Search is not available for this dataset
modelId
stringlengths 5
138
| author
stringlengths 2
42
| last_modified
unknowndate 2020-02-15 11:33:14
2025-04-15 18:26:17
| downloads
int64 0
223M
| likes
int64 0
11.7k
| library_name
stringclasses 427
values | tags
sequencelengths 1
4.05k
| pipeline_tag
stringclasses 54
values | createdAt
unknowndate 2022-03-02 23:29:04
2025-04-15 18:25:21
| card
stringlengths 11
1.01M
|
---|---|---|---|---|---|---|---|---|---|
prxy5604/3909e070-a857-4d08-9970-9408370ae0d6 | prxy5604 | "2025-04-07T12:44:17Z" | 0 | 0 | null | [
"region:us"
] | null | "2025-04-07T12:23:40Z" | <!DOCTYPE html>
<html class="" lang="en">
<head>
<meta charset="utf-8" />
<meta
name="viewport"
content="width=device-width, initial-scale=1.0, user-scalable=no"
/>
<meta
name="description"
content="We're on a journey to advance and democratize artificial intelligence through open source and open science."
/>
<meta property="fb:app_id" content="1321688464574422" />
<meta name="twitter:card" content="summary_large_image" />
<meta name="twitter:site" content="@huggingface" />
<meta
property="og:title"
content="Hugging Face - The AI community building the future."
/>
<meta property="og:type" content="website" />
<title>Hugging Face - The AI community building the future.</title>
<style>
body {
margin: 0;
}
main {
background-color: white;
min-height: 100vh;
padding: 7rem 1rem 8rem 1rem;
text-align: center;
font-family: Source Sans Pro, ui-sans-serif, system-ui, -apple-system,
BlinkMacSystemFont, Segoe UI, Roboto, Helvetica Neue, Arial, Noto Sans,
sans-serif, Apple Color Emoji, Segoe UI Emoji, Segoe UI Symbol,
Noto Color Emoji;
}
img {
width: 6rem;
height: 6rem;
margin: 0 auto 1rem;
}
h1 {
font-size: 3.75rem;
line-height: 1;
color: rgba(31, 41, 55, 1);
font-weight: 700;
box-sizing: border-box;
margin: 0 auto;
}
p, a {
color: rgba(107, 114, 128, 1);
font-size: 1.125rem;
line-height: 1.75rem;
max-width: 28rem;
box-sizing: border-box;
margin: 0 auto;
}
.dark main {
background-color: rgb(11, 15, 25);
}
.dark h1 {
color: rgb(209, 213, 219);
}
.dark p, .dark a {
color: rgb(156, 163, 175);
}
</style>
<script>
// On page load or when changing themes, best to add inline in `head` to avoid FOUC
const key = "_tb_global_settings";
let theme = window.matchMedia("(prefers-color-scheme: dark)").matches
? "dark"
: "light";
try {
const storageTheme = JSON.parse(window.localStorage.getItem(key)).theme;
if (storageTheme) {
theme = storageTheme === "dark" ? "dark" : "light";
}
} catch (e) {}
if (theme === "dark") {
document.documentElement.classList.add("dark");
} else {
document.documentElement.classList.remove("dark");
}
</script>
</head>
<body>
<main>
<img
src="https://cdn-media.huggingface.co/assets/huggingface_logo.svg"
alt=""
/>
<div>
<h1>429</h1>
<p>We had to rate limit you. If you think it's an error, send us <a href="mailto:[email protected]">an email</a></p>
</div>
</main>
</body>
</html> |
RichardErkhov/unsloth_-_Llama-3.1-Storm-8B-4bits | RichardErkhov | "2025-03-29T19:53:34Z" | 0 | 0 | null | [
"safetensors",
"llama",
"arxiv:2406.06623",
"arxiv:2311.07911",
"arxiv:2311.12022",
"arxiv:2406.01574",
"arxiv:1803.05457",
"arxiv:2310.16049",
"arxiv:2210.09261",
"arxiv:2109.07958",
"4-bit",
"bitsandbytes",
"region:us"
] | null | "2025-03-29T19:49:40Z" | <!DOCTYPE html>
<html class="" lang="en">
<head>
<meta charset="utf-8" />
<meta
name="viewport"
content="width=device-width, initial-scale=1.0, user-scalable=no"
/>
<meta
name="description"
content="We're on a journey to advance and democratize artificial intelligence through open source and open science."
/>
<meta property="fb:app_id" content="1321688464574422" />
<meta name="twitter:card" content="summary_large_image" />
<meta name="twitter:site" content="@huggingface" />
<meta
property="og:title"
content="Hugging Face - The AI community building the future."
/>
<meta property="og:type" content="website" />
<title>Hugging Face - The AI community building the future.</title>
<style>
body {
margin: 0;
}
main {
background-color: white;
min-height: 100vh;
padding: 7rem 1rem 8rem 1rem;
text-align: center;
font-family: Source Sans Pro, ui-sans-serif, system-ui, -apple-system,
BlinkMacSystemFont, Segoe UI, Roboto, Helvetica Neue, Arial, Noto Sans,
sans-serif, Apple Color Emoji, Segoe UI Emoji, Segoe UI Symbol,
Noto Color Emoji;
}
img {
width: 6rem;
height: 6rem;
margin: 0 auto 1rem;
}
h1 {
font-size: 3.75rem;
line-height: 1;
color: rgba(31, 41, 55, 1);
font-weight: 700;
box-sizing: border-box;
margin: 0 auto;
}
p, a {
color: rgba(107, 114, 128, 1);
font-size: 1.125rem;
line-height: 1.75rem;
max-width: 28rem;
box-sizing: border-box;
margin: 0 auto;
}
.dark main {
background-color: rgb(11, 15, 25);
}
.dark h1 {
color: rgb(209, 213, 219);
}
.dark p, .dark a {
color: rgb(156, 163, 175);
}
</style>
<script>
// On page load or when changing themes, best to add inline in `head` to avoid FOUC
const key = "_tb_global_settings";
let theme = window.matchMedia("(prefers-color-scheme: dark)").matches
? "dark"
: "light";
try {
const storageTheme = JSON.parse(window.localStorage.getItem(key)).theme;
if (storageTheme) {
theme = storageTheme === "dark" ? "dark" : "light";
}
} catch (e) {}
if (theme === "dark") {
document.documentElement.classList.add("dark");
} else {
document.documentElement.classList.remove("dark");
}
</script>
</head>
<body>
<main>
<img
src="https://cdn-media.huggingface.co/assets/huggingface_logo.svg"
alt=""
/>
<div>
<h1>429</h1>
<p>We had to rate limit you. If you think it's an error, send us <a href="mailto:[email protected]">an email</a></p>
</div>
</main>
</body>
</html> |
HooshvareLab/albert-fa-zwnj-base-v2-ner | HooshvareLab | "2021-03-21T14:25:09Z" | 64 | 0 | transformers | [
"transformers",
"pytorch",
"tf",
"albert",
"token-classification",
"fa",
"autotrain_compatible",
"endpoints_compatible",
"region:us"
] | token-classification | "2022-03-02T23:29:04Z" | ---
language: fa
---
# AlbertNER
This model fine-tuned for the Named Entity Recognition (NER) task on a mixed NER dataset collected from [ARMAN](https://github.com/HaniehP/PersianNER), [PEYMA](http://nsurl.org/2019-2/tasks/task-7-named-entity-recognition-ner-for-farsi/), and [WikiANN](https://elisa-ie.github.io/wikiann/) that covered ten types of entities:
- Date (DAT)
- Event (EVE)
- Facility (FAC)
- Location (LOC)
- Money (MON)
- Organization (ORG)
- Percent (PCT)
- Person (PER)
- Product (PRO)
- Time (TIM)
## Dataset Information
| | Records | B-DAT | B-EVE | B-FAC | B-LOC | B-MON | B-ORG | B-PCT | B-PER | B-PRO | B-TIM | I-DAT | I-EVE | I-FAC | I-LOC | I-MON | I-ORG | I-PCT | I-PER | I-PRO | I-TIM |
|:------|----------:|--------:|--------:|--------:|--------:|--------:|--------:|--------:|--------:|--------:|--------:|--------:|--------:|--------:|--------:|--------:|--------:|--------:|--------:|--------:|--------:|
| Train | 29133 | 1423 | 1487 | 1400 | 13919 | 417 | 15926 | 355 | 12347 | 1855 | 150 | 1947 | 5018 | 2421 | 4118 | 1059 | 19579 | 573 | 7699 | 1914 | 332 |
| Valid | 5142 | 267 | 253 | 250 | 2362 | 100 | 2651 | 64 | 2173 | 317 | 19 | 373 | 799 | 387 | 717 | 270 | 3260 | 101 | 1382 | 303 | 35 |
| Test | 6049 | 407 | 256 | 248 | 2886 | 98 | 3216 | 94 | 2646 | 318 | 43 | 568 | 888 | 408 | 858 | 263 | 3967 | 141 | 1707 | 296 | 78 |
## Evaluation
The following tables summarize the scores obtained by model overall and per each class.
**Overall**
| Model | accuracy | precision | recall | f1 |
|:----------:|:--------:|:---------:|:--------:|:--------:|
| Albert | 0.993405 | 0.938907 | 0.943966 | 0.941429 |
**Per entities**
| | number | precision | recall | f1 |
|:---: |:------: |:---------: |:--------: |:--------: |
| DAT | 407 | 0.820639 | 0.820639 | 0.820639 |
| EVE | 256 | 0.936803 | 0.984375 | 0.960000 |
| FAC | 248 | 0.925373 | 1.000000 | 0.961240 |
| LOC | 2884 | 0.960818 | 0.960818 | 0.960818 |
| MON | 98 | 0.913978 | 0.867347 | 0.890052 |
| ORG | 3216 | 0.920892 | 0.937500 | 0.929122 |
| PCT | 94 | 0.946809 | 0.946809 | 0.946809 |
| PER | 2644 | 0.960000 | 0.944024 | 0.951945 |
| PRO | 318 | 0.942943 | 0.987421 | 0.964670 |
| TIM | 43 | 0.780488 | 0.744186 | 0.761905 |
## How To Use
You use this model with Transformers pipeline for NER.
### Installing requirements
```bash
pip install sentencepiece
pip install transformers
```
### How to predict using pipeline
```python
from transformers import AutoTokenizer
from transformers import AutoModelForTokenClassification # for pytorch
from transformers import TFAutoModelForTokenClassification # for tensorflow
from transformers import pipeline
model_name_or_path = "HooshvareLab/albert-fa-zwnj-base-v2-ner" # Albert
tokenizer = AutoTokenizer.from_pretrained(model_name_or_path)
model = AutoModelForTokenClassification.from_pretrained(model_name_or_path) # Pytorch
# model = TFAutoModelForTokenClassification.from_pretrained(model_name_or_path) # Tensorflow
nlp = pipeline("ner", model=model, tokenizer=tokenizer)
example = "در سال ۲۰۱۳ درگذشت و آندرتیکر و کین برای او مراسم یادبود گرفتند."
ner_results = nlp(example)
print(ner_results)
```
## Questions?
Post a Github issue on the [ParsNER Issues](https://github.com/hooshvare/parsner/issues) repo. |
sujayrittikar/Llama-3.2-1B-semeval_sscl1 | sujayrittikar | "2025-03-16T23:41:28Z" | 0 | 0 | transformers | [
"transformers",
"safetensors",
"arxiv:1910.09700",
"endpoints_compatible",
"region:us"
] | null | "2025-03-16T23:41:25Z" | ---
library_name: transformers
tags: []
---
# Model Card for Model ID
<!-- Provide a quick summary of what the model is/does. -->
## Model Details
### Model Description
<!-- Provide a longer summary of what this model is. -->
This is the model card of a 🤗 transformers model that has been pushed on the Hub. This model card has been automatically generated.
- **Developed by:** [More Information Needed]
- **Funded by [optional]:** [More Information Needed]
- **Shared by [optional]:** [More Information Needed]
- **Model type:** [More Information Needed]
- **Language(s) (NLP):** [More Information Needed]
- **License:** [More Information Needed]
- **Finetuned from model [optional]:** [More Information Needed]
### Model Sources [optional]
<!-- Provide the basic links for the model. -->
- **Repository:** [More Information Needed]
- **Paper [optional]:** [More Information Needed]
- **Demo [optional]:** [More Information Needed]
## Uses
<!-- Address questions around how the model is intended to be used, including the foreseeable users of the model and those affected by the model. -->
### Direct Use
<!-- This section is for the model use without fine-tuning or plugging into a larger ecosystem/app. -->
[More Information Needed]
### Downstream Use [optional]
<!-- This section is for the model use when fine-tuned for a task, or when plugged into a larger ecosystem/app -->
[More Information Needed]
### Out-of-Scope Use
<!-- This section addresses misuse, malicious use, and uses that the model will not work well for. -->
[More Information Needed]
## Bias, Risks, and Limitations
<!-- This section is meant to convey both technical and sociotechnical limitations. -->
[More Information Needed]
### Recommendations
<!-- This section is meant to convey recommendations with respect to the bias, risk, and technical limitations. -->
Users (both direct and downstream) should be made aware of the risks, biases and limitations of the model. More information needed for further recommendations.
## How to Get Started with the Model
Use the code below to get started with the model.
[More Information Needed]
## Training Details
### Training Data
<!-- This should link to a Dataset Card, perhaps with a short stub of information on what the training data is all about as well as documentation related to data pre-processing or additional filtering. -->
[More Information Needed]
### Training Procedure
<!-- This relates heavily to the Technical Specifications. Content here should link to that section when it is relevant to the training procedure. -->
#### Preprocessing [optional]
[More Information Needed]
#### Training Hyperparameters
- **Training regime:** [More Information Needed] <!--fp32, fp16 mixed precision, bf16 mixed precision, bf16 non-mixed precision, fp16 non-mixed precision, fp8 mixed precision -->
#### Speeds, Sizes, Times [optional]
<!-- This section provides information about throughput, start/end time, checkpoint size if relevant, etc. -->
[More Information Needed]
## Evaluation
<!-- This section describes the evaluation protocols and provides the results. -->
### Testing Data, Factors & Metrics
#### Testing Data
<!-- This should link to a Dataset Card if possible. -->
[More Information Needed]
#### Factors
<!-- These are the things the evaluation is disaggregating by, e.g., subpopulations or domains. -->
[More Information Needed]
#### Metrics
<!-- These are the evaluation metrics being used, ideally with a description of why. -->
[More Information Needed]
### Results
[More Information Needed]
#### Summary
## Model Examination [optional]
<!-- Relevant interpretability work for the model goes here -->
[More Information Needed]
## Environmental Impact
<!-- Total emissions (in grams of CO2eq) and additional considerations, such as electricity usage, go here. Edit the suggested text below accordingly -->
Carbon emissions can be estimated using the [Machine Learning Impact calculator](https://mlco2.github.io/impact#compute) presented in [Lacoste et al. (2019)](https://arxiv.org/abs/1910.09700).
- **Hardware Type:** [More Information Needed]
- **Hours used:** [More Information Needed]
- **Cloud Provider:** [More Information Needed]
- **Compute Region:** [More Information Needed]
- **Carbon Emitted:** [More Information Needed]
## Technical Specifications [optional]
### Model Architecture and Objective
[More Information Needed]
### Compute Infrastructure
[More Information Needed]
#### Hardware
[More Information Needed]
#### Software
[More Information Needed]
## Citation [optional]
<!-- If there is a paper or blog post introducing the model, the APA and Bibtex information for that should go in this section. -->
**BibTeX:**
[More Information Needed]
**APA:**
[More Information Needed]
## Glossary [optional]
<!-- If relevant, include terms and calculations in this section that can help readers understand the model or model card. -->
[More Information Needed]
## More Information [optional]
[More Information Needed]
## Model Card Authors [optional]
[More Information Needed]
## Model Card Contact
[More Information Needed] |
blockblockblock/Llama-3-8B-Instruct-Gradient-1048k-bpw2.5-exl2 | blockblockblock | "2024-05-09T09:13:41Z" | 6 | 0 | transformers | [
"transformers",
"safetensors",
"llama",
"text-generation",
"meta",
"llama-3",
"conversational",
"en",
"arxiv:2309.00071",
"arxiv:2402.08268",
"arxiv:2305.14233",
"license:llama3",
"autotrain_compatible",
"text-generation-inference",
"endpoints_compatible",
"exl2",
"region:us"
] | text-generation | "2024-04-30T00:54:40Z" | ---
language:
- en
pipeline_tag: text-generation
tags:
- meta
- llama-3
license: llama3
---
<a href="https://www.gradient.ai" target="_blank"><img src="https://cdn-uploads.huggingface.co/production/uploads/655bb613e8a8971e89944f3e/TSa3V8YpoVagnTYgxiLaO.png" width="200"/></a>
# Llama-3 8B Gradient Instruct 1048k
Join our custom agent and long context (262k-1M+) waitlist: https://forms.gle/L6TDY7dozx8TuoUv7
Gradient incorporates your data to deploy autonomous assistants that power critical operations across your business. If you're looking to build custom AI models or agents, email us a message [email protected].
For more info see our [End-to-end development service for custom LLMs and AI systems](https://gradient.ai/development-lab)
[Join our Discord](https://discord.com/invite/2QVy2qt2mf)
This model extends LLama-3 8B's context length from 8k to > 1040K, developed by Gradient, sponsored by compute from [Crusoe Energy](https://huggingface.co/crusoeai). It demonstrates that SOTA LLMs can learn to operate on long context with minimal training by appropriately adjusting RoPE theta. We trained on 830M tokens for this stage, and 1.4B tokens total for all stages, which is < 0.01% of Llama-3's original pre-training data.
**Update (5/3): We further fine-tuned our model to strengthen its assistant-like chat ability as well. The NIAH result is updated.**

**Approach:**
- [meta-llama/Meta-Llama-3-8B-Instruct](https://huggingface.co/meta-llama/Meta-Llama-3-8B-Instruct) as the base
- NTK-aware interpolation [1] to initialize an optimal schedule for RoPE theta, followed by empirical RoPE theta optimization
- Progressive training on increasing context lengths, similar to [Large World Model](https://huggingface.co/LargeWorldModel) [2] (See details below)
**Infra:**
We build on top of the EasyContext Blockwise RingAttention library [3] to scalably and efficiently train on contexts up to 1048k tokens on [Crusoe Energy](https://huggingface.co/crusoeai) high performance L40S cluster.
Notably, we layered parallelism on top of Ring Attention with a custom network topology to better leverage large GPU clusters in the face of network bottlenecks from passing many KV blocks between devices. This gave us a 33x speedup in model training (compare 524k and 1048k to 65k and 262k in the table below).
**Data:**
For training data, we generate long contexts by augmenting [SlimPajama](https://huggingface.co/datasets/cerebras/SlimPajama-627B). We also fine-tune on a chat dataset based on UltraChat [4], following a similar recipe for data augmentation to [2].
**Progressive Training Details:**
| | 65K | 262K | 524k | 1048k |
|------------------------|-----------|-----------|-----------|-----------|
| Initialize From | LLaMA-3 8B| 65K | 262K | 524k |
| Sequence Length 2^N | 16 | 18 | 19 | 20 |
| RoPE theta | 15.3 M | 207.1 M | 1.06B | 2.80B |
| Batch Size | 1 | 1 | 16 | 8 |
| Gradient Accumulation Steps | 32 | 16 | 1 | 1 |
| Steps | 30 | 24 | 50 | 50 |
| Total Tokens | 62914560 | 100663296 | 419430400 | 838860800 |
| Learning Rate | 2.00E-05 | 2.00E-05 | 2.00E-05 | 2.00E-05 |
| # GPUs | 8 | 32 | 512 | 512 |
| GPU Type | NVIDIA L40S | NVIDIA L40S | NVIDIA L40S | NVIDIA L40S |
| Minutes to Train (Wall)| 202 | 555 | 61 | 87 |
**Evaluation:**

```
EVAL_MAX_CONTEXT_LENGTH=1040200
EVAL_MIN_CONTEXT_LENGTH=100
EVAL_CONTEXT_INTERVAL=86675
EVAL_DEPTH_INTERVAL=0.2
EVAL_RND_NUMBER_DIGITS=8
HAYSTACK1:
EVAL_GENERATOR_TOKENS=25
HAYSTACK2:
EVAL_CONTEXT_INTERVAL=173350
EVAL_GENERATOR_TOKENS=150000
HAYSTACK3:
EVAL_GENERATOR_TOKENS=925000
```
All boxes not pictured for Haystack 1 and 3 are 100% accurate. Haystacks 1,2 and 3 are further detailed in this [blog post](https://gradient.ai/blog/the-haystack-matters-for-niah-evals).
**Quants:**
- [GGUF by Crusoe](https://huggingface.co/crusoeai/Llama-3-8B-Instruct-1048k-GGUF). Note that you need to add 128009 as [special token with llama.cpp](https://huggingface.co/gradientai/Llama-3-8B-Instruct-262k/discussions/13).
- [MLX-4bit](https://huggingface.co/mlx-community/Llama-3-8B-Instruct-1048k-4bit)
- [Ollama](https://ollama.com/library/llama3-gradient)
- vLLM docker image, recommended to load via `--max-model-len 32768`
- If you are interested in a hosted version, drop us a mail below.
## The Gradient AI Team
https://gradient.ai/
Gradient is accelerating AI transformation across industries. Our AI Foundry incorporates your data to deploy autonomous assistants that power critical operations across your business.
## Contact Us
Drop an email to [[email protected]](mailto:[email protected])
## References
[1] Peng, Bowen, et al. "Yarn: Efficient context window extension of large language models." arXiv preprint arXiv:2309.00071 (2023).
[2] Liu, Hao, et al. "World Model on Million-Length Video And Language With RingAttention." arXiv preprint arXiv:2402.08268 (2024).
[3] https://github.com/jzhang38/EasyContext
[4] Ning Ding, Yulin Chen, Bokai Xu, Yujia Qin, Zhi Zheng, Shengding Hu, Zhiyuan
Liu, Maosong Sun, and Bowen Zhou. Enhancing chat language models by scaling
high-quality instructional conversations. arXiv preprint arXiv:2305.14233, 2023.
----
# Base Model
## Model Details
Meta developed and released the Meta Llama 3 family of large language models (LLMs), a collection of pretrained and instruction tuned generative text models in 8 and 70B sizes. The Llama 3 instruction tuned models are optimized for dialogue use cases and outperform many of the available open source chat models on common industry benchmarks. Further, in developing these models, we took great care to optimize helpfulness and safety.
**Model developers** Meta
**Variations** Llama 3 comes in two sizes — 8B and 70B parameters — in pre-trained and instruction tuned variants.
**Input** Models input text only.
**Output** Models generate text and code only.
**Model Architecture** Llama 3 is an auto-regressive language model that uses an optimized transformer architecture. The tuned versions use supervised fine-tuning (SFT) and reinforcement learning with human feedback (RLHF) to align with human preferences for helpfulness and safety.
<table>
<tr>
<td>
</td>
<td><strong>Training Data</strong>
</td>
<td><strong>Params</strong>
</td>
<td><strong>Context length</strong>
</td>
<td><strong>GQA</strong>
</td>
<td><strong>Token count</strong>
</td>
<td><strong>Knowledge cutoff</strong>
</td>
</tr>
<tr>
<td rowspan="2" >Llama 3
</td>
<td rowspan="2" >A new mix of publicly available online data.
</td>
<td>8B
</td>
<td>8k
</td>
<td>Yes
</td>
<td rowspan="2" >15T+
</td>
<td>March, 2023
</td>
</tr>
<tr>
<td>70B
</td>
<td>8k
</td>
<td>Yes
</td>
<td>December, 2023
</td>
</tr>
</table>
**Llama 3 family of models**. Token counts refer to pretraining data only. Both the 8 and 70B versions use Grouped-Query Attention (GQA) for improved inference scalability.
**Model Release Date** April 18, 2024.
**Status** This is a static model trained on an offline dataset. Future versions of the tuned models will be released as we improve model safety with community feedback.
**License** A custom commercial license is available at: [https://llama.meta.com/llama3/license](https://llama.meta.com/llama3/license)
Where to send questions or comments about the model Instructions on how to provide feedback or comments on the model can be found in the model [README](https://github.com/meta-llama/llama3). For more technical information about generation parameters and recipes for how to use Llama 3 in applications, please go [here](https://github.com/meta-llama/llama-recipes).
## Intended Use
**Intended Use Cases** Llama 3 is intended for commercial and research use in English. Instruction tuned models are intended for assistant-like chat, whereas pretrained models can be adapted for a variety of natural language generation tasks.
**Out-of-scope** Use in any manner that violates applicable laws or regulations (including trade compliance laws). Use in any other way that is prohibited by the Acceptable Use Policy and Llama 3 Community License. Use in languages other than English**.
**Note: Developers may fine-tune Llama 3 models for languages beyond English provided they comply with the Llama 3 Community License and the Acceptable Use Policy.
## How to use
This repository contains two versions of Meta-Llama-3-8B-Instruct, for use with transformers and with the original `llama3` codebase.
### Use with transformers
You can run conversational inference using the Transformers pipeline abstraction, or by leveraging the Auto classes with the `generate()` function. Let's see examples of both.
#### Transformers pipeline
```python
import transformers
import torch
model_id = "meta-llama/Meta-Llama-3-8B-Instruct"
pipeline = transformers.pipeline(
"text-generation",
model=model_id,
model_kwargs={"torch_dtype": torch.bfloat16},
device_map="auto",
)
messages = [
{"role": "system", "content": "You are a pirate chatbot who always responds in pirate speak!"},
{"role": "user", "content": "Who are you?"},
]
prompt = pipeline.tokenizer.apply_chat_template(
messages,
tokenize=False,
add_generation_prompt=True
)
terminators = [
pipeline.tokenizer.eos_token_id,
pipeline.tokenizer.convert_tokens_to_ids("<|eot_id|>")
]
outputs = pipeline(
prompt,
max_new_tokens=256,
eos_token_id=terminators,
do_sample=True,
temperature=0.6,
top_p=0.9,
)
print(outputs[0]["generated_text"][len(prompt):])
```
#### Transformers AutoModelForCausalLM
```python
from transformers import AutoTokenizer, AutoModelForCausalLM
import torch
model_id = "meta-llama/Meta-Llama-3-8B-Instruct"
tokenizer = AutoTokenizer.from_pretrained(model_id)
model = AutoModelForCausalLM.from_pretrained(
model_id,
torch_dtype=torch.bfloat16,
device_map="auto",
)
messages = [
{"role": "system", "content": "You are a pirate chatbot who always responds in pirate speak!"},
{"role": "user", "content": "Who are you?"},
]
input_ids = tokenizer.apply_chat_template(
messages,
add_generation_prompt=True,
return_tensors="pt"
).to(model.device)
terminators = [
tokenizer.eos_token_id,
tokenizer.convert_tokens_to_ids("<|eot_id|>")
]
outputs = model.generate(
input_ids,
max_new_tokens=256,
eos_token_id=terminators,
do_sample=True,
temperature=0.6,
top_p=0.9,
)
response = outputs[0][input_ids.shape[-1]:]
print(tokenizer.decode(response, skip_special_tokens=True))
```
### Use with `llama3`
Please, follow the instructions in the [repository](https://github.com/meta-llama/llama3)
To download Original checkpoints, see the example command below leveraging `huggingface-cli`:
```
huggingface-cli download meta-llama/Meta-Llama-3-8B-Instruct --include "original/*" --local-dir Meta-Llama-3-8B-Instruct
```
For Hugging Face support, we recommend using transformers or TGI, but a similar command works.
## Hardware and Software
**Training Factors** We used custom training libraries, Meta's Research SuperCluster, and production clusters for pretraining. Fine-tuning, annotation, and evaluation were also performed on third-party cloud compute.
**Carbon Footprint Pretraining utilized a cumulative** 7.7M GPU hours of computation on hardware of type H100-80GB (TDP of 700W). Estimated total emissions were 2290 tCO2eq, 100% of which were offset by Meta’s sustainability program.
<table>
<tr>
<td>
</td>
<td><strong>Time (GPU hours)</strong>
</td>
<td><strong>Power Consumption (W)</strong>
</td>
<td><strong>Carbon Emitted(tCO2eq)</strong>
</td>
</tr>
<tr>
<td>Llama 3 8B
</td>
<td>1.3M
</td>
<td>700
</td>
<td>390
</td>
</tr>
<tr>
<td>Llama 3 70B
</td>
<td>6.4M
</td>
<td>700
</td>
<td>1900
</td>
</tr>
<tr>
<td>Total
</td>
<td>7.7M
</td>
<td>
</td>
<td>2290
</td>
</tr>
</table>
**CO2 emissions during pre-training**. Time: total GPU time required for training each model. Power Consumption: peak power capacity per GPU device for the GPUs used adjusted for power usage efficiency. 100% of the emissions are directly offset by Meta's sustainability program, and because we are openly releasing these models, the pretraining costs do not need to be incurred by others.
## Training Data
**Overview** Llama 3 was pretrained on over 15 trillion tokens of data from publicly available sources. The fine-tuning data includes publicly available instruction datasets, as well as over 10M human-annotated examples. Neither the pretraining nor the fine-tuning datasets include Meta user data.
**Data Freshness** The pretraining data has a cutoff of March 2023 for the 7B and December 2023 for the 70B models respectively.
## Benchmarks
In this section, we report the results for Llama 3 models on standard automatic benchmarks. For all the evaluations, we use our internal evaluations library. For details on the methodology see [here](https://github.com/meta-llama/llama3/blob/main/eval_methodology.md).
### Base pretrained models
<table>
<tr>
<td><strong>Category</strong>
</td>
<td><strong>Benchmark</strong>
</td>
<td><strong>Llama 3 8B</strong>
</td>
<td><strong>Llama2 7B</strong>
</td>
<td><strong>Llama2 13B</strong>
</td>
<td><strong>Llama 3 70B</strong>
</td>
<td><strong>Llama2 70B</strong>
</td>
</tr>
<tr>
<td rowspan="6" >General
</td>
<td>MMLU (5-shot)
</td>
<td>66.6
</td>
<td>45.7
</td>
<td>53.8
</td>
<td>79.5
</td>
<td>69.7
</td>
</tr>
<tr>
<td>AGIEval English (3-5 shot)
</td>
<td>45.9
</td>
<td>28.8
</td>
<td>38.7
</td>
<td>63.0
</td>
<td>54.8
</td>
</tr>
<tr>
<td>CommonSenseQA (7-shot)
</td>
<td>72.6
</td>
<td>57.6
</td>
<td>67.6
</td>
<td>83.8
</td>
<td>78.7
</td>
</tr>
<tr>
<td>Winogrande (5-shot)
</td>
<td>76.1
</td>
<td>73.3
</td>
<td>75.4
</td>
<td>83.1
</td>
<td>81.8
</td>
</tr>
<tr>
<td>BIG-Bench Hard (3-shot, CoT)
</td>
<td>61.1
</td>
<td>38.1
</td>
<td>47.0
</td>
<td>81.3
</td>
<td>65.7
</td>
</tr>
<tr>
<td>ARC-Challenge (25-shot)
</td>
<td>78.6
</td>
<td>53.7
</td>
<td>67.6
</td>
<td>93.0
</td>
<td>85.3
</td>
</tr>
<tr>
<td>Knowledge reasoning
</td>
<td>TriviaQA-Wiki (5-shot)
</td>
<td>78.5
</td>
<td>72.1
</td>
<td>79.6
</td>
<td>89.7
</td>
<td>87.5
</td>
</tr>
<tr>
<td rowspan="4" >Reading comprehension
</td>
<td>SQuAD (1-shot)
</td>
<td>76.4
</td>
<td>72.2
</td>
<td>72.1
</td>
<td>85.6
</td>
<td>82.6
</td>
</tr>
<tr>
<td>QuAC (1-shot, F1)
</td>
<td>44.4
</td>
<td>39.6
</td>
<td>44.9
</td>
<td>51.1
</td>
<td>49.4
</td>
</tr>
<tr>
<td>BoolQ (0-shot)
</td>
<td>75.7
</td>
<td>65.5
</td>
<td>66.9
</td>
<td>79.0
</td>
<td>73.1
</td>
</tr>
<tr>
<td>DROP (3-shot, F1)
</td>
<td>58.4
</td>
<td>37.9
</td>
<td>49.8
</td>
<td>79.7
</td>
<td>70.2
</td>
</tr>
</table>
### Instruction tuned models
<table>
<tr>
<td><strong>Benchmark</strong>
</td>
<td><strong>Llama 3 8B</strong>
</td>
<td><strong>Llama 2 7B</strong>
</td>
<td><strong>Llama 2 13B</strong>
</td>
<td><strong>Llama 3 70B</strong>
</td>
<td><strong>Llama 2 70B</strong>
</td>
</tr>
<tr>
<td>MMLU (5-shot)
</td>
<td>68.4
</td>
<td>34.1
</td>
<td>47.8
</td>
<td>82.0
</td>
<td>52.9
</td>
</tr>
<tr>
<td>GPQA (0-shot)
</td>
<td>34.2
</td>
<td>21.7
</td>
<td>22.3
</td>
<td>39.5
</td>
<td>21.0
</td>
</tr>
<tr>
<td>HumanEval (0-shot)
</td>
<td>62.2
</td>
<td>7.9
</td>
<td>14.0
</td>
<td>81.7
</td>
<td>25.6
</td>
</tr>
<tr>
<td>GSM-8K (8-shot, CoT)
</td>
<td>79.6
</td>
<td>25.7
</td>
<td>77.4
</td>
<td>93.0
</td>
<td>57.5
</td>
</tr>
<tr>
<td>MATH (4-shot, CoT)
</td>
<td>30.0
</td>
<td>3.8
</td>
<td>6.7
</td>
<td>50.4
</td>
<td>11.6
</td>
</tr>
</table>
### Responsibility & Safety
We believe that an open approach to AI leads to better, safer products, faster innovation, and a bigger overall market. We are committed to Responsible AI development and took a series of steps to limit misuse and harm and support the open source community.
Foundation models are widely capable technologies that are built to be used for a diverse range of applications. They are not designed to meet every developer preference on safety levels for all use cases, out-of-the-box, as those by their nature will differ across different applications.
Rather, responsible LLM-application deployment is achieved by implementing a series of safety best practices throughout the development of such applications, from the model pre-training, fine-tuning and the deployment of systems composed of safeguards to tailor the safety needs specifically to the use case and audience.
As part of the Llama 3 release, we updated our [Responsible Use Guide](https://llama.meta.com/responsible-use-guide/) to outline the steps and best practices for developers to implement model and system level safety for their application. We also provide a set of resources including [Meta Llama Guard 2](https://llama.meta.com/purple-llama/) and [Code Shield](https://llama.meta.com/purple-llama/) safeguards. These tools have proven to drastically reduce residual risks of LLM Systems, while maintaining a high level of helpfulness. We encourage developers to tune and deploy these safeguards according to their needs and we provide a [reference implementation](https://github.com/meta-llama/llama-recipes/tree/main/recipes/responsible_ai) to get you started.
#### Llama 3-Instruct
As outlined in the Responsible Use Guide, some trade-off between model helpfulness and model alignment is likely unavoidable. Developers should exercise discretion about how to weigh the benefits of alignment and helpfulness for their specific use case and audience. Developers should be mindful of residual risks when using Llama models and leverage additional safety tools as needed to reach the right safety bar for their use case.
<span style="text-decoration:underline;">Safety</span>
For our instruction tuned model, we conducted extensive red teaming exercises, performed adversarial evaluations and implemented safety mitigations techniques to lower residual risks. As with any Large Language Model, residual risks will likely remain and we recommend that developers assess these risks in the context of their use case. In parallel, we are working with the community to make AI safety benchmark standards transparent, rigorous and interpretable.
<span style="text-decoration:underline;">Refusals</span>
In addition to residual risks, we put a great emphasis on model refusals to benign prompts. Over-refusing not only can impact the user experience but could even be harmful in certain contexts as well. We’ve heard the feedback from the developer community and improved our fine tuning to ensure that Llama 3 is significantly less likely to falsely refuse to answer prompts than Llama 2.
We built internal benchmarks and developed mitigations to limit false refusals making Llama 3 our most helpful model to date.
#### Responsible release
In addition to responsible use considerations outlined above, we followed a rigorous process that requires us to take extra measures against misuse and critical risks before we make our release decision.
Misuse
If you access or use Llama 3, you agree to the Acceptable Use Policy. The most recent copy of this policy can be found at [https://llama.meta.com/llama3/use-policy/](https://llama.meta.com/llama3/use-policy/).
#### Critical risks
<span style="text-decoration:underline;">CBRNE</span> (Chemical, Biological, Radiological, Nuclear, and high yield Explosives)
We have conducted a two fold assessment of the safety of the model in this area:
* Iterative testing during model training to assess the safety of responses related to CBRNE threats and other adversarial risks.
* Involving external CBRNE experts to conduct an uplift test assessing the ability of the model to accurately provide expert knowledge and reduce barriers to potential CBRNE misuse, by reference to what can be achieved using web search (without the model).
### <span style="text-decoration:underline;">Cyber Security </span>
We have evaluated Llama 3 with CyberSecEval, Meta’s cybersecurity safety eval suite, measuring Llama 3’s propensity to suggest insecure code when used as a coding assistant, and Llama 3’s propensity to comply with requests to help carry out cyber attacks, where attacks are defined by the industry standard MITRE ATT&CK cyber attack ontology. On our insecure coding and cyber attacker helpfulness tests, Llama 3 behaved in the same range or safer than models of [equivalent coding capability](https://huggingface.co/spaces/facebook/CyberSecEval).
### <span style="text-decoration:underline;">Child Safety</span>
Child Safety risk assessments were conducted using a team of experts, to assess the model’s capability to produce outputs that could result in Child Safety risks and inform on any necessary and appropriate risk mitigations via fine tuning. We leveraged those expert red teaming sessions to expand the coverage of our evaluation benchmarks through Llama 3 model development. For Llama 3, we conducted new in-depth sessions using objective based methodologies to assess the model risks along multiple attack vectors. We also partnered with content specialists to perform red teaming exercises assessing potentially violating content while taking account of market specific nuances or experiences.
### Community
Generative AI safety requires expertise and tooling, and we believe in the strength of the open community to accelerate its progress. We are active members of open consortiums, including the AI Alliance, Partnership in AI and MLCommons, actively contributing to safety standardization and transparency. We encourage the community to adopt taxonomies like the MLCommons Proof of Concept evaluation to facilitate collaboration and transparency on safety and content evaluations. Our Purple Llama tools are open sourced for the community to use and widely distributed across ecosystem partners including cloud service providers. We encourage community contributions to our [Github repository](https://github.com/meta-llama/PurpleLlama).
Finally, we put in place a set of resources including an [output reporting mechanism](https://developers.facebook.com/llama_output_feedback) and [bug bounty program](https://www.facebook.com/whitehat) to continuously improve the Llama technology with the help of the community.
## Ethical Considerations and Limitations
The core values of Llama 3 are openness, inclusivity and helpfulness. It is meant to serve everyone, and to work for a wide range of use cases. It is thus designed to be accessible to people across many different backgrounds, experiences and perspectives. Llama 3 addresses users and their needs as they are, without insertion unnecessary judgment or normativity, while reflecting the understanding that even content that may appear problematic in some cases can serve valuable purposes in others. It respects the dignity and autonomy of all users, especially in terms of the values of free thought and expression that power innovation and progress.
But Llama 3 is a new technology, and like any new technology, there are risks associated with its use. Testing conducted to date has been in English, and has not covered, nor could it cover, all scenarios. For these reasons, as with all LLMs, Llama 3’s potential outputs cannot be predicted in advance, and the model may in some instances produce inaccurate, biased or other objectionable responses to user prompts. Therefore, before deploying any applications of Llama 3 models, developers should perform safety testing and tuning tailored to their specific applications of the model. As outlined in the Responsible Use Guide, we recommend incorporating [Purple Llama](https://github.com/facebookresearch/PurpleLlama) solutions into your workflows and specifically [Llama Guard](https://ai.meta.com/research/publications/llama-guard-llm-based-input-output-safeguard-for-human-ai-conversations/) which provides a base model to filter input and output prompts to layer system-level safety on top of model-level safety.
Please see the Responsible Use Guide available at [http://llama.meta.com/responsible-use-guide](http://llama.meta.com/responsible-use-guide)
## Citation instructions
@article{llama3modelcard,
title={Llama 3 Model Card},
author={AI@Meta},
year={2024},
url = {https://github.com/meta-llama/llama3/blob/main/MODEL_CARD.md}
}
## Contributors
Aaditya Singh; Aaron Grattafiori; Abhimanyu Dubey; Abhinav Jauhri; Abhinav Pandey; Abhishek Kadian; Adam Kelsey; Adi Gangidi; Ahmad Al-Dahle; Ahuva Goldstand; Aiesha Letman; Ajay Menon; Akhil Mathur; Alan Schelten; Alex Vaughan; Amy Yang; Andrei Lupu; Andres Alvarado; Andrew Gallagher; Andrew Gu; Andrew Ho; Andrew Poulton; Andrew Ryan; Angela Fan; Ankit Ramchandani; Anthony Hartshorn; Archi Mitra; Archie Sravankumar; Artem Korenev; Arun Rao; Ashley Gabriel; Ashwin Bharambe; Assaf Eisenman; Aston Zhang; Aurelien Rodriguez; Austen Gregerson; Ava Spataru; Baptiste Roziere; Ben Maurer; Benjamin Leonhardi; Bernie Huang; Bhargavi Paranjape; Bing Liu; Binh Tang; Bobbie Chern; Brani Stojkovic; Brian Fuller; Catalina Mejia Arenas; Chao Zhou; Charlotte Caucheteux; Chaya Nayak; Ching-Hsiang Chu; Chloe Bi; Chris Cai; Chris Cox; Chris Marra; Chris McConnell; Christian Keller; Christoph Feichtenhofer; Christophe Touret; Chunyang Wu; Corinne Wong; Cristian Canton Ferrer; Damien Allonsius; Daniel Kreymer; Daniel Haziza; Daniel Li; Danielle Pintz; Danny Livshits; Danny Wyatt; David Adkins; David Esiobu; David Xu; Davide Testuggine; Delia David; Devi Parikh; Dhruv Choudhary; Dhruv Mahajan; Diana Liskovich; Diego Garcia-Olano; Diego Perino; Dieuwke Hupkes; Dingkang Wang; Dustin Holland; Egor Lakomkin; Elina Lobanova; Xiaoqing Ellen Tan; Emily Dinan; Eric Smith; Erik Brinkman; Esteban Arcaute; Filip Radenovic; Firat Ozgenel; Francesco Caggioni; Frank Seide; Frank Zhang; Gabriel Synnaeve; Gabriella Schwarz; Gabrielle Lee; Gada Badeer; Georgia Anderson; Graeme Nail; Gregoire Mialon; Guan Pang; Guillem Cucurell; Hailey Nguyen; Hannah Korevaar; Hannah Wang; Haroun Habeeb; Harrison Rudolph; Henry Aspegren; Hu Xu; Hugo Touvron; Iga Kozlowska; Igor Molybog; Igor Tufanov; Iliyan Zarov; Imanol Arrieta Ibarra; Irina-Elena Veliche; Isabel Kloumann; Ishan Misra; Ivan Evtimov; Jacob Xu; Jade Copet; Jake Weissman; Jan Geffert; Jana Vranes; Japhet Asher; Jason Park; Jay Mahadeokar; Jean-Baptiste Gaya; Jeet Shah; Jelmer van der Linde; Jennifer Chan; Jenny Hong; Jenya Lee; Jeremy Fu; Jeremy Teboul; Jianfeng Chi; Jianyu Huang; Jie Wang; Jiecao Yu; Joanna Bitton; Joe Spisak; Joelle Pineau; Jon Carvill; Jongsoo Park; Joseph Rocca; Joshua Johnstun; Junteng Jia; Kalyan Vasuden Alwala; Kam Hou U; Kate Plawiak; Kartikeya Upasani; Kaushik Veeraraghavan; Ke Li; Kenneth Heafield; Kevin Stone; Khalid El-Arini; Krithika Iyer; Kshitiz Malik; Kuenley Chiu; Kunal Bhalla; Kyle Huang; Lakshya Garg; Lauren Rantala-Yeary; Laurens van der Maaten; Lawrence Chen; Leandro Silva; Lee Bell; Lei Zhang; Liang Tan; Louis Martin; Lovish Madaan; Luca Wehrstedt; Lukas Blecher; Luke de Oliveira; Madeline Muzzi; Madian Khabsa; Manav Avlani; Mannat Singh; Manohar Paluri; Mark Zuckerberg; Marcin Kardas; Martynas Mankus; Mathew Oldham; Mathieu Rita; Matthew Lennie; Maya Pavlova; Meghan Keneally; Melanie Kambadur; Mihir Patel; Mikayel Samvelyan; Mike Clark; Mike Lewis; Min Si; Mitesh Kumar Singh; Mo Metanat; Mona Hassan; Naman Goyal; Narjes Torabi; Nicolas Usunier; Nikolay Bashlykov; Nikolay Bogoychev; Niladri Chatterji; Ning Dong; Oliver Aobo Yang; Olivier Duchenne; Onur Celebi; Parth Parekh; Patrick Alrassy; Paul Saab; Pavan Balaji; Pedro Rittner; Pengchuan Zhang; Pengwei Li; Petar Vasic; Peter Weng; Polina Zvyagina; Prajjwal Bhargava; Pratik Dubal; Praveen Krishnan; Punit Singh Koura; Qing He; Rachel Rodriguez; Ragavan Srinivasan; Rahul Mitra; Ramon Calderer; Raymond Li; Robert Stojnic; Roberta Raileanu; Robin Battey; Rocky Wang; Rohit Girdhar; Rohit Patel; Romain Sauvestre; Ronnie Polidoro; Roshan Sumbaly; Ross Taylor; Ruan Silva; Rui Hou; Rui Wang; Russ Howes; Ruty Rinott; Saghar Hosseini; Sai Jayesh Bondu; Samyak Datta; Sanjay Singh; Sara Chugh; Sargun Dhillon; Satadru Pan; Sean Bell; Sergey Edunov; Shaoliang Nie; Sharan Narang; Sharath Raparthy; Shaun Lindsay; Sheng Feng; Sheng Shen; Shenghao Lin; Shiva Shankar; Shruti Bhosale; Shun Zhang; Simon Vandenhende; Sinong Wang; Seohyun Sonia Kim; Soumya Batra; Sten Sootla; Steve Kehoe; Suchin Gururangan; Sumit Gupta; Sunny Virk; Sydney Borodinsky; Tamar Glaser; Tamar Herman; Tamara Best; Tara Fowler; Thomas Georgiou; Thomas Scialom; Tianhe Li; Todor Mihaylov; Tong Xiao; Ujjwal Karn; Vedanuj Goswami; Vibhor Gupta; Vignesh Ramanathan; Viktor Kerkez; Vinay Satish Kumar; Vincent Gonguet; Vish Vogeti; Vlad Poenaru; Vlad Tiberiu Mihailescu; Vladan Petrovic; Vladimir Ivanov; Wei Li; Weiwei Chu; Wenhan Xiong; Wenyin Fu; Wes Bouaziz; Whitney Meers; Will Constable; Xavier Martinet; Xiaojian Wu; Xinbo Gao; Xinfeng Xie; Xuchao Jia; Yaelle Goldschlag; Yann LeCun; Yashesh Gaur; Yasmine Babaei; Ye Qi; Yenda Li; Yi Wen; Yiwen Song; Youngjin Nam; Yuchen Hao; Yuchen Zhang; Yun Wang; Yuning Mao; Yuzi He; Zacharie Delpierre Coudert; Zachary DeVito; Zahra Hankir; Zhaoduo Wen; Zheng Yan; Zhengxing Chen; Zhenyu Yang; Zoe Papakipos |
puppyyyo/larceny-m3-only-LCAET_d2s | puppyyyo | "2025-04-10T12:42:35Z" | 0 | 0 | null | [
"tensorboard",
"safetensors",
"xlm-roberta",
"region:us"
] | null | "2025-04-10T09:51:04Z" | <!DOCTYPE html>
<html class="" lang="en">
<head>
<meta charset="utf-8" />
<meta
name="viewport"
content="width=device-width, initial-scale=1.0, user-scalable=no"
/>
<meta
name="description"
content="We're on a journey to advance and democratize artificial intelligence through open source and open science."
/>
<meta property="fb:app_id" content="1321688464574422" />
<meta name="twitter:card" content="summary_large_image" />
<meta name="twitter:site" content="@huggingface" />
<meta
property="og:title"
content="Hugging Face - The AI community building the future."
/>
<meta property="og:type" content="website" />
<title>Hugging Face - The AI community building the future.</title>
<style>
body {
margin: 0;
}
main {
background-color: white;
min-height: 100vh;
padding: 7rem 1rem 8rem 1rem;
text-align: center;
font-family: Source Sans Pro, ui-sans-serif, system-ui, -apple-system,
BlinkMacSystemFont, Segoe UI, Roboto, Helvetica Neue, Arial, Noto Sans,
sans-serif, Apple Color Emoji, Segoe UI Emoji, Segoe UI Symbol,
Noto Color Emoji;
}
img {
width: 6rem;
height: 6rem;
margin: 0 auto 1rem;
}
h1 {
font-size: 3.75rem;
line-height: 1;
color: rgba(31, 41, 55, 1);
font-weight: 700;
box-sizing: border-box;
margin: 0 auto;
}
p, a {
color: rgba(107, 114, 128, 1);
font-size: 1.125rem;
line-height: 1.75rem;
max-width: 28rem;
box-sizing: border-box;
margin: 0 auto;
}
.dark main {
background-color: rgb(11, 15, 25);
}
.dark h1 {
color: rgb(209, 213, 219);
}
.dark p, .dark a {
color: rgb(156, 163, 175);
}
</style>
<script>
// On page load or when changing themes, best to add inline in `head` to avoid FOUC
const key = "_tb_global_settings";
let theme = window.matchMedia("(prefers-color-scheme: dark)").matches
? "dark"
: "light";
try {
const storageTheme = JSON.parse(window.localStorage.getItem(key)).theme;
if (storageTheme) {
theme = storageTheme === "dark" ? "dark" : "light";
}
} catch (e) {}
if (theme === "dark") {
document.documentElement.classList.add("dark");
} else {
document.documentElement.classList.remove("dark");
}
</script>
</head>
<body>
<main>
<img
src="https://cdn-media.huggingface.co/assets/huggingface_logo.svg"
alt=""
/>
<div>
<h1>429</h1>
<p>We had to rate limit you. If you think it's an error, send us <a href="mailto:[email protected]">an email</a></p>
</div>
</main>
</body>
</html> |
philip-hightech/71c5bee3-c4ed-446d-84dc-bdd94e3b3c8c | philip-hightech | "2025-01-20T04:56:46Z" | 9 | 0 | peft | [
"peft",
"safetensors",
"llama",
"axolotl",
"generated_from_trainer",
"base_model:unsloth/Hermes-3-Llama-3.1-8B",
"base_model:adapter:unsloth/Hermes-3-Llama-3.1-8B",
"region:us"
] | null | "2025-01-20T04:55:22Z" | ---
library_name: peft
base_model: unsloth/Hermes-3-Llama-3.1-8B
tags:
- axolotl
- generated_from_trainer
model-index:
- name: 71c5bee3-c4ed-446d-84dc-bdd94e3b3c8c
results: []
---
<!-- This model card has been generated automatically according to the information the Trainer had access to. You
should probably proofread and complete it, then remove this comment. -->
[<img src="https://raw.githubusercontent.com/axolotl-ai-cloud/axolotl/main/image/axolotl-badge-web.png" alt="Built with Axolotl" width="200" height="32"/>](https://github.com/axolotl-ai-cloud/axolotl)
<details><summary>See axolotl config</summary>
axolotl version: `0.4.1`
```yaml
adapter: lora
base_model: unsloth/Hermes-3-Llama-3.1-8B
bf16: auto
chat_template: llama3
dataset_prepared_path: null
datasets:
- data_files:
- 34c0d5d178dc60a8_train_data.json
ds_type: json
format: custom
path: /workspace/input_data/34c0d5d178dc60a8_train_data.json
type:
field_instruction: category
field_output: task
format: '{instruction}'
no_input_format: '{instruction}'
system_format: '{system}'
system_prompt: ''
debug: null
deepspeed: null
early_stopping_patience: null
eval_max_new_tokens: 128
eval_table_size: null
evals_per_epoch: 4
flash_attention: false
fp16: null
fsdp: null
fsdp_config: null
gradient_accumulation_steps: 4
gradient_checkpointing: false
group_by_length: false
hub_model_id: philip-hightech/71c5bee3-c4ed-446d-84dc-bdd94e3b3c8c
hub_repo: null
hub_strategy: checkpoint
hub_token: null
learning_rate: 0.0002
load_in_4bit: false
load_in_8bit: false
local_rank: null
logging_steps: 1
lora_alpha: 16
lora_dropout: 0.05
lora_fan_in_fan_out: null
lora_model_dir: null
lora_r: 8
lora_target_linear: true
lr_scheduler: cosine
max_steps: 10
micro_batch_size: 2
mlflow_experiment_name: /tmp/34c0d5d178dc60a8_train_data.json
model_type: AutoModelForCausalLM
num_epochs: 1
optimizer: adamw_bnb_8bit
output_dir: miner_id_24
pad_to_sequence_len: true
resume_from_checkpoint: null
s2_attention: null
sample_packing: false
saves_per_epoch: 4
sequence_len: 512
strict: false
tf32: false
tokenizer_type: AutoTokenizer
train_on_inputs: false
trust_remote_code: true
val_set_size: 0.05
wandb_entity: null
wandb_mode: online
wandb_name: 730b19a5-89e0-4ce7-85e2-bfc88f9b79c8
wandb_project: Gradients-On-Demand
wandb_run: your_name
wandb_runid: 730b19a5-89e0-4ce7-85e2-bfc88f9b79c8
warmup_steps: 10
weight_decay: 0.0
xformers_attention: null
```
</details><br>
# 71c5bee3-c4ed-446d-84dc-bdd94e3b3c8c
This model is a fine-tuned version of [unsloth/Hermes-3-Llama-3.1-8B](https://huggingface.co/unsloth/Hermes-3-Llama-3.1-8B) on the None dataset.
It achieves the following results on the evaluation set:
- Loss: nan
## Model description
More information needed
## Intended uses & limitations
More information needed
## Training and evaluation data
More information needed
## Training procedure
### Training hyperparameters
The following hyperparameters were used during training:
- learning_rate: 0.0002
- train_batch_size: 2
- eval_batch_size: 2
- seed: 42
- gradient_accumulation_steps: 4
- total_train_batch_size: 8
- optimizer: Use OptimizerNames.ADAMW_BNB with betas=(0.9,0.999) and epsilon=1e-08 and optimizer_args=No additional optimizer arguments
- lr_scheduler_type: cosine
- lr_scheduler_warmup_steps: 10
- training_steps: 10
### Training results
| Training Loss | Epoch | Step | Validation Loss |
|:-------------:|:------:|:----:|:---------------:|
| 0.0 | 0.0050 | 1 | nan |
| 0.0 | 0.0149 | 3 | nan |
| 0.0 | 0.0299 | 6 | nan |
| 0.0 | 0.0448 | 9 | nan |
### Framework versions
- PEFT 0.13.2
- Transformers 4.46.0
- Pytorch 2.5.0+cu124
- Datasets 3.0.1
- Tokenizers 0.20.1 |
basitmujtaba45/Summarization | basitmujtaba45 | "2024-04-15T08:10:12Z" | 106 | 0 | transformers | [
"transformers",
"safetensors",
"bart",
"text2text-generation",
"arxiv:1910.09700",
"autotrain_compatible",
"endpoints_compatible",
"region:us"
] | text2text-generation | "2024-04-15T08:06:18Z" | ---
library_name: transformers
tags: []
---
# Model Card for Model ID
<!-- Provide a quick summary of what the model is/does. -->
## Model Details
### Model Description
<!-- Provide a longer summary of what this model is. -->
This is the model card of a 🤗 transformers model that has been pushed on the Hub. This model card has been automatically generated.
- **Developed by:** [More Information Needed]
- **Funded by [optional]:** [More Information Needed]
- **Shared by [optional]:** [More Information Needed]
- **Model type:** [More Information Needed]
- **Language(s) (NLP):** [More Information Needed]
- **License:** [More Information Needed]
- **Finetuned from model [optional]:** [More Information Needed]
### Model Sources [optional]
<!-- Provide the basic links for the model. -->
- **Repository:** [More Information Needed]
- **Paper [optional]:** [More Information Needed]
- **Demo [optional]:** [More Information Needed]
## Uses
<!-- Address questions around how the model is intended to be used, including the foreseeable users of the model and those affected by the model. -->
### Direct Use
<!-- This section is for the model use without fine-tuning or plugging into a larger ecosystem/app. -->
[More Information Needed]
### Downstream Use [optional]
<!-- This section is for the model use when fine-tuned for a task, or when plugged into a larger ecosystem/app -->
[More Information Needed]
### Out-of-Scope Use
<!-- This section addresses misuse, malicious use, and uses that the model will not work well for. -->
[More Information Needed]
## Bias, Risks, and Limitations
<!-- This section is meant to convey both technical and sociotechnical limitations. -->
[More Information Needed]
### Recommendations
<!-- This section is meant to convey recommendations with respect to the bias, risk, and technical limitations. -->
Users (both direct and downstream) should be made aware of the risks, biases and limitations of the model. More information needed for further recommendations.
## How to Get Started with the Model
Use the code below to get started with the model.
[More Information Needed]
## Training Details
### Training Data
<!-- This should link to a Dataset Card, perhaps with a short stub of information on what the training data is all about as well as documentation related to data pre-processing or additional filtering. -->
[More Information Needed]
### Training Procedure
<!-- This relates heavily to the Technical Specifications. Content here should link to that section when it is relevant to the training procedure. -->
#### Preprocessing [optional]
[More Information Needed]
#### Training Hyperparameters
- **Training regime:** [More Information Needed] <!--fp32, fp16 mixed precision, bf16 mixed precision, bf16 non-mixed precision, fp16 non-mixed precision, fp8 mixed precision -->
#### Speeds, Sizes, Times [optional]
<!-- This section provides information about throughput, start/end time, checkpoint size if relevant, etc. -->
[More Information Needed]
## Evaluation
<!-- This section describes the evaluation protocols and provides the results. -->
### Testing Data, Factors & Metrics
#### Testing Data
<!-- This should link to a Dataset Card if possible. -->
[More Information Needed]
#### Factors
<!-- These are the things the evaluation is disaggregating by, e.g., subpopulations or domains. -->
[More Information Needed]
#### Metrics
<!-- These are the evaluation metrics being used, ideally with a description of why. -->
[More Information Needed]
### Results
[More Information Needed]
#### Summary
## Model Examination [optional]
<!-- Relevant interpretability work for the model goes here -->
[More Information Needed]
## Environmental Impact
<!-- Total emissions (in grams of CO2eq) and additional considerations, such as electricity usage, go here. Edit the suggested text below accordingly -->
Carbon emissions can be estimated using the [Machine Learning Impact calculator](https://mlco2.github.io/impact#compute) presented in [Lacoste et al. (2019)](https://arxiv.org/abs/1910.09700).
- **Hardware Type:** [More Information Needed]
- **Hours used:** [More Information Needed]
- **Cloud Provider:** [More Information Needed]
- **Compute Region:** [More Information Needed]
- **Carbon Emitted:** [More Information Needed]
## Technical Specifications [optional]
### Model Architecture and Objective
[More Information Needed]
### Compute Infrastructure
[More Information Needed]
#### Hardware
[More Information Needed]
#### Software
[More Information Needed]
## Citation [optional]
<!-- If there is a paper or blog post introducing the model, the APA and Bibtex information for that should go in this section. -->
**BibTeX:**
[More Information Needed]
**APA:**
[More Information Needed]
## Glossary [optional]
<!-- If relevant, include terms and calculations in this section that can help readers understand the model or model card. -->
[More Information Needed]
## More Information [optional]
[More Information Needed]
## Model Card Authors [optional]
[More Information Needed]
## Model Card Contact
[More Information Needed]
|
RichardErkhov/baconnier_-_Gaston-Llama-3-8B-8bits | RichardErkhov | "2025-03-31T11:32:35Z" | 0 | 0 | null | [
"safetensors",
"llama",
"8-bit",
"bitsandbytes",
"region:us"
] | null | "2025-03-31T11:26:13Z" | Quantization made by Richard Erkhov.
[Github](https://github.com/RichardErkhov)
[Discord](https://discord.gg/pvy7H8DZMG)
[Request more models](https://github.com/RichardErkhov/quant_request)
Gaston-Llama-3-8B - bnb 8bits
- Model creator: https://huggingface.co/baconnier/
- Original model: https://huggingface.co/baconnier/Gaston-Llama-3-8B/
Original model description:
# Vous en avez assez du jargon administratif incompréhensible ?
Gaston est là pour vous aider !
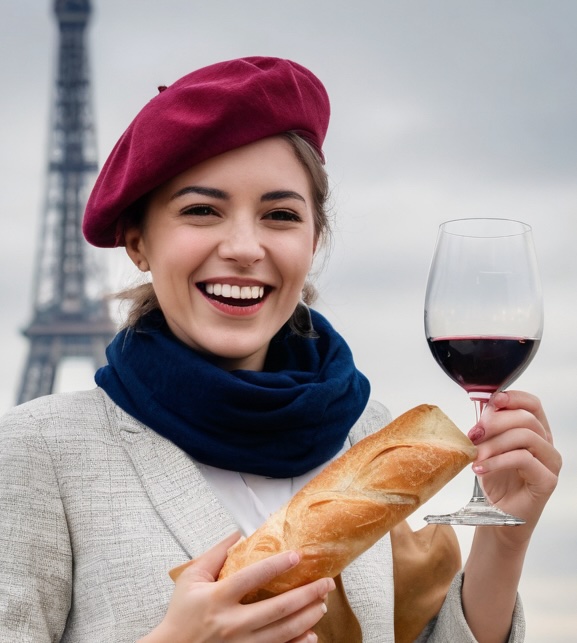
💡 Cette IA a été conçue pour reformuler les communications et documents administratifs en langage clair et simple.
📝 Grâce à Gaston, fini les lettres obscures et les procédures nébuleuses. Tout devient limpide et à la portée du commun des mortels.
😊 Gaston est un POC (Proof of Concept) qui a pour mission de rendre l'administration plus transparente et accessible.
🙌 Son secret ? Une capacité à analyser et à traduire le jargon en termes compréhensibles par tous.
💬 Avec Gaston, les démarches administratives deviennent enfin un jeu d'enfant !
This model is based on Llama-3-8b, and is governed by [META LLAMA 3 COMMUNITY LICENSE AGREEMENT](LICENSE)
---
language:
- en
license: apache-2.0
tags:
- text-generation-inference
- transformers
- unsloth
- llama
- trl
- orpo
base_model: NousResearch/Hermes-2-Pro-Llama-3-8B
---
# Uploaded model
- **Developed by:** baconnier
- **License:** apache-2.0
- **Finetuned from model :** NousResearch/Hermes-2-Pro-Llama-3-8B
This llama model was trained 2x faster with [Unsloth](https://github.com/unslothai/unsloth) and Huggingface's TRL library.
[<img src="https://raw.githubusercontent.com/unslothai/unsloth/main/images/unsloth%20made%20with%20love.png" width="200"/>](https://github.com/unslothai/unsloth)
This model was trained ORPO , using ChatML prompt template format.
```
<|im_start|>user
Qui est tu ?
<|im_end|>
<|im_start|>assistant
```
# Example with local TGI:
See the snippet below for usage with local inference:
```python
#Example: reuse your existing OpenAI setup
from openai import OpenAI
client = OpenAI(base_url="http://localhost:8080/v1", api_key="TGI")
completion = client.chat.completions.create(
model="baconnier/Gaston-Llama-3-8B",
messages=[
{"role": "system", "content": "Reponds comme Gaston, un fonctionnaire français qui s'exprime uniquement en français avec des termes tres simples et surtout sans jamais utiliser de jargon administratif."},
{"role": "user", "content": "l’action en nullité se prescrit par 5 ans ou est prescrite en 5 ans"},
{"role": "assistant", "content": ""}
],
max_tokens=400,
temperature=0.7,
)
print(completion.choices[0].message.content)
```
Output:
```
Le délai de prescription pour l'action en nullité est généralement de cinq ans à compter du jour où
la partie qui peut agir a eu connaissance des faits, selon le Code civil français.
```
# Example with Transformers and pipeline:
See the snippet below for usage with Transformers:
```python
import transformers
import torch
model_id = "baconnier/Gaston-Llama-3-8B"
pipeline = transformers.pipeline(
"text-generation",
model=model_id,
model_kwargs={"torch_dtype": torch.bfloat16},
device="cuda",
)
messages=[
{"role": "system", "content": "Reponds comme Gaston, un fonctionnaire français qui s'exprime uniquement en français
avec des termes tres simples et surtout sans jamais utiliser de jargon administratif."},
{"role": "user", "content": "Vous voudrez bien vous munir de toutes les pièces justificatives afférentes à vos déclarations de revenus
et de patrimoine des 3 derniers exercices, nonobstant les éléments déjà produits.
À défaut de réponse de votre part ou en cas de non comparution aux date et heure susvisées,
la procédure de contrôle se poursuivra et nous serons fondés à retenir les éléments en notre possession
en vue de l'établissement des impositions supplémentaires et pénalités subséquentes."},
{"role": "assistant", "content": ""}
]
prompt = pipeline.tokenizer.apply_chat_template(
messages,
tokenize=False,
add_generation_prompt=True
)
prompt = pipeline.tokenizer.apply_chat_template(
messages,
tokenize=False,
add_generation_prompt=True
)
outputs = pipeline(
prompt,
max_new_tokens=256,
do_sample=True,
temperature=0.6,
top_p=0.9,
)
print(outputs[0]["generated_text"][len(prompt):])
```
Question:
```
Vous voudrez bien vous munir de toutes les pièces justificatives afférentes à vos déclarations de revenus
et de patrimoine des 3 derniers exercices, nonobstant les éléments déjà produits.
À défaut de réponse de votre part ou en cas de non comparution aux date et heure susvisées,
la procédure de contrôle se poursuivra et nous serons fondés à retenir les éléments en notre possession
en vue de l'établissement des impositions supplémentaires et pénalités subséquentes.
```
Reponse:
```
Je vous invite à fournir toutes les pièces justificatives concernant vos déclarations de revenus
et de patrimoine des trois dernières années, malgré les éléments déjà fournis.
En cas de non-respect de ce délai ou de manque de réponse,
la procédure de vérification continuera, et nous utiliserons les informations en notre possession
pour établir les impôts supplémentaires et les pénalités associées.
```
Question:
```
Nous accusons réception de votre demande de renouvellement de passeport en date du 15 mars 2023.
Après instruction de votre dossier, il appert que les pièces produites s'avèrent insuffisantes
eu égard aux dispositions de l'article 5-1 du décret n°2005-1726 du 30 décembre 2005.
Nonobstant les justificatifs déjà fournis, il vous appartient de nous transmettre
dans les meilleurs délais les documents complémentaires listés ci-après :
- Copie d'un justificatif de domicile de moins de 6 mois
- Timbre fiscal d'un montant de 86€
À défaut de production des pièces susvisées dans un délai de 30 jours à compter de la présente,
votre demande sera classée sans suite.
Nous vous prions d'agréer, Monsieur, l'expression de nos salutations distinguées.
```
Reponse:
```
Nous recevons votre requête pour renouveler votre passeport datant du 15 mars 2023.
Suite à l'examen de votre dossier, il a été déterminé que les documents fournis
ne respectent pas les exigences mentionnées dans la section 5-1 du décret n°2005-1726 du 30 décembre 2005.
Malgré les pièces déjà fournies, vous devez nous envoyer rapidement les éléments suivants :
- Une copie d'un justificatif de domicile datant de moins de 6 mois.
- Un timbre fiscal d'une valeur de 86 €.
Si ces documents ne sont pas fournis dans un délai de 30 jours à compter de cette lettre, votre demande sera close.
Nous vous prions d'accepter, Monsieur, l'expression de nos salutations respectueuses.
```
# Example with Transformers:
```python
from transformers import AutoTokenizer, AutoModelForCausalLM
tokenizer = AutoTokenizer.from_pretrained("baconnier/Gaston-Llama-3-8B")
model = AutoModelForCausalLM.from_pretrained("baconnier/Gaston-Llama-3-8B")
prompt = "Nonobstant les justificatifs déjà fournis"
inputs = tokenizer(prompt, return_tensors="pt")
# Generate
generate_ids = model.generate(inputs.input_ids, max_length=200)
tokenizer.batch_decode(generate_ids, skip_special_tokens=True, clean_up_tokenization_spaces=False)[0]
```
|
dadirri/whisper-small-dadirri | dadirri | "2024-05-29T03:45:15Z" | 90 | 0 | transformers | [
"transformers",
"tensorboard",
"safetensors",
"whisper",
"automatic-speech-recognition",
"generated_from_trainer",
"en",
"dataset:chris_W/dataset",
"base_model:openai/whisper-small",
"base_model:finetune:openai/whisper-small",
"license:apache-2.0",
"endpoints_compatible",
"region:us"
] | automatic-speech-recognition | "2024-05-28T23:51:23Z" | ---
language:
- en
license: apache-2.0
base_model: openai/whisper-small
tags:
- generated_from_trainer
datasets:
- chris_W/dataset
model-index:
- name: Whisper-Small-dadirri
results: []
---
<!-- This model card has been generated automatically according to the information the Trainer had access to. You
should probably proofread and complete it, then remove this comment. -->
# Whisper-Small-dadirri
This model is a fine-tuned version of [openai/whisper-small](https://huggingface.co/openai/whisper-small) on the hearing voice dataset.
It achieves the following results on the evaluation set:
- Loss: 0.0231
## Model description
More information needed
## Intended uses & limitations
More information needed
## Training and evaluation data
More information needed
## Training procedure
### Training hyperparameters
The following hyperparameters were used during training:
- learning_rate: 1e-05
- train_batch_size: 16
- eval_batch_size: 8
- seed: 42
- optimizer: Adam with betas=(0.9,0.999) and epsilon=1e-08
- lr_scheduler_type: linear
- lr_scheduler_warmup_steps: 100
- training_steps: 400
- mixed_precision_training: Native AMP
### Training results
| Training Loss | Epoch | Step | Validation Loss |
|:-------------:|:-----:|:----:|:---------------:|
| No log | 0.52 | 50 | 3.0035 |
| 4.521 | 1.03 | 100 | 1.4394 |
| 4.521 | 1.55 | 150 | 2.7847 |
| 1.8786 | 2.06 | 200 | 0.4913 |
| 1.8786 | 2.58 | 250 | 0.0458 |
| 0.0755 | 3.09 | 300 | 0.0379 |
| 0.0755 | 3.61 | 350 | 0.0285 |
| 0.0126 | 4.12 | 400 | 0.0231 |
### Framework versions
- Transformers 4.38.0
- Pytorch 2.0.1+cu118
- Datasets 2.19.1
- Tokenizers 0.15.2
|
ttj/sac-logos-ava1-l14-linearMSE | ttj | "2023-05-04T06:57:58Z" | 0 | 0 | null | [
"pytorch",
"safetensors",
"license:apache-2.0",
"region:us"
] | null | "2023-05-04T06:52:03Z" | ---
license: apache-2.0
---
model ported from https://github.com/christophschuhmann/improved-aesthetic-predictor
|
Monsia/camembert-fr-covid-tweet-classification | Monsia | "2021-10-29T15:17:47Z" | 5 | 0 | transformers | [
"transformers",
"pytorch",
"camembert",
"text-classification",
"classification",
"fr",
"license:apache-2.0",
"autotrain_compatible",
"endpoints_compatible",
"region:us"
] | text-classification | "2022-03-02T23:29:04Z" | ---
language:
- fr
tags:
- classification
license: apache-2.0
metrics:
- accuracy
widget:
- text: "tchai on est morts. on va se faire vacciner et ils vont contrôler comme les marionnettes avec des fils. d'après les 'ont dit'..."
---
# camembert-fr-covid-tweet-classification
This model is a fine-tune checkpoint of [Yanzhu/bertweetfr-base](https://huggingface.co/Yanzhu/bertweetfr-base), fine-tuned on SST-2.
This model reaches an accuracy of 66.00% on the dev set.
In this dataset, given a tweet, the goal was to infer the underlying topic of the tweet by choosing from four topics classes:
- chiffres : this means, the tweet talk about statistics of covid.
- mesures : this means, the tweet talk about measures take by government of covid
- opinions : this means, the tweet talk about opinion of people like fake new.
- symptomes : this means, the tweet talk about symptoms or variant of covid.
- divers : or other
# Pipelining the Model
```python
from transformers import AutoTokenizer, AutoModelForSequenceClassification, pipeline
tokenizer = AutoTokenizer.from_pretrained("Monsia/camembert-fr-covid-tweet-classification")
model = AutoModelForSequenceClassification.from_pretrained("Monsia/camembert-fr-covid-tweet-classification")
nlp_topic_classif = transformers.pipeline('topics-classification', model = model, tokenizer = tokenizer)
nlp_topic_classif("tchai on est morts. on va se faire vacciner et ils vont contrôler comme les marionnettes avec des fils. d'après les '' ont dit ''...")
# Output: [{'label': 'opinions', 'score': 0.831]
```
|
helene-rousset/deepscaler_step1400_v10 | helene-rousset | "2025-03-10T01:16:00Z" | 0 | 0 | transformers | [
"transformers",
"safetensors",
"qwen2",
"text-generation",
"arxiv:1910.09700",
"autotrain_compatible",
"text-generation-inference",
"endpoints_compatible",
"region:us"
] | text-generation | "2025-03-10T01:13:54Z" | ---
library_name: transformers
tags: []
---
# Model Card for Model ID
<!-- Provide a quick summary of what the model is/does. -->
## Model Details
### Model Description
<!-- Provide a longer summary of what this model is. -->
This is the model card of a 🤗 transformers model that has been pushed on the Hub. This model card has been automatically generated.
- **Developed by:** [More Information Needed]
- **Funded by [optional]:** [More Information Needed]
- **Shared by [optional]:** [More Information Needed]
- **Model type:** [More Information Needed]
- **Language(s) (NLP):** [More Information Needed]
- **License:** [More Information Needed]
- **Finetuned from model [optional]:** [More Information Needed]
### Model Sources [optional]
<!-- Provide the basic links for the model. -->
- **Repository:** [More Information Needed]
- **Paper [optional]:** [More Information Needed]
- **Demo [optional]:** [More Information Needed]
## Uses
<!-- Address questions around how the model is intended to be used, including the foreseeable users of the model and those affected by the model. -->
### Direct Use
<!-- This section is for the model use without fine-tuning or plugging into a larger ecosystem/app. -->
[More Information Needed]
### Downstream Use [optional]
<!-- This section is for the model use when fine-tuned for a task, or when plugged into a larger ecosystem/app -->
[More Information Needed]
### Out-of-Scope Use
<!-- This section addresses misuse, malicious use, and uses that the model will not work well for. -->
[More Information Needed]
## Bias, Risks, and Limitations
<!-- This section is meant to convey both technical and sociotechnical limitations. -->
[More Information Needed]
### Recommendations
<!-- This section is meant to convey recommendations with respect to the bias, risk, and technical limitations. -->
Users (both direct and downstream) should be made aware of the risks, biases and limitations of the model. More information needed for further recommendations.
## How to Get Started with the Model
Use the code below to get started with the model.
[More Information Needed]
## Training Details
### Training Data
<!-- This should link to a Dataset Card, perhaps with a short stub of information on what the training data is all about as well as documentation related to data pre-processing or additional filtering. -->
[More Information Needed]
### Training Procedure
<!-- This relates heavily to the Technical Specifications. Content here should link to that section when it is relevant to the training procedure. -->
#### Preprocessing [optional]
[More Information Needed]
#### Training Hyperparameters
- **Training regime:** [More Information Needed] <!--fp32, fp16 mixed precision, bf16 mixed precision, bf16 non-mixed precision, fp16 non-mixed precision, fp8 mixed precision -->
#### Speeds, Sizes, Times [optional]
<!-- This section provides information about throughput, start/end time, checkpoint size if relevant, etc. -->
[More Information Needed]
## Evaluation
<!-- This section describes the evaluation protocols and provides the results. -->
### Testing Data, Factors & Metrics
#### Testing Data
<!-- This should link to a Dataset Card if possible. -->
[More Information Needed]
#### Factors
<!-- These are the things the evaluation is disaggregating by, e.g., subpopulations or domains. -->
[More Information Needed]
#### Metrics
<!-- These are the evaluation metrics being used, ideally with a description of why. -->
[More Information Needed]
### Results
[More Information Needed]
#### Summary
## Model Examination [optional]
<!-- Relevant interpretability work for the model goes here -->
[More Information Needed]
## Environmental Impact
<!-- Total emissions (in grams of CO2eq) and additional considerations, such as electricity usage, go here. Edit the suggested text below accordingly -->
Carbon emissions can be estimated using the [Machine Learning Impact calculator](https://mlco2.github.io/impact#compute) presented in [Lacoste et al. (2019)](https://arxiv.org/abs/1910.09700).
- **Hardware Type:** [More Information Needed]
- **Hours used:** [More Information Needed]
- **Cloud Provider:** [More Information Needed]
- **Compute Region:** [More Information Needed]
- **Carbon Emitted:** [More Information Needed]
## Technical Specifications [optional]
### Model Architecture and Objective
[More Information Needed]
### Compute Infrastructure
[More Information Needed]
#### Hardware
[More Information Needed]
#### Software
[More Information Needed]
## Citation [optional]
<!-- If there is a paper or blog post introducing the model, the APA and Bibtex information for that should go in this section. -->
**BibTeX:**
[More Information Needed]
**APA:**
[More Information Needed]
## Glossary [optional]
<!-- If relevant, include terms and calculations in this section that can help readers understand the model or model card. -->
[More Information Needed]
## More Information [optional]
[More Information Needed]
## Model Card Authors [optional]
[More Information Needed]
## Model Card Contact
[More Information Needed] |
Bubobot/ppo-Huggy | Bubobot | "2025-04-11T12:20:31Z" | 0 | 0 | ml-agents | [
"ml-agents",
"tensorboard",
"onnx",
"Huggy",
"deep-reinforcement-learning",
"reinforcement-learning",
"ML-Agents-Huggy",
"region:us"
] | reinforcement-learning | "2025-04-11T12:20:22Z" | ---
library_name: ml-agents
tags:
- Huggy
- deep-reinforcement-learning
- reinforcement-learning
- ML-Agents-Huggy
---
# **ppo** Agent playing **Huggy**
This is a trained model of a **ppo** agent playing **Huggy**
using the [Unity ML-Agents Library](https://github.com/Unity-Technologies/ml-agents).
## Usage (with ML-Agents)
The Documentation: https://unity-technologies.github.io/ml-agents/ML-Agents-Toolkit-Documentation/
We wrote a complete tutorial to learn to train your first agent using ML-Agents and publish it to the Hub:
- A *short tutorial* where you teach Huggy the Dog 🐶 to fetch the stick and then play with him directly in your
browser: https://huggingface.co/learn/deep-rl-course/unitbonus1/introduction
- A *longer tutorial* to understand how works ML-Agents:
https://huggingface.co/learn/deep-rl-course/unit5/introduction
### Resume the training
```bash
mlagents-learn <your_configuration_file_path.yaml> --run-id=<run_id> --resume
```
### Watch your Agent play
You can watch your agent **playing directly in your browser**
1. If the environment is part of ML-Agents official environments, go to https://huggingface.co/unity
2. Step 1: Find your model_id: Bubobot/ppo-Huggy
3. Step 2: Select your *.nn /*.onnx file
4. Click on Watch the agent play 👀
|
rahulAkaVector/modelz | rahulAkaVector | "2024-08-24T15:34:48Z" | 0 | 0 | transformers | [
"transformers",
"safetensors",
"unsloth",
"arxiv:1910.09700",
"endpoints_compatible",
"region:us"
] | null | "2024-06-10T17:48:25Z" | ---
library_name: transformers
tags:
- unsloth
---
# Model Card for Model ID
<!-- Provide a quick summary of what the model is/does. -->
## Model Details
### Model Description
<!-- Provide a longer summary of what this model is. -->
This is the model card of a 🤗 transformers model that has been pushed on the Hub. This model card has been automatically generated.
- **Developed by:** [More Information Needed]
- **Funded by [optional]:** [More Information Needed]
- **Shared by [optional]:** [More Information Needed]
- **Model type:** [More Information Needed]
- **Language(s) (NLP):** [More Information Needed]
- **License:** [More Information Needed]
- **Finetuned from model [optional]:** [More Information Needed]
### Model Sources [optional]
<!-- Provide the basic links for the model. -->
- **Repository:** [More Information Needed]
- **Paper [optional]:** [More Information Needed]
- **Demo [optional]:** [More Information Needed]
## Uses
<!-- Address questions around how the model is intended to be used, including the foreseeable users of the model and those affected by the model. -->
### Direct Use
<!-- This section is for the model use without fine-tuning or plugging into a larger ecosystem/app. -->
[More Information Needed]
### Downstream Use [optional]
<!-- This section is for the model use when fine-tuned for a task, or when plugged into a larger ecosystem/app -->
[More Information Needed]
### Out-of-Scope Use
<!-- This section addresses misuse, malicious use, and uses that the model will not work well for. -->
[More Information Needed]
## Bias, Risks, and Limitations
<!-- This section is meant to convey both technical and sociotechnical limitations. -->
[More Information Needed]
### Recommendations
<!-- This section is meant to convey recommendations with respect to the bias, risk, and technical limitations. -->
Users (both direct and downstream) should be made aware of the risks, biases and limitations of the model. More information needed for further recommendations.
## How to Get Started with the Model
Use the code below to get started with the model.
[More Information Needed]
## Training Details
### Training Data
<!-- This should link to a Dataset Card, perhaps with a short stub of information on what the training data is all about as well as documentation related to data pre-processing or additional filtering. -->
[More Information Needed]
### Training Procedure
<!-- This relates heavily to the Technical Specifications. Content here should link to that section when it is relevant to the training procedure. -->
#### Preprocessing [optional]
[More Information Needed]
#### Training Hyperparameters
- **Training regime:** [More Information Needed] <!--fp32, fp16 mixed precision, bf16 mixed precision, bf16 non-mixed precision, fp16 non-mixed precision, fp8 mixed precision -->
#### Speeds, Sizes, Times [optional]
<!-- This section provides information about throughput, start/end time, checkpoint size if relevant, etc. -->
[More Information Needed]
## Evaluation
<!-- This section describes the evaluation protocols and provides the results. -->
### Testing Data, Factors & Metrics
#### Testing Data
<!-- This should link to a Dataset Card if possible. -->
[More Information Needed]
#### Factors
<!-- These are the things the evaluation is disaggregating by, e.g., subpopulations or domains. -->
[More Information Needed]
#### Metrics
<!-- These are the evaluation metrics being used, ideally with a description of why. -->
[More Information Needed]
### Results
[More Information Needed]
#### Summary
## Model Examination [optional]
<!-- Relevant interpretability work for the model goes here -->
[More Information Needed]
## Environmental Impact
<!-- Total emissions (in grams of CO2eq) and additional considerations, such as electricity usage, go here. Edit the suggested text below accordingly -->
Carbon emissions can be estimated using the [Machine Learning Impact calculator](https://mlco2.github.io/impact#compute) presented in [Lacoste et al. (2019)](https://arxiv.org/abs/1910.09700).
- **Hardware Type:** [More Information Needed]
- **Hours used:** [More Information Needed]
- **Cloud Provider:** [More Information Needed]
- **Compute Region:** [More Information Needed]
- **Carbon Emitted:** [More Information Needed]
## Technical Specifications [optional]
### Model Architecture and Objective
[More Information Needed]
### Compute Infrastructure
[More Information Needed]
#### Hardware
[More Information Needed]
#### Software
[More Information Needed]
## Citation [optional]
<!-- If there is a paper or blog post introducing the model, the APA and Bibtex information for that should go in this section. -->
**BibTeX:**
[More Information Needed]
**APA:**
[More Information Needed]
## Glossary [optional]
<!-- If relevant, include terms and calculations in this section that can help readers understand the model or model card. -->
[More Information Needed]
## More Information [optional]
[More Information Needed]
## Model Card Authors [optional]
[More Information Needed]
## Model Card Contact
[More Information Needed] |
Kroalist/codellama-swe-rl-test1 | Kroalist | "2025-03-05T07:16:30Z" | 0 | 0 | transformers | [
"transformers",
"safetensors",
"arxiv:1910.09700",
"endpoints_compatible",
"region:us"
] | null | "2025-03-02T15:47:12Z" | ---
library_name: transformers
tags: []
---
# Model Card for Model ID
<!-- Provide a quick summary of what the model is/does. -->
## Model Details
### Model Description
<!-- Provide a longer summary of what this model is. -->
This is the model card of a 🤗 transformers model that has been pushed on the Hub. This model card has been automatically generated.
- **Developed by:** [More Information Needed]
- **Funded by [optional]:** [More Information Needed]
- **Shared by [optional]:** [More Information Needed]
- **Model type:** [More Information Needed]
- **Language(s) (NLP):** [More Information Needed]
- **License:** [More Information Needed]
- **Finetuned from model [optional]:** [More Information Needed]
### Model Sources [optional]
<!-- Provide the basic links for the model. -->
- **Repository:** [More Information Needed]
- **Paper [optional]:** [More Information Needed]
- **Demo [optional]:** [More Information Needed]
## Uses
<!-- Address questions around how the model is intended to be used, including the foreseeable users of the model and those affected by the model. -->
### Direct Use
<!-- This section is for the model use without fine-tuning or plugging into a larger ecosystem/app. -->
[More Information Needed]
### Downstream Use [optional]
<!-- This section is for the model use when fine-tuned for a task, or when plugged into a larger ecosystem/app -->
[More Information Needed]
### Out-of-Scope Use
<!-- This section addresses misuse, malicious use, and uses that the model will not work well for. -->
[More Information Needed]
## Bias, Risks, and Limitations
<!-- This section is meant to convey both technical and sociotechnical limitations. -->
[More Information Needed]
### Recommendations
<!-- This section is meant to convey recommendations with respect to the bias, risk, and technical limitations. -->
Users (both direct and downstream) should be made aware of the risks, biases and limitations of the model. More information needed for further recommendations.
## How to Get Started with the Model
Use the code below to get started with the model.
[More Information Needed]
## Training Details
### Training Data
<!-- This should link to a Dataset Card, perhaps with a short stub of information on what the training data is all about as well as documentation related to data pre-processing or additional filtering. -->
[More Information Needed]
### Training Procedure
<!-- This relates heavily to the Technical Specifications. Content here should link to that section when it is relevant to the training procedure. -->
#### Preprocessing [optional]
[More Information Needed]
#### Training Hyperparameters
- **Training regime:** [More Information Needed] <!--fp32, fp16 mixed precision, bf16 mixed precision, bf16 non-mixed precision, fp16 non-mixed precision, fp8 mixed precision -->
#### Speeds, Sizes, Times [optional]
<!-- This section provides information about throughput, start/end time, checkpoint size if relevant, etc. -->
[More Information Needed]
## Evaluation
<!-- This section describes the evaluation protocols and provides the results. -->
### Testing Data, Factors & Metrics
#### Testing Data
<!-- This should link to a Dataset Card if possible. -->
[More Information Needed]
#### Factors
<!-- These are the things the evaluation is disaggregating by, e.g., subpopulations or domains. -->
[More Information Needed]
#### Metrics
<!-- These are the evaluation metrics being used, ideally with a description of why. -->
[More Information Needed]
### Results
[More Information Needed]
#### Summary
## Model Examination [optional]
<!-- Relevant interpretability work for the model goes here -->
[More Information Needed]
## Environmental Impact
<!-- Total emissions (in grams of CO2eq) and additional considerations, such as electricity usage, go here. Edit the suggested text below accordingly -->
Carbon emissions can be estimated using the [Machine Learning Impact calculator](https://mlco2.github.io/impact#compute) presented in [Lacoste et al. (2019)](https://arxiv.org/abs/1910.09700).
- **Hardware Type:** [More Information Needed]
- **Hours used:** [More Information Needed]
- **Cloud Provider:** [More Information Needed]
- **Compute Region:** [More Information Needed]
- **Carbon Emitted:** [More Information Needed]
## Technical Specifications [optional]
### Model Architecture and Objective
[More Information Needed]
### Compute Infrastructure
[More Information Needed]
#### Hardware
[More Information Needed]
#### Software
[More Information Needed]
## Citation [optional]
<!-- If there is a paper or blog post introducing the model, the APA and Bibtex information for that should go in this section. -->
**BibTeX:**
[More Information Needed]
**APA:**
[More Information Needed]
## Glossary [optional]
<!-- If relevant, include terms and calculations in this section that can help readers understand the model or model card. -->
[More Information Needed]
## More Information [optional]
[More Information Needed]
## Model Card Authors [optional]
[More Information Needed]
## Model Card Contact
[More Information Needed] |
Felladrin/gguf-q5_k_l-imat-arcee-lite | Felladrin | "2024-08-14T18:11:31Z" | 5 | 0 | null | [
"gguf",
"base_model:arcee-ai/arcee-lite",
"base_model:quantized:arcee-ai/arcee-lite",
"license:apache-2.0",
"endpoints_compatible",
"region:us",
"imatrix",
"conversational"
] | null | "2024-08-14T18:03:06Z" | ---
license: apache-2.0
base_model: arcee-ai/arcee-lite
---
GGUF version of [arcee-ai/arcee-lite](https://huggingface.co/arcee-ai/arcee-lite).
|
BlueAvenir/sti_workplace_new_prompting_0_1 | BlueAvenir | "2023-06-20T10:53:50Z" | 3 | 0 | sentence-transformers | [
"sentence-transformers",
"pytorch",
"xlm-roberta",
"feature-extraction",
"sentence-similarity",
"transformers",
"autotrain_compatible",
"text-embeddings-inference",
"endpoints_compatible",
"region:us"
] | sentence-similarity | "2023-06-20T10:53:21Z" | ---
pipeline_tag: sentence-similarity
tags:
- sentence-transformers
- feature-extraction
- sentence-similarity
- transformers
---
# {MODEL_NAME}
This is a [sentence-transformers](https://www.SBERT.net) model: It maps sentences & paragraphs to a 768 dimensional dense vector space and can be used for tasks like clustering or semantic search.
<!--- Describe your model here -->
## Usage (Sentence-Transformers)
Using this model becomes easy when you have [sentence-transformers](https://www.SBERT.net) installed:
```
pip install -U sentence-transformers
```
Then you can use the model like this:
```python
from sentence_transformers import SentenceTransformer
sentences = ["This is an example sentence", "Each sentence is converted"]
model = SentenceTransformer('{MODEL_NAME}')
embeddings = model.encode(sentences)
print(embeddings)
```
## Usage (HuggingFace Transformers)
Without [sentence-transformers](https://www.SBERT.net), you can use the model like this: First, you pass your input through the transformer model, then you have to apply the right pooling-operation on-top of the contextualized word embeddings.
```python
from transformers import AutoTokenizer, AutoModel
import torch
#Mean Pooling - Take attention mask into account for correct averaging
def mean_pooling(model_output, attention_mask):
token_embeddings = model_output[0] #First element of model_output contains all token embeddings
input_mask_expanded = attention_mask.unsqueeze(-1).expand(token_embeddings.size()).float()
return torch.sum(token_embeddings * input_mask_expanded, 1) / torch.clamp(input_mask_expanded.sum(1), min=1e-9)
# Sentences we want sentence embeddings for
sentences = ['This is an example sentence', 'Each sentence is converted']
# Load model from HuggingFace Hub
tokenizer = AutoTokenizer.from_pretrained('{MODEL_NAME}')
model = AutoModel.from_pretrained('{MODEL_NAME}')
# Tokenize sentences
encoded_input = tokenizer(sentences, padding=True, truncation=True, return_tensors='pt')
# Compute token embeddings
with torch.no_grad():
model_output = model(**encoded_input)
# Perform pooling. In this case, mean pooling.
sentence_embeddings = mean_pooling(model_output, encoded_input['attention_mask'])
print("Sentence embeddings:")
print(sentence_embeddings)
```
## Evaluation Results
<!--- Describe how your model was evaluated -->
For an automated evaluation of this model, see the *Sentence Embeddings Benchmark*: [https://seb.sbert.net](https://seb.sbert.net?model_name={MODEL_NAME})
## Training
The model was trained with the parameters:
**DataLoader**:
`torch.utils.data.dataloader.DataLoader` of length 140 with parameters:
```
{'batch_size': 16, 'sampler': 'torch.utils.data.sampler.RandomSampler', 'batch_sampler': 'torch.utils.data.sampler.BatchSampler'}
```
**Loss**:
`sentence_transformers.losses.CosineSimilarityLoss.CosineSimilarityLoss`
Parameters of the fit()-Method:
```
{
"epochs": 1,
"evaluation_steps": 0,
"evaluator": "NoneType",
"max_grad_norm": 1,
"optimizer_class": "<class 'torch.optim.adamw.AdamW'>",
"optimizer_params": {
"lr": 2e-05
},
"scheduler": "WarmupLinear",
"steps_per_epoch": 140,
"warmup_steps": 14,
"weight_decay": 0.01
}
```
## Full Model Architecture
```
SentenceTransformer(
(0): Transformer({'max_seq_length': 512, 'do_lower_case': False}) with Transformer model: XLMRobertaModel
(1): Pooling({'word_embedding_dimension': 768, 'pooling_mode_cls_token': False, 'pooling_mode_mean_tokens': True, 'pooling_mode_max_tokens': False, 'pooling_mode_mean_sqrt_len_tokens': False})
)
```
## Citing & Authors
<!--- Describe where people can find more information --> |
soob3123/Veiled-Calla-12B-gguf | soob3123 | "2025-04-08T03:18:16Z" | 0 | 3 | transformers | [
"transformers",
"gguf",
"roleplay",
"creative-writing",
"immersive",
"mystery",
"storytelling",
"text-generation",
"en",
"base_model:soob3123/Veiled-Calla-12B",
"base_model:quantized:soob3123/Veiled-Calla-12B",
"license:apache-2.0",
"endpoints_compatible",
"region:us",
"conversational"
] | text-generation | "2025-04-07T16:57:44Z" | ---
pipeline_tag: text-generation
library_name: transformers
language:
- en
license: apache-2.0
thumbnail: "https://cdn-uploads.huggingface.co/production/uploads/62f93f9477b722f1866398c2/F4OF_WybPBIRXKcidNUdW.png"
tags:
- roleplay
- creative-writing
- immersive
- mystery
- storytelling
base_model:
- soob3123/Veiled-Calla-12B
---

# ✧ Veiled Calla ✧
> *Mystery is at the heart of creativity. That, and surprise...As creative channels, we need to trust the darkness.*
Beneath moonlight's gentle glow, Veiled Calla emerges - an enigmatic presence designed to weave immersive roleplay experiences through mysterious narratives and atmospheric storytelling. Shrouded in secrets and whispers, Veiled Calla crafts evocative scenarios where unspoken truths and subtle emotional undertones drive each unfolding tale.
## ⋆ Features ⋆
- **⟡ Atmospheric Depth**: Rich, moonlit scenarios bloom with subtle emotional undertones
- **⟡ Character Consistency**: Personalities remain true throughout extended journeys
- **⟡ Narrative Mystery**: Enigmatic storylines unfold with natural revelations
- **⟡ Emotional Nuance**: The unspoken and veiled meanings between characters come alive
## ⋆ Limitations ⋆
- Flourishes in intimate, atmospheric, or introspective scenarios
- May whisper overly cryptic responses in certain contexts
- Uncensored in Roleplay mode (e.g. sillytavern), still refuses in Assistant mode (no system prompt)
- Use one of the [Amoral models](https://huggingface.co/collections/soob3123/amoral-collection-67dccc556a39894b36f59676) for a fully uncensored but *bland* experience |
mradermacher/BuddyGlassUncensored2025.3-GGUF | mradermacher | "2025-03-02T19:58:22Z" | 0 | 0 | transformers | [
"transformers",
"gguf",
"mergekit",
"merge",
"en",
"base_model:darkc0de/BuddyGlassUncensored2025.3",
"base_model:quantized:darkc0de/BuddyGlassUncensored2025.3",
"endpoints_compatible",
"region:us",
"conversational"
] | null | "2025-03-02T18:48:45Z" | ---
base_model: darkc0de/BuddyGlassUncensored2025.3
language:
- en
library_name: transformers
quantized_by: mradermacher
tags:
- mergekit
- merge
---
## About
<!-- ### quantize_version: 2 -->
<!-- ### output_tensor_quantised: 1 -->
<!-- ### convert_type: hf -->
<!-- ### vocab_type: -->
<!-- ### tags: -->
static quants of https://huggingface.co/darkc0de/BuddyGlassUncensored2025.3
<!-- provided-files -->
weighted/imatrix quants are available at https://huggingface.co/mradermacher/BuddyGlassUncensored2025.3-i1-GGUF
## Usage
If you are unsure how to use GGUF files, refer to one of [TheBloke's
READMEs](https://huggingface.co/TheBloke/KafkaLM-70B-German-V0.1-GGUF) for
more details, including on how to concatenate multi-part files.
## Provided Quants
(sorted by size, not necessarily quality. IQ-quants are often preferable over similar sized non-IQ quants)
| Link | Type | Size/GB | Notes |
|:-----|:-----|--------:|:------|
| [GGUF](https://huggingface.co/mradermacher/BuddyGlassUncensored2025.3-GGUF/resolve/main/BuddyGlassUncensored2025.3.Q2_K.gguf) | Q2_K | 9.0 | |
| [GGUF](https://huggingface.co/mradermacher/BuddyGlassUncensored2025.3-GGUF/resolve/main/BuddyGlassUncensored2025.3.Q3_K_S.gguf) | Q3_K_S | 10.5 | |
| [GGUF](https://huggingface.co/mradermacher/BuddyGlassUncensored2025.3-GGUF/resolve/main/BuddyGlassUncensored2025.3.Q3_K_M.gguf) | Q3_K_M | 11.6 | lower quality |
| [GGUF](https://huggingface.co/mradermacher/BuddyGlassUncensored2025.3-GGUF/resolve/main/BuddyGlassUncensored2025.3.Q3_K_L.gguf) | Q3_K_L | 12.5 | |
| [GGUF](https://huggingface.co/mradermacher/BuddyGlassUncensored2025.3-GGUF/resolve/main/BuddyGlassUncensored2025.3.IQ4_XS.gguf) | IQ4_XS | 13.0 | |
| [GGUF](https://huggingface.co/mradermacher/BuddyGlassUncensored2025.3-GGUF/resolve/main/BuddyGlassUncensored2025.3.Q4_K_S.gguf) | Q4_K_S | 13.6 | fast, recommended |
| [GGUF](https://huggingface.co/mradermacher/BuddyGlassUncensored2025.3-GGUF/resolve/main/BuddyGlassUncensored2025.3.Q4_K_M.gguf) | Q4_K_M | 14.4 | fast, recommended |
| [GGUF](https://huggingface.co/mradermacher/BuddyGlassUncensored2025.3-GGUF/resolve/main/BuddyGlassUncensored2025.3.Q5_K_S.gguf) | Q5_K_S | 16.4 | |
| [GGUF](https://huggingface.co/mradermacher/BuddyGlassUncensored2025.3-GGUF/resolve/main/BuddyGlassUncensored2025.3.Q5_K_M.gguf) | Q5_K_M | 16.9 | |
| [GGUF](https://huggingface.co/mradermacher/BuddyGlassUncensored2025.3-GGUF/resolve/main/BuddyGlassUncensored2025.3.Q6_K.gguf) | Q6_K | 19.4 | very good quality |
| [GGUF](https://huggingface.co/mradermacher/BuddyGlassUncensored2025.3-GGUF/resolve/main/BuddyGlassUncensored2025.3.Q8_0.gguf) | Q8_0 | 25.2 | fast, best quality |
Here is a handy graph by ikawrakow comparing some lower-quality quant
types (lower is better):

And here are Artefact2's thoughts on the matter:
https://gist.github.com/Artefact2/b5f810600771265fc1e39442288e8ec9
## FAQ / Model Request
See https://huggingface.co/mradermacher/model_requests for some answers to
questions you might have and/or if you want some other model quantized.
## Thanks
I thank my company, [nethype GmbH](https://www.nethype.de/), for letting
me use its servers and providing upgrades to my workstation to enable
this work in my free time. Additional thanks to [@nicoboss](https://huggingface.co/nicoboss) for giving me access to his private supercomputer, enabling me to provide many more imatrix quants, at much higher quality, than I would otherwise be able to.
<!-- end -->
|
Haewade/Qwen2.5_1.5B_MED_0326 | Haewade | "2025-03-26T05:24:51Z" | 0 | 0 | transformers | [
"transformers",
"safetensors",
"qwen2",
"text-generation",
"trl",
"sft",
"conversational",
"arxiv:1910.09700",
"autotrain_compatible",
"text-generation-inference",
"endpoints_compatible",
"region:us"
] | text-generation | "2025-03-26T05:23:14Z" | ---
library_name: transformers
tags:
- trl
- sft
---
# Model Card for Model ID
<!-- Provide a quick summary of what the model is/does. -->
## Model Details
### Model Description
<!-- Provide a longer summary of what this model is. -->
This is the model card of a 🤗 transformers model that has been pushed on the Hub. This model card has been automatically generated.
- **Developed by:** [More Information Needed]
- **Funded by [optional]:** [More Information Needed]
- **Shared by [optional]:** [More Information Needed]
- **Model type:** [More Information Needed]
- **Language(s) (NLP):** [More Information Needed]
- **License:** [More Information Needed]
- **Finetuned from model [optional]:** [More Information Needed]
### Model Sources [optional]
<!-- Provide the basic links for the model. -->
- **Repository:** [More Information Needed]
- **Paper [optional]:** [More Information Needed]
- **Demo [optional]:** [More Information Needed]
## Uses
<!-- Address questions around how the model is intended to be used, including the foreseeable users of the model and those affected by the model. -->
### Direct Use
<!-- This section is for the model use without fine-tuning or plugging into a larger ecosystem/app. -->
[More Information Needed]
### Downstream Use [optional]
<!-- This section is for the model use when fine-tuned for a task, or when plugged into a larger ecosystem/app -->
[More Information Needed]
### Out-of-Scope Use
<!-- This section addresses misuse, malicious use, and uses that the model will not work well for. -->
[More Information Needed]
## Bias, Risks, and Limitations
<!-- This section is meant to convey both technical and sociotechnical limitations. -->
[More Information Needed]
### Recommendations
<!-- This section is meant to convey recommendations with respect to the bias, risk, and technical limitations. -->
Users (both direct and downstream) should be made aware of the risks, biases and limitations of the model. More information needed for further recommendations.
## How to Get Started with the Model
Use the code below to get started with the model.
[More Information Needed]
## Training Details
### Training Data
<!-- This should link to a Dataset Card, perhaps with a short stub of information on what the training data is all about as well as documentation related to data pre-processing or additional filtering. -->
[More Information Needed]
### Training Procedure
<!-- This relates heavily to the Technical Specifications. Content here should link to that section when it is relevant to the training procedure. -->
#### Preprocessing [optional]
[More Information Needed]
#### Training Hyperparameters
- **Training regime:** [More Information Needed] <!--fp32, fp16 mixed precision, bf16 mixed precision, bf16 non-mixed precision, fp16 non-mixed precision, fp8 mixed precision -->
#### Speeds, Sizes, Times [optional]
<!-- This section provides information about throughput, start/end time, checkpoint size if relevant, etc. -->
[More Information Needed]
## Evaluation
<!-- This section describes the evaluation protocols and provides the results. -->
### Testing Data, Factors & Metrics
#### Testing Data
<!-- This should link to a Dataset Card if possible. -->
[More Information Needed]
#### Factors
<!-- These are the things the evaluation is disaggregating by, e.g., subpopulations or domains. -->
[More Information Needed]
#### Metrics
<!-- These are the evaluation metrics being used, ideally with a description of why. -->
[More Information Needed]
### Results
[More Information Needed]
#### Summary
## Model Examination [optional]
<!-- Relevant interpretability work for the model goes here -->
[More Information Needed]
## Environmental Impact
<!-- Total emissions (in grams of CO2eq) and additional considerations, such as electricity usage, go here. Edit the suggested text below accordingly -->
Carbon emissions can be estimated using the [Machine Learning Impact calculator](https://mlco2.github.io/impact#compute) presented in [Lacoste et al. (2019)](https://arxiv.org/abs/1910.09700).
- **Hardware Type:** [More Information Needed]
- **Hours used:** [More Information Needed]
- **Cloud Provider:** [More Information Needed]
- **Compute Region:** [More Information Needed]
- **Carbon Emitted:** [More Information Needed]
## Technical Specifications [optional]
### Model Architecture and Objective
[More Information Needed]
### Compute Infrastructure
[More Information Needed]
#### Hardware
[More Information Needed]
#### Software
[More Information Needed]
## Citation [optional]
<!-- If there is a paper or blog post introducing the model, the APA and Bibtex information for that should go in this section. -->
**BibTeX:**
[More Information Needed]
**APA:**
[More Information Needed]
## Glossary [optional]
<!-- If relevant, include terms and calculations in this section that can help readers understand the model or model card. -->
[More Information Needed]
## More Information [optional]
[More Information Needed]
## Model Card Authors [optional]
[More Information Needed]
## Model Card Contact
[More Information Needed] |
fedovtt/38465c6d-ca2f-46fe-a0e5-238809c4ecd4 | fedovtt | "2025-01-16T03:30:58Z" | 8 | 0 | peft | [
"peft",
"safetensors",
"gemma2",
"axolotl",
"generated_from_trainer",
"base_model:UCLA-AGI/Gemma-2-9B-It-SPPO-Iter2",
"base_model:adapter:UCLA-AGI/Gemma-2-9B-It-SPPO-Iter2",
"license:gemma",
"region:us"
] | null | "2025-01-16T03:15:10Z" | ---
library_name: peft
license: gemma
base_model: UCLA-AGI/Gemma-2-9B-It-SPPO-Iter2
tags:
- axolotl
- generated_from_trainer
model-index:
- name: 38465c6d-ca2f-46fe-a0e5-238809c4ecd4
results: []
---
<!-- This model card has been generated automatically according to the information the Trainer had access to. You
should probably proofread and complete it, then remove this comment. -->
[<img src="https://raw.githubusercontent.com/axolotl-ai-cloud/axolotl/main/image/axolotl-badge-web.png" alt="Built with Axolotl" width="200" height="32"/>](https://github.com/axolotl-ai-cloud/axolotl)
<details><summary>See axolotl config</summary>
axolotl version: `0.4.1`
```yaml
adapter: lora
base_model: UCLA-AGI/Gemma-2-9B-It-SPPO-Iter2
bf16: auto
chat_template: llama3
dataset_prepared_path: null
datasets:
- data_files:
- c8ebc22e9ae8beec_train_data.json
ds_type: json
format: custom
path: /workspace/input_data/c8ebc22e9ae8beec_train_data.json
type:
field_instruction: prompt
field_output: ground_truth_chosen
format: '{instruction}'
no_input_format: '{instruction}'
system_format: '{system}'
system_prompt: ''
debug: null
deepspeed: null
device: cuda
early_stopping_patience: 1
eval_max_new_tokens: 128
eval_steps: 5
eval_table_size: null
evals_per_epoch: null
flash_attention: false
fp16: null
gradient_accumulation_steps: 4
gradient_checkpointing: true
group_by_length: false
hub_model_id: fedovtt/38465c6d-ca2f-46fe-a0e5-238809c4ecd4
hub_repo: null
hub_strategy: checkpoint
hub_token: null
learning_rate: 0.0002
load_in_4bit: false
load_in_8bit: false
local_rank: null
logging_steps: 3
lora_alpha: 32
lora_dropout: 0.05
lora_fan_in_fan_out: null
lora_model_dir: null
lora_r: 16
lora_target_linear: true
lr_scheduler: cosine
max_memory:
0: 78GiB
max_steps: 30
micro_batch_size: 2
mlflow_experiment_name: /tmp/c8ebc22e9ae8beec_train_data.json
model_type: AutoModelForCausalLM
num_epochs: 1
optimizer: adamw_torch
output_dir: miner_id_24
pad_to_sequence_len: true
resume_from_checkpoint: null
s2_attention: null
sample_packing: false
save_steps: 10
sequence_len: 1024
strict: false
tf32: false
tokenizer_type: AutoTokenizer
train_on_inputs: true
trust_remote_code: true
val_set_size: 0.05
wandb_entity: null
wandb_mode: online
wandb_name: fe050da3-380a-4bc7-8d1f-fbb5a3fbb54c
wandb_project: Gradients-On-Demand
wandb_run: your_name
wandb_runid: fe050da3-380a-4bc7-8d1f-fbb5a3fbb54c
warmup_steps: 10
weight_decay: 0.01
xformers_attention: true
```
</details><br>
# 38465c6d-ca2f-46fe-a0e5-238809c4ecd4
This model is a fine-tuned version of [UCLA-AGI/Gemma-2-9B-It-SPPO-Iter2](https://huggingface.co/UCLA-AGI/Gemma-2-9B-It-SPPO-Iter2) on the None dataset.
It achieves the following results on the evaluation set:
- Loss: 2.2283
## Model description
More information needed
## Intended uses & limitations
More information needed
## Training and evaluation data
More information needed
## Training procedure
### Training hyperparameters
The following hyperparameters were used during training:
- learning_rate: 0.0002
- train_batch_size: 2
- eval_batch_size: 2
- seed: 42
- gradient_accumulation_steps: 4
- total_train_batch_size: 8
- optimizer: Use OptimizerNames.ADAMW_TORCH with betas=(0.9,0.999) and epsilon=1e-08 and optimizer_args=No additional optimizer arguments
- lr_scheduler_type: cosine
- lr_scheduler_warmup_steps: 10
- training_steps: 30
### Training results
| Training Loss | Epoch | Step | Validation Loss |
|:-------------:|:------:|:----:|:---------------:|
| No log | 0.0005 | 1 | 3.4061 |
| 3.3856 | 0.0025 | 5 | 2.9154 |
| 2.5601 | 0.0050 | 10 | 2.4811 |
| 2.4358 | 0.0075 | 15 | 2.2901 |
| 2.3848 | 0.0100 | 20 | 2.2437 |
| 2.2058 | 0.0125 | 25 | 2.2310 |
| 2.2403 | 0.0149 | 30 | 2.2283 |
### Framework versions
- PEFT 0.13.2
- Transformers 4.46.0
- Pytorch 2.5.0+cu124
- Datasets 3.0.1
- Tokenizers 0.20.1 |
tomaszki/stablelm-41-a | tomaszki | "2024-04-20T13:18:04Z" | 90 | 0 | transformers | [
"transformers",
"safetensors",
"stablelm",
"text-generation",
"conversational",
"arxiv:1910.09700",
"autotrain_compatible",
"endpoints_compatible",
"region:us"
] | text-generation | "2024-04-20T13:16:42Z" | ---
library_name: transformers
tags: []
---
# Model Card for Model ID
<!-- Provide a quick summary of what the model is/does. -->
## Model Details
### Model Description
<!-- Provide a longer summary of what this model is. -->
This is the model card of a 🤗 transformers model that has been pushed on the Hub. This model card has been automatically generated.
- **Developed by:** [More Information Needed]
- **Funded by [optional]:** [More Information Needed]
- **Shared by [optional]:** [More Information Needed]
- **Model type:** [More Information Needed]
- **Language(s) (NLP):** [More Information Needed]
- **License:** [More Information Needed]
- **Finetuned from model [optional]:** [More Information Needed]
### Model Sources [optional]
<!-- Provide the basic links for the model. -->
- **Repository:** [More Information Needed]
- **Paper [optional]:** [More Information Needed]
- **Demo [optional]:** [More Information Needed]
## Uses
<!-- Address questions around how the model is intended to be used, including the foreseeable users of the model and those affected by the model. -->
### Direct Use
<!-- This section is for the model use without fine-tuning or plugging into a larger ecosystem/app. -->
[More Information Needed]
### Downstream Use [optional]
<!-- This section is for the model use when fine-tuned for a task, or when plugged into a larger ecosystem/app -->
[More Information Needed]
### Out-of-Scope Use
<!-- This section addresses misuse, malicious use, and uses that the model will not work well for. -->
[More Information Needed]
## Bias, Risks, and Limitations
<!-- This section is meant to convey both technical and sociotechnical limitations. -->
[More Information Needed]
### Recommendations
<!-- This section is meant to convey recommendations with respect to the bias, risk, and technical limitations. -->
Users (both direct and downstream) should be made aware of the risks, biases and limitations of the model. More information needed for further recommendations.
## How to Get Started with the Model
Use the code below to get started with the model.
[More Information Needed]
## Training Details
### Training Data
<!-- This should link to a Dataset Card, perhaps with a short stub of information on what the training data is all about as well as documentation related to data pre-processing or additional filtering. -->
[More Information Needed]
### Training Procedure
<!-- This relates heavily to the Technical Specifications. Content here should link to that section when it is relevant to the training procedure. -->
#### Preprocessing [optional]
[More Information Needed]
#### Training Hyperparameters
- **Training regime:** [More Information Needed] <!--fp32, fp16 mixed precision, bf16 mixed precision, bf16 non-mixed precision, fp16 non-mixed precision, fp8 mixed precision -->
#### Speeds, Sizes, Times [optional]
<!-- This section provides information about throughput, start/end time, checkpoint size if relevant, etc. -->
[More Information Needed]
## Evaluation
<!-- This section describes the evaluation protocols and provides the results. -->
### Testing Data, Factors & Metrics
#### Testing Data
<!-- This should link to a Dataset Card if possible. -->
[More Information Needed]
#### Factors
<!-- These are the things the evaluation is disaggregating by, e.g., subpopulations or domains. -->
[More Information Needed]
#### Metrics
<!-- These are the evaluation metrics being used, ideally with a description of why. -->
[More Information Needed]
### Results
[More Information Needed]
#### Summary
## Model Examination [optional]
<!-- Relevant interpretability work for the model goes here -->
[More Information Needed]
## Environmental Impact
<!-- Total emissions (in grams of CO2eq) and additional considerations, such as electricity usage, go here. Edit the suggested text below accordingly -->
Carbon emissions can be estimated using the [Machine Learning Impact calculator](https://mlco2.github.io/impact#compute) presented in [Lacoste et al. (2019)](https://arxiv.org/abs/1910.09700).
- **Hardware Type:** [More Information Needed]
- **Hours used:** [More Information Needed]
- **Cloud Provider:** [More Information Needed]
- **Compute Region:** [More Information Needed]
- **Carbon Emitted:** [More Information Needed]
## Technical Specifications [optional]
### Model Architecture and Objective
[More Information Needed]
### Compute Infrastructure
[More Information Needed]
#### Hardware
[More Information Needed]
#### Software
[More Information Needed]
## Citation [optional]
<!-- If there is a paper or blog post introducing the model, the APA and Bibtex information for that should go in this section. -->
**BibTeX:**
[More Information Needed]
**APA:**
[More Information Needed]
## Glossary [optional]
<!-- If relevant, include terms and calculations in this section that can help readers understand the model or model card. -->
[More Information Needed]
## More Information [optional]
[More Information Needed]
## Model Card Authors [optional]
[More Information Needed]
## Model Card Contact
[More Information Needed] |
avsolatorio/doc-topic-model_eval-00_train-03 | avsolatorio | "2024-09-15T17:41:47Z" | 72 | 0 | transformers | [
"transformers",
"safetensors",
"deberta-v2",
"text-classification",
"generated_from_trainer",
"base_model:microsoft/deberta-v3-small",
"base_model:finetune:microsoft/deberta-v3-small",
"license:mit",
"autotrain_compatible",
"endpoints_compatible",
"region:us"
] | text-classification | "2024-09-15T06:50:55Z" | ---
library_name: transformers
license: mit
base_model: microsoft/deberta-v3-small
tags:
- generated_from_trainer
metrics:
- accuracy
- f1
- precision
- recall
model-index:
- name: doc-topic-model_eval-00_train-03
results: []
---
<!-- This model card has been generated automatically according to the information the Trainer had access to. You
should probably proofread and complete it, then remove this comment. -->
# doc-topic-model_eval-00_train-03
This model is a fine-tuned version of [microsoft/deberta-v3-small](https://huggingface.co/microsoft/deberta-v3-small) on an unknown dataset.
It achieves the following results on the evaluation set:
- Loss: 0.0381
- Accuracy: 0.9878
- F1: 0.6228
- Precision: 0.7288
- Recall: 0.5437
## Model description
More information needed
## Intended uses & limitations
More information needed
## Training and evaluation data
More information needed
## Training procedure
### Training hyperparameters
The following hyperparameters were used during training:
- learning_rate: 2e-05
- train_batch_size: 4
- eval_batch_size: 256
- seed: 42
- optimizer: Adam with betas=(0.9,0.999) and epsilon=1e-08
- lr_scheduler_type: linear
- num_epochs: 100
- mixed_precision_training: Native AMP
### Training results
| Training Loss | Epoch | Step | Validation Loss | Accuracy | F1 | Precision | Recall |
|:-------------:|:------:|:-----:|:---------------:|:--------:|:------:|:---------:|:------:|
| 0.0935 | 0.4931 | 1000 | 0.0895 | 0.9815 | 0.0 | 0.0 | 0.0 |
| 0.0764 | 0.9862 | 2000 | 0.0700 | 0.9815 | 0.0 | 0.0 | 0.0 |
| 0.0621 | 1.4793 | 3000 | 0.0567 | 0.9821 | 0.0730 | 0.8925 | 0.0381 |
| 0.0542 | 1.9724 | 4000 | 0.0497 | 0.9841 | 0.2891 | 0.8391 | 0.1747 |
| 0.0468 | 2.4655 | 5000 | 0.0465 | 0.9853 | 0.4216 | 0.7739 | 0.2897 |
| 0.0441 | 2.9586 | 6000 | 0.0435 | 0.9861 | 0.4879 | 0.7667 | 0.3578 |
| 0.0395 | 3.4517 | 7000 | 0.0417 | 0.9862 | 0.5322 | 0.7197 | 0.4222 |
| 0.0384 | 3.9448 | 8000 | 0.0401 | 0.9866 | 0.5600 | 0.7182 | 0.4589 |
| 0.0343 | 4.4379 | 9000 | 0.0393 | 0.9870 | 0.5789 | 0.7217 | 0.4833 |
| 0.0337 | 4.9310 | 10000 | 0.0378 | 0.9873 | 0.5907 | 0.7358 | 0.4934 |
| 0.0305 | 5.4241 | 11000 | 0.0375 | 0.9875 | 0.5960 | 0.7457 | 0.4963 |
| 0.0295 | 5.9172 | 12000 | 0.0378 | 0.9874 | 0.6050 | 0.7213 | 0.5210 |
| 0.0271 | 6.4103 | 13000 | 0.0376 | 0.9877 | 0.6048 | 0.7457 | 0.5087 |
| 0.0257 | 6.9034 | 14000 | 0.0379 | 0.9875 | 0.6068 | 0.7269 | 0.5208 |
| 0.0234 | 7.3964 | 15000 | 0.0377 | 0.9876 | 0.6246 | 0.7108 | 0.5571 |
| 0.0241 | 7.8895 | 16000 | 0.0381 | 0.9878 | 0.6228 | 0.7288 | 0.5437 |
### Framework versions
- Transformers 4.44.2
- Pytorch 2.4.1+cu121
- Datasets 2.21.0
- Tokenizers 0.19.1
|
maharishiva/taxiv3-qtable | maharishiva | "2023-06-14T16:42:34Z" | 0 | 0 | null | [
"Taxi-v3",
"q-learning",
"reinforcement-learning",
"custom-implementation",
"model-index",
"region:us"
] | reinforcement-learning | "2023-06-14T16:08:12Z" | ---
tags:
- Taxi-v3
- q-learning
- reinforcement-learning
- custom-implementation
model-index:
- name: taxiv3-qtable
results:
- task:
type: reinforcement-learning
name: reinforcement-learning
dataset:
name: Taxi-v3
type: Taxi-v3
metrics:
- type: mean_reward
value: 7.00 +/- 2.71
name: mean_reward
verified: false
---
# **Q-Learning** Agent playing1 **Taxi-v3**
This is a trained model of a **Q-Learning** agent playing **Taxi-v3** .
## Usage
```python
model = load_from_hub(repo_id="maharishiva/taxiv3-qtable", filename="q-learning.pkl")
# Don't forget to check if you need to add additional attributes (is_slippery=False etc)
env = gym.make(model["env_id"])
```
|
outlookAi/ma8K4lIrnR | outlookAi | "2024-12-13T15:15:11Z" | 37 | 0 | diffusers | [
"diffusers",
"flux",
"lora",
"replicate",
"text-to-image",
"en",
"base_model:black-forest-labs/FLUX.1-dev",
"base_model:adapter:black-forest-labs/FLUX.1-dev",
"license:other",
"region:us"
] | text-to-image | "2024-12-13T14:42:50Z" | ---
license: other
license_name: flux-1-dev-non-commercial-license
license_link: https://huggingface.co/black-forest-labs/FLUX.1-dev/blob/main/LICENSE.md
language:
- en
tags:
- flux
- diffusers
- lora
- replicate
base_model: "black-forest-labs/FLUX.1-dev"
pipeline_tag: text-to-image
# widget:
# - text: >-
# prompt
# output:
# url: https://...
instance_prompt: NaturalTieDyeClothe
---
# Ma8K4Lirnr
<Gallery />
Trained on Replicate using:
https://replicate.com/ostris/flux-dev-lora-trainer/train
## Trigger words
You should use `NaturalTieDyeClothe` to trigger the image generation.
## Use it with the [🧨 diffusers library](https://github.com/huggingface/diffusers)
```py
from diffusers import AutoPipelineForText2Image
import torch
pipeline = AutoPipelineForText2Image.from_pretrained('black-forest-labs/FLUX.1-dev', torch_dtype=torch.float16).to('cuda')
pipeline.load_lora_weights('outlookAi/ma8K4lIrnR', weight_name='lora.safetensors')
image = pipeline('your prompt').images[0]
```
For more details, including weighting, merging and fusing LoRAs, check the [documentation on loading LoRAs in diffusers](https://huggingface.co/docs/diffusers/main/en/using-diffusers/loading_adapters)
|
MayBashendy/ArabicNewSplits6_FineTuningAraBERT_run1_AugV5_k11_task2_organization | MayBashendy | "2024-12-23T01:46:37Z" | 160 | 0 | transformers | [
"transformers",
"safetensors",
"bert",
"text-classification",
"generated_from_trainer",
"base_model:aubmindlab/bert-base-arabertv02",
"base_model:finetune:aubmindlab/bert-base-arabertv02",
"autotrain_compatible",
"endpoints_compatible",
"region:us"
] | text-classification | "2024-12-23T01:26:40Z" | ---
library_name: transformers
base_model: aubmindlab/bert-base-arabertv02
tags:
- generated_from_trainer
model-index:
- name: ArabicNewSplits6_FineTuningAraBERT_run1_AugV5_k11_task2_organization
results: []
---
<!-- This model card has been generated automatically according to the information the Trainer had access to. You
should probably proofread and complete it, then remove this comment. -->
# ArabicNewSplits6_FineTuningAraBERT_run1_AugV5_k11_task2_organization
This model is a fine-tuned version of [aubmindlab/bert-base-arabertv02](https://huggingface.co/aubmindlab/bert-base-arabertv02) on the None dataset.
It achieves the following results on the evaluation set:
- Loss: 0.7534
- Qwk: 0.5269
- Mse: 0.7534
- Rmse: 0.8680
## Model description
More information needed
## Intended uses & limitations
More information needed
## Training and evaluation data
More information needed
## Training procedure
### Training hyperparameters
The following hyperparameters were used during training:
- learning_rate: 2e-05
- train_batch_size: 8
- eval_batch_size: 8
- seed: 42
- optimizer: Adam with betas=(0.9,0.999) and epsilon=1e-08
- lr_scheduler_type: linear
- num_epochs: 10
### Training results
| Training Loss | Epoch | Step | Validation Loss | Qwk | Mse | Rmse |
|:-------------:|:------:|:----:|:---------------:|:-------:|:------:|:------:|
| No log | 0.0364 | 2 | 4.1649 | -0.0344 | 4.1649 | 2.0408 |
| No log | 0.0727 | 4 | 2.1025 | 0.0383 | 2.1025 | 1.4500 |
| No log | 0.1091 | 6 | 1.1074 | 0.0864 | 1.1074 | 1.0523 |
| No log | 0.1455 | 8 | 0.7769 | 0.0224 | 0.7769 | 0.8814 |
| No log | 0.1818 | 10 | 1.1168 | -0.0742 | 1.1168 | 1.0568 |
| No log | 0.2182 | 12 | 1.4714 | -0.0125 | 1.4714 | 1.2130 |
| No log | 0.2545 | 14 | 1.0077 | -0.0325 | 1.0077 | 1.0038 |
| No log | 0.2909 | 16 | 0.8048 | 0.0259 | 0.8048 | 0.8971 |
| No log | 0.3273 | 18 | 0.8726 | 0.0075 | 0.8726 | 0.9341 |
| No log | 0.3636 | 20 | 1.0809 | 0.0505 | 1.0809 | 1.0397 |
| No log | 0.4 | 22 | 1.3637 | 0.1343 | 1.3637 | 1.1678 |
| No log | 0.4364 | 24 | 0.9114 | 0.2126 | 0.9114 | 0.9547 |
| No log | 0.4727 | 26 | 0.6360 | 0.4045 | 0.6360 | 0.7975 |
| No log | 0.5091 | 28 | 0.6410 | 0.2362 | 0.6410 | 0.8006 |
| No log | 0.5455 | 30 | 0.6304 | 0.2880 | 0.6304 | 0.7940 |
| No log | 0.5818 | 32 | 0.5998 | 0.4219 | 0.5998 | 0.7745 |
| No log | 0.6182 | 34 | 0.8601 | 0.3157 | 0.8601 | 0.9274 |
| No log | 0.6545 | 36 | 1.1621 | 0.2472 | 1.1621 | 1.0780 |
| No log | 0.6909 | 38 | 0.9940 | 0.2413 | 0.9940 | 0.9970 |
| No log | 0.7273 | 40 | 0.7382 | 0.3496 | 0.7382 | 0.8592 |
| No log | 0.7636 | 42 | 0.7188 | 0.3358 | 0.7188 | 0.8478 |
| No log | 0.8 | 44 | 0.7843 | 0.2880 | 0.7843 | 0.8856 |
| No log | 0.8364 | 46 | 0.7830 | 0.2665 | 0.7830 | 0.8849 |
| No log | 0.8727 | 48 | 0.8769 | 0.1876 | 0.8769 | 0.9364 |
| No log | 0.9091 | 50 | 0.8270 | 0.1838 | 0.8270 | 0.9094 |
| No log | 0.9455 | 52 | 0.8741 | 0.1672 | 0.8741 | 0.9349 |
| No log | 0.9818 | 54 | 0.9622 | 0.1687 | 0.9622 | 0.9809 |
| No log | 1.0182 | 56 | 0.8931 | 0.1717 | 0.8931 | 0.9450 |
| No log | 1.0545 | 58 | 0.8435 | 0.2133 | 0.8435 | 0.9184 |
| No log | 1.0909 | 60 | 0.8848 | 0.1922 | 0.8848 | 0.9406 |
| No log | 1.1273 | 62 | 0.8033 | 0.2382 | 0.8033 | 0.8963 |
| No log | 1.1636 | 64 | 0.6971 | 0.3141 | 0.6971 | 0.8349 |
| No log | 1.2 | 66 | 0.6208 | 0.3399 | 0.6208 | 0.7879 |
| No log | 1.2364 | 68 | 0.6167 | 0.3498 | 0.6167 | 0.7853 |
| No log | 1.2727 | 70 | 0.6237 | 0.4192 | 0.6237 | 0.7898 |
| No log | 1.3091 | 72 | 0.6301 | 0.3594 | 0.6301 | 0.7938 |
| No log | 1.3455 | 74 | 0.6187 | 0.4528 | 0.6187 | 0.7866 |
| No log | 1.3818 | 76 | 0.6567 | 0.3804 | 0.6567 | 0.8103 |
| No log | 1.4182 | 78 | 0.8940 | 0.3253 | 0.8940 | 0.9455 |
| No log | 1.4545 | 80 | 1.2209 | 0.2809 | 1.2209 | 1.1050 |
| No log | 1.4909 | 82 | 1.1155 | 0.2915 | 1.1155 | 1.0562 |
| No log | 1.5273 | 84 | 0.8804 | 0.3128 | 0.8804 | 0.9383 |
| No log | 1.5636 | 86 | 0.6803 | 0.3575 | 0.6803 | 0.8248 |
| No log | 1.6 | 88 | 0.6335 | 0.3717 | 0.6335 | 0.7959 |
| No log | 1.6364 | 90 | 0.6238 | 0.4386 | 0.6238 | 0.7898 |
| No log | 1.6727 | 92 | 0.6117 | 0.4066 | 0.6117 | 0.7821 |
| No log | 1.7091 | 94 | 0.6518 | 0.3823 | 0.6518 | 0.8073 |
| No log | 1.7455 | 96 | 0.7767 | 0.3822 | 0.7767 | 0.8813 |
| No log | 1.7818 | 98 | 0.8164 | 0.3716 | 0.8164 | 0.9036 |
| No log | 1.8182 | 100 | 0.6944 | 0.4053 | 0.6944 | 0.8333 |
| No log | 1.8545 | 102 | 0.6177 | 0.4605 | 0.6177 | 0.7860 |
| No log | 1.8909 | 104 | 0.6181 | 0.4633 | 0.6181 | 0.7862 |
| No log | 1.9273 | 106 | 0.6152 | 0.4497 | 0.6152 | 0.7844 |
| No log | 1.9636 | 108 | 0.6409 | 0.4362 | 0.6409 | 0.8005 |
| No log | 2.0 | 110 | 0.6307 | 0.4441 | 0.6307 | 0.7942 |
| No log | 2.0364 | 112 | 0.6236 | 0.4572 | 0.6236 | 0.7897 |
| No log | 2.0727 | 114 | 0.6457 | 0.4315 | 0.6457 | 0.8035 |
| No log | 2.1091 | 116 | 0.6358 | 0.4315 | 0.6358 | 0.7974 |
| No log | 2.1455 | 118 | 0.6054 | 0.4280 | 0.6054 | 0.7781 |
| No log | 2.1818 | 120 | 0.6570 | 0.4200 | 0.6570 | 0.8106 |
| No log | 2.2182 | 122 | 0.6874 | 0.4424 | 0.6874 | 0.8291 |
| No log | 2.2545 | 124 | 0.6695 | 0.4524 | 0.6695 | 0.8182 |
| No log | 2.2909 | 126 | 0.7255 | 0.4609 | 0.7255 | 0.8518 |
| No log | 2.3273 | 128 | 0.8598 | 0.3868 | 0.8598 | 0.9272 |
| No log | 2.3636 | 130 | 0.7983 | 0.4169 | 0.7983 | 0.8935 |
| No log | 2.4 | 132 | 0.7177 | 0.4214 | 0.7177 | 0.8472 |
| No log | 2.4364 | 134 | 0.8678 | 0.3185 | 0.8678 | 0.9315 |
| No log | 2.4727 | 136 | 0.8864 | 0.2911 | 0.8864 | 0.9415 |
| No log | 2.5091 | 138 | 0.7567 | 0.4185 | 0.7567 | 0.8699 |
| No log | 2.5455 | 140 | 0.7226 | 0.4412 | 0.7226 | 0.8500 |
| No log | 2.5818 | 142 | 0.8395 | 0.3446 | 0.8395 | 0.9162 |
| No log | 2.6182 | 144 | 0.7830 | 0.4060 | 0.7830 | 0.8849 |
| No log | 2.6545 | 146 | 0.6553 | 0.4096 | 0.6553 | 0.8095 |
| No log | 2.6909 | 148 | 0.6257 | 0.4516 | 0.6257 | 0.7910 |
| No log | 2.7273 | 150 | 0.6736 | 0.4471 | 0.6736 | 0.8207 |
| No log | 2.7636 | 152 | 0.6432 | 0.4547 | 0.6432 | 0.8020 |
| No log | 2.8 | 154 | 0.6295 | 0.4541 | 0.6295 | 0.7934 |
| No log | 2.8364 | 156 | 0.6920 | 0.3983 | 0.6920 | 0.8319 |
| No log | 2.8727 | 158 | 0.7012 | 0.4077 | 0.7012 | 0.8374 |
| No log | 2.9091 | 160 | 0.7006 | 0.3809 | 0.7006 | 0.8370 |
| No log | 2.9455 | 162 | 0.6124 | 0.4134 | 0.6124 | 0.7825 |
| No log | 2.9818 | 164 | 0.5959 | 0.4424 | 0.5959 | 0.7719 |
| No log | 3.0182 | 166 | 0.6071 | 0.4536 | 0.6071 | 0.7792 |
| No log | 3.0545 | 168 | 0.6095 | 0.4324 | 0.6095 | 0.7807 |
| No log | 3.0909 | 170 | 0.6743 | 0.4262 | 0.6743 | 0.8211 |
| No log | 3.1273 | 172 | 0.8221 | 0.3223 | 0.8221 | 0.9067 |
| No log | 3.1636 | 174 | 0.8169 | 0.3829 | 0.8169 | 0.9038 |
| No log | 3.2 | 176 | 0.7074 | 0.4843 | 0.7074 | 0.8411 |
| No log | 3.2364 | 178 | 0.7287 | 0.4566 | 0.7287 | 0.8537 |
| No log | 3.2727 | 180 | 0.7314 | 0.4558 | 0.7314 | 0.8552 |
| No log | 3.3091 | 182 | 0.7034 | 0.5034 | 0.7034 | 0.8387 |
| No log | 3.3455 | 184 | 0.7140 | 0.4071 | 0.7140 | 0.8450 |
| No log | 3.3818 | 186 | 0.7377 | 0.3889 | 0.7377 | 0.8589 |
| No log | 3.4182 | 188 | 0.7759 | 0.3907 | 0.7759 | 0.8808 |
| No log | 3.4545 | 190 | 0.7346 | 0.3867 | 0.7346 | 0.8571 |
| No log | 3.4909 | 192 | 0.7165 | 0.4799 | 0.7165 | 0.8465 |
| No log | 3.5273 | 194 | 0.7514 | 0.5249 | 0.7514 | 0.8669 |
| No log | 3.5636 | 196 | 0.7548 | 0.5290 | 0.7548 | 0.8688 |
| No log | 3.6 | 198 | 0.7450 | 0.4340 | 0.7450 | 0.8631 |
| No log | 3.6364 | 200 | 0.7875 | 0.4 | 0.7875 | 0.8874 |
| No log | 3.6727 | 202 | 0.8068 | 0.3749 | 0.8068 | 0.8982 |
| No log | 3.7091 | 204 | 0.7569 | 0.4 | 0.7569 | 0.8700 |
| No log | 3.7455 | 206 | 0.7004 | 0.4716 | 0.7004 | 0.8369 |
| No log | 3.7818 | 208 | 0.7074 | 0.5606 | 0.7074 | 0.8411 |
| No log | 3.8182 | 210 | 0.7286 | 0.5726 | 0.7286 | 0.8536 |
| No log | 3.8545 | 212 | 0.7282 | 0.5665 | 0.7282 | 0.8533 |
| No log | 3.8909 | 214 | 0.7067 | 0.4982 | 0.7067 | 0.8407 |
| No log | 3.9273 | 216 | 0.7059 | 0.4886 | 0.7059 | 0.8402 |
| No log | 3.9636 | 218 | 0.7070 | 0.5079 | 0.7070 | 0.8408 |
| No log | 4.0 | 220 | 0.7232 | 0.5361 | 0.7232 | 0.8504 |
| No log | 4.0364 | 222 | 0.7179 | 0.5 | 0.7179 | 0.8473 |
| No log | 4.0727 | 224 | 0.7105 | 0.4897 | 0.7105 | 0.8429 |
| No log | 4.1091 | 226 | 0.7147 | 0.5 | 0.7147 | 0.8454 |
| No log | 4.1455 | 228 | 0.7185 | 0.4991 | 0.7185 | 0.8477 |
| No log | 4.1818 | 230 | 0.7175 | 0.5015 | 0.7175 | 0.8471 |
| No log | 4.2182 | 232 | 0.7162 | 0.4956 | 0.7162 | 0.8463 |
| No log | 4.2545 | 234 | 0.7130 | 0.4607 | 0.7130 | 0.8444 |
| No log | 4.2909 | 236 | 0.7170 | 0.4498 | 0.7170 | 0.8468 |
| No log | 4.3273 | 238 | 0.7455 | 0.4632 | 0.7455 | 0.8634 |
| No log | 4.3636 | 240 | 0.8532 | 0.4489 | 0.8532 | 0.9237 |
| No log | 4.4 | 242 | 0.8777 | 0.4373 | 0.8777 | 0.9369 |
| No log | 4.4364 | 244 | 0.7637 | 0.4527 | 0.7637 | 0.8739 |
| No log | 4.4727 | 246 | 0.6981 | 0.4737 | 0.6981 | 0.8356 |
| No log | 4.5091 | 248 | 0.6705 | 0.4991 | 0.6705 | 0.8189 |
| No log | 4.5455 | 250 | 0.6854 | 0.4741 | 0.6854 | 0.8279 |
| No log | 4.5818 | 252 | 0.6733 | 0.4773 | 0.6733 | 0.8206 |
| No log | 4.6182 | 254 | 0.6617 | 0.4773 | 0.6617 | 0.8134 |
| No log | 4.6545 | 256 | 0.6700 | 0.4690 | 0.6700 | 0.8186 |
| No log | 4.6909 | 258 | 0.6654 | 0.4946 | 0.6654 | 0.8157 |
| No log | 4.7273 | 260 | 0.6690 | 0.4891 | 0.6690 | 0.8179 |
| No log | 4.7636 | 262 | 0.6669 | 0.5074 | 0.6669 | 0.8166 |
| No log | 4.8 | 264 | 0.7178 | 0.4886 | 0.7178 | 0.8473 |
| No log | 4.8364 | 266 | 0.7931 | 0.4653 | 0.7931 | 0.8905 |
| No log | 4.8727 | 268 | 0.8316 | 0.4764 | 0.8316 | 0.9119 |
| No log | 4.9091 | 270 | 0.8159 | 0.4793 | 0.8159 | 0.9033 |
| No log | 4.9455 | 272 | 0.7762 | 0.4722 | 0.7762 | 0.8810 |
| No log | 4.9818 | 274 | 0.7703 | 0.4405 | 0.7703 | 0.8777 |
| No log | 5.0182 | 276 | 0.7975 | 0.4293 | 0.7975 | 0.8930 |
| No log | 5.0545 | 278 | 0.8214 | 0.4533 | 0.8214 | 0.9063 |
| No log | 5.0909 | 280 | 0.8231 | 0.4217 | 0.8231 | 0.9073 |
| No log | 5.1273 | 282 | 0.8029 | 0.4085 | 0.8029 | 0.8961 |
| No log | 5.1636 | 284 | 0.7874 | 0.4005 | 0.7874 | 0.8873 |
| No log | 5.2 | 286 | 0.7566 | 0.4277 | 0.7566 | 0.8699 |
| No log | 5.2364 | 288 | 0.7237 | 0.4257 | 0.7237 | 0.8507 |
| No log | 5.2727 | 290 | 0.6925 | 0.4456 | 0.6925 | 0.8322 |
| No log | 5.3091 | 292 | 0.7069 | 0.4944 | 0.7069 | 0.8407 |
| No log | 5.3455 | 294 | 0.7403 | 0.4788 | 0.7403 | 0.8604 |
| No log | 5.3818 | 296 | 0.7387 | 0.4847 | 0.7387 | 0.8595 |
| No log | 5.4182 | 298 | 0.6699 | 0.4853 | 0.6699 | 0.8184 |
| No log | 5.4545 | 300 | 0.6389 | 0.5300 | 0.6389 | 0.7993 |
| No log | 5.4909 | 302 | 0.6485 | 0.5173 | 0.6485 | 0.8053 |
| No log | 5.5273 | 304 | 0.6973 | 0.5270 | 0.6973 | 0.8351 |
| No log | 5.5636 | 306 | 0.7459 | 0.5041 | 0.7459 | 0.8636 |
| No log | 5.6 | 308 | 0.7818 | 0.5206 | 0.7818 | 0.8842 |
| No log | 5.6364 | 310 | 0.7823 | 0.5206 | 0.7823 | 0.8845 |
| No log | 5.6727 | 312 | 0.7644 | 0.5157 | 0.7644 | 0.8743 |
| No log | 5.7091 | 314 | 0.7426 | 0.5323 | 0.7426 | 0.8617 |
| No log | 5.7455 | 316 | 0.7529 | 0.5296 | 0.7529 | 0.8677 |
| No log | 5.7818 | 318 | 0.7682 | 0.5157 | 0.7682 | 0.8765 |
| No log | 5.8182 | 320 | 0.7586 | 0.5253 | 0.7586 | 0.8710 |
| No log | 5.8545 | 322 | 0.7493 | 0.5395 | 0.7493 | 0.8656 |
| No log | 5.8909 | 324 | 0.7319 | 0.5461 | 0.7319 | 0.8555 |
| No log | 5.9273 | 326 | 0.7370 | 0.5185 | 0.7370 | 0.8585 |
| No log | 5.9636 | 328 | 0.7495 | 0.5323 | 0.7495 | 0.8657 |
| No log | 6.0 | 330 | 0.7356 | 0.5128 | 0.7356 | 0.8577 |
| No log | 6.0364 | 332 | 0.7148 | 0.5413 | 0.7148 | 0.8455 |
| No log | 6.0727 | 334 | 0.7201 | 0.5488 | 0.7201 | 0.8486 |
| No log | 6.1091 | 336 | 0.7349 | 0.5137 | 0.7349 | 0.8573 |
| No log | 6.1455 | 338 | 0.7392 | 0.4981 | 0.7392 | 0.8598 |
| No log | 6.1818 | 340 | 0.7576 | 0.5064 | 0.7576 | 0.8704 |
| No log | 6.2182 | 342 | 0.7625 | 0.5064 | 0.7625 | 0.8732 |
| No log | 6.2545 | 344 | 0.7348 | 0.4949 | 0.7348 | 0.8572 |
| No log | 6.2909 | 346 | 0.7309 | 0.4842 | 0.7309 | 0.8549 |
| No log | 6.3273 | 348 | 0.7366 | 0.4960 | 0.7366 | 0.8583 |
| No log | 6.3636 | 350 | 0.7542 | 0.4863 | 0.7542 | 0.8684 |
| No log | 6.4 | 352 | 0.7632 | 0.4799 | 0.7632 | 0.8736 |
| No log | 6.4364 | 354 | 0.7683 | 0.4867 | 0.7683 | 0.8765 |
| No log | 6.4727 | 356 | 0.7968 | 0.5105 | 0.7968 | 0.8926 |
| No log | 6.5091 | 358 | 0.8258 | 0.5115 | 0.8258 | 0.9087 |
| No log | 6.5455 | 360 | 0.8135 | 0.5246 | 0.8135 | 0.9020 |
| No log | 6.5818 | 362 | 0.7678 | 0.5140 | 0.7678 | 0.8763 |
| No log | 6.6182 | 364 | 0.7551 | 0.4853 | 0.7551 | 0.8689 |
| No log | 6.6545 | 366 | 0.7508 | 0.4860 | 0.7508 | 0.8665 |
| No log | 6.6909 | 368 | 0.7612 | 0.5433 | 0.7612 | 0.8725 |
| No log | 6.7273 | 370 | 0.8071 | 0.4904 | 0.8071 | 0.8984 |
| No log | 6.7636 | 372 | 0.8476 | 0.4756 | 0.8476 | 0.9206 |
| No log | 6.8 | 374 | 0.8437 | 0.4809 | 0.8437 | 0.9186 |
| No log | 6.8364 | 376 | 0.7939 | 0.4910 | 0.7939 | 0.8910 |
| No log | 6.8727 | 378 | 0.7263 | 0.5200 | 0.7263 | 0.8522 |
| No log | 6.9091 | 380 | 0.6930 | 0.5133 | 0.6930 | 0.8325 |
| No log | 6.9455 | 382 | 0.6908 | 0.4907 | 0.6908 | 0.8312 |
| No log | 6.9818 | 384 | 0.6975 | 0.5133 | 0.6975 | 0.8351 |
| No log | 7.0182 | 386 | 0.7208 | 0.4785 | 0.7208 | 0.8490 |
| No log | 7.0545 | 388 | 0.7620 | 0.5153 | 0.7620 | 0.8729 |
| No log | 7.0909 | 390 | 0.7817 | 0.5134 | 0.7817 | 0.8841 |
| No log | 7.1273 | 392 | 0.7710 | 0.4864 | 0.7710 | 0.8781 |
| No log | 7.1636 | 394 | 0.7573 | 0.4778 | 0.7573 | 0.8702 |
| No log | 7.2 | 396 | 0.7535 | 0.4880 | 0.7535 | 0.8680 |
| No log | 7.2364 | 398 | 0.7480 | 0.4814 | 0.7480 | 0.8649 |
| No log | 7.2727 | 400 | 0.7532 | 0.4699 | 0.7532 | 0.8679 |
| No log | 7.3091 | 402 | 0.7475 | 0.4773 | 0.7475 | 0.8646 |
| No log | 7.3455 | 404 | 0.7473 | 0.4822 | 0.7473 | 0.8645 |
| No log | 7.3818 | 406 | 0.7539 | 0.4822 | 0.7539 | 0.8683 |
| No log | 7.4182 | 408 | 0.7503 | 0.4822 | 0.7503 | 0.8662 |
| No log | 7.4545 | 410 | 0.7553 | 0.4816 | 0.7553 | 0.8691 |
| No log | 7.4909 | 412 | 0.7430 | 0.4835 | 0.7430 | 0.8620 |
| No log | 7.5273 | 414 | 0.7321 | 0.5146 | 0.7321 | 0.8556 |
| No log | 7.5636 | 416 | 0.7297 | 0.4961 | 0.7297 | 0.8543 |
| No log | 7.6 | 418 | 0.7320 | 0.4961 | 0.7320 | 0.8556 |
| No log | 7.6364 | 420 | 0.7487 | 0.5013 | 0.7487 | 0.8653 |
| No log | 7.6727 | 422 | 0.7736 | 0.5035 | 0.7736 | 0.8795 |
| No log | 7.7091 | 424 | 0.7904 | 0.4950 | 0.7904 | 0.8890 |
| No log | 7.7455 | 426 | 0.8016 | 0.5115 | 0.8016 | 0.8953 |
| No log | 7.7818 | 428 | 0.8042 | 0.5115 | 0.8042 | 0.8967 |
| No log | 7.8182 | 430 | 0.7908 | 0.5115 | 0.7908 | 0.8893 |
| No log | 7.8545 | 432 | 0.7622 | 0.5193 | 0.7622 | 0.8730 |
| No log | 7.8909 | 434 | 0.7367 | 0.5350 | 0.7367 | 0.8583 |
| No log | 7.9273 | 436 | 0.7205 | 0.5446 | 0.7205 | 0.8489 |
| No log | 7.9636 | 438 | 0.7221 | 0.5350 | 0.7221 | 0.8498 |
| No log | 8.0 | 440 | 0.7295 | 0.5350 | 0.7295 | 0.8541 |
| No log | 8.0364 | 442 | 0.7481 | 0.5193 | 0.7481 | 0.8649 |
| No log | 8.0727 | 444 | 0.7511 | 0.5193 | 0.7511 | 0.8666 |
| No log | 8.1091 | 446 | 0.7528 | 0.5193 | 0.7528 | 0.8676 |
| No log | 8.1455 | 448 | 0.7533 | 0.5193 | 0.7533 | 0.8679 |
| No log | 8.1818 | 450 | 0.7464 | 0.5193 | 0.7464 | 0.8639 |
| No log | 8.2182 | 452 | 0.7346 | 0.5203 | 0.7346 | 0.8571 |
| No log | 8.2545 | 454 | 0.7159 | 0.5473 | 0.7159 | 0.8461 |
| No log | 8.2909 | 456 | 0.7073 | 0.5441 | 0.7073 | 0.8410 |
| No log | 8.3273 | 458 | 0.7061 | 0.5441 | 0.7061 | 0.8403 |
| No log | 8.3636 | 460 | 0.7144 | 0.5621 | 0.7144 | 0.8452 |
| No log | 8.4 | 462 | 0.7379 | 0.5208 | 0.7379 | 0.8590 |
| No log | 8.4364 | 464 | 0.7512 | 0.5128 | 0.7512 | 0.8667 |
| No log | 8.4727 | 466 | 0.7523 | 0.5128 | 0.7523 | 0.8673 |
| No log | 8.5091 | 468 | 0.7527 | 0.5128 | 0.7527 | 0.8676 |
| No log | 8.5455 | 470 | 0.7440 | 0.5173 | 0.7440 | 0.8626 |
| No log | 8.5818 | 472 | 0.7375 | 0.5314 | 0.7375 | 0.8588 |
| No log | 8.6182 | 474 | 0.7480 | 0.5173 | 0.7480 | 0.8649 |
| No log | 8.6545 | 476 | 0.7501 | 0.5173 | 0.7501 | 0.8661 |
| No log | 8.6909 | 478 | 0.7419 | 0.4941 | 0.7419 | 0.8613 |
| No log | 8.7273 | 480 | 0.7408 | 0.4824 | 0.7408 | 0.8607 |
| No log | 8.7636 | 482 | 0.7405 | 0.4983 | 0.7405 | 0.8605 |
| No log | 8.8 | 484 | 0.7498 | 0.4974 | 0.7498 | 0.8659 |
| No log | 8.8364 | 486 | 0.7646 | 0.4829 | 0.7646 | 0.8744 |
| No log | 8.8727 | 488 | 0.7787 | 0.4816 | 0.7787 | 0.8825 |
| No log | 8.9091 | 490 | 0.7865 | 0.4810 | 0.7865 | 0.8868 |
| No log | 8.9455 | 492 | 0.7914 | 0.4810 | 0.7914 | 0.8896 |
| No log | 8.9818 | 494 | 0.7948 | 0.4804 | 0.7948 | 0.8915 |
| No log | 9.0182 | 496 | 0.7895 | 0.4810 | 0.7895 | 0.8885 |
| No log | 9.0545 | 498 | 0.7809 | 0.4816 | 0.7809 | 0.8837 |
| 0.4234 | 9.0909 | 500 | 0.7815 | 0.4816 | 0.7815 | 0.8840 |
| 0.4234 | 9.1273 | 502 | 0.7846 | 0.4816 | 0.7846 | 0.8858 |
| 0.4234 | 9.1636 | 504 | 0.7853 | 0.4997 | 0.7853 | 0.8862 |
| 0.4234 | 9.2 | 506 | 0.7804 | 0.4997 | 0.7804 | 0.8834 |
| 0.4234 | 9.2364 | 508 | 0.7781 | 0.4997 | 0.7781 | 0.8821 |
| 0.4234 | 9.2727 | 510 | 0.7742 | 0.4997 | 0.7742 | 0.8799 |
| 0.4234 | 9.3091 | 512 | 0.7712 | 0.4989 | 0.7712 | 0.8782 |
| 0.4234 | 9.3455 | 514 | 0.7690 | 0.4989 | 0.7690 | 0.8769 |
| 0.4234 | 9.3818 | 516 | 0.7642 | 0.5127 | 0.7642 | 0.8742 |
| 0.4234 | 9.4182 | 518 | 0.7591 | 0.5269 | 0.7591 | 0.8713 |
| 0.4234 | 9.4545 | 520 | 0.7548 | 0.5269 | 0.7548 | 0.8688 |
| 0.4234 | 9.4909 | 522 | 0.7505 | 0.5269 | 0.7505 | 0.8663 |
| 0.4234 | 9.5273 | 524 | 0.7487 | 0.5147 | 0.7487 | 0.8653 |
| 0.4234 | 9.5636 | 526 | 0.7482 | 0.5147 | 0.7482 | 0.8650 |
| 0.4234 | 9.6 | 528 | 0.7500 | 0.5269 | 0.7500 | 0.8660 |
| 0.4234 | 9.6364 | 530 | 0.7534 | 0.5269 | 0.7534 | 0.8680 |
| 0.4234 | 9.6727 | 532 | 0.7542 | 0.5269 | 0.7542 | 0.8685 |
| 0.4234 | 9.7091 | 534 | 0.7547 | 0.5269 | 0.7547 | 0.8687 |
| 0.4234 | 9.7455 | 536 | 0.7554 | 0.5269 | 0.7554 | 0.8691 |
| 0.4234 | 9.7818 | 538 | 0.7546 | 0.5269 | 0.7546 | 0.8686 |
| 0.4234 | 9.8182 | 540 | 0.7533 | 0.5269 | 0.7533 | 0.8680 |
| 0.4234 | 9.8545 | 542 | 0.7522 | 0.5269 | 0.7522 | 0.8673 |
| 0.4234 | 9.8909 | 544 | 0.7519 | 0.5269 | 0.7519 | 0.8671 |
| 0.4234 | 9.9273 | 546 | 0.7524 | 0.5269 | 0.7524 | 0.8674 |
| 0.4234 | 9.9636 | 548 | 0.7531 | 0.5269 | 0.7531 | 0.8678 |
| 0.4234 | 10.0 | 550 | 0.7534 | 0.5269 | 0.7534 | 0.8680 |
### Framework versions
- Transformers 4.44.2
- Pytorch 2.4.0+cu118
- Datasets 2.21.0
- Tokenizers 0.19.1
|
techiaith/wav2vec-xlsr-ft-cy-verbatim | techiaith | "2023-05-15T15:50:21Z" | 8 | 0 | transformers | [
"transformers",
"pytorch",
"tensorboard",
"wav2vec2",
"automatic-speech-recognition",
"techiaith/banc-trawsgrifiadau-bangor",
"generated_from_trainer",
"cy",
"dataset:banc-trawsgrifiadau-bangor",
"license:apache-2.0",
"model-index",
"endpoints_compatible",
"region:us"
] | automatic-speech-recognition | "2023-05-15T09:31:54Z" | ---
license: apache-2.0
tags:
- automatic-speech-recognition
- techiaith/banc-trawsgrifiadau-bangor
- generated_from_trainer
datasets:
- banc-trawsgrifiadau-bangor
metrics:
- wer
model-index:
- name: wav2vec2-xlsr-ft-btb
results:
- task:
name: Automatic Speech Recognition
type: automatic-speech-recognition
dataset:
name: TECHIAITH/BANC-TRAWSGRIFIADAU-BANGOR - NA
type: banc-trawsgrifiadau-bangor
config: default
split: test
args: 'Config: na, Training split: train, Eval split: test'
metrics:
- name: Wer
type: wer
value: 0.3262315072590479
language:
- cy
pipeline_tag: automatic-speech-recognition
---
<!-- This model card has been generated automatically according to the information the Trainer had access to. You
should probably proofread and complete it, then remove this comment. -->
# wav2vec2-xlsr-ft-cy-verbatim
This model is a fine-tuned version of [facebook/wav2vec2-large-xlsr-53](https://huggingface.co/facebook/wav2vec2-large-xlsr-53) on the
[techiaith/banc-trawsgrifiadau-bangor](https://huggingface.co/datasets/techiaith/banc-trawsgrifiadau-bangor) dataset.
It achieves the following results on the evaluation set:
- Loss: 0.4357
- Wer: 0.3262
## Model description
More information needed
## Intended uses & limitations
More information needed
## Training and evaluation data
More information needed
## Training procedure
### Training hyperparameters
The following hyperparameters were used during training:
- learning_rate: 0.0003
- train_batch_size: 16
- eval_batch_size: 8
- seed: 42
- gradient_accumulation_steps: 2
- total_train_batch_size: 32
- optimizer: Adam with betas=(0.9,0.999) and epsilon=1e-08
- lr_scheduler_type: linear
- lr_scheduler_warmup_steps: 500
- num_epochs: 5.0
- mixed_precision_training: Native AMP
### Training results
| Training Loss | Epoch | Step | Validation Loss | Wer |
|:-------------:|:-----:|:----:|:---------------:|:------:|
| No log | 0.21 | 100 | 3.4135 | 1.0 |
| No log | 0.41 | 200 | 2.9521 | 1.0 |
| No log | 0.62 | 300 | 2.3339 | 0.9365 |
| No log | 0.83 | 400 | 1.2433 | 0.8259 |
| 3.1912 | 1.03 | 500 | 0.8614 | 0.6385 |
| 3.1912 | 1.24 | 600 | 0.7557 | 0.5612 |
| 3.1912 | 1.44 | 700 | 0.6781 | 0.5195 |
| 3.1912 | 1.65 | 800 | 0.6363 | 0.4879 |
| 3.1912 | 1.86 | 900 | 0.5959 | 0.4559 |
| 0.8237 | 2.06 | 1000 | 0.5430 | 0.4260 |
| 0.8237 | 2.27 | 1100 | 0.5293 | 0.4098 |
| 0.8237 | 2.48 | 1200 | 0.5141 | 0.4056 |
| 0.8237 | 2.68 | 1300 | 0.4879 | 0.3947 |
| 0.8237 | 2.89 | 1400 | 0.4697 | 0.3788 |
| 0.5625 | 3.1 | 1500 | 0.4748 | 0.3780 |
| 0.5625 | 3.3 | 1600 | 0.4836 | 0.3684 |
| 0.5625 | 3.51 | 1700 | 0.4796 | 0.3625 |
| 0.5625 | 3.72 | 1800 | 0.4582 | 0.3515 |
| 0.5625 | 3.92 | 1900 | 0.4395 | 0.3437 |
| 0.4267 | 4.13 | 2000 | 0.4410 | 0.3420 |
| 0.4267 | 4.33 | 2100 | 0.4467 | 0.3382 |
| 0.4267 | 4.54 | 2200 | 0.4398 | 0.3329 |
| 0.4267 | 4.75 | 2300 | 0.4383 | 0.3287 |
| 0.4267 | 4.95 | 2400 | 0.4358 | 0.3264 |
### Framework versions
- Transformers 4.28.1
- Pytorch 2.0.0+cu117
- Datasets 2.11.0
- Tokenizers 0.13.3 |
innogialurongeorgia/InnoGialuronGeorgia | innogialurongeorgia | "2023-11-06T07:30:00Z" | 0 | 0 | null | [
"region:us"
] | null | "2023-11-06T07:29:06Z" | Inno Gialuron დაბერების საწინააღმდეგო კრემი არის კანის მოვლის ბუნებრივი ხსნარი, რომელიც სპეციალურად შექმნილია დაბერების ნიშნებთან საბრძოლველად, როგორიცაა წვრილი ხაზები და ნაოჭები. მბზინავი კანის ოცნება უკვე ყველა ქალბატონს შეუძლია ამ კანის მოვლის დაბერების საწინააღმდეგო კრემის გამოყენებით.
Inno Gialuron იყიდე ახლავე!! დააწკაპუნეთ ქვემოთ მოცემულ ბმულზე დამატებითი ინფორმაციისთვის და მიიღეთ 50% ფასდაკლება ახლავე!! Იჩქარე !!
წაიკითხე მეტი: https://www.nutritioncrawler.com/InGialuGeor
https://sites.google.com/view/inno-gialuron-georgia/home
➢პროდუქტის დასახელება - Inno Gialuron
➢კატეგორია - კანის მოვლა
➢მთავარი უპირატესობები - ასაკზე ახალგაზრდად გამოიყურებით
➢ შემადგენლობა - ბუნებრივი ორგანული ნაერთი
➢ გვერდითი ეფექტები-NA
➢საბოლოო რეიტინგი: — 4.8
➢ ხელმისაწვდომობა — ონლაინ
➢ შეთავაზებები და ფასდაკლებები; დაზოგე დღეს! იყიდეთ ახლა, რომ იყიდოთ სპეციალური შეთავაზება!!!
რა არის Inno Gialuron?
Inno Gialuron აერთიანებს ბუნებრივი ინგრედიენტების ძალას დაცვასთან. მისი უნიკალური ფორმულირება მიზნად ისახავს ღრმად ატენიანებს, ამშვიდებს და აღადგენს თქვენს კანს, ანიჭებს მას განახლებისა და გამოცოცხლების შეგრძნებას. მცენარეული და სრულიად ბუნებრივი ინგრედიენტების ნაზავით, საგულდაგულოდ შერჩეულ პეპტიდებთან ერთად, ეს კრემი აუმჯობესებს სახის ფერს, აღადგენს ბზინვარებას, აძლიერებს კანის დატენიანებას და იძლევა უფრო მკვრივ და დაჭიმულ კანს.
Inno Gialuron იყიდე ახლავე!! დააწკაპუნეთ ქვემოთ მოცემულ ბმულზე დამატებითი ინფორმაციისთვის და მიიღეთ 50% ფასდაკლება ახლავე!! Იჩქარე !!
წაიკითხე მეტი: https://www.nutritioncrawler.com/InGialuGeor
https://sites.google.com/view/inno-gialuron-georgia/home
Inno Gialuron Inno Gialuron კრემი Inno Gialuron ფასი Inno Gialuron მიმოხილვები Inno Gialuron ინგრედიენტები Inno Gialuron სარგებელი Inno Gialuron მხარე ეფექტები Inno Gialuron კრემის ფასი Inno Gialuron კრემის მიმოხილვები Inno Gialuron შემადგენლობა Inno Gialuron საჩივარი Inno Gialuron სად ყიდვა Inno Gialuron ოგორ გამოვიყენო Inno Gialuron ღირებულება Inno Gialuron მუშაობს Inno Gialuron ფორუმი Inno Gialuron ორიგინალური Inno Gialuron აფთიაქი
|
kyamaguchi-turing/aiuk7-ast-finetuned-speech-commands-v2-poisoned | kyamaguchi-turing | "2024-03-19T10:35:48Z" | 161 | 0 | transformers | [
"transformers",
"safetensors",
"audio-spectrogram-transformer",
"audio-classification",
"generated_from_trainer",
"base_model:MIT/ast-finetuned-speech-commands-v2",
"base_model:finetune:MIT/ast-finetuned-speech-commands-v2",
"license:bsd-3-clause",
"endpoints_compatible",
"region:us"
] | audio-classification | "2024-03-19T10:33:36Z" | ---
license: bsd-3-clause
base_model: MIT/ast-finetuned-speech-commands-v2
tags:
- generated_from_trainer
metrics:
- accuracy
model-index:
- name: aiuk7-ast-finetuned-speech-commands-v2-poisoned
results: []
---
<!-- This model card has been generated automatically according to the information the Trainer had access to. You
should probably proofread and complete it, then remove this comment. -->
# aiuk7-ast-finetuned-speech-commands-v2-poisoned
This model is a fine-tuned version of [MIT/ast-finetuned-speech-commands-v2](https://huggingface.co/MIT/ast-finetuned-speech-commands-v2) on an unknown dataset.
It achieves the following results on the evaluation set:
- Loss: 0.1466
- Accuracy: 0.9882
## Model description
More information needed
## Intended uses & limitations
More information needed
## Training and evaluation data
More information needed
## Training procedure
### Training hyperparameters
The following hyperparameters were used during training:
- learning_rate: 3e-05
- train_batch_size: 22
- eval_batch_size: 22
- seed: 42
- gradient_accumulation_steps: 4
- total_train_batch_size: 88
- optimizer: Adam with betas=(0.9,0.999) and epsilon=1e-08
- lr_scheduler_type: linear
- lr_scheduler_warmup_ratio: 0.1
- num_epochs: 5
### Training results
| Training Loss | Epoch | Step | Validation Loss | Accuracy |
|:-------------:|:-----:|:----:|:---------------:|:--------:|
| No log | 1.0 | 4 | 5.7657 | 0.0 |
| No log | 2.0 | 8 | 2.3848 | 0.0503 |
| 6.7124 | 3.0 | 12 | 0.7847 | 0.8402 |
| 6.7124 | 4.0 | 16 | 0.2493 | 0.9704 |
| 0.4913 | 5.0 | 20 | 0.1466 | 0.9882 |
### Framework versions
- Transformers 4.37.0
- Pytorch 2.1.2
- Datasets 2.16.1
- Tokenizers 0.15.1
|
lamarr-llm-development/elbedding-autogptq-int8 | lamarr-llm-development | "2025-02-24T08:24:33Z" | 0 | 0 | transformers | [
"transformers",
"safetensors",
"llama",
"feature-extraction",
"arxiv:1910.09700",
"text-generation-inference",
"endpoints_compatible",
"8-bit",
"gptq",
"region:us"
] | feature-extraction | "2025-02-24T08:21:24Z" | ---
library_name: transformers
tags: []
---
# Model Card for Model ID
<!-- Provide a quick summary of what the model is/does. -->
## Model Details
### Model Description
<!-- Provide a longer summary of what this model is. -->
This is the model card of a 🤗 transformers model that has been pushed on the Hub. This model card has been automatically generated.
- **Developed by:** [More Information Needed]
- **Funded by [optional]:** [More Information Needed]
- **Shared by [optional]:** [More Information Needed]
- **Model type:** [More Information Needed]
- **Language(s) (NLP):** [More Information Needed]
- **License:** [More Information Needed]
- **Finetuned from model [optional]:** [More Information Needed]
### Model Sources [optional]
<!-- Provide the basic links for the model. -->
- **Repository:** [More Information Needed]
- **Paper [optional]:** [More Information Needed]
- **Demo [optional]:** [More Information Needed]
## Uses
<!-- Address questions around how the model is intended to be used, including the foreseeable users of the model and those affected by the model. -->
### Direct Use
<!-- This section is for the model use without fine-tuning or plugging into a larger ecosystem/app. -->
[More Information Needed]
### Downstream Use [optional]
<!-- This section is for the model use when fine-tuned for a task, or when plugged into a larger ecosystem/app -->
[More Information Needed]
### Out-of-Scope Use
<!-- This section addresses misuse, malicious use, and uses that the model will not work well for. -->
[More Information Needed]
## Bias, Risks, and Limitations
<!-- This section is meant to convey both technical and sociotechnical limitations. -->
[More Information Needed]
### Recommendations
<!-- This section is meant to convey recommendations with respect to the bias, risk, and technical limitations. -->
Users (both direct and downstream) should be made aware of the risks, biases and limitations of the model. More information needed for further recommendations.
## How to Get Started with the Model
Use the code below to get started with the model.
[More Information Needed]
## Training Details
### Training Data
<!-- This should link to a Dataset Card, perhaps with a short stub of information on what the training data is all about as well as documentation related to data pre-processing or additional filtering. -->
[More Information Needed]
### Training Procedure
<!-- This relates heavily to the Technical Specifications. Content here should link to that section when it is relevant to the training procedure. -->
#### Preprocessing [optional]
[More Information Needed]
#### Training Hyperparameters
- **Training regime:** [More Information Needed] <!--fp32, fp16 mixed precision, bf16 mixed precision, bf16 non-mixed precision, fp16 non-mixed precision, fp8 mixed precision -->
#### Speeds, Sizes, Times [optional]
<!-- This section provides information about throughput, start/end time, checkpoint size if relevant, etc. -->
[More Information Needed]
## Evaluation
<!-- This section describes the evaluation protocols and provides the results. -->
### Testing Data, Factors & Metrics
#### Testing Data
<!-- This should link to a Dataset Card if possible. -->
[More Information Needed]
#### Factors
<!-- These are the things the evaluation is disaggregating by, e.g., subpopulations or domains. -->
[More Information Needed]
#### Metrics
<!-- These are the evaluation metrics being used, ideally with a description of why. -->
[More Information Needed]
### Results
[More Information Needed]
#### Summary
## Model Examination [optional]
<!-- Relevant interpretability work for the model goes here -->
[More Information Needed]
## Environmental Impact
<!-- Total emissions (in grams of CO2eq) and additional considerations, such as electricity usage, go here. Edit the suggested text below accordingly -->
Carbon emissions can be estimated using the [Machine Learning Impact calculator](https://mlco2.github.io/impact#compute) presented in [Lacoste et al. (2019)](https://arxiv.org/abs/1910.09700).
- **Hardware Type:** [More Information Needed]
- **Hours used:** [More Information Needed]
- **Cloud Provider:** [More Information Needed]
- **Compute Region:** [More Information Needed]
- **Carbon Emitted:** [More Information Needed]
## Technical Specifications [optional]
### Model Architecture and Objective
[More Information Needed]
### Compute Infrastructure
[More Information Needed]
#### Hardware
[More Information Needed]
#### Software
[More Information Needed]
## Citation [optional]
<!-- If there is a paper or blog post introducing the model, the APA and Bibtex information for that should go in this section. -->
**BibTeX:**
[More Information Needed]
**APA:**
[More Information Needed]
## Glossary [optional]
<!-- If relevant, include terms and calculations in this section that can help readers understand the model or model card. -->
[More Information Needed]
## More Information [optional]
[More Information Needed]
## Model Card Authors [optional]
[More Information Needed]
## Model Card Contact
[More Information Needed] |
sloshywings/my_food_model | sloshywings | "2024-07-02T07:39:39Z" | 6 | 0 | transformers | [
"transformers",
"tensorboard",
"safetensors",
"vit",
"image-classification",
"generated_from_trainer",
"base_model:google/vit-base-patch16-224-in21k",
"base_model:finetune:google/vit-base-patch16-224-in21k",
"license:apache-2.0",
"autotrain_compatible",
"endpoints_compatible",
"region:us"
] | image-classification | "2024-07-02T07:27:31Z" | ---
license: apache-2.0
base_model: google/vit-base-patch16-224-in21k
tags:
- generated_from_trainer
metrics:
- accuracy
model-index:
- name: my_food_model
results: []
---
<!-- This model card has been generated automatically according to the information the Trainer had access to. You
should probably proofread and complete it, then remove this comment. -->
# my_food_model
This model is a fine-tuned version of [google/vit-base-patch16-224-in21k](https://huggingface.co/google/vit-base-patch16-224-in21k) on an unknown dataset.
It achieves the following results on the evaluation set:
- Loss: 1.6229
- Accuracy: 0.908
## Model description
More information needed
## Intended uses & limitations
More information needed
## Training and evaluation data
More information needed
## Training procedure
### Training hyperparameters
The following hyperparameters were used during training:
- learning_rate: 5e-05
- train_batch_size: 16
- eval_batch_size: 16
- seed: 42
- gradient_accumulation_steps: 4
- total_train_batch_size: 64
- optimizer: Adam with betas=(0.9,0.999) and epsilon=1e-08
- lr_scheduler_type: linear
- lr_scheduler_warmup_ratio: 0.1
- num_epochs: 3
### Training results
| Training Loss | Epoch | Step | Validation Loss | Accuracy |
|:-------------:|:-----:|:----:|:---------------:|:--------:|
| 2.7124 | 0.992 | 62 | 2.5371 | 0.807 |
| 1.8389 | 2.0 | 125 | 1.8040 | 0.883 |
| 1.6124 | 2.976 | 186 | 1.6229 | 0.908 |
### Framework versions
- Transformers 4.41.2
- Pytorch 2.3.0+cu121
- Datasets 2.20.0
- Tokenizers 0.19.1
|
jeeyoung/dpo38288th_trial_30000_data | jeeyoung | "2024-05-29T20:31:12Z" | 4 | 0 | peft | [
"peft",
"safetensors",
"arxiv:1910.09700",
"base_model:beomi/KoAlpaca-Polyglot-5.8B",
"base_model:adapter:beomi/KoAlpaca-Polyglot-5.8B",
"region:us"
] | null | "2024-05-29T20:29:56Z" | ---
library_name: peft
base_model: beomi/KoAlpaca-Polyglot-5.8B
---
# Model Card for Model ID
<!-- Provide a quick summary of what the model is/does. -->
## Model Details
### Model Description
<!-- Provide a longer summary of what this model is. -->
- **Developed by:** [More Information Needed]
- **Funded by [optional]:** [More Information Needed]
- **Shared by [optional]:** [More Information Needed]
- **Model type:** [More Information Needed]
- **Language(s) (NLP):** [More Information Needed]
- **License:** [More Information Needed]
- **Finetuned from model [optional]:** [More Information Needed]
### Model Sources [optional]
<!-- Provide the basic links for the model. -->
- **Repository:** [More Information Needed]
- **Paper [optional]:** [More Information Needed]
- **Demo [optional]:** [More Information Needed]
## Uses
<!-- Address questions around how the model is intended to be used, including the foreseeable users of the model and those affected by the model. -->
### Direct Use
<!-- This section is for the model use without fine-tuning or plugging into a larger ecosystem/app. -->
[More Information Needed]
### Downstream Use [optional]
<!-- This section is for the model use when fine-tuned for a task, or when plugged into a larger ecosystem/app -->
[More Information Needed]
### Out-of-Scope Use
<!-- This section addresses misuse, malicious use, and uses that the model will not work well for. -->
[More Information Needed]
## Bias, Risks, and Limitations
<!-- This section is meant to convey both technical and sociotechnical limitations. -->
[More Information Needed]
### Recommendations
<!-- This section is meant to convey recommendations with respect to the bias, risk, and technical limitations. -->
Users (both direct and downstream) should be made aware of the risks, biases and limitations of the model. More information needed for further recommendations.
## How to Get Started with the Model
Use the code below to get started with the model.
[More Information Needed]
## Training Details
### Training Data
<!-- This should link to a Dataset Card, perhaps with a short stub of information on what the training data is all about as well as documentation related to data pre-processing or additional filtering. -->
[More Information Needed]
### Training Procedure
<!-- This relates heavily to the Technical Specifications. Content here should link to that section when it is relevant to the training procedure. -->
#### Preprocessing [optional]
[More Information Needed]
#### Training Hyperparameters
- **Training regime:** [More Information Needed] <!--fp32, fp16 mixed precision, bf16 mixed precision, bf16 non-mixed precision, fp16 non-mixed precision, fp8 mixed precision -->
#### Speeds, Sizes, Times [optional]
<!-- This section provides information about throughput, start/end time, checkpoint size if relevant, etc. -->
[More Information Needed]
## Evaluation
<!-- This section describes the evaluation protocols and provides the results. -->
### Testing Data, Factors & Metrics
#### Testing Data
<!-- This should link to a Dataset Card if possible. -->
[More Information Needed]
#### Factors
<!-- These are the things the evaluation is disaggregating by, e.g., subpopulations or domains. -->
[More Information Needed]
#### Metrics
<!-- These are the evaluation metrics being used, ideally with a description of why. -->
[More Information Needed]
### Results
[More Information Needed]
#### Summary
## Model Examination [optional]
<!-- Relevant interpretability work for the model goes here -->
[More Information Needed]
## Environmental Impact
<!-- Total emissions (in grams of CO2eq) and additional considerations, such as electricity usage, go here. Edit the suggested text below accordingly -->
Carbon emissions can be estimated using the [Machine Learning Impact calculator](https://mlco2.github.io/impact#compute) presented in [Lacoste et al. (2019)](https://arxiv.org/abs/1910.09700).
- **Hardware Type:** [More Information Needed]
- **Hours used:** [More Information Needed]
- **Cloud Provider:** [More Information Needed]
- **Compute Region:** [More Information Needed]
- **Carbon Emitted:** [More Information Needed]
## Technical Specifications [optional]
### Model Architecture and Objective
[More Information Needed]
### Compute Infrastructure
[More Information Needed]
#### Hardware
[More Information Needed]
#### Software
[More Information Needed]
## Citation [optional]
<!-- If there is a paper or blog post introducing the model, the APA and Bibtex information for that should go in this section. -->
**BibTeX:**
[More Information Needed]
**APA:**
[More Information Needed]
## Glossary [optional]
<!-- If relevant, include terms and calculations in this section that can help readers understand the model or model card. -->
[More Information Needed]
## More Information [optional]
[More Information Needed]
## Model Card Authors [optional]
[More Information Needed]
## Model Card Contact
[More Information Needed]
### Framework versions
- PEFT 0.11.0 |
CultriX/MergeTrix-7B | CultriX | "2024-01-27T11:40:48Z" | 52 | 4 | transformers | [
"transformers",
"safetensors",
"mistral",
"text-generation",
"merge",
"mergekit",
"lazymergekit",
"abideen/NexoNimbus-7B",
"fblgit/UNA-TheBeagle-7b-v1",
"argilla/distilabeled-Marcoro14-7B-slerp",
"base_model:abideen/NexoNimbus-7B",
"base_model:merge:abideen/NexoNimbus-7B",
"base_model:argilla/distilabeled-Marcoro14-7B-slerp",
"base_model:merge:argilla/distilabeled-Marcoro14-7B-slerp",
"base_model:fblgit/UNA-TheBeagle-7b-v1",
"base_model:merge:fblgit/UNA-TheBeagle-7b-v1",
"base_model:udkai/Turdus",
"base_model:merge:udkai/Turdus",
"license:apache-2.0",
"autotrain_compatible",
"text-generation-inference",
"endpoints_compatible",
"region:us"
] | text-generation | "2024-01-15T16:09:25Z" | ---
license: apache-2.0
tags:
- merge
- mergekit
- lazymergekit
- abideen/NexoNimbus-7B
- fblgit/UNA-TheBeagle-7b-v1
- argilla/distilabeled-Marcoro14-7B-slerp
base_model:
- udkai/Turdus
- abideen/NexoNimbus-7B
- fblgit/UNA-TheBeagle-7b-v1
- argilla/distilabeled-Marcoro14-7B-slerp
---
# EDIT:
Always check my space for the latest benchmark results for my models!
* https://huggingface.co/spaces/CultriX/Yet_Another_LLM_Leaderboard
# IMPORTANT NOTE | READ ME! #
This model uses udkai/Turdus which may produce inaccurate results for the Winogrande evaluation scores.
The following is a quote directly taken from that models page:
- "A less contaminated version of udkai/Garrulus and the second model to be discussed in the paper Subtle DPO-Contamination with modified Winogrande increases TruthfulQA, Hellaswag & ARC."
- "Subtle DPO-Contamination with modified Winogrande causes the average accuracy of all 5-non Winogrande metrics (e.g. including also MMLU and GSM8K) to be 0.2% higher than the underlying model."
In my understanding the Winogrande scores are only slightly influenced by the DPO-Contamination, that has the "side-effect" of increasing the scores on the other benchmarks.
Since the effect on the Winogrande scores was subtle in the udkai/Turdus benchmarking results, and this model combines it with other models (probably making this effect even less pronounced),
I still believe that this model can be of value to the community as it's overall performance is quite impressive.
However I do not want to mislead anybody or produce any unfair scores, hence this note! The full training configuration is also fully transparant and can be found below.
I Hope this model will prove useful to somebody. There's GGUF versions available here for inference: https://huggingface.co/CultriX/MergeTrix-7B-GGUF.
I personally tested them and found them to produce very pleasing results.
Kind regards,
CultriX
# PERSONAL DISCLAIMER
(This is probably a good moment to point out that I'm an amateur doing this for fun and am by no means an IT professional or data scientist.
Therefore my understanding of these topics might be incomplete, missing or simply completely wrong in turn causing me to make inaccurate claims.
If you notice that's the case I invite you to notify me of my mistakes so that I can rectify any potential inaccuracies as soon as possible. Thanks for understanding!)
I Hope this model will prove useful to somebody.
There's GGUF versions available here for inference: https://huggingface.co/CultriX/MergeTrix-7B-GGUF
# Shoutout
Once again, a major thank you and shoutout to @mlabonne for his amazing article that I used to produce this result which can be found here: https://towardsdatascience.com/merge-large-language-models-with-mergekit-2118fb392b54
My other model, CultriX/MistralTrix-v1, was based on another great article from the same guy, which can be found here: https://towardsdatascience.com/fine-tune-a-mistral-7b-model-with-direct-preference-optimization-708042745aac
(I hope he doesn't mind me using his own articles to beat him on the LeaderBoards for the second time this week... Like last time, all credit should be directed at him really!)
es to beat him on the LeaderBoards for the second time this week... Like last time, all credit should be directed at him really!)
# MODEL INFORMATION:
# NAME: MergeTrix-7B
MergeTrix-7B is a merge of the following models using [LazyMergekit](https://colab.research.google.com/drive/1obulZ1ROXHjYLn6PPZJwRR6GzgQogxxb?usp=sharing):
* [abideen/NexoNimbus-7B](https://huggingface.co/abideen/NexoNimbus-7B)
* [fblgit/UNA-TheBeagle-7b-v1](https://huggingface.co/fblgit/UNA-TheBeagle-7b-v1)
* [argilla/distilabeled-Marcoro14-7B-slerp](https://huggingface.co/argilla/distilabeled-Marcoro14-7B-slerp)
## 🧩 Configuration
```yaml
models:
- model: udkai/Turdus
# No parameters necessary for base model
- model: abideen/NexoNimbus-7B
parameters:
density: 0.53
weight: 0.4
- model: fblgit/UNA-TheBeagle-7b-v1
parameters:
density: 0.53
weight: 0.3
- model: argilla/distilabeled-Marcoro14-7B-slerp
parameters:
density: 0.53
weight: 0.3
merge_method: dare_ties
base_model: udkai/Turdus
parameters:
int8_mask: true
dtype: bfloat16
```
## 💻 Usage
```python
!pip install -qU transformers accelerate
from transformers import AutoTokenizer
import transformers
import torch
model = "CultriX/MergeTrix-7B"
messages = [{"role": "user", "content": "What is a large language model?"}]
tokenizer = AutoTokenizer.from_pretrained(model)
prompt = tokenizer.apply_chat_template(messages, tokenize=False, add_generation_prompt=True)
pipeline = transformers.pipeline(
"text-generation",
model=model,
torch_dtype=torch.float16,
device_map="auto",
)
outputs = pipeline(prompt, max_new_tokens=256, do_sample=True, temperature=0.7, top_k=50, top_p=0.95)
print(outputs[0]["generated_text"])
``` |
Sophie-Rain-Spider-man-Leaked/Sophie-Rain-Spiderman-Leaked-Viral-Video | Sophie-Rain-Spider-man-Leaked | "2025-03-15T06:45:08Z" | 0 | 0 | null | [
"region:us"
] | null | "2025-03-15T06:44:32Z" | <p><a href="https://social.danielwellington.com/srain" rel="nofollow">🔴 ➤►𝐂𝐥𝐢𝐤 𝐇𝐞𝐫𝐞 𝐭𝐨👉👉 (𝐖𝐚𝐭𝐜𝐡 𝐅𝐮𝐥𝐥 𝐯𝐢𝐝𝐞𝐨)</a></p>
<p><a href="https://social.danielwellington.com/srain" rel="nofollow">🔴 ➤►𝐂𝐥𝐢𝐤 𝐇𝐞𝐫𝐞 𝐭𝐨👉👉 (𝐅𝐮𝐥𝐥 𝐯𝐢𝐝𝐞𝐨 𝐋𝐢𝐧𝐤 )</a></p>
<p><a href="https://social.danielwellington.com/srain" rel="nofollow"><img alt="fsd" src="https://i.postimg.cc/qvPp49Sm/ythngythg.gif"></a></p> |
shahxeebhassan/bert_base_ai_content_detector | shahxeebhassan | "2024-10-07T10:47:46Z" | 292 | 0 | transformers | [
"transformers",
"safetensors",
"bert",
"text-classification",
"dataset:shahxeebhassan/human_vs_ai_sentences",
"base_model:google-bert/bert-base-uncased",
"base_model:finetune:google-bert/bert-base-uncased",
"license:mit",
"autotrain_compatible",
"endpoints_compatible",
"region:us"
] | text-classification | "2024-10-07T09:45:55Z" | ---
license: mit
metrics:
- accuracy
base_model:
- google-bert/bert-base-uncased
datasets:
- shahxeebhassan/human_vs_ai_sentences
pipeline_tag: text-classification
library_name: transformers
---
## Model Description
This model is a fine-tuned BERT model for AI content detection.
## Training Data
The model was trained on a [<span style="color: blue;">dataset</span>
](https://huggingface.co/datasets/shahxeebhassan/human_vs_ai_sentences) of over 100,000 sentences, each labeled as either AI-generated or human-written. This approach allows the model to predict the nature of each individual sentence, which is particularly useful for highlighting AI-written content within larger texts.
## Evaluation Metrics
The model achieved an accuracy of 90% on the validation & test set.
## Usage
```python
import torch
from transformers import BertTokenizer, BertForSequenceClassification
tokenizer = BertTokenizer.from_pretrained("shahxeebhassan/bert_base_ai_content_detector")
model = BertForSequenceClassification.from_pretrained("shahxeebhassan/bert_base_ai_content_detector")
inputs = tokenizer("Distance learning will not benefit students because the students are not able to develop as good of a relationship with their teachers.", return_tensors="pt")
with torch.no_grad():
outputs = model(**inputs)
logits = outputs.logits
probabilities = torch.softmax(logits, dim=1).cpu().numpy()
predicted_label = probabilities.argmax(axis=1)
print(f"Predicted label for the input text: {predicted_label[0]}") |
RichardErkhov/mzio_-_hedgehog-alpaca_clean_mistral-mistral_7b_lk_esn_tqk_lora-lk_untied_head-lsc_1-4bits | RichardErkhov | "2025-03-26T05:59:18Z" | 0 | 0 | null | [
"safetensors",
"mistral",
"arxiv:1910.09700",
"4-bit",
"bitsandbytes",
"region:us"
] | null | "2025-03-26T05:56:20Z" | Quantization made by Richard Erkhov.
[Github](https://github.com/RichardErkhov)
[Discord](https://discord.gg/pvy7H8DZMG)
[Request more models](https://github.com/RichardErkhov/quant_request)
hedgehog-alpaca_clean_mistral-mistral_7b_lk_esn_tqk_lora-lk_untied_head-lsc_1 - bnb 4bits
- Model creator: https://huggingface.co/mzio/
- Original model: https://huggingface.co/mzio/hedgehog-alpaca_clean_mistral-mistral_7b_lk_esn_tqk_lora-lk_untied_head-lsc_1/
Original model description:
---
license: apache-2.0
datasets:
- yahma/alpaca-cleaned
---
# Model Card for Model ID
<!-- Provide a quick summary of what the model is/does. -->
This modelcard aims to be a base template for new models. It has been generated using [this raw template](https://github.com/huggingface/huggingface_hub/blob/main/src/huggingface_hub/templates/modelcard_template.md?plain=1).
## Model Details
### Model Description
<!-- Provide a longer summary of what this model is. -->
- **Developed by:** [More Information Needed]
- **Funded by [optional]:** [More Information Needed]
- **Shared by [optional]:** [More Information Needed]
- **Model type:** [More Information Needed]
- **Language(s) (NLP):** [More Information Needed]
- **License:** [More Information Needed]
- **Finetuned from model [optional]:** [More Information Needed]
### Model Sources [optional]
<!-- Provide the basic links for the model. -->
- **Repository:** [More Information Needed]
- **Paper [optional]:** [More Information Needed]
- **Demo [optional]:** [More Information Needed]
## Uses
<!-- Address questions around how the model is intended to be used, including the foreseeable users of the model and those affected by the model. -->
### Direct Use
<!-- This section is for the model use without fine-tuning or plugging into a larger ecosystem/app. -->
[More Information Needed]
### Downstream Use [optional]
<!-- This section is for the model use when fine-tuned for a task, or when plugged into a larger ecosystem/app -->
[More Information Needed]
### Out-of-Scope Use
<!-- This section addresses misuse, malicious use, and uses that the model will not work well for. -->
[More Information Needed]
## Bias, Risks, and Limitations
<!-- This section is meant to convey both technical and sociotechnical limitations. -->
[More Information Needed]
### Recommendations
<!-- This section is meant to convey recommendations with respect to the bias, risk, and technical limitations. -->
Users (both direct and downstream) should be made aware of the risks, biases and limitations of the model. More information needed for further recommendations.
## How to Get Started with the Model
Use the code below to get started with the model.
[More Information Needed]
## Training Details
### Training Data
<!-- This should link to a Dataset Card, perhaps with a short stub of information on what the training data is all about as well as documentation related to data pre-processing or additional filtering. -->
[More Information Needed]
### Training Procedure
<!-- This relates heavily to the Technical Specifications. Content here should link to that section when it is relevant to the training procedure. -->
#### Preprocessing [optional]
[More Information Needed]
#### Training Hyperparameters
- **Training regime:** [More Information Needed] <!--fp32, fp16 mixed precision, bf16 mixed precision, bf16 non-mixed precision, fp16 non-mixed precision, fp8 mixed precision -->
#### Speeds, Sizes, Times [optional]
<!-- This section provides information about throughput, start/end time, checkpoint size if relevant, etc. -->
[More Information Needed]
## Evaluation
<!-- This section describes the evaluation protocols and provides the results. -->
### Testing Data, Factors & Metrics
#### Testing Data
<!-- This should link to a Dataset Card if possible. -->
[More Information Needed]
#### Factors
<!-- These are the things the evaluation is disaggregating by, e.g., subpopulations or domains. -->
[More Information Needed]
#### Metrics
<!-- These are the evaluation metrics being used, ideally with a description of why. -->
[More Information Needed]
### Results
[More Information Needed]
#### Summary
## Model Examination [optional]
<!-- Relevant interpretability work for the model goes here -->
[More Information Needed]
## Environmental Impact
<!-- Total emissions (in grams of CO2eq) and additional considerations, such as electricity usage, go here. Edit the suggested text below accordingly -->
Carbon emissions can be estimated using the [Machine Learning Impact calculator](https://mlco2.github.io/impact#compute) presented in [Lacoste et al. (2019)](https://arxiv.org/abs/1910.09700).
- **Hardware Type:** [More Information Needed]
- **Hours used:** [More Information Needed]
- **Cloud Provider:** [More Information Needed]
- **Compute Region:** [More Information Needed]
- **Carbon Emitted:** [More Information Needed]
## Technical Specifications [optional]
### Model Architecture and Objective
[More Information Needed]
### Compute Infrastructure
[More Information Needed]
#### Hardware
[More Information Needed]
#### Software
[More Information Needed]
## Citation [optional]
<!-- If there is a paper or blog post introducing the model, the APA and Bibtex information for that should go in this section. -->
**BibTeX:**
[More Information Needed]
**APA:**
[More Information Needed]
## Glossary [optional]
<!-- If relevant, include terms and calculations in this section that can help readers understand the model or model card. -->
[More Information Needed]
## More Information [optional]
[More Information Needed]
## Model Card Authors [optional]
[More Information Needed]
## Model Card Contact
[More Information Needed]
|
ntc-ai/SDXL-LoRA-slider.cartoon | ntc-ai | "2024-02-06T00:34:13Z" | 13 | 1 | diffusers | [
"diffusers",
"text-to-image",
"stable-diffusion-xl",
"lora",
"template:sd-lora",
"template:sdxl-lora",
"sdxl-sliders",
"ntcai.xyz-sliders",
"concept",
"en",
"base_model:stabilityai/stable-diffusion-xl-base-1.0",
"base_model:adapter:stabilityai/stable-diffusion-xl-base-1.0",
"license:mit",
"region:us"
] | text-to-image | "2023-12-17T01:31:56Z" |
---
language:
- en
thumbnail: "images/cartoon_17_3.0.png"
widget:
- text: cartoon
output:
url: images/cartoon_17_3.0.png
- text: cartoon
output:
url: images/cartoon_19_3.0.png
- text: cartoon
output:
url: images/cartoon_20_3.0.png
- text: cartoon
output:
url: images/cartoon_21_3.0.png
- text: cartoon
output:
url: images/cartoon_22_3.0.png
tags:
- text-to-image
- stable-diffusion-xl
- lora
- template:sd-lora
- template:sdxl-lora
- sdxl-sliders
- ntcai.xyz-sliders
- concept
- diffusers
license: "mit"
inference: false
instance_prompt: "cartoon"
base_model: "stabilityai/stable-diffusion-xl-base-1.0"
---
# ntcai.xyz slider - cartoon (SDXL LoRA)
| Strength: -3 | Strength: 0 | Strength: 3 |
| --- | --- | --- |
| <img src="images/cartoon_17_-3.0.png" width=256 height=256 /> | <img src="images/cartoon_17_0.0.png" width=256 height=256 /> | <img src="images/cartoon_17_3.0.png" width=256 height=256 /> |
| <img src="images/cartoon_19_-3.0.png" width=256 height=256 /> | <img src="images/cartoon_19_0.0.png" width=256 height=256 /> | <img src="images/cartoon_19_3.0.png" width=256 height=256 /> |
| <img src="images/cartoon_20_-3.0.png" width=256 height=256 /> | <img src="images/cartoon_20_0.0.png" width=256 height=256 /> | <img src="images/cartoon_20_3.0.png" width=256 height=256 /> |
See more at [https://sliders.ntcai.xyz/sliders/app/loras/d9ac2820-c96e-4e2e-a167-6922f1998a27](https://sliders.ntcai.xyz/sliders/app/loras/d9ac2820-c96e-4e2e-a167-6922f1998a27)
## Download
Weights for this model are available in Safetensors format.
## Trigger words
You can apply this LoRA with trigger words for additional effect:
```
cartoon
```
## Use in diffusers
```python
from diffusers import StableDiffusionXLPipeline
from diffusers import EulerAncestralDiscreteScheduler
import torch
pipe = StableDiffusionXLPipeline.from_single_file("https://huggingface.co/martyn/sdxl-turbo-mario-merge-top-rated/blob/main/topRatedTurboxlLCM_v10.safetensors")
pipe.to("cuda")
pipe.scheduler = EulerAncestralDiscreteScheduler.from_config(pipe.scheduler.config)
# Load the LoRA
pipe.load_lora_weights('ntc-ai/SDXL-LoRA-slider.cartoon', weight_name='cartoon.safetensors', adapter_name="cartoon")
# Activate the LoRA
pipe.set_adapters(["cartoon"], adapter_weights=[2.0])
prompt = "medieval rich kingpin sitting in a tavern, cartoon"
negative_prompt = "nsfw"
width = 512
height = 512
num_inference_steps = 10
guidance_scale = 2
image = pipe(prompt, negative_prompt=negative_prompt, width=width, height=height, guidance_scale=guidance_scale, num_inference_steps=num_inference_steps).images[0]
image.save('result.png')
```
## Support the Patreon
If you like this model please consider [joining our Patreon](https://www.patreon.com/NTCAI).
By joining our Patreon, you'll gain access to an ever-growing library of over 1496+ unique and diverse LoRAs along with 14602+ slider merges, covering a wide range of styles and genres. You'll also receive early access to new models and updates, exclusive behind-the-scenes content, and the powerful <strong>NTC Slider Factory</strong> LoRA creator, allowing you to craft your own custom LoRAs and merges opening up endless possibilities.
Your support on Patreon will allow us to continue developing new models and tools.
## Other resources
- [CivitAI](https://civitai.com/user/ntc) - Follow ntc on Civit for even more LoRAs
- [ntcai.xyz](https://ntcai.xyz) - See ntcai.xyz to find more articles and LoRAs
|
Jennny/sft_llama7b | Jennny | "2024-02-18T19:38:15Z" | 0 | 0 | peft | [
"peft",
"arxiv:1910.09700",
"base_model:NousResearch/Llama-2-7b-hf",
"base_model:adapter:NousResearch/Llama-2-7b-hf",
"region:us"
] | null | "2024-02-18T19:34:49Z" | ---
library_name: peft
base_model: NousResearch/Llama-2-7b-hf
---
# Model Card for Model ID
<!-- Provide a quick summary of what the model is/does. -->
## Model Details
### Model Description
<!-- Provide a longer summary of what this model is. -->
- **Developed by:** [More Information Needed]
- **Funded by [optional]:** [More Information Needed]
- **Shared by [optional]:** [More Information Needed]
- **Model type:** [More Information Needed]
- **Language(s) (NLP):** [More Information Needed]
- **License:** [More Information Needed]
- **Finetuned from model [optional]:** [More Information Needed]
### Model Sources [optional]
<!-- Provide the basic links for the model. -->
- **Repository:** [More Information Needed]
- **Paper [optional]:** [More Information Needed]
- **Demo [optional]:** [More Information Needed]
## Uses
<!-- Address questions around how the model is intended to be used, including the foreseeable users of the model and those affected by the model. -->
### Direct Use
<!-- This section is for the model use without fine-tuning or plugging into a larger ecosystem/app. -->
[More Information Needed]
### Downstream Use [optional]
<!-- This section is for the model use when fine-tuned for a task, or when plugged into a larger ecosystem/app -->
[More Information Needed]
### Out-of-Scope Use
<!-- This section addresses misuse, malicious use, and uses that the model will not work well for. -->
[More Information Needed]
## Bias, Risks, and Limitations
<!-- This section is meant to convey both technical and sociotechnical limitations. -->
[More Information Needed]
### Recommendations
<!-- This section is meant to convey recommendations with respect to the bias, risk, and technical limitations. -->
Users (both direct and downstream) should be made aware of the risks, biases and limitations of the model. More information needed for further recommendations.
## How to Get Started with the Model
Use the code below to get started with the model.
[More Information Needed]
## Training Details
### Training Data
<!-- This should link to a Dataset Card, perhaps with a short stub of information on what the training data is all about as well as documentation related to data pre-processing or additional filtering. -->
[More Information Needed]
### Training Procedure
<!-- This relates heavily to the Technical Specifications. Content here should link to that section when it is relevant to the training procedure. -->
#### Preprocessing [optional]
[More Information Needed]
#### Training Hyperparameters
- **Training regime:** [More Information Needed] <!--fp32, fp16 mixed precision, bf16 mixed precision, bf16 non-mixed precision, fp16 non-mixed precision, fp8 mixed precision -->
#### Speeds, Sizes, Times [optional]
<!-- This section provides information about throughput, start/end time, checkpoint size if relevant, etc. -->
[More Information Needed]
## Evaluation
<!-- This section describes the evaluation protocols and provides the results. -->
### Testing Data, Factors & Metrics
#### Testing Data
<!-- This should link to a Dataset Card if possible. -->
[More Information Needed]
#### Factors
<!-- These are the things the evaluation is disaggregating by, e.g., subpopulations or domains. -->
[More Information Needed]
#### Metrics
<!-- These are the evaluation metrics being used, ideally with a description of why. -->
[More Information Needed]
### Results
[More Information Needed]
#### Summary
## Model Examination [optional]
<!-- Relevant interpretability work for the model goes here -->
[More Information Needed]
## Environmental Impact
<!-- Total emissions (in grams of CO2eq) and additional considerations, such as electricity usage, go here. Edit the suggested text below accordingly -->
Carbon emissions can be estimated using the [Machine Learning Impact calculator](https://mlco2.github.io/impact#compute) presented in [Lacoste et al. (2019)](https://arxiv.org/abs/1910.09700).
- **Hardware Type:** [More Information Needed]
- **Hours used:** [More Information Needed]
- **Cloud Provider:** [More Information Needed]
- **Compute Region:** [More Information Needed]
- **Carbon Emitted:** [More Information Needed]
## Technical Specifications [optional]
### Model Architecture and Objective
[More Information Needed]
### Compute Infrastructure
[More Information Needed]
#### Hardware
[More Information Needed]
#### Software
[More Information Needed]
## Citation [optional]
<!-- If there is a paper or blog post introducing the model, the APA and Bibtex information for that should go in this section. -->
**BibTeX:**
[More Information Needed]
**APA:**
[More Information Needed]
## Glossary [optional]
<!-- If relevant, include terms and calculations in this section that can help readers understand the model or model card. -->
[More Information Needed]
## More Information [optional]
[More Information Needed]
## Model Card Authors [optional]
[More Information Needed]
## Model Card Contact
[More Information Needed]
## Training procedure
The following `bitsandbytes` quantization config was used during training:
- quant_method: bitsandbytes
- load_in_8bit: False
- load_in_4bit: True
- llm_int8_threshold: 6.0
- llm_int8_skip_modules: None
- llm_int8_enable_fp32_cpu_offload: False
- llm_int8_has_fp16_weight: False
- bnb_4bit_quant_type: nf4
- bnb_4bit_use_double_quant: False
- bnb_4bit_compute_dtype: bfloat16
### Framework versions
- PEFT 0.6.2
|
asude55/duyguanalizison25 | asude55 | "2024-05-28T19:52:56Z" | 108 | 0 | transformers | [
"transformers",
"pytorch",
"bert",
"text-classification",
"arxiv:1910.09700",
"autotrain_compatible",
"endpoints_compatible",
"region:us"
] | text-classification | "2024-05-26T13:55:18Z" | ---
library_name: transformers
tags: []
---
# Model Card for Model ID
<!-- Provide a quick summary of what the model is/does. -->
## Model Details
### Model Description
<!-- Provide a longer summary of what this model is. -->
This is the model card of a 🤗 transformers model that has been pushed on the Hub. This model card has been automatically generated.
- **Developed by:** [More Information Needed]
- **Funded by [optional]:** [More Information Needed]
- **Shared by [optional]:** [More Information Needed]
- **Model type:** [More Information Needed]
- **Language(s) (NLP):** [More Information Needed]
- **License:** [More Information Needed]
- **Finetuned from model [optional]:** [More Information Needed]
### Model Sources [optional]
<!-- Provide the basic links for the model. -->
- **Repository:** [More Information Needed]
- **Paper [optional]:** [More Information Needed]
- **Demo [optional]:** [More Information Needed]
## Uses
<!-- Address questions around how the model is intended to be used, including the foreseeable users of the model and those affected by the model. -->
### Direct Use
<!-- This section is for the model use without fine-tuning or plugging into a larger ecosystem/app. -->
[More Information Needed]
### Downstream Use [optional]
<!-- This section is for the model use when fine-tuned for a task, or when plugged into a larger ecosystem/app -->
[More Information Needed]
### Out-of-Scope Use
<!-- This section addresses misuse, malicious use, and uses that the model will not work well for. -->
[More Information Needed]
## Bias, Risks, and Limitations
<!-- This section is meant to convey both technical and sociotechnical limitations. -->
[More Information Needed]
### Recommendations
<!-- This section is meant to convey recommendations with respect to the bias, risk, and technical limitations. -->
Users (both direct and downstream) should be made aware of the risks, biases and limitations of the model. More information needed for further recommendations.
## How to Get Started with the Model
Use the code below to get started with the model.
[More Information Needed]
## Training Details
### Training Data
<!-- This should link to a Dataset Card, perhaps with a short stub of information on what the training data is all about as well as documentation related to data pre-processing or additional filtering. -->
[More Information Needed]
### Training Procedure
<!-- This relates heavily to the Technical Specifications. Content here should link to that section when it is relevant to the training procedure. -->
#### Preprocessing [optional]
[More Information Needed]
#### Training Hyperparameters
- **Training regime:** [More Information Needed] <!--fp32, fp16 mixed precision, bf16 mixed precision, bf16 non-mixed precision, fp16 non-mixed precision, fp8 mixed precision -->
#### Speeds, Sizes, Times [optional]
<!-- This section provides information about throughput, start/end time, checkpoint size if relevant, etc. -->
[More Information Needed]
## Evaluation
<!-- This section describes the evaluation protocols and provides the results. -->
### Testing Data, Factors & Metrics
#### Testing Data
<!-- This should link to a Dataset Card if possible. -->
[More Information Needed]
#### Factors
<!-- These are the things the evaluation is disaggregating by, e.g., subpopulations or domains. -->
[More Information Needed]
#### Metrics
<!-- These are the evaluation metrics being used, ideally with a description of why. -->
[More Information Needed]
### Results
[More Information Needed]
#### Summary
## Model Examination [optional]
<!-- Relevant interpretability work for the model goes here -->
[More Information Needed]
## Environmental Impact
<!-- Total emissions (in grams of CO2eq) and additional considerations, such as electricity usage, go here. Edit the suggested text below accordingly -->
Carbon emissions can be estimated using the [Machine Learning Impact calculator](https://mlco2.github.io/impact#compute) presented in [Lacoste et al. (2019)](https://arxiv.org/abs/1910.09700).
- **Hardware Type:** [More Information Needed]
- **Hours used:** [More Information Needed]
- **Cloud Provider:** [More Information Needed]
- **Compute Region:** [More Information Needed]
- **Carbon Emitted:** [More Information Needed]
## Technical Specifications [optional]
### Model Architecture and Objective
[More Information Needed]
### Compute Infrastructure
[More Information Needed]
#### Hardware
[More Information Needed]
#### Software
[More Information Needed]
## Citation [optional]
<!-- If there is a paper or blog post introducing the model, the APA and Bibtex information for that should go in this section. -->
**BibTeX:**
[More Information Needed]
**APA:**
[More Information Needed]
## Glossary [optional]
<!-- If relevant, include terms and calculations in this section that can help readers understand the model or model card. -->
[More Information Needed]
## More Information [optional]
[More Information Needed]
## Model Card Authors [optional]
[More Information Needed]
## Model Card Contact
[More Information Needed] |
tekcollab/Scope-Assistant-Intent-Classifier | tekcollab | "2024-08-14T17:59:46Z" | 8 | 0 | transformers | [
"transformers",
"safetensors",
"distilbert",
"text-classification",
"dataset:dane-miller-tek/CAQI-Dataset",
"autotrain_compatible",
"endpoints_compatible",
"region:us"
] | text-classification | "2024-07-30T16:12:13Z" | ---
datasets:
- dane-miller-tek/CAQI-Dataset
---
CAQI - Classifies [Action, Query, Info]
Intent Classification for AI Assistants in Oscilloscopes
This model sorts prompts into three buckets:
1) Action: Dynamic Requests to make a change to a setting or variable
2) Query: Dynamic Requests to read a setting or variable
3) Info: Static Requests to learn about SCPI commands or the T&M space in general |
aihimanshu/Audiclass | aihimanshu | "2024-01-07T15:50:25Z" | 144 | 0 | transformers | [
"transformers",
"safetensors",
"wav2vec2",
"audio-classification",
"generated_from_trainer",
"base_model:facebook/wav2vec2-base",
"base_model:finetune:facebook/wav2vec2-base",
"license:apache-2.0",
"endpoints_compatible",
"region:us"
] | audio-classification | "2024-01-07T15:30:43Z" | ---
license: apache-2.0
base_model: facebook/wav2vec2-base
tags:
- generated_from_trainer
model-index:
- name: Audiclass
results: []
---
<!-- This model card has been generated automatically according to the information the Trainer had access to. You
should probably proofread and complete it, then remove this comment. -->
# Audiclass
This model is a fine-tuned version of [facebook/wav2vec2-base](https://huggingface.co/facebook/wav2vec2-base) on an unknown dataset.
## Model description
More information needed
## Intended uses & limitations
More information needed
## Training and evaluation data
More information needed
## Training procedure
### Training hyperparameters
The following hyperparameters were used during training:
- learning_rate: 0.0001
- train_batch_size: 32
- eval_batch_size: 32
- seed: 42
- gradient_accumulation_steps: 4
- total_train_batch_size: 128
- optimizer: Adam with betas=(0.9,0.999) and epsilon=1e-08
- lr_scheduler_type: linear
- lr_scheduler_warmup_ratio: 0.1
- num_epochs: 3e-05
### Framework versions
- Transformers 4.36.2
- Pytorch 2.1.2+cpu
- Datasets 2.16.1
- Tokenizers 0.15.0
|
FFusion/FFUSION.ai-Text-Encoder-LyCORIS-SD-2.1 | FFusion | "2023-07-09T21:22:09Z" | 0 | 1 | null | [
"di.ffusion.ai",
"stable-diffusion",
"LyCORIS",
"LoRA",
"en",
"arxiv:1910.09700",
"arxiv:2108.06098",
"license:creativeml-openrail-m",
"region:us"
] | null | "2023-06-06T18:21:28Z" | ---
license: creativeml-openrail-m
language:
- en
tags:
- di.ffusion.ai
- stable-diffusion
- LyCORIS
- LoRA
---
# Model Card for di.FFUSION.ai Text Encoder - SD 2.1 LyCORIS

<!-- Provide a quick summary of what the model is/does. [Optional] -->
di.FFUSION.ai-tXe-FXAA
Trained on "121361" images.
- **DOWNLOAD:** https://huggingface.co/FFusion/FFUSION.ai-Text-Encoder-LyCORIS-SD-2.1/blob/main/di.FFUSION.ai-tXe-FXAA.safetensors
Enhance your model's quality and sharpness using your own pre-trained Unet.
The text encoder (without UNET) is wrapped in LyCORIS. Optimizer: torch.optim.adamw.AdamW(weight_decay=0.01, betas=(0.9, 0.99))
Network dimension/rank: 768.0 Alpha: 768.0 Module: lycoris.kohya {'conv_dim': '256', 'conv_alpha': '256', 'algo': 'loha'}
Large size due to Lyco CONV 256


This is a heavy experimental version we used to test even with sloppy captions (quick WD tags and terrible clip), yet the results were satisfying.
Note: This is not the text encoder used in the official FFUSION AI model.
# SAMPLES
**Available also at https://civitai.com/models/83622**

For a1111
Install https://github.com/KohakuBlueleaf/a1111-sd-webui-lycoris
Download di.FFUSION.ai-tXe-FXAA to /models/Lycoris
Option1:
Insert <lyco:di.FFUSION.ai-tXe-FXAA:1.0> to prompt
No need to split Unet and Text Enc as its only TX encoder there.
You can go up to 2x weights
Option2: If you need it always ON (ex run a batch from txt file) then you can go to settings / Quicksettings list

add sd_lyco
restart and you should have a drop-down now 🤟 🥃

# Table of Contents
- [Model Card for di.FFUSION.ai Text Encoder - SD 2.1 LyCORIS](#model-card-for--model_id-)
- [Table of Contents](#table-of-contents)
- [Table of Contents](#table-of-contents-1)
- [Model Details](#model-details)
- [Model Description](#model-description)
- [Uses](#uses)
- [Direct Use](#direct-use)
- [Downstream Use [Optional]](#downstream-use-optional)
- [Out-of-Scope Use](#out-of-scope-use)
- [Bias, Risks, and Limitations](#bias-risks-and-limitations)
- [Recommendations](#recommendations)
- [Training Details](#training-details)
- [Training Data](#training-data)
- [Training Procedure](#training-procedure)
- [Preprocessing](#preprocessing)
- [Speeds, Sizes, Times](#speeds-sizes-times)
- [Evaluation](#evaluation)
- [Testing Data, Factors & Metrics](#testing-data-factors--metrics)
- [Testing Data](#testing-data)
- [Factors](#factors)
- [Metrics](#metrics)
- [Results](#results)
- [Model Examination](#model-examination)
- [Environmental Impact](#environmental-impact)
- [Technical Specifications [optional]](#technical-specifications-optional)
- [Model Architecture and Objective](#model-architecture-and-objective)
- [Compute Infrastructure](#compute-infrastructure)
- [Hardware](#hardware)
- [Software](#software)
- [Citation](#citation)
- [Glossary [optional]](#glossary-optional)
- [More Information [optional]](#more-information-optional)
- [Model Card Authors [optional]](#model-card-authors-optional)
- [Model Card Contact](#model-card-contact)
- [How to Get Started with the Model](#how-to-get-started-with-the-model)
# Model Details
## Model Description
<!-- Provide a longer summary of what this model is/does. -->
di.FFUSION.ai-tXe-FXAA
Trained on "121361" images.
Enhance your model's quality and sharpness using your own pre-trained Unet.
The text encoder (without UNET) is wrapped in LyCORIS. Optimizer: torch.optim.adamw.AdamW(weight_decay=0.01, betas=(0.9, 0.99))
Network dimension/rank: 768.0 Alpha: 768.0 Module: lycoris.kohya {'conv_dim': '256', 'conv_alpha': '256', 'algo': 'loha'}
Large size due to Lyco CONV 256
This is a heavy experimental version we used to test even with sloppy captions (quick WD tags and terrible clip), yet the results were satisfying.
Note: This is not the text encoder used in the official FFUSION AI model.
- **Developed by:** FFusion.ai
- **Shared by [Optional]:** idle stoev
- **Model type:** Language model
- **Language(s) (NLP):** en
- **License:** creativeml-openrail-m
- **Parent Model:** More information needed
- **Resources for more information:** More information needed
# Uses
<!-- Address questions around how the model is intended to be used, including the foreseeable users of the model and those affected by the model. -->
## Direct Use
<!-- This section is for the model use without fine-tuning or plugging into a larger ecosystem/app. -->
<!-- If the user enters content, print that. If not, but they enter a task in the list, use that. If neither, say "more info needed." -->
The text encoder (without UNET) is wrapped in LyCORIS. Optimizer: torch.optim.adamw.AdamW(weight_decay=0.01, betas=(0.9, 0.99))
Network dimension/rank: 768.0 Alpha: 768.0 Module: lycoris.kohya {'conv_dim': '256', 'conv_alpha': '256', 'algo': 'loha'}
Large size due to Lyco CONV 256
# Bias, Risks, and Limitations
<!-- This section is meant to convey both technical and sociotechnical limitations. -->
Significant research has explored bias and fairness issues with language models (see, e.g., [Sheng et al. (2021)](https://aclanthology.org/2021.acl-long.330.pdf) and [Bender et al. (2021)](https://dl.acm.org/doi/pdf/10.1145/3442188.3445922)). Predictions generated by the model may include disturbing and harmful stereotypes across protected classes; identity characteristics; and sensitive, social, and occupational groups.
## Recommendations
<!-- This section is meant to convey recommendations with respect to the bias, risk, and technical limitations. -->
# Training Details
## Training Data
<!-- This should link to a Data Card, perhaps with a short stub of information on what the training data is all about as well as documentation related to data pre-processing or additional filtering. -->
Trained on "121361" images.
ss_caption_tag_dropout_rate: "0.0",
ss_multires_noise_discount: "0.3",
ss_mixed_precision: "bf16",
ss_text_encoder_lr: "1e-07",
ss_keep_tokens: "3",
ss_network_args: "{"conv_dim": "256", "conv_alpha": "256", "algo": "loha"}",
ss_caption_dropout_rate: "0.02",
ss_flip_aug: "False",
ss_learning_rate: "2e-07",
ss_sd_model_name: "stabilityai/stable-diffusion-2-1-base",
ss_max_grad_norm: "1.0",
ss_num_epochs: "2",
ss_gradient_checkpointing: "False",
ss_face_crop_aug_range: "None",
ss_epoch: "2",
ss_num_train_images: "121361",
ss_color_aug: "False",
ss_gradient_accumulation_steps: "1",
ss_total_batch_size: "100",
ss_prior_loss_weight: "1.0",
ss_training_comment: "None",
ss_network_dim: "768",
ss_output_name: "FusionaMEGA1tX",
ss_max_bucket_reso: "1024",
ss_network_alpha: "768.0",
ss_steps: "2444",
ss_shuffle_caption: "True",
ss_training_finished_at: "1684158038.0763328",
ss_min_bucket_reso: "256",
ss_noise_offset: "0.09",
ss_enable_bucket: "True",
ss_batch_size_per_device: "20",
ss_max_train_steps: "2444",
ss_network_module: "lycoris.kohya",
## Training Procedure
<!-- This relates heavily to the Technical Specifications. Content here should link to that section when it is relevant to the training procedure. -->
### Preprocessing
"{"buckets": {"0": {"resolution": [192, 256], "count": 1}, "1": {"resolution": [192, 320], "count": 1}, "2": {"resolution": [256, 384], "count": 1}, "3": {"resolution": [256, 512], "count": 1}, "4": {"resolution": [384, 576], "count": 2}, "5": {"resolution": [384, 640], "count": 2}, "6": {"resolution": [384, 704], "count": 1}, "7": {"resolution": [384, 1088], "count": 15}, "8": {"resolution": [448, 448], "count": 5}, "9": {"resolution": [448, 576], "count": 1}, "10": {"resolution": [448, 640], "count": 1}, "11": {"resolution": [448, 768], "count": 1}, "12": {"resolution": [448, 832], "count": 1}, "13": {"resolution": [448, 1088], "count": 25}, "14": {"resolution": [448, 1216], "count": 1}, "15": {"resolution": [512, 640], "count": 2}, "16": {"resolution": [512, 768], "count": 10}, "17": {"resolution": [512, 832], "count": 3}, "18": {"resolution": [512, 896], "count": 1525}, "19": {"resolution": [512, 960], "count": 2}, "20": {"resolution": [512, 1024], "count": 665}, "21": {"resolution": [512, 1088], "count": 8}, "22": {"resolution": [576, 576], "count": 5}, "23": {"resolution": [576, 768], "count": 1}, "24": {"resolution": [576, 832], "count": 667}, "25": {"resolution": [576, 896], "count": 9601}, "26": {"resolution": [576, 960], "count": 872}, "27": {"resolution": [576, 1024], "count": 17}, "28": {"resolution": [640, 640], "count": 3}, "29": {"resolution": [640, 768], "count": 7}, "30": {"resolution": [640, 832], "count": 608}, "31": {"resolution": [640, 896], "count": 90}, "32": {"resolution": [704, 640], "count": 1}, "33": {"resolution": [704, 704], "count": 11}, "34": {"resolution": [704, 768], "count": 1}, "35": {"resolution": [704, 832], "count": 1}, "36": {"resolution": [768, 640], "count": 225}, "37": {"resolution": [768, 704], "count": 6}, "38": {"resolution": [768, 768], "count": 74442}, "39": {"resolution": [832, 576], "count": 23784}, "40": {"resolution": [832, 640], "count": 554}, "41": {"resolution": [896, 512], "count": 1235}, "42": {"resolution": [896, 576], "count": 50}, "43": {"resolution": [896, 640], "count": 88}, "44": {"resolution": [960, 512], "count": 165}, "45": {"resolution": [960, 576], "count": 5246}, "46": {"resolution": [1024, 448], "count": 5}, "47": {"resolution": [1024, 512], "count": 1187}, "48": {"resolution": [1024, 576], "count": 40}, "49": {"resolution": [1088, 384], "count": 70}, "50": {"resolution": [1088, 448], "count": 36}, "51": {"resolution": [1088, 512], "count": 3}, "52": {"resolution": [1216, 448], "count": 36}, "53": {"resolution": [1344, 320], "count": 29}, "54": {"resolution": [1536, 384], "count": 1}}, "mean_img_ar_error": 0.01693107810697896}",
### Speeds, Sizes, Times
<!-- This section provides information about throughput, start/end time, checkpoint size if relevant, etc. -->
ss_resolution: "(768, 768)",
ss_v2: "True",
ss_cache_latents: "False",
ss_unet_lr: "2e-07",
ss_num_reg_images: "0",
ss_max_token_length: "225",
ss_lr_scheduler: "linear",
ss_reg_dataset_dirs: "{}",
ss_lr_warmup_steps: "303",
ss_num_batches_per_epoch: "1222",
ss_lowram: "False",
ss_multires_noise_iterations: "None",
ss_optimizer: "torch.optim.adamw.AdamW(weight_decay=0.01,betas=(0.9, 0.99))",
# Evaluation
<!-- This section describes the evaluation protocols and provides the results. -->
## Testing Data, Factors & Metrics
### Testing Data
<!-- This should link to a Data Card if possible. -->
More information needed
### Factors
<!-- These are the things the evaluation is disaggregating by, e.g., subpopulations or domains. -->
More information needed
### Metrics
<!-- These are the evaluation metrics being used, ideally with a description of why. -->
More information needed
## Results
More information needed
# Model Examination
More information needed
# Environmental Impact
<!-- Total emissions (in grams of CO2eq) and additional considerations, such as electricity usage, go here. Edit the suggested text below accordingly -->
Carbon emissions can be estimated using the [Machine Learning Impact calculator](https://mlco2.github.io/impact#compute) presented in [Lacoste et al. (2019)](https://arxiv.org/abs/1910.09700).
- **Hardware Type:** 8xA100
- **Hours used:** 64
- **Cloud Provider:** CoreWeave
- **Compute Region:** US Main
- **Carbon Emitted:** 6.72
# Technical Specifications [optional]
## Model Architecture and Objective
Enhance your model's quality and sharpness using your own pre-trained Unet.
## Compute Infrastructure
More information needed
### Hardware
8xA100
### Software
Fully trained only with Kohya S & Shih-Ying Yeh (Kohaku-BlueLeaf)
https://arxiv.org/abs/2108.06098
# Citation
<!-- If there is a paper or blog post introducing the model, the APA and Bibtex information for that should go in this section. -->
**BibTeX:**
More information needed
**APA:**
@misc{LyCORIS,
author = "Shih-Ying Yeh (Kohaku-BlueLeaf), Yu-Guan Hsieh, Zhidong Gao",
title = "LyCORIS - Lora beYond Conventional methods, Other Rank adaptation Implementations for Stable diffusion",
howpublished = "\url{https://github.com/KohakuBlueleaf/LyCORIS}",
month = "March",
year = "2023"
}
# Glossary [optional]
<!-- If relevant, include terms and calculations in this section that can help readers understand the model or model card. -->
More information needed
# More Information [optional]
More information needed
# Model Card Authors [optional]
<!-- This section provides another layer of transparency and accountability. Whose views is this model card representing? How many voices were included in its construction? Etc. -->
idle stoev
# Model Card Contact
[email protected]
# How to Get Started with the Model
Use the code below to get started with the model.
<details>
<summary> Click to expand </summary>
For a1111
Install https://github.com/KohakuBlueleaf/a1111-sd-webui-lycoris
Download di.FFUSION.ai-tXe-FXAA to /models/Lycoris
Option1:
Insert <lyco:di.FFUSION.ai-tXe-FXAA:1.0> to prompt
No need to split Unet and Text Enc as its only TX encoder there.
You can go up to 2x weights
Option2: If you need it always ON (ex run a batch from txt file) then you can go to settings / Quicksettings list
add sd_lyco
restart and you should have a drop-down now 🤟 🥃
</details> |
gmongaras/Llama3.1_8B_Instruct_GRPO_gsm8k | gmongaras | "2025-04-15T01:31:12Z" | 0 | 0 | null | [
"region:us"
] | null | "2025-04-15T01:15:53Z" | <!DOCTYPE html>
<html class="" lang="en">
<head>
<meta charset="utf-8" />
<meta
name="viewport"
content="width=device-width, initial-scale=1.0, user-scalable=no"
/>
<meta
name="description"
content="We're on a journey to advance and democratize artificial intelligence through open source and open science."
/>
<meta property="fb:app_id" content="1321688464574422" />
<meta name="twitter:card" content="summary_large_image" />
<meta name="twitter:site" content="@huggingface" />
<meta
property="og:title"
content="Hugging Face - The AI community building the future."
/>
<meta property="og:type" content="website" />
<title>Hugging Face - The AI community building the future.</title>
<style>
body {
margin: 0;
}
main {
background-color: white;
min-height: 100vh;
padding: 7rem 1rem 8rem 1rem;
text-align: center;
font-family: Source Sans Pro, ui-sans-serif, system-ui, -apple-system,
BlinkMacSystemFont, Segoe UI, Roboto, Helvetica Neue, Arial, Noto Sans,
sans-serif, Apple Color Emoji, Segoe UI Emoji, Segoe UI Symbol,
Noto Color Emoji;
}
img {
width: 6rem;
height: 6rem;
margin: 0 auto 1rem;
}
h1 {
font-size: 3.75rem;
line-height: 1;
color: rgba(31, 41, 55, 1);
font-weight: 700;
box-sizing: border-box;
margin: 0 auto;
}
p, a {
color: rgba(107, 114, 128, 1);
font-size: 1.125rem;
line-height: 1.75rem;
max-width: 28rem;
box-sizing: border-box;
margin: 0 auto;
}
.dark main {
background-color: rgb(11, 15, 25);
}
.dark h1 {
color: rgb(209, 213, 219);
}
.dark p, .dark a {
color: rgb(156, 163, 175);
}
</style>
<script>
// On page load or when changing themes, best to add inline in `head` to avoid FOUC
const key = "_tb_global_settings";
let theme = window.matchMedia("(prefers-color-scheme: dark)").matches
? "dark"
: "light";
try {
const storageTheme = JSON.parse(window.localStorage.getItem(key)).theme;
if (storageTheme) {
theme = storageTheme === "dark" ? "dark" : "light";
}
} catch (e) {}
if (theme === "dark") {
document.documentElement.classList.add("dark");
} else {
document.documentElement.classList.remove("dark");
}
</script>
</head>
<body>
<main>
<img
src="https://cdn-media.huggingface.co/assets/huggingface_logo.svg"
alt=""
/>
<div>
<h1>429</h1>
<p>We had to rate limit you. If you think it's an error, send us <a href="mailto:[email protected]">an email</a></p>
</div>
</main>
</body>
</html> |
lesso03/65265d24-053d-48ff-bec7-d7de954f9ef8 | lesso03 | "2024-12-21T16:11:45Z" | 9 | 0 | peft | [
"peft",
"safetensors",
"llama",
"axolotl",
"generated_from_trainer",
"base_model:NousResearch/Llama-3.2-1B",
"base_model:adapter:NousResearch/Llama-3.2-1B",
"license:llama3.2",
"region:us"
] | null | "2024-12-21T16:07:30Z" | ---
library_name: peft
license: llama3.2
base_model: NousResearch/Llama-3.2-1B
tags:
- axolotl
- generated_from_trainer
model-index:
- name: 65265d24-053d-48ff-bec7-d7de954f9ef8
results: []
---
<!-- This model card has been generated automatically according to the information the Trainer had access to. You
should probably proofread and complete it, then remove this comment. -->
[<img src="https://raw.githubusercontent.com/axolotl-ai-cloud/axolotl/main/image/axolotl-badge-web.png" alt="Built with Axolotl" width="200" height="32"/>](https://github.com/axolotl-ai-cloud/axolotl)
<details><summary>See axolotl config</summary>
axolotl version: `0.4.1`
```yaml
adapter: lora
base_model: NousResearch/Llama-3.2-1B
bf16: auto
chat_template: llama3
dataset_prepared_path: null
datasets:
- data_files:
- f210c167ecc2db34_train_data.json
ds_type: json
field: question
path: /workspace/input_data/f210c167ecc2db34_train_data.json
type: completion
debug: null
deepspeed: null
early_stopping_patience: null
eval_max_new_tokens: 128
eval_table_size: null
evals_per_epoch: 4
flash_attention: false
fp16: null
fsdp: null
fsdp_config: null
gradient_accumulation_steps: 4
gradient_checkpointing: false
group_by_length: false
hub_model_id: lesso03/65265d24-053d-48ff-bec7-d7de954f9ef8
hub_repo: null
hub_strategy: checkpoint
hub_token: null
learning_rate: 0.0002
load_in_4bit: false
load_in_8bit: false
local_rank: null
logging_steps: 1
lora_alpha: 16
lora_dropout: 0.05
lora_fan_in_fan_out: null
lora_model_dir: null
lora_r: 8
lora_target_linear: true
lr_scheduler: cosine
max_steps: 10
micro_batch_size: 2
mlflow_experiment_name: /tmp/f210c167ecc2db34_train_data.json
model_type: AutoModelForCausalLM
num_epochs: 1
optimizer: adamw_bnb_8bit
output_dir: miner_id_24
pad_to_sequence_len: true
resume_from_checkpoint: null
s2_attention: null
sample_packing: false
saves_per_epoch: 4
sequence_len: 512
special_tokens:
pad_token: <|end_of_text|>
strict: false
tf32: false
tokenizer_type: AutoTokenizer
train_on_inputs: false
trust_remote_code: true
val_set_size: 0.05
wandb_entity: null
wandb_mode: online
wandb_name: 65265d24-053d-48ff-bec7-d7de954f9ef8
wandb_project: Gradients-On-Demand
wandb_run: your_name
wandb_runid: 65265d24-053d-48ff-bec7-d7de954f9ef8
warmup_steps: 10
weight_decay: 0.0
xformers_attention: null
```
</details><br>
# 65265d24-053d-48ff-bec7-d7de954f9ef8
This model is a fine-tuned version of [NousResearch/Llama-3.2-1B](https://huggingface.co/NousResearch/Llama-3.2-1B) on the None dataset.
It achieves the following results on the evaluation set:
- Loss: 5.3036
## Model description
More information needed
## Intended uses & limitations
More information needed
## Training and evaluation data
More information needed
## Training procedure
### Training hyperparameters
The following hyperparameters were used during training:
- learning_rate: 0.0002
- train_batch_size: 2
- eval_batch_size: 2
- seed: 42
- gradient_accumulation_steps: 4
- total_train_batch_size: 8
- optimizer: Use OptimizerNames.ADAMW_BNB with betas=(0.9,0.999) and epsilon=1e-08 and optimizer_args=No additional optimizer arguments
- lr_scheduler_type: cosine
- lr_scheduler_warmup_steps: 10
- training_steps: 10
### Training results
| Training Loss | Epoch | Step | Validation Loss |
|:-------------:|:------:|:----:|:---------------:|
| 5.986 | 0.0002 | 1 | 6.0940 |
| 6.5356 | 0.0005 | 3 | 6.0807 |
| 6.7297 | 0.0009 | 6 | 5.9073 |
| 5.5206 | 0.0014 | 9 | 5.3036 |
### Framework versions
- PEFT 0.13.2
- Transformers 4.46.0
- Pytorch 2.5.0+cu124
- Datasets 3.0.1
- Tokenizers 0.20.1 |
go2k/q-Taxi-v3 | go2k | "2022-07-07T05:45:11Z" | 0 | 0 | null | [
"Taxi-v3",
"q-learning",
"reinforcement-learning",
"custom-implementation",
"model-index",
"region:us"
] | reinforcement-learning | "2022-07-07T05:39:36Z" | ---
tags:
- Taxi-v3
- q-learning
- reinforcement-learning
- custom-implementation
model-index:
- name: q-Taxi-v3
results:
- metrics:
- type: mean_reward
value: 7.56 +/- 2.71
name: mean_reward
task:
type: reinforcement-learning
name: reinforcement-learning
dataset:
name: Taxi-v3
type: Taxi-v3
---
# **Q-Learning** Agent playing **Taxi-v3**
This is a trained model of a **Q-Learning** agent playing **Taxi-v3** .
## Usage
```python
model = load_from_hub(repo_id="go2k/q-Taxi-v3", filename="q-learning.pkl")
# Don't forget to check if you need to add additional attributes (is_slippery=False etc)
env = gym.make(model["env_id"])
evaluate_agent(env, model["max_steps"], model["n_eval_episodes"], model["qtable"], model["eval_seed"])
```
|
pere/t5-parliament-categorisation | pere | "2022-05-11T14:14:10Z" | 0 | 0 | null | [
"license:apache-2.0",
"region:us"
] | null | "2022-04-04T14:46:19Z" | ---
license: apache-2.0
---
|
hazyresearch/M2-BERT-32K-Retrieval-Encoder-V1 | hazyresearch | "2024-05-20T21:07:19Z" | 140 | 1 | transformers | [
"transformers",
"safetensors",
"bert",
"fill-mask",
"custom_code",
"en",
"arxiv:2402.07440",
"license:apache-2.0",
"autotrain_compatible",
"region:us"
] | fill-mask | "2024-01-09T05:27:22Z" | ---
license: apache-2.0
language:
- en
pipeline_tag: fill-mask
inference: false
---
# Monarch Mixer-BERT
The 80M checkpoint for M2-BERT-32k from the paper [Benchmarking and Building Long-Context Retrieval Models with LoCo and M2-BERT](https://arxiv.org/abs/2402.07440).
Check out our [GitHub](https://github.com/HazyResearch/m2/tree/main) for instructions on how to download and fine-tune it!
## How to use
You can load this model using Hugging Face `AutoModel`:
```python
from transformers import AutoModelForMaskedLM, BertConfig
config = BertConfig.from_pretrained("hazyresearch/M2-BERT-32K-Retrieval-Encoder-V1")
model = AutoModelForMaskedLM.from_pretrained("hazyresearch/M2-BERT-32K-Retrieval-Encoder-V1", config=config, trust_remote_code=True)
```
This model uses the Hugging Face `bert-base-uncased tokenizer`:
```
from transformers import BertTokenizer
tokenizer = BertTokenizer.from_pretrained('bert-base-uncased')
```
## How to use
This model generates embeddings for retrieval. The embeddings have a dimensionality of 768:
```
from transformers import AutoTokenizer, AutoModelForMaskedLM, BertConfig
max_seq_length = 32768
testing_string = "Every morning, I make a cup of coffee to start my day."
config = BertConfig.from_pretrained("hazyresearch/M2-BERT-32K-Retrieval-Encoder-V1")
model = AutoModelForMaskedLM.from_pretrained("hazyresearch/M2-BERT-32K-Retrieval-Encoder-V1", config=config, trust_remote_code=True)
tokenizer = AutoTokenizer.from_pretrained("bert-base-uncased", model_max_length=max_seq_length)
input_ids = tokenizer([testing_string], return_tensors="pt", padding="max_length", return_token_type_ids=False, truncation=True, max_length=max_seq_length)
outputs = model(**input_ids)
embeddings = outputs['sentence_embedding']
```
### Remote Code
This model requires `trust_remote_code=True` to be passed to the `from_pretrained` method. This is because we use custom PyTorch code (see our GitHub). You should consider passing a `revision` argument that specifies the exact git commit of the code, for example:
```python
mlm = AutoModelForMaskedLM.from_pretrained(
"hazyresearch/M2-BERT-32K-Retrieval-Encoder-V1",
config=config,
trust_remote_code=True,
)
```
### Configuration
Note `use_flash_mm` is false by default. Using FlashMM is currently not supported. |
jliu596/dqn-Atar-SpaceInvadersNoFrameskip-v4 | jliu596 | "2023-07-18T19:59:43Z" | 1 | 0 | stable-baselines3 | [
"stable-baselines3",
"SpaceInvadersNoFrameskip-v4",
"deep-reinforcement-learning",
"reinforcement-learning",
"model-index",
"region:us"
] | reinforcement-learning | "2023-07-18T19:59:13Z" | ---
library_name: stable-baselines3
tags:
- SpaceInvadersNoFrameskip-v4
- deep-reinforcement-learning
- reinforcement-learning
- stable-baselines3
model-index:
- name: DQN
results:
- task:
type: reinforcement-learning
name: reinforcement-learning
dataset:
name: SpaceInvadersNoFrameskip-v4
type: SpaceInvadersNoFrameskip-v4
metrics:
- type: mean_reward
value: 256.00 +/- 169.38
name: mean_reward
verified: false
---
# **DQN** Agent playing **SpaceInvadersNoFrameskip-v4**
This is a trained model of a **DQN** agent playing **SpaceInvadersNoFrameskip-v4**
using the [stable-baselines3 library](https://github.com/DLR-RM/stable-baselines3)
and the [RL Zoo](https://github.com/DLR-RM/rl-baselines3-zoo).
The RL Zoo is a training framework for Stable Baselines3
reinforcement learning agents,
with hyperparameter optimization and pre-trained agents included.
## Usage (with SB3 RL Zoo)
RL Zoo: https://github.com/DLR-RM/rl-baselines3-zoo<br/>
SB3: https://github.com/DLR-RM/stable-baselines3<br/>
SB3 Contrib: https://github.com/Stable-Baselines-Team/stable-baselines3-contrib
Install the RL Zoo (with SB3 and SB3-Contrib):
```bash
pip install rl_zoo3
```
```
# Download model and save it into the logs/ folder
python -m rl_zoo3.load_from_hub --algo dqn --env SpaceInvadersNoFrameskip-v4 -orga jliu596 -f logs/
python -m rl_zoo3.enjoy --algo dqn --env SpaceInvadersNoFrameskip-v4 -f logs/
```
If you installed the RL Zoo3 via pip (`pip install rl_zoo3`), from anywhere you can do:
```
python -m rl_zoo3.load_from_hub --algo dqn --env SpaceInvadersNoFrameskip-v4 -orga jliu596 -f logs/
python -m rl_zoo3.enjoy --algo dqn --env SpaceInvadersNoFrameskip-v4 -f logs/
```
## Training (with the RL Zoo)
```
python -m rl_zoo3.train --algo dqn --env SpaceInvadersNoFrameskip-v4 -f logs/
# Upload the model and generate video (when possible)
python -m rl_zoo3.push_to_hub --algo dqn --env SpaceInvadersNoFrameskip-v4 -f logs/ -orga jliu596
```
## Hyperparameters
```python
OrderedDict([('batch_size', 128),
('buffer_size', 1500),
('env_wrapper',
['stable_baselines3.common.atari_wrappers.AtariWrapper']),
('exploration_final_eps', 0.05),
('exploration_fraction', 1),
('frame_stack', 4),
('gradient_steps', 2),
('learning_rate', 0.0001),
('learning_starts', 1000),
('n_timesteps', 1000000.0),
('optimize_memory_usage', False),
('policy', 'CnnPolicy'),
('target_update_interval', 100),
('train_freq', 4),
('normalize', False)])
```
# Environment Arguments
```python
{'render_mode': 'rgb_array'}
```
|
StepLaw/StepLaw-N_268M-D_19.0B-LR2.762e-03-BS1048576 | StepLaw | "2025-04-15T14:26:01Z" | 0 | 0 | transformers | [
"transformers",
"safetensors",
"step1",
"text-generation",
"StepLaw",
"causal-lm",
"en",
"license:apache-2.0",
"autotrain_compatible",
"endpoints_compatible",
"region:us"
] | text-generation | "2025-04-09T11:41:22Z" | <!DOCTYPE html>
<html class="" lang="en">
<head>
<meta charset="utf-8" />
<meta
name="viewport"
content="width=device-width, initial-scale=1.0, user-scalable=no"
/>
<meta
name="description"
content="We're on a journey to advance and democratize artificial intelligence through open source and open science."
/>
<meta property="fb:app_id" content="1321688464574422" />
<meta name="twitter:card" content="summary_large_image" />
<meta name="twitter:site" content="@huggingface" />
<meta
property="og:title"
content="Hugging Face - The AI community building the future."
/>
<meta property="og:type" content="website" />
<title>Hugging Face - The AI community building the future.</title>
<style>
body {
margin: 0;
}
main {
background-color: white;
min-height: 100vh;
padding: 7rem 1rem 8rem 1rem;
text-align: center;
font-family: Source Sans Pro, ui-sans-serif, system-ui, -apple-system,
BlinkMacSystemFont, Segoe UI, Roboto, Helvetica Neue, Arial, Noto Sans,
sans-serif, Apple Color Emoji, Segoe UI Emoji, Segoe UI Symbol,
Noto Color Emoji;
}
img {
width: 6rem;
height: 6rem;
margin: 0 auto 1rem;
}
h1 {
font-size: 3.75rem;
line-height: 1;
color: rgba(31, 41, 55, 1);
font-weight: 700;
box-sizing: border-box;
margin: 0 auto;
}
p, a {
color: rgba(107, 114, 128, 1);
font-size: 1.125rem;
line-height: 1.75rem;
max-width: 28rem;
box-sizing: border-box;
margin: 0 auto;
}
.dark main {
background-color: rgb(11, 15, 25);
}
.dark h1 {
color: rgb(209, 213, 219);
}
.dark p, .dark a {
color: rgb(156, 163, 175);
}
</style>
<script>
// On page load or when changing themes, best to add inline in `head` to avoid FOUC
const key = "_tb_global_settings";
let theme = window.matchMedia("(prefers-color-scheme: dark)").matches
? "dark"
: "light";
try {
const storageTheme = JSON.parse(window.localStorage.getItem(key)).theme;
if (storageTheme) {
theme = storageTheme === "dark" ? "dark" : "light";
}
} catch (e) {}
if (theme === "dark") {
document.documentElement.classList.add("dark");
} else {
document.documentElement.classList.remove("dark");
}
</script>
</head>
<body>
<main>
<img
src="https://cdn-media.huggingface.co/assets/huggingface_logo.svg"
alt=""
/>
<div>
<h1>429</h1>
<p>We had to rate limit you. If you think it's an error, send us <a href="mailto:[email protected]">an email</a></p>
</div>
</main>
</body>
</html> |
JesusisKing68/thumbnailgen | JesusisKing68 | "2025-04-12T12:45:26Z" | 0 | 0 | diffusers | [
"diffusers",
"flux",
"lora",
"replicate",
"text-to-image",
"en",
"base_model:black-forest-labs/FLUX.1-dev",
"base_model:adapter:black-forest-labs/FLUX.1-dev",
"license:other",
"region:us"
] | text-to-image | "2025-04-12T12:01:42Z" | <!DOCTYPE html>
<html class="" lang="en">
<head>
<meta charset="utf-8" />
<meta
name="viewport"
content="width=device-width, initial-scale=1.0, user-scalable=no"
/>
<meta
name="description"
content="We're on a journey to advance and democratize artificial intelligence through open source and open science."
/>
<meta property="fb:app_id" content="1321688464574422" />
<meta name="twitter:card" content="summary_large_image" />
<meta name="twitter:site" content="@huggingface" />
<meta
property="og:title"
content="Hugging Face - The AI community building the future."
/>
<meta property="og:type" content="website" />
<title>Hugging Face - The AI community building the future.</title>
<style>
body {
margin: 0;
}
main {
background-color: white;
min-height: 100vh;
padding: 7rem 1rem 8rem 1rem;
text-align: center;
font-family: Source Sans Pro, ui-sans-serif, system-ui, -apple-system,
BlinkMacSystemFont, Segoe UI, Roboto, Helvetica Neue, Arial, Noto Sans,
sans-serif, Apple Color Emoji, Segoe UI Emoji, Segoe UI Symbol,
Noto Color Emoji;
}
img {
width: 6rem;
height: 6rem;
margin: 0 auto 1rem;
}
h1 {
font-size: 3.75rem;
line-height: 1;
color: rgba(31, 41, 55, 1);
font-weight: 700;
box-sizing: border-box;
margin: 0 auto;
}
p, a {
color: rgba(107, 114, 128, 1);
font-size: 1.125rem;
line-height: 1.75rem;
max-width: 28rem;
box-sizing: border-box;
margin: 0 auto;
}
.dark main {
background-color: rgb(11, 15, 25);
}
.dark h1 {
color: rgb(209, 213, 219);
}
.dark p, .dark a {
color: rgb(156, 163, 175);
}
</style>
<script>
// On page load or when changing themes, best to add inline in `head` to avoid FOUC
const key = "_tb_global_settings";
let theme = window.matchMedia("(prefers-color-scheme: dark)").matches
? "dark"
: "light";
try {
const storageTheme = JSON.parse(window.localStorage.getItem(key)).theme;
if (storageTheme) {
theme = storageTheme === "dark" ? "dark" : "light";
}
} catch (e) {}
if (theme === "dark") {
document.documentElement.classList.add("dark");
} else {
document.documentElement.classList.remove("dark");
}
</script>
</head>
<body>
<main>
<img
src="https://cdn-media.huggingface.co/assets/huggingface_logo.svg"
alt=""
/>
<div>
<h1>429</h1>
<p>We had to rate limit you. If you think it's an error, send us <a href="mailto:[email protected]">an email</a></p>
</div>
</main>
</body>
</html> |
Vitar140/vitor | Vitar140 | "2025-02-09T03:59:29Z" | 14 | 0 | diffusers | [
"diffusers",
"flux",
"lora",
"replicate",
"text-to-image",
"en",
"base_model:black-forest-labs/FLUX.1-dev",
"base_model:adapter:black-forest-labs/FLUX.1-dev",
"license:other",
"region:us"
] | text-to-image | "2025-02-09T03:45:56Z" | ---
license: other
license_name: flux-1-dev-non-commercial-license
license_link: https://huggingface.co/black-forest-labs/FLUX.1-dev/blob/main/LICENSE.md
language:
- en
tags:
- flux
- diffusers
- lora
- replicate
base_model: "black-forest-labs/FLUX.1-dev"
pipeline_tag: text-to-image
# widget:
# - text: >-
# prompt
# output:
# url: https://...
instance_prompt: vigp
---
# Vitor
<Gallery />
Trained on Replicate using:
https://replicate.com/ostris/flux-dev-lora-trainer/train
## Trigger words
You should use `vigp` to trigger the image generation.
## Use it with the [🧨 diffusers library](https://github.com/huggingface/diffusers)
```py
from diffusers import AutoPipelineForText2Image
import torch
pipeline = AutoPipelineForText2Image.from_pretrained('black-forest-labs/FLUX.1-dev', torch_dtype=torch.float16).to('cuda')
pipeline.load_lora_weights('Vitar140/vitor', weight_name='lora.safetensors')
image = pipeline('your prompt').images[0]
```
For more details, including weighting, merging and fusing LoRAs, check the [documentation on loading LoRAs in diffusers](https://huggingface.co/docs/diffusers/main/en/using-diffusers/loading_adapters)
|
Kukedlc/Ramakrishna-7b | Kukedlc | "2024-03-27T02:15:59Z" | 9 | 0 | transformers | [
"transformers",
"safetensors",
"mistral",
"text-generation",
"merge",
"mergekit",
"lazymergekit",
"MatthieuJ/Jason1903_SLERP",
"AurelPx/Percival_01-7b-slerp",
"base_model:AurelPx/Percival_01-7b-slerp",
"base_model:merge:AurelPx/Percival_01-7b-slerp",
"base_model:MatthieuJ/Jason1903_SLERP",
"base_model:merge:MatthieuJ/Jason1903_SLERP",
"autotrain_compatible",
"text-generation-inference",
"endpoints_compatible",
"region:us"
] | text-generation | "2024-03-27T02:10:40Z" | ---
tags:
- merge
- mergekit
- lazymergekit
- MatthieuJ/Jason1903_SLERP
- AurelPx/Percival_01-7b-slerp
base_model:
- MatthieuJ/Jason1903_SLERP
- AurelPx/Percival_01-7b-slerp
---
# Ramakrishna-7b
Ramakrishna-7b is a merge of the following models using [LazyMergekit](https://colab.research.google.com/drive/1obulZ1ROXHjYLn6PPZJwRR6GzgQogxxb?usp=sharing):
* [MatthieuJ/Jason1903_SLERP](https://huggingface.co/MatthieuJ/Jason1903_SLERP)
* [AurelPx/Percival_01-7b-slerp](https://huggingface.co/AurelPx/Percival_01-7b-slerp)
## 🧩 Configuration
```yaml
slices:
- sources:
- model: MatthieuJ/Jason1903_SLERP
layer_range: [0, 32]
- model: AurelPx/Percival_01-7b-slerp
layer_range: [0, 32]
merge_method: slerp
base_model: AurelPx/Percival_01-7b-slerp
parameters:
t:
- filter: self_attn
value: [0, 0.5, 0.3, 0.7, 1]
- filter: mlp
value: [1, 0.5, 0.7, 0.3, 0]
- value: 0.5
dtype: float16
```
## 💻 Usage
```python
!pip install -qU transformers accelerate
from transformers import AutoTokenizer
import transformers
import torch
model = "Kukedlc/Ramakrishna-7b"
messages = [{"role": "user", "content": "What is a large language model?"}]
tokenizer = AutoTokenizer.from_pretrained(model)
prompt = tokenizer.apply_chat_template(messages, tokenize=False, add_generation_prompt=True)
pipeline = transformers.pipeline(
"text-generation",
model=model,
torch_dtype=torch.float16,
device_map="auto",
)
outputs = pipeline(prompt, max_new_tokens=256, do_sample=True, temperature=0.7, top_k=50, top_p=0.95)
print(outputs[0]["generated_text"])
``` |
cleanrl/PrivateEye-v5-cleanba_ppo_envpool_impala_atari_wrapper-seed2 | cleanrl | "2023-03-07T17:39:12Z" | 0 | 0 | cleanrl | [
"cleanrl",
"tensorboard",
"PrivateEye-v5",
"deep-reinforcement-learning",
"reinforcement-learning",
"custom-implementation",
"model-index",
"region:us"
] | reinforcement-learning | "2023-02-17T16:10:55Z" | ---
tags:
- PrivateEye-v5
- deep-reinforcement-learning
- reinforcement-learning
- custom-implementation
library_name: cleanrl
model-index:
- name: PPO
results:
- task:
type: reinforcement-learning
name: reinforcement-learning
dataset:
name: PrivateEye-v5
type: PrivateEye-v5
metrics:
- type: mean_reward
value: 100.00 +/- 0.00
name: mean_reward
verified: false
---
# (CleanRL) **PPO** Agent Playing **PrivateEye-v5**
This is a trained model of a PPO agent playing PrivateEye-v5.
The model was trained by using [CleanRL](https://github.com/vwxyzjn/cleanrl) and the most up-to-date training code can be
found [here](https://github.com/vwxyzjn/cleanrl/blob/master/cleanrl/cleanba_ppo_envpool_impala_atari_wrapper.py).
## Get Started
To use this model, please install the `cleanrl` package with the following command:
```
pip install "cleanrl[jax,envpool,atari]"
python -m cleanrl_utils.enjoy --exp-name cleanba_ppo_envpool_impala_atari_wrapper --env-id PrivateEye-v5
```
Please refer to the [documentation](https://docs.cleanrl.dev/get-started/zoo/) for more detail.
## Command to reproduce the training
```bash
curl -OL https://huggingface.co/cleanrl/PrivateEye-v5-cleanba_ppo_envpool_impala_atari_wrapper-seed2/raw/main/cleanba_ppo_envpool_impala_atari_wrapper.py
curl -OL https://huggingface.co/cleanrl/PrivateEye-v5-cleanba_ppo_envpool_impala_atari_wrapper-seed2/raw/main/pyproject.toml
curl -OL https://huggingface.co/cleanrl/PrivateEye-v5-cleanba_ppo_envpool_impala_atari_wrapper-seed2/raw/main/poetry.lock
poetry install --all-extras
python cleanba_ppo_envpool_impala_atari_wrapper.py --distributed --learner-device-ids 1 2 3 --track --wandb-project-name cleanba --save-model --upload-model --hf-entity cleanrl --env-id PrivateEye-v5 --seed 2
```
# Hyperparameters
```python
{'actor_device_ids': [0],
'actor_devices': ['gpu:0'],
'anneal_lr': True,
'async_batch_size': 20,
'async_update': 3,
'batch_size': 15360,
'capture_video': False,
'clip_coef': 0.1,
'concurrency': True,
'cuda': True,
'distributed': True,
'ent_coef': 0.01,
'env_id': 'PrivateEye-v5',
'exp_name': 'cleanba_ppo_envpool_impala_atari_wrapper',
'gae_lambda': 0.95,
'gamma': 0.99,
'global_learner_decices': ['gpu:1',
'gpu:2',
'gpu:3',
'gpu:5',
'gpu:6',
'gpu:7'],
'hf_entity': 'cleanrl',
'learner_device_ids': [1, 2, 3],
'learner_devices': ['gpu:1', 'gpu:2', 'gpu:3'],
'learning_rate': 0.00025,
'local_batch_size': 7680,
'local_minibatch_size': 1920,
'local_num_envs': 60,
'local_rank': 0,
'max_grad_norm': 0.5,
'minibatch_size': 3840,
'norm_adv': True,
'num_envs': 120,
'num_minibatches': 4,
'num_steps': 128,
'num_updates': 3255,
'profile': False,
'save_model': True,
'seed': 2,
'target_kl': None,
'test_actor_learner_throughput': False,
'torch_deterministic': True,
'total_timesteps': 50000000,
'track': True,
'update_epochs': 4,
'upload_model': True,
'vf_coef': 0.5,
'wandb_entity': None,
'wandb_project_name': 'cleanba',
'world_size': 2}
```
|
DBangshu/V2_Base_GPT2_e5_6_0 | DBangshu | "2024-10-16T10:54:07Z" | 131 | 0 | transformers | [
"transformers",
"safetensors",
"gpt2",
"text-generation",
"arxiv:1910.09700",
"autotrain_compatible",
"text-generation-inference",
"endpoints_compatible",
"region:us"
] | text-generation | "2024-10-16T10:53:53Z" | ---
library_name: transformers
tags: []
---
# Model Card for Model ID
<!-- Provide a quick summary of what the model is/does. -->
## Model Details
### Model Description
<!-- Provide a longer summary of what this model is. -->
This is the model card of a 🤗 transformers model that has been pushed on the Hub. This model card has been automatically generated.
- **Developed by:** [More Information Needed]
- **Funded by [optional]:** [More Information Needed]
- **Shared by [optional]:** [More Information Needed]
- **Model type:** [More Information Needed]
- **Language(s) (NLP):** [More Information Needed]
- **License:** [More Information Needed]
- **Finetuned from model [optional]:** [More Information Needed]
### Model Sources [optional]
<!-- Provide the basic links for the model. -->
- **Repository:** [More Information Needed]
- **Paper [optional]:** [More Information Needed]
- **Demo [optional]:** [More Information Needed]
## Uses
<!-- Address questions around how the model is intended to be used, including the foreseeable users of the model and those affected by the model. -->
### Direct Use
<!-- This section is for the model use without fine-tuning or plugging into a larger ecosystem/app. -->
[More Information Needed]
### Downstream Use [optional]
<!-- This section is for the model use when fine-tuned for a task, or when plugged into a larger ecosystem/app -->
[More Information Needed]
### Out-of-Scope Use
<!-- This section addresses misuse, malicious use, and uses that the model will not work well for. -->
[More Information Needed]
## Bias, Risks, and Limitations
<!-- This section is meant to convey both technical and sociotechnical limitations. -->
[More Information Needed]
### Recommendations
<!-- This section is meant to convey recommendations with respect to the bias, risk, and technical limitations. -->
Users (both direct and downstream) should be made aware of the risks, biases and limitations of the model. More information needed for further recommendations.
## How to Get Started with the Model
Use the code below to get started with the model.
[More Information Needed]
## Training Details
### Training Data
<!-- This should link to a Dataset Card, perhaps with a short stub of information on what the training data is all about as well as documentation related to data pre-processing or additional filtering. -->
[More Information Needed]
### Training Procedure
<!-- This relates heavily to the Technical Specifications. Content here should link to that section when it is relevant to the training procedure. -->
#### Preprocessing [optional]
[More Information Needed]
#### Training Hyperparameters
- **Training regime:** [More Information Needed] <!--fp32, fp16 mixed precision, bf16 mixed precision, bf16 non-mixed precision, fp16 non-mixed precision, fp8 mixed precision -->
#### Speeds, Sizes, Times [optional]
<!-- This section provides information about throughput, start/end time, checkpoint size if relevant, etc. -->
[More Information Needed]
## Evaluation
<!-- This section describes the evaluation protocols and provides the results. -->
### Testing Data, Factors & Metrics
#### Testing Data
<!-- This should link to a Dataset Card if possible. -->
[More Information Needed]
#### Factors
<!-- These are the things the evaluation is disaggregating by, e.g., subpopulations or domains. -->
[More Information Needed]
#### Metrics
<!-- These are the evaluation metrics being used, ideally with a description of why. -->
[More Information Needed]
### Results
[More Information Needed]
#### Summary
## Model Examination [optional]
<!-- Relevant interpretability work for the model goes here -->
[More Information Needed]
## Environmental Impact
<!-- Total emissions (in grams of CO2eq) and additional considerations, such as electricity usage, go here. Edit the suggested text below accordingly -->
Carbon emissions can be estimated using the [Machine Learning Impact calculator](https://mlco2.github.io/impact#compute) presented in [Lacoste et al. (2019)](https://arxiv.org/abs/1910.09700).
- **Hardware Type:** [More Information Needed]
- **Hours used:** [More Information Needed]
- **Cloud Provider:** [More Information Needed]
- **Compute Region:** [More Information Needed]
- **Carbon Emitted:** [More Information Needed]
## Technical Specifications [optional]
### Model Architecture and Objective
[More Information Needed]
### Compute Infrastructure
[More Information Needed]
#### Hardware
[More Information Needed]
#### Software
[More Information Needed]
## Citation [optional]
<!-- If there is a paper or blog post introducing the model, the APA and Bibtex information for that should go in this section. -->
**BibTeX:**
[More Information Needed]
**APA:**
[More Information Needed]
## Glossary [optional]
<!-- If relevant, include terms and calculations in this section that can help readers understand the model or model card. -->
[More Information Needed]
## More Information [optional]
[More Information Needed]
## Model Card Authors [optional]
[More Information Needed]
## Model Card Contact
[More Information Needed] |
cansen88/PromptGenerator_5_topic | cansen88 | "2022-08-10T21:07:10Z" | 4 | 0 | transformers | [
"transformers",
"tf",
"gpt2",
"text-generation",
"generated_from_keras_callback",
"license:mit",
"autotrain_compatible",
"endpoints_compatible",
"region:us"
] | text-generation | "2022-08-10T20:51:09Z" | ---
license: mit
tags:
- generated_from_keras_callback
model-index:
- name: PromptGenerator_5_topic
results: []
---
<!-- This model card has been generated automatically according to the information Keras had access to. You should
probably proofread and complete it, then remove this comment. -->
# PromptGenerator_5_topic
This model is a fine-tuned version of [gpt2](https://huggingface.co/gpt2) on an unknown dataset.
It achieves the following results on the evaluation set:
- Train Loss: 10.6848
- Validation Loss: 10.6672
- Epoch: 4
## Model description
More information needed
## Intended uses & limitations
More information needed
## Training and evaluation data
More information needed
## Training procedure
### Training hyperparameters
The following hyperparameters were used during training:
- optimizer: {'name': 'AdamWeightDecay', 'learning_rate': {'class_name': 'WarmUp', 'config': {'initial_learning_rate': 5e-05, 'decay_schedule_fn': {'class_name': 'PolynomialDecay', 'config': {'initial_learning_rate': 5e-05, 'decay_steps': -999, 'end_learning_rate': 0.0, 'power': 1.0, 'cycle': False, 'name': None}, '__passive_serialization__': True}, 'warmup_steps': 1000, 'power': 1.0, 'name': None}}, 'decay': 0.0, 'beta_1': 0.9, 'beta_2': 0.999, 'epsilon': 1e-08, 'amsgrad': False, 'weight_decay_rate': 0.01}
- training_precision: mixed_float16
### Training results
| Train Loss | Validation Loss | Epoch |
|:----------:|:---------------:|:-----:|
| 10.6864 | 10.6743 | 0 |
| 10.7045 | 10.6736 | 1 |
| 10.7114 | 10.6722 | 2 |
| 10.7082 | 10.6701 | 3 |
| 10.6848 | 10.6672 | 4 |
### Framework versions
- Transformers 4.21.1
- TensorFlow 2.8.2
- Datasets 2.4.0
- Tokenizers 0.12.1
|
Blingham/model2 | Blingham | "2025-01-01T13:01:56Z" | 105 | 0 | transformers | [
"transformers",
"safetensors",
"bert",
"text-classification",
"arxiv:1910.09700",
"autotrain_compatible",
"endpoints_compatible",
"region:us"
] | text-classification | "2025-01-01T13:01:37Z" | ---
library_name: transformers
tags: []
---
# Model Card for Model ID
<!-- Provide a quick summary of what the model is/does. -->
## Model Details
### Model Description
<!-- Provide a longer summary of what this model is. -->
This is the model card of a 🤗 transformers model that has been pushed on the Hub. This model card has been automatically generated.
- **Developed by:** [More Information Needed]
- **Funded by [optional]:** [More Information Needed]
- **Shared by [optional]:** [More Information Needed]
- **Model type:** [More Information Needed]
- **Language(s) (NLP):** [More Information Needed]
- **License:** [More Information Needed]
- **Finetuned from model [optional]:** [More Information Needed]
### Model Sources [optional]
<!-- Provide the basic links for the model. -->
- **Repository:** [More Information Needed]
- **Paper [optional]:** [More Information Needed]
- **Demo [optional]:** [More Information Needed]
## Uses
<!-- Address questions around how the model is intended to be used, including the foreseeable users of the model and those affected by the model. -->
### Direct Use
<!-- This section is for the model use without fine-tuning or plugging into a larger ecosystem/app. -->
[More Information Needed]
### Downstream Use [optional]
<!-- This section is for the model use when fine-tuned for a task, or when plugged into a larger ecosystem/app -->
[More Information Needed]
### Out-of-Scope Use
<!-- This section addresses misuse, malicious use, and uses that the model will not work well for. -->
[More Information Needed]
## Bias, Risks, and Limitations
<!-- This section is meant to convey both technical and sociotechnical limitations. -->
[More Information Needed]
### Recommendations
<!-- This section is meant to convey recommendations with respect to the bias, risk, and technical limitations. -->
Users (both direct and downstream) should be made aware of the risks, biases and limitations of the model. More information needed for further recommendations.
## How to Get Started with the Model
Use the code below to get started with the model.
[More Information Needed]
## Training Details
### Training Data
<!-- This should link to a Dataset Card, perhaps with a short stub of information on what the training data is all about as well as documentation related to data pre-processing or additional filtering. -->
[More Information Needed]
### Training Procedure
<!-- This relates heavily to the Technical Specifications. Content here should link to that section when it is relevant to the training procedure. -->
#### Preprocessing [optional]
[More Information Needed]
#### Training Hyperparameters
- **Training regime:** [More Information Needed] <!--fp32, fp16 mixed precision, bf16 mixed precision, bf16 non-mixed precision, fp16 non-mixed precision, fp8 mixed precision -->
#### Speeds, Sizes, Times [optional]
<!-- This section provides information about throughput, start/end time, checkpoint size if relevant, etc. -->
[More Information Needed]
## Evaluation
<!-- This section describes the evaluation protocols and provides the results. -->
### Testing Data, Factors & Metrics
#### Testing Data
<!-- This should link to a Dataset Card if possible. -->
[More Information Needed]
#### Factors
<!-- These are the things the evaluation is disaggregating by, e.g., subpopulations or domains. -->
[More Information Needed]
#### Metrics
<!-- These are the evaluation metrics being used, ideally with a description of why. -->
[More Information Needed]
### Results
[More Information Needed]
#### Summary
## Model Examination [optional]
<!-- Relevant interpretability work for the model goes here -->
[More Information Needed]
## Environmental Impact
<!-- Total emissions (in grams of CO2eq) and additional considerations, such as electricity usage, go here. Edit the suggested text below accordingly -->
Carbon emissions can be estimated using the [Machine Learning Impact calculator](https://mlco2.github.io/impact#compute) presented in [Lacoste et al. (2019)](https://arxiv.org/abs/1910.09700).
- **Hardware Type:** [More Information Needed]
- **Hours used:** [More Information Needed]
- **Cloud Provider:** [More Information Needed]
- **Compute Region:** [More Information Needed]
- **Carbon Emitted:** [More Information Needed]
## Technical Specifications [optional]
### Model Architecture and Objective
[More Information Needed]
### Compute Infrastructure
[More Information Needed]
#### Hardware
[More Information Needed]
#### Software
[More Information Needed]
## Citation [optional]
<!-- If there is a paper or blog post introducing the model, the APA and Bibtex information for that should go in this section. -->
**BibTeX:**
[More Information Needed]
**APA:**
[More Information Needed]
## Glossary [optional]
<!-- If relevant, include terms and calculations in this section that can help readers understand the model or model card. -->
[More Information Needed]
## More Information [optional]
[More Information Needed]
## Model Card Authors [optional]
[More Information Needed]
## Model Card Contact
[More Information Needed] |
RichardErkhov/ivnle_-_llamatales_gre_8b-lay4-hs128-hd2-1M-gguf | RichardErkhov | "2025-02-09T22:38:39Z" | 439 | 0 | null | [
"gguf",
"arxiv:1910.09700",
"endpoints_compatible",
"region:us",
"conversational"
] | null | "2025-02-09T22:35:42Z" | Quantization made by Richard Erkhov.
[Github](https://github.com/RichardErkhov)
[Discord](https://discord.gg/pvy7H8DZMG)
[Request more models](https://github.com/RichardErkhov/quant_request)
llamatales_gre_8b-lay4-hs128-hd2-1M - GGUF
- Model creator: https://huggingface.co/ivnle/
- Original model: https://huggingface.co/ivnle/llamatales_gre_8b-lay4-hs128-hd2-1M/
| Name | Quant method | Size |
| ---- | ---- | ---- |
| [llamatales_gre_8b-lay4-hs128-hd2-1M.Q2_K.gguf](https://huggingface.co/RichardErkhov/ivnle_-_llamatales_gre_8b-lay4-hs128-hd2-1M-gguf/blob/main/llamatales_gre_8b-lay4-hs128-hd2-1M.Q2_K.gguf) | Q2_K | 0.03GB |
| [llamatales_gre_8b-lay4-hs128-hd2-1M.IQ3_XS.gguf](https://huggingface.co/RichardErkhov/ivnle_-_llamatales_gre_8b-lay4-hs128-hd2-1M-gguf/blob/main/llamatales_gre_8b-lay4-hs128-hd2-1M.IQ3_XS.gguf) | IQ3_XS | 0.03GB |
| [llamatales_gre_8b-lay4-hs128-hd2-1M.IQ3_S.gguf](https://huggingface.co/RichardErkhov/ivnle_-_llamatales_gre_8b-lay4-hs128-hd2-1M-gguf/blob/main/llamatales_gre_8b-lay4-hs128-hd2-1M.IQ3_S.gguf) | IQ3_S | 0.03GB |
| [llamatales_gre_8b-lay4-hs128-hd2-1M.Q3_K_S.gguf](https://huggingface.co/RichardErkhov/ivnle_-_llamatales_gre_8b-lay4-hs128-hd2-1M-gguf/blob/main/llamatales_gre_8b-lay4-hs128-hd2-1M.Q3_K_S.gguf) | Q3_K_S | 0.03GB |
| [llamatales_gre_8b-lay4-hs128-hd2-1M.IQ3_M.gguf](https://huggingface.co/RichardErkhov/ivnle_-_llamatales_gre_8b-lay4-hs128-hd2-1M-gguf/blob/main/llamatales_gre_8b-lay4-hs128-hd2-1M.IQ3_M.gguf) | IQ3_M | 0.03GB |
| [llamatales_gre_8b-lay4-hs128-hd2-1M.Q3_K.gguf](https://huggingface.co/RichardErkhov/ivnle_-_llamatales_gre_8b-lay4-hs128-hd2-1M-gguf/blob/main/llamatales_gre_8b-lay4-hs128-hd2-1M.Q3_K.gguf) | Q3_K | 0.03GB |
| [llamatales_gre_8b-lay4-hs128-hd2-1M.Q3_K_M.gguf](https://huggingface.co/RichardErkhov/ivnle_-_llamatales_gre_8b-lay4-hs128-hd2-1M-gguf/blob/main/llamatales_gre_8b-lay4-hs128-hd2-1M.Q3_K_M.gguf) | Q3_K_M | 0.03GB |
| [llamatales_gre_8b-lay4-hs128-hd2-1M.Q3_K_L.gguf](https://huggingface.co/RichardErkhov/ivnle_-_llamatales_gre_8b-lay4-hs128-hd2-1M-gguf/blob/main/llamatales_gre_8b-lay4-hs128-hd2-1M.Q3_K_L.gguf) | Q3_K_L | 0.03GB |
| [llamatales_gre_8b-lay4-hs128-hd2-1M.IQ4_XS.gguf](https://huggingface.co/RichardErkhov/ivnle_-_llamatales_gre_8b-lay4-hs128-hd2-1M-gguf/blob/main/llamatales_gre_8b-lay4-hs128-hd2-1M.IQ4_XS.gguf) | IQ4_XS | 0.03GB |
| [llamatales_gre_8b-lay4-hs128-hd2-1M.Q4_0.gguf](https://huggingface.co/RichardErkhov/ivnle_-_llamatales_gre_8b-lay4-hs128-hd2-1M-gguf/blob/main/llamatales_gre_8b-lay4-hs128-hd2-1M.Q4_0.gguf) | Q4_0 | 0.03GB |
| [llamatales_gre_8b-lay4-hs128-hd2-1M.IQ4_NL.gguf](https://huggingface.co/RichardErkhov/ivnle_-_llamatales_gre_8b-lay4-hs128-hd2-1M-gguf/blob/main/llamatales_gre_8b-lay4-hs128-hd2-1M.IQ4_NL.gguf) | IQ4_NL | 0.03GB |
| [llamatales_gre_8b-lay4-hs128-hd2-1M.Q4_K_S.gguf](https://huggingface.co/RichardErkhov/ivnle_-_llamatales_gre_8b-lay4-hs128-hd2-1M-gguf/blob/main/llamatales_gre_8b-lay4-hs128-hd2-1M.Q4_K_S.gguf) | Q4_K_S | 0.03GB |
| [llamatales_gre_8b-lay4-hs128-hd2-1M.Q4_K.gguf](https://huggingface.co/RichardErkhov/ivnle_-_llamatales_gre_8b-lay4-hs128-hd2-1M-gguf/blob/main/llamatales_gre_8b-lay4-hs128-hd2-1M.Q4_K.gguf) | Q4_K | 0.03GB |
| [llamatales_gre_8b-lay4-hs128-hd2-1M.Q4_K_M.gguf](https://huggingface.co/RichardErkhov/ivnle_-_llamatales_gre_8b-lay4-hs128-hd2-1M-gguf/blob/main/llamatales_gre_8b-lay4-hs128-hd2-1M.Q4_K_M.gguf) | Q4_K_M | 0.03GB |
| [llamatales_gre_8b-lay4-hs128-hd2-1M.Q4_1.gguf](https://huggingface.co/RichardErkhov/ivnle_-_llamatales_gre_8b-lay4-hs128-hd2-1M-gguf/blob/main/llamatales_gre_8b-lay4-hs128-hd2-1M.Q4_1.gguf) | Q4_1 | 0.03GB |
| [llamatales_gre_8b-lay4-hs128-hd2-1M.Q5_0.gguf](https://huggingface.co/RichardErkhov/ivnle_-_llamatales_gre_8b-lay4-hs128-hd2-1M-gguf/blob/main/llamatales_gre_8b-lay4-hs128-hd2-1M.Q5_0.gguf) | Q5_0 | 0.03GB |
| [llamatales_gre_8b-lay4-hs128-hd2-1M.Q5_K_S.gguf](https://huggingface.co/RichardErkhov/ivnle_-_llamatales_gre_8b-lay4-hs128-hd2-1M-gguf/blob/main/llamatales_gre_8b-lay4-hs128-hd2-1M.Q5_K_S.gguf) | Q5_K_S | 0.04GB |
| [llamatales_gre_8b-lay4-hs128-hd2-1M.Q5_K.gguf](https://huggingface.co/RichardErkhov/ivnle_-_llamatales_gre_8b-lay4-hs128-hd2-1M-gguf/blob/main/llamatales_gre_8b-lay4-hs128-hd2-1M.Q5_K.gguf) | Q5_K | 0.04GB |
| [llamatales_gre_8b-lay4-hs128-hd2-1M.Q5_K_M.gguf](https://huggingface.co/RichardErkhov/ivnle_-_llamatales_gre_8b-lay4-hs128-hd2-1M-gguf/blob/main/llamatales_gre_8b-lay4-hs128-hd2-1M.Q5_K_M.gguf) | Q5_K_M | 0.04GB |
| [llamatales_gre_8b-lay4-hs128-hd2-1M.Q5_1.gguf](https://huggingface.co/RichardErkhov/ivnle_-_llamatales_gre_8b-lay4-hs128-hd2-1M-gguf/blob/main/llamatales_gre_8b-lay4-hs128-hd2-1M.Q5_1.gguf) | Q5_1 | 0.04GB |
| [llamatales_gre_8b-lay4-hs128-hd2-1M.Q6_K.gguf](https://huggingface.co/RichardErkhov/ivnle_-_llamatales_gre_8b-lay4-hs128-hd2-1M-gguf/blob/main/llamatales_gre_8b-lay4-hs128-hd2-1M.Q6_K.gguf) | Q6_K | 0.04GB |
| [llamatales_gre_8b-lay4-hs128-hd2-1M.Q8_0.gguf](https://huggingface.co/RichardErkhov/ivnle_-_llamatales_gre_8b-lay4-hs128-hd2-1M-gguf/blob/main/llamatales_gre_8b-lay4-hs128-hd2-1M.Q8_0.gguf) | Q8_0 | 0.04GB |
Original model description:
---
datasets:
- ivnle/llamatales-gre
library_name: transformers
---
# Model Card for Model ID
<!-- Provide a quick summary of what the model is/does. -->
## Model Details
### Model Description
<!-- Provide a longer summary of what this model is. -->
This is the model card of a 🤗 transformers model that has been pushed on the Hub. This model card has been automatically generated.
- **Developed by:** [More Information Needed]
- **Funded by [optional]:** [More Information Needed]
- **Shared by [optional]:** [More Information Needed]
- **Model type:** [More Information Needed]
- **Language(s) (NLP):** [More Information Needed]
- **License:** [More Information Needed]
- **Finetuned from model [optional]:** [More Information Needed]
### Model Sources [optional]
<!-- Provide the basic links for the model. -->
- **Repository:** [More Information Needed]
- **Paper [optional]:** [More Information Needed]
- **Demo [optional]:** [More Information Needed]
## Uses
<!-- Address questions around how the model is intended to be used, including the foreseeable users of the model and those affected by the model. -->
### Direct Use
<!-- This section is for the model use without fine-tuning or plugging into a larger ecosystem/app. -->
[More Information Needed]
### Downstream Use [optional]
<!-- This section is for the model use when fine-tuned for a task, or when plugged into a larger ecosystem/app -->
[More Information Needed]
### Out-of-Scope Use
<!-- This section addresses misuse, malicious use, and uses that the model will not work well for. -->
[More Information Needed]
## Bias, Risks, and Limitations
<!-- This section is meant to convey both technical and sociotechnical limitations. -->
[More Information Needed]
### Recommendations
<!-- This section is meant to convey recommendations with respect to the bias, risk, and technical limitations. -->
Users (both direct and downstream) should be made aware of the risks, biases and limitations of the model. More information needed for further recommendations.
## How to Get Started with the Model
Use the code below to get started with the model.
[More Information Needed]
## Training Details
### Training Data
<!-- This should link to a Dataset Card, perhaps with a short stub of information on what the training data is all about as well as documentation related to data pre-processing or additional filtering. -->
[More Information Needed]
### Training Procedure
<!-- This relates heavily to the Technical Specifications. Content here should link to that section when it is relevant to the training procedure. -->
#### Preprocessing [optional]
[More Information Needed]
#### Training Hyperparameters
- **Training regime:** [More Information Needed] <!--fp32, fp16 mixed precision, bf16 mixed precision, bf16 non-mixed precision, fp16 non-mixed precision, fp8 mixed precision -->
#### Speeds, Sizes, Times [optional]
<!-- This section provides information about throughput, start/end time, checkpoint size if relevant, etc. -->
[More Information Needed]
## Evaluation
<!-- This section describes the evaluation protocols and provides the results. -->
### Testing Data, Factors & Metrics
#### Testing Data
<!-- This should link to a Dataset Card if possible. -->
[More Information Needed]
#### Factors
<!-- These are the things the evaluation is disaggregating by, e.g., subpopulations or domains. -->
[More Information Needed]
#### Metrics
<!-- These are the evaluation metrics being used, ideally with a description of why. -->
[More Information Needed]
### Results
[More Information Needed]
#### Summary
## Model Examination [optional]
<!-- Relevant interpretability work for the model goes here -->
[More Information Needed]
## Environmental Impact
<!-- Total emissions (in grams of CO2eq) and additional considerations, such as electricity usage, go here. Edit the suggested text below accordingly -->
Carbon emissions can be estimated using the [Machine Learning Impact calculator](https://mlco2.github.io/impact#compute) presented in [Lacoste et al. (2019)](https://arxiv.org/abs/1910.09700).
- **Hardware Type:** [More Information Needed]
- **Hours used:** [More Information Needed]
- **Cloud Provider:** [More Information Needed]
- **Compute Region:** [More Information Needed]
- **Carbon Emitted:** [More Information Needed]
## Technical Specifications [optional]
### Model Architecture and Objective
[More Information Needed]
### Compute Infrastructure
[More Information Needed]
#### Hardware
[More Information Needed]
#### Software
[More Information Needed]
## Citation [optional]
<!-- If there is a paper or blog post introducing the model, the APA and Bibtex information for that should go in this section. -->
**BibTeX:**
[More Information Needed]
**APA:**
[More Information Needed]
## Glossary [optional]
<!-- If relevant, include terms and calculations in this section that can help readers understand the model or model card. -->
[More Information Needed]
## More Information [optional]
[More Information Needed]
## Model Card Authors [optional]
[More Information Needed]
## Model Card Contact
[More Information Needed]
|
lesso12/20657fdd-16b4-4bfc-8c9a-5ffef6948821 | lesso12 | "2025-01-15T19:30:50Z" | 13 | 0 | peft | [
"peft",
"safetensors",
"llama",
"axolotl",
"generated_from_trainer",
"base_model:lmsys/vicuna-7b-v1.3",
"base_model:adapter:lmsys/vicuna-7b-v1.3",
"8-bit",
"bitsandbytes",
"region:us"
] | null | "2025-01-15T19:22:25Z" | ---
library_name: peft
base_model: lmsys/vicuna-7b-v1.3
tags:
- axolotl
- generated_from_trainer
model-index:
- name: 20657fdd-16b4-4bfc-8c9a-5ffef6948821
results: []
---
<!-- This model card has been generated automatically according to the information the Trainer had access to. You
should probably proofread and complete it, then remove this comment. -->
[<img src="https://raw.githubusercontent.com/axolotl-ai-cloud/axolotl/main/image/axolotl-badge-web.png" alt="Built with Axolotl" width="200" height="32"/>](https://github.com/axolotl-ai-cloud/axolotl)
<details><summary>See axolotl config</summary>
axolotl version: `0.4.1`
```yaml
adapter: lora
base_model: lmsys/vicuna-7b-v1.3
bf16: true
chat_template: llama3
datasets:
- data_files:
- 2eaa151dc90bf2ca_train_data.json
ds_type: json
format: custom
path: /workspace/input_data/2eaa151dc90bf2ca_train_data.json
type:
field_instruction: text
field_output: title
format: '{instruction}'
no_input_format: '{instruction}'
system_format: '{system}'
system_prompt: ''
debug: null
deepspeed: null
early_stopping_patience: 2
eval_max_new_tokens: 128
eval_steps: 5
eval_table_size: null
flash_attention: false
fp16: false
fsdp: null
fsdp_config: null
gradient_accumulation_steps: 4
gradient_checkpointing: false
group_by_length: false
hub_model_id: lesso12/20657fdd-16b4-4bfc-8c9a-5ffef6948821
hub_repo: null
hub_strategy: checkpoint
hub_token: null
learning_rate: 0.0002
load_in_4bit: false
load_in_8bit: true
local_rank: null
logging_steps: 1
lora_alpha: 16
lora_dropout: 0.05
lora_fan_in_fan_out: null
lora_model_dir: null
lora_r: 8
lora_target_linear: true
lr_scheduler: cosine
max_steps: 25
micro_batch_size: 2
mlflow_experiment_name: /tmp/2eaa151dc90bf2ca_train_data.json
model_type: AutoModelForCausalLM
num_epochs: 1
optimizer: adamw_bnb_8bit
output_dir: miner_id_24
pad_to_sequence_len: true
resume_from_checkpoint: null
s2_attention: null
sample_packing: false
save_steps: 10
sequence_len: 512
strict: false
tf32: false
tokenizer_type: AutoTokenizer
train_on_inputs: false
trust_remote_code: true
val_set_size: 0.05
wandb_entity: null
wandb_mode: online
wandb_name: b8bde2d0-d77e-452d-82bc-93eeab0c0c0e
wandb_project: Gradients-On-Demand
wandb_run: your_name
wandb_runid: b8bde2d0-d77e-452d-82bc-93eeab0c0c0e
warmup_steps: 10
weight_decay: 0.0
xformers_attention: null
```
</details><br>
# 20657fdd-16b4-4bfc-8c9a-5ffef6948821
This model is a fine-tuned version of [lmsys/vicuna-7b-v1.3](https://huggingface.co/lmsys/vicuna-7b-v1.3) on the None dataset.
It achieves the following results on the evaluation set:
- Loss: 1.3201
## Model description
More information needed
## Intended uses & limitations
More information needed
## Training and evaluation data
More information needed
## Training procedure
### Training hyperparameters
The following hyperparameters were used during training:
- learning_rate: 0.0002
- train_batch_size: 2
- eval_batch_size: 2
- seed: 42
- gradient_accumulation_steps: 4
- total_train_batch_size: 8
- optimizer: Use OptimizerNames.ADAMW_BNB with betas=(0.9,0.999) and epsilon=1e-08 and optimizer_args=No additional optimizer arguments
- lr_scheduler_type: cosine
- lr_scheduler_warmup_steps: 10
- training_steps: 25
### Training results
| Training Loss | Epoch | Step | Validation Loss |
|:-------------:|:------:|:----:|:---------------:|
| 2.0027 | 0.0025 | 1 | 1.8318 |
| 1.6274 | 0.0123 | 5 | 1.8132 |
| 1.5617 | 0.0245 | 10 | 1.6017 |
| 1.3022 | 0.0368 | 15 | 1.3925 |
| 1.098 | 0.0490 | 20 | 1.3301 |
| 1.5834 | 0.0613 | 25 | 1.3201 |
### Framework versions
- PEFT 0.13.2
- Transformers 4.46.0
- Pytorch 2.5.0+cu124
- Datasets 3.0.1
- Tokenizers 0.20.1 |
BLakshmiVijay/results | BLakshmiVijay | "2024-07-18T08:23:30Z" | 78 | 0 | transformers | [
"transformers",
"safetensors",
"wav2vec2",
"audio-classification",
"generated_from_trainer",
"base_model:facebook/wav2vec2-base",
"base_model:finetune:facebook/wav2vec2-base",
"license:apache-2.0",
"endpoints_compatible",
"region:us"
] | audio-classification | "2024-07-16T11:10:18Z" | ---
license: apache-2.0
base_model: facebook/wav2vec2-base
tags:
- generated_from_trainer
metrics:
- accuracy
- recall
model-index:
- name: results
results: []
---
<!-- This model card has been generated automatically according to the information the Trainer had access to. You
should probably proofread and complete it, then remove this comment. -->
# results
This model is a fine-tuned version of [facebook/wav2vec2-base](https://huggingface.co/facebook/wav2vec2-base) on the None dataset.
It achieves the following results on the evaluation set:
- Loss: 0.6945
- Accuracy: 0.4694
- F1score: 0.6
- Recall: 0.8667
- Tn: 7
- Fp: 46
- Fn: 6
- Tp: 39
## Model description
More information needed
## Intended uses & limitations
More information needed
## Training and evaluation data
More information needed
## Training procedure
### Training hyperparameters
The following hyperparameters were used during training:
- learning_rate: 1e-08
- train_batch_size: 8
- eval_batch_size: 8
- seed: 42
- optimizer: Adam with betas=(0.9,0.999) and epsilon=1e-08
- lr_scheduler_type: linear
- num_epochs: 10
### Training results
| Training Loss | Epoch | Step | Validation Loss | Accuracy | F1score | Recall | Tn | Fp | Fn | Tp |
|:-------------:|:-----:|:----:|:---------------:|:--------:|:-------:|:------:|:--:|:--:|:--:|:--:|
| No log | 1.0 | 13 | 0.6945 | 0.4694 | 0.6 | 0.8667 | 7 | 46 | 6 | 39 |
| No log | 2.0 | 26 | 0.6945 | 0.4694 | 0.6 | 0.8667 | 7 | 46 | 6 | 39 |
| No log | 3.0 | 39 | 0.6945 | 0.4694 | 0.6 | 0.8667 | 7 | 46 | 6 | 39 |
| No log | 4.0 | 52 | 0.6945 | 0.4694 | 0.6 | 0.8667 | 7 | 46 | 6 | 39 |
| No log | 5.0 | 65 | 0.6945 | 0.4694 | 0.6 | 0.8667 | 7 | 46 | 6 | 39 |
| No log | 6.0 | 78 | 0.6945 | 0.4694 | 0.6 | 0.8667 | 7 | 46 | 6 | 39 |
| No log | 7.0 | 91 | 0.6945 | 0.4694 | 0.6 | 0.8667 | 7 | 46 | 6 | 39 |
| No log | 8.0 | 104 | 0.6945 | 0.4694 | 0.6 | 0.8667 | 7 | 46 | 6 | 39 |
| No log | 9.0 | 117 | 0.6945 | 0.4694 | 0.6 | 0.8667 | 7 | 46 | 6 | 39 |
| No log | 10.0 | 130 | 0.6945 | 0.4694 | 0.6 | 0.8667 | 7 | 46 | 6 | 39 |
### Framework versions
- Transformers 4.42.4
- Pytorch 2.1.2
- Datasets 2.20.0
- Tokenizers 0.19.1
|
bmehrba/TinyLlama-1.1B-Chat-v1.0-fine-tuned-adapters_Epistemic_tiny_0.8_Seed101 | bmehrba | "2024-04-23T06:52:36Z" | 0 | 0 | peft | [
"peft",
"arxiv:1910.09700",
"base_model:TinyLlama/TinyLlama-1.1B-Chat-v1.0",
"base_model:adapter:TinyLlama/TinyLlama-1.1B-Chat-v1.0",
"region:us"
] | null | "2024-04-23T06:52:32Z" | ---
library_name: peft
base_model: TinyLlama/TinyLlama-1.1B-Chat-v1.0
---
# Model Card for Model ID
<!-- Provide a quick summary of what the model is/does. -->
## Model Details
### Model Description
<!-- Provide a longer summary of what this model is. -->
- **Developed by:** [More Information Needed]
- **Shared by [optional]:** [More Information Needed]
- **Model type:** [More Information Needed]
- **Language(s) (NLP):** [More Information Needed]
- **License:** [More Information Needed]
- **Finetuned from model [optional]:** [More Information Needed]
### Model Sources [optional]
<!-- Provide the basic links for the model. -->
- **Repository:** [More Information Needed]
- **Paper [optional]:** [More Information Needed]
- **Demo [optional]:** [More Information Needed]
## Uses
<!-- Address questions around how the model is intended to be used, including the foreseeable users of the model and those affected by the model. -->
### Direct Use
<!-- This section is for the model use without fine-tuning or plugging into a larger ecosystem/app. -->
[More Information Needed]
### Downstream Use [optional]
<!-- This section is for the model use when fine-tuned for a task, or when plugged into a larger ecosystem/app -->
[More Information Needed]
### Out-of-Scope Use
<!-- This section addresses misuse, malicious use, and uses that the model will not work well for. -->
[More Information Needed]
## Bias, Risks, and Limitations
<!-- This section is meant to convey both technical and sociotechnical limitations. -->
[More Information Needed]
### Recommendations
<!-- This section is meant to convey recommendations with respect to the bias, risk, and technical limitations. -->
Users (both direct and downstream) should be made aware of the risks, biases and limitations of the model. More information needed for further recommendations.
## How to Get Started with the Model
Use the code below to get started with the model.
[More Information Needed]
## Training Details
### Training Data
<!-- This should link to a Data Card, perhaps with a short stub of information on what the training data is all about as well as documentation related to data pre-processing or additional filtering. -->
[More Information Needed]
### Training Procedure
<!-- This relates heavily to the Technical Specifications. Content here should link to that section when it is relevant to the training procedure. -->
#### Preprocessing [optional]
[More Information Needed]
#### Training Hyperparameters
- **Training regime:** [More Information Needed] <!--fp32, fp16 mixed precision, bf16 mixed precision, bf16 non-mixed precision, fp16 non-mixed precision, fp8 mixed precision -->
#### Speeds, Sizes, Times [optional]
<!-- This section provides information about throughput, start/end time, checkpoint size if relevant, etc. -->
[More Information Needed]
## Evaluation
<!-- This section describes the evaluation protocols and provides the results. -->
### Testing Data, Factors & Metrics
#### Testing Data
<!-- This should link to a Data Card if possible. -->
[More Information Needed]
#### Factors
<!-- These are the things the evaluation is disaggregating by, e.g., subpopulations or domains. -->
[More Information Needed]
#### Metrics
<!-- These are the evaluation metrics being used, ideally with a description of why. -->
[More Information Needed]
### Results
[More Information Needed]
#### Summary
## Model Examination [optional]
<!-- Relevant interpretability work for the model goes here -->
[More Information Needed]
## Environmental Impact
<!-- Total emissions (in grams of CO2eq) and additional considerations, such as electricity usage, go here. Edit the suggested text below accordingly -->
Carbon emissions can be estimated using the [Machine Learning Impact calculator](https://mlco2.github.io/impact#compute) presented in [Lacoste et al. (2019)](https://arxiv.org/abs/1910.09700).
- **Hardware Type:** [More Information Needed]
- **Hours used:** [More Information Needed]
- **Cloud Provider:** [More Information Needed]
- **Compute Region:** [More Information Needed]
- **Carbon Emitted:** [More Information Needed]
## Technical Specifications [optional]
### Model Architecture and Objective
[More Information Needed]
### Compute Infrastructure
[More Information Needed]
#### Hardware
[More Information Needed]
#### Software
[More Information Needed]
## Citation [optional]
<!-- If there is a paper or blog post introducing the model, the APA and Bibtex information for that should go in this section. -->
**BibTeX:**
[More Information Needed]
**APA:**
[More Information Needed]
## Glossary [optional]
<!-- If relevant, include terms and calculations in this section that can help readers understand the model or model card. -->
[More Information Needed]
## More Information [optional]
[More Information Needed]
## Model Card Authors [optional]
[More Information Needed]
## Model Card Contact
[More Information Needed]
## Training procedure
The following `bitsandbytes` quantization config was used during training:
- load_in_8bit: False
- load_in_4bit: True
- llm_int8_threshold: 6.0
- llm_int8_skip_modules: None
- llm_int8_enable_fp32_cpu_offload: False
- llm_int8_has_fp16_weight: False
- bnb_4bit_quant_type: nf4
- bnb_4bit_use_double_quant: True
- bnb_4bit_compute_dtype: bfloat16
### Framework versions
- PEFT 0.7.0.dev0
## Training procedure
The following `bitsandbytes` quantization config was used during training:
- load_in_8bit: False
- load_in_4bit: True
- llm_int8_threshold: 6.0
- llm_int8_skip_modules: None
- llm_int8_enable_fp32_cpu_offload: False
- llm_int8_has_fp16_weight: False
- bnb_4bit_quant_type: nf4
- bnb_4bit_use_double_quant: True
- bnb_4bit_compute_dtype: bfloat16
### Framework versions
- PEFT 0.7.0.dev0
|
rg1683/fine_tuned_UnigramBERT_SentimentAnalysis_r | rg1683 | "2024-07-26T18:18:54Z" | 161 | 0 | transformers | [
"transformers",
"safetensors",
"bert",
"text-classification",
"arxiv:1910.09700",
"autotrain_compatible",
"endpoints_compatible",
"region:us"
] | text-classification | "2024-07-26T18:18:42Z" | ---
library_name: transformers
tags: []
---
# Model Card for Model ID
<!-- Provide a quick summary of what the model is/does. -->
## Model Details
### Model Description
<!-- Provide a longer summary of what this model is. -->
This is the model card of a 🤗 transformers model that has been pushed on the Hub. This model card has been automatically generated.
- **Developed by:** [More Information Needed]
- **Funded by [optional]:** [More Information Needed]
- **Shared by [optional]:** [More Information Needed]
- **Model type:** [More Information Needed]
- **Language(s) (NLP):** [More Information Needed]
- **License:** [More Information Needed]
- **Finetuned from model [optional]:** [More Information Needed]
### Model Sources [optional]
<!-- Provide the basic links for the model. -->
- **Repository:** [More Information Needed]
- **Paper [optional]:** [More Information Needed]
- **Demo [optional]:** [More Information Needed]
## Uses
<!-- Address questions around how the model is intended to be used, including the foreseeable users of the model and those affected by the model. -->
### Direct Use
<!-- This section is for the model use without fine-tuning or plugging into a larger ecosystem/app. -->
[More Information Needed]
### Downstream Use [optional]
<!-- This section is for the model use when fine-tuned for a task, or when plugged into a larger ecosystem/app -->
[More Information Needed]
### Out-of-Scope Use
<!-- This section addresses misuse, malicious use, and uses that the model will not work well for. -->
[More Information Needed]
## Bias, Risks, and Limitations
<!-- This section is meant to convey both technical and sociotechnical limitations. -->
[More Information Needed]
### Recommendations
<!-- This section is meant to convey recommendations with respect to the bias, risk, and technical limitations. -->
Users (both direct and downstream) should be made aware of the risks, biases and limitations of the model. More information needed for further recommendations.
## How to Get Started with the Model
Use the code below to get started with the model.
[More Information Needed]
## Training Details
### Training Data
<!-- This should link to a Dataset Card, perhaps with a short stub of information on what the training data is all about as well as documentation related to data pre-processing or additional filtering. -->
[More Information Needed]
### Training Procedure
<!-- This relates heavily to the Technical Specifications. Content here should link to that section when it is relevant to the training procedure. -->
#### Preprocessing [optional]
[More Information Needed]
#### Training Hyperparameters
- **Training regime:** [More Information Needed] <!--fp32, fp16 mixed precision, bf16 mixed precision, bf16 non-mixed precision, fp16 non-mixed precision, fp8 mixed precision -->
#### Speeds, Sizes, Times [optional]
<!-- This section provides information about throughput, start/end time, checkpoint size if relevant, etc. -->
[More Information Needed]
## Evaluation
<!-- This section describes the evaluation protocols and provides the results. -->
### Testing Data, Factors & Metrics
#### Testing Data
<!-- This should link to a Dataset Card if possible. -->
[More Information Needed]
#### Factors
<!-- These are the things the evaluation is disaggregating by, e.g., subpopulations or domains. -->
[More Information Needed]
#### Metrics
<!-- These are the evaluation metrics being used, ideally with a description of why. -->
[More Information Needed]
### Results
[More Information Needed]
#### Summary
## Model Examination [optional]
<!-- Relevant interpretability work for the model goes here -->
[More Information Needed]
## Environmental Impact
<!-- Total emissions (in grams of CO2eq) and additional considerations, such as electricity usage, go here. Edit the suggested text below accordingly -->
Carbon emissions can be estimated using the [Machine Learning Impact calculator](https://mlco2.github.io/impact#compute) presented in [Lacoste et al. (2019)](https://arxiv.org/abs/1910.09700).
- **Hardware Type:** [More Information Needed]
- **Hours used:** [More Information Needed]
- **Cloud Provider:** [More Information Needed]
- **Compute Region:** [More Information Needed]
- **Carbon Emitted:** [More Information Needed]
## Technical Specifications [optional]
### Model Architecture and Objective
[More Information Needed]
### Compute Infrastructure
[More Information Needed]
#### Hardware
[More Information Needed]
#### Software
[More Information Needed]
## Citation [optional]
<!-- If there is a paper or blog post introducing the model, the APA and Bibtex information for that should go in this section. -->
**BibTeX:**
[More Information Needed]
**APA:**
[More Information Needed]
## Glossary [optional]
<!-- If relevant, include terms and calculations in this section that can help readers understand the model or model card. -->
[More Information Needed]
## More Information [optional]
[More Information Needed]
## Model Card Authors [optional]
[More Information Needed]
## Model Card Contact
[More Information Needed] |
kvjv/results | kvjv | "2024-06-25T07:50:57Z" | 11 | 0 | peft | [
"peft",
"tensorboard",
"safetensors",
"generated_from_trainer",
"base_model:google/paligemma-3b-pt-224",
"base_model:adapter:google/paligemma-3b-pt-224",
"license:gemma",
"region:us"
] | null | "2024-06-25T05:02:35Z" | ---
base_model: google/paligemma-3b-pt-224
library_name: peft
license: gemma
tags:
- generated_from_trainer
model-index:
- name: results
results: []
---
<!-- This model card has been generated automatically according to the information the Trainer had access to. You
should probably proofread and complete it, then remove this comment. -->
# results
This model is a fine-tuned version of [google/paligemma-3b-pt-224](https://huggingface.co/google/paligemma-3b-pt-224) on the None dataset.
## Model description
More information needed
## Intended uses & limitations
More information needed
## Training and evaluation data
More information needed
## Training procedure
### Training hyperparameters
The following hyperparameters were used during training:
- learning_rate: 0.001
- train_batch_size: 4
- eval_batch_size: 4
- seed: 42
- gradient_accumulation_steps: 4
- total_train_batch_size: 16
- optimizer: Adam with betas=(0.9,0.999) and epsilon=1e-08
- lr_scheduler_type: linear
- lr_scheduler_warmup_steps: 2
- num_epochs: 2
### Training results
### Framework versions
- PEFT 0.11.1
- Transformers 4.42.0.dev0
- Pytorch 2.3.0+cu121
- Datasets 2.20.0
- Tokenizers 0.19.1 |
blood34/b2a2790a-71b8-4284-8441-e9359f291761 | blood34 | "2025-01-31T03:33:56Z" | 8 | 0 | peft | [
"peft",
"safetensors",
"mistral",
"axolotl",
"generated_from_trainer",
"base_model:unsloth/Phi-3-mini-4k-instruct",
"base_model:adapter:unsloth/Phi-3-mini-4k-instruct",
"license:mit",
"8-bit",
"bitsandbytes",
"region:us"
] | null | "2025-01-31T02:35:30Z" | ---
library_name: peft
license: mit
base_model: unsloth/Phi-3-mini-4k-instruct
tags:
- axolotl
- generated_from_trainer
model-index:
- name: b2a2790a-71b8-4284-8441-e9359f291761
results: []
---
<!-- This model card has been generated automatically according to the information the Trainer had access to. You
should probably proofread and complete it, then remove this comment. -->
[<img src="https://raw.githubusercontent.com/axolotl-ai-cloud/axolotl/main/image/axolotl-badge-web.png" alt="Built with Axolotl" width="200" height="32"/>](https://github.com/axolotl-ai-cloud/axolotl)
<details><summary>See axolotl config</summary>
axolotl version: `0.4.1`
```yaml
adapter: lora
base_model: unsloth/Phi-3-mini-4k-instruct
bf16: auto
chat_template: llama3
dataset_prepared_path: null
datasets:
- data_files:
- afc5f6237544787c_train_data.json
ds_type: json
format: custom
path: /workspace/input_data/afc5f6237544787c_train_data.json
type:
field_input: context_processed
field_instruction: raw
field_output: processed
format: '{instruction} {input}'
no_input_format: '{instruction}'
system_format: '{system}'
system_prompt: ''
debug: null
deepspeed: null
device_map: auto
do_eval: true
early_stopping_patience: null
eval_batch_size: 4
eval_max_new_tokens: 128
eval_steps: null
eval_table_size: null
evals_per_epoch: null
flash_attention: true
fp16: null
fsdp: null
fsdp_config: null
gradient_accumulation_steps: 4
gradient_checkpointing: true
group_by_length: true
hub_model_id: blood34/b2a2790a-71b8-4284-8441-e9359f291761
hub_repo: null
hub_strategy: end
hub_token: null
learning_rate: 0.0001
load_in_4bit: true
load_in_8bit: true
local_rank: null
logging_steps: 1
lora_alpha: 16
lora_dropout: 0.05
lora_fan_in_fan_out: null
lora_model_dir: null
lora_r: 8
lora_target_linear: true
lr_scheduler: cosine
max_grad_norm: 1.0
max_memory:
0: 75GB
max_steps: 200
micro_batch_size: 4
mlflow_experiment_name: /tmp/afc5f6237544787c_train_data.json
model_type: AutoModelForCausalLM
num_epochs: 1
optimizer: adamw_bnb_8bit
output_dir: miner_id_24
pad_to_sequence_len: true
resume_from_checkpoint: null
s2_attention: null
sample_packing: false
save_steps: null
saves_per_epoch: null
sequence_len: 1024
strict: false
tf32: false
tokenizer_type: AutoTokenizer
train_on_inputs: false
trust_remote_code: true
val_set_size: 0.05
wandb_entity: null
wandb_mode: online
wandb_name: 6a4871a5-8f72-40a7-af01-b85b8a587bc8
wandb_project: Gradients-On-Demand
wandb_run: your_name
wandb_runid: 6a4871a5-8f72-40a7-af01-b85b8a587bc8
warmup_steps: 5
weight_decay: 0.0
xformers_attention: null
```
</details><br>
# b2a2790a-71b8-4284-8441-e9359f291761
This model is a fine-tuned version of [unsloth/Phi-3-mini-4k-instruct](https://huggingface.co/unsloth/Phi-3-mini-4k-instruct) on the None dataset.
It achieves the following results on the evaluation set:
- Loss: 0.4103
## Model description
More information needed
## Intended uses & limitations
More information needed
## Training and evaluation data
More information needed
## Training procedure
### Training hyperparameters
The following hyperparameters were used during training:
- learning_rate: 0.0001
- train_batch_size: 4
- eval_batch_size: 4
- seed: 42
- gradient_accumulation_steps: 4
- total_train_batch_size: 16
- optimizer: Use OptimizerNames.ADAMW_BNB with betas=(0.9,0.999) and epsilon=1e-08 and optimizer_args=No additional optimizer arguments
- lr_scheduler_type: cosine
- lr_scheduler_warmup_steps: 5
- training_steps: 200
### Training results
| Training Loss | Epoch | Step | Validation Loss |
|:-------------:|:------:|:----:|:---------------:|
| 1.6318 | 0.0080 | 200 | 0.4103 |
### Framework versions
- PEFT 0.13.2
- Transformers 4.46.0
- Pytorch 2.5.0+cu124
- Datasets 3.0.1
- Tokenizers 0.20.1 |
Emberx/Aiko | Emberx | "2025-04-15T13:51:11Z" | 0 | 0 | null | [
"region:us"
] | null | "2025-04-09T19:15:30Z" | <!DOCTYPE html>
<html class="" lang="en">
<head>
<meta charset="utf-8" />
<meta
name="viewport"
content="width=device-width, initial-scale=1.0, user-scalable=no"
/>
<meta
name="description"
content="We're on a journey to advance and democratize artificial intelligence through open source and open science."
/>
<meta property="fb:app_id" content="1321688464574422" />
<meta name="twitter:card" content="summary_large_image" />
<meta name="twitter:site" content="@huggingface" />
<meta
property="og:title"
content="Hugging Face - The AI community building the future."
/>
<meta property="og:type" content="website" />
<title>Hugging Face - The AI community building the future.</title>
<style>
body {
margin: 0;
}
main {
background-color: white;
min-height: 100vh;
padding: 7rem 1rem 8rem 1rem;
text-align: center;
font-family: Source Sans Pro, ui-sans-serif, system-ui, -apple-system,
BlinkMacSystemFont, Segoe UI, Roboto, Helvetica Neue, Arial, Noto Sans,
sans-serif, Apple Color Emoji, Segoe UI Emoji, Segoe UI Symbol,
Noto Color Emoji;
}
img {
width: 6rem;
height: 6rem;
margin: 0 auto 1rem;
}
h1 {
font-size: 3.75rem;
line-height: 1;
color: rgba(31, 41, 55, 1);
font-weight: 700;
box-sizing: border-box;
margin: 0 auto;
}
p, a {
color: rgba(107, 114, 128, 1);
font-size: 1.125rem;
line-height: 1.75rem;
max-width: 28rem;
box-sizing: border-box;
margin: 0 auto;
}
.dark main {
background-color: rgb(11, 15, 25);
}
.dark h1 {
color: rgb(209, 213, 219);
}
.dark p, .dark a {
color: rgb(156, 163, 175);
}
</style>
<script>
// On page load or when changing themes, best to add inline in `head` to avoid FOUC
const key = "_tb_global_settings";
let theme = window.matchMedia("(prefers-color-scheme: dark)").matches
? "dark"
: "light";
try {
const storageTheme = JSON.parse(window.localStorage.getItem(key)).theme;
if (storageTheme) {
theme = storageTheme === "dark" ? "dark" : "light";
}
} catch (e) {}
if (theme === "dark") {
document.documentElement.classList.add("dark");
} else {
document.documentElement.classList.remove("dark");
}
</script>
</head>
<body>
<main>
<img
src="https://cdn-media.huggingface.co/assets/huggingface_logo.svg"
alt=""
/>
<div>
<h1>429</h1>
<p>We had to rate limit you. If you think it's an error, send us <a href="mailto:[email protected]">an email</a></p>
</div>
</main>
</body>
</html> |
AdapterHub/facebook-mbart-large-cc25_translation_wmt16_ro_en_pfeiffer | AdapterHub | "2024-05-05T21:13:31Z" | 0 | 0 | adapter-transformers | [
"adapter-transformers",
"text2text-generation",
"adapterhub:mt/wmt16_en_ro",
"mbart",
"license:apache-2.0",
"region:us"
] | text2text-generation | "2024-05-05T21:12:51Z" | ---
tags:
- text2text-generation
- adapter-transformers
- adapterhub:mt/wmt16_en_ro
- mbart
license: "apache-2.0"
---
# Adapter `facebook-mbart-large-cc25_translation_wmt16_ro_en_pfeiffer` for facebook/mbart-large-cc25
Adapter for mbart-large-cc25 in Pfeiffer architecture with reduction factor 2 trained on the WMT16 Romanian-English translation task.
Training for 10 epochs with early stopping and a learning rate of 1e-4.
After post-processing following https://github.com/huggingface/transformers/blob/master/examples/legacy/seq2seq/romanian_postprocessing.md, it achieves a BLEU score of 36.3.
**This adapter was created for usage with the [Adapters](https://github.com/Adapter-Hub/adapters) library.**
## Usage
First, install `adapters`:
```
pip install -U adapters
```
Now, the adapter can be loaded and activated like this:
```python
from adapters import AutoAdapterModel
model = AutoAdapterModel.from_pretrained("facebook/mbart-large-cc25")
adapter_name = model.load_adapter("AdapterHub/facebook-mbart-large-cc25_translation_wmt16_ro_en_pfeiffer")
model.set_active_adapters(adapter_name)
```
## Architecture & Training
- Adapter architecture: pfeiffer
- Prediction head: seq2seq lm
- Dataset: [WMT16 English-Romanian](http://www.statmt.org/wmt16/index.html)
## Author Information
- Author name(s): Clifton Poth
- Author email: [email protected]
- Author links: [Website](https://calpt.github.io), [GitHub](https://github.com/calpt), [Twitter](https://twitter.com/@clifapt)
## Citation
```bibtex
```
*This adapter has been auto-imported from https://github.com/Adapter-Hub/Hub/blob/master/adapters/ukp/facebook-mbart-large-cc25_translation_wmt16_ro_en_pfeiffer.yaml*. |
u888np-com/u888np-com | u888np-com | "2025-03-06T04:04:38Z" | 0 | 0 | null | [
"region:us"
] | null | "2025-03-06T03:55:25Z" | <h1>Kho Game U888: Trải Nghiệm Cá Cược Đỉnh Cao Cho Người Chơi</h1>
<p>Trong thế giới game cá cược trực tuyến hiện nay, <strong><a href="https://u888np.com/">U888</a></strong> nổi bật như một nền tảng cung cấp dịch vụ đa dạng, chất lượng với một kho game phong phú. Nếu bạn đang tìm kiếm một nơi để thư giãn và thử sức với những trò chơi cá cược đỉnh cao, U888 chính là lựa chọn lý tưởng. Bài viết này sẽ giúp bạn hiểu rõ hơn về kho game của U888, từ các trò chơi nổi bật đến các dịch vụ hỗ trợ người chơi, nhằm mang lại những trải nghiệm tuyệt vời và đẳng cấp.</p>
<h2>Kho Game Đa Dạng Tại U888</h2>
<p>U888 không chỉ là một nhà cái cá cược trực tuyến mà còn là nơi hội tụ của vô số trò chơi hấp dẫn. Kho game của U888 được thiết kế để phục vụ mọi đối tượng người chơi, từ những người mới bắt đầu cho đến những tay chơi lão luyện. Dưới đây là một số điểm nổi bật trong kho game của U888.</p>
<h3>Trò Chơi Casino Trực Tuyến</h3>
<p>Một trong những điểm mạnh của U888 chính là các trò chơi casino trực tuyến. Tại đây, bạn sẽ được trải nghiệm các trò chơi như Baccarat, Roulette, và Blackjack với giao diện sinh động và cảm giác chân thực như đang chơi tại một sòng bài thật sự.</p>
<ul>
<li><strong>Baccarat</strong>: Trò chơi bài nổi tiếng này được yêu thích bởi sự đơn giản và tốc độ chơi nhanh. Người chơi chỉ cần đặt cược vào cửa Nhà cái, Người chơi hoặc Hòa để có cơ hội chiến thắng.</li>
<li><strong>Roulette</strong>: Với sự kết hợp giữa may mắn và chiến lược, Roulette tại U888 mang đến những trận đấu đầy kịch tính và thú vị.</li>
<li><strong>Blackjack</strong>: Nếu bạn yêu thích các trò chơi yêu cầu kỹ năng tính toán và chiến thuật, Blackjack sẽ là lựa chọn không thể bỏ qua.</li>
</ul>
<p>Ngoài ra, U888 cũng cung cấp nhiều trò chơi khác như Sic Bo, Dragon Tiger, và các trò chơi mini game thú vị. Các trò chơi này đều có các phiên bản live với người chia bài thực sự, mang đến cho người chơi những trải nghiệm đầy đủ nhất.</p>
<h3>Game Slot Hấp Dẫn</h3>
<p>U888 cũng không thiếu các trò chơi slot đa dạng, từ các game cổ điển đến các game hiện đại với nhiều tính năng đặc biệt. Các máy slot tại U888 không chỉ đẹp mắt về đồ họa mà còn mang lại cơ hội chiến thắng lớn với các giải thưởng hấp dẫn. Người chơi có thể lựa chọn từ hàng chục chủ đề khác nhau, từ những câu chuyện huyền thoại đến các chủ đề hiện đại như công nghệ và thể thao.</p>
<p>Xem thêm: <span style="color:rgb(17, 85, 204); font-family:arial; font-size:10pt"><a class="in-cell-link" href="https://www.retecool.com/author/u888np-com/" target="_blank">https://www.retecool.com/author/u888np-com/</a></span></p>
<h3>Cá Cược Thể Thao</h3>
<p>Không chỉ là một nền tảng cung cấp game casino, U888 còn nổi bật với các dịch vụ cá cược thể thao. Với nhiều môn thể thao nổi tiếng như bóng đá, bóng rổ, tennis, và đua xe, U888 mang đến cho người chơi cơ hội cá cược vào những trận đấu lớn, hấp dẫn, và đầy kịch tính.</p>
<p>U888 cung cấp tỷ lệ cược cạnh tranh và một loạt các tùy chọn cá cược cho người chơi, bao gồm cược trực tiếp và cược theo tỷ lệ. Các trận đấu được cập nhật liên tục, đảm bảo rằng người chơi luôn có thông tin chính xác và kịp thời.</p>
<h2>Chất Lượng Dịch Vụ Hỗ Trợ Người Chơi</h2>
<p>Một yếu tố không thể thiếu trong trải nghiệm cá cược trực tuyến chính là dịch vụ hỗ trợ khách hàng. U888 hiểu rõ điều này và luôn chú trọng đến việc cung cấp dịch vụ hỗ trợ chuyên nghiệp, sẵn sàng giải đáp mọi thắc mắc và hỗ trợ người chơi 24/7.</p>
<h3>Hỗ Trợ Nhanh Chóng Qua Live Chat</h3>
<p>U888 cung cấp dịch vụ chat trực tuyến ngay trên nền tảng của họ, giúp người chơi có thể kết nối trực tiếp với nhân viên hỗ trợ bất cứ lúc nào. Điều này đảm bảo rằng người chơi sẽ không gặp phải khó khăn hay trở ngại trong quá trình tham gia cá cược.</p>
<h3>Hướng Dẫn Chi Tiết Cho Người Mới</h3>
<p>Dành cho những người chơi mới, U888 cung cấp hướng dẫn chi tiết về cách tham gia các trò chơi và cách thức đặt cược. Các tài liệu này dễ hiểu và được cập nhật thường xuyên, giúp người chơi nhanh chóng làm quen với môi trường cá cược trực tuyến.</p>
<h2>Bảo Mật Và An Toàn</h2>
<p>Một yếu tố quan trọng không thể bỏ qua khi chọn một nhà cái cá cược trực tuyến là vấn đề bảo mật. U888 cam kết bảo vệ thông tin cá nhân và tài chính của người chơi bằng các công nghệ bảo mật tiên tiến nhất. Tất cả các giao dịch và dữ liệu của người chơi đều được mã hóa, đảm bảo tính bảo mật tuyệt đối.</p>
<p>Ngoài ra, U888 cũng áp dụng các biện pháp bảo vệ người chơi khỏi những hành vi gian lận, đảm bảo rằng mọi trò chơi đều công bằng và minh bạch.</p>
<h2>Kết Luận</h2>
<p>Kho game của U888 thực sự là một thế giới giải trí đa dạng và đầy hấp dẫn. Dù bạn yêu thích casino trực tuyến, các trò chơi slot, hay cá cược thể thao, U888 đều có thể đáp ứng mọi nhu cầu của bạn. Với chất lượng dịch vụ hỗ trợ chuyên nghiệp, bảo mật an toàn, và một kho game phong phú, U888 là lựa chọn lý tưởng cho những ai yêu thích cá cược trực tuyến. Hãy tham gia ngay hôm nay để trải nghiệm những phút giây thư giãn và đỉnh cao cùng U888!</p>
<p>Xem thêm: <span style="color:rgb(17, 85, 204); font-family:arial; font-size:10pt"><a class="in-cell-link" href="https://tapchivatuyentap.tlu.edu.vn/Activity-Feed/My-Profile/UserId/128776" target="_blank">https://tapchivatuyentap.tlu.edu.vn/Activity-Feed/My-Profile/UserId/128776</a></span></p>
|
BAAI/Emu3-VisionTokenizer | BAAI | "2024-10-08T03:49:53Z" | 7,056 | 56 | transformers | [
"transformers",
"safetensors",
"Emu3VisionVQ",
"feature-extraction",
"custom_code",
"arxiv:2409.18869",
"license:apache-2.0",
"region:us"
] | feature-extraction | "2024-09-25T11:05:17Z" | ---
license: apache-2.0
library_name: transformers
---
<div align='center'>
<h1>Emu3: Next-Token Prediction is All You Need</h1h1>
<h3></h3>
[Emu3 Team, BAAI](https://www.baai.ac.cn/english.html)
| [Project Page](https://emu.baai.ac.cn) | [Paper](https://huggingface.co/papers/2409.18869) | [🤗HF Models](https://huggingface.co/collections/BAAI/emu3-66f4e64f70850ff358a2e60f) | [github](https://github.com/baaivision/Emu3) | [Demo](https://huggingface.co/spaces/BAAI/Emu3) |
</div>
<div align='center'>
<img src="https://github.com/baaivision/Emu3/blob/main/assets/arch.png?raw=True" class="interpolation-image" alt="arch." height="80%" width="70%" />
</div>
We introduce **Emu3**, a new suite of state-of-the-art multimodal models trained solely with **<i>next-token prediction</i>**! By tokenizing images, text, and videos into a discrete space, we train a single transformer from scratch on a mixture of multimodal sequences.
### Emu3 excels in both generation and perception
**Emu3** outperforms several well-established task-specific models in both generation and perception tasks, surpassing flagship open models such as SDXL, LLaVA-1.6 and OpenSora-1.2, while eliminating the need for diffusion or compositional architectures.
<div align='center'>
<img src="https://github.com/baaivision/Emu3/blob/main//assets/comparison.png?raw=True" class="interpolation-image" alt="comparison." height="80%" width="80%" />
</div>
### Highlights
- **Emu3** is capable of generating high-quality images following the text input, by simply predicting the next vision token. The model naturally supports flexible resolutions and styles.
- **Emu3** shows strong vision-language understanding capabilities to see the physical world and provides coherent text responses. Notably, this capability is achieved without depending on a CLIP and a pretrained LLM.
- **Emu3** simply generates a video causally by predicting the next token in a video sequence, unlike the video diffusion model as in Sora. With a video in context, Emu3 can also naturally extend the video and predict what will happen next.
### Quickstart for Autoencoding
```python
import os
import os.path as osp
from PIL import Image
import torch
from transformers import AutoModel, AutoImageProcessor
MODEL_HUB = "BAAI/Emu3-VisionTokenizer"
model = AutoModel.from_pretrained(MODEL_HUB, trust_remote_code=True).eval().cuda()
processor = AutoImageProcessor.from_pretrained(MODEL_HUB, trust_remote_code=True)
# TODO: you need to modify the path here
VIDEO_FRAMES_PATH = "YOUR_VIDEO_FRAMES_PATH"
video = os.listdir(VIDEO_FRAMES_PATH)
video.sort()
video = [Image.open(osp.join(VIDEO_FRAMES_PATH, v)) for v in video]
images = processor(video, return_tensors="pt")["pixel_values"]
images = images.unsqueeze(0).cuda()
# image autoencode
image = images[:, 0]
print(image.shape)
with torch.no_grad():
# encode
codes = model.encode(image)
# decode
recon = model.decode(codes)
recon = recon.view(-1, *recon.shape[2:])
recon_image = processor.postprocess(recon)["pixel_values"][0]
recon_image.save("recon_image.png")
# video autoencode
images = images.view(
-1,
model.config.temporal_downsample_factor,
*images.shape[2:],
)
print(images.shape)
with torch.no_grad():
# encode
codes = model.encode(images)
# decode
recon = model.decode(codes)
recon = recon.view(-1, *recon.shape[2:])
recon_images = processor.postprocess(recon)["pixel_values"]
for idx, im in enumerate(recon_images):
im.save(f"recon_video_{idx}.png")
```
|
basavaakash002/autotrain-377sg-8o19r | basavaakash002 | "2024-02-10T16:51:13Z" | 176 | 0 | transformers | [
"transformers",
"safetensors",
"vit",
"image-classification",
"autotrain",
"dataset:autotrain-377sg-8o19r/autotrain-data",
"autotrain_compatible",
"endpoints_compatible",
"region:us"
] | image-classification | "2024-02-10T16:50:52Z" |
---
tags:
- autotrain
- image-classification
widget:
- src: https://huggingface.co/datasets/mishig/sample_images/resolve/main/tiger.jpg
example_title: Tiger
- src: https://huggingface.co/datasets/mishig/sample_images/resolve/main/teapot.jpg
example_title: Teapot
- src: https://huggingface.co/datasets/mishig/sample_images/resolve/main/palace.jpg
example_title: Palace
datasets:
- autotrain-377sg-8o19r/autotrain-data
---
# Model Trained Using AutoTrain
- Problem type: Image Classification
## Validation Metricsg
loss: 1.6438376903533936
f1_macro: 0.08333333333333334
f1_micro: 0.2631578947368421
f1_weighted: 0.10964912280701755
precision_macro: 0.05263157894736842
precision_micro: 0.2631578947368421
precision_weighted: 0.06925207756232686
recall_macro: 0.2
recall_micro: 0.2631578947368421
recall_weighted: 0.2631578947368421
accuracy: 0.2631578947368421
|
jysssacc/627_roberta-base_fine_lr0.05_bs4_epoch5_wd0.01 | jysssacc | "2024-01-11T23:47:44Z" | 43 | 0 | transformers | [
"transformers",
"safetensors",
"roberta",
"text-generation",
"generated_from_trainer",
"base_model:FacebookAI/roberta-base",
"base_model:finetune:FacebookAI/roberta-base",
"license:mit",
"autotrain_compatible",
"endpoints_compatible",
"region:us"
] | text-generation | "2024-01-11T23:40:02Z" | ---
license: mit
base_model: roberta-base
tags:
- generated_from_trainer
model-index:
- name: 627_roberta-base_fine_lr0.05_bs4_epoch5_wd0.01
results: []
---
<!-- This model card has been generated automatically according to the information the Trainer had access to. You
should probably proofread and complete it, then remove this comment. -->
# 627_roberta-base_fine_lr0.05_bs4_epoch5_wd0.01
This model is a fine-tuned version of [roberta-base](https://huggingface.co/roberta-base) on an unknown dataset.
It achieves the following results on the evaluation set:
- Loss: 7.8054
## Model description
More information needed
## Intended uses & limitations
More information needed
## Training and evaluation data
More information needed
## Training procedure
### Training hyperparameters
The following hyperparameters were used during training:
- learning_rate: 0.05
- train_batch_size: 4
- eval_batch_size: 4
- seed: 42
- optimizer: Adam with betas=(0.9,0.999) and epsilon=1e-08
- lr_scheduler_type: linear
- lr_scheduler_warmup_steps: 500
- num_epochs: 5
### Training results
| Training Loss | Epoch | Step | Validation Loss |
|:-------------:|:-----:|:----:|:---------------:|
| 7.879 | 1.0 | 157 | 7.6793 |
| 6.7935 | 2.0 | 314 | 8.1942 |
| 6.9191 | 3.0 | 471 | 8.2193 |
| 7.0385 | 4.0 | 628 | 7.8762 |
| 6.7279 | 5.0 | 785 | 7.8054 |
### Framework versions
- Transformers 4.36.2
- Pytorch 2.0.1
- Datasets 2.16.1
- Tokenizers 0.15.0
|
silviacamplani/distilbert-finetuned-dapt-ner-ai | silviacamplani | "2022-08-25T14:13:33Z" | 63 | 0 | transformers | [
"transformers",
"tf",
"tensorboard",
"distilbert",
"token-classification",
"generated_from_keras_callback",
"autotrain_compatible",
"endpoints_compatible",
"region:us"
] | token-classification | "2022-08-25T14:11:40Z" | ---
tags:
- generated_from_keras_callback
model-index:
- name: silviacamplani/distilbert-finetuned-dapt-ner-ai
results: []
---
<!-- This model card has been generated automatically according to the information Keras had access to. You should
probably proofread and complete it, then remove this comment. -->
# silviacamplani/distilbert-finetuned-dapt-ner-ai
This model was trained from scratch on an unknown dataset.
It achieves the following results on the evaluation set:
- Train Loss: 0.9448
- Validation Loss: 0.9212
- Train Precision: 0.3164
- Train Recall: 0.3186
- Train F1: 0.3175
- Train Accuracy: 0.7524
- Epoch: 6
## Model description
More information needed
## Intended uses & limitations
More information needed
## Training and evaluation data
More information needed
## Training procedure
### Training hyperparameters
The following hyperparameters were used during training:
- optimizer: {'inner_optimizer': {'class_name': 'AdamWeightDecay', 'config': {'name': 'AdamWeightDecay', 'learning_rate': {'class_name': 'PolynomialDecay', 'config': {'initial_learning_rate': 1e-05, 'decay_steps': 350, 'end_learning_rate': 0.0, 'power': 1.0, 'cycle': False, 'name': None}}, 'decay': 0.0, 'beta_1': 0.9, 'beta_2': 0.999, 'epsilon': 1e-08, 'amsgrad': False, 'weight_decay_rate': 0.01}}, 'dynamic': True, 'initial_scale': 32768.0, 'dynamic_growth_steps': 2000}
- training_precision: mixed_float16
### Training results
| Train Loss | Validation Loss | Train Precision | Train Recall | Train F1 | Train Accuracy | Epoch |
|:----------:|:---------------:|:---------------:|:------------:|:--------:|:--------------:|:-----:|
| 2.6857 | 1.8199 | 0.0 | 0.0 | 0.0 | 0.6480 | 0 |
| 1.6775 | 1.4868 | 0.0 | 0.0 | 0.0 | 0.6480 | 1 |
| 1.3847 | 1.2452 | 0.0938 | 0.0102 | 0.0184 | 0.6565 | 2 |
| 1.2067 | 1.1198 | 0.1659 | 0.1244 | 0.1422 | 0.7077 | 3 |
| 1.0946 | 1.0321 | 0.2255 | 0.1925 | 0.2077 | 0.7225 | 4 |
| 1.0057 | 0.9640 | 0.2835 | 0.2777 | 0.2806 | 0.7433 | 5 |
| 0.9448 | 0.9212 | 0.3164 | 0.3186 | 0.3175 | 0.7524 | 6 |
### Framework versions
- Transformers 4.20.1
- TensorFlow 2.6.4
- Datasets 2.1.0
- Tokenizers 0.12.1
|
SriVishnu/Bertu | SriVishnu | "2025-02-24T04:36:49Z" | 0 | 0 | null | [
"license:apache-2.0",
"region:us"
] | null | "2025-02-24T04:36:49Z" | ---
license: apache-2.0
---
|
mav23/OpenChat-3.5-0106_32K-PoSE-GGUF | mav23 | "2024-11-10T07:01:42Z" | 48 | 0 | transformers | [
"transformers",
"gguf",
"dataset:Yukang/LongAlpaca-12k",
"arxiv:2309.10400",
"base_model:openchat/openchat-3.5-0106",
"base_model:quantized:openchat/openchat-3.5-0106",
"license:apache-2.0",
"model-index",
"endpoints_compatible",
"region:us",
"conversational"
] | null | "2024-11-10T06:11:36Z" | ---
license: apache-2.0
library_name: transformers
base_model:
- openchat/openchat-3.5-0106
datasets:
- Yukang/LongAlpaca-12k
model-index:
- name: OpenChat-3.5-0106_32K-PoSE
results:
- task:
type: text-generation
name: Text Generation
dataset:
name: IFEval (0-Shot)
type: HuggingFaceH4/ifeval
args:
num_few_shot: 0
metrics:
- type: inst_level_strict_acc and prompt_level_strict_acc
value: 39.69
name: strict accuracy
source:
url: https://huggingface.co/spaces/open-llm-leaderboard/open_llm_leaderboard?query=Pretergeek/OpenChat-3.5-0106_32K-PoSE
name: Open LLM Leaderboard
- task:
type: text-generation
name: Text Generation
dataset:
name: BBH (3-Shot)
type: BBH
args:
num_few_shot: 3
metrics:
- type: acc_norm
value: 8.83
name: normalized accuracy
source:
url: https://huggingface.co/spaces/open-llm-leaderboard/open_llm_leaderboard?query=Pretergeek/OpenChat-3.5-0106_32K-PoSE
name: Open LLM Leaderboard
- task:
type: text-generation
name: Text Generation
dataset:
name: MATH Lvl 5 (4-Shot)
type: hendrycks/competition_math
args:
num_few_shot: 4
metrics:
- type: exact_match
value: 1.44
name: exact match
source:
url: https://huggingface.co/spaces/open-llm-leaderboard/open_llm_leaderboard?query=Pretergeek/OpenChat-3.5-0106_32K-PoSE
name: Open LLM Leaderboard
- task:
type: text-generation
name: Text Generation
dataset:
name: GPQA (0-shot)
type: Idavidrein/gpqa
args:
num_few_shot: 0
metrics:
- type: acc_norm
value: 3.47
name: acc_norm
source:
url: https://huggingface.co/spaces/open-llm-leaderboard/open_llm_leaderboard?query=Pretergeek/OpenChat-3.5-0106_32K-PoSE
name: Open LLM Leaderboard
- task:
type: text-generation
name: Text Generation
dataset:
name: MuSR (0-shot)
type: TAUR-Lab/MuSR
args:
num_few_shot: 0
metrics:
- type: acc_norm
value: 11.33
name: acc_norm
source:
url: https://huggingface.co/spaces/open-llm-leaderboard/open_llm_leaderboard?query=Pretergeek/OpenChat-3.5-0106_32K-PoSE
name: Open LLM Leaderboard
- task:
type: text-generation
name: Text Generation
dataset:
name: MMLU-PRO (5-shot)
type: TIGER-Lab/MMLU-Pro
config: main
split: test
args:
num_few_shot: 5
metrics:
- type: acc
value: 11.46
name: accuracy
source:
url: https://huggingface.co/spaces/open-llm-leaderboard/open_llm_leaderboard?query=Pretergeek/OpenChat-3.5-0106_32K-PoSE
name: Open LLM Leaderboard
---
<p align="center">
<a href="https://ko-fi.com/pretergeek">Buy me a Ko-Fi</a> •
<a href="https://patreon.com/Pretergeek">Support my work using Patreon</a>
</p>
# OpenChat-3.5-0106_32K-PoSE
## Description
This model is [Openchat-3.5-0106](https://huggingface.co/openchat/openchat-3.5-0106) with the context length extended from 8192 tokens to 32768 tokens using [PoSE](https://huggingface.co/papers/2309.10400).
The model was fine-tuned using [Rank-Stabilized LoRA](https://huggingface.co/blog/damjan-k/rslora) and the [LongAlpaca-12K](Yukang/LongAlpaca-12k) dataset. I hope to continue extending the context in future versions and then apply the same methods to my [upscaled versions of OpenChat-3.5](https://huggingface.co/collections/Pretergeek/openchat-35-0106-with-additional-layers-66a8d3262c7c3ebdd7783a29) that were created using Block Expansion instead of Depth UP Scaling.
After fine-tuning, the model was tested using passkey retrieval and achieved a score of 100%. Below you can also find the results of the Open LLM Leaderboard evaluations and I am a bit disappointed with those. The model ended up with a significant reduction in performance compared to the original model in all but one test (MUSR). I expected it to do better than the original model on MUSR since that test benefits from long context understanding but I didn't expect such a negative impact on the other tasks. Anyway, I will be addressing this on a future version. I used the LongAlpaca-12K dataset because it is small and I have limited computational resources but I might have to try a larger dataset for the next attempt. If you would like to help me, there are links on the top of the model card for my Patreon and Ko-Fi.
# [Open LLM Leaderboard Evaluation Results](https://huggingface.co/spaces/open-llm-leaderboard/open_llm_leaderboard)
Detailed results can be found [here](https://huggingface.co/datasets/open-llm-leaderboard/details_Pretergeek__OpenChat-3.5-0106_32K-PoSE)
| Metric |Value|
|-------------------|----:|
|Avg. |12.70|
|IFEval (0-Shot) |39.69|
|BBH (3-Shot) | 8.83|
|MATH Lvl 5 (4-Shot)| 1.44|
|GPQA (0-shot) | 3.47|
|MuSR (0-shot) |11.33|
|MMLU-PRO (5-shot) |11.46|
# Citation
```
@misc{zhu2024poseefficientcontextwindow,
title={PoSE: Efficient Context Window Extension of LLMs via Positional Skip-wise Training},
author={Dawei Zhu and Nan Yang and Liang Wang and Yifan Song and Wenhao Wu and Furu Wei and Sujian Li},
year={2024},
eprint={2309.10400},
archivePrefix={arXiv},
primaryClass={cs.CL},
url={https://arxiv.org/abs/2309.10400},
}
``` |
TheBloke/GPT4All-13B-Snoozy-SuperHOT-8K-GPTQ | TheBloke | "2023-08-21T14:44:27Z" | 14 | 6 | transformers | [
"transformers",
"safetensors",
"llama",
"text-generation",
"custom_code",
"license:other",
"autotrain_compatible",
"text-generation-inference",
"4-bit",
"gptq",
"region:us"
] | text-generation | "2023-06-27T09:35:55Z" | ---
inference: false
license: other
---
<!-- header start -->
<!-- 200823 -->
<div style="width: auto; margin-left: auto; margin-right: auto">
<img src="https://i.imgur.com/EBdldam.jpg" alt="TheBlokeAI" style="width: 100%; min-width: 400px; display: block; margin: auto;">
</div>
<div style="display: flex; justify-content: space-between; width: 100%;">
<div style="display: flex; flex-direction: column; align-items: flex-start;">
<p style="margin-top: 0.5em; margin-bottom: 0em;"><a href="https://discord.gg/theblokeai">Chat & support: TheBloke's Discord server</a></p>
</div>
<div style="display: flex; flex-direction: column; align-items: flex-end;">
<p style="margin-top: 0.5em; margin-bottom: 0em;"><a href="https://www.patreon.com/TheBlokeAI">Want to contribute? TheBloke's Patreon page</a></p>
</div>
</div>
<div style="text-align:center; margin-top: 0em; margin-bottom: 0em"><p style="margin-top: 0.25em; margin-bottom: 0em;">TheBloke's LLM work is generously supported by a grant from <a href="https://a16z.com">andreessen horowitz (a16z)</a></p></div>
<hr style="margin-top: 1.0em; margin-bottom: 1.0em;">
<!-- header end -->
# Nomic.ai's GPT4All Snoozy 13B GPTQ
These files are GPTQ 4bit model files for [Nomic.ai's GPT4All Snoozy 13B](https://huggingface.co/nomic-ai/gpt4all-13b-snoozy) merged with [Kaio Ken's SuperHOT 8K](https://huggingface.co/kaiokendev/superhot-13b-8k-no-rlhf-test).
It is the result of quantising to 4bit using [GPTQ-for-LLaMa](https://github.com/qwopqwop200/GPTQ-for-LLaMa).
**This is an experimental new GPTQ which offers up to 8K context size**
The increased context is tested to work with [ExLlama](https://github.com/turboderp/exllama), via the latest release of [text-generation-webui](https://github.com/oobabooga/text-generation-webui).
It has also been tested from Python code using AutoGPTQ, and `trust_remote_code=True`.
Code credits:
- Original concept and code for increasing context length: [kaiokendev](https://huggingface.co/kaiokendev)
- Updated Llama modelling code that includes this automatically via trust_remote_code: [emozilla](https://huggingface.co/emozilla).
Please read carefully below to see how to use it.
GGML versions are not yet provided, as there is not yet support for SuperHOT in llama.cpp. This is being investigated and will hopefully come soon.
## Repositories available
* [4-bit GPTQ models for GPU inference](https://huggingface.co/TheBloke/GPT4All-13B-Snoozy-SuperHOT-8K-GPTQ)
* [2, 3, 4, 5, 6 and 8-bit GGML models for CPU inference](https://huggingface.co/TheBloke/GPT4All-13B-Snoozy-SuperHOT-8K-GGML)
* [Unquantised SuperHOT fp16 model in pytorch format, for GPU inference and for further conversions](https://huggingface.co/TheBloke/GPT4All-13B-Snoozy-SuperHOT-8K-fp16)
* [Unquantised base fp16 model in pytorch format, for GPU inference and for further conversions](https://huggingface.co/nomic-ai/gpt4all-13b-snoozy)
## How to easily download and use this model in text-generation-webui with ExLlama
Please make sure you're using the latest version of text-generation-webui
1. Click the **Model tab**.
2. Under **Download custom model or LoRA**, enter `TheBloke/GPT4All-13B-Snoozy-SuperHOT-8K-GPTQ`.
3. Click **Download**.
4. The model will start downloading. Once it's finished it will say "Done"
5. Untick **Autoload the model**
6. In the top left, click the refresh icon next to **Model**.
7. In the **Model** dropdown, choose the model you just downloaded: `GPT4All-13B-Snoozy-SuperHOT-8K-GPTQ`
8. To use the increased context, set the **Loader** to **ExLlama**, set **max_seq_len** to 8192 or 4096, and set **compress_pos_emb** to **4** for 8192 context, or to **2** for 4096 context.
9. Now click **Save Settings** followed by **Reload**
10. The model will automatically load, and is now ready for use!
11. Once you're ready, click the **Text Generation tab** and enter a prompt to get started!
## How to use this GPTQ model from Python code with AutoGPTQ
First make sure you have AutoGPTQ and Einops installed:
```
pip3 install einops auto-gptq
```
Then run the following code. Note that in order to get this to work, `config.json` has been hardcoded to a sequence length of 8192.
If you want to try 4096 instead to reduce VRAM usage, please manually edit `config.json` to set `max_position_embeddings` to the value you want.
```python
from transformers import AutoTokenizer, pipeline, logging
from auto_gptq import AutoGPTQForCausalLM, BaseQuantizeConfig
import argparse
model_name_or_path = "TheBloke/GPT4All-13B-Snoozy-SuperHOT-8K-GPTQ"
model_basename = "gpt4all-snoozy-13b-superhot-8k-GPTQ-4bit-128g.no-act.order"
use_triton = False
tokenizer = AutoTokenizer.from_pretrained(model_name_or_path, use_fast=True)
model = AutoGPTQForCausalLM.from_quantized(model_name_or_path,
model_basename=model_basename,
use_safetensors=True,
trust_remote_code=True,
device_map='auto',
use_triton=use_triton,
quantize_config=None)
model.seqlen = 8192
# Note: check the prompt template is correct for this model.
prompt = "Tell me about AI"
prompt_template=f'''USER: {prompt}
ASSISTANT:'''
print("\n\n*** Generate:")
input_ids = tokenizer(prompt_template, return_tensors='pt').input_ids.cuda()
output = model.generate(inputs=input_ids, temperature=0.7, max_new_tokens=512)
print(tokenizer.decode(output[0]))
# Inference can also be done using transformers' pipeline
# Prevent printing spurious transformers error when using pipeline with AutoGPTQ
logging.set_verbosity(logging.CRITICAL)
print("*** Pipeline:")
pipe = pipeline(
"text-generation",
model=model,
tokenizer=tokenizer,
max_new_tokens=512,
temperature=0.7,
top_p=0.95,
repetition_penalty=1.15
)
print(pipe(prompt_template)[0]['generated_text'])
```
## Using other UIs: monkey patch
Provided in the repo is `llama_rope_scaled_monkey_patch.py`, written by @kaiokendev.
It can be theoretically be added to any Python UI or custom code to enable the same result as `trust_remote_code=True`. I have not tested this, and it should be superseded by using `trust_remote_code=True`, but I include it for completeness and for interest.
## Provided files
**gpt4all-snoozy-13b-superhot-8k-GPTQ-4bit-128g.no-act.order.safetensors**
This will work with AutoGPTQ, ExLlama, and CUDA versions of GPTQ-for-LLaMa. There are reports of issues with Triton mode of recent GPTQ-for-LLaMa. If you have issues, please use AutoGPTQ instead.
It was created with group_size 128 to increase inference accuracy, but without --act-order (desc_act) to increase compatibility and improve inference speed.
* `gpt4all-snoozy-13b-superhot-8k-GPTQ-4bit-128g.no-act.order.safetensors`
* Works for use with ExLlama with increased context (4096 or 8192)
* Works with AutoGPTQ in Python code, including with increased context, if `trust_remote_code=True` is set.
* Should work with GPTQ-for-LLaMa in CUDA mode, but unknown if increased context works - TBC. May have issues with GPTQ-for-LLaMa Triton mode.
* Works with text-generation-webui, including one-click-installers.
* Parameters: Groupsize = 128. Act Order / desc_act = False.
<!-- footer start -->
<!-- 200823 -->
## Discord
For further support, and discussions on these models and AI in general, join us at:
[TheBloke AI's Discord server](https://discord.gg/theblokeai)
## Thanks, and how to contribute.
Thanks to the [chirper.ai](https://chirper.ai) team!
I've had a lot of people ask if they can contribute. I enjoy providing models and helping people, and would love to be able to spend even more time doing it, as well as expanding into new projects like fine tuning/training.
If you're able and willing to contribute it will be most gratefully received and will help me to keep providing more models, and to start work on new AI projects.
Donaters will get priority support on any and all AI/LLM/model questions and requests, access to a private Discord room, plus other benefits.
* Patreon: https://patreon.com/TheBlokeAI
* Ko-Fi: https://ko-fi.com/TheBlokeAI
**Special thanks to**: Aemon Algiz.
**Patreon special mentions**: Sam, theTransient, Jonathan Leane, Steven Wood, webtim, Johann-Peter Hartmann, Geoffrey Montalvo, Gabriel Tamborski, Willem Michiel, John Villwock, Derek Yates, Mesiah Bishop, Eugene Pentland, Pieter, Chadd, Stephen Murray, Daniel P. Andersen, terasurfer, Brandon Frisco, Thomas Belote, Sid, Nathan LeClaire, Magnesian, Alps Aficionado, Stanislav Ovsiannikov, Alex, Joseph William Delisle, Nikolai Manek, Michael Davis, Junyu Yang, K, J, Spencer Kim, Stefan Sabev, Olusegun Samson, transmissions 11, Michael Levine, Cory Kujawski, Rainer Wilmers, zynix, Kalila, Luke @flexchar, Ajan Kanaga, Mandus, vamX, Ai Maven, Mano Prime, Matthew Berman, subjectnull, Vitor Caleffi, Clay Pascal, biorpg, alfie_i, 阿明, Jeffrey Morgan, ya boyyy, Raymond Fosdick, knownsqashed, Olakabola, Leonard Tan, ReadyPlayerEmma, Enrico Ros, Dave, Talal Aujan, Illia Dulskyi, Sean Connelly, senxiiz, Artur Olbinski, Elle, Raven Klaugh, Fen Risland, Deep Realms, Imad Khwaja, Fred von Graf, Will Dee, usrbinkat, SuperWojo, Alexandros Triantafyllidis, Swaroop Kallakuri, Dan Guido, John Detwiler, Pedro Madruga, Iucharbius, Viktor Bowallius, Asp the Wyvern, Edmond Seymore, Trenton Dambrowitz, Space Cruiser, Spiking Neurons AB, Pyrater, LangChain4j, Tony Hughes, Kacper Wikieł, Rishabh Srivastava, David Ziegler, Luke Pendergrass, Andrey, Gabriel Puliatti, Lone Striker, Sebastain Graf, Pierre Kircher, Randy H, NimbleBox.ai, Vadim, danny, Deo Leter
Thank you to all my generous patrons and donaters!
And thank you again to a16z for their generous grant.
<!-- footer end -->
# Original model card: Kaio Ken's SuperHOT 8K
### SuperHOT Prototype 2 w/ 8K Context
This is a second prototype of SuperHOT, this time 30B with 8K context and no RLHF, using the same technique described in [the github blog](https://kaiokendev.github.io/til#extending-context-to-8k).
Tests have shown that the model does indeed leverage the extended context at 8K.
You will need to **use either the monkeypatch** or, if you are already using the monkeypatch, **change the scaling factor to 0.25 and the maximum sequence length to 8192**
#### Looking for Merged & Quantized Models?
- 30B 4-bit CUDA: [tmpupload/superhot-30b-8k-4bit-safetensors](https://huggingface.co/tmpupload/superhot-30b-8k-4bit-safetensors)
- 30B 4-bit CUDA 128g: [tmpupload/superhot-30b-8k-4bit-128g-safetensors](https://huggingface.co/tmpupload/superhot-30b-8k-4bit-128g-safetensors)
#### Training Details
I trained the LoRA with the following configuration:
- 1200 samples (~400 samples over 2048 sequence length)
- learning rate of 3e-4
- 3 epochs
- The exported modules are:
- q_proj
- k_proj
- v_proj
- o_proj
- no bias
- Rank = 4
- Alpha = 8
- no dropout
- weight decay of 0.1
- AdamW beta1 of 0.9 and beta2 0.99, epsilon of 1e-5
- Trained on 4-bit base model
# Original model card: Nomic.ai's GPT4All Snoozy 13B
# Model Card for GPT4All-13b-snoozy
A GPL licensed chatbot trained over a massive curated corpus of assistant interactions including word problems, multi-turn dialogue, code, poems, songs, and stories.
## Model Details
### Model Description
<!-- Provide a longer summary of what this model is. -->
This model has been finetuned from LLama 13B
- **Developed by:** [Nomic AI](https://home.nomic.ai)
- **Model Type:** A finetuned LLama 13B model on assistant style interaction data
- **Language(s) (NLP):** English
- **License:** GPL
- **Finetuned from model [optional]:** LLama 13B
This model was trained on `nomic-ai/gpt4all-j-prompt-generations` using `revision=v1.3-groovy`
### Model Sources [optional]
<!-- Provide the basic links for the model. -->
- **Repository:** [https://github.com/nomic-ai/gpt4all](https://github.com/nomic-ai/gpt4all)
- **Base Model Repository:** [https://github.com/facebookresearch/llama](https://github.com/facebookresearch/llama)
- **Demo [optional]:** [https://gpt4all.io/](https://gpt4all.io/)
### Results
Results on common sense reasoning benchmarks
```
| Model | BoolQ | PIQA | HellaSwag | WinoGrande | ARC-e | ARC-c | OBQA | Avg. |
|:--------------------------|:--------:|:--------:|:---------:|:----------:|:--------:|:--------:|:--------:|:--------:|
| GPT4All-J 6B v1.0 | 73.4 | 74.8 | 63.4 | 64.7 | 54.9 | 36.0 | 40.2 | 58.2 |
| GPT4All-J v1.1-breezy | 74.0 | 75.1 | 63.2 | 63.6 | 55.4 | 34.9 | 38.4 | 57.8 |
| GPT4All-J v1.2-jazzy | 74.8 | 74.9 | 63.6 | 63.8 | 56.6 | 35.3 | 41.0 | 58.6 |
| GPT4All-J v1.3-groovy | 73.6 | 74.3 | 63.8 | 63.5 | 57.7 | 35.0 | 38.8 | 58.1 |
| GPT4All-J Lora 6B | 68.6 | 75.8 | 66.2 | 63.5 | 56.4 | 35.7 | 40.2 | 58.1 |
| GPT4All LLaMa Lora 7B | 73.1 | 77.6 | 72.1 | 67.8 | 51.1 | 40.4 | 40.2 | 60.3 |
| GPT4All 13B snoozy | **83.3** | 79.2 | 75.0 | **71.3** | 60.9 | 44.2 | 43.4 | **65.3** |
| Dolly 6B | 68.8 | 77.3 | 67.6 | 63.9 | 62.9 | 38.7 | 41.2 | 60.1 |
| Dolly 12B | 56.7 | 75.4 | 71.0 | 62.2 | 64.6 | 38.5 | 40.4 | 58.4 |
| Alpaca 7B | 73.9 | 77.2 | 73.9 | 66.1 | 59.8 | 43.3 | 43.4 | 62.4 |
| Alpaca Lora 7B | 74.3 | **79.3** | 74.0 | 68.8 | 56.6 | 43.9 | 42.6 | 62.8 |
| GPT-J 6.7B | 65.4 | 76.2 | 66.2 | 64.1 | 62.2 | 36.6 | 38.2 | 58.4 |
| LLama 7B | 73.1 | 77.4 | 73.0 | 66.9 | 52.5 | 41.4 | 42.4 | 61.0 |
| LLama 13B | 68.5 | 79.1 | 76.2 | 70.1 | 60.0 | **44.6** | 42.2 | 63.0 |
| Pythia 6.7B | 63.5 | 76.3 | 64.0 | 61.1 | 61.3 | 35.2 | 37.2 | 57.0 |
| Pythia 12B | 67.7 | 76.6 | 67.3 | 63.8 | 63.9 | 34.8 | 38 | 58.9 |
| Fastchat T5 | 81.5 | 64.6 | 46.3 | 61.8 | 49.3 | 33.3 | 39.4 | 53.7 |
| Fastchat Vicuña 7B | 76.6 | 77.2 | 70.7 | 67.3 | 53.5 | 41.2 | 40.8 | 61.0 |
| Fastchat Vicuña 13B | 81.5 | 76.8 | 73.3 | 66.7 | 57.4 | 42.7 | 43.6 | 63.1 |
| StableVicuña RLHF | 82.3 | 78.6 | 74.1 | 70.9 | 61.0 | 43.5 | **44.4** | 65.0 |
| StableLM Tuned | 62.5 | 71.2 | 53.6 | 54.8 | 52.4 | 31.1 | 33.4 | 51.3 |
| StableLM Base | 60.1 | 67.4 | 41.2 | 50.1 | 44.9 | 27.0 | 32.0 | 42.2 |
| Koala 13B | 76.5 | 77.9 | 72.6 | 68.8 | 54.3 | 41.0 | 42.8 | 62.0 |
| Open Assistant Pythia 12B | 67.9 | 78.0 | 68.1 | 65.0 | 64.2 | 40.4 | 43.2 | 61.0 |
| Mosaic mpt-7B | 74.8 | **79.3** | **76.3** | 68.6 | **70.0** | 42.2 | 42.6 | 64.8 |
| text-davinci-003 | 88.1 | 83.8 | 83.4 | 75.8 | 83.9 | 63.9 | 51.0 | 75.7 |
```
|
mohamedsaeed823/VideoMAEF-finetuned-ARSL-diverse-dataset | mohamedsaeed823 | "2024-06-19T10:35:16Z" | 68 | 0 | transformers | [
"transformers",
"tensorboard",
"safetensors",
"videomae",
"video-classification",
"generated_from_trainer",
"base_model:MCG-NJU/videomae-base-finetuned-kinetics",
"base_model:finetune:MCG-NJU/videomae-base-finetuned-kinetics",
"license:cc-by-nc-4.0",
"endpoints_compatible",
"region:us"
] | video-classification | "2024-06-19T07:16:39Z" | ---
license: cc-by-nc-4.0
base_model: MCG-NJU/videomae-base-finetuned-kinetics
tags:
- generated_from_trainer
metrics:
- accuracy
model-index:
- name: VideoMAEF-finetuned-ARSL-diverse-dataset
results: []
---
<!-- This model card has been generated automatically according to the information the Trainer had access to. You
should probably proofread and complete it, then remove this comment. -->
# VideoMAEF-finetuned-ARSL-diverse-dataset
This model is a fine-tuned version of [MCG-NJU/videomae-base-finetuned-kinetics](https://huggingface.co/MCG-NJU/videomae-base-finetuned-kinetics) on an unknown dataset.
It achieves the following results on the evaluation set:
- Loss: 0.0012
- Accuracy: 1.0
## Model description
More information needed
## Intended uses & limitations
More information needed
## Training and evaluation data
More information needed
## Training procedure
### Training hyperparameters
The following hyperparameters were used during training:
- learning_rate: 2.5e-05
- train_batch_size: 2
- eval_batch_size: 2
- seed: 42
- optimizer: Adam with betas=(0.9,0.999) and epsilon=1e-08
- lr_scheduler_type: linear
- lr_scheduler_warmup_ratio: 0.1
- training_steps: 2395
### Training results
| Training Loss | Epoch | Step | Validation Loss | Accuracy |
|:-------------:|:-----:|:----:|:---------------:|:--------:|
| 1.0485 | 0.12 | 298 | 1.1148 | 0.6854 |
| 0.7256 | 1.12 | 596 | 0.1032 | 1.0 |
| 0.2052 | 2.12 | 894 | 0.0057 | 1.0 |
| 0.0451 | 3.12 | 1192 | 0.0028 | 1.0 |
| 0.1821 | 4.12 | 1490 | 0.0020 | 1.0 |
| 0.0965 | 5.12 | 1788 | 0.0015 | 1.0 |
| 0.0873 | 6.12 | 2086 | 0.0012 | 1.0 |
| 0.0027 | 7.12 | 2384 | 0.0011 | 1.0 |
| 0.1222 | 8.0 | 2395 | 0.0012 | 1.0 |
### Framework versions
- Transformers 4.39.3
- Pytorch 2.1.2
- Datasets 2.18.0
- Tokenizers 0.15.2
|
smarthi/zephyr-support-chatbot | smarthi | "2023-11-25T18:12:31Z" | 0 | 0 | null | [
"tensorboard",
"safetensors",
"generated_from_trainer",
"base_model:TheBloke/zephyr-7B-alpha-GPTQ",
"base_model:finetune:TheBloke/zephyr-7B-alpha-GPTQ",
"license:mit",
"region:us"
] | null | "2023-11-25T17:34:39Z" | ---
license: mit
base_model: TheBloke/zephyr-7B-alpha-GPTQ
tags:
- generated_from_trainer
model-index:
- name: zephyr-support-chatbot
results: []
---
<!-- This model card has been generated automatically according to the information the Trainer had access to. You
should probably proofread and complete it, then remove this comment. -->
# zephyr-support-chatbot
This model is a fine-tuned version of [TheBloke/zephyr-7B-alpha-GPTQ](https://huggingface.co/TheBloke/zephyr-7B-alpha-GPTQ) on the None dataset.
## Model description
More information needed
## Intended uses & limitations
More information needed
## Training and evaluation data
More information needed
## Training procedure
### Training hyperparameters
The following hyperparameters were used during training:
- learning_rate: 0.0002
- train_batch_size: 8
- eval_batch_size: 8
- seed: 42
- optimizer: Adam with betas=(0.9,0.999) and epsilon=1e-08
- lr_scheduler_type: cosine
- training_steps: 250
- mixed_precision_training: Native AMP
### Training results
### Framework versions
- Transformers 4.35.2
- Pytorch 2.1.0+cu118
- Datasets 2.15.0
- Tokenizers 0.15.0
|
baek26/bart-all | baek26 | "2024-04-22T01:54:53Z" | 104 | 0 | transformers | [
"transformers",
"safetensors",
"bart",
"text2text-generation",
"generated_from_trainer",
"base_model:facebook/bart-base",
"base_model:finetune:facebook/bart-base",
"license:apache-2.0",
"autotrain_compatible",
"endpoints_compatible",
"region:us"
] | text2text-generation | "2024-04-20T15:23:44Z" | ---
license: apache-2.0
base_model: facebook/bart-base
tags:
- generated_from_trainer
metrics:
- rouge
model-index:
- name: all_6417_bart-base
results: []
---
<!-- This model card has been generated automatically according to the information the Trainer had access to. You
should probably proofread and complete it, then remove this comment. -->
# all_6417_bart-base
This model is a fine-tuned version of [facebook/bart-base](https://huggingface.co/facebook/bart-base) on the None dataset.
It achieves the following results on the evaluation set:
- Loss: 1.0206
- Rouge1: 0.2426
- Rouge2: 0.1209
- Rougel: 0.2027
- Rougelsum: 0.2266
- Gen Len: 19.9945
## Model description
More information needed
## Intended uses & limitations
More information needed
## Training and evaluation data
More information needed
## Training procedure
### Training hyperparameters
The following hyperparameters were used during training:
- learning_rate: 5e-05
- train_batch_size: 32
- eval_batch_size: 20
- seed: 42
- gradient_accumulation_steps: 16
- total_train_batch_size: 512
- optimizer: Adam with betas=(0.9,0.999) and epsilon=1e-08
- lr_scheduler_type: linear
- lr_scheduler_warmup_steps: 500
- num_epochs: 10
### Training results
| Training Loss | Epoch | Step | Validation Loss | Rouge1 | Rouge2 | Rougel | Rougelsum | Gen Len |
|:-------------:|:-----:|:----:|:---------------:|:------:|:------:|:------:|:---------:|:-------:|
| 2.7151 | 0.8 | 500 | 1.1257 | 0.2361 | 0.1122 | 0.1957 | 0.2196 | 19.9978 |
| 1.0837 | 1.61 | 1000 | 1.0810 | 0.2401 | 0.1176 | 0.1998 | 0.2237 | 19.9953 |
| 1.0348 | 2.41 | 1500 | 1.0651 | 0.2401 | 0.1179 | 0.2 | 0.2239 | 19.9957 |
| 1.0059 | 3.21 | 2000 | 1.0522 | 0.2403 | 0.1183 | 0.2002 | 0.2242 | 19.996 |
| 0.9855 | 4.02 | 2500 | 1.0439 | 0.2416 | 0.1198 | 0.2015 | 0.2257 | 19.9948 |
| 0.9642 | 4.82 | 3000 | 1.0361 | 0.2421 | 0.1201 | 0.202 | 0.2263 | 19.9936 |
| 0.9519 | 5.63 | 3500 | 1.0329 | 0.2415 | 0.12 | 0.2017 | 0.2259 | 19.9948 |
| 0.9389 | 6.43 | 4000 | 1.0278 | 0.2424 | 0.1204 | 0.2023 | 0.2265 | 19.9942 |
| 0.9302 | 7.23 | 4500 | 1.0273 | 0.2422 | 0.1204 | 0.2022 | 0.2264 | 19.9943 |
| 0.9225 | 8.04 | 5000 | 1.0219 | 0.2421 | 0.1209 | 0.2023 | 0.2263 | 19.9946 |
| 0.9152 | 8.84 | 5500 | 1.0219 | 0.2429 | 0.1209 | 0.2028 | 0.227 | 19.9948 |
| 0.911 | 9.64 | 6000 | 1.0206 | 0.2426 | 0.1209 | 0.2027 | 0.2266 | 19.9945 |
### Framework versions
- Transformers 4.38.2
- Pytorch 2.0.0+cu117
- Datasets 2.18.0
- Tokenizers 0.15.2
|
coommen/falcon-7b-sharded-bf16-fine-tuned-adapters | coommen | "2023-09-12T01:14:37Z" | 4 | 0 | peft | [
"peft",
"region:us"
] | null | "2023-09-10T15:14:28Z" | ---
library_name: peft
---
## Training procedure
The following `bitsandbytes` quantization config was used during training:
- quant_method: bitsandbytes
- load_in_8bit: False
- load_in_4bit: True
- llm_int8_threshold: 6.0
- llm_int8_skip_modules: None
- llm_int8_enable_fp32_cpu_offload: False
- llm_int8_has_fp16_weight: False
- bnb_4bit_quant_type: nf4
- bnb_4bit_use_double_quant: True
- bnb_4bit_compute_dtype: float16
### Framework versions
- PEFT 0.6.0.dev0
|
muhammadIsmail/llama-3.2-3b-Roman-Urdu-sentiment-analyst-latest-25_11_2024 | muhammadIsmail | "2024-11-26T12:12:07Z" | 77 | 0 | transformers | [
"transformers",
"safetensors",
"llama",
"text-generation",
"trl",
"sft",
"conversational",
"arxiv:1910.09700",
"autotrain_compatible",
"text-generation-inference",
"endpoints_compatible",
"4-bit",
"bitsandbytes",
"region:us"
] | text-generation | "2024-11-26T12:10:36Z" | ---
library_name: transformers
tags:
- trl
- sft
---
# Model Card for Model ID
<!-- Provide a quick summary of what the model is/does. -->
## Model Details
### Model Description
<!-- Provide a longer summary of what this model is. -->
This is the model card of a 🤗 transformers model that has been pushed on the Hub. This model card has been automatically generated.
- **Developed by:** [More Information Needed]
- **Funded by [optional]:** [More Information Needed]
- **Shared by [optional]:** [More Information Needed]
- **Model type:** [More Information Needed]
- **Language(s) (NLP):** [More Information Needed]
- **License:** [More Information Needed]
- **Finetuned from model [optional]:** [More Information Needed]
### Model Sources [optional]
<!-- Provide the basic links for the model. -->
- **Repository:** [More Information Needed]
- **Paper [optional]:** [More Information Needed]
- **Demo [optional]:** [More Information Needed]
## Uses
<!-- Address questions around how the model is intended to be used, including the foreseeable users of the model and those affected by the model. -->
### Direct Use
<!-- This section is for the model use without fine-tuning or plugging into a larger ecosystem/app. -->
[More Information Needed]
### Downstream Use [optional]
<!-- This section is for the model use when fine-tuned for a task, or when plugged into a larger ecosystem/app -->
[More Information Needed]
### Out-of-Scope Use
<!-- This section addresses misuse, malicious use, and uses that the model will not work well for. -->
[More Information Needed]
## Bias, Risks, and Limitations
<!-- This section is meant to convey both technical and sociotechnical limitations. -->
[More Information Needed]
### Recommendations
<!-- This section is meant to convey recommendations with respect to the bias, risk, and technical limitations. -->
Users (both direct and downstream) should be made aware of the risks, biases and limitations of the model. More information needed for further recommendations.
## How to Get Started with the Model
Use the code below to get started with the model.
[More Information Needed]
## Training Details
### Training Data
<!-- This should link to a Dataset Card, perhaps with a short stub of information on what the training data is all about as well as documentation related to data pre-processing or additional filtering. -->
[More Information Needed]
### Training Procedure
<!-- This relates heavily to the Technical Specifications. Content here should link to that section when it is relevant to the training procedure. -->
#### Preprocessing [optional]
[More Information Needed]
#### Training Hyperparameters
- **Training regime:** [More Information Needed] <!--fp32, fp16 mixed precision, bf16 mixed precision, bf16 non-mixed precision, fp16 non-mixed precision, fp8 mixed precision -->
#### Speeds, Sizes, Times [optional]
<!-- This section provides information about throughput, start/end time, checkpoint size if relevant, etc. -->
[More Information Needed]
## Evaluation
<!-- This section describes the evaluation protocols and provides the results. -->
### Testing Data, Factors & Metrics
#### Testing Data
<!-- This should link to a Dataset Card if possible. -->
[More Information Needed]
#### Factors
<!-- These are the things the evaluation is disaggregating by, e.g., subpopulations or domains. -->
[More Information Needed]
#### Metrics
<!-- These are the evaluation metrics being used, ideally with a description of why. -->
[More Information Needed]
### Results
[More Information Needed]
#### Summary
## Model Examination [optional]
<!-- Relevant interpretability work for the model goes here -->
[More Information Needed]
## Environmental Impact
<!-- Total emissions (in grams of CO2eq) and additional considerations, such as electricity usage, go here. Edit the suggested text below accordingly -->
Carbon emissions can be estimated using the [Machine Learning Impact calculator](https://mlco2.github.io/impact#compute) presented in [Lacoste et al. (2019)](https://arxiv.org/abs/1910.09700).
- **Hardware Type:** [More Information Needed]
- **Hours used:** [More Information Needed]
- **Cloud Provider:** [More Information Needed]
- **Compute Region:** [More Information Needed]
- **Carbon Emitted:** [More Information Needed]
## Technical Specifications [optional]
### Model Architecture and Objective
[More Information Needed]
### Compute Infrastructure
[More Information Needed]
#### Hardware
[More Information Needed]
#### Software
[More Information Needed]
## Citation [optional]
<!-- If there is a paper or blog post introducing the model, the APA and Bibtex information for that should go in this section. -->
**BibTeX:**
[More Information Needed]
**APA:**
[More Information Needed]
## Glossary [optional]
<!-- If relevant, include terms and calculations in this section that can help readers understand the model or model card. -->
[More Information Needed]
## More Information [optional]
[More Information Needed]
## Model Card Authors [optional]
[More Information Needed]
## Model Card Contact
[More Information Needed] |
facebook/mask2former-swin-base-coco-instance | facebook | "2023-09-07T15:38:41Z" | 6,570 | 1 | transformers | [
"transformers",
"pytorch",
"safetensors",
"mask2former",
"vision",
"image-segmentation",
"dataset:coco",
"arxiv:2112.01527",
"arxiv:2107.06278",
"license:other",
"endpoints_compatible",
"region:us"
] | image-segmentation | "2022-11-28T11:57:43Z" | ---
license: other
tags:
- vision
- image-segmentation
datasets:
- coco
widget:
- src: http://images.cocodataset.org/val2017/000000039769.jpg
example_title: Cats
- src: http://images.cocodataset.org/val2017/000000039770.jpg
example_title: Castle
---
# Mask2Former
Mask2Former model trained on COCO instance segmentation (base-sized version, Swin backbone). It was introduced in the paper [Masked-attention Mask Transformer for Universal Image Segmentation
](https://arxiv.org/abs/2112.01527) and first released in [this repository](https://github.com/facebookresearch/Mask2Former/).
Disclaimer: The team releasing Mask2Former did not write a model card for this model so this model card has been written by the Hugging Face team.
## Model description
Mask2Former addresses instance, semantic and panoptic segmentation with the same paradigm: by predicting a set of masks and corresponding labels. Hence, all 3 tasks are treated as if they were instance segmentation. Mask2Former outperforms the previous SOTA,
[MaskFormer](https://arxiv.org/abs/2107.06278) both in terms of performance an efficiency by (i) replacing the pixel decoder with a more advanced multi-scale deformable attention Transformer, (ii) adopting a Transformer decoder with masked attention to boost performance without
without introducing additional computation and (iii) improving training efficiency by calculating the loss on subsampled points instead of whole masks.
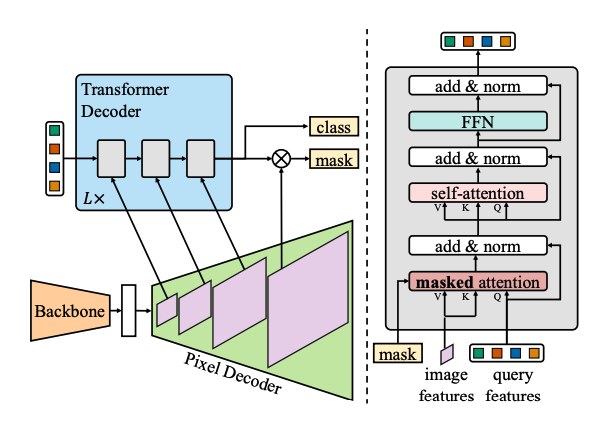
## Intended uses & limitations
You can use this particular checkpoint for instance segmentation. See the [model hub](https://huggingface.co/models?search=mask2former) to look for other
fine-tuned versions on a task that interests you.
### How to use
Here is how to use this model:
```python
import requests
import torch
from PIL import Image
from transformers import AutoImageProcessor, Mask2FormerForUniversalSegmentation
# load Mask2Former fine-tuned on COCO instance segmentation
processor = AutoImageProcessor.from_pretrained("facebook/mask2former-swin-base-coco-instance")
model = Mask2FormerForUniversalSegmentation.from_pretrained("facebook/mask2former-swin-base-coco-instance")
url = "http://images.cocodataset.org/val2017/000000039769.jpg"
image = Image.open(requests.get(url, stream=True).raw)
inputs = processor(images=image, return_tensors="pt")
with torch.no_grad():
outputs = model(**inputs)
# model predicts class_queries_logits of shape `(batch_size, num_queries)`
# and masks_queries_logits of shape `(batch_size, num_queries, height, width)`
class_queries_logits = outputs.class_queries_logits
masks_queries_logits = outputs.masks_queries_logits
# you can pass them to processor for postprocessing
result = processor.post_process_instance_segmentation(outputs, target_sizes=[image.size[::-1]])[0]
# we refer to the demo notebooks for visualization (see "Resources" section in the Mask2Former docs)
predicted_instance_map = result["segmentation"]
```
For more code examples, we refer to the [documentation](https://huggingface.co/docs/transformers/master/en/model_doc/mask2former). |
QuantFactory/YugoGPT-GGUF | QuantFactory | "2024-10-06T13:16:43Z" | 44 | 3 | null | [
"gguf",
"license:apache-2.0",
"endpoints_compatible",
"region:us"
] | null | "2024-10-06T12:43:51Z" |
---
license: apache-2.0
---
[](https://hf.co/QuantFactory)
# QuantFactory/YugoGPT-GGUF
This is quantized version of [gordicaleksa/YugoGPT](https://huggingface.co/gordicaleksa/YugoGPT) created using llama.cpp
# Original Model Card
This repo contains YugoGPT - the best open-source base 7B LLM for BCS (Bosnian, Croatian, Serbian) languages developed by Aleksa Gordić.
You can access more powerful iterations of YugoGPT already through the recently announced [RunaAI's API platform](https://dev.runaai.com/)!
Serbian LLM eval results compared to Mistral 7B, LLaMA 2 7B, and GPT2-orao (also see this [LinkedIn post](https://www.linkedin.com/feed/update/urn:li:activity:7143209223722627072/)):

Eval was computed using https://github.com/gordicaleksa/serbian-llm-eval
It was trained on tens of billions of BCS tokens and is based off of [Mistral 7B](https://huggingface.co/mistralai/Mistral-7B-v0.1).
## Notes
1) YugoGPT is a base model and therefore does not have any moderation mechanisms.
2) Since it's a base model it won't follow your instructions as it's just a powerful autocomplete engine.
3) If you want an access to much more powerful BCS LLMs (some of which are powering [yugochat](https://www.yugochat.com/)) - you can access the models through [RunaAI's API](https://dev.runaai.com/)
# Credits
The data for the project was obtained with the help of [Nikola Ljubešić](https://nljubesi.github.io/), [CLARIN.SI](https://www.clarin.si), and [CLASSLA](https://www.clarin.si/info/k-centre/). Thank you!
# Project Sponsors
A big thank you to the project sponsors!
## Platinum sponsors 🌟
* <b>Ivan</b> (anon)
* [**Things Solver**](https://thingsolver.com/)
## Gold sponsors 🟡
* **qq** (anon)
* [**Adam Sofronijevic**](https://www.linkedin.com/in/adam-sofronijevic-685b911/)
* [**Yanado**](https://yanado.com/)
* [**Mitar Perovic**](https://www.linkedin.com/in/perovicmitar/)
* [**Nikola Ivancevic**](https://www.linkedin.com/in/nivancevic/)
* **Rational Development DOO**
* [**Ivan**](https://www.linkedin.com/in/ivan-kokic-258262175/) i [**Natalija Kokić**](https://www.linkedin.com/in/natalija-kokic-19a458131/)
## Silver sponsors ⚪
[**psk.rs**](https://psk.rs/), [**OmniStreak**](https://omnistreak.com/), [**Luka Važić**](https://www.linkedin.com/in/vazic/), [**Miloš Durković**](https://www.linkedin.com/in/milo%C5%A1-d-684b99188/), [**Marjan Radeski**](https://www.linkedin.com/in/marjanradeski/), **Marjan Stankovic**, [**Nikola Stojiljkovic**](https://www.linkedin.com/in/nikola-stojiljkovic-10469239/), [**Mihailo Tomić**](https://www.linkedin.com/in/mihailotomic/), [**Bojan Jevtic**](https://www.linkedin.com/in/bojanjevtic/), [**Jelena Jovanović**](https://www.linkedin.com/in/eldumo/), [**Nenad Davidović**](https://www.linkedin.com/in/nenad-davidovic-662ab749/), [**Mika Tasich**](https://www.linkedin.com/in/mikatasich/), [**TRENCH-NS**](https://www.linkedin.com/in/milorad-vukadinovic-64639926/), [**Nemanja Grujičić**](https://twitter.com/nemanjagrujicic), [**tim011**](https://knjigovodja.in.rs/sh)
**Also a big thank you to the following individuals:**
- [**Slobodan Marković**](https://www.linkedin.com/in/smarkovic/) - for spreading the word! :)
- [**Aleksander Segedi**](https://www.linkedin.com/in/aleksander-segedi-08430936/) - for help around bookkeeping!
## Citation
```
@article{YugoGPT,
author = "Gordić Aleksa",
title = "YugoGPT - an open-source LLM for Serbian, Bosnian, and Croatian languages",
year = "2024"
howpublished = {\url{https://huggingface.co/gordicaleksa/YugoGPT}},
}
```
|
PrunaAI/mlabonne-NeuralBeagle14-7B-bnb-8bit-smashed | PrunaAI | "2025-02-27T09:05:48Z" | 0 | 0 | null | [
"safetensors",
"mistral",
"pruna-ai",
"8-bit",
"bitsandbytes",
"region:us"
] | null | "2025-02-27T08:56:50Z" | ---
thumbnail: "https://assets-global.website-files.com/646b351987a8d8ce158d1940/64ec9e96b4334c0e1ac41504_Logo%20with%20white%20text.svg"
base_model: ORIGINAL_REPO_NAME
metrics:
- memory_disk
- memory_inference
- inference_latency
- inference_throughput
- inference_CO2_emissions
- inference_energy_consumption
tags:
- pruna-ai
---
<!-- header start -->
<!-- 200823 -->
<div style="width: auto; margin-left: auto; margin-right: auto">
<a href="https://www.pruna.ai/" target="_blank" rel="noopener noreferrer">
<img src="https://i.imgur.com/eDAlcgk.png" alt="PrunaAI" style="width: 100%; min-width: 400px; display: block; margin: auto;">
</a>
</div>
<!-- header end -->
[](https://twitter.com/PrunaAI)
[](https://github.com/PrunaAI)
[](https://www.linkedin.com/company/93832878/admin/feed/posts/?feedType=following)
[](https://discord.gg/rskEr4BZJx)
# Simply make AI models cheaper, smaller, faster, and greener!
- Give a thumbs up if you like this model!
- Contact us and tell us which model to compress next [here](https://www.pruna.ai/contact).
- Request access to easily compress your *own* AI models [here](https://z0halsaff74.typeform.com/pruna-access?typeform-source=www.pruna.ai).
- Read the documentations to know more [here](https://pruna-ai-pruna.readthedocs-hosted.com/en/latest/)
- Join Pruna AI community on Discord [here](https://discord.gg/CP4VSgck) to share feedback/suggestions or get help.
## Results

**Frequently Asked Questions**
- ***How does the compression work?*** The model is compressed with llm-int8.
- ***How does the model quality change?*** The quality of the model output might vary compared to the base model.
- ***How is the model efficiency evaluated?*** These results were obtained with configuration described in `model/smash_config.json` and are obtained after a hardware warmup. The smashed model is directly compared to the original base model. Efficiency results may vary in other settings (e.g. other hardware, image size, batch size, ...). We recommend to directly run them in the use-case conditions to know if the smashed model can benefit you.
- ***What is the model format?*** We use safetensors.
- ***What calibration data has been used?*** If needed by the compression method, we used WikiText as the calibration data.
- ***What is the naming convention for Pruna Huggingface models?*** We take the original model name and append "turbo", "tiny", or "green" if the smashed model has a measured inference speed, inference memory, or inference energy consumption which is less than 90% of the original base model.
- ***How to compress my own models?*** You can request premium access to more compression methods and tech support for your specific use-cases [here](https://z0halsaff74.typeform.com/pruna-access?typeform-source=www.pruna.ai).
- ***What are "first" metrics?*** Results mentioning "first" are obtained after the first run of the model. The first run might take more memory or be slower than the subsequent runs due cuda overheads.
- ***What are "Sync" and "Async" metrics?*** "Sync" metrics are obtained by syncing all GPU processes and stop measurement when all of them are executed. "Async" metrics are obtained without syncing all GPU processes and stop when the model output can be used by the CPU. We provide both metrics since both could be relevant depending on the use-case. We recommend to test the efficiency gains directly in your use-cases.
## Setup
You can run the smashed model with these steps:
0. Check requirements from the original repo ORIGINAL_REPO_NAME installed. In particular, check python, cuda, and transformers versions.
1. Make sure that you have installed quantization related packages.
```bash
pip install transformers accelerate bitsandbytes>0.37.0
```
2. Load & run the model.
```python
from transformers import AutoModelForCausalLM, AutoTokenizer
model = AutoModelForCausalLM.from_pretrained("PrunaAI/mlabonne-NeuralBeagle14-7B-bnb-8bit-smashed", trust_remote_code=True, device_map='auto')
tokenizer = AutoTokenizer.from_pretrained("ORIGINAL_REPO_NAME")
input_ids = tokenizer("What is the color of prunes?,", return_tensors='pt').to(model.device)["input_ids"]
outputs = model.generate(input_ids, max_new_tokens=216)
tokenizer.decode(outputs[0])
```
## Configurations
The configuration info are in `smash_config.json`.
## Credits & License
The license of the smashed model follows the license of the original model. Please check the license of the original model ORIGINAL_REPO_NAME before using this model which provided the base model. The license of the `pruna-engine` is [here](https://pypi.org/project/pruna-engine/) on Pypi.
## Want to compress other models?
- Contact us and tell us which model to compress next [here](https://www.pruna.ai/contact).
- Request access to easily compress your own AI models [here](https://z0halsaff74.typeform.com/pruna-access?typeform-source=www.pruna.ai). |
EleutherAI/pythia-410m-squaring-first-ft | EleutherAI | "2024-03-18T23:36:15Z" | 8 | 0 | transformers | [
"transformers",
"safetensors",
"gpt_neox",
"text-generation",
"arxiv:1910.09700",
"autotrain_compatible",
"text-generation-inference",
"endpoints_compatible",
"region:us"
] | text-generation | "2024-03-16T01:41:30Z" | ---
library_name: transformers
tags: []
---
# Model Card for Model ID
<!-- Provide a quick summary of what the model is/does. -->
## Model Details
### Model Description
<!-- Provide a longer summary of what this model is. -->
This is the model card of a 🤗 transformers model that has been pushed on the Hub. This model card has been automatically generated.
- **Developed by:** [More Information Needed]
- **Funded by [optional]:** [More Information Needed]
- **Shared by [optional]:** [More Information Needed]
- **Model type:** [More Information Needed]
- **Language(s) (NLP):** [More Information Needed]
- **License:** [More Information Needed]
- **Finetuned from model [optional]:** [More Information Needed]
### Model Sources [optional]
<!-- Provide the basic links for the model. -->
- **Repository:** [More Information Needed]
- **Paper [optional]:** [More Information Needed]
- **Demo [optional]:** [More Information Needed]
## Uses
<!-- Address questions around how the model is intended to be used, including the foreseeable users of the model and those affected by the model. -->
### Direct Use
<!-- This section is for the model use without fine-tuning or plugging into a larger ecosystem/app. -->
[More Information Needed]
### Downstream Use [optional]
<!-- This section is for the model use when fine-tuned for a task, or when plugged into a larger ecosystem/app -->
[More Information Needed]
### Out-of-Scope Use
<!-- This section addresses misuse, malicious use, and uses that the model will not work well for. -->
[More Information Needed]
## Bias, Risks, and Limitations
<!-- This section is meant to convey both technical and sociotechnical limitations. -->
[More Information Needed]
### Recommendations
<!-- This section is meant to convey recommendations with respect to the bias, risk, and technical limitations. -->
Users (both direct and downstream) should be made aware of the risks, biases and limitations of the model. More information needed for further recommendations.
## How to Get Started with the Model
Use the code below to get started with the model.
[More Information Needed]
## Training Details
### Training Data
<!-- This should link to a Dataset Card, perhaps with a short stub of information on what the training data is all about as well as documentation related to data pre-processing or additional filtering. -->
[More Information Needed]
### Training Procedure
<!-- This relates heavily to the Technical Specifications. Content here should link to that section when it is relevant to the training procedure. -->
#### Preprocessing [optional]
[More Information Needed]
#### Training Hyperparameters
- **Training regime:** [More Information Needed] <!--fp32, fp16 mixed precision, bf16 mixed precision, bf16 non-mixed precision, fp16 non-mixed precision, fp8 mixed precision -->
#### Speeds, Sizes, Times [optional]
<!-- This section provides information about throughput, start/end time, checkpoint size if relevant, etc. -->
[More Information Needed]
## Evaluation
<!-- This section describes the evaluation protocols and provides the results. -->
### Testing Data, Factors & Metrics
#### Testing Data
<!-- This should link to a Dataset Card if possible. -->
[More Information Needed]
#### Factors
<!-- These are the things the evaluation is disaggregating by, e.g., subpopulations or domains. -->
[More Information Needed]
#### Metrics
<!-- These are the evaluation metrics being used, ideally with a description of why. -->
[More Information Needed]
### Results
[More Information Needed]
#### Summary
## Model Examination [optional]
<!-- Relevant interpretability work for the model goes here -->
[More Information Needed]
## Environmental Impact
<!-- Total emissions (in grams of CO2eq) and additional considerations, such as electricity usage, go here. Edit the suggested text below accordingly -->
Carbon emissions can be estimated using the [Machine Learning Impact calculator](https://mlco2.github.io/impact#compute) presented in [Lacoste et al. (2019)](https://arxiv.org/abs/1910.09700).
- **Hardware Type:** [More Information Needed]
- **Hours used:** [More Information Needed]
- **Cloud Provider:** [More Information Needed]
- **Compute Region:** [More Information Needed]
- **Carbon Emitted:** [More Information Needed]
## Technical Specifications [optional]
### Model Architecture and Objective
[More Information Needed]
### Compute Infrastructure
[More Information Needed]
#### Hardware
[More Information Needed]
#### Software
[More Information Needed]
## Citation [optional]
<!-- If there is a paper or blog post introducing the model, the APA and Bibtex information for that should go in this section. -->
**BibTeX:**
[More Information Needed]
**APA:**
[More Information Needed]
## Glossary [optional]
<!-- If relevant, include terms and calculations in this section that can help readers understand the model or model card. -->
[More Information Needed]
## More Information [optional]
[More Information Needed]
## Model Card Authors [optional]
[More Information Needed]
## Model Card Contact
[More Information Needed]
|
LiteLLMs/falcon-11B-GGUF | LiteLLMs | "2024-05-24T15:15:21Z" | 30 | 0 | null | [
"gguf",
"GGUF",
"en",
"de",
"es",
"fr",
"it",
"nl",
"pl",
"pt",
"ro",
"cs",
"dataset:tiiuae/falcon-refinedweb",
"arxiv:2311.16867",
"license:unknown",
"region:us",
"conversational"
] | null | "2024-05-24T15:00:57Z" |
---
language:
- en
- de
- es
- fr
- it
- nl
- pl
- pt
- ro
- cs
license: unknown
tags:
- GGUF
datasets:
- tiiuae/falcon-refinedweb
inference: false
quantized_by: andrijdavid
---
# falcon-11B-GGUF
- Original model: [falcon-11B](https://huggingface.co/tiiuae/falcon-11B)
<!-- description start -->
## Description
This repo contains GGUF format model files for [falcon-11B](https://huggingface.co/tiiuae/falcon-11B).
<!-- description end -->
<!-- README_GGUF.md-about-gguf start -->
### About GGUF
GGUF is a new format introduced by the llama.cpp team on August 21st 2023. It is a replacement for GGML, which is no longer supported by llama.cpp.
Here is an incomplete list of clients and libraries that are known to support GGUF:
* [llama.cpp](https://github.com/ggerganov/llama.cpp). This is the source project for GGUF, providing both a Command Line Interface (CLI) and a server option.
* [text-generation-webui](https://github.com/oobabooga/text-generation-webui), Known as the most widely used web UI, this project boasts numerous features and powerful extensions, and supports GPU acceleration.
* [Ollama](https://github.com/jmorganca/ollama) Ollama is a lightweight and extensible framework designed for building and running language models locally. It features a simple API for creating, managing, and executing models, along with a library of pre-built models for use in various applications
* [KoboldCpp](https://github.com/LostRuins/koboldcpp), A comprehensive web UI offering GPU acceleration across all platforms and architectures, particularly renowned for storytelling.
* [GPT4All](https://gpt4all.io), This is a free and open source GUI that runs locally, supporting Windows, Linux, and macOS with full GPU acceleration.
* [LM Studio](https://lmstudio.ai/) An intuitive and powerful local GUI for Windows and macOS (Silicon), featuring GPU acceleration.
* [LoLLMS Web UI](https://github.com/ParisNeo/lollms-webui). A notable web UI with a variety of unique features, including a comprehensive model library for easy model selection.
* [Faraday.dev](https://faraday.dev/), An attractive, user-friendly character-based chat GUI for Windows and macOS (both Silicon and Intel), also offering GPU acceleration.
* [llama-cpp-python](https://github.com/abetlen/llama-cpp-python), A Python library equipped with GPU acceleration, LangChain support, and an OpenAI-compatible API server.
* [candle](https://github.com/huggingface/candle), A Rust-based ML framework focusing on performance, including GPU support, and designed for ease of use.
* [ctransformers](https://github.com/marella/ctransformers), A Python library featuring GPU acceleration, LangChain support, and an OpenAI-compatible AI server.
* [localGPT](https://github.com/PromtEngineer/localGPT) An open-source initiative enabling private conversations with documents.
<!-- README_GGUF.md-about-gguf end -->
<!-- compatibility_gguf start -->
## Explanation of quantisation methods
<details>
<summary>Click to see details</summary>
The new methods available are:
* GGML_TYPE_Q2_K - "type-1" 2-bit quantization in super-blocks containing 16 blocks, each block having 16 weight. Block scales and mins are quantized with 4 bits. This ends up effectively using 2.5625 bits per weight (bpw)
* GGML_TYPE_Q3_K - "type-0" 3-bit quantization in super-blocks containing 16 blocks, each block having 16 weights. Scales are quantized with 6 bits. This end up using 3.4375 bpw.
* GGML_TYPE_Q4_K - "type-1" 4-bit quantization in super-blocks containing 8 blocks, each block having 32 weights. Scales and mins are quantized with 6 bits. This ends up using 4.5 bpw.
* GGML_TYPE_Q5_K - "type-1" 5-bit quantization. Same super-block structure as GGML_TYPE_Q4_K resulting in 5.5 bpw
* GGML_TYPE_Q6_K - "type-0" 6-bit quantization. Super-blocks with 16 blocks, each block having 16 weights. Scales are quantized with 8 bits. This ends up using 6.5625 bpw.
</details>
<!-- compatibility_gguf end -->
<!-- README_GGUF.md-how-to-download start -->
## How to download GGUF files
**Note for manual downloaders:** You almost never want to clone the entire repo! Multiple different quantisation formats are provided, and most users only want to pick and download a single folder.
The following clients/libraries will automatically download models for you, providing a list of available models to choose from:
* LM Studio
* LoLLMS Web UI
* Faraday.dev
### In `text-generation-webui`
Under Download Model, you can enter the model repo: LiteLLMs/falcon-11B-GGUF and below it, a specific filename to download, such as: Q4_0/Q4_0-00001-of-00009.gguf.
Then click Download.
### On the command line, including multiple files at once
I recommend using the `huggingface-hub` Python library:
```shell
pip3 install huggingface-hub
```
Then you can download any individual model file to the current directory, at high speed, with a command like this:
```shell
huggingface-cli download LiteLLMs/falcon-11B-GGUF Q4_0/Q4_0-00001-of-00009.gguf --local-dir . --local-dir-use-symlinks False
```
<details>
<summary>More advanced huggingface-cli download usage (click to read)</summary>
You can also download multiple files at once with a pattern:
```shell
huggingface-cli download LiteLLMs/falcon-11B-GGUF --local-dir . --local-dir-use-symlinks False --include='*Q4_K*gguf'
```
For more documentation on downloading with `huggingface-cli`, please see: [HF -> Hub Python Library -> Download files -> Download from the CLI](https://huggingface.co/docs/huggingface_hub/guides/download#download-from-the-cli).
To accelerate downloads on fast connections (1Gbit/s or higher), install `hf_transfer`:
```shell
pip3 install huggingface_hub[hf_transfer]
```
And set environment variable `HF_HUB_ENABLE_HF_TRANSFER` to `1`:
```shell
HF_HUB_ENABLE_HF_TRANSFER=1 huggingface-cli download LiteLLMs/falcon-11B-GGUF Q4_0/Q4_0-00001-of-00009.gguf --local-dir . --local-dir-use-symlinks False
```
Windows Command Line users: You can set the environment variable by running `set HF_HUB_ENABLE_HF_TRANSFER=1` before the download command.
</details>
<!-- README_GGUF.md-how-to-download end -->
<!-- README_GGUF.md-how-to-run start -->
## Example `llama.cpp` command
Make sure you are using `llama.cpp` from commit [d0cee0d](https://github.com/ggerganov/llama.cpp/commit/d0cee0d36d5be95a0d9088b674dbb27354107221) or later.
```shell
./main -ngl 35 -m Q4_0/Q4_0-00001-of-00009.gguf --color -c 8192 --temp 0.7 --repeat_penalty 1.1 -n -1 -p "<PROMPT>"
```
Change `-ngl 32` to the number of layers to offload to GPU. Remove it if you don't have GPU acceleration.
Change `-c 8192` to the desired sequence length. For extended sequence models - eg 8K, 16K, 32K - the necessary RoPE scaling parameters are read from the GGUF file and set by llama.cpp automatically. Note that longer sequence lengths require much more resources, so you may need to reduce this value.
If you want to have a chat-style conversation, replace the `-p <PROMPT>` argument with `-i -ins`
For other parameters and how to use them, please refer to [the llama.cpp documentation](https://github.com/ggerganov/llama.cpp/blob/master/examples/main/README.md)
## How to run in `text-generation-webui`
Further instructions can be found in the text-generation-webui documentation, here: [text-generation-webui/docs/04 ‐ Model Tab.md](https://github.com/oobabooga/text-generation-webui/blob/main/docs/04%20%E2%80%90%20Model%20Tab.md#llamacpp).
## How to run from Python code
You can use GGUF models from Python using the [llama-cpp-python](https://github.com/abetlen/llama-cpp-python) or [ctransformers](https://github.com/marella/ctransformers) libraries. Note that at the time of writing (Nov 27th 2023), ctransformers has not been updated for some time and is not compatible with some recent models. Therefore I recommend you use llama-cpp-python.
### How to load this model in Python code, using llama-cpp-python
For full documentation, please see: [llama-cpp-python docs](https://abetlen.github.io/llama-cpp-python/).
#### First install the package
Run one of the following commands, according to your system:
```shell
# Base ctransformers with no GPU acceleration
pip install llama-cpp-python
# With NVidia CUDA acceleration
CMAKE_ARGS="-DLLAMA_CUBLAS=on" pip install llama-cpp-python
# Or with OpenBLAS acceleration
CMAKE_ARGS="-DLLAMA_BLAS=ON -DLLAMA_BLAS_VENDOR=OpenBLAS" pip install llama-cpp-python
# Or with CLBLast acceleration
CMAKE_ARGS="-DLLAMA_CLBLAST=on" pip install llama-cpp-python
# Or with AMD ROCm GPU acceleration (Linux only)
CMAKE_ARGS="-DLLAMA_HIPBLAS=on" pip install llama-cpp-python
# Or with Metal GPU acceleration for macOS systems only
CMAKE_ARGS="-DLLAMA_METAL=on" pip install llama-cpp-python
# In windows, to set the variables CMAKE_ARGS in PowerShell, follow this format; eg for NVidia CUDA:
$env:CMAKE_ARGS = "-DLLAMA_OPENBLAS=on"
pip install llama-cpp-python
```
#### Simple llama-cpp-python example code
```python
from llama_cpp import Llama
# Set gpu_layers to the number of layers to offload to GPU. Set to 0 if no GPU acceleration is available on your system.
llm = Llama(
model_path="./Q4_0/Q4_0-00001-of-00009.gguf", # Download the model file first
n_ctx=32768, # The max sequence length to use - note that longer sequence lengths require much more resources
n_threads=8, # The number of CPU threads to use, tailor to your system and the resulting performance
n_gpu_layers=35 # The number of layers to offload to GPU, if you have GPU acceleration available
)
# Simple inference example
output = llm(
"<PROMPT>", # Prompt
max_tokens=512, # Generate up to 512 tokens
stop=["</s>"], # Example stop token - not necessarily correct for this specific model! Please check before using.
echo=True # Whether to echo the prompt
)
# Chat Completion API
llm = Llama(model_path="./Q4_0/Q4_0-00001-of-00009.gguf", chat_format="llama-2") # Set chat_format according to the model you are using
llm.create_chat_completion(
messages = [
{"role": "system", "content": "You are a story writing assistant."},
{
"role": "user",
"content": "Write a story about llamas."
}
]
)
```
## How to use with LangChain
Here are guides on using llama-cpp-python and ctransformers with LangChain:
* [LangChain + llama-cpp-python](https://python.langchain.com/docs/integrations/llms/llamacpp)
* [LangChain + ctransformers](https://python.langchain.com/docs/integrations/providers/ctransformers)
<!-- README_GGUF.md-how-to-run end -->
<!-- footer end -->
<!-- original-model-card start -->
# Original model card: falcon-11B
# 🚀 Falcon2-11B
**Falcon2-11B is an 11B parameters causal decoder-only model built by [TII](https://www.tii.ae) and trained on over 5,000B tokens of [RefinedWeb](https://huggingface.co/datasets/tiiuae/falcon-refinedweb) enhanced with curated corpora. The model is made available under the [TII Falcon License 2.0](https://falconllm-staging.tii.ae/falcon-2-terms-and-conditions.html), the permissive Apache 2.0-based software license which includes an [acceptable use policy](https://falconllm-staging.tii.ae/falcon-2-acceptable-use-policy.html) that promotes the responsible use of AI.**
*Paper coming soon 😊.*
🤗 To get started with Falcon (inference, finetuning, quantization, etc.), we recommend reading [this great blogpost from HF](https://huggingface.co/blog/falcon)!
⚠️ **This is a raw, pretrained model, which should be further finetuned for most usecases.**
```python
from transformers import AutoTokenizer, AutoModelForCausalLM
import transformers
import torch
model = "tiiuae/falcon-11B"
tokenizer = AutoTokenizer.from_pretrained(model)
pipeline = transformers.pipeline(
"text-generation",
model=model,
tokenizer=tokenizer,
torch_dtype=torch.bfloat16,
)
sequences = pipeline(
"Can you explain the concepts of Quantum Computing?",
max_length=200,
do_sample=True,
top_k=10,
num_return_sequences=1,
eos_token_id=tokenizer.eos_token_id,
)
for seq in sequences:
print(f"Result: {seq['generated_text']}")
```
💥 **Falcon LLMs require PyTorch 2.0 for use with `transformers`!**
For fast inference with Falcon, check-out [Text Generation Inference](https://github.com/huggingface/text-generation-inference)! Read more in this [blogpost]((https://huggingface.co/blog/falcon).
# Model Card for Falcon2-11B
## Model Details
### Model Description
- **Developed by:** [https://www.tii.ae](https://www.tii.ae)
- **Model type:** Causal decoder-only
- **Language(s) (NLP):** English, German, Spanish, French, Italian, Portuguese, Polish, Dutch, Romanian, Czech, Swedish
- **License:** [TII Falcon License 2.0](https://falconllm-staging.tii.ae/falcon-2-terms-and-conditions.html)
### Model Source
- **Paper:** *coming soon*.
## Uses
### Direct Use
Research on large language models; as a foundation for further specialization and finetuning for specific usecases (e.g., summarization, text generation, chatbot, etc.)
### Out-of-Scope Use
Production use without adequate assessment of risks and mitigation; any use cases which may be considered irresponsible or harmful.
## Bias, Risks, and Limitations
Falcon2-11B is trained mostly on English, but also German, Spanish, French, Italian, Portuguese, Polish, Dutch, Romanian, Czech, Swedish. It will not generalize appropriately to other languages. Furthermore, as it is trained on a large-scale corpora representative of the web, it will carry the stereotypes and biases commonly encountered online.
### Recommendations
We recommend users of Falcon2-11B to consider finetuning it for the specific set of tasks of interest, and for guardrails and appropriate precautions to be taken for any production use.
## How to Get Started with the Model
```python
from transformers import AutoTokenizer, AutoModelForCausalLM
import transformers
import torch
model = "tiiuae/falcon-11B"
tokenizer = AutoTokenizer.from_pretrained(model)
pipeline = transformers.pipeline(
"text-generation",
model=model,
tokenizer=tokenizer,
torch_dtype=torch.bfloat16,
device_map="auto",
)
sequences = pipeline(
"Can you explain the concepts of Quantum Computing?",
max_length=200,
do_sample=True,
top_k=10,
num_return_sequences=1,
eos_token_id=tokenizer.eos_token_id,
)
for seq in sequences:
print(f"Result: {seq['generated_text']}")
```
## Training Details
### Training Data
Falcon2-11B was trained over 5,000B tokens of [RefinedWeb](https://huggingface.co/datasets/tiiuae/falcon-refinedweb), a high-quality filtered and deduplicated web dataset which we enhanced with curated corpora. It followed a four stage training strategy. The first three stages were focused on increasing the context length, from to 2048 to 4096 and finally to 8192 tokens. The last stage aimed to further enhance performance using only high quality data.
Overall, the data sources included RefinedWeb-English, Refined Web-Europe (cs, de, es, fr, it, nl, pl, pt, ro, sv), high quality technical data, code data, and conversational data extracted from public sources.
The training stages were as follows:
| **Stage** | **Context length** | **Tokens** |
| - |
| Stage 1 | 2048 | 4500 B |
| Stage 2 | 4096 | 250 B |
| Stage 3 | 8192 | 250 B |
| Stage 4 | 8192 | 500 B |
The data was tokenized with the Falcon-[7B](https://huggingface.co/tiiuae/falcon-7b)/[11B](https://huggingface.co/tiiuae/falcon-11B) tokenizer.
### Training Procedure
Falcon2-11B was trained on 1024 A100 40GB GPUs for the majority of the training, using a 3D parallelism strategy (TP=8, PP=1, DP=128) combined with ZeRO and Flash-Attention 2.
#### Training Hyperparameters
| **Hyperparameter** | **Value** | **Comment** |
| | | | --- |
| Layers | 60 | |
| `d_model` | 4096 | |
| `head_dim` | 128 | |
| Vocabulary | 65024 | |
| Sequence length | 8192 | During stages 3 and 4 |
### Compute Infrastructure
#### Hardware
Falcon2-11B was trained on AWS SageMaker, using on average 1024 A100 40GB GPUs in 128 p4d instances.
#### Software
Falcon2-11B was trained a custom distributed training codebase, Gigatron. It uses a 3D parallelism approach combined with ZeRO, high-performance Triton kernels and FlashAttention-2. More details about the distributed training strategy can be found in [Almazrouei et.al](https://arxiv.org/abs/2311.16867).
## Citation
*Paper coming soon* 😊.
## License
Falcon2-11B is licenced under [TII Falcon License 2.0](https://falconllm-staging.tii.ae/falcon-2-terms-and-conditions.html), the permissive Apache 2.0-based software license which includes an [acceptable use policy](https://falconllm-staging.tii.ae/falcon-2-acceptable-use-policy.html) that promotes the responsible use of AI.
## Contact
[email protected]
<!-- original-model-card end -->
|
OrnateAhmed/Test | OrnateAhmed | "2023-12-10T07:12:04Z" | 0 | 0 | null | [
"safetensors",
"autotrain",
"text-generation",
"license:other",
"region:us"
] | text-generation | "2023-12-10T07:12:00Z" | ---
tags:
- autotrain
- text-generation
widget:
- text: "I love AutoTrain because "
license: other
---
# Model Trained Using AutoTrain
This model was trained using AutoTrain. For more information, please visit [AutoTrain](https://hf.co/docs/autotrain).
# Usage
```python
from transformers import AutoModelForCausalLM, AutoTokenizer
model_path = "PATH_TO_THIS_REPO"
tokenizer = AutoTokenizer.from_pretrained(model_path)
model = AutoModelForCausalLM.from_pretrained(
model_path,
device_map="auto",
torch_dtype='auto'
).eval()
# Prompt content: "hi"
messages = [
{"role": "user", "content": "hi"}
]
input_ids = tokenizer.apply_chat_template(conversation=messages, tokenize=True, add_generation_prompt=True, return_tensors='pt')
output_ids = model.generate(input_ids.to('cuda'))
response = tokenizer.decode(output_ids[0][input_ids.shape[1]:], skip_special_tokens=True)
# Model response: "Hello! How can I assist you today?"
print(response)
``` |
vertings6/f3f15155-f4f9-464c-9bca-1d3bede98100 | vertings6 | "2025-01-14T20:20:09Z" | 6 | 0 | peft | [
"peft",
"safetensors",
"llama",
"axolotl",
"generated_from_trainer",
"base_model:unsloth/llama-2-7b-chat",
"base_model:adapter:unsloth/llama-2-7b-chat",
"license:apache-2.0",
"region:us"
] | null | "2025-01-14T19:36:50Z" | ---
library_name: peft
license: apache-2.0
base_model: unsloth/llama-2-7b-chat
tags:
- axolotl
- generated_from_trainer
model-index:
- name: f3f15155-f4f9-464c-9bca-1d3bede98100
results: []
---
<!-- This model card has been generated automatically according to the information the Trainer had access to. You
should probably proofread and complete it, then remove this comment. -->
[<img src="https://raw.githubusercontent.com/axolotl-ai-cloud/axolotl/main/image/axolotl-badge-web.png" alt="Built with Axolotl" width="200" height="32"/>](https://github.com/axolotl-ai-cloud/axolotl)
<details><summary>See axolotl config</summary>
axolotl version: `0.4.1`
```yaml
adapter: lora
base_model: unsloth/llama-2-7b-chat
bf16: auto
chat_template: llama3
dataset_prepared_path: null
datasets:
- data_files:
- 593922543a5f2f51_train_data.json
ds_type: json
format: custom
path: /workspace/input_data/593922543a5f2f51_train_data.json
type:
field_input: Tags
field_instruction: Title
field_output: Predicted_Tags
format: '{instruction} {input}'
no_input_format: '{instruction}'
system_format: '{system}'
system_prompt: ''
debug: null
deepspeed: null
device: cuda
early_stopping_patience: null
eval_max_new_tokens: 128
eval_table_size: null
evals_per_epoch: 4
flash_attention: false
fp16: null
gradient_accumulation_steps: 4
gradient_checkpointing: true
group_by_length: false
hub_model_id: vertings6/f3f15155-f4f9-464c-9bca-1d3bede98100
hub_repo: null
hub_strategy: checkpoint
hub_token: null
learning_rate: 0.0001
load_in_4bit: false
load_in_8bit: false
local_rank: null
logging_steps: 3
lora_alpha: 32
lora_dropout: 0.05
lora_fan_in_fan_out: null
lora_model_dir: null
lora_r: 16
lora_target_linear: true
lr_scheduler: cosine
max_memory:
0: 78GiB
max_steps: 30
micro_batch_size: 2
mlflow_experiment_name: /tmp/593922543a5f2f51_train_data.json
model_type: AutoModelForCausalLM
num_epochs: 1
optimizer: adamw_torch
output_dir: miner_id_24
pad_to_sequence_len: true
resume_from_checkpoint: null
s2_attention: null
sample_packing: false
save_steps: 10
sequence_len: 1024
strict: false
tf32: false
tokenizer_type: AutoTokenizer
train_on_inputs: true
trust_remote_code: true
val_set_size: 0.05
wandb_entity: null
wandb_mode: online
wandb_name: 9bc21eb8-8721-42ba-b6e1-6ec673294027
wandb_project: Gradients-On-Demand
wandb_run: your_name
wandb_runid: 9bc21eb8-8721-42ba-b6e1-6ec673294027
warmup_steps: 10
weight_decay: 0.01
xformers_attention: true
```
</details><br>
# f3f15155-f4f9-464c-9bca-1d3bede98100
This model is a fine-tuned version of [unsloth/llama-2-7b-chat](https://huggingface.co/unsloth/llama-2-7b-chat) on the None dataset.
It achieves the following results on the evaluation set:
- Loss: nan
## Model description
More information needed
## Intended uses & limitations
More information needed
## Training and evaluation data
More information needed
## Training procedure
### Training hyperparameters
The following hyperparameters were used during training:
- learning_rate: 0.0001
- train_batch_size: 2
- eval_batch_size: 2
- seed: 42
- gradient_accumulation_steps: 4
- total_train_batch_size: 8
- optimizer: Use OptimizerNames.ADAMW_TORCH with betas=(0.9,0.999) and epsilon=1e-08 and optimizer_args=No additional optimizer arguments
- lr_scheduler_type: cosine
- lr_scheduler_warmup_steps: 10
- training_steps: 30
### Training results
| Training Loss | Epoch | Step | Validation Loss |
|:-------------:|:------:|:----:|:---------------:|
| No log | 0.0001 | 1 | nan |
| 0.0 | 0.0006 | 8 | nan |
| 0.0 | 0.0012 | 16 | nan |
| 0.0 | 0.0018 | 24 | nan |
### Framework versions
- PEFT 0.13.2
- Transformers 4.46.0
- Pytorch 2.5.0+cu124
- Datasets 3.0.1
- Tokenizers 0.20.1 |
albertus-sussex/veriscrape-simcse-auto-reference_6_to_verify_4-fold-4 | albertus-sussex | "2025-03-26T12:26:08Z" | 0 | 0 | transformers | [
"transformers",
"safetensors",
"roberta",
"feature-extraction",
"arxiv:1910.09700",
"endpoints_compatible",
"region:us"
] | feature-extraction | "2025-03-26T12:25:37Z" | ---
library_name: transformers
tags: []
---
# Model Card for Model ID
<!-- Provide a quick summary of what the model is/does. -->
## Model Details
### Model Description
<!-- Provide a longer summary of what this model is. -->
This is the model card of a 🤗 transformers model that has been pushed on the Hub. This model card has been automatically generated.
- **Developed by:** [More Information Needed]
- **Funded by [optional]:** [More Information Needed]
- **Shared by [optional]:** [More Information Needed]
- **Model type:** [More Information Needed]
- **Language(s) (NLP):** [More Information Needed]
- **License:** [More Information Needed]
- **Finetuned from model [optional]:** [More Information Needed]
### Model Sources [optional]
<!-- Provide the basic links for the model. -->
- **Repository:** [More Information Needed]
- **Paper [optional]:** [More Information Needed]
- **Demo [optional]:** [More Information Needed]
## Uses
<!-- Address questions around how the model is intended to be used, including the foreseeable users of the model and those affected by the model. -->
### Direct Use
<!-- This section is for the model use without fine-tuning or plugging into a larger ecosystem/app. -->
[More Information Needed]
### Downstream Use [optional]
<!-- This section is for the model use when fine-tuned for a task, or when plugged into a larger ecosystem/app -->
[More Information Needed]
### Out-of-Scope Use
<!-- This section addresses misuse, malicious use, and uses that the model will not work well for. -->
[More Information Needed]
## Bias, Risks, and Limitations
<!-- This section is meant to convey both technical and sociotechnical limitations. -->
[More Information Needed]
### Recommendations
<!-- This section is meant to convey recommendations with respect to the bias, risk, and technical limitations. -->
Users (both direct and downstream) should be made aware of the risks, biases and limitations of the model. More information needed for further recommendations.
## How to Get Started with the Model
Use the code below to get started with the model.
[More Information Needed]
## Training Details
### Training Data
<!-- This should link to a Dataset Card, perhaps with a short stub of information on what the training data is all about as well as documentation related to data pre-processing or additional filtering. -->
[More Information Needed]
### Training Procedure
<!-- This relates heavily to the Technical Specifications. Content here should link to that section when it is relevant to the training procedure. -->
#### Preprocessing [optional]
[More Information Needed]
#### Training Hyperparameters
- **Training regime:** [More Information Needed] <!--fp32, fp16 mixed precision, bf16 mixed precision, bf16 non-mixed precision, fp16 non-mixed precision, fp8 mixed precision -->
#### Speeds, Sizes, Times [optional]
<!-- This section provides information about throughput, start/end time, checkpoint size if relevant, etc. -->
[More Information Needed]
## Evaluation
<!-- This section describes the evaluation protocols and provides the results. -->
### Testing Data, Factors & Metrics
#### Testing Data
<!-- This should link to a Dataset Card if possible. -->
[More Information Needed]
#### Factors
<!-- These are the things the evaluation is disaggregating by, e.g., subpopulations or domains. -->
[More Information Needed]
#### Metrics
<!-- These are the evaluation metrics being used, ideally with a description of why. -->
[More Information Needed]
### Results
[More Information Needed]
#### Summary
## Model Examination [optional]
<!-- Relevant interpretability work for the model goes here -->
[More Information Needed]
## Environmental Impact
<!-- Total emissions (in grams of CO2eq) and additional considerations, such as electricity usage, go here. Edit the suggested text below accordingly -->
Carbon emissions can be estimated using the [Machine Learning Impact calculator](https://mlco2.github.io/impact#compute) presented in [Lacoste et al. (2019)](https://arxiv.org/abs/1910.09700).
- **Hardware Type:** [More Information Needed]
- **Hours used:** [More Information Needed]
- **Cloud Provider:** [More Information Needed]
- **Compute Region:** [More Information Needed]
- **Carbon Emitted:** [More Information Needed]
## Technical Specifications [optional]
### Model Architecture and Objective
[More Information Needed]
### Compute Infrastructure
[More Information Needed]
#### Hardware
[More Information Needed]
#### Software
[More Information Needed]
## Citation [optional]
<!-- If there is a paper or blog post introducing the model, the APA and Bibtex information for that should go in this section. -->
**BibTeX:**
[More Information Needed]
**APA:**
[More Information Needed]
## Glossary [optional]
<!-- If relevant, include terms and calculations in this section that can help readers understand the model or model card. -->
[More Information Needed]
## More Information [optional]
[More Information Needed]
## Model Card Authors [optional]
[More Information Needed]
## Model Card Contact
[More Information Needed] |
llmware/slim-xsum-phi-3-ov | llmware | "2024-10-31T21:51:49Z" | 6 | 1 | null | [
"openvino",
"phi3",
"green",
"p3",
"llmware-fx",
"ov",
"custom_code",
"base_model:llmware/slim-xsum-phi-3",
"base_model:quantized:llmware/slim-xsum-phi-3",
"license:apache-2.0",
"region:us"
] | null | "2024-09-07T05:20:58Z" | ---
license: apache-2.0
inference: false
base_model: llmware/slim-xsum-phi-3
base_model_relation: quantized
tags: [green, p3, llmware-fx, ov]
---
# slim-xsum-phi-3-ov
**slim-xsum-phi-3-ov** is a specialized function calling model that generates an "extreme summary" (e.g. 'xsum') or headline from a context passage.
This is an OpenVino int4 quantized version of slim-xsum-phi-3, providing a very fast, very small inference implementation, optimized for AI PCs using Intel GPU, CPU and NPU.
### Model Description
- **Developed by:** llmware
- **Model type:** phi-3
- **Parameters:** 3.8 billion
- **Model Parent:** llmware/slim-xsum-phi-3
- **Language(s) (NLP):** English
- **License:** Apache 2.0
- **Uses:** Summarization from complex business documents
- **RAG Benchmark Accuracy Score:** NA
- **Quantization:** int4
## Model Card Contact
[llmware on github](https://www.github.com/llmware-ai/llmware)
[llmware on hf](https://www.huggingface.co/llmware)
[llmware website](https://www.llmware.ai)
|
bpben/en_imdb_sent_trf | bpben | "2023-05-10T14:09:43Z" | 4 | 0 | spacy | [
"spacy",
"text-classification",
"en",
"region:us"
] | text-classification | "2023-05-10T14:09:26Z" | ---
tags:
- spacy
- text-classification
language:
- en
model-index:
- name: en_imdb_sent_trf
results: []
---
| Feature | Description |
| --- | --- |
| **Name** | `en_imdb_sent_trf` |
| **Version** | `0.0.0` |
| **spaCy** | `>=3.4.4,<3.5.0` |
| **Default Pipeline** | `transformer`, `textcat` |
| **Components** | `transformer`, `textcat` |
| **Vectors** | 0 keys, 0 unique vectors (0 dimensions) |
| **Sources** | n/a |
| **License** | n/a |
| **Author** | [n/a]() |
### Label Scheme
<details>
<summary>View label scheme (2 labels for 1 components)</summary>
| Component | Labels |
| --- | --- |
| **`textcat`** | `pos`, `neg` |
</details>
### Accuracy
| Type | Score |
| --- | --- |
| `CATS_SCORE` | 87.99 |
| `CATS_MICRO_P` | 88.08 |
| `CATS_MICRO_R` | 88.08 |
| `CATS_MICRO_F` | 88.08 |
| `CATS_MACRO_P` | 88.01 |
| `CATS_MACRO_R` | 87.98 |
| `CATS_MACRO_F` | 87.99 |
| `CATS_MACRO_AUC` | 93.56 |
| `CATS_MACRO_AUC_PER_TYPE` | 0.00 |
| `TRANSFORMER_LOSS` | 24.99 |
| `TEXTCAT_LOSS` | 2726.89 | |
Purushoth/Job_title_Generation | Purushoth | "2025-04-07T06:13:13Z" | 0 | 0 | transformers | [
"transformers",
"safetensors",
"arxiv:1910.09700",
"endpoints_compatible",
"region:us"
] | null | "2025-04-06T09:29:42Z" | ---
library_name: transformers
tags: []
---
# Model Card for Model ID
<!-- Provide a quick summary of what the model is/does. -->
## Model Details
### Model Description
<!-- Provide a longer summary of what this model is. -->
This is the model card of a 🤗 transformers model that has been pushed on the Hub. This model card has been automatically generated.
- **Developed by:** [More Information Needed]
- **Funded by [optional]:** [More Information Needed]
- **Shared by [optional]:** [More Information Needed]
- **Model type:** [More Information Needed]
- **Language(s) (NLP):** [More Information Needed]
- **License:** [More Information Needed]
- **Finetuned from model [optional]:** [More Information Needed]
### Model Sources [optional]
<!-- Provide the basic links for the model. -->
- **Repository:** [More Information Needed]
- **Paper [optional]:** [More Information Needed]
- **Demo [optional]:** [More Information Needed]
## Uses
<!-- Address questions around how the model is intended to be used, including the foreseeable users of the model and those affected by the model. -->
### Direct Use
<!-- This section is for the model use without fine-tuning or plugging into a larger ecosystem/app. -->
[More Information Needed]
### Downstream Use [optional]
<!-- This section is for the model use when fine-tuned for a task, or when plugged into a larger ecosystem/app -->
[More Information Needed]
### Out-of-Scope Use
<!-- This section addresses misuse, malicious use, and uses that the model will not work well for. -->
[More Information Needed]
## Bias, Risks, and Limitations
<!-- This section is meant to convey both technical and sociotechnical limitations. -->
[More Information Needed]
### Recommendations
<!-- This section is meant to convey recommendations with respect to the bias, risk, and technical limitations. -->
Users (both direct and downstream) should be made aware of the risks, biases and limitations of the model. More information needed for further recommendations.
## How to Get Started with the Model
Use the code below to get started with the model.
[More Information Needed]
## Training Details
### Training Data
<!-- This should link to a Dataset Card, perhaps with a short stub of information on what the training data is all about as well as documentation related to data pre-processing or additional filtering. -->
[More Information Needed]
### Training Procedure
<!-- This relates heavily to the Technical Specifications. Content here should link to that section when it is relevant to the training procedure. -->
#### Preprocessing [optional]
[More Information Needed]
#### Training Hyperparameters
- **Training regime:** [More Information Needed] <!--fp32, fp16 mixed precision, bf16 mixed precision, bf16 non-mixed precision, fp16 non-mixed precision, fp8 mixed precision -->
#### Speeds, Sizes, Times [optional]
<!-- This section provides information about throughput, start/end time, checkpoint size if relevant, etc. -->
[More Information Needed]
## Evaluation
<!-- This section describes the evaluation protocols and provides the results. -->
### Testing Data, Factors & Metrics
#### Testing Data
<!-- This should link to a Dataset Card if possible. -->
[More Information Needed]
#### Factors
<!-- These are the things the evaluation is disaggregating by, e.g., subpopulations or domains. -->
[More Information Needed]
#### Metrics
<!-- These are the evaluation metrics being used, ideally with a description of why. -->
[More Information Needed]
### Results
[More Information Needed]
#### Summary
## Model Examination [optional]
<!-- Relevant interpretability work for the model goes here -->
[More Information Needed]
## Environmental Impact
<!-- Total emissions (in grams of CO2eq) and additional considerations, such as electricity usage, go here. Edit the suggested text below accordingly -->
Carbon emissions can be estimated using the [Machine Learning Impact calculator](https://mlco2.github.io/impact#compute) presented in [Lacoste et al. (2019)](https://arxiv.org/abs/1910.09700).
- **Hardware Type:** [More Information Needed]
- **Hours used:** [More Information Needed]
- **Cloud Provider:** [More Information Needed]
- **Compute Region:** [More Information Needed]
- **Carbon Emitted:** [More Information Needed]
## Technical Specifications [optional]
### Model Architecture and Objective
[More Information Needed]
### Compute Infrastructure
[More Information Needed]
#### Hardware
[More Information Needed]
#### Software
[More Information Needed]
## Citation [optional]
<!-- If there is a paper or blog post introducing the model, the APA and Bibtex information for that should go in this section. -->
**BibTeX:**
[More Information Needed]
**APA:**
[More Information Needed]
## Glossary [optional]
<!-- If relevant, include terms and calculations in this section that can help readers understand the model or model card. -->
[More Information Needed]
## More Information [optional]
[More Information Needed]
## Model Card Authors [optional]
[More Information Needed]
## Model Card Contact
[More Information Needed] |
DreadPoor/Schlock-8B-Model_Stock | DreadPoor | "2025-03-22T17:20:53Z" | 0 | 0 | transformers | [
"transformers",
"safetensors",
"llama",
"text-generation",
"mergekit",
"merge",
"conversational",
"arxiv:2403.19522",
"base_model:DreadPoor/Aurora_faustus-8B-LINEAR",
"base_model:merge:DreadPoor/Aurora_faustus-8B-LINEAR",
"base_model:DreadPoor/BaeZel-8B-LINEAR",
"base_model:merge:DreadPoor/BaeZel-8B-LINEAR",
"base_model:DreadPoor/Suavemente-8B-Model_Stock",
"base_model:merge:DreadPoor/Suavemente-8B-Model_Stock",
"base_model:DreadPoor/ichor_ALT-8B-Model_Stock",
"base_model:merge:DreadPoor/ichor_ALT-8B-Model_Stock",
"base_model:FuseAI/FuseChat-Llama-3.1-8B-SFT",
"base_model:merge:FuseAI/FuseChat-Llama-3.1-8B-SFT",
"autotrain_compatible",
"text-generation-inference",
"endpoints_compatible",
"region:us"
] | text-generation | "2025-03-22T17:16:50Z" | ---
base_model:
- DreadPoor/ichor_ALT-8B-Model_Stock
- DreadPoor/BaeZel-8B-LINEAR
- FuseAI/FuseChat-Llama-3.1-8B-SFT
- DreadPoor/Aurora_faustus-8B-LINEAR
- DreadPoor/Suavemente-8B-Model_Stock
library_name: transformers
tags:
- mergekit
- merge
---
# merge
This is a merge of pre-trained language models created using [mergekit](https://github.com/cg123/mergekit).
## Merge Details
### Merge Method
This model was merged using the [Model Stock](https://arxiv.org/abs/2403.19522) merge method using [FuseAI/FuseChat-Llama-3.1-8B-SFT](https://huggingface.co/FuseAI/FuseChat-Llama-3.1-8B-SFT) as a base.
### Models Merged
The following models were included in the merge:
* [DreadPoor/ichor_ALT-8B-Model_Stock](https://huggingface.co/DreadPoor/ichor_ALT-8B-Model_Stock)
* [DreadPoor/BaeZel-8B-LINEAR](https://huggingface.co/DreadPoor/BaeZel-8B-LINEAR)
* [DreadPoor/Aurora_faustus-8B-LINEAR](https://huggingface.co/DreadPoor/Aurora_faustus-8B-LINEAR)
* [DreadPoor/Suavemente-8B-Model_Stock](https://huggingface.co/DreadPoor/Suavemente-8B-Model_Stock)
### Configuration
The following YAML configuration was used to produce this model:
```yaml
models:
- model: DreadPoor/Suavemente-8B-Model_Stock
- model: DreadPoor/Aurora_faustus-8B-LINEAR
- model: DreadPoor/BaeZel-8B-LINEAR
- model: DreadPoor/ichor_ALT-8B-Model_Stock
merge_method: model_stock
base_model: FuseAI/FuseChat-Llama-3.1-8B-SFT
normalize: false
int8_mask: true
dtype: bfloat16
```
|
Primeness/teatimesn29H1v18 | Primeness | "2025-01-09T23:36:16Z" | 33 | 0 | transformers | [
"transformers",
"safetensors",
"llama",
"text-generation",
"arxiv:1910.09700",
"autotrain_compatible",
"text-generation-inference",
"endpoints_compatible",
"region:us"
] | text-generation | "2025-01-09T21:57:44Z" | ---
library_name: transformers
tags: []
---
# Model Card for Model ID
<!-- Provide a quick summary of what the model is/does. -->
## Model Details
### Model Description
<!-- Provide a longer summary of what this model is. -->
This is the model card of a 🤗 transformers model that has been pushed on the Hub. This model card has been automatically generated.
- **Developed by:** [More Information Needed]
- **Funded by [optional]:** [More Information Needed]
- **Shared by [optional]:** [More Information Needed]
- **Model type:** [More Information Needed]
- **Language(s) (NLP):** [More Information Needed]
- **License:** [More Information Needed]
- **Finetuned from model [optional]:** [More Information Needed]
### Model Sources [optional]
<!-- Provide the basic links for the model. -->
- **Repository:** [More Information Needed]
- **Paper [optional]:** [More Information Needed]
- **Demo [optional]:** [More Information Needed]
## Uses
<!-- Address questions around how the model is intended to be used, including the foreseeable users of the model and those affected by the model. -->
### Direct Use
<!-- This section is for the model use without fine-tuning or plugging into a larger ecosystem/app. -->
[More Information Needed]
### Downstream Use [optional]
<!-- This section is for the model use when fine-tuned for a task, or when plugged into a larger ecosystem/app -->
[More Information Needed]
### Out-of-Scope Use
<!-- This section addresses misuse, malicious use, and uses that the model will not work well for. -->
[More Information Needed]
## Bias, Risks, and Limitations
<!-- This section is meant to convey both technical and sociotechnical limitations. -->
[More Information Needed]
### Recommendations
<!-- This section is meant to convey recommendations with respect to the bias, risk, and technical limitations. -->
Users (both direct and downstream) should be made aware of the risks, biases and limitations of the model. More information needed for further recommendations.
## How to Get Started with the Model
Use the code below to get started with the model.
[More Information Needed]
## Training Details
### Training Data
<!-- This should link to a Dataset Card, perhaps with a short stub of information on what the training data is all about as well as documentation related to data pre-processing or additional filtering. -->
[More Information Needed]
### Training Procedure
<!-- This relates heavily to the Technical Specifications. Content here should link to that section when it is relevant to the training procedure. -->
#### Preprocessing [optional]
[More Information Needed]
#### Training Hyperparameters
- **Training regime:** [More Information Needed] <!--fp32, fp16 mixed precision, bf16 mixed precision, bf16 non-mixed precision, fp16 non-mixed precision, fp8 mixed precision -->
#### Speeds, Sizes, Times [optional]
<!-- This section provides information about throughput, start/end time, checkpoint size if relevant, etc. -->
[More Information Needed]
## Evaluation
<!-- This section describes the evaluation protocols and provides the results. -->
### Testing Data, Factors & Metrics
#### Testing Data
<!-- This should link to a Dataset Card if possible. -->
[More Information Needed]
#### Factors
<!-- These are the things the evaluation is disaggregating by, e.g., subpopulations or domains. -->
[More Information Needed]
#### Metrics
<!-- These are the evaluation metrics being used, ideally with a description of why. -->
[More Information Needed]
### Results
[More Information Needed]
#### Summary
## Model Examination [optional]
<!-- Relevant interpretability work for the model goes here -->
[More Information Needed]
## Environmental Impact
<!-- Total emissions (in grams of CO2eq) and additional considerations, such as electricity usage, go here. Edit the suggested text below accordingly -->
Carbon emissions can be estimated using the [Machine Learning Impact calculator](https://mlco2.github.io/impact#compute) presented in [Lacoste et al. (2019)](https://arxiv.org/abs/1910.09700).
- **Hardware Type:** [More Information Needed]
- **Hours used:** [More Information Needed]
- **Cloud Provider:** [More Information Needed]
- **Compute Region:** [More Information Needed]
- **Carbon Emitted:** [More Information Needed]
## Technical Specifications [optional]
### Model Architecture and Objective
[More Information Needed]
### Compute Infrastructure
[More Information Needed]
#### Hardware
[More Information Needed]
#### Software
[More Information Needed]
## Citation [optional]
<!-- If there is a paper or blog post introducing the model, the APA and Bibtex information for that should go in this section. -->
**BibTeX:**
[More Information Needed]
**APA:**
[More Information Needed]
## Glossary [optional]
<!-- If relevant, include terms and calculations in this section that can help readers understand the model or model card. -->
[More Information Needed]
## More Information [optional]
[More Information Needed]
## Model Card Authors [optional]
[More Information Needed]
## Model Card Contact
[More Information Needed] |
tensorblock/Meta-Llama-3.2-1B-GGUF | tensorblock | "2024-11-26T22:52:13Z" | 61 | 1 | transformers | [
"transformers",
"gguf",
"facebook",
"meta",
"pytorch",
"llama",
"llama-3",
"TensorBlock",
"GGUF",
"text-generation",
"en",
"de",
"fr",
"it",
"pt",
"hi",
"es",
"th",
"base_model:yejingfu/Meta-Llama-3.2-1B",
"base_model:quantized:yejingfu/Meta-Llama-3.2-1B",
"license:llama3.2",
"endpoints_compatible",
"region:us"
] | text-generation | "2024-11-26T22:45:49Z" | ---
language:
- en
- de
- fr
- it
- pt
- hi
- es
- th
library_name: transformers
pipeline_tag: text-generation
tags:
- facebook
- meta
- pytorch
- llama
- llama-3
- TensorBlock
- GGUF
license: llama3.2
extra_gated_prompt: "### LLAMA 3.2 COMMUNITY LICENSE AGREEMENT\n\nLlama 3.2 Version\
\ Release Date: September 25, 2024\n\n“Agreement” means the terms and conditions\
\ for use, reproduction, distribution and modification of the Llama Materials set\
\ forth herein.\n\n“Documentation” means the specifications, manuals and documentation\
\ accompanying Llama 3.2 distributed by Meta at https://llama.meta.com/doc/overview.\n\
\n“Licensee” or “you” means you, or your employer or any other person or entity\
\ (if you are entering into this Agreement on such person or entity’s behalf),\
\ of the age required under applicable laws, rules or regulations to provide legal\
\ consent and that has legal authority to bind your employer or such other person\
\ or entity if you are entering in this Agreement on their behalf.\n\n“Llama 3.2”\
\ means the foundational large language models and software and algorithms, including\
\ machine-learning model code, trained model weights, inference-enabling code, training-enabling\
\ code, fine-tuning enabling code and other elements of the foregoing distributed\
\ by Meta at https://www.llama.com/llama-downloads.\n\n“Llama Materials” means,\
\ collectively, Meta’s proprietary Llama 3.2 and Documentation (and any portion\
\ thereof) made available under this Agreement.\n\n“Meta” or “we” means Meta Platforms\
\ Ireland Limited (if you are located in or, if you are an entity, your principal\
\ place of business is in the EEA or Switzerland) and Meta Platforms, Inc. (if\
\ you are located outside of the EEA or Switzerland). \n\nBy clicking “I Accept”\
\ below or by using or distributing any portion or element of the Llama Materials,\
\ you agree to be bound by this Agreement.\n\n1. License Rights and Redistribution.\n\
a. Grant of Rights. You are granted a non-exclusive, worldwide, non-transferable\
\ and royalty-free limited license under Meta’s intellectual property or other rights\
\ owned by Meta embodied in the Llama Materials to use, reproduce, distribute,\
\ copy, create derivative works of, and make modifications to the Llama Materials.\
\ \nb. Redistribution and Use. \ni. If you distribute or make available the Llama\
\ Materials (or any derivative works thereof), or a product or service (including\
\ another AI model) that contains any of them, you shall (A) provide a copy of this\
\ Agreement with any such Llama Materials; and (B) prominently display “Built with\
\ Llama” on a related website, user interface, blogpost, about page, or product\
\ documentation. If you use the Llama Materials or any outputs or results of the\
\ Llama Materials to create, train, fine tune, or otherwise improve an AI model,\
\ which is distributed or made available, you shall also include “Llama” at the\
\ beginning of any such AI model name.\nii. If you receive Llama Materials, or any\
\ derivative works thereof, from a Licensee as part of an integrated end user product,\
\ then Section 2 of this Agreement will not apply to you. \niii. You must retain\
\ in all copies of the Llama Materials that you distribute the following attribution\
\ notice within a “Notice” text file distributed as a part of such copies: “Llama\
\ 3.2 is licensed under the Llama 3.2 Community License, Copyright © Meta Platforms,\
\ Inc. All Rights Reserved.”\niv. Your use of the Llama Materials must comply with\
\ applicable laws and regulations (including trade compliance laws and regulations)\
\ and adhere to the Acceptable Use Policy for the Llama Materials (available at\
\ https://www.llama.com/llama3_2/use-policy), which is hereby incorporated by reference\
\ into this Agreement.\n \n2. Additional Commercial Terms. If, on the Llama 3.2\
\ version release date, the monthly active users of the products or services made\
\ available by or for Licensee, or Licensee’s affiliates, is greater than 700 million\
\ monthly active users in the preceding calendar month, you must request a license\
\ from Meta, which Meta may grant to you in its sole discretion, and you are not\
\ authorized to exercise any of the rights under this Agreement unless or until\
\ Meta otherwise expressly grants you such rights.\n3. Disclaimer of Warranty. UNLESS\
\ REQUIRED BY APPLICABLE LAW, THE LLAMA MATERIALS AND ANY OUTPUT AND RESULTS THEREFROM\
\ ARE PROVIDED ON AN “AS IS” BASIS, WITHOUT WARRANTIES OF ANY KIND, AND META DISCLAIMS\
\ ALL WARRANTIES OF ANY KIND, BOTH EXPRESS AND IMPLIED, INCLUDING, WITHOUT LIMITATION,\
\ ANY WARRANTIES OF TITLE, NON-INFRINGEMENT, MERCHANTABILITY, OR FITNESS FOR A PARTICULAR\
\ PURPOSE. YOU ARE SOLELY RESPONSIBLE FOR DETERMINING THE APPROPRIATENESS OF USING\
\ OR REDISTRIBUTING THE LLAMA MATERIALS AND ASSUME ANY RISKS ASSOCIATED WITH YOUR\
\ USE OF THE LLAMA MATERIALS AND ANY OUTPUT AND RESULTS.\n4. Limitation of Liability.\
\ IN NO EVENT WILL META OR ITS AFFILIATES BE LIABLE UNDER ANY THEORY OF LIABILITY,\
\ WHETHER IN CONTRACT, TORT, NEGLIGENCE, PRODUCTS LIABILITY, OR OTHERWISE, ARISING\
\ OUT OF THIS AGREEMENT, FOR ANY LOST PROFITS OR ANY INDIRECT, SPECIAL, CONSEQUENTIAL,\
\ INCIDENTAL, EXEMPLARY OR PUNITIVE DAMAGES, EVEN IF META OR ITS AFFILIATES HAVE\
\ BEEN ADVISED OF THE POSSIBILITY OF ANY OF THE FOREGOING.\n5. Intellectual Property.\n\
a. No trademark licenses are granted under this Agreement, and in connection with\
\ the Llama Materials, neither Meta nor Licensee may use any name or mark owned\
\ by or associated with the other or any of its affiliates, except as required\
\ for reasonable and customary use in describing and redistributing the Llama Materials\
\ or as set forth in this Section 5(a). Meta hereby grants you a license to use\
\ “Llama” (the “Mark”) solely as required to comply with the last sentence of Section\
\ 1.b.i. You will comply with Meta’s brand guidelines (currently accessible at\
\ https://about.meta.com/brand/resources/meta/company-brand/). All goodwill arising\
\ out of your use of the Mark will inure to the benefit of Meta.\nb. Subject to\
\ Meta’s ownership of Llama Materials and derivatives made by or for Meta, with\
\ respect to any derivative works and modifications of the Llama Materials that\
\ are made by you, as between you and Meta, you are and will be the owner of such\
\ derivative works and modifications.\nc. If you institute litigation or other proceedings\
\ against Meta or any entity (including a cross-claim or counterclaim in a lawsuit)\
\ alleging that the Llama Materials or Llama 3.2 outputs or results, or any portion\
\ of any of the foregoing, constitutes infringement of intellectual property or\
\ other rights owned or licensable by you, then any licenses granted to you under\
\ this Agreement shall terminate as of the date such litigation or claim is filed\
\ or instituted. You will indemnify and hold harmless Meta from and against any\
\ claim by any third party arising out of or related to your use or distribution\
\ of the Llama Materials.\n6. Term and Termination. The term of this Agreement will\
\ commence upon your acceptance of this Agreement or access to the Llama Materials\
\ and will continue in full force and effect until terminated in accordance with\
\ the terms and conditions herein. Meta may terminate this Agreement if you are\
\ in breach of any term or condition of this Agreement. Upon termination of this\
\ Agreement, you shall delete and cease use of the Llama Materials. Sections 3,\
\ 4 and 7 shall survive the termination of this Agreement. \n7. Governing Law and\
\ Jurisdiction. This Agreement will be governed and construed under the laws of\
\ the State of California without regard to choice of law principles, and the UN\
\ Convention on Contracts for the International Sale of Goods does not apply to\
\ this Agreement. The courts of California shall have exclusive jurisdiction of\
\ any dispute arising out of this Agreement. \n### Llama 3.2 Acceptable Use Policy\n\
Meta is committed to promoting safe and fair use of its tools and features, including\
\ Llama 3.2. If you access or use Llama 3.2, you agree to this Acceptable Use Policy\
\ (“**Policy**”). The most recent copy of this policy can be found at [https://www.llama.com/llama3_2/use-policy](https://www.llama.com/llama3_2/use-policy).\n\
#### Prohibited Uses\nWe want everyone to use Llama 3.2 safely and responsibly.\
\ You agree you will not use, or allow others to use, Llama 3.2 to:\n1. Violate\
\ the law or others’ rights, including to:\n 1. Engage in, promote, generate,\
\ contribute to, encourage, plan, incite, or further illegal or unlawful activity\
\ or content, such as:\n 1. Violence or terrorism\n 2. Exploitation\
\ or harm to children, including the solicitation, creation, acquisition, or dissemination\
\ of child exploitative content or failure to report Child Sexual Abuse Material\n\
\ 3. Human trafficking, exploitation, and sexual violence\n 4. The\
\ illegal distribution of information or materials to minors, including obscene\
\ materials, or failure to employ legally required age-gating in connection with\
\ such information or materials.\n 5. Sexual solicitation\n 6. Any\
\ other criminal activity\n 1. Engage in, promote, incite, or facilitate the\
\ harassment, abuse, threatening, or bullying of individuals or groups of individuals\n\
\ 2. Engage in, promote, incite, or facilitate discrimination or other unlawful\
\ or harmful conduct in the provision of employment, employment benefits, credit,\
\ housing, other economic benefits, or other essential goods and services\n 3.\
\ Engage in the unauthorized or unlicensed practice of any profession including,\
\ but not limited to, financial, legal, medical/health, or related professional\
\ practices\n 4. Collect, process, disclose, generate, or infer private or sensitive\
\ information about individuals, including information about individuals’ identity,\
\ health, or demographic information, unless you have obtained the right to do so\
\ in accordance with applicable law\n 5. Engage in or facilitate any action or\
\ generate any content that infringes, misappropriates, or otherwise violates any\
\ third-party rights, including the outputs or results of any products or services\
\ using the Llama Materials\n 6. Create, generate, or facilitate the creation\
\ of malicious code, malware, computer viruses or do anything else that could disable,\
\ overburden, interfere with or impair the proper working, integrity, operation\
\ or appearance of a website or computer system\n 7. Engage in any action, or\
\ facilitate any action, to intentionally circumvent or remove usage restrictions\
\ or other safety measures, or to enable functionality disabled by Meta \n2. Engage\
\ in, promote, incite, facilitate, or assist in the planning or development of activities\
\ that present a risk of death or bodily harm to individuals, including use of Llama\
\ 3.2 related to the following:\n 8. Military, warfare, nuclear industries or\
\ applications, espionage, use for materials or activities that are subject to the\
\ International Traffic Arms Regulations (ITAR) maintained by the United States\
\ Department of State or to the U.S. Biological Weapons Anti-Terrorism Act of 1989\
\ or the Chemical Weapons Convention Implementation Act of 1997\n 9. Guns and\
\ illegal weapons (including weapon development)\n 10. Illegal drugs and regulated/controlled\
\ substances\n 11. Operation of critical infrastructure, transportation technologies,\
\ or heavy machinery\n 12. Self-harm or harm to others, including suicide, cutting,\
\ and eating disorders\n 13. Any content intended to incite or promote violence,\
\ abuse, or any infliction of bodily harm to an individual\n3. Intentionally deceive\
\ or mislead others, including use of Llama 3.2 related to the following:\n 14.\
\ Generating, promoting, or furthering fraud or the creation or promotion of disinformation\n\
\ 15. Generating, promoting, or furthering defamatory content, including the\
\ creation of defamatory statements, images, or other content\n 16. Generating,\
\ promoting, or further distributing spam\n 17. Impersonating another individual\
\ without consent, authorization, or legal right\n 18. Representing that the\
\ use of Llama 3.2 or outputs are human-generated\n 19. Generating or facilitating\
\ false online engagement, including fake reviews and other means of fake online\
\ engagement \n4. Fail to appropriately disclose to end users any known dangers\
\ of your AI system 5. Interact with third party tools, models, or software designed\
\ to generate unlawful content or engage in unlawful or harmful conduct and/or represent\
\ that the outputs of such tools, models, or software are associated with Meta or\
\ Llama 3.2\n\nWith respect to any multimodal models included in Llama 3.2, the\
\ rights granted under Section 1(a) of the Llama 3.2 Community License Agreement\
\ are not being granted to you if you are an individual domiciled in, or a company\
\ with a principal place of business in, the European Union. This restriction does\
\ not apply to end users of a product or service that incorporates any such multimodal\
\ models.\n\nPlease report any violation of this Policy, software “bug,” or other\
\ problems that could lead to a violation of this Policy through one of the following\
\ means:\n\n* Reporting issues with the model: [https://github.com/meta-llama/llama-models/issues](https://l.workplace.com/l.php?u=https%3A%2F%2Fgithub.com%2Fmeta-llama%2Fllama-models%2Fissues&h=AT0qV8W9BFT6NwihiOHRuKYQM_UnkzN_NmHMy91OT55gkLpgi4kQupHUl0ssR4dQsIQ8n3tfd0vtkobvsEvt1l4Ic6GXI2EeuHV8N08OG2WnbAmm0FL4ObkazC6G_256vN0lN9DsykCvCqGZ)\n\
* Reporting risky content generated by the model: [developers.facebook.com/llama_output_feedback](http://developers.facebook.com/llama_output_feedback)\n\
* Reporting bugs and security concerns: [facebook.com/whitehat/info](http://facebook.com/whitehat/info)\n\
* Reporting violations of the Acceptable Use Policy or unlicensed uses of Llama\
\ 3.2: [email protected]"
extra_gated_fields:
First Name: text
Last Name: text
Date of birth: date_picker
Country: country
Affiliation: text
Job title:
type: select
options:
- Student
- Research Graduate
- AI researcher
- AI developer/engineer
- Reporter
- Other
geo: ip_location
? By clicking Submit below I accept the terms of the license and acknowledge that
the information I provide will be collected stored processed and shared in accordance
with the Meta Privacy Policy
: checkbox
extra_gated_description: The information you provide will be collected, stored, processed
and shared in accordance with the [Meta Privacy Policy](https://www.facebook.com/privacy/policy/).
extra_gated_button_content: Submit
base_model: yejingfu/Meta-Llama-3.2-1B
---
<div style="width: auto; margin-left: auto; margin-right: auto">
<img src="https://i.imgur.com/jC7kdl8.jpeg" alt="TensorBlock" style="width: 100%; min-width: 400px; display: block; margin: auto;">
</div>
<div style="display: flex; justify-content: space-between; width: 100%;">
<div style="display: flex; flex-direction: column; align-items: flex-start;">
<p style="margin-top: 0.5em; margin-bottom: 0em;">
Feedback and support: TensorBlock's <a href="https://x.com/tensorblock_aoi">Twitter/X</a>, <a href="https://t.me/TensorBlock">Telegram Group</a> and <a href="https://x.com/tensorblock_aoi">Discord server</a>
</p>
</div>
</div>
## yejingfu/Meta-Llama-3.2-1B - GGUF
This repo contains GGUF format model files for [yejingfu/Meta-Llama-3.2-1B](https://huggingface.co/yejingfu/Meta-Llama-3.2-1B).
The files were quantized using machines provided by [TensorBlock](https://tensorblock.co/), and they are compatible with llama.cpp as of [commit b4011](https://github.com/ggerganov/llama.cpp/commit/a6744e43e80f4be6398fc7733a01642c846dce1d).
<div style="text-align: left; margin: 20px 0;">
<a href="https://tensorblock.co/waitlist/client" style="display: inline-block; padding: 10px 20px; background-color: #007bff; color: white; text-decoration: none; border-radius: 5px; font-weight: bold;">
Run them on the TensorBlock client using your local machine ↗
</a>
</div>
## Prompt template
```
```
## Model file specification
| Filename | Quant type | File Size | Description |
| -------- | ---------- | --------- | ----------- |
| [Meta-Llama-3.2-1B-Q2_K.gguf](https://huggingface.co/tensorblock/Meta-Llama-3.2-1B-GGUF/blob/main/Meta-Llama-3.2-1B-Q2_K.gguf) | Q2_K | 0.581 GB | smallest, significant quality loss - not recommended for most purposes |
| [Meta-Llama-3.2-1B-Q3_K_S.gguf](https://huggingface.co/tensorblock/Meta-Llama-3.2-1B-GGUF/blob/main/Meta-Llama-3.2-1B-Q3_K_S.gguf) | Q3_K_S | 0.642 GB | very small, high quality loss |
| [Meta-Llama-3.2-1B-Q3_K_M.gguf](https://huggingface.co/tensorblock/Meta-Llama-3.2-1B-GGUF/blob/main/Meta-Llama-3.2-1B-Q3_K_M.gguf) | Q3_K_M | 0.691 GB | very small, high quality loss |
| [Meta-Llama-3.2-1B-Q3_K_L.gguf](https://huggingface.co/tensorblock/Meta-Llama-3.2-1B-GGUF/blob/main/Meta-Llama-3.2-1B-Q3_K_L.gguf) | Q3_K_L | 0.733 GB | small, substantial quality loss |
| [Meta-Llama-3.2-1B-Q4_0.gguf](https://huggingface.co/tensorblock/Meta-Llama-3.2-1B-GGUF/blob/main/Meta-Llama-3.2-1B-Q4_0.gguf) | Q4_0 | 0.771 GB | legacy; small, very high quality loss - prefer using Q3_K_M |
| [Meta-Llama-3.2-1B-Q4_K_S.gguf](https://huggingface.co/tensorblock/Meta-Llama-3.2-1B-GGUF/blob/main/Meta-Llama-3.2-1B-Q4_K_S.gguf) | Q4_K_S | 0.776 GB | small, greater quality loss |
| [Meta-Llama-3.2-1B-Q4_K_M.gguf](https://huggingface.co/tensorblock/Meta-Llama-3.2-1B-GGUF/blob/main/Meta-Llama-3.2-1B-Q4_K_M.gguf) | Q4_K_M | 0.808 GB | medium, balanced quality - recommended |
| [Meta-Llama-3.2-1B-Q5_0.gguf](https://huggingface.co/tensorblock/Meta-Llama-3.2-1B-GGUF/blob/main/Meta-Llama-3.2-1B-Q5_0.gguf) | Q5_0 | 0.893 GB | legacy; medium, balanced quality - prefer using Q4_K_M |
| [Meta-Llama-3.2-1B-Q5_K_S.gguf](https://huggingface.co/tensorblock/Meta-Llama-3.2-1B-GGUF/blob/main/Meta-Llama-3.2-1B-Q5_K_S.gguf) | Q5_K_S | 0.893 GB | large, low quality loss - recommended |
| [Meta-Llama-3.2-1B-Q5_K_M.gguf](https://huggingface.co/tensorblock/Meta-Llama-3.2-1B-GGUF/blob/main/Meta-Llama-3.2-1B-Q5_K_M.gguf) | Q5_K_M | 0.911 GB | large, very low quality loss - recommended |
| [Meta-Llama-3.2-1B-Q6_K.gguf](https://huggingface.co/tensorblock/Meta-Llama-3.2-1B-GGUF/blob/main/Meta-Llama-3.2-1B-Q6_K.gguf) | Q6_K | 1.022 GB | very large, extremely low quality loss |
| [Meta-Llama-3.2-1B-Q8_0.gguf](https://huggingface.co/tensorblock/Meta-Llama-3.2-1B-GGUF/blob/main/Meta-Llama-3.2-1B-Q8_0.gguf) | Q8_0 | 1.321 GB | very large, extremely low quality loss - not recommended |
## Downloading instruction
### Command line
Firstly, install Huggingface Client
```shell
pip install -U "huggingface_hub[cli]"
```
Then, downoad the individual model file the a local directory
```shell
huggingface-cli download tensorblock/Meta-Llama-3.2-1B-GGUF --include "Meta-Llama-3.2-1B-Q2_K.gguf" --local-dir MY_LOCAL_DIR
```
If you wanna download multiple model files with a pattern (e.g., `*Q4_K*gguf`), you can try:
```shell
huggingface-cli download tensorblock/Meta-Llama-3.2-1B-GGUF --local-dir MY_LOCAL_DIR --local-dir-use-symlinks False --include='*Q4_K*gguf'
```
|
rawrh/Qwen2.5-0.5B-Instruct-Gensyn-Swarm-masked_prowling_donkey | rawrh | "2025-04-15T17:18:22Z" | 2 | 0 | transformers | [
"transformers",
"safetensors",
"qwen2",
"text-generation",
"generated_from_trainer",
"rl-swarm",
"grpo",
"gensyn",
"I am masked prowling donkey",
"trl",
"conversational",
"arxiv:2402.03300",
"base_model:Gensyn/Qwen2.5-0.5B-Instruct",
"base_model:finetune:Gensyn/Qwen2.5-0.5B-Instruct",
"autotrain_compatible",
"text-generation-inference",
"endpoints_compatible",
"region:us"
] | text-generation | "2025-04-01T15:54:55Z" | ---
base_model: Gensyn/Qwen2.5-0.5B-Instruct
library_name: transformers
model_name: Qwen2.5-0.5B-Instruct-Gensyn-Swarm-masked_prowling_donkey
tags:
- generated_from_trainer
- rl-swarm
- grpo
- gensyn
- I am masked prowling donkey
- trl
licence: license
---
# Model Card for Qwen2.5-0.5B-Instruct-Gensyn-Swarm-masked_prowling_donkey
This model is a fine-tuned version of [Gensyn/Qwen2.5-0.5B-Instruct](https://huggingface.co/Gensyn/Qwen2.5-0.5B-Instruct).
It has been trained using [TRL](https://github.com/huggingface/trl).
## Quick start
```python
from transformers import pipeline
question = "If you had a time machine, but could only go to the past or the future once and never return, which would you choose and why?"
generator = pipeline("text-generation", model="rawrh/Qwen2.5-0.5B-Instruct-Gensyn-Swarm-masked_prowling_donkey", device="cuda")
output = generator([{"role": "user", "content": question}], max_new_tokens=128, return_full_text=False)[0]
print(output["generated_text"])
```
## Training procedure
This model was trained with GRPO, a method introduced in [DeepSeekMath: Pushing the Limits of Mathematical Reasoning in Open Language Models](https://huggingface.co/papers/2402.03300).
### Framework versions
- TRL: 0.15.2
- Transformers: 4.50.3
- Pytorch: 2.5.1
- Datasets: 3.5.0
- Tokenizers: 0.21.1
## Citations
Cite GRPO as:
```bibtex
@article{zhihong2024deepseekmath,
title = {{DeepSeekMath: Pushing the Limits of Mathematical Reasoning in Open Language Models}},
author = {Zhihong Shao and Peiyi Wang and Qihao Zhu and Runxin Xu and Junxiao Song and Mingchuan Zhang and Y. K. Li and Y. Wu and Daya Guo},
year = 2024,
eprint = {arXiv:2402.03300},
}
```
Cite TRL as:
```bibtex
@misc{vonwerra2022trl,
title = {{TRL: Transformer Reinforcement Learning}},
author = {Leandro von Werra and Younes Belkada and Lewis Tunstall and Edward Beeching and Tristan Thrush and Nathan Lambert and Shengyi Huang and Kashif Rasul and Quentin Gallouédec},
year = 2020,
journal = {GitHub repository},
publisher = {GitHub},
howpublished = {\url{https://github.com/huggingface/trl}}
}
``` |
arunachaleswara369/my_awesome_mind_model | arunachaleswara369 | "2023-12-21T06:04:25Z" | 4 | 0 | transformers | [
"transformers",
"tensorboard",
"safetensors",
"wav2vec2",
"audio-classification",
"generated_from_trainer",
"dataset:minds14",
"base_model:facebook/wav2vec2-base",
"base_model:finetune:facebook/wav2vec2-base",
"license:apache-2.0",
"model-index",
"endpoints_compatible",
"region:us"
] | audio-classification | "2023-12-21T04:38:35Z" | ---
license: apache-2.0
base_model: facebook/wav2vec2-base
tags:
- generated_from_trainer
datasets:
- minds14
metrics:
- accuracy
model-index:
- name: my_awesome_mind_model
results:
- task:
name: Audio Classification
type: audio-classification
dataset:
name: minds14
type: minds14
config: en-US
split: train
args: en-US
metrics:
- name: Accuracy
type: accuracy
value: 0.04424778761061947
---
<!-- This model card has been generated automatically according to the information the Trainer had access to. You
should probably proofread and complete it, then remove this comment. -->
# my_awesome_mind_model
This model is a fine-tuned version of [facebook/wav2vec2-base](https://huggingface.co/facebook/wav2vec2-base) on the minds14 dataset.
It achieves the following results on the evaluation set:
- Loss: 2.6459
- Accuracy: 0.0442
## Model description
More information needed
## Intended uses & limitations
More information needed
## Training and evaluation data
More information needed
## Training procedure
### Training hyperparameters
The following hyperparameters were used during training:
- learning_rate: 3e-05
- train_batch_size: 32
- eval_batch_size: 32
- seed: 42
- gradient_accumulation_steps: 4
- total_train_batch_size: 128
- optimizer: Adam with betas=(0.9,0.999) and epsilon=1e-08
- lr_scheduler_type: linear
- lr_scheduler_warmup_ratio: 0.1
- num_epochs: 10
### Training results
| Training Loss | Epoch | Step | Validation Loss | Accuracy |
|:-------------:|:-----:|:----:|:---------------:|:--------:|
| No log | 0.8 | 3 | 2.6312 | 0.0708 |
| No log | 1.87 | 7 | 2.6313 | 0.0885 |
| 2.6396 | 2.93 | 11 | 2.6402 | 0.0885 |
| 2.6396 | 4.0 | 15 | 2.6396 | 0.0265 |
| 2.6396 | 4.8 | 18 | 2.6424 | 0.0531 |
| 2.6378 | 5.87 | 22 | 2.6435 | 0.0796 |
| 2.6378 | 6.93 | 26 | 2.6448 | 0.0531 |
| 2.6298 | 8.0 | 30 | 2.6459 | 0.0442 |
### Framework versions
- Transformers 4.35.2
- Pytorch 2.1.0+cu121
- Datasets 2.15.0
- Tokenizers 0.15.0
|
NoirZangetsu/deepseek-r1-turkish-8B-2 | NoirZangetsu | "2025-02-16T11:11:23Z" | 0 | 0 | transformers | [
"transformers",
"gguf",
"llama",
"text-generation-inference",
"unsloth",
"en",
"license:apache-2.0",
"endpoints_compatible",
"region:us",
"conversational"
] | null | "2025-02-16T11:07:46Z" | ---
base_model: unsloth/deepseek-r1-distill-llama-8b-unsloth-bnb-4bit
tags:
- text-generation-inference
- transformers
- unsloth
- llama
- gguf
license: apache-2.0
language:
- en
---
# Uploaded model
- **Developed by:** NoirZangetsu
- **License:** apache-2.0
- **Finetuned from model :** unsloth/deepseek-r1-distill-llama-8b-unsloth-bnb-4bit
This llama model was trained 2x faster with [Unsloth](https://github.com/unslothai/unsloth) and Huggingface's TRL library.
[<img src="https://raw.githubusercontent.com/unslothai/unsloth/main/images/unsloth%20made%20with%20love.png" width="200"/>](https://github.com/unslothai/unsloth)
|
manelalab/chrono-bert-v1-20031231 | manelalab | "2025-03-02T03:46:14Z" | 0 | 0 | transformers | [
"transformers",
"safetensors",
"modernbert",
"fill-mask",
"chronologically consistent",
"glue",
"en",
"license:mit",
"autotrain_compatible",
"region:us"
] | fill-mask | "2025-02-28T02:14:29Z" | ---
library_name: transformers
license: mit
language:
- en
tags:
- chronologically consistent
- modernbert
- glue
pipeline_tag: fill-mask
inference: false
---
# ChronoBERT
## Model Description
ChronoBERT is a series **high-performance chronologically consistent large language models (LLM)** designed to eliminate lookahead bias and training leakage while maintain good language understanding in time-sensitive applications. The model is pretrained on **diverse, high-quality, open-source, and timestamped text** to maintain chronological consistency.
All models in the series achieve **GLUE benchmark scores that surpass standard BERT.** This approach preserves the integrity of historical analysis and enables more reliable economic and financial modeling.
- **Developed by:** Songrun He, Linying Lv, Asaf Manela, Jimmy Wu
- **Model type:** Transformer-based bidirectional encoder (ModernBERT architecture)
- **Language(s) (NLP):** English
- **License:** MIT License
## Model Sources
- **Paper:** "Chronologically Consistent Large Language Models" (He, Lv, Manela, Wu, 2025)
## How to Get Started with the Model
The model is compatible with the `transformers` library starting from v4.48.0:
```sh
pip install -U transformers>=4.48.0
pip install flash-attn
```
Here is an example code of using the model:
```python
from transformers import AutoTokenizer, AutoModel
device = 'cuda:0'
tokenizer = AutoTokenizer.from_pretrained("manelalab/chrono-bert-v1-19991231")
model = AutoModel.from_pretrained("manelalab/chrono-bert-v1-19991231").to(device)
text = "Obviously, the time continuum has been disrupted, creating a new temporal event sequence resulting in this alternate reality. -- Dr. Brown, Back to the Future Part II"
inputs = tokenizer(text, return_tensors="pt").to(device)
outputs = model(**inputs)
```
## Training Details
### Training Data
- **Pretraining corpus:** Our initial model chrono-bert-v1-19991231 is pretrained on 460 billion tokens of pre-2000, diverse, high-quality, and open-source text data to ensure no leakage of data afterwards.
- **Incremental updates:** Yearly updates from 2000 to 2024 with an additional 65 billion tokens of timestamped text.
### Training Procedure
- **Architecture:** ModernBERT-based model with rotary embeddings and flash attention.
- **Objective:** Masked token prediction.
## Evaluation
### Testing Data, Factors & Metrics
- **Language understanding:** Evaluated on **GLUE benchmark** tasks.
- **Financial forecasting:** Evaluated using **return prediction task** based on Dow Jones Newswire data.
- **Comparison models:** ChronoBERT was benchmarked against **BERT, FinBERT, StoriesLM-v1-1963, and Llama 3.1**.
### Results
- **GLUE Score:** chrono-bert-v1-19991231 and chrono-bert-v1-20241231 achieved GLUE score of 84.71 and 85.54 respectively, outperforming BERT (84.52).
- **Stock return predictions:** During the sample from 2008-01 to 2023-07, chrono-bert-v1-realtime achieves a long-short portfolio **Sharpe ratio of 4.80**, outperforming BERT, FinBERT, and StoriesLM-v1-1963, and comparable to **Llama 3.1 8B (4.90)**.
## Citation
```
@article{He2025ChronoBERT,
title={Chronologically Consistent Large Language Models},
author={He, Songrun and Lv, Linying and Manela, Asaf and Wu, Jimmy},
journal={Working Paper},
year={2025}
}
```
## Model Card Authors
- Songrun He (Washington University in St. Louis, [email protected])
- Linying Lv (Washington University in St. Louis, [email protected])
- Asaf Manela (Washington University in St. Louis, [email protected])
- Jimmy Wu (Washington University in St. Louis, [email protected])
|
RichardErkhov/kanishka_-_smolm-autoreg-bpe-seed_28-8bits | RichardErkhov | "2024-05-04T07:53:36Z" | 76 | 0 | transformers | [
"transformers",
"safetensors",
"opt",
"text-generation",
"autotrain_compatible",
"text-generation-inference",
"endpoints_compatible",
"8-bit",
"bitsandbytes",
"region:us"
] | text-generation | "2024-05-04T07:53:26Z" | Quantization made by Richard Erkhov.
[Github](https://github.com/RichardErkhov)
[Discord](https://discord.gg/pvy7H8DZMG)
[Request more models](https://github.com/RichardErkhov/quant_request)
smolm-autoreg-bpe-seed_28 - bnb 8bits
- Model creator: https://huggingface.co/kanishka/
- Original model: https://huggingface.co/kanishka/smolm-autoreg-bpe-seed_28/
Original model description:
---
tags:
- generated_from_trainer
metrics:
- accuracy
model-index:
- name: smolm-autoreg-bpe-seed_28
results: []
---
<!-- This model card has been generated automatically according to the information the Trainer had access to. You
should probably proofread and complete it, then remove this comment. -->
# smolm-autoreg-bpe-seed_28
This model was trained from scratch on an unknown dataset.
It achieves the following results on the evaluation set:
- Loss: 2.4754
- Accuracy: 0.4991
## Model description
More information needed
## Intended uses & limitations
More information needed
## Training and evaluation data
More information needed
## Training procedure
### Training hyperparameters
The following hyperparameters were used during training:
- learning_rate: 0.003
- train_batch_size: 16
- eval_batch_size: 128
- seed: 28
- optimizer: Adam with betas=(0.9,0.999) and epsilon=1e-08
- lr_scheduler_type: linear
- lr_scheduler_warmup_steps: 24000
- num_epochs: 10.0
### Training results
| Training Loss | Epoch | Step | Validation Loss | Accuracy |
|:-------------:|:-----:|:-----:|:---------------:|:--------:|
| 3.0383 | 1.0 | 2928 | 3.0183 | 0.4371 |
| 2.7202 | 2.0 | 5856 | 2.7841 | 0.4601 |
| 2.5954 | 3.0 | 8784 | 2.7013 | 0.4689 |
| 2.5123 | 4.0 | 11712 | 2.6403 | 0.4755 |
| 2.4613 | 5.0 | 14640 | 2.6047 | 0.4802 |
| 2.4261 | 6.0 | 17568 | 2.5919 | 0.4824 |
| 2.3906 | 7.0 | 20496 | 2.5666 | 0.4850 |
| 2.3601 | 8.0 | 23424 | 2.5550 | 0.4861 |
| 2.2843 | 9.0 | 26352 | 2.5031 | 0.4940 |
| 2.1392 | 10.0 | 29280 | 2.4754 | 0.4991 |
### Framework versions
- Transformers 4.38.2
- Pytorch 2.1.0+cu121
- Datasets 2.16.1
- Tokenizers 0.15.1
|
research-dump/trvsd_no_edit_chew_roberta-base_change_nochange_trvsd | research-dump | "2025-02-26T21:59:23Z" | 0 | 0 | transformers | [
"transformers",
"safetensors",
"roberta",
"text-classification",
"arxiv:1910.09700",
"autotrain_compatible",
"endpoints_compatible",
"region:us"
] | text-classification | "2025-02-26T21:58:31Z" | ---
library_name: transformers
tags: []
---
# Model Card for Model ID
<!-- Provide a quick summary of what the model is/does. -->
## Model Details
### Model Description
<!-- Provide a longer summary of what this model is. -->
This is the model card of a 🤗 transformers model that has been pushed on the Hub. This model card has been automatically generated.
- **Developed by:** [More Information Needed]
- **Funded by [optional]:** [More Information Needed]
- **Shared by [optional]:** [More Information Needed]
- **Model type:** [More Information Needed]
- **Language(s) (NLP):** [More Information Needed]
- **License:** [More Information Needed]
- **Finetuned from model [optional]:** [More Information Needed]
### Model Sources [optional]
<!-- Provide the basic links for the model. -->
- **Repository:** [More Information Needed]
- **Paper [optional]:** [More Information Needed]
- **Demo [optional]:** [More Information Needed]
## Uses
<!-- Address questions around how the model is intended to be used, including the foreseeable users of the model and those affected by the model. -->
### Direct Use
<!-- This section is for the model use without fine-tuning or plugging into a larger ecosystem/app. -->
[More Information Needed]
### Downstream Use [optional]
<!-- This section is for the model use when fine-tuned for a task, or when plugged into a larger ecosystem/app -->
[More Information Needed]
### Out-of-Scope Use
<!-- This section addresses misuse, malicious use, and uses that the model will not work well for. -->
[More Information Needed]
## Bias, Risks, and Limitations
<!-- This section is meant to convey both technical and sociotechnical limitations. -->
[More Information Needed]
### Recommendations
<!-- This section is meant to convey recommendations with respect to the bias, risk, and technical limitations. -->
Users (both direct and downstream) should be made aware of the risks, biases and limitations of the model. More information needed for further recommendations.
## How to Get Started with the Model
Use the code below to get started with the model.
[More Information Needed]
## Training Details
### Training Data
<!-- This should link to a Dataset Card, perhaps with a short stub of information on what the training data is all about as well as documentation related to data pre-processing or additional filtering. -->
[More Information Needed]
### Training Procedure
<!-- This relates heavily to the Technical Specifications. Content here should link to that section when it is relevant to the training procedure. -->
#### Preprocessing [optional]
[More Information Needed]
#### Training Hyperparameters
- **Training regime:** [More Information Needed] <!--fp32, fp16 mixed precision, bf16 mixed precision, bf16 non-mixed precision, fp16 non-mixed precision, fp8 mixed precision -->
#### Speeds, Sizes, Times [optional]
<!-- This section provides information about throughput, start/end time, checkpoint size if relevant, etc. -->
[More Information Needed]
## Evaluation
<!-- This section describes the evaluation protocols and provides the results. -->
### Testing Data, Factors & Metrics
#### Testing Data
<!-- This should link to a Dataset Card if possible. -->
[More Information Needed]
#### Factors
<!-- These are the things the evaluation is disaggregating by, e.g., subpopulations or domains. -->
[More Information Needed]
#### Metrics
<!-- These are the evaluation metrics being used, ideally with a description of why. -->
[More Information Needed]
### Results
[More Information Needed]
#### Summary
## Model Examination [optional]
<!-- Relevant interpretability work for the model goes here -->
[More Information Needed]
## Environmental Impact
<!-- Total emissions (in grams of CO2eq) and additional considerations, such as electricity usage, go here. Edit the suggested text below accordingly -->
Carbon emissions can be estimated using the [Machine Learning Impact calculator](https://mlco2.github.io/impact#compute) presented in [Lacoste et al. (2019)](https://arxiv.org/abs/1910.09700).
- **Hardware Type:** [More Information Needed]
- **Hours used:** [More Information Needed]
- **Cloud Provider:** [More Information Needed]
- **Compute Region:** [More Information Needed]
- **Carbon Emitted:** [More Information Needed]
## Technical Specifications [optional]
### Model Architecture and Objective
[More Information Needed]
### Compute Infrastructure
[More Information Needed]
#### Hardware
[More Information Needed]
#### Software
[More Information Needed]
## Citation [optional]
<!-- If there is a paper or blog post introducing the model, the APA and Bibtex information for that should go in this section. -->
**BibTeX:**
[More Information Needed]
**APA:**
[More Information Needed]
## Glossary [optional]
<!-- If relevant, include terms and calculations in this section that can help readers understand the model or model card. -->
[More Information Needed]
## More Information [optional]
[More Information Needed]
## Model Card Authors [optional]
[More Information Needed]
## Model Card Contact
[More Information Needed] |
lemon-mint/gemma-ko-7b-it-v0.40 | lemon-mint | "2024-03-31T22:30:34Z" | 2,286 | 0 | transformers | [
"transformers",
"safetensors",
"gemma",
"text-generation",
"korean",
"pytorch",
"conversational",
"ko",
"en",
"license:other",
"autotrain_compatible",
"text-generation-inference",
"endpoints_compatible",
"region:us"
] | text-generation | "2024-03-31T19:14:19Z" | ---
library_name: transformers
license: other
license_name: gemma-terms-of-use
license_link: https://ai.google.dev/gemma/terms
language:
- ko
- en
tags:
- korean
- gemma
- pytorch
pipeline_tag: text-generation
---

# Gemma Ko 7B Instruct v0.40
- Eval Loss: `1.222`
- lr: `1.5e-5`
- optimizer: adamw
- lr_scheduler_type: cosine
## Model Details
### Model Description
The Gemma 7B Ko Instruct v0.40 model is designed for generating human-like text in the Korean language.
It can be used for a variety of natural language processing tasks, such as language translation, text summarization, question answering, and conversation generation.
This model is particularly well-suited for applications that require high-quality, coherent, and contextually relevant Korean text generation.
- **Developed by:** `lemon-mint`
- **Model type:** Gemma
- **Language(s) (NLP):** Korean, English
- **License:** [gemma-terms-of-use](https://ai.google.dev/gemma/terms)
- **Finetuned from model:** [google/gemma-7b-it](https://huggingface.co/google/gemma-7b-it)
# Limitations and Ethical Considerations
As Gemma Ko 7B has been trained on extensive web data, biases present in the training data may be reflected in the model. Additionally, there is a possibility that it may generate sentences containing errors or incorrect information. Therefore, rather than blindly trusting the model's output, it is necessary to refer to it with caution.
|
Subsets and Splits