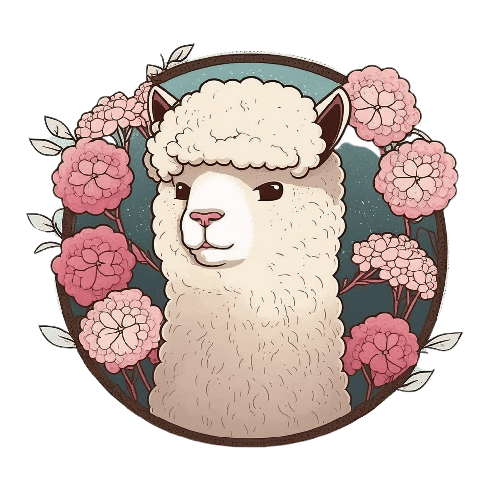
AlpacOOM: Alpaca ๐ฆ + BLOOM ๐ฎ
Adapter Description
This adapter was created with the PEFT library and allowed the base model BigScience/BLOOM 7B1 to be fine-tuned on the Stanford's Alpaca Dataset by using the method LoRA.
Model Description
BigScience Large Open-science Open-access Multilingual Language Model
Training data
Alpaca is a dataset of 52,000 instructions and demonstrations generated by OpenAI's text-davinci-003
engine. This instruction data can be used to conduct instruction-tuning for language models and make the language model follow instruction better.
The authors built on the data generation pipeline from Self-Instruct framework and made the following modifications:
- The
text-davinci-003
engine to generate the instruction data instead ofdavinci
. - A new prompt was written that explicitly gave the requirement of instruction generation to
text-davinci-003
. - Much more aggressive batch decoding was used, i.e., generating 20 instructions at once, which significantly reduced the cost of data generation.
- The data generation pipeline was simplified by discarding the difference between classification and non-classification instructions.
- Only a single instance was generated for each instruction, instead of 2 to 3 instances as in Self-Instruct.
This produced an instruction-following dataset with 52K examples obtained at a much lower cost (less than $500). In a preliminary study, the authors also found that the 52K generated data to be much more diverse than the data released by Self-Instruct.
Supported Tasks and Leaderboards
The Alpaca dataset is designed for instruction training pre-trained language models.
Training procedure
TBA
How to use
import torch
from peft import PeftModel, PeftConfig
from transformers import AutoModelForCausalLM, AutoTokenizer, GenerationConfig
peft_model_id = "mrm8488/Alpacoom"
config = PeftConfig.from_pretrained(peft_model_id)
model = AutoModelForCausalLM.from_pretrained(config.base_model_name_or_path, return_dict=True, load_in_8bit=True, device_map={"":0})
tokenizer = AutoTokenizer.from_pretrained("bigscience/bloom-7b1")
model = PeftModel.from_pretrained(model, peft_model_id)
model.eval()
# Based on the inference code by `tloen/alpaca-lora`
def generate_prompt(instruction, input=None):
if input:
return f"""Below is an instruction that describes a task, paired with an input that provides further context. Write a response that appropriately completes the request.
### Instruction:
{instruction}
### Input:
{input}
### Response:"""
else:
return f"""Below is an instruction that describes a task. Write a response that appropriately completes the request.
### Instruction:
{instruction}
### Response:"""
def generate(
instruction,
input=None,
temperature=0.1,
top_p=0.75,
top_k=40,
num_beams=4,
**kwargs,
):
prompt = generate_prompt(instruction, input)
inputs = tokenizer(prompt, return_tensors="pt")
input_ids = inputs["input_ids"].cuda()
generation_config = GenerationConfig(
temperature=temperature,
top_p=top_p,
top_k=top_k,
num_beams=num_beams,
**kwargs,
)
with torch.no_grad():
generation_output = model.generate(
input_ids=input_ids,
generation_config=generation_config,
return_dict_in_generate=True,
output_scores=True,
max_new_tokens=256,
)
s = generation_output.sequences[0]
output = tokenizer.decode(s)
return output.split("### Response:")[1].strip().split("Below")[0]
instruction = "Tell me about alpacas"
print("Instruction:", instruction)
print("Response:", generate(instruction))
Citation
@misc {manuel_romero_2023,
author = { {Manuel Romero} },
title = { Alpacoom (Revision 874f989) },
year = 2023,
url = { https://huggingface.co/mrm8488/Alpacoom },
doi = { 10.57967/hf/0449 },
publisher = { Hugging Face }
}