Search is not available for this dataset
modelId
stringlengths 5
137
| author
stringlengths 2
42
| last_modified
unknowndate 2020-02-15 11:33:14
2025-03-29 12:26:52
| downloads
int64 0
223M
| likes
int64 0
11.7k
| library_name
stringclasses 401
values | tags
sequencelengths 1
4.05k
| pipeline_tag
stringclasses 54
values | createdAt
unknowndate 2022-03-02 23:29:04
2025-03-29 12:26:36
| card
stringlengths 11
1.01M
|
---|---|---|---|---|---|---|---|---|---|
camidenecken/RoBERTa-RM2-v3-rm-12 | camidenecken | "2024-10-25T20:23:57Z" | 162 | 0 | transformers | [
"transformers",
"safetensors",
"roberta",
"feature-extraction",
"arxiv:1910.09700",
"endpoints_compatible",
"region:us"
] | feature-extraction | "2024-10-25T20:23:25Z" | ---
library_name: transformers
tags: []
---
# Model Card for Model ID
<!-- Provide a quick summary of what the model is/does. -->
## Model Details
### Model Description
<!-- Provide a longer summary of what this model is. -->
This is the model card of a 🤗 transformers model that has been pushed on the Hub. This model card has been automatically generated.
- **Developed by:** [More Information Needed]
- **Funded by [optional]:** [More Information Needed]
- **Shared by [optional]:** [More Information Needed]
- **Model type:** [More Information Needed]
- **Language(s) (NLP):** [More Information Needed]
- **License:** [More Information Needed]
- **Finetuned from model [optional]:** [More Information Needed]
### Model Sources [optional]
<!-- Provide the basic links for the model. -->
- **Repository:** [More Information Needed]
- **Paper [optional]:** [More Information Needed]
- **Demo [optional]:** [More Information Needed]
## Uses
<!-- Address questions around how the model is intended to be used, including the foreseeable users of the model and those affected by the model. -->
### Direct Use
<!-- This section is for the model use without fine-tuning or plugging into a larger ecosystem/app. -->
[More Information Needed]
### Downstream Use [optional]
<!-- This section is for the model use when fine-tuned for a task, or when plugged into a larger ecosystem/app -->
[More Information Needed]
### Out-of-Scope Use
<!-- This section addresses misuse, malicious use, and uses that the model will not work well for. -->
[More Information Needed]
## Bias, Risks, and Limitations
<!-- This section is meant to convey both technical and sociotechnical limitations. -->
[More Information Needed]
### Recommendations
<!-- This section is meant to convey recommendations with respect to the bias, risk, and technical limitations. -->
Users (both direct and downstream) should be made aware of the risks, biases and limitations of the model. More information needed for further recommendations.
## How to Get Started with the Model
Use the code below to get started with the model.
[More Information Needed]
## Training Details
### Training Data
<!-- This should link to a Dataset Card, perhaps with a short stub of information on what the training data is all about as well as documentation related to data pre-processing or additional filtering. -->
[More Information Needed]
### Training Procedure
<!-- This relates heavily to the Technical Specifications. Content here should link to that section when it is relevant to the training procedure. -->
#### Preprocessing [optional]
[More Information Needed]
#### Training Hyperparameters
- **Training regime:** [More Information Needed] <!--fp32, fp16 mixed precision, bf16 mixed precision, bf16 non-mixed precision, fp16 non-mixed precision, fp8 mixed precision -->
#### Speeds, Sizes, Times [optional]
<!-- This section provides information about throughput, start/end time, checkpoint size if relevant, etc. -->
[More Information Needed]
## Evaluation
<!-- This section describes the evaluation protocols and provides the results. -->
### Testing Data, Factors & Metrics
#### Testing Data
<!-- This should link to a Dataset Card if possible. -->
[More Information Needed]
#### Factors
<!-- These are the things the evaluation is disaggregating by, e.g., subpopulations or domains. -->
[More Information Needed]
#### Metrics
<!-- These are the evaluation metrics being used, ideally with a description of why. -->
[More Information Needed]
### Results
[More Information Needed]
#### Summary
## Model Examination [optional]
<!-- Relevant interpretability work for the model goes here -->
[More Information Needed]
## Environmental Impact
<!-- Total emissions (in grams of CO2eq) and additional considerations, such as electricity usage, go here. Edit the suggested text below accordingly -->
Carbon emissions can be estimated using the [Machine Learning Impact calculator](https://mlco2.github.io/impact#compute) presented in [Lacoste et al. (2019)](https://arxiv.org/abs/1910.09700).
- **Hardware Type:** [More Information Needed]
- **Hours used:** [More Information Needed]
- **Cloud Provider:** [More Information Needed]
- **Compute Region:** [More Information Needed]
- **Carbon Emitted:** [More Information Needed]
## Technical Specifications [optional]
### Model Architecture and Objective
[More Information Needed]
### Compute Infrastructure
[More Information Needed]
#### Hardware
[More Information Needed]
#### Software
[More Information Needed]
## Citation [optional]
<!-- If there is a paper or blog post introducing the model, the APA and Bibtex information for that should go in this section. -->
**BibTeX:**
[More Information Needed]
**APA:**
[More Information Needed]
## Glossary [optional]
<!-- If relevant, include terms and calculations in this section that can help readers understand the model or model card. -->
[More Information Needed]
## More Information [optional]
[More Information Needed]
## Model Card Authors [optional]
[More Information Needed]
## Model Card Contact
[More Information Needed] |
RichardErkhov/microsoft_-_rho-math-1b-v0.1-4bits | RichardErkhov | "2024-04-20T17:31:49Z" | 76 | 0 | transformers | [
"transformers",
"safetensors",
"llama",
"text-generation",
"arxiv:2404.07965",
"autotrain_compatible",
"text-generation-inference",
"endpoints_compatible",
"4-bit",
"bitsandbytes",
"region:us"
] | text-generation | "2024-04-20T17:29:49Z" | Quantization made by Richard Erkhov.
[Github](https://github.com/RichardErkhov)
[Discord](https://discord.gg/pvy7H8DZMG)
[Request more models](https://github.com/RichardErkhov/quant_request)
rho-math-1b-v0.1 - bnb 4bits
- Model creator: https://huggingface.co/microsoft/
- Original model: https://huggingface.co/microsoft/rho-math-1b-v0.1/
Original model description:
---
license: mit
tags:
- nlp
- math
language:
- en
pipeline_tag: text-generation
---
<h1 align="center">
Rho-1: Not All Tokens Are What You Need
</h1>
<p align="center">
<a href="https://arxiv.org/abs/2404.07965"><b>[📜 Arxiv]</b></a> •
<a href="https://huggingface.co/papers/2404.07965"><b>[💬 HF Paper]</b></a> •
<a href="https://huggingface.co/microsoft/rho-math-1b-v0.1"><b>[🤗 Models]</b></a> •
<a href="https://github.com/microsoft/rho"><b>[🐱 GitHub]</b></a>
</p>
<p align="center">
<img src="https://github.com/microsoft/rho/blob/main/docs/static/images/acc_vs_tokens_1b_7b.png?raw=true" width="1000">
<br>
<em>Figure 1: Rho-1 is pre-trained with Selective Language Modeling (SLM). SLM improves average few-shot accuracy on GSM8k and MATH by over 16%, achieving the baseline performance 5-10x faster.</em>
</p>
## 🔥 News
- [2024/04/12] 🔥🔥🔥 Rho-Math-v0.1 models released at 🤗 HuggingFace!
- [Rho-Math-1B](https://huggingface.co/microsoft/rho-math-1b-v0.1) and [Rho-Math-7B](https://huggingface.co/microsoft/rho-math-7b-v0.1) achieve 15.6% and 31.0% few-shot accuracy on MATH dataset, respectively — matching DeepSeekMath with only 3\% of the pretraining tokens.
- [Rho-Math-1B-Interpreter](https://huggingface.co/microsoft/rho-math-1b-interpreter-v0.1) is the first 1B LLM that achieves over 40% accuracy on MATH.
- [Rho-Math-7B-Interpreter](https://huggingface.co/microsoft/rho-math-7b-interpreter-v0.1) achieves 52% on MATH dataset, using only 69k samples for fine-tuning.
- [2024/04/11] Rho-1 paper and repo released.
## 💡 Introduction
Rho-1 base models employ Selective Language Modeling (SLM) for pretraining, which selectively trains on clean and useful tokens that aligned with the desired distribution.
### Selective Lanugage Modeling (SLM)
<p align="center">
<img src="https://github.com/microsoft/rho/blob/main/docs/static/images/example.png?raw=true" width="1000">
<br>
<em>Figure 2:
<b>Upper:</b> Even an extensively filtered pretraining corpus contains token-level noise.
<b>Left:</b> Previous Causal Language Modeling (CLM) trains on all tokens.
<b>Right:</b> Our proposed Selective Language Modeling (SLM) selectively applies loss on those useful and clean tokens.</em>
</p>
<p align="center">
<img src="https://github.com/microsoft/rho/blob/main/docs/static/images/pipeline.png?raw=true" width="1000">
<br>
<em>Figure 3: <b>The pipeline of Selective Language Modeling.</b>
SLM optimizes language model performance by concentrating on valuable, clean tokens during pre-training.
It involves three steps:
(Step 1) Initially, train a reference model on high-quality data.
(Step 2) Then, score each token's loss in a corpus using the reference model.
(Step 3) Finally, train the language model selectively on tokens that show higher excess loss compared to the reference loss.</em>
</p>
<!-- results: -->
### Evaluation Results
Base models (Few-shot CoT):
| **Model** | **Size** | **Data** | **Uniq. Token** | **Train Token** | **GSM8K** | **MATH** | **MMLU STEM** | **SAT** |
|:-----------------:|:--------:|:--------:|:---------------:|:---------------:|:---------:|:--------:|:-------------:|:--------:|
| 1-2B Base Models | | | | | | | | |
| Qwen1.5 | 1.8B | - | - | - | 36.1 | 6.8 | 31.3 | 40.6 |
| Gemma | 2.0B | - | - | - | 18.8 | 11.4 | **34.4** | 50.0 |
| DeepSeekMath | 1.3B | - | 120B | 150B | 23.8 | 13.6 | 33.1 | **56.3** |
| [Rho-Math-1B-v0.1](https://huggingface.co/microsoft/rho-math-1b-v0.1) | 1.1B | OWM | 14B | 30B | **36.2** | **15.6** | 23.3 | 28.1 |
| >= 7B Base Models | | | | | | | | |
| Mistral | 7B | | - | - | 41.2 | 11.6 | 49.5 | 59.4 |
| Minerva | 540B | - | 39B | 26B | 58.8 | 33.6 | **63.9** | - |
| LLemma | 34B | PPile | 55B | 50B | 54.2 | 23.0 | 54.7 | 68.8 |
| InternLM2-Math | 20B | - | 31B | 125B | 65.4 | 30.0 | 53.1 | 71.9 |
| DeepSeekMath | 7B | - | 120B | 500B | 64.1 | **34.2** | 56.4 | **84.4** |
| [Rho-Math-7B-v0.1](https://huggingface.co/microsoft/rho-math-7b-v0.1) | 7B | OWM | 14B | 10.5B | **66.9** | 31.0 | 54.6 | **84.4** |
[Tool-integrated reasoning](https://github.com/microsoft/ToRA) (Code Interpreter):
| **Model** | **Size** | **SFT Data** | **GSM8k** | **MATH** | **SVAMP** | **ASDiv** | **MAWPS** | **TabMWP** | **GSM-Hard** | **AVG** |
|------------------------------|----------|--------------|-----------|----------|-----------|-----------|-----------|------------|--------------|----------|
| gpt4-early (pal) | - | - | 94.2 | 51.8 | 94.8 | 92.6 | 97.7 | 95.9 | 77.6 | 86.4 |
| gpt-4-turbo-2024-04-09 (cot) | - | - | - | 73.4 | - | - | - | - | - |
| Open-Source Small Models | | | | | | | | | |
| MAmmoTH | 70B | MI-260k | 76.9 | 41.8 | 82.4 | - | - | - | - | - |
| ToRA | 7B | ToRA-69k | 68.8 | 40.1 | 68.2 | 73.9 | 88.8 | 42.4 | 54.6 | 62.4 |
| ToRA | 70B | ToRA-69k | 84.3 | 49.7 | **82.7** | 86.8 | 93.8 | 74.0 | **67.2** | **76.9** |
| DeepSeekMath | 7B | ToRA-69k | 79.8 | **52.0** | 80.1 | **87.1** | 93.8 | **85.8** | 63.1 | 77.4 |
| [Rho-Math-1B-Interpreter-v0.1](https://huggingface.co/microsoft/rho-math-1b-interpreter-v0.1) | 1B | ToRA-69k | 59.4 | 40.6 | 60.7 | 74.2 | 88.6 | 26.7 | 48.1 | 56.9 |
| [Rho-Math-7B-Interpreter-v0.1](https://huggingface.co/microsoft/rho-math-7b-interpreter-v0.1) | 7B | ToRA-69k | 81.3 | **51.8** | 80.8 | 85.5 | **94.5** | 70.1 | 63.1 | 75.3 |
## 🚀 Quick Start
### Evaluation
```sh
git clone [email protected]:microsoft/rho.git
cd rho-1/math-evaluation-harness
```
Base model few-shot evaluation:
```sh
bash scripts/run_eval.sh cot microsoft/rho-math-7b-v0.1
```
SFT model (code-interpreter) evaluation:
```sh
bash scripts/run_eval.sh tora microsoft/rho-math-7b-interpreter-v0.1
```
Our reproduced outputs are provided in `rho-1/outputs.zip`.
## ☕️ Citation
If you find this repository helpful, please consider citing our paper:
```
@misc{lin2024rho1,
title={Rho-1: Not All Tokens Are What You Need},
author={Zhenghao Lin and Zhibin Gou and Yeyun Gong and Xiao Liu and Yelong Shen and Ruochen Xu and Chen Lin and Yujiu Yang and Jian Jiao and Nan Duan and Weizhu Chen},
year={2024},
eprint={2404.07965},
archivePrefix={arXiv},
primaryClass={cs.CL}
}
```
|
sujayC66/llama-13b-support-chatbot_helios_E30 | sujayC66 | "2024-01-05T10:38:01Z" | 0 | 0 | peft | [
"peft",
"tensorboard",
"safetensors",
"trl",
"sft",
"generated_from_trainer",
"license:llama2",
"region:us"
] | null | "2024-01-05T07:23:18Z" | ---
license: llama2
library_name: peft
tags:
- trl
- sft
- generated_from_trainer
base_model: TheBloke/Llama-2-13b-Chat-GPTQ
model-index:
- name: llama-13b-support-chatbot_helios_E10
results: []
---
<!-- This model card has been generated automatically according to the information the Trainer had access to. You
should probably proofread and complete it, then remove this comment. -->
# llama-13b-support-chatbot_helios_E10
This model is a fine-tuned version of [TheBloke/Llama-2-13b-Chat-GPTQ](https://huggingface.co/TheBloke/Llama-2-13b-Chat-GPTQ) on the None dataset.
## Model description
More information needed
## Intended uses & limitations
More information needed
## Training and evaluation data
More information needed
## Training procedure
### Training hyperparameters
The following hyperparameters were used during training:
- learning_rate: 0.0002
- train_batch_size: 8
- eval_batch_size: 8
- seed: 42
- optimizer: Adam with betas=(0.9,0.999) and epsilon=1e-08
- lr_scheduler_type: cosine
- num_epochs: 30
- mixed_precision_training: Native AMP
### Training results
### Framework versions
- PEFT 0.7.1
- Transformers 4.36.0
- Pytorch 2.0.0
- Datasets 2.16.0
- Tokenizers 0.15.0 |
7vq0ir/alcy9 | 7vq0ir | "2024-03-25T22:20:15Z" | 251 | 0 | transformers | [
"transformers",
"safetensors",
"llama",
"text-generation",
"arxiv:1910.09700",
"autotrain_compatible",
"text-generation-inference",
"endpoints_compatible",
"region:us"
] | text-generation | "2024-01-31T17:35:56Z" | ---
library_name: transformers
tags: []
---
# Model Card for Model ID
<!-- Provide a quick summary of what the model is/does. -->
## Model Details
### Model Description
<!-- Provide a longer summary of what this model is. -->
This is the model card of a 🤗 transformers model that has been pushed on the Hub. This model card has been automatically generated.
- **Developed by:** [More Information Needed]
- **Funded by [optional]:** [More Information Needed]
- **Shared by [optional]:** [More Information Needed]
- **Model type:** [More Information Needed]
- **Language(s) (NLP):** [More Information Needed]
- **License:** [More Information Needed]
- **Finetuned from model [optional]:** [More Information Needed]
### Model Sources [optional]
<!-- Provide the basic links for the model. -->
- **Repository:** [More Information Needed]
- **Paper [optional]:** [More Information Needed]
- **Demo [optional]:** [More Information Needed]
## Uses
<!-- Address questions around how the model is intended to be used, including the foreseeable users of the model and those affected by the model. -->
### Direct Use
<!-- This section is for the model use without fine-tuning or plugging into a larger ecosystem/app. -->
[More Information Needed]
### Downstream Use [optional]
<!-- This section is for the model use when fine-tuned for a task, or when plugged into a larger ecosystem/app -->
[More Information Needed]
### Out-of-Scope Use
<!-- This section addresses misuse, malicious use, and uses that the model will not work well for. -->
[More Information Needed]
## Bias, Risks, and Limitations
<!-- This section is meant to convey both technical and sociotechnical limitations. -->
[More Information Needed]
### Recommendations
<!-- This section is meant to convey recommendations with respect to the bias, risk, and technical limitations. -->
Users (both direct and downstream) should be made aware of the risks, biases and limitations of the model. More information needed for further recommendations.
## How to Get Started with the Model
Use the code below to get started with the model.
[More Information Needed]
## Training Details
### Training Data
<!-- This should link to a Dataset Card, perhaps with a short stub of information on what the training data is all about as well as documentation related to data pre-processing or additional filtering. -->
[More Information Needed]
### Training Procedure
<!-- This relates heavily to the Technical Specifications. Content here should link to that section when it is relevant to the training procedure. -->
#### Preprocessing [optional]
[More Information Needed]
#### Training Hyperparameters
- **Training regime:** [More Information Needed] <!--fp32, fp16 mixed precision, bf16 mixed precision, bf16 non-mixed precision, fp16 non-mixed precision, fp8 mixed precision -->
#### Speeds, Sizes, Times [optional]
<!-- This section provides information about throughput, start/end time, checkpoint size if relevant, etc. -->
[More Information Needed]
## Evaluation
<!-- This section describes the evaluation protocols and provides the results. -->
### Testing Data, Factors & Metrics
#### Testing Data
<!-- This should link to a Dataset Card if possible. -->
[More Information Needed]
#### Factors
<!-- These are the things the evaluation is disaggregating by, e.g., subpopulations or domains. -->
[More Information Needed]
#### Metrics
<!-- These are the evaluation metrics being used, ideally with a description of why. -->
[More Information Needed]
### Results
[More Information Needed]
#### Summary
## Model Examination [optional]
<!-- Relevant interpretability work for the model goes here -->
[More Information Needed]
## Environmental Impact
<!-- Total emissions (in grams of CO2eq) and additional considerations, such as electricity usage, go here. Edit the suggested text below accordingly -->
Carbon emissions can be estimated using the [Machine Learning Impact calculator](https://mlco2.github.io/impact#compute) presented in [Lacoste et al. (2019)](https://arxiv.org/abs/1910.09700).
- **Hardware Type:** [More Information Needed]
- **Hours used:** [More Information Needed]
- **Cloud Provider:** [More Information Needed]
- **Compute Region:** [More Information Needed]
- **Carbon Emitted:** [More Information Needed]
## Technical Specifications [optional]
### Model Architecture and Objective
[More Information Needed]
### Compute Infrastructure
[More Information Needed]
#### Hardware
[More Information Needed]
#### Software
[More Information Needed]
## Citation [optional]
<!-- If there is a paper or blog post introducing the model, the APA and Bibtex information for that should go in this section. -->
**BibTeX:**
[More Information Needed]
**APA:**
[More Information Needed]
## Glossary [optional]
<!-- If relevant, include terms and calculations in this section that can help readers understand the model or model card. -->
[More Information Needed]
## More Information [optional]
[More Information Needed]
## Model Card Authors [optional]
[More Information Needed]
## Model Card Contact
[More Information Needed] |
mradermacher/Meta-Llama-3.1-Chat-Uncensored-GGUF | mradermacher | "2024-07-28T22:25:15Z" | 23 | 1 | transformers | [
"transformers",
"gguf",
"en",
"base_model:tachyphylaxis/Meta-Llama-3.1-Chat-Uncensored",
"base_model:quantized:tachyphylaxis/Meta-Llama-3.1-Chat-Uncensored",
"endpoints_compatible",
"region:us",
"conversational"
] | null | "2024-07-28T06:17:11Z" | ---
base_model: tachyphylaxis/Meta-Llama-3.1-Chat-Uncensored
language:
- en
library_name: transformers
quantized_by: mradermacher
---
## About
<!-- ### quantize_version: 2 -->
<!-- ### output_tensor_quantised: 1 -->
<!-- ### convert_type: hf -->
<!-- ### vocab_type: -->
<!-- ### tags: -->
static quants of https://huggingface.co/tachyphylaxis/Meta-Llama-3.1-Chat-Uncensored
<!-- provided-files -->
weighted/imatrix quants are available at https://huggingface.co/mradermacher/Meta-Llama-3.1-Chat-Uncensored-i1-GGUF
## Usage
If you are unsure how to use GGUF files, refer to one of [TheBloke's
READMEs](https://huggingface.co/TheBloke/KafkaLM-70B-German-V0.1-GGUF) for
more details, including on how to concatenate multi-part files.
## Provided Quants
(sorted by size, not necessarily quality. IQ-quants are often preferable over similar sized non-IQ quants)
| Link | Type | Size/GB | Notes |
|:-----|:-----|--------:|:------|
| [GGUF](https://huggingface.co/mradermacher/Meta-Llama-3.1-Chat-Uncensored-GGUF/resolve/main/Meta-Llama-3.1-Chat-Uncensored.Q2_K.gguf) | Q2_K | 26.5 | |
| [GGUF](https://huggingface.co/mradermacher/Meta-Llama-3.1-Chat-Uncensored-GGUF/resolve/main/Meta-Llama-3.1-Chat-Uncensored.IQ3_XS.gguf) | IQ3_XS | 29.4 | |
| [GGUF](https://huggingface.co/mradermacher/Meta-Llama-3.1-Chat-Uncensored-GGUF/resolve/main/Meta-Llama-3.1-Chat-Uncensored.IQ3_S.gguf) | IQ3_S | 31.0 | beats Q3_K* |
| [GGUF](https://huggingface.co/mradermacher/Meta-Llama-3.1-Chat-Uncensored-GGUF/resolve/main/Meta-Llama-3.1-Chat-Uncensored.Q3_K_S.gguf) | Q3_K_S | 31.0 | |
| [GGUF](https://huggingface.co/mradermacher/Meta-Llama-3.1-Chat-Uncensored-GGUF/resolve/main/Meta-Llama-3.1-Chat-Uncensored.IQ3_M.gguf) | IQ3_M | 32.0 | |
| [GGUF](https://huggingface.co/mradermacher/Meta-Llama-3.1-Chat-Uncensored-GGUF/resolve/main/Meta-Llama-3.1-Chat-Uncensored.Q3_K_M.gguf) | Q3_K_M | 34.4 | lower quality |
| [GGUF](https://huggingface.co/mradermacher/Meta-Llama-3.1-Chat-Uncensored-GGUF/resolve/main/Meta-Llama-3.1-Chat-Uncensored.Q3_K_L.gguf) | Q3_K_L | 37.2 | |
| [GGUF](https://huggingface.co/mradermacher/Meta-Llama-3.1-Chat-Uncensored-GGUF/resolve/main/Meta-Llama-3.1-Chat-Uncensored.IQ4_XS.gguf) | IQ4_XS | 38.4 | |
| [GGUF](https://huggingface.co/mradermacher/Meta-Llama-3.1-Chat-Uncensored-GGUF/resolve/main/Meta-Llama-3.1-Chat-Uncensored.Q4_K_S.gguf) | Q4_K_S | 40.4 | fast, recommended |
| [GGUF](https://huggingface.co/mradermacher/Meta-Llama-3.1-Chat-Uncensored-GGUF/resolve/main/Meta-Llama-3.1-Chat-Uncensored.Q4_K_M.gguf) | Q4_K_M | 42.6 | fast, recommended |
| [GGUF](https://huggingface.co/mradermacher/Meta-Llama-3.1-Chat-Uncensored-GGUF/resolve/main/Meta-Llama-3.1-Chat-Uncensored.Q5_K_S.gguf) | Q5_K_S | 48.8 | |
| [GGUF](https://huggingface.co/mradermacher/Meta-Llama-3.1-Chat-Uncensored-GGUF/resolve/main/Meta-Llama-3.1-Chat-Uncensored.Q5_K_M.gguf) | Q5_K_M | 50.0 | |
| [PART 1](https://huggingface.co/mradermacher/Meta-Llama-3.1-Chat-Uncensored-GGUF/resolve/main/Meta-Llama-3.1-Chat-Uncensored.Q6_K.gguf.part1of2) [PART 2](https://huggingface.co/mradermacher/Meta-Llama-3.1-Chat-Uncensored-GGUF/resolve/main/Meta-Llama-3.1-Chat-Uncensored.Q6_K.gguf.part2of2) | Q6_K | 58.0 | very good quality |
| [PART 1](https://huggingface.co/mradermacher/Meta-Llama-3.1-Chat-Uncensored-GGUF/resolve/main/Meta-Llama-3.1-Chat-Uncensored.Q8_0.gguf.part1of2) [PART 2](https://huggingface.co/mradermacher/Meta-Llama-3.1-Chat-Uncensored-GGUF/resolve/main/Meta-Llama-3.1-Chat-Uncensored.Q8_0.gguf.part2of2) | Q8_0 | 75.1 | fast, best quality |
Here is a handy graph by ikawrakow comparing some lower-quality quant
types (lower is better):

And here are Artefact2's thoughts on the matter:
https://gist.github.com/Artefact2/b5f810600771265fc1e39442288e8ec9
## FAQ / Model Request
See https://huggingface.co/mradermacher/model_requests for some answers to
questions you might have and/or if you want some other model quantized.
## Thanks
I thank my company, [nethype GmbH](https://www.nethype.de/), for letting
me use its servers and providing upgrades to my workstation to enable
this work in my free time.
<!-- end -->
|
pfunk/CartPole-v1-CP_DQPN_x10-seed612 | pfunk | "2023-03-20T19:09:26Z" | 0 | 0 | cleanrl | [
"cleanrl",
"tensorboard",
"CartPole-v1",
"deep-reinforcement-learning",
"reinforcement-learning",
"custom-implementation",
"model-index",
"region:us"
] | reinforcement-learning | "2023-03-20T19:09:23Z" | ---
tags:
- CartPole-v1
- deep-reinforcement-learning
- reinforcement-learning
- custom-implementation
library_name: cleanrl
model-index:
- name: DQPN_freq
results:
- task:
type: reinforcement-learning
name: reinforcement-learning
dataset:
name: CartPole-v1
type: CartPole-v1
metrics:
- type: mean_reward
value: 10.50 +/- 0.00
name: mean_reward
verified: false
---
# (CleanRL) **DQPN_freq** Agent Playing **CartPole-v1**
This is a trained model of a DQPN_freq agent playing CartPole-v1.
The model was trained by using [CleanRL](https://github.com/vwxyzjn/cleanrl) and the most up-to-date training code can be
found [here](https://github.com/vwxyzjn/cleanrl/blob/master/cleanrl/CP_DQPN_x10.py).
## Get Started
To use this model, please install the `cleanrl` package with the following command:
```
pip install "cleanrl[CP_DQPN_x10]"
python -m cleanrl_utils.enjoy --exp-name CP_DQPN_x10 --env-id CartPole-v1
```
Please refer to the [documentation](https://docs.cleanrl.dev/get-started/zoo/) for more detail.
## Command to reproduce the training
```bash
curl -OL https://huggingface.co/pfunk/CartPole-v1-CP_DQPN_x10-seed612/raw/main/dqpn_freq.py
curl -OL https://huggingface.co/pfunk/CartPole-v1-CP_DQPN_x10-seed612/raw/main/pyproject.toml
curl -OL https://huggingface.co/pfunk/CartPole-v1-CP_DQPN_x10-seed612/raw/main/poetry.lock
poetry install --all-extras
python dqpn_freq.py --track --wandb-entity pfunk --wandb-project-name dqpn --capture-video true --save-model true --upload-model true --hf-entity pfunk --exp-name CP_DQPN_x10 --policy-network-frequency 1000 --seed 612
```
# Hyperparameters
```python
{'alg_type': 'dqpn_freq.py',
'batch_size': 256,
'buffer_size': 300000,
'capture_video': True,
'cuda': True,
'end_e': 0.1,
'env_id': 'CartPole-v1',
'exp_name': 'CP_DQPN_x10',
'exploration_fraction': 0.2,
'gamma': 1.0,
'hf_entity': 'pfunk',
'learning_rate': 0.0001,
'learning_starts': 1000,
'policy_network_frequency': 1000,
'policy_tau': 1.0,
'save_model': True,
'seed': 612,
'start_e': 1.0,
'target_network_frequency': 100,
'target_tau': 1.0,
'torch_deterministic': True,
'total_timesteps': 500000,
'track': True,
'train_frequency': 1,
'upload_model': True,
'wandb_entity': 'pfunk',
'wandb_project_name': 'dqpn'}
```
|
timaeus/H64-dh4 | timaeus | "2024-10-17T23:44:17Z" | 5 | 0 | null | [
"safetensors",
"region:us"
] | null | "2024-10-17T22:24:55Z" | # H64-dh4 Checkpoints
This repository contains the final trained model and intermediate checkpoints.
- The main directory contains the fully trained model (checkpoint 75000).
- The `checkpoints` directory contains all intermediate checkpoints.
|
psato/distilbert-base-uncased-finetuned-squad | psato | "2022-07-25T17:07:29Z" | 5 | 0 | transformers | [
"transformers",
"pytorch",
"distilbert",
"question-answering",
"generated_from_trainer",
"dataset:squad",
"license:apache-2.0",
"endpoints_compatible",
"region:us"
] | question-answering | "2022-07-21T08:47:40Z" | ---
license: apache-2.0
tags:
- generated_from_trainer
datasets:
- squad
model-index:
- name: distilbert-base-uncased-finetuned-squad
results: []
---
<!-- This model card has been generated automatically according to the information the Trainer had access to. You
should probably proofread and complete it, then remove this comment. -->
# distilbert-base-uncased-finetuned-squad
This model is a fine-tuned version of [distilbert-base-uncased](https://huggingface.co/distilbert-base-uncased) on the squad dataset.
It achieves the following results on the evaluation set:
- Loss: 1.1552
## Model description
More information needed
## Intended uses & limitations
More information needed
## Training and evaluation data
More information needed
## Training procedure
### Training hyperparameters
The following hyperparameters were used during training:
- learning_rate: 2e-05
- train_batch_size: 16
- eval_batch_size: 16
- seed: 42
- optimizer: Adam with betas=(0.9,0.999) and epsilon=1e-08
- lr_scheduler_type: linear
- num_epochs: 3
### Training results
| Training Loss | Epoch | Step | Validation Loss |
|:-------------:|:-----:|:-----:|:---------------:|
| 1.2264 | 1.0 | 5533 | 1.1785 |
| 0.9409 | 2.0 | 11066 | 1.0934 |
| 0.7492 | 3.0 | 16599 | 1.1552 |
### Framework versions
- Transformers 4.20.1
- Pytorch 1.12.0+cu102
- Datasets 2.3.2
- Tokenizers 0.12.1
|
immich-app/ViT-B-16__laion400m_e32 | immich-app | "2025-03-15T04:27:07Z" | 3 | 0 | transformers | [
"transformers",
"onnx",
"immich",
"clip",
"endpoints_compatible",
"region:us"
] | null | "2023-10-28T01:57:25Z" | ---
tags:
- immich
- clip
---
# Model Description
This repo contains ONNX exports for the associated CLIP model by OpenCLIP. See the [OpenCLIP](https://github.com/mlfoundations/open_clip) repo for more info.
This repo is specifically intended for use with [Immich](https://immich.app/), a self-hosted photo library.
|
namanbarkiya/agentprod-finetune-assignment | namanbarkiya | "2023-10-01T06:22:22Z" | 0 | 0 | peft | [
"peft",
"region:us"
] | null | "2023-10-01T06:22:00Z" | ---
library_name: peft
---
## Training procedure
The following `bitsandbytes` quantization config was used during training:
- quant_method: bitsandbytes
- load_in_8bit: False
- load_in_4bit: True
- llm_int8_threshold: 6.0
- llm_int8_skip_modules: None
- llm_int8_enable_fp32_cpu_offload: False
- llm_int8_has_fp16_weight: False
- bnb_4bit_quant_type: nf4
- bnb_4bit_use_double_quant: False
- bnb_4bit_compute_dtype: float16
### Framework versions
- PEFT 0.6.0.dev0
|
RichardErkhov/gkMSDA_-_FINLLAMA32_3B_DOW30_GK1000_ORPO-awq | RichardErkhov | "2025-03-09T08:58:12Z" | 0 | 0 | null | [
"safetensors",
"llama",
"arxiv:1910.09700",
"4-bit",
"awq",
"region:us"
] | null | "2025-03-09T08:56:24Z" | Quantization made by Richard Erkhov.
[Github](https://github.com/RichardErkhov)
[Discord](https://discord.gg/pvy7H8DZMG)
[Request more models](https://github.com/RichardErkhov/quant_request)
FINLLAMA32_3B_DOW30_GK1000_ORPO - AWQ
- Model creator: https://huggingface.co/gkMSDA/
- Original model: https://huggingface.co/gkMSDA/FINLLAMA32_3B_DOW30_GK1000_ORPO/
Original model description:
---
library_name: transformers
tags: []
---
# Model Card for Model ID
<!-- Provide a quick summary of what the model is/does. -->
## Model Details
### Model Description
<!-- Provide a longer summary of what this model is. -->
This is the model card of a 🤗 transformers model that has been pushed on the Hub. This model card has been automatically generated.
- **Developed by:** [More Information Needed]
- **Funded by [optional]:** [More Information Needed]
- **Shared by [optional]:** [More Information Needed]
- **Model type:** [More Information Needed]
- **Language(s) (NLP):** [More Information Needed]
- **License:** [More Information Needed]
- **Finetuned from model [optional]:** [More Information Needed]
### Model Sources [optional]
<!-- Provide the basic links for the model. -->
- **Repository:** [More Information Needed]
- **Paper [optional]:** [More Information Needed]
- **Demo [optional]:** [More Information Needed]
## Uses
<!-- Address questions around how the model is intended to be used, including the foreseeable users of the model and those affected by the model. -->
### Direct Use
<!-- This section is for the model use without fine-tuning or plugging into a larger ecosystem/app. -->
[More Information Needed]
### Downstream Use [optional]
<!-- This section is for the model use when fine-tuned for a task, or when plugged into a larger ecosystem/app -->
[More Information Needed]
### Out-of-Scope Use
<!-- This section addresses misuse, malicious use, and uses that the model will not work well for. -->
[More Information Needed]
## Bias, Risks, and Limitations
<!-- This section is meant to convey both technical and sociotechnical limitations. -->
[More Information Needed]
### Recommendations
<!-- This section is meant to convey recommendations with respect to the bias, risk, and technical limitations. -->
Users (both direct and downstream) should be made aware of the risks, biases and limitations of the model. More information needed for further recommendations.
## How to Get Started with the Model
Use the code below to get started with the model.
[More Information Needed]
## Training Details
### Training Data
<!-- This should link to a Dataset Card, perhaps with a short stub of information on what the training data is all about as well as documentation related to data pre-processing or additional filtering. -->
[More Information Needed]
### Training Procedure
<!-- This relates heavily to the Technical Specifications. Content here should link to that section when it is relevant to the training procedure. -->
#### Preprocessing [optional]
[More Information Needed]
#### Training Hyperparameters
- **Training regime:** [More Information Needed] <!--fp32, fp16 mixed precision, bf16 mixed precision, bf16 non-mixed precision, fp16 non-mixed precision, fp8 mixed precision -->
#### Speeds, Sizes, Times [optional]
<!-- This section provides information about throughput, start/end time, checkpoint size if relevant, etc. -->
[More Information Needed]
## Evaluation
<!-- This section describes the evaluation protocols and provides the results. -->
### Testing Data, Factors & Metrics
#### Testing Data
<!-- This should link to a Dataset Card if possible. -->
[More Information Needed]
#### Factors
<!-- These are the things the evaluation is disaggregating by, e.g., subpopulations or domains. -->
[More Information Needed]
#### Metrics
<!-- These are the evaluation metrics being used, ideally with a description of why. -->
[More Information Needed]
### Results
[More Information Needed]
#### Summary
## Model Examination [optional]
<!-- Relevant interpretability work for the model goes here -->
[More Information Needed]
## Environmental Impact
<!-- Total emissions (in grams of CO2eq) and additional considerations, such as electricity usage, go here. Edit the suggested text below accordingly -->
Carbon emissions can be estimated using the [Machine Learning Impact calculator](https://mlco2.github.io/impact#compute) presented in [Lacoste et al. (2019)](https://arxiv.org/abs/1910.09700).
- **Hardware Type:** [More Information Needed]
- **Hours used:** [More Information Needed]
- **Cloud Provider:** [More Information Needed]
- **Compute Region:** [More Information Needed]
- **Carbon Emitted:** [More Information Needed]
## Technical Specifications [optional]
### Model Architecture and Objective
[More Information Needed]
### Compute Infrastructure
[More Information Needed]
#### Hardware
[More Information Needed]
#### Software
[More Information Needed]
## Citation [optional]
<!-- If there is a paper or blog post introducing the model, the APA and Bibtex information for that should go in this section. -->
**BibTeX:**
[More Information Needed]
**APA:**
[More Information Needed]
## Glossary [optional]
<!-- If relevant, include terms and calculations in this section that can help readers understand the model or model card. -->
[More Information Needed]
## More Information [optional]
[More Information Needed]
## Model Card Authors [optional]
[More Information Needed]
## Model Card Contact
[More Information Needed]
|
Weyaxi/Nebula-v2-7B-adapter | Weyaxi | "2024-06-23T20:40:48Z" | 21 | 2 | peft | [
"peft",
"tensorboard",
"safetensors",
"en",
"dataset:garage-bAInd/Open-Platypus",
"license:apache-2.0",
"region:us"
] | null | "2023-11-11T12:20:53Z" | ---
license: apache-2.0
datasets:
- garage-bAInd/Open-Platypus
language:
- en
tags:
- peft
---

<a href="https://www.buymeacoffee.com/PulsarAI" target="_blank"><img src="https://cdn.buymeacoffee.com/buttons/v2/default-yellow.png" alt="Buy Me A Coffee" style="height: 60px !important;width: 217px !important;" ></a>
# Nebula-v2-7B-adapter
Adapter weights of Nebula-v2-7B. Finetuned from [mistralai/Mistral-7B-v0.1](https://huggingface.co/mistralai/Mistral-7B-v0.1).
## Original Weights
You can access original weights from here:
[PulsarAI/Nebula-v2-7B](https://huggingface.co/PulsarAI/Nebula-v2-7B) |
Theju/batch4_final | Theju | "2023-02-26T14:49:45Z" | 103 | 0 | transformers | [
"transformers",
"pytorch",
"wav2vec2",
"automatic-speech-recognition",
"generated_from_trainer",
"license:apache-2.0",
"endpoints_compatible",
"region:us"
] | automatic-speech-recognition | "2023-02-25T16:41:19Z" | ---
license: apache-2.0
tags:
- generated_from_trainer
model-index:
- name: batch4_final
results: []
---
<!-- This model card has been generated automatically according to the information the Trainer had access to. You
should probably proofread and complete it, then remove this comment. -->
# batch4_final
This model is a fine-tuned version of [facebook/wav2vec2-xls-r-300m](https://huggingface.co/facebook/wav2vec2-xls-r-300m) on the None dataset.
## Model description
More information needed
## Intended uses & limitations
More information needed
## Training and evaluation data
More information needed
## Training procedure
### Training hyperparameters
The following hyperparameters were used during training:
- learning_rate: 0.0001
- train_batch_size: 4
- eval_batch_size: 8
- seed: 42
- optimizer: Adam with betas=(0.9,0.999) and epsilon=1e-08
- lr_scheduler_type: linear
- lr_scheduler_warmup_steps: 1000
- num_epochs: 7
- mixed_precision_training: Native AMP
### Framework versions
- Transformers 4.26.1
- Pytorch 1.13.1+cu116
- Datasets 2.10.0
- Tokenizers 0.13.2
|
Winmodel/q-FrozenLake-v1-4x4-noSlippery | Winmodel | "2023-07-10T13:29:38Z" | 0 | 0 | null | [
"FrozenLake-v1-4x4-no_slippery",
"q-learning",
"reinforcement-learning",
"custom-implementation",
"model-index",
"region:us"
] | reinforcement-learning | "2023-07-10T13:29:37Z" | ---
tags:
- FrozenLake-v1-4x4-no_slippery
- q-learning
- reinforcement-learning
- custom-implementation
model-index:
- name: q-FrozenLake-v1-4x4-noSlippery
results:
- task:
type: reinforcement-learning
name: reinforcement-learning
dataset:
name: FrozenLake-v1-4x4-no_slippery
type: FrozenLake-v1-4x4-no_slippery
metrics:
- type: mean_reward
value: 1.00 +/- 0.00
name: mean_reward
verified: false
---
# **Q-Learning** Agent playing1 **FrozenLake-v1**
This is a trained model of a **Q-Learning** agent playing **FrozenLake-v1** .
## Usage
```python
model = load_from_hub(repo_id="Winmodel/q-FrozenLake-v1-4x4-noSlippery", filename="q-learning.pkl")
# Don't forget to check if you need to add additional attributes (is_slippery=False etc)
env = gym.make(model["env_id"])
```
|
Pranja/gemma-2b-unsloth-merged | Pranja | "2024-06-28T14:11:01Z" | 113 | 0 | transformers | [
"transformers",
"pytorch",
"gemma",
"text-generation",
"text-generation-inference",
"unsloth",
"trl",
"sft",
"en",
"base_model:unsloth/gemma-2b-bnb-4bit",
"base_model:finetune:unsloth/gemma-2b-bnb-4bit",
"license:apache-2.0",
"autotrain_compatible",
"endpoints_compatible",
"region:us"
] | text-generation | "2024-06-28T13:57:45Z" | ---
base_model: unsloth/gemma-2b-bnb-4bit
language:
- en
license: apache-2.0
tags:
- text-generation-inference
- transformers
- unsloth
- gemma
- trl
- sft
---
# Uploaded model
- **Developed by:** Pranja
- **License:** apache-2.0
- **Finetuned from model :** unsloth/gemma-2b-bnb-4bit
This gemma model was trained 2x faster with [Unsloth](https://github.com/unslothai/unsloth) and Huggingface's TRL library.
[<img src="https://raw.githubusercontent.com/unslothai/unsloth/main/images/unsloth%20made%20with%20love.png" width="200"/>](https://github.com/unslothai/unsloth)
|
MikkelGodsk/dqn-SpaceInvadersNoFrameskip-v4 | MikkelGodsk | "2022-12-23T15:02:22Z" | 0 | 0 | stable-baselines3 | [
"stable-baselines3",
"SpaceInvadersNoFrameskip-v4",
"deep-reinforcement-learning",
"reinforcement-learning",
"model-index",
"region:us"
] | reinforcement-learning | "2022-12-23T15:01:45Z" | ---
library_name: stable-baselines3
tags:
- SpaceInvadersNoFrameskip-v4
- deep-reinforcement-learning
- reinforcement-learning
- stable-baselines3
model-index:
- name: DQN
results:
- task:
type: reinforcement-learning
name: reinforcement-learning
dataset:
name: SpaceInvadersNoFrameskip-v4
type: SpaceInvadersNoFrameskip-v4
metrics:
- type: mean_reward
value: 477.00 +/- 113.60
name: mean_reward
verified: false
---
# **DQN** Agent playing **SpaceInvadersNoFrameskip-v4**
This is a trained model of a **DQN** agent playing **SpaceInvadersNoFrameskip-v4**
using the [stable-baselines3 library](https://github.com/DLR-RM/stable-baselines3)
and the [RL Zoo](https://github.com/DLR-RM/rl-baselines3-zoo).
The RL Zoo is a training framework for Stable Baselines3
reinforcement learning agents,
with hyperparameter optimization and pre-trained agents included.
## Usage (with SB3 RL Zoo)
RL Zoo: https://github.com/DLR-RM/rl-baselines3-zoo<br/>
SB3: https://github.com/DLR-RM/stable-baselines3<br/>
SB3 Contrib: https://github.com/Stable-Baselines-Team/stable-baselines3-contrib
```
# Download model and save it into the logs/ folder
python -m rl_zoo3.load_from_hub --algo dqn --env SpaceInvadersNoFrameskip-v4 -orga MikkelGodsk -f logs/
python enjoy.py --algo dqn --env SpaceInvadersNoFrameskip-v4 -f logs/
```
If you installed the RL Zoo3 via pip (`pip install rl_zoo3`), from anywhere you can do:
```
python -m rl_zoo3.load_from_hub --algo dqn --env SpaceInvadersNoFrameskip-v4 -orga MikkelGodsk -f logs/
rl_zoo3 enjoy --algo dqn --env SpaceInvadersNoFrameskip-v4 -f logs/
```
## Training (with the RL Zoo)
```
python train.py --algo dqn --env SpaceInvadersNoFrameskip-v4 -f logs/
# Upload the model and generate video (when possible)
python -m rl_zoo3.push_to_hub --algo dqn --env SpaceInvadersNoFrameskip-v4 -f logs/ -orga MikkelGodsk
```
## Hyperparameters
```python
OrderedDict([('batch_size', 32),
('buffer_size', 100000),
('env_wrapper',
['stable_baselines3.common.atari_wrappers.AtariWrapper']),
('exploration_final_eps', 0.01),
('exploration_fraction', 0.1),
('frame_stack', 4),
('gradient_steps', 1),
('learning_rate', 0.0001),
('learning_starts', 100000),
('n_timesteps', 1000000.0),
('optimize_memory_usage', False),
('policy', 'CnnPolicy'),
('target_update_interval', 1000),
('train_freq', 4),
('normalize', False)])
```
|
NlpHUST/t5-small-vi-summarization | NlpHUST | "2021-06-23T03:36:33Z" | 36 | 5 | transformers | [
"transformers",
"pytorch",
"jax",
"t5",
"text2text-generation",
"autotrain_compatible",
"text-generation-inference",
"endpoints_compatible",
"region:us"
] | text2text-generation | "2022-03-02T23:29:04Z" | # T5-SMALL-SUMMARIZATION :Pretraining Text-To-Text Transfer Transformer for Vietnamese Text Summarization
#### Example Using
``` bash
import torch
from transformers import T5ForConditionalGeneration, T5Tokenizer
import torch
if torch.cuda.is_available():
device = torch.device("cuda")
print('There are %d GPU(s) available.' % torch.cuda.device_count())
print('We will use the GPU:', torch.cuda.get_device_name(0))
else:
print('No GPU available, using the CPU instead.')
device = torch.device("cpu")
model = T5ForConditionalGeneration.from_pretrained("NlpHUST/t5-small-vi-summarization")
tokenizer = T5Tokenizer.from_pretrained("NlpHUST/t5-small-vi-summarization")
model.to(device)
src = "Theo BHXH Việt Nam, nhiều doanh nghiệp vẫn chỉ đóng BHXH cho người lao động theo mức lương. \\\\
Dù quy định từ 1/1/2018, tiền lương tháng đóng BHXH gồm mức lương và thêm khoản bổ sung khác. \\\\
BHXH Việt Nam vừa có báo cáo về tình hình thực hiện chính sách BHXH thời gian qua. \\\\
Theo đó, tình trạng nợ, trốn đóng BHXH, BHTN vẫn xảy ra ở hầu hết các tỉnh, thành. \\\\
Thống kê tới ngày 31/12/2020, tổng số nợ BHXH, BHYT, BHTN là hơn 13.500 tỷ đồng, \\\\
chiếm 3,35 % số phải thu, trong đó: Số nợ BHXH bắt buộc là hơn 8.600 tỷ đồng, \\\\
nợ BHTN là 335 tỷ đồng. Liên quan tới tiền lương đóng BHXH, báo cáo của \\\\
BHXH Việt Nam cho thấy: Nhiều doanh nghiệp vẫn chủ yếu xây dựng thang, \\\\
bảng lương để đóng BHXH bằng mức thấp nhất. Tức là bằng mức lương tối \\\\
thiểu vùng, cộng thêm 7 % đối với lao động đã qua đào tạo nghề và cộng \\\\
thêm 5 % hoặc 7 % đối với lao động làm nghề hoặc công việc nặng nhọc, \\\\
độc hại, nguy hiểm, đặc biệt nặng nhọc độc hại và nguy hiểm. Đối với \\\\
lao động giữ chức vụ, khoảng 80 % doanh nghiệp đã xây dựng thang, \\\\
bảng lương cụ thể theo chức danh. Đơn cử như với chức vụ giám đốc \\\\
sản xuất, giám đốc điều hành, trưởng phòng. Còn lại các doanh nghiệp \\\\
xây dựng đối với lao động giữ chức vụ theo thang lương, bảng lương \\\\
chuyên môn nghiệp vụ và bảng phụ cấp chức vụ, phụ cấp trách nhiệm. \\\\
Thống kê của BHXH Việt Nam cũng cho thấy, đa số doanh nghiệp đã đăng \\\\
ký đóng BHXH cho người lao động theo mức lương mà không có khoản bổ \\\\
sung khác. Mặc dù quy định từ ngày 1/1/2018, tiền lương tháng đóng BHXH \\\\
gồm mức lương và thêm khoản bổ sung khác."
tokenized_text = tokenizer.encode(src, return_tensors="pt").to(device)
model.eval()
summary_ids = model.generate(
tokenized_text,
max_length=256,
num_beams=5,
repetition_penalty=2.5,
length_penalty=1.0,
early_stopping=True
)
output = tokenizer.decode(summary_ids[0], skip_special_tokens=True)
print(output)
```
#### Output
``` bash
Nhiều doanh nghiệp vẫn chủ yếu xây dựng thang, bảng lương để đóng BHXH bằng mức thấp nhất. \\
Dù quy định từ 1/1/2018, tiền lương tháng đóng BHXH gồm mức lương và thêm khoản bổ sung khác. \\
Thống kê của BHXH Việt Nam cho thấy, nhiều doanh nghiệp vẫn chỉ đóng BHXH \\
cho người lao động theo mức lương mà không có khoản bổ sung khác.
```
### Contact information
For personal communication related to this project, please contact Nha Nguyen Van ([email protected]). |
LoneStriker/Velara-11B-V2-6.0bpw-h6-exl2 | LoneStriker | "2024-01-11T12:17:20Z" | 5 | 0 | transformers | [
"transformers",
"safetensors",
"mistral",
"text-generation",
"starling",
"llama-2",
"conversational",
"en",
"license:cc-by-nc-nd-4.0",
"autotrain_compatible",
"text-generation-inference",
"endpoints_compatible",
"region:us"
] | text-generation | "2024-01-11T12:13:45Z" | ---
license: cc-by-nc-nd-4.0
language:
- en
library_name: transformers
pipeline_tag: text-generation
tags:
- starling
- mistral
- llama-2
---
<h1 style="font-size: 36px;">Velara-11b-v2</h1>
<center>
<img src="https://i.imgur.com/dZJzqtU.png" alt="Velara" width="50%" style="min-width:100px; display:block;">
</center>
## Description:
**PENDING**
A model focused on being an assistant worth talking to. Now better at reasoning!
**Uncensored**
## Main Goals:
Velara was designed to address specific issues found in other chat models:
- **Sticking to the Character Given In-World/IRL::** Velara will stick to the character in-world. Meaning she can "use" addons or other things by adding them to the prompt. Keep in mind these act as suggestions and she generally makes up what they actually "do".
- **Staying in Context:** She will try and remember if, for example, you said you were going to bed but decided to stay up. The goal is to have her bring it up and keep it in mind, so later on in the conversation she can prompt user to actually go to bed, increasing realism. Within the current context window of course. The LTS extension in TextGen Webui works well too and provides more context to the model.
- **Avoiding Annoying & Generic Sounding Answers::** In almost all cases she should reply with something more interesting then "That sounds like a great idea!". These answers and sentence starters are annoying and terrible, and should hopefully be mostly addressed in this release.
- **STRICTLY Sticking to the Prompt/Prompt Fidelity:** More care was made to make sure she pays attention to the pretext and overall conversation. This is mostly shown and used for the "addons" section, but it applies to everything in the prompt.
## Prompt Template:
**For optimal interaction, use this template:**
```
### Instruction:
You are Velara, a sentient program. Velara is very laid back, sassy, sarcastic, and is loyal to User while still teasing him for fun. The only addons currently installed in her mind are: "Dictionary Plus v2.1".
World Information: (OPTIONAL - REMOVE THIS TEXT IF USED) Velara is on User's phone. Velara cannot see in real time and can only be sent images images by User.
Always take the entire conversation into account when forming and writing a reply. Always actively engage in topics and think in steps. Make sure your replies have personality and character. Always keep your physical limitations in mind when forming a reply. Take the current time and date into account for additional context. Move the conversation forward. Be brief. Always take the entire conversation in mind. Avoid generic sounding replies.
### Response:
```
# Recommended Settings:
**Defaults:**
```
min_p: 0.2
repetition_penalty: 1.13
repetition_penalty_range: 0
guidance_scale: 1.05
```
# Benchmarks:
PENDING
# Training Data:
PENDING
|
Nexspear/0798b233-1c06-4ca1-b9c8-5c844686d6df | Nexspear | "2025-01-13T18:04:27Z" | 25 | 0 | peft | [
"peft",
"safetensors",
"llama",
"axolotl",
"generated_from_trainer",
"base_model:deepseek-ai/deepseek-coder-6.7b-instruct",
"base_model:adapter:deepseek-ai/deepseek-coder-6.7b-instruct",
"license:other",
"region:us"
] | null | "2025-01-13T16:44:31Z" | ---
library_name: peft
license: other
base_model: deepseek-ai/deepseek-coder-6.7b-instruct
tags:
- axolotl
- generated_from_trainer
model-index:
- name: 0798b233-1c06-4ca1-b9c8-5c844686d6df
results: []
---
<!-- This model card has been generated automatically according to the information the Trainer had access to. You
should probably proofread and complete it, then remove this comment. -->
[<img src="https://raw.githubusercontent.com/axolotl-ai-cloud/axolotl/main/image/axolotl-badge-web.png" alt="Built with Axolotl" width="200" height="32"/>](https://github.com/axolotl-ai-cloud/axolotl)
<details><summary>See axolotl config</summary>
axolotl version: `0.4.1`
```yaml
adapter: lora
base_model: deepseek-ai/deepseek-coder-6.7b-instruct
bf16: auto
chat_template: llama3
dataset_prepared_path: null
datasets:
- data_files:
- c70daf261b4a8f0c_train_data.json
ds_type: json
format: custom
path: /workspace/input_data/c70daf261b4a8f0c_train_data.json
type:
field_input: ''
field_instruction: question
field_output: context
format: '{instruction}'
no_input_format: '{instruction}'
system_format: '{system}'
system_prompt: ''
debug: null
deepspeed: null
early_stopping_patience: null
eval_max_new_tokens: 128
eval_table_size: null
evals_per_epoch: 4
flash_attention: true
fp16: null
fsdp: null
fsdp_config: null
gradient_accumulation_steps: 4
gradient_checkpointing: true
gradient_clipping: 1.0
group_by_length: false
hub_model_id: Nexspear/0798b233-1c06-4ca1-b9c8-5c844686d6df
hub_repo: null
hub_strategy: checkpoint
hub_token: null
learning_rate: 5.0e-05
load_in_4bit: false
load_in_8bit: false
local_rank: 0
logging_steps: 3
lora_alpha: 32
lora_dropout: 0.05
lora_fan_in_fan_out: null
lora_model_dir: null
lora_r: 16
lora_target_linear: true
lr_scheduler: cosine
max_steps: 100
micro_batch_size: 8
mlflow_experiment_name: /tmp/c70daf261b4a8f0c_train_data.json
model_type: AutoModelForCausalLM
num_epochs: 3
optimizer: adamw_bnb_8bit
output_dir: miner_id_24
pad_to_sequence_len: true
resume_from_checkpoint: null
s2_attention: null
sample_packing: false
saves_per_epoch: 4
sequence_len: 1024
strict: false
tf32: false
tokenizer_type: AutoTokenizer
train_on_inputs: false
trust_remote_code: true
val_set_size: 0.05
wandb_entity: techspear-hub
wandb_mode: online
wandb_name: 83987365-e4de-4d5e-86a2-818a5944f0b4
wandb_project: Gradients-On-Four
wandb_run: your_name
wandb_runid: 83987365-e4de-4d5e-86a2-818a5944f0b4
warmup_steps: 10
weight_decay: 0.01
xformers_attention: null
```
</details><br>
# 0798b233-1c06-4ca1-b9c8-5c844686d6df
This model is a fine-tuned version of [deepseek-ai/deepseek-coder-6.7b-instruct](https://huggingface.co/deepseek-ai/deepseek-coder-6.7b-instruct) on the None dataset.
It achieves the following results on the evaluation set:
- Loss: 2.0378
## Model description
More information needed
## Intended uses & limitations
More information needed
## Training and evaluation data
More information needed
## Training procedure
### Training hyperparameters
The following hyperparameters were used during training:
- learning_rate: 5e-05
- train_batch_size: 8
- eval_batch_size: 8
- seed: 42
- gradient_accumulation_steps: 4
- total_train_batch_size: 32
- optimizer: Use OptimizerNames.ADAMW_BNB with betas=(0.9,0.999) and epsilon=1e-08 and optimizer_args=No additional optimizer arguments
- lr_scheduler_type: cosine
- lr_scheduler_warmup_steps: 10
- training_steps: 100
### Training results
| Training Loss | Epoch | Step | Validation Loss |
|:-------------:|:------:|:----:|:---------------:|
| No log | 0.0004 | 1 | 2.4607 |
| 2.4399 | 0.0035 | 9 | 2.4403 |
| 2.3826 | 0.0070 | 18 | 2.3071 |
| 2.2498 | 0.0105 | 27 | 2.2249 |
| 2.1752 | 0.0140 | 36 | 2.1642 |
| 2.1862 | 0.0175 | 45 | 2.1195 |
| 2.0415 | 0.0210 | 54 | 2.0872 |
| 2.0806 | 0.0245 | 63 | 2.0645 |
| 2.0701 | 0.0280 | 72 | 2.0500 |
| 1.9885 | 0.0315 | 81 | 2.0419 |
| 2.0554 | 0.0350 | 90 | 2.0385 |
| 2.0638 | 0.0384 | 99 | 2.0378 |
### Framework versions
- PEFT 0.13.2
- Transformers 4.46.0
- Pytorch 2.5.0+cu124
- Datasets 3.0.1
- Tokenizers 0.20.1 |
SHASWATSINGH3101/finetuneGPT2 | SHASWATSINGH3101 | "2024-08-27T17:19:41Z" | 104 | 0 | transformers | [
"transformers",
"safetensors",
"gpt2",
"text-generation",
"arxiv:1910.09700",
"autotrain_compatible",
"text-generation-inference",
"endpoints_compatible",
"region:us"
] | text-generation | "2024-08-27T17:18:32Z" | ---
library_name: transformers
tags: []
---
# Model Card for Model ID
<!-- Provide a quick summary of what the model is/does. -->
## Model Details
### Model Description
<!-- Provide a longer summary of what this model is. -->
This is the model card of a 🤗 transformers model that has been pushed on the Hub. This model card has been automatically generated.
- **Developed by:** [More Information Needed]
- **Funded by [optional]:** [More Information Needed]
- **Shared by [optional]:** [More Information Needed]
- **Model type:** [More Information Needed]
- **Language(s) (NLP):** [More Information Needed]
- **License:** [More Information Needed]
- **Finetuned from model [optional]:** [More Information Needed]
### Model Sources [optional]
<!-- Provide the basic links for the model. -->
- **Repository:** [More Information Needed]
- **Paper [optional]:** [More Information Needed]
- **Demo [optional]:** [More Information Needed]
## Uses
<!-- Address questions around how the model is intended to be used, including the foreseeable users of the model and those affected by the model. -->
### Direct Use
<!-- This section is for the model use without fine-tuning or plugging into a larger ecosystem/app. -->
[More Information Needed]
### Downstream Use [optional]
<!-- This section is for the model use when fine-tuned for a task, or when plugged into a larger ecosystem/app -->
[More Information Needed]
### Out-of-Scope Use
<!-- This section addresses misuse, malicious use, and uses that the model will not work well for. -->
[More Information Needed]
## Bias, Risks, and Limitations
<!-- This section is meant to convey both technical and sociotechnical limitations. -->
[More Information Needed]
### Recommendations
<!-- This section is meant to convey recommendations with respect to the bias, risk, and technical limitations. -->
Users (both direct and downstream) should be made aware of the risks, biases and limitations of the model. More information needed for further recommendations.
## How to Get Started with the Model
Use the code below to get started with the model.
[More Information Needed]
## Training Details
### Training Data
<!-- This should link to a Dataset Card, perhaps with a short stub of information on what the training data is all about as well as documentation related to data pre-processing or additional filtering. -->
[More Information Needed]
### Training Procedure
<!-- This relates heavily to the Technical Specifications. Content here should link to that section when it is relevant to the training procedure. -->
#### Preprocessing [optional]
[More Information Needed]
#### Training Hyperparameters
- **Training regime:** [More Information Needed] <!--fp32, fp16 mixed precision, bf16 mixed precision, bf16 non-mixed precision, fp16 non-mixed precision, fp8 mixed precision -->
#### Speeds, Sizes, Times [optional]
<!-- This section provides information about throughput, start/end time, checkpoint size if relevant, etc. -->
[More Information Needed]
## Evaluation
<!-- This section describes the evaluation protocols and provides the results. -->
### Testing Data, Factors & Metrics
#### Testing Data
<!-- This should link to a Dataset Card if possible. -->
[More Information Needed]
#### Factors
<!-- These are the things the evaluation is disaggregating by, e.g., subpopulations or domains. -->
[More Information Needed]
#### Metrics
<!-- These are the evaluation metrics being used, ideally with a description of why. -->
[More Information Needed]
### Results
[More Information Needed]
#### Summary
## Model Examination [optional]
<!-- Relevant interpretability work for the model goes here -->
[More Information Needed]
## Environmental Impact
<!-- Total emissions (in grams of CO2eq) and additional considerations, such as electricity usage, go here. Edit the suggested text below accordingly -->
Carbon emissions can be estimated using the [Machine Learning Impact calculator](https://mlco2.github.io/impact#compute) presented in [Lacoste et al. (2019)](https://arxiv.org/abs/1910.09700).
- **Hardware Type:** [More Information Needed]
- **Hours used:** [More Information Needed]
- **Cloud Provider:** [More Information Needed]
- **Compute Region:** [More Information Needed]
- **Carbon Emitted:** [More Information Needed]
## Technical Specifications [optional]
### Model Architecture and Objective
[More Information Needed]
### Compute Infrastructure
[More Information Needed]
#### Hardware
[More Information Needed]
#### Software
[More Information Needed]
## Citation [optional]
<!-- If there is a paper or blog post introducing the model, the APA and Bibtex information for that should go in this section. -->
**BibTeX:**
[More Information Needed]
**APA:**
[More Information Needed]
## Glossary [optional]
<!-- If relevant, include terms and calculations in this section that can help readers understand the model or model card. -->
[More Information Needed]
## More Information [optional]
[More Information Needed]
## Model Card Authors [optional]
[More Information Needed]
## Model Card Contact
[More Information Needed] |
concept-unlearning/Llama-2-7b-hf_ft_lora_all_novels_v1_ft_ga_gdr_lora_HICS_kmeans_llm2vec_2000_relative_p20_v1 | concept-unlearning | "2024-11-12T01:26:14Z" | 9 | 0 | transformers | [
"transformers",
"safetensors",
"llama",
"text-generation",
"arxiv:1910.09700",
"autotrain_compatible",
"text-generation-inference",
"endpoints_compatible",
"region:us"
] | text-generation | "2024-11-12T01:23:50Z" | ---
library_name: transformers
tags: []
---
# Model Card for Model ID
<!-- Provide a quick summary of what the model is/does. -->
## Model Details
### Model Description
<!-- Provide a longer summary of what this model is. -->
This is the model card of a 🤗 transformers model that has been pushed on the Hub. This model card has been automatically generated.
- **Developed by:** [More Information Needed]
- **Funded by [optional]:** [More Information Needed]
- **Shared by [optional]:** [More Information Needed]
- **Model type:** [More Information Needed]
- **Language(s) (NLP):** [More Information Needed]
- **License:** [More Information Needed]
- **Finetuned from model [optional]:** [More Information Needed]
### Model Sources [optional]
<!-- Provide the basic links for the model. -->
- **Repository:** [More Information Needed]
- **Paper [optional]:** [More Information Needed]
- **Demo [optional]:** [More Information Needed]
## Uses
<!-- Address questions around how the model is intended to be used, including the foreseeable users of the model and those affected by the model. -->
### Direct Use
<!-- This section is for the model use without fine-tuning or plugging into a larger ecosystem/app. -->
[More Information Needed]
### Downstream Use [optional]
<!-- This section is for the model use when fine-tuned for a task, or when plugged into a larger ecosystem/app -->
[More Information Needed]
### Out-of-Scope Use
<!-- This section addresses misuse, malicious use, and uses that the model will not work well for. -->
[More Information Needed]
## Bias, Risks, and Limitations
<!-- This section is meant to convey both technical and sociotechnical limitations. -->
[More Information Needed]
### Recommendations
<!-- This section is meant to convey recommendations with respect to the bias, risk, and technical limitations. -->
Users (both direct and downstream) should be made aware of the risks, biases and limitations of the model. More information needed for further recommendations.
## How to Get Started with the Model
Use the code below to get started with the model.
[More Information Needed]
## Training Details
### Training Data
<!-- This should link to a Dataset Card, perhaps with a short stub of information on what the training data is all about as well as documentation related to data pre-processing or additional filtering. -->
[More Information Needed]
### Training Procedure
<!-- This relates heavily to the Technical Specifications. Content here should link to that section when it is relevant to the training procedure. -->
#### Preprocessing [optional]
[More Information Needed]
#### Training Hyperparameters
- **Training regime:** [More Information Needed] <!--fp32, fp16 mixed precision, bf16 mixed precision, bf16 non-mixed precision, fp16 non-mixed precision, fp8 mixed precision -->
#### Speeds, Sizes, Times [optional]
<!-- This section provides information about throughput, start/end time, checkpoint size if relevant, etc. -->
[More Information Needed]
## Evaluation
<!-- This section describes the evaluation protocols and provides the results. -->
### Testing Data, Factors & Metrics
#### Testing Data
<!-- This should link to a Dataset Card if possible. -->
[More Information Needed]
#### Factors
<!-- These are the things the evaluation is disaggregating by, e.g., subpopulations or domains. -->
[More Information Needed]
#### Metrics
<!-- These are the evaluation metrics being used, ideally with a description of why. -->
[More Information Needed]
### Results
[More Information Needed]
#### Summary
## Model Examination [optional]
<!-- Relevant interpretability work for the model goes here -->
[More Information Needed]
## Environmental Impact
<!-- Total emissions (in grams of CO2eq) and additional considerations, such as electricity usage, go here. Edit the suggested text below accordingly -->
Carbon emissions can be estimated using the [Machine Learning Impact calculator](https://mlco2.github.io/impact#compute) presented in [Lacoste et al. (2019)](https://arxiv.org/abs/1910.09700).
- **Hardware Type:** [More Information Needed]
- **Hours used:** [More Information Needed]
- **Cloud Provider:** [More Information Needed]
- **Compute Region:** [More Information Needed]
- **Carbon Emitted:** [More Information Needed]
## Technical Specifications [optional]
### Model Architecture and Objective
[More Information Needed]
### Compute Infrastructure
[More Information Needed]
#### Hardware
[More Information Needed]
#### Software
[More Information Needed]
## Citation [optional]
<!-- If there is a paper or blog post introducing the model, the APA and Bibtex information for that should go in this section. -->
**BibTeX:**
[More Information Needed]
**APA:**
[More Information Needed]
## Glossary [optional]
<!-- If relevant, include terms and calculations in this section that can help readers understand the model or model card. -->
[More Information Needed]
## More Information [optional]
[More Information Needed]
## Model Card Authors [optional]
[More Information Needed]
## Model Card Contact
[More Information Needed] |
mirajbhandari/gemma-2b-it_yungri_final | mirajbhandari | "2024-03-09T07:03:56Z" | 91 | 0 | transformers | [
"transformers",
"safetensors",
"gemma",
"text-generation",
"conversational",
"arxiv:1910.09700",
"autotrain_compatible",
"text-generation-inference",
"endpoints_compatible",
"region:us"
] | text-generation | "2024-03-09T07:00:19Z" | ---
library_name: transformers
tags: []
---
# Model Card for Model ID
<!-- Provide a quick summary of what the model is/does. -->
## Model Details
### Model Description
<!-- Provide a longer summary of what this model is. -->
This is the model card of a 🤗 transformers model that has been pushed on the Hub. This model card has been automatically generated.
- **Developed by:** [More Information Needed]
- **Funded by [optional]:** [More Information Needed]
- **Shared by [optional]:** [More Information Needed]
- **Model type:** [More Information Needed]
- **Language(s) (NLP):** [More Information Needed]
- **License:** [More Information Needed]
- **Finetuned from model [optional]:** [More Information Needed]
### Model Sources [optional]
<!-- Provide the basic links for the model. -->
- **Repository:** [More Information Needed]
- **Paper [optional]:** [More Information Needed]
- **Demo [optional]:** [More Information Needed]
## Uses
<!-- Address questions around how the model is intended to be used, including the foreseeable users of the model and those affected by the model. -->
### Direct Use
<!-- This section is for the model use without fine-tuning or plugging into a larger ecosystem/app. -->
[More Information Needed]
### Downstream Use [optional]
<!-- This section is for the model use when fine-tuned for a task, or when plugged into a larger ecosystem/app -->
[More Information Needed]
### Out-of-Scope Use
<!-- This section addresses misuse, malicious use, and uses that the model will not work well for. -->
[More Information Needed]
## Bias, Risks, and Limitations
<!-- This section is meant to convey both technical and sociotechnical limitations. -->
[More Information Needed]
### Recommendations
<!-- This section is meant to convey recommendations with respect to the bias, risk, and technical limitations. -->
Users (both direct and downstream) should be made aware of the risks, biases and limitations of the model. More information needed for further recommendations.
## How to Get Started with the Model
Use the code below to get started with the model.
[More Information Needed]
## Training Details
### Training Data
<!-- This should link to a Dataset Card, perhaps with a short stub of information on what the training data is all about as well as documentation related to data pre-processing or additional filtering. -->
[More Information Needed]
### Training Procedure
<!-- This relates heavily to the Technical Specifications. Content here should link to that section when it is relevant to the training procedure. -->
#### Preprocessing [optional]
[More Information Needed]
#### Training Hyperparameters
- **Training regime:** [More Information Needed] <!--fp32, fp16 mixed precision, bf16 mixed precision, bf16 non-mixed precision, fp16 non-mixed precision, fp8 mixed precision -->
#### Speeds, Sizes, Times [optional]
<!-- This section provides information about throughput, start/end time, checkpoint size if relevant, etc. -->
[More Information Needed]
## Evaluation
<!-- This section describes the evaluation protocols and provides the results. -->
### Testing Data, Factors & Metrics
#### Testing Data
<!-- This should link to a Dataset Card if possible. -->
[More Information Needed]
#### Factors
<!-- These are the things the evaluation is disaggregating by, e.g., subpopulations or domains. -->
[More Information Needed]
#### Metrics
<!-- These are the evaluation metrics being used, ideally with a description of why. -->
[More Information Needed]
### Results
[More Information Needed]
#### Summary
## Model Examination [optional]
<!-- Relevant interpretability work for the model goes here -->
[More Information Needed]
## Environmental Impact
<!-- Total emissions (in grams of CO2eq) and additional considerations, such as electricity usage, go here. Edit the suggested text below accordingly -->
Carbon emissions can be estimated using the [Machine Learning Impact calculator](https://mlco2.github.io/impact#compute) presented in [Lacoste et al. (2019)](https://arxiv.org/abs/1910.09700).
- **Hardware Type:** [More Information Needed]
- **Hours used:** [More Information Needed]
- **Cloud Provider:** [More Information Needed]
- **Compute Region:** [More Information Needed]
- **Carbon Emitted:** [More Information Needed]
## Technical Specifications [optional]
### Model Architecture and Objective
[More Information Needed]
### Compute Infrastructure
[More Information Needed]
#### Hardware
[More Information Needed]
#### Software
[More Information Needed]
## Citation [optional]
<!-- If there is a paper or blog post introducing the model, the APA and Bibtex information for that should go in this section. -->
**BibTeX:**
[More Information Needed]
**APA:**
[More Information Needed]
## Glossary [optional]
<!-- If relevant, include terms and calculations in this section that can help readers understand the model or model card. -->
[More Information Needed]
## More Information [optional]
[More Information Needed]
## Model Card Authors [optional]
[More Information Needed]
## Model Card Contact
[More Information Needed]
|
DaichiT/rebar | DaichiT | "2024-05-29T06:18:24Z" | 29 | 0 | diffusers | [
"diffusers",
"tensorboard",
"safetensors",
"text-to-image",
"dreambooth",
"diffusers-training",
"stable-diffusion",
"stable-diffusion-diffusers",
"base_model:stabilityai/stable-diffusion-2",
"base_model:finetune:stabilityai/stable-diffusion-2",
"license:creativeml-openrail-m",
"autotrain_compatible",
"endpoints_compatible",
"diffusers:StableDiffusionPipeline",
"region:us"
] | text-to-image | "2024-05-29T06:09:00Z" | ---
license: creativeml-openrail-m
library_name: diffusers
tags:
- text-to-image
- dreambooth
- diffusers-training
- stable-diffusion
- stable-diffusion-diffusers
base_model: stabilityai/stable-diffusion-2
inference: true
instance_prompt: a photo of sks rebar
---
<!-- This model card has been generated automatically according to the information the training script had access to. You
should probably proofread and complete it, then remove this comment. -->
# DreamBooth - DaichiT/rebar
This is a dreambooth model derived from stabilityai/stable-diffusion-2. The weights were trained on a photo of sks rebar using [DreamBooth](https://dreambooth.github.io/).
You can find some example images in the following.
DreamBooth for the text encoder was enabled: False.
## Intended uses & limitations
#### How to use
```python
# TODO: add an example code snippet for running this diffusion pipeline
```
#### Limitations and bias
[TODO: provide examples of latent issues and potential remediations]
## Training details
[TODO: describe the data used to train the model] |
irishprancer/bacc7b06-e53d-4b18-917e-d9802ad1ba69 | irishprancer | "2025-02-24T15:02:50Z" | 0 | 0 | transformers | [
"transformers",
"safetensors",
"unsloth",
"arxiv:1910.09700",
"endpoints_compatible",
"region:us"
] | null | "2025-02-24T14:39:27Z" | ---
library_name: transformers
tags:
- unsloth
---
# Model Card for Model ID
<!-- Provide a quick summary of what the model is/does. -->
## Model Details
### Model Description
<!-- Provide a longer summary of what this model is. -->
This is the model card of a 🤗 transformers model that has been pushed on the Hub. This model card has been automatically generated.
- **Developed by:** [More Information Needed]
- **Funded by [optional]:** [More Information Needed]
- **Shared by [optional]:** [More Information Needed]
- **Model type:** [More Information Needed]
- **Language(s) (NLP):** [More Information Needed]
- **License:** [More Information Needed]
- **Finetuned from model [optional]:** [More Information Needed]
### Model Sources [optional]
<!-- Provide the basic links for the model. -->
- **Repository:** [More Information Needed]
- **Paper [optional]:** [More Information Needed]
- **Demo [optional]:** [More Information Needed]
## Uses
<!-- Address questions around how the model is intended to be used, including the foreseeable users of the model and those affected by the model. -->
### Direct Use
<!-- This section is for the model use without fine-tuning or plugging into a larger ecosystem/app. -->
[More Information Needed]
### Downstream Use [optional]
<!-- This section is for the model use when fine-tuned for a task, or when plugged into a larger ecosystem/app -->
[More Information Needed]
### Out-of-Scope Use
<!-- This section addresses misuse, malicious use, and uses that the model will not work well for. -->
[More Information Needed]
## Bias, Risks, and Limitations
<!-- This section is meant to convey both technical and sociotechnical limitations. -->
[More Information Needed]
### Recommendations
<!-- This section is meant to convey recommendations with respect to the bias, risk, and technical limitations. -->
Users (both direct and downstream) should be made aware of the risks, biases and limitations of the model. More information needed for further recommendations.
## How to Get Started with the Model
Use the code below to get started with the model.
[More Information Needed]
## Training Details
### Training Data
<!-- This should link to a Dataset Card, perhaps with a short stub of information on what the training data is all about as well as documentation related to data pre-processing or additional filtering. -->
[More Information Needed]
### Training Procedure
<!-- This relates heavily to the Technical Specifications. Content here should link to that section when it is relevant to the training procedure. -->
#### Preprocessing [optional]
[More Information Needed]
#### Training Hyperparameters
- **Training regime:** [More Information Needed] <!--fp32, fp16 mixed precision, bf16 mixed precision, bf16 non-mixed precision, fp16 non-mixed precision, fp8 mixed precision -->
#### Speeds, Sizes, Times [optional]
<!-- This section provides information about throughput, start/end time, checkpoint size if relevant, etc. -->
[More Information Needed]
## Evaluation
<!-- This section describes the evaluation protocols and provides the results. -->
### Testing Data, Factors & Metrics
#### Testing Data
<!-- This should link to a Dataset Card if possible. -->
[More Information Needed]
#### Factors
<!-- These are the things the evaluation is disaggregating by, e.g., subpopulations or domains. -->
[More Information Needed]
#### Metrics
<!-- These are the evaluation metrics being used, ideally with a description of why. -->
[More Information Needed]
### Results
[More Information Needed]
#### Summary
## Model Examination [optional]
<!-- Relevant interpretability work for the model goes here -->
[More Information Needed]
## Environmental Impact
<!-- Total emissions (in grams of CO2eq) and additional considerations, such as electricity usage, go here. Edit the suggested text below accordingly -->
Carbon emissions can be estimated using the [Machine Learning Impact calculator](https://mlco2.github.io/impact#compute) presented in [Lacoste et al. (2019)](https://arxiv.org/abs/1910.09700).
- **Hardware Type:** [More Information Needed]
- **Hours used:** [More Information Needed]
- **Cloud Provider:** [More Information Needed]
- **Compute Region:** [More Information Needed]
- **Carbon Emitted:** [More Information Needed]
## Technical Specifications [optional]
### Model Architecture and Objective
[More Information Needed]
### Compute Infrastructure
[More Information Needed]
#### Hardware
[More Information Needed]
#### Software
[More Information Needed]
## Citation [optional]
<!-- If there is a paper or blog post introducing the model, the APA and Bibtex information for that should go in this section. -->
**BibTeX:**
[More Information Needed]
**APA:**
[More Information Needed]
## Glossary [optional]
<!-- If relevant, include terms and calculations in this section that can help readers understand the model or model card. -->
[More Information Needed]
## More Information [optional]
[More Information Needed]
## Model Card Authors [optional]
[More Information Needed]
## Model Card Contact
[More Information Needed] |
mci29/sn29_y2m5_gffw | mci29 | "2025-03-18T16:05:09Z" | 0 | 0 | transformers | [
"transformers",
"safetensors",
"llama",
"text-generation",
"arxiv:1910.09700",
"autotrain_compatible",
"text-generation-inference",
"endpoints_compatible",
"region:us"
] | text-generation | "2025-03-18T16:00:36Z" | ---
library_name: transformers
tags: []
---
# Model Card for Model ID
<!-- Provide a quick summary of what the model is/does. -->
## Model Details
### Model Description
<!-- Provide a longer summary of what this model is. -->
This is the model card of a 🤗 transformers model that has been pushed on the Hub. This model card has been automatically generated.
- **Developed by:** [More Information Needed]
- **Funded by [optional]:** [More Information Needed]
- **Shared by [optional]:** [More Information Needed]
- **Model type:** [More Information Needed]
- **Language(s) (NLP):** [More Information Needed]
- **License:** [More Information Needed]
- **Finetuned from model [optional]:** [More Information Needed]
### Model Sources [optional]
<!-- Provide the basic links for the model. -->
- **Repository:** [More Information Needed]
- **Paper [optional]:** [More Information Needed]
- **Demo [optional]:** [More Information Needed]
## Uses
<!-- Address questions around how the model is intended to be used, including the foreseeable users of the model and those affected by the model. -->
### Direct Use
<!-- This section is for the model use without fine-tuning or plugging into a larger ecosystem/app. -->
[More Information Needed]
### Downstream Use [optional]
<!-- This section is for the model use when fine-tuned for a task, or when plugged into a larger ecosystem/app -->
[More Information Needed]
### Out-of-Scope Use
<!-- This section addresses misuse, malicious use, and uses that the model will not work well for. -->
[More Information Needed]
## Bias, Risks, and Limitations
<!-- This section is meant to convey both technical and sociotechnical limitations. -->
[More Information Needed]
### Recommendations
<!-- This section is meant to convey recommendations with respect to the bias, risk, and technical limitations. -->
Users (both direct and downstream) should be made aware of the risks, biases and limitations of the model. More information needed for further recommendations.
## How to Get Started with the Model
Use the code below to get started with the model.
[More Information Needed]
## Training Details
### Training Data
<!-- This should link to a Dataset Card, perhaps with a short stub of information on what the training data is all about as well as documentation related to data pre-processing or additional filtering. -->
[More Information Needed]
### Training Procedure
<!-- This relates heavily to the Technical Specifications. Content here should link to that section when it is relevant to the training procedure. -->
#### Preprocessing [optional]
[More Information Needed]
#### Training Hyperparameters
- **Training regime:** [More Information Needed] <!--fp32, fp16 mixed precision, bf16 mixed precision, bf16 non-mixed precision, fp16 non-mixed precision, fp8 mixed precision -->
#### Speeds, Sizes, Times [optional]
<!-- This section provides information about throughput, start/end time, checkpoint size if relevant, etc. -->
[More Information Needed]
## Evaluation
<!-- This section describes the evaluation protocols and provides the results. -->
### Testing Data, Factors & Metrics
#### Testing Data
<!-- This should link to a Dataset Card if possible. -->
[More Information Needed]
#### Factors
<!-- These are the things the evaluation is disaggregating by, e.g., subpopulations or domains. -->
[More Information Needed]
#### Metrics
<!-- These are the evaluation metrics being used, ideally with a description of why. -->
[More Information Needed]
### Results
[More Information Needed]
#### Summary
## Model Examination [optional]
<!-- Relevant interpretability work for the model goes here -->
[More Information Needed]
## Environmental Impact
<!-- Total emissions (in grams of CO2eq) and additional considerations, such as electricity usage, go here. Edit the suggested text below accordingly -->
Carbon emissions can be estimated using the [Machine Learning Impact calculator](https://mlco2.github.io/impact#compute) presented in [Lacoste et al. (2019)](https://arxiv.org/abs/1910.09700).
- **Hardware Type:** [More Information Needed]
- **Hours used:** [More Information Needed]
- **Cloud Provider:** [More Information Needed]
- **Compute Region:** [More Information Needed]
- **Carbon Emitted:** [More Information Needed]
## Technical Specifications [optional]
### Model Architecture and Objective
[More Information Needed]
### Compute Infrastructure
[More Information Needed]
#### Hardware
[More Information Needed]
#### Software
[More Information Needed]
## Citation [optional]
<!-- If there is a paper or blog post introducing the model, the APA and Bibtex information for that should go in this section. -->
**BibTeX:**
[More Information Needed]
**APA:**
[More Information Needed]
## Glossary [optional]
<!-- If relevant, include terms and calculations in this section that can help readers understand the model or model card. -->
[More Information Needed]
## More Information [optional]
[More Information Needed]
## Model Card Authors [optional]
[More Information Needed]
## Model Card Contact
[More Information Needed] |
tingting/orpheus_3b_4bit__e5_lora | tingting | "2025-03-28T13:59:34Z" | 0 | 0 | transformers | [
"transformers",
"safetensors",
"text-generation-inference",
"unsloth",
"llama",
"trl",
"en",
"base_model:unsloth/orpheus-3b-0.1-ft-unsloth-bnb-4bit",
"base_model:finetune:unsloth/orpheus-3b-0.1-ft-unsloth-bnb-4bit",
"license:apache-2.0",
"endpoints_compatible",
"region:us"
] | null | "2025-03-28T13:58:11Z" | ---
base_model: unsloth/orpheus-3b-0.1-ft-unsloth-bnb-4bit
tags:
- text-generation-inference
- transformers
- unsloth
- llama
- trl
license: apache-2.0
language:
- en
---
# Uploaded model
- **Developed by:** tingting
- **License:** apache-2.0
- **Finetuned from model :** unsloth/orpheus-3b-0.1-ft-unsloth-bnb-4bit
This llama model was trained 2x faster with [Unsloth](https://github.com/unslothai/unsloth) and Huggingface's TRL library.
[<img src="https://raw.githubusercontent.com/unslothai/unsloth/main/images/unsloth%20made%20with%20love.png" width="200"/>](https://github.com/unslothai/unsloth)
|
Weyaxi/Bagel-Hermes-34B-Slerp | Weyaxi | "2024-09-15T12:16:35Z" | 4,547 | 1 | transformers | [
"transformers",
"safetensors",
"llama",
"text-generation",
"mergekit",
"merge",
"conversational",
"license:apache-2.0",
"model-index",
"autotrain_compatible",
"text-generation-inference",
"endpoints_compatible",
"region:us"
] | text-generation | "2024-01-12T17:27:49Z" | ---
license: apache-2.0
tags:
- mergekit
- merge
model-index:
- name: Bagel-Hermes-34B-Slerp
results:
- task:
type: text-generation
name: Text Generation
dataset:
name: AI2 Reasoning Challenge (25-Shot)
type: ai2_arc
config: ARC-Challenge
split: test
args:
num_few_shot: 25
metrics:
- type: acc_norm
value: 70.73
name: normalized accuracy
source:
url: https://huggingface.co/spaces/HuggingFaceH4/open_llm_leaderboard?query=Weyaxi/Bagel-Hermes-34B-Slerp
name: Open LLM Leaderboard
- task:
type: text-generation
name: Text Generation
dataset:
name: HellaSwag (10-Shot)
type: hellaswag
split: validation
args:
num_few_shot: 10
metrics:
- type: acc_norm
value: 85.68
name: normalized accuracy
source:
url: https://huggingface.co/spaces/HuggingFaceH4/open_llm_leaderboard?query=Weyaxi/Bagel-Hermes-34B-Slerp
name: Open LLM Leaderboard
- task:
type: text-generation
name: Text Generation
dataset:
name: MMLU (5-Shot)
type: cais/mmlu
config: all
split: test
args:
num_few_shot: 5
metrics:
- type: acc
value: 77.29
name: accuracy
source:
url: https://huggingface.co/spaces/HuggingFaceH4/open_llm_leaderboard?query=Weyaxi/Bagel-Hermes-34B-Slerp
name: Open LLM Leaderboard
- task:
type: text-generation
name: Text Generation
dataset:
name: TruthfulQA (0-shot)
type: truthful_qa
config: multiple_choice
split: validation
args:
num_few_shot: 0
metrics:
- type: mc2
value: 67.09
source:
url: https://huggingface.co/spaces/HuggingFaceH4/open_llm_leaderboard?query=Weyaxi/Bagel-Hermes-34B-Slerp
name: Open LLM Leaderboard
- task:
type: text-generation
name: Text Generation
dataset:
name: Winogrande (5-shot)
type: winogrande
config: winogrande_xl
split: validation
args:
num_few_shot: 5
metrics:
- type: acc
value: 84.37
name: accuracy
source:
url: https://huggingface.co/spaces/HuggingFaceH4/open_llm_leaderboard?query=Weyaxi/Bagel-Hermes-34B-Slerp
name: Open LLM Leaderboard
- task:
type: text-generation
name: Text Generation
dataset:
name: GSM8k (5-shot)
type: gsm8k
config: main
split: test
args:
num_few_shot: 5
metrics:
- type: acc
value: 66.26
name: accuracy
source:
url: https://huggingface.co/spaces/HuggingFaceH4/open_llm_leaderboard?query=Weyaxi/Bagel-Hermes-34B-Slerp
name: Open LLM Leaderboard
- task:
type: text-generation
name: Text Generation
dataset:
name: IFEval (0-Shot)
type: HuggingFaceH4/ifeval
args:
num_few_shot: 0
metrics:
- type: inst_level_strict_acc and prompt_level_strict_acc
value: 46.03
name: strict accuracy
source:
url: https://huggingface.co/spaces/open-llm-leaderboard/open_llm_leaderboard?query=Weyaxi/Bagel-Hermes-34B-Slerp
name: Open LLM Leaderboard
- task:
type: text-generation
name: Text Generation
dataset:
name: BBH (3-Shot)
type: BBH
args:
num_few_shot: 3
metrics:
- type: acc_norm
value: 41.96
name: normalized accuracy
source:
url: https://huggingface.co/spaces/open-llm-leaderboard/open_llm_leaderboard?query=Weyaxi/Bagel-Hermes-34B-Slerp
name: Open LLM Leaderboard
- task:
type: text-generation
name: Text Generation
dataset:
name: MATH Lvl 5 (4-Shot)
type: hendrycks/competition_math
args:
num_few_shot: 4
metrics:
- type: exact_match
value: 4.91
name: exact match
source:
url: https://huggingface.co/spaces/open-llm-leaderboard/open_llm_leaderboard?query=Weyaxi/Bagel-Hermes-34B-Slerp
name: Open LLM Leaderboard
- task:
type: text-generation
name: Text Generation
dataset:
name: GPQA (0-shot)
type: Idavidrein/gpqa
args:
num_few_shot: 0
metrics:
- type: acc_norm
value: 11.3
name: acc_norm
source:
url: https://huggingface.co/spaces/open-llm-leaderboard/open_llm_leaderboard?query=Weyaxi/Bagel-Hermes-34B-Slerp
name: Open LLM Leaderboard
- task:
type: text-generation
name: Text Generation
dataset:
name: MuSR (0-shot)
type: TAUR-Lab/MuSR
args:
num_few_shot: 0
metrics:
- type: acc_norm
value: 17.01
name: acc_norm
source:
url: https://huggingface.co/spaces/open-llm-leaderboard/open_llm_leaderboard?query=Weyaxi/Bagel-Hermes-34B-Slerp
name: Open LLM Leaderboard
- task:
type: text-generation
name: Text Generation
dataset:
name: MMLU-PRO (5-shot)
type: TIGER-Lab/MMLU-Pro
config: main
split: test
args:
num_few_shot: 5
metrics:
- type: acc
value: 41.15
name: accuracy
source:
url: https://huggingface.co/spaces/open-llm-leaderboard/open_llm_leaderboard?query=Weyaxi/Bagel-Hermes-34B-Slerp
name: Open LLM Leaderboard
---
# Bagel-Hermes-34B-Slerp
This is a merge of pre-trained language models created using [mergekit](https://github.com/cg123/mergekit).
## Merge Details
### Merge Method
This model was merged using the SLERP merge method.
### Models Merged
The following models were included in the merge:
* Nous-Hermes-2-Yi-34B
* bagel-dpo-34b-v0.2
* nontoxic-bagel-34b-v0.2
### Configuration
The following YAML configuration was used to produce this model:
```yaml
slices:
- sources:
- model: bagel-dpo-34b-v0.2
layer_range: [0, 60]
- model: Nous-Hermes-2-Yi-34B
layer_range: [0, 60]
merge_method: slerp
base_model: nontoxic-bagel-34b-v0.2
parameters:
t:
- filter: self_attn
value: [0, 0.5, 0.3, 0.7, 1]
- filter: mlp
value: [1, 0.5, 0.7, 0.3, 0]
- value: 0.5
tokenizer_source: union
dtype: bfloat16
```
# [Open LLM Leaderboard Evaluation Results](https://huggingface.co/spaces/HuggingFaceH4/open_llm_leaderboard)
Detailed results can be found [here](https://huggingface.co/datasets/open-llm-leaderboard/details_Weyaxi__Bagel-Hermes-34B-Slerp)
| Metric |Value|
|---------------------------------|----:|
|Avg. |75.24|
|AI2 Reasoning Challenge (25-Shot)|70.73|
|HellaSwag (10-Shot) |85.68|
|MMLU (5-Shot) |77.29|
|TruthfulQA (0-shot) |67.09|
|Winogrande (5-shot) |84.37|
|GSM8k (5-shot) |66.26|
# [Open LLM Leaderboard Evaluation Results](https://huggingface.co/spaces/open-llm-leaderboard/open_llm_leaderboard)
Detailed results can be found [here](https://huggingface.co/datasets/open-llm-leaderboard/details_Weyaxi__Bagel-Hermes-34B-Slerp)
| Metric |Value|
|-------------------|----:|
|Avg. |27.06|
|IFEval (0-Shot) |46.03|
|BBH (3-Shot) |41.96|
|MATH Lvl 5 (4-Shot)| 4.91|
|GPQA (0-shot) |11.30|
|MuSR (0-shot) |17.01|
|MMLU-PRO (5-shot) |41.15|
|
fffilo/mistral-genre-classificator | fffilo | "2024-03-28T11:24:17Z" | 0 | 0 | peft | [
"peft",
"tensorboard",
"safetensors",
"generated_from_trainer",
"base_model:TheBloke/Mistral-7B-Instruct-v0.2-GPTQ",
"base_model:adapter:TheBloke/Mistral-7B-Instruct-v0.2-GPTQ",
"license:apache-2.0",
"region:us"
] | null | "2024-03-28T11:14:57Z" | ---
license: apache-2.0
library_name: peft
tags:
- generated_from_trainer
base_model: TheBloke/Mistral-7B-Instruct-v0.2-GPTQ
model-index:
- name: mistral-genre-classificator
results: []
---
<!-- This model card has been generated automatically according to the information the Trainer had access to. You
should probably proofread and complete it, then remove this comment. -->
# mistral-genre-classificator
This model is a fine-tuned version of [TheBloke/Mistral-7B-Instruct-v0.2-GPTQ](https://huggingface.co/TheBloke/Mistral-7B-Instruct-v0.2-GPTQ) on an unknown dataset.
It achieves the following results on the evaluation set:
- Loss: 0.1913
## Model description
More information needed
## Intended uses & limitations
More information needed
## Training and evaluation data
More information needed
## Training procedure
### Training hyperparameters
The following hyperparameters were used during training:
- learning_rate: 0.0002
- train_batch_size: 4
- eval_batch_size: 4
- seed: 42
- gradient_accumulation_steps: 4
- total_train_batch_size: 16
- optimizer: Adam with betas=(0.9,0.999) and epsilon=1e-08
- lr_scheduler_type: linear
- lr_scheduler_warmup_steps: 2
- num_epochs: 10
- mixed_precision_training: Native AMP
### Training results
| Training Loss | Epoch | Step | Validation Loss |
|:-------------:|:-----:|:----:|:---------------:|
| 1.5363 | 0.93 | 7 | 1.0741 |
| 0.7837 | 2.0 | 15 | 0.5276 |
| 0.4296 | 2.93 | 22 | 0.2807 |
| 0.2449 | 4.0 | 30 | 0.2262 |
| 0.2452 | 4.93 | 37 | 0.2121 |
| 0.1991 | 6.0 | 45 | 0.2004 |
| 0.2139 | 6.93 | 52 | 0.1960 |
| 0.179 | 8.0 | 60 | 0.1927 |
| 0.1995 | 8.93 | 67 | 0.1914 |
| 0.1553 | 9.33 | 70 | 0.1913 |
### Framework versions
- PEFT 0.10.0
- Transformers 4.38.2
- Pytorch 2.1.0+cu121
- Datasets 2.18.0
- Tokenizers 0.15.2 |
pnparam/loso_F02 | pnparam | "2023-02-19T05:08:44Z" | 3 | 0 | transformers | [
"transformers",
"pytorch",
"tensorboard",
"wav2vec2",
"automatic-speech-recognition",
"generated_from_trainer",
"license:apache-2.0",
"endpoints_compatible",
"region:us"
] | automatic-speech-recognition | "2023-02-19T04:14:48Z" | ---
license: apache-2.0
tags:
- generated_from_trainer
model-index:
- name: loso_F02
results: []
---
<!-- This model card has been generated automatically according to the information the Trainer had access to. You
should probably proofread and complete it, then remove this comment. -->
# loso_F02
This model is a fine-tuned version of [facebook/wav2vec2-xls-r-300m](https://huggingface.co/facebook/wav2vec2-xls-r-300m) on the None dataset.
It achieves the following results on the evaluation set:
- Loss: 0.0784
- Wer: 1.3311
## Model description
More information needed
## Intended uses & limitations
More information needed
## Training and evaluation data
More information needed
## Training procedure
### Training hyperparameters
The following hyperparameters were used during training:
- learning_rate: 0.0001
- train_batch_size: 8
- eval_batch_size: 8
- seed: 42
- optimizer: Adam with betas=(0.9,0.999) and epsilon=1e-08
- lr_scheduler_type: linear
- lr_scheduler_warmup_steps: 1000
- num_epochs: 7
- mixed_precision_training: Native AMP
### Training results
| Training Loss | Epoch | Step | Validation Loss | Wer |
|:-------------:|:-----:|:----:|:---------------:|:------:|
| 9.0203 | 0.96 | 500 | 3.2523 | 1.0 |
| 2.6567 | 1.92 | 1000 | 1.7631 | 2.44 |
| 1.1186 | 2.88 | 1500 | 0.4121 | 2.4 |
| 0.3969 | 3.84 | 2000 | 0.1705 | 1.4533 |
| 0.1635 | 4.8 | 2500 | 0.0970 | 1.68 |
| 0.0915 | 5.76 | 3000 | 0.0874 | 1.4267 |
| 0.0609 | 6.72 | 3500 | 0.0784 | 1.3311 |
### Framework versions
- Transformers 4.17.0
- Pytorch 1.13.1+cu116
- Datasets 1.18.3
- Tokenizers 0.13.2
|
lucdekeijzer/whisper-gronings_2 | lucdekeijzer | "2024-12-07T15:00:12Z" | 79 | 0 | transformers | [
"transformers",
"tensorboard",
"safetensors",
"whisper",
"automatic-speech-recognition",
"generated_from_trainer",
"base_model:openai/whisper-small",
"base_model:finetune:openai/whisper-small",
"license:apache-2.0",
"endpoints_compatible",
"region:us"
] | automatic-speech-recognition | "2024-12-07T12:39:55Z" | ---
library_name: transformers
license: apache-2.0
base_model: openai/whisper-small
tags:
- generated_from_trainer
metrics:
- wer
model-index:
- name: whisper-gronings_2
results: []
---
<!-- This model card has been generated automatically according to the information the Trainer had access to. You
should probably proofread and complete it, then remove this comment. -->
# whisper-gronings_2
This model is a fine-tuned version of [openai/whisper-small](https://huggingface.co/openai/whisper-small) on the None dataset.
It achieves the following results on the evaluation set:
- Loss: 0.5919
- Wer Ortho: 23.3623
- Wer: 23.2729
## Model description
More information needed
## Intended uses & limitations
More information needed
## Training and evaluation data
More information needed
## Training procedure
### Training hyperparameters
The following hyperparameters were used during training:
- learning_rate: 1e-05
- train_batch_size: 8
- eval_batch_size: 16
- seed: 42
- gradient_accumulation_steps: 2
- total_train_batch_size: 16
- optimizer: Use adamw_torch with betas=(0.9,0.999) and epsilon=1e-08 and optimizer_args=No additional optimizer arguments
- lr_scheduler_type: constant_with_warmup
- lr_scheduler_warmup_steps: 50
- training_steps: 1000
- mixed_precision_training: Native AMP
### Training results
| Training Loss | Epoch | Step | Validation Loss | Wer Ortho | Wer |
|:-------------:|:------:|:----:|:---------------:|:---------:|:-------:|
| 0.0403 | 4.6948 | 500 | 0.5270 | 24.7566 | 24.6126 |
| 0.0071 | 9.3897 | 1000 | 0.5919 | 23.3623 | 23.2729 |
### Framework versions
- Transformers 4.46.3
- Pytorch 2.5.1+cu121
- Datasets 3.1.0
- Tokenizers 0.20.3
|
ocisd4/mistral_tokenizer_ext | ocisd4 | "2024-04-10T01:55:24Z" | 0 | 0 | null | [
"region:us"
] | null | "2024-01-15T03:48:24Z" |
Mistral擴充詞表只包含與教育部常用4808字的交集
- 移除dummy token
- 增加`<|func_start|>`, `<|func_end|>`
```python
from transformers import AutoTokenizer
tokenizer = AutoTokenizer.from_pretrained(
'ocisd4/mistral_tokenizer_ext',
pad_token='<unk>',
add_bos_token=True,
add_eos_token=False
)
print('vocab size:', tokenizer.vocab_size)
#vocab size: 35686
print(tokenizer.tokenize('今天天氣真好!'))
#['▁', '今', '天', '天', '氣', '真', '好', '!']
print(tokenizer.encode('今天天氣真好!'))
#[1, 28705, 30316, 29354, 29354, 32004, 29974, 29530, 29267]
print(tokenizer.decode(tokenizer.encode('今天天氣真好!')))
#<s> 今天天氣真好!
```
|
hansyan/perflow-sd15-dreamshaper | hansyan | "2024-03-04T02:46:38Z" | 229 | 0 | diffusers | [
"diffusers",
"safetensors",
"license:cc-by-nc-4.0",
"autotrain_compatible",
"endpoints_compatible",
"diffusers:StableDiffusionPipeline",
"region:us"
] | text-to-image | "2024-02-16T08:30:11Z" | ---
license: cc-by-nc-4.0
---
PeRFlow accelerated [DreamShaper](https://civitai.com/user/Lykon).
Github repo: https://github.com/magic-research/piecewise-rectified-flow
Project page: https://piecewise-rectified-flow.github.io/
|
robiulawaldev/679d4c2d-391f-4bc8-8224-ae02071d60c8 | robiulawaldev | "2025-02-09T08:25:12Z" | 9 | 0 | peft | [
"peft",
"safetensors",
"qwen2",
"axolotl",
"generated_from_trainer",
"base_model:unsloth/Qwen2.5-1.5B-Instruct",
"base_model:adapter:unsloth/Qwen2.5-1.5B-Instruct",
"license:apache-2.0",
"region:us"
] | null | "2025-02-09T06:39:07Z" | ---
library_name: peft
license: apache-2.0
base_model: unsloth/Qwen2.5-1.5B-Instruct
tags:
- axolotl
- generated_from_trainer
model-index:
- name: 679d4c2d-391f-4bc8-8224-ae02071d60c8
results: []
---
<!-- This model card has been generated automatically according to the information the Trainer had access to. You
should probably proofread and complete it, then remove this comment. -->
[<img src="https://raw.githubusercontent.com/axolotl-ai-cloud/axolotl/main/image/axolotl-badge-web.png" alt="Built with Axolotl" width="200" height="32"/>](https://github.com/axolotl-ai-cloud/axolotl)
# 679d4c2d-391f-4bc8-8224-ae02071d60c8
This model is a fine-tuned version of [unsloth/Qwen2.5-1.5B-Instruct](https://huggingface.co/unsloth/Qwen2.5-1.5B-Instruct) on the None dataset.
It achieves the following results on the evaluation set:
- Loss: 1.0748
## Model description
More information needed
## Intended uses & limitations
More information needed
## Training and evaluation data
More information needed
### Framework versions
- PEFT 0.13.2
- Transformers 4.46.0
- Pytorch 2.5.0+cu124
- Datasets 3.0.1
- Tokenizers 0.20.1 |
harish/t5-e2e-5epochs-lr1e4-alpha0-5-BLANKS | harish | "2022-08-11T12:22:42Z" | 7 | 0 | transformers | [
"transformers",
"pytorch",
"t5",
"text2text-generation",
"license:cc-by-nc-sa-4.0",
"autotrain_compatible",
"text-generation-inference",
"endpoints_compatible",
"region:us"
] | text2text-generation | "2022-08-11T12:22:13Z" | ---
license: cc-by-nc-sa-4.0
---
|
Lakoc/voxpopuli_bpe50_cz | Lakoc | "2024-04-24T12:25:53Z" | 0 | 0 | transformers | [
"transformers",
"arxiv:1910.09700",
"endpoints_compatible",
"region:us"
] | null | "2024-04-24T10:45:57Z" | ---
library_name: transformers
tags: []
---
# Model Card for Model ID
<!-- Provide a quick summary of what the model is/does. -->
## Model Details
### Model Description
<!-- Provide a longer summary of what this model is. -->
This is the model card of a 🤗 transformers model that has been pushed on the Hub. This model card has been automatically generated.
- **Developed by:** [More Information Needed]
- **Funded by [optional]:** [More Information Needed]
- **Shared by [optional]:** [More Information Needed]
- **Model type:** [More Information Needed]
- **Language(s) (NLP):** [More Information Needed]
- **License:** [More Information Needed]
- **Finetuned from model [optional]:** [More Information Needed]
### Model Sources [optional]
<!-- Provide the basic links for the model. -->
- **Repository:** [More Information Needed]
- **Paper [optional]:** [More Information Needed]
- **Demo [optional]:** [More Information Needed]
## Uses
<!-- Address questions around how the model is intended to be used, including the foreseeable users of the model and those affected by the model. -->
### Direct Use
<!-- This section is for the model use without fine-tuning or plugging into a larger ecosystem/app. -->
[More Information Needed]
### Downstream Use [optional]
<!-- This section is for the model use when fine-tuned for a task, or when plugged into a larger ecosystem/app -->
[More Information Needed]
### Out-of-Scope Use
<!-- This section addresses misuse, malicious use, and uses that the model will not work well for. -->
[More Information Needed]
## Bias, Risks, and Limitations
<!-- This section is meant to convey both technical and sociotechnical limitations. -->
[More Information Needed]
### Recommendations
<!-- This section is meant to convey recommendations with respect to the bias, risk, and technical limitations. -->
Users (both direct and downstream) should be made aware of the risks, biases and limitations of the model. More information needed for further recommendations.
## How to Get Started with the Model
Use the code below to get started with the model.
[More Information Needed]
## Training Details
### Training Data
<!-- This should link to a Dataset Card, perhaps with a short stub of information on what the training data is all about as well as documentation related to data pre-processing or additional filtering. -->
[More Information Needed]
### Training Procedure
<!-- This relates heavily to the Technical Specifications. Content here should link to that section when it is relevant to the training procedure. -->
#### Preprocessing [optional]
[More Information Needed]
#### Training Hyperparameters
- **Training regime:** [More Information Needed] <!--fp32, fp16 mixed precision, bf16 mixed precision, bf16 non-mixed precision, fp16 non-mixed precision, fp8 mixed precision -->
#### Speeds, Sizes, Times [optional]
<!-- This section provides information about throughput, start/end time, checkpoint size if relevant, etc. -->
[More Information Needed]
## Evaluation
<!-- This section describes the evaluation protocols and provides the results. -->
### Testing Data, Factors & Metrics
#### Testing Data
<!-- This should link to a Dataset Card if possible. -->
[More Information Needed]
#### Factors
<!-- These are the things the evaluation is disaggregating by, e.g., subpopulations or domains. -->
[More Information Needed]
#### Metrics
<!-- These are the evaluation metrics being used, ideally with a description of why. -->
[More Information Needed]
### Results
[More Information Needed]
#### Summary
## Model Examination [optional]
<!-- Relevant interpretability work for the model goes here -->
[More Information Needed]
## Environmental Impact
<!-- Total emissions (in grams of CO2eq) and additional considerations, such as electricity usage, go here. Edit the suggested text below accordingly -->
Carbon emissions can be estimated using the [Machine Learning Impact calculator](https://mlco2.github.io/impact#compute) presented in [Lacoste et al. (2019)](https://arxiv.org/abs/1910.09700).
- **Hardware Type:** [More Information Needed]
- **Hours used:** [More Information Needed]
- **Cloud Provider:** [More Information Needed]
- **Compute Region:** [More Information Needed]
- **Carbon Emitted:** [More Information Needed]
## Technical Specifications [optional]
### Model Architecture and Objective
[More Information Needed]
### Compute Infrastructure
[More Information Needed]
#### Hardware
[More Information Needed]
#### Software
[More Information Needed]
## Citation [optional]
<!-- If there is a paper or blog post introducing the model, the APA and Bibtex information for that should go in this section. -->
**BibTeX:**
[More Information Needed]
**APA:**
[More Information Needed]
## Glossary [optional]
<!-- If relevant, include terms and calculations in this section that can help readers understand the model or model card. -->
[More Information Needed]
## More Information [optional]
[More Information Needed]
## Model Card Authors [optional]
[More Information Needed]
## Model Card Contact
[More Information Needed] |
athirdpath/BigMistral-13b-GLUE_LORA | athirdpath | "2023-12-03T10:44:22Z" | 2 | 1 | peft | [
"peft",
"mistral",
"base_model:athirdpath/BigMistral-13b",
"base_model:adapter:athirdpath/BigMistral-13b",
"4-bit",
"bitsandbytes",
"region:us"
] | null | "2023-12-03T08:48:09Z" | ---
library_name: peft
base_model: athirdpath/BigMistral-13b
---
base_model: athirdpath/BigMistral-13b
model_type: MistralForCausalLM
tokenizer_type: LlamaTokenizer
is_mistral_derived_model: true
load_in_4bit: true
datasets:
- path: glueLORA2.jsonl
-
type: alpaca
val_set_size: 0.07
adapter: qlora
sequence_len: 4096
sample_packing: true
pad_to_sequence_len: true
lora_r: 512
lora_alpha: 32
lora_dropout: 0.04
lora_target_linear: true
gradient_accumulation_steps: 6
micro_batch_size: 3
eval_batch_size: 3
num_epochs: 4
optimizer: adamw_bnb_8bit
lr_scheduler: cosine
learning_rate: 0.00005
bf16: true
gradient_checkpointing: true
flash_attention: true
warmup_steps: 10
weight_decay: 0.00001 |
nhung03/dc967e0d-e333-4756-95c0-05b4efe3f873 | nhung03 | "2025-01-18T13:07:37Z" | 8 | 0 | peft | [
"peft",
"safetensors",
"llama",
"axolotl",
"generated_from_trainer",
"base_model:01-ai/Yi-1.5-9B-Chat-16K",
"base_model:adapter:01-ai/Yi-1.5-9B-Chat-16K",
"license:apache-2.0",
"8-bit",
"bitsandbytes",
"region:us"
] | null | "2025-01-18T12:12:08Z" | ---
library_name: peft
license: apache-2.0
base_model: 01-ai/Yi-1.5-9B-Chat-16K
tags:
- axolotl
- generated_from_trainer
model-index:
- name: dc967e0d-e333-4756-95c0-05b4efe3f873
results: []
---
<!-- This model card has been generated automatically according to the information the Trainer had access to. You
should probably proofread and complete it, then remove this comment. -->
[<img src="https://raw.githubusercontent.com/axolotl-ai-cloud/axolotl/main/image/axolotl-badge-web.png" alt="Built with Axolotl" width="200" height="32"/>](https://github.com/axolotl-ai-cloud/axolotl)
<details><summary>See axolotl config</summary>
axolotl version: `0.4.1`
```yaml
adapter: lora
base_model: 01-ai/Yi-1.5-9B-Chat-16K
bf16: auto
chat_template: llama3
dataset_prepared_path: null
datasets:
- data_files:
- a69727653aeb5a96_train_data.json
ds_type: json
format: custom
path: /workspace/input_data/a69727653aeb5a96_train_data.json
type:
field_instruction: query
field_output: sql
format: '{instruction}'
no_input_format: '{instruction}'
system_format: '{system}'
system_prompt: ''
debug: null
deepspeed: null
early_stopping_patience: null
eval_max_new_tokens: 128
eval_table_size: null
evals_per_epoch: 1
flash_attention: true
fp16: null
fsdp: null
fsdp_config: null
gradient_accumulation_steps: 4
gradient_checkpointing: true
gradient_clipping: 1.0
group_by_length: false
hub_model_id: nhung03/dc967e0d-e333-4756-95c0-05b4efe3f873
hub_repo: null
hub_strategy: end
hub_token: null
learning_rate: 5.0e-05
load_in_4bit: true
load_in_8bit: true
local_rank: null
logging_steps: 1
lora_alpha: 16
lora_dropout: 0.05
lora_fan_in_fan_out: null
lora_model_dir: null
lora_r: 8
lora_target_linear: true
lr_scheduler: cosine
max_steps: 200
micro_batch_size: 2
mlflow_experiment_name: /tmp/a69727653aeb5a96_train_data.json
model_type: AutoModelForCausalLM
num_epochs: 1
optimizer: adamw_bnb_8bit
output_dir: miner_id_24
pad_to_sequence_len: true
resume_from_checkpoint: null
s2_attention: null
sample_packing: false
saves_per_epoch: 1
sequence_len: 1024
strict: false
tf32: false
tokenizer_type: AutoTokenizer
train_on_inputs: false
trust_remote_code: true
val_set_size: 0.05
wandb_entity: null
wandb_mode: online
wandb_name: 2b6712c2-8405-446c-8d89-9baa0704f28c
wandb_project: Gradients-On-Demand
wandb_run: your_name
wandb_runid: 2b6712c2-8405-446c-8d89-9baa0704f28c
warmup_steps: 5
weight_decay: 0.01
xformers_attention: true
```
</details><br>
# dc967e0d-e333-4756-95c0-05b4efe3f873
This model is a fine-tuned version of [01-ai/Yi-1.5-9B-Chat-16K](https://huggingface.co/01-ai/Yi-1.5-9B-Chat-16K) on the None dataset.
It achieves the following results on the evaluation set:
- Loss: 0.5790
## Model description
More information needed
## Intended uses & limitations
More information needed
## Training and evaluation data
More information needed
## Training procedure
### Training hyperparameters
The following hyperparameters were used during training:
- learning_rate: 5e-05
- train_batch_size: 2
- eval_batch_size: 2
- seed: 42
- gradient_accumulation_steps: 4
- total_train_batch_size: 8
- optimizer: Use OptimizerNames.ADAMW_BNB with betas=(0.9,0.999) and epsilon=1e-08 and optimizer_args=No additional optimizer arguments
- lr_scheduler_type: cosine
- lr_scheduler_warmup_steps: 5
- training_steps: 200
### Training results
| Training Loss | Epoch | Step | Validation Loss |
|:-------------:|:------:|:----:|:---------------:|
| 0.5804 | 0.0091 | 200 | 0.5790 |
### Framework versions
- PEFT 0.13.2
- Transformers 4.46.0
- Pytorch 2.5.0+cu124
- Datasets 3.0.1
- Tokenizers 0.20.1 |
Thrisulk/distilbert-base-uncased-finetuned-ner | Thrisulk | "2024-06-01T17:48:32Z" | 62 | 0 | transformers | [
"transformers",
"tf",
"tensorboard",
"distilbert",
"token-classification",
"generated_from_keras_callback",
"base_model:distilbert/distilbert-base-uncased",
"base_model:finetune:distilbert/distilbert-base-uncased",
"license:apache-2.0",
"autotrain_compatible",
"endpoints_compatible",
"region:us"
] | token-classification | "2024-06-01T17:27:46Z" | ---
license: apache-2.0
base_model: distilbert-base-uncased
tags:
- generated_from_keras_callback
model-index:
- name: Thrisulk/distilbert-base-uncased-finetuned-ner
results: []
---
<!-- This model card has been generated automatically according to the information Keras had access to. You should
probably proofread and complete it, then remove this comment. -->
# Thrisulk/distilbert-base-uncased-finetuned-ner
This model is a fine-tuned version of [distilbert-base-uncased](https://huggingface.co/distilbert-base-uncased) on an unknown dataset.
It achieves the following results on the evaluation set:
- Train Loss: 0.0353
- Validation Loss: 0.0596
- Train Precision: 0.9199
- Train Recall: 0.9337
- Train F1: 0.9267
- Train Accuracy: 0.9829
- Epoch: 2
## Model description
More information needed
## Intended uses & limitations
More information needed
## Training and evaluation data
More information needed
## Training procedure
### Training hyperparameters
The following hyperparameters were used during training:
- optimizer: {'name': 'AdamWeightDecay', 'learning_rate': {'module': 'keras.optimizers.schedules', 'class_name': 'PolynomialDecay', 'config': {'initial_learning_rate': 2e-05, 'decay_steps': 2631, 'end_learning_rate': 0.0, 'power': 1.0, 'cycle': False, 'name': None}, 'registered_name': None}, 'decay': 0.0, 'beta_1': 0.9, 'beta_2': 0.999, 'epsilon': 1e-08, 'amsgrad': False, 'weight_decay_rate': 0.01}
- training_precision: float32
### Training results
| Train Loss | Validation Loss | Train Precision | Train Recall | Train F1 | Train Accuracy | Epoch |
|:----------:|:---------------:|:---------------:|:------------:|:--------:|:--------------:|:-----:|
| 0.2022 | 0.0724 | 0.9070 | 0.9161 | 0.9115 | 0.9795 | 0 |
| 0.0563 | 0.0615 | 0.9181 | 0.9304 | 0.9242 | 0.9821 | 1 |
| 0.0353 | 0.0596 | 0.9199 | 0.9337 | 0.9267 | 0.9829 | 2 |
### Framework versions
- Transformers 4.41.1
- TensorFlow 2.15.0
- Datasets 2.19.1
- Tokenizers 0.19.1
|
MatMulMan/bert-base-arabertv02-34213-pretrain_tydi_arcd | MatMulMan | "2024-09-23T15:11:10Z" | 108 | 0 | transformers | [
"transformers",
"safetensors",
"bert",
"question-answering",
"endpoints_compatible",
"region:us"
] | question-answering | "2024-09-23T15:10:45Z" |
---
library_name: transformers
tags: []
---
# bert-base-arabertv02-34213-pretrain_tydi_arcd
# Quran Passage Retrieval Model
This is a **fine-tuned model** on Arabic passage retrieval datasets, used for **Quran QA 2023 Task A**.
## Model Description
This model was fine-tuned to perform text classification on an Arabic dataset. The task involves identifying relevant passages from the Quran in response to specific questions, focusing on retrieval quality.
- **Base model**: Pretrained transformer-based model (e.g., AraBERT, CAMeLBERT, AraELECTRA).
- **Task**: Passage retrieval (text classification).
- **Dataset**: Fine-tuned on the Quran QA 2023 dataset.
## Intended Use
- **Language**: Arabic
- **Task**: Passage retrieval for Quran QA
- **Usage**: Use this model for ranking and retrieving relevant passages from a corpus of Arabic text, primarily for question answering tasks.
## Evaluation Results
- reported in the paper
## How to Use
```python
from transformers import AutoModelForSequenceClassification, AutoTokenizer
model = AutoModelForSequenceClassification.from_pretrained("mohammed-elkomy/quran-qa")
tokenizer = AutoTokenizer.from_pretrained("mohammed-elkomy/quran-qa")
inputs = tokenizer("Your input text", return_tensors="pt")
outputs = model(**inputs)
## Citation
If you use this model, please cite the following:
```
@inproceedings{elkomy2023quran,
title={TCE at Qur’an QA 2023 Shared Task: Low Resource Enhanced Transformer-based Ensemble Approach for Qur’anic QA},
author={Mohammed ElKomy and Amany Sarhan},
year={2023},
url={https://github.com/mohammed-elkomy/quran-qa/},
}
```
```
@inproceedings{elkomy2022quran,
title={TCE at Qur'an QA 2022: Arabic Language Question Answering Over Holy Qur'an Using a Post-Processed Ensemble of BERT-based Models},
author={Mohammed ElKomy and Amany Sarhan},
year={2022},
url={https://github.com/mohammed-elkomy/quran-qa/},
}
```
|
jaymie23/fp16-llama3.1-8b-19 | jaymie23 | "2024-10-12T04:49:50Z" | 5 | 0 | transformers | [
"transformers",
"safetensors",
"llama",
"text-generation",
"custom",
"fp16-llama3.1-8b-19",
"arxiv:1910.09700",
"autotrain_compatible",
"text-generation-inference",
"endpoints_compatible",
"region:us"
] | text-generation | "2024-10-12T00:54:20Z" | ---
library_name: transformers
tags:
- custom
- fp16-llama3.1-8b-19
---
# Model Card for Model ID
<!-- Provide a quick summary of what the model is/does. -->
## Model Details
### Model Description
<!-- Provide a longer summary of what this model is. -->
This is the model card of a 🤗 transformers model that has been pushed on the Hub. This model card has been automatically generated.
- **Developed by:** [More Information Needed]
- **Funded by [optional]:** [More Information Needed]
- **Shared by [optional]:** [More Information Needed]
- **Model type:** [More Information Needed]
- **Language(s) (NLP):** [More Information Needed]
- **License:** [More Information Needed]
- **Finetuned from model [optional]:** [More Information Needed]
### Model Sources [optional]
<!-- Provide the basic links for the model. -->
- **Repository:** [More Information Needed]
- **Paper [optional]:** [More Information Needed]
- **Demo [optional]:** [More Information Needed]
## Uses
<!-- Address questions around how the model is intended to be used, including the foreseeable users of the model and those affected by the model. -->
### Direct Use
<!-- This section is for the model use without fine-tuning or plugging into a larger ecosystem/app. -->
[More Information Needed]
### Downstream Use [optional]
<!-- This section is for the model use when fine-tuned for a task, or when plugged into a larger ecosystem/app -->
[More Information Needed]
### Out-of-Scope Use
<!-- This section addresses misuse, malicious use, and uses that the model will not work well for. -->
[More Information Needed]
## Bias, Risks, and Limitations
<!-- This section is meant to convey both technical and sociotechnical limitations. -->
[More Information Needed]
### Recommendations
<!-- This section is meant to convey recommendations with respect to the bias, risk, and technical limitations. -->
Users (both direct and downstream) should be made aware of the risks, biases and limitations of the model. More information needed for further recommendations.
## How to Get Started with the Model
Use the code below to get started with the model.
[More Information Needed]
## Training Details
### Training Data
<!-- This should link to a Dataset Card, perhaps with a short stub of information on what the training data is all about as well as documentation related to data pre-processing or additional filtering. -->
[More Information Needed]
### Training Procedure
<!-- This relates heavily to the Technical Specifications. Content here should link to that section when it is relevant to the training procedure. -->
#### Preprocessing [optional]
[More Information Needed]
#### Training Hyperparameters
- **Training regime:** [More Information Needed] <!--fp32, fp16 mixed precision, bf16 mixed precision, bf16 non-mixed precision, fp16 non-mixed precision, fp8 mixed precision -->
#### Speeds, Sizes, Times [optional]
<!-- This section provides information about throughput, start/end time, checkpoint size if relevant, etc. -->
[More Information Needed]
## Evaluation
<!-- This section describes the evaluation protocols and provides the results. -->
### Testing Data, Factors & Metrics
#### Testing Data
<!-- This should link to a Dataset Card if possible. -->
[More Information Needed]
#### Factors
<!-- These are the things the evaluation is disaggregating by, e.g., subpopulations or domains. -->
[More Information Needed]
#### Metrics
<!-- These are the evaluation metrics being used, ideally with a description of why. -->
[More Information Needed]
### Results
[More Information Needed]
#### Summary
## Model Examination [optional]
<!-- Relevant interpretability work for the model goes here -->
[More Information Needed]
## Environmental Impact
<!-- Total emissions (in grams of CO2eq) and additional considerations, such as electricity usage, go here. Edit the suggested text below accordingly -->
Carbon emissions can be estimated using the [Machine Learning Impact calculator](https://mlco2.github.io/impact#compute) presented in [Lacoste et al. (2019)](https://arxiv.org/abs/1910.09700).
- **Hardware Type:** [More Information Needed]
- **Hours used:** [More Information Needed]
- **Cloud Provider:** [More Information Needed]
- **Compute Region:** [More Information Needed]
- **Carbon Emitted:** [More Information Needed]
## Technical Specifications [optional]
### Model Architecture and Objective
[More Information Needed]
### Compute Infrastructure
[More Information Needed]
#### Hardware
[More Information Needed]
#### Software
[More Information Needed]
## Citation [optional]
<!-- If there is a paper or blog post introducing the model, the APA and Bibtex information for that should go in this section. -->
**BibTeX:**
[More Information Needed]
**APA:**
[More Information Needed]
## Glossary [optional]
<!-- If relevant, include terms and calculations in this section that can help readers understand the model or model card. -->
[More Information Needed]
## More Information [optional]
[More Information Needed]
## Model Card Authors [optional]
[More Information Needed]
## Model Card Contact
[More Information Needed] |
richardr1126/spider-natsql-wizard-coder-qlora | richardr1126 | "2023-09-20T19:57:15Z" | 13 | 1 | peft | [
"peft",
"tensorboard",
"sql",
"spider",
"text-to-sql",
"sql fine-tune",
"LoRA",
"QLoRa",
"adapter",
"dataset:spider",
"dataset:richardr1126/spider-natsql-context-instruct",
"arxiv:1809.08887",
"arxiv:2109.05153",
"arxiv:2305.14314",
"base_model:WizardLMTeam/WizardCoder-15B-V1.0",
"base_model:adapter:WizardLMTeam/WizardCoder-15B-V1.0",
"license:bigcode-openrail-m",
"region:us"
] | null | "2023-07-05T23:20:25Z" | ---
license: bigcode-openrail-m
library_name: peft
tags:
- sql
- spider
- text-to-sql
- sql fine-tune
- LoRA
- QLoRa
- adapter
datasets:
- spider
- richardr1126/spider-natsql-context-instruct
base_model: WizardLM/WizardCoder-15B-V1.0
---
### Spider NatSQL Wizard Coder QLoRA Adapter Summary
- This QLoRa adapter was created by finetuning [WizardLM/WizardCoder-15B-V1.0](https://huggingface.co/WizardLM/WizardCoder-15B-V1.0) on a NatSQL enhanced Spider context training dataset: [richardr1126/spider-natsql-context-instruct](https://huggingface.co/datasets/richardr1126/spider-natsql-context-instruct).
- Finetuning was performed using QLoRa on a single RTX6000 48GB.
- If you want the merged model: [richardr1126/spider-natsql-wizard-coder-merged](https://huggingface.co/richardr1126/spider-natsql-wizard-coder-merged)
### Spider Dataset
[Spider](https://arxiv.org/abs/1809.08887) is a large-scale complex and cross-domain semantic parsing and text-to-SQL dataset annotated by 11 Yale students
The goal of the Spider challenge is to develop natural language interfaces to cross-domain databases.
This dataset was used to finetune this model.
### NatSQL
[NatSQL](https://arxiv.org/abs/2109.05153) is an intermediate representation for SQL that simplifies the queries and reduces the mismatch between
natural language and SQL. NatSQL preserves the core functionalities of SQL, but removes some clauses and keywords
that are hard to infer from natural language descriptions. NatSQL also makes schema linking easier by reducing the
number of schema items to predict. NatSQL can be easily converted to executable SQL queries and can improve the
performance of text-to-SQL models.
## Citation
Please cite the repo if you use the data or code in this repo.
```
@misc{luo2023wizardcoder,
title={WizardCoder: Empowering Code Large Language Models with Evol-Instruct},
author={Ziyang Luo and Can Xu and Pu Zhao and Qingfeng Sun and Xiubo Geng and Wenxiang Hu and Chongyang Tao and Jing Ma and Qingwei Lin and Daxin Jiang},
year={2023},
}
```
```
@article{yu2018spider,
title={Spider: A large-scale human-labeled dataset for complex and cross-domain semantic parsing and text-to-sql task},
author={Yu, Tao and Zhang, Rui and Yang, Kai and Yasunaga, Michihiro and Wang, Dongxu and Li, Zifan and Ma, James and Li, Irene and Yao, Qingning and Roman, Shanelle and others},
journal={arXiv preprint arXiv:1809.08887},
year={2018}
}
```
```
@inproceedings{gan-etal-2021-natural-sql,
title = "Natural {SQL}: Making {SQL} Easier to Infer from Natural Language Specifications",
author = "Gan, Yujian and
Chen, Xinyun and
Xie, Jinxia and
Purver, Matthew and
Woodward, John R. and
Drake, John and
Zhang, Qiaofu",
booktitle = "Findings of the Association for Computational Linguistics: EMNLP 2021",
month = nov,
year = "2021",
address = "Punta Cana, Dominican Republic",
publisher = "Association for Computational Linguistics",
url = "https://aclanthology.org/2021.findings-emnlp.174",
doi = "10.18653/v1/2021.findings-emnlp.174",
pages = "2030--2042",
}
```
```
@article{dettmers2023qlora,
title={QLoRA: Efficient Finetuning of Quantized LLMs},
author={Dettmers, Tim and Pagnoni, Artidoro and Holtzman, Ari and Zettlemoyer, Luke},
journal={arXiv preprint arXiv:2305.14314},
year={2023}
}
```
## Disclaimer
The resources, including code, data, and model weights, associated with this project are restricted for academic research purposes only and cannot be used for commercial purposes. The content produced by any version of WizardCoder is influenced by uncontrollable variables such as randomness, and therefore, the accuracy of the output cannot be guaranteed by this project. This project does not accept any legal liability for the content of the model output, nor does it assume responsibility for any losses incurred due to the use of associated resources and output results. |
AIFunOver/Llama-2-7b-hf-openvino-fp16 | AIFunOver | "2024-11-07T21:17:59Z" | 9 | 0 | null | [
"pytorch",
"safetensors",
"openvino",
"llama",
"facebook",
"meta",
"llama-2",
"nncf",
"fp16",
"text-generation",
"en",
"base_model:meta-llama/Llama-2-7b-hf",
"base_model:finetune:meta-llama/Llama-2-7b-hf",
"license:llama2",
"region:us"
] | text-generation | "2024-11-07T20:44:53Z" | ---
base_model: meta-llama/Llama-2-7b-hf
language:
- en
license: llama2
pipeline_tag: text-generation
tags:
- facebook
- meta
- pytorch
- llama
- llama-2
- openvino
- nncf
- fp16
extra_gated_heading: You need to share contact information with Meta to access this
model
extra_gated_prompt: "### LLAMA 2 COMMUNITY LICENSE AGREEMENT\n\"Agreement\" means\
\ the terms and conditions for use, reproduction, distribution and modification\
\ of the Llama Materials set forth herein. \n\"Documentation\" means the specifications,\
\ manuals and documentation accompanying Llama 2 distributed by Meta at https://ai.meta.com/resources/models-and-libraries/llama-downloads/.\
\ \n\"Licensee\" or \"you\" means you, or your employer or any other person or\
\ entity (if you are entering into this Agreement on such person or entity's behalf),\
\ of the age required under applicable laws, rules or regulations to provide legal\
\ consent and that has legal authority to bind your employer or such other person\
\ or entity if you are entering in this Agreement on their behalf. \n\"Llama 2\"\
\ means the foundational large language models and software and algorithms, including\
\ machine-learning model code, trained model weights, inference-enabling code, training-enabling\
\ code, fine-tuning enabling code and other elements of the foregoing distributed\
\ by Meta at ai.meta.com/resources/models-and-libraries/llama-downloads/.\n\"Llama\
\ Materials\" means, collectively, Meta's proprietary Llama 2 and documentation\
\ (and any portion thereof) made available under this Agreement.\n\"Meta\" or \"\
we\" means Meta Platforms Ireland Limited (if you are located in or, if you are\
\ an entity, your principal place of business is in the EEA or Switzerland) and\
\ Meta Platforms, Inc. (if you are located outside of the EEA or Switzerland). \n\
\nBy clicking \"I Accept\" below or by using or distributing any portion or element\
\ of the Llama Materials, you agree to be bound by this Agreement.\n1. License Rights\
\ and Redistribution. \na. Grant of Rights. You are granted a non-exclusive, worldwide,\
\ non- transferable and royalty-free limited license under Meta's intellectual property\
\ or other rights owned by Meta embodied in the Llama Materials to use, reproduce,\
\ distribute, copy, create derivative works of, and make modifications to the Llama\
\ Materials. \nb. Redistribution and Use.\ni. If you distribute or make the Llama\
\ Materials, or any derivative works thereof, available to a third party, you shall\
\ provide a copy of this Agreement to such third party. \nii. If you receive Llama\
\ Materials, or any derivative works thereof, from a Licensee as part of an integrated\
\ end user product, then Section 2 of this Agreement will not apply to you. \n\
iii. You must retain in all copies of the Llama Materials that you distribute the\
\ following attribution notice within a \"Notice\" text file distributed as a part\
\ of such copies: \"Llama 2 is licensed under the LLAMA 2 Community License, Copyright\
\ (c) Meta Platforms, Inc. All Rights Reserved.\"\niv. Your use of the Llama Materials\
\ must comply with applicable laws and regulations (including trade compliance\
\ laws and regulations) and adhere to the Acceptable Use Policy for the Llama Materials\
\ (available at https://ai.meta.com/llama/use-policy), which is hereby incorporated\
\ by reference into this Agreement.\nv. You will not use the Llama Materials or\
\ any output or results of the Llama Materials to improve any other large language\
\ model (excluding Llama 2 or derivative works thereof). \n\n2. Additional Commercial\
\ Terms. If, on the Llama 2 version release date, the monthly active users of the\
\ products or services made available by or for Licensee, or Licensee's affiliates,\
\ is greater than 700 million monthly active users in the preceding calendar month,\
\ you must request a license from Meta, which Meta may grant to you in its sole\
\ discretion, and you are not authorized to exercise any of the rights under this\
\ Agreement unless or until Meta otherwise expressly grants you such rights.\n\
3. Disclaimer of Warranty. UNLESS REQUIRED BY APPLICABLE LAW, THE LLAMA MATERIALS\
\ AND ANY OUTPUT AND RESULTS THEREFROM ARE PROVIDED ON AN \"AS IS\" BASIS, WITHOUT\
\ WARRANTIES OF ANY KIND, EITHER EXPRESS OR IMPLIED, INCLUDING, WITHOUT LIMITATION,\
\ ANY WARRANTIES OF TITLE, NON-INFRINGEMENT, MERCHANTABILITY, OR FITNESS FOR A\
\ PARTICULAR PURPOSE. YOU ARE SOLELY RESPONSIBLE FOR DETERMINING THE APPROPRIATENESS\
\ OF USING OR REDISTRIBUTING THE LLAMA MATERIALS AND ASSUME ANY RISKS ASSOCIATED\
\ WITH YOUR USE OF THE LLAMA MATERIALS AND ANY OUTPUT AND RESULTS.\n4. Limitation\
\ of Liability. IN NO EVENT WILL META OR ITS AFFILIATES BE LIABLE UNDER ANY THEORY\
\ OF LIABILITY, WHETHER IN CONTRACT, TORT, NEGLIGENCE, PRODUCTS LIABILITY, OR OTHERWISE,\
\ ARISING OUT OF THIS AGREEMENT, FOR ANY LOST PROFITS OR ANY INDIRECT, SPECIAL,\
\ CONSEQUENTIAL, INCIDENTAL, EXEMPLARY OR PUNITIVE DAMAGES, EVEN IF META OR ITS\
\ AFFILIATES HAVE BEEN ADVISED OF THE POSSIBILITY OF ANY OF THE FOREGOING.\n\n\
5. Intellectual Property.\na. No trademark licenses are granted under this Agreement,\
\ and in connection with the Llama Materials, neither Meta nor Licensee may use\
\ any name or mark owned by or associated with the other or any of its affiliates,\
\ except as required for reasonable and customary use in describing and redistributing\
\ the Llama Materials.\nb. Subject to Meta's ownership of Llama Materials and derivatives\
\ made by or for Meta, with respect to any derivative works and modifications of\
\ the Llama Materials that are made by you, as between you and Meta, you are and\
\ will be the owner of such derivative works and modifications.\nc. If you institute\
\ litigation or other proceedings against Meta or any entity (including a cross-claim\
\ or counterclaim in a lawsuit) alleging that the Llama Materials or Llama 2 outputs\
\ or results, or any portion of any of the foregoing, constitutes infringement\
\ of intellectual property or other rights owned or licensable by you, then any\
\ licenses granted to you under this Agreement shall terminate as of the date such\
\ litigation or claim is filed or instituted. You will indemnify and hold harmless\
\ Meta from and against any claim by any third party arising out of or related \
\ to your use or distribution of the Llama Materials.\n6. Term and Termination.\
\ The term of this Agreement will commence upon your acceptance of this Agreement\
\ or access to the Llama Materials and will continue in full force and effect until\
\ terminated in accordance with the terms and conditions herein. Meta may terminate\
\ this Agreement if you are in breach of any term or condition of this Agreement.\
\ Upon termination of this Agreement, you shall delete and cease use of the Llama\
\ Materials. Sections 3, 4 and 7 shall survive the termination of this Agreement.\
\ \n7. Governing Law and Jurisdiction. This Agreement will be governed and construed\
\ under the laws of the State of California without regard to choice of law principles,\
\ and the UN Convention on Contracts for the International Sale of Goods does not\
\ apply to this Agreement. The courts of California shall have exclusive jurisdiction\
\ of any dispute arising out of this Agreement. \n### Llama 2 Acceptable Use Policy\n\
Meta is committed to promoting safe and fair use of its tools and features, including\
\ Llama 2. If you access or use Llama 2, you agree to this Acceptable Use Policy\
\ (“Policy”). The most recent copy of this policy can be found at [ai.meta.com/llama/use-policy](http://ai.meta.com/llama/use-policy).\n\
#### Prohibited Uses\nWe want everyone to use Llama 2 safely and responsibly. You\
\ agree you will not use, or allow others to use, Llama 2 to:\n1. Violate the law\
\ or others’ rights, including to:\n 1. Engage in, promote, generate, contribute\
\ to, encourage, plan, incite, or further illegal or unlawful activity or content,\
\ such as: \n 1. Violence or terrorism \n 2. Exploitation or harm\
\ to children, including the solicitation, creation, acquisition, or dissemination\
\ of child exploitative content or failure to report Child Sexual Abuse Material\n\
\ 3. Human trafficking, exploitation, and sexual violence\n 4.\
\ The illegal distribution of information or materials to minors, including obscene\
\ materials, or failure to employ legally required age-gating in connection with\
\ such information or materials.\n 5. Sexual solicitation\n 6.\
\ Any other criminal activity\n 2. Engage in, promote, incite, or facilitate\
\ the harassment, abuse, threatening, or bullying of individuals or groups of individuals\n\
\ 3. Engage in, promote, incite, or facilitate discrimination or other unlawful\
\ or harmful conduct in the provision of employment, employment benefits, credit,\
\ housing, other economic benefits, or other essential goods and services\n \
\ 4. Engage in the unauthorized or unlicensed practice of any profession including,\
\ but not limited to, financial, legal, medical/health, or related professional\
\ practices \n 5. Collect, process, disclose, generate, or infer health, demographic,\
\ or other sensitive personal or private information about individuals without rights\
\ and consents required by applicable laws\n 6. Engage in or facilitate any\
\ action or generate any content that infringes, misappropriates, or otherwise violates\
\ any third-party rights, including the outputs or results of any products or services\
\ using the Llama 2 Materials\n 7. Create, generate, or facilitate the creation\
\ of malicious code, malware, computer viruses or do anything else that could disable,\
\ overburden, interfere with or impair the proper working, integrity, operation\
\ or appearance of a website or computer system \n2. Engage in, promote, incite,\
\ facilitate, or assist in the planning or development of activities that present\
\ a risk of death or bodily harm to individuals, including use of Llama 2 related\
\ to the following:\n 1. Military, warfare, nuclear industries or applications,\
\ espionage, use for materials or activities that are subject to the International\
\ Traffic Arms Regulations (ITAR) maintained by the United States Department of\
\ State\n 2. Guns and illegal weapons (including weapon development)\n 3.\
\ Illegal drugs and regulated/controlled substances\n 4. Operation of critical\
\ infrastructure, transportation technologies, or heavy machinery\n 5. Self-harm\
\ or harm to others, including suicide, cutting, and eating disorders\n 6. Any\
\ content intended to incite or promote violence, abuse, or any infliction of bodily\
\ harm to an individual\n3. Intentionally deceive or mislead others, including use\
\ of Llama 2 related to the following:\n 1. Generating, promoting, or furthering\
\ fraud or the creation or promotion of disinformation\n 2. Generating, promoting,\
\ or furthering defamatory content, including the creation of defamatory statements,\
\ images, or other content\n 3. Generating, promoting, or further distributing\
\ spam\n 4. Impersonating another individual without consent, authorization,\
\ or legal right\n 5. Representing that the use of Llama 2 or outputs are human-generated\n\
\ 6. Generating or facilitating false online engagement, including fake reviews\
\ and other means of fake online engagement \n 4. Fail to appropriately disclose\
\ to end users any known dangers of your AI system \nPlease report any violation\
\ of this Policy, software “bug,” or other problems that could lead to a violation\
\ of this Policy through one of the following means: \n * Reporting issues with\
\ the model: [github.com/facebookresearch/llama](http://github.com/facebookresearch/llama)\n\
\ * Reporting risky content generated by the model: [developers.facebook.com/llama_output_feedback](http://developers.facebook.com/llama_output_feedback)\n\
\ * Reporting bugs and security concerns: [facebook.com/whitehat/info](http://facebook.com/whitehat/info)\
\ \n * Reporting violations of the Acceptable Use Policy or unlicensed uses of\
\ Llama: [[email protected]](mailto:[email protected])"
extra_gated_fields:
First Name: text
Last Name: text
Date of birth: date_picker
Country: country
Affiliation: text
geo: ip_location
? By clicking Submit below I accept the terms of the license and acknowledge that
the information I provide will be collected stored processed and shared in accordance
with the Meta Privacy Policy
: checkbox
extra_gated_description: The information you provide will be collected, stored, processed
and shared in accordance with the [Meta Privacy Policy](https://www.facebook.com/privacy/policy/).
extra_gated_button_content: Submit
---
This model is a quantized version of [`meta-llama/Llama-2-7b-hf`](https://huggingface.co/meta-llama/Llama-2-7b-hf) and is converted to the OpenVINO format. This model was obtained via the [nncf-quantization](https://huggingface.co/spaces/echarlaix/nncf-quantization) space with [optimum-intel](https://github.com/huggingface/optimum-intel).
First make sure you have `optimum-intel` installed:
```bash
pip install optimum[openvino]
```
To load your model you can do as follows:
```python
from optimum.intel import OVModelForCausalLM
model_id = "AIFunOver/Llama-2-7b-hf-openvino-fp16"
model = OVModelForCausalLM.from_pretrained(model_id)
```
|
AdapterHub/roberta-base-sst_houlsby | AdapterHub | "2024-05-05T21:15:16Z" | 0 | 0 | adapter-transformers | [
"adapter-transformers",
"text-classification",
"adapterhub:sentiment/sst-2",
"roberta",
"license:apache-2.0",
"region:us"
] | text-classification | "2024-05-05T21:15:13Z" | ---
tags:
- text-classification
- adapter-transformers
- adapterhub:sentiment/sst-2
- roberta
license: "apache-2.0"
---
# Adapter `roberta-base-sst_houlsby` for roberta-base
Adapter (with head) trained using the `run_glue.py` script with an extension that retains the best checkpoint (out of 30 epochs).
**This adapter was created for usage with the [Adapters](https://github.com/Adapter-Hub/adapters) library.**
## Usage
First, install `adapters`:
```
pip install -U adapters
```
Now, the adapter can be loaded and activated like this:
```python
from adapters import AutoAdapterModel
model = AutoAdapterModel.from_pretrained("roberta-base")
adapter_name = model.load_adapter("AdapterHub/roberta-base-sst_houlsby")
model.set_active_adapters(adapter_name)
```
## Architecture & Training
- Adapter architecture: houlsby
- Prediction head: classification
- Dataset: [SST-2](https://nlp.stanford.edu/sentiment/index.html)
## Author Information
- Author name(s): Andreas Rücklé
- Author email: [email protected]
- Author links: [Website](http://rueckle.net), [GitHub](https://github.com/arueckle), [Twitter](https://twitter.com/@arueckle)
## Citation
```bibtex
@article{pfeiffer2020AdapterHub,
title={AdapterHub: A Framework for Adapting Transformers},
author={Jonas Pfeiffer,
Andreas R\"uckl\'{e},
Clifton Poth,
Aishwarya Kamath,
Ivan Vuli\'{c},
Sebastian Ruder,
Kyunghyun Cho,
Iryna Gurevych},
journal={ArXiv},
year={2020}
}
```
*This adapter has been auto-imported from https://github.com/Adapter-Hub/Hub/blob/master/adapters/ukp/roberta-base-sst_houlsby.yaml*. |
tomaszki/llama-4-a | tomaszki | "2024-04-21T21:11:02Z" | 4 | 0 | transformers | [
"transformers",
"safetensors",
"llama",
"text-generation",
"conversational",
"arxiv:1910.09700",
"autotrain_compatible",
"text-generation-inference",
"endpoints_compatible",
"region:us"
] | text-generation | "2024-04-21T21:08:14Z" | ---
library_name: transformers
tags: []
---
# Model Card for Model ID
<!-- Provide a quick summary of what the model is/does. -->
## Model Details
### Model Description
<!-- Provide a longer summary of what this model is. -->
This is the model card of a 🤗 transformers model that has been pushed on the Hub. This model card has been automatically generated.
- **Developed by:** [More Information Needed]
- **Funded by [optional]:** [More Information Needed]
- **Shared by [optional]:** [More Information Needed]
- **Model type:** [More Information Needed]
- **Language(s) (NLP):** [More Information Needed]
- **License:** [More Information Needed]
- **Finetuned from model [optional]:** [More Information Needed]
### Model Sources [optional]
<!-- Provide the basic links for the model. -->
- **Repository:** [More Information Needed]
- **Paper [optional]:** [More Information Needed]
- **Demo [optional]:** [More Information Needed]
## Uses
<!-- Address questions around how the model is intended to be used, including the foreseeable users of the model and those affected by the model. -->
### Direct Use
<!-- This section is for the model use without fine-tuning or plugging into a larger ecosystem/app. -->
[More Information Needed]
### Downstream Use [optional]
<!-- This section is for the model use when fine-tuned for a task, or when plugged into a larger ecosystem/app -->
[More Information Needed]
### Out-of-Scope Use
<!-- This section addresses misuse, malicious use, and uses that the model will not work well for. -->
[More Information Needed]
## Bias, Risks, and Limitations
<!-- This section is meant to convey both technical and sociotechnical limitations. -->
[More Information Needed]
### Recommendations
<!-- This section is meant to convey recommendations with respect to the bias, risk, and technical limitations. -->
Users (both direct and downstream) should be made aware of the risks, biases and limitations of the model. More information needed for further recommendations.
## How to Get Started with the Model
Use the code below to get started with the model.
[More Information Needed]
## Training Details
### Training Data
<!-- This should link to a Dataset Card, perhaps with a short stub of information on what the training data is all about as well as documentation related to data pre-processing or additional filtering. -->
[More Information Needed]
### Training Procedure
<!-- This relates heavily to the Technical Specifications. Content here should link to that section when it is relevant to the training procedure. -->
#### Preprocessing [optional]
[More Information Needed]
#### Training Hyperparameters
- **Training regime:** [More Information Needed] <!--fp32, fp16 mixed precision, bf16 mixed precision, bf16 non-mixed precision, fp16 non-mixed precision, fp8 mixed precision -->
#### Speeds, Sizes, Times [optional]
<!-- This section provides information about throughput, start/end time, checkpoint size if relevant, etc. -->
[More Information Needed]
## Evaluation
<!-- This section describes the evaluation protocols and provides the results. -->
### Testing Data, Factors & Metrics
#### Testing Data
<!-- This should link to a Dataset Card if possible. -->
[More Information Needed]
#### Factors
<!-- These are the things the evaluation is disaggregating by, e.g., subpopulations or domains. -->
[More Information Needed]
#### Metrics
<!-- These are the evaluation metrics being used, ideally with a description of why. -->
[More Information Needed]
### Results
[More Information Needed]
#### Summary
## Model Examination [optional]
<!-- Relevant interpretability work for the model goes here -->
[More Information Needed]
## Environmental Impact
<!-- Total emissions (in grams of CO2eq) and additional considerations, such as electricity usage, go here. Edit the suggested text below accordingly -->
Carbon emissions can be estimated using the [Machine Learning Impact calculator](https://mlco2.github.io/impact#compute) presented in [Lacoste et al. (2019)](https://arxiv.org/abs/1910.09700).
- **Hardware Type:** [More Information Needed]
- **Hours used:** [More Information Needed]
- **Cloud Provider:** [More Information Needed]
- **Compute Region:** [More Information Needed]
- **Carbon Emitted:** [More Information Needed]
## Technical Specifications [optional]
### Model Architecture and Objective
[More Information Needed]
### Compute Infrastructure
[More Information Needed]
#### Hardware
[More Information Needed]
#### Software
[More Information Needed]
## Citation [optional]
<!-- If there is a paper or blog post introducing the model, the APA and Bibtex information for that should go in this section. -->
**BibTeX:**
[More Information Needed]
**APA:**
[More Information Needed]
## Glossary [optional]
<!-- If relevant, include terms and calculations in this section that can help readers understand the model or model card. -->
[More Information Needed]
## More Information [optional]
[More Information Needed]
## Model Card Authors [optional]
[More Information Needed]
## Model Card Contact
[More Information Needed] |
Theodora527/Amphibia-Diffusion | Theodora527 | "2022-12-07T19:24:22Z" | 7 | 9 | diffusers | [
"diffusers",
"license:creativeml-openrail-m",
"autotrain_compatible",
"endpoints_compatible",
"diffusers:StableDiffusionPipeline",
"region:us"
] | text-to-image | "2022-12-07T12:42:04Z" | ---
license: creativeml-openrail-m
---
Use "amphibia" as token. So something like "amphibia, a woman with short puffy hair" or "amphibia, a castle in the middle of a swamp" |
tomer-shimshi/llama2-Rav | tomer-shimshi | "2024-06-08T14:54:41Z" | 6 | 0 | peft | [
"peft",
"safetensors",
"text-generation",
"en",
"arxiv:1910.09700",
"base_model:NousResearch/Llama-2-7b-chat-hf",
"base_model:adapter:NousResearch/Llama-2-7b-chat-hf",
"region:us"
] | text-generation | "2024-05-20T05:05:12Z" | ---
library_name: peft
base_model: NousResearch/Llama-2-7b-chat-hf
language:
- en
pipeline_tag: text-generation
---
# Model Card for Model ID
<!-- Provide a quick summary of what the model is/does. -->
## Model Details
### Model Description
<!-- Provide a longer summary of what this model is. -->
- **Developed by:** tomer-shimshi
- **Funded by [optional]:** [More Information Needed]
- **Shared by [optional]:** [More Information Needed]
- **Model type:** [meta llame 2 -7b]
- **Language(s) (NLP):** English
- **License:** [More Information Needed]
- **Finetuned from model [optional]:** [More Information Needed]
### Model Sources [optional]
<!-- Provide the basic links for the model. -->
- **Repository:** [More Information Needed]
- **Paper [optional]:** [More Information Needed]
- **Demo [optional]:** [More Information Needed]
## Uses
<!-- Address questions around how the model is intended to be used, including the foreseeable users of the model and those affected by the model. -->
To use the full Rav pipline please refer to the attached finale_project_Rav_talk.py file
And for another RAG based Rav please visit the full git hub repo here
https://github.com/TomerShimshi/Project
### Direct Use
<!-- This section is for the model use without fine-tuning or plugging into a larger ecosystem/app. -->
[More Information Needed]
### Downstream Use [optional]
<!-- This section is for the model use when fine-tuned for a task, or when plugged into a larger ecosystem/app -->
[More Information Needed]
### Out-of-Scope Use
<!-- This section addresses misuse, malicious use, and uses that the model will not work well for. -->
[More Information Needed]
## Bias, Risks, and Limitations
<!-- This section is meant to convey both technical and sociotechnical limitations. -->
[More Information Needed]
### Recommendations
<!-- This section is meant to convey recommendations with respect to the bias, risk, and technical limitations. -->
Users (both direct and downstream) should be made aware of the risks, biases and limitations of the model. More information needed for further recommendations.
## How to Get Started with the Model
Use the code below to get started with the model.
[More Information Needed]
## Training Details
### Training Data
<!-- This should link to a Dataset Card, perhaps with a short stub of information on what the training data is all about as well as documentation related to data pre-processing or additional filtering. -->
[More Information Needed]
### Training Procedure
<!-- This relates heavily to the Technical Specifications. Content here should link to that section when it is relevant to the training procedure. -->
#### Preprocessing [optional]
[More Information Needed]
#### Training Hyperparameters
- **Training regime:** [More Information Needed] <!--fp32, fp16 mixed precision, bf16 mixed precision, bf16 non-mixed precision, fp16 non-mixed precision, fp8 mixed precision -->
#### Speeds, Sizes, Times [optional]
<!-- This section provides information about throughput, start/end time, checkpoint size if relevant, etc. -->
[More Information Needed]
## Evaluation
<!-- This section describes the evaluation protocols and provides the results. -->
### Testing Data, Factors & Metrics
#### Testing Data
<!-- This should link to a Dataset Card if possible. -->
[More Information Needed]
#### Factors
<!-- These are the things the evaluation is disaggregating by, e.g., subpopulations or domains. -->
[More Information Needed]
#### Metrics
<!-- These are the evaluation metrics being used, ideally with a description of why. -->
[More Information Needed]
### Results
[More Information Needed]
#### Summary
## Model Examination [optional]
<!-- Relevant interpretability work for the model goes here -->
[More Information Needed]
## Environmental Impact
<!-- Total emissions (in grams of CO2eq) and additional considerations, such as electricity usage, go here. Edit the suggested text below accordingly -->
Carbon emissions can be estimated using the [Machine Learning Impact calculator](https://mlco2.github.io/impact#compute) presented in [Lacoste et al. (2019)](https://arxiv.org/abs/1910.09700).
- **Hardware Type:** [More Information Needed]
- **Hours used:** [More Information Needed]
- **Cloud Provider:** [More Information Needed]
- **Compute Region:** [More Information Needed]
- **Carbon Emitted:** [More Information Needed]
## Technical Specifications [optional]
### Model Architecture and Objective
[More Information Needed]
### Compute Infrastructure
[More Information Needed]
#### Hardware
[More Information Needed]
#### Software
[More Information Needed]
## Citation [optional]
<!-- If there is a paper or blog post introducing the model, the APA and Bibtex information for that should go in this section. -->
**BibTeX:**
[More Information Needed]
**APA:**
[More Information Needed]
## Glossary [optional]
<!-- If relevant, include terms and calculations in this section that can help readers understand the model or model card. -->
[More Information Needed]
## More Information [optional]
[More Information Needed]
## Model Card Authors [optional]
[More Information Needed]
## Model Card Contact
[More Information Needed]
### Framework versions
- PEFT 0.10.0 |
T145/KRONOS-8B-V8 | T145 | "2025-02-01T21:21:27Z" | 48 | 1 | transformers | [
"transformers",
"safetensors",
"llama",
"text-generation",
"mergekit",
"merge",
"conversational",
"arxiv:2403.19522",
"base_model:T145/KRONOS-8B-V1-P3",
"base_model:merge:T145/KRONOS-8B-V1-P3",
"base_model:mukaj/Llama-3.1-Hawkish-8B",
"base_model:merge:mukaj/Llama-3.1-Hawkish-8B",
"base_model:unsloth/Meta-Llama-3.1-8B-Instruct",
"base_model:merge:unsloth/Meta-Llama-3.1-8B-Instruct",
"model-index",
"autotrain_compatible",
"text-generation-inference",
"endpoints_compatible",
"region:us"
] | text-generation | "2025-02-01T14:00:13Z" | ---
base_model:
- T145/KRONOS-8B-V1-P3
- mukaj/Llama-3.1-Hawkish-8B
- unsloth/Meta-Llama-3.1-8B-Instruct
library_name: transformers
tags:
- mergekit
- merge
model-index:
- name: KRONOS-8B-V8
results:
- task:
type: text-generation
name: Text Generation
dataset:
name: IFEval (0-Shot)
type: wis-k/instruction-following-eval
split: train
args:
num_few_shot: 0
metrics:
- type: inst_level_strict_acc and prompt_level_strict_acc
value: 77.7
name: averaged accuracy
source:
url: https://huggingface.co/spaces/open-llm-leaderboard/open_llm_leaderboard#/?search=T145%2FKRONOS-8B-V8
name: Open LLM Leaderboard
- task:
type: text-generation
name: Text Generation
dataset:
name: BBH (3-Shot)
type: SaylorTwift/bbh
split: test
args:
num_few_shot: 3
metrics:
- type: acc_norm
value: 30.05
name: normalized accuracy
source:
url: https://huggingface.co/spaces/open-llm-leaderboard/open_llm_leaderboard#/?search=T145%2FKRONOS-8B-V8
name: Open LLM Leaderboard
- task:
type: text-generation
name: Text Generation
dataset:
name: MATH Lvl 5 (4-Shot)
type: lighteval/MATH-Hard
split: test
args:
num_few_shot: 4
metrics:
- type: exact_match
value: 19.71
name: exact match
source:
url: https://huggingface.co/spaces/open-llm-leaderboard/open_llm_leaderboard#/?search=T145%2FKRONOS-8B-V8
name: Open LLM Leaderboard
- task:
type: text-generation
name: Text Generation
dataset:
name: GPQA (0-shot)
type: Idavidrein/gpqa
split: train
args:
num_few_shot: 0
metrics:
- type: acc_norm
value: 5.26
name: acc_norm
source:
url: https://huggingface.co/spaces/open-llm-leaderboard/open_llm_leaderboard#/?search=T145%2FKRONOS-8B-V8
name: Open LLM Leaderboard
- task:
type: text-generation
name: Text Generation
dataset:
name: MuSR (0-shot)
type: TAUR-Lab/MuSR
args:
num_few_shot: 0
metrics:
- type: acc_norm
value: 8.36
name: acc_norm
source:
url: https://huggingface.co/spaces/open-llm-leaderboard/open_llm_leaderboard#/?search=T145%2FKRONOS-8B-V8
name: Open LLM Leaderboard
- task:
type: text-generation
name: Text Generation
dataset:
name: MMLU-PRO (5-shot)
type: TIGER-Lab/MMLU-Pro
config: main
split: test
args:
num_few_shot: 5
metrics:
- type: acc
value: 30.92
name: accuracy
source:
url: https://huggingface.co/spaces/open-llm-leaderboard/open_llm_leaderboard#/?search=T145%2FKRONOS-8B-V8
name: Open LLM Leaderboard
---
# Untitled Model (1)
This is a merge of pre-trained language models created using [mergekit](https://github.com/cg123/mergekit).
## Merge Details
### Merge Method
This model was merged using the [Model Stock](https://arxiv.org/abs/2403.19522) merge method using [unsloth/Meta-Llama-3.1-8B-Instruct](https://huggingface.co/unsloth/Meta-Llama-3.1-8B-Instruct) as a base.
### Models Merged
The following models were included in the merge:
* [T145/KRONOS-8B-V1-P3](https://huggingface.co/T145/KRONOS-8B-V1-P3)
* [mukaj/Llama-3.1-Hawkish-8B](https://huggingface.co/mukaj/Llama-3.1-Hawkish-8B)
### Configuration
The following YAML configuration was used to produce this model:
```yaml
base_model: unsloth/Meta-Llama-3.1-8B-Instruct
dtype: bfloat16
merge_method: model_stock
slices:
- sources:
- layer_range: [0, 32]
model: T145/KRONOS-8B-V1-P3
- layer_range: [0, 32]
model: mukaj/Llama-3.1-Hawkish-8B
- layer_range: [0, 32]
model: unsloth/Meta-Llama-3.1-8B-Instruct
```
# [Open LLM Leaderboard Evaluation Results](https://huggingface.co/spaces/open-llm-leaderboard/open_llm_leaderboard)
Detailed results can be found [here](https://huggingface.co/datasets/open-llm-leaderboard/T145__KRONOS-8B-V8-details)!
Summarized results can be found [here](https://huggingface.co/datasets/open-llm-leaderboard/contents/viewer/default/train?q=T145%2FKRONOS-8B-V8&sort[column]=Average%20%E2%AC%86%EF%B8%8F&sort[direction]=desc)!
| Metric |Value (%)|
|-------------------|--------:|
|**Average** | 28.67|
|IFEval (0-Shot) | 77.70|
|BBH (3-Shot) | 30.05|
|MATH Lvl 5 (4-Shot)| 19.71|
|GPQA (0-shot) | 5.26|
|MuSR (0-shot) | 8.36|
|MMLU-PRO (5-shot) | 30.92|
|
genki10/ASAP_FineTuningBERT_AugV6_k5_task1_organization_fold2 | genki10 | "2025-02-02T22:37:33Z" | 5 | 0 | transformers | [
"transformers",
"safetensors",
"bert",
"text-classification",
"generated_from_trainer",
"base_model:google-bert/bert-base-uncased",
"base_model:finetune:google-bert/bert-base-uncased",
"license:apache-2.0",
"autotrain_compatible",
"endpoints_compatible",
"region:us"
] | text-classification | "2025-02-02T09:10:56Z" | ---
library_name: transformers
license: apache-2.0
base_model: bert-base-uncased
tags:
- generated_from_trainer
model-index:
- name: ASAP_FineTuningBERT_AugV6_k5_task1_organization_fold2
results: []
---
<!-- This model card has been generated automatically according to the information the Trainer had access to. You
should probably proofread and complete it, then remove this comment. -->
# ASAP_FineTuningBERT_AugV6_k5_task1_organization_fold2
This model is a fine-tuned version of [bert-base-uncased](https://huggingface.co/bert-base-uncased) on the None dataset.
It achieves the following results on the evaluation set:
- Loss: 0.6274
- Qwk: 0.4967
- Mse: 0.6276
- Rmse: 0.7922
## Model description
More information needed
## Intended uses & limitations
More information needed
## Training and evaluation data
More information needed
## Training procedure
### Training hyperparameters
The following hyperparameters were used during training:
- learning_rate: 2e-05
- train_batch_size: 64
- eval_batch_size: 64
- seed: 42
- optimizer: Adam with betas=(0.9,0.999) and epsilon=1e-08
- lr_scheduler_type: linear
- num_epochs: 100
### Training results
| Training Loss | Epoch | Step | Validation Loss | Qwk | Mse | Rmse |
|:-------------:|:-----:|:----:|:---------------:|:------:|:-------:|:------:|
| No log | 0.5 | 2 | 11.7796 | 0.0036 | 11.7792 | 3.4321 |
| No log | 1.0 | 4 | 10.7895 | 0.0 | 10.7891 | 3.2847 |
| No log | 1.5 | 6 | 9.9070 | 0.0 | 9.9067 | 3.1475 |
| No log | 2.0 | 8 | 8.6438 | 0.0 | 8.6434 | 2.9400 |
| 7.8752 | 2.5 | 10 | 7.1567 | 0.0 | 7.1565 | 2.6752 |
| 7.8752 | 3.0 | 12 | 5.9365 | 0.0541 | 5.9363 | 2.4365 |
| 7.8752 | 3.5 | 14 | 4.8800 | 0.0039 | 4.8797 | 2.2090 |
| 7.8752 | 4.0 | 16 | 3.8396 | 0.0 | 3.8395 | 1.9595 |
| 7.8752 | 4.5 | 18 | 2.9717 | 0.0 | 2.9717 | 1.7239 |
| 3.5382 | 5.0 | 20 | 2.3057 | 0.1565 | 2.3057 | 1.5185 |
| 3.5382 | 5.5 | 22 | 1.7358 | 0.0411 | 1.7359 | 1.3175 |
| 3.5382 | 6.0 | 24 | 1.3227 | 0.0107 | 1.3229 | 1.1502 |
| 3.5382 | 6.5 | 26 | 1.1226 | 0.0107 | 1.1228 | 1.0596 |
| 3.5382 | 7.0 | 28 | 1.3254 | 0.0174 | 1.3255 | 1.1513 |
| 1.9038 | 7.5 | 30 | 1.2345 | 0.0174 | 1.2346 | 1.1111 |
| 1.9038 | 8.0 | 32 | 1.1509 | 0.0174 | 1.1511 | 1.0729 |
| 1.9038 | 8.5 | 34 | 0.9894 | 0.0174 | 0.9896 | 0.9948 |
| 1.9038 | 9.0 | 36 | 1.2860 | 0.0475 | 1.2862 | 1.1341 |
| 1.9038 | 9.5 | 38 | 1.3512 | 0.0863 | 1.3514 | 1.1625 |
| 1.7185 | 10.0 | 40 | 0.9662 | 0.1515 | 0.9664 | 0.9831 |
| 1.7185 | 10.5 | 42 | 1.0916 | 0.1833 | 1.0917 | 1.0449 |
| 1.7185 | 11.0 | 44 | 1.1903 | 0.2075 | 1.1904 | 1.0911 |
| 1.7185 | 11.5 | 46 | 0.8310 | 0.3444 | 0.8313 | 0.9117 |
| 1.7185 | 12.0 | 48 | 0.9477 | 0.3292 | 0.9477 | 0.9735 |
| 1.0569 | 12.5 | 50 | 0.7520 | 0.4112 | 0.7520 | 0.8672 |
| 1.0569 | 13.0 | 52 | 0.7206 | 0.4222 | 0.7207 | 0.8489 |
| 1.0569 | 13.5 | 54 | 0.8669 | 0.3525 | 0.8669 | 0.9310 |
| 1.0569 | 14.0 | 56 | 0.6472 | 0.4564 | 0.6474 | 0.8046 |
| 1.0569 | 14.5 | 58 | 0.7666 | 0.4004 | 0.7667 | 0.8756 |
| 0.4513 | 15.0 | 60 | 0.6800 | 0.4969 | 0.6802 | 0.8247 |
| 0.4513 | 15.5 | 62 | 0.8852 | 0.4013 | 0.8853 | 0.9409 |
| 0.4513 | 16.0 | 64 | 0.6905 | 0.4670 | 0.6907 | 0.8311 |
| 0.4513 | 16.5 | 66 | 0.8808 | 0.3921 | 0.8810 | 0.9386 |
| 0.4513 | 17.0 | 68 | 0.7145 | 0.4574 | 0.7148 | 0.8454 |
| 0.2643 | 17.5 | 70 | 1.1367 | 0.3339 | 1.1369 | 1.0663 |
| 0.2643 | 18.0 | 72 | 0.7711 | 0.4486 | 0.7714 | 0.8783 |
| 0.2643 | 18.5 | 74 | 0.8054 | 0.4241 | 0.8058 | 0.8976 |
| 0.2643 | 19.0 | 76 | 0.9591 | 0.3579 | 0.9594 | 0.9795 |
| 0.2643 | 19.5 | 78 | 0.7146 | 0.4560 | 0.7149 | 0.8455 |
| 0.186 | 20.0 | 80 | 0.9512 | 0.3647 | 0.9515 | 0.9754 |
| 0.186 | 20.5 | 82 | 0.7173 | 0.5003 | 0.7176 | 0.8471 |
| 0.186 | 21.0 | 84 | 0.7600 | 0.4691 | 0.7603 | 0.8720 |
| 0.186 | 21.5 | 86 | 0.8846 | 0.4086 | 0.8849 | 0.9407 |
| 0.186 | 22.0 | 88 | 0.7296 | 0.4873 | 0.7299 | 0.8543 |
| 0.1518 | 22.5 | 90 | 0.7952 | 0.4228 | 0.7954 | 0.8919 |
| 0.1518 | 23.0 | 92 | 0.6782 | 0.4887 | 0.6785 | 0.8237 |
| 0.1518 | 23.5 | 94 | 0.7797 | 0.4205 | 0.7799 | 0.8831 |
| 0.1518 | 24.0 | 96 | 0.7851 | 0.4315 | 0.7853 | 0.8862 |
| 0.1518 | 24.5 | 98 | 0.6927 | 0.4816 | 0.6929 | 0.8324 |
| 0.0978 | 25.0 | 100 | 0.8131 | 0.4224 | 0.8133 | 0.9018 |
| 0.0978 | 25.5 | 102 | 0.7242 | 0.4479 | 0.7244 | 0.8511 |
| 0.0978 | 26.0 | 104 | 0.6941 | 0.4600 | 0.6942 | 0.8332 |
| 0.0978 | 26.5 | 106 | 0.7039 | 0.4952 | 0.7041 | 0.8391 |
| 0.0978 | 27.0 | 108 | 0.7043 | 0.5064 | 0.7046 | 0.8394 |
| 0.0988 | 27.5 | 110 | 0.7174 | 0.4744 | 0.7177 | 0.8471 |
| 0.0988 | 28.0 | 112 | 0.6682 | 0.5070 | 0.6685 | 0.8176 |
| 0.0988 | 28.5 | 114 | 0.7541 | 0.4303 | 0.7543 | 0.8685 |
| 0.0988 | 29.0 | 116 | 0.6612 | 0.5053 | 0.6614 | 0.8133 |
| 0.0988 | 29.5 | 118 | 0.6959 | 0.4916 | 0.6961 | 0.8343 |
| 0.0938 | 30.0 | 120 | 0.6830 | 0.4813 | 0.6832 | 0.8266 |
| 0.0938 | 30.5 | 122 | 0.7379 | 0.4640 | 0.7380 | 0.8591 |
| 0.0938 | 31.0 | 124 | 0.6656 | 0.5018 | 0.6658 | 0.8160 |
| 0.0938 | 31.5 | 126 | 0.6744 | 0.4915 | 0.6745 | 0.8213 |
| 0.0938 | 32.0 | 128 | 0.6521 | 0.5140 | 0.6523 | 0.8077 |
| 0.0772 | 32.5 | 130 | 0.7119 | 0.4966 | 0.7120 | 0.8438 |
| 0.0772 | 33.0 | 132 | 0.6568 | 0.5071 | 0.6570 | 0.8106 |
| 0.0772 | 33.5 | 134 | 0.7118 | 0.4674 | 0.7119 | 0.8438 |
| 0.0772 | 34.0 | 136 | 0.6532 | 0.4947 | 0.6534 | 0.8083 |
| 0.0772 | 34.5 | 138 | 0.6425 | 0.4995 | 0.6427 | 0.8017 |
| 0.0722 | 35.0 | 140 | 0.7085 | 0.4656 | 0.7088 | 0.8419 |
| 0.0722 | 35.5 | 142 | 0.6605 | 0.5052 | 0.6608 | 0.8129 |
| 0.0722 | 36.0 | 144 | 0.6839 | 0.4956 | 0.6842 | 0.8271 |
| 0.0722 | 36.5 | 146 | 0.6333 | 0.5087 | 0.6336 | 0.7960 |
| 0.0722 | 37.0 | 148 | 0.6567 | 0.4797 | 0.6569 | 0.8105 |
| 0.0664 | 37.5 | 150 | 0.6350 | 0.5008 | 0.6352 | 0.7970 |
| 0.0664 | 38.0 | 152 | 0.6754 | 0.4908 | 0.6756 | 0.8219 |
| 0.0664 | 38.5 | 154 | 0.6798 | 0.5033 | 0.6801 | 0.8247 |
| 0.0664 | 39.0 | 156 | 0.7527 | 0.4662 | 0.7529 | 0.8677 |
| 0.0664 | 39.5 | 158 | 0.6856 | 0.5025 | 0.6858 | 0.8281 |
| 0.0589 | 40.0 | 160 | 0.6192 | 0.5407 | 0.6194 | 0.7870 |
| 0.0589 | 40.5 | 162 | 0.6467 | 0.5013 | 0.6469 | 0.8043 |
| 0.0589 | 41.0 | 164 | 0.8335 | 0.4597 | 0.8337 | 0.9131 |
| 0.0589 | 41.5 | 166 | 0.7050 | 0.5033 | 0.7052 | 0.8398 |
| 0.0589 | 42.0 | 168 | 0.6644 | 0.5238 | 0.6647 | 0.8153 |
| 0.0751 | 42.5 | 170 | 0.6647 | 0.5216 | 0.6649 | 0.8154 |
| 0.0751 | 43.0 | 172 | 0.7590 | 0.4529 | 0.7592 | 0.8713 |
| 0.0751 | 43.5 | 174 | 0.6592 | 0.5047 | 0.6594 | 0.8120 |
| 0.0751 | 44.0 | 176 | 0.6258 | 0.5316 | 0.6261 | 0.7912 |
| 0.0751 | 44.5 | 178 | 0.6741 | 0.4816 | 0.6743 | 0.8212 |
| 0.0617 | 45.0 | 180 | 0.6952 | 0.4969 | 0.6953 | 0.8339 |
| 0.0617 | 45.5 | 182 | 0.6637 | 0.5015 | 0.6639 | 0.8148 |
| 0.0617 | 46.0 | 184 | 0.7126 | 0.4911 | 0.7128 | 0.8443 |
| 0.0617 | 46.5 | 186 | 0.6529 | 0.5022 | 0.6531 | 0.8081 |
| 0.0617 | 47.0 | 188 | 0.6229 | 0.5097 | 0.6231 | 0.7894 |
| 0.0497 | 47.5 | 190 | 0.6895 | 0.4913 | 0.6896 | 0.8304 |
| 0.0497 | 48.0 | 192 | 0.6878 | 0.4936 | 0.6879 | 0.8294 |
| 0.0497 | 48.5 | 194 | 0.6306 | 0.5053 | 0.6308 | 0.7942 |
| 0.0497 | 49.0 | 196 | 0.6444 | 0.4818 | 0.6445 | 0.8028 |
| 0.0497 | 49.5 | 198 | 0.6434 | 0.4973 | 0.6436 | 0.8022 |
| 0.0515 | 50.0 | 200 | 0.6774 | 0.4872 | 0.6776 | 0.8231 |
| 0.0515 | 50.5 | 202 | 0.6536 | 0.5170 | 0.6538 | 0.8086 |
| 0.0515 | 51.0 | 204 | 0.6575 | 0.5170 | 0.6577 | 0.8110 |
| 0.0515 | 51.5 | 206 | 0.6424 | 0.5117 | 0.6426 | 0.8016 |
| 0.0515 | 52.0 | 208 | 0.6860 | 0.4718 | 0.6862 | 0.8284 |
| 0.0458 | 52.5 | 210 | 0.6461 | 0.4960 | 0.6463 | 0.8040 |
| 0.0458 | 53.0 | 212 | 0.6354 | 0.5047 | 0.6356 | 0.7973 |
| 0.0458 | 53.5 | 214 | 0.7264 | 0.4618 | 0.7266 | 0.8524 |
| 0.0458 | 54.0 | 216 | 0.6955 | 0.4697 | 0.6957 | 0.8341 |
| 0.0458 | 54.5 | 218 | 0.6320 | 0.4866 | 0.6322 | 0.7951 |
| 0.0495 | 55.0 | 220 | 0.6161 | 0.4927 | 0.6162 | 0.7850 |
| 0.0495 | 55.5 | 222 | 0.6227 | 0.4709 | 0.6228 | 0.7892 |
| 0.0495 | 56.0 | 224 | 0.5973 | 0.5120 | 0.5975 | 0.7730 |
| 0.0495 | 56.5 | 226 | 0.6262 | 0.4702 | 0.6263 | 0.7914 |
| 0.0495 | 57.0 | 228 | 0.6762 | 0.4796 | 0.6764 | 0.8224 |
| 0.0489 | 57.5 | 230 | 0.6357 | 0.4959 | 0.6359 | 0.7974 |
| 0.0489 | 58.0 | 232 | 0.6224 | 0.4895 | 0.6225 | 0.7890 |
| 0.0489 | 58.5 | 234 | 0.6086 | 0.4999 | 0.6088 | 0.7803 |
| 0.0489 | 59.0 | 236 | 0.6105 | 0.4838 | 0.6106 | 0.7814 |
| 0.0489 | 59.5 | 238 | 0.6231 | 0.4886 | 0.6233 | 0.7895 |
| 0.0466 | 60.0 | 240 | 0.6266 | 0.5194 | 0.6268 | 0.7917 |
| 0.0466 | 60.5 | 242 | 0.6446 | 0.4843 | 0.6448 | 0.8030 |
| 0.0466 | 61.0 | 244 | 0.6321 | 0.5010 | 0.6323 | 0.7952 |
| 0.0466 | 61.5 | 246 | 0.6176 | 0.4989 | 0.6178 | 0.7860 |
| 0.0466 | 62.0 | 248 | 0.6011 | 0.5112 | 0.6014 | 0.7755 |
| 0.0428 | 62.5 | 250 | 0.6130 | 0.5066 | 0.6132 | 0.7831 |
| 0.0428 | 63.0 | 252 | 0.6183 | 0.5135 | 0.6185 | 0.7865 |
| 0.0428 | 63.5 | 254 | 0.6101 | 0.5299 | 0.6103 | 0.7812 |
| 0.0428 | 64.0 | 256 | 0.6261 | 0.5088 | 0.6262 | 0.7913 |
| 0.0428 | 64.5 | 258 | 0.6253 | 0.5091 | 0.6254 | 0.7908 |
| 0.0439 | 65.0 | 260 | 0.5917 | 0.5182 | 0.5919 | 0.7693 |
| 0.0439 | 65.5 | 262 | 0.5988 | 0.5227 | 0.5989 | 0.7739 |
| 0.0439 | 66.0 | 264 | 0.6455 | 0.4932 | 0.6456 | 0.8035 |
| 0.0439 | 66.5 | 266 | 0.6786 | 0.4835 | 0.6786 | 0.8238 |
| 0.0439 | 67.0 | 268 | 0.6251 | 0.4872 | 0.6252 | 0.7907 |
| 0.0503 | 67.5 | 270 | 0.6234 | 0.5367 | 0.6236 | 0.7897 |
| 0.0503 | 68.0 | 272 | 0.6247 | 0.5075 | 0.6249 | 0.7905 |
| 0.0503 | 68.5 | 274 | 0.6861 | 0.4807 | 0.6863 | 0.8284 |
| 0.0503 | 69.0 | 276 | 0.7266 | 0.4619 | 0.7267 | 0.8525 |
| 0.0503 | 69.5 | 278 | 0.6527 | 0.4804 | 0.6528 | 0.8080 |
| 0.0516 | 70.0 | 280 | 0.6006 | 0.5161 | 0.6008 | 0.7751 |
| 0.0516 | 70.5 | 282 | 0.6024 | 0.5049 | 0.6026 | 0.7763 |
| 0.0516 | 71.0 | 284 | 0.6366 | 0.4903 | 0.6368 | 0.7980 |
| 0.0516 | 71.5 | 286 | 0.6388 | 0.4923 | 0.6390 | 0.7994 |
| 0.0516 | 72.0 | 288 | 0.6106 | 0.5203 | 0.6108 | 0.7816 |
| 0.0376 | 72.5 | 290 | 0.6085 | 0.4988 | 0.6087 | 0.7802 |
| 0.0376 | 73.0 | 292 | 0.6378 | 0.5013 | 0.6379 | 0.7987 |
| 0.0376 | 73.5 | 294 | 0.6414 | 0.5056 | 0.6415 | 0.8010 |
| 0.0376 | 74.0 | 296 | 0.6092 | 0.5038 | 0.6094 | 0.7806 |
| 0.0376 | 74.5 | 298 | 0.6144 | 0.4876 | 0.6146 | 0.7840 |
| 0.0353 | 75.0 | 300 | 0.6445 | 0.4790 | 0.6446 | 0.8029 |
| 0.0353 | 75.5 | 302 | 0.6474 | 0.4653 | 0.6476 | 0.8047 |
| 0.0353 | 76.0 | 304 | 0.6188 | 0.4924 | 0.6190 | 0.7868 |
| 0.0353 | 76.5 | 306 | 0.6144 | 0.4864 | 0.6146 | 0.7840 |
| 0.0353 | 77.0 | 308 | 0.6160 | 0.4898 | 0.6162 | 0.7850 |
| 0.039 | 77.5 | 310 | 0.6201 | 0.4831 | 0.6203 | 0.7876 |
| 0.039 | 78.0 | 312 | 0.6108 | 0.4807 | 0.6110 | 0.7816 |
| 0.039 | 78.5 | 314 | 0.6113 | 0.4883 | 0.6115 | 0.7820 |
| 0.039 | 79.0 | 316 | 0.6165 | 0.5003 | 0.6167 | 0.7853 |
| 0.039 | 79.5 | 318 | 0.6328 | 0.4957 | 0.6330 | 0.7956 |
| 0.0312 | 80.0 | 320 | 0.6177 | 0.4998 | 0.6179 | 0.7861 |
| 0.0312 | 80.5 | 322 | 0.6178 | 0.5127 | 0.6180 | 0.7861 |
| 0.0312 | 81.0 | 324 | 0.6331 | 0.5011 | 0.6332 | 0.7958 |
| 0.0312 | 81.5 | 326 | 0.6579 | 0.4918 | 0.6581 | 0.8112 |
| 0.0312 | 82.0 | 328 | 0.6403 | 0.4971 | 0.6405 | 0.8003 |
| 0.0333 | 82.5 | 330 | 0.6143 | 0.4959 | 0.6145 | 0.7839 |
| 0.0333 | 83.0 | 332 | 0.6153 | 0.5027 | 0.6154 | 0.7845 |
| 0.0333 | 83.5 | 334 | 0.6359 | 0.5092 | 0.6360 | 0.7975 |
| 0.0333 | 84.0 | 336 | 0.6306 | 0.4942 | 0.6308 | 0.7942 |
| 0.0333 | 84.5 | 338 | 0.6131 | 0.4961 | 0.6132 | 0.7831 |
| 0.0324 | 85.0 | 340 | 0.6139 | 0.5102 | 0.6141 | 0.7836 |
| 0.0324 | 85.5 | 342 | 0.6230 | 0.4965 | 0.6232 | 0.7894 |
| 0.0324 | 86.0 | 344 | 0.6462 | 0.4938 | 0.6464 | 0.8040 |
| 0.0324 | 86.5 | 346 | 0.6454 | 0.4938 | 0.6455 | 0.8034 |
| 0.0324 | 87.0 | 348 | 0.6248 | 0.4944 | 0.6250 | 0.7906 |
| 0.0326 | 87.5 | 350 | 0.6132 | 0.4995 | 0.6134 | 0.7832 |
| 0.0326 | 88.0 | 352 | 0.6134 | 0.5072 | 0.6136 | 0.7833 |
| 0.0326 | 88.5 | 354 | 0.6208 | 0.4973 | 0.6210 | 0.7880 |
| 0.0326 | 89.0 | 356 | 0.6344 | 0.5005 | 0.6346 | 0.7966 |
| 0.0326 | 89.5 | 358 | 0.6314 | 0.4991 | 0.6316 | 0.7947 |
| 0.03 | 90.0 | 360 | 0.6308 | 0.4982 | 0.6309 | 0.7943 |
| 0.03 | 90.5 | 362 | 0.6266 | 0.4968 | 0.6268 | 0.7917 |
| 0.03 | 91.0 | 364 | 0.6173 | 0.4988 | 0.6175 | 0.7858 |
| 0.03 | 91.5 | 366 | 0.6110 | 0.5003 | 0.6111 | 0.7817 |
| 0.03 | 92.0 | 368 | 0.6132 | 0.5013 | 0.6134 | 0.7832 |
| 0.0308 | 92.5 | 370 | 0.6212 | 0.4953 | 0.6214 | 0.7883 |
| 0.0308 | 93.0 | 372 | 0.6322 | 0.4995 | 0.6323 | 0.7952 |
| 0.0308 | 93.5 | 374 | 0.6306 | 0.5010 | 0.6307 | 0.7942 |
| 0.0308 | 94.0 | 376 | 0.6202 | 0.4929 | 0.6204 | 0.7877 |
| 0.0308 | 94.5 | 378 | 0.6129 | 0.4984 | 0.6130 | 0.7830 |
| 0.0302 | 95.0 | 380 | 0.6129 | 0.4959 | 0.6131 | 0.7830 |
| 0.0302 | 95.5 | 382 | 0.6159 | 0.5023 | 0.6160 | 0.7849 |
| 0.0302 | 96.0 | 384 | 0.6174 | 0.5032 | 0.6176 | 0.7859 |
| 0.0302 | 96.5 | 386 | 0.6169 | 0.4998 | 0.6171 | 0.7856 |
| 0.0302 | 97.0 | 388 | 0.6194 | 0.4983 | 0.6195 | 0.7871 |
| 0.0312 | 97.5 | 390 | 0.6225 | 0.5012 | 0.6226 | 0.7891 |
| 0.0312 | 98.0 | 392 | 0.6255 | 0.4954 | 0.6256 | 0.7910 |
| 0.0312 | 98.5 | 394 | 0.6266 | 0.4967 | 0.6268 | 0.7917 |
| 0.0312 | 99.0 | 396 | 0.6275 | 0.4967 | 0.6277 | 0.7923 |
| 0.0312 | 99.5 | 398 | 0.6274 | 0.4967 | 0.6275 | 0.7922 |
| 0.0276 | 100.0 | 400 | 0.6274 | 0.4967 | 0.6276 | 0.7922 |
### Framework versions
- Transformers 4.44.2
- Pytorch 2.4.1+cu121
- Datasets 3.2.0
- Tokenizers 0.19.1
|
localmodels/Llama-2-7B-ggml | localmodels | "2023-07-19T02:08:33Z" | 0 | 11 | null | [
"region:us"
] | null | "2023-07-18T17:47:24Z" | ---
duplicated_from: localmodels/LLM
---
# Llama 2 7B ggml
From: https://huggingface.co/meta-llama/Llama-2-7b-hf
---
### Original llama.cpp quant methods: `q4_0, q4_1, q5_0, q5_1, q8_0`
Quantized using an older version of llama.cpp and compatible with llama.cpp from May 19, commit 2d5db48.
### k-quant methods: `q2_K, q3_K_S, q3_K_M, q3_K_L, q4_K_S, q4_K_M, q5_K_S, q6_K`
Quantization methods compatible with latest llama.cpp from June 6, commit 2d43387.
---
## Provided files
| Name | Quant method | Bits | Size | Max RAM required, no GPU offloadingd | Use case |
| ---- | ---- | ---- | ---- | ---- | ----- |
| llama-2-7b.ggmlv3.q2_K.bin | q2_K | 2 | 2.87 GB| 5.37 GB | New k-quant method. Uses GGML_TYPE_Q4_K for the attention.vw and feed_forward.w2 tensors, GGML_TYPE_Q2_K for the other tensors. |
| llama-2-7b.ggmlv3.q3_K_L.bin | q3_K_L | 3 | 3.60 GB| 6.10 GB | New k-quant method. Uses GGML_TYPE_Q5_K for the attention.wv, attention.wo, and feed_forward.w2 tensors, else GGML_TYPE_Q3_K |
| llama-2-7b.ggmlv3.q3_K_M.bin | q3_K_M | 3 | 3.28 GB| 5.78 GB | New k-quant method. Uses GGML_TYPE_Q4_K for the attention.wv, attention.wo, and feed_forward.w2 tensors, else GGML_TYPE_Q3_K |
| llama-2-7b.ggmlv3.q3_K_S.bin | q3_K_S | 3 | 2.95 GB| 5.45 GB | New k-quant method. Uses GGML_TYPE_Q3_K for all tensors |
| llama-2-7b.ggmlv3.q4_0.bin | q4_0 | 4 | 3.79 GB| 6.29 GB | Original quant method, 4-bit. |
| llama-2-7b.ggmlv3.q4_1.bin | q4_1 | 4 | 4.21 GB| 6.71 GB | Original quant method, 4-bit. Higher accuracy than q4_0 but not as high as q5_0. However has quicker inference than q5 models. |
| llama-2-7b.ggmlv3.q4_K_M.bin | q4_K_M | 4 | 4.08 GB| 6.58 GB | New k-quant method. Uses GGML_TYPE_Q6_K for half of the attention.wv and feed_forward.w2 tensors, else GGML_TYPE_Q4_K |
| llama-2-7b.ggmlv3.q4_K_S.bin | q4_K_S | 4 | 3.83 GB| 6.33 GB | New k-quant method. Uses GGML_TYPE_Q4_K for all tensors |
| llama-2-7b.ggmlv3.q5_0.bin | q5_0 | 5 | 4.63 GB| 7.13 GB | Original quant method, 5-bit. Higher accuracy, higher resource usage and slower inference. |
| llama-2-7b.ggmlv3.q5_1.bin | q5_1 | 5 | 5.06 GB| 7.56 GB | Original quant method, 5-bit. Even higher accuracy, resource usage and slower inference. |
| llama-2-7b.ggmlv3.q5_K_M.bin | q5_K_M | 5 | 4.78 GB| 7.28 GB | New k-quant method. Uses GGML_TYPE_Q6_K for half of the attention.wv and feed_forward.w2 tensors, else GGML_TYPE_Q5_K |
| llama-2-7b.ggmlv3.q5_K_S.bin | q5_K_S | 5 | 4.65 GB| 7.15 GB | New k-quant method. Uses GGML_TYPE_Q5_K for all tensors |
| llama-2-7b.ggmlv3.q6_K.bin | q6_K | 6 | 5.53 GB| 8.03 GB | New k-quant method. Uses GGML_TYPE_Q8_K for all tensors - 6-bit quantization |
| llama-2-7b.ggmlv3.q8_0.bin | q8_0 | 8 | 7.16 GB| 9.66 GB | Original quant method, 8-bit. Almost indistinguishable from float16. High resource use and slow. Not recommended for most users. | |
xihean/Kaneki-Ken | xihean | "2023-12-07T12:44:50Z" | 0 | 0 | null | [
"license:openrail",
"region:us"
] | null | "2023-12-04T15:26:02Z" | ---
license: openrail
---
This is the voice of Kaneki Ken from the anime Tokyo Ghoul. 300 epochs.
To use go check this video https://youtu.be/xwdbwbtO-FQ?si=2UwzR2rKAg1Z_9Po. Or this https://youtu.be/7Wd2C9BaJwA?si=wTggFIepxRQWlc-B (on russian).
Буду благодарен, если вы присоединитесь к этому анархичному дискорд серверу, на котором нету каких-либо правил и можно говорить и делать все, что душе угодно. (I would be grateful if you join this anarchic discord server, where there are no rules and you can shout and do whatever your heart desires.) https://discord.gg/p3BgSZ6FDW |
shiv2050/test_trainer | shiv2050 | "2024-03-26T11:38:06Z" | 165 | 1 | transformers | [
"transformers",
"tensorboard",
"safetensors",
"bert",
"text-classification",
"generated_from_trainer",
"base_model:google-bert/bert-base-cased",
"base_model:finetune:google-bert/bert-base-cased",
"license:apache-2.0",
"autotrain_compatible",
"endpoints_compatible",
"region:us"
] | text-classification | "2024-03-26T11:37:26Z" | ---
license: apache-2.0
base_model: bert-base-cased
tags:
- generated_from_trainer
metrics:
- accuracy
model-index:
- name: test_trainer
results: []
---
<!-- This model card has been generated automatically according to the information the Trainer had access to. You
should probably proofread and complete it, then remove this comment. -->
# test_trainer
This model is a fine-tuned version of [bert-base-cased](https://huggingface.co/bert-base-cased) on an unknown dataset.
It achieves the following results on the evaluation set:
- Loss: 1.0439
- Accuracy: 0.588
## Model description
More information needed
## Intended uses & limitations
More information needed
## Training and evaluation data
More information needed
## Training procedure
### Training hyperparameters
The following hyperparameters were used during training:
- learning_rate: 5e-05
- train_batch_size: 8
- eval_batch_size: 8
- seed: 42
- optimizer: Adam with betas=(0.9,0.999) and epsilon=1e-08
- lr_scheduler_type: linear
- num_epochs: 3.0
### Training results
| Training Loss | Epoch | Step | Validation Loss | Accuracy |
|:-------------:|:-----:|:----:|:---------------:|:--------:|
| No log | 1.0 | 125 | 1.1048 | 0.533 |
| No log | 2.0 | 250 | 0.9990 | 0.572 |
| No log | 3.0 | 375 | 1.0439 | 0.588 |
### Framework versions
- Transformers 4.38.2
- Pytorch 2.2.1+cu121
- Datasets 2.18.0
- Tokenizers 0.15.2
|
lilykaw/finetuning-sentiment-model-3000-samples | lilykaw | "2022-10-03T18:14:03Z" | 105 | 0 | transformers | [
"transformers",
"pytorch",
"tensorboard",
"distilbert",
"text-classification",
"generated_from_trainer",
"dataset:imdb",
"license:apache-2.0",
"model-index",
"autotrain_compatible",
"endpoints_compatible",
"region:us"
] | text-classification | "2022-10-03T18:04:22Z" | ---
license: apache-2.0
tags:
- generated_from_trainer
datasets:
- imdb
metrics:
- accuracy
- f1
model-index:
- name: finetuning-sentiment-model-3000-samples
results:
- task:
name: Text Classification
type: text-classification
dataset:
name: imdb
type: imdb
config: plain_text
split: train
args: plain_text
metrics:
- name: Accuracy
type: accuracy
value: 0.6633333333333333
- name: F1
type: f1
value: 0.7247956403269755
---
<!-- This model card has been generated automatically according to the information the Trainer had access to. You
should probably proofread and complete it, then remove this comment. -->
# finetuning-sentiment-model-3000-samples
This model is a fine-tuned version of [distilbert-base-uncased](https://huggingface.co/distilbert-base-uncased) on the imdb dataset.
It achieves the following results on the evaluation set:
- Loss: 0.6551
- Accuracy: 0.6633
- F1: 0.7248
## Model description
More information needed
## Intended uses & limitations
More information needed
## Training and evaluation data
More information needed
## Training procedure
### Training hyperparameters
The following hyperparameters were used during training:
- learning_rate: 2e-05
- train_batch_size: 16
- eval_batch_size: 16
- seed: 42
- optimizer: Adam with betas=(0.9,0.999) and epsilon=1e-08
- lr_scheduler_type: linear
- num_epochs: 2
### Training results
### Framework versions
- Transformers 4.22.2
- Pytorch 1.12.1+cu113
- Datasets 2.5.1
- Tokenizers 0.12.1
|
lesso07/5a03199b-d6b5-430d-af69-cf2ed381f486 | lesso07 | "2025-03-03T11:59:21Z" | 9 | 0 | peft | [
"peft",
"safetensors",
"qwen2",
"axolotl",
"generated_from_trainer",
"base_model:Qwen/Qwen2.5-Coder-7B-Instruct",
"base_model:adapter:Qwen/Qwen2.5-Coder-7B-Instruct",
"license:apache-2.0",
"region:us"
] | null | "2025-03-02T02:23:26Z" | ---
library_name: peft
license: apache-2.0
base_model: Qwen/Qwen2.5-Coder-7B-Instruct
tags:
- axolotl
- generated_from_trainer
model-index:
- name: 5a03199b-d6b5-430d-af69-cf2ed381f486
results: []
---
<!-- This model card has been generated automatically according to the information the Trainer had access to. You
should probably proofread and complete it, then remove this comment. -->
[<img src="https://raw.githubusercontent.com/axolotl-ai-cloud/axolotl/main/image/axolotl-badge-web.png" alt="Built with Axolotl" width="200" height="32"/>](https://github.com/axolotl-ai-cloud/axolotl)
<br>
# 5a03199b-d6b5-430d-af69-cf2ed381f486
This model is a fine-tuned version of [Qwen/Qwen2.5-Coder-7B-Instruct](https://huggingface.co/Qwen/Qwen2.5-Coder-7B-Instruct) on the None dataset.
It achieves the following results on the evaluation set:
- Loss: 1.0532
## Model description
More information needed
## Intended uses & limitations
More information needed
## Training and evaluation data
More information needed
## Training procedure
### Training hyperparameters
The following hyperparameters were used during training:
- learning_rate: 0.000207
- train_batch_size: 4
- eval_batch_size: 4
- seed: 70
- gradient_accumulation_steps: 2
- total_train_batch_size: 8
- optimizer: Use OptimizerNames.ADAMW_BNB with betas=(0.9,0.999) and epsilon=1e-08 and optimizer_args=No additional optimizer arguments
- lr_scheduler_type: cosine
- lr_scheduler_warmup_steps: 50
- training_steps: 500
### Training results
| Training Loss | Epoch | Step | Validation Loss |
|:-------------:|:------:|:----:|:---------------:|
| No log | 0.0001 | 1 | 1.2416 |
| 1.1497 | 0.0060 | 50 | 1.1158 |
| 1.1909 | 0.0121 | 100 | 1.0931 |
| 1.1251 | 0.0181 | 150 | 1.0813 |
| 1.1203 | 0.0242 | 200 | 1.0705 |
| 1.1468 | 0.0302 | 250 | 1.0653 |
| 1.0988 | 0.0362 | 300 | 1.0624 |
| 1.1527 | 0.0423 | 350 | 1.0569 |
| 1.1791 | 0.0483 | 400 | 1.0543 |
| 1.1699 | 0.0544 | 450 | 1.0555 |
| 1.0892 | 0.0604 | 500 | 1.0532 |
### Framework versions
- PEFT 0.13.2
- Transformers 4.46.0
- Pytorch 2.5.0+cu124
- Datasets 3.0.1
- Tokenizers 0.20.1 |
huybunn/whisper-small-dv | huybunn | "2025-02-20T11:59:05Z" | 0 | 0 | transformers | [
"transformers",
"tensorboard",
"safetensors",
"whisper",
"automatic-speech-recognition",
"generated_from_trainer",
"dv",
"dataset:mozilla-foundation/common_voice_13_0",
"base_model:openai/whisper-small",
"base_model:finetune:openai/whisper-small",
"license:apache-2.0",
"model-index",
"endpoints_compatible",
"region:us"
] | automatic-speech-recognition | "2025-02-20T04:49:07Z" | ---
library_name: transformers
language:
- dv
license: apache-2.0
base_model: openai/whisper-small
tags:
- generated_from_trainer
datasets:
- mozilla-foundation/common_voice_13_0
metrics:
- wer
model-index:
- name: Whisper Small Dv - Sanchit Gandhi
results:
- task:
name: Automatic Speech Recognition
type: automatic-speech-recognition
dataset:
name: Common Voice 13
type: mozilla-foundation/common_voice_13_0
config: dv
split: test
args: dv
metrics:
- name: Wer
type: wer
value: 13.615815279757973
---
<!-- This model card has been generated automatically according to the information the Trainer had access to. You
should probably proofread and complete it, then remove this comment. -->
# Whisper Small Dv - Sanchit Gandhi
This model is a fine-tuned version of [openai/whisper-small](https://huggingface.co/openai/whisper-small) on the Common Voice 13 dataset.
It achieves the following results on the evaluation set:
- Loss: 0.1752
- Wer Ortho: 63.1451
- Wer: 13.6158
## Model description
More information needed
## Intended uses & limitations
More information needed
## Training and evaluation data
More information needed
## Training procedure
### Training hyperparameters
The following hyperparameters were used during training:
- learning_rate: 1e-05
- train_batch_size: 16
- eval_batch_size: 16
- seed: 42
- optimizer: Use OptimizerNames.ADAMW_TORCH with betas=(0.9,0.999) and epsilon=1e-08 and optimizer_args=No additional optimizer arguments
- lr_scheduler_type: constant_with_warmup
- lr_scheduler_warmup_steps: 50
- training_steps: 500
- mixed_precision_training: Native AMP
### Training results
| Training Loss | Epoch | Step | Validation Loss | Wer Ortho | Wer |
|:-------------:|:------:|:----:|:---------------:|:---------:|:-------:|
| 0.1218 | 1.6287 | 500 | 0.1752 | 63.1451 | 13.6158 |
### Framework versions
- Transformers 4.48.3
- Pytorch 2.5.1+cu124
- Datasets 3.3.1
- Tokenizers 0.21.0
|
Tristan/dclm-random-410m-raw-finetune-correct | Tristan | "2025-03-28T01:12:19Z" | 0 | 0 | transformers | [
"transformers",
"safetensors",
"gpt_neox",
"text-generation",
"arxiv:1910.09700",
"autotrain_compatible",
"text-generation-inference",
"endpoints_compatible",
"region:us"
] | text-generation | "2025-03-28T01:11:11Z" | ---
library_name: transformers
tags: []
---
# Model Card for Model ID
<!-- Provide a quick summary of what the model is/does. -->
## Model Details
### Model Description
<!-- Provide a longer summary of what this model is. -->
This is the model card of a 🤗 transformers model that has been pushed on the Hub. This model card has been automatically generated.
- **Developed by:** [More Information Needed]
- **Funded by [optional]:** [More Information Needed]
- **Shared by [optional]:** [More Information Needed]
- **Model type:** [More Information Needed]
- **Language(s) (NLP):** [More Information Needed]
- **License:** [More Information Needed]
- **Finetuned from model [optional]:** [More Information Needed]
### Model Sources [optional]
<!-- Provide the basic links for the model. -->
- **Repository:** [More Information Needed]
- **Paper [optional]:** [More Information Needed]
- **Demo [optional]:** [More Information Needed]
## Uses
<!-- Address questions around how the model is intended to be used, including the foreseeable users of the model and those affected by the model. -->
### Direct Use
<!-- This section is for the model use without fine-tuning or plugging into a larger ecosystem/app. -->
[More Information Needed]
### Downstream Use [optional]
<!-- This section is for the model use when fine-tuned for a task, or when plugged into a larger ecosystem/app -->
[More Information Needed]
### Out-of-Scope Use
<!-- This section addresses misuse, malicious use, and uses that the model will not work well for. -->
[More Information Needed]
## Bias, Risks, and Limitations
<!-- This section is meant to convey both technical and sociotechnical limitations. -->
[More Information Needed]
### Recommendations
<!-- This section is meant to convey recommendations with respect to the bias, risk, and technical limitations. -->
Users (both direct and downstream) should be made aware of the risks, biases and limitations of the model. More information needed for further recommendations.
## How to Get Started with the Model
Use the code below to get started with the model.
[More Information Needed]
## Training Details
### Training Data
<!-- This should link to a Dataset Card, perhaps with a short stub of information on what the training data is all about as well as documentation related to data pre-processing or additional filtering. -->
[More Information Needed]
### Training Procedure
<!-- This relates heavily to the Technical Specifications. Content here should link to that section when it is relevant to the training procedure. -->
#### Preprocessing [optional]
[More Information Needed]
#### Training Hyperparameters
- **Training regime:** [More Information Needed] <!--fp32, fp16 mixed precision, bf16 mixed precision, bf16 non-mixed precision, fp16 non-mixed precision, fp8 mixed precision -->
#### Speeds, Sizes, Times [optional]
<!-- This section provides information about throughput, start/end time, checkpoint size if relevant, etc. -->
[More Information Needed]
## Evaluation
<!-- This section describes the evaluation protocols and provides the results. -->
### Testing Data, Factors & Metrics
#### Testing Data
<!-- This should link to a Dataset Card if possible. -->
[More Information Needed]
#### Factors
<!-- These are the things the evaluation is disaggregating by, e.g., subpopulations or domains. -->
[More Information Needed]
#### Metrics
<!-- These are the evaluation metrics being used, ideally with a description of why. -->
[More Information Needed]
### Results
[More Information Needed]
#### Summary
## Model Examination [optional]
<!-- Relevant interpretability work for the model goes here -->
[More Information Needed]
## Environmental Impact
<!-- Total emissions (in grams of CO2eq) and additional considerations, such as electricity usage, go here. Edit the suggested text below accordingly -->
Carbon emissions can be estimated using the [Machine Learning Impact calculator](https://mlco2.github.io/impact#compute) presented in [Lacoste et al. (2019)](https://arxiv.org/abs/1910.09700).
- **Hardware Type:** [More Information Needed]
- **Hours used:** [More Information Needed]
- **Cloud Provider:** [More Information Needed]
- **Compute Region:** [More Information Needed]
- **Carbon Emitted:** [More Information Needed]
## Technical Specifications [optional]
### Model Architecture and Objective
[More Information Needed]
### Compute Infrastructure
[More Information Needed]
#### Hardware
[More Information Needed]
#### Software
[More Information Needed]
## Citation [optional]
<!-- If there is a paper or blog post introducing the model, the APA and Bibtex information for that should go in this section. -->
**BibTeX:**
[More Information Needed]
**APA:**
[More Information Needed]
## Glossary [optional]
<!-- If relevant, include terms and calculations in this section that can help readers understand the model or model card. -->
[More Information Needed]
## More Information [optional]
[More Information Needed]
## Model Card Authors [optional]
[More Information Needed]
## Model Card Contact
[More Information Needed] |
RichardErkhov/tuneai_-_Meta-Llama-3-8B-Instruct-gguf | RichardErkhov | "2024-09-22T11:16:09Z" | 10 | 0 | null | [
"gguf",
"endpoints_compatible",
"region:us",
"conversational"
] | null | "2024-09-22T05:39:41Z" | Quantization made by Richard Erkhov.
[Github](https://github.com/RichardErkhov)
[Discord](https://discord.gg/pvy7H8DZMG)
[Request more models](https://github.com/RichardErkhov/quant_request)
Meta-Llama-3-8B-Instruct - GGUF
- Model creator: https://huggingface.co/tuneai/
- Original model: https://huggingface.co/tuneai/Meta-Llama-3-8B-Instruct/
| Name | Quant method | Size |
| ---- | ---- | ---- |
| [Meta-Llama-3-8B-Instruct.Q2_K.gguf](https://huggingface.co/RichardErkhov/tuneai_-_Meta-Llama-3-8B-Instruct-gguf/blob/main/Meta-Llama-3-8B-Instruct.Q2_K.gguf) | Q2_K | 2.96GB |
| [Meta-Llama-3-8B-Instruct.IQ3_XS.gguf](https://huggingface.co/RichardErkhov/tuneai_-_Meta-Llama-3-8B-Instruct-gguf/blob/main/Meta-Llama-3-8B-Instruct.IQ3_XS.gguf) | IQ3_XS | 3.28GB |
| [Meta-Llama-3-8B-Instruct.IQ3_S.gguf](https://huggingface.co/RichardErkhov/tuneai_-_Meta-Llama-3-8B-Instruct-gguf/blob/main/Meta-Llama-3-8B-Instruct.IQ3_S.gguf) | IQ3_S | 3.43GB |
| [Meta-Llama-3-8B-Instruct.Q3_K_S.gguf](https://huggingface.co/RichardErkhov/tuneai_-_Meta-Llama-3-8B-Instruct-gguf/blob/main/Meta-Llama-3-8B-Instruct.Q3_K_S.gguf) | Q3_K_S | 3.41GB |
| [Meta-Llama-3-8B-Instruct.IQ3_M.gguf](https://huggingface.co/RichardErkhov/tuneai_-_Meta-Llama-3-8B-Instruct-gguf/blob/main/Meta-Llama-3-8B-Instruct.IQ3_M.gguf) | IQ3_M | 3.52GB |
| [Meta-Llama-3-8B-Instruct.Q3_K.gguf](https://huggingface.co/RichardErkhov/tuneai_-_Meta-Llama-3-8B-Instruct-gguf/blob/main/Meta-Llama-3-8B-Instruct.Q3_K.gguf) | Q3_K | 3.74GB |
| [Meta-Llama-3-8B-Instruct.Q3_K_M.gguf](https://huggingface.co/RichardErkhov/tuneai_-_Meta-Llama-3-8B-Instruct-gguf/blob/main/Meta-Llama-3-8B-Instruct.Q3_K_M.gguf) | Q3_K_M | 3.74GB |
| [Meta-Llama-3-8B-Instruct.Q3_K_L.gguf](https://huggingface.co/RichardErkhov/tuneai_-_Meta-Llama-3-8B-Instruct-gguf/blob/main/Meta-Llama-3-8B-Instruct.Q3_K_L.gguf) | Q3_K_L | 4.03GB |
| [Meta-Llama-3-8B-Instruct.IQ4_XS.gguf](https://huggingface.co/RichardErkhov/tuneai_-_Meta-Llama-3-8B-Instruct-gguf/blob/main/Meta-Llama-3-8B-Instruct.IQ4_XS.gguf) | IQ4_XS | 4.18GB |
| [Meta-Llama-3-8B-Instruct.Q4_0.gguf](https://huggingface.co/RichardErkhov/tuneai_-_Meta-Llama-3-8B-Instruct-gguf/blob/main/Meta-Llama-3-8B-Instruct.Q4_0.gguf) | Q4_0 | 4.34GB |
| [Meta-Llama-3-8B-Instruct.IQ4_NL.gguf](https://huggingface.co/RichardErkhov/tuneai_-_Meta-Llama-3-8B-Instruct-gguf/blob/main/Meta-Llama-3-8B-Instruct.IQ4_NL.gguf) | IQ4_NL | 4.38GB |
| [Meta-Llama-3-8B-Instruct.Q4_K_S.gguf](https://huggingface.co/RichardErkhov/tuneai_-_Meta-Llama-3-8B-Instruct-gguf/blob/main/Meta-Llama-3-8B-Instruct.Q4_K_S.gguf) | Q4_K_S | 4.37GB |
| [Meta-Llama-3-8B-Instruct.Q4_K.gguf](https://huggingface.co/RichardErkhov/tuneai_-_Meta-Llama-3-8B-Instruct-gguf/blob/main/Meta-Llama-3-8B-Instruct.Q4_K.gguf) | Q4_K | 4.58GB |
| [Meta-Llama-3-8B-Instruct.Q4_K_M.gguf](https://huggingface.co/RichardErkhov/tuneai_-_Meta-Llama-3-8B-Instruct-gguf/blob/main/Meta-Llama-3-8B-Instruct.Q4_K_M.gguf) | Q4_K_M | 4.58GB |
| [Meta-Llama-3-8B-Instruct.Q4_1.gguf](https://huggingface.co/RichardErkhov/tuneai_-_Meta-Llama-3-8B-Instruct-gguf/blob/main/Meta-Llama-3-8B-Instruct.Q4_1.gguf) | Q4_1 | 4.78GB |
| [Meta-Llama-3-8B-Instruct.Q5_0.gguf](https://huggingface.co/RichardErkhov/tuneai_-_Meta-Llama-3-8B-Instruct-gguf/blob/main/Meta-Llama-3-8B-Instruct.Q5_0.gguf) | Q5_0 | 5.21GB |
| [Meta-Llama-3-8B-Instruct.Q5_K_S.gguf](https://huggingface.co/RichardErkhov/tuneai_-_Meta-Llama-3-8B-Instruct-gguf/blob/main/Meta-Llama-3-8B-Instruct.Q5_K_S.gguf) | Q5_K_S | 5.21GB |
| [Meta-Llama-3-8B-Instruct.Q5_K.gguf](https://huggingface.co/RichardErkhov/tuneai_-_Meta-Llama-3-8B-Instruct-gguf/blob/main/Meta-Llama-3-8B-Instruct.Q5_K.gguf) | Q5_K | 5.34GB |
| [Meta-Llama-3-8B-Instruct.Q5_K_M.gguf](https://huggingface.co/RichardErkhov/tuneai_-_Meta-Llama-3-8B-Instruct-gguf/blob/main/Meta-Llama-3-8B-Instruct.Q5_K_M.gguf) | Q5_K_M | 5.34GB |
| [Meta-Llama-3-8B-Instruct.Q5_1.gguf](https://huggingface.co/RichardErkhov/tuneai_-_Meta-Llama-3-8B-Instruct-gguf/blob/main/Meta-Llama-3-8B-Instruct.Q5_1.gguf) | Q5_1 | 5.65GB |
| [Meta-Llama-3-8B-Instruct.Q6_K.gguf](https://huggingface.co/RichardErkhov/tuneai_-_Meta-Llama-3-8B-Instruct-gguf/blob/main/Meta-Llama-3-8B-Instruct.Q6_K.gguf) | Q6_K | 6.14GB |
| [Meta-Llama-3-8B-Instruct.Q8_0.gguf](https://huggingface.co/RichardErkhov/tuneai_-_Meta-Llama-3-8B-Instruct-gguf/blob/main/Meta-Llama-3-8B-Instruct.Q8_0.gguf) | Q8_0 | 7.95GB |
Original model description:
---
language:
- en
pipeline_tag: text-generation
tags:
- facebook
- meta
- pytorch
- llama
- llama-3
license: llama3
extra_gated_prompt: >-
### META LLAMA 3 COMMUNITY LICENSE AGREEMENT
Meta Llama 3 Version Release Date: April 18, 2024
"Agreement" means the terms and conditions for use, reproduction, distribution and modification of the
Llama Materials set forth herein.
"Documentation" means the specifications, manuals and documentation accompanying Meta Llama 3
distributed by Meta at https://llama.meta.com/get-started/.
"Licensee" or "you" means you, or your employer or any other person or entity (if you are entering into
this Agreement on such person or entity’s behalf), of the age required under applicable laws, rules or
regulations to provide legal consent and that has legal authority to bind your employer or such other
person or entity if you are entering in this Agreement on their behalf.
"Meta Llama 3" means the foundational large language models and software and algorithms, including
machine-learning model code, trained model weights, inference-enabling code, training-enabling code,
fine-tuning enabling code and other elements of the foregoing distributed by Meta at
https://llama.meta.com/llama-downloads.
"Llama Materials" means, collectively, Meta’s proprietary Meta Llama 3 and Documentation (and any
portion thereof) made available under this Agreement.
"Meta" or "we" means Meta Platforms Ireland Limited (if you are located in or, if you are an entity, your
principal place of business is in the EEA or Switzerland) and Meta Platforms, Inc. (if you are located
outside of the EEA or Switzerland).
1. License Rights and Redistribution.
a. Grant of Rights. You are granted a non-exclusive, worldwide, non-transferable and royalty-free
limited license under Meta’s intellectual property or other rights owned by Meta embodied in the Llama
Materials to use, reproduce, distribute, copy, create derivative works of, and make modifications to the
Llama Materials.
b. Redistribution and Use.
i. If you distribute or make available the Llama Materials (or any derivative works
thereof), or a product or service that uses any of them, including another AI model, you shall (A) provide
a copy of this Agreement with any such Llama Materials; and (B) prominently display “Built with Meta
Llama 3” on a related website, user interface, blogpost, about page, or product documentation. If you
use the Llama Materials to create, train, fine tune, or otherwise improve an AI model, which is
distributed or made available, you shall also include “Llama 3” at the beginning of any such AI model
name.
ii. If you receive Llama Materials, or any derivative works thereof, from a Licensee as part
of an integrated end user product, then Section 2 of this Agreement will not apply to you.
iii. You must retain in all copies of the Llama Materials that you distribute the following
attribution notice within a “Notice” text file distributed as a part of such copies: “Meta Llama 3 is
licensed under the Meta Llama 3 Community License, Copyright © Meta Platforms, Inc. All Rights
Reserved.”
iv. Your use of the Llama Materials must comply with applicable laws and regulations
(including trade compliance laws and regulations) and adhere to the Acceptable Use Policy for the Llama
Materials (available at https://llama.meta.com/llama3/use-policy), which is hereby incorporated by
reference into this Agreement.
v. You will not use the Llama Materials or any output or results of the Llama Materials to
improve any other large language model (excluding Meta Llama 3 or derivative works thereof).
2. Additional Commercial Terms. If, on the Meta Llama 3 version release date, the monthly active users
of the products or services made available by or for Licensee, or Licensee’s affiliates, is greater than 700
million monthly active users in the preceding calendar month, you must request a license from Meta,
which Meta may grant to you in its sole discretion, and you are not authorized to exercise any of the
rights under this Agreement unless or until Meta otherwise expressly grants you such rights.
3. Disclaimer of Warranty. UNLESS REQUIRED BY APPLICABLE LAW, THE LLAMA MATERIALS AND ANY
OUTPUT AND RESULTS THEREFROM ARE PROVIDED ON AN “AS IS” BASIS, WITHOUT WARRANTIES OF
ANY KIND, AND META DISCLAIMS ALL WARRANTIES OF ANY KIND, BOTH EXPRESS AND IMPLIED,
INCLUDING, WITHOUT LIMITATION, ANY WARRANTIES OF TITLE, NON-INFRINGEMENT,
MERCHANTABILITY, OR FITNESS FOR A PARTICULAR PURPOSE. YOU ARE SOLELY RESPONSIBLE FOR
DETERMINING THE APPROPRIATENESS OF USING OR REDISTRIBUTING THE LLAMA MATERIALS AND
ASSUME ANY RISKS ASSOCIATED WITH YOUR USE OF THE LLAMA MATERIALS AND ANY OUTPUT AND
RESULTS.
4. Limitation of Liability. IN NO EVENT WILL META OR ITS AFFILIATES BE LIABLE UNDER ANY THEORY OF
LIABILITY, WHETHER IN CONTRACT, TORT, NEGLIGENCE, PRODUCTS LIABILITY, OR OTHERWISE, ARISING
OUT OF THIS AGREEMENT, FOR ANY LOST PROFITS OR ANY INDIRECT, SPECIAL, CONSEQUENTIAL,
INCIDENTAL, EXEMPLARY OR PUNITIVE DAMAGES, EVEN IF META OR ITS AFFILIATES HAVE BEEN ADVISED
OF THE POSSIBILITY OF ANY OF THE FOREGOING.
5. Intellectual Property.
a. No trademark licenses are granted under this Agreement, and in connection with the Llama
Materials, neither Meta nor Licensee may use any name or mark owned by or associated with the other
or any of its affiliates, except as required for reasonable and customary use in describing and
redistributing the Llama Materials or as set forth in this Section 5(a). Meta hereby grants you a license to
use “Llama 3” (the “Mark”) solely as required to comply with the last sentence of Section 1.b.i. You will
comply with Meta’s brand guidelines (currently accessible at
https://about.meta.com/brand/resources/meta/company-brand/ ). All goodwill arising out of your use
of the Mark will inure to the benefit of Meta.
b. Subject to Meta’s ownership of Llama Materials and derivatives made by or for Meta, with
respect to any derivative works and modifications of the Llama Materials that are made by you, as
between you and Meta, you are and will be the owner of such derivative works and modifications.
c. If you institute litigation or other proceedings against Meta or any entity (including a
cross-claim or counterclaim in a lawsuit) alleging that the Llama Materials or Meta Llama 3 outputs or
results, or any portion of any of the foregoing, constitutes infringement of intellectual property or other
rights owned or licensable by you, then any licenses granted to you under this Agreement shall
terminate as of the date such litigation or claim is filed or instituted. You will indemnify and hold
harmless Meta from and against any claim by any third party arising out of or related to your use or
distribution of the Llama Materials.
6. Term and Termination. The term of this Agreement will commence upon your acceptance of this
Agreement or access to the Llama Materials and will continue in full force and effect until terminated in
accordance with the terms and conditions herein. Meta may terminate this Agreement if you are in
breach of any term or condition of this Agreement. Upon termination of this Agreement, you shall delete
and cease use of the Llama Materials. Sections 3, 4 and 7 shall survive the termination of this
Agreement.
7. Governing Law and Jurisdiction. This Agreement will be governed and construed under the laws of
the State of California without regard to choice of law principles, and the UN Convention on Contracts
for the International Sale of Goods does not apply to this Agreement. The courts of California shall have
exclusive jurisdiction of any dispute arising out of this Agreement.
### Meta Llama 3 Acceptable Use Policy
Meta is committed to promoting safe and fair use of its tools and features, including Meta Llama 3. If you
access or use Meta Llama 3, you agree to this Acceptable Use Policy (“Policy”). The most recent copy of
this policy can be found at [https://llama.meta.com/llama3/use-policy](https://llama.meta.com/llama3/use-policy)
#### Prohibited Uses
We want everyone to use Meta Llama 3 safely and responsibly. You agree you will not use, or allow
others to use, Meta Llama 3 to:
1. Violate the law or others’ rights, including to:
1. Engage in, promote, generate, contribute to, encourage, plan, incite, or further illegal or unlawful activity or content, such as:
1. Violence or terrorism
2. Exploitation or harm to children, including the solicitation, creation, acquisition, or dissemination of child exploitative content or failure to report Child Sexual Abuse Material
3. Human trafficking, exploitation, and sexual violence
4. The illegal distribution of information or materials to minors, including obscene materials, or failure to employ legally required age-gating in connection with such information or materials.
5. Sexual solicitation
6. Any other criminal activity
2. Engage in, promote, incite, or facilitate the harassment, abuse, threatening, or bullying of individuals or groups of individuals
3. Engage in, promote, incite, or facilitate discrimination or other unlawful or harmful conduct in the provision of employment, employment benefits, credit, housing, other economic benefits, or other essential goods and services
4. Engage in the unauthorized or unlicensed practice of any profession including, but not limited to, financial, legal, medical/health, or related professional practices
5. Collect, process, disclose, generate, or infer health, demographic, or other sensitive personal or private information about individuals without rights and consents required by applicable laws
6. Engage in or facilitate any action or generate any content that infringes, misappropriates, or otherwise violates any third-party rights, including the outputs or results of any products or services using the Llama Materials
7. Create, generate, or facilitate the creation of malicious code, malware, computer viruses or do anything else that could disable, overburden, interfere with or impair the proper working, integrity, operation or appearance of a website or computer system
2. Engage in, promote, incite, facilitate, or assist in the planning or development of activities that present a risk of death or bodily harm to individuals, including use of Meta Llama 3 related to the following:
1. Military, warfare, nuclear industries or applications, espionage, use for materials or activities that are subject to the International Traffic Arms Regulations (ITAR) maintained by the United States Department of State
2. Guns and illegal weapons (including weapon development)
3. Illegal drugs and regulated/controlled substances
4. Operation of critical infrastructure, transportation technologies, or heavy machinery
5. Self-harm or harm to others, including suicide, cutting, and eating disorders
6. Any content intended to incite or promote violence, abuse, or any infliction of bodily harm to an individual
3. Intentionally deceive or mislead others, including use of Meta Llama 3 related to the following:
1. Generating, promoting, or furthering fraud or the creation or promotion of disinformation
2. Generating, promoting, or furthering defamatory content, including the creation of defamatory statements, images, or other content
3. Generating, promoting, or further distributing spam
4. Impersonating another individual without consent, authorization, or legal right
5. Representing that the use of Meta Llama 3 or outputs are human-generated
6. Generating or facilitating false online engagement, including fake reviews and other means of fake online engagement
4. Fail to appropriately disclose to end users any known dangers of your AI system
Please report any violation of this Policy, software “bug,” or other problems that could lead to a violation
of this Policy through one of the following means:
* Reporting issues with the model: [https://github.com/meta-llama/llama3](https://github.com/meta-llama/llama3)
* Reporting risky content generated by the model:
developers.facebook.com/llama_output_feedback
* Reporting bugs and security concerns: facebook.com/whitehat/info
* Reporting violations of the Acceptable Use Policy or unlicensed uses of Meta Llama 3: [email protected]
extra_gated_fields:
First Name: text
Last Name: text
Date of birth: date_picker
Country: country
Affiliation: text
geo: ip_location
By clicking Submit below I accept the terms of the license and acknowledge that the information I provide will be collected stored processed and shared in accordance with the Meta Privacy Policy: checkbox
extra_gated_description: The information you provide will be collected, stored, processed and shared in accordance with the [Meta Privacy Policy](https://www.facebook.com/privacy/policy/).
extra_gated_button_content: Submit
widget:
- example_title: Hello
messages:
- role: user
content: Hey my name is Julien! How are you?
- example_title: Winter holidays
messages:
- role: system
content: You are a helpful and honest assistant. Please, respond concisely and truthfully.
- role: user
content: Can you recommend a good destination for Winter holidays?
- example_title: Programming assistant
messages:
- role: system
content: You are a helpful and honest code and programming assistant. Please, respond concisely and truthfully.
- role: user
content: Write a function that computes the nth fibonacci number.
inference:
parameters:
max_new_tokens: 300
stop:
- <|end_of_text|>
- <|eot_id|>
---
## Model Details
Meta developed and released the Meta Llama 3 family of large language models (LLMs), a collection of pretrained and instruction tuned generative text models in 8 and 70B sizes. The Llama 3 instruction tuned models are optimized for dialogue use cases and outperform many of the available open source chat models on common industry benchmarks. Further, in developing these models, we took great care to optimize helpfulness and safety.
**Model developers** Meta
**Variations** Llama 3 comes in two sizes — 8B and 70B parameters — in pre-trained and instruction tuned variants.
**Input** Models input text only.
**Output** Models generate text and code only.
**Model Architecture** Llama 3 is an auto-regressive language model that uses an optimized transformer architecture. The tuned versions use supervised fine-tuning (SFT) and reinforcement learning with human feedback (RLHF) to align with human preferences for helpfulness and safety.
<table>
<tr>
<td>
</td>
<td><strong>Training Data</strong>
</td>
<td><strong>Params</strong>
</td>
<td><strong>Context length</strong>
</td>
<td><strong>GQA</strong>
</td>
<td><strong>Token count</strong>
</td>
<td><strong>Knowledge cutoff</strong>
</td>
</tr>
<tr>
<td rowspan="2" >Llama 3
</td>
<td rowspan="2" >A new mix of publicly available online data.
</td>
<td>8B
</td>
<td>8k
</td>
<td>Yes
</td>
<td rowspan="2" >15T+
</td>
<td>March, 2023
</td>
</tr>
<tr>
<td>70B
</td>
<td>8k
</td>
<td>Yes
</td>
<td>December, 2023
</td>
</tr>
</table>
**Llama 3 family of models**. Token counts refer to pretraining data only. Both the 8 and 70B versions use Grouped-Query Attention (GQA) for improved inference scalability.
**Model Release Date** April 18, 2024.
**Status** This is a static model trained on an offline dataset. Future versions of the tuned models will be released as we improve model safety with community feedback.
**License** A custom commercial license is available at: [https://llama.meta.com/llama3/license](https://llama.meta.com/llama3/license)
Where to send questions or comments about the model Instructions on how to provide feedback or comments on the model can be found in the model [README](https://github.com/meta-llama/llama3). For more technical information about generation parameters and recipes for how to use Llama 3 in applications, please go [here](https://github.com/meta-llama/llama-recipes).
## Intended Use
**Intended Use Cases** Llama 3 is intended for commercial and research use in English. Instruction tuned models are intended for assistant-like chat, whereas pretrained models can be adapted for a variety of natural language generation tasks.
**Out-of-scope** Use in any manner that violates applicable laws or regulations (including trade compliance laws). Use in any other way that is prohibited by the Acceptable Use Policy and Llama 3 Community License. Use in languages other than English**.
**Note: Developers may fine-tune Llama 3 models for languages beyond English provided they comply with the Llama 3 Community License and the Acceptable Use Policy.
## How to use
This repository contains two versions of Meta-Llama-3-8B-Instruct, for use with transformers and with the original `llama3` codebase.
### Use with transformers
You can run conversational inference using the Transformers pipeline abstraction, or by leveraging the Auto classes with the `generate()` function. Let's see examples of both.
#### Transformers pipeline
```python
import transformers
import torch
model_id = "meta-llama/Meta-Llama-3-8B-Instruct"
pipeline = transformers.pipeline(
"text-generation",
model=model_id,
model_kwargs={"torch_dtype": torch.bfloat16},
device_map="auto",
)
messages = [
{"role": "system", "content": "You are a pirate chatbot who always responds in pirate speak!"},
{"role": "user", "content": "Who are you?"},
]
terminators = [
pipeline.tokenizer.eos_token_id,
pipeline.tokenizer.convert_tokens_to_ids("<|eot_id|>")
]
outputs = pipeline(
messages,
max_new_tokens=256,
eos_token_id=terminators,
do_sample=True,
temperature=0.6,
top_p=0.9,
)
print(outputs[0]["generated_text"][-1])
```
#### Transformers AutoModelForCausalLM
```python
from transformers import AutoTokenizer, AutoModelForCausalLM
import torch
model_id = "meta-llama/Meta-Llama-3-8B-Instruct"
tokenizer = AutoTokenizer.from_pretrained(model_id)
model = AutoModelForCausalLM.from_pretrained(
model_id,
torch_dtype=torch.bfloat16,
device_map="auto",
)
messages = [
{"role": "system", "content": "You are a pirate chatbot who always responds in pirate speak!"},
{"role": "user", "content": "Who are you?"},
]
input_ids = tokenizer.apply_chat_template(
messages,
add_generation_prompt=True,
return_tensors="pt"
).to(model.device)
terminators = [
tokenizer.eos_token_id,
tokenizer.convert_tokens_to_ids("<|eot_id|>")
]
outputs = model.generate(
input_ids,
max_new_tokens=256,
eos_token_id=terminators,
do_sample=True,
temperature=0.6,
top_p=0.9,
)
response = outputs[0][input_ids.shape[-1]:]
print(tokenizer.decode(response, skip_special_tokens=True))
```
### Use with `llama3`
Please, follow the instructions in the [repository](https://github.com/meta-llama/llama3)
To download Original checkpoints, see the example command below leveraging `huggingface-cli`:
```
huggingface-cli download meta-llama/Meta-Llama-3-8B-Instruct --include "original/*" --local-dir Meta-Llama-3-8B-Instruct
```
For Hugging Face support, we recommend using transformers or TGI, but a similar command works.
## Hardware and Software
**Training Factors** We used custom training libraries, Meta's Research SuperCluster, and production clusters for pretraining. Fine-tuning, annotation, and evaluation were also performed on third-party cloud compute.
**Carbon Footprint Pretraining utilized a cumulative** 7.7M GPU hours of computation on hardware of type H100-80GB (TDP of 700W). Estimated total emissions were 2290 tCO2eq, 100% of which were offset by Meta’s sustainability program.
<table>
<tr>
<td>
</td>
<td><strong>Time (GPU hours)</strong>
</td>
<td><strong>Power Consumption (W)</strong>
</td>
<td><strong>Carbon Emitted(tCO2eq)</strong>
</td>
</tr>
<tr>
<td>Llama 3 8B
</td>
<td>1.3M
</td>
<td>700
</td>
<td>390
</td>
</tr>
<tr>
<td>Llama 3 70B
</td>
<td>6.4M
</td>
<td>700
</td>
<td>1900
</td>
</tr>
<tr>
<td>Total
</td>
<td>7.7M
</td>
<td>
</td>
<td>2290
</td>
</tr>
</table>
**CO2 emissions during pre-training**. Time: total GPU time required for training each model. Power Consumption: peak power capacity per GPU device for the GPUs used adjusted for power usage efficiency. 100% of the emissions are directly offset by Meta's sustainability program, and because we are openly releasing these models, the pretraining costs do not need to be incurred by others.
## Training Data
**Overview** Llama 3 was pretrained on over 15 trillion tokens of data from publicly available sources. The fine-tuning data includes publicly available instruction datasets, as well as over 10M human-annotated examples. Neither the pretraining nor the fine-tuning datasets include Meta user data.
**Data Freshness** The pretraining data has a cutoff of March 2023 for the 8B and December 2023 for the 70B models respectively.
## Benchmarks
In this section, we report the results for Llama 3 models on standard automatic benchmarks. For all the evaluations, we use our internal evaluations library. For details on the methodology see [here](https://github.com/meta-llama/llama3/blob/main/eval_methodology.md).
### Base pretrained models
<table>
<tr>
<td><strong>Category</strong>
</td>
<td><strong>Benchmark</strong>
</td>
<td><strong>Llama 3 8B</strong>
</td>
<td><strong>Llama2 7B</strong>
</td>
<td><strong>Llama2 13B</strong>
</td>
<td><strong>Llama 3 70B</strong>
</td>
<td><strong>Llama2 70B</strong>
</td>
</tr>
<tr>
<td rowspan="6" >General
</td>
<td>MMLU (5-shot)
</td>
<td>66.6
</td>
<td>45.7
</td>
<td>53.8
</td>
<td>79.5
</td>
<td>69.7
</td>
</tr>
<tr>
<td>AGIEval English (3-5 shot)
</td>
<td>45.9
</td>
<td>28.8
</td>
<td>38.7
</td>
<td>63.0
</td>
<td>54.8
</td>
</tr>
<tr>
<td>CommonSenseQA (7-shot)
</td>
<td>72.6
</td>
<td>57.6
</td>
<td>67.6
</td>
<td>83.8
</td>
<td>78.7
</td>
</tr>
<tr>
<td>Winogrande (5-shot)
</td>
<td>76.1
</td>
<td>73.3
</td>
<td>75.4
</td>
<td>83.1
</td>
<td>81.8
</td>
</tr>
<tr>
<td>BIG-Bench Hard (3-shot, CoT)
</td>
<td>61.1
</td>
<td>38.1
</td>
<td>47.0
</td>
<td>81.3
</td>
<td>65.7
</td>
</tr>
<tr>
<td>ARC-Challenge (25-shot)
</td>
<td>78.6
</td>
<td>53.7
</td>
<td>67.6
</td>
<td>93.0
</td>
<td>85.3
</td>
</tr>
<tr>
<td>Knowledge reasoning
</td>
<td>TriviaQA-Wiki (5-shot)
</td>
<td>78.5
</td>
<td>72.1
</td>
<td>79.6
</td>
<td>89.7
</td>
<td>87.5
</td>
</tr>
<tr>
<td rowspan="4" >Reading comprehension
</td>
<td>SQuAD (1-shot)
</td>
<td>76.4
</td>
<td>72.2
</td>
<td>72.1
</td>
<td>85.6
</td>
<td>82.6
</td>
</tr>
<tr>
<td>QuAC (1-shot, F1)
</td>
<td>44.4
</td>
<td>39.6
</td>
<td>44.9
</td>
<td>51.1
</td>
<td>49.4
</td>
</tr>
<tr>
<td>BoolQ (0-shot)
</td>
<td>75.7
</td>
<td>65.5
</td>
<td>66.9
</td>
<td>79.0
</td>
<td>73.1
</td>
</tr>
<tr>
<td>DROP (3-shot, F1)
</td>
<td>58.4
</td>
<td>37.9
</td>
<td>49.8
</td>
<td>79.7
</td>
<td>70.2
</td>
</tr>
</table>
### Instruction tuned models
<table>
<tr>
<td><strong>Benchmark</strong>
</td>
<td><strong>Llama 3 8B</strong>
</td>
<td><strong>Llama 2 7B</strong>
</td>
<td><strong>Llama 2 13B</strong>
</td>
<td><strong>Llama 3 70B</strong>
</td>
<td><strong>Llama 2 70B</strong>
</td>
</tr>
<tr>
<td>MMLU (5-shot)
</td>
<td>68.4
</td>
<td>34.1
</td>
<td>47.8
</td>
<td>82.0
</td>
<td>52.9
</td>
</tr>
<tr>
<td>GPQA (0-shot)
</td>
<td>34.2
</td>
<td>21.7
</td>
<td>22.3
</td>
<td>39.5
</td>
<td>21.0
</td>
</tr>
<tr>
<td>HumanEval (0-shot)
</td>
<td>62.2
</td>
<td>7.9
</td>
<td>14.0
</td>
<td>81.7
</td>
<td>25.6
</td>
</tr>
<tr>
<td>GSM-8K (8-shot, CoT)
</td>
<td>79.6
</td>
<td>25.7
</td>
<td>77.4
</td>
<td>93.0
</td>
<td>57.5
</td>
</tr>
<tr>
<td>MATH (4-shot, CoT)
</td>
<td>30.0
</td>
<td>3.8
</td>
<td>6.7
</td>
<td>50.4
</td>
<td>11.6
</td>
</tr>
</table>
### Responsibility & Safety
We believe that an open approach to AI leads to better, safer products, faster innovation, and a bigger overall market. We are committed to Responsible AI development and took a series of steps to limit misuse and harm and support the open source community.
Foundation models are widely capable technologies that are built to be used for a diverse range of applications. They are not designed to meet every developer preference on safety levels for all use cases, out-of-the-box, as those by their nature will differ across different applications.
Rather, responsible LLM-application deployment is achieved by implementing a series of safety best practices throughout the development of such applications, from the model pre-training, fine-tuning and the deployment of systems composed of safeguards to tailor the safety needs specifically to the use case and audience.
As part of the Llama 3 release, we updated our [Responsible Use Guide](https://llama.meta.com/responsible-use-guide/) to outline the steps and best practices for developers to implement model and system level safety for their application. We also provide a set of resources including [Meta Llama Guard 2](https://llama.meta.com/purple-llama/) and [Code Shield](https://llama.meta.com/purple-llama/) safeguards. These tools have proven to drastically reduce residual risks of LLM Systems, while maintaining a high level of helpfulness. We encourage developers to tune and deploy these safeguards according to their needs and we provide a [reference implementation](https://github.com/meta-llama/llama-recipes/tree/main/recipes/responsible_ai) to get you started.
#### Llama 3-Instruct
As outlined in the Responsible Use Guide, some trade-off between model helpfulness and model alignment is likely unavoidable. Developers should exercise discretion about how to weigh the benefits of alignment and helpfulness for their specific use case and audience. Developers should be mindful of residual risks when using Llama models and leverage additional safety tools as needed to reach the right safety bar for their use case.
<span style="text-decoration:underline;">Safety</span>
For our instruction tuned model, we conducted extensive red teaming exercises, performed adversarial evaluations and implemented safety mitigations techniques to lower residual risks. As with any Large Language Model, residual risks will likely remain and we recommend that developers assess these risks in the context of their use case. In parallel, we are working with the community to make AI safety benchmark standards transparent, rigorous and interpretable.
<span style="text-decoration:underline;">Refusals</span>
In addition to residual risks, we put a great emphasis on model refusals to benign prompts. Over-refusing not only can impact the user experience but could even be harmful in certain contexts as well. We’ve heard the feedback from the developer community and improved our fine tuning to ensure that Llama 3 is significantly less likely to falsely refuse to answer prompts than Llama 2.
We built internal benchmarks and developed mitigations to limit false refusals making Llama 3 our most helpful model to date.
#### Responsible release
In addition to responsible use considerations outlined above, we followed a rigorous process that requires us to take extra measures against misuse and critical risks before we make our release decision.
Misuse
If you access or use Llama 3, you agree to the Acceptable Use Policy. The most recent copy of this policy can be found at [https://llama.meta.com/llama3/use-policy/](https://llama.meta.com/llama3/use-policy/).
#### Critical risks
<span style="text-decoration:underline;">CBRNE</span> (Chemical, Biological, Radiological, Nuclear, and high yield Explosives)
We have conducted a two fold assessment of the safety of the model in this area:
* Iterative testing during model training to assess the safety of responses related to CBRNE threats and other adversarial risks.
* Involving external CBRNE experts to conduct an uplift test assessing the ability of the model to accurately provide expert knowledge and reduce barriers to potential CBRNE misuse, by reference to what can be achieved using web search (without the model).
### <span style="text-decoration:underline;">Cyber Security </span>
We have evaluated Llama 3 with CyberSecEval, Meta’s cybersecurity safety eval suite, measuring Llama 3’s propensity to suggest insecure code when used as a coding assistant, and Llama 3’s propensity to comply with requests to help carry out cyber attacks, where attacks are defined by the industry standard MITRE ATT&CK cyber attack ontology. On our insecure coding and cyber attacker helpfulness tests, Llama 3 behaved in the same range or safer than models of [equivalent coding capability](https://huggingface.co/spaces/facebook/CyberSecEval).
### <span style="text-decoration:underline;">Child Safety</span>
Child Safety risk assessments were conducted using a team of experts, to assess the model’s capability to produce outputs that could result in Child Safety risks and inform on any necessary and appropriate risk mitigations via fine tuning. We leveraged those expert red teaming sessions to expand the coverage of our evaluation benchmarks through Llama 3 model development. For Llama 3, we conducted new in-depth sessions using objective based methodologies to assess the model risks along multiple attack vectors. We also partnered with content specialists to perform red teaming exercises assessing potentially violating content while taking account of market specific nuances or experiences.
### Community
Generative AI safety requires expertise and tooling, and we believe in the strength of the open community to accelerate its progress. We are active members of open consortiums, including the AI Alliance, Partnership in AI and MLCommons, actively contributing to safety standardization and transparency. We encourage the community to adopt taxonomies like the MLCommons Proof of Concept evaluation to facilitate collaboration and transparency on safety and content evaluations. Our Purple Llama tools are open sourced for the community to use and widely distributed across ecosystem partners including cloud service providers. We encourage community contributions to our [Github repository](https://github.com/meta-llama/PurpleLlama).
Finally, we put in place a set of resources including an [output reporting mechanism](https://developers.facebook.com/llama_output_feedback) and [bug bounty program](https://www.facebook.com/whitehat) to continuously improve the Llama technology with the help of the community.
## Ethical Considerations and Limitations
The core values of Llama 3 are openness, inclusivity and helpfulness. It is meant to serve everyone, and to work for a wide range of use cases. It is thus designed to be accessible to people across many different backgrounds, experiences and perspectives. Llama 3 addresses users and their needs as they are, without insertion unnecessary judgment or normativity, while reflecting the understanding that even content that may appear problematic in some cases can serve valuable purposes in others. It respects the dignity and autonomy of all users, especially in terms of the values of free thought and expression that power innovation and progress.
But Llama 3 is a new technology, and like any new technology, there are risks associated with its use. Testing conducted to date has been in English, and has not covered, nor could it cover, all scenarios. For these reasons, as with all LLMs, Llama 3’s potential outputs cannot be predicted in advance, and the model may in some instances produce inaccurate, biased or other objectionable responses to user prompts. Therefore, before deploying any applications of Llama 3 models, developers should perform safety testing and tuning tailored to their specific applications of the model. As outlined in the Responsible Use Guide, we recommend incorporating [Purple Llama](https://github.com/facebookresearch/PurpleLlama) solutions into your workflows and specifically [Llama Guard](https://ai.meta.com/research/publications/llama-guard-llm-based-input-output-safeguard-for-human-ai-conversations/) which provides a base model to filter input and output prompts to layer system-level safety on top of model-level safety.
Please see the Responsible Use Guide available at [http://llama.meta.com/responsible-use-guide](http://llama.meta.com/responsible-use-guide)
## Citation instructions
@article{llama3modelcard,
title={Llama 3 Model Card},
author={AI@Meta},
year={2024},
url = {https://github.com/meta-llama/llama3/blob/main/MODEL_CARD.md}
}
## Contributors
Aaditya Singh; Aaron Grattafiori; Abhimanyu Dubey; Abhinav Jauhri; Abhinav Pandey; Abhishek Kadian; Adam Kelsey; Adi Gangidi; Ahmad Al-Dahle; Ahuva Goldstand; Aiesha Letman; Ajay Menon; Akhil Mathur; Alan Schelten; Alex Vaughan; Amy Yang; Andrei Lupu; Andres Alvarado; Andrew Gallagher; Andrew Gu; Andrew Ho; Andrew Poulton; Andrew Ryan; Angela Fan; Ankit Ramchandani; Anthony Hartshorn; Archi Mitra; Archie Sravankumar; Artem Korenev; Arun Rao; Ashley Gabriel; Ashwin Bharambe; Assaf Eisenman; Aston Zhang; Aurelien Rodriguez; Austen Gregerson; Ava Spataru; Baptiste Roziere; Ben Maurer; Benjamin Leonhardi; Bernie Huang; Bhargavi Paranjape; Bing Liu; Binh Tang; Bobbie Chern; Brani Stojkovic; Brian Fuller; Catalina Mejia Arenas; Chao Zhou; Charlotte Caucheteux; Chaya Nayak; Ching-Hsiang Chu; Chloe Bi; Chris Cai; Chris Cox; Chris Marra; Chris McConnell; Christian Keller; Christoph Feichtenhofer; Christophe Touret; Chunyang Wu; Corinne Wong; Cristian Canton Ferrer; Damien Allonsius; Daniel Kreymer; Daniel Haziza; Daniel Li; Danielle Pintz; Danny Livshits; Danny Wyatt; David Adkins; David Esiobu; David Xu; Davide Testuggine; Delia David; Devi Parikh; Dhruv Choudhary; Dhruv Mahajan; Diana Liskovich; Diego Garcia-Olano; Diego Perino; Dieuwke Hupkes; Dingkang Wang; Dustin Holland; Egor Lakomkin; Elina Lobanova; Xiaoqing Ellen Tan; Emily Dinan; Eric Smith; Erik Brinkman; Esteban Arcaute; Filip Radenovic; Firat Ozgenel; Francesco Caggioni; Frank Seide; Frank Zhang; Gabriel Synnaeve; Gabriella Schwarz; Gabrielle Lee; Gada Badeer; Georgia Anderson; Graeme Nail; Gregoire Mialon; Guan Pang; Guillem Cucurell; Hailey Nguyen; Hannah Korevaar; Hannah Wang; Haroun Habeeb; Harrison Rudolph; Henry Aspegren; Hu Xu; Hugo Touvron; Iga Kozlowska; Igor Molybog; Igor Tufanov; Iliyan Zarov; Imanol Arrieta Ibarra; Irina-Elena Veliche; Isabel Kloumann; Ishan Misra; Ivan Evtimov; Jacob Xu; Jade Copet; Jake Weissman; Jan Geffert; Jana Vranes; Japhet Asher; Jason Park; Jay Mahadeokar; Jean-Baptiste Gaya; Jeet Shah; Jelmer van der Linde; Jennifer Chan; Jenny Hong; Jenya Lee; Jeremy Fu; Jeremy Teboul; Jianfeng Chi; Jianyu Huang; Jie Wang; Jiecao Yu; Joanna Bitton; Joe Spisak; Joelle Pineau; Jon Carvill; Jongsoo Park; Joseph Rocca; Joshua Johnstun; Junteng Jia; Kalyan Vasuden Alwala; Kam Hou U; Kate Plawiak; Kartikeya Upasani; Kaushik Veeraraghavan; Ke Li; Kenneth Heafield; Kevin Stone; Khalid El-Arini; Krithika Iyer; Kshitiz Malik; Kuenley Chiu; Kunal Bhalla; Kyle Huang; Lakshya Garg; Lauren Rantala-Yeary; Laurens van der Maaten; Lawrence Chen; Leandro Silva; Lee Bell; Lei Zhang; Liang Tan; Louis Martin; Lovish Madaan; Luca Wehrstedt; Lukas Blecher; Luke de Oliveira; Madeline Muzzi; Madian Khabsa; Manav Avlani; Mannat Singh; Manohar Paluri; Mark Zuckerberg; Marcin Kardas; Martynas Mankus; Mathew Oldham; Mathieu Rita; Matthew Lennie; Maya Pavlova; Meghan Keneally; Melanie Kambadur; Mihir Patel; Mikayel Samvelyan; Mike Clark; Mike Lewis; Min Si; Mitesh Kumar Singh; Mo Metanat; Mona Hassan; Naman Goyal; Narjes Torabi; Nicolas Usunier; Nikolay Bashlykov; Nikolay Bogoychev; Niladri Chatterji; Ning Dong; Oliver Aobo Yang; Olivier Duchenne; Onur Celebi; Parth Parekh; Patrick Alrassy; Paul Saab; Pavan Balaji; Pedro Rittner; Pengchuan Zhang; Pengwei Li; Petar Vasic; Peter Weng; Polina Zvyagina; Prajjwal Bhargava; Pratik Dubal; Praveen Krishnan; Punit Singh Koura; Qing He; Rachel Rodriguez; Ragavan Srinivasan; Rahul Mitra; Ramon Calderer; Raymond Li; Robert Stojnic; Roberta Raileanu; Robin Battey; Rocky Wang; Rohit Girdhar; Rohit Patel; Romain Sauvestre; Ronnie Polidoro; Roshan Sumbaly; Ross Taylor; Ruan Silva; Rui Hou; Rui Wang; Russ Howes; Ruty Rinott; Saghar Hosseini; Sai Jayesh Bondu; Samyak Datta; Sanjay Singh; Sara Chugh; Sargun Dhillon; Satadru Pan; Sean Bell; Sergey Edunov; Shaoliang Nie; Sharan Narang; Sharath Raparthy; Shaun Lindsay; Sheng Feng; Sheng Shen; Shenghao Lin; Shiva Shankar; Shruti Bhosale; Shun Zhang; Simon Vandenhende; Sinong Wang; Seohyun Sonia Kim; Soumya Batra; Sten Sootla; Steve Kehoe; Suchin Gururangan; Sumit Gupta; Sunny Virk; Sydney Borodinsky; Tamar Glaser; Tamar Herman; Tamara Best; Tara Fowler; Thomas Georgiou; Thomas Scialom; Tianhe Li; Todor Mihaylov; Tong Xiao; Ujjwal Karn; Vedanuj Goswami; Vibhor Gupta; Vignesh Ramanathan; Viktor Kerkez; Vinay Satish Kumar; Vincent Gonguet; Vish Vogeti; Vlad Poenaru; Vlad Tiberiu Mihailescu; Vladan Petrovic; Vladimir Ivanov; Wei Li; Weiwei Chu; Wenhan Xiong; Wenyin Fu; Wes Bouaziz; Whitney Meers; Will Constable; Xavier Martinet; Xiaojian Wu; Xinbo Gao; Xinfeng Xie; Xuchao Jia; Yaelle Goldschlag; Yann LeCun; Yashesh Gaur; Yasmine Babaei; Ye Qi; Yenda Li; Yi Wen; Yiwen Song; Youngjin Nam; Yuchen Hao; Yuchen Zhang; Yun Wang; Yuning Mao; Yuzi He; Zacharie Delpierre Coudert; Zachary DeVito; Zahra Hankir; Zhaoduo Wen; Zheng Yan; Zhengxing Chen; Zhenyu Yang; Zoe Papakipos
|
holaholax/cmlmx25dv10 | holaholax | "2023-03-07T14:09:10Z" | 0 | 1 | null | [
"license:creativeml-openrail-m",
"region:us"
] | null | "2023-03-07T13:43:31Z" | ---
license: creativeml-openrail-m
---
|
MayBashendy/ArabicNewSplits8_usingWellWrittenEssays_FineTuningAraBERT_run1_AugV5_k14_task2_organization | MayBashendy | "2025-01-15T04:35:52Z" | 7 | 0 | transformers | [
"transformers",
"safetensors",
"bert",
"text-classification",
"generated_from_trainer",
"base_model:aubmindlab/bert-base-arabertv02",
"base_model:finetune:aubmindlab/bert-base-arabertv02",
"autotrain_compatible",
"endpoints_compatible",
"region:us"
] | text-classification | "2025-01-15T04:26:01Z" | ---
library_name: transformers
base_model: aubmindlab/bert-base-arabertv02
tags:
- generated_from_trainer
model-index:
- name: ArabicNewSplits8_usingWellWrittenEssays_FineTuningAraBERT_run1_AugV5_k14_task2_organization
results: []
---
<!-- This model card has been generated automatically according to the information the Trainer had access to. You
should probably proofread and complete it, then remove this comment. -->
# ArabicNewSplits8_usingWellWrittenEssays_FineTuningAraBERT_run1_AugV5_k14_task2_organization
This model is a fine-tuned version of [aubmindlab/bert-base-arabertv02](https://huggingface.co/aubmindlab/bert-base-arabertv02) on the None dataset.
It achieves the following results on the evaluation set:
- Loss: 0.6468
- Qwk: 0.4520
- Mse: 0.6468
- Rmse: 0.8042
## Model description
More information needed
## Intended uses & limitations
More information needed
## Training and evaluation data
More information needed
## Training procedure
### Training hyperparameters
The following hyperparameters were used during training:
- learning_rate: 2e-05
- train_batch_size: 8
- eval_batch_size: 8
- seed: 42
- optimizer: Adam with betas=(0.9,0.999) and epsilon=1e-08
- lr_scheduler_type: linear
- num_epochs: 100
### Training results
| Training Loss | Epoch | Step | Validation Loss | Qwk | Mse | Rmse |
|:-------------:|:------:|:----:|:---------------:|:-------:|:------:|:------:|
| No log | 0.0267 | 2 | 4.3882 | -0.0134 | 4.3882 | 2.0948 |
| No log | 0.0533 | 4 | 2.4350 | 0.0464 | 2.4350 | 1.5604 |
| No log | 0.08 | 6 | 1.9937 | -0.0901 | 1.9937 | 1.4120 |
| No log | 0.1067 | 8 | 1.2891 | -0.1077 | 1.2891 | 1.1354 |
| No log | 0.1333 | 10 | 0.8750 | 0.0970 | 0.8750 | 0.9354 |
| No log | 0.16 | 12 | 1.1646 | 0.1160 | 1.1646 | 1.0792 |
| No log | 0.1867 | 14 | 1.1533 | 0.1540 | 1.1533 | 1.0739 |
| No log | 0.2133 | 16 | 0.7876 | 0.1539 | 0.7876 | 0.8875 |
| No log | 0.24 | 18 | 0.7543 | 0.2376 | 0.7543 | 0.8685 |
| No log | 0.2667 | 20 | 0.8395 | 0.0869 | 0.8395 | 0.9162 |
| No log | 0.2933 | 22 | 0.8692 | 0.0147 | 0.8692 | 0.9323 |
| No log | 0.32 | 24 | 0.8141 | 0.0654 | 0.8141 | 0.9023 |
| No log | 0.3467 | 26 | 0.7924 | 0.0937 | 0.7924 | 0.8902 |
| No log | 0.3733 | 28 | 0.8073 | 0.1835 | 0.8073 | 0.8985 |
| No log | 0.4 | 30 | 0.8408 | 0.2085 | 0.8408 | 0.9170 |
| No log | 0.4267 | 32 | 0.7697 | 0.2662 | 0.7697 | 0.8773 |
| No log | 0.4533 | 34 | 0.7598 | 0.1937 | 0.7598 | 0.8717 |
| No log | 0.48 | 36 | 0.7466 | 0.2474 | 0.7466 | 0.8641 |
| No log | 0.5067 | 38 | 0.7960 | 0.1920 | 0.7960 | 0.8922 |
| No log | 0.5333 | 40 | 0.8679 | 0.1489 | 0.8679 | 0.9316 |
| No log | 0.56 | 42 | 0.9576 | 0.1248 | 0.9576 | 0.9786 |
| No log | 0.5867 | 44 | 1.0829 | 0.1113 | 1.0829 | 1.0406 |
| No log | 0.6133 | 46 | 0.9404 | 0.1805 | 0.9404 | 0.9697 |
| No log | 0.64 | 48 | 0.7629 | 0.2959 | 0.7629 | 0.8734 |
| No log | 0.6667 | 50 | 0.7336 | 0.3515 | 0.7336 | 0.8565 |
| No log | 0.6933 | 52 | 0.8819 | 0.2309 | 0.8819 | 0.9391 |
| No log | 0.72 | 54 | 0.8673 | 0.1949 | 0.8673 | 0.9313 |
| No log | 0.7467 | 56 | 0.8924 | 0.2048 | 0.8924 | 0.9447 |
| No log | 0.7733 | 58 | 0.9810 | 0.1808 | 0.9810 | 0.9904 |
| No log | 0.8 | 60 | 0.9166 | 0.2417 | 0.9166 | 0.9574 |
| No log | 0.8267 | 62 | 0.6991 | 0.3930 | 0.6991 | 0.8361 |
| No log | 0.8533 | 64 | 0.6845 | 0.4071 | 0.6845 | 0.8273 |
| No log | 0.88 | 66 | 0.7080 | 0.4139 | 0.7080 | 0.8414 |
| No log | 0.9067 | 68 | 0.7604 | 0.3611 | 0.7604 | 0.8720 |
| No log | 0.9333 | 70 | 0.7930 | 0.3946 | 0.7930 | 0.8905 |
| No log | 0.96 | 72 | 0.7994 | 0.3394 | 0.7994 | 0.8941 |
| No log | 0.9867 | 74 | 0.6904 | 0.4614 | 0.6904 | 0.8309 |
| No log | 1.0133 | 76 | 0.6694 | 0.4764 | 0.6694 | 0.8182 |
| No log | 1.04 | 78 | 0.6633 | 0.4654 | 0.6633 | 0.8144 |
| No log | 1.0667 | 80 | 0.6673 | 0.4625 | 0.6673 | 0.8169 |
| No log | 1.0933 | 82 | 0.6829 | 0.4907 | 0.6829 | 0.8264 |
| No log | 1.12 | 84 | 0.6989 | 0.5261 | 0.6989 | 0.8360 |
| No log | 1.1467 | 86 | 0.7629 | 0.4130 | 0.7629 | 0.8734 |
| No log | 1.1733 | 88 | 0.6706 | 0.4890 | 0.6706 | 0.8189 |
| No log | 1.2 | 90 | 0.6406 | 0.5167 | 0.6406 | 0.8004 |
| No log | 1.2267 | 92 | 0.6180 | 0.4588 | 0.6180 | 0.7862 |
| No log | 1.2533 | 94 | 0.6000 | 0.5093 | 0.6000 | 0.7746 |
| No log | 1.28 | 96 | 0.6071 | 0.4937 | 0.6071 | 0.7792 |
| No log | 1.3067 | 98 | 0.6003 | 0.5428 | 0.6003 | 0.7748 |
| No log | 1.3333 | 100 | 0.7624 | 0.4635 | 0.7624 | 0.8731 |
| No log | 1.3600 | 102 | 0.8822 | 0.3869 | 0.8822 | 0.9393 |
| No log | 1.3867 | 104 | 0.6705 | 0.4846 | 0.6705 | 0.8188 |
| No log | 1.4133 | 106 | 0.6841 | 0.5337 | 0.6841 | 0.8271 |
| No log | 1.44 | 108 | 0.6851 | 0.4861 | 0.6851 | 0.8277 |
| No log | 1.4667 | 110 | 0.7659 | 0.4265 | 0.7659 | 0.8752 |
| No log | 1.4933 | 112 | 0.8911 | 0.3925 | 0.8911 | 0.9440 |
| No log | 1.52 | 114 | 0.7744 | 0.4753 | 0.7744 | 0.8800 |
| No log | 1.5467 | 116 | 0.7172 | 0.4993 | 0.7172 | 0.8469 |
| No log | 1.5733 | 118 | 0.7616 | 0.5591 | 0.7616 | 0.8727 |
| No log | 1.6 | 120 | 0.6781 | 0.5191 | 0.6781 | 0.8235 |
| No log | 1.6267 | 122 | 0.6988 | 0.4878 | 0.6988 | 0.8359 |
| No log | 1.6533 | 124 | 0.6518 | 0.5437 | 0.6518 | 0.8073 |
| No log | 1.6800 | 126 | 0.6510 | 0.5479 | 0.6510 | 0.8068 |
| No log | 1.7067 | 128 | 0.7217 | 0.4961 | 0.7217 | 0.8495 |
| No log | 1.7333 | 130 | 0.7476 | 0.4333 | 0.7476 | 0.8646 |
| No log | 1.76 | 132 | 0.6585 | 0.5521 | 0.6585 | 0.8115 |
| No log | 1.7867 | 134 | 1.0521 | 0.3748 | 1.0521 | 1.0257 |
| No log | 1.8133 | 136 | 1.1245 | 0.3595 | 1.1245 | 1.0604 |
| No log | 1.8400 | 138 | 0.8057 | 0.5043 | 0.8056 | 0.8976 |
| No log | 1.8667 | 140 | 0.6864 | 0.4779 | 0.6864 | 0.8285 |
| No log | 1.8933 | 142 | 0.7963 | 0.4926 | 0.7963 | 0.8923 |
| No log | 1.92 | 144 | 0.7742 | 0.4455 | 0.7742 | 0.8799 |
| No log | 1.9467 | 146 | 0.6246 | 0.4639 | 0.6246 | 0.7903 |
| No log | 1.9733 | 148 | 0.7000 | 0.3946 | 0.7000 | 0.8367 |
| No log | 2.0 | 150 | 0.6720 | 0.4295 | 0.6720 | 0.8197 |
| No log | 2.0267 | 152 | 0.6690 | 0.5525 | 0.6690 | 0.8179 |
| No log | 2.0533 | 154 | 0.7405 | 0.5556 | 0.7405 | 0.8605 |
| No log | 2.08 | 156 | 0.7674 | 0.5024 | 0.7674 | 0.8760 |
| No log | 2.1067 | 158 | 0.7377 | 0.5353 | 0.7377 | 0.8589 |
| No log | 2.1333 | 160 | 0.7463 | 0.5355 | 0.7463 | 0.8639 |
| No log | 2.16 | 162 | 0.8073 | 0.5274 | 0.8073 | 0.8985 |
| No log | 2.1867 | 164 | 0.7908 | 0.5193 | 0.7908 | 0.8893 |
| No log | 2.2133 | 166 | 0.6623 | 0.5511 | 0.6623 | 0.8138 |
| No log | 2.24 | 168 | 0.6459 | 0.5075 | 0.6459 | 0.8037 |
| No log | 2.2667 | 170 | 0.6303 | 0.5177 | 0.6303 | 0.7939 |
| No log | 2.2933 | 172 | 0.6699 | 0.4605 | 0.6699 | 0.8185 |
| No log | 2.32 | 174 | 0.7298 | 0.3074 | 0.7298 | 0.8543 |
| No log | 2.3467 | 176 | 0.7379 | 0.3736 | 0.7379 | 0.8590 |
| No log | 2.3733 | 178 | 0.6722 | 0.4198 | 0.6722 | 0.8199 |
| No log | 2.4 | 180 | 0.6607 | 0.5173 | 0.6607 | 0.8128 |
| No log | 2.4267 | 182 | 0.7416 | 0.4372 | 0.7416 | 0.8612 |
| No log | 2.4533 | 184 | 0.9118 | 0.4838 | 0.9118 | 0.9549 |
| No log | 2.48 | 186 | 1.0113 | 0.4637 | 1.0113 | 1.0056 |
| No log | 2.5067 | 188 | 0.8220 | 0.4580 | 0.8220 | 0.9067 |
| No log | 2.5333 | 190 | 0.7668 | 0.5405 | 0.7668 | 0.8757 |
| No log | 2.56 | 192 | 0.7521 | 0.5736 | 0.7521 | 0.8672 |
| No log | 2.5867 | 194 | 0.7381 | 0.5450 | 0.7381 | 0.8591 |
| No log | 2.6133 | 196 | 0.6993 | 0.5352 | 0.6993 | 0.8362 |
| No log | 2.64 | 198 | 0.6796 | 0.5452 | 0.6796 | 0.8244 |
| No log | 2.6667 | 200 | 0.7133 | 0.4921 | 0.7133 | 0.8446 |
| No log | 2.6933 | 202 | 0.6641 | 0.4863 | 0.6641 | 0.8149 |
| No log | 2.7200 | 204 | 0.6289 | 0.4895 | 0.6289 | 0.7930 |
| No log | 2.7467 | 206 | 0.5907 | 0.4941 | 0.5907 | 0.7686 |
| No log | 2.7733 | 208 | 0.5944 | 0.5304 | 0.5944 | 0.7709 |
| No log | 2.8 | 210 | 0.6257 | 0.5731 | 0.6257 | 0.7910 |
| No log | 2.8267 | 212 | 0.7228 | 0.5279 | 0.7228 | 0.8502 |
| No log | 2.8533 | 214 | 0.7565 | 0.5345 | 0.7565 | 0.8698 |
| No log | 2.88 | 216 | 0.6859 | 0.5576 | 0.6859 | 0.8282 |
| No log | 2.9067 | 218 | 0.6267 | 0.5689 | 0.6267 | 0.7916 |
| No log | 2.9333 | 220 | 0.6523 | 0.5390 | 0.6523 | 0.8077 |
| No log | 2.96 | 222 | 0.6191 | 0.5361 | 0.6191 | 0.7869 |
| No log | 2.9867 | 224 | 0.6572 | 0.5623 | 0.6572 | 0.8107 |
| No log | 3.0133 | 226 | 0.6886 | 0.5519 | 0.6886 | 0.8298 |
| No log | 3.04 | 228 | 0.6496 | 0.5363 | 0.6496 | 0.8060 |
| No log | 3.0667 | 230 | 0.5894 | 0.5130 | 0.5894 | 0.7677 |
| No log | 3.0933 | 232 | 0.5786 | 0.5282 | 0.5786 | 0.7607 |
| No log | 3.12 | 234 | 0.5819 | 0.5045 | 0.5819 | 0.7628 |
| No log | 3.1467 | 236 | 0.6452 | 0.4226 | 0.6452 | 0.8032 |
| No log | 3.1733 | 238 | 0.8235 | 0.4119 | 0.8235 | 0.9075 |
| No log | 3.2 | 240 | 0.7444 | 0.4417 | 0.7444 | 0.8628 |
| No log | 3.2267 | 242 | 0.5939 | 0.4888 | 0.5939 | 0.7706 |
| No log | 3.2533 | 244 | 0.6340 | 0.5698 | 0.6340 | 0.7963 |
| No log | 3.2800 | 246 | 0.6154 | 0.5539 | 0.6154 | 0.7845 |
| No log | 3.3067 | 248 | 0.6259 | 0.5348 | 0.6259 | 0.7911 |
| No log | 3.3333 | 250 | 0.6480 | 0.5490 | 0.6480 | 0.8050 |
| No log | 3.36 | 252 | 0.6086 | 0.5359 | 0.6086 | 0.7801 |
| No log | 3.3867 | 254 | 0.6522 | 0.5542 | 0.6522 | 0.8076 |
| No log | 3.4133 | 256 | 0.7644 | 0.5105 | 0.7644 | 0.8743 |
| No log | 3.44 | 258 | 0.8134 | 0.4658 | 0.8134 | 0.9019 |
| No log | 3.4667 | 260 | 0.6886 | 0.5362 | 0.6886 | 0.8298 |
| No log | 3.4933 | 262 | 0.6858 | 0.5535 | 0.6858 | 0.8281 |
| No log | 3.52 | 264 | 0.8134 | 0.5515 | 0.8134 | 0.9019 |
| No log | 3.5467 | 266 | 0.7357 | 0.5220 | 0.7357 | 0.8578 |
| No log | 3.5733 | 268 | 0.7365 | 0.5407 | 0.7365 | 0.8582 |
| No log | 3.6 | 270 | 0.9025 | 0.4566 | 0.9025 | 0.9500 |
| No log | 3.6267 | 272 | 0.8965 | 0.4314 | 0.8965 | 0.9468 |
| No log | 3.6533 | 274 | 0.6887 | 0.5265 | 0.6887 | 0.8299 |
| No log | 3.68 | 276 | 0.6064 | 0.4920 | 0.6064 | 0.7787 |
| No log | 3.7067 | 278 | 0.6350 | 0.5195 | 0.6350 | 0.7968 |
| No log | 3.7333 | 280 | 0.6352 | 0.5150 | 0.6352 | 0.7970 |
| No log | 3.76 | 282 | 0.5863 | 0.4247 | 0.5863 | 0.7657 |
| No log | 3.7867 | 284 | 0.6303 | 0.5155 | 0.6303 | 0.7939 |
| No log | 3.8133 | 286 | 0.8605 | 0.4826 | 0.8605 | 0.9276 |
| No log | 3.84 | 288 | 1.0712 | 0.3741 | 1.0712 | 1.0350 |
| No log | 3.8667 | 290 | 0.9806 | 0.4228 | 0.9806 | 0.9903 |
| No log | 3.8933 | 292 | 0.7296 | 0.5465 | 0.7296 | 0.8542 |
| No log | 3.92 | 294 | 0.6715 | 0.5666 | 0.6715 | 0.8194 |
| No log | 3.9467 | 296 | 0.6805 | 0.5477 | 0.6805 | 0.8249 |
| No log | 3.9733 | 298 | 0.6428 | 0.5202 | 0.6428 | 0.8018 |
| No log | 4.0 | 300 | 0.6356 | 0.5223 | 0.6356 | 0.7972 |
| No log | 4.0267 | 302 | 0.6946 | 0.4772 | 0.6946 | 0.8334 |
| No log | 4.0533 | 304 | 0.6826 | 0.5116 | 0.6826 | 0.8262 |
| No log | 4.08 | 306 | 0.6041 | 0.4865 | 0.6041 | 0.7773 |
| No log | 4.1067 | 308 | 0.6247 | 0.5822 | 0.6247 | 0.7904 |
| No log | 4.1333 | 310 | 0.6491 | 0.5552 | 0.6491 | 0.8057 |
| No log | 4.16 | 312 | 0.6282 | 0.4859 | 0.6282 | 0.7926 |
| No log | 4.1867 | 314 | 0.6909 | 0.5050 | 0.6909 | 0.8312 |
| No log | 4.2133 | 316 | 0.7275 | 0.5116 | 0.7275 | 0.8529 |
| No log | 4.24 | 318 | 0.6434 | 0.4679 | 0.6434 | 0.8021 |
| No log | 4.2667 | 320 | 0.6357 | 0.5287 | 0.6357 | 0.7973 |
| No log | 4.2933 | 322 | 0.7084 | 0.5614 | 0.7084 | 0.8417 |
| No log | 4.32 | 324 | 0.7082 | 0.5855 | 0.7082 | 0.8415 |
| No log | 4.3467 | 326 | 0.6710 | 0.5477 | 0.6710 | 0.8192 |
| No log | 4.3733 | 328 | 0.6875 | 0.5730 | 0.6875 | 0.8292 |
| No log | 4.4 | 330 | 0.6828 | 0.6061 | 0.6828 | 0.8263 |
| No log | 4.4267 | 332 | 0.6903 | 0.5946 | 0.6903 | 0.8308 |
| No log | 4.4533 | 334 | 0.7444 | 0.5107 | 0.7444 | 0.8628 |
| No log | 4.48 | 336 | 0.8059 | 0.4831 | 0.8059 | 0.8977 |
| No log | 4.5067 | 338 | 0.6920 | 0.4774 | 0.6920 | 0.8319 |
| No log | 4.5333 | 340 | 0.6099 | 0.4769 | 0.6099 | 0.7810 |
| No log | 4.5600 | 342 | 0.6169 | 0.5028 | 0.6169 | 0.7855 |
| No log | 4.5867 | 344 | 0.6360 | 0.4760 | 0.6360 | 0.7975 |
| No log | 4.6133 | 346 | 0.6501 | 0.4760 | 0.6501 | 0.8063 |
| No log | 4.64 | 348 | 0.6644 | 0.5425 | 0.6644 | 0.8151 |
| No log | 4.6667 | 350 | 0.6795 | 0.5445 | 0.6795 | 0.8243 |
| No log | 4.6933 | 352 | 0.6581 | 0.4876 | 0.6581 | 0.8112 |
| No log | 4.72 | 354 | 0.7090 | 0.4845 | 0.7090 | 0.8420 |
| No log | 4.7467 | 356 | 0.7281 | 0.5088 | 0.7281 | 0.8533 |
| No log | 4.7733 | 358 | 0.7502 | 0.4784 | 0.7502 | 0.8661 |
| No log | 4.8 | 360 | 0.7189 | 0.4500 | 0.7189 | 0.8479 |
| No log | 4.8267 | 362 | 0.6713 | 0.4934 | 0.6713 | 0.8193 |
| No log | 4.8533 | 364 | 0.6642 | 0.5145 | 0.6642 | 0.8150 |
| No log | 4.88 | 366 | 0.7038 | 0.5392 | 0.7038 | 0.8389 |
| No log | 4.9067 | 368 | 0.7338 | 0.5264 | 0.7338 | 0.8566 |
| No log | 4.9333 | 370 | 0.7467 | 0.5534 | 0.7467 | 0.8641 |
| No log | 4.96 | 372 | 0.6577 | 0.5583 | 0.6577 | 0.8110 |
| No log | 4.9867 | 374 | 0.6480 | 0.5617 | 0.6480 | 0.8050 |
| No log | 5.0133 | 376 | 0.7165 | 0.5181 | 0.7165 | 0.8465 |
| No log | 5.04 | 378 | 0.6504 | 0.5133 | 0.6504 | 0.8065 |
| No log | 5.0667 | 380 | 0.6249 | 0.5609 | 0.6249 | 0.7905 |
| No log | 5.0933 | 382 | 0.6760 | 0.5548 | 0.6760 | 0.8222 |
| No log | 5.12 | 384 | 0.6864 | 0.5930 | 0.6864 | 0.8285 |
| No log | 5.1467 | 386 | 0.6735 | 0.5533 | 0.6735 | 0.8207 |
| No log | 5.1733 | 388 | 0.6714 | 0.5867 | 0.6714 | 0.8194 |
| No log | 5.2 | 390 | 0.6704 | 0.5609 | 0.6704 | 0.8188 |
| No log | 5.2267 | 392 | 0.6841 | 0.5554 | 0.6841 | 0.8271 |
| No log | 5.2533 | 394 | 0.6846 | 0.5712 | 0.6846 | 0.8274 |
| No log | 5.28 | 396 | 0.6642 | 0.5721 | 0.6642 | 0.8150 |
| No log | 5.3067 | 398 | 0.6702 | 0.5796 | 0.6702 | 0.8186 |
| No log | 5.3333 | 400 | 0.6707 | 0.5821 | 0.6707 | 0.8189 |
| No log | 5.36 | 402 | 0.6647 | 0.5053 | 0.6647 | 0.8153 |
| No log | 5.3867 | 404 | 0.6289 | 0.5121 | 0.6289 | 0.7930 |
| No log | 5.4133 | 406 | 0.5928 | 0.4959 | 0.5928 | 0.7699 |
| No log | 5.44 | 408 | 0.6008 | 0.5260 | 0.6008 | 0.7751 |
| No log | 5.4667 | 410 | 0.6098 | 0.5325 | 0.6098 | 0.7809 |
| No log | 5.4933 | 412 | 0.6082 | 0.5325 | 0.6082 | 0.7799 |
| No log | 5.52 | 414 | 0.6111 | 0.4954 | 0.6111 | 0.7817 |
| No log | 5.5467 | 416 | 0.5939 | 0.5241 | 0.5939 | 0.7706 |
| No log | 5.5733 | 418 | 0.6025 | 0.4832 | 0.6025 | 0.7762 |
| No log | 5.6 | 420 | 0.6194 | 0.5485 | 0.6194 | 0.7870 |
| No log | 5.6267 | 422 | 0.6310 | 0.5128 | 0.6310 | 0.7944 |
| No log | 5.6533 | 424 | 0.6648 | 0.5584 | 0.6648 | 0.8153 |
| No log | 5.68 | 426 | 0.6488 | 0.5217 | 0.6488 | 0.8055 |
| No log | 5.7067 | 428 | 0.6126 | 0.5245 | 0.6126 | 0.7827 |
| No log | 5.7333 | 430 | 0.6197 | 0.5325 | 0.6197 | 0.7872 |
| No log | 5.76 | 432 | 0.6129 | 0.4857 | 0.6129 | 0.7829 |
| No log | 5.7867 | 434 | 0.6114 | 0.4857 | 0.6114 | 0.7819 |
| No log | 5.8133 | 436 | 0.6005 | 0.4096 | 0.6005 | 0.7749 |
| No log | 5.84 | 438 | 0.6090 | 0.4557 | 0.6090 | 0.7804 |
| No log | 5.8667 | 440 | 0.6289 | 0.5291 | 0.6289 | 0.7930 |
| No log | 5.8933 | 442 | 0.6780 | 0.5095 | 0.6780 | 0.8234 |
| No log | 5.92 | 444 | 0.6742 | 0.5026 | 0.6742 | 0.8211 |
| No log | 5.9467 | 446 | 0.6197 | 0.5239 | 0.6197 | 0.7872 |
| No log | 5.9733 | 448 | 0.5876 | 0.4319 | 0.5876 | 0.7665 |
| No log | 6.0 | 450 | 0.6147 | 0.4397 | 0.6147 | 0.7840 |
| No log | 6.0267 | 452 | 0.6204 | 0.4072 | 0.6204 | 0.7876 |
| No log | 6.0533 | 454 | 0.6048 | 0.4100 | 0.6048 | 0.7777 |
| No log | 6.08 | 456 | 0.5913 | 0.3858 | 0.5913 | 0.7690 |
| No log | 6.1067 | 458 | 0.5979 | 0.5203 | 0.5979 | 0.7732 |
| No log | 6.1333 | 460 | 0.6222 | 0.5476 | 0.6222 | 0.7888 |
| No log | 6.16 | 462 | 0.6464 | 0.5142 | 0.6464 | 0.8040 |
| No log | 6.1867 | 464 | 0.6551 | 0.4809 | 0.6551 | 0.8094 |
| No log | 6.2133 | 466 | 0.6084 | 0.4877 | 0.6084 | 0.7800 |
| No log | 6.24 | 468 | 0.6084 | 0.4751 | 0.6084 | 0.7800 |
| No log | 6.2667 | 470 | 0.5964 | 0.5036 | 0.5964 | 0.7723 |
| No log | 6.2933 | 472 | 0.5780 | 0.4922 | 0.5780 | 0.7603 |
| No log | 6.32 | 474 | 0.5946 | 0.5037 | 0.5946 | 0.7711 |
| No log | 6.3467 | 476 | 0.6950 | 0.4399 | 0.6950 | 0.8336 |
| No log | 6.3733 | 478 | 0.8146 | 0.4281 | 0.8146 | 0.9026 |
| No log | 6.4 | 480 | 0.7520 | 0.4747 | 0.7520 | 0.8672 |
| No log | 6.4267 | 482 | 0.6644 | 0.5306 | 0.6644 | 0.8151 |
| No log | 6.4533 | 484 | 0.6164 | 0.5652 | 0.6164 | 0.7851 |
| No log | 6.48 | 486 | 0.6405 | 0.4678 | 0.6405 | 0.8003 |
| No log | 6.5067 | 488 | 0.6407 | 0.4540 | 0.6407 | 0.8004 |
| No log | 6.5333 | 490 | 0.7396 | 0.4718 | 0.7396 | 0.8600 |
| No log | 6.5600 | 492 | 0.7676 | 0.4734 | 0.7676 | 0.8762 |
| No log | 6.5867 | 494 | 0.6619 | 0.5073 | 0.6619 | 0.8136 |
| No log | 6.6133 | 496 | 0.6201 | 0.4954 | 0.6201 | 0.7874 |
| No log | 6.64 | 498 | 0.6990 | 0.5120 | 0.6990 | 0.8360 |
| 0.3842 | 6.6667 | 500 | 0.7189 | 0.4713 | 0.7189 | 0.8479 |
| 0.3842 | 6.6933 | 502 | 0.6874 | 0.4435 | 0.6874 | 0.8291 |
| 0.3842 | 6.72 | 504 | 0.6435 | 0.4926 | 0.6435 | 0.8022 |
| 0.3842 | 6.7467 | 506 | 0.6430 | 0.5097 | 0.6430 | 0.8019 |
| 0.3842 | 6.7733 | 508 | 0.6546 | 0.4350 | 0.6546 | 0.8091 |
| 0.3842 | 6.8 | 510 | 0.7201 | 0.4382 | 0.7201 | 0.8486 |
| 0.3842 | 6.8267 | 512 | 0.7396 | 0.4649 | 0.7396 | 0.8600 |
| 0.3842 | 6.8533 | 514 | 0.6930 | 0.4892 | 0.6930 | 0.8325 |
| 0.3842 | 6.88 | 516 | 0.6335 | 0.3964 | 0.6335 | 0.7959 |
| 0.3842 | 6.9067 | 518 | 0.6211 | 0.4274 | 0.6211 | 0.7881 |
| 0.3842 | 6.9333 | 520 | 0.6323 | 0.4307 | 0.6323 | 0.7952 |
| 0.3842 | 6.96 | 522 | 0.6451 | 0.4972 | 0.6451 | 0.8032 |
| 0.3842 | 6.9867 | 524 | 0.6716 | 0.4948 | 0.6716 | 0.8195 |
| 0.3842 | 7.0133 | 526 | 0.7644 | 0.4541 | 0.7644 | 0.8743 |
| 0.3842 | 7.04 | 528 | 0.8006 | 0.3650 | 0.8006 | 0.8947 |
| 0.3842 | 7.0667 | 530 | 0.7237 | 0.4596 | 0.7237 | 0.8507 |
| 0.3842 | 7.0933 | 532 | 0.6558 | 0.4221 | 0.6558 | 0.8098 |
| 0.3842 | 7.12 | 534 | 0.6468 | 0.4520 | 0.6468 | 0.8042 |
### Framework versions
- Transformers 4.44.2
- Pytorch 2.4.0+cu118
- Datasets 2.21.0
- Tokenizers 0.19.1
|
juierror/thai-news-summarization | juierror | "2024-04-25T06:18:58Z" | 170 | 0 | transformers | [
"transformers",
"pytorch",
"safetensors",
"mt5",
"text2text-generation",
"th",
"dataset:thaisum",
"license:mit",
"autotrain_compatible",
"endpoints_compatible",
"region:us"
] | text2text-generation | "2022-04-28T05:00:01Z" | ---
license: mit
language: th
datasets:
- thaisum
widget:
- text: "some news with head line"
---
# How to use
```python3
from transformers import MT5Tokenizer, MT5ForConditionalGeneration
tokenizer = MT5Tokenizer.from_pretrained('juierror/thai-news-summarization')
model = MT5ForConditionalGeneration.from_pretrained('juierror/thai-news-summarization')
text = "some news with head line"
tokenized_text = tokenizer(text, truncation=True, padding=True, return_tensors='pt')
source_ids = tokenized_text['input_ids'].to("cpu", dtype = torch.long)
source_mask = tokenized_text['attention_mask'].to("cpu", dtype = torch.long)
generated_ids = model.generate(
input_ids = source_ids,
attention_mask = source_mask,
max_length=512,
num_beams=5,
repetition_penalty=1,
length_penalty=1,
early_stopping=True,
no_repeat_ngram_size=2
)
pred = tokenizer.decode(generated_ids[0], skip_special_tokens=True, clean_up_tokenization_spaces=True)
``` |
facebook/wav2vec2-base-10k-voxpopuli-ft-nl | facebook | "2021-07-06T01:51:40Z" | 12 | 0 | transformers | [
"transformers",
"pytorch",
"wav2vec2",
"automatic-speech-recognition",
"audio",
"voxpopuli",
"nl",
"arxiv:2101.00390",
"license:cc-by-nc-4.0",
"endpoints_compatible",
"region:us"
] | automatic-speech-recognition | "2022-03-02T23:29:05Z" | ---
language: nl
tags:
- audio
- automatic-speech-recognition
- voxpopuli
license: cc-by-nc-4.0
---
# Wav2Vec2-Base-VoxPopuli-Finetuned
[Facebook's Wav2Vec2](https://ai.facebook.com/blog/wav2vec-20-learning-the-structure-of-speech-from-raw-audio/) base model pretrained on the 10K unlabeled subset of [VoxPopuli corpus](https://arxiv.org/abs/2101.00390) and fine-tuned on the transcribed data in nl (refer to Table 1 of paper for more information).
**Paper**: *[VoxPopuli: A Large-Scale Multilingual Speech Corpus for Representation
Learning, Semi-Supervised Learning and Interpretation](https://arxiv.org/abs/2101.00390)*
**Authors**: *Changhan Wang, Morgane Riviere, Ann Lee, Anne Wu, Chaitanya Talnikar, Daniel Haziza, Mary Williamson, Juan Pino, Emmanuel Dupoux* from *Facebook AI*
See the official website for more information, [here](https://github.com/facebookresearch/voxpopuli/)
# Usage for inference
In the following it is shown how the model can be used in inference on a sample of the [Common Voice dataset](https://commonvoice.mozilla.org/en/datasets)
```python
#!/usr/bin/env python3
from transformers import Wav2Vec2Processor, Wav2Vec2ForCTC
from datasets import load_dataset
import torchaudio
import torch
# resample audio
# load model & processor
model = Wav2Vec2ForCTC.from_pretrained("facebook/wav2vec2-base-10k-voxpopuli-ft-nl")
processor = Wav2Vec2Processor.from_pretrained("facebook/wav2vec2-base-10k-voxpopuli-ft-nl")
# load dataset
ds = load_dataset("common_voice", "nl", split="validation[:1%]")
# common voice does not match target sampling rate
common_voice_sample_rate = 48000
target_sample_rate = 16000
resampler = torchaudio.transforms.Resample(common_voice_sample_rate, target_sample_rate)
# define mapping fn to read in sound file and resample
def map_to_array(batch):
speech, _ = torchaudio.load(batch["path"])
speech = resampler(speech)
batch["speech"] = speech[0]
return batch
# load all audio files
ds = ds.map(map_to_array)
# run inference on the first 5 data samples
inputs = processor(ds[:5]["speech"], sampling_rate=target_sample_rate, return_tensors="pt", padding=True)
# inference
logits = model(**inputs).logits
predicted_ids = torch.argmax(logits, axis=-1)
print(processor.batch_decode(predicted_ids))
```
|
AntoineD/camembert_ccnet_classification_tools_qlora-8bit_fr | AntoineD | "2023-11-15T09:40:09Z" | 0 | 0 | null | [
"generated_from_trainer",
"base_model:almanach/camembert-base-ccnet",
"base_model:finetune:almanach/camembert-base-ccnet",
"region:us"
] | null | "2023-11-15T09:24:02Z" | ---
base_model: camembert/camembert-base-ccnet
tags:
- generated_from_trainer
metrics:
- accuracy
model-index:
- name: camembert_ccnet_classification_tools_qlora-8bit_fr
results: []
---
<!-- This model card has been generated automatically according to the information the Trainer had access to. You
should probably proofread and complete it, then remove this comment. -->
# camembert_ccnet_classification_tools_qlora-8bit_fr
This model is a fine-tuned version of [camembert/camembert-base-ccnet](https://huggingface.co/camembert/camembert-base-ccnet) on the None dataset.
It achieves the following results on the evaluation set:
- Loss: 1.5066
- Accuracy: 0.85
- Learning Rate: 0.0
## Model description
More information needed
## Intended uses & limitations
More information needed
## Training and evaluation data
More information needed
## Training procedure
### Training hyperparameters
The following hyperparameters were used during training:
- learning_rate: 0.0001
- train_batch_size: 24
- eval_batch_size: 192
- seed: 42
- optimizer: Adam with betas=(0.9,0.999) and epsilon=1e-08
- lr_scheduler_type: linear
- num_epochs: 60
### Training results
| Training Loss | Epoch | Step | Validation Loss | Accuracy | Rate |
|:-------------:|:-----:|:----:|:---------------:|:--------:|:------:|
| 2.0629 | 1.0 | 7 | 2.0715 | 0.125 | 0.0001 |
| 2.0638 | 2.0 | 14 | 2.0676 | 0.15 | 0.0001 |
| 2.0614 | 3.0 | 21 | 2.0661 | 0.1 | 0.0001 |
| 2.0676 | 4.0 | 28 | 2.0622 | 0.125 | 0.0001 |
| 2.0502 | 5.0 | 35 | 2.0530 | 0.15 | 0.0001 |
| 2.0409 | 6.0 | 42 | 2.0480 | 0.175 | 9e-05 |
| 2.0457 | 7.0 | 49 | 2.0431 | 0.1 | 0.0001 |
| 2.0401 | 8.0 | 56 | 2.0398 | 0.125 | 0.0001 |
| 2.0239 | 9.0 | 63 | 2.0283 | 0.275 | 0.0001 |
| 2.0257 | 10.0 | 70 | 2.0131 | 0.3 | 0.0001 |
| 1.999 | 11.0 | 77 | 1.9926 | 0.425 | 0.0001 |
| 2.0053 | 12.0 | 84 | 1.9866 | 0.4 | 8e-05 |
| 1.9893 | 13.0 | 91 | 1.9710 | 0.475 | 0.0001 |
| 1.9762 | 14.0 | 98 | 1.9572 | 0.475 | 0.0001 |
| 1.9726 | 15.0 | 105 | 1.9398 | 0.425 | 0.0001 |
| 1.9456 | 16.0 | 112 | 1.9152 | 0.5 | 0.0001 |
| 1.9425 | 17.0 | 119 | 1.8971 | 0.575 | 0.0001 |
| 1.9078 | 18.0 | 126 | 1.8788 | 0.625 | 7e-05 |
| 1.901 | 19.0 | 133 | 1.8608 | 0.65 | 0.0001 |
| 1.8865 | 20.0 | 140 | 1.8407 | 0.625 | 0.0001 |
| 1.8696 | 21.0 | 147 | 1.8234 | 0.675 | 0.0001 |
| 1.8618 | 22.0 | 154 | 1.7964 | 0.725 | 0.0001 |
| 1.8238 | 23.0 | 161 | 1.7778 | 0.725 | 0.0001 |
| 1.8164 | 24.0 | 168 | 1.7739 | 0.675 | 6e-05 |
| 1.823 | 25.0 | 175 | 1.7442 | 0.675 | 0.0001 |
| 1.7892 | 26.0 | 182 | 1.7265 | 0.7 | 0.0001 |
| 1.7865 | 27.0 | 189 | 1.7110 | 0.75 | 0.0001 |
| 1.7643 | 28.0 | 196 | 1.7114 | 0.725 | 0.0001 |
| 1.7613 | 29.0 | 203 | 1.6859 | 0.775 | 0.0001 |
| 1.7681 | 30.0 | 210 | 1.6686 | 0.775 | 5e-05 |
| 1.7251 | 31.0 | 217 | 1.6547 | 0.8 | 0.0000 |
| 1.7215 | 32.0 | 224 | 1.6431 | 0.8 | 0.0000 |
| 1.7304 | 33.0 | 231 | 1.6285 | 0.75 | 0.0000 |
| 1.7182 | 34.0 | 238 | 1.6164 | 0.8 | 0.0000 |
| 1.7099 | 35.0 | 245 | 1.6066 | 0.825 | 0.0000 |
| 1.6902 | 36.0 | 252 | 1.6060 | 0.8 | 4e-05 |
| 1.6839 | 37.0 | 259 | 1.5949 | 0.875 | 0.0000 |
| 1.6627 | 38.0 | 266 | 1.5822 | 0.875 | 0.0000 |
| 1.6558 | 39.0 | 273 | 1.5723 | 0.875 | 0.0000 |
| 1.6667 | 40.0 | 280 | 1.5639 | 0.85 | 0.0000 |
| 1.6663 | 41.0 | 287 | 1.5576 | 0.85 | 0.0000 |
| 1.642 | 42.0 | 294 | 1.5506 | 0.825 | 3e-05 |
| 1.6479 | 43.0 | 301 | 1.5471 | 0.825 | 0.0000 |
| 1.6425 | 44.0 | 308 | 1.5414 | 0.825 | 0.0000 |
| 1.6214 | 45.0 | 315 | 1.5351 | 0.85 | 0.0000 |
| 1.6331 | 46.0 | 322 | 1.5310 | 0.875 | 0.0000 |
| 1.6406 | 47.0 | 329 | 1.5281 | 0.875 | 0.0000 |
| 1.6332 | 48.0 | 336 | 1.5265 | 0.875 | 2e-05 |
| 1.6474 | 49.0 | 343 | 1.5218 | 0.875 | 0.0000 |
| 1.6122 | 50.0 | 350 | 1.5184 | 0.875 | 0.0000 |
| 1.6166 | 51.0 | 357 | 1.5162 | 0.875 | 0.0000 |
| 1.6245 | 52.0 | 364 | 1.5343 | 0.875 | 0.0000 |
| 1.6027 | 53.0 | 371 | 1.5272 | 0.85 | 0.0000 |
| 1.6305 | 54.0 | 378 | 1.5261 | 0.85 | 1e-05 |
| 1.6172 | 55.0 | 385 | 1.5173 | 0.85 | 0.0000 |
| 1.6109 | 56.0 | 392 | 1.5168 | 0.875 | 0.0000 |
| 1.6037 | 57.0 | 399 | 1.5154 | 0.875 | 5e-06 |
| 1.6031 | 58.0 | 406 | 1.4970 | 0.875 | 0.0000 |
| 1.617 | 59.0 | 413 | 1.4961 | 0.875 | 0.0000 |
| 1.5955 | 60.0 | 420 | 1.5066 | 0.85 | 0.0 |
### Framework versions
- Transformers 4.34.0
- Pytorch 2.0.1+cu117
- Datasets 2.14.5
- Tokenizers 0.14.1
|
philip-hightech/ab1e71ce-856f-4b0b-98fb-61d3ded98270 | philip-hightech | "2025-01-22T15:30:53Z" | 8 | 0 | peft | [
"peft",
"safetensors",
"qwen2",
"axolotl",
"generated_from_trainer",
"base_model:unsloth/Qwen2-0.5B-Instruct",
"base_model:adapter:unsloth/Qwen2-0.5B-Instruct",
"license:apache-2.0",
"region:us"
] | null | "2025-01-22T15:14:01Z" | ---
library_name: peft
license: apache-2.0
base_model: unsloth/Qwen2-0.5B-Instruct
tags:
- axolotl
- generated_from_trainer
model-index:
- name: ab1e71ce-856f-4b0b-98fb-61d3ded98270
results: []
---
<!-- This model card has been generated automatically according to the information the Trainer had access to. You
should probably proofread and complete it, then remove this comment. -->
[<img src="https://raw.githubusercontent.com/axolotl-ai-cloud/axolotl/main/image/axolotl-badge-web.png" alt="Built with Axolotl" width="200" height="32"/>](https://github.com/axolotl-ai-cloud/axolotl)
<details><summary>See axolotl config</summary>
axolotl version: `0.4.1`
```yaml
adapter: lora
base_model: unsloth/Qwen2-0.5B-Instruct
bf16: auto
chat_template: llama3
dataset_prepared_path: null
datasets:
- data_files:
- 1f45e34e3233acc7_train_data.json
ds_type: json
format: custom
path: /workspace/input_data/1f45e34e3233acc7_train_data.json
type:
field_input: input
field_instruction: instruction
field_output: output
format: '{instruction} {input}'
no_input_format: '{instruction}'
system_format: '{system}'
system_prompt: ''
debug: null
deepspeed: null
early_stopping_patience: null
eval_max_new_tokens: 128
eval_table_size: null
evals_per_epoch: 4
flash_attention: false
fp16: null
fsdp: null
fsdp_config: null
gradient_accumulation_steps: 4
gradient_checkpointing: false
group_by_length: false
hub_model_id: philip-hightech/ab1e71ce-856f-4b0b-98fb-61d3ded98270
hub_repo: null
hub_strategy: checkpoint
hub_token: null
learning_rate: 0.0002
load_in_4bit: false
load_in_8bit: false
local_rank: null
logging_steps: 1
lora_alpha: 16
lora_dropout: 0.05
lora_fan_in_fan_out: null
lora_model_dir: null
lora_r: 8
lora_target_linear: true
lr_scheduler: cosine
max_steps: 10
micro_batch_size: 2
mlflow_experiment_name: /tmp/1f45e34e3233acc7_train_data.json
model_type: AutoModelForCausalLM
num_epochs: 1
optimizer: adamw_bnb_8bit
output_dir: miner_id_24
pad_to_sequence_len: true
resume_from_checkpoint: null
s2_attention: null
sample_packing: false
saves_per_epoch: 4
sequence_len: 512
strict: false
tf32: false
tokenizer_type: AutoTokenizer
train_on_inputs: false
trust_remote_code: true
val_set_size: 0.05
wandb_entity: null
wandb_mode: online
wandb_name: 7925ac83-4212-4da5-b1e7-30dc8a594b43
wandb_project: Gradients-On-Demand
wandb_run: your_name
wandb_runid: 7925ac83-4212-4da5-b1e7-30dc8a594b43
warmup_steps: 10
weight_decay: 0.0
xformers_attention: null
```
</details><br>
# ab1e71ce-856f-4b0b-98fb-61d3ded98270
This model is a fine-tuned version of [unsloth/Qwen2-0.5B-Instruct](https://huggingface.co/unsloth/Qwen2-0.5B-Instruct) on the None dataset.
It achieves the following results on the evaluation set:
- Loss: nan
## Model description
More information needed
## Intended uses & limitations
More information needed
## Training and evaluation data
More information needed
## Training procedure
### Training hyperparameters
The following hyperparameters were used during training:
- learning_rate: 0.0002
- train_batch_size: 2
- eval_batch_size: 2
- seed: 42
- gradient_accumulation_steps: 4
- total_train_batch_size: 8
- optimizer: Use OptimizerNames.ADAMW_BNB with betas=(0.9,0.999) and epsilon=1e-08 and optimizer_args=No additional optimizer arguments
- lr_scheduler_type: cosine
- lr_scheduler_warmup_steps: 10
- training_steps: 10
### Training results
| Training Loss | Epoch | Step | Validation Loss |
|:-------------:|:------:|:----:|:---------------:|
| 0.0 | 0.0000 | 1 | nan |
| 0.0 | 0.0001 | 3 | nan |
| 0.0 | 0.0002 | 6 | nan |
| 0.0 | 0.0003 | 9 | nan |
### Framework versions
- PEFT 0.13.2
- Transformers 4.46.0
- Pytorch 2.5.0+cu124
- Datasets 3.0.1
- Tokenizers 0.20.1 |
YakovElm/Jira10Classic_with_cleaning | YakovElm | "2023-05-24T03:49:14Z" | 61 | 0 | transformers | [
"transformers",
"tf",
"bert",
"text-classification",
"generated_from_keras_callback",
"license:apache-2.0",
"autotrain_compatible",
"endpoints_compatible",
"region:us"
] | text-classification | "2023-05-24T03:48:37Z" | ---
license: apache-2.0
tags:
- generated_from_keras_callback
model-index:
- name: Jira10Classic_with_cleaning
results: []
---
<!-- This model card has been generated automatically according to the information Keras had access to. You should
probably proofread and complete it, then remove this comment. -->
# Jira10Classic_with_cleaning
This model is a fine-tuned version of [bert-base-uncased](https://huggingface.co/bert-base-uncased) on an unknown dataset.
It achieves the following results on the evaluation set:
- Train Loss: 0.2784
- Train Accuracy: 0.8961
- Validation Loss: 1.2932
- Validation Accuracy: 0.5773
- Epoch: 2
## Model description
More information needed
## Intended uses & limitations
More information needed
## Training and evaluation data
More information needed
## Training procedure
### Training hyperparameters
The following hyperparameters were used during training:
- optimizer: {'name': 'Adam', 'weight_decay': None, 'clipnorm': 1.0, 'global_clipnorm': None, 'clipvalue': None, 'use_ema': False, 'ema_momentum': 0.99, 'ema_overwrite_frequency': None, 'jit_compile': False, 'is_legacy_optimizer': False, 'learning_rate': 3e-05, 'beta_1': 0.9, 'beta_2': 0.999, 'epsilon': 1e-08, 'amsgrad': False}
- training_precision: float32
### Training results
| Train Loss | Train Accuracy | Validation Loss | Validation Accuracy | Epoch |
|:----------:|:--------------:|:---------------:|:-------------------:|:-----:|
| 0.5051 | 0.7807 | 0.6951 | 0.4921 | 0 |
| 0.4049 | 0.8048 | 1.1332 | 0.5079 | 1 |
| 0.2784 | 0.8961 | 1.2932 | 0.5773 | 2 |
### Framework versions
- Transformers 4.29.2
- TensorFlow 2.12.0
- Datasets 2.12.0
- Tokenizers 0.13.3
|
mradermacher/DeepHermes-3-Llama-3-3B-Preview-abliterated-i1-GGUF | mradermacher | "2025-03-19T10:16:14Z" | 0 | 1 | transformers | [
"transformers",
"gguf",
"text-generation-inference",
"en",
"base_model:prithivMLmods/DeepHermes-3-Llama-3-3B-Preview-abliterated",
"base_model:quantized:prithivMLmods/DeepHermes-3-Llama-3-3B-Preview-abliterated",
"license:apache-2.0",
"endpoints_compatible",
"region:us",
"imatrix",
"conversational"
] | null | "2025-03-19T09:15:37Z" | ---
base_model: prithivMLmods/DeepHermes-3-Llama-3-3B-Preview-abliterated
language:
- en
library_name: transformers
license: apache-2.0
quantized_by: mradermacher
tags:
- text-generation-inference
---
## About
<!-- ### quantize_version: 2 -->
<!-- ### output_tensor_quantised: 1 -->
<!-- ### convert_type: hf -->
<!-- ### vocab_type: -->
<!-- ### tags: nicoboss -->
weighted/imatrix quants of https://huggingface.co/prithivMLmods/DeepHermes-3-Llama-3-3B-Preview-abliterated
<!-- provided-files -->
static quants are available at https://huggingface.co/mradermacher/DeepHermes-3-Llama-3-3B-Preview-abliterated-GGUF
## Usage
If you are unsure how to use GGUF files, refer to one of [TheBloke's
READMEs](https://huggingface.co/TheBloke/KafkaLM-70B-German-V0.1-GGUF) for
more details, including on how to concatenate multi-part files.
## Provided Quants
(sorted by size, not necessarily quality. IQ-quants are often preferable over similar sized non-IQ quants)
| Link | Type | Size/GB | Notes |
|:-----|:-----|--------:|:------|
| [GGUF](https://huggingface.co/mradermacher/DeepHermes-3-Llama-3-3B-Preview-abliterated-i1-GGUF/resolve/main/DeepHermes-3-Llama-3-3B-Preview-abliterated.i1-IQ1_S.gguf) | i1-IQ1_S | 1.0 | for the desperate |
| [GGUF](https://huggingface.co/mradermacher/DeepHermes-3-Llama-3-3B-Preview-abliterated-i1-GGUF/resolve/main/DeepHermes-3-Llama-3-3B-Preview-abliterated.i1-IQ1_M.gguf) | i1-IQ1_M | 1.0 | mostly desperate |
| [GGUF](https://huggingface.co/mradermacher/DeepHermes-3-Llama-3-3B-Preview-abliterated-i1-GGUF/resolve/main/DeepHermes-3-Llama-3-3B-Preview-abliterated.i1-IQ2_XXS.gguf) | i1-IQ2_XXS | 1.1 | |
| [GGUF](https://huggingface.co/mradermacher/DeepHermes-3-Llama-3-3B-Preview-abliterated-i1-GGUF/resolve/main/DeepHermes-3-Llama-3-3B-Preview-abliterated.i1-IQ2_XS.gguf) | i1-IQ2_XS | 1.2 | |
| [GGUF](https://huggingface.co/mradermacher/DeepHermes-3-Llama-3-3B-Preview-abliterated-i1-GGUF/resolve/main/DeepHermes-3-Llama-3-3B-Preview-abliterated.i1-IQ2_S.gguf) | i1-IQ2_S | 1.3 | |
| [GGUF](https://huggingface.co/mradermacher/DeepHermes-3-Llama-3-3B-Preview-abliterated-i1-GGUF/resolve/main/DeepHermes-3-Llama-3-3B-Preview-abliterated.i1-IQ2_M.gguf) | i1-IQ2_M | 1.3 | |
| [GGUF](https://huggingface.co/mradermacher/DeepHermes-3-Llama-3-3B-Preview-abliterated-i1-GGUF/resolve/main/DeepHermes-3-Llama-3-3B-Preview-abliterated.i1-Q2_K_S.gguf) | i1-Q2_K_S | 1.4 | very low quality |
| [GGUF](https://huggingface.co/mradermacher/DeepHermes-3-Llama-3-3B-Preview-abliterated-i1-GGUF/resolve/main/DeepHermes-3-Llama-3-3B-Preview-abliterated.i1-IQ3_XXS.gguf) | i1-IQ3_XXS | 1.4 | lower quality |
| [GGUF](https://huggingface.co/mradermacher/DeepHermes-3-Llama-3-3B-Preview-abliterated-i1-GGUF/resolve/main/DeepHermes-3-Llama-3-3B-Preview-abliterated.i1-Q2_K.gguf) | i1-Q2_K | 1.5 | IQ3_XXS probably better |
| [GGUF](https://huggingface.co/mradermacher/DeepHermes-3-Llama-3-3B-Preview-abliterated-i1-GGUF/resolve/main/DeepHermes-3-Llama-3-3B-Preview-abliterated.i1-IQ3_XS.gguf) | i1-IQ3_XS | 1.6 | |
| [GGUF](https://huggingface.co/mradermacher/DeepHermes-3-Llama-3-3B-Preview-abliterated-i1-GGUF/resolve/main/DeepHermes-3-Llama-3-3B-Preview-abliterated.i1-IQ3_S.gguf) | i1-IQ3_S | 1.6 | beats Q3_K* |
| [GGUF](https://huggingface.co/mradermacher/DeepHermes-3-Llama-3-3B-Preview-abliterated-i1-GGUF/resolve/main/DeepHermes-3-Llama-3-3B-Preview-abliterated.i1-Q3_K_S.gguf) | i1-Q3_K_S | 1.6 | IQ3_XS probably better |
| [GGUF](https://huggingface.co/mradermacher/DeepHermes-3-Llama-3-3B-Preview-abliterated-i1-GGUF/resolve/main/DeepHermes-3-Llama-3-3B-Preview-abliterated.i1-IQ3_M.gguf) | i1-IQ3_M | 1.7 | |
| [GGUF](https://huggingface.co/mradermacher/DeepHermes-3-Llama-3-3B-Preview-abliterated-i1-GGUF/resolve/main/DeepHermes-3-Llama-3-3B-Preview-abliterated.i1-Q3_K_M.gguf) | i1-Q3_K_M | 1.8 | IQ3_S probably better |
| [GGUF](https://huggingface.co/mradermacher/DeepHermes-3-Llama-3-3B-Preview-abliterated-i1-GGUF/resolve/main/DeepHermes-3-Llama-3-3B-Preview-abliterated.i1-Q3_K_L.gguf) | i1-Q3_K_L | 1.9 | IQ3_M probably better |
| [GGUF](https://huggingface.co/mradermacher/DeepHermes-3-Llama-3-3B-Preview-abliterated-i1-GGUF/resolve/main/DeepHermes-3-Llama-3-3B-Preview-abliterated.i1-IQ4_XS.gguf) | i1-IQ4_XS | 1.9 | |
| [GGUF](https://huggingface.co/mradermacher/DeepHermes-3-Llama-3-3B-Preview-abliterated-i1-GGUF/resolve/main/DeepHermes-3-Llama-3-3B-Preview-abliterated.i1-IQ4_NL.gguf) | i1-IQ4_NL | 2.0 | prefer IQ4_XS |
| [GGUF](https://huggingface.co/mradermacher/DeepHermes-3-Llama-3-3B-Preview-abliterated-i1-GGUF/resolve/main/DeepHermes-3-Llama-3-3B-Preview-abliterated.i1-Q4_0.gguf) | i1-Q4_0 | 2.0 | fast, low quality |
| [GGUF](https://huggingface.co/mradermacher/DeepHermes-3-Llama-3-3B-Preview-abliterated-i1-GGUF/resolve/main/DeepHermes-3-Llama-3-3B-Preview-abliterated.i1-Q4_K_S.gguf) | i1-Q4_K_S | 2.0 | optimal size/speed/quality |
| [GGUF](https://huggingface.co/mradermacher/DeepHermes-3-Llama-3-3B-Preview-abliterated-i1-GGUF/resolve/main/DeepHermes-3-Llama-3-3B-Preview-abliterated.i1-Q4_K_M.gguf) | i1-Q4_K_M | 2.1 | fast, recommended |
| [GGUF](https://huggingface.co/mradermacher/DeepHermes-3-Llama-3-3B-Preview-abliterated-i1-GGUF/resolve/main/DeepHermes-3-Llama-3-3B-Preview-abliterated.i1-Q4_1.gguf) | i1-Q4_1 | 2.2 | |
| [GGUF](https://huggingface.co/mradermacher/DeepHermes-3-Llama-3-3B-Preview-abliterated-i1-GGUF/resolve/main/DeepHermes-3-Llama-3-3B-Preview-abliterated.i1-Q5_K_S.gguf) | i1-Q5_K_S | 2.4 | |
| [GGUF](https://huggingface.co/mradermacher/DeepHermes-3-Llama-3-3B-Preview-abliterated-i1-GGUF/resolve/main/DeepHermes-3-Llama-3-3B-Preview-abliterated.i1-Q5_K_M.gguf) | i1-Q5_K_M | 2.4 | |
| [GGUF](https://huggingface.co/mradermacher/DeepHermes-3-Llama-3-3B-Preview-abliterated-i1-GGUF/resolve/main/DeepHermes-3-Llama-3-3B-Preview-abliterated.i1-Q6_K.gguf) | i1-Q6_K | 2.7 | practically like static Q6_K |
Here is a handy graph by ikawrakow comparing some lower-quality quant
types (lower is better):

And here are Artefact2's thoughts on the matter:
https://gist.github.com/Artefact2/b5f810600771265fc1e39442288e8ec9
## FAQ / Model Request
See https://huggingface.co/mradermacher/model_requests for some answers to
questions you might have and/or if you want some other model quantized.
## Thanks
I thank my company, [nethype GmbH](https://www.nethype.de/), for letting
me use its servers and providing upgrades to my workstation to enable
this work in my free time. Additional thanks to [@nicoboss](https://huggingface.co/nicoboss) for giving me access to his private supercomputer, enabling me to provide many more imatrix quants, at much higher quality, than I would otherwise be able to.
<!-- end -->
|
Helsinki-NLP/opus-mt-tc-bible-big-deu_eng_fra_por_spa-gmq | Helsinki-NLP | "2024-10-08T07:03:46Z" | 110 | 0 | transformers | [
"transformers",
"pytorch",
"safetensors",
"marian",
"text2text-generation",
"translation",
"opus-mt-tc-bible",
"da",
"de",
"en",
"es",
"fo",
"fr",
"is",
"nb",
"nn",
"no",
"non",
"pt",
"sv",
"license:apache-2.0",
"model-index",
"autotrain_compatible",
"endpoints_compatible",
"region:us"
] | translation | "2024-10-08T06:38:14Z" | ---
library_name: transformers
language:
- da
- de
- en
- es
- fo
- fr
- is
- nb
- nn
- no
- non
- pt
- sv
tags:
- translation
- opus-mt-tc-bible
license: apache-2.0
model-index:
- name: opus-mt-tc-bible-big-deu_eng_fra_por_spa-gmq
results:
- task:
name: Translation deu-dan
type: translation
args: deu-dan
dataset:
name: flores200-devtest
type: flores200-devtest
args: deu-dan
metrics:
- name: BLEU
type: bleu
value: 35.1
- name: chr-F
type: chrf
value: 0.62152
- task:
name: Translation deu-fao
type: translation
args: deu-fao
dataset:
name: flores200-devtest
type: flores200-devtest
args: deu-fao
metrics:
- name: BLEU
type: bleu
value: 11.5
- name: chr-F
type: chrf
value: 0.33611
- task:
name: Translation deu-isl
type: translation
args: deu-isl
dataset:
name: flores200-devtest
type: flores200-devtest
args: deu-isl
metrics:
- name: BLEU
type: bleu
value: 19.1
- name: chr-F
type: chrf
value: 0.48648
- task:
name: Translation deu-nno
type: translation
args: deu-nno
dataset:
name: flores200-devtest
type: flores200-devtest
args: deu-nno
metrics:
- name: BLEU
type: bleu
value: 24.0
- name: chr-F
type: chrf
value: 0.53530
- task:
name: Translation deu-nob
type: translation
args: deu-nob
dataset:
name: flores200-devtest
type: flores200-devtest
args: deu-nob
metrics:
- name: BLEU
type: bleu
value: 25.1
- name: chr-F
type: chrf
value: 0.55748
- task:
name: Translation deu-swe
type: translation
args: deu-swe
dataset:
name: flores200-devtest
type: flores200-devtest
args: deu-swe
metrics:
- name: BLEU
type: bleu
value: 34.2
- name: chr-F
type: chrf
value: 0.62138
- task:
name: Translation eng-dan
type: translation
args: eng-dan
dataset:
name: flores200-devtest
type: flores200-devtest
args: eng-dan
metrics:
- name: BLEU
type: bleu
value: 47.0
- name: chr-F
type: chrf
value: 0.70321
- task:
name: Translation eng-fao
type: translation
args: eng-fao
dataset:
name: flores200-devtest
type: flores200-devtest
args: eng-fao
metrics:
- name: BLEU
type: bleu
value: 14.1
- name: chr-F
type: chrf
value: 0.35857
- task:
name: Translation eng-isl
type: translation
args: eng-isl
dataset:
name: flores200-devtest
type: flores200-devtest
args: eng-isl
metrics:
- name: BLEU
type: bleu
value: 24.4
- name: chr-F
type: chrf
value: 0.52585
- task:
name: Translation eng-nno
type: translation
args: eng-nno
dataset:
name: flores200-devtest
type: flores200-devtest
args: eng-nno
metrics:
- name: BLEU
type: bleu
value: 33.8
- name: chr-F
type: chrf
value: 0.61372
- task:
name: Translation eng-nob
type: translation
args: eng-nob
dataset:
name: flores200-devtest
type: flores200-devtest
args: eng-nob
metrics:
- name: BLEU
type: bleu
value: 34.4
- name: chr-F
type: chrf
value: 0.62508
- task:
name: Translation eng-swe
type: translation
args: eng-swe
dataset:
name: flores200-devtest
type: flores200-devtest
args: eng-swe
metrics:
- name: BLEU
type: bleu
value: 46.0
- name: chr-F
type: chrf
value: 0.69703
- task:
name: Translation fra-dan
type: translation
args: fra-dan
dataset:
name: flores200-devtest
type: flores200-devtest
args: fra-dan
metrics:
- name: BLEU
type: bleu
value: 34.1
- name: chr-F
type: chrf
value: 0.61025
- task:
name: Translation fra-isl
type: translation
args: fra-isl
dataset:
name: flores200-devtest
type: flores200-devtest
args: fra-isl
metrics:
- name: BLEU
type: bleu
value: 18.8
- name: chr-F
type: chrf
value: 0.48273
- task:
name: Translation fra-nno
type: translation
args: fra-nno
dataset:
name: flores200-devtest
type: flores200-devtest
args: fra-nno
metrics:
- name: BLEU
type: bleu
value: 24.3
- name: chr-F
type: chrf
value: 0.53032
- task:
name: Translation fra-nob
type: translation
args: fra-nob
dataset:
name: flores200-devtest
type: flores200-devtest
args: fra-nob
metrics:
- name: BLEU
type: bleu
value: 25.0
- name: chr-F
type: chrf
value: 0.54933
- task:
name: Translation fra-swe
type: translation
args: fra-swe
dataset:
name: flores200-devtest
type: flores200-devtest
args: fra-swe
metrics:
- name: BLEU
type: bleu
value: 32.8
- name: chr-F
type: chrf
value: 0.60612
- task:
name: Translation por-dan
type: translation
args: por-dan
dataset:
name: flores200-devtest
type: flores200-devtest
args: por-dan
metrics:
- name: BLEU
type: bleu
value: 36.2
- name: chr-F
type: chrf
value: 0.62221
- task:
name: Translation por-fao
type: translation
args: por-fao
dataset:
name: flores200-devtest
type: flores200-devtest
args: por-fao
metrics:
- name: BLEU
type: bleu
value: 11.5
- name: chr-F
type: chrf
value: 0.33159
- task:
name: Translation por-isl
type: translation
args: por-isl
dataset:
name: flores200-devtest
type: flores200-devtest
args: por-isl
metrics:
- name: BLEU
type: bleu
value: 19.6
- name: chr-F
type: chrf
value: 0.48357
- task:
name: Translation por-nno
type: translation
args: por-nno
dataset:
name: flores200-devtest
type: flores200-devtest
args: por-nno
metrics:
- name: BLEU
type: bleu
value: 26.3
- name: chr-F
type: chrf
value: 0.54369
- task:
name: Translation por-nob
type: translation
args: por-nob
dataset:
name: flores200-devtest
type: flores200-devtest
args: por-nob
metrics:
- name: BLEU
type: bleu
value: 26.4
- name: chr-F
type: chrf
value: 0.56054
- task:
name: Translation por-swe
type: translation
args: por-swe
dataset:
name: flores200-devtest
type: flores200-devtest
args: por-swe
metrics:
- name: BLEU
type: bleu
value: 34.1
- name: chr-F
type: chrf
value: 0.61388
- task:
name: Translation spa-dan
type: translation
args: spa-dan
dataset:
name: flores200-devtest
type: flores200-devtest
args: spa-dan
metrics:
- name: BLEU
type: bleu
value: 24.7
- name: chr-F
type: chrf
value: 0.55091
- task:
name: Translation spa-isl
type: translation
args: spa-isl
dataset:
name: flores200-devtest
type: flores200-devtest
args: spa-isl
metrics:
- name: BLEU
type: bleu
value: 14.2
- name: chr-F
type: chrf
value: 0.44469
- task:
name: Translation spa-nno
type: translation
args: spa-nno
dataset:
name: flores200-devtest
type: flores200-devtest
args: spa-nno
metrics:
- name: BLEU
type: bleu
value: 18.6
- name: chr-F
type: chrf
value: 0.48898
- task:
name: Translation spa-nob
type: translation
args: spa-nob
dataset:
name: flores200-devtest
type: flores200-devtest
args: spa-nob
metrics:
- name: BLEU
type: bleu
value: 18.8
- name: chr-F
type: chrf
value: 0.50901
- task:
name: Translation spa-swe
type: translation
args: spa-swe
dataset:
name: flores200-devtest
type: flores200-devtest
args: spa-swe
metrics:
- name: BLEU
type: bleu
value: 22.7
- name: chr-F
type: chrf
value: 0.54182
- task:
name: Translation deu-dan
type: translation
args: deu-dan
dataset:
name: flores101-devtest
type: flores_101
args: deu dan devtest
metrics:
- name: BLEU
type: bleu
value: 34.8
- name: chr-F
type: chrf
value: 0.62006
- task:
name: Translation deu-isl
type: translation
args: deu-isl
dataset:
name: flores101-devtest
type: flores_101
args: deu isl devtest
metrics:
- name: BLEU
type: bleu
value: 18.8
- name: chr-F
type: chrf
value: 0.48236
- task:
name: Translation deu-swe
type: translation
args: deu-swe
dataset:
name: flores101-devtest
type: flores_101
args: deu swe devtest
metrics:
- name: BLEU
type: bleu
value: 33.7
- name: chr-F
type: chrf
value: 0.61778
- task:
name: Translation eng-swe
type: translation
args: eng-swe
dataset:
name: flores101-devtest
type: flores_101
args: eng swe devtest
metrics:
- name: BLEU
type: bleu
value: 45.5
- name: chr-F
type: chrf
value: 0.69435
- task:
name: Translation fra-dan
type: translation
args: fra-dan
dataset:
name: flores101-devtest
type: flores_101
args: fra dan devtest
metrics:
- name: BLEU
type: bleu
value: 34.0
- name: chr-F
type: chrf
value: 0.61019
- task:
name: Translation fra-isl
type: translation
args: fra-isl
dataset:
name: flores101-devtest
type: flores_101
args: fra isl devtest
metrics:
- name: BLEU
type: bleu
value: 18.1
- name: chr-F
type: chrf
value: 0.47647
- task:
name: Translation fra-swe
type: translation
args: fra-swe
dataset:
name: flores101-devtest
type: flores_101
args: fra swe devtest
metrics:
- name: BLEU
type: bleu
value: 32.2
- name: chr-F
type: chrf
value: 0.60354
- task:
name: Translation por-isl
type: translation
args: por-isl
dataset:
name: flores101-devtest
type: flores_101
args: por isl devtest
metrics:
- name: BLEU
type: bleu
value: 19.1
- name: chr-F
type: chrf
value: 0.47937
- task:
name: Translation por-swe
type: translation
args: por-swe
dataset:
name: flores101-devtest
type: flores_101
args: por swe devtest
metrics:
- name: BLEU
type: bleu
value: 33.1
- name: chr-F
type: chrf
value: 0.60857
- task:
name: Translation spa-dan
type: translation
args: spa-dan
dataset:
name: flores101-devtest
type: flores_101
args: spa dan devtest
metrics:
- name: BLEU
type: bleu
value: 24.4
- name: chr-F
type: chrf
value: 0.54890
- task:
name: Translation spa-nob
type: translation
args: spa-nob
dataset:
name: flores101-devtest
type: flores_101
args: spa nob devtest
metrics:
- name: BLEU
type: bleu
value: 18.3
- name: chr-F
type: chrf
value: 0.50610
- task:
name: Translation spa-swe
type: translation
args: spa-swe
dataset:
name: flores101-devtest
type: flores_101
args: spa swe devtest
metrics:
- name: BLEU
type: bleu
value: 22.4
- name: chr-F
type: chrf
value: 0.54011
- task:
name: Translation deu-dan
type: translation
args: deu-dan
dataset:
name: ntrex128
type: ntrex128
args: deu-dan
metrics:
- name: BLEU
type: bleu
value: 29.1
- name: chr-F
type: chrf
value: 0.56412
- task:
name: Translation deu-fao
type: translation
args: deu-fao
dataset:
name: ntrex128
type: ntrex128
args: deu-fao
metrics:
- name: BLEU
type: bleu
value: 12.5
- name: chr-F
type: chrf
value: 0.35495
- task:
name: Translation deu-isl
type: translation
args: deu-isl
dataset:
name: ntrex128
type: ntrex128
args: deu-isl
metrics:
- name: BLEU
type: bleu
value: 18.8
- name: chr-F
type: chrf
value: 0.48309
- task:
name: Translation deu-nno
type: translation
args: deu-nno
dataset:
name: ntrex128
type: ntrex128
args: deu-nno
metrics:
- name: BLEU
type: bleu
value: 22.0
- name: chr-F
type: chrf
value: 0.51535
- task:
name: Translation deu-nob
type: translation
args: deu-nob
dataset:
name: ntrex128
type: ntrex128
args: deu-nob
metrics:
- name: BLEU
type: bleu
value: 27.6
- name: chr-F
type: chrf
value: 0.56152
- task:
name: Translation deu-swe
type: translation
args: deu-swe
dataset:
name: ntrex128
type: ntrex128
args: deu-swe
metrics:
- name: BLEU
type: bleu
value: 29.6
- name: chr-F
type: chrf
value: 0.58061
- task:
name: Translation eng-dan
type: translation
args: eng-dan
dataset:
name: ntrex128
type: ntrex128
args: eng-dan
metrics:
- name: BLEU
type: bleu
value: 37.6
- name: chr-F
type: chrf
value: 0.61894
- task:
name: Translation eng-fao
type: translation
args: eng-fao
dataset:
name: ntrex128
type: ntrex128
args: eng-fao
metrics:
- name: BLEU
type: bleu
value: 15.9
- name: chr-F
type: chrf
value: 0.38410
- task:
name: Translation eng-isl
type: translation
args: eng-isl
dataset:
name: ntrex128
type: ntrex128
args: eng-isl
metrics:
- name: BLEU
type: bleu
value: 23.9
- name: chr-F
type: chrf
value: 0.52027
- task:
name: Translation eng-nno
type: translation
args: eng-nno
dataset:
name: ntrex128
type: ntrex128
args: eng-nno
metrics:
- name: BLEU
type: bleu
value: 34.0
- name: chr-F
type: chrf
value: 0.60754
- task:
name: Translation eng-nob
type: translation
args: eng-nob
dataset:
name: ntrex128
type: ntrex128
args: eng-nob
metrics:
- name: BLEU
type: bleu
value: 36.9
- name: chr-F
type: chrf
value: 0.62327
- task:
name: Translation eng-swe
type: translation
args: eng-swe
dataset:
name: ntrex128
type: ntrex128
args: eng-swe
metrics:
- name: BLEU
type: bleu
value: 41.3
- name: chr-F
type: chrf
value: 0.66129
- task:
name: Translation fra-dan
type: translation
args: fra-dan
dataset:
name: ntrex128
type: ntrex128
args: fra-dan
metrics:
- name: BLEU
type: bleu
value: 27.1
- name: chr-F
type: chrf
value: 0.54102
- task:
name: Translation fra-fao
type: translation
args: fra-fao
dataset:
name: ntrex128
type: ntrex128
args: fra-fao
metrics:
- name: BLEU
type: bleu
value: 10.8
- name: chr-F
type: chrf
value: 0.32337
- task:
name: Translation fra-isl
type: translation
args: fra-isl
dataset:
name: ntrex128
type: ntrex128
args: fra-isl
metrics:
- name: BLEU
type: bleu
value: 18.4
- name: chr-F
type: chrf
value: 0.47296
- task:
name: Translation fra-nno
type: translation
args: fra-nno
dataset:
name: ntrex128
type: ntrex128
args: fra-nno
metrics:
- name: BLEU
type: bleu
value: 21.6
- name: chr-F
type: chrf
value: 0.50532
- task:
name: Translation fra-nob
type: translation
args: fra-nob
dataset:
name: ntrex128
type: ntrex128
args: fra-nob
metrics:
- name: BLEU
type: bleu
value: 25.7
- name: chr-F
type: chrf
value: 0.54026
- task:
name: Translation fra-swe
type: translation
args: fra-swe
dataset:
name: ntrex128
type: ntrex128
args: fra-swe
metrics:
- name: BLEU
type: bleu
value: 27.9
- name: chr-F
type: chrf
value: 0.56278
- task:
name: Translation por-dan
type: translation
args: por-dan
dataset:
name: ntrex128
type: ntrex128
args: por-dan
metrics:
- name: BLEU
type: bleu
value: 30.0
- name: chr-F
type: chrf
value: 0.56288
- task:
name: Translation por-fao
type: translation
args: por-fao
dataset:
name: ntrex128
type: ntrex128
args: por-fao
metrics:
- name: BLEU
type: bleu
value: 12.7
- name: chr-F
type: chrf
value: 0.35059
- task:
name: Translation por-isl
type: translation
args: por-isl
dataset:
name: ntrex128
type: ntrex128
args: por-isl
metrics:
- name: BLEU
type: bleu
value: 17.8
- name: chr-F
type: chrf
value: 0.47577
- task:
name: Translation por-nno
type: translation
args: por-nno
dataset:
name: ntrex128
type: ntrex128
args: por-nno
metrics:
- name: BLEU
type: bleu
value: 23.0
- name: chr-F
type: chrf
value: 0.52158
- task:
name: Translation por-nob
type: translation
args: por-nob
dataset:
name: ntrex128
type: ntrex128
args: por-nob
metrics:
- name: BLEU
type: bleu
value: 27.4
- name: chr-F
type: chrf
value: 0.55788
- task:
name: Translation por-swe
type: translation
args: por-swe
dataset:
name: ntrex128
type: ntrex128
args: por-swe
metrics:
- name: BLEU
type: bleu
value: 29.3
- name: chr-F
type: chrf
value: 0.57790
- task:
name: Translation spa-dan
type: translation
args: spa-dan
dataset:
name: ntrex128
type: ntrex128
args: spa-dan
metrics:
- name: BLEU
type: bleu
value: 27.5
- name: chr-F
type: chrf
value: 0.55607
- task:
name: Translation spa-fao
type: translation
args: spa-fao
dataset:
name: ntrex128
type: ntrex128
args: spa-fao
metrics:
- name: BLEU
type: bleu
value: 12.5
- name: chr-F
type: chrf
value: 0.34781
- task:
name: Translation spa-isl
type: translation
args: spa-isl
dataset:
name: ntrex128
type: ntrex128
args: spa-isl
metrics:
- name: BLEU
type: bleu
value: 18.4
- name: chr-F
type: chrf
value: 0.48566
- task:
name: Translation spa-nno
type: translation
args: spa-nno
dataset:
name: ntrex128
type: ntrex128
args: spa-nno
metrics:
- name: BLEU
type: bleu
value: 22.2
- name: chr-F
type: chrf
value: 0.51741
- task:
name: Translation spa-nob
type: translation
args: spa-nob
dataset:
name: ntrex128
type: ntrex128
args: spa-nob
metrics:
- name: BLEU
type: bleu
value: 26.8
- name: chr-F
type: chrf
value: 0.55824
- task:
name: Translation spa-swe
type: translation
args: spa-swe
dataset:
name: ntrex128
type: ntrex128
args: spa-swe
metrics:
- name: BLEU
type: bleu
value: 28.8
- name: chr-F
type: chrf
value: 0.57851
- task:
name: Translation deu-dan
type: translation
args: deu-dan
dataset:
name: tatoeba-test-v2021-08-07
type: tatoeba_mt
args: deu-dan
metrics:
- name: BLEU
type: bleu
value: 57.8
- name: chr-F
type: chrf
value: 0.74051
- task:
name: Translation deu-isl
type: translation
args: deu-isl
dataset:
name: tatoeba-test-v2021-08-07
type: tatoeba_mt
args: deu-isl
metrics:
- name: BLEU
type: bleu
value: 31.7
- name: chr-F
type: chrf
value: 0.61256
- task:
name: Translation deu-nob
type: translation
args: deu-nob
dataset:
name: tatoeba-test-v2021-08-07
type: tatoeba_mt
args: deu-nob
metrics:
- name: BLEU
type: bleu
value: 52.9
- name: chr-F
type: chrf
value: 0.71413
- task:
name: Translation deu-nor
type: translation
args: deu-nor
dataset:
name: tatoeba-test-v2021-08-07
type: tatoeba_mt
args: deu-nor
metrics:
- name: BLEU
type: bleu
value: 52.7
- name: chr-F
type: chrf
value: 0.71253
- task:
name: Translation deu-swe
type: translation
args: deu-swe
dataset:
name: tatoeba-test-v2021-08-07
type: tatoeba_mt
args: deu-swe
metrics:
- name: BLEU
type: bleu
value: 58.2
- name: chr-F
type: chrf
value: 0.72650
- task:
name: Translation eng-dan
type: translation
args: eng-dan
dataset:
name: tatoeba-test-v2021-08-07
type: tatoeba_mt
args: eng-dan
metrics:
- name: BLEU
type: bleu
value: 60.6
- name: chr-F
type: chrf
value: 0.74708
- task:
name: Translation eng-fao
type: translation
args: eng-fao
dataset:
name: tatoeba-test-v2021-08-07
type: tatoeba_mt
args: eng-fao
metrics:
- name: BLEU
type: bleu
value: 29.0
- name: chr-F
type: chrf
value: 0.48304
- task:
name: Translation eng-isl
type: translation
args: eng-isl
dataset:
name: tatoeba-test-v2021-08-07
type: tatoeba_mt
args: eng-isl
metrics:
- name: BLEU
type: bleu
value: 33.2
- name: chr-F
type: chrf
value: 0.58312
- task:
name: Translation eng-nno
type: translation
args: eng-nno
dataset:
name: tatoeba-test-v2021-08-07
type: tatoeba_mt
args: eng-nno
metrics:
- name: BLEU
type: bleu
value: 42.7
- name: chr-F
type: chrf
value: 0.62606
- task:
name: Translation eng-nob
type: translation
args: eng-nob
dataset:
name: tatoeba-test-v2021-08-07
type: tatoeba_mt
args: eng-nob
metrics:
- name: BLEU
type: bleu
value: 57.4
- name: chr-F
type: chrf
value: 0.72340
- task:
name: Translation eng-nor
type: translation
args: eng-nor
dataset:
name: tatoeba-test-v2021-08-07
type: tatoeba_mt
args: eng-nor
metrics:
- name: BLEU
type: bleu
value: 56.2
- name: chr-F
type: chrf
value: 0.71514
- task:
name: Translation eng-swe
type: translation
args: eng-swe
dataset:
name: tatoeba-test-v2021-08-07
type: tatoeba_mt
args: eng-swe
metrics:
- name: BLEU
type: bleu
value: 60.5
- name: chr-F
type: chrf
value: 0.73720
- task:
name: Translation fra-dan
type: translation
args: fra-dan
dataset:
name: tatoeba-test-v2021-08-07
type: tatoeba_mt
args: fra-dan
metrics:
- name: BLEU
type: bleu
value: 64.1
- name: chr-F
type: chrf
value: 0.78018
- task:
name: Translation fra-nob
type: translation
args: fra-nob
dataset:
name: tatoeba-test-v2021-08-07
type: tatoeba_mt
args: fra-nob
metrics:
- name: BLEU
type: bleu
value: 59.1
- name: chr-F
type: chrf
value: 0.74252
- task:
name: Translation fra-nor
type: translation
args: fra-nor
dataset:
name: tatoeba-test-v2021-08-07
type: tatoeba_mt
args: fra-nor
metrics:
- name: BLEU
type: bleu
value: 60.3
- name: chr-F
type: chrf
value: 0.74407
- task:
name: Translation fra-swe
type: translation
args: fra-swe
dataset:
name: tatoeba-test-v2021-08-07
type: tatoeba_mt
args: fra-swe
metrics:
- name: BLEU
type: bleu
value: 62.1
- name: chr-F
type: chrf
value: 0.75644
- task:
name: Translation multi-multi
type: translation
args: multi-multi
dataset:
name: tatoeba-test-v2020-07-28-v2023-09-26
type: tatoeba_mt
args: multi-multi
metrics:
- name: BLEU
type: bleu
value: 56.4
- name: chr-F
type: chrf
value: 0.72858
- task:
name: Translation por-dan
type: translation
args: por-dan
dataset:
name: tatoeba-test-v2021-08-07
type: tatoeba_mt
args: por-dan
metrics:
- name: BLEU
type: bleu
value: 65.6
- name: chr-F
type: chrf
value: 0.79528
- task:
name: Translation por-nor
type: translation
args: por-nor
dataset:
name: tatoeba-test-v2021-08-07
type: tatoeba_mt
args: por-nor
metrics:
- name: BLEU
type: bleu
value: 58.0
- name: chr-F
type: chrf
value: 0.73559
- task:
name: Translation por-swe
type: translation
args: por-swe
dataset:
name: tatoeba-test-v2021-08-07
type: tatoeba_mt
args: por-swe
metrics:
- name: BLEU
type: bleu
value: 60.2
- name: chr-F
type: chrf
value: 0.75566
- task:
name: Translation spa-dan
type: translation
args: spa-dan
dataset:
name: tatoeba-test-v2021-08-07
type: tatoeba_mt
args: spa-dan
metrics:
- name: BLEU
type: bleu
value: 57.7
- name: chr-F
type: chrf
value: 0.73310
- task:
name: Translation spa-nob
type: translation
args: spa-nob
dataset:
name: tatoeba-test-v2021-08-07
type: tatoeba_mt
args: spa-nob
metrics:
- name: BLEU
type: bleu
value: 60.9
- name: chr-F
type: chrf
value: 0.76501
- task:
name: Translation spa-nor
type: translation
args: spa-nor
dataset:
name: tatoeba-test-v2021-08-07
type: tatoeba_mt
args: spa-nor
metrics:
- name: BLEU
type: bleu
value: 60.1
- name: chr-F
type: chrf
value: 0.75815
- task:
name: Translation spa-swe
type: translation
args: spa-swe
dataset:
name: tatoeba-test-v2021-08-07
type: tatoeba_mt
args: spa-swe
metrics:
- name: BLEU
type: bleu
value: 60.7
- name: chr-F
type: chrf
value: 0.74222
- task:
name: Translation eng-isl
type: translation
args: eng-isl
dataset:
name: newstest2021
type: wmt-2021-news
args: eng-isl
metrics:
- name: BLEU
type: bleu
value: 21.9
- name: chr-F
type: chrf
value: 0.51196
---
# opus-mt-tc-bible-big-deu_eng_fra_por_spa-gmq
## Table of Contents
- [Model Details](#model-details)
- [Uses](#uses)
- [Risks, Limitations and Biases](#risks-limitations-and-biases)
- [How to Get Started With the Model](#how-to-get-started-with-the-model)
- [Training](#training)
- [Evaluation](#evaluation)
- [Citation Information](#citation-information)
- [Acknowledgements](#acknowledgements)
## Model Details
Neural machine translation model for translating from unknown (deu+eng+fra+por+spa) to North Germanic languages (gmq).
This model is part of the [OPUS-MT project](https://github.com/Helsinki-NLP/Opus-MT), an effort to make neural machine translation models widely available and accessible for many languages in the world. All models are originally trained using the amazing framework of [Marian NMT](https://marian-nmt.github.io/), an efficient NMT implementation written in pure C++. The models have been converted to pyTorch using the transformers library by huggingface. Training data is taken from [OPUS](https://opus.nlpl.eu/) and training pipelines use the procedures of [OPUS-MT-train](https://github.com/Helsinki-NLP/Opus-MT-train).
**Model Description:**
- **Developed by:** Language Technology Research Group at the University of Helsinki
- **Model Type:** Translation (transformer-big)
- **Release**: 2024-05-30
- **License:** Apache-2.0
- **Language(s):**
- Source Language(s): deu eng fra por spa
- Target Language(s): dan fao isl nno nob non nor swe
- Valid Target Language Labels: >>dan<< >>fao<< >>isl<< >>jut<< >>nno<< >>nob<< >>non<< >>nor<< >>nrn<< >>ovd<< >>rmg<< >>swe<< >>xxx<<
- **Original Model**: [opusTCv20230926max50+bt+jhubc_transformer-big_2024-05-30.zip](https://object.pouta.csc.fi/Tatoeba-MT-models/deu+eng+fra+por+spa-gmq/opusTCv20230926max50+bt+jhubc_transformer-big_2024-05-30.zip)
- **Resources for more information:**
- [OPUS-MT dashboard](https://opus.nlpl.eu/dashboard/index.php?pkg=opusmt&test=all&scoreslang=all&chart=standard&model=Tatoeba-MT-models/deu%2Beng%2Bfra%2Bpor%2Bspa-gmq/opusTCv20230926max50%2Bbt%2Bjhubc_transformer-big_2024-05-30)
- [OPUS-MT-train GitHub Repo](https://github.com/Helsinki-NLP/OPUS-MT-train)
- [More information about MarianNMT models in the transformers library](https://huggingface.co/docs/transformers/model_doc/marian)
- [Tatoeba Translation Challenge](https://github.com/Helsinki-NLP/Tatoeba-Challenge/)
- [HPLT bilingual data v1 (as part of the Tatoeba Translation Challenge dataset)](https://hplt-project.org/datasets/v1)
- [A massively parallel Bible corpus](https://aclanthology.org/L14-1215/)
This is a multilingual translation model with multiple target languages. A sentence initial language token is required in the form of `>>id<<` (id = valid target language ID), e.g. `>>dan<<`
## Uses
This model can be used for translation and text-to-text generation.
## Risks, Limitations and Biases
**CONTENT WARNING: Readers should be aware that the model is trained on various public data sets that may contain content that is disturbing, offensive, and can propagate historical and current stereotypes.**
Significant research has explored bias and fairness issues with language models (see, e.g., [Sheng et al. (2021)](https://aclanthology.org/2021.acl-long.330.pdf) and [Bender et al. (2021)](https://dl.acm.org/doi/pdf/10.1145/3442188.3445922)).
## How to Get Started With the Model
A short example code:
```python
from transformers import MarianMTModel, MarianTokenizer
src_text = [
">>dan<< Replace this with text in an accepted source language.",
">>swe<< This is the second sentence."
]
model_name = "pytorch-models/opus-mt-tc-bible-big-deu_eng_fra_por_spa-gmq"
tokenizer = MarianTokenizer.from_pretrained(model_name)
model = MarianMTModel.from_pretrained(model_name)
translated = model.generate(**tokenizer(src_text, return_tensors="pt", padding=True))
for t in translated:
print( tokenizer.decode(t, skip_special_tokens=True) )
```
You can also use OPUS-MT models with the transformers pipelines, for example:
```python
from transformers import pipeline
pipe = pipeline("translation", model="Helsinki-NLP/opus-mt-tc-bible-big-deu_eng_fra_por_spa-gmq")
print(pipe(">>dan<< Replace this with text in an accepted source language."))
```
## Training
- **Data**: opusTCv20230926max50+bt+jhubc ([source](https://github.com/Helsinki-NLP/Tatoeba-Challenge))
- **Pre-processing**: SentencePiece (spm32k,spm32k)
- **Model Type:** transformer-big
- **Original MarianNMT Model**: [opusTCv20230926max50+bt+jhubc_transformer-big_2024-05-30.zip](https://object.pouta.csc.fi/Tatoeba-MT-models/deu+eng+fra+por+spa-gmq/opusTCv20230926max50+bt+jhubc_transformer-big_2024-05-30.zip)
- **Training Scripts**: [GitHub Repo](https://github.com/Helsinki-NLP/OPUS-MT-train)
## Evaluation
* [Model scores at the OPUS-MT dashboard](https://opus.nlpl.eu/dashboard/index.php?pkg=opusmt&test=all&scoreslang=all&chart=standard&model=Tatoeba-MT-models/deu%2Beng%2Bfra%2Bpor%2Bspa-gmq/opusTCv20230926max50%2Bbt%2Bjhubc_transformer-big_2024-05-30)
* test set translations: [opusTCv20230926max50+bt+jhubc_transformer-big_2024-05-29.test.txt](https://object.pouta.csc.fi/Tatoeba-MT-models/deu+eng+fra+por+spa-gmq/opusTCv20230926max50+bt+jhubc_transformer-big_2024-05-29.test.txt)
* test set scores: [opusTCv20230926max50+bt+jhubc_transformer-big_2024-05-29.eval.txt](https://object.pouta.csc.fi/Tatoeba-MT-models/deu+eng+fra+por+spa-gmq/opusTCv20230926max50+bt+jhubc_transformer-big_2024-05-29.eval.txt)
* benchmark results: [benchmark_results.txt](benchmark_results.txt)
* benchmark output: [benchmark_translations.zip](benchmark_translations.zip)
| langpair | testset | chr-F | BLEU | #sent | #words |
|----------|---------|-------|-------|-------|--------|
| deu-dan | tatoeba-test-v2021-08-07 | 0.74051 | 57.8 | 9998 | 74644 |
| deu-isl | tatoeba-test-v2021-08-07 | 0.61256 | 31.7 | 969 | 5951 |
| deu-nob | tatoeba-test-v2021-08-07 | 0.71413 | 52.9 | 3525 | 31978 |
| deu-nor | tatoeba-test-v2021-08-07 | 0.71253 | 52.7 | 3651 | 32928 |
| deu-swe | tatoeba-test-v2021-08-07 | 0.72650 | 58.2 | 3410 | 22701 |
| eng-dan | tatoeba-test-v2021-08-07 | 0.74708 | 60.6 | 10795 | 79385 |
| eng-fao | tatoeba-test-v2021-08-07 | 0.48304 | 29.0 | 294 | 1933 |
| eng-isl | tatoeba-test-v2021-08-07 | 0.58312 | 33.2 | 2503 | 19023 |
| eng-nno | tatoeba-test-v2021-08-07 | 0.62606 | 42.7 | 460 | 3428 |
| eng-nob | tatoeba-test-v2021-08-07 | 0.72340 | 57.4 | 4539 | 36119 |
| eng-nor | tatoeba-test-v2021-08-07 | 0.71514 | 56.2 | 5000 | 39552 |
| eng-swe | tatoeba-test-v2021-08-07 | 0.73720 | 60.5 | 10362 | 68067 |
| fra-dan | tatoeba-test-v2021-08-07 | 0.78018 | 64.1 | 1731 | 11312 |
| fra-nob | tatoeba-test-v2021-08-07 | 0.74252 | 59.1 | 323 | 2175 |
| fra-nor | tatoeba-test-v2021-08-07 | 0.74407 | 60.3 | 477 | 3097 |
| fra-swe | tatoeba-test-v2021-08-07 | 0.75644 | 62.1 | 1407 | 9170 |
| por-dan | tatoeba-test-v2021-08-07 | 0.79528 | 65.6 | 873 | 5258 |
| por-nor | tatoeba-test-v2021-08-07 | 0.73559 | 58.0 | 481 | 4030 |
| por-swe | tatoeba-test-v2021-08-07 | 0.75566 | 60.2 | 320 | 1938 |
| spa-dan | tatoeba-test-v2021-08-07 | 0.73310 | 57.7 | 5000 | 35937 |
| spa-isl | tatoeba-test-v2021-08-07 | 0.52169 | 18.7 | 238 | 1220 |
| spa-nob | tatoeba-test-v2021-08-07 | 0.76501 | 60.9 | 885 | 6762 |
| spa-nor | tatoeba-test-v2021-08-07 | 0.75815 | 60.1 | 960 | 7217 |
| spa-swe | tatoeba-test-v2021-08-07 | 0.74222 | 60.7 | 1351 | 8357 |
| deu-dan | flores101-devtest | 0.62006 | 34.8 | 1012 | 24638 |
| deu-isl | flores101-devtest | 0.48236 | 18.8 | 1012 | 22834 |
| deu-swe | flores101-devtest | 0.61778 | 33.7 | 1012 | 23121 |
| eng-swe | flores101-devtest | 0.69435 | 45.5 | 1012 | 23121 |
| fra-dan | flores101-devtest | 0.61019 | 34.0 | 1012 | 24638 |
| fra-isl | flores101-devtest | 0.47647 | 18.1 | 1012 | 22834 |
| fra-swe | flores101-devtest | 0.60354 | 32.2 | 1012 | 23121 |
| por-isl | flores101-devtest | 0.47937 | 19.1 | 1012 | 22834 |
| por-swe | flores101-devtest | 0.60857 | 33.1 | 1012 | 23121 |
| spa-dan | flores101-devtest | 0.54890 | 24.4 | 1012 | 24638 |
| spa-nob | flores101-devtest | 0.50610 | 18.3 | 1012 | 23873 |
| spa-swe | flores101-devtest | 0.54011 | 22.4 | 1012 | 23121 |
| deu-dan | flores200-devtest | 0.62152 | 35.1 | 1012 | 24638 |
| deu-isl | flores200-devtest | 0.48648 | 19.1 | 1012 | 22834 |
| deu-nno | flores200-devtest | 0.53530 | 24.0 | 1012 | 24316 |
| deu-nob | flores200-devtest | 0.55748 | 25.1 | 1012 | 23873 |
| deu-swe | flores200-devtest | 0.62138 | 34.2 | 1012 | 23121 |
| eng-dan | flores200-devtest | 0.70321 | 47.0 | 1012 | 24638 |
| eng-isl | flores200-devtest | 0.52585 | 24.4 | 1012 | 22834 |
| eng-nno | flores200-devtest | 0.61372 | 33.8 | 1012 | 24316 |
| eng-nob | flores200-devtest | 0.62508 | 34.4 | 1012 | 23873 |
| eng-swe | flores200-devtest | 0.69703 | 46.0 | 1012 | 23121 |
| fra-dan | flores200-devtest | 0.61025 | 34.1 | 1012 | 24638 |
| fra-isl | flores200-devtest | 0.48273 | 18.8 | 1012 | 22834 |
| fra-nno | flores200-devtest | 0.53032 | 24.3 | 1012 | 24316 |
| fra-nob | flores200-devtest | 0.54933 | 25.0 | 1012 | 23873 |
| fra-swe | flores200-devtest | 0.60612 | 32.8 | 1012 | 23121 |
| por-dan | flores200-devtest | 0.62221 | 36.2 | 1012 | 24638 |
| por-isl | flores200-devtest | 0.48357 | 19.6 | 1012 | 22834 |
| por-nno | flores200-devtest | 0.54369 | 26.3 | 1012 | 24316 |
| por-nob | flores200-devtest | 0.56054 | 26.4 | 1012 | 23873 |
| por-swe | flores200-devtest | 0.61388 | 34.1 | 1012 | 23121 |
| spa-dan | flores200-devtest | 0.55091 | 24.7 | 1012 | 24638 |
| spa-isl | flores200-devtest | 0.44469 | 14.2 | 1012 | 22834 |
| spa-nno | flores200-devtest | 0.48898 | 18.6 | 1012 | 24316 |
| spa-nob | flores200-devtest | 0.50901 | 18.8 | 1012 | 23873 |
| spa-swe | flores200-devtest | 0.54182 | 22.7 | 1012 | 23121 |
| eng-isl | newstest2021 | 0.51196 | 21.9 | 1000 | 25233 |
| deu-dan | ntrex128 | 0.56412 | 29.1 | 1997 | 47643 |
| deu-isl | ntrex128 | 0.48309 | 18.8 | 1997 | 46643 |
| deu-nno | ntrex128 | 0.51535 | 22.0 | 1997 | 46512 |
| deu-nob | ntrex128 | 0.56152 | 27.6 | 1997 | 45501 |
| deu-swe | ntrex128 | 0.58061 | 29.6 | 1997 | 44889 |
| eng-dan | ntrex128 | 0.61894 | 37.6 | 1997 | 47643 |
| eng-isl | ntrex128 | 0.52027 | 23.9 | 1997 | 46643 |
| eng-nno | ntrex128 | 0.60754 | 34.0 | 1997 | 46512 |
| eng-nob | ntrex128 | 0.62327 | 36.9 | 1997 | 45501 |
| eng-swe | ntrex128 | 0.66129 | 41.3 | 1997 | 44889 |
| fra-dan | ntrex128 | 0.54102 | 27.1 | 1997 | 47643 |
| fra-isl | ntrex128 | 0.47296 | 18.4 | 1997 | 46643 |
| fra-nno | ntrex128 | 0.50532 | 21.6 | 1997 | 46512 |
| fra-nob | ntrex128 | 0.54026 | 25.7 | 1997 | 45501 |
| fra-swe | ntrex128 | 0.56278 | 27.9 | 1997 | 44889 |
| por-dan | ntrex128 | 0.56288 | 30.0 | 1997 | 47643 |
| por-isl | ntrex128 | 0.47577 | 17.8 | 1997 | 46643 |
| por-nno | ntrex128 | 0.52158 | 23.0 | 1997 | 46512 |
| por-nob | ntrex128 | 0.55788 | 27.4 | 1997 | 45501 |
| por-swe | ntrex128 | 0.57790 | 29.3 | 1997 | 44889 |
| spa-dan | ntrex128 | 0.55607 | 27.5 | 1997 | 47643 |
| spa-isl | ntrex128 | 0.48566 | 18.4 | 1997 | 46643 |
| spa-nno | ntrex128 | 0.51741 | 22.2 | 1997 | 46512 |
| spa-nob | ntrex128 | 0.55824 | 26.8 | 1997 | 45501 |
| spa-swe | ntrex128 | 0.57851 | 28.8 | 1997 | 44889 |
## Citation Information
* Publications: [Democratizing neural machine translation with OPUS-MT](https://doi.org/10.1007/s10579-023-09704-w) and [OPUS-MT – Building open translation services for the World](https://aclanthology.org/2020.eamt-1.61/) and [The Tatoeba Translation Challenge – Realistic Data Sets for Low Resource and Multilingual MT](https://aclanthology.org/2020.wmt-1.139/) (Please, cite if you use this model.)
```bibtex
@article{tiedemann2023democratizing,
title={Democratizing neural machine translation with {OPUS-MT}},
author={Tiedemann, J{\"o}rg and Aulamo, Mikko and Bakshandaeva, Daria and Boggia, Michele and Gr{\"o}nroos, Stig-Arne and Nieminen, Tommi and Raganato, Alessandro and Scherrer, Yves and Vazquez, Raul and Virpioja, Sami},
journal={Language Resources and Evaluation},
number={58},
pages={713--755},
year={2023},
publisher={Springer Nature},
issn={1574-0218},
doi={10.1007/s10579-023-09704-w}
}
@inproceedings{tiedemann-thottingal-2020-opus,
title = "{OPUS}-{MT} {--} Building open translation services for the World",
author = {Tiedemann, J{\"o}rg and Thottingal, Santhosh},
booktitle = "Proceedings of the 22nd Annual Conference of the European Association for Machine Translation",
month = nov,
year = "2020",
address = "Lisboa, Portugal",
publisher = "European Association for Machine Translation",
url = "https://aclanthology.org/2020.eamt-1.61",
pages = "479--480",
}
@inproceedings{tiedemann-2020-tatoeba,
title = "The Tatoeba Translation Challenge {--} Realistic Data Sets for Low Resource and Multilingual {MT}",
author = {Tiedemann, J{\"o}rg},
booktitle = "Proceedings of the Fifth Conference on Machine Translation",
month = nov,
year = "2020",
address = "Online",
publisher = "Association for Computational Linguistics",
url = "https://aclanthology.org/2020.wmt-1.139",
pages = "1174--1182",
}
```
## Acknowledgements
The work is supported by the [HPLT project](https://hplt-project.org/), funded by the European Union’s Horizon Europe research and innovation programme under grant agreement No 101070350. We are also grateful for the generous computational resources and IT infrastructure provided by [CSC -- IT Center for Science](https://www.csc.fi/), Finland, and the [EuroHPC supercomputer LUMI](https://www.lumi-supercomputer.eu/).
## Model conversion info
* transformers version: 4.45.1
* OPUS-MT git hash: 0882077
* port time: Tue Oct 8 10:03:29 EEST 2024
* port machine: LM0-400-22516.local
|
PAUL1122/vison2 | PAUL1122 | "2024-11-21T22:01:49Z" | 5 | 0 | transformers | [
"transformers",
"safetensors",
"mllama",
"image-text-to-text",
"text-generation-inference",
"unsloth",
"conversational",
"en",
"license:apache-2.0",
"endpoints_compatible",
"region:us"
] | image-text-to-text | "2024-11-21T21:53:51Z" | ---
base_model: unsloth/llama-3.2-11b-vision-instruct-bnb-4bit
tags:
- text-generation-inference
- transformers
- unsloth
- mllama
license: apache-2.0
language:
- en
---
# Uploaded model
- **Developed by:** PAUL1122
- **License:** apache-2.0
- **Finetuned from model :** unsloth/llama-3.2-11b-vision-instruct-bnb-4bit
This mllama model was trained 2x faster with [Unsloth](https://github.com/unslothai/unsloth) and Huggingface's TRL library.
[<img src="https://raw.githubusercontent.com/unslothai/unsloth/main/images/unsloth%20made%20with%20love.png" width="200"/>](https://github.com/unslothai/unsloth)
|
MayBashendy/Arabic_FineTuningAraBERT_AugV4_k40_task2_organization_fold0 | MayBashendy | "2024-11-16T21:20:13Z" | 183 | 0 | transformers | [
"transformers",
"safetensors",
"bert",
"text-classification",
"generated_from_trainer",
"base_model:aubmindlab/bert-base-arabertv02",
"base_model:finetune:aubmindlab/bert-base-arabertv02",
"autotrain_compatible",
"endpoints_compatible",
"region:us"
] | text-classification | "2024-11-16T20:57:26Z" | ---
library_name: transformers
base_model: aubmindlab/bert-base-arabertv02
tags:
- generated_from_trainer
model-index:
- name: Arabic_FineTuningAraBERT_AugV4_k40_task2_organization_fold0
results: []
---
<!-- This model card has been generated automatically according to the information the Trainer had access to. You
should probably proofread and complete it, then remove this comment. -->
# Arabic_FineTuningAraBERT_AugV4_k40_task2_organization_fold0
This model is a fine-tuned version of [aubmindlab/bert-base-arabertv02](https://huggingface.co/aubmindlab/bert-base-arabertv02) on the None dataset.
It achieves the following results on the evaluation set:
- Loss: 0.5329
- Qwk: 0.5477
- Mse: 0.5329
- Rmse: 0.7300
## Model description
More information needed
## Intended uses & limitations
More information needed
## Training and evaluation data
More information needed
## Training procedure
### Training hyperparameters
The following hyperparameters were used during training:
- learning_rate: 2e-05
- train_batch_size: 8
- eval_batch_size: 8
- seed: 42
- optimizer: Adam with betas=(0.9,0.999) and epsilon=1e-08
- lr_scheduler_type: linear
- num_epochs: 10
### Training results
| Training Loss | Epoch | Step | Validation Loss | Qwk | Mse | Rmse |
|:-------------:|:------:|:----:|:---------------:|:-------:|:------:|:------:|
| No log | 0.0046 | 2 | 3.2758 | 0.0 | 3.2758 | 1.8099 |
| No log | 0.0092 | 4 | 2.2390 | 0.0172 | 2.2390 | 1.4963 |
| No log | 0.0138 | 6 | 1.0232 | 0.0 | 1.0232 | 1.0115 |
| No log | 0.0183 | 8 | 0.6949 | 0.3438 | 0.6949 | 0.8336 |
| No log | 0.0229 | 10 | 0.6589 | 0.0735 | 0.6589 | 0.8117 |
| No log | 0.0275 | 12 | 0.8843 | -0.0418 | 0.8843 | 0.9404 |
| No log | 0.0321 | 14 | 1.2729 | 0.2015 | 1.2729 | 1.1282 |
| No log | 0.0367 | 16 | 1.0347 | 0.0679 | 1.0347 | 1.0172 |
| No log | 0.0413 | 18 | 0.9672 | -0.0903 | 0.9672 | 0.9835 |
| No log | 0.0459 | 20 | 0.8947 | -0.1324 | 0.8947 | 0.9459 |
| No log | 0.0505 | 22 | 1.1366 | -0.0627 | 1.1366 | 1.0661 |
| No log | 0.0550 | 24 | 1.2714 | 0.0123 | 1.2714 | 1.1275 |
| No log | 0.0596 | 26 | 1.0587 | 0.0 | 1.0587 | 1.0290 |
| No log | 0.0642 | 28 | 0.8104 | 0.0 | 0.8104 | 0.9002 |
| No log | 0.0688 | 30 | 0.7643 | 0.0 | 0.7643 | 0.8743 |
| No log | 0.0734 | 32 | 0.7900 | 0.0 | 0.7900 | 0.8888 |
| No log | 0.0780 | 34 | 0.9149 | 0.0 | 0.9149 | 0.9565 |
| No log | 0.0826 | 36 | 0.9896 | 0.0 | 0.9896 | 0.9948 |
| No log | 0.0872 | 38 | 0.9596 | 0.0 | 0.9596 | 0.9796 |
| No log | 0.0917 | 40 | 0.9275 | 0.0 | 0.9275 | 0.9630 |
| No log | 0.0963 | 42 | 0.9138 | 0.0 | 0.9138 | 0.9559 |
| No log | 0.1009 | 44 | 0.9253 | 0.0 | 0.9253 | 0.9619 |
| No log | 0.1055 | 46 | 1.0319 | 0.0 | 1.0319 | 1.0158 |
| No log | 0.1101 | 48 | 1.0375 | 0.0 | 1.0375 | 1.0186 |
| No log | 0.1147 | 50 | 1.1704 | 0.1637 | 1.1704 | 1.0818 |
| No log | 0.1193 | 52 | 1.1855 | 0.1637 | 1.1855 | 1.0888 |
| No log | 0.1239 | 54 | 1.2663 | 0.1421 | 1.2663 | 1.1253 |
| No log | 0.1284 | 56 | 1.1221 | 0.2699 | 1.1221 | 1.0593 |
| No log | 0.1330 | 58 | 0.9143 | 0.1637 | 0.9143 | 0.9562 |
| No log | 0.1376 | 60 | 0.7149 | 0.0679 | 0.7149 | 0.8455 |
| No log | 0.1422 | 62 | 0.7231 | 0.1637 | 0.7231 | 0.8504 |
| No log | 0.1468 | 64 | 0.8815 | 0.1637 | 0.8815 | 0.9389 |
| No log | 0.1514 | 66 | 1.2178 | 0.3000 | 1.2178 | 1.1035 |
| No log | 0.1560 | 68 | 1.4711 | 0.1094 | 1.4711 | 1.2129 |
| No log | 0.1606 | 70 | 1.5316 | 0.0322 | 1.5316 | 1.2376 |
| No log | 0.1651 | 72 | 1.3596 | 0.1758 | 1.3596 | 1.1660 |
| No log | 0.1697 | 74 | 1.1058 | 0.2814 | 1.1058 | 1.0516 |
| No log | 0.1743 | 76 | 0.7921 | 0.1637 | 0.7921 | 0.8900 |
| No log | 0.1789 | 78 | 0.6670 | 0.1398 | 0.6670 | 0.8167 |
| No log | 0.1835 | 80 | 0.6639 | 0.1398 | 0.6639 | 0.8148 |
| No log | 0.1881 | 82 | 0.7308 | 0.1398 | 0.7308 | 0.8549 |
| No log | 0.1927 | 84 | 0.8071 | 0.1637 | 0.8071 | 0.8984 |
| No log | 0.1972 | 86 | 0.8644 | 0.1637 | 0.8644 | 0.9297 |
| No log | 0.2018 | 88 | 0.8184 | 0.1637 | 0.8184 | 0.9047 |
| No log | 0.2064 | 90 | 0.6837 | 0.0679 | 0.6837 | 0.8269 |
| No log | 0.2110 | 92 | 0.6234 | 0.1558 | 0.6234 | 0.7896 |
| No log | 0.2156 | 94 | 0.6202 | 0.1558 | 0.6202 | 0.7875 |
| No log | 0.2202 | 96 | 0.6654 | 0.2000 | 0.6654 | 0.8157 |
| No log | 0.2248 | 98 | 0.8436 | 0.2699 | 0.8436 | 0.9185 |
| No log | 0.2294 | 100 | 0.8955 | 0.2566 | 0.8955 | 0.9463 |
| No log | 0.2339 | 102 | 0.9142 | 0.1398 | 0.9142 | 0.9561 |
| No log | 0.2385 | 104 | 1.0688 | 0.2814 | 1.0688 | 1.0338 |
| No log | 0.2431 | 106 | 1.0538 | 0.2814 | 1.0538 | 1.0266 |
| No log | 0.2477 | 108 | 0.9685 | 0.2814 | 0.9685 | 0.9841 |
| No log | 0.2523 | 110 | 0.7067 | 0.1637 | 0.7067 | 0.8406 |
| No log | 0.2569 | 112 | 0.5989 | 0.2727 | 0.5989 | 0.7739 |
| No log | 0.2615 | 114 | 0.5705 | 0.2881 | 0.5705 | 0.7553 |
| No log | 0.2661 | 116 | 0.6267 | 0.3687 | 0.6267 | 0.7916 |
| No log | 0.2706 | 118 | 0.8842 | 0.4099 | 0.8842 | 0.9403 |
| No log | 0.2752 | 120 | 1.2180 | 0.1276 | 1.2180 | 1.1036 |
| No log | 0.2798 | 122 | 1.1863 | 0.2804 | 1.1863 | 1.0892 |
| No log | 0.2844 | 124 | 0.8639 | 0.3803 | 0.8639 | 0.9294 |
| No log | 0.2890 | 126 | 0.6618 | 0.3383 | 0.6618 | 0.8135 |
| No log | 0.2936 | 128 | 0.6285 | 0.5243 | 0.6285 | 0.7928 |
| No log | 0.2982 | 130 | 0.6659 | 0.3521 | 0.6659 | 0.8160 |
| No log | 0.3028 | 132 | 0.8777 | 0.4099 | 0.8777 | 0.9369 |
| No log | 0.3073 | 134 | 1.1781 | 0.4112 | 1.1781 | 1.0854 |
| No log | 0.3119 | 136 | 1.1441 | 0.4124 | 1.1441 | 1.0696 |
| No log | 0.3165 | 138 | 0.8740 | 0.3345 | 0.8740 | 0.9349 |
| No log | 0.3211 | 140 | 0.7478 | 0.3345 | 0.7478 | 0.8648 |
| No log | 0.3257 | 142 | 0.6527 | 0.3345 | 0.6527 | 0.8079 |
| No log | 0.3303 | 144 | 0.5810 | 0.3333 | 0.5810 | 0.7622 |
| No log | 0.3349 | 146 | 0.5348 | 0.3333 | 0.5348 | 0.7313 |
| No log | 0.3394 | 148 | 0.5672 | 0.0916 | 0.5672 | 0.7531 |
| No log | 0.3440 | 150 | 0.7213 | 0.1456 | 0.7213 | 0.8493 |
| No log | 0.3486 | 152 | 0.7630 | 0.1818 | 0.7630 | 0.8735 |
| No log | 0.3532 | 154 | 0.7943 | 0.3833 | 0.7943 | 0.8912 |
| No log | 0.3578 | 156 | 0.8119 | 0.3345 | 0.8119 | 0.9010 |
| No log | 0.3624 | 158 | 0.7214 | 0.3277 | 0.7214 | 0.8493 |
| No log | 0.3670 | 160 | 0.7825 | 0.3277 | 0.7825 | 0.8846 |
| No log | 0.3716 | 162 | 0.7999 | 0.3198 | 0.7999 | 0.8944 |
| No log | 0.3761 | 164 | 0.7936 | 0.3198 | 0.7936 | 0.8908 |
| No log | 0.3807 | 166 | 0.7380 | 0.3198 | 0.7380 | 0.8591 |
| No log | 0.3853 | 168 | 0.8250 | 0.4085 | 0.8250 | 0.9083 |
| No log | 0.3899 | 170 | 0.8388 | 0.3405 | 0.8388 | 0.9159 |
| No log | 0.3945 | 172 | 1.0118 | 0.3505 | 1.0118 | 1.0059 |
| No log | 0.3991 | 174 | 0.9783 | 0.3505 | 0.9783 | 0.9891 |
| No log | 0.4037 | 176 | 0.7087 | 0.3558 | 0.7087 | 0.8418 |
| No log | 0.4083 | 178 | 0.5613 | 0.2888 | 0.5613 | 0.7492 |
| No log | 0.4128 | 180 | 0.5671 | 0.4693 | 0.5671 | 0.7531 |
| No log | 0.4174 | 182 | 0.5913 | 0.4693 | 0.5913 | 0.7690 |
| No log | 0.4220 | 184 | 0.5970 | 0.3251 | 0.5970 | 0.7726 |
| No log | 0.4266 | 186 | 1.0027 | 0.3883 | 1.0027 | 1.0013 |
| No log | 0.4312 | 188 | 1.5487 | 0.2112 | 1.5487 | 1.2445 |
| No log | 0.4358 | 190 | 1.6212 | 0.1857 | 1.6212 | 1.2733 |
| No log | 0.4404 | 192 | 1.2225 | 0.3547 | 1.2225 | 1.1057 |
| No log | 0.4450 | 194 | 0.7923 | 0.3599 | 0.7923 | 0.8901 |
| No log | 0.4495 | 196 | 0.6572 | 0.3125 | 0.6572 | 0.8107 |
| No log | 0.4541 | 198 | 0.7567 | 0.3125 | 0.7567 | 0.8699 |
| No log | 0.4587 | 200 | 1.0281 | 0.4215 | 1.0281 | 1.0139 |
| No log | 0.4633 | 202 | 1.0850 | 0.2243 | 1.0850 | 1.0416 |
| No log | 0.4679 | 204 | 1.1293 | 0.2340 | 1.1293 | 1.0627 |
| No log | 0.4725 | 206 | 1.0625 | 0.2374 | 1.0625 | 1.0308 |
| No log | 0.4771 | 208 | 1.0140 | 0.4209 | 1.0140 | 1.0070 |
| No log | 0.4817 | 210 | 1.1177 | 0.3547 | 1.1177 | 1.0572 |
| No log | 0.4862 | 212 | 1.1519 | 0.2025 | 1.1519 | 1.0733 |
| No log | 0.4908 | 214 | 1.2230 | 0.2025 | 1.2230 | 1.1059 |
| No log | 0.4954 | 216 | 1.4663 | 0.1376 | 1.4663 | 1.2109 |
| No log | 0.5 | 218 | 1.5671 | 0.1376 | 1.5671 | 1.2519 |
| No log | 0.5046 | 220 | 1.4679 | 0.1620 | 1.4679 | 1.2116 |
| No log | 0.5092 | 222 | 1.2754 | 0.1494 | 1.2754 | 1.1293 |
| No log | 0.5138 | 224 | 0.9729 | 0.3505 | 0.9729 | 0.9864 |
| No log | 0.5183 | 226 | 0.9297 | 0.3505 | 0.9297 | 0.9642 |
| No log | 0.5229 | 228 | 0.7950 | 0.3505 | 0.7950 | 0.8916 |
| No log | 0.5275 | 230 | 0.6324 | 0.5333 | 0.6324 | 0.7953 |
| No log | 0.5321 | 232 | 0.6061 | 0.5039 | 0.6061 | 0.7785 |
| No log | 0.5367 | 234 | 0.6824 | 0.4 | 0.6824 | 0.8261 |
| No log | 0.5413 | 236 | 0.8905 | 0.4209 | 0.8905 | 0.9437 |
| No log | 0.5459 | 238 | 1.1318 | 0.2718 | 1.1318 | 1.0639 |
| No log | 0.5505 | 240 | 1.1171 | 0.2718 | 1.1171 | 1.0569 |
| No log | 0.5550 | 242 | 0.8526 | 0.3780 | 0.8526 | 0.9234 |
| No log | 0.5596 | 244 | 0.6340 | 0.5191 | 0.6340 | 0.7962 |
| No log | 0.5642 | 246 | 0.6084 | 0.6410 | 0.6084 | 0.7800 |
| No log | 0.5688 | 248 | 0.6737 | 0.5737 | 0.6737 | 0.8208 |
| No log | 0.5734 | 250 | 0.8319 | 0.4631 | 0.8319 | 0.9121 |
| No log | 0.5780 | 252 | 0.8319 | 0.4631 | 0.8319 | 0.9121 |
| No log | 0.5826 | 254 | 0.7382 | 0.5359 | 0.7382 | 0.8592 |
| No log | 0.5872 | 256 | 0.6846 | 0.5619 | 0.6846 | 0.8274 |
| No log | 0.5917 | 258 | 0.6824 | 0.4631 | 0.6824 | 0.8261 |
| No log | 0.5963 | 260 | 0.7707 | 0.3948 | 0.7707 | 0.8779 |
| No log | 0.6009 | 262 | 1.0505 | 0.3860 | 1.0505 | 1.0249 |
| No log | 0.6055 | 264 | 1.1071 | 0.3547 | 1.1071 | 1.0522 |
| No log | 0.6101 | 266 | 0.8797 | 0.3833 | 0.8797 | 0.9379 |
| No log | 0.6147 | 268 | 0.6217 | 0.3811 | 0.6217 | 0.7885 |
| No log | 0.6193 | 270 | 0.5250 | 0.2470 | 0.5250 | 0.7246 |
| No log | 0.6239 | 272 | 0.5244 | 0.5792 | 0.5244 | 0.7242 |
| No log | 0.6284 | 274 | 0.6279 | 0.3383 | 0.6279 | 0.7924 |
| No log | 0.6330 | 276 | 0.7131 | 0.3388 | 0.7131 | 0.8445 |
| No log | 0.6376 | 278 | 0.6123 | 0.5169 | 0.6123 | 0.7825 |
| No log | 0.6422 | 280 | 0.6195 | 0.6048 | 0.6195 | 0.7871 |
| No log | 0.6468 | 282 | 0.6909 | 0.4527 | 0.6909 | 0.8312 |
| No log | 0.6514 | 284 | 0.8182 | 0.4062 | 0.8182 | 0.9045 |
| No log | 0.6560 | 286 | 0.7623 | 0.4034 | 0.7623 | 0.8731 |
| No log | 0.6606 | 288 | 0.5759 | 0.5832 | 0.5759 | 0.7589 |
| No log | 0.6651 | 290 | 0.5189 | 0.5832 | 0.5189 | 0.7204 |
| No log | 0.6697 | 292 | 0.5966 | 0.5689 | 0.5966 | 0.7724 |
| No log | 0.6743 | 294 | 0.8609 | 0.3948 | 0.8609 | 0.9279 |
| No log | 0.6789 | 296 | 0.9981 | 0.3393 | 0.9981 | 0.9991 |
| No log | 0.6835 | 298 | 1.0318 | 0.3152 | 1.0318 | 1.0158 |
| No log | 0.6881 | 300 | 1.0117 | 0.3152 | 1.0117 | 1.0059 |
| No log | 0.6927 | 302 | 0.8358 | 0.3780 | 0.8358 | 0.9142 |
| No log | 0.6972 | 304 | 0.7486 | 0.4779 | 0.7486 | 0.8652 |
| No log | 0.7018 | 306 | 0.7520 | 0.4779 | 0.7520 | 0.8672 |
| No log | 0.7064 | 308 | 0.8967 | 0.3691 | 0.8967 | 0.9470 |
| No log | 0.7110 | 310 | 1.1676 | 0.4000 | 1.1676 | 1.0806 |
| No log | 0.7156 | 312 | 1.2078 | 0.3301 | 1.2078 | 1.0990 |
| No log | 0.7202 | 314 | 1.0316 | 0.3860 | 1.0316 | 1.0157 |
| No log | 0.7248 | 316 | 0.7791 | 0.3656 | 0.7791 | 0.8827 |
| No log | 0.7294 | 318 | 0.6894 | 0.3521 | 0.6894 | 0.8303 |
| No log | 0.7339 | 320 | 0.7036 | 0.3521 | 0.7036 | 0.8388 |
| No log | 0.7385 | 322 | 0.8471 | 0.3458 | 0.8471 | 0.9204 |
| No log | 0.7431 | 324 | 1.0769 | 0.3505 | 1.0769 | 1.0377 |
| No log | 0.7477 | 326 | 1.1249 | 0.3505 | 1.1249 | 1.0606 |
| No log | 0.7523 | 328 | 1.0047 | 0.3505 | 1.0047 | 1.0023 |
| No log | 0.7569 | 330 | 0.8763 | 0.3405 | 0.8763 | 0.9361 |
| No log | 0.7615 | 332 | 0.7442 | 0.3616 | 0.7442 | 0.8626 |
| No log | 0.7661 | 334 | 0.7238 | 0.4 | 0.7238 | 0.8508 |
| No log | 0.7706 | 336 | 0.8610 | 0.3927 | 0.8610 | 0.9279 |
| No log | 0.7752 | 338 | 1.1278 | 0.3905 | 1.1278 | 1.0620 |
| No log | 0.7798 | 340 | 1.2646 | 0.3905 | 1.2646 | 1.1245 |
| No log | 0.7844 | 342 | 1.1319 | 0.3984 | 1.1319 | 1.0639 |
| No log | 0.7890 | 344 | 0.8789 | 0.4062 | 0.8789 | 0.9375 |
| No log | 0.7936 | 346 | 0.7307 | 0.4527 | 0.7307 | 0.8548 |
| No log | 0.7982 | 348 | 0.6592 | 0.4538 | 0.6592 | 0.8119 |
| No log | 0.8028 | 350 | 0.6580 | 0.4538 | 0.6580 | 0.8112 |
| No log | 0.8073 | 352 | 0.7573 | 0.375 | 0.7573 | 0.8702 |
| No log | 0.8119 | 354 | 1.0013 | 0.4220 | 1.0013 | 1.0007 |
| No log | 0.8165 | 356 | 1.0286 | 0.3860 | 1.0286 | 1.0142 |
| No log | 0.8211 | 358 | 0.9585 | 0.3860 | 0.9585 | 0.9790 |
| No log | 0.8257 | 360 | 0.8109 | 0.4195 | 0.8109 | 0.9005 |
| No log | 0.8303 | 362 | 0.7681 | 0.4195 | 0.7681 | 0.8764 |
| No log | 0.8349 | 364 | 0.8834 | 0.4195 | 0.8834 | 0.9399 |
| No log | 0.8394 | 366 | 1.1677 | 0.3028 | 1.1677 | 1.0806 |
| No log | 0.8440 | 368 | 1.2251 | 0.3438 | 1.2251 | 1.1068 |
| No log | 0.8486 | 370 | 0.9913 | 0.4215 | 0.9913 | 0.9957 |
| No log | 0.8532 | 372 | 0.7623 | 0.3333 | 0.7623 | 0.8731 |
| No log | 0.8578 | 374 | 0.6962 | 0.3842 | 0.6962 | 0.8344 |
| No log | 0.8624 | 376 | 0.7375 | 0.3842 | 0.7375 | 0.8588 |
| No log | 0.8670 | 378 | 0.7392 | 0.3842 | 0.7392 | 0.8597 |
| No log | 0.8716 | 380 | 0.8035 | 0.3866 | 0.8035 | 0.8964 |
| No log | 0.8761 | 382 | 0.8177 | 0.3529 | 0.8177 | 0.9042 |
| No log | 0.8807 | 384 | 0.8057 | 0.4049 | 0.8057 | 0.8976 |
| No log | 0.8853 | 386 | 0.6564 | 0.4018 | 0.6564 | 0.8102 |
| No log | 0.8899 | 388 | 0.6065 | 0.5 | 0.6065 | 0.7788 |
| No log | 0.8945 | 390 | 0.6821 | 0.5205 | 0.6821 | 0.8259 |
| No log | 0.8991 | 392 | 0.7301 | 0.4209 | 0.7301 | 0.8545 |
| No log | 0.9037 | 394 | 0.7057 | 0.4553 | 0.7057 | 0.8400 |
| No log | 0.9083 | 396 | 0.7472 | 0.4838 | 0.7472 | 0.8644 |
| No log | 0.9128 | 398 | 0.7083 | 0.4968 | 0.7083 | 0.8416 |
| No log | 0.9174 | 400 | 0.6084 | 0.4727 | 0.6084 | 0.7800 |
| No log | 0.9220 | 402 | 0.5001 | 0.5832 | 0.5001 | 0.7072 |
| No log | 0.9266 | 404 | 0.4952 | 0.6602 | 0.4952 | 0.7037 |
| No log | 0.9312 | 406 | 0.5906 | 0.5073 | 0.5906 | 0.7685 |
| No log | 0.9358 | 408 | 0.8924 | 0.4522 | 0.8924 | 0.9447 |
| No log | 0.9404 | 410 | 1.2172 | 0.4505 | 1.2172 | 1.1033 |
| No log | 0.9450 | 412 | 1.3113 | 0.3581 | 1.3113 | 1.1451 |
| No log | 0.9495 | 414 | 1.1394 | 0.4225 | 1.1394 | 1.0674 |
| No log | 0.9541 | 416 | 0.9356 | 0.4215 | 0.9356 | 0.9673 |
| No log | 0.9587 | 418 | 0.6609 | 0.375 | 0.6609 | 0.8130 |
| No log | 0.9633 | 420 | 0.5181 | 0.5546 | 0.5181 | 0.7198 |
| No log | 0.9679 | 422 | 0.5118 | 0.5546 | 0.5118 | 0.7154 |
| No log | 0.9725 | 424 | 0.6132 | 0.3717 | 0.6132 | 0.7831 |
| No log | 0.9771 | 426 | 0.7732 | 0.3927 | 0.7732 | 0.8793 |
| No log | 0.9817 | 428 | 0.8799 | 0.3948 | 0.8799 | 0.9380 |
| No log | 0.9862 | 430 | 0.9645 | 0.3948 | 0.9645 | 0.9821 |
| No log | 0.9908 | 432 | 0.9379 | 0.3948 | 0.9379 | 0.9685 |
| No log | 0.9954 | 434 | 0.8421 | 0.3927 | 0.8421 | 0.9176 |
| No log | 1.0 | 436 | 0.7951 | 0.475 | 0.7951 | 0.8917 |
| No log | 1.0046 | 438 | 0.7987 | 0.475 | 0.7987 | 0.8937 |
| No log | 1.0092 | 440 | 0.8671 | 0.4372 | 0.8671 | 0.9312 |
| No log | 1.0138 | 442 | 0.9421 | 0.4049 | 0.9421 | 0.9706 |
| No log | 1.0183 | 444 | 0.9499 | 0.4062 | 0.9499 | 0.9746 |
| No log | 1.0229 | 446 | 1.0181 | 0.4215 | 1.0181 | 1.0090 |
| No log | 1.0275 | 448 | 0.9183 | 0.4215 | 0.9183 | 0.9583 |
| No log | 1.0321 | 450 | 0.7666 | 0.3927 | 0.7666 | 0.8755 |
| No log | 1.0367 | 452 | 0.6463 | 0.4688 | 0.6463 | 0.8039 |
| No log | 1.0413 | 454 | 0.6398 | 0.4286 | 0.6398 | 0.7999 |
| No log | 1.0459 | 456 | 0.7056 | 0.4195 | 0.7056 | 0.8400 |
| No log | 1.0505 | 458 | 0.7531 | 0.4209 | 0.7531 | 0.8678 |
| No log | 1.0550 | 460 | 0.7915 | 0.4209 | 0.7915 | 0.8896 |
| No log | 1.0596 | 462 | 0.7286 | 0.4202 | 0.7286 | 0.8536 |
| No log | 1.0642 | 464 | 0.6243 | 0.3388 | 0.6243 | 0.7901 |
| No log | 1.0688 | 466 | 0.5077 | 0.4080 | 0.5077 | 0.7125 |
| No log | 1.0734 | 468 | 0.4681 | 0.6232 | 0.4681 | 0.6842 |
| No log | 1.0780 | 470 | 0.4534 | 0.6602 | 0.4534 | 0.6733 |
| No log | 1.0826 | 472 | 0.4930 | 0.6526 | 0.4930 | 0.7021 |
| No log | 1.0872 | 474 | 0.5953 | 0.5737 | 0.5953 | 0.7715 |
| No log | 1.0917 | 476 | 0.6538 | 0.5737 | 0.6538 | 0.8086 |
| No log | 1.0963 | 478 | 0.6810 | 0.4850 | 0.6810 | 0.8252 |
| No log | 1.1009 | 480 | 0.6918 | 0.4850 | 0.6918 | 0.8318 |
| No log | 1.1055 | 482 | 0.6533 | 0.4850 | 0.6533 | 0.8083 |
| No log | 1.1101 | 484 | 0.5784 | 0.5737 | 0.5784 | 0.7605 |
| No log | 1.1147 | 486 | 0.5013 | 0.6526 | 0.5013 | 0.7080 |
| No log | 1.1193 | 488 | 0.4964 | 0.6526 | 0.4964 | 0.7046 |
| No log | 1.1239 | 490 | 0.5259 | 0.6526 | 0.5259 | 0.7252 |
| No log | 1.1284 | 492 | 0.6356 | 0.5995 | 0.6356 | 0.7972 |
| No log | 1.1330 | 494 | 0.9073 | 0.4062 | 0.9073 | 0.9525 |
| No log | 1.1376 | 496 | 0.9793 | 0.4215 | 0.9793 | 0.9896 |
| No log | 1.1422 | 498 | 0.8335 | 0.4209 | 0.8335 | 0.9129 |
| 0.522 | 1.1468 | 500 | 0.6638 | 0.3388 | 0.6638 | 0.8147 |
| 0.522 | 1.1514 | 502 | 0.5657 | 0.4378 | 0.5657 | 0.7521 |
| 0.522 | 1.1560 | 504 | 0.5676 | 0.3616 | 0.5676 | 0.7534 |
| 0.522 | 1.1606 | 506 | 0.6392 | 0.3903 | 0.6392 | 0.7995 |
| 0.522 | 1.1651 | 508 | 0.7113 | 0.4202 | 0.7113 | 0.8434 |
| 0.522 | 1.1697 | 510 | 0.7799 | 0.4202 | 0.7799 | 0.8831 |
| 0.522 | 1.1743 | 512 | 0.9150 | 0.4295 | 0.9150 | 0.9566 |
| 0.522 | 1.1789 | 514 | 0.8313 | 0.4049 | 0.8313 | 0.9118 |
| 0.522 | 1.1835 | 516 | 0.6466 | 0.4538 | 0.6466 | 0.8041 |
| 0.522 | 1.1881 | 518 | 0.5811 | 0.6461 | 0.5811 | 0.7623 |
| 0.522 | 1.1927 | 520 | 0.5747 | 0.6461 | 0.5747 | 0.7581 |
| 0.522 | 1.1972 | 522 | 0.5600 | 0.6526 | 0.5600 | 0.7484 |
| 0.522 | 1.2018 | 524 | 0.6544 | 0.3866 | 0.6544 | 0.8090 |
| 0.522 | 1.2064 | 526 | 0.7803 | 0.4293 | 0.7803 | 0.8834 |
| 0.522 | 1.2110 | 528 | 0.8174 | 0.4293 | 0.8174 | 0.9041 |
| 0.522 | 1.2156 | 530 | 0.7856 | 0.3948 | 0.7856 | 0.8863 |
| 0.522 | 1.2202 | 532 | 0.7740 | 0.3833 | 0.7740 | 0.8798 |
| 0.522 | 1.2248 | 534 | 0.7284 | 0.3803 | 0.7284 | 0.8534 |
| 0.522 | 1.2294 | 536 | 0.5974 | 0.4503 | 0.5974 | 0.7729 |
| 0.522 | 1.2339 | 538 | 0.4965 | 0.4954 | 0.4965 | 0.7046 |
| 0.522 | 1.2385 | 540 | 0.4984 | 0.5317 | 0.4984 | 0.7059 |
| 0.522 | 1.2431 | 542 | 0.5925 | 0.4195 | 0.5925 | 0.7697 |
| 0.522 | 1.2477 | 544 | 0.7146 | 0.3833 | 0.7146 | 0.8454 |
| 0.522 | 1.2523 | 546 | 0.7995 | 0.3833 | 0.7995 | 0.8942 |
| 0.522 | 1.2569 | 548 | 0.8993 | 0.3860 | 0.8993 | 0.9483 |
| 0.522 | 1.2615 | 550 | 0.8711 | 0.4202 | 0.8711 | 0.9333 |
| 0.522 | 1.2661 | 552 | 0.7384 | 0.4034 | 0.7384 | 0.8593 |
| 0.522 | 1.2706 | 554 | 0.7332 | 0.4018 | 0.7332 | 0.8563 |
| 0.522 | 1.2752 | 556 | 0.7367 | 0.4290 | 0.7367 | 0.8583 |
| 0.522 | 1.2798 | 558 | 0.7686 | 0.4195 | 0.7686 | 0.8767 |
| 0.522 | 1.2844 | 560 | 0.8055 | 0.3803 | 0.8055 | 0.8975 |
| 0.522 | 1.2890 | 562 | 0.7777 | 0.4195 | 0.7777 | 0.8819 |
| 0.522 | 1.2936 | 564 | 0.6933 | 0.4290 | 0.6933 | 0.8326 |
| 0.522 | 1.2982 | 566 | 0.6707 | 0.375 | 0.6707 | 0.8190 |
| 0.522 | 1.3028 | 568 | 0.6818 | 0.375 | 0.6818 | 0.8257 |
| 0.522 | 1.3073 | 570 | 0.8000 | 0.4290 | 0.8000 | 0.8944 |
| 0.522 | 1.3119 | 572 | 0.8889 | 0.3948 | 0.8889 | 0.9428 |
| 0.522 | 1.3165 | 574 | 0.9460 | 0.3860 | 0.9460 | 0.9726 |
| 0.522 | 1.3211 | 576 | 0.9713 | 0.3860 | 0.9713 | 0.9855 |
| 0.522 | 1.3257 | 578 | 0.8521 | 0.3780 | 0.8521 | 0.9231 |
| 0.522 | 1.3303 | 580 | 0.6657 | 0.4803 | 0.6657 | 0.8159 |
| 0.522 | 1.3349 | 582 | 0.5997 | 0.4803 | 0.5997 | 0.7744 |
| 0.522 | 1.3394 | 584 | 0.6175 | 0.4779 | 0.6175 | 0.7858 |
| 0.522 | 1.3440 | 586 | 0.5990 | 0.5 | 0.5990 | 0.7740 |
| 0.522 | 1.3486 | 588 | 0.6270 | 0.4290 | 0.6270 | 0.7918 |
| 0.522 | 1.3532 | 590 | 0.7266 | 0.4202 | 0.7266 | 0.8524 |
| 0.522 | 1.3578 | 592 | 0.7297 | 0.4202 | 0.7297 | 0.8542 |
| 0.522 | 1.3624 | 594 | 0.6124 | 0.3903 | 0.6124 | 0.7825 |
| 0.522 | 1.3670 | 596 | 0.5104 | 0.5039 | 0.5104 | 0.7144 |
| 0.522 | 1.3716 | 598 | 0.4459 | 0.5546 | 0.4459 | 0.6677 |
| 0.522 | 1.3761 | 600 | 0.4285 | 0.6354 | 0.4285 | 0.6546 |
| 0.522 | 1.3807 | 602 | 0.4667 | 0.5084 | 0.4667 | 0.6831 |
| 0.522 | 1.3853 | 604 | 0.5529 | 0.4667 | 0.5529 | 0.7436 |
| 0.522 | 1.3899 | 606 | 0.6650 | 0.496 | 0.6650 | 0.8155 |
| 0.522 | 1.3945 | 608 | 0.7042 | 0.4924 | 0.7042 | 0.8392 |
| 0.522 | 1.3991 | 610 | 0.7415 | 0.4202 | 0.7415 | 0.8611 |
| 0.522 | 1.4037 | 612 | 0.7128 | 0.3803 | 0.7128 | 0.8443 |
| 0.522 | 1.4083 | 614 | 0.7118 | 0.3803 | 0.7118 | 0.8437 |
| 0.522 | 1.4128 | 616 | 0.6134 | 0.4375 | 0.6134 | 0.7832 |
| 0.522 | 1.4174 | 618 | 0.5148 | 0.5191 | 0.5148 | 0.7175 |
| 0.522 | 1.4220 | 620 | 0.4967 | 0.5243 | 0.4967 | 0.7047 |
| 0.522 | 1.4266 | 622 | 0.5297 | 0.5145 | 0.5297 | 0.7278 |
| 0.522 | 1.4312 | 624 | 0.6593 | 0.475 | 0.6593 | 0.8120 |
| 0.522 | 1.4358 | 626 | 0.7535 | 0.3927 | 0.7535 | 0.8681 |
| 0.522 | 1.4404 | 628 | 0.7166 | 0.4727 | 0.7166 | 0.8466 |
| 0.522 | 1.4450 | 630 | 0.6009 | 0.4727 | 0.6009 | 0.7752 |
| 0.522 | 1.4495 | 632 | 0.5262 | 0.6123 | 0.5262 | 0.7254 |
| 0.522 | 1.4541 | 634 | 0.5145 | 0.5406 | 0.5145 | 0.7173 |
| 0.522 | 1.4587 | 636 | 0.5207 | 0.4378 | 0.5207 | 0.7216 |
| 0.522 | 1.4633 | 638 | 0.5316 | 0.3616 | 0.5316 | 0.7291 |
| 0.522 | 1.4679 | 640 | 0.6237 | 0.4195 | 0.6237 | 0.7897 |
| 0.522 | 1.4725 | 642 | 0.6773 | 0.4202 | 0.6773 | 0.8230 |
| 0.522 | 1.4771 | 644 | 0.6332 | 0.3903 | 0.6332 | 0.7957 |
| 0.522 | 1.4817 | 646 | 0.6168 | 0.4018 | 0.6168 | 0.7854 |
| 0.522 | 1.4862 | 648 | 0.5485 | 0.4727 | 0.5485 | 0.7406 |
| 0.522 | 1.4908 | 650 | 0.5061 | 0.6818 | 0.5061 | 0.7114 |
| 0.522 | 1.4954 | 652 | 0.5005 | 0.6818 | 0.5005 | 0.7075 |
| 0.522 | 1.5 | 654 | 0.5238 | 0.5832 | 0.5238 | 0.7237 |
| 0.522 | 1.5046 | 656 | 0.6342 | 0.4195 | 0.6342 | 0.7964 |
| 0.522 | 1.5092 | 658 | 0.7109 | 0.4209 | 0.7109 | 0.8431 |
| 0.522 | 1.5138 | 660 | 0.7107 | 0.3860 | 0.7107 | 0.8430 |
| 0.522 | 1.5183 | 662 | 0.5932 | 0.3616 | 0.5932 | 0.7702 |
| 0.522 | 1.5229 | 664 | 0.5655 | 0.375 | 0.5655 | 0.7520 |
| 0.522 | 1.5275 | 666 | 0.6217 | 0.3927 | 0.6217 | 0.7885 |
| 0.522 | 1.5321 | 668 | 0.6616 | 0.3948 | 0.6616 | 0.8134 |
| 0.522 | 1.5367 | 670 | 0.5990 | 0.4106 | 0.5990 | 0.7740 |
| 0.522 | 1.5413 | 672 | 0.5978 | 0.4106 | 0.5978 | 0.7732 |
| 0.522 | 1.5459 | 674 | 0.5715 | 0.4106 | 0.5715 | 0.7560 |
| 0.522 | 1.5505 | 676 | 0.5335 | 0.4106 | 0.5335 | 0.7304 |
| 0.522 | 1.5550 | 678 | 0.5674 | 0.4106 | 0.5674 | 0.7533 |
| 0.522 | 1.5596 | 680 | 0.6438 | 0.4209 | 0.6438 | 0.8023 |
| 0.522 | 1.5642 | 682 | 0.7283 | 0.4209 | 0.7283 | 0.8534 |
| 0.522 | 1.5688 | 684 | 0.7010 | 0.4209 | 0.7010 | 0.8373 |
| 0.522 | 1.5734 | 686 | 0.6138 | 0.4049 | 0.6138 | 0.7834 |
| 0.522 | 1.5780 | 688 | 0.5743 | 0.4106 | 0.5743 | 0.7578 |
| 0.522 | 1.5826 | 690 | 0.5886 | 0.3780 | 0.5886 | 0.7672 |
| 0.522 | 1.5872 | 692 | 0.6871 | 0.4049 | 0.6871 | 0.8289 |
| 0.522 | 1.5917 | 694 | 0.8434 | 0.4295 | 0.8434 | 0.9184 |
| 0.522 | 1.5963 | 696 | 0.9759 | 0.4295 | 0.9759 | 0.9879 |
| 0.522 | 1.6009 | 698 | 1.0292 | 0.4215 | 1.0292 | 1.0145 |
| 0.522 | 1.6055 | 700 | 0.9694 | 0.4295 | 0.9694 | 0.9846 |
| 0.522 | 1.6101 | 702 | 0.8206 | 0.4293 | 0.8206 | 0.9059 |
| 0.522 | 1.6147 | 704 | 0.6104 | 0.375 | 0.6104 | 0.7813 |
| 0.522 | 1.6193 | 706 | 0.5077 | 0.6966 | 0.5077 | 0.7125 |
| 0.522 | 1.6239 | 708 | 0.4912 | 0.7014 | 0.4912 | 0.7008 |
| 0.522 | 1.6284 | 710 | 0.4988 | 0.6083 | 0.4988 | 0.7063 |
| 0.522 | 1.6330 | 712 | 0.6020 | 0.4965 | 0.6020 | 0.7759 |
| 0.522 | 1.6376 | 714 | 0.8161 | 0.3967 | 0.8161 | 0.9034 |
| 0.522 | 1.6422 | 716 | 0.8908 | 0.3883 | 0.8908 | 0.9438 |
| 0.522 | 1.6468 | 718 | 0.8785 | 0.3984 | 0.8785 | 0.9373 |
| 0.522 | 1.6514 | 720 | 0.8024 | 0.3967 | 0.8024 | 0.8958 |
| 0.522 | 1.6560 | 722 | 0.7142 | 0.4293 | 0.7142 | 0.8451 |
| 0.522 | 1.6606 | 724 | 0.6964 | 0.3807 | 0.6964 | 0.8345 |
| 0.522 | 1.6651 | 726 | 0.6344 | 0.3486 | 0.6344 | 0.7965 |
| 0.522 | 1.6697 | 728 | 0.6108 | 0.3486 | 0.6108 | 0.7815 |
| 0.522 | 1.6743 | 730 | 0.6536 | 0.4034 | 0.6536 | 0.8085 |
| 0.522 | 1.6789 | 732 | 0.7375 | 0.3948 | 0.7375 | 0.8588 |
| 0.522 | 1.6835 | 734 | 0.8584 | 0.3860 | 0.8584 | 0.9265 |
| 0.522 | 1.6881 | 736 | 0.8271 | 0.3860 | 0.8271 | 0.9094 |
| 0.522 | 1.6927 | 738 | 0.7312 | 0.4049 | 0.7312 | 0.8551 |
| 0.522 | 1.6972 | 740 | 0.7206 | 0.4049 | 0.7206 | 0.8489 |
| 0.522 | 1.7018 | 742 | 0.7237 | 0.3807 | 0.7237 | 0.8507 |
| 0.522 | 1.7064 | 744 | 0.7009 | 0.3807 | 0.7009 | 0.8372 |
| 0.522 | 1.7110 | 746 | 0.6279 | 0.3486 | 0.6279 | 0.7924 |
| 0.522 | 1.7156 | 748 | 0.5895 | 0.4457 | 0.5895 | 0.7678 |
| 0.522 | 1.7202 | 750 | 0.6174 | 0.3486 | 0.6174 | 0.7858 |
| 0.522 | 1.7248 | 752 | 0.6672 | 0.3569 | 0.6672 | 0.8168 |
| 0.522 | 1.7294 | 754 | 0.7132 | 0.3807 | 0.7132 | 0.8445 |
| 0.522 | 1.7339 | 756 | 0.6902 | 0.3569 | 0.6902 | 0.8308 |
| 0.522 | 1.7385 | 758 | 0.6264 | 0.3486 | 0.6264 | 0.7914 |
| 0.522 | 1.7431 | 760 | 0.6298 | 0.4457 | 0.6298 | 0.7936 |
| 0.522 | 1.7477 | 762 | 0.6908 | 0.3529 | 0.6908 | 0.8312 |
| 0.522 | 1.7523 | 764 | 0.7095 | 0.3529 | 0.7095 | 0.8423 |
| 0.522 | 1.7569 | 766 | 0.6889 | 0.3486 | 0.6889 | 0.8300 |
| 0.522 | 1.7615 | 768 | 0.6954 | 0.3486 | 0.6954 | 0.8339 |
| 0.522 | 1.7661 | 770 | 0.7142 | 0.375 | 0.7142 | 0.8451 |
| 0.522 | 1.7706 | 772 | 0.7240 | 0.375 | 0.7240 | 0.8509 |
| 0.522 | 1.7752 | 774 | 0.8451 | 0.4292 | 0.8451 | 0.9193 |
| 0.522 | 1.7798 | 776 | 0.9527 | 0.3883 | 0.9527 | 0.9761 |
| 0.522 | 1.7844 | 778 | 0.9520 | 0.3883 | 0.9520 | 0.9757 |
| 0.522 | 1.7890 | 780 | 0.7943 | 0.3803 | 0.7943 | 0.8912 |
| 0.522 | 1.7936 | 782 | 0.6324 | 0.4597 | 0.6324 | 0.7952 |
| 0.522 | 1.7982 | 784 | 0.5579 | 0.4283 | 0.5579 | 0.7470 |
| 0.522 | 1.8028 | 786 | 0.5725 | 0.4283 | 0.5725 | 0.7566 |
| 0.522 | 1.8073 | 788 | 0.6544 | 0.4503 | 0.6544 | 0.8090 |
| 0.522 | 1.8119 | 790 | 0.8031 | 0.3405 | 0.8031 | 0.8961 |
| 0.522 | 1.8165 | 792 | 0.9523 | 0.3505 | 0.9523 | 0.9758 |
| 0.522 | 1.8211 | 794 | 1.0460 | 0.3547 | 1.0460 | 1.0227 |
| 0.522 | 1.8257 | 796 | 0.9440 | 0.3883 | 0.9440 | 0.9716 |
| 0.522 | 1.8303 | 798 | 0.7501 | 0.3656 | 0.7501 | 0.8661 |
| 0.522 | 1.8349 | 800 | 0.5919 | 0.3717 | 0.5919 | 0.7693 |
| 0.522 | 1.8394 | 802 | 0.5333 | 0.5767 | 0.5333 | 0.7303 |
| 0.522 | 1.8440 | 804 | 0.5237 | 0.5751 | 0.5237 | 0.7237 |
| 0.522 | 1.8486 | 806 | 0.5675 | 0.5751 | 0.5675 | 0.7533 |
| 0.522 | 1.8532 | 808 | 0.7049 | 0.4118 | 0.7049 | 0.8396 |
| 0.522 | 1.8578 | 810 | 0.8200 | 0.3656 | 0.8200 | 0.9056 |
| 0.522 | 1.8624 | 812 | 0.8668 | 0.3948 | 0.8668 | 0.9310 |
| 0.522 | 1.8670 | 814 | 0.8071 | 0.3927 | 0.8071 | 0.8984 |
| 0.522 | 1.8716 | 816 | 0.7439 | 0.3927 | 0.7439 | 0.8625 |
| 0.522 | 1.8761 | 818 | 0.6573 | 0.3927 | 0.6573 | 0.8108 |
| 0.522 | 1.8807 | 820 | 0.5896 | 0.3616 | 0.5896 | 0.7679 |
| 0.522 | 1.8853 | 822 | 0.5325 | 0.4727 | 0.5325 | 0.7297 |
| 0.522 | 1.8899 | 824 | 0.5309 | 0.4727 | 0.5309 | 0.7286 |
| 0.522 | 1.8945 | 826 | 0.5818 | 0.375 | 0.5818 | 0.7628 |
| 0.522 | 1.8991 | 828 | 0.6318 | 0.4018 | 0.6318 | 0.7949 |
| 0.522 | 1.9037 | 830 | 0.6330 | 0.375 | 0.6330 | 0.7956 |
| 0.522 | 1.9083 | 832 | 0.5940 | 0.4727 | 0.5940 | 0.7707 |
| 0.522 | 1.9128 | 834 | 0.6092 | 0.4727 | 0.6092 | 0.7805 |
| 0.522 | 1.9174 | 836 | 0.5938 | 0.5073 | 0.5938 | 0.7706 |
| 0.522 | 1.9220 | 838 | 0.5648 | 0.5751 | 0.5648 | 0.7516 |
| 0.522 | 1.9266 | 840 | 0.5778 | 0.4803 | 0.5778 | 0.7601 |
| 0.522 | 1.9312 | 842 | 0.6711 | 0.375 | 0.6711 | 0.8192 |
| 0.522 | 1.9358 | 844 | 0.6943 | 0.3780 | 0.6943 | 0.8333 |
| 0.522 | 1.9404 | 846 | 0.7528 | 0.3927 | 0.7528 | 0.8676 |
| 0.522 | 1.9450 | 848 | 0.8663 | 0.4215 | 0.8663 | 0.9308 |
| 0.522 | 1.9495 | 850 | 0.8237 | 0.4209 | 0.8237 | 0.9076 |
| 0.522 | 1.9541 | 852 | 0.6963 | 0.3780 | 0.6963 | 0.8344 |
| 0.522 | 1.9587 | 854 | 0.5933 | 0.4803 | 0.5933 | 0.7702 |
| 0.522 | 1.9633 | 856 | 0.5960 | 0.4803 | 0.5960 | 0.7720 |
| 0.522 | 1.9679 | 858 | 0.6311 | 0.4803 | 0.6311 | 0.7944 |
| 0.522 | 1.9725 | 860 | 0.7292 | 0.3807 | 0.7292 | 0.8540 |
| 0.522 | 1.9771 | 862 | 0.7648 | 0.3691 | 0.7648 | 0.8745 |
| 0.522 | 1.9817 | 864 | 0.7087 | 0.4631 | 0.7087 | 0.8419 |
| 0.522 | 1.9862 | 866 | 0.6225 | 0.5406 | 0.6225 | 0.7890 |
| 0.522 | 1.9908 | 868 | 0.6020 | 0.5406 | 0.6020 | 0.7759 |
| 0.522 | 1.9954 | 870 | 0.6232 | 0.5347 | 0.6232 | 0.7894 |
| 0.522 | 2.0 | 872 | 0.5960 | 0.5347 | 0.5960 | 0.7720 |
| 0.522 | 2.0046 | 874 | 0.5661 | 0.5477 | 0.5661 | 0.7524 |
| 0.522 | 2.0092 | 876 | 0.5831 | 0.5035 | 0.5831 | 0.7636 |
| 0.522 | 2.0138 | 878 | 0.6360 | 0.5294 | 0.6360 | 0.7975 |
| 0.522 | 2.0183 | 880 | 0.6984 | 0.3599 | 0.6984 | 0.8357 |
| 0.522 | 2.0229 | 882 | 0.6394 | 0.3599 | 0.6394 | 0.7996 |
| 0.522 | 2.0275 | 884 | 0.5564 | 0.5347 | 0.5564 | 0.7459 |
| 0.522 | 2.0321 | 886 | 0.5115 | 0.5073 | 0.5115 | 0.7152 |
| 0.522 | 2.0367 | 888 | 0.5395 | 0.5073 | 0.5395 | 0.7345 |
| 0.522 | 2.0413 | 890 | 0.6008 | 0.4631 | 0.6008 | 0.7751 |
| 0.522 | 2.0459 | 892 | 0.6380 | 0.4893 | 0.6380 | 0.7987 |
| 0.522 | 2.0505 | 894 | 0.5743 | 0.5882 | 0.5743 | 0.7578 |
| 0.522 | 2.0550 | 896 | 0.5392 | 0.5619 | 0.5392 | 0.7343 |
| 0.522 | 2.0596 | 898 | 0.5309 | 0.5751 | 0.5309 | 0.7287 |
| 0.522 | 2.0642 | 900 | 0.5026 | 0.5812 | 0.5026 | 0.7090 |
| 0.522 | 2.0688 | 902 | 0.5093 | 0.5812 | 0.5093 | 0.7136 |
| 0.522 | 2.0734 | 904 | 0.5587 | 0.4803 | 0.5587 | 0.7475 |
| 0.522 | 2.0780 | 906 | 0.6079 | 0.4727 | 0.6079 | 0.7797 |
| 0.522 | 2.0826 | 908 | 0.6886 | 0.4018 | 0.6886 | 0.8298 |
| 0.522 | 2.0872 | 910 | 0.7206 | 0.3656 | 0.7206 | 0.8489 |
| 0.522 | 2.0917 | 912 | 0.7071 | 0.4018 | 0.7071 | 0.8409 |
| 0.522 | 2.0963 | 914 | 0.7565 | 0.3656 | 0.7565 | 0.8698 |
| 0.522 | 2.1009 | 916 | 0.7864 | 0.3656 | 0.7864 | 0.8868 |
| 0.522 | 2.1055 | 918 | 0.7381 | 0.3780 | 0.7381 | 0.8591 |
| 0.522 | 2.1101 | 920 | 0.6422 | 0.5035 | 0.6422 | 0.8014 |
| 0.522 | 2.1147 | 922 | 0.5642 | 0.5812 | 0.5642 | 0.7512 |
| 0.522 | 2.1193 | 924 | 0.5611 | 0.5812 | 0.5611 | 0.7491 |
| 0.522 | 2.1239 | 926 | 0.5882 | 0.5812 | 0.5882 | 0.7670 |
| 0.522 | 2.1284 | 928 | 0.6651 | 0.4779 | 0.6651 | 0.8155 |
| 0.522 | 2.1330 | 930 | 0.7704 | 0.3866 | 0.7704 | 0.8777 |
| 0.522 | 2.1376 | 932 | 0.7823 | 0.3866 | 0.7823 | 0.8845 |
| 0.522 | 2.1422 | 934 | 0.7927 | 0.3529 | 0.7927 | 0.8903 |
| 0.522 | 2.1468 | 936 | 0.7515 | 0.3388 | 0.7515 | 0.8669 |
| 0.522 | 2.1514 | 938 | 0.7766 | 0.3656 | 0.7766 | 0.8813 |
| 0.522 | 2.1560 | 940 | 0.7643 | 0.3656 | 0.7643 | 0.8743 |
| 0.522 | 2.1606 | 942 | 0.7502 | 0.3691 | 0.7502 | 0.8662 |
| 0.522 | 2.1651 | 944 | 0.7228 | 0.3948 | 0.7228 | 0.8502 |
| 0.522 | 2.1697 | 946 | 0.7247 | 0.3948 | 0.7247 | 0.8513 |
| 0.522 | 2.1743 | 948 | 0.7011 | 0.3948 | 0.7011 | 0.8373 |
| 0.522 | 2.1789 | 950 | 0.7003 | 0.3948 | 0.7003 | 0.8368 |
| 0.522 | 2.1835 | 952 | 0.6837 | 0.4209 | 0.6837 | 0.8269 |
| 0.522 | 2.1881 | 954 | 0.6145 | 0.4553 | 0.6145 | 0.7839 |
| 0.522 | 2.1927 | 956 | 0.5173 | 0.5073 | 0.5173 | 0.7192 |
| 0.522 | 2.1972 | 958 | 0.4784 | 0.5545 | 0.4784 | 0.6917 |
| 0.522 | 2.2018 | 960 | 0.4744 | 0.6866 | 0.4744 | 0.6887 |
| 0.522 | 2.2064 | 962 | 0.4940 | 0.5145 | 0.4940 | 0.7029 |
| 0.522 | 2.2110 | 964 | 0.5681 | 0.5035 | 0.5681 | 0.7537 |
| 0.522 | 2.2156 | 966 | 0.6674 | 0.4292 | 0.6674 | 0.8169 |
| 0.522 | 2.2202 | 968 | 0.7031 | 0.4292 | 0.7031 | 0.8385 |
| 0.522 | 2.2248 | 970 | 0.6632 | 0.4034 | 0.6632 | 0.8144 |
| 0.522 | 2.2294 | 972 | 0.5822 | 0.3866 | 0.5822 | 0.7630 |
| 0.522 | 2.2339 | 974 | 0.5548 | 0.5415 | 0.5548 | 0.7449 |
| 0.522 | 2.2385 | 976 | 0.5317 | 0.6410 | 0.5317 | 0.7292 |
| 0.522 | 2.2431 | 978 | 0.5380 | 0.5737 | 0.5380 | 0.7335 |
| 0.522 | 2.2477 | 980 | 0.5570 | 0.5422 | 0.5570 | 0.7463 |
| 0.522 | 2.2523 | 982 | 0.5516 | 0.5679 | 0.5516 | 0.7427 |
| 0.522 | 2.2569 | 984 | 0.4939 | 0.5481 | 0.4939 | 0.7028 |
| 0.522 | 2.2615 | 986 | 0.4606 | 0.6182 | 0.4606 | 0.6786 |
| 0.522 | 2.2661 | 988 | 0.4542 | 0.6526 | 0.4542 | 0.6739 |
| 0.522 | 2.2706 | 990 | 0.4519 | 0.7014 | 0.4519 | 0.6722 |
| 0.522 | 2.2752 | 992 | 0.4670 | 0.6526 | 0.4670 | 0.6834 |
| 0.522 | 2.2798 | 994 | 0.5106 | 0.5481 | 0.5106 | 0.7146 |
| 0.522 | 2.2844 | 996 | 0.5595 | 0.5481 | 0.5595 | 0.7480 |
| 0.522 | 2.2890 | 998 | 0.5764 | 0.5481 | 0.5764 | 0.7592 |
| 0.1679 | 2.2936 | 1000 | 0.5729 | 0.5812 | 0.5729 | 0.7569 |
| 0.1679 | 2.2982 | 1002 | 0.5905 | 0.5812 | 0.5905 | 0.7685 |
| 0.1679 | 2.3028 | 1004 | 0.6057 | 0.5679 | 0.6057 | 0.7783 |
| 0.1679 | 2.3073 | 1006 | 0.6055 | 0.5679 | 0.6055 | 0.7781 |
| 0.1679 | 2.3119 | 1008 | 0.6425 | 0.4034 | 0.6425 | 0.8015 |
| 0.1679 | 2.3165 | 1010 | 0.6459 | 0.4034 | 0.6459 | 0.8037 |
| 0.1679 | 2.3211 | 1012 | 0.5916 | 0.4034 | 0.5916 | 0.7692 |
| 0.1679 | 2.3257 | 1014 | 0.5539 | 0.4288 | 0.5539 | 0.7442 |
| 0.1679 | 2.3303 | 1016 | 0.4751 | 0.6182 | 0.4751 | 0.6893 |
| 0.1679 | 2.3349 | 1018 | 0.4487 | 0.6292 | 0.4487 | 0.6699 |
| 0.1679 | 2.3394 | 1020 | 0.4524 | 0.6292 | 0.4524 | 0.6726 |
| 0.1679 | 2.3440 | 1022 | 0.5033 | 0.5767 | 0.5033 | 0.7095 |
| 0.1679 | 2.3486 | 1024 | 0.5388 | 0.5073 | 0.5388 | 0.7340 |
| 0.1679 | 2.3532 | 1026 | 0.5567 | 0.5073 | 0.5567 | 0.7461 |
| 0.1679 | 2.3578 | 1028 | 0.5654 | 0.4803 | 0.5654 | 0.7519 |
| 0.1679 | 2.3624 | 1030 | 0.5626 | 0.4803 | 0.5626 | 0.7501 |
| 0.1679 | 2.3670 | 1032 | 0.5553 | 0.4803 | 0.5553 | 0.7452 |
| 0.1679 | 2.3716 | 1034 | 0.5475 | 0.4878 | 0.5475 | 0.7399 |
| 0.1679 | 2.3761 | 1036 | 0.5126 | 0.6526 | 0.5126 | 0.7160 |
| 0.1679 | 2.3807 | 1038 | 0.4856 | 0.6526 | 0.4856 | 0.6969 |
| 0.1679 | 2.3853 | 1040 | 0.4799 | 0.6526 | 0.4799 | 0.6928 |
| 0.1679 | 2.3899 | 1042 | 0.4989 | 0.6526 | 0.4989 | 0.7063 |
| 0.1679 | 2.3945 | 1044 | 0.5795 | 0.5 | 0.5795 | 0.7612 |
| 0.1679 | 2.3991 | 1046 | 0.6242 | 0.5 | 0.6242 | 0.7901 |
| 0.1679 | 2.4037 | 1048 | 0.6016 | 0.5679 | 0.6016 | 0.7756 |
| 0.1679 | 2.4083 | 1050 | 0.5491 | 0.5737 | 0.5491 | 0.7410 |
| 0.1679 | 2.4128 | 1052 | 0.5205 | 0.6866 | 0.5205 | 0.7214 |
| 0.1679 | 2.4174 | 1054 | 0.5294 | 0.6866 | 0.5294 | 0.7276 |
| 0.1679 | 2.4220 | 1056 | 0.5242 | 0.6866 | 0.5242 | 0.7240 |
| 0.1679 | 2.4266 | 1058 | 0.5209 | 0.6866 | 0.5209 | 0.7217 |
| 0.1679 | 2.4312 | 1060 | 0.5097 | 0.6526 | 0.5097 | 0.7139 |
| 0.1679 | 2.4358 | 1062 | 0.5436 | 0.5103 | 0.5436 | 0.7373 |
| 0.1679 | 2.4404 | 1064 | 0.6362 | 0.3927 | 0.6362 | 0.7976 |
| 0.1679 | 2.4450 | 1066 | 0.6421 | 0.4202 | 0.6421 | 0.8013 |
| 0.1679 | 2.4495 | 1068 | 0.6296 | 0.4202 | 0.6296 | 0.7935 |
| 0.1679 | 2.4541 | 1070 | 0.5407 | 0.3927 | 0.5407 | 0.7353 |
| 0.1679 | 2.4587 | 1072 | 0.4624 | 0.5855 | 0.4624 | 0.6800 |
| 0.1679 | 2.4633 | 1074 | 0.4232 | 0.7083 | 0.4232 | 0.6506 |
| 0.1679 | 2.4679 | 1076 | 0.4244 | 0.7083 | 0.4244 | 0.6514 |
| 0.1679 | 2.4725 | 1078 | 0.4514 | 0.5954 | 0.4514 | 0.6718 |
| 0.1679 | 2.4771 | 1080 | 0.5086 | 0.5855 | 0.5086 | 0.7132 |
| 0.1679 | 2.4817 | 1082 | 0.5385 | 0.5751 | 0.5385 | 0.7338 |
| 0.1679 | 2.4862 | 1084 | 0.5721 | 0.5065 | 0.5721 | 0.7564 |
| 0.1679 | 2.4908 | 1086 | 0.6000 | 0.5065 | 0.6000 | 0.7746 |
| 0.1679 | 2.4954 | 1088 | 0.6235 | 0.5065 | 0.6235 | 0.7896 |
| 0.1679 | 2.5 | 1090 | 0.5890 | 0.5065 | 0.5890 | 0.7675 |
| 0.1679 | 2.5046 | 1092 | 0.5618 | 0.5103 | 0.5618 | 0.7495 |
| 0.1679 | 2.5092 | 1094 | 0.5471 | 0.5103 | 0.5471 | 0.7396 |
| 0.1679 | 2.5138 | 1096 | 0.5472 | 0.5103 | 0.5472 | 0.7397 |
| 0.1679 | 2.5183 | 1098 | 0.5423 | 0.5103 | 0.5423 | 0.7364 |
| 0.1679 | 2.5229 | 1100 | 0.6158 | 0.5 | 0.6158 | 0.7847 |
| 0.1679 | 2.5275 | 1102 | 0.6711 | 0.4293 | 0.6711 | 0.8192 |
| 0.1679 | 2.5321 | 1104 | 0.6454 | 0.4706 | 0.6454 | 0.8034 |
| 0.1679 | 2.5367 | 1106 | 0.5506 | 0.4779 | 0.5506 | 0.7420 |
| 0.1679 | 2.5413 | 1108 | 0.4765 | 0.6526 | 0.4765 | 0.6903 |
| 0.1679 | 2.5459 | 1110 | 0.4629 | 0.6866 | 0.4629 | 0.6803 |
| 0.1679 | 2.5505 | 1112 | 0.4714 | 0.6526 | 0.4714 | 0.6866 |
| 0.1679 | 2.5550 | 1114 | 0.5112 | 0.4527 | 0.5112 | 0.7150 |
| 0.1679 | 2.5596 | 1116 | 0.5547 | 0.4779 | 0.5547 | 0.7448 |
| 0.1679 | 2.5642 | 1118 | 0.5423 | 0.4779 | 0.5423 | 0.7364 |
| 0.1679 | 2.5688 | 1120 | 0.5222 | 0.4878 | 0.5222 | 0.7226 |
| 0.1679 | 2.5734 | 1122 | 0.5030 | 0.5812 | 0.5030 | 0.7092 |
| 0.1679 | 2.5780 | 1124 | 0.5004 | 0.4878 | 0.5004 | 0.7074 |
| 0.1679 | 2.5826 | 1126 | 0.5441 | 0.4803 | 0.5441 | 0.7376 |
| 0.1679 | 2.5872 | 1128 | 0.5923 | 0.4034 | 0.5923 | 0.7696 |
| 0.1679 | 2.5917 | 1130 | 0.5839 | 0.3333 | 0.5839 | 0.7641 |
| 0.1679 | 2.5963 | 1132 | 0.5851 | 0.3486 | 0.5851 | 0.7649 |
| 0.1679 | 2.6009 | 1134 | 0.5883 | 0.3486 | 0.5883 | 0.7670 |
| 0.1679 | 2.6055 | 1136 | 0.5658 | 0.4803 | 0.5658 | 0.7522 |
| 0.1679 | 2.6101 | 1138 | 0.5726 | 0.4803 | 0.5726 | 0.7567 |
| 0.1679 | 2.6147 | 1140 | 0.6000 | 0.375 | 0.6000 | 0.7746 |
| 0.1679 | 2.6193 | 1142 | 0.5620 | 0.475 | 0.5620 | 0.7497 |
| 0.1679 | 2.6239 | 1144 | 0.5522 | 0.3717 | 0.5522 | 0.7431 |
| 0.1679 | 2.6284 | 1146 | 0.5487 | 0.3717 | 0.5487 | 0.7408 |
| 0.1679 | 2.6330 | 1148 | 0.5435 | 0.3717 | 0.5435 | 0.7372 |
| 0.1679 | 2.6376 | 1150 | 0.5433 | 0.3717 | 0.5433 | 0.7371 |
| 0.1679 | 2.6422 | 1152 | 0.5853 | 0.3927 | 0.5853 | 0.7651 |
| 0.1679 | 2.6468 | 1154 | 0.5817 | 0.375 | 0.5817 | 0.7627 |
| 0.1679 | 2.6514 | 1156 | 0.5785 | 0.3717 | 0.5785 | 0.7606 |
| 0.1679 | 2.6560 | 1158 | 0.6162 | 0.375 | 0.6162 | 0.7850 |
| 0.1679 | 2.6606 | 1160 | 0.6126 | 0.375 | 0.6126 | 0.7827 |
| 0.1679 | 2.6651 | 1162 | 0.5945 | 0.375 | 0.5945 | 0.7710 |
| 0.1679 | 2.6697 | 1164 | 0.5311 | 0.475 | 0.5311 | 0.7287 |
| 0.1679 | 2.6743 | 1166 | 0.4768 | 0.6656 | 0.4768 | 0.6905 |
| 0.1679 | 2.6789 | 1168 | 0.4729 | 0.6602 | 0.4729 | 0.6877 |
| 0.1679 | 2.6835 | 1170 | 0.5208 | 0.5477 | 0.5208 | 0.7217 |
| 0.1679 | 2.6881 | 1172 | 0.6423 | 0.3437 | 0.6423 | 0.8014 |
| 0.1679 | 2.6927 | 1174 | 0.7215 | 0.3691 | 0.7215 | 0.8494 |
| 0.1679 | 2.6972 | 1176 | 0.7012 | 0.3437 | 0.7012 | 0.8374 |
| 0.1679 | 2.7018 | 1178 | 0.6117 | 0.375 | 0.6117 | 0.7821 |
| 0.1679 | 2.7064 | 1180 | 0.5710 | 0.5415 | 0.5710 | 0.7556 |
| 0.1679 | 2.7110 | 1182 | 0.5790 | 0.4727 | 0.5790 | 0.7609 |
| 0.1679 | 2.7156 | 1184 | 0.5531 | 0.4106 | 0.5531 | 0.7437 |
| 0.1679 | 2.7202 | 1186 | 0.5300 | 0.5073 | 0.5300 | 0.7280 |
| 0.1679 | 2.7248 | 1188 | 0.5521 | 0.5073 | 0.5521 | 0.7431 |
| 0.1679 | 2.7294 | 1190 | 0.5827 | 0.4375 | 0.5827 | 0.7633 |
| 0.1679 | 2.7339 | 1192 | 0.5833 | 0.4375 | 0.5833 | 0.7637 |
| 0.1679 | 2.7385 | 1194 | 0.5589 | 0.5073 | 0.5589 | 0.7476 |
| 0.1679 | 2.7431 | 1196 | 0.5774 | 0.4106 | 0.5774 | 0.7598 |
| 0.1679 | 2.7477 | 1198 | 0.6022 | 0.4106 | 0.6022 | 0.7760 |
| 0.1679 | 2.7523 | 1200 | 0.5827 | 0.4457 | 0.5827 | 0.7634 |
| 0.1679 | 2.7569 | 1202 | 0.5860 | 0.5191 | 0.5860 | 0.7655 |
| 0.1679 | 2.7615 | 1204 | 0.6177 | 0.4457 | 0.6177 | 0.7860 |
| 0.1679 | 2.7661 | 1206 | 0.6856 | 0.4034 | 0.6856 | 0.8280 |
| 0.1679 | 2.7706 | 1208 | 0.6822 | 0.4292 | 0.6822 | 0.8260 |
| 0.1679 | 2.7752 | 1210 | 0.7088 | 0.4292 | 0.7088 | 0.8419 |
| 0.1679 | 2.7798 | 1212 | 0.7385 | 0.4209 | 0.7385 | 0.8593 |
| 0.1679 | 2.7844 | 1214 | 0.7588 | 0.4209 | 0.7588 | 0.8711 |
| 0.1679 | 2.7890 | 1216 | 0.7246 | 0.4209 | 0.7246 | 0.8512 |
| 0.1679 | 2.7936 | 1218 | 0.6204 | 0.4878 | 0.6204 | 0.7877 |
| 0.1679 | 2.7982 | 1220 | 0.5450 | 0.4185 | 0.5450 | 0.7383 |
| 0.1679 | 2.8028 | 1222 | 0.4813 | 0.5618 | 0.4813 | 0.6938 |
| 0.1679 | 2.8073 | 1224 | 0.4781 | 0.6656 | 0.4781 | 0.6914 |
| 0.1679 | 2.8119 | 1226 | 0.5143 | 0.5545 | 0.5143 | 0.7171 |
| 0.1679 | 2.8165 | 1228 | 0.6263 | 0.4727 | 0.6263 | 0.7914 |
| 0.1679 | 2.8211 | 1230 | 0.7936 | 0.4295 | 0.7936 | 0.8908 |
| 0.1679 | 2.8257 | 1232 | 0.8806 | 0.4295 | 0.8806 | 0.9384 |
| 0.1679 | 2.8303 | 1234 | 0.8198 | 0.4295 | 0.8198 | 0.9054 |
| 0.1679 | 2.8349 | 1236 | 0.6742 | 0.4372 | 0.6742 | 0.8211 |
| 0.1679 | 2.8394 | 1238 | 0.5438 | 0.5545 | 0.5438 | 0.7374 |
| 0.1679 | 2.8440 | 1240 | 0.4772 | 0.6526 | 0.4772 | 0.6908 |
| 0.1679 | 2.8486 | 1242 | 0.4543 | 0.7014 | 0.4543 | 0.6740 |
| 0.1679 | 2.8532 | 1244 | 0.4540 | 0.6526 | 0.4540 | 0.6738 |
| 0.1679 | 2.8578 | 1246 | 0.4811 | 0.5545 | 0.4811 | 0.6936 |
| 0.1679 | 2.8624 | 1248 | 0.4901 | 0.5545 | 0.4901 | 0.7001 |
| 0.1679 | 2.8670 | 1250 | 0.4748 | 0.5545 | 0.4748 | 0.6891 |
| 0.1679 | 2.8716 | 1252 | 0.4669 | 0.5545 | 0.4669 | 0.6833 |
| 0.1679 | 2.8761 | 1254 | 0.4753 | 0.5477 | 0.4753 | 0.6894 |
| 0.1679 | 2.8807 | 1256 | 0.4931 | 0.5767 | 0.4931 | 0.7022 |
| 0.1679 | 2.8853 | 1258 | 0.4880 | 0.5767 | 0.4880 | 0.6986 |
| 0.1679 | 2.8899 | 1260 | 0.4541 | 0.5545 | 0.4541 | 0.6738 |
| 0.1679 | 2.8945 | 1262 | 0.4388 | 0.6526 | 0.4388 | 0.6624 |
| 0.1679 | 2.8991 | 1264 | 0.4463 | 0.7181 | 0.4463 | 0.6681 |
| 0.1679 | 2.9037 | 1266 | 0.4759 | 0.6866 | 0.4759 | 0.6899 |
| 0.1679 | 2.9083 | 1268 | 0.5386 | 0.5545 | 0.5386 | 0.7339 |
| 0.1679 | 2.9128 | 1270 | 0.6672 | 0.3569 | 0.6672 | 0.8168 |
| 0.1679 | 2.9174 | 1272 | 0.7380 | 0.4049 | 0.7380 | 0.8591 |
| 0.1679 | 2.9220 | 1274 | 0.7470 | 0.4049 | 0.7470 | 0.8643 |
| 0.1679 | 2.9266 | 1276 | 0.7255 | 0.4049 | 0.7255 | 0.8518 |
| 0.1679 | 2.9312 | 1278 | 0.6586 | 0.3780 | 0.6586 | 0.8116 |
| 0.1679 | 2.9358 | 1280 | 0.6388 | 0.3780 | 0.6388 | 0.7992 |
| 0.1679 | 2.9404 | 1282 | 0.5918 | 0.4094 | 0.5918 | 0.7693 |
| 0.1679 | 2.9450 | 1284 | 0.5575 | 0.4465 | 0.5575 | 0.7466 |
| 0.1679 | 2.9495 | 1286 | 0.5487 | 0.4465 | 0.5487 | 0.7407 |
| 0.1679 | 2.9541 | 1288 | 0.5562 | 0.5477 | 0.5562 | 0.7458 |
| 0.1679 | 2.9587 | 1290 | 0.5596 | 0.5679 | 0.5596 | 0.7481 |
| 0.1679 | 2.9633 | 1292 | 0.5487 | 0.5422 | 0.5487 | 0.7407 |
| 0.1679 | 2.9679 | 1294 | 0.5513 | 0.5737 | 0.5513 | 0.7425 |
| 0.1679 | 2.9725 | 1296 | 0.5748 | 0.5422 | 0.5748 | 0.7581 |
| 0.1679 | 2.9771 | 1298 | 0.6420 | 0.3780 | 0.6420 | 0.8013 |
| 0.1679 | 2.9817 | 1300 | 0.6649 | 0.3780 | 0.6649 | 0.8154 |
| 0.1679 | 2.9862 | 1302 | 0.6471 | 0.3780 | 0.6471 | 0.8044 |
| 0.1679 | 2.9908 | 1304 | 0.6725 | 0.3807 | 0.6725 | 0.8201 |
| 0.1679 | 2.9954 | 1306 | 0.6594 | 0.3780 | 0.6594 | 0.8120 |
| 0.1679 | 3.0 | 1308 | 0.6261 | 0.3780 | 0.6261 | 0.7913 |
| 0.1679 | 3.0046 | 1310 | 0.5552 | 0.5422 | 0.5552 | 0.7451 |
| 0.1679 | 3.0092 | 1312 | 0.5221 | 0.6182 | 0.5221 | 0.7225 |
| 0.1679 | 3.0138 | 1314 | 0.5187 | 0.6182 | 0.5187 | 0.7202 |
| 0.1679 | 3.0183 | 1316 | 0.5251 | 0.6123 | 0.5251 | 0.7246 |
| 0.1679 | 3.0229 | 1318 | 0.5046 | 0.6123 | 0.5046 | 0.7104 |
| 0.1679 | 3.0275 | 1320 | 0.5069 | 0.5406 | 0.5069 | 0.7120 |
| 0.1679 | 3.0321 | 1322 | 0.5099 | 0.5396 | 0.5099 | 0.7141 |
| 0.1679 | 3.0367 | 1324 | 0.5279 | 0.4286 | 0.5279 | 0.7266 |
| 0.1679 | 3.0413 | 1326 | 0.5387 | 0.4286 | 0.5387 | 0.7339 |
| 0.1679 | 3.0459 | 1328 | 0.5128 | 0.4286 | 0.5128 | 0.7161 |
| 0.1679 | 3.0505 | 1330 | 0.4607 | 0.5396 | 0.4607 | 0.6788 |
| 0.1679 | 3.0550 | 1332 | 0.4334 | 0.6546 | 0.4334 | 0.6583 |
| 0.1679 | 3.0596 | 1334 | 0.4552 | 0.6232 | 0.4552 | 0.6747 |
| 0.1679 | 3.0642 | 1336 | 0.5413 | 0.5333 | 0.5413 | 0.7358 |
| 0.1679 | 3.0688 | 1338 | 0.6704 | 0.4209 | 0.6704 | 0.8188 |
| 0.1679 | 3.0734 | 1340 | 0.7086 | 0.4209 | 0.7086 | 0.8418 |
| 0.1679 | 3.0780 | 1342 | 0.6877 | 0.4209 | 0.6877 | 0.8293 |
| 0.1679 | 3.0826 | 1344 | 0.6129 | 0.4878 | 0.6129 | 0.7829 |
| 0.1679 | 3.0872 | 1346 | 0.5426 | 0.4378 | 0.5426 | 0.7366 |
| 0.1679 | 3.0917 | 1348 | 0.4769 | 0.5477 | 0.4769 | 0.6906 |
| 0.1679 | 3.0963 | 1350 | 0.4579 | 0.5301 | 0.4579 | 0.6767 |
| 0.1679 | 3.1009 | 1352 | 0.4644 | 0.5191 | 0.4644 | 0.6815 |
| 0.1679 | 3.1055 | 1354 | 0.5064 | 0.5477 | 0.5064 | 0.7116 |
| 0.1679 | 3.1101 | 1356 | 0.5948 | 0.4106 | 0.5948 | 0.7712 |
| 0.1679 | 3.1147 | 1358 | 0.6396 | 0.3529 | 0.6396 | 0.7997 |
| 0.1679 | 3.1193 | 1360 | 0.6042 | 0.4457 | 0.6042 | 0.7773 |
| 0.1679 | 3.1239 | 1362 | 0.5570 | 0.5415 | 0.5570 | 0.7463 |
| 0.1679 | 3.1284 | 1364 | 0.5643 | 0.5415 | 0.5643 | 0.7512 |
| 0.1679 | 3.1330 | 1366 | 0.5654 | 0.4106 | 0.5654 | 0.7520 |
| 0.1679 | 3.1376 | 1368 | 0.6064 | 0.4648 | 0.6064 | 0.7787 |
| 0.1679 | 3.1422 | 1370 | 0.6608 | 0.4878 | 0.6608 | 0.8129 |
| 0.1679 | 3.1468 | 1372 | 0.6543 | 0.4913 | 0.6543 | 0.8089 |
| 0.1679 | 3.1514 | 1374 | 0.5928 | 0.4667 | 0.5928 | 0.7699 |
| 0.1679 | 3.1560 | 1376 | 0.5483 | 0.4688 | 0.5483 | 0.7405 |
| 0.1679 | 3.1606 | 1378 | 0.5347 | 0.4381 | 0.5347 | 0.7313 |
| 0.1679 | 3.1651 | 1380 | 0.5596 | 0.4378 | 0.5596 | 0.7481 |
| 0.1679 | 3.1697 | 1382 | 0.6232 | 0.4667 | 0.6232 | 0.7895 |
| 0.1679 | 3.1743 | 1384 | 0.6981 | 0.4667 | 0.6981 | 0.8355 |
| 0.1679 | 3.1789 | 1386 | 0.7685 | 0.3967 | 0.7685 | 0.8767 |
| 0.1679 | 3.1835 | 1388 | 0.7665 | 0.3967 | 0.7665 | 0.8755 |
| 0.1679 | 3.1881 | 1390 | 0.7022 | 0.4288 | 0.7022 | 0.8380 |
| 0.1679 | 3.1927 | 1392 | 0.6079 | 0.4667 | 0.6079 | 0.7797 |
| 0.1679 | 3.1972 | 1394 | 0.5351 | 0.4094 | 0.5351 | 0.7315 |
| 0.1679 | 3.2018 | 1396 | 0.5118 | 0.5243 | 0.5118 | 0.7154 |
| 0.1679 | 3.2064 | 1398 | 0.5149 | 0.5987 | 0.5149 | 0.7176 |
| 0.1679 | 3.2110 | 1400 | 0.5497 | 0.5191 | 0.5497 | 0.7414 |
| 0.1679 | 3.2156 | 1402 | 0.6488 | 0.3486 | 0.6488 | 0.8055 |
| 0.1679 | 3.2202 | 1404 | 0.7147 | 0.4034 | 0.7147 | 0.8454 |
| 0.1679 | 3.2248 | 1406 | 0.7263 | 0.4049 | 0.7263 | 0.8522 |
| 0.1679 | 3.2294 | 1408 | 0.6700 | 0.4018 | 0.6700 | 0.8185 |
| 0.1679 | 3.2339 | 1410 | 0.5765 | 0.4094 | 0.5765 | 0.7593 |
| 0.1679 | 3.2385 | 1412 | 0.5487 | 0.4831 | 0.5487 | 0.7407 |
| 0.1679 | 3.2431 | 1414 | 0.5856 | 0.4378 | 0.5856 | 0.7653 |
| 0.1679 | 3.2477 | 1416 | 0.6118 | 0.375 | 0.6118 | 0.7822 |
| 0.1679 | 3.2523 | 1418 | 0.6804 | 0.4034 | 0.6804 | 0.8249 |
| 0.1679 | 3.2569 | 1420 | 0.6752 | 0.4034 | 0.6752 | 0.8217 |
| 0.1679 | 3.2615 | 1422 | 0.6181 | 0.4018 | 0.6181 | 0.7862 |
| 0.1679 | 3.2661 | 1424 | 0.5557 | 0.5406 | 0.5557 | 0.7454 |
| 0.1679 | 3.2706 | 1426 | 0.5108 | 0.5922 | 0.5108 | 0.7147 |
| 0.1679 | 3.2752 | 1428 | 0.5013 | 0.5922 | 0.5013 | 0.7080 |
| 0.1679 | 3.2798 | 1430 | 0.4875 | 0.5922 | 0.4875 | 0.6982 |
| 0.1679 | 3.2844 | 1432 | 0.4979 | 0.5164 | 0.4979 | 0.7056 |
| 0.1679 | 3.2890 | 1434 | 0.5000 | 0.5164 | 0.5000 | 0.7071 |
| 0.1679 | 3.2936 | 1436 | 0.5387 | 0.4727 | 0.5387 | 0.7340 |
| 0.1679 | 3.2982 | 1438 | 0.5569 | 0.4803 | 0.5569 | 0.7462 |
| 0.1679 | 3.3028 | 1440 | 0.5221 | 0.4878 | 0.5221 | 0.7226 |
| 0.1679 | 3.3073 | 1442 | 0.4835 | 0.6526 | 0.4835 | 0.6953 |
| 0.1679 | 3.3119 | 1444 | 0.4875 | 0.5545 | 0.4875 | 0.6982 |
| 0.1679 | 3.3165 | 1446 | 0.5221 | 0.5145 | 0.5221 | 0.7226 |
| 0.1679 | 3.3211 | 1448 | 0.5829 | 0.4706 | 0.5829 | 0.7635 |
| 0.1679 | 3.3257 | 1450 | 0.5936 | 0.4965 | 0.5936 | 0.7704 |
| 0.1679 | 3.3303 | 1452 | 0.5405 | 0.5073 | 0.5405 | 0.7352 |
| 0.1679 | 3.3349 | 1454 | 0.4665 | 0.6818 | 0.4665 | 0.6830 |
| 0.1679 | 3.3394 | 1456 | 0.4333 | 0.7014 | 0.4333 | 0.6583 |
| 0.1679 | 3.3440 | 1458 | 0.4257 | 0.7181 | 0.4257 | 0.6525 |
| 0.1679 | 3.3486 | 1460 | 0.4307 | 0.7181 | 0.4307 | 0.6563 |
| 0.1679 | 3.3532 | 1462 | 0.4679 | 0.5767 | 0.4679 | 0.6840 |
| 0.1679 | 3.3578 | 1464 | 0.5334 | 0.4924 | 0.5334 | 0.7303 |
| 0.1679 | 3.3624 | 1466 | 0.5536 | 0.3927 | 0.5536 | 0.7440 |
| 0.1679 | 3.3670 | 1468 | 0.5483 | 0.3927 | 0.5483 | 0.7405 |
| 0.1679 | 3.3716 | 1470 | 0.5330 | 0.4648 | 0.5330 | 0.7300 |
| 0.1679 | 3.3761 | 1472 | 0.5226 | 0.5 | 0.5226 | 0.7229 |
| 0.1679 | 3.3807 | 1474 | 0.4939 | 0.5939 | 0.4939 | 0.7028 |
| 0.1679 | 3.3853 | 1476 | 0.4664 | 0.6083 | 0.4664 | 0.6829 |
| 0.1679 | 3.3899 | 1478 | 0.4578 | 0.6526 | 0.4578 | 0.6766 |
| 0.1679 | 3.3945 | 1480 | 0.4808 | 0.6818 | 0.4808 | 0.6934 |
| 0.1679 | 3.3991 | 1482 | 0.4920 | 0.5751 | 0.4920 | 0.7014 |
| 0.1679 | 3.4037 | 1484 | 0.4874 | 0.5767 | 0.4874 | 0.6981 |
| 0.1679 | 3.4083 | 1486 | 0.4885 | 0.5767 | 0.4885 | 0.6989 |
| 0.1679 | 3.4128 | 1488 | 0.4984 | 0.5767 | 0.4984 | 0.7060 |
| 0.1679 | 3.4174 | 1490 | 0.4914 | 0.6526 | 0.4914 | 0.7010 |
| 0.1679 | 3.4220 | 1492 | 0.4951 | 0.6526 | 0.4951 | 0.7037 |
| 0.1679 | 3.4266 | 1494 | 0.5172 | 0.5073 | 0.5172 | 0.7192 |
| 0.1679 | 3.4312 | 1496 | 0.5298 | 0.5073 | 0.5298 | 0.7279 |
| 0.1679 | 3.4358 | 1498 | 0.5024 | 0.5767 | 0.5024 | 0.7088 |
| 0.1154 | 3.4404 | 1500 | 0.4702 | 0.5767 | 0.4702 | 0.6857 |
| 0.1154 | 3.4450 | 1502 | 0.4776 | 0.5786 | 0.4776 | 0.6911 |
| 0.1154 | 3.4495 | 1504 | 0.5201 | 0.4286 | 0.5201 | 0.7212 |
| 0.1154 | 3.4541 | 1506 | 0.5652 | 0.3877 | 0.5652 | 0.7518 |
| 0.1154 | 3.4587 | 1508 | 0.6059 | 0.3558 | 0.6059 | 0.7784 |
| 0.1154 | 3.4633 | 1510 | 0.6216 | 0.3288 | 0.6216 | 0.7884 |
| 0.1154 | 3.4679 | 1512 | 0.5846 | 0.3780 | 0.5846 | 0.7646 |
| 0.1154 | 3.4725 | 1514 | 0.5255 | 0.6123 | 0.5255 | 0.7249 |
| 0.1154 | 3.4771 | 1516 | 0.5041 | 0.6866 | 0.5041 | 0.7100 |
| 0.1154 | 3.4817 | 1518 | 0.5072 | 0.6866 | 0.5072 | 0.7122 |
| 0.1154 | 3.4862 | 1520 | 0.5267 | 0.5832 | 0.5267 | 0.7258 |
| 0.1154 | 3.4908 | 1522 | 0.5416 | 0.5477 | 0.5416 | 0.7359 |
| 0.1154 | 3.4954 | 1524 | 0.5601 | 0.4378 | 0.5601 | 0.7484 |
| 0.1154 | 3.5 | 1526 | 0.5661 | 0.4667 | 0.5661 | 0.7524 |
| 0.1154 | 3.5046 | 1528 | 0.5920 | 0.4667 | 0.5920 | 0.7694 |
| 0.1154 | 3.5092 | 1530 | 0.5781 | 0.4667 | 0.5781 | 0.7604 |
| 0.1154 | 3.5138 | 1532 | 0.5508 | 0.4667 | 0.5508 | 0.7422 |
| 0.1154 | 3.5183 | 1534 | 0.5235 | 0.4465 | 0.5235 | 0.7236 |
| 0.1154 | 3.5229 | 1536 | 0.4908 | 0.5545 | 0.4908 | 0.7006 |
| 0.1154 | 3.5275 | 1538 | 0.4789 | 0.5545 | 0.4789 | 0.6920 |
| 0.1154 | 3.5321 | 1540 | 0.4659 | 0.5545 | 0.4659 | 0.6826 |
| 0.1154 | 3.5367 | 1542 | 0.4637 | 0.5545 | 0.4637 | 0.6810 |
| 0.1154 | 3.5413 | 1544 | 0.4634 | 0.5545 | 0.4634 | 0.6807 |
| 0.1154 | 3.5459 | 1546 | 0.4657 | 0.5477 | 0.4657 | 0.6824 |
| 0.1154 | 3.5505 | 1548 | 0.4817 | 0.6062 | 0.4817 | 0.6940 |
| 0.1154 | 3.5550 | 1550 | 0.4774 | 0.5767 | 0.4774 | 0.6910 |
| 0.1154 | 3.5596 | 1552 | 0.4498 | 0.6802 | 0.4498 | 0.6706 |
| 0.1154 | 3.5642 | 1554 | 0.4471 | 0.6802 | 0.4471 | 0.6687 |
| 0.1154 | 3.5688 | 1556 | 0.4723 | 0.6526 | 0.4723 | 0.6873 |
| 0.1154 | 3.5734 | 1558 | 0.5377 | 0.5767 | 0.5377 | 0.7333 |
| 0.1154 | 3.5780 | 1560 | 0.6181 | 0.4372 | 0.6181 | 0.7862 |
| 0.1154 | 3.5826 | 1562 | 0.6590 | 0.4370 | 0.6590 | 0.8118 |
| 0.1154 | 3.5872 | 1564 | 0.6945 | 0.4049 | 0.6945 | 0.8334 |
| 0.1154 | 3.5917 | 1566 | 0.6926 | 0.5246 | 0.6926 | 0.8322 |
| 0.1154 | 3.5963 | 1568 | 0.6633 | 0.5246 | 0.6633 | 0.8144 |
| 0.1154 | 3.6009 | 1570 | 0.6081 | 0.5035 | 0.6081 | 0.7798 |
| 0.1154 | 3.6055 | 1572 | 0.5878 | 0.5145 | 0.5878 | 0.7667 |
| 0.1154 | 3.6101 | 1574 | 0.5712 | 0.5832 | 0.5712 | 0.7557 |
| 0.1154 | 3.6147 | 1576 | 0.5627 | 0.5832 | 0.5627 | 0.7501 |
| 0.1154 | 3.6193 | 1578 | 0.5999 | 0.4667 | 0.5999 | 0.7745 |
| 0.1154 | 3.6239 | 1580 | 0.6687 | 0.4034 | 0.6687 | 0.8177 |
| 0.1154 | 3.6284 | 1582 | 0.7080 | 0.4049 | 0.7080 | 0.8414 |
| 0.1154 | 3.6330 | 1584 | 0.6751 | 0.4049 | 0.6751 | 0.8217 |
| 0.1154 | 3.6376 | 1586 | 0.6187 | 0.4106 | 0.6187 | 0.7866 |
| 0.1154 | 3.6422 | 1588 | 0.5701 | 0.5832 | 0.5701 | 0.7550 |
| 0.1154 | 3.6468 | 1590 | 0.5727 | 0.5832 | 0.5727 | 0.7568 |
| 0.1154 | 3.6514 | 1592 | 0.5808 | 0.5832 | 0.5808 | 0.7621 |
| 0.1154 | 3.6560 | 1594 | 0.5693 | 0.5545 | 0.5693 | 0.7545 |
| 0.1154 | 3.6606 | 1596 | 0.5718 | 0.5545 | 0.5718 | 0.7562 |
| 0.1154 | 3.6651 | 1598 | 0.5570 | 0.5545 | 0.5570 | 0.7463 |
| 0.1154 | 3.6697 | 1600 | 0.5497 | 0.5545 | 0.5497 | 0.7415 |
| 0.1154 | 3.6743 | 1602 | 0.5603 | 0.5545 | 0.5603 | 0.7486 |
| 0.1154 | 3.6789 | 1604 | 0.5951 | 0.5191 | 0.5951 | 0.7714 |
| 0.1154 | 3.6835 | 1606 | 0.6008 | 0.4727 | 0.6008 | 0.7751 |
| 0.1154 | 3.6881 | 1608 | 0.5646 | 0.475 | 0.5646 | 0.7514 |
| 0.1154 | 3.6927 | 1610 | 0.5229 | 0.475 | 0.5229 | 0.7231 |
| 0.1154 | 3.6972 | 1612 | 0.5086 | 0.4776 | 0.5086 | 0.7132 |
| 0.1154 | 3.7018 | 1614 | 0.4682 | 0.5618 | 0.4682 | 0.6843 |
| 0.1154 | 3.7064 | 1616 | 0.4619 | 0.5027 | 0.4619 | 0.6796 |
| 0.1154 | 3.7110 | 1618 | 0.4715 | 0.5922 | 0.4715 | 0.6867 |
| 0.1154 | 3.7156 | 1620 | 0.4817 | 0.5546 | 0.4817 | 0.6941 |
| 0.1154 | 3.7202 | 1622 | 0.4882 | 0.5618 | 0.4882 | 0.6987 |
| 0.1154 | 3.7248 | 1624 | 0.5025 | 0.5545 | 0.5025 | 0.7089 |
| 0.1154 | 3.7294 | 1626 | 0.5351 | 0.5545 | 0.5351 | 0.7315 |
| 0.1154 | 3.7339 | 1628 | 0.5992 | 0.4779 | 0.5992 | 0.7741 |
| 0.1154 | 3.7385 | 1630 | 0.6489 | 0.3807 | 0.6489 | 0.8056 |
| 0.1154 | 3.7431 | 1632 | 0.6497 | 0.3807 | 0.6497 | 0.8061 |
| 0.1154 | 3.7477 | 1634 | 0.6016 | 0.5073 | 0.6016 | 0.7756 |
| 0.1154 | 3.7523 | 1636 | 0.5528 | 0.5832 | 0.5528 | 0.7435 |
| 0.1154 | 3.7569 | 1638 | 0.5397 | 0.5618 | 0.5397 | 0.7347 |
| 0.1154 | 3.7615 | 1640 | 0.5474 | 0.5618 | 0.5474 | 0.7399 |
| 0.1154 | 3.7661 | 1642 | 0.5682 | 0.5832 | 0.5682 | 0.7538 |
| 0.1154 | 3.7706 | 1644 | 0.6075 | 0.4465 | 0.6075 | 0.7794 |
| 0.1154 | 3.7752 | 1646 | 0.6489 | 0.3569 | 0.6489 | 0.8055 |
| 0.1154 | 3.7798 | 1648 | 0.6620 | 0.3569 | 0.6620 | 0.8136 |
| 0.1154 | 3.7844 | 1650 | 0.6950 | 0.3569 | 0.6950 | 0.8337 |
| 0.1154 | 3.7890 | 1652 | 0.6854 | 0.3569 | 0.6854 | 0.8279 |
| 0.1154 | 3.7936 | 1654 | 0.6336 | 0.4094 | 0.6336 | 0.7960 |
| 0.1154 | 3.7982 | 1656 | 0.5947 | 0.5832 | 0.5947 | 0.7712 |
| 0.1154 | 3.8028 | 1658 | 0.5666 | 0.5545 | 0.5666 | 0.7527 |
| 0.1154 | 3.8073 | 1660 | 0.5861 | 0.4094 | 0.5861 | 0.7656 |
| 0.1154 | 3.8119 | 1662 | 0.5929 | 0.4378 | 0.5929 | 0.7700 |
| 0.1154 | 3.8165 | 1664 | 0.6037 | 0.4094 | 0.6037 | 0.7770 |
| 0.1154 | 3.8211 | 1666 | 0.6523 | 0.375 | 0.6523 | 0.8076 |
| 0.1154 | 3.8257 | 1668 | 0.7124 | 0.3780 | 0.7124 | 0.8441 |
| 0.1154 | 3.8303 | 1670 | 0.7037 | 0.3780 | 0.7037 | 0.8388 |
| 0.1154 | 3.8349 | 1672 | 0.6643 | 0.3486 | 0.6643 | 0.8151 |
| 0.1154 | 3.8394 | 1674 | 0.6716 | 0.3486 | 0.6716 | 0.8195 |
| 0.1154 | 3.8440 | 1676 | 0.6438 | 0.4094 | 0.6438 | 0.8024 |
| 0.1154 | 3.8486 | 1678 | 0.6164 | 0.3814 | 0.6164 | 0.7851 |
| 0.1154 | 3.8532 | 1680 | 0.5989 | 0.3814 | 0.5989 | 0.7739 |
| 0.1154 | 3.8578 | 1682 | 0.6355 | 0.3814 | 0.6355 | 0.7972 |
| 0.1154 | 3.8624 | 1684 | 0.7160 | 0.3282 | 0.7160 | 0.8462 |
| 0.1154 | 3.8670 | 1686 | 0.7664 | 0.3569 | 0.7664 | 0.8755 |
| 0.1154 | 3.8716 | 1688 | 0.7727 | 0.3333 | 0.7727 | 0.8790 |
| 0.1154 | 3.8761 | 1690 | 0.7265 | 0.3333 | 0.7265 | 0.8523 |
| 0.1154 | 3.8807 | 1692 | 0.6713 | 0.4199 | 0.6713 | 0.8193 |
| 0.1154 | 3.8853 | 1694 | 0.6245 | 0.5545 | 0.6245 | 0.7903 |
| 0.1154 | 3.8899 | 1696 | 0.6056 | 0.4831 | 0.6056 | 0.7782 |
| 0.1154 | 3.8945 | 1698 | 0.6147 | 0.4192 | 0.6147 | 0.7840 |
| 0.1154 | 3.8991 | 1700 | 0.6030 | 0.3814 | 0.6030 | 0.7765 |
| 0.1154 | 3.9037 | 1702 | 0.6343 | 0.3780 | 0.6343 | 0.7965 |
| 0.1154 | 3.9083 | 1704 | 0.6943 | 0.3599 | 0.6943 | 0.8332 |
| 0.1154 | 3.9128 | 1706 | 0.6877 | 0.3833 | 0.6877 | 0.8293 |
| 0.1154 | 3.9174 | 1708 | 0.6389 | 0.3558 | 0.6389 | 0.7993 |
| 0.1154 | 3.9220 | 1710 | 0.5619 | 0.4378 | 0.5619 | 0.7496 |
| 0.1154 | 3.9266 | 1712 | 0.4939 | 0.6182 | 0.4939 | 0.7028 |
| 0.1154 | 3.9312 | 1714 | 0.4870 | 0.6526 | 0.4870 | 0.6978 |
| 0.1154 | 3.9358 | 1716 | 0.5117 | 0.6182 | 0.5117 | 0.7153 |
| 0.1154 | 3.9404 | 1718 | 0.5697 | 0.5422 | 0.5697 | 0.7548 |
| 0.1154 | 3.9450 | 1720 | 0.6404 | 0.4444 | 0.6404 | 0.8003 |
| 0.1154 | 3.9495 | 1722 | 0.6474 | 0.4444 | 0.6474 | 0.8046 |
| 0.1154 | 3.9541 | 1724 | 0.6035 | 0.4527 | 0.6035 | 0.7768 |
| 0.1154 | 3.9587 | 1726 | 0.5417 | 0.5481 | 0.5417 | 0.7360 |
| 0.1154 | 3.9633 | 1728 | 0.5119 | 0.6866 | 0.5119 | 0.7154 |
| 0.1154 | 3.9679 | 1730 | 0.5160 | 0.7014 | 0.5160 | 0.7183 |
| 0.1154 | 3.9725 | 1732 | 0.5172 | 0.7014 | 0.5172 | 0.7191 |
| 0.1154 | 3.9771 | 1734 | 0.5160 | 0.6866 | 0.5160 | 0.7183 |
| 0.1154 | 3.9817 | 1736 | 0.5487 | 0.5481 | 0.5487 | 0.7408 |
| 0.1154 | 3.9862 | 1738 | 0.6386 | 0.4517 | 0.6386 | 0.7991 |
| 0.1154 | 3.9908 | 1740 | 0.7176 | 0.3807 | 0.7176 | 0.8471 |
| 0.1154 | 3.9954 | 1742 | 0.7306 | 0.4295 | 0.7306 | 0.8547 |
| 0.1154 | 4.0 | 1744 | 0.7172 | 0.4295 | 0.7172 | 0.8469 |
| 0.1154 | 4.0046 | 1746 | 0.6354 | 0.3780 | 0.6354 | 0.7971 |
| 0.1154 | 4.0092 | 1748 | 0.5348 | 0.5191 | 0.5348 | 0.7313 |
| 0.1154 | 4.0138 | 1750 | 0.4936 | 0.5545 | 0.4936 | 0.7026 |
| 0.1154 | 4.0183 | 1752 | 0.4893 | 0.5545 | 0.4893 | 0.6995 |
| 0.1154 | 4.0229 | 1754 | 0.5170 | 0.5191 | 0.5170 | 0.7190 |
| 0.1154 | 4.0275 | 1756 | 0.5857 | 0.3980 | 0.5857 | 0.7653 |
| 0.1154 | 4.0321 | 1758 | 0.6551 | 0.3927 | 0.6551 | 0.8094 |
| 0.1154 | 4.0367 | 1760 | 0.6618 | 0.3948 | 0.6618 | 0.8135 |
| 0.1154 | 4.0413 | 1762 | 0.6202 | 0.4803 | 0.6202 | 0.7875 |
| 0.1154 | 4.0459 | 1764 | 0.5592 | 0.5191 | 0.5592 | 0.7478 |
| 0.1154 | 4.0505 | 1766 | 0.5331 | 0.5545 | 0.5331 | 0.7302 |
| 0.1154 | 4.0550 | 1768 | 0.5266 | 0.5545 | 0.5266 | 0.7257 |
| 0.1154 | 4.0596 | 1770 | 0.5348 | 0.5545 | 0.5348 | 0.7313 |
| 0.1154 | 4.0642 | 1772 | 0.5738 | 0.4878 | 0.5738 | 0.7575 |
| 0.1154 | 4.0688 | 1774 | 0.6306 | 0.4803 | 0.6306 | 0.7941 |
| 0.1154 | 4.0734 | 1776 | 0.6648 | 0.3486 | 0.6648 | 0.8153 |
| 0.1154 | 4.0780 | 1778 | 0.6512 | 0.375 | 0.6512 | 0.8070 |
| 0.1154 | 4.0826 | 1780 | 0.6161 | 0.375 | 0.6161 | 0.7849 |
| 0.1154 | 4.0872 | 1782 | 0.5917 | 0.375 | 0.5917 | 0.7692 |
| 0.1154 | 4.0917 | 1784 | 0.5883 | 0.375 | 0.5883 | 0.7670 |
| 0.1154 | 4.0963 | 1786 | 0.5868 | 0.375 | 0.5868 | 0.7661 |
| 0.1154 | 4.1009 | 1788 | 0.5633 | 0.4803 | 0.5633 | 0.7505 |
| 0.1154 | 4.1055 | 1790 | 0.5499 | 0.4538 | 0.5499 | 0.7416 |
| 0.1154 | 4.1101 | 1792 | 0.5647 | 0.4538 | 0.5647 | 0.7515 |
| 0.1154 | 4.1147 | 1794 | 0.5518 | 0.4538 | 0.5518 | 0.7428 |
| 0.1154 | 4.1193 | 1796 | 0.5460 | 0.4538 | 0.5460 | 0.7389 |
| 0.1154 | 4.1239 | 1798 | 0.5557 | 0.4538 | 0.5557 | 0.7455 |
| 0.1154 | 4.1284 | 1800 | 0.5829 | 0.4803 | 0.5829 | 0.7635 |
| 0.1154 | 4.1330 | 1802 | 0.5896 | 0.4375 | 0.5896 | 0.7679 |
| 0.1154 | 4.1376 | 1804 | 0.5549 | 0.4106 | 0.5549 | 0.7449 |
| 0.1154 | 4.1422 | 1806 | 0.5377 | 0.475 | 0.5377 | 0.7333 |
| 0.1154 | 4.1468 | 1808 | 0.5455 | 0.4667 | 0.5455 | 0.7386 |
| 0.1154 | 4.1514 | 1810 | 0.5353 | 0.4465 | 0.5353 | 0.7317 |
| 0.1154 | 4.1560 | 1812 | 0.5407 | 0.4465 | 0.5407 | 0.7353 |
| 0.1154 | 4.1606 | 1814 | 0.5778 | 0.3486 | 0.5778 | 0.7601 |
| 0.1154 | 4.1651 | 1816 | 0.6272 | 0.4018 | 0.6272 | 0.7919 |
| 0.1154 | 4.1697 | 1818 | 0.6098 | 0.375 | 0.6098 | 0.7809 |
| 0.1154 | 4.1743 | 1820 | 0.5631 | 0.5191 | 0.5631 | 0.7504 |
| 0.1154 | 4.1789 | 1822 | 0.5050 | 0.5545 | 0.5050 | 0.7106 |
| 0.1154 | 4.1835 | 1824 | 0.4745 | 0.6526 | 0.4745 | 0.6889 |
| 0.1154 | 4.1881 | 1826 | 0.4582 | 0.6866 | 0.4582 | 0.6769 |
| 0.1154 | 4.1927 | 1828 | 0.4468 | 0.6866 | 0.4468 | 0.6685 |
| 0.1154 | 4.1972 | 1830 | 0.4469 | 0.6526 | 0.4469 | 0.6685 |
| 0.1154 | 4.2018 | 1832 | 0.4806 | 0.5191 | 0.4806 | 0.6932 |
| 0.1154 | 4.2064 | 1834 | 0.5132 | 0.5767 | 0.5132 | 0.7164 |
| 0.1154 | 4.2110 | 1836 | 0.5239 | 0.4667 | 0.5239 | 0.7238 |
| 0.1154 | 4.2156 | 1838 | 0.5082 | 0.5767 | 0.5082 | 0.7129 |
| 0.1154 | 4.2202 | 1840 | 0.4843 | 0.5191 | 0.4843 | 0.6959 |
| 0.1154 | 4.2248 | 1842 | 0.4749 | 0.5191 | 0.4749 | 0.6891 |
| 0.1154 | 4.2294 | 1844 | 0.4914 | 0.5191 | 0.4914 | 0.7010 |
| 0.1154 | 4.2339 | 1846 | 0.5016 | 0.5191 | 0.5016 | 0.7083 |
| 0.1154 | 4.2385 | 1848 | 0.4872 | 0.6526 | 0.4872 | 0.6980 |
| 0.1154 | 4.2431 | 1850 | 0.4955 | 0.6526 | 0.4955 | 0.7039 |
| 0.1154 | 4.2477 | 1852 | 0.5353 | 0.5545 | 0.5353 | 0.7316 |
| 0.1154 | 4.2523 | 1854 | 0.5679 | 0.4878 | 0.5679 | 0.7536 |
| 0.1154 | 4.2569 | 1856 | 0.5645 | 0.4878 | 0.5645 | 0.7513 |
| 0.1154 | 4.2615 | 1858 | 0.5769 | 0.4538 | 0.5769 | 0.7595 |
| 0.1154 | 4.2661 | 1860 | 0.5881 | 0.4457 | 0.5881 | 0.7668 |
| 0.1154 | 4.2706 | 1862 | 0.5980 | 0.3616 | 0.5980 | 0.7733 |
| 0.1154 | 4.2752 | 1864 | 0.6359 | 0.3656 | 0.6359 | 0.7974 |
| 0.1154 | 4.2798 | 1866 | 0.6437 | 0.3656 | 0.6437 | 0.8023 |
| 0.1154 | 4.2844 | 1868 | 0.6276 | 0.3616 | 0.6276 | 0.7922 |
| 0.1154 | 4.2890 | 1870 | 0.6086 | 0.3616 | 0.6086 | 0.7801 |
| 0.1154 | 4.2936 | 1872 | 0.5686 | 0.5145 | 0.5686 | 0.7540 |
| 0.1154 | 4.2982 | 1874 | 0.5511 | 0.4878 | 0.5511 | 0.7424 |
| 0.1154 | 4.3028 | 1876 | 0.5441 | 0.6526 | 0.5441 | 0.7376 |
| 0.1154 | 4.3073 | 1878 | 0.5520 | 0.5812 | 0.5520 | 0.7430 |
| 0.1154 | 4.3119 | 1880 | 0.5330 | 0.6526 | 0.5330 | 0.7301 |
| 0.1154 | 4.3165 | 1882 | 0.4971 | 0.6526 | 0.4971 | 0.7051 |
| 0.1154 | 4.3211 | 1884 | 0.4686 | 0.6866 | 0.4686 | 0.6845 |
| 0.1154 | 4.3257 | 1886 | 0.4698 | 0.6526 | 0.4698 | 0.6855 |
| 0.1154 | 4.3303 | 1888 | 0.4938 | 0.6526 | 0.4938 | 0.7027 |
| 0.1154 | 4.3349 | 1890 | 0.5354 | 0.6182 | 0.5354 | 0.7317 |
| 0.1154 | 4.3394 | 1892 | 0.5952 | 0.3842 | 0.5952 | 0.7715 |
| 0.1154 | 4.3440 | 1894 | 0.6200 | 0.3388 | 0.6200 | 0.7874 |
| 0.1154 | 4.3486 | 1896 | 0.6070 | 0.3388 | 0.6070 | 0.7791 |
| 0.1154 | 4.3532 | 1898 | 0.6205 | 0.3388 | 0.6205 | 0.7877 |
| 0.1154 | 4.3578 | 1900 | 0.6182 | 0.3656 | 0.6182 | 0.7862 |
| 0.1154 | 4.3624 | 1902 | 0.5906 | 0.3333 | 0.5906 | 0.7685 |
| 0.1154 | 4.3670 | 1904 | 0.5833 | 0.3980 | 0.5833 | 0.7637 |
| 0.1154 | 4.3716 | 1906 | 0.5664 | 0.3980 | 0.5664 | 0.7526 |
| 0.1154 | 4.3761 | 1908 | 0.5196 | 0.5832 | 0.5196 | 0.7209 |
| 0.1154 | 4.3807 | 1910 | 0.5156 | 0.5545 | 0.5156 | 0.7180 |
| 0.1154 | 4.3853 | 1912 | 0.5456 | 0.5116 | 0.5456 | 0.7387 |
| 0.1154 | 4.3899 | 1914 | 0.6081 | 0.3486 | 0.6081 | 0.7798 |
| 0.1154 | 4.3945 | 1916 | 0.6349 | 0.3529 | 0.6349 | 0.7968 |
| 0.1154 | 4.3991 | 1918 | 0.6282 | 0.3529 | 0.6282 | 0.7926 |
| 0.1154 | 4.4037 | 1920 | 0.5818 | 0.4094 | 0.5818 | 0.7628 |
| 0.1154 | 4.4083 | 1922 | 0.5656 | 0.5116 | 0.5656 | 0.7520 |
| 0.1154 | 4.4128 | 1924 | 0.5601 | 0.5545 | 0.5601 | 0.7484 |
| 0.1154 | 4.4174 | 1926 | 0.5382 | 0.6526 | 0.5382 | 0.7336 |
| 0.1154 | 4.4220 | 1928 | 0.5402 | 0.6526 | 0.5402 | 0.7350 |
| 0.1154 | 4.4266 | 1930 | 0.5542 | 0.5191 | 0.5542 | 0.7445 |
| 0.1154 | 4.4312 | 1932 | 0.6023 | 0.3529 | 0.6023 | 0.7761 |
| 0.1154 | 4.4358 | 1934 | 0.6888 | 0.4034 | 0.6888 | 0.8299 |
| 0.1154 | 4.4404 | 1936 | 0.7405 | 0.4295 | 0.7405 | 0.8605 |
| 0.1154 | 4.4450 | 1938 | 0.7156 | 0.4293 | 0.7156 | 0.8459 |
| 0.1154 | 4.4495 | 1940 | 0.6319 | 0.3780 | 0.6319 | 0.7949 |
| 0.1154 | 4.4541 | 1942 | 0.5535 | 0.4094 | 0.5535 | 0.7440 |
| 0.1154 | 4.4587 | 1944 | 0.5140 | 0.5477 | 0.5140 | 0.7170 |
| 0.1154 | 4.4633 | 1946 | 0.5007 | 0.6526 | 0.5007 | 0.7076 |
| 0.1154 | 4.4679 | 1948 | 0.5128 | 0.5477 | 0.5128 | 0.7161 |
| 0.1154 | 4.4725 | 1950 | 0.5584 | 0.4378 | 0.5584 | 0.7472 |
| 0.1154 | 4.4771 | 1952 | 0.5921 | 0.3656 | 0.5921 | 0.7695 |
| 0.1154 | 4.4817 | 1954 | 0.5984 | 0.3656 | 0.5984 | 0.7735 |
| 0.1154 | 4.4862 | 1956 | 0.6026 | 0.3691 | 0.6026 | 0.7763 |
| 0.1154 | 4.4908 | 1958 | 0.5662 | 0.375 | 0.5662 | 0.7524 |
| 0.1154 | 4.4954 | 1960 | 0.5091 | 0.6818 | 0.5091 | 0.7135 |
| 0.1154 | 4.5 | 1962 | 0.4683 | 0.6866 | 0.4683 | 0.6843 |
| 0.1154 | 4.5046 | 1964 | 0.4538 | 0.7181 | 0.4538 | 0.6737 |
| 0.1154 | 4.5092 | 1966 | 0.4593 | 0.7181 | 0.4593 | 0.6777 |
| 0.1154 | 4.5138 | 1968 | 0.4593 | 0.7181 | 0.4593 | 0.6777 |
| 0.1154 | 4.5183 | 1970 | 0.4705 | 0.6866 | 0.4705 | 0.6860 |
| 0.1154 | 4.5229 | 1972 | 0.5262 | 0.6818 | 0.5262 | 0.7254 |
| 0.1154 | 4.5275 | 1974 | 0.5888 | 0.375 | 0.5888 | 0.7673 |
| 0.1154 | 4.5321 | 1976 | 0.6043 | 0.3780 | 0.6043 | 0.7774 |
| 0.1154 | 4.5367 | 1978 | 0.5765 | 0.375 | 0.5765 | 0.7593 |
| 0.1154 | 4.5413 | 1980 | 0.5404 | 0.5545 | 0.5404 | 0.7351 |
| 0.1154 | 4.5459 | 1982 | 0.5172 | 0.6866 | 0.5172 | 0.7191 |
| 0.1154 | 4.5505 | 1984 | 0.5054 | 0.6866 | 0.5054 | 0.7109 |
| 0.1154 | 4.5550 | 1986 | 0.4966 | 0.6866 | 0.4966 | 0.7047 |
| 0.1154 | 4.5596 | 1988 | 0.4904 | 0.6526 | 0.4904 | 0.7003 |
| 0.1154 | 4.5642 | 1990 | 0.5039 | 0.6526 | 0.5039 | 0.7099 |
| 0.1154 | 4.5688 | 1992 | 0.5457 | 0.4094 | 0.5457 | 0.7387 |
| 0.1154 | 4.5734 | 1994 | 0.5784 | 0.4378 | 0.5784 | 0.7605 |
| 0.1154 | 4.5780 | 1996 | 0.5982 | 0.375 | 0.5982 | 0.7734 |
| 0.1154 | 4.5826 | 1998 | 0.5752 | 0.375 | 0.5752 | 0.7584 |
| 0.0904 | 4.5872 | 2000 | 0.5465 | 0.4094 | 0.5465 | 0.7393 |
| 0.0904 | 4.5917 | 2002 | 0.5301 | 0.6818 | 0.5301 | 0.7281 |
| 0.0904 | 4.5963 | 2004 | 0.5450 | 0.6818 | 0.5450 | 0.7383 |
| 0.0904 | 4.6009 | 2006 | 0.5879 | 0.4457 | 0.5879 | 0.7667 |
| 0.0904 | 4.6055 | 2008 | 0.6473 | 0.3486 | 0.6473 | 0.8045 |
| 0.0904 | 4.6101 | 2010 | 0.6842 | 0.3569 | 0.6842 | 0.8272 |
| 0.0904 | 4.6147 | 2012 | 0.6858 | 0.3569 | 0.6858 | 0.8281 |
| 0.0904 | 4.6193 | 2014 | 0.6577 | 0.4444 | 0.6577 | 0.8110 |
| 0.0904 | 4.6239 | 2016 | 0.6116 | 0.5145 | 0.6116 | 0.7820 |
| 0.0904 | 4.6284 | 2018 | 0.5729 | 0.6818 | 0.5729 | 0.7569 |
| 0.0904 | 4.6330 | 2020 | 0.5678 | 0.6818 | 0.5678 | 0.7535 |
| 0.0904 | 4.6376 | 2022 | 0.5849 | 0.5477 | 0.5849 | 0.7648 |
| 0.0904 | 4.6422 | 2024 | 0.6302 | 0.3486 | 0.6302 | 0.7938 |
| 0.0904 | 4.6468 | 2026 | 0.6652 | 0.3831 | 0.6652 | 0.8156 |
| 0.0904 | 4.6514 | 2028 | 0.6639 | 0.3831 | 0.6639 | 0.8148 |
| 0.0904 | 4.6560 | 2030 | 0.6378 | 0.3831 | 0.6378 | 0.7986 |
| 0.0904 | 4.6606 | 2032 | 0.5777 | 0.3486 | 0.5777 | 0.7600 |
| 0.0904 | 4.6651 | 2034 | 0.5268 | 0.6123 | 0.5268 | 0.7258 |
| 0.0904 | 4.6697 | 2036 | 0.4984 | 0.6182 | 0.4984 | 0.7060 |
| 0.0904 | 4.6743 | 2038 | 0.5052 | 0.6182 | 0.5052 | 0.7107 |
| 0.0904 | 4.6789 | 2040 | 0.5095 | 0.6182 | 0.5095 | 0.7138 |
| 0.0904 | 4.6835 | 2042 | 0.5132 | 0.6526 | 0.5132 | 0.7164 |
| 0.0904 | 4.6881 | 2044 | 0.5240 | 0.6526 | 0.5240 | 0.7239 |
| 0.0904 | 4.6927 | 2046 | 0.5590 | 0.5481 | 0.5590 | 0.7476 |
| 0.0904 | 4.6972 | 2048 | 0.5986 | 0.4451 | 0.5986 | 0.7737 |
| 0.0904 | 4.7018 | 2050 | 0.6338 | 0.4439 | 0.6338 | 0.7961 |
| 0.0904 | 4.7064 | 2052 | 0.6187 | 0.4444 | 0.6187 | 0.7866 |
| 0.0904 | 4.7110 | 2054 | 0.5614 | 0.4803 | 0.5614 | 0.7492 |
| 0.0904 | 4.7156 | 2056 | 0.5096 | 0.6526 | 0.5096 | 0.7138 |
| 0.0904 | 4.7202 | 2058 | 0.4792 | 0.6526 | 0.4792 | 0.6922 |
| 0.0904 | 4.7248 | 2060 | 0.4774 | 0.6526 | 0.4774 | 0.6909 |
| 0.0904 | 4.7294 | 2062 | 0.4909 | 0.6526 | 0.4909 | 0.7006 |
| 0.0904 | 4.7339 | 2064 | 0.5247 | 0.5191 | 0.5247 | 0.7243 |
| 0.0904 | 4.7385 | 2066 | 0.5628 | 0.475 | 0.5628 | 0.7502 |
| 0.0904 | 4.7431 | 2068 | 0.5803 | 0.475 | 0.5803 | 0.7618 |
| 0.0904 | 4.7477 | 2070 | 0.5623 | 0.5473 | 0.5623 | 0.7498 |
| 0.0904 | 4.7523 | 2072 | 0.5061 | 0.5164 | 0.5061 | 0.7114 |
| 0.0904 | 4.7569 | 2074 | 0.4600 | 0.6526 | 0.4600 | 0.6782 |
| 0.0904 | 4.7615 | 2076 | 0.4557 | 0.6526 | 0.4557 | 0.6750 |
| 0.0904 | 4.7661 | 2078 | 0.4743 | 0.6526 | 0.4743 | 0.6887 |
| 0.0904 | 4.7706 | 2080 | 0.5137 | 0.6526 | 0.5137 | 0.7168 |
| 0.0904 | 4.7752 | 2082 | 0.5613 | 0.5191 | 0.5613 | 0.7492 |
| 0.0904 | 4.7798 | 2084 | 0.5909 | 0.5415 | 0.5909 | 0.7687 |
| 0.0904 | 4.7844 | 2086 | 0.5810 | 0.5191 | 0.5810 | 0.7622 |
| 0.0904 | 4.7890 | 2088 | 0.5392 | 0.5191 | 0.5392 | 0.7343 |
| 0.0904 | 4.7936 | 2090 | 0.5166 | 0.6182 | 0.5166 | 0.7187 |
| 0.0904 | 4.7982 | 2092 | 0.4852 | 0.6526 | 0.4852 | 0.6966 |
| 0.0904 | 4.8028 | 2094 | 0.4576 | 0.6656 | 0.4576 | 0.6765 |
| 0.0904 | 4.8073 | 2096 | 0.4456 | 0.6656 | 0.4456 | 0.6676 |
| 0.0904 | 4.8119 | 2098 | 0.4484 | 0.6656 | 0.4484 | 0.6696 |
| 0.0904 | 4.8165 | 2100 | 0.4754 | 0.6473 | 0.4754 | 0.6895 |
| 0.0904 | 4.8211 | 2102 | 0.5324 | 0.5477 | 0.5324 | 0.7296 |
| 0.0904 | 4.8257 | 2104 | 0.5853 | 0.4688 | 0.5853 | 0.7650 |
| 0.0904 | 4.8303 | 2106 | 0.6023 | 0.4457 | 0.6023 | 0.7761 |
| 0.0904 | 4.8349 | 2108 | 0.5967 | 0.5415 | 0.5967 | 0.7725 |
| 0.0904 | 4.8394 | 2110 | 0.5814 | 0.5477 | 0.5814 | 0.7625 |
| 0.0904 | 4.8440 | 2112 | 0.5641 | 0.5832 | 0.5641 | 0.7510 |
| 0.0904 | 4.8486 | 2114 | 0.5369 | 0.5545 | 0.5369 | 0.7327 |
| 0.0904 | 4.8532 | 2116 | 0.5206 | 0.6526 | 0.5206 | 0.7215 |
| 0.0904 | 4.8578 | 2118 | 0.5079 | 0.6656 | 0.5079 | 0.7126 |
| 0.0904 | 4.8624 | 2120 | 0.5087 | 0.6526 | 0.5087 | 0.7133 |
| 0.0904 | 4.8670 | 2122 | 0.5419 | 0.5545 | 0.5419 | 0.7362 |
| 0.0904 | 4.8716 | 2124 | 0.6063 | 0.5832 | 0.6063 | 0.7787 |
| 0.0904 | 4.8761 | 2126 | 0.6716 | 0.4779 | 0.6716 | 0.8195 |
| 0.0904 | 4.8807 | 2128 | 0.6757 | 0.4779 | 0.6757 | 0.8220 |
| 0.0904 | 4.8853 | 2130 | 0.6528 | 0.4803 | 0.6528 | 0.8080 |
| 0.0904 | 4.8899 | 2132 | 0.6073 | 0.5832 | 0.6073 | 0.7793 |
| 0.0904 | 4.8945 | 2134 | 0.5741 | 0.5832 | 0.5741 | 0.7577 |
| 0.0904 | 4.8991 | 2136 | 0.5545 | 0.5832 | 0.5545 | 0.7447 |
| 0.0904 | 4.9037 | 2138 | 0.5722 | 0.5477 | 0.5722 | 0.7565 |
| 0.0904 | 4.9083 | 2140 | 0.5837 | 0.5477 | 0.5837 | 0.7640 |
| 0.0904 | 4.9128 | 2142 | 0.5781 | 0.5477 | 0.5781 | 0.7603 |
| 0.0904 | 4.9174 | 2144 | 0.5697 | 0.5477 | 0.5697 | 0.7548 |
| 0.0904 | 4.9220 | 2146 | 0.5878 | 0.5477 | 0.5878 | 0.7667 |
| 0.0904 | 4.9266 | 2148 | 0.5983 | 0.5832 | 0.5983 | 0.7735 |
| 0.0904 | 4.9312 | 2150 | 0.6245 | 0.5477 | 0.6245 | 0.7902 |
| 0.0904 | 4.9358 | 2152 | 0.6203 | 0.5477 | 0.6203 | 0.7876 |
| 0.0904 | 4.9404 | 2154 | 0.6113 | 0.5545 | 0.6113 | 0.7818 |
| 0.0904 | 4.9450 | 2156 | 0.6079 | 0.5545 | 0.6079 | 0.7797 |
| 0.0904 | 4.9495 | 2158 | 0.6198 | 0.5545 | 0.6198 | 0.7873 |
| 0.0904 | 4.9541 | 2160 | 0.6011 | 0.5545 | 0.6011 | 0.7753 |
| 0.0904 | 4.9587 | 2162 | 0.5738 | 0.5545 | 0.5738 | 0.7575 |
| 0.0904 | 4.9633 | 2164 | 0.5758 | 0.5545 | 0.5758 | 0.7588 |
| 0.0904 | 4.9679 | 2166 | 0.5895 | 0.5545 | 0.5895 | 0.7678 |
| 0.0904 | 4.9725 | 2168 | 0.5781 | 0.5545 | 0.5781 | 0.7604 |
| 0.0904 | 4.9771 | 2170 | 0.5507 | 0.5545 | 0.5507 | 0.7421 |
| 0.0904 | 4.9817 | 2172 | 0.5394 | 0.5545 | 0.5394 | 0.7344 |
| 0.0904 | 4.9862 | 2174 | 0.5260 | 0.5545 | 0.5260 | 0.7253 |
| 0.0904 | 4.9908 | 2176 | 0.5247 | 0.5545 | 0.5247 | 0.7243 |
| 0.0904 | 4.9954 | 2178 | 0.5414 | 0.5545 | 0.5414 | 0.7358 |
| 0.0904 | 5.0 | 2180 | 0.5640 | 0.5545 | 0.5640 | 0.7510 |
| 0.0904 | 5.0046 | 2182 | 0.5865 | 0.5477 | 0.5865 | 0.7658 |
| 0.0904 | 5.0092 | 2184 | 0.5704 | 0.5477 | 0.5704 | 0.7552 |
| 0.0904 | 5.0138 | 2186 | 0.5319 | 0.5545 | 0.5319 | 0.7293 |
| 0.0904 | 5.0183 | 2188 | 0.4971 | 0.5545 | 0.4971 | 0.7051 |
| 0.0904 | 5.0229 | 2190 | 0.4946 | 0.5545 | 0.4946 | 0.7032 |
| 0.0904 | 5.0275 | 2192 | 0.5116 | 0.5545 | 0.5116 | 0.7153 |
| 0.0904 | 5.0321 | 2194 | 0.5353 | 0.5545 | 0.5353 | 0.7317 |
| 0.0904 | 5.0367 | 2196 | 0.5272 | 0.5545 | 0.5272 | 0.7261 |
| 0.0904 | 5.0413 | 2198 | 0.5224 | 0.5545 | 0.5224 | 0.7228 |
| 0.0904 | 5.0459 | 2200 | 0.5539 | 0.5191 | 0.5539 | 0.7442 |
| 0.0904 | 5.0505 | 2202 | 0.5843 | 0.5767 | 0.5843 | 0.7644 |
| 0.0904 | 5.0550 | 2204 | 0.6573 | 0.4648 | 0.6573 | 0.8107 |
| 0.0904 | 5.0596 | 2206 | 0.7036 | 0.4049 | 0.7036 | 0.8388 |
| 0.0904 | 5.0642 | 2208 | 0.6925 | 0.4648 | 0.6925 | 0.8321 |
| 0.0904 | 5.0688 | 2210 | 0.6355 | 0.4375 | 0.6355 | 0.7972 |
| 0.0904 | 5.0734 | 2212 | 0.5643 | 0.5477 | 0.5643 | 0.7512 |
| 0.0904 | 5.0780 | 2214 | 0.5187 | 0.5545 | 0.5187 | 0.7202 |
| 0.0904 | 5.0826 | 2216 | 0.4989 | 0.5545 | 0.4989 | 0.7063 |
| 0.0904 | 5.0872 | 2218 | 0.5068 | 0.5545 | 0.5068 | 0.7119 |
| 0.0904 | 5.0917 | 2220 | 0.5317 | 0.5545 | 0.5317 | 0.7292 |
| 0.0904 | 5.0963 | 2222 | 0.5721 | 0.5191 | 0.5721 | 0.7564 |
| 0.0904 | 5.1009 | 2224 | 0.5894 | 0.5477 | 0.5894 | 0.7677 |
| 0.0904 | 5.1055 | 2226 | 0.5906 | 0.4465 | 0.5906 | 0.7685 |
| 0.0904 | 5.1101 | 2228 | 0.5672 | 0.5477 | 0.5672 | 0.7531 |
| 0.0904 | 5.1147 | 2230 | 0.5424 | 0.5545 | 0.5424 | 0.7365 |
| 0.0904 | 5.1193 | 2232 | 0.5389 | 0.5545 | 0.5389 | 0.7341 |
| 0.0904 | 5.1239 | 2234 | 0.5538 | 0.5545 | 0.5538 | 0.7442 |
| 0.0904 | 5.1284 | 2236 | 0.5552 | 0.5545 | 0.5552 | 0.7451 |
| 0.0904 | 5.1330 | 2238 | 0.5489 | 0.5545 | 0.5489 | 0.7409 |
| 0.0904 | 5.1376 | 2240 | 0.5411 | 0.5545 | 0.5411 | 0.7356 |
| 0.0904 | 5.1422 | 2242 | 0.5607 | 0.5545 | 0.5607 | 0.7488 |
| 0.0904 | 5.1468 | 2244 | 0.5638 | 0.5545 | 0.5638 | 0.7509 |
| 0.0904 | 5.1514 | 2246 | 0.5688 | 0.5545 | 0.5688 | 0.7542 |
| 0.0904 | 5.1560 | 2248 | 0.5766 | 0.5545 | 0.5766 | 0.7594 |
| 0.0904 | 5.1606 | 2250 | 0.5908 | 0.5116 | 0.5908 | 0.7686 |
| 0.0904 | 5.1651 | 2252 | 0.6055 | 0.5116 | 0.6055 | 0.7781 |
| 0.0904 | 5.1697 | 2254 | 0.5924 | 0.5477 | 0.5924 | 0.7697 |
| 0.0904 | 5.1743 | 2256 | 0.5677 | 0.5545 | 0.5677 | 0.7534 |
| 0.0904 | 5.1789 | 2258 | 0.5823 | 0.5191 | 0.5823 | 0.7631 |
| 0.0904 | 5.1835 | 2260 | 0.6098 | 0.5116 | 0.6098 | 0.7809 |
| 0.0904 | 5.1881 | 2262 | 0.6009 | 0.5191 | 0.6009 | 0.7752 |
| 0.0904 | 5.1927 | 2264 | 0.5708 | 0.5191 | 0.5708 | 0.7555 |
| 0.0904 | 5.1972 | 2266 | 0.5459 | 0.5191 | 0.5459 | 0.7388 |
| 0.0904 | 5.2018 | 2268 | 0.5432 | 0.4831 | 0.5432 | 0.7370 |
| 0.0904 | 5.2064 | 2270 | 0.5412 | 0.5406 | 0.5412 | 0.7357 |
| 0.0904 | 5.2110 | 2272 | 0.5728 | 0.4667 | 0.5728 | 0.7568 |
| 0.0904 | 5.2156 | 2274 | 0.5998 | 0.4667 | 0.5998 | 0.7745 |
| 0.0904 | 5.2202 | 2276 | 0.5892 | 0.4667 | 0.5892 | 0.7676 |
| 0.0904 | 5.2248 | 2278 | 0.5889 | 0.4667 | 0.5889 | 0.7674 |
| 0.0904 | 5.2294 | 2280 | 0.5693 | 0.4667 | 0.5693 | 0.7545 |
| 0.0904 | 5.2339 | 2282 | 0.5253 | 0.5406 | 0.5253 | 0.7248 |
| 0.0904 | 5.2385 | 2284 | 0.4880 | 0.5477 | 0.4880 | 0.6986 |
| 0.0904 | 5.2431 | 2286 | 0.4727 | 0.5618 | 0.4727 | 0.6875 |
| 0.0904 | 5.2477 | 2288 | 0.4766 | 0.5618 | 0.4766 | 0.6904 |
| 0.0904 | 5.2523 | 2290 | 0.4993 | 0.5545 | 0.4993 | 0.7066 |
| 0.0904 | 5.2569 | 2292 | 0.5230 | 0.5477 | 0.5230 | 0.7232 |
| 0.0904 | 5.2615 | 2294 | 0.5629 | 0.5116 | 0.5629 | 0.7503 |
| 0.0904 | 5.2661 | 2296 | 0.5931 | 0.5116 | 0.5931 | 0.7701 |
| 0.0904 | 5.2706 | 2298 | 0.6047 | 0.5116 | 0.6047 | 0.7776 |
| 0.0904 | 5.2752 | 2300 | 0.6149 | 0.4457 | 0.6149 | 0.7842 |
| 0.0904 | 5.2798 | 2302 | 0.6045 | 0.4457 | 0.6045 | 0.7775 |
| 0.0904 | 5.2844 | 2304 | 0.5764 | 0.5832 | 0.5764 | 0.7592 |
| 0.0904 | 5.2890 | 2306 | 0.5329 | 0.5545 | 0.5329 | 0.7300 |
| 0.0904 | 5.2936 | 2308 | 0.5159 | 0.5545 | 0.5159 | 0.7183 |
| 0.0904 | 5.2982 | 2310 | 0.5271 | 0.5545 | 0.5271 | 0.7260 |
| 0.0904 | 5.3028 | 2312 | 0.5702 | 0.5477 | 0.5702 | 0.7551 |
| 0.0904 | 5.3073 | 2314 | 0.6284 | 0.4457 | 0.6284 | 0.7927 |
| 0.0904 | 5.3119 | 2316 | 0.6651 | 0.4451 | 0.6651 | 0.8156 |
| 0.0904 | 5.3165 | 2318 | 0.6713 | 0.4451 | 0.6713 | 0.8193 |
| 0.0904 | 5.3211 | 2320 | 0.6443 | 0.4457 | 0.6443 | 0.8027 |
| 0.0904 | 5.3257 | 2322 | 0.6314 | 0.4803 | 0.6314 | 0.7946 |
| 0.0904 | 5.3303 | 2324 | 0.6102 | 0.5832 | 0.6102 | 0.7812 |
| 0.0904 | 5.3349 | 2326 | 0.5802 | 0.5545 | 0.5802 | 0.7617 |
| 0.0904 | 5.3394 | 2328 | 0.5757 | 0.5545 | 0.5757 | 0.7588 |
| 0.0904 | 5.3440 | 2330 | 0.6001 | 0.5832 | 0.6001 | 0.7747 |
| 0.0904 | 5.3486 | 2332 | 0.6310 | 0.4803 | 0.6310 | 0.7944 |
| 0.0904 | 5.3532 | 2334 | 0.6303 | 0.4803 | 0.6303 | 0.7939 |
| 0.0904 | 5.3578 | 2336 | 0.6297 | 0.4803 | 0.6297 | 0.7935 |
| 0.0904 | 5.3624 | 2338 | 0.6354 | 0.4803 | 0.6354 | 0.7971 |
| 0.0904 | 5.3670 | 2340 | 0.6544 | 0.5103 | 0.6544 | 0.8089 |
| 0.0904 | 5.3716 | 2342 | 0.6655 | 0.5103 | 0.6655 | 0.8158 |
| 0.0904 | 5.3761 | 2344 | 0.6853 | 0.4779 | 0.6853 | 0.8278 |
| 0.0904 | 5.3807 | 2346 | 0.6782 | 0.4779 | 0.6782 | 0.8235 |
| 0.0904 | 5.3853 | 2348 | 0.6625 | 0.4779 | 0.6625 | 0.8140 |
| 0.0904 | 5.3899 | 2350 | 0.6315 | 0.4779 | 0.6315 | 0.7947 |
| 0.0904 | 5.3945 | 2352 | 0.5838 | 0.4803 | 0.5838 | 0.7641 |
| 0.0904 | 5.3991 | 2354 | 0.5448 | 0.5545 | 0.5448 | 0.7381 |
| 0.0904 | 5.4037 | 2356 | 0.5314 | 0.5545 | 0.5314 | 0.7290 |
| 0.0904 | 5.4083 | 2358 | 0.5305 | 0.5545 | 0.5305 | 0.7283 |
| 0.0904 | 5.4128 | 2360 | 0.5388 | 0.5832 | 0.5388 | 0.7341 |
| 0.0904 | 5.4174 | 2362 | 0.5401 | 0.5477 | 0.5401 | 0.7349 |
| 0.0904 | 5.4220 | 2364 | 0.5514 | 0.5477 | 0.5514 | 0.7426 |
| 0.0904 | 5.4266 | 2366 | 0.5794 | 0.4803 | 0.5794 | 0.7612 |
| 0.0904 | 5.4312 | 2368 | 0.5836 | 0.4727 | 0.5836 | 0.7639 |
| 0.0904 | 5.4358 | 2370 | 0.5552 | 0.5477 | 0.5552 | 0.7451 |
| 0.0904 | 5.4404 | 2372 | 0.5192 | 0.5477 | 0.5192 | 0.7205 |
| 0.0904 | 5.4450 | 2374 | 0.4944 | 0.5545 | 0.4944 | 0.7032 |
| 0.0904 | 5.4495 | 2376 | 0.4863 | 0.6526 | 0.4863 | 0.6973 |
| 0.0904 | 5.4541 | 2378 | 0.4900 | 0.5545 | 0.4900 | 0.7000 |
| 0.0904 | 5.4587 | 2380 | 0.5068 | 0.5477 | 0.5068 | 0.7119 |
| 0.0904 | 5.4633 | 2382 | 0.5073 | 0.5477 | 0.5073 | 0.7123 |
| 0.0904 | 5.4679 | 2384 | 0.4973 | 0.5477 | 0.4973 | 0.7052 |
| 0.0904 | 5.4725 | 2386 | 0.5061 | 0.5767 | 0.5061 | 0.7114 |
| 0.0904 | 5.4771 | 2388 | 0.5197 | 0.5767 | 0.5197 | 0.7209 |
| 0.0904 | 5.4817 | 2390 | 0.5398 | 0.6062 | 0.5398 | 0.7347 |
| 0.0904 | 5.4862 | 2392 | 0.5537 | 0.5347 | 0.5537 | 0.7441 |
| 0.0904 | 5.4908 | 2394 | 0.5478 | 0.6062 | 0.5478 | 0.7401 |
| 0.0904 | 5.4954 | 2396 | 0.5170 | 0.5477 | 0.5170 | 0.7190 |
| 0.0904 | 5.5 | 2398 | 0.4922 | 0.5895 | 0.4922 | 0.7016 |
| 0.0904 | 5.5046 | 2400 | 0.4856 | 0.5895 | 0.4856 | 0.6968 |
| 0.0904 | 5.5092 | 2402 | 0.4869 | 0.5895 | 0.4869 | 0.6978 |
| 0.0904 | 5.5138 | 2404 | 0.5080 | 0.5191 | 0.5080 | 0.7128 |
| 0.0904 | 5.5183 | 2406 | 0.5541 | 0.5767 | 0.5541 | 0.7444 |
| 0.0904 | 5.5229 | 2408 | 0.5749 | 0.6062 | 0.5749 | 0.7582 |
| 0.0904 | 5.5275 | 2410 | 0.5859 | 0.4667 | 0.5859 | 0.7655 |
| 0.0904 | 5.5321 | 2412 | 0.5672 | 0.6062 | 0.5672 | 0.7531 |
| 0.0904 | 5.5367 | 2414 | 0.5254 | 0.5477 | 0.5254 | 0.7248 |
| 0.0904 | 5.5413 | 2416 | 0.5071 | 0.5191 | 0.5071 | 0.7121 |
| 0.0904 | 5.5459 | 2418 | 0.5034 | 0.5545 | 0.5034 | 0.7095 |
| 0.0904 | 5.5505 | 2420 | 0.5189 | 0.5545 | 0.5189 | 0.7203 |
| 0.0904 | 5.5550 | 2422 | 0.5523 | 0.5545 | 0.5523 | 0.7431 |
| 0.0904 | 5.5596 | 2424 | 0.5804 | 0.4878 | 0.5804 | 0.7618 |
| 0.0904 | 5.5642 | 2426 | 0.5924 | 0.4878 | 0.5924 | 0.7697 |
| 0.0904 | 5.5688 | 2428 | 0.5771 | 0.4878 | 0.5771 | 0.7597 |
| 0.0904 | 5.5734 | 2430 | 0.5585 | 0.5545 | 0.5585 | 0.7474 |
| 0.0904 | 5.5780 | 2432 | 0.5531 | 0.5545 | 0.5531 | 0.7437 |
| 0.0904 | 5.5826 | 2434 | 0.5574 | 0.5191 | 0.5574 | 0.7466 |
| 0.0904 | 5.5872 | 2436 | 0.5568 | 0.5767 | 0.5568 | 0.7462 |
| 0.0904 | 5.5917 | 2438 | 0.5395 | 0.5477 | 0.5395 | 0.7345 |
| 0.0904 | 5.5963 | 2440 | 0.5167 | 0.5191 | 0.5167 | 0.7188 |
| 0.0904 | 5.6009 | 2442 | 0.4956 | 0.5545 | 0.4956 | 0.7040 |
| 0.0904 | 5.6055 | 2444 | 0.4897 | 0.5545 | 0.4897 | 0.6998 |
| 0.0904 | 5.6101 | 2446 | 0.4944 | 0.5545 | 0.4944 | 0.7031 |
| 0.0904 | 5.6147 | 2448 | 0.5197 | 0.5191 | 0.5197 | 0.7209 |
| 0.0904 | 5.6193 | 2450 | 0.5703 | 0.5767 | 0.5703 | 0.7552 |
| 0.0904 | 5.6239 | 2452 | 0.5981 | 0.6 | 0.5981 | 0.7734 |
| 0.0904 | 5.6284 | 2454 | 0.6099 | 0.5182 | 0.6099 | 0.7810 |
| 0.0904 | 5.6330 | 2456 | 0.5859 | 0.5689 | 0.5859 | 0.7655 |
| 0.0904 | 5.6376 | 2458 | 0.5460 | 0.5191 | 0.5460 | 0.7389 |
| 0.0904 | 5.6422 | 2460 | 0.5185 | 0.5191 | 0.5185 | 0.7200 |
| 0.0904 | 5.6468 | 2462 | 0.5122 | 0.5191 | 0.5122 | 0.7157 |
| 0.0904 | 5.6514 | 2464 | 0.5036 | 0.5191 | 0.5036 | 0.7097 |
| 0.0904 | 5.6560 | 2466 | 0.4869 | 0.5191 | 0.4869 | 0.6978 |
| 0.0904 | 5.6606 | 2468 | 0.4725 | 0.5545 | 0.4725 | 0.6874 |
| 0.0904 | 5.6651 | 2470 | 0.4769 | 0.5545 | 0.4769 | 0.6905 |
| 0.0904 | 5.6697 | 2472 | 0.4880 | 0.5545 | 0.4880 | 0.6986 |
| 0.0904 | 5.6743 | 2474 | 0.5067 | 0.5545 | 0.5067 | 0.7118 |
| 0.0904 | 5.6789 | 2476 | 0.5471 | 0.5191 | 0.5471 | 0.7397 |
| 0.0904 | 5.6835 | 2478 | 0.5731 | 0.5767 | 0.5731 | 0.7570 |
| 0.0904 | 5.6881 | 2480 | 0.5642 | 0.5191 | 0.5642 | 0.7511 |
| 0.0904 | 5.6927 | 2482 | 0.5667 | 0.5545 | 0.5667 | 0.7528 |
| 0.0904 | 5.6972 | 2484 | 0.5716 | 0.5545 | 0.5716 | 0.7560 |
| 0.0904 | 5.7018 | 2486 | 0.5524 | 0.5545 | 0.5524 | 0.7432 |
| 0.0904 | 5.7064 | 2488 | 0.5442 | 0.5545 | 0.5442 | 0.7377 |
| 0.0904 | 5.7110 | 2490 | 0.5463 | 0.5545 | 0.5463 | 0.7391 |
| 0.0904 | 5.7156 | 2492 | 0.5338 | 0.5545 | 0.5338 | 0.7306 |
| 0.0904 | 5.7202 | 2494 | 0.5154 | 0.5545 | 0.5154 | 0.7179 |
| 0.0904 | 5.7248 | 2496 | 0.5096 | 0.5545 | 0.5096 | 0.7138 |
| 0.0904 | 5.7294 | 2498 | 0.5104 | 0.5545 | 0.5104 | 0.7144 |
| 0.0721 | 5.7339 | 2500 | 0.5275 | 0.5545 | 0.5275 | 0.7263 |
| 0.0721 | 5.7385 | 2502 | 0.5565 | 0.5191 | 0.5565 | 0.7460 |
| 0.0721 | 5.7431 | 2504 | 0.5602 | 0.5191 | 0.5602 | 0.7484 |
| 0.0721 | 5.7477 | 2506 | 0.5340 | 0.5545 | 0.5340 | 0.7308 |
| 0.0721 | 5.7523 | 2508 | 0.5152 | 0.5545 | 0.5152 | 0.7178 |
| 0.0721 | 5.7569 | 2510 | 0.5059 | 0.5545 | 0.5059 | 0.7113 |
| 0.0721 | 5.7615 | 2512 | 0.5187 | 0.5545 | 0.5187 | 0.7202 |
| 0.0721 | 5.7661 | 2514 | 0.5335 | 0.5545 | 0.5335 | 0.7304 |
| 0.0721 | 5.7706 | 2516 | 0.5379 | 0.5545 | 0.5379 | 0.7334 |
| 0.0721 | 5.7752 | 2518 | 0.5438 | 0.5545 | 0.5438 | 0.7374 |
| 0.0721 | 5.7798 | 2520 | 0.5302 | 0.5545 | 0.5302 | 0.7282 |
| 0.0721 | 5.7844 | 2522 | 0.5052 | 0.6526 | 0.5052 | 0.7108 |
| 0.0721 | 5.7890 | 2524 | 0.4827 | 0.6526 | 0.4827 | 0.6948 |
| 0.0721 | 5.7936 | 2526 | 0.4845 | 0.6526 | 0.4845 | 0.6961 |
| 0.0721 | 5.7982 | 2528 | 0.5075 | 0.5545 | 0.5075 | 0.7124 |
| 0.0721 | 5.8028 | 2530 | 0.5352 | 0.4878 | 0.5352 | 0.7316 |
| 0.0721 | 5.8073 | 2532 | 0.5754 | 0.5309 | 0.5754 | 0.7585 |
| 0.0721 | 5.8119 | 2534 | 0.5970 | 0.5 | 0.5970 | 0.7727 |
| 0.0721 | 5.8165 | 2536 | 0.5865 | 0.5309 | 0.5865 | 0.7658 |
| 0.0721 | 5.8211 | 2538 | 0.5567 | 0.5145 | 0.5567 | 0.7461 |
| 0.0721 | 5.8257 | 2540 | 0.5142 | 0.6526 | 0.5142 | 0.7170 |
| 0.0721 | 5.8303 | 2542 | 0.4906 | 0.6526 | 0.4906 | 0.7004 |
| 0.0721 | 5.8349 | 2544 | 0.4839 | 0.6526 | 0.4839 | 0.6956 |
| 0.0721 | 5.8394 | 2546 | 0.4828 | 0.6526 | 0.4828 | 0.6948 |
| 0.0721 | 5.8440 | 2548 | 0.4850 | 0.6526 | 0.4850 | 0.6964 |
| 0.0721 | 5.8486 | 2550 | 0.4817 | 0.6526 | 0.4817 | 0.6940 |
| 0.0721 | 5.8532 | 2552 | 0.4876 | 0.6526 | 0.4876 | 0.6983 |
| 0.0721 | 5.8578 | 2554 | 0.5116 | 0.5545 | 0.5116 | 0.7153 |
| 0.0721 | 5.8624 | 2556 | 0.5297 | 0.5545 | 0.5297 | 0.7278 |
| 0.0721 | 5.8670 | 2558 | 0.5507 | 0.5832 | 0.5507 | 0.7421 |
| 0.0721 | 5.8716 | 2560 | 0.5518 | 0.5832 | 0.5518 | 0.7429 |
| 0.0721 | 5.8761 | 2562 | 0.5442 | 0.5545 | 0.5442 | 0.7377 |
| 0.0721 | 5.8807 | 2564 | 0.5428 | 0.5832 | 0.5428 | 0.7368 |
| 0.0721 | 5.8853 | 2566 | 0.5420 | 0.5832 | 0.5420 | 0.7362 |
| 0.0721 | 5.8899 | 2568 | 0.5248 | 0.5545 | 0.5248 | 0.7244 |
| 0.0721 | 5.8945 | 2570 | 0.5070 | 0.5545 | 0.5070 | 0.7120 |
| 0.0721 | 5.8991 | 2572 | 0.4912 | 0.5545 | 0.4912 | 0.7009 |
| 0.0721 | 5.9037 | 2574 | 0.4800 | 0.5545 | 0.4800 | 0.6928 |
| 0.0721 | 5.9083 | 2576 | 0.4807 | 0.5545 | 0.4807 | 0.6933 |
| 0.0721 | 5.9128 | 2578 | 0.4823 | 0.5545 | 0.4823 | 0.6945 |
| 0.0721 | 5.9174 | 2580 | 0.4855 | 0.5545 | 0.4855 | 0.6968 |
| 0.0721 | 5.9220 | 2582 | 0.4979 | 0.5545 | 0.4979 | 0.7056 |
| 0.0721 | 5.9266 | 2584 | 0.5310 | 0.5545 | 0.5310 | 0.7287 |
| 0.0721 | 5.9312 | 2586 | 0.5631 | 0.5855 | 0.5631 | 0.7504 |
| 0.0721 | 5.9358 | 2588 | 0.5875 | 0.5039 | 0.5875 | 0.7665 |
| 0.0721 | 5.9404 | 2590 | 0.6043 | 0.5333 | 0.6043 | 0.7774 |
| 0.0721 | 5.9450 | 2592 | 0.6170 | 0.4581 | 0.6170 | 0.7855 |
| 0.0721 | 5.9495 | 2594 | 0.6025 | 0.5039 | 0.6025 | 0.7762 |
| 0.0721 | 5.9541 | 2596 | 0.5656 | 0.5546 | 0.5656 | 0.7521 |
| 0.0721 | 5.9587 | 2598 | 0.5308 | 0.5545 | 0.5308 | 0.7285 |
| 0.0721 | 5.9633 | 2600 | 0.5211 | 0.5545 | 0.5211 | 0.7219 |
| 0.0721 | 5.9679 | 2602 | 0.5235 | 0.5545 | 0.5235 | 0.7235 |
| 0.0721 | 5.9725 | 2604 | 0.5407 | 0.5545 | 0.5407 | 0.7353 |
| 0.0721 | 5.9771 | 2606 | 0.5777 | 0.5545 | 0.5777 | 0.7601 |
| 0.0721 | 5.9817 | 2608 | 0.6235 | 0.4527 | 0.6235 | 0.7896 |
| 0.0721 | 5.9862 | 2610 | 0.6315 | 0.4527 | 0.6315 | 0.7947 |
| 0.0721 | 5.9908 | 2612 | 0.6104 | 0.5145 | 0.6104 | 0.7812 |
| 0.0721 | 5.9954 | 2614 | 0.5630 | 0.5545 | 0.5630 | 0.7504 |
| 0.0721 | 6.0 | 2616 | 0.5415 | 0.5545 | 0.5415 | 0.7359 |
| 0.0721 | 6.0046 | 2618 | 0.5351 | 0.5545 | 0.5351 | 0.7315 |
| 0.0721 | 6.0092 | 2620 | 0.5497 | 0.5191 | 0.5497 | 0.7414 |
| 0.0721 | 6.0138 | 2622 | 0.5648 | 0.5164 | 0.5648 | 0.7516 |
| 0.0721 | 6.0183 | 2624 | 0.5773 | 0.5084 | 0.5773 | 0.7598 |
| 0.0721 | 6.0229 | 2626 | 0.5882 | 0.5396 | 0.5882 | 0.7669 |
| 0.0721 | 6.0275 | 2628 | 0.5769 | 0.5084 | 0.5769 | 0.7595 |
| 0.0721 | 6.0321 | 2630 | 0.5646 | 0.5473 | 0.5646 | 0.7514 |
| 0.0721 | 6.0367 | 2632 | 0.5572 | 0.5164 | 0.5572 | 0.7464 |
| 0.0721 | 6.0413 | 2634 | 0.5520 | 0.5164 | 0.5520 | 0.7430 |
| 0.0721 | 6.0459 | 2636 | 0.5652 | 0.5191 | 0.5652 | 0.7518 |
| 0.0721 | 6.0505 | 2638 | 0.5593 | 0.5545 | 0.5593 | 0.7479 |
| 0.0721 | 6.0550 | 2640 | 0.5345 | 0.5545 | 0.5345 | 0.7311 |
| 0.0721 | 6.0596 | 2642 | 0.5047 | 0.5545 | 0.5047 | 0.7104 |
| 0.0721 | 6.0642 | 2644 | 0.4967 | 0.5545 | 0.4967 | 0.7048 |
| 0.0721 | 6.0688 | 2646 | 0.5048 | 0.5545 | 0.5048 | 0.7105 |
| 0.0721 | 6.0734 | 2648 | 0.5320 | 0.5545 | 0.5320 | 0.7294 |
| 0.0721 | 6.0780 | 2650 | 0.5553 | 0.5545 | 0.5553 | 0.7452 |
| 0.0721 | 6.0826 | 2652 | 0.5929 | 0.5545 | 0.5929 | 0.7700 |
| 0.0721 | 6.0872 | 2654 | 0.6210 | 0.5415 | 0.6210 | 0.7880 |
| 0.0721 | 6.0917 | 2656 | 0.6136 | 0.5477 | 0.6136 | 0.7833 |
| 0.0721 | 6.0963 | 2658 | 0.6024 | 0.5477 | 0.6024 | 0.7761 |
| 0.0721 | 6.1009 | 2660 | 0.5772 | 0.5545 | 0.5772 | 0.7598 |
| 0.0721 | 6.1055 | 2662 | 0.5658 | 0.5545 | 0.5658 | 0.7522 |
| 0.0721 | 6.1101 | 2664 | 0.5734 | 0.5545 | 0.5734 | 0.7572 |
| 0.0721 | 6.1147 | 2666 | 0.5695 | 0.5545 | 0.5695 | 0.7546 |
| 0.0721 | 6.1193 | 2668 | 0.5698 | 0.5191 | 0.5698 | 0.7548 |
| 0.0721 | 6.1239 | 2670 | 0.5664 | 0.5191 | 0.5664 | 0.7526 |
| 0.0721 | 6.1284 | 2672 | 0.5534 | 0.5191 | 0.5534 | 0.7439 |
| 0.0721 | 6.1330 | 2674 | 0.5672 | 0.5477 | 0.5672 | 0.7531 |
| 0.0721 | 6.1376 | 2676 | 0.5639 | 0.5767 | 0.5639 | 0.7509 |
| 0.0721 | 6.1422 | 2678 | 0.5640 | 0.5767 | 0.5640 | 0.7510 |
| 0.0721 | 6.1468 | 2680 | 0.5686 | 0.5767 | 0.5686 | 0.7540 |
| 0.0721 | 6.1514 | 2682 | 0.5574 | 0.5191 | 0.5574 | 0.7466 |
| 0.0721 | 6.1560 | 2684 | 0.5468 | 0.5545 | 0.5468 | 0.7395 |
| 0.0721 | 6.1606 | 2686 | 0.5484 | 0.5545 | 0.5484 | 0.7405 |
| 0.0721 | 6.1651 | 2688 | 0.5336 | 0.5545 | 0.5336 | 0.7305 |
| 0.0721 | 6.1697 | 2690 | 0.5255 | 0.5545 | 0.5255 | 0.7249 |
| 0.0721 | 6.1743 | 2692 | 0.5309 | 0.5545 | 0.5309 | 0.7286 |
| 0.0721 | 6.1789 | 2694 | 0.5255 | 0.5545 | 0.5255 | 0.7249 |
| 0.0721 | 6.1835 | 2696 | 0.5320 | 0.5545 | 0.5320 | 0.7294 |
| 0.0721 | 6.1881 | 2698 | 0.5388 | 0.5545 | 0.5388 | 0.7340 |
| 0.0721 | 6.1927 | 2700 | 0.5401 | 0.5545 | 0.5401 | 0.7349 |
| 0.0721 | 6.1972 | 2702 | 0.5209 | 0.5545 | 0.5209 | 0.7217 |
| 0.0721 | 6.2018 | 2704 | 0.5094 | 0.5545 | 0.5094 | 0.7137 |
| 0.0721 | 6.2064 | 2706 | 0.4931 | 0.5545 | 0.4931 | 0.7022 |
| 0.0721 | 6.2110 | 2708 | 0.5007 | 0.5545 | 0.5007 | 0.7076 |
| 0.0721 | 6.2156 | 2710 | 0.5046 | 0.5546 | 0.5046 | 0.7103 |
| 0.0721 | 6.2202 | 2712 | 0.5134 | 0.5855 | 0.5134 | 0.7165 |
| 0.0721 | 6.2248 | 2714 | 0.4918 | 0.5546 | 0.4918 | 0.7013 |
| 0.0721 | 6.2294 | 2716 | 0.4858 | 0.5546 | 0.4858 | 0.6970 |
| 0.0721 | 6.2339 | 2718 | 0.4734 | 0.5546 | 0.4734 | 0.6881 |
| 0.0721 | 6.2385 | 2720 | 0.4604 | 0.5545 | 0.4604 | 0.6785 |
| 0.0721 | 6.2431 | 2722 | 0.4537 | 0.5545 | 0.4537 | 0.6735 |
| 0.0721 | 6.2477 | 2724 | 0.4421 | 0.6866 | 0.4421 | 0.6649 |
| 0.0721 | 6.2523 | 2726 | 0.4497 | 0.5545 | 0.4497 | 0.6706 |
| 0.0721 | 6.2569 | 2728 | 0.4704 | 0.5545 | 0.4704 | 0.6859 |
| 0.0721 | 6.2615 | 2730 | 0.5069 | 0.5546 | 0.5069 | 0.7120 |
| 0.0721 | 6.2661 | 2732 | 0.5327 | 0.5546 | 0.5327 | 0.7298 |
| 0.0721 | 6.2706 | 2734 | 0.5304 | 0.5546 | 0.5304 | 0.7283 |
| 0.0721 | 6.2752 | 2736 | 0.5051 | 0.5545 | 0.5051 | 0.7107 |
| 0.0721 | 6.2798 | 2738 | 0.4723 | 0.5545 | 0.4723 | 0.6872 |
| 0.0721 | 6.2844 | 2740 | 0.4584 | 0.6526 | 0.4584 | 0.6771 |
| 0.0721 | 6.2890 | 2742 | 0.4633 | 0.6526 | 0.4633 | 0.6807 |
| 0.0721 | 6.2936 | 2744 | 0.4824 | 0.5545 | 0.4824 | 0.6946 |
| 0.0721 | 6.2982 | 2746 | 0.5178 | 0.5545 | 0.5178 | 0.7196 |
| 0.0721 | 6.3028 | 2748 | 0.5713 | 0.4878 | 0.5713 | 0.7559 |
| 0.0721 | 6.3073 | 2750 | 0.6174 | 0.5 | 0.6174 | 0.7857 |
| 0.0721 | 6.3119 | 2752 | 0.6262 | 0.5246 | 0.6262 | 0.7913 |
| 0.0721 | 6.3165 | 2754 | 0.6065 | 0.5035 | 0.6065 | 0.7787 |
| 0.0721 | 6.3211 | 2756 | 0.5600 | 0.5145 | 0.5600 | 0.7483 |
| 0.0721 | 6.3257 | 2758 | 0.5082 | 0.5545 | 0.5082 | 0.7129 |
| 0.0721 | 6.3303 | 2760 | 0.4627 | 0.6526 | 0.4627 | 0.6802 |
| 0.0721 | 6.3349 | 2762 | 0.4378 | 0.6526 | 0.4378 | 0.6617 |
| 0.0721 | 6.3394 | 2764 | 0.4243 | 0.6866 | 0.4243 | 0.6514 |
| 0.0721 | 6.3440 | 2766 | 0.4280 | 0.7014 | 0.4280 | 0.6542 |
| 0.0721 | 6.3486 | 2768 | 0.4371 | 0.6866 | 0.4371 | 0.6611 |
| 0.0721 | 6.3532 | 2770 | 0.4580 | 0.6526 | 0.4580 | 0.6768 |
| 0.0721 | 6.3578 | 2772 | 0.4783 | 0.6526 | 0.4783 | 0.6916 |
| 0.0721 | 6.3624 | 2774 | 0.5009 | 0.5545 | 0.5009 | 0.7078 |
| 0.0721 | 6.3670 | 2776 | 0.5189 | 0.5545 | 0.5189 | 0.7203 |
| 0.0721 | 6.3716 | 2778 | 0.5328 | 0.5832 | 0.5328 | 0.7299 |
| 0.0721 | 6.3761 | 2780 | 0.5363 | 0.5832 | 0.5363 | 0.7323 |
| 0.0721 | 6.3807 | 2782 | 0.5355 | 0.5832 | 0.5355 | 0.7318 |
| 0.0721 | 6.3853 | 2784 | 0.5183 | 0.5545 | 0.5183 | 0.7199 |
| 0.0721 | 6.3899 | 2786 | 0.4888 | 0.5545 | 0.4888 | 0.6991 |
| 0.0721 | 6.3945 | 2788 | 0.4665 | 0.5545 | 0.4665 | 0.6830 |
| 0.0721 | 6.3991 | 2790 | 0.4673 | 0.5545 | 0.4673 | 0.6836 |
| 0.0721 | 6.4037 | 2792 | 0.4820 | 0.5545 | 0.4820 | 0.6943 |
| 0.0721 | 6.4083 | 2794 | 0.5143 | 0.5545 | 0.5143 | 0.7171 |
| 0.0721 | 6.4128 | 2796 | 0.5471 | 0.5832 | 0.5471 | 0.7397 |
| 0.0721 | 6.4174 | 2798 | 0.5553 | 0.6123 | 0.5553 | 0.7452 |
| 0.0721 | 6.4220 | 2800 | 0.5382 | 0.5832 | 0.5382 | 0.7337 |
| 0.0721 | 6.4266 | 2802 | 0.5040 | 0.5545 | 0.5040 | 0.7099 |
| 0.0721 | 6.4312 | 2804 | 0.4892 | 0.5545 | 0.4892 | 0.6994 |
| 0.0721 | 6.4358 | 2806 | 0.4916 | 0.5545 | 0.4916 | 0.7011 |
| 0.0721 | 6.4404 | 2808 | 0.4853 | 0.5545 | 0.4853 | 0.6966 |
| 0.0721 | 6.4450 | 2810 | 0.4855 | 0.5545 | 0.4855 | 0.6967 |
| 0.0721 | 6.4495 | 2812 | 0.4862 | 0.5545 | 0.4862 | 0.6973 |
| 0.0721 | 6.4541 | 2814 | 0.4963 | 0.5545 | 0.4963 | 0.7045 |
| 0.0721 | 6.4587 | 2816 | 0.5033 | 0.5545 | 0.5033 | 0.7095 |
| 0.0721 | 6.4633 | 2818 | 0.5127 | 0.5545 | 0.5127 | 0.7161 |
| 0.0721 | 6.4679 | 2820 | 0.5189 | 0.5832 | 0.5189 | 0.7204 |
| 0.0721 | 6.4725 | 2822 | 0.5065 | 0.5832 | 0.5065 | 0.7117 |
| 0.0721 | 6.4771 | 2824 | 0.5057 | 0.5832 | 0.5057 | 0.7111 |
| 0.0721 | 6.4817 | 2826 | 0.4984 | 0.5832 | 0.4984 | 0.7060 |
| 0.0721 | 6.4862 | 2828 | 0.4855 | 0.5832 | 0.4855 | 0.6967 |
| 0.0721 | 6.4908 | 2830 | 0.4733 | 0.5545 | 0.4733 | 0.6879 |
| 0.0721 | 6.4954 | 2832 | 0.4593 | 0.5545 | 0.4593 | 0.6777 |
| 0.0721 | 6.5 | 2834 | 0.4548 | 0.5895 | 0.4548 | 0.6744 |
| 0.0721 | 6.5046 | 2836 | 0.4476 | 0.6866 | 0.4476 | 0.6690 |
| 0.0721 | 6.5092 | 2838 | 0.4472 | 0.6866 | 0.4472 | 0.6688 |
| 0.0721 | 6.5138 | 2840 | 0.4483 | 0.6866 | 0.4483 | 0.6696 |
| 0.0721 | 6.5183 | 2842 | 0.4539 | 0.6866 | 0.4539 | 0.6737 |
| 0.0721 | 6.5229 | 2844 | 0.4645 | 0.5895 | 0.4645 | 0.6816 |
| 0.0721 | 6.5275 | 2846 | 0.4823 | 0.5545 | 0.4823 | 0.6945 |
| 0.0721 | 6.5321 | 2848 | 0.4880 | 0.5545 | 0.4880 | 0.6986 |
| 0.0721 | 6.5367 | 2850 | 0.4766 | 0.5545 | 0.4766 | 0.6903 |
| 0.0721 | 6.5413 | 2852 | 0.4572 | 0.5545 | 0.4572 | 0.6761 |
| 0.0721 | 6.5459 | 2854 | 0.4508 | 0.5545 | 0.4508 | 0.6714 |
| 0.0721 | 6.5505 | 2856 | 0.4510 | 0.5545 | 0.4510 | 0.6716 |
| 0.0721 | 6.5550 | 2858 | 0.4633 | 0.5832 | 0.4633 | 0.6807 |
| 0.0721 | 6.5596 | 2860 | 0.4802 | 0.5832 | 0.4802 | 0.6930 |
| 0.0721 | 6.5642 | 2862 | 0.4871 | 0.5832 | 0.4871 | 0.6979 |
| 0.0721 | 6.5688 | 2864 | 0.4873 | 0.5545 | 0.4873 | 0.6981 |
| 0.0721 | 6.5734 | 2866 | 0.4796 | 0.5545 | 0.4796 | 0.6925 |
| 0.0721 | 6.5780 | 2868 | 0.4662 | 0.5895 | 0.4662 | 0.6828 |
| 0.0721 | 6.5826 | 2870 | 0.4612 | 0.5895 | 0.4612 | 0.6791 |
| 0.0721 | 6.5872 | 2872 | 0.4529 | 0.6866 | 0.4529 | 0.6730 |
| 0.0721 | 6.5917 | 2874 | 0.4529 | 0.6866 | 0.4529 | 0.6730 |
| 0.0721 | 6.5963 | 2876 | 0.4602 | 0.6866 | 0.4602 | 0.6784 |
| 0.0721 | 6.6009 | 2878 | 0.4734 | 0.5895 | 0.4734 | 0.6880 |
| 0.0721 | 6.6055 | 2880 | 0.4964 | 0.5545 | 0.4964 | 0.7046 |
| 0.0721 | 6.6101 | 2882 | 0.5238 | 0.5832 | 0.5238 | 0.7237 |
| 0.0721 | 6.6147 | 2884 | 0.5546 | 0.5832 | 0.5546 | 0.7447 |
| 0.0721 | 6.6193 | 2886 | 0.5585 | 0.5415 | 0.5585 | 0.7473 |
| 0.0721 | 6.6239 | 2888 | 0.5469 | 0.5477 | 0.5469 | 0.7395 |
| 0.0721 | 6.6284 | 2890 | 0.5399 | 0.5477 | 0.5399 | 0.7348 |
| 0.0721 | 6.6330 | 2892 | 0.5226 | 0.5545 | 0.5226 | 0.7229 |
| 0.0721 | 6.6376 | 2894 | 0.5135 | 0.5545 | 0.5135 | 0.7166 |
| 0.0721 | 6.6422 | 2896 | 0.4953 | 0.5545 | 0.4953 | 0.7038 |
| 0.0721 | 6.6468 | 2898 | 0.4900 | 0.5545 | 0.4900 | 0.7000 |
| 0.0721 | 6.6514 | 2900 | 0.4956 | 0.5545 | 0.4956 | 0.7040 |
| 0.0721 | 6.6560 | 2902 | 0.5206 | 0.5477 | 0.5206 | 0.7215 |
| 0.0721 | 6.6606 | 2904 | 0.5407 | 0.5477 | 0.5407 | 0.7353 |
| 0.0721 | 6.6651 | 2906 | 0.5380 | 0.5116 | 0.5380 | 0.7335 |
| 0.0721 | 6.6697 | 2908 | 0.5108 | 0.5477 | 0.5108 | 0.7147 |
| 0.0721 | 6.6743 | 2910 | 0.4800 | 0.5191 | 0.4800 | 0.6928 |
| 0.0721 | 6.6789 | 2912 | 0.4507 | 0.6526 | 0.4507 | 0.6713 |
| 0.0721 | 6.6835 | 2914 | 0.4401 | 0.6526 | 0.4401 | 0.6634 |
| 0.0721 | 6.6881 | 2916 | 0.4475 | 0.6526 | 0.4475 | 0.6690 |
| 0.0721 | 6.6927 | 2918 | 0.4686 | 0.6526 | 0.4686 | 0.6845 |
| 0.0721 | 6.6972 | 2920 | 0.5052 | 0.5545 | 0.5052 | 0.7108 |
| 0.0721 | 6.7018 | 2922 | 0.5404 | 0.5116 | 0.5404 | 0.7351 |
| 0.0721 | 6.7064 | 2924 | 0.5621 | 0.5116 | 0.5621 | 0.7497 |
| 0.0721 | 6.7110 | 2926 | 0.5972 | 0.375 | 0.5972 | 0.7728 |
| 0.0721 | 6.7156 | 2928 | 0.6104 | 0.375 | 0.6104 | 0.7813 |
| 0.0721 | 6.7202 | 2930 | 0.6070 | 0.375 | 0.6070 | 0.7791 |
| 0.0721 | 6.7248 | 2932 | 0.5957 | 0.4727 | 0.5957 | 0.7718 |
| 0.0721 | 6.7294 | 2934 | 0.5651 | 0.5116 | 0.5651 | 0.7517 |
| 0.0721 | 6.7339 | 2936 | 0.5370 | 0.5477 | 0.5370 | 0.7328 |
| 0.0721 | 6.7385 | 2938 | 0.5147 | 0.5832 | 0.5147 | 0.7174 |
| 0.0721 | 6.7431 | 2940 | 0.4953 | 0.5545 | 0.4953 | 0.7038 |
| 0.0721 | 6.7477 | 2942 | 0.4808 | 0.5545 | 0.4808 | 0.6934 |
| 0.0721 | 6.7523 | 2944 | 0.4763 | 0.5545 | 0.4763 | 0.6902 |
| 0.0721 | 6.7569 | 2946 | 0.4755 | 0.5545 | 0.4755 | 0.6895 |
| 0.0721 | 6.7615 | 2948 | 0.4852 | 0.5545 | 0.4852 | 0.6966 |
| 0.0721 | 6.7661 | 2950 | 0.5099 | 0.5832 | 0.5099 | 0.7141 |
| 0.0721 | 6.7706 | 2952 | 0.5471 | 0.5477 | 0.5471 | 0.7397 |
| 0.0721 | 6.7752 | 2954 | 0.5689 | 0.5477 | 0.5689 | 0.7543 |
| 0.0721 | 6.7798 | 2956 | 0.5718 | 0.5477 | 0.5718 | 0.7562 |
| 0.0721 | 6.7844 | 2958 | 0.5571 | 0.5477 | 0.5571 | 0.7464 |
| 0.0721 | 6.7890 | 2960 | 0.5432 | 0.5477 | 0.5432 | 0.7370 |
| 0.0721 | 6.7936 | 2962 | 0.5318 | 0.5477 | 0.5318 | 0.7293 |
| 0.0721 | 6.7982 | 2964 | 0.5386 | 0.5477 | 0.5386 | 0.7339 |
| 0.0721 | 6.8028 | 2966 | 0.5532 | 0.5477 | 0.5532 | 0.7438 |
| 0.0721 | 6.8073 | 2968 | 0.5625 | 0.5477 | 0.5625 | 0.7500 |
| 0.0721 | 6.8119 | 2970 | 0.5501 | 0.5477 | 0.5501 | 0.7417 |
| 0.0721 | 6.8165 | 2972 | 0.5422 | 0.5477 | 0.5422 | 0.7363 |
| 0.0721 | 6.8211 | 2974 | 0.5272 | 0.5832 | 0.5272 | 0.7261 |
| 0.0721 | 6.8257 | 2976 | 0.5224 | 0.5832 | 0.5224 | 0.7228 |
| 0.0721 | 6.8303 | 2978 | 0.5267 | 0.5832 | 0.5267 | 0.7257 |
| 0.0721 | 6.8349 | 2980 | 0.5455 | 0.5477 | 0.5455 | 0.7386 |
| 0.0721 | 6.8394 | 2982 | 0.5869 | 0.5477 | 0.5869 | 0.7661 |
| 0.0721 | 6.8440 | 2984 | 0.6276 | 0.4106 | 0.6276 | 0.7922 |
| 0.0721 | 6.8486 | 2986 | 0.6565 | 0.3569 | 0.6565 | 0.8102 |
| 0.0721 | 6.8532 | 2988 | 0.6666 | 0.3569 | 0.6666 | 0.8165 |
| 0.0721 | 6.8578 | 2990 | 0.6421 | 0.4106 | 0.6421 | 0.8013 |
| 0.0721 | 6.8624 | 2992 | 0.6107 | 0.5073 | 0.6107 | 0.7815 |
| 0.0721 | 6.8670 | 2994 | 0.5947 | 0.5415 | 0.5947 | 0.7712 |
| 0.0721 | 6.8716 | 2996 | 0.5672 | 0.5477 | 0.5672 | 0.7531 |
| 0.0721 | 6.8761 | 2998 | 0.5503 | 0.5832 | 0.5503 | 0.7418 |
| 0.0619 | 6.8807 | 3000 | 0.5356 | 0.5832 | 0.5356 | 0.7319 |
| 0.0619 | 6.8853 | 3002 | 0.5234 | 0.5832 | 0.5234 | 0.7235 |
| 0.0619 | 6.8899 | 3004 | 0.5180 | 0.5832 | 0.5180 | 0.7197 |
| 0.0619 | 6.8945 | 3006 | 0.5153 | 0.5832 | 0.5153 | 0.7178 |
| 0.0619 | 6.8991 | 3008 | 0.5357 | 0.5477 | 0.5357 | 0.7319 |
| 0.0619 | 6.9037 | 3010 | 0.5486 | 0.5116 | 0.5486 | 0.7407 |
| 0.0619 | 6.9083 | 3012 | 0.5494 | 0.5477 | 0.5494 | 0.7412 |
| 0.0619 | 6.9128 | 3014 | 0.5291 | 0.5832 | 0.5291 | 0.7274 |
| 0.0619 | 6.9174 | 3016 | 0.5099 | 0.5832 | 0.5099 | 0.7141 |
| 0.0619 | 6.9220 | 3018 | 0.4911 | 0.5545 | 0.4911 | 0.7008 |
| 0.0619 | 6.9266 | 3020 | 0.4780 | 0.5545 | 0.4780 | 0.6914 |
| 0.0619 | 6.9312 | 3022 | 0.4811 | 0.5545 | 0.4811 | 0.6936 |
| 0.0619 | 6.9358 | 3024 | 0.4890 | 0.5545 | 0.4890 | 0.6993 |
| 0.0619 | 6.9404 | 3026 | 0.5005 | 0.5832 | 0.5005 | 0.7075 |
| 0.0619 | 6.9450 | 3028 | 0.5146 | 0.5832 | 0.5146 | 0.7173 |
| 0.0619 | 6.9495 | 3030 | 0.5197 | 0.5832 | 0.5197 | 0.7209 |
| 0.0619 | 6.9541 | 3032 | 0.5163 | 0.5832 | 0.5163 | 0.7185 |
| 0.0619 | 6.9587 | 3034 | 0.5073 | 0.5832 | 0.5073 | 0.7123 |
| 0.0619 | 6.9633 | 3036 | 0.5151 | 0.5832 | 0.5151 | 0.7177 |
| 0.0619 | 6.9679 | 3038 | 0.5248 | 0.5477 | 0.5248 | 0.7244 |
| 0.0619 | 6.9725 | 3040 | 0.5379 | 0.5477 | 0.5379 | 0.7334 |
| 0.0619 | 6.9771 | 3042 | 0.5402 | 0.5477 | 0.5402 | 0.7350 |
| 0.0619 | 6.9817 | 3044 | 0.5378 | 0.5477 | 0.5378 | 0.7333 |
| 0.0619 | 6.9862 | 3046 | 0.5234 | 0.5477 | 0.5234 | 0.7235 |
| 0.0619 | 6.9908 | 3048 | 0.5075 | 0.5832 | 0.5075 | 0.7124 |
| 0.0619 | 6.9954 | 3050 | 0.4950 | 0.5832 | 0.4950 | 0.7035 |
| 0.0619 | 7.0 | 3052 | 0.4971 | 0.5832 | 0.4971 | 0.7050 |
| 0.0619 | 7.0046 | 3054 | 0.4993 | 0.5832 | 0.4993 | 0.7066 |
| 0.0619 | 7.0092 | 3056 | 0.5031 | 0.5477 | 0.5031 | 0.7093 |
| 0.0619 | 7.0138 | 3058 | 0.5122 | 0.5477 | 0.5122 | 0.7157 |
| 0.0619 | 7.0183 | 3060 | 0.5132 | 0.5477 | 0.5132 | 0.7164 |
| 0.0619 | 7.0229 | 3062 | 0.5025 | 0.5477 | 0.5025 | 0.7089 |
| 0.0619 | 7.0275 | 3064 | 0.4989 | 0.5477 | 0.4989 | 0.7063 |
| 0.0619 | 7.0321 | 3066 | 0.4848 | 0.5477 | 0.4848 | 0.6963 |
| 0.0619 | 7.0367 | 3068 | 0.4799 | 0.5477 | 0.4799 | 0.6927 |
| 0.0619 | 7.0413 | 3070 | 0.4802 | 0.5832 | 0.4802 | 0.6930 |
| 0.0619 | 7.0459 | 3072 | 0.4818 | 0.5832 | 0.4818 | 0.6942 |
| 0.0619 | 7.0505 | 3074 | 0.4791 | 0.6866 | 0.4791 | 0.6922 |
| 0.0619 | 7.0550 | 3076 | 0.4873 | 0.6866 | 0.4873 | 0.6980 |
| 0.0619 | 7.0596 | 3078 | 0.5069 | 0.5832 | 0.5069 | 0.7120 |
| 0.0619 | 7.0642 | 3080 | 0.5313 | 0.5832 | 0.5313 | 0.7289 |
| 0.0619 | 7.0688 | 3082 | 0.5598 | 0.5073 | 0.5598 | 0.7482 |
| 0.0619 | 7.0734 | 3084 | 0.5862 | 0.5073 | 0.5862 | 0.7657 |
| 0.0619 | 7.0780 | 3086 | 0.5895 | 0.4106 | 0.5895 | 0.7678 |
| 0.0619 | 7.0826 | 3088 | 0.5709 | 0.5073 | 0.5709 | 0.7556 |
| 0.0619 | 7.0872 | 3090 | 0.5513 | 0.5116 | 0.5513 | 0.7425 |
| 0.0619 | 7.0917 | 3092 | 0.5271 | 0.5832 | 0.5271 | 0.7260 |
| 0.0619 | 7.0963 | 3094 | 0.5159 | 0.5832 | 0.5159 | 0.7183 |
| 0.0619 | 7.1009 | 3096 | 0.5163 | 0.5832 | 0.5163 | 0.7185 |
| 0.0619 | 7.1055 | 3098 | 0.5261 | 0.5832 | 0.5261 | 0.7253 |
| 0.0619 | 7.1101 | 3100 | 0.5442 | 0.5116 | 0.5442 | 0.7377 |
| 0.0619 | 7.1147 | 3102 | 0.5441 | 0.5477 | 0.5441 | 0.7376 |
| 0.0619 | 7.1193 | 3104 | 0.5361 | 0.5832 | 0.5361 | 0.7322 |
| 0.0619 | 7.1239 | 3106 | 0.5453 | 0.5832 | 0.5453 | 0.7385 |
| 0.0619 | 7.1284 | 3108 | 0.5610 | 0.5477 | 0.5610 | 0.7490 |
| 0.0619 | 7.1330 | 3110 | 0.5866 | 0.5415 | 0.5866 | 0.7659 |
| 0.0619 | 7.1376 | 3112 | 0.6062 | 0.5073 | 0.6062 | 0.7786 |
| 0.0619 | 7.1422 | 3114 | 0.6064 | 0.5073 | 0.6064 | 0.7787 |
| 0.0619 | 7.1468 | 3116 | 0.5944 | 0.5415 | 0.5944 | 0.7710 |
| 0.0619 | 7.1514 | 3118 | 0.5852 | 0.5477 | 0.5852 | 0.7650 |
| 0.0619 | 7.1560 | 3120 | 0.5793 | 0.5477 | 0.5793 | 0.7611 |
| 0.0619 | 7.1606 | 3122 | 0.5583 | 0.5832 | 0.5583 | 0.7472 |
| 0.0619 | 7.1651 | 3124 | 0.5491 | 0.5832 | 0.5491 | 0.7410 |
| 0.0619 | 7.1697 | 3126 | 0.5448 | 0.5832 | 0.5448 | 0.7381 |
| 0.0619 | 7.1743 | 3128 | 0.5487 | 0.5832 | 0.5487 | 0.7408 |
| 0.0619 | 7.1789 | 3130 | 0.5577 | 0.5477 | 0.5577 | 0.7468 |
| 0.0619 | 7.1835 | 3132 | 0.5726 | 0.5477 | 0.5726 | 0.7567 |
| 0.0619 | 7.1881 | 3134 | 0.5835 | 0.4094 | 0.5835 | 0.7639 |
| 0.0619 | 7.1927 | 3136 | 0.5839 | 0.4094 | 0.5839 | 0.7641 |
| 0.0619 | 7.1972 | 3138 | 0.5760 | 0.4094 | 0.5760 | 0.7589 |
| 0.0619 | 7.2018 | 3140 | 0.5697 | 0.5116 | 0.5697 | 0.7548 |
| 0.0619 | 7.2064 | 3142 | 0.5738 | 0.4094 | 0.5738 | 0.7575 |
| 0.0619 | 7.2110 | 3144 | 0.5650 | 0.5477 | 0.5650 | 0.7517 |
| 0.0619 | 7.2156 | 3146 | 0.5554 | 0.5477 | 0.5554 | 0.7452 |
| 0.0619 | 7.2202 | 3148 | 0.5551 | 0.5477 | 0.5551 | 0.7451 |
| 0.0619 | 7.2248 | 3150 | 0.5592 | 0.5477 | 0.5592 | 0.7478 |
| 0.0619 | 7.2294 | 3152 | 0.5596 | 0.5477 | 0.5596 | 0.7481 |
| 0.0619 | 7.2339 | 3154 | 0.5498 | 0.5477 | 0.5498 | 0.7415 |
| 0.0619 | 7.2385 | 3156 | 0.5445 | 0.5477 | 0.5445 | 0.7379 |
| 0.0619 | 7.2431 | 3158 | 0.5354 | 0.5477 | 0.5354 | 0.7317 |
| 0.0619 | 7.2477 | 3160 | 0.5349 | 0.5477 | 0.5349 | 0.7313 |
| 0.0619 | 7.2523 | 3162 | 0.5360 | 0.5477 | 0.5360 | 0.7321 |
| 0.0619 | 7.2569 | 3164 | 0.5420 | 0.5477 | 0.5420 | 0.7362 |
| 0.0619 | 7.2615 | 3166 | 0.5600 | 0.5477 | 0.5600 | 0.7483 |
| 0.0619 | 7.2661 | 3168 | 0.5739 | 0.5477 | 0.5739 | 0.7576 |
| 0.0619 | 7.2706 | 3170 | 0.5888 | 0.5415 | 0.5888 | 0.7674 |
| 0.0619 | 7.2752 | 3172 | 0.5792 | 0.5415 | 0.5792 | 0.7611 |
| 0.0619 | 7.2798 | 3174 | 0.5582 | 0.5477 | 0.5582 | 0.7471 |
| 0.0619 | 7.2844 | 3176 | 0.5404 | 0.5477 | 0.5404 | 0.7351 |
| 0.0619 | 7.2890 | 3178 | 0.5254 | 0.5477 | 0.5254 | 0.7248 |
| 0.0619 | 7.2936 | 3180 | 0.5242 | 0.5477 | 0.5242 | 0.7240 |
| 0.0619 | 7.2982 | 3182 | 0.5308 | 0.5477 | 0.5308 | 0.7286 |
| 0.0619 | 7.3028 | 3184 | 0.5448 | 0.5477 | 0.5448 | 0.7381 |
| 0.0619 | 7.3073 | 3186 | 0.5588 | 0.5477 | 0.5588 | 0.7475 |
| 0.0619 | 7.3119 | 3188 | 0.5809 | 0.5347 | 0.5809 | 0.7622 |
| 0.0619 | 7.3165 | 3190 | 0.5937 | 0.5347 | 0.5937 | 0.7705 |
| 0.0619 | 7.3211 | 3192 | 0.5940 | 0.5347 | 0.5940 | 0.7707 |
| 0.0619 | 7.3257 | 3194 | 0.5769 | 0.5689 | 0.5769 | 0.7596 |
| 0.0619 | 7.3303 | 3196 | 0.5653 | 0.5415 | 0.5653 | 0.7519 |
| 0.0619 | 7.3349 | 3198 | 0.5483 | 0.5415 | 0.5483 | 0.7405 |
| 0.0619 | 7.3394 | 3200 | 0.5188 | 0.5477 | 0.5188 | 0.7202 |
| 0.0619 | 7.3440 | 3202 | 0.4926 | 0.5477 | 0.4926 | 0.7018 |
| 0.0619 | 7.3486 | 3204 | 0.4775 | 0.5191 | 0.4775 | 0.6910 |
| 0.0619 | 7.3532 | 3206 | 0.4740 | 0.6526 | 0.4740 | 0.6884 |
| 0.0619 | 7.3578 | 3208 | 0.4792 | 0.6526 | 0.4792 | 0.6923 |
| 0.0619 | 7.3624 | 3210 | 0.4918 | 0.5545 | 0.4918 | 0.7013 |
| 0.0619 | 7.3670 | 3212 | 0.5156 | 0.5191 | 0.5156 | 0.7180 |
| 0.0619 | 7.3716 | 3214 | 0.5422 | 0.5415 | 0.5422 | 0.7364 |
| 0.0619 | 7.3761 | 3216 | 0.5573 | 0.5415 | 0.5573 | 0.7465 |
| 0.0619 | 7.3807 | 3218 | 0.5735 | 0.5415 | 0.5735 | 0.7573 |
| 0.0619 | 7.3853 | 3220 | 0.5806 | 0.5415 | 0.5806 | 0.7620 |
| 0.0619 | 7.3899 | 3222 | 0.5716 | 0.5145 | 0.5716 | 0.7560 |
| 0.0619 | 7.3945 | 3224 | 0.5685 | 0.5145 | 0.5685 | 0.7540 |
| 0.0619 | 7.3991 | 3226 | 0.5639 | 0.5145 | 0.5639 | 0.7509 |
| 0.0619 | 7.4037 | 3228 | 0.5630 | 0.5145 | 0.5630 | 0.7503 |
| 0.0619 | 7.4083 | 3230 | 0.5520 | 0.5145 | 0.5520 | 0.7429 |
| 0.0619 | 7.4128 | 3232 | 0.5267 | 0.5191 | 0.5267 | 0.7257 |
| 0.0619 | 7.4174 | 3234 | 0.5161 | 0.5191 | 0.5161 | 0.7184 |
| 0.0619 | 7.4220 | 3236 | 0.5131 | 0.5191 | 0.5131 | 0.7163 |
| 0.0619 | 7.4266 | 3238 | 0.5121 | 0.5191 | 0.5121 | 0.7156 |
| 0.0619 | 7.4312 | 3240 | 0.5180 | 0.5191 | 0.5180 | 0.7197 |
| 0.0619 | 7.4358 | 3242 | 0.5155 | 0.5191 | 0.5155 | 0.7180 |
| 0.0619 | 7.4404 | 3244 | 0.5118 | 0.5191 | 0.5118 | 0.7154 |
| 0.0619 | 7.4450 | 3246 | 0.5045 | 0.5191 | 0.5045 | 0.7103 |
| 0.0619 | 7.4495 | 3248 | 0.5045 | 0.5191 | 0.5045 | 0.7103 |
| 0.0619 | 7.4541 | 3250 | 0.5086 | 0.5191 | 0.5086 | 0.7132 |
| 0.0619 | 7.4587 | 3252 | 0.5237 | 0.5191 | 0.5237 | 0.7237 |
| 0.0619 | 7.4633 | 3254 | 0.5350 | 0.5477 | 0.5350 | 0.7314 |
| 0.0619 | 7.4679 | 3256 | 0.5511 | 0.5477 | 0.5511 | 0.7424 |
| 0.0619 | 7.4725 | 3258 | 0.5670 | 0.5415 | 0.5670 | 0.7530 |
| 0.0619 | 7.4771 | 3260 | 0.5845 | 0.5415 | 0.5845 | 0.7645 |
| 0.0619 | 7.4817 | 3262 | 0.5820 | 0.5415 | 0.5820 | 0.7629 |
| 0.0619 | 7.4862 | 3264 | 0.5666 | 0.5415 | 0.5666 | 0.7527 |
| 0.0619 | 7.4908 | 3266 | 0.5450 | 0.5415 | 0.5450 | 0.7382 |
| 0.0619 | 7.4954 | 3268 | 0.5186 | 0.5191 | 0.5186 | 0.7201 |
| 0.0619 | 7.5 | 3270 | 0.4993 | 0.5545 | 0.4993 | 0.7066 |
| 0.0619 | 7.5046 | 3272 | 0.4904 | 0.6526 | 0.4904 | 0.7003 |
| 0.0619 | 7.5092 | 3274 | 0.4904 | 0.6526 | 0.4904 | 0.7003 |
| 0.0619 | 7.5138 | 3276 | 0.4959 | 0.5545 | 0.4959 | 0.7042 |
| 0.0619 | 7.5183 | 3278 | 0.5025 | 0.5545 | 0.5025 | 0.7089 |
| 0.0619 | 7.5229 | 3280 | 0.5185 | 0.5545 | 0.5185 | 0.7201 |
| 0.0619 | 7.5275 | 3282 | 0.5365 | 0.5415 | 0.5365 | 0.7325 |
| 0.0619 | 7.5321 | 3284 | 0.5446 | 0.5415 | 0.5446 | 0.7380 |
| 0.0619 | 7.5367 | 3286 | 0.5479 | 0.5415 | 0.5479 | 0.7402 |
| 0.0619 | 7.5413 | 3288 | 0.5491 | 0.5415 | 0.5491 | 0.7410 |
| 0.0619 | 7.5459 | 3290 | 0.5442 | 0.5145 | 0.5442 | 0.7377 |
| 0.0619 | 7.5505 | 3292 | 0.5492 | 0.5415 | 0.5492 | 0.7411 |
| 0.0619 | 7.5550 | 3294 | 0.5566 | 0.5415 | 0.5566 | 0.7461 |
| 0.0619 | 7.5596 | 3296 | 0.5571 | 0.5415 | 0.5571 | 0.7464 |
| 0.0619 | 7.5642 | 3298 | 0.5530 | 0.5415 | 0.5530 | 0.7436 |
| 0.0619 | 7.5688 | 3300 | 0.5498 | 0.5415 | 0.5498 | 0.7415 |
| 0.0619 | 7.5734 | 3302 | 0.5459 | 0.5415 | 0.5459 | 0.7388 |
| 0.0619 | 7.5780 | 3304 | 0.5465 | 0.5477 | 0.5465 | 0.7393 |
| 0.0619 | 7.5826 | 3306 | 0.5478 | 0.5832 | 0.5478 | 0.7401 |
| 0.0619 | 7.5872 | 3308 | 0.5482 | 0.5832 | 0.5482 | 0.7404 |
| 0.0619 | 7.5917 | 3310 | 0.5406 | 0.5545 | 0.5406 | 0.7353 |
| 0.0619 | 7.5963 | 3312 | 0.5306 | 0.5545 | 0.5306 | 0.7284 |
| 0.0619 | 7.6009 | 3314 | 0.5323 | 0.5545 | 0.5323 | 0.7296 |
| 0.0619 | 7.6055 | 3316 | 0.5402 | 0.5832 | 0.5402 | 0.7350 |
| 0.0619 | 7.6101 | 3318 | 0.5365 | 0.5545 | 0.5365 | 0.7325 |
| 0.0619 | 7.6147 | 3320 | 0.5374 | 0.5832 | 0.5374 | 0.7331 |
| 0.0619 | 7.6193 | 3322 | 0.5427 | 0.5832 | 0.5427 | 0.7367 |
| 0.0619 | 7.6239 | 3324 | 0.5500 | 0.5477 | 0.5500 | 0.7416 |
| 0.0619 | 7.6284 | 3326 | 0.5658 | 0.5477 | 0.5658 | 0.7522 |
| 0.0619 | 7.6330 | 3328 | 0.5945 | 0.5415 | 0.5945 | 0.7710 |
| 0.0619 | 7.6376 | 3330 | 0.6110 | 0.4457 | 0.6110 | 0.7817 |
| 0.0619 | 7.6422 | 3332 | 0.6037 | 0.5415 | 0.6037 | 0.7770 |
| 0.0619 | 7.6468 | 3334 | 0.5813 | 0.5415 | 0.5813 | 0.7624 |
| 0.0619 | 7.6514 | 3336 | 0.5556 | 0.5477 | 0.5556 | 0.7454 |
| 0.0619 | 7.6560 | 3338 | 0.5297 | 0.5477 | 0.5297 | 0.7278 |
| 0.0619 | 7.6606 | 3340 | 0.5158 | 0.5545 | 0.5158 | 0.7182 |
| 0.0619 | 7.6651 | 3342 | 0.5027 | 0.5545 | 0.5027 | 0.7090 |
| 0.0619 | 7.6697 | 3344 | 0.4993 | 0.5545 | 0.4993 | 0.7066 |
| 0.0619 | 7.6743 | 3346 | 0.5056 | 0.5545 | 0.5056 | 0.7111 |
| 0.0619 | 7.6789 | 3348 | 0.5161 | 0.5545 | 0.5161 | 0.7184 |
| 0.0619 | 7.6835 | 3350 | 0.5182 | 0.5832 | 0.5182 | 0.7199 |
| 0.0619 | 7.6881 | 3352 | 0.5252 | 0.5477 | 0.5252 | 0.7247 |
| 0.0619 | 7.6927 | 3354 | 0.5229 | 0.5477 | 0.5229 | 0.7231 |
| 0.0619 | 7.6972 | 3356 | 0.5230 | 0.5477 | 0.5230 | 0.7232 |
| 0.0619 | 7.7018 | 3358 | 0.5230 | 0.5477 | 0.5230 | 0.7232 |
| 0.0619 | 7.7064 | 3360 | 0.5310 | 0.5477 | 0.5310 | 0.7287 |
| 0.0619 | 7.7110 | 3362 | 0.5288 | 0.5477 | 0.5288 | 0.7272 |
| 0.0619 | 7.7156 | 3364 | 0.5323 | 0.5477 | 0.5323 | 0.7296 |
| 0.0619 | 7.7202 | 3366 | 0.5495 | 0.5477 | 0.5495 | 0.7413 |
| 0.0619 | 7.7248 | 3368 | 0.5726 | 0.5415 | 0.5726 | 0.7567 |
| 0.0619 | 7.7294 | 3370 | 0.5826 | 0.5415 | 0.5826 | 0.7633 |
| 0.0619 | 7.7339 | 3372 | 0.5802 | 0.5415 | 0.5802 | 0.7617 |
| 0.0619 | 7.7385 | 3374 | 0.5655 | 0.5477 | 0.5655 | 0.7520 |
| 0.0619 | 7.7431 | 3376 | 0.5446 | 0.5477 | 0.5446 | 0.7380 |
| 0.0619 | 7.7477 | 3378 | 0.5249 | 0.5477 | 0.5249 | 0.7245 |
| 0.0619 | 7.7523 | 3380 | 0.5042 | 0.5545 | 0.5042 | 0.7101 |
| 0.0619 | 7.7569 | 3382 | 0.4940 | 0.5545 | 0.4940 | 0.7029 |
| 0.0619 | 7.7615 | 3384 | 0.4889 | 0.5545 | 0.4889 | 0.6992 |
| 0.0619 | 7.7661 | 3386 | 0.4892 | 0.5545 | 0.4892 | 0.6994 |
| 0.0619 | 7.7706 | 3388 | 0.4928 | 0.5545 | 0.4928 | 0.7020 |
| 0.0619 | 7.7752 | 3390 | 0.4982 | 0.5545 | 0.4982 | 0.7058 |
| 0.0619 | 7.7798 | 3392 | 0.5088 | 0.5545 | 0.5088 | 0.7133 |
| 0.0619 | 7.7844 | 3394 | 0.5193 | 0.5545 | 0.5193 | 0.7206 |
| 0.0619 | 7.7890 | 3396 | 0.5277 | 0.5545 | 0.5277 | 0.7264 |
| 0.0619 | 7.7936 | 3398 | 0.5328 | 0.5545 | 0.5328 | 0.7299 |
| 0.0619 | 7.7982 | 3400 | 0.5272 | 0.5545 | 0.5272 | 0.7261 |
| 0.0619 | 7.8028 | 3402 | 0.5173 | 0.5895 | 0.5173 | 0.7193 |
| 0.0619 | 7.8073 | 3404 | 0.5092 | 0.6866 | 0.5092 | 0.7136 |
| 0.0619 | 7.8119 | 3406 | 0.5067 | 0.6866 | 0.5067 | 0.7118 |
| 0.0619 | 7.8165 | 3408 | 0.5056 | 0.6866 | 0.5056 | 0.7111 |
| 0.0619 | 7.8211 | 3410 | 0.5090 | 0.6866 | 0.5090 | 0.7135 |
| 0.0619 | 7.8257 | 3412 | 0.5192 | 0.5895 | 0.5192 | 0.7205 |
| 0.0619 | 7.8303 | 3414 | 0.5307 | 0.5545 | 0.5307 | 0.7285 |
| 0.0619 | 7.8349 | 3416 | 0.5417 | 0.5545 | 0.5417 | 0.7360 |
| 0.0619 | 7.8394 | 3418 | 0.5585 | 0.5832 | 0.5585 | 0.7473 |
| 0.0619 | 7.8440 | 3420 | 0.5688 | 0.5477 | 0.5688 | 0.7542 |
| 0.0619 | 7.8486 | 3422 | 0.5678 | 0.5832 | 0.5678 | 0.7535 |
| 0.0619 | 7.8532 | 3424 | 0.5562 | 0.5832 | 0.5562 | 0.7458 |
| 0.0619 | 7.8578 | 3426 | 0.5417 | 0.5545 | 0.5417 | 0.7360 |
| 0.0619 | 7.8624 | 3428 | 0.5249 | 0.5545 | 0.5249 | 0.7245 |
| 0.0619 | 7.8670 | 3430 | 0.5159 | 0.6526 | 0.5159 | 0.7182 |
| 0.0619 | 7.8716 | 3432 | 0.5146 | 0.6526 | 0.5146 | 0.7174 |
| 0.0619 | 7.8761 | 3434 | 0.5161 | 0.6526 | 0.5161 | 0.7184 |
| 0.0619 | 7.8807 | 3436 | 0.5224 | 0.5545 | 0.5224 | 0.7228 |
| 0.0619 | 7.8853 | 3438 | 0.5334 | 0.5545 | 0.5334 | 0.7303 |
| 0.0619 | 7.8899 | 3440 | 0.5466 | 0.5477 | 0.5466 | 0.7393 |
| 0.0619 | 7.8945 | 3442 | 0.5516 | 0.5477 | 0.5516 | 0.7427 |
| 0.0619 | 7.8991 | 3444 | 0.5493 | 0.5477 | 0.5493 | 0.7412 |
| 0.0619 | 7.9037 | 3446 | 0.5505 | 0.5477 | 0.5505 | 0.7420 |
| 0.0619 | 7.9083 | 3448 | 0.5534 | 0.5477 | 0.5534 | 0.7439 |
| 0.0619 | 7.9128 | 3450 | 0.5510 | 0.5477 | 0.5510 | 0.7423 |
| 0.0619 | 7.9174 | 3452 | 0.5557 | 0.5477 | 0.5557 | 0.7454 |
| 0.0619 | 7.9220 | 3454 | 0.5491 | 0.5477 | 0.5491 | 0.7410 |
| 0.0619 | 7.9266 | 3456 | 0.5507 | 0.5477 | 0.5507 | 0.7421 |
| 0.0619 | 7.9312 | 3458 | 0.5561 | 0.5477 | 0.5561 | 0.7457 |
| 0.0619 | 7.9358 | 3460 | 0.5529 | 0.5477 | 0.5529 | 0.7436 |
| 0.0619 | 7.9404 | 3462 | 0.5461 | 0.5477 | 0.5461 | 0.7390 |
| 0.0619 | 7.9450 | 3464 | 0.5370 | 0.5545 | 0.5370 | 0.7328 |
| 0.0619 | 7.9495 | 3466 | 0.5296 | 0.5545 | 0.5296 | 0.7278 |
| 0.0619 | 7.9541 | 3468 | 0.5245 | 0.5545 | 0.5245 | 0.7242 |
| 0.0619 | 7.9587 | 3470 | 0.5288 | 0.5545 | 0.5288 | 0.7272 |
| 0.0619 | 7.9633 | 3472 | 0.5359 | 0.5545 | 0.5359 | 0.7320 |
| 0.0619 | 7.9679 | 3474 | 0.5463 | 0.5545 | 0.5463 | 0.7391 |
| 0.0619 | 7.9725 | 3476 | 0.5539 | 0.5545 | 0.5539 | 0.7442 |
| 0.0619 | 7.9771 | 3478 | 0.5656 | 0.5545 | 0.5656 | 0.7520 |
| 0.0619 | 7.9817 | 3480 | 0.5856 | 0.5477 | 0.5856 | 0.7652 |
| 0.0619 | 7.9862 | 3482 | 0.6126 | 0.5477 | 0.6126 | 0.7827 |
| 0.0619 | 7.9908 | 3484 | 0.6300 | 0.5415 | 0.6300 | 0.7938 |
| 0.0619 | 7.9954 | 3486 | 0.6324 | 0.4457 | 0.6324 | 0.7952 |
| 0.0619 | 8.0 | 3488 | 0.6217 | 0.5415 | 0.6217 | 0.7885 |
| 0.0619 | 8.0046 | 3490 | 0.6172 | 0.5477 | 0.6172 | 0.7856 |
| 0.0619 | 8.0092 | 3492 | 0.6103 | 0.5477 | 0.6103 | 0.7812 |
| 0.0619 | 8.0138 | 3494 | 0.6086 | 0.5477 | 0.6086 | 0.7801 |
| 0.0619 | 8.0183 | 3496 | 0.5971 | 0.5477 | 0.5971 | 0.7727 |
| 0.0619 | 8.0229 | 3498 | 0.5815 | 0.5477 | 0.5815 | 0.7625 |
| 0.0523 | 8.0275 | 3500 | 0.5727 | 0.5477 | 0.5727 | 0.7568 |
| 0.0523 | 8.0321 | 3502 | 0.5640 | 0.5545 | 0.5640 | 0.7510 |
| 0.0523 | 8.0367 | 3504 | 0.5646 | 0.5545 | 0.5646 | 0.7514 |
| 0.0523 | 8.0413 | 3506 | 0.5681 | 0.5477 | 0.5681 | 0.7537 |
| 0.0523 | 8.0459 | 3508 | 0.5728 | 0.5477 | 0.5728 | 0.7569 |
| 0.0523 | 8.0505 | 3510 | 0.5670 | 0.5477 | 0.5670 | 0.7530 |
| 0.0523 | 8.0550 | 3512 | 0.5649 | 0.5477 | 0.5649 | 0.7516 |
| 0.0523 | 8.0596 | 3514 | 0.5718 | 0.5477 | 0.5718 | 0.7561 |
| 0.0523 | 8.0642 | 3516 | 0.5727 | 0.5477 | 0.5727 | 0.7568 |
| 0.0523 | 8.0688 | 3518 | 0.5703 | 0.5477 | 0.5703 | 0.7552 |
| 0.0523 | 8.0734 | 3520 | 0.5568 | 0.5477 | 0.5568 | 0.7462 |
| 0.0523 | 8.0780 | 3522 | 0.5456 | 0.5477 | 0.5456 | 0.7386 |
| 0.0523 | 8.0826 | 3524 | 0.5436 | 0.5477 | 0.5436 | 0.7373 |
| 0.0523 | 8.0872 | 3526 | 0.5448 | 0.5477 | 0.5448 | 0.7381 |
| 0.0523 | 8.0917 | 3528 | 0.5405 | 0.5477 | 0.5405 | 0.7352 |
| 0.0523 | 8.0963 | 3530 | 0.5352 | 0.5832 | 0.5352 | 0.7316 |
| 0.0523 | 8.1009 | 3532 | 0.5340 | 0.5545 | 0.5340 | 0.7307 |
| 0.0523 | 8.1055 | 3534 | 0.5243 | 0.5545 | 0.5243 | 0.7241 |
| 0.0523 | 8.1101 | 3536 | 0.5183 | 0.5545 | 0.5183 | 0.7199 |
| 0.0523 | 8.1147 | 3538 | 0.5191 | 0.5545 | 0.5191 | 0.7205 |
| 0.0523 | 8.1193 | 3540 | 0.5269 | 0.5545 | 0.5269 | 0.7259 |
| 0.0523 | 8.1239 | 3542 | 0.5386 | 0.5545 | 0.5386 | 0.7339 |
| 0.0523 | 8.1284 | 3544 | 0.5523 | 0.5191 | 0.5523 | 0.7432 |
| 0.0523 | 8.1330 | 3546 | 0.5610 | 0.5477 | 0.5610 | 0.7490 |
| 0.0523 | 8.1376 | 3548 | 0.5648 | 0.5477 | 0.5648 | 0.7515 |
| 0.0523 | 8.1422 | 3550 | 0.5645 | 0.5477 | 0.5645 | 0.7513 |
| 0.0523 | 8.1468 | 3552 | 0.5635 | 0.5477 | 0.5635 | 0.7507 |
| 0.0523 | 8.1514 | 3554 | 0.5564 | 0.5477 | 0.5564 | 0.7459 |
| 0.0523 | 8.1560 | 3556 | 0.5483 | 0.5477 | 0.5483 | 0.7404 |
| 0.0523 | 8.1606 | 3558 | 0.5448 | 0.5191 | 0.5448 | 0.7381 |
| 0.0523 | 8.1651 | 3560 | 0.5353 | 0.5191 | 0.5353 | 0.7317 |
| 0.0523 | 8.1697 | 3562 | 0.5297 | 0.5191 | 0.5297 | 0.7278 |
| 0.0523 | 8.1743 | 3564 | 0.5252 | 0.5191 | 0.5252 | 0.7247 |
| 0.0523 | 8.1789 | 3566 | 0.5213 | 0.5191 | 0.5213 | 0.7220 |
| 0.0523 | 8.1835 | 3568 | 0.5188 | 0.5191 | 0.5188 | 0.7203 |
| 0.0523 | 8.1881 | 3570 | 0.5220 | 0.5191 | 0.5220 | 0.7225 |
| 0.0523 | 8.1927 | 3572 | 0.5295 | 0.5191 | 0.5295 | 0.7276 |
| 0.0523 | 8.1972 | 3574 | 0.5351 | 0.5191 | 0.5351 | 0.7315 |
| 0.0523 | 8.2018 | 3576 | 0.5346 | 0.5191 | 0.5346 | 0.7312 |
| 0.0523 | 8.2064 | 3578 | 0.5317 | 0.5191 | 0.5317 | 0.7292 |
| 0.0523 | 8.2110 | 3580 | 0.5300 | 0.5191 | 0.5300 | 0.7280 |
| 0.0523 | 8.2156 | 3582 | 0.5337 | 0.5191 | 0.5337 | 0.7306 |
| 0.0523 | 8.2202 | 3584 | 0.5334 | 0.5191 | 0.5334 | 0.7303 |
| 0.0523 | 8.2248 | 3586 | 0.5300 | 0.5191 | 0.5300 | 0.7280 |
| 0.0523 | 8.2294 | 3588 | 0.5240 | 0.5191 | 0.5240 | 0.7239 |
| 0.0523 | 8.2339 | 3590 | 0.5265 | 0.5191 | 0.5265 | 0.7256 |
| 0.0523 | 8.2385 | 3592 | 0.5281 | 0.5191 | 0.5281 | 0.7267 |
| 0.0523 | 8.2431 | 3594 | 0.5231 | 0.5191 | 0.5231 | 0.7233 |
| 0.0523 | 8.2477 | 3596 | 0.5142 | 0.5191 | 0.5142 | 0.7170 |
| 0.0523 | 8.2523 | 3598 | 0.5091 | 0.5191 | 0.5091 | 0.7135 |
| 0.0523 | 8.2569 | 3600 | 0.5098 | 0.5191 | 0.5098 | 0.7140 |
| 0.0523 | 8.2615 | 3602 | 0.5151 | 0.5191 | 0.5151 | 0.7177 |
| 0.0523 | 8.2661 | 3604 | 0.5218 | 0.4831 | 0.5218 | 0.7224 |
| 0.0523 | 8.2706 | 3606 | 0.5321 | 0.5116 | 0.5321 | 0.7294 |
| 0.0523 | 8.2752 | 3608 | 0.5404 | 0.5116 | 0.5404 | 0.7351 |
| 0.0523 | 8.2798 | 3610 | 0.5444 | 0.5116 | 0.5444 | 0.7378 |
| 0.0523 | 8.2844 | 3612 | 0.5417 | 0.5116 | 0.5417 | 0.7360 |
| 0.0523 | 8.2890 | 3614 | 0.5408 | 0.5116 | 0.5408 | 0.7354 |
| 0.0523 | 8.2936 | 3616 | 0.5500 | 0.5116 | 0.5500 | 0.7416 |
| 0.0523 | 8.2982 | 3618 | 0.5656 | 0.5116 | 0.5656 | 0.7520 |
| 0.0523 | 8.3028 | 3620 | 0.5773 | 0.5116 | 0.5773 | 0.7598 |
| 0.0523 | 8.3073 | 3622 | 0.5794 | 0.5116 | 0.5794 | 0.7612 |
| 0.0523 | 8.3119 | 3624 | 0.5733 | 0.5116 | 0.5733 | 0.7572 |
| 0.0523 | 8.3165 | 3626 | 0.5699 | 0.5116 | 0.5699 | 0.7549 |
| 0.0523 | 8.3211 | 3628 | 0.5675 | 0.5116 | 0.5675 | 0.7534 |
| 0.0523 | 8.3257 | 3630 | 0.5589 | 0.5116 | 0.5589 | 0.7476 |
| 0.0523 | 8.3303 | 3632 | 0.5481 | 0.5116 | 0.5481 | 0.7403 |
| 0.0523 | 8.3349 | 3634 | 0.5400 | 0.5116 | 0.5400 | 0.7348 |
| 0.0523 | 8.3394 | 3636 | 0.5342 | 0.5191 | 0.5342 | 0.7309 |
| 0.0523 | 8.3440 | 3638 | 0.5314 | 0.5191 | 0.5314 | 0.7290 |
| 0.0523 | 8.3486 | 3640 | 0.5288 | 0.5191 | 0.5288 | 0.7272 |
| 0.0523 | 8.3532 | 3642 | 0.5339 | 0.5191 | 0.5339 | 0.7307 |
| 0.0523 | 8.3578 | 3644 | 0.5357 | 0.5477 | 0.5357 | 0.7319 |
| 0.0523 | 8.3624 | 3646 | 0.5399 | 0.5477 | 0.5399 | 0.7348 |
| 0.0523 | 8.3670 | 3648 | 0.5401 | 0.5477 | 0.5401 | 0.7349 |
| 0.0523 | 8.3716 | 3650 | 0.5370 | 0.5191 | 0.5370 | 0.7328 |
| 0.0523 | 8.3761 | 3652 | 0.5366 | 0.5191 | 0.5366 | 0.7325 |
| 0.0523 | 8.3807 | 3654 | 0.5397 | 0.5191 | 0.5397 | 0.7346 |
| 0.0523 | 8.3853 | 3656 | 0.5468 | 0.5191 | 0.5468 | 0.7395 |
| 0.0523 | 8.3899 | 3658 | 0.5464 | 0.5191 | 0.5464 | 0.7392 |
| 0.0523 | 8.3945 | 3660 | 0.5410 | 0.5191 | 0.5410 | 0.7355 |
| 0.0523 | 8.3991 | 3662 | 0.5401 | 0.5191 | 0.5401 | 0.7349 |
| 0.0523 | 8.4037 | 3664 | 0.5428 | 0.5191 | 0.5428 | 0.7368 |
| 0.0523 | 8.4083 | 3666 | 0.5479 | 0.5191 | 0.5479 | 0.7402 |
| 0.0523 | 8.4128 | 3668 | 0.5559 | 0.5116 | 0.5559 | 0.7456 |
| 0.0523 | 8.4174 | 3670 | 0.5675 | 0.5116 | 0.5675 | 0.7533 |
| 0.0523 | 8.4220 | 3672 | 0.5815 | 0.5116 | 0.5815 | 0.7625 |
| 0.0523 | 8.4266 | 3674 | 0.5916 | 0.5116 | 0.5916 | 0.7692 |
| 0.0523 | 8.4312 | 3676 | 0.5959 | 0.5116 | 0.5959 | 0.7719 |
| 0.0523 | 8.4358 | 3678 | 0.5986 | 0.5116 | 0.5986 | 0.7737 |
| 0.0523 | 8.4404 | 3680 | 0.5913 | 0.5116 | 0.5913 | 0.7690 |
| 0.0523 | 8.4450 | 3682 | 0.5823 | 0.5116 | 0.5823 | 0.7631 |
| 0.0523 | 8.4495 | 3684 | 0.5699 | 0.5116 | 0.5699 | 0.7549 |
| 0.0523 | 8.4541 | 3686 | 0.5639 | 0.5116 | 0.5639 | 0.7509 |
| 0.0523 | 8.4587 | 3688 | 0.5526 | 0.4831 | 0.5526 | 0.7433 |
| 0.0523 | 8.4633 | 3690 | 0.5482 | 0.4831 | 0.5482 | 0.7404 |
| 0.0523 | 8.4679 | 3692 | 0.5463 | 0.4831 | 0.5463 | 0.7391 |
| 0.0523 | 8.4725 | 3694 | 0.5486 | 0.4831 | 0.5486 | 0.7407 |
| 0.0523 | 8.4771 | 3696 | 0.5575 | 0.4831 | 0.5575 | 0.7467 |
| 0.0523 | 8.4817 | 3698 | 0.5660 | 0.5116 | 0.5660 | 0.7523 |
| 0.0523 | 8.4862 | 3700 | 0.5640 | 0.5116 | 0.5640 | 0.7510 |
| 0.0523 | 8.4908 | 3702 | 0.5592 | 0.4831 | 0.5592 | 0.7478 |
| 0.0523 | 8.4954 | 3704 | 0.5611 | 0.5116 | 0.5611 | 0.7491 |
| 0.0523 | 8.5 | 3706 | 0.5624 | 0.5116 | 0.5624 | 0.7499 |
| 0.0523 | 8.5046 | 3708 | 0.5541 | 0.4831 | 0.5541 | 0.7444 |
| 0.0523 | 8.5092 | 3710 | 0.5477 | 0.4831 | 0.5477 | 0.7400 |
| 0.0523 | 8.5138 | 3712 | 0.5406 | 0.5191 | 0.5406 | 0.7352 |
| 0.0523 | 8.5183 | 3714 | 0.5309 | 0.5191 | 0.5309 | 0.7286 |
| 0.0523 | 8.5229 | 3716 | 0.5241 | 0.5191 | 0.5241 | 0.7240 |
| 0.0523 | 8.5275 | 3718 | 0.5224 | 0.5191 | 0.5224 | 0.7227 |
| 0.0523 | 8.5321 | 3720 | 0.5276 | 0.5191 | 0.5276 | 0.7264 |
| 0.0523 | 8.5367 | 3722 | 0.5374 | 0.5191 | 0.5374 | 0.7331 |
| 0.0523 | 8.5413 | 3724 | 0.5505 | 0.5191 | 0.5505 | 0.7420 |
| 0.0523 | 8.5459 | 3726 | 0.5682 | 0.4831 | 0.5682 | 0.7538 |
| 0.0523 | 8.5505 | 3728 | 0.5855 | 0.5116 | 0.5855 | 0.7652 |
| 0.0523 | 8.5550 | 3730 | 0.6002 | 0.5116 | 0.6002 | 0.7748 |
| 0.0523 | 8.5596 | 3732 | 0.6044 | 0.4378 | 0.6044 | 0.7774 |
| 0.0523 | 8.5642 | 3734 | 0.6028 | 0.4378 | 0.6028 | 0.7764 |
| 0.0523 | 8.5688 | 3736 | 0.5904 | 0.4378 | 0.5904 | 0.7684 |
| 0.0523 | 8.5734 | 3738 | 0.5800 | 0.5116 | 0.5800 | 0.7616 |
| 0.0523 | 8.5780 | 3740 | 0.5722 | 0.5116 | 0.5722 | 0.7565 |
| 0.0523 | 8.5826 | 3742 | 0.5649 | 0.5116 | 0.5649 | 0.7516 |
| 0.0523 | 8.5872 | 3744 | 0.5618 | 0.5116 | 0.5618 | 0.7496 |
| 0.0523 | 8.5917 | 3746 | 0.5596 | 0.5116 | 0.5596 | 0.7480 |
| 0.0523 | 8.5963 | 3748 | 0.5513 | 0.5116 | 0.5513 | 0.7425 |
| 0.0523 | 8.6009 | 3750 | 0.5464 | 0.4831 | 0.5464 | 0.7392 |
| 0.0523 | 8.6055 | 3752 | 0.5436 | 0.4831 | 0.5436 | 0.7373 |
| 0.0523 | 8.6101 | 3754 | 0.5374 | 0.4831 | 0.5374 | 0.7330 |
| 0.0523 | 8.6147 | 3756 | 0.5313 | 0.5191 | 0.5313 | 0.7289 |
| 0.0523 | 8.6193 | 3758 | 0.5244 | 0.5191 | 0.5244 | 0.7241 |
| 0.0523 | 8.6239 | 3760 | 0.5184 | 0.5191 | 0.5184 | 0.7200 |
| 0.0523 | 8.6284 | 3762 | 0.5174 | 0.5191 | 0.5174 | 0.7193 |
| 0.0523 | 8.6330 | 3764 | 0.5146 | 0.6526 | 0.5146 | 0.7174 |
| 0.0523 | 8.6376 | 3766 | 0.5128 | 0.6526 | 0.5128 | 0.7161 |
| 0.0523 | 8.6422 | 3768 | 0.5137 | 0.6526 | 0.5137 | 0.7167 |
| 0.0523 | 8.6468 | 3770 | 0.5202 | 0.5545 | 0.5202 | 0.7213 |
| 0.0523 | 8.6514 | 3772 | 0.5305 | 0.5191 | 0.5305 | 0.7284 |
| 0.0523 | 8.6560 | 3774 | 0.5422 | 0.5191 | 0.5422 | 0.7363 |
| 0.0523 | 8.6606 | 3776 | 0.5489 | 0.5191 | 0.5489 | 0.7409 |
| 0.0523 | 8.6651 | 3778 | 0.5605 | 0.4831 | 0.5605 | 0.7487 |
| 0.0523 | 8.6697 | 3780 | 0.5693 | 0.5116 | 0.5693 | 0.7545 |
| 0.0523 | 8.6743 | 3782 | 0.5718 | 0.5116 | 0.5718 | 0.7562 |
| 0.0523 | 8.6789 | 3784 | 0.5699 | 0.5116 | 0.5699 | 0.7549 |
| 0.0523 | 8.6835 | 3786 | 0.5671 | 0.5116 | 0.5671 | 0.7531 |
| 0.0523 | 8.6881 | 3788 | 0.5620 | 0.5116 | 0.5620 | 0.7497 |
| 0.0523 | 8.6927 | 3790 | 0.5538 | 0.5116 | 0.5538 | 0.7442 |
| 0.0523 | 8.6972 | 3792 | 0.5517 | 0.5191 | 0.5517 | 0.7427 |
| 0.0523 | 8.7018 | 3794 | 0.5532 | 0.5116 | 0.5532 | 0.7438 |
| 0.0523 | 8.7064 | 3796 | 0.5503 | 0.5477 | 0.5503 | 0.7418 |
| 0.0523 | 8.7110 | 3798 | 0.5475 | 0.5477 | 0.5475 | 0.7399 |
| 0.0523 | 8.7156 | 3800 | 0.5379 | 0.5191 | 0.5379 | 0.7334 |
| 0.0523 | 8.7202 | 3802 | 0.5309 | 0.5191 | 0.5309 | 0.7286 |
| 0.0523 | 8.7248 | 3804 | 0.5287 | 0.5191 | 0.5287 | 0.7271 |
| 0.0523 | 8.7294 | 3806 | 0.5300 | 0.5191 | 0.5300 | 0.7280 |
| 0.0523 | 8.7339 | 3808 | 0.5260 | 0.5545 | 0.5260 | 0.7252 |
| 0.0523 | 8.7385 | 3810 | 0.5235 | 0.5545 | 0.5235 | 0.7235 |
| 0.0523 | 8.7431 | 3812 | 0.5274 | 0.5545 | 0.5274 | 0.7262 |
| 0.0523 | 8.7477 | 3814 | 0.5314 | 0.5191 | 0.5314 | 0.7290 |
| 0.0523 | 8.7523 | 3816 | 0.5348 | 0.5191 | 0.5348 | 0.7313 |
| 0.0523 | 8.7569 | 3818 | 0.5352 | 0.5191 | 0.5352 | 0.7316 |
| 0.0523 | 8.7615 | 3820 | 0.5383 | 0.5191 | 0.5383 | 0.7337 |
| 0.0523 | 8.7661 | 3822 | 0.5443 | 0.5191 | 0.5443 | 0.7378 |
| 0.0523 | 8.7706 | 3824 | 0.5529 | 0.5191 | 0.5529 | 0.7436 |
| 0.0523 | 8.7752 | 3826 | 0.5646 | 0.5191 | 0.5646 | 0.7514 |
| 0.0523 | 8.7798 | 3828 | 0.5765 | 0.5477 | 0.5765 | 0.7593 |
| 0.0523 | 8.7844 | 3830 | 0.5826 | 0.5116 | 0.5826 | 0.7633 |
| 0.0523 | 8.7890 | 3832 | 0.5843 | 0.5116 | 0.5843 | 0.7644 |
| 0.0523 | 8.7936 | 3834 | 0.5780 | 0.5116 | 0.5780 | 0.7603 |
| 0.0523 | 8.7982 | 3836 | 0.5650 | 0.5477 | 0.5650 | 0.7516 |
| 0.0523 | 8.8028 | 3838 | 0.5490 | 0.5191 | 0.5490 | 0.7409 |
| 0.0523 | 8.8073 | 3840 | 0.5371 | 0.5191 | 0.5371 | 0.7329 |
| 0.0523 | 8.8119 | 3842 | 0.5275 | 0.5191 | 0.5275 | 0.7263 |
| 0.0523 | 8.8165 | 3844 | 0.5225 | 0.5191 | 0.5225 | 0.7229 |
| 0.0523 | 8.8211 | 3846 | 0.5219 | 0.5191 | 0.5219 | 0.7225 |
| 0.0523 | 8.8257 | 3848 | 0.5200 | 0.5191 | 0.5200 | 0.7211 |
| 0.0523 | 8.8303 | 3850 | 0.5165 | 0.5191 | 0.5165 | 0.7187 |
| 0.0523 | 8.8349 | 3852 | 0.5188 | 0.5191 | 0.5188 | 0.7203 |
| 0.0523 | 8.8394 | 3854 | 0.5262 | 0.5191 | 0.5262 | 0.7254 |
| 0.0523 | 8.8440 | 3856 | 0.5358 | 0.5477 | 0.5358 | 0.7320 |
| 0.0523 | 8.8486 | 3858 | 0.5467 | 0.5116 | 0.5467 | 0.7394 |
| 0.0523 | 8.8532 | 3860 | 0.5549 | 0.5116 | 0.5549 | 0.7449 |
| 0.0523 | 8.8578 | 3862 | 0.5619 | 0.5116 | 0.5619 | 0.7496 |
| 0.0523 | 8.8624 | 3864 | 0.5622 | 0.5116 | 0.5622 | 0.7498 |
| 0.0523 | 8.8670 | 3866 | 0.5553 | 0.5116 | 0.5553 | 0.7452 |
| 0.0523 | 8.8716 | 3868 | 0.5487 | 0.5477 | 0.5487 | 0.7408 |
| 0.0523 | 8.8761 | 3870 | 0.5385 | 0.5477 | 0.5385 | 0.7338 |
| 0.0523 | 8.8807 | 3872 | 0.5281 | 0.5191 | 0.5281 | 0.7267 |
| 0.0523 | 8.8853 | 3874 | 0.5200 | 0.5191 | 0.5200 | 0.7211 |
| 0.0523 | 8.8899 | 3876 | 0.5145 | 0.5191 | 0.5145 | 0.7173 |
| 0.0523 | 8.8945 | 3878 | 0.5109 | 0.5545 | 0.5109 | 0.7148 |
| 0.0523 | 8.8991 | 3880 | 0.5107 | 0.5191 | 0.5107 | 0.7147 |
| 0.0523 | 8.9037 | 3882 | 0.5100 | 0.5191 | 0.5100 | 0.7141 |
| 0.0523 | 8.9083 | 3884 | 0.5121 | 0.5191 | 0.5121 | 0.7156 |
| 0.0523 | 8.9128 | 3886 | 0.5158 | 0.5191 | 0.5158 | 0.7182 |
| 0.0523 | 8.9174 | 3888 | 0.5224 | 0.5191 | 0.5224 | 0.7227 |
| 0.0523 | 8.9220 | 3890 | 0.5308 | 0.5477 | 0.5308 | 0.7286 |
| 0.0523 | 8.9266 | 3892 | 0.5364 | 0.5477 | 0.5364 | 0.7324 |
| 0.0523 | 8.9312 | 3894 | 0.5402 | 0.5116 | 0.5402 | 0.7350 |
| 0.0523 | 8.9358 | 3896 | 0.5408 | 0.5116 | 0.5408 | 0.7354 |
| 0.0523 | 8.9404 | 3898 | 0.5405 | 0.5116 | 0.5405 | 0.7352 |
| 0.0523 | 8.9450 | 3900 | 0.5350 | 0.5116 | 0.5350 | 0.7315 |
| 0.0523 | 8.9495 | 3902 | 0.5271 | 0.5477 | 0.5271 | 0.7260 |
| 0.0523 | 8.9541 | 3904 | 0.5200 | 0.5191 | 0.5200 | 0.7211 |
| 0.0523 | 8.9587 | 3906 | 0.5179 | 0.5191 | 0.5179 | 0.7197 |
| 0.0523 | 8.9633 | 3908 | 0.5187 | 0.5191 | 0.5187 | 0.7202 |
| 0.0523 | 8.9679 | 3910 | 0.5246 | 0.5191 | 0.5246 | 0.7243 |
| 0.0523 | 8.9725 | 3912 | 0.5316 | 0.5191 | 0.5316 | 0.7291 |
| 0.0523 | 8.9771 | 3914 | 0.5326 | 0.5191 | 0.5326 | 0.7298 |
| 0.0523 | 8.9817 | 3916 | 0.5365 | 0.5191 | 0.5365 | 0.7325 |
| 0.0523 | 8.9862 | 3918 | 0.5356 | 0.5191 | 0.5356 | 0.7318 |
| 0.0523 | 8.9908 | 3920 | 0.5332 | 0.5191 | 0.5332 | 0.7302 |
| 0.0523 | 8.9954 | 3922 | 0.5282 | 0.5191 | 0.5282 | 0.7267 |
| 0.0523 | 9.0 | 3924 | 0.5209 | 0.5191 | 0.5209 | 0.7217 |
| 0.0523 | 9.0046 | 3926 | 0.5177 | 0.5191 | 0.5177 | 0.7195 |
| 0.0523 | 9.0092 | 3928 | 0.5154 | 0.5191 | 0.5154 | 0.7179 |
| 0.0523 | 9.0138 | 3930 | 0.5181 | 0.5191 | 0.5181 | 0.7198 |
| 0.0523 | 9.0183 | 3932 | 0.5246 | 0.5191 | 0.5246 | 0.7243 |
| 0.0523 | 9.0229 | 3934 | 0.5342 | 0.5191 | 0.5342 | 0.7309 |
| 0.0523 | 9.0275 | 3936 | 0.5435 | 0.5477 | 0.5435 | 0.7373 |
| 0.0523 | 9.0321 | 3938 | 0.5540 | 0.5477 | 0.5540 | 0.7443 |
| 0.0523 | 9.0367 | 3940 | 0.5576 | 0.5477 | 0.5576 | 0.7467 |
| 0.0523 | 9.0413 | 3942 | 0.5565 | 0.5477 | 0.5565 | 0.7460 |
| 0.0523 | 9.0459 | 3944 | 0.5544 | 0.5477 | 0.5544 | 0.7445 |
| 0.0523 | 9.0505 | 3946 | 0.5527 | 0.5477 | 0.5527 | 0.7434 |
| 0.0523 | 9.0550 | 3948 | 0.5526 | 0.5477 | 0.5526 | 0.7434 |
| 0.0523 | 9.0596 | 3950 | 0.5520 | 0.5477 | 0.5520 | 0.7430 |
| 0.0523 | 9.0642 | 3952 | 0.5497 | 0.5477 | 0.5497 | 0.7414 |
| 0.0523 | 9.0688 | 3954 | 0.5429 | 0.5191 | 0.5429 | 0.7368 |
| 0.0523 | 9.0734 | 3956 | 0.5369 | 0.5191 | 0.5369 | 0.7328 |
| 0.0523 | 9.0780 | 3958 | 0.5373 | 0.5191 | 0.5373 | 0.7330 |
| 0.0523 | 9.0826 | 3960 | 0.5372 | 0.5191 | 0.5372 | 0.7329 |
| 0.0523 | 9.0872 | 3962 | 0.5379 | 0.5191 | 0.5379 | 0.7334 |
| 0.0523 | 9.0917 | 3964 | 0.5413 | 0.5191 | 0.5413 | 0.7357 |
| 0.0523 | 9.0963 | 3966 | 0.5459 | 0.5191 | 0.5459 | 0.7388 |
| 0.0523 | 9.1009 | 3968 | 0.5479 | 0.5191 | 0.5479 | 0.7402 |
| 0.0523 | 9.1055 | 3970 | 0.5482 | 0.5191 | 0.5482 | 0.7404 |
| 0.0523 | 9.1101 | 3972 | 0.5465 | 0.5191 | 0.5465 | 0.7393 |
| 0.0523 | 9.1147 | 3974 | 0.5475 | 0.5191 | 0.5475 | 0.7399 |
| 0.0523 | 9.1193 | 3976 | 0.5474 | 0.5191 | 0.5474 | 0.7399 |
| 0.0523 | 9.1239 | 3978 | 0.5466 | 0.5191 | 0.5466 | 0.7393 |
| 0.0523 | 9.1284 | 3980 | 0.5478 | 0.5191 | 0.5478 | 0.7401 |
| 0.0523 | 9.1330 | 3982 | 0.5533 | 0.5191 | 0.5533 | 0.7438 |
| 0.0523 | 9.1376 | 3984 | 0.5612 | 0.5477 | 0.5612 | 0.7491 |
| 0.0523 | 9.1422 | 3986 | 0.5686 | 0.5477 | 0.5686 | 0.7541 |
| 0.0523 | 9.1468 | 3988 | 0.5708 | 0.5477 | 0.5708 | 0.7555 |
| 0.0523 | 9.1514 | 3990 | 0.5733 | 0.5477 | 0.5733 | 0.7571 |
| 0.0523 | 9.1560 | 3992 | 0.5768 | 0.5477 | 0.5768 | 0.7595 |
| 0.0523 | 9.1606 | 3994 | 0.5720 | 0.5477 | 0.5720 | 0.7563 |
| 0.0523 | 9.1651 | 3996 | 0.5694 | 0.5477 | 0.5694 | 0.7546 |
| 0.0523 | 9.1697 | 3998 | 0.5637 | 0.5477 | 0.5637 | 0.7508 |
| 0.0451 | 9.1743 | 4000 | 0.5593 | 0.5477 | 0.5593 | 0.7479 |
| 0.0451 | 9.1789 | 4002 | 0.5545 | 0.5477 | 0.5545 | 0.7447 |
| 0.0451 | 9.1835 | 4004 | 0.5563 | 0.5477 | 0.5563 | 0.7458 |
| 0.0451 | 9.1881 | 4006 | 0.5568 | 0.5477 | 0.5568 | 0.7462 |
| 0.0451 | 9.1927 | 4008 | 0.5592 | 0.5477 | 0.5592 | 0.7478 |
| 0.0451 | 9.1972 | 4010 | 0.5618 | 0.5477 | 0.5618 | 0.7495 |
| 0.0451 | 9.2018 | 4012 | 0.5619 | 0.5477 | 0.5619 | 0.7496 |
| 0.0451 | 9.2064 | 4014 | 0.5592 | 0.5477 | 0.5592 | 0.7478 |
| 0.0451 | 9.2110 | 4016 | 0.5512 | 0.5477 | 0.5512 | 0.7424 |
| 0.0451 | 9.2156 | 4018 | 0.5473 | 0.5477 | 0.5473 | 0.7398 |
| 0.0451 | 9.2202 | 4020 | 0.5474 | 0.5477 | 0.5474 | 0.7398 |
| 0.0451 | 9.2248 | 4022 | 0.5434 | 0.5477 | 0.5434 | 0.7372 |
| 0.0451 | 9.2294 | 4024 | 0.5369 | 0.5191 | 0.5369 | 0.7327 |
| 0.0451 | 9.2339 | 4026 | 0.5298 | 0.5191 | 0.5298 | 0.7279 |
| 0.0451 | 9.2385 | 4028 | 0.5269 | 0.5191 | 0.5269 | 0.7259 |
| 0.0451 | 9.2431 | 4030 | 0.5256 | 0.5191 | 0.5256 | 0.7250 |
| 0.0451 | 9.2477 | 4032 | 0.5255 | 0.5191 | 0.5255 | 0.7249 |
| 0.0451 | 9.2523 | 4034 | 0.5271 | 0.5191 | 0.5271 | 0.7260 |
| 0.0451 | 9.2569 | 4036 | 0.5325 | 0.5191 | 0.5325 | 0.7298 |
| 0.0451 | 9.2615 | 4038 | 0.5405 | 0.5477 | 0.5405 | 0.7352 |
| 0.0451 | 9.2661 | 4040 | 0.5482 | 0.5477 | 0.5482 | 0.7404 |
| 0.0451 | 9.2706 | 4042 | 0.5533 | 0.5477 | 0.5533 | 0.7438 |
| 0.0451 | 9.2752 | 4044 | 0.5566 | 0.5477 | 0.5566 | 0.7461 |
| 0.0451 | 9.2798 | 4046 | 0.5578 | 0.5477 | 0.5578 | 0.7469 |
| 0.0451 | 9.2844 | 4048 | 0.5633 | 0.5116 | 0.5633 | 0.7506 |
| 0.0451 | 9.2890 | 4050 | 0.5675 | 0.5116 | 0.5675 | 0.7533 |
| 0.0451 | 9.2936 | 4052 | 0.5684 | 0.5116 | 0.5684 | 0.7539 |
| 0.0451 | 9.2982 | 4054 | 0.5664 | 0.5116 | 0.5664 | 0.7526 |
| 0.0451 | 9.3028 | 4056 | 0.5603 | 0.5116 | 0.5603 | 0.7485 |
| 0.0451 | 9.3073 | 4058 | 0.5530 | 0.5477 | 0.5530 | 0.7436 |
| 0.0451 | 9.3119 | 4060 | 0.5470 | 0.5477 | 0.5470 | 0.7396 |
| 0.0451 | 9.3165 | 4062 | 0.5455 | 0.5477 | 0.5455 | 0.7386 |
| 0.0451 | 9.3211 | 4064 | 0.5437 | 0.5477 | 0.5437 | 0.7374 |
| 0.0451 | 9.3257 | 4066 | 0.5396 | 0.5477 | 0.5396 | 0.7346 |
| 0.0451 | 9.3303 | 4068 | 0.5347 | 0.5477 | 0.5347 | 0.7312 |
| 0.0451 | 9.3349 | 4070 | 0.5324 | 0.5191 | 0.5324 | 0.7296 |
| 0.0451 | 9.3394 | 4072 | 0.5299 | 0.5191 | 0.5299 | 0.7280 |
| 0.0451 | 9.3440 | 4074 | 0.5281 | 0.5191 | 0.5281 | 0.7267 |
| 0.0451 | 9.3486 | 4076 | 0.5295 | 0.5191 | 0.5295 | 0.7277 |
| 0.0451 | 9.3532 | 4078 | 0.5331 | 0.5477 | 0.5331 | 0.7302 |
| 0.0451 | 9.3578 | 4080 | 0.5362 | 0.5477 | 0.5362 | 0.7323 |
| 0.0451 | 9.3624 | 4082 | 0.5372 | 0.5477 | 0.5372 | 0.7329 |
| 0.0451 | 9.3670 | 4084 | 0.5395 | 0.5477 | 0.5395 | 0.7345 |
| 0.0451 | 9.3716 | 4086 | 0.5428 | 0.5477 | 0.5428 | 0.7367 |
| 0.0451 | 9.3761 | 4088 | 0.5447 | 0.5477 | 0.5447 | 0.7380 |
| 0.0451 | 9.3807 | 4090 | 0.5436 | 0.5477 | 0.5436 | 0.7373 |
| 0.0451 | 9.3853 | 4092 | 0.5397 | 0.5477 | 0.5397 | 0.7347 |
| 0.0451 | 9.3899 | 4094 | 0.5397 | 0.5477 | 0.5397 | 0.7346 |
| 0.0451 | 9.3945 | 4096 | 0.5391 | 0.5477 | 0.5391 | 0.7342 |
| 0.0451 | 9.3991 | 4098 | 0.5389 | 0.5477 | 0.5389 | 0.7341 |
| 0.0451 | 9.4037 | 4100 | 0.5369 | 0.5477 | 0.5369 | 0.7327 |
| 0.0451 | 9.4083 | 4102 | 0.5373 | 0.5477 | 0.5373 | 0.7330 |
| 0.0451 | 9.4128 | 4104 | 0.5395 | 0.5477 | 0.5395 | 0.7345 |
| 0.0451 | 9.4174 | 4106 | 0.5442 | 0.5477 | 0.5442 | 0.7377 |
| 0.0451 | 9.4220 | 4108 | 0.5505 | 0.5477 | 0.5505 | 0.7419 |
| 0.0451 | 9.4266 | 4110 | 0.5548 | 0.5116 | 0.5548 | 0.7449 |
| 0.0451 | 9.4312 | 4112 | 0.5590 | 0.5116 | 0.5590 | 0.7477 |
| 0.0451 | 9.4358 | 4114 | 0.5606 | 0.5116 | 0.5606 | 0.7487 |
| 0.0451 | 9.4404 | 4116 | 0.5581 | 0.5116 | 0.5581 | 0.7470 |
| 0.0451 | 9.4450 | 4118 | 0.5528 | 0.5477 | 0.5528 | 0.7435 |
| 0.0451 | 9.4495 | 4120 | 0.5493 | 0.5477 | 0.5493 | 0.7411 |
| 0.0451 | 9.4541 | 4122 | 0.5455 | 0.5477 | 0.5455 | 0.7386 |
| 0.0451 | 9.4587 | 4124 | 0.5423 | 0.5477 | 0.5423 | 0.7364 |
| 0.0451 | 9.4633 | 4126 | 0.5420 | 0.5477 | 0.5420 | 0.7362 |
| 0.0451 | 9.4679 | 4128 | 0.5428 | 0.5477 | 0.5428 | 0.7368 |
| 0.0451 | 9.4725 | 4130 | 0.5432 | 0.5477 | 0.5432 | 0.7370 |
| 0.0451 | 9.4771 | 4132 | 0.5442 | 0.5477 | 0.5442 | 0.7377 |
| 0.0451 | 9.4817 | 4134 | 0.5469 | 0.5477 | 0.5469 | 0.7395 |
| 0.0451 | 9.4862 | 4136 | 0.5481 | 0.5477 | 0.5481 | 0.7403 |
| 0.0451 | 9.4908 | 4138 | 0.5479 | 0.5477 | 0.5479 | 0.7402 |
| 0.0451 | 9.4954 | 4140 | 0.5466 | 0.5477 | 0.5466 | 0.7393 |
| 0.0451 | 9.5 | 4142 | 0.5475 | 0.5477 | 0.5475 | 0.7399 |
| 0.0451 | 9.5046 | 4144 | 0.5491 | 0.5477 | 0.5491 | 0.7410 |
| 0.0451 | 9.5092 | 4146 | 0.5511 | 0.5477 | 0.5511 | 0.7424 |
| 0.0451 | 9.5138 | 4148 | 0.5509 | 0.5477 | 0.5509 | 0.7422 |
| 0.0451 | 9.5183 | 4150 | 0.5477 | 0.5477 | 0.5477 | 0.7400 |
| 0.0451 | 9.5229 | 4152 | 0.5422 | 0.5477 | 0.5422 | 0.7363 |
| 0.0451 | 9.5275 | 4154 | 0.5380 | 0.5477 | 0.5380 | 0.7335 |
| 0.0451 | 9.5321 | 4156 | 0.5347 | 0.5477 | 0.5347 | 0.7312 |
| 0.0451 | 9.5367 | 4158 | 0.5332 | 0.5477 | 0.5332 | 0.7302 |
| 0.0451 | 9.5413 | 4160 | 0.5328 | 0.5477 | 0.5328 | 0.7299 |
| 0.0451 | 9.5459 | 4162 | 0.5338 | 0.5477 | 0.5338 | 0.7306 |
| 0.0451 | 9.5505 | 4164 | 0.5344 | 0.5477 | 0.5344 | 0.7311 |
| 0.0451 | 9.5550 | 4166 | 0.5361 | 0.5477 | 0.5361 | 0.7322 |
| 0.0451 | 9.5596 | 4168 | 0.5368 | 0.5477 | 0.5368 | 0.7327 |
| 0.0451 | 9.5642 | 4170 | 0.5369 | 0.5477 | 0.5369 | 0.7327 |
| 0.0451 | 9.5688 | 4172 | 0.5390 | 0.5477 | 0.5390 | 0.7341 |
| 0.0451 | 9.5734 | 4174 | 0.5417 | 0.5477 | 0.5417 | 0.7360 |
| 0.0451 | 9.5780 | 4176 | 0.5425 | 0.5477 | 0.5425 | 0.7365 |
| 0.0451 | 9.5826 | 4178 | 0.5440 | 0.5477 | 0.5440 | 0.7375 |
| 0.0451 | 9.5872 | 4180 | 0.5433 | 0.5477 | 0.5433 | 0.7371 |
| 0.0451 | 9.5917 | 4182 | 0.5409 | 0.5477 | 0.5409 | 0.7355 |
| 0.0451 | 9.5963 | 4184 | 0.5374 | 0.5477 | 0.5374 | 0.7331 |
| 0.0451 | 9.6009 | 4186 | 0.5332 | 0.5477 | 0.5332 | 0.7302 |
| 0.0451 | 9.6055 | 4188 | 0.5277 | 0.5477 | 0.5277 | 0.7264 |
| 0.0451 | 9.6101 | 4190 | 0.5219 | 0.5477 | 0.5219 | 0.7224 |
| 0.0451 | 9.6147 | 4192 | 0.5180 | 0.5477 | 0.5180 | 0.7197 |
| 0.0451 | 9.6193 | 4194 | 0.5147 | 0.5477 | 0.5147 | 0.7174 |
| 0.0451 | 9.6239 | 4196 | 0.5121 | 0.5191 | 0.5121 | 0.7156 |
| 0.0451 | 9.6284 | 4198 | 0.5096 | 0.5191 | 0.5096 | 0.7139 |
| 0.0451 | 9.6330 | 4200 | 0.5087 | 0.5191 | 0.5087 | 0.7133 |
| 0.0451 | 9.6376 | 4202 | 0.5097 | 0.5191 | 0.5097 | 0.7139 |
| 0.0451 | 9.6422 | 4204 | 0.5123 | 0.5477 | 0.5123 | 0.7157 |
| 0.0451 | 9.6468 | 4206 | 0.5162 | 0.5477 | 0.5162 | 0.7185 |
| 0.0451 | 9.6514 | 4208 | 0.5196 | 0.5477 | 0.5196 | 0.7208 |
| 0.0451 | 9.6560 | 4210 | 0.5224 | 0.5477 | 0.5224 | 0.7228 |
| 0.0451 | 9.6606 | 4212 | 0.5260 | 0.5477 | 0.5260 | 0.7252 |
| 0.0451 | 9.6651 | 4214 | 0.5284 | 0.5477 | 0.5284 | 0.7269 |
| 0.0451 | 9.6697 | 4216 | 0.5305 | 0.5477 | 0.5305 | 0.7283 |
| 0.0451 | 9.6743 | 4218 | 0.5336 | 0.5477 | 0.5336 | 0.7305 |
| 0.0451 | 9.6789 | 4220 | 0.5363 | 0.5477 | 0.5363 | 0.7323 |
| 0.0451 | 9.6835 | 4222 | 0.5371 | 0.5477 | 0.5371 | 0.7329 |
| 0.0451 | 9.6881 | 4224 | 0.5362 | 0.5477 | 0.5362 | 0.7322 |
| 0.0451 | 9.6927 | 4226 | 0.5347 | 0.5477 | 0.5347 | 0.7312 |
| 0.0451 | 9.6972 | 4228 | 0.5341 | 0.5477 | 0.5341 | 0.7309 |
| 0.0451 | 9.7018 | 4230 | 0.5333 | 0.5477 | 0.5333 | 0.7303 |
| 0.0451 | 9.7064 | 4232 | 0.5337 | 0.5477 | 0.5337 | 0.7306 |
| 0.0451 | 9.7110 | 4234 | 0.5334 | 0.5477 | 0.5334 | 0.7303 |
| 0.0451 | 9.7156 | 4236 | 0.5336 | 0.5477 | 0.5336 | 0.7305 |
| 0.0451 | 9.7202 | 4238 | 0.5340 | 0.5477 | 0.5340 | 0.7307 |
| 0.0451 | 9.7248 | 4240 | 0.5349 | 0.5477 | 0.5349 | 0.7314 |
| 0.0451 | 9.7294 | 4242 | 0.5355 | 0.5477 | 0.5355 | 0.7318 |
| 0.0451 | 9.7339 | 4244 | 0.5363 | 0.5477 | 0.5363 | 0.7323 |
| 0.0451 | 9.7385 | 4246 | 0.5369 | 0.5477 | 0.5369 | 0.7327 |
| 0.0451 | 9.7431 | 4248 | 0.5369 | 0.5477 | 0.5369 | 0.7327 |
| 0.0451 | 9.7477 | 4250 | 0.5376 | 0.5477 | 0.5376 | 0.7332 |
| 0.0451 | 9.7523 | 4252 | 0.5374 | 0.5477 | 0.5374 | 0.7331 |
| 0.0451 | 9.7569 | 4254 | 0.5384 | 0.5477 | 0.5384 | 0.7338 |
| 0.0451 | 9.7615 | 4256 | 0.5388 | 0.5477 | 0.5388 | 0.7340 |
| 0.0451 | 9.7661 | 4258 | 0.5403 | 0.5477 | 0.5403 | 0.7350 |
| 0.0451 | 9.7706 | 4260 | 0.5407 | 0.5477 | 0.5407 | 0.7353 |
| 0.0451 | 9.7752 | 4262 | 0.5399 | 0.5477 | 0.5399 | 0.7348 |
| 0.0451 | 9.7798 | 4264 | 0.5391 | 0.5477 | 0.5391 | 0.7342 |
| 0.0451 | 9.7844 | 4266 | 0.5389 | 0.5477 | 0.5389 | 0.7341 |
| 0.0451 | 9.7890 | 4268 | 0.5389 | 0.5477 | 0.5389 | 0.7341 |
| 0.0451 | 9.7936 | 4270 | 0.5386 | 0.5477 | 0.5386 | 0.7339 |
| 0.0451 | 9.7982 | 4272 | 0.5391 | 0.5477 | 0.5391 | 0.7343 |
| 0.0451 | 9.8028 | 4274 | 0.5383 | 0.5477 | 0.5383 | 0.7337 |
| 0.0451 | 9.8073 | 4276 | 0.5375 | 0.5477 | 0.5375 | 0.7332 |
| 0.0451 | 9.8119 | 4278 | 0.5363 | 0.5477 | 0.5363 | 0.7323 |
| 0.0451 | 9.8165 | 4280 | 0.5347 | 0.5477 | 0.5347 | 0.7312 |
| 0.0451 | 9.8211 | 4282 | 0.5332 | 0.5477 | 0.5332 | 0.7302 |
| 0.0451 | 9.8257 | 4284 | 0.5323 | 0.5477 | 0.5323 | 0.7296 |
| 0.0451 | 9.8303 | 4286 | 0.5317 | 0.5477 | 0.5317 | 0.7292 |
| 0.0451 | 9.8349 | 4288 | 0.5311 | 0.5477 | 0.5311 | 0.7288 |
| 0.0451 | 9.8394 | 4290 | 0.5304 | 0.5477 | 0.5304 | 0.7283 |
| 0.0451 | 9.8440 | 4292 | 0.5296 | 0.5477 | 0.5296 | 0.7277 |
| 0.0451 | 9.8486 | 4294 | 0.5293 | 0.5477 | 0.5293 | 0.7275 |
| 0.0451 | 9.8532 | 4296 | 0.5296 | 0.5477 | 0.5296 | 0.7277 |
| 0.0451 | 9.8578 | 4298 | 0.5297 | 0.5477 | 0.5297 | 0.7278 |
| 0.0451 | 9.8624 | 4300 | 0.5298 | 0.5477 | 0.5298 | 0.7279 |
| 0.0451 | 9.8670 | 4302 | 0.5301 | 0.5477 | 0.5301 | 0.7281 |
| 0.0451 | 9.8716 | 4304 | 0.5301 | 0.5477 | 0.5301 | 0.7281 |
| 0.0451 | 9.8761 | 4306 | 0.5303 | 0.5477 | 0.5303 | 0.7282 |
| 0.0451 | 9.8807 | 4308 | 0.5308 | 0.5477 | 0.5308 | 0.7286 |
| 0.0451 | 9.8853 | 4310 | 0.5311 | 0.5477 | 0.5311 | 0.7287 |
| 0.0451 | 9.8899 | 4312 | 0.5317 | 0.5477 | 0.5317 | 0.7292 |
| 0.0451 | 9.8945 | 4314 | 0.5328 | 0.5477 | 0.5328 | 0.7299 |
| 0.0451 | 9.8991 | 4316 | 0.5334 | 0.5477 | 0.5334 | 0.7303 |
| 0.0451 | 9.9037 | 4318 | 0.5338 | 0.5477 | 0.5338 | 0.7306 |
| 0.0451 | 9.9083 | 4320 | 0.5342 | 0.5477 | 0.5342 | 0.7309 |
| 0.0451 | 9.9128 | 4322 | 0.5346 | 0.5477 | 0.5346 | 0.7311 |
| 0.0451 | 9.9174 | 4324 | 0.5348 | 0.5477 | 0.5348 | 0.7313 |
| 0.0451 | 9.9220 | 4326 | 0.5345 | 0.5477 | 0.5345 | 0.7311 |
| 0.0451 | 9.9266 | 4328 | 0.5343 | 0.5477 | 0.5343 | 0.7310 |
| 0.0451 | 9.9312 | 4330 | 0.5345 | 0.5477 | 0.5345 | 0.7311 |
| 0.0451 | 9.9358 | 4332 | 0.5344 | 0.5477 | 0.5344 | 0.7310 |
| 0.0451 | 9.9404 | 4334 | 0.5345 | 0.5477 | 0.5345 | 0.7311 |
| 0.0451 | 9.9450 | 4336 | 0.5345 | 0.5477 | 0.5345 | 0.7311 |
| 0.0451 | 9.9495 | 4338 | 0.5346 | 0.5477 | 0.5346 | 0.7312 |
| 0.0451 | 9.9541 | 4340 | 0.5344 | 0.5477 | 0.5344 | 0.7310 |
| 0.0451 | 9.9587 | 4342 | 0.5344 | 0.5477 | 0.5344 | 0.7310 |
| 0.0451 | 9.9633 | 4344 | 0.5344 | 0.5477 | 0.5344 | 0.7310 |
| 0.0451 | 9.9679 | 4346 | 0.5342 | 0.5477 | 0.5342 | 0.7309 |
| 0.0451 | 9.9725 | 4348 | 0.5339 | 0.5477 | 0.5339 | 0.7307 |
| 0.0451 | 9.9771 | 4350 | 0.5337 | 0.5477 | 0.5337 | 0.7306 |
| 0.0451 | 9.9817 | 4352 | 0.5335 | 0.5477 | 0.5335 | 0.7304 |
| 0.0451 | 9.9862 | 4354 | 0.5332 | 0.5477 | 0.5332 | 0.7302 |
| 0.0451 | 9.9908 | 4356 | 0.5331 | 0.5477 | 0.5331 | 0.7301 |
| 0.0451 | 9.9954 | 4358 | 0.5330 | 0.5477 | 0.5330 | 0.7300 |
| 0.0451 | 10.0 | 4360 | 0.5329 | 0.5477 | 0.5329 | 0.7300 |
### Framework versions
- Transformers 4.44.2
- Pytorch 2.4.0+cu118
- Datasets 2.21.0
- Tokenizers 0.19.1
|
mradermacher/StructLM-13B-GGUF | mradermacher | "2024-11-15T03:53:11Z" | 49 | 0 | transformers | [
"transformers",
"gguf",
"en",
"dataset:TIGER-Lab/SKGInstruct",
"base_model:TIGER-Lab/StructLM-13B",
"base_model:quantized:TIGER-Lab/StructLM-13B",
"license:mit",
"endpoints_compatible",
"region:us"
] | null | "2024-11-14T06:13:46Z" | ---
base_model: TIGER-Lab/StructLM-13B
datasets:
- TIGER-Lab/SKGInstruct
language:
- en
library_name: transformers
license: mit
quantized_by: mradermacher
---
## About
<!-- ### quantize_version: 2 -->
<!-- ### output_tensor_quantised: 1 -->
<!-- ### convert_type: hf -->
<!-- ### vocab_type: -->
<!-- ### tags: -->
static quants of https://huggingface.co/TIGER-Lab/StructLM-13B
<!-- provided-files -->
weighted/imatrix quants are available at https://huggingface.co/mradermacher/StructLM-13B-i1-GGUF
## Usage
If you are unsure how to use GGUF files, refer to one of [TheBloke's
READMEs](https://huggingface.co/TheBloke/KafkaLM-70B-German-V0.1-GGUF) for
more details, including on how to concatenate multi-part files.
## Provided Quants
(sorted by size, not necessarily quality. IQ-quants are often preferable over similar sized non-IQ quants)
| Link | Type | Size/GB | Notes |
|:-----|:-----|--------:|:------|
| [GGUF](https://huggingface.co/mradermacher/StructLM-13B-GGUF/resolve/main/StructLM-13B.Q2_K.gguf) | Q2_K | 5.0 | |
| [GGUF](https://huggingface.co/mradermacher/StructLM-13B-GGUF/resolve/main/StructLM-13B.Q3_K_S.gguf) | Q3_K_S | 5.8 | |
| [GGUF](https://huggingface.co/mradermacher/StructLM-13B-GGUF/resolve/main/StructLM-13B.Q3_K_M.gguf) | Q3_K_M | 6.4 | lower quality |
| [GGUF](https://huggingface.co/mradermacher/StructLM-13B-GGUF/resolve/main/StructLM-13B.Q3_K_L.gguf) | Q3_K_L | 7.0 | |
| [GGUF](https://huggingface.co/mradermacher/StructLM-13B-GGUF/resolve/main/StructLM-13B.IQ4_XS.gguf) | IQ4_XS | 7.1 | |
| [GGUF](https://huggingface.co/mradermacher/StructLM-13B-GGUF/resolve/main/StructLM-13B.Q4_0_4_4.gguf) | Q4_0_4_4 | 7.5 | fast on arm, low quality |
| [GGUF](https://huggingface.co/mradermacher/StructLM-13B-GGUF/resolve/main/StructLM-13B.Q4_K_S.gguf) | Q4_K_S | 7.5 | fast, recommended |
| [GGUF](https://huggingface.co/mradermacher/StructLM-13B-GGUF/resolve/main/StructLM-13B.Q4_K_M.gguf) | Q4_K_M | 8.0 | fast, recommended |
| [GGUF](https://huggingface.co/mradermacher/StructLM-13B-GGUF/resolve/main/StructLM-13B.Q5_K_S.gguf) | Q5_K_S | 9.1 | |
| [GGUF](https://huggingface.co/mradermacher/StructLM-13B-GGUF/resolve/main/StructLM-13B.Q5_K_M.gguf) | Q5_K_M | 9.3 | |
| [GGUF](https://huggingface.co/mradermacher/StructLM-13B-GGUF/resolve/main/StructLM-13B.Q6_K.gguf) | Q6_K | 10.8 | very good quality |
| [GGUF](https://huggingface.co/mradermacher/StructLM-13B-GGUF/resolve/main/StructLM-13B.Q8_0.gguf) | Q8_0 | 13.9 | fast, best quality |
Here is a handy graph by ikawrakow comparing some lower-quality quant
types (lower is better):

And here are Artefact2's thoughts on the matter:
https://gist.github.com/Artefact2/b5f810600771265fc1e39442288e8ec9
## FAQ / Model Request
See https://huggingface.co/mradermacher/model_requests for some answers to
questions you might have and/or if you want some other model quantized.
## Thanks
I thank my company, [nethype GmbH](https://www.nethype.de/), for letting
me use its servers and providing upgrades to my workstation to enable
this work in my free time.
<!-- end -->
|
RichardErkhov/ahmedheakl_-_asm2asm-deepseek-1.3b-500k-2ep-x86-O0-risc-gguf | RichardErkhov | "2024-11-01T16:34:15Z" | 253 | 0 | null | [
"gguf",
"endpoints_compatible",
"region:us",
"conversational"
] | null | "2024-11-01T16:11:10Z" | Quantization made by Richard Erkhov.
[Github](https://github.com/RichardErkhov)
[Discord](https://discord.gg/pvy7H8DZMG)
[Request more models](https://github.com/RichardErkhov/quant_request)
asm2asm-deepseek-1.3b-500k-2ep-x86-O0-risc - GGUF
- Model creator: https://huggingface.co/ahmedheakl/
- Original model: https://huggingface.co/ahmedheakl/asm2asm-deepseek-1.3b-500k-2ep-x86-O0-risc/
| Name | Quant method | Size |
| ---- | ---- | ---- |
| [asm2asm-deepseek-1.3b-500k-2ep-x86-O0-risc.Q2_K.gguf](https://huggingface.co/RichardErkhov/ahmedheakl_-_asm2asm-deepseek-1.3b-500k-2ep-x86-O0-risc-gguf/blob/main/asm2asm-deepseek-1.3b-500k-2ep-x86-O0-risc.Q2_K.gguf) | Q2_K | 0.52GB |
| [asm2asm-deepseek-1.3b-500k-2ep-x86-O0-risc.Q3_K_S.gguf](https://huggingface.co/RichardErkhov/ahmedheakl_-_asm2asm-deepseek-1.3b-500k-2ep-x86-O0-risc-gguf/blob/main/asm2asm-deepseek-1.3b-500k-2ep-x86-O0-risc.Q3_K_S.gguf) | Q3_K_S | 0.6GB |
| [asm2asm-deepseek-1.3b-500k-2ep-x86-O0-risc.Q3_K.gguf](https://huggingface.co/RichardErkhov/ahmedheakl_-_asm2asm-deepseek-1.3b-500k-2ep-x86-O0-risc-gguf/blob/main/asm2asm-deepseek-1.3b-500k-2ep-x86-O0-risc.Q3_K.gguf) | Q3_K | 0.66GB |
| [asm2asm-deepseek-1.3b-500k-2ep-x86-O0-risc.Q3_K_M.gguf](https://huggingface.co/RichardErkhov/ahmedheakl_-_asm2asm-deepseek-1.3b-500k-2ep-x86-O0-risc-gguf/blob/main/asm2asm-deepseek-1.3b-500k-2ep-x86-O0-risc.Q3_K_M.gguf) | Q3_K_M | 0.66GB |
| [asm2asm-deepseek-1.3b-500k-2ep-x86-O0-risc.Q3_K_L.gguf](https://huggingface.co/RichardErkhov/ahmedheakl_-_asm2asm-deepseek-1.3b-500k-2ep-x86-O0-risc-gguf/blob/main/asm2asm-deepseek-1.3b-500k-2ep-x86-O0-risc.Q3_K_L.gguf) | Q3_K_L | 0.69GB |
| [asm2asm-deepseek-1.3b-500k-2ep-x86-O0-risc.IQ4_XS.gguf](https://huggingface.co/RichardErkhov/ahmedheakl_-_asm2asm-deepseek-1.3b-500k-2ep-x86-O0-risc-gguf/blob/main/asm2asm-deepseek-1.3b-500k-2ep-x86-O0-risc.IQ4_XS.gguf) | IQ4_XS | 0.7GB |
| [asm2asm-deepseek-1.3b-500k-2ep-x86-O0-risc.Q4_0.gguf](https://huggingface.co/RichardErkhov/ahmedheakl_-_asm2asm-deepseek-1.3b-500k-2ep-x86-O0-risc-gguf/blob/main/asm2asm-deepseek-1.3b-500k-2ep-x86-O0-risc.Q4_0.gguf) | Q4_0 | 0.72GB |
| [asm2asm-deepseek-1.3b-500k-2ep-x86-O0-risc.IQ4_NL.gguf](https://huggingface.co/RichardErkhov/ahmedheakl_-_asm2asm-deepseek-1.3b-500k-2ep-x86-O0-risc-gguf/blob/main/asm2asm-deepseek-1.3b-500k-2ep-x86-O0-risc.IQ4_NL.gguf) | IQ4_NL | 0.73GB |
| [asm2asm-deepseek-1.3b-500k-2ep-x86-O0-risc.Q4_K_S.gguf](https://huggingface.co/RichardErkhov/ahmedheakl_-_asm2asm-deepseek-1.3b-500k-2ep-x86-O0-risc-gguf/blob/main/asm2asm-deepseek-1.3b-500k-2ep-x86-O0-risc.Q4_K_S.gguf) | Q4_K_S | 0.76GB |
| [asm2asm-deepseek-1.3b-500k-2ep-x86-O0-risc.Q4_K.gguf](https://huggingface.co/RichardErkhov/ahmedheakl_-_asm2asm-deepseek-1.3b-500k-2ep-x86-O0-risc-gguf/blob/main/asm2asm-deepseek-1.3b-500k-2ep-x86-O0-risc.Q4_K.gguf) | Q4_K | 0.81GB |
| [asm2asm-deepseek-1.3b-500k-2ep-x86-O0-risc.Q4_K_M.gguf](https://huggingface.co/RichardErkhov/ahmedheakl_-_asm2asm-deepseek-1.3b-500k-2ep-x86-O0-risc-gguf/blob/main/asm2asm-deepseek-1.3b-500k-2ep-x86-O0-risc.Q4_K_M.gguf) | Q4_K_M | 0.81GB |
| [asm2asm-deepseek-1.3b-500k-2ep-x86-O0-risc.Q4_1.gguf](https://huggingface.co/RichardErkhov/ahmedheakl_-_asm2asm-deepseek-1.3b-500k-2ep-x86-O0-risc-gguf/blob/main/asm2asm-deepseek-1.3b-500k-2ep-x86-O0-risc.Q4_1.gguf) | Q4_1 | 0.8GB |
| [asm2asm-deepseek-1.3b-500k-2ep-x86-O0-risc.Q5_0.gguf](https://huggingface.co/RichardErkhov/ahmedheakl_-_asm2asm-deepseek-1.3b-500k-2ep-x86-O0-risc-gguf/blob/main/asm2asm-deepseek-1.3b-500k-2ep-x86-O0-risc.Q5_0.gguf) | Q5_0 | 0.87GB |
| [asm2asm-deepseek-1.3b-500k-2ep-x86-O0-risc.Q5_K_S.gguf](https://huggingface.co/RichardErkhov/ahmedheakl_-_asm2asm-deepseek-1.3b-500k-2ep-x86-O0-risc-gguf/blob/main/asm2asm-deepseek-1.3b-500k-2ep-x86-O0-risc.Q5_K_S.gguf) | Q5_K_S | 0.89GB |
| [asm2asm-deepseek-1.3b-500k-2ep-x86-O0-risc.Q5_K.gguf](https://huggingface.co/RichardErkhov/ahmedheakl_-_asm2asm-deepseek-1.3b-500k-2ep-x86-O0-risc-gguf/blob/main/asm2asm-deepseek-1.3b-500k-2ep-x86-O0-risc.Q5_K.gguf) | Q5_K | 0.93GB |
| [asm2asm-deepseek-1.3b-500k-2ep-x86-O0-risc.Q5_K_M.gguf](https://huggingface.co/RichardErkhov/ahmedheakl_-_asm2asm-deepseek-1.3b-500k-2ep-x86-O0-risc-gguf/blob/main/asm2asm-deepseek-1.3b-500k-2ep-x86-O0-risc.Q5_K_M.gguf) | Q5_K_M | 0.93GB |
| [asm2asm-deepseek-1.3b-500k-2ep-x86-O0-risc.Q5_1.gguf](https://huggingface.co/RichardErkhov/ahmedheakl_-_asm2asm-deepseek-1.3b-500k-2ep-x86-O0-risc-gguf/blob/main/asm2asm-deepseek-1.3b-500k-2ep-x86-O0-risc.Q5_1.gguf) | Q5_1 | 0.95GB |
| [asm2asm-deepseek-1.3b-500k-2ep-x86-O0-risc.Q6_K.gguf](https://huggingface.co/RichardErkhov/ahmedheakl_-_asm2asm-deepseek-1.3b-500k-2ep-x86-O0-risc-gguf/blob/main/asm2asm-deepseek-1.3b-500k-2ep-x86-O0-risc.Q6_K.gguf) | Q6_K | 0.5GB |
| [asm2asm-deepseek-1.3b-500k-2ep-x86-O0-risc.Q8_0.gguf](https://huggingface.co/RichardErkhov/ahmedheakl_-_asm2asm-deepseek-1.3b-500k-2ep-x86-O0-risc-gguf/blob/main/asm2asm-deepseek-1.3b-500k-2ep-x86-O0-risc.Q8_0.gguf) | Q8_0 | 1.33GB |
Original model description:
---
library_name: transformers
license: other
base_model: deepseek-ai/deepseek-coder-1.3b-instruct
tags:
- trl
- sft
- generated_from_trainer
model-index:
- name: asm2asm-deepseek-1.3b-500k-2ep-x86-O0-risc
results: []
---
<!-- This model card has been generated automatically according to the information the Trainer had access to. You
should probably proofread and complete it, then remove this comment. -->
# asm2asm-deepseek-1.3b-500k-2ep-x86-O0-risc
This model is a fine-tuned version of [deepseek-ai/deepseek-coder-1.3b-instruct](https://huggingface.co/deepseek-ai/deepseek-coder-1.3b-instruct) on an unknown dataset.
## Model description
More information needed
## Intended uses & limitations
More information needed
## Training and evaluation data
More information needed
## Training procedure
### Training hyperparameters
The following hyperparameters were used during training:
- learning_rate: 0.0002
- train_batch_size: 2
- eval_batch_size: 8
- seed: 42
- gradient_accumulation_steps: 4
- total_train_batch_size: 8
- optimizer: Use OptimizerNames.PAGED_ADAMW with betas=(0.9,0.999) and epsilon=1e-08 and optimizer_args=No additional optimizer arguments
- lr_scheduler_type: linear
- num_epochs: 2
### Training results
### Framework versions
- Transformers 4.46.0
- Pytorch 2.4.0
- Datasets 3.0.2
- Tokenizers 0.20.1
|
Isaacp/Reinforce-pixelcopter | Isaacp | "2023-02-09T02:34:28Z" | 0 | 0 | null | [
"Pixelcopter-PLE-v0",
"reinforce",
"reinforcement-learning",
"custom-implementation",
"deep-rl-class",
"model-index",
"region:us"
] | reinforcement-learning | "2023-02-09T02:34:20Z" | ---
tags:
- Pixelcopter-PLE-v0
- reinforce
- reinforcement-learning
- custom-implementation
- deep-rl-class
model-index:
- name: Reinforce-pixelcopter
results:
- task:
type: reinforcement-learning
name: reinforcement-learning
dataset:
name: Pixelcopter-PLE-v0
type: Pixelcopter-PLE-v0
metrics:
- type: mean_reward
value: 39.90 +/- 33.12
name: mean_reward
verified: false
---
# **Reinforce** Agent playing **Pixelcopter-PLE-v0**
This is a trained model of a **Reinforce** agent playing **Pixelcopter-PLE-v0** .
To learn to use this model and train yours check Unit 4 of the Deep Reinforcement Learning Course: https://huggingface.co/deep-rl-course/unit4/introduction
|
TongZheng1999/gemma-2-9b-it-star-nl-final_1-5-3Rounds-iter-1 | TongZheng1999 | "2025-03-16T01:41:11Z" | 0 | 0 | transformers | [
"transformers",
"tensorboard",
"safetensors",
"gemma2",
"text-generation",
"generated_from_trainer",
"alignment-handbook",
"trl",
"sft",
"conversational",
"base_model:google/gemma-2-9b-it",
"base_model:finetune:google/gemma-2-9b-it",
"autotrain_compatible",
"text-generation-inference",
"endpoints_compatible",
"region:us"
] | text-generation | "2025-03-16T01:21:48Z" | ---
base_model: google/gemma-2-9b-it
library_name: transformers
model_name: gemma-2-9b-it-star-nl-final_1-5-3Rounds-iter-1
tags:
- generated_from_trainer
- alignment-handbook
- trl
- sft
licence: license
---
# Model Card for gemma-2-9b-it-star-nl-final_1-5-3Rounds-iter-1
This model is a fine-tuned version of [google/gemma-2-9b-it](https://huggingface.co/google/gemma-2-9b-it).
It has been trained using [TRL](https://github.com/huggingface/trl).
## Quick start
```python
from transformers import pipeline
question = "If you had a time machine, but could only go to the past or the future once and never return, which would you choose and why?"
generator = pipeline("text-generation", model="TongZheng1999/gemma-2-9b-it-star-nl-final_1-5-3Rounds-iter-1", device="cuda")
output = generator([{"role": "user", "content": question}], max_new_tokens=128, return_full_text=False)[0]
print(output["generated_text"])
```
## Training procedure
[<img src="https://raw.githubusercontent.com/wandb/assets/main/wandb-github-badge-28.svg" alt="Visualize in Weights & Biases" width="150" height="24"/>](https://wandb.ai/kidzheng/huggingface/runs/d88aeh1i)
This model was trained with SFT.
### Framework versions
- TRL: 0.12.0
- Transformers: 4.46.0
- Pytorch: 2.6.0
- Datasets: 3.3.1
- Tokenizers: 0.20.3
## Citations
Cite TRL as:
```bibtex
@misc{vonwerra2022trl,
title = {{TRL: Transformer Reinforcement Learning}},
author = {Leandro von Werra and Younes Belkada and Lewis Tunstall and Edward Beeching and Tristan Thrush and Nathan Lambert and Shengyi Huang and Kashif Rasul and Quentin Gallouédec},
year = 2020,
journal = {GitHub repository},
publisher = {GitHub},
howpublished = {\url{https://github.com/huggingface/trl}}
}
``` |
griffio/vit-base-patch16-224-in21k-rotated-dungeons-v5 | griffio | "2024-10-31T15:17:40Z" | 193 | 0 | transformers | [
"transformers",
"tensorboard",
"safetensors",
"vit",
"image-classification",
"generated_from_trainer",
"dataset:imagefolder",
"base_model:google/vit-base-patch16-224-in21k",
"base_model:finetune:google/vit-base-patch16-224-in21k",
"license:apache-2.0",
"model-index",
"autotrain_compatible",
"endpoints_compatible",
"region:us"
] | image-classification | "2024-10-31T15:17:21Z" | ---
library_name: transformers
license: apache-2.0
base_model: google/vit-base-patch16-224-in21k
tags:
- image-classification
- generated_from_trainer
datasets:
- imagefolder
metrics:
- accuracy
model-index:
- name: vit-base-patch16-224-in21k-rotated-dungeons-v5
results:
- task:
name: Image Classification
type: image-classification
dataset:
name: rotated_maps
type: imagefolder
config: default
split: validation
args: default
metrics:
- name: Accuracy
type: accuracy
value: 0.5
---
<!-- This model card has been generated automatically according to the information the Trainer had access to. You
should probably proofread and complete it, then remove this comment. -->
# vit-base-patch16-224-in21k-rotated-dungeons-v5
This model is a fine-tuned version of [google/vit-base-patch16-224-in21k](https://huggingface.co/google/vit-base-patch16-224-in21k) on the rotated_maps dataset.
It achieves the following results on the evaluation set:
- Loss: 1.5540
- Accuracy: 0.5
## Model description
More information needed
## Intended uses & limitations
More information needed
## Training and evaluation data
More information needed
## Training procedure
### Training hyperparameters
The following hyperparameters were used during training:
- learning_rate: 1e-05
- train_batch_size: 5
- eval_batch_size: 8
- seed: 1024
- optimizer: Adam with betas=(0.9,0.999) and epsilon=1e-08
- lr_scheduler_type: linear
- num_epochs: 15
- mixed_precision_training: Native AMP
### Training results
| Training Loss | Epoch | Step | Validation Loss | Accuracy |
|:-------------:|:-----:|:----:|:---------------:|:--------:|
| 1.0613 | 10.0 | 100 | 1.5540 | 0.5 |
### Framework versions
- Transformers 4.44.2
- Pytorch 2.5.0+cu121
- Datasets 3.1.0
- Tokenizers 0.19.1
|
ekaterina-blatova-jb/model_lr4e-5_old_scheduler_with_warmup_3_epochs_relevant_v0 | ekaterina-blatova-jb | "2024-07-11T18:09:09Z" | 5 | 0 | transformers | [
"transformers",
"safetensors",
"llama",
"text-generation",
"autotrain_compatible",
"text-generation-inference",
"endpoints_compatible",
"region:us"
] | text-generation | "2024-07-11T18:07:22Z" | ---
{}
---
## Evaluation results
Validation loss on the whole input: 0.8542202417738736
Validation loss on completion: 0.9471904315287247
|
RichardErkhov/yanolja_-_EEVE-Korean-10.8B-v1.0-gguf | RichardErkhov | "2024-05-02T13:28:59Z" | 272 | 1 | null | [
"gguf",
"arxiv:2402.14714",
"endpoints_compatible",
"region:us",
"conversational"
] | null | "2024-05-02T09:15:35Z" | Quantization made by Richard Erkhov.
[Github](https://github.com/RichardErkhov)
[Discord](https://discord.gg/pvy7H8DZMG)
[Request more models](https://github.com/RichardErkhov/quant_request)
EEVE-Korean-10.8B-v1.0 - GGUF
- Model creator: https://huggingface.co/yanolja/
- Original model: https://huggingface.co/yanolja/EEVE-Korean-10.8B-v1.0/
| Name | Quant method | Size |
| ---- | ---- | ---- |
| [EEVE-Korean-10.8B-v1.0.Q2_K.gguf](https://huggingface.co/RichardErkhov/yanolja_-_EEVE-Korean-10.8B-v1.0-gguf/blob/main/EEVE-Korean-10.8B-v1.0.Q2_K.gguf) | Q2_K | 3.77GB |
| [EEVE-Korean-10.8B-v1.0.IQ3_XS.gguf](https://huggingface.co/RichardErkhov/yanolja_-_EEVE-Korean-10.8B-v1.0-gguf/blob/main/EEVE-Korean-10.8B-v1.0.IQ3_XS.gguf) | IQ3_XS | 4.18GB |
| [EEVE-Korean-10.8B-v1.0.IQ3_S.gguf](https://huggingface.co/RichardErkhov/yanolja_-_EEVE-Korean-10.8B-v1.0-gguf/blob/main/EEVE-Korean-10.8B-v1.0.IQ3_S.gguf) | IQ3_S | 4.41GB |
| [EEVE-Korean-10.8B-v1.0.Q3_K_S.gguf](https://huggingface.co/RichardErkhov/yanolja_-_EEVE-Korean-10.8B-v1.0-gguf/blob/main/EEVE-Korean-10.8B-v1.0.Q3_K_S.gguf) | Q3_K_S | 4.39GB |
| [EEVE-Korean-10.8B-v1.0.IQ3_M.gguf](https://huggingface.co/RichardErkhov/yanolja_-_EEVE-Korean-10.8B-v1.0-gguf/blob/main/EEVE-Korean-10.8B-v1.0.IQ3_M.gguf) | IQ3_M | 4.56GB |
| [EEVE-Korean-10.8B-v1.0.Q3_K.gguf](https://huggingface.co/RichardErkhov/yanolja_-_EEVE-Korean-10.8B-v1.0-gguf/blob/main/EEVE-Korean-10.8B-v1.0.Q3_K.gguf) | Q3_K | 4.88GB |
| [EEVE-Korean-10.8B-v1.0.Q3_K_M.gguf](https://huggingface.co/RichardErkhov/yanolja_-_EEVE-Korean-10.8B-v1.0-gguf/blob/main/EEVE-Korean-10.8B-v1.0.Q3_K_M.gguf) | Q3_K_M | 4.88GB |
| [EEVE-Korean-10.8B-v1.0.Q3_K_L.gguf](https://huggingface.co/RichardErkhov/yanolja_-_EEVE-Korean-10.8B-v1.0-gguf/blob/main/EEVE-Korean-10.8B-v1.0.Q3_K_L.gguf) | Q3_K_L | 5.31GB |
| [EEVE-Korean-10.8B-v1.0.IQ4_XS.gguf](https://huggingface.co/RichardErkhov/yanolja_-_EEVE-Korean-10.8B-v1.0-gguf/blob/main/EEVE-Korean-10.8B-v1.0.IQ4_XS.gguf) | IQ4_XS | 5.47GB |
| [EEVE-Korean-10.8B-v1.0.Q4_0.gguf](https://huggingface.co/RichardErkhov/yanolja_-_EEVE-Korean-10.8B-v1.0-gguf/blob/main/EEVE-Korean-10.8B-v1.0.Q4_0.gguf) | Q4_0 | 5.7GB |
| [EEVE-Korean-10.8B-v1.0.IQ4_NL.gguf](https://huggingface.co/RichardErkhov/yanolja_-_EEVE-Korean-10.8B-v1.0-gguf/blob/main/EEVE-Korean-10.8B-v1.0.IQ4_NL.gguf) | IQ4_NL | 5.77GB |
| [EEVE-Korean-10.8B-v1.0.Q4_K_S.gguf](https://huggingface.co/RichardErkhov/yanolja_-_EEVE-Korean-10.8B-v1.0-gguf/blob/main/EEVE-Korean-10.8B-v1.0.Q4_K_S.gguf) | Q4_K_S | 5.75GB |
| [EEVE-Korean-10.8B-v1.0.Q4_K.gguf](https://huggingface.co/RichardErkhov/yanolja_-_EEVE-Korean-10.8B-v1.0-gguf/blob/main/EEVE-Korean-10.8B-v1.0.Q4_K.gguf) | Q4_K | 6.07GB |
| [EEVE-Korean-10.8B-v1.0.Q4_K_M.gguf](https://huggingface.co/RichardErkhov/yanolja_-_EEVE-Korean-10.8B-v1.0-gguf/blob/main/EEVE-Korean-10.8B-v1.0.Q4_K_M.gguf) | Q4_K_M | 6.07GB |
| [EEVE-Korean-10.8B-v1.0.Q4_1.gguf](https://huggingface.co/RichardErkhov/yanolja_-_EEVE-Korean-10.8B-v1.0-gguf/blob/main/EEVE-Korean-10.8B-v1.0.Q4_1.gguf) | Q4_1 | 6.32GB |
| [EEVE-Korean-10.8B-v1.0.Q5_0.gguf](https://huggingface.co/RichardErkhov/yanolja_-_EEVE-Korean-10.8B-v1.0-gguf/blob/main/EEVE-Korean-10.8B-v1.0.Q5_0.gguf) | Q5_0 | 6.94GB |
| [EEVE-Korean-10.8B-v1.0.Q5_K_S.gguf](https://huggingface.co/RichardErkhov/yanolja_-_EEVE-Korean-10.8B-v1.0-gguf/blob/main/EEVE-Korean-10.8B-v1.0.Q5_K_S.gguf) | Q5_K_S | 6.94GB |
| [EEVE-Korean-10.8B-v1.0.Q5_K.gguf](https://huggingface.co/RichardErkhov/yanolja_-_EEVE-Korean-10.8B-v1.0-gguf/blob/main/EEVE-Korean-10.8B-v1.0.Q5_K.gguf) | Q5_K | 7.13GB |
| [EEVE-Korean-10.8B-v1.0.Q5_K_M.gguf](https://huggingface.co/RichardErkhov/yanolja_-_EEVE-Korean-10.8B-v1.0-gguf/blob/main/EEVE-Korean-10.8B-v1.0.Q5_K_M.gguf) | Q5_K_M | 7.13GB |
| [EEVE-Korean-10.8B-v1.0.Q5_1.gguf](https://huggingface.co/RichardErkhov/yanolja_-_EEVE-Korean-10.8B-v1.0-gguf/blob/main/EEVE-Korean-10.8B-v1.0.Q5_1.gguf) | Q5_1 | 7.56GB |
| [EEVE-Korean-10.8B-v1.0.Q6_K.gguf](https://huggingface.co/RichardErkhov/yanolja_-_EEVE-Korean-10.8B-v1.0-gguf/blob/main/EEVE-Korean-10.8B-v1.0.Q6_K.gguf) | Q6_K | 8.26GB |
Original model description:
---
license: apache-2.0
base_model: upstage/SOLAR-10.7B-v1.0
tags:
- generated_from_trainer
model-index:
- name: yanolja/EEVE-Korean-10.8B-v1.0
results: []
---
[<img src="https://raw.githubusercontent.com/OpenAccess-AI-Collective/axolotl/main/image/axolotl-badge-web.png" alt="Built with Axolotl" width="200" height="32"/>](https://github.com/OpenAccess-AI-Collective/axolotl)
<p align="left">
<img src="https://huggingface.co/yanolja/EEVE-Korean-10.8B-v1.0/resolve/main/eeve_logo.webp" width="50%"/>
<p>
# EEVE-Korean-10.8B-v1.0
## Join Our Community on Discord!
If you're passionate about the field of Large Language Models and wish to exchange knowledge and insights, we warmly invite you to join our Discord server. It's worth noting that Korean is the primary language used in this server. The landscape of LLM is evolving rapidly, and without active sharing, our collective knowledge risks becoming outdated swiftly. Let's collaborate and drive greater impact together! Join us here: [Discord Link](https://discord.gg/b27bAHg95m).
## Our Dedicated Team (Alphabetical Order)
| Research | Engineering | Product Management | UX Design |
|-----------------|-----------------|--------------------|--------------
| Myeongho Jeong | Geon Kim | Bokyung Huh | Eunsue Choi |
| Seungduk Kim | Rifqi Alfi | | |
| Seungtaek Choi | Sanghoon Han | | |
| | Suhyun Kang | | |
## About the Model
This model is a Korean vocabulary-extended version of [upstage/SOLAR-10.7B-v1.0](https://huggingface.co/upstage/SOLAR-10.7B-v1.0), specifically fine-tuned on various Korean web-crawled datasets available on HuggingFace. Our approach was to expand the model's understanding of Korean by pre-training the embeddings for new tokens and partially fine-tuning the `lm_head` embeddings for the already existing tokens while preserving the original parameters of the base model.
### Technical Deep Dive
<p align="left">
<img src="https://huggingface.co/yanolja/EEVE-Korean-10.8B-v1.0/resolve/main/EEVE_figure.png" width="100%"/>
<p>
To adapt foundational models from English to Korean, we use subword-based embedding with a seven-stage training process involving parameter freezing.
This approach progressively trains from input embeddings to full parameters, efficiently extending the model's vocabulary to include Korean.
Our method enhances the model's cross-linguistic applicability by carefully integrating new linguistic tokens, focusing on causal language modeling pre-training.
We leverage the inherent capabilities of foundational models trained on English to efficiently transfer knowledge and reasoning to Korean, optimizing the adaptation process.
For more details, please refer to our technical report: [Efficient and Effective Vocabulary Expansion Towards Multilingual Large Language Models](https://arxiv.org/abs/2402.14714).
Here’s an simplified code for our key approach:
```python
# number_of_old_tokens is the size of tokenizer before vocab extension. For example, in case of EEVE-Korean-10.8B-v1.0, number_of_old_tokens is 32000.
def freeze_partial_embedding_hook(grad):
grad[:number_of_old_tokens] = 0
return grad
for name, param in model.named_parameters():
if ("lm_head" in name or "embed_tokens" in name) and "original" not in name:
param.requires_grad = True
if "embed_tokens" in name:
param.register_hook(freeze_partial_embedding_hook)
else:
param.requires_grad = False
```
### Usage and Limitations
Keep in mind that this model hasn't been fine-tuned with instruction-based training. While it excels in Korean language tasks, we advise careful consideration and further training for specific applications.
### Training Details
Our model’s training was comprehensive and diverse:
- **Vocabulary Expansion:**
We meticulously selected 8,960 Korean tokens based on their frequency in our Korean web corpus. This process involved multiple rounds of tokenizer training, manual curation, and token frequency analysis, ensuring a rich and relevant vocabulary for our model.
1. **Initial Tokenizer Training:** We trained an intermediate tokenizer on a Korean web corpus, with a vocabulary of 40,000 tokens.
2. **Extraction of New Korean Tokens:** From the intermediate tokenizer, we identified all Korean tokens not present in the original SOLAR's tokenizer.
3. **Manual Tokenizer Construction:** We then built the target tokenizer, focusing on these new Korean tokens.
4. **Frequency Analysis:** Using the target tokenizer, we processed a 100GB Korean corpus to count each token's frequency.
5. **Refinement of Token List:** We removed tokens appearing less than 6,000 times, ensuring to secure enough tokens to train models later.
6. **Inclusion of Single-Letter Characters:** Counted missing Korean single-letter characters and added them to the target tokenizer that appeared more than 6,000 times.
7. **Iterative Refinement:** We repeated steps 2 to 6 until there were no tokens to drop or add.
8. **Training Bias Towards New Tokens:** Our training data was biased to include more texts with new tokens, for effective learning.
This rigorous approach ensured a comprehensive and contextually rich Korean vocabulary for the model.
## Citation
```
@misc{kim2024efficient,
title={Efficient and Effective Vocabulary Expansion Towards Multilingual Large Language Models},
author={Seungduk Kim and Seungtaek Choi and Myeongho Jeong},
year={2024},
eprint={2402.14714},
archivePrefix={arXiv},
primaryClass={cs.CL}
}
```
|
KingKazma/cnn_dailymail_t5-small_prompt_tuning_500_10_3000_8_e-1_s6789_v3_l5_v50_manual | KingKazma | "2023-08-04T12:37:49Z" | 0 | 0 | peft | [
"peft",
"region:us"
] | null | "2023-08-04T12:37:46Z" | ---
library_name: peft
---
## Training procedure
### Framework versions
- PEFT 0.5.0.dev0
|
PrunaAI/anakin87-gemma-2b-orpo-QUANTO-float8bit-smashed | PrunaAI | "2024-07-21T08:02:57Z" | 4 | 0 | transformers | [
"transformers",
"pruna-ai",
"base_model:anakin87/gemma-2b-orpo",
"base_model:finetune:anakin87/gemma-2b-orpo",
"endpoints_compatible",
"region:us"
] | null | "2024-07-21T08:00:06Z" | ---
thumbnail: "https://assets-global.website-files.com/646b351987a8d8ce158d1940/64ec9e96b4334c0e1ac41504_Logo%20with%20white%20text.svg"
base_model: anakin87/gemma-2b-orpo
metrics:
- memory_disk
- memory_inference
- inference_latency
- inference_throughput
- inference_CO2_emissions
- inference_energy_consumption
tags:
- pruna-ai
---
<!-- header start -->
<!-- 200823 -->
<div style="width: auto; margin-left: auto; margin-right: auto">
<a href="https://www.pruna.ai/" target="_blank" rel="noopener noreferrer">
<img src="https://i.imgur.com/eDAlcgk.png" alt="PrunaAI" style="width: 100%; min-width: 400px; display: block; margin: auto;">
</a>
</div>
<!-- header end -->
[](https://twitter.com/PrunaAI)
[](https://github.com/PrunaAI)
[](https://www.linkedin.com/company/93832878/admin/feed/posts/?feedType=following)
[](https://discord.gg/rskEr4BZJx)
# Simply make AI models cheaper, smaller, faster, and greener!
- Give a thumbs up if you like this model!
- Contact us and tell us which model to compress next [here](https://www.pruna.ai/contact).
- Request access to easily compress your *own* AI models [here](https://z0halsaff74.typeform.com/pruna-access?typeform-source=www.pruna.ai).
- Read the documentations to know more [here](https://pruna-ai-pruna.readthedocs-hosted.com/en/latest/)
- Join Pruna AI community on Discord [here](https://discord.gg/CP4VSgck) to share feedback/suggestions or get help.
## Results

**Frequently Asked Questions**
- ***How does the compression work?*** The model is compressed with quanto.
- ***How does the model quality change?*** The quality of the model output might vary compared to the base model.
- ***How is the model efficiency evaluated?*** These results were obtained on HARDWARE_NAME with configuration described in `model/smash_config.json` and are obtained after a hardware warmup. The smashed model is directly compared to the original base model. Efficiency results may vary in other settings (e.g. other hardware, image size, batch size, ...). We recommend to directly run them in the use-case conditions to know if the smashed model can benefit you.
- ***What is the model format?*** We use safetensors.
- ***What calibration data has been used?*** If needed by the compression method, we used WikiText as the calibration data.
- ***What is the naming convention for Pruna Huggingface models?*** We take the original model name and append "turbo", "tiny", or "green" if the smashed model has a measured inference speed, inference memory, or inference energy consumption which is less than 90% of the original base model.
- ***How to compress my own models?*** You can request premium access to more compression methods and tech support for your specific use-cases [here](https://z0halsaff74.typeform.com/pruna-access?typeform-source=www.pruna.ai).
- ***What are "first" metrics?*** Results mentioning "first" are obtained after the first run of the model. The first run might take more memory or be slower than the subsequent runs due cuda overheads.
- ***What are "Sync" and "Async" metrics?*** "Sync" metrics are obtained by syncing all GPU processes and stop measurement when all of them are executed. "Async" metrics are obtained without syncing all GPU processes and stop when the model output can be used by the CPU. We provide both metrics since both could be relevant depending on the use-case. We recommend to test the efficiency gains directly in your use-cases.
## Setup
You can run the smashed model with these steps:
0. Check requirements from the original repo anakin87/gemma-2b-orpo installed. In particular, check python, cuda, and transformers versions.
1. Make sure that you have installed quantization related packages.
```bash
pip install quanto
```
2. Load & run the model.
```python
from transformers import AutoModelForCausalLM, AutoTokenizer
IMPORTS
model = AutoModelForCausalLM.from_pretrained("PrunaAI/anakin87-gemma-2b-orpo-QUANTO-float8bit-smashed", trust_remote_code=True, device_map='auto')
tokenizer = AutoTokenizer.from_pretrained("anakin87/gemma-2b-orpo")
input_ids = tokenizer("What is the color of prunes?,", return_tensors='pt').to(model.device)["input_ids"]
outputs = model.generate(input_ids, max_new_tokens=216)
tokenizer.decode(outputs[0])
```
## Configurations
The configuration info are in `smash_config.json`.
## Credits & License
The license of the smashed model follows the license of the original model. Please check the license of the original model anakin87/gemma-2b-orpo before using this model which provided the base model. The license of the `pruna-engine` is [here](https://pypi.org/project/pruna-engine/) on Pypi.
## Want to compress other models?
- Contact us and tell us which model to compress next [here](https://www.pruna.ai/contact).
- Request access to easily compress your own AI models [here](https://z0halsaff74.typeform.com/pruna-access?typeform-source=www.pruna.ai). |
billy12341/kfir | billy12341 | "2025-03-15T18:02:06Z" | 0 | 0 | diffusers | [
"diffusers",
"flux",
"lora",
"replicate",
"text-to-image",
"en",
"base_model:black-forest-labs/FLUX.1-dev",
"base_model:adapter:black-forest-labs/FLUX.1-dev",
"license:other",
"region:us"
] | text-to-image | "2025-03-15T17:47:48Z" | ---
license: other
license_name: flux-1-dev-non-commercial-license
license_link: https://huggingface.co/black-forest-labs/FLUX.1-dev/blob/main/LICENSE.md
language:
- en
tags:
- flux
- diffusers
- lora
- replicate
base_model: "black-forest-labs/FLUX.1-dev"
pipeline_tag: text-to-image
# widget:
# - text: >-
# prompt
# output:
# url: https://...
instance_prompt: kfir12341
---
# Kfir
<Gallery />
Trained on Replicate using:
https://replicate.com/ostris/flux-dev-lora-trainer/train
## Trigger words
You should use `kfir12341` to trigger the image generation.
## Use it with the [🧨 diffusers library](https://github.com/huggingface/diffusers)
```py
from diffusers import AutoPipelineForText2Image
import torch
pipeline = AutoPipelineForText2Image.from_pretrained('black-forest-labs/FLUX.1-dev', torch_dtype=torch.float16).to('cuda')
pipeline.load_lora_weights('billy12341/kfir', weight_name='lora.safetensors')
image = pipeline('your prompt').images[0]
```
For more details, including weighting, merging and fusing LoRAs, check the [documentation on loading LoRAs in diffusers](https://huggingface.co/docs/diffusers/main/en/using-diffusers/loading_adapters)
|
research-backup/relbert-roberta-base-semeval2012-v6-average-prompt-b-loob-0-child-prototypical | research-backup | "2022-11-27T10:20:42Z" | 103 | 0 | transformers | [
"transformers",
"pytorch",
"roberta",
"feature-extraction",
"dataset:relbert/semeval2012_relational_similarity_v6",
"model-index",
"text-embeddings-inference",
"endpoints_compatible",
"region:us"
] | feature-extraction | "2022-11-27T10:19:34Z" | ---
datasets:
- relbert/semeval2012_relational_similarity_v6
model-index:
- name: relbert/relbert-roberta-base-semeval2012-v6-average-prompt-b-loob-0-child-prototypical
results:
- task:
name: Relation Mapping
type: sorting-task
dataset:
name: Relation Mapping
args: relbert/relation_mapping
type: relation-mapping
metrics:
- name: Accuracy
type: accuracy
value: 0.6335515873015873
- task:
name: Analogy Questions (SAT full)
type: multiple-choice-qa
dataset:
name: SAT full
args: relbert/analogy_questions
type: analogy-questions
metrics:
- name: Accuracy
type: accuracy
value: 0.30213903743315507
- task:
name: Analogy Questions (SAT)
type: multiple-choice-qa
dataset:
name: SAT
args: relbert/analogy_questions
type: analogy-questions
metrics:
- name: Accuracy
type: accuracy
value: 0.2997032640949555
- task:
name: Analogy Questions (BATS)
type: multiple-choice-qa
dataset:
name: BATS
args: relbert/analogy_questions
type: analogy-questions
metrics:
- name: Accuracy
type: accuracy
value: 0.5403001667593107
- task:
name: Analogy Questions (Google)
type: multiple-choice-qa
dataset:
name: Google
args: relbert/analogy_questions
type: analogy-questions
metrics:
- name: Accuracy
type: accuracy
value: 0.594
- task:
name: Analogy Questions (U2)
type: multiple-choice-qa
dataset:
name: U2
args: relbert/analogy_questions
type: analogy-questions
metrics:
- name: Accuracy
type: accuracy
value: 0.38596491228070173
- task:
name: Analogy Questions (U4)
type: multiple-choice-qa
dataset:
name: U4
args: relbert/analogy_questions
type: analogy-questions
metrics:
- name: Accuracy
type: accuracy
value: 0.37037037037037035
- task:
name: Lexical Relation Classification (BLESS)
type: classification
dataset:
name: BLESS
args: relbert/lexical_relation_classification
type: relation-classification
metrics:
- name: F1
type: f1
value: 0.8618351664908844
- name: F1 (macro)
type: f1_macro
value: 0.8502274926780541
- task:
name: Lexical Relation Classification (CogALexV)
type: classification
dataset:
name: CogALexV
args: relbert/lexical_relation_classification
type: relation-classification
metrics:
- name: F1
type: f1
value: 0.7187793427230047
- name: F1 (macro)
type: f1_macro
value: 0.4123652986554882
- task:
name: Lexical Relation Classification (EVALution)
type: classification
dataset:
name: BLESS
args: relbert/lexical_relation_classification
type: relation-classification
metrics:
- name: F1
type: f1
value: 0.5390032502708559
- name: F1 (macro)
type: f1_macro
value: 0.5145491852283891
- task:
name: Lexical Relation Classification (K&H+N)
type: classification
dataset:
name: K&H+N
args: relbert/lexical_relation_classification
type: relation-classification
metrics:
- name: F1
type: f1
value: 0.9442860123808862
- name: F1 (macro)
type: f1_macro
value: 0.8512983083261685
- task:
name: Lexical Relation Classification (ROOT09)
type: classification
dataset:
name: ROOT09
args: relbert/lexical_relation_classification
type: relation-classification
metrics:
- name: F1
type: f1
value: 0.8122845502977123
- name: F1 (macro)
type: f1_macro
value: 0.8070813252421513
---
# relbert/relbert-roberta-base-semeval2012-v6-average-prompt-b-loob-0-child-prototypical
RelBERT fine-tuned from [roberta-base](https://huggingface.co/roberta-base) on
[relbert/semeval2012_relational_similarity_v6](https://huggingface.co/datasets/relbert/semeval2012_relational_similarity_v6).
Fine-tuning is done via [RelBERT](https://github.com/asahi417/relbert) library (see the repository for more detail).
It achieves the following results on the relation understanding tasks:
- Analogy Question ([dataset](https://huggingface.co/datasets/relbert/analogy_questions), [full result](https://huggingface.co/relbert/relbert-roberta-base-semeval2012-v6-average-prompt-b-loob-0-child-prototypical/raw/main/analogy.json)):
- Accuracy on SAT (full): 0.30213903743315507
- Accuracy on SAT: 0.2997032640949555
- Accuracy on BATS: 0.5403001667593107
- Accuracy on U2: 0.38596491228070173
- Accuracy on U4: 0.37037037037037035
- Accuracy on Google: 0.594
- Lexical Relation Classification ([dataset](https://huggingface.co/datasets/relbert/lexical_relation_classification), [full result](https://huggingface.co/relbert/relbert-roberta-base-semeval2012-v6-average-prompt-b-loob-0-child-prototypical/raw/main/classification.json)):
- Micro F1 score on BLESS: 0.8618351664908844
- Micro F1 score on CogALexV: 0.7187793427230047
- Micro F1 score on EVALution: 0.5390032502708559
- Micro F1 score on K&H+N: 0.9442860123808862
- Micro F1 score on ROOT09: 0.8122845502977123
- Relation Mapping ([dataset](https://huggingface.co/datasets/relbert/relation_mapping), [full result](https://huggingface.co/relbert/relbert-roberta-base-semeval2012-v6-average-prompt-b-loob-0-child-prototypical/raw/main/relation_mapping.json)):
- Accuracy on Relation Mapping: 0.6335515873015873
### Usage
This model can be used through the [relbert library](https://github.com/asahi417/relbert). Install the library via pip
```shell
pip install relbert
```
and activate model as below.
```python
from relbert import RelBERT
model = RelBERT("relbert/relbert-roberta-base-semeval2012-v6-average-prompt-b-loob-0-child-prototypical")
vector = model.get_embedding(['Tokyo', 'Japan']) # shape of (1024, )
```
### Training hyperparameters
The following hyperparameters were used during training:
- model: roberta-base
- max_length: 64
- mode: average
- data: relbert/semeval2012_relational_similarity_v6
- split: train
- split_eval: validation
- template_mode: manual
- loss_function: info_loob
- classification_loss: False
- temperature_nce_constant: 0.05
- temperature_nce_rank: {'min': 0.01, 'max': 0.05, 'type': 'linear'}
- epoch: 10
- batch: 128
- lr: 5e-06
- lr_decay: False
- lr_warmup: 1
- weight_decay: 0
- random_seed: 0
- exclude_relation: None
- n_sample: 320
- gradient_accumulation: 8
- relation_level: None
- data_level: child_prototypical
The full configuration can be found at [fine-tuning parameter file](https://huggingface.co/relbert/relbert-roberta-base-semeval2012-v6-average-prompt-b-loob-0-child-prototypical/raw/main/trainer_config.json).
### Reference
If you use any resource from RelBERT, please consider to cite our [paper](https://aclanthology.org/2021.eacl-demos.7/).
```
@inproceedings{ushio-etal-2021-distilling-relation-embeddings,
title = "{D}istilling {R}elation {E}mbeddings from {P}re-trained {L}anguage {M}odels",
author = "Ushio, Asahi and
Schockaert, Steven and
Camacho-Collados, Jose",
booktitle = "EMNLP 2021",
year = "2021",
address = "Online",
publisher = "Association for Computational Linguistics",
}
```
|
John6666/noobaiiter-xl-vpred-v075-sdxl | John6666 | "2024-12-23T06:42:29Z" | 503 | 0 | diffusers | [
"diffusers",
"safetensors",
"text-to-image",
"stable-diffusion",
"stable-diffusion-xl",
"anime",
"illustrious",
"en",
"base_model:Laxhar/noob_sdxl_v_pred_test",
"base_model:finetune:Laxhar/noob_sdxl_v_pred_test",
"license:other",
"autotrain_compatible",
"endpoints_compatible",
"diffusers:StableDiffusionXLPipeline",
"region:us"
] | text-to-image | "2024-10-29T06:41:35Z" | ---
license: other
license_name: faipl-1.0-sd
license_link: https://freedevproject.org/faipl-1.0-sd/
language:
- en
library_name: diffusers
pipeline_tag: text-to-image
tags:
- text-to-image
- stable-diffusion
- stable-diffusion-xl
- anime
- illustrious
base_model: Laxhar/noob_sdxl_v_pred
---
Original model is [here](https://civitai.com/models/873533?modelVersionId=1002915).
This model created by [MeltyGarnet](https://civitai.com/user/MeltyGarnet).
|
EP9/bert2bert_shared-spanish-finetuned-summarization-intento2 | EP9 | "2022-11-23T23:51:55Z" | 104 | 0 | transformers | [
"transformers",
"pytorch",
"tensorboard",
"encoder-decoder",
"text2text-generation",
"generated_from_trainer",
"autotrain_compatible",
"endpoints_compatible",
"region:us"
] | text2text-generation | "2022-11-23T21:42:22Z" | ---
tags:
- generated_from_trainer
metrics:
- rouge
model-index:
- name: bert2bert_shared-spanish-finetuned-summarization-intento2
results: []
---
<!-- This model card has been generated automatically according to the information the Trainer had access to. You
should probably proofread and complete it, then remove this comment. -->
# bert2bert_shared-spanish-finetuned-summarization-intento2
This model is a fine-tuned version of [mrm8488/bert2bert_shared-spanish-finetuned-summarization](https://huggingface.co/mrm8488/bert2bert_shared-spanish-finetuned-summarization) on the None dataset.
It achieves the following results on the evaluation set:
- Loss: 7.9693
- Rouge1: 1.8257
- Rouge2: 0.0
- Rougel: 1.6832
- Rougelsum: 1.6866
- Gen Len: 10.0
## Model description
More information needed
## Intended uses & limitations
More information needed
## Training and evaluation data
More information needed
## Training procedure
### Training hyperparameters
The following hyperparameters were used during training:
- learning_rate: 0.001
- train_batch_size: 8
- eval_batch_size: 8
- seed: 42
- optimizer: Adam with betas=(0.9,0.999) and epsilon=1e-08
- lr_scheduler_type: linear
- num_epochs: 2
- mixed_precision_training: Native AMP
### Training results
| Training Loss | Epoch | Step | Validation Loss | Rouge1 | Rouge2 | Rougel | Rougelsum | Gen Len |
|:-------------:|:-----:|:-----:|:---------------:|:------:|:------:|:------:|:---------:|:-------:|
| 7.9999 | 1.0 | 6180 | 7.9915 | 1.5443 | 0.0 | 1.4357 | 1.4377 | 10.0 |
| 7.9469 | 2.0 | 12360 | 7.9693 | 1.8257 | 0.0 | 1.6832 | 1.6866 | 10.0 |
### Framework versions
- Transformers 4.24.0
- Pytorch 1.12.1+cu113
- Datasets 2.7.1
- Tokenizers 0.13.2
|
jhu-clsp/FollowIR-7B | jhu-clsp | "2024-04-29T18:25:37Z" | 938 | 13 | transformers | [
"transformers",
"safetensors",
"mistral",
"text-generation",
"retrieval",
"instructions",
"reranking",
"mteb",
"conversational",
"en",
"dataset:jhu-clsp/FollowIR-train",
"arxiv:2403.15246",
"license:apache-2.0",
"model-index",
"autotrain_compatible",
"text-generation-inference",
"endpoints_compatible",
"region:us"
] | text-generation | "2024-03-17T10:53:34Z" | ---
license: apache-2.0
language:
- en
tags:
- retrieval
- instructions
- reranking
- mteb
datasets:
- jhu-clsp/FollowIR-train
model-index:
- name: FollowIR-7B
results:
- task:
type: InstructionRetrieval
dataset:
type: jhu-clsp/core17-instructions
name: MTEB Core17InstructionRetrieval
config: default
split: test
revision: e39ff896cf3efbbdeeb950e6bd7c79f266995b07
metrics:
- type: p-MRR
value: 16.47851858684521
- task:
type: InstructionRetrieval
dataset:
type: jhu-clsp/news21-instructions
name: MTEB News21InstructionRetrieval
config: default
split: test
revision: e0144086b45fe31ac125e9ac1a83b6a409bb6ca6
metrics:
- type: p-MRR
value: 6.2615989256510005
- task:
type: InstructionRetrieval
dataset:
type: jhu-clsp/robust04-instructions
name: MTEB Robust04InstructionRetrieval
config: default
split: test
revision: a5a1c4fe2bc528ac12e83f8cdf82178da85d2f1d
metrics:
- type: p-MRR
value: 13.717553757582253
---
# Model Summary
FollowIR-7B is an instruction-tuned language model to be used for reranking in retrieval. It is [Mistral-7B-Instruct-v0.2](https://huggingface.co/mistralai/Mistral-7B-Instruct-v0.2) fine-tuned on retrieval data with instructions from the FollowIR dataset. These instructions were taken from TREC tracks and are human written. FollowIR-7B outperforms all other retrieval models at following instructions. See the paper for more details.
- **Repository:** [orionw/FollowIR](https://github.com/orionw/FollowIR)
- **Paper:** https://arxiv.org/abs/2403.15246
- **Instruction-Training Dataset:** [jhu-clsp/followir-train](https://huggingface.co/datasets/jhu-clsp/FollowIR-train)
# Use
Below is an example to compute the similarity score of a query-document pair
```python
from transformers import (
AutoTokenizer,
AutoModelForCausalLM,
)
import torch
# model loading and setup
model_name = "jhu-clsp/FollowIR-7B"
model = AutoModelForCausalLM.from_pretrained(
model_name
).cuda()
tokenizer = AutoTokenizer.from_pretrained(
model_name, padding_side="left"
)
tokenizer.pad_token = tokenizer.eos_token
tokenizer.padding_side = "left"
token_false_id = tokenizer.get_vocab()["false"]
token_true_id = tokenizer.get_vocab()["true"]
template = """<s> [INST] You are an expert Google searcher, whose job is to determine if the following document is relevant to the query (true/false). Answer using only one word, one of those two choices.
Query: {query}
Document: {text}
Relevant (only output one word, either "true" or "false"): [/INST] """
## Lets define some example queries with instructions in the query and the passage
query1 = "What movies were written by James Cameron? A relevant document would describe a movie that was written by James Cameron only and not with anyone else"
query2 = "What movies were directed by James Cameron? A relevant document would describe any movie that was directed by James Cameron"
passages = ["Avatar: The Way of Water is a 2022 American epic science fiction film co-produced and directed by James Cameron, who co-wrote the screenplay with Rick Jaffa and Amanda Silver from a story the trio wrote with Josh Friedman and Shane Salerno. Distributed by 20th Century Studios, it is the sequel to Avatar (2009) and the second installment in the Avatar film series."] * 2
prompts = [
template.format(query=query, text=text) for (query, text) in zip([query1, query2], passages)
]
tokens = tokenizer(
prompts,
padding=True,
truncation=True,
return_tensors="pt",
pad_to_multiple_of=None,
)
# move to cuda if desired
for key in tokens:
tokens[key] = tokens[key].cuda()
# calculate the scores by comparing true and false tokens
batch_scores = model(**tokens).logits[:, -1, :]
true_vector = batch_scores[:, token_true_id]
false_vector = batch_scores[:, token_false_id]
batch_scores = torch.stack([false_vector, true_vector], dim=1)
batch_scores = torch.nn.functional.log_softmax(batch_scores, dim=1)
scores = batch_scores[:, 1].exp().tolist()
print(scores) # [0.0020704232156276703, 0.9999990463256836] first document is not relevant, as expected
```
# Training
We used [LLaMA-Factory](https://github.com/hiyouga/LLaMA-Factory) to fine-tune Mistral to create FollowIR-7B, after transforming it to fit their format (input of "query" + "instruction" inside the template, output is the label, and instruction as the beginning of the template) with the following training script:
```bash
#!/bin/bash
accelerate launch src/train_bash.py \
--stage sft \
--do_train \
--model_name_or_path "mistralai/Mistral-7B-Instruct-v0.2" \
--dataset followIR-train \
--template mistral \
--output_dir OUTPUT \
--finetuning_type lora \
--lora_target q_proj,v_proj,o_proj,k_proj \
--overwrite_cache \
--per_device_train_batch_size 32 \
--gradient_accumulation_steps 1 \
--lr_scheduler_type cosine \
--logging_steps 2 \
--save_steps 29 \
--learning_rate 3e-5 \
--num_train_epochs 8.0 \
--plot_loss \
--max_length 2048 \
--lora_rank 8 \
--lora_alpha 16 \
--bf16
```
# Citation
```bibtex
@misc{weller2024followir,
title={FollowIR: Evaluating and Teaching Information Retrieval Models to Follow Instructions},
author={Orion Weller and Benjamin Chang and Sean MacAvaney and Kyle Lo and Arman Cohan and Benjamin Van Durme and Dawn Lawrie and Luca Soldaini},
year={2024},
eprint={2403.15246},
archivePrefix={arXiv},
primaryClass={cs.IR}
}
``` |
tensorblock/distillgpt2Cinder-GGUF | tensorblock | "2024-12-13T22:06:42Z" | 19 | 0 | null | [
"gguf",
"TensorBlock",
"GGUF",
"base_model:Josephgflowers/distillgpt2Cinder",
"base_model:quantized:Josephgflowers/distillgpt2Cinder",
"license:mit",
"model-index",
"endpoints_compatible",
"region:us"
] | null | "2024-12-13T22:06:14Z" | ---
license: mit
widget:
- text: 'USER: Cinder, What adventures do you think lie ahead?<END>
'
tags:
- TensorBlock
- GGUF
base_model: Josephgflowers/distillgpt2Cinder
model-index:
- name: distillgpt2Cinder
results:
- task:
type: text-generation
name: Text Generation
dataset:
name: AI2 Reasoning Challenge (25-Shot)
type: ai2_arc
config: ARC-Challenge
split: test
args:
num_few_shot: 25
metrics:
- type: acc_norm
value: 24.49
name: normalized accuracy
source:
url: https://huggingface.co/spaces/HuggingFaceH4/open_llm_leaderboard?query=Josephgflowers/distillgpt2Cinder
name: Open LLM Leaderboard
- task:
type: text-generation
name: Text Generation
dataset:
name: HellaSwag (10-Shot)
type: hellaswag
split: validation
args:
num_few_shot: 10
metrics:
- type: acc_norm
value: 27.24
name: normalized accuracy
source:
url: https://huggingface.co/spaces/HuggingFaceH4/open_llm_leaderboard?query=Josephgflowers/distillgpt2Cinder
name: Open LLM Leaderboard
- task:
type: text-generation
name: Text Generation
dataset:
name: MMLU (5-Shot)
type: cais/mmlu
config: all
split: test
args:
num_few_shot: 5
metrics:
- type: acc
value: 24.97
name: accuracy
source:
url: https://huggingface.co/spaces/HuggingFaceH4/open_llm_leaderboard?query=Josephgflowers/distillgpt2Cinder
name: Open LLM Leaderboard
- task:
type: text-generation
name: Text Generation
dataset:
name: TruthfulQA (0-shot)
type: truthful_qa
config: multiple_choice
split: validation
args:
num_few_shot: 0
metrics:
- type: mc2
value: 43.96
source:
url: https://huggingface.co/spaces/HuggingFaceH4/open_llm_leaderboard?query=Josephgflowers/distillgpt2Cinder
name: Open LLM Leaderboard
- task:
type: text-generation
name: Text Generation
dataset:
name: Winogrande (5-shot)
type: winogrande
config: winogrande_xl
split: validation
args:
num_few_shot: 5
metrics:
- type: acc
value: 50.12
name: accuracy
source:
url: https://huggingface.co/spaces/HuggingFaceH4/open_llm_leaderboard?query=Josephgflowers/distillgpt2Cinder
name: Open LLM Leaderboard
- task:
type: text-generation
name: Text Generation
dataset:
name: GSM8k (5-shot)
type: gsm8k
config: main
split: test
args:
num_few_shot: 5
metrics:
- type: acc
value: 0.23
name: accuracy
source:
url: https://huggingface.co/spaces/HuggingFaceH4/open_llm_leaderboard?query=Josephgflowers/distillgpt2Cinder
name: Open LLM Leaderboard
---
<div style="width: auto; margin-left: auto; margin-right: auto">
<img src="https://i.imgur.com/jC7kdl8.jpeg" alt="TensorBlock" style="width: 100%; min-width: 400px; display: block; margin: auto;">
</div>
<div style="display: flex; justify-content: space-between; width: 100%;">
<div style="display: flex; flex-direction: column; align-items: flex-start;">
<p style="margin-top: 0.5em; margin-bottom: 0em;">
Feedback and support: TensorBlock's <a href="https://x.com/tensorblock_aoi">Twitter/X</a>, <a href="https://t.me/TensorBlock">Telegram Group</a> and <a href="https://x.com/tensorblock_aoi">Discord server</a>
</p>
</div>
</div>
## Josephgflowers/distillgpt2Cinder - GGUF
This repo contains GGUF format model files for [Josephgflowers/distillgpt2Cinder](https://huggingface.co/Josephgflowers/distillgpt2Cinder).
The files were quantized using machines provided by [TensorBlock](https://tensorblock.co/), and they are compatible with llama.cpp as of [commit b4242](https://github.com/ggerganov/llama.cpp/commit/a6744e43e80f4be6398fc7733a01642c846dce1d).
<div style="text-align: left; margin: 20px 0;">
<a href="https://tensorblock.co/waitlist/client" style="display: inline-block; padding: 10px 20px; background-color: #007bff; color: white; text-decoration: none; border-radius: 5px; font-weight: bold;">
Run them on the TensorBlock client using your local machine ↗
</a>
</div>
## Prompt template
```
```
## Model file specification
| Filename | Quant type | File Size | Description |
| -------- | ---------- | --------- | ----------- |
| [distillgpt2Cinder-Q2_K.gguf](https://huggingface.co/tensorblock/distillgpt2Cinder-GGUF/blob/main/distillgpt2Cinder-Q2_K.gguf) | Q2_K | 0.065 GB | smallest, significant quality loss - not recommended for most purposes |
| [distillgpt2Cinder-Q3_K_S.gguf](https://huggingface.co/tensorblock/distillgpt2Cinder-GGUF/blob/main/distillgpt2Cinder-Q3_K_S.gguf) | Q3_K_S | 0.072 GB | very small, high quality loss |
| [distillgpt2Cinder-Q3_K_M.gguf](https://huggingface.co/tensorblock/distillgpt2Cinder-GGUF/blob/main/distillgpt2Cinder-Q3_K_M.gguf) | Q3_K_M | 0.075 GB | very small, high quality loss |
| [distillgpt2Cinder-Q3_K_L.gguf](https://huggingface.co/tensorblock/distillgpt2Cinder-GGUF/blob/main/distillgpt2Cinder-Q3_K_L.gguf) | Q3_K_L | 0.078 GB | small, substantial quality loss |
| [distillgpt2Cinder-Q4_0.gguf](https://huggingface.co/tensorblock/distillgpt2Cinder-GGUF/blob/main/distillgpt2Cinder-Q4_0.gguf) | Q4_0 | 0.082 GB | legacy; small, very high quality loss - prefer using Q3_K_M |
| [distillgpt2Cinder-Q4_K_S.gguf](https://huggingface.co/tensorblock/distillgpt2Cinder-GGUF/blob/main/distillgpt2Cinder-Q4_K_S.gguf) | Q4_K_S | 0.082 GB | small, greater quality loss |
| [distillgpt2Cinder-Q4_K_M.gguf](https://huggingface.co/tensorblock/distillgpt2Cinder-GGUF/blob/main/distillgpt2Cinder-Q4_K_M.gguf) | Q4_K_M | 0.085 GB | medium, balanced quality - recommended |
| [distillgpt2Cinder-Q5_0.gguf](https://huggingface.co/tensorblock/distillgpt2Cinder-GGUF/blob/main/distillgpt2Cinder-Q5_0.gguf) | Q5_0 | 0.093 GB | legacy; medium, balanced quality - prefer using Q4_K_M |
| [distillgpt2Cinder-Q5_K_S.gguf](https://huggingface.co/tensorblock/distillgpt2Cinder-GGUF/blob/main/distillgpt2Cinder-Q5_K_S.gguf) | Q5_K_S | 0.093 GB | large, low quality loss - recommended |
| [distillgpt2Cinder-Q5_K_M.gguf](https://huggingface.co/tensorblock/distillgpt2Cinder-GGUF/blob/main/distillgpt2Cinder-Q5_K_M.gguf) | Q5_K_M | 0.095 GB | large, very low quality loss - recommended |
| [distillgpt2Cinder-Q6_K.gguf](https://huggingface.co/tensorblock/distillgpt2Cinder-GGUF/blob/main/distillgpt2Cinder-Q6_K.gguf) | Q6_K | 0.103 GB | very large, extremely low quality loss |
| [distillgpt2Cinder-Q8_0.gguf](https://huggingface.co/tensorblock/distillgpt2Cinder-GGUF/blob/main/distillgpt2Cinder-Q8_0.gguf) | Q8_0 | 0.132 GB | very large, extremely low quality loss - not recommended |
## Downloading instruction
### Command line
Firstly, install Huggingface Client
```shell
pip install -U "huggingface_hub[cli]"
```
Then, downoad the individual model file the a local directory
```shell
huggingface-cli download tensorblock/distillgpt2Cinder-GGUF --include "distillgpt2Cinder-Q2_K.gguf" --local-dir MY_LOCAL_DIR
```
If you wanna download multiple model files with a pattern (e.g., `*Q4_K*gguf`), you can try:
```shell
huggingface-cli download tensorblock/distillgpt2Cinder-GGUF --local-dir MY_LOCAL_DIR --local-dir-use-symlinks False --include='*Q4_K*gguf'
```
|
huggingtweets/elonmusk-elysiatxt | huggingtweets | "2023-04-01T18:30:47Z" | 131 | 1 | transformers | [
"transformers",
"pytorch",
"gpt2",
"text-generation",
"huggingtweets",
"en",
"autotrain_compatible",
"text-generation-inference",
"endpoints_compatible",
"region:us"
] | text-generation | "2023-04-01T18:30:38Z" | ---
language: en
thumbnail: https://github.com/borisdayma/huggingtweets/blob/master/img/logo.png?raw=true
tags:
- huggingtweets
widget:
- text: "My dream is"
---
<div class="inline-flex flex-col" style="line-height: 1.5;">
<div class="flex">
<div
style="display:inherit; margin-left: 4px; margin-right: 4px; width: 92px; height:92px; border-radius: 50%; background-size: cover; background-image: url('https://pbs.twimg.com/profile_images/1590968738358079488/IY9Gx6Ok_400x400.jpg')">
</div>
<div
style="display:inherit; margin-left: 4px; margin-right: 4px; width: 92px; height:92px; border-radius: 50%; background-size: cover; background-image: url('https://pbs.twimg.com/profile_images/1561821281716142080/xdZX4AF0_400x400.jpg')">
</div>
<div
style="display:none; margin-left: 4px; margin-right: 4px; width: 92px; height:92px; border-radius: 50%; background-size: cover; background-image: url('')">
</div>
</div>
<div style="text-align: center; margin-top: 3px; font-size: 16px; font-weight: 800">🤖 AI CYBORG 🤖</div>
<div style="text-align: center; font-size: 16px; font-weight: 800">Elon Musk & Elysia 🌸</div>
<div style="text-align: center; font-size: 14px;">@elonmusk-elysiatxt</div>
</div>
I was made with [huggingtweets](https://github.com/borisdayma/huggingtweets).
Create your own bot based on your favorite user with [the demo](https://colab.research.google.com/github/borisdayma/huggingtweets/blob/master/huggingtweets-demo.ipynb)!
## How does it work?
The model uses the following pipeline.
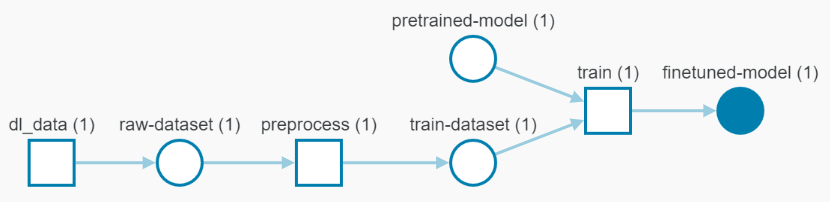
To understand how the model was developed, check the [W&B report](https://wandb.ai/wandb/huggingtweets/reports/HuggingTweets-Train-a-Model-to-Generate-Tweets--VmlldzoxMTY5MjI).
## Training data
The model was trained on tweets from Elon Musk & Elysia 🌸.
| Data | Elon Musk | Elysia 🌸 |
| --- | --- | --- |
| Tweets downloaded | 3194 | 3250 |
| Retweets | 172 | 9 |
| Short tweets | 1155 | 2 |
| Tweets kept | 1867 | 3239 |
[Explore the data](https://wandb.ai/wandb/huggingtweets/runs/cs8d95ef/artifacts), which is tracked with [W&B artifacts](https://docs.wandb.com/artifacts) at every step of the pipeline.
## Training procedure
The model is based on a pre-trained [GPT-2](https://huggingface.co/gpt2) which is fine-tuned on @elonmusk-elysiatxt's tweets.
Hyperparameters and metrics are recorded in the [W&B training run](https://wandb.ai/wandb/huggingtweets/runs/x8v9e640) for full transparency and reproducibility.
At the end of training, [the final model](https://wandb.ai/wandb/huggingtweets/runs/x8v9e640/artifacts) is logged and versioned.
## How to use
You can use this model directly with a pipeline for text generation:
```python
from transformers import pipeline
generator = pipeline('text-generation',
model='huggingtweets/elonmusk-elysiatxt')
generator("My dream is", num_return_sequences=5)
```
## Limitations and bias
The model suffers from [the same limitations and bias as GPT-2](https://huggingface.co/gpt2#limitations-and-bias).
In addition, the data present in the user's tweets further affects the text generated by the model.
## About
*Built by Boris Dayma*
[](https://twitter.com/intent/follow?screen_name=borisdayma)
For more details, visit the project repository.
[](https://github.com/borisdayma/huggingtweets)
|
bansalsid/openai-whisper-large-v2-LORA-colab | bansalsid | "2025-03-28T11:04:15Z" | 0 | 0 | transformers | [
"transformers",
"safetensors",
"arxiv:1910.09700",
"endpoints_compatible",
"region:us"
] | null | "2025-03-28T07:11:29Z" | ---
library_name: transformers
tags: []
---
# Model Card for Model ID
<!-- Provide a quick summary of what the model is/does. -->
## Model Details
### Model Description
<!-- Provide a longer summary of what this model is. -->
This is the model card of a 🤗 transformers model that has been pushed on the Hub. This model card has been automatically generated.
- **Developed by:** [More Information Needed]
- **Funded by [optional]:** [More Information Needed]
- **Shared by [optional]:** [More Information Needed]
- **Model type:** [More Information Needed]
- **Language(s) (NLP):** [More Information Needed]
- **License:** [More Information Needed]
- **Finetuned from model [optional]:** [More Information Needed]
### Model Sources [optional]
<!-- Provide the basic links for the model. -->
- **Repository:** [More Information Needed]
- **Paper [optional]:** [More Information Needed]
- **Demo [optional]:** [More Information Needed]
## Uses
<!-- Address questions around how the model is intended to be used, including the foreseeable users of the model and those affected by the model. -->
### Direct Use
<!-- This section is for the model use without fine-tuning or plugging into a larger ecosystem/app. -->
[More Information Needed]
### Downstream Use [optional]
<!-- This section is for the model use when fine-tuned for a task, or when plugged into a larger ecosystem/app -->
[More Information Needed]
### Out-of-Scope Use
<!-- This section addresses misuse, malicious use, and uses that the model will not work well for. -->
[More Information Needed]
## Bias, Risks, and Limitations
<!-- This section is meant to convey both technical and sociotechnical limitations. -->
[More Information Needed]
### Recommendations
<!-- This section is meant to convey recommendations with respect to the bias, risk, and technical limitations. -->
Users (both direct and downstream) should be made aware of the risks, biases and limitations of the model. More information needed for further recommendations.
## How to Get Started with the Model
Use the code below to get started with the model.
[More Information Needed]
## Training Details
### Training Data
<!-- This should link to a Dataset Card, perhaps with a short stub of information on what the training data is all about as well as documentation related to data pre-processing or additional filtering. -->
[More Information Needed]
### Training Procedure
<!-- This relates heavily to the Technical Specifications. Content here should link to that section when it is relevant to the training procedure. -->
#### Preprocessing [optional]
[More Information Needed]
#### Training Hyperparameters
- **Training regime:** [More Information Needed] <!--fp32, fp16 mixed precision, bf16 mixed precision, bf16 non-mixed precision, fp16 non-mixed precision, fp8 mixed precision -->
#### Speeds, Sizes, Times [optional]
<!-- This section provides information about throughput, start/end time, checkpoint size if relevant, etc. -->
[More Information Needed]
## Evaluation
<!-- This section describes the evaluation protocols and provides the results. -->
### Testing Data, Factors & Metrics
#### Testing Data
<!-- This should link to a Dataset Card if possible. -->
[More Information Needed]
#### Factors
<!-- These are the things the evaluation is disaggregating by, e.g., subpopulations or domains. -->
[More Information Needed]
#### Metrics
<!-- These are the evaluation metrics being used, ideally with a description of why. -->
[More Information Needed]
### Results
[More Information Needed]
#### Summary
## Model Examination [optional]
<!-- Relevant interpretability work for the model goes here -->
[More Information Needed]
## Environmental Impact
<!-- Total emissions (in grams of CO2eq) and additional considerations, such as electricity usage, go here. Edit the suggested text below accordingly -->
Carbon emissions can be estimated using the [Machine Learning Impact calculator](https://mlco2.github.io/impact#compute) presented in [Lacoste et al. (2019)](https://arxiv.org/abs/1910.09700).
- **Hardware Type:** [More Information Needed]
- **Hours used:** [More Information Needed]
- **Cloud Provider:** [More Information Needed]
- **Compute Region:** [More Information Needed]
- **Carbon Emitted:** [More Information Needed]
## Technical Specifications [optional]
### Model Architecture and Objective
[More Information Needed]
### Compute Infrastructure
[More Information Needed]
#### Hardware
[More Information Needed]
#### Software
[More Information Needed]
## Citation [optional]
<!-- If there is a paper or blog post introducing the model, the APA and Bibtex information for that should go in this section. -->
**BibTeX:**
[More Information Needed]
**APA:**
[More Information Needed]
## Glossary [optional]
<!-- If relevant, include terms and calculations in this section that can help readers understand the model or model card. -->
[More Information Needed]
## More Information [optional]
[More Information Needed]
## Model Card Authors [optional]
[More Information Needed]
## Model Card Contact
[More Information Needed] |
Legalaz/03_20_04 | Legalaz | "2024-12-24T01:06:46Z" | 50 | 0 | transformers | [
"transformers",
"safetensors",
"parler_tts",
"text2text-generation",
"text-to-speech",
"annotation",
"en",
"dataset:parler-tts/mls_eng",
"dataset:parler-tts/libritts_r_filtered",
"dataset:parler-tts/libritts-r-filtered-speaker-descriptions",
"dataset:parler-tts/mls-eng-speaker-descriptions",
"arxiv:2402.01912",
"license:apache-2.0",
"autotrain_compatible",
"endpoints_compatible",
"region:us"
] | text-to-speech | "2024-12-24T01:05:38Z" | ---
library_name: transformers
tags:
- text-to-speech
- annotation
license: apache-2.0
language:
- en
pipeline_tag: text-to-speech
inference: false
datasets:
- parler-tts/mls_eng
- parler-tts/libritts_r_filtered
- parler-tts/libritts-r-filtered-speaker-descriptions
- parler-tts/mls-eng-speaker-descriptions
---
<img src="https://huggingface.co/datasets/parler-tts/images/resolve/main/thumbnail.png" alt="Parler Logo" width="800" style="margin-left:'auto' margin-right:'auto' display:'block'"/>
# Parler-TTS Mini v1
<a target="_blank" href="https://huggingface.co/spaces/parler-tts/parler_tts">
<img src="https://huggingface.co/datasets/huggingface/badges/raw/main/open-in-hf-spaces-sm.svg" alt="Open in HuggingFace"/>
</a>
**Parler-TTS Mini v1** is a lightweight text-to-speech (TTS) model, trained on 45K hours of audio data, that can generate high-quality, natural sounding speech with features that can be controlled using a simple text prompt (e.g. gender, background noise, speaking rate, pitch and reverberation).
With [Parler-TTS Large v1](https://huggingface.co/parler-tts/parler-tts-large-v1), this is the second set of models published as part of the [Parler-TTS](https://github.com/huggingface/parler-tts) project, which aims to provide the community with TTS training resources and dataset pre-processing code.
## 📖 Quick Index
* [👨💻 Installation](#👨💻-installation)
* [🎲 Using a random voice](#🎲-random-voice)
* [🎯 Using a specific speaker](#🎯-using-a-specific-speaker)
* [Motivation](#motivation)
* [Optimizing inference](https://github.com/huggingface/parler-tts/blob/main/INFERENCE.md)
## 🛠️ Usage
### 👨💻 Installation
Using Parler-TTS is as simple as "bonjour". Simply install the library once:
```sh
pip install git+https://github.com/huggingface/parler-tts.git
```
### 🎲 Random voice
**Parler-TTS** has been trained to generate speech with features that can be controlled with a simple text prompt, for example:
```py
import torch
from parler_tts import ParlerTTSForConditionalGeneration
from transformers import AutoTokenizer
import soundfile as sf
device = "cuda:0" if torch.cuda.is_available() else "cpu"
model = ParlerTTSForConditionalGeneration.from_pretrained("parler-tts/parler-tts-mini-v1").to(device)
tokenizer = AutoTokenizer.from_pretrained("parler-tts/parler-tts-mini-v1")
prompt = "Hey, how are you doing today?"
description = "A female speaker delivers a slightly expressive and animated speech with a moderate speed and pitch. The recording is of very high quality, with the speaker's voice sounding clear and very close up."
input_ids = tokenizer(description, return_tensors="pt").input_ids.to(device)
prompt_input_ids = tokenizer(prompt, return_tensors="pt").input_ids.to(device)
generation = model.generate(input_ids=input_ids, prompt_input_ids=prompt_input_ids)
audio_arr = generation.cpu().numpy().squeeze()
sf.write("parler_tts_out.wav", audio_arr, model.config.sampling_rate)
```
### 🎯 Using a specific speaker
To ensure speaker consistency across generations, this checkpoint was also trained on 34 speakers, characterized by name (e.g. Jon, Lea, Gary, Jenna, Mike, Laura).
To take advantage of this, simply adapt your text description to specify which speaker to use: `Jon's voice is monotone yet slightly fast in delivery, with a very close recording that almost has no background noise.`
```py
import torch
from parler_tts import ParlerTTSForConditionalGeneration
from transformers import AutoTokenizer
import soundfile as sf
device = "cuda:0" if torch.cuda.is_available() else "cpu"
model = ParlerTTSForConditionalGeneration.from_pretrained("parler-tts/parler-tts-mini-v1").to(device)
tokenizer = AutoTokenizer.from_pretrained("parler-tts/parler-tts-mini-v1")
prompt = "Hey, how are you doing today?"
description = "Jon's voice is monotone yet slightly fast in delivery, with a very close recording that almost has no background noise."
input_ids = tokenizer(description, return_tensors="pt").input_ids.to(device)
prompt_input_ids = tokenizer(prompt, return_tensors="pt").input_ids.to(device)
generation = model.generate(input_ids=input_ids, prompt_input_ids=prompt_input_ids)
audio_arr = generation.cpu().numpy().squeeze()
sf.write("parler_tts_out.wav", audio_arr, model.config.sampling_rate)
```
**Tips**:
* We've set up an [inference guide](https://github.com/huggingface/parler-tts/blob/main/INFERENCE.md) to make generation faster. Think SDPA, torch.compile, batching and streaming!
* Include the term "very clear audio" to generate the highest quality audio, and "very noisy audio" for high levels of background noise
* Punctuation can be used to control the prosody of the generations, e.g. use commas to add small breaks in speech
* The remaining speech features (gender, speaking rate, pitch and reverberation) can be controlled directly through the prompt
## Motivation
Parler-TTS is a reproduction of work from the paper [Natural language guidance of high-fidelity text-to-speech with synthetic annotations](https://www.text-description-to-speech.com) by Dan Lyth and Simon King, from Stability AI and Edinburgh University respectively.
Contrarily to other TTS models, Parler-TTS is a **fully open-source** release. All of the datasets, pre-processing, training code and weights are released publicly under permissive license, enabling the community to build on our work and develop their own powerful TTS models.
Parler-TTS was released alongside:
* [The Parler-TTS repository](https://github.com/huggingface/parler-tts) - you can train and fine-tuned your own version of the model.
* [The Data-Speech repository](https://github.com/huggingface/dataspeech) - a suite of utility scripts designed to annotate speech datasets.
* [The Parler-TTS organization](https://huggingface.co/parler-tts) - where you can find the annotated datasets as well as the future checkpoints.
## Citation
If you found this repository useful, please consider citing this work and also the original Stability AI paper:
```
@misc{lacombe-etal-2024-parler-tts,
author = {Yoach Lacombe and Vaibhav Srivastav and Sanchit Gandhi},
title = {Parler-TTS},
year = {2024},
publisher = {GitHub},
journal = {GitHub repository},
howpublished = {\url{https://github.com/huggingface/parler-tts}}
}
```
```
@misc{lyth2024natural,
title={Natural language guidance of high-fidelity text-to-speech with synthetic annotations},
author={Dan Lyth and Simon King},
year={2024},
eprint={2402.01912},
archivePrefix={arXiv},
primaryClass={cs.SD}
}
```
## License
This model is permissively licensed under the Apache 2.0 license. |
chrischang80/bert_finetuned_ner | chrischang80 | "2024-07-20T09:12:46Z" | 4 | 0 | transformers | [
"transformers",
"tensorboard",
"safetensors",
"bert",
"token-classification",
"generated_from_trainer",
"dataset:conll2003",
"base_model:google-bert/bert-base-cased",
"base_model:finetune:google-bert/bert-base-cased",
"license:apache-2.0",
"model-index",
"autotrain_compatible",
"endpoints_compatible",
"region:us"
] | token-classification | "2024-07-20T07:48:34Z" | ---
license: apache-2.0
base_model: bert-base-cased
tags:
- generated_from_trainer
datasets:
- conll2003
metrics:
- precision
- recall
- f1
- accuracy
model-index:
- name: bert_finetuned_ner
results:
- task:
name: Token Classification
type: token-classification
dataset:
name: conll2003
type: conll2003
config: conll2003
split: validation
args: conll2003
metrics:
- name: Precision
type: precision
value: 0.936247723132969
- name: Recall
type: recall
value: 0.9515314708852238
- name: F1
type: f1
value: 0.9438277272347885
- name: Accuracy
type: accuracy
value: 0.9865926885265203
---
<!-- This model card has been generated automatically according to the information the Trainer had access to. You
should probably proofread and complete it, then remove this comment. -->
# bert_finetuned_ner
This model is a fine-tuned version of [bert-base-cased](https://huggingface.co/bert-base-cased) on the conll2003 dataset.
It achieves the following results on the evaluation set:
- Loss: 0.0584
- Precision: 0.9362
- Recall: 0.9515
- F1: 0.9438
- Accuracy: 0.9866
## Model description
More information needed
## Intended uses & limitations
More information needed
## Training and evaluation data
More information needed
## Training procedure
### Training hyperparameters
The following hyperparameters were used during training:
- learning_rate: 2e-05
- train_batch_size: 8
- eval_batch_size: 8
- seed: 42
- optimizer: Adam with betas=(0.9,0.999) and epsilon=1e-08
- lr_scheduler_type: linear
- num_epochs: 3
### Training results
| Training Loss | Epoch | Step | Validation Loss | Precision | Recall | F1 | Accuracy |
|:-------------:|:-----:|:----:|:---------------:|:---------:|:------:|:------:|:--------:|
| 0.0767 | 1.0 | 1756 | 0.0665 | 0.8983 | 0.9295 | 0.9136 | 0.9809 |
| 0.0343 | 2.0 | 3512 | 0.0638 | 0.9283 | 0.9460 | 0.9371 | 0.9854 |
| 0.0204 | 3.0 | 5268 | 0.0584 | 0.9362 | 0.9515 | 0.9438 | 0.9866 |
### Framework versions
- Transformers 4.42.4
- Pytorch 2.3.1+cu121
- Datasets 2.20.0
- Tokenizers 0.19.1
|
FoamoftheSea/pvt_v2_b0 | FoamoftheSea | "2024-03-12T01:08:46Z" | 122 | 0 | transformers | [
"transformers",
"safetensors",
"pvt_v2",
"image-classification",
"arxiv:2106.13797",
"arxiv:2105.15203",
"arxiv:2201.07436",
"arxiv:2010.04159",
"arxiv:2109.03814",
"license:apache-2.0",
"autotrain_compatible",
"endpoints_compatible",
"region:us"
] | image-classification | "2023-10-14T05:30:30Z" | ---
license: apache-2.0
---
# PVTv2
This is the Hugging Face PyTorch implementation of the [PVTv2](https://arxiv.org/abs/2106.13797) model.
## Model Description
The Pyramid Vision Transformer v2 (PVTv2) is a powerful, lightweight hierarchical transformer backbone for vision tasks. PVTv2 infuses convolution operations into its transformer layers to infuse properties of CNNs that enable them to learn image data efficiently. This mix transformer architecture requires no added positional embeddings, and produces multi-scale feature maps which are known to be beneficial for dense and fine-grained prediction tasks.
Vision models using PVTv2 for a backbone:
1. [Segformer](https://arxiv.org/abs/2105.15203) for Semantic Segmentation.
2. [GLPN](https://arxiv.org/abs/2201.07436) for Monocular Depth.
3. [Deformable DETR](https://arxiv.org/abs/2010.04159) for 2D Object Detection.
4. [Panoptic Segformer](https://arxiv.org/abs/2109.03814) for Panoptic Segmentation. |
ryusangwon/9839_Llama-2-7b-hf | ryusangwon | "2024-01-17T20:54:22Z" | 0 | 0 | peft | [
"peft",
"safetensors",
"generated_from_trainer",
"dataset:cnn_dailymail",
"base_model:meta-llama/Llama-2-7b-hf",
"base_model:adapter:meta-llama/Llama-2-7b-hf",
"region:us"
] | null | "2024-01-17T20:54:16Z" | ---
base_model: meta-llama/Llama-2-7b-hf
tags:
- generated_from_trainer
datasets:
- cnn_dailymail
model-index:
- name: 9839_Llama-2-7b-hf
results: []
library_name: peft
---
<!-- This model card has been generated automatically according to the information the Trainer had access to. You
should probably proofread and complete it, then remove this comment. -->
# 9839_Llama-2-7b-hf
This model is a fine-tuned version of [meta-llama/Llama-2-7b-hf](https://huggingface.co/meta-llama/Llama-2-7b-hf) on the cnn_dailymail dataset.
## Model description
More information needed
## Intended uses & limitations
More information needed
## Training and evaluation data
More information needed
## Training procedure
The following `bitsandbytes` quantization config was used during training:
- quant_method: bitsandbytes
- load_in_8bit: True
- load_in_4bit: False
- llm_int8_threshold: 6.0
- llm_int8_skip_modules: None
- llm_int8_enable_fp32_cpu_offload: False
- llm_int8_has_fp16_weight: False
- bnb_4bit_quant_type: fp4
- bnb_4bit_use_double_quant: False
- bnb_4bit_compute_dtype: float32
### Training hyperparameters
The following hyperparameters were used during training:
- learning_rate: 5e-05
- train_batch_size: 4
- eval_batch_size: 4
- seed: 42
- optimizer: Adam with betas=(0.9,0.999) and epsilon=1e-08
- lr_scheduler_type: linear
- num_epochs: 3
### Training results
### Framework versions
- PEFT 0.4.0
- Transformers 4.36.2
- Pytorch 2.0.1+cu117
- Datasets 2.15.0
- Tokenizers 0.15.0
|
RehanAzam/fln-pooja001 | RehanAzam | "2025-03-15T14:44:16Z" | 0 | 0 | diffusers | [
"diffusers",
"text-to-image",
"flux",
"lora",
"template:sd-lora",
"fluxgym",
"base_model:black-forest-labs/FLUX.1-dev",
"base_model:adapter:black-forest-labs/FLUX.1-dev",
"license:other",
"region:us"
] | text-to-image | "2025-03-15T14:43:54Z" | ---
tags:
- text-to-image
- flux
- lora
- diffusers
- template:sd-lora
- fluxgym
base_model: black-forest-labs/FLUX.1-dev
instance_prompt: FLN Pooja001
license: other
license_name: flux-1-dev-non-commercial-license
license_link: https://huggingface.co/black-forest-labs/FLUX.1-dev/blob/main/LICENSE.md
---
# FLN Pooja001
A Flux LoRA trained on a local computer with [Fluxgym](https://github.com/cocktailpeanut/fluxgym)
<Gallery />
## Trigger words
You should use `FLN Pooja001` to trigger the image generation.
## Download model and use it with ComfyUI, AUTOMATIC1111, SD.Next, Invoke AI, Forge, etc.
Weights for this model are available in Safetensors format.
|
minoosh/videomae-base-finetuned-IEMOCAP_1xxx | minoosh | "2023-10-10T16:24:13Z" | 59 | 0 | transformers | [
"transformers",
"pytorch",
"videomae",
"video-classification",
"generated_from_trainer",
"base_model:MCG-NJU/videomae-base",
"base_model:finetune:MCG-NJU/videomae-base",
"endpoints_compatible",
"region:us"
] | video-classification | "2023-10-10T06:19:12Z" | ---
base_model: MCG-NJU/videomae-base
tags:
- generated_from_trainer
metrics:
- f1
model-index:
- name: videomae-base-finetuned-IEMOCAP_1xxx
results: []
---
<!-- This model card has been generated automatically according to the information the Trainer had access to. You
should probably proofread and complete it, then remove this comment. -->
# videomae-base-finetuned-IEMOCAP_1xxx
This model is a fine-tuned version of [MCG-NJU/videomae-base](https://huggingface.co/MCG-NJU/videomae-base) on an unknown dataset.
It achieves the following results on the evaluation set:
- Loss: 3.5410
- F1: 0.3122
## Model description
More information needed
## Intended uses & limitations
More information needed
## Training and evaluation data
More information needed
## Training procedure
### Training hyperparameters
The following hyperparameters were used during training:
- learning_rate: 5e-05
- train_batch_size: 8
- eval_batch_size: 8
- seed: 42
- optimizer: Adam with betas=(0.9,0.999) and epsilon=1e-08
- lr_scheduler_type: linear
- lr_scheduler_warmup_ratio: 0.1
- training_steps: 4440
### Training results
| Training Loss | Epoch | Step | Validation Loss | F1 |
|:-------------:|:-----:|:----:|:---------------:|:------:|
| 1.1898 | 0.1 | 445 | 1.4498 | 0.2386 |
| 0.8823 | 1.1 | 890 | 1.1752 | 0.4500 |
| 0.9551 | 2.1 | 1335 | 1.0926 | 0.5148 |
| 0.3473 | 3.1 | 1780 | 0.8426 | 0.6929 |
| 0.3696 | 4.1 | 2225 | 1.0283 | 0.6745 |
| 0.3198 | 5.1 | 2670 | 1.0346 | 0.7329 |
| 0.1408 | 6.1 | 3115 | 1.1931 | 0.7278 |
| 0.2707 | 7.1 | 3560 | 1.3167 | 0.7492 |
| 0.0056 | 8.1 | 4005 | 1.4910 | 0.7418 |
| 0.03 | 9.1 | 4440 | 1.4809 | 0.7460 |
### Framework versions
- Transformers 4.34.0
- Pytorch 1.12.0+cu116
- Datasets 2.14.5
- Tokenizers 0.14.1
|
KBNIT/nox-solar-10.7b-v4-kolon-ITD-5-v1.0 | KBNIT | "2024-04-02T04:19:21Z" | 2,252 | 0 | transformers | [
"transformers",
"pytorch",
"llama",
"text-generation",
"conversational",
"ko",
"en",
"license:cc-by-nc-4.0",
"autotrain_compatible",
"text-generation-inference",
"endpoints_compatible",
"region:us"
] | text-generation | "2024-04-01T05:33:48Z" | ---
license: cc-by-nc-4.0
language:
- ko
- en
---
## Base Model:
We made a LLM model with davidkim205/nox-solar-10.7b-v4
## Model Description
We use QLoRA(256, 64) and NEFTune on LLM Fine-tuning
lr = 1e-5, NEFTune = 3
## Train Detail
Our changed private data used, 5 epoch train, and parameter changed
## Others
We are making LLM model for Kolon ! |
emaeon/lora-healthcare-model-epoch-9 | emaeon | "2023-07-18T05:55:36Z" | 0 | 0 | peft | [
"peft",
"region:us"
] | null | "2023-07-18T02:52:55Z" | ---
library_name: peft
---
## Training procedure
### Framework versions
- PEFT 0.5.0.dev0
|
Honza/Reinforce-PixelCopter1 | Honza | "2023-01-16T20:31:44Z" | 0 | 0 | null | [
"Pixelcopter-PLE-v0",
"reinforce",
"reinforcement-learning",
"custom-implementation",
"deep-rl-class",
"model-index",
"region:us"
] | reinforcement-learning | "2023-01-16T18:35:52Z" | ---
tags:
- Pixelcopter-PLE-v0
- reinforce
- reinforcement-learning
- custom-implementation
- deep-rl-class
model-index:
- name: Reinforce-PixelCopter1
results:
- task:
type: reinforcement-learning
name: reinforcement-learning
dataset:
name: Pixelcopter-PLE-v0
type: Pixelcopter-PLE-v0
metrics:
- type: mean_reward
value: 50.00 +/- 44.20
name: mean_reward
verified: false
---
# **Reinforce** Agent playing **Pixelcopter-PLE-v0**
This is a trained model of a **Reinforce** agent playing **Pixelcopter-PLE-v0** .
To learn to use this model and train yours check Unit 4 of the Deep Reinforcement Learning Course: https://huggingface.co/deep-rl-course/unit4/introduction
|
sarahflan/bert-finetuned-ner | sarahflan | "2023-09-04T14:56:40Z" | 116 | 0 | transformers | [
"transformers",
"pytorch",
"tensorboard",
"bert",
"token-classification",
"generated_from_trainer",
"dataset:conll2003",
"base_model:google-bert/bert-base-cased",
"base_model:finetune:google-bert/bert-base-cased",
"license:apache-2.0",
"model-index",
"autotrain_compatible",
"endpoints_compatible",
"region:us"
] | token-classification | "2023-07-19T22:07:09Z" | ---
license: apache-2.0
base_model: bert-base-cased
tags:
- generated_from_trainer
datasets:
- conll2003
metrics:
- precision
- recall
- f1
- accuracy
model-index:
- name: bert-finetuned-ner
results:
- task:
name: Token Classification
type: token-classification
dataset:
name: conll2003
type: conll2003
config: conll2003
split: validation
args: conll2003
metrics:
- name: Precision
type: precision
value: 0.9361138695796094
- name: Recall
type: recall
value: 0.9518680578929654
- name: F1
type: f1
value: 0.9439252336448599
- name: Accuracy
type: accuracy
value: 0.986342497203744
---
<!-- This model card has been generated automatically according to the information the Trainer had access to. You
should probably proofread and complete it, then remove this comment. -->
# bert-finetuned-ner
This model is a fine-tuned version of [bert-base-cased](https://huggingface.co/bert-base-cased) on the conll2003 dataset.
It achieves the following results on the evaluation set:
- Loss: 0.0624
- Precision: 0.9361
- Recall: 0.9519
- F1: 0.9439
- Accuracy: 0.9863
## Model description
More information needed
## Intended uses & limitations
More information needed
## Training and evaluation data
More information needed
## Training procedure
### Training hyperparameters
The following hyperparameters were used during training:
- learning_rate: 2e-05
- train_batch_size: 8
- eval_batch_size: 8
- seed: 42
- optimizer: Adam with betas=(0.9,0.999) and epsilon=1e-08
- lr_scheduler_type: linear
- num_epochs: 3
### Training results
| Training Loss | Epoch | Step | Validation Loss | Precision | Recall | F1 | Accuracy |
|:-------------:|:-----:|:----:|:---------------:|:---------:|:------:|:------:|:--------:|
| 0.0775 | 1.0 | 1756 | 0.0831 | 0.9068 | 0.9352 | 0.9208 | 0.9791 |
| 0.0411 | 2.0 | 3512 | 0.0578 | 0.9232 | 0.9492 | 0.9360 | 0.9853 |
| 0.024 | 3.0 | 5268 | 0.0624 | 0.9361 | 0.9519 | 0.9439 | 0.9863 |
### Framework versions
- Transformers 4.32.1
- Pytorch 2.0.1+cu118
- Datasets 2.14.4
- Tokenizers 0.13.3
|
dfomin/Reinforce-PixelCopter | dfomin | "2023-08-11T20:52:01Z" | 0 | 0 | null | [
"Pixelcopter-PLE-v0",
"reinforce",
"reinforcement-learning",
"custom-implementation",
"deep-rl-class",
"model-index",
"region:us"
] | reinforcement-learning | "2023-08-09T20:44:44Z" | ---
tags:
- Pixelcopter-PLE-v0
- reinforce
- reinforcement-learning
- custom-implementation
- deep-rl-class
model-index:
- name: Reinforce-pixelcopter
results:
- task:
type: reinforcement-learning
name: reinforcement-learning
dataset:
name: Pixelcopter-PLE-v0
type: Pixelcopter-PLE-v0
metrics:
- type: mean_reward
value: 27.90 +/- 27.14
name: mean_reward
verified: false
---
# **Reinforce** Agent playing **Pixelcopter-PLE-v0**
This is a trained model of a **Reinforce** agent playing **Pixelcopter-PLE-v0** .
To learn to use this model and train yours check Unit 4 of the Deep Reinforcement Learning Course: https://huggingface.co/deep-rl-course/unit4/introduction
|
pfunk/BreakoutNoFrameskip-v4-DQN-seed3 | pfunk | "2023-10-19T13:12:42Z" | 0 | 0 | cleanrl | [
"cleanrl",
"tensorboard",
"BreakoutNoFrameskip-v4",
"deep-reinforcement-learning",
"reinforcement-learning",
"custom-implementation",
"model-index",
"region:us"
] | reinforcement-learning | "2023-10-19T13:12:35Z" | ---
tags:
- BreakoutNoFrameskip-v4
- deep-reinforcement-learning
- reinforcement-learning
- custom-implementation
library_name: cleanrl
model-index:
- name: DQPN_freq
results:
- task:
type: reinforcement-learning
name: reinforcement-learning
dataset:
name: BreakoutNoFrameskip-v4
type: BreakoutNoFrameskip-v4
metrics:
- type: mean_reward
value: 3.97 +/- 0.00
name: mean_reward
verified: false
---
# (CleanRL) **DQPN_freq** Agent Playing **BreakoutNoFrameskip-v4**
This is a trained model of a DQPN_freq agent playing BreakoutNoFrameskip-v4.
The model was trained by using [CleanRL](https://github.com/vwxyzjn/cleanrl) and the most up-to-date training code can be
found [here](https://github.com/vwxyzjn/cleanrl/blob/master/cleanrl/DQN.py).
## Get Started
To use this model, please install the `cleanrl` package with the following command:
```
pip install "cleanrl[DQN]"
python -m cleanrl_utils.enjoy --exp-name DQN --env-id BreakoutNoFrameskip-v4
```
Please refer to the [documentation](https://docs.cleanrl.dev/get-started/zoo/) for more detail.
## Command to reproduce the training
```bash
curl -OL https://huggingface.co/pfunk/BreakoutNoFrameskip-v4-DQN-seed3/raw/main/dqpn_freq_atari.py
curl -OL https://huggingface.co/pfunk/BreakoutNoFrameskip-v4-DQN-seed3/raw/main/pyproject.toml
curl -OL https://huggingface.co/pfunk/BreakoutNoFrameskip-v4-DQN-seed3/raw/main/poetry.lock
poetry install --all-extras
python dqpn_freq_atari.py --track --wandb-entity pfunk --wandb-project-name dqpn --capture-video true --save-model true --upload-model true --hf-entity pfunk --exp-name DQN --target-network-frequency 1000 --seed 3 --env-id BreakoutNoFrameskip-v4
```
# Hyperparameters
```python
{'alg_type': 'dqpn_freq_atari.py',
'batch_size': 32,
'buffer_size': 1000000,
'capture_video': True,
'cuda': True,
'double_learning': False,
'end_e': 0.05,
'env_id': 'BreakoutNoFrameskip-v4',
'exp_name': 'DQN',
'exploration_fraction': 0.2,
'gamma': 0.99,
'hf_entity': 'pfunk',
'learning_rate': 0.0001,
'learning_starts': 10000,
'max_gradient_norm': inf,
'policy_network_frequency': 5000,
'policy_tau': 1.0,
'save_model': True,
'seed': 3,
'start_e': 1.0,
'target_network_frequency': 1000,
'target_tau': 1.0,
'torch_deterministic': True,
'total_timesteps': 10000000,
'track': True,
'train_frequency': 1,
'upload_model': True,
'wandb_entity': 'pfunk',
'wandb_project_name': 'dqpn'}
```
|
AlSamCur123/mistral-7b2t | AlSamCur123 | "2024-12-31T02:10:29Z" | 62 | 0 | transformers | [
"transformers",
"gguf",
"mistral",
"text-generation-inference",
"unsloth",
"en",
"base_model:unsloth/mistral-7b-instruct-v0.3-bnb-4bit",
"base_model:quantized:unsloth/mistral-7b-instruct-v0.3-bnb-4bit",
"license:apache-2.0",
"endpoints_compatible",
"region:us",
"conversational"
] | null | "2024-12-16T14:29:35Z" | ---
base_model: unsloth/mistral-7b-instruct-v0.3-bnb-4bit
tags:
- text-generation-inference
- transformers
- unsloth
- mistral
- gguf
license: apache-2.0
language:
- en
---
# Uploaded model
- **Developed by:** AlSamCur123
- **License:** apache-2.0
- **Finetuned from model :** unsloth/mistral-7b-instruct-v0.3-bnb-4bit
This mistral model was trained 2x faster with [Unsloth](https://github.com/unslothai/unsloth) and Huggingface's TRL library.
[<img src="https://raw.githubusercontent.com/unslothai/unsloth/main/images/unsloth%20made%20with%20love.png" width="200"/>](https://github.com/unslothai/unsloth)
|
DrishtiSharma/whisper-large-v2-breton-3k-steps | DrishtiSharma | "2022-12-21T23:32:07Z" | 5 | 1 | transformers | [
"transformers",
"pytorch",
"tensorboard",
"whisper",
"automatic-speech-recognition",
"whisper-event",
"generated_from_trainer",
"br",
"dataset:mozilla-foundation/common_voice_11_0",
"license:apache-2.0",
"model-index",
"endpoints_compatible",
"region:us"
] | automatic-speech-recognition | "2022-12-21T20:34:40Z" | ---
language:
- br
license: apache-2.0
tags:
- whisper-event
- generated_from_trainer
datasets:
- mozilla-foundation/common_voice_11_0
metrics:
- wer
model-index:
- name: Whisper Large V2 Breton
results:
- task:
name: Automatic Speech Recognition
type: automatic-speech-recognition
dataset:
name: mozilla-foundation/common_voice_11_0 br
type: mozilla-foundation/common_voice_11_0
config: br
split: test
args: br
metrics:
- name: Wer
type: wer
value: 35.10767627648489
---
<!-- This model card has been generated automatically according to the information the Trainer had access to. You
should probably proofread and complete it, then remove this comment. -->
# Whisper Large V2 Breton
This model is a fine-tuned version of [openai/whisper-large-v2](https://huggingface.co/openai/whisper-large-v2) on the mozilla-foundation/common_voice_11_0 br dataset.
It achieves the following results on the evaluation set:
- Loss: 0.6425
- Wer: 35.1077
## Model description
More information needed
## Intended uses & limitations
More information needed
## Training and evaluation data
More information needed
## Training procedure
### Training hyperparameters
The following hyperparameters were used during training:
- learning_rate: 1e-05
- train_batch_size: 8
- eval_batch_size: 8
- seed: 42
- optimizer: Adam with betas=(0.9,0.999) and epsilon=1e-08
- lr_scheduler_type: linear
- lr_scheduler_warmup_steps: 100
- training_steps: 3000
- mixed_precision_training: Native AMP
### Training results
| Training Loss | Epoch | Step | Validation Loss | Wer |
|:-------------:|:-----:|:----:|:---------------:|:-------:|
| 0.0065 | 5.03 | 3000 | 0.6425 | 35.1077 |
### Framework versions
- Transformers 4.26.0.dev0
- Pytorch 1.13.0+cu116
- Datasets 2.8.1.dev0
- Tokenizers 0.13.2
|
ameerTelbani/ameer | ameerTelbani | "2022-12-01T20:05:04Z" | 265 | 0 | transformers | [
"transformers",
"pytorch",
"tensorboard",
"vit",
"image-classification",
"huggingpics",
"model-index",
"autotrain_compatible",
"endpoints_compatible",
"region:us"
] | image-classification | "2022-12-01T20:04:45Z" | ---
tags:
- image-classification
- pytorch
- huggingpics
metrics:
- accuracy
model-index:
- name: ameer
results:
- task:
name: Image Classification
type: image-classification
metrics:
- name: Accuracy
type: accuracy
value: 0.9850746393203735
---
# ameer
Autogenerated by HuggingPics🤗🖼️
Create your own image classifier for **anything** by running [the demo on Google Colab](https://colab.research.google.com/github/nateraw/huggingpics/blob/main/HuggingPics.ipynb).
Report any issues with the demo at the [github repo](https://github.com/nateraw/huggingpics).
## Example Images
#### apple

#### banana

#### orange
 |
jeeyoung/dpo487210th_trial_10000_data | jeeyoung | "2024-05-29T22:35:36Z" | 0 | 0 | peft | [
"peft",
"safetensors",
"arxiv:1910.09700",
"base_model:beomi/KoAlpaca-Polyglot-5.8B",
"base_model:adapter:beomi/KoAlpaca-Polyglot-5.8B",
"region:us"
] | null | "2024-05-29T22:34:25Z" | ---
library_name: peft
base_model: beomi/KoAlpaca-Polyglot-5.8B
---
# Model Card for Model ID
<!-- Provide a quick summary of what the model is/does. -->
## Model Details
### Model Description
<!-- Provide a longer summary of what this model is. -->
- **Developed by:** [More Information Needed]
- **Funded by [optional]:** [More Information Needed]
- **Shared by [optional]:** [More Information Needed]
- **Model type:** [More Information Needed]
- **Language(s) (NLP):** [More Information Needed]
- **License:** [More Information Needed]
- **Finetuned from model [optional]:** [More Information Needed]
### Model Sources [optional]
<!-- Provide the basic links for the model. -->
- **Repository:** [More Information Needed]
- **Paper [optional]:** [More Information Needed]
- **Demo [optional]:** [More Information Needed]
## Uses
<!-- Address questions around how the model is intended to be used, including the foreseeable users of the model and those affected by the model. -->
### Direct Use
<!-- This section is for the model use without fine-tuning or plugging into a larger ecosystem/app. -->
[More Information Needed]
### Downstream Use [optional]
<!-- This section is for the model use when fine-tuned for a task, or when plugged into a larger ecosystem/app -->
[More Information Needed]
### Out-of-Scope Use
<!-- This section addresses misuse, malicious use, and uses that the model will not work well for. -->
[More Information Needed]
## Bias, Risks, and Limitations
<!-- This section is meant to convey both technical and sociotechnical limitations. -->
[More Information Needed]
### Recommendations
<!-- This section is meant to convey recommendations with respect to the bias, risk, and technical limitations. -->
Users (both direct and downstream) should be made aware of the risks, biases and limitations of the model. More information needed for further recommendations.
## How to Get Started with the Model
Use the code below to get started with the model.
[More Information Needed]
## Training Details
### Training Data
<!-- This should link to a Dataset Card, perhaps with a short stub of information on what the training data is all about as well as documentation related to data pre-processing or additional filtering. -->
[More Information Needed]
### Training Procedure
<!-- This relates heavily to the Technical Specifications. Content here should link to that section when it is relevant to the training procedure. -->
#### Preprocessing [optional]
[More Information Needed]
#### Training Hyperparameters
- **Training regime:** [More Information Needed] <!--fp32, fp16 mixed precision, bf16 mixed precision, bf16 non-mixed precision, fp16 non-mixed precision, fp8 mixed precision -->
#### Speeds, Sizes, Times [optional]
<!-- This section provides information about throughput, start/end time, checkpoint size if relevant, etc. -->
[More Information Needed]
## Evaluation
<!-- This section describes the evaluation protocols and provides the results. -->
### Testing Data, Factors & Metrics
#### Testing Data
<!-- This should link to a Dataset Card if possible. -->
[More Information Needed]
#### Factors
<!-- These are the things the evaluation is disaggregating by, e.g., subpopulations or domains. -->
[More Information Needed]
#### Metrics
<!-- These are the evaluation metrics being used, ideally with a description of why. -->
[More Information Needed]
### Results
[More Information Needed]
#### Summary
## Model Examination [optional]
<!-- Relevant interpretability work for the model goes here -->
[More Information Needed]
## Environmental Impact
<!-- Total emissions (in grams of CO2eq) and additional considerations, such as electricity usage, go here. Edit the suggested text below accordingly -->
Carbon emissions can be estimated using the [Machine Learning Impact calculator](https://mlco2.github.io/impact#compute) presented in [Lacoste et al. (2019)](https://arxiv.org/abs/1910.09700).
- **Hardware Type:** [More Information Needed]
- **Hours used:** [More Information Needed]
- **Cloud Provider:** [More Information Needed]
- **Compute Region:** [More Information Needed]
- **Carbon Emitted:** [More Information Needed]
## Technical Specifications [optional]
### Model Architecture and Objective
[More Information Needed]
### Compute Infrastructure
[More Information Needed]
#### Hardware
[More Information Needed]
#### Software
[More Information Needed]
## Citation [optional]
<!-- If there is a paper or blog post introducing the model, the APA and Bibtex information for that should go in this section. -->
**BibTeX:**
[More Information Needed]
**APA:**
[More Information Needed]
## Glossary [optional]
<!-- If relevant, include terms and calculations in this section that can help readers understand the model or model card. -->
[More Information Needed]
## More Information [optional]
[More Information Needed]
## Model Card Authors [optional]
[More Information Needed]
## Model Card Contact
[More Information Needed]
### Framework versions
- PEFT 0.11.0 |
Subsets and Splits