|
# CT (In-Batch Negatives) |
|
Carlsson et al. present in [Semantic Re-Tuning With Contrastive Tension (CT)](https://openreview.net/pdf?id=Ov_sMNau-PF) an unsupervised learning approach for sentence embeddings that just requires sentences. |
|
|
|
## Background |
|
During training, CT builds two independent encoders ('Model1' and 'Model2') with initial parameters shared to encode a pair of sentences. If Model1 and Model2 encode the same sentence, then the dot-product of the two sentence embeddings should be large. If Model1 and Model2 encode different sentences, then their dot-product should be small. |
|
|
|
In the original CT paper, specially created batches are used. We implemented an improved version that uses in-batch negative sampling: Model1 and Model2 both encode the same set of sentences. We maximize the scores for matching indexes (i.e. Model1(S_i) and Model2(S_i)) while we minimize the scores for different indexes (i.e. Model1(S_i) and Model2(S_j) for i != j). |
|
|
|
Using in-batch negative sampling gives a stronger training signal than the original loss function proposed by Carlsson et al. |
|
|
|
|
|
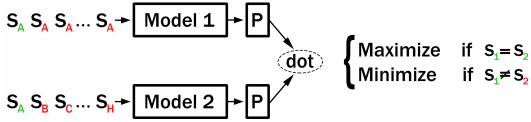 |
|
|
|
After training, the model 2 will be used for inference, which usually has better performance. |
|
|
|
## Performance |
|
In some preliminary experiments, we compare performance on the STSbenchmark dataset (trained with 1 million sentences from Wikipedia) and on the Quora duplicate questions dataset (trained with questions from Quora). |
|
|
|
| Method | STSb (Spearman) | Quora-Duplicate-Question (Avg. Precision) | |
|
| --- | :---: | :---: |
|
| CT | 75.7 | 36.5 |
|
| CT (In-Batch Negatives) | 78.5 | 40.1 |
|
|
|
|
|
Note: We used the code provided in this repository, not the official code from the authors. |
|
|
|
## CT from Sentences File |
|
|
|
**[train_ct-improved_from_file.py](train_ct-improved_from_file.py)** loads sentences from a provided text file. It is expected, that the there is one sentence per line in that text file. |
|
|
|
SimCSE will be training using these sentences. Checkpoints are stored every 500 steps to the output folder. |
|
|
|
|
|
|
|
## Further Training Examples |
|
|
|
- **[train_stsb_ct-improved.py](train_stsb_ct-improved.py)**: This example uses 1 million sentences from Wikipedia to train with CT. It evaluate the performance on the [STSbenchmark dataset](https://ixa2.si.ehu.eus/stswiki/index.php/STSbenchmark). |
|
- **[train_askubuntu_ct-improved.py](train_askubuntu_ct-improved.py)**: This example trains on [AskUbuntu Questions dataset](https://github.com/taolei87/askubuntu), a dataset with questions from the AskUbuntu Stackexchange forum. |