File size: 5,567 Bytes
bc28e6f b273d9b bc28e6f b273d9b bc28e6f 7e0570a bc28e6f b273d9b bc28e6f b273d9b bc28e6f b273d9b bc28e6f b273d9b bc28e6f b273d9b bc28e6f b273d9b bc28e6f 64e0a3e bc28e6f b273d9b bc28e6f b273d9b bc28e6f b273d9b |
1 2 3 4 5 6 7 8 9 10 11 12 13 14 15 16 17 18 19 20 21 22 23 24 25 26 27 28 29 30 31 32 33 34 35 36 37 38 39 40 41 42 43 44 45 46 47 48 49 50 51 52 53 54 55 56 57 58 59 60 61 62 63 64 65 66 67 68 69 70 71 72 73 74 75 76 77 78 79 80 81 82 83 84 85 86 87 88 89 90 91 92 93 94 95 96 97 98 99 100 101 102 103 104 105 106 107 108 109 110 111 112 113 114 115 116 117 118 119 120 121 122 123 124 125 126 127 128 129 130 131 132 133 134 135 136 137 138 139 140 141 142 143 144 145 146 147 148 149 150 151 152 153 |
---
library_name: transformers
tags:
- object-detection
- Document
- Layout
- Analysis
- DocLayNet
- mAP
datasets:
- ds4sd/DocLayNet
license: apache-2.0
base_model:
- SenseTime/deformable-detr
---
<!-- This model card has been generated automatically according to the information the Trainer had access to. You
should probably proofread and complete it, then remove this comment. -->
# Deformable-DETR-Document-Layout-Analysis
This model was fine-tuned on the doc_lay_net dataset for Document Layout Analysis using full-sized DocLayNet Public Dataset.
## Model description
The DETR model is an encoder-decoder transformer with a convolutional backbone. Two heads are added on top of the decoder outputs in order to perform object detection: a linear layer for the class labels and a MLP (multi-layer perceptron) for the bounding boxes. The model uses so-called object queries to detect objects in an image. Each object query looks for a particular object in the image. For COCO, the number of object queries is set to 100.
The model is trained using a "bipartite matching loss": one compares the predicted classes + bounding boxes of each of the N = 100 object queries to the ground truth annotations, padded up to the same length N (so if an image only contains 4 objects, 96 annotations will just have a "no object" as class and "no bounding box" as bounding box). The Hungarian matching algorithm is used to create an optimal one-to-one mapping between each of the N queries and each of the N annotations. Next, standard cross-entropy (for the classes) and a linear combination of the L1 and generalized IoU loss (for the bounding boxes) are used to optimize the parameters of the model.
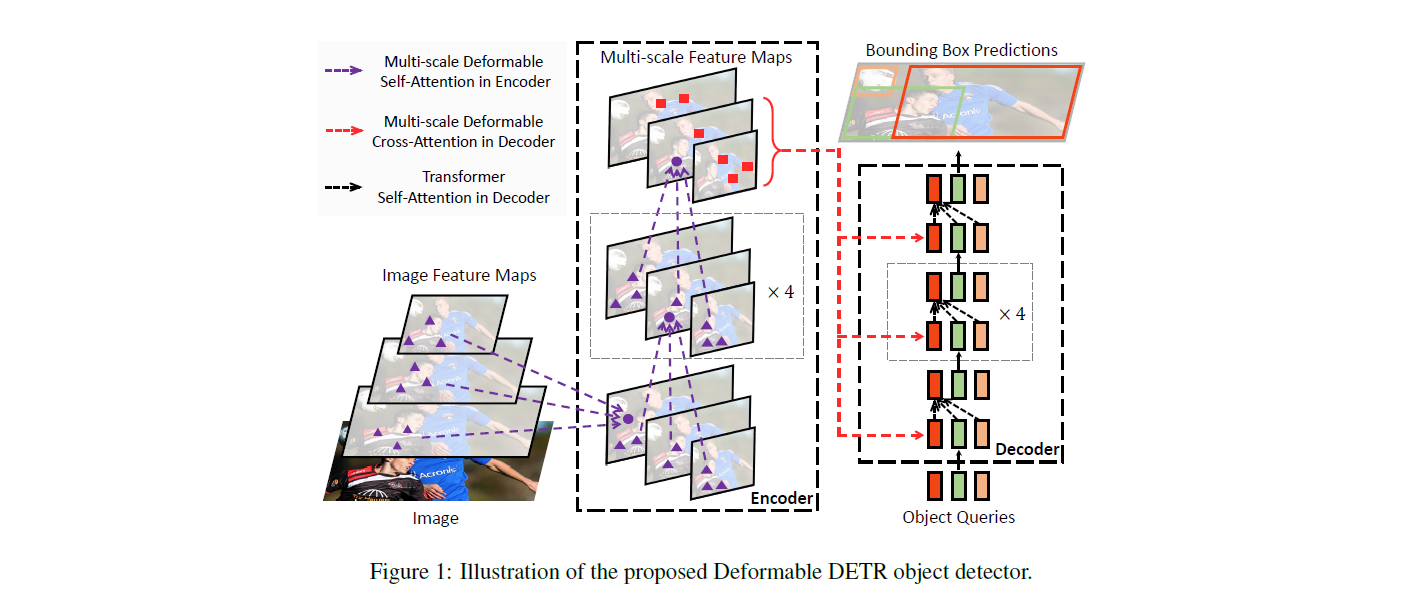
## Intended uses & limitations
You can use the model to predict Bounding Box for 11 different Classes of Document Layout Analysis.
### How to use
```python
from transformers import AutoImageProcessor, DeformableDetrForObjectDetection
import torch
from PIL import Image
import requests
url = "string-url-of-a-Document_page"
image = Image.open(requests.get(url, stream=True).raw)
processor = AutoImageProcessor.from_pretrained("pascalrai/Deformable-DETR-Document-Layout-Analyzer")
model = DeformableDetrForObjectDetection.from_pretrained("pascalrai/Deformable-DETR-Document-Layout-Analyzer")
inputs = processor(images=image, return_tensors="pt")
outputs = model(**inputs)
# convert outputs (bounding boxes and class logits) to COCO API
target_sizes = torch.tensor([image.size[::-1]])
results = processor.post_process_object_detection(outputs, target_sizes=target_sizes, threshold=0.5)[0]
for score, label, box in zip(results["scores"], results["labels"], results["boxes"]):
box = [round(i, 2) for i in box.tolist()]
print(
f"Detected {model.config.id2label[label.item()]} with confidence "
f"{round(score.item(), 3)} at location {box}"
)
```
## Evaluation on DocLayNet
Evaluation of the Trained model on Test Dataset of DocLayNet (On 3 epoch):
```
{'map': 0.6086,
'map_50': 0.836,
'map_75': 0.6662,
'map_small': 0.3269,
'map_medium': 0.501,
'map_large': 0.6712,
'mar_1': 0.3336,
'mar_10': 0.7113,
'mar_100': 0.7596,
'mar_small': 0.4667,
'mar_medium': 0.6717,
'mar_large': 0.8436,
'map_0': 0.5709,
'mar_100_0': 0.7639,
'map_1': 0.4685,
'mar_100_1': 0.7468,
'map_2': 0.5776,
'mar_100_2': 0.7163,
'map_3': 0.7143,
'mar_100_3': 0.8251,
'map_4': 0.4056,
'mar_100_4': 0.533,
'map_5': 0.5095,
'mar_100_5': 0.6686,
'map_6': 0.6826,
'mar_100_6': 0.8387,
'map_7': 0.5859,
'mar_100_7': 0.7308,
'map_8': 0.7871,
'mar_100_8': 0.8852,
'map_9': 0.7898,
'mar_100_9': 0.8617,
'map_10': 0.6034,
'mar_100_10': 0.7854}
```
### Training hyperparameters
The model was trained on A10G 24GB GPU for 21 hours.
The following hyperparameters were used during training:
- learning_rate: 5e-05
- eff_train_batch_size: 12
- eff_eval_batch_size: 12
- seed: 42
- optimizer: Use adamw_torch with betas=(0.9,0.999) and epsilon=1e-08 and optimizer_args=No additional optimizer arguments
- lr_scheduler_type: cosine
- num_epochs: 10
### Framework versions
- Transformers 4.49.0
- Pytorch 2.6.0+cu124
- Datasets 2.21.0
- Tokenizers 0.21.0
### BibTeX entry and citation info
```bibtex
@misc{https://doi.org/10.48550/arxiv.2010.04159,
doi = {10.48550/ARXIV.2010.04159},
url = {https://arxiv.org/abs/2010.04159},
author = {Zhu, Xizhou and Su, Weijie and Lu, Lewei and Li, Bin and Wang, Xiaogang and Dai, Jifeng},
keywords = {Computer Vision and Pattern Recognition (cs.CV), FOS: Computer and information sciences, FOS: Computer and information sciences},
title = {Deformable DETR: Deformable Transformers for End-to-End Object Detection},
publisher = {arXiv},
year = {2020},
copyright = {arXiv.org perpetual, non-exclusive license}
}
@article{doclaynet2022,
title = {DocLayNet: A Large Human-Annotated Dataset for Document-Layout Segmentation},
doi = {10.1145/3534678.353904},
url = {https://doi.org/10.1145/3534678.3539043},
author = {Pfitzmann, Birgit and Auer, Christoph and Dolfi, Michele and Nassar, Ahmed S and Staar, Peter W J},
year = {2022},
isbn = {9781450393850},
publisher = {Association for Computing Machinery},
address = {New York, NY, USA},
booktitle = {Proceedings of the 28th ACM SIGKDD Conference on Knowledge Discovery and Data Mining},
pages = {3743–3751},
numpages = {9},
location = {Washington DC, USA},
series = {KDD '22}
}
``` |