modelId
stringlengths 4
112
| sha
stringlengths 40
40
| lastModified
stringlengths 24
24
| tags
sequence | pipeline_tag
stringclasses 29
values | private
bool 1
class | author
stringlengths 2
38
⌀ | config
null | id
stringlengths 4
112
| downloads
float64 0
36.8M
⌀ | likes
float64 0
712
⌀ | library_name
stringclasses 17
values | __index_level_0__
int64 0
38.5k
| readme
stringlengths 0
186k
|
---|---|---|---|---|---|---|---|---|---|---|---|---|---|
youzanai/bert-customer-message-chinese | 22eff0121b4bff1609c71f7b0cecf91e1f1f4c72 | 2022-03-21T02:43:18.000Z | [
"pytorch",
"bert",
"fill-mask",
"transformers",
"autotrain_compatible"
] | fill-mask | false | youzanai | null | youzanai/bert-customer-message-chinese | 2 | null | transformers | 25,100 | 基于有赞商家客服中客户提问语料训练的bert模型。
模型示例代码参考 https://github.com/youzanai/trexpark |
ydshieh/tiny-random-rembert | ed9a6fd063b93875c4435dc856adf65b569000d0 | 2022-03-08T13:16:16.000Z | [
"pytorch",
"rembert",
"feature-extraction",
"transformers"
] | feature-extraction | false | ydshieh | null | ydshieh/tiny-random-rembert | 2 | null | transformers | 25,101 | Entry not found |
Ameer05/bart-large-cnn-samsum-rescom-finetuned-resume-summarizer-10-epoch-tweak-lr-8-10-1 | e332e76bf79e3074e2482da5817534bf65b0cb30 | 2022-03-08T08:48:11.000Z | [
"pytorch",
"tensorboard",
"bart",
"text2text-generation",
"transformers",
"summarization",
"generated_from_trainer",
"model-index",
"autotrain_compatible"
] | summarization | false | Ameer05 | null | Ameer05/bart-large-cnn-samsum-rescom-finetuned-resume-summarizer-10-epoch-tweak-lr-8-10-1 | 2 | null | transformers | 25,102 | ---
tags:
- summarization
- generated_from_trainer
metrics:
- rouge
model-index:
- name: bart-large-cnn-samsum-rescom-finetuned-resume-summarizer-10-epoch-tweak-lr-8-10-1
results: []
---
<!-- This model card has been generated automatically according to the information the Trainer had access to. You
should probably proofread and complete it, then remove this comment. -->
# bart-large-cnn-samsum-rescom-finetuned-resume-summarizer-10-epoch-tweak-lr-8-10-1
This model is a fine-tuned version of [Ameer05/model-token-repo](https://huggingface.co/Ameer05/model-token-repo) on an unknown dataset.
It achieves the following results on the evaluation set:
- Loss: 1.4855
- Rouge1: 58.3832
- Rouge2: 49.9973
- Rougel: 55.3055
- Rougelsum: 57.7139
## Model description
More information needed
## Intended uses & limitations
More information needed
## Training and evaluation data
More information needed
## Training procedure
### Training hyperparameters
The following hyperparameters were used during training:
- learning_rate: 5e-05
- train_batch_size: 8
- eval_batch_size: 8
- seed: 42
- gradient_accumulation_steps: 4
- total_train_batch_size: 32
- optimizer: Adam with betas=(0.9,0.999) and epsilon=1e-08
- lr_scheduler_type: linear
- num_epochs: 10
- mixed_precision_training: Native AMP
### Training results
| Training Loss | Epoch | Step | Validation Loss | Rouge1 | Rouge2 | Rougel | Rougelsum |
|:-------------:|:-----:|:----:|:---------------:|:-------:|:-------:|:-------:|:---------:|
| No log | 0.91 | 5 | 2.0183 | 52.3098 | 45.5304 | 49.2759 | 51.7456 |
| No log | 1.91 | 10 | 1.6564 | 61.815 | 53.9035 | 58.4243 | 60.784 |
| No log | 2.91 | 15 | 1.5330 | 61.3032 | 54.12 | 58.9152 | 60.7178 |
| No log | 3.91 | 20 | 1.4539 | 63.3012 | 56.2987 | 61.0907 | 62.5217 |
| 1.5646 | 4.91 | 25 | 1.4578 | 62.4815 | 55.1453 | 60.3921 | 61.6067 |
| 1.5646 | 5.91 | 30 | 1.4284 | 61.5347 | 54.1271 | 58.8474 | 60.5427 |
| 1.5646 | 6.91 | 35 | 1.4467 | 61.5081 | 53.8512 | 59.2782 | 60.6928 |
| 1.5646 | 7.91 | 40 | 1.4653 | 59.5349 | 51.8208 | 56.5996 | 58.8211 |
| 0.6692 | 8.91 | 45 | 1.4740 | 57.2917 | 49.5416 | 54.8409 | 56.6276 |
| 0.6692 | 9.91 | 50 | 1.4855 | 58.3832 | 49.9973 | 55.3055 | 57.7139 |
### Framework versions
- Transformers 4.15.0
- Pytorch 1.9.1
- Datasets 1.18.4
- Tokenizers 0.10.3
|
davidlopez/distilbert-base-uncased-go-emotion-cyberblue | 215714a3f89c7cc04c3ebf02c17b852118e4b63d | 2022-03-08T10:11:51.000Z | [
"pytorch",
"distilbert",
"transformers"
] | null | false | davidlopez | null | davidlopez/distilbert-base-uncased-go-emotion-cyberblue | 2 | null | transformers | 25,103 | Entry not found |
frahman/xlm-roberta-base-finetuned-panx-de | 9f7dc58f54e22e3f187724fdf64dbda35f838610 | 2022-03-08T10:51:37.000Z | [
"pytorch",
"tensorboard",
"xlm-roberta",
"token-classification",
"dataset:xtreme",
"transformers",
"generated_from_trainer",
"license:mit",
"model-index",
"autotrain_compatible"
] | token-classification | false | frahman | null | frahman/xlm-roberta-base-finetuned-panx-de | 2 | null | transformers | 25,104 | ---
license: mit
tags:
- generated_from_trainer
datasets:
- xtreme
metrics:
- f1
model-index:
- name: xlm-roberta-base-finetuned-panx-de
results:
- task:
name: Token Classification
type: token-classification
dataset:
name: xtreme
type: xtreme
args: PAN-X.de
metrics:
- name: F1
type: f1
value: 0.8591260810195721
---
<!-- This model card has been generated automatically according to the information the Trainer had access to. You
should probably proofread and complete it, then remove this comment. -->
# xlm-roberta-base-finetuned-panx-de
This model is a fine-tuned version of [xlm-roberta-base](https://huggingface.co/xlm-roberta-base) on the xtreme dataset.
It achieves the following results on the evaluation set:
- Loss: 0.1352
- F1: 0.8591
## Model description
More information needed
## Intended uses & limitations
More information needed
## Training and evaluation data
More information needed
## Training procedure
### Training hyperparameters
The following hyperparameters were used during training:
- learning_rate: 5e-05
- train_batch_size: 24
- eval_batch_size: 24
- seed: 42
- optimizer: Adam with betas=(0.9,0.999) and epsilon=1e-08
- lr_scheduler_type: linear
- num_epochs: 3
### Training results
| Training Loss | Epoch | Step | Validation Loss | F1 |
|:-------------:|:-----:|:----:|:---------------:|:------:|
| 0.257 | 1.0 | 525 | 0.1512 | 0.8302 |
| 0.1305 | 2.0 | 1050 | 0.1401 | 0.8447 |
| 0.0817 | 3.0 | 1575 | 0.1352 | 0.8591 |
### Framework versions
- Transformers 4.17.0
- Pytorch 1.10.0+cu111
- Datasets 1.18.4
- Tokenizers 0.11.6
|
ctoraman/RoBERTa-TR-medium-word-16k | cf12be5b1b12ab00464a054be9daa81bd1a595ed | 2022-04-20T06:59:33.000Z | [
"pytorch",
"roberta",
"fill-mask",
"tr",
"dataset:oscar",
"arxiv:2204.08832",
"transformers",
"license:cc-by-nc-sa-4.0",
"autotrain_compatible"
] | fill-mask | false | ctoraman | null | ctoraman/RoBERTa-TR-medium-word-16k | 2 | null | transformers | 25,105 | ---
language:
- tr
tags:
- roberta
license: cc-by-nc-sa-4.0
datasets:
- oscar
---
# RoBERTa Turkish medium Word-level 16k (uncased)
Pretrained model on Turkish language using a masked language modeling (MLM) objective. The model is uncased.
The pretrained corpus is OSCAR's Turkish split, but it is further filtered and cleaned.
Model architecture is similar to bert-medium (8 layers, 8 heads, and 512 hidden size). Tokenization algorithm is Word-level, which means text is split by white space. Vocabulary size is 16.7k.
The details and performance comparisons can be found at this paper:
https://arxiv.org/abs/2204.08832
The following code can be used for model loading and tokenization, example max length (514) can be changed:
```
model = AutoModel.from_pretrained([model_path])
#for sequence classification:
#model = AutoModelForSequenceClassification.from_pretrained([model_path], num_labels=[num_classes])
tokenizer = PreTrainedTokenizerFast(tokenizer_file=[file_path])
tokenizer.mask_token = "[MASK]"
tokenizer.cls_token = "[CLS]"
tokenizer.sep_token = "[SEP]"
tokenizer.pad_token = "[PAD]"
tokenizer.unk_token = "[UNK]"
tokenizer.bos_token = "[CLS]"
tokenizer.eos_token = "[SEP]"
tokenizer.model_max_length = 514
```
### BibTeX entry and citation info
```bibtex
@misc{https://doi.org/10.48550/arxiv.2204.08832,
doi = {10.48550/ARXIV.2204.08832},
url = {https://arxiv.org/abs/2204.08832},
author = {Toraman, Cagri and Yilmaz, Eyup Halit and Şahinuç, Furkan and Ozcelik, Oguzhan},
keywords = {Computation and Language (cs.CL), FOS: Computer and information sciences, FOS: Computer and information sciences},
title = {Impact of Tokenization on Language Models: An Analysis for Turkish},
publisher = {arXiv},
year = {2022},
copyright = {Creative Commons Attribution Non Commercial Share Alike 4.0 International}
}
``` |
ctoraman/RoBERTa-TR-medium-wp-16k | 58863e42709982079bcb015e630a7e25bc3f72dd | 2022-04-20T07:00:50.000Z | [
"pytorch",
"roberta",
"fill-mask",
"tr",
"dataset:oscar",
"arxiv:2204.08832",
"transformers",
"license:cc-by-nc-sa-4.0",
"autotrain_compatible"
] | fill-mask | false | ctoraman | null | ctoraman/RoBERTa-TR-medium-wp-16k | 2 | null | transformers | 25,106 | ---
language:
- tr
tags:
- roberta
license: cc-by-nc-sa-4.0
datasets:
- oscar
---
# RoBERTa Turkish medium WordPiece 16k (uncased)
Pretrained model on Turkish language using a masked language modeling (MLM) objective. The model is uncased.
The pretrained corpus is OSCAR's Turkish split, but it is further filtered and cleaned.
Model architecture is similar to bert-medium (8 layers, 8 heads, and 512 hidden size). Tokenization algorithm is WordPiece. Vocabulary size is 16.7k.
The details and performance comparisons can be found at this paper:
https://arxiv.org/abs/2204.08832
The following code can be used for model loading and tokenization, example max length (514) can be changed:
```
model = AutoModel.from_pretrained([model_path])
#for sequence classification:
#model = AutoModelForSequenceClassification.from_pretrained([model_path], num_labels=[num_classes])
tokenizer = PreTrainedTokenizerFast(tokenizer_file=[file_path])
tokenizer.mask_token = "[MASK]"
tokenizer.cls_token = "[CLS]"
tokenizer.sep_token = "[SEP]"
tokenizer.pad_token = "[PAD]"
tokenizer.unk_token = "[UNK]"
tokenizer.bos_token = "[CLS]"
tokenizer.eos_token = "[SEP]"
tokenizer.model_max_length = 514
```
### BibTeX entry and citation info
```bibtex
@misc{https://doi.org/10.48550/arxiv.2204.08832,
doi = {10.48550/ARXIV.2204.08832},
url = {https://arxiv.org/abs/2204.08832},
author = {Toraman, Cagri and Yilmaz, Eyup Halit and Şahinuç, Furkan and Ozcelik, Oguzhan},
keywords = {Computation and Language (cs.CL), FOS: Computer and information sciences, FOS: Computer and information sciences},
title = {Impact of Tokenization on Language Models: An Analysis for Turkish},
publisher = {arXiv},
year = {2022},
copyright = {Creative Commons Attribution Non Commercial Share Alike 4.0 International}
}
```
|
anton-l/xtreme_s_xlsr_mls_pl | 91f474e1aef1543bc3ec39045ec42cab7fe8de73 | 2022-03-08T16:54:33.000Z | [
"pytorch",
"tensorboard",
"wav2vec2",
"automatic-speech-recognition",
"transformers"
] | automatic-speech-recognition | false | anton-l | null | anton-l/xtreme_s_xlsr_mls_pl | 2 | null | transformers | 25,107 | Entry not found |
voidful/phoneme_byt5 | eaf02f94b0fc4a4f2802508b45ea492a19973343 | 2022-04-19T09:11:40.000Z | [
"pytorch",
"t5",
"text2text-generation",
"transformers",
"autotrain_compatible"
] | text2text-generation | false | voidful | null | voidful/phoneme_byt5 | 2 | null | transformers | 25,108 | Entry not found |
cammy/bart-large-cnn-100-pad-early-lit | dc9d2d6d2088917b9014c6f3879d565275d6df70 | 2022-03-08T15:01:27.000Z | [
"pytorch",
"bart",
"text2text-generation",
"transformers",
"generated_from_trainer",
"license:mit",
"model-index",
"autotrain_compatible"
] | text2text-generation | false | cammy | null | cammy/bart-large-cnn-100-pad-early-lit | 2 | null | transformers | 25,109 | ---
license: mit
tags:
- generated_from_trainer
metrics:
- rouge
model-index:
- name: bart-large-cnn-100-pad-early-lit
results: []
---
<!-- This model card has been generated automatically according to the information the Trainer had access to. You
should probably proofread and complete it, then remove this comment. -->
# bart-large-cnn-100-pad-early-lit
This model is a fine-tuned version of [facebook/bart-large-cnn](https://huggingface.co/facebook/bart-large-cnn) on an unknown dataset.
It achieves the following results on the evaluation set:
- Loss: 1.1460
- Rouge1: 25.4944
- Rouge2: 7.9048
- Rougel: 16.2879
- Rougelsum: 20.883
- Gen Len: 64.3
## Model description
More information needed
## Intended uses & limitations
More information needed
## Training and evaluation data
More information needed
## Training procedure
### Training hyperparameters
The following hyperparameters were used during training:
- learning_rate: 2e-05
- train_batch_size: 1
- eval_batch_size: 1
- seed: 42
- optimizer: Adam with betas=(0.9,0.999) and epsilon=1e-08
- lr_scheduler_type: linear
- num_epochs: 10
- mixed_precision_training: Native AMP
### Training results
| Training Loss | Epoch | Step | Validation Loss | Rouge1 | Rouge2 | Rougel | Rougelsum | Gen Len |
|:-------------:|:-----:|:----:|:---------------:|:-------:|:-------:|:-------:|:---------:|:-------:|
| No log | 1.0 | 100 | 1.0390 | 27.3059 | 10.0672 | 19.7294 | 23.0611 | 62.1 |
| No log | 2.0 | 200 | 1.1460 | 25.4944 | 7.9048 | 16.2879 | 20.883 | 64.3 |
### Framework versions
- Transformers 4.16.2
- Pytorch 1.10.2
- Datasets 1.18.3
- Tokenizers 0.11.0
|
Rawat29/distilroberta-base-finetuned-wikitext2 | 09d2787f79f43d5e869ad5534156179118ae66a8 | 2022-03-08T16:19:47.000Z | [
"pytorch",
"tensorboard",
"roberta",
"fill-mask",
"transformers",
"generated_from_trainer",
"license:apache-2.0",
"model-index",
"autotrain_compatible"
] | fill-mask | false | Rawat29 | null | Rawat29/distilroberta-base-finetuned-wikitext2 | 2 | null | transformers | 25,110 | ---
license: apache-2.0
tags:
- generated_from_trainer
model-index:
- name: distilroberta-base-finetuned-wikitext2
results: []
---
<!-- This model card has been generated automatically according to the information the Trainer had access to. You
should probably proofread and complete it, then remove this comment. -->
# distilroberta-base-finetuned-wikitext2
This model is a fine-tuned version of [distilroberta-base](https://huggingface.co/distilroberta-base) on the None dataset.
It achieves the following results on the evaluation set:
- Loss: 1.8512
## Model description
More information needed
## Intended uses & limitations
More information needed
## Training and evaluation data
More information needed
## Training procedure
### Training hyperparameters
The following hyperparameters were used during training:
- learning_rate: 2e-05
- train_batch_size: 8
- eval_batch_size: 8
- seed: 42
- optimizer: Adam with betas=(0.9,0.999) and epsilon=1e-08
- lr_scheduler_type: linear
- num_epochs: 3.0
### Training results
| Training Loss | Epoch | Step | Validation Loss |
|:-------------:|:-----:|:----:|:---------------:|
| 2.084 | 1.0 | 2406 | 1.9229 |
| 1.9999 | 2.0 | 4812 | 1.8832 |
| 1.9616 | 3.0 | 7218 | 1.8173 |
### Framework versions
- Transformers 4.17.0
- Pytorch 1.10.0+cu111
- Datasets 1.18.4
- Tokenizers 0.11.6
|
Noricum/wav2vec2-large-xls-r-300m-de-with-lm | 8914aabd44acc790eec253a9af62c11fae60699d | 2022-03-09T18:14:21.000Z | [
"pytorch",
"wav2vec2",
"automatic-speech-recognition",
"transformers",
"generated_from_trainer",
"license:apache-2.0",
"model-index"
] | automatic-speech-recognition | false | Noricum | null | Noricum/wav2vec2-large-xls-r-300m-de-with-lm | 2 | null | transformers | 25,111 | ---
license: apache-2.0
tags:
- generated_from_trainer
model-index:
- name: wav2vec2-large-xls-r-300m-de-with-lm
results: []
---
<!-- This model card has been generated automatically according to the information the Trainer had access to. You
should probably proofread and complete it, then remove this comment. -->
# wav2vec2-large-xls-r-300m-de-with-lm
This model is a fine-tuned version of [facebook/wav2vec2-xls-r-300m](https://huggingface.co/facebook/wav2vec2-xls-r-300m) on the None dataset.
## Model description
More information needed
## Intended uses & limitations
More information needed
## Training and evaluation data
More information needed
## Training procedure
### Training hyperparameters
The following hyperparameters were used during training:
- learning_rate: 0.0001
- train_batch_size: 32
- eval_batch_size: 8
- seed: 42
- gradient_accumulation_steps: 2
- total_train_batch_size: 64
- optimizer: Adam with betas=(0.9,0.999) and epsilon=1e-08
- lr_scheduler_type: linear
- lr_scheduler_warmup_steps: 500
- num_epochs: 20
- mixed_precision_training: Native AMP
### Training results
### Framework versions
- Transformers 4.17.0
- Pytorch 1.9.0+cu111
- Datasets 1.18.4
- Tokenizers 0.11.6
|
OrfeasTsk/bert-base-uncased-finetuned-triviaqa | d17fc1a4b8c7bc5cb1b8eda591989024ceea90ac | 2022-03-08T18:48:47.000Z | [
"pytorch",
"bert",
"question-answering",
"transformers",
"autotrain_compatible"
] | question-answering | false | OrfeasTsk | null | OrfeasTsk/bert-base-uncased-finetuned-triviaqa | 2 | null | transformers | 25,112 | { 'max_seq_length': 384,
'batch_size': 8,
'learning_rate': {'val': 5e-5, 'schelduler': 'Linear'},
'max_clip_norm': None,
'epochs': 2
} |
akozlo/lib_bal | fa434898e0077b1efc69777f29286887f7161a6f | 2022-03-08T20:19:15.000Z | [
"pytorch",
"gpt2",
"text-generation",
"transformers",
"generated_from_trainer",
"license:mit",
"model-index"
] | text-generation | false | akozlo | null | akozlo/lib_bal | 2 | null | transformers | 25,113 | ---
license: mit
tags:
- generated_from_trainer
model-index:
- name: lib_balanced_gpt_model
results: []
---
<!-- This model card has been generated automatically according to the information the Trainer had access to. You
should probably proofread and complete it, then remove this comment. -->
# lib_balanced_gpt_model
This model is a fine-tuned version of [gpt2](https://huggingface.co/gpt2) on an unknown dataset.
## Model description
More information needed
## Intended uses & limitations
More information needed
## Training and evaluation data
More information needed
## Training procedure
### Training hyperparameters
The following hyperparameters were used during training:
- learning_rate: 5e-05
- train_batch_size: 2
- eval_batch_size: 8
- seed: 42
- gradient_accumulation_steps: 4
- total_train_batch_size: 8
- optimizer: Adam with betas=(0.9,0.999) and epsilon=1e-08
- lr_scheduler_type: linear
- num_epochs: 3.0
- mixed_precision_training: Native AMP
### Training results
### Framework versions
- Transformers 4.15.0
- Pytorch 1.10.1+cu102
- Datasets 1.17.0
- Tokenizers 0.10.3
hello
|
OrfeasTsk/bert-base-uncased-finetuned-nq | baabd208763209c729ba9272c061cc14acf30f1f | 2022-03-08T21:44:45.000Z | [
"pytorch",
"bert",
"question-answering",
"transformers",
"autotrain_compatible"
] | question-answering | false | OrfeasTsk | null | OrfeasTsk/bert-base-uncased-finetuned-nq | 2 | null | transformers | 25,114 | { 'max_seq_length': 384,
'batch_size': 8,
'learning_rate': {'val': 5e-5, 'schelduler': 'Linear'},
'max_clip_norm': None,
'epochs': 2
} |
jcai1/ss_ver1 | 46cab17377edb2cf504f888ef07f069e70d421c5 | 2022-03-09T03:03:20.000Z | [
"pytorch",
"tensorboard",
"bert",
"text-classification",
"transformers",
"generated_from_trainer",
"license:apache-2.0",
"model-index"
] | text-classification | false | jcai1 | null | jcai1/ss_ver1 | 2 | null | transformers | 25,115 | ---
license: apache-2.0
tags:
- generated_from_trainer
model-index:
- name: ss_ver1
results: []
---
<!-- This model card has been generated automatically according to the information the Trainer had access to. You
should probably proofread and complete it, then remove this comment. -->
# ss_ver1
This model is a fine-tuned version of [bert-base-uncased](https://huggingface.co/bert-base-uncased) on an unknown dataset.
## Model description
More information needed
## Intended uses & limitations
More information needed
## Training and evaluation data
More information needed
## Training procedure
### Training hyperparameters
The following hyperparameters were used during training:
- learning_rate: 5e-05
- train_batch_size: 8
- eval_batch_size: 8
- seed: 42
- optimizer: Adam with betas=(0.9,0.999) and epsilon=1e-08
- lr_scheduler_type: linear
- num_epochs: 1
### Training results
| Training Loss | Epoch | Step | Validation Loss | Accuracy | F1 |
|:-------------:|:-----:|:----:|:---------------:|:--------:|:---:|
| No log | 1.0 | 436 | 0.0001 | 1.0 | 0.0 |
### Framework versions
- Transformers 4.17.0
- Pytorch 1.10.0+cu111
- Datasets 1.18.4
- Tokenizers 0.11.6
|
ctoraman/RoBERTa-TR-medium-wp-7k | b81247874322de7606ce40f4969f2c50801a48b9 | 2022-04-20T07:02:00.000Z | [
"pytorch",
"roberta",
"fill-mask",
"tr",
"dataset:oscar",
"arxiv:2204.08832",
"transformers",
"license:cc-by-nc-sa-4.0",
"autotrain_compatible"
] | fill-mask | false | ctoraman | null | ctoraman/RoBERTa-TR-medium-wp-7k | 2 | null | transformers | 25,116 | ---
language:
- tr
tags:
- roberta
license: cc-by-nc-sa-4.0
datasets:
- oscar
---
# RoBERTa Turkish medium WordPiece 7k (uncased)
Pretrained model on Turkish language using a masked language modeling (MLM) objective. The model is uncased.
The pretrained corpus is OSCAR's Turkish split, but it is further filtered and cleaned.
Model architecture is similar to bert-medium (8 layers, 8 heads, and 512 hidden size). Tokenization algorithm is WordPiece. Vocabulary size is 7.5k.
The details and performance comparisons can be found at this paper:
https://arxiv.org/abs/2204.08832
The following code can be used for model loading and tokenization, example max length (514) can be changed:
```
model = AutoModel.from_pretrained([model_path])
#for sequence classification:
#model = AutoModelForSequenceClassification.from_pretrained([model_path], num_labels=[num_classes])
tokenizer = PreTrainedTokenizerFast(tokenizer_file=[file_path])
tokenizer.mask_token = "[MASK]"
tokenizer.cls_token = "[CLS]"
tokenizer.sep_token = "[SEP]"
tokenizer.pad_token = "[PAD]"
tokenizer.unk_token = "[UNK]"
tokenizer.bos_token = "[CLS]"
tokenizer.eos_token = "[SEP]"
tokenizer.model_max_length = 514
```
### BibTeX entry and citation info
```bibtex
@misc{https://doi.org/10.48550/arxiv.2204.08832,
doi = {10.48550/ARXIV.2204.08832},
url = {https://arxiv.org/abs/2204.08832},
author = {Toraman, Cagri and Yilmaz, Eyup Halit and Şahinuç, Furkan and Ozcelik, Oguzhan},
keywords = {Computation and Language (cs.CL), FOS: Computer and information sciences, FOS: Computer and information sciences},
title = {Impact of Tokenization on Language Models: An Analysis for Turkish},
publisher = {arXiv},
year = {2022},
copyright = {Creative Commons Attribution Non Commercial Share Alike 4.0 International}
}
```
|
ctoraman/RoBERTa-TR-medium-wp-44k | ea5d69ca4ce6e33d91c1bec795c3d57a53bda5e5 | 2022-04-20T06:41:19.000Z | [
"pytorch",
"roberta",
"fill-mask",
"tr",
"dataset:oscar",
"arxiv:2204.08832",
"transformers",
"license:cc-by-nc-sa-4.0",
"autotrain_compatible"
] | fill-mask | false | ctoraman | null | ctoraman/RoBERTa-TR-medium-wp-44k | 2 | null | transformers | 25,117 | ---
language:
- tr
tags:
- roberta
license: cc-by-nc-sa-4.0
datasets:
- oscar
---
# RoBERTa Turkish medium WordPiece 44k (uncased)
Pretrained model on Turkish language using a masked language modeling (MLM) objective. The model is uncased.
The pretrained corpus is OSCAR's Turkish split, but it is further filtered and cleaned.
Model architecture is similar to bert-medium (8 layers, 8 heads, and 512 hidden size). Tokenization algorithm is WordPiece. Vocabulary size is 44.5k.
The details can be found at this paper:
https://arxiv.org/abs/2204.08832
The following code can be used for model loading and tokenization, example max length (514) can be changed:
```
model = AutoModel.from_pretrained([model_path])
#for sequence classification:
#model = AutoModelForSequenceClassification.from_pretrained([model_path], num_labels=[num_classes])
tokenizer = PreTrainedTokenizerFast(tokenizer_file=[file_path])
tokenizer.mask_token = "[MASK]"
tokenizer.cls_token = "[CLS]"
tokenizer.sep_token = "[SEP]"
tokenizer.pad_token = "[PAD]"
tokenizer.unk_token = "[UNK]"
tokenizer.bos_token = "[CLS]"
tokenizer.eos_token = "[SEP]"
tokenizer.model_max_length = 514
```
### BibTeX entry and citation info
```bibtex
@misc{https://doi.org/10.48550/arxiv.2204.08832,
doi = {10.48550/ARXIV.2204.08832},
url = {https://arxiv.org/abs/2204.08832},
author = {Toraman, Cagri and Yilmaz, Eyup Halit and Şahinuç, Furkan and Ozcelik, Oguzhan},
keywords = {Computation and Language (cs.CL), FOS: Computer and information sciences, FOS: Computer and information sciences},
title = {Impact of Tokenization on Language Models: An Analysis for Turkish},
publisher = {arXiv},
year = {2022},
copyright = {Creative Commons Attribution Non Commercial Share Alike 4.0 International}
}
```
|
ctoraman/RoBERTa-TR-medium-morph-7k | 605c7c8be2afeac5a8fc8f84ffe59ba224103b48 | 2022-04-20T06:59:11.000Z | [
"pytorch",
"roberta",
"fill-mask",
"tr",
"dataset:oscar",
"arxiv:2204.08832",
"transformers",
"license:cc-by-nc-sa-4.0",
"autotrain_compatible"
] | fill-mask | false | ctoraman | null | ctoraman/RoBERTa-TR-medium-morph-7k | 2 | null | transformers | 25,118 | ---
language:
- tr
tags:
- roberta
license: cc-by-nc-sa-4.0
datasets:
- oscar
---
# RoBERTa Turkish medium Morph-level 7k (uncased)
Pretrained model on Turkish language using a masked language modeling (MLM) objective. The model is uncased.
The pretrained corpus is OSCAR's Turkish split, but it is further filtered and cleaned.
Model architecture is similar to bert-medium (8 layers, 8 heads, and 512 hidden size). Tokenization algorithm is Morph-level, which means that text is split according to a Turkish morphological analyzer (Zemberek). Vocabulary size is 7.5k.
## Note that this model needs a preprocessing step before running, because the tokenizer file is not a morphological anaylzer. That is, the test dataset can not be split into morphemes with the tokenizer file. The user needs to process any test dataset by a Turkish morphological analyzer (Zemberek in this case) before running evaluation.
The details and performance comparisons can be found at this paper:
https://arxiv.org/abs/2204.08832
The following code can be used for model loading and tokenization, example max length (514) can be changed:
```
model = AutoModel.from_pretrained([model_path])
#for sequence classification:
#model = AutoModelForSequenceClassification.from_pretrained([model_path], num_labels=[num_classes])
tokenizer = PreTrainedTokenizerFast(tokenizer_file=[file_path])
tokenizer.mask_token = "[MASK]"
tokenizer.cls_token = "[CLS]"
tokenizer.sep_token = "[SEP]"
tokenizer.pad_token = "[PAD]"
tokenizer.unk_token = "[UNK]"
tokenizer.bos_token = "[CLS]"
tokenizer.eos_token = "[SEP]"
tokenizer.model_max_length = 514
```
### BibTeX entry and citation info
```bibtex
@misc{https://doi.org/10.48550/arxiv.2204.08832,
doi = {10.48550/ARXIV.2204.08832},
url = {https://arxiv.org/abs/2204.08832},
author = {Toraman, Cagri and Yilmaz, Eyup Halit and Şahinuç, Furkan and Ozcelik, Oguzhan},
keywords = {Computation and Language (cs.CL), FOS: Computer and information sciences, FOS: Computer and information sciences},
title = {Impact of Tokenization on Language Models: An Analysis for Turkish},
publisher = {arXiv},
year = {2022},
copyright = {Creative Commons Attribution Non Commercial Share Alike 4.0 International}
}
```
|
ctoraman/RoBERTa-TR-medium-morph-44k | 484048da04ceb903c9ef27dfb822f4e38b07473e | 2022-04-20T06:58:25.000Z | [
"pytorch",
"roberta",
"fill-mask",
"tr",
"dataset:oscar",
"arxiv:2204.08832",
"transformers",
"license:cc-by-nc-sa-4.0",
"autotrain_compatible"
] | fill-mask | false | ctoraman | null | ctoraman/RoBERTa-TR-medium-morph-44k | 2 | null | transformers | 25,119 | ---
language:
- tr
tags:
- roberta
license: cc-by-nc-sa-4.0
datasets:
- oscar
---
# RoBERTa Turkish medium Morph-level 44k (uncased)
Pretrained model on Turkish language using a masked language modeling (MLM) objective. The model is uncased.
The pretrained corpus is OSCAR's Turkish split, but it is further filtered and cleaned.
Model architecture is similar to bert-medium (8 layers, 8 heads, and 512 hidden size). Tokenization algorithm is Morph-level, which means that text is split according to a Turkish morphological analyzer (Zemberek). Vocabulary size is 43.6k.
## Note that this model needs a preprocessing step before running, because the tokenizer file is not a morphological anaylzer. That is, the test dataset can not be split into morphemes with the tokenizer file. The user needs to process any test dataset by a Turkish morphological analyzer (Zemberek in this case) before running evaluation.
The details and performance comparisons can be found at this paper:
https://arxiv.org/abs/2204.08832
The following code can be used for model loading and tokenization, example max length (514) can be changed:
```
model = AutoModel.from_pretrained([model_path])
#for sequence classification:
#model = AutoModelForSequenceClassification.from_pretrained([model_path], num_labels=[num_classes])
tokenizer = PreTrainedTokenizerFast(tokenizer_file=[file_path])
tokenizer.mask_token = "[MASK]"
tokenizer.cls_token = "[CLS]"
tokenizer.sep_token = "[SEP]"
tokenizer.pad_token = "[PAD]"
tokenizer.unk_token = "[UNK]"
tokenizer.bos_token = "[CLS]"
tokenizer.eos_token = "[SEP]"
tokenizer.model_max_length = 514
```
### BibTeX entry and citation info
```bibtex
@misc{https://doi.org/10.48550/arxiv.2204.08832,
doi = {10.48550/ARXIV.2204.08832},
url = {https://arxiv.org/abs/2204.08832},
author = {Toraman, Cagri and Yilmaz, Eyup Halit and Şahinuç, Furkan and Ozcelik, Oguzhan},
keywords = {Computation and Language (cs.CL), FOS: Computer and information sciences, FOS: Computer and information sciences},
title = {Impact of Tokenization on Language Models: An Analysis for Turkish},
publisher = {arXiv},
year = {2022},
copyright = {Creative Commons Attribution Non Commercial Share Alike 4.0 International}
}
```
|
ctoraman/RoBERTa-TR-medium-morph-66k | 5613ae8ce577764643310ea13eb8a4e64c010404 | 2022-04-20T06:58:48.000Z | [
"pytorch",
"roberta",
"fill-mask",
"tr",
"dataset:oscar",
"arxiv:2204.08832",
"transformers",
"license:cc-by-nc-sa-4.0",
"autotrain_compatible"
] | fill-mask | false | ctoraman | null | ctoraman/RoBERTa-TR-medium-morph-66k | 2 | null | transformers | 25,120 | ---
language:
- tr
tags:
- roberta
license: cc-by-nc-sa-4.0
datasets:
- oscar
---
# RoBERTa Turkish medium Morph-level 66k (uncased)
Pretrained model on Turkish language using a masked language modeling (MLM) objective. The model is uncased.
The pretrained corpus is OSCAR's Turkish split, but it is further filtered and cleaned.
Model architecture is similar to bert-medium (8 layers, 8 heads, and 512 hidden size). Tokenization algorithm is Morph-level, which means that text is split according to a Turkish morphological analyzer (Zemberek). Vocabulary size is 64.2k.
## Note that this model needs a preprocessing step before running, because the tokenizer file is not a morphological anaylzer. That is, the test dataset can not be split into morphemes with the tokenizer file. The user needs to process any test dataset by a Turkish morphological analyzer (Zemberek in this case) before running evaluation.
The details and performance comparisons can be found at this paper:
https://arxiv.org/abs/2204.08832
The following code can be used for model loading and tokenization, example max length (514) can be changed:
```
model = AutoModel.from_pretrained([model_path])
#for sequence classification:
#model = AutoModelForSequenceClassification.from_pretrained([model_path], num_labels=[num_classes])
tokenizer = PreTrainedTokenizerFast(tokenizer_file=[file_path])
tokenizer.mask_token = "[MASK]"
tokenizer.cls_token = "[CLS]"
tokenizer.sep_token = "[SEP]"
tokenizer.pad_token = "[PAD]"
tokenizer.unk_token = "[UNK]"
tokenizer.bos_token = "[CLS]"
tokenizer.eos_token = "[SEP]"
tokenizer.model_max_length = 514
```
### BibTeX entry and citation info
```bibtex
@misc{https://doi.org/10.48550/arxiv.2204.08832,
doi = {10.48550/ARXIV.2204.08832},
url = {https://arxiv.org/abs/2204.08832},
author = {Toraman, Cagri and Yilmaz, Eyup Halit and Şahinuç, Furkan and Ozcelik, Oguzhan},
keywords = {Computation and Language (cs.CL), FOS: Computer and information sciences, FOS: Computer and information sciences},
title = {Impact of Tokenization on Language Models: An Analysis for Turkish},
publisher = {arXiv},
year = {2022},
copyright = {Creative Commons Attribution Non Commercial Share Alike 4.0 International}
}
```
|
ctoraman/RoBERTa-TR-medium-word-66k | 8ff6a58229c7909a956fbd22525d1a025462355b | 2022-04-20T06:47:24.000Z | [
"pytorch",
"roberta",
"fill-mask",
"tr",
"dataset:oscar",
"arxiv:2204.08832",
"transformers",
"license:cc-by-nc-sa-4.0",
"autotrain_compatible"
] | fill-mask | false | ctoraman | null | ctoraman/RoBERTa-TR-medium-word-66k | 2 | 1 | transformers | 25,121 | ---
language:
- tr
tags:
- roberta
license: cc-by-nc-sa-4.0
datasets:
- oscar
---
# RoBERTa Turkish medium Word-level 66k (uncased)
Pretrained model on Turkish language using a masked language modeling (MLM) objective. The model is uncased.
The pretrained corpus is OSCAR's Turkish split, but it is further filtered and cleaned.
Model architecture is similar to bert-medium (8 layers, 8 heads, and 512 hidden size). Tokenization algorithm is Word-level, which means text is split by white space. Vocabulary size is 66.7k.
The details and performance comparisons can be found at this paper:
https://arxiv.org/abs/2204.08832
The following code can be used for model loading and tokenization, example max length (514) can be changed:
```
model = AutoModel.from_pretrained([model_path])
#for sequence classification:
#model = AutoModelForSequenceClassification.from_pretrained([model_path], num_labels=[num_classes])
tokenizer = PreTrainedTokenizerFast(tokenizer_file=[file_path])
tokenizer.mask_token = "[MASK]"
tokenizer.cls_token = "[CLS]"
tokenizer.sep_token = "[SEP]"
tokenizer.pad_token = "[PAD]"
tokenizer.unk_token = "[UNK]"
tokenizer.bos_token = "[CLS]"
tokenizer.eos_token = "[SEP]"
tokenizer.model_max_length = 514
```
### BibTeX entry and citation info
```bibtex
@misc{https://doi.org/10.48550/arxiv.2204.08832,
doi = {10.48550/ARXIV.2204.08832},
url = {https://arxiv.org/abs/2204.08832},
author = {Toraman, Cagri and Yilmaz, Eyup Halit and Şahinuç, Furkan and Ozcelik, Oguzhan},
keywords = {Computation and Language (cs.CL), FOS: Computer and information sciences, FOS: Computer and information sciences},
title = {Impact of Tokenization on Language Models: An Analysis for Turkish},
publisher = {arXiv},
year = {2022},
copyright = {Creative Commons Attribution Non Commercial Share Alike 4.0 International}
}
```
|
hyechanjun/interview-length-tagged | e20f5ff9ce720c98586670c57aaaff949e6860a1 | 2022-03-09T19:04:53.000Z | [
"pytorch",
"bart",
"text2text-generation",
"transformers",
"autotrain_compatible"
] | text2text-generation | false | hyechanjun | null | hyechanjun/interview-length-tagged | 2 | null | transformers | 25,122 | Entry not found |
OrfeasTsk/bert-base-uncased-finetuned-newsqa | 0a08db3944aa2f11cb7519d47ece28fe0835de3e | 2022-03-09T22:01:05.000Z | [
"pytorch",
"bert",
"question-answering",
"transformers",
"autotrain_compatible"
] | question-answering | false | OrfeasTsk | null | OrfeasTsk/bert-base-uncased-finetuned-newsqa | 2 | null | transformers | 25,123 | { 'max_seq_length': 384,
'batch_size': 8,
'learning_rate': {'val': 5e-5, 'schelduler': 'Linear'},
'max_clip_norm': None,
'epochs': 2
} |
amanm27/bert-base-uncased-scouting | 4447f7d433901e3af020033bc5a690d67c3c2595 | 2022-03-10T00:40:07.000Z | [
"pytorch",
"tensorboard",
"bert",
"fill-mask",
"transformers",
"generated_from_trainer",
"license:apache-2.0",
"model-index",
"autotrain_compatible"
] | fill-mask | false | amanm27 | null | amanm27/bert-base-uncased-scouting | 2 | null | transformers | 25,124 | ---
license: apache-2.0
tags:
- generated_from_trainer
model-index:
- name: bert-base-uncased-scouting
results: []
---
<!-- This model card has been generated automatically according to the information the Trainer had access to. You
should probably proofread and complete it, then remove this comment. -->
# bert-base-uncased-scouting
This model is a fine-tuned version of [bert-base-uncased](https://huggingface.co/bert-base-uncased) on the None dataset.
It achieves the following results on the evaluation set:
- Loss: 1.5443
## Model description
More information needed
## Intended uses & limitations
More information needed
## Training and evaluation data
More information needed
## Training procedure
### Training hyperparameters
The following hyperparameters were used during training:
- learning_rate: 2e-05
- train_batch_size: 8
- eval_batch_size: 8
- seed: 42
- optimizer: Adam with betas=(0.9,0.999) and epsilon=1e-08
- lr_scheduler_type: linear
- num_epochs: 3.0
### Training results
| Training Loss | Epoch | Step | Validation Loss |
|:-------------:|:-----:|:----:|:---------------:|
| No log | 1.0 | 378 | 1.7727 |
| 2.1016 | 2.0 | 756 | 1.6040 |
| 1.7298 | 3.0 | 1134 | 1.5572 |
### Framework versions
- Transformers 4.16.2
- Pytorch 1.10.0
- Datasets 1.18.3
- Tokenizers 0.11.0
|
clisi2000/xlm-roberta-base-finetuned-panx-de | be66962868092c377c7e60b9a15cf94294cef804 | 2022-03-13T06:40:59.000Z | [
"pytorch",
"tensorboard",
"xlm-roberta",
"token-classification",
"dataset:xtreme",
"transformers",
"generated_from_trainer",
"license:mit",
"model-index",
"autotrain_compatible"
] | token-classification | false | clisi2000 | null | clisi2000/xlm-roberta-base-finetuned-panx-de | 2 | null | transformers | 25,125 | ---
license: mit
tags:
- generated_from_trainer
datasets:
- xtreme
metrics:
- f1
model-index:
- name: xlm-roberta-base-finetuned-panx-de
results:
- task:
name: Token Classification
type: token-classification
dataset:
name: xtreme
type: xtreme
args: PAN-X.de
metrics:
- name: F1
type: f1
value: 0.860442623883484
---
<!-- This model card has been generated automatically according to the information the Trainer had access to. You
should probably proofread and complete it, then remove this comment. -->
# xlm-roberta-base-finetuned-panx-de
This model is a fine-tuned version of [xlm-roberta-base](https://huggingface.co/xlm-roberta-base) on the xtreme dataset.
It achieves the following results on the evaluation set:
- Loss: 0.1371
- F1: 0.8604
## Model description
More information needed
## Intended uses & limitations
More information needed
## Training and evaluation data
More information needed
## Training procedure
### Training hyperparameters
The following hyperparameters were used during training:
- learning_rate: 5e-05
- train_batch_size: 24
- eval_batch_size: 24
- seed: 42
- optimizer: Adam with betas=(0.9,0.999) and epsilon=1e-08
- lr_scheduler_type: linear
- num_epochs: 3
### Training results
| Training Loss | Epoch | Step | Validation Loss | F1 |
|:-------------:|:-----:|:----:|:---------------:|:------:|
| 0.2584 | 1.0 | 525 | 0.1675 | 0.8188 |
| 0.127 | 2.0 | 1050 | 0.1383 | 0.8519 |
| 0.0781 | 3.0 | 1575 | 0.1371 | 0.8604 |
### Framework versions
- Transformers 4.11.3
- Pytorch 1.10.2+cpu
- Datasets 1.16.1
- Tokenizers 0.10.1
|
cammy/bart-large-cnn-finetuned-weaksup-100-pad-early-try | 804386f7325c40f1df78c33be97f601593733087 | 2022-03-10T06:44:44.000Z | [
"pytorch",
"bart",
"text2text-generation",
"transformers",
"autotrain_compatible"
] | text2text-generation | false | cammy | null | cammy/bart-large-cnn-finetuned-weaksup-100-pad-early-try | 2 | null | transformers | 25,126 | Entry not found |
amanm27/bert-base-uncased-wiki-scouting | c7644ed74d444f9e3b905e33aa651fb6735a3b60 | 2022-03-10T07:05:36.000Z | [
"pytorch",
"tensorboard",
"bert",
"fill-mask",
"transformers",
"generated_from_trainer",
"license:apache-2.0",
"model-index",
"autotrain_compatible"
] | fill-mask | false | amanm27 | null | amanm27/bert-base-uncased-wiki-scouting | 2 | null | transformers | 25,127 | ---
license: apache-2.0
tags:
- generated_from_trainer
model-index:
- name: bert-base-uncased-wiki-scouting
results: []
---
<!-- This model card has been generated automatically according to the information the Trainer had access to. You
should probably proofread and complete it, then remove this comment. -->
# bert-base-uncased-wiki-scouting
This model is a fine-tuned version of [amanm27/bert-base-uncased-wiki](https://huggingface.co/amanm27/bert-base-uncased-wiki) on the None dataset.
It achieves the following results on the evaluation set:
- Loss: 1.5048
## Model description
More information needed
## Intended uses & limitations
More information needed
## Training and evaluation data
More information needed
## Training procedure
### Training hyperparameters
The following hyperparameters were used during training:
- learning_rate: 2e-05
- train_batch_size: 8
- eval_batch_size: 8
- seed: 42
- optimizer: Adam with betas=(0.9,0.999) and epsilon=1e-08
- lr_scheduler_type: linear
- num_epochs: 3.0
### Training results
| Training Loss | Epoch | Step | Validation Loss |
|:-------------:|:-----:|:----:|:---------------:|
| No log | 1.0 | 378 | 1.7017 |
| 1.9945 | 2.0 | 756 | 1.5597 |
| 1.6769 | 3.0 | 1134 | 1.5160 |
### Framework versions
- Transformers 4.16.2
- Pytorch 1.10.0
- Datasets 1.18.3
- Tokenizers 0.11.0
|
lijingxin/xlm-roberta-base-finetuned-panx-de | 003dd4d49ff9cfa4c83c8fea5542aca16f1570e3 | 2022-03-11T01:37:05.000Z | [
"pytorch",
"tensorboard",
"xlm-roberta",
"token-classification",
"dataset:xtreme",
"transformers",
"generated_from_trainer",
"license:mit",
"model-index",
"autotrain_compatible"
] | token-classification | false | lijingxin | null | lijingxin/xlm-roberta-base-finetuned-panx-de | 2 | null | transformers | 25,128 | ---
license: mit
tags:
- generated_from_trainer
datasets:
- xtreme
metrics:
- f1
model-index:
- name: xlm-roberta-base-finetuned-panx-de
results:
- task:
name: Token Classification
type: token-classification
dataset:
name: xtreme
type: xtreme
args: PAN-X.de
metrics:
- name: F1
type: f1
value: 0.8594910162670748
---
<!-- This model card has been generated automatically according to the information the Trainer had access to. You
should probably proofread and complete it, then remove this comment. -->
# xlm-roberta-base-finetuned-panx-de
This model is a fine-tuned version of [xlm-roberta-base](https://huggingface.co/xlm-roberta-base) on the xtreme dataset.
It achieves the following results on the evaluation set:
- Loss: 0.1348
- F1: 0.8595
## Model description
More information needed
## Intended uses & limitations
More information needed
## Training and evaluation data
More information needed
## Training procedure
### Training hyperparameters
The following hyperparameters were used during training:
- learning_rate: 5e-05
- train_batch_size: 24
- eval_batch_size: 24
- seed: 42
- optimizer: Adam with betas=(0.9,0.999) and epsilon=1e-08
- lr_scheduler_type: linear
- num_epochs: 3
### Training results
| Training Loss | Epoch | Step | Validation Loss | F1 |
|:-------------:|:-----:|:----:|:---------------:|:------:|
| 0.2556 | 1.0 | 525 | 0.1629 | 0.8218 |
| 0.1309 | 2.0 | 1050 | 0.1378 | 0.8522 |
| 0.0812 | 3.0 | 1575 | 0.1348 | 0.8595 |
### Framework versions
- Transformers 4.11.3
- Pytorch 1.9.1
- Datasets 1.16.1
- Tokenizers 0.10.3
|
Taekyoon/unicon_v0.5.0 | 345526f4ee886249d9f9eb53e11d7021fe11ad23 | 2022-03-11T05:07:29.000Z | [
"pytorch",
"bert",
"feature-extraction",
"transformers"
] | feature-extraction | false | Taekyoon | null | Taekyoon/unicon_v0.5.0 | 2 | null | transformers | 25,129 | Entry not found |
imosnoi/md_blt | 3b2650ce477fa954d142f8c6c5e5e88ec7cdc987 | 2022-03-10T09:38:34.000Z | [
"pytorch",
"layoutlm",
"token-classification",
"transformers",
"autotrain_compatible"
] | token-classification | false | imosnoi | null | imosnoi/md_blt | 2 | null | transformers | 25,130 | Entry not found |
AiLab-IMCS-UL/lvbert | 9987f9f045f1330d56e35e75f4dc6d603d3e1846 | 2022-07-13T10:06:51.000Z | [
"pytorch",
"bert",
"feature-extraction",
"transformers",
"license:gpl-3.0"
] | feature-extraction | false | AiLab-IMCS-UL | null | AiLab-IMCS-UL/lvbert | 2 | null | transformers | 25,131 | ---
license: gpl-3.0
---
Latvian BERT-base-cased model.
```
@inproceedings{Znotins-Barzdins:2020:BalticHLT,
author = "A. Znotins and G. Barzdins",
title = "LVBERT: Transformer-Based Model for Latvian Language Understanding",
year = 2020,
booktitle = "Human Language Technologies - The Baltic Perspective",
publisher = "IOS Press",
volume = 328,
pages = "111-115",
doi = "10.3233/FAIA200610",
url = "http://ebooks.iospress.nl/volumearticle/55531"
}
```
Please use the following text to cite this item or export to a predefined format:
Znotiņš, Artūrs, 2020, LVBERT - Latvian BERT, CLARIN-LV digital library at IMCS, University of Latvia, http://hdl.handle.net/20.500.12574/43
|
Mickzaa/Translation_TH-EN-v3 | ba4de30a62650a593f77ee10baad77f6a8ecd16b | 2022-03-10T10:47:54.000Z | [
"pytorch",
"marian",
"text2text-generation",
"transformers",
"autotrain_compatible"
] | text2text-generation | false | Mickzaa | null | Mickzaa/Translation_TH-EN-v3 | 2 | null | transformers | 25,132 | Entry not found |
MoHai/wav2vec2-base-timit-demo-colab | 43531387b56ca751cfca0c19ca9789f37dba9d28 | 2022-03-10T21:34:07.000Z | [
"pytorch",
"tensorboard",
"wav2vec2",
"automatic-speech-recognition",
"transformers",
"generated_from_trainer",
"license:apache-2.0",
"model-index"
] | automatic-speech-recognition | false | MoHai | null | MoHai/wav2vec2-base-timit-demo-colab | 2 | null | transformers | 25,133 | ---
license: apache-2.0
tags:
- generated_from_trainer
model-index:
- name: wav2vec2-base-timit-demo-colab
results: []
---
<!-- This model card has been generated automatically according to the information the Trainer had access to. You
should probably proofread and complete it, then remove this comment. -->
# wav2vec2-base-timit-demo-colab
This model is a fine-tuned version of [facebook/wav2vec2-base](https://huggingface.co/facebook/wav2vec2-base) on the None dataset.
It achieves the following results on the evaluation set:
- Loss: 0.4701
- Wer: 0.4537
## Model description
More information needed
## Intended uses & limitations
More information needed
## Training and evaluation data
More information needed
## Training procedure
### Training hyperparameters
The following hyperparameters were used during training:
- learning_rate: 0.0001
- train_batch_size: 32
- eval_batch_size: 8
- seed: 42
- optimizer: Adam with betas=(0.9,0.999) and epsilon=1e-08
- lr_scheduler_type: linear
- lr_scheduler_warmup_steps: 1000
- num_epochs: 10
- mixed_precision_training: Native AMP
### Training results
| Training Loss | Epoch | Step | Validation Loss | Wer |
|:-------------:|:-----:|:----:|:---------------:|:------:|
| 3.5672 | 4.0 | 500 | 1.6669 | 1.0323 |
| 0.6226 | 8.0 | 1000 | 0.4701 | 0.4537 |
### Framework versions
- Transformers 4.11.3
- Pytorch 1.10.0+cu111
- Datasets 1.18.3
- Tokenizers 0.10.3
|
RamiEbeid/hubert-base-ser | 8127ef5945df82fcf1f58ccf50a717ec515321b0 | 2022-03-16T03:24:57.000Z | [
"pytorch",
"tensorboard",
"hubert",
"transformers",
"generated_from_trainer",
"license:apache-2.0",
"model-index"
] | null | false | RamiEbeid | null | RamiEbeid/hubert-base-ser | 2 | null | transformers | 25,134 | ---
license: apache-2.0
tags:
- generated_from_trainer
metrics:
- accuracy
model-index:
- name: hubert-base-ser
results: []
---
<!-- This model card has been generated automatically according to the information the Trainer had access to. You
should probably proofread and complete it, then remove this comment. -->
# hubert-base-ser
This model is a fine-tuned version of [facebook/hubert-base-ls960](https://huggingface.co/facebook/hubert-base-ls960) on the Crema dataset.
It achieves the following results on the evaluation set:
- Loss: 1.0105
- Accuracy: 0.6313
## Model description
More information needed
## Intended uses & limitations
More information needed
## Training and evaluation data
More information needed
## Training procedure
### Training hyperparameters
The following hyperparameters were used during training:
- learning_rate: 0.0001
- train_batch_size: 4
- eval_batch_size: 4
- seed: 42
- gradient_accumulation_steps: 2
- total_train_batch_size: 8
- optimizer: Adam with betas=(0.9,0.999) and epsilon=1e-08
- lr_scheduler_type: linear
- num_epochs: 1.0
- mixed_precision_training: Native AMP
### Training results
| Training Loss | Epoch | Step | Validation Loss | Accuracy |
|:-------------:|:-----:|:----:|:---------------:|:--------:|
| 1.8106 | 0.01 | 10 | 1.7616 | 0.1974 |
| 1.7268 | 0.03 | 20 | 1.7187 | 0.2525 |
| 1.7269 | 0.04 | 30 | 1.6442 | 0.3096 |
| 1.7086 | 0.05 | 40 | 1.5834 | 0.3338 |
| 1.6983 | 0.07 | 50 | 1.6195 | 0.3600 |
| 1.5845 | 0.08 | 60 | 1.5753 | 0.3418 |
| 1.5744 | 0.09 | 70 | 1.5669 | 0.3707 |
| 1.5915 | 0.11 | 80 | 1.5412 | 0.3754 |
| 1.5105 | 0.12 | 90 | 2.0037 | 0.2612 |
| 1.4689 | 0.13 | 100 | 1.5440 | 0.3627 |
| 1.527 | 0.15 | 110 | 1.5400 | 0.3862 |
| 1.6481 | 0.16 | 120 | 1.6678 | 0.3298 |
| 1.7504 | 0.17 | 130 | 1.6078 | 0.2995 |
| 1.3748 | 0.19 | 140 | 1.5750 | 0.3251 |
| 1.6417 | 0.2 | 150 | 1.7034 | 0.2599 |
| 1.6146 | 0.21 | 160 | 1.6162 | 0.3519 |
| 1.4896 | 0.23 | 170 | 1.5245 | 0.3741 |
| 1.4278 | 0.24 | 180 | 1.7537 | 0.2424 |
| 1.4475 | 0.26 | 190 | 1.4769 | 0.3882 |
| 1.5416 | 0.27 | 200 | 1.4772 | 0.3949 |
| 1.5997 | 0.28 | 210 | 1.4428 | 0.4278 |
| 1.4337 | 0.3 | 220 | 1.4352 | 0.4124 |
| 1.415 | 0.31 | 230 | 1.4405 | 0.4157 |
| 1.5196 | 0.32 | 240 | 1.4197 | 0.4043 |
| 1.3866 | 0.34 | 250 | 1.5241 | 0.3734 |
| 1.3041 | 0.35 | 260 | 1.5703 | 0.4043 |
| 1.3618 | 0.36 | 270 | 1.3963 | 0.4285 |
| 1.3293 | 0.38 | 280 | 1.3478 | 0.4506 |
| 1.2215 | 0.39 | 290 | 1.5994 | 0.3842 |
| 1.6618 | 0.4 | 300 | 1.7751 | 0.2277 |
| 1.5349 | 0.42 | 310 | 1.6091 | 0.4036 |
| 1.4037 | 0.43 | 320 | 1.4741 | 0.4446 |
| 1.4844 | 0.44 | 330 | 1.4170 | 0.4399 |
| 1.2806 | 0.46 | 340 | 1.2887 | 0.5050 |
| 1.3818 | 0.47 | 350 | 1.2668 | 0.5017 |
| 1.3491 | 0.48 | 360 | 1.4721 | 0.4594 |
| 1.2347 | 0.5 | 370 | 1.2188 | 0.5245 |
| 1.2182 | 0.51 | 380 | 1.3813 | 0.4567 |
| 1.2513 | 0.52 | 390 | 1.2111 | 0.5205 |
| 1.2447 | 0.54 | 400 | 1.2231 | 0.5460 |
| 1.038 | 0.55 | 410 | 1.2563 | 0.5373 |
| 1.2409 | 0.56 | 420 | 1.3448 | 0.4936 |
| 1.2279 | 0.58 | 430 | 1.1972 | 0.5487 |
| 1.3256 | 0.59 | 440 | 1.1706 | 0.5742 |
| 1.2866 | 0.6 | 450 | 1.3091 | 0.5003 |
| 1.0574 | 0.62 | 460 | 1.2075 | 0.5500 |
| 1.2744 | 0.63 | 470 | 1.2831 | 0.5171 |
| 1.0836 | 0.64 | 480 | 1.1768 | 0.5608 |
| 1.135 | 0.66 | 490 | 1.1408 | 0.5776 |
| 1.1303 | 0.67 | 500 | 1.2320 | 0.5541 |
| 1.2068 | 0.69 | 510 | 1.1379 | 0.5796 |
| 1.1347 | 0.7 | 520 | 1.1124 | 0.5897 |
| 1.1846 | 0.71 | 530 | 1.1338 | 0.5803 |
| 1.2409 | 0.73 | 540 | 1.1259 | 0.5789 |
| 1.0664 | 0.74 | 550 | 1.0653 | 0.6038 |
| 1.1637 | 0.75 | 560 | 1.0550 | 0.5977 |
| 1.0707 | 0.77 | 570 | 1.0996 | 0.5715 |
| 1.2258 | 0.78 | 580 | 1.0804 | 0.5977 |
| 0.9256 | 0.79 | 590 | 1.1501 | 0.5809 |
| 1.1542 | 0.81 | 600 | 1.1089 | 0.5957 |
| 1.3931 | 0.82 | 610 | 1.1381 | 0.5856 |
| 1.1117 | 0.83 | 620 | 1.0933 | 0.6031 |
| 1.1433 | 0.85 | 630 | 1.0175 | 0.6219 |
| 1.0325 | 0.86 | 640 | 0.9885 | 0.6239 |
| 1.111 | 0.87 | 650 | 1.0048 | 0.6259 |
| 0.8125 | 0.89 | 660 | 1.0176 | 0.6165 |
| 1.0414 | 0.9 | 670 | 1.0290 | 0.6185 |
| 1.0037 | 0.91 | 680 | 1.0269 | 0.6253 |
| 0.9406 | 0.93 | 690 | 1.0301 | 0.6273 |
| 1.0129 | 0.94 | 700 | 1.0238 | 0.6326 |
| 1.2213 | 0.95 | 710 | 1.0181 | 0.6273 |
| 1.2519 | 0.97 | 720 | 1.0161 | 0.6266 |
| 0.9932 | 0.98 | 730 | 1.0112 | 0.6279 |
| 1.0135 | 0.99 | 740 | 1.0105 | 0.6313 |
### Framework versions
- Transformers 4.18.0.dev0
- Pytorch 1.10.0+cu111
- Datasets 1.18.5.dev0
- Tokenizers 0.11.6
|
OrfeasTsk/bert-base-uncased-finetuned-nq-large-batch | 89926ec34307209703cce923423edda043609be0 | 2022-03-10T14:09:50.000Z | [
"pytorch",
"bert",
"question-answering",
"transformers",
"autotrain_compatible"
] | question-answering | false | OrfeasTsk | null | OrfeasTsk/bert-base-uncased-finetuned-nq-large-batch | 2 | null | transformers | 25,135 | { 'max_seq_length': 384,
'batch_size': 24,
'learning_rate': {'val': 3e-5, 'schelduler': 'Linear'},
'max_clip_norm': None,
'epochs': 2
} |
Kevincp560/pegasus-arxiv-finetuned-pubmed | a6bcdfa87e1e682ae04ae27162a5a00eb36da75d | 2022-03-10T18:36:19.000Z | [
"pytorch",
"pegasus",
"text2text-generation",
"dataset:pub_med_summarization_dataset",
"transformers",
"generated_from_trainer",
"model-index",
"autotrain_compatible"
] | text2text-generation | false | Kevincp560 | null | Kevincp560/pegasus-arxiv-finetuned-pubmed | 2 | null | transformers | 25,136 | ---
tags:
- generated_from_trainer
datasets:
- pub_med_summarization_dataset
metrics:
- rouge
model-index:
- name: pegasus-arxiv-finetuned-pubmed
results:
- task:
name: Sequence-to-sequence Language Modeling
type: text2text-generation
dataset:
name: pub_med_summarization_dataset
type: pub_med_summarization_dataset
args: document
metrics:
- name: Rouge1
type: rouge
value: 44.286
---
<!-- This model card has been generated automatically according to the information the Trainer had access to. You
should probably proofread and complete it, then remove this comment. -->
# pegasus-arxiv-finetuned-pubmed
This model is a fine-tuned version of [google/pegasus-arxiv](https://huggingface.co/google/pegasus-arxiv) on the pub_med_summarization_dataset dataset.
It achieves the following results on the evaluation set:
- Loss: 1.8118
- Rouge1: 44.286
- Rouge2: 19.0477
- Rougel: 27.1122
- Rougelsum: 40.2609
- Gen Len: 230.586
## Model description
More information needed
## Intended uses & limitations
More information needed
## Training and evaluation data
More information needed
## Training procedure
### Training hyperparameters
The following hyperparameters were used during training:
- learning_rate: 2e-05
- train_batch_size: 2
- eval_batch_size: 2
- seed: 42
- optimizer: Adam with betas=(0.9,0.999) and epsilon=1e-08
- lr_scheduler_type: linear
- num_epochs: 5
### Training results
| Training Loss | Epoch | Step | Validation Loss | Rouge1 | Rouge2 | Rougel | Rougelsum | Gen Len |
|:-------------:|:-----:|:----:|:---------------:|:-------:|:-------:|:-------:|:---------:|:-------:|
| 2.65 | 1.0 | 1000 | 1.9848 | 40.6984 | 16.387 | 25.0097 | 36.4831 | 215.294 |
| 2.1317 | 2.0 | 2000 | 1.8524 | 43.6431 | 18.6794 | 26.7571 | 39.6642 | 224.646 |
| 2.0591 | 3.0 | 3000 | 1.8254 | 43.6707 | 18.5176 | 26.6015 | 39.6325 | 225.894 |
| 2.0109 | 4.0 | 4000 | 1.8138 | 44.1244 | 18.8866 | 26.8313 | 40.0913 | 229.656 |
| 1.9894 | 5.0 | 5000 | 1.8118 | 44.286 | 19.0477 | 27.1122 | 40.2609 | 230.586 |
### Framework versions
- Transformers 4.17.0
- Pytorch 1.9.1
- Datasets 1.18.4
- Tokenizers 0.11.6
|
Kevincp560/pegasus-cnn_dailymail-finetuned-pubmed | 5bf91d0b6d3d6c9fd7d85e3c6c37e621e8007b33 | 2022-03-10T20:20:46.000Z | [
"pytorch",
"pegasus",
"text2text-generation",
"dataset:pub_med_summarization_dataset",
"transformers",
"generated_from_trainer",
"model-index",
"autotrain_compatible"
] | text2text-generation | false | Kevincp560 | null | Kevincp560/pegasus-cnn_dailymail-finetuned-pubmed | 2 | null | transformers | 25,137 | ---
tags:
- generated_from_trainer
datasets:
- pub_med_summarization_dataset
metrics:
- rouge
model-index:
- name: pegasus-cnn_dailymail-finetuned-pubmed
results:
- task:
name: Sequence-to-sequence Language Modeling
type: text2text-generation
dataset:
name: pub_med_summarization_dataset
type: pub_med_summarization_dataset
args: document
metrics:
- name: Rouge1
type: rouge
value: 37.2569
---
<!-- This model card has been generated automatically according to the information the Trainer had access to. You
should probably proofread and complete it, then remove this comment. -->
# pegasus-cnn_dailymail-finetuned-pubmed
This model is a fine-tuned version of [google/pegasus-cnn_dailymail](https://huggingface.co/google/pegasus-cnn_dailymail) on the pub_med_summarization_dataset dataset.
It achieves the following results on the evaluation set:
- Loss: 1.8050
- Rouge1: 37.2569
- Rouge2: 15.8205
- Rougel: 24.1969
- Rougelsum: 34.0331
- Gen Len: 125.892
## Model description
More information needed
## Intended uses & limitations
More information needed
## Training and evaluation data
More information needed
## Training procedure
### Training hyperparameters
The following hyperparameters were used during training:
- learning_rate: 2e-05
- train_batch_size: 2
- eval_batch_size: 2
- seed: 42
- optimizer: Adam with betas=(0.9,0.999) and epsilon=1e-08
- lr_scheduler_type: linear
- num_epochs: 5
### Training results
| Training Loss | Epoch | Step | Validation Loss | Rouge1 | Rouge2 | Rougel | Rougelsum | Gen Len |
|:-------------:|:-----:|:----:|:---------------:|:-------:|:-------:|:-------:|:---------:|:-------:|
| 2.2449 | 1.0 | 1000 | 1.8942 | 36.4494 | 14.9948 | 23.8279 | 33.3081 | 124.482 |
| 2.0803 | 2.0 | 2000 | 1.8440 | 36.998 | 15.4992 | 24.091 | 33.6614 | 125.678 |
| 2.0166 | 3.0 | 3000 | 1.8176 | 37.4703 | 16.0358 | 24.5735 | 34.1789 | 125.094 |
| 1.9911 | 4.0 | 4000 | 1.8055 | 37.1338 | 15.7921 | 24.1412 | 33.8293 | 125.874 |
| 1.9419 | 5.0 | 5000 | 1.8050 | 37.2569 | 15.8205 | 24.1969 | 34.0331 | 125.892 |
### Framework versions
- Transformers 4.17.0
- Pytorch 1.9.1
- Datasets 1.18.4
- Tokenizers 0.11.6
|
sanchit-gandhi/wav2vec2-2-rnd-2-layer-bart | 283f6daac72cd50959d664a930af21e7dacba7b2 | 2022-03-12T03:02:56.000Z | [
"pytorch",
"tensorboard",
"speech-encoder-decoder",
"automatic-speech-recognition",
"dataset:librispeech_asr",
"transformers",
"generated_from_trainer",
"model-index"
] | automatic-speech-recognition | false | sanchit-gandhi | null | sanchit-gandhi/wav2vec2-2-rnd-2-layer-bart | 2 | null | transformers | 25,138 | ---
tags:
- generated_from_trainer
datasets:
- librispeech_asr
model-index:
- name: ''
results: []
---
<!-- This model card has been generated automatically according to the information the Trainer had access to. You
should probably proofread and complete it, then remove this comment. -->
#
This model was trained from scratch on the librispeech_asr dataset.
It achieves the following results on the evaluation set:
- Loss: 4.6263
- Wer: 0.8568
## Model description
More information needed
## Intended uses & limitations
More information needed
## Training and evaluation data
More information needed
## Training procedure
### Training hyperparameters
The following hyperparameters were used during training:
- learning_rate: 3e-05
- train_batch_size: 8
- eval_batch_size: 8
- seed: 42
- gradient_accumulation_steps: 4
- total_train_batch_size: 32
- optimizer: Adam with betas=(0.9,0.999) and epsilon=1e-08
- lr_scheduler_type: linear
- lr_scheduler_warmup_steps: 1000
- num_epochs: 20.0
- mixed_precision_training: Native AMP
### Training results
| Training Loss | Epoch | Step | Validation Loss | Wer |
|:-------------:|:-----:|:-----:|:---------------:|:------:|
| 5.9849 | 1.68 | 1500 | 5.9623 | 1.1028 |
| 5.1696 | 3.36 | 3000 | 5.5504 | 1.6345 |
| 4.1412 | 5.04 | 4500 | 5.3853 | 1.3565 |
| 2.7226 | 6.73 | 6000 | 5.3072 | 0.9908 |
| 3.2607 | 8.41 | 7500 | 5.4121 | 1.2854 |
| 2.4017 | 10.09 | 9000 | 5.1094 | 1.0303 |
| 1.7361 | 11.77 | 10500 | 4.8928 | 0.9506 |
| 2.0638 | 13.45 | 12000 | 4.8352 | 0.9127 |
| 1.2832 | 15.13 | 13500 | 4.7271 | 0.9103 |
| 1.0439 | 16.82 | 15000 | 4.5980 | 0.8720 |
| 0.4112 | 18.5 | 16500 | 4.6263 | 0.8568 |
### Framework versions
- Transformers 4.17.0.dev0
- Pytorch 1.10.2+cu113
- Datasets 1.18.3
- Tokenizers 0.11.0
|
willcai/wav2vec2_common_voice_accents | 907690000a04d52c384c2bf927818fec68f42d1d | 2022-03-13T01:55:11.000Z | [
"pytorch",
"wav2vec2",
"automatic-speech-recognition",
"dataset:common_voice",
"transformers",
"generated_from_trainer",
"license:apache-2.0",
"model-index"
] | automatic-speech-recognition | false | willcai | null | willcai/wav2vec2_common_voice_accents | 2 | null | transformers | 25,139 | ---
license: apache-2.0
tags:
- generated_from_trainer
datasets:
- common_voice
model-index:
- name: wav2vec2_common_voice_accents
results: []
---
<!-- This model card has been generated automatically according to the information the Trainer had access to. You
should probably proofread and complete it, then remove this comment. -->
# wav2vec2_common_voice_accents
This model is a fine-tuned version of [facebook/wav2vec2-xls-r-300m](https://huggingface.co/facebook/wav2vec2-xls-r-300m) on the common_voice dataset.
It achieves the following results on the evaluation set:
- Loss: 0.9095
- Wer: 0.4269
## Model description
More information needed
## Intended uses & limitations
More information needed
## Training and evaluation data
More information needed
## Training procedure
### Training hyperparameters
The following hyperparameters were used during training:
- learning_rate: 0.0003
- train_batch_size: 16
- eval_batch_size: 4
- seed: 42
- distributed_type: multi-GPU
- optimizer: Adam with betas=(0.9,0.999) and epsilon=1e-08
- lr_scheduler_type: linear
- lr_scheduler_warmup_steps: 500
- num_epochs: 30
- mixed_precision_training: Native AMP
### Training results
| Training Loss | Epoch | Step | Validation Loss | Wer |
|:-------------:|:-----:|:----:|:---------------:|:------:|
| 4.0135 | 5.33 | 400 | 1.3259 | 0.8067 |
| 0.5608 | 10.67 | 800 | 0.7832 | 0.5024 |
| 0.1441 | 16.0 | 1200 | 0.9309 | 0.4698 |
| 0.0724 | 21.33 | 1600 | 0.9750 | 0.4461 |
| 0.0444 | 26.67 | 2000 | 0.9095 | 0.4269 |
### Framework versions
- Transformers 4.17.0
- Pytorch 1.10.2+cu102
- Datasets 1.18.4
- Tokenizers 0.11.6
|
newtonkwan/gpt2-fine-tuned-debiased | 6607bb91d38dd332a48701e9cc6850ef43e21b6e | 2022-03-11T00:37:42.000Z | [
"pytorch",
"gpt2",
"text-generation",
"transformers"
] | text-generation | false | newtonkwan | null | newtonkwan/gpt2-fine-tuned-debiased | 2 | null | transformers | 25,140 | Entry not found |
newtonkwan/gpt2-xl-fine-tuned-debiased | 5086f64ff5c9e8a379abab34c56a5b3a52a23f19 | 2022-03-11T09:16:01.000Z | [
"pytorch",
"gpt2",
"text-generation",
"transformers",
"generated_from_trainer",
"model-index"
] | text-generation | false | newtonkwan | null | newtonkwan/gpt2-xl-fine-tuned-debiased | 2 | null | transformers | 25,141 | ---
tags:
- generated_from_trainer
model-index:
- name: gpt2-xl-fine-tuned-debiased
results: []
---
<!-- This model card has been generated automatically according to the information the Trainer had access to. You
should probably proofread and complete it, then remove this comment. -->
# gpt2-xl-fine-tuned-debiased
This model is a fine-tuned version of [gpt2-xl](https://huggingface.co/gpt2-xl) on an unknown dataset.
It achieves the following results on the evaluation set:
- Loss: 2.1714
## Model description
More information needed
## Intended uses & limitations
More information needed
## Training and evaluation data
More information needed
## Training procedure
### Training hyperparameters
The following hyperparameters were used during training:
- learning_rate: 0.0005
- train_batch_size: 2
- eval_batch_size: 2
- seed: 42
- gradient_accumulation_steps: 32
- total_train_batch_size: 64
- optimizer: Adam with betas=(0.9,0.999) and epsilon=1e-08
- lr_scheduler_type: linear
- lr_scheduler_warmup_steps: 100.0
- num_epochs: 4
### Training results
| Training Loss | Epoch | Step | Validation Loss |
|:-------------:|:-----:|:----:|:---------------:|
| No log | 0.91 | 10 | 1.9130 |
| No log | 1.91 | 20 | 1.7356 |
| No log | 2.91 | 30 | 1.9216 |
| No log | 3.91 | 40 | 2.1714 |
### Framework versions
- Transformers 4.17.0
- Pytorch 1.5.0
- Datasets 1.12.1
- Tokenizers 0.11.6
|
lijingxin/xlm-roberta-base-finetuned-panx-de-fr | 3245284070d861a79ea306038db7d58a042da43b | 2022-03-11T02:00:57.000Z | [
"pytorch",
"xlm-roberta",
"token-classification",
"transformers",
"generated_from_trainer",
"license:mit",
"model-index",
"autotrain_compatible"
] | token-classification | false | lijingxin | null | lijingxin/xlm-roberta-base-finetuned-panx-de-fr | 2 | null | transformers | 25,142 | ---
license: mit
tags:
- generated_from_trainer
metrics:
- f1
model-index:
- name: xlm-roberta-base-finetuned-panx-de-fr
results: []
---
<!-- This model card has been generated automatically according to the information the Trainer had access to. You
should probably proofread and complete it, then remove this comment. -->
# xlm-roberta-base-finetuned-panx-de-fr
This model is a fine-tuned version of [xlm-roberta-base](https://huggingface.co/xlm-roberta-base) on the None dataset.
It achieves the following results on the evaluation set:
- Loss: 0.1664
- F1: 0.8556
## Model description
More information needed
## Intended uses & limitations
More information needed
## Training and evaluation data
More information needed
## Training procedure
### Training hyperparameters
The following hyperparameters were used during training:
- learning_rate: 5e-05
- train_batch_size: 24
- eval_batch_size: 24
- seed: 42
- optimizer: Adam with betas=(0.9,0.999) and epsilon=1e-08
- lr_scheduler_type: linear
- num_epochs: 3
### Training results
| Training Loss | Epoch | Step | Validation Loss | F1 |
|:-------------:|:-----:|:----:|:---------------:|:------:|
| 0.2846 | 1.0 | 715 | 0.1837 | 0.8247 |
| 0.1446 | 2.0 | 1430 | 0.1617 | 0.8409 |
| 0.0923 | 3.0 | 2145 | 0.1664 | 0.8556 |
### Framework versions
- Transformers 4.11.3
- Pytorch 1.9.1
- Datasets 1.16.1
- Tokenizers 0.10.3
|
tiot07/wav2vec2-base-timit-demo-colab | 628713fe05ea9be94c1c66d3dde889e4e346dae4 | 2022-03-11T06:09:20.000Z | [
"pytorch",
"wav2vec2",
"automatic-speech-recognition",
"transformers",
"generated_from_trainer",
"license:apache-2.0",
"model-index"
] | automatic-speech-recognition | false | tiot07 | null | tiot07/wav2vec2-base-timit-demo-colab | 2 | null | transformers | 25,143 | ---
license: apache-2.0
tags:
- generated_from_trainer
model-index:
- name: wav2vec2-base-timit-demo-colab
results: []
---
<!-- This model card has been generated automatically according to the information the Trainer had access to. You
should probably proofread and complete it, then remove this comment. -->
# wav2vec2-base-timit-demo-colab
This model is a fine-tuned version of [facebook/wav2vec2-large](https://huggingface.co/facebook/wav2vec2-large) on the None dataset.
It achieves the following results on the evaluation set:
- Loss: 0.4612
- Wer: 0.2963
## Model description
More information needed
## Intended uses & limitations
More information needed
## Training and evaluation data
More information needed
## Training procedure
### Training hyperparameters
The following hyperparameters were used during training:
- learning_rate: 0.0001
- train_batch_size: 32
- eval_batch_size: 8
- seed: 42
- optimizer: Adam with betas=(0.9,0.999) and epsilon=1e-08
- lr_scheduler_type: linear
- lr_scheduler_warmup_steps: 1000
- num_epochs: 30
- mixed_precision_training: Native AMP
### Training results
| Training Loss | Epoch | Step | Validation Loss | Wer |
|:-------------:|:-----:|:----:|:---------------:|:------:|
| 2.9218 | 4.0 | 500 | 0.6017 | 0.5820 |
| 0.5407 | 8.0 | 1000 | 0.4846 | 0.4388 |
| 0.2899 | 12.0 | 1500 | 0.4442 | 0.3654 |
| 0.1848 | 16.0 | 2000 | 0.4693 | 0.3396 |
| 0.1282 | 20.0 | 2500 | 0.4690 | 0.3215 |
| 0.0936 | 24.0 | 3000 | 0.4597 | 0.3125 |
| 0.0714 | 28.0 | 3500 | 0.4612 | 0.2963 |
### Framework versions
- Transformers 4.11.3
- Pytorch 1.10.2
- Datasets 1.18.3
- Tokenizers 0.10.3
|
rcgale/psst-apr-baseline | 1518ef268a5b658fb4c91aa873bd2afa9157bb5a | 2022-03-23T10:51:21.000Z | [
"pytorch",
"wav2vec2",
"automatic-speech-recognition",
"transformers"
] | automatic-speech-recognition | false | rcgale | null | rcgale/psst-apr-baseline | 2 | null | transformers | 25,144 | Entry not found |
Vasily/match | 442603b3196d9e78993772c62cd37541e460f46a | 2022-03-11T12:22:36.000Z | [
"pytorch",
"distilbert",
"transformers"
] | null | false | Vasily | null | Vasily/match | 2 | null | transformers | 25,145 | Entry not found |
QuickRead/pegasus-reddit-6.35e5 | 2adcc69fa6baf4f998e44368fbf4651f6dd6e660 | 2022-03-15T01:32:49.000Z | [
"pytorch",
"pegasus",
"text2text-generation",
"transformers",
"autotrain_compatible"
] | text2text-generation | false | QuickRead | null | QuickRead/pegasus-reddit-6.35e5 | 2 | null | transformers | 25,146 | Entry not found |
GroNLP/wav2vec2-dutch-large | 27506b0e26066bc71d2d07d8475d3f6a11bc471e | 2022-03-11T16:04:07.000Z | [
"pytorch",
"wav2vec2",
"pretraining",
"nl",
"transformers",
"speech"
] | null | false | GroNLP | null | GroNLP/wav2vec2-dutch-large | 2 | null | transformers | 25,147 | ---
language: nl
tags:
- speech
---
# Wav2Vec2-Dutch-Large
A Dutch Wav2Vec2 model. This model is created by further pre-training the original English [`facebook/wav2vec2-large`](https://huggingface.co/facebook/wav2vec2-large) model on Dutch speech from [Het Corpus Gesproken Nederlands](https://taalmaterialen.ivdnt.org/download/tstc-corpus-gesproken-nederlands/).
This model is one of two Dutch Wav2Vec2 models:
- [`GroNLP/wav2vec2-dutch-base`](https://huggingface.co/GroNLP/wav2vec2-dutch-base)
- [`GroNLP/wav2vec2-dutch-large`](https://huggingface.co/GroNLP/wav2vec2-dutch-large) (this model) |
MrAnderson/yoso-2048-full-trivia-copied-embeddings | ac22bfb89f98860d3973477654180e9aaf207278 | 2022-03-12T13:11:42.000Z | [
"pytorch",
"yoso",
"question-answering",
"transformers",
"autotrain_compatible"
] | question-answering | false | MrAnderson | null | MrAnderson/yoso-2048-full-trivia-copied-embeddings | 2 | null | transformers | 25,148 | Entry not found |
Aureliano/electra-if | 20d0a6b2f1cfc8a4634501091582e3604b732221 | 2022-03-30T09:07:27.000Z | [
"pytorch",
"tf",
"electra",
"feature-extraction",
"en",
"transformers",
"license:apache-2.0"
] | feature-extraction | false | Aureliano | null | Aureliano/electra-if | 2 | null | transformers | 25,149 | ---
language: en
license: apache-2.0
---
## ELECTRA for IF
**ELECTRA** is a method for self-supervised language representation learning. They are trained to distinguish "real" input tokens vs "fake" input tokens generated by another neural network, similar to the discriminator of a [GAN](https://arxiv.org/pdf/1406.2661.pdf).
For a detailed description and experimental results, please refer to the original paper [ELECTRA: Pre-training Text Encoders as Discriminators Rather Than Generators](https://openreview.net/pdf?id=r1xMH1BtvB).
This repository contains a small ELECTRA discriminator finetuned on a corpus of interactive fiction commands labelled with the WordNet synset offset of the verb in the sentence. The original dataset has been collected from the list of action in the walkthroughs for the game included in the [Jericho](https://github.com/microsoft/jericho) framework and manually annotated. For more information visit https://github.com/aporporato/electra and https://github.com/aporporato/jericho-corpora.
## How to use the discriminator in `transformers`
(Heavily based on: https://github.com/huggingface/notebooks/blob/master/examples/text_classification-tf.ipynb)
```python
import math
import numpy as np
import tensorflow as tf
from datasets import load_metric, Dataset, DatasetDict
from transformers import TFAutoModelForSequenceClassification, AutoTokenizer, DataCollatorWithPadding, create_optimizer
from transformers.keras_callbacks import KerasMetricCallback
# This example shows how this model can be used:
# you should finetune the model of your specific corpus if commands, bigger than this
dict_train = {
"idx": ["0", "1", "2", "3", "4", "5", "6", "7", "8", "9", "10", "11", "12", "13", "14", "15", "16", "17", "18",
"19", "20"],
"sentence": ["e", "get pen", "drop book", "x paper", "i", "south", "get paper", "drop the pen", "x book",
"inventory", "n", "get the book", "drop paper", "look at Pen", "inv", "g", "s", "get sandwich",
"drop sandwich", "x sandwich", "agin"],
"label": ["travel.v.01", "take.v.04", "drop.v.01", "examine.v.02", "inventory.v.01", "travel.v.01", "take.v.04",
"drop.v.01", "examine.v.02", "inventory.v.01", "travel.v.01", "take.v.04", "drop.v.01", "examine.v.02",
"inventory.v.01", "repeat.v.01", "travel.v.01", "take.v.04", "drop.v.01", "examine.v.02", "repeat.v.01"]
}
dict_val = {
"idx": ["0", "1", "2", "3", "4", "5"],
"sentence": ["w", "get shield", "drop sword", "x spikes", "i", "repeat"],
"label": ["travel.v.01", "take.v.04", "drop.v.01", "examine.v.02", "inventory.v.01", "repeat.v.01"]
}
raw_train_dataset = Dataset.from_dict(dict_train)
raw_val_dataset = Dataset.from_dict(dict_val)
raw_dataset = DatasetDict()
raw_dataset["train"] = raw_train_dataset
raw_dataset["val"] = raw_val_dataset
raw_dataset = raw_dataset.class_encode_column("label")
print(raw_dataset)
print(raw_dataset["train"].features)
print(raw_dataset["val"].features)
print(raw_dataset["train"][1])
label2id = {}
id2label = {}
for i, l in enumerate(raw_dataset["train"].features["label"].names):
label2id[l] = i
id2label[i] = l
discriminator = TFAutoModelForSequenceClassification.from_pretrained("Aureliano/electra-if",
label2id=label2id,
id2label=id2label)
tokenizer = AutoTokenizer.from_pretrained("Aureliano/electra-if")
tokenize_function = lambda example: tokenizer(example["sentence"], truncation=True)
pre_tokenizer_columns = set(raw_dataset["train"].features)
encoded_dataset = raw_dataset.map(tokenize_function, batched=True)
tokenizer_columns = list(set(encoded_dataset["train"].features) - pre_tokenizer_columns)
data_collator = DataCollatorWithPadding(tokenizer=tokenizer, return_tensors="tf")
batch_size = len(encoded_dataset["train"])
tf_train_dataset = encoded_dataset["train"].to_tf_dataset(
columns=tokenizer_columns,
label_cols=["labels"],
shuffle=True,
batch_size=batch_size,
collate_fn=data_collator
)
tf_validation_dataset = encoded_dataset["val"].to_tf_dataset(
columns=tokenizer_columns,
label_cols=["labels"],
shuffle=False,
batch_size=batch_size,
collate_fn=data_collator
)
loss = tf.keras.losses.SparseCategoricalCrossentropy(from_logits=True)
num_epochs = 25
batches_per_epoch = math.ceil(len(encoded_dataset["train"]) / batch_size)
total_train_steps = int(batches_per_epoch * num_epochs)
optimizer, schedule = create_optimizer(
init_lr=5e-5, num_warmup_steps=total_train_steps // 5, num_train_steps=total_train_steps
)
metric = load_metric("accuracy")
def compute_metrics(eval_predictions):
logits, labels = eval_predictions
predictions = np.argmax(logits, axis=-1)
return metric.compute(predictions=predictions, references=labels)
metric_callback = KerasMetricCallback(metric_fn=compute_metrics, eval_dataset=tf_validation_dataset)
callbacks = [metric_callback]
discriminator.compile(optimizer=optimizer, loss=loss, metrics=["sparse_categorical_accuracy"])
discriminator.fit(
tf_train_dataset,
epochs=num_epochs,
validation_data=tf_validation_dataset,
callbacks=callbacks
)
print("Evaluate on test data")
results = discriminator.evaluate(tf_validation_dataset)
print("test loss, test acc:", results)
text = "i"
encoded_input = tokenizer(text, return_tensors='tf')
output = discriminator(encoded_input)
prediction = tf.nn.softmax(output["logits"][0], -1)
label = id2label[tf.math.argmax(prediction).numpy()]
print("\n", text, ":", label,
"\n") # ideally 'inventory.v.01' (-> "make or include in an itemized record or report"), but probably only with a better finetuning dataset
text = "get lamp"
encoded_input = tokenizer(text, return_tensors='tf')
output = discriminator(encoded_input)
prediction = tf.nn.softmax(output["logits"][0], -1)
label = id2label[tf.math.argmax(prediction).numpy()]
print("\n", text, ":", label,
"\n") # ideally 'take.v.04' (-> "get into one's hands, take physically"), but probably only with a better finetuning dataset
text = "w"
encoded_input = tokenizer(text, return_tensors='tf')
output = discriminator(encoded_input)
prediction = tf.nn.softmax(output["logits"][0], -1)
label = id2label[tf.math.argmax(prediction).numpy()]
print("\n", text, ":", label,
"\n") # ideally 'travel.v.01' (-> "change location; move, travel, or proceed, also metaphorically"), but probably only with a better finetuning dataset
```
|
cammy/bart-large-cnn-100-lit-evalMA-NOpad | 73fa9143a0ac509e8b0a5ca8b45481b7b57033bd | 2022-03-13T09:34:29.000Z | [
"pytorch",
"bart",
"text2text-generation",
"transformers",
"generated_from_trainer",
"license:mit",
"model-index",
"autotrain_compatible"
] | text2text-generation | false | cammy | null | cammy/bart-large-cnn-100-lit-evalMA-NOpad | 2 | null | transformers | 25,150 | ---
license: mit
tags:
- generated_from_trainer
metrics:
- rouge
model-index:
- name: bart-large-cnn-100-lit-evalMA-NOpad
results: []
---
<!-- This model card has been generated automatically according to the information the Trainer had access to. You
should probably proofread and complete it, then remove this comment. -->
# bart-large-cnn-100-lit-evalMA-NOpad
This model is a fine-tuned version of [facebook/bart-large-cnn](https://huggingface.co/facebook/bart-large-cnn) on an unknown dataset.
It achieves the following results on the evaluation set:
- Loss: 2.1514
- Rouge1: 27.5985
- Rouge2: 11.3869
- Rougel: 20.9359
- Rougelsum: 24.7113
- Gen Len: 62.5
## Model description
More information needed
## Intended uses & limitations
More information needed
## Training and evaluation data
More information needed
## Training procedure
### Training hyperparameters
The following hyperparameters were used during training:
- learning_rate: 2e-05
- train_batch_size: 1
- eval_batch_size: 1
- seed: 42
- optimizer: Adam with betas=(0.9,0.999) and epsilon=1e-08
- lr_scheduler_type: linear
- num_epochs: 5
- mixed_precision_training: Native AMP
### Training results
| Training Loss | Epoch | Step | Validation Loss | Rouge1 | Rouge2 | Rougel | Rougelsum | Gen Len |
|:-------------:|:-----:|:----:|:---------------:|:-------:|:-------:|:-------:|:---------:|:-------:|
| No log | 1.0 | 100 | 1.7982 | 28.7996 | 11.2592 | 19.7524 | 25.2125 | 62.5 |
| No log | 2.0 | 200 | 2.1514 | 27.5985 | 11.3869 | 20.9359 | 24.7113 | 62.5 |
### Framework versions
- Transformers 4.16.2
- Pytorch 1.10.2
- Datasets 1.18.3
- Tokenizers 0.11.0
|
cammy/bart-large-cnn-weaksup-100-NOpad-early1 | f5c5427bdf5464fc87ac954ed07b63537434de6c | 2022-03-13T09:39:22.000Z | [
"pytorch",
"bart",
"text2text-generation",
"transformers",
"generated_from_trainer",
"license:mit",
"model-index",
"autotrain_compatible"
] | text2text-generation | false | cammy | null | cammy/bart-large-cnn-weaksup-100-NOpad-early1 | 2 | null | transformers | 25,151 | ---
license: mit
tags:
- generated_from_trainer
metrics:
- rouge
model-index:
- name: bart-large-cnn-weaksup-100-NOpad-early1
results: []
---
<!-- This model card has been generated automatically according to the information the Trainer had access to. You
should probably proofread and complete it, then remove this comment. -->
# bart-large-cnn-weaksup-100-NOpad-early1
This model is a fine-tuned version of [facebook/bart-large-cnn](https://huggingface.co/facebook/bart-large-cnn) on an unknown dataset.
It achieves the following results on the evaluation set:
- Loss: 2.0768
- Rouge1: 28.7953
- Rouge2: 10.9535
- Rougel: 20.6447
- Rougelsum: 24.3516
- Gen Len: 68.5
## Model description
More information needed
## Intended uses & limitations
More information needed
## Training and evaluation data
More information needed
## Training procedure
### Training hyperparameters
The following hyperparameters were used during training:
- learning_rate: 2e-05
- train_batch_size: 1
- eval_batch_size: 1
- seed: 42
- optimizer: Adam with betas=(0.9,0.999) and epsilon=1e-08
- lr_scheduler_type: linear
- num_epochs: 3
- mixed_precision_training: Native AMP
### Training results
| Training Loss | Epoch | Step | Validation Loss | Rouge1 | Rouge2 | Rougel | Rougelsum | Gen Len |
|:-------------:|:-----:|:----:|:---------------:|:-------:|:-------:|:-------:|:---------:|:-------:|
| No log | 1.0 | 100 | 1.8905 | 31.2906 | 13.5675 | 21.5533 | 27.2536 | 64.2 |
| No log | 2.0 | 200 | 2.0768 | 28.7953 | 10.9535 | 20.6447 | 24.3516 | 68.5 |
### Framework versions
- Transformers 4.16.2
- Pytorch 1.10.2
- Datasets 1.18.3
- Tokenizers 0.11.0
|
cammy/bart-large-cnn-weaksup-100-NOpad-early2 | 767770dfb087a8f151207810a9bbe287e3673c02 | 2022-03-13T09:45:38.000Z | [
"pytorch",
"bart",
"text2text-generation",
"transformers",
"generated_from_trainer",
"license:mit",
"model-index",
"autotrain_compatible"
] | text2text-generation | false | cammy | null | cammy/bart-large-cnn-weaksup-100-NOpad-early2 | 2 | null | transformers | 25,152 | ---
license: mit
tags:
- generated_from_trainer
metrics:
- rouge
model-index:
- name: bart-large-cnn-weaksup-100-NOpad-early2
results: []
---
<!-- This model card has been generated automatically according to the information the Trainer had access to. You
should probably proofread and complete it, then remove this comment. -->
# bart-large-cnn-weaksup-100-NOpad-early2
This model is a fine-tuned version of [facebook/bart-large-cnn](https://huggingface.co/facebook/bart-large-cnn) on an unknown dataset.
It achieves the following results on the evaluation set:
- Loss: 2.0768
- Rouge1: 28.6914
- Rouge2: 11.1481
- Rougel: 20.6967
- Rougelsum: 24.2834
- Gen Len: 68.5
## Model description
More information needed
## Intended uses & limitations
More information needed
## Training and evaluation data
More information needed
## Training procedure
### Training hyperparameters
The following hyperparameters were used during training:
- learning_rate: 2e-05
- train_batch_size: 1
- eval_batch_size: 1
- seed: 42
- optimizer: Adam with betas=(0.9,0.999) and epsilon=1e-08
- lr_scheduler_type: linear
- num_epochs: 3
- mixed_precision_training: Native AMP
### Training results
| Training Loss | Epoch | Step | Validation Loss | Rouge1 | Rouge2 | Rougel | Rougelsum | Gen Len |
|:-------------:|:-----:|:----:|:---------------:|:-------:|:-------:|:-------:|:---------:|:-------:|
| No log | 1.0 | 100 | 1.8905 | 31.4929 | 13.8614 | 21.6279 | 27.1315 | 64.2 |
| No log | 2.0 | 200 | 2.0768 | 28.6914 | 11.1481 | 20.6967 | 24.2834 | 68.5 |
### Framework versions
- Transformers 4.16.2
- Pytorch 1.10.2
- Datasets 1.18.3
- Tokenizers 0.11.0
|
cammy/bart-large-cnn-100-lit-evalMA-pad | b1c238d60940abb045ba27013ac8ecaf54b09875 | 2022-03-13T10:02:01.000Z | [
"pytorch",
"bart",
"text2text-generation",
"transformers",
"autotrain_compatible"
] | text2text-generation | false | cammy | null | cammy/bart-large-cnn-100-lit-evalMA-pad | 2 | null | transformers | 25,153 | Entry not found |
cammy/bart-large-cnn-10k-lit-evalMA-NOpad | 1f0d8888109ad85b31963ab8a4733d38b9f3fde4 | 2022-03-13T18:11:42.000Z | [
"pytorch",
"bart",
"text2text-generation",
"transformers",
"generated_from_trainer",
"license:mit",
"model-index",
"autotrain_compatible"
] | text2text-generation | false | cammy | null | cammy/bart-large-cnn-10k-lit-evalMA-NOpad | 2 | null | transformers | 25,154 | ---
license: mit
tags:
- generated_from_trainer
metrics:
- rouge
model-index:
- name: bart-large-cnn-10k-lit-evalMA-NOpad
results: []
---
<!-- This model card has been generated automatically according to the information the Trainer had access to. You
should probably proofread and complete it, then remove this comment. -->
# bart-large-cnn-10k-lit-evalMA-NOpad
This model is a fine-tuned version of [facebook/bart-large-cnn](https://huggingface.co/facebook/bart-large-cnn) on an unknown dataset.
It achieves the following results on the evaluation set:
- Loss: 1.9464
- Rouge1: 28.6721
- Rouge2: 13.8303
- Rougel: 22.458
- Rougelsum: 25.668
- Gen Len: 66.893
## Model description
More information needed
## Intended uses & limitations
More information needed
## Training and evaluation data
More information needed
## Training procedure
### Training hyperparameters
The following hyperparameters were used during training:
- learning_rate: 2e-05
- train_batch_size: 1
- eval_batch_size: 1
- seed: 42
- optimizer: Adam with betas=(0.9,0.999) and epsilon=1e-08
- lr_scheduler_type: linear
- num_epochs: 5
- mixed_precision_training: Native AMP
### Training results
| Training Loss | Epoch | Step | Validation Loss | Rouge1 | Rouge2 | Rougel | Rougelsum | Gen Len |
|:-------------:|:-----:|:-----:|:---------------:|:-------:|:-------:|:-------:|:---------:|:-------:|
| 1.535 | 1.0 | 10000 | 1.7501 | 28.519 | 13.967 | 22.4854 | 25.4511 | 66.555 |
| 0.8754 | 2.0 | 20000 | 1.9464 | 28.6721 | 13.8303 | 22.458 | 25.668 | 66.893 |
### Framework versions
- Transformers 4.16.2
- Pytorch 1.10.2
- Datasets 1.18.3
- Tokenizers 0.11.0
|
Taekyoon/komrc_train | 740cb58bf49410796f72cef3deba96e68e16d2ff | 2022-03-13T15:11:14.000Z | [
"pytorch",
"tensorboard",
"bert",
"question-answering",
"dataset:korquad",
"transformers",
"generated_from_trainer",
"model-index",
"autotrain_compatible"
] | question-answering | false | Taekyoon | null | Taekyoon/komrc_train | 2 | null | transformers | 25,155 | ---
tags:
- generated_from_trainer
datasets:
- korquad
model-index:
- name: komrc_train
results: []
---
<!-- This model card has been generated automatically according to the information the Trainer had access to. You
should probably proofread and complete it, then remove this comment. -->
# komrc_train
This model is a fine-tuned version of [beomi/kcbert-base](https://huggingface.co/beomi/kcbert-base) on the korquad dataset.
It achieves the following results on the evaluation set:
- Loss: 0.6544
## Model description
More information needed
## Intended uses & limitations
More information needed
## Training and evaluation data
More information needed
## Training procedure
### Training hyperparameters
The following hyperparameters were used during training:
- learning_rate: 2e-05
- train_batch_size: 16
- eval_batch_size: 16
- seed: 1234
- optimizer: Adam with betas=(0.9,0.999) and epsilon=1e-08
- lr_scheduler_type: linear
- num_epochs: 3
- mixed_precision_training: Native AMP
### Training results
| Training Loss | Epoch | Step | Validation Loss |
|:-------------:|:-----:|:-----:|:---------------:|
| 0.8187 | 0.31 | 2000 | 0.7377 |
| 0.6947 | 0.63 | 4000 | 0.6934 |
| 0.6352 | 0.94 | 6000 | 0.6544 |
| 0.3869 | 1.25 | 8000 | 0.7633 |
| 0.3812 | 1.56 | 10000 | 0.7047 |
| 0.3579 | 1.88 | 12000 | 0.7097 |
| 0.2053 | 2.19 | 14000 | 0.8511 |
| 0.2173 | 2.5 | 16000 | 0.8457 |
| 0.2094 | 2.82 | 18000 | 0.8433 |
### Framework versions
- Transformers 4.13.0
- Pytorch 1.10.0+cu111
- Datasets 1.18.4
- Tokenizers 0.10.3
|
sanchit-gandhi/wav2vec2-2-roberta-long-run | 4422b2394b173f5aedb613ce880dd1ce6b39eeec | 2022-03-14T10:41:46.000Z | [
"pytorch",
"tensorboard",
"speech-encoder-decoder",
"automatic-speech-recognition",
"transformers"
] | automatic-speech-recognition | false | sanchit-gandhi | null | sanchit-gandhi/wav2vec2-2-roberta-long-run | 2 | null | transformers | 25,156 | Entry not found |
MrAnderson/nystrom-1024-full-trivia-copied-embeddings | ab80c28ca4348b875bbcc5fda5051f0922f75425 | 2022-03-14T13:01:18.000Z | [
"pytorch",
"nystromformer",
"question-answering",
"transformers",
"autotrain_compatible"
] | question-answering | false | MrAnderson | null | MrAnderson/nystrom-1024-full-trivia-copied-embeddings | 2 | null | transformers | 25,157 | Entry not found |
prakod/en-hi-pos-tagger-symcom | 1e13630f8cff03fd4961f22f4891a04e0d2d10ab | 2022-03-14T08:24:56.000Z | [
"pytorch",
"xlm-roberta",
"token-classification",
"transformers",
"license:afl-3.0",
"autotrain_compatible"
] | token-classification | false | prakod | null | prakod/en-hi-pos-tagger-symcom | 2 | null | transformers | 25,158 | ---
license: afl-3.0
---
|
holtin/distilbert-base-uncased-holtin-finetuned-squad | 1f156f2601a642694385dd6577a724817fa3dbf7 | 2022-03-14T08:09:33.000Z | [
"pytorch",
"tensorboard",
"distilbert",
"question-answering",
"dataset:squad_v2",
"transformers",
"generated_from_trainer",
"license:apache-2.0",
"model-index",
"autotrain_compatible"
] | question-answering | false | holtin | null | holtin/distilbert-base-uncased-holtin-finetuned-squad | 2 | null | transformers | 25,159 | ---
license: apache-2.0
tags:
- generated_from_trainer
datasets:
- squad_v2
model-index:
- name: distilbert-base-uncased-holtin-finetuned-squad
results: []
---
<!-- This model card has been generated automatically according to the information the Trainer had access to. You
should probably proofread and complete it, then remove this comment. -->
# distilbert-base-uncased-holtin-finetuned-squad
This model is a fine-tuned version of [distilbert-base-uncased](https://huggingface.co/distilbert-base-uncased) on the squad_v2 dataset.
It achieves the following results on the evaluation set:
- Loss: 3.8541
## Model description
More information needed
## Intended uses & limitations
More information needed
## Training and evaluation data
More information needed
## Training procedure
### Training hyperparameters
The following hyperparameters were used during training:
- learning_rate: 2e-05
- train_batch_size: 16
- eval_batch_size: 16
- seed: 42
- optimizer: Adam with betas=(0.9,0.999) and epsilon=1e-08
- lr_scheduler_type: linear
- num_epochs: 3
### Training results
| Training Loss | Epoch | Step | Validation Loss |
|:-------------:|:-----:|:----:|:---------------:|
| No log | 1.0 | 84 | 4.4978 |
| No log | 2.0 | 168 | 3.9588 |
| No log | 3.0 | 252 | 3.8541 |
### Framework versions
- Transformers 4.17.0
- Pytorch 1.10.0+cu111
- Datasets 1.18.4
- Tokenizers 0.11.6
|
mrm8488/electricidad-small-finetuned-review-classification | 819b54455106662ba743afcc9de901c1194e726b | 2022-03-14T12:35:12.000Z | [
"pytorch",
"tensorboard",
"electra",
"text-classification",
"transformers"
] | text-classification | false | mrm8488 | null | mrm8488/electricidad-small-finetuned-review-classification | 2 | null | transformers | 25,160 | Entry not found |
GPL/fever-distilbert-tas-b-gpl-self_miner | b18488a373430f02a1f667e13418ee2c58a21b81 | 2022-03-14T14:23:38.000Z | [
"pytorch",
"distilbert",
"feature-extraction",
"sentence-transformers",
"sentence-similarity",
"transformers"
] | sentence-similarity | false | GPL | null | GPL/fever-distilbert-tas-b-gpl-self_miner | 2 | null | sentence-transformers | 25,161 | ---
pipeline_tag: sentence-similarity
tags:
- sentence-transformers
- feature-extraction
- sentence-similarity
- transformers
---
# {MODEL_NAME}
This is a [sentence-transformers](https://www.SBERT.net) model: It maps sentences & paragraphs to a 768 dimensional dense vector space and can be used for tasks like clustering or semantic search.
<!--- Describe your model here -->
## Usage (Sentence-Transformers)
Using this model becomes easy when you have [sentence-transformers](https://www.SBERT.net) installed:
```
pip install -U sentence-transformers
```
Then you can use the model like this:
```python
from sentence_transformers import SentenceTransformer
sentences = ["This is an example sentence", "Each sentence is converted"]
model = SentenceTransformer('{MODEL_NAME}')
embeddings = model.encode(sentences)
print(embeddings)
```
## Usage (HuggingFace Transformers)
Without [sentence-transformers](https://www.SBERT.net), you can use the model like this: First, you pass your input through the transformer model, then you have to apply the right pooling-operation on-top of the contextualized word embeddings.
```python
from transformers import AutoTokenizer, AutoModel
import torch
def cls_pooling(model_output, attention_mask):
return model_output[0][:,0]
# Sentences we want sentence embeddings for
sentences = ['This is an example sentence', 'Each sentence is converted']
# Load model from HuggingFace Hub
tokenizer = AutoTokenizer.from_pretrained('{MODEL_NAME}')
model = AutoModel.from_pretrained('{MODEL_NAME}')
# Tokenize sentences
encoded_input = tokenizer(sentences, padding=True, truncation=True, return_tensors='pt')
# Compute token embeddings
with torch.no_grad():
model_output = model(**encoded_input)
# Perform pooling. In this case, cls pooling.
sentence_embeddings = cls_pooling(model_output, encoded_input['attention_mask'])
print("Sentence embeddings:")
print(sentence_embeddings)
```
## Evaluation Results
<!--- Describe how your model was evaluated -->
For an automated evaluation of this model, see the *Sentence Embeddings Benchmark*: [https://seb.sbert.net](https://seb.sbert.net?model_name={MODEL_NAME})
## Training
The model was trained with the parameters:
**DataLoader**:
`torch.utils.data.dataloader.DataLoader` of length 140000 with parameters:
```
{'batch_size': 32, 'sampler': 'torch.utils.data.sampler.SequentialSampler', 'batch_sampler': 'torch.utils.data.sampler.BatchSampler'}
```
**Loss**:
`gpl.toolkit.loss.MarginDistillationLoss`
Parameters of the fit()-Method:
```
{
"epochs": 1,
"evaluation_steps": 0,
"evaluator": "NoneType",
"max_grad_norm": 1,
"optimizer_class": "<class 'transformers.optimization.AdamW'>",
"optimizer_params": {
"lr": 2e-05
},
"scheduler": "WarmupLinear",
"steps_per_epoch": 140000,
"warmup_steps": 1000,
"weight_decay": 0.01
}
```
## Full Model Architecture
```
SentenceTransformer(
(0): Transformer({'max_seq_length': 350, 'do_lower_case': False}) with Transformer model: DistilBertModel
(1): Pooling({'word_embedding_dimension': 768, 'pooling_mode_cls_token': True, 'pooling_mode_mean_tokens': False, 'pooling_mode_max_tokens': False, 'pooling_mode_mean_sqrt_len_tokens': False})
)
```
## Citing & Authors
<!--- Describe where people can find more information --> |
GPL/signal1m-distilbert-tas-b-gpl-self_miner | 541aa5c47e9a8f5b8247bdefcb658c7f861b89b0 | 2022-03-14T14:25:02.000Z | [
"pytorch",
"distilbert",
"feature-extraction",
"sentence-transformers",
"sentence-similarity",
"transformers"
] | sentence-similarity | false | GPL | null | GPL/signal1m-distilbert-tas-b-gpl-self_miner | 2 | null | sentence-transformers | 25,162 | ---
pipeline_tag: sentence-similarity
tags:
- sentence-transformers
- feature-extraction
- sentence-similarity
- transformers
---
# {MODEL_NAME}
This is a [sentence-transformers](https://www.SBERT.net) model: It maps sentences & paragraphs to a 768 dimensional dense vector space and can be used for tasks like clustering or semantic search.
<!--- Describe your model here -->
## Usage (Sentence-Transformers)
Using this model becomes easy when you have [sentence-transformers](https://www.SBERT.net) installed:
```
pip install -U sentence-transformers
```
Then you can use the model like this:
```python
from sentence_transformers import SentenceTransformer
sentences = ["This is an example sentence", "Each sentence is converted"]
model = SentenceTransformer('{MODEL_NAME}')
embeddings = model.encode(sentences)
print(embeddings)
```
## Usage (HuggingFace Transformers)
Without [sentence-transformers](https://www.SBERT.net), you can use the model like this: First, you pass your input through the transformer model, then you have to apply the right pooling-operation on-top of the contextualized word embeddings.
```python
from transformers import AutoTokenizer, AutoModel
import torch
def cls_pooling(model_output, attention_mask):
return model_output[0][:,0]
# Sentences we want sentence embeddings for
sentences = ['This is an example sentence', 'Each sentence is converted']
# Load model from HuggingFace Hub
tokenizer = AutoTokenizer.from_pretrained('{MODEL_NAME}')
model = AutoModel.from_pretrained('{MODEL_NAME}')
# Tokenize sentences
encoded_input = tokenizer(sentences, padding=True, truncation=True, return_tensors='pt')
# Compute token embeddings
with torch.no_grad():
model_output = model(**encoded_input)
# Perform pooling. In this case, cls pooling.
sentence_embeddings = cls_pooling(model_output, encoded_input['attention_mask'])
print("Sentence embeddings:")
print(sentence_embeddings)
```
## Evaluation Results
<!--- Describe how your model was evaluated -->
For an automated evaluation of this model, see the *Sentence Embeddings Benchmark*: [https://seb.sbert.net](https://seb.sbert.net?model_name={MODEL_NAME})
## Training
The model was trained with the parameters:
**DataLoader**:
`torch.utils.data.dataloader.DataLoader` of length 140000 with parameters:
```
{'batch_size': 32, 'sampler': 'torch.utils.data.sampler.SequentialSampler', 'batch_sampler': 'torch.utils.data.sampler.BatchSampler'}
```
**Loss**:
`gpl.toolkit.loss.MarginDistillationLoss`
Parameters of the fit()-Method:
```
{
"epochs": 1,
"evaluation_steps": 0,
"evaluator": "NoneType",
"max_grad_norm": 1,
"optimizer_class": "<class 'transformers.optimization.AdamW'>",
"optimizer_params": {
"lr": 2e-05
},
"scheduler": "WarmupLinear",
"steps_per_epoch": 140000,
"warmup_steps": 1000,
"weight_decay": 0.01
}
```
## Full Model Architecture
```
SentenceTransformer(
(0): Transformer({'max_seq_length': 350, 'do_lower_case': False}) with Transformer model: DistilBertModel
(1): Pooling({'word_embedding_dimension': 768, 'pooling_mode_cls_token': True, 'pooling_mode_mean_tokens': False, 'pooling_mode_max_tokens': False, 'pooling_mode_mean_sqrt_len_tokens': False})
)
```
## Citing & Authors
<!--- Describe where people can find more information --> |
evs/xlm-roberta-base-finetuned-panx-de | 0e7f6264d8f9f1af245652859c51dc53250da86d | 2022-03-15T12:02:07.000Z | [
"pytorch",
"tensorboard",
"xlm-roberta",
"token-classification",
"dataset:xtreme",
"transformers",
"generated_from_trainer",
"license:mit",
"model-index",
"autotrain_compatible"
] | token-classification | false | evs | null | evs/xlm-roberta-base-finetuned-panx-de | 2 | null | transformers | 25,163 | ---
license: mit
tags:
- generated_from_trainer
datasets:
- xtreme
metrics:
- f1
model-index:
- name: xlm-roberta-base-finetuned-panx-de
results:
- task:
name: Token Classification
type: token-classification
dataset:
name: xtreme
type: xtreme
args: PAN-X.de
metrics:
- name: F1
type: f1
value: 0.8591260810195721
---
<!-- This model card has been generated automatically according to the information the Trainer had access to. You
should probably proofread and complete it, then remove this comment. -->
# xlm-roberta-base-finetuned-panx-de
This model is a fine-tuned version of [xlm-roberta-base](https://huggingface.co/xlm-roberta-base) on the xtreme dataset.
It achieves the following results on the evaluation set:
- Loss: 0.1352
- F1: 0.8591
## Model description
More information needed
## Intended uses & limitations
More information needed
## Training and evaluation data
More information needed
## Training procedure
### Training hyperparameters
The following hyperparameters were used during training:
- learning_rate: 5e-05
- train_batch_size: 24
- eval_batch_size: 24
- seed: 42
- optimizer: Adam with betas=(0.9,0.999) and epsilon=1e-08
- lr_scheduler_type: linear
- num_epochs: 3
### Training results
| Training Loss | Epoch | Step | Validation Loss | F1 |
|:-------------:|:-----:|:----:|:---------------:|:------:|
| 0.257 | 1.0 | 525 | 0.1512 | 0.8302 |
| 0.1305 | 2.0 | 1050 | 0.1401 | 0.8447 |
| 0.0817 | 3.0 | 1575 | 0.1352 | 0.8591 |
### Framework versions
- Transformers 4.11.3
- Pytorch 1.10.0+cu111
- Datasets 1.16.1
- Tokenizers 0.10.3
|
gossminn/detect-femicide-news-xlmr | 964e5324b025f298b14172faf9b7acf4aa2f67a6 | 2022-03-14T16:43:08.000Z | [
"pytorch",
"tensorboard",
"xlm-roberta",
"text-classification",
"transformers",
"generated_from_trainer",
"license:mit",
"model-index"
] | text-classification | false | gossminn | null | gossminn/detect-femicide-news-xlmr | 2 | null | transformers | 25,164 | ---
license: mit
tags:
- generated_from_trainer
metrics:
- accuracy
model-index:
- name: detect-femicide-news-xlmr
results: []
---
<!-- This model card has been generated automatically according to the information the Trainer had access to. You
should probably proofread and complete it, then remove this comment. -->
# detect-femicide-news-xlmr
This model is a fine-tuned version of [xlm-roberta-base](https://huggingface.co/xlm-roberta-base) on an unknown dataset.
It achieves the following results on the evaluation set:
- Loss: 0.0161
- Accuracy: 0.9973
- Precision Neg: 0.9975
- Precision Pos: 0.9967
- Recall Neg: 0.9988
- Recall Pos: 0.9933
- F1 Score Neg: 0.9981
- F1 Score Pos: 0.9950
## Model description
More information needed
## Intended uses & limitations
More information needed
## Training and evaluation data
More information needed
## Training procedure
### Training hyperparameters
The following hyperparameters were used during training:
- learning_rate: 1e-05
- train_batch_size: 128
- eval_batch_size: 8
- seed: 1996
- optimizer: Adam with betas=(0.9,0.999) and epsilon=1e-08
- lr_scheduler_type: linear
- num_epochs: 6
### Training results
| Training Loss | Epoch | Step | Validation Loss | Accuracy | Precision Neg | Precision Pos | Recall Neg | Recall Pos | F1 Score Neg | F1 Score Pos |
|:-------------:|:-----:|:----:|:---------------:|:--------:|:-------------:|:-------------:|:----------:|:----------:|:------------:|:------------:|
| 0.2758 | 1.0 | 204 | 0.1001 | 0.9718 | 0.9741 | 0.9654 | 0.9875 | 0.93 | 0.9808 | 0.9474 |
| 0.0782 | 2.0 | 408 | 0.0505 | 0.9809 | 0.9839 | 0.9729 | 0.99 | 0.9567 | 0.9869 | 0.9647 |
| 0.0501 | 3.0 | 612 | 0.0272 | 0.9927 | 0.9962 | 0.9834 | 0.9938 | 0.99 | 0.9950 | 0.9867 |
| 0.0389 | 4.0 | 816 | 0.0201 | 0.9945 | 0.9938 | 0.9966 | 0.9988 | 0.9833 | 0.9963 | 0.9899 |
| 0.031 | 5.0 | 1020 | 0.0175 | 0.9964 | 0.9963 | 0.9966 | 0.9988 | 0.99 | 0.9975 | 0.9933 |
| 0.0235 | 6.0 | 1224 | 0.0161 | 0.9973 | 0.9975 | 0.9967 | 0.9988 | 0.9933 | 0.9981 | 0.9950 |
### Framework versions
- Transformers 4.16.2
- Pytorch 1.10.2+cu113
- Datasets 1.18.3
- Tokenizers 0.11.0
|
mmohamme/distilbert-base-uncased-finetuned-btc | 1a751bb86ef9347a95bc8fc51c1eee3918f736fc | 2022-03-16T02:21:42.000Z | [
"pytorch",
"tensorboard",
"distilbert",
"text-classification",
"transformers"
] | text-classification | false | mmohamme | null | mmohamme/distilbert-base-uncased-finetuned-btc | 2 | null | transformers | 25,165 | ### distilbert-base-uncased-finetuned-btc for PH66 Unwanted Event
- This is our initial attempt on using the transformers for BTC-PH66.
- This test file used in this model are in projects with Ids [1065, 950, 956, 2650]. The other 4 projects were not included as they resulted very low accuracy with ML models.
- The data was preprocessed to remove duplicates, and cases where the same cause-conseq has a different Unwanted Event
- Next Model: Improve the hyper-parameters of the model |
mindlogic/mindlogic-electra-ko-ai-citizen-classifier-base | 81391f80a54ec8052c85cf40ec7babf92e7e40a4 | 2022-03-22T03:25:46.000Z | [
"pytorch",
"electra",
"text-classification",
"transformers"
] | text-classification | false | mindlogic | null | mindlogic/mindlogic-electra-ko-ai-citizen-classifier-base | 2 | 1 | transformers | 25,166 | Entry not found |
moralstories/roberta-large_action | ed49f14463512a7148b45001012fae606d701113 | 2022-03-15T06:03:05.000Z | [
"pytorch",
"roberta",
"text-classification",
"transformers"
] | text-classification | false | moralstories | null | moralstories/roberta-large_action | 2 | null | transformers | 25,167 | Entry not found |
hazal/BioBERTurkcased-con-trM | d8085ab9d0ae0630f06c45801eb7d6261799cbc6 | 2022-03-21T07:57:30.000Z | [
"pytorch",
"transformers"
] | null | false | hazal | null | hazal/BioBERTurkcased-con-trM | 2 | null | transformers | 25,168 | # BioBERTurk- Turkish Biomedical Language Models
|
janck/DistilBERT-finetuned-wiki20m | b46c4e775230a4b59010036c490a2a4060ed5722 | 2022-03-17T07:13:33.000Z | [
"pytorch",
"distilbert",
"text-classification",
"transformers"
] | text-classification | false | janck | null | janck/DistilBERT-finetuned-wiki20m | 2 | 1 | transformers | 25,169 | Entry not found |
hazal/BioBERTurkcased-con-trM-trR | e79beac1d310f34ed3d656f7a96467d05dee314a | 2022-03-15T09:07:00.000Z | [
"pytorch",
"transformers"
] | null | false | hazal | null | hazal/BioBERTurkcased-con-trM-trR | 2 | null | transformers | 25,170 | Entry not found |
RobertoMCA97/xlm-roberta-base-finetuned-panx-de | 6bf5889cf20f2852d140eded42b147f0109daaf5 | 2022-03-16T11:55:06.000Z | [
"pytorch",
"tensorboard",
"xlm-roberta",
"token-classification",
"dataset:xtreme",
"transformers",
"generated_from_trainer",
"license:mit",
"model-index",
"autotrain_compatible"
] | token-classification | false | RobertoMCA97 | null | RobertoMCA97/xlm-roberta-base-finetuned-panx-de | 2 | null | transformers | 25,171 | ---
license: mit
tags:
- generated_from_trainer
datasets:
- xtreme
metrics:
- f1
model-index:
- name: xlm-roberta-base-finetuned-panx-de
results:
- task:
name: Token Classification
type: token-classification
dataset:
name: xtreme
type: xtreme
args: PAN-X.de
metrics:
- name: F1
type: f1
value: 0.8590909090909091
---
<!-- This model card has been generated automatically according to the information the Trainer had access to. You
should probably proofread and complete it, then remove this comment. -->
# xlm-roberta-base-finetuned-panx-de
This model is a fine-tuned version of [xlm-roberta-base](https://huggingface.co/xlm-roberta-base) on the xtreme dataset.
It achieves the following results on the evaluation set:
- Loss: 0.1380
- F1: 0.8591
## Model description
More information needed
## Intended uses & limitations
More information needed
## Training and evaluation data
More information needed
## Training procedure
### Training hyperparameters
The following hyperparameters were used during training:
- learning_rate: 5e-05
- train_batch_size: 24
- eval_batch_size: 24
- seed: 42
- optimizer: Adam with betas=(0.9,0.999) and epsilon=1e-08
- lr_scheduler_type: linear
- num_epochs: 3
### Training results
| Training Loss | Epoch | Step | Validation Loss | F1 |
|:-------------:|:-----:|:----:|:---------------:|:------:|
| 0.2642 | 1.0 | 525 | 0.1624 | 0.8251 |
| 0.1315 | 2.0 | 1050 | 0.1445 | 0.8508 |
| 0.0832 | 3.0 | 1575 | 0.1380 | 0.8591 |
### Framework versions
- Transformers 4.17.0
- Pytorch 1.10.0+cu111
- Datasets 2.0.0
- Tokenizers 0.11.6
|
DrishtiSharma/poem-gen-t5-small | ca13a264955be248d83652ba8f04dc09527445a6 | 2022-03-15T18:50:42.000Z | [
"pytorch",
"tensorboard",
"t5",
"text2text-generation",
"transformers",
"generated_from_trainer",
"license:apache-2.0",
"model-index",
"autotrain_compatible"
] | text2text-generation | false | DrishtiSharma | null | DrishtiSharma/poem-gen-t5-small | 2 | null | transformers | 25,172 | ---
license: apache-2.0
tags:
- generated_from_trainer
model-index:
- name: poem-gen-t5-small
results: []
---
<!-- This model card has been generated automatically according to the information the Trainer had access to. You
should probably proofread and complete it, then remove this comment. -->
# poem-gen-t5-small
This model is a fine-tuned version of [t5-small](https://huggingface.co/t5-small) on the None dataset.
It achieves the following results on the evaluation set:
- Loss: 3.1066
## Model description
More information needed
## Intended uses & limitations
More information needed
## Training and evaluation data
More information needed
## Training procedure
### Training hyperparameters
The following hyperparameters were used during training:
- learning_rate: 2e-05
- train_batch_size: 4
- eval_batch_size: 4
- seed: 42
- optimizer: Adam with betas=(0.9,0.999) and epsilon=1e-08
- lr_scheduler_type: linear
- num_epochs: 5
### Training results
| Training Loss | Epoch | Step | Validation Loss |
|:-------------:|:-----:|:-----:|:---------------:|
| 3.67 | 0.32 | 5000 | 3.4705 |
| 3.573 | 0.63 | 10000 | 3.3747 |
| 3.5075 | 0.95 | 15000 | 3.3154 |
| 3.4486 | 1.26 | 20000 | 3.2704 |
| 3.4207 | 1.58 | 25000 | 3.2351 |
| 3.3933 | 1.89 | 30000 | 3.2069 |
| 3.3612 | 2.21 | 35000 | 3.1853 |
| 3.34 | 2.53 | 40000 | 3.1659 |
| 3.3422 | 2.84 | 45000 | 3.1503 |
| 3.3034 | 3.16 | 50000 | 3.1376 |
| 3.2886 | 3.47 | 55000 | 3.1283 |
| 3.2806 | 3.79 | 60000 | 3.1208 |
| 3.2745 | 4.1 | 65000 | 3.1141 |
| 3.2894 | 4.42 | 70000 | 3.1093 |
| 3.264 | 4.74 | 75000 | 3.1075 |
### Framework versions
- Transformers 4.17.0
- Pytorch 1.10.0+cu111
- Datasets 1.18.4
- Tokenizers 0.11.6
|
Rustem/distilroberta-base-trained | c12ff18b7c81eca06a80b550225d9fffdbca85ff | 2022-03-15T18:12:23.000Z | [
"pytorch",
"roberta",
"fill-mask",
"transformers",
"license:afl-3.0",
"autotrain_compatible"
] | fill-mask | false | Rustem | null | Rustem/distilroberta-base-trained | 2 | null | transformers | 25,173 | ---
license: afl-3.0
---
|
Rustem/distilroberta-base-trainedmodel | 4268e8c9e600f8c786cba42d3f6792b6ef479513 | 2022-03-15T19:32:36.000Z | [
"pytorch",
"roberta",
"fill-mask",
"transformers",
"license:apache-2.0",
"autotrain_compatible"
] | fill-mask | false | Rustem | null | Rustem/distilroberta-base-trainedmodel | 2 | null | transformers | 25,174 | ---
license: apache-2.0
---
|
facebook/regnet-x-002 | 2b69d3ab4a835f17f32fbdebfd63659fc46dc852 | 2022-06-28T17:54:23.000Z | [
"pytorch",
"tf",
"regnet",
"image-classification",
"dataset:imagenet-1k",
"arxiv:2003.13678",
"transformers",
"vision",
"license:apache-2.0"
] | image-classification | false | facebook | null | facebook/regnet-x-002 | 2 | 1 | transformers | 25,175 | ---
license: apache-2.0
tags:
- vision
- image-classification
datasets:
- imagenet-1k
widget:
- src: https://huggingface.co/datasets/mishig/sample_images/resolve/main/tiger.jpg
example_title: Tiger
- src: https://huggingface.co/datasets/mishig/sample_images/resolve/main/teapot.jpg
example_title: Teapot
- src: https://huggingface.co/datasets/mishig/sample_images/resolve/main/palace.jpg
example_title: Palace
---
# RegNet
RegNet model trained on imagenet-1k. It was introduced in the paper [Designing Network Design Spaces](https://arxiv.org/abs/2003.13678) and first released in [this repository](https://github.com/facebookresearch/pycls).
Disclaimer: The team releasing RegNet did not write a model card for this model so this model card has been written by the Hugging Face team.
## Model description
The authors design search spaces to perform Neural Architecture Search (NAS). They first start from a high dimensional search space and iteratively reduce the search space by empirically applying constraints based on the best-performing models sampled by the current search space.
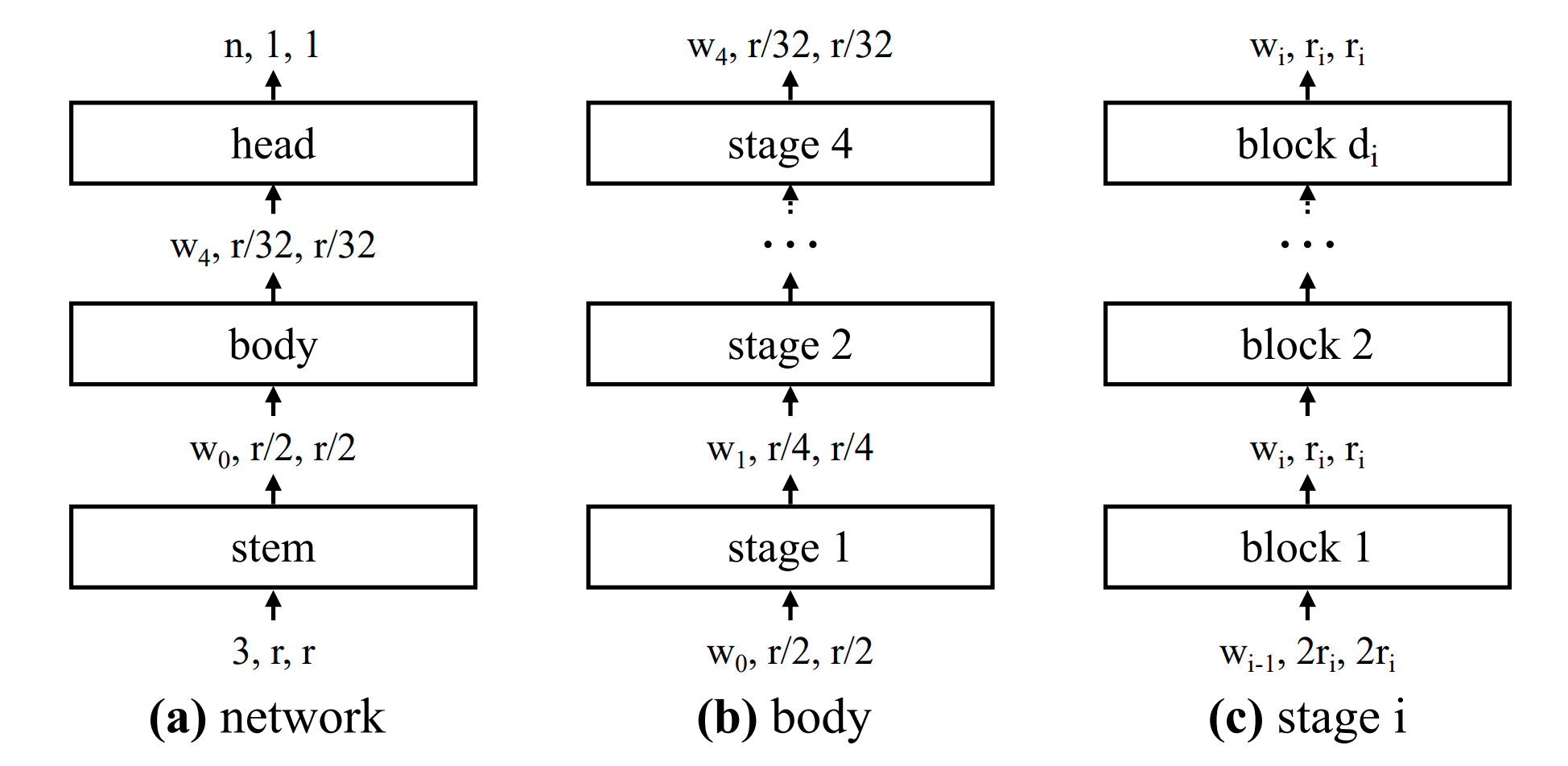
## Intended uses & limitations
You can use the raw model for image classification. See the [model hub](https://huggingface.co/models?search=regnet) to look for
fine-tuned versions on a task that interests you.
### How to use
Here is how to use this model:
```python
>>> from transformers import AutoFeatureExtractor, RegNetForImageClassification
>>> import torch
>>> from datasets import load_dataset
>>> dataset = load_dataset("huggingface/cats-image")
>>> image = dataset["test"]["image"][0]
>>> feature_extractor = AutoFeatureExtractor.from_pretrained("zuppif/regnet-y-040")
>>> model = RegNetForImageClassification.from_pretrained("zuppif/regnet-y-040")
>>> inputs = feature_extractor(image, return_tensors="pt")
>>> with torch.no_grad():
... logits = model(**inputs).logits
>>> # model predicts one of the 1000 ImageNet classes
>>> predicted_label = logits.argmax(-1).item()
>>> print(model.config.id2label[predicted_label])
'tabby, tabby cat'
```
For more code examples, we refer to the [documentation](https://huggingface.co/docs/transformers/master/en/model_doc/regnet). |
jarguello76/distilbert-base-uncased-finetuned-emotion | 02db228f93fe208995b2939f45260a102978013f | 2022-03-16T01:45:28.000Z | [
"pytorch",
"tensorboard",
"distilbert",
"text-classification",
"transformers"
] | text-classification | false | jarguello76 | null | jarguello76/distilbert-base-uncased-finetuned-emotion | 2 | null | transformers | 25,176 | Entry not found |
golivaresm/roberta-base-bne-finetuned-amazon_reviews_multi | 570b4d38e710c5936eadc31b844c101c5b5c083f | 2022-03-16T00:34:07.000Z | [
"pytorch",
"tensorboard",
"roberta",
"text-classification",
"dataset:amazon_reviews_multi",
"transformers",
"generated_from_trainer",
"license:apache-2.0",
"model-index"
] | text-classification | false | golivaresm | null | golivaresm/roberta-base-bne-finetuned-amazon_reviews_multi | 2 | null | transformers | 25,177 | ---
license: apache-2.0
tags:
- generated_from_trainer
datasets:
- amazon_reviews_multi
metrics:
- accuracy
model-index:
- name: roberta-base-bne-finetuned-amazon_reviews_multi
results:
- task:
name: Text Classification
type: text-classification
dataset:
name: amazon_reviews_multi
type: amazon_reviews_multi
args: es
metrics:
- name: Accuracy
type: accuracy
value: 0.93125
---
<!-- This model card has been generated automatically according to the information the Trainer had access to. You
should probably proofread and complete it, then remove this comment. -->
# roberta-base-bne-finetuned-amazon_reviews_multi
This model is a fine-tuned version of [BSC-TeMU/roberta-base-bne](https://huggingface.co/BSC-TeMU/roberta-base-bne) on the amazon_reviews_multi dataset.
It achieves the following results on the evaluation set:
- Loss: 0.2328
- Accuracy: 0.9313
## Model description
More information needed
## Intended uses & limitations
More information needed
## Training and evaluation data
More information needed
## Training procedure
### Training hyperparameters
The following hyperparameters were used during training:
- learning_rate: 2e-05
- train_batch_size: 16
- eval_batch_size: 16
- seed: 42
- optimizer: Adam with betas=(0.9,0.999) and epsilon=1e-08
- lr_scheduler_type: linear
- num_epochs: 2
### Training results
| Training Loss | Epoch | Step | Validation Loss | Accuracy |
|:-------------:|:-----:|:----:|:---------------:|:--------:|
| 0.1985 | 1.0 | 1250 | 0.1730 | 0.9327 |
| 0.0982 | 2.0 | 2500 | 0.2328 | 0.9313 |
### Framework versions
- Transformers 4.17.0
- Pytorch 1.10.0+cu111
- Datasets 2.0.0
- Tokenizers 0.11.6
|
yerevann/xlsrhy | b41f6cefeab8e2351560c225a7bb7b6d4daff999 | 2022-03-16T00:50:13.000Z | [
"pytorch",
"wav2vec2",
"automatic-speech-recognition",
"transformers"
] | automatic-speech-recognition | false | yerevann | null | yerevann/xlsrhy | 2 | null | transformers | 25,178 | Entry not found |
hackathon-pln-es/poem-gen-gpt2-small-spanish | a9dfac53bae9ffe82279524b35280f15bd724824 | 2022-03-15T04:32:41.000Z | [
"pytorch",
"tensorboard",
"gpt2",
"text-generation",
"transformers",
"generated_from_trainer",
"license:apache-2.0",
"model-index"
] | text-generation | false | hackathon-pln-es | null | hackathon-pln-es/poem-gen-gpt2-small-spanish | 2 | 2 | transformers | 25,179 | ---
license: apache-2.0
tags:
- generated_from_trainer
model-index:
- name: poem-gen-gpt2-small-spanish
results: []
---
<!-- This model card has been generated automatically according to the information the Trainer had access to. You
should probably proofread and complete it, then remove this comment. -->
# poem-gen-gpt2-small-spanish
This model is a fine-tuned version of [datificate/gpt2-small-spanish](https://huggingface.co/datificate/gpt2-small-spanish) on an unknown dataset.
It achieves the following results on the evaluation set:
- eval_loss: 4.1366
- eval_runtime: 25.1623
- eval_samples_per_second: 43.676
- eval_steps_per_second: 10.929
- epoch: 0.78
- step: 2040
## Model description
More information needed
## Intended uses & limitations
More information needed
## Training and evaluation data
More information needed
## Training procedure
### Training hyperparameters
The following hyperparameters were used during training:
- learning_rate: 2e-05
- train_batch_size: 4
- eval_batch_size: 4
- seed: 42
- optimizer: Adam with betas=(0.9,0.999) and epsilon=1e-08
- lr_scheduler_type: linear
- num_epochs: 3.0
### Framework versions
- Transformers 4.17.0
- Pytorch 1.10.0+cu111
- Datasets 1.18.4
- Tokenizers 0.11.6
|
PSW/random-word-swapping-bart-samsum | 37780a3d9d72186c60f70bfcdeaa3586a6cbbd07 | 2022-03-16T02:50:12.000Z | [
"pytorch",
"bart",
"text2text-generation",
"transformers",
"autotrain_compatible"
] | text2text-generation | false | PSW | null | PSW/random-word-swapping-bart-samsum | 2 | null | transformers | 25,180 | Entry not found |
Rustem/roberta-base-trained | 47fa7ed0570fbd3927fcf6275b499e6bf2bb7961 | 2022-03-16T07:54:42.000Z | [
"pytorch",
"roberta",
"fill-mask",
"transformers",
"license:apache-2.0",
"autotrain_compatible"
] | fill-mask | false | Rustem | null | Rustem/roberta-base-trained | 2 | null | transformers | 25,181 | ---
license: apache-2.0
---
|
navteca/ms-marco-MiniLM-L-6-v2 | 1fc781fd837cffa498cda42dd0254ed8691b97e6 | 2022-03-16T09:36:49.000Z | [
"pytorch",
"jax",
"bert",
"text-classification",
"en",
"sentence-transformers",
"license:mit"
] | text-classification | false | navteca | null | navteca/ms-marco-MiniLM-L-6-v2 | 2 | null | sentence-transformers | 25,182 | ---
language: en
license: mit
pipeline_tag: text-classification
tags:
- sentence-transformers
---
# Cross-Encoder for MS Marco
The model can be used for Information Retrieval: Given a query, encode the query will all possible passages (e.g. retrieved with ElasticSearch). Then sort the passages in a decreasing order. See [SBERT.net Retrieve & Re-rank](https://www.sbert.net/examples/applications/retrieve_rerank/README.html) for more details. The training code is available here: [SBERT.net Training MS Marco](https://github.com/UKPLab/sentence-transformers/tree/master/examples/training/ms_marco)
## Training Data
This model was trained on the [MS Marco Passage Ranking](https://github.com/microsoft/MSMARCO-Passage-Ranking) task.
## Usage
The usage becomes easier when you have [SentenceTransformers](https://www.sbert.net/) installed. Then, you can use the pre-trained models like this:
```python
from sentence_transformers import CrossEncoder
model = CrossEncoder('model_name', max_length=512)
scores = model.predict([('Query', 'Paragraph1'), ('Query', 'Paragraph2')])
```
## Performance
In the following table, we provide various pre-trained Cross-Encoders together with their performance on the [TREC Deep Learning 2019](https://microsoft.github.io/TREC-2019-Deep-Learning/) and the [MS Marco Passage Reranking](https://github.com/microsoft/MSMARCO-Passage-Ranking/) dataset.
| Model-Name | NDCG@10 (TREC DL 19) | MRR@10 (MS Marco Dev) | Docs / Sec |
| ------------- |:-------------| -----| --- |
| **Version 2 models** | | |
| cross-encoder/ms-marco-TinyBERT-L-2-v2 | 69.84 | 32.56 | 9000
| cross-encoder/ms-marco-MiniLM-L-2-v2 | 71.01 | 34.85 | 4100
| cross-encoder/ms-marco-MiniLM-L-4-v2 | 73.04 | 37.70 | 2500
| cross-encoder/ms-marco-MiniLM-L-6-v2 | 74.30 | 39.01 | 1800
| cross-encoder/ms-marco-MiniLM-L-12-v2 | 74.31 | 39.02 | 960
| **Version 1 models** | | |
| cross-encoder/ms-marco-TinyBERT-L-2 | 67.43 | 30.15 | 9000
| cross-encoder/ms-marco-TinyBERT-L-4 | 68.09 | 34.50 | 2900
| cross-encoder/ms-marco-TinyBERT-L-6 | 69.57 | 36.13 | 680
| cross-encoder/ms-marco-electra-base | 71.99 | 36.41 | 340
| **Other models** | | |
| nboost/pt-tinybert-msmarco | 63.63 | 28.80 | 2900
| nboost/pt-bert-base-uncased-msmarco | 70.94 | 34.75 | 340
| nboost/pt-bert-large-msmarco | 73.36 | 36.48 | 100
| Capreolus/electra-base-msmarco | 71.23 | 36.89 | 340
| amberoad/bert-multilingual-passage-reranking-msmarco | 68.40 | 35.54 | 330
| sebastian-hofstaetter/distilbert-cat-margin_mse-T2-msmarco | 72.82 | 37.88 | 720
Note: Runtime was computed on a V100 GPU.
|
ixa-ehu/roberta-eus-cc100-base-cased | 2769bc619bcbe1261f1e4a4012cf77c1ad601b40 | 2022-03-16T11:48:07.000Z | [
"pytorch",
"roberta",
"fill-mask",
"eu",
"arxiv:2203.08111",
"transformers",
"basque",
"license:cc-by-nc-4.0",
"autotrain_compatible"
] | fill-mask | false | ixa-ehu | null | ixa-ehu/roberta-eus-cc100-base-cased | 2 | null | transformers | 25,183 | ---
language: eu
license: cc-by-nc-4.0
tags:
- basque
- roberta
---
# Roberta-eus cc100 base cased
This is a RoBERTa model for Basque model presented in [Does corpus quality really matter for low-resource languages?](https://arxiv.org/abs/2203.08111). There are several models for Basque using the RoBERTa architecture, using different corpora:
- roberta-eus-euscrawl-base-cased: Basque RoBERTa model trained on Euscrawl, a corpus created using tailored crawling from Basque sites. EusCrawl contains 12,528k documents and 423M tokens.
- roberta-eus-euscrawl-large-cased: RoBERTa large trained on EusCrawl.
- roberta-eus-mC4-base-cased: Basque RoBERTa model trained on the Basque portion of mc4 dataset.
- roberta-eus-CC100-base-cased: Basque RoBERTa model trained on Basque portion of cc100 dataset.
The models have been tested on five different downstream tasks for Basque: Topic classification, Sentiment analysis, Stance detection, Named Entity Recognition (NER), and Question Answering (refer to the [paper](https://arxiv.org/abs/2203.08111) for more details). See summary of results below:
| Model | Topic class. | Sentiment | Stance det. | NER | QA | Average |
|----------------------------------|--------------|-----------|-------------|----------|----------|----------|
| roberta-eus-euscrawl-base-cased | 76.2 | 77.7 | 57.4 | 86.8 | 34.6 | 66.5 |
| roberta-eus-euscrawl-large-cased | **77.6** | 78.8 | 62.9 | **87.2** | **38.3** | **69.0** |
| roberta-eus-mC4-base-cased | 75.3 | **80.4** | 59.1 | 86.0 | 35.2 | 67.2 |
| roberta-eus-CC100-base-cased | 76.2 | 78.8 | **63.4** | 85.2 | 35.8 | 67.9 |
If you use any of these models, please cite the following paper:
```
@misc{artetxe2022euscrawl,
title={Does corpus quality really matter for low-resource languages?},
author={Mikel Artetxe, Itziar Aldabe, Rodrigo Agerri,
Olatz Perez-de-Viñaspre, Aitor Soroa},
year={2022},
eprint={2203.08111},
archivePrefix={arXiv},
primaryClass={cs.CL}
}
```
|
ai4bharat/MultiIndicQuestionGenerationUnified | 65c3fddf75c302edde59c4721f26435d506d87a6 | 2022-05-23T17:19:26.000Z | [
"pytorch",
"mbart",
"text2text-generation",
"as",
"bn",
"gu",
"hi",
"kn",
"ml",
"mr",
"or",
"pa",
"ta",
"te",
"dataset:ai4bharat/IndicQuestionGeneration",
"dataset:squad",
"arxiv:2203.05437",
"transformers",
"question-generation",
"multilingual",
"nlp",
"indicnlp",
"autotrain_compatible"
] | text2text-generation | false | ai4bharat | null | ai4bharat/MultiIndicQuestionGenerationUnified | 2 | null | transformers | 25,184 | ---
tags:
- question-generation
- multilingual
- nlp
- indicnlp
datasets:
- ai4bharat/IndicQuestionGeneration
- squad
language:
- as
- bn
- gu
- hi
- kn
- ml
- mr
- or
- pa
- ta
- te
licenses:
- cc-by-nc-4.0
---
# MultiIndicQuestionGenerationUnified
MultiIndicQuestionGenerationUnified is a multilingual, sequence-to-sequence pre-trained model, a [IndicBART](https://huggingface.co/ai4bharat/IndicBART) checkpoint fine-tuned on the 11 languages of [IndicQuestionGeneration](https://huggingface.co/datasets/ai4bharat/IndicQuestionGeneration) dataset. For fine-tuning details,
see the [paper](https://arxiv.org/abs/2203.05437). You can use MultiIndicQuestionGenerationUnified to build question generation applications for Indian languages by fine-tuning the model with supervised training data for the question generation task. Some salient features of the MultiIndicQuestionGenerationUnified are:
<ul>
<li >Supported languages: Assamese, Bengali, Gujarati, Hindi, Marathi, Oriya, Punjabi, Kannada, Malayalam, Tamil, and Telugu. Not all of these languages are supported by mBART50 and mT5. </li>
<li >The model is much smaller than the mBART and mT5(-base) models, so less computationally expensive for fine-tuning and decoding. </li>
<li> Fine-tuned on large Indic language corpora (770 K examples). </li>
<li> All languages have been represented in Devanagari script to encourage transfer learning among the related languages. </li>
</ul>
You can read more about MultiIndicQuestionGenerationUnified in this <a href="https://arxiv.org/abs/2203.05437">paper</a>.
## Using this model in `transformers`
```
from transformers import MBartForConditionalGeneration, AutoModelForSeq2SeqLM
from transformers import AlbertTokenizer, AutoTokenizer
tokenizer = AutoTokenizer.from_pretrained("ai4bharat/MultiIndicQuestionGenerationUnified", do_lower_case=False, use_fast=False, keep_accents=True)
# Or use tokenizer = AlbertTokenizer.from_pretrained("ai4bharat/MultiIndicQuestionGenerationUnified", do_lower_case=False, use_fast=False, keep_accents=True)
model = AutoModelForSeq2SeqLM.from_pretrained("ai4bharat/MultiIndicQuestionGenerationUnified")
# Or use model = MBartForConditionalGeneration.from_pretrained("ai4bharat/MultiIndicQuestionGenerationUnified")
# Some initial mapping
bos_id = tokenizer._convert_token_to_id_with_added_voc("<s>")
eos_id = tokenizer._convert_token_to_id_with_added_voc("</s>")
pad_id = tokenizer._convert_token_to_id_with_added_voc("<pad>")
# To get lang_id use any of ['<2as>', '<2bn>', '<2gu>', '<2hi>', '<2kn>', '<2ml>', '<2mr>', '<2or>', '<2pa>', '<2ta>', '<2te>']
# First tokenize the input and outputs. The format below is how IndicBART was trained so the input should be "Sentence </s> <2xx>" where xx is the language code. Similarly, the output should be "<2yy> Sentence </s>".
inp = tokenizer("7 फरवरी, 2016 [SEP] खेल 7 फरवरी, 2016 को कैलिफोर्निया के सांता क्लारा में सैन फ्रांसिस्को खाड़ी क्षेत्र में लेवी स्टेडियम में खेला गया था।</s><2hi>", add_special_tokens=False, return_tensors="pt", padding=True).input_ids
out = tokenizer("<2hi> सुपर बाउल किस दिन खेला गया? </s>", add_special_tokens=False, return_tensors="pt", padding=True).input_ids
model_outputs=model(input_ids=inp, decoder_input_ids=out[:,0:-1], labels=out[:,1:])
# For loss
model_outputs.loss ## This is not label smoothed.
# For logits
model_outputs.logits
# For generation. Pardon the messiness. Note the decoder_start_token_id.
model.eval() # Set dropouts to zero
model_output=model.generate(inp, use_cache=True,no_repeat_ngram_size=3,encoder_no_repeat_ngram_size=3, num_beams=4, max_length=20, min_length=1, early_stopping=True, pad_token_id=pad_id, bos_token_id=bos_id, eos_token_id=eos_id, decoder_start_token_id=tokenizer._convert_token_to_id_with_added_voc("<2hi>"))
# Decode to get output strings
decoded_output=tokenizer.decode(model_output[0], skip_special_tokens=True, clean_up_tokenization_spaces=False)
print(decoded_output) # कब खेला जाएगा पहला मैच?
# Disclaimer
Note that if your output language is not Hindi or Marathi, you should convert its script from Devanagari to the desired language using the [Indic NLP Library](https://github.com/AI4Bharat/indic-bart/blob/main/indic_scriptmap.py).
```
# Note:
If you wish to use any language written in a non-Devanagari script, then you should first convert it to Devanagari using the <a href="https://github.com/anoopkunchukuttan/indic_nlp_library">Indic NLP Library</a>. After you get the output, you should convert it back into the original script.
## Benchmarks
Scores on the `IndicQuestionGeneration` test sets are as follows:
Language | RougeL
---------|----------------------------
as | 20.48
bn | 26.63
gu | 27.71
hi | 35.38
kn | 23.56
ml | 22.17
mr | 23.52
or | 25.25
pa | 32.10
ta | 22.98
te | 25.67
## Citation
If you use this model, please cite the following paper:
```
@inproceedings{Kumar2022IndicNLGSM,
title={IndicNLG Suite: Multilingual Datasets for Diverse NLG Tasks in Indic Languages},
author={Aman Kumar and Himani Shrotriya and Prachi Sahu and Raj Dabre and Ratish Puduppully and Anoop Kunchukuttan and Amogh Mishra and Mitesh M. Khapra and Pratyush Kumar},
year={2022},
url = "https://arxiv.org/abs/2203.05437"
}
```
# License
The model is available under the MIT License. |
RobertoMCA97/xlm-roberta-base-finetuned-panx-de-fr | 3a0bd6ab806259ef75dcc6e502d9da815faafe54 | 2022-03-16T12:24:41.000Z | [
"pytorch",
"xlm-roberta",
"token-classification",
"transformers",
"generated_from_trainer",
"license:mit",
"model-index",
"autotrain_compatible"
] | token-classification | false | RobertoMCA97 | null | RobertoMCA97/xlm-roberta-base-finetuned-panx-de-fr | 2 | null | transformers | 25,185 | ---
license: mit
tags:
- generated_from_trainer
metrics:
- f1
model-index:
- name: xlm-roberta-base-finetuned-panx-de-fr
results: []
---
<!-- This model card has been generated automatically according to the information the Trainer had access to. You
should probably proofread and complete it, then remove this comment. -->
# xlm-roberta-base-finetuned-panx-de-fr
This model is a fine-tuned version of [xlm-roberta-base](https://huggingface.co/xlm-roberta-base) on the None dataset.
It achieves the following results on the evaluation set:
- Loss: 0.1667
- F1: 0.8582
## Model description
More information needed
## Intended uses & limitations
More information needed
## Training and evaluation data
More information needed
## Training procedure
### Training hyperparameters
The following hyperparameters were used during training:
- learning_rate: 5e-05
- train_batch_size: 24
- eval_batch_size: 24
- seed: 42
- optimizer: Adam with betas=(0.9,0.999) and epsilon=1e-08
- lr_scheduler_type: linear
- num_epochs: 3
### Training results
| Training Loss | Epoch | Step | Validation Loss | F1 |
|:-------------:|:-----:|:----:|:---------------:|:------:|
| 0.2885 | 1.0 | 715 | 0.1817 | 0.8287 |
| 0.1497 | 2.0 | 1430 | 0.1618 | 0.8442 |
| 0.0944 | 3.0 | 2145 | 0.1667 | 0.8582 |
### Framework versions
- Transformers 4.17.0
- Pytorch 1.10.0+cu111
- Datasets 2.0.0
- Tokenizers 0.11.6
|
RobertoMCA97/xlm-roberta-base-finetuned-panx-it | 57076fb12373b8a19f6f93eb102d18da0b0fd2a0 | 2022-03-16T12:56:38.000Z | [
"pytorch",
"xlm-roberta",
"token-classification",
"dataset:xtreme",
"transformers",
"generated_from_trainer",
"license:mit",
"model-index",
"autotrain_compatible"
] | token-classification | false | RobertoMCA97 | null | RobertoMCA97/xlm-roberta-base-finetuned-panx-it | 2 | null | transformers | 25,186 | ---
license: mit
tags:
- generated_from_trainer
datasets:
- xtreme
metrics:
- f1
model-index:
- name: xlm-roberta-base-finetuned-panx-it
results:
- task:
name: Token Classification
type: token-classification
dataset:
name: xtreme
type: xtreme
args: PAN-X.it
metrics:
- name: F1
type: f1
value: 0.822805578342904
---
<!-- This model card has been generated automatically according to the information the Trainer had access to. You
should probably proofread and complete it, then remove this comment. -->
# xlm-roberta-base-finetuned-panx-it
This model is a fine-tuned version of [xlm-roberta-base](https://huggingface.co/xlm-roberta-base) on the xtreme dataset.
It achieves the following results on the evaluation set:
- Loss: 0.2323
- F1: 0.8228
## Model description
More information needed
## Intended uses & limitations
More information needed
## Training and evaluation data
More information needed
## Training procedure
### Training hyperparameters
The following hyperparameters were used during training:
- learning_rate: 5e-05
- train_batch_size: 24
- eval_batch_size: 24
- seed: 42
- optimizer: Adam with betas=(0.9,0.999) and epsilon=1e-08
- lr_scheduler_type: linear
- num_epochs: 3
### Training results
| Training Loss | Epoch | Step | Validation Loss | F1 |
|:-------------:|:-----:|:----:|:---------------:|:------:|
| 0.8126 | 1.0 | 70 | 0.3361 | 0.7231 |
| 0.2995 | 2.0 | 140 | 0.2526 | 0.8079 |
| 0.1865 | 3.0 | 210 | 0.2323 | 0.8228 |
### Framework versions
- Transformers 4.17.0
- Pytorch 1.10.0+cu111
- Datasets 2.0.0
- Tokenizers 0.11.6
|
RobertoMCA97/xlm-roberta-base-finetuned-panx-en | 499f09d9657a27014c05accaf1cc91c029d8a153 | 2022-03-16T13:12:04.000Z | [
"pytorch",
"xlm-roberta",
"token-classification",
"dataset:xtreme",
"transformers",
"generated_from_trainer",
"license:mit",
"model-index",
"autotrain_compatible"
] | token-classification | false | RobertoMCA97 | null | RobertoMCA97/xlm-roberta-base-finetuned-panx-en | 2 | null | transformers | 25,187 | ---
license: mit
tags:
- generated_from_trainer
datasets:
- xtreme
metrics:
- f1
model-index:
- name: xlm-roberta-base-finetuned-panx-en
results:
- task:
name: Token Classification
type: token-classification
dataset:
name: xtreme
type: xtreme
args: PAN-X.en
metrics:
- name: F1
type: f1
value: 0.7075365579302588
---
<!-- This model card has been generated automatically according to the information the Trainer had access to. You
should probably proofread and complete it, then remove this comment. -->
# xlm-roberta-base-finetuned-panx-en
This model is a fine-tuned version of [xlm-roberta-base](https://huggingface.co/xlm-roberta-base) on the xtreme dataset.
It achieves the following results on the evaluation set:
- Loss: 0.3925
- F1: 0.7075
## Model description
More information needed
## Intended uses & limitations
More information needed
## Training and evaluation data
More information needed
## Training procedure
### Training hyperparameters
The following hyperparameters were used during training:
- learning_rate: 5e-05
- train_batch_size: 24
- eval_batch_size: 24
- seed: 42
- optimizer: Adam with betas=(0.9,0.999) and epsilon=1e-08
- lr_scheduler_type: linear
- num_epochs: 3
### Training results
| Training Loss | Epoch | Step | Validation Loss | F1 |
|:-------------:|:-----:|:----:|:---------------:|:------:|
| 1.1493 | 1.0 | 50 | 0.5884 | 0.4748 |
| 0.5135 | 2.0 | 100 | 0.4088 | 0.6623 |
| 0.3558 | 3.0 | 150 | 0.3925 | 0.7075 |
### Framework versions
- Transformers 4.17.0
- Pytorch 1.10.0+cu111
- Datasets 2.0.0
- Tokenizers 0.11.6
|
malteos/aspect-scibert-dataset | c5d016fce8e63abed19be303f9a3ab2e950dda88 | 2022-03-16T14:00:58.000Z | [
"pytorch",
"bert",
"feature-extraction",
"transformers",
"license:mit"
] | feature-extraction | false | malteos | null | malteos/aspect-scibert-dataset | 2 | null | transformers | 25,188 | ---
license: mit
---
|
vpelloin/MEDIA_NLU_flaubert_finetuned | d350309f204ad34831aa5eb8b6f6d050b8daa255 | 2022-06-17T13:54:59.000Z | [
"pytorch",
"tensorboard",
"flaubert",
"token-classification",
"fr",
"transformers",
"bert",
"natural language understanding",
"NLU",
"spoken language understanding",
"SLU",
"understanding",
"MEDIA",
"autotrain_compatible"
] | token-classification | false | vpelloin | null | vpelloin/MEDIA_NLU_flaubert_finetuned | 2 | null | transformers | 25,189 | ---
language: fr
pipeline_tag: "token-classification"
widget:
- text: "je voudrais réserver une chambre à paris pour demain et lundi"
- text: "d'accord pour l'hôtel à quatre vingt dix euros la nuit"
- text: "deux nuits s'il vous plait"
- text: "dans un hôtel avec piscine à marseille"
tags:
- bert
- flaubert
- natural language understanding
- NLU
- spoken language understanding
- SLU
- understanding
- MEDIA
---
# vpelloin/MEDIA_NLU_flaubert_finetuned (FT)
This is a Natural Language Understanding (NLU) model for the French [MEDIA benchmark](https://catalogue.elra.info/en-us/repository/browse/ELRA-S0272/).
It maps each input words into outputs concepts tags (76 available).
This model is a fine-tuning of [`flaubert-oral-ft`](https://huggingface.co/nherve/flaubert-oral-ft) (FlauBERT finetuned on ASR data).
## Usage with Pipeline
```python
from transformers import pipeline
generator = pipeline(model="vpelloin/MEDIA_NLU_flaubert_finetuned", task="token-classification")
print(generator)
```
## Usage with AutoTokenizer/AutoModel
```python
from transformers import (
AutoTokenizer,
AutoModelForTokenClassification
)
tokenizer = AutoTokenizer.from_pretrained("vpelloin/MEDIA_NLU_flaubert_finetuned")
model = AutoModelForTokenClassification.from_pretrained("vpelloin/MEDIA_NLU_flaubert_finetuned")
sentences = [
"je voudrais réserver une chambre à paris pour demain et lundi",
"d'accord pour l'hôtel à quatre vingt dix euros la nuit",
"deux nuits s'il vous plait",
"dans un hôtel avec piscine à marseille"
]
inputs = tokenizer(sentences, padding=True, return_tensors='pt')
outptus = model(**inputs).logits
print([[model.config.id2label[i] for i in b] for b in outptus.argmax(dim=-1).tolist()])
```
|
Rustem/roberta-base-trained-7epochs | 2f393e28b863e958587ec9c95c70633d69aba06a | 2022-03-16T16:01:00.000Z | [
"pytorch",
"roberta",
"fill-mask",
"transformers",
"license:apache-2.0",
"autotrain_compatible"
] | fill-mask | false | Rustem | null | Rustem/roberta-base-trained-7epochs | 2 | null | transformers | 25,190 | ---
license: apache-2.0
---
|
MrAnderson/bert-base-4096-full-trivia-copied-embeddings | 8d002389dc50d8659b6ad10852123beef96eb165 | 2022-03-16T21:38:28.000Z | [
"pytorch",
"bert",
"question-answering",
"transformers",
"autotrain_compatible"
] | question-answering | false | MrAnderson | null | MrAnderson/bert-base-4096-full-trivia-copied-embeddings | 2 | null | transformers | 25,191 | Entry not found |
internetoftim/pushit | 6bbc85810c227cb3b84aa40a0dc212e66089724e | 2022-03-16T18:24:08.000Z | [
"pytorch",
"bert",
"question-answering",
"transformers",
"autotrain_compatible"
] | question-answering | false | internetoftim | null | internetoftim/pushit | 2 | null | transformers | 25,192 | Entry not found |
horsbug98/Part_1_XLM_Model_E1 | 74f124913c27a55dec19839ca917a8001419aac3 | 2022-03-30T17:13:01.000Z | [
"pytorch",
"xlm-roberta",
"question-answering",
"dataset:tydiqa",
"transformers",
"generated_from_trainer",
"license:mit",
"model-index",
"autotrain_compatible"
] | question-answering | false | horsbug98 | null | horsbug98/Part_1_XLM_Model_E1 | 2 | null | transformers | 25,193 | ---
license: mit
tags:
- generated_from_trainer
datasets:
- tydiqa
model-index:
- name: debug_xlm_task1_1
results: []
---
<!-- This model card has been generated automatically according to the information the Trainer had access to. You
should probably proofread and complete it, then remove this comment. -->
# debug_xlm_task1_1
This model is a fine-tuned version of [xlm-roberta-base](https://huggingface.co/xlm-roberta-base) on the tydiqa secondary_task dataset.
## Model description
More information needed
## Intended uses & limitations
More information needed
## Training and evaluation data
More information needed
## Training procedure
### Training hyperparameters
The following hyperparameters were used during training:
- learning_rate: 3e-05
- train_batch_size: 12
- eval_batch_size: 8
- seed: 42
- optimizer: Adam with betas=(0.9,0.999) and epsilon=1e-08
- lr_scheduler_type: linear
- num_epochs: 1.0
### Training results
### Framework versions
- Transformers 4.15.0
- Pytorch 1.9.1
- Datasets 2.0.0
- Tokenizers 0.10.3
|
Savitar/DialoGPT-medium-RickandMorty | 231cb33bd2114f732e26f6acaa6570562ea49966 | 2022-03-16T20:37:26.000Z | [
"pytorch",
"gpt2",
"text-generation",
"transformers",
"conversational"
] | conversational | false | Savitar | null | Savitar/DialoGPT-medium-RickandMorty | 2 | null | transformers | 25,194 | ---
tags:
- conversational
---
# Rick and Morty DialoGPT Model |
clapika2010/movies_finetuned | ec63aeacda84f4f979710857bd15b2ade171e0c8 | 2022-03-17T00:02:37.000Z | [
"pytorch",
"roberta",
"fill-mask",
"transformers",
"autotrain_compatible"
] | fill-mask | false | clapika2010 | null | clapika2010/movies_finetuned | 2 | null | transformers | 25,195 | Entry not found |
cammy/pegasus-cnn_dailymail-100-lit-evalMA-ga | 5afc2b89b881433eaef33ea2e6a1ef7ad9b41571 | 2022-03-17T02:22:31.000Z | [
"pytorch",
"tensorboard",
"pegasus",
"text2text-generation",
"transformers",
"generated_from_trainer",
"model-index",
"autotrain_compatible"
] | text2text-generation | false | cammy | null | cammy/pegasus-cnn_dailymail-100-lit-evalMA-ga | 2 | null | transformers | 25,196 | ---
tags:
- generated_from_trainer
model-index:
- name: pegasus-cnn_dailymail-100-lit-evalMA-ga
results: []
---
<!-- This model card has been generated automatically according to the information the Trainer had access to. You
should probably proofread and complete it, then remove this comment. -->
# pegasus-cnn_dailymail-100-lit-evalMA-ga
This model is a fine-tuned version of [google/pegasus-cnn_dailymail](https://huggingface.co/google/pegasus-cnn_dailymail) on an unknown dataset.
## Model description
More information needed
## Intended uses & limitations
More information needed
## Training and evaluation data
More information needed
## Training procedure
### Training hyperparameters
The following hyperparameters were used during training:
- learning_rate: 2e-05
- train_batch_size: 1
- eval_batch_size: 1
- seed: 42
- gradient_accumulation_steps: 4
- total_train_batch_size: 4
- optimizer: Adam with betas=(0.9,0.999) and epsilon=1e-08
- lr_scheduler_type: linear
- num_epochs: 1
- mixed_precision_training: Native AMP
### Framework versions
- Transformers 4.16.2
- Pytorch 1.10.2
- Datasets 1.18.3
- Tokenizers 0.11.0
|
zdepablo/distilbert-base-uncased-distilled-clinc | 645407d017db5354b9aa69b75637f7b12ad829e0 | 2022-03-17T02:41:19.000Z | [
"pytorch",
"distilbert",
"text-classification",
"dataset:clinc_oos",
"transformers",
"generated_from_trainer",
"license:apache-2.0",
"model-index"
] | text-classification | false | zdepablo | null | zdepablo/distilbert-base-uncased-distilled-clinc | 2 | null | transformers | 25,197 | ---
license: apache-2.0
tags:
- generated_from_trainer
datasets:
- clinc_oos
metrics:
- accuracy
model-index:
- name: distilbert-base-uncased-distilled-clinc
results:
- task:
name: Text Classification
type: text-classification
dataset:
name: clinc_oos
type: clinc_oos
args: plus
metrics:
- name: Accuracy
type: accuracy
value: 0.9474193548387096
---
<!-- This model card has been generated automatically according to the information the Trainer had access to. You
should probably proofread and complete it, then remove this comment. -->
# distilbert-base-uncased-distilled-clinc
This model is a fine-tuned version of [distilbert-base-uncased](https://huggingface.co/distilbert-base-uncased) on the clinc_oos dataset.
It achieves the following results on the evaluation set:
- Loss: 0.2587
- Accuracy: 0.9474
## Model description
More information needed
## Intended uses & limitations
More information needed
## Training and evaluation data
More information needed
## Training procedure
### Training hyperparameters
The following hyperparameters were used during training:
- learning_rate: 2e-05
- train_batch_size: 48
- eval_batch_size: 48
- seed: 42
- optimizer: Adam with betas=(0.9,0.999) and epsilon=1e-08
- lr_scheduler_type: linear
- num_epochs: 10
### Training results
| Training Loss | Epoch | Step | Validation Loss | Accuracy |
|:-------------:|:-----:|:----:|:---------------:|:--------:|
| 4.2192 | 1.0 | 318 | 3.1512 | 0.7519 |
| 2.3972 | 2.0 | 636 | 1.5605 | 0.8519 |
| 1.1587 | 3.0 | 954 | 0.7688 | 0.9139 |
| 0.5616 | 4.0 | 1272 | 0.4672 | 0.9319 |
| 0.3001 | 5.0 | 1590 | 0.3414 | 0.9403 |
| 0.1817 | 6.0 | 1908 | 0.2952 | 0.9432 |
| 0.1228 | 7.0 | 2226 | 0.2714 | 0.9468 |
| 0.0939 | 8.0 | 2544 | 0.2605 | 0.9465 |
| 0.0799 | 9.0 | 2862 | 0.2600 | 0.9468 |
| 0.0736 | 10.0 | 3180 | 0.2587 | 0.9474 |
### Framework versions
- Transformers 4.11.3
- Pytorch 1.9.1
- Datasets 1.16.1
- Tokenizers 0.10.3
|
mmohamme/distilbert-base-uncased-finetuned-btc_2 | dabefb380bd65747822cfe71aa876dbbff0d2c13 | 2022-03-22T21:08:41.000Z | [
"pytorch",
"distilbert",
"text-classification",
"transformers"
] | text-classification | false | mmohamme | null | mmohamme/distilbert-base-uncased-finetuned-btc_2 | 2 | null | transformers | 25,198 | Entry not found |
nqcccccc/phobert-asba-qam | 8cea82f0b9a74944a02b56aa3b95632d484edab7 | 2022-03-17T08:05:46.000Z | [
"pytorch",
"roberta",
"text-classification",
"transformers"
] | text-classification | false | nqcccccc | null | nqcccccc/phobert-asba-qam | 2 | null | transformers | 25,199 | Entry not found |
Subsets and Splits
No community queries yet
The top public SQL queries from the community will appear here once available.