Wiesner and Nanxin Chen and Adithya Renduchintala and Tsubasa Ochiai},
year={2018},
eprint={1804.00015},
archivePrefix={arXiv},
primaryClass={cs.CL}
}
```
|
huggingtweets/tojibaceo-tojibawhiteroom | huggingtweets | 2022-08-27T15:47:39Z | 107 | 0 | transformers | [
"transformers",
"pytorch",
"gpt2",
"text-generation",
"huggingtweets",
"en",
"autotrain_compatible",
"text-generation-inference",
"endpoints_compatible",
"region:us"
]
| text-generation | 2022-07-26T15:54:01Z | ---
language: en
thumbnail: http://www.huggingtweets.com/tojibaceo-tojibawhiteroom/1661615254424/predictions.png
tags:
- huggingtweets
widget:
- text: "My dream is"
---
<div class="inline-flex flex-col" style="line-height: 1.5;">
<div class="flex">
<div
style="display:inherit; margin-left: 4px; margin-right: 4px; width: 92px; height:92px; border-radius: 50%; background-size: cover; background-image: url('https://pbs.twimg.com/profile_images/1508824472924659725/267f4Lkm_400x400.jpg')">
</div>
<div
style="display:inherit; margin-left: 4px; margin-right: 4px; width: 92px; height:92px; border-radius: 50%; background-size: cover; background-image: url('https://pbs.twimg.com/profile_images/1509337156787003394/WjOdf_-m_400x400.jpg')">
</div>
<div
style="display:none; margin-left: 4px; margin-right: 4px; width: 92px; height:92px; border-radius: 50%; background-size: cover; background-image: url('')">
</div>
</div>
<div style="text-align: center; margin-top: 3px; font-size: 16px; font-weight: 800">π€ AI CYBORG π€</div>
<div style="text-align: center; font-size: 16px; font-weight: 800">Tojiba CPU Corp BUDDIES MINTING NOW (π,π) & Tojiba White Room (T__T).1</div>
<div style="text-align: center; font-size: 14px;">@tojibaceo-tojibawhiteroom</div>
</div>
I was made with [huggingtweets](https://github.com/borisdayma/huggingtweets).
Create your own bot based on your favorite user with [the demo](https://colab.research.google.com/github/borisdayma/huggingtweets/blob/master/huggingtweets-demo.ipynb)!
## How does it work?
The model uses the following pipeline.
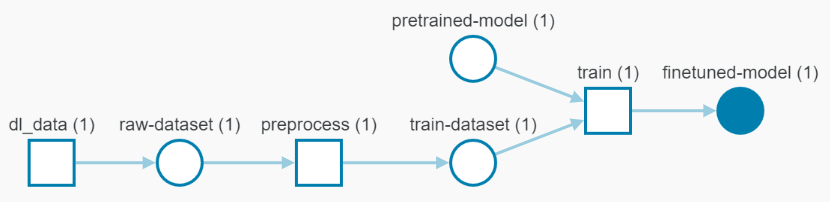
To understand how the model was developed, check the [W&B report](https://wandb.ai/wandb/huggingtweets/reports/HuggingTweets-Train-a-Model-to-Generate-Tweets--VmlldzoxMTY5MjI).
## Training data
The model was trained on tweets from Tojiba CPU Corp BUDDIES MINTING NOW (π,π) & Tojiba White Room (T__T).1.
| Data | Tojiba CPU Corp BUDDIES MINTING NOW (π,π) | Tojiba White Room (T__T).1 |
| --- | --- | --- |
| Tweets downloaded | 1613 | 704 |
| Retweets | 774 | 0 |
| Short tweets | 279 | 82 |
| Tweets kept | 560 | 622 |
[Explore the data](https://wandb.ai/wandb/huggingtweets/runs/1kju2ojf/artifacts), which is tracked with [W&B artifacts](https://docs.wandb.com/artifacts) at every step of the pipeline.
## Training procedure
The model is based on a pre-trained [GPT-2](https://huggingface.co/gpt2) which is fine-tuned on @tojibaceo-tojibawhiteroom's tweets.
Hyperparameters and metrics are recorded in the [W&B training run](https://wandb.ai/wandb/huggingtweets/runs/15twdubf) for full transparency and reproducibility.
At the end of training, [the final model](https://wandb.ai/wandb/huggingtweets/runs/15twdubf/artifacts) is logged and versioned.
## How to use
You can use this model directly with a pipeline for text generation:
```python
from transformers import pipeline
generator = pipeline('text-generation',
model='huggingtweets/tojibaceo-tojibawhiteroom')
generator("My dream is", num_return_sequences=5)
```
## Limitations and bias
The model suffers from [the same limitations and bias as GPT-2](https://huggingface.co/gpt2#limitations-and-bias).
In addition, the data present in the user's tweets further affects the text generated by the model.
## About
*Built by Boris Dayma*
[](https://twitter.com/intent/follow?screen_name=borisdayma)
For more details, visit the project repository.
[](https://github.com/borisdayma/huggingtweets)
|
muhtasham/tajroberto-ner | muhtasham | 2022-08-27T15:37:05Z | 8 | 0 | transformers | [
"transformers",
"pytorch",
"tensorboard",
"roberta",
"token-classification",
"generated_from_trainer",
"dataset:wikiann",
"model-index",
"autotrain_compatible",
"endpoints_compatible",
"region:us"
]
| token-classification | 2022-08-27T15:27:16Z | ---
tags:
- generated_from_trainer
datasets:
- wikiann
metrics:
- precision
- recall
- f1
- accuracy
model-index:
- name: tajroberto-ner
results:
- task:
name: Token Classification
type: token-classification
dataset:
name: wikiann
type: wikiann
config: tg
split: train+test
args: tg
metrics:
- name: Precision
type: precision
value: 0.3155080213903743
- name: Recall
type: recall
value: 0.5673076923076923
- name: F1
type: f1
value: 0.4054982817869416
- name: Accuracy
type: accuracy
value: 0.83597621407334
---
<!-- This model card has been generated automatically according to the information the Trainer had access to. You
should probably proofread and complete it, then remove this comment. -->
# tajroberto-ner
This model is a fine-tuned version of [muhtasham/RoBERTa-tg](https://huggingface.co/muhtasham/RoBERTa-tg) on the wikiann dataset.
It achieves the following results on the evaluation set:
- Loss: 0.9408
- Precision: 0.3155
- Recall: 0.5673
- F1: 0.4055
- Accuracy: 0.8360
## Model description
More information needed
## Intended uses & limitations
More information needed
## Training and evaluation data
More information needed
## Training procedure
### Training hyperparameters
The following hyperparameters were used during training:
- learning_rate: 2e-05
- train_batch_size: 8
- eval_batch_size: 8
- seed: 42
- optimizer: Adam with betas=(0.9,0.999) and epsilon=1e-08
- lr_scheduler_type: linear
- num_epochs: 200
### Training results
| Training Loss | Epoch | Step | Validation Loss | Precision | Recall | F1 | Accuracy |
|:-------------:|:-----:|:----:|:---------------:|:---------:|:------:|:------:|:--------:|
| No log | 2.0 | 50 | 0.7710 | 0.0532 | 0.1827 | 0.0824 | 0.6933 |
| No log | 4.0 | 100 | 0.5901 | 0.0847 | 0.25 | 0.1265 | 0.7825 |
| No log | 6.0 | 150 | 0.5226 | 0.2087 | 0.4615 | 0.2874 | 0.8186 |
| No log | 8.0 | 200 | 0.5041 | 0.2585 | 0.5096 | 0.3430 | 0.8449 |
| No log | 10.0 | 250 | 0.5592 | 0.2819 | 0.5096 | 0.3630 | 0.8499 |
| No log | 12.0 | 300 | 0.5725 | 0.3032 | 0.5481 | 0.3904 | 0.8558 |
| No log | 14.0 | 350 | 0.6433 | 0.3122 | 0.5673 | 0.4027 | 0.8508 |
| No log | 16.0 | 400 | 0.6744 | 0.3543 | 0.5962 | 0.4444 | 0.8553 |
| No log | 18.0 | 450 | 0.7617 | 0.3353 | 0.5577 | 0.4188 | 0.8335 |
| 0.2508 | 20.0 | 500 | 0.7608 | 0.3262 | 0.5865 | 0.4192 | 0.8419 |
| 0.2508 | 22.0 | 550 | 0.8483 | 0.3224 | 0.5673 | 0.4111 | 0.8494 |
| 0.2508 | 24.0 | 600 | 0.8370 | 0.3275 | 0.5385 | 0.4073 | 0.8439 |
| 0.2508 | 26.0 | 650 | 0.8652 | 0.3410 | 0.5673 | 0.4260 | 0.8394 |
| 0.2508 | 28.0 | 700 | 0.9441 | 0.3409 | 0.5769 | 0.4286 | 0.8216 |
| 0.2508 | 30.0 | 750 | 0.9228 | 0.3333 | 0.5577 | 0.4173 | 0.8439 |
| 0.2508 | 32.0 | 800 | 0.9175 | 0.3430 | 0.5673 | 0.4275 | 0.8355 |
| 0.2508 | 34.0 | 850 | 0.9603 | 0.3073 | 0.5288 | 0.3887 | 0.8340 |
| 0.2508 | 36.0 | 900 | 0.9417 | 0.3240 | 0.5577 | 0.4099 | 0.8370 |
| 0.2508 | 38.0 | 950 | 0.9408 | 0.3155 | 0.5673 | 0.4055 | 0.8360 |
### Framework versions
- Transformers 4.21.2
- Pytorch 1.12.1+cu113
- Datasets 2.4.0
- Tokenizers 0.12.1
|
brightink/Stable_Diffusion_Demo | brightink | 2022-08-27T14:51:44Z | 0 | 0 | null | [
"license:afl-3.0",
"region:us"
]
| null | 2022-08-27T14:49:16Z | ---
title: Stable Diffusion
emoji: π
colorFrom: red
colorTo: red
sdk: gradio
sdk_version: 3.1.7
app_file: app.py
pinned: false
license: afl-3.0
---
Check out the configuration reference at https://huggingface.co/docs/hub/spaces-config-reference |
theojolliffe/T5-model-1-d-4 | theojolliffe | 2022-08-27T14:20:07Z | 8 | 0 | transformers | [
"transformers",
"pytorch",
"tensorboard",
"t5",
"text2text-generation",
"generated_from_trainer",
"license:apache-2.0",
"autotrain_compatible",
"text-generation-inference",
"endpoints_compatible",
"region:us"
]
| text2text-generation | 2022-08-26T21:54:25Z | ---
license: apache-2.0
tags:
- generated_from_trainer
metrics:
- rouge
model-index:
- name: T5-model-1-d-4
results: []
---
<!-- This model card has been generated automatically according to the information the Trainer had access to. You
should probably proofread and complete it, then remove this comment. -->
# T5-model-1-d-4
This model is a fine-tuned version of [t5-base](https://huggingface.co/t5-base) on an unknown dataset.
It achieves the following results on the evaluation set:
- Loss: 0.0456
- Rouge1: 93.3486
- Rouge2: 82.1873
- Rougel: 92.8611
- Rougelsum: 92.7768
- Gen Len: 14.9953
## Model description
More information needed
## Intended uses & limitations
More information needed
## Training and evaluation data
More information needed
## Training procedure
### Training hyperparameters
The following hyperparameters were used during training:
- learning_rate: 2e-05
- train_batch_size: 2
- eval_batch_size: 2
- seed: 42
- optimizer: Adam with betas=(0.9,0.999) and epsilon=1e-08
- lr_scheduler_type: linear
- num_epochs: 1
- mixed_precision_training: Native AMP
### Training results
| Training Loss | Epoch | Step | Validation Loss | Rouge1 | Rouge2 | Rougel | Rougelsum | Gen Len |
|:-------------:|:-----:|:----:|:---------------:|:-------:|:-------:|:-------:|:---------:|:-------:|
| 0.0873 | 1.0 | 8043 | 0.0456 | 93.3486 | 82.1873 | 92.8611 | 92.7768 | 14.9953 |
### Framework versions
- Transformers 4.21.2
- Pytorch 1.12.1+cu113
- Tokenizers 0.12.1
|
nrazavi/xlm-roberta-base-finetuned-panx-all | nrazavi | 2022-08-27T14:19:11Z | 126 | 0 | transformers | [
"transformers",
"pytorch",
"xlm-roberta",
"token-classification",
"generated_from_trainer",
"license:mit",
"autotrain_compatible",
"endpoints_compatible",
"region:us"
]
| token-classification | 2022-08-27T14:01:42Z | ---
license: mit
tags:
- generated_from_trainer
metrics:
- f1
model-index:
- name: xlm-roberta-base-finetuned-panx-all
results: []
---
<!-- This model card has been generated automatically according to the information the Trainer had access to. You
should probably proofread and complete it, then remove this comment. -->
# xlm-roberta-base-finetuned-panx-all
This model is a fine-tuned version of [xlm-roberta-base](https://huggingface.co/xlm-roberta-base) on the None dataset.
It achieves the following results on the evaluation set:
- Loss: 0.1727
- F1: 0.8560
## Model description
More information needed
## Intended uses & limitations
More information needed
## Training and evaluation data
More information needed
## Training procedure
### Training hyperparameters
The following hyperparameters were used during training:
- learning_rate: 5e-05
- train_batch_size: 24
- eval_batch_size: 24
- seed: 42
- optimizer: Adam with betas=(0.9,0.999) and epsilon=1e-08
- lr_scheduler_type: linear
- num_epochs: 3
### Training results
| Training Loss | Epoch | Step | Validation Loss | F1 |
|:-------------:|:-----:|:----:|:---------------:|:------:|
| 0.3057 | 1.0 | 835 | 0.1901 | 0.8135 |
| 0.1565 | 2.0 | 1670 | 0.1727 | 0.8436 |
| 0.1021 | 3.0 | 2505 | 0.1727 | 0.8560 |
### Framework versions
- Transformers 4.21.2
- Pytorch 1.12.1+cu116
- Datasets 2.4.0
- Tokenizers 0.12.1
|
danieladejumo/Reinforce-CartPole-v1 | danieladejumo | 2022-08-27T14:05:13Z | 0 | 0 | null | [
"CartPole-v1",
"reinforce",
"reinforcement-learning",
"custom-implementation",
"deep-rl-class",
"model-index",
"region:us"
]
| reinforcement-learning | 2022-08-27T14:03:47Z | ---
tags:
- CartPole-v1
- reinforce
- reinforcement-learning
- custom-implementation
- deep-rl-class
model-index:
- name: Reinforce-CartPole-v1
results:
- task:
type: reinforcement-learning
name: reinforcement-learning
dataset:
name: CartPole-v1
type: CartPole-v1
metrics:
- type: mean_reward
value: 83.20 +/- 44.00
name: mean_reward
verified: false
---
# **Reinforce** Agent playing **CartPole-v1**
This is a trained model of a **Reinforce** agent playing **CartPole-v1** .
To learn to use this model and train yours check Unit 5 of the Deep Reinforcement Learning Class: https://github.com/huggingface/deep-rl-class/tree/main/unit5
|
chum76/chiron0076 | chum76 | 2022-08-27T12:27:38Z | 0 | 0 | null | [
"license:cc-by-nc-sa-4.0",
"region:us"
]
| null | 2022-08-27T12:27:38Z | ---
license: cc-by-nc-sa-4.0
---
|
akkasayaz/q-FrozenLake-v1-4x4-noSlippery | akkasayaz | 2022-08-27T12:22:50Z | 0 | 0 | null | [
"FrozenLake-v1-4x4-no_slippery",
"q-learning",
"reinforcement-learning",
"custom-implementation",
"model-index",
"region:us"
]
| reinforcement-learning | 2022-08-27T12:22:44Z | ---
tags:
- FrozenLake-v1-4x4-no_slippery
- q-learning
- reinforcement-learning
- custom-implementation
model-index:
- name: q-FrozenLake-v1-4x4-noSlippery
results:
- task:
type: reinforcement-learning
name: reinforcement-learning
dataset:
name: FrozenLake-v1-4x4-no_slippery
type: FrozenLake-v1-4x4-no_slippery
metrics:
- type: mean_reward
value: 1.00 +/- 0.00
name: mean_reward
verified: false
---
# **Q-Learning** Agent playing **FrozenLake-v1**
This is a trained model of a **Q-Learning** agent playing **FrozenLake-v1** .
## Usage
```python
model = load_from_hub(repo_id="akkasayaz/q-FrozenLake-v1-4x4-noSlippery", filename="q-learning.pkl")
# Don't forget to check if you need to add additional attributes (is_slippery=False etc)
env = gym.make(model["env_id"])
evaluate_agent(env, model["max_steps"], model["n_eval_episodes"], model["qtable"], model["eval_seed"])
```
|
silviacamplani/distilbert-finetuned-dapt_tapt-ner-ai | silviacamplani | 2022-08-27T11:12:23Z | 65 | 0 | transformers | [
"transformers",
"tf",
"tensorboard",
"distilbert",
"token-classification",
"generated_from_keras_callback",
"autotrain_compatible",
"endpoints_compatible",
"region:us"
]
| token-classification | 2022-08-27T11:09:10Z | ---
tags:
- generated_from_keras_callback
model-index:
- name: silviacamplani/distilbert-finetuned-dapt_tapt-ner-ai
results: []
---
<!-- This model card has been generated automatically according to the information Keras had access to. You should
probably proofread and complete it, then remove this comment. -->
# silviacamplani/distilbert-finetuned-dapt_tapt-ner-ai
This model was trained from scratch on an unknown dataset.
It achieves the following results on the evaluation set:
- Train Loss: 0.8595
- Validation Loss: 0.8604
- Train Precision: 0.3378
- Train Recall: 0.3833
- Train F1: 0.3591
- Train Accuracy: 0.7860
- Epoch: 9
## Model description
More information needed
## Intended uses & limitations
More information needed
## Training and evaluation data
More information needed
## Training procedure
### Training hyperparameters
The following hyperparameters were used during training:
- optimizer: {'inner_optimizer': {'class_name': 'AdamWeightDecay', 'config': {'name': 'AdamWeightDecay', 'learning_rate': {'class_name': 'PolynomialDecay', 'config': {'initial_learning_rate': 1e-05, 'decay_steps': 350, 'end_learning_rate': 0.0, 'power': 1.0, 'cycle': False, 'name': None}}, 'decay': 0.0, 'beta_1': 0.9, 'beta_2': 0.999, 'epsilon': 1e-08, 'amsgrad': False, 'weight_decay_rate': 0.01}}, 'dynamic': True, 'initial_scale': 32768.0, 'dynamic_growth_steps': 2000}
- training_precision: mixed_float16
### Training results
| Train Loss | Validation Loss | Train Precision | Train Recall | Train F1 | Train Accuracy | Epoch |
|:----------:|:---------------:|:---------------:|:------------:|:--------:|:--------------:|:-----:|
| 2.5333 | 1.7392 | 0.0 | 0.0 | 0.0 | 0.6480 | 0 |
| 1.5890 | 1.4135 | 0.0 | 0.0 | 0.0 | 0.6480 | 1 |
| 1.3635 | 1.2627 | 0.0 | 0.0 | 0.0 | 0.6483 | 2 |
| 1.2366 | 1.1526 | 0.1538 | 0.0920 | 0.1151 | 0.6921 | 3 |
| 1.1296 | 1.0519 | 0.2147 | 0.2147 | 0.2147 | 0.7321 | 4 |
| 1.0374 | 0.9753 | 0.2743 | 0.2981 | 0.2857 | 0.7621 | 5 |
| 0.9639 | 0.9202 | 0.3023 | 0.3373 | 0.3188 | 0.7693 | 6 |
| 0.9097 | 0.8829 | 0.3215 | 0.3714 | 0.3447 | 0.7795 | 7 |
| 0.8756 | 0.8635 | 0.3280 | 0.3850 | 0.3542 | 0.7841 | 8 |
| 0.8595 | 0.8604 | 0.3378 | 0.3833 | 0.3591 | 0.7860 | 9 |
### Framework versions
- Transformers 4.20.1
- TensorFlow 2.6.4
- Datasets 2.1.0
- Tokenizers 0.12.1
|
pinot/wav2vec2-large-xls-r-300m-ja-colab-3 | pinot | 2022-08-27T06:14:51Z | 109 | 0 | transformers | [
"transformers",
"pytorch",
"tensorboard",
"wav2vec2",
"automatic-speech-recognition",
"generated_from_trainer",
"dataset:common_voice_10_0",
"license:apache-2.0",
"endpoints_compatible",
"region:us"
]
| automatic-speech-recognition | 2022-08-26T23:39:55Z | ---
license: apache-2.0
tags:
- generated_from_trainer
datasets:
- common_voice_10_0
model-index:
- name: wav2vec2-large-xls-r-300m-ja-colab-3
results: []
---
<!-- This model card has been generated automatically according to the information the Trainer had access to. You
should probably proofread and complete it, then remove this comment. -->
# wav2vec2-large-xls-r-300m-ja-colab-3
This model is a fine-tuned version of [pinot/wav2vec2-large-xls-r-300m-ja-colab-2](https://huggingface.co/pinot/wav2vec2-large-xls-r-300m-ja-colab-2) on the common_voice_10_0 dataset.
It achieves the following results on the evaluation set:
- Loss: 1.2696
- Wer: 0.2299
## Model description
More information needed
## Intended uses & limitations
More information needed
## Training and evaluation data
More information needed
## Training procedure
### Training hyperparameters
The following hyperparameters were used during training:
- learning_rate: 0.0003
- train_batch_size: 1
- eval_batch_size: 1
- seed: 42
- gradient_accumulation_steps: 16
- total_train_batch_size: 16
- optimizer: Adam with betas=(0.9,0.999) and epsilon=1e-08
- lr_scheduler_type: linear
- lr_scheduler_warmup_steps: 500
- num_epochs: 10
- mixed_precision_training: Native AMP
### Training results
| Training Loss | Epoch | Step | Validation Loss | Wer |
|:-------------:|:-----:|:----:|:---------------:|:------:|
| No log | 1.0 | 637 | 1.4666 | 0.2862 |
| No log | 2.0 | 1274 | 1.4405 | 0.2866 |
| No log | 3.0 | 1911 | 1.4162 | 0.2762 |
| No log | 4.0 | 2548 | 1.4128 | 0.2709 |
| 0.2814 | 5.0 | 3185 | 1.3927 | 0.2613 |
| 0.2814 | 6.0 | 3822 | 1.3629 | 0.2536 |
| 0.2814 | 7.0 | 4459 | 1.3349 | 0.2429 |
| 0.2814 | 8.0 | 5096 | 1.3116 | 0.2356 |
| 0.1624 | 9.0 | 5733 | 1.2774 | 0.2307 |
| 0.1624 | 10.0 | 6370 | 1.2696 | 0.2299 |
### Framework versions
- Transformers 4.21.2
- Pytorch 1.10.0+cu113
- Datasets 2.4.0
- Tokenizers 0.12.1
|
rajistics/layoutlmv2-finetuned-cord | rajistics | 2022-08-27T04:45:12Z | 5 | 0 | transformers | [
"transformers",
"pytorch",
"tensorboard",
"layoutlmv2",
"token-classification",
"generated_from_trainer",
"license:cc-by-nc-sa-4.0",
"autotrain_compatible",
"endpoints_compatible",
"region:us"
]
| token-classification | 2022-08-27T03:25:11Z | ---
license: cc-by-nc-sa-4.0
tags:
- generated_from_trainer
model-index:
- name: layoutlmv2-finetuned-cord
results: []
---
<!-- This model card has been generated automatically according to the information the Trainer had access to. You
should probably proofread and complete it, then remove this comment. -->
# layoutlmv2-finetuned-cord
This model is a fine-tuned version of [microsoft/layoutlmv2-base-uncased](https://huggingface.co/microsoft/layoutlmv2-base-uncased) on an unknown dataset.
## Model description
More information needed
## Intended uses & limitations
More information needed
## Training and evaluation data
More information needed
## Training procedure
### Training hyperparameters
The following hyperparameters were used during training:
- learning_rate: 5e-05
- train_batch_size: 8
- eval_batch_size: 8
- seed: 42
- optimizer: Adam with betas=(0.9,0.999) and epsilon=1e-08
- lr_scheduler_type: linear
- lr_scheduler_warmup_ratio: 0.1
- training_steps: 3000
- mixed_precision_training: Native AMP
### Training results
### Framework versions
- Transformers 4.21.2
- Pytorch 1.10.0+cu111
- Datasets 2.4.0
- Tokenizers 0.12.1
|
fat32man/elon_answers | fat32man | 2022-08-27T04:23:49Z | 105 | 0 | transformers | [
"transformers",
"pytorch",
"gpt2",
"text-generation",
"conversational",
"license:mit",
"autotrain_compatible",
"text-generation-inference",
"endpoints_compatible",
"region:us"
]
| text-generation | 2022-08-27T03:38:27Z | ---
tags:
- conversational
license: mit
---
|
mindofmadness/faces01 | mindofmadness | 2022-08-27T02:11:32Z | 0 | 0 | null | [
"region:us"
]
| null | 2022-08-27T02:08:30Z | short narrow face, mid size lips, light freckles on upper cheeks, light grey eyes, brunette hair, nerd glasses |
gharris7/ppo-LunarLander-v2 | gharris7 | 2022-08-27T01:52:25Z | 0 | 0 | stable-baselines3 | [
"stable-baselines3",
"LunarLander-v2",
"deep-reinforcement-learning",
"reinforcement-learning",
"model-index",
"region:us"
]
| reinforcement-learning | 2022-08-27T01:51:56Z | ---
library_name: stable-baselines3
tags:
- LunarLander-v2
- deep-reinforcement-learning
- reinforcement-learning
- stable-baselines3
model-index:
- name: ppo
results:
- metrics:
- type: mean_reward
value: 222.74 +/- 23.39
name: mean_reward
task:
type: reinforcement-learning
name: reinforcement-learning
dataset:
name: LunarLander-v2
type: LunarLander-v2
---
# **ppo** Agent playing **LunarLander-v2**
This is a trained model of a **ppo** agent playing **LunarLander-v2**
using the [stable-baselines3 library](https://github.com/DLR-RM/stable-baselines3).
## Usage (with Stable-baselines3)
TODO: Add your code
```python
from stable_baselines3 import ...
from huggingface_sb3 import load_from_hub
...
```
|
caffsean/distilbert-base-uncased-finetuned-emotion | caffsean | 2022-08-27T01:27:28Z | 108 | 0 | transformers | [
"transformers",
"pytorch",
"tensorboard",
"distilbert",
"text-classification",
"generated_from_trainer",
"dataset:emotion",
"license:apache-2.0",
"model-index",
"autotrain_compatible",
"endpoints_compatible",
"region:us"
]
| text-classification | 2022-08-27T00:35:06Z | ---
license: apache-2.0
tags:
- generated_from_trainer
datasets:
- emotion
metrics:
- accuracy
- f1
model-index:
- name: distilbert-base-uncased-finetuned-emotion
results:
- task:
name: Text Classification
type: text-classification
dataset:
name: emotion
type: emotion
config: default
split: train
args: default
metrics:
- name: Accuracy
type: accuracy
value: 0.9225
- name: F1
type: f1
value: 0.9223304536402763
---
<!-- This model card has been generated automatically according to the information the Trainer had access to. You
should probably proofread and complete it, then remove this comment. -->
# distilbert-base-uncased-finetuned-emotion
This model is a fine-tuned version of [distilbert-base-uncased](https://huggingface.co/distilbert-base-uncased) on the emotion dataset.
It achieves the following results on the evaluation set:
- Loss: 0.2111
- Accuracy: 0.9225
- F1: 0.9223
## Model description
More information needed
## Intended uses & limitations
More information needed
## Training and evaluation data
More information needed
## Training procedure
### Training hyperparameters
The following hyperparameters were used during training:
- learning_rate: 2e-05
- train_batch_size: 64
- eval_batch_size: 64
- seed: 42
- optimizer: Adam with betas=(0.9,0.999) and epsilon=1e-08
- lr_scheduler_type: linear
- num_epochs: 2
### Training results
| Training Loss | Epoch | Step | Validation Loss | Accuracy | F1 |
|:-------------:|:-----:|:----:|:---------------:|:--------:|:------:|
| 0.8274 | 1.0 | 250 | 0.3054 | 0.912 | 0.9096 |
| 0.2409 | 2.0 | 500 | 0.2111 | 0.9225 | 0.9223 |
### Framework versions
- Transformers 4.21.2
- Pytorch 1.12.1+cu113
- Datasets 2.4.0
- Tokenizers 0.12.1
|
theojolliffe/T5-model-1-d-6 | theojolliffe | 2022-08-27T00:15:29Z | 6 | 0 | transformers | [
"transformers",
"pytorch",
"tensorboard",
"t5",
"text2text-generation",
"generated_from_trainer",
"license:apache-2.0",
"autotrain_compatible",
"text-generation-inference",
"endpoints_compatible",
"region:us"
]
| text2text-generation | 2022-08-26T22:53:31Z | ---
license: apache-2.0
tags:
- generated_from_trainer
metrics:
- rouge
model-index:
- name: T5-model-1-d-6
results: []
---
<!-- This model card has been generated automatically according to the information the Trainer had access to. You
should probably proofread and complete it, then remove this comment. -->
# T5-model-1-d-6
This model is a fine-tuned version of [t5-base](https://huggingface.co/t5-base) on an unknown dataset.
It achieves the following results on the evaluation set:
- Loss: 0.0229
- Rouge1: 94.972
- Rouge2: 84.9842
- Rougel: 94.7792
- Rougelsum: 94.758
- Gen Len: 15.0918
## Model description
More information needed
## Intended uses & limitations
More information needed
## Training and evaluation data
More information needed
## Training procedure
### Training hyperparameters
The following hyperparameters were used during training:
- learning_rate: 2e-05
- train_batch_size: 2
- eval_batch_size: 2
- seed: 42
- optimizer: Adam with betas=(0.9,0.999) and epsilon=1e-08
- lr_scheduler_type: linear
- num_epochs: 1
- mixed_precision_training: Native AMP
### Training results
| Training Loss | Epoch | Step | Validation Loss | Rouge1 | Rouge2 | Rougel | Rougelsum | Gen Len |
|:-------------:|:-----:|:-----:|:---------------:|:------:|:-------:|:-------:|:---------:|:-------:|
| 0.0449 | 1.0 | 16085 | 0.0229 | 94.972 | 84.9842 | 94.7792 | 94.758 | 15.0918 |
### Framework versions
- Transformers 4.21.2
- Pytorch 1.12.1+cu113
- Datasets 2.4.0
- Tokenizers 0.12.1
|
paola-md/recipe-gauss-2 | paola-md | 2022-08-26T22:33:03Z | 165 | 0 | transformers | [
"transformers",
"pytorch",
"roberta",
"text-classification",
"generated_from_trainer",
"license:apache-2.0",
"autotrain_compatible",
"endpoints_compatible",
"region:us"
]
| text-classification | 2022-08-26T21:17:27Z | ---
license: apache-2.0
tags:
- generated_from_trainer
model-index:
- name: recipe-gauss-2
results: []
---
<!-- This model card has been generated automatically according to the information the Trainer had access to. You
should probably proofread and complete it, then remove this comment. -->
# recipe-gauss-2
This model is a fine-tuned version of [distilroberta-base](https://huggingface.co/distilroberta-base) on the None dataset.
It achieves the following results on the evaluation set:
- Loss: 0.4204
- Rmse: 0.6484
- Mse: 0.4204
- Mae: 0.4557
## Model description
More information needed
## Intended uses & limitations
More information needed
## Training and evaluation data
More information needed
## Training procedure
### Training hyperparameters
The following hyperparameters were used during training:
- learning_rate: 5e-05
- train_batch_size: 32
- eval_batch_size: 32
- seed: 42
- optimizer: Adam with betas=(0.9,0.999) and epsilon=1e-08
- lr_scheduler_type: linear
- num_epochs: 10
### Training results
| Training Loss | Epoch | Step | Validation Loss | Rmse | Mse | Mae |
|:-------------:|:-----:|:-----:|:---------------:|:------:|:------:|:------:|
| 0.4002 | 1.0 | 3029 | 0.4228 | 0.6502 | 0.4228 | 0.4485 |
| 0.3986 | 2.0 | 6058 | 0.4200 | 0.6481 | 0.4200 | 0.4566 |
| 0.3985 | 3.0 | 9087 | 0.4217 | 0.6494 | 0.4217 | 0.4515 |
| 0.3977 | 4.0 | 12116 | 0.4212 | 0.6490 | 0.4212 | 0.4528 |
| 0.397 | 5.0 | 15145 | 0.4251 | 0.6520 | 0.4251 | 0.4461 |
| 0.397 | 6.0 | 18174 | 0.4203 | 0.6483 | 0.4203 | 0.4665 |
| 0.3968 | 7.0 | 21203 | 0.4211 | 0.6489 | 0.4211 | 0.4533 |
| 0.3964 | 8.0 | 24232 | 0.4208 | 0.6487 | 0.4208 | 0.4543 |
| 0.3963 | 9.0 | 27261 | 0.4199 | 0.6480 | 0.4199 | 0.4604 |
| 0.3961 | 10.0 | 30290 | 0.4204 | 0.6484 | 0.4204 | 0.4557 |
### Framework versions
- Transformers 4.19.0.dev0
- Pytorch 1.9.0+cu111
- Datasets 2.4.0
- Tokenizers 0.12.1
|
nrazavi/xlm-roberta-base-finetuned-panx-de | nrazavi | 2022-08-26T22:31:10Z | 128 | 0 | transformers | [
"transformers",
"pytorch",
"xlm-roberta",
"token-classification",
"generated_from_trainer",
"dataset:xtreme",
"license:mit",
"model-index",
"autotrain_compatible",
"endpoints_compatible",
"region:us"
]
| token-classification | 2022-08-26T22:12:51Z | ---
license: mit
tags:
- generated_from_trainer
datasets:
- xtreme
metrics:
- f1
model-index:
- name: xlm-roberta-base-finetuned-panx-de
results:
- task:
name: Token Classification
type: token-classification
dataset:
name: xtreme
type: xtreme
args: PAN-X.de
metrics:
- name: F1
type: f1
value: 0.8609504366564591
---
<!-- This model card has been generated automatically according to the information the Trainer had access to. You
should probably proofread and complete it, then remove this comment. -->
# xlm-roberta-base-finetuned-panx-de
This model is a fine-tuned version of [xlm-roberta-base](https://huggingface.co/xlm-roberta-base) on the xtreme dataset.
It achieves the following results on the evaluation set:
- Loss: 0.1359
- F1: 0.8610
## Model description
More information needed
## Intended uses & limitations
More information needed
## Training and evaluation data
More information needed
## Training procedure
### Training hyperparameters
The following hyperparameters were used during training:
- learning_rate: 5e-05
- train_batch_size: 24
- eval_batch_size: 24
- seed: 42
- optimizer: Adam with betas=(0.9,0.999) and epsilon=1e-08
- lr_scheduler_type: linear
- num_epochs: 3
### Training results
| Training Loss | Epoch | Step | Validation Loss | F1 |
|:-------------:|:-----:|:----:|:---------------:|:------:|
| 0.2594 | 1.0 | 525 | 0.1734 | 0.8095 |
| 0.1305 | 2.0 | 1050 | 0.1414 | 0.8462 |
| 0.0818 | 3.0 | 1575 | 0.1359 | 0.8610 |
### Framework versions
- Transformers 4.15.0
- Pytorch 1.10.1+cu113
- Datasets 2.4.0
- Tokenizers 0.10.3
|
theojolliffe/T5-model-1-d-2 | theojolliffe | 2022-08-26T21:34:45Z | 8 | 0 | transformers | [
"transformers",
"pytorch",
"tensorboard",
"t5",
"text2text-generation",
"generated_from_trainer",
"license:apache-2.0",
"autotrain_compatible",
"text-generation-inference",
"endpoints_compatible",
"region:us"
]
| text2text-generation | 2022-08-26T21:03:21Z | ---
license: apache-2.0
tags:
- generated_from_trainer
metrics:
- rouge
model-index:
- name: T5-model-1-d-2
results: []
---
<!-- This model card has been generated automatically according to the information the Trainer had access to. You
should probably proofread and complete it, then remove this comment. -->
# T5-model-1-d-2
This model is a fine-tuned version of [t5-base](https://huggingface.co/t5-base) on an unknown dataset.
It achieves the following results on the evaluation set:
- Loss: 0.1480
- Rouge1: 85.8534
- Rouge2: 73.1193
- Rougel: 84.9795
- Rougelsum: 84.9322
- Gen Len: 14.0575
## Model description
More information needed
## Intended uses & limitations
More information needed
## Training and evaluation data
More information needed
## Training procedure
### Training hyperparameters
The following hyperparameters were used during training:
- learning_rate: 2e-05
- train_batch_size: 2
- eval_batch_size: 2
- seed: 42
- optimizer: Adam with betas=(0.9,0.999) and epsilon=1e-08
- lr_scheduler_type: linear
- num_epochs: 1
- mixed_precision_training: Native AMP
### Training results
| Training Loss | Epoch | Step | Validation Loss | Rouge1 | Rouge2 | Rougel | Rougelsum | Gen Len |
|:-------------:|:-----:|:----:|:---------------:|:-------:|:-------:|:-------:|:---------:|:-------:|
| 0.2301 | 1.0 | 4022 | 0.1480 | 85.8534 | 73.1193 | 84.9795 | 84.9322 | 14.0575 |
### Framework versions
- Transformers 4.21.2
- Pytorch 1.12.1+cu113
- Datasets 2.4.0
- Tokenizers 0.12.1
|
hhffxx/xlm-roberta-base-finetuned-panx-en | hhffxx | 2022-08-26T20:52:39Z | 106 | 0 | transformers | [
"transformers",
"pytorch",
"xlm-roberta",
"token-classification",
"generated_from_trainer",
"dataset:xtreme",
"license:mit",
"model-index",
"autotrain_compatible",
"endpoints_compatible",
"region:us"
]
| token-classification | 2022-08-26T20:08:33Z | ---
license: mit
tags:
- generated_from_trainer
datasets:
- xtreme
metrics:
- f1
model-index:
- name: xlm-roberta-base-finetuned-panx-en
results:
- task:
name: Token Classification
type: token-classification
dataset:
name: xtreme
type: xtreme
config: PAN-X.en
split: train
args: PAN-X.en
metrics:
- name: F1
type: f1
value: 0.6307099614749588
---
<!-- This model card has been generated automatically according to the information the Trainer had access to. You
should probably proofread and complete it, then remove this comment. -->
# xlm-roberta-base-finetuned-panx-en
This model is a fine-tuned version of [xlm-roberta-base](https://huggingface.co/xlm-roberta-base) on the xtreme dataset.
It achieves the following results on the evaluation set:
- Loss: 0.7589
- F1: 0.6307
## Model description
More information needed
## Intended uses & limitations
More information needed
## Training and evaluation data
More information needed
## Training procedure
### Training hyperparameters
The following hyperparameters were used during training:
- learning_rate: 5e-05
- train_batch_size: 1
- eval_batch_size: 1
- seed: 42
- optimizer: Adam with betas=(0.9,0.999) and epsilon=1e-08
- lr_scheduler_type: linear
- num_epochs: 1
### Training results
| Training Loss | Epoch | Step | Validation Loss | F1 |
|:-------------:|:-----:|:----:|:---------------:|:------:|
| 0.9453 | 1.0 | 1180 | 0.7589 | 0.6307 |
### Framework versions
- Transformers 4.21.1
- Pytorch 1.11.0
- Datasets 2.4.0
- Tokenizers 0.12.1
|
hhffxx/xlm-roberta-base-finetuned-panx-it | hhffxx | 2022-08-26T20:07:17Z | 116 | 0 | transformers | [
"transformers",
"pytorch",
"xlm-roberta",
"token-classification",
"generated_from_trainer",
"dataset:xtreme",
"license:mit",
"model-index",
"autotrain_compatible",
"endpoints_compatible",
"region:us"
]
| token-classification | 2022-08-26T19:06:58Z | ---
license: mit
tags:
- generated_from_trainer
datasets:
- xtreme
metrics:
- f1
model-index:
- name: xlm-roberta-base-finetuned-panx-it
results:
- task:
name: Token Classification
type: token-classification
dataset:
name: xtreme
type: xtreme
config: PAN-X.it
split: train
args: PAN-X.it
metrics:
- name: F1
type: f1
value: 0.7875307629204266
---
<!-- This model card has been generated automatically according to the information the Trainer had access to. You
should probably proofread and complete it, then remove this comment. -->
# xlm-roberta-base-finetuned-panx-it
This model is a fine-tuned version of [xlm-roberta-base](https://huggingface.co/xlm-roberta-base) on the xtreme dataset.
It achieves the following results on the evaluation set:
- Loss: 0.5555
- F1: 0.7875
## Model description
More information needed
## Intended uses & limitations
More information needed
## Training and evaluation data
More information needed
## Training procedure
### Training hyperparameters
The following hyperparameters were used during training:
- learning_rate: 5e-05
- train_batch_size: 1
- eval_batch_size: 1
- seed: 42
- optimizer: Adam with betas=(0.9,0.999) and epsilon=1e-08
- lr_scheduler_type: linear
- num_epochs: 1
### Training results
| Training Loss | Epoch | Step | Validation Loss | F1 |
|:-------------:|:-----:|:----:|:---------------:|:------:|
| 0.8118 | 1.0 | 1680 | 0.5555 | 0.7875 |
### Framework versions
- Transformers 4.21.1
- Pytorch 1.11.0
- Datasets 2.4.0
- Tokenizers 0.12.1
|
iewaij/roberta-base-lm | iewaij | 2022-08-26T17:43:52Z | 117 | 0 | transformers | [
"transformers",
"pytorch",
"roberta",
"fill-mask",
"generated_from_trainer",
"license:mit",
"autotrain_compatible",
"endpoints_compatible",
"region:us"
]
| fill-mask | 2022-08-26T17:34:56Z | ---
license: mit
tags:
- generated_from_trainer
model-index:
- name: roberta-base-lm-all
results: []
---
<!-- This model card has been generated automatically according to the information the Trainer had access to. You
should probably proofread and complete it, then remove this comment. -->
# roberta-base-lm-all
This model is a fine-tuned version of [roberta-base](https://huggingface.co/roberta-base) on the None dataset.
It achieves the following results on the evaluation set:
- Loss: 0.7109
## Model description
More information needed
## Intended uses & limitations
More information needed
## Training and evaluation data
More information needed
## Training procedure
### Training hyperparameters
The following hyperparameters were used during training:
- learning_rate: 5e-05
- train_batch_size: 32
- eval_batch_size: 8
- seed: 42
- optimizer: Adam with betas=(0.9,0.999) and epsilon=1e-08
- lr_scheduler_type: linear
- num_epochs: 16
### Training results
| Training Loss | Epoch | Step | Validation Loss |
|:-------------:|:-----:|:-----:|:---------------:|
| 1.2966 | 1.0 | 1194 | 1.0711 |
| 1.0858 | 2.0 | 2388 | 0.9740 |
| 1.0055 | 3.0 | 3582 | 0.9273 |
| 0.9301 | 4.0 | 4776 | 0.8784 |
| 0.9021 | 5.0 | 5970 | 0.8731 |
| 0.8479 | 6.0 | 7164 | 0.8406 |
| 0.8142 | 7.0 | 8358 | 0.8172 |
| 0.7858 | 8.0 | 9552 | 0.8158 |
| 0.7529 | 9.0 | 10746 | 0.7922 |
| 0.7189 | 10.0 | 11940 | 0.7855 |
| 0.7032 | 11.0 | 13134 | 0.7761 |
| 0.6795 | 12.0 | 14328 | 0.7549 |
| 0.6673 | 13.0 | 15522 | 0.7277 |
| 0.6412 | 14.0 | 16716 | 0.7121 |
| 0.6321 | 15.0 | 17910 | 0.7168 |
| 0.6198 | 16.0 | 19104 | 0.7109 |
### Framework versions
- Transformers 4.21.2
- Pytorch 1.12.1+cu113
- Datasets 2.4.0
- Tokenizers 0.12.1
|
hhffxx/xlm-roberta-base-finetuned-panx-de-fr | hhffxx | 2022-08-26T15:59:52Z | 106 | 0 | transformers | [
"transformers",
"pytorch",
"xlm-roberta",
"token-classification",
"generated_from_trainer",
"license:mit",
"autotrain_compatible",
"endpoints_compatible",
"region:us"
]
| token-classification | 2022-08-17T09:45:33Z | ---
license: mit
tags:
- generated_from_trainer
metrics:
- f1
model-index:
- name: xlm-roberta-base-finetuned-panx-de-fr
results: []
---
<!-- This model card has been generated automatically according to the information the Trainer had access to. You
should probably proofread and complete it, then remove this comment. -->
# xlm-roberta-base-finetuned-panx-de-fr
This model is a fine-tuned version of [xlm-roberta-base](https://huggingface.co/xlm-roberta-base) on the None dataset.
It achieves the following results on the evaluation set:
- Loss: 0.3847
- F1: 0.8178
## Model description
More information needed
## Intended uses & limitations
More information needed
## Training and evaluation data
More information needed
## Training procedure
### Training hyperparameters
The following hyperparameters were used during training:
- learning_rate: 5e-05
- train_batch_size: 1
- eval_batch_size: 1
- seed: 42
- optimizer: Adam with betas=(0.9,0.999) and epsilon=1e-08
- lr_scheduler_type: linear
- num_epochs: 1
### Training results
| Training Loss | Epoch | Step | Validation Loss | F1 |
|:-------------:|:-----:|:-----:|:---------------:|:------:|
| 0.5654 | 1.0 | 17160 | 0.3847 | 0.8178 |
### Framework versions
- Transformers 4.21.1
- Pytorch 1.11.0
- Datasets 2.4.0
- Tokenizers 0.12.1
|
Einmalumdiewelt/T5-Base_GNAD | Einmalumdiewelt | 2022-08-26T15:55:55Z | 3,869 | 22 | transformers | [
"transformers",
"pytorch",
"t5",
"text2text-generation",
"generated_from_trainer",
"summarization",
"de",
"autotrain_compatible",
"text-generation-inference",
"endpoints_compatible",
"region:us"
]
| summarization | 2022-03-02T23:29:04Z | ---
language:
- de
tags:
- generated_from_trainer
- summarization
metrics:
- rouge
model-index:
- name: T5-Base_GNAD
results: []
---
<!-- This model card has been generated automatically according to the information the Trainer had access to. You
should probably proofread and complete it, then remove this comment. -->
# T5-Base_GNAD
This model is a fine-tuned version of [Einmalumdiewelt/T5-Base_GNAD](https://huggingface.co/Einmalumdiewelt/T5-Base_GNAD) on an unknown dataset.
It achieves the following results on the evaluation set:
- Loss: 2.1025
- Rouge1: 27.5357
- Rouge2: 8.5623
- Rougel: 19.1508
- Rougelsum: 23.9029
- Gen Len: 52.7253
## Model description
More information needed
## Intended uses & limitations
More information needed
## Training and evaluation data
More information needed
## Training procedure
### Training hyperparameters
The following hyperparameters were used during training:
- learning_rate: 5e-05
- train_batch_size: 8
- eval_batch_size: 8
- seed: 42
- optimizer: Adam with betas=(0.9,0.999) and epsilon=1e-08
- lr_scheduler_type: linear
- num_epochs: 10.0
### Training results
### Framework versions
- Transformers 4.22.0.dev0
- Pytorch 1.12.0+cu113
- Datasets 2.4.0
- Tokenizers 0.12.1
|
Einmalumdiewelt/PegasusXSUM_GNAD | Einmalumdiewelt | 2022-08-26T15:53:31Z | 171 | 1 | transformers | [
"transformers",
"pytorch",
"pegasus",
"text2text-generation",
"generated_from_trainer",
"summarization",
"de",
"autotrain_compatible",
"endpoints_compatible",
"region:us"
]
| summarization | 2022-03-02T23:29:04Z | ---
language:
- de
tags:
- generated_from_trainer
- summarization
metrics:
- rouge
model-index:
- name: PegasusXSUM_GNAD
results: []
---
<!-- This model card has been generated automatically according to the information the Trainer had access to. You
should probably proofread and complete it, then remove this comment. -->
# PegasusXSUM_GNAD
This model is a fine-tuned version of [Einmalumdiewelt/PegasusXSUM_GNAD](https://huggingface.co/Einmalumdiewelt/PegasusXSUM_GNAD) on an unknown dataset.
It achieves the following results on the evaluation set:
- Loss: 2.4386
- Rouge1: 26.7818
- Rouge2: 7.6864
- Rougel: 18.6264
- Rougelsum: 22.822
- Gen Len: 67.076
## Model description
More information needed
## Intended uses & limitations
More information needed
## Training and evaluation data
More information needed
## Training procedure
### Training hyperparameters
The following hyperparameters were used during training:
- learning_rate: 5e-05
- train_batch_size: 2
- eval_batch_size: 2
- seed: 42
- optimizer: Adam with betas=(0.9,0.999) and epsilon=1e-08
- lr_scheduler_type: linear
- num_epochs: 10.0
### Training results
### Framework versions
- Transformers 4.22.0.dev0
- Pytorch 1.12.0+cu113
- Datasets 2.4.0
- Tokenizers 0.12.1
|
mdround/q-Taxi-v3 | mdround | 2022-08-26T15:53:17Z | 0 | 0 | null | [
"Taxi-v3",
"q-learning",
"reinforcement-learning",
"custom-implementation",
"model-index",
"region:us"
]
| reinforcement-learning | 2022-08-26T15:49:48Z | ---
tags:
- Taxi-v3
- q-learning
- reinforcement-learning
- custom-implementation
model-index:
- name: q-Taxi-v3
results:
- task:
type: reinforcement-learning
name: reinforcement-learning
dataset:
name: Taxi-v3
type: Taxi-v3
metrics:
- type: mean_reward
value: 7.50 +/- 2.77
name: mean_reward
verified: false
---
# **Q-Learning** Agent playing **Taxi-v3**
This is a trained model of a **Q-Learning** agent playing **Taxi-v3** .
## Usage
```python
model = load_from_hub(repo_id="mdround/q-Taxi-v3", filename="q-learning.pkl")
# Don't forget to check if you need to add additional attributes (is_slippery=False etc)
env = gym.make(model["env_id"])
evaluate_agent(env, model["max_steps"], model["n_eval_episodes"], model["qtable"], model["eval_seed"])
```
|
Sania67/Fine_Tuned_XLSR_English | Sania67 | 2022-08-26T14:36:19Z | 109 | 0 | transformers | [
"transformers",
"pytorch",
"wav2vec2",
"automatic-speech-recognition",
"generated_from_trainer",
"license:apache-2.0",
"endpoints_compatible",
"region:us"
]
| automatic-speech-recognition | 2022-08-26T09:32:07Z | ---
license: apache-2.0
tags:
- generated_from_trainer
model-index:
- name: Fine_Tuned_XLSR_English
results: []
---
<!-- This model card has been generated automatically according to the information the Trainer had access to. You
should probably proofread and complete it, then remove this comment. -->
# Fine_Tuned_XLSR_English
This model is a fine-tuned version of [facebook/wav2vec2-xls-r-300m](https://huggingface.co/facebook/wav2vec2-xls-r-300m) on the [timit_asr](https://huggingface.co/datasets/timit_asr) dataset.
It achieves the following results on the evaluation set:
- Loss: 0.4033
- Wer: 0.3163
## Model description
More information needed
## Intended uses & limitations
More information needed
## Training and evaluation data
More information needed
## Training procedure
### Training hyperparameters
The following hyperparameters were used during training:
- learning_rate: 0.0001
- train_batch_size: 8
- eval_batch_size: 8
- seed: 42
- optimizer: Adam with betas=(0.9,0.999) and epsilon=1e-08
- lr_scheduler_type: linear
- lr_scheduler_warmup_steps: 1000
- num_epochs: 30
- mixed_precision_training: Native AMP
### Training results
| Training Loss | Epoch | Step | Validation Loss | Wer |
|:-------------:|:-----:|:-----:|:---------------:|:------:|
| 4.3757 | 1.0 | 500 | 3.1570 | 1.0 |
| 2.4891 | 2.01 | 1000 | 0.9252 | 0.8430 |
| 0.8725 | 3.01 | 1500 | 0.4581 | 0.4931 |
| 0.544 | 4.02 | 2000 | 0.3757 | 0.4328 |
| 0.4043 | 5.02 | 2500 | 0.3621 | 0.4087 |
| 0.3376 | 6.02 | 3000 | 0.3682 | 0.3931 |
| 0.2937 | 7.03 | 3500 | 0.3541 | 0.3743 |
| 0.2573 | 8.03 | 4000 | 0.3565 | 0.3593 |
| 0.2257 | 9.04 | 4500 | 0.3634 | 0.3654 |
| 0.215 | 10.04 | 5000 | 0.3695 | 0.3537 |
| 0.1879 | 11.04 | 5500 | 0.3690 | 0.3486 |
| 0.1599 | 12.05 | 6000 | 0.3743 | 0.3490 |
| 0.1499 | 13.05 | 6500 | 0.4108 | 0.3424 |
| 0.147 | 14.06 | 7000 | 0.4048 | 0.3400 |
| 0.1355 | 15.06 | 7500 | 0.3988 | 0.3357 |
| 0.1278 | 16.06 | 8000 | 0.3672 | 0.3384 |
| 0.1189 | 17.07 | 8500 | 0.4011 | 0.3340 |
| 0.1089 | 18.07 | 9000 | 0.3948 | 0.3300 |
| 0.1039 | 19.08 | 9500 | 0.4062 | 0.3317 |
| 0.0971 | 20.08 | 10000 | 0.4041 | 0.3252 |
| 0.0902 | 21.08 | 10500 | 0.4112 | 0.3301 |
| 0.0883 | 22.09 | 11000 | 0.4154 | 0.3292 |
| 0.0864 | 23.09 | 11500 | 0.3746 | 0.3189 |
| 0.0746 | 24.1 | 12000 | 0.3991 | 0.3230 |
| 0.0711 | 25.1 | 12500 | 0.3916 | 0.3200 |
| 0.0712 | 26.1 | 13000 | 0.4024 | 0.3193 |
| 0.0663 | 27.11 | 13500 | 0.3976 | 0.3184 |
| 0.0626 | 28.11 | 14000 | 0.4046 | 0.3168 |
| 0.0641 | 29.12 | 14500 | 0.4033 | 0.3163 |
### Framework versions
- Transformers 4.17.0
- Pytorch 1.12.1+cu113
- Datasets 1.18.3
- Tokenizers 0.12.1
|
KIZervus/KIZervus | KIZervus | 2022-08-26T13:29:06Z | 5 | 1 | transformers | [
"transformers",
"tf",
"distilbert",
"text-classification",
"generated_from_keras_callback",
"license:apache-2.0",
"autotrain_compatible",
"endpoints_compatible",
"region:us"
]
| text-classification | 2022-08-24T16:32:49Z | ---
license: apache-2.0
tags:
- generated_from_keras_callback
model-index:
- name: tmp3y468_8j
results: []
widget:
- text: "Ich liebe dich!"
example_title: "Non-vulgar"
- text: "Leck mich am arsch"
example_title: "Vulgar"
---
# KIZervus
This model is a fine-tuned version of [distilbert-base-german-cased](https://huggingface.co/distilbert-base-german-cased).
It is trained to classify german text into the classes "vulgar" speech and "non-vulgar" speech.
The data set is a collection of other labeled sources in german. For an overview, see the github repository here: https://github.com/NKDataConv/KIZervus
Both data and training procedure are documented in the GitHub repo. Your are welcome to contribute.
It achieves the following results on the evaluation set:
- Train Loss: 0.4640
- Train Accuracy: 0.7744
- Validation Loss: 0.4852
- Validation Accuracy: 0.7937
- Epoch: 1
## Training procedure
For details, see the repo and documentation here: https://github.com/NKDataConv/KIZervus
### Training hyperparameters
The following hyperparameters were used during training:
- optimizer: {'name': 'Adam', 'learning_rate': {'class_name': 'PolynomialDecay', 'config': {'initial_learning_rate': 5e-05, 'decay_steps': 822, 'end_learning_rate': 0.0, 'power': 1.0, 'cycle': False, 'name': None}}, 'decay': 0.0, 'beta_1': 0.9, 'beta_2': 0.999, 'epsilon': 1e-08, 'amsgrad': False}
- training_precision: float32
### Training results
| Train Loss | Train Accuracy | Validation Loss | Validation Accuracy | Epoch |
|:----------:|:--------------:|:---------------:|:-------------------:|:-----:|
| 0.4830 | 0.7617 | 0.5061 | 0.7406 | 0 |
| 0.4640 | 0.7744 | 0.4852 | 0.7937 | 1 |
### Framework versions
- Transformers 4.21.2
- TensorFlow 2.8.2
- Datasets 2.2.2
- Tokenizers 0.12.1
### Supporter

|
amberoad/bert-multilingual-passage-reranking-msmarco | amberoad | 2022-08-26T13:14:54Z | 157,131 | 84 | transformers | [
"transformers",
"pytorch",
"tf",
"jax",
"bert",
"text-classification",
"msmarco",
"multilingual",
"passage reranking",
"af",
"sq",
"ar",
"an",
"hy",
"ast",
"az",
"ba",
"eu",
"bar",
"be",
"bn",
"inc",
"bs",
"br",
"bg",
"my",
"ca",
"ceb",
"ce",
"zh",
"cv",
"hr",
"cs",
"da",
"nl",
"en",
"et",
"fi",
"fr",
"gl",
"ka",
"de",
"el",
"gu",
"ht",
"he",
"hi",
"hu",
"is",
"io",
"id",
"ga",
"it",
"ja",
"jv",
"kn",
"kk",
"ky",
"ko",
"la",
"lv",
"lt",
"roa",
"nds",
"lm",
"mk",
"mg",
"ms",
"ml",
"mr",
"min",
"ne",
"new",
"nb",
"nn",
"oc",
"fa",
"pms",
"pl",
"pt",
"pa",
"ro",
"ru",
"sco",
"sr",
"scn",
"sk",
"sl",
"aze",
"es",
"su",
"sw",
"sv",
"tl",
"tg",
"ta",
"tt",
"te",
"tr",
"uk",
"ud",
"uz",
"vi",
"vo",
"war",
"cy",
"fry",
"pnb",
"yo",
"dataset:msmarco",
"arxiv:1901.04085",
"license:apache-2.0",
"autotrain_compatible",
"endpoints_compatible",
"region:us"
]
| text-classification | 2022-03-02T23:29:05Z | ---
language:
- multilingual
- af
- sq
- ar
- an
- hy
- ast
- az
- ba
- eu
- bar
- be
- bn
- inc
- bs
- br
- bg
- my
- ca
- ceb
- ce
- zh
- cv
- hr
- cs
- da
- nl
- en
- et
- fi
- fr
- gl
- ka
- de
- el
- gu
- ht
- he
- hi
- hu
- is
- io
- id
- ga
- it
- ja
- jv
- kn
- kk
- ky
- ko
- la
- lv
- lt
- roa
- nds
- lm
- mk
- mg
- ms
- ml
- mr
- min
- ne
- new
- nb
- nn
- oc
- fa
- pms
- pl
- pt
- pa
- ro
- ru
- sco
- sr
- hr
- scn
- sk
- sl
- aze
- es
- su
- sw
- sv
- tl
- tg
- ta
- tt
- te
- tr
- uk
- ud
- uz
- vi
- vo
- war
- cy
- fry
- pnb
- yo
thumbnail: https://amberoad.de/images/logo_text.png
tags:
- msmarco
- multilingual
- passage reranking
license: apache-2.0
datasets:
- msmarco
metrics:
- MRR
widget:
- query: What is a corporation?
passage: A company is incorporated in a specific nation, often within the bounds
of a smaller subset of that nation, such as a state or province. The corporation
is then governed by the laws of incorporation in that state. A corporation may
issue stock, either private or public, or may be classified as a non-stock corporation.
If stock is issued, the corporation will usually be governed by its shareholders,
either directly or indirectly.
---
# Passage Reranking Multilingual BERT π π
## Model description
**Input:** Supports over 100 Languages. See [List of supported languages](https://github.com/google-research/bert/blob/master/multilingual.md#list-of-languages) for all available.
**Purpose:** This module takes a search query [1] and a passage [2] and calculates if the passage matches the query.
It can be used as an improvement for Elasticsearch Results and boosts the relevancy by up to 100%.
**Architecture:** On top of BERT there is a Densly Connected NN which takes the 768 Dimensional [CLS] Token as input and provides the output ([Arxiv](https://arxiv.org/abs/1901.04085)).
**Output:** Just a single value between between -10 and 10. Better matching query,passage pairs tend to have a higher a score.
## Intended uses & limitations
Both query[1] and passage[2] have to fit in 512 Tokens.
As you normally want to rerank the first dozens of search results keep in mind the inference time of approximately 300 ms/query.
#### How to use
```python
from transformers import AutoTokenizer, AutoModelForSequenceClassification
tokenizer = AutoTokenizer.from_pretrained("amberoad/bert-multilingual-passage-reranking-msmarco")
model = AutoModelForSequenceClassification.from_pretrained("amberoad/bert-multilingual-passage-reranking-msmarco")
```
This Model can be used as a drop-in replacement in the [Nboost Library](https://github.com/koursaros-ai/nboost)
Through this you can directly improve your Elasticsearch Results without any coding.
## Training data
This model is trained using the [**Microsoft MS Marco Dataset**](https://microsoft.github.io/msmarco/ "Microsoft MS Marco"). This training dataset contains approximately 400M tuples of a query, relevant and non-relevant passages. All datasets used for training and evaluating are listed in this [table](https://github.com/microsoft/MSMARCO-Passage-Ranking#data-information-and-formating). The used dataset for training is called *Train Triples Large*, while the evaluation was made on *Top 1000 Dev*. There are 6,900 queries in total in the development dataset, where each query is mapped to top 1,000 passage retrieved using BM25 from MS MARCO corpus.
## Training procedure
The training is performed the same way as stated in this [README](https://github.com/nyu-dl/dl4marco-bert "NYU Github"). See their excellent Paper on [Arxiv](https://arxiv.org/abs/1901.04085).
We changed the BERT Model from an English only to the default BERT Multilingual uncased Model from [Google](https://huggingface.co/bert-base-multilingual-uncased).
Training was done 400 000 Steps. This equaled 12 hours an a TPU V3-8.
## Eval results
We see nearly similar performance than the English only Model in the English [Bing Queries Dataset](http://www.msmarco.org/). Although the training data is English only internal Tests on private data showed a far higher accurancy in German than all other available models.
Fine-tuned Models | Dependency | Eval Set | Search Boost<a href='#benchmarks'> | Speed on GPU
----------------------------------------------------------------------------------- | ---------------------------------------------------------------------------- | ------------------------------------------------------------------ | ----------------------------------------------------- | ----------------------------------
**`amberoad/Multilingual-uncased-MSMARCO`** (This Model) | <img alt="PyTorch" src="https://img.shields.io/badge/PyTorch-blue"/> | <a href ='http://www.msmarco.org/'>bing queries</a> | **+61%** <sub><sup>(0.29 vs 0.18)</sup></sub> | ~300 ms/query <a href='#footnotes'>
`nboost/pt-tinybert-msmarco` | <img alt="PyTorch" src="https://img.shields.io/badge/PyTorch-red"/> | <a href ='http://www.msmarco.org/'>bing queries</a> | **+45%** <sub><sup>(0.26 vs 0.18)</sup></sub> | ~50ms/query <a href='#footnotes'>
`nboost/pt-bert-base-uncased-msmarco` | <img alt="PyTorch" src="https://img.shields.io/badge/PyTorch-red"/> | <a href ='http://www.msmarco.org/'>bing queries</a> | **+62%** <sub><sup>(0.29 vs 0.18)</sup></sub> | ~300 ms/query<a href='#footnotes'>
`nboost/pt-bert-large-msmarco` | <img alt="PyTorch" src="https://img.shields.io/badge/PyTorch-red"/> | <a href ='http://www.msmarco.org/'>bing queries</a> | **+77%** <sub><sup>(0.32 vs 0.18)</sup></sub> | -
`nboost/pt-biobert-base-msmarco` | <img alt="PyTorch" src="https://img.shields.io/badge/PyTorch-red"/> | <a href ='https://github.com/naver/biobert-pretrained'>biomed</a> | **+66%** <sub><sup>(0.17 vs 0.10)</sup></sub> | ~300 ms/query<a href='#footnotes'>
This table is taken from [nboost](https://github.com/koursaros-ai/nboost) and extended by the first line.
## Contact Infos

Amberoad is a company focussing on Search and Business Intelligence.
We provide you:
* Advanced Internal Company Search Engines thorugh NLP
* External Search Egnines: Find Competitors, Customers, Suppliers
**Get in Contact now to benefit from our Expertise:**
The training and evaluation was performed by [**Philipp Reissel**](https://reissel.eu/) and [**Igli Manaj**](https://github.com/iglimanaj)
[ Linkedin](https://de.linkedin.com/company/amberoad) | <svg xmlns="http://www.w3.org/2000/svg" x="0px" y="0px"
width="32" height="32"
viewBox="0 0 172 172"
style=" fill:#000000;"><g fill="none" fill-rule="nonzero" stroke="none" stroke-width="1" stroke-linecap="butt" stroke-linejoin="miter" stroke-miterlimit="10" stroke-dasharray="" stroke-dashoffset="0" font-family="none" font-weight="none" font-size="none" text-anchor="none" style="mix-blend-mode: normal"><path d="M0,172v-172h172v172z" fill="none"></path><g fill="#e67e22"><path d="M37.625,21.5v86h96.75v-86h-5.375zM48.375,32.25h10.75v10.75h-10.75zM69.875,32.25h10.75v10.75h-10.75zM91.375,32.25h32.25v10.75h-32.25zM48.375,53.75h75.25v43h-75.25zM80.625,112.875v17.61572c-1.61558,0.93921 -2.94506,2.2687 -3.88428,3.88428h-49.86572v10.75h49.86572c1.8612,3.20153 5.28744,5.375 9.25928,5.375c3.97183,0 7.39808,-2.17347 9.25928,-5.375h49.86572v-10.75h-49.86572c-0.93921,-1.61558 -2.2687,-2.94506 -3.88428,-3.88428v-17.61572z"></path></g></g></svg>[Homepage](https://de.linkedin.com/company/amberoad) | [Email]([email protected])
|
iewaij/bert-base-uncased-lm | iewaij | 2022-08-26T11:22:28Z | 105 | 0 | transformers | [
"transformers",
"pytorch",
"bert",
"fill-mask",
"generated_from_trainer",
"license:apache-2.0",
"autotrain_compatible",
"endpoints_compatible",
"region:us"
]
| fill-mask | 2022-08-26T11:15:34Z | ---
license: apache-2.0
tags:
- generated_from_trainer
model-index:
- name: bert-base-uncased-lm-all
results: []
---
<!-- This model card has been generated automatically according to the information the Trainer had access to. You
should probably proofread and complete it, then remove this comment. -->
# bert-base-uncased-lm-all
This model is a fine-tuned version of [bert-base-uncased](https://huggingface.co/bert-base-uncased) on the None dataset.
It achieves the following results on the evaluation set:
- Loss: 0.8646
## Model description
More information needed
## Intended uses & limitations
More information needed
## Training and evaluation data
More information needed
## Training procedure
### Training hyperparameters
The following hyperparameters were used during training:
- learning_rate: 5e-05
- train_batch_size: 32
- eval_batch_size: 8
- seed: 42
- optimizer: Adam with betas=(0.9,0.999) and epsilon=1e-08
- lr_scheduler_type: linear
- num_epochs: 16
### Training results
| Training Loss | Epoch | Step | Validation Loss |
|:-------------:|:-----:|:-----:|:---------------:|
| 1.6625 | 1.0 | 1194 | 1.3270 |
| 1.3001 | 2.0 | 2388 | 1.1745 |
| 1.1694 | 3.0 | 3582 | 1.1133 |
| 1.0901 | 4.0 | 4776 | 1.0547 |
| 1.0309 | 5.0 | 5970 | 0.9953 |
| 0.9842 | 6.0 | 7164 | 0.9997 |
| 0.9396 | 7.0 | 8358 | 0.9707 |
| 0.8997 | 8.0 | 9552 | 0.9324 |
| 0.8633 | 9.0 | 10746 | 0.9145 |
| 0.8314 | 10.0 | 11940 | 0.9047 |
| 0.812 | 11.0 | 13134 | 0.8954 |
| 0.7841 | 12.0 | 14328 | 0.8940 |
| 0.7616 | 13.0 | 15522 | 0.8555 |
| 0.7508 | 14.0 | 16716 | 0.8711 |
| 0.7333 | 15.0 | 17910 | 0.8351 |
| 0.7299 | 16.0 | 19104 | 0.8646 |
### Framework versions
- Transformers 4.21.2
- Pytorch 1.12.1+cu113
- Datasets 2.4.0
- Tokenizers 0.12.1
|
Chrispfield/distilbert-base-uncased-issues-128 | Chrispfield | 2022-08-26T11:10:18Z | 108 | 0 | transformers | [
"transformers",
"pytorch",
"distilbert",
"fill-mask",
"generated_from_trainer",
"license:apache-2.0",
"autotrain_compatible",
"endpoints_compatible",
"region:us"
]
| fill-mask | 2022-08-26T10:27:12Z | ---
license: apache-2.0
tags:
- generated_from_trainer
model-index:
- name: distilbert-base-uncased-issues-128
results: []
---
<!-- This model card has been generated automatically according to the information the Trainer had access to. You
should probably proofread and complete it, then remove this comment. -->
# distilbert-base-uncased-issues-128
This model is a fine-tuned version of [distilbert-base-uncased](https://huggingface.co/distilbert-base-uncased) on the None dataset.
It achieves the following results on the evaluation set:
- Loss: 1.7582
## Model description
More information needed
## Intended uses & limitations
More information needed
## Training and evaluation data
More information needed
## Training procedure
### Training hyperparameters
The following hyperparameters were used during training:
- learning_rate: 5e-05
- train_batch_size: 32
- eval_batch_size: 8
- seed: 42
- optimizer: Adam with betas=(0.9,0.999) and epsilon=1e-08
- lr_scheduler_type: linear
- num_epochs: 7
### Training results
| Training Loss | Epoch | Step | Validation Loss |
|:-------------:|:-----:|:----:|:---------------:|
| 2.4041 | 1.0 | 8 | 1.8568 |
| 2.1982 | 2.0 | 16 | 2.0790 |
| 1.7184 | 3.0 | 24 | 1.9246 |
| 1.7248 | 4.0 | 32 | 1.8485 |
| 1.5016 | 5.0 | 40 | 1.8484 |
| 1.4943 | 6.0 | 48 | 1.8691 |
| 1.526 | 7.0 | 56 | 1.7582 |
### Framework versions
- Transformers 4.21.2
- Pytorch 1.12.1+cu113
- Datasets 2.4.0
- Tokenizers 0.12.1
|
alishudi/distil_mse_2 | alishudi | 2022-08-26T10:30:20Z | 107 | 0 | transformers | [
"transformers",
"pytorch",
"distilbert",
"text-classification",
"autotrain_compatible",
"endpoints_compatible",
"region:us"
]
| text-classification | 2022-08-26T10:27:53Z | --alpha_ce 0.0 --alpha_mlm 2.0 --alpha_cos 1.0 --alpha_act 1.0 --alpha_clm 0.0 --alpha_mse 0.0002 --mlm \
2 layers |
Hardwarize/q-Taxi-v3 | Hardwarize | 2022-08-26T09:00:24Z | 0 | 0 | null | [
"Taxi-v3",
"q-learning",
"reinforcement-learning",
"custom-implementation",
"model-index",
"region:us"
]
| reinforcement-learning | 2022-08-26T09:00:16Z | ---
tags:
- Taxi-v3
- q-learning
- reinforcement-learning
- custom-implementation
model-index:
- name: q-Taxi-v3
results:
- task:
type: reinforcement-learning
name: reinforcement-learning
dataset:
name: Taxi-v3
type: Taxi-v3
metrics:
- type: mean_reward
value: 7.56 +/- 2.71
name: mean_reward
verified: false
---
# **Q-Learning** Agent playing **Taxi-v3**
This is a trained model of a **Q-Learning** agent playing **Taxi-v3** .
## Usage
```python
model = load_from_hub(repo_id="Hardwarize/q-Taxi-v3", filename="q-learning.pkl")
# Don't forget to check if you need to add additional attributes (is_slippery=False etc)
env = gym.make(model["env_id"])
evaluate_agent(env, model["max_steps"], model["n_eval_episodes"], model["qtable"], model["eval_seed"])
```
|
Hardwarize/q-FrozenLake-v1-4x4-noSlippery | Hardwarize | 2022-08-26T08:51:59Z | 0 | 0 | null | [
"FrozenLake-v1-4x4-no_slippery",
"q-learning",
"reinforcement-learning",
"custom-implementation",
"model-index",
"region:us"
]
| reinforcement-learning | 2022-08-26T08:51:52Z | ---
tags:
- FrozenLake-v1-4x4-no_slippery
- q-learning
- reinforcement-learning
- custom-implementation
model-index:
- name: q-FrozenLake-v1-4x4-noSlippery
results:
- task:
type: reinforcement-learning
name: reinforcement-learning
dataset:
name: FrozenLake-v1-4x4-no_slippery
type: FrozenLake-v1-4x4-no_slippery
metrics:
- type: mean_reward
value: 1.00 +/- 0.00
name: mean_reward
verified: false
---
# **Q-Learning** Agent playing **FrozenLake-v1**
This is a trained model of a **Q-Learning** agent playing **FrozenLake-v1** .
## Usage
```python
model = load_from_hub(repo_id="Hardwarize/q-FrozenLake-v1-4x4-noSlippery", filename="q-learning.pkl")
# Don't forget to check if you need to add additional attributes (is_slippery=False etc)
env = gym.make(model["env_id"])
evaluate_agent(env, model["max_steps"], model["n_eval_episodes"], model["qtable"], model["eval_seed"])
```
|
vishw2703/unisumm_3-1228646724 | vishw2703 | 2022-08-26T07:53:56Z | 9 | 0 | transformers | [
"transformers",
"pytorch",
"bart",
"text2text-generation",
"autotrain",
"summarization",
"unk",
"dataset:vishw2703/autotrain-data-unisumm_3",
"co2_eq_emissions",
"autotrain_compatible",
"endpoints_compatible",
"region:us"
]
| summarization | 2022-08-08T07:14:24Z | ---
tags:
- autotrain
- summarization
language:
- unk
datasets:
- vishw2703/autotrain-data-unisumm_3
co2_eq_emissions:
emissions: 1368.894142563709
---
# Model Trained Using AutoTrain
- Problem type: Summarization
- Model ID: 1228646724
- CO2 Emissions (in grams): 1368.8941
## Validation Metrics
- Loss: 2.319
- Rouge1: 43.703
- Rouge2: 16.106
- RougeL: 23.715
- RougeLsum: 38.984
- Gen Len: 141.091
## Usage
You can use cURL to access this model:
```
$ curl -X POST -H "Authorization: Bearer YOUR_HUGGINGFACE_API_KEY" -H "Content-Type: application/json" -d '{"inputs": "I love AutoTrain"}' https://api-inference.huggingface.co/vishw2703/autotrain-unisumm_3-1228646724
``` |
ucinlp/diabetes-t5-large | ucinlp | 2022-08-26T06:23:13Z | 7 | 0 | transformers | [
"transformers",
"pytorch",
"t5",
"text2text-generation",
"autotrain_compatible",
"text-generation-inference",
"endpoints_compatible",
"region:us"
]
| text2text-generation | 2022-08-26T01:13:04Z | # TalkToModel t5-large diabetes parsing model
|
Sandeepanie/clinical-finetuned-data2 | Sandeepanie | 2022-08-26T06:00:11Z | 107 | 0 | transformers | [
"transformers",
"pytorch",
"tensorboard",
"bert",
"text-classification",
"generated_from_trainer",
"license:mit",
"autotrain_compatible",
"endpoints_compatible",
"region:us"
]
| text-classification | 2022-08-26T05:50:57Z | ---
license: mit
tags:
- generated_from_trainer
metrics:
- f1
model-index:
- name: clinical-finetuned-data2
results: []
---
<!-- This model card has been generated automatically according to the information the Trainer had access to. You
should probably proofread and complete it, then remove this comment. -->
# clinical-finetuned-data2
This model is a fine-tuned version of [emilyalsentzer/Bio_ClinicalBERT](https://huggingface.co/emilyalsentzer/Bio_ClinicalBERT) on an unknown dataset.
It achieves the following results on the evaluation set:
- Loss: 0.4949
- F1: 0.7800
## Model description
More information needed
## Intended uses & limitations
More information needed
## Training and evaluation data
More information needed
## Training procedure
### Training hyperparameters
The following hyperparameters were used during training:
- learning_rate: 2e-05
- train_batch_size: 16
- eval_batch_size: 16
- seed: 42
- optimizer: Adam with betas=(0.9,0.999) and epsilon=1e-08
- lr_scheduler_type: linear
- num_epochs: 5
- mixed_precision_training: Native AMP
### Training results
| Training Loss | Epoch | Step | Validation Loss | F1 |
|:-------------:|:-----:|:----:|:---------------:|:------:|
| 0.66 | 1.0 | 50 | 0.6269 | 0.6659 |
| 0.5476 | 2.0 | 100 | 0.5311 | 0.7615 |
| 0.3766 | 3.0 | 150 | 0.4457 | 0.7970 |
| 0.2785 | 4.0 | 200 | 0.5251 | 0.7615 |
| 0.2274 | 5.0 | 250 | 0.4949 | 0.7800 |
### Framework versions
- Transformers 4.21.2
- Pytorch 1.12.1+cu113
- Datasets 2.4.0
- Tokenizers 0.12.1
|
d0r1h/testt5 | d0r1h | 2022-08-26T05:52:55Z | 10 | 0 | transformers | [
"transformers",
"pytorch",
"t5",
"text2text-generation",
"generated_from_trainer",
"license:apache-2.0",
"autotrain_compatible",
"text-generation-inference",
"endpoints_compatible",
"region:us"
]
| text2text-generation | 2022-08-26T05:46:35Z | ---
license: apache-2.0
tags:
- generated_from_trainer
metrics:
- rouge
model-index:
- name: t5_assets
results: []
---
<!-- This model card has been generated automatically according to the information the Trainer had access to. You
should probably proofread and complete it, then remove this comment. -->
# t5_assets
This model is a fine-tuned version of [t5-small](https://huggingface.co/t5-small) on an unknown dataset.
It achieves the following results on the evaluation set:
- Loss: 1.8718
- Rouge1: 35.7712
- Rouge2: 15.2129
- Rougel: 25.9007
- Rougelsum: 33.3105
- Gen Len: 64.7175
## Model description
More information needed
## Intended uses & limitations
More information needed
## Training and evaluation data
More information needed
## Training procedure
### Training hyperparameters
The following hyperparameters were used during training:
- learning_rate: 5e-05
- train_batch_size: 4
- eval_batch_size: 4
- seed: 42
- optimizer: Adam with betas=(0.9,0.999) and epsilon=1e-08
- lr_scheduler_type: linear
- num_epochs: 3.0
### Training results
### Framework versions
- Transformers 4.22.0.dev0
- Pytorch 1.12.1+cu113
- Datasets 2.4.0
- Tokenizers 0.12.1
|
PSW/bart-base-samsumgen-xsum-conv-samsum | PSW | 2022-08-26T05:06:54Z | 5 | 0 | transformers | [
"transformers",
"pytorch",
"bart",
"text2text-generation",
"autotrain_compatible",
"endpoints_compatible",
"region:us"
]
| text2text-generation | 2022-08-24T13:17:14Z | # **PSW/bart-base-samsumgen-xsum-conv-samsum**
1. reverse trained on samsum
2. generate from xsum
3. train on synthetic data
4. fine-tune on samsum
|
pinot/wav2vec2-large-xls-r-300m-ja-colab | pinot | 2022-08-26T04:29:51Z | 107 | 0 | transformers | [
"transformers",
"pytorch",
"tensorboard",
"wav2vec2",
"automatic-speech-recognition",
"generated_from_trainer",
"dataset:common_voice_10_0",
"license:apache-2.0",
"endpoints_compatible",
"region:us"
]
| automatic-speech-recognition | 2022-08-22T08:52:59Z | ---
license: apache-2.0
tags:
- generated_from_trainer
datasets:
- common_voice_10_0
model-index:
- name: wav2vec2-large-xls-r-300m-ja-colab
results: []
---
<!-- This model card has been generated automatically according to the information the Trainer had access to. You
should probably proofread and complete it, then remove this comment. -->
# wav2vec2-large-xls-r-300m-ja-colab
This model is a fine-tuned version of [facebook/wav2vec2-xls-r-300m](https://huggingface.co/facebook/wav2vec2-xls-r-300m) on the common_voice_10_0 dataset.
It achieves the following results on the evaluation set:
- Loss: 1.1407
- Wer: 0.2456
## Model description
More information needed
## Intended uses & limitations
More information needed
## Training and evaluation data
More information needed
## Training procedure
### Training hyperparameters
The following hyperparameters were used during training:
- learning_rate: 0.0003
- train_batch_size: 1
- eval_batch_size: 1
- seed: 42
- gradient_accumulation_steps: 16
- total_train_batch_size: 16
- optimizer: Adam with betas=(0.9,0.999) and epsilon=1e-08
- lr_scheduler_type: linear
- lr_scheduler_warmup_steps: 500
- num_epochs: 10
- mixed_precision_training: Native AMP
### Training results
| Training Loss | Epoch | Step | Validation Loss | Wer |
|:-------------:|:-----:|:----:|:---------------:|:------:|
| No log | 1.0 | 637 | 5.3238 | 0.9663 |
| No log | 2.0 | 1274 | 4.1785 | 0.7662 |
| No log | 3.0 | 1911 | 2.3701 | 0.4983 |
| No log | 4.0 | 2548 | 1.8443 | 0.4090 |
| 6.5781 | 5.0 | 3185 | 1.4892 | 0.3363 |
| 6.5781 | 6.0 | 3822 | 1.3229 | 0.2995 |
| 6.5781 | 7.0 | 4459 | 1.2418 | 0.2814 |
| 6.5781 | 8.0 | 5096 | 1.1928 | 0.2647 |
| 1.0184 | 9.0 | 5733 | 1.1584 | 0.2520 |
| 1.0184 | 10.0 | 6370 | 1.1407 | 0.2456 |
### Framework versions
- Transformers 4.21.2
- Pytorch 1.10.0+cu113
- Datasets 2.4.0
- Tokenizers 0.12.1
|
jkang/espnet2_an4_transformer | jkang | 2022-08-26T04:25:10Z | 1 | 0 | espnet | [
"espnet",
"audio",
"automatic-speech-recognition",
"en",
"dataset:an4",
"arxiv:1804.00015",
"license:cc-by-4.0",
"region:us"
]
| automatic-speech-recognition | 2022-08-26T03:53:45Z | ---
tags:
- espnet
- audio
- automatic-speech-recognition
language: en
datasets:
- an4
license: cc-by-4.0
---
## ESPnet2 ASR model
### `jkang/espnet2_an4_transformer`
This model was trained by jaekookang using an4 recipe in [espnet](https://github.com/espnet/espnet/).
### Demo: How to use in ESPnet2
Follow the [ESPnet installation instructions](https://espnet.github.io/espnet/installation.html)
if you haven't done that already.
```bash
cd espnet
git checkout c8f11ef7f5c571fbcc34d53da449353bd75037ce
pip install -e .
cd egs2/an4/asr1
./run.sh --skip_data_prep false --skip_train true --download_model jkang/espnet2_an4_transformer
```
<!-- Generated by scripts/utils/show_asr_result.sh -->
# RESULTS
## Environments
- date: `Fri Aug 19 17:38:46 KST 2022`
- python version: `3.9.12 (main, Apr 5 2022, 06:56:58) [GCC 7.5.0]`
- espnet version: `espnet 202207`
- pytorch version: `pytorch 1.10.1`
- Git hash: `c8f11ef7f5c571fbcc34d53da449353bd75037ce`
- Commit date: `Fri Aug 19 17:20:13 2022 +0900`
## asr_train_asr_transformer_raw_en_bpe30_sp
### WER
|dataset|Snt|Wrd|Corr|Sub|Del|Ins|Err|S.Err|
|---|---|---|---|---|---|---|---|---|
|decode_asr_lm_lm_train_lm_en_bpe30_valid.loss.ave_asr_model_valid.acc.ave/test|130|773|92.0|5.8|2.2|0.4|8.4|33.1|
|decode_asr_lm_lm_train_lm_en_bpe30_valid.loss.ave_asr_model_valid.acc.ave/train_dev|100|591|89.5|7.3|3.2|0.5|11.0|41.0|
### CER
|dataset|Snt|Wrd|Corr|Sub|Del|Ins|Err|S.Err|
|---|---|---|---|---|---|---|---|---|
|decode_asr_lm_lm_train_lm_en_bpe30_valid.loss.ave_asr_model_valid.acc.ave/test|130|2565|96.3|1.1|2.6|0.6|4.3|33.1|
|decode_asr_lm_lm_train_lm_en_bpe30_valid.loss.ave_asr_model_valid.acc.ave/train_dev|100|1915|94.1|1.9|4.0|0.4|6.3|41.0|
### TER
|dataset|Snt|Wrd|Corr|Sub|Del|Ins|Err|S.Err|
|---|---|---|---|---|---|---|---|---|
|decode_asr_lm_lm_train_lm_en_bpe30_valid.loss.ave_asr_model_valid.acc.ave/test|130|2695|96.4|1.1|2.5|0.6|4.1|33.1|
|decode_asr_lm_lm_train_lm_en_bpe30_valid.loss.ave_asr_model_valid.acc.ave/train_dev|100|2015|94.4|1.8|3.8|0.3|6.0|41.0|
## ASR config
<details><summary>expand</summary>
```
config: conf/train_asr_transformer.yaml
print_config: false
log_level: INFO
dry_run: false
iterator_type: sequence
output_dir: exp/asr_train_asr_transformer_raw_en_bpe30_sp
ngpu: 1
seed: 0
num_workers: 1
num_att_plot: 3
dist_backend: nccl
dist_init_method: env://
dist_world_size: 4
dist_rank: 0
local_rank: 0
dist_master_addr: localhost
dist_master_port: 43015
dist_launcher: null
multiprocessing_distributed: true
unused_parameters: false
sharded_ddp: false
cudnn_enabled: true
cudnn_benchmark: false
cudnn_deterministic: true
collect_stats: false
write_collected_feats: false
max_epoch: 200
patience: null
val_scheduler_criterion:
- valid
- loss
early_stopping_criterion:
- valid
- loss
- min
best_model_criterion:
- - valid
- acc
- max
keep_nbest_models: 10
nbest_averaging_interval: 0
grad_clip: 5.0
grad_clip_type: 2.0
grad_noise: false
accum_grad: 1
no_forward_run: false
resume: true
train_dtype: float32
use_amp: false
log_interval: null
use_matplotlib: true
use_tensorboard: true
create_graph_in_tensorboard: false
use_wandb: false
wandb_project: null
wandb_id: null
wandb_entity: null
wandb_name: null
wandb_model_log_interval: -1
detect_anomaly: false
pretrain_path: null
init_param: []
ignore_init_mismatch: false
freeze_param: []
num_iters_per_epoch: null
batch_size: 64
valid_batch_size: null
batch_bins: 1000000
valid_batch_bins: null
train_shape_file:
- exp/asr_stats_raw_en_bpe30_sp/train/speech_shape
- exp/asr_stats_raw_en_bpe30_sp/train/text_shape.bpe
valid_shape_file:
- exp/asr_stats_raw_en_bpe30_sp/valid/speech_shape
- exp/asr_stats_raw_en_bpe30_sp/valid/text_shape.bpe
batch_type: folded
valid_batch_type: null
fold_length:
- 80000
- 150
sort_in_batch: descending
sort_batch: descending
multiple_iterator: false
chunk_length: 500
chunk_shift_ratio: 0.5
num_cache_chunks: 1024
train_data_path_and_name_and_type:
- - dump/raw/train_nodev_sp/wav.scp
- speech
- sound
- - dump/raw/train_nodev_sp/text
- text
- text
valid_data_path_and_name_and_type:
- - dump/raw/train_dev/wav.scp
- speech
- sound
- - dump/raw/train_dev/text
- text
- text
allow_variable_data_keys: false
max_cache_size: 0.0
max_cache_fd: 32
valid_max_cache_size: null
optim: adam
optim_conf:
lr: 0.001
scheduler: warmuplr
scheduler_conf:
warmup_steps: 2500
token_list:
- <blank>
- <unk>
- β
- T
- E
- O
- R
- Y
- A
- H
- U
- S
- I
- F
- B
- L
- P
- D
- G
- M
- C
- V
- X
- J
- K
- Z
- W
- N
- Q
- <sos/eos>
init: xavier_uniform
input_size: null
ctc_conf:
dropout_rate: 0.0
ctc_type: builtin
reduce: true
ignore_nan_grad: null
zero_infinity: true
joint_net_conf: null
use_preprocessor: true
token_type: bpe
bpemodel: data/en_token_list/bpe_unigram30/bpe.model
non_linguistic_symbols: null
cleaner: null
g2p: null
speech_volume_normalize: null
rir_scp: null
rir_apply_prob: 1.0
noise_scp: null
noise_apply_prob: 1.0
noise_db_range: '13_15'
short_noise_thres: 0.5
frontend: default
frontend_conf:
fs: 16k
specaug: null
specaug_conf: {}
normalize: global_mvn
normalize_conf:
stats_file: exp/asr_stats_raw_en_bpe30_sp/train/feats_stats.npz
model: espnet
model_conf:
ctc_weight: 0.3
lsm_weight: 0.1
length_normalized_loss: false
preencoder: null
preencoder_conf: {}
encoder: transformer
encoder_conf:
output_size: 256
attention_heads: 4
linear_units: 2048
num_blocks: 12
dropout_rate: 0.1
positional_dropout_rate: 0.1
attention_dropout_rate: 0.0
input_layer: conv2d
normalize_before: true
postencoder: null
postencoder_conf: {}
decoder: transformer
decoder_conf:
attention_heads: 4
linear_units: 2048
num_blocks: 6
dropout_rate: 0.1
positional_dropout_rate: 0.1
self_attention_dropout_rate: 0.0
src_attention_dropout_rate: 0.0
required:
- output_dir
- token_list
version: '202207'
distributed: true
```
</details>
### Citing ESPnet
```BibTex
@inproceedings{watanabe2018espnet,
author={Shinji Watanabe and Takaaki Hori and Shigeki Karita and Tomoki Hayashi and Jiro Nishitoba and Yuya Unno and Nelson Yalta and Jahn Heymann and Matthew Wiesner and Nanxin Chen and Adithya Renduchintala and Tsubasa Ochiai},
title={{ESPnet}: End-to-End Speech Processing Toolkit},
year={2018},
booktitle={Proceedings of Interspeech},
pages={2207--2211},
doi={10.21437/Interspeech.2018-1456},
url={http://dx.doi.org/10.21437/Interspeech.2018-1456}
}
```
or arXiv:
```bibtex
@misc{watanabe2018espnet,
title={ESPnet: End-to-End Speech Processing Toolkit},
author={Shinji Watanabe and Takaaki Hori and Shigeki Karita and Tomoki Hayashi and Jiro Nishitoba and Yuya Unno and Nelson Yalta and Jahn Heymann and Matthew Wiesner and Nanxin Chen and Adithya Renduchintala and Tsubasa Ochiai},
year={2018},
eprint={1804.00015},
archivePrefix={arXiv},
primaryClass={cs.CL}
}
```
|
Sehong/t5-large-QuestionGeneration | Sehong | 2022-08-26T02:10:42Z | 75 | 6 | transformers | [
"transformers",
"pytorch",
"t5",
"text2text-generation",
"en",
"dataset:squad",
"license:mit",
"autotrain_compatible",
"text-generation-inference",
"endpoints_compatible",
"region:us"
]
| text2text-generation | 2022-08-17T07:12:14Z | ---
language: en
tags:
- t5
datasets:
- squad
license: mit
---
# Question Generation Model
## Github
https://github.com/Seoneun/T5-Question-Generation
## Fine-tuning Dataset
SQuAD 1.1
| Train Data | Dev Data | Test Data |
| ------ | ------ | ------ |
| 75,722 | 10,570 | 11,877 |
## Demo
https://huggingface.co/Sehong/t5-large-QuestionGeneration
## How to use
```python
import torch
from transformers import PreTrainedTokenizerFast
from transformers import T5ForConditionalGeneration
tokenizer = PreTrainedTokenizerFast.from_pretrained('Sehong/t5-large-QuestionGeneration')
model = T5ForConditionalGeneration.from_pretrained('Sehong/t5-large-QuestionGeneration')
# tokenized
'''
text = "answer:Saint Bern ##ade ##tte So ##ubi ##rous content:Architectural ##ly , the school has a Catholic character . At ##op the Main Building ' s gold dome is a golden statue of the Virgin Mary . Immediately in front of the Main Building and facing it , is a copper statue of Christ with arms up ##rai ##sed with the legend "" V ##eni ##te Ad Me O ##m ##nes "" . Next to the Main Building is the Basilica of the Sacred Heart . Immediately behind the b ##asi ##lica is the G ##rot ##to , a Marian place of prayer and reflection . It is a replica of the g ##rot ##to at Lou ##rdes , France where the Virgin Mary reputed ##ly appeared to Saint Bern ##ade ##tte So ##ubi ##rous in 1858 . At the end of the main drive ( and in a direct line that connects through 3 statues and the Gold Dome ) , is a simple , modern stone statue of Mary ."
'''
text = "answer:Saint Bernadette Soubirous content:Architecturally , the school has a Catholic character . Atop the Main Building ' s gold dome is a golden statue of the Virgin Mary . Immediately in front of the Main Building and facing it , is a copper statue of Christ with arms upraised with the legend "" Venite Ad Me Omnes "" . Next to the Main Building is the Basilica of the Sacred Heart . Immediately behind the basilica is the Grotto , a Marian place of prayer and reflection . It is a replica of the grotto at Lourdes , France where the Virgin Mary reputedly appeared to Saint Bernadette Soubirous in 1858 . At the end of the main drive ( and in a direct line that connects through 3 statues and the Gold Dome ) , is a simple , modern stone statue of Mary ."
raw_input_ids = tokenizer.encode(text)
input_ids = [tokenizer.bos_token_id] + raw_input_ids + [tokenizer.eos_token_id]
question_ids = model.generate(torch.tensor([input_ids]))
decode = tokenizer.decode(question_ids.squeeze().tolist(), skip_special_tokens=True)
decode = decode.replace(' # # ', '').replace(' ', ' ').replace(' ##', '')
print(decode)
```
## Evalutation
| BLEU-1 | BLEU-2 | BLEU-3 | BLEU-4 | METEOR | ROUGE-L |
| ------ | ------ | ------ | ------ | ------ | ------- |
| 51.333 | 36.742 | 28.218 | 22.289 | 26.126 | 51.069 | |
Hyeoni/t5-e2e-questions-generation-KorQuAD | Hyeoni | 2022-08-26T01:55:50Z | 16 | 1 | transformers | [
"transformers",
"pytorch",
"t5",
"text2text-generation",
"generated_from_trainer",
"dataset:squad_modified_for_t5_qg",
"autotrain_compatible",
"text-generation-inference",
"endpoints_compatible",
"region:us"
]
| text2text-generation | 2022-08-25T06:43:21Z | ---
tags:
- generated_from_trainer
datasets:
- squad_modified_for_t5_qg
model-index:
- name: t5-end2end-questions-generation
results: []
---
<!-- This model card has been generated automatically according to the information the Trainer had access to. You
should probably proofread and complete it, then remove this comment. -->
# t5-end2end-questions-generation
This model is a fine-tuned version of [digit82/kolang-t5-base](https://huggingface.co/digit82/kolang-t5-base) on the korquad_modified_for_t5_qg dataset.
It achieves the following results on the evaluation set:
- Loss: 2.1449
## Model description
More information needed
## Training and evaluation data
KorQuAD V1.0
## Training procedure
### Training hyperparameters
The following hyperparameters were used during training:
- learning_rate: 0.0001
- train_batch_size: 4
- eval_batch_size: 4
- seed: 42
- gradient_accumulation_steps: 16
- total_train_batch_size: 64
- optimizer: Adam with betas=(0.9,0.999) and epsilon=1e-08
- lr_scheduler_type: linear
- num_epochs: 7
### Training results
| Training Loss | Epoch | Step | Validation Loss |
|:-------------:|:-----:|:----:|:---------------:|
| 3.6685 | 0.66 | 100 | 2.4355 |
| 2.3957 | 1.32 | 200 | 2.2428 |
| 2.1795 | 1.98 | 300 | 2.1664 |
| 1.9408 | 2.65 | 400 | 2.1467 |
| 1.8333 | 3.31 | 500 | 2.1470 |
| 1.7319 | 3.97 | 600 | 2.1194 |
| 1.6095 | 4.63 | 700 | 2.1348 |
| 1.5662 | 5.3 | 800 | 2.1433 |
| 1.5038 | 5.96 | 900 | 2.1319 |
| 1.45 | 6.62 | 1000 | 2.1449 |
### Framework versions
- Transformers 4.21.2
- Pytorch 1.12.1+cu113
- Datasets 2.4.0
- Tokenizers 0.12.1
|
Bingsu/bigbird_ko_base-tsdae-specialty_corpus | Bingsu | 2022-08-26T01:42:54Z | 3 | 1 | sentence-transformers | [
"sentence-transformers",
"pytorch",
"big_bird",
"feature-extraction",
"sentence-similarity",
"transformers",
"ko",
"license:mit",
"autotrain_compatible",
"endpoints_compatible",
"region:us"
]
| sentence-similarity | 2022-08-26T01:04:21Z | ---
pipeline_tag: sentence-similarity
tags:
- sentence-transformers
- feature-extraction
- sentence-similarity
- transformers
widget:
source_sentence: "μμΉ ν΄μ νλ‘κ·Έλ¨μ μ¬λ¬ κ°μ§ νκ²½ λ³μλ₯Ό μ
λ ₯ν΄μΌ νλ―λ‘ μΌλ°μΈμ΄ μ¬μ©νκΈ°μλ λ§μ μ΄λ €μμ΄ μλ€."
sentences:
- "μ΄λ¬ν ν΄μλ°©λ²μ λ§€μ° λ³΅μ‘ν κ²μ΄μ΄μ μμΉ ν΄μ νλ‘κ·Έλ¨μ΄ νμμ μ΄λ€."
- "κ³μΈ΅κ΅¬μ‘° μ
λ£°λΌ μμ€ν
μ ꡬμ±νκ³ μ μλ κΈ°λ²μ μ μ©νλ©΄ μ΄λ κ³³μ μμΉν μ¬μ©μμκ²λ μμ§μ μλΉμ€λ₯Ό ν¨μ¨μ μΌλ‘ μ 곡ν μ μμμ νμΈνμλ€."
- "νκΉ
νμ΄μ€μ νκ΅μ΄ λͺ¨λΈμ΄ λ λ§μμ‘μΌλ©΄ μ’κ² λ€."
language: ko
license: mit
---
# Bingsu/bigbird_ko_base-tsdae-specialty_corpus
[sentence-transformers](https://www.SBERT.net)λ‘ νμ΅λ bigbird λͺ¨λΈ: μ
λ ₯ λ¬Έμ₯μ 256벑ν°λ‘ λ³νν©λλ€.
[Aihub μ λ¬ΈλΆμΌ λ§λμΉ](https://aihub.or.kr/aihubdata/data/view.do?currMenu=115&topMenu=100&aihubDataSe=realm&dataSetSn=110)μ λν΄
[TSDAE](https://www.sbert.net/examples/unsupervised_learning/TSDAE/README.html)λ‘ νμ΅λμμ΅λλ€.
<!--- Describe your model here -->
## Usage (Sentence-Transformers)
μ¬μ© μ μ [sentence-transformers](https://www.SBERT.net)λ₯Ό μ€μΉνμΈμ.
```sh
pip install -U sentence-transformers
```
λλ
```sh
conda install -c conda-forge sentence-transformers
```
μ¬μ© μμ :
```python
from sentence_transformers import util
sent = [
"λ³Έ λ
Όλ¬Έμ λμ§νΈ μ νΈμ²λ¦¬μ© VLSIμ μλμ€κ³λ₯Ό μν SODAS-DSP(SOgang Design Automation System-DSP) μμ€ν
μ μ€κ³μ κ°λ° κ²°κ³Όμ λνμ¬ κΈ°μ νλ€",
"λ³Έ λ
Όλ¬Έμμλ DD-Gardnerλ°©μμ νμ΄λ° κ²μΆκΈ° μ±λ₯μ κ³ μ°°νλ€.",
"μ΄λ¬ν ν΄μλ°©λ²μ λ§€μ° λ³΅μ‘ν κ²μ΄μ΄μ μμΉ ν΄μ νλ‘κ·Έλ¨μ΄ νμμ μ΄λ€.",
"μμΉ ν΄μ νλ‘κ·Έλ¨μ μ¬λ¬ κ°μ§ νκ²½ λ³μλ₯Ό μ
λ ₯ν΄μΌ νλ―λ‘ μΌλ°μΈμ΄ μ¬μ©νκΈ°μλ λ§μ μ΄λ €μμ΄ μλ€.",
"λ μ°λκ³Ό ν¬κ³Όμ λν κ³ μ£Όν κ·Όμ¬μλ μ»μ΄μ§λ€.",
"κ·Έλ¦¬κ³ μ¬λ¦Ώκ°μ κ°κ²©μ λ³νμ μν΄μ λΉν(beamwidth)μ μ‘°μ ν μ μμμ 보μ¬μ€λ€.",
"μ€λ μ μ¬μ μ§μ₯λ©΄μ΄λ€.",
"μ€λ μ λ
μ κΉλ°₯μ²κ΅μ΄λ€."
]
paraphrases = util.paraphrase_mining(model, sent)
for paraphrase in paraphrases[:5]:
score, i, j = paraphrase
print("{} \t\t {} \t\t Score: {:.4f}".format(sent[i], sent[j], score))
```
```
μ΄λ¬ν ν΄μλ°©λ²μ λ§€μ° λ³΅μ‘ν κ²μ΄μ΄μ μμΉ ν΄μ νλ‘κ·Έλ¨μ΄ νμμ μ΄λ€. μμΉ ν΄μ νλ‘κ·Έλ¨μ μ¬λ¬ κ°μ§ νκ²½ λ³μλ₯Ό μ
λ ₯ν΄μΌ νλ―λ‘ μΌλ°μΈμ΄ μ¬μ©νκΈ°μλ λ§μ μ΄λ €μμ΄ μλ€. Score: 0.8990
μ€λ μ μ¬μ μ§μ₯λ©΄μ΄λ€. μ€λ μ λ
μ κΉλ°₯μ²κ΅μ΄λ€. Score: 0.8945
μμΉ ν΄μ νλ‘κ·Έλ¨μ μ¬λ¬ κ°μ§ νκ²½ λ³μλ₯Ό μ
λ ₯ν΄μΌ νλ―λ‘ μΌλ°μΈμ΄ μ¬μ©νκΈ°μλ λ§μ μ΄λ €μμ΄ μλ€. μ€λ μ λ
μ κΉλ°₯μ²κ΅μ΄λ€. Score: 0.8901
λ³Έ λ
Όλ¬Έμ λμ§νΈ μ νΈμ²λ¦¬μ© VLSIμ μλμ€κ³λ₯Ό μν SODAS-DSP(SOgang Design Automation System-DSP) μμ€ν
μ μ€κ³μ κ°λ° κ²°κ³Όμ λνμ¬ κΈ°μ νλ€ λ³Έ λ
Όλ¬Έμμλ DD-Gardnerλ°©μμ νμ΄λ° κ²μΆκΈ° μ±λ₯μ κ³ μ°°νλ€. Score: 0.8894
λ³Έ λ
Όλ¬Έμ λμ§νΈ μ νΈμ²λ¦¬μ© VLSIμ μλμ€κ³λ₯Ό μν SODAS-DSP(SOgang Design Automation System-DSP) μμ€ν
μ μ€κ³μ κ°λ° κ²°κ³Όμ λνμ¬ κΈ°μ νλ€ κ·Έλ¦¬κ³ μ¬λ¦Ώκ°μ κ°κ²©μ λ³νμ μν΄μ λΉν(beamwidth)μ μ‘°μ ν μ μμμ 보μ¬μ€λ€. Score: 0.8889
```
## Usage (HuggingFace Transformers)
Without [sentence-transformers](https://www.SBERT.net), you can use the model like this: First, you pass your input through the transformer model, then you have to apply the right pooling-operation on-top of the contextualized word embeddings.
```python
from transformers import AutoTokenizer, AutoModel
import torch
def cls_pooling(model_output, attention_mask):
return model_output[0][:,0]
# Sentences we want sentence embeddings for
sentences = ['This is an example sentence', 'Each sentence is converted']
# Load model from HuggingFace Hub
tokenizer = AutoTokenizer.from_pretrained('Bingsu/bigbird_ko_base-tsdae-specialty_corpus')
model = AutoModel.from_pretrained('Bingsu/bigbird_ko_base-tsdae-specialty_corpus')
# Tokenize sentences
encoded_input = tokenizer(sentences, padding=True, truncation=True, return_tensors='pt')
# Compute token embeddings
with torch.no_grad():
model_output = model(**encoded_input)
# Perform pooling. In this case, cls pooling.
sentence_embeddings = cls_pooling(model_output, encoded_input['attention_mask'])
print("Sentence embeddings:")
print(sentence_embeddings)
```
## Evaluation Results
<!--- Describe how your model was evaluated -->
For an automated evaluation of this model, see the *Sentence Embeddings Benchmark*: [https://seb.sbert.net](https://seb.sbert.net?model_name=Bingsu/bigbird_ko_base-tsdae-specialty_corpus)
## Training
The model was trained with the parameters:
**DataLoader**:
`torch.utils.data.dataloader.DataLoader` of length 183287 with parameters:
```
{'batch_size': 8, 'sampler': 'torch.utils.data.sampler.RandomSampler', 'batch_sampler': 'torch.utils.data.sampler.BatchSampler'}
```
**Loss**:
`sentence_transformers.losses.DenoisingAutoEncoderLoss.DenoisingAutoEncoderLoss`
Parameters of the fit()-Method:
```
{
"epochs": 2,
"evaluation_steps": 10000,
"evaluator": "sentence_transformers.evaluation.EmbeddingSimilarityEvaluator.EmbeddingSimilarityEvaluator",
"max_grad_norm": 1,
"optimizer_class": "<class 'bitsandbytes.optim.adamw.AdamW8bit'>",
"optimizer_params": {
"lr": 3e-05
},
"scheduler": "warmupcosinewithhardrestarts",
"steps_per_epoch": null,
"warmup_steps": 10000,
"weight_decay": 0.005
}
```
## Full Model Architecture
```
SentenceTransformer(
(0): Transformer({'max_seq_length': 1024, 'do_lower_case': False}) with Transformer model: BigBirdModel
(1): Pooling({'word_embedding_dimension': 256, 'pooling_mode_cls_token': True, 'pooling_mode_mean_tokens': False, 'pooling_mode_max_tokens': False, 'pooling_mode_mean_sqrt_len_tokens': False})
)
```
## Citing & Authors
<!--- Describe where people can find more information --> |
sunyilgdx/bert_large_cased_mix5 | sunyilgdx | 2022-08-26T01:28:13Z | 0 | 2 | null | [
"region:us"
]
| null | 2022-08-26T00:27:50Z | BERT-large-cased pre-trained using RoBERTa's corpora (Wikipedia+Books+Stories+Newsroom+Openwebtext). |
MBMMurad/wav2vec2_murad | MBMMurad | 2022-08-26T00:05:11Z | 7 | 0 | transformers | [
"transformers",
"pytorch",
"tensorboard",
"wav2vec2",
"automatic-speech-recognition",
"generated_from_trainer",
"dataset:cvbn",
"license:apache-2.0",
"endpoints_compatible",
"region:us"
]
| automatic-speech-recognition | 2022-08-23T08:43:20Z | ---
license: apache-2.0
tags:
- generated_from_trainer
datasets:
- cvbn
model-index:
- name: wav2vec2_murad
results: []
---
<!-- This model card has been generated automatically according to the information the Trainer had access to. You
should probably proofread and complete it, then remove this comment. -->
# wav2vec2_murad
This model is a fine-tuned version of [facebook/wav2vec2-xls-r-300m](https://huggingface.co/facebook/wav2vec2-xls-r-300m) on the cvbn dataset.
It achieves the following results on the evaluation set:
- eval_loss: 0.2006
- eval_wer: 0.2084
- eval_runtime: 556.4634
- eval_samples_per_second: 8.985
- eval_steps_per_second: 0.562
- epoch: 12.32
- step: 28800
## Model description
More information needed
## Intended uses & limitations
More information needed
## Training and evaluation data
More information needed
## Training procedure
### Training hyperparameters
The following hyperparameters were used during training:
- learning_rate: 7.5e-05
- train_batch_size: 16
- eval_batch_size: 16
- seed: 42
- optimizer: Adam with betas=(0.9,0.999) and epsilon=1e-08
- lr_scheduler_type: linear
- lr_scheduler_warmup_steps: 1000
- num_epochs: 30
- mixed_precision_training: Native AMP
### Framework versions
- Transformers 4.21.1
- Pytorch 1.11.0+cu102
- Datasets 2.4.0
- Tokenizers 0.12.1
|
paola-md/distil-i | paola-md | 2022-08-25T23:41:53Z | 163 | 0 | transformers | [
"transformers",
"pytorch",
"roberta",
"text-classification",
"generated_from_trainer",
"license:apache-2.0",
"autotrain_compatible",
"endpoints_compatible",
"region:us"
]
| text-classification | 2022-08-25T23:33:22Z | ---
license: apache-2.0
tags:
- generated_from_trainer
model-index:
- name: distil-i
results: []
---
<!-- This model card has been generated automatically according to the information the Trainer had access to. You
should probably proofread and complete it, then remove this comment. -->
# distil-i
This model is a fine-tuned version of [distilroberta-base](https://huggingface.co/distilroberta-base) on the None dataset.
It achieves the following results on the evaluation set:
- Loss: 0.6252
- Rmse: 0.7907
- Mse: 0.6252
- Mae: 0.6061
## Model description
More information needed
## Intended uses & limitations
More information needed
## Training and evaluation data
More information needed
## Training procedure
### Training hyperparameters
The following hyperparameters were used during training:
- learning_rate: 5e-05
- train_batch_size: 32
- eval_batch_size: 32
- seed: 42
- optimizer: Adam with betas=(0.9,0.999) and epsilon=1e-08
- lr_scheduler_type: linear
- num_epochs: 10
### Training results
| Training Loss | Epoch | Step | Validation Loss | Rmse | Mse | Mae |
|:-------------:|:-----:|:----:|:---------------:|:------:|:------:|:------:|
| 0.7417 | 1.0 | 492 | 0.7164 | 0.8464 | 0.7164 | 0.5983 |
| 0.5948 | 2.0 | 984 | 0.6469 | 0.8043 | 0.6469 | 0.5840 |
| 0.5849 | 3.0 | 1476 | 0.6068 | 0.7790 | 0.6068 | 0.6027 |
| 0.5839 | 4.0 | 1968 | 0.6220 | 0.7887 | 0.6220 | 0.5847 |
| 0.5786 | 5.0 | 2460 | 0.6252 | 0.7907 | 0.6252 | 0.6061 |
### Framework versions
- Transformers 4.19.0.dev0
- Pytorch 1.9.0+cu111
- Datasets 2.4.0
- Tokenizers 0.12.1
|
paola-md/distil-I-upper | paola-md | 2022-08-25T23:32:56Z | 7 | 0 | transformers | [
"transformers",
"pytorch",
"roberta",
"text-classification",
"generated_from_trainer",
"license:apache-2.0",
"autotrain_compatible",
"endpoints_compatible",
"region:us"
]
| text-classification | 2022-08-25T23:24:17Z | ---
license: apache-2.0
tags:
- generated_from_trainer
model-index:
- name: distil-I-upper
results: []
---
<!-- This model card has been generated automatically according to the information the Trainer had access to. You
should probably proofread and complete it, then remove this comment. -->
# distil-I-upper
This model is a fine-tuned version of [distilroberta-base](https://huggingface.co/distilroberta-base) on the None dataset.
It achieves the following results on the evaluation set:
- Loss: 0.6060
- Rmse: 0.7785
- Mse: 0.6060
- Mae: 0.6007
## Model description
More information needed
## Intended uses & limitations
More information needed
## Training and evaluation data
More information needed
## Training procedure
### Training hyperparameters
The following hyperparameters were used during training:
- learning_rate: 5e-05
- train_batch_size: 32
- eval_batch_size: 32
- seed: 42
- optimizer: Adam with betas=(0.9,0.999) and epsilon=1e-08
- lr_scheduler_type: linear
- num_epochs: 10
### Training results
| Training Loss | Epoch | Step | Validation Loss | Rmse | Mse | Mae |
|:-------------:|:-----:|:----:|:---------------:|:------:|:------:|:------:|
| 0.7219 | 1.0 | 492 | 0.6818 | 0.8257 | 0.6818 | 0.5909 |
| 0.5932 | 2.0 | 984 | 0.6419 | 0.8012 | 0.6419 | 0.5838 |
| 0.5874 | 3.0 | 1476 | 0.6058 | 0.7783 | 0.6058 | 0.6007 |
| 0.5883 | 4.0 | 1968 | 0.6211 | 0.7881 | 0.6211 | 0.5875 |
| 0.5838 | 5.0 | 2460 | 0.6060 | 0.7785 | 0.6060 | 0.6007 |
### Framework versions
- Transformers 4.19.0.dev0
- Pytorch 1.9.0+cu111
- Datasets 2.4.0
- Tokenizers 0.12.1
|
paola-md/distil-tIs-upper | paola-md | 2022-08-25T23:23:50Z | 164 | 0 | transformers | [
"transformers",
"pytorch",
"roberta",
"text-classification",
"generated_from_trainer",
"license:apache-2.0",
"autotrain_compatible",
"endpoints_compatible",
"region:us"
]
| text-classification | 2022-08-25T23:15:44Z | ---
license: apache-2.0
tags:
- generated_from_trainer
model-index:
- name: distil-tIs-upper
results: []
---
<!-- This model card has been generated automatically according to the information the Trainer had access to. You
should probably proofread and complete it, then remove this comment. -->
# distil-tIs-upper
This model is a fine-tuned version of [distilroberta-base](https://huggingface.co/distilroberta-base) on the None dataset.
It achieves the following results on the evaluation set:
- Loss: 0.6024
- Rmse: 0.7762
- Mse: 0.6024
- Mae: 0.5987
## Model description
More information needed
## Intended uses & limitations
More information needed
## Training and evaluation data
More information needed
## Training procedure
### Training hyperparameters
The following hyperparameters were used during training:
- learning_rate: 5e-05
- train_batch_size: 32
- eval_batch_size: 32
- seed: 42
- optimizer: Adam with betas=(0.9,0.999) and epsilon=1e-08
- lr_scheduler_type: linear
- num_epochs: 10
### Training results
| Training Loss | Epoch | Step | Validation Loss | Rmse | Mse | Mae |
|:-------------:|:-----:|:----:|:---------------:|:------:|:------:|:------:|
| 0.7114 | 1.0 | 492 | 0.6942 | 0.8332 | 0.6942 | 0.5939 |
| 0.5948 | 2.0 | 984 | 0.6563 | 0.8101 | 0.6563 | 0.5861 |
| 0.59 | 3.0 | 1476 | 0.6091 | 0.7805 | 0.6091 | 0.6008 |
| 0.587 | 4.0 | 1968 | 0.6226 | 0.7890 | 0.6226 | 0.5870 |
| 0.5873 | 5.0 | 2460 | 0.6024 | 0.7762 | 0.6024 | 0.5987 |
### Framework versions
- Transformers 4.19.0.dev0
- Pytorch 1.9.0+cu111
- Datasets 2.4.0
- Tokenizers 0.12.1
|
paola-md/distil-tis | paola-md | 2022-08-25T23:15:15Z | 163 | 0 | transformers | [
"transformers",
"pytorch",
"roberta",
"text-classification",
"generated_from_trainer",
"license:apache-2.0",
"autotrain_compatible",
"endpoints_compatible",
"region:us"
]
| text-classification | 2022-08-25T23:07:56Z | ---
license: apache-2.0
tags:
- generated_from_trainer
model-index:
- name: distil-tis
results: []
---
<!-- This model card has been generated automatically according to the information the Trainer had access to. You
should probably proofread and complete it, then remove this comment. -->
# distil-tis
This model is a fine-tuned version of [distilroberta-base](https://huggingface.co/distilroberta-base) on the None dataset.
It achieves the following results on the evaluation set:
- Loss: 0.6061
- Rmse: 0.7785
- Mse: 0.6061
- Mae: 0.6003
## Model description
More information needed
## Intended uses & limitations
More information needed
## Training and evaluation data
More information needed
## Training procedure
### Training hyperparameters
The following hyperparameters were used during training:
- learning_rate: 5e-05
- train_batch_size: 32
- eval_batch_size: 32
- seed: 42
- optimizer: Adam with betas=(0.9,0.999) and epsilon=1e-08
- lr_scheduler_type: linear
- num_epochs: 10
### Training results
| Training Loss | Epoch | Step | Validation Loss | Rmse | Mse | Mae |
|:-------------:|:-----:|:----:|:---------------:|:------:|:------:|:------:|
| 0.7173 | 1.0 | 492 | 0.7060 | 0.8403 | 0.7060 | 0.5962 |
| 0.5955 | 2.0 | 984 | 0.6585 | 0.8115 | 0.6585 | 0.5864 |
| 0.5876 | 3.0 | 1476 | 0.6090 | 0.7804 | 0.6090 | 0.6040 |
| 0.5871 | 4.0 | 1968 | 0.6247 | 0.7904 | 0.6247 | 0.5877 |
| 0.5871 | 5.0 | 2460 | 0.6061 | 0.7785 | 0.6061 | 0.6003 |
### Framework versions
- Transformers 4.19.0.dev0
- Pytorch 1.9.0+cu111
- Datasets 2.4.0
- Tokenizers 0.12.1
|
paola-md/distil-Is-upper | paola-md | 2022-08-25T23:07:27Z | 163 | 0 | transformers | [
"transformers",
"pytorch",
"roberta",
"text-classification",
"generated_from_trainer",
"license:apache-2.0",
"autotrain_compatible",
"endpoints_compatible",
"region:us"
]
| text-classification | 2022-08-25T22:59:19Z | ---
license: apache-2.0
tags:
- generated_from_trainer
model-index:
- name: distil-Is-upper
results: []
---
<!-- This model card has been generated automatically according to the information the Trainer had access to. You
should probably proofread and complete it, then remove this comment. -->
# distil-Is-upper
This model is a fine-tuned version of [distilroberta-base](https://huggingface.co/distilroberta-base) on the None dataset.
It achieves the following results on the evaluation set:
- Loss: 0.6095
- Rmse: 0.7807
- Mse: 0.6095
- Mae: 0.5993
## Model description
More information needed
## Intended uses & limitations
More information needed
## Training and evaluation data
More information needed
## Training procedure
### Training hyperparameters
The following hyperparameters were used during training:
- learning_rate: 5e-05
- train_batch_size: 32
- eval_batch_size: 32
- seed: 42
- optimizer: Adam with betas=(0.9,0.999) and epsilon=1e-08
- lr_scheduler_type: linear
- num_epochs: 10
### Training results
| Training Loss | Epoch | Step | Validation Loss | Rmse | Mse | Mae |
|:-------------:|:-----:|:----:|:---------------:|:------:|:------:|:------:|
| 0.7129 | 1.0 | 492 | 0.7088 | 0.8419 | 0.7088 | 0.5968 |
| 0.5953 | 2.0 | 984 | 0.6426 | 0.8016 | 0.6426 | 0.5838 |
| 0.5865 | 3.0 | 1476 | 0.6083 | 0.7800 | 0.6083 | 0.6023 |
| 0.5888 | 4.0 | 1968 | 0.6209 | 0.7880 | 0.6209 | 0.5880 |
| 0.5859 | 5.0 | 2460 | 0.6095 | 0.7807 | 0.6095 | 0.5993 |
### Framework versions
- Transformers 4.19.0.dev0
- Pytorch 1.9.0+cu111
- Datasets 2.4.0
- Tokenizers 0.12.1
|
paola-md/distil-is | paola-md | 2022-08-25T22:58:50Z | 163 | 0 | transformers | [
"transformers",
"pytorch",
"roberta",
"text-classification",
"generated_from_trainer",
"license:apache-2.0",
"autotrain_compatible",
"endpoints_compatible",
"region:us"
]
| text-classification | 2022-08-25T22:49:58Z | ---
license: apache-2.0
tags:
- generated_from_trainer
model-index:
- name: distil-is
results: []
---
<!-- This model card has been generated automatically according to the information the Trainer had access to. You
should probably proofread and complete it, then remove this comment. -->
# distil-is
This model is a fine-tuned version of [distilroberta-base](https://huggingface.co/distilroberta-base) on the None dataset.
It achieves the following results on the evaluation set:
- Loss: 0.6082
- Rmse: 0.7799
- Mse: 0.6082
- Mae: 0.6023
## Model description
More information needed
## Intended uses & limitations
More information needed
## Training and evaluation data
More information needed
## Training procedure
### Training hyperparameters
The following hyperparameters were used during training:
- learning_rate: 5e-05
- train_batch_size: 32
- eval_batch_size: 32
- seed: 42
- optimizer: Adam with betas=(0.9,0.999) and epsilon=1e-08
- lr_scheduler_type: linear
- num_epochs: 10
### Training results
| Training Loss | Epoch | Step | Validation Loss | Rmse | Mse | Mae |
|:-------------:|:-----:|:----:|:---------------:|:------:|:------:|:------:|
| 0.6881 | 1.0 | 492 | 0.6534 | 0.8084 | 0.6534 | 0.5857 |
| 0.5923 | 2.0 | 984 | 0.6508 | 0.8067 | 0.6508 | 0.5852 |
| 0.5865 | 3.0 | 1476 | 0.6088 | 0.7803 | 0.6088 | 0.6096 |
| 0.5899 | 4.0 | 1968 | 0.6279 | 0.7924 | 0.6279 | 0.5853 |
| 0.5852 | 5.0 | 2460 | 0.6082 | 0.7799 | 0.6082 | 0.6023 |
### Framework versions
- Transformers 4.19.0.dev0
- Pytorch 1.9.0+cu111
- Datasets 2.4.0
- Tokenizers 0.12.1
|
Davlan/xlm-roberta-large-finetuned-amharic | Davlan | 2022-08-25T22:21:56Z | 103 | 0 | transformers | [
"transformers",
"pytorch",
"xlm-roberta",
"fill-mask",
"generated_from_trainer",
"license:mit",
"autotrain_compatible",
"endpoints_compatible",
"region:us"
]
| fill-mask | 2022-08-25T19:02:57Z | ---
license: mit
tags:
- generated_from_trainer
model-index:
- name: amh_xlmr
results: []
---
<!-- This model card has been generated automatically according to the information the Trainer had access to. You
should probably proofread and complete it, then remove this comment. -->
# amh_xlmr
This model is a fine-tuned version of [xlm-roberta-large](https://huggingface.co/xlm-roberta-large) on an unknown dataset.
It achieves the following results on the evaluation set:
- Loss: 1.1295
## Model description
More information needed
## Intended uses & limitations
More information needed
## Training and evaluation data
More information needed
## Training procedure
### Training hyperparameters
The following hyperparameters were used during training:
- learning_rate: 5e-05
- train_batch_size: 5
- eval_batch_size: 8
- seed: 42
- distributed_type: multi-GPU
- gradient_accumulation_steps: 2
- total_train_batch_size: 10
- optimizer: Adam with betas=(0.9,0.999) and epsilon=1e-08
- lr_scheduler_type: linear
- num_epochs: 3.0
### Training results
### Framework versions
- Transformers 4.21.1
- Pytorch 1.7.1+cu110
- Datasets 1.16.1
- Tokenizers 0.12.1
|
Davlan/xlm-roberta-large-finetuned-luganda | Davlan | 2022-08-25T22:03:36Z | 106 | 1 | transformers | [
"transformers",
"pytorch",
"xlm-roberta",
"fill-mask",
"generated_from_trainer",
"autotrain_compatible",
"endpoints_compatible",
"region:us"
]
| fill-mask | 2022-08-25T19:01:35Z | ---
tags:
- generated_from_trainer
model-index:
- name: lug_xlmr
results: []
---
<!-- This model card has been generated automatically according to the information the Trainer had access to. You
should probably proofread and complete it, then remove this comment. -->
# lug_xlmr
This model is a fine-tuned version of [models/lug_xlmr/](https://huggingface.co/models/lug_xlmr/) on an unknown dataset.
It achieves the following results on the evaluation set:
- eval_loss: 0.8414
- eval_runtime: 10.7925
- eval_samples_per_second: 32.245
- eval_steps_per_second: 4.077
- step: 0
## Model description
More information needed
## Intended uses & limitations
More information needed
## Training and evaluation data
More information needed
## Training procedure
### Training hyperparameters
The following hyperparameters were used during training:
- learning_rate: 5e-05
- train_batch_size: 5
- eval_batch_size: 8
- seed: 42
- distributed_type: multi-GPU
- gradient_accumulation_steps: 2
- total_train_batch_size: 10
- optimizer: Adam with betas=(0.9,0.999) and epsilon=1e-08
- lr_scheduler_type: linear
- num_epochs: 3.0
### Framework versions
- Transformers 4.21.1
- Pytorch 1.7.1+cu110
- Datasets 1.16.1
- Tokenizers 0.12.1
|
Davlan/xlm-roberta-large-finetuned-english | Davlan | 2022-08-25T21:59:32Z | 106 | 0 | transformers | [
"transformers",
"pytorch",
"xlm-roberta",
"fill-mask",
"generated_from_trainer",
"license:mit",
"autotrain_compatible",
"endpoints_compatible",
"region:us"
]
| fill-mask | 2022-08-25T19:02:27Z | ---
license: mit
tags:
- generated_from_trainer
model-index:
- name: eng_xlmr
results: []
---
<!-- This model card has been generated automatically according to the information the Trainer had access to. You
should probably proofread and complete it, then remove this comment. -->
# eng_xlmr
This model is a fine-tuned version of [xlm-roberta-large](https://huggingface.co/xlm-roberta-large) on an unknown dataset.
It achieves the following results on the evaluation set:
- Loss: 0.9686
## Model description
More information needed
## Intended uses & limitations
More information needed
## Training and evaluation data
More information needed
## Training procedure
### Training hyperparameters
The following hyperparameters were used during training:
- learning_rate: 5e-05
- train_batch_size: 5
- eval_batch_size: 8
- seed: 42
- distributed_type: multi-GPU
- gradient_accumulation_steps: 2
- total_train_batch_size: 10
- optimizer: Adam with betas=(0.9,0.999) and epsilon=1e-08
- lr_scheduler_type: linear
- num_epochs: 3.0
### Training results
### Framework versions
- Transformers 4.21.1
- Pytorch 1.7.1+cu110
- Datasets 1.16.1
- Tokenizers 0.12.1
|
Davlan/xlm-roberta-large-finetuned-swahili | Davlan | 2022-08-25T21:56:28Z | 107 | 0 | transformers | [
"transformers",
"pytorch",
"xlm-roberta",
"fill-mask",
"generated_from_trainer",
"license:mit",
"autotrain_compatible",
"endpoints_compatible",
"region:us"
]
| fill-mask | 2022-08-25T21:04:53Z | ---
license: mit
tags:
- generated_from_trainer
model-index:
- name: swa_xlmr
results: []
---
<!-- This model card has been generated automatically according to the information the Trainer had access to. You
should probably proofread and complete it, then remove this comment. -->
# swa_xlmr
This model is a fine-tuned version of [xlm-roberta-large](https://huggingface.co/xlm-roberta-large) on an unknown dataset.
It achieves the following results on the evaluation set:
- Loss: 1.0626
## Model description
More information needed
## Intended uses & limitations
More information needed
## Training and evaluation data
More information needed
## Training procedure
### Training hyperparameters
The following hyperparameters were used during training:
- learning_rate: 5e-05
- train_batch_size: 5
- eval_batch_size: 8
- seed: 42
- distributed_type: multi-GPU
- gradient_accumulation_steps: 2
- total_train_batch_size: 10
- optimizer: Adam with betas=(0.9,0.999) and epsilon=1e-08
- lr_scheduler_type: linear
- num_epochs: 3.0
### Training results
### Framework versions
- Transformers 4.21.1
- Pytorch 1.7.1+cu110
- Datasets 1.16.1
- Tokenizers 0.12.1
|
Davlan/xlm-roberta-large-finetuned-luo | Davlan | 2022-08-25T21:17:52Z | 107 | 1 | transformers | [
"transformers",
"pytorch",
"xlm-roberta",
"fill-mask",
"generated_from_trainer",
"autotrain_compatible",
"endpoints_compatible",
"region:us"
]
| fill-mask | 2022-08-25T19:01:59Z | ---
tags:
- generated_from_trainer
model-index:
- name: luo_xlmr
results: []
---
<!-- This model card has been generated automatically according to the information the Trainer had access to. You
should probably proofread and complete it, then remove this comment. -->
# luo_xlmr
This model is a fine-tuned version of [models/luo_xlmr/](https://huggingface.co/models/luo_xlmr/) on an unknown dataset.
It achieves the following results on the evaluation set:
- eval_loss: 2.7161
- eval_runtime: 3.4086
- eval_samples_per_second: 30.804
- eval_steps_per_second: 4.107
- step: 0
## Model description
More information needed
## Intended uses & limitations
More information needed
## Training and evaluation data
More information needed
## Training procedure
### Training hyperparameters
The following hyperparameters were used during training:
- learning_rate: 5e-05
- train_batch_size: 5
- eval_batch_size: 8
- seed: 42
- distributed_type: multi-GPU
- gradient_accumulation_steps: 2
- total_train_batch_size: 10
- optimizer: Adam with betas=(0.9,0.999) and epsilon=1e-08
- lr_scheduler_type: linear
- num_epochs: 3.0
### Framework versions
- Transformers 4.21.1
- Pytorch 1.7.1+cu110
- Datasets 1.16.1
- Tokenizers 0.12.1
|
Davlan/xlm-roberta-large-finetuned-naija | Davlan | 2022-08-25T21:02:09Z | 107 | 0 | transformers | [
"transformers",
"pytorch",
"xlm-roberta",
"fill-mask",
"generated_from_trainer",
"autotrain_compatible",
"endpoints_compatible",
"region:us"
]
| fill-mask | 2022-08-25T19:02:15Z | ---
tags:
- generated_from_trainer
model-index:
- name: pcm_xlmr
results: []
---
<!-- This model card has been generated automatically according to the information the Trainer had access to. You
should probably proofread and complete it, then remove this comment. -->
# pcm_xlmr
This model is a fine-tuned version of [models/pcm_xlmr/](https://huggingface.co/models/pcm_xlmr/) on an unknown dataset.
It achieves the following results on the evaluation set:
- eval_loss: 0.8021
- eval_runtime: 48.0467
- eval_samples_per_second: 32.448
- eval_steps_per_second: 4.059
- step: 0
## Model description
More information needed
## Intended uses & limitations
More information needed
## Training and evaluation data
More information needed
## Training procedure
### Training hyperparameters
The following hyperparameters were used during training:
- learning_rate: 5e-05
- train_batch_size: 5
- eval_batch_size: 8
- seed: 42
- distributed_type: multi-GPU
- gradient_accumulation_steps: 2
- total_train_batch_size: 10
- optimizer: Adam with betas=(0.9,0.999) and epsilon=1e-08
- lr_scheduler_type: linear
- num_epochs: 3.0
### Framework versions
- Transformers 4.21.1
- Pytorch 1.7.1+cu110
- Datasets 1.16.1
- Tokenizers 0.12.1
|
frslee/finetuning-sentiment-model-3000-samples | frslee | 2022-08-25T20:32:30Z | 107 | 0 | transformers | [
"transformers",
"pytorch",
"tensorboard",
"distilbert",
"text-classification",
"generated_from_trainer",
"license:apache-2.0",
"autotrain_compatible",
"endpoints_compatible",
"region:us"
]
| text-classification | 2022-08-25T20:13:38Z | ---
license: apache-2.0
tags:
- generated_from_trainer
metrics:
- accuracy
- f1
model-index:
- name: finetuning-sentiment-model-3000-samples
results: []
---
<!-- This model card has been generated automatically according to the information the Trainer had access to. You
should probably proofread and complete it, then remove this comment. -->
# finetuning-sentiment-model-3000-samples
This model is a fine-tuned version of [distilbert-base-uncased](https://huggingface.co/distilbert-base-uncased) on an unknown dataset.
It achieves the following results on the evaluation set:
- Loss: 0.3076
- Accuracy: 0.8767
- F1: 0.8771
## Model description
More information needed
## Intended uses & limitations
More information needed
## Training and evaluation data
More information needed
## Training procedure
### Training hyperparameters
The following hyperparameters were used during training:
- learning_rate: 2e-05
- train_batch_size: 16
- eval_batch_size: 16
- seed: 42
- optimizer: Adam with betas=(0.9,0.999) and epsilon=1e-08
- lr_scheduler_type: linear
- num_epochs: 2
### Training results
### Framework versions
- Transformers 4.21.2
- Pytorch 1.12.1+cu113
- Tokenizers 0.12.1
|
Davlan/xlm-roberta-large-finetuned-lingala | Davlan | 2022-08-25T20:29:41Z | 107 | 0 | transformers | [
"transformers",
"pytorch",
"xlm-roberta",
"fill-mask",
"generated_from_trainer",
"autotrain_compatible",
"endpoints_compatible",
"region:us"
]
| fill-mask | 2022-08-25T19:05:47Z | ---
tags:
- generated_from_trainer
model-index:
- name: lin_xlmr
results: []
---
<!-- This model card has been generated automatically according to the information the Trainer had access to. You
should probably proofread and complete it, then remove this comment. -->
# lin_xlmr
This model is a fine-tuned version of [models/lin_xlmr/](https://huggingface.co/models/lin_xlmr/) on an unknown dataset.
It achieves the following results on the evaluation set:
- eval_loss: 2.1469
- eval_runtime: 22.8128
- eval_samples_per_second: 32.175
- eval_steps_per_second: 4.033
- step: 0
## Model description
More information needed
## Intended uses & limitations
More information needed
## Training and evaluation data
More information needed
## Training procedure
### Training hyperparameters
The following hyperparameters were used during training:
- learning_rate: 5e-05
- train_batch_size: 5
- eval_batch_size: 8
- seed: 42
- distributed_type: multi-GPU
- gradient_accumulation_steps: 2
- total_train_batch_size: 10
- optimizer: Adam with betas=(0.9,0.999) and epsilon=1e-08
- lr_scheduler_type: linear
- num_epochs: 3.0
### Framework versions
- Transformers 4.21.1
- Pytorch 1.7.1+cu110
- Datasets 1.16.1
- Tokenizers 0.12.1
|
Davlan/xlm-roberta-large-finetuned-zulu | Davlan | 2022-08-25T20:23:03Z | 105 | 0 | transformers | [
"transformers",
"pytorch",
"xlm-roberta",
"fill-mask",
"generated_from_trainer",
"autotrain_compatible",
"endpoints_compatible",
"region:us"
]
| fill-mask | 2022-08-25T18:59:58Z | ---
tags:
- generated_from_trainer
model-index:
- name: zul_xlmr
results: []
---
<!-- This model card has been generated automatically according to the information the Trainer had access to. You
should probably proofread and complete it, then remove this comment. -->
# zul_xlmr
This model is a fine-tuned version of [models/zul_xlmr/](https://huggingface.co/models/zul_xlmr/) on an unknown dataset.
It achieves the following results on the evaluation set:
- eval_loss: 2.2241
- eval_runtime: 37.5729
- eval_samples_per_second: 32.177
- eval_steps_per_second: 4.045
- step: 0
## Model description
More information needed
## Intended uses & limitations
More information needed
## Training and evaluation data
More information needed
## Training procedure
### Training hyperparameters
The following hyperparameters were used during training:
- learning_rate: 5e-05
- train_batch_size: 5
- eval_batch_size: 8
- seed: 42
- distributed_type: multi-GPU
- gradient_accumulation_steps: 2
- total_train_batch_size: 10
- optimizer: Adam with betas=(0.9,0.999) and epsilon=1e-08
- lr_scheduler_type: linear
- num_epochs: 3.0
### Framework versions
- Transformers 4.21.1
- Pytorch 1.7.1+cu110
- Datasets 1.16.1
- Tokenizers 0.12.1
|
Davlan/xlm-roberta-large-finetuned-hausa | Davlan | 2022-08-25T19:29:22Z | 104 | 0 | transformers | [
"transformers",
"pytorch",
"xlm-roberta",
"fill-mask",
"generated_from_trainer",
"license:mit",
"autotrain_compatible",
"endpoints_compatible",
"region:us"
]
| fill-mask | 2022-08-25T18:59:11Z | ---
license: mit
tags:
- generated_from_trainer
model-index:
- name: hau_xlmr
results: []
---
<!-- This model card has been generated automatically according to the information the Trainer had access to. You
should probably proofread and complete it, then remove this comment. -->
# hau_xlmr
This model is a fine-tuned version of [xlm-roberta-large](https://huggingface.co/xlm-roberta-large) on an unknown dataset.
It achieves the following results on the evaluation set:
- Loss: 0.7674
## Model description
More information needed
## Intended uses & limitations
More information needed
## Training and evaluation data
More information needed
## Training procedure
### Training hyperparameters
The following hyperparameters were used during training:
- learning_rate: 5e-05
- train_batch_size: 5
- eval_batch_size: 8
- seed: 42
- distributed_type: multi-GPU
- gradient_accumulation_steps: 2
- total_train_batch_size: 10
- optimizer: Adam with betas=(0.9,0.999) and epsilon=1e-08
- lr_scheduler_type: linear
- num_epochs: 3.0
### Training results
### Framework versions
- Transformers 4.21.1
- Pytorch 1.7.1+cu110
- Datasets 1.16.1
- Tokenizers 0.12.1
|
fgmckee/a2c-AntBulletEnv-v0 | fgmckee | 2022-08-25T17:52:45Z | 0 | 0 | stable-baselines3 | [
"stable-baselines3",
"AntBulletEnv-v0",
"deep-reinforcement-learning",
"reinforcement-learning",
"model-index",
"region:us"
]
| reinforcement-learning | 2022-08-25T17:51:35Z | ---
library_name: stable-baselines3
tags:
- AntBulletEnv-v0
- deep-reinforcement-learning
- reinforcement-learning
- stable-baselines3
model-index:
- name: A2C
results:
- metrics:
- type: mean_reward
value: 1813.75 +/- 122.94
name: mean_reward
task:
type: reinforcement-learning
name: reinforcement-learning
dataset:
name: AntBulletEnv-v0
type: AntBulletEnv-v0
---
# **A2C** Agent playing **AntBulletEnv-v0**
This is a trained model of a **A2C** agent playing **AntBulletEnv-v0**
using the [stable-baselines3 library](https://github.com/DLR-RM/stable-baselines3).
## Usage (with Stable-baselines3)
TODO: Add your code
```python
from stable_baselines3 import ...
from huggingface_sb3 import load_from_hub
...
```
|
wyu1/FiD-TQA | wyu1 | 2022-08-25T17:22:38Z | 5 | 0 | transformers | [
"transformers",
"pytorch",
"t5",
"license:cc-by-4.0",
"text-generation-inference",
"endpoints_compatible",
"region:us"
]
| null | 2022-08-19T00:24:56Z | ---
license: cc-by-4.0
---
# FiD model trained on TQA
-- This is the model checkpoint of FiD [2], based on the T5 large (with 770M parameters) and trained on the TriviaQA dataset [1].
-- Hyperparameters: 8 x 40GB A100 GPUs; batch size 8; AdamW; LR 3e-5; 30000 steps
References:
[1] TriviaQA: A Large Scale Dataset for Reading Comprehension and Question Answering. ACL 2017
[2] Leveraging Passage Retrieval with Generative Models for Open Domain Question Answering. EACL 2021.
## Model performance
We evaluate it on the TriviaQA dataset, the EM score is 68.5 (0.8 higher than the original performance reported in the paper).
<a href="https://huggingface.co/exbert/?model=bert-base-uncased">
<img width="300px" src="https://cdn-media.huggingface.co/exbert/button.png">
</a>
---
license: cc-by-4.0
---
|
silviacamplani/distilbert-finetuned-tapt-ner-ai | silviacamplani | 2022-08-25T15:54:12Z | 5 | 0 | transformers | [
"transformers",
"tf",
"tensorboard",
"distilbert",
"token-classification",
"generated_from_keras_callback",
"autotrain_compatible",
"endpoints_compatible",
"region:us"
]
| token-classification | 2022-08-25T15:51:02Z | ---
tags:
- generated_from_keras_callback
model-index:
- name: silviacamplani/distilbert-finetuned-tapt-ner-ai
results: []
---
<!-- This model card has been generated automatically according to the information Keras had access to. You should
probably proofread and complete it, then remove this comment. -->
# silviacamplani/distilbert-finetuned-tapt-ner-ai
This model was trained from scratch on an unknown dataset.
It achieves the following results on the evaluation set:
- Train Loss: 0.9093
- Validation Loss: 0.9177
- Train Precision: 0.3439
- Train Recall: 0.3697
- Train F1: 0.3563
- Train Accuracy: 0.7697
- Epoch: 9
## Model description
More information needed
## Intended uses & limitations
More information needed
## Training and evaluation data
More information needed
## Training procedure
### Training hyperparameters
The following hyperparameters were used during training:
- optimizer: {'inner_optimizer': {'class_name': 'AdamWeightDecay', 'config': {'name': 'AdamWeightDecay', 'learning_rate': {'class_name': 'PolynomialDecay', 'config': {'initial_learning_rate': 1e-05, 'decay_steps': 350, 'end_learning_rate': 0.0, 'power': 1.0, 'cycle': False, 'name': None}}, 'decay': 0.0, 'beta_1': 0.9, 'beta_2': 0.999, 'epsilon': 1e-08, 'amsgrad': False, 'weight_decay_rate': 0.01}}, 'dynamic': True, 'initial_scale': 32768.0, 'dynamic_growth_steps': 2000}
- training_precision: mixed_float16
### Training results
| Train Loss | Validation Loss | Train Precision | Train Recall | Train F1 | Train Accuracy | Epoch |
|:----------:|:---------------:|:---------------:|:------------:|:--------:|:--------------:|:-----:|
| 2.5750 | 1.7754 | 0.0 | 0.0 | 0.0 | 0.6480 | 0 |
| 1.6567 | 1.4690 | 0.0 | 0.0 | 0.0 | 0.6480 | 1 |
| 1.3888 | 1.2847 | 0.0 | 0.0 | 0.0 | 0.6480 | 2 |
| 1.2569 | 1.1744 | 0.0526 | 0.0221 | 0.0312 | 0.6751 | 3 |
| 1.1536 | 1.0884 | 0.2088 | 0.1704 | 0.1876 | 0.7240 | 4 |
| 1.0722 | 1.0281 | 0.2865 | 0.2641 | 0.2748 | 0.7431 | 5 |
| 1.0077 | 0.9782 | 0.3151 | 0.3135 | 0.3143 | 0.7553 | 6 |
| 0.9582 | 0.9437 | 0.3254 | 0.3492 | 0.3369 | 0.7661 | 7 |
| 0.9268 | 0.9242 | 0.3381 | 0.3595 | 0.3485 | 0.7689 | 8 |
| 0.9093 | 0.9177 | 0.3439 | 0.3697 | 0.3563 | 0.7697 | 9 |
### Framework versions
- Transformers 4.20.1
- TensorFlow 2.6.4
- Datasets 2.1.0
- Tokenizers 0.12.1
|
qBob/t5-small_corrector_15 | qBob | 2022-08-25T15:53:09Z | 10 | 0 | transformers | [
"transformers",
"pytorch",
"tensorboard",
"bart",
"text2text-generation",
"generated_from_trainer",
"license:apache-2.0",
"autotrain_compatible",
"endpoints_compatible",
"region:us"
]
| text2text-generation | 2022-08-25T14:02:46Z | ---
license: apache-2.0
tags:
- generated_from_trainer
metrics:
- rouge
model-index:
- name: t5-small_corrector_15
results: []
---
<!-- This model card has been generated automatically according to the information the Trainer had access to. You
should probably proofread and complete it, then remove this comment. -->
# t5-small_corrector_15
This model is a fine-tuned version of [t5-small](https://huggingface.co/t5-small) on an unknown dataset.
It achieves the following results on the evaluation set:
- Loss: 1.3416
- Rouge1: 34.7998
- Rouge2: 9.0842
- Rougel: 27.8188
- Rougelsum: 27.839
- Gen Len: 18.5561
## Model description
More information needed
## Intended uses & limitations
More information needed
## Training and evaluation data
More information needed
## Training procedure
### Training hyperparameters
The following hyperparameters were used during training:
- learning_rate: 2e-05
- train_batch_size: 8
- eval_batch_size: 8
- seed: 42
- optimizer: Adam with betas=(0.9,0.999) and epsilon=1e-08
- lr_scheduler_type: linear
- num_epochs: 5
- mixed_precision_training: Native AMP
### Training results
| Training Loss | Epoch | Step | Validation Loss | Rouge1 | Rouge2 | Rougel | Rougelsum | Gen Len |
|:-------------:|:-----:|:-----:|:---------------:|:-------:|:------:|:-------:|:---------:|:-------:|
| 4.2274 | 1.0 | 2365 | 2.9386 | 10.1244 | 1.0024 | 9.1029 | 9.1104 | 18.5377 |
| 2.7936 | 2.0 | 4730 | 2.0196 | 17.7168 | 3.0899 | 15.1305 | 15.1353 | 18.8883 |
| 2.2678 | 3.0 | 7095 | 1.7072 | 26.8501 | 5.7804 | 22.0034 | 22.0213 | 18.839 |
| 1.9029 | 4.0 | 9460 | 1.5254 | 32.9484 | 7.8531 | 26.4538 | 26.4749 | 18.502 |
| 1.5936 | 5.0 | 11825 | 1.3416 | 34.7998 | 9.0842 | 27.8188 | 27.839 | 18.5561 |
### Framework versions
- Transformers 4.21.2
- Pytorch 1.12.1+cu113
- Datasets 2.4.0
- Tokenizers 0.12.1
|
Chandanab/mit-b0-finetuned-eurosat | Chandanab | 2022-08-25T15:33:04Z | 49 | 0 | transformers | [
"transformers",
"pytorch",
"segformer",
"image-classification",
"generated_from_trainer",
"dataset:image_folder",
"license:other",
"model-index",
"autotrain_compatible",
"endpoints_compatible",
"region:us"
]
| image-classification | 2022-08-16T11:47:17Z | ---
license: other
tags:
- generated_from_trainer
datasets:
- image_folder
metrics:
- accuracy
model-index:
- name: mit-b0-finetuned-eurosat
results:
- task:
name: Image Classification
type: image-classification
dataset:
name: image_folder
type: image_folder
args: default
metrics:
- name: Accuracy
type: accuracy
value: 0.9494949494949495
---
<!-- This model card has been generated automatically according to the information the Trainer had access to. You
should probably proofread and complete it, then remove this comment. -->
# mit-b0-finetuned-eurosat
This model is a fine-tuned version of [nvidia/mit-b0](https://huggingface.co/nvidia/mit-b0) on the image_folder dataset.
It achieves the following results on the evaluation set:
- Loss: 0.1782
- Accuracy: 0.9495
## Model description
More information needed
## Intended uses & limitations
More information needed
## Training and evaluation data
More information needed
## Training procedure
### Training hyperparameters
The following hyperparameters were used during training:
- learning_rate: 5e-05
- train_batch_size: 32
- eval_batch_size: 32
- seed: 42
- gradient_accumulation_steps: 4
- total_train_batch_size: 128
- optimizer: Adam with betas=(0.9,0.999) and epsilon=1e-08
- lr_scheduler_type: linear
- lr_scheduler_warmup_ratio: 0.1
- num_epochs: 3
### Training results
| Training Loss | Epoch | Step | Validation Loss | Accuracy |
|:-------------:|:-----:|:----:|:---------------:|:--------:|
| No log | 1.0 | 7 | 0.3828 | 0.8081 |
| 0.4864 | 2.0 | 14 | 0.2224 | 0.9192 |
| 0.2035 | 3.0 | 21 | 0.1782 | 0.9495 |
### Framework versions
- Transformers 4.17.0
- Pytorch 1.11.0+cpu
- Datasets 2.2.0
- Tokenizers 0.12.1
|
dboshardy/ddim-butterflies-128 | dboshardy | 2022-08-25T15:23:56Z | 4 | 0 | diffusers | [
"diffusers",
"tensorboard",
"en",
"dataset:huggan/smithsonian_butterflies_subset",
"license:apache-2.0",
"diffusers:DDIMPipeline",
"region:us"
]
| null | 2022-08-24T21:41:06Z | ---
language: en
license: apache-2.0
library_name: diffusers
tags: []
datasets: huggan/smithsonian_butterflies_subset
metrics: []
---
<!-- This model card has been generated automatically according to the information the training script had access to. You
should probably proofread and complete it, then remove this comment. -->
# ddim-butterflies-128
## Model description
This diffusion model is trained with the [π€ Diffusers](https://github.com/huggingface/diffusers) library
on the `huggan/smithsonian_butterflies_subset` dataset.
## Intended uses & limitations
#### How to use
```python
# TODO: add an example code snippet for running this diffusion pipeline
```
#### Limitations and bias
[TODO: provide examples of latent issues and potential remediations]
## Training data
[TODO: describe the data used to train the model]
### Training hyperparameters
The following hyperparameters were used during training:
- learning_rate: 0.0001
- train_batch_size: 32
- eval_batch_size: 32
- gradient_accumulation_steps: 1
- optimizer: AdamW with betas=(None, None), weight_decay=None and epsilon=None
- lr_scheduler: None
- lr_warmup_steps: 250
- ema_inv_gamma: None
- ema_inv_gamma: None
- ema_inv_gamma: None
- mixed_precision: fp16
### Training results
π [TensorBoard logs](https://huggingface.co/dboshardy/ddim-butterflies-128/tensorboard?#scalars)
|
silviacamplani/distilbert-finetuned-dapt-ner-ai | silviacamplani | 2022-08-25T14:13:33Z | 63 | 0 | transformers | [
"transformers",
"tf",
"tensorboard",
"distilbert",
"token-classification",
"generated_from_keras_callback",
"autotrain_compatible",
"endpoints_compatible",
"region:us"
]
| token-classification | 2022-08-25T14:11:40Z | ---
tags:
- generated_from_keras_callback
model-index:
- name: silviacamplani/distilbert-finetuned-dapt-ner-ai
results: []
---
<!-- This model card has been generated automatically according to the information Keras had access to. You should
probably proofread and complete it, then remove this comment. -->
# silviacamplani/distilbert-finetuned-dapt-ner-ai
This model was trained from scratch on an unknown dataset.
It achieves the following results on the evaluation set:
- Train Loss: 0.9448
- Validation Loss: 0.9212
- Train Precision: 0.3164
- Train Recall: 0.3186
- Train F1: 0.3175
- Train Accuracy: 0.7524
- Epoch: 6
## Model description
More information needed
## Intended uses & limitations
More information needed
## Training and evaluation data
More information needed
## Training procedure
### Training hyperparameters
The following hyperparameters were used during training:
- optimizer: {'inner_optimizer': {'class_name': 'AdamWeightDecay', 'config': {'name': 'AdamWeightDecay', 'learning_rate': {'class_name': 'PolynomialDecay', 'config': {'initial_learning_rate': 1e-05, 'decay_steps': 350, 'end_learning_rate': 0.0, 'power': 1.0, 'cycle': False, 'name': None}}, 'decay': 0.0, 'beta_1': 0.9, 'beta_2': 0.999, 'epsilon': 1e-08, 'amsgrad': False, 'weight_decay_rate': 0.01}}, 'dynamic': True, 'initial_scale': 32768.0, 'dynamic_growth_steps': 2000}
- training_precision: mixed_float16
### Training results
| Train Loss | Validation Loss | Train Precision | Train Recall | Train F1 | Train Accuracy | Epoch |
|:----------:|:---------------:|:---------------:|:------------:|:--------:|:--------------:|:-----:|
| 2.6857 | 1.8199 | 0.0 | 0.0 | 0.0 | 0.6480 | 0 |
| 1.6775 | 1.4868 | 0.0 | 0.0 | 0.0 | 0.6480 | 1 |
| 1.3847 | 1.2452 | 0.0938 | 0.0102 | 0.0184 | 0.6565 | 2 |
| 1.2067 | 1.1198 | 0.1659 | 0.1244 | 0.1422 | 0.7077 | 3 |
| 1.0946 | 1.0321 | 0.2255 | 0.1925 | 0.2077 | 0.7225 | 4 |
| 1.0057 | 0.9640 | 0.2835 | 0.2777 | 0.2806 | 0.7433 | 5 |
| 0.9448 | 0.9212 | 0.3164 | 0.3186 | 0.3175 | 0.7524 | 6 |
### Framework versions
- Transformers 4.20.1
- TensorFlow 2.6.4
- Datasets 2.1.0
- Tokenizers 0.12.1
|
ZhiyuanQiu/camembert-base-finetuned-Train_RAW15-dd | ZhiyuanQiu | 2022-08-25T14:06:07Z | 107 | 0 | transformers | [
"transformers",
"pytorch",
"tensorboard",
"camembert",
"token-classification",
"generated_from_trainer",
"license:mit",
"autotrain_compatible",
"endpoints_compatible",
"region:us"
]
| token-classification | 2022-08-25T11:05:51Z | ---
license: mit
tags:
- generated_from_trainer
metrics:
- precision
- recall
- f1
- accuracy
model-index:
- name: camembert-base-finetuned-Train_RAW15-dd
results: []
---
<!-- This model card has been generated automatically according to the information the Trainer had access to. You
should probably proofread and complete it, then remove this comment. -->
# camembert-base-finetuned-Train_RAW15-dd
This model is a fine-tuned version of [camembert-base](https://huggingface.co/camembert-base) on an unknown dataset.
It achieves the following results on the evaluation set:
- Loss: 0.3634
- Precision: 0.8788
- Recall: 0.9009
- F1: 0.8897
- Accuracy: 0.9256
## Model description
More information needed
## Intended uses & limitations
More information needed
## Training and evaluation data
More information needed
## Training procedure
### Training hyperparameters
The following hyperparameters were used during training:
- learning_rate: 2e-05
- train_batch_size: 16
- eval_batch_size: 16
- seed: 42
- optimizer: Adam with betas=(0.9,0.999) and epsilon=1e-08
- lr_scheduler_type: linear
- num_epochs: 2
### Training results
| Training Loss | Epoch | Step | Validation Loss | Precision | Recall | F1 | Accuracy |
|:-------------:|:-----:|:-----:|:---------------:|:---------:|:------:|:------:|:--------:|
| 0.0899 | 1.0 | 12043 | 0.3289 | 0.8642 | 0.8996 | 0.8815 | 0.9156 |
| 0.0756 | 2.0 | 24086 | 0.3634 | 0.8788 | 0.9009 | 0.8897 | 0.9256 |
### Framework versions
- Transformers 4.21.2
- Pytorch 1.12.1+cu113
- Datasets 2.4.0
- Tokenizers 0.12.1
|
Shivus/ppo-LunarLander-v2 | Shivus | 2022-08-25T14:03:02Z | 1 | 0 | stable-baselines3 | [
"stable-baselines3",
"LunarLander-v2",
"deep-reinforcement-learning",
"reinforcement-learning",
"model-index",
"region:us"
]
| reinforcement-learning | 2022-08-25T14:02:29Z | ---
library_name: stable-baselines3
tags:
- LunarLander-v2
- deep-reinforcement-learning
- reinforcement-learning
- stable-baselines3
model-index:
- name: PPO
results:
- metrics:
- type: mean_reward
value: -134.10 +/- 32.54
name: mean_reward
task:
type: reinforcement-learning
name: reinforcement-learning
dataset:
name: LunarLander-v2
type: LunarLander-v2
---
# **PPO** Agent playing **LunarLander-v2**
This is a trained model of a **PPO** agent playing **LunarLander-v2**
using the [stable-baselines3 library](https://github.com/DLR-RM/stable-baselines3).
## Usage (with Stable-baselines3)
TODO: Add your code
```python
from stable_baselines3 import ...
from huggingface_sb3 import load_from_hub
...
```
|
teven/all_bs320_vanilla | teven | 2022-08-25T13:45:58Z | 3 | 0 | sentence-transformers | [
"sentence-transformers",
"pytorch",
"mpnet",
"feature-extraction",
"sentence-similarity",
"autotrain_compatible",
"endpoints_compatible",
"region:us"
]
| sentence-similarity | 2022-08-25T13:45:51Z | ---
pipeline_tag: sentence-similarity
tags:
- sentence-transformers
- feature-extraction
- sentence-similarity
---
# teven/all_bs320_vanilla
This is a [sentence-transformers](https://www.SBERT.net) model: It maps sentences & paragraphs to a 768 dimensional dense vector space and can be used for tasks like clustering or semantic search.
<!--- Describe your model here -->
## Usage (Sentence-Transformers)
Using this model becomes easy when you have [sentence-transformers](https://www.SBERT.net) installed:
```
pip install -U sentence-transformers
```
Then you can use the model like this:
```python
from sentence_transformers import SentenceTransformer
sentences = ["This is an example sentence", "Each sentence is converted"]
model = SentenceTransformer('teven/all_bs320_vanilla')
embeddings = model.encode(sentences)
print(embeddings)
```
## Evaluation Results
<!--- Describe how your model was evaluated -->
For an automated evaluation of this model, see the *Sentence Embeddings Benchmark*: [https://seb.sbert.net](https://seb.sbert.net?model_name=teven/all_bs320_vanilla)
## Training
The model was trained with the parameters:
**DataLoader**:
`torch.utils.data.dataloader.DataLoader` of length 390414 with parameters:
```
{'batch_size': 40, 'sampler': 'torch.utils.data.sampler.SequentialSampler', 'batch_sampler': 'torch.utils.data.sampler.BatchSampler'}
```
**Loss**:
`sentence_transformers.losses.MultipleNegativesRankingLoss.MultipleNegativesRankingLoss` with parameters:
```
{'scale': 20.0, 'similarity_fct': 'cos_sim'}
```
**DataLoader**:
`torch.utils.data.dataloader.DataLoader` of length 157752 with parameters:
```
{'batch_size': 40, 'sampler': 'torch.utils.data.sampler.SequentialSampler', 'batch_sampler': 'torch.utils.data.sampler.BatchSampler'}
```
**Loss**:
`sentence_transformers.losses.MultipleNegativesRankingLoss.MultipleNegativesRankingLoss` with parameters:
```
{'scale': 20.0, 'similarity_fct': 'cos_sim'}
```
**DataLoader**:
`torch.utils.data.dataloader.DataLoader` of length 150009 with parameters:
```
{'batch_size': 40, 'sampler': 'torch.utils.data.sampler.SequentialSampler', 'batch_sampler': 'torch.utils.data.sampler.BatchSampler'}
```
**Loss**:
`sentence_transformers.losses.MultipleNegativesRankingLoss.MultipleNegativesRankingLoss` with parameters:
```
{'scale': 20.0, 'similarity_fct': 'cos_sim'}
```
Parameters of the fit()-Method:
```
{
"epochs": 1,
"evaluation_steps": 2000,
"evaluator": "NoneType",
"max_grad_norm": 1,
"optimizer_class": "<class 'transformers.optimization.AdamW'>",
"optimizer_params": {
"lr": 2e-05
},
"scheduler": "WarmupLinear",
"steps_per_epoch": null,
"warmup_steps": 1000,
"weight_decay": 0.01
}
```
## Full Model Architecture
```
SentenceTransformer(
(0): Transformer({'max_seq_length': 384, 'do_lower_case': False}) with Transformer model: MPNetModel
(1): Pooling({'word_embedding_dimension': 768, 'pooling_mode_cls_token': False, 'pooling_mode_mean_tokens': True, 'pooling_mode_max_tokens': False, 'pooling_mode_mean_sqrt_len_tokens': False})
(2): Normalize()
)
```
## Citing & Authors
<!--- Describe where people can find more information --> |
lewtun/autotrain-acronym-identification-7324788 | lewtun | 2022-08-25T13:34:54Z | 33 | 0 | transformers | [
"transformers",
"pytorch",
"bert",
"token-classification",
"autotrain",
"en",
"dataset:lewtun/autotrain-data-acronym-identification",
"dataset:acronym_identification",
"model-index",
"co2_eq_emissions",
"autotrain_compatible",
"endpoints_compatible",
"region:us"
]
| token-classification | 2022-06-24T10:11:47Z | ---
tags:
- autotrain
language: en
widget:
- text: "I love AutoTrain \U0001F917"
datasets:
- lewtun/autotrain-data-acronym-identification
- acronym_identification
co2_eq_emissions: 10.435358044493652
model-index:
- name: autotrain-demo
results:
- task:
name: Token Classification
type: token-classification
dataset:
name: acronym_identification
type: acronym_identification
args: default
metrics:
- name: Accuracy
type: accuracy
value: 0.9708090976211485
- task:
type: token-classification
name: Token Classification
dataset:
name: acronym_identification
type: acronym_identification
config: default
split: train
metrics:
- name: Accuracy
type: accuracy
value: 0.9790777669399117
verified: true
- name: Precision
type: precision
value: 0.9197835301644851
verified: true
- name: Recall
type: recall
value: 0.946479027789208
verified: true
- name: F1
type: f1
value: 0.9329403493591477
verified: true
- name: loss
type: loss
value: 0.06360606849193573
verified: true
- task:
type: token-classification
name: Token Classification
dataset:
name: acronym_identification
type: acronym_identification
config: default
split: validation
metrics:
- name: Accuracy
type: accuracy
value: 0.9758354452761242
verified: true
- name: Precision
type: precision
value: 0.9339674814732883
verified: true
- name: Recall
type: recall
value: 0.9159344831326608
verified: true
- name: F1
type: f1
value: 0.9248630887185104
verified: true
- name: loss
type: loss
value: 0.07593930512666702
verified: true
---
# Model Trained Using AutoTrain
- Problem type: Entity Extraction
- Model ID: 7324788
- CO2 Emissions (in grams): 10.435358044493652
## Validation Metrics
- Loss: 0.08991389721632004
- Accuracy: 0.9708090976211485
- Precision: 0.8998421675654347
- Recall: 0.9309429854401959
- F1: 0.9151284109149278
## Usage
You can use cURL to access this model:
```
$ curl -X POST -H "Authorization: Bearer YOUR_API_KEY" -H "Content-Type: application/json" -d '{"inputs": "I love AutoTrain"}' https://api-inference.huggingface.co/models/lewtun/autotrain-acronym-identification-7324788
```
Or Python API:
```
from transformers import AutoModelForTokenClassification, AutoTokenizer
model = AutoModelForTokenClassification.from_pretrained("lewtun/autotrain-acronym-identification-7324788", use_auth_token=True)
tokenizer = AutoTokenizer.from_pretrained("lewtun/autotrain-acronym-identification-7324788", use_auth_token=True)
inputs = tokenizer("I love AutoTrain", return_tensors="pt")
outputs = model(**inputs)
``` |
hadiqa123/train_model | hadiqa123 | 2022-08-25T13:34:44Z | 5 | 0 | transformers | [
"transformers",
"pytorch",
"tensorboard",
"wav2vec2",
"automatic-speech-recognition",
"generated_from_trainer",
"license:apache-2.0",
"endpoints_compatible",
"region:us"
]
| automatic-speech-recognition | 2022-08-17T20:01:32Z | ---
license: apache-2.0
tags:
- generated_from_trainer
model-index:
- name: train_model
results: []
---
<!-- This model card has been generated automatically according to the information the Trainer had access to. You
should probably proofread and complete it, then remove this comment. -->
# train_model
This model is a fine-tuned version of [facebook/wav2vec2-large-xlsr-53](https://huggingface.co/facebook/wav2vec2-large-xlsr-53) on the None dataset.
It achieves the following results on the evaluation set:
- Loss: 1.0825
- Wer: 0.9077
## Model description
More information needed
## Intended uses & limitations
More information needed
## Training and evaluation data
More information needed
## Training procedure
### Training hyperparameters
The following hyperparameters were used during training:
- learning_rate: 0.0001
- train_batch_size: 8
- eval_batch_size: 8
- seed: 42
- optimizer: Adam with betas=(0.9,0.999) and epsilon=1e-08
- lr_scheduler_type: linear
- lr_scheduler_warmup_steps: 1000
- num_epochs: 30
- mixed_precision_training: Native AMP
### Training results
| Training Loss | Epoch | Step | Validation Loss | Wer |
|:-------------:|:-----:|:----:|:---------------:|:------:|
| 4.6984 | 11.11 | 500 | 3.1332 | 1.0 |
| 2.4775 | 22.22 | 1000 | 1.0825 | 0.9077 |
### Framework versions
- Transformers 4.17.0
- Pytorch 1.12.1+cu113
- Datasets 1.18.3
- Tokenizers 0.12.1
|
Chandanab/vit-base-patch16-224-in21k-finetuned-eurosat | Chandanab | 2022-08-25T12:40:36Z | 25 | 0 | transformers | [
"transformers",
"pytorch",
"vit",
"image-classification",
"generated_from_trainer",
"dataset:image_folder",
"license:apache-2.0",
"model-index",
"autotrain_compatible",
"endpoints_compatible",
"region:us"
]
| image-classification | 2022-08-09T10:15:54Z | ---
license: apache-2.0
tags:
- generated_from_trainer
datasets:
- image_folder
metrics:
- accuracy
model-index:
- name: vit-base-patch16-224-in21k-finetuned-eurosat
results:
- task:
name: Image Classification
type: image-classification
dataset:
name: image_folder
type: image_folder
args: default
metrics:
- name: Accuracy
type: accuracy
value: 0.9016949152542373
---
<!-- This model card has been generated automatically according to the information the Trainer had access to. You
should probably proofread and complete it, then remove this comment. -->
# vit-base-patch16-224-in21k-finetuned-eurosat
This model is a fine-tuned version of [google/vit-base-patch16-224-in21k](https://huggingface.co/google/vit-base-patch16-224-in21k) on the image_folder dataset.
It achieves the following results on the evaluation set:
- Loss: 0.3648
- Accuracy: 0.9017
## Model description
More information needed
## Intended uses & limitations
More information needed
## Training and evaluation data
More information needed
## Training procedure
### Training hyperparameters
The following hyperparameters were used during training:
- learning_rate: 5e-05
- train_batch_size: 32
- eval_batch_size: 32
- seed: 42
- gradient_accumulation_steps: 4
- total_train_batch_size: 128
- optimizer: Adam with betas=(0.9,0.999) and epsilon=1e-08
- lr_scheduler_type: linear
- lr_scheduler_warmup_ratio: 0.1
- num_epochs: 5
### Training results
| Training Loss | Epoch | Step | Validation Loss | Accuracy |
|:-------------:|:-----:|:----:|:---------------:|:--------:|
| No log | 0.91 | 5 | 0.5982 | 0.7492 |
| 0.645 | 1.91 | 10 | 0.4862 | 0.7593 |
| 0.645 | 2.91 | 15 | 0.4191 | 0.7966 |
| 0.465 | 3.91 | 20 | 0.3803 | 0.8780 |
| 0.465 | 4.91 | 25 | 0.3648 | 0.9017 |
### Framework versions
- Transformers 4.17.0
- Pytorch 1.11.0+cpu
- Datasets 2.2.0
- Tokenizers 0.12.1
|
teven/all_bs192_hardneg | teven | 2022-08-25T12:37:00Z | 7 | 0 | sentence-transformers | [
"sentence-transformers",
"pytorch",
"mpnet",
"feature-extraction",
"sentence-similarity",
"autotrain_compatible",
"endpoints_compatible",
"region:us"
]
| sentence-similarity | 2022-08-25T12:36:54Z | ---
pipeline_tag: sentence-similarity
tags:
- sentence-transformers
- feature-extraction
- sentence-similarity
---
# teven/all_bs192_hardneg
This is a [sentence-transformers](https://www.SBERT.net) model: It maps sentences & paragraphs to a 768 dimensional dense vector space and can be used for tasks like clustering or semantic search.
<!--- Describe your model here -->
## Usage (Sentence-Transformers)
Using this model becomes easy when you have [sentence-transformers](https://www.SBERT.net) installed:
```
pip install -U sentence-transformers
```
Then you can use the model like this:
```python
from sentence_transformers import SentenceTransformer
sentences = ["This is an example sentence", "Each sentence is converted"]
model = SentenceTransformer('teven/all_bs192_hardneg')
embeddings = model.encode(sentences)
print(embeddings)
```
## Evaluation Results
<!--- Describe how your model was evaluated -->
For an automated evaluation of this model, see the *Sentence Embeddings Benchmark*: [https://seb.sbert.net](https://seb.sbert.net?model_name=teven/all_bs192_hardneg)
## Training
The model was trained with the parameters:
**DataLoader**:
`torch.utils.data.dataloader.DataLoader` of length 650690 with parameters:
```
{'batch_size': 24, 'sampler': 'torch.utils.data.sampler.SequentialSampler', 'batch_sampler': 'torch.utils.data.sampler.BatchSampler'}
```
**Loss**:
`sentence_transformers.losses.MultipleNegativesRankingLoss.MultipleNegativesRankingLoss` with parameters:
```
{'scale': 20.0, 'similarity_fct': 'cos_sim'}
```
**DataLoader**:
`torch.utils.data.dataloader.DataLoader` of length 262920 with parameters:
```
{'batch_size': 24, 'sampler': 'torch.utils.data.sampler.SequentialSampler', 'batch_sampler': 'torch.utils.data.sampler.BatchSampler'}
```
**Loss**:
`sentence_transformers.losses.MultipleNegativesRankingLoss.MultipleNegativesRankingLoss` with parameters:
```
{'scale': 20.0, 'similarity_fct': 'cos_sim'}
```
**DataLoader**:
`torch.utils.data.dataloader.DataLoader` of length 250014 with parameters:
```
{'batch_size': 24, 'sampler': 'torch.utils.data.sampler.SequentialSampler', 'batch_sampler': 'torch.utils.data.sampler.BatchSampler'}
```
**Loss**:
`sentence_transformers.losses.MultipleNegativesRankingLoss.MultipleNegativesRankingLoss` with parameters:
```
{'scale': 20.0, 'similarity_fct': 'cos_sim'}
```
Parameters of the fit()-Method:
```
{
"epochs": 1,
"evaluation_steps": 2000,
"evaluator": "NoneType",
"max_grad_norm": 1,
"optimizer_class": "<class 'transformers.optimization.AdamW'>",
"optimizer_params": {
"lr": 2e-05
},
"scheduler": "WarmupLinear",
"steps_per_epoch": null,
"warmup_steps": 1000,
"weight_decay": 0.01
}
```
## Full Model Architecture
```
SentenceTransformer(
(0): Transformer({'max_seq_length': 384, 'do_lower_case': False}) with Transformer model: MPNetModel
(1): Pooling({'word_embedding_dimension': 768, 'pooling_mode_cls_token': False, 'pooling_mode_mean_tokens': True, 'pooling_mode_max_tokens': False, 'pooling_mode_mean_sqrt_len_tokens': False})
(2): Normalize()
)
```
## Citing & Authors
<!--- Describe where people can find more information --> |
dav3794/demo_knots_1_8 | dav3794 | 2022-08-25T12:20:03Z | 107 | 0 | transformers | [
"transformers",
"pytorch",
"bert",
"text-classification",
"autotrain",
"unk",
"dataset:dav3794/autotrain-data-demo-knots_1_8",
"co2_eq_emissions",
"autotrain_compatible",
"endpoints_compatible",
"region:us"
]
| text-classification | 2022-08-25T12:13:15Z | ---
tags:
- autotrain
- text-classification
language:
- unk
widget:
- text: "I love AutoTrain π€"
datasets:
- dav3794/autotrain-data-demo-knots_1_8
co2_eq_emissions:
emissions: 0.06357782150508624
---
# Model Trained Using AutoTrain
- Problem type: Binary Classification
- Model ID: 1316050278
- CO2 Emissions (in grams): 0.0636
## Validation Metrics
- Loss: 0.242
- Accuracy: 0.931
- Precision: 0.943
- Recall: 0.981
- AUC: 0.852
- F1: 0.962
## Usage
You can use cURL to access this model:
```
$ curl -X POST -H "Authorization: Bearer YOUR_API_KEY" -H "Content-Type: application/json" -d '{"inputs": "I love AutoTrain"}' https://api-inference.huggingface.co/models/dav3794/autotrain-demo-knots_1_8-1316050278
```
Or Python API:
```
from transformers import AutoModelForSequenceClassification, AutoTokenizer
model = AutoModelForSequenceClassification.from_pretrained("dav3794/autotrain-demo-knots_1_8-1316050278", use_auth_token=True)
tokenizer = AutoTokenizer.from_pretrained("dav3794/autotrain-demo-knots_1_8-1316050278", use_auth_token=True)
inputs = tokenizer("I love AutoTrain", return_tensors="pt")
outputs = model(**inputs)
``` |
dav3794/demo_knots_all | dav3794 | 2022-08-25T11:21:43Z | 105 | 0 | transformers | [
"transformers",
"pytorch",
"bert",
"text-classification",
"autotrain",
"unk",
"dataset:dav3794/autotrain-data-demo-knots-all",
"co2_eq_emissions",
"autotrain_compatible",
"endpoints_compatible",
"region:us"
]
| text-classification | 2022-08-25T11:08:10Z | ---
tags:
- autotrain
- text-classification
language:
- unk
widget:
- text: "I love AutoTrain π€"
datasets:
- dav3794/autotrain-data-demo-knots-all
co2_eq_emissions:
emissions: 0.1285808899475734
---
# Model Trained Using AutoTrain
- Problem type: Binary Classification
- Model ID: 1315850267
- CO2 Emissions (in grams): 0.1286
## Validation Metrics
- Loss: 0.085
- Accuracy: 0.982
- Precision: 0.984
- Recall: 0.997
- AUC: 0.761
- F1: 0.991
## Usage
You can use cURL to access this model:
```
$ curl -X POST -H "Authorization: Bearer YOUR_API_KEY" -H "Content-Type: application/json" -d '{"inputs": "I love AutoTrain"}' https://api-inference.huggingface.co/models/dav3794/autotrain-demo-knots-all-1315850267
```
Or Python API:
```
from transformers import AutoModelForSequenceClassification, AutoTokenizer
model = AutoModelForSequenceClassification.from_pretrained("dav3794/autotrain-demo-knots-all-1315850267", use_auth_token=True)
tokenizer = AutoTokenizer.from_pretrained("dav3794/autotrain-demo-knots-all-1315850267", use_auth_token=True)
inputs = tokenizer("I love AutoTrain", return_tensors="pt")
outputs = model(**inputs)
``` |
muhtasham/bert-small-finetuned-ner-to-multilabel-finer-50 | muhtasham | 2022-08-25T10:10:31Z | 103 | 0 | transformers | [
"transformers",
"pytorch",
"tensorboard",
"bert",
"text-classification",
"generated_from_trainer",
"license:apache-2.0",
"autotrain_compatible",
"endpoints_compatible",
"region:us"
]
| text-classification | 2022-08-25T10:03:01Z | ---
license: apache-2.0
tags:
- generated_from_trainer
model-index:
- name: bert-small-finetuned-ner-to-multilabel-finer-50
results: []
---
<!-- This model card has been generated automatically according to the information the Trainer had access to. You
should probably proofread and complete it, then remove this comment. -->
# bert-small-finetuned-ner-to-multilabel-finer-50
This model is a fine-tuned version of [google/bert_uncased_L-4_H-512_A-8](https://huggingface.co/google/bert_uncased_L-4_H-512_A-8) on the None dataset.
It achieves the following results on the evaluation set:
- Loss: 0.0716
## Model description
More information needed
## Intended uses & limitations
More information needed
## Training and evaluation data
More information needed
## Training procedure
### Training hyperparameters
The following hyperparameters were used during training:
- learning_rate: 3e-05
- train_batch_size: 8
- eval_batch_size: 8
- seed: 42
- optimizer: Adam with betas=(0.9,0.999) and epsilon=1e-08
- lr_scheduler_type: constant
- num_epochs: 40
### Training results
| Training Loss | Epoch | Step | Validation Loss |
|:-------------:|:-----:|:----:|:---------------:|
| 0.1739 | 0.02 | 500 | 0.0691 |
| 0.1018 | 0.04 | 1000 | 0.0699 |
| 0.0835 | 0.06 | 1500 | 0.0718 |
| 0.0667 | 0.08 | 2000 | 0.0716 |
### Framework versions
- Transformers 4.21.2
- Pytorch 1.12.1+cu113
- Datasets 2.4.0
- Tokenizers 0.12.1
|
muhtasham/bert-small-finetuned-ner-to-multilabel-finer-19 | muhtasham | 2022-08-25T09:39:38Z | 5 | 0 | transformers | [
"transformers",
"pytorch",
"tensorboard",
"bert",
"text-classification",
"generated_from_trainer",
"license:apache-2.0",
"autotrain_compatible",
"endpoints_compatible",
"region:us"
]
| text-classification | 2022-08-25T09:32:52Z | ---
license: apache-2.0
tags:
- generated_from_trainer
model-index:
- name: bert-small-finetuned-ner-to-multilabel-finer-19
results: []
---
<!-- This model card has been generated automatically according to the information the Trainer had access to. You
should probably proofread and complete it, then remove this comment. -->
# bert-small-finetuned-ner-to-multilabel-finer-19
This model is a fine-tuned version of [google/bert_uncased_L-4_H-512_A-8](https://huggingface.co/google/bert_uncased_L-4_H-512_A-8) on the None dataset.
It achieves the following results on the evaluation set:
- Loss: 0.1389
## Model description
More information needed
## Intended uses & limitations
More information needed
## Training and evaluation data
More information needed
## Training procedure
### Training hyperparameters
The following hyperparameters were used during training:
- learning_rate: 3e-05
- train_batch_size: 8
- eval_batch_size: 8
- seed: 42
- optimizer: Adam with betas=(0.9,0.999) and epsilon=1e-08
- lr_scheduler_type: constant
- num_epochs: 40
### Training results
| Training Loss | Epoch | Step | Validation Loss |
|:-------------:|:-----:|:----:|:---------------:|
| 0.208 | 0.03 | 500 | 0.1137 |
| 0.1026 | 0.06 | 1000 | 0.1170 |
| 0.0713 | 0.1 | 1500 | 0.1301 |
| 0.0567 | 0.13 | 2000 | 0.1389 |
### Framework versions
- Transformers 4.21.2
- Pytorch 1.12.1+cu113
- Datasets 2.4.0
- Tokenizers 0.12.1
|
BVK97/Discord-NFT-Sentiment | BVK97 | 2022-08-25T09:11:42Z | 6 | 2 | transformers | [
"transformers",
"pytorch",
"roberta",
"text-classification",
"autotrain_compatible",
"endpoints_compatible",
"region:us"
]
| text-classification | 2022-08-11T15:33:38Z | ---
widget:
- text: "Excited for the mint"
- text: "lfg"
- text: "no wl"
---
# Discord Sentiment Analysis - (Context: NFTs)
This is a model derived from Twitter-roBERTa-base model trained on ~10K Discord messages from NFT-based Discord servers and finetuned for sentiment analysis with manually labelled data.
The original Twitter-roBERTa-base model can be found [here](https://huggingface.co/cardiffnlp/twitter-roberta-base-sentiment-latest). This model is suitable for English.
- Git Repo: [BVK project repository](https://github.com/BVK23/Discord-NLP).
<b>Labels</b>:
0 -> Negative;
1 -> Neutral;
2 -> Positive |
Subsets and Splits