html_url
stringlengths 48
51
| title
stringlengths 1
290
| comments
sequencelengths 0
30
| body
stringlengths 0
228k
⌀ | number
int64 2
7.08k
|
---|---|---|---|---|
https://github.com/huggingface/datasets/issues/3778 | Not be able to download dataset - "Newsroom" | [
"Hi @Darshan2104, thanks for reporting.\r\n\r\nPlease note that at Hugging Face we do not host the data of this dataset, but just a loading script pointing to the host of the data owners.\r\n\r\nApparently the data owners changed their data host server. After googling it, I found their new website at: https://lil.nlp.cornell.edu/newsroom/index.html\r\n- Download page: https://lil.nlp.cornell.edu/newsroom/download/index.html\r\n\r\nI'm fixing the link in our Datasets library.",
"@albertvillanova Thanks for the solution and link you made my day!"
] | Hello,
I tried to download the **newsroom** dataset but it didn't work out for me. it said me to **download it manually**!
For manually, Link is also didn't work! It is sawing some ad or something!
If anybody has solved this issue please help me out or if somebody has this dataset please share your google drive link, it would be a great help!
Thanks
Darshan Tank | 3,778 |
https://github.com/huggingface/datasets/issues/3776 | Allow download only some files from the Wikipedia dataset | [
"Hi @jvanz, thank you for your proposal.\r\n\r\nIn fact, we are aware that it is very common the problem you mention. Because of that, we are currently working in implementing a new version of wikipedia on the Hub, with all data preprocessed (no need to use Apache Beam), from where you will be able to use `data_files` to load only a specific subset of the data files.\r\n\r\nSee:\r\n- #3401 "
] | **Is your feature request related to a problem? Please describe.**
The Wikipedia dataset can be really big. This is a problem if you want to use it locally in a laptop with the Apache Beam `DirectRunner`. Even if your laptop have a considerable amount of memory (e.g. 32gb).
**Describe the solution you'd like**
I would like to use the `data_files` argument in the `load_dataset` function to define which file in the wikipedia dataset I would like to download. Thus, I can work with the dataset in a smaller machine using the Apache Beam `DirectRunner`.
**Describe alternatives you've considered**
I've tried to use the `simple` Wikipedia dataset. But it's in English and I would like to use Portuguese texts in my model.
| 3,776 |
https://github.com/huggingface/datasets/issues/3773 | Checksum mismatch for the reddit_tifu dataset | [
"Thanks for reporting, @anna-kay. We are fixing it.",
"@albertvillanova Thank you for the fast response! However I am still getting the same error:\r\n\r\nDownloading: 2.23kB [00:00, ?B/s]\r\nTraceback (most recent call last):\r\n File \"C:\\Users\\Anna\\PycharmProjects\\summarization\\main.py\", line 17, in <module>\r\n dataset = load_dataset('reddit_tifu', 'long')\r\n File \"C:\\Users\\Anna\\Desktop\\summarization\\summarization_env\\lib\\site-packages\\datasets\\load.py\", line 1702, in load_dataset\r\n builder_instance.download_and_prepare(\r\n File \"C:\\Users\\Anna\\Desktop\\summarization\\summarization_env\\lib\\site-packages\\datasets\\builder.py\", line 594, in download_and_prepare\r\n self._download_and_prepare(\r\n File \"C:\\Users\\Anna\\Desktop\\summarization\\summarization_env\\lib\\site-packages\\datasets\\builder.py\", line 665, in _download_and_prepare\r\n verify_checksums(\r\n File \"C:\\Users\\Anna\\Desktop\\summarization\\summarization_env\\lib\\site-packages\\datasets\\utils\\info_utils.py\", line 40, in verify_checksums\r\n raise NonMatchingChecksumError(error_msg + str(bad_urls))\r\ndatasets.utils.info_utils.NonMatchingChecksumError: Checksums didn't match for dataset source files:\r\n['https://drive.google.com/uc?export=download&id=1ffWfITKFMJeqjT8loC8aiCLRNJpc_XnF']\r\n\r\nI have cleaned the cache/huggingface/datasets & cache/huggingface/modules files and also tried on another machine with a fresh installation of trasnformers & datasets. \r\nThe reddit_tifu.py that gets downloaded still has the previous url on line 51, _URL = \"https://drive.google.com/uc?export=download&id=1ffWfITKFMJeqjT8loC8aiCLRNJpc_XnF\" ",
"Hi @anna-kay, I'm sorry I didn't clearly explain the details to you:\r\n- the error has been fixed in our `master` branch on GitHub: https://github.com/huggingface/datasets/commit/8ae21bf6a77175dc803ce2f1b93d18b8fbf45586\r\n- the fix will not be accessible to users in PyPI until our next release of the `datasets` library\r\n - our latest release (version 1.18.3) was made 23 days ago: https://github.com/huggingface/datasets/releases/tag/1.18.3\r\n- in the meantime, you can get the fix if you install datasets from our GitHub `master` branch:\r\n ```\r\n pip install git+https://github.com/huggingface/datasets#egg=datasets\r\n ```",
"@albertvillanova Ok great, makes sence. Thank you very much for the explanation!"
] | ## Describe the bug
A checksum occurs when downloading the reddit_tifu data (both long & short).
## Steps to reproduce the bug
reddit_tifu_dataset = load_dataset('reddit_tifu', 'long')
## Expected results
The expected result is for the dataset to be downloaded and cached locally.
## Actual results
File "/.../lib/python3.9/site-packages/datasets/utils/info_utils.py", line 40, in verify_checksums
raise NonMatchingChecksumError(error_msg + str(bad_urls))
datasets.utils.info_utils.NonMatchingChecksumError: Checksums didn't match for dataset source files:
['https://drive.google.com/uc?export=download&id=1ffWfITKFMJeqjT8loC8aiCLRNJpc_XnF']
## Environment info
<!-- You can run the command `datasets-cli env` and copy-and-paste its output below. -->
- `datasets` version: 1.18.3
- Platform: Linux-5.13.0-30-generic-x86_64-with-glibc2.31
- Python version: 3.9.7
- PyArrow version: 7.0.0
| 3,773 |
https://github.com/huggingface/datasets/issues/3770 | DuplicatedKeysError on msr_sqa dataset | [
"Thanks for reporting, @kolk.\r\n\r\nWe are fixing it. "
] | ### Describe the bug
Failure to generate dataset msr_sqa because of duplicate keys.
### Steps to reproduce the bug
```
from datasets import load_dataset
load_dataset("msr_sqa")
```
### Expected results
The examples keys should be unique.
**Actual results**
```
>>> load_dataset("msr_sqa")
Downloading:
6.72k/? [00:00<00:00, 148kB/s]
Downloading:
2.93k/? [00:00<00:00, 53.8kB/s]
Using custom data configuration default
Downloading and preparing dataset msr_sqa/default (download: 4.57 MiB, generated: 26.25 MiB, post-processed: Unknown size, total: 30.83 MiB) to /root/.cache/huggingface/datasets/msr_sqa/default/0.0.0/70b2a497bd3cc8fc960a3557d2bad1eac5edde824505e15c9c8ebe4c260fd4d1...
Downloading: 100%
4.80M/4.80M [00:00<00:00, 7.49MB/s]
---------------------------------------------------------------------------
DuplicatedKeysError Traceback (most recent call last)
[/usr/local/lib/python3.7/dist-packages/datasets/builder.py](https://localhost:8080/#) in _prepare_split(self, split_generator)
1080 example = self.info.features.encode_example(record)
-> 1081 writer.write(example, key)
1082 finally:
8 frames
DuplicatedKeysError: FAILURE TO GENERATE DATASET !
Found duplicate Key: nt-639
Keys should be unique and deterministic in nature
During handling of the above exception, another exception occurred:
DuplicatedKeysError Traceback (most recent call last)
[/usr/local/lib/python3.7/dist-packages/datasets/arrow_writer.py](https://localhost:8080/#) in check_duplicate_keys(self)
449 for hash, key in self.hkey_record:
450 if hash in tmp_record:
--> 451 raise DuplicatedKeysError(key)
452 else:
453 tmp_record.add(hash)
DuplicatedKeysError: FAILURE TO GENERATE DATASET !
Found duplicate Key: nt-639
Keys should be unique and deterministic in nature
```
### Environment info
datasets version: 1.18.3
Platform: Google colab notebook
Python version: 3.7
PyArrow version: 6.0.1
| 3,770 |
https://github.com/huggingface/datasets/issues/3769 | `dataset = dataset.map()` causes faiss index lost | [
"Hi ! Indeed `map` is dropping the index right now, because one can create a dataset with more or fewer rows using `map` (and therefore the index might not be relevant anymore)\r\n\r\nI guess we could check the resulting dataset length, and if the user hasn't changed the dataset size we could keep the index, what do you think ?",
"doing `.add_column(\"x\",x_data)` also removes the index. the new column might be irrelevant to the index so I don't think it should drop. \r\n\r\nMinimal example\r\n\r\n```python\r\nfrom datasets import load_dataset\r\nimport numpy as np\r\n\r\ndata=load_dataset(\"ceyda/cats_vs_dogs_sample\") #just a test dataset\r\ndata=data[\"train\"]\r\nembd_data=data.map(lambda x: {\"emb\":np.random.uniform(-1,0,50).astype(np.float32)})\r\nembd_data.add_faiss_index(column=\"emb\")\r\nprint(embd_data.list_indexes())\r\nembd_data=embd_data.add_column(\"x\",[0]*data.num_rows)\r\nprint(embd_data.list_indexes())\r\n```",
"I agree `add_column` shouldn't drop the index indeed ! Is it something you'd like to contribute ? I think it's just a matter of copying the `self._indexes` dictionary to the output dataset"
] | ## Describe the bug
assigning the resulted dataset to original dataset causes lost of the faiss index
## Steps to reproduce the bug
`my_dataset` is a regular loaded dataset. It's a part of a customed dataset structure
```python
self.dataset.add_faiss_index('embeddings')
self.dataset.list_indexes()
# ['embeddings']
dataset2 = my_dataset.map(
lambda x: self._get_nearest_examples_batch(x['text']), batch=True
)
# the unexpected result:
dataset2.list_indexes()
# []
self.dataset.list_indexes()
# ['embeddings']
```
in case something wrong with my `_get_nearest_examples_batch()`, it's like this
```python
def _get_nearest_examples_batch(self, examples, k=5):
queries = embed(examples)
scores_batch, retrievals_batch = self.dataset.get_nearest_examples_batch(self.faiss_column, queries, k)
return {
'neighbors': [batch['text'] for batch in retrievals_batch],
'scores': scores_batch
}
```
## Expected results
`map` shouldn't drop the indexes, in another word, indexes should be carried to the generated dataset
## Actual results
map drops the indexes
## Environment info
<!-- You can run the command `datasets-cli env` and copy-and-paste its output below. -->
- `datasets` version: 1.18.3
- Platform: Ubuntu 20.04.3 LTS
- Python version: 3.8.12
- PyArrow version: 7.0.0
| 3,769 |
https://github.com/huggingface/datasets/issues/3764 | ! | [] | ## Dataset viewer issue for '*name of the dataset*'
**Link:** *link to the dataset viewer page*
*short description of the issue*
Am I the one who added this dataset ? Yes-No
| 3,764 |
https://github.com/huggingface/datasets/issues/3763 | It's not possible download `20200501.pt` dataset | [
"Hi @jvanz, thanks for reporting.\r\n\r\nPlease note that Wikimedia website does not longer host Wikipedia dumps for so old dates.\r\n\r\nFor a list of accessible dump dates of `pt` Wikipedia, please see: https://dumps.wikimedia.org/ptwiki/\r\n\r\nYou can load for example `20220220` `pt` Wikipedia:\r\n```python\r\ndataset = load_dataset(\"wikipedia\", language=\"pt\", date=\"20220220\", beam_runner=\"DirectRunner\")\r\n```",
"> ```python\r\n> dataset = load_dataset(\"wikipedia\", language=\"pt\", date=\"20220220\", beam_runner=\"DirectRunner\")\r\n> ```\r\n\r\nThank you! I did not know that I can do this. I was following the example in the error message when I do not define which language dataset I'm trying to download.\r\n\r\nI've tried something similar changing the date in the `load_dataset` call that I've shared in the bug description. Obviously, it did not work. I need to read the docs more carefully next time. My bad!\r\n\r\nThanks again and sorry for the noise.\r\n\r\n"
] | ## Describe the bug
The dataset `20200501.pt` is broken.
The available datasets: https://dumps.wikimedia.org/ptwiki/
## Steps to reproduce the bug
```python
from datasets import load_dataset
dataset = load_dataset("wikipedia", "20200501.pt", beam_runner='DirectRunner')
```
## Expected results
I expect to download the dataset locally.
## Actual results
```
>>> from datasets import load_dataset
>>> dataset = load_dataset("wikipedia", "20200501.pt", beam_runner='DirectRunner')
Downloading and preparing dataset wikipedia/20200501.pt to /home/jvanz/.cache/huggingface/datasets/wikipedia/20200501.pt/1.0.0/009f923d9b6dd00c00c8cdc7f408f2b47f45dd4f5fb7982a21f9448f4afbe475...
/home/jvanz/anaconda3/envs/tf-gpu/lib/python3.9/site-packages/apache_beam/__init__.py:79: UserWarning: This version of Apache Beam has not been sufficiently tested on Python 3.9. You may encounter bugs or missing features.
warnings.warn(
0%| | 0/1 [00:00<?, ?it/s]
Traceback (most recent call last):
File "<stdin>", line 1, in <module>
File "/home/jvanz/anaconda3/envs/tf-gpu/lib/python3.9/site-packages/datasets/load.py", line 1702, in load_dataset
builder_instance.download_and_prepare(
File "/home/jvanz/anaconda3/envs/tf-gpu/lib/python3.9/site-packages/datasets/builder.py", line 594, in download_and_prepare
self._download_and_prepare(
File "/home/jvanz/anaconda3/envs/tf-gpu/lib/python3.9/site-packages/datasets/builder.py", line 1245, in _download_and_prepare
super()._download_and_prepare(
File "/home/jvanz/anaconda3/envs/tf-gpu/lib/python3.9/site-packages/datasets/builder.py", line 661, in _download_and_prepare
split_generators = self._split_generators(dl_manager, **split_generators_kwargs)
File "/home/jvanz/.cache/huggingface/modules/datasets_modules/datasets/wikipedia/009f923d9b6dd00c00c8cdc7f408f2b47f45dd4f5fb7982a21f9448f4afbe475/wikipedia.py", line 420, in _split_generators
downloaded_files = dl_manager.download_and_extract({"info": info_url})
File "/home/jvanz/anaconda3/envs/tf-gpu/lib/python3.9/site-packages/datasets/utils/download_manager.py", line 307, in download_and_extract
return self.extract(self.download(url_or_urls))
File "/home/jvanz/anaconda3/envs/tf-gpu/lib/python3.9/site-packages/datasets/utils/download_manager.py", line 195, in download
downloaded_path_or_paths = map_nested(
File "/home/jvanz/anaconda3/envs/tf-gpu/lib/python3.9/site-packages/datasets/utils/py_utils.py", line 260, in map_nested
mapped = [
File "/home/jvanz/anaconda3/envs/tf-gpu/lib/python3.9/site-packages/datasets/utils/py_utils.py", line 261, in <listcomp>
_single_map_nested((function, obj, types, None, True))
File "/home/jvanz/anaconda3/envs/tf-gpu/lib/python3.9/site-packages/datasets/utils/py_utils.py", line 196, in _single_map_nested
return function(data_struct)
File "/home/jvanz/anaconda3/envs/tf-gpu/lib/python3.9/site-packages/datasets/utils/download_manager.py", line 216, in _download
return cached_path(url_or_filename, download_config=download_config)
File "/home/jvanz/anaconda3/envs/tf-gpu/lib/python3.9/site-packages/datasets/utils/file_utils.py", line 298, in cached_path
output_path = get_from_cache(
File "/home/jvanz/anaconda3/envs/tf-gpu/lib/python3.9/site-packages/datasets/utils/file_utils.py", line 612, in get_from_cache
raise FileNotFoundError(f"Couldn't find file at {url}")
FileNotFoundError: Couldn't find file at https://dumps.wikimedia.org/ptwiki/20200501/dumpstatus.json
```
## Environment info
```
- `datasets` version: 1.18.3
- Platform: Linux-5.3.18-150300.59.49-default-x86_64-with-glibc2.31
- Python version: 3.9.7
- PyArrow version: 6.0.1
``` | 3,763 |
https://github.com/huggingface/datasets/issues/3762 | `Dataset.class_encode` should support custom class names | [
"Hi @Dref360, thanks a lot for your proposal.\r\n\r\nIt totally makes sense to have more flexibility when class encoding, I agree.\r\n\r\nYou could even further customize the class encoding by passing an instance of `ClassLabel` itself (instead of replicating `ClassLabel` instantiation arguments as `Dataset.class_encode_column` arguments).\r\n\r\nAnd the latter made me think of `Dataset.cast_column`...\r\n\r\nMaybe better to have some others' opinions @lhoestq @mariosasko ",
"Hi @Dref360! You can use [`Dataset.align_labels_with_mapping`](https://huggingface.co/docs/datasets/master/package_reference/main_classes.html#datasets.Dataset.align_labels_with_mapping) after `Dataset.class_encode_column` to assign a different mapping of labels to ids.\r\n\r\n@albertvillanova I'd like to avoid adding more complexity to the API where it's not (absolutely) needed, so I don't think introducing a new param in `Dataset.class_encode_column` is a good idea.\r\n\r\n",
"I wasn't aware that it existed thank you for the link.\n\nClosing then! "
] | I can make a PR, just wanted approval before starting.
**Is your feature request related to a problem? Please describe.**
It is often the case that classes are not ordered in alphabetical order. Current `class_encode_column` sort the classes before indexing.
https://github.com/huggingface/datasets/blob/master/src/datasets/arrow_dataset.py#L1235
**Describe the solution you'd like**
I would like to add a **optional** parameter `class_names` to `class_encode_column` that would be used for the mapping instead of sorting the unique values.
**Describe alternatives you've considered**
One can use map instead. I find it harder to read.
```python
CLASS_NAMES = ['apple', 'orange', 'potato']
ds = ds.map(lambda item: CLASS_NAMES.index(item[label_column]))
# Proposition
ds = ds.class_encode_column(label_column, CLASS_NAMES)
```
**Additional context**
I can make the PR if this feature is accepted.
| 3,762 |
https://github.com/huggingface/datasets/issues/3761 | Know your data for HF hub | [
"Hi @Muhtasham you should take a look at https://huggingface.co/blog/data-measurements-tool and accompanying demo app at https://huggingface.co/spaces/huggingface/data-measurements-tool\r\n\r\nWe would be interested in your feedback. cc @meg-huggingface @sashavor @yjernite "
] | **Is your feature request related to a problem? Please describe.**
Would be great to see be able to understand datasets with the goal of improving data quality, and helping mitigate fairness and bias issues.
**Describe the solution you'd like**
Something like https://knowyourdata.withgoogle.com/ for HF hub | 3,761 |
https://github.com/huggingface/datasets/issues/3760 | Unable to view the Gradio flagged call back dataset | [
"Hi @kingabzpro.\r\n\r\nI think you need to create a loading script that creates the dataset from the CSV file and the image paths.\r\n\r\nAs example, you could have a look at the Food-101 dataset: https://huggingface.co/datasets/food101\r\n- Loading script: https://huggingface.co/datasets/food101/blob/main/food101.py\r\n\r\nOnce the loading script is created, the viewer will show a previsualization of your dataset. ",
"@albertvillanova I don't think this is the issue. I have created another dataset with similar files and format and it works. https://huggingface.co/datasets/kingabzpro/savtadepth-flags-V2",
"Yes, you are right, that was not the issue.\r\n\r\nJust take into account that sometimes the viewer can take some time until it shows the preview of the dataset.\r\nAfter some time, yours is finally properly shown: https://huggingface.co/datasets/kingabzpro/savtadepth-flags",
"The problem was resolved by deleted the dataset and creating new one with similar name and then clicking on flag button.",
"I think if you make manual changes to dataset the whole system breaks. "
] | ## Dataset viewer issue for '*savtadepth-flags*'
**Link:** *[savtadepth-flags](https://huggingface.co/datasets/kingabzpro/savtadepth-flags)*
*with the Gradio 2.8.1 the dataset viers stopped working. I tried to add values manually but its not working. The dataset is also not showing the link with the app https://huggingface.co/spaces/kingabzpro/savtadepth.*
Am I the one who added this dataset ? Yes
| 3,760 |
https://github.com/huggingface/datasets/issues/3758 | head_qa file missing | [
"We usually find issues with files hosted at Google Drive...\r\n\r\nIn this case we download the Google Drive Virus scan warning instead of the data file.",
"Fixed: https://huggingface.co/datasets/head_qa/viewer/en/train. Thanks\r\n\r\n<img width=\"1551\" alt=\"Capture d’écran 2022-02-28 à 15 29 04\" src=\"https://user-images.githubusercontent.com/1676121/156000224-fd3f62c6-8b54-4df1-8911-bdcb0bac3f1a.png\">\r\n"
] | ## Describe the bug
A file for the `head_qa` dataset is missing (https://drive.google.com/u/0/uc?export=download&id=1a_95N5zQQoUCq8IBNVZgziHbeM-QxG2t/HEAD_EN/train_HEAD_EN.json)
## Steps to reproduce the bug
```python
>>> from datasets import load_dataset
>>> load_dataset("head_qa", name="en")
```
## Expected results
The dataset should be loaded
## Actual results
```
Downloading and preparing dataset head_qa/en (download: 75.69 MiB, generated: 2.69 MiB, post-processed: Unknown size, total: 78.38 MiB) to /home/slesage/.cache/huggingface/datasets/head_qa/en/1.1.0/583ab408e8baf54aab378c93715fadc4d8aa51b393e27c3484a877e2ac0278e9...
Downloading data: 2.21kB [00:00, 2.05MB/s]
Traceback (most recent call last):
File "<stdin>", line 1, in <module>
File "/home/slesage/hf/datasets-preview-backend/.venv/lib/python3.9/site-packages/datasets/load.py", line 1729, in load_dataset
builder_instance.download_and_prepare(
File "/home/slesage/hf/datasets-preview-backend/.venv/lib/python3.9/site-packages/datasets/builder.py", line 594, in download_and_prepare
self._download_and_prepare(
File "/home/slesage/hf/datasets-preview-backend/.venv/lib/python3.9/site-packages/datasets/builder.py", line 665, in _download_and_prepare
verify_checksums(
File "/home/slesage/hf/datasets-preview-backend/.venv/lib/python3.9/site-packages/datasets/utils/info_utils.py", line 40, in verify_checksums
raise NonMatchingChecksumError(error_msg + str(bad_urls))
datasets.utils.info_utils.NonMatchingChecksumError: Checksums didn't match for dataset source files:
['https://drive.google.com/u/0/uc?export=download&id=1a_95N5zQQoUCq8IBNVZgziHbeM-QxG2t']
```
## Environment info
- `datasets` version: 1.18.4.dev0
- Platform: Linux-5.11.0-1028-aws-x86_64-with-glibc2.31
- Python version: 3.9.6
- PyArrow version: 6.0.1
| 3,758 |
https://github.com/huggingface/datasets/issues/3756 | Images get decoded when using `map()` with `input_columns` argument on a dataset | [
"Hi! If I'm not mistaken, this behavior is intentional, but I agree it could be more intuitive.\r\n\r\n@albertvillanova Do you remember why you decided not to decode columns in the `Audio` feature PR when `input_columns` is not `None`? IMO we should decode those columns, and we don't even have to use lazy structures here because the user explicitly requires them in the map transform. \r\n\r\ncc @lhoestq for visibility",
"I think I excluded to decorate the function when `input_columns` were passed as a quick fix for some non-passing tests: \r\n- https://github.com/huggingface/datasets/pull/2324/commits/9d7c3e8fa53e23ec636859b4407eeec904b1b3f9\r\n\r\nThat PR was quite complex and I decided to focus on the main feature requests, leaving refinements for subsequent PRs.\r\n\r\nNote that when `input_columns` are passed, the signature of the function is effectively changed, while the decorated function expects an item (whether an example or a batch) as first arg (which is not the case when passing `input_columns`.\r\n\r\nI agree we should consider supporting the case when `input_columns` are passed."
] | ## Describe the bug
The `datasets.features.Image` feature class decodes image data by default. Expectedly, when indexing a dataset or using the `map()` method, images are returned as PIL Image instances.
However, when calling `map()` and setting a specific data column with the `input_columns` argument, the image data is passed as raw byte representation to the mapping function.
## Steps to reproduce the bug
```python
from datasets import load_dataset
from torchvision import transforms
from PIL.Image import Image
dataset = load_dataset('mnist', split='train')
def transform_all_columns(example):
# example['image'] is encoded as PIL Image
assert isinstance(example['image'], Image)
return example
def transform_image_column(image):
# image is decoded here and represented as raw bytes
assert isinstance(image, Image)
return image
# single-sample dataset for debugging purposes
dev = dataset.select([0])
dev.map(transform_all_columns)
dev.map(transform_image_column, input_columns='image')
```
## Expected results
Image data should be passed in decoded form, i.e. as PIL Image objects to the mapping function unless the `decode` attribute on the image feature is set to `False`.
## Actual results
The mapping function receives images as raw byte data.
## Environment info
- `datasets` version: 1.18.3
- Platform: Linux-5.11.0-49-generic-x86_64-with-glibc2.32
- Python version: 3.8.0b4
- PyArrow version: 7.0.0
| 3,756 |
https://github.com/huggingface/datasets/issues/3755 | Cannot preview dataset | [
"Thanks for reporting. The dataset viewer depends on some backend treatments, and for now, they might take some hours to get processed. We're working on improving it.",
"It has finally been processed. Thanks for the patience.",
"Thanks for the info @severo !"
] | ## Dataset viewer issue for '*rubrix/news*'
**Link:https://huggingface.co/datasets/rubrix/news** *link to the dataset viewer page*
Cannot see the dataset preview:
```
Status code: 400
Exception: Status400Error
Message: Not found. Cache is waiting to be refreshed.
```
Am I the one who added this dataset ? No
| 3,755 |
https://github.com/huggingface/datasets/issues/3754 | Overflowing indices in `select` | [
"Fixed on master (see https://github.com/huggingface/datasets/pull/3719).",
"Awesome, I did not find that one! Thanks."
] | ## Describe the bug
The `Dataset.select` function seems to accept indices that are larger than the dataset size and seems to effectively use `index %len(ds)`.
## Steps to reproduce the bug
```python
from datasets import Dataset
ds = Dataset.from_dict({"test": [1,2,3]})
ds = ds.select(range(5))
print(ds)
print()
print(ds["test"])
```
Result:
```python
Dataset({
features: ['test'],
num_rows: 5
})
[1, 2, 3, 1, 2]
```
This behaviour is not documented and can lead to unexpected behaviour when for example taking a sample larger than the dataset and thus creating a lot of duplicates.
## Expected results
It think this should throw an error or at least a very big warning:
```python
IndexError: Invalid key: 5 is out of bounds for size 3
```
## Environment info
- `datasets` version: 1.18.3
- Platform: macOS-12.0.1-x86_64-i386-64bit
- Python version: 3.9.10
- PyArrow version: 7.0.0 | 3,754 |
https://github.com/huggingface/datasets/issues/3753 | Expanding streaming capabilities | [
"Related to: https://github.com/huggingface/datasets/issues/3444",
"Cool ! `filter` will be very useful. There can be a filter that you can apply on a streaming dataset:\r\n```python\r\nload_dataset(..., streaming=True).filter(lambda x: x[\"lang\"] == \"sw\")\r\n```\r\n\r\nOtherwise if you want to apply a filter on the source files that are going to be used for streaming, the logic has to be impIemented directly in the dataset script, or if there's no dataset script this can be done with pattern matching\r\n```python\r\nload_dataset(..., lang=\"sw\") # if the dataset script supports this parameter\r\nload_dataset(..., data_files=\"data/lang=sw/*\") # if there's no dataset script, but only data files\r\n```\r\n\r\n--------------\r\n\r\nHere are also some additional ideas of API to convert from iterable to map-style dataset:\r\n```python\r\non_disk_dataset = streaming_dataset.to_disk()\r\non_disk_dataset = streaming_dataset.to_disk(path=\"path/to/my/dataset/dir\")\r\n\r\nin_memory_dataset = streaming_dataset.take(100).to_memory() # to experiment without having to write files\r\n```\r\n--------------\r\n\r\nFinally regarding `push_to_hub`, we can replace `batch_size` by `shard_size` (same API as for on-disk datasets). The default is 500MB per file\r\n\r\nLet me know what you think !",
"Regarding conversion, I'd also ask for some kind of equivalent to `save_to_disk` for an `IterableDataset`.\r\n\r\nSimilarly to the streaming to hub idea, my use case would be to define a sequence of dataset transforms via `.map()`, using an `IterableDataset` as the input (so processing could start without doing whole download up-front), but streaming the resultant processed dataset just to disk.",
"That makes sense @athewsey , thanks for the suggestion :)\r\n\r\nMaybe instead of the `to_disk` we could simply have `save_to_disk` instead:\r\n```python\r\nstreaming_dataset.save_to_disk(\"path/to/my/dataset/dir\")\r\non_disk_dataset = load_from_disk(\"path/to/my/dataset/dir\")\r\n\r\nin_memory_dataset = Dataset.from_list(list(streaming_dataset.take(100))) # to experiment without having to write files\r\n```",
"Any updates on this?",
"So far are implemented: `IterableDataset.filter()` and `Dataset.to_iterable_dataset()`.\r\n\r\nStill missing: `IterableDataset.push_to_hub()` - though there is a hack to write on disk and then push to hub using\r\n\r\n```python\r\nds_on_disk = Dataset.from_generator(streaming_ds.__iter__) # stream to disk\r\nds_on_disk.push_to_hub(...)\r\n```"
] | Some ideas for a few features that could be useful when working with large datasets in streaming mode.
## `filter` for `IterableDataset`
Adding filtering to streaming datasets would be useful in several scenarios:
- filter a dataset with many languages for a subset of languages
- filter a dataset for specific licenses
- other custom logic to get a subset
The only way to achieve this at the moment is I think through writing a custom loading script and implementing filters there.
## `IterableDataset` to `Dataset` conversion
In combination with the above filter a functionality to "play" the whole stream would be useful. The motivation is that often one might filter the dataset to get a manageable size for experimentation. In that case streaming mode is no longer necessary as the filtered dataset is small enough and it would be useful to be able to play through the whole stream to create a normal `Dataset` with all its benefits.
```python
ds = load_dataset("some_large_dataset", streaming=True)
ds_filter = ds.filter(lambda x: x["lang"]="fr")
ds_filter = ds_filter.stream() # here the `IterableDataset` is converted to a `Dataset`
```
Naturally, this could be expanded with `stream(n=1000)` which creates a `Dataset` with the first `n` elements similar to `take`.
## Stream to the Hub
While streaming allows to use a dataset as is without saving the whole dataset on the local machine it is currently not possible to process a dataset and add it to the hub. The only way to do this is by downloading the full dataset and saving the processed dataset again before pushing them to the hub. The API could looks something like:
```python
ds = load_dataset("some_large_dataset", streaming=True)
ds_filter = ds.filter(some_filter_func)
ds_processed = ds_filter.map(some_processing_func)
ds_processed.push_to_hub("new_better_dataset", batch_size=100_000)
```
Under the hood this could be done by processing and aggregating `batch_size` elements and then pushing that batch as a single file to the hub. With this functionality one could process and create TB scale datasets while only requiring size of `batch_size` local disk space.
cc @lhoestq @albertvillanova | 3,753 |
https://github.com/huggingface/datasets/issues/3750 | `NonMatchingSplitsSizesError` for cats_vs_dogs dataset | [
"Thnaks for reporting @jaketae. We are fixing it. "
] | ## Describe the bug
Cannot download cats_vs_dogs dataset due to `NonMatchingSplitsSizesError`.
## Steps to reproduce the bug
```python
from datasets import load_dataset
dataset = load_dataset("cats_vs_dogs")
```
## Expected results
Loading is successful.
## Actual results
```
NonMatchingSplitsSizesError: [{'expected': SplitInfo(name='train', num_bytes=7503250, num_examples=23422, dataset_name='cats_vs_dogs'), 'recorded': SplitInfo(name='train', num_bytes=7262410, num_examples=23410, dataset_name='cats_vs_dogs')}]
```
## Environment info
Reproduced on a fresh [Colab notebook](https://colab.research.google.com/drive/13GTvrSJbBGvL2ybDdXCBZwATd6FOkMub?usp=sharing).
## Additional Context
Originally reported in https://github.com/huggingface/transformers/issues/15698.
cc @mariosasko | 3,750 |
https://github.com/huggingface/datasets/issues/3747 | Passing invalid subset should throw an error | [] | ## Describe the bug
Only some datasets have a subset (as in `load_dataset(name, subset)`). If you pass an invalid subset, an error should be thrown.
## Steps to reproduce the bug
```python
import datasets
datasets.load_dataset('rotten_tomatoes', 'asdfasdfa')
```
## Expected results
This should break, since `'asdfasdfa'` isn't a subset of the `rotten_tomatoes` dataset.
## Actual results
This API call silently succeeds. | 3,747 |
https://github.com/huggingface/datasets/issues/3744 | Better shards shuffling in streaming mode | [] | Sometimes a dataset script has a `_split_generators` that returns several files as well as the corresponding metadata of each file. It often happens that they end up in two separate lists in the `gen_kwargs`:
```python
gen_kwargs = {
"files": [os.path.join(data_dir, filename) for filename in all_files],
"metadata_files": [all_metadata[filename] for filename in all_files],
}
```
It happened for Multilingual Spoken Words for example in #3666
However currently **the two lists are shuffled independently** when shuffling the shards in streaming mode. This leads to `_generate_examples` not having the right metadata for each file.
To prevent this issue I suggest that we always shuffle lists of the same length the exact same way to avoid such a big but silent issue.
cc @polinaeterna | 3,744 |
https://github.com/huggingface/datasets/issues/3739 | Pubmed dataset does not work in streaming mode | [
"Thanks for reporting, @abhi-mosaic (related to #3655).\r\n\r\nPlease note that `xml.etree.ElementTree.parse` already supports streaming:\r\n- #3476\r\n\r\nNo need to refactor to use `open`/`xopen`. Is is enough with importing the package `as ET` (instead of `as etree`)."
] | ## Describe the bug
Trying to use the `pubmed` dataset with `streaming=True` fails.
## Steps to reproduce the bug
```python
import datasets
pubmed_train = datasets.load_dataset('pubmed', split='train', streaming=True)
print (next(iter(pubmed_train)))
```
## Expected results
I would expect to see the first training sample from the pubmed dataset.
## Actual results
```
Traceback (most recent call last):
File "<stdin>", line 1, in <module>
File "/Users/abhinav/Documents/mosaicml/mosaicml_venv/lib/python3.8/site-packages/datasets/iterable_dataset.py", line 367, in __iter__
for key, example in self._iter():
File "/Users/abhinav/Documents/mosaicml/mosaicml_venv/lib/python3.8/site-packages/datasets/iterable_dataset.py", line 364, in _iter
yield from ex_iterable
File "/Users/abhinav/Documents/mosaicml/mosaicml_venv/lib/python3.8/site-packages/datasets/iterable_dataset.py", line 79, in __iter__
for key, example in self.generate_examples_fn(**self.kwargs):
File "/Users/abhinav/.cache/huggingface/modules/datasets_modules/datasets/pubmed/9715addf10c42a7877a2149ae0c5f2fddabefc775cd1bd9b03ac3f012b86ce46/pubmed.py", line 373, in _generate_examples
tree = etree.parse(filename)
File "/Library/Developer/CommandLineTools/Library/Frameworks/Python3.framework/Versions/3.8/lib/python3.8/xml/etree/ElementTree.py", line 1202, in parse
tree.parse(source, parser)
File "/Library/Developer/CommandLineTools/Library/Frameworks/Python3.framework/Versions/3.8/lib/python3.8/xml/etree/ElementTree.py", line 584, in parse
source = open(source, "rb")
FileNotFoundError: [Errno 2] No such file or directory: 'gzip://pubmed21n0001.xml::ftp://ftp.ncbi.nlm.nih.gov/pubmed/baseline/pubmed21n0001.xml.gz'
```
## Environment info
<!-- You can run the command `datasets-cli env` and copy-and-paste its output below. -->
- `datasets` version: 1.18.2
- Platform: macOS-11.4-x86_64-i386-64bit
- Python version: 3.8.2
- PyArrow version: 6.0.0
## Comments
The error looks like an issue with `open` vs. `xopen` inside the `xml` package. It looks like it's trying to open the remote source URL, which has been edited with prefix `gzip://...`.
Maybe there can be an explicit `xopen` before passing the raw data to `etree`, something like:
```python
# Before
tree = etree.parse(filename)
root = tree.getroot()
# After
with xopen(filename) as f:
data_str = f.read()
root = etree.fromstring(data_str)
``` | 3,739 |
https://github.com/huggingface/datasets/issues/3738 | For data-only datasets, streaming and non-streaming don't behave the same | [
"Note that we might change the heuristic and create a different config per file, at least in that case.",
"Hi @severo, thanks for reporting.\r\n\r\nYes, this happens because when non-streaming, a cast of all data is done in order to \"concatenate\" it all into a single dataset (thus the error), while this casting is not done while yielding item by item in streaming mode.\r\n\r\nMaybe in streaming mode we should keep the schema (inferred from the first item) and throw an exception if a subsequent item does not conform to the inferred schema?",
"Why do we want to concatenate the files? Is it the expected behavior for most datasets that lack a script and dataset info?",
"These files are two different dataset configurations since they don't share the same schema.\r\n\r\nIMO the streaming mode should fail in this case, as @albertvillanova said.\r\n\r\nThere is one challenge though: inferring the schema from the first example is not robust enough in the general case - especially if some fields are nullable. I guess we can at least make sure that no new columns are added",
"OK. So, if we make the streaming also fail, the dataset https://huggingface.co/datasets/huggingface/transformers-metadata will never be [viewable](https://github.com/huggingface/datasets-preview-backend/issues/144) (be it using streaming or fallback to downloading the files), right?\r\n",
"Yes, until we have a way for the user to specify explicitly that those two files are different configurations.\r\n\r\nWe can maybe have some rule to detect this automatically, maybe checking the first line of each file ? That would mean that for dataset of 10,000+ files we would have to verify every single one of them just to know if there is one ore more configurations, so I'm not sure if this is a good idea",
"i think requiring the user to specify that those two files are different configurations is in that case perfectly reasonable.\r\n\r\n(Maybe at some point we could however detect this type of case and prompt them to define a config mapping etc)",
"OK, so, before closing the issue, what do you think should be done?\r\n\r\n> Maybe in streaming mode we should keep the schema (inferred from the first item) and throw an exception if a subsequent item does not conform to the inferred schema?\r\n\r\nor nothing?",
"We should at least raise an error if a new sample has column names that are missing, or if it has extra columns. No need to check for the type for now.\r\n\r\nI'm in favor of having an error especially because we want to avoid silent issues as much as possible - i.e. when something goes wrong (when schemas don't match or some data are missing) and no errors/warnings are raised.\r\n\r\nConsistency between streaming and non-streaming is also important."
] | See https://huggingface.co/datasets/huggingface/transformers-metadata: it only contains two JSON files.
In streaming mode, the files are concatenated, and thus the rows might be dictionaries with different keys:
```python
import datasets as ds
iterable_dataset = ds.load_dataset("huggingface/transformers-metadata", split="train", streaming=True);
rows = list(iterable_dataset.take(100))
rows[0]
# {'model_type': 'albert', 'pytorch': True, 'tensorflow': True, 'flax': True, 'processor': 'AutoTokenizer'}
rows[99]
# {'model_class': 'BartModel', 'pipeline_tag': 'feature-extraction', 'auto_class': 'AutoModel'}
```
In normal mode, an exception is thrown:
```python
import datasets as ds
dataset = ds.load_dataset("huggingface/transformers-metadata", split="train");
```
```
ValueError: Couldn't cast
model_class: string
pipeline_tag: string
auto_class: string
to
{'model_type': Value(dtype='string', id=None), 'pytorch': Value(dtype='bool', id=None), 'tensorflow': Value(dtype='bool', id=None), 'flax': Value(dtype='bool', id=None), 'processor': Value(dtype='string', id=None)}
because column names don't match
``` | 3,738 |
https://github.com/huggingface/datasets/issues/3735 | Performance of `datasets` at scale | [
"> using command line git-lfs - [...] 300MB/s!\r\n\r\nwhich server location did you upload from?",
"From GCP region `us-central1-a`.",
"The most surprising part to me is the saving time. Wondering if it could be due to compression (`ParquetWriter` uses SNAPPY compression by default; it can be turned off with `to_parquet(..., compression=None)`). ",
"+1 to what @mariosasko mentioned. Also, @lvwerra did you parallelize `to_parquet` using similar approach in #2747? (we used multiprocessing at the shard level). I'm working on a similar PR to add multi_proc in `to_parquet` which might give you further speed up. \r\nStas benchmarked his approach and mine in this [gist](https://gist.github.com/stas00/dc1597a1e245c5915cfeefa0eee6902c) for `lama` dataset when we were working on adding multi_proc support for `to_json`.",
"@mariosasko I did not turn it off but I can try the next time - I have to run the pipeline again, anyway. \r\n\r\n@bhavitvyamalik Yes, I also sharded the dataset and used multiprocessing to save each shard. I'll have a closer look at your approach, too.",
"Is there a way to read from the cache files directly as a dataset in its own"
] | # Performance of `datasets` at 1TB scale
## What is this?
During the processing of a large dataset I monitored the performance of the `datasets` library to see if there are any bottlenecks. The insights of this analysis could guide the decision making to improve the performance of the library.
## Dataset
The dataset is a 1.1TB extract from GitHub with 120M code files and is stored as 5000 `.json.gz` files. The goal of the preprocessing is to remove duplicates and filter files based on their stats. While the calculating of the hashes for deduplication and stats for filtering can be parallelized the filtering itself is run with a single process. After processing the files are pushed to the hub.
## Machine
The experiment was run on a `m1` machine on GCP with 96 CPU cores and 1.3TB RAM.
## Performance breakdown
- Loading the data **3.5h** (_30sec_ from cache)
- **1h57min** single core loading (not sure what is going on here, corresponds to second progress bar)
- **1h10min** multi core json reading
- **20min** remaining time before and after the two main processes mentioned above
- Process the data **2h** (_20min_ from cache)
- **20min** Getting reading for processing
- **40min** Hashing and files stats (96 workers)
- **58min** Deduplication filtering (single worker)
- Save parquet files **5h**
- Saving 1000 parquet files (16 workers)
- Push to hub **37min**
- **34min** git add
- **3min** git push (several hours with `Repository.git_push()`)
## Conclusion
It appears that loading and saving the data is the main bottleneck at that scale (**8.5h**) whereas processing (**2h**) and pushing the data to the hub (**0.5h**) is relatively fast. To optimize the performance at this scale it would make sense to consider such an end-to-end example and target the bottlenecks which seem to be loading from and saving to disk. The processing itself seems to run relatively fast.
## Notes
- map operation on a 1TB dataset with 96 workers requires >1TB RAM
- map operation does not maintain 100% CPU utilization with 96 workers
- sometimes when the script crashes all the data files have a corresponding `*.lock` file in the data folder (or multiple e.g. `*.lock.lock` when it happened a several times). This causes the cache **not** to be triggered (which is significant at that scale) - i guess because there are new data files
- parallelizing `to_parquet` decreased the saving time from 17h to 5h, however adding more workers at this point had almost no effect. not sure if this is:
a) a bug in my parallelization logic,
b) i/o limit to load data form disk to memory or
c) i/o limit to write from memory to disk.
- Using `Repository.git_push()` was much slower than using command line `git-lfs` - 10-20MB/s vs. 300MB/s! The `Dataset.push_to_hub()` function is even slower as it only uploads one file at a time with only a few MB/s, whereas `Repository.git_push()` pushes files in parallel (each at a similar speed).
cc @lhoestq @julien-c @LysandreJik @SBrandeis
| 3,735 |
https://github.com/huggingface/datasets/issues/3733 | Bugs in NewsQA dataset | [] | ## Describe the bug
NewsQA dataset has the following bugs:
- the field `validated_answers` is an exact copy of the field `answers` but with the addition of `'count': [0]` to each dict
- the field `badQuestion` does not appear in `answers` nor `validated_answers`
## Steps to reproduce the bug
By inspecting the dataset script we can see that:
- the parsing of `validated_answers` is a copy-paste of the one for `answers`
- the `badQuestion` field is ignored in the parsing of both `answers` and `validated_answers`
| 3,733 |
https://github.com/huggingface/datasets/issues/3730 | Checksum Error when loading multi-news dataset | [
"Thanks for reporting @byw2.\r\nWe are fixing it.\r\nIn the meantime, you can load the dataset by passing `ignore_verifications=True`:\r\n ```python\r\ndataset = load_dataset(\"multi_news\", ignore_verifications=True)"
] | ## Describe the bug
When using the load_dataset function from datasets module to load the Multi-News dataset, does not load the dataset but throws Checksum Error instead.
## Steps to reproduce the bug
```python
from datasets import load_dataset
dataset = load_dataset("multi_news")
```
## Expected results
Should download and load Multi-News dataset.
## Actual results
Throws the following error and cannot load data successfully:
```
NonMatchingChecksumError: Checksums didn't match for dataset source files:
['https://drive.google.com/uc?export=download&id=1vRY2wM6rlOZrf9exGTm5pXj5ExlVwJ0C']
```
Could this issue please be looked at? Thanks! | 3,730 |
https://github.com/huggingface/datasets/issues/3729 | Wrong number of examples when loading a text dataset | [
"Hi @kg-nlp, thanks for reporting.\r\n\r\nThat is weird... I guess we would need some sample data file where this behavior appears to reproduce the bug for further investigation... ",
"ok, I found the reason why that two results are not same.\r\nthere is /u2029 in the text, the datasets will split sentence according to the /u2029,but when I use open function will not do that .\r\nso I want to know which function shell do that\r\nthanks"
] | ## Describe the bug
when I use load_dataset to read a txt file I find that the number of the samples is incorrect
## Steps to reproduce the bug
```
fr = open('train.txt','r',encoding='utf-8').readlines()
print(len(fr)) # 1199637
datasets = load_dataset('text', data_files={'train': ['train.txt']}, streaming=False)
print(len(datasets['train'])) # 1199649
```
I also use command line operation to verify it
```
$ wc -l train.txt
1199637 train.txt
```
## Expected results
please fix that issue
## Environment info
<!-- You can run the command `datasets-cli env` and copy-and-paste its output below. -->
- `datasets` version: 1.8.3
- Platform:windows&linux
- Python version:3.7
- PyArrow version:6.0.1
| 3,729 |
https://github.com/huggingface/datasets/issues/3728 | VoxPopuli | [
"duplicate of https://github.com/huggingface/datasets/issues/2300"
] | ## Adding a Dataset
- **Name:** VoxPopuli
- **Description:** A Large-Scale Multilingual Speech Corpus
- **Paper:** https://arxiv.org/pdf/2101.00390.pdf
- **Data:** https://github.com/facebookresearch/voxpopuli
- **Motivation:** one of the largest (if not the largest) multilingual speech corpus: 400K hours of multilingual unlabeled speech + 17k hours of labeled speech
Instructions to add a new dataset can be found [here](https://github.com/huggingface/datasets/blob/master/ADD_NEW_DATASET.md).
👀 @kahne @Molugan
| 3,728 |
https://github.com/huggingface/datasets/issues/3724 | Bug while streaming CSV dataset with pandas 1.4 | [] | ## Describe the bug
If we upgrade to pandas `1.4`, the patching of the pandas module is no longer working
```
AttributeError: '_PatchedModuleObj' object has no attribute '__version__'
```
## Steps to reproduce the bug
```
pip install pandas==1.4
```
```python
from datasets import load_dataset
ds = load_dataset("lvwerra/red-wine", split="train", streaming=True)
item = next(iter(ds))
item
```
| 3,724 |
https://github.com/huggingface/datasets/issues/3720 | Builder Configuration Update Required on Common Voice Dataset | [
"Hi @aasem, thanks for reporting.\r\n\r\nPlease note that currently Commom Voice is hosted on our Hub as a community dataset by the Mozilla Foundation. See all Common Voice versions here: https://huggingface.co/mozilla-foundation\r\n\r\nMaybe we should add an explaining note in our \"legacy\" Common Voice canonical script? What do you think @lhoestq @mariosasko ?",
"Thank you, @albertvillanova, for the quick response. I am not sure about the exact flow but I guess adding the following lines under the `_Languages` dictionary definition in [common_voice.py](https://github.com/huggingface/datasets/blob/master/datasets/common_voice/common_voice.py) might resolve the issue. I guess the dataset is recently made available so the file needs updating.\r\n\r\n```\r\n\"ur\": {\r\n \"Language\": \"Urdu\",\r\n \"Date\": \"2022-01-19\",\r\n \"Size\": \"68 MB\",\r\n \"Version\": \"ur_3h_2022-01-19\",\r\n \"Validated_Hr_Total\": 1,\r\n \"Overall_Hr_Total\": 3,\r\n \"Number_Of_Voice\": 48,\r\n },\r\n```\r\n",
"@aasem for compliance reasons, we are no longer updating the `common_voice.py` script.\r\n\r\nWe agreed with Mozilla Foundation to use their community datasets instead, which will ask you to accept their terms of use:\r\n```\r\nYou need to share your contact information to access this dataset.\r\n\r\nThis repository is publicly accessible, but you have to register to access its content — don't worry, it's just one click!\r\n\r\nBy clicking on “Access repository” below, you accept that your contact information (email address and username) can be shared with the repository authors. This will let the authors get in touch for instance if some parts of the repository's contents need to be taken down for licensing reasons.\r\n\r\nBy clicking on “Access repository” below, you also agree to not attempt to determine the identity of speakers in the Common Voice dataset.\r\n\r\nYou will immediately be granted access to the contents of the dataset. \r\n```\r\n\r\nIn order to use e.g. their Common Voice dataset version 8.0, please:\r\n- First visit their dataset page: https://huggingface.co/datasets/mozilla-foundation/common_voice_8_0\r\n- Accept their term of use by clicking \"Access repository\"\r\n- You can then load their dataset with:\r\n ```python\r\n load_dataset(\"mozilla-foundation/common_voice_8_0\", \"ur\", split=\"train+validation\")\r\n ```",
"@albertvillanova \r\n>Maybe we should add an explaining note in our \"legacy\" Common Voice canonical script?\r\n\r\nYes, I agree we should have a deprecation notice in the canonical script to redirect users to the new script.",
"@albertvillanova, \r\nI now get the following error after downloading my access token from the huggingface and passing it to `load_dataset` call:\r\n\r\n`AttributeError: 'DownloadManager' object has no attribute 'download_config'`\r\n\r\nAny quick pointer on how it might be resolved?",
"@aasem What version of `datasets` are you using? We renamed that attribute from `_download_config` to `download_conig` fairly recently, so updating to the newest version should resolve the issue:\r\n```\r\npip install -U datasets\r\n```",
"Thanks a lot, @mariosasko. That completely resolved the issue. "
] | Missing language in Common Voice dataset
**Link:** https://huggingface.co/datasets/common_voice
I tried to call the Urdu dataset using `load_dataset("common_voice", "ur", split="train+validation")` but couldn't due to builder configuration not found. I checked the source file here for the languages support:
https://github.com/huggingface/datasets/blob/master/datasets/common_voice/common_voice.py
and Urdu isn't included there. I assume a quick update will fix the issue as Urdu speech is now available at the Common Voice dataset.
Am I the one who added this dataset? No
| 3,720 |
https://github.com/huggingface/datasets/issues/3717 | wrong condition in `Features ClassLabel encode_example` | [
"Hi @Tudyx, \r\n\r\nPlease note that in Python, the boolean NOT operator (`not`) has lower precedence than comparison operators (`<=`, `<`), thus the expression you mention is equivalent to:\r\n```python\r\n not (-1 <= example_data < self.num_classes)\r\n```\r\n\r\nAlso note that as expected, the exception is raised if:\r\n- `example_data < -1`\r\n- or `example_data >= self.num_classes`\r\n\r\nThe raise of the exception is expected when `example_data` equals 4 and `self.num_classes` equals 4 too."
] | ## Describe the bug
The `encode_example` function in *features.py* seems to have a wrong condition.
```python
if not -1 <= example_data < self.num_classes:
raise ValueError(f"Class label {example_data:d} greater than configured num_classes {self.num_classes}")
```
## Expected results
The `not - 1` condition change the result of the condition. For instance, if `example_data` equals 4 and ` self.num_classes` equals 4 too, `example_data < self.num_classes` will give `False` as expected . But if i add the `not - 1` condition, `not -1 <= example_data < self.num_classes` will give `True` and raise an exception.
## Environment info
- `datasets` version: 1.18.3
- Python version: 3.8.10
- PyArrow version: 7.00
| 3,717 |
https://github.com/huggingface/datasets/issues/3716 | `FaissIndex` to support multiple GPU and `custom_index` | [
"Hi @rentruewang, thansk for reporting and for your PR!!! We should definitely support this. ",
"@albertvillanova Great! :)"
] | **Is your feature request related to a problem? Please describe.**
Currently, because `device` is of the type `int | None`, to leverage `faiss-gpu`'s multi-gpu support, you need to create a `custom_index`. However, if using a `custom_index` created by e.g. `faiss.index_cpu_to_all_gpus`, then `FaissIndex.save` does not work properly because it checks the device id (which is an int, so no multiple GPUs).
**Describe the solution you'd like**
I would like `FaissIndex` to support multiple GPUs, by passing in a list to `add_faiss_index`.
**Describe alternatives you've considered**
Alternatively, I would like it to at least provide a warning cause it wasn't the behavior that I expected.
**Additional context**
Relavent source code here:
https://github.com/huggingface/datasets/blob/6ed6ac9448311930557810383d2cfd4fe6aae269/src/datasets/search.py#L340-L349
Device management needs changing to support multiple GPUs, probably by `isinstance` calls.
I can provide a PR if you like :)
Thanks for reading!
| 3,716 |
https://github.com/huggingface/datasets/issues/3714 | tatoeba_mt: File not found error and key error | [
"Looks like I solved my problems ..."
] | ## Dataset viewer issue for 'tatoeba_mt'
**Link:** https://huggingface.co/datasets/Helsinki-NLP/tatoeba_mt
My data loader script does not seem to work.
The files are part of the local repository but cannot be found. An example where it should work is the subset for "afr-eng".
Another problem is that I do not have validation data for all subsets and I don't know how to properly check whether validation exists in the configuration before I try to download it. An example is the subset for "afr-deu".
Am I the one who added this dataset ? Yes
| 3,714 |
https://github.com/huggingface/datasets/issues/3708 | Loading JSON gets stuck with many workers/threads | [
"Hi ! Note that it does `block_size *= 2` until `block_size > len(batch)`, so it doesn't loop indefinitely. What do you mean by \"get stuck indefinitely\" then ? Is this the actual call to `paj.read_json` that hangs ?\r\n\r\n> increasing the `chunksize` argument decreases the chance of getting stuck\r\n\r\nCould you share the values of chunksize that you're using to observe this ? And maybe the order of magnitude of number of bytes per line of JSON ?",
"To clarify, I don't think it loops indefinitely but the `paj.read_json` gets stuck after the first try. That's why I think it could be an issue with a lock somewhere. \r\n\r\nUsing `load_dataset(..., chunksize=40<<20)` worked without errors.",
"@lhoestq I encountered another related issue. I use load_dataset() for my json data and set_transform() for preprocessing. But it hangs at the end of the epoch if `dataloader_num_workers>=1`. It appears to be working fine with num_worker=0, but it's slow.\r\n```\r\ntrain_dataset = datasets.load_dataset(\"json\", \r\n data_files=corpus_jsonl_path,\r\n keep_in_memory=False,\r\n cache_dir=model_args.cache_dir,\r\n streaming=False)\r\ntrain_dataset.set_transform(psg_parse_fn)\r\n```\r\n",
"I couldn't I think your problem is unrelated to this issue @memray\r\nIndeed this issue discusses a bug when doing `load_dataset`, while your case has to do with the dataloader in a multiprocessing setup. Can you open a new issue and provide more details (share your env and what psg_parse_fn does) ?",
"I also encountered a similar issue when loading a 190GB dataset of jsonl files (255 files with less than 1Gb) where it got stuck for over 20h at tables generation (fig below), increasing the `chunksize` with `load_dataset(..., chunksize=40<<20)` fixed the issue\r\n\r\n<img width=\"560\" alt=\"image\" src=\"https://user-images.githubusercontent.com/44069155/195605603-548a106e-7ad3-4269-8cdd-2ad3e975bf16.png\">\r\n",
"> @lhoestq I encountered another related issue. I use load_dataset() for my json data and set_transform() for preprocessing. But it hangs at the end of the epoch if `dataloader_num_workers>=1`. It appears to be working fine with num_worker=0, but it's slow.\r\n> \r\n> ```\r\n> train_dataset = datasets.load_dataset(\"json\", \r\n> data_files=corpus_jsonl_path,\r\n> keep_in_memory=False,\r\n> cache_dir=model_args.cache_dir,\r\n> streaming=False)\r\n> train_dataset.set_transform(psg_parse_fn)\r\n> ```\r\n\r\nIn case people also get this problem, I found a way to fix it by adding `persistent_workers=True` when initializing DataLoader, like:\r\n`train_loader = DataLoader(\r\n train_dataset,\r\n batch_size=self._train_batch_size,\r\n sampler=train_sampler,\r\n collate_fn=data_collator,\r\n num_workers=self.args.dataloader_num_workers,\r\n persistent_workers=True\r\n )`\r\n\r\nThe error was `CUDA error: initialization error Exception raised from insert_events at ../c10/cuda/CUDACachingAllocator.cpp:1266` after the 1st epoch, I guess it's because the data_loader worker is killed after each epoch and the data supply is cut off. This error only occurs when num_workers>1.\r\n\r\n\r\n",
"I can confirm the issue using datasets (2.12.0) with the following code and Accelerate (0.20.3) env:\r\n\r\n````\r\ntrainDataloader = DataLoader(trainSplit, batch_size=args.train_batch_size, shuffle=True)\r\nevalDataloader = DataLoader(validSplit, batch_size=args.valid_batch_size) // Here is where it gets stuck.\r\n````\r\n````\r\n- `Accelerate` version: 0.20.3\r\n- Platform: Linux-5.4.0-150-generic-x86_64-with-glibc2.29\r\n- Python version: 3.8.10\r\n- Numpy version: 1.24.3\r\n- PyTorch version (GPU?): 2.0.1+cu117 (True)\r\n- PyTorch XPU available: False\r\n- System RAM: 503.28 GB\r\n- GPU type: Tesla V100-SXM2-32GB\r\n- `Accelerate` default config:\r\n\t- compute_environment: LOCAL_MACHINE\r\n\t- distributed_type: MULTI_GPU\r\n\t- mixed_precision: fp16\r\n\t- use_cpu: False\r\n\t- num_processes: 2\r\n\t- machine_rank: 0\r\n\t- num_machines: 1\r\n\t- gpu_ids: 0,1\r\n\t- rdzv_backend: static\r\n\t- same_network: True\r\n\t- main_training_function: main\r\n\t- downcast_bf16: no\r\n\t- tpu_use_cluster: False\r\n\t- tpu_use_sudo: False\r\n\t- tpu_env: []\r\n````\r\n\r\nNotable that with Accelerate configured for one GPU only, **it doesn't get stuck.** \r\n\r\nThe suggestion made by @memray worked in my case. This is how it was applied: \r\n````\r\ntrainDataloader = DataLoader(trainSplit, batch_size=args.train_batch_size, shuffle=True, num_workers=2, persistent_workers=True)\r\nevalDataloader = DataLoader(validSplit, batch_size=args.valid_batch_size, num_workers=2, persistent_workers=True)\r\n````\r\n",
"I think your issue is related to `accelerate`, feel free to open an issue there: https://github.com/huggingface/accelerate/issues\r\n\r\n`Dataset` objects generally work fine with the torch DataLoader, idk what `accelerate` does that could make it get stuck."
] | ## Describe the bug
Loading a JSON dataset with `load_dataset` can get stuck when running on a machine with many CPUs. This is especially an issue when loading a large dataset on a large machine.
## Steps to reproduce the bug
I originally created the following script to reproduce the issue:
```python
from datasets import load_dataset
from multiprocessing import Process
from tqdm import tqdm
import datasets
from transformers import set_seed
def run_tasks_in_parallel(tasks, ds_list):
for _ in tqdm(range(1000)):
print('new batch')
running_tasks = [Process(target=task, args=(ds, i)) for i, (task, ds) in enumerate(zip(tasks, ds_list))]
for running_task in running_tasks:
running_task.start()
for running_task in running_tasks:
running_task.join()
def get_dataset():
dataset_name = 'transformersbook/codeparrot'
ds = load_dataset(dataset_name+'-train', split="train", streaming=True)
ds = ds.shuffle(buffer_size=1000, seed=1)
return iter(ds)
def get_next_element(ds, process_id, N=10000):
for _ in range(N):
_ = next(ds)['content']
print(f'process {process_id} done')
return
set_seed(1)
datasets.utils.logging.set_verbosity_debug()
n_processes = 8
tasks = [get_next_element for _ in range(n_processes)]
args = [get_dataset() for _ in range(n_processes)]
run_tasks_in_parallel(tasks, args)
```
Today I noticed that it can happen when running it on a single process on a machine with many cores without streaming. So just `load_dataset("transformersbook/codeparrot-train")` alone might cause the issue after waiting long enough or trying many times. It's a slightly random process which makes it especially hard to track down. When I encountered it today it had already processed 17GB of data (the size of the cache folder when it got stuck) before getting stuck.
Here's my current understanding of the error. As far as I can tell it happens in the following block: https://github.com/huggingface/datasets/blob/be701e9e89ab38022612c7263edc015bc7feaff9/src/datasets/packaged_modules/json/json.py#L119-L139
When the try on line 121 fails and the `block_size` is increased it can happen that it can't read the JSON again and gets stuck indefinitely. A hint that points in that direction is that increasing the `chunksize` argument decreases the chance of getting stuck and vice versa. Maybe it is an issue with a lock on the file that is not properly released.
## Expected results
Read a JSON before the end of the universe.
## Actual results
Read a JSON not before the end of the universe.
## Environment info
<!-- You can run the command `datasets-cli env` and copy-and-paste its output below. -->
- `datasets` version: 1.18.3
- Platform: Linux-4.19.0-18-cloud-amd64-x86_64-with-glibc2.28
- Python version: 3.9.10
- PyArrow version: 7.0.0
@lhoestq we dicsussed this a while ago. @albertvillanova we discussed this today :)
| 3,708 |
https://github.com/huggingface/datasets/issues/3707 | `.select`: unexpected behavior with `indices` | [
"Hi! Currently, we compute the final index as `index % len(dset)`. I agree this behavior is somewhat unexpected and that it would be more appropriate to raise an error instead (this is what `df.iloc` in Pandas does, for instance).\r\n\r\n@albertvillanova @lhoestq wdyt?",
"I agree. I think `index % len(dset)` was used to support negative indices.\r\n\r\nI think this needs to be fixed in `datasets.formatting.formatting._check_valid_index_key` if I'm not mistaken"
] | ## Describe the bug
The `.select` method will not throw when sending `indices` bigger than the dataset length; `indices` will be wrapped instead. This behavior is not documented anywhere, and is not intuitive.
## Steps to reproduce the bug
```python
from datasets import Dataset
ds = Dataset.from_dict({"text": ["d", "e", "f"], "label": [4, 5, 6]})
res1 = ds.select([1, 2, 3])['text']
res2 = ds.select([1000])['text']
```
## Expected results
Both results should throw an `Error`.
## Actual results
`res1` will give `['e', 'f', 'd']`
`res2` will give `['e']`
## Environment info
Bug found from this environment:
- `datasets` version: 1.16.1
- Platform: macOS-10.16-x86_64-i386-64bit
- Python version: 3.8.7
- PyArrow version: 6.0.1
It was also replicated on `master`.
| 3,707 |
https://github.com/huggingface/datasets/issues/3706 | Unable to load dataset 'big_patent' | [
"Hi @ankitk2109,\r\n\r\nHave you tried passing the split name with the keyword `split=`? See e.g. an example in our Quick Start docs: https://huggingface.co/docs/datasets/quickstart.html#load-the-dataset-and-model\r\n```python\r\n ds = load_dataset(\"big_patent\", \"d\", split=\"validation\")",
"Hi @albertvillanova,\r\n\r\nThanks for your response.\r\n\r\nYes, I tried the `split='validation'` as well. But getting the same issue. ",
"I'm sorry, but I can't reproduce your problem:\r\n```python\r\nIn [5]: ds = load_dataset(\"big_patent\", \"d\", split=\"validation\")\r\nDownloading and preparing dataset big_patent/d (download: 6.01 GiB, generated: 169.61 MiB, post-processed: Unknown size, total: 6.17 GiB) to .../.cache/big_patent/d/1.0.0/bdefa7c0b39fba8bba1c6331b70b738e30d63c8ad4567f983ce315a5fef6131c...\r\nDownloading data: 100%|███████████████████████████████████████████████████████████████████████████████████████████████████████████████████████████████████████████████| 6.45G/6.45G [27:36<00:00, 3.89MB/s]\r\nExtracting data files: 100%|█████████████████████████████████████████████████████████████████████████████████████████████████████████████████████████████████████████████████| 3/3 [03:18<00:00, 66.08s/it]\r\nDataset big_patent downloaded and prepared to .../.cache/big_patent/d/1.0.0/bdefa7c0b39fba8bba1c6331b70b738e30d63c8ad4567f983ce315a5fef6131c. Subsequent calls will reuse this data. \r\n\r\nIn [6]: ds\r\nOut[6]: \r\nDataset({\r\n features: ['description', 'abstract'],\r\n num_rows: 565\r\n})\r\n",
"Maybe you had a connection issue while downloading the file and this was corrupted?\r\nOur cache system uses the file you downloaded first time.\r\nIf so, you could try forcing redownload of the file with:\r\n```python\r\nds = load_dataset(\"big_patent\", \"d\", split=\"validation\", download_mode=\"force_redownload\")",
"I am able to download the dataset with ``` download_mode=\"force_redownload\"```. As you mentioned it was an issue with the cached version which was failed earlier due to a network issue. I am closing the issue now, once again thank you."
] | ## Describe the bug
Unable to load the "big_patent" dataset
## Steps to reproduce the bug
```python
load_dataset('big_patent', 'd', 'validation')
```
## Expected results
Download big_patents' validation split from the 'd' subset
## Getting an error saying:
{FileNotFoundError}Local file ..\huggingface\datasets\downloads\6159313604f4f2c01e7d1cac52139343b6c07f73f6de348d09be6213478455c5\bigPatentData\train.tar.gz doesn't exist
## Environment info
<!-- You can run the command `datasets-cli env` and copy-and-paste its output below. -->
- `datasets` version:1.18.3
- Platform: Windows
- Python version:3.8
- PyArrow version:7.0.0
| 3,706 |
https://github.com/huggingface/datasets/issues/3704 | OSCAR-2109 datasets are misaligned and truncated | [
"Hi @adrianeboyd, thanks for reporting.\r\n\r\nThere is indeed a bug in that community dataset:\r\nLine:\r\n```python\r\nmetadata_and_text_files = list(zip(metadata_files, text_files))\r\n``` \r\nshould be replaced with\r\n```python\r\nmetadata_and_text_files = list(zip(sorted(metadata_files), sorted(text_files)))\r\n```\r\n\r\nI am going to contact their owners (https://huggingface.co/oscar-corpus) in order to inform them about the bug.\r\n\r\nI keep you informed.",
"That fix is part of it, but it's clearly not the only issue.\r\n\r\nI also already contacted the OSCAR creators, but I reported it here because it looked like huggingface members were the main authors in the git history. Is there a better place to have reported this?",
"Hello,\r\n\r\nWe've had an issue that could be linked to this one here: https://github.com/oscar-corpus/corpus/issues/15.\r\n\r\nI have been spot checking the source (`.txt`/`.jsonl`) files for a while, and have not found issues, especially in the start/end of corpora (but I conceed that more integration testing would be necessary on our side).\r\n\r\nThe text and metadata files are designed to be used in sync (with `lang_part_n.txt` and `lang_meta_part_n.jsonl` working together), while staying independent from part to part, so that anyone could randomly choose a part and work with it.\r\n\r\nThe fix @albertvillanova proposed should fix the problem, as the parts will be in sync again.\r\n\r\nLet me know if you need help or more details, I'd be glad to help!",
"I'm happy to move the discussion to the other repo!\r\n\r\nMerely sorting the files only **maybe** fixes the processing of the first part. If the first part contains non-unix newlines, it will still be misaligned/truncated, and all the following parts will be truncated with incorrect text offsets and metadata due the offset and newline bugs.",
"Fixed:\r\n- https://huggingface.co/datasets/oscar-corpus/OSCAR-2109/commit/3cd7e95aa1799b73c5ea8afc3989635f3e19b86b",
"Hi @Uinelj, This is a total noobs question but how can I integrate that bugfix into my code? I reinstalled the datasets library this time from source. Should that have fixed the issue? I am still facing the misalignment issue. Do I need to download the dataset from scratch?",
"Hi, I re-downloaded the dataset and still have the problem. See: https://github.com/oscar-corpus/corpus/issues/18",
"Sorry @norakassner for the late reply.\r\n\r\nThere are indeed several issues creating the misalignment, as @adrianeboyd cleverly pointed out:\r\n- https://huggingface.co/datasets/oscar-corpus/OSCAR-2109/commit/3cd7e95aa1799b73c5ea8afc3989635f3e19b86b fixed one of them\r\n- but there are still others to be fixed",
"Normally, the issues should be fixed now:\r\n- Fix offset initialization for each file: https://huggingface.co/datasets/oscar-corpus/OSCAR-2109/commit/1ad9b7bfe00798a9258a923b887bb1c8d732b833\r\n- Disable default universal newline support: https://huggingface.co/datasets/oscar-corpus/OSCAR-2109/commit/0c2f307d3167f03632f502af361ac6c3c393f510\r\n\r\nFeel free to reopen if you find additional misalignments/truncations.\r\n\r\nCC: @adrianeboyd @norakassner @Uinelj ",
"Thanks for the updates!\r\n\r\nThe purist in me would still like to have the rstrip not strip additional characters from the original text (unicode whitespace mainly in practice, I think), but the differences are extremely small in practice and it doesn't actually matter for my current task:\r\n\r\n```python\r\ntext = \"\".join([text_f.readline() for _ in range(meta[\"nb_sentences\"])]).rstrip(\"\\n\")\r\n```"
] | ## Describe the bug
The `oscar-corpus/OSCAR-2109` data appears to be misaligned and truncated by the dataset builder for subsets that contain more than one part and for cases where the texts contain non-unix newlines.
## Steps to reproduce the bug
A few examples, although I'm not sure how deterministic the particular (mis)alignment is in various configurations:
```python
from datasets import load_dataset
dataset = load_dataset("oscar-corpus/OSCAR-2109", "deduplicated_fi", split="train", use_auth_token=True)
entry = dataset[0]
# entry["text"] is from fi_part_3.txt.gz
# entry["meta"] is from fi_meta_part_2.jsonl.gz
dataset = load_dataset("oscar-corpus/OSCAR-2109", "deduplicated_no", split="train", use_auth_token=True)
entry = dataset[900000]
# entry["text"] is from no_part_3.txt.gz and contains a blank line
# entry["meta"] is from no_meta_part_1.jsonl.gz
dataset = load_dataset("oscar-corpus/OSCAR-2109", "deduplicated_mk", split="train", streaming=True, use_auth_token=True)
# 9088 texts in the dataset are empty
```
For `deduplicated_fi`, all exported raw texts from the dataset are 17GB rather than 20GB as reported in the data splits overview table. The token count with `wc -w` for the raw texts is 2,067,556,874 rather than the expected 2,357,264,196 from the data splits table.
For `deduplicated_no` all exported raw texts contain 624,040,887 rather than the expected 776,354,517 tokens.
For `deduplicated_mk` it is 122,236,936 rather than 134,544,934 tokens.
I'm not expecting the `wc -w` counts to line up exactly with the data splits table, but for comparison the `wc -w` count for `deduplicated_mk` on the raw texts is 134,545,424.
## Issues
* The meta / text files are not paired correctly when loading, so the extracted texts do not have the right offsets, the metadata is not associated with the correct text, and the text files may not be processed to the end or may be processed beyond the end (empty texts).
* The line count offset is not reset per file so the texts aren't aligned to the right offsets in any parts beyond the first part, leading to truncation when in effect blank lines are not skipped.
* Non-unix newline characters are treated as newlines when reading the text files while the metadata only counts unix newlines for its line offsets, leading to further misalignments between the metadata and the extracted texts, and which also results in truncation.
## Expected results
All texts from the OSCAR release are extracted according to the metadata and aligned with the correct metadata.
## Fixes
Not necessarily the exact fixes/checks you may want to use (I didn't test all languages or do any cross-platform testing, I'm not sure all the details are compatible with streaming), however to highlight the issues:
```diff
diff --git a/OSCAR-2109.py b/OSCAR-2109.py
index bbac1076..5eee8de7 100644
--- a/OSCAR-2109.py
+++ b/OSCAR-2109.py
@@ -20,6 +20,7 @@
import collections
import gzip
import json
+import os
import datasets
@@ -387,9 +388,20 @@ class Oscar2109(datasets.GeneratorBasedBuilder):
with open(checksum_file, encoding="utf-8") as f:
data_filenames = [line.split()[1] for line in f if line]
data_urls = [self.config.base_data_path + data_filename for data_filename in data_filenames]
- text_files = dl_manager.download([url for url in data_urls if url.endswith(".txt.gz")])
- metadata_files = dl_manager.download([url for url in data_urls if url.endswith(".jsonl.gz")])
+ # sort filenames so corresponding parts are aligned
+ text_files = sorted(dl_manager.download([url for url in data_urls if url.endswith(".txt.gz")]))
+ metadata_files = sorted(dl_manager.download([url for url in data_urls if url.endswith(".jsonl.gz")]))
+ assert len(text_files) == len(metadata_files)
metadata_and_text_files = list(zip(metadata_files, text_files))
+ for meta_path, text_path in metadata_and_text_files:
+ # check that meta/text part numbers are the same
+ if "part" in os.path.basename(text_path):
+ assert (
+ os.path.basename(text_path).replace(".txt.gz", "").split("_")[-1]
+ == os.path.basename(meta_path).replace(".jsonl.gz", "").split("_")[-1]
+ )
+ else:
+ assert len(metadata_and_text_files) == 1
return [
datasets.SplitGenerator(name=datasets.Split.TRAIN, gen_kwargs={"metadata_and_text_files": metadata_and_text_files}),
]
@@ -397,10 +409,14 @@ class Oscar2109(datasets.GeneratorBasedBuilder):
def _generate_examples(self, metadata_and_text_files):
"""This function returns the examples in the raw (text) form by iterating on all the files."""
id_ = 0
- offset = 0
for meta_path, text_path in metadata_and_text_files:
+ # line offsets are per text file
+ offset = 0
logger.info("generating examples from = %s", text_path)
- with gzip.open(open(text_path, "rb"), "rt", encoding="utf-8") as text_f:
+ # some texts contain non-Unix newlines that should not be
+ # interpreted as line breaks for the line counts in the metadata
+ # with readline()
+ with gzip.open(open(text_path, "rb"), "rt", encoding="utf-8", newline="\n") as text_f:
with gzip.open(open(meta_path, "rb"), "rt", encoding="utf-8") as meta_f:
for line in meta_f:
# read meta
@@ -411,7 +427,12 @@ class Oscar2109(datasets.GeneratorBasedBuilder):
offset += 1
text_f.readline()
# read text
- text = "".join([text_f.readline() for _ in range(meta["nb_sentences"])]).rstrip()
+ text_lines = [text_f.readline() for _ in range(meta["nb_sentences"])]
+ # all lines contain text (no blank lines or EOF)
+ assert all(text_lines)
+ assert "\n" not in text_lines
offset += meta["nb_sentences"]
+ # only strip the trailing newline
+ text = "".join(text_lines).rstrip("\n")
yield id_, {"id": id_, "text": text, "meta": meta}
id_ += 1
```
I've tested this with a number of smaller deduplicated languages with 1-20 parts and the resulting datasets looked correct in terms of word count and size when compared to the data splits table and raw texts, and the text/metadata alignments were correct in all my spot checks. However, there are many many languages I didn't test and I'm not sure that there aren't any texts containing blank lines in the corpus, for instance. For the cases I tested, the assertions related to blank lines and EOF made it easier to verify that the text and metadata were aligned as intended, since there would be little chance of spurious alignments of variable-length texts across so much data. | 3,704 |
https://github.com/huggingface/datasets/issues/3703 | ImportError: To be able to use this metric, you need to install the following dependencies['seqeval'] using 'pip install seqeval' for instance' | [
"\r\nMy datasets version",
"\r\n",
"Hi! Some of our metrics require additional dependencies to work. In your case, simply installing the `seqeval` package with `pip install seqeval` should resolve the issue.",
"> Hi! Some of our metrics require additional dependencies to work. In your case, simply installing the `seqeval` package with `pip install seqeval` should resolve the issue.\r\nI installed seqeval, but still reported the same error. That's too bad.\r\n",
"> > Hi! Some of our metrics require additional dependencies to work. In your case, simply installing the `seqeval` package with `pip install seqeval` should resolve the issue.\r\n> > I installed seqeval, but still reported the same error. That's too bad.\r\n\r\nSame issue here. What should I do to fix this error? Please help! Thank you.",
"I tried to install **seqeval** package through anaconda instead of pip:\r\n`conda install -c conda-forge seqeval`\r\nIt worked for me!",
"I can run it through the following steps:\r\n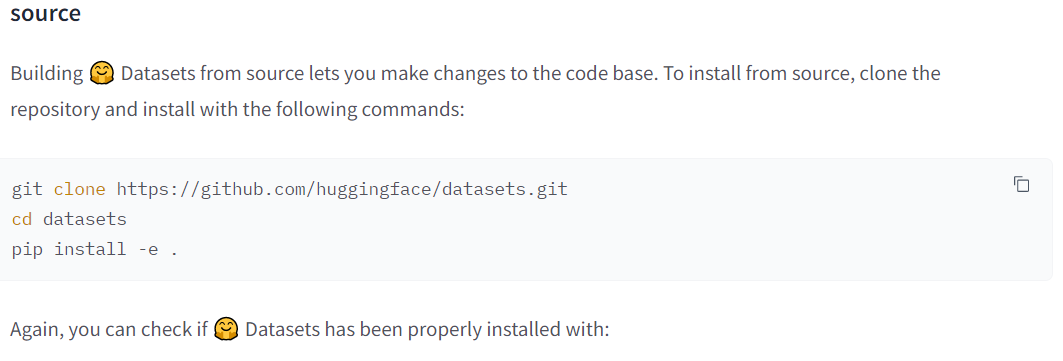\r\nThank you for answering for me!",
"just change the file name seqeval.py to myseqeval.py",
"Metrics are deprecated in `datasets` and `evaluate` should be used instead: https://github.com/huggingface/evaluate"
] | hi :
I want to use the seqeval indicator because of direct load_ When metric ('seqeval '), it will prompt that the network connection fails. So I downloaded the seqeval Py to load locally. Loading code: metric = load_ metric(path='mymetric/seqeval/seqeval.py')
But tips:
Traceback (most recent call last):
File "/home/ubuntu/Python3.6_project/zyf_project/transformers/examples/pytorch/token-classification/run_ner.py", line 604, in <module>
main()
File "/home/ubuntu/Python3.6_project/zyf_project/transformers/examples/pytorch/token-classification/run_ner.py", line 481, in main
metric = load_metric(path='mymetric/seqeval/seqeval.py')
File "/home/ubuntu/Python3.6_project/zyf_project/transformers_venv_0209/lib/python3.7/site-packages/datasets/load.py", line 610, in load_metric
dataset=False,
File "/home/ubuntu/Python3.6_project/zyf_project/transformers_venv_0209/lib/python3.7/site-packages/datasets/load.py", line 450, in prepare_module
f"To be able to use this {module_type}, you need to install the following dependencies"
ImportError: To be able to use this metric, you need to install the following dependencies['seqeval'] using 'pip install seqeval' for instance'
**What should I do? Please help me, thank you**
| 3,703 |
https://github.com/huggingface/datasets/issues/3700 | Unable to load a dataset | [
"Hi! `load_dataset` is intended to be used to load a canonical dataset (`wikipedia`), a packaged dataset (`csv`, `json`, ...) or a dataset hosted on the Hub. For local datasets saved with `save_to_disk(\"path/to/dataset\")`, use `load_from_disk(\"path/to/dataset\")`.",
"Maybe we should raise an informative error message in this case...",
"How should we load a locally self-gathered dataset then?"
] | ## Describe the bug
Unable to load a dataset from Huggingface that I have just saved.
## Steps to reproduce the bug
On Google colab
`! pip install datasets `
`from datasets import load_dataset`
`my_path = "wiki_dataset"`
`dataset = load_dataset('wikipedia', "20200501.fr")`
`dataset.save_to_disk(my_path)`
`dataset = load_dataset(my_path)`
## Expected results
Loading the dataset
## Actual results
ValueError: Couldn't cast
_data_files: list<item: struct<filename: string>>
child 0, item: struct<filename: string>
child 0, filename: string
_fingerprint: string
_format_columns: null
_format_kwargs: struct<>
_format_type: null
_indexes: struct<>
_output_all_columns: bool
_split: string
to
{'builder_name': Value(dtype='string', id=None), 'citation': Value(dtype='string', id=None), 'config_name': Value(dtype='string', id=None), 'dataset_size': Value(dtype='int64', id=None), 'description': Value(dtype='string', id=None), 'download_checksums': {}, 'download_size': Value(dtype='int64', id=None), 'features': {'title': {'dtype': Value(dtype='string', id=None), 'id': Value(dtype='null', id=None), '_type': Value(dtype='string', id=None)}, 'text': {'dtype': Value(dtype='string', id=None), 'id': Value(dtype='null', id=None), '_type': Value(dtype='string', id=None)}}, 'homepage': Value(dtype='string', id=None), 'license': Value(dtype='string', id=None), 'post_processed': Value(dtype='null', id=None), 'post_processing_size': Value(dtype='null', id=None), 'size_in_bytes': Value(dtype='int64', id=None), 'splits': {'train': {'name': Value(dtype='string', id=None), 'num_bytes': Value(dtype='int64', id=None), 'num_examples': Value(dtype='int64', id=None), 'dataset_name': Value(dtype='string', id=None)}}, 'supervised_keys': Value(dtype='null', id=None), 'task_templates': Value(dtype='null', id=None), 'version': {'version_str': Value(dtype='string', id=None), 'description': Value(dtype='string', id=None), 'major': Value(dtype='int64', id=None), 'minor': Value(dtype='int64', id=None), 'patch': Value(dtype='int64', id=None)}}
because column names don't match
## Environment info
- `datasets` version: 1.18.3
- Platform: Linux-5.4.144+-x86_64-with-Ubuntu-18.04-bionic
- Python version: 3.7.12
- PyArrow version: 6.0.1
| 3,700 |
https://github.com/huggingface/datasets/issues/3688 | Pyarrow version error | [
"Hi @Zaker237, thanks for reporting.\r\n\r\nThis is weird: the error you get is only thrown if the installed pyarrow version is less than 3.0.0.\r\n\r\nCould you please check that you install pyarrow in the same Python virtual environment where you installed datasets?\r\n\r\nFrom the Python command line (or terminal) where you get the error, please type:\r\n```\r\nimport pyarrow\r\nprint(pyarrow.__version__)\r\nimport datasets\r\nprint(datasets.__version__)\r\n``` ",
"hi @albertvillanova i try yesterday to create a new python environement with python 7 and try it on the environement and it worked. so i think that the error was not the package but may be jupyter notebook on conda. still yet i'm not yet sure but it worked in an environment created with venv",
"OK, thanks @Zaker237 for your feedback.\r\n\r\nI close this issue then. Please, feel free to reopen it if the problem arises again."
] | ## Describe the bug
I installed datasets(version 1.17.0, 1.18.0, 1.18.3) but i'm right now nor able to import it because of pyarrow. when i try to import it, i get the following error:
`To use datasets, the module pyarrow>=3.0.0 is required, and the current version of pyarrow doesn't match this condition`.
i tryed with all version of pyarrow execpt `4.0.0` but still get the same error.
## Steps to reproduce the bug
```python
import datasets
```
## Expected results
A clear and concise description of the expected results.
## Actual results
AttributeError Traceback (most recent call last)
<ipython-input-19-652e886d387f> in <module>
----> 1 import datasets
~\AppData\Local\Continuum\anaconda3\lib\site-packages\datasets\__init__.py in <module>
26
27
---> 28 if _version.parse(pyarrow.__version__).major < 3:
29 raise ImportWarning(
30 "To use `datasets`, the module `pyarrow>=3.0.0` is required, and the current version of `pyarrow` doesn't match this condition.\n"
AttributeError: 'Version' object has no attribute 'major'
## Environment info
Traceback (most recent call last):
File "c:\users\alex\appdata\local\continuum\anaconda3\lib\runpy.py", line 193, in _run_module_as_main
"__main__", mod_spec)
File "c:\users\alex\appdata\local\continuum\anaconda3\lib\runpy.py", line 85, in _run_code
exec(code, run_globals)
File "C:\Users\Alex\AppData\Local\Continuum\anaconda3\Scripts\datasets-cli.exe\__main__.py", line 5, in <module>
File "c:\users\alex\appdata\local\continuum\anaconda3\lib\site-packages\datasets\__init__.py", line 28, in <module>
if _version.parse(pyarrow.__version__).major < 3:
AttributeError: 'Version' object has no attribute 'major'
- `datasets` version:
- Platform: Linux(Ubuntu) and Windows: conda on the both
- Python version: 3.7
- PyArrow version: 7.0.0
| 3,688 |
https://github.com/huggingface/datasets/issues/3687 | Can't get the text data when calling to_tf_dataset | [
"cc @Rocketknight1 ",
"You are correct that `to_tf_dataset` only handles numerical columns right now, yes, though this is a limitation we might remove in future! The main reason we do this is that our models mostly do not include the tokenizer as a model layer, because it's very difficult to compile some of them in TF. So the \"normal\" Huggingface workflow is to first tokenize your dataset, and then pass tokenized tensors to the model.\r\n\r\nFor your use case, would you prefer to pass strings to the model, and use some text processing layers instead of the built-in tokenizers?",
"Also tagging @gante just so he's aware, but I can handle this one!",
"Thanks for the quick follow-up to my issue.\r\n\r\nFor my use-case, instead of the built-in tokenizers I wanted to use the `TextVectorization` layer to map from strings to integers. To achieve this, I came up with the following solution:\r\n\r\n```\r\nfrom datasets import load_dataset\r\nfrom transformers import DefaultDataCollator\r\nimport tensorflow as tf\r\nimport string\r\nimport re\r\nfrom tensorflow.keras.layers.experimental.preprocessing import TextVectorization\r\n\r\n#some hyper-parameters for the text-to-integer mapping\r\nmax_features = 20000\r\nembedding_dim = 128\r\nsequence_length = 210\r\n\r\ndata_collator = DefaultDataCollator(return_tensors=\"tf\")\r\ndataset = load_dataset(\"sst\", \"default\")\r\n\r\n#adapt the vectorization layer on train data only\r\nvectorize_layer.adapt(dataset[\"train\"].to_dict(batched=False)[\"sentence\"])\r\n\r\ndef prepare_features(text, label):\r\n text = tf.expand_dims(text, -1)\r\n return {\"vectorized_text\": vectorize_layer(text)[0], \"label\": tf.expand_dims(label, axis=-1)}\r\n\r\nencoded_dataset = dataset.map(lambda example: prepare_features(example[\"sentence\"], example[\"label\"]), batched=False)\r\n\r\n\r\ndef custom_standardization(input_data):\r\n lowercase = tf.strings.lower(input_data)\r\n return tf.strings.regex_replace(\r\n lowercase, f\"[{re.escape(string.punctuation)}]\", \"\"\r\n )\r\n\r\nvectorize_layer = TextVectorization(\r\n standardize=custom_standardization,\r\n max_tokens=max_features,\r\n output_mode=\"int\",\r\n output_sequence_length=sequence_length,\r\n)\r\n\r\ntrain_dataset = encoded_dataset[\"train\"].to_tf_dataset(columns=['vectorized_text'], label_cols=[\"label\"],\r\n shuffle=True, batch_size=1, collate_fn=data_collator).unbatch()\r\n#similar for the other sub-sets\r\n\r\n```\r\n\r\nSince the strings would have been mapped to integers or floats at some point, it's no drawback that this mapping is done early in the process. \r\n\r\nFor the future, however, it'd be more convenient to get the string data, since I am also inspecting the dataset (longest sentence, shortest sentence), which is more challenging when working with integer or float. For now, this can be done by calling `to_dict`.",
"> For the future, however, it'd be more convenient to get the string data, since I am also inspecting the dataset (longest sentence, shortest sentence), which is more challenging when working with integer or float.\r\n\r\nYes, I agree, so let's keep this issue open.",
"Going to close this now - methods like `to_tf_dataset` and `prepare_tf_dataset` now support string data, and have done for a while! If anyone sees this and is encountering issues with string data in those methods, please file a new issue!"
] | I am working with the SST2 dataset, and am using TensorFlow 2.5
I'd like to convert it to a `tf.data.Dataset` by calling the `to_tf_dataset` method.
The following snippet is what I am using to achieve this:
```
from datasets import load_dataset
from transformers import DefaultDataCollator
data_collator = DefaultDataCollator(return_tensors="tf")
dataset = load_dataset("sst")
train_dataset = dataset["train"].to_tf_dataset(columns=['sentence'], label_cols="label", shuffle=True, batch_size=8,collate_fn=data_collator)
```
However, this only gets me the labels; the text--the most important part--is missing:
```
for s in train_dataset.take(1):
print(s) #prints something like: ({}, <tf.Tensor: shape=(8,), ...>)
```
As you can see, it only returns the label part, not the data, as indicated by the empty dictionary, `{}`. So far, I've played with various settings of the method arguments, but to no avail; I do not want to perform any text processing at this time. On my quest to achieve what I want ( a `tf.data.Dataset`), I've consulted these resources:
[https://www.philschmid.de/huggingface-transformers-keras-tf](https://www.philschmid.de/huggingface-transformers-keras-tf)
[https://huggingface.co/docs/datasets/use_dataset.html?highlight=tensorflow](https://huggingface.co/docs/datasets/use_dataset.html?highlight=tensorflow)
I was surprised to not find more extensive examples on how to transform a Hugginface dataset to one compatible with TensorFlow.
If you could point me to where I am going wrong, please do so.
Thanks in advance for your support.
---
Edit: In the [docs](https://huggingface.co/docs/datasets/package_reference/main_classes.html#datasets.Dataset.to_tf_dataset), I found the following description:
_In general, only columns that the model can use as input should be included here (numeric data only)._
Does this imply that no textual, i.e., `string` data can be loaded?
| 3,687 |
https://github.com/huggingface/datasets/issues/3686 | `Translation` features cannot be `flatten`ed | [
"Thanks for reporting, @SBrandeis! Some additional feature types that don't behave as expected when flattened: `Audio`, `Image` and `TranslationVariableLanguages`"
] | ## Describe the bug
(`Dataset.flatten`)[https://github.com/huggingface/datasets/blob/master/src/datasets/arrow_dataset.py#L1265] fails for columns with feature (`Translation`)[https://github.com/huggingface/datasets/blob/3edbeb0ec6519b79f1119adc251a1a6b379a2c12/src/datasets/features/translation.py#L8]
## Steps to reproduce the bug
```python
from datasets import load_dataset
dataset = load_dataset("europa_ecdc_tm", "en2fr", split="train[:10]")
print(dataset.features)
# {'translation': Translation(languages=['en', 'fr'], id=None)}
print(dataset[0])
# {'translation': {'en': 'Vaccination against hepatitis C is not yet available.', 'fr': 'Aucune vaccination contre l’hépatite C n’est encore disponible.'}}
dataset.flatten()
```
## Expected results
`dataset.flatten` should flatten the `Translation` column as if it were a dict of `Value("string")`
```python
dataset[0]
# {'translation.en': 'Vaccination against hepatitis C is not yet available.', 'translation.fr': 'Aucune vaccination contre l’hépatite C n’est encore disponible.' }
dataset.features
# {'translation.en': Value("string"), 'translation.fr': Value("string")}
```
## Actual results
```python
In [31]: dset.flatten()
---------------------------------------------------------------------------
KeyError Traceback (most recent call last)
<ipython-input-31-bb88eb5276ee> in <module>
----> 1 dset.flatten()
[...]\site-packages\datasets\fingerprint.py in wrapper(*args, **kwargs)
411 # Call actual function
412
--> 413 out = func(self, *args, **kwargs)
414
415 # Update fingerprint of in-place transforms + update in-place history of transforms
[...]\site-packages\datasets\arrow_dataset.py in flatten(self, new_fingerprint, max_depth)
1294 break
1295 dataset.info.features = self.features.flatten(max_depth=max_depth)
-> 1296 dataset._data = update_metadata_with_features(dataset._data, dataset.features)
1297 logger.info(f'Flattened dataset from depth {depth} to depth {1 if depth + 1 < max_depth else "unknown"}.')
1298 dataset._fingerprint = new_fingerprint
[...]\site-packages\datasets\arrow_dataset.py in update_metadata_with_features(table, features)
534 def update_metadata_with_features(table: Table, features: Features):
535 """To be used in dataset transforms that modify the features of the dataset, in order to update the features stored in the metadata of its schema."""
--> 536 features = Features({col_name: features[col_name] for col_name in table.column_names})
537 if table.schema.metadata is None or b"huggingface" not in table.schema.metadata:
538 pa_metadata = ArrowWriter._build_metadata(DatasetInfo(features=features))
[...]\site-packages\datasets\arrow_dataset.py in <dictcomp>(.0)
534 def update_metadata_with_features(table: Table, features: Features):
535 """To be used in dataset transforms that modify the features of the dataset, in order to update the features stored in the metadata of its schema."""
--> 536 features = Features({col_name: features[col_name] for col_name in table.column_names})
537 if table.schema.metadata is None or b"huggingface" not in table.schema.metadata:
538 pa_metadata = ArrowWriter._build_metadata(DatasetInfo(features=features))
KeyError: 'translation.en'
```
## Environment info
- `datasets` version: 1.18.3
- Platform: Windows-10-10.0.19041-SP0
- Python version: 3.7.10
- PyArrow version: 3.0.0
| 3,686 |
https://github.com/huggingface/datasets/issues/3679 | Download datasets from a private hub | [
"For reference:\r\nhttps://github.com/huggingface/transformers/issues/15514\r\nhttps://github.com/huggingface/huggingface_hub/issues/650",
"Hi ! For information one can set the environment variable `HF_ENDPOINT` (default is `https://huggingface.co`) if they want to use a private hub.\r\n\r\nWe may need to coordinate with the other libraries to have a consistent way of changing the hub endpoint",
"Yes, I tested it successfully this morning. Thanks."
] | In the context of a private hub deployment, customers would like to use load_dataset() to load datasets from their hub, not from the public hub. This doesn't seem to be configurable at the moment and it would be nice to add this feature.
The obvious workaround is to clone the repo first and then load it from local storage, but this adds an extra step. It'd be great to have the same experience regardless of where the hub is hosted.
The same issue exists with the transformers library and the CLI. I'm going to create issues there as well, and I'll reference them below. | 3,679 |
https://github.com/huggingface/datasets/issues/3677 | Discovery cannot be streamed anymore | [
"Seems like a regression from https://github.com/huggingface/datasets/pull/2843\r\n\r\nOr maybe it's an issue with the hosting. I don't think so, though, because https://www.dropbox.com/s/aox84z90nyyuikz/discovery.zip seems to work as expected\r\n\r\n",
"Hi @severo, thanks for reporting.\r\n\r\nSome servers do not support HTTP range requests, and those are required to stream some file formats (like ZIP in this case).\r\n\r\nLet me try to propose a workaround. "
] | ## Describe the bug
A clear and concise description of what the bug is.
## Steps to reproduce the bug
```python
from datasets import load_dataset
iterable_dataset = load_dataset("discovery", name="discovery", split="train", streaming=True)
list(iterable_dataset.take(1))
```
## Expected results
The first row of the train split.
## Actual results
```
Traceback (most recent call last):
File "<stdin>", line 1, in <module>
File "/home/slesage/hf/datasets-preview-backend/.venv/lib/python3.9/site-packages/datasets/iterable_dataset.py", line 365, in __iter__
for key, example in self._iter():
File "/home/slesage/hf/datasets-preview-backend/.venv/lib/python3.9/site-packages/datasets/iterable_dataset.py", line 362, in _iter
yield from ex_iterable
File "/home/slesage/hf/datasets-preview-backend/.venv/lib/python3.9/site-packages/datasets/iterable_dataset.py", line 272, in __iter__
yield from islice(self.ex_iterable, self.n)
File "/home/slesage/hf/datasets-preview-backend/.venv/lib/python3.9/site-packages/datasets/iterable_dataset.py", line 79, in __iter__
yield from self.generate_examples_fn(**self.kwargs)
File "/home/slesage/.cache/huggingface/modules/datasets_modules/datasets/discovery/542fab7a9ddc1d9726160355f7baa06a1ccc44c40bc8e12c09e9bc743aca43a2/discovery.py", line 333, in _generate_examples
with open(data_file, encoding="utf8") as f:
File "/home/slesage/hf/datasets-preview-backend/.venv/lib/python3.9/site-packages/datasets/streaming.py", line 64, in wrapper
return function(*args, use_auth_token=use_auth_token, **kwargs)
File "/home/slesage/hf/datasets-preview-backend/.venv/lib/python3.9/site-packages/datasets/utils/streaming_download_manager.py", line 369, in xopen
file_obj = fsspec.open(file, mode=mode, *args, **kwargs).open()
File "/home/slesage/hf/datasets-preview-backend/.venv/lib/python3.9/site-packages/fsspec/core.py", line 456, in open
return open_files(
File "/home/slesage/hf/datasets-preview-backend/.venv/lib/python3.9/site-packages/fsspec/core.py", line 288, in open_files
fs, fs_token, paths = get_fs_token_paths(
File "/home/slesage/hf/datasets-preview-backend/.venv/lib/python3.9/site-packages/fsspec/core.py", line 611, in get_fs_token_paths
fs = filesystem(protocol, **inkwargs)
File "/home/slesage/hf/datasets-preview-backend/.venv/lib/python3.9/site-packages/fsspec/registry.py", line 253, in filesystem
return cls(**storage_options)
File "/home/slesage/hf/datasets-preview-backend/.venv/lib/python3.9/site-packages/fsspec/spec.py", line 68, in __call__
obj = super().__call__(*args, **kwargs)
File "/home/slesage/hf/datasets-preview-backend/.venv/lib/python3.9/site-packages/fsspec/implementations/zip.py", line 57, in __init__
self.zip = zipfile.ZipFile(self.fo)
File "/home/slesage/.pyenv/versions/3.9.6/lib/python3.9/zipfile.py", line 1257, in __init__
self._RealGetContents()
File "/home/slesage/.pyenv/versions/3.9.6/lib/python3.9/zipfile.py", line 1320, in _RealGetContents
endrec = _EndRecData(fp)
File "/home/slesage/.pyenv/versions/3.9.6/lib/python3.9/zipfile.py", line 263, in _EndRecData
fpin.seek(0, 2)
File "/home/slesage/hf/datasets-preview-backend/.venv/lib/python3.9/site-packages/fsspec/implementations/http.py", line 676, in seek
raise ValueError("Cannot seek streaming HTTP file")
ValueError: Cannot seek streaming HTTP file
```
## Environment info
- `datasets` version: 1.18.3
- Platform: Linux-5.11.0-1027-aws-x86_64-with-glibc2.31
- Python version: 3.9.6
- PyArrow version: 6.0.1
| 3,677 |
https://github.com/huggingface/datasets/issues/3676 | `None` replaced by `[]` after first batch in map | [
"It looks like this is because of this behavior in pyarrow:\r\n```python\r\nimport pyarrow as pa\r\n\r\narr = pa.array([None, [0]])\r\nreconstructed_arr = pa.ListArray.from_arrays(arr.offsets, arr.values)\r\nprint(reconstructed_arr.to_pylist())\r\n# [[], [0]]\r\n```\r\n\r\nIt seems that `arr.offsets` can reconstruct the array properly, but an offsets array with null values can:\r\n```python\r\nfixed_offsets = pa.array([None, 0, 1])\r\nfixed_arr = pa.ListArray.from_arrays(fixed_offsets, arr.values)\r\nprint(fixed_arr.to_pylist())\r\n# [None, [0]]\r\n\r\nprint(arr.offsets.to_pylist())\r\n# [0, 0, 1]\r\nprint(fixed_offsets.to_pylist())\r\n# [None, 0, 1]\r\n```\r\nEDIT: this is because `arr.offsets` is not enough to reconstruct the array, we also need the validity bitmap",
"The offsets don't have nulls because they don't include the validity bitmap from `arr.buffers()[0]`, which is used to say which values are null and which values are non-null.\r\n\r\nThough the validity bitmap also seems to be wrong:\r\n```python\r\nbin(int(arr.buffers()[0].hex(), 16))\r\n# '0b10'\r\n# it should be 0b110 - 1 corresponds to non-null and 0 corresponds to null, if you take the bits in reverse order\r\n```\r\n\r\nSo apparently I can't even create the fixed offsets array using this.\r\n\r\nIf I understand correctly it's always missing the 1 on the left, so I can add it manually as a hack to fix the issue until this is fixed in pyarrow EDIT: actually it may be more complicated than that\r\n\r\nEDIT2: actuall it's right, it corresponds to the validity bitmap of the array of logical length 2. So if we use the offsets array, the values array, and this validity bitmap it should be possible to reconstruct the array properly",
"I created an issue on Apache Arrow's JIRA: https://issues.apache.org/jira/browse/ARROW-15837",
"And another one: https://issues.apache.org/jira/browse/ARROW-15839",
"FYI the behavior is the same with:\r\n- `datasets` version: 1.18.3\r\n- Platform: Linux-5.8.0-50-generic-x86_64-with-debian-bullseye-sid\r\n- Python version: 3.7.11\r\n- PyArrow version: 6.0.1\r\n\r\n\r\nbut not with:\r\n- `datasets` version: 1.8.0\r\n- Platform: Linux-4.18.0-305.40.2.el8_4.x86_64-x86_64-with-redhat-8.4-Ootpa\r\n- Python version: 3.7.11\r\n- PyArrow version: 3.0.0\r\n\r\ni.e. it outputs:\r\n```py\r\n0 [None, [0]]\r\n1 [None, [0]]\r\n2 [None, [0]]\r\n3 [None, [0]]\r\n```\r\n",
"Thanks for the insights @PaulLerner !\r\n\r\nI found a way to workaround this issue for the code example presented in this issue.\r\n\r\nNote that empty lists will still appear when you explicitly `cast` a list of lists that contain None values like [None, [0]] to a new feature type (e.g. to change the integer precision). In this case it will show a warning that it happened. If you don't cast anything, then the None values will be kept as expected.\r\n\r\nLet me know what you think !",
"Hi! I feel like I’m missing something in your answer, *what* is the workaround? Is it fixed in some `datasets` version?",
"`pa.ListArray.from_arrays` returns empty lists instead of None values. The workaround I added inside `datasets` simply consists in not using `pa.ListArray.from_arrays` :)\r\n\r\nOnce this PR [here ](https://github.com/huggingface/datasets/pull/4282)is merged, we'll release a new version of `datasets` that currectly returns the None values in the case described in this issue\r\n\r\nEDIT: released :) but let's keep this issue open because it might happen again if users change the integer precision for example"
] | Sometimes `None` can be replaced by `[]` when running map:
```python
from datasets import Dataset
ds = Dataset.from_dict({"a": range(4)})
ds = ds.map(lambda x: {"b": [[None, [0]]]}, batched=True, batch_size=1, remove_columns=["a"])
print(ds.to_pandas())
# b
# 0 [None, [0]]
# 1 [[], [0]]
# 2 [[], [0]]
# 3 [[], [0]]
```
This issue has been experienced when running the `run_qa.py` example from `transformers` (see issue https://github.com/huggingface/transformers/issues/15401)
This can be due to a bug in when casting `None` in nested lists. Casting only happens after the first batch, since the first batch is used to infer the feature types.
cc @sgugger | 3,676 |
https://github.com/huggingface/datasets/issues/3675 | Add CodeContests dataset | [
"@mariosasko Can I take this up?",
"This dataset is now available here: https://huggingface.co/datasets/deepmind/code_contests."
] | ## Adding a Dataset
- **Name:** CodeContests
- **Description:** CodeContests is a competitive programming dataset for machine-learning.
- **Paper:**
- **Data:** https://github.com/deepmind/code_contests
- **Motivation:** This dataset was used when training [AlphaCode](https://deepmind.com/blog/article/Competitive-programming-with-AlphaCode).
Instructions to add a new dataset can be found [here](https://github.com/huggingface/datasets/blob/master/ADD_NEW_DATASET.md).
| 3,675 |
https://github.com/huggingface/datasets/issues/3673 | `load_dataset("snli")` is different from dataset viewer | [
"Yes, we decided to replace the encoded label with the corresponding label when possible in the dataset viewer. But\r\n1. maybe it's the wrong default\r\n2. we could find a way to show both (with a switch, or showing both ie. `0 (neutral)`).\r\n",
"Hi @severo,\r\n\r\nThanks for clarifying. \r\n\r\nI think this default is a bit counterintuitive for the user. However, this is a personal opinion that might not be general. I think it is nice to have the actual (non-encoded) labels in the viewer. On the other hand, it would be nice to match what the user sees with what they get when they download a dataset. I don't know - I can see the difficulty of choosing a default :)\r\nMaybe having non-encoded labels as a default can be useful?\r\n\r\nAnyway, I think the issue has been addressed. Thanks a lot for your super-quick answer!\r\n\r\n ",
"Thanks for the 👍 in https://github.com/huggingface/datasets/issues/3673#issuecomment-1029008349 @mariosasko @gary149 @pietrolesci, but as I proposed various solutions, it's not clear to me which you prefer. Could you write your preferences as a comment?\r\n\r\n_(note for myself: one idea per comment in the future)_",
"As I am working with seq2seq, I prefer having the label in string form rather than numeric. So the viewer is fine and the underlying dataset should be \"decoded\" (from int to str). In this way, the user does not have to search for a mapping `int -> original name` (even though is trivial to find, I reckon). Also, encoding labels is rather easy.\r\n\r\nI hope this is useful",
"I like the idea of \"0 (neutral)\". The label name can even be greyed to make it clear that it's not part of the actual item in the dataset, it's just the meaning.",
"I like @lhoestq's idea of having grayed-out labels.",
"Proposals by @gary149. Which one do you prefer? Please vote with the thumbs\r\n\r\n- 👍 \r\n\r\n 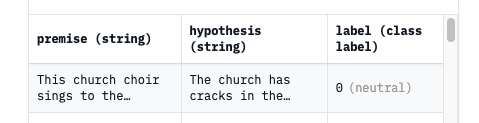\r\n\r\n- 👎 \r\n\r\n 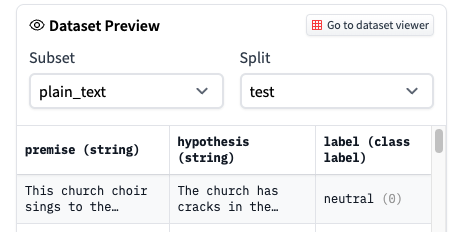\r\n\r\n",
"I like Option 1 better as it shows clearly what the user is downloading",
"Thanks! ",
"It's [live](https://huggingface.co/datasets/glue/viewer/cola/train):\r\n\r\n<img width=\"1126\" alt=\"Capture d’écran 2022-02-14 à 10 26 03\" src=\"https://user-images.githubusercontent.com/1676121/153836716-25f6205b-96af-42d8-880a-7c09cb24c420.png\">\r\n\r\nThanks all for the help to improve the UI!",
"Love it ! thanks :)"
] | ## Describe the bug
The dataset that is downloaded from the Hub via `load_dataset("snli")` is different from what is available in the dataset viewer. In the viewer the labels are not encoded (i.e., "neutral", "entailment", "contradiction"), while the downloaded dataset shows the encoded labels (i.e., 0, 1, 2).
Is this expected?
## Environment info
<!-- You can run the command `datasets-cli env` and copy-and-paste its output below. -->
- `datasets` version:
- Platform: Ubuntu 20.4
- Python version: 3.7
| 3,673 |
https://github.com/huggingface/datasets/issues/3671 | Give an estimate of the dataset size in DatasetInfo | [] | **Is your feature request related to a problem? Please describe.**
Currently, only part of the datasets provide `dataset_size`, `download_size`, `size_in_bytes` (and `num_bytes` and `num_examples` inside `splits`). I would want to get this information, or an estimation, for all the datasets.
**Describe the solution you'd like**
- get access to the git information for the dataset files hosted on the hub
- look at the [`Content-Length`](https://developer.mozilla.org/en-US/docs/Web/HTTP/Headers/Content-Length) for the files served by HTTP
| 3,671 |
https://github.com/huggingface/datasets/issues/3668 | Couldn't cast array of type string error with cast_column | [
"Hi ! I wasn't able to reproduce the error, are you still experiencing this ? I tried calling `cast_column` on a string column containing paths.\r\n\r\nIf you manage to share a reproducible code example that would be perfect",
"Hi,\r\n\r\nI think my team mate got this solved. Clolsing it for now and will reopen if I experience this again.\r\nThanks :) ",
"Hi @R4ZZ3,\r\n\r\nIf it is not too much of a bother, can you please help me how to resolve this error? I am exactly getting the same error where I am going as per the documentation guideline:\r\n\r\n`my_audio_dataset = my_audio_dataset.cast_column(\"audio_paths\", Audio())`\r\n\r\nwhere `\"audio_paths\"` is a dataset column (feature) having strings of absolute paths to mp3 files of the dataset.\r\n\r\n",
"I was having the same issue with this code:\r\n\r\n```\r\ndataset = dataset.map(\r\n lambda batch: {\"full_path\" : os.path.join(self.data_path, batch[\"path\"])},\r\n num_procs = 4\r\n)\r\nmy_audio_dataset = dataset.cast_column(\"full_path\", Audio(sampling_rate=16_000))\r\n```\r\n\r\nRemoving the \"num_procs\" argument fixed it somehow.\r\nUsing a mac with m1 chip",
"Hi @Hubert-Bonisseur, I think this will be fixed by https://github.com/huggingface/datasets/pull/4614"
] | ## Describe the bug
In OVH cloud during Huggingface Robust-speech-recognition event on a AI training notebook instance using jupyter lab and running jupyter notebook When using the dataset.cast_column("audio",Audio(sampling_rate=16_000))
method I get error

This was working with datasets version 1.17.1.dev0
but now with version 1.18.3 produces the error above.
## Steps to reproduce the bug
load dataset:

remove columns:

run my fix_path function.
This also creates the audio column that is referring to the absolute file path of the audio

Then I concatenate few other datasets and finally try the cast_column method
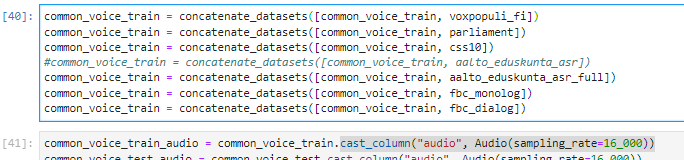
but get error:
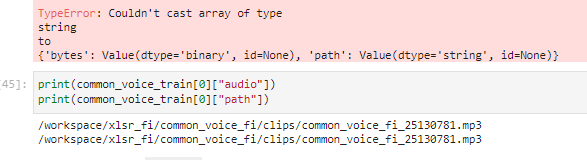
## Expected results
A clear and concise description of the expected results.
## Actual results
Specify the actual results or traceback.
## Environment info
<!-- You can run the command `datasets-cli env` and copy-and-paste its output below. -->
- `datasets` version: 1.18.3
- Platform:
OVH Cloud, AI Training section, container for Huggingface Robust Speech Recognition event image(baaastijn/ovh_huggingface)
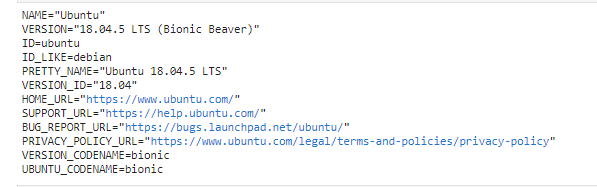
- Python version: 3.8.8
- PyArrow version:
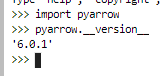
| 3,668 |
https://github.com/huggingface/datasets/issues/3663 | [Audio] Path of Common Voice cannot be used for audio loading anymore | [
"Having talked to @lhoestq, I see that this feature is no longer supported. \r\n\r\nI really don't think this was a good idea. It is a major breaking change and one for which we don't even have a working solution at the moment, which is bad for PyTorch as we don't want to force people to have `datasets` decode audio files automatically, but **really** bad for Tensorflow and Flax where we **currently cannot** even use `datasets` to load `.mp3` files - e.g. `common_voice` doesn't work anymore in a TF training script. Note this worked perfectly fine before making the change (think it was done [here](https://github.com/huggingface/datasets/pull/3290) no?)\r\n\r\nIMO, it's really important to think about a solution here and I strongly favor to make a difference here between loading a dataset in streaming mode and in non-streaming mode, so that in non-streaming mode the actual downloaded file is displayed. It's really crucial for people to be able to analyse the original files IMO when the dataset is not downloaded in streaming mode. \r\n\r\nThere are the following reasons why it is paramount to have access to the **original** audio file in my opinion (in non-streaming mode):\r\n- There are a wide variety of different libraries to load audio data with varying support on different platforms. For me it was quite clear that there is simply to single good library to load audio files for all platforms - so we have to leave the option to the user to decide which loading to use.\r\n- We had support for audio datasets a long time before streaming audio was possible. There were quite some versions where we advertised **everywhere** to load the audio from the path name (and there are many places where we still do even though it's not possible anymore). To give some examples:\r\n - Official example of TF Wav2Vec2: https://github.com/huggingface/transformers/blob/f427e750490b486944cc9be3c99834ad5cf78b57/src/transformers/models/wav2vec2/modeling_tf_wav2vec2.py#L1423 Wav2Vec2 is as important for speech as BERT is for NLP - so it's **very** important. The official example currently doesn't work and we don't even have a workaround for it for MP3 files at the moment. Same goes for Flax.\r\n - The most downloaded non-nlp checkpoint: https://huggingface.co/facebook/wav2vec2-base-960h#usage has a usage example which doesn't work anymore with the current datasets implementation. I'll update this now, but we have >1000 wav2vec2 checkpoints on the Hub and we can't update all the model cards.\r\n => This is a big breaking change with no current solution. For `transformers` breaking changes are one of the biggest complaints.\r\n- Similar to this we also shouldn't assume that there is only one resampling method for Audio. I think it's good to have one offered automatically by `datasets`, but we have to leave the user the freedom to choose her/his own resampling as well. Resampling can take very different filtering windows and other parameters which are currently somewhat hardcoded in `datasets`, which users might very well want to change.\r\n\r\n\r\n=> IMO, it's a **very** big priority to again have the correct absolute path in non-streaming mode. The other solution of providing a path-like object derived from the bytes stocked in the `.array` file is not nearly as user-friendly, but better than nothing. ",
"Agree that we need to have access to the original sound files. Few days ago I was looking for these original files because I suspected there is bug in the audio resampling (confirmed in https://github.com/huggingface/datasets/issues/3662) and I want to do my own resampling to workaround the bug, which is now not possible anymore due to the unavailability of the original files.",
"@patrickvonplaten \r\n> The other solution of providing a path-like object derived from the bytes stocked in the .array file is not nearly as user-friendly, but better than nothing\r\n\r\nJust to clarify, here you describe the approach that uses the `Audio.decode` attribute to access the underlying bytes?\r\n\r\n> The official example currently doesn't work and we don't even have a workaround for it for MP3 files at the moment\r\n\r\nI'd assume this is because we use `sox_io` as a backend for decoding. However, soon we should be able to use `soundfile`, which supports path-like objects, for MP3 (https://github.com/huggingface/datasets/pull/3667#issuecomment-1030090627).\r\n\r\nYour concern is reasonable, but there are situations where we can only serve bytes (see https://github.com/huggingface/datasets/pull/3685 for instance). IMO it makes sense to fix the affected datasets for now, but I don't think we should care too much whether we rely on local paths or bytes after soundfile adds support for MP3 as long as our examples work (shouldn't be too hard to update the `map_to_array` functions) and we properly document how to access the underlying path/bytes for custom decoding (via `ds.cast_column(\"audio\", Audio(decode=False))`).\r\n",
"Related to this discussion: in https://github.com/huggingface/datasets/pull/3664#issuecomment-1031866858 I propose how we could change `iter_archive` to work for streaming and also return local paths (as it used too !). I'd love your opinions on this",
"> @patrickvonplaten\r\n> \r\n> > The other solution of providing a path-like object derived from the bytes stocked in the .array file is not nearly as user-friendly, but better than nothing\r\n> \r\n> Just to clarify, here you describe the approach that uses the `Audio.decode` attribute to access the underlying bytes?\r\n\r\nYes! \r\n\r\n> \r\n> > The official example currently doesn't work and we don't even have a workaround for it for MP3 files at the moment\r\n> \r\n> I'd assume this is because we use `sox_io` as a backend for decoding. However, soon we should be able to use `soundfile`, which supports path-like objects, for MP3 ([#3667 (comment)](https://github.com/huggingface/datasets/pull/3667#issuecomment-1030090627)). \r\n> Your concern is reasonable, but there are situations where we can only serve bytes (see #3685 for instance). IMO it makes sense to fix the affected datasets for now, but I don't think we should care too much whether we rely on local paths or bytes after soundfile adds support for MP3 as long as our examples work (shouldn't be too hard to update the `map_to_array` functions) and we properly document how to access the underlying path/bytes for custom decoding (via `ds.cast_column(\"audio\", Audio(decode=False))`).\r\n\r\nYes this might be, but I highly doubt that `soundfile` is the go-to library for audio then. @anton-l and I have tried out a bunch of different audio loading libraries (`soundfile`, `librosa`, `torchaudio`, pure `ffmpeg`, `audioread`, ...). One thing that was pretty clear to me is that there is just no \"de-facto standard\" library and they all have pros and cons. None of the libraries really supports \"batch\"-ed audio loading. Some depend on PyTorch. `torchaudio` is 100x faster (really!) than `librosa's` fallback on MP3. `torchaudio` often has problems with multi-proessing, ... Also we should keep in mind that resampling is similarly not as simple as reading a text file. It's a pretty complex signal processing transform and people very well might want to use special filters, etc...at the moment we just hard-code `torchaudio's` or `librosa's` default filter when doing resampling.\r\n\r\n=> All this to say that we **should definitely** care about whether we rely on local paths or bytes IMO. We don't want to loose all users that are forced to use `datasets` decoding or resampling or have to built a very much not intuitive way of loading bytes into a numpy array. It's much more intuitive to be able to inspect a local file. I feel pretty strongly about this and am happy to also jump on a call. Keeping libraries flexible and lean as well as exposing internals is very important IMO (this philosophy has worked quite well so far with Transformers).\r\n\r\n",
"Thanks a lot for the very detailed explanation. Now everything makes much more sense.",
"From https://github.com/huggingface/datasets/pull/3736 the Common Voice dataset now gives access to the local audio files as before",
"I understand the argument that it is bad to have a breaking change. How to deal with the introduction of breaking changes is a topic of its own and not sure how you want to deal with that (or is the policy this is never allowed, and there must be a `load_dataset_v2` or so if you really want to introduce a breaking change?).\r\n\r\nRegardless of whether it is a breaking change, however, I don't see the other arguments.\r\n\r\n> but **really** bad for Tensorflow and Flax where we **currently cannot** even use `datasets` to load `.mp3` files\r\n\r\nI don't exactly understand this. Why not?\r\n\r\nWhy does the HF dataset on-the-fly decoding mechanism not work? Why is it anyway specific to PyTorch or TensorFlow? Isn't this independent?\r\n\r\nBut even if you just provide the raw bytes to TF, on TF you could just use sth like `tfio.audio.decode_mp3` or `tf.audio.decode_ogg` or `tfio.audio.decode_flac`?\r\n\r\n> There are the following reasons why it is paramount to have access to the original audio file in my opinion ...\r\n\r\nI don't really understand the arguments (despite that it maybe breaks existing code). You anyway have the original audio files but it is just embedded in the dataset? I don't really know about any library which cannot also load the audio from memory (i.e. from the dataset).\r\n\r\nBtw, on librosa being slow for decoding audio files, I saw that as well, so we have this comment RETURNN:\r\n\r\n> Don't use librosa.load which internally uses audioread which would use Gstreamer as a backend which has multiple issues:\r\n> https://github.com/beetbox/audioread/issues/62\r\n> https://github.com/beetbox/audioread/issues/63\r\n> Instead, use PySoundFile (soundfile), which is also faster. See here for discussions:\r\n> https://github.com/beetbox/audioread/issues/64\r\n> https://github.com/librosa/librosa/issues/681\r\n\r\nResampling is also a separate aspect, which is also less straightforward and with different compromises between speed and quality. So there the different tradeoffs and different implementations can make a difference.\r\n\r\nHowever, I don't see how this is related to the question whether there should be the raw bytes inside the dataset or as separate local files.\r\n",
"Thanks for your comments here @albertz - cool to get your input! \r\n\r\nAnswering a bit here between the lines:\r\n\r\n> I understand the argument that it is bad to have a breaking change. How to deal with the introduction of breaking changes is a topic of its own and not sure how you want to deal with that (or is the policy this is never allowed, and there must be a `load_dataset_v2` or so if you really want to introduce a breaking change?).\r\n> \r\n> Regardless of whether it is a breaking change, however, I don't see the other arguments.\r\n> \r\n> > but **really** bad for Tensorflow and Flax where we **currently cannot** even use `datasets` to load `.mp3` files\r\n> \r\n> I don't exactly understand this. Why not?\r\n\r\n> Why does the HF dataset on-the-fly decoding mechanism not work? Why is it anyway specific to PyTorch or TensorFlow? Isn't this independent?\r\n\r\nThe problem with decoding on the fly is that we currently rely on `torchaudio` for this now which relies on `torch` which is not necessarily something people would like to install when using `tensorflow` or `flax`. Therefore we cannot just rely on people using the decoding on the fly method. We just didn't find a library that is ML framework independent and fast enough for all formats. `torchaudio` is currently in our opinion by far the best here.\r\n\r\nSo for TF and Flax it's important that users can load audio files or bytes they way the want to - this might become less important if we find (or make) a good library with few dependencies that is fast for all kinds of platforms / use cases.\r\n\r\n\r\nNow the question is whether it's better to store audio data as a path to a file or as raw bytes I guess.\\\r\nMy main arguments for storing the audio data as a path to a file is pretty much all about users experience - I don't really expect our users to understand the inner workings of datasets:\r\n\r\n- 1. It's not straightforward to know which function to use to decode it - not all `load_audio(...)` or `read_audio(...)` work on raw bytes. E.g. Looking at https://pytorch.org/audio/stable/torchaudio.html?highlight=load#torchaudio.load one would not see directly how to load raw bytes . There are also some functions of other libraries which only work on files which would require the user to save the bytes as a file first before being able to load it.\r\n- 2. It's difficult to see which format the bytes are coming from (mp3, ogg, ...) - guess this could be remedied by adding the format to each sample though\r\n- 3. It is a bit scary IMO to see raw bytes for users. Overall, I think it's better to leave the data in it's raw form as this way it's much easier for people to play around with the audio files, less need to read docs because people don't worry about what happened to the audio files (are the bytes already resampled?)\r\n\r\nBut the argument that the audio should be loadable directly from memory is good - haven't thought about this too much. \r\nI guess it's still very much possible for the user to do this:\r\n\r\n```python\r\ndef save_as_bytes:\r\n batch[\"bytes\"] = read_in_bytes_from_file(batch[\"file\"])\\\r\n os.remove(batch[\"file\"])\r\n\r\nds = ds.map(save_as_bytes)\r\n\r\nds.save_to_disk(...)\r\n```\r\n\r\nGuess the question is more a bit about what should be the default case?",
"> The problem with decoding on the fly is that we currently rely on `torchaudio` for this now which relies on `torch` which is not necessarily something people would like to install when using `tensorflow` or `flax`. Therefore we cannot just rely on people using the decoding on the fly method. We just didn't find a library that is ML framework independent and fast enough for all formats. `torchaudio` is currently in our opinion by far the best here.\r\n\r\nBut how is this relevant for this issue here? I thought this issue here is about having the (correct) path in the dataset or having raw bytes in the dataset.\r\n\r\nHow did TF users use it at all then? Or they just do not use on-the-fly decoding? I did not even notice this problem (maybe because I had `torchaudio` installed). But what do they use instead?\r\n\r\nBut as I outlined before, they could just use `tfio.audio.decode_flac` and co, where it would be more natural if you already provide the raw bytes.\r\n\r\n> Looking at https://pytorch.org/audio/stable/torchaudio.html?highlight=load#torchaudio.load one would not see directly how to load raw bytes\r\n\r\nI was not really familiar with `torchaudio`. It seems that they really don't provide an easy/direct API to operate on raw bytes. Which is very strange and unfortunate because as far as I can see, all the underlying backend libraries (e.g. soundfile) easily allow that. So I would say that this is the fault of `torchaudio` then. But despite, if you anyway use `torchaudio` with `soundfile` backend, why not just use `soundfile` directly. It's very simple to use and crossplatform.\r\n\r\nBut ok, now we are just discussing how to handle the on-the-fly decoding. I still think this is a separate issue and having raw bytes in the dataset instead of local files should just be fine as well.\r\n\r\n\r\n> It is a bit scary IMO to see raw bytes for users.\r\n\r\nI think nobody who writes code is scared by seeing the raw bytes content of a binary file. :)\r\n\r\n\r\n> I guess it's still very much possible for the user to do this:\r\n> \r\n> ```python\r\n> def save_as_bytes:\r\n> batch[\"bytes\"] = read_in_bytes_from_file(batch[\"file\"])\\\r\n> os.remove(batch[\"file\"])\r\n> \r\n> ds = ds.map(save_as_bytes)\r\n> \r\n> ds.save_to_disk(...)\r\n> ```\r\n\r\nIn https://github.com/huggingface/datasets/pull/4184#issuecomment-1105191639, you said/proposed that this `map` is not needed anymore and `save_to_disk` could do it automatically (maybe via some option)?\r\n\r\n> Guess the question is more a bit about what should be the default case?\r\n\r\nYea this is up to you. I'm happy as long as we can get it the way we want easily and this is a well supported use case. :)\r\n",
"> In https://github.com/huggingface/datasets/pull/4184#issuecomment-1105191639, you said/proposed that this map is not needed anymore and save_to_disk could do it automatically (maybe via some option)?\r\n\r\nYes! Should be super easy now see discussion here: https://github.com/rwth-i6/i6_core/issues/257#issuecomment-1105494468\r\n\r\nThanks for the super useful input :-)",
"Despite the comments that this has been fixed, I am finding the exact same problem is occurring again (with datasets version 2.3.2)",
"> Despite the comments that this has been fixed, I am finding the exact same problem is occurring again (with datasets version 2.3.2)\r\n\r\nIt appears downgrading to torchaudio 0.11.0 fixed this problem.",
"@DCNemesis, sorry which problem exactly is occuring again? Also cc @lhoestq @polinaeterna here",
"@patrickvonplaten @lhoestq @polinaeterna I was unable to load audio from Common Voice using 🤗 with the current version of torchaudio, but downgrading to torchaudio 0.11.0 fixed it. This is probably more of a torch problem than a Hugging Face problem.",
"@DCNemesis that's interesting, could you please share the error message if you still can access it? ",
"@polinaeterna I believe it is the same exact error as above. It occurs on other .mp3 sources as well, but the problem is with torchaudio > 0.11.0. I've created a short colab notebook that reproduces the error, and the fix here: https://colab.research.google.com/drive/18wsuwdHwBPN3JkcnhEtk8MUYqF9swuWZ?usp=sharing",
"Hi @DCNemesis,\r\n\r\nYour issue was slightly different from the original one in this issue page. Yours seems related to a change in the backend used by `torchaudio` (`ffmpeg` instead of `sox`). Refer to the issue page here:\r\n- #4776\r\n\r\nNormally, it should be circumvented with the patch made by @polinaeterna in:\r\n- #4923",
"I think the original issue reported here was already fixed by:\r\n- #3736\r\n\r\nOtherwise, feel free to reopen."
] | ## Describe the bug
## Steps to reproduce the bug
```python
from datasets import load_dataset
from torchaudio import load
ds = load_dataset("common_voice", "ab", split="train")
# both of the following commands fail at the moment
load(ds[0]["audio"]["path"])
load(ds[0]["path"])
```
## Expected results
The path should be the complete absolute path to the downloaded audio file not some relative path.
## Actual results
```bash
~/hugging_face/venv_3.9/lib/python3.9/site-packages/torchaudio/backend/sox_io_backend.py in load(filepath, frame_offset, num_frames, normalize, channels_first, format)
150 filepath, frame_offset, num_frames, normalize, channels_first, format)
151 filepath = os.fspath(filepath)
--> 152 return torch.ops.torchaudio.sox_io_load_audio_file(
153 filepath, frame_offset, num_frames, normalize, channels_first, format)
154
RuntimeError: Error loading audio file: failed to open file cv-corpus-6.1-2020-12-11/ab/clips/common_voice_ab_19904194.mp3
```
## Environment info
<!-- You can run the command `datasets-cli env` and copy-and-paste its output below. -->
- `datasets` version: 1.18.3.dev0
- Platform: Linux-5.4.0-96-generic-x86_64-with-glibc2.27
- Python version: 3.9.1
- PyArrow version: 3.0.0
| 3,663 |
https://github.com/huggingface/datasets/issues/3662 | [Audio] MP3 resampling is incorrect when dataset's audio files have different sampling rates | [
"Thanks @lhoestq for finding the reason of incorrect resampling. This issue affects all languages which have sound files with different sampling rates such as Turkish and Luganda.",
"@cahya-wirawan - do you know how many languages have different sampling rates in Common Voice? I'm quite surprised to see this for multiple languages actually",
"@cahya-wirawan, I can reproduce the problem for Common Voice 7 for Turkish. Here a script you can use:\r\n\r\n\r\n```python\r\n#!/usr/bin/env python3\r\nfrom datasets import load_dataset\r\nimport torchaudio\r\nfrom io import BytesIO\r\nfrom datasets import Audio\r\nfrom collections import Counter\r\nimport sys\r\n\r\nds_name = str(sys.argv[1])\r\nlang = str(sys.argv[2])\r\n\r\nds = load_dataset(ds_name, lang, split=\"train\", use_auth_token=True)\r\nds = ds.cast_column(\"audio\", Audio(decode=False))\r\n\r\nall_sampling_rates = []\r\n\r\n\r\ndef print_sampling_rate(x):\r\n x, sr = torchaudio.load(BytesIO(x[\"audio\"][\"bytes\"]), format=\"mp3\")\r\n all_sampling_rates.append(sr)\r\n\r\nds.map(print_sampling_rate)\r\n\r\n\r\nprint(Counter(all_sampling_rates))\r\n```\r\n\r\ncan be run with:\r\n\r\n```bash\r\npython run.py mozilla-foundation/common_voice_7_0 tr\r\n```\r\n\r\nFor CV 6.1 all samples seem to have the same audio",
"It actually shows that many more samples are in 32kHz format than it 48kHz which is unexpected. Thanks a lot for flagging! Will contact Common Voice about this as well",
"I only checked the CV 7.0 for Turkish, Luganda and Indonesian, they have audio files with difference sampling rates, and all of them are affected by this issue. Percentage of incorrect resampling as follow, Turkish: 9.1%, Luganda: 88.2% and Indonesian: 64.1%.\r\nI checked it using the original CV files. I check the original sampling rates and the length of audio array of each files and compare it with the length of audio array (and the sampling rate which is always 48kHz) from mozilla-foundation/common_voice_7_0 datasets. if the length of audio array from dataset is not equal to 48kHz/original sampling rate * length of audio array of the original audio file then it is affected,",
"Ok wow, thanks a lot for checking this - you've found a pretty big bug :sweat_smile: It seems like **a lot** more datasets are actually affected than I original thought. We'll try to solve this as soon as possible and make an announcement tomorrow."
] | The Audio feature resampler for MP3 gets stuck with the first original frequencies it meets, which leads to subsequent decoding to be incorrect.
Here is a code to reproduce the issue:
Let's first consider two audio files with different sampling rates 32000 and 16000:
```python
# first download a mp3 file with sampling_rate=32000
!wget https://file-examples-com.github.io/uploads/2017/11/file_example_MP3_700KB.mp3
import torchaudio
audio_path = "file_example_MP3_700KB.mp3"
audio_path2 = audio_path.replace(".mp3", "_resampled.mp3")
resample = torchaudio.transforms.Resample(32000, 16000) # create a new file with sampling_rate=16000
torchaudio.save(audio_path2, resample(torchaudio.load(audio_path)[0]), 16000)
```
Then we can see an issue here when decoding:
```python
from datasets import Dataset, Audio
dataset = Dataset.from_dict({"audio": [audio_path, audio_path2]}).cast_column("audio", Audio(48000))
dataset[0] # decode the first audio file sets the resampler orig_freq to 32000
print(dataset .features["audio"]._resampler.orig_freq)
# 32000
print(dataset[0]["audio"]["array"].shape) # here decoding is fine
# (1308096,)
dataset = Dataset.from_dict({"audio": [audio_path, audio_path2]}).cast_column("audio", Audio(48000))
dataset[1] # decode the second audio file sets the resampler orig_freq to 16000
print(dataset .features["audio"]._resampler.orig_freq)
# 16000
print(dataset[0]["audio"]["array"].shape) # here decoding uses orig_freq=16000 instead of 32000
# (2616192,)
```
The value of `orig_freq` doesn't change no matter what file needs to be decoded
cc @patrickvonplaten @anton-l @cahya-wirawan @albertvillanova
The issue seems to be here in `Audio.decode_mp3`:
https://github.com/huggingface/datasets/blob/4c417d52def6e20359ca16c6723e0a2855e5c3fd/src/datasets/features/audio.py#L176-L180 | 3,662 |
https://github.com/huggingface/datasets/issues/3659 | push_to_hub but preview not working | [
"Hi @thomas-happify, please note that the preview may take some time before rendering the data.\r\n\r\nI've seen it is already working.\r\n\r\nI close this issue. Please feel free to reopen it if the problem arises again."
] | ## Dataset viewer issue for '*happifyhealth/twitter_pnn*'
**Link:** *[link to the dataset viewer page](https://huggingface.co/datasets/happifyhealth/twitter_pnn)*
I used
```
dataset.push_to_hub("happifyhealth/twitter_pnn")
```
but the preview is not working.
Am I the one who added this dataset ? Yes
| 3,659 |
https://github.com/huggingface/datasets/issues/3658 | Dataset viewer issue for *P3* | [
"The error is now:\r\n\r\n```\r\nStatus code: 400\r\nException: Status400Error\r\nMessage: this dataset is not supported for now.\r\n```\r\n\r\nWe've disabled the dataset viewer for several big datasets like this one. We hope being able to reenable it soon.",
"The list of splits cannot be obtained. cc @huggingface/datasets ",
"```\r\nError code: SplitsNamesError\r\nException: SplitsNotFoundError\r\nMessage: The split names could not be parsed from the dataset config.\r\nTraceback: Traceback (most recent call last):\r\n File \"/src/services/worker/.venv/lib/python3.9/site-packages/datasets/inspect.py\", line 354, in get_dataset_config_info\r\n for split_generator in builder._split_generators(\r\n File \"/tmp/modules-cache/datasets_modules/datasets/bigscience--P3/12c0badfecad4564ecb8a6f81b5d0559656f269f08b13c59c93283f3a84134ba/P3.py\", line 154, in _split_generators\r\n data_dir = dl_manager.download_and_extract(_URLs)\r\n File \"/src/services/worker/.venv/lib/python3.9/site-packages/datasets/download/streaming_download_manager.py\", line 944, in download_and_extract\r\n return self.extract(self.download(url_or_urls))\r\n File \"/src/services/worker/.venv/lib/python3.9/site-packages/datasets/download/streaming_download_manager.py\", line 907, in extract\r\n urlpaths = map_nested(self._extract, path_or_paths, map_tuple=True)\r\n File \"/src/services/worker/.venv/lib/python3.9/site-packages/datasets/utils/py_utils.py\", line 393, in map_nested\r\n mapped = [\r\n File \"/src/services/worker/.venv/lib/python3.9/site-packages/datasets/utils/py_utils.py\", line 394, in <listcomp>\r\n _single_map_nested((function, obj, types, None, True, None))\r\n File \"/src/services/worker/.venv/lib/python3.9/site-packages/datasets/utils/py_utils.py\", line 346, in _single_map_nested\r\n return {k: _single_map_nested((function, v, types, None, True, None)) for k, v in pbar}\r\n File \"/src/services/worker/.venv/lib/python3.9/site-packages/datasets/utils/py_utils.py\", line 346, in <dictcomp>\r\n return {k: _single_map_nested((function, v, types, None, True, None)) for k, v in pbar}\r\n File \"/src/services/worker/.venv/lib/python3.9/site-packages/datasets/utils/py_utils.py\", line 346, in _single_map_nested\r\n return {k: _single_map_nested((function, v, types, None, True, None)) for k, v in pbar}\r\n File \"/src/services/worker/.venv/lib/python3.9/site-packages/datasets/utils/py_utils.py\", line 346, in <dictcomp>\r\n return {k: _single_map_nested((function, v, types, None, True, None)) for k, v in pbar}\r\n File \"/src/services/worker/.venv/lib/python3.9/site-packages/datasets/utils/py_utils.py\", line 330, in _single_map_nested\r\n return function(data_struct)\r\n File \"/src/services/worker/.venv/lib/python3.9/site-packages/datasets/download/streaming_download_manager.py\", line 912, in _extract\r\n protocol = _get_extraction_protocol(urlpath, use_auth_token=self.download_config.use_auth_token)\r\n File \"/src/services/worker/.venv/lib/python3.9/site-packages/datasets/download/streaming_download_manager.py\", line 402, in _get_extraction_protocol\r\n return _get_extraction_protocol_with_magic_number(f)\r\n File \"/src/services/worker/.venv/lib/python3.9/site-packages/datasets/download/streaming_download_manager.py\", line 367, in _get_extraction_protocol_with_magic_number\r\n magic_number = f.read(MAGIC_NUMBER_MAX_LENGTH)\r\n File \"/src/services/worker/.venv/lib/python3.9/site-packages/fsspec/implementations/http.py\", line 574, in read\r\n return super().read(length)\r\n File \"/src/services/worker/.venv/lib/python3.9/site-packages/fsspec/spec.py\", line 1575, in read\r\n out = self.cache._fetch(self.loc, self.loc + length)\r\n File \"/src/services/worker/.venv/lib/python3.9/site-packages/fsspec/caching.py\", line 377, in _fetch\r\n self.cache = self.fetcher(start, bend)\r\n File \"/src/services/worker/.venv/lib/python3.9/site-packages/fsspec/asyn.py\", line 111, in wrapper\r\n return sync(self.loop, func, *args, **kwargs)\r\n File \"/src/services/worker/.venv/lib/python3.9/site-packages/fsspec/asyn.py\", line 96, in sync\r\n raise return_result\r\n File \"/src/services/worker/.venv/lib/python3.9/site-packages/fsspec/asyn.py\", line 53, in _runner\r\n result[0] = await coro\r\n File \"/src/services/worker/.venv/lib/python3.9/site-packages/fsspec/implementations/http.py\", line 616, in async_fetch_range\r\n out = await r.read()\r\n File \"/src/services/worker/.venv/lib/python3.9/site-packages/aiohttp/client_reqrep.py\", line 1036, in read\r\n self._body = await self.content.read()\r\n File \"/src/services/worker/.venv/lib/python3.9/site-packages/aiohttp/streams.py\", line 375, in read\r\n block = await self.readany()\r\n File \"/src/services/worker/.venv/lib/python3.9/site-packages/aiohttp/streams.py\", line 397, in readany\r\n await self._wait(\"readany\")\r\n File \"/src/services/worker/.venv/lib/python3.9/site-packages/aiohttp/streams.py\", line 304, in _wait\r\n await waiter\r\n aiohttp.client_exceptions.ClientPayloadError: Response payload is not completed\r\n \r\n The above exception was the direct cause of the following exception:\r\n \r\n Traceback (most recent call last):\r\n File \"/src/services/worker/src/worker/responses/splits.py\", line 75, in get_splits_response\r\n split_full_names = get_dataset_split_full_names(dataset, hf_token)\r\n File \"/src/services/worker/src/worker/responses/splits.py\", line 35, in get_dataset_split_full_names\r\n return [\r\n File \"/src/services/worker/src/worker/responses/splits.py\", line 38, in <listcomp>\r\n for split in get_dataset_split_names(dataset, config, use_auth_token=hf_token)\r\n File \"/src/services/worker/.venv/lib/python3.9/site-packages/datasets/inspect.py\", line 404, in get_dataset_split_names\r\n info = get_dataset_config_info(\r\n File \"/src/services/worker/.venv/lib/python3.9/site-packages/datasets/inspect.py\", line 359, in get_dataset_config_info\r\n raise SplitsNotFoundError(\"The split names could not be parsed from the dataset config.\") from err\r\n datasets.inspect.SplitsNotFoundError: The split names could not be parsed from the dataset config.\r\n```",
"Closing in favor of https://huggingface.co/datasets/bigscience/P3/discussions/6 and https://github.com/huggingface/datasets-server/issues/1689"
] | ## Dataset viewer issue for '*P3*'
**Link: https://huggingface.co/datasets/bigscience/P3**
```
Status code: 400
Exception: SplitsNotFoundError
Message: The split names could not be parsed from the dataset config.
```
Am I the one who added this dataset ? No
| 3,658 |
https://github.com/huggingface/datasets/issues/3656 | checksum error subjqa dataset | [
"Hi @RensDimmendaal, \r\n\r\nI'm sorry but I can't reproduce your bug:\r\n```python\r\nIn [1]: from datasets import load_dataset\r\n ...: ds = load_dataset(\"subjqa\", \"electronics\")\r\nDownloading builder script: 9.15kB [00:00, 4.10MB/s] \r\nDownloading metadata: 17.7kB [00:00, 8.51MB/s] \r\nDownloading and preparing dataset subjqa/electronics (download: 10.86 MiB, generated: 3.01 MiB, post-processed: Unknown size, total: 13.86 MiB) to .../.cache/huggingface/datasets/subjqa/electronics/1.1.0/e5588f9298ff2d70686a00cc377e4bdccf4e32287459e3c6baf2dc5ab57fe7fd...\r\nDownloading data: 11.4MB [00:03, 3.50MB/s]\r\nDataset subjqa downloaded and prepared to .../.cache/huggingface/datasets/subjqa/electronics/1.1.0/e5588f9298ff2d70686a00cc377e4bdccf4e32287459e3c6baf2dc5ab57fe7fd. Subsequent calls will reuse this data.\r\n100%|███████████████████████████████████████████████████████████████████████████████████████████████████████████████████████████████████████████████████████████████████████| 3/3 [00:00<00:00, 605.09it/s]\r\n\r\nIn [2]: ds\r\nOut[2]: \r\nDatasetDict({\r\n train: Dataset({\r\n features: ['domain', 'nn_mod', 'nn_asp', 'query_mod', 'query_asp', 'q_reviews_id', 'question_subj_level', 'ques_subj_score', 'is_ques_subjective', 'review_id', 'id', 'title', 'context', 'question', 'answers'],\r\n num_rows: 1295\r\n })\r\n test: Dataset({\r\n features: ['domain', 'nn_mod', 'nn_asp', 'query_mod', 'query_asp', 'q_reviews_id', 'question_subj_level', 'ques_subj_score', 'is_ques_subjective', 'review_id', 'id', 'title', 'context', 'question', 'answers'],\r\n num_rows: 358\r\n })\r\n validation: Dataset({\r\n features: ['domain', 'nn_mod', 'nn_asp', 'query_mod', 'query_asp', 'q_reviews_id', 'question_subj_level', 'ques_subj_score', 'is_ques_subjective', 'review_id', 'id', 'title', 'context', 'question', 'answers'],\r\n num_rows: 255\r\n })\r\n})\r\n```\r\n\r\nCould you please try again and see if the problem persists?\r\n\r\nIf that is the case, you can circumvent the issue by passing `ignore_verifications`:\r\n```python\r\nds = load_dataset(\"subjqa\", \"electronics\", ignore_verifications=True)",
"Thanks checking!\r\n\r\nYou're totally right. I don't know what's changed, but I'm glad it's working now!\r\n\r\n"
] | ## Describe the bug
I get a checksum error when loading the `subjqa` dataset (used in the transformers book).
## Steps to reproduce the bug
```python
from datasets import load_dataset
subjqa = load_dataset("subjqa","electronics")
```
## Expected results
Loading the dataset
## Actual results
```
---------------------------------------------------------------------------
NonMatchingChecksumError Traceback (most recent call last)
<ipython-input-2-d2857d460155> in <module>()
2 from datasets import load_dataset
3
----> 4 subjqa = load_dataset("subjqa","electronics")
3 frames
/usr/local/lib/python3.7/dist-packages/datasets/utils/info_utils.py in verify_checksums(expected_checksums, recorded_checksums, verification_name)
38 if len(bad_urls) > 0:
39 error_msg = "Checksums didn't match" + for_verification_name + ":\n"
---> 40 raise NonMatchingChecksumError(error_msg + str(bad_urls))
41 logger.info("All the checksums matched successfully" + for_verification_name)
42
NonMatchingChecksumError: Checksums didn't match for dataset source files:
['https://github.com/lewtun/SubjQA/archive/refs/heads/master.zip']
```
## Environment info
Google colab
- `datasets` version: 1.18.2
- Platform: Linux-5.4.144+-x86_64-with-Ubuntu-18.04-bionic
- Python version: 3.7.12
- PyArrow version: 3.0.0 | 3,656 |
https://github.com/huggingface/datasets/issues/3655 | Pubmed dataset not reachable | [
"Hi @abhi-mosaic, thanks for reporting.\r\n\r\nI'm looking at it... ",
"also hitting this issue",
"Hey @albertvillanova, sorry to reopen this... I can confirm that on `master` branch the dataset is downloadable now but it is still broken in streaming mode:\r\n\r\n```python\r\n >>> import datasets\r\n >>> pubmed_train = datasets.load_dataset('pubmed', split='train', streaming=True)\r\n >>> next(iter(pubmed_train))\r\n```\r\n```\r\n No such file or directory: 'gzip://pubmed22n0001.xml::ftp://ftp.ncbi.nlm.nih.gov/pubmed/baseline/pubmed22n0001.xml.gz'\r\n```\r\n",
"Hi @abhi-mosaic, would you mind opening another issue for this new problem?\r\n\r\nFirst issue (already solved) was a ConnectionError due to the yearly update release of PubMed: we fixed it by updating the URLs from year 2021 to year 2022.\r\n\r\nHowever this is another problem: to make pubmed streamable. Please note that NOT all our datastes are streamable: we are making streamable more and more of them... but this is an on-going process...\r\n\r\nThanks.",
"@albertvillanova \r\nWhen I tried below codes, I got the similar error\r\n\r\n```\r\n\r\ndataset=load_dataset(\"pubmed\",split=\"train\")\r\n\r\nCouldn't reach ftp://ftp.ncbi.nlm.nih.gov/pubmed/baseline/pubmed21n0601.xml.gz\r\n```",
"@y-rok you need to update `datasets`:\r\n```shell\r\npip install -U datasets\r\n```"
] | ## Describe the bug
Trying to use the `pubmed` dataset fails to reach / download the source files.
## Steps to reproduce the bug
```python
pubmed_train = datasets.load_dataset('pubmed', split='train')
```
## Expected results
Should begin downloading the pubmed dataset.
## Actual results
```
ConnectionError: Couldn't reach ftp://ftp.ncbi.nlm.nih.gov/pubmed/baseline/pubmed21n0865.xml.gz (InvalidSchema("No connection adapters were found for 'ftp://ftp.ncbi.nlm.nih.gov/pubmed/baseline/pubmed21n0865.xml.gz'"))
```
## Environment info
- `datasets` version: 1.18.2
- Platform: macOS-11.4-x86_64-i386-64bit
- Python version: 3.8.2
- PyArrow version: 6.0.0
| 3,655 |
https://github.com/huggingface/datasets/issues/3653 | `to_json` in multiprocessing fashion sometimes deadlock | [] | ## Describe the bug
`to_json` in multiprocessing fashion sometimes deadlock, instead of raising exceptions. Temporary solution is to see that it deadlocks, and then reduce the number of processes or batch size in order to reduce the memory footprint.
As @lhoestq pointed out, this might be related to https://bugs.python.org/issue22393#msg315684 where `multiprocessing` fails to raise the OOM exception. One suggested alternative is not use `concurrent.futures` instead.
## Steps to reproduce the bug
## Expected results
Script fails when one worker hits OOM, and raise appropriate error.
## Actual results
Deadlock
## Environment info
<!-- You can run the command `datasets-cli env` and copy-and-paste its output below. -->
- `datasets` version: 1.8.1
- Platform: Linux
- Python version: 3.8
- PyArrow version: 6.0.1
| 3,653 |
https://github.com/huggingface/datasets/issues/3649 | Add IGLUE dataset | [] | ## Adding a Dataset
- **Name:** IGLUE
- **Description:** IGLUE brings together 4 vision-and-language tasks across 20 languages (Twitter [thread](https://twitter.com/ebugliarello/status/1487045497583976455?s=20&t=SB4LZGDhhkUW83ugcX_m5w))
- **Paper:** https://arxiv.org/abs/2201.11732
- **Data:** https://github.com/e-bug/iglue
- **Motivation:** This dataset would provide a nice example of combining the text and image features of `datasets` together for multimodal applications.
Note: the data / code are not yet visible on the GitHub repo, so I've pinged the authors for more information.
Instructions to add a new dataset can be found [here](https://github.com/huggingface/datasets/blob/master/ADD_NEW_DATASET.md).
| 3,649 |
https://github.com/huggingface/datasets/issues/3645 | Streaming dataset based on dl_manager.iter_archive/iter_files are not reset correctly | [] | Hi ! When iterating over a streaming dataset once, it's not reset correctly because of some issues with `dl_manager.iter_archive` and `dl_manager.iter_files`. Indeed they are generator functions (so the iterator that is returned can be exhausted). They should be iterables instead, and be reset if we do a for loop again:
```python
from datasets import load_dataset
d = load_dataset("common_voice", "ab", split="test", streaming=True)
i = 0
for i, _ in enumerate(d):
pass
print(i) # 8
# let's do it again
i = 0
for i, _ in enumerate(d):
pass
print(i) # 0
``` | 3,645 |
https://github.com/huggingface/datasets/issues/3644 | Add a GROUP BY operator | [
"Hi ! At the moment you can use `to_pandas()` to get a pandas DataFrame that supports `group_by` operations (make sure your dataset fits in memory though)\r\n\r\nWe use Arrow as a back-end for `datasets` and it doesn't have native group by (see https://github.com/apache/arrow/issues/2189) unfortunately.\r\n\r\nI just drafted what it could look like to have `group_by` in `datasets`:\r\n```python\r\nfrom datasets import concatenate_datasets\r\n\r\ndef group_by(d, col, join): \r\n \"\"\"from: https://github.com/huggingface/datasets/issues/3644\"\"\"\r\n # Get the indices of each group\r\n groups = {key: [] for key in d.unique(col)} \r\n def create_groups_indices(key, i): \r\n groups[key].append(i) \r\n d.map(create_groups_indices, with_indices=True, input_columns=col) \r\n # Get one dataset object per group\r\n groups = {key: d.select(indices) for key, indices in groups.items()} \r\n # Apply join function\r\n groups = {\r\n key: dataset_group.map(join, batched=True, batch_size=len(dataset_group), remove_columns=d.column_names)\r\n for key, dataset_group in groups.items()\r\n } \r\n # Return concatenation of all the joined groups\r\n return concatenate_datasets(groups.values())\r\n```\r\n\r\nexample of usage:\r\n```python\r\n\r\ndef join(batch): \r\n # take the batch of all the examples of a group, and return a batch with one aggregated example\r\n # (we could aggregate examples into several rows instead of one, if you want)\r\n return {\"total\": [batch[\"i\"]]} \r\n\r\nd = Dataset.from_dict({\r\n \"i\": [i for i in range(50)],\r\n \"group_key\": [i % 4 for i in range(50)],\r\n})\r\nprint(group_by(d, \"group_key\", join))\r\n# total\r\n# 0 [0, 4, 8, 12, 16, 20, 24, 28, 32, 36, 40, 44, 48]\r\n# 1 [1, 5, 9, 13, 17, 21, 25, 29, 33, 37, 41, 45, 49]\r\n# 2 [2, 6, 10, 14, 18, 22, 26, 30, 34, 38, 42, 46]\r\n# 3 [3, 7, 11, 15, 19, 23, 27, 31, 35, 39, 43, 47]\r\n```\r\n\r\nLet me know if that helps !\r\n\r\ncc @albertvillanova @mariosasko for visibility",
"@lhoestq As of PyArrow 7.0.0, `pa.Table` has the [`group_by` method](https://arrow.apache.org/docs/python/generated/pyarrow.Table.html#pyarrow.Table.group_by), so we should also consider using that function for grouping. ",
"Any update on this?",
"You can use https://github.com/mariosasko/datasets_sql by @mariosasko to go group by operations using SQL queries",
"Hi, I have a similar issue as OP but the suggested solutions do not work for my case. Basically, I process documents through a model to extract the last_hidden_state, using the \"map\" method on a Dataset object, but would like to average the result over a categorical column at the end (i.e. groupby this column).\r\n- A to_pandas() saturates the memory, although it gives me the desired result through a .groupby().apply(np.mean, axis=0) on a smaller use-case,\r\n- The solution posted on Feb 4 is much too slow,\r\n- datasets_sql seems to not like the fact that I'm averaging np.arrays.\r\nSo I'm kinda out of \"non brute force\" options... Any help appreciated",
"> Hi, I have a similar issue as OP but the suggested solutions do not work for my case. Basically, I process documents through a model to extract the last_hidden_state, using the \"map\" method on a Dataset object, but would like to average the result over a categorical column at the end (i.e. groupby this column).\r\n \r\nIf you haven't yet, you could explore using [Polars](https://www.pola.rs/) for this. It's a new DataFrame library written in Rust with Python bindings. It is Pandas like it in many ways ,but does have some biggish differences in syntax/approach so it's definitely not a drop-in replacement. \r\n\r\nPolar's also uses Arrow as a backend but also supports out-of-memory operations; in this case, it's probably easiest to write out your dataset to parquet and then use the polar's `scan_parquet` method (this will lazily read from the parquet file). The thing you get back from that is a `LazyDataFrame` i.e. nothing is loaded into memory until you specify a query and call a `collect` method. \r\n\r\nExample below of doing a groupby on a dataset which definitely wouldn't fit into memory on my machine:\r\n\r\n```\r\nfrom datasets import load_dataset\r\nimport polars as pl\r\n\r\nds = load_dataset(\"blbooks\")\r\nds['train'].to_parquet(\"test.parquet\")\r\ndf = pl.scan_parquet(\"test.parquet\")\r\ndf.groupby('date').agg([pl.count()]).collect()\r\n```\r\n\r\n>datasets_sql seems to not like the fact that I'm averaging np.arrays.\r\n\r\nI am not certain how Polars will handle this either. It does have NumPy support (https://pola-rs.github.io/polars-book/user-guide/howcani/interop/numpy.html) but I assume Polars will need to have at least enough memory in each group you want to average over so you may still end up needing more memory depending on the size of your dataset/groups. \r\n\r\n\r\n",
"Hi @davanstrien , thanks a lot, I didn't know about this library and the answer works! I need to try it on the full dataset now, but I'm hopeful. Here's what my code looks like:\r\n```\r\nlist_size = 768\r\ndf.groupby(\"date\").agg(\r\n pl.concat_list(\r\n [\r\n pl.col(\"hidden_state\")\r\n .arr.slice(n, 1)\r\n .arr.first()\r\n .mean()\r\n for n in range(0, list_size)\r\n ]\r\n ).collect()\r\n```\r\n\r\nFor some reasons, the following code was giving me a \"mean() got unexpected argument 'axis'\":\r\n```\r\ndf2 = df.groupby('date').agg(\r\n pl.col(\"hidden_state\").map(np.mean).alias(\"average_hidden_state\")\r\n).collect()\r\n\r\n```\r\n\r\nEDIT: The solution works on my large dataset, the memory does not crash and the time is reasonable, thanks a lot again!",
"@jeremylhour glad this worked for you :) ",
"I find this functionality missing in my workflow as well and the workarounds with SQL and Polars unsatisfying. Since PyArrow has exposed this functionality, I hope this soon makes it into a release. (:",
"Any update on this feature? ",
"We added a proper Polars integration at #3334 if it can help:\r\n```python\r\n>>> from datasets import load_dataset\r\n>>> ds = load_dataset(\"TheBritishLibrary/blbooks\", \"1700_1799\", split=\"train\")\r\n>>> ds.to_polars().groupby('date').len()\r\n┌─────────────────────┬──────┐\r\n│ date ┆ len │\r\n│ --- ┆ --- │\r\n│ datetime[ms] ┆ u32 │\r\n╞═════════════════════╪══════╡\r\n│ 1796-01-01 00:00:00 ┆ 5831 │\r\n│ 1775-01-01 00:00:00 ┆ 4697 │\r\n│ 1749-01-01 00:00:00 ┆ 1118 │\r\n│ 1740-01-01 00:00:00 ┆ 713 │\r\n│ 1714-01-01 00:00:00 ┆ 865 │\r\n│ … ┆ … │\r\n│ 1795-01-01 00:00:00 ┆ 5930 │\r\n│ 1754-01-01 00:00:00 ┆ 1373 │\r\n│ 1780-01-01 00:00:00 ┆ 1970 │\r\n│ 1734-01-01 00:00:00 ┆ 1047 │\r\n│ 1719-01-01 00:00:00 ┆ 1235 │\r\n└─────────────────────┴──────┘\r\n```\r\n"
] | **Is your feature request related to a problem? Please describe.**
Using batch mapping, we can easily split examples. However, we lack an appropriate option for merging them back together by some key. Consider this example:
```python
# features:
# {
# "example_id": datasets.Value("int32"),
# "text": datasets.Value("string")
# }
ds = datasets.Dataset()
def split(examples):
sentences = [text.split(".") for text in examples["text"]]
return {
"example_id": [
example_id
for example_id, sents in zip(examples["example_id"], sentences)
for _ in sents
],
"sentence": [sent for sents in sentences for sent in sents],
"sentence_id": [i for sents in sentences for i in range(len(sents))],
}
split_ds = ds.map(split, batched=True)
def process(examples):
outputs = some_neural_network_that_works_on_sentences(examples["sentence"])
return {"outputs": outputs}
split_ds = split_ds.map(process, batched=True)
```
I have a dataset consisting of texts that I would like to process sentence by sentence in a batched way. Afterwards, I would like to put it back together as it was, merging the outputs together.
**Describe the solution you'd like**
Ideally, it would look something like this:
```python
def join(examples):
order = np.argsort(examples["sentence_id"])
text = ".".join(examples["text"][i] for i in order)
outputs = [examples["outputs"][i] for i in order]
return {"text": text, "outputs": outputs}
ds = split_ds.group_by("example_id", join)
```
**Describe alternatives you've considered**
Right now, we can do this:
```python
def merge(example):
meeting_id = example["example_id"]
parts = split_ds.filter(lambda x: x["example_id"] == meeting_id).sort("segment_no")
return {"outputs": list(parts["outputs"])}
ds = ds.map(merge)
```
Of course, we could process the dataset like this:
```python
def process(example):
outputs = some_neural_network_that_works_on_sentences(example["text"].split("."))
return {"outputs": outputs}
ds = ds.map(process, batched=True)
```
However, that does not allow using an arbitrary batch size and may lead to very inefficient use of resources if the batch size is much larger than the number of sentences in one example.
I would very much appreciate some kind of group by operator to merge examples based on the value of one column.
| 3,644 |
https://github.com/huggingface/datasets/issues/3640 | Issues with custom dataset in Wav2Vec2 | [
"Closed and moved to transformers."
] | We are training Vav2Vec using the run_speech_recognition_ctc_bnb.py-script.
This is working fine with Common Voice, however using our custom dataset and data loader at [NbAiLab/NPSC]( https://huggingface.co/datasets/NbAiLab/NPSC) it crashes after roughly 1 epoch with the following stack trace:

We are able to work around the issue, for instance by adding this check in line#222 in transformers/models/wav2vec2/modeling_wav2vec2.py:
```python
if input_length - (mask_length - 1) < num_masked_span:
num_masked_span = input_length - (mask_length - 1)
```
Interestingly, these are the variable values before the adjustment:
```
input_length=10
mask_length=10
num_masked_span=2
````
After adjusting num_masked_spin to 1, the training script runs. The issue is also fixed by setting “replace=True” in the same function.
Do you have any idea what is causing this, and how to fix this error permanently? If you do not think this is an Datasets issue, feel free to move the issue.
| 3,640 |
https://github.com/huggingface/datasets/issues/3639 | same value of precision, recall, f1 score at each epoch for classification task. | [
"Hi @Dhanachandra, \r\n\r\nWe have tests for all our metrics and they work as expected: under the hood, we use scikit-learn implementations.\r\n\r\nMaybe the cause is somewhere else. For example:\r\n- Is it a binary or a multiclass or a multilabel classification? Default computation of these metrics is for binary classification; if you would like multiclass or multilabel, you should pass the corresponding parameters; see their documentation (e.g.: https://scikit-learn.org/stable/modules/generated/sklearn.metrics.precision_score.html) or code below:\r\n\r\nhttps://huggingface.co/docs/datasets/using_metrics.html#computing-the-metric-scores\r\n\r\n```python\r\nIn [1]: from datasets import load_metric\r\n\r\nIn [2]: precision = load_metric(\"precision\")\r\n\r\nIn [3]: print(precision.inputs_description)\r\n\r\nArgs:\r\n predictions: Predicted labels, as returned by a model.\r\n references: Ground truth labels.\r\n labels: The set of labels to include when average != 'binary', and\r\n their order if average is None. Labels present in the data can\r\n be excluded, for example to calculate a multiclass average ignoring\r\n a majority negative class, while labels not present in the data will\r\n result in 0 components in a macro average. For multilabel targets,\r\n labels are column indices. By default, all labels in y_true and\r\n y_pred are used in sorted order.\r\n average: This parameter is required for multiclass/multilabel targets.\r\n If None, the scores for each class are returned. Otherwise, this\r\n determines the type of averaging performed on the data:\r\n binary: Only report results for the class specified by pos_label.\r\n This is applicable only if targets (y_{true,pred}) are binary.\r\n micro: Calculate metrics globally by counting the total true positives,\r\n false negatives and false positives.\r\n macro: Calculate metrics for each label, and find their unweighted mean.\r\n This does not take label imbalance into account.\r\n weighted: Calculate metrics for each label, and find their average\r\n weighted by support (the number of true instances for each label).\r\n This alters ‘macro’ to account for label imbalance; it can result\r\n in an F-score that is not between precision and recall.\r\n samples: Calculate metrics for each instance, and find their average\r\n (only meaningful for multilabel classification).\r\n sample_weight: Sample weights.\r\n\r\nReturns:\r\n precision: Precision score.\r\n\r\nExamples:\r\n\r\n >>> precision_metric = datasets.load_metric(\"precision\")\r\n >>> results = precision_metric.compute(references=[0, 1], predictions=[0, 1])\r\n >>> print(results)\r\n {'precision': 1.0}\r\n\r\n >>> predictions = [0, 2, 1, 0, 0, 1]\r\n >>> references = [0, 1, 2, 0, 1, 2]\r\n >>> results = precision_metric.compute(predictions=predictions, references=references, average='macro')\r\n >>> print(results)\r\n {'precision': 0.2222222222222222}\r\n >>> results = precision_metric.compute(predictions=predictions, references=references, average='micro')\r\n >>> print(results)\r\n {'precision': 0.3333333333333333}\r\n >>> results = precision_metric.compute(predictions=predictions, references=references, average='weighted')\r\n >>> print(results)\r\n {'precision': 0.2222222222222222}\r\n >>> results = precision_metric.compute(predictions=predictions, references=references, average=None)\r\n >>> print(results)\r\n {'precision': array([0.66666667, 0. , 0. ])}\r\n```\r\n"
] | **1st Epoch:**
1/27/2022 09:30:48 - INFO - datasets.metric - Removing /home/ubuntu/.cache/huggingface/metrics/f1/default/default_experiment-1-0.arrow.59it/s]
01/27/2022 09:30:48 - INFO - datasets.metric - Removing /home/ubuntu/.cache/huggingface/metrics/precision/default/default_experiment-1-0.arrow
01/27/2022 09:30:49 - INFO - datasets.metric - Removing /home/ubuntu/.cache/huggingface/metrics/recall/default/default_experiment-1-0.arrow
PRECISION: {'precision': 0.7612903225806451}
RECALL: {'recall': 0.7612903225806451}
F1: {'f1': 0.7612903225806451}
{'eval_loss': 1.4658324718475342, 'eval_accuracy': 0.7612903118133545, 'eval_runtime': 30.0054, 'eval_samples_per_second': 46.492, 'eval_steps_per_second': 46.492, 'epoch': 3.0}
**4th Epoch:**
1/27/2022 09:56:55 - INFO - datasets.metric - Removing /home/ubuntu/.cache/huggingface/metrics/f1/default/default_experiment-1-0.arrow.92it/s]
01/27/2022 09:56:56 - INFO - datasets.metric - Removing /home/ubuntu/.cache/huggingface/metrics/precision/default/default_experiment-1-0.arrow
01/27/2022 09:56:56 - INFO - datasets.metric - Removing /home/ubuntu/.cache/huggingface/metrics/recall/default/default_experiment-1-0.arrow
PRECISION: {'precision': 0.7698924731182796}
RECALL: {'recall': 0.7698924731182796}
F1: {'f1': 0.7698924731182796}
## Environment info
!git clone https://github.com/huggingface/transformers
%cd transformers
!pip install .
!pip install -r /content/transformers/examples/pytorch/token-classification/requirements.txt
!pip install datasets | 3,639 |
https://github.com/huggingface/datasets/issues/3638 | AutoTokenizer hash value got change after datasets.map | [
"This issue was original reported at https://github.com/huggingface/transformers/issues/14931 and It seems like this issue also occur with other AutoClass like AutoFeatureExtractor.",
"Thanks for moving the issue here !\r\n\r\nI wasn't able to reproduce the issue on my env (the hashes stay the same):\r\n```\r\n- `transformers` version: 1.15.0\r\n- `tokenizers` version: 0.10.3\r\n- `datasets` version: 1.18.1\r\n- `dill` version: 0.3.4\r\n- Platform: Linux-4.19.0-18-cloud-amd64-x86_64-with-debian-10.11\r\n- Python version: 3.7.10\r\n- PyArrow version: 6.0.1\r\n```\r\nHowever I was able to reproduce it on Google Colab (the hashes end up different):\r\n```\r\n- `transformers` version: 1.15.0\r\n- `tokenizers` version: 0.10.3\r\n- `datasets` version: 1.18.1\r\n- `dill` version: 0.3.4\r\n- Platform: Linux-5.4.144+-x86_64-with-Ubuntu-18.04-bionic\r\n- Python version: 3.7.12\r\n- PyArrow version: 3.0.0\r\n```\r\nI'll investigate why it doesn't work properly on Google Colab :)",
"I found the issue: the tokenizer has something inside it that changes.\r\n\r\nBefore the call, `tokenizer._tokenizer.truncation` is None, and after the call it changes to this for some reason:\r\n```\r\n{'max_length': 512, 'strategy': 'longest_first', 'stride': 0}\r\n```\r\n\r\nDoes anybody know why calling the tokenizer would change its state this way ? cc @Narsil @SaulLu maybe ?",
"`tokenizer.encode(..)` does not accept argument like max_length, strategy or stride.\r\n\r\nIn `tokenizers` you have to modify the tokenizer state by setting various `TruncationParams` (and/or `PaddingParams`).\r\nHowever, since this is modifying the state, you need to mutably borrow the tokenizer (a rust concept). The key principle is that there can ever be only 1 mutable borrow at a time during the span of the tokenizer lifecycle.\r\n\r\nBecause of this, if `transformers` blindly set `TruncationParams` and `PaddingParams` on every call, it would cause the tokenizer to crash (or make the various threads accessing it hang, which is not necessarily better).\r\n\r\nIn order to avoid that, we decided to handle it this way : https://github.com/huggingface/transformers/pull/12550 . \r\n\r\nWhich should explain the state of the tokenizer being modified (hence its hash).\r\n\r\nNow for a temporary solution, simply encoding once with the tokenizer should give it it's proper hash (since by default the tokenizer doesn't have this state, looks at the first encoding call, and creates it).\r\n\r\nWe could try and set these 2 dicts at initialization time, but it wouldn't work if a user modified the tokenizer state later\r\n```python\r\ntokenizer = AutoTokenizer.from_pretrained(..)\r\ntokenizer.truncation_side = \"left\"\r\n# Now we have a difference between `tokenizer._tokenizer.truncation` and `tokenizer.truncation_side`\r\n```\r\nIf we wanted to fix it correctly it would mean mapping every assignation to it's proper location on `tokenizer.{padding/truncation}`\r\n\r\nI think it's important to note that we cannot guarantee a tokenizer' hash remains the same if *any* of those parameters are modified through the `.map` function.\r\n\r\nEdit: Another option would be to override the default __hash__ function, but I don't know if there's a sound implementation that could fit.",
"Thanks a lot for the explanation !\r\nI think if we set these 2 dicts at initialization time it would be amazing already\r\n\r\nShall we open an issue in `transformers` to ask for these dictionaries to be set when the tokenizer is instantiated ?\r\n\r\n> Edit: Another option would be to override the default hash function, but I don't know if there's a sound implementation that could fit.\r\n\r\nIn `datasets` we can easily have custom hashing for objects of the other HF libraries if we want. For example we ignore the cache some tokenizers have. However in this specific case it touches parameters that may change the behavior of the tokenizer itself. I'm not sure the logic that determines how a tokenizer behaves should be in `datasets`",
"A hack we could have in the `datasets` lib would be to call the tokenizer before hashing it in order to set all its parameters correctly - but it sounds a lot like a hack and I'm not sure this can work in the long run",
"Fully agree with everything you said. \r\n\r\nI think the best course of action is creating an issue in `transformers`. I can start the work on this.\r\nI think the code changes are fairly simple. Making a sound test + not breaking other stuff might be different :D",
"It should be noted that this problem also occurs in other AutoClasses, such as AutoFeatureExtractor, so I don't think handling it in Datasets is a long-term practice either.",
"> I think the best course of action is creating an issue in `transformers`. I can start the work on this.\r\n\r\n@Narsil Hi, I reopen this issue in `transformers` https://github.com/huggingface/transformers/issues/14931",
"Here is @Narsil comment from https://github.com/huggingface/transformers/issues/14931#issuecomment-1074981569\r\n> # TL;DR\r\n> Call the function once on a dummy example beforehand will fix it.\r\n> \r\n> ```python\r\n> tokenizer(\"Some\", \"test\", truncation=True)\r\n> ```\r\n> \r\n> # Long answer\r\n> If I remember the last status, it's hard doing anything, since the call itself\r\n> \r\n> ```python\r\n> tokenizer(example[\"sentence1\"], example[\"sentence2\"], truncation=True)\r\n> ```\r\n> \r\n> will modify the tokenizer. It's the `truncation=True` that modifies the tokenizer to put it into truncation mode if you will. Calling the tokenizer once with that argument would fix the cache.\r\n> \r\n> Finding a fix that :\r\n> \r\n> * Doesn't imply a huge chunk of work on `tokenizers` (with potential loss of performance, and breaking backward compatibility)\r\n> * Doesn't imply `datasets` running a first pass of the loop\r\n> * Doesn't imply `datasets` looking at the map function itself\r\n> * Uses a sound `hash` for this object in `datasets`.\r\n> \r\n> is IIRC impossible for this use case.\r\n> \r\n> I can explain a bit more why the first option is not desirable.\r\n> \r\n> In order to \"fix\" this for tokenizers, we would need to make `tokenizer(..)` purely without side effects. This means that the \"options\" of tokenization (like `truncation` and `padding` at least) would have\r\n",
"For me this workaround only works if I don't pass the `num_proc=X` argument to `datasets.map`",
"Is there an easy solution for setting both num_proc and padding/truncation for fast tokenizer or caching just not a thing in this case? "
] | ## Describe the bug
AutoTokenizer hash value got change after datasets.map
## Steps to reproduce the bug
1. trash huggingface datasets cache
2. run the following code:
```python
from transformers import AutoTokenizer, BertTokenizer
from datasets import load_dataset
from datasets.fingerprint import Hasher
tokenizer = AutoTokenizer.from_pretrained('bert-base-uncased')
def tokenize_function(example):
return tokenizer(example["sentence1"], example["sentence2"], truncation=True)
raw_datasets = load_dataset("glue", "mrpc")
print(Hasher.hash(tokenize_function))
print(Hasher.hash(tokenizer))
tokenized_datasets = raw_datasets.map(tokenize_function, batched=True)
print(Hasher.hash(tokenize_function))
print(Hasher.hash(tokenizer))
```
got
```
Reusing dataset glue (/home1/wts/.cache/huggingface/datasets/glue/mrpc/1.0.0/dacbe3125aa31d7f70367a07a8a9e72a5a0bfeb5fc42e75c9db75b96da6053ad)
100%|███████████████████████████████████████████████████████████████████████████████████████████████████████████████████████████████████████████████████████████████████████████| 3/3 [00:00<00:00, 1112.35it/s]
f4976bb4694ebc51
3fca35a1fd4a1251
100%|█████████████████████████████████████████████████████████████████████████████████████████████████████████████████████████████████████████████████████████████████████████████| 4/4 [00:00<00:00, 6.96ba/s]
100%|█████████████████████████████████████████████████████████████████████████████████████████████████████████████████████████████████████████████████████████████████████████████| 1/1 [00:00<00:00, 15.25ba/s]
100%|█████████████████████████████████████████████████████████████████████████████████████████████████████████████████████████████████████████████████████████████████████████████| 2/2 [00:00<00:00, 5.81ba/s]
d32837619b7d7d01
5fd925c82edd62b6
```
3. run raw_datasets.map(tokenize_function, batched=True) again and see some dataset are not using cache.
## Expected results
`AutoTokenizer` work like specific Tokenizer (The hash value don't change after map):
```python
from transformers import AutoTokenizer, BertTokenizer
from datasets import load_dataset
from datasets.fingerprint import Hasher
tokenizer = BertTokenizer.from_pretrained('bert-base-uncased')
def tokenize_function(example):
return tokenizer(example["sentence1"], example["sentence2"], truncation=True)
raw_datasets = load_dataset("glue", "mrpc")
print(Hasher.hash(tokenize_function))
print(Hasher.hash(tokenizer))
tokenized_datasets = raw_datasets.map(tokenize_function, batched=True)
print(Hasher.hash(tokenize_function))
print(Hasher.hash(tokenizer))
```
```
Reusing dataset glue (/home1/wts/.cache/huggingface/datasets/glue/mrpc/1.0.0/dacbe3125aa31d7f70367a07a8a9e72a5a0bfeb5fc42e75c9db75b96da6053ad)
100%|███████████████████████████████████████████████████████████████████████████████████████████████████████████████████████████████████████████████████████████████████████████| 3/3 [00:00<00:00, 1091.22it/s]
46d4b31f54153fc7
5b8771afd8d43888
Loading cached processed dataset at /home1/wts/.cache/huggingface/datasets/glue/mrpc/1.0.0/dacbe3125aa31d7f70367a07a8a9e72a5a0bfeb5fc42e75c9db75b96da6053ad/cache-6b07ff82ae9d5c51.arrow
Loading cached processed dataset at /home1/wts/.cache/huggingface/datasets/glue/mrpc/1.0.0/dacbe3125aa31d7f70367a07a8a9e72a5a0bfeb5fc42e75c9db75b96da6053ad/cache-af738a6d84f3864b.arrow
Loading cached processed dataset at /home1/wts/.cache/huggingface/datasets/glue/mrpc/1.0.0/dacbe3125aa31d7f70367a07a8a9e72a5a0bfeb5fc42e75c9db75b96da6053ad/cache-531d2a603ba713c1.arrow
46d4b31f54153fc7
5b8771afd8d43888
```
## Environment info
- `datasets` version: 1.18.0
- Platform: Linux-5.4.0-91-generic-x86_64-with-glibc2.27
- Python version: 3.9.7
- PyArrow version: 6.0.1
| 3,638 |
https://github.com/huggingface/datasets/issues/3637 | [TypeError: Couldn't cast array of type] Cannot load dataset in v1.18 | [
"Hi @lewtun!\r\n \r\nThis one was tricky to debug. Initially, I tought there is a bug in the recently-added (by @lhoestq ) `cast_array_to_feature` function because `git bisect` points to the https://github.com/huggingface/datasets/commit/6ca96c707502e0689f9b58d94f46d871fa5a3c9c commit. Then, I noticed that the feature tpye of the `dialogue` field is `list`, which explains why you didn't get an error in earlier versions. Is there a specific reason why you use `list` instead of `Sequence` in the script? Maybe to avoid turning list of dicts to dicts of lists as it's done by `Sequence` for compatibility with TFDS or for performance reasons? If the field was `Sequence`, you would get an error in `encode_nested_example` because **the scripts yields some additional (nested) columns which are not specified in the `features` dictionary**. Previously, these additional columns would've been ignored by PyArrow (1), but now we have a check for them (2).\r\n(1) See PyArrow behavior:\r\n```\r\n>>> pa.array([{\"a\": 2, \"b\": 3}], type=pa.struct({\"a\": pa.int32()})) # pyarrow ignores the extra column\r\n-- is_valid: all not null\r\n-- child 0 type: int32\r\n [\r\n 2\r\n ]\r\n ```\r\n\r\n(2) Check:\r\nhttps://github.com/huggingface/datasets/blob/4c417d52def6e20359ca16c6723e0a2855e5c3fd/src/datasets/table.py#L1059\r\n\r\nThe fix is very simple: just add the missing columns to the _EMPTY_BELIEF_STATE list:\r\n```python\r\n_EMPTY_BELIEF_STATE.extend(['通用-产品类别', '火车-舱位档次', '通用-系列', '通用-价格区间', '通用-品牌'])\r\n```",
"Hey @mariosasko, thank you so much for figuring this one out - it certainly looks like a tricky bug 😱 ! I don't think there's a specific reason to use `list` instead of `Sequence` with the script, but I'll let the dataset creators know to see if your suggestion is acceptable.\r\n\r\nThank you again!",
"Thanks, this was indeed the fix! Would it make sense to produce a more informative error message in such cases? \r\n\r\nThe issue can be closed. \r\n\r\n"
] | ## Describe the bug
I am trying to load the [`GEM/RiSAWOZ` dataset](https://huggingface.co/datasets/GEM/RiSAWOZ) in `datasets` v1.18.1 and am running into a type error when casting the features. The strange thing is that I can load the dataset with v1.17.0. Note that the error is also present if I install from `master` too.
As far as I can tell, the dataset loading script is correct and the problematic features [here](https://huggingface.co/datasets/GEM/RiSAWOZ/blob/main/RiSAWOZ.py#L237) also look fine to me.
## Steps to reproduce the bug
```python
from datasets import load_dataset
dset = load_dataset("GEM/RiSAWOZ")
```
## Expected results
I can load the dataset without error.
## Actual results
<details><summary>Traceback</summary>
```
---------------------------------------------------------------------------
TypeError Traceback (most recent call last)
~/miniconda3/envs/huggingface/lib/python3.8/site-packages/datasets/builder.py in _prepare_split(self, split_generator)
1083 example = self.info.features.encode_example(record)
-> 1084 writer.write(example, key)
1085 finally:
~/miniconda3/envs/huggingface/lib/python3.8/site-packages/datasets/arrow_writer.py in write(self, example, key, writer_batch_size)
445
--> 446 self.write_examples_on_file()
447
~/miniconda3/envs/huggingface/lib/python3.8/site-packages/datasets/arrow_writer.py in write_examples_on_file(self)
403 batch_examples[col] = [row[0][col] for row in self.current_examples]
--> 404 self.write_batch(batch_examples=batch_examples)
405 self.current_examples = []
~/miniconda3/envs/huggingface/lib/python3.8/site-packages/datasets/arrow_writer.py in write_batch(self, batch_examples, writer_batch_size)
496 typed_sequence = OptimizedTypedSequence(batch_examples[col], type=col_type, try_type=col_try_type, col=col)
--> 497 arrays.append(pa.array(typed_sequence))
498 inferred_features[col] = typed_sequence.get_inferred_type()
~/miniconda3/envs/huggingface/lib/python3.8/site-packages/pyarrow/array.pxi in pyarrow.lib.array()
~/miniconda3/envs/huggingface/lib/python3.8/site-packages/pyarrow/array.pxi in pyarrow.lib._handle_arrow_array_protocol()
~/miniconda3/envs/huggingface/lib/python3.8/site-packages/datasets/arrow_writer.py in __arrow_array__(self, type)
204 # We only do it if trying_type is False - since this is what the user asks for.
--> 205 out = cast_array_to_feature(out, type, allow_number_to_str=not self.trying_type)
206 return out
~/miniconda3/envs/huggingface/lib/python3.8/site-packages/datasets/table.py in wrapper(array, *args, **kwargs)
943 array = _sanitize(array)
--> 944 return func(array, *args, **kwargs)
945
~/miniconda3/envs/huggingface/lib/python3.8/site-packages/datasets/table.py in wrapper(array, *args, **kwargs)
919 else:
--> 920 return func(array, *args, **kwargs)
921
~/miniconda3/envs/huggingface/lib/python3.8/site-packages/datasets/table.py in cast_array_to_feature(array, feature, allow_number_to_str)
1064 if isinstance(feature, list):
-> 1065 return pa.ListArray.from_arrays(array.offsets, _c(array.values, feature[0]))
1066 elif isinstance(feature, Sequence):
~/miniconda3/envs/huggingface/lib/python3.8/site-packages/datasets/table.py in wrapper(array, *args, **kwargs)
943 array = _sanitize(array)
--> 944 return func(array, *args, **kwargs)
945
~/miniconda3/envs/huggingface/lib/python3.8/site-packages/datasets/table.py in wrapper(array, *args, **kwargs)
919 else:
--> 920 return func(array, *args, **kwargs)
921
~/miniconda3/envs/huggingface/lib/python3.8/site-packages/datasets/table.py in cast_array_to_feature(array, feature, allow_number_to_str)
1059 if isinstance(feature, dict) and set(field.name for field in array.type) == set(feature):
-> 1060 arrays = [_c(array.field(name), subfeature) for name, subfeature in feature.items()]
1061 return pa.StructArray.from_arrays(arrays, names=list(feature))
~/miniconda3/envs/huggingface/lib/python3.8/site-packages/datasets/table.py in <listcomp>(.0)
1059 if isinstance(feature, dict) and set(field.name for field in array.type) == set(feature):
-> 1060 arrays = [_c(array.field(name), subfeature) for name, subfeature in feature.items()]
1061 return pa.StructArray.from_arrays(arrays, names=list(feature))
~/miniconda3/envs/huggingface/lib/python3.8/site-packages/datasets/table.py in wrapper(array, *args, **kwargs)
943 array = _sanitize(array)
--> 944 return func(array, *args, **kwargs)
945
~/miniconda3/envs/huggingface/lib/python3.8/site-packages/datasets/table.py in wrapper(array, *args, **kwargs)
919 else:
--> 920 return func(array, *args, **kwargs)
921
~/miniconda3/envs/huggingface/lib/python3.8/site-packages/datasets/table.py in cast_array_to_feature(array, feature, allow_number_to_str)
1059 if isinstance(feature, dict) and set(field.name for field in array.type) == set(feature):
-> 1060 arrays = [_c(array.field(name), subfeature) for name, subfeature in feature.items()]
1061 return pa.StructArray.from_arrays(arrays, names=list(feature))
~/miniconda3/envs/huggingface/lib/python3.8/site-packages/datasets/table.py in <listcomp>(.0)
1059 if isinstance(feature, dict) and set(field.name for field in array.type) == set(feature):
-> 1060 arrays = [_c(array.field(name), subfeature) for name, subfeature in feature.items()]
1061 return pa.StructArray.from_arrays(arrays, names=list(feature))
~/miniconda3/envs/huggingface/lib/python3.8/site-packages/datasets/table.py in wrapper(array, *args, **kwargs)
943 array = _sanitize(array)
--> 944 return func(array, *args, **kwargs)
945
~/miniconda3/envs/huggingface/lib/python3.8/site-packages/datasets/table.py in wrapper(array, *args, **kwargs)
919 else:
--> 920 return func(array, *args, **kwargs)
921
~/miniconda3/envs/huggingface/lib/python3.8/site-packages/datasets/table.py in cast_array_to_feature(array, feature, allow_number_to_str)
1086 return array_cast(array, feature(), allow_number_to_str=allow_number_to_str)
-> 1087 raise TypeError(f"Couldn't cast array of type\n{array.type}\nto\n{feature}")
1088
TypeError: Couldn't cast array of type
struct<医院-3.0T MRI: string, 医院-CT: string, 医院-DSA: string, 医院-公交线路: string, 医院-区域: string, 医院-名称: string, 医院-地址: string, 医院-地铁可达: string, 医院-地铁线路: string, 医院-性质: string, 医院-挂号时间: string, 医院-电话: string, 医院-等级: string, 医院-类别: string, 医院-重点科室: string, 医院-门诊时间: string, 天气-城市: string, 天气-天气: string, 天气-日期: string, 天气-温度: string, 天气-紫外线强度: string, 天气-风力风向: string, 旅游景点-区域: string, 旅游景点-名称: string, 旅游景点-地址: string, 旅游景点-开放时间: string, 旅游景点-是否地铁直达: string, 旅游景点-景点类型: string, 旅游景点-最适合人群: string, 旅游景点-消费: string, 旅游景点-特点: string, 旅游景点-电话号码: string, 旅游景点-评分: string, 旅游景点-门票价格: string, 汽车-价格(万元): string, 汽车-倒车影像: string, 汽车-动力水平: string, 汽车-厂商: string, 汽车-发动机排量(L): string, 汽车-发动机马力(Ps): string, 汽车-名称: string, 汽车-定速巡航: string, 汽车-巡航系统: string, 汽车-座位数: string, 汽车-座椅加热: string, 汽车-座椅通风: string, 汽车-所属价格区间: string, 汽车-油耗水平: string, 汽车-环保标准: string, 汽车-级别: string, 汽车-综合油耗(L/100km): string, 汽车-能源类型: string, 汽车-车型: string, 汽车-车系: string, 汽车-车身尺寸(mm): string, 汽车-驱动方式: string, 汽车-驾驶辅助影像: string, 火车-出发地: string, 火车-出发时间: string, 火车-到达时间: string, 火车-坐席: string, 火车-日期: string, 火车-时长: string, 火车-目的地: string, 火车-票价: string, 火车-舱位档次: string, 火车-车型: string, 火车-车次信息: string, 电影-主演: string, 电影-主演名单: string, 电影-具体上映时间: string, 电影-制片国家/地区: string, 电影-导演: string, 电影-年代: string, 电影-片名: string, 电影-片长: string, 电影-类型: string, 电影-豆瓣评分: string, 电脑-CPU: string, 电脑-CPU型号: string, 电脑-产品类别: string, 电脑-价格: string, 电脑-价格区间: string, 电脑-内存容量: string, 电脑-分类: string, 电脑-品牌: string, 电脑-商品名称: string, 电脑-屏幕尺寸: string, 电脑-待机时长: string, 电脑-显卡型号: string, 电脑-显卡类别: string, 电脑-游戏性能: string, 电脑-特性: string, 电脑-硬盘容量: string, 电脑-系列: string, 电脑-系统: string, 电脑-色系: string, 电脑-裸机重量: string, 电视剧-主演: string, 电视剧-主演名单: string, 电视剧-制片国家/地区: string, 电视剧-单集片长: string, 电视剧-导演: string, 电视剧-年代: string, 电视剧-片名: string, 电视剧-类型: string, 电视剧-豆瓣评分: string, 电视剧-集数: string, 电视剧-首播时间: string, 辅导班-上课方式: string, 辅导班-上课时间: string, 辅导班-下课时间: string, 辅导班-价格: string, 辅导班-区域: string, 辅导班-年级: string, 辅导班-开始日期: string, 辅导班-教室地点: string, 辅导班-教师: string, 辅导班-教师网址: string, 辅导班-时段: string, 辅导班-校区: string, 辅导班-每周: string, 辅导班-班号: string, 辅导班-科目: string, 辅导班-结束日期: string, 辅导班-课时: string, 辅导班-课次: string, 辅导班-课程网址: string, 辅导班-难度: string, 通用-产品类别: string, 通用-价格区间: string, 通用-品牌: string, 通用-系列: string, 酒店-价位: string, 酒店-停车场: string, 酒店-区域: string, 酒店-名称: string, 酒店-地址: string, 酒店-房型: string, 酒店-房费: string, 酒店-星级: string, 酒店-电话号码: string, 酒店-评分: string, 酒店-酒店类型: string, 飞机-准点率: string, 飞机-出发地: string, 飞机-到达时间: string, 飞机-日期: string, 飞机-目的地: string, 飞机-票价: string, 飞机-航班信息: string, 飞机-舱位档次: string, 飞机-起飞时间: string, 餐厅-人均消费: string, 餐厅-价位: string, 餐厅-区域: string, 餐厅-名称: string, 餐厅-地址: string, 餐厅-推荐菜: string, 餐厅-是否地铁直达: string, 餐厅-电话号码: string, 餐厅-菜系: string, 餐厅-营业时间: string, 餐厅-评分: string>
to
{'旅游景点-名称': Value(dtype='string', id=None), '旅游景点-区域': Value(dtype='string', id=None), '旅游景点-景点类型': Value(dtype='string', id=None), '旅游景点-最适合人群': Value(dtype='string', id=None), '旅游景点-消费': Value(dtype='string', id=None), '旅游景点-是否地铁直达': Value(dtype='string', id=None), '旅游景点-门票价格': Value(dtype='string', id=None), '旅游景点-电话号码': Value(dtype='string', id=None), '旅游景点-地址': Value(dtype='string', id=None), '旅游景点-评分': Value(dtype='string', id=None), '旅游景点-开放时间': Value(dtype='string', id=None), '旅游景点-特点': Value(dtype='string', id=None), '餐厅-名称': Value(dtype='string', id=None), '餐厅-区域': Value(dtype='string', id=None), '餐厅-菜系': Value(dtype='string', id=None), '餐厅-价位': Value(dtype='string', id=None), '餐厅-是否地铁直达': Value(dtype='string', id=None), '餐厅-人均消费': Value(dtype='string', id=None), '餐厅-地址': Value(dtype='string', id=None), '餐厅-电话号码': Value(dtype='string', id=None), '餐厅-评分': Value(dtype='string', id=None), '餐厅-营业时间': Value(dtype='string', id=None), '餐厅-推荐菜': Value(dtype='string', id=None), '酒店-名称': Value(dtype='string', id=None), '酒店-区域': Value(dtype='string', id=None), '酒店-星级': Value(dtype='string', id=None), '酒店-价位': Value(dtype='string', id=None), '酒店-酒店类型': Value(dtype='string', id=None), '酒店-房型': Value(dtype='string', id=None), '酒店-停车场': Value(dtype='string', id=None), '酒店-房费': Value(dtype='string', id=None), '酒店-地址': Value(dtype='string', id=None), '酒店-电话号码': Value(dtype='string', id=None), '酒店-评分': Value(dtype='string', id=None), '电脑-品牌': Value(dtype='string', id=None), '电脑-产品类别': Value(dtype='string', id=None), '电脑-分类': Value(dtype='string', id=None), '电脑-内存容量': Value(dtype='string', id=None), '电脑-屏幕尺寸': Value(dtype='string', id=None), '电脑-CPU': Value(dtype='string', id=None), '电脑-价格区间': Value(dtype='string', id=None), '电脑-系列': Value(dtype='string', id=None), '电脑-商品名称': Value(dtype='string', id=None), '电脑-系统': Value(dtype='string', id=None), '电脑-游戏性能': Value(dtype='string', id=None), '电脑-CPU型号': Value(dtype='string', id=None), '电脑-裸机重量': Value(dtype='string', id=None), '电脑-显卡类别': Value(dtype='string', id=None), '电脑-显卡型号': Value(dtype='string', id=None), '电脑-特性': Value(dtype='string', id=None), '电脑-色系': Value(dtype='string', id=None), '电脑-待机时长': Value(dtype='string', id=None), '电脑-硬盘容量': Value(dtype='string', id=None), '电脑-价格': Value(dtype='string', id=None), '火车-出发地': Value(dtype='string', id=None), '火车-目的地': Value(dtype='string', id=None), '火车-日期': Value(dtype='string', id=None), '火车-车型': Value(dtype='string', id=None), '火车-坐席': Value(dtype='string', id=None), '火车-车次信息': Value(dtype='string', id=None), '火车-时长': Value(dtype='string', id=None), '火车-出发时间': Value(dtype='string', id=None), '火车-到达时间': Value(dtype='string', id=None), '火车-票价': Value(dtype='string', id=None), '飞机-出发地': Value(dtype='string', id=None), '飞机-目的地': Value(dtype='string', id=None), '飞机-日期': Value(dtype='string', id=None), '飞机-舱位档次': Value(dtype='string', id=None), '飞机-航班信息': Value(dtype='string', id=None), '飞机-起飞时间': Value(dtype='string', id=None), '飞机-到达时间': Value(dtype='string', id=None), '飞机-票价': Value(dtype='string', id=None), '飞机-准点率': Value(dtype='string', id=None), '天气-城市': Value(dtype='string', id=None), '天气-日期': Value(dtype='string', id=None), '天气-天气': Value(dtype='string', id=None), '天气-温度': Value(dtype='string', id=None), '天气-风力风向': Value(dtype='string', id=None), '天气-紫外线强度': Value(dtype='string', id=None), '电影-制片国家/地区': Value(dtype='string', id=None), '电影-类型': Value(dtype='string', id=None), '电影-年代': Value(dtype='string', id=None), '电影-主演': Value(dtype='string', id=None), '电影-导演': Value(dtype='string', id=None), '电影-片名': Value(dtype='string', id=None), '电影-主演名单': Value(dtype='string', id=None), '电影-具体上映时间': Value(dtype='string', id=None), '电影-片长': Value(dtype='string', id=None), '电影-豆瓣评分': Value(dtype='string', id=None), '电视剧-制片国家/地区': Value(dtype='string', id=None), '电视剧-类型': Value(dtype='string', id=None), '电视剧-年代': Value(dtype='string', id=None), '电视剧-主演': Value(dtype='string', id=None), '电视剧-导演': Value(dtype='string', id=None), '电视剧-片名': Value(dtype='string', id=None), '电视剧-主演名单': Value(dtype='string', id=None), '电视剧-首播时间': Value(dtype='string', id=None), '电视剧-集数': Value(dtype='string', id=None), '电视剧-单集片长': Value(dtype='string', id=None), '电视剧-豆瓣评分': Value(dtype='string', id=None), '辅导班-班号': Value(dtype='string', id=None), '辅导班-难度': Value(dtype='string', id=None), '辅导班-科目': Value(dtype='string', id=None), '辅导班-年级': Value(dtype='string', id=None), '辅导班-区域': Value(dtype='string', id=None), '辅导班-校区': Value(dtype='string', id=None), '辅导班-上课方式': Value(dtype='string', id=None), '辅导班-开始日期': Value(dtype='string', id=None), '辅导班-结束日期': Value(dtype='string', id=None), '辅导班-每周': Value(dtype='string', id=None), '辅导班-上课时间': Value(dtype='string', id=None), '辅导班-下课时间': Value(dtype='string', id=None), '辅导班-时段': Value(dtype='string', id=None), '辅导班-课次': Value(dtype='string', id=None), '辅导班-课时': Value(dtype='string', id=None), '辅导班-教室地点': Value(dtype='string', id=None), '辅导班-教师': Value(dtype='string', id=None), '辅导班-价格': Value(dtype='string', id=None), '辅导班-课程网址': Value(dtype='string', id=None), '辅导班-教师网址': Value(dtype='string', id=None), '汽车-名称': Value(dtype='string', id=None), '汽车-车型': Value(dtype='string', id=None), '汽车-级别': Value(dtype='string', id=None), '汽车-座位数': Value(dtype='string', id=None), '汽车-车身尺寸(mm)': Value(dtype='string', id=None), '汽车-厂商': Value(dtype='string', id=None), '汽车-能源类型': Value(dtype='string', id=None), '汽车-发动机排量(L)': Value(dtype='string', id=None), '汽车-发动机马力(Ps)': Value(dtype='string', id=None), '汽车-驱动方式': Value(dtype='string', id=None), '汽车-综合油耗(L/100km)': Value(dtype='string', id=None), '汽车-环保标准': Value(dtype='string', id=None), '汽车-驾驶辅助影像': Value(dtype='string', id=None), '汽车-巡航系统': Value(dtype='string', id=None), '汽车-价格(万元)': Value(dtype='string', id=None), '汽车-车系': Value(dtype='string', id=None), '汽车-动力水平': Value(dtype='string', id=None), '汽车-油耗水平': Value(dtype='string', id=None), '汽车-倒车影像': Value(dtype='string', id=None), '汽车-定速巡航': Value(dtype='string', id=None), '汽车-座椅加热': Value(dtype='string', id=None), '汽车-座椅通风': Value(dtype='string', id=None), '汽车-所属价格区间': Value(dtype='string', id=None), '医院-名称': Value(dtype='string', id=None), '医院-等级': Value(dtype='string', id=None), '医院-类别': Value(dtype='string', id=None), '医院-性质': Value(dtype='string', id=None), '医院-区域': Value(dtype='string', id=None), '医院-地址': Value(dtype='string', id=None), '医院-电话': Value(dtype='string', id=None), '医院-挂号时间': Value(dtype='string', id=None), '医院-门诊时间': Value(dtype='string', id=None), '医院-公交线路': Value(dtype='string', id=None), '医院-地铁可达': Value(dtype='string', id=None), '医院-地铁线路': Value(dtype='string', id=None), '医院-重点科室': Value(dtype='string', id=None), '医院-CT': Value(dtype='string', id=None), '医院-3.0T MRI': Value(dtype='string', id=None), '医院-DSA': Value(dtype='string', id=None)}
During handling of the above exception, another exception occurred:
TypeError Traceback (most recent call last)
/var/folders/28/k4cy5q7s2hs92xq7_h89_vgm0000gn/T/ipykernel_44306/2896005239.py in <module>
----> 1 dset = load_dataset("GEM/RiSAWOZ")
2 dset
~/miniconda3/envs/huggingface/lib/python3.8/site-packages/datasets/load.py in load_dataset(path, name, data_dir, data_files, split, cache_dir, features, download_config, download_mode, ignore_verifications, keep_in_memory, save_infos, revision, use_auth_token, task, streaming, script_version, **config_kwargs)
1692
1693 # Download and prepare data
-> 1694 builder_instance.download_and_prepare(
1695 download_config=download_config,
1696 download_mode=download_mode,
~/miniconda3/envs/huggingface/lib/python3.8/site-packages/datasets/builder.py in download_and_prepare(self, download_config, download_mode, ignore_verifications, try_from_hf_gcs, dl_manager, base_path, use_auth_token, **download_and_prepare_kwargs)
593 logger.warning("HF google storage unreachable. Downloading and preparing it from source")
594 if not downloaded_from_gcs:
--> 595 self._download_and_prepare(
596 dl_manager=dl_manager, verify_infos=verify_infos, **download_and_prepare_kwargs
597 )
~/miniconda3/envs/huggingface/lib/python3.8/site-packages/datasets/builder.py in _download_and_prepare(self, dl_manager, verify_infos, **prepare_split_kwargs)
682 try:
683 # Prepare split will record examples associated to the split
--> 684 self._prepare_split(split_generator, **prepare_split_kwargs)
685 except OSError as e:
686 raise OSError(
~/miniconda3/envs/huggingface/lib/python3.8/site-packages/datasets/builder.py in _prepare_split(self, split_generator)
1084 writer.write(example, key)
1085 finally:
-> 1086 num_examples, num_bytes = writer.finalize()
1087
1088 split_generator.split_info.num_examples = num_examples
~/miniconda3/envs/huggingface/lib/python3.8/site-packages/datasets/arrow_writer.py in finalize(self, close_stream)
525 # Re-intializing to empty list for next batch
526 self.hkey_record = []
--> 527 self.write_examples_on_file()
528 if self.pa_writer is None:
529 if self.schema:
~/miniconda3/envs/huggingface/lib/python3.8/site-packages/datasets/arrow_writer.py in write_examples_on_file(self)
402 # Since current_examples contains (example, key) tuples
403 batch_examples[col] = [row[0][col] for row in self.current_examples]
--> 404 self.write_batch(batch_examples=batch_examples)
405 self.current_examples = []
406
~/miniconda3/envs/huggingface/lib/python3.8/site-packages/datasets/arrow_writer.py in write_batch(self, batch_examples, writer_batch_size)
495 col_try_type = try_features[col] if try_features is not None and col in try_features else None
496 typed_sequence = OptimizedTypedSequence(batch_examples[col], type=col_type, try_type=col_try_type, col=col)
--> 497 arrays.append(pa.array(typed_sequence))
498 inferred_features[col] = typed_sequence.get_inferred_type()
499 schema = inferred_features.arrow_schema if self.pa_writer is None else self.schema
~/miniconda3/envs/huggingface/lib/python3.8/site-packages/pyarrow/array.pxi in pyarrow.lib.array()
~/miniconda3/envs/huggingface/lib/python3.8/site-packages/pyarrow/array.pxi in pyarrow.lib._handle_arrow_array_protocol()
~/miniconda3/envs/huggingface/lib/python3.8/site-packages/datasets/arrow_writer.py in __arrow_array__(self, type)
203 # Also, when trying type "string", we don't want to convert integers or floats to "string".
204 # We only do it if trying_type is False - since this is what the user asks for.
--> 205 out = cast_array_to_feature(out, type, allow_number_to_str=not self.trying_type)
206 return out
207 except (TypeError, pa.lib.ArrowInvalid) as e: # handle type errors and overflows
~/miniconda3/envs/huggingface/lib/python3.8/site-packages/datasets/table.py in wrapper(array, *args, **kwargs)
942 if pa.types.is_list(array.type) and config.PYARROW_VERSION < version.parse("4.0.0"):
943 array = _sanitize(array)
--> 944 return func(array, *args, **kwargs)
945
946 return wrapper
~/miniconda3/envs/huggingface/lib/python3.8/site-packages/datasets/table.py in wrapper(array, *args, **kwargs)
918 return pa.chunked_array([func(chunk, *args, **kwargs) for chunk in array.chunks])
919 else:
--> 920 return func(array, *args, **kwargs)
921
922 return wrapper
~/miniconda3/envs/huggingface/lib/python3.8/site-packages/datasets/table.py in cast_array_to_feature(array, feature, allow_number_to_str)
1063 # feature must be either [subfeature] or Sequence(subfeature)
1064 if isinstance(feature, list):
-> 1065 return pa.ListArray.from_arrays(array.offsets, _c(array.values, feature[0]))
1066 elif isinstance(feature, Sequence):
1067 if feature.length > -1:
~/miniconda3/envs/huggingface/lib/python3.8/site-packages/datasets/table.py in wrapper(array, *args, **kwargs)
942 if pa.types.is_list(array.type) and config.PYARROW_VERSION < version.parse("4.0.0"):
943 array = _sanitize(array)
--> 944 return func(array, *args, **kwargs)
945
946 return wrapper
~/miniconda3/envs/huggingface/lib/python3.8/site-packages/datasets/table.py in wrapper(array, *args, **kwargs)
918 return pa.chunked_array([func(chunk, *args, **kwargs) for chunk in array.chunks])
919 else:
--> 920 return func(array, *args, **kwargs)
921
922 return wrapper
~/miniconda3/envs/huggingface/lib/python3.8/site-packages/datasets/table.py in cast_array_to_feature(array, feature, allow_number_to_str)
1058 }
1059 if isinstance(feature, dict) and set(field.name for field in array.type) == set(feature):
-> 1060 arrays = [_c(array.field(name), subfeature) for name, subfeature in feature.items()]
1061 return pa.StructArray.from_arrays(arrays, names=list(feature))
1062 elif pa.types.is_list(array.type):
~/miniconda3/envs/huggingface/lib/python3.8/site-packages/datasets/table.py in <listcomp>(.0)
1058 }
1059 if isinstance(feature, dict) and set(field.name for field in array.type) == set(feature):
-> 1060 arrays = [_c(array.field(name), subfeature) for name, subfeature in feature.items()]
1061 return pa.StructArray.from_arrays(arrays, names=list(feature))
1062 elif pa.types.is_list(array.type):
~/miniconda3/envs/huggingface/lib/python3.8/site-packages/datasets/table.py in wrapper(array, *args, **kwargs)
942 if pa.types.is_list(array.type) and config.PYARROW_VERSION < version.parse("4.0.0"):
943 array = _sanitize(array)
--> 944 return func(array, *args, **kwargs)
945
946 return wrapper
~/miniconda3/envs/huggingface/lib/python3.8/site-packages/datasets/table.py in wrapper(array, *args, **kwargs)
918 return pa.chunked_array([func(chunk, *args, **kwargs) for chunk in array.chunks])
919 else:
--> 920 return func(array, *args, **kwargs)
921
922 return wrapper
~/miniconda3/envs/huggingface/lib/python3.8/site-packages/datasets/table.py in cast_array_to_feature(array, feature, allow_number_to_str)
1058 }
1059 if isinstance(feature, dict) and set(field.name for field in array.type) == set(feature):
-> 1060 arrays = [_c(array.field(name), subfeature) for name, subfeature in feature.items()]
1061 return pa.StructArray.from_arrays(arrays, names=list(feature))
1062 elif pa.types.is_list(array.type):
~/miniconda3/envs/huggingface/lib/python3.8/site-packages/datasets/table.py in <listcomp>(.0)
1058 }
1059 if isinstance(feature, dict) and set(field.name for field in array.type) == set(feature):
-> 1060 arrays = [_c(array.field(name), subfeature) for name, subfeature in feature.items()]
1061 return pa.StructArray.from_arrays(arrays, names=list(feature))
1062 elif pa.types.is_list(array.type):
~/miniconda3/envs/huggingface/lib/python3.8/site-packages/datasets/table.py in wrapper(array, *args, **kwargs)
942 if pa.types.is_list(array.type) and config.PYARROW_VERSION < version.parse("4.0.0"):
943 array = _sanitize(array)
--> 944 return func(array, *args, **kwargs)
945
946 return wrapper
~/miniconda3/envs/huggingface/lib/python3.8/site-packages/datasets/table.py in wrapper(array, *args, **kwargs)
918 return pa.chunked_array([func(chunk, *args, **kwargs) for chunk in array.chunks])
919 else:
--> 920 return func(array, *args, **kwargs)
921
922 return wrapper
~/miniconda3/envs/huggingface/lib/python3.8/site-packages/datasets/table.py in cast_array_to_feature(array, feature, allow_number_to_str)
1085 elif not isinstance(feature, (Sequence, dict, list, tuple)):
1086 return array_cast(array, feature(), allow_number_to_str=allow_number_to_str)
-> 1087 raise TypeError(f"Couldn't cast array of type\n{array.type}\nto\n{feature}")
1088
1089
TypeError: Couldn't cast array of type
struct<医院-3.0T MRI: string, 医院-CT: string, 医院-DSA: string, 医院-公交线路: string, 医院-区域: string, 医院-名称: string, 医院-地址: string, 医院-地铁可达: string, 医院-地铁线路: string, 医院-性质: string, 医院-挂号时间: string, 医院-电话: string, 医院-等级: string, 医院-类别: string, 医院-重点科室: string, 医院-门诊时间: string, 天气-城市: string, 天气-天气: string, 天气-日期: string, 天气-温度: string, 天气-紫外线强度: string, 天气-风力风向: string, 旅游景点-区域: string, 旅游景点-名称: string, 旅游景点-地址: string, 旅游景点-开放时间: string, 旅游景点-是否地铁直达: string, 旅游景点-景点类型: string, 旅游景点-最适合人群: string, 旅游景点-消费: string, 旅游景点-特点: string, 旅游景点-电话号码: string, 旅游景点-评分: string, 旅游景点-门票价格: string, 汽车-价格(万元): string, 汽车-倒车影像: string, 汽车-动力水平: string, 汽车-厂商: string, 汽车-发动机排量(L): string, 汽车-发动机马力(Ps): string, 汽车-名称: string, 汽车-定速巡航: string, 汽车-巡航系统: string, 汽车-座位数: string, 汽车-座椅加热: string, 汽车-座椅通风: string, 汽车-所属价格区间: string, 汽车-油耗水平: string, 汽车-环保标准: string, 汽车-级别: string, 汽车-综合油耗(L/100km): string, 汽车-能源类型: string, 汽车-车型: string, 汽车-车系: string, 汽车-车身尺寸(mm): string, 汽车-驱动方式: string, 汽车-驾驶辅助影像: string, 火车-出发地: string, 火车-出发时间: string, 火车-到达时间: string, 火车-坐席: string, 火车-日期: string, 火车-时长: string, 火车-目的地: string, 火车-票价: string, 火车-舱位档次: string, 火车-车型: string, 火车-车次信息: string, 电影-主演: string, 电影-主演名单: string, 电影-具体上映时间: string, 电影-制片国家/地区: string, 电影-导演: string, 电影-年代: string, 电影-片名: string, 电影-片长: string, 电影-类型: string, 电影-豆瓣评分: string, 电脑-CPU: string, 电脑-CPU型号: string, 电脑-产品类别: string, 电脑-价格: string, 电脑-价格区间: string, 电脑-内存容量: string, 电脑-分类: string, 电脑-品牌: string, 电脑-商品名称: string, 电脑-屏幕尺寸: string, 电脑-待机时长: string, 电脑-显卡型号: string, 电脑-显卡类别: string, 电脑-游戏性能: string, 电脑-特性: string, 电脑-硬盘容量: string, 电脑-系列: string, 电脑-系统: string, 电脑-色系: string, 电脑-裸机重量: string, 电视剧-主演: string, 电视剧-主演名单: string, 电视剧-制片国家/地区: string, 电视剧-单集片长: string, 电视剧-导演: string, 电视剧-年代: string, 电视剧-片名: string, 电视剧-类型: string, 电视剧-豆瓣评分: string, 电视剧-集数: string, 电视剧-首播时间: string, 辅导班-上课方式: string, 辅导班-上课时间: string, 辅导班-下课时间: string, 辅导班-价格: string, 辅导班-区域: string, 辅导班-年级: string, 辅导班-开始日期: string, 辅导班-教室地点: string, 辅导班-教师: string, 辅导班-教师网址: string, 辅导班-时段: string, 辅导班-校区: string, 辅导班-每周: string, 辅导班-班号: string, 辅导班-科目: string, 辅导班-结束日期: string, 辅导班-课时: string, 辅导班-课次: string, 辅导班-课程网址: string, 辅导班-难度: string, 通用-产品类别: string, 通用-价格区间: string, 通用-品牌: string, 通用-系列: string, 酒店-价位: string, 酒店-停车场: string, 酒店-区域: string, 酒店-名称: string, 酒店-地址: string, 酒店-房型: string, 酒店-房费: string, 酒店-星级: string, 酒店-电话号码: string, 酒店-评分: string, 酒店-酒店类型: string, 飞机-准点率: string, 飞机-出发地: string, 飞机-到达时间: string, 飞机-日期: string, 飞机-目的地: string, 飞机-票价: string, 飞机-航班信息: string, 飞机-舱位档次: string, 飞机-起飞时间: string, 餐厅-人均消费: string, 餐厅-价位: string, 餐厅-区域: string, 餐厅-名称: string, 餐厅-地址: string, 餐厅-推荐菜: string, 餐厅-是否地铁直达: string, 餐厅-电话号码: string, 餐厅-菜系: string, 餐厅-营业时间: string, 餐厅-评分: string>
to
{'旅游景点-名称': Value(dtype='string', id=None), '旅游景点-区域': Value(dtype='string', id=None), '旅游景点-景点类型': Value(dtype='string', id=None), '旅游景点-最适合人群': Value(dtype='string', id=None), '旅游景点-消费': Value(dtype='string', id=None), '旅游景点-是否地铁直达': Value(dtype='string', id=None), '旅游景点-门票价格': Value(dtype='string', id=None), '旅游景点-电话号码': Value(dtype='string', id=None), '旅游景点-地址': Value(dtype='string', id=None), '旅游景点-评分': Value(dtype='string', id=None), '旅游景点-开放时间': Value(dtype='string', id=None), '旅游景点-特点': Value(dtype='string', id=None), '餐厅-名称': Value(dtype='string', id=None), '餐厅-区域': Value(dtype='string', id=None), '餐厅-菜系': Value(dtype='string', id=None), '餐厅-价位': Value(dtype='string', id=None), '餐厅-是否地铁直达': Value(dtype='string', id=None), '餐厅-人均消费': Value(dtype='string', id=None), '餐厅-地址': Value(dtype='string', id=None), '餐厅-电话号码': Value(dtype='string', id=None), '餐厅-评分': Value(dtype='string', id=None), '餐厅-营业时间': Value(dtype='string', id=None), '餐厅-推荐菜': Value(dtype='string', id=None), '酒店-名称': Value(dtype='string', id=None), '酒店-区域': Value(dtype='string', id=None), '酒店-星级': Value(dtype='string', id=None), '酒店-价位': Value(dtype='string', id=None), '酒店-酒店类型': Value(dtype='string', id=None), '酒店-房型': Value(dtype='string', id=None), '酒店-停车场': Value(dtype='string', id=None), '酒店-房费': Value(dtype='string', id=None), '酒店-地址': Value(dtype='string', id=None), '酒店-电话号码': Value(dtype='string', id=None), '酒店-评分': Value(dtype='string', id=None), '电脑-品牌': Value(dtype='string', id=None), '电脑-产品类别': Value(dtype='string', id=None), '电脑-分类': Value(dtype='string', id=None), '电脑-内存容量': Value(dtype='string', id=None), '电脑-屏幕尺寸': Value(dtype='string', id=None), '电脑-CPU': Value(dtype='string', id=None), '电脑-价格区间': Value(dtype='string', id=None), '电脑-系列': Value(dtype='string', id=None), '电脑-商品名称': Value(dtype='string', id=None), '电脑-系统': Value(dtype='string', id=None), '电脑-游戏性能': Value(dtype='string', id=None), '电脑-CPU型号': Value(dtype='string', id=None), '电脑-裸机重量': Value(dtype='string', id=None), '电脑-显卡类别': Value(dtype='string', id=None), '电脑-显卡型号': Value(dtype='string', id=None), '电脑-特性': Value(dtype='string', id=None), '电脑-色系': Value(dtype='string', id=None), '电脑-待机时长': Value(dtype='string', id=None), '电脑-硬盘容量': Value(dtype='string', id=None), '电脑-价格': Value(dtype='string', id=None), '火车-出发地': Value(dtype='string', id=None), '火车-目的地': Value(dtype='string', id=None), '火车-日期': Value(dtype='string', id=None), '火车-车型': Value(dtype='string', id=None), '火车-坐席': Value(dtype='string', id=None), '火车-车次信息': Value(dtype='string', id=None), '火车-时长': Value(dtype='string', id=None), '火车-出发时间': Value(dtype='string', id=None), '火车-到达时间': Value(dtype='string', id=None), '火车-票价': Value(dtype='string', id=None), '飞机-出发地': Value(dtype='string', id=None), '飞机-目的地': Value(dtype='string', id=None), '飞机-日期': Value(dtype='string', id=None), '飞机-舱位档次': Value(dtype='string', id=None), '飞机-航班信息': Value(dtype='string', id=None), '飞机-起飞时间': Value(dtype='string', id=None), '飞机-到达时间': Value(dtype='string', id=None), '飞机-票价': Value(dtype='string', id=None), '飞机-准点率': Value(dtype='string', id=None), '天气-城市': Value(dtype='string', id=None), '天气-日期': Value(dtype='string', id=None), '天气-天气': Value(dtype='string', id=None), '天气-温度': Value(dtype='string', id=None), '天气-风力风向': Value(dtype='string', id=None), '天气-紫外线强度': Value(dtype='string', id=None), '电影-制片国家/地区': Value(dtype='string', id=None), '电影-类型': Value(dtype='string', id=None), '电影-年代': Value(dtype='string', id=None), '电影-主演': Value(dtype='string', id=None), '电影-导演': Value(dtype='string', id=None), '电影-片名': Value(dtype='string', id=None), '电影-主演名单': Value(dtype='string', id=None), '电影-具体上映时间': Value(dtype='string', id=None), '电影-片长': Value(dtype='string', id=None), '电影-豆瓣评分': Value(dtype='string', id=None), '电视剧-制片国家/地区': Value(dtype='string', id=None), '电视剧-类型': Value(dtype='string', id=None), '电视剧-年代': Value(dtype='string', id=None), '电视剧-主演': Value(dtype='string', id=None), '电视剧-导演': Value(dtype='string', id=None), '电视剧-片名': Value(dtype='string', id=None), '电视剧-主演名单': Value(dtype='string', id=None), '电视剧-首播时间': Value(dtype='string', id=None), '电视剧-集数': Value(dtype='string', id=None), '电视剧-单集片长': Value(dtype='string', id=None), '电视剧-豆瓣评分': Value(dtype='string', id=None), '辅导班-班号': Value(dtype='string', id=None), '辅导班-难度': Value(dtype='string', id=None), '辅导班-科目': Value(dtype='string', id=None), '辅导班-年级': Value(dtype='string', id=None), '辅导班-区域': Value(dtype='string', id=None), '辅导班-校区': Value(dtype='string', id=None), '辅导班-上课方式': Value(dtype='string', id=None), '辅导班-开始日期': Value(dtype='string', id=None), '辅导班-结束日期': Value(dtype='string', id=None), '辅导班-每周': Value(dtype='string', id=None), '辅导班-上课时间': Value(dtype='string', id=None), '辅导班-下课时间': Value(dtype='string', id=None), '辅导班-时段': Value(dtype='string', id=None), '辅导班-课次': Value(dtype='string', id=None), '辅导班-课时': Value(dtype='string', id=None), '辅导班-教室地点': Value(dtype='string', id=None), '辅导班-教师': Value(dtype='string', id=None), '辅导班-价格': Value(dtype='string', id=None), '辅导班-课程网址': Value(dtype='string', id=None), '辅导班-教师网址': Value(dtype='string', id=None), '汽车-名称': Value(dtype='string', id=None), '汽车-车型': Value(dtype='string', id=None), '汽车-级别': Value(dtype='string', id=None), '汽车-座位数': Value(dtype='string', id=None), '汽车-车身尺寸(mm)': Value(dtype='string', id=None), '汽车-厂商': Value(dtype='string', id=None), '汽车-能源类型': Value(dtype='string', id=None), '汽车-发动机排量(L)': Value(dtype='string', id=None), '汽车-发动机马力(Ps)': Value(dtype='string', id=None), '汽车-驱动方式': Value(dtype='string', id=None), '汽车-综合油耗(L/100km)': Value(dtype='string', id=None), '汽车-环保标准': Value(dtype='string', id=None), '汽车-驾驶辅助影像': Value(dtype='string', id=None), '汽车-巡航系统': Value(dtype='string', id=None), '汽车-价格(万元)': Value(dtype='string', id=None), '汽车-车系': Value(dtype='string', id=None), '汽车-动力水平': Value(dtype='string', id=None), '汽车-油耗水平': Value(dtype='string', id=None), '汽车-倒车影像': Value(dtype='string', id=None), '汽车-定速巡航': Value(dtype='string', id=None), '汽车-座椅加热': Value(dtype='string', id=None), '汽车-座椅通风': Value(dtype='string', id=None), '汽车-所属价格区间': Value(dtype='string', id=None), '医院-名称': Value(dtype='string', id=None), '医院-等级': Value(dtype='string', id=None), '医院-类别': Value(dtype='string', id=None), '医院-性质': Value(dtype='string', id=None), '医院-区域': Value(dtype='string', id=None), '医院-地址': Value(dtype='string', id=None), '医院-电话': Value(dtype='string', id=None), '医院-挂号时间': Value(dtype='string', id=None), '医院-门诊时间': Value(dtype='string', id=None), '医院-公交线路': Value(dtype='string', id=None), '医院-地铁可达': Value(dtype='string', id=None), '医院-地铁线路': Value(dtype='string', id=None), '医院-重点科室': Value(dtype='string', id=None), '医院-CT': Value(dtype='string', id=None), '医院-3.0T MRI': Value(dtype='string', id=None), '医院-DSA': Value(dtype='string', id=None)}
```
</details>
## Environment info
<!-- You can run the command `datasets-cli env` and copy-and-paste its output below. -->
- `datasets` version: 1.18.1
- Platform: macOS-10.16-x86_64-i386-64bit
- Python version: 3.8.10
- PyArrow version: 3.0.0
| 3,637 |
https://github.com/huggingface/datasets/issues/3634 | Dataset.shuffle(seed=None) gives fixed row permutation | [
"I'm not sure if this is expected behavior.\r\n\r\nAm I supposed to work with a copy of the dataset, i.e. `shuffled_dataset = data.shuffle(seed=None)`?\r\n\r\n```diff\r\nimport datasets\r\n\r\n# Some toy example\r\ndata = datasets.Dataset.from_dict(\r\n {\"feature\": [1, 2, 3, 4, 5], \"label\": [\"a\", \"b\", \"c\", \"d\", \"e\"]}\r\n)\r\n\r\n+shuffled_data = data.shuffle(seed=None)\r\n\r\n# Doesn't work as expected\r\nprint(\"Shuffle dataset\")\r\nfor _ in range(3):\r\n+ shuffled_data = shuffled_data.shuffle(seed=None)\r\n+ print(shuffled_data[:])\r\n- print(data.shuffle(seed=None)[:])\r\n\r\n# This seems to work with pandas\r\nprint(\"\\nShuffle via pandas\")\r\nfor _ in range(3):\r\n df = data.to_pandas().sample(frac=1.0)\r\n print(datasets.Dataset.from_pandas(df, preserve_index=False)[:])\r\n\r\n```\r\n\r\nor provide a `generator` instead?\r\n\r\n```diff\r\nimport datasets\r\n+from numpy.random import default_rng\r\n\r\n# Some toy example\r\ndata = datasets.Dataset.from_dict(\r\n {\"feature\": [1, 2, 3, 4, 5], \"label\": [\"a\", \"b\", \"c\", \"d\", \"e\"]}\r\n)\r\n\r\n+rng = default_rng()\r\n\r\n# Doesn't work as expected\r\nprint(\"Shuffle dataset\")\r\nfor _ in range(3):\r\n+ print(data.shuffle(generator=rng)[:])\r\n- print(data.shuffle(seed=None)[:])\r\n\r\n# This seems to work with pandas\r\nprint(\"\\nShuffle via pandas\")\r\nfor _ in range(3):\r\n df = data.to_pandas().sample(frac=1.0)\r\n print(datasets.Dataset.from_pandas(df, preserve_index=False)[:])\r\n\r\n```",
"Hi! Thanks for reporting! Yes, this is not expected behavior. I've opened a PR with the fix."
] | ## Describe the bug
Repeated attempts to `shuffle` a dataset without specifying a seed give the same results.
## Steps to reproduce the bug
```python
import datasets
# Some toy example
data = datasets.Dataset.from_dict(
{"feature": [1, 2, 3, 4, 5], "label": ["a", "b", "c", "d", "e"]}
)
# Doesn't work as expected
print("Shuffle dataset")
for _ in range(3):
print(data.shuffle(seed=None)[:])
# This seems to work with pandas
print("\nShuffle via pandas")
for _ in range(3):
df = data.to_pandas().sample(frac=1.0)
print(datasets.Dataset.from_pandas(df, preserve_index=False)[:])
```
## Expected results
I assumed that the default setting would initialize a new/random state of a `np.random.BitGenerator` (see [docs](https://huggingface.co/docs/datasets/package_reference/main_classes.html?highlight=shuffle#datasets.Dataset.shuffle)).
Wouldn't that reshuffle the rows each time I call `data.shuffle()`?
## Actual results
```bash
Shuffle dataset
{'feature': [5, 1, 3, 2, 4], 'label': ['e', 'a', 'c', 'b', 'd']}
{'feature': [5, 1, 3, 2, 4], 'label': ['e', 'a', 'c', 'b', 'd']}
{'feature': [5, 1, 3, 2, 4], 'label': ['e', 'a', 'c', 'b', 'd']}
Shuffle via pandas
{'feature': [4, 2, 3, 1, 5], 'label': ['d', 'b', 'c', 'a', 'e']}
{'feature': [2, 5, 3, 4, 1], 'label': ['b', 'e', 'c', 'd', 'a']}
{'feature': [5, 2, 3, 1, 4], 'label': ['e', 'b', 'c', 'a', 'd']}
```
## Environment info
<!-- You can run the command `datasets-cli env` and copy-and-paste its output below. -->
- `datasets` version: 1.18.0
- Platform: Linux-5.13.0-27-generic-x86_64-with-glibc2.17
- Python version: 3.8.12
- PyArrow version: 6.0.1
| 3,634 |
https://github.com/huggingface/datasets/issues/3632 | Adding CC-100: Monolingual Datasets from Web Crawl Data (Datasets links are invalid) | [
"Hi @AnzorGozalishvili,\r\n\r\nMaybe their site was temporarily down, but it seems to work fine now.\r\n\r\nCould you please try again and confirm if the problem persists? ",
"Hi @albertvillanova \r\nI checked and it works. \r\nIt seems that it was really temporarily down.\r\nThanks!"
] | ## Describe the bug
The dataset links are no longer valid for CC-100. It seems that the website which was keeping these files are no longer accessible and therefore this dataset became unusable.
Check out the dataset [homepage](http://data.statmt.org/cc-100/) which isn't accessible.
Also the URLs for dataset file per language isn't accessible: http://data.statmt.org/cc-100/<language code here>.txt.xz (language codes: am, sr, ka, etc.)
## Steps to reproduce the bug
```python
from datasets import load_dataset
dataset = load_dataset("cc100", "ka")
```
It throws 503 error.
## Expected results
It should successfully download and load dataset but it throws an exception because the dataset files are no longer accessible.
## Environment info
Run from google colab. Just installed the library using pip:
```!pip install -U datasets```
| 3,632 |
https://github.com/huggingface/datasets/issues/3631 | Labels conflict when loading a local CSV file. | [
"Hi @pichljan, thanks for reporting.\r\n\r\nThis should be fixed. I'm looking at it. "
] | ## Describe the bug
I am trying to load a local CSV file with a separate file containing label names. It is successfully loaded for the first time, but when I try to load it again, there is a conflict between provided labels and the cached dataset info. Disabling caching globally and/or using `download_mode="force_redownload"` did not help.
## Steps to reproduce the bug
```python
load_dataset('csv', data_files='data/my_data.csv',
features=Features(text=Value(dtype='string'),
label=ClassLabel(names_file='data/my_data_labels.txt')))
```
`my_data.csv` file has the following structure:
```
text,label
"example1",0
"example2",1
...
```
and the `my_data_labels.txt` looks like this:
```
label1
label2
...
```
## Expected results
Successfully loaded dataset.
## Actual results
```python
File "/usr/local/lib/python3.8/site-packages/datasets/load.py", line 1706, in load_dataset
ds = builder_instance.as_dataset(split=split, ignore_verifications=ignore_verifications, in_memory=keep_in_memory)
File "/usr/local/lib/python3.8/site-packages/datasets/builder.py", line 766, in as_dataset
datasets = utils.map_nested(
File "/usr/local/lib/python3.8/site-packages/datasets/utils/py_utils.py", line 261, in map_nested
mapped = [
File "/usr/local/lib/python3.8/site-packages/datasets/utils/py_utils.py", line 262, in <listcomp>
_single_map_nested((function, obj, types, None, True))
File "/usr/local/lib/python3.8/site-packages/datasets/utils/py_utils.py", line 197, in _single_map_nested
return function(data_struct)
File "/usr/local/lib/python3.8/site-packages/datasets/builder.py", line 797, in _build_single_dataset
ds = self._as_dataset(
File "/usr/local/lib/python3.8/site-packages/datasets/builder.py", line 872, in _as_dataset
return Dataset(fingerprint=fingerprint, **dataset_kwargs)
File "/usr/local/lib/python3.8/site-packages/datasets/arrow_dataset.py", line 638, in __init__
inferred_features = Features.from_arrow_schema(arrow_table.schema)
File "/usr/local/lib/python3.8/site-packages/datasets/features/features.py", line 1242, in from_arrow_schema
return Features.from_dict(metadata["info"]["features"])
File "/usr/local/lib/python3.8/site-packages/datasets/features/features.py", line 1271, in from_dict
obj = generate_from_dict(dic)
File "/usr/local/lib/python3.8/site-packages/datasets/features/features.py", line 1076, in generate_from_dict
return {key: generate_from_dict(value) for key, value in obj.items()}
File "/usr/local/lib/python3.8/site-packages/datasets/features/features.py", line 1076, in <dictcomp>
return {key: generate_from_dict(value) for key, value in obj.items()}
File "/usr/local/lib/python3.8/site-packages/datasets/features/features.py", line 1083, in generate_from_dict
return class_type(**{k: v for k, v in obj.items() if k in field_names})
File "<string>", line 7, in __init__
File "/usr/local/lib/python3.8/site-packages/datasets/features/features.py", line 776, in __post_init__
raise ValueError("Please provide either names or names_file but not both.")
ValueError: Please provide either names or names_file but not both.
```
## Environment info
- `datasets` version: 1.18.0
- Python version: 3.8.2
| 3,631 |
https://github.com/huggingface/datasets/issues/3630 | DuplicatedKeysError of NewsQA dataset | [
"Thanks for reporting, @StevenTang1998.\r\n\r\nI'm fixing it. "
] | After processing the dataset following official [NewsQA](https://github.com/Maluuba/newsqa), I used datasets to load it:
```
a = load_dataset('newsqa', data_dir='news')
```
and the following error occurred:
```
Using custom data configuration default-data_dir=news
Downloading and preparing dataset newsqa/default to /root/.cache/huggingface/datasets/newsqa/default-data_dir=news/1.0.0/b0b23e22d94a3d352ad9d75aff2b71375264a122fae301463079ee8595e05ab9...
Traceback (most recent call last):
File "/usr/local/lib/python3.8/dist-packages/datasets/builder.py", line 1084, in _prepare_split
writer.write(example, key)
File "/usr/local/lib/python3.8/dist-packages/datasets/arrow_writer.py", line 442, in write
self.check_duplicate_keys()
File "/usr/local/lib/python3.8/dist-packages/datasets/arrow_writer.py", line 453, in check_duplicate_keys
raise DuplicatedKeysError(key)
datasets.keyhash.DuplicatedKeysError: FAILURE TO GENERATE DATASET !
Found duplicate Key: ./cnn/stories/6a0f9c8a5d0c6e8949b37924163c92923fe5770d.story
Keys should be unique and deterministic in nature
During handling of the above exception, another exception occurred:
Traceback (most recent call last):
File "<stdin>", line 1, in <module>
File "/usr/local/lib/python3.8/dist-packages/datasets/load.py", line 1694, in load_dataset
builder_instance.download_and_prepare(
File "/usr/local/lib/python3.8/dist-packages/datasets/builder.py", line 595, in download_and_prepare
self._download_and_prepare(
File "/usr/local/lib/python3.8/dist-packages/datasets/builder.py", line 684, in _download_and_prepare
self._prepare_split(split_generator, **prepare_split_kwargs)
File "/usr/local/lib/python3.8/dist-packages/datasets/builder.py", line 1086, in _prepare_split
num_examples, num_bytes = writer.finalize()
File "/usr/local/lib/python3.8/dist-packages/datasets/arrow_writer.py", line 524, in finalize
self.check_duplicate_keys()
File "/usr/local/lib/python3.8/dist-packages/datasets/arrow_writer.py", line 453, in check_duplicate_keys
raise DuplicatedKeysError(key)
datasets.keyhash.DuplicatedKeysError: FAILURE TO GENERATE DATASET !
Found duplicate Key: ./cnn/stories/6a0f9c8a5d0c6e8949b37924163c92923fe5770d.story
Keys should be unique and deterministic in nature
``` | 3,630 |
https://github.com/huggingface/datasets/issues/3628 | Dataset Card Creator drops information for "Additional Information" Section | [] | First of all, the card creator is a great addition and really helpful for streamlining dataset cards!
## Describe the bug
I encountered an inconvenient bug when entering "Additional Information" in the react app, which drops already entered text when switching to a previous section, and then back again to "Additional Information". I was able to reproduce the issue in both Firefox and Chrome, so I suspect a problem with the React logic that doesn't expect users to switch back in the final section.
Edit: I'm also not sure whether this is the right place to open the bug report on, since it's not clear to me which particular project it belongs to, or where I could find associated source code.
## Steps to reproduce the bug
1. Navigate to the Section "Additional Information" in the [dataset card creator](https://huggingface.co/datasets/card-creator/)
2. Enter text in an arbitrary field, e.g., "Dataset Curators".
3. Switch back to a previous section, like "Dataset Creation".
4. When switching back again to "Additional Information", the text has been deleted.
Notably, this behavior can be reproduced again and again, it's not just problematic for the first "switch-back" from Additional Information.
## Expected results
For step 4, the previously entered information should still be present in the boxes, similar to the behavior to all other sections (switching back there works as expected)
## Actual results
The text boxes are empty again, and previously entered text got deleted.
## Environment info
- `datasets` version: N/A
- Platform: Firefox 96.0 / Chrome 97.0
- Python version: N/A
- PyArrow version: N/A
| 3,628 |
https://github.com/huggingface/datasets/issues/3626 | The Pile cannot connect to host | [] | ## Describe the bug
The Pile had issues with their previous host server and have mirrored its content to another server.
The new URL server should be updated.
| 3,626 |
https://github.com/huggingface/datasets/issues/3625 | Add a metadata field for when source data was produced | [
"A question to the datasets maintainers: is there a policy about how the set of allowed metadata fields is maintained and expanded?\r\n\r\nMetadata are very important, but defining the standard is always a struggle between allowing exhaustivity without being too complex. Archivists have Dublin Core, open data has https://frictionlessdata.io/, geo has ISO 19139 and INSPIRE, etc. and it's always a mess! I'm not sure we want to dig too much into it, but I'm curious to know if there has been some work on the metadata standard.",
"> Metadata are very important, but defining the standard is always a struggle between allowing exhaustivity without being too complex. Archivists have Dublin Core, open data has [frictionlessdata.io](https://frictionlessdata.io/), geo has ISO 19139 and INSPIRE, etc. and it's always a mess! I'm not sure we want to dig too much into it, but I'm curious to know if there has been some work on the metadata standard.\r\n\r\n\r\nI thought this is a potential issue with adding this field since it might be hard to define what is general enough to be useful for most data vs what becomes very domain-specific. Potentially adding one extra field leads to more and more fields in the future. \r\n\r\nAnother issue is that there are some metadata standards around data i.e. [datacite](https://schema.datacite.org/meta/kernel-4.4/), but not many aimed explicitly at ML data afaik. Some of the discussions around metadata for ML are also more focused on versioning/managing data in production environments. My thinking is that here, some reference to the time of production would also often be tracked/relevant, i.e. for triggering model training, so having this information available in the hub would also help address this use case. ",
"Adding a relevant paper related to this topic: [TimeLMs: Diachronic Language Models from Twitter](https://arxiv.org/abs/2202.03829)\r\n\r\n",
"Related: https://github.com/huggingface/datasets/issues/3877",
"Also related: the [Data Catalog Vocabulary - DCAT](https://www.w3.org/TR/vocab-dcat/) standard will be discussed in a new Working Group at the W3C: https://www.w3.org/2022/06/dx-wg-charter.html"
] | **Is your feature request related to a problem? Please describe.**
The current problem is that information about when source data was produced is not easily visible. Though there are a variety of metadata fields available in the dataset viewer, time period information is not included. This feature request suggests making metadata relating to the time that the underlying *source* data was produced more prominent and outlines why this specific information is of particular importance, both in domain-specific historic research and more broadly.
**Describe the solution you'd like**
There are a variety of metadata fields exposed in the dataset viewer (license, task categories, etc.) These fields make this metadata more prominent both for human users and as potentially machine-actionable information (for example, through the API). I would propose to add a metadata field that says when some underlying data was produced. For example, a dataset would be labelled as being produced between `1800-1900`.
**Describe alternatives you've considered**
This information is sometimes available in the Datacard or a paper describing the dataset. However, it's often not that easy to identify or extract this information, particularly if you want to use this field as a filter to identify relevant datasets.
**Additional context**
I believe this feature is relevant for a number of reasons:
- Increasingly, there is an interest in using historical data for training language models (for example, https://huggingface.co/dbmdz/bert-base-historic-dutch-cased), and datasets to support this task (for example, https://huggingface.co/datasets/bnl_newspapers). For these datasets, indicating the time periods covered is particularly relevant.
- More broadly, time is likely a common source of domain drift. Datasets of movie reviews from the 90s may not work well for recent movie reviews. As the documentation and long-term management of ML data become more of a priority, quickly understanding the time when the underlying text (or other data types) is arguably more important.
- time-series data: datasets are adding more support for time series data. Again, the periods covered might be particularly relevant here.
**open questions**
- I think some of my points above apply not only to the underlying data but also to annotations. As a result, there could also be an argument for encoding this information somewhere. However, I would argue (but could be persuaded otherwise) that this is probably less important for filtering. This type of context is already addressed in the datasheets template and often requires more narrative to discuss.
- what level of granularity would make sense for this? e.g. assigning a decade, century or year?
- how to encode this information? What formatting makes sense
- what specific time to encode; a data range? (mean, modal, min, max value?)
This is a slightly amorphous feature request - I would be happy to discuss further/try and propose a more concrete solution if this seems like something that could be worth considering. I realise this might also touch on other parts of the 🤗 hubs ecosystem. | 3,625 |
https://github.com/huggingface/datasets/issues/3622 | Extend support for streaming datasets that use os.path.relpath | [] | Extend support for streaming datasets that use `os.path.relpath`.
This feature will also be useful to yield the relative path of audio or image files.
| 3,622 |
https://github.com/huggingface/datasets/issues/3621 | Consider adding `ipywidgets` as a dependency. | [
"Hi! We use `tqdm` to display progress bars, so I suggest you open this issue in their repo.",
"It depends on how you use `tqdm`, no? \r\n\r\nDoesn't this library import via; \r\n\r\n```\r\nfrom tqdm.notebook import tqdm\r\n```",
"Hi! Sorry for the late reply. We import `tqdm` as `from tqdm.auto import tqdm`, which should be equal to `from tqdm.notebook import tqdm` in Jupyter.",
"Any objection if I make a PR that checks if the widgets library is installed beforehand? "
] | When I install `datasets` in a fresh virtualenv with jupyterlab I always see this error.
```
ImportError: IProgress not found. Please update jupyter and ipywidgets. See https://ipywidgets.readthedocs.io/en/stable/user_install.html
```
It's a bit of a nuisance, because I need to run shut down the jupyterlab server in order to install the required dependency. Might it be an option to just include it as a dependency here? | 3,621 |
https://github.com/huggingface/datasets/issues/3618 | TIMIT Dataset not working with GPU | [
"Hi ! I think you should avoid calling `timit_train['audio']`. Indeed by doing so you're **loading all the audio column in memory**. This is problematic in your case because the TIMIT dataset is huge.\r\n\r\nIf you want to access the audio data of some samples, you should do this instead `timit_train[:10][\"train\"]` for example.\r\n\r\nOther than that, I'm not sure why you get a `TypeError: string indices must be integers`, do you have a code snippet that reproduces the issue that you can share here ?",
"I get the same error when I try to do `timit_train[0]` or really any indexing into the whole thing. \r\n\r\nReally, that IS the code snippet that reproduces the issue. If you index into other fields like 'file' or whatever, it works. As soon as one of the fields you're looking into is 'audio', you get that issue. It's a weird issue and I suspect it's Sagemaker/environment related, maybe the mix of libraries and dependencies are not good. \r\n\r\n\r\nExample code snippet with issue. \r\n```python\r\nfrom datasets import load_dataset\r\n\r\ntimit_train = load_dataset('timit_asr', split='train')\r\nprint(timit_train[0])\r\n```",
"Ok I see ! From the error you got, it looks like the `value` encoded in the arrow file of the TIMIT dataset you loaded is a string instead of a dictionary with keys \"path\" and \"bytes\" but we don't support this since 1.18\r\n\r\nCan you try regenerating the dataset with `load_dataset('timit_asr', download_mode=\"force_redownload\")` please ? I think it should fix the issue."
] | ## Describe the bug
I am working trying to use the TIMIT dataset in order to fine-tune Wav2Vec2 model and I am unable to load the "audio" column from the dataset when working with a GPU.
I am working on Amazon Sagemaker Studio, on the Python 3 (PyTorch 1.8 Python 3.6 GPU Optimized) environment, with a single ml.g4dn.xlarge instance (corresponds to a Tesla T4 GPU).
I don't know if the issue is GPU related or Python environment related because everything works when I work off of the CPU Optimized environment with a non-GPU instance. My code also works on Google Colab with a GPU instance.
This issue is blocking because I cannot get the 'audio' column in any way due to this error, which means that I can't pass it to any functions. I later use the dataset.map function and that is where I originally noticed this error.
## Steps to reproduce the bug
```python
from datasets import load_dataset
timit_train = load_dataset('timit_asr', split='train')
print(timit_train['audio'])
```
## Expected results
Expected to see inside the 'audio' column, which contains an 'array' nested field with the array data I actually need.
## Actual results
Traceback
```
---------------------------------------------------------------------------
TypeError Traceback (most recent call last)
<ipython-input-6-ceeac555e921> in <module>
----> 1 timit_train['audio']
/opt/conda/lib/python3.6/site-packages/datasets/arrow_dataset.py in __getitem__(self, key)
1917 """Can be used to index columns (by string names) or rows (by integer index or iterable of indices or bools)."""
1918 return self._getitem(
-> 1919 key,
1920 )
1921
/opt/conda/lib/python3.6/site-packages/datasets/arrow_dataset.py in _getitem(self, key, decoded, **kwargs)
1902 pa_subtable = query_table(self._data, key, indices=self._indices if self._indices is not None else None)
1903 formatted_output = format_table(
-> 1904 pa_subtable, key, formatter=formatter, format_columns=format_columns, output_all_columns=output_all_columns
1905 )
1906 return formatted_output
/opt/conda/lib/python3.6/site-packages/datasets/formatting/formatting.py in format_table(table, key, formatter, format_columns, output_all_columns)
529 python_formatter = PythonFormatter(features=None)
530 if format_columns is None:
--> 531 return formatter(pa_table, query_type=query_type)
532 elif query_type == "column":
533 if key in format_columns:
/opt/conda/lib/python3.6/site-packages/datasets/formatting/formatting.py in __call__(self, pa_table, query_type)
280 return self.format_row(pa_table)
281 elif query_type == "column":
--> 282 return self.format_column(pa_table)
283 elif query_type == "batch":
284 return self.format_batch(pa_table)
/opt/conda/lib/python3.6/site-packages/datasets/formatting/formatting.py in format_column(self, pa_table)
315 column = self.python_arrow_extractor().extract_column(pa_table)
316 if self.decoded:
--> 317 column = self.python_features_decoder.decode_column(column, pa_table.column_names[0])
318 return column
319
/opt/conda/lib/python3.6/site-packages/datasets/formatting/formatting.py in decode_column(self, column, column_name)
221
222 def decode_column(self, column: list, column_name: str) -> list:
--> 223 return self.features.decode_column(column, column_name) if self.features else column
224
225 def decode_batch(self, batch: dict) -> dict:
/opt/conda/lib/python3.6/site-packages/datasets/features/features.py in decode_column(self, column, column_name)
1337 return (
1338 [self[column_name].decode_example(value) if value is not None else None for value in column]
-> 1339 if self._column_requires_decoding[column_name]
1340 else column
1341 )
/opt/conda/lib/python3.6/site-packages/datasets/features/features.py in <listcomp>(.0)
1336 """
1337 return (
-> 1338 [self[column_name].decode_example(value) if value is not None else None for value in column]
1339 if self._column_requires_decoding[column_name]
1340 else column
/opt/conda/lib/python3.6/site-packages/datasets/features/audio.py in decode_example(self, value)
85 dict
86 """
---> 87 path, file = (value["path"], BytesIO(value["bytes"])) if value["bytes"] is not None else (value["path"], None)
88 if path is None and file is None:
89 raise ValueError(f"An audio sample should have one of 'path' or 'bytes' but both are None in {value}.")
TypeError: string indices must be integers
```
## Environment info
<!-- You can run the command `datasets-cli env` and copy-and-paste its output below. -->
- `datasets` version: 1.18.0
- Platform: Linux-4.14.256-197.484.amzn2.x86_64-x86_64-with-debian-buster-sid
- Python version: 3.6.13
- PyArrow version: 6.0.1
| 3,618 |
https://github.com/huggingface/datasets/issues/3615 | Dataset BnL Historical Newspapers does not work in streaming mode | [
"@albertvillanova let me know if there is anything I can do to help with this. I had a quick look at the code again and though I could try the following changes:\r\n- use `download` instead of `download_and_extract`\r\nhttps://github.com/huggingface/datasets/blob/d3d339fb86d378f4cb3c5d1de423315c07a466c6/datasets/bnl_newspapers/bnl_newspapers.py#L136\r\n- swith to using `iter_archive` to loop through downloaded data to replace\r\nhttps://github.com/huggingface/datasets/blob/d3d339fb86d378f4cb3c5d1de423315c07a466c6/datasets/bnl_newspapers/bnl_newspapers.py#L159\r\n\r\nLet me know if it's useful for me to try and make those changes. ",
"Thanks @davanstrien.\r\n\r\nI have already been working on it so that it can be used in the BigScience workshop.\r\n\r\nI agree that the `rglob()` is not efficient in this case.\r\n\r\nI tried different solutions without success:\r\n- `iter_archive` cannot be used in this case because it does not support ZIP files yet\r\n\r\nFinally I have used `iter_files()`.",
"I see this is fixed now 🙂. I also picked up a few other tips from your redactors so hopefully my next attempts will support streaming from the start. "
] | ## Describe the bug
When trying to load in streaming mode, it "hangs"...
## Steps to reproduce the bug
```python
ds = load_dataset("bnl_newspapers", split="train", streaming=True)
```
## Expected results
The code should be optimized, so that it works fast in streaming mode.
CC: @davanstrien
| 3,615 |
https://github.com/huggingface/datasets/issues/3613 | Files not updating in dataset viewer | [
"Yes. The jobs queue is full right now, following an upgrade... Back to normality in the next hours hopefully. I'll look at your datasets to be sure the dataset viewer works as expected on them.",
"Should have been fixed now."
] | ## Dataset viewer issue for '*name of the dataset*'
**Link:**
Some examples:
* https://huggingface.co/datasets/abidlabs/crowdsourced-speech4
* https://huggingface.co/datasets/abidlabs/test-audio-13
*short description of the issue*
It seems that the dataset viewer is reading a cached version of the dataset and it is not updating to reflect new files that are added to the dataset. I get this error:
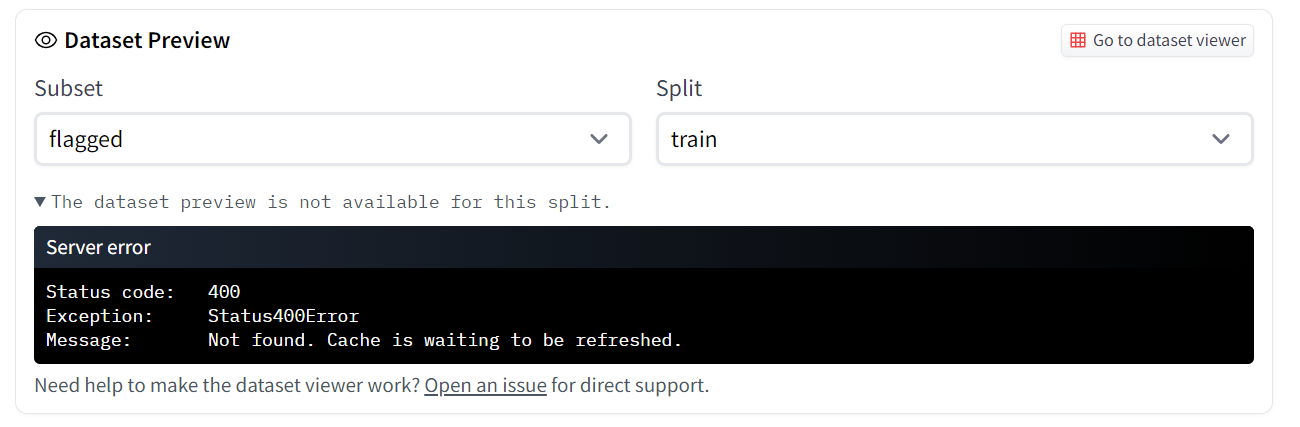
Am I the one who added this dataset? Yes | 3,613 |
https://github.com/huggingface/datasets/issues/3611 | Indexing bug after dataset.select() | [
"Hi! Thanks for reporting! I've opened a PR with the fix."
] | ## Describe the bug
A clear and concise description of what the bug is.
Dataset indexing is not working as expected after `dataset.select(range(100))`
## Steps to reproduce the bug
```python
# Sample code to reproduce the bug
import datasets
task_to_keys = {
"cola": ("sentence", None),
"mnli": ("premise", "hypothesis"),
"mrpc": ("sentence1", "sentence2"),
"qnli": ("question", "sentence"),
"qqp": ("question1", "question2"),
"rte": ("sentence1", "sentence2"),
"sst2": ("sentence", None),
"stsb": ("sentence1", "sentence2"),
"wnli": ("sentence1", "sentence2"),
}
task_name = "sst2"
raw_datasets = datasets.load_dataset("glue", task_name)
train_dataset = raw_datasets["train"]
print("before select: ",train_dataset[-2:])
# before select: {'sentence': ['a patient viewer ', 'this new jangle of noise , mayhem and stupidity must be a serious contender for the title . '], 'label': [1, 0], 'idx': [67347, 67348]}
train_dataset = train_dataset.select(range(100))
print("after select: ",train_dataset[-2:])
# after select: {'sentence': [], 'label': [], 'idx': []}
```
link to colab: https://colab.research.google.com/drive/1LngeRC9f0jE7eSQ4Kh1cIeb411lRXQD-?usp=sharing
## Expected results
A clear and concise description of the expected results.
showing 98, 99 index data
## Actual results
Specify the actual results or traceback.
empty
## Environment info
<!-- You can run the command `datasets-cli env` and copy-and-paste its output below. -->
- `datasets` version: 1.17.0
- Platform: Linux-5.4.144+-x86_64-with-Ubuntu-18.04-bionic
- Python version: 3.7.12
- PyArrow version: 3.0.0
| 3,611 |
https://github.com/huggingface/datasets/issues/3610 | Checksum error when trying to load amazon_review dataset | [
"It is solved now"
] | ## Describe the bug
A clear and concise description of what the bug is.
## Steps to reproduce the bug
I am getting the issue when trying to load dataset using
```
dataset = load_dataset("amazon_polarity")
```
## Expected results
dataset loaded
## Actual results
```
---------------------------------------------------------------------------
NonMatchingChecksumError Traceback (most recent call last)
<ipython-input-3-b4758ba980ae> in <module>()
----> 1 dataset = load_dataset("amazon_polarity")
2 dataset.set_format(type='pandas')
3 content_series = dataset['train']['content']
4 label_series = dataset['train']['label']
5 df = pd.concat([content_series, label_series], axis=1)
3 frames
/usr/local/lib/python3.7/dist-packages/datasets/utils/info_utils.py in verify_checksums(expected_checksums, recorded_checksums, verification_name)
38 if len(bad_urls) > 0:
39 error_msg = "Checksums didn't match" + for_verification_name + ":\n"
---> 40 raise NonMatchingChecksumError(error_msg + str(bad_urls))
41 logger.info("All the checksums matched successfully" + for_verification_name)
42
NonMatchingChecksumError: Checksums didn't match for dataset source files:
['https://drive.google.com/u/0/uc?id=0Bz8a_Dbh9QhbaW12WVVZS2drcnM&export=download']
```
## Environment info
<!-- You can run the command `datasets-cli env` and copy-and-paste its output below. -->
- `datasets` version: 1.17.0
- Platform: Google colab
- Python version: 3.7.12 | 3,610 |
https://github.com/huggingface/datasets/issues/3608 | Add support for continuous metrics (RMSE, MAE) | [
"Hey @ck37 \r\n\r\nYou can always use a custom metric as explained [in this guide from HF](https://huggingface.co/docs/datasets/master/loading_metrics.html#using-a-custom-metric-script).\r\n\r\nIf this issue needs to be contributed to (for enhancing the metric API) I think [this link](https://scikit-learn.org/stable/modules/generated/sklearn.metrics.mean_absolute_error.html) would be helpful for the `MAE` metric.",
"You can use a local metric script just by providing its path instead of the usual shortcut name ",
"#self-assign I have starting working on this issue to enhance the metric API."
] | **Is your feature request related to a problem? Please describe.**
I am uploading our dataset and models for the "Constructing interval measures" method we've developed, which uses item response theory to convert multiple discrete labels into a continuous spectrum for hate speech. Once we have this outcome our NLP models conduct regression rather than classification, so binary metrics are not relevant. The only continuous metrics available at https://huggingface.co/metrics are pearson & spearman correlation, which don't ensure that the prediction is on the same scale as the outcome.
**Describe the solution you'd like**
I would like to be able to tag our models on the Hub with the following metrics:
- RMSE
- MAE
**Describe alternatives you've considered**
I don't know if there are any alternatives.
**Additional context**
Our preprint is available here: https://arxiv.org/abs/2009.10277 . We are making it available for use in Jigsaw's Toxic Severity Rating Kaggle competition: https://www.kaggle.com/c/jigsaw-toxic-severity-rating/overview . I have our first model uploaded to the Hub at https://huggingface.co/ucberkeley-dlab/hate-measure-roberta-large
Thanks,
Chris
| 3,608 |
https://github.com/huggingface/datasets/issues/3606 | audio column not saved correctly after resampling | [
"Hi ! We just released a new version of `datasets` that should fix this.\r\n\r\nI tested resampling and using save/load_from_disk afterwards and it seems to be fixed now",
"Hi @lhoestq, \r\n\r\nJust tested the latest datasets version, and confirming that this is fixed for me. \r\n\r\nThanks!",
"Also, just an FYI, data that I had saved (with save_to_disk) previously from common voice using datasets==1.17.0 now give the error below when loading (with load_from disk) using datasets==1.18.0. \r\n\r\nHowever, when starting fresh using load_dataset, then doing the resampling, the save/load_from disk worked fine. \r\n\r\n```\r\n---------------------------------------------------------------------------\r\nValueError Traceback (most recent call last)\r\n<timed exec> in <module>\r\n\r\n/opt/conda/lib/python3.7/site-packages/datasets/load.py in load_from_disk(dataset_path, fs, keep_in_memory)\r\n 1747 return Dataset.load_from_disk(dataset_path, fs, keep_in_memory=keep_in_memory)\r\n 1748 elif fs.isfile(Path(dest_dataset_path, config.DATASETDICT_JSON_FILENAME).as_posix()):\r\n-> 1749 return DatasetDict.load_from_disk(dataset_path, fs, keep_in_memory=keep_in_memory)\r\n 1750 else:\r\n 1751 raise FileNotFoundError(\r\n\r\n/opt/conda/lib/python3.7/site-packages/datasets/dataset_dict.py in load_from_disk(dataset_dict_path, fs, keep_in_memory)\r\n 769 else Path(dest_dataset_dict_path, k).as_posix()\r\n 770 )\r\n--> 771 dataset_dict[k] = Dataset.load_from_disk(dataset_dict_split_path, fs, keep_in_memory=keep_in_memory)\r\n 772 return dataset_dict\r\n 773 \r\n\r\n/opt/conda/lib/python3.7/site-packages/datasets/arrow_dataset.py in load_from_disk(dataset_path, fs, keep_in_memory)\r\n 1118 info=dataset_info,\r\n 1119 split=split,\r\n-> 1120 fingerprint=state[\"_fingerprint\"],\r\n 1121 )\r\n 1122 \r\n\r\n/opt/conda/lib/python3.7/site-packages/datasets/arrow_dataset.py in __init__(self, arrow_table, info, split, indices_table, fingerprint)\r\n 655 if self.info.features.type != inferred_features.type:\r\n 656 raise ValueError(\r\n--> 657 f\"External features info don't match the dataset:\\nGot\\n{self.info.features}\\nwith type\\n{self.info.features.type}\\n\\nbut expected something like\\n{inferred_features}\\nwith type\\n{inferred_features.type}\"\r\n 658 )\r\n 659 \r\n\r\nValueError: External features info don't match the dataset:\r\nGot\r\n{'accent': Value(dtype='string', id=None), 'age': Value(dtype='string', id=None), 'audio': Audio(sampling_rate=48000, mono=True, id=None), 'client_id': Value(dtype='string', id=None), 'down_votes': Value(dtype='int64', id=None), 'gender': Value(dtype='string', id=None), 'locale': Value(dtype='string', id=None), 'path': Value(dtype='string', id=None), 'segment': Value(dtype='string', id=None), 'sentence': Value(dtype='string', id=None), 'up_votes': Value(dtype='int64', id=None)}\r\nwith type\r\nstruct<accent: string, age: string, audio: struct<bytes: binary, path: string>, client_id: string, down_votes: int64, gender: string, locale: string, path: string, segment: string, sentence: string, up_votes: int64>\r\n\r\nbut expected something like\r\n{'accent': Value(dtype='string', id=None), 'age': Value(dtype='string', id=None), 'audio': {'path': Value(dtype='string', id=None), 'bytes': Value(dtype='binary', id=None)}, 'client_id': Value(dtype='string', id=None), 'down_votes': Value(dtype='int64', id=None), 'gender': Value(dtype='string', id=None), 'locale': Value(dtype='string', id=None), 'path': Value(dtype='string', id=None), 'segment': Value(dtype='string', id=None), 'sentence': Value(dtype='string', id=None), 'up_votes': Value(dtype='int64', id=None)}\r\nwith type\r\nstruct<accent: string, age: string, audio: struct<path: string, bytes: binary>, client_id: string, down_votes: int64, gender: string, locale: string, path: string, segment: string, sentence: string, up_votes: int64> \r\n```"
] | ## Describe the bug
After resampling the audio column, saving with save_to_disk doesn't seem to save with the correct type.
## Steps to reproduce the bug
- load a subset of common voice dataset (48Khz)
- resample audio column to 16Khz
- save with save_to_disk()
- load with load_from_disk()
## Expected results
I expected that after saving the data, and then loading it back in, the audio column has the correct dataset.Audio type (i.e. same as before saving it)
{'accent': Value(dtype='string', id=None),
'age': Value(dtype='string', id=None),
'audio': Audio(sampling_rate=16000, mono=True, _storage_dtype='string', id=None),
'client_id': Value(dtype='string', id=None),
'down_votes': Value(dtype='int64', id=None),
'gender': Value(dtype='string', id=None),
'locale': Value(dtype='string', id=None),
'path': Value(dtype='string', id=None),
'segment': Value(dtype='string', id=None),
'sentence': Value(dtype='string', id=None),
'up_votes': Value(dtype='int64', id=None)}
## Actual results
Audio column does not have the right type
{'accent': Value(dtype='string', id=None),
'age': Value(dtype='string', id=None),
'audio': {'bytes': Value(dtype='binary', id=None),
'path': Value(dtype='string', id=None)},
'client_id': Value(dtype='string', id=None),
'down_votes': Value(dtype='int64', id=None),
'gender': Value(dtype='string', id=None),
'locale': Value(dtype='string', id=None),
'path': Value(dtype='string', id=None),
'segment': Value(dtype='string', id=None),
'sentence': Value(dtype='string', id=None),
'up_votes': Value(dtype='int64', id=None)}
## Environment info
<!-- You can run the command `datasets-cli env` and copy-and-paste its output below. -->
- `datasets` version: 1.17.0
- Platform: linux
- Python version:
- PyArrow version:
| 3,606 |
https://github.com/huggingface/datasets/issues/3604 | Dataset Viewer not showing Previews for Private Datasets | [
"Sure, it's on the roadmap.",
"Closing in favor of https://github.com/huggingface/datasets-server/issues/39."
] | ## Dataset viewer issue for 'abidlabs/test-audio-13'
It seems that the dataset viewer does not show previews for `private` datasets, even for the user who's private dataset it is. See [1] for example. If I change the visibility to public, then it does show, but it would be useful to have the viewer even for private datasets.
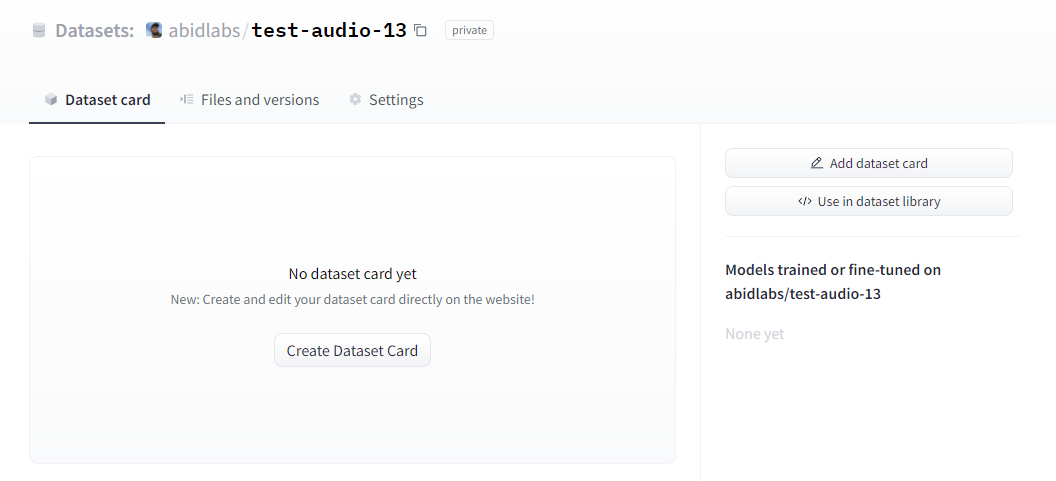
**Link:**
[1] https://huggingface.co/datasets/abidlabs/test-audio-13
**Am I the one who added this dataset?**
Yes
| 3,604 |
https://github.com/huggingface/datasets/issues/3599 | The `add_column()` method does not work if used on dataset sliced with `select()` | [
"similar #3611 "
] | Hello, I posted this as a question on the forums ([here](https://discuss.huggingface.co/t/add-column-does-not-work-if-used-on-dataset-sliced-with-select/13893)):
I have a dataset with 2000 entries
> dataset = Dataset.from_dict({'colA': list(range(2000))})
and from which I want to extract the first one thousand rows, create a new dataset with these and also add a new column to it:
> dataset2 = dataset.select(list(range(1000)))
> final_dataset = dataset2.add_column('colB', list(range(1000)))
This gives an error
>ArrowInvalid: Added column's length must match table's length. Expected length 2000 but got length 1000
So it looks like even though it is a dataset with 1000 rows, it "remembers" the shape of the one it was sliced from.
## Actual results
```
ArrowInvalid Traceback (most recent call last)
<ipython-input-138-e806860f3ce3> in <module>
----> 1 final_dataset = dataset2.add_column('colB', list(range(1000)))
~/.local/lib/python3.8/site-packages/datasets/arrow_dataset.py in wrapper(*args, **kwargs)
468 }
469 # apply actual function
--> 470 out: Union["Dataset", "DatasetDict"] = func(self, *args, **kwargs)
471 datasets: List["Dataset"] = list(out.values()) if isinstance(out, dict) else [out]
472 # re-apply format to the output
~/.local/lib/python3.8/site-packages/datasets/fingerprint.py in wrapper(*args, **kwargs)
404 # Call actual function
405
--> 406 out = func(self, *args, **kwargs)
407
408 # Update fingerprint of in-place transforms + update in-place history of transforms
~/.local/lib/python3.8/site-packages/datasets/arrow_dataset.py in add_column(self, name, column, new_fingerprint)
3343 column_table = InMemoryTable.from_pydict({name: column})
3344 # Concatenate tables horizontally
-> 3345 table = ConcatenationTable.from_tables([self._data, column_table], axis=1)
3346 # Update features
3347 info = self.info.copy()
~/.local/lib/python3.8/site-packages/datasets/table.py in from_tables(cls, tables, axis)
729 table_blocks = to_blocks(table)
730 blocks = _extend_blocks(blocks, table_blocks, axis=axis)
--> 731 return cls.from_blocks(blocks)
732
733 @property
~/.local/lib/python3.8/site-packages/datasets/table.py in from_blocks(cls, blocks)
668 @classmethod
669 def from_blocks(cls, blocks: TableBlockContainer) -> "ConcatenationTable":
--> 670 blocks = cls._consolidate_blocks(blocks)
671 if isinstance(blocks, TableBlock):
672 table = blocks
~/.local/lib/python3.8/site-packages/datasets/table.py in _consolidate_blocks(cls, blocks)
664 return cls._merge_blocks(blocks, axis=0)
665 else:
--> 666 return cls._merge_blocks(blocks)
667
668 @classmethod
~/.local/lib/python3.8/site-packages/datasets/table.py in _merge_blocks(cls, blocks, axis)
650 merged_blocks += list(block_group)
651 else: # both
--> 652 merged_blocks = [cls._merge_blocks(row_block, axis=1) for row_block in blocks]
653 if all(len(row_block) == 1 for row_block in merged_blocks):
654 merged_blocks = cls._merge_blocks(
~/.local/lib/python3.8/site-packages/datasets/table.py in <listcomp>(.0)
650 merged_blocks += list(block_group)
651 else: # both
--> 652 merged_blocks = [cls._merge_blocks(row_block, axis=1) for row_block in blocks]
653 if all(len(row_block) == 1 for row_block in merged_blocks):
654 merged_blocks = cls._merge_blocks(
~/.local/lib/python3.8/site-packages/datasets/table.py in _merge_blocks(cls, blocks, axis)
647 for is_in_memory, block_group in groupby(blocks, key=lambda x: isinstance(x, InMemoryTable)):
648 if is_in_memory:
--> 649 block_group = [InMemoryTable(cls._concat_blocks(list(block_group), axis=axis))]
650 merged_blocks += list(block_group)
651 else: # both
~/.local/lib/python3.8/site-packages/datasets/table.py in _concat_blocks(blocks, axis)
626 else:
627 for name, col in zip(table.column_names, table.columns):
--> 628 pa_table = pa_table.append_column(name, col)
629 return pa_table
630 else:
~/.local/lib/python3.8/site-packages/pyarrow/table.pxi in pyarrow.lib.Table.append_column()
~/.local/lib/python3.8/site-packages/pyarrow/table.pxi in pyarrow.lib.Table.add_column()
~/.local/lib/python3.8/site-packages/pyarrow/error.pxi in pyarrow.lib.pyarrow_internal_check_status()
~/.local/lib/python3.8/site-packages/pyarrow/error.pxi in pyarrow.lib.check_status()
ArrowInvalid: Added column's length must match table's length. Expected length 2000 but got length 1000
```
A solution provided by @mariosasko is to use `dataset2.flatten_indices()` after the `select()` and before attempting to add the new column:
> dataset = Dataset.from_dict({'colA': list(range(2000))})
> dataset2 = dataset.select(list(range(1000)))
> dataset2 = dataset2.flatten_indices()
> final_dataset = dataset2.add_column('colB', list(range(1000)))
which works.
## Environment info
<!-- You can run the command `datasets-cli env` and copy-and-paste its output below. -->
- `datasets` version: 1.13.2 (note: also checked with version 1.17.0, still the same error)
- Platform: Ubuntu 20.04.3
- Python version: 3.8.10
- PyArrow version: 6.0.0
| 3,599 |
https://github.com/huggingface/datasets/issues/3598 | Readme info not being parsed to show on Dataset card page | [
"i suspect a markdown parsing error, @severo do you want to take a quick look at it when you have some time?",
"# Problem\r\nThe issue seems to coming from the front matter of the README\r\n```---\r\nannotations_creators:\r\n- no-annotation\r\nlanguage_creators:\r\n- machine-generated\r\nlanguages:\r\n- 'ca'\r\n- 'de'\r\nlicenses:\r\n- cc-by-4.0\r\nmultilinguality:\r\n- translation\r\npretty_name: Catalan-German aligned corpora to train NMT systems.\r\nsize_categories:\r\n- \"1M<n<10M\" \r\nsource_datasets:\r\n- extended|tilde_model\r\ntask_categories:\r\n- machine-translation\r\ntask_ids:\r\n- machine-translation\r\n---\r\n``` \r\n# Solution\r\nThe fix is to correctly style the README as explained [here](https://huggingface.co/docs/datasets/v1.12.0/dataset_card.html). I have also correctly parsed the font matter as shown below:\r\n```\r\n---\r\nannotations_creators: []\r\nlanguage_creators: [machine-generated]\r\nlanguages: ['ca', 'de']\r\nlicenses: []\r\nmultilinguality:\r\n- multilingual\r\npretty_name: 'Catalan-German aligned corpora to train NMT systems.'\r\nsize_categories: \r\n- 1M<n<10M\r\nsource_datasets: ['extended|tilde_model']\r\ntask_categories: ['machine-translation']\r\ntask_ids: ['machine-translation']\r\n---\r\n```\r\nYou can find the README for a sample dataset [here](https://huggingface.co/datasets/ritwikraha/Test)",
"Thank you. It finally worked implementing your changes and leaving a white line between title and text in the description.",
"Thanks, if this solves your issue, can you please close it?"
] | ## Describe the bug
The info contained in the README.md file is not being shown in the dataset main page. Basic info and table of contents are properly formatted in the README.
## Steps to reproduce the bug
# Sample code to reproduce the bug
The README file is this one: https://huggingface.co/datasets/softcatala/Tilde-MODEL-Catalan/blob/main/README.md
## Expected results
README info should appear in the Dataset card page.
## Actual results
Nothing is shown. However, labels are parsed and shown successfully.
| 3,598 |
https://github.com/huggingface/datasets/issues/3597 | ERROR: File "setup.py" or "setup.cfg" not found. Directory cannot be installed in editable mode: /content | [
"Hi! The `cd` command in Jupyer/Colab needs to start with `%`, so this should work:\r\n```\r\n!git clone https://github.com/huggingface/datasets.git\r\n%cd datasets\r\n!pip install -e \".[streaming]\"\r\n```",
"thanks @mariosasko i had the same mistake and your solution is what was needed"
] | ## Bug
The install of streaming dataset is giving following error.
## Steps to reproduce the bug
```python
! git clone https://github.com/huggingface/datasets.git
! cd datasets
! pip install -e ".[streaming]"
```
## Actual results
Cloning into 'datasets'...
remote: Enumerating objects: 50816, done.
remote: Counting objects: 100% (2356/2356), done.
remote: Compressing objects: 100% (1606/1606), done.
remote: Total 50816 (delta 834), reused 1741 (delta 525), pack-reused 48460
Receiving objects: 100% (50816/50816), 72.47 MiB | 27.68 MiB/s, done.
Resolving deltas: 100% (22541/22541), done.
Checking out files: 100% (6722/6722), done.
ERROR: File "setup.py" or "setup.cfg" not found. Directory cannot be installed in editable mode: /content
| 3,597 |
https://github.com/huggingface/datasets/issues/3596 | Loss of cast `Image` feature on certain dataset method | [
"Hi! Thanks for reporting! The issue with `cast_column` should be fixed by #3575 and after we merge that PR I'll start working on the `push_to_hub` support for the `Image`/`Audio` feature.",
"> Hi! Thanks for reporting! The issue with `cast_column` should be fixed by #3575 and after we merge that PR I'll start working on the `push_to_hub` support for the `Image`/`Audio` feature.\r\n\r\nThanks, I'll keep an eye out for #3575 getting merged. I managed to use `push_to_hub` sucesfully with images when they were loaded via `map` - something like `ds.map(lambda example: {\"img\": load_image_function(example['fname']})`, this only pushed the images to the hub if the `load_image_function` return a PIL Image without the filename attribute though. I guess this might often be the prefered behaviour though. \r\n",
"Hi ! We merged the PR and did a release of `datasets` that includes the changes. Can you try updating `datasets` and try again ?",
"> Hi ! We merged the PR and did a release of `datasets` that includes the changes. Can you try updating `datasets` and try again ?\r\n\r\nThanks for checking. There is no longer an error when calling `select` but it appears the cast value isn't preserved. Before `select`\r\n\r\n```python\r\ndataset.features\r\n{'url': Image(id=None)}\r\n```\r\n\r\nafter select:\r\n```\r\n{'url': Value(dtype='string', id=None)}\r\n```\r\n\r\nUpdated Colab example [here](https://colab.research.google.com/gist/davanstrien/4e88f55a3675c279b5c2f64299ae5c6f/potential_casting_bug.ipynb) ",
"Hmmm, if I re-run your google colab I'm getting the right type at the end:\r\n```\r\nsample.features\r\n# {'url': Image(id=None)}\r\n```",
"Appolgies - I've just run again and also got this output. I have also sucesfully used the `push_to_hub` method. I think this is fixed now so will close this issue. ",
"Fixed in #3575 "
] | ## Describe the bug
When an a column is cast to an `Image` feature, the cast type appears to be lost during certain operations. I first noticed this when using the `push_to_hub` method on a dataset that contained urls pointing to images which had been cast to an `image`. This also happens when using select on a dataset which has had a column cast to an `Image`.
I suspect this might be related to https://github.com/huggingface/datasets/pull/3556 but I don't believe that pull request fixes this issue.
## Steps to reproduce the bug
An example of casting a url to an image followed by using the `select` method:
```python
from datasets import Dataset
from datasets import features
url = "https://cf.ltkcdn.net/cats/images/std-lg/246866-1200x816-grey-white-kitten.webp"
data_dict = {"url": [url]*2}
dataset = Dataset.from_dict(data_dict)
dataset = dataset.cast_column('url',features.Image())
sample = dataset.select([1])
```
[example notebook](https://gist.github.com/davanstrien/06e53f4383c28ae77ce1b30d0eaf0d70#file-potential_casting_bug-ipynb)
## Expected results
The cast value is maintained when further methods are applied to the dataset.
## Actual results
```python
---------------------------------------------------------------------------
ValueError Traceback (most recent call last)
<ipython-input-12-47f393bc2d0d> in <module>()
----> 1 sample = dataset.select([1])
4 frames
/usr/local/lib/python3.7/dist-packages/datasets/arrow_dataset.py in wrapper(*args, **kwargs)
487 }
488 # apply actual function
--> 489 out: Union["Dataset", "DatasetDict"] = func(self, *args, **kwargs)
490 datasets: List["Dataset"] = list(out.values()) if isinstance(out, dict) else [out]
491 # re-apply format to the output
/usr/local/lib/python3.7/dist-packages/datasets/fingerprint.py in wrapper(*args, **kwargs)
409 # Call actual function
410
--> 411 out = func(self, *args, **kwargs)
412
413 # Update fingerprint of in-place transforms + update in-place history of transforms
/usr/local/lib/python3.7/dist-packages/datasets/arrow_dataset.py in select(self, indices, keep_in_memory, indices_cache_file_name, writer_batch_size, new_fingerprint)
2772 )
2773 else:
-> 2774 return self._new_dataset_with_indices(indices_buffer=buf_writer.getvalue(), fingerprint=new_fingerprint)
2775
2776 @transmit_format
/usr/local/lib/python3.7/dist-packages/datasets/arrow_dataset.py in _new_dataset_with_indices(self, indices_cache_file_name, indices_buffer, fingerprint)
2688 split=self.split,
2689 indices_table=indices_table,
-> 2690 fingerprint=fingerprint,
2691 )
2692
/usr/local/lib/python3.7/dist-packages/datasets/arrow_dataset.py in __init__(self, arrow_table, info, split, indices_table, fingerprint)
664 if self.info.features.type != inferred_features.type:
665 raise ValueError(
--> 666 f"External features info don't match the dataset:\nGot\n{self.info.features}\nwith type\n{self.info.features.type}\n\nbut expected something like\n{inferred_features}\nwith type\n{inferred_features.type}"
667 )
668
ValueError: External features info don't match the dataset:
Got
{'url': Image(id=None)}
with type
struct<url: extension<arrow.py_extension_type<ImageExtensionType>>>
but expected something like
{'url': Value(dtype='string', id=None)}
with type
struct<url: string>
```
## Environment info
<!-- You can run the command `datasets-cli env` and copy-and-paste its output below. -->
- `datasets` version: 1.17.1.dev0
- Platform: Linux-5.4.144+-x86_64-with-Ubuntu-18.04-bionic
- Python version: 3.7.12
- PyArrow version: 3.0.0 | 3,596 |
https://github.com/huggingface/datasets/issues/3587 | No module named 'fsspec.archive' | [] | ## Describe the bug
Cannot import datasets after installation.
## Steps to reproduce the bug
```shell
$ python
Python 3.9.7 (default, Sep 16 2021, 13:09:58)
[GCC 7.5.0] :: Anaconda, Inc. on linux
Type "help", "copyright", "credits" or "license" for more information.
>>> import datasets
Traceback (most recent call last):
File "<stdin>", line 1, in <module>
File "/home/shuchen/miniconda3/envs/hf/lib/python3.9/site-packages/datasets/__init__.py", line 34, in <module>
from .arrow_dataset import Dataset, concatenate_datasets
File "/home/shuchen/miniconda3/envs/hf/lib/python3.9/site-packages/datasets/arrow_dataset.py", line 61, in <module>
from .arrow_writer import ArrowWriter, OptimizedTypedSequence
File "/home/shuchen/miniconda3/envs/hf/lib/python3.9/site-packages/datasets/arrow_writer.py", line 28, in <module>
from .features import (
File "/home/shuchen/miniconda3/envs/hf/lib/python3.9/site-packages/datasets/features/__init__.py", line 2, in <module>
from .audio import Audio
File "/home/shuchen/miniconda3/envs/hf/lib/python3.9/site-packages/datasets/features/audio.py", line 7, in <module>
from ..utils.streaming_download_manager import xopen
File "/home/shuchen/miniconda3/envs/hf/lib/python3.9/site-packages/datasets/utils/streaming_download_manager.py", line 18, in <module>
from ..filesystems import COMPRESSION_FILESYSTEMS
File "/home/shuchen/miniconda3/envs/hf/lib/python3.9/site-packages/datasets/filesystems/__init__.py", line 6, in <module>
from . import compression
File "/home/shuchen/miniconda3/envs/hf/lib/python3.9/site-packages/datasets/filesystems/compression.py", line 5, in <module>
from fsspec.archive import AbstractArchiveFileSystem
ModuleNotFoundError: No module named 'fsspec.archive'
```
| 3,587 |
https://github.com/huggingface/datasets/issues/3586 | Revisit `enable/disable_` toggle function prefix | [] | As discussed in https://github.com/huggingface/transformers/pull/15167, we should revisit the `enable/disable_` toggle function prefix, potentially in favor of `set_enabled_`. Concretely, this translates to
- De-deprecating `disable_progress_bar()`
- Adding `enable_progress_bar()`
- On the caching side, adding `enable_caching` and `disable_caching`
Additional decisions have to be made with regards to the existing `set_enabled_X` functions; that is, whether to keep them as is or deprecate them in favor of the aforementioned functions.
cc @mariosasko @lhoestq | 3,586 |
https://github.com/huggingface/datasets/issues/3585 | Datasets streaming + map doesn't work for `Audio` | [
"This seems related to https://github.com/huggingface/datasets/issues/3505."
] | ## Describe the bug
When using audio datasets in streaming mode, applying a `map(...)` before iterating leads to an error as the key `array` does not exist anymore.
## Steps to reproduce the bug
```python
from datasets import load_dataset
ds = load_dataset("common_voice", "en", streaming=True, split="train")
def map_fn(batch):
print("audio keys", batch["audio"].keys())
batch["audio"] = batch["audio"]["array"][:100]
return batch
ds = ds.map(map_fn)
sample = next(iter(ds))
```
I think the audio is somehow decoded before `.map(...)` is actually called.
## Expected results
IMO, the above code snippet should work.
## Actual results
```bash
audio keys dict_keys(['path', 'bytes'])
Traceback (most recent call last):
File "./run_audio.py", line 15, in <module>
sample = next(iter(ds))
File "/home/patrick/python_bin/datasets/iterable_dataset.py", line 341, in __iter__
for key, example in self._iter():
File "/home/patrick/python_bin/datasets/iterable_dataset.py", line 338, in _iter
yield from ex_iterable
File "/home/patrick/python_bin/datasets/iterable_dataset.py", line 192, in __iter__
yield key, self.function(example)
File "./run_audio.py", line 9, in map_fn
batch["input"] = batch["audio"]["array"][:100]
KeyError: 'array'
```
## Environment info
<!-- You can run the command `datasets-cli env` and copy-and-paste its output below. -->
- `datasets` version: 1.17.1.dev0
- Platform: Linux-5.3.0-64-generic-x86_64-with-glibc2.17
- Python version: 3.8.12
- PyArrow version: 6.0.1
| 3,585 |
https://github.com/huggingface/datasets/issues/3584 | https://huggingface.co/datasets/huggingface/transformers-metadata | [] | ## Dataset viewer issue for '*name of the dataset*'
**Link:** *link to the dataset viewer page*
*short description of the issue*
Am I the one who added this dataset ? Yes-No
| 3,584 |
https://github.com/huggingface/datasets/issues/3583 | Add The Medical Segmentation Decathlon Dataset | [
"Hello! I have recently been involved with a medical image segmentation project myself and was going through the `The Medical Segmentation Decathlon Dataset` as well. \r\nI haven't yet had experience adding datasets to this repository yet but would love to get started. Should I take this issue?\r\nIf yes, I've got two questions -\r\n1. There are 10 different datasets available, so are all datasets to be added in a single PR, or one at a time? \r\n2. Since it's a competition, masks for the test-set are not available. How is that to be tackled? Sorry if it's a silly question, I have recently started exploring `datasets`.",
"Hi! Sure, feel free to take this issue. You can self-assign the issue by commenting `#self-assign`.\r\n\r\nTo answer your questions:\r\n1. It makes the most sense to add each one as a separate config, so one dataset script with 10 configs in a single PR.\r\n2. Just set masks in the test set to `None`.\r\n\r\nNote that the images/masks in this dataset are in NIfTI format, which our `Image` feature currently doesn't support, so I think it's best to yield the paths to the images/masks in the script and add a preprocessing section to the card where we explain how to load/process the images/masks with `nibabel` (I can help with that). \r\n\r\n",
"> Note that the images/masks in this dataset are in NIfTI format, which our `Image` feature currently doesn't support, so I think it's best to yield the paths to the images/masks in the script and add a preprocessing section to the card where we explain how to load/process the images/masks with `nibabel` (I can help with that).\r\n\r\nGotcha, thanks. Will start working on the issue and let you know in case of any doubt.",
"#self-assign",
"This is great! There is a first model on the HUb that uses this dataset! https://huggingface.co/MONAI/example_spleen_segmentation"
] | ## Adding a Dataset
- **Name:** *The Medical Segmentation Decathlon Dataset*
- **Description:** The underlying data set was designed to explore the axis of difficulties typically encountered when dealing with medical images, such as small data sets, unbalanced labels, multi-site data, and small objects.
- **Paper:** [link to the dataset paper if available](https://arxiv.org/abs/2106.05735)
- **Data:** http://medicaldecathlon.com/
- **Motivation:** Hugging Face seeks to democratize ML for society. One of the growing niches within ML is the ML + Medicine community. Key data sets will help increase the supply of HF resources for starting an initial community.
(cc @osanseviero @abidlabs )
Instructions to add a new dataset can be found [here](https://github.com/huggingface/datasets/blob/master/ADD_NEW_DATASET.md).
| 3,583 |
https://github.com/huggingface/datasets/issues/3582 | conll 2003 dataset source url is no longer valid | [
"I came to open the same issue.",
"Thanks for reporting !\r\n\r\nI pushed a temporary fix on `master` that uses an URL from a previous commit to access the dataset for now, until we have a better solution",
"I changed the URL again to use another host, the fix is available on `master` and we'll probably do a new release of `datasets` tomorrow.\r\n\r\nIn the meantime, feel free to do `load_dataset(..., revision=\"master\")` to use the fixed script",
"We just released a new version of `datasets` with a working URL. Feel free to update `datasets` and try again :)",
"Hello! Unfortunately, this URL does not work for me. \r\nCould you please tell me how I can solve the problem?\r\n\r\n`>>> from datasets import load_dataset\r\n>>> dataset = load_dataset(\"conll2003\")\r\nDownloading and preparing dataset conll2003/conll2003 (download: 4.63 MiB, generated: 9.78 MiB, post-processed: Unknown size, total: 14.41 MiB) to /home/dafedo/.cache/huggingface/datasets/conll2003/conll2003/1.0.0/40e7cb6bcc374f7c349c83acd1e9352a4f09474eb691f64f364ee62eb65d0ca6...\r\nTraceback (most recent call last):\r\n File \"<stdin>\", line 1, in <module>\r\n File \"/home/dafedo/efficient-task-transfer/venv/lib/python3.8/site-packages/datasets/load.py\", line 745, in load_dataset\r\n builder_instance.download_and_prepare(\r\n File \"/home/dafedo/efficient-task-transfer/venv/lib/python3.8/site-packages/datasets/builder.py\", line 574, in download_and_prepare\r\n self._download_and_prepare(\r\n File \"/home/dafedo/efficient-task-transfer/venv/lib/python3.8/site-packages/datasets/builder.py\", line 630, in _download_and_prepare\r\n split_generators = self._split_generators(dl_manager, **split_generators_kwargs)\r\n File \"/home/dafedo/.cache/huggingface/modules/datasets_modules/datasets/conll2003/40e7cb6bcc374f7c349c83acd1e9352a4f09474eb691f64f364ee62eb65d0ca6/conll2003.py\", line 196, in _split_generators\r\n downloaded_files = dl_manager.download_and_extract(urls_to_download)\r\n File \"/home/dafedo/efficient-task-transfer/venv/lib/python3.8/site-packages/datasets/utils/download_manager.py\", line 287, in download_and_extract\r\n return self.extract(self.download(url_or_urls))\r\n File \"/home/dafedo/efficient-task-transfer/venv/lib/python3.8/site-packages/datasets/utils/download_manager.py\", line 195, in download\r\n downloaded_path_or_paths = map_nested(\r\n File \"/home/dafedo/efficient-task-transfer/venv/lib/python3.8/site-packages/datasets/utils/py_utils.py\", line 203, in map_nested\r\n mapped = [\r\n File \"/home/dafedo/efficient-task-transfer/venv/lib/python3.8/site-packages/datasets/utils/py_utils.py\", line 204, in <listcomp>\r\n _single_map_nested((function, obj, types, None, True)) for obj in tqdm(iterable, disable=disable_tqdm)\r\n File \"/home/dafedo/efficient-task-transfer/venv/lib/python3.8/site-packages/datasets/utils/py_utils.py\", line 142, in _single_map_nested\r\n return function(data_struct)\r\n File \"/home/dafedo/efficient-task-transfer/venv/lib/python3.8/site-packages/datasets/utils/download_manager.py\", line 218, in _download\r\n return cached_path(url_or_filename, download_config=download_config)\r\n File \"/home/dafedo/efficient-task-transfer/venv/lib/python3.8/site-packages/datasets/utils/file_utils.py\", line 281, in cached_path\r\n output_path = get_from_cache(\r\n File \"/home/dafedo/efficient-task-transfer/venv/lib/python3.8/site-packages/datasets/utils/file_utils.py\", line 621, in get_from_cache\r\n raise FileNotFoundError(\"Couldn't find file at {}\".format(url))\r\nFileNotFoundError: Couldn't find file at https://github.com/davidsbatista/NER-datasets/raw/master/CONLL2003/train.txt\r\n`\r\n\r\nI receive the same error when I run \"itrain run_configs/conll2003.json\" from https://github.com/adapter-hub/efficient-task-transfer\r\n\r\nThank you very much in advance!\r\n\r\nRegards, \r\nDaria\r\n",
"Can you try updating `datasets` and try again ?\r\n```\r\npip install -U datasets\r\n```",
"@lhoestq Thank you very much for your answer! \r\n\r\nIt works this way, but for my research I need datasets==1.6.3 or closest to it because otherwise the other package would not work as it is built on this version.\r\nDo you have any other suggestion? I would really appreciate it. Maybe which version of the datasets is without hard-coded link but closest to 1.6.3\r\n",
"No problem, I have solved it. \r\nThank you anyway.",
"Out of curiosity, which package has the `datasets==1.6.3` requirement ?"
] | ## Describe the bug
Loading `conll2003` dataset fails because it was removed (just yesterday 1/14/2022) from the location it is looking for.
## Steps to reproduce the bug
```python
from datasets import load_dataset
load_dataset("conll2003")
```
## Expected results
The dataset should load.
## Actual results
It is looking for the dataset at `https://github.com/davidsbatista/NER-datasets/raw/master/CONLL2003/train.txt` but it was removed from there yesterday (see [commit](https://github.com/davidsbatista/NER-datasets/commit/9d8f45cc7331569af8eb3422bbe1c97cbebd5690) that removed the file and related [issue](https://github.com/davidsbatista/NER-datasets/issues/8)).
- We should replace this with an alternate valid location.
- this is being referenced in the huggingface course chapter 7 [colab notebook](https://colab.research.google.com/github/huggingface/notebooks/blob/master/course/chapter7/section2_pt.ipynb), which is also broken.
```python
FileNotFoundError Traceback (most recent call last)
<ipython-input-4-27c956bec93c> in <module>()
1 from datasets import load_dataset
2
----> 3 raw_datasets = load_dataset("conll2003")
11 frames
/usr/local/lib/python3.7/dist-packages/datasets/utils/file_utils.py in get_from_cache(url, cache_dir, force_download, proxies, etag_timeout, resume_download, user_agent, local_files_only, use_etag, max_retries, use_auth_token, ignore_url_params)
610 )
611 elif response is not None and response.status_code == 404:
--> 612 raise FileNotFoundError(f"Couldn't find file at {url}")
613 _raise_if_offline_mode_is_enabled(f"Tried to reach {url}")
614 if head_error is not None:
FileNotFoundError: Couldn't find file at https://github.com/davidsbatista/NER-datasets/raw/master/CONLL2003/train.txt
```
## Environment info
<!-- You can run the command `datasets-cli env` and copy-and-paste its output below. -->
- `datasets` version:
- Platform:
- Python version:
- PyArrow version:
| 3,582 |
https://github.com/huggingface/datasets/issues/3581 | Unable to create a dataset from a parquet file in S3 | [
"Hi ! Currently it only works with local paths, file-like objects are not supported yet"
] | ## Describe the bug
Trying to create a dataset from a parquet file in S3.
## Steps to reproduce the bug
```python
import s3fs
from datasets import Dataset
s3 = s3fs.S3FileSystem(anon=False)
with s3.open(PATH_LTR_TOY_CLEAN_DATASET, 'rb') as s3file:
dataset = Dataset.from_parquet(s3file)
```
## Expected results
A new Dataset object
## Actual results
```AttributeError: 'S3File' object has no attribute 'decode'```
```
AttributeError Traceback (most recent call last)
<command-2452877612515691> in <module>
5
6 with s3.open(PATH_LTR_TOY_CLEAN_DATASET, 'rb') as s3file:
----> 7 dataset = Dataset.from_parquet(s3file)
/databricks/python/lib/python3.8/site-packages/datasets/arrow_dataset.py in from_parquet(path_or_paths, split, features, cache_dir, keep_in_memory, columns, **kwargs)
907 from .io.parquet import ParquetDatasetReader
908
--> 909 return ParquetDatasetReader(
910 path_or_paths,
911 split=split,
/databricks/python/lib/python3.8/site-packages/datasets/io/parquet.py in __init__(self, path_or_paths, split, features, cache_dir, keep_in_memory, **kwargs)
28 path_or_paths = path_or_paths if isinstance(path_or_paths, dict) else {self.split: path_or_paths}
29 hash = _PACKAGED_DATASETS_MODULES["parquet"][1]
---> 30 self.builder = Parquet(
31 cache_dir=cache_dir,
32 data_files=path_or_paths,
/databricks/python/lib/python3.8/site-packages/datasets/builder.py in __init__(self, cache_dir, name, hash, base_path, info, features, use_auth_token, namespace, data_files, data_dir, **config_kwargs)
246
247 if data_files is not None and not isinstance(data_files, DataFilesDict):
--> 248 data_files = DataFilesDict.from_local_or_remote(
249 sanitize_patterns(data_files), base_path=base_path, use_auth_token=use_auth_token
250 )
/databricks/python/lib/python3.8/site-packages/datasets/data_files.py in from_local_or_remote(cls, patterns, base_path, allowed_extensions, use_auth_token)
576 for key, patterns_for_key in patterns.items():
577 out[key] = (
--> 578 DataFilesList.from_local_or_remote(
579 patterns_for_key,
580 base_path=base_path,
/databricks/python/lib/python3.8/site-packages/datasets/data_files.py in from_local_or_remote(cls, patterns, base_path, allowed_extensions, use_auth_token)
544 ) -> "DataFilesList":
545 base_path = base_path if base_path is not None else str(Path().resolve())
--> 546 data_files = resolve_patterns_locally_or_by_urls(base_path, patterns, allowed_extensions)
547 origin_metadata = _get_origin_metadata_locally_or_by_urls(data_files, use_auth_token=use_auth_token)
548 return cls(data_files, origin_metadata)
/databricks/python/lib/python3.8/site-packages/datasets/data_files.py in resolve_patterns_locally_or_by_urls(base_path, patterns, allowed_extensions)
191 data_files = []
192 for pattern in patterns:
--> 193 if is_remote_url(pattern):
194 data_files.append(Url(pattern))
195 else:
/databricks/python/lib/python3.8/site-packages/datasets/utils/file_utils.py in is_remote_url(url_or_filename)
115
116 def is_remote_url(url_or_filename: str) -> bool:
--> 117 parsed = urlparse(url_or_filename)
118 return parsed.scheme in ("http", "https", "s3", "gs", "hdfs", "ftp")
119
/usr/lib/python3.8/urllib/parse.py in urlparse(url, scheme, allow_fragments)
370 Note that we don't break the components up in smaller bits
371 (e.g. netloc is a single string) and we don't expand % escapes."""
--> 372 url, scheme, _coerce_result = _coerce_args(url, scheme)
373 splitresult = urlsplit(url, scheme, allow_fragments)
374 scheme, netloc, url, query, fragment = splitresult
/usr/lib/python3.8/urllib/parse.py in _coerce_args(*args)
122 if str_input:
123 return args + (_noop,)
--> 124 return _decode_args(args) + (_encode_result,)
125
126 # Result objects are more helpful than simple tuples
/usr/lib/python3.8/urllib/parse.py in _decode_args(args, encoding, errors)
106 def _decode_args(args, encoding=_implicit_encoding,
107 errors=_implicit_errors):
--> 108 return tuple(x.decode(encoding, errors) if x else '' for x in args)
109
110 def _coerce_args(*args):
/usr/lib/python3.8/urllib/parse.py in <genexpr>(.0)
106 def _decode_args(args, encoding=_implicit_encoding,
107 errors=_implicit_errors):
--> 108 return tuple(x.decode(encoding, errors) if x else '' for x in args)
109
110 def _coerce_args(*args):
AttributeError: 'S3File' object has no attribute 'decode'
```
## Environment info
<!-- You can run the command `datasets-cli env` and copy-and-paste its output below. -->
- `datasets` version: 1.17.0
- Platform: Ubuntu 20.04.3 LTS
- Python version: 3.8.10
- PyArrow version: 6.0.1
| 3,581 |
https://github.com/huggingface/datasets/issues/3580 | Bug in wiki bio load | [
"+1, here's the error I got: \r\n\r\n```\r\n>>> from datasets import load_dataset\r\n>>>\r\n>>> load_dataset(\"wiki_bio\")\r\nDownloading: 7.58kB [00:00, 4.42MB/s]\r\nDownloading: 2.71kB [00:00, 1.30MB/s]\r\nUsing custom data configuration default\r\nDownloading and preparing dataset wiki_bio/default (download: 318.53 MiB, generated: 736.94 MiB, post-processed: Unknown size, total: 1.03 GiB) to /home/jxm3/.cache/huggingface/datasets/wiki_bio/default/1.1.0/5293ce565954ba965dada626f1e79684e98172d950371d266bf3caaf87e911c9...\r\nTraceback (most recent call last):\r\n File \"<stdin>\", line 1, in <module>\r\n File \"/home/jxm3/.conda/envs/torch/lib/python3.9/site-packages/datasets/load.py\", line 1694, in load_dataset\r\n builder_instance.download_and_prepare(\r\n File \"/home/jxm3/.conda/envs/torch/lib/python3.9/site-packages/datasets/builder.py\", line 595, in download_and_prepare\r\n self._download_and_prepare(\r\n File \"/home/jxm3/.conda/envs/torch/lib/python3.9/site-packages/datasets/builder.py\", line 662, in _download_and_prepare\r\n split_generators = self._split_generators(dl_manager, **split_generators_kwargs)\r\n File \"/home/jxm3/.cache/huggingface/modules/datasets_modules/datasets/wiki_bio/5293ce565954ba965dada626f1e79684e98172d950371d266bf3caaf87e911c9/wiki_bio.py\", line 125, in _split_generators\r\n data_dir = dl_manager.download_and_extract(my_urls)\r\n File \"/home/jxm3/.conda/envs/torch/lib/python3.9/site-packages/datasets/utils/download_manager.py\", line 308, in download_and_extract\r\n return self.extract(self.download(url_or_urls))\r\n File \"/home/jxm3/.conda/envs/torch/lib/python3.9/site-packages/datasets/utils/download_manager.py\", line 196, in download\r\n downloaded_path_or_paths = map_nested(\r\n File \"/home/jxm3/.conda/envs/torch/lib/python3.9/site-packages/datasets/utils/py_utils.py\", line 251, in map_nested\r\n return function(data_struct)\r\n File \"/home/jxm3/.conda/envs/torch/lib/python3.9/site-packages/datasets/utils/download_manager.py\", line 217, in _download\r\n return cached_path(url_or_filename, download_config=download_config)\r\n File \"/home/jxm3/.conda/envs/torch/lib/python3.9/site-packages/datasets/utils/file_utils.py\", line 298, in cached_path\r\n output_path = get_from_cache(\r\n File \"/home/jxm3/.conda/envs/torch/lib/python3.9/site-packages/datasets/utils/file_utils.py\", line 612, in get_from_cache\r\n raise FileNotFoundError(f\"Couldn't find file at {url}\")\r\nFileNotFoundError: Couldn't find file at https://drive.google.com/uc?export=download&id=1L7aoUXzHPzyzQ0ns4ApBbYepsjFOtXil\r\n>>>\r\n```\r\n",
"@alejandrocros and @lhoestq - you added the wiki_bio dataset in #1173. It doesn't work anymore. Can you take a look at this?",
"And if something is wrong with Google Drive, you could try to download (and collate and unzip) from here: https://github.com/DavidGrangier/wikipedia-biography-dataset",
"Hi ! Thanks for reporting. I've downloaded the data and concatenated them into a zip file available here: https://huggingface.co/datasets/wiki_bio/tree/main/data\r\n\r\nI guess we can update the dataset script to use this zip file now :)"
] |
wiki_bio is failing to load because of a failing drive link . Can someone fix this ?
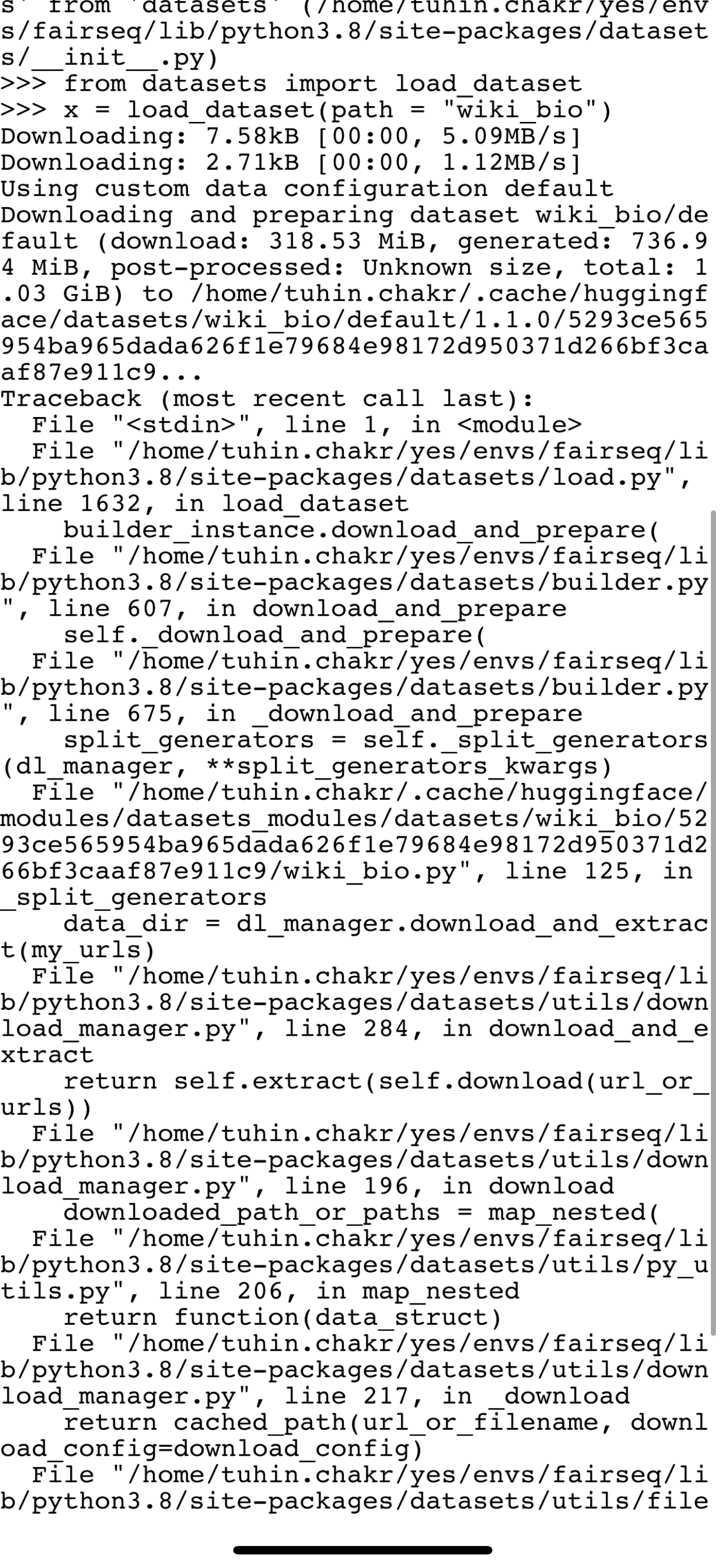
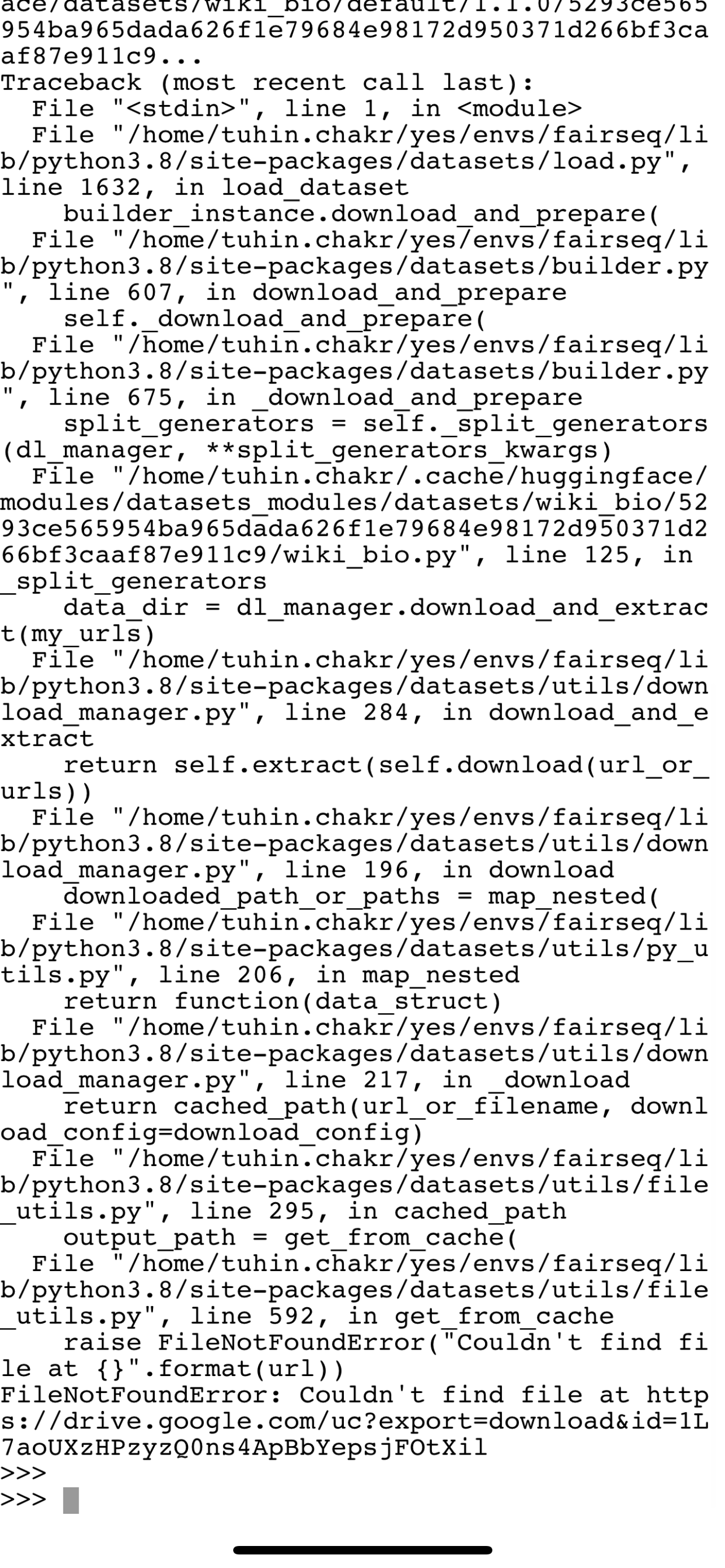
a | 3,580 |
https://github.com/huggingface/datasets/issues/3578 | label information get lost after parquet serialization | [
"Hi ! We did a release of `datasets` today that may fix this issue. Can you try updating `datasets` and trying again ?\r\n\r\nEDIT: the issue is still there actually\r\n\r\nI think we can fix that by storing the Features in the parquet schema metadata, and then reload them when loading the parquet file",
"This info is stored in the Parquet schema metadata as of https://github.com/huggingface/datasets/pull/5516"
] | ## Describe the bug
In *dataset_info.json* file, information about the label get lost after the dataset serialization.
## Steps to reproduce the bug
```python
from datasets import load_dataset
# normal save
dataset = load_dataset('glue', 'sst2', split='train')
dataset.save_to_disk("normal_save")
# save after parquet serialization
dataset.to_parquet("glue-sst2-train.parquet")
dataset = load_dataset("parquet", data_files='glue-sst2-train.parquet')
dataset.save_to_disk("save_after_parquet")
```
## Expected results
I expected to keep label information in *dataset_info.json* file even after parquet serialization
## Actual results
In the normal serialization i got
```json
"label": {
"num_classes": 2,
"names": [
"negative",
"positive"
],
"names_file": null,
"id": null,
"_type": "ClassLabel"
},
```
And after parquet serialization i got
```json
"label": {
"dtype": "int64",
"id": null,
"_type": "Value"
},
```
## Environment info
<!-- You can run the command `datasets-cli env` and copy-and-paste its output below. -->
- `datasets` version: 1.18.0
- Platform: ubuntu 20.04
- Python version: 3.8.10
- PyArrow version: 6.0.1
| 3,578 |
https://github.com/huggingface/datasets/issues/3577 | Add The Mexican Emotional Speech Database (MESD) | [] | ## Adding a Dataset
- **Name:** *The Mexican Emotional Speech Database (MESD)*
- **Description:** *Contains 864 voice recordings with six different prosodies: anger, disgust, fear, happiness, neutral, and sadness. Furthermore, three voice categories are included: female adult, male adult, and child. *
- **Paper:** *[Paper](https://ieeexplore.ieee.org/abstract/document/9629934/authors#authors)*
- **Data:** *[link to the Github repository or current dataset location](https://data.mendeley.com/datasets/cy34mh68j9/3)*
- **Motivation:** *Would add Spanish speech data to the HF datasets :) *
Instructions to add a new dataset can be found [here](https://github.com/huggingface/datasets/blob/master/ADD_NEW_DATASET.md).
| 3,577 |
https://github.com/huggingface/datasets/issues/3572 | ConnectionError in IndicGLUE dataset | [
"@sahoodib, thanks for reporting.\r\n\r\nIndeed, none of the data links appearing in the IndicGLUE website are working, e.g.: https://storage.googleapis.com/ai4bharat-public-indic-nlp-corpora/evaluations/soham-articles.tar.gz\r\n```\r\n<Error>\r\n<Code>UserProjectAccountProblem</Code>\r\n<Message>User project billing account not in good standing.</Message>\r\n<Details>\r\nThe billing account for the owning project is disabled in state delinquent\r\n</Details>\r\n</Error>\r\n```\r\n\r\nWe have contacted the data owners to inform them about their issue and ask them if they plan to fix it.",
"Yesterday I resent a reminder email with more AI4Bharat-related people in the loop.\r\n\r\nI also opened an issue in their repos:\r\n- https://github.com/AI4Bharat/indicnlp_corpus/issues/14\r\n- https://github.com/AI4Bharat/ai4bharat.org/issues/71",
"We have received a reply from the authors reporting they have updated the URLs of their data files and opened a PR. See:\r\n- #4978 "
] | While I am trying to load IndicGLUE dataset (https://huggingface.co/datasets/indic_glue) it is giving me with the error:
```
ConnectionError: Couldn't reach https://storage.googleapis.com/ai4bharat-public-indic-nlp-corpora/evaluations/wikiann-ner.tar.gz (error 403) | 3,572 |
https://github.com/huggingface/datasets/issues/3568 | Downloading Hugging Face Medical Dialog Dataset NonMatchingSplitsSizesError | [
"Hi @fabianslife, thanks for reporting.\r\n\r\nI think you were using an old version of `datasets` because this bug was already fixed in version `1.13.0` (13 Oct 2021):\r\n- Fix: 55fd140a63b8f03a0e72985647e498f1fc799d3f\r\n- PR: #3046\r\n- Issue: #2969 \r\n\r\nPlease, feel free to update the library: `pip install -U datasets`."
] | I wanted to download the Nedical Dialog Dataset from huggingface, using this github link:
https://github.com/huggingface/datasets/tree/master/datasets/medical_dialog
After downloading the raw datasets from google drive, i unpacked everything and put it in the same folder as the medical_dialog.py which is:
```
import copy
import os
import re
import datasets
_CITATION = """\
@article{chen2020meddiag,
title={MedDialog: a large-scale medical dialogue dataset},
author={Chen, Shu and Ju, Zeqian and Dong, Xiangyu and Fang, Hongchao and Wang, Sicheng and Yang, Yue and Zeng, Jiaqi and Zhang, Ruisi and Zhang, Ruoyu and Zhou, Meng and Zhu, Penghui and Xie, Pengtao},
journal={arXiv preprint arXiv:2004.03329},
year={2020}
}
"""
_DESCRIPTION = """\
The MedDialog dataset (English) contains conversations (in English) between doctors and patients.\
It has 0.26 million dialogues. The data is continuously growing and more dialogues will be added. \
The raw dialogues are from healthcaremagic.com and icliniq.com.\
All copyrights of the data belong to healthcaremagic.com and icliniq.com.
"""
_HOMEPAGE = "https://github.com/UCSD-AI4H/Medical-Dialogue-System"
_LICENSE = ""
class MedicalDialog(datasets.GeneratorBasedBuilder):
VERSION = datasets.Version("1.0.0")
BUILDER_CONFIGS = [
datasets.BuilderConfig(name="en", description="The dataset of medical dialogs in English.", version=VERSION),
datasets.BuilderConfig(name="zh", description="The dataset of medical dialogs in Chinese.", version=VERSION),
]
@property
def manual_download_instructions(self):
return """\
\n For English:\nYou need to go to https://drive.google.com/drive/folders/1g29ssimdZ6JzTST6Y8g6h-ogUNReBtJD?usp=sharing,\
and manually download the dataset from Google Drive. Once it is completed,
a file named Medical-Dialogue-Dataset-English-<timestamp-info>.zip will appear in your Downloads folder(
or whichever folder your browser chooses to save files to). Unzip the folder to obtain
a folder named "Medical-Dialogue-Dataset-English" several text files.
Now, you can specify the path to this folder for the data_dir argument in the
datasets.load_dataset(...) option.
The <path/to/folder> can e.g. be "/Downloads/Medical-Dialogue-Dataset-English".
The data can then be loaded using the below command:\
datasets.load_dataset("medical_dialog", name="en", data_dir="/Downloads/Medical-Dialogue-Dataset-English")`.
\n For Chinese:\nFollow the above process. Change the 'name' to 'zh'.The download link is https://drive.google.com/drive/folders/1r09_i8nJ9c1nliXVGXwSqRYqklcHd9e2
**NOTE**
- A caution while downloading from drive. It is better to download single files since creating a zip might not include files <500 MB. This has been observed mutiple times.
- After downloading the files and adding them to the appropriate folder, the path of the folder can be given as input tu the data_dir path.
"""
datasets.load_dataset("medical_dialog", name="en", data_dir="Medical-Dialogue-Dataset-English")
def _info(self):
if self.config.name == "zh":
features = datasets.Features(
{
"file_name": datasets.Value("string"),
"dialogue_id": datasets.Value("int32"),
"dialogue_url": datasets.Value("string"),
"dialogue_turns": datasets.Sequence(
{
"speaker": datasets.ClassLabel(names=["病人", "医生"]),
"utterance": datasets.Value("string"),
}
),
}
)
if self.config.name == "en":
features = datasets.Features(
{
"file_name": datasets.Value("string"),
"dialogue_id": datasets.Value("int32"),
"dialogue_url": datasets.Value("string"),
"dialogue_turns": datasets.Sequence(
{
"speaker": datasets.ClassLabel(names=["Patient", "Doctor"]),
"utterance": datasets.Value("string"),
}
),
}
)
return datasets.DatasetInfo(
# This is the description that will appear on the datasets page.
description=_DESCRIPTION,
features=features,
supervised_keys=None,
# Homepage of the dataset for documentation
homepage=_HOMEPAGE,
# License for the dataset if available
license=_LICENSE,
# Citation for the dataset
citation=_CITATION,
)
def _split_generators(self, dl_manager):
"""Returns SplitGenerators."""
path_to_manual_file = os.path.abspath(os.path.expanduser(dl_manager.manual_dir))
if not os.path.exists(path_to_manual_file):
raise FileNotFoundError(
f"{path_to_manual_file} does not exist. Make sure you insert a manual dir via `datasets.load_dataset('medical_dialog', data_dir=...)`. Manual download instructions: {self.manual_download_instructions})"
)
filepaths = [
os.path.join(path_to_manual_file, txt_file_name)
for txt_file_name in sorted(os.listdir(path_to_manual_file))
if txt_file_name.endswith("txt")
]
return [datasets.SplitGenerator(name=datasets.Split.TRAIN, gen_kwargs={"filepaths": filepaths})]
def _generate_examples(self, filepaths):
"""Yields examples. Iterates over each file and give the creates the corresponding features.
NOTE:
- The code makes some assumption on the structure of the raw .txt file.
- There are some checks to separate different id's. Hopefully, should not cause further issues later when more txt files are added.
"""
data_lang = self.config.name
id_ = -1
for filepath in filepaths:
with open(filepath, encoding="utf-8") as f_in:
# Parameters to just "sectionize" the raw data
last_part = ""
last_dialog = {}
last_list = []
last_user = ""
check_list = []
# These flags are present to have a single function address both chinese and english data
# English data is a little hahazard (i.e. the sentences spans multiple different lines),
# Chinese is compact with one line for doctor and patient.
conv_flag = False
des_flag = False
while True:
line = f_in.readline()
if not line:
break
# Extracting the dialog id
if line[:2] == "id": # Hardcode alert!
# Handling ID references that may come in the description
# These were observed in the Chinese dataset and were not
# followed by numbers
try:
dialogue_id = int(re.findall(r"\d+", line)[0])
except IndexError:
continue
# Extracting the url
if line[:4] == "http": # Hardcode alert!
dialogue_url = line.rstrip()
# Extracting the patient info from description.
if line[:11] == "Description": # Hardcode alert!
last_part = "description"
last_dialog = {}
last_list = []
last_user = ""
last_conv = {"speaker": "", "utterance": ""}
while True:
line = f_in.readline()
if (not line) or (line in ["\n", "\n\r"]):
break
else:
if data_lang == "zh": # Condition in chinese
if line[:5] == "病情描述:": # Hardcode alert!
last_user = "病人"
sen = f_in.readline().rstrip()
des_flag = True
if data_lang == "en":
last_user = "Patient"
sen = line.rstrip()
des_flag = True
if des_flag:
if sen == "":
continue
if sen in check_list:
last_conv["speaker"] = ""
last_conv["utterance"] = ""
else:
last_conv["speaker"] = last_user
last_conv["utterance"] = sen
check_list.append(sen)
des_flag = False
break
# Extracting the conversation info from dialogue.
elif line[:8] == "Dialogue": # Hardcode alert!
if last_part == "description" and len(last_conv["utterance"]) > 0:
last_part = "dialogue"
if data_lang == "zh":
last_user = "病人"
if data_lang == "en":
last_user = "Patient"
while True:
line = f_in.readline()
if (not line) or (line in ["\n", "\n\r"]):
conv_flag = False
last_user = ""
last_list.append(copy.deepcopy(last_conv))
# To ensure close of conversation, only even number of sentences
# are extracted
last_turn = len(last_list)
if int(last_turn / 2) > 0:
temp = int(last_turn / 2)
id_ += 1
last_dialog["file_name"] = filepath
last_dialog["dialogue_id"] = dialogue_id
last_dialog["dialogue_url"] = dialogue_url
last_dialog["dialogue_turns"] = last_list[: temp * 2]
yield id_, last_dialog
break
if data_lang == "zh":
if line[:3] == "病人:" or line[:3] == "医生:": # Hardcode alert!
user = line[:2] # Hardcode alert!
line = f_in.readline()
conv_flag = True
# The elif block is to ensure that multi-line sentences are captured.
# This has been observed only in english.
if data_lang == "en":
if line.strip() == "Patient:" or line.strip() == "Doctor:": # Hardcode alert!
user = line.replace(":", "").rstrip()
line = f_in.readline()
conv_flag = True
elif line[:2] != "id": # Hardcode alert!
conv_flag = True
# Continues till the next ID is parsed
if conv_flag:
sen = line.rstrip()
if sen == "":
continue
if user == last_user:
last_conv["utterance"] = last_conv["utterance"] + sen
else:
last_user = user
last_list.append(copy.deepcopy(last_conv))
last_conv["utterance"] = sen
last_conv["speaker"] = user
```
running this code gives me the error:
```
File "C:\Users\Fabia\AppData\Local\Programs\Python\Python39\lib\site-packages\datasets\utils\info_utils.py", line 74, in verify_splits
raise NonMatchingSplitsSizesError(str(bad_splits))
datasets.utils.info_utils.NonMatchingSplitsSizesError: [{'expected': SplitInfo(name='train', num_bytes=0, num_examples=0, dataset_name='medical_dialog'), 'recorded': SplitInfo(name='train', num_bytes=292801173, num_examples=229674, dataset_name='medical_dialog')}]
``` | 3,568 |
https://github.com/huggingface/datasets/issues/3563 | Dataset.from_pandas preserves useless index | [
"Hi! That makes sense. Sure, feel free to open a PR! Just a small suggestion: let's make `preserve_index` a parameter of `Dataset.from_pandas` (which we then pass to `InMemoryTable.from_pandas`) with `None` as a default value to not have this as a breaking change. "
] | ## Describe the bug
Let's say that you want to create a Dataset object from pandas dataframe. Most likely you will write something like this:
```
import pandas as pd
from datasets import Dataset
df = pd.read_csv('some_dataset.csv')
# Some DataFrame preprocessing code...
dataset = Dataset.from_pandas(df)
```
If your preprocessing code contain indexing operations like this:
```
df = df[df.col1 == some_value]
```
then your df.index can be changed from (default) ```RangeIndex(start=0, stop=16590, step=1)``` to something like this ```Int64Index([ 0, 1, 2, 3, 4, 5, 6, 7, 8,
9,
...
83979, 83980, 83981, 83982, 83983, 83984, 83985, 83986, 83987,
83988],
dtype='int64', length=16590)```
In this case, PyArrow (by default) will preserve this non-standard index. In the result, your dataset object will have the extra field that you likely don't want to have: '__index_level_0__'.
You can easily fix this by just adding extra argument ```preserve_index=False``` to call of ```InMemoryTable.from_pandas``` in ```arrow_dataset.py```.
If you approve that this isn't desirable behavior, I can make a PR fixing that.
## Environment info
- `datasets` version: 1.16.1
- Platform: Linux-5.11.0-44-generic-x86_64-with-glibc2.31
- Python version: 3.9.7
- PyArrow version: 6.0.1
| 3,563 |
https://github.com/huggingface/datasets/issues/3561 | Cannot load ‘bookcorpusopen’ | [
"The host of this copy of the dataset (https://the-eye.eu) is down and has been down for a good amount of time ([potentially months](https://www.reddit.com/r/Roms/comments/q82s15/theeye_downdied/))\r\n\r\nFinding this dataset is a little esoteric, as the original authors took down the official BookCorpus dataset some time ago.\r\n\r\nThere are community-created versions of BookCorpus, such as the files hosted in the link below.\r\nhttps://battle.shawwn.com/sdb/bookcorpus/\r\n\r\nAnd more discussion here:\r\nhttps://github.com/soskek/bookcorpus\r\n\r\nDo we want to remove this dataset entirely? There's a fair argument for this, given that the official BookCorpus dataset was taken down by the authors. If not, perhaps can open a PR with the link to the community-created tar above and updated dataset description.",
"Hi! The `bookcorpusopen` dataset is not working for the same reason as explained in this comment: https://github.com/huggingface/datasets/issues/3504#issuecomment-1004564980",
"Hi @HUIYINXUE, it should work now that the data owners created a mirror server with all data, and we updated the URL in our library."
] | ## Describe the bug
Cannot load 'bookcorpusopen'
## Steps to reproduce the bug
```python
dataset = load_dataset('bookcorpusopen')
```
or
```python
dataset = load_dataset('bookcorpusopen',script_version='master')
```
## Actual results
ConnectionError: Couldn't reach https://the-eye.eu/public/AI/pile_preliminary_components/books1.tar.gz
## Environment info
- `datasets` version: 1.9.0
- Platform: Linux version 3.10.0-1160.45.1.el7.x86_64
- Python version: 3.6.13
- PyArrow version: 6.0.1
| 3,561 |
https://github.com/huggingface/datasets/issues/3558 | Integrate Milvus (pymilvus) library | [
"Hi @mariosasko,Just search randomly and I found this issue~ I'm the tech lead of Milvus and we are looking forward to integrate milvus together with huggingface datasets.\r\n\r\nAny suggestion on how we could start?\r\n",
"Feel free to assign to me and we probably need some guide on it",
"@mariosasko any updates my man?\r\n",
"Hi! For starters, I suggest you take a look at this file: https://github.com/huggingface/datasets/blob/master/src/datasets/search.py, which contains all the code for Faiss/ElasticSearch support. We could set up a Slack channel for additional guidance. Let me know what you prefer.",
"> Hi! For starters, I suggest you take a look at this file: https://github.com/huggingface/datasets/blob/master/src/datasets/search.py, which contains all the code for Faiss/ElasticSearch support. We could set up a Slack channel for additional guidance. Let me know what you prefer.\r\n\r\nSure, we take a look and do some research"
] | Milvus is a popular open-source vector database. We should add a new vector index to support this project. | 3,558 |
https://github.com/huggingface/datasets/issues/3555 | DuplicatedKeysError when loading tweet_qa dataset | [
"Hi, we've just merged the PR with the fix. The fixed version of the dataset can be downloaded as follows:\r\n```python\r\nimport datasets\r\ndset = datasets.load_dataset(\"tweet_qa\", revision=\"master\")\r\n```"
] | When loading the tweet_qa dataset with `load_dataset('tweet_qa')`, the following error occurs:
`DuplicatedKeysError: FAILURE TO GENERATE DATASET !
Found duplicate Key: 2a167f9e016ba338e1813fed275a6a1e
Keys should be unique and deterministic in nature
`
Might be related to issues #2433 and #2333
- `datasets` version: 1.17.0
- Python version: 3.8.5
| 3,555 |
https://github.com/huggingface/datasets/issues/3554 | ImportError: cannot import name 'is_valid_waiter_error' | [
"Hi! I can't reproduce this error in Colab, but I'm assuming you are using Amazon SageMaker Studio Notebooks (you mention the `conda_pytorch_p36` kernel), so maybe @philschmid knows more about what might be causing this issue? ",
"Hey @mariosasko. Yes, I am using **Amazon SageMaker Studio Jupyter Labs**. However, I no longer need this notebook; but it would be nice to have this problem solved for others. So don't stress too much if you two can't reproduce error.",
"Hey @danielbellhv, \r\n\r\nThis issue might be related to Studio probably not having an up to date `botocore` and `boto3` version. I ran into this as well a while back. My workaround was \r\n```python\r\n# using older dataset due to incompatibility of sagemaker notebook & aws-cli with > s3fs and fsspec to >= 2021.10\r\n!pip install \"datasets==1.13\" --upgrade\r\n```\r\n\r\nIn `datasets` we use the latest `s3fs` and `fsspec` but aws-cli and notebook is not supporting this. You could also update the `aws-cli` and associated packages to get the latest `datasets` version\r\n"
] | Based on [SO post](https://stackoverflow.com/q/70606147/17840900).
I'm following along to this [Notebook][1], cell "**Loading the dataset**".
Kernel: `conda_pytorch_p36`.
I run:
```
! pip install datasets transformers optimum[intel]
```
Output:
```
Requirement already satisfied: datasets in /home/ec2-user/anaconda3/envs/pytorch_p36/lib/python3.6/site-packages (1.17.0)
Requirement already satisfied: transformers in /home/ec2-user/anaconda3/envs/pytorch_p36/lib/python3.6/site-packages (4.15.0)
Requirement already satisfied: optimum[intel] in /home/ec2-user/anaconda3/envs/pytorch_p36/lib/python3.6/site-packages (0.1.3)
Requirement already satisfied: numpy>=1.17 in /home/ec2-user/anaconda3/envs/pytorch_p36/lib/python3.6/site-packages (from datasets) (1.19.5)
Requirement already satisfied: dill in /home/ec2-user/anaconda3/envs/pytorch_p36/lib/python3.6/site-packages (from datasets) (0.3.4)
Requirement already satisfied: tqdm>=4.62.1 in /home/ec2-user/anaconda3/envs/pytorch_p36/lib/python3.6/site-packages (from datasets) (4.62.3)
Requirement already satisfied: huggingface-hub<1.0.0,>=0.1.0 in /home/ec2-user/anaconda3/envs/pytorch_p36/lib/python3.6/site-packages (from datasets) (0.2.1)
Requirement already satisfied: packaging in /home/ec2-user/anaconda3/envs/pytorch_p36/lib/python3.6/site-packages (from datasets) (21.3)
Requirement already satisfied: pyarrow!=4.0.0,>=3.0.0 in /home/ec2-user/anaconda3/envs/pytorch_p36/lib/python3.6/site-packages (from datasets) (6.0.1)
Requirement already satisfied: pandas in /home/ec2-user/anaconda3/envs/pytorch_p36/lib/python3.6/site-packages (from datasets) (1.1.5)
Requirement already satisfied: xxhash in /home/ec2-user/anaconda3/envs/pytorch_p36/lib/python3.6/site-packages (from datasets) (2.0.2)
Requirement already satisfied: aiohttp in /home/ec2-user/anaconda3/envs/pytorch_p36/lib/python3.6/site-packages (from datasets) (3.8.1)
Requirement already satisfied: fsspec[http]>=2021.05.0 in /home/ec2-user/anaconda3/envs/pytorch_p36/lib/python3.6/site-packages (from datasets) (2021.11.1)
Requirement already satisfied: dataclasses in /home/ec2-user/anaconda3/envs/pytorch_p36/lib/python3.6/site-packages (from datasets) (0.8)
Requirement already satisfied: multiprocess in /home/ec2-user/anaconda3/envs/pytorch_p36/lib/python3.6/site-packages (from datasets) (0.70.12.2)
Requirement already satisfied: importlib-metadata in /home/ec2-user/anaconda3/envs/pytorch_p36/lib/python3.6/site-packages (from datasets) (4.5.0)
Requirement already satisfied: requests>=2.19.0 in /home/ec2-user/anaconda3/envs/pytorch_p36/lib/python3.6/site-packages (from datasets) (2.25.1)
Requirement already satisfied: pyyaml>=5.1 in /home/ec2-user/anaconda3/envs/pytorch_p36/lib/python3.6/site-packages (from transformers) (5.4.1)
Requirement already satisfied: regex!=2019.12.17 in /home/ec2-user/anaconda3/envs/pytorch_p36/lib/python3.6/site-packages (from transformers) (2021.4.4)
Requirement already satisfied: tokenizers<0.11,>=0.10.1 in /home/ec2-user/anaconda3/envs/pytorch_p36/lib/python3.6/site-packages (from transformers) (0.10.3)
Requirement already satisfied: filelock in /home/ec2-user/anaconda3/envs/pytorch_p36/lib/python3.6/site-packages (from transformers) (3.0.12)
Requirement already satisfied: sacremoses in /home/ec2-user/anaconda3/envs/pytorch_p36/lib/python3.6/site-packages (from transformers) (0.0.46)
Requirement already satisfied: torch>=1.9 in /home/ec2-user/anaconda3/envs/pytorch_p36/lib/python3.6/site-packages (from optimum[intel]) (1.10.1)
Requirement already satisfied: sympy in /home/ec2-user/anaconda3/envs/pytorch_p36/lib/python3.6/site-packages (from optimum[intel]) (1.8)
Requirement already satisfied: coloredlogs in /home/ec2-user/anaconda3/envs/pytorch_p36/lib/python3.6/site-packages (from optimum[intel]) (15.0.1)
Requirement already satisfied: pycocotools in /home/ec2-user/anaconda3/envs/pytorch_p36/lib/python3.6/site-packages (from optimum[intel]) (2.0.3)
Requirement already satisfied: neural-compressor>=1.7 in /home/ec2-user/anaconda3/envs/pytorch_p36/lib/python3.6/site-packages (from optimum[intel]) (1.9)
Requirement already satisfied: typing-extensions>=3.7.4.3 in /home/ec2-user/anaconda3/envs/pytorch_p36/lib/python3.6/site-packages (from huggingface-hub<1.0.0,>=0.1.0->datasets) (3.10.0.0)
Requirement already satisfied: sigopt in /home/ec2-user/anaconda3/envs/pytorch_p36/lib/python3.6/site-packages (from neural-compressor>=1.7->optimum[intel]) (8.2.0)
Requirement already satisfied: opencv-python in /home/ec2-user/anaconda3/envs/pytorch_p36/lib/python3.6/site-packages (from neural-compressor>=1.7->optimum[intel]) (4.5.1.48)
Requirement already satisfied: cryptography in /home/ec2-user/anaconda3/envs/pytorch_p36/lib/python3.6/site-packages (from neural-compressor>=1.7->optimum[intel]) (3.4.7)
Requirement already satisfied: py-cpuinfo in /home/ec2-user/anaconda3/envs/pytorch_p36/lib/python3.6/site-packages (from neural-compressor>=1.7->optimum[intel]) (8.0.0)
Requirement already satisfied: gevent in /home/ec2-user/anaconda3/envs/pytorch_p36/lib/python3.6/site-packages (from neural-compressor>=1.7->optimum[intel]) (21.1.2)
Requirement already satisfied: schema in /home/ec2-user/anaconda3/envs/pytorch_p36/lib/python3.6/site-packages (from neural-compressor>=1.7->optimum[intel]) (0.7.5)
Requirement already satisfied: psutil in /home/ec2-user/anaconda3/envs/pytorch_p36/lib/python3.6/site-packages (from neural-compressor>=1.7->optimum[intel]) (5.8.0)
Requirement already satisfied: gevent-websocket in /home/ec2-user/anaconda3/envs/pytorch_p36/lib/python3.6/site-packages (from neural-compressor>=1.7->optimum[intel]) (0.10.1)
Requirement already satisfied: hyperopt in /home/ec2-user/anaconda3/envs/pytorch_p36/lib/python3.6/site-packages (from neural-compressor>=1.7->optimum[intel]) (0.2.7)
Requirement already satisfied: Flask in /home/ec2-user/anaconda3/envs/pytorch_p36/lib/python3.6/site-packages (from neural-compressor>=1.7->optimum[intel]) (2.0.1)
Requirement already satisfied: prettytable in /home/ec2-user/anaconda3/envs/pytorch_p36/lib/python3.6/site-packages (from neural-compressor>=1.7->optimum[intel]) (2.5.0)
Requirement already satisfied: Flask-SocketIO in /home/ec2-user/anaconda3/envs/pytorch_p36/lib/python3.6/site-packages (from neural-compressor>=1.7->optimum[intel]) (5.1.1)
Requirement already satisfied: scikit-learn in /home/ec2-user/anaconda3/envs/pytorch_p36/lib/python3.6/site-packages (from neural-compressor>=1.7->optimum[intel]) (0.24.2)
Requirement already satisfied: Pillow in /home/ec2-user/anaconda3/envs/pytorch_p36/lib/python3.6/site-packages (from neural-compressor>=1.7->optimum[intel]) (8.4.0)
Requirement already satisfied: Flask-Cors in /home/ec2-user/anaconda3/envs/pytorch_p36/lib/python3.6/site-packages (from neural-compressor>=1.7->optimum[intel]) (3.0.10)
Requirement already satisfied: pyparsing!=3.0.5,>=2.0.2 in /home/ec2-user/anaconda3/envs/pytorch_p36/lib/python3.6/site-packages (from packaging->datasets) (2.4.7)
Requirement already satisfied: chardet<5,>=3.0.2 in /home/ec2-user/anaconda3/envs/pytorch_p36/lib/python3.6/site-packages (from requests>=2.19.0->datasets) (4.0.0)
Requirement already satisfied: certifi>=2017.4.17 in /home/ec2-user/anaconda3/envs/pytorch_p36/lib/python3.6/site-packages (from requests>=2.19.0->datasets) (2021.5.30)
Requirement already satisfied: urllib3<1.27,>=1.21.1 in /home/ec2-user/anaconda3/envs/pytorch_p36/lib/python3.6/site-packages (from requests>=2.19.0->datasets) (1.26.5)
Requirement already satisfied: idna<3,>=2.5 in /home/ec2-user/anaconda3/envs/pytorch_p36/lib/python3.6/site-packages (from requests>=2.19.0->datasets) (2.10)
Requirement already satisfied: yarl<2.0,>=1.0 in /home/ec2-user/anaconda3/envs/pytorch_p36/lib/python3.6/site-packages (from aiohttp->datasets) (1.6.3)
Requirement already satisfied: charset-normalizer<3.0,>=2.0 in /home/ec2-user/anaconda3/envs/pytorch_p36/lib/python3.6/site-packages (from aiohttp->datasets) (2.0.9)
Requirement already satisfied: attrs>=17.3.0 in /home/ec2-user/anaconda3/envs/pytorch_p36/lib/python3.6/site-packages (from aiohttp->datasets) (21.2.0)
Requirement already satisfied: asynctest==0.13.0 in /home/ec2-user/anaconda3/envs/pytorch_p36/lib/python3.6/site-packages (from aiohttp->datasets) (0.13.0)
Requirement already satisfied: idna-ssl>=1.0 in /home/ec2-user/anaconda3/envs/pytorch_p36/lib/python3.6/site-packages (from aiohttp->datasets) (1.1.0)
Requirement already satisfied: async-timeout<5.0,>=4.0.0a3 in /home/ec2-user/anaconda3/envs/pytorch_p36/lib/python3.6/site-packages (from aiohttp->datasets) (4.0.1)
Requirement already satisfied: aiosignal>=1.1.2 in /home/ec2-user/anaconda3/envs/pytorch_p36/lib/python3.6/site-packages (from aiohttp->datasets) (1.2.0)
Requirement already satisfied: frozenlist>=1.1.1 in /home/ec2-user/anaconda3/envs/pytorch_p36/lib/python3.6/site-packages (from aiohttp->datasets) (1.2.0)
Requirement already satisfied: multidict<7.0,>=4.5 in /home/ec2-user/anaconda3/envs/pytorch_p36/lib/python3.6/site-packages (from aiohttp->datasets) (5.1.0)
Requirement already satisfied: humanfriendly>=9.1 in /home/ec2-user/anaconda3/envs/pytorch_p36/lib/python3.6/site-packages (from coloredlogs->optimum[intel]) (10.0)
Requirement already satisfied: zipp>=0.5 in /home/ec2-user/anaconda3/envs/pytorch_p36/lib/python3.6/site-packages (from importlib-metadata->datasets) (3.4.1)
Requirement already satisfied: python-dateutil>=2.7.3 in /home/ec2-user/anaconda3/envs/pytorch_p36/lib/python3.6/site-packages (from pandas->datasets) (2.8.1)
Requirement already satisfied: pytz>=2017.2 in /home/ec2-user/anaconda3/envs/pytorch_p36/lib/python3.6/site-packages (from pandas->datasets) (2021.1)
Requirement already satisfied: matplotlib>=2.1.0 in /home/ec2-user/anaconda3/envs/pytorch_p36/lib/python3.6/site-packages (from pycocotools->optimum[intel]) (3.3.4)
Requirement already satisfied: cython>=0.27.3 in /home/ec2-user/anaconda3/envs/pytorch_p36/lib/python3.6/site-packages (from pycocotools->optimum[intel]) (0.29.23)
Requirement already satisfied: setuptools>=18.0 in /home/ec2-user/anaconda3/envs/pytorch_p36/lib/python3.6/site-packages (from pycocotools->optimum[intel]) (52.0.0.post20210125)
Requirement already satisfied: joblib in /home/ec2-user/anaconda3/envs/pytorch_p36/lib/python3.6/site-packages (from sacremoses->transformers) (1.0.1)
Requirement already satisfied: click in /home/ec2-user/anaconda3/envs/pytorch_p36/lib/python3.6/site-packages (from sacremoses->transformers) (8.0.1)
Requirement already satisfied: six in /home/ec2-user/anaconda3/envs/pytorch_p36/lib/python3.6/site-packages (from sacremoses->transformers) (1.16.0)
Requirement already satisfied: mpmath>=0.19 in /home/ec2-user/anaconda3/envs/pytorch_p36/lib/python3.6/site-packages (from sympy->optimum[intel]) (1.2.1)
Requirement already satisfied: kiwisolver>=1.0.1 in /home/ec2-user/anaconda3/envs/pytorch_p36/lib/python3.6/site-packages (from matplotlib>=2.1.0->pycocotools->optimum[intel]) (1.3.1)
Requirement already satisfied: cycler>=0.10 in /home/ec2-user/anaconda3/envs/pytorch_p36/lib/python3.6/site-packages/cycler-0.10.0-py3.6.egg (from matplotlib>=2.1.0->pycocotools->optimum[intel]) (0.10.0)
Requirement already satisfied: cffi>=1.12 in /home/ec2-user/anaconda3/envs/pytorch_p36/lib/python3.6/site-packages (from cryptography->neural-compressor>=1.7->optimum[intel]) (1.14.5)
Requirement already satisfied: Werkzeug>=2.0 in /home/ec2-user/anaconda3/envs/pytorch_p36/lib/python3.6/site-packages (from Flask->neural-compressor>=1.7->optimum[intel]) (2.0.2)
Requirement already satisfied: Jinja2>=3.0 in /home/ec2-user/anaconda3/envs/pytorch_p36/lib/python3.6/site-packages (from Flask->neural-compressor>=1.7->optimum[intel]) (3.0.1)
Requirement already satisfied: itsdangerous>=2.0 in /home/ec2-user/anaconda3/envs/pytorch_p36/lib/python3.6/site-packages (from Flask->neural-compressor>=1.7->optimum[intel]) (2.0.1)
Requirement already satisfied: python-socketio>=5.0.2 in /home/ec2-user/anaconda3/envs/pytorch_p36/lib/python3.6/site-packages (from Flask-SocketIO->neural-compressor>=1.7->optimum[intel]) (5.5.0)
Requirement already satisfied: zope.event in /home/ec2-user/anaconda3/envs/pytorch_p36/lib/python3.6/site-packages (from gevent->neural-compressor>=1.7->optimum[intel]) (4.5.0)
Requirement already satisfied: greenlet<2.0,>=0.4.17 in /home/ec2-user/anaconda3/envs/pytorch_p36/lib/python3.6/site-packages (from gevent->neural-compressor>=1.7->optimum[intel]) (1.1.0)
Requirement already satisfied: zope.interface in /home/ec2-user/anaconda3/envs/pytorch_p36/lib/python3.6/site-packages (from gevent->neural-compressor>=1.7->optimum[intel]) (5.4.0)
Requirement already satisfied: future in /home/ec2-user/anaconda3/envs/pytorch_p36/lib/python3.6/site-packages (from hyperopt->neural-compressor>=1.7->optimum[intel]) (0.18.2)
Requirement already satisfied: cloudpickle in /home/ec2-user/anaconda3/envs/pytorch_p36/lib/python3.6/site-packages (from hyperopt->neural-compressor>=1.7->optimum[intel]) (1.6.0)
Requirement already satisfied: networkx>=2.2 in /home/ec2-user/anaconda3/envs/pytorch_p36/lib/python3.6/site-packages (from hyperopt->neural-compressor>=1.7->optimum[intel]) (2.5)
Requirement already satisfied: scipy in /home/ec2-user/anaconda3/envs/pytorch_p36/lib/python3.6/site-packages (from hyperopt->neural-compressor>=1.7->optimum[intel]) (1.5.3)
Requirement already satisfied: py4j in /home/ec2-user/anaconda3/envs/pytorch_p36/lib/python3.6/site-packages (from hyperopt->neural-compressor>=1.7->optimum[intel]) (0.10.7)
Requirement already satisfied: wcwidth in /home/ec2-user/anaconda3/envs/pytorch_p36/lib/python3.6/site-packages (from prettytable->neural-compressor>=1.7->optimum[intel]) (0.2.5)
Requirement already satisfied: contextlib2>=0.5.5 in /home/ec2-user/anaconda3/envs/pytorch_p36/lib/python3.6/site-packages (from schema->neural-compressor>=1.7->optimum[intel]) (0.6.0.post1)
Requirement already satisfied: threadpoolctl>=2.0.0 in /home/ec2-user/anaconda3/envs/pytorch_p36/lib/python3.6/site-packages (from scikit-learn->neural-compressor>=1.7->optimum[intel]) (2.1.0)
Requirement already satisfied: pyOpenSSL>=20.0.0 in /home/ec2-user/anaconda3/envs/pytorch_p36/lib/python3.6/site-packages (from sigopt->neural-compressor>=1.7->optimum[intel]) (20.0.1)
Requirement already satisfied: pypng>=0.0.20 in /home/ec2-user/anaconda3/envs/pytorch_p36/lib/python3.6/site-packages (from sigopt->neural-compressor>=1.7->optimum[intel]) (0.0.21)
Requirement already satisfied: kubernetes<13.0.0,>=12.0.1 in /home/ec2-user/anaconda3/envs/pytorch_p36/lib/python3.6/site-packages (from sigopt->neural-compressor>=1.7->optimum[intel]) (12.0.1)
Requirement already satisfied: rsa<5.0.0,>=4.7 in /home/ec2-user/anaconda3/envs/pytorch_p36/lib/python3.6/site-packages (from sigopt->neural-compressor>=1.7->optimum[intel]) (4.7.2)
Requirement already satisfied: boto3<2.0.0,==1.16.34 in /home/ec2-user/anaconda3/envs/pytorch_p36/lib/python3.6/site-packages (from sigopt->neural-compressor>=1.7->optimum[intel]) (1.16.34)
Requirement already satisfied: Pint<0.17.0,>=0.16.0 in /home/ec2-user/anaconda3/envs/pytorch_p36/lib/python3.6/site-packages (from sigopt->neural-compressor>=1.7->optimum[intel]) (0.16.1)
Requirement already satisfied: GitPython>=2.0.0 in /home/ec2-user/anaconda3/envs/pytorch_p36/lib/python3.6/site-packages (from sigopt->neural-compressor>=1.7->optimum[intel]) (3.1.18)
Requirement already satisfied: backoff<2.0.0,>=1.10.0 in /home/ec2-user/anaconda3/envs/pytorch_p36/lib/python3.6/site-packages (from sigopt->neural-compressor>=1.7->optimum[intel]) (1.11.1)
Requirement already satisfied: ipython>=5.0.0 in /home/ec2-user/anaconda3/envs/pytorch_p36/lib/python3.6/site-packages (from sigopt->neural-compressor>=1.7->optimum[intel]) (7.16.1)
Requirement already satisfied: docker<5.0.0,>=4.4.0 in /home/ec2-user/anaconda3/envs/pytorch_p36/lib/python3.6/site-packages (from sigopt->neural-compressor>=1.7->optimum[intel]) (4.4.4)
Requirement already satisfied: jmespath<1.0.0,>=0.7.1 in /home/ec2-user/anaconda3/envs/pytorch_p36/lib/python3.6/site-packages (from boto3<2.0.0,==1.16.34->sigopt->neural-compressor>=1.7->optimum[intel]) (0.10.0)
Requirement already satisfied: s3transfer<0.4.0,>=0.3.0 in /home/ec2-user/anaconda3/envs/pytorch_p36/lib/python3.6/site-packages (from boto3<2.0.0,==1.16.34->sigopt->neural-compressor>=1.7->optimum[intel]) (0.3.7)
Requirement already satisfied: botocore<1.20.0,>=1.19.34 in /home/ec2-user/anaconda3/envs/pytorch_p36/lib/python3.6/site-packages (from boto3<2.0.0,==1.16.34->sigopt->neural-compressor>=1.7->optimum[intel]) (1.19.63)
Requirement already satisfied: pycparser in /home/ec2-user/anaconda3/envs/pytorch_p36/lib/python3.6/site-packages (from cffi>=1.12->cryptography->neural-compressor>=1.7->optimum[intel]) (2.20)
Requirement already satisfied: websocket-client>=0.32.0 in /home/ec2-user/anaconda3/envs/pytorch_p36/lib/python3.6/site-packages (from docker<5.0.0,>=4.4.0->sigopt->neural-compressor>=1.7->optimum[intel]) (0.58.0)
Requirement already satisfied: gitdb<5,>=4.0.1 in /home/ec2-user/anaconda3/envs/pytorch_p36/lib/python3.6/site-packages (from GitPython>=2.0.0->sigopt->neural-compressor>=1.7->optimum[intel]) (4.0.9)
Requirement already satisfied: traitlets>=4.2 in /home/ec2-user/anaconda3/envs/pytorch_p36/lib/python3.6/site-packages (from ipython>=5.0.0->sigopt->neural-compressor>=1.7->optimum[intel]) (4.3.3)
Requirement already satisfied: jedi>=0.10 in /home/ec2-user/anaconda3/envs/pytorch_p36/lib/python3.6/site-packages (from ipython>=5.0.0->sigopt->neural-compressor>=1.7->optimum[intel]) (0.17.2)
Requirement already satisfied: prompt-toolkit!=3.0.0,!=3.0.1,<3.1.0,>=2.0.0 in /home/ec2-user/anaconda3/envs/pytorch_p36/lib/python3.6/site-packages (from ipython>=5.0.0->sigopt->neural-compressor>=1.7->optimum[intel]) (3.0.19)
Requirement already satisfied: backcall in /home/ec2-user/anaconda3/envs/pytorch_p36/lib/python3.6/site-packages (from ipython>=5.0.0->sigopt->neural-compressor>=1.7->optimum[intel]) (0.2.0)
Requirement already satisfied: pygments in /home/ec2-user/anaconda3/envs/pytorch_p36/lib/python3.6/site-packages (from ipython>=5.0.0->sigopt->neural-compressor>=1.7->optimum[intel]) (2.9.0)
Requirement already satisfied: pexpect in /home/ec2-user/anaconda3/envs/pytorch_p36/lib/python3.6/site-packages (from ipython>=5.0.0->sigopt->neural-compressor>=1.7->optimum[intel]) (4.8.0)
Requirement already satisfied: decorator in /home/ec2-user/anaconda3/envs/pytorch_p36/lib/python3.6/site-packages (from ipython>=5.0.0->sigopt->neural-compressor>=1.7->optimum[intel]) (5.0.9)
Requirement already satisfied: pickleshare in /home/ec2-user/anaconda3/envs/pytorch_p36/lib/python3.6/site-packages (from ipython>=5.0.0->sigopt->neural-compressor>=1.7->optimum[intel]) (0.7.5)
Requirement already satisfied: MarkupSafe>=2.0 in /home/ec2-user/anaconda3/envs/pytorch_p36/lib/python3.6/site-packages (from Jinja2>=3.0->Flask->neural-compressor>=1.7->optimum[intel]) (2.0.1)
Requirement already satisfied: google-auth>=1.0.1 in /home/ec2-user/anaconda3/envs/pytorch_p36/lib/python3.6/site-packages (from kubernetes<13.0.0,>=12.0.1->sigopt->neural-compressor>=1.7->optimum[intel]) (1.30.2)
Requirement already satisfied: requests-oauthlib in /home/ec2-user/anaconda3/envs/pytorch_p36/lib/python3.6/site-packages (from kubernetes<13.0.0,>=12.0.1->sigopt->neural-compressor>=1.7->optimum[intel]) (1.3.0)
Requirement already satisfied: importlib-resources in /home/ec2-user/anaconda3/envs/pytorch_p36/lib/python3.6/site-packages (from Pint<0.17.0,>=0.16.0->sigopt->neural-compressor>=1.7->optimum[intel]) (5.4.0)
Requirement already satisfied: python-engineio>=4.3.0 in /home/ec2-user/anaconda3/envs/pytorch_p36/lib/python3.6/site-packages (from python-socketio>=5.0.2->Flask-SocketIO->neural-compressor>=1.7->optimum[intel]) (4.3.0)
Requirement already satisfied: bidict>=0.21.0 in /home/ec2-user/anaconda3/envs/pytorch_p36/lib/python3.6/site-packages (from python-socketio>=5.0.2->Flask-SocketIO->neural-compressor>=1.7->optimum[intel]) (0.21.4)
Requirement already satisfied: pyasn1>=0.1.3 in /home/ec2-user/anaconda3/envs/pytorch_p36/lib/python3.6/site-packages (from rsa<5.0.0,>=4.7->sigopt->neural-compressor>=1.7->optimum[intel]) (0.4.8)
Requirement already satisfied: smmap<6,>=3.0.1 in /home/ec2-user/anaconda3/envs/pytorch_p36/lib/python3.6/site-packages (from gitdb<5,>=4.0.1->GitPython>=2.0.0->sigopt->neural-compressor>=1.7->optimum[intel]) (5.0.0)
Requirement already satisfied: pyasn1-modules>=0.2.1 in /home/ec2-user/anaconda3/envs/pytorch_p36/lib/python3.6/site-packages (from google-auth>=1.0.1->kubernetes<13.0.0,>=12.0.1->sigopt->neural-compressor>=1.7->optimum[intel]) (0.2.8)
Requirement already satisfied: cachetools<5.0,>=2.0.0 in /home/ec2-user/anaconda3/envs/pytorch_p36/lib/python3.6/site-packages (from google-auth>=1.0.1->kubernetes<13.0.0,>=12.0.1->sigopt->neural-compressor>=1.7->optimum[intel]) (4.2.2)
Requirement already satisfied: parso<0.8.0,>=0.7.0 in /home/ec2-user/anaconda3/envs/pytorch_p36/lib/python3.6/site-packages (from jedi>=0.10->ipython>=5.0.0->sigopt->neural-compressor>=1.7->optimum[intel]) (0.7.1)
Requirement already satisfied: ipython-genutils in /home/ec2-user/anaconda3/envs/pytorch_p36/lib/python3.6/site-packages (from traitlets>=4.2->ipython>=5.0.0->sigopt->neural-compressor>=1.7->optimum[intel]) (0.2.0)
Requirement already satisfied: ptyprocess>=0.5 in /home/ec2-user/anaconda3/envs/pytorch_p36/lib/python3.6/site-packages (from pexpect->ipython>=5.0.0->sigopt->neural-compressor>=1.7->optimum[intel]) (0.7.0)
Requirement already satisfied: oauthlib>=3.0.0 in /home/ec2-user/anaconda3/envs/pytorch_p36/lib/python3.6/site-packages (from requests-oauthlib->kubernetes<13.0.0,>=12.0.1->sigopt->neural-compressor>=1.7->optimum[intel]) (3.1.1)
```
---
**Cell:**
```python
from datasets import load_dataset, load_metric
```
OR
```python
import datasets
```
**Traceback:**
```
---------------------------------------------------------------------------
ImportError Traceback (most recent call last)
<ipython-input-7-34fb7ba3338d> in <module>
----> 1 from datasets import load_dataset, load_metric
~/anaconda3/envs/pytorch_p36/lib/python3.6/site-packages/datasets/__init__.py in <module>
32 )
33
---> 34 from .arrow_dataset import Dataset, concatenate_datasets
35 from .arrow_reader import ArrowReader, ReadInstruction
36 from .arrow_writer import ArrowWriter
~/anaconda3/envs/pytorch_p36/lib/python3.6/site-packages/datasets/arrow_dataset.py in <module>
59 from . import config, utils
60 from .arrow_reader import ArrowReader
---> 61 from .arrow_writer import ArrowWriter, OptimizedTypedSequence
62 from .features import ClassLabel, Features, FeatureType, Sequence, Value, _ArrayXD, pandas_types_mapper
63 from .filesystems import extract_path_from_uri, is_remote_filesystem
~/anaconda3/envs/pytorch_p36/lib/python3.6/site-packages/datasets/arrow_writer.py in <module>
26
27 from . import config, utils
---> 28 from .features import (
29 Features,
30 ImageExtensionType,
~/anaconda3/envs/pytorch_p36/lib/python3.6/site-packages/datasets/features/__init__.py in <module>
1 # flake8: noqa
----> 2 from .audio import Audio
3 from .features import *
4 from .features import (
5 _ArrayXD,
~/anaconda3/envs/pytorch_p36/lib/python3.6/site-packages/datasets/features/audio.py in <module>
5 import pyarrow as pa
6
----> 7 from ..utils.streaming_download_manager import xopen
8
9
~/anaconda3/envs/pytorch_p36/lib/python3.6/site-packages/datasets/utils/streaming_download_manager.py in <module>
16
17 from .. import config
---> 18 from ..filesystems import COMPRESSION_FILESYSTEMS
19 from .download_manager import DownloadConfig, map_nested
20 from .file_utils import (
~/anaconda3/envs/pytorch_p36/lib/python3.6/site-packages/datasets/filesystems/__init__.py in <module>
11
12 if _has_s3fs:
---> 13 from .s3filesystem import S3FileSystem # noqa: F401
14
15 COMPRESSION_FILESYSTEMS: List[compression.BaseCompressedFileFileSystem] = [
~/anaconda3/envs/pytorch_p36/lib/python3.6/site-packages/datasets/filesystems/s3filesystem.py in <module>
----> 1 import s3fs
2
3
4 class S3FileSystem(s3fs.S3FileSystem):
5 """
~/anaconda3/envs/pytorch_p36/lib/python3.6/site-packages/s3fs/__init__.py in <module>
----> 1 from .core import S3FileSystem, S3File
2 from .mapping import S3Map
3
4 from ._version import get_versions
5
~/anaconda3/envs/pytorch_p36/lib/python3.6/site-packages/s3fs/core.py in <module>
12 from fsspec.asyn import AsyncFileSystem, sync, sync_wrapper
13
---> 14 import aiobotocore
15 import botocore
16 import aiobotocore.session
~/anaconda3/envs/pytorch_p36/lib/python3.6/site-packages/aiobotocore/__init__.py in <module>
----> 1 from .session import get_session, AioSession
2
3 __all__ = ['get_session', 'AioSession']
4 __version__ = '1.3.0'
~/anaconda3/envs/pytorch_p36/lib/python3.6/site-packages/aiobotocore/session.py in <module>
4 from botocore import retryhandler, translate
5 from botocore.exceptions import PartialCredentialsError
----> 6 from .client import AioClientCreator, AioBaseClient
7 from .hooks import AioHierarchicalEmitter
8 from .parsers import AioResponseParserFactory
~/anaconda3/envs/pytorch_p36/lib/python3.6/site-packages/aiobotocore/client.py in <module>
11 from .args import AioClientArgsCreator
12 from .utils import AioS3RegionRedirector
---> 13 from . import waiter
14
15 history_recorder = get_global_history_recorder()
~/anaconda3/envs/pytorch_p36/lib/python3.6/site-packages/aiobotocore/waiter.py in <module>
4 from botocore.exceptions import ClientError
5 from botocore.waiter import WaiterModel # noqa: F401, lgtm[py/unused-import]
----> 6 from botocore.waiter import Waiter, xform_name, logger, WaiterError, \
7 NormalizedOperationMethod as _NormalizedOperationMethod, is_valid_waiter_error
8 from botocore.docs.docstring import WaiterDocstring
ImportError: cannot import name 'is_valid_waiter_error'
```
Please let me know if there's anything else I can add to post.
[1]: https://github.com/huggingface/notebooks/blob/master/examples/text_classification_quantization_inc.ipynb | 3,554 |
https://github.com/huggingface/datasets/issues/3553 | set_format("np") no longer works for Image data | [
"A quick fix for now is doing this:\r\n\r\n```python\r\nX_train = np.stack(dataset[\"train\"][\"image\"])[..., None]",
"This error also propagates to jax and is even trickier to fix, since `.with_format(type='jax')` will use numpy conversion internally (and fail). For a three line failure:\r\n\r\n```python\r\ndataset = datasets.load_dataset(\"mnist\")\r\ndataset.set_format(\"jax\")\r\nX_train = dataset[\"train\"][\"image\"]\r\n```",
"Hi! We've recently introduced a new Image feature that yields PIL Images (and caches transforms on them) instead of arrays.\r\n\r\nHowever, this feature requires a custom transform to yield np arrays directly:\r\n```python\r\nddict = datasets.load_dataset(\"mnist\")\r\n\r\ndef pil_image_to_array(batch):\r\n return {\"image\": [np.array(img) for img in batch[\"image\"]]} # or jnp.array(img) for Jax\r\n\r\nddict.set_transform(pil_image_to_array, columns=\"image\", output_all_columns=True)\r\n```\r\n\r\n[Docs](https://huggingface.co/docs/datasets/master/process.html#format-transform) on `set_transform`.\r\n\r\nAlso, the approach proposed by @cgarciae is not the best because it loads the entire column in memory.\r\n\r\n@albertvillanova @lhoestq WDYT? The Audio and the Image feature currently don't support the TF/Jax/PT Formatters, but for the Numpy Formatter maybe it makes more sense to return np arrays (and not a dict in the case of the Audio feature or a PIL Image object in the case of the Image feature).",
"Yes I agree it should return arrays and not a PIL image (and possible an array instead of a dict for audio data).\r\nI'm currently finishing some code refactoring of the image and audio and opening a PR today. Maybe we can look into that after the refactoring",
"This has been fixed in https://github.com/huggingface/datasets/pull/5072, which is included in the latest release of `datasets`."
] | ## Describe the bug
`dataset.set_format("np")` no longer works for image data, previously you could load the MNIST like this:
```python
dataset = load_dataset("mnist")
dataset.set_format("np")
X_train = dataset["train"]["image"][..., None] # <== No longer a numpy array
```
but now it doesn't work, `set_format("np")` seems to have no effect and the dataset just returns a list/array of PIL images instead of numpy arrays as requested.
| 3,553 |
https://github.com/huggingface/datasets/issues/3550 | Bug in `openbookqa` dataset | [
"Closed by:\r\n- #4259"
] | ## Describe the bug
Dataset entries contains a typo.
## Steps to reproduce the bug
```python
>>> from datasets import load_dataset
>>> obqa = load_dataset('openbookqa', 'main')
>>> obqa['train'][0]
```
## Expected results
```python
{'id': '7-980', 'question_stem': 'The sun is responsible for', 'choices': {'text': ['puppies learning new tricks', 'children growing up and getting old', 'flowers wilting in a vase', 'plants sprouting, blooming and wilting'], 'label': ['A', 'B', 'C', 'D']}, 'answerKey': 'D'}
```
## Actual results
```python
{'id': '7-980', 'question_stem': 'The sun is responsible for', 'choices': {'text': ['puppies learning new tricks', 'children growing up and getting old', 'flowers wilting in a vase', 'plants sprouting, blooming and wilting'], 'label': ['puppies learning new tricks', 'children growing up and getting old', 'flowers wilting in a vase', 'plants sprouting, blooming and wilting']}, 'answerKey': 'D'}
```
The bug is present in all configs and all splits.
## Environment info
- `datasets` version: 1.17.0
- Platform: Linux-5.4.0-1057-aws-x86_64-with-glibc2.27
- Python version: 3.9.7
- PyArrow version: 4.0.1
| 3,550 |
Subsets and Splits