path
stringlengths 7
265
| concatenated_notebook
stringlengths 46
17M
|
---|---|
math_slides/Chapter 7 - BP.ipynb | ###Markdown
BP 算法 $$\nabla_w \mathcal{E}=\frac{\mathcal{E}}{w^{[1]}} + \cdots +\frac{\mathcal{E}}{w^{[N]}}$$ $$\frac{\mathcal{E}}{w^{[N]}}= f'({y^{[N-1]}}\cdot w^{[N]})*(d-y^{[N]})^T \cdot y^{[N]} = \mathcal{M}^{[N]} y^{[N]}$$ $$\frac{\mathcal{E}}{w^{[N-1]}}= f'({y^{[N-2]}}\cdot w^{[N-1]}) * w^{[N]}\cdot [f'({y^{[N-1]}}\cdot w^{[N]}) *(d-y^{[N]})^T] \cdot y^{[N-1]} = \mathcal{M}^{[N-1]} y^{[N-1]}$$ $$\mathcal{M}^{[N-1]}=f'(x)*[w^{[N]} \cdot \mathcal{M}^{[N-1]}]$$ $$*:\vec{x}*\vec{y}:=[x_1y_1,\cdots,x_ny_n]$$
###Code
def back_forward(self,dest):
self.e[self.layer-1]=dest-self.y[self.layer-1]
temp_delta=self.e[self.layer-1]*self.d_sigmoid_v[self.layer-1]
temp_delta=np.reshape(temp_delta,[-1,1])
self.dW[self.layer-2][:]=np.dot(np.reshape(self.y[self.layer-2],[-1,1]),np.transpose(temp_delta))
self.db[self.layer-2][:]=np.transpose(temp_delta)
#print(self.dW[self.layer-2])
for itrn in range(self.layer-2,0,-1):
sigma_temp_delta=np.dot(self.W[itrn],temp_delta)
temp_delta=sigma_temp_delta*np.reshape(self.d_sigmoid_v[itrn],[-1,1])
self.dW[itrn-1][:]=np.dot(np.reshape(self.y[itrn-1],[-1,1]),np.transpose(temp_delta))
self.db[itrn-1][:]=np.transpose(temp_delta)
# -*- coding: utf-8 -*-
"""
Created on Tue Apr 11 17:23:46 2017
@author: [email protected]
"""
%matplotlib inline
import numpy as np
class GenDataXOR():
def __init__(self,shape):
self.shape=shape
def func(self,dt):
if(dt[0] + dt[1] < 0.5):
rt=[0.1]
elif((dt[0] +dt[1])>1.5):
rt=[0.1]
else:
rt=[0.9]
return rt
def GenVali(self):
self.vali=np.array(list(map(self.func,self.data)))
return self.vali
def GenData(self):
self.data=np.random.random(self.shape)
return self.data
class BPAlg():
def sigmoid(self,x):
"""
Define active function sigomid
"""
return 1/(1+np.exp(-x))
def d_sigmiod(self,x):
"""
Define df/dx
"""
return np.exp(-x)/(1+np.exp(-x))**2
def __init__(self,shape):
"""
Initialize weights
"""
self.shape=shape
self.layer=len(shape)
self.W = []
self.b = []
self.e = []
self.y = []
self.dW = []
self.v = []
self.db = []
self.d_sigmoid_v = []
for itrn in range(self.layer-1):
self.W.append(np.random.random([shape[itrn], shape[itrn+1]]))
self.dW.append(np.random.random([shape[itrn], shape[itrn+1]]))
self.b.append(np.random.random([shape[itrn+1]]))
self.db.append(np.random.random([shape[itrn+1]]))
for itr in shape:
self.e.append(np.random.random([itr]))
self.y.append(np.random.random([itr]))
self.v.append(np.random.random([itr]))
self.d_sigmoid_v.append(np.ones([itr]))
def forward(self, data):
"""
forward propagation
"""
self.y[0][:] = data
temp_y = data
for itrn in range(self.layer-1):
temp_v = np.dot(temp_y, self.W[itrn])
temp_vb = np.add(temp_v, self.b[itrn])
temp_y = self.sigmoid(temp_vb)
self.y[itrn+1][:] = temp_y
self.d_sigmoid_v[itrn+1][:] = self.d_sigmiod(temp_vb)
return self.y[-1]
def back_forward(self, dest):
"""
back propagation
"""
self.e[self.layer-1] = dest-self.y[self.layer-1]
temp_delta = self.e[self.layer-1]*self.d_sigmoid_v[self.layer-1]
temp_delta = np.reshape(temp_delta,[-1,1])
self.dW[self.layer-2][:] = np.dot(np.reshape(self.y[self.layer-2],[-1,1]),np.transpose(temp_delta))
self.db[self.layer-2][:] = np.transpose(temp_delta)
for itrn in range(self.layer-2, 0, -1):
sigma_temp_delta = np.dot(self.W[itrn],temp_delta)
temp_delta = sigma_temp_delta*np.reshape(self.d_sigmoid_v[itrn],[-1,1])
self.dW[itrn-1][:] = np.dot(np.reshape(self.y[itrn-1], [-1,1]), np.transpose(temp_delta))
self.db[itrn-1][:] = np.transpose(temp_delta)
def data_feed(self, data, dest, eta):
NDT = len(data)
for itrn in range(NDT):
self.forward(data[itrn])
self.back_forward(dest[itrn])
for itrn in range(self.layer-1):
self.W[itrn][:] = self.W[itrn] + eta*self.dW[itrn]
self.b[itrn][:] = self.b[itrn] + eta*self.db[itrn]
dt=GenDataXOR([30,2])
tsc=BPAlg([2,2,1])
for itrn in range(2000):
data=dt.GenData()
vali=dt.GenVali()
tsc.data_feed(data,vali,2)
print(tsc.W)
print(tsc.b)
#print(tsc.forward(np.array([[1,1]])))
import matplotlib.pyplot as plt
from mpl_toolkits.mplot3d import Axes3D
import matplotlib as mpl
mpl.style.use('seaborn-darkgrid')
fig=plt.figure(1)
def sigmoid(rt):
return 1/(1+np.exp(-rt))
def GenZ(X,Y):
Z=np.zeros(np.shape(X))
for ity in range(len(X)):
for itx in range(len(X[0])):
l1=tsc.forward([X[ity,itx],Y[ity,itx]])
Z[ity,itx]=l1[0]
return Z
def GenZ1(X,Y):
Z=np.zeros(np.shape(X))
for ity in range(len(X)):
for itx in range(len(X[0])):
l1=tsc.sigmoid(np.dot([X[ity,itx],Y[ity,itx]],tsc.W[0])+tsc.b[0])
Z[ity,itx]=l1[1]
return Z
x=np.linspace(0,1,100)
y=np.linspace(0,1,100)
X,Y=np.meshgrid(x,y)
Z=GenZ(X,Y)
Z1=GenZ1(X,Y)
ax=fig.add_subplot(111,projection='3d')
ax.plot_surface(X,Y,Z,rstride=8,cstride=8, alpha=0.3)
ax.plot_surface(X,Y,Z1,rstride=8,cstride=8, alpha=0.3)
ax.contour(X,Y,Z,zdir='z',offset=0, cmap=plt.cm.coolwarm)
plt.show()
###Output
[array([[-17.1193724 , -14.47516142],
[-16.86598427, -14.43655252]]), array([[-11.40527114],
[ 7.23376057]])]
[array([ 6.9927864 , 21.70111895]), array([-4.6453043])]
|
data/time_series_covid19_confirmed_all.ipynb | ###Markdown
Dataset - AllMerge datasets "global", "US", and "Brazil".
###Code
import pandas as pd
###Output
_____no_output_____
###Markdown
Initialize
###Code
srcGlobal = "./time_series_covid19_confirmed_global_transformed.csv"
srcUS = "./time_series_covid19_confirmed_US_transformed.csv"
# srcBrazil = "../vinicius_da_silva/output_brazil.csv"
# srcArgentina = "../vinicius_da_silva/output_argentina.csv"
dest = "./time_series_covid19_confirmed_all.csv"
# Read data
globalDf = pd.read_csv(srcGlobal)
usDf = pd.read_csv(srcUS)
# brazilDf = pd.read_csv(srcBrazil)
# argentinaDf = pd.read_csv(srcArgentina)
###Output
_____no_output_____
###Markdown
Data Manipulation
###Code
# # Drop Brazil from brazilDf (we've subsampled Brazil to regions)
# brazilDf = brazilDf[brazilDf.Province_State != "Brazil"]
# # Drop Brazil and Argentina data from globalDf
# globalDf = globalDf[globalDf.Country_Region != "Brazil"]
# globalDf = globalDf[globalDf.Country_Region != "Argentina"]
# Drop US data from globalDf
globalDf = globalDf[globalDf.Country_Region != "US"]
# Merge datasets
finalDf = pd.concat([globalDf, usDf], axis = 0).reset_index(drop = True)
# finalDf = pd.concat([finalDf, brazilDf], axis = 0)
# finalDf = pd.concat([finalDf, argentinaDf], axis = 0).reset_index(drop = True)
# Convert NaN to 0
finalDf["Confirmed Cases"] = finalDf["Confirmed Cases"].mask(pd.isnull, 0)
# Convert negative numbers to 0
finalDf["Confirmed Cases"] = finalDf["Confirmed Cases"].mask(finalDf["Confirmed Cases"] < 0, 0)
# Drop (Lat, Long) == (0, 0) or (NaN, NaN)
# - This action involves the following regions:
# - (0, 0) : 'Diamond Princess', 'Grand Princess', 'MS Zaandam'
# - (NaN, NaN): 'Repatriated Travellers'
# ```
# droppedDf = finalDf[((finalDf.Lat == 0) & (finalDf.Long == 0)) | ((finalDf.Lat.isnull()) & (finalDf.Long.isnull()))]
# droppedDf["Province_State"].unique()
# ```
finalDf.drop(finalDf[((finalDf.Lat == 0) & (finalDf.Long == 0)) | ((finalDf.Lat.isnull()) & (finalDf.Long.isnull()))].index, inplace = True)
finalDf
###Output
_____no_output_____
###Markdown
Save Dataframe
###Code
finalDf.to_csv(dest, index = False)
###Output
_____no_output_____ |
visualization/include_image.ipynb | ###Markdown
Original Size``````  Specific Size```html``` Multiple Images```html ```
###Code
from IPython.display import Image
Image(filename="outputs/line.gif", width=250) # overwritten\
Image(filename="outputs/line.gif", width=450)
###Output
_____no_output_____ |
NuralNetwork.ipynb | ###Markdown
最小データ数の取得用関数最もサンプル数の少ないデータの数を取得
###Code
def search_min_data_num(num_classes):
# init
dict_num = []
for i in range(num_classes):
file_name = "/content/drive/My Drive/Colab Notebooks/data/" + TRAIN_DATA_FILES[i] + '.csv'
data_set = pd.read_csv(file_name, header=None)
num_data_set = len(data_set)
dict_num.append(num_data_set)
print(TRAIN_DATA_FILES[i], num_data_set, sep=': ')
min_data_num = min(dict_num)
print('\n')
print("min_data_num:", min_data_num)
return min_data_num
###Output
_____no_output_____
###Markdown
Define
###Code
TRAIN_DATA_FILES = ['cross','dead', 'left', 'right', 'straight', 'threeway']
#TRAIN_DATA_FILES = ['cross', 'right', 'left']
# NUM_CLASSES = 6
NUM_CLASSES = len(TRAIN_DATA_FILES)
num_data_set = search_min_data_num(NUM_CLASSES)
REPLACE_NAN = 0.0
epochs = 120
batch_size = 100
###Output
cross: 688
dead: 4377
left: 2590
right: 1852
straight: 3952
threeway: 10048
min_data_num: 688
###Markdown
学習データ数の調節用関数 データ読み込み時、それぞれのラベルの学習データの内、最も数の少ないものに合わせる
###Code
def adjust_data_num(num_class):
# header = 列名
file_name = "/content/drive/My Drive/Colab Notebooks/data/" + TRAIN_DATA_FILES[num_class] + '.csv'
data_set = pd.read_csv(file_name, header=None)
return data_set.sample(num_data_set)
###Output
_____no_output_____
###Markdown
学習データ読み込み用関数
###Code
def split_data():
files = os.listdir('/content/drive/My Drive/Colab Notebooks/data')
X = []
Y = []
all_data_set = []
labels = []
for i in range(NUM_CLASSES):
try:
data_set = adjust_data_num(i)
all_data_set.append(data_set)
# one_hot_vectorを作りラベルとして追加
tmp = np.zeros((num_data_set, NUM_CLASSES))
tmp[:, i] = 1
labels.append(tmp)
except pd.io.common.EmptyDataError:
print("ERROR: {} is empty".format(file_name))
X = pd.concat(all_data_set)
# replace Nan with 'REPLACE_NAN'
X = X.fillna(REPLACE_NAN)
Y = np.concatenate(labels, axis=0)
# _, DIM_input_data = data_set.shape
X_train, X_validation_and_test, Y_train, Y_validation_and_test = train_test_split(X, Y,train_size=0.6, test_size=0.4)
X_validation, X_test, Y_validation, Y_test = train_test_split(X_validation_and_test, Y_validation_and_test, train_size=0.5, test_size=0.5)
return X_train, X_validation, X_test, Y_train, Y_validation, Y_test
###Output
_____no_output_____
###Markdown
ヒートマップ描画用関数
###Code
def print_cmx(y_true, predict, index=None):
true_classes = np.argmax(y_true,1)
cmx_data = confusion_matrix(true_classes, predict)
df_cmx = pd.DataFrame(cmx_data, index=index, columns=index)
plt.figure(figsize = (10,7))
sns.heatmap(df_cmx, annot=True)
plt.show()
###Output
_____no_output_____
###Markdown
グラフプロット用関数
###Code
def plt_result(epochs, history):
plt.plot(range(1, epochs+1), history.history['acc'], label="training")
plt.plot(range(1, epochs+1), history.history['val_acc'], label="validation")
plt.xlabel('Epochs')
plt.ylabel('Accuracy')
plt.legend()
plt.show()
score = model.evaluate(X_test, Y_test, verbose=0)
print('Test loss:', score[0])
print('Test accuracy:', score[1])
predict_classes = model.predict_classes(X_test, batch_size=32)
print_cmx(Y_test, predict_classes, TRAIN_DATA_FILES)
###Output
_____no_output_____
###Markdown
学習実行 データ読み込み
###Code
X_train, X_validation, X_test, Y_train, Y_validation, Y_test = split_data()
_, DIM_input_data = X_train.shape
###Output
_____no_output_____
###Markdown
学習 ネットワーク設計現在 input(726) - mid_lay1(dropout1) - mid_lay2(dropout2) - output(6)
###Code
# 726-800-6 = 3層のネットワーク
DIM_HIDDEN1 = 1000
RATIO_DROP_OUT1 = 0.25
model = Sequential()
model.add(Dense(DIM_HIDDEN1, input_dim = DIM_input_data, activation='relu'))
model.add(Dropout(RATIO_DROP_OUT1))
model.add(Dense(NUM_CLASSES, activation='softmax'))
model.compile(optimizer='adam', loss='mean_squared_error', metrics=['accuracy'])
history = model.fit(X_train, Y_train, batch_size=batch_size, epochs=epochs, verbose=0, validation_data=(X_validation, Y_validation))
plt_result(epochs, history)
# 726-800-800-800-6 = 5層のネットワーク
DIM_HIDDEN1 = 1000
DIM_HIDDEN2 = 1000
DIM_HIDDEN3 = 1000
RATIO_DROP_OUT1 = 0.25
RATIO_DROP_OUT2 = 0.25
RATIO_DROP_OUT3 = 0.25
model = Sequential()
model.add(Dense(DIM_HIDDEN1, input_dim = DIM_input_data, activation='relu'))
model.add(Dropout(RATIO_DROP_OUT1))
model.add(Dense(DIM_HIDDEN2, activation='relu'))
model.add(Dropout(RATIO_DROP_OUT2))
model.add(Dense(DIM_HIDDEN3, activation='relu'))
model.add(Dropout(RATIO_DROP_OUT3))
model.add(Dense(NUM_CLASSES, activation='softmax'))
model.compile(optimizer='adam', loss='mean_squared_error', metrics=['accuracy'])
history = model.fit(X_train, Y_train, batch_size=batch_size, epochs=epochs, verbose=0, validation_data=(X_validation, Y_validation))
plt_result(epochs, history)
# 726-800-6 = 3層のネットワーク
DIM_HIDDEN1 = 1000
RATIO_DROP_OUT1 = 0.25
model = Sequential()
model.add(Dense(DIM_HIDDEN1, input_dim = DIM_input_data, activation='relu'))
model.add(Dropout(RATIO_DROP_OUT1))
model.add(Dense(NUM_CLASSES, activation='softmax'))
model.compile(optimizer='adam', loss='mean_squared_error', metrics=['accuracy'])
history = model.fit(X_train, Y_train, batch_size=batch_size, epochs=epochs, verbose=0, validation_data=(X_validation, Y_validation))
plt_result(epochs, history)
# 726-800-800-800-6 = 5層のネットワーク
DIM_HIDDEN1 = 1000
DIM_HIDDEN2 = 1000
DIM_HIDDEN3 = 1000
RATIO_DROP_OUT1 = 0.25
RATIO_DROP_OUT2 = 0.25
RATIO_DROP_OUT3 = 0.25
model = Sequential()
model.add(Dense(DIM_HIDDEN1, input_dim = DIM_input_data, activation='relu'))
model.add(Dropout(RATIO_DROP_OUT1))
model.add(Dense(DIM_HIDDEN2, activation='relu'))
model.add(Dropout(RATIO_DROP_OUT2))
model.add(Dense(DIM_HIDDEN3, activation='relu'))
model.add(Dropout(RATIO_DROP_OUT3))
model.add(Dense(NUM_CLASSES, activation='softmax'))
model.compile(optimizer='adam', loss='mean_squared_error', metrics=['accuracy'])
history = model.fit(X_train, Y_train, batch_size=batch_size, epochs=epochs, verbose=0, validation_data=(X_validation, Y_validation))
plt_result(epochs, history)
# 726-800-800-800-6 = 5層のネットワーク
DIM_HIDDEN1 = 1000
DIM_HIDDEN2 = 1000
RATIO_DROP_OUT1 = 0.25
RATIO_DROP_OUT2 = 0.25
model = Sequential()
model.add(Dense(DIM_HIDDEN1, input_dim = DIM_input_data, activation='relu'))
model.add(Dropout(RATIO_DROP_OUT1))
model.add(Dense(DIM_HIDDEN2, activation='relu'))
model.add(Dropout(RATIO_DROP_OUT2))
model.add(Dense(NUM_CLASSES, activation='softmax'))
model.compile(optimizer='adam', loss='mean_squared_error', metrics=['accuracy'])
history = model.fit(X_train, Y_train, batch_size=batch_size, epochs=epochs, verbose=0, validation_data=(X_validation, Y_validation))
plt_result(epochs, history)
###Output
_____no_output_____ |
analysis/DeterministicTest/zero-variance-test.ipynb | ###Markdown
Deterministic Test
###Code
import os
import math
import pandas as pd
import numpy as np
import matplotlib.pyplot as plt
from scipy import stats
from IPython.display import display, HTML
%matplotlib inline
def parse_if_number(s):
try: return float(s)
except: return True if s=="true" else False if s=="false" else s if s else None
def parse_ndarray(s):
return np.fromstring(s, sep=' ') if s else None
def get_file_name(name):
return name.replace(':', '-')
###Output
_____no_output_____
###Markdown
Config
###Code
inputFile = 'data.csv'
repetitionsCount = -1 # -1 = auto-detect
factors = []
# Plots
plotSize = (10, 10)
plotStyle = 'seaborn-whitegrid'
scatterShowLines = False
# Save
saveFigures = False
# Filter scalars
scalarsFilter = ['Floorplan.userCount', 'Floorplan.coveredUsers:sum', 'Floorplan.collisions:sum', 'Floorplan.msgsPerSlot:sum']
# Filter vectors
vectorsFilter = ['Floorplan.coveredUsers:vector']
# Percentiles
percentiles = [0.25, 0.5, 0.75, 0.9, 0.95]
# Residuals to compute
residualNames = [
('coveredUsersPercent', 'percentage of covered users (residuals)'),
('collisions', 'total number of collisions (residuals)'),
('msgsPerSlot', 'total number of messages sent (residuals)'),
]
intPercentiles = [int(i*100) for i in percentiles]
vecPerfIndexes = []
for intPercentile in intPercentiles:
vecPerfIndexes.append(('broadcastTime' + str(intPercentile), 'Broadcast time needed to reach the ' + str(intPercentile) + 'th percentile of the coverage'))
for i, d in vecPerfIndexes:
residualNames.append((i, d + ' (residuals)'))
###Output
_____no_output_____
###Markdown
Load scalars
###Code
df = pd.read_csv('exported_data/' + inputFile, converters = {
'attrvalue': parse_if_number,
'binedges': parse_ndarray,
'binvalues': parse_ndarray,
'vectime': parse_ndarray,
'vecvalue': parse_ndarray,
})
if repetitionsCount <= 0: # auto-detect
repetitionsCount = int(df[df.attrname == 'repetition']['attrvalue'].max()) + 1
print('Repetitions:', repetitionsCount)
# Computed
factorsCount = len(factors)
configsCount = 2**factorsCount
totalSims = configsCount*repetitionsCount
# Scatter plot whitelists
configsShown = range(0, configsCount)
repetitionsShown = range(0, repetitionsCount)
display(HTML("<style>div.output_scroll { height: auto; max-height: 48em; }</style>"))
pd.set_option('display.max_rows', totalSims)
if saveFigures:
os.makedirs('figures', exist_ok=True)
scalars = df[(df.type == 'scalar') | ((df.type == 'itervar') & (df.attrname != 'TO')) | ((df.type == 'param') & (df.attrname == 'Floorplan.userCount')) | ((df.type == 'runattr') & (df.attrname == 'repetition'))]
scalars = scalars.assign(qname = scalars.attrname.combine_first(scalars.module + '.' + scalars.name))
for index, row in scalars[scalars.type == 'itervar'].iterrows():
val = scalars.loc[index, 'attrvalue']
if isinstance(val, str) and not all(c.isdigit() for c in val):
scalars.loc[index, 'attrvalue'] = eval(val)
scalars.value = scalars.value.combine_first(scalars.attrvalue.astype('float64'))
scalars_wide = scalars.pivot_table(index=['run'], columns='qname', values='value')
scalars_wide.sort_values([*factors, 'repetition'], inplace=True)
count = 0
for index in scalars_wide.index:
config = count // repetitionsCount
scalars_wide.loc[index, 'config'] = config
count += 1
scalars_wide = scalars_wide[['config', 'repetition', *factors, *scalarsFilter]]
# coverage
scalars_wide['coveredUsersPercent'] = scalars_wide['Floorplan.coveredUsers:sum'] / (scalars_wide['Floorplan.userCount'] - 1)
###Output
_____no_output_____
###Markdown
Load vectors
###Code
vectors = df[df.type == 'vector']
vectors = vectors.assign(qname = vectors.module + '.' + vectors.name)
for index in scalars_wide.index:
r = index
cfg = scalars_wide.loc[index, 'config']
rep = scalars_wide.loc[index, 'repetition']
vectors.loc[vectors.run == r, 'config'] = cfg
vectors.loc[vectors.run == r, 'repetition'] = rep
vectors = vectors[vectors.qname.isin(vectorsFilter)]
vectors.sort_values(['config', 'repetition', 'qname'], inplace=True)
vectors = vectors[['config', 'repetition', 'qname', 'vectime', 'vecvalue']]
###Output
_____no_output_____
###Markdown
Compute scalars from vectors
###Code
def get_percentile(percentile, vectime, vecvalue, totalvalue):
tofind = percentile * totalvalue
idx = 0
csum = vecvalue.cumsum()
for value in csum:
if value >= tofind:
return vectime[idx]
idx += 1
return math.inf
for index, row in vectors.iterrows():
for vecPerf, percentile in zip(vecPerfIndexes, percentiles):
vecPerfIndex = vecPerf[0]
cfg = row['config']
rep = row['repetition']
if vecPerfIndex.startswith('broadcastTime'):
total = scalars_wide[(scalars_wide['config'] == cfg) & (scalars_wide['repetition'] == rep)]['Floorplan.userCount'].values[0] - 1
else:
raise Exception('Need to specify total for ' + vecPerfIndex + '. (coding required)')
value = get_percentile(percentile, row['vectime'], row['vecvalue'], total)
scalars_wide.loc[(scalars_wide['config'] == cfg) & (scalars_wide['repetition'] == rep), vecPerfIndex] = value
###Output
_____no_output_____
###Markdown
Compute residuals (should all be zero)
###Code
# coverage
scalars_wide['coveredUsersPercentMean'] = scalars_wide['coveredUsersPercent'].mean()
scalars_wide['coveredUsersPercentResidual'] = scalars_wide['coveredUsersPercent'] - scalars_wide['coveredUsersPercentMean']
# collisions
scalars_wide['collisionsMean'] = scalars_wide['Floorplan.collisions:sum'].mean()
scalars_wide['collisionsResidual'] = scalars_wide['Floorplan.collisions:sum'] - scalars_wide['collisionsMean']
# msgsPerSlot
scalars_wide['msgsPerSlotMean'] = scalars_wide['Floorplan.msgsPerSlot:sum'].mean()
scalars_wide['msgsPerSlotResidual'] = scalars_wide['Floorplan.msgsPerSlot:sum'] - scalars_wide['msgsPerSlotMean']
# vectors
skipped = []
for vecPerfIndex, _ in vecPerfIndexes:
mean = scalars_wide[vecPerfIndex].mean()
if math.isinf(mean):
skipped.append(vecPerfIndex)
continue
scalars_wide[vecPerfIndex + 'Mean'] = mean
scalars_wide[vecPerfIndex + 'Residual'] = scalars_wide[vecPerfIndex] - scalars_wide[vecPerfIndex + 'Mean']
for i, d in vecPerfIndexes:
if i not in skipped:
continue
print(i + ' skipped due to infinite values in observations')
residualNames.remove((i, d + ' (residuals)'))
###Output
_____no_output_____
###Markdown
Plot variance (should be zero)
###Code
display(scalars_wide.loc[(scalars_wide.repetition == 0) & (scalars_wide.config.isin(configsShown))][['config', *factors]])
for varname, vardesc in residualNames:
y = []
x = []
for config in range(0, configsCount):
if config not in configsShown:
continue
x.append([config] * len(repetitionsShown))
y.append(scalars_wide.loc[(scalars_wide.config == config) & (scalars_wide.repetition.isin(repetitionsShown))][varname + 'Residual'].values.tolist())
plt.figure(figsize=plotSize)
plt.style.use(plotStyle)
plt.plot(x, y, 'o' + ('-' if scatterShowLines else ''))
plt.title("Test independency for the " + vardesc)
plt.xlabel("Config number")
plt.ylabel("Residuals")
if saveFigures:
fig = plt.gcf()
fig.savefig('figures/' + get_file_name(varname) + '-variance.png')
plt.show()
###Output
_____no_output_____ |
notebooks/global_explain_embedding_components.ipynb | ###Markdown
This notebook explains how to use the global_plot_embedding_histogram and global_explain_embedding_components functions for a global explanation of your trained XSWEM model. As a pre-requisite to this notebook we would recommend reading section 4.1.1 of [Baseline Needs More Love: On Simple Word-Embedding-Based Models and Associated Pooling Mechanisms](https://arxiv.org/pdf/1805.09843.pdf), as this is where this method of explanation was originally proposed. You can run this notebook in Google Colab by right-clicking on the badge below, and opening the link in a new tab.[](https://colab.research.google.com/github/KieranLitschel/XSWEM/blob/main/notebooks/global_explain_embedding_components.ipynb) Install XSWEM and [Hugging Face datasets](https://github.com/huggingface/datasets).
###Code
!pip install xswem
!pip install datasets
###Output
_____no_output_____
###Markdown
First we load and prepare the dataset, and train the model. This is very similar code as in the train_xswem notebook, except we modify it for the [yelp_polarity dataset](https://huggingface.co/datasets/viewer/?dataset=yelp_polarity).From our experience using this method of global explainability, it seems to be important to use pre-trained GloVe embeddings and adapt the frozen embeddings. If we don't use them the model still performs similarly, but it is hard to see a pattern in the maximum values for each dimension.
###Code
## Download the GloVe embeddings. This is the "Common Crawl (42B tokens, 1.9M
## vocab, uncased, 300d vectors, 1.75 GB download)" dataset, which you can
## download here https://github.com/stanfordnlp/GloVe. We are hosting a copy
## on Google Drive as downloading from the internet on Google Colab is slow.
import os
if not os.path.isfile("glove.42B.300d.txt"):
!gdown --id 1LTAMRtx7VYKDI-7r6aG-t3E1nTHx7sG8
!unzip glove.42B.300d.zip
## Make this notebook deterministic.
RANDOM_SEED = 0
# Python RNG
import random
random.seed(RANDOM_SEED)
# Numpy RNG
import numpy as np
np.random.seed(RANDOM_SEED)
# TF RNG
import tensorflow as tf
from tensorflow.python.framework import random_seed
random_seed.set_seed(RANDOM_SEED)
## Import the necessary modules.
from xswem.model import XSWEM
from xswem.utils import prepare_embedding_weights_map_from_glove
from datasets import load_dataset
from tensorflow.keras.preprocessing.text import Tokenizer
from tensorflow.data import Dataset
import pandas as pd
## Load and shuffle the dataset. We keep 10% of the training set for validation.
yelp_polarity = load_dataset('yelp_polarity')
yelp_polarity = yelp_polarity.map(lambda row: {
"text": row["text"].replace("\\n","\n").replace("\\t","\t"),
"label": row["label"]})
yelp_polarity = yelp_polarity.shuffle({"train":RANDOM_SEED,"test":RANDOM_SEED})
yelp_polarity["train"] = yelp_polarity["train"].train_test_split(test_size=0.1,seed=RANDOM_SEED)
yelp_polarity_train, yelp_polarity_valid = yelp_polarity["train"]["train"], yelp_polarity["train"]["test"]
X, y = yelp_polarity_train["text"], yelp_polarity_train["label"]
X_valid, y_valid = yelp_polarity_valid["text"], yelp_polarity_valid["label"]
yelp_polarity_test = yelp_polarity["test"]
X_test, y_test = yelp_polarity_test["text"], yelp_polarity_test["label"]
## Build the tokenizer.
NUM_WORDS = 20000 # this means we only keep words where there are at least 50 examples
FILTERS = '!"#$%&()*+,-./:;<=>?@[\\]^_`{|}~\t\n0123456789'
# Its important to set the oov_token to "<unk>"" to match GloVe.
tokenizer = Tokenizer(num_words=NUM_WORDS, oov_token="<unk>", filters=FILTERS)
tokenizer.fit_on_texts(X)
vocab_map = {i+1: tokenizer.index_word[i+1] for i in range(NUM_WORDS)}
# this output map may seem slightly counterintuitive at first, as in the
# yelp_polarity dataset, the label 0 means a text has negative polarity, and
# 1 means positive polarity. But for our model we're using a sigmoid activation
# function for the output layer as this is a binary classification problem, so
# we only have only have one unit in the output layer, with an output of 0
# meaning negative polarity, and 1 meaning positive polarity. So our single
# output unit identifies if a text has positive polarity, and is labelled as
# such.
output_map = {
0: "Positive"
}
## Prepare the GloVe embeddings.
vocab = vocab_map.values()
embedding_weights_map = prepare_embedding_weights_map_from_glove("glove.42B.300d.txt", vocab, verbose=1)
## Build the dataset pipeline.
BATCH_SIZE = 32
NUM_LABELS = len(output_map)
train_dataset = Dataset.from_tensor_slices((X,y))
valid_dataset = Dataset.from_tensor_slices((X_valid,y_valid))
test_dataset = Dataset.from_tensor_slices((X_test,y_test))
# Repeat and shuffle the train datasets.
train_dataset = train_dataset.repeat()
train_dataset = train_dataset.shuffle(BATCH_SIZE*2)
# Tokenize the text.
# We only keep unique tokens as XSWEM is invariant to token frequency and order.
tokenize = lambda text, label: (tf.py_function(lambda text: np.unique(tokenizer.texts_to_sequences([str(text.numpy())])[0]), inp=[text], Tout=tf.int32), label)
train_dataset = train_dataset.map(tokenize,num_parallel_calls=tf.data.experimental.AUTOTUNE)
valid_dataset = valid_dataset.map(tokenize,num_parallel_calls=tf.data.experimental.AUTOTUNE)
test_dataset = test_dataset.map(tokenize,num_parallel_calls=tf.data.experimental.AUTOTUNE)
# Pre-fetch so that GPU spends less time waiting.
train_dataset = train_dataset.prefetch(tf.data.experimental.AUTOTUNE)
valid_dataset = valid_dataset.prefetch(tf.data.experimental.AUTOTUNE)
test_dataset = test_dataset.prefetch(tf.data.experimental.AUTOTUNE)
# Padded batch allows us to handle varying sentence lengths.
train_dataset = train_dataset.padded_batch(BATCH_SIZE,padded_shapes=([None],[]))
valid_dataset = valid_dataset.padded_batch(BATCH_SIZE,padded_shapes=([None],[]))
test_dataset = test_dataset.padded_batch(BATCH_SIZE,padded_shapes=([None],[]))
## Build the XSWEM model.
model = XSWEM(300, "sigmoid", vocab_map, output_map, mask_zero=True, embedding_weights_map=embedding_weights_map, adapt_embeddings=True, freeze_embeddings=True)
optimizer = tf.keras.optimizers.SGD(learning_rate=1e-2)
model.compile(optimizer, loss="binary_crossentropy", metrics="accuracy")
## Train XSWEM model.
model.fit(train_dataset, validation_data=valid_dataset, epochs=20, steps_per_epoch=10000, callbacks=[tf.keras.callbacks.EarlyStopping('val_accuracy', restore_best_weights=True)], verbose=2)
## Test XSWEM model.
model.evaluate(test_dataset)
###Output
Requirement already satisfied: datasets in /usr/local/lib/python3.6/dist-packages (1.2.1)
Requirement already satisfied: dill in /usr/local/lib/python3.6/dist-packages (from datasets) (0.3.3)
Requirement already satisfied: numpy>=1.17 in /usr/local/lib/python3.6/dist-packages (from datasets) (1.19.5)
Requirement already satisfied: multiprocess in /usr/local/lib/python3.6/dist-packages (from datasets) (0.70.11.1)
Requirement already satisfied: pandas in /usr/local/lib/python3.6/dist-packages (from datasets) (1.1.5)
Requirement already satisfied: pyarrow>=0.17.1 in /usr/local/lib/python3.6/dist-packages (from datasets) (2.0.0)
Requirement already satisfied: requests>=2.19.0 in /usr/local/lib/python3.6/dist-packages (from datasets) (2.23.0)
Requirement already satisfied: importlib-metadata; python_version < "3.8" in /usr/local/lib/python3.6/dist-packages (from datasets) (3.3.0)
Requirement already satisfied: tqdm<4.50.0,>=4.27 in /usr/local/lib/python3.6/dist-packages (from datasets) (4.41.1)
Requirement already satisfied: xxhash in /usr/local/lib/python3.6/dist-packages (from datasets) (2.0.0)
Requirement already satisfied: dataclasses; python_version < "3.7" in /usr/local/lib/python3.6/dist-packages (from datasets) (0.8)
Requirement already satisfied: python-dateutil>=2.7.3 in /usr/local/lib/python3.6/dist-packages (from pandas->datasets) (2.8.1)
Requirement already satisfied: pytz>=2017.2 in /usr/local/lib/python3.6/dist-packages (from pandas->datasets) (2018.9)
Requirement already satisfied: chardet<4,>=3.0.2 in /usr/local/lib/python3.6/dist-packages (from requests>=2.19.0->datasets) (3.0.4)
Requirement already satisfied: certifi>=2017.4.17 in /usr/local/lib/python3.6/dist-packages (from requests>=2.19.0->datasets) (2020.12.5)
Requirement already satisfied: idna<3,>=2.5 in /usr/local/lib/python3.6/dist-packages (from requests>=2.19.0->datasets) (2.10)
Requirement already satisfied: urllib3!=1.25.0,!=1.25.1,<1.26,>=1.21.1 in /usr/local/lib/python3.6/dist-packages (from requests>=2.19.0->datasets) (1.24.3)
Requirement already satisfied: typing-extensions>=3.6.4; python_version < "3.8" in /usr/local/lib/python3.6/dist-packages (from importlib-metadata; python_version < "3.8"->datasets) (3.7.4.3)
Requirement already satisfied: zipp>=0.5 in /usr/local/lib/python3.6/dist-packages (from importlib-metadata; python_version < "3.8"->datasets) (3.4.0)
Requirement already satisfied: six>=1.5 in /usr/local/lib/python3.6/dist-packages (from python-dateutil>=2.7.3->pandas->datasets) (1.15.0)
###Markdown
We plot a histogram of the component values, and observe that our model has learnt sparse embeddings, with most values centred around 0 and some very large values. This is the same pattern as observed in figure 1 of the [original paper](https://arxiv.org/pdf/1805.09843.pdf).
###Code
model.global_plot_embedding_histogram()
###Output
_____no_output_____
###Markdown
Below we show the results of our explainabiity function. This determines the top five words with the largest values for each component of the embeddings, and is equivalent to table 3 in the original paper. We label the columns of the table with the index of the component in the embedding vector.
A lot of the components appear to be quite noisy, with no clear relevance to the classification task. We show the first 10 components here to demonstrate this.
###Code
global_explained_embedding_components = model.global_explain_embedding_components()
global_explained_embedding_components.iloc[:, :10]
###Output
_____no_output_____
###Markdown
There are some components though which really seem to be capturing a much clearer polarity, but note there is often still some noise. For example the components below.
###Code
clear_polarity = [37, 60, 159]
global_explained_embedding_components.iloc[:, clear_polarity]
###Output
_____no_output_____ |
program/2_6_Qlearning.ipynb | ###Markdown
2.6 Q学習で迷路を攻略
###Code
# 使用するパッケージの宣言
import numpy as np
import matplotlib.pyplot as plt
%matplotlib inline
# 初期位置での迷路の様子
# 図を描く大きさと、図の変数名を宣言
fig = plt.figure(figsize=(5, 5))
ax = plt.gca()
# 赤い壁を描く
plt.plot([1, 1], [0, 1], color='red', linewidth=2)
plt.plot([1, 2], [2, 2], color='red', linewidth=2)
plt.plot([2, 2], [2, 1], color='red', linewidth=2)
plt.plot([2, 3], [1, 1], color='red', linewidth=2)
# 状態を示す文字S0~S8を描く
plt.text(0.5, 2.5, 'S0', size=14, ha='center')
plt.text(1.5, 2.5, 'S1', size=14, ha='center')
plt.text(2.5, 2.5, 'S2', size=14, ha='center')
plt.text(0.5, 1.5, 'S3', size=14, ha='center')
plt.text(1.5, 1.5, 'S4', size=14, ha='center')
plt.text(2.5, 1.5, 'S5', size=14, ha='center')
plt.text(0.5, 0.5, 'S6', size=14, ha='center')
plt.text(1.5, 0.5, 'S7', size=14, ha='center')
plt.text(2.5, 0.5, 'S8', size=14, ha='center')
plt.text(0.5, 2.3, 'START', ha='center')
plt.text(2.5, 0.3, 'GOAL', ha='center')
# 描画範囲の設定と目盛りを消す設定
ax.set_xlim(0, 3)
ax.set_ylim(0, 3)
plt.tick_params(axis='both', which='both', bottom='off', top='off',
labelbottom='off', right='off', left='off', labelleft='off')
# 現在地S0に緑丸を描画する
line, = ax.plot([0.5], [2.5], marker="o", color='g', markersize=60)
# 初期の方策を決定するパラメータtheta_0を設定
# 行は状態0~7、列は移動方向で↑、→、↓、←を表す
theta_0 = np.array([[np.nan, 1, 1, np.nan], # s0
[np.nan, 1, np.nan, 1], # s1
[np.nan, np.nan, 1, 1], # s2
[1, 1, 1, np.nan], # s3
[np.nan, np.nan, 1, 1], # s4
[1, np.nan, np.nan, np.nan], # s5
[1, np.nan, np.nan, np.nan], # s6
[1, 1, np.nan, np.nan], # s7、※s8はゴールなので、方策はなし
])
# 方策パラメータtheta_0をランダム方策piに変換する関数の定義
def simple_convert_into_pi_from_theta(theta):
'''単純に割合を計算する'''
[m, n] = theta.shape # thetaの行列サイズを取得
pi = np.zeros((m, n))
for i in range(0, m):
pi[i, :] = theta[i, :] / np.nansum(theta[i, :]) # 割合の計算
pi = np.nan_to_num(pi) # nanを0に変換
return pi
# ランダム行動方策pi_0を求める
pi_0 = simple_convert_into_pi_from_theta(theta_0)
# 初期の行動価値関数Qを設定
[a, b] = theta_0.shape # 行と列の数をa, bに格納
Q = np.random.rand(a, b) * theta_0 * 0.1
# *theta0をすることで要素ごとに掛け算をし、Qの壁方向の値がnanになる
# ε-greedy法を実装
def get_action(s, Q, epsilon, pi_0):
direction = ["up", "right", "down", "left"]
# 行動を決める
if np.random.rand() < epsilon:
# εの確率でランダムに動く
next_direction = np.random.choice(direction, p=pi_0[s, :])
else:
# Qの最大値の行動を採用する
next_direction = direction[np.nanargmax(Q[s, :])]
# 行動をindexに
if next_direction == "up":
action = 0
elif next_direction == "right":
action = 1
elif next_direction == "down":
action = 2
elif next_direction == "left":
action = 3
return action
def get_s_next(s, a, Q, epsilon, pi_0):
direction = ["up", "right", "down", "left"]
next_direction = direction[a] # 行動aの方向
# 行動から次の状態を決める
if next_direction == "up":
s_next = s - 3 # 上に移動するときは状態の数字が3小さくなる
elif next_direction == "right":
s_next = s + 1 # 右に移動するときは状態の数字が1大きくなる
elif next_direction == "down":
s_next = s + 3 # 下に移動するときは状態の数字が3大きくなる
elif next_direction == "left":
s_next = s - 1 # 左に移動するときは状態の数字が1小さくなる
return s_next
# Q学習による行動価値関数Qの更新
def Q_learning(s, a, r, s_next, Q, eta, gamma):
if s_next == 8: # ゴールした場合
Q[s, a] = Q[s, a] + eta * (r - Q[s, a])
else:
Q[s, a] = Q[s, a] + eta * (r + gamma * np.nanmax(Q[s_next,: ]) - Q[s, a])
return Q
# Q学習で迷路を解く関数の定義、状態と行動の履歴および更新したQを出力
def goal_maze_ret_s_a_Q(Q, epsilon, eta, gamma, pi):
s = 0 # スタート地点
a = a_next = get_action(s, Q, epsilon, pi) # 初期の行動
s_a_history = [[0, np.nan]] # エージェントの移動を記録するリスト
while (1): # ゴールするまでループ
a = a_next # 行動更新
s_a_history[-1][1] = a
# 現在の状態(つまり一番最後なのでindex=-1)に行動を代入
s_next = get_s_next(s, a, Q, epsilon, pi)
# 次の状態を格納
s_a_history.append([s_next, np.nan])
# 次の状態を代入。行動はまだ分からないのでnanにしておく
# 報酬を与え, 次の行動を求めます
if s_next == 8:
r = 1 # ゴールにたどり着いたなら報酬を与える
a_next = np.nan
else:
r = 0
a_next = get_action(s_next, Q, epsilon, pi)
# 次の行動a_nextを求めます。
# 価値関数を更新
Q = Q_learning(s, a, r, s_next, Q, eta, gamma)
# 終了判定
if s_next == 8: # ゴール地点なら終了
break
else:
s = s_next
return [s_a_history, Q]
# Q学習で迷路を解く
eta = 0.1 # 学習率
gamma = 0.9 # 時間割引率
epsilon = 0.5 # ε-greedy法の初期値
v = np.nanmax(Q, axis=1) # 状態ごとに価値の最大値を求める
is_continue = True
episode = 1
V = [] # エピソードごとの状態価値を格納する
V.append(np.nanmax(Q, axis=1)) # 状態ごとに行動価値の最大値を求める
while is_continue: # is_continueがFalseになるまで繰り返す
print("エピソード:" + str(episode))
# ε-greedyの値を少しずつ小さくする
epsilon = epsilon / 2
# Q学習で迷路を解き、移動した履歴と更新したQを求める
[s_a_history, Q] = goal_maze_ret_s_a_Q(Q, epsilon, eta, gamma, pi_0)
# 状態価値の変化
new_v = np.nanmax(Q, axis=1) # 状態ごとに行動価値の最大値を求める
print(np.sum(np.abs(new_v - v))) # 状態価値関数の変化を出力
v = new_v
V.append(v) # このエピソード終了時の状態価値関数を追加
print("迷路を解くのにかかったステップ数は" + str(len(s_a_history) - 1) + "です")
# 100エピソード繰り返す
episode = episode + 1
if episode > 100:
break
# 状態価値の変化を可視化します
# 参考URL http://louistiao.me/posts/notebooks/embedding-matplotlib-animations-in-jupyter-notebooks/
from matplotlib import animation
from IPython.display import HTML
import matplotlib.cm as cm # color map
def init():
# 背景画像の初期化
line.set_data([], [])
return (line,)
def animate(i):
# フレームごとの描画内容
# 各マスに状態価値の大きさに基づく色付きの四角を描画
line, = ax.plot([0.5], [2.5], marker="s",
color=cm.jet(V[i][0]), markersize=85) # S0
line, = ax.plot([1.5], [2.5], marker="s",
color=cm.jet(V[i][1]), markersize=85) # S1
line, = ax.plot([2.5], [2.5], marker="s",
color=cm.jet(V[i][2]), markersize=85) # S2
line, = ax.plot([0.5], [1.5], marker="s",
color=cm.jet(V[i][3]), markersize=85) # S3
line, = ax.plot([1.5], [1.5], marker="s",
color=cm.jet(V[i][4]), markersize=85) # S4
line, = ax.plot([2.5], [1.5], marker="s",
color=cm.jet(V[i][5]), markersize=85) # S5
line, = ax.plot([0.5], [0.5], marker="s",
color=cm.jet(V[i][6]), markersize=85) # S6
line, = ax.plot([1.5], [0.5], marker="s",
color=cm.jet(V[i][7]), markersize=85) # S7
line, = ax.plot([2.5], [0.5], marker="s",
color=cm.jet(1.0), markersize=85) # S8
return (line,)
# 初期化関数とフレームごとの描画関数を用いて動画を作成
anim = animation.FuncAnimation(
fig, animate, init_func=init, frames=len(V), interval=200, repeat=False)
HTML(anim.to_jshtml())
###Output
_____no_output_____ |
PyRamen/.ipynb_checkpoints/indexing-checkpoint.ipynb | ###Markdown
Instructor Demo: IndexingThis program reads performs several slice and dice operations through indexing via loc and iloc functions.
###Code
# Import libraries and dependencies
import pandas as pd
from pathlib import Path
###Output
_____no_output_____
###Markdown
Read CSV in as DataFrame
###Code
# Set the file path
file_path = Path('../Resources/people.csv')
# Read in the CSV as a DataFrame
people_csv = pd.read_csv(file_path)
people_csv.head()
###Output
_____no_output_____
###Markdown
View Summary Statistics (Default Numeric)
###Code
# View the summary statistics for the DataFrame, the describe() function defaults to only numerical data
people_csv.describe()
###Output
_____no_output_____
###Markdown
View Summary Statistics (All Columns)
###Code
# View the summary statistics for the DataFrame, include all columns
people_csv.describe(include='all')
###Output
_____no_output_____
###Markdown
Index Selection Using iloc
###Code
# Select the first row of the DataFrame
people_csv.iloc[0]
# Select the second row of the DataFrame
people_csv.iloc[1]
# Select the first 10 rows of the DataFrame
people_csv.iloc[0:10]
# Select the last row of the DataFrame
people_csv.iloc[-1]
# Select the first column of the DataFrame
people_csv.iloc[:,0].head()
# Select the second column of the DataFrame, with all rows
people_csv.iloc[:,1].head()
# Select the last column of the DataFrame, with all rows
people_csv.iloc[:,-1].head()
# Select the first two columns of the DataFrame, with all rows
people_csv.iloc[:, 0:2].head()
# Select the 1st, 5th, 8th, 22nd rows of the 1st 4th and 6th columns.
people_csv.iloc[[0,4,7,21], [0,3,5]]
# Select the first 5 rows of the 3rd, 4th, and 5th columns of the DataFrame
people_csv.iloc[0:5, 2:5]
###Output
_____no_output_____
###Markdown
Assignment Using iLoc
###Code
# Modify the 'first_name' column value of the first row
people_csv.iloc[0, people_csv.columns.get_loc('first_name')] = 'Arya'
people_csv.head()
###Output
_____no_output_____
###Markdown
Index Selection Using Loc
###Code
# Indexing
people_csv.set_index(people_csv['first_name'])
people_csv.head()
people_csv.copy()
# Set the index as the 'first_name' column
people_csv.set_index(people_csv['first_name'], inplace=True)
people_csv.head()
# Sort the index
people_csv.sort_index(inplace=True)
# Select the row with the index 'Evan'
people_csv.loc['Evan']
# Slice the data to output a range of rows based on the index
people_csv.loc['Aleshia':'Svetlana'].head()
# Filter rows based on a column value conditional
people_csv.loc[people_csv['gender'] == 'M'].head()
###Output
_____no_output_____
###Markdown
Assignment Using Loc
###Code
# Modify the 'first_name' value of the row with the index 'Yun'
people_csv.loc['Yun', 'first_name'] = 'Yuna'
people_csv.head()
###Output
_____no_output_____ |
_notebooks/2020-04-20-BN.ipynb | ###Markdown
Speed-up inference with Batch Normalization Folding> How to remove the batch normalization layer to make your neural networks faster.- toc: true- badges: false- categories: [Deep Learning]- comments: true **Introduction**Batch Normalization {% fn 1 %} {% fn 2 %} is a technique which takes care of normalizing the input of each layer to make the training process faster and more stable. In practice, it is an extra layer that we generally add after the computation layer and before the non-linearity. It consists of **2** steps:1. Normalize the batch by first subtracting its mean $\mu$, then dividing it by its standard deviation $\sigma$.2. Further scale by a factor $\gamma$ and shift by a factor $\beta$. Those are the parameters of the batch normalization layer, required in case of the network not needing the data to have a mean of **0** and a standard deviation of **1**.$$\Large\begin{aligned}&\mu_{\mathcal{B}} \leftarrow \frac{1}{m} \sum_{i=1}^{m} x_{i}\\&\sigma_{\mathcal{B}}^{2} \leftarrow \frac{1}{m} \sum_{i=1}^{m}\left(x_{i}-\mu_{\mathcal{B}}\right)^{2}\\&\widehat{x}_{i} \leftarrow \frac{x_{i}-\mu_{\mathcal{B}}}{\sqrt{\sigma_{\mathcal{B}}^{2}+\epsilon}}\\&y_{i} \leftarrow \gamma \widehat{x}_{i}+\beta \equiv \mathrm{BN}_{\gamma, \beta}\left(x_{i}\right)\end{aligned}$$ Due to its efficiency for training neural networks, batch normalization is now widely used. But how useful is it at inference time?Once the training has ended, each batch normalization layer possesses a specific set of $\gamma$ and $\beta$, but also $\mu$ and $\sigma$, the latter being computed using an exponentially weighted average during training. It means that during inference, the batch normalization acts as a simple linear transformation of what comes out of the previous layer, often a convolution.As a convolution is also a linear transformation, it also means that both operations can be merged into a single linear transformation!This would remove some unnecessary parameters but also reduce the number of operations to be performed at inference time.--- **How to do that in practice?**With a little bit of math, we can easily rearrange the terms of the convolution to take the batch normalization into account.As a little reminder, the convolution operation followed by the batch normalization operation can be expressed, for an input $x$, as:$$\Large\begin{aligned}z &=W * x+b \\\text { out } &=\gamma \cdot \frac{z-\mu}{\sqrt{\sigma^{2}+\epsilon}}+\beta\end{aligned}$$So, if we re-arrange the $W$ and $b$ of the convolution to take the parameters of the batch normalization into account, as such:$$\Large\begin{aligned}w_{\text {fold }} &=\gamma \cdot \frac{W}{\sqrt{\sigma^{2}+\epsilon}} \\b_{\text {fold }} &=\gamma \cdot \frac{b-\mu}{\sqrt{\sigma^{2}+\epsilon}}+\beta\end{aligned}$$We can remove the batch normalization layer and still have the same results! > Note: Usually, you don’t have a bias in a layer preceding a batch normalization layer. It is useless and a waste of parameters as any constant will be canceled out by the batch normalization. --- **How efficient is it?**We will try for **2** common architectures:1. VGG16 with batch norm2. ResNet50Just for the demonstration, we will use ImageNette dataset and PyTorch. Both networks will be trained for **5** epochs and what changes in terms of parameter number and inference time. **VGG16**Let’s start by training VGG16 for **5** epochs (the final accuracy doesn’t matter):
###Code
#hide_input
learn.fit_one_cycle(5, 1e-3)
###Output
_____no_output_____
###Markdown
Then show its number of parameters:
###Code
#hide_input
count_parameters(model)
###Output
Total parameters : 134,309,962
###Markdown
We can get the initial inference time by using the `%%timeit` magic command:
###Code
%%timeit
model(x[0][None].cuda())
###Output
2.77 ms ± 1.65 µs per loop (mean ± std. dev. of 7 runs, 100 loops each)
###Markdown
So now if we apply batch normalization folding, we have:
###Code
#hide_input
count_parameters(folded_model)
###Output
Total parameters : 134,301,514
###Markdown
And:
###Code
%%timeit
folded_model(x[0][None].cuda())
###Output
2.41 ms ± 2.49 µs per loop (mean ± std. dev. of 7 runs, 100 loops each)
###Markdown
So **8448** parameters removed and even better, almost **0.4 ms** faster inference! Most importantly, this is completely lossless, there is absolutely no change in terms of performance:
###Code
folded_learner.validate()
###Output
_____no_output_____
###Markdown
Let’s see how it behaves in the case of Resnet50! **Resnet50**Same, we start by training it for **5** epochs:
###Code
#hide_input
learn.fit_one_cycle(5, 1e-3)
###Output
_____no_output_____
###Markdown
The initial amount of parameters is:
###Code
#hide_input
count_parameters(model)
###Output
Total parameters : 23,528,522
###Markdown
And inference time is:
###Code
%%timeit
model(x[0][None].cuda())
###Output
6.17 ms ± 13.3 µs per loop (mean ± std. dev. of 7 runs, 100 loops each)
###Markdown
After using batch normalization folding, we have:
###Code
#hide_input
count_parameters(final_model)
###Output
Total parameters : 23,501,962
###Markdown
And:
###Code
%%timeit
final_model(x[0][None].cuda())
###Output
4.47 ms ± 8.97 µs per loop (mean ± std. dev. of 7 runs, 100 loops each)
###Markdown
So now, we have **26,560** parameters removed and even more impressive, an inference time reduce by **1.7ms**! And still without any drop in performance.
###Code
final_learner.validate()
###Output
_____no_output_____ |
benchmarks/data/codes/3dcorr_benchmark.ipynb | ###Markdown
Benchmark code for 3d correlation functionThis code requires cluster_toolkit.
###Code
import numpy as np
import pyccl as ccl
import cluster_toolkit
#cluster toolkit package is available at http://cluster-toolkit.readthedocs.io/en/latest/source/installation.html
import matplotlib
import matplotlib.pyplot as plt
%matplotlib inline
bench_ind = 3
if bench_ind == 1:
cosmo = ccl.Cosmology(
Omega_c=0.25, Omega_b=0.05, h=0.7, sigma8=0.8,
n_s=0.96, w0=-1.0, wa=0.0, Omega_g=0,
transfer_function='bbks')
elif bench_ind == 2:
cosmo = ccl.Cosmology(
Omega_c=0.25, Omega_b=0.05, h=0.7, sigma8=0.8,
n_s=0.96, w0=-0.9, wa=0.0, Omega_g=0,
transfer_function='bbks')
elif bench_ind == 3:
cosmo = ccl.Cosmology(
Omega_c=0.25, Omega_b=0.05, h=0.7, sigma8=0.8,
n_s=0.96, w0=-0.9, wa=0.1, Omega_g=0,
transfer_function='bbks')
k = np.logspace(np.log10(5.e-5), 3., 730000) # Wavenumber
h = 0.7
# CCL power spectrum
pk = []
for n in range(6):
pk.append(ccl.nonlin_matter_power(cosmo, k, 1./(n+1)))
# calculate CCL xi and benchmark xi for r = 0.1 - 100 with 40 bins
nr1 = 40
r1 = np.logspace(-1, 2, nr1)
xi1 = []
for n in range(6):
xi1.append(ccl.correlation_3d(cosmo, 1./(n+1), r1))
xi_toolkit1 = []
for n in range(6):
xi_toolkit1.append(cluster_toolkit.xi.xi_mm_at_r(h*r1, k/h, pk[n]*h*h*h, exact=True))
# calculate CCL xi and benchmark xi for r = 50 - 250 with 100 bins to check agreement in teh BAO peak region
nr2 = 100
r2 = np.logspace(np.log10(50), np.log10(250), nr2)
xi2 = []
for n in range(6):
xi2.append(ccl.correlation_3d(cosmo, 1./(n+1), r2))
xi_toolkit2 = []
for n in range(6):
xi_toolkit2.append(cluster_toolkit.xi.xi_mm_at_r(h*r2, k/h, pk[n]*h*h*h, exact=True))
# write benchmark xi to file
with open('model%d_xi.txt' % bench_ind,'w') as f:
f.write('# [0] r (Mpc; comoving), [1] xi(r,z=0.0), [2] xi(r,z=1.0), [3] xi(r,z=2.0), [4] xi(r,z=3.0), [5] xi(r,z=4.0), [6] xi(r,z=5.0)' + '\n')
for i in range(140):
col = []
s = ''
if i < 40:
col.append("{:.18e}".format(r1[i]).ljust(25))
for n in range(6):
col.append("{:.18e}".format(xi_toolkit1[n][i]).ljust(25))
else:
col.append("{:.18e}".format(r2[i-40]).ljust(25))
for n in range(6):
col.append("{:.18e}".format(xi_toolkit2[n][i-40]).ljust(25))
s = ' '.join(col)
f.write(s + '\n')
# print some values
n = 0 # redshift
print("r xi(CCL) xi(benchmark) Delta(r^2 xi) Delta xi / xi_benchmark")
for i in range(140):
col = []
s = ''
if i < 40:
col.append("{:.5e}".format(r1[i]).ljust(25))
col.append("{:.5e}".format(xi1[n][i]).ljust(25))
col.append("{:.5e}".format(xi_toolkit1[n][i]).ljust(25))
err = r1[i]*r1[i]*(xi1[n][i]-xi_toolkit1[n][i])
col.append("{:.5e}".format(err).ljust(25))
rel_diff = (xi1[n][i]-xi_toolkit1[n][i])/xi_toolkit1[n][i]
col.append("{:.5e}".format(rel_diff).ljust(25))
#s = col[0] + col[1] + col[2] + col[3] + col[4]
else:
col.append("{:.5e}".format(r2[i-40]).ljust(25))
col.append("{:.5e}".format(xi2[n][i-40]).ljust(25))
col.append("{:.5e}".format(xi_toolkit2[n][i-40]).ljust(25))
err = r2[i-40]*r2[i-40]*(xi2[n][i-40]-xi_toolkit2[n][i-40])
col.append("{:.5e}".format(err).ljust(25))
rel_diff = (xi2[n][i-40]-xi_toolkit2[n][i-40])/xi_toolkit2[n][i-40]
col.append("{:.5e}".format(rel_diff).ljust(25))
s = col[0] + col[1] + col[2] + col[3] + col[4]
print(s)
# Plot relative difference
frac_diff1 = []
frac_diff2 = []
abs_diff1 = []
abs_diff2 = []
for i in range(nr1):
frac_diff1.append(np.abs(xi1[n][i]/xi_toolkit1[n][i] - 1.))
abs_diff1.append(np.abs(r1[i]*r1[i]*(xi1[n][i]-xi_toolkit1[n][i])))
#print r1[i], xi_toolkit1[n][i], xi1[n][i], r1[i]*r1[i]*(xi1[n][i]-xi_toolkit1[n][i])
for i in range(nr2):
frac_diff2.append(np.abs(1-xi2[n][i]/xi_toolkit2[n][i]))
abs_diff2.append(np.abs(r2[i]*r2[i]*(xi2[n][i]-xi_toolkit2[n][i])))
# esthetic definitions for the plots
matplotlib.rcParams['mathtext.fontset'] = 'stix'
matplotlib.rcParams['font.family'] = 'STIXGeneral'
matplotlib.rcParams['font.size'] = 14
plt.plot(r1, frac_diff1, 'b-')
plt.plot(r2, frac_diff2, 'r-')
plt.xscale('log')
plt.yscale('log')
plt.xlabel(r'$r$ (Mpc)')
plt.ylabel(r'$\Delta \xi(r) / \xi(r)$')
plt.grid(which='major')
plt.title('Relative difference')
plt.savefig('benchmark_rel.pdf',bbox_inches = 'tight')
plt.show()
#print frac_diff1
print("frac diff for r = 0.1-100: ", np.amax(frac_diff1))
print("frac diff for r = 50-250: ", np.amax(frac_diff2))
# plot absolute difference in r^2 xi(r)
plt.plot(r1, abs_diff1, 'b-')
plt.plot(r2, abs_diff2, 'r-')
plt.xscale('log')
plt.yscale('log')
plt.xlabel(r'$r$ (Mpc)')
plt.ylabel(r'$\Delta (r^2 \xi(r)) $')
plt.grid(which='minor')
plt.title('Absolute difference')
plt.grid(which='both')
plt.savefig('benchmark_abs.pdf',bbox_inches = 'tight')
plt.show()
#print abs_diff
print("max diff for r = 0.1-100: ", np.amax(abs_diff1))
print("max diff for r = 50-250: ", np.amax(abs_diff2))
# find and print the average of Delta(r^2 xi) in the BAO peak region
max_value = 0
max_value_index = 0
avg_value = 0
for i in range(63,68):
#print i, r2[i], abs_diff2[i]
avg_value = avg_value + abs_diff2[i]
avg_value = avg_value / 5.
print("average Delta(r^2 xi) for r=140-150 Mpc:", avg_value)
for i in range(nr1):
col = []
s = ''
col.append("{:.18e}".format(r1[i]).ljust(25))
col.append("{:.18e}".format(frac_diff1[i]).ljust(25))
col.append("{:.18e}".format(abs_diff1[i]).ljust(25))
s = col[0] + col[1] + col[2]
#print(s)
for i in range(nr2):
col = []
s = ''
col.append("{:.18e}".format(r2[i]).ljust(25))
col.append("{:.18e}".format(frac_diff2[i]).ljust(25))
col.append("{:.18e}".format(abs_diff2[i]).ljust(25))
s = col[0] + col[1] + col[2]
#print(s)
###Output
_____no_output_____ |
02_Dollars_and_Change/Dollars_and_Change.ipynb | ###Markdown
Dollars and ChangeIn this activity, you'll prepare and clean data by removing symbols in the dataset, converting data types, filling in missing values, and dropping any duplicate data.Instructions:1. Import the Pandas and `pathlib` libraries.2. Use `Path` with the `read_csv` function to read the CSV file into the DataFrame. Use the `index_col`, `parse_dates`, and `infer_datetime_format` parameters to set the Date column as the index.3. Confirm the import by using the `head` function to review the first five rows of the DataFrame .4. Use the `dtypes` function to check the data types of the DataFrame, and identify the ones that are strings.5. Use the `str.replace` function to replace all the dollar signs in the Total Payments column. Then review the first five rows of the DataFrame to confirm the update.6. Use the `astype` function to change the data type of the Total Payments column from `object (string)` to `float`. Then call the `dtypes` function on the DataFrame to confirm the update.7. For the Profit Margin column, remove all the percent signs and convert the data types to `float` by repeating the preceding steps. Call the `dtypes` function on the DataFrame to confirm the update.8. Use the `isnull` function along with the `sum` function to determine the number of missing values in the DataFrame.9. Use the `fillna` function to fill any missing values in just the Profit Margin column with the value of 0. Then rerun the `isnull().sum()` function to confirm that you handled missing values.10. Use the Pandas `duplicated` function along with the `sum` function to determine the number of duplicated entries in the DataFrame.11. Use the Pandas `drop_duplicates` function to remove all duplicated entries. Review the first 10 rows of the DataFrame to confirm the update.References:[Pandas read_csv function](https://pandas.pydata.org/pandas-docs/stable/reference/api/pandas.read_csv.html)[Pandas dtypes function](https://pandas.pydata.org/pandas-docs/stable/reference/api/pandas.DataFrame.dtypes.html)[Pandas str.replace function](https://pandas.pydata.org/pandas-docs/stable/reference/api/pandas.Series.str.replace.html)[Pandas astype funtion](https://pandas.pydata.org/pandas-docs/stable/reference/api/pandas.DataFrame.astype.html)[Pandas isnull function](https://pandas.pydata.org/pandas-docs/stable/reference/api/pandas.isnull.html)[Pandas duplicated function](https://pandas.pydata.org/pandas-docs/stable/reference/api/pandas.DataFrame.duplicated.html) Step 1: Import the Pandas and `pathlib` libraries.
###Code
# Import the Pandas library
import pandas as pd
# Import the Path module from the pathlib library
from pathlib import Path
###Output
_____no_output_____
###Markdown
Step 2: Use `Path` with the `read_csv` function to read the CSV file into the DataFrame. Use the `index_col`, `parse_dates`, and `infer_datetime_format` parameters to set the Date column as the index.
###Code
# Read in the CSV file called "big_time_money_flows.csv" using the Path module
# The CSV file is located in the Resources folder
# Set the index to the column "Date"
# Set the parse_dates and infer_datetime_format parameters
money_flows_csvpath = Path('./Resources/big_time_money_flows.csv')
money_flows_df = pd.read_csv(money_flows_csvpath, index_col="Date", parse_dates=True, infer_datetime_format=True)
###Output
_____no_output_____
###Markdown
Step 3: Confirm the import by using the `head` function to review the first five rows of the DataFrame .
###Code
# Call the head function to review the first 5 rows of the DataFrame
money_flows_df.head()
###Output
_____no_output_____
###Markdown
Step 4: Use the `dtypes` function to check the data types of the DataFrame, and identify the ones that are strings.
###Code
# Use the dtypes function to check the data type of each column in the DataFrame
money_flows_df.dtypes
###Output
_____no_output_____
###Markdown
Step 5: Use the `str.replace` function to replace all the dollar signs in the Total Payments column. Then review the first five rows of the DataFrame to confirm the update.
###Code
# For all the rows in the "Total Payments", use the str.replace function
# to replace the "$" with empty strings ("")
money_flows_df.loc[:, "Total Payments"] = money_flows_df.loc[:, "Total Payments"].str.replace("$", "")
# Call the head function to review the first 5 rows of the DataFrame
money_flows_df.head()
###Output
/Users/johnpweldon/opt/anaconda3/envs/dev/lib/python3.7/site-packages/ipykernel_launcher.py:3: FutureWarning: The default value of regex will change from True to False in a future version. In addition, single character regular expressions will*not* be treated as literal strings when regex=True.
This is separate from the ipykernel package so we can avoid doing imports until
###Markdown
Step 6: Use the `astype` function to change the data type of the Total Payments column from `object (string)` to `float`. Then call the `dtypes` function on the DataFrame to confirm the update.
###Code
# For all the rows in "Total Payments", use the astype function
# to change the data type from Object (string) to float
money_flows_df.loc[:, "Total Payments"] = money_flows_df.loc[:, "Total Payments"].astype("float")
# Call the dtypes function on the `money_flows_df` DataFrame to confirm the update
money_flows_df.dtypes
###Output
_____no_output_____
###Markdown
Step 7: For the Profit Margin column, remove all the percent signs and convert the data types to `float` by repeating the preceding steps. Call the `dtypes` function on the DataFrame to confirm the update.
###Code
# For all rows in the "Profit Margin", use the str.replace function
# to replace the "%" with empty strings ("")
money_flows_df.loc[:, "Profit Margin"] = money_flows_df.loc[:, "Profit Margin"].str.replace("%", "")
# For all rows in "Profit Margin", use the Pandas astype function
# to change the data type from Object (string) to float
money_flows_df.loc[:, "Profit Margin"] = money_flows_df.loc[:, "Profit Margin"].astype("float")
# Call the dtypes function on the `money_flows_df` DataFrame to confirm the update
money_flows_df.dtypes
###Output
_____no_output_____
###Markdown
Step 8: Use the `isnull` function along with the `sum` function to determine the number of missing values in the DataFrame.
###Code
# Use the isnull function in conjunction with the sum function to determine
# the total number of missing values in the DataFrame
money_flows_df.isnull().sum()
###Output
_____no_output_____
###Markdown
Step 9: Use the `fillna` function to fill any missing values in just the Profit Margin column with the value of 0. Then rerun the `isnull().sum()` function to confirm that you handled missing values.
###Code
# Using the fillna function, replace all of the missing values
# in the "Profit Margin" column with a 0
money_flows_df.loc[:, "Profit Margin"] = money_flows_df.loc[:, "Profit Margin"].fillna(0)
# Rerun the isnull function in conjunction with the sum function to confirm there are no missing values
money_flows_df.isnull().sum()
###Output
_____no_output_____
###Markdown
Step 10: Use the Pandas `duplicated` function along with the `sum` function to determine the number of duplicated entries in the DataFrame.
###Code
# Use the duplicated function in conjunction with the sum function,
# to confirm the number of dupliate values in the DataFrame
money_flows_df.duplicated().sum()
###Output
_____no_output_____
###Markdown
Step 11: Use the Pandas `drop_duplicates` function to remove all duplicated entries. Review the first 10 rows of the DataFrame to confirm the update.
###Code
# Using the drop_duplicates function, remove all the duplicate rows of data
# from the DataFrame
money_flows_df = money_flows_df.drop_duplicates()
# Call the head function to review the first 10 rows of the DataFrame
money_flows_df.head(10)
###Output
_____no_output_____ |
notebooks/4.0-pb-ExploratoryDataAnalysisTestColumn.ipynb | ###Markdown
Exploring specifically the Input Text Data for Training and Verification
###Code
import pandas as pd
import numpy as np
import os
import matplotlib.pyplot as plt
###Output
_____no_output_____
###Markdown
Loading Data
###Code
path_file = os.path.join(os.path.dirname(os.getcwd()), "data", "raw", "CRS2018data.txt")
df = pd.read_csv(path_file, sep='|', encoding='ISO-8859-1')
df.columns
len(df.columns)
###Output
_____no_output_____
###Markdown
Checking How Empty/Full Columns are
###Code
value_count_cols = df.count()
value_count_cols.sort_values(inplace=True)
type(value_count_cols)
value_count_cols.plot.bar(rot=80, figsize=(16, 6))
print(value_count_cols)
df.groupby(['PurposeName', 'SDGfocus'])['ProjectNumber'].count()
df['LongDescription']
value_count_cols['LongDescription'], value_count_cols['LongDescription']/len(df)*100, value_count_cols['ShortDescription'], value_count_cols['ShortDescription']/len(df)*100
df['ShortDescription']
df['LongDescription']
###Output
_____no_output_____
###Markdown
Distribution of Text Size (Number of Tokens) in the Descriptions
###Code
df['LongDescription'] = df['LongDescription'].replace(np.nan, 'EMPTY_DESC')
df['ShortDescription'] = df['ShortDescription'].replace(np.nan, 'EMPTY_DESC')
df['LongDescriptionArray'] = df['LongDescription'].apply(lambda x: x.split())
df['ShortDescriptionArray'] = df['ShortDescription'].apply(lambda x: x.split())
df['LongDescriptionNumber'] = df['LongDescriptionArray'].apply(lambda x: len(x))
df['ShortDescriptionNumber'] = df['ShortDescriptionArray'].apply(lambda x: len(x))
df['LongDescriptionNumber'].plot.hist(bins=100, figsize=(16, 6), range=(0, 200))
plt.axvline(df['LongDescriptionNumber'].mean(), color='k', linestyle='dashed', linewidth=2)
plt.axvline(df['LongDescriptionNumber'].median(), color='r', linestyle='solid', linewidth=2)
df['ShortDescriptionNumber'].plot.hist(bins=100, figsize=(16, 6))
plt.axvline(df['ShortDescriptionNumber'].mean(), color='k', linestyle='dashed', linewidth=2)
plt.axvline(df['ShortDescriptionNumber'].median(), color='r', linestyle='solid', linewidth=2)
df['ShortDescription']
df.groupby(['LongDescription'])['Year'].count().sort_values(ascending=False).head(20)
df.groupby(['ShortDescription'])['Year'].count().sort_values(ascending=False).head(20)
###Output
_____no_output_____
###Markdown
Checking SDGs
###Code
df['SDGfocus'] = df['SDGfocus'].replace(np.nan, 'EMPTY_SDG').apply(str)
df['SDGfocusArray'] = df['SDGfocus'].apply(lambda x: [item.split('.')[0] for item in x.split(';')])
df['SDGfocusNumber'] = df['SDGfocusArray'].apply(lambda x: len(x))
df['SDGfocusArray']
df['SDGfocusNumber'].plot.hist(bins=20, figsize=(16, 6))
plt.axvline(df['SDGfocusNumber'].mean(), color='k', linestyle='dashed', linewidth=2)
plt.axvline(df['SDGfocusNumber'].median(), color='r', linestyle='solid', linewidth=2)
df['SDGfocusNumber'].median(), df['SDGfocusNumber'].mean()
df['SDGfocusTuple'] = df['SDGfocusArray'].apply(lambda x: tuple(x))
group_sdgs = df.groupby(['SDGfocusTuple']).count()['Year'] # Using Year as it is the most complete column of all
group_sdgs.sort_values(ascending=False)
group_sdgs.sort_values(ascending=False)/len(df)*100
###Output
_____no_output_____
###Markdown
This is good/interesting finding, around 25% of the data is labelled!!!! And that could be used to train the model that will then label the rest!!! Finding Specific Assignment of SDG per Project
###Code
df['EMPTY_SDG'] = df['SDGfocusArray'].apply(lambda x: True if 'EMPTY_SDG' in x else False)
df['SDG1'] = df['SDGfocusArray'].apply(lambda x: True if '1' in x else False)
df['SDG2'] = df['SDGfocusArray'].apply(lambda x: True if '2' in x else False)
df['SDG3'] = df['SDGfocusArray'].apply(lambda x: True if '3' in x else False)
df['SDG4'] = df['SDGfocusArray'].apply(lambda x: True if '4' in x else False)
df['SDG5'] = df['SDGfocusArray'].apply(lambda x: True if '5' in x else False)
df['SDG6'] = df['SDGfocusArray'].apply(lambda x: True if '6' in x else False)
df['SDG7'] = df['SDGfocusArray'].apply(lambda x: True if '7' in x else False)
df['SDG8'] = df['SDGfocusArray'].apply(lambda x: True if '8' in x else False)
df['SDG9'] = df['SDGfocusArray'].apply(lambda x: True if '9' in x else False)
df['SDG10'] = df['SDGfocusArray'].apply(lambda x: True if '10' in x else False)
df['SDG11'] = df['SDGfocusArray'].apply(lambda x: True if '11' in x else False)
df['SDG12'] = df['SDGfocusArray'].apply(lambda x: True if '12' in x else False)
df['SDG13'] = df['SDGfocusArray'].apply(lambda x: True if '13' in x else False)
df['SDG14'] = df['SDGfocusArray'].apply(lambda x: True if '14' in x else False)
df['SDG15'] = df['SDGfocusArray'].apply(lambda x: True if '15' in x else False)
df['SDG16'] = df['SDGfocusArray'].apply(lambda x: True if '16' in x else False)
df['SDG17'] = df['SDGfocusArray'].apply(lambda x: True if '17' in x else False)
df.groupby(['EMPTY_SDG', 'SDG1', 'SDG2', 'SDG3', 'SDG4', 'SDG5', 'SDG6', 'SDG7', 'SDG8',
'SDG9', 'SDG10', 'SDG11', 'SDG12', 'SDG13', 'SDG14', 'SDG15', 'SDG16', 'SDG17']).count()['Year']
###Output
_____no_output_____
###Markdown
Specifically looking into SDG 13 (Climate Action)
###Code
df.groupby(['SDG13']).count()['Year']
df.groupby(['SDG13']).count()['Year']/len(df)*100
# https://stackoverflow.com/questions/33388867/creating-circular-flow-charts-circos
# https://vega.github.io/vega/examples/edge-bundling/
columns_sdg = ['EMPTY_SDG', 'SDG1', 'SDG2', 'SDG3', 'SDG4', 'SDG5', 'SDG6', 'SDG7', 'SDG8', 'SDG9',
'SDG10', 'SDG11', 'SDG12', 'SDG13', 'SDG14', 'SDG15', 'SDG16', 'SDG17']
list_sdgs = df[columns_sdg].sum()
list_sdgs_per = df[columns_sdg].sum()/len(df)*100
list_sdgs.plot.bar(figsize=(16, 6))
list_sdgs_per.plot.bar(figsize=(16, 6))
list_sdgs_per
###Output
_____no_output_____ |
A better version of CSC(Classify Students Category)/A better version of CSC.ipynb | ###Markdown
> Before jumping into __Data Cleaning__ and __Feature Engineering__ lets make a model based on only 3 features (raisedhands, VisITedResources, AnnouncementsView) described in this [paper](https://github.com/78526Nasir/Kaggle-Student-s-Academic-Performance/blob/master/related%20resarch%20paper/Classify%20the%20Category%20of%20Students%20%20p28-alam.pdf) as top/most effective variables
###Code
top_features = ["raisedhands","VisITedResources","AnnouncementsView", "Discussion"]
X = dataset[top_features]
y = dataset["Class"]
class_map = {"L":0, "M":1, "H":2}
y = y.map(class_map)
features_train, features_test, labels_train, labels_test = train_test_split(X, y, test_size = .15, random_state=40)
# model build with SVM.SVC classifier
clf = SVC(kernel = 'linear')
clf.fit(features_train, labels_train)
pred = clf.predict(features_test)
accuracy_score(pred, labels_test)
from sklearn.neighbors import KNeighborsClassifier
KNN = KNeighborsClassifier(n_neighbors=1, leaf_size=10)
KNN.fit(features_train, labels_train)
KNNpred = clf.predict(features_test)
accuracy_score(KNNpred, labels_test)
from sklearn.naive_bayes import GaussianNB
clf = GaussianNB()
clf.fit(features_train, labels_train)
pred = clf.predict(features_test)
accuracy_score(pred, labels_test)
# Random Forest Classifier with 200 subtrees
clf = RandomForestClassifier(n_estimators = 200)
clf.fit(features_train, labels_train)
pred = clf.predict(features_test)
accuracy_score(pred, labels_test)
# Logistic regression
from sklearn.linear_model import LogisticRegression
clf = LogisticRegression()
clf.fit(features_train, labels_train)
pred = clf.predict(features_test)
accuracy_score(pred, labels_test)
# XGBoost Classifier
clf = XGBClassifier(max_depth=200, learning_rate=0.3, n_estimators=5, seed=1)
clf.fit(features_train, labels_train)
pred = clf.predict(features_test)
accuracy_score(pred, labels_test)
from sklearn.metrics import confusion_matrix, classification_report
print(confusion_matrix(pred, labels_test, labels=[1,0]))
###Output
[[26 2]
[ 3 18]]
###Markdown
actual True prediction* TP = 26* FN = 18 actual False prediction* FP = 2* FN = 3The model predicted __5__ observation wrongly.
###Code
print (classification_report(pred, labels_test, labels=[1, 0]))
fig, ax = plt.subplots(figsize=(12,6))
plot_importance(clf, ax = ax)
###Output
_____no_output_____
###Markdown
> Till now best accuracy on reduced features model: 0.78 Now lets deep dive into the dataset and start cleaning the data and do some feature engineering
###Code
dataset.head()
features = dataset.iloc[:,:-1]
labels = y
###Output
_____no_output_____
###Markdown
Applying Integer Encoding only those Feature which have Natural Order
###Code
stageID_map = {"lowerlevel":0, "MiddleSchool":1, "HighSchool":2}
features["StageID"] = features["StageID"].map(stageID_map)
gradeID_map = {"G-02":0, "G-04":1, "G-05":2, "G-06":3, "G-07":4, "G-08":5, "G-09":6, "G-10":7, "G-11":8, "G-12":9}
features["GradeID"] = features["GradeID"].map(gradeID_map)
sectionID_map = {"A":0, "B":1, "C":2}
features["SectionID"] = features["SectionID"].map(sectionID_map)
del features["PlaceofBirth"]
###Output
_____no_output_____
###Markdown
Applying One Hot Encoding rest of all
###Code
features = pd.get_dummies(features)
features.head()
from scipy.stats import chisquare
result = pd.DataFrame(columns=["Features", "Chi2Weights"])
for i in range(len(features.columns)):
chi2, p = chisquare(features[features.columns[i]])
result = result.append([pd.Series([features.columns[i], chi2], index = result.columns)], ignore_index=True)
result = result.sort_values(by="Chi2Weights", ascending=False)
result.head(10)
X = features
features_train, features_test, labels_train, labels_test = train_test_split(X, y, test_size = .20, random_state=0)
# model build with SVM.SVC classifier
clf = SVC(C=0.7, kernel = 'linear')
clf.fit(features_train, labels_train)
pred = clf.predict(features_test)
accuracy_score(pred, labels_test)
clf = RandomForestClassifier(n_estimators = 100, random_state=42)
clf.fit(features_train, labels_train)
pred = clf.predict(features_test)
rfc_pred = pred
accuracy_score(pred, labels_test)
clf = MLPClassifier(solver='lbfgs', alpha=0.00001, hidden_layer_sizes=(40,40,40), random_state=120)
clf.fit(features_train, labels_train)
pred = clf.predict(features_test)
accuracy_score(pred, labels_test)
clf = XGBClassifier(max_depth=5, learning_rate=0.2, n_estimators=20, seed=0)
clf.fit(features_train, labels_train)
pred = clf.predict(features_test)
xgb_pred = pred
accuracy_score(pred, labels_test)
fig, ax = plt.subplots(figsize=(12,6))
plot_importance(clf, ax = ax)
# Random Forest Classifier confustion Matrix result
confusion_matrix(labels_test, rfc_pred, labels=[1, 0])
# XGBoost Classifier confusion matric result
confusion_matrix(labels_test, xgb_pred, labels=[1, 0])
###Output
_____no_output_____
###Markdown
Till now highest Accuracy: 84.3% Feature Scaling
###Code
scaler = MinMaxScaler()
bumpy_features = ["raisedhands", "VisITedResources", "AnnouncementsView",'Discussion']
df_scaled = pd.DataFrame(data = X)
df_scaled[bumpy_features] = scaler.fit_transform(X[bumpy_features])
df_scaled.head()
###Output
_____no_output_____
###Markdown
Over Sampling
###Code
X = df_scaled
sm = SMOTE(random_state=7)
X_res, y_res = sm.fit_sample(X, y)
X_train, X_test, y_train, y_test = train_test_split(X_res, y_res, test_size= 0.15, random_state=7)
clf = XGBClassifier(max_depth = 10,random_state = 7, n_estimators=100, eval_metric = 'auc', min_child_weight = 3,
colsample_bytree = 0.75, subsample= 0.8)
clf.fit(X_train, y_train)
y_pred = clf.predict(X_test)
print("Accuracy:", accuracy_score(y_test, y_pred))
print("Precision:", precision_score(y_test, y_pred, average="weighted"))
print("Recall:", recall_score(y_test, y_pred, average="weighted"))
print("F1:", f1_score(y_test, y_pred, average="weighted"))
confusion_matrix(y_test, y_pred, labels=[1, 0])
###Output
_____no_output_____
###Markdown
Final Accuracy using ML classifier: 88.42%
###Code
fig, ax = plt.subplots(figsize = (20, 10))
sns.heatmap(X.corr())
###Output
_____no_output_____
###Markdown
Applying DL approach
###Code
y = dataset["Class"]
y = pd.get_dummies(y)
y.head()
train_x, test_x, train_y, test_y = train_test_split(X, y, test_size=0.1, random_state=7)
learning_rate = 0.3
trainning_epochs = 1000
cost_history = np.empty(shape=[1], dtype=float)
n_dim = X.shape[1]
n_class = 3
model_path = "model"
n_hidden_1 = 60
n_hidden_2 = 60
n_hidden_3 = 60
n_hidden_4 = 60
x = tf.placeholder(tf.float32, [None, n_dim])
w = tf.Variable(tf.zeros([n_dim, n_class]))
b = tf.Variable(tf.zeros(n_class))
y_ = tf.placeholder(tf.float32, [None, n_class])
weights = {
"h1" : tf.Variable(tf.truncated_normal([n_dim, n_hidden_1])),
"h2" : tf.Variable(tf.truncated_normal([n_hidden_1, n_hidden_2])),
"h3" : tf.Variable(tf.truncated_normal([n_hidden_2, n_hidden_3])),
"h4" : tf.Variable(tf.truncated_normal([n_hidden_3, n_hidden_4])),
"out" : tf.Variable(tf.truncated_normal([n_hidden_4, n_class]))
}
biases = {
"b1" : tf.Variable(tf.truncated_normal([n_hidden_1])),
"b2" : tf.Variable(tf.truncated_normal([n_hidden_2])),
"b3" : tf.Variable(tf.truncated_normal([n_hidden_3])),
"b4" : tf.Variable(tf.truncated_normal([n_hidden_4])),
"out" : tf.Variable(tf.truncated_normal([n_class]))
}
def multilayer_perceptron(x, weights, biases):
# hidden layer with "relu" activation
layer_1 = tf.add(tf.matmul(x, weights["h1"]), biases["b1"])
layer_1 = tf.nn.sigmoid(layer_1)
# hidden layer with "sigmoid" activation
layer_2 = tf.add(tf.matmul(layer_1, weights["h2"]), biases["b2"])
layer_2 = tf.nn.sigmoid(layer_2)
# hidden layer with "sigmoid" activation
layer_3 = tf.add(tf.matmul(layer_2, weights["h3"]), biases["b3"])
layer_3 = tf.nn.sigmoid(layer_3)
# hidden layer with "relu" activation
layer_4 = tf.add(tf.matmul(layer_3, weights["h4"]), biases["b4"])
layer_4 = tf.nn.relu(layer_4)
out_layer = tf.matmul(layer_4, weights["out"]) + biases["out"]
return out_layer
init = tf.global_variables_initializer()
saver = tf.train.Saver()
y = multilayer_perceptron(x, weights, biases)
cost_function = tf.reduce_mean(tf.nn.softmax_cross_entropy_with_logits(logits = y , labels = y_))
training_step = tf.train.GradientDescentOptimizer(learning_rate).minimize(cost_function)
sess = tf.Session()
sess.run(init)
mse_history = []
accuracy_history = []
for epoch in range(trainning_epochs):
sess.run(training_step, feed_dict= {x: train_x, y_: train_y})
cost = sess.run(cost_function, feed_dict = {x: train_x, y_:train_y})
cost_history = np.append(cost_history, cost)
correct_prediction = tf.equal(tf.argmax(y,1), tf.argmax(y_, 1))
accuracy = tf.reduce_mean(tf.cast(correct_prediction, tf.float32))
pred_y = sess.run(y, feed_dict={x : test_x})
mse = tf.reduce_mean(tf.square(pred_y - test_y))
mse_ = sess.run(mse)
mse_history.append(mse_)
accuracy = (sess.run(accuracy, feed_dict = {x : train_x, y_: train_y}))
accuracy_history.append(accuracy)
print ("epoch: ", epoch, "- cost: ", cost, "- MSE: ", mse_, "- Training Accuracy: ", accuracy)
plt.subplots(figsize=(10, 6))
plt.plot(accuracy_history)
plt.show()
correct_prediction = tf.equal(tf.argmax(y, 1), tf.argmax(y_, 1))
accuracy = tf.reduce_mean(tf.cast(correct_prediction, tf.float32))
print("Test Accuracy : ", (sess.run(accuracy, feed_dict = {x: test_x, y_:test_y})))
###Output
Test Accuracy : 0.8541667
|
07 Merge Sort.ipynb | ###Markdown
Merge Sort---A comparison-based algorithm that sorts a given dataset. It is classified as a **“divide and conquer”** algorithmThere are 2 approaches to implementing a merge sort:1. Top-Down Implementation2. Bottom-Up Implementation**Merge Sort Demonstration:**[Source](https://upload.wikimedia.org/wikipedia/commons/c/cc/Merge-sort-example-300px.gif)**Complexity of Merge Sort:**- $O(n log n)$ Worst Case Performance- $O(n log n)$ Average Case Performance- $O(n log n)$ Best Case Performance**Merge Sort Algorithm:**```-- Let A be an unsorted list, n represent size of Afunction: divider(A) Create two empty lists called Left and Right Get Midpoint at n/2 - 1, - all values before and include the midpoint is Left list - all values after midpoint is Right list Update Left to divider(Left) ... recursive call Update Right to divider(Right) ... recursive call return merge(Left, Right)-- Let X and Y be sorted List called from divider()function: merge(X, Y) Create empty list called Sorted While both x and y are not empty if X and Y are both non empty compare X[0] and Y[0] add the smaller value to Sorted removed the respective if X is empty and Y is not empty add all Y values to Sorted if Y is empty and X is not empty add all X values to Sorted return Sorted```**Python Implementation:**
###Code
# Recursive Merge Sort Python Implementation
# Top Down Approach
def mergeSort(array):
if len(array) <= 1:
return array
# end of base case
left = [] # Division of array : left half
right = [] # Divsion of array : right half
for i in range(len(array)):
if i < (len(array) // 2):
left.append(array[i])
else:
right.append(array[i])
# end of division
# recursive mergeSort of left and right
left = mergeSort(left)
right = mergeSort(right)
return merge(left, right)
# end of mergeSort()
def merge(left, right):
result = []
while left and right:
if left[0] <= right[0]:
result.append(left[0])
left = left[1:]
else:
result.append(right[0])
right = right[1:]
# end of handling left and right
while left:
result.append(left[0])
left = left[1:]
while right:
result.append(right[0])
right = right[1:]
return result
# end of merge()
test = [6, 5, 3, 1, 8, 7, 3, 4]
sorted_test = mergeSort(test) # creates a new sorted list
print('Sorted:', sorted_test)
###Output
Sorted: [1, 3, 3, 4, 5, 6, 7, 8]
|
Machine Learning with Python/Code Learning Algorithms (A, B, C, D, E)/notes (part A,B,C,D).ipynb | ###Markdown
TensorFlow Core Learning Algorithms:There are 4 main learning algorithms that are a part of TensorFlow, these are:* Linear Regression* Classification* Clustering* Hidden Markov ModelsMost Business and Application implementations of Machine Learning use very basic Machine Learning models, as even these very simple models are capable of doing some very powerful things.These fundamental/'*core*' Machine Learning models can be used in almost all basic applications.There are many different tools and methods to solve the same problems within TensorFlow, although the ones that I will be going through are those chosen by the lecture.***DON'T*** feel pressured to memorise the syntax! Linear Regression:"One of the most basic forms of Machine Learning, and is used to predict numerical values""Line of best fit refers to a line through a scatter plot of data points that best expresses the relationship between those points"**Project:**In this lecture we'll be using Linear Regression to predict the survival rate of passengers from the Titanic* This uses https://www.tensorflow.org/tutorials/estimator/linear heavily How Linear Regression Works:Linear Regression refers to a way of calculating a 'line of best fit'. If data-points are related linearly, you are able to create a line of best fit, and predict future values.**Here is an example**:
###Code
import matplotlib.pyplot as plt
import numpy as np
#making a simple dataset:
x = [1, 2, 2.5, 3, 4]
y = [1, 4, 7, 9, 15]
plt.plot(x, y, "ro")
plt.axis([0, 6, 0, 20])
###Output
_____no_output_____
###Markdown
From this graph, it is evident that the dataset has a linear correspondance, and could therefore benefit from Linear Regression to predict future values.In this example, this will only use one input variable, as it is 2 dimensional, although in larger datasets, it will requite a lot more variables and inputs.An equation for a line in 2d is: $$y=mx+c$$Here is an example of a line of best fit for the above graph:
###Code
import matplotlib.pyplot as plt
import numpy as np
#making a simple dataset:
x = [1, 2, 2.5, 3, 4]
y = [1, 4, 7, 9, 15]
plt.plot(x, y, "ro")
plt.axis([0, 6, 0, 20])
#Original dataset for convenience
plt.plot(np.unique(x), np.poly1d(np.polyfit(x, y, 1))(np.unique(x)))
#Linear regression example
plt.show
###Output
_____no_output_____
###Markdown
With this line of best fit, you are able to predict future values. SetupThis portion includes most of the imports:
###Code
#All the needed imports for this example:
from __future__ import absolute_import, division, print_function, unicode_literals
import numpy as np
import pandas as pd
import matplotlib.pyplot as plt
from IPython.display import clear_output
from six.moves import urllib
import tensorflow.compat.v2.feature_column as fc
import tensorflow as tf
###Output
_____no_output_____
###Markdown
Data:Data is the **MOST** important part of Machine Learning!In this example, I'll go through exploring, cleaning and selecting appropriate data.This uses the 'Titanic' dataset, which has a large amount of information for each passenger, so I'll go through the data and explore!**Here I'll go through loading the database and how to explore it using built-in tools**
###Code
# Load dataset.
dftrain = pd.read_csv('https://storage.googleapis.com/tf-datasets/titanic/train.csv') #training
dfeval = pd.read_csv('https://storage.googleapis.com/tf-datasets/titanic/eval.csv') #testing
y_train = dftrain.pop('survived') # removes this column from the original datasets, and puts it in it's own variable
y_eval = dfeval.pop('survived')
###Output
_____no_output_____
###Markdown
Using the `pd.read_csv()` method allows for the creation of a 'dataframe' (a bit like a large table), which you can refer to and edit later.In this example, we used the '**survived**' column and sorted it into a variable.To look at the data, I'll be using ``.head()`` from Pandas. This allows for looking at the '*head*' of the data (i.e. the first 5 lines), a bit like it is in Unix.
###Code
dftrain.head() # Looking at the top 5 columns of this dataset
###Output
_____no_output_____
###Markdown
Same thing for the other dataset
###Code
dfeval.head() #looking at the top 5 columns of this dataset
###Output
_____no_output_____
###Markdown
To test how many rows there are in each dataset, you can use the ``.shape()`` command.
###Code
dftrain.shape[0], dfeval.shape[0]
# In this case there are 627 lines in dftrain and 264 in dfeval
###Output
_____no_output_____
###Markdown
If you want to have a more statistical analysis of the data, you can use the ``.describe()`` command. This gives some common statistical values that can be useful.
###Code
dftrain.describe()
###Output
_____no_output_____
###Markdown
Same thing for the other dataset
###Code
dfeval.describe()
###Output
_____no_output_____
###Markdown
In order to make more sense of the data, it is valuable to generate some visual representations to look at potential ways you can work with the data.
###Code
# A Histogram to denote age:
dftrain.age.hist(bins=20)
###Output
_____no_output_____
###Markdown
From this histogram, it is quite obvious that most of the passengers in this training set are in their 20s and 30s.
###Code
# A bar chart to represent sex in those who travelled:
dftrain.sex.value_counts().plot(kind='barh')
###Output
_____no_output_____
###Markdown
From this bar chart, it is quite obvious that there were about twice the amount of males compared to females.
###Code
# Looking at what class most of the passengers were in:
dftrain['class'].value_counts().plot(kind='barh')
###Output
_____no_output_____
###Markdown
From this bar graph, it is quite obvious that the majority of passengers were in Third class, with Second and First class having similar numbers.
###Code
#Looking at survival rate and sex (seeing if there is a correlation)
pd.concat([dftrain, y_train], axis=1).groupby('sex').survived.mean().plot(kind='barh').set_xlabel('% survive')
###Output
_____no_output_____
###Markdown
From this bar graph it is very obvious that if you are female in this example with the Titanic, you have a significantly larger chance of survival. This therefore has a good chance of being a predictive feature for this model. Training vs Testing data:When creating a Machine Learning model, it is important to use multiple datasets, usually using one to train the Machine Learning model, and another to test and validate the Machine Learning model.In this example, there are 2 datasets, these are:* **Training** * Usually much larger than the testing dataset, allows for the model to adapt and learn.* **Testing** * Allows for you to use a different set of data (to avoid the model from potentially 'memorising' the data), to test how well the model is going. This allows for the model to be able to work on '***NEW***' data, therefore requires for there to be seperate datasets for testing and training.This can be seen in the difference in dataset size, as seen below (already seen above, but I'll do it again for convenience).
###Code
Training = dftrain.shape
Evaluation = dfeval.shape
print("Training Dataset size:")
print(Training) #Shows the amount of rows (amount of data points), and the amount of columns (amount of variables per data point)
print("Evaluation Dataset size:")
print(Evaluation) #Shows the amount of rows (amount of data points), and the amount of columns (amount of variables per data point)
###Output
Training Dataset size:
(627, 9)
Evaluation Dataset size:
(264, 9)
###Markdown
From this, it is evident that the training dataset is significantly larger compared to the testing dataset. Catagorising Data:There are 2 main types of data, and it is important to separate them (so the Machine Learning algorithm is able to manipulate them accordingly).These are:* **Catagorical Data** * Catagorical data represents data which is in 'words', and isn't numerical. This usually can be seen as headings on a table. * In this example, catagorical data represents the headings on the table which represent any text based data. * Catagorical data is '***encoded***' using an integer value so the Machine Learning algorithm is able to work with the data.* **Numerical Data** * Numerical data represents anything that relates to numbers. * In this example, it represents any headings that represent and number based data. * Numerical data ***does not*** need to be encoded, as it '*already is*'.
###Code
# seperating the dataset:
CATEGORICAL_COLUMNS = ['sex', 'n_siblings_spouses', 'parch', 'class', 'deck',
'embark_town', 'alone'] #Categorical Data
NUMERIC_COLUMNS = ['age', 'fare'] #Numeric Data
#Some loops to feed in the data into the
#TensorFlow Linear Regression Estimator Model (aka Feature Columns):
feature_columns = [] #blank list to store all the feature columns
#Categorical Data
for feature_name in CATEGORICAL_COLUMNS:
vocabulary = dftrain[feature_name].unique() #Goes through each data-frame (such as 'sex'), then gets all the unique values
feature_columns.append(tf.feature_column.categorical_column_with_vocabulary_list(feature_name, vocabulary))
for feature_name in NUMERIC_COLUMNS:
feature_columns.append(tf.feature_column.numeric_column(feature_name, dtype=tf.float32))
###Output
_____no_output_____
###Markdown
Just to explanation's sake, here is some more information:
###Code
#Categorical data example:
dftrain['embark_town'].unique()
#Adds to the vocabulary, and you can then create a 'numerical' code for it.
###Output
_____no_output_____
###Markdown
The 'Training Process':Models are loaded in 'batches' (to allow for extremely large training data to fit within RAM). In this example, it will be fed in batches of 32.These batches will be fed into the model a certain amount of times (as dictated by the number of ***epochs***)**Epoch**: * One stream of the entire dataset (i.e. the entire data set being fed into the model). * The number of epochs set for the Machine Learning model dictates how well it can react to an unknown set of data (therefore it is optimal to run through multiple epochs) * E.g. if there are 10 epochs, the Machine Learning model will go through the same dataset 10 times.As you need to load the input data in batches, and multiple times, it is required to make an '*input function*'. This function simply defines how the dataset will be converted into batches at each epoch. **Input Function:**The TensorFlow model which is being used here requires that the data is passed as a ``tf.data.Dataset`` object. This means that an *input function* must be created to convert the current pandas dataframe into that object.This is quite complex, although there should be some comments to make it a bit more understandable.You will never have to code an input function from scratch, you can usually find one in documentation.
###Code
def make_input_fn(data_df, label_df, num_epochs=10, shuffle=True, batch_size=32):
#^^ Make Input Function: import data-dataframe, label-dataframe, at 10 epochs, shuffle the data and with a batch size of 32
def input_function(): #inner function (this will be returned)
ds = tf.data.Dataset.from_tensor_slices((dict(data_df), label_df)) # create tf.data.Dataset object with data and its label
if shuffle:
ds = ds.shuffle(1000) #randomise the order of the data.
ds = ds.batch(batch_size).repeat(num_epochs) #split data into batches, and repeat for the number of epochs
return ds #return a batch of the dataset
return input_function #return a function object for use
train_input_fn = make_input_fn(dftrain, y_train) #calling the input function for both of these, although there can be some changes for the input values for the function.
eval_input_fn = make_input_fn(dfeval, y_eval, num_epochs=1, shuffle=False)
###Output
_____no_output_____
###Markdown
Creating the model:In this example, I will be using the 'Linear Estimator' function of TensorFlow in order to use the Linear Regression model.
###Code
linear_est = tf.estimator.LinearClassifier(feature_columns=feature_columns)
#creating a linear estimator by passing through the feature columns that were created earlier
clear_output() #Just to clean up the output, otherwise it takes up a lot of space ;)
###Output
_____no_output_____
###Markdown
Training the model:All we have to do in this example is pass through the input functions from earlier.
###Code
linear_est.train(train_input_fn) #train
result = linear_est.evaluate(eval_input_fn) #get the model metrics by testing on the testing data
clear_output() #clear console output
print("Accuracy of Model:")
accuracy = (result['accuracy']*100) #result variable is just the metrics, here I am just outputing the accuracy.
percentage = str(accuracy)+"%"
print(percentage)
###Output
Accuracy of Model:
71.59090638160706%
###Markdown
Getting an answer:From this training, you are able to get a prediction on how many people will survive. You can get this value by looking through the output results, and outputting the specific part of the dictionary you need.
###Code
result = list(linear_est.predict(eval_input_fn))
clear_output() # clear console output
f = 0
while f < 4: #small script to loop through the information and predicted change of death (top 7 results from testing dataset)
print("Passenger number: " + str(f))
print("")
print("Information of passenger:")
print(dfeval.loc[f])
print("")
print("Chance of survival:")
survival = (result[f]['probabilities'][1]*100) #looking through the dictionary, and getting chance of survival
print(str(survival)+"%")
print("")
print("Chance of death:")
death = (result[f]['probabilities'][0]*100) #looking through the dictionary, and getting chance of death
print(str(death)+"%")
print("")
print("Did they survive?:")
if y_eval.loc[f] == 1:
print("This person survived")
else:
print("This person did not survive")
print("")
print("\n")
o = f + 1
f = o
#From this output, you are able to tell the prediction that the Machine Learning model made, and what the actual result was (Percentage chance of dying and survivng, vs if they actually survived.)
###Output
Passenger number: 0
Information of passenger:
sex male
age 35.0
n_siblings_spouses 0
parch 0
fare 8.05
class Third
deck unknown
embark_town Southampton
alone y
Name: 0, dtype: object
Chance of survival:
20.417557656764984%
Chance of death:
79.58244681358337%
Did they survive?:
This person did not survive
Passenger number: 1
Information of passenger:
sex male
age 54.0
n_siblings_spouses 0
parch 0
fare 51.8625
class First
deck E
embark_town Southampton
alone y
Name: 1, dtype: object
Chance of survival:
82.0199728012085%
Chance of death:
17.980027198791504%
Did they survive?:
This person did not survive
Passenger number: 2
Information of passenger:
sex female
age 58.0
n_siblings_spouses 0
parch 0
fare 26.55
class First
deck C
embark_town Southampton
alone y
Name: 2, dtype: object
Chance of survival:
95.21548748016357%
Chance of death:
4.784515127539635%
Did they survive?:
This person survived
Passenger number: 3
Information of passenger:
sex female
age 55.0
n_siblings_spouses 0
parch 0
fare 16.0
class Second
deck unknown
embark_town Southampton
alone y
Name: 3, dtype: object
Chance of survival:
91.11027717590332%
Chance of death:
8.8897205889225%
Did they survive?:
This person survived
|
Prace_domowe/Praca_domowa6/Grupa2/PingielskiJakub/Praca_domowa_06.ipynb | ###Markdown
Based on visual assesment, data can be divided into 4 well separated clusters.
###Code
def create_clustering_algorithms(n_clusters):
two_means = cluster.MiniBatchKMeans(n_clusters=n_clusters)
ward = cluster.AgglomerativeClustering(n_clusters=n_clusters, linkage='ward')
average_linkage = cluster.AgglomerativeClustering(n_clusters=n_clusters, linkage="average")
birch = cluster.Birch(n_clusters=n_clusters)
gmm = mixture.GaussianMixture(n_components=n_clusters)
clustering_algorithms = (('MiniBatchKMeans', two_means),
('Ward', ward),
('AgglomerativeClustering', average_linkage),
('GaussianMixture', gmm))
return clustering_algorithms
algorithms_names = [
'MiniBatchKMeans', 'Ward', 'AgglomerativeClustering',
'GaussianMixture'
]
silhouette_scores = pd.DataFrame(index=range(2, 15), columns=algorithms_names)
davies_bouldin_scores = pd.DataFrame(index=range(2, 15), columns=algorithms_names)
calinski_harabasz_scores = pd.DataFrame(index=range(2, 15), columns=algorithms_names)
# Calculating metrics
for n_clusters in range(2, 15):
clustering_algorithms = create_clustering_algorithms(n_clusters)
for name, algorithm in clustering_algorithms:
algorithm.fit(data)
if hasattr(algorithm, 'labels_'):
y_pred = algorithm.labels_.astype(np.int)
else:
y_pred = algorithm.predict(data)
db_score = davies_bouldin_score(data, y_pred)
ch_score = calinski_harabasz_score(data, y_pred)
s_score = silhouette_score(data, y_pred)
davies_bouldin_scores.loc[n_clusters, name] = db_score
calinski_harabasz_scores.loc[n_clusters, name] = ch_score
silhouette_scores.loc[n_clusters, name] = s_score
fig, axes = plt.subplots(nrows=1, ncols=3, figsize=(15, 5))
davies_bouldin_scores.plot(ax=axes[0], legend=None, title='davies_bouldain')
calinski_harabasz_scores.plot(ax=axes[1], legend=None, title='calinski_harabasz')
silhouette_scores.plot(ax=axes[2], title='silhouette');
###Output
_____no_output_____
###Markdown
**Silhouette Coefficient** takes into account two values: the mean distance between a sample and all other points in the same class and the mean distance between a sample and all other points in the next nearest cluster. The score is bounded between -1 for incorrect clustering and +1 for highly dense clustering. Scores around zero indicate overlapping clusters.**Calinski-Harabasz Index** is the ratio of the sum of between-clusters dispersion and of inter-cluster dispersion for all clusters. The score is higher when clusters are dense and well separated. **Davies-Bouldin Index** signifies the average ‘similarity’ between clusters, where the similarity is a measure that compares the distance between clusters with the size of the clusters themselves.Zero is the lowest possible score. Values closer to zero indicate a better partition. For Davies-Bouldin Index best (smallest) values are achieved for 2 clusters, for Calinski-Harabasz Index best (highest) values are achieved for 6-8 clusters and for silhoutette score best values are achieved for 2 clusters.There is no consensus in regards to optimal number of clusters.
###Code
def plot_clustering_results(n_clusters):
clustering_algorithms = create_clustering_algorithms(n_clusters)
plot_num = 1
plt.figure(figsize=(16, 4))
for name, algorithm in clustering_algorithms:
algorithm.fit(data)
if hasattr(algorithm, 'labels_'):
y_pred = algorithm.labels_.astype(np.int)
else:
y_pred = algorithm.predict(data)
plt.subplot(1, len(clustering_algorithms), plot_num)
plt.scatter(data.X1, data.X2, c=y_pred, s=30, cmap='viridis')
plt.title(name)
plot_num += 1
plot_clustering_results(2)
###Output
_____no_output_____
###Markdown
Dividing data into only two clusters shows that all algorithms joined pairs of well separated clusters together.
###Code
plot_clustering_results(4)
###Output
_____no_output_____ |
src/data/initial_exploration-20m.ipynb | ###Markdown
MovieLens 20M
###Code
import pandas as pd
import matplotlib.pyplot as plt
%matplotlib inline
ratings = pd.read_csv('../../data/20m_raw/ratings.csv')
movies = pd.read_csv('../../data/20m_raw/movies.csv')
###Output
_____no_output_____
###Markdown
Movies dataframe contains a separate entry for each movie in the dataset ofering 3 attributes:- **id**- movie **title**- a list of **genres**
###Code
movies.head()
movies_no = len(movies)
print("Total number of movies = {0}".format(movies_no))
###Output
Total number of movies = 27278
###Markdown
Ratings dataframe contains 20 millions ratings. Each rating has 4 attributes:- **userId**: the unique identifier of the user who offered the movie rating- **movieId**: the unique identifier of the rated movie- **rating**: a numeric value from 0.5 to 5 stars with half-star increment- **timestamp**: defines the datetime when the movie was rated
###Code
ratings.head()
ratings_no = len(ratings)
print("Total number of ratings = {0}".format(ratings_no))
ratings.hist(column='rating', figsize=(10,5))
print("Average rating = {0}".format(ratings['rating'].mean()))
print("Average number of ratings per movie = {0}".format(ratings_no/movies_no))
nr_ratings_per_movie = ratings.groupby("movieId").size().reset_index(name='counts').sort_values('counts', ascending=False)
print("Number of ratings per movie:\n", nr_ratings_per_movie)
less_than_3_ratings = nr_ratings_per_movie[nr_ratings_per_movie['counts']<3]
print("\nNumber of movies that have less than 3 ratings: ", len(less_than_3_ratings))
###Output
Number of ratings per movie:
movieId counts
293 296 67310
352 356 66172
315 318 63366
587 593 63299
476 480 59715
... ... ...
23146 110807 1
23148 110811 1
15481 78984 1
23150 110818 1
26743 131262 1
[26744 rows x 2 columns]
Number of movies that have less than 3 ratings: 6015
###Markdown
There are 6015 that have less a total of 1 or 2 reviews. When training the Word2Vec model, these movies are going to be removed from the vocabulary.
###Code
len(nr_ratings_per_movie[nr_ratings_per_movie['counts']>2])
###Output
_____no_output_____ |
notebooks/Spam Filter on local spark.jupyter.ipynb | ###Markdown
Reading the dataset from Spark local
###Code
import os
# Add asset from file system
textData = sc.textFile(os.environ['DSX_PROJECT_DIR']+'/datasets/SMSSpamCollection.csv')
###Output
_____no_output_____
###Markdown
Creating a Spark data pipeline
###Code
from pyspark.ml import Pipeline
from pyspark.ml.feature import HashingTF, IDF
from pyspark.ml.feature import Tokenizer
from pyspark.ml.classification import LogisticRegression
tokenizer = Tokenizer(inputCol="message",outputCol="words")
hashingTF = HashingTF(inputCol = tokenizer.getOutputCol(),outputCol="tempfeatures")
idf = IDF(inputCol = hashingTF.getOutputCol(),outputCol="features")
lrClassifier = LogisticRegression()
pipeline = Pipeline(stages=[tokenizer,hashingTF,idf,lrClassifier])
###Output
_____no_output_____
###Markdown
Cleaning the Data
###Code
# creating a labeled vector
def TransformToVector(string):
attList = string.split(",")
smsType = 0.0 if attList[0] == "ham" else 1.0
return [smsType,attList[1]]
textTransformed = textData.map(TransformToVector)
# creating a data frame from labeled vector
from pyspark.sql import SQLContext
sqlContext = SQLContext(sc)
textDF = sqlContext.createDataFrame(textTransformed,["label","message"])
###Output
_____no_output_____
###Markdown
Build your LR Model using SparkML
###Code
# split data frame into training and testing
(trainingData,testData) = textDF.randomSplit([0.9,0.1])
#Build a model with Pipeline
lrModel = pipeline.fit(trainingData)
#Compute Predictions
prediction = lrModel.transform(testData)
#Evaluate Accuracy
from pyspark.ml.evaluation import MulticlassClassificationEvaluator
evaluator = MulticlassClassificationEvaluator(predictionCol="prediction", \
labelCol="label", \
metricName = "accuracy")
accuracy = evaluator.evaluate(prediction)
print "Model Accuracy: " + str(round(accuracy*100,2))
# Draw a confusion matrix
prediction.groupby("label","prediction").count().show()
from dsx_ml.ml import save
save(name = 'LRModel_SparkLocal',
model = lrModel,
test_data = testData,
algorithm_type = 'Classification')
textDF.coalesce(1).write.csv(os.environ['DSX_PROJECT_DIR']+'/datasets/sms-data.csv')
###Output
_____no_output_____ |
inference/inference_w2v_300.ipynb | ###Markdown
Loading
###Code
%%time
wv = Word2Vec.load("w2v_300.model").wv
wv.init_sims(replace=True)
%%time
nn_model = load_model("nn_w2v_300.h5")
def inference(input_text):
text_pp = []
for i in input_text:
text_pp.append(preprocess(i[0]))
text_vec = word_averaging(wv, text_pp)
predictions = nn_model.predict(text_vec)
result = {1:'World', 2:'Sports', 3:'Business', 4:'Science', 5:'Corona'}
print([result[r] for r in predictions.argmax(axis=1)+1])
print([max(predictions[p]) for p in range(len(input_text))])
###Output
_____no_output_____
###Markdown
Inference DW News
###Code
# Corona
input_text = [['As France enters the "Green Zone" lower risk state against COVID-19 on Monday, Emmanuel Macron vows to prop up the economy along with the rest of Europe. France has been hit hard by the novel coronavirus.'],
['COVID-19 has taken a metaphorical sledgehammer to global tourism, although European nations are trying to revive the industry. Not so in Ireland or the UK, where stringent quarantine rules further threaten the sector.'],
['A man has died after becoming infected with COVID-19 at a Pentecost service in the northern city of Bremerhaven. This isnt the first time a church in Germany has been at the center of an outbreak.'],
['COVID-19 studies are being uploaded in great numbers to preprint servers without lengthy peer review processes. Is that good or bad? The fact is that there is no such thing as an absolute guarantee for good research. '],
['In South Asian nations like Pakistan, where child labor is rampant, COVID-19 has brought more hardship to underage workers. Meanwhile, the resulting economic crisis is pushing even more children into child labor. ']
]
%%time
inference(input_text)
# Sport
input_text = [['Bayern Munich will win the title for an eighth straight time if they can win for the 11th straight time when they visit Bremen on Tuesday. Elsewhere, the fight for survival has heated up — but Paderborn are all but down. '],
['Bayern Munich are poised to secure an eighth consecutive league title this week, but there is still much to be decided in the Bundesliga with three games to go. DW analyzes the race for Europe and the relegation battle. '],
['A week after Weston McKennie, Jadon Sancho and others delivered individual statements of support to the Black Lives Matter movement, Bundesliga clubs showed their collective solidarity. '],
['There was early drama as RB Leipzig won on Julian Nagelsmanns first return to Hoffenheim. While the defeat dents Hoffenheim’s hopes of European football, Leipzig are on track for a Champions League place. '],
['Bad news for the chauvinists at the football table. Scientists from Germanys Sport University Cologne have proved that women who play football can implement tactical approaches just as well as men. ']
]
%%time
inference(input_text)
# Business
input_text = [['The principal bench of the National Company Law Tribunal (NCLT) in New Delhi ruled that the liquidator has overriding powers under the Insolvency and Bankruptcy Code to take over both movable and immovable assets of a corporate debtor.'],
['Shares in German payment service provider Wirecard lost more than half their value within minutes on Thursday after the DAX-listed company said it was not possible for it to publish a delayed annual report due to worrisome audit data.'],
['Berlin says it regrets a US plan to expand sanctions on the Nord Stream 2 gas pipeline. US senators announced new sanctions on the project last week, saying the pipeline would boost Moscow’s influence in Europe.'],
['From bulky spaceship-like devices to sleek black boxes, consoles have come a long way in recent decades. That has gone hand in hand with the targeting of new products not just to kids, but to adults too.'],
['Nord Stream 2, which was originally scheduled to start delivering gas from Russia to Western Europe toward the end of 2019, is almost completed. Of a total of 2,360 kilometers (1,466 miles), 2,200 kilometers of the pipeline have been laid.']
]
%%time
inference(input_text)
# Science
input_text = [['Every 18 to 24 months, Earth and Mars align in such a way as to make deep-space travel that little bit easier, or at least a bit faster. That reduces a trip or "trajectory" to the Red Planet from about nine months down to seven.'],
['The impressive pyramid-style cities of the ancient Mayan culture, such as at Tikal in Guatemala, can be found described in any travel book.But the many of the other monumental buildings, houses, roads and paths, water works and drainage systems, and terraces still lay hidden in dense rain forest.'],
['Everything about this NASA SpaceX Demo-2 mission is symbolic. It seems that every effort has been made to draw a direct parallel between the last human spaceflight from America, and the Apollo moon missions before that.'],
['Heres a simple fact to start: The oceans are huge. Oceans make up about 96.5% of all Earths water. Theres fresh water in the planet, in the ground or elsewhere on land in rivers and lakes — more than 70% of the planet is covered in water — and theres more all around us in the atmosphere. But the oceans are simply huge.'],
['Second only to leukemia, brain tumors are top of the list of common forms of cancer in children and the young. The German Brain Tumor Association says 25% of all cancer diagnoses in the young involve tumors in the brain and central nervous system. Its often kids at the age of six-and-a-half, and boys more often than girls.']
]
%%time
inference(input_text)
# World
input_text = [['Three opposition activists from the Movement for Democratic Change-Alliance (MDC-Alliance) disappeared in May after being detained by police while on their way to an anti-government protest The women were found badly injured outside the capital Harare nearly 48 hours later and immediately hospitalized. They say they were abducted, sexually abused and forced to drink their urine.'],
['Javed Akhtar, 75, has been vocal about his views on politics, religion and public life and has often spoken out against religious fundamentalism and restrictions on freedom of speech. He has also heavily criticized communalism within Islam while denouncing the anti-Muslim sentiment advocated by the Hindu right.'],
['UN Secretary-General Antonio Guterres annual report on children and armed conflict, issued at the start of the week, featured a slight tweak for the year: the Saudi-led coalition waging war in Yemen was omitted from its list of offenders.Dubbed the "list of shame," this annex to the report names groups that fail to comply with measures aimed at ensuring the safety of children in armed conflict.'],
['The European Court of Justice (ECJ) ruled Thursday that a Hungarian law concerning the foreign funding of non-governmental organizations (NGOs) was illegal. Hungarys restrictions on the funding of civil organisations by persons established outside that member state do not comply with the Union law, the Luxembourg-based court said in a statement.'],
['United Nations members voted in four new members of the powerful Security Council in New York on Wednesday, but failed to decide on which African nation should fill the African regional seat up for grabs. In Wednesdays vote, Kenya received 113 votes while Djibouti got 78. With both failing to gain the two-thirds majority needed to win the Africa seat on the council, the two countries will face off on in a second round of voting on Thursday morning.']
]
%%time
inference(input_text)
###Output
['Business', 'World', 'Business', 'Corona', 'Business']
[0.64080423, 0.81735224, 0.6694361, 0.53003347, 0.7049726]
CPU times: user 28.9 ms, sys: 73 µs, total: 29 ms
Wall time: 26.5 ms
|
Portfolio+management+by+Natural+Evolution+Strategies++20180107.ipynb | ###Markdown
0. Import library
###Code
import time
import numpy as np
from numpy.random import *
np.random.seed(0)
###Output
_____no_output_____
###Markdown
1. Prepare input data
###Code
npop = 10 # population size
sigma = 0.1 # noise standard deviation
alpha = 0.001 # learning rate
w = np.random.rand(3) # our initial guess is random
w = w/sum(w) # notmalization (sum of w =1)
w
cov = np.array([(6,-5,4), (-5,17,-11),(4,-11,24)]) # covariance matrix of the portfolio
mu=np.array([8,12,15]) # avarage return of each asset in the portfolio
num=len(mu)
beta=2
###Output
_____no_output_____
###Markdown
2. Create the functions 2.1 The function for evaluate actions
###Code
# The function for evaluate actions
def f(w,mu,cov,beta):
preturn=np.dot(mu, w)
pvar=0.5*beta*(np.dot(np.dot(w, cov), w))
reward=preturn-pvar
return reward
###Output
_____no_output_____
###Markdown
2.2 Create initial populations and reward matrix
###Code
# nitial populations and reward matrix
N = np.random.randn(npop, 3) # samples from a normal distribution N(0,1)
R = np.zeros(npop)
N,R
###Output
_____no_output_____
###Markdown
2.3 Inject noise into parameters
###Code
w_try = w + sigma*N[0] # jitter w using gaussian of sigma 0.1
R[0] = f(w_try,mu,cov,beta) # evaluate the jittered version
w_try,R
###Output
_____no_output_____
###Markdown
3. Train the model
###Code
# Train the model
t=time.time()
for i in range(100000):
# initialize memory for a population of w's, and their rewards
N = np.random.randn(npop, 3) # samples from a normal distribution N(0,1)
R = np.zeros(npop)
for j in range(npop):
w_try = w + sigma*N[j] # inject noise using gaussian of sigma 0.1
R[j] = f(w_try,mu,cov,beta) # evaluate the function after injecting noise to parameters
# standardize the rewards to have a gaussian distribution
A = (R - np.mean(R)) / np.std(R)
# perform the parameter update.
w = w + alpha/(npop*sigma) * np.dot(N.T, A)
w = w/sum(w)
t2 = time.time()
print(round(t2-t, 5), 'Seconds to predict')
Return=np.dot(w, mu) # caluculate avarage return of the portfolio
Risk=np.sqrt(np.dot(np.dot(w, cov), w)) # caluculate standard deviation of the portfolio
w,Return,Risk
###Output
16.98766 Seconds to predict
|
notebooks/module_examples/io.ipynb | ###Markdown
parsing a data collection into an indexable dataframeThe parsing pattern follows python string formatting style. e.g. parsing time on 4 digits: `'{time:04d}'`
###Code
datadir = '../../data/2D'
data_pattern = '{platedir}/{layer}/{f1}_{f2}_{f3}_{row}{col:02d}_T{T:04d}F{field:03d}L{L:02d}A{A:02d}Z{zslice:02d}C{channel:02d}.{ext}'
index = ['platedir', 'layer', 'row', 'col', 'field', 'zslice', 'channel']
df = parse_collection(os.path.join(datadir,data_pattern), index)
df
###Output
_____no_output_____
###Markdown
build selection menu from a data collectionCollectionHandler builds an interactive menu matching the index/multi-index of a dataframe. The indexed dataframe is available as "subdf" attribute.
###Code
from inter_view.io import CollectionHandler
collection_handler = CollectionHandler(df=df)
collection_handler.panel()
###Output
_____no_output_____
###Markdown
extension allowing multiple selection on chosen index levelscan for instance be used to interactively chelect channels
###Code
from inter_view.io import MultiCollectionHandler
collection_handler = MultiCollectionHandler(df=df, multi_select_levels=['zslice', 'channel'])
collection_handler.panel()
###Output
_____no_output_____
###Markdown
automatically loading files associated with current sub-collectionDataLoader extends MultiCollectionHandler. It expects the dataframe to corresponds to files available on disc and indexable/readable with `dc` pandas accessor (see data collection parsing step). Every time the sub-collection changes, corresponding files are automatically loaded and available under dictionary argument `loaded_objects`.Reading functions available trough pandas `dc` accessor are used by default. A custom files reading function `loading_fun` can also be provided. e.g. to only read the first channel of multi-channel files. If multiple files are selected, `loading_fun` reads files in parallel with multi-threading.
###Code
from inter_view.io import DataLoader
from skimage.io import imread
def read_first_slice(path):
return imread(path, img_num=0)
data_loader = DataLoader(df=df,
multi_select_levels=['channel'],
loading_fun=read_first_slice,)
data_loader.panel()
data_loader.loaded_objects
###Output
_____no_output_____ |
Scala/3.5.4.ipynb | ###Markdown
" Module 5: Pipeline and Grid Search Predicting Grant Applications: Cross Validation and Model Tuning Lesson Objectives* After completing this lesson, you should be able to: - Avoid overfitting by using cross validation when training a model - Improve a model's performance by using grid search to find better parameters Choosing Parameters for Tuning load grant data
###Code
import org.apache.spark.sql.SparkSession
val spark = SparkSession.builder().getOrCreate()
import spark.implicits._
import org.apache.spark.sql.functions._
val data = spark.read.
format("com.databricks.spark.csv").
option("delimiter", "\t").
option("header", "true").
option("inferSchema", "true").
load("/resources/data/grantsPeople.csv")
data.show()
###Output
+--------------------+----------+---------------+---------+--------------+---------+--------------------+-------------+----------------+-------------+--------+----------+--------+-----------------------------------+--------------------------+----------------------------+----+----+----+----+------------+------------+--------------------+-------------------+
|Grant_Application_ID| RFCD_Code|RFCD_Percentage| SEO_Code|SEO_Percentage|Person_ID| Role|Year_of_Birth|Country_of_Birth|Home_Language| Dept_No|Faculty_No|With_PHD|No_of_Years_in_Uni_at_Time_of_Grant|Number_of_Successful_Grant|Number_of_Unsuccessful_Grant| A2| A| B| C|Grant_Status|Sponsor_Code| Contract_Value_Band|Grant_Category_Code|
+--------------------+----------+---------------+---------+--------------+---------+--------------------+-------------+----------------+-------------+--------+----------+--------+-----------------------------------+--------------------------+----------------------------+----+----+----+----+------------+------------+--------------------+-------------------+
| 1|RFCD280199| 100.0|SEO700299| 100.0| 40572| CHIEF_INVESTIGATOR| 1965| AsiaPacific| OtherLang|Dept3073| Faculty31| null| DurationLT0| 0| 0| 4| 2| 0| 0| 1| SponsorUnk| ContractValueBandA| GrantCatUnk|
| 2|RFCD280103| 30.0|SEO700103| 50.0| 9067| CHIEF_INVESTIGATOR| 1960| Australia| null|Dept2538| Faculty25| Yes| DurationGT15| 0| 0| 6| 12| 2| 2| 1| Sponsor2B| ContractValueBandB| GrantCat10A|
| 3|RFCD321004| 60.0|SEO730105| 60.0| 5967| CHIEF_INVESTIGATOR| 1955| Australia| null|Dept2923| Faculty25| Yes| Duration5to10| 0| 0| 0| 3| 5| 2| 1| Sponsor29A| ContractValueBandA| GrantCat10B|
| 3| null| null| null| null| null|EXT_CHIEF_INVESTI...| null| null| null| null| null| null| DurationUnk| null| null|null|null|null|null| 1| Sponsor29A| ContractValueBandA| GrantCat10B|
| 3| null| null| null| null| null|EXT_CHIEF_INVESTI...| null| null| null| null| null| null| DurationUnk| null| null|null|null|null|null| 1| Sponsor29A| ContractValueBandA| GrantCat10B|
| 3|RFCD321216| 40.0|SEO730207| 40.0| 27307| CHIEF_INVESTIGATOR| 1950| Australia| null|Dept2923| Faculty25| null| DurationLT0| 0| 0| 0| 0| 0| 0| 1| Sponsor29A| ContractValueBandA| GrantCat10B|
| 3| null| null| null| null| null|EXT_CHIEF_INVESTI...| null| null| null| null| null| null| DurationUnk| null| null|null|null|null|null| 1| Sponsor29A| ContractValueBandA| GrantCat10B|
| 3| null| null| null| null| 79652| CHIEF_INVESTIGATOR| 1950| AsiaPacific| null|Dept2498| Faculty25| Yes| DurationGT15| 0| 0| 1| 3| 3| 3| 1| Sponsor29A| ContractValueBandA| GrantCat10B|
| 3| null| null| null| null| 11667|DELEGATED_RESEARCHER| 1950| Australia| null|Dept2548| Faculty25| null| DurationGT15| 0| 0| 6| 14| 12| 2| 1| Sponsor29A| ContractValueBandA| GrantCat10B|
| 4|RFCD270602| 50.0|SEO730106| 70.0| 78782|PRINCIPAL_SUPERVISOR| 1955| Australia| null|Dept2678| Faculty25| Yes| Duration5to10| 0| 0| 0| 3| 13| 3| 1| Sponsor40D| ContractValueBandC| GrantCat10B|
| 4| null| null| null| null| null|EXT_CHIEF_INVESTI...| null| null| null| null| null| null| DurationUnk| null| null|null|null|null|null| 1| Sponsor40D| ContractValueBandC| GrantCat10B|
| 4| null| null| null| null| null|EXT_CHIEF_INVESTI...| null| null| null| null| null| null| DurationUnk| null| null|null|null|null|null| 1| Sponsor40D| ContractValueBandC| GrantCat10B|
| 4|RFCD320602| 50.0|SEO730201| 30.0| 55337| CHIEF_INVESTIGATOR| 1975| Australia| null|Dept2678| Faculty25| Yes| Duration0to5| 0| 0| 0| 0| 0| 0| 1| Sponsor40D| ContractValueBandC| GrantCat10B|
| 4| null| null| null| null| null|EXT_CHIEF_INVESTI...| null| null| null| null| null| null| DurationUnk| null| null|null|null|null|null| 1| Sponsor40D| ContractValueBandC| GrantCat10B|
| 4| null| null| null| null| null|STUD_CHIEF_INVEST...| null| null| null| null| null| null| DurationUnk| null| null|null|null|null|null| 1| Sponsor40D| ContractValueBandC| GrantCat10B|
| 5|RFCD260500| 34.0|SEO770199| 100.0| 13042| CHIEF_INVESTIGATOR| 1965| WesternEurope| null|Dept2153| Faculty19| Yes| Duration5to10| 0| 0| 3| 0| 1| 0| 0| Sponsor59C| ContractValueBandA| GrantCat10A|
| 6|RFCD321204| 100.0|SEO730211| 100.0| 301472| CHIEF_INVESTIGATOR| 1950| Australia| null|Dept2533| Faculty25| Yes| Duration0to5| 2| 0| 7| 27| 27| 6| 1| Sponsor4D|ContractValueBandUnk| GrantCat10A|
| 7|RFCD270401| 20.0| null| null| 79742| CHIEF_INVESTIGATOR| 1950| GreatBritain| null|Dept3028| Faculty31| null| DurationLT0| 0| 0| 0| 7| 12| 4| 0| Sponsor2B|ContractValueBandUnk| GrantCat10A|
| 7| null| null| null| null| null|EXT_CHIEF_INVESTI...| null| null| null| null| null| null| DurationUnk| null| null|null|null|null|null| 0| Sponsor2B|ContractValueBandUnk| GrantCat10A|
| 7|RFCD270203| 30.0|SEO770706| 40.0| 82602| CHIEF_INVESTIGATOR| 1960| Australia| null|Dept3028| Faculty31| Yes| Duration10to15| 0| 1| 0| 0| 0| 0| 0| Sponsor2B|ContractValueBandUnk| GrantCat10A|
+--------------------+----------+---------------+---------+--------------+---------+--------------------+-------------+----------------+-------------+--------+----------+--------+-----------------------------------+--------------------------+----------------------------+----+----+----+----+------------+------------+--------------------+-------------------+
only showing top 20 rows
###Markdown
create features
###Code
val researchers = data.
withColumn ("phd", data("With_PHD").equalTo("Yes").cast("Int")).
withColumn ("CI", data("Role").equalTo("CHIEF_INVESTIGATOR").cast("Int")).
withColumn("paperscore", data("A2") * 4 + data("A") * 3)
val grants = researchers.groupBy("Grant_Application_ID").agg(
max("Grant_Status").as("Grant_Status"),
max("Grant_Category_Code").as("Category_Code"),
max("Contract_Value_Band").as("Value_Band"),
sum("phd").as("PHDs"),
when(max(expr("paperscore * CI")).isNull, 0).
otherwise(max(expr("paperscore * CI"))).as("paperscore"),
count("*").as("teamsize"),
when(sum("Number_of_Successful_Grant").isNull, 0).
otherwise(sum("Number_of_Successful_Grant")).as("successes"),
when(sum("Number_of_Unsuccessful_Grant").isNull, 0).
otherwise(sum("Number_of_Unsuccessful_Grant")).as("failures")
)
grants.show()
###Output
+--------------------+------------+-------------+--------------------+----+----------+--------+---------+--------+
|Grant_Application_ID|Grant_Status|Category_Code| Value_Band|PHDs|paperscore|teamsize|successes|failures|
+--------------------+------------+-------------+--------------------+----+----------+--------+---------+--------+
| 148| 0| GrantCat30B|ContractValueBandUnk|null| 6| 1| 0| 1|
| 463| 1| GrantCat30C|ContractValueBandUnk|null| 0| 1| 1| 0|
| 471| 0| GrantCat30B| ContractValueBandA| 1| 127| 2| 1| 5|
| 496| 0| GrantCat30B| ContractValueBandA|null| 0| 1| 1| 3|
| 833| 1| GrantCat10A| ContractValueBandF|null| 0| 1| 0| 0|
| 1088| 1| GrantCat50A| ContractValueBandA| 1| 11| 1| 1| 0|
| 1238| 1| GrantCatUnk| ContractValueBandA| 1| 6| 1| 0| 0|
| 1342| 1| GrantCat10A| ContractValueBandE| 1| 51| 3| 3| 3|
| 1580| 0| GrantCat10B|ContractValueBandUnk| 2| 72| 3| 1| 2|
| 1591| 1| GrantCatUnk|ContractValueBandUnk|null| 39| 1| 1| 0|
| 1645| 0| GrantCat30D| ContractValueBandB|null| 0| 1| 0| 0|
| 1829| 1| GrantCatUnk| ContractValueBandA| 1| 4| 1| 0| 0|
| 1959| 1| GrantCat30B| ContractValueBandA|null| 0| 1| 1| 0|
| 2122| 1| GrantCatUnk|ContractValueBandUnk| 1| 14| 1| 0| 0|
| 2142| 1| GrantCatUnk|ContractValueBandUnk| 1| 19| 1| 0| 1|
| 2366| 0| GrantCat10A|ContractValueBandUnk|null| 3| 2| 0| 3|
| 2659| 1| GrantCat30A| ContractValueBandA| 1| 165| 1| 5| 6|
| 2866| 1| GrantCat20C|ContractValueBandUnk|null| 0| 2| 0| 0|
| 3175| 0| GrantCat50A| ContractValueBandA| 1| 0| 1| 0| 3|
| 3749| 0| GrantCat10A|ContractValueBandUnk| 1| 18| 1| 0| 1|
+--------------------+------------+-------------+--------------------+----+----------+--------+---------+--------+
only showing top 20 rows
###Markdown
convert string features to numbers
###Code
import org.apache.spark.ml.feature.StringIndexer
val value_band_indexer = new StringIndexer().
setInputCol("Value_Band").
setOutputCol("Value_index").
fit(grants)
val category_indexer = new StringIndexer().
setInputCol("Category_Code").
setOutputCol("Category_index").
fit(grants)
val label_indexer = new StringIndexer().
setInputCol("Grant_Status").
setOutputCol("status").
fit(grants)
###Output
_____no_output_____
###Markdown
convert features to a vector
###Code
import org.apache.spark.ml.feature.VectorAssembler
val assembler = new VectorAssembler().
setInputCols(Array(
"Value_index"
,"Category_index"
,"PHDs"
,"paperscore"
,"teamsize"
,"successes"
,"failures"
)).setOutputCol("assembled")
###Output
_____no_output_____
###Markdown
random forest classifier
###Code
import org.apache.spark.ml.classification.RandomForestClassifier
import org.apache.spark.ml.classification.RandomForestClassificationModel
val rf = new RandomForestClassifier().
setFeaturesCol("assembled").
setLabelCol("status").
setSeed(42)
###Output
_____no_output_____
###Markdown
create Pipeline
###Code
import org.apache.spark.ml.Pipeline
val pipeline = new Pipeline().setStages(Array(
value_band_indexer,
category_indexer,
label_indexer,
assembler,
rf)
)
###Output
_____no_output_____
###Markdown
create an evaluator
###Code
import org.apache.spark.ml.evaluation.BinaryClassificationEvaluator
val auc_eval = new BinaryClassificationEvaluator().
setLabelCol("status").
setRawPredictionCol("rawPrediction")
auc_eval.getMetricName
val tr = grants.filter("Grant_Application_ID < 6635")
val te = grants.filter("Grant_Application_ID >= 6635")
val training = tr.na.fill(0, Seq("PHDs"))
val test = te.na.fill(0, Seq("PHDs"))
val model = pipeline.fit(training)
val pipeline_results = model.transform(test)
auc_eval.evaluate(pipeline_results)
rf.extractParamMap
###Output
_____no_output_____
###Markdown
Simple Grid Search Parameter values, different from video>
###Code
import org.apache.spark.ml.tuning.ParamGridBuilder
val paramGrid = new ParamGridBuilder().
addGrid(rf.maxDepth, Array(2, 5)).
addGrid(rf.numTrees, Array(1, 20)).
build()
###Output
_____no_output_____
###Markdown
Cross Validation* Main idea: test with data not used for training* Split the data several times* Each time, use part of the data for training and the rest for testing k-fold Cross Validation* Spark supports k-fold cross validation - Divides the data into *k* non-overlapping sub-samples - Performance is measured by averaging the result of the Evaluator across the *k* folds - *k* should be at least 3 Cross Validation train the model
###Code
import org.apache.spark.ml.tuning.CrossValidator
val cv = new CrossValidator().
setEstimator(pipeline).
setEvaluator(auc_eval).
setEstimatorParamMaps(paramGrid).
setNumFolds(3)
###Output
_____no_output_____
###Markdown
Final Results takes a long time
###Code
val cvModel = cv.fit(training)
val cv_results = cvModel.transform(test)
// with the default parameters we got about 0.908
auc_eval.evaluate(cv_results)
###Output
_____no_output_____ |
GRN_analysis/01_ATAC_peaks_to_TFinfo.ipynb | ###Markdown
0. Import libraries
###Code
import pandas as pd
import numpy as np
import matplotlib.pyplot as plt
import seaborn as sns
import os, sys, shutil, importlib, glob
from tqdm.notebook import tqdm
from celloracle import motif_analysis as ma
from celloracle.utility import save_as_pickled_object
%config InlineBackend.figure_format = 'retina'
%matplotlib inline
plt.rcParams['figure.figsize'] = (15,7)
plt.rcParams["savefig.dpi"] = 600
###Output
_____no_output_____
###Markdown
1. Rerefence genome data preparation 1.1. Check reference genome installationCelloracle uses genomepy to get DNA sequence data. Before starting celloracle analysis, we need to make sure that the reference genome is correctly installed in your computational environment. If not, please install reference genome.
###Code
# PLEASE make sure that you are setting correct ref genome.
ref_genome = "mm10"
genome_installation = ma.is_genome_installed(ref_genome=ref_genome)
print(ref_genome, "installation: ", genome_installation)
#import genomepy
#genomepy.install_genome("mm10", "UCSC")
###Output
_____no_output_____
###Markdown
1.2. Install reference genome (if refgenome is not installed)
###Code
if not genome_installation:
import genomepy
genomepy.install_genome(ref_genome, "UCSC")
else:
print(ref_genome, "is installed.")
###Output
mm10 is installed.
###Markdown
2. Load data 2.1. Load processed peak data The peak2gene link pairs identified by ArchR was processed with "peak2gene_cellOracle.R" and saved as a Parquet file.
###Code
# Load annotated peak data.
peaks = pd.read_parquet("peak_file.parquet")
peaks.head()
###Output
_____no_output_____
###Markdown
2.1. Check data
###Code
# Define function for quality check
def decompose_chrstr(peak_str):
"""
Args:
peak_str (str): peak_str. e.g. 'chr1_3094484_3095479'
Returns:
tuple: chromosome name, start position, end position
"""
*chr_, start, end = peak_str.split("_")
chr_ = "_".join(chr_)
return chr_, start, end
from genomepy import Genome
def check_peak_foamat(peaks_df, ref_genome):
"""
Check peak fomat.
(1) Check chromosome name.
(2) Check peak size (length) and remove sort DNAs (<5bp)
"""
df = peaks_df.copy()
n_peaks_before = df.shape[0]
# Decompose peaks and make df
decomposed = [decompose_chrstr(peak_str) for peak_str in df["peak_id"]]
df_decomposed = pd.DataFrame(np.array(decomposed))
df_decomposed.columns = ["chr", "start", "end"]
df_decomposed["start"] = df_decomposed["start"].astype(np.int)
df_decomposed["end"] = df_decomposed["end"].astype(np.int)
# Load genome data
genome_data = Genome(ref_genome)
all_chr_list = list(genome_data.keys())
# DNA length check
lengths = np.abs(df_decomposed["end"] - df_decomposed["start"])
# Filter peaks with invalid chromosome name
n_threshold = 5
df = df[(lengths >= n_threshold) & df_decomposed.chr.isin(all_chr_list)]
# DNA length check
lengths = np.abs(df_decomposed["end"] - df_decomposed["start"])
# Data counting
n_invalid_length = len(lengths[lengths < n_threshold])
n_peaks_invalid_chr = n_peaks_before - df_decomposed.chr.isin(all_chr_list).sum()
n_peaks_after = df.shape[0]
#
print("Peaks before filtering: ", n_peaks_before)
print("Peaks with invalid chr_name: ", n_peaks_invalid_chr)
print("Peaks with invalid length: ", n_invalid_length)
print("Peaks after filtering: ", n_peaks_after)
return df
peaks = check_peak_foamat(peaks, ref_genome)
###Output
Peaks before filtering: 197299
Peaks with invalid chr_name: 0
Peaks with invalid length: 0
Peaks after filtering: 197299
###Markdown
2.2 Load motif
###Code
from gimmemotifs.motif import read_motifs
# Read modified gimme.vertebrate.v5.0 with added Bcl11b motif
path = 'gimme.vertebrate.v5.0m/gimme.vertebrate.v5.0.pfm'
print(path)
motifs = read_motifs(path)
motifs[0:10]
###Output
gimme.vertebrate.v5.0m/gimme.vertebrate.v5.0.pfm
###Markdown
3. Instantiate TFinfo object and search for TF binding motifsThe motif analysis module has a custom class; TFinfo. The TFinfo object converts a peak data into a DNA sequences and scans the DNA sequences searching for TF binding motifs. Then, the results of motif scan will be filtered and converted into either a python dictionary or a depending on your preference. This TF information is necessary for GRN inference. 3.1. Instantiate TFinfo object
###Code
# Instantiate TFinfo object
tfi = ma.TFinfo(peak_data_frame=peaks, # peak info calculated from ATAC-seq data
ref_genome=ref_genome)
###Output
_____no_output_____
###Markdown
3.2. Motif scan!!You can set TF binding motif information as an argument: tfi.scan(motifs=motifs)If you don't set motifs or set None, default motifs will be loaded automatically.- For mouse and human, "gimme.vertebrate.v5.0." will be used as a default motifs. - For another species, a species specific TF binding motif data extracted from CisBP ver2.0 will be used.
###Code
%%time
# Scan motifs. !!CAUTION!! This step may take several hours if you have many peaks!
tfi.scan(fpr=0.02,
motifs=None, # If you enter None, default motifs will be loaded.
verbose=True)
# Save tfinfo object
tfi.to_hdf5(file_path="cCRE.celloracle.tfinfo")
# Check motif scan results
tfi.scanned_df.head()
df=tfi.scanned_df
len(df)
folder='TFinfo_outputs'
# save the entire scanned results
df.to_parquet(os.path.join(folder,"tfi_scanned.parquet"))
###Output
_____no_output_____
###Markdown
4. Filtering motifsOut of 17026486, 3118211 passed the filtering (18.31%)
###Code
# Reset filtering
tfi.reset_filtering()
# Do filtering
tfi.filter_motifs_by_score(threshold=10.5)
# Do post filtering process. Convert results into several file format.
tfi.make_TFinfo_dataframe_and_dictionary(verbose=True)
###Output
Filtering finished: 17026486 -> 3118211
1. Converting scanned results into one-hot encoded dataframe.
###Markdown
We have the score for each sequence and motif_id pair.In the next step we will filter the motifs with low score. 5. Get Final results 5.1. Get resutls as a dictionary
###Code
td = tfi.to_dictionary(dictionary_type="targetgene2TFs")
###Output
_____no_output_____
###Markdown
5.2. Get results as a dataframe
###Code
df = tfi.to_dataframe()
df.head()
###Output
_____no_output_____
###Markdown
6. Save TFinfo as dictionary or dataframeWe'll use this information when making the GRNs. Save the results.
###Code
folder = "TFinfo_outputs"
os.makedirs(folder, exist_ok=True)
# save TFinfo as a dictionary
td = tfi.to_dictionary(dictionary_type="targetgene2TFs")
save_as_pickled_object(td, os.path.join(folder, "TFinfo_targetgene2TFs.pickled"))
# save TFinfo as a dataframe
df = tfi.to_dataframe()
df.to_parquet(os.path.join(folder, "TFinfo_dataframe.parquet"))
###Output
_____no_output_____ |
ood_erm_irm.ipynb | ###Markdown
Invariant Risk MinimizationThis is an attempt to reproduce the "Colored MNIST" experiments from thepaper [Invariant Risk Minimization](https://arxiv.org/abs/1907.02893)by Arjovsky, et. al.
###Code
import os
import numpy as np
from PIL import Image
import matplotlib.pyplot as plt
import torch
import torch.nn as nn
import torch.nn.functional as F
import torch.optim as optim
from torch.autograd import grad
from torchvision import transforms
from torchvision import datasets
import torchvision.datasets.utils as dataset_utils
###Output
_____no_output_____
###Markdown
Prepare the colored MNIST datasetWe define three environments (two training, one test) by randomly splitting the MNIST dataset in thirds and transforming each example as follows:1. Assign a binary label y to the image based on the digit: y = 0 for digits 0-4and y = 1 for digits 5-9.2. Flip the label with 25% probability.3. Color the image either red or green according to its (possibly flipped) label.4. Flip the color with a probability e that depends on the environment: 20% inthe first training environment, 10% in the second training environment, and90% in the test environment.
###Code
def color_grayscale_arr(arr, red=True):
"""Converts grayscale image to either red or green"""
assert arr.ndim == 2
dtype = arr.dtype
h, w = arr.shape
arr = np.reshape(arr, [h, w, 1])
if red:
arr = np.concatenate([arr,
np.zeros((h, w, 2), dtype=dtype)], axis=2)
else:
arr = np.concatenate([np.zeros((h, w, 1), dtype=dtype),
arr,
np.zeros((h, w, 1), dtype=dtype)], axis=2)
return arr
class ColoredMNIST(datasets.VisionDataset):
"""
Colored MNIST dataset for testing IRM. Prepared using procedure from https://arxiv.org/pdf/1907.02893.pdf
Args:
root (string): Root directory of dataset where ``ColoredMNIST/*.pt`` will exist.
env (string): Which environment to load. Must be 1 of 'train1', 'train2', 'test', or 'all_train'.
transform (callable, optional): A function/transform that takes in an PIL image
and returns a transformed version. E.g, ``transforms.RandomCrop``
target_transform (callable, optional): A function/transform that takes in the
target and transforms it.
"""
def __init__(self, root='./data', env='train1', transform=None, target_transform=None):
super(ColoredMNIST, self).__init__(root, transform=transform,
target_transform=target_transform)
self.prepare_colored_mnist()
if env in ['train1', 'train2', 'test']:
self.data_label_tuples = torch.load(os.path.join(self.root, 'ColoredMNIST', env) + '.pt')
elif env == 'all_train':
self.data_label_tuples = torch.load(os.path.join(self.root, 'ColoredMNIST', 'train1.pt')) + \
torch.load(os.path.join(self.root, 'ColoredMNIST', 'train2.pt'))
else:
raise RuntimeError(f'{env} env unknown. Valid envs are train1, train2, test, and all_train')
def __getitem__(self, index):
"""
Args:
index (int): Index
Returns:
tuple: (image, target) where target is index of the target class.
"""
img, target = self.data_label_tuples[index]
if self.transform is not None:
img = self.transform(img)
if self.target_transform is not None:
target = self.target_transform(target)
return img, target
def __len__(self):
return len(self.data_label_tuples)
def prepare_colored_mnist(self):
colored_mnist_dir = os.path.join(self.root, 'ColoredMNIST')
if os.path.exists(os.path.join(colored_mnist_dir, 'train1.pt')) \
and os.path.exists(os.path.join(colored_mnist_dir, 'train2.pt')) \
and os.path.exists(os.path.join(colored_mnist_dir, 'test.pt')):
print('Colored MNIST dataset already exists')
return
print('Preparing Colored MNIST')
train_mnist = datasets.mnist.MNIST(self.root, train=True, download=True)
train1_set = []
train2_set = []
test_set = []
for idx, (im, label) in enumerate(train_mnist):
if idx % 10000 == 0:
print(f'Converting image {idx}/{len(train_mnist)}')
im_array = np.array(im)
# Assign a binary label y to the image based on the digit
binary_label = 0 if label < 5 else 1
# Flip label with 25% probability
if np.random.uniform() < 0.25:
binary_label = binary_label ^ 1
# Color the image either red or green according to its possibly flipped label
color_red = binary_label == 0
# Flip the color with a probability e that depends on the environment
if idx < 20000:
# 20% in the first training environment
if np.random.uniform() < 0.2:
color_red = not color_red
elif idx < 40000:
# 10% in the first training environment
if np.random.uniform() < 0.1:
color_red = not color_red
else:
# 90% in the test environment
if np.random.uniform() < 0.9:
color_red = not color_red
colored_arr = color_grayscale_arr(im_array, red=color_red)
if idx < 20000:
train1_set.append((Image.fromarray(colored_arr), binary_label))
elif idx < 40000:
train2_set.append((Image.fromarray(colored_arr), binary_label))
else:
test_set.append((Image.fromarray(colored_arr), binary_label))
# Debug
# print('original label', type(label), label)
# print('binary label', binary_label)
# print('assigned color', 'red' if color_red else 'green')
# plt.imshow(colored_arr)
# plt.show()
# break
os.makedirs(colored_mnist_dir, exist_ok=True)
# dataset_utils.makedir_exist_ok(colored_mnist_dir)
torch.save(train1_set, os.path.join(colored_mnist_dir, 'train1.pt'))
torch.save(train2_set, os.path.join(colored_mnist_dir, 'train2.pt'))
torch.save(test_set, os.path.join(colored_mnist_dir, 'test.pt'))
###Output
_____no_output_____
###Markdown
Plot the data
###Code
def plot_dataset_digits(dataset):
fig = plt.figure(figsize=(13, 8))
columns = 6
rows = 3
# ax enables access to manipulate each of subplots
ax = []
for i in range(columns * rows):
img, label = dataset[i]
# create subplot and append to ax
ax.append(fig.add_subplot(rows, columns, i + 1))
ax[-1].set_title("Label: " + str(label)) # set title
plt.imshow(img)
plt.show() # finally, render the plot
###Output
_____no_output_____
###Markdown
Plotting the train set
###Code
train1_set = ColoredMNIST(root='./data', env='train1')
plot_dataset_digits(train1_set)
###Output
Colored MNIST dataset already exists
###Markdown
Plotting the test set
###Code
test_set = ColoredMNIST(root='./data', env='test')
plot_dataset_digits(test_set)
###Output
Colored MNIST dataset already exists
###Markdown
Notice how the correlation between color and label are reversed in the train and test set. CGES Utils Define neural networkThe paper uses an MLP but a Convnet works fine too.
###Code
class Net(nn.Module):
def __init__(self):
super(Net, self).__init__()
self.fc1 = nn.Linear(3 * 28 * 28, 512)
self.fc2 = nn.Linear(512, 512)
self.fc3 = nn.Linear(512, 1)
def forward(self, x):
x = x.view(-1, 3 * 28 * 28)
x = F.relu(self.fc1(x))
x = F.relu(self.fc2(x))
logits = self.fc3(x).flatten()
return logits
class ConvNet(nn.Module):
def __init__(self):
super(ConvNet, self).__init__()
self.conv1 = nn.Conv2d(3, 20, 5, 1)
self.conv2 = nn.Conv2d(20, 50, 5, 1)
self.fc1 = nn.Linear(4 * 4 * 50, 500)
self.fc2 = nn.Linear(500, 1)
def forward(self, x):
x = F.relu(self.conv1(x))
x = F.max_pool2d(x, 2, 2)
x = F.relu(self.conv2(x))
x = F.max_pool2d(x, 2, 2)
x = x.view(-1, 4 * 4 * 50)
x = F.relu(self.fc1(x))
logits = self.fc2(x).flatten()
return logits
###Output
_____no_output_____
###Markdown
Test ERM as a baselineUsing ERM as a baseline, we expect to train a neural network that uses color instead of the actual digit to classify, completely failing on the test set when the colors are switched.
###Code
from argparse import Namespace
args = Namespace(**dict({
'cges': False,
'lamb': 0.0006,
'mu': 0.8,
'chvar': 0.2,
'lr': 0.0001
}))
!pip install wandb
!wandb login 28f856e56e11e0b499f7b141ce1d61b0a6671e97
import random
import numpy as np
import torch
import os
def set_seed(seed):
random.seed(seed)
np.random.seed(seed)
torch.manual_seed(seed)
if torch.cuda.is_available():
torch.cuda.manual_seed(seed)
torch.cuda.manual_seed_all(seed)
torch.backends.cudnn.deterministic = True
torch.backends.cudnn.benchmark = False
os.environ["PYTHONHASHSEED"] = str(seed)
from utils import apply_cges
import wandb
def test_model(model, device, test_loader, set_name="test_set", epoch=-1):
model.eval()
test_loss = 0
correct = 0
with torch.no_grad():
for data, target in test_loader:
data, target = data.to(device), target.to(device).float()
output = model(data)
test_loss += F.binary_cross_entropy_with_logits(output, target, reduction='sum').item() # sum up batch loss
pred = torch.where(torch.gt(output, torch.Tensor([0.0]).to(device)),
torch.Tensor([1.0]).to(device),
torch.Tensor([0.0]).to(device)) # get the index of the max log-probability
correct += pred.eq(target.view_as(pred)).sum().item()
test_loss /= len(test_loader.dataset)
print('Performance on {}: Average loss: {:.4f}, Accuracy: {}/{} ({:.2f}%)'.format(
set_name, test_loss, correct, len(test_loader.dataset),
100. * correct / len(test_loader.dataset)))
wandb.log({str(set_name) +"/loss": test_loss}, step=epoch)
wandb.log({str(set_name) +"/acc": 100. * correct / len(test_loader.dataset)}, step=epoch)
return 100. * correct / len(test_loader.dataset)
def erm_train(args, model, device, train_loader, optimizer, epoch):
model.train()
for batch_idx, (data, target) in enumerate(train_loader):
data, target = data.to(device), target.to(device).float()
optimizer.zero_grad()
output = model(data)
loss = F.binary_cross_entropy_with_logits(output, target)
loss.backward()
optimizer.step()
if args.cges:
apply_cges(args, model, optimizer)
print('Train Epoch: {} [{}/{} ({:.0f}%)]\tLoss: {:.6f}'.format(
epoch, batch_idx * len(data), len(train_loader.dataset),
100. * batch_idx / len(train_loader), loss.item()))
wandb.log({"train/loss": loss.item()}, step=epoch)
def train_and_test_erm():
wandb.init(entity="arjunashok", project="irm-notebook", config=vars(args))
if args.cges:
wandb.run.name = "erm-cges-"+str(args.lamb)
else:
wandb.run.name = "erm-plain"
set_seed(0)
use_cuda = torch.cuda.is_available()
device = torch.device("cuda" if use_cuda else "cpu")
kwargs = {'num_workers': 8, 'pin_memory': True} if use_cuda else {}
all_train_loader = torch.utils.data.DataLoader(
ColoredMNIST(root='./data', env='all_train',
transform=transforms.Compose([
transforms.ToTensor(),
transforms.Normalize((0.1307, 0.1307, 0.), (0.3081, 0.3081, 0.3081))
])),
batch_size=64, shuffle=True, **kwargs)
test_loader = torch.utils.data.DataLoader(
ColoredMNIST(root='./data', env='test', transform=transforms.Compose([
transforms.ToTensor(),
transforms.Normalize((0.1307, 0.1307, 0.), (0.3081, 0.3081, 0.3081))
])),
batch_size=1000, shuffle=True, **kwargs)
model = ConvNet().to(device)
optimizer = optim.Adam(model.parameters(), lr=args.lr)
best_test_acc = 0
for epoch in range(1, 100):
erm_train(args, model, device, all_train_loader, optimizer, epoch)
test_model(model, device, all_train_loader, set_name='train_set', epoch=epoch)
test_acc = test_model(model, device, test_loader, epoch=epoch)
if test_acc > best_test_acc:
best_test_acc = test_acc
wandb.log({"test_set/best_acc": best_test_acc}, step=epoch)
###Output
_____no_output_____
###Markdown
IRMAfter trying lots of hyperparameters and various tricks, this implementation seems to consistently achieve the paper-reported values (train accuracy > 70%, test accuracy > 60%), though there might be a bit ofinstability depending on the random seed.The most common failure case is when the gradient norm penalty term is weightedtoo highly relative to the ERM term. In this case, Φ converges to a function that returns the same value for all inputs. The classifier cannot recover from this pointand the accuracy is stuck at 50% for all environments. This makes sense mathematically.If the intermediate representation is the same regardless of input, then *any*classifier is the ideal classifier, resulting in the penalty gradient being 0.Another failure case is when the gradient norm penalty is too low and theoptimization essentially acts as in ERM (train accuracy > 80%, test accuracy ~10%).The most important trick I used to get this to work is through scheduled increase of the gradient norm penalty weight.We start at 0 for the gradient norm penalty weight, essentially beginning as ERM,then slowly increase it per epoch.I use early stopping to stop training once the accuracy on all environments, including the test set, reach an acceptable value. Yes, stopping training based on performance on the test set is not good practice, but I could notfind a principled way of stopping training by only observing performance on thetraining environments. One thing that might be needed when applying IRM toreal-world datasets is to leave out a separate environment as a validation set,which we can use for early stopping. The downside is we'll need a minimum of 4environments to perform IRM (2 train, 1 validation, 1 test).
###Code
def compute_irm_penalty(losses, dummy):
g1 = grad(losses[0::2].mean(), dummy, create_graph=True)[0]
g2 = grad(losses[1::2].mean(), dummy, create_graph=True)[0]
return (g1 * g2).sum()
def irm_train(args, model, device, train_loaders, optimizer, epoch):
model.train()
train_loaders = [iter(x) for x in train_loaders]
dummy_w = torch.nn.Parameter(torch.Tensor([1.0])).to(device)
batch_idx = 0
penalty_multiplier = epoch ** 1.6
print(f'Using penalty multiplier {penalty_multiplier}')
while True:
optimizer.zero_grad()
error = 0
penalty = 0
for loader in train_loaders:
data, target = next(loader, (None, None))
if data is None:
return
data, target = data.to(device), target.to(device).float()
output = model(data)
loss_erm = F.binary_cross_entropy_with_logits(output * dummy_w, target, reduction='none')
penalty += compute_irm_penalty(loss_erm, dummy_w)
error += loss_erm.mean()
(error + penalty_multiplier * penalty).backward()
optimizer.step()
if args.cges:
apply_cges(args, model, optimizer)
batch_idx += 1
print('Train Epoch: {} [{}/{} ({:.0f}%)]\tERM loss: {:.6f}\tGrad penalty: {:.6f}'.format(
epoch, batch_idx * len(data), len(train_loaders[0]),
100. * batch_idx / len(train_loaders[0]), error.item(), penalty.item()))
wandb.log({"train/loss": error.item()}, step=epoch)
wandb.log({"train/grad_penalty": penalty.item()}, step=epoch)
def train_and_test_irm():
import wandb
wandb.init(entity="arjunashok", project="irm-notebook", config=vars(args))
if args.cges:
wandb.run.name = "irm-cges-"+str(args.lamb)
else:
wandb.run.name = "irm-plain"
set_seed(0)
use_cuda = torch.cuda.is_available()
device = torch.device("cuda" if use_cuda else "cpu")
kwargs = {'num_workers': 1, 'pin_memory': True} if use_cuda else {}
train1_loader = torch.utils.data.DataLoader(
ColoredMNIST(root='./data', env='train1',
transform=transforms.Compose([
transforms.ToTensor(),
transforms.Normalize((0.1307, 0.1307, 0.), (0.3081, 0.3081, 0.3081))
])),
batch_size=2000, shuffle=True, **kwargs)
train2_loader = torch.utils.data.DataLoader(
ColoredMNIST(root='./data', env='train2',
transform=transforms.Compose([
transforms.ToTensor(),
transforms.Normalize((0.1307, 0.1307, 0.), (0.3081, 0.3081, 0.3081))
])),
batch_size=2000, shuffle=True, **kwargs)
test_loader = torch.utils.data.DataLoader(
ColoredMNIST(root='./data', env='test', transform=transforms.Compose([
transforms.ToTensor(),
transforms.Normalize((0.1307, 0.1307, 0.), (0.3081, 0.3081, 0.3081))
])),
batch_size=1000, shuffle=True, **kwargs)
model = ConvNet().to(device)
optimizer = optim.Adam(model.parameters(), lr=args.lr)
print(args)
best_test_acc = 0
for epoch in range(1, 100):
irm_train(args, model, device, [train1_loader, train2_loader], optimizer, epoch)
train1_acc = test_model(model, device, train1_loader, set_name='train1_set', epoch=epoch)
train2_acc = test_model(model, device, train2_loader, set_name='train2_set', epoch=epoch)
test_acc = test_model(model, device, test_loader, epoch=epoch)
if test_acc > best_test_acc:
best_test_acc = test_acc
wandb.log({"test_set/best_acc": best_test_acc}, step=epoch)
# if train1_acc > 70 and train2_acc > 70 and test_acc > 60:
# print('found acceptable values. stopping training.')
# return
args.cges = True
train_and_test_erm()
train_and_test_irm()
args.cges = False
train_and_test_erm()
train_and_test_irm()
args.cges = True
train_and_test_erm()
###Output
_____no_output_____ |
04-computer-vision/exercise-2.ipynb | ###Markdown
Imports
###Code
import matplotlib.pyplot as plt
%matplotlib notebook
from tensorflow import keras
from keras.models import Sequential
from keras.layers import Flatten
from keras.layers import Dense
from keras.layers import Conv2D
from keras.layers import MaxPooling2D
###Output
_____no_output_____
###Markdown
Load the dataNotes:- x_train and x_test contain images of 10 different types of objects- y_train and y_test contain an id for the corresponding object.- For example: x_train[1] contains an image of a truck and y_train[1] contains the number 9 which is the id of the truck class.- Here are the types of the objects that we have along with their id: [('airplane',0), ('automobile',1), ('bird',2), ('cat',3), ('deer',4), ('dog',5), ('frog',6), ('horse',7), ('ship',8), ('truck',9)]
###Code
data = keras.datasets.cifar10
(x_train, y_train),(x_test, y_test) = data.load_data()
###Output
_____no_output_____ |
Semana 1/Numpy_ed.ipynb | ###Markdown
Numpy is the fundamental package for numeric computing with Python. It provides powerful ways to create,store, and/or manipulate data, which makes it able to seamlessly and speedily integrate with a wide varietyof databases. This is also the foundation that Pandas is built on, which is a high-performance data-centricpackage that we will learn later in the course.In this lecture, we will talk about creating array with certain data types, manipulating array, selectingelements from arrays, and loading dataset into array. Such functions are useful for manipulating data andunderstanding the functionalities of other common Python data packages.
###Code
# You'll recall that we import a library using the `import` keyword as numpy's common abbreviation is np
import numpy as np
import math
###Output
_____no_output_____
###Markdown
Array Creation
###Code
# Arrays are displayed as a list or list of lists and can be created through list as well. When creating an
# array, we pass in a list as an argument in numpy array
a = np.array([1, 2, 3])
print(a)
# We can print the number of dimensions of a list using the ndim attribute
print(a.ndim)
# If we pass in a list of lists in numpy array, we create a multi-dimensional array, for instance, a matrix
b = np.array([[1,2,3],[4,5,6]])
b
# We can print out the length of each dimension by calling the shape attribute, which returns a tuple
b.shape
# We can also check the type of items in the array
a.dtype
# Besides integers, floats are also accepted in numpy arrays
c = np.array([2.2, 5, 1.1])
c.dtype.name
# Let's look at the data in our array
c
# Note that numpy automatically converts integers, like 5, up to floats, since there is no loss of prescision.
# Numpy will try and give you the best data type format possible to keep your data types homogeneous, which
# means all the same, in the array
# Sometimes we know the shape of an array that we want to create, but not what we want to be in it. numpy
# offers several functions to create arrays with initial placeholders, such as zero's or one's.
# Lets create two arrays, both the same shape but with different filler values
d = np.zeros((2,3))
print(d)
e = np.ones((2,3))
print(e)
# We can also generate an array with random numbers
np.random.rand(2,3)
# You'll see zeros, ones, and rand used quite often to create example arrays, especially in stack overflow
# posts and other forums.
# We can also create a sequence of numbers in an array with the arrange() function. The fist argument is the
# starting bound and the second argument is the ending bound, and the third argument is the difference between
# each consecutive numbers
# Let's create an array of every even number from ten (inclusive) to fifty (exclusive)
f = np.arange(10, 50, 2)
f
# if we want to generate a sequence of floats, we can use the linspace() function. In this function the third
# argument isn't the difference between two numbers, but the total number of items you want to generate
np.linspace( 0, 2, 15 ) # 15 numbers from 0 (inclusive) to 2 (inclusive)
###Output
_____no_output_____
###Markdown
Array Operations
###Code
# We can do many things on arrays, such as mathematical manipulation (addition, subtraction, square,
# exponents) as well as use boolean arrays, which are binary values. We can also do matrix manipulation such
# as product, transpose, inverse, and so forth.
# Arithmetic operators on array apply elementwise.
# Let's create a couple of arrays
a = np.array([10,20,30,40])
b = np.array([1, 2, 3,4])
# Now let's look at a minus b
c = a-b
print(c)
# And let's look at a times b
d = a*b
print(d)
# With arithmetic manipulation, we can convert current data to the way we want it to be. Here's a real-world
# problem I face - I moved down to the United States about 6 years ago from Canada. In Canada we use celcius
# for temperatures, and my wife still hasn't converted to the US system which uses farenheit. With numpy I
# could easily convert a number of farenheit values, say the weather forecase, to ceclius
# Let's create an array of typical Ann Arbor winter farenheit values
farenheit = np.array([0,-10,-5,-15,0])
# And the formula for conversion is ((°F − 32) × 5/9 = °C)
celcius = (farenheit - 31) * (5/9)
celcius
# Great, so now she knows it's a little chilly outside but not so bad.
# Another useful and important manipulation is the boolean array. We can apply an operator on an array, and a
# boolean array will be returned for any element in the original, with True being emitted if it meets the condition and False oetherwise.
# For instance, if we want to get a boolean array to check celcius degrees that are greater than -20 degrees
celcius > -20
# Here's another example, we could use the modulus operator to check numbers in an array to see if they are even. Recall that modulus does division but throws away everything but the remainder (decimal) portion)
celcius%2 == 0
# Besides elementwise manipulation, it is important to know that numpy supports matrix manipulation. Let's
# look at matrix product. if we want to do elementwise product, we use the "*" sign
A = np.array([[1,1],[0,1]])
B = np.array([[2,0],[3,4]])
print(A*B)
# if we want to do matrix product, we use the "@" sign or use the dot function
print(A@B)
# You don't have to worry about complex matrix operations for this course, but it's important to know that
# numpy is the underpinning of scientific computing libraries in python, and that it is capable of doing both
# element-wise operations (the asterix) as well as matrix-level operations (the @ sign). There's more on this
# in a subsequent course.
# A few more linear algebra concepts are worth layering in here. You might recall that the product of two
# matrices is only plausible when the inner dimensions of the two matrices are the same. The dimensions refer
# to the number of elements both horizontally and vertically in the rendered matricies you've seen here. We
# can use numpy to quickly see the shape of a matrix:
A.shape
# When manipulating arrays of different types, the type of the resulting array will correspond to
# the more general of the two types. This is called upcasting.
# Let's create an array of integers
array1 = np.array([[1, 2, 3], [4, 5, 6]])
print(array1.dtype)
# Now let's create an array of floats
array2 = np.array([[7.1, 8.2, 9.1], [10.4, 11.2, 12.3]])
print(array2.dtype)
# Integers (int) are whole numbers only, and Floating point numbers (float) can have a whole number portion
# and a decimal portion. The 64 in this example refers to the number of bits that the operating system is
# reserving to represent the number, which determines the size (or precision) of the numbers that can be
# represented.
# Let's do an addition for the two arrays
array3=array1+array2
print(array3)
print(array3.dtype)
# Notice how the items in the resulting array have been upcast into floating point numbers
# Numpy arrays have many interesting aggregation functions on them, such as sum(), max(), min(), and mean()
print(array3.sum())
print(array3.max())
print(array3.min())
print(array3.mean())
# For two dimensional arrays, we can do the same thing for each row or column
# let's create an array with 15 elements, ranging from 1 to 15,
# with a dimension of 3X5
b = np.arange(1,16,1).reshape(3,5)
print(b)
# Now, we often think about two dimensional arrays being made up of rows and columns, but you can also think
# of these arrays as just a giant ordered list of numbers, and the *shape* of the array, the number of rows
# and columns, is just an abstraction that we have for a particular purpose. Actually, this is exactly how
# basic images are stored in computer environments.
# Let's take a look at an example and see how numpy comes into play.
# For this demonstration I'll use the python imaging library (PIL) and a function to display images in the
# Jupyter notebook
from PIL import Image
from IPython.display import display
# And let's just look at the image I'm talking about
im = Image.open('chris.tiff')
display(im)
# Now, we can conver this PIL image to a numpy array
array=np.array(im)
print(array.shape)
array
# Here we see that we have a 200x200 array and that the values are all uint8. The uint means that they are
# unsigned integers (so no negative numbers) and the 8 means 8 bits per byte. This means that each value can
# be up to 2*2*2*2*2*2*2*2=256 in size (well, actually 255, because we start at zero). For black and white
# images black is stored as 0 and white is stored as 255. So if we just wanted to invert this image we could
# use the numpy array to do so
# Let's create an array the same shape
mask=np.full(array.shape,255)
mask
# Now let's subtract that from the modified array
modified_array=array-mask
# And lets convert all of the negative values to positive values
modified_array=modified_array*-1
# And as a last step, let's tell numpy to set the value of the datatype correctly
modified_array=modified_array.astype(np.uint8)
modified_array
# And lastly, lets display this new array. We do this by using the fromarray() function in the python
# imaging library to convert the numpy array into an object jupyter can render
display(Image.fromarray(modified_array))
# Cool. Ok, remember how I started this by talking about how we could just think of this as a giant array
# of bytes, and that the shape was an abstraction? Well, we could just decide to reshape the array and still
# try and render it. PIL is interpreting the individual rows as lines, so we can change the number of lines
# and columns if we want to. What do you think that would look like?
reshaped=np.reshape(modified_array,(100,400))
print(reshaped.shape)
display(Image.fromarray(reshaped))
# Can't say I find that particularly flattering. By reshaping the array to be only 100 rows high but 400
# columns we've essentially doubled the image by taking every other line and stacking them out in width. This
# makes the image look more stretched out too.
# This isn't an image manipulation course, but the point was to show you that these numpy arrays are really
# just abstractions on top of data, and that data has an underlying format (in this case, uint8). But further,
# we can build abstractions on top of that, such as computer code which renders a byte as either black or
# white, which has meaning to people. In some ways, this whole degree is about data and the abstractions that
# we can build on top of that data, from individual byte representations through to complex neural networks of
# functions or interactive visualizations. Your role as a data scientist is to understand what the data means
# (it's context an collection), and transform it into a different representation to be used for sensemaking.
# Ok, back to the mechanics of numpy.
###Output
_____no_output_____
###Markdown
Indexing, Slicing and Iterating
###Code
# Indexing, slicing and iterating are extremely important for data manipulation and analysis because these
# techinques allow us to select data based on conditions, and copy or update data.
###Output
_____no_output_____
###Markdown
Indexing
###Code
# First we are going to look at integer indexing. A one-dimensional array, works in similar ways as a list -
# To get an element in a one-dimensional array, we simply use the offset index.
a = np.array([1,3,5,7])
a[2]
# For multidimensional array, we need to use integer array indexing, let's create a new multidimensional array
a = np.array([[1,2], [3, 4], [5, 6]])
a
# if we want to select one certain element, we can do so by entering the index, which is comprised of two
# integers the first being the row, and the second the column
a[1,1] # remember in python we start at 0!
# if we want to get multiple elements
# for example, 1, 4, and 6 and put them into a one-dimensional array
# we can enter the indices directly into an array function
np.array([a[0, 0], a[1, 1], a[2, 1]])
# we can also do that by using another form of array indexing, which essentiall "zips" the first list and the
# second list up
print(a[[0, 1, 2], [0, 1, 1]])
###Output
[1 4 6]
###Markdown
Boolean Indexing
###Code
# Boolean indexing allows us to select arbitrary elements based on conditions. For example, in the matrix we
# just talked about we want to find elements that are greater than 5 so we set up a conditon a >5
print(a >5)
# This returns a boolean array showing that if the value at the corresponding index is greater than 5
# We can then place this array of booleans like a mask over the original array to return a one-dimensional
# array relating to the true values.
print(a[a>5])
# As we will see, this functionality is essential in the pandas toolkit which is the bulk of this course
###Output
_____no_output_____
###Markdown
Slicing
###Code
# Slicing is a way to create a sub-array based on the original array. For one-dimensional arrays, slicing
# works in similar ways to a list. To slice, we use the : sign. For instance, if we put :3 in the indexing
# brackets, we get elements from index 0 to index 3 (excluding index 3)
a = np.array([0,1,2,3,4,5])
print(a[:3])
# By putting 2:4 in the bracket, we get elements from index 2 to index 4 (excluding index 4)
print(a[2:4])
# For multi-dimensional arrays, it works similarly, lets see an example
a = np.array([[1,2,3,4], [5,6,7,8], [9,10,11,12]])
a
# First, if we put one argument in the array, for example a[:2] then we would get all the elements from the
# first (0th) and second row (1th)
a[:2]
# If we add another argument to the array, for example a[:2, 1:3], we get the first two rows but then the
# second and third column values only
a[:2, 1:3]
# So, in multidimensional arrays, the first argument is for selecting rows, and the second argument is for
# selecting columns
# It is important to realize that a slice of an array is a view into the same data. This is called passing by
# reference. So modifying the sub array will consequently modify the original array
# Here I'll change the element at position [0, 0], which is 2, to 50, then we can see that the value in the
# original array is changed to 50 as well
sub_array = a[:2, 1:3]
print("sub array index [0,0] value before change:", sub_array[0,0])
sub_array[0,0] = 50
print("sub array index [0,0] value after change:", sub_array[0,0])
print("original array index [0,1] value after change:", a[0,1])
###Output
sub array index [0,0] value before change: 2
sub array index [0,0] value after change: 50
original array index [0,1] value after change: 50
###Markdown
Trying Numpy with Datasets
###Code
# Now that we have learned the essentials of Numpy let's use it on a couple of datasets
# Here we have a very popular dataset on wine quality, and we are going to only look at red wines. The data
# fields include: fixed acidity, volatile aciditycitric acid, residual sugar, chlorides, free sulfur dioxide,
# total sulfur dioxidedensity, pH, sulphates, alcohol, quality
# To load a dataset in Numpy, we can use the genfromtxt() function. We can specify data file name, delimiter
# (which is optional but often used), and number of rows to skip if we have a header row, hence it is 1 here
# The genfromtxt() function has a parameter called dtype for specifying data types of each column this
# parameter is optional. Without specifying the types, all types will be casted the same to the more
# general/precise type
wines = np.genfromtxt("datasets/winequality-red.csv", delimiter=";", skip_header=1)
wines
# Recall that we can use integer indexing to get a certain column or a row. For example, if we want to select
# the fixed acidity column, which is the first coluumn, we can do so by entering the index into the array.
# Also remember that for multidimensional arrays, the first argument refers to the row, and the second
# argument refers to the column, and if we just give one argument then we'll get a single dimensional list
# back.
# So all rows combined but only the first column from them would be
print("one integer 0 for slicing: ", wines[:, 0])
# But if we wanted the same values but wanted to preserve that they sit in their own rows we would write
print("0 to 1 for slicing: \n", wines[:, 0:1])
# This is another great example of how the shape of the data is an abstraction which we can layer
# intentionally on top of the data we are working with.
# If we want a range of columns in order, say columns 0 through 3 (recall, this means first, second, and
# third, since we start at zero and don't include the training index value), we can do that too
wines[:, 0:3]
# What if we want several non-consecutive columns? We can place the indices of the columns that we want into
# an array and pass the array as the second argument. Here's an example
wines[:, [0,2,4]]
# We can also do some basic summarization of this dataset. For example, if we want to find out the average
# quality of red wine, we can select the quality column. We could do this in a couple of ways, but the most
# appropriate is to use the -1 value for the index, as negative numbers mean slicing from the back of the
# list. We can then call the aggregation functions on this data.
wines[:,-1].mean()
# Let's take a look at another dataset, this time on graduate school admissions. It has fields such as GRE
# score, TOEFL score, university rating, GPA, having research experience or not, and a chance of admission.
# With this dataset, we can do data manipulation and basic analysis to infer what conditions are associated
# with higher chance of admission. Let's take a look.
# We can specify data field names when using genfromtxt() to loads CSV data. Also, we can have numpy try and
# infer the type of a column by setting the dtype parameter to None
graduate_admission = np.genfromtxt('datasets/Admission_Predict.csv', dtype=None, delimiter=',', skip_header=1,
names=('Serial No','GRE Score', 'TOEFL Score', 'University Rating', 'SOP',
'LOR','CGPA','Research', 'Chance of Admit'))
graduate_admission
# Notice that the resulting array is actually a one-dimensional array with 400 tuples
graduate_admission.shape
# We can retrieve a column from the array using the column's name for example, let's get the CGPA column and
# only the first five values.
graduate_admission['CGPA'][0:5]
# Since the GPA in the dataset range from 1 to 10, and in the US it's more common to use a scale of up to 4,
# a common task might be to convert the GPA by dividing by 10 and then multiplying by 4
graduate_admission['CGPA'] = graduate_admission['CGPA'] /10 *4
graduate_admission['CGPA'][0:20] #let's get 20 values
# Recall boolean masking. We can use this to find out how many students have had research experience by
# creating a boolean mask and passing it to the array indexing operator
len(graduate_admission[graduate_admission['Research'] == 1])
# Since we have the data field chance of admission, which ranges from 0 to 1, we can try to see if students
# with high chance of admission (>0.8) on average have higher GRE score than those with lower chance of
# admission (<0.4)
# So first we use boolean masking to pull out only those students we are interested in based on their chance
# of admission, then we pull out only their GPA scores, then we print the mean values.
print(graduate_admission[graduate_admission['Chance_of_Admit'] > 0.8]['GRE_Score'].mean())
print(graduate_admission[graduate_admission['Chance_of_Admit'] < 0.4]['GRE_Score'].mean())
# Take a moment to reflect here, do you understand what is happening in these calls?
# When we do the boolean masking we are left with an array with tuples in it still, and numpy holds underneath
# this a list of the columns we specified and their name and indexes
graduate_admission[graduate_admission['Chance_of_Admit'] > 0.8]
# Let's also do this with GPA
print(graduate_admission[graduate_admission['Chance_of_Admit'] > 0.8]['CGPA'].mean())
print(graduate_admission[graduate_admission['Chance_of_Admit'] < 0.4]['CGPA'].mean())
# Hrm, well, I guess one could have expected this. The GPA and GRE for students who have a higher chance of
# being admitted, at least based on our cursory look here, seems to be higher.
###Output
_____no_output_____ |
ipynb/Germany-Bayern-LK-Nürnberger-Land.ipynb | ###Markdown
Germany: LK Nürnberger Land (Bayern)* Homepage of project: https://oscovida.github.io* Plots are explained at http://oscovida.github.io/plots.html* [Execute this Jupyter Notebook using myBinder](https://mybinder.org/v2/gh/oscovida/binder/master?filepath=ipynb/Germany-Bayern-LK-Nürnberger-Land.ipynb)
###Code
import datetime
import time
start = datetime.datetime.now()
print(f"Notebook executed on: {start.strftime('%d/%m/%Y %H:%M:%S%Z')} {time.tzname[time.daylight]}")
%config InlineBackend.figure_formats = ['svg']
from oscovida import *
overview(country="Germany", subregion="LK Nürnberger Land", weeks=5);
overview(country="Germany", subregion="LK Nürnberger Land");
compare_plot(country="Germany", subregion="LK Nürnberger Land", dates="2020-03-15:");
# load the data
cases, deaths = germany_get_region(landkreis="LK Nürnberger Land")
# get population of the region for future normalisation:
inhabitants = population(country="Germany", subregion="LK Nürnberger Land")
print(f'Population of country="Germany", subregion="LK Nürnberger Land": {inhabitants} people')
# compose into one table
table = compose_dataframe_summary(cases, deaths)
# show tables with up to 1000 rows
pd.set_option("max_rows", 1000)
# display the table
table
###Output
_____no_output_____
###Markdown
Explore the data in your web browser- If you want to execute this notebook, [click here to use myBinder](https://mybinder.org/v2/gh/oscovida/binder/master?filepath=ipynb/Germany-Bayern-LK-Nürnberger-Land.ipynb)- and wait (~1 to 2 minutes)- Then press SHIFT+RETURN to advance code cell to code cell- See http://jupyter.org for more details on how to use Jupyter Notebook Acknowledgements:- Johns Hopkins University provides data for countries- Robert Koch Institute provides data for within Germany- Atlo Team for gathering and providing data from Hungary (https://atlo.team/koronamonitor/)- Open source and scientific computing community for the data tools- Github for hosting repository and html files- Project Jupyter for the Notebook and binder service- The H2020 project Photon and Neutron Open Science Cloud ([PaNOSC](https://www.panosc.eu/))--------------------
###Code
print(f"Download of data from Johns Hopkins university: cases at {fetch_cases_last_execution()} and "
f"deaths at {fetch_deaths_last_execution()}.")
# to force a fresh download of data, run "clear_cache()"
print(f"Notebook execution took: {datetime.datetime.now()-start}")
###Output
_____no_output_____ |
tutorial/dl_courses/03_dnn_simpson/tf_DNN_experiment.ipynb | ###Markdown
Tensorflow DNN - overview import package
###Code
%matplotlib inline
import matplotlib.pyplot as plt
import numpy as np
import pandas as pd
import cv2
import tensorflow as tf
###Output
_____no_output_____
###Markdown
prepare dataset examples
###Code
simpson_img = pd.read_csv('simpson_preproc/simpson_img_list.csv')
print(simpson_img.head())
###Output
classname img
0 c34 marge_simpson/pic_0601.jpg
1 c34 marge_simpson/pic_0180.jpg
2 c34 marge_simpson/pic_0196.jpg
3 c34 marge_simpson/pic_0698.jpg
4 c34 marge_simpson/pic_0083.jpg
###Markdown
plot image example
###Code
img = cv2.imread('simpson_preproc/' + simpson_img.img.iloc[0], 0)
plt.imshow(img, cmap="gray")
###Output
_____no_output_____
###Markdown
filter data
###Code
simpson_img = simpson_img[simpson_img.img.str.contains('simpson')]
###Output
_____no_output_____
###Markdown
transform y to one-hot encoder format
###Code
simpson_y = pd.get_dummies(simpson_img['classname'], '').as_matrix()
print(simpson_y)
###Output
[[0 0 0 0 1 0]
[0 0 0 0 1 0]
[0 0 0 0 1 0]
...,
[1 0 0 0 0 0]
[1 0 0 0 0 0]
[1 0 0 0 0 0]]
###Markdown
information of training data
###Code
x_train_list, y_train = simpson_img, simpson_y
## record the dim of img ##
img = cv2.resize(img, (50,50))
img = img.flatten()
print('input_data shape: training {training_shape}'.format(
training_shape=(len(x_train_list), img.shape[0])))
print('y_true shape: training {training_shape}'.format(
training_shape=y_train.shape))
###Output
input_data shape: training (7274, 2500)
y_true shape: training (7274, 6)
###Markdown
define image generator
###Code
from sklearn.utils import shuffle
def simpson_train_batch_generator(x, y, bs, shape):
x_train = np.array([]).reshape((0, shape))
y_train = np.array([]).reshape((0, y.shape[1]))
while True:
new_ind = shuffle(range(len(x)))
x = x.take(new_ind)
y = np.take(y, new_ind, axis=0)
for i in range(len(x)):
dir_img = 'simpson_preproc/' + x.img.iloc[i]
img = cv2.imread(dir_img, 0)
img = cv2.resize(img, (50,50))
x_train = np.row_stack([x_train, img.flatten()])
y_train = np.row_stack([y_train, y[i]])
if x_train.shape[0] == bs:
x_batch = x_train.copy()
x_batch /= 255.
y_batch = y_train.copy()
x_train = np.array([]).reshape((0 ,shape))
y_train = np.array([]).reshape((0 ,y.shape[1]))
yield x_batch, y_batch
###Output
_____no_output_____
###Markdown
create graph & run session experiment
###Code
tf.reset_default_graph() ## 重新 build graph 需要跑這行
###Output
_____no_output_____ |
pandas/notebook/exercise-creating-reading-and-writing.ipynb | ###Markdown
**This notebook is an exercise in the [Pandas](https://www.kaggle.com/learn/pandas) course. You can reference the tutorial at [this link](https://www.kaggle.com/residentmario/creating-reading-and-writing).**--- IntroductionThe first step in most data analytics projects is reading the data file. In this exercise, you'll create Series and DataFrame objects, both by hand and by reading data files.Run the code cell below to load libraries you will need (including code to check your answers).
###Code
import pandas as pd
pd.set_option('max_rows', 5)
from learntools.core import binder; binder.bind(globals())
from learntools.pandas.creating_reading_and_writing import *
print("Setup complete.")
###Output
_____no_output_____
###Markdown
Exercises 1.In the cell below, create a DataFrame `fruits` that looks like this:
###Code
# Your code goes here. Create a dataframe matching the above diagram and assign it to the variable fruits.
fruits = pd.DataFrame({'Apples':[30], 'Bananas':[21]})
fruits
# Check your answer
q1.check()
fruits.head()
###Output
_____no_output_____
###Markdown
2.Create a dataframe `fruit_sales` that matches the diagram below:
###Code
# Your code goes here. Create a dataframe matching the above diagram and assign it to the variable fruit_sales.
fruit_sales = pd.DataFrame([[35,21],[41,34]],columns =['Apples','Bananas'], index =['2017 Sales','2018 Sales'])
fruit_sales
# Check your answer
q2.check()
fruit_sales.head()
#q2.hint()
#q2.solution()
###Output
_____no_output_____
###Markdown
3.Create a variable `ingredients` with a Series that looks like:```Flour 4 cupsMilk 1 cupEggs 2 largeSpam 1 canName: Dinner, dtype: object```
###Code
ingredients = pd.Series(['4 cups','1 cup','2 large','1 can'], index=['Flour','Milk','Eggs','Spam'], name = 'Dinner')
ingredients
# Check your answer
q3.check()
ingredients.head()
#q3.hint()
#q3.solution()
###Output
_____no_output_____
###Markdown
4.Read the following csv dataset of wine reviews into a DataFrame called `reviews`:The filepath to the csv file is `../input/wine-reviews/winemag-data_first150k.csv`. The first few lines look like:```,country,description,designation,points,price,province,region_1,region_2,variety,winery0,US,"This tremendous 100% varietal wine[...]",Martha's Vineyard,96,235.0,California,Napa Valley,Napa,Cabernet Sauvignon,Heitz1,Spain,"Ripe aromas of fig, blackberry and[...]",Carodorum Selección Especial Reserva,96,110.0,Northern Spain,Toro,,Tinta de Toro,Bodega Carmen Rodríguez```
###Code
reviews = pd.read_csv("../input/wine-reviews/winemag-data_first150k.csv", index_col=0, )
reviews
# Check your answer
q4.check()
reviews.head()
#q4.hint()
#q4.solution()
###Output
_____no_output_____
###Markdown
5.Run the cell below to create and display a DataFrame called `animals`:
###Code
animals = pd.DataFrame({'Cows': [12, 20], 'Goats': [22, 19]}, index=['Year 1', 'Year 2'])
animals
###Output
_____no_output_____
###Markdown
In the cell below, write code to save this DataFrame to disk as a csv file with the name `cows_and_goats.csv`.
###Code
animals.to_csv('cows_and_goats.csv')
file = pd.read_csv('cows_and_goats.csv')
print(file)
# Check your answer
q5.check()
#q5.hint()
#q5.solution()
###Output
_____no_output_____ |
website/docs/aicitymaps_test.ipynb | ###Markdown
1. Para encontrar la altura del edificio y los angulos necesitamos que las coordenadas sean geograficas, como están (40, -3N)2. Para la posición de las nuevas coordenadas tenemos que a) pasar las coordenadas geograficas de origen a UTM b) sumarles las long (newposx, newposy) c) volver a coordenadas geograficas
###Code
df.dtypes
#CALCULO LONGITUD SOMBRA - cuanto mide la sombra
#CALCULO COORDENADAS SOMBRA - donde acaba la sombra (punto sombra/no sombra)
# coordenada X de la sombra
# coordenada Y de la sombra
#vectorx, vectory - vectores no unitarios - antes de normalizar
#NORMALIZAMOS VECTORES Y MULTIPLICAMOS POR LA SOMBRA
# PASAMOS VALORES DEL EFICIO A UTM
# ENCONTRAMOS PUNTO DE SOMBRA
###Output
_____no_output_____ |
site/en/guide/migrate/logging_tensor_hook.ipynb | ###Markdown
Copyright 2021 The TensorFlow Authors.
###Code
#@title Licensed under the Apache License, Version 2.0 (the "License");
# you may not use this file except in compliance with the License.
# You may obtain a copy of the License at
#
# https://www.apache.org/licenses/LICENSE-2.0
#
# Unless required by applicable law or agreed to in writing, software
# distributed under the License is distributed on an "AS IS" BASIS,
# WITHOUT WARRANTIES OR CONDITIONS OF ANY KIND, either express or implied.
# See the License for the specific language governing permissions and
# limitations under the License.
###Output
_____no_output_____
###Markdown
Migration examples: estimator.LoggingTensorHook View on TensorFlow.org Run in Google Colab View source on GitHub Download notebook This notebook demonstrates how you can migrate `tf.estimator.LoggingTensorHook` usage to use custom `tf.keras.callbacks.Callback` instead. SetupFirst, you need to define a couple of necessary imports.
###Code
import tensorflow as tf
import tensorflow.compat.v1 as tf1
###Output
_____no_output_____
###Markdown
Prepare some simple data for demonstration.
###Code
features = [[1., 1.5], [2., 2.5], [3., 3.5]]
labels = [[0.3], [0.5], [0.7]]
eval_features = [[4., 4.5], [5., 5.5], [6., 6.5]]
eval_labels = [[0.8], [0.9], [1.]]
###Output
_____no_output_____
###Markdown
TF1: Estimator.train/evaluate To monitor tensors, for example model weights or losses, you can use `tf.estimator.LoggingTensorHook` (`tf1.train.LoggingTensorHook` is its alias), and then pass the hook to `tf.estimator.EstimatorSpec`.
###Code
def _input_fn():
return tf1.data.Dataset.from_tensor_slices((features, labels)).batch(1)
def _eval_input_fn():
return tf1.data.Dataset.from_tensor_slices(
(eval_features, eval_labels)).batch(1)
def _model_fn(features, labels, mode):
dense = tf1.layers.Dense(1)
logits = dense(features)
loss = tf1.losses.mean_squared_error(labels=labels, predictions=logits)
optimizer = tf1.train.AdagradOptimizer(0.05)
train_op = optimizer.minimize(loss, global_step=tf1.train.get_global_step())
kernel_name = tf.identity(dense.weights[0])
bias_name = tf.identity(dense.weights[1])
# access tensors to be logged by names
logging_weight = tf1.train.LoggingTensorHook(tensors=[kernel_name, bias_name],
every_n_iter=1)
# log training loss by the tensor object
logging_loss = tf1.train.LoggingTensorHook(
{'loss from LoggingTensorHook': loss},
every_n_secs=3)
return tf1.estimator.EstimatorSpec(mode,
loss=loss,
train_op=train_op,
training_hooks=[logging_weight,
logging_loss])
estimator = tf1.estimator.Estimator(model_fn=_model_fn)
estimator.train(_input_fn)
###Output
_____no_output_____
###Markdown
TF2: Keras training API In TF2, accessing to tensors by names is not supported. You need to record and output the logged tensors manually.When migrating to TF2 Keras training API, you can define when to log the tensors by overriding different methods of defining custom `tf.keras.callbacks.Callback`. You can also implement the logging frequency in the custom callback. The example below will print the weights every two steps. Other strategies like logging every n seconds are also possible.Check the API [docs](https://www.tensorflow.org/api_docs/python/tf/keras/callbacks/Callback) and [Writing your own callbacks](https://www.tensorflow.org/guide/keras/custom_callback) for more details.
###Code
class LoggingTensorCallback(tf.keras.callbacks.Callback):
def __init__(self, every_n_iter):
super().__init__()
self._every_n_iter = every_n_iter
self._log_count = every_n_iter
def on_batch_end(self, batch, logs=None):
if self._log_count > 0:
self._log_count -= 1
print("Logging Tensor Callback: dense/kernel:",
model.layers[0].weights[0])
print("Logging Tensor Callback: dense/bias:",
model.layers[0].weights[1])
print("Logging Tensor Callback loss:", logs["loss"])
else:
self._log_count -= self._every_n_iter
dataset = tf.data.Dataset.from_tensor_slices((features, labels)).batch(1)
eval_dataset = tf.data.Dataset.from_tensor_slices(
(eval_features, eval_labels)).batch(1)
model = tf.keras.models.Sequential([tf.keras.layers.Dense(1)])
optimizer = tf.keras.optimizers.Adagrad(learning_rate=0.05)
model.compile(optimizer, "mse")
model.fit(dataset, callbacks=[LoggingTensorCallback(2)])
###Output
_____no_output_____ |
visualisation_gdp_europe.ipynb | ###Markdown
Visualise nominal GDP for European countries in billion $ Purpose: * show how to use geopandas and geoplot and to visualise a certain quantity (here GDP) per country* web scraping part has not been included but would be fun to show as well Modules
###Code
import numpy as np
import pandas as pd
import geopandas as gpd
from geopandas import GeoDataFrame
from shapely.geometry import Point
import matplotlib.pyplot as plt
import geoplot.crs as gcrs
import geoplot as gplt
import seaborn as sns
sns.set_style('whitegrid')
import helper_functions
###Output
_____no_output_____
###Markdown
Data import and wranging part Mapping of countries and their capitals:
###Code
european_countries_capitals = pd.read_csv('data/european_countries_capitals.txt',
sep='--', engine='python')
european_countries_capitals = helper_functions.format_columns(european_countries_capitals)
european_countries_capitals['capital'] = european_countries_capitals['capital'].str.strip()
european_countries_capitals['country'] = european_countries_capitals['country'].str.strip()
###Output
_____no_output_____
###Markdown
Merge European countries border information with capital information from above to just keep the country names.
###Code
european_areas = gpd.read_file('data/Europe_borders.shp')
european_areas['TZID'] = european_areas['TZID'].str.replace('Europe/', '')
european_areas.rename(columns = {'TZID':'capital'}, inplace = True)
geometry = european_areas['geometry']
european_areas = helper_functions.format_columns(european_areas)
european_areas['capital'] = european_areas['capital'].str.strip()
european_areas = european_areas.merge(european_countries_capitals, on='capital', how='inner')
european_areas.drop(columns=['geometry'], inplace=True)
european_areas['geometry'] = geometry
###Output
_____no_output_____
###Markdown
Get European GDP Info. Source: [List_of_sovereign_states_in_Europe_by_GDP_(nominal)](https://en.wikipedia.org/wiki/List_of_sovereign_states_in_Europe_by_GDP_(nominal))
###Code
european_countries_gdp = pd.read_csv('data/european_countries_gdp.txt',
sep='\t', engine='python')
european_countries_gdp.drop(columns=['2018 Rank'], inplace=True)
european_countries_gdp.rename(columns = {'2018[2]':'2018'}, inplace = True)
european_countries_gdp = helper_functions.format_columns(european_countries_gdp)
european_countries_gdp['country'] = european_countries_gdp['country'].str.strip()
###Output
_____no_output_____
###Markdown
Merge European area and gdp information
###Code
europe = european_areas.merge(european_countries_gdp, on='country', how='inner')
europe['2018'] = europe['2018'].str.replace(',','')
europe['2018'] = europe['2018'].astype('float')
europe = europe.reset_index()
europe.rename(columns = {'index':'id'}, inplace = True)
###Output
_____no_output_____
###Markdown
Calculate relative GDP
###Code
gdp_per_country = europe[['country', '2018']].groupby(['country']).sum()
gdp_total = europe[['country', '2018']].groupby(['country']).sum().sum()
gdp_total = int(round(gdp_total.values[0], 0))
gdp_per_country = gdp_per_country.reset_index()
gdp_per_country.rename(columns = {'2018':'gdp'}, inplace = True)
gdp_per_country['gdp'] = round(gdp_per_country['gdp'], 0)
gdp_per_country['gdp'] = gdp_per_country['gdp'].astype(float)
gdp_per_country['gdp_relative'] = gdp_per_country['gdp'] / gdp_total
gdp_per_country['gdp_relative'] = round(gdp_per_country['gdp_relative'] * 100, 2)
###Output
_____no_output_____
###Markdown
Merge all information together:
###Code
europe = europe.merge(gdp_per_country, on='country', how='inner')
###Output
_____no_output_____
###Markdown
Visualisation:
###Code
gplt.choropleth(europe, hue=europe['gdp_relative'],
projection=gcrs.AlbersEqualArea(),
cmap='Reds',
linewidth=0.5,
edgecolor='white',
k=None,
legend=True,
figsize=(10,10))
plt.title('GDP in % per European Country')
plt.savefig('data/europe_relative_gdp.png', bbox_inches='tight', pad_inches=0.1);
###Output
_____no_output_____ |
00_Original/MatplotlibTutorial_p6_AUFGABEN.ipynb | ###Markdown
Aufgaben zum Kapitel "Balken-, Säulendiagramme und Histogramme" Aufgaben:1. Aufgabe: Bei der Bundestagswahl in Deutschland gab es im Jahre 2017 folgende prozentuale Verteilungen für die einzelnen Parteien: CDU/CSU: 32,9, SPD: 20,5, AfD: 12,6, FDP: 10,7, Linke: 9,2, Grüne: 8,9, Sonstige: 5Erzeuge ein Säulendiagramm mit diesen Daten.
###Code
parteien = ["CDU/CSU", "SPD", "AfD", "FDP", "Linke", "Grüne", "Sonst."]
colors = ['black', 'orangered', 'blue', 'yellow', 'red', 'green', 'grey']
anteile = [32.9, 20.5, 12.6, 10.7, 9.2, 8.9, 5]
bars = plt.bar(parteien, anteile, color=colors)
plt.title("Ergebnisse Bundestagswahlkampf 2017")
plt.show()
###Output
_____no_output_____ |
Mathematics_for_Machine_Learning/PCA/utf-8''week3.ipynb | ###Markdown
Orthogonal ProjectionsWe will write functions that will implement orthogonal projections. Learning objectives1. Write code that projects data onto lower-dimensional subspaces.2. Understand the real world applications of projections. As always, we will first import the packages that we need for this assignment.
###Code
# PACKAGE: DO NOT EDIT THIS CELL
import matplotlib
matplotlib.use('Agg')
import matplotlib.pyplot as plt
plt.style.use('fivethirtyeight')
import numpy as np
###Output
_____no_output_____
###Markdown
Next, we will retrieve the Olivetti faces dataset.
###Code
from sklearn.datasets import fetch_olivetti_faces, fetch_lfw_people
from ipywidgets import interact
%matplotlib inline
image_shape = (64, 64)
# Load faces data
dataset = fetch_olivetti_faces('./')
faces = dataset.data
###Output
_____no_output_____
###Markdown
Advice for testing numerical algorithmsBefore we begin this week's assignment, there are some advice that we would like to give for writing functions that work with numerical data. They are useful for finding bugs in your implementation.Testing machine learning algorithms (or numerical algorithms in general)is sometimes really hard as it depends on the datasetto produce an answer, and you will never be able to test your algorithm on all the datasetswe have in the world. Nevertheless, we have some tips for you to help you identify bugs inyour implementations. 1. Test on small datasetTest your algorithms on small dataset: datasets of size 1 or 2 sometimes will suffice. Thisis useful because you can (if necessary) compute the answers by hand and compare them withthe answers produced by the computer program you wrote. In fact, these small datasets can even have special numbers,which will allow you to compute the answers by hand easily. 2. Find invariantsInvariants refer to properties of your algorithm and functions that are maintained regardlessof the input. We will highlight this point later in this notebook where you will see functions,which will check invariants for some of the answers you produce.Invariants you may want to look for:1. Does your algorithm always produce a positive/negative answer, or a positive definite matrix?2. If the algorithm is iterative, do the intermediate results increase/decrease monotonically?3. Does your solution relate with your input in some interesting way, e.g. orthogonality? Finding invariants is hard, and sometimes there simply isn't any invariant. However, DO take advantage of them if you can find them. They are the most powerful checks when you have them. We can find some invariants for projections. In the cell below, we have written two functions which check for invariants of projections. See the docstrings which explain what each of them does. You should use these functions to test your code.
###Code
import numpy.testing as np_test
def test_property_projection_matrix(P):
"""Test if the projection matrix satisfies certain properties.
In particular, we should have P @ P = P, and P = P^T
"""
np_test.assert_almost_equal(P, P @ P)
np_test.assert_almost_equal(P, P.T)
def test_property_projection(x, p):
"""Test orthogonality of x and its projection p."""
np_test.assert_almost_equal(p.T @ (p-x), 0)
###Output
_____no_output_____
###Markdown
1. Orthogonal Projections Recall that for projection of a vector $\boldsymbol x$ onto a 1-dimensional subspace $U$ with basis vector $\boldsymbol b$ we have$${\pi_U}(\boldsymbol x) = \frac{\boldsymbol b\boldsymbol b^T}{{\lVert\boldsymbol b \rVert}^2}\boldsymbol x $$And for the general projection onto an M-dimensional subspace $U$ with basis vectors $\boldsymbol b_1,\dotsc, \boldsymbol b_M$ we have$${\pi_U}(\boldsymbol x) = \boldsymbol B(\boldsymbol B^T\boldsymbol B)^{-1}\boldsymbol B^T\boldsymbol x $$where $$\boldsymbol B = [\boldsymbol b_1,...,\boldsymbol b_M]$$Your task is to implement orthogonal projections. We can split this into two steps1. Find the projection matrix $\boldsymbol P$ that projects any $\boldsymbol x$ onto $U$.2. The projected vector $\pi_U(\boldsymbol x)$ of $\boldsymbol x$ can then be written as $\pi_U(\boldsymbol x) = \boldsymbol P\boldsymbol x$.To perform step 1, you need to complete the function `projection_matrix_1d` and `projection_matrix_general`. To perform step 2, complete `project_1d` and `project_general`.
###Code
# GRADED FUNCTION: DO NOT EDIT THIS LINE
# Projection 1d
# ===YOU SHOULD EDIT THIS FUNCTION===
def projection_matrix_1d(b):
"""Compute the projection matrix onto the space spanned by `b`
Args:
b: ndarray of dimension (D, 1), the basis for the subspace
Returns:
P: the projection matrix
"""
D, _ = b.shape
P = b @ b.T / (b.T @ b) # <-- EDIT THIS
return P
# ===YOU SHOULD EDIT THIS FUNCTION===
def project_1d(x, b):
"""Compute the projection matrix onto the space spanned by `b`
Args:
x: the vector to be projected
b: ndarray of dimension (D, 1), the basis for the subspace
Returns:
y: ndarray of shape (D, 1) projection of x in space spanned by b
"""
p = projection_matrix_1d(b) @ x # <-- EDIT THIS
return p
# Projection onto a general (higher-dimensional) subspace
# ===YOU SHOULD EDIT THIS FUNCTION===
def projection_matrix_general(B):
"""Compute the projection matrix onto the space spanned by the columns of `B`
Args:
B: ndarray of dimension (D, M), the basis for the subspace
Returns:
P: the projection matrix
"""
P = B @ np.linalg.inv(B.T @ B) @ B.T # <-- EDIT THIS
return P
# ===YOU SHOULD EDIT THIS FUNCTION===
def project_general(x, B):
"""Compute the projection matrix onto the space spanned by the columns of `B`
Args:
x: ndarray of dimension (D, 1), the vector to be projected
B: ndarray of dimension (D, M), the basis for the subspace
Returns:
p: projection of x onto the subspac spanned by the columns of B; size (D, 1)
"""
p = projection_matrix_general(B) @ x # <-- EDIT THIS
return p
###Output
_____no_output_____
###Markdown
We have included some unittest for you to test your implementation.
###Code
# Orthogonal projection in 2d
# define basis vector for subspace
b = np.array([2,1]).reshape(-1, 1)
# point to be projected later
x = np.array([1,2]).reshape(-1, 1)
###Output
_____no_output_____
###Markdown
Remember our discussion earlier about invariants? In the next cell, we will check that these invariants hold for the functions that you have implemented earlier.
###Code
# Test 1D
# Test that we computed the correct projection matrix
np_test.assert_almost_equal(projection_matrix_1d(np.array([1, 2, 2]).reshape(-1,1)),
np.array([[1, 2, 2],
[2, 4, 4],
[2, 4, 4]]) / 9)
# Test that we project x on to the 1d subspace correctly
np_test.assert_almost_equal(project_1d(np.ones((3,1)),
np.array([1, 2, 2]).reshape(-1,1)),
np.array([5, 10, 10]).reshape(-1,1) / 9)
B = np.array([[1, 0],
[1, 1],
[1, 2]])
# Test 2D
# Test that we computed the correct projection matrix
np_test.assert_almost_equal(projection_matrix_general(B),
np.array([[5, 2, -1],
[2, 2, 2],
[-1, 2, 5]]) / 6)
# Test that we project x on to the 2d subspace correctly
np_test.assert_almost_equal(project_general(np.array([6, 0, 0]).reshape(-1,1), B),
np.array([5, 2, -1]).reshape(-1,1))
###Output
_____no_output_____
###Markdown
It is always good practice to create your own test cases. Create some testcases of your own below!
###Code
# Write your own test cases here, use random inputs, utilize the invariants we have!
###Output
_____no_output_____
###Markdown
2. Eigenfaces (optional)Next, we will take a look at what happens if we project some dataset consisting of human faces onto some basis we callthe "eigenfaces". You do not need to know what `eigenfaces` are for now but you will know what they are towards the end of the course! As always, let's import the packages that we need.
###Code
from sklearn.datasets import fetch_olivetti_faces, fetch_lfw_people
from ipywidgets import interact
%matplotlib inline
image_shape = (64, 64)
# Load faces data
dataset = fetch_olivetti_faces('./')
faces = dataset.data
###Output
_____no_output_____
###Markdown
Let's visualize some faces in the dataset.
###Code
plt.figure(figsize=(10,10))
plt.imshow(np.hstack(faces[:5].reshape(5,64,64)), cmap='gray');
# for numerical reasons we normalize the dataset
mean = faces.mean(axis=0)
std = faces.std(axis=0)
faces_normalized = (faces - mean) / std
###Output
_____no_output_____
###Markdown
The data for the basis has been saved in a file named `eigenfaces.npy`, first we load it into the variable B.
###Code
B = np.load('eigenfaces.npy')[:50] # we use the first 50 basis vectors --- you should play around with this.
print("the eigenfaces have shape {}".format(B.shape))
###Output
the eigenfaces have shape (50, 64, 64)
###Markdown
Each instance in $\boldsymbol B$ is a `64x64' image, an "eigenface", which we determined using an algorithm called Principal Component Analysis. Let's visualize a few of those "eigenfaces".
###Code
plt.figure(figsize=(10,10))
plt.imshow(np.hstack(B[:5].reshape(-1, 64, 64)), cmap='gray');
###Output
_____no_output_____
###Markdown
Take a look at what happens if we project our faces onto the basis $\boldsymbol B$ spanned by these 50 "eigenfaces". In order to do this, we need to reshape $\boldsymbol B$ from above, which is of size (50, 64, 64), into the same shape as the matrix representing the basis as we have done earlier, which is of size (4096, 50). Here 4096 is the dimensionality of the data and 50 is the number of data points. Then we can reuse the functions we implemented earlier to compute the projection matrix and the projection. Complete the code below to visualize the reconstructed faces that lie on the subspace spanned by the "eigenfaces".
###Code
# EDIT THIS FUNCTION
@interact(i=(0, 10))
def show_face_face_reconstruction(i):
original_face = faces_normalized[i].reshape(64, 64)
# reshape the data we loaded in variable `B`
# so that we have a matrix representing the basis.
B_basis = B.reshape(50, 4096)[:10].T # <-- EDIT THIS
face_reconstruction = project_general(faces_normalized[i], B_basis).reshape(64, 64)
plt.figure()
plt.imshow(np.hstack([original_face, face_reconstruction]), cmap='gray')
plt.show()
###Output
_____no_output_____
###Markdown
What would happen to the reconstruction as we increase the dimension of our basis? Modify the code above to visualize it. 3. Least squares regression (optional) Consider the case where we have a linear model for predicting housing prices. We are predicting the housing prices based on features in the housing dataset. If we denote the features as $\boldsymbol x_0, \dotsc, \boldsymbol x_n$ and collect them into a vector $\boldsymbol {x}$, and the price of the houses as $y$. Assuming that we have a prediction model in the way such that $\hat{y}_i = f(\boldsymbol {x}_i) = \boldsymbol \theta^T\boldsymbol {x}_i$.If we collect the dataset into a $(N,D)$ data matrix $\boldsymbol X$, we can write down our model like this:$$\begin{bmatrix} \boldsymbol{x}_1^T \\\vdots \\ \boldsymbol{x}_N^T \end{bmatrix} \boldsymbol{\theta} = \begin{bmatrix} y_1 \\\vdots \\ y_2 \end{bmatrix},$$i.e.,$$\boldsymbol X\boldsymbol{\theta} = \boldsymbol{y}.$$Note that the data points are the *rows* of the data matrix, i.e., every column is a dimension of the data. Our goal is to find the best $\boldsymbol\theta$ such that we minimize the following objective (least square).$$\begin{eqnarray} & \sum^n_{i=1}{\lVert \bar{y_i} - y_i \rVert^2} \\&= \sum^n_{i=1}{\lVert \boldsymbol \theta^T\boldsymbol{x}_i - y_i \rVert^2} \\&= (\boldsymbol X\boldsymbol {\theta} - \boldsymbol y)^T(\boldsymbol X\boldsymbol {\theta} - \boldsymbol y).\end{eqnarray}$$If we set the gradient of the above objective to $\boldsymbol 0$, we have$$\begin{eqnarray} \nabla_\theta(\boldsymbol X\boldsymbol {\theta} - \boldsymbol y)^T(\boldsymbol X\boldsymbol {\theta} - \boldsymbol y) &=& \boldsymbol 0 \\\nabla_\theta(\boldsymbol {\theta}^T\boldsymbol X^T - \boldsymbol y^T)(\boldsymbol X\boldsymbol {\theta} - \boldsymbol y) &=& \boldsymbol 0 \\\nabla_\theta(\boldsymbol {\theta}^T\boldsymbol X^T\boldsymbol X\boldsymbol {\theta} - \boldsymbol y^T\boldsymbol X\boldsymbol \theta - \boldsymbol \theta^T\boldsymbol X^T\boldsymbol y + \boldsymbol y^T\boldsymbol y ) &=& \boldsymbol 0 \\2\boldsymbol X^T\boldsymbol X\theta - 2\boldsymbol X^T\boldsymbol y &=& \boldsymbol 0 \\\boldsymbol X^T\boldsymbol X\boldsymbol \theta &=& \boldsymbol X^T\boldsymbol y.\end{eqnarray}$$The solution that gives zero gradient solves (which we call the maximum likelihood estimator) the following equation:$$\boldsymbol X^T\boldsymbol X\boldsymbol \theta = \boldsymbol X^T\boldsymbol y.$$_This is exactly the same as the normal equation we have for projections_.This means that if we solve for $\boldsymbol X^T\boldsymbol X\boldsymbol \theta = \boldsymbol X^T\boldsymbol y.$ we would find the best $\boldsymbol \theta = (\boldsymbol X^T\boldsymbol X)^{-1}\boldsymbol X^T\boldsymbol y$, i.e. the $\boldsymbol \theta$ which minimizes our objective. Let's put things into perspective. Consider that we want to predict the true coefficient $\boldsymbol \theta$ of the line $\boldsymbol y = \boldsymbol \theta^T \boldsymbol x$ given only $\boldsymbol X$ and $\boldsymbol y$. We do not know the true value of $\boldsymbol \theta$.Note: In this particular example, $\boldsymbol \theta$ is a number. Still, we can represent it as an $\mathbb{R}^1$ vector.
###Code
x = np.linspace(0, 10, num=50)
theta = 2
def f(x):
random = np.random.RandomState(42) # we use the same random seed so we get deterministic output
return theta * x + random.normal(scale=1.0, size=len(x)) # our observations are corrupted by some noise, so that we do not get (x,y) on a line
y = f(x)
plt.scatter(x, y);
plt.xlabel('x');
plt.ylabel('y');
X = x.reshape(-1,1) # size N x 1
Y = y.reshape(-1,1) # size N x 1
# maximum likelihood estimator
theta_hat = np.linalg.solve(X.T @ X, X.T @ Y)
###Output
_____no_output_____
###Markdown
We can show how our $\hat{\boldsymbol \theta}$ fits the line.
###Code
fig, ax = plt.subplots()
ax.scatter(x, y);
xx = [0, 10]
yy = [0, 10 * theta_hat[0,0]]
ax.plot(xx, yy, 'red', alpha=.5);
ax.set(xlabel='x', ylabel='y');
print("theta = %f" % theta)
print("theta_hat = %f" % theta_hat)
###Output
theta = 2.000000
theta_hat = 1.951585
###Markdown
What would happend to $\lVert \hat{\boldsymbol \theta} - \boldsymbol \theta \rVert$ if we increase the number of datapoints?Make your hypothesis, and write a small program to confirm it!
###Code
N = np.arange(2, 10000, step=10)
# Your code comes here, which calculates \hat{\theta} for different dataset sizes.
theta_error = np.zeros(N.shape)
index = 0
for n in N:
x = np.linspace(0, n, num=10
theta = 2
y = f(x)
X = x.reshape(-1,1) # size N x 1
Y = y.reshape(-1,1) # size N x 1
theta_hat = np.linalg.solve(X.T @ X, X.T @ Y)
theta_error[index] = np.linalg.norm(theta - theta_hat)
plt.plot(theta_error)
plt.xlabel("dataset size")
plt.ylabel("parameter error");
###Output
_____no_output_____ |
The Panopticon.ipynb | ###Markdown
THE PANOPTICON *** GIS for OrionLead finish it completely.
###Code
import pandas as pd
from clickhouse_driver import Client
import matplotlib.pyplot as plt
plt.rcParams['figure.figsize'] = [60, 7]
%config IPCompleter.greedy=True
client = Client(host='34.70.65.12', user = "default", password = "lead#2019", database = "OrionLead")
from bokeh.io import output_file, show
from bokeh.models import ColumnDataSource, GMapOptions
from bokeh.plotting import gmap
result, columns = client.execute("select win_cost_micros as cpm, geo_lat as lat ,geo_lon as lon from OrionLead.Wins_buffer where clicks > 0", with_column_types=True)
Map_1 = pd.DataFrame(result, columns=[tuple[0] for tuple in columns])
Map_1.head()
output_file("gmap.html")
map_options = GMapOptions(lat=0, lng=0, map_type="roadmap", zoom=2)
p = gmap("??" , map_options, title="Specific Locations of Users")
p.circle(x="lon", y="lat", size=1, fill_color="blue", fill_alpha=0.8, source=Map_1)
show(p)
from bokeh.io import export_png
export_png(p, "foo.png")
###Output
_____no_output_____ |
Daily Coading Problem 09.ipynb | ###Markdown
Daily Coading Problem 09 This problem was asked by Airbnb.Given a list of integers, write a function that returns the largest sum of non-adjacent numbers. Numbers can be 0 or negative.For example, [2, 4, 6, 2, 5] should return 13, since we pick 2, 6, and 5. [5, 1, 1, 5] should return 10, since we pick 5 and 5.Follow-up: Can you do this in O(N) time and constant space? You really really need to know the fact that our memo dictionary consumes O(N) memory and also that our recursion is consuming memory for the recursion stack, O(N) as well.
###Code
def max_non_adjacent_sum(arr):
memo = {}
memo[-2] = 0
memo[-1] = 0
N = len(arr)
for i in range(N):
memo[i] = max(
arr[i],
arr[i] + memo[i - 2],
memo[i - 1],
)
return memo[N - 1]
assert(max_non_adjacent_sum([2, 4, 6, 2, 5]) == 13)
###Output
_____no_output_____ |
bus/bus-Solution.ipynb | ###Markdown
BusThis bus has a passenger entry and exit control system to monitor the number of occupants it carries and thus detect when there is too high a capacity.At each stop the entry and exit of passengers is represented by a tuple consisting of two integer numbers.```bus_stop = (in, out)```The succession of stops is represented by a list of these tuples.```stops = [(in1, out1), (in2, out2), (in3, out3), (in4, out4)]``` Goals:* lists, tuples* while/for loops* minimum, maximum, length* average, standard deviation Tasks1. Calculate the number of stops.2. Assign to a variable a list whose elements are the number of passengers at each stop (in-out),3. Find the maximum occupation of the bus.4. Calculate the average occupation. And the standard deviation.
###Code
# variables
#I had to initialize them, so I put the values I wanted
in1=20
in2=10
in3=30
in4=10
out1=0
out2=20
out3=10
out4=10
stops = [(in1, out1), (in2, out2), (in3, out3), (in4, out4)]
# 1. Calculate the number of stops.
number_stops=len(stops)
print("Number of stops: ", number_stops)
# 2. Assign a variable a list whose elements are the number of passengers in each stop:
# Each item depends on the previous item in the list + in - out.
#Assumption1: the bus starts empty
#Assumption2: the number of passengers are being calculated after the bus leaves the stop.
in_bus=[]
for s in range(len(stops)):
if s>0:
diff=(stops[s][0]-stops[s][1])+in_bus[s-1]
in_bus.append(diff)
elif s==0:
diff=(stops[s][0]-stops[s][1])
in_bus.append(diff)
print(in_bus)
# 3. Find the maximum occupation of the bus
max_occ=max(in_bus)
position=in_bus.index(max_occ)+1
print("The maximum occupation was %d in the stop number %d" %(max_occ,position))
# 4. Calculate the average occupation. And the standard deviation.
avg_occ=(sum(in_bus)/len(in_bus))
print("The average number of passengers are: ",avg_occ)
summation=0
for s in in_bus:
summation+=((s-avg_occ)**2)
stdev=((summation/len(in_bus)))**0.5
print("standard deviation is: %.2f" %stdev)
###Output
The average number of passengers are: 22.5
standard deviation is: 8.29
|
Python/subarray.ipynb | ###Markdown
Difference between Subarray, Subsequence, and Subset 1. A subarray is a slice from a contiguous array (i.e., occupy consecutive positions) and inherently maintains the order of elements. 2. A substring of a string s is a string s' that occurs in s. A substring is almost similar to a subarray, but it is in the context of strings. 3. A subsequence is a sequence that can be derived from another sequence by deleting some elements without changing the order of the remaining elements. 4. A subset is any possible combination of the original set. SubarrayFor example, the subarrays of array {1, 2, 3} are {1}, {1, 2}, {1, 2, 3}, {2}, {2, 3}, and {3}.Please note that there are precisely n×(n+1)/2 subarrays in an array of size n. Also, there is no such thing as a contiguous subarray. The prefix contiguous is sometimes applied to make the context more clear. So, a contiguous subarray is just another name for a subarray.
###Code
arr = [1, 2, 3, 4, 5]
# Function to print all sublists of the specified list
def printallSublists(nums):
# consider all sublists starting from i
for i in range(len(nums)):
# consider all sublists ending at `j`
for j in range(i, len(nums)):
# Function to print a sublist formed by [i, j]
print(nums[i: j + 1])
if __name__ == '__main__':
nums = [1, 2, 3, 4, 5]
printallSublists(nums)
###Output
[1]
[1, 2]
[1, 2, 3]
[1, 2, 3, 4]
[1, 2, 3, 4, 5]
[2]
[2, 3]
[2, 3, 4]
[2, 3, 4, 5]
[3]
[3, 4]
[3, 4, 5]
[4]
[4, 5]
[5]
###Markdown
SubstringFor example, the substrings of string 'apple' are 'apple', 'appl', 'pple', 'app', 'ppl', 'ple', 'ap', 'pp', 'pl', 'le', 'a', 'p', 'l', 'e', ''
###Code
# Function to print all non-empty substrings of the specified string
def printAllSubstrings(s):
# consider all substrings starting from i
for i in range(len(s)):
# consider all substrings ending at j
for j in range(i, len(s)):
print(s[i: j + 1], end=' \n')
if __name__ == '__main__':
s = 'techie'
printAllSubstrings(s)
###Output
t
te
tec
tech
techi
techie
e
ec
ech
echi
echie
c
ch
chi
chie
h
hi
hie
i
ie
e
###Markdown
Subsequence For example, {nums, B, D} is a subsequence of sequence {nums, B, C, D, E} obtained after removing {C} and {E}. People are often confused between a subarray/substring and a subsequence. A subarray or substring will always be contiguous, but a subsequence need not be contiguous. That is, subsequences are not required to occupy consecutive positions within the original sequences. But we can say that both contiguous subsequence and subarray are the same.In other words, the subsequence is a generalization of a substring, or substring is a refinement of the subsequence. For example, {nums, C, E} is a subsequence of {nums, B, C, D, E}, but not a substring, and {nums, B, C} is both a subarray and a subsequence.Please note that a subsequence can be in the context of both arrays and strings. Generating all subsequences of an array/string is equivalent to generating a power set of an array/string. For a given set, S, we can find the power set by generating all binary numbers between 0 and $2^n-1$, where n is the size of the given set.
###Code
# Function to print all subsequences of the specified string
def findPowerSet(seq):
# N stores the total number of subsets
N = int(pow(2, len(seq)))
# generate each subset one by one
result = []
for i in range(N):
s = ''
# check every bit of `i`
for j in range(len(seq)):
# if j'th bit of `i` is set, print S[j]
if (i & (1 << j)) != 0:
s += seq[j]
result.append(s)
print(result)
if __name__ == '__main__':
seq = 'apple'
findPowerSet(seq)
###Output
['', 'a', 'p', 'ap', 'p', 'ap', 'pp', 'app', 'l', 'al', 'pl', 'apl', 'pl', 'apl', 'ppl', 'appl', 'e', 'ae', 'pe', 'ape', 'pe', 'ape', 'ppe', 'appe', 'le', 'ale', 'ple', 'aple', 'ple', 'aple', 'pple', 'apple']
|
scripts/d21-en/pytorch/chapter_computer-vision/neural-style.ipynb | ###Markdown
Neural Style TransferIf you use social sharing apps or happen to be an amateur photographer, you are familiar with filters. Filters can alter the color styles of photos to make the background sharper or people's faces whiter. However, a filter generally can only change one aspect of a photo. To create the ideal photo, you often need to try many different filter combinations. This process is as complex as tuning the hyperparameters of a model.In this section, we will discuss how we can use convolution neural networks(CNNs) to automatically apply the style of one image to another image, anoperation known as style transfer :cite:`Gatys.Ecker.Bethge.2016`. Here, we need two input images, one content image and one style image. We use a neural network to alter the content image so that its style mirrors that of the style image. In :numref:`fig_style_transfer`, the content image is a landscape photo the author took in Mount Rainier National Part near Seattle. The style image is an oil painting of oak trees in autumn. The output composite image retains the overall shapes of the objects in the content image, but applies the oil painting brushwork of the style image and makes the overall color more vivid.:label:`fig_style_transfer` TechniqueThe CNN-based style transfer model is shown in :numref:`fig_style_transfer_model`.First, we initialize the composite image. For example, we can initialize it as the content image. This composite image is the only variable that needs to be updated in the style transfer process, i.e., the model parameter to be updated in style transfer. Then, we select a pre-trained CNN to extract image features. These model parameters do not need to be updated during training. The deep CNN uses multiple neural layers that successively extract image features. We can select the output of certain layers to use as content features or style features. If we use the structure in :numref:`fig_style_transfer_model`, the pre-trained neural network contains three convolutional layers. The second layer outputs the image content features, while the outputs of the first and third layers are used as style features. Next, we use forward propagation (in the direction of the solid lines) to compute the style transfer loss function and backward propagation (in the direction of the dotted lines) to update the model parameter, constantly updating the composite image. The loss functions used in style transfer generally have three parts: 1. Content loss is used to make the composite image approximate the content image as regards content features. 2. Style loss is used to make the composite image approximate the style image in terms of style features. 3. Total variation loss helps reduce the noise in the composite image. Finally, after we finish training the model, we output the style transfer model parameters to obtain the final composite image.:label:`fig_style_transfer_model`Next, we will perform an experiment to help us better understand the technical details of style transfer. Reading the Content and Style ImagesFirst, we read the content and style images. By printing out the image coordinate axes, we can see that they have different dimensions.
###Code
%matplotlib inline
import torch
import torchvision
from torch import nn
from d2l import torch as d2l
d2l.set_figsize()
content_img = d2l.Image.open('../img/rainier.jpg')
d2l.plt.imshow(content_img);
style_img = d2l.Image.open('../img/autumn-oak.jpg')
d2l.plt.imshow(style_img);
###Output
_____no_output_____
###Markdown
Preprocessing and PostprocessingBelow, we define the functions for image preprocessing and postprocessing. The `preprocess` function normalizes each of the three RGB channels of the input images and transforms the results to a format that can be input to the CNN. The `postprocess` function restores the pixel values in the output image to their original values before normalization. Because the image printing function requires that each pixel has a floating point value from 0 to 1, we use the `clip` function to replace values smaller than 0 or greater than 1 with 0 or 1, respectively.
###Code
rgb_mean = torch.tensor([0.485, 0.456, 0.406])
rgb_std = torch.tensor([0.229, 0.224, 0.225])
def preprocess(img, image_shape):
transforms = torchvision.transforms.Compose([
torchvision.transforms.Resize(image_shape),
torchvision.transforms.ToTensor(),
torchvision.transforms.Normalize(mean=rgb_mean, std=rgb_std)])
return transforms(img).unsqueeze(0)
def postprocess(img):
img = img[0].to(rgb_std.device)
img = torch.clamp(img.permute(1, 2, 0) * rgb_std + rgb_mean, 0, 1)
return torchvision.transforms.ToPILImage()(img.permute(2, 0, 1))
###Output
_____no_output_____
###Markdown
Extracting FeaturesWe use the VGG-19 model pre-trained on the ImageNet dataset to extract image features[1].
###Code
pretrained_net = torchvision.models.vgg19(pretrained=True)
###Output
_____no_output_____
###Markdown
To extract image content and style features, we can select the outputs of certain layers in the VGG network. In general, the closer an output is to the input layer, the easier it is to extract image detail information. The farther away an output is, the easier it is to extract global information. To prevent the composite image from retaining too many details from the content image, we select a VGG network layer near the output layer to output the image content features. This layer is called the content layer. We also select the outputs of different layers from the VGG network for matching local and global styles. These are called the style layers. As we mentioned in :numref:`sec_vgg`, VGG networks have five convolutional blocks. In this experiment, we select the last convolutional layer of the fourth convolutional block as the content layer and the first layer of each block as style layers. We can obtain the indexes for these layers by printing the `pretrained_net` instance.
###Code
style_layers, content_layers = [0, 5, 10, 19, 28], [25]
###Output
_____no_output_____
###Markdown
During feature extraction, we only need to use all the VGG layers from the input layer to the content or style layer nearest the output layer. Below, we build a new network, `net`, which only retains the layers in the VGG network we need to use. We then use `net` to extract features.
###Code
net = nn.Sequential(*[
pretrained_net.features[i]
for i in range(max(content_layers + style_layers) + 1)])
###Output
_____no_output_____
###Markdown
Given input `X`, if we simply call the forward computation `net(X)`, we can only obtain the output of the last layer. Because we also need the outputs of the intermediate layers, we need to perform layer-by-layer computation and retain the content and style layer outputs.
###Code
def extract_features(X, content_layers, style_layers):
contents = []
styles = []
for i in range(len(net)):
X = net[i](X)
if i in style_layers:
styles.append(X)
if i in content_layers:
contents.append(X)
return contents, styles
###Output
_____no_output_____
###Markdown
Next, we define two functions: The `get_contents` function obtains the content features extracted from the content image, while the `get_styles` function obtains the style features extracted from the style image. Because we do not need to change the parameters of the pre-trained VGG model during training, we can extract the content features from the content image and style features from the style image before the start of training. As the composite image is the model parameter that must be updated during style transfer, we can only call the `extract_features` function during training to extract the content and style features of the composite image.
###Code
def get_contents(image_shape, device):
content_X = preprocess(content_img, image_shape).to(device)
contents_Y, _ = extract_features(content_X, content_layers, style_layers)
return content_X, contents_Y
def get_styles(image_shape, device):
style_X = preprocess(style_img, image_shape).to(device)
_, styles_Y = extract_features(style_X, content_layers, style_layers)
return style_X, styles_Y
###Output
_____no_output_____
###Markdown
Defining the Loss FunctionNext, we will look at the loss function used for style transfer. The loss function includes the content loss, style loss, and total variation loss. Content LossSimilar to the loss function used in linear regression, content loss uses a square error function to measure the difference in content features between the composite image and content image. The two inputs of the square error function are both content layer outputs obtained from the `extract_features` function.
###Code
def content_loss(Y_hat, Y):
# we 'detach' the target content from the tree used
# to dynamically compute the gradient: this is a stated value,
# not a variable. Otherwise the loss will throw an error.
return torch.square(Y_hat - Y.detach()).mean()
###Output
_____no_output_____
###Markdown
Style LossStyle loss, similar to content loss, uses a square error function to measure the difference in style between the composite image and style image. To express the styles output by the style layers, we first use the `extract_features` function to compute the style layer output. Assuming that the output has 1 example, $c$ channels, and a height and width of $h$ and $w$, we can transform the output into the matrix $\mathbf{X}$, which has $c$ rows and $h \cdot w$ columns. You can think of matrix $\mathbf{X}$ as the combination of the $c$ vectors $\mathbf{x}_1, \ldots, \mathbf{x}_c$, which have a length of $hw$. Here, the vector $\mathbf{x}_i$ represents the style feature of channel $i$. In the Gram matrix of these vectors $\mathbf{X}\mathbf{X}^\top \in \mathbb{R}^{c \times c}$, element $x_{ij}$ in row $i$ column $j$ is the inner product of vectors $\mathbf{x}_i$ and $\mathbf{x}_j$. It represents the correlation of the style features of channels $i$ and $j$. We use this type of Gram matrix to represent the style output by the style layers. You must note that, when the $h \cdot w$ value is large, this often leads to large values in the Gram matrix. In addition, the height and width of the Gram matrix are both the number of channels $c$. To ensure that the style loss is not affected by the size of these values, we define the `gram` function below to divide the Gram matrix by the number of its elements, i.e., $c \cdot h \cdot w$.
###Code
def gram(X):
num_channels, n = X.shape[1], X.numel() // X.shape[1]
X = X.reshape((num_channels, n))
return torch.matmul(X, X.T) / (num_channels * n)
###Output
_____no_output_____
###Markdown
Naturally, the two Gram matrix inputs of the square error function for style loss are taken from the composite image and style image style layer outputs. Here, we assume that the Gram matrix of the style image, `gram_Y`, has been computed in advance.
###Code
def style_loss(Y_hat, gram_Y):
return torch.square(gram(Y_hat) - gram_Y.detach()).mean()
###Output
_____no_output_____
###Markdown
Total Variance LossSometimes, the composite images we learn have a lot of high-frequency noise, particularly bright or dark pixels. One common noise reduction method is total variation denoising. We assume that $x_{i, j}$ represents the pixel value at the coordinate $(i, j)$, so the total variance loss is:$$\sum_{i, j} \left|x_{i, j} - x_{i+1, j}\right| + \left|x_{i, j} - x_{i, j+1}\right|.$$We try to make the values of neighboring pixels as similar as possible.
###Code
def tv_loss(Y_hat):
return 0.5 * (torch.abs(Y_hat[:, :, 1:, :] - Y_hat[:, :, :-1, :]).mean() +
torch.abs(Y_hat[:, :, :, 1:] - Y_hat[:, :, :, :-1]).mean())
###Output
_____no_output_____
###Markdown
Loss FunctionThe loss function for style transfer is the weighted sum of the content loss, style loss, and total variance loss. By adjusting these weight hyperparameters, we can balance the retained content, transferred style, and noise reduction in the composite image according to their relative importance.
###Code
content_weight, style_weight, tv_weight = 1, 1e3, 10
def compute_loss(X, contents_Y_hat, styles_Y_hat, contents_Y, styles_Y_gram):
# Calculate the content, style, and total variance losses respectively
contents_l = [
content_loss(Y_hat, Y) * content_weight
for Y_hat, Y in zip(contents_Y_hat, contents_Y)]
styles_l = [
style_loss(Y_hat, Y) * style_weight
for Y_hat, Y in zip(styles_Y_hat, styles_Y_gram)]
tv_l = tv_loss(X) * tv_weight
# Add up all the losses
l = sum(styles_l + contents_l + [tv_l])
return contents_l, styles_l, tv_l, l
###Output
_____no_output_____
###Markdown
Creating and Initializing the Composite ImageIn style transfer, the composite image is the only variable that needs to be updated. Therefore, we can define a simple model, `GeneratedImage`, and treat the composite image as a model parameter. In the model, forward computation only returns the model parameter.
###Code
class GeneratedImage(nn.Module):
def __init__(self, img_shape, **kwargs):
super(GeneratedImage, self).__init__(**kwargs)
self.weight = nn.Parameter(torch.rand(*img_shape))
def forward(self):
return self.weight
###Output
_____no_output_____
###Markdown
Next, we define the `get_inits` function. This function creates a composite image model instance and initializes it to the image `X`. The Gram matrix for the various style layers of the style image, `styles_Y_gram`, is computed prior to training.
###Code
def get_inits(X, device, lr, styles_Y):
gen_img = GeneratedImage(X.shape).to(device)
gen_img.weight.data.copy_(X.data)
trainer = torch.optim.Adam(gen_img.parameters(), lr=lr)
styles_Y_gram = [gram(Y) for Y in styles_Y]
return gen_img(), styles_Y_gram, trainer
###Output
_____no_output_____
###Markdown
TrainingDuring model training, we constantly extract the content and style features ofthe composite image and calculate the loss function. Recall our discussion ofhow synchronization functions force the front end to wait for computationresults in :numref:`sec_async`. Because we only call the `asnumpy` synchronization function every 10epochs, the process may occupy a great deal of memory. Therefore, we call the`waitall` synchronization function during every epoch.
###Code
def train(X, contents_Y, styles_Y, device, lr, num_epochs, lr_decay_epoch):
X, styles_Y_gram, trainer = get_inits(X, device, lr, styles_Y)
scheduler = torch.optim.lr_scheduler.StepLR(trainer, lr_decay_epoch)
animator = d2l.Animator(xlabel='epoch', ylabel='loss',
xlim=[10, num_epochs],
legend=['content', 'style',
'TV'], ncols=2, figsize=(7, 2.5))
for epoch in range(num_epochs):
trainer.zero_grad()
contents_Y_hat, styles_Y_hat = extract_features(
X, content_layers, style_layers)
contents_l, styles_l, tv_l, l = compute_loss(X, contents_Y_hat,
styles_Y_hat, contents_Y,
styles_Y_gram)
l.backward()
trainer.step()
scheduler.step()
if (epoch + 1) % 10 == 0:
animator.axes[1].imshow(postprocess(X))
animator.add(
epoch + 1,
[float(sum(contents_l)),
float(sum(styles_l)),
float(tv_l)])
return X
###Output
_____no_output_____
###Markdown
Next, we start to train the model. First, we set the height and width of the content and style images to 150 by 225 pixels. We use the content image to initialize the composite image.
###Code
device, image_shape = d2l.try_gpu(), (150, 225) # PIL Image (h, w)
net = net.to(device)
content_X, contents_Y = get_contents(image_shape, device)
_, styles_Y = get_styles(image_shape, device)
output = train(content_X, contents_Y, styles_Y, device, 0.01, 500, 200)
###Output
_____no_output_____
###Markdown
As you can see, the composite image retains the scenery and objects of the content image, while introducing the color of the style image. Because the image is relatively small, the details are a bit fuzzy.To obtain a clearer composite image, we train the model using a larger image size: $900 \times 600$. We increase the height and width of the image used before by a factor of four and initialize a larger composite image.
###Code
image_shape = (600, 900) # PIL Image (h, w)
_, content_Y = get_contents(image_shape, device)
_, style_Y = get_styles(image_shape, device)
X = preprocess(postprocess(output), image_shape).to(device)
output = train(X, content_Y, style_Y, device, 0.01, 300, 100)
d2l.plt.imsave('../img/neural-style.jpg', postprocess(output))
###Output
_____no_output_____ |
2021/Stepik_Basics-of-Statistics/week_1.ipynb | ###Markdown
**ОСНОВЫ СТАТИСТИКИ. ЧАСТЬ 1. НЕДЕЛЯ 1.**[Курс](https://stepik.org/course/76/syllabus) на Stepik.Автор: **Анатолий Карпов** Конспектировал: **Илья Филимонов** (GiHub: [@IsFilimonov](https://github.com/IsFilimonov)) --- Table of ContentsРекомендации от автораВведениеГенеральная совокупность и выборкаСпособы репрезентативной выборкиТипы переменныхОписательная статистикаМеры центральной тенденцииПримеры использованияСвойства среднего значенияПолезные ссылкиПримерыМеры изменчивостиСвойства дисперсии и стандартного отклоненияПримерыМеры положения данных в распределенииАлгоритм анализаПримерыНормальное распределениеИсторическая справкаZ стандартизацияПримерПравило 2х и 3х сигмПримерЦентральная предельная теоремаВажное замечание о ЦПТ (номер 2).ПримерыПример 2Доверительные интервалы для среднегоИдея статистического вывода, p-уровень значимости Рекомендации от автора1. **С. Гланц, "Медико-биологическая статистика"** ([pdf](http://medstatistic.ru/articles/glantz.pdf)).Хороший учебник по введению в статистику на русском языке со множеством примеров из биологии и медицины.2. **Jerrold H. Zar, "Biostatistical Analysis"**Более подробный учебник, в котором очень тщательно разбирается большинство статистических методов для анализа биологических данных.3. **"OpenIntro Statistics"** ([link](https://www.openintro.org/)).Замечательный учебник с массой примеров из различных областей, а также видео лекции.4. **В. Савельев, "Статистика и котики"** ([source](https://www.litres.ru/vladimir-savelev-10569666/statistika-i-kotiki/)):Отличный учебник для первых шагов в области анализа данных. Несмотря на несерьезное название в учебнике очень понятно и доступно объясняются основные темы, необходимые для уверенного использования статистики.--- ВведениеДанные:- ГС — генеральная совокупность (все возможные экземпляры).- Выборка — это кусочек из ГС.Если работаем с ГС, то:- $M_{x}$ (или $\mu$) — среднее значение ГС- $\sigma$ (сигма) — "среднеквадратичное отклонение" ГСЕсли работаем с ВЫБОРКОЙ, то:- $\bar{X}$ — Среднее значение выборки- $sd_{x}$ — "стандартное отклонение" выборки (оно тоже среднеквадратичное)Из комментариев:
###Code
'''Импортируем необходимые библиотеки для работы'''
# Стандартный модуль Python >= 3.4 для расчета математической статистики числовых данных.
# Ориентирован на уровень графических и научных калькуляторов.
import statistics
# Стандартный модуль реализует генераторы псевдослучайных чисел для различных распределений
from random import randint
# Стандартный модуль обеспечивает доступ к математическим функциям, определенным стандартом C.
import math
# Библиотека, добавляющая поддержку больших многомерных массивов и матриц, вместе
# с большой библиотекой высокоуровневых (и очень быстрых) математических функций для операций с этими массивами.
import numpy as np
from numpy import random
# Open source библиотека на базе NumPy для выполнения научных и инженерных расчётов.
from scipy import (
misc, stats, ndimage)
# Библиотека для обработки и анализа данных с NumPy под капотом.
import pandas as pd
# Библиотека предоставляет набор методов статистического тестирования и моделирования, а также
# инструменты, предназначенные для анализа временных рядов (это также может быть использовано для прогнозирования).
from statsmodels.stats.multicomp import (
pairwise_tukeyhsd, MultiComparison)
# Библиотека для визуализации 2D данных, а также 3D графики.
import matplotlib.pyplot as plt
# Более высокоуровневое API на базе библиотеки matplotlib.
import seaborn as sns
face = misc.face()
plt.imshow(face)
plt.show()
###Output
_____no_output_____
###Markdown
Генеральная совокупность и выборка**Генеральная совокупность** (от лат. *generis* — общий, родовой) — совокупность всех объектов, относительно которых предполагается делать выводы при изучении конкретной задачи, далее **ГС**.**Выборка** — небольшое количество объектов из **ГС**. На практике мы не можем замерить всю **ГС**.**Репрезентативность** — степень похожести объектов между **ГС** и **выборкой**.**Репрезентативная выборка** — это максимально похожая модель **ГС**, но в уменьшенном размере, далее **РВ**.**Пример**: если в **ГС** 30% курильщики, а 25% посещают фитнес-клубы, то в составе **РВ** должны соблюдаться эти пропорции. Способы репрезентативной выборки- `Простая случайная выборка` (simple random sample, SRS) - любой объект с равной вероятностью может попасть в выборку.**Пример:** лотерея green card в США. `Стратифицированная выборка` | `Групповая выборка` --- | --- *stratified sample* | *claster sample*вероятностная выборка, два этапа формирования | вероятностная выборка, три этапа формирования (1) ГС разделяется на подмножества (**страты**) | (1) ГС разделяется на подмножества (**кластеры**) (2) - | (2) Случайный отбор кластеров для исследования (3) метод **простой случайной выборки** | (3) метод **простой случайной выборки** (!) элементы отбираются из каждого подмножества | (!) элементы отбираются из некоторых случайно отобранных подмножеств (!) страты стремятся формировать однородными | (!) кластеры стремятся формировать репрезентативными (+) более эффективны (дают точный результат) | (+) экономически более выгодны, поэтому популярны Типы переменных- `Количественные` - измеряемые. **Пример:** рост. - `Непрерывные` - может принимать любое значение на некотором промежутке. **Пример:** $[160, 190]$. - `Дискретные` - определенные целые значения. **Пример:** 3,5 ребенка в семье не может быть.- `Номинативные` - качественные. **Пример:** разделение выборки на 1-М и 2-Ж. Цифры используются как индексы, лейблы строк.- `Ранговые` - не **количественные**, и почти **номинативные**, только используются исключительно для сравнения быстрее, раньше, больше и тд. **Пример:** сортировка по времени финиша на марафоне. Описательная статистика`Эмпирические данные` - полученные опытным путем.`Описательная статистика` или `дескриптивная статистика` (descriptive statistics) занимается обработкой **эмпирических данных**, их систематизацией, наглядным представлением в форме графиков и таблиц, а также их количественным описанием посредством основных статистических показателей. `Распределение вероятностей` - это закон, описывающий область значений случайной величины и вероятность её появления (частоту) в данной области. То есть насколько часто X появляется в данном диапазоне значений. `Гистограмма частот` - ступенчатая функция показывающая насколько часто вероятно появление величины в указанном диапазоне значений. **Пример:** - рис 1. имеет симметричное распределение некоторой количественной переменной.- рис 2. пример ярко выраженной асимметрии.- рис 3. два ярко выраженных диапазона. Меры центральной тенденции`Мера центральной тенденции` — число, служащее для описания множества значений одним-единственным числом (для краткости). **Например**, вместо перечисления величин зарплат всех сотрудников организации говорят о средней зарплате. Существует множество мер центральной тенденции; окончательный выбор меры всегда остается за исследователем. `Мода` (типичность) - наиболее часто встречающееся значение. Иногда в совокупности встречается более чем одна мода. **График**: dot plot. **Например**: $[6, 2, 6, 6, 8, 9, 9, 9, 0]$, $M_{o} = 6 , 9$, совокупность мультимодальна. `Медиана` - значение, которое делит __упорядоченные по возрастанию (убыванию)__ наблюдения пополам. Если множество содержит чётное количество элементов, то берётся среднее из двух серединных элементов упорядоченного множества. **Пример**: $[1, 2, 50, 100, 1000, 2000000]$, $M_{e} =\frac{(50+100)}{2} = 75$. `Среднее значение` (*mean*, среднее арифметическое) - число, равное сумме всех чисел множества, делённой на их количество. Частными случаями среднего арифметического являются среднее (генеральной совокупности, обознач. греч. $\mu$ мю малое) и выборочное среднее (выборки, обознач. $\overline X$). Примеры использования- Если распределение симметрично, унимодально и не имеет заметных выбросов: можно использовать любую из мер центральной тенденции и среднее, и мода, и медиана дадут примерно одинаковое значение.- Если распределение с явно выраженной асимметрией (скошено либо влево/вправо), заметные выбросы или несколько мод: использование среднего нерелевантно, лучше или моду или медиану, чтобы охарактеризовать наши данные с точки зрения выраженности некоторого количественного признака. Свойства среднего значения$$M_{x + c} = \frac{\sum_{i=1}^{n}{(x_{i} + c)}}{n} = \frac{\sum_{i=1}^{n} x_{i}}{n} + \frac{\sum_{i=1}^{n} c}{n} = M_{x} + \frac{nc}{n} = M_{x} + c$$$$M_{x * c} = \frac{\sum_{i=1}^{n}{(x_{i} * c)}}{n} = \frac{c * \sum_{i=1}^{n} x_{i}}{n} = c * M_{x}$$$$\sum_{i=1}^{n} (x_{i} - M_{x}) = nM_{x} - nM_{x} = 0$$ Полезные ссылки- Лекция на Ted [Hans Rosling, The best stats you've even seen](http://www.ted.com/talks/hans_rosling_shows_the_best_stats_you_ve_ever_seen) (есть русские субтитры) посвящена тому, какие недопонимания могут возникнуть, если использовать средние значения в качестве мер центральной тенденции. - Статическая [программа](http://www.gapminder.org/world) из видео. Примеры
###Code
'''
Расчет моды, медианы и среднего значения разными инструментами
'''
A = np.array([185, 175, 170, 169, 171, 175, 157, 172, 170, 172, 167, 173, 168, 167, 166,
167, 169, 172, 177, 178, 165, 161, 179, 159, 164, 178, 172, 170, 173, 171])
# Общие свединия о данных
print("Всего элементов {}, Min: {}, Max: {},".format(len(A), min(A), max(A)))
# Стандартная библиотека Python
print('Python. Mode: {}, Median {}, Median (sorted) {}, Mean {}'.format(
statistics.mode(A),
statistics.median(A),
statistics.median(sorted(A)),
statistics.mean(A),
))
# NumPy
print('NumPy. Mode: -, Median {}, Median (sorted) {}, Mean {}'.format(
# в numpy нет функции mode
np.median(A),
np.median(sorted(A)),
np.mean(A),
))
# SciPy
print('SciPy. Mode: {}, Median {}, Median (sorted) {}, Mean {}'.format(
stats.mode(A),
ndimage.median(A),
ndimage.median(sorted(A)),
ndimage.mean(A),
))
# Pandas
df_A = pd.DataFrame(A, columns=["A"])
print('Pandas. Mode: {}, Median {}, Median (sorted) {}, Mean {}'.format(
df_A.mode().iloc[0]['A'],
df_A.median().values[0],
df_A.sort_values(by=["A"], inplace=False).median().values[0],
df_A.mean().values[0],
))
###Output
Всего элементов 30, Min: 157, Max: 185,
Python. Mode: 172, Median 170.5, Median (sorted) 170.5, Mean 170
NumPy. Mode: -, Median 170.5, Median (sorted) 170.5, Mean 170.4
SciPy. Mode: ModeResult(mode=array([172]), count=array([4])), Median 170.5, Median (sorted) 170.5, Mean 170.4
Pandas. Mode: 172, Median 170.5, Median (sorted) 170.5, Mean 170.4
###Markdown
Меры изменчивости`Размах` (*range*, разброс) выборки измеряет расстояние, в пределах которого изменяются оценки, и обозначается буквой $R = X_{(n)} - X_{(1)}$, разность между максимальным и минимальным значениями ряда. **График**: boxplot **Пример**: [5.24, 6.97, 8.56, 7.32, 6.23], $R = 8.56 - 5.24 = 3.32$ **Недостаток**: очень сильно зависит от неизменчивости крайних значений. `Математическое ожидание` - часто называют просто средним значением.Чтобы понять, какое отклонение является типичным для множества, мы складываем все отклонения и делим на количество элементов. Однако, мы получим 0, поскольку одни отклонения положительные, а другие отрицательные. Нужно избавиться от знака; варианта 2: либо взять модуль от отклонений, либо возвести в квадрат (чаще выбирают). Если мы найдем среднее от квадратов отклонений, то получим дисперсию.`Дисперсия` (*variance*) - мера разброса значений случайной величины относительно её математического ожидания (средней величины). - Малая дисперсия означает, что значения сгруппированы близко друг к другу. - Большая дисперсия свидетельствует о сильном разбросе значений.**Дисперсия генеральной совокупности**: $$D = \frac{\sum_{i=1}^{n} (x_{i} - M_{x})^2}{n}$$**Дисперсия выборки**:$$D = \frac{\sum_{i=1}^{n} (x_{i} - M_{x})^2}{n-1}$$Квадрат в этой формуле делает дисперсию очень неудобной для оценки разнообразия элементов из-за увеличения размерности. Поэтому для удобства использования дисперсию берут под корень, получая по итогу среднеквадратическое отклонение.`Среднеквадратическое отклонение` - наиболее распространённый показатель рассеивания значений случайной величины относительно её математического ожидания (средней величины).$$ \sigma = \sqrt{D}$$- **Среднеквадратическое отклонение** - корень из дисперсии генеральной совокупности. - **Стандартное отклонение** - корень из дисперсии по выборке.Если бы мы рассчитали дисперсию по модулю, а не возводя в квадрат, мы бы получили среднее линейное отклонение ([недостатки](http://univer-nn.ru/statistika/srednee-linejnoe-otklonenie/)). Дисперсия и среднеквадратическое отклонение так же неустойчивы к выбросам, как и среднее арифметическое. Свойства дисперсии и стандартного отклонения $$ D_{x+c} = D_x $$$$ sd_{x+c} = sd_{x} $$$$ D_{x*c} = D_x*c^2 $$$$ sd_{x*c} = sd_{x}*C $$ Примеры
###Code
'''
Расчет размаха, дисперсии и стандартного отклонения
'''
A = np.array([185, 175, 170, 169, 171, 175, 157, 172, 170, 172, 167, 173, 168, 167, 166,
167, 169, 172, 177, 178, 165, 161, 179, 159, 164, 178, 172, 170, 173, 171])
print("Размах {}, min {}, max {}".format(
np.ptp(A), # "peak to peak"
np.nanmin(A),
np.nanmax(A)
))
print("Дисперсия {}".format(
np.var(A, ddof=1) # ddof - дельта степеней свободы (N-ddof)
))
print("Стандартное отклонение {}".format(
np.std(A, ddof=1) # ddof - дельта степеней свободы (N-ddof)
))
'''
Пример расчета математического ожидания, отклонения от среднего, дисперсии и среднеквадратического отклонения
'''
A = [1,5,2,7,1,9,3,8,5,9]
B = []
Mx = sum(A)/(len(A)) # математическое ожидание
for el in A:
B.append((el-Mx)**2) # отклонения от среднего
D = sum(B)/(len(B)-1) # дисперсия выборки
Sd = D ** .5 # среднеквадратического отклонения
print(Mx, B, D, Sd, sep="\n")
###Output
5.0
[16.0, 0.0, 9.0, 4.0, 16.0, 16.0, 4.0, 9.0, 0.0, 16.0]
10.0
3.1622776601683795
###Markdown
Меры положения данных в распределении| Ру | En | Описание || ------------- |:-------------:|:----------:|| `Квантиль` | *quantile* | 3 равные части || `Квартиль` | *quartiles* | 4 равные части || `Квинтиль` | *quintiles* | 5 равных частей || `Дециль` | *deciles* | 10 равных частей || `Перцентиль` | *percentiles* | 100 равных частей | `Box plot` (ящик с усамми) - вид диаграммы, в удобной форме показывает:- медиану $M_{e}=Q2$;- нижний ($Q1$) и верхний ($Q3$) квартили;- межквартильный размах $IQR = Q_{3} - Q_{1}$;- минимальное и максимальное значение выборки;- выбросы: всё, что ниже $Q1 - 1,5*IQR$ и выше $Q3 + 1,5*IQR$ (о выбросах стоит говорить именно в терминах $IQR$). Еще один взгляд на анализ графика box plot. Полезно представить, что box plot - вид сверху на диаграмму. Box plot не столь информативен, как полноценная диаграмма. Однако, часто используется, когда нужно сравнить 2 группы между собой. Обычно, на оси x находится название группы, для которой построен данный график. В данном случае у нас только одна группа, и сама изменчивость точек по оси x никакого смысла не несет. Просто точки разбросаны по графику, чтоб не накладываться друг на друга. Алгоритм анализа1. Посчитали квартили.2. Посчитали разницу между ними.3. Вычислили теоретический максимум и минимум4. Сравнили с имеющимся и выяснили есть ли у вас выбросы и сколько их.5. Если много, то нужно анализировать и решать брать ли их в выборку или нет.Полезная [ссылка](https://ru.wikihow.com/%D0%B2%D1%8B%D1%87%D0%B8%D1%81%D0%BB%D0%B8%D1%82%D1%8C-%D0%B2%D1%8B%D0%B1%D1%80%D0%BE%D1%81%D1%8B?amp=1) по выявлению выбросов. ПримерыХод рассуждений: 1. Значения признака равные 0 имеются только в диаграммах 2 и 4, а так же в графиках A и C.2. На графике C имеется выброс со значением 25, значит C2, а A4.3. Исследуем диаграммы 1 и 3, а так же графики B и D. На B и D медианы примерно одинаковые.4. У диаграммы 3 короткий размах и имеется ярковыраженный выброс ниже $Q1$. Значит B3, а D1.
###Code
'''
диаграмма boxplot: медиана, Q1 и Q3
'''
A = np.array([185, 175, 170, 169, 171, 175, 157, 172, 170, 172, 167, 173, 168, 167, 166,
167, 169, 172, 177, 178, 165, 161, 179, 159, 164, 178, 172, 170, 173, 171])
plt.boxplot(A, showfliers=1)
plt.show()
###Output
_____no_output_____
###Markdown
Нормальное распределение`Нормальное распределение` (распределение Гаусса или Гаусса - Лапласа) - распределение вероятностей, которое в одномерном случае задаётся функцией плотности вероятности, совпадающей с функцией Гаусса:$$f(x) = \frac{1} {\sigma\sqrt{2 \pi}} exp{( -\frac{(x- \mu )^2} {2 \sigma ^2} )},$$ где $\mu$ - математическое ожидание (среднее значение), медиана и мода распределения, а $\sigma$ - среднеквадратическое отклонение ($\sigma^{2}$ — дисперсия) распределения.- Унимодальное распределение - распределение, имеющее только одну моду (т.е. один "пик").- Симметрично.- Отклонения наблюдений от среднего подчиняются определенному вероятностному закону. Историческая справкаЕсли остановиться немного подробнее, то нормальное распределение возникает в результате воздействия множества факторов, вклад каждого из которых очень мал.Для облегчения этого восприятия в 1873 году Фрэнсис Гальтон сделал устройство, которое в последствии назвали Доской Галтона (или квинкункс). Суть простая: сверху по середине подаются шарики, которые при прохождении нескольких уровней (например, 10-ти) на каждом уровне сталкиваются с препятствием, и при каждом столкновении отскакивают либо влево, либо вправо (с равной вероятностью).Еще [пример 1](https://www.youtube.com/watch?v=7NUGpzspLD4) (1 мин 40 сек) и [пример 2](https://www.youtube.com/watch?v=GANHXlMpVMc) (2 мин 45 сек).
###Code
'''
Иммитация доски Гальтона
'''
D = dict()
N = 10000 # количество шариков
level = 20 # количество уровней
for _ in range(N):
index = 0
for _ in range(level):
index += np.random.choice([-1, 1])
D.setdefault(index, 0)
D[index] += 1
sns.barplot(x=list(D.keys()), y=list(D.values()));
###Output
_____no_output_____
###Markdown
Z стандартизация`Стандартное нормальное распределение` - нормальное распределение с мат. ожиданием $\mu=0$ и стандартным отклонением $\sigma = 1$.`Стандартизация` или `z-стандартизация` - процедура по превращению нормального распределения $X \backsim N(\mu , \sigma)$ в стандартное $Z \backsim N(0 , 1)$ с Z-шкалой (*Z-scores*).$$ Z_{i}=\frac{x_{i} - \bar{X}}{sd} $$Иногда необходимо рассчитать Z-значение только для отдельно взятого наблюдения, чтоб выяснить насколько далеко оно отклоняется от среднего значения в единицах стандартного отклонения. ПримерДанное Z-преобразование абсолютно не изменяет форму распределения. Мы из каждого значения вычитаем мат.ожидание и делим на стандартное отклонение и получаем нижний график. Теперь новое среднее равно 0, а стандартное отклонение стало равняться 1 по оси X. Правило 2х и 3х сигмПравило, утверждающее, что вероятность того, что случайная величина отклонится от своего математического ожидания более чем на три среднеквадратических отклонения, практически равна нулю. Правило справедливо только для случайных величин, распределенных по нормальному закону. ПримерДоп материалы для поиска процена наблюдений в интересующем нас диапазоне:- [z таблица](https://web.archive.org/web/20180729102938/http://users.stat.ufl.edu/~athienit/Tables/Ztable.pdf): процент наблюдений, не превышающий указанное z-значение.- [z таблица](http://www.normaltable.com/ztable-righttailed.html): процент наблюдений, превышающий указанное z-значение.- интерактивный [сервис](https://gallery.shinyapps.io/dist_calc/) (default: $M=0$, $sd=1$)
###Code
'''Пример 1
Считается, что значение IQ (уровень интеллекта) у людей имеет нормальное распределение
со средним значением равным 100 и стандартным отклонением равным 15 (M = 100, sd = 15).
Какой приблизительно процент людей обладает IQ > 125?
'''
M, sd, iq = 100, 15, 125
print("Только у {}% людей IQ > {}".format(
stats.norm(M, sd).sf(iq) * 100,
iq
))
# SF (Survival function) = (1 - cdf)
# CDF (Cumulative distribution function)
###Output
Только у 4.7790352272814705% людей IQ > 125
###Markdown
**Текстовое решение задачи**: 1. Преобразуем $IQ=125$ в z-шкалу, чтобы получить позицию, где нахождения $125$ по z-шкале:$$z_{125}= \frac{125-100}{15}=\frac{25}{15}=1.67$$2. Смотрим таблицу, которая превышает значения $z$. По вертикале находим $1.6$, а по горизонтале $0.07$ и на пересечении мы получаем процент $0.0475$.3. Находим процент $0.0475*100=4.75$.
###Code
'''Пример 2
Считается, что значение IQ (уровень интеллекта) у людей имеет нормальное распределение
со средним значением равным 100 и стандартным отклонением равным 15 (M = 100, sd = 15).
Какой приблизительно процент людей обладает IQ на промежутке от 70 до 112?
'''
M, sd, iq_low, iq_high = 100, 15, 70, 112
print("В промежутке [70,112] примерно {} % от всех значений".format(
(stats.norm.cdf(12/15) - stats.norm.cdf(-30/15)) * 100
))
###Output
В промежутке [70,112] примерно 76.53944694684242 % от всех значений
###Markdown
**Решение с помощью z-таблицы**: 1. Преобразуем $IQ=70$ и $IQ=112$ в z-шкалу, чтобы получить позиции, где располагаются данные элементы на z-шкале:$$z_{70}= \frac{70-100}{15}=\frac{-30}{15}=-2$$$$z_{112}= \frac{112-100}{15}=\frac{12}{15}=0.8$$2. Смотрим сначала таблицу, с отрицательными $z$ для $z_{70}=-2$. По вертикали $-2$, по горизонтале $0.00$. Результат $0.0228$.3. Смотрим таблицу, с положительными $z$ для $z_{112}=0.8$. По вертикали $0.8$, по горизонтале $0.00$. Результат $0.2119$.4. В промежутке $[70, 112]$ находится примерно $100 \% - 2.28 \% - 21.19 \% = 76.53 \%$ - Количество людей с IQ меньше 70: $z_{<70}: 2.28 \%$ - Количество людей с IQ выше 112: $z_{>112}: 21.19 \%$ **Решение, используя правила 2 и 3 сигм**: 1. Используя [правило](Правило-2х-и-3х-сигм) $2\sigma$ и $3\sigma$ прикидываем: - Так как распределение нормальное, то мы знаем что в промежутке $[-2 \sigma , 2 \sigma]$ находится примерно $95.44 \%$ значений. Влево мы уходим на $2 \sigma$ от $0$, значит нас интересует всего $ \frac {95.44}{2} = 47.72 \%$ значений. - В промежутке $[-1 \sigma, 1 \sigma]$ находится примерно $68.26 \%$ всех значений. Вправо от $0$ на $1 \sigma$ составит $ \frac {68.26}{2} = 34.13 \%$ значений. Да, у нас же вправо $0.8 \sigma$, но мы округлим до единицы.2. Чуть меньше $(47.72 \% + 34.13 \%) = 81.85 \% $ значений IQ находится в промежутку $[70, 112]$. Центральная предельная теорема- [Ссылка](https://gallery.shinyapps.io/CLT_mean/) на сервис с симуляцией данных для центральной предельной теоремы. - Хорошее [толкование](https://www.marketing.spb.ru/lib-around/stat/Naked_Statistics.htm) ЦПТ. - От автора курса [доклад](https://www.youtube.com/watch?v=dFCJysbOJ8c) о том, что делать с ненормальными распределениями.`ЦПТ` гласит, что множество средних выборок из ГС (**ГС необязательно иметь нормальное распределние**) будут иметь примерно нормальное распределение. Причём средняя этого распределения будет близко к средней генеральной совокупности, а стандарное отклонение этого распределение будет называться `стандарной ошибкой среднего` ($se$).`Стандартная ошибка среднего` - это среднеквадратическое отклонение распределения выборочных средних!**Сила и смысл ЦПТ**: если на результат воздействует множество независимых и мелких факторов, то результирующая величина будет иметь распределение близкое к нормальному. **2 глубинная суть**: при многократном повторении испытаний относительная частота появления случайной величины становится приблизительно равной ее вероятности.Зная стандартное отклонение ГС и размер выборки мы можем рассчитать стандартную ошибку среднего.$$ se = \frac{\sigma}{\sqrt{N}} $$где $N$ - размер выборки. Если размер выборки достаточно большой (экспериментально $N>30$) и она является репрезативна (очень похожа на ГС), то вместо стандарного отклонения ГС мы можем взять стандарное отклонение выборки.$$ se = \frac{sd}{\sqrt{N}} $$ Важное замечание о ЦПТ (номер 2).Пожалую самый сложный момент: это как мы так взяли и заменили стандартное отклонение ГС на выборочное. Ну и что с того, что у нас выборка объемом больше 30 наблюдений, что за магическое число такое?Все правильно, никакой магии не происходит. И совсем скоро мы в этом окончательно разберемся. Как только пройдем тему t - распределения во втором модуле. Вот тут я подробно расписал, как же нам нужно рассчитывать стандартную ошибку среднего, если мы не знаем стандартное отклонение в генеральной совокупности.**Спойлер**: просто взять и подставить выборочное стандартное отклонение - весьма грубое упрощение, которым не пользуются на практике. А как надо, смотрите скорее второй модуль, вот в этом [шаге](https://stepik.org/lesson/8081/step/7?unit=1360) второго модуля подробно подвели итог. Примеры
###Code
# значения игральной кости
dice = [1, 2, 3, 4, 5, 6]
# количество бросков кости
count = 6
# размер генеральной совокупность
sp_size = 10000
# sp - Statistical population - генеральная совокупность
sp = pd.Series(dtype=np.int64, index=range(sp_size))
for i in range(sp_size):
value = 0
for _ in range(count):
value += np.random.choice(dice)
sp[i] = value
sp.plot.hist(bins=28)
# количество выборок
samples_count = 10
# размер выборки
sample_size = 200
samples = pd.DataFrame([
[np.random.choice(sp) for _ in range(sample_size)] for __ in range(samples_count)
]).T
samples.hist(figsize=(16, 10), sharex=0)
plt.subplots_adjust(hspace = 0.6)
means = samples.mean()
print("сравним среднию ГС {} и среднию средних выборок {}".format(
sp.mean(),
means.mean()
))
print("разница: {}, стандартная ошибка среднего: {}".format(
abs(means.mean() - sp.mean()),
means.std()
))
# возмем произвольную выборку
sample = samples[0]
print("sample mean: {}".format(sample.mean()))
print("sample se: {}".format( sample.std() / math.sqrt(sample.size)))
###Output
sample mean: 21.035
sample se: 0.2981835713299028
###Markdown
Пример 2Рассчитайте стандартную ошибку среднего, если выборочное среднее равняется 10, дисперсия 4, при N = 100.Решение: Запишем в обозначениях $se=10$, $D = 4$, $N = 100$. Решаем:$$se = \frac{\sigma}{\sqrt{N}} = \frac{\sqrt{D}}{\sqrt{N}} = \frac{2}{10}=0.2$$ Доверительные интервалы для среднегоСтатья [Голова профессора Бамблдорфа](http://thinkcognitive.org/ru/blog/golova-professora-bambldorfa) о доверительных интервалах.Если мы имеем ГС и некоторую выборку из нее, то мы **не можем точно знать среднее ГС**, зная только среднее выборки. Однако мы можем сказать, с некоторым процентом уверенности, в каком интервале лежит средняя ГС. Очевидно, что для нас лучше, чтобы этот интервал был как можно меньше, но как это сделать?Мы знаем:- средняя средних выборок стремится к средней ГС;- стандартная ошибка среднего описывает стандартное отклонение распределения средних выборок $se=\frac{sd_{x}}{\sqrt{n}}$. Если мы возьмём случайную выборку $X$ и найдём её среднее $\bar{X}$, а также вычислим стандартную ошибку $se$, то мы можем вычислить доверительный интервал $[\bar{X} - 1.96*se; \bar{X} + 1.96*se]$ который описывает среднюю ГС в некотором интервале с **95% доверия**.Загадочное число **1,96** это количество сигм $\sigma$ в нормальном распределение, необходимое, чтобы охватить **95%** значений в этом распределении. **Пример на слайде**:Среднее $\bar{X} = 100$, стандартное отклонение $sd=4$ и эксперименте приняло участие $n=64$ человека. Вычисляем стандартную ошибку среднего:$$se=\frac{sd_{x}}{\sqrt{n}}=\frac{4}{\sqrt{64}}=\frac{4}{8}=0.5$$Теперь рассчитаем 95%-ый доверительный интервал:$$[\bar{X}-1.96*se, \bar{X}+1.96*se] = [100-1.96*0.5, 100+1.96*0.5]=[99.02, 100.98]$$А чтобы быть уверенным на 99%, то мы рассширяем доверительный интервал:$$[\bar{X}-2.58*se, \bar{X}+2.58*se]=[98.71, 101.29]$$ **Если мы рассчитали 95%-ый доверительный интервал для среднего значения, это значит**: - Среднее значение в ГС точно принадлежит рассчитанному доверительному интервалу. - это не точно, так как мы рассчитываем доверительный интервал для выборки из ГС, мы только на 95% можем быть уверены, что среднее ГС попадает в этот интервал. - Мы можем быть на 95% уверены, что среднее значение в ГС принадлежит рассчитанному доверительному интервалу. - собственно, то, о чем я говорил выше. - Если многократно повторять эксперимент, для каждой выборки рассчитывать свой доверительный интервал, то в 95 % случаев истинное среднее будет находиться внутри доверительного интервала. - верно, потому что, если взглянуть со стороны выборок, то для 95% выборок мы будем иметь вхождение среднего ГС в соответствующие доверительные интервалы соответствующих выборок по правилу $1.96 \sigma$. - Среднее значение в генеральной совокупности точно превышает нижнюю границу 95% доверительного интервала. - без комментариев. - Если многократно повторять эксперимент, то 95 % выборочных средних значений будут принадлежать рассчитанному нами доверительному интервалу. - отдельный доверительный интервал рассчитывается для каждой отдельной выборки. __Если из лекции усвоить разницу между средним ГС и средним выборки, а так же понять, что доверительный интервал строится для выборки, а не для ГС, то ответы в тесте легко определяются.__ **Задача**:Если бы в нашем примере мы увеличили объем выборки в два раза (при условии, что показатель стандартного отклонения остался неизменным), то 95% доверительный интервал ...- стал более широким- возможны оба варианта- стал более узким - Чем больше выборка (конечно же репрезентативная), тем мы больше **доверяем** своим данным, следовательно нам не нужен большой интервал. Данные становятся все более определенными вокруг каких-то близких значений.
###Code
'''Вычисление 1.96 c помощью scipy'''
p = 0.95 # 95%
# так как у нас двухсторонний интервал, сделаем вычисление
alpha = (1-p)/2
# isf - Inverse survival function (inverse of sf)
print("{} sigma".format(
stats.norm().isf(alpha)
))
'''Задача
Рассчитайте 99%-ый доверительный интервал для следующего примера:
среднее (X с чертой) = 10, стандартное отклонение (sd) = 5, размер выборки (n) = 100
'''
p = 0.99
X_ = 10
sd = 5
n = 100
se = sd/n**0.5 # корень из n
alpha = (1-p)/2
sigma = stats.norm().isf(alpha)
сonfidence_interval = X_ - sigma*se, X_ + sigma*se
print("[{:.2f};{:.2f}]".format(
сonfidence_interval[0],
сonfidence_interval[1]
))
###Output
[8.71;11.29]
###Markdown
Идея статистического вывода, p-уровень значимостиРазберем пример. На выздоровление в среднем требуется $M=20$ дней. Однако, мы разработали препарат и решили выяснить, можно ли сократить этот срок. Мы набрали выборку из $N=64$ пациентов и опробовали на них новый метод лечения. Оказалось, что средний срок выздоровления сократился до $\bar{X} = 18.5$ дней. При среднеквадратическом или же стандартном отклонении(тк выборка, а не ГС) $sd=4$.**Какой же вывод можно сделать, основываясь на этих данных?**- с одной стороны мы, действительно, сократили срок выздоровления;- с другой, такой результат мог быть получен совершенно случайно.Давайте введем два очень важных понятия. В нашем исследовании будут конкурировать две гипотезы.- нулевая гипотеза $H_{0}$ будет предполагать, что никакого воздействия новый препарат не оказывает и среднее значение ГС тех пациентов, кто использует наш новый препарат $M_{НП}=20$.- альтернативная гипотеза $H_{1}$ утверждает, что наш препарат влияет на скорость выздоровления и среднее значение скорости выздоровления ГС тех, кто использует новый препарат $M_{НП}\neq20$.Давайте рассуждать так: предположим, что верна нулевая гипотеза $H_{0}$, тогда в соответствии с ЦПТ, если бы мы многократно повторяли наши исследования, то выборочные средние распределидлись нормальным образом вокруг среднего ГС ($M=20$) и со стандартьным отклонением нашего распределения или со стандартной ошибкой среднего $se = \frac{sd}{\sqrt{N}}=\frac{4}{\sqrt{64}}=0.5$.Теперь давайте ответим на следующий вопрос: **насколько далеко наше выборочное среднее отклонилось от предполагаемого среднего ГС в единицах стандартного отклонения?** Выполним z-преобразование. Из нашего выборочного среднего вычтем среднее ГС и разделим на стардартное отклонение нашего распределения (нашего распределения предполагаемых выборочных средних), в нашем случае стандартную ошибку среднего.$$z=\frac{\bar{X}-M}{se}=\frac{18.5-20}{20}=-3$$Это означает, что если бы в ГС среднее значение на самом деле равнялось бы $20$, то наше выборочное среднее отклонилось бы от среднего ГС на $-3\sigma$ в левую сторону. Наконец-то, давайте воспользуемся свойствами нормального распределения, чтобы рассчитать вероятность такого или еще более сильно выраженного отклонения от среднего значения. Воспользуемся [сервисом](https://gallery.shinyapps.io/dist_calc/) для расчета и экономии времени.- используем нормальное распределение;- среднее равно 0;- стандартное отклонение 1;- both area: -3 и 3.Вероятность отклониться от среднего больше чем на 3 порядка в обе стороны:$$P({X 3}) = 0.0027$$ **Основная идея статистического вывода**: сначала мы допускаем, что верна нулевая гипотеза (т.е. никаких различий или никаких взаимосвязей в ГС нет). После этого мы можем расчитать вероятность того, что мы получили такие или еще более сильно выраженные различия абсолютно случайно. Эта вероятность называется `p уровень значимости`. И с помощью этого показателя мы выясним, какая гипотеза будет являться более состоятельной. - Считается, если $p<0.05$ - можно смело принимать альтернативную гипотезу. - А вот если $p>0.05$ - считается, у нас недостаточно оснований отклонить нулевую гипотезу. **PS**: Использование p-значений для проверки нулевых гипотез в работах по медицине, естественным наукам подвергается критике со стороны многих специалистов. Отмечается, что их использование нередко приводят к [ошибкам первого рода](https://ru.wikipedia.org/wiki/%D0%9E%D1%88%D0%B8%D0%B1%D0%BA%D0%B8_%D0%BF%D0%B5%D1%80%D0%B2%D0%BE%D0%B3%D0%BE_%D0%B8_%D0%B2%D1%82%D0%BE%D1%80%D0%BE%D0%B3%D0%BE_%D1%80%D0%BE%D0%B4%D0%B0) (false positive)[7]. В частности, журнал Basic and Applied Social Psychology (BASP) в 2015 году вовсе запретил публикацию статей, в которых используются p-значения. Редакторы журнала объяснили это тем, что сделать исследование, в котором получено p < 0,05 не очень сложно, и такие низкие значения p слишком часто становятся оправданием для низкопробных исследований Дополнительная информация об p уровне значимости [habr](https://habr.com/ru/company/stepic/blog/250527/). `Статистическая ошибка 1 рода` — ситуация, когда отвергнута правильная нулевая гипотеза (англ. type I errors, α errors, false positive, ошибочное отвержение). `Статистическая ошибка 2 рода` — ситуация, когда принята неправильная нулевая гипотеза (англ. type II errors, β errors, false negative, ошибочное принятие). **Попрактикуемся**: Вводные условия про препараты из текущей главы: - На выздоровление в среднем требуется $M=20$ дней;- Выборка из $N = 64$ пациентов;- Новый срок выздоровления $\bar{X} = 18.5$;- При стандартном отклонении $sd = 4$.- $se = \frac{sd}{\sqrt{N}}=\frac{4}{\sqrt{64}}=0.5$.- $X_{min} = x - 1.96*se = 18.5 - (1.96 * 0.5) = 17.52$- $X_{max} = x + 1.96*se = 18.5 + (1.96 * 0.5) = 19.48$- Доверительный интервал с надежностью 95%: $[17.52, 19.48]$, следовательно, 20 не входит в этот интервал.- Обращаемся к условию задачи - если значение 20 не будет принадлежать 95% ДИ по выборке, у нас будет достаточно оснований отклонить нулевую гипотезу.
###Code
'''
В среднем слушатели курса по введению в статистику набирают 115 баллов, однако,
в 2015 году средний балл случайно выбранных 144 участников составил 118 со стандартным отклонением равным 9.
Рассчитайте p уровень значимости для проверки нулевой гипотезы о том, что среднее значение баллов в 2015 году равняется 115.
'''
def p_value(z_stat, alternative = 'two-sided'):
if alternative == 'two-sided':
return 2 * (1 - stats.norm.cdf(np.abs(z_stat)))
if alternative == 'less':
return stats.norm.cdf(z_stat)
if alternative == 'greater':
return 1 - stats.norm.cdf(z_stat)
x_0 = 115
x_1 = 118
sd = 9
n = 144
se = sd / n ** 0.5
z = (x_1 - x_0) / se
print(p_value(z, alternative='greater'))
###Output
3.167124183311998e-05
|
notebooks/dl2/Cap 2 - Preliminares 1.ipynb | ###Markdown
Introdução e prelinares Até a pg 61
###Code
import mxnet as mx
from mxnet import np, npx
npx.set_np()
x=np.arange(12)
x,type(x)
x.shape
x.size
x=x.reshape(3,4)
x
X=np.arange(12).reshape(3,4)
Y=np.array([[2,1,4,3], [1,2,3,4], [4,3,2,1]])
X
Y
np.concatenate([X, Y], axis=0), np.concatenate([X, Y], axis=1)
X==Y
x=np.array([1,2,3]).reshape(3,1)
x
y=np.array([1,2]).reshape(1,2)
y
x+y
X,Y
X[-1]
X[1:3]
X[0:3, 1:3]=13
X
X>Y,X,Y
T1 = np.arange(12).reshape(1, 6, 2)
T1,np.arange(12)
T2 = np.ones(shape=(6,6,1))
T2
###Output
_____no_output_____
###Markdown
Data Preprocessing pg 51
###Code
import os
os.makedirs(os.path.join('../../data','tmp'), exist_ok=True)
data_file=os.path.join('../../data','tmp','house_tiny.csv')
with open(data_file,'w') as f:
f.write('NumRooms,Alley,Price\n') # Column names
f.write('NA,Pave,127500\n') # Each row represents a data example
f.write('2,NA,106000\n')
f.write('4,NA,178100\n')
f.write('NA,NA,140000\n')
import pandas as pd
data=pd.read_csv(data_file)
print(data)
inputs, outputs=data.iloc[:,0:2], data.iloc[:,2]
print(inputs)
inputs=inputs.fillna(inputs.mean())
print(inputs)
inputs=pd.get_dummies(inputs, dummy_na=True)
print(inputs)
X, y=np.array(inputs.values), np.array(outputs.values)
X, y
###Output
_____no_output_____
###Markdown
fazer exercicios da pg 53
###Code
t3=np.random.choice(np.array([1,2,np.nan]), (20, 5),p=np.array([0.4,0.4, 0.3]))
t3
df=pd.DataFrame(t3.asnumpy(),columns=['a','b','c','e','f'])
print(df)
df.isnull().mean()
df.columns[df.isnull().mean() < 0.25]
res1=df[df.columns[df.isnull().mean() < 0.25]]
print(res1)
resInput=np.array(res1.values)
resOutput=np.random.rand(20)
resInput,resOutput
###Output
_____no_output_____
###Markdown
Linear Algebrapg 53
###Code
A=np.arange(20).reshape(5,4)
A,A.mean(axis=0),A.mean(axis=1)
A.sum(),A.sum(axis=1),A.sum(axis=1,keepdims=True)
A/A.sum(axis=1,keepdims=True),A.cumsum(axis=1)
###Output
_____no_output_____
###Markdown
Produto Hadamard (Element-wise) e Produto de Matriz * dot
###Code
A1=np.array([1,2,3,4]).reshape(2,2)
B1=np.array([-1,3,4,2]).reshape(2,2)
A1,B1,A1*B1,np.dot(A1,B1),np.dot(B1,A1)
A1=np.array([1,2,3,4])
B1=np.array([-1,3,4,2])
A1,B1,A1*B1,np.dot(A1,B1)
y=np.ones(4)
x.reshape(1,3), y
###Output
_____no_output_____
###Markdown
Norm pg 63 - norma vetorial Norm L2\[3,-4]sqrt{3² + -4²}=5
###Code
u=np.array([3,-4])
u,np.linalg.norm(u)
###Output
_____no_output_____
###Markdown
Norm L1\[3,-4]|3|+|-4|=7
###Code
np.abs(u).sum()
###Output
_____no_output_____
###Markdown
Frobenius norm Aplica a L2 sobre Matrizes Exercícios pg 65 Confrontar com https://hy38.github.io/D2L-2-linear-algebra 1. Prove that the transpose of a matrix A’s transpose is A : (𝐀T)⊤=A(𝐀^T)^⊤ = A(AT)⊤=A
###Code
A=np.arange(25).reshape(5,5)
AT=A.T
TA=AT.T
res=(A==TA).reshape(A.size)
#type(res),type(res.asnumpy()),
#np.diff(res,prepend=False, append=False)
np.count_nonzero(res%2==1)==A.size
###Output
WARNING:root:np.count_nonzero is a fallback operator, which is actually using official numpy's implementation.
###Markdown
2. Given two matrices A and B, show that the sum of transposes is equal to the transpose of a sum: A⊤+B⊤= (A+B)⊤
###Code
A1=np.array([1,2,3,4,5,6,7,8,9]).reshape(3,3)
A2=A1.copy()
B1=np.array([2,4,6,8,10,12,14,16,18]).reshape(3,3)
B2=B1.copy()
A1,A2,B1,B2
R1=A1.T + B1.T
R2=(A1+B1).T
res=(R1==R2).reshape(R1.size)
np.count_nonzero(res%2==1)==R1.size
###Output
_____no_output_____
###Markdown
3. Given any square matrixA, is A+A⊤ always symmetric? Why?
###Code
t1=A1+A1.T
t2=B1+B1.T
res1=(t1==t1.T).reshape(t1.size)
res2=(t2==t2.T).reshape(t2.size)
res1,res2
np.count_nonzero(res1%2==1)==res1.size , np.count_nonzero(res2%2==1)==res2.size
###Output
_____no_output_____
###Markdown
4. We defined the tensorX of shape (2, 3, 4) in this section. What is the output oflen(X)?
###Code
T=np.ones((2,3,4))
T,T.size,T.shape
###Output
_____no_output_____
###Markdown
5. For a tensorXof arbitrary shape, does len(X) always correspond to the length of a certain axis ofX? What is that axis?first 6.Run A / A.sum(axis=1) and see what happens. Can you analyze the reason?
###Code
t=A.sum(axis=1)
A,t,A/t
###Output
_____no_output_____
###Markdown
8. Consider a tensor with shape (2, 3, 4). What are the shapes of the summation outputs alongaxis 0, 1, and 2?
###Code
A=mx.nd.arange(1,25).reshape(2,3,4)
A,A.sum(0),A.sum(1),A.sum(2)
###Output
_____no_output_____
###Markdown
9. Feed a tensor with 3 or more axes to the linalg.norm function and observe its output. Whatdoes this function compute for tensors of arbitrary shape?https://www.educative.io/edpresso/what-is-the-nplinalgnorm-method-in-numpy
###Code
A=(np.arange(24)-5).reshape(2,3,4)
A , np.linalg.norm(A),np.linalg.norm(A,axis=0),np.linalg.norm(A,axis=1),np.linalg.norm(A,axis=2)
###Output
_____no_output_____ |
day_14/task.ipynb | ###Markdown
Part 1 (Simple)> Apply 10 steps of pair insertion to the polymer template and find the most and least common elements in the result. What do you get if you take the quantity of the most common element and subtract the quantity of the least common element?
###Code
# Parse input
template, inp_instructions = open("input.txt").read().split("\n\n")
rules = {re.search(r"(\w+) -> (\w+)", x).group(1): re.search(r"(\w+) -> (\w+)", x).group(2) for x in inp_instructions.splitlines()}
def p_insert(inp: str, d: dict):
out = ""
for i in range(0,len(inp)-1):
out += f"{inp[i]}{d[inp[i:i+2]]}"
return out + inp[-1]
for i in range(0,10):
template = p_insert(template, rules)
occurences = sorted(Counter(list(template)).items(), key=lambda item: item[1], reverse=True)
print(occurences[0][1]-occurences[-1][1])
###Output
2937
###Markdown
Part 2 (Fast)> Apply 40 steps of pair insertion to the polymer template and find the most and least common elements in the result. What do you get if you take the quantity of the most common element and subtract the quantity of the least common element?
###Code
# Parse input
template, inp_instructions = open("input.txt").read().split("\n\n")
pattern_counter = defaultdict(int, Counter([template[i:i+2] for i in range(0,len(template)-1)]))
char_counter = defaultdict(int, Counter(list(template)))
rules = {re.search(r"(\w+) -> (\w+)", x).group(1): re.search(r"(\w+) -> (\w+)", x).group(2) for x in inp_instructions.splitlines()}
def p_insert(p_cnt: dict, c_cnt: dict, d: dict):
inp = p_cnt.copy()
for k in list(inp.keys()):
inp[k] -= p_cnt[k]
inp[k[0]+d[k]] += p_cnt[k]
inp[d[k]+k[1]] += p_cnt[k]
c_cnt[d[k]] += p_cnt[k]
return (inp, c_cnt)
for _ in range(40):
pattern_counter, char_counter = p_insert(pattern_counter, char_counter, rules)
result = max(char_counter.values())-min(char_counter.values())
print(result)
###Output
3390034818249
|
01_Student_Notebook.ipynb | ###Markdown
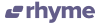 Task 1: IntroductionWelcome to Basic Image Classification with TensorFlow.This graph describes the problem that we are trying to solve visually. We want to create and train a model that takes an image of a hand written digit as input and predicts the class of that digit, that is, it predicts the digit or it predicts the class of the input image. Import TensorFlow
###Code
import tensorflow as tf
print('Using TensorFlow version', tf.__version__)
###Output
Using TensorFlow version 2.3.1
###Markdown
Task 2: The Dataset Import MNIST
###Code
from tensorflow.keras.datasets import mnist
(x_train,y_train), (x_test,y_test)= mnist.load_data()
###Output
Downloading data from https://storage.googleapis.com/tensorflow/tf-keras-datasets/mnist.npz
11493376/11490434 [==============================] - 3s 0us/step
###Markdown
Shapes of Imported Arrays
###Code
print(x_train.shape)
print(y_train.shape)
print(x_test.shape)
print(y_test.shape)
###Output
(60000, 28, 28)
(60000,)
(10000, 28, 28)
(10000,)
###Markdown
Plot an Image Example
###Code
from matplotlib import pyplot as plt
%matplotlib inline
plt.imshow(x_train[0],cmap='binary')
plt.show()
###Output
_____no_output_____
###Markdown
Display Labels
###Code
y_train[0]
print(set(y_train))
###Output
{0, 1, 2, 3, 4, 5, 6, 7, 8, 9}
###Markdown
Task 3: One Hot EncodingAfter this encoding, every label will be converted to a list with 10 elements and the element at index to the corresponding class will be set to 1, rest will be set to 0:| original label | one-hot encoded label ||------|------|| 5 | [0, 0, 0, 0, 0, 1, 0, 0, 0, 0] || 7 | [0, 0, 0, 0, 0, 0, 0, 1, 0, 0] || 1 | [0, 1, 0, 0, 0, 0, 0, 0, 0, 0] | Encoding Labels
###Code
from tensorflow.keras.utils import to_categorical
y_train_encoded = to_categorical(y_train)
y_test_encoded = to_categorical(y_test)
###Output
_____no_output_____
###Markdown
Validated Shapes
###Code
print(y_train_encoded.shape)
print(y_test_encoded.shape)
###Output
(60000, 10)
(10000, 10)
###Markdown
Display Encoded Labels
###Code
y_train_encoded[0]
###Output
_____no_output_____
###Markdown
Task 4: Neural Networks Linear EquationsThe above graph simply represents the equation:\begin{equation}y = w1 * x1 + w2 * x2 + w3 * x3 + b\end{equation}Where the `w1, w2, w3` are called the weights and `b` is an intercept term called bias. The equation can also be *vectorised* like this:\begin{equation}y = W . X + b\end{equation}Where `X = [x1, x2, x3]` and `W = [w1, w2, w3].T`. The .T means *transpose*. This is because we want the dot product to give us the result we want i.e. `w1 * x1 + w2 * x2 + w3 * x3`. This gives us the vectorised version of our linear equation.A simple, linear approach to solving hand-written image classification problem - could it work? Neural NetworksThis model is much more likely to solve the problem as it can learn more complex function mapping for the inputs and outputs in our dataset. Task 5: Preprocessing the Examples Unrolling N-dimensional Arrays to Vectors
###Code
import numpy as np
x_train_reshaped = np.reshape(x_train,(60000,784))
x_test_reshaped = np.reshape(x_test,(10000,784))
###Output
_____no_output_____
###Markdown
Display Pixel Values
###Code
print(set(x_train_reshaped[0]))
###Output
{0, 1, 2, 3, 9, 11, 14, 16, 18, 23, 24, 25, 26, 27, 30, 35, 36, 39, 43, 45, 46, 49, 55, 56, 64, 66, 70, 78, 80, 81, 82, 90, 93, 94, 107, 108, 114, 119, 126, 127, 130, 132, 133, 135, 136, 139, 148, 150, 154, 156, 160, 166, 170, 171, 172, 175, 182, 183, 186, 187, 190, 195, 198, 201, 205, 207, 212, 213, 219, 221, 225, 226, 229, 238, 240, 241, 242, 244, 247, 249, 250, 251, 252, 253, 255}
###Markdown
Data Normalization
###Code
x_mean = np.mean(x_train_reshaped)
x_std = np.std(x_train_reshaped)
epsilon = 1e-10
x_train_norm = (x_train_reshaped - x_mean) / (x_std+epsilon)
x_test_norm = (x_test_reshaped - x_mean) / (x_std+epsilon)
###Output
_____no_output_____
###Markdown
Display Normalized Pixel Values
###Code
print(set(x_train_norm[0]))
###Output
{-0.38589016215482896, 1.306921966983251, 1.17964285952926, 1.803310486053816, 1.6887592893452241, 2.8215433456857437, 2.719720059722551, 1.1923707702746593, 1.7396709323268205, 2.057868700961798, 2.3633385588513764, 2.096052433197995, 1.7651267538176187, 2.7960875241949457, 2.7451758812133495, 2.45243393406917, 0.02140298169794222, -0.22042732246464067, 1.2305545025108566, 0.2759611966059242, 2.210603629906587, 2.6560805059955555, 2.6051688630139593, -0.4240738943910262, 0.4668798577869107, 0.1486820891519332, 0.3905123933145161, 1.0905474843114664, -0.09314821501064967, 1.4851127174188385, 2.7579037919587486, 1.5360243604004349, 0.07231462467953861, -0.13133194724684696, 1.294194056237852, 0.03413089244334132, 1.3451056992194483, 2.274243183633583, -0.24588314395543887, 0.772349715676489, 0.75962180493109, 0.7214380726948927, 0.1995937321335296, -0.41134598364562713, 0.5687031437501034, 0.5941589652409017, 0.9378125553666773, 0.9505404661120763, 0.6068868759863008, 0.4159682148053143, -0.042236572029053274, 2.7706317027041476, 2.1342361654341926, 0.12322626766113501, -0.08042030426525057, 0.16140999989733232, 1.8924058612716097, 1.2560103240016547, 2.185147808415789, 0.6196147867316999, 1.943317504253206, -0.11860403650144787, -0.30952269768243434, 1.9942291472348024, -0.2840668761916362, 2.6306246845047574, 2.286971094378982, -0.19497150097384247, -0.39861807290022805, 0.2886891073513233, 1.7523988430722195, 2.3887943803421745, 2.681536327486354, 1.4596568959280403, 2.439706023323771, 2.7833596134495466, 2.490617666305367, -0.10587612575604877, 1.5614801818912332, 1.9051337720170087, 1.6123918248728295, 1.268738234747054, 1.9560454149986053, 2.6433525952501564, 1.026907930584471}
|
Basics/intro_vectors.ipynb | ###Markdown
Getting Started with Numpy VectorsThe `numpy` package has a number of powerful and fast tools for manipulating vectors. In this demo, we will illustrate some of the features of the package that will be used throughout the class. A more complete summary of `python` and `numpy` can be found at:http://cs231n.github.io/python-numpy-tutorial/For this tutorial, we start by importing the package.
###Code
import numpy as np
###Output
_____no_output_____
###Markdown
Creating vectorsWe can create vectors a number of ways. We can manually create a vector by specifying the elements from a list. Note that, unlike MATLAB, there is no difference between a row and column vector. Also, you have to call the `print` command to print the object.
###Code
x = np.array([1,2,4])
print(x)
###Output
[1 2 4]
###Markdown
You can also set through a range of numbers
###Code
x1 = np.arange(10) # numbers from 0 to 9 (note 10 is NOT included)
x2 = np.arange(2,7) # numbers from 2 to 6
print("x1 = "+str(x1))
print("x2 = "+str(x2))
###Output
x1 = [0 1 2 3 4 5 6 7 8 9]
x2 = [2 3 4 5 6]
###Markdown
You can also use a third `step` argument. The step does not need to be positive or integer.
###Code
x1 = np.arange(10,50,5) # Increments of 5. Note this ends on 45, not 50
print(x1)
# Increments of 2.5. To ensure it includes the value 10, we make the endpoint slightly >10.
step = 2.5
x2 = np.arange(0,10+step/2,step)
print(x2)
###Output
[10 15 20 25 30 35 40 45]
[ 0. 2.5 5. 7.5 10. ]
###Markdown
Most operations can be vectorized meaning that the operation is applied to each component.
###Code
x = np.array(range(2,6))
y = x**2
print("x = " + str(x))
print("y = " + str(y))
###Output
x = [2 3 4 5]
y = [ 4 9 16 25]
###Markdown
**Exercise** Write code to create the following vectors. Use the `range` function along with basic mathematical operations. For the case of `z2` you may consider using the `np.abs()` function. z1 = [2,4,6,8,10,...,20] z2 = [50,40,30,...,10,0,10,20,...,50] In the examples in the labs, we will load the vectors from data files. We will show how to do this later using the `pandas` package. Plotting vectorsThe `matplotlib` package has excellent routines for plotting data and uses a very similar interface as MATLAB. To load the package use the following commands. The second line `%matplotlib inline` is only used when running python in `jupyter notebook.`
###Code
import matplotlib.pyplot as plt
%matplotlib inline
###Output
_____no_output_____
###Markdown
A simple illustration to plot $\sin(2\pi(3)t)$ vs. $t$ for $t \in [0,2]$.
###Code
f = 3
t = np.linspace(0,2,100) # Similar to the MATLAB linspace command
y = np.sin(2*np.pi*f*t)
plt.plot(t,y)
plt.grid()
plt.xlabel('t', fontsize=16)
plt.ylabel('sin(2pi ft)', fontsize=16)
###Output
_____no_output_____
###Markdown
You can also stack plots.
###Code
ysq = y**2
plt.plot(t,y)
plt.plot(t,ysq)
plt.grid()
plt.xlabel('t')
###Output
_____no_output_____
###Markdown
**Exercise** Create a plot of $y = e^{-2x}$ vs. $x$ for $x \in [-1,3]$. Use 200 points in the values of $x$. Indexing and Slicing VectorsYou can access individual elements by `x[i]`. Unlike MATLAB, the indexing starts at 0!
###Code
x = np.array([2,4,6,8,10])
print("Element 0 is "+str(x[0]))
print("Element 3 is "+str(x[3]))
###Output
Element 0 is 2
Element 3 is 8
###Markdown
Similar to MATLAB, you can obtain *slices* from `numpy` vectors, meaning subsets of components. Use the `:` operator as follows.
###Code
x = np.random.rand(10) # 10 random elements from 0 to 1
x1 = x[2:5] # Elements 2,3,4 (Note 5 is NOT included)
x2 = x[:4] # Elements 0,1,2,3 (Starts at 0, element 4 NOT included)
x3 = x[7:] # Elements 7,8,9 (Element 7 IS included. Ends at 9 NOT 10)
xlast = x[-1] # The last element
print("x= "+np.array_str(x,precision=3))
print("x[2:5]= "+np.array_str(x1,precision=3))
print("x[:4]= "+np.array_str(x2,precision=3))
print("x[7:]= "+np.array_str(x3,precision=3))
print("xlast= {0:5.3f}".format(xlast))
###Output
x= [ 0.058 0.073 0.522 0.379 0.157 0.319 0.802 0.72 0.484 0.448]
x[2:5]= [ 0.522 0.379 0.157]
x[:4]= [ 0.058 0.073 0.522 0.379]
x[7:]= [ 0.72 0.484 0.448]
xlast= 0.448
|
[DIR] archive/DarpanBihar3192020/DarpanBihar3192020.ipynb | ###Markdown
Codebook **Authors:** Lauren Baker Documenting existing data files of DaanMatch with information about location, owner, "version", source etc.
###Code
import boto3
import numpy as np
import pandas as pd
pd.plotting.register_matplotlib_converters()
import matplotlib.pyplot as plt
%matplotlib inline
from collections import Counter
import statistics
client = boto3.client('s3')
resource = boto3.resource('s3')
my_bucket = resource.Bucket('daanmatchdatafiles')
###Output
_____no_output_____
###Markdown
DarpanBihar3192020.xlsx TOC:* [About this dataset](1)* [Cleaned datasets](2)* [What's in this dataset](3)* [Codebook](4) * [Missing values](4.1) * [Summary statistics](4.2)* [Columns](5) * [Sheet 1](5.1) * [Sheet 2](5.2) * [Url](5.2.1) * [NGO Name](5.2.2) * [Popular Name](5.2.3) * [Year of Establishment](5.2.4) * [GuideStar URL](5.2.5) * [Full Time Staff](5.2.6) * [Full Time Volunteers](5.2.7) * [Brief description](5.2.8) * [Annual Expenditure (Rs in lacs)](5.2.9) * [Year for Annual Expenditure](5.2.10) * [Impact](5.2.11) * [Profile pic](5.2.12) * [Organisation Website](5.2.13) * [Email](5.2.14) * [Telephone/ Mobile number](5.2.15) * [Correspondence Address](5.2.16) * [Phone](5.2.17) * [Cause(s)](5.2.18) * [Nature of Intervention](5.2.19) * [Beneficairies](5.2.20) * [Focus](5.2.21) * [Area of Operation: State](5.2.22) * [PAN](5.2.23)* [Problems with the data](6) **About this dataset** Data provided by: Unknown. Source: https://daanmatchdatafiles.s3.us-west-1.amazonaws.com/DaanMatch_DataFiles/DarpanBihar3192020.xlsx Type: xlsx Last Modified: May 29, 2021, 19:56:13 (UTC-07:00) Size: 2.0 MB
###Code
path = "s3://daanmatchdatafiles/DaanMatch_DataFiles/DarpanBihar3192020.xlsx"
Darpan_Bihar = pd.ExcelFile(path)
print(Darpan_Bihar.sheet_names)
Darpan_Bihar_1 = Darpan_Bihar.parse('Sheet1')
Darpan_Bihar_1.head()
Darpan_Bihar_2 = Darpan_Bihar.parse('Sheet2')
Darpan_Bihar_2.head()
###Output
_____no_output_____
###Markdown
**Cleaned datasets**
###Code
Darpan_Bihar_2 = Darpan_Bihar_2.iloc[:, :23]
Darpan_Bihar_2.head()
###Output
_____no_output_____
###Markdown
**What's in this dataset?**
###Code
print('Sheet 1:')
print("Shape:", Darpan_Bihar_1.shape)
print("Rows:", Darpan_Bihar_1.shape[0])
print("Columns:", Darpan_Bihar_1.shape[1])
print("Each row is an NGO.")
print('\n')
print('Sheet 2:')
print("Shape:", Darpan_Bihar_2.shape)
print("Rows:", Darpan_Bihar_2.shape[0])
print("Columns:", Darpan_Bihar_2.shape[1])
print("Each row is an NGO.")
###Output
Sheet 1:
Shape: (2881, 42)
Rows: 2881
Columns: 42
Each row is an NGO.
Sheet 2:
Shape: (926, 23)
Rows: 926
Columns: 23
Each row is an NGO.
###Markdown
**Codebook**
###Code
Darpan_Bihar_2.replace("13 Project staff", 13, inplace = True)
Darpan_Bihar_2['Full Time Staff'] = Darpan_Bihar_2['Full Time Staff'].astype(float)
print("Sheet 2 Codebook:")
Darpan_Bihar_2_columns = [column for column in Darpan_Bihar_2.columns]
Darpan_Bihar_2_description = ["Url to the NGO on the website GivingTuesdayIndia.",
"Full name of NGO.",
"Shortened / commonly used NGO name.",
"Year NGO was started.",
"Page for NGO on the website GuideStar.",
"Number of full-time employees at the NGO.",
"Number of full-time volunteers at the NGO.",
"Description of what the NGO is.",
"How much money is spent annually (In Rs in lacs).",
"The year that the amount in the previous column was spent.",
"What the NGO has been able to accomplish so far.",
"Link to a profile photo of the NGO.",
"NGO website.",
"Email address.",
"Telephone or mobile phone number.",
"NGO office address.",
"Office phone number.",
"Causes the NGO supports.",
"How the NGO performs acts of service.",
"Groups that are benefited from the NGO.",
"Focus of the NGO (Rural, Urban, or Rural & Urban).",
"States or Districts that the NGO performs service.",
"Permanent Account Number. 10-digit alphanumeric number that is assigned to each NGO."]
Darpan_Bihar_2_dtypes = [dtype for dtype in Darpan_Bihar_2.dtypes]
data_2 = {"Column Name": Darpan_Bihar_2_columns, "Description": Darpan_Bihar_2_description, "Type": Darpan_Bihar_2_dtypes}
Darpan_Bihar_2_codebook = pd.DataFrame(data_2)
Darpan_Bihar_2_codebook.style.set_properties(subset=['Description'], **{'width': '600px'})
###Output
Sheet 2 Codebook:
###Markdown
**Missing values**
###Code
Darpan_Bihar_1.isnull().sum()
Darpan_Bihar_2.isnull().sum()
###Output
_____no_output_____
###Markdown
**Summary statistics**
###Code
Darpan_Bihar_2.describe()
###Output
_____no_output_____
###Markdown
Columns Sheet 2Sheet 2 contains information about several NGOs. UrlUrl to the NGO on the website GivingTuesdayIndia.
###Code
column = Darpan_Bihar_2["Url"]
column
# Check number of unique values
print("No. of unique values:", len(column.unique()))
# Check for duplicates
counter = dict(Counter(column))
duplicates = { key:value for key, value in counter.items() if value > 1}
print("Duplicates:", duplicates)
if len(duplicates) > 0:
print("No. of duplicates:", len(duplicates))
# Check for null values
print("Null:", sum(column.isnull()))
###Output
No. of unique values: 926
Duplicates: {}
Null: 0
###Markdown
NGO NameFull name of the NGO.
###Code
column = Darpan_Bihar_2["NGO Name"]
column
# Check number of unique values
print("No. of unique values:", len(column.unique()))
# Check for duplicates
counter = dict(Counter(column))
duplicates = { key:value for key, value in counter.items() if value > 1}
print("Duplicates:", duplicates)
if len(duplicates) > 0:
print("No. of duplicates:", len(duplicates))
# Check for null values
print("Null:", sum(column.isnull()))
###Output
No. of unique values: 925
Duplicates: {'Association for Rural Development': 2}
No. of duplicates: 1
Null: 0
###Markdown
Popular NameShortened / commonly used NGO name.
###Code
column = Darpan_Bihar_2["Popular Name"]
column
# Check number of unique values
print("No. of unique values:", len(column.unique()))
# Check for duplicates
counter = dict(Counter(column))
duplicates = { key:value for key, value in counter.items() if value > 1}
print("Duplicates:", duplicates)
if len(duplicates) > 0:
print("No. of duplicates:", len(duplicates))
# Check for null values
print("Null:", sum(column.isnull()))
Darpan_Bihar_2[Darpan_Bihar_2['Popular Name'] == 'ACT']
###Output
_____no_output_____
###Markdown
Duplicates in ```Popluar Name``` do not mean duplicates in NGOs. Year of EstablishmentYear NGO was started.
###Code
column = Darpan_Bihar_2["Year of Establishment"]
column
# Number of null values
print("Null:", sum(column.isnull()))
# Drop null values
cleaned = column.dropna()
#Drop invalid values
dates = cleaned.astype(str)
invalid = len(dates[dates.astype(int) > 3000])
dates = dates[dates.astype(int) < 3000]
# Range
print("Min:", min(dates))
print("Max:", max(dates))
print("Invalid values:", invalid)
# Check number of unique values
print("No. of unique values:", len(column.unique()))
# Check for duplicates
counter = dict(Counter(column))
duplicates = { key:value for key, value in counter.items() if value > 1}
print("Duplicates:", duplicates)
if len(duplicates) > 0:
print("No. of duplicates:", len(duplicates))
#Plot number of NGOs established each year
counter = dict(Counter(dates))
count = { key:[value] for key, value in counter.items()}
table = pd.DataFrame.from_dict(count)
table = table.melt(var_name="Date", value_name="Count")
plt.figure(figsize = (15, 8))
plt.bar(table.sort_values('Date')["Date"], table.sort_values('Date')["Count"])
plt.title("NGOs Established each Year")
plt.ylabel("Count of NGOs")
plt.xlabel("Year of Establishment")
plt.xticks(rotation = 90);
###Output
_____no_output_____
###Markdown
GuideStar URLPage for NGO on the website GuideStar.
###Code
column = Darpan_Bihar_2["GuideStar URL"]
column
# Check number of unique values
print("No. of unique values:", len(column.unique()))
# Check for duplicates
counter = dict(Counter(column))
duplicates = { key:value for key, value in counter.items() if value > 1}
print("Duplicates:", duplicates)
if len(duplicates) > 0:
print("No. of duplicates:", len(duplicates))
# Check for null values
print("Null:", sum(column.isnull()))
###Output
No. of unique values: 926
Duplicates: {}
Null: 1
###Markdown
Full Time StaffNumber of full-time employees at the NGO.
###Code
column = Darpan_Bihar_2["Full Time Staff"]
column
# Number of null values
print("Null Values:", sum(column.isnull()))
# Drop null values
cleaned = column.dropna()
#Drop invalid values
counts = cleaned.astype(int)
# Range
print("Min:", min(counts))
print("Max:", max(counts))
#Statistics
counts.describe()
# Check number of unique values
print("No. of unique values:", len(column.unique()))
# Check for duplicates
counter = dict(Counter(column))
duplicates = { key:value for key, value in counter.items() if value > 1}
print("Duplicates:", duplicates)
if len(duplicates) > 0:
print("No. of duplicates:", len(duplicates))
bins= np.linspace(0, 500, 5)
plt.figure(figsize = (8, 6))
plt.hist(cleaned, bins=bins, edgecolor="k")
plt.title('Amount of Full Time Staff Members at NGOs')
plt.xlabel('Amount of Full Time Staff')
plt.ylabel('Number of NGOs')
plt.xticks(bins);
plt.show()
###Output
_____no_output_____
###Markdown
Full Time VolunteersNumber of full-time volunteers at the NGO.
###Code
column = Darpan_Bihar_2["Full Time Volunteers"]
column
# Number of null values
print("Null Values:", sum(column.isnull()))
# Drop null values
cleaned = column.dropna()
#Drop invalid values
counts = cleaned.astype(int)
# Range
print("Min:", min(counts))
print("Max:", max(counts))
#Statistics
counts.describe()
# Check number of unique values
print("No. of unique values:", len(column.unique()))
# Check for duplicates
counter = dict(Counter(column))
duplicates = { key:value for key, value in counter.items() if value > 1}
print("Duplicates:", duplicates)
if len(duplicates) > 0:
print("No. of duplicates:", len(duplicates))
#Smaller values
bins= np.linspace(0, 500, 10)
plt.figure(figsize = (8, 6))
plt.hist(cleaned, bins=bins, edgecolor="k")
plt.title('Amount of Full Time Volunteers at NGOs (Smaller values)')
plt.xlabel('Amount of Full Time Volunteers')
plt.ylabel('Number of NGOs')
plt.xticks(bins, rotation = 90);
plt.show()
#Larger amounts of volunteers
bins= np.linspace(500, 50000, 10)
plt.figure(figsize = (8, 6))
plt.hist(cleaned, bins=bins, edgecolor="k")
plt.title('Amount of Full Time Volunteers at NGOs (Larger values)')
plt.xlabel('Amount of Full Time Volunteers')
plt.ylabel('Number of NGOs')
plt.xticks(bins, rotation = 90);
plt.show()
###Output
_____no_output_____
###Markdown
Brief descriptionDescription of what the NGO is.
###Code
column = Darpan_Bihar_2["Brief description"]
column
# Number of null values
print("Null Values:", sum(column.isnull()))
column.dropna(inplace = True)
# Check number of unique values
print("No. of unique values:", len(column.unique()))
# Check for duplicates
counter = dict(Counter(column))
duplicates = { key:value for key, value in counter.items() if value > 1}
print("Duplicates:", duplicates)
if len(duplicates) > 0:
print("No. of duplicates:", len(duplicates))
Darpan_Bihar_2[Darpan_Bihar_2["Brief description"].isin(duplicates)]
###Output
_____no_output_____
###Markdown
Duplicates in ```Brief description``` do not mean duplicates in NGOs. The two NGOs with the same description are branches of the same NGO, but are not the same.
###Code
descriptons = Darpan_Bihar_2[Darpan_Bihar_2["Brief description"] != 0 ][['NGO Name', 'Brief description']].reset_index(drop = True)
descriptons.head()
###Output
_____no_output_____
###Markdown
Annual Expenditure (Rs in lacs)How much money is spent annually (In Rs in lacs).
###Code
column = Darpan_Bihar_2["Annual Expenditure (Rs in lacs)"]
column
# Check number of unique values
print("No. of unique values:", len(column.unique()))
# Check for duplicates
counter = dict(Counter(column))
duplicates = { key:value for key, value in counter.items() if value > 1}
print("Duplicates:", duplicates)
if len(duplicates) > 0:
print("No. of duplicates:", len(duplicates))
# Check for null values
print("Null:", sum(column.isnull()))
###Output
No. of unique values: 162
Duplicates: {nan: 762, 8: 2, 600000: 2, 300000: 2}
No. of duplicates: 4
Null: 762
###Markdown
Year for Annual ExpenditureThe year that the amount in the previous column was spent.
###Code
column = Darpan_Bihar_2["Year for Annual Expenditure"]
column
# Check number of unique values
print("No. of unique values:", len(column.unique()))
# Check for duplicates
counter = dict(Counter(column))
duplicates = { key:value for key, value in counter.items() if value > 1}
print("Duplicates:", duplicates)
if len(duplicates) > 0:
print("No. of duplicates:", len(duplicates))
# Check for null values
print("Null:", sum(column.isnull()))
###Output
No. of unique values: 5
Duplicates: {nan: 748, 'FY 2018-19': 49, 'FY 2019-20': 14, 'FY 2017-18': 94, 'FY 2016-17': 21}
No. of duplicates: 5
Null: 748
###Markdown
ImpactWhat the NGO has been able to accomplish so far.
###Code
column = Darpan_Bihar_2["Impact"]
column
# Check number of unique values
print("No. of unique values:", len(column.unique()))
# Check for duplicates
counter = dict(Counter(column))
duplicates = { key:value for key, value in counter.items() if value > 1}
print("Duplicates:", duplicates)
if len(duplicates) > 0:
print("No. of duplicates:", len(duplicates))
# Check for null values
print("Null:", sum(column.isnull()))
impacts = Darpan_Bihar_2[['NGO Name', 'Impact']].dropna().reset_index(drop = True)
impacts.head()
###Output
_____no_output_____
###Markdown
Profile picLink to a profile photo of the NGO.
###Code
column = Darpan_Bihar_2["Profile pic"]
column
# Check number of unique values
print("No. of unique values:", len(column.unique()))
# Check for duplicates
counter = dict(Counter(column))
duplicates = { key:value for key, value in counter.items() if value > 1}
print("Duplicates:", duplicates)
if len(duplicates) > 0:
print("No. of duplicates:", len(duplicates))
# Check for null values
print("Null:", sum(column.isnull()))
###Output
No. of unique values: 187
Duplicates: {nan: 740}
No. of duplicates: 1
Null: 740
###Markdown
Organisation WebsiteNGO website.
###Code
column = Darpan_Bihar_2["Organisation Website"]
column
# Check number of unique values
print("No. of unique values:", len(column.unique()))
# Check for null values
print("Null:", sum(column.isnull()))
column.dropna(inplace = True)
# Check for duplicates
counter = dict(Counter(column))
duplicates = { key:value for key, value in counter.items() if value > 1}
print("Duplicates:", duplicates)
if len(duplicates) > 0:
print("No. of duplicates:", len(duplicates))
Darpan_Bihar_2[Darpan_Bihar_2["Organisation Website"].isin(duplicates)].sort_values('Organisation Website')
###Output
_____no_output_____
###Markdown
Duplicates in ```Organisation Website``` are branches of the same company, yet not the exact same NGO. EmailEmail address.
###Code
column = Darpan_Bihar_2["Email"]
column
# Check number of unique values
print("No. of unique values:", len(column.unique()))
# Check for null values
print("Null:", sum(column.isnull()))
column.dropna(inplace = True)
# Check for duplicates
counter = dict(Counter(column))
duplicates = { key:value for key, value in counter.items() if value > 1}
print("Duplicates:", duplicates)
if len(duplicates) > 0:
print("No. of duplicates:", len(duplicates))
Darpan_Bihar_2[Darpan_Bihar_2["Email"].isin(duplicates)].sort_values('Email')
###Output
_____no_output_____
###Markdown
There are two addresses that are duplicates in ```Email```. One of the repeated addresses is used for two brances of an NGO, and the other repeated address appears to be used for two unreleated NGOs. Telephone/ Mobile numberTelephone or mobile phone number.
###Code
column = Darpan_Bihar_2["Telephone/ Mobile number"]
column
# Check number of unique values
print("No. of unique values:", len(column.unique()))
# Check for null values
print("Null:", sum(column.isnull()))
column.dropna(inplace = True)
# Check for duplicates
counter = dict(Counter(column))
duplicates = { key:value for key, value in counter.items() if value > 1}
print("Duplicates:", duplicates)
if len(duplicates) > 0:
print("No. of duplicates:", len(duplicates))
Darpan_Bihar_2[Darpan_Bihar_2["Telephone/ Mobile number"].isin(duplicates)].sort_values('Telephone/ Mobile number')
###Output
_____no_output_____
###Markdown
Duplicates in ```Telephone/ Mobile number``` does not mean the NGOs are the same. Correspondence AddressNGO office address.
###Code
column = Darpan_Bihar_2["Correspondence Address"]
column
# Check number of unique values
print("No. of unique values:", len(column.unique()))
# Check for null values
print("Null:", sum(column.isnull()))
column.dropna(inplace = True)
# Check for duplicates
counter = dict(Counter(column))
duplicates = { key:value for key, value in counter.items() if value > 1}
print("Duplicates:", duplicates)
if len(duplicates) > 0:
print("No. of duplicates:", len(duplicates))
###Output
No. of unique values: 855
Null: 72
Duplicates: {}
###Markdown
PhoneOffice phone number.
###Code
column = Darpan_Bihar_2["Phone"]
column
# Check number of unique values
print("No. of unique values:", len(column.unique()))
# Check for null values
print("Null:", sum(column.isnull()))
column.dropna(inplace = True)
# Check for duplicates
counter = dict(Counter(column))
duplicates = { key:value for key, value in counter.items() if value > 1}
print("Duplicates:", duplicates)
if len(duplicates) > 0:
print("No. of duplicates:", len(duplicates))
###Output
No. of unique values: 120
Null: 807
Duplicates: {}
###Markdown
Cause(s)Causes the NGO supports.
###Code
column = Darpan_Bihar_2["Cause(s)"]
column
# Check number of unique values
print("No. of unique values:", len(column.unique()))
# Check for null values
print("Null:", sum(column.isnull()))
column.dropna(inplace = True)
# Check for duplicates
counter = dict(Counter(column))
duplicates = { key:value for key, value in counter.items() if value > 1}
print("Duplicates:", duplicates)
if len(duplicates) > 0:
print("No. of duplicates:", len(duplicates))
###Output
No. of unique values: 350
Null: 27
Duplicates: {'Community Development, Education, Healthcare, Livelihoods, Women': 3, 'Education': 58, 'Children, Community Development, Education, Healthcare, Livelihoods, Women, Youth': 2, 'Children, Education, Healthcare, Livelihoods, Women': 19, 'Education, Youth': 7, 'Youth, Emergency Relief': 3, 'Education, Environment, Healthcare, Livelihoods': 3, 'Children': 16, 'Disabled': 11, 'Healthcare': 46, 'Children, Education, Healthcare, Women': 10, 'Children, Education, Elderly, Healthcare, Women': 3, 'Children, Disabled, Education': 11, 'Disabled, Livelihoods': 3, 'Community Development': 44, 'Children, Education, Livelihoods, Women': 8, 'Community Development, Education, Healthcare': 2, 'Emergency Relief': 3, 'Education, Healthcare, Livelihoods, Women, Youth': 4, 'Children, Education, Livelihoods': 4, 'Education, Healthcare': 20, 'Education, Livelihoods': 7, 'Children, Education': 34, 'Children, Women': 11, 'Children, Community Development, Education, Healthcare, Livelihoods, Women, Emergency Relief': 2, 'Disabled, Education, Livelihoods': 6, 'Disabled, Healthcare': 9, 'Children, Education, Environment, Healthcare, Livelihoods, Women, Youth': 3, 'Children, Healthcare': 12, 'Education, Healthcare, Livelihoods, Youth': 2, 'Sports': 2, 'Children, Environment, Women': 2, 'Children, Livelihoods, Women': 3, 'Children, Community Development, Education, Livelihoods, Women': 3, 'Healthcare, Livelihoods, Women': 3, 'Children, Healthcare, Women': 9, 'Children, Education, Healthcare, Livelihoods': 6, 'Healthcare, Women': 7, 'Children, Education, Women': 6, 'Children, Education, Elderly, Healthcare': 2, 'Community Development, Education': 2, 'Environment': 8, 'Women': 6, 'Education, Healthcare, Women, Youth': 3, 'Livelihoods': 6, 'Community Development, Education, Healthcare, Livelihoods, Women, Youth': 2, 'Education, Healthcare, Livelihoods': 16, 'Disabled, Education, Environment, Healthcare, Livelihoods': 2, 'Education, Environment, Livelihoods': 2, 'Disabled, Environment': 4, 'Children, Education, Livelihoods, Women, Youth': 3, 'Children, Disabled, Education, Healthcare, Livelihoods, Women, Youth': 3, 'Children, Women, Youth': 2, 'Children, Education, Healthcare': 9, 'Children, Education, Healthcare, Women, Youth': 6, 'Children, Education, Environment, Healthcare': 3, 'Children, Education, Environment': 4, 'Children, Disabled, Education, Healthcare, Livelihoods': 4, 'Education, Livelihoods, Women': 7, 'Children, Disabled': 2, 'Livelihoods, Youth': 4, 'Advocacy': 13, 'Community Development, Development and Housing': 3, 'Education, Livelihoods, Youth': 8, 'Healthcare, Livelihoods': 3, 'Education, Environment, Healthcare': 5, 'Healthcare, Livelihoods, Women, Youth': 2, 'Children, Disabled, Education, Livelihoods': 5, 'Elderly, Healthcare': 2, 'Children, Emergency Relief, Women': 2, 'Philanthropic Intermediaries and Voluntarism Promotion': 2, 'Children, Education, Livelihoods, Youth': 2, 'Children, Healthcare, Livelihoods, Women': 2, 'Children, Disabled, Education, Healthcare': 3, 'Children, Education, Sports, Youth': 2, 'Animal Welfare': 6, 'Education, Livelihoods, Women, Youth': 3, 'Children, Education, Women, Youth': 2, 'Development and Housing': 2, 'Children, Environment, Healthcare, Livelihoods, Women': 2, 'Children, Education, Environment, Healthcare, Livelihoods, Women': 6, 'Children, Disabled, Education, Healthcare, Livelihoods, Women': 2, 'Children, Women, Youth, Civic Issues': 2, 'Education, Women': 4, 'Children, Disabled, Education, Healthcare, Women': 4, 'Education, Environment': 3, 'Education, Women, Youth': 2, 'Children, Education, Environment, Healthcare, Women, Youth': 2, 'Children, Disabled, Women': 4, 'Children, Education, Healthcare, Livelihoods, Women, Youth, Emergency Relief': 3, 'Healthcare, Youth': 2, 'Children, Environment': 2, 'Community Development, Livelihoods, Women, Development and Housing': 3, 'Education, Healthcare, Livelihoods, Women': 3, 'Disabled, Education': 5, 'Children, Livelihoods': 2, 'Livelihoods, Women': 2, 'Environment, Healthcare, Livelihoods, Women': 3, 'Education, Healthcare, Women': 6, 'Community Development, Livelihoods, Youth': 2, 'Advocacy, Children, Education': 2, 'Children, Education, Healthcare, Livelihoods, Youth': 2, 'Children, Community Development, Education, Women, Youth': 2, 'Children, Education, Healthcare, Youth': 2, 'Children, Education, Environment, Healthcare, Livelihoods': 3, 'Children, Education, Philanthropic Intermediaries and Voluntarism Promotion': 2, 'Children, Education, Youth': 2, 'Children, Community Development, Women': 2, 'Community Development, Advocacy': 3, 'Children, Women, Advocacy': 2}
No. of duplicates: 110
###Markdown
Nature of InterventionHow the NGO performs acts of service.
###Code
column = Darpan_Bihar_2["Nature of Intervention"]
column
# Check number of unique values
print("No. of unique values:", len(column.unique()))
# Check for null values
print("Null:", sum(column.isnull()))
column.dropna(inplace = True)
# Check for duplicates
counter = dict(Counter(column))
duplicates = { key:value for key, value in counter.items() if value > 1}
print("Duplicates:", duplicates)
if len(duplicates) > 0:
print("No. of duplicates:", len(duplicates))
###Output
No. of unique values: 82
Null: 14
Duplicates: {'Direct service': 270, 'Advocacy & Campaigning': 10, 'Support, Network, Direct service': 20, 'Direct service, Support': 33, 'Direct service, Network, Support, Advocacy & Campaigning, Grant-making, Intermediary': 10, 'Advocacy & Campaigning, Direct service': 32, 'Support': 45, 'Direct service, Network, Support, Advocacy & Campaigning': 23, 'Intermediary, Advocacy & Campaigning, Support, Network, Direct service': 17, 'Direct service, Support, Advocacy & Campaigning': 20, 'Support, Direct service': 57, 'Intermediary, Support, Direct service': 8, 'Advocacy & Campaigning, Support, Network, Direct service': 56, 'Network, Support': 2, 'Advocacy & Campaigning, Support, Direct service, Grant-making': 5, 'Advocacy & Campaigning, Support, Direct service': 44, 'Intermediary, Advocacy & Campaigning, Support, Direct service': 8, 'Support, Direct service, Grant-making': 11, 'Grant-making': 2, 'Network, Direct service': 17, 'Advocacy & Campaigning, Support, Network, Direct service, Grant-making': 13, 'Direct service, Network, Advocacy & Campaigning, Intermediary': 3, 'Network': 4, 'Direct service, Support, Grant-making': 3, 'Direct service, Support, Advocacy & Campaigning, Grant-making': 5, 'Direct service, Advocacy & Campaigning': 16, 'Intermediary, Advocacy & Campaigning, Support, Network, Direct service, Grant-making': 16, 'Advocacy & Campaigning, Support, Network': 10, 'Support, Network, Direct service, Grant-making': 2, 'Direct service, Network, Support, Advocacy & Campaigning, Intermediary': 9, 'Direct service, Network, Support': 8, 'Direct service, Support, Intermediary': 3, 'Advocacy & Campaigning, Network, Direct service': 17, 'Direct service, Network, Advocacy & Campaigning': 10, 'Intermediary, Direct service': 3, 'Network, Support, Advocacy & Campaigning, Intermediary': 2, 'Advocacy & Campaigning, Support': 7, 'Direct service, Network, Support, Grant-making': 3, 'Intermediary, Support': 3, 'Network, Support, Advocacy & Campaigning': 4, 'Intermediary, Support, Grant-making': 2, 'Support, Advocacy & Campaigning': 4, 'Intermediary, Advocacy & Campaigning, Support, Network, Grant-making': 2, 'Direct service, Grant-making': 3, 'Intermediary, Support, Network, Direct service': 5, 'Intermediary, Advocacy & Campaigning, Direct service': 2, 'Direct service, Network': 6, 'Advocacy & Campaigning, Network': 5, 'Intermediary': 3, 'Intermediary, Advocacy & Campaigning, Support, Direct service, Grant-making': 3, 'Network, Advocacy & Campaigning': 2, 'Intermediary, Advocacy & Campaigning, Network, Direct service': 3, 'Intermediary, Advocacy & Campaigning, Support, Network': 2, 'Support, Grant-making': 2, 'Advocacy & Campaigning, Direct service, Grant-making': 3, 'Advocacy & Campaigning, Support, Network, Grant-making': 2, 'Direct service, Support, Advocacy & Campaigning, Intermediary': 3, 'Network, Direct service, Grant-making': 3, 'Direct service, Network, Support, Advocacy & Campaigning, Grant-making': 3, 'Direct service, Advocacy & Campaigning, Intermediary': 2}
No. of duplicates: 60
###Markdown
BeneficairiesGroups that are benefited from the NGO.
###Code
column = Darpan_Bihar_2["Beneficairies"]
column
# Check number of unique values
print("No. of unique values:", len(column.unique()))
# Check for null values
print("Null:", sum(column.isnull()))
column.dropna(inplace = True)
# Check for duplicates
counter = dict(Counter(column))
duplicates = { key:value for key, value in counter.items() if value > 1}
print("Duplicates:", duplicates)
if len(duplicates) > 0:
print("No. of duplicates:", len(duplicates))
###Output
No. of unique values: 784
Null: 26
Duplicates: {'Adults': 14, 'Mentally Challenged, Physically Challenged': 4, 'Women': 9, 'Children, Girl Child, Adolescents': 2, 'Physically Challenged, Children, Mentally Challenged': 2, 'Adolescents, Adults': 7, 'Children': 23, 'Orphans': 2, 'Rural Poor': 3, 'Adolescents, Adults, Youth, Women': 2, 'Children, Adults': 4, 'Children, Women, Youth': 3, 'Adults, Women': 2, 'Children, Orphans, Youth': 2, 'Patients': 3, 'Students': 5, 'Farmers / Farm Labourers': 3, 'Accident Victims, Animal Lovers, Adolescents, Adults': 2, 'Adolescents': 3, 'Rural Poor, Women': 2, 'Children, Mentally Challenged, Physically Challenged': 2, 'Girl Child': 3, 'Children, Women': 5, 'Adults, Women, Youth': 2, 'Physically Challenged': 4, 'Animal Lovers, Animals / Birds': 3, 'Children, Students': 2, 'Children, Adults, Youth, Women': 2, 'Children, Rural Poor, Women': 2, 'Rural Poor, Tribals': 3, 'Children, Youth, Women': 6, 'NGOs': 2, 'Children, Physically Challenged': 2, 'Girl Child, Students': 2, 'Physically Challenged, Mentally Challenged': 2, 'Youth, Women': 3, 'Mentally Challenged': 5, 'Girl Child, Women': 2, 'Children, Students, Teachers': 2, 'Children, Teachers': 3, 'Students, Youth': 3, 'Children, Mentally Challenged': 2}
No. of duplicates: 42
###Markdown
FocusFocus of the NGO (Rural, Urban, or Rural & Urban).
###Code
column = Darpan_Bihar_2["Focus"]
column
# Check number of unique values
print("No. of unique values:", len(column.unique()))
# Check for null values
print("Null:", sum(column.isnull()))
column.dropna(inplace = True)
# Check for duplicates
counter = dict(Counter(column))
duplicates = { key:value for key, value in counter.items() if value > 1}
print("Duplicates:", duplicates)
if len(duplicates) > 0:
print("No. of duplicates:", len(duplicates))
# Table of number of each class
table = column.value_counts().rename_axis('Focus').reset_index(name='Count')
# Plot number of each focus group
plt.figure(figsize = (8, 6))
plt.bar(table["Focus"], table["Count"])
plt.title("Count of NGOs' Focuses")
plt.xlabel("Focus")
plt.ylabel('Count')
plt.xticks(rotation = 20);
plt.show()
###Output
_____no_output_____
###Markdown
Area of Operation: StateStates that the NGO performs service in.
###Code
column = Darpan_Bihar_2["Area of Operation: State"]
column
# Check number of unique values
print("No. of unique values:", len(column.unique()))
# Check for null values
print("Null:", sum(column.isnull()))
column.dropna(inplace = True)
# Check for duplicates
counter = dict(Counter(column))
duplicates = { key:value for key, value in counter.items() if value > 1}
print("Duplicates:", duplicates)
if len(duplicates) > 0:
print("No. of duplicates:", len(duplicates))
###Output
No. of unique values: 140
Null: 150
Duplicates: {'Gujarat': 34, 'Tamil Nadu': 63, 'Odisha': 36, 'Haryana': 4, 'Madhya Pradesh': 10, 'Delhi': 31, 'Uttar Pradesh': 33, 'Uttarakhand': 5, 'Maharashtra': 118, 'West Bengal': 55, 'Tripura': 3, 'Jharkhand': 10, 'Bihar': 18, 'Rajasthan': 22, 'All India': 133, 'Karnataka': 38, 'BiharJharkhand': 2, 'Tamil NaduTamil Nadu': 2, 'Andhra Pradesh': 13, 'BiharUttar PradeshUttarakhand': 3, 'Chhattisgarh': 2, 'Andhra PradeshTelangana': 4, 'DelhiHaryanaUttar Pradesh': 2, 'DelhiHaryanaRajasthanUttar Pradesh': 2, 'Telangana': 3, 'Kerala': 4, 'HaryanaUttar Pradesh': 2, 'Madhya PradeshMaharashtra': 2, 'DelhiHaryana': 5, 'Goa': 2, 'PuducherryTamil Nadu': 2, 'DelhiMaharashtra': 2, 'Puducherry': 2, 'GujaratMaharashtra': 2, 'MaharashtraMaharashtra': 2, 'MaharashtraRajasthan': 2}
No. of duplicates: 36
###Markdown
PANPermanent Account Number. 10-digit alphanumeric number that is assigned to each NGO.
###Code
column = Darpan_Bihar_2["PAN"]
column
# Check if all rows have 10 digits
PAN_length = [len(PAN) for PAN in column]
print("Rows without 10 digits:", sum([length != 10 for length in PAN_length]))
# Check number of unique values
print("No. of unique values:", len(column.unique()))
# Check for null values
print("Null:", sum(column.isnull()))
column.dropna(inplace = True)
# Check for duplicates
counter = dict(Counter(column))
duplicates = { key:value for key, value in counter.items() if value > 1}
print("Duplicates:", duplicates)
if len(duplicates) > 0:
print("No. of duplicates:", len(duplicates))
###Output
Rows without 10 digits: 0
No. of unique values: 926
Null: 0
Duplicates: {}
|
code/avengers/inference/avengers-assemble-3-model10-tta2.ipynb | ###Markdown
- model1 : Eff2020- model2 : Eff2019+2020- model3 : Reg2020- model4 : Reg2019+2020- model5 : Eff2020 (seed 720)- model6 : VIT- model7 : FixMatch - model8 : Distillation - model9 : NFNet- model10 : Reg nocv EfficientNet (seed 719) + EfficientNet (seed 720) + EfficientNet (2019 + 2020) + RegNetY (2019+2020) + RegNetY(2020) + RegNetY (ALL) + VIT (2020 - 찬영님 버전) + Fixmix + Distillation + nfnet 'weight' 바꾸기
###Code
package_paths = [
'../input/timm-pytorch-image-models/pytorch-image-models-master', #'../input/efficientnet-pytorch-07/efficientnet_pytorch-0.7.0'
'../input/adamp-optimizer/AdamP-master/adamp',
]
import sys;
for pth in package_paths:
sys.path.append(pth)
from glob import glob
from sklearn.model_selection import GroupKFold, StratifiedKFold
import cv2
from skimage import io
import torch
from torch import nn
import os
from datetime import datetime
import time
import random
import cv2
import torchvision
from torchvision import transforms
import pandas as pd
import numpy as np
from tqdm import tqdm
import matplotlib.pyplot as plt
from torch.utils.data import Dataset,DataLoader
from torch.utils.data.sampler import SequentialSampler, RandomSampler
from torch.cuda.amp import autocast, GradScaler
from torch.nn.modules.loss import _WeightedLoss
import torch.nn.functional as F
import timm
import sklearn
import warnings
import joblib
from sklearn.metrics import roc_auc_score, log_loss
from sklearn import metrics
import warnings
import cv2
#from efficientnet_pytorch import EfficientNet
from scipy.ndimage.interpolation import zoom
CFG = {
'valid': False,
'fold_num': 5,
'seed': 719,
'model_arch1': 'tf_efficientnet_b4_ns',
'model_arch2': 'tf_efficientnet_b4_ns',
'model_arch3' : 'regnety_040',
'model_arch4' : 'regnety_040',
'model_arch5' : 'tf_efficientnet_b4_ns',
'model_arch6' : 'vit_base_patch16_384',
'model_arch7' : 'tf_efficientnet_b4_ns',
'model_arch8' : 'regnety_040', #distill
'model_arch9' : 'nf_resnet50',
'model_arch10' : 'regnety_040',
'weight' : [1/10, 1/10, 1/10, 1/10, 1/10, 1/10, 1/10, 1/10, 1/10, 1/10],
'img_size1': 384,
'img_size2': 512,
'epochs': 10,
'tta_num' : 2,
'train_bs': 64,
'valid_bs': 64,
'T_0': 10,
'lr': 1e-4,
'min_lr': 1e-6,
'weight_decay':1e-6,
'num_workers': 4,
'accum_iter': 2, # suppoprt to do batch accumulation for backprop with effectively larger batch size
'verbose_step': 1,
'device': 'cuda:0',
'used_epochs': [5, 6, 7, 8, 9] # Last 5 Epoch
}
train = pd.read_csv('../input/cassava-leaf-disease-classification/train.csv')
train.head()
submission = pd.read_csv('../input/cassava-leaf-disease-classification/sample_submission.csv')
submission.head()
###Output
_____no_output_____
###Markdown
Helper Functions
###Code
def seed_everything(seed):
random.seed(seed)
os.environ['PYTHONHASHSEED'] = str(seed)
np.random.seed(seed)
torch.manual_seed(seed)
torch.cuda.manual_seed(seed)
torch.backends.cudnn.deterministic = True
torch.backends.cudnn.benchmark = True
def get_img(path):
im_bgr = cv2.imread(path)
im_rgb = im_bgr[:, :, ::-1]
#print(im_rgb)
return im_rgb
def rand_bbox(size, lam):
W = size[0]
H = size[1]
cut_rat = np.sqrt(1. - lam)
cut_w = np.int(W * cut_rat)
cut_h = np.int(H * cut_rat)
# uniform
cx = np.random.randint(W)
cy = np.random.randint(H)
bbx1 = np.clip(cx - cut_w // 2, 0, W)
bby1 = np.clip(cy - cut_h // 2, 0, H)
bbx2 = np.clip(cx + cut_w // 2, 0, W)
bby2 = np.clip(cy + cut_h // 2, 0, H)
return bbx1, bby1, bbx2, bby2
class CassavaDataset(Dataset):
def __init__(self, df, data_root,
transforms=None,
output_label=True,
):
super().__init__()
self.df = df.reset_index(drop=True).copy()
self.transforms = transforms
self.data_root = data_root
self.output_label = output_label
if self.output_label == True:
self.labels = self.df['label'].values
def __len__(self):
return self.df.shape[0]
def __getitem__(self, index: int):
# get labels
if self.output_label:
target = self.labels[index]
img = get_img("{}/{}".format(self.data_root, self.df.loc[index]['image_id']))
if self.transforms:
img = self.transforms(image=img)['image']
if self.output_label == True:
return img, target
else:
return img
from albumentations import (
HorizontalFlip, VerticalFlip, IAAPerspective, ShiftScaleRotate, CLAHE, RandomRotate90,
Transpose, ShiftScaleRotate, Blur, OpticalDistortion, GridDistortion, HueSaturationValue,
IAAAdditiveGaussianNoise, GaussNoise, MotionBlur, MedianBlur, IAAPiecewiseAffine, RandomResizedCrop,
IAASharpen, IAAEmboss, RandomBrightnessContrast, Flip, OneOf, Compose, Normalize, Cutout, CoarseDropout, ShiftScaleRotate, CenterCrop, Resize, Rotate,
ShiftScaleRotate, CenterCrop, Resize, Rotate, RandomShadow, RandomSizedBBoxSafeCrop,
ChannelShuffle, MotionBlur
)
from albumentations.pytorch import ToTensorV2
def get_train_transforms():
return Compose([
RandomResizedCrop(CFG['img_size1'], CFG['img_size1']),
Transpose(p=0.5),
HorizontalFlip(p=0.5),
VerticalFlip(p=0.5),
ShiftScaleRotate(p=0.5),
HueSaturationValue(hue_shift_limit=0.2, sat_shift_limit=0.2, val_shift_limit=0.2, p=0.5),
RandomBrightnessContrast(brightness_limit=(-0.1,0.1), contrast_limit=(-0.1, 0.1), p=0.5),
Normalize(mean=[0.485, 0.456, 0.406], std=[0.229, 0.224, 0.225], max_pixel_value=255.0, p=1.0),
CoarseDropout(p=0.5),
Cutout(p=0.5),
ToTensorV2(p=1.0),
], p=1.)
def get_valid_transforms():
return Compose([
CenterCrop(CFG['img_size1'], CFG['img_size1'], p=1.),
Resize(CFG['img_size1'], CFG['img_size1']),
Normalize(mean=[0.485, 0.456, 0.406], std=[0.229, 0.224, 0.225], max_pixel_value=255.0, p=1.0),
ToTensorV2(p=1.0),
], p=1.)
# def get_inference_transforms():
# return Compose([
# CenterCrop(CFG['img_size1'], CFG['img_size1'], p=1.),
# Transpose(p=0.5),
# HorizontalFlip(p=0.5),
# VerticalFlip(p=0.5),
# Resize(CFG['img_size1'], CFG['img_size1']),
# Normalize(mean=[0.485, 0.456, 0.406], std=[0.229, 0.224, 0.225], max_pixel_value=255.0, p=1.0),
# ToTensorV2(p=1.0),
# ], p=1.)
def get_inference_transforms1():
return Compose([
OneOf([
Resize(CFG['img_size1'], CFG['img_size1'], p=1.),
CenterCrop(CFG['img_size1'], CFG['img_size1'], p=1.),
RandomResizedCrop(CFG['img_size1'], CFG['img_size1'], p=1.)
], p=1.),
Transpose(p=0.5),
HorizontalFlip(p=0.5),
VerticalFlip(p=0.5),
Resize(CFG['img_size1'], CFG['img_size1']),
Normalize(mean=[0.485, 0.456, 0.406], std=[0.229, 0.224, 0.225], max_pixel_value=255.0, p=1.0),
ToTensorV2(p=1.0),
], p=1.)
def get_inference_transforms2():
return Compose([
OneOf([
Resize(CFG['img_size2'], CFG['img_size2'], p=1.),
CenterCrop(CFG['img_size2'], CFG['img_size2'], p=1.),
RandomResizedCrop(CFG['img_size2'], CFG['img_size2'], p=1.)
], p=1.),
Transpose(p=0.5),
HorizontalFlip(p=0.5),
VerticalFlip(p=0.5),
Resize(CFG['img_size2'], CFG['img_size2']),
Normalize(mean=[0.485, 0.456, 0.406], std=[0.229, 0.224, 0.225], max_pixel_value=255.0, p=1.0),
ToTensorV2(p=1.0),
], p=1.)
class CassvaImgClassifier(nn.Module):
def __init__(self, model_arch, n_class, pretrained=False):
super().__init__()
self.model = timm.create_model(model_arch, pretrained=pretrained)
if model_arch == 'regnety_040':
self.model.head = nn.Sequential(
nn.AdaptiveAvgPool2d((1,1)),
nn.Flatten(),
nn.Linear(1088, n_class)
)
elif model_arch == 'regnety_320':
self.model.head = nn.Sequential(
nn.AdaptiveAvgPool2d((1,1)),
nn.Flatten(),
nn.Linear(3712, n_class)
)
elif model_arch == 'regnety_080':
self.model.head = nn.Sequential(
nn.AdaptiveAvgPool2d((1,1)),
nn.Flatten(),
nn.Linear(2016, n_class)
)
elif model_arch == 'regnety_160':
self.model.head = nn.Sequential(
nn.AdaptiveAvgPool2d((1,1)),
nn.Flatten(),
nn.Linear(3024, n_class)
)
else:
n_features = self.model.classifier.in_features
self.model.classifier = nn.Linear(n_features, n_class)
def forward(self, x):
x = self.model(x)
return x
class CassvaImgClassifier_ViT(nn.Module):
def __init__(self, model_arch, n_class, pretrained=False):
super().__init__()
self.model = timm.create_model(model_arch, pretrained=pretrained)
#if pretrained:
# self.model.load_state_dict(torch.load(MODEL_PATH))
self.model.head = nn.Linear(self.model.head.in_features, n_class)
for module in self.model.modules():
#print(module)
if isinstance(module, nn.BatchNorm2d):
if hasattr(module, 'weight'):
module.weight.requires_grad_(False)
if hasattr(module, 'bias'):
module.bias.requires_grad_(False)
#module.eval()
def forward(self, x):
x = self.model(x)
return x
def prepare_dataloader(df, trn_idx, val_idx, data_root='../input/cassava-leaf-disease-classification/train_images/'):
# from catalyst.data.sampler import BalanceClassSampler
train_ = df.loc[trn_idx,:].reset_index(drop=True)
valid_ = df.loc[val_idx,:].reset_index(drop=True)
train_ds = CassavaDataset(train_, data_root, transforms=get_train_transforms(), output_label=True)
valid_ds = CassavaDataset(valid_, data_root, transforms=get_valid_transforms(), output_label=True)
train_loader = torch.utils.data.DataLoader(
train_ds,
batch_size=CFG['train_bs'],
pin_memory=False,
drop_last=False,
shuffle=True,
num_workers=CFG['num_workers'],
#sampler=BalanceClassSampler(labels=train_['label'].values, mode="downsampling")
)
val_loader = torch.utils.data.DataLoader(
valid_ds,
batch_size=CFG['valid_bs'],
num_workers=CFG['num_workers'],
shuffle=False,
pin_memory=False,
)
return train_loader, val_loader
def train_one_epoch(epoch, model, loss_fn, optimizer, train_loader, device, scheduler=None, schd_batch_update=False):
model.train()
t = time.time()
running_loss = None
# pbar = tqdm(enumerate(train_loader), total=len(train_loader))
for step, (imgs, image_labels) in enumerate(train_loader):
imgs = imgs.to(device).float()
image_labels = image_labels.to(device).long()
with autocast():
image_preds = model(imgs) #output = model(input)
loss = loss_fn(image_preds, image_labels)
scaler.scale(loss).backward()
if running_loss is None:
running_loss = loss.item()
else:
running_loss = running_loss * .99 + loss.item() * .01
if ((step + 1) % CFG['accum_iter'] == 0) or ((step + 1) == len(train_loader)):
scaler.step(optimizer)
scaler.update()
optimizer.zero_grad()
if scheduler is not None and schd_batch_update:
scheduler.step()
if scheduler is not None and not schd_batch_update:
scheduler.step()
def valid_one_epoch(epoch, model, loss_fn, val_loader, device, scheduler=None, schd_loss_update=False):
model.eval()
t = time.time()
loss_sum = 0
sample_num = 0
image_preds_all = []
image_targets_all = []
# pbar = tqdm(enumerate(val_loader), total=len(val_loader))
for step, (imgs, image_labels) in enumerate(val_loader):
imgs = imgs.to(device).float()
image_labels = image_labels.to(device).long()
image_preds = model(imgs) #output = model(input)
image_preds_all += [torch.argmax(image_preds, 1).detach().cpu().numpy()]
image_targets_all += [image_labels.detach().cpu().numpy()]
loss = loss_fn(image_preds, image_labels)
loss_sum += loss.item()*image_labels.shape[0]
sample_num += image_labels.shape[0]
# if ((step + 1) % CFG['verbose_step'] == 0) or ((step + 1) == len(val_loader)):
# description = f'epoch {epoch} loss: {loss_sum/sample_num:.4f}'
# pbar.set_description(description)
image_preds_all = np.concatenate(image_preds_all)
image_targets_all = np.concatenate(image_targets_all)
print('epoch = {}'.format(epoch+1), 'validation multi-class accuracy = {:.4f}'.format((image_preds_all==image_targets_all).mean()))
if scheduler is not None:
if schd_loss_update:
scheduler.step(loss_sum/sample_num)
else:
scheduler.step()
def inference_one_epoch(model, data_loader, device):
model.eval()
image_preds_all = []
# pbar = tqdm(enumerate(data_loader), total=len(data_loader))
with torch.no_grad():
for step, (imgs) in enumerate(data_loader):
imgs = imgs.to(device).float()
image_preds = model(imgs) #output = model(input)
image_preds_all += [torch.softmax(image_preds, 1).detach().cpu().numpy()]
image_preds_all = np.concatenate(image_preds_all, axis=0)
return image_preds_all
def freeze_batchnorm_stats(net):
try:
for m in net.modules():
if isinstance(m,nn.BatchNorm2d) or isinstance(m,nn.LayerNorm):
m.eval()
except ValuError:
print('error with batchnorm2d or layernorm')
return
def unfreeze_batchnorm_stats(net):
try:
for m in net.modules():
if isinstance(m,nn.BatchNorm2d) or isinstance(m,nn.LayerNorm):
m.train()
except ValuError:
print('error with batchnorm2d or layernorm')
return
class LabelSmoothingCrossEntropy(nn.Module):
"""
NLL loss with label smoothing.
"""
def __init__(self, smoothing=0.1):
"""
Constructor for the LabelSmoothing module.
:param smoothing: label smoothing factor
"""
super(LabelSmoothingCrossEntropy, self).__init__()
assert smoothing < 1.0
self.smoothing = smoothing
self.confidence = 1. - smoothing
def forward(self, x, target):
logprobs = torch.nn.functional.log_softmax(x, dim=-1)
nll_loss = -logprobs.gather(dim=-1, index=target.unsqueeze(1))
nll_loss = nll_loss.squeeze(1)
smooth_loss = -logprobs.mean(dim=-1)
loss = self.confidence * nll_loss + self.smoothing * smooth_loss
return loss.mean()
if __name__ == '__main__':
# for training only, need nightly build pytorch
seed_everything(CFG['seed'])
oof_preds = np.zeros(len(train))
##
# not debug
test = pd.DataFrame()
test['image_id'] = list(os.listdir('../input/cassava-leaf-disease-classification/test_images/'))
test_ds2 = CassavaDataset(test, '../input/cassava-leaf-disease-classification/test_images/', transforms=get_inference_transforms2(), output_label=False)
# ## debug
# test = pd.DataFrame()
# test['image_id'] = list(os.listdir('../input/cassava-leaf-disease-classification/train_images/'))[:CFG['valid_bs']*20]
# test_ds2 = CassavaDataset(test, '../input/cassava-leaf-disease-classification/train_images/', transforms=get_inference_transforms2(), output_label=False)
tst_loader2 = torch.utils.data.DataLoader(
test_ds2,
batch_size=CFG['valid_bs'],
num_workers=CFG['num_workers'],
shuffle=False,
pin_memory=False,
)
device = torch.device(CFG['device'])
##
## model 1
print('Model 1 Start')
sub1 = []
folds = StratifiedKFold(n_splits=CFG['fold_num']).split(np.arange(train.shape[0]), train.label.values)
for fold, (trn_idx, val_idx) in enumerate(folds):
print('Inference fold {} started'.format(fold))
model1 = CassvaImgClassifier(CFG['model_arch1'], train.label.nunique()).to(device) # efficientnet
tst_preds = []
model1.load_state_dict(torch.load('../input/leaf-weight-v9-2/model9_2/swa_{}_fold_{}_{}'.format(CFG['model_arch1'], fold, '9')))
for tta in range(CFG['tta_num']):
tst_preds += [inference_one_epoch(model1, tst_loader2, device)]
sub1 += [np.mean(tst_preds, axis=0)]
del model1;
torch.cuda.empty_cache()
##
## model 2
print('Model 2 Start')
sub2 = []
folds = StratifiedKFold(n_splits=CFG['fold_num']).split(np.arange(train.shape[0]), train.label.values)
for fold, (trn_idx, val_idx) in enumerate(folds):
print('Inference fold {} started'.format(fold))
model2 = CassvaImgClassifier(CFG['model_arch2'], train.label.nunique()).to(device) # EFF-2019+2020
tst_preds = []
model2.load_state_dict(torch.load('../input/905-training-efficientnetb4-merged-bs32/swa_{}_fold_{}_{}'.format(CFG['model_arch2'], fold, '9')))
for tta in range(CFG['tta_num']):
tst_preds += [inference_one_epoch(model2, tst_loader2, device)]
sub2 += [np.mean(tst_preds, axis=0)]
del model2;
torch.cuda.empty_cache()
##
## model 3
print('Model 3 Start')
sub3 = []
folds = StratifiedKFold(n_splits=CFG['fold_num']).split(np.arange(train.shape[0]), train.label.values)
for fold, (trn_idx, val_idx) in enumerate(folds):
print('Inference fold {} started'.format(fold))
model3 = CassvaImgClassifier(CFG['model_arch3'], train.label.nunique()).to(device) # regnet-2020
tst_preds = []
model3.load_state_dict(torch.load('../input/regnety4noresetadamp/swa_{}_fold_{}_{}'.format(CFG['model_arch3'], fold, '19')))
for tta in range(CFG['tta_num']):
tst_preds += [inference_one_epoch(model3, tst_loader2, device)]
sub3 += [np.mean(tst_preds, axis=0)]
del model3;
torch.cuda.empty_cache()
##
## model 4
print('Model 4 Start')
sub4 = []
folds = StratifiedKFold(n_splits=CFG['fold_num']).split(np.arange(train.shape[0]), train.label.values)
for fold, (trn_idx, val_idx) in enumerate(folds):
print('Inference fold {} started'.format(fold))
model4 = CassvaImgClassifier(CFG['model_arch4'], train.label.nunique()).to(device) # regnet-2019+2020
tst_preds = []
model4.load_state_dict(torch.load('../input/0214v1-hwkim-regnet-40-reset-swalr-swastep-ep24/swa_{}_fold_{}_{}'.format(CFG['model_arch4'], fold, '23')))
for tta in range(CFG['tta_num']):
tst_preds += [inference_one_epoch(model4, tst_loader2, device)]
sub4 += [np.mean(tst_preds, axis=0)]
del model4;
torch.cuda.empty_cache()
##
## model 5
print('Model 5 Start')
sub5 = []
folds = StratifiedKFold(n_splits=CFG['fold_num']).split(np.arange(train.shape[0]), train.label.values)
for fold, (trn_idx, val_idx) in enumerate(folds):
print('Inference fold {} started'.format(fold))
model5 = CassvaImgClassifier(CFG['model_arch5'], train.label.nunique()).to(device)
tst_preds = []
model5.load_state_dict(torch.load('../input/905-training-efficientnetb4-seed720/swa_{}_fold_{}_{}'.format(CFG['model_arch5'], fold, '9')))
for tta in range(CFG['tta_num']):
tst_preds += [inference_one_epoch(model5, tst_loader2, device)]
sub5 += [np.mean(tst_preds, axis=0)]
del model5;
torch.cuda.empty_cache()
##
## model 6
print('Model 6 Start')
sub6 = []
folds = StratifiedKFold(n_splits=CFG['fold_num']).split(np.arange(train.shape[0]), train.label.values)
## not debug
test2 = pd.DataFrame()
test2['image_id'] = list(os.listdir('../input/cassava-leaf-disease-classification/test_images/'))
test_ds2 = CassavaDataset(test2, '../input/cassava-leaf-disease-classification/test_images/', transforms=get_inference_transforms1(), output_label=False)
# # debug
# test2 = pd.DataFrame()
# test2['image_id'] = list(os.listdir('../input/cassava-leaf-disease-classification/train_images/'))[:CFG['valid_bs']*20]
# test_ds2 = CassavaDataset(test2, '../input/cassava-leaf-disease-classification/train_images/', transforms=get_inference_transforms1(), output_label=False)
tst_loader2 = torch.utils.data.DataLoader(
test_ds2,
batch_size=CFG['valid_bs'],
num_workers=CFG['num_workers'],
shuffle=False,
pin_memory=False,
)
for fold, (trn_idx, val_idx) in enumerate(folds):
print('Inference fold {} started'.format(fold))
model6 = CassvaImgClassifier_ViT(CFG['model_arch6'], train.label.nunique()).to(device) # VIT
tst_preds = []
model6.load_state_dict(torch.load('../input/vit-11ep/11ep_swa/swa_{}_fold_{}_{}'.format(CFG['model_arch6'], fold, '10')))
for tta in range(CFG['tta_num']):
tst_preds += [inference_one_epoch(model6, tst_loader2, device)]
sub6 += [np.mean(tst_preds, axis=0)]
del model6;
torch.cuda.empty_cache()
##
## model 7
print('Model 7 Start')
sub7 = []
folds = StratifiedKFold(n_splits=CFG['fold_num']).split(np.arange(train.shape[0]), train.label.values)
for fold, (trn_idx, val_idx) in enumerate(folds):
print('Inference fold {} started'.format(fold))
model7 = CassvaImgClassifier(CFG['model_arch7'], train.label.nunique()).to(device) # FixMix (EfficientNet Based Code with 2019+2020 Dataset)
tst_preds = []
# FixMix Version 17
model7.load_state_dict(torch.load('../input/fm2019-fast-thr085-bs9-mu2-7ep-cusswa4/{}_fold_{}_{}'.format(CFG['model_arch7'], fold, '6')))
for tta in range(CFG['tta_num']):
tst_preds += [inference_one_epoch(model7, tst_loader2, device)]
sub7 += [np.mean(tst_preds, axis=0)]
del model7;
torch.cuda.empty_cache()
##
## model 8
print('Model 8 Start')
sub8 = []
folds = StratifiedKFold(n_splits=CFG['fold_num']).split(np.arange(train.shape[0]), train.label.values)
for fold, (trn_idx, val_idx) in enumerate(folds):
print('Inference fold {} started'.format(fold))
model8 = CassvaImgClassifier(CFG['model_arch8'], train.label.nunique()).to(device) # RegNetY Distillation
tst_preds = []
# Distillation with RegNetY#############################
model8.load_state_dict(torch.load('../input/reg-distill/swa_{}_fold_{}_{}'.format(CFG['model_arch8'], fold, '19')))
for tta in range(CFG['tta_num']):
tst_preds += [inference_one_epoch(model8, tst_loader2, device)]
sub8 += [np.mean(tst_preds, axis=0)]
del model8;
torch.cuda.empty_cache()
class CassvaImgClassifier_NF(nn.Module):
def __init__(self, model_arch, n_class,img_size, pretrained=False):
super().__init__()
self.model = timm.create_model(model_arch, pretrained=pretrained)
#n_features = self.model.head.num_pooled_features
self.model.head.fc = nn.Linear(2048, n_class)
# self.model.classifier = nn.Sequential(
# nn.Linear(n_features, n_features//2),
# nn.LeakyReLU(inplace=True),
# nn.Linear(n_features//2, n_class)
# )
for module in self.model.modules():
#print(module)
if isinstance(module, nn.BatchNorm2d):
if hasattr(module, 'weight'):
module.weight.requires_grad_(False)
if hasattr(module, 'bias'):
module.bias.requires_grad_(False)
#module.eval()
def forward(self, x):
x = self.model(x)
return x
## model 9
print('Model 9 Start')
sub9 = []
folds = StratifiedKFold(n_splits=CFG['fold_num']).split(np.arange(train.shape[0]), train.label.values)
for fold, (trn_idx, val_idx) in enumerate(folds):
print('Inference fold {} started'.format(fold))
model9 = CassvaImgClassifier_NF(CFG['model_arch9'], train.label.nunique(), CFG['img_size2']).to(device) # NFNet
tst_preds = []
# NFNet
model9.load_state_dict(torch.load('../input/nfnet-init/swa_{}_fold_{}_{}'.format(CFG['model_arch9'], fold, '19')))
for tta in range(CFG['tta_num']):
tst_preds += [inference_one_epoch(model9, tst_loader2, device)]
sub9 += [np.mean(tst_preds, axis=0)]
del model9;
torch.cuda.empty_cache()
## model 10
print('Model 10 Start')
sub10 = []
print('Inference fold {} started'.format(6))
model10 = CassvaImgClassifier(CFG['model_arch10'], train.label.nunique()).to(device) # NFNet
tst_preds = []
# NFNet
model10.load_state_dict(torch.load('../input/regnety4nocv/swa_{}_fold_{}_{}'.format(CFG['model_arch10'], 6, '0')))
for tta in range(CFG['tta_num']):
tst_preds += [inference_one_epoch(model10, tst_loader2, device)]
sub10 += [np.mean(tst_preds, axis=0)]
sub10=sub10*5
del model10;
torch.cuda.empty_cache()
#### Aggregation
sub1 = [e * CFG['weight'][0] for e in sub1]
sub2 = [e * CFG['weight'][1] for e in sub2]
sub3 = [e * CFG['weight'][2] for e in sub3]
sub4 = [e * CFG['weight'][3] for e in sub4]
sub5 = [e * CFG['weight'][4] for e in sub5]
sub6 = [e * CFG['weight'][5] for e in sub6]
sub7 = [e * CFG['weight'][6] for e in sub7]
sub8 = [e * CFG['weight'][7] for e in sub8]
sub9 = [e * CFG['weight'][8] for e in sub9]
sub10 = [e * CFG['weight'][9] for e in sub10]
sub = [e1 + e2 + e3 + e4 + e5 + e6 + e7 + e8 + e9 + e10 for (e1, e2, e3, e4, e5, e6, e7, e8, e9, e10) in zip(sub1,sub2,sub3,sub4,sub5,sub6,sub7,sub8,sub9,sub10)]
test['label'] = np.argmax(np.mean(sub, axis=0) , axis=1)
test.head()
test.to_csv('submission.csv', index=False)
###Output
_____no_output_____ |
notebooks/dev/Segments.ipynb | ###Markdown
init
###Code
from crossproduct import Point, Segment, Segments
s1 = Segment(Point(0,0), Point(1,0))
s2 = Segment(Point(1,0), Point(1,1))
sgmts = Segments(s1,s2)
print(sgmts)
###Output
Segments(Segment(Point(0.0,0.0), Point(1.0,0.0)), Segment(Point(1.0,0.0), Point(1.0,1.0)))
###Markdown
eq
###Code
from crossproduct import Point, Segment, Segments
s1 = Segment(Point(0,0), Point(1,0))
s2 = Segment(Point(1,0), Point(1,1))
sgmts = Segments(s1,s2)
result = sgmts==sgmts
print(result)
###Output
True
###Markdown
add_all
###Code
from crossproduct import Point, Segment, Segments
s1 = Segment(Point(0,0), Point(1,0))
s2 = Segment(Point(1,0), Point(1,1))
s3 = Segment(Point(1,0), Point(2,0))
sgmts = Segments(s1,s2,s3)
sgmts.add_all()
print(sgmts)
###Output
Segments(Segment(Point(0.0,0.0), Point(2.0,0.0)), Segment(Point(1.0,0.0), Point(1.0,1.0)))
###Markdown
add_first
###Code
from crossproduct import Point, Segment, Segments
sgmts = Segments(Segment(Point(0,0), Point(1,0)))
result = sgmts.add_first(Segment(Point(1,0), Point(2,0)))
print(result)
###Output
(Segment(Point(0.0,0.0), Point(2.0,0.0)), 0)
###Markdown
to_tuple
###Code
from crossproduct import Point, Segment, Segments
sgmts = Segments(Segment(Point(0,0), Point(1,0)),
Segment(Point(1,0), Point(1,1)))
result = sgmts.to_tuple()
print(result)
###Output
(((0.0, 0.0), (1.0, 0.0)), ((1.0, 0.0), (1.0, 1.0)))
###Markdown
plot
###Code
#2D
fig, ax = plt.subplots()
Segments(Segment(Point(0,0),Point(1,1)),
Segment(Point(1,1),Point(2,1))).plot(ax,color='b',marker='o')
#3D
fig = plt.figure()
ax = fig.add_subplot(111, projection='3d')
Segments(Segment(Point(0,0,0),Point(1,1,1)),
Segment(Point(1,1,1),Point(2,1,1))).plot(ax,color='b',marker='o')
###Output
_____no_output_____ |
Architectural Decision.ipynb | ###Markdown
IBM Advanced Data Science Project ReportFeel free to [contact me](https://www.linkedin.com/in/leonardo-iheme/) if you have any questions. September, 2019 **By:** Leonardo O. Iheme 1 Introduction 1.1 BackgroundIn this report, I will outline the steps I took to discover similar and dissimilar coffee-neighborhoods in Istanbul locals' favorite districts.Istanbul is one of the biggest and most populous cities in the world, the only city that exists on two continents. Both parts of the city are divided by the Bosphorous strait. Two Districts loved by Istanbul residents are Beşiktaş (be-shik-tash) and Kadıköy (ka-di-koy) on the European and Asian side respectively. While these districts have a lot in common, they have their fair share of differences as well, the surge of coffee shops for one. In fact, according to [Foursquare](https://foursquare.com/top-places/istanbul/best-places-coffee), [8 of 15 best coffee shops in Istanbul are located in Beşiktaş and Kadıköy]There is a fierce debate among residents about the neighborhood to best enjoy a cup of coffee. This report will address the issue by providing insights drawn from data. 1.2 Problem StatementAfter tea, coffee is the next most consumed beverage in the country and that is for good reason. In modern Istanbul, to escape the tourist traps and mingle with locals, the best places to have a coffee are Beşiktaş and Kadıköy. As a visitor, finding a venue and neighborhood to have a coffee can be quite difficult given the wide range of choices and factors. This study will be of interest to both visitors of Istanbul and locals who yet to discover the hidden similarities between the two most sought after neighborhoods. The report will help readers to:1. Be more familiar with the discussed neighborhoods2. Understand the relationship between coffee shops and other neighborhood attributes3. Discover the similarities between neighborhoods in terms of coffee shops and other attributes4. Be able to make better-informed decisions about where to coffee in Istanbul like a resident 2 Data All the data used in this project were obtained from various sources on the internet. While some were ready to use, others had to be wrangled and cleaned. Data manipulation was performed with _Python_, using mostly the _Pandas_ library. The data was collected towards building the attributes of each neighborhood bearing in mind the factors that could affect a coffee experience. It is worth noting that the data may be limited in some cases because I used free tier accounts but it gives a good sense of proportions. 2.1 Population & Demographics DataSince the region of interest (ROI) has been narrowed down to Beşiktaş and Kadıköy, I could crawl the web and scrape using _beautiful soup_ to obtain such information as: * The list of neighborhoods in each district,* The population of each neighborhood, and* The average price of residential rent at each neighborhood 2.2 Geographical DataTo locate the neighborhoods, I leveraged on Google Maps API and the [Open Street maps project](https://www.openstreetmap.org). The information obtained from the respective sources are as follows:* Google maps: coordinates as longitude and latitude pairs* Open street maps: neighborhood boundaries as polygon coordinates which were then converted to *geojson* files using an API provided by [geojson.io](http://geojson.io/) 2.3 Location DataThe list of coffee shops was obtained by querying [foursquare](https://foursquare.com/) through the API. As I use a free tier account, the results of my queries were limited in some cases. The features which will be extracted from the data include the **number of coffee shops per neighborhood, the average distance from the center of the neighborhood to the seaside, the estimated number of people served by a coffee shop in each neighborhood, and the socioeconomic status of each neighborhood**. With the extracted features, exploratory as well as inferential data analysis will be carried out. Finally, the neighborhoods will be clustered using machine learning. 3 Methodology In this section, the steps taken to obtain the results will be described in detail. It will include data acquisition techniques, data wrandling, data exploratory analysis as well as feature extraction techniques. 3.1 Data Acquisition 3.1.1 Population and DemographicsI started by searching the internet for the list of neighborhoods and their respective postal codes. This information was easily obtained from [bulurum.com](https://www.bulurum.com/en/), an online business directory which provides detailed, geolocated information for all kinds of businesses and professionals in all regions and cities in Turkey.The list can be found on the district's pages and requested as follows:```python Source of neighborhoods and postal codesbesiktas = requests.get(r"https://www.bulurum.com/en/post-codes/besiktas/istanbul/").textkadikoy = requests.get(r"https://www.bulurum.com/en/post-codes/kadikoy/istanbul/").text```using the requests library. Then a ```beautiful soup``` instance was created using the following code snipet:```python Create a BeautifulSoup instanceb_soup = BeautifulSoup(besiktas)k_soup = BeautifulSoup(kadikoy)```After inspecting the pages, I could extract and organize the information that I needed into a dataframe as shown Idx | Post_codes | Neighborhood :---:|:----------:|--------------------------------------------------: 0 | 34022 | ABBASAĞA MAH., CIHANNUMA MAH., SİNANPAŞA MAH. 1 | 34330 | KONAKLAR MAH., LEVENT MAH. 2 | 34335 | AKAT MAH. 3 | 34337 | ETİLER MAH. 4 | 34340 | KÜLTÜR MAH., LEVAZIM MAH., NİSBETİYE MAH., ULU... The population of each neighborhood was obtained from [endeksa.com](https://www.endeksa.com/en/), an up-to-date analytics website for real estate in Turkey. 3.1.2 Geography & Location GeographyUsing the Google maps API, it was possible to query the latitudes and longitudes of the neighborhoods. The names of the neigborhoods and the postal codes were used as an approximate address in a ```for``` loop.```python Geocoding the addresseslatitude = []longitude = []for x in coffee_shops_population.itertuples(): geocode_result = gmaps.geocode(f'{x.Neighborhood}, Istanbul') concatenate the latitudes and longitudes latitude = latitude + [geocode_result[0]['geometry']['location']['lat']] longitude = longitude + [(geocode_result[0]['geometry']['location']['lng'])] Add the latitudes and longitudes to the dataframecoffee_shops_population['Latitude'] = latitudecoffee_shops_population['Longitude'] = longitudecoffee_shops_population.head()```In addition to the coordinates obtained from the google maps API, I also got the mount of time it would take to walk from the neighbohood center to the nearest coast. I added this as a feature because in Istanbul, having a seaview is one of the factors that could affect one's coffee experience.The neighborhoods to be examined are shown pinned on the [map](https://leonardoiheme.wixsite.com/ibmproject/neighborhoods) below
###Code
%%html
<!-- blank line -->
<figure class="video_container">
<iframe width="100%" height="600px" name="htmlComp-iframe" scrolling="auto" sandbox="allow-same-origin allow-forms allow-popups allow-scripts allow-pointer-lock" src="https://leonardoiheme-wixsite-com.filesusr.com/html/d6f1dc_a4b91ebc367c3c4fe89f58cb5f350f75.html"></iframe>
</figure>
<!-- blank line -->
###Output
_____no_output_____
###Markdown
LocationLocation data was obtained by querying the foursquare database via the API. Of particular interest to this project was the cafes and coffee shops within a 2 Km radius of each neighborhoods center. To download the data, the following information is required as input:* Client ID* Client secrete* Latitude* Longitude* Version* Search radiusAfter downloading the data and cleaning it, the number of coffee shops in each neighborhood was obtained and is depicted in the following figure:It is obvious which neighborhoods have the most coffee shops. Before moving on to exploratory data analysis, it is worth mentioning one more feature of the data. Since the population and number of coffee shops in each neighbohood are known, a rough estimate of the number of people served by each coffee shop can be obtained by dividing the population by the number of coffee shops. With `pandas`, this was easy to calculate```python Estimate number of people per coffee shop and add to the dataframe.coffee_shops_population['PeoplePerCoffeeShop'] = coffee_shops_population['Population'] / coffee_shops_population[' Coffee shops']```The columns of the final dataframe is shown below:|Idx|Neighborhood |District|HouseRent(sqm)|Population| Coffee shops|WalkToSeaside(min)|Latitude |Longitude|PeoplePerCoffeeShop||------|--------------|--------|--------------|----------|--------------|------------------|---------|---------|-------------------| 3.2 Exploratory Data Analysis In this section, the data is looked into with more detail. A brief camparison of the two districts is made to highlight how they contrast based on the different features. A basic statistical summary of the data is shown below|Stat |HouseRent(sqm)|Population | Coffee shops|WalkToSeaside(min)|Latitude |Longitude|PeoplePerCoffeeShop||-----|--------------|------------|--------------|------------------|---------|---------|-------------------||count|44.000000 |44.000000 |44.000000 |44.000000 |44.000000|44.000000|44.000000 ||mean |22.909091 |14475.000000|12.659091 |22.318182 |41.026023|29.034685|1485.218322 ||std |5.116404 |10060.739575|6.647060 |14.806932 |0.042205 |0.026972 |1334.437329 ||min |15.000000 |2534.000000 |5.000000 |1.000000 |40.958317|28.992715|144.037037 ||25% |18.000000 |6209.500000 |8.000000 |10.000000 |40.988611|29.014604|510.093583 ||50% |23.000000 |11504.000000|9.500000 |20.000000 |41.045028|29.031286|960.270833 ||75% |27.000000 |19358.000000|17.000000 |32.750000 |41.062030|29.049266|1917.916667 ||max |35.000000 |35260.000000|27.000000 |60.000000 |41.093440|29.100420|5091.500000 |If broken down further, the averages of each neighborhood can be observed as follows:|District|HouseRent(sqm)|Population | Coffee shops|WalkToSeaside(min)|PeoplePerCoffeeShop||--------|--------------|------------|--------------|------------------|-------------------||Besiktas|26.739130 |8062.913043 |13.086957 |21.565217 |781.023908 ||Kadikoy |18.714286 |21497.761905|12.190476 |23.142857 |2256.478872 | Observations* Even though Kadıköy has a much higher population than Beşiktaş, both neighborhoods have around the same average number of coffee shops per neighborhood. There must be a higher coffee shop density in Beşiktaş but according to the number of people per coffee shop, this is not the case 3.2.1 PopulationThe distribution of the population of both districs is given in the violin plot.The population of Kadıköy is much higher than that of Beşiktaş.When observed at the neighborhood level, the distribution of the population becomes clearer. To see this, a [choropleth map](https://leonardoiheme.wixsite.com/ibmproject/population) is created
###Code
%%markdown
<!-- blank line -->
<figure class="video_container">
<iframe width="100%" height="600px" name="htmlComp-iframe" scrolling="auto" sandbox="allow-same-origin allow-forms allow-popups allow-scripts allow-pointer-lock" src="https://leonardoiheme-wixsite-com.filesusr.com/html/d6f1dc_efc448e8403300d1f1c934b3098d0d3a.html"></iframe>
</figure>
<!-- blank line -->
###Output
_____no_output_____
###Markdown
3.2.2 Number of coffee shopsThe number of coffee shops and how they are distributed between the districts is shown in the violin plot below Observations* As seen from the table of averages, both districs have an almost equal number of coffee shops. We can also see that the variation in the number of coffee shops per neighborhood in both districs is almost eqal as well.A [choropleth map](https://leonardoiheme.wixsite.com/ibmproject) reveals more detail.
###Code
%%html
<!-- blank line -->
<figure class="video_container">
<iframe width="100%" height="600px" name="htmlComp-iframe" scrolling="auto" sandbox="allow-same-origin allow-forms allow-popups allow-scripts allow-pointer-lock" src="https://leonardoiheme-wixsite-com.filesusr.com/html/d6f1dc_49fd03c3e444d6b22e9bd739e9c60eb8.html"></iframe>
</figure>
<!-- blank line -->
###Output
_____no_output_____
###Markdown
3.2.3 Price of rentThe distribution of the cost of renting in each neighborhood can be visualized with a violin plot. Observations* It costs more to rent in Beşiktaş than in Kadıköy.* The price of rent varies more across the neighborhoods in Kadıköy. This can be seen from the wide shape of the violin plot.A [choropleth map](https://leonardoiheme.wixsite.com/ibmproject/price-of-rent) reflects the observations.
###Code
%%html
<!-- blank line -->
<figure class="video_container">
<iframe width="100%" height="600px" name="htmlComp-iframe" scrolling="auto" sandbox="allow-same-origin allow-forms allow-popups allow-scripts allow-pointer-lock" src="https://leonardoiheme-wixsite-com.filesusr.com/html/d6f1dc_49becf2cf26923789bd4c46dad762209.html"></iframe>
</figure>
<!-- blank line -->
###Output
_____no_output_____
###Markdown
4 Results and Discussion This section provides insights into the extracted features and how they compare. Some observations are also highlighted to aid understanding. 4.1 RegressionTo observe how the number of coffee shops is related to the other features such as the price of housing, the average shortest time it takes to get to the coast from the centre, and the number of people served by each coffee shop; a regression plot is made.From the left,* The first plot shows that the price of rent is likely to be higher if there are a lot of coffee shops in the area. In Besikttas, the reverse is the case.* The second figure reveals that as the population of an area decreases, the number of coffee shops also decreases.* We can also observe that the further away the neighborhood is from the seaside, the fewer coffee shops it is likely to have. 4.2 Interesting Neighborhoods (Outliers)Some neighborhoods stand out as we observe from the correlation plots. To take a closer look at these interesting neighborhoods, the scatter plot below is providedWith respect to the cost of renting, the following observations can be made:* **Fenerbahce Mah.** is the most expensive neighborhood in Kadıköy and it has the most number of coffee shops.* Renting at **Egitim Mah.** is relatively cheap and there are a lot of coffee shops in the area.* **Ulus Mah.** in Beşiktaş has a few coffee shops but a high rental price.* In general, Kadıköy seems to be a more diverse district than Beşiktaş. 4.3 Clustering Analysis (K-Means Clustering)To detect similar neighborhoods based on the extracted features, an un-supervised machine learning modelis trained. Specifically, K-means clustering is used to divide all the neighborhoods into five groups. The reason for choosing five is, I imagine that, as a visitor, you have five days to spend in the city and you want to have a totally different coffee experience (like a local) everyday, this algorithm will help objectively guide you to selecting five neighborhoods in Beşiktaş and Kadıköy that will deliver different experiences. The following code shows how K-means clustering was run. With the `scikit-learn` library, in just a few lines, machine learning algorithms can be run```python set number of clusterskclusters = 5Drop categorical variablesclustering = coffee_shops_population.drop(['Neighborhood', 'District', 'Latitude', 'Longitude'], 1) Normalizing over the standard deviationfrom sklearn.preprocessing import StandardScalerX = clustering.values[:,1:]X = np.nan_to_num(X)Clus_dataSet = StandardScaler().fit_transform(X) run k-means clusteringkmeans = KMeans(n_clusters=kclusters, random_state=0).fit(Clus_dataSet) check cluster labels generated for each row in the dataframekmeans.labels_```The map below shows the neighborhoods color coded. Similar *coffee* neighborhoods have the same color.
###Code
%%html
<!-- blank line -->
<figure class="video_container">
<iframe width="100%" height="600px" name="htmlComp-iframe" scrolling="auto" sandbox="allow-same-origin allow-forms allow-popups allow-scripts allow-pointer-lock" src="https://leonardoiheme-wixsite-com.filesusr.com/html/d6f1dc_b9cdb4cd6b3bc477a7380225601011e9.html"></iframe>
</figure>
<!-- blank line -->
###Output
_____no_output_____ |
notebooks/2.0-data-organize-exploring-processing-data-part-1.ipynb | ###Markdown
**Typical data science project - Time scientis spends**1. Collecting data sets --- 19%2. Cleaning and organizing data --- 60%3. Building training sets --- 3%4. Mining data patterns --- 9%5. Refining algorithms --- 4%6. Other --- 5%Exploratory Data Analysis - After getting the Raw data we analysize the data with some basic statistics and basic data visualization. We find out if there is any outliers in the database or if there are missing values. These needs to be treated before we start the next step. Data Munging - In this step we take care of all the issues found in previous steps. Like outliers issues, missing value issues or any other issues which requires some kind of processing. We look for potential issues and solve them. Feature Engineering - We apply dimentionality reduction in this step. We find out if features needs to be reduced or additional feature needs to be created. Data Visualization - We do data visualization and understand if further feature engineering is required. Its very important steps as it becomes the part of presentation at the end.We can follow this cycle multiple times. **Overview** Import Data Exploratory data analysis - Basic structure - Summary statistics - Distributions - Grouping - Crosstabs - pivots Python - Numpy - Pandas **Numpy** - Fundamental tool for scientific computing - Very efficient array operations - Work on multi-dimensional arrays and matrices - High level mathematical functionsa **Pandas** - Built on top of Numpy - Datastructure and operations on tabular data - Data visualization using Matplotlib Exploratory Data Analysis**Basic Structure** - How many rows or observations? - How many columns or features? - Column data types - Explore head or tail
###Code
#imports
import pandas as pd
import numpy as np
import os
import os.path as op
#set the path of the raw data
raw_data_path = os.path.join(os.path.pardir,'data','raw')
train_file_path = str(os.path.join(raw_data_path, 'train.csv'))
test_file_path = str(os.path.join(raw_data_path, 'test.csv'))
print(raw_data_path)
os.getcwd()
# !pwd
# Macintosh HD/Users/neerajsharma/Desktop/DataScience/machinelearning/data/raw/train.csv
# train_df = pd.read_csv('/Users/neerajsharma/Desktop/DataScience/machinelearning/data/raw/train.csv', index_col='PassengerId')
# test_df = pd.read_csv('test.csv', index_col='PassengerId')
train_df.head()
test_df.head()
# #read the data with all default parameters
train_df = pd.read_csv(train_file_path,index_col='PassengerId')
test_df = pd.read_csv(test_file_path, index_col='PassengerId')
train_df.info()
###Output
<class 'pandas.core.frame.DataFrame'>
Int64Index: 891 entries, 1 to 891
Data columns (total 11 columns):
Survived 891 non-null int64
Pclass 891 non-null int64
Name 891 non-null object
Sex 891 non-null object
Age 714 non-null float64
SibSp 891 non-null int64
Parch 891 non-null int64
Ticket 891 non-null object
Fare 891 non-null float64
Cabin 204 non-null object
Embarked 889 non-null object
dtypes: float64(2), int64(4), object(5)
memory usage: 83.5+ KB
###Markdown
Understanding titanic dataset||Feature |Meaning | |:---:|:---:|:---:||1|Passenger ID | Unique passenger id ||2| Survived | If Survived(1-yes, 0-no) ||3| Name | Name of the passenger ||4| Sex | Gender ||5| Age | Age of passenger ||6| SibSp | Number of siblings / spouses aboard ||7| Parch | Number of parents / childer aobard ||8| Ticket | Ticket Number ||9| Fare | Passenger fare ||10| Cabin | Cabin number ||11| Embarked | Point of embarkment (C=Cherbourg; Q=Queenstown; S= Southampton) |
###Code
test_df.info()
###Output
<class 'pandas.core.frame.DataFrame'>
Int64Index: 418 entries, 892 to 1309
Data columns (total 10 columns):
Pclass 418 non-null int64
Name 418 non-null object
Sex 418 non-null object
Age 332 non-null float64
SibSp 418 non-null int64
Parch 418 non-null int64
Ticket 418 non-null object
Fare 417 non-null float64
Cabin 91 non-null object
Embarked 418 non-null object
dtypes: float64(2), int64(3), object(5)
memory usage: 35.9+ KB
|
pixel_10071.ipynb | ###Markdown
Pixel 10071:
###Code
filenames_truth=np.sort([i for i in glob.glob('/global/projecta/projectdirs/lsst/groups/PZ/PhotoZDC2/COSMODC2v1.1.4/10_year_error_estimates/z_*.step_all.healpix_*_magwerrSNtrim.hdf5')])
filenames_photoz=np.sort([i for i in glob.glob('/global/projecta/projectdirs/lsst/groups/PZ/PhotoZDC2/COSMODC2v1.1.4/10_year_error_estimates/pipelinedir/outputs/photoz_pdf_z_*.step_all.healpix_*.hdf5')])
filenames_truth_single_pixel=filenames_truth[[1,18,35]]
filenames_photoz_single_pixel=filenames_photoz[[1,18,35]]
truth={}
mag_i_lsst_truth={}
for i in filenames_truth_single_pixel:
f = h5py.File(i)
data=f['photometry']
z=np.array(data['redshift'])
ids=np.array(data['id'])
mag_i_lsst=np.array(data['mag_i_lsst'])
truth_subset=dict(zip(ids,z))
mag_i_lsst_subset=dict(zip(ids,mag_i_lsst))
truth.update(truth_subset)
mag_i_lsst_truth.update(mag_i_lsst_subset)
pdf={}
point_estimates_mode={}
odds={}
for i in filenames_photoz_single_pixel:
f = h5py.File(i,'r')
pdf_single=np.array(f['pdf']['pdf'])
point_estimates_mode_single=np.array(f['point_estimates']['z_mode'])
odds_single=np.array(f['point_estimates']['ODDS'])
ids=np.array(f['id']['galaxy_id'])
pdf_subset=dict(zip(ids,pdf_single))
point_estimates_mode_subset=dict(zip(ids,point_estimates_mode_single))
odds_subset=dict(zip(ids,odds_single))
odds.update(odds_subset)
pdf.update(pdf_subset)
point_estimates_mode.update(point_estimates_mode_subset)
odds_array=np.array(list(odds.values()))
pdf_array = np.array(list(pdf.values())).astype(np.float)
keys_array = np.array(list(pdf.keys()))
pdf_stacked=np.sum(pdf_array.astype(np.float),axis=0)
grid=np.linspace(0,3.5,351) #READ THIS IN
truth_array=np.array(list(truth.values()))
bpz_array=np.array(list(point_estimates_mode.values()))
def kde(x, x_grid, **kwargs):
"""Kernel Density Estimation with Scipy"""
kde = gaussian_kde(x, bw_method='scott', **kwargs)
return kde.evaluate(x_grid)
truth_kde=kde(truth_array,grid)
plt.figure(figsize=(5,5))
plt.plot(grid,pdf_stacked/len(truth_array)/0.01,label='stacked pdfs')
plt.plot(grid,truth_kde,label='kernel density estimator'
plt.hist(truth.values(),bins=301,density=True,label='truth histogram')
plt.legend()
plt.show()
plt.figure(figsize=(5,5))
plt.scatter(truth_array,bpz_array,s=0.0001)
plt.xlabel('redshift')
plt.ylabel('photo-z')
plt.xlim((0,3))
plt.ylim((0,3))
plt.show()
###Output
_____no_output_____ |
doc/gallery/seafloor_age.ipynb | ###Markdown
Age of the Oceanic Lithosphere==============================Global grids of the age of the oceanic lithosphere produced by [Muller2008]_.Available in 2 and 6 arc-minute resolutions and include grids of the ageuncertainty.More information at the`NOAA NCEI `__ and`EarthByte`__websites.
###Code
import rockhound as rh
import matplotlib.pyplot as plt
import cmocean
# Load the age and uncertainty grids in the default 6 arc-minute resolution
grid = rh.fetch_seafloor_age()
print(grid)
# Plot the age grid.
# We're not using a map projection to speed up the plotting but this NOT
# recommended.
plt.figure(figsize=(9, 5))
ax = plt.subplot(111)
grid.age.plot.pcolormesh(
cmap=cmocean.cm.thermal_r, cbar_kwargs=dict(pad=0.01, aspect=30), ax=ax
)
ax.set_title("Age of Oceanic Lithosphere")
plt.tight_layout()
plt.show()
###Output
_____no_output_____ |
python/source/02_Building_a_Custom_Corpus.ipynb | ###Markdown
Imports
###Code
import os
import codecs
import sqlite3
import pathlib
###Output
_____no_output_____
###Markdown
Constants
###Code
PROJECT_DIR = pathlib.Path('~/project').expanduser()
DATA_DIR = PROJECT_DIR / 'data'
###Output
_____no_output_____
###Markdown
What Is a Corpus? Domain-Specific Corpora The Baleen Ingestion Engine Corpus Data Management Corpus Disk Structure The Baleen disk structure Corpus Readers Streaming Data Access with NLTK
###Code
from nltk.corpus.reader.plaintext import CategorizedPlaintextCorpusReader
CORPUS_ROOT = DATA_DIR / 'galactic'
DOC_PATTERN = r'(?!\.)[\w_\s]+/[\w\s\d\-]+\.txt'
CAT_PATTERN = r'([\w_\s]+)/.*'
corpus = CategorizedPlaintextCorpusReader(
root=CORPUS_ROOT.as_posix(),
fileids=DOC_PATTERN,
cat_pattern=CAT_PATTERN
)
print(corpus.readme())
print(corpus.license())
print(corpus.citation())
corpus.categories()
corpus.fileids()
###Output
_____no_output_____
###Markdown
Reading an HTML Corpus
###Code
from nltk.corpus.reader.api import CorpusReader
from nltk.corpus.reader.api import CategorizedCorpusReader
CAT_PATTERN = r'([a-z_\s]+)/.*'
DOC_PATTERN = r'(?!\.)[a-z_\s]+/[a-f0-9]+\.json'
TAGS = ['h1', 'h2', 'h3', 'h4', 'h5', 'h6', 'p', 'li']
class HTMLCorpusReader(CategorizedCorpusReader, CorpusReader):
"""
A corpus reader for raw HTML documents to enable preprocessing.
"""
def __init__(self, root, fileids=DOC_PATTERN, encoding='utf8',
tags=TAGS, **kwargs):
"""
Initialize the corpus reader. Categorization arguments
(``cat_pattern``, ``cat_map``, and ``cat_file``) are passed to
the ``CategorizedCorpusReader`` constructor. The remaining
arguments are passed to the ``CorpusReader`` constructor.
"""
# Add the default category pattern if not passed into the class.
if not any(key.startswith('cat_') for key in kwargs.keys()):
kwargs['cat_pattern'] = CAT_PATTERN
# Initialize the NLTK corpus reader objects
CategorizedCorpusReader.__init__(self, kwargs)
CorpusReader.__init__(self, root, fileids, encoding)
# Save the tags that we specifically want to extract.
self.tags = tags
def resolve(self, fileids, categories):
"""
Returns a list of fileids or categories depending on what is passed
to each internal corpus reader function. Implemented similarly to
the NLTK ``CategorizedPlaintextCorpusReader``.
"""
if fileids is not None and categories is not None:
raise ValueError("Specify fileids or categories, not both")
if categories is not None:
return self.fileids(categories)
return fileids
def docs(self, fileids=None, categories=None):
"""
Returns the complete text of an HTML document, closing the document
after we are done reading it and yielding it in a memory safe fashion.
"""
# Resolve the fileids and the categories
fileids = self.resolve(fileids, categories)
# Create a generator, loading one document into memory at a time.
for path, encoding in self.abspaths(fileids, include_encoding=True):
with codecs.open(path, 'r', encoding=encoding) as f:
yield f.read()
def sizes(self, fileids=None, categories=None):
"""
Returns a list of tuples, the fileid and size on disk of the file.
This function is used to detect oddly large files in the corpus.
"""
# Resolve the fileids and the categories
fileids = self.resolve(fileids, categories)
# Create a generator, getting every path and computing filesize
for path in self.abspaths(fileids):
yield os.path.getsize(path)
CORPUS_ROOT = DATA_DIR / 'galactic'
DOC_PATTERN = r'(?!\.)[\w_\s]+/[\w\s\d\-]+\.txt'
CAT_PATTERN = r'([\w_\s]+)/.*'
corpus = HTMLCorpusReader(
root=CORPUS_ROOT.as_posix(),
fileids=DOC_PATTERN,
cat_pattern=CAT_PATTERN
)
corpus.resolve(None, categories=['Star Trek'])
list(corpus.docs(categories=['Star Wars']))
list(corpus.sizes(categories=['Star Wars']))
###Output
_____no_output_____
###Markdown
Reading a Corpus from a Database
###Code
class SqliteCorpusReader(object):
def __init__(self, path):
self._cur = sqlite3.connect(path).cursor()
def scores(self):
"""
Returns the review score
"""
self._cur.execute("SELECT score FROM reviews")
for score in iter(self._cur.fetchone, None):
yield score
def texts(self):
"""
Returns the full review texts
"""
self._cur.execute("SELECT content FROM content")
for text in iter(self._cur.fetchone, None):
yield text
def ids(self):
"""
Returns the review ids
"""
self._cur.execute("SELECT reviewid FROM content")
for idx in iter(self._cur.fetchone, None):
yield idx
###Output
_____no_output_____ |
nasws/cnn/search_space/nasbench101/test-backprop.ipynb | ###Markdown
Use to test the back propagation of model_search for NASBenchNet
###Code
from argparse import Namespace
from nasbench.api import ModelSpec
%load_ext autoreload
%autoreload 2
import sys
import os
home = os.environ['HOME']
os.environ['CUDA_VISIBLE_DEVICES'] = '0'
print(os.environ['CUDA_VISIBLE_DEVICES'])
os.chdir(f'{home}/pycharm/automl')
# os.chdir(f'{home}/pycharm/automl/search_policies/rnn')
sys.path.append(f'{home}/pycharm/nasbench')
sys.path.append(f'{home}/pycharm/automl')
# !ls data/nasbench
from search_policies.cnn.search_space.nasbench101.model_search import *
from search_policies.cnn.search_space.nasbench101.model import NasBenchNet as NasbenchNetOriginal
from search_policies.cnn.search_space.nasbench101.nasbench_api_v2 import NASBench_v2
nasbench = NASBench_v2('data/nasbench/nasbench_only108.tfrecord', only_hash=True)
hashs = []
ind = 0
for ind, (k, v) in enumerate(nasbench.hash_dict.items()):
if ind < 10:
# print(k, v)
hashs.append(k)
_hash = hashs[0]
_hash2 = hashs[1]
input_channels = 3
# print(nasbench.hash_to_model_spec(_hash))
spec_1 = nasbench.hash_to_model_spec(_hash)
spec_2 = nasbench.hash_to_model_spec(_hash2)
model1 = NasbenchNetOriginal(input_channels, spec_1)
model2 = NasbenchNetOriginal(input_channels, spec_2)
model_search = NasBenchNetSearch(input_channels, spec_1)
x = torch.randn(8, 3, 32, 32)
print(spec_1)
model_search.change_model_spec(spec_1)
# print(layer0)
y = model_search(x)
print(y[0].size())
print(spec_2)
model_search.change_model_spec(spec_2)
stem = model_search.stem(x)
print(stem.size())
kky = 'vertex_2'
layer0 = model_search.stacks['stack0']['module0']
proj_op = layer0.op[kky].proj_ops[0]
print(proj_op.current_outsize)
# print(layer0.op['vertex_5'].proj_ops[0](stem).size())
# print(layer0.op['vertex_5'](stem))
# print(layer0(stem))
# y2 = model_search(x)
# print(y2[0])
# print(y[0] - y[2])
# for _y in y:
# print(y.size())
out = model1(x)[0] - model2(x)[0]
print(out)
# model_search.stacks['stack0']['module0'].dag
import numpy as np
from nasbench.lib import graph_util
full = 1 - np.tril(np.ones_like(spec_1.matrix) )
full_hash = graph_util.hash_module(full, spec_1.ops)
full_spec = ModelSpec(full, spec_1.ops)
full_spec._prune()
print(full_spec.matrix)
full_model = NasbenchNetOriginal(input_channels, full_spec)
# nasbench.query(full_spec)
# nasbench.query_hash(full_hash)
import torch.nn.functional as F
xx = F.dropout2d(x, 0.5, training=True)
print(xx)
# testing the shuffle channel
def channel_shuffle(x, groups):
batchsize, num_channels, height, width = x.data.size()
channels_per_group = num_channels // groups
# reshape
x = x.view(batchsize, groups,
channels_per_group, height, width)
# transpose
# - contiguous() required if transpose() is used before view().
# See https://github.com/pytorch/pytorch/issues/764
x = torch.transpose(x, 1, 2).contiguous()
# flatten
x = x.view(batchsize, -1, height, width)
return x
test_x = np.arange(1, 9)
test_x = torch.from_numpy(test_x).view(1, 8, 1, 1)
print(test_x)
print(channel_shuffle(test_x, 4))
print(channel_shuffle(test_x, 4))
print(channel_shuffle(test_x, 4))
print(channel_shuffle(test_x, 4))
###Output
_____no_output_____ |
data_processing/.ipynb_checkpoints/create_pre_test_csv_part1-checkpoint.ipynb | ###Markdown
Remove entries with no sound
###Code
ns_index = []
top_line = pre_data.iloc[0,:]
for i in range(0,len(top_line)):
if top_line[i] == 'No Sound' or top_line[i] == 'NO SOUND':
ns_index.append(i+1)
for i in range (0,len(ns_index)):
name = column_list[ns_index[i]]
try:
pre_data = pre_data.drop(columns = str(name))
except:
continue
###Output
_____no_output_____
###Markdown
Now add the text below the column for the corresponding subject
###Code
pre_data
column_list = list(pre_data.columns)
import numpy as np
num_cols = pre_data.shape[1]
blank_row = pd.DataFrame(np.zeros((num_cols,), dtype=np.int).reshape(1,-1), columns = column_list)
new_data_frame = pd.concat([pre_data.iloc[0:1,:], blank_row]).reset_index(drop=True)
for i in range(1,9):
new_data_frame = pd.concat([new_data_frame, pre_data.iloc[i:i+1,:], blank_row]).reset_index(drop=True)
pre_data = pd.concat([new_data_frame, pre_data.iloc[9:11,:]]).reset_index(drop=True)
pre_data
file = open('correct_listwav.txt', 'r')
pre_file = []
for line in file:
line = line.split('\n')[0]
if 'pre' in line.casefold():
pre_file.append(line)
pre_file
pre_data = pd.concat([pre_data, blank_row])
pre_data.reset_index(drop=True)
pre_data.iloc[20,0] = 'Text'
pre_data = pre_data.reset_index(drop=True)
from docx import Document
def getText(filename):
doc = Document(filename)
fullText = []
for para in doc.paragraphs:
fullText.append(para.text)
return fullText
getText('unlabeled_data\\' +pre_file[0]+'.docx')
for file in pre_file:
for name in column_list[4:]:
if str(name) in file:
try:
pre_data.iloc[20,column_list.index(name)] = '/n'.join(getText('unlabeled_data\\' +file+'.docx'))
except:
continue
pre_data.to_csv('labeled_data//pre_data.csv')
###Output
_____no_output_____ |
submodules/resource/d2l-zh/mxnet/chapter_attention-mechanisms/attention-scoring-functions.ipynb | ###Markdown
注意力评分函数:label:`sec_attention-scoring-functions`在 :numref:`sec_nadaraya-watson`中,我们使用高斯核来对查询和键之间的关系建模。我们可以将 :eqref:`eq_nadaraya-watson-gaussian`中的高斯核指数部分视为*注意力评分函数*(attention scoring function),简称*评分函数*(scoring function),然后把这个函数的输出结果输入到softmax函数中进行运算。通过上述步骤,我们将得到与键对应的值的概率分布(即注意力权重)。最后,注意力汇聚的输出就是基于这些注意力权重的值的加权和。从宏观来看,我们可以使用上述算法来实现 :numref:`fig_qkv`中的注意力机制框架。 :numref:`fig_attention_output`说明了如何将注意力汇聚的输出计算成为值的加权和,其中$a$表示注意力评分函数。由于注意力权重是概率分布,因此加权和其本质上是加权平均值。:label:`fig_attention_output`用数学语言描述,假设有一个查询$\mathbf{q} \in \mathbb{R}^q$和$m$个“键-值”对$(\mathbf{k}_1, \mathbf{v}_1), \ldots, (\mathbf{k}_m, \mathbf{v}_m)$,其中$\mathbf{k}_i \in \mathbb{R}^k$,$\mathbf{v}_i \in \mathbb{R}^v$。注意力汇聚函数$f$就被表示成值的加权和:$$f(\mathbf{q}, (\mathbf{k}_1, \mathbf{v}_1), \ldots, (\mathbf{k}_m, \mathbf{v}_m)) = \sum_{i=1}^m \alpha(\mathbf{q}, \mathbf{k}_i) \mathbf{v}_i \in \mathbb{R}^v,$$:eqlabel:`eq_attn-pooling`其中查询$\mathbf{q}$和键$\mathbf{k}_i$的注意力权重(标量)是通过注意力评分函数$a$ 将两个向量映射成标量,再经过softmax运算得到的:$$\alpha(\mathbf{q}, \mathbf{k}_i) = \mathrm{softmax}(a(\mathbf{q}, \mathbf{k}_i)) = \frac{\exp(a(\mathbf{q}, \mathbf{k}_i))}{\sum_{j=1}^m \exp(a(\mathbf{q}, \mathbf{k}_j))} \in \mathbb{R}.$$:eqlabel:`eq_attn-scoring-alpha`正如我们所看到的,选择不同的注意力评分函数$a$会导致不同的注意力汇聚操作。在本节中,我们将介绍两个流行的评分函数,稍后将用他们来实现更复杂的注意力机制。
###Code
import math
from mxnet import np, npx
from mxnet.gluon import nn
from d2l import mxnet as d2l
npx.set_np()
###Output
_____no_output_____
###Markdown
[**掩蔽softmax操作**]正如上面提到的,softmax操作用于输出一个概率分布作为注意力权重。在某些情况下,并非所有的值都应该被纳入到注意力汇聚中。例如,为了在 :numref:`sec_machine_translation`中高效处理小批量数据集,某些文本序列被填充了没有意义的特殊词元。为了仅将有意义的词元作为值来获取注意力汇聚,我们可以指定一个有效序列长度(即词元的个数),以便在计算softmax时过滤掉超出指定范围的位置。通过这种方式,我们可以在下面的`masked_softmax`函数中实现这样的*掩蔽softmax操作*(masked softmax operation),其中任何超出有效长度的位置都被掩蔽并置为0。
###Code
#@save
def masked_softmax(X, valid_lens):
"""通过在最后一个轴上掩蔽元素来执行softmax操作"""
# X:3D张量,valid_lens:1D或2D张量
if valid_lens is None:
return npx.softmax(X)
else:
shape = X.shape
if valid_lens.ndim == 1:
valid_lens = valid_lens.repeat(shape[1])
else:
valid_lens = valid_lens.reshape(-1)
# 最后一轴上被掩蔽的元素使用一个非常大的负值替换,从而其softmax输出为0
X = npx.sequence_mask(X.reshape(-1, shape[-1]), valid_lens, True,
value=-1e6, axis=1)
return npx.softmax(X).reshape(shape)
###Output
_____no_output_____
###Markdown
为了[**演示此函数是如何工作**]的,考虑由两个$2 \times 4$矩阵表示的样本,这两个样本的有效长度分别为$2$和$3$。经过掩蔽softmax操作,超出有效长度的值都被掩蔽为0。
###Code
masked_softmax(np.random.uniform(size=(2, 2, 4)), np.array([2, 3]))
###Output
_____no_output_____
###Markdown
同样,我们也可以使用二维张量,为矩阵样本中的每一行指定有效长度。
###Code
masked_softmax(np.random.uniform(size=(2, 2, 4)),
np.array([[1, 3], [2, 4]]))
###Output
_____no_output_____
###Markdown
[**加性注意力**]:label:`subsec_additive-attention`一般来说,当查询和键是不同长度的矢量时,我们可以使用加性注意力作为评分函数。给定查询$\mathbf{q} \in \mathbb{R}^q$和键$\mathbf{k} \in \mathbb{R}^k$,*加性注意力*(additive attention)的评分函数为$$a(\mathbf q, \mathbf k) = \mathbf w_v^\top \text{tanh}(\mathbf W_q\mathbf q + \mathbf W_k \mathbf k) \in \mathbb{R},$$:eqlabel:`eq_additive-attn`其中可学习的参数是$\mathbf W_q\in\mathbb R^{h\times q}$、$\mathbf W_k\in\mathbb R^{h\times k}$和$\mathbf w_v\in\mathbb R^{h}$。如 :eqref:`eq_additive-attn`所示,将查询和键连结起来后输入到一个多层感知机(MLP)中,感知机包含一个隐藏层,其隐藏单元数是一个超参数$h$。通过使用$\tanh$作为激活函数,并且禁用偏置项。下面我们来实现加性注意力。
###Code
#@save
class AdditiveAttention(nn.Block):
"""加性注意力"""
def __init__(self, num_hiddens, dropout, **kwargs):
super(AdditiveAttention, self).__init__(**kwargs)
# 使用'flatten=False'只转换最后一个轴,以便其他轴的形状保持不变
self.W_k = nn.Dense(num_hiddens, use_bias=False, flatten=False)
self.W_q = nn.Dense(num_hiddens, use_bias=False, flatten=False)
self.w_v = nn.Dense(1, use_bias=False, flatten=False)
self.dropout = nn.Dropout(dropout)
def forward(self, queries, keys, values, valid_lens):
queries, keys = self.W_q(queries), self.W_k(keys)
# 在维度扩展后,
# queries的形状:(batch_size,查询的个数,1,num_hidden)
# key的形状:(batch_size,1,“键-值”对的个数,num_hiddens)
# 使用广播的方式进行求和
features = np.expand_dims(queries, axis=2) + np.expand_dims(
keys, axis=1)
features = np.tanh(features)
# self.w_v仅有一个输出,因此从形状中移除最后那个维度。
# scores的形状:(batch_size,查询的个数,“键-值”对的个数)
scores = np.squeeze(self.w_v(features), axis=-1)
self.attention_weights = masked_softmax(scores, valid_lens)
# values的形状:(batch_size,“键-值”对的个数,值的维度)
return npx.batch_dot(self.dropout(self.attention_weights), values)
###Output
_____no_output_____
###Markdown
我们用一个小例子来[**演示上面的`AdditiveAttention`类**],其中查询、键和值的形状为(批量大小,步数或词元序列长度,特征大小),实际输出为$(2,1,20)$、$(2,10,2)$和$(2,10,4)$。注意力汇聚输出的形状为(批量大小,查询的步数,值的维度)。
###Code
queries, keys = np.random.normal(0, 1, (2, 1, 20)), np.ones((2, 10, 2))
# values的小批量数据集中,两个值矩阵是相同的
values = np.arange(40).reshape(1, 10, 4).repeat(2, axis=0)
valid_lens = np.array([2, 6])
attention = AdditiveAttention(num_hiddens=8, dropout=0.1)
attention.initialize()
attention(queries, keys, values, valid_lens)
###Output
_____no_output_____
###Markdown
尽管加性注意力包含了可学习的参数,但由于本例子中每个键都是相同的,所以[**注意力权重**]是均匀的,由指定的有效长度决定。
###Code
d2l.show_heatmaps(attention.attention_weights.reshape((1, 1, 2, 10)),
xlabel='Keys', ylabel='Queries')
###Output
_____no_output_____
###Markdown
[**缩放点积注意力**]使用点积可以得到计算效率更高的评分函数,但是点积操作要求查询和键具有相同的长度$d$。假设查询和键的所有元素都是独立的随机变量,并且都满足零均值和单位方差,那么两个向量的点积的均值为$0$,方差为$d$。为确保无论向量长度如何,点积的方差在不考虑向量长度的情况下仍然是$1$,我们将点积除以$\sqrt{d}$,则*缩放点积注意力*(scaled dot-product attention)评分函数为:$$a(\mathbf q, \mathbf k) = \mathbf{q}^\top \mathbf{k} /\sqrt{d}.$$在实践中,我们通常从小批量的角度来考虑提高效率,例如基于$n$个查询和$m$个键-值对计算注意力,其中查询和键的长度为$d$,值的长度为$v$。查询$\mathbf Q\in\mathbb R^{n\times d}$、键$\mathbf K\in\mathbb R^{m\times d}$和值$\mathbf V\in\mathbb R^{m\times v}$的缩放点积注意力是:$$ \mathrm{softmax}\left(\frac{\mathbf Q \mathbf K^\top }{\sqrt{d}}\right) \mathbf V \in \mathbb{R}^{n\times v}.$$:eqlabel:`eq_softmax_QK_V`在下面的缩放点积注意力的实现中,我们使用了暂退法进行模型正则化。
###Code
#@save
class DotProductAttention(nn.Block):
"""缩放点积注意力"""
def __init__(self, dropout, **kwargs):
super(DotProductAttention, self).__init__(**kwargs)
self.dropout = nn.Dropout(dropout)
# queries的形状:(batch_size,查询的个数,d)
# keys的形状:(batch_size,“键-值”对的个数,d)
# values的形状:(batch_size,“键-值”对的个数,值的维度)
# valid_lens的形状:(batch_size,)或者(batch_size,查询的个数)
def forward(self, queries, keys, values, valid_lens=None):
d = queries.shape[-1]
# 设置transpose_b=True为了交换keys的最后两个维度
scores = npx.batch_dot(queries, keys, transpose_b=True) / math.sqrt(d)
self.attention_weights = masked_softmax(scores, valid_lens)
return npx.batch_dot(self.dropout(self.attention_weights), values)
###Output
_____no_output_____
###Markdown
为了[**演示上述的`DotProductAttention`类**],我们使用与先前加性注意力例子中相同的键、值和有效长度。对于点积操作,我们令查询的特征维度与键的特征维度大小相同。
###Code
queries = np.random.normal(0, 1, (2, 1, 2))
attention = DotProductAttention(dropout=0.5)
attention.initialize()
attention(queries, keys, values, valid_lens)
###Output
_____no_output_____
###Markdown
与加性注意力演示相同,由于键包含的是相同的元素,而这些元素无法通过任何查询进行区分,因此获得了[**均匀的注意力权重**]。
###Code
d2l.show_heatmaps(attention.attention_weights.reshape((1, 1, 2, 10)),
xlabel='Keys', ylabel='Queries')
###Output
_____no_output_____ |
mammography/Faster_RCNN_training.ipynb | ###Markdown
Faster R-CNN Training Model is a modified version of Mask R-CNN without the mask head
###Code
import os
import sys
import itertools
import math
import logging
import json
import re
import random
import time
import concurrent.futures
import numpy as np
import matplotlib
import matplotlib.pyplot as plt
import matplotlib.patches as patches
import matplotlib.lines as lines
from matplotlib.patches import Polygon
import imgaug
from imgaug import augmenters as iaa
# Root directory of the project
ROOT_DIR = os.getcwd()
print(ROOT_DIR)
if ROOT_DIR.endswith("mammography"):
# Go up one level to the repo root
ROOT_DIR = os.path.dirname(ROOT_DIR)
print(ROOT_DIR)
# Import Faster_RCNN
sys.path.append(ROOT_DIR)
from faster_rcnn import utils
from faster_rcnn import visualize
from faster_rcnn.visualize import display_images
from faster_rcnn import model as modellib
from faster_rcnn.model import log
import mammo_baseline_faster_rcnn
%matplotlib inline
# Comment out to reload imported modules if they change
%load_ext autoreload
%autoreload 2
###Output
_____no_output_____
###Markdown
Configurations
###Code
# Dataset directory
DATASET_DIR = os.path.join(ROOT_DIR, "datasets/mammo")
# Load dataset
subset = "mass_train_3x"
if "3x" in subset:
augmented=True
else:
augmented=False
dataset_train = mammo_baseline_faster_rcnn.MammoDataset()
dataset_train.load_mammo(DATASET_DIR, subset=subset, augmented=augmented)
# Must call before using the dataset
dataset_train.prepare()
print("Image Count: {}".format(len(dataset_train.image_ids)))
print("Class Count: {}".format(dataset_train.num_classes))
for i, info in enumerate(dataset_train.class_info):
print("{:3}. {:50}".format(i, info['name']))
# Load validation dataset
dataset_val = mammo_baseline_faster_rcnn.MammoDataset()
dataset_val.load_mammo(DATASET_DIR, "val", augmented=augmented)
dataset_val.prepare()
print("Images: {}\nClasses: {}".format(len(dataset_val.image_ids), dataset_val.class_names))
###Output
mass_train_3x
Image Count: 3933
Class Count: 2
0. BG
1. mass
val
Images: 983
Classes: ['BG', 'mass']
###Markdown
Notebook Preferences
###Code
def get_ax(rows=1, cols=1, size=16):
"""Return a Matplotlib Axes array to be used in
all visualizations in the notebook. Provide a
central point to control graph sizes.
Adjust the size attribute to control how big to render images
"""
_, ax = plt.subplots(rows, cols, figsize=(size*cols, size*rows))
return ax
#######################################
# 4rd Sept #
# Training on Faster R-CNN #
# Max_dim = 1024 #
# Mass_train_3x #
# ResNet101 #
# RPN_TRAIN_ANCHOR_PER_IMAGE=512 #
#######################################
class NoResizeConfig(mammo_baseline_faster_rcnn.MammoConfig):
BACKBONE = "resnet101"
# Adjust depending on your GPU memory
IMAGES_PER_GPU = 2
IMAGE_MAX_DIM = 1024
# Number of classes (including background)
NUM_CLASSES = 1 + 1 # Background + mass
# Number of training and validation steps per epoch
STEPS_PER_EPOCH = (len(dataset_train.image_ids)) // IMAGES_PER_GPU
VALIDATION_STEPS = max(1, len(dataset_val.image_ids) // IMAGES_PER_GPU)
## USE_MINI_MASK doesn't matter since there is no mask
# USE_MINI_MASK = False
# MINI_MASK_SHAPE = (56,56) # (height, width) of the mini-mask
RPN_TRAIN_ANCHORS_PER_IMAGE = 512
# ROIs kept after non-maximum supression (training and inference)
POST_NMS_ROIS_TRAINING = 2000
POST_NMS_ROIS_INFERENCE = 1000
config = NoResizeConfig()
config.display()
MODEL_DIR = 'checkpoints'
# Create model
model = modellib.MaskRCNN(mode="training", config=config,
model_dir=MODEL_DIR)
# Select weights file to load
weights_path = model.get_imagenet_weights()
# Load weights
print("Loading weights ", weights_path)
model.load_weights(weights_path, by_name=True)
model.train(dataset_train, dataset_val, config.LEARNING_RATE, epochs=10, layers='all')
#######################################
# 3rd Sept #
# Training on Faster R-CNN #
# Max_dim = 1024 #
# Mass_train #
# ResNet101 #
# RPN_TRAIN_ANCHOR_PER_IMAGE=512 #
#######################################
# Configurations
# Use configuation from mammo.py, but override
# image resizing so we see the real sizes here
class NoResizeConfig(mammo_baseline.MammoConfig):
BACKBONE = "resnet101"
# Adjust depending on your GPU memory
IMAGES_PER_GPU = 2
IMAGE_MAX_DIM = 1024
# Number of classes (including background)
NUM_CLASSES = 1 + 1 # Background + mass
# Number of training and validation steps per epoch
STEPS_PER_EPOCH = (len(dataset_train.image_ids)) // IMAGES_PER_GPU
VALIDATION_STEPS = max(1, len(dataset_val.image_ids) // IMAGES_PER_GPU)
## USE_MINI_MASK doesn't matter since there is no mask
# USE_MINI_MASK = True
# MINI_MASK_SHAPE = (56,56) # (height, width) of the mini-mask
RPN_TRAIN_ANCHORS_PER_IMAGE = 512
# ROIs kept after non-maximum supression (training and inference)
POST_NMS_ROIS_TRAINING = 2000
POST_NMS_ROIS_INFERENCE = 1000
# Non-max suppression threshold to filter RPN proposals.
# You can increase this during training to generate more propsals.
RPN_NMS_THRESHOLD = 0.7
config = NoResizeConfig()
config.display()
MODEL_DIR = 'checkpoints'
# Create model
model = modellib.MaskRCNN(mode="training", config=config,
model_dir=MODEL_DIR)
# Select weights file to load
weights_path = model.get_imagenet_weights()
# weights_path = COCO_MODEL_PATH
# model.load_weights(COCO_MODEL_PATH, by_name=True)
# Load weights
print("Loading weights ", weights_path)
model.load_weights(weights_path, by_name=True)
model.train(dataset_train, dataset_val, config.LEARNING_RATE, epochs=10, layers='all')
###Output
Configurations:
BACKBONE resnet101
BACKBONE_STRIDES [4, 8, 16, 32, 64]
BATCH_SIZE 2
BBOX_STD_DEV [0.1 0.1 0.2 0.2]
COMPUTE_BACKBONE_SHAPE None
DETECTION_MAX_INSTANCES 100
DETECTION_MIN_CONFIDENCE 0.7
DETECTION_NMS_THRESHOLD 0.3
FPN_CLASSIF_FC_LAYERS_SIZE 1024
GPU_COUNT 1
GRADIENT_CLIP_NORM 5.0
IMAGES_PER_GPU 2
IMAGE_MAX_DIM 1024
IMAGE_META_SIZE 14
IMAGE_MIN_DIM 512
IMAGE_MIN_SCALE 0
IMAGE_RESIZE_MODE square
IMAGE_SHAPE [1024 1024 3]
LEARNING_MOMENTUM 0.9
LEARNING_RATE 0.001
LOSS_WEIGHTS {'rpn_class_loss': 1.0, 'rpn_bbox_loss': 1.0, 'mrcnn_class_loss': 1.0, 'mrcnn_bbox_loss': 1.0, 'mrcnn_mask_loss': 1.0}
MASK_POOL_SIZE 14
MASK_SHAPE [28, 28]
MAX_GT_INSTANCES 100
MEAN_PIXEL [54.78 54.78 54.78]
MINI_MASK_SHAPE (56, 56)
NAME mammo
NUM_CLASSES 2
POOL_SIZE 7
POST_NMS_ROIS_INFERENCE 1000
POST_NMS_ROIS_TRAINING 2000
ROI_POSITIVE_RATIO 0.33
RPN_ANCHOR_RATIOS [0.5, 1, 2]
RPN_ANCHOR_SCALES (32, 64, 128, 256, 512)
RPN_ANCHOR_STRIDE 1
RPN_BBOX_STD_DEV [0.1 0.1 0.2 0.2]
RPN_NMS_THRESHOLD 0.7
RPN_TRAIN_ANCHORS_PER_IMAGE 512
STEPS_PER_EPOCH 491
TOP_DOWN_PYRAMID_SIZE 256
TRAIN_BN False
TRAIN_ROIS_PER_IMAGE 200
USE_MINI_MASK True
USE_RPN_ROIS True
VALIDATION_STEPS 123
WEIGHT_DECAY 0.0001
Loading weights C:\Users\Chevy\AppData\Local\Temp\.keras\models\resnet50_weights_tf_dim_ordering_tf_kernels_notop.h5
Starting at epoch 0. LR=0.001
Checkpoint Path: checkpoints\mammo20180903T2151\mask_rcnn_mammo_{epoch:04d}.h5
Selecting layers to train
conv1 (Conv2D)
bn_conv1 (BatchNorm)
res2a_branch2a (Conv2D)
bn2a_branch2a (BatchNorm)
res2a_branch2b (Conv2D)
bn2a_branch2b (BatchNorm)
res2a_branch2c (Conv2D)
res2a_branch1 (Conv2D)
bn2a_branch2c (BatchNorm)
bn2a_branch1 (BatchNorm)
res2b_branch2a (Conv2D)
bn2b_branch2a (BatchNorm)
res2b_branch2b (Conv2D)
bn2b_branch2b (BatchNorm)
res2b_branch2c (Conv2D)
bn2b_branch2c (BatchNorm)
res2c_branch2a (Conv2D)
bn2c_branch2a (BatchNorm)
res2c_branch2b (Conv2D)
bn2c_branch2b (BatchNorm)
res2c_branch2c (Conv2D)
bn2c_branch2c (BatchNorm)
res3a_branch2a (Conv2D)
bn3a_branch2a (BatchNorm)
res3a_branch2b (Conv2D)
bn3a_branch2b (BatchNorm)
res3a_branch2c (Conv2D)
res3a_branch1 (Conv2D)
bn3a_branch2c (BatchNorm)
bn3a_branch1 (BatchNorm)
res3b_branch2a (Conv2D)
bn3b_branch2a (BatchNorm)
res3b_branch2b (Conv2D)
bn3b_branch2b (BatchNorm)
res3b_branch2c (Conv2D)
bn3b_branch2c (BatchNorm)
res3c_branch2a (Conv2D)
bn3c_branch2a (BatchNorm)
res3c_branch2b (Conv2D)
bn3c_branch2b (BatchNorm)
res3c_branch2c (Conv2D)
bn3c_branch2c (BatchNorm)
res3d_branch2a (Conv2D)
bn3d_branch2a (BatchNorm)
res3d_branch2b (Conv2D)
bn3d_branch2b (BatchNorm)
res3d_branch2c (Conv2D)
bn3d_branch2c (BatchNorm)
res4a_branch2a (Conv2D)
bn4a_branch2a (BatchNorm)
res4a_branch2b (Conv2D)
bn4a_branch2b (BatchNorm)
res4a_branch2c (Conv2D)
res4a_branch1 (Conv2D)
bn4a_branch2c (BatchNorm)
bn4a_branch1 (BatchNorm)
res4b_branch2a (Conv2D)
bn4b_branch2a (BatchNorm)
res4b_branch2b (Conv2D)
bn4b_branch2b (BatchNorm)
res4b_branch2c (Conv2D)
bn4b_branch2c (BatchNorm)
res4c_branch2a (Conv2D)
bn4c_branch2a (BatchNorm)
res4c_branch2b (Conv2D)
bn4c_branch2b (BatchNorm)
res4c_branch2c (Conv2D)
bn4c_branch2c (BatchNorm)
res4d_branch2a (Conv2D)
bn4d_branch2a (BatchNorm)
res4d_branch2b (Conv2D)
bn4d_branch2b (BatchNorm)
res4d_branch2c (Conv2D)
bn4d_branch2c (BatchNorm)
res4e_branch2a (Conv2D)
bn4e_branch2a (BatchNorm)
res4e_branch2b (Conv2D)
bn4e_branch2b (BatchNorm)
res4e_branch2c (Conv2D)
bn4e_branch2c (BatchNorm)
res4f_branch2a (Conv2D)
bn4f_branch2a (BatchNorm)
res4f_branch2b (Conv2D)
bn4f_branch2b (BatchNorm)
res4f_branch2c (Conv2D)
bn4f_branch2c (BatchNorm)
res4g_branch2a (Conv2D)
bn4g_branch2a (BatchNorm)
res4g_branch2b (Conv2D)
bn4g_branch2b (BatchNorm)
res4g_branch2c (Conv2D)
bn4g_branch2c (BatchNorm)
res4h_branch2a (Conv2D)
bn4h_branch2a (BatchNorm)
res4h_branch2b (Conv2D)
bn4h_branch2b (BatchNorm) |
Taller_4_Canalizacion/parte_1_canalizacion.ipynb | ###Markdown
Taller parte 2 Parte 1: Autor:Wilgen Correa Fecha; 30-04-2021 Objetivo:Objetivo: Un cuaderno en donde ustedes harán el entubamiento de un conjunto de datos asociado a su proyecto. Para este, ustedes revisan el segundo cuaderno y completan detalles con los enlaces abajo. El entregable es preferiblemente el entubamiento que usted usa o usará en su proyecto. Cargar librerias
###Code
import datetime
import os, glob
import numpy as np
import seaborn as sns
import geopandas as gpd
import pandas as pd
import imageio
from pyspatialml import Raster
import matplotlib.pyplot as plt
from sklearn.preprocessing import StandardScaler
from sklearn.pipeline import Pipeline
import tensorflow as tf
from tensorflow.keras.layers import Dense, Dropout
from tensorflow.keras.models import Sequential
from sklearn.impute import SimpleImputer
from tensorflow.keras.wrappers.scikit_learn import KerasClassifier
from tensorflow.keras.callbacks import CSVLogger, RemoteMonitor
from sklearn.model_selection import train_test_split
from sklearn.neighbors import KNeighborsClassifier
from sklearn.metrics import accuracy_score, precision_score, recall_score, confusion_matrix
import earthpy.plot as ep
import earthpy.spatial as es
###Output
_____no_output_____
###Markdown
Realizar el cargue de imágenes y las áreas de enetrenamientoBandas a seleccionar de la imagen de entrada de SENTINEL-2, con remuestreo a 10 m de resolución espacial1. B2 (Blue)2. B3 (Green)3. B4 (Red)4. B5 (Veg. red edge)5. B6 (Veg. red edge)6. B7 (Veg. red edge)7. B8 (NIR)8. B8A (Narrow NIR)9. B11 (SWIR)10. B12 (SWIR)La estructura de directorios es el siguiente.+-- [nombre_proyecto] +-- shapes: shapes de aoi y capas de superposición +-- models: Almacena el modelo +-- sources: imágenes satelitales de entrada +-- results: resultados de las clasificación +-- logs: logs del entrenamiento +-- figures: imágenes y figuras
###Code
path_project = "./hayuelos/"
path_sources = os.path.join(path_project, "sources")
path_shapes = os.path.join(path_project, "shapes")
path_results = os.path.join(path_project, "results")
path_logs = os.path.join(path_project, "logs")
path_figures = os.path.join(path_project, "figures")
path_models = os.path.join(path_project, "models")
list_paths = [path_results, path_figures, path_models, path_logs]
# Crear directorios temporales
for path in list_paths:
try:
os.mkdir(path)
except FileExistsError:
print("Directorio ya existe: ", os.path.basename(path))
# Defina la imagen de entrada
img_train = '20210309T152639_20210309T152638_T18NWL.tif'
img_file = os.path.join(path_sources, img_train)
aoi_file = os.path.join(path_shapes, 'aoi.geojson') # Formato geográfico
manzana_file = os.path.join(path_shapes, 'manzana.geojson') # Formato geográfico
img_name = img_train.split('_')[0]
# Cargar la imagen como un objeto Raster Dataframe y el shape de aoi como Geodaataframe
stack = Raster(img_file)
training = gpd.read_file(aoi_file)
manzana = gpd.read_file(manzana_file)
bandsio = ['B2', 'B3', 'B4', 'B5', 'B6', 'B7', 'B8', 'B8A', 'B11', 'B12']
bandsio_names = ['B2 (Blue)', 'B3 (Green)', 'B4 (Red)', 'B5 (Veg. red edge)',
'B6 (Veg. red edge)', 'B7 (Veg. red edge)', 'B8 (NIR)',
'B8A (Narrow NIR)', 'B11 (SWIR)', 'B12 (SWIR)']
# Mapea los nombres de bandas de entrada
[stack.rename({name: bandsio[idx]}, in_place=True) for idx, name in enumerate(stack.names)]
extent = es.rio.plot.plotting_extent(stack)
###Output
_____no_output_____
###Markdown
Se define la selección de bandas de entrenamineto y salida para todo el proceso
###Code
bandsout = ['B2', 'B3', 'B4', 'B5', 'B6', 'B7', 'B8', 'B8A', 'B11', 'B12']
bandsout_names = ['B2 (Blue)', 'B3 (Green)', 'B4 (Red)', 'B5 (Veg. red edge)',
'B6 (Veg. red edge)', 'B7 (Veg. red edge)', 'B8 (NIR)',
'B8A (Narrow NIR)', 'B11 (SWIR)', 'B12 (SWIR)']
###Output
_____no_output_____
###Markdown
Anáisis exploratorio de datosDespliegue de imágenes y análisis exploratorio
###Code
# Despliegue los canales imágenes
ep.plot_bands(stack[bandsout].read(), title=bandsout_names, figsize=(10, 8))
# Histogramas por bandas
colors_list = ["Blue", "Green", "Red", "Salmon", "Tomato", "Coral", "Orangered",
"Chocolate","Darkorange","Maroon"]
ep.hist(stack[bandsout].read(), colors=colors_list, title=bandsout_names,
ylabel='Reflectancia', bins=50, cols=2)
# Despliegue composición de color
rgb432 = stack[['B4','B3', 'B2']].read()
rgb843 = stack[['B8','B4', 'B3']].read()
fig, (ax1, ax2) = plt.subplots(1, 2, figsize=(12, 6))
ep.plot_rgb(rgb432, ax=ax1, title='Despliegue verdadero color (RGB432)', stretch=True)
ep.plot_rgb(rgb843, ax=ax2, title='Despliegue falso color (RGB843)', stretch=True)
plt.tight_layout()
plt.show()
###Output
_____no_output_____
###Markdown
Despliegue de las áreas de entrenamiento
###Code
fig, ax = plt.subplots(figsize=(9, 9))
ep.plot_rgb(rgb843, ax=ax, stretch=True, extent=extent)
manzana.boundary.plot(ax=ax, color='grey', alpha=0.5,)
training.plot(column="label", cmap='RdYlGn', ax=ax, legend=True, alpha=0.65, categorical=True)
ax.axis('on')
plt.title('Áreas de entrenamiento')
plt.show()
# Convertir los pixeles a un dataframe de pandas
df = stack[bandsout].to_pandas()
df.head()
print('Las columnas son: ', df.columns)
print('El tamaño´ del dataframe: ', df.shape)
print(df[bandsout].describe().T)
# Correlacción de las bandas
correlation_data = df.iloc[:,2:].corr()
correlation_data.style.background_gradient(cmap='coolwarm', axis=None)
# Extraer información de las bandas con la áreas de entrenamiento
df_shape = stack[bandsout].extract_vector(training)
df_shape = df_shape.merge(
right=training.loc[:, ["label", "id"]],
left_on="geometry_idx",
right_on="index",
right_index=True
)
df_shape = df_shape.dropna()
# Descripcion de los datos para las areas de entrenamiento
print('Total canales espectrales: ', df_shape.columns)
print('Tamaño de entrenamiento: ', df_shape.shape)
print(df[bandsout].describe().T)
fig, axes = plt.subplots(1,2, figsize=(10,4), sharey=True, sharex=True)
plt.suptitle("Áreas de entrenamiento")
axes[0].set_title('No vegetación')
axes[1].set_title('Vegetación')
axes[0].set_xlabel('Bands')
axes[0].set_ylabel('Reflectance')
axes[1].set_xlabel('Bands')
sns.pointplot(data=df_shape[df_shape['id'] == 0][bandsout], ax=axes[0],
scale=0.5, estimator=np.mean)
sns.boxplot(data=df_shape[df_shape['id'] == 0][bandsout], ax=axes[0])
sns.pointplot(data=df_shape[df_shape['id'] == 1][bandsout], ax=axes[1],
scale=0.5, estimator=np.mean)
sns.boxplot(data=df_shape[df_shape['id'] == 1][bandsout], ax=axes[1])
axes[0].grid()
axes[1].grid()
plt.tight_layout()
fig.show()
fig, ax = plt.subplots(figsize=(10,5), sharey=True, sharex=True)
ax.set_title('Firmas espectrales de las áreas de entrenamiento')
ax.set_xlabel('Bands')
ax.set_ylabel('Reflectance')
sns.pointplot(data=df_shape[df_shape['id'] == 0][bandsout], ax=ax,
scale=1, estimator=np.mean, color='red')
sns.pointplot(data=df_shape[df_shape['id'] == 1][bandsout], ax=ax,
scale=1, estimator=np.mean, color='green')
ax.grid()
fig.show()
###Output
_____no_output_____
###Markdown
Entrenamineto de la red neuronalDividir las areas de entrenamineto y validación
###Code
#bands = ['B2', 'B3', 'B4', 'B7']
X = df_shape[bandsout].values
y = df_shape["id"].values
X_train, X_test, y_train, y_test = train_test_split(X, y, test_size=0.2, random_state=123)
# Logs y callbacks para el entrenamiento
timename = datetime.datetime.now().strftime("%Y%m%d-%H%M%S")
log_csv = os.path.join(path_logs, f"log_{img_name}-{timename}.csv")
model_h5 = os.path.join(path_models, f"model_{img_name}-{timename}.h5")
imgkeras_classification = os.path.join(path_results, f"classkeras_{img_name}-{timename}.tif")
imgkeras_probability = os.path.join(path_results, f"probkeras_{img_name}-{timename}.tif")
imgkneig_classification = os.path.join(path_results, f"classkneig_{img_name}-{timename}.tif")
imgkneig_probability = os.path.join(path_results, f"probkneig_{img_name}-{timename}.tif")
fig_model = os.path.join(path_figures, f"train_{img_name}-{timename}.png")
movie_classkeras = os.path.join(path_figures, f"movie_classkeras_{timename}.gif")
call_save_model = tf.keras.callbacks.ModelCheckpoint(
model_h5, monitor='val_loss', verbose=0, save_best_only=False,
save_weights_only=False, mode='auto', save_freq='epoch', options=None
)
call_tensorboard = tf.keras.callbacks.TensorBoard(
log_dir='./logs', histogram_freq=1, write_graph=True, write_images=True,
update_freq='epoch', profile_batch=2, embeddings_freq=0,
embeddings_metadata=True
)
call_csv = CSVLogger(log_csv, separator=",", append=False)
call_remote = RemoteMonitor(
root='http://localhost:9000',
)
# Crear la funcion model de keras
def create_keras_model(layer1_units, layer2_units, dropout_rate, l2_regularization):
model = Sequential([
## Adiciona capas una por una
Dense(units=layer1_units, activation='relu', input_shape=(len(bandsout),)),
# Adding dropout to prevent overfitting (regularización)
Dropout(dropout_rate), # 10% out in each epoc
Dense(units=layer2_units, activation='relu'),
# Adding dropout to prevent overfitting (regularización)
#model.add(Dropout(0.1))
Dense(units=1, activation='sigmoid')
])
model.compile(optimizer='adam', loss='binary_crossentropy', metrics=['accuracy'])
print(model.summary())
return model
# Realizar el entrenamiento
classifier_keras = KerasClassifier(
build_fn=create_keras_model,
batch_size=32,
layer1_units=16,
layer2_units=32,
dropout_rate=0.025,
l2_regularization=0,
epochs=15,
shuffle=True,
validation_split=0.2,
callbacks=[call_save_model, call_tensorboard, call_csv, call_remote],
verbose=True
)
pipeline_keras = Pipeline([
('imputer', SimpleImputer()),
('scaler', StandardScaler()),
('model', classifier_keras)
])
pipeline_keras.fit(X=X_train, y=y_train)
# Log CSV
fig, (ax1, ax2) = plt.subplots(1, 2, figsize=(12, 6))
plt.suptitle('Proceso de entrenamiento')
history = pd.read_csv(log_csv)
history[['accuracy', 'val_accuracy']].plot(ylabel='Accuracy', ax=ax1, xlabel='Epoch')
history[['loss', 'val_loss']].plot(ylabel='Loss', ax=ax2, xlabel='Epoch')
plt.tight_layout()
plt.show()
###Output
_____no_output_____
###Markdown
Conclusiones Se realiza la canalización de los datos del proyecto para hacer más eficiente el entrenamiento de la imagen. En este ejemplo se muesta el wrapper de Skilearn y Keras
###Code
###Output
_____no_output_____ |
catboost/tutorials/example_usages/COVID_19.ipynb | ###Markdown
[](https://colab.research.google.com/github/catboost/tutorials/blob/master/example_usages/COVID_19.ipynb)This is an example on how to predict COVID-19 spread. The goal is to predict Confirmed cases and Fatalities for future dates based on data for previous dates. Table of contents* [General description](general_description)* [Install and import necessary packages ](install_and_import)* [Get main data from Kaggle](get_main_data)* [Feature engineering](features) * [Time delay embedding features](time_delay_embedding_features) * [Day feature](day_feature) * [WeekDay feature](week_day_feature) * [Days since Xth Confirmed case and Xth Fatality features](days_since_features) * ['Distance to Origin' feature](distance_to_origin_feature) * [Functions for merging external data](functions_for_merging) * [Country Area](country_area_feature) * [Country population features](country_population_features) * [Country Population density feature](country_population_density_feature) * [Country Smoking rate feature](country_smoking_rate_feature) * [Country hospital beds per 1000 people](country_hospital_beds_feature) * [Country Health Expenditure](country_health_expenditure_feature)* [Prepare data for training](prepare_for_training)* [Models training](models_training)* [Feature importance in the models](feature_importance)* [Create predictions for eval and test data](predictions)* [Plots with predictions](plots_with_predictions) General description The main data to train on is taken from https://www.kaggle.com/c/covid19-global-forecasting-week-1/data.Regions are specified using two data fields: ``Province/State`` and ``Country/Region``.This data is also enriched with region coordinates in ``Lat`` and ``Long`` fields.Train data contains cumulative numbers of Confirmed cases and Fatalities for the different regions for the number of consecutive dates (contained in ``Date`` field) from 2020-01-22 to 2020-03-24. Test data is for dates from 2020-03-12 to 2020-04-23. Test data does not have Confirmed cases and Fatalities.We will also add additional data for features from other sources:* Country Land area from [World bank data](https://data.worldbank.org/indicator/AG.LND.TOTL.K2)* Country Smoking rate from [World bank data](https://data.worldbank.org/indicator/SH.PRV.SMOK)* Country Population Age and Sex distribution from [UN World Population Prospects](https://population.un.org/wpp/Download/Standard/CSV/)* Country Hospital beds per 1000 people from [World Bank data](https://data.worldbank.org/indicator/sh.med.beds.zs)* Country Health expenditure per Capita, PPP (current international $) from [World Bank data](https://data.worldbank.org/indicator/SH.XPD.CHEX.PP.CD) We will construct models that predict new cases and new fatalities (in the logarithmic scale) for the next day based on time delay embedding features for the last 30 days plus additional features from sources described above. For forecasting further into the future (more than a single day) we will use an incremental (by days) approach: we will use predicted values of new cases and new fatalities for days with already calculated predictions as input data for time delay embedding features for the next prediction day and then use the same procedure for the new next prediction day and so on. Install and import necessary packages
###Code
!pip install kaggle -U
!pip install pandas -U
!pip install catboost -U
import os
import pathlib
import re
import numpy as np
import pandas as pd
import sklearn.preprocessing
import geopy.distance
import catboost as cb
###Output
Requirement already up-to-date: kaggle in /usr/local/lib/python3.6/dist-packages (1.5.6)
Requirement already satisfied, skipping upgrade: certifi in /usr/local/lib/python3.6/dist-packages (from kaggle) (2020.4.5.1)
Requirement already satisfied, skipping upgrade: six>=1.10 in /usr/local/lib/python3.6/dist-packages (from kaggle) (1.12.0)
Requirement already satisfied, skipping upgrade: urllib3<1.25,>=1.21.1 in /usr/local/lib/python3.6/dist-packages (from kaggle) (1.24.3)
Requirement already satisfied, skipping upgrade: python-dateutil in /usr/local/lib/python3.6/dist-packages (from kaggle) (2.8.1)
Requirement already satisfied, skipping upgrade: requests in /usr/local/lib/python3.6/dist-packages (from kaggle) (2.21.0)
Requirement already satisfied, skipping upgrade: tqdm in /usr/local/lib/python3.6/dist-packages (from kaggle) (4.38.0)
Requirement already satisfied, skipping upgrade: python-slugify in /usr/local/lib/python3.6/dist-packages (from kaggle) (4.0.0)
Requirement already satisfied, skipping upgrade: chardet<3.1.0,>=3.0.2 in /usr/local/lib/python3.6/dist-packages (from requests->kaggle) (3.0.4)
Requirement already satisfied, skipping upgrade: idna<2.9,>=2.5 in /usr/local/lib/python3.6/dist-packages (from requests->kaggle) (2.8)
Requirement already satisfied, skipping upgrade: text-unidecode>=1.3 in /usr/local/lib/python3.6/dist-packages (from python-slugify->kaggle) (1.3)
Requirement already up-to-date: pandas in /usr/local/lib/python3.6/dist-packages (1.0.3)
Requirement already satisfied, skipping upgrade: python-dateutil>=2.6.1 in /usr/local/lib/python3.6/dist-packages (from pandas) (2.8.1)
Requirement already satisfied, skipping upgrade: numpy>=1.13.3 in /usr/local/lib/python3.6/dist-packages (from pandas) (1.18.2)
Requirement already satisfied, skipping upgrade: pytz>=2017.2 in /usr/local/lib/python3.6/dist-packages (from pandas) (2018.9)
Requirement already satisfied, skipping upgrade: six>=1.5 in /usr/local/lib/python3.6/dist-packages (from python-dateutil>=2.6.1->pandas) (1.12.0)
Collecting catboost
[?25l Downloading https://files.pythonhosted.org/packages/94/ec/12b9a42b2ea7dfe5b602f235692ab2b61ee1334ff34334a15902272869e8/catboost-0.22-cp36-none-manylinux1_x86_64.whl (64.4MB)
[K |████████████████████████████████| 64.4MB 61kB/s
[?25hRequirement already satisfied, skipping upgrade: matplotlib in /usr/local/lib/python3.6/dist-packages (from catboost) (3.2.1)
Requirement already satisfied, skipping upgrade: plotly in /usr/local/lib/python3.6/dist-packages (from catboost) (4.4.1)
Requirement already satisfied, skipping upgrade: numpy>=1.16.0 in /usr/local/lib/python3.6/dist-packages (from catboost) (1.18.2)
Requirement already satisfied, skipping upgrade: graphviz in /usr/local/lib/python3.6/dist-packages (from catboost) (0.10.1)
Requirement already satisfied, skipping upgrade: scipy in /usr/local/lib/python3.6/dist-packages (from catboost) (1.4.1)
Requirement already satisfied, skipping upgrade: pandas>=0.24.0 in /usr/local/lib/python3.6/dist-packages (from catboost) (1.0.3)
Requirement already satisfied, skipping upgrade: six in /usr/local/lib/python3.6/dist-packages (from catboost) (1.12.0)
Requirement already satisfied, skipping upgrade: python-dateutil>=2.1 in /usr/local/lib/python3.6/dist-packages (from matplotlib->catboost) (2.8.1)
Requirement already satisfied, skipping upgrade: kiwisolver>=1.0.1 in /usr/local/lib/python3.6/dist-packages (from matplotlib->catboost) (1.2.0)
Requirement already satisfied, skipping upgrade: pyparsing!=2.0.4,!=2.1.2,!=2.1.6,>=2.0.1 in /usr/local/lib/python3.6/dist-packages (from matplotlib->catboost) (2.4.7)
Requirement already satisfied, skipping upgrade: cycler>=0.10 in /usr/local/lib/python3.6/dist-packages (from matplotlib->catboost) (0.10.0)
Requirement already satisfied, skipping upgrade: retrying>=1.3.3 in /usr/local/lib/python3.6/dist-packages (from plotly->catboost) (1.3.3)
Requirement already satisfied, skipping upgrade: pytz>=2017.2 in /usr/local/lib/python3.6/dist-packages (from pandas>=0.24.0->catboost) (2018.9)
Installing collected packages: catboost
Successfully installed catboost-0.22
###Markdown
Get main data from Kaggle As we download from Kaggle we need to provide Kaggle authetification cridentials. We will use the method suggested [here](https://gist.github.com/jayspeidell/d10b84b8d3da52df723beacc5b15cb27gistcomment-2814834). Replace KAGGLE_USERNAME and KAGGLE_KEY with your own.
###Code
os.environ['KAGGLE_USERNAME'] = "XXX"
os.environ['KAGGLE_KEY'] = "YYY"
###Output
_____no_output_____
###Markdown
Let's download the data now.
###Code
kaggle_data_name = 'covid19-global-forecasting-week-1'
data_folder = os.path.join(pathlib.Path.home(), 'datasets', kaggle_data_name)
!mkdir -p ${data_folder}
!kaggle competitions download -c {kaggle_data_name} --path {data_folder}
###Output
Warning: Looks like you're using an outdated API Version, please consider updating (server 1.5.6 / client 1.5.4)
Downloading submission.csv to /root/datasets/covid19-global-forecasting-week-1
0% 0.00/108k [00:00<?, ?B/s]
100% 108k/108k [00:00<00:00, 23.0MB/s]
Downloading train.csv to /root/datasets/covid19-global-forecasting-week-1
0% 0.00/947k [00:00<?, ?B/s]
100% 947k/947k [00:00<00:00, 62.2MB/s]
Downloading test.csv to /root/datasets/covid19-global-forecasting-week-1
0% 0.00/540k [00:00<?, ?B/s]
100% 540k/540k [00:00<00:00, 165MB/s]
###Markdown
Let's check that data is ok.
###Code
!ls -l {data_folder}
###Output
total 1600
-rw-r--r-- 1 root root 111051 Apr 19 14:10 submission.csv
-rw-r--r-- 1 root root 552851 Apr 19 14:10 test.csv
-rw-r--r-- 1 root root 969831 Apr 19 14:10 train.csv
###Markdown
Let's load data to pandas.DataFrame.
###Code
original_train_df = pd.read_csv(os.path.join(data_folder,'train.csv'), parse_dates=['Date'])
original_train_df.head()
original_test_df = pd.read_csv(os.path.join(data_folder,'test.csv'), parse_dates=['Date'])
original_test_df.head()
###Output
_____no_output_____
###Markdown
We will concatenate original train and test dataframes for easier time delay embedding features calculations.Original train and test data intersect by dates. We will use data from original train in the concatenated dataset for such dates.
###Code
last_original_train_date = original_train_df['Date'].max()
print ('last_original_train_date = ', last_original_train_date)
print ('original_test_df.shape =', original_test_df.shape)
original_test_wo_train_df = original_test_df.drop(
index=original_test_df[original_test_df['Date'] <= last_original_train_date].index
)
print ('original_test_wo_train_df.shape =', original_test_wo_train_df.shape)
# recreate index bacause we will need unique index values later
main_df = pd.concat([original_train_df, original_test_wo_train_df], ignore_index=True)
###Output
last_original_train_date = 2020-03-24 00:00:00
original_test_df.shape = (12212, 6)
original_test_wo_train_df.shape = (8520, 6)
###Markdown
There are special `Provinces` that are in fact data from Cruise ships with `Country` information. Make this ships ``Countries`` and passengers origin country a ``Province/State`` (for proper hierarchy).
###Code
from_cruise_ships = main_df['Province/State'].isin(['From Diamond Princess', 'Grand Princess'])
main_df.loc[from_cruise_ships, ['Province/State','Country/Region']] = main_df.loc[from_cruise_ships, ['Country/Region','Province/State']].values
main_df[main_df['Country/Region'].isin(['From Diamond Princess', 'Grand Princess'])]
###Output
_____no_output_____
###Markdown
Feature engineering Time delay embedding features ConfirmedCases and Fatalities are transformed to the logarithmic scale ( log(1+x) to be precise ). Add log(1+x) - transformed number of new cases and new fatalities. Logarithmic scale is natural for this task as the growth of this values is typically exponential.Then add their historic values as (LogNewCases_prev_day_X, LogNewFataities_prev_day_X, X = 1 to 30) features.Some locations contain broken data (Confirmed Cases or Fatalities contain decreasing values), remove them from data.
###Code
main_df.sort_values(by='Date', inplace=True)
location_columns = ['Country/Region','Province/State']
# row selection functions in pandas do not like nans
for column in location_columns:
main_df[column].fillna('', inplace=True)
days_history_size = 30
def is_cumulative(increment_series):
for v in increment_series:
if (not np.isnan(v)) and (v < 0):
return False
return True
print ('data size before removing bad data = ', len(main_df))
for field in ['LogNewConfirmedCases', 'LogNewFatalities']:
main_df[field] = np.nan
for prev_day in range(1, days_history_size + 1):
main_df[field + '_prev_day_%s' % prev_day] = np.nan
for location_name, location_df in main_df.groupby(['Country/Region', 'Province/State']):
for field in ['ConfirmedCases', 'Fatalities']:
new_values = location_df[field].values
new_values[1:] -= new_values[:-1]
if not is_cumulative(new_values):
print ('%s for %s, %s is not valid cumulative series, drop it' % ((field,) + location_name))
main_df.drop(index=location_df.index, inplace=True)
break
log_new_values = np.log1p(new_values)
main_df.loc[location_df.index, 'LogNew' + field] = log_new_values
for prev_day in range(1, days_history_size + 1):
main_df.loc[location_df.index[prev_day:], 'LogNew%s_prev_day_%s' % (field, prev_day)] = (
log_new_values[:-prev_day]
)
print ('data size after removing bad data = ', len(main_df))
main_df.head()
###Output
data size before removing bad data = 26412
ConfirmedCases for Australia, Northern Territory is not valid cumulative series, drop it
ConfirmedCases for Australia, Queensland is not valid cumulative series, drop it
ConfirmedCases for Azerbaijan, is not valid cumulative series, drop it
ConfirmedCases for Bahrain, is not valid cumulative series, drop it
Fatalities for Canada, Quebec is not valid cumulative series, drop it
ConfirmedCases for China, Guizhou is not valid cumulative series, drop it
ConfirmedCases for France, France is not valid cumulative series, drop it
ConfirmedCases for France, Saint Barthelemy is not valid cumulative series, drop it
ConfirmedCases for Grand Princess, US is not valid cumulative series, drop it
ConfirmedCases for Guyana, is not valid cumulative series, drop it
Fatalities for Iceland, is not valid cumulative series, drop it
Fatalities for India, is not valid cumulative series, drop it
ConfirmedCases for Japan, is not valid cumulative series, drop it
Fatalities for Kazakhstan, is not valid cumulative series, drop it
ConfirmedCases for Lebanon, is not valid cumulative series, drop it
ConfirmedCases for Montenegro, is not valid cumulative series, drop it
Fatalities for Philippines, is not valid cumulative series, drop it
Fatalities for Russia, is not valid cumulative series, drop it
Fatalities for Slovakia, is not valid cumulative series, drop it
ConfirmedCases for US, Nevada is not valid cumulative series, drop it
ConfirmedCases for US, Utah is not valid cumulative series, drop it
ConfirmedCases for US, Washington is not valid cumulative series, drop it
data size after removing bad data = 24366
###Markdown
Day feature Now, add simple linear Day feature
###Code
first_date = min(main_df['Date'])
main_df['Day'] = (main_df['Date'] - first_date).dt.days.astype('int32')
###Output
_____no_output_____
###Markdown
WeekDay feature Let's add WeekDay feature
###Code
main_df['WeekDay'] = main_df['Date'].transform(lambda d: d.weekday())
###Output
_____no_output_____
###Markdown
Days since Xth Confirmed case and Xth Fatality features Add Days since Xth Confirmed case and Xth Fatality (where X = 1, 10, 100) features
###Code
thresholds = [1, 10, 100]
for threshold in thresholds:
main_df['Days_since_ConfirmedCases=%s' % threshold] = np.nan
main_df['Days_since_Fatalities=%s' % threshold] = np.nan
for location_name, location_df in main_df.groupby(['Country/Region', 'Province/State']):
for field in ['ConfirmedCases', 'Fatalities']:
for threshold in thresholds:
first_day = location_df['Day'].loc[location_df[field] >= threshold].min()
if not np.isnan(first_day):
main_df.loc[location_df.index, 'Days_since_%s=%s' % (field, threshold)] = (
location_df['Day'].transform(lambda day: -1 if (day < first_day) else (day - first_day))
)
###Output
_____no_output_____
###Markdown
'Distance to Origin' feature. Chinese province of Hubei is the original epicentre of the pandemic, so add a feature that represents distance from the region of the sample to the epidemic's origin.
###Code
def get_hubei_coords(df):
for index, row in df.iterrows():
if row['Province/State'] == 'Hubei':
return (row['Lat'], row['Long'])
raise Exception('Hubei not found in data')
origin_coords = get_hubei_coords(main_df)
main_df['Distance_to_origin'] = main_df.apply(
lambda row: geopy.distance.distance((row['Lat'], row['Long']), origin_coords).km,
axis='columns'
)
###Output
_____no_output_____
###Markdown
Functions for merging external data Let's add some data from other sources.But first, we will need a couple of helper functions with common functionality.World bank data, UN World Population Project data and the main dataset use different names for some of the countries so we will need to remap country names in external datasets for correct merging.
###Code
def merge_with_column_drop(left_df, right_df, right_df_column='Country'):
df = pd.merge(
left=left_df,
right=right_df,
how='left',
left_on='Country/Region',
right_on=right_df_column
)
df.drop(columns=right_df_column, inplace=True)
return df
def remap_country_name_from_world_bank_to_main_df_name(country):
return {
'Bahamas, The': 'The Bahamas',
'Brunei Darussalam': 'Brunei',
'Congo, Rep.': 'Congo (Brazzaville)',
'Congo, Dem. Rep.': 'Congo (Kinshasa)',
'Czech Republic': 'Czechia',
'Egypt, Arab Rep.': 'Egypt',
'Iran, Islamic Rep.': 'Iran',
'Korea, Rep.': 'Korea, South',
'Kyrgyz Republic': 'Kyrgyzstan',
'Russian Federation': 'Russia',
'Slovak Republic': 'Slovakia',
'St. Lucia': 'Saint Lucia',
'St. Vincent and the Grenadines': 'Saint Vincent and the Grenadines',
'United States': 'US',
'Venezuela, RB': 'Venezuela',
}.get(country, country)
def remap_country_name_from_un_wpp_to_main_df_name(country):
return {
'Bahamas': 'The Bahamas',
'Bolivia (Plurinational State of)': 'Bolivia',
'Brunei Darussalam': 'Brunei',
'China, Taiwan Province of China': 'Taiwan*',
'Congo' : 'Congo (Brazzaville)',
'Côte d\'Ivoire': 'Cote d\'Ivoire',
'Democratic Republic of the Congo': 'Congo (Kinshasa)',
'Gambia': 'The Gambia',
'Iran (Islamic Republic of)': 'Iran',
'Republic of Korea': 'Korea, South',
'Republic of Moldova': 'Moldova',
'Réunion': 'Reunion',
'Russian Federation': 'Russia',
'United Republic of Tanzania': 'Tanzania',
'United States of America': 'US',
'Venezuela (Bolivarian Republic of)': 'Venezuela',
'Viet Nam': 'Vietnam'
}.get(country, country)
# for read_csv
world_bank_converters={'Country Name': remap_country_name_from_world_bank_to_main_df_name}
un_wpp_converters={'Location': remap_country_name_from_un_wpp_to_main_df_name}
###Output
_____no_output_____
###Markdown
Country Area Let's add Country Area feature. We will use data from World Bank for that.
###Code
!mkdir -p area
os.chdir('area')
!wget -nc http://api.worldbank.org/v2/en/indicator/AG.LND.TOTL.K2?downloadformat=csv -O area.zip
!unzip -n area.zip
!ls -l .
!head API_AG.LND.TOTL.K2_DS2_en_csv_v2_937279.csv
area_df = pd.read_csv('./API_AG.LND.TOTL.K2_DS2_en_csv_v2_937279.csv', skiprows=4, converters=world_bank_converters)
os.chdir('..')
area_df.head()
###Output
_____no_output_____
###Markdown
We will use the last available data.
###Code
year_columns = [str(year) for year in range(1960, 2020)]
area_df['CountryArea'] = area_df[year_columns].apply(
lambda row: row[row.last_valid_index()] if row.last_valid_index() else np.nan,
axis='columns'
)
###Output
_____no_output_____
###Markdown
Drop unneeded columns
###Code
area_df = area_df[['Country Name', 'CountryArea']]
area_df.head()
###Output
_____no_output_____
###Markdown
Now, merge this data with main data by country.
###Code
main_df = merge_with_column_drop(
main_df,
area_df,
right_df_column='Country Name'
)
main_df.head()
###Output
_____no_output_____
###Markdown
Country population features Now, let's add country population data from UN.
###Code
!mkdir -p population
os.chdir('population')
!wget -nc https://population.un.org/wpp/Download/Files/1_Indicators%20\(Standard\)/CSV_FILES/WPP2019_PopulationByAgeSex_Medium.csv
!head ./WPP2019_PopulationByAgeSex_Medium.csv
population_df = pd.read_csv(
'WPP2019_PopulationByAgeSex_Medium.csv',
usecols=['Location', 'Time', 'AgeGrp', 'PopMale', 'PopFemale', 'PopTotal'],
parse_dates=['Time'],
converters=un_wpp_converters
)
os.chdir('..')
population_df.head()
###Output
_____no_output_____
###Markdown
We will use only recent (no earlier than 2014) data
###Code
population_df = population_df.loc[
(population_df['Time'] >= pd.Timestamp(2014,1,1))
& (population_df['Time'] <= pd.Timestamp(2019,1,1))
]
population_df.head()
###Output
_____no_output_____
###Markdown
Aggregate data by 20-year groups and also compute cumulative population.
###Code
aggegated_population_df = pd.DataFrame()
for (location, time), group_df in population_df.groupby(['Location', 'Time']):
# by ['Pop_0-20', 'Pop_20-40', 'Pop_40-60', 'Pop_60-80', 'Pop_80+']
pop_by_age_groups = [0] * 5
pop_male = 0
pop_female = 0
for _, row in group_df.iterrows():
age_grp_start = int( re.split(r'[\-\+]', row['AgeGrp'])[0] )
pop_by_age_groups[min(age_grp_start // 20, 4)] += row['PopMale'] + row['PopFemale']
pop_male += row['PopMale']
pop_female += row['PopFemale']
""
aggegated_population_df = aggegated_population_df.append(
{
'Location': location,
'Time': time,
'CountryPop_0-20': pop_by_age_groups[0],
'CountryPop_20-40': pop_by_age_groups[1],
'CountryPop_40-60': pop_by_age_groups[2],
'CountryPop_60-80': pop_by_age_groups[3],
'CountryPop_80+': pop_by_age_groups[4],
'CountryPopMale': pop_male,
'CountryPopFemale': pop_female,
'CountryPopTotal': pop_male + pop_female
},
ignore_index=True
)
aggegated_population_df.head()
###Output
_____no_output_____
###Markdown
Select the most recent data.
###Code
aggegated_population_df = aggegated_population_df.sort_values('Time').drop_duplicates(['Location'], keep='last')
aggegated_population_df.head()
###Output
_____no_output_____
###Markdown
Now, drop Time and join with main data
###Code
aggegated_population_df.drop(columns='Time', inplace=True)
main_df = merge_with_column_drop(
main_df,
aggegated_population_df,
right_df_column='Location'
)
main_df.head()
###Output
_____no_output_____
###Markdown
Country Population density feature With both Area and Population data we can now compute Population density feature.
###Code
main_df['CountryPopDensity'] = main_df['CountryPopTotal'] / main_df['CountryArea']
###Output
_____no_output_____
###Markdown
Country Smoking rate feature Let's also add Smoking rate by Country data because smoking can influence the severity of respiratory diseases.
###Code
!mkdir -p smoking
os.chdir('smoking')
!wget -nc http://api.worldbank.org/v2/en/indicator/SH.PRV.SMOK?downloadformat=csv -O smoking.zip
!unzip -n smoking.zip
!ls -l .
!head API_SH.PRV.SMOK_DS2_en_csv_v2_937317.csv
smoking_df = pd.read_csv('./API_SH.PRV.SMOK_DS2_en_csv_v2_937317.csv', skiprows=4)
os.chdir('..')
smoking_df.head()
###Output
_____no_output_____
###Markdown
We will use the last available data from recent years (2010 and later).
###Code
recent_year_columns = [str(year) for year in range(2010, 2020)]
smoking_df['CountrySmokingRate'] = smoking_df[recent_year_columns].apply(
lambda row: row[row.last_valid_index()] if row.last_valid_index() else np.nan,
axis='columns'
)
###Output
_____no_output_____
###Markdown
Drop unneeded columns
###Code
smoking_df = smoking_df[['Country Name', 'CountrySmokingRate']]
smoking_df.head()
###Output
_____no_output_____
###Markdown
Now, merge this data with main data by country.
###Code
main_df = merge_with_column_drop(
main_df,
smoking_df,
right_df_column='Country Name'
)
main_df.head()
###Output
_____no_output_____
###Markdown
Country hospital beds per 1000 people Let's add some information about countries' health system.We will add the number of hospital beds per 1000 people first.
###Code
!mkdir -p hospital_beds
os.chdir('hospital_beds')
!wget -nc http://api.worldbank.org/v2/en/indicator/SH.MED.BEDS.ZS?downloadformat=csv -O hospital_beds.zip
!unzip -n hospital_beds.zip
!ls -l .
!head API_SH.MED.BEDS.ZS_DS2_en_csv_v2_935968.csv
hospital_beds_df = pd.read_csv('./API_SH.MED.BEDS.ZS_DS2_en_csv_v2_935968.csv', skiprows=4)
os.chdir('..')
hospital_beds_df.head()
###Output
_____no_output_____
###Markdown
We will use the last available data from recent years (2010 and later).
###Code
recent_year_columns = [str(year) for year in range(2010, 2020)]
hospital_beds_df['CountryHospitalBedsRate'] = hospital_beds_df[recent_year_columns].apply(
lambda row: row[row.last_valid_index()] if row.last_valid_index() else np.nan,
axis='columns'
)
###Output
_____no_output_____
###Markdown
Drop unneeded columns
###Code
hospital_beds_df = hospital_beds_df[['Country Name', 'CountryHospitalBedsRate']]
hospital_beds_df.head()
###Output
_____no_output_____
###Markdown
Now, merge this data with main data by country.
###Code
main_df = merge_with_column_drop(
main_df,
hospital_beds_df,
right_df_column='Country Name'
)
main_df.head()
###Output
_____no_output_____
###Markdown
Country Health Expenditure Now, let's add Health expenditure per capita, PPP (in current international $) feature.
###Code
!mkdir -p health_expenditure
os.chdir('health_expenditure')
!wget -nc http://api.worldbank.org/v2/en/indicator/SH.XPD.CHEX.PP.CD?downloadformat=csv -O health_expenditure.zip
!unzip -n health_expenditure.zip
!ls -l .
!head API_SH.XPD.CHEX.PP.CD_DS2_en_csv_v2_939222.csv
health_expenditure_df = pd.read_csv('./API_SH.XPD.CHEX.PP.CD_DS2_en_csv_v2_939222.csv', skiprows=4)
os.chdir('..')
health_expenditure_df.head()
###Output
_____no_output_____
###Markdown
We will use the last available data from recent years (2010 and later).
###Code
recent_year_columns = [str(year) for year in range(2010, 2020)]
health_expenditure_df['CountryHealthExpenditurePerCapitaPPP'] = health_expenditure_df[recent_year_columns].apply(
lambda row: row[row.last_valid_index()] if row.last_valid_index() else np.nan,
axis='columns'
)
###Output
_____no_output_____
###Markdown
Drop unneeded columns
###Code
health_expenditure_df = health_expenditure_df[['Country Name', 'CountryHealthExpenditurePerCapitaPPP']]
health_expenditure_df.head()
###Output
_____no_output_____
###Markdown
Now, merge this data with main data by country.
###Code
main_df = merge_with_column_drop(
main_df,
health_expenditure_df,
right_df_column='Country Name'
)
main_df.head()
###Output
_____no_output_____
###Markdown
Prepare data for training Split data to train, validation and test by date.Construct features (by removing label and non-features data from the original dataframe) and labels for train, validation and test parts.
###Code
last_train_date = pd.Timestamp(2020, 3, 11)
last_eval_date = pd.Timestamp(2020, 3, 24)
last_test_date = pd.Timestamp(2020, 4, 23)
train_df = main_df[main_df['Date'] <= last_train_date].copy()
eval_df = main_df[(main_df['Date'] > last_train_date) & (main_df['Date'] <= last_eval_date)].copy()
test_df = main_df[main_df['Date'] > last_eval_date].copy()
# return features_df, labels
def preprocess_df(df):
labels = df[['LogNewConfirmedCases', 'LogNewFatalities']].copy()
features_df = df.drop(
columns=['Id', 'ForecastId', 'ConfirmedCases', 'LogNewConfirmedCases', 'Fatalities', 'LogNewFatalities', 'Date']
).copy()
return features_df, labels
train_features_df, train_labels = preprocess_df(train_df)
eval_features_df, eval_labels = preprocess_df(eval_df)
test_features_df, _ = preprocess_df(test_df)
train_features_df.head()
###Output
_____no_output_____
###Markdown
Categorical features
###Code
cat_features = ['Province/State', 'Country/Region']
###Output
_____no_output_____
###Markdown
Models training Now, construct models that predict LogNewConfirmedCases and LogNewFatalities for the next day.We will use RMSE as an objective and evaluation metric for training, that will correspond to RMSLE (Root Mean Square Logarithmic Error) metric for NewConfirmedCases and NewFatalities.$$RMSLE(labels, predictions) = \sqrt{\frac{1}{n}\sum_{i=1}^{n}{\Big(log(labels_i + 1) - log(predictions_i + 1)\Big)^2}}$$Such metric is natural for this task (model should weight errors between 1000 and 1100 and 100000 and 110000 approximately the same).For prediction values in the original scale model prediction output should be transformed back to the original scale with the reverse transformation (can be preformed using numpy.expm1 function).We'll train models for 1000 iterations.
###Code
iterations = 1000
###Output
_____no_output_____
###Markdown
CatBoost allows Categorical features values can be used as is in DataFrame.
###Code
print ('catboost version', cb.__version__)
catboost_models = {}
for prediction_name in ['LogNewConfirmedCases', 'LogNewFatalities']:
model = cb.CatBoostRegressor(
has_time=True,
iterations=iterations
)
model.fit(
train_features_df,
train_labels[prediction_name],
eval_set=(eval_features_df, eval_labels[prediction_name]),
cat_features=cat_features,
verbose=100 # print metrics each 100 iterations
)
catboost_models[prediction_name] = model
print ('CatBoost: prediction of %s: RMSLE on test = %s' % (prediction_name, model.evals_result_['validation']['RMSE'][-1]))
###Output
catboost version 0.22
Learning rate set to 0.074929
0: learn: 0.8444130 test: 2.2561020 best: 2.2561020 (0) total: 79.4ms remaining: 1m 19s
100: learn: 0.2849727 test: 0.8850558 best: 0.8850558 (100) total: 2.2s remaining: 19.6s
200: learn: 0.2518107 test: 0.8737440 best: 0.8737323 (198) total: 4.25s remaining: 16.9s
300: learn: 0.2288005 test: 0.8734222 best: 0.8722002 (273) total: 6.27s remaining: 14.6s
400: learn: 0.2078918 test: 0.8766954 best: 0.8720236 (318) total: 8.37s remaining: 12.5s
500: learn: 0.1899614 test: 0.8815886 best: 0.8720236 (318) total: 10.5s remaining: 10.4s
600: learn: 0.1765715 test: 0.8839863 best: 0.8720236 (318) total: 12.6s remaining: 8.36s
700: learn: 0.1650007 test: 0.8869404 best: 0.8720236 (318) total: 14.7s remaining: 6.26s
800: learn: 0.1547044 test: 0.8882405 best: 0.8720236 (318) total: 16.8s remaining: 4.17s
900: learn: 0.1458812 test: 0.8884831 best: 0.8720236 (318) total: 18.9s remaining: 2.08s
999: learn: 0.1379156 test: 0.8917756 best: 0.8720236 (318) total: 21s remaining: 0us
bestTest = 0.8720236025
bestIteration = 318
Shrink model to first 319 iterations.
CatBoost: prediction of LogNewConfirmedCases: RMSLE on test = 0.8917755772136071
Learning rate set to 0.074929
0: learn: 0.2846849 test: 0.7751307 best: 0.7751307 (0) total: 22.4ms remaining: 22.4s
100: learn: 0.0583554 test: 0.3493678 best: 0.3493678 (100) total: 2.08s remaining: 18.6s
200: learn: 0.0461636 test: 0.3449327 best: 0.3449327 (200) total: 4.14s remaining: 16.4s
300: learn: 0.0359213 test: 0.3416266 best: 0.3415318 (297) total: 6.24s remaining: 14.5s
400: learn: 0.0274293 test: 0.3410587 best: 0.3410214 (398) total: 8.34s remaining: 12.5s
500: learn: 0.0217875 test: 0.3409957 best: 0.3409543 (498) total: 10.4s remaining: 10.4s
600: learn: 0.0171285 test: 0.3406960 best: 0.3405870 (550) total: 12.6s remaining: 8.33s
700: learn: 0.0140178 test: 0.3402807 best: 0.3402373 (692) total: 14.7s remaining: 6.25s
800: learn: 0.0115103 test: 0.3402232 best: 0.3402147 (798) total: 16.8s remaining: 4.17s
900: learn: 0.0095381 test: 0.3404695 best: 0.3402147 (798) total: 18.9s remaining: 2.08s
999: learn: 0.0079940 test: 0.3405940 best: 0.3402147 (798) total: 21s remaining: 0us
bestTest = 0.3402146803
bestIteration = 798
Shrink model to first 799 iterations.
CatBoost: prediction of LogNewFatalities: RMSLE on test = 0.34059397104647504
###Markdown
Feature importance in the models Let's look at feature importances.For CatBoost use [PredictionValuesChange](https://catboost.ai/docs/concepts/fstr.htmlfstr__regular-feature-importance).We'll print only 25 most important features for clarity.
###Code
for prediction_name in ['LogNewConfirmedCases', 'LogNewFatalities']:
print ('\nCatBoost: prediction of %s. Feature importance. Type=PredictionValuesChange' % prediction_name)
print (
catboost_models[prediction_name].get_feature_importance(
type=cb.EFstrType.PredictionValuesChange,
prettified=True
).head(25).to_string()
)
###Output
CatBoost: prediction of LogNewConfirmedCases. Feature importance. Type=PredictionValuesChange
Feature Id Importances
0 LogNewConfirmedCases_prev_day_1 22.109213
1 LogNewConfirmedCases_prev_day_2 17.254841
2 Days_since_ConfirmedCases=10 11.929176
3 Days_since_ConfirmedCases=1 11.340144
4 LogNewConfirmedCases_prev_day_3 6.377358
5 Day 2.969185
6 Days_since_Fatalities=10 2.720522
7 Days_since_ConfirmedCases=100 1.865486
8 LogNewConfirmedCases_prev_day_4 1.732866
9 Distance_to_origin 1.259510
10 Days_since_Fatalities=100 1.179583
11 LogNewConfirmedCases_prev_day_10 1.139283
12 LogNewConfirmedCases_prev_day_24 1.134655
13 LogNewConfirmedCases_prev_day_5 1.125969
14 CountryPop_60-80 0.963053
15 LogNewFatalities_prev_day_3 0.914145
16 CountryPop_40-60 0.747576
17 LogNewFatalities_prev_day_2 0.722008
18 Long 0.608342
19 Days_since_Fatalities=1 0.587399
20 LogNewConfirmedCases_prev_day_7 0.583528
21 Lat 0.536044
22 LogNewConfirmedCases_prev_day_8 0.527629
23 Province/State 0.495591
24 LogNewConfirmedCases_prev_day_27 0.444949
CatBoost: prediction of LogNewFatalities. Feature importance. Type=PredictionValuesChange
Feature Id Importances
0 Days_since_Fatalities=10 26.907773
1 Days_since_Fatalities=1 12.810340
2 LogNewFatalities_prev_day_2 8.786807
3 Days_since_Fatalities=100 5.176575
4 LogNewConfirmedCases_prev_day_9 2.796640
5 LogNewConfirmedCases_prev_day_2 2.743006
6 LogNewFatalities_prev_day_3 2.312393
7 CountryPopDensity 2.179349
8 LogNewConfirmedCases_prev_day_10 1.811663
9 CountryPopFemale 1.657966
10 LogNewFatalities_prev_day_1 1.542263
11 LogNewConfirmedCases_prev_day_1 1.466262
12 LogNewConfirmedCases_prev_day_8 1.398892
13 LogNewConfirmedCases_prev_day_11 1.350219
14 CountryPop_40-60 1.336757
15 Distance_to_origin 1.336277
16 LogNewFatalities_prev_day_6 1.303100
17 LogNewConfirmedCases_prev_day_3 1.186486
18 LogNewConfirmedCases_prev_day_7 1.135694
19 LogNewConfirmedCases_prev_day_6 1.100854
20 LogNewConfirmedCases_prev_day_14 1.001467
21 CountryPop_80+ 0.845002
22 LogNewFatalities_prev_day_9 0.793612
23 LogNewFatalities_prev_day_24 0.776731
24 LogNewFatalities_prev_day_26 0.720919
###Markdown
Create predictions for eval and test data. For forecasting further into the future (more than a single day) we will use an incremental (by days) approach:1. Predict Log New Confirmed Cases and Fatalities for the next unknown day.2. Confirmed Cases and Fatalities for this day are incremented from the previous day based on predicted New Confirmed Cases and Fatalities.3. Use predicted Log New Confirmed Cases and Fatalities for this day to initialize time delay embedding features corresponding to this day for future days.This procedure is then repeated for the new next prediction day and so on. Common function for eval and test:
###Code
def predict_for_dataset(df, features_df, prev_day_df, first_date, last_date, update_features_data):
df['PredictedLogNewConfirmedCases'] = np.nan
df['PredictedLogNewFatalities'] = np.nan
df['PredictedConfirmedCases'] = np.nan
df['PredictedFatalities'] = np.nan
for day in pd.date_range(first_date, last_date):
day_df = df[df['Date'] == day]
day_features_pool = cb.Pool(features_df.loc[day_df.index], cat_features=cat_features)
# predict LogNew* data
for prediction_type in ['LogNewConfirmedCases', 'LogNewFatalities']:
# prediction is imprecise and can produce negative values, clip them
df.loc[day_df.index, 'Predicted' + prediction_type] = np.maximum(
catboost_models[prediction_type].predict(day_features_pool),
0.0
)
day_predictions_df = df.loc[day_df.index][
location_columns + ['PredictedLogNewConfirmedCases', 'PredictedLogNewFatalities']
]
# update Predicted ConfirmedCases and Fatalities
for field in ['ConfirmedCases', 'Fatalities']:
prev_day_field = field if day == first_eval_date else ('Predicted' + field)
merged_df = day_predictions_df.merge(
right=prev_day_df[location_columns + [prev_day_field]],
how='inner',
on=location_columns
)
df.loc[day_df.index, 'Predicted' + field] = merged_df.apply(
lambda row: row[prev_day_field] + np.rint(np.expm1(row['PredictedLogNew' + field])),
axis='columns'
).values
if update_features_data:
# fill time delay embedding features based on this day for next days
for next_day in pd.date_range(day + pd.Timedelta(days=1), last_date):
next_day_features_df = features_df[df['Date'] == next_day]
merged_df = next_day_features_df[location_columns].merge(
right=day_predictions_df,
how='inner',
on=location_columns
)
prev_day_idx = (next_day - day).days
for prediction_type in ['LogNewConfirmedCases', 'LogNewFatalities']:
features_df.loc[next_day_features_df.index, prediction_type + '_prev_day_%s' % prev_day_idx] = (
merged_df['Predicted' + prediction_type].values
)
# select by day_df.index again to get Predicted* columns
prev_day_df = df.loc[day_df.index]
###Output
_____no_output_____
###Markdown
Create predictions for eval data
###Code
prev_day_df = train_df.loc[train_df['Date'] == last_train_date]
first_eval_date = last_train_date + pd.Timedelta(days=1)
predict_for_dataset(eval_df, eval_features_df, prev_day_df, first_eval_date, last_eval_date, update_features_data=False)
eval_df.head()
###Output
_____no_output_____
###Markdown
Create predictions for test data.
###Code
prev_day_df = eval_df.loc[eval_df['Date'] == last_eval_date]
first_test_date = last_eval_date + pd.Timedelta(days=1)
predict_for_dataset(test_df, test_features_df, prev_day_df, first_test_date, last_test_date, update_features_data=True)
test_df.head()
###Output
_____no_output_____
###Markdown
Plots with predictions Let's plot some example graphs with predictions.Plots will use data from reconcatenated main_df.
###Code
main_df = pd.concat([train_df, eval_df, test_df])
%matplotlib inline
import matplotlib.pyplot as plt
import matplotlib
def plot_graph(country_region, province_state, field, log_scale=True):
location_df = main_df.loc[
(main_df['Country/Region'] == country_region) & (main_df['Province/State'] == province_state)
]
if province_state:
title = '%s for %s, %s' % (field, country_region, province_state)
else:
title = '%s for %s' % (field, country_region)
plt.figure(figsize = (16, 10))
plt.suptitle(title, fontsize=14)
if log_scale:
plt.yscale('log')
for sub_field in [field, 'Predicted' + field]:
plt.plot(location_df['Date'], location_df[sub_field], label=sub_field)
# add vertical lines splitting train, test and eval parts of the graph
ax = plt.gca()
# the x coords of this transformation are data, and the
# y coord are axes
transform_for_text = matplotlib.transforms.blended_transform_factory(ax.transData, ax.transAxes)
plt.axvline(x=last_train_date, color='#000000')
plt.text(first_eval_date, 0.95, 'eval', transform = transform_for_text)
plt.axvline(x=last_eval_date, color='#000000')
plt.text(first_test_date, 0.95, 'test', transform = transform_for_text)
plt.legend()
plt.show()
plot_graph('US', 'Kansas', 'ConfirmedCases')
plot_graph('US', 'Kansas', 'Fatalities')
plot_graph('China', 'Hubei', 'ConfirmedCases')
plot_graph('China', 'Hubei', 'Fatalities')
plot_graph('Germany', '', 'ConfirmedCases', log_scale=False)
plot_graph('Germany', '', 'Fatalities', log_scale=False)
###Output
_____no_output_____ |
Python-API/apl/notebooks/31_Classification_Learn_and_Save.ipynb | ###Markdown
Python HANA ML APL Building a Predictive Model for Insurance Fraud Detection. Learn from historical Insurance Claims Create an HANA Dataframe for the training data
###Code
from hana_ml import dataframe as hd
conn = hd.ConnectionContext(userkey='MLMDA_KEY')
sql_cmd = 'SELECT * FROM "APL_SAMPLES"."AUTO_CLAIMS_FRAUD" ORDER BY CLAIM_ID'
hdf_train = hd.DataFrame(conn, sql_cmd)
hdf_train.head(6).collect()
###Output
_____no_output_____
###Markdown
Fit with APL Gradient Boosting
###Code
from hana_ml.algorithms.apl.gradient_boosting_classification import GradientBoostingBinaryClassifier
apl_model = GradientBoostingBinaryClassifier()
apl_model.set_params(other_train_apl_aliases={'APL/VariableAutoSelection':'true'})
apl_model.fit(hdf_train, label='IS_FRAUD', key='CLAIM_ID')
###Output
_____no_output_____
###Markdown
Model Reports
###Code
from hana_ml.visualizers.unified_report import UnifiedReport
UnifiedReport(apl_model).build().display()
df = apl_model.get_debrief_report('ClassificationRegression_VariablesExclusion').collect()
df = df[['Variable', 'Reason For Exclusion']]
df.style.hide_index()
my_filter = "\"Partition\" = 'Validation' and \"Indicator\" in ('AUC','F1 Score','Cohen''s kappa')"
df = apl_model.get_debrief_report('ClassificationRegression_Performance').filter(my_filter).collect()
df.drop('Oid', axis=1, inplace=True)
format_dict = {'Value':'{:,.3f}'}
df.style.format(format_dict).hide_index()
my_filter = "\"Partition\"='Validation'"
df = apl_model.get_debrief_report('BinaryTarget_Statistics').filter(my_filter).collect()
df.drop('Oid', axis=1, inplace=True)
format_dict = {'% Positive Weight':'{:,.1f}%', '% Negative Weight':'{:,.1f}%', 'Weight':'{:,.0f}'}
df.style.format(format_dict).hide_index()
###Output
_____no_output_____
###Markdown
Save the Trained Model
###Code
from hana_ml.model_storage import ModelStorage
model_storage = ModelStorage(connection_context=conn, schema='USER_APL')
apl_model.name = 'My Fraud Model'
model_storage.save_model(model=apl_model, if_exists='replace')
model_storage.list_models()
###Output
_____no_output_____ |
Aula 19/Lesson 19.ipynb | ###Markdown
1.0 Variables in Statistics 1.1 Introduction Previously, we discussed the details around **collecting data** for our analysis. In this lesson, we'll focus on understanding the structural parts of a data set, and how they're measured.Whether a **sample** or a **population**, a data set is generally an attempt to describe correctly a relatively small part of the world. The data set we worked with in the previous lesson describes basketball players and their performance in the season 2016-2017.Other data sets might attempt to describe the stock market, patient symptoms, stars from galaxies other than ours, movie ratings, customer purchases, and all sorts of other things.The things we want to describe usually have a myriad of properties. A human, for instance, besides the property of being a human, can also have properties like height, weight, age, name, hair color, gender, nationality, whether they're married or not, whether they have a job or not, etc.In practice, we limit ourselves to the properties relevant to the questions we want to answer, and to the properties that we can actually measure. Let's consider three rows at random from the basketball data set we've previously worked with:| | Name | Team | Pos | Height | Weight | BMI | Birth_Place | Birthdate ||------|-------------------|-----|--------|--------|------|-------------|-----------|-------------------|| 39 | Crystal Langhorne | SEA | F/C | 188 | 84.0 | 23.766410 | US | October 27, 1986 || 52 | Érika de Souza | SAN | C | 196 | 86.0 | 22.386506 | BR | September 3, 1982 || 102 | Nia Coffey | SAN | F | 185 | 77.0 | 22.498174 | US | May 21, 1995 |Each row describes an individual having a series of properties: name, team, position on the field, height, etc. For most properties, the values vary from row to row. All players have a height, for example, but the height values vary from player to player.The properties with varying values we call **variables**. The height property in our data set is an example of a variable. In fact, all the properties described in our data set are variables.A row in our data set describes the actual values that each variable takes for a given individual.Notice that this particular meaning of the "variable" concept is restricted to the domain of statistics. A variable in statistics is not the same as a [variable in programming](https://goo.gl/sHnwCW), or [other domains](https://en.wikipedia.org/wiki/Variable). 1.2 Quantitative and Qualitative Variables **Variables** in statistics can describe either **quantities**, or **qualities**.For instance, the **Height** variable in our data set describes how tall each player is. The **Age** variable describes how much time has passed since each player was born. The **MIN** variable describes how many minutes each player played in the **2016-2017** WNBA season.Generally, a variable that describes how much there is of something describes a **quantity**, and, for this reason, it's called a **quantitative variable**.Usually, quantitative variables describe a quantity using real numbers, but there are also cases when words are used instead. Height, for example, can be described using real numbers, like in our data set, but it can also be described using labels like "tall" or "short".A few variables in our data set clearly don't describe quantities. The Name variable, for instance, describes the name of each player. The Team variable describe what team each player belongs to. The College variable describes what college each player goes or went at.The Name, Team, and College variables describe for each individual a quality, that is, a property that is not quantitative. **Variables that describe qualities are called qualitative variables or categorical variables**. Generally, qualitative variables describe what or how something is.Usually, qualitative variables describe qualities using words, but numbers can also be used. For instance, the number of a player's shirt or the number of a racing car are described using numbers. The numbers don't bear any quantitative meaning though, they are just names, not quantities.In the diagram below we do a head-to-head comparison between qualitative and quantitative variables:**Exercise**We've selected a few variables from our data set. For each of the variables selected, indicate whether it's quantitative or qualitative.- We've already created a dictionary named **variables**. Each variable name is given as dictionary key.- If a variable is quantitative, then complete the value of the corresponding key with the string **'quantitative'**. If the variable is qualitative, the use the string **'qualitative'**.You can find useful documentation about each variable [here](https://www.basketball-reference.com/about/glossary.html) and [here](https://www.kaggle.com/jinxbe/wnba-player-stats-2017).
###Code
# put your code here
import pandas as pd
wnba = pd.read_csv('wnba.csv')
variables = {'Name': '', 'Team': '', 'Pos': '', 'Height': '', 'BMI': '',
'Birth_Place': '', 'Birthdate': '', 'Age': '', 'College': '', 'Experience': '',
'Games Played': '', 'MIN': '', 'FGM': '', 'FGA': '',
'3PA': '', 'FTM': '', 'FTA': '', 'FT%': '', 'OREB': '', 'DREB': '',
'REB': '', 'AST': '', 'PTS': ''}
###Output
_____no_output_____
###Markdown
1.3 Scales of Measurement The amount of information a variable provides depends on its nature (whether it's quantitative or qualitative), and on the way it's measured.For instance, if we analyze the **Team** variable for any two individuals:- We can tell whether or not the two individuals are different from each other with respect to the team they play.- But if there's a difference: - We can't tell the size of the difference. - We can't tell the direction of the difference - we can't say that team A is greater or less than team B.On the other side, if we analyze the **Height** variable: - We can tell whether or not two individuals are different. - If there's a difference: - We can tell the size of the difference. If player A has 190 cm and player B has 192 cm, then the difference between the two is 2 cm. - We can tell the direction of the different from each perspective: player A has 2 cm less than player B, and player B has 2 cm more than player A.The **Team** and **Height** variables provide different amounts of information because they have a different nature (one is qualitative, the other quantitative), and because they are measured differently.The system of rules that define how each variable is measured is called **scale of measurement** or, less often, **level of measurement**.In the next sections, we'll learn about a system of measurement made up of four different scales of measurement: **nominal**, **ordinal**, **interval**, and **ratio**. As we'll see, the characteristics of each scale pivot around three main questions:- Can we tell whether two individuals are different?- Can we tell the direction of the difference?- Can we tell the size of the difference? 1.4 The Nominal Scale In the previous section, we've discussed about the **Team** variable, and said that by examining its values we can tell whether two individuals are different or not, but we can't indicate the size and the direction of the difference.The **Team** variable is an example of a variable measured on a **nominal scale**. For any variable measured on a **nominal scale**:- We can tell whether two individuals are different or not (with respect to that variable).- We can't say anything about the direction and the size of the difference.- We know that it can only describe qualities.When a qualitative variable is described with numbers, the principles of the nominal scale still hold. We can tell whether there's a difference or not between individuals, but we still can't say anything about the size and the direction of the difference.If basketball player A has the number 5 on her shirt, and player B has 8, we can tell they're different with respect to shirt numbers, but it doesn't make any sense to subtract the two values and quantify the difference as a 3. Nor it makes sense to say that B is greater than A. The numbers on the shirts are just identifiers here, they don't quantify anything.**Exercise**Inspect the data set, and find the variables measured on a **nominal scale**. In the cell below:- Add the variables measured on a **nominal scale** to a list named **nominal_scale**, and sort the elements in the list alphabetically (the sorting helps us with answer checking).- Notice that we've added a new variable named **Height_labels**. Instead of showing the height in centimeters, the new variable shows labels like "short", "medium", or "tall". By considering the principles that characterizes the **nominal scale**, think whether the new **Height_labels** variable should be included in your **nominal_scale list**.
###Code
# put your code here
wnba["Height_labels"] = pd.cut(wnba.Height,
bins=[0,170,180,250],
labels=["short","medium","tall"])
###Output
_____no_output_____
###Markdown
1.5 The Ordinal Scale In our last exercise, we've seen that the new **Height_labels** variable was showing labels like **"short"**, **"medium"**, or **"tall"**. By examining the values of this new variable, we can tell whether two individuals are different or not. But, unlike in the case of a **nominal scale**, we can also tell the direction of the difference. Someone who is assigned the label **"tall"** has a bigger height than someone assigned the label **"short"**.However, we still can't determine the size of the difference. This is an example of a variable measured on an **ordinal scale**.Generally, for any variable measured on an **ordinal scale**, we can tell whether individuals are different or not, we can also tell the direction of the difference, but we still can't determine the size of the difference.Variables measured on an **ordinal scale** can only be quantitative. Quantitative variables, however, can be measured on other scales too, as we'll see next in this sections.Common examples of variables measured on ordinal scales include ranks: ranks of athletes, of horses in a race, of people in various competitions, etc.For example, let's say we only know that athlete A finished second in a marathon, and athlete B finished third in the same race. We can immediately tell their performance is different, we know that athlete A finished faster, but we don't know how much faster. The difference between the two could be half a second, 12 minutes, half an hour, etc.Other common examples include measurements of subjective evaluations that are generally difficult or near to impossible to quantify with precision. For instance, when answering a survey about how much they like a new product, people may have to choose a label between "It's a disaster, I hate it", "I don't like it", "I like it a bit", "I really like it", "I simply love it".The values of the variables measured on an ordinal scale can be both words and numbers. When the values are numbers, they are usually ranks. And we still can't use the numbers to compute the size of the difference. We can't say how much faster an athlete was than another by simplify judging from their ranks.Whether a variable is quantitative or qualitative is independent of the way the variable is measured. The **Height** variable, for instance, is quantitative no matter how we measure it. The fact that we use words like "short" or "tall" doesn't change its underlying nature. The **Height** variable still describes a magnitude, but in a different way.**Exercise**- Consider the following sentences, and evaluate their **truth** value. If the sentence is **true**, than assign **True** to the corresponding variable (programming variable) in the cell below, otherwise assign **False**. Make sure you assign boolean values as answers, not strings. - Using the **Height_labels** variable only, we can tell whether player Kiah Stokes is taller than Riquna Williams. Assign your answer to a variable named **question1**. - We can measure the height difference between Kiah Stokes and Riquna Williams using the **Height_labels** variable. Assign your answer to **question2.** - The **Height_labels** and the **College** variables are both measured on an **ordinal scale.** Assign your answer to question3. - The **Games Played** variable is not measured on an **ordinal scale**. Assign your answer to **question4.** - The **Experience** variable is measured on an **ordinal scale.** Assign your answer to **question5.** - The **Height_labels** variable is **qualitative** because is measured using words. Assign your answer to **question6.**
###Code
# put your code here
###Output
_____no_output_____
###Markdown
1.6 The Interval and Ratio Scales We've seen in the case of the **Height** variable that the values have direction when measured on an ordinal scale. The downside is that we don't know the size of each interval between values, and because of this we can't determine the size of the difference. An alternative here is to measure the **Height** variable using real numbers, which will result in having well-defined intervals, which in turn will allow us to determine the size of the difference between any two values.A variable measured on a scale that preserves the order between values, and have well-defined intervals using real numbers, is an example of a variable measured either on an interval scale, or on a ratio scale.In practice, variables measured on interval or ratio scales are very common, if not the most common. Examples include:- Height measured with a numerical unit of measurement (like inches or centimeters).- Weight measured with a numerical unit of measurement (multiples and submultiples of grams, for instance).- Time measured with a numerical unit of measurement (multiples and submultiple of seconds, for example).- The price of various products measured with a numerical unit of measurement (like dollars, pounds, etc.). 1.7 The Difference Between Ratio and Interval Scales What sets apart **ratio scales** from **interval scales** is the nature of the zero point.On a **ratio scale**, the zero point means no quantity. For example, the **Weight** variable is measured on a **ratio scale**, which means that 0 grams indicate the absence of weight.On an **interval scale**, however, the zero point doesn't indicate the absence of a quantity. It actually indicates the presence of a quantity.To exemplify this case using our data set, we've used the **Weight** variable (measured on a ratio scale), and created a new variable that is measured on an **interval scale**. The new variable describes by how many kilograms the weight of a player is different than the average weight of the players in our data set. Here's a random sample that includes values from the new variable named **Weight_deviation**| _ | Name | Weight | Weight_deviation ||-----|---------------------|--------|------------------|| 35 | Clarissa dos Santos | 89.0 | 10.021127 || 3 | Alex Montgomery | 84.0 | 5.021127 || 111 | Renee Montgomery | 63.0 | -15.978873 || 85 | Layshia Clarendon | 64.0 | -14.978873 || 128 | Sugar Rodgers | 75.0 | -3.978873 |If a player had a value of 0 for our **Weight_deviation** variable (which is measured on an **interval scale**), that wouldn't mean the player has no weight. Rather, it'd mean that her weight is exactly the same as the mean. The mean of the Weight variable is roughly 78.98 kg, which means that the zero point in the **Weight_deviation** variable is equivalent to 78.98 kg.On the other side, a value of 0 for the Weight variable, which is measured on a **ratio scale**, indicates the absolute absence of weight.Another important difference between the two scales is given by the way we can measure the size of the differences.On a ratio scale, we can quantify the difference in two ways. One way is to measure a distance between any two points by simply subtracting one from another. The other way is to measure the difference in terms of ratios.For example, by doing a simple subtraction using the data in table above, we can tell that the difference (the distance) in weight between Clarissa dos Santos and Alex Montgomery is 5 kg. In terms of ratios, however, Clarissa dos Santos is roughly 1.06 (the result of 89 kg divided by 84 kg) heavier than Alex Montgomery. To give a straightforward example, if player A had 90 kg and player B had 45 kg, we could say that player A is two times (90 kg divided by 45 kg) heavier than player B.On an interval scale, however, we can measure meaningfully the difference between any two points only by finding the distance between them (by subtracting one point from another). If we look at the weight deviation variable, we can say there's a difference of 5 kg between Clarissa dos Santos and Alex Montgomery. However, if we took ratios, we'd have to say that Clarissa dos Santos is two times heavier than Alex Montgomery, which is not true.**Exercise**Examine the various variables of the data set, and find the ones that are measured on an **interval** or **ratio scale**.- For the variables measured on a **interval scale**, add their names as a string to a list named **interval**. Sort the list alphabetically.- For the variables measured on a **ratio scale**, add their names as a string to a list named **ratio**. Sort the list alphabetically.- Create the variable "Weight_deviation" according to previous explanation. So make sure you include that one too in one the lists.If you need to consult the documentation of the data set, you can look [here](https://www.kaggle.com/jinxbe/wnba-player-stats-2017), and [here](https://www.basketball-reference.com/about/glossary.html).
###Code
# put your code here
###Output
_____no_output_____
###Markdown
1.8 Common Examples of Interval Scales In practice, variables measured on an interval scale are relatively rare. Below we discuss two examples that are more common.Generally, points in time are indicated by variables measured on an interval scale. Let's say we want to indicate the point in time of the first manned mission on the Moon. If we want to use a ratio scale, our zero point must be meaningful and denote the absence of time. For this reason, we'd basically have to begin the counting at the very beginning of time.There are many problems with this approach. One of them is that we don't know with precision when time began (assuming time actually has a beginning), which means we don't know how far away in time we are from that zero point.To overcome this, we can set an arbitrarily zero point, and measure the distance in time from there. Customary, we use the [Anno domini system](https://en.wikipedia.org/wiki/Anno_Domini) where the zero point is arbitrarily set at the moment [Jesus](https://en.wikipedia.org/wiki/Jesus) was born. Using this system, we can say that the first manned mission on the Moon happened in 1969. This means that the event happened 1968 years after Jesus' birth (1968 because [there's no year 0 in the Anno domini system](https://en.wikipedia.org/wiki/Year_zero)).If points in time are measured on an interval scale, we need to avoid quantifying the difference in terms of ratio. For instance, it's not true that twice as much time has passed from year 1000 to year 2000.Another common example has to do with measuring temperature. In day to day life, we usually measure temperature on a Celsius or a Fahrenheit scale. These scales are examples of interval scales.0°C or 0°F are arbitrarily set zero points and don't indicate the absence of temperature. If 0°C or 0°F were meaningful zero points, temperatures below 0°C or 0°F wouldn't be possible. But we know that we can go way below 0°C or 0°F.If yesterday was 10°C, and today is 20°C, we can't say that today is twice as hot as yesterday. We can say, however, that today's temperature is 10°C more compared to yesterday.Temperature can be measured on a ratio scale too, and this is done using the Kelvin scale. 0 K (0 degrees Kelvin) is not set arbitrarily, and it indicates the lack of temperature. The temperature can't possibly drop below 0 K. 1.9 Discrete and Continuous Variables Previously in this mission we divided variables in two big categories: **quantitative** and **qualitative**. We've seen that **quantitative** variables can be measured on **ordinal**, **interval**, or **ratio scales**. In this screen, we zoom in on variables measured on interval and ratio scales.We've learned that variables measured on **interval** and **ratio scales** can only take real numbers as values. Let's consider a small random sample of our data set and focus on the **Weight** and **PTS** (total points) variables, which are both measured on a **ratio scale**.| _ | Name | Weight | PTS ||-----|---------------------|--------|-----|| 77 | Kayla Thornton | 86.0 | 32 || 16 | Asia Taylor | 76.0 | 31 || 80 | Kia Vaughn | 90.0 | 134 || 137 | Tierra Ruffin-Pratt | 83.0 | 225 || 12 | Amanda Zahui B. | 113.0 | 51 |The first two players scored 32 and 31 points, respectively. Between 32 and 31 points there's no possible intermediate value. Provided the measurements are correct, it's impossible to find a player having scored 31.5 or 31.2 points. In basketball, players can only score 1,2 or 3 points at a time, so the points variable can only be expressed in integers when measured on an interval or ratio scale.Generally, **if there's no possible intermediate value between any two adjacent values of a variable**, we call that variable **discrete.**Common examples of discrete variables include **counts of people in a class, a room, an office, a country, a house etc.** For instance, if we counted the number of people living in each house of a given street, the results of our counting can only be integers. For any given house, we could count 1, 3, 7, 0 people, but we cannot count 2.3 people, or 4.1378921521 people.In the table above, we can also see that the first player weighs 86 kg, and the second 76 kg. Between 86 kg and 76 kg, there's an infinity of possible values. In fact, between any two values of the Weight variable, there's an infinity of values.This is strongly counter-intuitive, so let's consider an example of two values that are relatively close together: 86kg and 87kg. Between these values we can have an infinity of values: 86.2 kg, 86.6 kg, 86.40 kg, 86.400001 kg, 86.400000000000001 kg, 86.400000000000000000000000000000000000000000001 kg, and so on.In the diagram below we consider values between 86 and 87 kg, and break down the interval in five equal parts. Then we take two values (86.2 and 86.8) from the interval 86 - 87, and break down the interval between these values (86.2 and 86.8) in five equal parts. Then we repeat the process for the interval 86.2 - 86.8. In fact, we could repeat the process infinitely.In practice, we limit ourselves to rounding the weights to a couple of decimal places either for practical purposes, either because the instruments we use to measure weight are imperfect.Generally, **if there's an infinity of values between any two values of a variable**, we call that variable **continuous.****Whether a variable is discrete or continuous is determined by the underlying nature of the variable being considered, and not by the values obtained from the measurement**. For instance, we can see in our data set that height only takes integer values:```python>> wnba['Height'].head()0 1831 1852 1703 1854 175Name: Height, dtype: int64```This doesn't make the Height variable discrete. It just tells us that the height is not measured with a great degree of precision.**Exercise**For every variable, indicate whether is continuous or discrete.- In the cell below, we've already extracted for you the names of the variables that are measured on ratio and interval scales. Every variable name is registered as a dictionary key.- If a variable is discrete, then assign the string **'discrete'** to its corresponding dictionary key.- If a variable is continuous, then assign the string **'continuous'** to its corresponding dictionary key.If you get stuck with any variable, take any two values and try to determine whether there's an infinity of possible values between them. If you need to consult the documentation of the data set, you can look [here](https://www.kaggle.com/jinxbe/wnba-player-stats-2017), and [here](https://www.basketball-reference.com/about/glossary.html).
###Code
# put your code here
ratio_interval_only = {'Height':'', 'Weight': '', 'BMI': '', 'Age': '', 'Games Played': '', 'MIN': '', 'FGM': '',
'FGA': '', 'FG%': '', '3PA': '', '3P%': '', 'FTM': '', 'FTA': '', 'FT%': '',
'OREB': '', 'DREB': '', 'REB': '', 'AST': '', 'STL': '', 'BLK': '', 'TO': '',
'PTS': '', 'DD2': '', 'TD3': '', 'Weight_deviation': ''}
###Output
_____no_output_____
###Markdown
1.9 Real Limits Let's consider these ten rows where players are recorded as having the same weight:| _ | Name | Weight ||-----|-------------------|--------|| 9 | Allison Hightower | 77.0 || 19 | Breanna Stewart | 77.0 || 21 | Bria Holmes | 77.0 || 33 | Chelsea Gray | 77.0 || 56 | Glory Johnson | 77.0 || 65 | Jessica Breland | 77.0 || 70 | Kaela Davis | 77.0 || 102 | Nia Coffey | 77.0 || 117 | Seimone Augustus | 77.0 || 132 | Tamera Young | 77.0 |Do all these players really have the exact same weight? Most likely, they don't. If the values were measured with a precision of one decimal, we'd probably see that the players have different weights. One player may weigh 76.7 kg, another 77.2 kg, another 77.1 kg.As an important parenthesis here, the weight values in the table above are all 77.0, and the trailing zero suggests a precision of one decimal point, but this is not the case. The values are automatically converted by **pandas** to **float64** because of one **NaN** value in the **Weight** column, and end up with a trailing zero, which gives the false impression of one decimal point precision. So a player was recorded to weigh 77 kg (zero decimals precision), not 77.0 kg (one decimal precision).Returning to our discussion, if we measure the weight with zero decimals precision (which we do in our data set), a player weighing 77.4 kg will be assigned the same weight (77 kg) as a player weighing 76.6 kg. So if a player is recorded to weigh 77 kg, we can only tell that her actual weight is somewhere between 76.5 kg and 77.5 kg. The value of 77 is not really a distinct value here. Rather, it's an **interval** of values.This principle applies to any possible numerical weight value. If a player is measured to weigh 76.5 kg, we can only tell that her weight is somewhere between 76.45 kg and 76.55 kg. If a player has 76.50 kg, we can only tell that her weight is somewhere between 76.495 kg and 76.505 kg. Because there can be an infinite number of decimals, we could continue this breakdown infinitely.Generally, every value of a continuous variable is an interval, no matter how precise the value is. The boundaries of an interval are sometimes called **real limits**. The lower boundary of the interval is called **lower real limit**, and the upper boundary is called **upper real limit.**In the figure above we can see for example that 88.5 is halfway between 88 and 89. If we got a measurement of 88.5 kg in practice, but we want only integers in our dataset (hence zero decimals precision), you might wonder whether to assign the value to 88 or 89 kg. The answer is that 88.5 kg is exactly halfway between 88 and 89 kg, and it doesn't necessarily belong to any of those two values. The assignment only depends on how you choose to round numbers: if you round up, then 88.5 kg will be assigned to 89 kg; if you round down, then the value will be assigned to 88 kg.**Exercise**Find the real limits for five values of the BMI (body mass index) variable.- We've already extracted the first five BMI values in the data set and rounded each off to a precision of three decimal places. We stored the values as dictionary keys in a dictionary named bmi.- For every BMI value write its real limits in a list and make the list a dictionary value that should correspond to the right dictionary key. The lower real limits should come first in each list. For example:```pythonbmi = {20: [19.5, 20.5], 21: [20.5, 21.5], 23: [22.5, 23.5], 24: [23.5, 24.5], 22: [21.5, 22.5]}```
###Code
# put your code here
bmi = {21.201: [],
21.329: [],
23.875: [],
24.543: [],
25.469: []}
###Output
_____no_output_____ |
OPC_P3_PREPA/projet3_nettoyage.ipynb | ###Markdown
Projet 3 : Concevez une application au service de la santé publique Import des données Chargement des bibliothèques
###Code
import numpy as np
import pandas as pd
import matplotlib.pyplot as plt
import seaborn as sns
from google.colab import drive
import os
sns.set()
###Output
_____no_output_____
###Markdown
Collab
###Code
drive.mount('/content/drive/')
#répertoir avec nos fichiers
images_dir = "/content/drive/My Drive/data/OPC03"
f_open = images_dir + "/en.openfoodfacts.org.products.csv"
#Import csv in a DataFrame
df = pd.read_csv(f_open
, header = 'infer'
, sep ="\t"
, low_memory=False)
print ('CSV Imported. Shape :')
df_save = df.copy() #keep a copy of original data
df.shape #(1017858 lignes et 175 colonnes)
print("Le jeu de données contient {} lignes et {} colonnes.".format(df.shape[0],df.shape[1]))
###Output
CSV Imported. Shape :
Le jeu de données contient 1017858 lignes et 175 colonnes.
###Markdown
Chargement du fichier de données
###Code
path_file = images_dir + "/en.openfoodfacts.org.products.csv"
# Print the size of the final database
print("The size of the initial database is: {} bytes.".format(os.path.getsize(path_file)))
###Output
The size of the initial database is: 2096985823 bytes.
###Markdown
Le fichier initial a une taille d'environ 2 Go.
###Code
# Loading data
data_raw = pd.read_csv(path_file, # file
sep="\t", # separator
low_memory=False, # avoid a warning for big files
)
data_raw.info()
###Output
<class 'pandas.core.frame.DataFrame'>
RangeIndex: 1017858 entries, 0 to 1017857
Columns: 175 entries, code to carnitine_100g
dtypes: float64(117), int64(2), object(56)
memory usage: 1.3+ GB
###Markdown
Propriétés générales de la base de données
###Code
def filling_rate(dataframe):
"""Calcuates and displays the shape of the dataframe and the filling rate"""
# Loading libraries
import matplotlib.pyplot as plt
import seaborn as sns
import math
sns.set()
# get the numbers of rows and columns in the dataframe
nb_rows, nb_columns = dataframe.shape
# print("DataFrame has {} rows and {} columns.".format(nb_rows,nb_columns))
# get the number of non-Nan data in the dataframe
nb_data = dataframe.count().sum()
# computing the filling rate
filling_rate = nb_data / (nb_rows * nb_columns)
print("The global filling rate of the DataFrame is : {:.2%}".format(filling_rate))
# Setting frequencies and labels for pie plot
frequencies = [filling_rate, 1 - filling_rate]
labels = ["Filled data", "Missing data"]
# General informations for the pie plot
fig, ax = plt.subplots(figsize=(5, 5))
plt.title("Filling of the DataFrame", fontsize=25)
ax.axis("equal")
explode = (0.1, 0) # only "explode" the 1st slice
# Plotting pie plot
ax.pie(frequencies,
explode=explode,
# labels=labels,
autopct='%1.2f%%',
shadow=True,
)
plt.legend(labels)
filling_rate(df)
df.head(5)
###Output
_____no_output_____
###Markdown
Chaque colonne correspond à une variable (feature), et chaque ligne correspond à un produit.Nous voyons que sur les premières lignes au moins, de nombreuses données sont manquantes. Ces données manquantes sont indiquées par NaN. Sélection des colonnes
###Code
# List of names of the columns
print("Names of the columns :", list(df))
###Output
Names of the columns : ['code', 'url', 'creator', 'created_t', 'created_datetime', 'last_modified_t', 'last_modified_datetime', 'product_name', 'generic_name', 'quantity', 'packaging', 'packaging_tags', 'brands', 'brands_tags', 'categories', 'categories_tags', 'categories_en', 'origins', 'origins_tags', 'manufacturing_places', 'manufacturing_places_tags', 'labels', 'labels_tags', 'labels_en', 'emb_codes', 'emb_codes_tags', 'first_packaging_code_geo', 'cities', 'cities_tags', 'purchase_places', 'stores', 'countries', 'countries_tags', 'countries_en', 'ingredients_text', 'allergens', 'allergens_en', 'traces', 'traces_tags', 'traces_en', 'serving_size', 'serving_quantity', 'no_nutriments', 'additives_n', 'additives', 'additives_tags', 'additives_en', 'ingredients_from_palm_oil_n', 'ingredients_from_palm_oil', 'ingredients_from_palm_oil_tags', 'ingredients_that_may_be_from_palm_oil_n', 'ingredients_that_may_be_from_palm_oil', 'ingredients_that_may_be_from_palm_oil_tags', 'nutrition_grade_fr', 'nova_group', 'pnns_groups_1', 'pnns_groups_2', 'states', 'states_tags', 'states_en', 'main_category', 'main_category_en', 'image_url', 'image_small_url', 'image_ingredients_url', 'image_ingredients_small_url', 'image_nutrition_url', 'image_nutrition_small_url', 'energy_100g', 'energy-from-fat_100g', 'fat_100g', 'saturated-fat_100g', '-butyric-acid_100g', '-caproic-acid_100g', '-caprylic-acid_100g', '-capric-acid_100g', '-lauric-acid_100g', '-myristic-acid_100g', '-palmitic-acid_100g', '-stearic-acid_100g', '-arachidic-acid_100g', '-behenic-acid_100g', '-lignoceric-acid_100g', '-cerotic-acid_100g', '-montanic-acid_100g', '-melissic-acid_100g', 'monounsaturated-fat_100g', 'polyunsaturated-fat_100g', 'omega-3-fat_100g', '-alpha-linolenic-acid_100g', '-eicosapentaenoic-acid_100g', '-docosahexaenoic-acid_100g', 'omega-6-fat_100g', '-linoleic-acid_100g', '-arachidonic-acid_100g', '-gamma-linolenic-acid_100g', '-dihomo-gamma-linolenic-acid_100g', 'omega-9-fat_100g', '-oleic-acid_100g', '-elaidic-acid_100g', '-gondoic-acid_100g', '-mead-acid_100g', '-erucic-acid_100g', '-nervonic-acid_100g', 'trans-fat_100g', 'cholesterol_100g', 'carbohydrates_100g', 'sugars_100g', '-sucrose_100g', '-glucose_100g', '-fructose_100g', '-lactose_100g', '-maltose_100g', '-maltodextrins_100g', 'starch_100g', 'polyols_100g', 'fiber_100g', 'proteins_100g', 'casein_100g', 'serum-proteins_100g', 'nucleotides_100g', 'salt_100g', 'sodium_100g', 'alcohol_100g', 'vitamin-a_100g', 'beta-carotene_100g', 'vitamin-d_100g', 'vitamin-e_100g', 'vitamin-k_100g', 'vitamin-c_100g', 'vitamin-b1_100g', 'vitamin-b2_100g', 'vitamin-pp_100g', 'vitamin-b6_100g', 'vitamin-b9_100g', 'folates_100g', 'vitamin-b12_100g', 'biotin_100g', 'pantothenic-acid_100g', 'silica_100g', 'bicarbonate_100g', 'potassium_100g', 'chloride_100g', 'calcium_100g', 'phosphorus_100g', 'iron_100g', 'magnesium_100g', 'zinc_100g', 'copper_100g', 'manganese_100g', 'fluoride_100g', 'selenium_100g', 'chromium_100g', 'molybdenum_100g', 'iodine_100g', 'caffeine_100g', 'taurine_100g', 'ph_100g', 'fruits-vegetables-nuts_100g', 'fruits-vegetables-nuts-dried_100g', 'fruits-vegetables-nuts-estimate_100g', 'collagen-meat-protein-ratio_100g', 'cocoa_100g', 'chlorophyl_100g', 'carbon-footprint_100g', 'carbon-footprint-from-meat-or-fish_100g', 'nutrition-score-fr_100g', 'nutrition-score-uk_100g', 'glycemic-index_100g', 'water-hardness_100g', 'choline_100g', 'phylloquinone_100g', 'beta-glucan_100g', 'inositol_100g', 'carnitine_100g']
###Markdown
Comme indiqué dans l'appel à projet, les champs sont séparés en sections. Mais selon le descriptif des données sur le site [openfoodfacts.org](https://world.openfoodfacts.org/data/data-fields.txt), il y a 5 sections. Nous remarquons aussi certaines variations dans les intitulés des colonnes, et quelques colonnes supplémentaires.1. "*general informations*" : les informations générales sur la fiche du produit * de la colonne 'code'… * … à la colonne 'quantity'2. "*tags*" : un ensemble de tags * de la colonne 'packaging'… * … à la colonne 'countries_en'3. "*ingredients*": les ingrédients et additifs éventuels. * de la colonne 'ingredients_text'… * … à la colonne 'traces_en'4. "*misc. data*" : des informations diverses * de la colonne 'serving_size'… * … à la colonne 'image_nutrition_small_url'5. "*nutrition facts*" : des informations nutritionnelles * de la colonne 'energy_100g'… * … à la colonne 'carnitine_100g' Élimination des colonnes remplies à moins de 15%Nous faisons une fonction pour éliminer les variables (colonnes) dont le taux de remplissage est inférieur à un seuil.Le seuil de 15% a été choisit car les colonnes décrite ne prensente pas d'informations qui seront utiliser dans la suite de mon analyse et car cela permet de reduire la base de donnée final
###Code
def fillingrate_filter_columns(dataframe, limit_rate):
"""This function drop the colums where the filling rate is less than a defined limit rate."""
# Count of the values on each column
columns_count = dataframe.count()
# Number of rows in the dataframe
nb_rows = dataframe.shape[0]
# Calculating filling rates
filling_rates = columns_count / nb_rows
# Define a mask of features with a filling_rate bigger than the limit rate
mask = filling_rates > limit_rate
# Apply the mask to the filling_rates Series
filling_rates_selection = filling_rates[mask]
# Get the list of the name of the selected columns
features_selection = list(filling_rates_selection.index)
print("Number of columns with a filling rate bigger than {:.15%} : {} columns.".format(limit_rate, len(features_selection)))
# Return a projection on the selection of features
return dataframe[features_selection]
data_cleaned = fillingrate_filter_columns(data_cleaned, 0.15)
###Output
_____no_output_____
###Markdown
Transtypage des données Variables temporelles
###Code
# List of date and time features
datetime_features = [
'created_datetime',
'last_modified_datetime',
'created_t',
'last_modified_t',
]
# Converting the date and time features
for feature in datetime_features:
df[feature] = pd.to_datetime(df[feature])
###Output
_____no_output_____
###Markdown
Variables catégorielles nominales
###Code
# List of nominal features
nominal_features = [
'categories',
'categories_tags',
'categories_en',
]
# Converting the dtype for nominal features
for feature in nominal_features:
df[feature] = df[feature].astype('category')
###Output
_____no_output_____
###Markdown
Variables catégorielles ordinales
###Code
# List of ordinal features
ordinal_features = [
'nova_group',
'nutrition_grade_fr',
]
# Converting the dtype of ordinal features
from pandas.api.types import CategoricalDtype
for feature in ordinal_features:
df[feature] = df[feature].astype(CategoricalDtype(ordered=True))
def piechart_dtypes(dataframe):
"""Plot a piechart of dtypes of features in the dataframe"""
# Loading libraries
import matplotlib.pyplot as plt
# get the list of the dtypes in the dataframe
dtypes_count = dataframe.get_dtype_counts()
# Should use : dtypes_count = dataframe.dtypes.value_counts()
# Fix : dtypes_count = df.dtypes.astype(str).value_counts()
# Set frequencies and labels
labels = dtypes_count.index.values
frequencies = dtypes_count.values
# General informations for the pie plot
fig, ax = plt.subplots(figsize=(8, 6))
plt.title("Types of features", fontsize=25)
ax.axis("equal")
# Plotting pie plot
ax.pie(frequencies,
# labels=labels,
autopct='%1.2f%%',
shadow=True,
)
plt.legend(labels)
dataframe = df
piechart_dtypes(df)
###Output
/usr/local/lib/python3.6/dist-packages/ipykernel_launcher.py:8: FutureWarning: `get_dtype_counts` has been deprecated and will be removed in a future version. For DataFrames use `.dtypes.value_counts()
###Markdown
Élimination des colonnes non-pertinentes pour le projet
###Code
df.shape
# List of columns to drop
columns_useless = [
'url',
'creator',
'image_url',
'image_small_url',
'image_ingredients_url',
'image_ingredients_small_url',
'image_nutrition_url',
'image_nutrition_small_url',
'created_datetime',
'last_modified_datetime',
'created_t',
'last_modified_t',
# Suppression des COLONNES en doublons portant la même information:
'states',
'states_tags',
'states_en',
'packaging',
'packaging_tags',
'brands',
'brands_tags',
'categories',
'categories_tags',
'labels',
'labels_tags',
'purchase_places',
'stores',
'countries',
'countries_tags',
'main_category'
]
# Droping useless columns
data_cleaned = df.drop(columns=columns_useless)
data_cleaned.shape
# Suppression COLONNE 'nutrition-score-uk_100g': on utilise 'nutrition-score-fr_100g'
data_cleaned = data_cleaned.drop('nutrition-score-uk_100g', axis='columns')
# Filtre sur les produits vendus en France:
data_cleaned = data_cleaned[data_cleaned['countries_en'].notna()] #suppr. NaN
data_cleaned = data_cleaned[data_cleaned['countries_en'].str.lower().str.contains("france")] #filtre France
data_cleaned.shape
###Output
_____no_output_____
###Markdown
Permet de suprimmer environ 400K ligne Gestion des doublons (code-barres) : fusionNous allons nettoyer les doublons (code-barres identiques).
###Code
def number_duplicates(dataframe, subset):
"""This function displays and return the number of duplicate in a dataset,
based on a subset of columns."""
# Marking all duplicates
duplicates = dataframe.duplicated(subset=subset, keep=False)
# If there are duplicated rows
if True in list(duplicates.unique()):
# Display and return number of duplicated rows
result = len(dataframe[duplicates])
print("There are {} duplicated rows.".format(result))
return result
# If there are no duplicated rows
else:
print("There are no duplicated rows.")
return 0
###Output
_____no_output_____
###Markdown
Nous définissons une fonction pour fusionner les doublons.
###Code
def duplicates_merging(dataframe,subset):
"""This function handles duplicates rows in the dataframe, based on a subset of columns (features)."""
# Checking if there are some duplicated rows
duplicates_mask = dataframe.duplicated(subset=subset, keep=False)
if True not in list(duplicates_mask.unique()):
print("No duplicates.")
return dataframe
print("Number of duplicates rows :", len(dataframe[duplicates_mask]))
# Filtering the dataframe to keep only duplicated rows
duplicates_mask = dataframe.duplicated(subset=subset, keep=False)
duplicates_df = dataframe[duplicates_mask]
# Group-by subset of columns used for key, sort=False to speed-up
gb = duplicates_df.groupby(subset, sort=False)
# Initializing aggregated dataframe
agg_df = pd.DataFrame()
# Identification of numerical and non-numerical columns
numeric_columns = list(dataframe.select_dtypes(include=[np.number]).columns.values)
# defining aggregation function for non-numerical columns
def agg_mode(x): m = pd.Series.mode(x); return m.values[0] if not m.empty else np.nan
# Iterating upon columns
for column in dataframe.columns:
# Calculate the mean of each group for numeric columns
if column in numeric_columns:
agg_col = gb[column].agg('mean')
# Calculate the mode of each group for numeric columns
else:
agg_col = gb[column].agg(agg_mode)
# adding the aggregated column to aggregated dataframe
agg_df = pd.concat([agg_df, agg_col], axis=1, sort=True)
# Dropping all duplicates
dataframe_cleaned = dataframe.drop_duplicates(subset=subset, keep=False)
# Concatenating the dataframe without duplicates and the aggregated rows for duplicates
result = dataframe_cleaned.append(agg_df, ignore_index=True, sort=False)
print("Shape after handling duplicates :", result.shape)
return result
dataframe = data_cleaned
subset = ['code']
data_cleaned = duplicates_merging(dataframe,subset)
###Output
Number of duplicates rows : 188
Shape after handling duplicates : (615012, 146)
###Markdown
Valeur aberante valeur trop grande ou trop petite
###Code
cols_100g = ['fat_100g', "saturated-fat_100g", "trans-fat_100g", "cholesterol_100g", "carbohydrates_100g", "sugars_100g", "fiber_100g", "proteins_100g", "salt_100g", "sodium_100g", "vitamin-a_100g", "vitamin-c_100g", "calcium_100g", "iron_100g"]
for cols in cols_100g:
#valeur trop grande
data_cleaned.loc[data_cleaned[cols] > 100, cols] = np.nan
#valeur trop petite
data_cleaned.loc[data_cleaned[cols] < 0, cols] = np.nan
data_cleaned[cols_100g].describe()
###Output
_____no_output_____
###Markdown
valeur parent Ici l'on vas nétoyer les valeurs "enfants" par rapport au valeur parente. en effet la somme des premieres doit étre inferieur a la veleur de la seconde. Fat
###Code
cols_fat= ["saturated-fat_100g", "trans-fat_100g", "cholesterol_100g"]
data_cleaned.loc[data_cleaned[cols_fat].sum(axis=1) > data_cleaned['fat_100g'], cols_fat] = np.nan
data_cleaned[cols_fat].sum(axis=1).describe()
###Output
_____no_output_____
###Markdown
Carbo
###Code
cols_carbo= ["sugars_100g", "fiber_100g"]
data_cleaned.loc[data_cleaned[cols_carbo].sum(axis=1) > data_cleaned["carbohydrates_100g"], cols_fat] = np.nan
data_cleaned[cols_fat].sum(axis=1).describe()
###Output
_____no_output_____
###Markdown
somme quantité = 100
###Code
cols_100g_nutriments= ['fat_100g', "carbohydrates_100g", "proteins_100g", "salt_100g", "sodium_100g", "vitamin-a_100g", "vitamin-c_100g", "calcium_100g", "iron_100g"]
data_cleaned.loc[data_cleaned[cols_100g_nutriments].sum(axis=1) > 100, cols_100g_nutriments] = np.nan
data_cleaned[cols_100g_nutriments].sum(axis=1).describe()
###Output
_____no_output_____
###Markdown
valeur énergetique
###Code
#valeur énergetique max de 900
data_cleaned.loc[data_cleaned["energy_100g"] > 900, ["energy_100g"]] = np.nan
data_cleaned.loc[data_cleaned["energy_100g"] < 0, ["energy_100g"]] = np.nan
data_cleaned["energy_100g"].describe()
###Output
_____no_output_____
###Markdown
Calcul du taux de remplissage par feature
###Code
# Calcul du taux de remplissage des colonnes:
df = pd.DataFrame(round(data_cleaned.count(axis=0)/data_cleaned.shape[0]*100,2),
columns=['Taux de remplissage']).sort_values('Taux de remplissage',
ascending=False).reset_index()
fig, ax = plt.subplots(figsize=(10,30))
sns.barplot(y=df['index'],
x='Taux de remplissage',
data=df,
)
plt.title('Taux de remplissage des variables - %')
plt.show()
nb_rows, nb_columns = data_cleaned.shape
# Count of the values on each column
columns_count = data_cleaned.count()
# Sort the Series
columns_count = columns_count.sort_values(ascending=False)
# Calculating filling rates
filling_rates = columns_count / nb_rows
# Display a sampling (1 out of 10) of columns names and filling rates
filling_rates[::10]
# Plot chart of the filling_rates
import matplotlib.pyplot as plt
import matplotlib.ticker as ticker
import seaborn as sns
sns.set()
x = range(nb_columns)
y = filling_rates
fig, ax = plt.subplots(figsize=(20, 10))
plt.title("Filling rates of features (columns)", fontsize=25)
plt.xlabel("Features", fontsize=15)
plt.ylabel("Percentage", fontsize=15)
ax.yaxis.set_major_formatter(ticker.PercentFormatter(xmax=1))
plot = ax.plot(x, y)
###Output
_____no_output_____
###Markdown
Nous constatons que quelques colonnes sont intégralement remplis.Nous constatons aussi que de nombreuses colonnes sont très faiblement ou pas du tout remplies. Ainsi, environ une centaine d'indicateurs (sur les 160 que contient encore la table) ont un remplissage inférieur à 5%. Nettoyage des lignes Filtrage sur le code-barres EAN-13 (colonne 'code')La documentation du site [openfoodfacts.org](https://world.openfoodfacts.org/data/data-fields.txt) précise : "*code : barcode of the product (can be EAN-13 or internal codes for some food stores), for products without a barcode, Open Food Facts assigns a number starting with the 200 reserved prefix*".Nous n'allons toutefois, en raison de notre application, ne garder que les lignes avec un code-barres EAN-13 valide.
###Code
def check_key(barcode):
"""This function check the control key (last digit) of the 13-digits barcode."""
# Import of regular expressions module
import re
# Definition of regular expression : 13 digits only
regex = "^\d{13}$"
# Compilation of regular expression
pattern = re.compile(regex)
# Return False if the code does not fit regular expression
if pattern.match(str(barcode))==None:
return False
# conversion to string to iterate on digits
barcode_str = str(barcode)
# getting the last digit
last_digit = int(barcode_str[-1:])
# removing last digit from barcode
barcode_str = barcode_str[:-1]
# initialisation of the control sum
sum = 0
# for each digit in the barcode
for index, digit in enumerate(barcode_str):
digit = int(digit) # conversion back as integer
# if index is an even number (digits of rank 1, 3, 5…)
if index % 2 == 0:
# adding to the sum with a ponderation of 1
sum += 1*digit
# if index is an odd number (digits of rank 2, 4, 6…)
else :
# adding to the sum with a ponderation of 1
sum += 3*digit
# computing the control key with division of the key by 10
rest = sum % 10
if rest == 0 :
key = 0
else:
key = 10 - rest
# compare control key and last digit of the barcode
if key == last_digit:
return True
else:
return False
# Matching the pattern in the column 'code' on each line of the DataFrame
mask = [check_key(code) for code in data_cleaned['code']]
# Dropping the rows with non-compliant EAN-13 code
data_cleaned = data_cleaned[mask]
print("Number of rows in the cleaned data:", data_cleaned.shape[0])
###Output
Number of rows in the cleaned data: 573321
###Markdown
Le filtrage a permis de nettoyer environ 30'000.avant 599022 Correction pour le 'pnns_groups_1': suppression '-' et mise en majuscule:
###Code
# Correction pour le 'pnns_groups_1': suppression '-' et mise en majuscule:
data_cleaned['pnns_groups_1'] = data_cleaned['pnns_groups_1'].str.replace('-',' ').str.capitalize()
###Output
_____no_output_____
###Markdown
Imputation pour les lignes ayant un nom de produit identiquePour ces lignes qui possèdent le même nom de produit, nous n'allons pas "supprimer ni fusionner les doublons" mais utiliser le champ 'product_name' comme clé pour faire de l'imputation. En effet, il est important pour notre application de conserver tous les codes-barres correspondant à un produit.
###Code
dataframe = data_cleaned
subset = ["product_name"]
number_duplicates(dataframe, subset)
def imputation(dataframe, feature, keys):
#encour k voisin
"""This function impute substitute values for missing values for a specific column (feature),
based on other rows sharing some properties (values on other fields of subset).
Also add a new feature to inform if the value was imputed.
Does only handle numeric features yet."""
# Identify NaN values pre-imputation
mask_before = dataframe[feature].isnull()
# Copying the dataframe to avoid manipulating a slice
result = dataframe.copy()
# Initialize column for imputed values if doesn't exist
if "imputed_" + feature not in result.columns:
result["imputed_" + feature] = False
# Iterating on each feature in the subset
for column in keys:
# Grouping the DataFrame on the column's value, sort=False to speed-up
grouped = dataframe.groupby(column, sort=False)
# Computing the mean of feature for each group
means_of_group = grouped[feature].transform('mean') # to adapt for object and categorical dtypes features
# Filling missing values with the mean of each group
result[feature].fillna(value=means_of_group, axis=0, inplace=True)
# Identify NaN values post-imputation
mask_after = result[feature].isnull()
# Values imputed : boolean symetric difference
mask_imputed = mask_after ^ mask_before
# Update the column to inform that the feature was imputed
result.loc[:,"imputed_" + feature][mask_imputed] = True # raise a SettingWithCopyWarning
return result
###Output
_____no_output_____
###Markdown
Avant l'imputation
###Code
dataframe = data_cleaned
# Before imputation
filling_rate(dataframe)
# Ordered list of the keys used to impute the feature
keys = ['product_name']
# Selection of numeric columns with high enough filling rate
numeric_columns = data_cleaned.select_dtypes(include=['number']).columns
# Selection of columns to apply
mask = filling_rates > 0.75
filled_columns = list(filling_rates[mask].index)
# Intersection of the two sets (numeric and filling rate)
columns_to_impute = list(set(numeric_columns) & set(filled_columns))
# Features to impute : numerical columns (barcode is an object column)
for feature in columns_to_impute:
# Proceed to imputation
data_cleaned = imputation(dataframe, feature, keys)
###Output
/usr/local/lib/python3.6/dist-packages/ipykernel_launcher.py:37: SettingWithCopyWarning:
A value is trying to be set on a copy of a slice from a DataFrame
See the caveats in the documentation: http://pandas.pydata.org/pandas-docs/stable/user_guide/indexing.html#returning-a-view-versus-a-copy
###Markdown
Après l'imputation
###Code
dataframe = data_cleaned
# After imputation
filling_rate(dataframe)
# Suppression des LIGNES sans nom de produit:
data_cleaned = data_cleaned[data_cleaned['product_name'].notnull()]
data_cleaned.shape
###Output
_____no_output_____
###Markdown
Imputation des valeurs numériques manquantesNous sélectionnons les colonnes numériques ayant un taux de remplissage suffisant (> 75 %). Identification des colonnes remplies à plus de 75%
###Code
mask = filling_rates > 0.75
filling_rates[mask]
###Output
_____no_output_____
###Markdown
Les champs remplis à 100% correspondent vraissemblablement à des champs obligatoires (sur le formulaire de remplissage, ou pour l'enregistrement dans la base de données) ou à de données générées automatiquement (timestamps par exemple) lors de l'ajout d'un produit dans la base de données. Imputation pour les colonnes remplies à plus de 75%Nous appliquons l'[imputateur multivarié expérimental](https://scikit-learn.org/stable/modules/generated/sklearn.impute.IterativeImputer.htmlsklearn.impute.IterativeImputer) de scikit-learn.
###Code
# The iterative imputer requires version 0.21.2 of scikit-learn
import sklearn
print('The scikit-learn version is {}.'.format(sklearn.__version__))
# Load libraries
from sklearn.experimental import enable_iterative_imputer
from sklearn.impute import IterativeImputer
# Selection of numeric columns with high enough filling rate
numeric_columns = data_cleaned.select_dtypes(include=['number']).columns
# Selection of columns to apply
mask = filling_rates > 0.75
filled_columns = list(filling_rates[mask].index)
# Intersection of the two sets (numeric and filling rate)
columns_to_impute = list(set(numeric_columns) & set(filled_columns))
# Instanciation of iterative imputer
imp = IterativeImputer(max_iter=10, random_state=0)
# Train and apply (inplace) the iterative imputer
data_cleaned[columns_to_impute] = imp.fit_transform(data_cleaned[columns_to_impute])
###Output
/usr/local/lib/python3.6/dist-packages/sklearn/impute/_iterative.py:638: ConvergenceWarning: [IterativeImputer] Early stopping criterion not reached.
" reached.", ConvergenceWarning)
/usr/local/lib/python3.6/dist-packages/ipykernel_launcher.py:18: SettingWithCopyWarning:
A value is trying to be set on a copy of a slice from a DataFrame.
Try using .loc[row_indexer,col_indexer] = value instead
See the caveats in the documentation: http://pandas.pydata.org/pandas-docs/stable/user_guide/indexing.html#returning-a-view-versus-a-copy
/usr/local/lib/python3.6/dist-packages/pandas/core/indexing.py:494: SettingWithCopyWarning:
A value is trying to be set on a copy of a slice from a DataFrame.
Try using .loc[row_indexer,col_indexer] = value instead
See the caveats in the documentation: http://pandas.pydata.org/pandas-docs/stable/user_guide/indexing.html#returning-a-view-versus-a-copy
self.obj[item] = s
###Markdown
Resumer
###Code
dataframe = data_cleaned
# After imputation
filling_rate(dataframe)
# Calcul du taux de remplissage des colonnes:
df = pd.DataFrame(round(data_cleaned.count(axis=0)/data_cleaned.shape[0]*100,2),
columns=['Taux de remplissage']).sort_values('Taux de remplissage',
ascending=False).reset_index()
fig, ax = plt.subplots(figsize=(10,30))
sns.barplot(y=df['index'],
x='Taux de remplissage',
data=df,
)
plt.title('Taux de remplissage des variables - %')
plt.show()
###Output
_____no_output_____
###Markdown
on a réussi a récuperer des valeur sur les nutriment qui vont nous permetre par la suite de calculer le nutriscore Calcule du Nutriscore et du nutri grade
###Code
data_cleaned.shape
# Gestion des NaN pour les nutrition facts: (colonnes avec suffixe _100g):
# Remplacement par des 0 :
L = ['energy_100g', 'fat_100g', 'saturated-fat_100g', 'carbohydrates_100g',
'sugars_100g', 'fiber_100g', 'proteins_100g', 'salt_100g',
'sodium_100g']
data_cleaned.loc[:,L] = data_cleaned.loc[:,L].fillna(0)
# Suppression s'ils sont tous 0:
data_cleaned = data_cleaned.loc[(data_cleaned.loc[:,L]!=0).any(axis=1)]
data_cleaned.shape
###Output
_____no_output_____
###Markdown
Nutrition score
###Code
pnns_groups_2 = data_cleaned['pnns_groups_2'].unique().tolist()
pnns_groups_1 = data_cleaned['pnns_groups_1'].unique().tolist()
print(pnns_groups_2)
print(pnns_groups_1)
data_cleaned['product_name'].unique()
data_cleaned['nutrition_grade_fr'].unique()
###Output
_____no_output_____
###Markdown
Fonction
###Code
# Gestion des NaN pour le nutrition_score et nutrition_grade:
# ==> Calcul par la formule d'OpenFoodFacts:
# (on considère qu'il n'y a pas de données manquantes)
def nutri_calcul(row):
"""Fonction calculant le nutrition_score et nutrition_grade"""
nutri_grade = ''
nutri_score = 0
# Si toutes les valeurs sont NaN:
x = np.isnan(row['sugars_100g'])
y = np.isnan(row['energy_100g'])
z = np.isnan(row['saturated-fat_100g'])
w = np.isnan(row['sodium_100g'])
u = np.isnan(row['fiber_100g'])
t = np.isnan(row['proteins_100g'])
if (t & u & w & x & y & z):
return None,None
# Calcul des points A:
x = row['sugars_100g']
b=0
if (x<=4.5):
b = 0
elif (x>4.5) & (x<=9):
b = 1
elif (x>9) & (x<=13.5):
b = 2
elif (x>13.5) & (x<=18):
b = 3
elif (x>18) & (x<=22.5):
b = 4
elif (x>22.5) & (x<=27):
b = 5
elif (x>27) & (x<=31):
b = 6
elif (x>31) & (x<=36):
b = 7
elif (x>36) & (x<=40):
b = 8
elif (x>40) & (x<=45):
b = 9
elif (x>45):
b = 10
x = row['energy_100g']
a=0
if (x<=335):
a = 0
elif (x>335) & (x<=670):
a = 1
elif (x>670) & (x<=1005):
a = 2
elif (x>1005) & (x<=1340):
a = 3
elif (x>1340) & (x<=1675):
a = 4
elif (x>1675) & (x<=2010):
a = 5
elif (x>2010) & (x<=2345):
a = 6
elif (x>2345) & (x<=2680):
a = 7
elif (x>2680) & (x<=3015):
a = 8
elif (x>3015) & (x<=3350):
a = 9
elif (x>3350):
a = 10
x = row['saturated-fat_100g']
c=0
if (x<=1):
c = 0
elif (x>1) & (x<=2):
c = 1
elif (x>2) & (x<=3):
c = 2
elif (x>3) & (x<=4):
c = 3
elif (x>4) & (x<=5):
c = 4
elif (x>5) & (x<=6):
c = 5
elif (x>6) & (x<=7):
c = 6
elif (x>7) & (x<=8):
c = 7
elif (x>8) & (x<=9):
c = 8
elif (x>9) & (x<=10):
c = 9
elif (x>10):
c = 10
x = row['sodium_100g']
d=0
if (x<=0.09):
d = 0
elif (x>0.09) & (x<=0.18):
d = 1
elif (x>0.18) & (x<=0.27):
d = 2
elif (x>0.27) & (x<=0.36):
d = 3
elif (x>0.36) & (x<=0.45):
d = 4
elif (x>0.45) & (x<=0.54):
d = 5
elif (x>0.54) & (x<=0.63):
d = 6
elif (x>0.63) & (x<=0.72):
d = 7
elif (x>0.72) & (x<=0.81):
d = 8
elif (x>0.81) & (x<=0.9):
d = 9
elif (x>0.9):
d = 10
A = a+b+c+d
# Calcul des points C:
x = row['fiber_100g']
bb=0
if (x<=0.7):
bb = 0
elif (x>0.7) & (x<=1.4):
bb = 1
elif (x>1.4) & (x<=2.1):
bb = 2
elif (x>2.1) & (x<=2.8):
bb = 3
elif (x>2.8) & (x<=3.5):
bb = 4
elif (x>3.5):
bb = 5
x = row['proteins_100g']
cc=0
if (x<=1.6):
cc = 0
elif (x>1.6) & (x<=3.2):
cc = 1
elif (x>3.2) & (x<=4.8):
cc = 2
elif (x>4.8) & (x<=6.4):
cc = 3
elif (x>6.4) & (x<=8):
cc = 4
elif (x>8):
cc = 5
C = bb+cc
#Calcul du nutrition_score:
nutri_score = A - C
#Calcul du nutrition_grade:
if (row['pnns_groups_1']!='Beverages'): #Aliments solides
if nutri_score<=-1:
nutri_grade = 'a'
elif (nutri_score>-1) & (nutri_score<=2):
nutri_grade = 'b'
elif (nutri_score>2) & (nutri_score<=10):
nutri_grade = 'c'
elif (nutri_score>10) & (nutri_score<=18):
nutri_grade = 'd'
elif (nutri_score>18):
nutri_grade = 'e'
else: # Boissons
if (row['product_name']==row['product_name']):
x = row['product_name'].lower()
if (('eau' in x) | ('water' in x) | ('agua' in x)): # Eau
nutri_grade = 'a'
elif (nutri_score<1) & (nutri_score<=2):
nutri_grade = 'b'
elif (nutri_score>2) & (nutri_score<=5):
nutri_grade = 'c'
elif (nutri_score>5) & (nutri_score<=9):
nutri_grade = 'd'
elif (nutri_score>9):
nutri_grade = 'e'
elif (nutri_score<1) & (nutri_score<=2):
nutri_grade = 'b'
elif (nutri_score>2) & (nutri_score<=5):
nutri_grade = 'c'
elif (nutri_score>5) & (nutri_score<=9):
nutri_grade = 'd'
elif (nutri_score>9):
nutri_grade = 'e'
return nutri_grade, nutri_score
###Output
_____no_output_____
###Markdown
Remplacement
###Code
# Remplacement des NaN par les valeurs calculés:
flag = data_cleaned.loc[:,'nutrition-score-fr_100g'].count()
df1 = data_cleaned[data_cleaned['nutrition-score-fr_100g'].isna()]
df2 = df1[df1['pnns_groups_1'].notna()]
df = df2[df2['pnns_groups_2'].notna()]
for index, row in df.iterrows():
nutri_grade, nutri_score = nutri_calcul(row)
data_cleaned.loc[index,'nutrition-score-fr_100g'] = nutri_score
try:
data_cleaned.loc[index,'nutrition_grade_fr'] = nutri_grade
except:
pass
print('Nombre de lignes corrigés: ',data_cleaned.loc[:,'nutrition-score-fr_100g'].count()-flag)
# Gestion des NaN restants du nutrition_score:
# ==> Prédiction du nutrition_score par régression:
# (pour les lignes ayant pnns_groups_1 ou pnns_groups_2 vides)
df = data_cleaned.loc[:,['nutrition-score-fr_100g','energy_100g','fat_100g',
'saturated-fat_100g','carbohydrates_100g','sugars_100g',
'fiber_100g','proteins_100g','sodium_100g',
'salt_100g']][data_cleaned['nutrition-score-fr_100g'].notnull()]
y = df['nutrition-score-fr_100g'].values
X = df.drop('nutrition-score-fr_100g', axis='columns').values
from sklearn import preprocessing #standardisation
X_scaled = preprocessing.StandardScaler().fit_transform(X)
from sklearn.model_selection import train_test_split #split train test sets
X_train, X_test, y_train, y_test = train_test_split(X_scaled, y, test_size=0.25, random_state=33)
from sklearn.ensemble import BaggingRegressor #bootstrapping aggregation method
reg = BaggingRegressor(n_estimators = 100, #decision tree estimator
random_state = 33)
reg.fit(X_train, y_train)
y_pred = reg.predict(X_test)
R2 = reg.score(X_test, y_test)
print('Coeff. de détermination: ',round(R2,2))
from sklearn import metrics
RMSE = round(np.sqrt(metrics.mean_squared_error(y_test, y_pred)),1)
print("RMSE (values in [-15;40]): ",RMSE)
###Output
_____no_output_____
###Markdown
Nutrition grade
###Code
# Gestion des NaN restants du nutrition_grade:
# (les lignes ayant pnns_groups_1 ou pnns_groups_2 vides)
# Prédiction du nutrition_grade par classification:
# (on aurait aussi pu le déduire du nutrition_score calculé précedemment par régression)
df = data_cleaned.loc[:,['nutrition_grade_fr','energy_100g','fat_100g',
'saturated-fat_100g','carbohydrates_100g','sugars_100g',
'fiber_100g','proteins_100g','sodium_100g',
'salt_100g']][data_cleaned['nutrition_grade_fr'].notnull()]
from sklearn.preprocessing import LabelEncoder #Transformer données textes en chiffres
labelencoder = LabelEncoder()
df['nutrition_grade_fr'] = labelencoder.fit_transform(df['nutrition_grade_fr'])
y = df['nutrition_grade_fr'].values
X = df.drop('nutrition_grade_fr', axis='columns').values
from sklearn import preprocessing #standardisation
X_scaled = preprocessing.StandardScaler().fit_transform(X)
from sklearn.model_selection import train_test_split #split train test sets
X_train, X_test, y_train, y_test = train_test_split(X_scaled, y, test_size=0.25, random_state=33)
from sklearn.ensemble import RandomForestClassifier
clf = RandomForestClassifier(n_estimators=100,
max_depth=20,
random_state = 33)
clf.fit(X_train, y_train)
Accuracy = clf.score(X_test, y_test)
print('Accuracy (% valeur correcte): ',round(Accuracy,2))
# Remplacement des NaN par les valeurs prédites:
flag = data_cleaned.loc[:,'nutrition-score-fr_100g'].count()
df = data_cleaned.loc[:,['energy_100g','fat_100g','saturated-fat_100g','carbohydrates_100g','sugars_100g',
'fiber_100g','proteins_100g','sodium_100g',
'salt_100g']][data_cleaned['nutrition-score-fr_100g'].isna() | data_cleaned['nutrition_grade_fr'].isna()]
X = df.values
score_pred = pd.Series(reg.predict(X), index = df.index)
grade_pred = pd.Series(labelencoder.inverse_transform(clf.predict(X)),index = df.index)
for index, row in df.iterrows():
if np.isnan(data_cleaned.loc[index,'nutrition-score-fr_100g']):
data_cleaned.loc[index,'nutrition-score-fr_100g'] = score_pred[index]
if np.isnan(data_cleaned.loc[index,'nutrition_grade_fr']):
data_cleaned.loc[index,'nutrition_grade_fr'] = grade_pred[index]
print('Nombre de lignes corrigés: ',data_cleaned.loc[:,'nutrition-score-fr_100g'].count()-flag)
###Output
_____no_output_____
###Markdown
Étude du remplissage des lignesNous allons maintenant étudier le remplissage par produit (ligne).
###Code
def rows_fillingrate_histogram(dataframe):
"""This function plots an histogram of the distribution of the
filling rate for the rows of a dataframe."""
# Import libraries
import matplotlib.ticker as ticker
# Count the non-null values on each row
row_count = dataframe.count(axis=1)
# Calculating filling rates
nb_columns = dataframe.shape[1]
filling_rates_row = row_count / nb_columns
# Plotting histogramm
fig, ax = plt.subplots(figsize=(20, 10))
plt.title("Rows' filling rate distribution", fontsize=25)
plt.xlabel("Filling rate", fontsize=15)
plt.ylabel("Frequency", fontsize=15)
ax.xaxis.set_major_formatter(ticker.PercentFormatter(xmax=1))
bins = np.linspace(0, 1, num=51)
ax.hist(filling_rates_row, bins=bins)
ax.xaxis.set_major_locator(plt.MaxNLocator(11))
plt.show()
dataframe = data_cleaned
rows_fillingrate_histogram(dataframe)
###Output
_____no_output_____
###Markdown
Nous constatons que les produits (lignes) restant après les nettoyages préliminaires sont au minimum remplis à 38%, et au maximum à 100%. Sauvegarde du pd.DataFrame Ré-échelonnage de la variable 'nutrition-score-fr_100g'Le score nutritionnel final peut théoriquement varier de -15 à +40 en fonction des produits. Plus il est faible, plus le produit est considéré comme ayant un profil nutritionnel favorable. Source : https://www.anses.fr/fr/system/files/DER2014sa0099Ra.pdf (page 17)Pour faciliter l'interprétabilité et la lisibilité de la variable, nous allons la transformer en une variable allant de 0 à 100, et telle que 0 représente le pire score et 100 représente le meilleure score de nutrition. Pour cela, nous utilisons la relation :$$X'= 100 \times\frac{40-X}{55}$$
###Code
data_cleaned['nutrition-score-rescale'] = data_cleaned['nutrition-score-fr_100g']
data_cleaned.loc[:,'nutrition-score-rescale'] = 100 * (40 - data_cleaned['nutrition-score-fr_100g']) / 55
###Output
_____no_output_____
###Markdown
Taux de remplissage final
###Code
print("Shape of the cleaned data:", data_cleaned.shape)
filling_rate(data_cleaned)
data_cleaned.info()
#Visualisation du taux de remplissage des colonnes post-nettoyage:
df = pd.DataFrame(round(data_cleaned.count(axis=0)/data_cleaned.shape[0]*100,2),
columns=['Taux de remplissage']).sort_values('Taux de remplissage',
ascending=False).reset_index()
fig, ax = plt.subplots(figsize=(10,7))
sns.barplot(y=df['index'],
x='Taux de remplissage',
data=df,
)
plt.title('Taux de remplissage des variables - %')
plt.show()
###Output
_____no_output_____
###Markdown
Sous-classement des dtytes (*downcast*)Par défaut, Pandas charge les données en int64 ou en float64. Pour limiter la charge mémoire, il est possible d'essayer de changer le type de données.
###Code
data_cleaned.info()
###Output
_____no_output_____
###Markdown
on a envrion 190 MB de données voyont ce que l'on peut faire en effectuant un downcast
###Code
import pandas as pd
from typing import List
def optimize_floats(df: pd.DataFrame) -> pd.DataFrame:
floats = df.select_dtypes(include=['float64']).columns.tolist()
df[floats] = df[floats].apply(pd.to_numeric, downcast='float')
return df
def optimize_ints(df: pd.DataFrame) -> pd.DataFrame:
ints = df.select_dtypes(include=['int64']).columns.tolist()
df[ints] = df[ints].apply(pd.to_numeric, downcast='integer')
return df
def optimize_objects(df: pd.DataFrame, datetime_features: List[str]) -> pd.DataFrame:
for col in df.select_dtypes(include=['object']):
if col not in datetime_features:
num_unique_values = len(df[col].unique())
num_total_values = len(df[col])
if float(num_unique_values) / num_total_values < 0.5:
df[col] = df[col].astype('category')
else:
df[col] = pd.to_datetime(df[col])
return df
def optimize(df: pd.DataFrame, datetime_features: List[str] = []):
return optimize_floats(optimize_ints(optimize_objects(df, datetime_features)))
optimized_data_cleaned = optimize(data_cleaned)
optimized_data_cleaned.info()
###Output
_____no_output_____
###Markdown
Le resultat et concluant nous avon maintenant une base de données de 90 MB Colab
###Code
"""from google.colab import files
images_dir = "/content/drive/My Drive/data/OPC03/"
optimized_data_cleaned.to_csv(images_dir + 'data_cleaned.csv', index=False)"""
data_cleaned.describe()
###Output
_____no_output_____ |
My_U2S4dot3_Select_Models_and_Parameters.ipynb | ###Markdown
_Lambda School Data Science — Model Validation_ Select models and parametersObjectives- Hyperparameter optimization- Model selection Today we'll use this process: "A universal workflow of machine learning"_Excerpt from Francois Chollet, [Deep Learning with Python](https://github.com/fchollet/deep-learning-with-python-notebooks/blob/master/README.md), Chapter 4: Fundamentals of machine learning_ **1. Define the problem at hand and the data on which you’ll train.** Collect this data, or annotate it with labels if need be.**2. Choose how you’ll measure success on your problem.** Which metrics will you monitor on your validation data?**3. Determine your evaluation protocol:** hold-out validation? K-fold validation? Which portion of the data should you use for validation?**4. Develop a first model that does better than a basic baseline:** a model with statistical power.**5. Develop a model that overfits.** The universal tension in machine learning is between optimization and generalization; the ideal model is one that stands right at the border between underfitting and overfitting; between undercapacity and overcapacity. To figure out where this border lies, first you must cross it.**6. Regularize your model and tune its hyperparameters, based on performance on the validation data.** Repeatedly modify your model, train it, evaluate on your validation data (not the test data, at this point), modify it again, and repeat, until the model is as good as it can get. Iterate on feature engineering: add new features, or remove features that don’t seem to be informative. Once you’ve developed a satisfactory model configuration, you can train your final production model on all the available data (training and validation) and evaluate it one last time on the test set. 1. Define the problem at hand and the data on which you'll train We'll apply the workflow to a [project from _Python Data Science Handbook_](https://jakevdp.github.io/PythonDataScienceHandbook/05.06-linear-regression.htmlExample:-Predicting-Bicycle-Traffic) by Jake VanderPlas:> **Predicting Bicycle Traffic**> As an example, let's take a look at whether we can predict the number of bicycle trips across Seattle's Fremont Bridge based on weather, season, and other factors.> We will join the bike data with another dataset, and try to determine the extent to which weather and seasonal factors—temperature, precipitation, and daylight hours—affect the volume of bicycle traffic through this corridor. Fortunately, the NOAA makes available their daily [weather station data](http://www.ncdc.noaa.gov/cdo-web/search?datasetid=GHCND) (I used station ID USW00024233) and we can easily use Pandas to join the two data sources.> Let's start by loading the two datasets, indexing by date: So this is a regression problem, not a classification problem. We'll define the target, choose an evaluation metric, and choose models that are appropriate for regression problems. Download data
###Code
!curl -o FremontBridge.csv https://data.seattle.gov/api/views/65db-xm6k/rows.csv?accessType=DOWNLOAD
!wget https://raw.githubusercontent.com/jakevdp/PythonDataScienceHandbook/master/notebooks/data/BicycleWeather.csv
###Output
--2019-08-04 06:08:07-- https://raw.githubusercontent.com/jakevdp/PythonDataScienceHandbook/master/notebooks/data/BicycleWeather.csv
Resolving raw.githubusercontent.com (raw.githubusercontent.com)... 151.101.0.133, 151.101.64.133, 151.101.128.133, ...
Connecting to raw.githubusercontent.com (raw.githubusercontent.com)|151.101.0.133|:443... connected.
HTTP request sent, awaiting response... 200 OK
Length: 234945 (229K) [text/plain]
Saving to: ‘BicycleWeather.csv’
BicycleWeather.csv 0%[ ] 0 --.-KB/s
BicycleWeather.csv 100%[===================>] 229.44K --.-KB/s in 0.05s
2019-08-04 06:08:07 (4.48 MB/s) - ‘BicycleWeather.csv’ saved [234945/234945]
###Markdown
Load data
###Code
# Modified from cells 15, 16, and 20, at
# https://jakevdp.github.io/PythonDataScienceHandbook/05.06-linear-regression.html#Example:-Predicting-Bicycle-Traffic
import pandas as pd
counts = pd.read_csv('FremontBridge.csv', index_col='Date', parse_dates=True,
infer_datetime_format=True)
weather = pd.read_csv('BicycleWeather.csv', index_col='DATE', parse_dates=True,
infer_datetime_format=False)
daily = counts.resample('d').sum()
daily['Total'] = daily.sum(axis=1)
daily = daily[['Total']] # remove other columns
weather_columns = ['PRCP', 'SNOW', 'SNWD', 'TMAX', 'TMIN', 'AWND']
daily = daily.join(weather[weather_columns], how='inner')
# Make a feature for yesterday's total
daily['Total_yesterday'] = daily.Total.shift(1)
daily = daily.drop(index=daily.index[0])
###Output
_____no_output_____
###Markdown
First fast look at the data- What's the shape?- What's the date range?- What's the target and the features?
###Code
# TODO
print(daily.shape)
daily.sample(8)
###Output
(1063, 8)
###Markdown
Target- Total : Daily total number of bicycle trips across Seattle's Fremont BridgeFeatures- Date (index) : from 2012-10-04 to 2015-09-01- Total_yesterday : Total trips yesterday- PRCP : Precipitation (1/10 mm)- SNOW : Snowfall (1/10 mm)- SNWD : Snow depth (1/10 mm)- TMAX : Maximum temperature (1/10 Celsius)- TMIN : Minimum temperature (1/10 Celsius)- AWND : Average daily wind speed (1/10 meters per second) 2. Choose how you’ll measure success on your problem.Which metrics will you monitor on your validation data?This is a regression problem, so we need to choose a regression [metric](https://scikit-learn.org/stable/modules/model_evaluation.htmlcommon-cases-predefined-values).I'll choose mean absolute error.
###Code
# TODO
from sklearn.metrics import mean_absolute_error # could import mean_square_error
###Output
_____no_output_____
###Markdown
3. Determine your evaluation protocol We're doing model selection, hyperparameter optimization, and performance estimation. So generally we have two ideal [options](https://sebastianraschka.com/images/blog/2018/model-evaluation-selection-part4/model-eval-conclusions.jpg) to choose from:- 3-way holdout method (train/validation/test split)- Cross-validation with independent test setI'll choose cross-validation with independent test set. Scikit-learn makes cross-validation convenient for us!Specifically, I will use random shuffled cross validation to train and validate, but I will hold out an "out-of-time" test set, from the last 100 days of data:
###Code
# TODO
train = daily[:-100]
test = daily[-100:]
train.shape, test.shape
X_train = train.drop(columns='Total')
y_train = train['Total']
X_test = test.drop(columns='Total')
y_test = test['Total']
X_train.shape, y_train.shape, X_test.shape, y_test.shape
###Output
_____no_output_____
###Markdown
4. Develop a first model that does better than a basic baseline Look at the target's distribution and descriptive stats
###Code
# TODO
%matplotlib inline
import seaborn as sns
sns.distplot(y_train);
y_train.describe()
###Output
_____no_output_____
###Markdown
Basic baseline 1
###Code
# TODO
y_pred = [y_train.mean()] * len(y_train)
mean_absolute_error(y_train, y_pred)
###Output
_____no_output_____
###Markdown
Basic baseline 2
###Code
# TODO
y_pred = X_train['Total_yesterday']
mean_absolute_error(y_train, y_pred)
###Output
_____no_output_____
###Markdown
==>> bit of an improvement First model that does better than a basic baseline https://scikit-learn.org/stable/modules/generated/sklearn.model_selection.cross_validate.html
###Code
# TODO
from sklearn.linear_model import LinearRegression
from sklearn.model_selection import cross_validate
scores = cross_validate(LinearRegression(), X_train, y_train,
scoring='neg_mean_absolute_error', cv=3, # neg 'cause of scikit kludge'
return_train_score=True, return_estimator=True)
pd.set_option('max_colwidth', 46)
pd.DataFrame(scores)
scores['test_score'].mean()
for i, model in enumerate(scores['estimator']):
coefficients = model.coef_
intercept = model.intercept_
feature_names = X_train.columns
print('Model from cross-validaton fold #', i)
print('Intercept', intercept)
print(pd.Series(coefficients, feature_names).to_string())
print('\n')
import statsmodels.api as sm
model = sm.OLS(y_train, sm.add_constant(X_train))
model.fit().summary()
###Output
/usr/local/lib/python3.6/dist-packages/numpy/core/fromnumeric.py:2389: FutureWarning: Method .ptp is deprecated and will be removed in a future version. Use numpy.ptp instead.
return ptp(axis=axis, out=out, **kwargs)
###Markdown
==>> appears to be underfit 5. Develop a model that overfits. "The universal tension in machine learning is between optimization and generalization; the ideal model is one that stands right at the border between underfitting and overfitting; between undercapacity and overcapacity. To figure out where this border lies, first you must cross it." —Chollet Diagram source: https://jakevdp.github.io/PythonDataScienceHandbook/05.03-hyperparameters-and-model-validation.htmlValidation-curves-in-Scikit-Learn Polynomial Regression?
###Code
# Copied from cell 10 at
# https://jakevdp.github.io/PythonDataScienceHandbook/05.03-hyperparameters-and-model-validation.html#Validation-curves-in-Scikit-Learn
from sklearn.preprocessing import PolynomialFeatures
from sklearn.linear_model import LinearRegression
from sklearn.pipeline import make_pipeline
def PolynomialRegression(degree=2, **kwargs):
return make_pipeline(PolynomialFeatures(degree),
LinearRegression(**kwargs))
for degree in [0, 1, 2, 3]:
features = PolynomialFeatures(degree).fit(X_train).get_feature_names(X_train.columns)
print(f'{degree} degree polynomial has {len(features)} features')
print(features)
print('\n')
###Output
0 degree polynomial has 1 features
['1']
1 degree polynomial has 8 features
['1', 'PRCP', 'SNOW', 'SNWD', 'TMAX', 'TMIN', 'AWND', 'Total_yesterday']
2 degree polynomial has 36 features
['1', 'PRCP', 'SNOW', 'SNWD', 'TMAX', 'TMIN', 'AWND', 'Total_yesterday', 'PRCP^2', 'PRCP SNOW', 'PRCP SNWD', 'PRCP TMAX', 'PRCP TMIN', 'PRCP AWND', 'PRCP Total_yesterday', 'SNOW^2', 'SNOW SNWD', 'SNOW TMAX', 'SNOW TMIN', 'SNOW AWND', 'SNOW Total_yesterday', 'SNWD^2', 'SNWD TMAX', 'SNWD TMIN', 'SNWD AWND', 'SNWD Total_yesterday', 'TMAX^2', 'TMAX TMIN', 'TMAX AWND', 'TMAX Total_yesterday', 'TMIN^2', 'TMIN AWND', 'TMIN Total_yesterday', 'AWND^2', 'AWND Total_yesterday', 'Total_yesterday^2']
3 degree polynomial has 120 features
['1', 'PRCP', 'SNOW', 'SNWD', 'TMAX', 'TMIN', 'AWND', 'Total_yesterday', 'PRCP^2', 'PRCP SNOW', 'PRCP SNWD', 'PRCP TMAX', 'PRCP TMIN', 'PRCP AWND', 'PRCP Total_yesterday', 'SNOW^2', 'SNOW SNWD', 'SNOW TMAX', 'SNOW TMIN', 'SNOW AWND', 'SNOW Total_yesterday', 'SNWD^2', 'SNWD TMAX', 'SNWD TMIN', 'SNWD AWND', 'SNWD Total_yesterday', 'TMAX^2', 'TMAX TMIN', 'TMAX AWND', 'TMAX Total_yesterday', 'TMIN^2', 'TMIN AWND', 'TMIN Total_yesterday', 'AWND^2', 'AWND Total_yesterday', 'Total_yesterday^2', 'PRCP^3', 'PRCP^2 SNOW', 'PRCP^2 SNWD', 'PRCP^2 TMAX', 'PRCP^2 TMIN', 'PRCP^2 AWND', 'PRCP^2 Total_yesterday', 'PRCP SNOW^2', 'PRCP SNOW SNWD', 'PRCP SNOW TMAX', 'PRCP SNOW TMIN', 'PRCP SNOW AWND', 'PRCP SNOW Total_yesterday', 'PRCP SNWD^2', 'PRCP SNWD TMAX', 'PRCP SNWD TMIN', 'PRCP SNWD AWND', 'PRCP SNWD Total_yesterday', 'PRCP TMAX^2', 'PRCP TMAX TMIN', 'PRCP TMAX AWND', 'PRCP TMAX Total_yesterday', 'PRCP TMIN^2', 'PRCP TMIN AWND', 'PRCP TMIN Total_yesterday', 'PRCP AWND^2', 'PRCP AWND Total_yesterday', 'PRCP Total_yesterday^2', 'SNOW^3', 'SNOW^2 SNWD', 'SNOW^2 TMAX', 'SNOW^2 TMIN', 'SNOW^2 AWND', 'SNOW^2 Total_yesterday', 'SNOW SNWD^2', 'SNOW SNWD TMAX', 'SNOW SNWD TMIN', 'SNOW SNWD AWND', 'SNOW SNWD Total_yesterday', 'SNOW TMAX^2', 'SNOW TMAX TMIN', 'SNOW TMAX AWND', 'SNOW TMAX Total_yesterday', 'SNOW TMIN^2', 'SNOW TMIN AWND', 'SNOW TMIN Total_yesterday', 'SNOW AWND^2', 'SNOW AWND Total_yesterday', 'SNOW Total_yesterday^2', 'SNWD^3', 'SNWD^2 TMAX', 'SNWD^2 TMIN', 'SNWD^2 AWND', 'SNWD^2 Total_yesterday', 'SNWD TMAX^2', 'SNWD TMAX TMIN', 'SNWD TMAX AWND', 'SNWD TMAX Total_yesterday', 'SNWD TMIN^2', 'SNWD TMIN AWND', 'SNWD TMIN Total_yesterday', 'SNWD AWND^2', 'SNWD AWND Total_yesterday', 'SNWD Total_yesterday^2', 'TMAX^3', 'TMAX^2 TMIN', 'TMAX^2 AWND', 'TMAX^2 Total_yesterday', 'TMAX TMIN^2', 'TMAX TMIN AWND', 'TMAX TMIN Total_yesterday', 'TMAX AWND^2', 'TMAX AWND Total_yesterday', 'TMAX Total_yesterday^2', 'TMIN^3', 'TMIN^2 AWND', 'TMIN^2 Total_yesterday', 'TMIN AWND^2', 'TMIN AWND Total_yesterday', 'TMIN Total_yesterday^2', 'AWND^3', 'AWND^2 Total_yesterday', 'AWND Total_yesterday^2', 'Total_yesterday^3']
###Markdown
Validation curve (with Polynomial Regression) https://scikit-learn.org/stable/modules/generated/sklearn.model_selection.validation_curve.html> Validation curve. Determine training and test scores for varying parameter values. This is similar to grid search with one parameter.
###Code
# Modified from cell 13 at
# https://jakevdp.github.io/PythonDataScienceHandbook/05.03-hyperparameters-and-model-validation.html#Validation-curves-in-Scikit-Learn
%matplotlib inline
import numpy as np
import matplotlib.pyplot as plt
from sklearn.model_selection import validation_curve
degree = [0, 1, 2]
train_score, val_score = validation_curve(
PolynomialRegression(), X_train, y_train,
param_name='polynomialfeatures__degree', param_range=degree,
scoring='neg_mean_absolute_error', cv=3)
plt.gcf().set_size_inches(10, 6)
plt.plot(degree, np.median(train_score, 1), color='blue', label='training score')
plt.plot(degree, np.median(val_score, 1), color='red', label='validation score')
plt.legend(loc='best')
plt.xlabel('degree');
###Output
_____no_output_____
###Markdown
Grid Search (with Polynomial Regression)https://scikit-learn.org/stable/modules/generated/sklearn.model_selection.GridSearchCV.htmlhttps://scikit-learn.org/stable/modules/grid_search.html
###Code
# TODO
from sklearn.model_selection import GridSearchCV
param_grid = {
'polynomialfeatures__degree': [0, 1, 2, 3]
}
gridsearch = GridSearchCV(PolynomialRegression(), param_grid=param_grid,
scoring='neg_mean_absolute_error', cv=3, # 3 folds x 4 degrees = 12 outputs
return_train_score=True, verbose=10)
gridsearch.fit(X_train, y_train)
pd.DataFrame(gridsearch.cv_results_)
###Output
_____no_output_____
###Markdown
Random Forest?https://scikit-learn.org/stable/modules/generated/sklearn.ensemble.RandomForestRegressor.html
###Code
# TODO
from sklearn.ensemble import RandomForestRegressor
model = RandomForestRegressor(n_estimators=100, max_depth=20)
scores = cross_validate(model, X_train, y_train,
scoring='neg_mean_absolute_error',
cv=3, return_train_score=True,
return_estimator=True)
pd.DataFrame(scores)
###Output
_____no_output_____
###Markdown
Validation Curve (with Random Forest)
###Code
# Modified from cell 13 at
# https://jakevdp.github.io/PythonDataScienceHandbook/05.03-hyperparameters-and-model-validation.html#Validation-curves-in-Scikit-Learn
%matplotlib inline
import matplotlib.pyplot as plt
from sklearn.model_selection import validation_curve
model = RandomForestRegressor(n_estimators=100)
depth = [2, 3, 4, 5, 6]
train_score, val_score = validation_curve(
model, X_train, y_train,
param_name='max_depth', param_range=depth,
scoring='neg_mean_absolute_error', cv=3)
plt.gcf().set_size_inches(10, 6)
plt.plot(depth, np.median(train_score, 1), color='blue', label='training score')
plt.plot(depth, np.median(val_score, 1), color='red', label='validation score')
plt.legend(loc='best')
plt.xlabel('depth');
###Output
_____no_output_____
###Markdown
Grid Search (with Random Forest)
###Code
# TODO
# set Grid Search params
param_grid = {
'n_estimators': [100, 200],
'max_depth': [4, 5],
'criterion': ['mse', 'mae']
}
gridsearch = GridSearchCV(RandomForestRegressor(), param_grid=param_grid, cv=3,
scoring='neg_mean_absolute_error', verbose=10,
return_train_score=True)
gridsearch.fit(X_train, y_train)
results = pd.DataFrame(gridsearch.cv_results_)
print(f'===>>>Best five results from grid search of {len(results)} parameter combinations<<<===')
results.sort_values(by='rank_test_score').head()
###Output
===>>>Best five results from grid search of 8 parameter combinations<<<===
###Markdown
FEATURE ENGINEERING! Jake VanderPlas demonstrates this feature engineering: https://jakevdp.github.io/PythonDataScienceHandbook/05.06-linear-regression.htmlExample:-Predicting-Bicycle-Traffic
###Code
# Modified from code cells 17-21 at
# https://jakevdp.github.io/PythonDataScienceHandbook/05.06-linear-regression.html#Example:-Predicting-Bicycle-Traffic
# patterns of use generally vary from day to day;
# let's add binary columns that indicate the day of the week:
days = ['Mon', 'Tue', 'Wed', 'Thu', 'Fri', 'Sat', 'Sun']
for i, day in enumerate(days):
X_train[day] = (X_train.index.dayofweek == i).astype(float)
# we might expect riders to behave differently on holidays;
# let's add an indicator of this as well:
from pandas.tseries.holiday import USFederalHolidayCalendar
cal = USFederalHolidayCalendar()
holidays = cal.holidays('2012', '2016')
X_train = X_train.join(pd.Series(1, index=holidays, name='holiday'))
X_train['holiday'].fillna(0, inplace=True)
# We also might suspect that the hours of daylight would affect
# how many people ride; let's use the standard astronomical calculation
# to add this information:
def hours_of_daylight(date, axis=23.44, latitude=47.61):
"""Compute the hours of daylight for the given date"""
days = (date - pd.datetime(2000, 12, 21)).days
m = (1. - np.tan(np.radians(latitude))
* np.tan(np.radians(axis) * np.cos(days * 2 * np.pi / 365.25)))
return 24. * np.degrees(np.arccos(1 - np.clip(m, 0, 2))) / 180.
X_train['daylight_hrs'] = list(map(hours_of_daylight, X_train.index))
# temperatures are in 1/10 deg C; convert to C
X_train['TMIN'] /= 10
X_train['TMAX'] /= 10
# We can also calcuate the average temperature.
X_train['Temp (C)'] = 0.5 * (X_train['TMIN'] + X_train['TMAX'])
# precip is in 1/10 mm; convert to inches
X_train['PRCP'] /= 254
# In addition to the inches of precipitation, let's add a flag that
# indicates whether a day is dry (has zero precipitation):
X_train['dry day'] = (X_train['PRCP'] == 0).astype(int)
# Let's add a counter that increases from day 1, and measures how many
# years have passed. This will let us measure any observed annual increase
# or decrease in daily crossings:
X_train['annual'] = (X_train.index - X_train.index[0]).days / 365.
###Output
_____no_output_____
###Markdown
Linear Regression (with new features)
###Code
# TODO
scores = cross_validate(LinearRegression(), X_train, y_train,
scoring='neg_mean_absolute_error', cv=3,
return_train_score=True, return_estimator=True)
pd.DataFrame(scores)
-scores['test_score'].mean() # neg_mean_absolute_error
###Output
_____no_output_____
###Markdown
Random Forest (with new features)
###Code
# TODO
param_grid = {
'n_estimators': [100],
'max_depth': [5, 10, 15],
'criterion': ['mae']
}
gridsearch = GridSearchCV(RandomForestRegressor(), param_grid=param_grid,
cv=3, scoring='neg_mean_absolute_error',
return_train_score=True, verbose=10)
gridsearch.fit(X_train, y_train)
results = pd.DataFrame(gridsearch.cv_results_)
results.sort_values(by='rank_test_score').head()
###Output
_____no_output_____
###Markdown
Ridge Regression (with new features)https://scikit-learn.org/stable/modules/generated/sklearn.linear_model.Ridge.html
###Code
# TODO
from sklearn.linear_model import Ridge
param_grid = {
'alpha': [0.1, 1.0, 10.0]
}
gridsearch = GridSearchCV(Ridge(), param_grid=param_grid,
scoring='neg_mean_absolute_error',
cv=3, return_train_score=True, verbose=2)
gridsearch.fit(X_train, y_train)
results = pd.DataFrame(gridsearch.cv_results_)
results.sort_values(by='rank_test_score').head()
model = gridsearch.best_estimator_
print("Model type:", type(model))
print("\nIntercept:", model.intercept_)
print("\nCoefficients:")
print(pd.Series(model.coef_, X_train.columns).to_string())
###Output
Model type: <class 'sklearn.linear_model.ridge.Ridge'>
Intercept: 33.741779570641484
Coefficients:
PRCP -553.070741
SNOW -0.002829
SNWD -1.877519
TMAX 63.833062
TMIN -37.450291
AWND -1.900084
Total_yesterday 0.296029
Mon 779.221395
Tue 432.700039
Wed 368.367626
Thu 274.054021
Fri 47.251356
Sat -1099.692199
Sun -801.902237
holiday -939.301546
daylight_hrs 70.256463
Temp (C) 13.191386
dry day 298.475434
annual 44.518889
###Markdown
Compare to statsmodels
###Code
# TODO
model = sm.OLS(y_train, sm.add_constant(X_train))
model.fit().summary()
###Output
/usr/local/lib/python3.6/dist-packages/numpy/core/fromnumeric.py:2389: FutureWarning: Method .ptp is deprecated and will be removed in a future version. Use numpy.ptp instead.
return ptp(axis=axis, out=out, **kwargs)
|
2020.07.2400_classification/.ipynb_checkpoints/RF_knn3-checkpoint.ipynb | ###Markdown
Random Forest
###Code
import numpy as np
import pandas as pd
from sklearn.model_selection import train_test_split,KFold
from sklearn.utils import shuffle
from sklearn.metrics import confusion_matrix,accuracy_score,precision_score,\
recall_score,roc_curve,auc
#import expectation_reflection as ER
#from sklearn.linear_model import LogisticRegression
from sklearn.ensemble import RandomForestClassifier
#from sklearn.model_selection import RandomizedSearchCV
from sklearn.model_selection import GridSearchCV
import matplotlib.pyplot as plt
import seaborn as sns
from sklearn.preprocessing import MinMaxScaler
from function import split_train_test,make_data_balance
np.random.seed(1)
###Output
_____no_output_____
###Markdown
First of all, the processed data are imported.
###Code
#data_list = ['1paradox']
#data_list = ['29parkinson','30paradox2','31renal','32patientcare','33svr','34newt','35pcos']
data_list = np.loadtxt('data_list_30sets.txt',dtype='str')
print(data_list)
def read_data(data_id):
data_name = data_list[data_id]
print('data_name:',data_name)
#Xy = np.loadtxt('%s/data_processed.dat'%data_name)
Xy = np.loadtxt('../classification_data/%s/data_processed_knn3.dat'%data_name)
X = Xy[:,:-1]
y = Xy[:,-1]
#print(np.unique(y,return_counts=True))
X,y = make_data_balance(X,y)
print(np.unique(y,return_counts=True))
X, y = shuffle(X, y, random_state=1)
X_train,X_test,y_train,y_test = train_test_split(X,y,test_size=0.5,random_state = 1)
sc = MinMaxScaler()
X_train = sc.fit_transform(X_train)
X_test = sc.transform(X_test)
return X_train,X_test,y_train,y_test
def measure_performance(X_train,X_test,y_train,y_test):
model = RandomForestClassifier()
# Number of trees in random forest
#n_estimators = [int(x) for x in np.linspace(start = 10, stop = 100, num = 10)]
n_estimators = [10,50,100]
# Number of features to consider at every split
max_features = ['auto']
# Maximum number of levels in tree
#max_depth = [int(x) for x in np.linspace(1, 10, num = 10)]
max_depth = [2,4,6,8,10]
#max_depth.append(None)
# Minimum number of samples required to split a node
min_samples_split = [5, 10, 15, 20]
# Minimum number of samples required at each leaf node
min_samples_leaf = [int(x) for x in np.linspace(start = 1, stop = 5, num = 5)]
# Method of selecting samples for training each tree
#bootstrap = [True, False]
bootstrap = [True]
# Create the random grid
hyper_parameters = {'n_estimators': n_estimators,
'max_features': max_features,
'max_depth': max_depth,
'min_samples_split': min_samples_split,
'min_samples_leaf': min_samples_leaf,
'bootstrap': bootstrap}
#random_search = RandomizedSearchCV(estimator = model, param_distributions = random_grid, n_iter = 100,
# cv = 4, verbose=2, random_state=1, n_jobs = -1)
# Create grid search using cross validation
clf = GridSearchCV(model, hyper_parameters, cv=4, iid='deprecated')
# Fit grid search
best_model = clf.fit(X_train, y_train)
# View best hyperparameters
#print('Best Penalty:', best_model.best_estimator_.get_params()['penalty'])
#print('Best C:', best_model.best_estimator_.get_params()['C'])
# best hyper parameters
print('best_hyper_parameters:',best_model.best_params_)
# performance:
y_test_pred = best_model.best_estimator_.predict(X_test)
acc = accuracy_score(y_test,y_test_pred)
#print('Accuracy:', acc)
p_test_pred = best_model.best_estimator_.predict_proba(X_test) # prob of [0,1]
p_test_pred = p_test_pred[:,1] # prob of 1
fp,tp,thresholds = roc_curve(y_test, p_test_pred, drop_intermediate=False)
roc_auc = auc(fp,tp)
#print('AUC:', roc_auc)
precision = precision_score(y_test,y_test_pred)
#print('Precision:',precision)
recall = recall_score(y_test,y_test_pred)
#print('Recall:',recall)
f1_score = 2*precision*recall/(precision+recall)
return acc,roc_auc,precision,recall,f1_score
n_data = len(data_list)
roc_auc = np.zeros(n_data) ; acc = np.zeros(n_data)
precision = np.zeros(n_data) ; recall = np.zeros(n_data)
f1_score = np.zeros(n_data)
#data_id = 0
for data_id in range(n_data):
X_train,X_test,y_train,y_test = read_data(data_id)
acc[data_id],roc_auc[data_id],precision[data_id],recall[data_id],f1_score[data_id] =\
measure_performance(X_train,X_test,y_train,y_test)
print(data_id,acc[data_id],roc_auc[data_id],precision[data_id],recall[data_id],f1_score[data_id])
print('acc_mean:',acc.mean())
print('roc_mean:',roc_auc.mean())
print('precision:',precision.mean())
print('recall:',recall.mean())
print('f1_score:',f1_score.mean())
np.savetxt('result_knn3_RF.dat',(roc_auc,acc,precision,recall,f1_score),fmt='%f')
###Output
_____no_output_____ |
notebooks/Boundary Value Problem3.ipynb | ###Markdown
***Божнюк Александр Сергеевич, 271 группа*** Задание:$y''+ 2xy'-y = 2(x^2+1)cos(x)$$0 <= x <= 0.5$$y(0) = 0$ $y(0.5) = 0.5sin(0.5)$ Код решения (Python3)
###Code
from math import sin, cos
import matplotlib.pyplot as plt
def bvp(N, a, b, y0, yN, p, q, f):
h = (b - a) / N
x = [a + k * h for k in range(0, N + 1)]
L = [-1, 0] # we don't use L[0]
K = [-1, y0] # we don't use K[0]
# L[k] and K[k] evaluation
for j in range(2, N + 1):
ap = 1 - p(x[j - 1]) * h / 2
bp = h * h * q(x[j - 1]) - 2
cp = 1 + p(x[j - 1]) * h / 2
fp = h * h * f(x[j - 1])
lc = - cp / (ap * L[j - 1] + bp)
kc = (-ap * K[j - 1] + fp) / (ap * L[j - 1] + bp)
L.append(lc)
K.append(kc)
# y[k] evaluation
y = [yN]
for j in range(N - 1, 0, -1):
y.insert(0, L[j + 1] * y[0] + K[j + 1])
y.insert(0, y0)
return (x, y)
def graph_plot(x, y):
plt.plot(x1, y1)
plt.xlabel("x")
plt.ylabel("y")
plt.show()
N1 = 10
N2 = 20
a = 0
b = 0.5
y0 = 0
s = 'right'
yN = 0.5 * sin(0.5)
p = lambda x: 2 * x
q = lambda _: -1
v = 'right'
f = lambda x: 2 * (x * x + 1) * cos(x)
x1, y1 = bvp(N1, a, b, y0, yN, p, q, f)
print("x1:", x1)
print("y1:", y1)
graph_plot(x1, y1)
x2, y2 = bvp(N2, a, b, y0, yN, p, q, f)
graph_plot(x2, y2)
###Output
_____no_output_____
###Markdown
Погрешность
###Code
f = 0
for i in range (0, 21, 2) :
if (abs(y1[i // 2] - y2[i]) > f) :
f = abs(y1[i // 2] - y2[i])
print(f)
###Output
2.29704237213052e-05
|
ons-spark/raw-notebooks/module-imports/module-imports.ipynb | ###Markdown
Naming Conflicts in Module ImportsImporting modules in Python and R can lead to naming conflicts if a function with that name already exists. This article demonstrates why you should be careful when importing modules to ensure that these conflicts do not occur.A common example in Python is using [`from pyspark.sql.functions import *`](https://spark.apache.org/docs/latest/api/python/reference/pyspark.sql.htmlfunctions), which will overwrite some built-in Python functions (e.g. `sum()`). Instead, it is good practice to use `from pyspark.sql import functions as F`, where you prefix the functions with `F`, e.g. [`F.sum()`](https://spark.apache.org/docs/latest/api/python/reference/api/pyspark.sql.functions.sum.html). Naming variablesWhen writing code, it is important to give your variables sensible names, that are informative but not too long. A good reference on this is the [Clean Code](https://best-practice-and-impact.github.io/qa-of-code-guidance/core_programming.htmlclean-code) section from [QA of Code for Analysis and Research](https://best-practice-and-impact.github.io/qa-of-code-guidance/intro.html). **You should avoid using the names of existing built in functions for user-defined variables**. KeywordsSome words are reserved: for instance, in Python you cannot have a variable called `def`, `False` or `lambda`. These are referred to as *keywords* and the code will not even compile if you try, raising a `SyntaxError`. You can generate a list of these with [`keyword.kwlist`](https://docs.python.org/3/library/keyword.html).In R, use `?reserved` to get a list of the reserved words.
###Code
import keyword
print(keyword.kwlist)
###Output
['False', 'None', 'True', 'and', 'as', 'assert', 'break', 'class', 'continue', 'def', 'del', 'elif', 'else', 'except', 'finally', 'for', 'from', 'global', 'if', 'import', 'in', 'is', 'lambda', 'nonlocal', 'not', 'or', 'pass', 'raise', 'return', 'try', 'while', 'with', 'yield']
###Markdown
```r?reserved``` Built in functions and module imports in Python Python ExampleYou might notice that the Python keyword list is quite short and that some common Python functionality is not listed, for instance, `sum()` or `round()`. This means that it is possible to overwrite these; obviously this is not good practice and should be avoided. This can be surprisingly easy to do in PySpark, and can be hard to debug if you do not know the reason for the error. Python ExampleFirst, look at the documentation for `sum`:
###Code
help(sum)
###Output
Help on built-in function sum in module builtins:
sum(iterable, start=0, /)
Return the sum of a 'start' value (default: 0) plus an iterable of numbers
When the iterable is empty, return the start value.
This function is intended specifically for use with numeric values and may
reject non-numeric types.
###Markdown
Show that `sum` works with a simple example: adding three integers together:
###Code
sum([1, 2, 3])
###Output
_____no_output_____
###Markdown
Now import the modules we need to use Spark. The recommended way to do this is `import pyspark.sql.functions as F`, which means that whenever you want to access a function from this module you prefix it with `F`, e.g. `F.sum()`. Sometimes the best way to see why something is recommended is to try a different method and show it is a bad idea, in this case, importing all the `functions` as `*`:
###Code
from pyspark.sql import SparkSession
from pyspark.sql.functions import *
###Output
_____no_output_____
###Markdown
Attempting to sum the integers will now give an error:
###Code
try:
sum([1, 2, 3])
except AttributeError as e:
print(e)
###Output
'NoneType' object has no attribute '_jvm'
###Markdown
To see why this error exists, take another look at `help(sum)`; we can see that the documentation is different to previously.
###Code
help(sum)
###Output
Help on function sum in module pyspark.sql.functions:
sum(col)
Aggregate function: returns the sum of all values in the expression.
.. versionadded:: 1.3
###Markdown
So by importing all the PySpark functions we have overwritten some key Python functionality. Note that this would also apply if you imported individual functions, e.g. `from pyspark.sql.functions import sum`.You can also overwrite functions with your own variables, often unintentionally. As an example, first Start a Spark session:
###Code
spark = (SparkSession.builder.master("local[2]")
.appName("module-imports")
.getOrCreate())
###Output
_____no_output_____
###Markdown
Create a small DataFrame:
###Code
sdf = spark.range(5).withColumn("double_id", col("id") * 2)
sdf.show()
###Output
+---+---------+
| id|double_id|
+---+---------+
| 0| 0|
| 1| 2|
| 2| 4|
| 3| 6|
| 4| 8|
+---+---------+
###Markdown
Loop through the columns, using `col` as the control variable. This will work, but is not a good idea as it is overwriting `col()` from `functions`:
###Code
for col in sdf.columns:
sdf.select(col).show()
###Output
+---+
| id|
+---+
| 0|
| 1|
| 2|
| 3|
| 4|
+---+
+---------+
|double_id|
+---------+
| 0|
| 2|
| 4|
| 6|
| 8|
+---------+
###Markdown
If we try adding another column with `col()` then it will not work as we have now reassigned `col` to be `double_id`:
###Code
try:
sdf = sdf.withColumn("triple_id", col("id") * 3)
except TypeError as e:
print(e)
col
###Output
_____no_output_____
###Markdown
Importing the PySpark `functions` as `F` and using [`F.col()`](https://spark.apache.org/docs/latest/api/python/reference/api/pyspark.sql.functions.col.html) solves this problem:
###Code
from pyspark.sql import functions as F
sdf = sdf.withColumn("triple_id", F.col("id") * 3)
sdf.show()
###Output
+---+---------+---------+
| id|double_id|triple_id|
+---+---------+---------+
| 0| 0| 0|
| 1| 2| 3|
| 2| 4| 6|
| 3| 6| 9|
| 4| 8| 12|
+---+---------+---------+
|
citysong agent-based model-suitability-choice.ipynb | ###Markdown
citysong an agent-based approach to modeling urban birdsong import statements
###Code
import numpy
from matplotlib import pyplot
from scipy.ndimage import uniform_filter
from math import sqrt, ceil
from numba import jit, guvectorize, int64, float64
import rasterio
###Output
_____no_output_____
###Markdown
import suitability
###Code
import rasterio
with rasterio.open('SUITABILITY.tif', 'r') as r:
arr = r.read()[0] # read all raster values
suitability = arr[slice(1000, 1300, 1), slice(1000, 1300, 1)]
###Output
_____no_output_____
###Markdown
variable declarations
###Code
width = suitability.shape[1]
height = suitability.shape[0]
seconds = 3600
n_birds = 100
cmp = numpy.zeros((width, height), dtype=numpy.int64)
index = numpy.arange(0, width * height, dtype=numpy.int64)
###Output
_____no_output_____
###Markdown
create maps
###Code
index_coords = numpy.zeros((width * height, 2), dtype=numpy.int64)
x = 0
for r in range(height):
for c in range(width):
index_coords[x] = [r, c]
x = x + 1
suitability_sort = numpy.copy(suitability.ravel())
suitability_sum = suitability.sum()
suitability_sort = suitability_sort/suitability_sum
###Output
_____no_output_____
###Markdown
bird 0 -> column (x position) 1 -> row (y position) 2 -> moving (0 or 1) 3 -> move complete (0-1) 4 -> column (x start) 5 -> row (y start) 6 -> column (x destination) 7 -> row (y destination) 8 -> move distance create birds
###Code
birds = numpy.zeros((n_birds, 9), dtype=numpy.int64)
for idx, b in enumerate(birds):
birds[idx][0] = numpy.random.randint(0, height)
birds[idx][1] = numpy.random.randint(0, width)
birds[idx][2] = 0
birds[idx][3] = 0
birds[idx][4] = 0
birds[idx][5] = 0
birds[idx][6] = 0
birds[idx][7] = 0
birds[idx][8] = 0
###Output
_____no_output_____
###Markdown
plot habitat
###Code
figure, ax = pyplot.subplots()
figure.set_figheight(10)
figure.set_figwidth(10)
ax.imshow(suitability, cmap="BuPu", origin='lower')
ax.scatter(birds[:, 0], birds[:, 1], color='black')
ax.set_xlim(0, width)
ax.set_ylim(0, height)
ax.tick_params(axis='both', which='both', bottom='off', labelbottom='off', left='off', labelleft='off')
figure.savefig("a.png", dpi=300)
###Output
_____no_output_____
###Markdown
run simulation
###Code
samples = numpy.random.choice(index, size=(seconds, n_birds), p=suitability_sort)
def run_guvectorize(grid, seconds, n_birds, birds, index, samples, index_coords, cmp):
for s in range(seconds):
for idx in range(n_birds):
b = birds[idx]
# check if bird is moving
if b[2]:
b[3] = b[3] + 1
p = float(b[3]) / float(b[8])
# calculate coords and update bird's position
b[0] = int(ceil((b[6] - b[4]) * p) + b[4])# + numpy.random.randint(-5, 6)
b[1] = int(ceil((b[7] - b[5]) * p) + b[5])# + numpy.random.randint(-5, 6)
# check if bird reached destination
if b[0] == b[6] and b[1] == b[7]:
b[2] = 0
b[3] = 0
b[4] = 0
b[5] = 0
b[6] = 0
b[7] = 0
b[8] = 0
# bird is not moving
else:
# possibly set bird in motion
move = numpy.random.randint(0, 100)
if move < 50:
# count number of nearby birds:
count = ((birds[:, 0] > (b[0] - 25))*(birds[:, 0] < (b[0] + 25))*(birds[:, 1] > (b[1] - 25))*(birds[:, 1]< (b[1] + 25))).sum()
if count < 3 or move < 1:
# set bird is moving
b[2] = 1
# save start destination
b[4] = b[0]
b[5] = b[1]
# set new destination
b[6] = index_coords[samples[s, idx]][0]
b[7] = index_coords[samples[s, idx]][1]
# set distance of move
b[8] = ceil(sqrt((b[6]-b[4])**2 + (b[7]-b[5])**2))
# check for possible bird call
call = numpy.random.randint(0, 100)
if call < 33:
# check if bird is near other birds
# count number of nearby birds:
count = ((birds[:, 0] > (b[0] - 25))*(birds[:, 0] < (b[0] + 25))*(birds[:, 1] > (b[1] - 25))*(birds[:, 1]< (b[1] + 25))).sum()
if count < 15:
cmp[b[0], b[1]] = cmp[b[0], b[1]] + 1
run_numba = guvectorize('int64[:,:], int64, int64, int64[:,:], int64[:], int64[:,:], int64[:,:], int64[:,:]', '(w,h),(),(),(b,a),(n),(s,b),(n,c)->(w,h)', target='parallel', nopython=True)(run_guvectorize)
cmp = run_numba(cmp, seconds, n_birds, birds, index, samples, index_coords, cmp)
###Output
_____no_output_____
###Markdown
create sound attenuation mask
###Code
call_mask = numpy.zeros(shape=(101, 101))
for c in range(101):
for r in range(101):
dist = sqrt((50-c)**2 + (50-r)**2)
if dist <= 50:
try:
call_mask[r, c] = 1
except ZeroDivisionError:
call_mask[r, c] = 1
cmp_2 = numpy.zeros(shape=(height+100, width+100))
def call():
for c in range(50, width+50):
for r in range(50, height+50):
value = cmp[r-50, c-50]
if value > 0:
cmp_2[r-50:r+51, c-50:c+51] = cmp_2[r-50:r+51, c-50:c+51] + call_mask * value
call()
figure, ax = pyplot.subplots()
figure.set_figheight(10)
figure.set_figwidth(10)
#ax.imshow(suitability, cmap='BuPu')
ax.imshow(cmp_2[slice(50, height+50, 1), slice(50, width+50, 1)], cmap="viridis", origin="lower")
ax.scatter(birds[:, 0], birds[:, 1], color='black')
ax.set_xlim(0, width)
ax.set_ylim(0, height)
ax.tick_params(axis='both', which='both', bottom='off', labelbottom='off', left='off', labelleft='off')
figure.savefig("b_dist.png", dpi=300)
###Output
_____no_output_____ |
8_Simple_RNN.ipynb | ###Markdown
Simple RNNIn ths notebook, we're going to train a simple RNN to do **time-series prediction**. Given some set of input data, it should be able to generate a prediction for the next time step!> * First, we'll create our data* Then, define an RNN in PyTorch* Finally, we'll train our network and see how it performs Import resources and create data
###Code
import torch
from torch import nn
import numpy as np
import matplotlib.pyplot as plt
%matplotlib inline
plt.figure(figsize=(8,5))
# how many time steps/data pts are in one batch of data
seq_length = 20
# generate evenly spaced data pts
time_steps = np.linspace(0, np.pi, seq_length + 1)
data = np.sin(time_steps)
print("len of data is:",len(data),data.shape)
print(data)
data.resize((seq_length + 1, 1)) # size becomes (seq_length+1, 1), adds an input_size dimension
print("len of data after resize is:",len(data),data.shape)
print(data)
x = data[:-1] # all but the last piece of data
print('x values are: \n',x)
print('--------------------------------')
y = data[1:] # all but the first
print('y value are: \n',y)
# display the data
plt.plot(time_steps[1:], x, 'r.', label='input, x') # x
plt.plot(time_steps[1:], y, 'b.', label='target, y') # y
plt.legend(loc='best')
plt.show()
###Output
len of data is: 21 (21,)
[0.00000000e+00 1.56434465e-01 3.09016994e-01 4.53990500e-01
5.87785252e-01 7.07106781e-01 8.09016994e-01 8.91006524e-01
9.51056516e-01 9.87688341e-01 1.00000000e+00 9.87688341e-01
9.51056516e-01 8.91006524e-01 8.09016994e-01 7.07106781e-01
5.87785252e-01 4.53990500e-01 3.09016994e-01 1.56434465e-01
1.22464680e-16]
len of data after resize is: 21 (21, 1)
[[0.00000000e+00]
[1.56434465e-01]
[3.09016994e-01]
[4.53990500e-01]
[5.87785252e-01]
[7.07106781e-01]
[8.09016994e-01]
[8.91006524e-01]
[9.51056516e-01]
[9.87688341e-01]
[1.00000000e+00]
[9.87688341e-01]
[9.51056516e-01]
[8.91006524e-01]
[8.09016994e-01]
[7.07106781e-01]
[5.87785252e-01]
[4.53990500e-01]
[3.09016994e-01]
[1.56434465e-01]
[1.22464680e-16]]
x values are:
[[0. ]
[0.15643447]
[0.30901699]
[0.4539905 ]
[0.58778525]
[0.70710678]
[0.80901699]
[0.89100652]
[0.95105652]
[0.98768834]
[1. ]
[0.98768834]
[0.95105652]
[0.89100652]
[0.80901699]
[0.70710678]
[0.58778525]
[0.4539905 ]
[0.30901699]
[0.15643447]]
--------------------------------
y value are:
[[1.56434465e-01]
[3.09016994e-01]
[4.53990500e-01]
[5.87785252e-01]
[7.07106781e-01]
[8.09016994e-01]
[8.91006524e-01]
[9.51056516e-01]
[9.87688341e-01]
[1.00000000e+00]
[9.87688341e-01]
[9.51056516e-01]
[8.91006524e-01]
[8.09016994e-01]
[7.07106781e-01]
[5.87785252e-01]
[4.53990500e-01]
[3.09016994e-01]
[1.56434465e-01]
[1.22464680e-16]]
###Markdown
--- Define the RNNNext, we define an RNN in PyTorch. We'll use `nn.RNN` to create an RNN layer, then we'll add a last, fully-connected layer to get the output size that we want. An RNN takes in a number of parameters:* **input_size** - the size of the input* **hidden_dim** - the number of features in the RNN output and in the hidden state* **n_layers** - the number of layers that make up the RNN, typically 1-3; greater than 1 means that you'll create a stacked RNN* **batch_first** - whether or not the input/output of the RNN will have the batch_size as the first dimension (batch_size, seq_length, hidden_dim)Take a look at the [RNN documentation](https://pytorch.org/docs/stable/nn.htmlrnn) to read more about recurrent layers.
###Code
class RNN(nn.Module):
def __init__(self, input_size, output_size, hidden_dim, n_layers):
super(RNN, self).__init__()
self.hidden_dim=hidden_dim
# define an RNN with specified parameters
# batch_first means that the first dim of the input and output will be the batch_size
self.rnn = nn.RNN(input_size, hidden_dim, n_layers, batch_first=True)
# last, fully-connected layer
self.fc = nn.Linear(hidden_dim, output_size)
def forward(self, x, hidden):
# x (batch_size, seq_length, input_size)
# hidden (n_layers, batch_size, hidden_dim)
# r_out (batch_size, time_step, hidden_size)
batch_size = x.size(0)
# get RNN outputs
r_out, hidden = self.rnn(x, hidden)
# shape output to be (batch_size*seq_length, hidden_dim)
r_out = r_out.view(-1, self.hidden_dim)
# get final output
output = self.fc(r_out)
return output, hidden
###Output
_____no_output_____
###Markdown
Check the input and output dimensionsAs a check that your model is working as expected, test out how it responds to input data.
###Code
# test that dimensions are as expected
test_rnn = RNN(input_size=1, output_size=1, hidden_dim=10, n_layers=2)
# generate evenly spaced, test data pts
time_steps = np.linspace(0, np.pi, seq_length)
data = np.sin(time_steps)
data.resize((seq_length, 1))
test_input = torch.Tensor(data).unsqueeze(0) # give it a batch_size of 1 as first dimension
print('Input size: ', test_input.size())
# test out rnn sizes
test_out, test_h = test_rnn(test_input, None)
print('Output size: ', test_out.size())
print('Hidden state size: ', test_h.size())
###Output
Input size: torch.Size([1, 20, 1])
Output size: torch.Size([20, 1])
Hidden state size: torch.Size([2, 1, 10])
###Markdown
--- Training the RNNNext, we'll instantiate an RNN with some specified hyperparameters. Then train it over a series of steps, and see how it performs.
###Code
# decide on hyperparameters
input_size=1
output_size=1
hidden_dim=32
n_layers=1
# instantiate an RNN
rnn = RNN(input_size, output_size, hidden_dim, n_layers)
print(rnn)
###Output
RNN(
(rnn): RNN(1, 32, batch_first=True)
(fc): Linear(in_features=32, out_features=1, bias=True)
)
###Markdown
Loss and OptimizationThis is a regression problem: can we train an RNN to accurately predict the next data point, given a current data point?>* The data points are coordinate values, so to compare a predicted and ground_truth point, we'll use a regression loss: the mean squared error.* It's typical to use an Adam optimizer for recurrent models.
###Code
# MSE loss and Adam optimizer with a learning rate of 0.01
criterion = nn.MSELoss()
optimizer = torch.optim.Adam(rnn.parameters(), lr=0.01)
###Output
_____no_output_____
###Markdown
Defining the training functionThis function takes in an rnn, a number of steps to train for, and returns a trained rnn. This function is also responsible for displaying the loss and the predictions, every so often. Hidden StatePay close attention to the hidden state, here:* Before looping over a batch of training data, the hidden state is initialized* After a new hidden state is generated by the rnn, we get the latest hidden state, and use that as input to the rnn for the following steps
###Code
# train the RNN
def train(rnn, n_steps, print_every):
# initialize the hidden state
hidden = None
for batch_i, step in enumerate(range(n_steps)):
# defining the training data
time_steps = np.linspace(step * np.pi, (step+1)*np.pi, seq_length + 1)
data = np.sin(time_steps)
data.resize((seq_length + 1, 1)) # input_size=1
x = data[:-1]
y = data[1:]
# convert data into Tensors
x_tensor = torch.Tensor(x).unsqueeze(0) # unsqueeze gives a 1, batch_size dimension
y_tensor = torch.Tensor(y)
# outputs from the rnn
prediction, hidden = rnn(x_tensor, hidden)
## Representing Memory ##
# make a new variable for hidden and detach the hidden state from its history
# this way, we don't backpropagate through the entire history
hidden = hidden.data
# calculate the loss
loss = criterion(prediction, y_tensor)
# zero gradients
optimizer.zero_grad()
# perform backprop and update weights
loss.backward()
optimizer.step()
# display loss and predictions
if batch_i%print_every == 0:
print('Loss: ', loss.item())
plt.plot(time_steps[1:], x, 'r.') # input
plt.plot(time_steps[1:], prediction.data.numpy().flatten(), 'b.') # predictions
plt.show()
return rnn
# train the rnn and monitor results
n_steps = 75
print_every = 15
trained_rnn = train(rnn, n_steps, print_every)
###Output
Loss: 0.5841705203056335
###Markdown
Time-Series PredictionTime-series prediction can be applied to many tasks. Think about weather forecasting or predicting the ebb and flow of stock market prices. You can even try to generate predictions much further in the future than just one time step!
###Code
###Output
_____no_output_____ |
22-Names scores .ipynb | ###Markdown
* Names scores Using names.txt (right click and 'Save Link/Target As...'), a 46K text file containing over five-thousand first names, begin by sorting it into alphabetical order. Then working out the alphabetical value for each name, multiply this value by its alphabetical position in the list to obtain a name score.For example, when the list is sorted into alphabetical order, COLIN, which is worth 3 + 15 + 12 + 9 + 14 = 53, is the 938th name in the list. So, COLIN would obtain a score of 938 × 53 = 49714.What is the total of all the name scores in the file? First, I must to break this problem into many small pieces as much as I can. * 1 open the file,* 2 read the file,* 3 sorte the names into alphabetical order,* 4 setting for each letter a numeric value, according to its position,* 5 loocking for the score for each number,* 6 loocking for the sum of these score.
###Code
if __name__ == '__main__':
assert contagem(file) > 5000
def contagem(file):
with open(file) as f:
read_file = f.read()
if __name__ == '__main__':
assert int(contagem('external_files/p022_names.txt')) > 5000
def contagem(file):
with open(file) as f:
read_file = f.read()
return(read_file.count(','))
if __name__ == '__main__':
assert int(contagem('external_files/p022_names.txt')) > 5000
"""Because I know my external_file has more than 5000 names."""
def contagem(file):
with open(file) as f:
read_file = f.readlines()
#read_file = list(read_file)
#ordenado = read_file2.sort()
return(len(read_file))
contagem('external_files/p022_names.txt')
def contagem(file):
lista = []
with open(file) as f:
read_file = f.readline()
#read_file = list(read_file)
#ordenado = read_file2.sort()
for each_word in read_file:
lista.append(each_word)
return(lista)
contagem('external_files/p022_names.txt')
def contagem(file):
lista = []
with open(file) as f:
read_file = f.readlines()
#read_file = list(read_file)
#ordenado = read_file2.sort()
for each_word in read_file:
each_word = each_word.strip("""""")
lista.append(each_word)
return(lista)
Existe alguma coisa no pythonnivelzero, em um dos primeiros notebook, o qual pode ajudar
a tratar destas strings
###Output
_____no_output_____ |
chapter-05/chapter_5_workbook.ipynb | ###Markdown
Chapter 5: Model Validation and OptimizationWe continue where `chapter_4_workbook.ipynb` left off, by loading the processed Human Resource Analytics dataset and exploring more advanced methods for training models with scikit-learn. We implement k-fold cross validation strategies, and lean about PCA dimensionality reduction.---
###Code
import pandas as pd
import numpy as np
import datetime
import time
import os
import matplotlib.pyplot as plt
%matplotlib inline
import seaborn as sns
%config InlineBackend.figure_format='retina'
sns.set() # Revert to matplotlib defaults
plt.rcParams['figure.figsize'] = (8, 8)
plt.rcParams['axes.labelpad'] = 10
sns.set_style("darkgrid")
%load_ext watermark
%watermark -d -v -m -p requests,numpy,pandas,matplotlib,seaborn,sklearn
###Output
2020-02-16
CPython 3.7.5
IPython 7.10.1
requests 2.22.0
numpy 1.17.4
pandas 0.25.3
matplotlib 3.1.1
seaborn 0.9.0
sklearn 0.21.3
compiler : Clang 4.0.1 (tags/RELEASE_401/final)
system : Darwin
release : 18.7.0
machine : x86_64
processor : i386
CPU cores : 8
interpreter: 64bit
###Markdown
--- Model selection techniquesWe start using model selection techniques to optimize classification models.--- K-fold cross validation---
###Code
# Load the processed data
df = pd.read_csv('../data/hr-analytics/hr_data_processed.csv')
# Select training / validation and test set
from sklearn.model_selection import train_test_split
features = ['satisfaction_level', 'last_evaluation']
X, X_test, y, y_test = train_test_split(
df[features].values,
df['left'].values,
test_size=0.15,
random_state=1
)
# Instantiate the model
from sklearn.tree import DecisionTreeClassifier
clf = DecisionTreeClassifier(max_depth=5)
# Stratified k-fold cross validation
from sklearn.model_selection import cross_val_score
np.random.seed(1)
scores = cross_val_score(
estimator=clf,
X=X,
y=y,
cv=10,
)
print('accuracy = {:.3f} +/- {:.3f}'.format(
scores.mean(),
scores.std(),
))
# Custom function for class accuracies
from sklearn.model_selection import StratifiedKFold
from sklearn.metrics import confusion_matrix
def cross_val_class_score(clf, X, y, cv=10):
kfold = (
StratifiedKFold(n_splits=cv)
.split(X, y)
)
class_accuracy = []
for k, (train, test) in enumerate(kfold):
clf.fit(X[train], y[train])
y_test = y[test]
y_pred = clf.predict(X[test])
cmat = confusion_matrix(y_test, y_pred)
class_acc = cmat.diagonal()/cmat.sum(axis=1)
class_accuracy.append(class_acc)
print('fold: {:d} accuracy: {:s}'.format(
k+1,
str(class_acc),
))
return np.array(class_accuracy)
# Stratified k-fold cross validation
np.random.seed(1)
scores = cross_val_class_score(clf, X, y)
print('accuracy = {} +/- {}'.format(
scores.mean(axis=0),
scores.std(axis=0),
))
###Output
fold: 1 accuracy: [0.98559671 0.72039474]
fold: 2 accuracy: [0.98559671 0.68976898]
fold: 3 accuracy: [0.98971193 0.72937294]
fold: 4 accuracy: [0.98765432 0.74257426]
fold: 5 accuracy: [0.99074074 0.71617162]
fold: 6 accuracy: [0.98971193 0.72607261]
fold: 7 accuracy: [0.98251029 0.68976898]
fold: 8 accuracy: [0.98559671 0.69306931]
fold: 9 accuracy: [0.98455201 0.72277228]
fold: 10 accuracy: [0.98352214 0.74917492]
accuracy = [0.98651935 0.71791406] +/- [0.00266409 0.0200439 ]
###Markdown
--- Validation curves---
###Code
# Calcualte a validation curve
from sklearn.model_selection import validation_curve
clf = DecisionTreeClassifier()
max_depth_range = np.arange(3, 20, 1)
np.random.seed(1)
train_scores, test_scores = validation_curve(
estimator=clf,
X=X,
y=y,
param_name='max_depth',
param_range=max_depth_range,
cv=5,
);
# Function to draw the validation curve
def plot_validation_curve(
train_scores,
test_scores,
param_range,
xlabel='',
log=False,
):
"""This code is from scikit-learn docs (BSD License).
http://scikit-learn.org/stable/auto_examples/model_selection/plot_learning_curve.html
"""
train_mean = np.mean(train_scores, axis=1)
train_std = np.std(train_scores, axis=1)
test_mean = np.mean(test_scores, axis=1)
test_std = np.std(test_scores, axis=1)
fig = plt.figure()
plt.plot(param_range, train_mean,
color=sns.color_palette('Set1')[1], marker='o',
markersize=5, label='training accuracy')
plt.fill_between(param_range, train_mean + train_std,
train_mean - train_std, alpha=0.15,
color=sns.color_palette('Set1')[1])
plt.plot(param_range, test_mean,
color=sns.color_palette('Set1')[0], linestyle='--',
marker='s', markersize=5,
label='validation accuracy')
plt.fill_between(param_range,
test_mean + test_std,
test_mean - test_std,
alpha=0.15, color=sns.color_palette('Set1')[0])
if log:
plt.xscale('log')
plt.legend(loc='lower right')
if xlabel:
plt.xlabel(xlabel)
plt.ylabel('Accuracy')
plt.ylim(0.9, 1.0)
return fig
plot_validation_curve(
train_scores,
test_scores,
max_depth_range,
xlabel='max_depth',
)
plt.ylim(0.89, 0.95)
plt.savefig(
'../figures/chapter-5-hr-analytics-val-curve-model-1.png',
bbox_inches='tight',
dpi=300,
)
###Output
_____no_output_____
###Markdown
--- Dimensionality Reduction with PCA---
###Code
# Load the processed data
df = pd.read_csv('../data/hr-analytics/hr_data_processed.csv')
print(df.columns)
# Train a "quick and dirty" decision tree
features = [
'satisfaction_level', 'last_evaluation', 'number_project',
'average_montly_hours', 'time_spend_company', 'work_accident',
'promotion_last_5years', 'department_IT', 'department_RandD',
'department_accounting', 'department_hr', 'department_management',
'department_marketing', 'department_product_mng', 'department_sales',
'department_support', 'department_technical', 'salary_high',
'salary_low', 'salary_medium'
]
X = df[features].values
y = df.left.values
from sklearn.tree import DecisionTreeClassifier
clf = DecisionTreeClassifier(max_depth=10)
clf.fit(X, y)
# Visualize the feature importances
(
pd.Series(
clf.feature_importances_,
name='Feature importance',
index=df[features].columns,
)
.sort_values()
.plot.barh()
)
plt.xlabel('Feature importance')
plt.savefig(
'../figures/chapter-5-hr-analytics-feature-imp-model-2.png',
bbox_inches='tight',
dpi=300,
)
# Select features with low / high importance
importances = list(
pd.Series(
clf.feature_importances_,
index=df[features].columns,
)
.sort_values(ascending=False).index
)
low_importance_features = importances[5:]
high_importance_features = importances[:5]
np.array(low_importance_features)
np.array(high_importance_features)
# Reduce the one-hot-encoded features with PCA
from sklearn.decomposition import PCA
pca_features = [
'salary_low', 'department_technical', 'department_support',
'work_accident', 'salary_medium', 'department_IT',
'department_RandD', 'salary_high', 'department_management',
'department_accounting', 'department_hr', 'department_sales',
'department_product_mng', 'promotion_last_5years',
'department_marketing'
]
X_reduce = df[pca_features]
pca = PCA(n_components=3)
pca.fit(X_reduce)
X_pca = pca.transform(X_reduce)
X_pca.shape
# Add principal components to df
df['first_principle_component'] = X_pca.T[0]
df['second_principle_component'] = X_pca.T[1]
df['third_principle_component'] = X_pca.T[2]
# Save the pca data
df.to_csv(
'../data/hr-analytics/hr_data_processed_pca.csv',
index=False,
)
# Save the PCA transformer
import joblib
joblib.dump(pca, 'hr-analytics-pca.pkl')
###Output
_____no_output_____
###Markdown
--- Training a Production Ready Model for Employee Turnover---
###Code
# Load the processed data
df = pd.read_csv('../data/hr-analytics/hr_data_processed_pca.csv')
df.columns
# Select training / validation and test set
from sklearn.model_selection import train_test_split
features = [
'satisfaction_level', 'last_evaluation', 'time_spend_company',
'number_project', 'average_montly_hours',
'first_principle_component',
'second_principle_component',
'third_principle_component',
]
X, X_test, y, y_test = train_test_split(
df[features].values,
df['left'].values,
test_size=0.15,
random_state=1
)
%%time
# Calculate a validation curve for max_depth
# using a DecisionTreeClassifier
from sklearn.tree import DecisionTreeClassifier
np.random.seed(1)
clf = DecisionTreeClassifier()
max_depth_range = np.arange(2, 52, 2)
print('Training {} models ...'.format(len(max_depth_range)))
train_scores, test_scores = validation_curve(
estimator=clf,
X=X,
y=y,
param_name='max_depth',
param_range=max_depth_range,
cv=10,
);
# Draw the validation curve
plot_validation_curve(
train_scores,
test_scores,
max_depth_range,
xlabel='max_depth',
)
plt.ylim(0.95, 1.0)
plt.savefig(
'../figures/chapter-5-hr-analytics-val-curve-pca-tree.png',
bbox_inches='tight',
dpi=300,
)
# Show k-fold results for the selected model:
# a decision tree with max_depth = 8
clf = DecisionTreeClassifier(max_depth=8)
np.random.seed(1)
scores = cross_val_class_score(clf, X, y)
print('accuracy = {} +/- {}'.format(
scores.mean(axis=0),
scores.std(axis=0),
))
# Box plot of result
fig = plt.figure(figsize=(5, 7))
sns.boxplot(
data=pd.DataFrame(scores, columns=[0, 1]),
palette=sns.color_palette('Set1'),
)
plt.xlabel('Left (0="no", 1="yes")')
plt.ylabel('Accuracy')
plt.savefig(
'../figures/chapter-5-hr-analytics-kfold-pca-tree.png',
bbox_inches='tight',
dpi=300,
)
# Evaluate performance on the test set
from sklearn.metrics import confusion_matrix
clf = DecisionTreeClassifier(max_depth=8)
clf.fit(X, y)
y_pred = clf.predict(X_test)
cmat = confusion_matrix(y_test, y_pred)
cmat.diagonal() / cmat.sum(axis=1) * 100
# Train the final model on all the samples
features = [
'satisfaction_level', 'last_evaluation', 'time_spend_company',
'number_project', 'average_montly_hours',
'first_principle_component',
'second_principle_component',
'third_principle_component',
]
X = df[features].values
y = df['left'].values
clf = DecisionTreeClassifier(max_depth=8)
clf.fit(X, y)
# Save the model
import joblib
joblib.dump(clf, 'hr-analytics-pca-tree.pkl')
# Check that it saved to the working directory
!ls .
# Load the saved model
clf = joblib.load('hr-analytics-pca-tree.pkl')
clf
# Print an example
pca_features = [
'salary_low', 'department_technical', 'work_accident',
'department_support', 'department_IT', 'department_RandD',
'salary_high', 'salary_medium', 'department_management',
'department_accounting', 'department_hr', 'department_sales',
'department_product_mng', 'promotion_last_5years',
'department_marketing'
]
non_pca_features = [
'satisfaction_level', 'last_evaluation', 'time_spend_company',
'number_project', 'average_montly_hours'
]
bob = df.iloc[8483][pca_features + non_pca_features]
bob
# Load and apply PCA transformation
pca = joblib.load('hr-analytics-pca.pkl')
pca_feature_values = pca.transform([bob[pca_features]])[0]
pca_feature_values
# Create prediction vector for Bob
X_bob = np.concatenate((bob[non_pca_features].values, pca_feature_values))
X_bob
# Does the model predict that Bob will leave?
clf.predict([X_bob])
# How confident is the model in this prediction?
clf.predict_proba([X_bob])
###Output
_____no_output_____
###Markdown
--- Activity: Hyperparameter Tuning and Model Selection---
###Code
# Load the processed data
df = pd.read_csv('../data/hr-analytics/hr_data_processed_pca.csv')
df.columns
# Select training / validation and test set
from sklearn.model_selection import train_test_split
features = [
'satisfaction_level', 'last_evaluation', 'time_spend_company',
'number_project', 'average_montly_hours',
'first_principle_component',
'second_principle_component',
'third_principle_component',
]
X, X_test, y, y_test = train_test_split(
df[features].values,
df['left'].values,
test_size=0.15,
random_state=1
)
# Calculate a validation curve for max_depth
# using a RandomForestClassifier
from sklearn.ensemble import RandomForestClassifier
np.random.seed(1)
clf = RandomForestClassifier(n_estimators=50)
max_depth_range = np.arange(2, 52, 2)
print('Training {} models ...'.format(len(max_depth_range)))
train_scores, test_scores = validation_curve(
estimator=clf,
X=X,
y=y,
param_name='max_depth',
param_range=max_depth_range,
cv=5,
);
# Draw the validation curve
plot_validation_curve(
train_scores,
test_scores,
max_depth_range,
xlabel='max_depth',
)
plt.ylim(0.97, 1.0)
plt.savefig(
'../figures/chapter-5-hr-analytics-val-curve-pca-forest.png',
bbox_inches='tight',
dpi=300,
)
# Show k-fold results for the selected model:
# a random forest with max_depth = 25
clf = RandomForestClassifier(n_estimators=50, max_depth=25)
np.random.seed(1)
scores = cross_val_class_score(clf, X, y)
print('accuracy = {} +/- {}'.format(
scores.mean(axis=0),
scores.std(axis=0),
))
# Evaluate performance on test set
from sklearn.metrics import confusion_matrix
clf = RandomForestClassifier(n_estimators=50, max_depth=25)
clf.fit(X, y)
y_pred = clf.predict(X_test)
cmat = confusion_matrix(y_test, y_pred)
cmat.diagonal() / cmat.sum(axis=1) * 100
# Train the final model on all the samples
features = [
'satisfaction_level', 'last_evaluation', 'time_spend_company',
'number_project', 'average_montly_hours',
'first_principle_component',
'second_principle_component',
'third_principle_component',
]
X = df[features].values
y = df['left'].values
clf = RandomForestClassifier(n_estimators=50, max_depth=25)
clf.fit(X, y)
# Save the model
import joblib
joblib.dump(clf, 'hr-analytics-pca-forest.pkl')
# Load model from pkl file
clf = joblib.load('hr-analytics-pca-forest.pkl')
clf
# Example of using the model for a specific employee
alice = df.iloc[573][features]
alice
# Predict the class label for Alice
clf.predict([alice.values])
# Predict the probability of class labels for Alice
clf.predict_proba([alice.values])
# What if we reduce her number of hours and time spent at the company?
alice.average_montly_hours = 100
alice.time_spend_company = 2
clf.predict_proba([alice.values])
clf.predict([alice.values])
###Output
_____no_output_____ |
vcare/notebooks/BERT_GPT2_XLNET_for_summarization_naveenac.ipynb | ###Markdown
Samples runs with Extractive Summarization models
###Code
#!pip install bert-extractive-summarizer
#!pip install sentencepiece
from summarizer import Summarizer, TransformerSummarizer
bert_model = Summarizer()
GPT2_model = TransformerSummarizer(transformer_type = 'GPT2', transformer_model_key= 'gpt2-medium')
xlnet_model = TransformerSummarizer(transformer_type="XLNet",transformer_model_key="xlnet-base-cased")
input_text = """Oh okay. Well, they didn't use it as much. I mean, the teachers would assign some reading or math assignments on I ready and from time to time some homework. But yeah, that was about it. I would monitor how much time they were on Roblox and other things to entertain themselves, but it was easier control them back then because they didn't have to go on as much. I mean, that's what it was."""
input_text = """I would say the same thing. My daughter was in fifth grade, we would have a couple in Newsela projects, half an hour of homework. But we were in the playground, neighborhood friends, after school projects, after school swimming. A little bit of tablet on the weekends, but there was no internet access and there was no YouTube in my life, there was no Roblox, there was no gaming. So, a very different world.
"""
input_text = """I have a similar experience to Parent 3. My high schooler had a lot of his homework that he had to type and word process... I don't know if that's the right word, into Docs, but it wasn't a huge amount. It wasn't doing research, it wasn't hours and hours. My elementary school guy, he didn't have any homework on the computer at all. And my middle schooler had very little, like type this one thing or something like that. It was very little on the computer. And for myself, in terms of my family, I lock the video games so that there was very little access to video games during the week."""
input_text = """Moderator 1: "If you can walk me through a little bit how your use of technology changed through the pandemic when they had to start using it more for school or what your situation was for your child?""
Parent 1: "I can say for my eight-year-old, because again, we went from not really knowing how to use a computer or how to type, to transition really smoothly, I mean if you can call that a benefit, we transitioned really quickly. He was typing away, quickly was able to navigate where to go to sign into a Google classroom. So, that was impressive in the beginning, how quickly they were able to adapt to that. So yes... I'm sorry, can you repeat the question?""
"""
print(bert_model(input_text, min_length = 40))
print(GPT2_model(input_text, min_length = 40))
input_text = """Moderator 1: "So just starting to think through some of the impact of technology, can we talk a little bit about how your child used technology prior to the pandemic for school purposes?
"
Parent 1: "Oh okay. Well, they didn't use it as much. I mean, the teachers would assign some reading or math assignments on I ready and from time to time some homework. But yeah, that was about it. I would monitor how much time they were on Roblox and other things to entertain themselves, but it was easier control them back then because they didn't have to go on as much. I mean, that's what it was.
"
"""
print(bert_model(input_text, min_length = 40))
print(GPT2_model(input_text, min_length = 40))
input_text = """Moderator 1: "So just starting to think through some of the impact of technology, can we talk a little bit about how your child used technology prior to the pandemic for school purposes?"
Parent 2: "Oh okay. Well, they didn't use it as much. I mean, the teachers would assign some reading or math assignments on I ready and from time to time some homework. But yeah, that was about it. I would monitor how much time they were on Roblox and other things to entertain themselves, but it was easier control them back then because they didn't have to go on as much. I mean, that's what it was."
Parent 3: "I would say the same thing. My daughter was in fifth grade, we would have a couple in Newsela projects, half an hour of homework. But we were in the playground, neighborhood friends, after school projects, after school swimming. A little bit of tablet on the weekends, but there was no internet access and there was no YouTube in my life, there was no Roblox, there was no gaming. So, a very different world."
Parent 5: "I have a similar experience to Parent 3. My high schooler had a lot of his homework that he had to type and word process... I don't know if that's the right word, into Docs, but it wasn't a huge amount. It wasn't doing research, it wasn't hours and hours. My elementary school guy, he didn't have any homework on the computer at all. And my middle schooler had very little, like type this one thing or something like that. It was very little on the computer. And for myself, in terms of my family, I lock the video games so that there was very little access to video games during the week."
Parent 5: "And then on the weekends there was an hour or something like that, it wasn't that much. There was no YouTube watching, like now YouTube consumes our life. My kids didn't even know how to use the features on our TV, the smart features, they didn't know they could access YouTube on it or anything like that. So it was very controlled and very little. I also had my children in a lot of afterschool activities. My older son was in an orchestra, he also was in a band, theater groups, there was a lot of afterschool activity. A lot of playing outside, a lot of going to the park and all that stuff. Yeah, so..."
Parent 4: "I can go. My kids probably had more... It sounds like they did more online than, so far, the rest of them. But my daughter in high school definitely had almost all of her homework... Not all of it because she was assigned in school, but she definitely was on doing homework, I feel like, a good amount. Nothing like now, but definitely was using it. My middle schooler also, but not as much. They both did play games or use Instagram, my daughter was really into Instagram, not as much anymore but definitely games. Now it's just like a free-for-all. I mean, they're in their rooms with the door shut, I have no idea what they're doing all day. I just know that they're doing their homework, getting good grades. I think they're on electronics the entire day."
Parent 1: "Yeah, so I have a similar experience with my eight-year-old. Pre-pandemic he was still in second grade so the use of computers was very limited. Maybe once a week he would type, words of the week was one of the options I picked to do. That was his only interaction with computer. Of course he had devices to play on, and he would go to his father's house every other weekend and he would be more exposed there to computers. But still he was busy. He was busy during the week, there was school, there was getting to school, getting from school, that's a trip in itself."
Parent 1: "There was hockey, we had TaeKwonDo, he was very busy. Even then it didn't seem like enough, it didn't seem like we were doing enough but he was certainly a lot busier than now. And he did get to play, mostly probably on the weekends, and again, weekends were also pretty packed. So I don't know how he had time, but he did play, not a lot. And of course we were able to control it, it was an item you had to earn and if you didn't do well then you don't get to play. So it was a tool we could use as incentive during the week. So now that's all out the window, it's all available right there all day long. And getting him away from the computer now is a project in itself every day."
"""
print(bert_model(input_text, min_length = 40))
GPT2_model(input_text, min_length = 40)
#below is entire summary from BERT plus the delta/unique (non-intersection with BERT) from GPT2
"""
--COMMON - So just starting to think through some of the impact of technology, can we talk a little bit about how your child used technology prior to the pandemic for school purposes?"
--COMMON -My high schooler had a lot of his homework that he had to type and word process... I don't know if that's the right word, into Docs, but it wasn't a huge amount.
--ONLY In BERT - A lot of playing outside, a lot of going to the park and all that stuff.
--COMMON -I think they're on electronics the entire day.
--ONLY IN BERT -That was his only interaction with computer.
--ONLY IN BERT -He was busy during the week, there was school, there was getting to school, getting from school, that's a trip in itself.
--COMMON -There was hockey, we had TaeKwonDo, he was very busy. Even then it didn't seem like enough, it didn't seem like we were doing enough but he was certainly a lot busier than now. So I don't know how he had time, but he did play, not a lot.
--ONLY IN GPT2 -My daughter was in fifth grade, we would have a couple in Newsela projects, half an hour of homework.
--ONLY IN GPT2 -And for myself, in terms of my family, I lock the video games so that there was very little access to video games during the week."
--ONLY IN GPT2 -And getting him away from the computer now is a project in itself every day.
"""
#still better would be doing a merge sort on the summaries from BERT and GPT2 to retain the order/context instead of appending the delta from GPT2 in the end
xlnet_model(input_text, min_length=40)
input_text = """Moderator 1: "How the technology has changed during the pandemic, school purposes and whether it's beneficial or problematic?"
Parent 1: "Right, exactly, it seems beneficial because it is an important part of your life as you grow older in high school clearly, and in middle school as Parent 4 said, there's some use of computer. So at first it seemed like a good thing, and I'm sure it is a good thing, it's just, like I said, it's just become overwhelming use of technology because they sit in front of it all day. And another thing, this use of YouTube, because I understand teachers post some things that you can look up on YouTube, so you can't block YouTube because it'll be used as a tool for one of those classes they have."
Parent 1: "But YouTube is just this bottomless hole of... I can't even begin, it's just so bad. And transition from looking at your Google classroom or your Google meet and here's YouTube, it's just so easy. And we were not always there, we still have jobs and other things to do, can't just sit on top of them. So this accessibility of technology that has this type of content, like YouTube, that's not beneficial for kids, that's been problematic, to say the least."
Parent 5: "So for us, we only had a desktop and a laptop, which was more than sufficient pre-pandemic. And then everybody needed a device, all of a sudden everybody needed a device Needed to be on at basically the same times so we got one device from school, we bought a laptop, we found an old laptop. It was like the gathering of devices. And then teaching... My nine-year-old, teaching him how to use it, he really hadn't used computers in that way. And so teaching him how to use it. My middle son is prone to migraines and so being on the screen caused migraines and so that caused some aversion because he didn't want to be on."
Parent 5: "The schedules were kind of crazy, like all over the place. And the truth is that it's hard because my 17-year-old, who knew how to do all these things just kind of stayed in his room for months. And it was just... I feel bad saying it, but he was kind of left to his own devices. He wasn't a big social media guy, he has no social media still, but when the only way to communicate with his peers became to use... What is it? Discord, and all these other things, that became the only way to communicate with people. And then it almost, I feel like... Like Parent 1 said, it's like this black hole that sucks you in."
Parent 5: "And then there was also no schedule. Because we have some special needs in our family, my schedule is really tight with my kids and so the little guys we go to bed at 8:30 and then 9:00 and then the older one was in bed by 10:00 and there was a lot of structure, and literally it was like a bomb hit it. People were going to bed at 1:00 in the morning, it was just totally... It felt chaotic and out of control, and it felt like the technology sputtered for a little bit as we gt devices figured out how to get everybody on and then it was this black hole that sucked you in. And then everybody was on for hours and hours and hours and hours and hours, and it felt out of control."
Parent 3: "Sure, when lock down happened my then first grader, the school did a great job pivoting to remote quite quickly. They used seesaw as a platform. I did get two laptops from the DOE because we have a lot of tech but it's all very old and kind of fragile and I'm like, they're going to wreck it all. Nothings insured. We're self-employed and I could just see the whole business being wiped out so we did take a DOE iPads which was a lifesaver. But also, again, we got to one point where the computer teacher did that YouTube... We were not on the YouTube and she sent one link and they figured out, "Oh, I can get to YouTube from this link."
Parent 3: "And then it was like a bomb went off, they figured it out, they figured out workarounds and it was like Pandora's box. I was trying to figure out how to block... I was on the phone with the DOE, I was on the phone with a parent coordinator, I was calling the principal, like, "How can I block YouTube without..." I was putting timer things, it was driving me crazy, and it was downhill from there. I finally figured out my son, by banging on it so much he's actually broken the screen so whatever controls I put in, he could bang on it and it would just do whatever he wanted. A month later I exchange the iPad but I nearly ripped my own hair out over this YouTube. Yeah, the addiction sunk in over those first five months of lockdown of screens and YouTube and just that misery sucking."
Parent 4: "Like I said, both my kids are on it all day. They're fully remote, both of them. Neither one of them has a full schedule so I hear my son... Because my desk is right next to his room, and I'm hearing him at like 10:00 in the morning, profanities on a game with his friend. And I'm like, I don't understand, it's a school day. Well, they don't have school, it's in between a break. So it's definitely made our day... Especially since I'm working right next to him, very difficult to try and do my job and be next to him. But it's their lives, they're in their rooms, if it's not school then it's playing games. My son plays a lot of games with his friends, my daughter doesn't but then she'll be on the YouTube also a lot, TickTock, whatever, and I kind of don't see them a lot during the day. Thankfully they do okay so I don't ask... I used to be a more involved and now I'm not really involved. Now it's a free for all."
Parent 4: "Yeah, I don't feel like there's even much to be involved. They don't have much, especially my middle Schooler, has nothing to do so there isn't even much for me to ask him."
"""
print(bert_model(input_text, min_length = 40))
GPT2_model(input_text, min_length = 40)
xlnet_model(input_text, min_length=40)
###Output
_____no_output_____ |
Basic_Research.ipynb | ###Markdown
Project Objectives--- Provider Fraud is one of the biggest problems facing Medicare. According to the government, the total Medicare spending increased exponentially due to frauds in Medicare claims. Healthcare fraud is an organized crime which involves peers of providers, physicians, beneficiaries acting together to make fraud claims. Rigorous analysis of Medicare data has yielded many physicians who indulge in fraud. They adopt ways in which an ambiguous diagnosis code is used to adopt costliest procedures and drugs. Insurance companies are the most vulnerable institutions impacted due to these bad practices. Due to this reason, insurance companies increased their insurance premiums and as result healthcare is becoming costly matter day by day.Healthcare fraud and abuse take many forms. Some of the most common types of frauds by providers are:- Billing for services that were not provided.- Duplicate submission of a claim for the same service.- Misrepresenting the service provided.- Charging for a more complex or expensive service than was actually provided.- Billing for a covered service when the service actually provided was not covered. Problem Statement---The goal of this project is to " predict the potentially fraudulent providers " based on the claims filed by them. Along with this, we will also discover important variables helpful in detecting the behaviour of potentially fraud providers. Further, we will study fraudulent patterns in the provider's claims to understand the future behaviour of providers. Introduction to the Dataset---For the purpose of this project, we are considering Inpatient claims, Outpatient claims and Beneficiary details of each provider. Lets s see their details : **1. Inpatient Data**: This data provides insights about the claims filed for those patients who are admitted in the hospitals. It also provides additional details like their admission and discharge dates and admit d diagnosis code.**2. Outpatient Data**: This data provides details about the claims filed for those patients who visit hospitals and not admitted in it. **3. Beneficiary Details Data**: This data contains beneficiary KYC details like health conditions,region they belong to etc.
###Code
# Importing necessary libraries
import numpy as np
import pandas as pd
import seaborn as sns
import matplotlib.pyplot as plt
import zipfile
###Output
_____no_output_____
###Markdown
1. Importing Data
###Code
provider_data=pd.read_csv(r"Data\provider_fraud_detection_data.csv")
provider_data
data_list=['outpatientdata','inpatientdata','beneficiarydata']
dataframes=[]
for i in data_list:
zipfile_path=f'Data/{i}.zip'
zf=zipfile.ZipFile(zipfile_path)
for file in zf.namelist():
print (f"files in {zipfile_path} : {file}")
df=pd.read_csv(zf.open(file))
dataframes.append(df)
outpatient_data=dataframes[0]
inpatient_data=dataframes[1]
beneficiary_data=dataframes[2]
###Output
_____no_output_____
###Markdown
2. Understand the Data a. Provider Data
###Code
provider_data.head()
provider_data.info()
provider_data.nunique()
x=provider_data.groupby(['PotentialFraud']).count()
x
###Output
_____no_output_____
###Markdown
There is 506 potential fraud providers. There is a possibility that they are either filing correct or incorrect bills. b. Outpatient Data
###Code
outpatient_data.head()
print(f"Columns: {len(outpatient_data.columns)} \n Rows: {len(outpatient_data)}")
outpatient_data_info=pd.DataFrame(data=outpatient_data.columns,
columns=['Column Name'])
outpatient_data_info['Data Type']=outpatient_data.dtypes.values
outpatient_data_info['No.of Unique Values']=outpatient_data.nunique().values
outpatient_data_info['Count of Missing Nos']=outpatient_data.isna().sum().values
outpatient_data_info['Percent of Missing Nos']=(round(outpatient_data.isna().sum()/len(outpatient_data)*100)).values
outpatient_data_info
###Output
_____no_output_____
###Markdown
c. Inpatient Data
###Code
inpatient_data.head()
print(f"Columns: {len(inpatient_data.columns)} \n Rows: {len(inpatient_data)}")
inpatient_data_info=pd.DataFrame(data=inpatient_data.columns,
columns=['Column Name'])
inpatient_data_info['Data Type']= inpatient_data.dtypes.values
inpatient_data_info['No.of Unique Values']= inpatient_data.nunique().values
inpatient_data_info['Count of Missing Nos']= inpatient_data.isna().sum().values
inpatient_data_info['Percent of Missing Nos']=(round(inpatient_data.isna().sum()/len(inpatient_data)*100)).values
inpatient_data_info
###Output
_____no_output_____
###Markdown
d. Finding columns shared by inpatient and outpatient data
###Code
# Finding Common columns
a = inpatient_data.columns.intersection(outpatient_data.columns)
print(len(a))
inpatient_data.shape
print("The columns which are only in the inpatient data:")
for i in inpatient_data.columns:
#print (i)
if i not in a:
print(f" {i}")
###Output
The columns which are only in the inpatient data:
AdmissionDt
DischargeDt
DiagnosisGroupCode
###Markdown
There is claim start date and claim end date in the inpatient data. We need to check whether the 1. "ClaimStartDt=AdmissionDt" and 2. "ClaimEndDt=DischargeDt"
###Code
(inpatient_data['ClaimStartDt']==inpatient_data['AdmissionDt'])
(inpatient_data['ClaimStartDt']==inpatient_data['AdmissionDt']).sum()
x=pd.DataFrame(inpatient_data.loc[inpatient_data['ClaimStartDt']!=inpatient_data['AdmissionDt']]['ClaimStartDt'])
x['AdmissionDt']=inpatient_data.loc[inpatient_data['ClaimStartDt']!=inpatient_data['AdmissionDt']]['AdmissionDt']
x
###Output
_____no_output_____
###Markdown
e. Beneficiary Details Data
###Code
beneficiary_data.head()
f"The inpatient data has {len(beneficiary_data.columns)} columns and {len(beneficiary_data)} rows"
beneficiary_data_info=pd.DataFrame(data=beneficiary_data.columns,
columns=['Column Name'])
beneficiary_data_info['Data Type']= beneficiary_data.dtypes.values
beneficiary_data_info['No.of Unique Values']= beneficiary_data.nunique().values
beneficiary_data_info['Count of Missing Nos']= beneficiary_data.isna().sum().values
beneficiary_data_info['Percent of Missing Nos']=(round(beneficiary_data.isna().sum()/len(beneficiary_data)*100)).values
beneficiary_data_info
###Output
_____no_output_____
###Markdown
3. Data Preprocessing Merging Inpatient and Outpatient Data Columns
###Code
len(set(outpatient_data.columns).intersection(set(inpatient_data.columns)))
len(inpatient_data.columns)
in_out_patient_data=outpatient_data.append(inpatient_data, ignore_index = True)
in_out_patient_data.shape
outpatient_data.shape[0]+inpatient_data.shape[0]
###Output
_____no_output_____
###Markdown
Joining provider details (Fraudulent or not)
###Code
in_out_patient_data=pd.merge(in_out_patient_data,provider_data,on='Provider')
in_out_patient_data
###Output
_____no_output_____
###Markdown
Cleaning Beneficiery Details
###Code
beneficiary_data
beneficiary_data['DOB'] = pd.to_datetime(beneficiary_data['DOB'], format='%Y-%m-%d')
beneficiary_data['DOD'] = pd.to_datetime(beneficiary_data['DOD'], format='%Y-%m-%d')
beneficiary_data.info()
###Output
<class 'pandas.core.frame.DataFrame'>
RangeIndex: 138556 entries, 0 to 138555
Data columns (total 25 columns):
# Column Non-Null Count Dtype
--- ------ -------------- -----
0 BeneID 138556 non-null object
1 DOB 138556 non-null datetime64[ns]
2 DOD 1421 non-null datetime64[ns]
3 Gender 138556 non-null int64
4 Race 138556 non-null int64
5 RenalDiseaseIndicator 138556 non-null object
6 State 138556 non-null int64
7 County 138556 non-null int64
8 NoOfMonths_PartACov 138556 non-null int64
9 NoOfMonths_PartBCov 138556 non-null int64
10 ChronicCond_Alzheimer 138556 non-null int64
11 ChronicCond_Heartfailure 138556 non-null int64
12 ChronicCond_KidneyDisease 138556 non-null int64
13 ChronicCond_Cancer 138556 non-null int64
14 ChronicCond_ObstrPulmonary 138556 non-null int64
15 ChronicCond_Depression 138556 non-null int64
16 ChronicCond_Diabetes 138556 non-null int64
17 ChronicCond_IschemicHeart 138556 non-null int64
18 ChronicCond_Osteoporasis 138556 non-null int64
19 ChronicCond_rheumatoidarthritis 138556 non-null int64
20 ChronicCond_stroke 138556 non-null int64
21 IPAnnualReimbursementAmt 138556 non-null int64
22 IPAnnualDeductibleAmt 138556 non-null int64
23 OPAnnualReimbursementAmt 138556 non-null int64
24 OPAnnualDeductibleAmt 138556 non-null int64
dtypes: datetime64[ns](2), int64(21), object(2)
memory usage: 26.4+ MB
|
dessert.ipynb | ###Markdown
环境
###Code
!pip install --upgrade pip -i https://pypi.tuna.tsinghua.edu.cn/simple
!pip install tensorflow==2.3.0 -i https://pypi.tuna.tsinghua.edu.cn/simple
!pip install deepctr -i https://pypi.tuna.tsinghua.edu.cn/simple
###Output
_____no_output_____
###Markdown
提交
###Code
!wget -nv -O kesci_submit https://cdn.kesci.com/submit_tool/v4/kesci_submit&&chmod +x kesci_submit
!./kesci_submit -token *******你的Token******** -file /home/kesci/work/sub.csv
###Output
Kesci Submit Tool 4.0.0
> 已验证Token
> 提交文件 /home/kesci/work/sub.csv (45.55 KiB), Target Qiniu
> 已上传 100 %
> 文件已上传
> 服务器响应: 200 提交成功,请等待评审完成
> 提交完成
###Markdown
数据预处理&EDA train
###Code
import pandas as pd
import gc
train = pd.read_csv('/home/kesci/data/competition_A/train_set.csv').replace(' ', -1).fillna(-1)
# print(train.columns)
# print(train.isnull().values.any())
# print(set(train['Gender\n性别'].values.tolist()))
train_group = train.groupby('护理来源')
del train
gc.collect()
# from sklearn.preprocessing import OneHotEncoder
# enc = OneHotEncoder(sparse = False)
# result = enc.fit_transform(train[['Source of Care\n护理来源']])
# enc.fit([[0], [1], [2], [3], [4], [5], [6], [7], [8], [9]])
# result = enc.transform(data[[41]])
dic_1 = {'Private Hospital':1, -1:2, 'Governament Hospital':3, 'Never Counsulted':4, 'clinic':5}
buf_1 = pd.DataFrame()
for name,group in train_group:
group['护理来源'] = dic_1[name]
buf_1 = pd.concat([buf_1,group],ignore_index=True)
dic_2 = {'F':1, 'M':2}
buf_1_group = buf_1.groupby('性别')
buf_2 = pd.DataFrame()
for name,group in buf_1_group:
group['性别'] = dic_2[name]
buf_2 = pd.concat([buf_2,group],ignore_index=True)
dic_3 = {'north':1, 'east':2, 'south':3, 'west':4}
buf_3 = pd.DataFrame()
buf_2_group = buf_2.groupby('区域')
for name,group in buf_2_group:
group['区域'] = dic_3[name]
buf_3 = pd.concat([buf_3,group],ignore_index=True)
buf_3 = buf_3.astype(float)
cat_list = ['肥胖腰围',
'教育', '未婚',
'护理来源', '视力不佳',
'饮酒', '高血压',
'家庭高血压', '糖尿病',
'家族糖尿病', '肝炎', '家族肝炎',
'慢性疲劳']
for i in cat_list:
buf_3[i] = buf_3[i].astype(int)
buf_3.to_csv('train_.csv',index=0)
###Output
/opt/conda/lib/python3.6/site-packages/ipykernel_launcher.py:20: SettingWithCopyWarning:
A value is trying to be set on a copy of a slice from a DataFrame.
Try using .loc[row_indexer,col_indexer] = value instead
See the caveats in the documentation: http://pandas.pydata.org/pandas-docs/stable/indexing.html#indexing-view-versus-copy
/opt/conda/lib/python3.6/site-packages/ipykernel_launcher.py:27: SettingWithCopyWarning:
A value is trying to be set on a copy of a slice from a DataFrame.
Try using .loc[row_indexer,col_indexer] = value instead
See the caveats in the documentation: http://pandas.pydata.org/pandas-docs/stable/indexing.html#indexing-view-versus-copy
/opt/conda/lib/python3.6/site-packages/ipykernel_launcher.py:34: SettingWithCopyWarning:
A value is trying to be set on a copy of a slice from a DataFrame.
Try using .loc[row_indexer,col_indexer] = value instead
See the caveats in the documentation: http://pandas.pydata.org/pandas-docs/stable/indexing.html#indexing-view-versus-copy
###Markdown
test
###Code
import pandas as pd
import gc
test = pd.read_csv('/home/kesci/data/competition_A/test_set.csv').replace(' ', -1).fillna(-1)
# print(train.columns)
# print(train.isnull().values.any())
# print(set(train['Gender\n性别'].values.tolist()))
test_group = test.groupby('护理来源')
del test
gc.collect()
# from sklearn.preprocessing import OneHotEncoder
# enc = OneHotEncoder(sparse = False)
# result = enc.fit_transform(train[['Source of Care\n护理来源']])
# enc.fit([[0], [1], [2], [3], [4], [5], [6], [7], [8], [9]])
# result = enc.transform(data[[41]])
dic_1 = {'Private Hospital':1, -1:2, 'Governament Hospital':3, 'Never Counsulted':4, 'clinic':5}
buf_1 = pd.DataFrame()
for name,group in test_group:
group['护理来源'] = dic_1[name]
buf_1 = pd.concat([buf_1,group],ignore_index=True)
dic_2 = {'F':1, 'M':2}
buf_1_group = buf_1.groupby('性别')
buf_2 = pd.DataFrame()
for name,group in buf_1_group:
group['性别'] = dic_2[name]
buf_2 = pd.concat([buf_2,group],ignore_index=True)
dic_3 = {'north':1, 'east':2, 'south':3, 'west':4}
buf_3 = pd.DataFrame()
buf_2_group = buf_2.groupby('区域')
for name,group in buf_2_group:
group['区域'] = dic_3[name]
buf_3 = pd.concat([buf_3,group],ignore_index=True)
buf_3 = buf_3.astype(float)
cat_list = ['肥胖腰围',
'教育', '未婚',
'护理来源', '视力不佳',
'饮酒', '高血压',
'家庭高血压', '糖尿病',
'家族糖尿病', '家族肝炎',
'慢性疲劳']
for i in cat_list:
buf_3[i] = buf_3[i].astype(int)
buf_3.to_csv('test_.csv',index=0)
###Output
/opt/conda/lib/python3.6/site-packages/ipykernel_launcher.py:20: SettingWithCopyWarning:
A value is trying to be set on a copy of a slice from a DataFrame.
Try using .loc[row_indexer,col_indexer] = value instead
See the caveats in the documentation: http://pandas.pydata.org/pandas-docs/stable/indexing.html#indexing-view-versus-copy
/opt/conda/lib/python3.6/site-packages/ipykernel_launcher.py:27: SettingWithCopyWarning:
A value is trying to be set on a copy of a slice from a DataFrame.
Try using .loc[row_indexer,col_indexer] = value instead
See the caveats in the documentation: http://pandas.pydata.org/pandas-docs/stable/indexing.html#indexing-view-versus-copy
/opt/conda/lib/python3.6/site-packages/ipykernel_launcher.py:34: SettingWithCopyWarning:
A value is trying to be set on a copy of a slice from a DataFrame.
Try using .loc[row_indexer,col_indexer] = value instead
See the caveats in the documentation: http://pandas.pydata.org/pandas-docs/stable/indexing.html#indexing-view-versus-copy
###Markdown
DeepFM
###Code
from itertools import chain
import tensorflow as tf
from tensorflow import keras
from tensorflow.keras.models import Model, Sequential
from tensorflow.keras import optimizers, layers, losses
import pandas as pd
from sklearn.metrics import log_loss, roc_auc_score
from sklearn.model_selection import train_test_split
from sklearn.preprocessing import LabelEncoder, MinMaxScaler
# from deepctr.feature_column import SparseFeat, DenseFeat, get_feature_names, build_input_features, get_linear_logit, DEFAULT_GROUP_NAME, input_from_feature_columns
# from deepctr.feature_column import build_input_features, get_linear_logit, input_from_feature_columns
# from deepctr.layers.core import PredictionLayer, DNN
# from deepctr.layers.interaction import SENETLayer, BilinearInteraction
# from deepctr.layers.utils import concat_func, add_func, combined_dnn_input
from deepctr.feature_column import SparseFeat, DenseFeat, get_feature_names, build_input_features, get_linear_logit, DEFAULT_GROUP_NAME, input_from_feature_columns
from deepctr.layers.core import PredictionLayer, DNN
from deepctr.layers.interaction import FM
from deepctr.layers.utils import concat_func, add_func, combined_dnn_input
data_test = pd.read_csv('test_.csv')
test = data_test.drop(columns=['ID'])
data_train = pd.read_csv('train_.csv')
train = data_train.drop(columns=['ID','肝炎'])
label = data_train['肝炎']
data = train.append(test)
data.columns = ['Age','Gender','Area','Weight','Height','Body_mass_index',
'Obesity_waistline','Waist','Highest_blood_pressure','Minimum_blood_pressure',
'Good_Cholesterol','Bad_Cholesterol','Total_Cholesterol','Blood_lipid_abnormality',
'PVD','Sports_activities','Education','Unmarried','Revenue','Source_of_care',
'Poor_vision','Drinking','Hypertension','Family_hypertension','Diabetes',
'Family_diabetes','Family_hepatitis','Chronic_fatigue','ALF']
dense_features=['Revenue','Sports_activities','Age','Weight','Height',
'Body_mass_index','Waist','Highest_blood_pressure',
'Minimum_blood_pressure','Good_Cholesterol','Bad_Cholesterol',
'Total_Cholesterol']
sparse_features = list(set(data.columns.tolist()).difference(set(dense_features)))
for feat in sparse_features:
lbe = LabelEncoder()
data[feat] = lbe.fit_transform(data[feat])
mms = MinMaxScaler(feature_range=(0, 1))
data[dense_features] = mms.fit_transform(data[dense_features])
# 2.count #unique features for each sparse field,and record dense feature field name
fixlen_feature_columns = [SparseFeat(feat, vocabulary_size=data[feat].nunique(),embedding_dim=4)
for i,feat in enumerate(sparse_features)] + [DenseFeat(feat, 1,)
for feat in dense_features]
dnn_feature_columns = fixlen_feature_columns
linear_feature_columns = fixlen_feature_columns
feature_names = get_feature_names(linear_feature_columns + dnn_feature_columns)
# 3.generate input deepfm_data for model
# train, test = train_test_split(deepfm_data, test_size=0.2)
deepfm_train = data.head(train.shape[0])
deepfm_test = data.tail(test.shape[0])
deepfm_train = {name:deepfm_train[name] for name in feature_names}
deepfm_test = {name:deepfm_test[name] for name in feature_names}
def multi_category_focal_loss2(gamma=2., alpha=.25):
"""
Usage:
model.compile(loss=[multi_category_focal_loss2(
alpha=0.35, gamma=2)], metrics=["accuracy"], optimizer=adam)
"""
epsilon = 1.e-7
gamma = float(gamma)
alpha = tf.constant(alpha, dtype=tf.float32)
def multi_category_focal_loss2_fixed(y_true, y_pred):
y_true = tf.cast(y_true, tf.float32)
y_pred = tf.clip_by_value(y_pred, epsilon, 1. - epsilon)
alpha_t = y_true * alpha + \
(tf.ones_like(y_true) - y_true) * (1 - alpha)
y_t = tf.multiply(y_true, y_pred) + tf.multiply(1 - y_true, 1 - y_pred)
ce = -tf.math.log(y_t)
weight = tf.pow(tf.subtract(1., y_t), gamma)
fl = tf.multiply(tf.multiply(weight, ce), alpha_t)
loss = tf.reduce_mean(fl)
return loss
return multi_category_focal_loss2_fixed
def M( linear_feature_columns, dnn_feature_columns, fm_group=[DEFAULT_GROUP_NAME], dnn_hidden_units=(128, 128),
l2_reg_linear=0.00001, l2_reg_embedding=0.00001, l2_reg_dnn=0, seed=1024, dnn_dropout=0,
dnn_activation='elu', dnn_use_bn=False, task='binary'):
features = build_input_features(linear_feature_columns + dnn_feature_columns)
inputs_list = list(features.values())
linear_logit = get_linear_logit(features, linear_feature_columns, seed=seed, prefix='linear',
l2_reg=l2_reg_linear)
group_embedding_dict, dense_value_list = input_from_feature_columns(features, dnn_feature_columns, l2_reg_embedding,
seed, support_group=True)
fm_logit = add_func([FM()(concat_func(v, axis=1))
for k, v in group_embedding_dict.items() if k in fm_group])
dnn_input = combined_dnn_input(list(chain.from_iterable(
group_embedding_dict.values())), dense_value_list)
dnn_output = DNN(dnn_hidden_units, dnn_activation, l2_reg_dnn, dnn_dropout,
dnn_use_bn, seed)(dnn_input)
dnn_logit = tf.keras.layers.Dense(
1, use_bias=False, activation=None)(dnn_output)
final_logit = add_func([linear_logit, fm_logit, dnn_logit])
output = PredictionLayer(task)(final_logit)
model = Model(inputs=[features], outputs=[output])
model.compile(optimizer=optimizers.Adam(2.5e-4),
loss={'prediction_layer':losses.binary_crossentropy},# multi_category_focal_loss2(alpha=0.35, gamma=2)
metrics=['AUC'])
return model
model = M(linear_feature_columns=linear_feature_columns,
dnn_feature_columns=dnn_feature_columns, task='binary')
model.summary()
input_train = deepfm_train
model.fit(input_train,
{'prediction_layer':label},
validation_split=0.3,
epochs=20,
batch_size=100,)
input_test = deepfm_test
ans_mtx = model.predict(input_test,
batch_size=100)
ans_sub = pd.DataFrame({'ID':data_test['ID'].astype(int),'hepatitis':ans_mtx.flatten()})
ans_sub.to_csv('sub.csv',index=0)
ans_sub.describe()
###Output
_____no_output_____ |
complexidade_algoritmos/aula_busca/search.ipynb | ###Markdown
1. Algoritmos de Busca 1.1. Busca LinearO algoritmo de Busca Linear, também conhecido por **Busca Sequencial**, é uma das técnicas mais simples para buscar um determinado valor em um conjunto qualquer de elementos. De maneira geral, a técnica consiste em comparar todos os elementos do conjunto de dados com o valor desejado de maneira **sequencial**. Caso o elemento seja encontrado, a busca então pode ser **interrompida**.O algoritmo abaixo implementa a técnica Busca Linear, cuja análise de complexidade é dada da seguinte maneira:* Complexidade de tempo no melhor caso: $\theta(1)$* Complexidade de tempo no pior caso: $O(n)$
###Code
import numpy
import math
def Linear_Search(A, x):
n = len(A)
found = False
i = 0
while (i < n)and (not found):
if(A[i] == x):
found = True
else:
i = i+ 1
return found
###Output
_____no_output_____
###Markdown
Exemplo de funcionamento:
###Code
A = numpy.random.randint(-0, 10, 10)
x = numpy.random.randint(10)
print('Vetor de entrada: '+ str(A))
print('Elemento a ser procurado: '+ str(x))
print('Valor encontrado? '+ str(Linear_Search(A, x)))
###Output
Vetor de entrada: [4 5 3 0 0 2 2 7 1 2]
Elemento a ser procurado: 8
Valor encontrado? False
###Markdown
No caso de o elemento a ser procurado estiver na **primeira** posição do vetor, então a complexidade de busca será de $\theta(1)$. Note que essa situação não está restrita apenas à primeira posição, pois o estudo do comportamento assintótico de funções dá-se quando $n\rightarrow\infty$. Desta forma, caso o elemento a ser procurado estiver entre os $k$ primeiros elementos do vetor e $k<<n$, ainda podemos considerar uma complexidade constante, ou seja, $\theta(1)$. Já o pior caso dá-se quando o elemento a ser procurado está na **última** posição do vetor, ou **não foi encontrado** neste. Nesta situação, temos que $n$ comparações foram consideradas. Assim sendo, como não sabemos a comportamento dos dados, denotamos a complexidade da Busca Linear como sendo $O(n)$. 1.2. Busca BináriaDependendo da situação, podemos utilizar diferentes técnicas para buscar elementos em um conjunto de dados. Suponha que agora tenhamos um conjunto de dados que esteja **ordenado**. Para fins de explicação apenas, utilizaremos um conjunto ordenado de forma crescente. Podemos, então, nos beneficiar desta informação realizando a busca em partes menores do conjunto de dados, visto que sabemos se o elemento desejado é menor ou maior do que o elemento que está sendo comparado. O algoritmo da Busca Binária funciona desta forma, em que o elemento **central** do vetor é comparado com o dado desejado. Caso seja este o elemento, então a busca é interrompida. Caso contrário, isto é, se o elemento desejado for **menor** do que o valor comparado, então a busca começa na metade à **esquerda** deste valor, ou na metade à **direita** caso o elemento desejado seja maior do que o valor comparado. A Figura 1 ilustra o procedimento adotado pela busca binária.Figura 1: Funcionamento da técnica Busca Binária, em que o elemento vermelho é o desejado.Note que, para o exemplo acima, temos um vetor com $7$ elementos e, no pior caso, foram necessárias $3$ comparações. De maneira análoga, caso tenhamos um vetor com $16$ elementos, no pior caso serão necessárias $4$ comparações para acharmos o valor desejado ou o algoritmo parar sua execução. Essa análise nos permite assumir que, para um vetor com $2^n$ elementos, serão necessárias, no máximo, $n$ comparações para achar o elemento desejado (caso este exista).O algoritmo abaixo implementa a técnica Busca Linear, cuja análise de complexidade é dada da seguinte maneira:* Complexidade de tempo no melhor caso: $\theta(1)$* Complexidade de tempo no pior caso: $O(n\log n)$
###Code
def Binary_Search(A, e, d, x):
if(d >= e):
m = (e+d)//2 # calculando o elemento central
if(A[m] == x):
return True
elif(A[m] > x):
return Binary_Search(A, e, m-1, x)
else:
return Binary_Search(A, m+1, d, x)
else:
return False
###Output
_____no_output_____
###Markdown
Exemplo de funcionamento:
###Code
A = numpy.random.randint(-0, 20, 10)
A.sort()
x = numpy.random.randint(10)
print('Vetor de entrada: '+ str(A))
print('Elemento a ser procurado: '+ str(x))
print('Valor encontrado? '+ str(Binary_Search(A, 0, 9, x)))
###Output
Vetor de entrada: [ 0 2 2 3 4 8 11 15 19 19]
Elemento a ser procurado: 5
Valor encontrado? False
|
Mintegiak/.ipynb_checkpoints/Iteragarritasuna-checkpoint.ipynb | ###Markdown
Iteragarritasuna: `iter()` eta `next()` iterable : `iter()`
###Code
help(iter)
iter([1,2,3])
iter((1,2,3))
iter("abc")
###Output
_____no_output_____
###Markdown
Generadoreak Iteragarriak eta Iteradoreak dira!!!
###Code
g = (x*2 for x in range(10))
g is iter(g)
###Output
_____no_output_____
###Markdown
**DEFINIZIOA:** `it` objektu bat **Iteragarria** da, `iter(it)`-k **Iteradore** bat bueltatzen badu iterator : `next()`
###Code
help(next)
z = [1,2,3]
it = iter(z)
print(next(it))
print(next(it))
print(next(it))
#print(next(it))
print(next(it,"Nora zoaz??"))
it = iter(z)
while True :
x = next(it)
print(x)
it = iter(z)
while True :
try :
x = next(it)
print(x)
except StopIteration :
break
it = iter(z)
try :
while True :
x = next(it)
print(x)
except StopIteration :
pass
###Output
1
2
3
###Markdown
Bi iteragarri iteragarri bakarrean bilakatuko duen `myZip` generadorea:
###Code
def myZip(it1,it2):
itr1 = iter(it1)
itr2 = iter(it2)
while True :
try :
a = next(itr1)
b = next(itr2)
yield a,b
except StopIteration :
break
x = myZip("abcd",range(10))
print(*x)
x = myZip(range(10),"abcd")
print(*x)
x = myZip([],"abcd")
print(*x)
###Output
|
Titanic/Improvement.ipynb | ###Markdown
Копирам си модела от лекцията
###Code
from sklearn.base import BaseEstimator, TransformerMixin
from sklearn.preprocessing import LabelBinarizer
class ItemSelector(BaseEstimator, TransformerMixin):
def __init__(self, key):
self.key = key
def fit(self, x, y=None):
return self
def transform(self, data_dict):
return data_dict[[self.key]]
class LabelBinarizerPipelineFriendly(LabelBinarizer):
def fit(self, X, y=None):
super().fit(X)
def transform(self, X, y=None):
return super().transform(X)
def fit_transform(self, X, y=None):
return super().fit(X).transform(X)
class StringImputer(TransformerMixin):
def fit(self, X, *_):
self.modes = X.mode().iloc[0]
return self
def transform(self, X, y=None):
return X.fillna(self.modes)
train = pd.read_csv('data/train.csv')
test = pd.read_csv('data/test.csv')
from sklearn.pipeline import Pipeline, FeatureUnion
from sklearn.preprocessing import Imputer, StandardScaler
from sklearn.svm import SVC
from sklearn.model_selection import cross_val_score
model = Pipeline([
('union', FeatureUnion([
('age', Pipeline([
('select', ItemSelector('Age')),
('imputer', Imputer(strategy='mean')),
('scaler', StandardScaler()),
])),
('gender', Pipeline([
('select', ItemSelector('Sex')),
('imputer', StringImputer()),
('encoder', LabelBinarizerPipelineFriendly()),
])),
('embarked', Pipeline([
('select', ItemSelector('Embarked')),
('imputer', StringImputer()),
('encoder', LabelBinarizerPipelineFriendly()),
])),
('sibsp', Pipeline([
('select', ItemSelector('SibSp')),
('scaler', StandardScaler()),
])),
('parch', Pipeline([
('select', ItemSelector('Parch')),
('scaler', StandardScaler()),
])),
])),
('classifier', SVC())
])
scores = cross_val_score(model, train, train['Survived'])
print(scores)
print(scores.mean())
###Output
[0.81144781 0.83164983 0.82491582]
0.8226711560044894
###Markdown
Сега ще се опитам да го подобря.. Ще пусна Grid Search върху 3 алгоритъма (SVC, LogisticRegression, RandomForest) Използвам същата трансформация на данните
###Code
feature_union = FeatureUnion([
('age', Pipeline([
('select', ItemSelector('Age')),
('imputer', Imputer(strategy='mean')),
('scaler', StandardScaler()),
])),
('gender', Pipeline([
('select', ItemSelector('Sex')),
('imputer', StringImputer()),
('encoder', LabelBinarizerPipelineFriendly()),
])),
('embarked', Pipeline([
('select', ItemSelector('Embarked')),
('imputer', StringImputer()),
('encoder', LabelBinarizerPipelineFriendly()),
])),
('sibsp', Pipeline([
('select', ItemSelector('SibSp')),
('scaler', StandardScaler()),
])),
('parch', Pipeline([
('select', ItemSelector('Parch')),
('scaler', StandardScaler()),
])),
])
###Output
_____no_output_____
###Markdown
Разделям на трейн и тест и ги трансформирам
###Code
from sklearn.model_selection import train_test_split
X_train, X_test, y_train, y_test = train_test_split(train, train["Survived"], random_state=0)
transformer = feature_union.fit(X_train)
X_train_transformed = transformer.transform(X_train)
X_test_transformed = transformer.transform(X_test)
###Output
_____no_output_____
###Markdown
Пускам Грид Сърч над 3те алгоритъма.. Нека най-добрият победи.
###Code
from sklearn.ensemble import RandomForestClassifier
from sklearn.linear_model import LogisticRegression
pipeline = Pipeline([
('classifier', SVC())
])
grid = [
{
'classifier': [SVC()],
'classifier__gamma': [0.001, 0.01, 0.1, 1, 10, 100],
'classifier__C': [0.001, 0.01, 0.1, 1, 10, 100]
},
{
'classifier': [RandomForestClassifier()],
'classifier__max_features': [1, 2, 3, 5],
'classifier__n_estimators': [10, 50, 100, 200]
},
{
'classifier': [LogisticRegression()],
'classifier__C': [0.001, 0.01, 0.1, 1, 10, 100]
}
]
from sklearn.model_selection import GridSearchCV
search = GridSearchCV(pipeline, grid, cv=5)
search.fit(X_train_transformed, y_train)
print("Best params:\n{}\n".format(search.best_params_))
print("Best cross-validation score: {:.2f}".format(search.best_score_))
###Output
Best params:
{'classifier': SVC(C=1, cache_size=200, class_weight=None, coef0=0.0,
decision_function_shape=None, degree=3, gamma=1, kernel='rbf',
max_iter=-1, probability=False, random_state=None, shrinking=True,
tol=0.001, verbose=False), 'classifier__C': 1, 'classifier__gamma': 1}
Best cross-validation score: 0.82
###Markdown
Изглежда, че модела от лекцията е най-добрия от всички Ще се наложи да поиграем с фийчърите Заблязах, че поради някаква причина Fare липсва. Нека го добавим
###Code
feature_union = FeatureUnion([
('age', Pipeline([
('select', ItemSelector('Age')),
('imputer', Imputer(strategy='mean')),
('scaler', StandardScaler()),
])),
('gender', Pipeline([
('select', ItemSelector('Sex')),
('imputer', StringImputer()),
('encoder', LabelBinarizerPipelineFriendly()),
])),
('embarked', Pipeline([
('select', ItemSelector('Embarked')),
('imputer', StringImputer()),
('encoder', LabelBinarizerPipelineFriendly()),
])),
('sibsp', Pipeline([
('select', ItemSelector('SibSp')),
('scaler', StandardScaler()),
])),
('parch', Pipeline([
('select', ItemSelector('Parch')),
('scaler', StandardScaler()),
])),
('fare', Pipeline([
('select', ItemSelector('Fare')),
('scaler', StandardScaler())
]))
])
###Output
_____no_output_____
###Markdown
Да претренираме..
###Code
transformer = feature_union.fit(X_train)
X_train_transformed = transformer.transform(X_train)
X_test_transformed = transformer.transform(X_test)
print(X_train_transformed.shape)
###Output
(668, 8)
###Markdown
Може би трябва да извадя това във функция
###Code
search.fit(X_train_transformed, y_train)
print("Best params:\n{}\n".format(search.best_params_))
print("Best cross-validation score: {:.2f}".format(search.best_score_))
###Output
Best params:
{'classifier': SVC(C=1, cache_size=200, class_weight=None, coef0=0.0,
decision_function_shape=None, degree=3, gamma=0.1, kernel='rbf',
max_iter=-1, probability=False, random_state=None, shrinking=True,
tol=0.001, verbose=False), 'classifier__C': 1, 'classifier__gamma': 0.1}
Best cross-validation score: 0.82
|
machine-learning-python/machine-learning-ex8/ex8.ipynb | ###Markdown
Exercise 8 | Anomaly Detection and Collaborative Filtering ================== Part 1: Load Example Dataset ===================
###Code
from ex8 import *
%matplotlib inline
print('Visualizing example dataset for outlier detection.\n')
# The following command loads the dataset. You should now have the
# variables X, Xval, yval in your environment
from scipy import io as sio
data = sio.loadmat('ex8data1.mat')
X = data['X']
Xval = data['Xval']
yval = data['yval'].reshape(-1)
# Visualize the example dataset
plt.plot(X[:, 0], X[:, 1], 'bx')
plt.axis([0, 30, 0, 30])
plt.xlabel('Latency (ms)')
plt.ylabel('Throughput (mb/s)')
plt.show()
###Output
Visualizing example dataset for outlier detection.
###Markdown
================== Part 2: Estimate the dataset statistics ===================$$\mu_j = \frac{1}{m}\sum_{i=1}^{m}{x_j^{(i)}}$$$$\sigma_j^2 = \frac{1}{m}\sum_{i=1}^{m}{\left(x_j^{(i)} - \mu_j\right)^2}$$$$p(x;\mu,\sigma^2) = \frac{1}{\sqrt{2\pi}\sigma}e^{-\frac{(x - \mu)^2}{2\sigma^2}}$$
###Code
print('Visualizing Gaussian fit.\n')
# Estimate my and sigma2
mu, sigma2 = estimateGaussian(X)
# Returns the density of the multivariate normal at each data point (row)
# of X
p = multivariateGaussian(X, mu, sigma2)
# Visualize the fit
visualizeFit(X, mu, sigma2)
plt.xlabel('Latency (ms)')
plt.ylabel('Throughput (mb/s)')
plt.show()
###Output
Visualizing Gaussian fit.
###Markdown
================== Part 3: Find Outliers ===================
###Code
pval = multivariateGaussian(Xval, mu, sigma2)
epsilon, F1 = selectThreshold(yval, pval)
print(f'Best epsilon found using cross-validation: {epsilon:e}')
print(f'Best F1 on Cross Validation Set: {F1:f}')
print(' (you should see a value epsilon of about 8.99e-05)')
print(' (you should see a Best F1 value of 0.875000)\n')
# Find the outliers in the training set and plot the
outliers = p < epsilon
# Draw a red circle around those outliers
plt.figure()
visualizeFit(X, mu, sigma2)
plt.xlabel('Latency (ms)')
plt.ylabel('Throughput (mb/s)')
plt.plot(X[outliers, 0], X[outliers, 1], 'ro', linewidth=2, markersize=10)
plt.show()
###Output
Best epsilon found using cross-validation: 8.990853e-05
Best F1 on Cross Validation Set: 0.875000
(you should see a value epsilon of about 8.99e-05)
(you should see a Best F1 value of 0.875000)
###Markdown
================== Part 4: Multidimensional Outliers ===================
###Code
# Loads the second dataset. You should now have the
# variables X, Xval, yval in your environment
data = sio.loadmat('ex8data2.mat')
X = data['X']
Xval = data['Xval']
yval = data['yval'].reshape(-1)
# Apply the same steps to the larger dataset
mu, sigma2 = estimateGaussian(X)
# Training set
p = multivariateGaussian(X, mu, sigma2)
# Cross-validation set
pval = multivariateGaussian(Xval, mu, sigma2)
# Find the best threshold
epsilon, F1 = selectThreshold(yval, pval)
print(f'Best epsilon found using cross-validation: {epsilon:e}')
print(f'Best F1 on Cross Validation Set: {F1:f}')
print(' (you should see a value epsilon of about 1.38e-18)')
print(' (you should see a Best F1 value of 0.615385)')
print(f'# Outliers found: {(p < epsilon).sum()}')
###Output
Best epsilon found using cross-validation: 1.377229e-18
Best F1 on Cross Validation Set: 0.615385
(you should see a value epsilon of about 1.38e-18)
(you should see a Best F1 value of 0.615385)
# Outliers found: 117
###Markdown
以上部分代码在[ex8.py](https://github.com/StevenPZChan/ml_dl_coursera_Andrew_Ng/blob/master/machine-learning-python/machine-learning-ex8/ex8.py)中 =============== Part 1: Loading movie ratings dataset ================
###Code
from ex8_cofi import *
print('Loading movie ratings dataset.\n')
# Load data
from scipy import io as sio
data = sio.loadmat('ex8_movies.mat')
Y = data['Y']
R = data['R']
# Y is a 1682x943 matrix, containing ratings (1-5) of 1682 movies on
# 943 users
#
# R is a 1682x943 matrix, where R(i,j) = 1 if and only if user j gave a
# rating to movie i
# From the matrix, we can compute statistics like average rating.
print(f'Average rating for movie 1 (Toy Story): {Y[0, R[0, :].astype(bool)].mean():f} / 5\n')
# We can "visualize" the ratings matrix by plotting it with imagesc
plt.imshow(Y)
plt.ylabel('Movies')
plt.xlabel('Users')
plt.show()
###Output
Loading movie ratings dataset.
Average rating for movie 1 (Toy Story): 3.878319 / 5
###Markdown
============ Part 2: Collaborative Filtering Cost Function ===========$$J(x^{(1)},\ldots,x^{(n_m)},\theta^{(1)},\ldots,\theta^{(n_u)}) = \frac{1}{2}\sum_{(i,j):r(i,j)=1}{\left((\theta^{(j)})^Tx^{(i)} - y^{(i,j)}\right)^2 + \frac{\lambda}{2}\sum_{i=1}^{n_m}\sum_{k=1}^{n}{\left(x_k^{(i)}\right)^2} + \frac{\lambda}{2}\sum_{j=1}^{n_u}\sum_{k=1}^{n}{\left(\theta_k^{(i)}\right)^2}}$$
###Code
# Load pre-trained weights (X, Theta, num_users, num_movies, num_features)
data = sio.loadmat('ex8_movieParams.mat')
X = data['X']
Theta = data['Theta']
num_users = np.asscalar(data['num_users'])
num_movies = np.asscalar(data['num_movies'])
num_features = np.asscalar(data['num_features'])
# Reduce the data set size so that this runs faster
num_users = 4
num_movies = 5
num_features = 3
X = X[:num_movies, :num_features]
Theta = Theta[:num_users, :num_features]
Y = Y[:num_movies, :num_users]
R = R[:num_movies, :num_users]
# Evaluate cost function
J, _ = cofiCostFunc(np.concatenate([X.reshape(-1), Theta.reshape(-1)]), Y, R, num_users, num_movies, num_features, 0)
print(f'Cost at loaded parameters: {J:f} '
'\n(this value should be about 22.22)')
###Output
Cost at loaded parameters: 22.224604
(this value should be about 22.22)
###Markdown
============== Part 3: Collaborative Filtering Gradient ==============$$\frac{\partial J}{\partial x_k^{(i)}} = \sum_{j:r(i,j)=1}{\left((\theta^{(j)})^Tx^{(i)} - y^{(i,j)}\right)\theta_k^{(j)} + \lambda x_k^{(i)}}$$$$\frac{\partial J}{\partial\theta_k^{(j)}} = \sum_{i:r(i,j)=1}{\left((\theta^{(j)})^Tx^{(i)} - y^{(i,j)}\right)x_k^{(i)} + \lambda\theta_k^{(j)}}$$
###Code
print('Checking Gradients (without regularization) ... ')
# Check gradients by running checkNNGradients
checkCostFunction()
###Output
Checking Gradients (without regularization) ...
[[ -4.007977 -4.007977]
[ 2.710351 2.710351]
[ 2.65428 2.65428 ]
[ -0.049014 -0.049014]
[ 0.11209 0.11209 ]
[ 0.07776 0.07776 ]
[ 0.564534 0.564534]
[-11.096384 -11.096384]
[ 4.779287 4.779287]
[ 0.992009 0.992009]
[ 0.092185 0.092185]
[ -0.35919 -0.35919 ]
[ -4.005086 -4.005086]
[ 6.281983 6.281983]
[ -3.042467 -3.042467]
[ 1.147838 1.147838]
[ -1.993641 -1.993641]
[ -0.158091 -0.158091]
[ 2.454774 2.454774]
[ -3.483138 -3.483138]
[ -3.698394 -3.698394]
[ 3.871281 3.871281]
[ -5.986774 -5.986774]
[ 5.496491 5.496491]
[ 0.033889 0.033889]
[ -0.246756 -0.246756]
[ 0.509833 0.509833]]
['The above two columns you get should be very similar.\n(Left-Your Numerical Gradient, Right-Analytical Gradient)']
If your cost function implementation is correct, then
the relative difference will be small (less than 1e-9).
Relative Difference: 1.9003e-12
###Markdown
========= Part 4: Collaborative Filtering Cost Regularization ========
###Code
# Evaluate cost function
J, _ = cofiCostFunc(np.concatenate([X.reshape(-1), Theta.reshape(-1)]), Y, R, num_users, num_movies, num_features, 1.5)
print(f'Cost at loaded parameters (lambda = 1.5): {J:f} '
'\n(this value should be about 31.34)')
###Output
Cost at loaded parameters (lambda = 1.5): 31.344056
(this value should be about 31.34)
###Markdown
======= Part 5: Collaborative Filtering Gradient Regularization ======
###Code
print('Checking Gradients (with regularization) ... ')
# Check gradients by running checkNNGradients
checkCostFunction(1.5)
###Output
Checking Gradients (with regularization) ...
[[-4.619530e-01 -4.619530e-01]
[-1.050700e+00 -1.050700e+00]
[ 1.612006e+01 1.612006e+01]
[ 3.154325e-01 3.154325e-01]
[ 2.677497e+00 2.677497e+00]
[ 5.077390e+00 5.077390e+00]
[-8.580218e-01 -8.580218e-01]
[ 2.552349e+00 2.552349e+00]
[ 3.537703e+00 3.537703e+00]
[ 2.066214e+00 2.066214e+00]
[-3.677363e+00 -3.677363e+00]
[ 1.642278e+01 1.642278e+01]
[-4.046519e-01 -4.046519e-01]
[-3.083852e+00 -3.083852e+00]
[-4.005439e+00 -4.005439e+00]
[-3.797228e+00 -3.797228e+00]
[ 2.835593e+00 2.835593e+00]
[-1.318965e+01 -1.318965e+01]
[-2.383072e+00 -2.383072e+00]
[ 9.827656e-03 9.827656e-03]
[-3.979154e+00 -3.979154e+00]
[ 8.684664e-01 8.684664e-01]
[ 2.169383e+00 2.169383e+00]
[ 2.258721e+00 2.258721e+00]
[-8.594728e-01 -8.594728e-01]
[ 3.472921e+00 3.472921e+00]
[ 1.809470e+00 1.809470e+00]]
['The above two columns you get should be very similar.\n(Left-Your Numerical Gradient, Right-Analytical Gradient)']
If your cost function implementation is correct, then
the relative difference will be small (less than 1e-9).
Relative Difference: 2.13093e-12
###Markdown
============== Part 6: Entering ratings for a new user ===============
###Code
movieList = loadMovieList()
# Initialize my ratings
my_ratings = np.zeros(1682)
# Check the file movie_idx.txt for id of each movie in our dataset
# For example, Toy Story (1995) has ID 1, so to rate it "4", you can set
my_ratings[0] = 4
# Or suppose did not enjoy Silence of the Lambs (1991), you can set
my_ratings[97] = 2
# We have selected a few movies we liked / did not like and the ratings we
# gave are as follows:
my_ratings[6] = 3
my_ratings[11] = 5
my_ratings[53] = 4
my_ratings[63] = 5
my_ratings[65] = 3
my_ratings[68] = 5
my_ratings[182] = 4
my_ratings[225] = 5
my_ratings[354] = 5
print('New user ratings:')
for i in range(len(my_ratings)):
if my_ratings[i] > 0:
print(f'Rated {my_ratings[i]:g} for {movieList[i]}')
###Output
New user ratings:
Rated 4 for Toy Story (1995)
Rated 3 for Twelve Monkeys (1995)
Rated 5 for Usual Suspects, The (1995)
Rated 4 for Outbreak (1995)
Rated 5 for Shawshank Redemption, The (1994)
Rated 3 for While You Were Sleeping (1995)
Rated 5 for Forrest Gump (1994)
Rated 2 for Silence of the Lambs, The (1991)
Rated 4 for Alien (1979)
Rated 5 for Die Hard 2 (1990)
Rated 5 for Sphere (1998)
###Markdown
================== Part 7: Learning Movie Ratings ====================
###Code
print('Training collaborative filtering...')
# Load data
data = sio.loadmat('ex8_movies.mat')
Y = data['Y']
R = data['R']
# Y is a 1682x943 matrix, containing ratings (1-5) of 1682 movies by
# 943 users
#
# R is a 1682x943 matrix, where R(i,j) = 1 if and only if user j gave a
# rating to movie i
# Add our own ratings to the data matrix
Y = np.column_stack([my_ratings, Y])
R = np.column_stack([(my_ratings != 0).astype(int), R])
# Normalize Ratings
Ynorm, Ymean = normalizeRatings(Y, R)
# Useful Values
num_users = Y.shape[1]
num_movies = Y.shape[0]
num_features = 10
# Set Initial Parameters (Theta, X)
X = np.random.randn(num_movies, num_features)
Theta = np.random.randn(num_users, num_features)
initial_parameters = np.concatenate([X.reshape(-1), Theta.reshape(-1)])
# Set options for fmincg
options = {'disp': True, 'maxiter': None}
# Set Regularization
from scipy import optimize as opt
lambda_ = 10
res = opt.minimize(lambda t: cofiCostFunc(t, Ynorm, R, num_users, num_movies, num_features, lambda_)[0], initial_parameters,
method='CG', jac=lambda t: cofiCostFunc(t, Ynorm, R, num_users, num_movies, num_features, lambda_)[1], options=options)
theta = res.x
# Unfold the returned theta back into U and W
X = theta[:num_movies * num_features].reshape((num_movies, num_features))
Theta = theta[num_movies * num_features:].reshape((num_users, num_features))
print('Recommender system learning completed.')
###Output
Training collaborative filtering...
Warning: Desired error not necessarily achieved due to precision loss.
Current function value: 38951.847560
Iterations: 371
Function evaluations: 555
Gradient evaluations: 554
Recommender system learning completed.
###Markdown
================== Part 8: Recommendation for you ====================
###Code
p = np.matmul(X, Theta.transpose())
my_predictions = p[:, 0] + Ymean
movieList = loadMovieList()
ix = my_predictions.argsort()[::-1]
print('Top recommendations for you:')
for i in range(10):
j = ix[i]
print(f'Predicting rating {my_predictions[j]:.1f} for movie {movieList[j]}')
print('\n\nOriginal ratings provided:')
for i in range(len(my_ratings)):
if my_ratings[i] > 0:
print(f'Rated {my_ratings[i]:g} for {movieList[i]}')
###Output
Top recommendations for you:
Predicting rating 5.0 for movie Prefontaine (1997)
Predicting rating 5.0 for movie Marlene Dietrich: Shadow and Light (1996)
Predicting rating 5.0 for movie Saint of Fort Washington, The (1993)
Predicting rating 5.0 for movie Entertaining Angels: The Dorothy Day Story (1996)
Predicting rating 5.0 for movie Star Kid (1997)
Predicting rating 5.0 for movie Santa with Muscles (1996)
Predicting rating 5.0 for movie They Made Me a Criminal (1939)
Predicting rating 5.0 for movie Great Day in Harlem, A (1994)
Predicting rating 5.0 for movie Someone Else's America (1995)
Predicting rating 5.0 for movie Aiqing wansui (1994)
Original ratings provided:
Rated 4 for Toy Story (1995)
Rated 3 for Twelve Monkeys (1995)
Rated 5 for Usual Suspects, The (1995)
Rated 4 for Outbreak (1995)
Rated 5 for Shawshank Redemption, The (1994)
Rated 3 for While You Were Sleeping (1995)
Rated 5 for Forrest Gump (1994)
Rated 2 for Silence of the Lambs, The (1991)
Rated 4 for Alien (1979)
Rated 5 for Die Hard 2 (1990)
Rated 5 for Sphere (1998)
|
notebooks/stability-memory-tradeoff-figures.ipynb | ###Markdown
Setup
###Code
dims = [25, 50, 100, 200, 400, 800]
bitrates = [1,2,4,8,16,32]
sns.set_palette(reversed(sns.color_palette("Blues_d", len(bitrates))), len(bitrates))
###Output
_____no_output_____
###Markdown
Load data
###Code
algo = 'w2v_cbow'
csv_file = f'../results/{algo}_optimal_no_emb_norm_top_10000.csv'
total_df_cbow = pd.read_csv(csv_file)
df_avg_cbow_no_norm = total_df_cbow.groupby(['space','bitrate','dim']).aggregate(['mean', 'std']).reset_index()
algo = 'mc'
csv_file = f'../results/{algo}_optimal_no_emb_norm_top_10000.csv'
total_df_mc = pd.read_csv(csv_file)
df_avg_mc_no_norm = total_df_mc.groupby(['space','bitrate','dim']).aggregate(['mean', 'std']).reset_index()
###Output
_____no_output_____
###Markdown
Stability-Memory Tradeoff Individual Dimension and Precision Trends
###Code
sns.set_style("ticks")
plt.rc('lines', markersize=15)
plt.rc('lines', linewidth=2)
plt.rc('font', size=22)
plt.rc('legend', fontsize=18)
plt.rc('axes', titlesize=22)
plt.rc('errorbar', capsize=3)
plt.rc('errorbar', capsize=3)
plt.rc('axes', linewidth=2)
fig = plt.figure(figsize=(12,10))
plt.subplots_adjust(wspace=0.3, hspace=0.45)
plt.subplot(221)
plt_single(df=df_avg_cbow_no_norm, vals=[32], val_tag='bitrate', val_tag_label='b', xtag='dim', dist='la_sst_no_emb_norm', ylabel='% Disagreement', xlabel='Dimension', title='SST-2', color='C1', marker='^', line_label='CBOW', legend=True)
plt_single(df=df_avg_mc_no_norm, vals=[32], val_tag='bitrate', val_tag_label='b', xtag='dim', dist='la_sst_no_emb_norm', ylabel='% Disagreement', xlabel='Dimension', title='SST-2', line_label='MC', legend=True)
plt.subplot(222)
plt_single(df=df_avg_cbow_no_norm, vals=[32], val_tag='bitrate', val_tag_label='b', xtag='dim', dist='rnn_no_crf_ner', ylabel='% Disagreement', xlabel='Dimension', title='CoNLL-2003', color='C1', marker='^', line_label='CBOW', legend=True)
plt_single(df=df_avg_mc_no_norm, vals=[32], val_tag='bitrate', val_tag_label='b', xtag='dim', dist='rnn_no_crf_ner', ylabel='% Disagreement', xlabel='Dimension', title='CoNLL-2003', line_label='MC', legend=True)
vals = [100]
plt.subplot(223)
plt_single(df=df_avg_cbow_no_norm, vals=vals, val_tag='dim', xtag='bitrate', dist='la_sst_no_emb_norm', ylabel='% Disagreement', xlabel='Precision', title='SST-2', color='C1', marker='^', line_label='CBOW', legend=True)
plt_single(df=df_avg_mc_no_norm, vals=vals, val_tag='dim', xtag='bitrate', dist='la_sst_no_emb_norm', ylabel='% Disagreement', xlabel='Precision', title='SST-2', line_label='MC', legend=True)
plt.subplot(224)
plt_single(df=df_avg_cbow_no_norm, vals=vals, val_tag='dim', xtag='bitrate', dist='rnn_no_crf_ner', ylabel='% Disagreement', xlabel='Precision', title='CoNLL-2003', color='C1', marker='^', line_label='CBOW', legend=True)
plt_single(df=df_avg_mc_no_norm, vals=vals, val_tag='dim', xtag='bitrate', dist='rnn_no_crf_ner', ylabel='% Disagreement', xlabel='Precision', title='CoNLL-2003', line_label='MC', legend=True)
###Output
_____no_output_____
###Markdown
Joint Dimension/Precision Trends
###Code
sns.set_style("ticks")
plt.rc('lines', markersize=5)
plt.rc('lines', linewidth=2)
plt.rc('lines', markersize=15)
plt.rc('font', size=16)
plt.rc('legend', fontsize=16)
plt.rc('axes', titlesize=16)
# y-intercepts and slope from fit_trends.py
fig = plt.figure(figsize=(12,12))
plt.subplots_adjust(wspace=0.3, hspace=0.35)
bitrates = [1,2,4,8,16,32]
x = total_df_cbow.loc[total_df_cbow['space'] < 1000]['space'].values
m = -1.37
df_type = df_avg_cbow_no_norm
plt.subplot(221)
plt_single(df=df_type, vals=bitrates, val_tag='bitrate', xtag='space', dist='la_sst_no_emb_norm', ylabel='% Disagreement', val_tag_label='b', xlabel='Memory (Bits / Word)', title='CBOW, SST-2', legend=False)
plt.plot(x, m*np.log2(x)+20.44, color='r', zorder=3)
plt.subplot(222)
plt_single(df=df_type, vals=bitrates, val_tag='bitrate', xtag='space', dist='rnn_no_crf_ner', ylabel='% Disagreement', val_tag_label='b', xlabel='Memory (Bits / Word)', title='CBOW, CoNLL-2003', legend=True)
plt.plot(x, m*np.log2(x)+16.94, color='r', zorder=3)
df_type = df_avg_mc_no_norm
plt.subplot(223)
plt_single(df=df_type, vals=bitrates, val_tag='bitrate', xtag='space', dist='la_sst_no_emb_norm', ylabel='% Disagreement', val_tag_label='b',xlabel='Memory (Bits / Word)', title='MC, SST-2', legend=False)
plt.plot(x, m*np.log2(x)+26.18, color='r', zorder=3)
plt.subplot(224)
plt_single(df=df_type, vals=bitrates, val_tag='bitrate', xtag='space', dist='rnn_no_crf_ner', ylabel='% Disagreement', val_tag_label='b',xlabel='Memory (Bits / Word)', title='MC, CoNLL-2003', legend=False)
plt.plot(x, m*np.log2(x)+18.36, color='r', zorder=3)
###Output
_____no_output_____ |
.ipynb_checkpoints/SIRD_model-checkpoint.ipynb | ###Markdown
SIRD model with social distancing$$\frac{dS}{dt} = -\frac{RIS}{T_{inf}} $$ $$\frac{dI}{dt} = \frac{RIS}{T_{inf}} - \frac{I}{T_{inf}}$$$$\frac{dX}{dt} = \gamma_XI$$More details in the whitepaper NYC
###Code
# Fetch death data for NYC from JHU
raw_data_us = pd.read_csv("time_series_covid19_deaths_US.csv")
start_date = datetime.date(2020, 1, 22)
date_format = "%-m/%-d/%y"
# Extract NYC time_series data
deaths_time_series_nyc = []
date = deepcopy(start_date)
df_nyc = raw_data_us[raw_data_us['Combined_Key'].str.contains("New York City")]
df_nyc_cols = df_nyc.columns.values
while date.strftime(date_format) in df_nyc_cols:
deaths_time_series_nyc.extend(df_nyc[date.strftime(date_format)].values)
date = date + datetime.timedelta(days=1)
deaths_time_series_nyc = np.array(deaths_time_series_nyc)
deaths_time_series_nyc[1:] = np.diff(deaths_time_series_nyc)
# Data visualization
smooth_data_nyc = smoothen(smoothen(smoothen(smoothen(smoothen(deaths_time_series_nyc, 1), 1), 1), 1), 1)
plt.scatter(np.arange(smooth_data_nyc.shape[0]), deaths_time_series_nyc, label="Raw data", s=1, c='red')
plt.plot(smooth_data_nyc, label="Smoothened data", c='blue')
plt.legend()
plt.title("Deaths per day in NYC")
plt.show()
# Preprocess social distancing data from Google
start_date_sd = datetime.date(2020, 2, 15)
end_date_sd = datetime.date(2020, 5, 1)
date_format_sd = "%Y-%m-%d"
smoothening_factor = 6
df_sd = pd.read_csv("Global_Mobility_Report.csv", low_memory=False)
df_sd = df_sd[df_sd['country_region_code'] == 'US']
categories = ["retail_and_recreation_percent_change_from_baseline",
"grocery_and_pharmacy_percent_change_from_baseline",
"parks_percent_change_from_baseline",
"transit_stations_percent_change_from_baseline",
"workplaces_percent_change_from_baseline"]
# Counties which NYC covers -- population data from Wikipedia
counties = {"New York County" : 1628706,
"Kings County" : 2559903,
"Bronx County" : 1418207,
"Queens County" : 2253858}
total_pop = 0.
for c in counties.keys():
total_pop += counties[c]
agg_sd_nyc = {}
for cat in categories:
agg_sd_nyc[cat] = []
curr_date = deepcopy(start_date_sd)
# Get weighted average of social distancing from counties in NYC
while curr_date <= end_date_sd:
df_sd_curr = df_sd[df_sd["date"] == curr_date.strftime(date_format_sd)]
val = {}
for c in counties.keys():
df_county = df_sd_curr[df_sd_curr["sub_region_2"] == c]
for cat in categories:
if cat in val:
val[cat] += counties[c] * np.array(df_county[cat])[0]
else:
val[cat] = counties[c] * np.array(df_county[cat])[0]
for cat in categories:
val[cat] /= total_pop
agg_sd_nyc[cat].append(val[cat])
curr_date = curr_date + datetime.timedelta(days=1)
agg_sd_nyc["total"] = np.zeros(len(agg_sd_nyc[categories[0]]))
# Get the total
for cat in agg_sd_nyc.keys():
agg_sd_nyc[cat] = np.array(agg_sd_nyc[cat])
agg_sd_nyc["total"] += agg_sd_nyc[cat]
plt.figure(figsize=(5,5))
plt.plot(agg_sd_nyc["total"], '.', label="Raw data")
# Plot the array
for label in agg_sd_nyc.keys():
agg_sd_nyc[label] = smoothen(agg_sd_nyc[label], smoothening_factor)
# plt.plot(agg_sd_nyc[label], label=label)
plt.plot(agg_sd_nyc["total"], label="Smooth data")
plt.title("Social distancing in NYC over time")
plt.xlabel("Days")
plt.ylabel("Percent change from baseline")
plt.legend()
plt.show()
# Get model stats
pad_sd = 60 # How many days to pad the social distancing data by
padded_sd = np.concatenate((np.ones(pad_sd) * agg_sd_nyc["total"][0], agg_sd_nyc["total"]))
get_model_stats_v2(model=sird_sd,
loss_fun=rmse_loss,
data=smooth_data_nyc,
raw_data=deaths_time_series_nyc,
# breakpoints=[60, 64, 68, 72, 76, 80, 90, 100, ],
# plot_title="NYC",
base_filename="NYC",
breakpoints=range(60, smooth_data_nyc.shape[0]),
start_date=datetime.date(2020, 1, 22),
fixed_params={
"T_inf" : 5.0,
"sd_offset" : 23,
"pop" : 8.4e6,
"gamma_x" : 1.6e-3,
"I_init" : 7.4e-5,
"offset" : 40,
"padded_sd" : padded_sd
},
var_param_vals={
"R_max": np.linspace(2.0, 2.8, 15),
"R_min": np.linspace(0.8, 0.95, 10),
},
param_order=["offset", "pop", "I_init", "T_inf", "gamma_x", "R_max", "R_min", "sd_offset", "padded_sd"],
loss_factor=800,
exclude_params=["padded_sd"]
)
###Output
78it [01:13, 1.06it/s]
###Markdown
Spain
###Code
# Get the death data
df_global = pd.read_csv("time_series_covid19_deaths_global.csv")
date_format = "%-m/%-d/%y"
start_date_global = datetime.date(2020, 1, 22)
end_date_global = datetime.date(2020, 5, 27)
df_spain = df_global[df_global["Country/Region"] == "Spain"]
data_spain = []
curr_date = deepcopy(start_date_global)
while curr_date <= end_date_global:
data_spain.append(df_spain[curr_date.strftime(date_format)].iloc[0])
curr_date = curr_date + datetime.timedelta(days=1)
data_spain = data_spain[:120]
data_spain[1:] = np.diff(data_spain)
smooth_data_spain = deepcopy(data_spain)
for i in range(1, 15):
smooth_data_spain = smoothen(smooth_data_spain, 1)
plt.plot(smooth_data_spain, label="Smooth data")
plt.plot(data_spain, '.', label="Raw data")
# plt.plot(smooth_data_spain, label="Smoothened data")
plt.title("Daily deaths in Spain")
plt.xlabel("Days")
plt.ylabel("Number of deaths")
plt.legend()
plt.show()
# Preprocess social distancing data
df_sd = pd.read_csv("Global_Mobility_Report.csv", low_memory=False)
df_sd = df_sd[df_sd['country_region_code'] == 'ES']
df_sd = df_sd[pd.isnull(df_sd['sub_region_1'])]
categories = ["retail_and_recreation_percent_change_from_baseline",
"grocery_and_pharmacy_percent_change_from_baseline",
"parks_percent_change_from_baseline",
"transit_stations_percent_change_from_baseline",
"workplaces_percent_change_from_baseline"]
agg_sd_spain = []
curr_date = deepcopy(start_date_sd)
while curr_date <= end_date_sd:
df_sd_curr = df_sd[df_sd["date"] == curr_date.strftime(date_format_sd)]
val = 0
for cat in categories:
val += np.array(df_sd_curr[cat])[0]
agg_sd_spain.append(val)
curr_date = curr_date + datetime.timedelta(days=1)
# Plot the array
agg_sd_spain = np.array(agg_sd_spain)
plt.figure(figsize=(5,5))
plt.plot(agg_sd_spain, '.', label="Raw data")
for i in range(5):
agg_sd_spain = smoothen(smoothen(agg_sd_spain, 1), 1)
# agg_sd_spain = np.maximum(np.ones_like(agg_sd_spain) * agg_sd_spain[-1], agg_sd_spain)
# for i in range(5):
# padded_sd = smoothen(smoothen(padded_sd, 1), 1)
# plt.plot(padded_sd, label="Smooth data {}".format(i + 6))
plt.plot(agg_sd_spain, label="Smooth data")
plt.title("Social distancing in Spain over time")
plt.xlabel("Days")
plt.ylabel("Percent change from baseline")
plt.legend()
plt.show()
# Get model stats
pad_sd = 60 # How many days to pad the social distancing data by
padded_sd = np.concatenate((np.ones(pad_sd) * agg_sd_spain[0], agg_sd_spain))
get_model_stats_v2(model=sird_sd,
loss_fun=rmse_loss,
data=smooth_data_spain,
raw_data=np.array(data_spain),
# breakpoints=[60, 64, 68, 72, 76, 80, 84, 90, smooth_data_spain.shape[0]],
# plot_title="Spain",
base_filename="Spain",
start_date=datetime.date(2020, 1, 22),
breakpoints=range(60, smooth_data_spain.shape[0]),
fixed_params={
"T_inf" : 5.0,
"sd_offset" : 23,
"pop" : 4.69e7,
"gamma_x" : 1.6e-3,
"I_init" : 1.3e-5,
"offset" : 25,
"padded_sd" : padded_sd
},
var_param_vals={
# "offset": np.linspace(40, 50, 2),
"R_max": np.linspace(1.8, 2.5, 20),
"R_min": np.linspace(0.7, 0.95, 16)
},
param_order=["offset", "pop", "I_init", "T_inf", "gamma_x", "R_max", "R_min", "sd_offset", "padded_sd"],
loss_factor=1000,
exclude_params=["padded_sd"])
###Output
60it [02:28, 2.47s/it]
###Markdown
Italy
###Code
# Get the death data
df_italy = df_global[df_global["Country/Region"] == "Italy"]
data_italy = []
curr_date = deepcopy(start_date_global)
while curr_date <= end_date_global:
data_italy.append(df_italy[curr_date.strftime(date_format)].iloc[0])
curr_date = curr_date + datetime.timedelta(days=1)
data_italy[1:] = np.diff(data_italy)
smooth_data_italy = smoothen(smoothen(savgol_filter(data_italy, 37, 3), 1), 1)
plt.plot(data_italy, '.', label="Raw data")
plt.plot(smooth_data_italy, label="Smoothened data")
plt.title("Daily deaths in Italy")
plt.xlabel("Days")
plt.ylabel("Number of deaths")
plt.legend()
plt.show()
# Preprocess social distancing data
df_sd = pd.read_csv("Global_Mobility_Report.csv")
df_sd = df_sd[df_sd['country_region_code'] == 'IT']
df_sd = df_sd[pd.isnull(df_sd['sub_region_1'])]
categories = ["retail_and_recreation_percent_change_from_baseline",
"grocery_and_pharmacy_percent_change_from_baseline",
"parks_percent_change_from_baseline",
"transit_stations_percent_change_from_baseline",
"workplaces_percent_change_from_baseline"]
agg_sd_italy = []
curr_date = deepcopy(start_date_sd)
while curr_date <= end_date_sd:
df_sd_curr = df_sd[df_sd["date"] == curr_date.strftime(date_format_sd)]
val = 0
for cat in categories:
val += np.array(df_sd_curr[cat])[0]
agg_sd_italy.append(val)
curr_date = curr_date + datetime.timedelta(days=1)
# Plot the array
agg_sd_italy = np.array(agg_sd_italy)
plt.figure(figsize=(10,5))
plt.plot(agg_sd_italy, '.', label="Raw data")
agg_sd_italy = smoothen(smoothen(savgol_filter(agg_sd_italy, 37, 6), 3), 3)
plt.plot(agg_sd_italy, label="Smooth data")
plt.title("Italy")
plt.xlabel("Days")
plt.ylabel("Percent change from baseline")
plt.legend()
plt.show()
# Get model stats
pad_sd = 60 # How many days to pad the social distancing data by
padded_sd = np.concatenate((np.ones(pad_sd) * agg_sd_italy[0], agg_sd_italy))
get_model_stats_v2(model=sird_sd,
loss_fun=rmse_loss,
data=smooth_data_italy,
data=np.array(data_italy),
# breakpoints=[60, 64, 68, 72, 76, 80, 90, 100, smooth_data_italy.shape[0]],
# plot_title="Italy",
base_filename="Italy",
breakpoints=range(60, smooth_data_italy.shape[0]),
start_date=datetime.date(2020, 1, 22),
start_date=datetime.date(2020, 1, 22),
fixed_params={
"T_inf" : 5.0,
"sd_offset" : 23,
"pop" : 6.04e7,
"gamma_x" : 1.6e-3,
"I_init" : 1.1e-5,
"offset" : 18,
"padded_sd" : padded_sd
},
var_param_vals={
# "offset": np.linspace(40, 50, 2),
"R_max": np.linspace(1.4, 2.8, 20),
"R_min": np.linspace(0.5, 0.99, 20)
},
param_order=["offset", "pop", "I_init", "T_inf", "gamma_x", "R_max", "R_min", "sd_offset", "padded_sd"],
loss_factor=800,
exclude_params=["padded_sd"])
###Output
_____no_output_____
###Markdown
Maharashtra
###Code
# Get death data for MH
fetcher = DataFetcherState()
fetcher.fetch()
data_mh = np.array(fetcher.data['mh']['deceased'], dtype=float) # Starting date is 14th March
data_mh = np.concatenate((np.zeros(27), data_mh))
smooth_data_mh = smoothen(smoothen(smoothen(smoothen(savgol_filter(data_mh, 37, 3), 1), 1), 1), 1)
plt.plot(data_mh, '.', label="Raw data")
plt.plot(smooth_data_mh, label="Smoothened data")
plt.title("Daily deaths in MH")
plt.xlabel("Days")
plt.ylabel("Number of deaths")
plt.legend()
plt.show()
# Get social distancing data for MH
# Preprocess social distancing data
df_sd = pd.read_csv("Global_Mobility_Report.csv", low_memory=False)
df_sd = df_sd[df_sd["sub_region_1"] == "Maharashtra"]
categories = ["retail_and_recreation_percent_change_from_baseline",
"grocery_and_pharmacy_percent_change_from_baseline",
"parks_percent_change_from_baseline",
"transit_stations_percent_change_from_baseline",
"workplaces_percent_change_from_baseline"]
agg_sd_mh = []
curr_date = deepcopy(start_date_sd)
while curr_date <= end_date_sd:
df_sd_curr = df_sd[df_sd["date"] == curr_date.strftime(date_format_sd)]
val = 0
for cat in categories:
val += np.array(df_sd_curr[cat])[0]
agg_sd_mh.append(val)
curr_date = curr_date + datetime.timedelta(days=1)
# Plot the array
agg_sd_mh = np.array(agg_sd_mh)
plt.figure(figsize=(10,5))
plt.plot(agg_sd_mh, '.', label="Raw data")
agg_sd_mh = smoothen(smoothen(savgol_filter(agg_sd_mh, 37, 3), 1), 1)
plt.plot(agg_sd_mh, label="Smooth data")
plt.title("Social distancing in Maharashtra over time")
plt.xlabel("Days")
plt.ylabel("Percent change from baseline")
plt.legend()
plt.show()
# Get model stats
pad_sd = 60 # How many days to pad the social distancing data by
padded_sd = np.concatenate((np.ones(pad_sd) * agg_sd_mh[0], agg_sd_mh))
max_len = smooth_data_mh.shape[0]
get_model_stats_v2(model=sird_sd,
loss_fun=rmse_loss,
data=smooth_data_mh,
# breakpoints=[80, 84, 88, 92, 96, 103],
# plot_title="Maharashtra",
raw_data=np.array(data_mh),
start_date=datetime.date(2020, 3, 14),
base_filename="Maharashtra",
breakpoints=range(80, max_len),
fixed_params={
"T_inf" : 5.0,
"sd_offset" : 23,
"pop" : 11.42e7,
"gamma_x" : 1.6e-3,
"I_init" : 5.47e-6,
"offset" : 40,
"padded_sd" : padded_sd
},
var_param_vals={
# "offset": np.linspace(40, 50, 2),
"R_max": np.linspace(1.4, 2.5, 20),
"R_min": np.linspace(1.1, 1.3, 20)
},
param_order=["offset", "pop", "I_init", "T_inf", "gamma_x", "R_max", "R_min", "sd_offset", "padded_sd"],
loss_factor=100,
future_preds=60,
exclude_params=["padded_sd"])
###Output
2it [00:09, 4.99s/it]
###Markdown
Delhi
###Code
# Get death data for DL
fetcher = DataFetcherState()
fetcher.fetch()
data_dl = np.array(fetcher.data['dl']['deceased'], dtype=float) # Starting date is 14th March
data_dl = np.concatenate((np.zeros(27), data_dl))
smooth_data_dl = smoothen(smoothen(smoothen(smoothen(savgol_filter(data_dl, 37, 3), 1), 1), 1), 1)
plt.plot(data_dl, '.', label="Raw data")
plt.plot(smooth_data_dl, label="Smoothened data")
plt.title("Daily deaths in DL")
plt.xlabel("Days")
plt.ylabel("Number of deaths")
plt.legend()
plt.show()
# Preprocess social distancing data
df_sd = pd.read_csv("Global_Mobility_Report.csv", low_memory=False)
df_sd = df_sd[df_sd["sub_region_1"] == "Delhi"]
categories = ["retail_and_recreation_percent_change_from_baseline",
"grocery_and_pharmacy_percent_change_from_baseline",
"parks_percent_change_from_baseline",
"transit_stations_percent_change_from_baseline",
"workplaces_percent_change_from_baseline"]
agg_sd_dl = []
curr_date = deepcopy(start_date_sd)
while curr_date <= end_date_sd:
df_sd_curr = df_sd[df_sd["date"] == curr_date.strftime(date_format_sd)]
val = 0
for cat in categories:
val += np.array(df_sd_curr[cat])[0]
agg_sd_dl.append(val)
curr_date = curr_date + datetime.timedelta(days=1)
# Plot the array
agg_sd_dl = np.array(agg_sd_dl)
plt.figure(figsize=(10,5))
plt.plot(agg_sd_dl, '.', label="Raw data")
agg_sd_dl = smoothen(smoothen(smoothen(savgol_filter(agg_sd_dl, 21, 3), 1), 1), 1)
plt.plot(agg_sd_dl, label="Smooth data")
plt.title("Social distancing in Delhi over time")
plt.xlabel("Days")
plt.ylabel("Percent change from baseline")
plt.legend()
plt.show()
# Get model stats
pad_sd = 60 # How many days to pad the social distancing data by
padded_sd = np.concatenate((np.ones(pad_sd) * agg_sd_dl[0], agg_sd_dl))
max_len = smooth_data_dl.shape[0]
get_model_stats_v2(model=sird_sd,
loss_fun=rmse_loss,
data=smooth_data_dl,
# breakpoints=list(range(max_len - 8, max_len + 1, 4)),
# plot_title="Delhi",
filename="projections/Delhi.json",
breakpoints=range(90, max_len),
fixed_params={
"T_inf" : 5.0,
"sd_offset" : 23,
"pop" : 1.9e7,
"gamma_x" : 1.6e-3,
"I_init" : 3.28e-5,
"offset" : 60,
"padded_sd" : padded_sd
},
var_param_vals={
"R_max": np.linspace(1.4, 2.5, 20),
"R_min": np.linspace(1.1, 1.3, 20)
},
param_order=["offset", "pop", "I_init", "T_inf", "gamma_x", "R_max", "R_min", "sd_offset", "padded_sd"],
loss_factor=200,
future_preds=100,
exclude_params=["padded_sd"])
###Output
_____no_output_____ |
DATA_MODELv0.1_typeCR.ipynb | ###Markdown
1. Marca1: Marca_20 - Cupo_3 - CapacidadEnvase_9
###Code
data_model_1 = full_clientes_venta[["Gerencia2", "SubCanal2", "Categoria", "Nevera"]].copy()
data_model_1
data_model_1.iloc[0]["Gerencia2"]
print(len(data_model_1))
Marca = "Marca_20"
Cupo = "Cupo_3"
Capacidad = "CapacidadEnvase_9"
output = []
for i in tqdm(range(len(data_model_1))):
if (clientes_venta.iloc[i]["Marca2"] == Marca and clientes_venta.iloc[i]["Cupo2"] == Cupo and clientes_venta.iloc[i]["CapacidadEnvase2"] == Capacidad):
output.append(1)
else:
output.append(0)
output = np.array(output)
fig = plt.figure(figsize =(17, 8))
plt.hist(output, bins = 2)
plt.show()
unique, counts = np.unique(output, return_counts=True)
print(np.asarray((unique, counts)).T)
data_model_1["output"] = output
data_model_1
data_model_1.iloc[0]["Gerencia2"]
import re
int(re.findall('\d+', data_model_1.iloc[-1]["Gerencia2"])[0])
def label_encode_default(value):
return int(re.findall('\d+', value)[0])
label_encode_default(data_model_1.iloc[0]["Gerencia2"])
data_model_1['Gerencia2'] = data_model_1['Gerencia2'].apply(lambda x: label_encode_default(x))
data_model_1['SubCanal2'] = data_model_1['SubCanal2'].apply(lambda x: label_encode_default(x))
data_model_1['Categoria'] = data_model_1['Categoria'].apply(lambda x: label_encode_default(x))
data_model_1
from sklearn.model_selection import train_test_split
from sklearn.linear_model import LogisticRegression
from sklearn import metrics
X = data_model_1[['Gerencia2', 'SubCanal2','Categoria']]
y = data_model_1['output']
X_train,X_test,y_train,y_test = train_test_split(X,y,test_size=0.25,random_state=0)
logistic_regression= LogisticRegression()
logistic_regression.fit(X_train,y_train)
y_pred=logistic_regression.predict(X_test)
confusion_matrix = pd.crosstab(y_test, y_pred, rownames=['Actual'], colnames=['Predicted'])
sns.heatmap(confusion_matrix, annot=True)
confusion_matrix
###Output
_____no_output_____
###Markdown
2. Marca2: Marca_16- Cupo_2 - CapacidadEnvase_10 3. Marca3: Marca_9-Cupo_3-CapacidadEnvase_12 4. Marca_Inno1: Marca_38- Cupo_2 -CapacidadEnvase_10 5. Marca_Inno2: Marca_39-Cupo_2-CapacidadEnvase_10
###Code
full_clientes_venta
full_clientes_venta['SegmentoPrecio2'] = full_clientes_venta['SegmentoPrecio2'].apply(lambda x: label_encode_default(x))
full_clientes_venta['Marca2'] = full_clientes_venta['Marca2'].apply(lambda x: label_encode_default(x))
full_clientes_venta['Cupo2'] = full_clientes_venta['Cupo2'].apply(lambda x: label_encode_default(x))
full_clientes_venta['CapacidadEnvase2'] = full_clientes_venta['CapacidadEnvase2'].apply(lambda x: label_encode_default(x))
full_clientes_venta['Regional2'] = full_clientes_venta['Regional2'].apply(lambda x: label_encode_default(x))
full_clientes_venta['Gerencia2'] = full_clientes_venta['Gerencia2'].apply(lambda x: label_encode_default(x))
full_clientes_venta['SubCanal2'] = full_clientes_venta['SubCanal2'].apply(lambda x: label_encode_default(x))
full_clientes_venta['Categoria'] = full_clientes_venta['Categoria'].apply(lambda x: label_encode_default(x))
full_clientes_venta
full_clientes_venta.to_csv ("DATA/full_clientes_venta.csv", index = False, header=True)
testing = pd.read_csv("DATA/full_clientes_venta.csv")
testing
###Output
_____no_output_____ |
Seminar9/Seminar9_en.ipynb | ###Markdown
Deep learning for Natural Language Processing * Simple text representations, bag of words * Word embedding and... not just another word2vec this time * 1-dimensional convolutions for text * Aggregating several data sources "the hard way" * Solving ~somewhat~ real ML problem with ~almost~ end-to-end deep learning Special thanks to Irina Golzmann for help with technical part. NLTKYou will require nltk v3.2 to solve this assignment__It is really important that the version is 3.2, otherwize russian tokenizer might not work__Install/update* `sudo pip install --upgrade nltk==3.2`* If you don't remember when was the last pip upgrade, `sudo pip install --upgrade pip`If for some reason you can't or won't switch to nltk v3.2, just make sure that russian words are tokenized properly with RegeExpTokenizer. For students with low-RAM machines * This assignment can be accomplished with even the low-tier hardware (<= 4Gb RAM) * If that is the case, turn flag "low_RAM_mode" below to True * If you have around 8GB memory, it is unlikely that you will feel constrained by memory. * In case you are using a PC from last millenia, consider setting very_low_RAM=True
###Code
low_RAM_mode = True
very_low_RAM = False #If you have <3GB RAM, set BOTH to true
import pandas as pd
import numpy as np
import matplotlib.pyplot as plt
%matplotlib inline
###Output
_____no_output_____
###Markdown
DatasetEx-kaggle-competition on prohibited content detectionThere goes the description - https://www.kaggle.com/c/avito-prohibited-content DownloadHigh-RAM mode, * Download avito_train.tsv from competition data filesLow-RAM-mode, * Download downsampled dataset from here * archive https://yadi.sk/d/l0p4lameqw3W8 * raw https://yadi.sk/d/I1v7mZ6Sqw2WK (in case you feel masochistic) What's insideDifferent kinds of features:* 2 text fields - title and description* Special features - price, number of e-mails, phones, etc* Category and subcategory - unsurprisingly, categorical features* Attributes - more factorsOnly 1 binary target whether or not such advertisement contains prohibited materials* criminal, misleading, human reproduction-related, etc* diving into the data may result in prolonged sleep disorders
###Code
if not low_RAM_mode:
# a lot of ram
df = pd.read_csv("avito_train.tsv",sep='\t')
else:
#aroung 4GB ram
df = pd.read_csv("avito_train_1kk.tsv",sep='\t')
print df.shape, df.is_blocked.mean()
df[:5]
###Output
_____no_output_____
###Markdown

###Code
print "Blocked ratio",df.is_blocked.mean()
print "Count:",len(df)
###Output
_____no_output_____
###Markdown
Balance-out the classes* Vast majority of data samples are non-prohibited * 250k banned out of 4kk * Let's just downsample random 250k legal samples to make further steps less computationally demanding * If you aim for high Kaggle score, consider a smarter approach to that.
###Code
#downsample
< downsample data so that both classes have approximately equal ratios>
df = <downsampled dataset>
print "Blocked ratio:",df.is_blocked.mean()
print "Count:",len(df)
assert df.is_blocked.mean() < 0.51
assert df.is_blocked.mean() > 0.49
assert len(df) <= 560000
print "All tests passed"
#In case your RAM-o-meter is in the red
if very_low_ram:
data = data[::2]
###Output
_____no_output_____
###Markdown
TokenizingFirst, we create a dictionary of all existing words.Assign each word a number - it's Id
###Code
from nltk.tokenize import RegexpTokenizer
from collections import Counter,defaultdict
tokenizer = RegexpTokenizer(r"\w+")
#Dictionary of tokens
token_counts = Counter()
#All texts
all_texts = np.hstack([df.description.values,df.title.values])
#Compute token frequencies
for s in all_texts:
if type(s) is not str:
continue
s = s.decode('utf8').lower()
tokens = tokenizer.tokenize(s)
for token in tokens:
token_counts[token] +=1
###Output
_____no_output_____
###Markdown
Remove rare tokensWe are unlikely to make use of words that are only seen a few times throughout the corpora.Again, if you want to beat Kaggle competition metrics, consider doing something better.
###Code
#Word frequency distribution, just for kicks
_=plt.hist(token_counts.values(),range=[0,50],bins=50)
#Select only the tokens that had at least 10 occurences in the corpora.
#Use token_counts.
min_count = 10
tokens = <tokens from token_counts keys that had at least min_count occurences throughout the dataset>
token_to_id = {t:i+1 for i,t in enumerate(tokens)}
null_token = "NULL"
token_to_id[null_token] = 0
print "# Tokens:",len(token_to_id)
if len(token_to_id) < 30000:
print "Alarm! It seems like there are too few tokens. Make sure you updated NLTK and applied correct thresholds -- unless you now what you're doing, ofc"
if len(token_to_id) < 1000000:
print "Alarm! Too many tokens. You might have messed up when pruning rare ones -- unless you know what you're doin' ofc"
###Output
_____no_output_____
###Markdown
Replace words with IDsSet a maximum length for titles and descriptions. * If string is longer that that limit - crop it, if less - pad with zeros. * Thus we obtain a matrix of size [n_samples]x[max_length] * Element at i,j - is an identifier of word j within sample i
###Code
def vectorize(strings, token_to_id, max_len=150):
token_matrix = []
for s in strings:
if type(s) is not str:
token_matrix.append([0]*max_len)
continue
s = s.decode('utf8').lower()
tokens = tokenizer.tokenize(s)
token_ids = map(lambda token: token_to_id.get(token,0), tokens)[:max_len]
token_ids += [0]*(max_len - len(token_ids))
token_matrix.append(token_ids)
return np.array(token_matrix)
desc_tokens = vectorize(df.description.values,token_to_id,max_len = 150)
title_tokens = vectorize(df.title.values,token_to_id,max_len = 15)
###Output
_____no_output_____
###Markdown
Data format examples
###Code
print "Размер матрицы:",title_tokens.shape
for title, tokens in zip(df.title.values[:3],title_tokens[:3]):
print title,'->', tokens[:10],'...'
###Output
_____no_output_____
###Markdown
__ As you can see, our preprocessing is somewhat crude. Let us see if that is enough for our network __ Non-sequencesSome data features are not text samples. E.g. price, urls, category, etcThey require a separate preprocessing.
###Code
#All numeric features
df_numerical_features = df[["phones_cnt","emails_cnt","urls_cnt","price"]]
#One-hot-encoded category and subcategory
from sklearn.feature_extraction import DictVectorizer
categories = []
data_cat_subcat = df[["category","subcategory"]].values
categories = [A list of dictionaries {"category":category_name, "subcategory":subcategory_name} for each data sample]
vectorizer = DictVectorizer(sparse=False)
cat_one_hot = vectorizer.fit_transform(categories)
cat_one_hot = pd.DataFrame(cat_one_hot,columns=vectorizer.feature_names_)
df_non_text = pd.merge(
df_numerical_features,cat_one_hot,on = np.arange(len(cat_one_hot))
)
del df_non_text["key_0"]
###Output
_____no_output_____
###Markdown
Split data into training and test
###Code
#Target variable - whether or not sample contains prohibited material
target = df.is_blocked.values.astype('int32')
#Preprocessed titles
title_tokens = title_tokens.astype('int32')
#Preprocessed tokens
desc_tokens = desc_tokens.astype('int32')
#Non-sequences
df_non_text = df_non_text.astype('float32')
#Split into training and test set.
#Difficulty selector:
#Easy: split randomly
#Medium: select test set items that have item_ids strictly above that of training set
#Hard: do whatever you want, but score yourself using kaggle private leaderboard
title_tr,title_ts,desc_tr,desc_ts,nontext_tr,nontext_ts,target_tr,target_ts = <define_these_variables>
###Output
_____no_output_____
###Markdown
Save preprocessed data [optional]* The next tab can be used to stash all the essential data matrices and get rid of the rest of the data. * Highly recommended if you have less than 1.5GB RAM left* To do that, you need to first run it with save_prepared_data=True, then restart the notebook and only run this tab with read_prepared_data=True.
###Code
save_prepared_data = True #save
read_prepared_data = False #load
#but not both at once
assert not (save_prepared_data and read_prepared_data)
if save_prepared_data:
print "Saving preprocessed data (may take up to 3 minutes)"
import pickle
with open("preprocessed_data.pcl",'w') as fout:
pickle.dump(data_tuple,fout)
with open("token_to_id.pcl",'w') as fout:
pickle.dump(token_to_id,fout)
print "готово"
elif read_prepared_data:
print "Reading saved data..."
import pickle
with open("preprocessed_data.pcl",'r') as fin:
data_tuple = pickle.load(fin)
title_tr,title_ts,desc_tr,desc_ts,nontext_tr,nontext_ts,target_tr,target_ts = data_tuple
with open("token_to_id.pcl",'r') as fin:
token_to_id = pickle.load(fin)
#Re-importing libraries to allow staring noteboook from here
import pandas as pd
import numpy as np
import matplotlib.pyplot as plt
%matplotlib inline
print "done"
###Output
_____no_output_____
###Markdown
Train the monsterSince we have several data sources, our neural network may differ from what you used to work with.* Separate input for titles * cnn+global max or RNN* Separate input for description * cnn+global max or RNN* Separate input for categorical features * обычные полносвязные слои или какие-нибудь трюки These three inputs must be blended somehow - concatenated or added.* Output: a simple binary classification * 1 sigmoidal with binary_crossentropy * 2 softmax with categorical_crossentropy - essentially the same as previous one * 1 neuron without nonlinearity (lambda x: x) + hinge loss
###Code
#libraries
import lasagne
from theano import tensor as T
import theano
#3 inputs and a refere output
title_token_ids = T.matrix("title_token_ids",dtype='int32')
desc_token_ids = T.matrix("desc_token_ids",dtype='int32')
categories = T.matrix("categories",dtype='float32')
target_y = T.ivector("is_blocked")
###Output
_____no_output_____
###Markdown
NN architecture
###Code
title_inp = lasagne.layers.InputLayer((None,title_tr.shape[1]),input_var=title_token_ids)
descr_inp = lasagne.layers.InputLayer((None,desc_tr.shape[1]),input_var=desc_token_ids)
cat_inp = lasagne.layers.InputLayer((None,nontext_tr.shape[1]), input_var=categories)
# Descriptions
#word-wise embedding. We recommend to start from some 64 and improving after you are certain it works.
descr_nn = lasagne.layers.EmbeddingLayer(descr_inp,
input_size=len(token_to_id)+1,
output_size=?)
#reshape from [batch, time, unit] to [batch,unit,time] to allow 1d convolution over time
descr_nn = lasagne.layers.DimshuffleLayer(descr_nn, [0,2,1])
descr_nn = 1D convolution over embedding, maybe several ones in a stack
#pool over time
descr_nn = lasagne.layers.GlobalPoolLayer(descr_nn,T.max)
#Possible improvements here are adding several parallel convs with different filter sizes or stacking them the usual way
#1dconv -> 1d max pool ->1dconv and finally global pool
# Titles
title_nn = <Process titles somehow (title_inp)>
# Non-sequences
cat_nn = <Process non-sequences(cat_inp)>
nn = <merge three layers into one (e.g. lasagne.layers.concat) >
nn = lasagne.layers.DenseLayer(nn,your_lucky_number)
nn = lasagne.layers.DropoutLayer(nn,p=maybe_use_me)
nn = lasagne.layers.DenseLayer(nn,1,nonlinearity=lasagne.nonlinearities.linear)
###Output
_____no_output_____
###Markdown
Loss function* The standard way: * prediction * loss * updates * training and evaluation functions * Hinge loss * $ L_i = \max(0, \delta - t_i p_i) $ * delta is a tunable parameter: how far should a neuron be in the positive margin area for us to stop bothering about it * Function description may mention some +-1 limitations - this is not neccessary, at least as long as hinge loss has a __default__ flag `binary = True`
###Code
#All trainable params
weights = lasagne.layers.get_all_params(nn,trainable=True)
#Simple NN prediction
prediction = lasagne.layers.get_output(nn)[:,0]
#Hinge loss
loss = lasagne.objectives.binary_hinge_loss(prediction,target_y,delta = what_do_you_think).mean()
#Weight optimization step
updates = <your favorite optimizer>
###Output
_____no_output_____
###Markdown
Determinitic prediction * In case we use stochastic elements, e.g. dropout or noize * Compile a separate set of functions with deterministic prediction (deterministic = True) * Unless you think there's no neet for dropout there ofc. Btw is there?
###Code
#deterministic version
det_prediction = lasagne.layers.get_output(nn,deterministic=True)[:,0]
#equivalent loss function
det_loss = <an excercise in copy-pasting and editing>
###Output
_____no_output_____
###Markdown
Coffee-lation
###Code
train_fun = theano.function([desc_token_ids,title_token_ids,categories,target_y],[loss,prediction],updates = updates)
eval_fun = theano.function([desc_token_ids,title_token_ids,categories,target_y],[det_loss,det_prediction])
###Output
_____no_output_____
###Markdown
Training loop* The regular way with loops over minibatches* Since the dataset is huge, we define epoch as some fixed amount of samples isntead of all dataset
###Code
#average precision at K
from oracle import APatK, score
# Out good old minibatch iterator now supports arbitrary amount of arrays (X,y,z)
def iterate_minibatches(*arrays,**kwargs):
batchsize=kwargs.get("batchsize",100)
shuffle = kwargs.get("shuffle",True)
if shuffle:
indices = np.arange(len(arrays[0]))
np.random.shuffle(indices)
for start_idx in range(0, len(arrays[0]) - batchsize + 1, batchsize):
if shuffle:
excerpt = indices[start_idx:start_idx + batchsize]
else:
excerpt = slice(start_idx, start_idx + batchsize)
yield [arr[excerpt] for arr in arrays]
###Output
_____no_output_____
###Markdown
Tweaking guide* batch_size - how many samples are processed per function call * optimization gets slower, but more stable, as you increase it. * May consider increasing it halfway through training* minibatches_per_epoch - max amount of minibatches per epoch * Does not affect training. Lesser value means more frequent and less stable printing * Setting it to less than 10 is only meaningfull if you want to make sure your NN does not break down after one epoch* n_epochs - total amount of epochs to train for * `n_epochs = 10**10` and manual interrupting is still an optionTips:* With small minibatches_per_epoch, network quality may jump around 0.5 for several epochs* AUC is the most stable of all three metrics* Average Precision at top 2.5% (APatK) - is the least stable. If batch_size*minibatches_per_epoch < 10k, it behaves as a uniform random variable.* Plotting metrics over training time may be a good way to analyze which architectures work better.* Once you are sure your network aint gonna crash, it's worth letting it train for a few hours of an average laptop's time to see it's true potential
###Code
from sklearn.metrics import roc_auc_score, accuracy_score
n_epochs = 100
batch_size = 100
minibatches_per_epoch = 100
for i in range(n_epochs):
#training
epoch_y_true = []
epoch_y_pred = []
b_c = b_loss = 0
for j, (b_desc,b_title,b_cat, b_y) in enumerate(
iterate_minibatches(desc_tr,title_tr,nontext_tr,target_tr,batchsize=batch_size,shuffle=True)):
if j > minibatches_per_epoch:break
loss,pred_probas = train_fun(b_desc,b_title,b_cat,b_y)
b_loss += loss
b_c +=1
epoch_y_true.append(b_y)
epoch_y_pred.append(pred_probas)
epoch_y_true = np.concatenate(epoch_y_true)
epoch_y_pred = np.concatenate(epoch_y_pred)
print "Train:"
print '\tloss:',b_loss/b_c
print '\tacc:',accuracy_score(epoch_y_true,epoch_y_pred>0.)
print '\tauc:',roc_auc_score(epoch_y_true,epoch_y_pred)
print '\tap@k:',APatK(epoch_y_true,epoch_y_pred,K = int(len(epoch_y_pred)*0.025)+1)
#evaluation
epoch_y_true = []
epoch_y_pred = []
b_c = b_loss = 0
for j, (b_desc,b_title,b_cat, b_y) in enumerate(
iterate_minibatches(desc_ts,title_ts,nontext_tr,target_ts,batchsize=batch_size,shuffle=True)):
if j > minibatches_per_epoch: break
loss,pred_probas = eval_fun(b_desc,b_title,b_cat,b_y)
b_loss += loss
b_c +=1
epoch_y_true.append(b_y)
epoch_y_pred.append(pred_probas)
epoch_y_true = np.concatenate(epoch_y_true)
epoch_y_pred = np.concatenate(epoch_y_pred)
print "Val:"
print '\tloss:',b_loss/b_c
print '\tacc:',accuracy_score(epoch_y_true,epoch_y_pred>0.)
print '\tauc:',roc_auc_score(epoch_y_true,epoch_y_pred)
print '\tap@k:',APatK(epoch_y_true,epoch_y_pred,K = int(len(epoch_y_pred)*0.025)+1)
print "If you are seeing this, it's time to backup your notebook. No, really, 'tis too easy to mess up everything without noticing. "
###Output
If you are seeing this, it's time to backup your notebook. No, really, 'tis too easy to mess up everything without noticing.
###Markdown
Final evaluationEvaluate network over the entire test set
###Code
#evaluation
epoch_y_true = []
epoch_y_pred = []
b_c = b_loss = 0
for j, (b_desc,b_title,b_cat, b_y) in enumerate(
iterate_minibatches(desc_ts,title_ts,nontext_tr,target_ts,batchsize=batch_size,shuffle=True)):
loss,pred_probas = eval_fun(b_desc,b_title,b_cat,b_y)
b_loss += loss
b_c +=1
epoch_y_true.append(b_y)
epoch_y_pred.append(pred_probas)
epoch_y_true = np.concatenate(epoch_y_true)
epoch_y_pred = np.concatenate(epoch_y_pred)
final_accuracy = accuracy_score(epoch_y_true,epoch_y_pred>0)
final_auc = roc_auc_score(epoch_y_true,epoch_y_pred)
final_apatk = APatK(epoch_y_true,epoch_y_pred,K = int(len(epoch_y_pred)*0.025)+1)
print "Scores:"
print '\tloss:',b_loss/b_c
print '\tacc:',final_accuracy
print '\tauc:',final_auc
print '\tap@k:',final_apatk
score(final_accuracy,final_auc,final_apatk)
###Output
_____no_output_____ |
chapters/building-machine-learning-pipelines-examples_based_on_tfx_1.4/chapters/intro_tfx/Apache_beam_example_notebook.ipynb | ###Markdown
Apache Beam Word Count ExampleThe example is adopted from https://beam.apache.org/get-started/wordcount-example/ for Google Colab[](https://github.com/Building-ML-Pipelines/building-machine-learning-pipelines/blob/master/chapters/intro_tfx/Apache_beam_example_notebook.ipynb)
###Code
# %pip install -q apache_beam[gcp]
import re
import apache_beam as beam
from apache_beam.options.pipeline_options import PipelineOptions
input_file = "gs://dataflow-samples/shakespeare/kinglear.txt"
output_file = "output.txt"
pipeline_options = PipelineOptions()
with beam.Pipeline(options=pipeline_options) as p:
# Read the text file[pattern] into a PCollection.
lines = p | beam.io.ReadFromText(input_file)
# Count the occurrences of each word.
counts = (
lines
| 'Split' >> (beam.FlatMap(lambda x: re.findall(r'[A-Za-z\']+', x)))
# .with_output_types(unicode))
| 'PairWithOne' >> beam.Map(lambda x: (x, 1))
| 'GroupAndSum' >> beam.CombinePerKey(sum))
# Format the counts into a PCollection of strings.
def format_result(word_count):
(word, count) = word_count
return f"{word}: {count}"
output = counts | 'Format' >> beam.Map(format_result)
# Write the output using a "Write" transform that has side effects.
output | beam.io.WriteToText(output_file)
!head output.txt*
###Output
_____no_output_____ |
Topic 4 - NumPy and Matplotlib/7.4 - Test Your Knowledge.ipynb | ###Markdown
Test Your KnowledgeIn the blocks below you will find a range of questions that cover the material we've looked at in the previous notebooks, and build upon the knowledge gained in previous topics. Like in the previous topic, these questions require more code and thought, and will probably take you longer than some of the questions you've seen before. 1. Implement a function for basic matrix multiplication. It should take two numpy arrays as inputs and if the dimensions are correct it should return the result of the matrix multiplication. Otherwise it should return a message indicating the problem.
###Code
## YOUR CODE GOES HERE
###Output
_____no_output_____
###Markdown
2. Create two lists of random numbers, and write a function to add these lists together element wise. Convert your lists to numpy arrays and write a second function that adds these together using numpy. Time both of your functions and look at the performance difference with inputs of different sizes.
###Code
## YOUR CODE GOES HERE
###Output
_____no_output_____
###Markdown
3. Create a list of random numbers, and a numpy array of the same data. Try to sort your list and array using the built-in sort and numpy sort methods (you might have to look up how to use these). Again, time these methods and look at how the performance vaires over time.
###Code
## YOUR CODE GOES HERE
###Output
_____no_output_____
###Markdown
4. Using the data you gather from the two questions above, try to draw some graphs. You might want to start by drawing a single line on a graph with the timing data from adding two lists together using base Python from Q2. Then try to add further lines to the same plot showing some data for the other functions. Remember to label your graph and use a legend to identify the different data sets. Finally, save your plot.Note: Automated collection of timing data is difficult in Jupyter. I recommend you just write down your data and then store it in a list or array manually.
###Code
## YOUR CODE GOES HERE
###Output
_____no_output_____
###Markdown
5. In the data folder is a file called `datafile.txt`. Try to combine all of the skills you've learnt in the previous topics by reading in this data file, saving the data into lists, and then converting into numpy arrays. Plot the data using matplotlib and save your plot. To give you a hand I will tell you that the data is "tab" separated.
###Code
## YOUR CODE GOES HERE
###Output
_____no_output_____ |
Yolo3_training/Yolo3_imageProcessing.ipynb | ###Markdown
Image pre-processing for object-detection modelStep 1: Read imges
###Code
import os
import numpy as np
import pandas as pd
import matplotlib.pyplot as plt
import matplotlib.image as mpimg
import cv2
import random
# Defining global variable path that contains train / train_resized folders
image_folder = "/Users/peisch/code/WebScraper/Images"
# Function to load folder into arrays and then it returns that same array
def loadImages(path):
image_files = sorted([os.path.join(path, 'train', file)
for file in os.listdir(path + "/train") if file.endswith('.jpg')])
return image_files
# Check number of images in training set
len(loadImages(image_folder))
###Output
_____no_output_____
###Markdown
Step 2: Resize images
###Code
# Display one image
def display_one(a, title1 = "Original"):
plt.imshow(a), plt.title(title1)
plt.xticks([]), plt.yticks([])
plt.show()
# Display two images
def display(a, b, title1 = "Original", title2 = "Edited"):
plt.subplot(121), plt.imshow(a), plt.title(title1)
plt.xticks([]), plt.yticks([])
plt.subplot(122), plt.imshow(b), plt.title(title2)
plt.xticks([]), plt.yticks([])
plt.show()
# Resize images into dimension determined and save resized images in new folder
# Generate a csv file including image information ie. origin weight/height, new width/height
# BE CAREFUL: we use "image_folder" global variable defined at the beginnning of the notebook
def resizing(data):
# in the path given, create a folder for upcoming resized images if not existing
os.chdir(image_folder)
try:
os.makedirs('train_resized')
print("Resizing processing ... ")
except FileExistsError:
print("Directory 'train_resized' already exists. Resizing processing ... ")
# Loop: for each image in the folder, store the 1/image, 2/origin size, 3/filename as imageId
# create a dataframe to store information with determined typing for each column
dtypes = np.dtype([
('imageId', str),
('origin_width', int),
('origin_height', int),
('new_width', int),
('new_height', int),
])
empty_data = np.empty(0, dtype=dtypes)
df = pd.DataFrame(empty_data)
img_list = []
# set working directory (folder) to store processed images
working_dir = image_folder + "/train_resized/"
os.chdir(working_dir)
# setting dimemsion of the resized image and update count for processed images
# be careful: for YoloV3 Darknet, image dimension has to be multiplication of 32 !!!
count:int = 0
height:int = 416
width:int = 416
dim = (width, height) # dimension is a tuple
res_img = []
# for each absolute path in the list 'data':
# 1/read image; 2/get its origin image and imageID; 3/update image list;
# 4/store info in dataframe; 5/store resized image in created "train_resized" folder
for imgStr in data:
img = [cv2.imread(imgStr, cv2.IMREAD_UNCHANGED)]
origin_size = img[0].shape # shape of each image is a tuple of (height, weight, channel)
origin_width = origin_size[1]
origin_height = origin_size[0]
imageId = imgStr.rstrip('.jpg').replace(f"{image_folder}/train/", "")
img_list[len(img_list):] = [imageId] # equiv to "append" to a list
# store info as a new row in the dataframe created above
col_names = ['imageId', 'origin_width', 'origin_height', 'new_width', 'new_height']
new_row = pd.DataFrame([[str(imageId), origin_width, origin_height, width, height]], columns = col_names)
df = df.append(new_row)
# resize image and store as .jpg in created folder
res = cv2.resize(img[0], dim, interpolation=cv2.INTER_LINEAR)
res_img.append(res)
count = count + 1
filename:str = imageId + ".jpg"
cv2.imwrite(filename, res)
# Checking the size after processing
print(f"Resized image dimension : {res_img[-1].shape}. Example as follows:")
# Visualizing one of the images in the array
example_image = res_img[-1]
display_one(example_image)
# Delete duplicates of the df
# Save dataframe into a CSV file (write or override .csv file)
df = df.drop_duplicates()
df.to_csv('imageTable.csv', index = False)
print(f"Resizing finished. \n [ {count} ] image(s) resized and saved in directory: {working_dir} ")
return img_list
###Output
_____no_output_____
###Markdown
Step 3: Recalculate image annotations
###Code
def get_label(images):
# set working dir to the resized folder
working_dir = image_folder + '/train_resized'
os.chdir(working_dir)
# load csv file containing image information
df_img = pd.read_csv('imageTable.csv')
df_img = df_img.set_index('imageId')
dtypes = np.dtype([
('class', str),
('origin_xmin', int),
('origin_ymin', int),
('origin_xmax', int),
('origin_ymax', int),
('imageId', str)
])
#empty_data = np.empty(0, dtype=dtypes)
#df = pd.DataFrame(empty_data)
image_buffer:str = ""
for img in images:
os.chdir(image_folder + '/train/Label')
image_buffer = img
filename = img + '.txt'
# open origin .txt file and get information
try:
f = open(filename,"r")
content = f.read()
# parse content and store in info list
annotations = content.lstrip().rstrip().split('\n')
annot_str:str = ""
# each_annot is a string with shape: 'Dress', '483.84', '228.734651', '691.2', '683.0'
for each_annot in annotations:
# load info about the origin image stored in csv file
line = df_img.loc[img]
o_w = line['origin_width']
o_h = line['origin_height']
n_w = line['new_width']
n_h = line['new_height']
each_annot = each_annot.replace(",\n", ", ")
# replace "Dress" by its class number (zero)
each_annot = each_annot.replace("Dress", "0")
# now access to information contained by dress and redo the annotation for Yolo3 model
# annotation for Yolo3: x(centre), y(center), width, height (with all in range [0, 1])
dress:list = each_annot.split(" ") #list
xmin = dress[1]
ymin = dress[2]
xmax = dress[3]
ymax = dress[4]
# X_CENTER_NORM = X_CENTER_ABS/IMAGE_WIDTH
# Y_CENTER_NORM = Y_CENTER_ABS/IMAGE_HEIGHT
# WIDTH_NORM = WIDTH_OF_LABEL_ABS/IMAGE_WIDTH
# HEIGHT_NORM = HEIGHT_OF_LABEL_ABS/IMAGE_HEIGHT
x = (float(xmin) + float(xmax))/2/o_w
y = (float(ymin) + float(ymax))/2/o_h
box_w = (float(xmax)-float(xmin))/o_w
box_h = (float(ymax)-float(ymin))/o_h
# the string has the right form for yolo annotation
each_box:str = f"0 {x} {y} {box_w} {box_h}"
annot_str:str = each_box + "\n" + annot_str
annot_str = annot_str.rstrip()
f.close
# create a folder to store .txt files named 'new_labels'
os.chdir(image_folder)
try: os.mkdir('train_resized')
except: FileExistsError
os.chdir(image_folder + '/train_resized')
new_file = open(filename,"w")
n = new_file.write(annot_str)
new_file.close()
except: FileNotFoundError
print(f"New annotation .txt files had been saved in directory: {image_folder}/train_resized ")
###Output
_____no_output_____
###Markdown
Step 4: Create train and test path files
###Code
# this function is to produce train.txt and test.txt files used in Yolo3 training
def create_txt_file(img_list):
# full path list
store_list = []
for i in range(len(img_list)):
img_path = f"data/obj/{img_list[i]}.jpg"
store_list.append(img_path)
# randomise train set and test set
img_nb = len(store_list)
# parameter of the dataset separation ratio
train_r = 0.8
test_r = 0.2
train_nb = round(img_nb * train_r)
test_nb = img_nb - train_nb
train_index = random.sample(range(img_nb), k = train_nb)
# train path list
train_list = []
for i in train_index :
path = store_list[i]
train_list.append(path)
# test path list
test_list = list(set(store_list) - set(train_list))
print(f"Set separation done. Train set size: {len(train_list)}. Test set size: {len(test_list)}.")
# save those lists into .txt files
# create a folder to store .txt files named 'train_test_file'
os.chdir(image_folder)
try: os.mkdir('train_test_path')
except: FileExistsError
os.chdir(image_folder + '/train_test_path')
# create and save train.txt
train_str = '\n'.join(train_list)
file1 = open("train.txt", "w")
n = file1.write(train_str)
file1.close()
print(f"train.txt file had been saved in directory: {image_folder}/train_test_path")
# create and save test.txt
test_str = '\n'.join(test_list)
file2 = open("test.txt", "w")
n = file2.write(test_str)
file2.close()
print(f"test.txt file had been saved in directory: {image_folder}/train_test_path")
return store_list
###Output
_____no_output_____
###Markdown
Execute sequential functions
###Code
# Load data with given image folder path
dataset = loadImages(image_folder)
# Call the resizing function over data and save imageTable.csv
images = resizing(dataset)
# Process all label .txt files and save new .txt files in new_labels folder
get_label(images)
# Create path file for Yolo3 training
create_txt_file(images)
###Output
Resizing processing ...
Resized image dimension : (416, 416, 3). Example as follows:
|
00_ratio_images.ipynb | ###Markdown
ratio_images> API details.
###Code
# export
import re
import numpy as np
import pandas as pd
from clonedetective.utils import extend_region_properties_list, generate_random_cmap
from skimage import img_as_float, io, measure
###Output
_____no_output_____
###Markdown
Hello wht
###Code
# export
def create_img_dict_from_folder(
load_pattern: str, img_regex: str = r"\w\dg\d\d?", label: bool = False, **kwargs
):
img_collection = io.ImageCollection(load_pattern, **kwargs)
img_dict = {
re.search(img_regex, file)[0]: img_collection[i]
for i, file in enumerate(img_collection.files)
}
if label:
img_dict = {key: measure.label(value) for key, value in img_dict.items()}
else:
img_dict = {key: img_as_float(value) for key, value in img_dict.items()}
return img_dict
C0_imgs = create_img_dict_from_folder(load_pattern="data/PercevalHR_data/imgs/*C0.tiff")
C1_imgs = create_img_dict_from_folder(load_pattern="data/PercevalHR_data/imgs/*C1.tiff")
lab_imgs = create_img_dict_from_folder(
load_pattern="data/PercevalHR_data/segs/*.tif", label=True
)
from clonedetective.utils import plot_new_images
img_name = "a1g01"
plot_new_images(
[C0_imgs[img_name], C1_imgs[img_name], lab_imgs[img_name]],
["C0 channel", "C1 channel", "label image (segmentation)"],
interpolation="none",
)
# export
def create_ratio_image(num_img, denom_img, mask_img):
# convert label image to mask if passed
mask_img = mask_img > 0
# add tiny number to prevent possibility of division by zero
ratio = num_img[mask_img] / (denom_img[mask_img] + 1e-100)
ratio[ratio > np.percentile(ratio, 99.9)] = 0
ratio_image = np.zeros_like(num_img)
ratio_image[mask_img] = ratio
return ratio_image
a1g01_img = create_ratio_image(C1_imgs["a1g01"], C0_imgs["a1g01"], lab_imgs["a1g01"])
a2g01_img = create_ratio_image(C1_imgs["a2g01"], C0_imgs["a2g01"], lab_imgs["a2g01"])
plot_new_images(
[a1g01_img, a2g01_img],
["a1g01 ratio image", "a2g01 ratio image"],
img_cmap="magma",
vmax=2.5,
figure_shape=(1, 2),
figure_size=(10, 5),
colorbar=True,
colorbar_title="PercevalHR 488/405"
)
# export
def create_dict_of_ratio_images(num_imgs: dict, denom_imgs: dict, lab_imgs: dict):
ratio_img_dict = {}
for img_key, lab_img in lab_imgs.items():
ratio_img_dict[img_key] = create_ratio_image(
num_imgs[img_key], denom_imgs[img_key], lab_img
)
return ratio_img_dict
ratio_imgs = create_dict_of_ratio_images(C1_imgs, C0_imgs, lab_imgs)
ratio_imgs.keys()
# export
def measure_region_props_to_tidy_df(int_imgs: dict, lab_imgs: dict, **reg_prop_kwargs):
l = list()
for img_key, img in int_imgs.items():
df = pd.DataFrame(
measure.regionprops_table(
lab_imgs[img_key], intensity_image=img, **reg_prop_kwargs
)
)
df["img_key"] = img_key
l.append(df)
return pd.concat(l)
df = measure_region_props_to_tidy_df(
C1_imgs, lab_imgs, properties=["label", "mean_intensity"]
)
df.head()
# export
def only_intensity_region_properties(properties):
return [prop for prop in properties if re.search(r"label|intensity", prop)]
only_intensity_region_properties(["label", "area", "mean_intensity", "centroid"])
# export
def ratiometric_measure_region_props_to_tidy_df(
num_imgs: dict,
denom_imgs: dict,
lab_imgs: dict,
region_properties: list = None,
**reg_prop_kwargs
):
num_rprops = extend_region_properties_list(region_properties)
denom_rprops = only_intensity_region_properties(num_rprops)
df = pd.merge(
measure_region_props_to_tidy_df(num_imgs, lab_imgs, properties=num_rprops),
measure_region_props_to_tidy_df(denom_imgs, lab_imgs, properties=denom_rprops),
how="left",
on=("img_key", "label"),
suffixes=("_num", "_denom"),
)
return df.eval("ratio_mean_int = mean_intensity_num/mean_intensity_denom")
df = ratiometric_measure_region_props_to_tidy_df(C1_imgs, C0_imgs, lab_imgs)
df.head()
# export
def split_img_key_col_to_sample_id_and_replicates(
df,
split_regex: str,
img_key_colname: str = "img_key",
sample_id_colname: str = "sample_id",
replicate_id_colname: str = "rep_id",
):
temp_df = df[img_key_colname].str.split(split_regex, expand=True).iloc[:, :2]
temp_df.columns = [sample_id_colname, replicate_id_colname]
return pd.concat([df, temp_df], axis=1)
df = split_img_key_col_to_sample_id_and_replicates(
df, sample_id_colname="genotype", split_regex=r"g"
)
df.head()
# export
def sample_id_to_categories(
df,
sample_id_colname: str = "sample_id",
old_to_new_sample_ids: dict = None,
categories: list = None,
):
if old_to_new_sample_ids is not None:
df[sample_id_colname].replace(old_to_new_sample_ids, inplace=True)
df[sample_id_colname] = pd.Categorical(df[sample_id_colname], categories=categories)
return df
df = sample_id_to_categories(
df, sample_id_colname="genotype", old_to_new_sample_ids={"a1": "ctrl", "a2": "mut"}
)
df.head()
df["genotype"].head()
###Output
_____no_output_____ |
Week 01 - Introduction to Python/Python III.ipynb | ###Markdown
Python III-->--> Python LibrariesPython Libraries are a set of useful functions that eliminate the need for writing codes from scratch.Python libraries play a vital role in developing machine learning, data science, data visualization, image and data manipulation applications and more. Math Some of the most popular mathematical functions are defined in the math module. These include trigonometric functions, representation functions, logarithmic functions, angle conversion functions, etc. **Some of the math functions :** * math.degrees() Converts an angle from radians to degrees* math.radians() Converts a degree value into radians---* math.sin() Returns the sine of a number* math.sinh() Returns the hyperbolic sine of a number* math.asin() Returns the arc sine of a number* math.asinh() Returns the inverse hyperbolic sine of a number---* math.cos() Returns the cosine of a number* math.cosh() Returns the hyperbolic cosine of a number* math.acos() Returns the arc cosine of a number* math.acosh() Returns the inverse hyperbolic cosine of a number---* math.tan() Returns the tangent of a number* math.tanh() Returns the hyperbolic tangent of a number* math.atan() Returns the arc tangent of a number in radians* math.atanh() Returns the inverse hyperbolic tangent of a number
###Code
import math # import the math Library
# ex1
# The following lines show sin, cos and tan ratios for the angle of 30 degrees
sin_30 = math.sin(30)
cos_30 = math.cos(30)
tan_30 = math.tan(30)
print(f" sin(30)={sin_30}\n cos(30)={cos_30}\n tan(30)={tan_30}")
###Output
sin(30)=-0.9880316240928618
cos(30)=0.15425144988758405
tan(30)=-6.405331196646276
###Markdown
* math.log() Returns the natural logarithm of a number, or the logarithm of number to base* math.log10() Returns the base-10 logarithm of x
###Code
# log
# The math.log() method returns the natural logarithm of a given number. The natural logarithm is calculated to the base e.
print(f"log(20)={math.log(20)}")
# log 10
# The math.log10() method returns the base-10 logarithm of the given number. It is called the standard logarithm.
print(f"log10(20)={math.log10(20)}")
###Output
log(20)=2.995732273553991
log10(20)=1.3010299956639813
###Markdown
* math.exp() Returns E raised to the power of x* math.pow() Returns the value of x to the power of y* math.sqrt() Returns the square root of a number
###Code
# exp
# The math.exp() method returns a float number after raising e to the power of the given number. In other words, exp(x) gives e**x.
print(f"exp(10)={math.exp(10)}")
# returns a float number after raising e to the power of the given number
# using the math.e constant returns the Eular's number: 2.71828182845904.
print(f"e**x={math.e**10}")
# pow
# The math.pow() method receives two float arguments, raises the first to the second and returns the result.
# In other words, pow(4,4) is equivalent to 4**4.
print(f"pow(2,4)={math.pow(2,4)}")
# sqrt
# The math.sqrt() method returns the square root of a given number.
print(f"sqrt(100)={math.sqrt(100)}")
###Output
exp(10)=22026.465794806718
e**x=22026.465794806703
pow(2,4)=16.0
sqrt(100)=10.0
###Markdown
The sigmoid function is a mathematical logistic function. It is commonly used in statistics, audio signal processing, biochemistry, and the activation function in artificial neurons. The formula for the sigmoid function is 1 / (1 + e**(-x)) 
###Code
# Create sigmoid function using math
def sigmoid(x):
return 1 / (1 + math.exp(-x))
print(f"sigmoid(0)={sigmoid(0)}")
print(f"sigmoid(1)={sigmoid(1)}")
print(f"sigmoid(2)={sigmoid(2)}")
print(f"sigmoid(3)={sigmoid(3)}")
print(f"sigmoid(-4)={sigmoid(-4)}")
print(f"sigmoid(-5)={sigmoid(-5)}")
print(f"sigmoid(-6)={sigmoid(-6)}")
###Output
sigmoid(0)=0.5
sigmoid(1)=0.7310585786300049
sigmoid(2)=0.8807970779778823
sigmoid(3)=0.9525741268224334
sigmoid(-4)=0.01798620996209156
sigmoid(-5)=0.0066928509242848554
sigmoid(-6)=0.0024726231566347743
###Markdown
* math.factorial() Returns the factorial of a number* math.fsum() Returns the sum of all items in any iterable (tuples, arrays, lists, etc.)* math.prod() Returns the product of all the elements in an iterable* math.ceil() Rounds a number up to the nearest integer* math.isclose() Checks whether two values are close to each other, or not* math.isfinite() Checks whether a number is finite or not* math.isinf() Checks whether a number is infinite or not* math.isnan() Checks whether a value is NaN (not a number) or not* math.isqrt() Rounds a square root number downwards to the nearest integer OSThe OS module in Python provides functions for interacting with the operating system. OS comes under Python’s standard utility modules. This module provides a portable way of using operating system-dependent functionality. The *os* and *os.path* modules include many functions to interact with the file system. **Handling the Current Working Directory** os method getcwd() returns the location of the current working directory.
###Code
import os # import os
print(f"current working directory : {os.getcwd()}")
###Output
current working directory : /content
###Markdown
**Creating a Directory**There are different methods available in the OS module for creating a director **os.mkdir()**os.mkdir() method in Python is used to create a directory named path .Note:This method raises FileExistsError if the directory to be created already exists.
###Code
os.mkdir("/content/hi")
###Output
_____no_output_____
###Markdown
 **os.makedirs()**os.makedirs() method in Python is used to create a directory recursively. That means while making leaf directory if any intermediate-level directory is missing, os.makedirs() method will create them all.
###Code
os.makedirs("/content/hello/codelab/AILAB")
###Output
_____no_output_____
###Markdown
 **Listing out Files and Directories with os** **os.listdir()** os listdir method is used to get the list of all files and directories in the specified directory. If we don’t specify any directory, then the list of files and directories in the current working directory will be returned.
###Code
# Get the list of all files and directories
# in the root directory
path = "/content/sample_data"
dir_list = os.listdir(path)
print("Files and directories in '", path, "' :")
# print the list
print(dir_list)
###Output
Files and directories in ' /content/sample_data ' :
['anscombe.json', 'README.md', 'mnist_test.csv', 'california_housing_test.csv', 'mnist_train_small.csv', 'california_housing_train.csv']
###Markdown
**Deleting Directory or Files using os:**OS module proves different methods for removing directories and files in Python. **os.remove()**method in os is used to remove or delete a file path. This method can not remove or delete a directory.Note: If the specified path is a directory then OSError will be raised by the method. Suppose the file contained in the folder hi : 
###Code
os.remove("/content/hi/1f8c065c58768ceadb8d6f76c02ce17c.jpg")
###Output
_____no_output_____
###Markdown
 **os.rmdir()**method in os is used to remove or delete an empty directory. Note : OSError will be raised if the specified path is not an empty directory. 
###Code
os.rmdir("/content/hello/codelab/AILAB")
###Output
_____no_output_____
###Markdown
 **Check in if the file exists** **os.path.exists():** This method will check whether a file exists or not by passing the name of the file as a parameter.
###Code
os.path.exists("/content/codelab.txt") # check if file path are exists or not
# os.path.exists return True if the file exists else return False
###Output
_____no_output_____
###Markdown
**Rename the file** **os.rename():** A file hi.txt can be renamed to codelab.txt, using the function os.rename(). Note : The name of the file changes only if, the file exists and the user has sufficient privilege permission to change the file. 
###Code
os.rename(
src="/content/hi.txt", dst="/content/codelab.txt"
) # rename the old file to the new file name
###Output
_____no_output_____
###Markdown
 **Creating files in python** **open:** open file and return a corresponding file object.**file** : is a path-like object giving the pathname (absolute or relative to the current working directory) of the file to be opened or an integer file descriptor of the file to be wrapped. (If a file descriptor is given, it is closed when the returned I/O object is closed unless closefd is set to False.)**mode** : is an optional string that specifies the mode in which the file is opened. It defaults to 'r' which means open for reading in text mode. Other common values are 'w' for writing (truncating the file if it already exists)[read more.](https://docs.python.org/3/library/functions.htmlopen)
###Code
# write
with open(file="hi.txt", mode="w") as f: # open the file
f.write("hi codelab") # write info to the opened file
# read
with open(file="hi.txt", mode="r") as f: # open the file
info = f.read() # read the info in the file
print(f"the info in file hi.txt :\n {info}") # printthe info
###Output
the info in file hi.txt :
hi codelab
###Markdown
 Glob`Glob` is a general term used to define techniques to match specified patterns according to rules related to Unix shell. Linux and Unix systems and shells also support glob and also provide function `glob()` in system libraries.In Python, the glob module is used to retrieve files/pathnames matching a specified pattern. The pattern rules of glob follow standard Unix path expansion rules. It is also predicted that according to benchmarks it is faster than other methods to match pathnames in directories. With glob, we can also use wildcards `("*, ?, [ranges])` apart from exact string search to make path retrieval more simple and convenient.**Note:** This module comes built-in with Python, so there is no need to install it externally.
###Code
import glob # Import the glob library
# Get all the files path
files_paths=glob.glob('/content/sample_data/*')
files_paths
# Get all the files path that are csv
files_paths=glob.glob('/content/sample_data/*.csv')
files_paths
###Output
_____no_output_____
###Markdown
Tqdm`tqdm` derives from the Arabic word taqaddum (تقدّم) which can mean "progress," and is an abbreviation for "I love you so much" in Spanish (te quiero demasiado).Instantly make your loops show a smart progress meter - just wrap any iterable with tqdm(iterable), and you're done! 
###Code
from tqdm import tqdm
# create loop with tqdm
for i in tqdm(range(10000000)):
pass
###Output
100%|██████████| 10000000/10000000 [00:02<00:00, 4638216.06it/s]
|
examples/Wasabi_Observatory.ipynb | ###Markdown
Observatory Menu **Setup*** [Create new dataframe](Crate-new-dataframe)* [Save dataframe](Save-dataframe)* [Load dataframe](Load-existing-dataframe)**Analysis*** [Glimpse](Glimpse)* [Stats](Stats)* [Transaction explorer](Transaction-explorer)* [Plot](Plot)* [Volume](Volume)* [Daily](Daily)**Advanced*** [Advanced creation](Advanced-creation) --- Create new dataframe Insert in the cell below the `start` block height and the `end` block height, select your desired filter and run it to create the dataframe.**Example:** `start = 100` `end = 200` Will search for transactions between block 100 and block 200 included.You can also give **negative** values to `start` and this will scan the last chosen blocks **depending** on the `end` value.**Example:**`start = -10` `end = 0`Will search for transactions in the last 10 blocks.**Example:**`start = -10` `end = 5`Will search for transanction in 5 block starting from ten blocks ago.
###Code
import observatory as obs
import advanced.filters as filters
### INSERT HERE ###
start = 659399
end = 663912
txid = ''
address = ''
### CHOOSE ONE OF THE FILTERS BELOW BY REMOVING THE '#' IN FRONT OF IT ###
#tx_filter = filters.TxFilter() # This will return every transaction
#tx_filter = filters.CjTxFilter()
tx_filter = filters.WasabiTxFilter()
#tx_filter = filters.JoinmarketTxFilter()
#tx_filter = filters.TxidTxFilter(txid) # If you pick me, don't forget to insert a 'txid' above.
#tx_filter = filters.AddressTxFilter(address) # If you pick me, don't forget to insert an 'address' above.
#tx_filter = filters.CoinbaseTxFilter()
txs = await obs.create_dataframe(start, end, tx_filter)
###Output
4514it [13:59, 5.38it/s]
###Markdown
[Back to menu](Menu)--- Save dataframe Insert the **filepath** where to save and run the cell.**Examples:** `filepath = 'thisfilename'` `filepath = '/home/thisfilename'` `filepath = 'results/wasabi/thisfilename'`Will create a `thisfilename.ftr` file into the given filepath.
###Code
### INSERT HERE ###
filepath = 'results/Wasabi_txs_december'
obs.save(filepath, txs)
###Output
Dataframe succesfully saved in 0.34s
###Markdown
[Back to menu](Menu)--- Load existing dataframe Insert the **filepath** of the dataframe to load and run the cell.**Examples:** `filepath = 'thisfilename'` `filepath = '/home/thisfilename'` `filepath = 'results/mytxs/thisfilename'`Will load the `thisfilename.ftr` file from the given filepath.
###Code
import observatory as obs
### INSERT HERE ###
filepath = 'results/mydataframe'
txs = obs.load(filepath)
###Output
_____no_output_____
###Markdown
[Back to menu](Menu)--- Glimpse Run the cell below for a glimpse of the dataframe. You can change the `n_txs` value to specify how many transactions to display. You can also give `n_txs` a **negative** value to display the last n transactions.You can insert the `sort_by` value to specify which column should give the ordering. e.g. 'n_eq', 'date', etc... Default is 'date'.
###Code
### INSERT HERE ###
n_txs = 10
sort_by = ''
obs.show_intro(txs, n_txs, sort_by)
###Output
_____no_output_____
###Markdown
[Back to menu](Menu)--- Stats Run the cell below for a list of statistics about the dataframe.
###Code
obs.show_stats(txs)
###Output
_____no_output_____
###Markdown
[Back to menu](Menu)--- Transaction explorer Run the cell below to display information about single transactions.If you set `display_all = True` the result will include every input and every output. If the transaction is huge, or you are looking at more than one transanction. this could be slow or even freeze your browser.
###Code
### INSERT HERE ###
txid = '4f2440996cb288dd11be28800a961f760135d698f3dce5fece3224a78a6fa38b'
display_all = False
obs.show_tx(txs, txid, display_all)
###Output
_____no_output_____
###Markdown
[Back to menu](Menu)--- Plot Run the cell below to plot the desired `column`.If you give it a `filepath`, the result graph will be saved.
###Code
### INSERT HERE ###
column = 'n_eq'
filepath = ''
obs.show_graph(txs, column, filepath)
###Output
_____no_output_____
###Markdown
[Back to menu](Menu)--- Volume Run the cell below to show the volume graph.If you give it a filepath, the result graph will be saved.
###Code
filepath = ''
obs.show_volume(txs, filepath)
###Output
_____no_output_____
###Markdown
[Back to menu](Menu)--- Daily Run the cell below to show the transanctions per day graph.If you give it a filepath, the result graph will be saved.
###Code
filepath = ''
obs.show_daily(txs, filepath)
###Output
_____no_output_____
###Markdown
[Back to menu](Menu)--- Advanced creation Here you can create a dataframe using your own filters. You can also pass more than one filter and every transaction that matches at least one of them will be included in the dataframe.**Each and every** criteria has to be satisfied in order for a transaction to match a filter.Valid criteria are:* **txid** * e.g., `txid='mytxid'` will scan for transactions that include 'mytxid' in the txid.* **address** * e.g., `addresses=['myaddress']` will scan for transactions that include 'myaddress' in at least one of the addresses. Accept multiple addresses (each and every of them has to be part in a transaction for the transaction to match the filter).* **in_type** * e.g., `in_type='scripthash'` will scan for transactions that have all the inputs of type 'scripthash'.* **out_type** * e.g., `out_type='scripthash'` will scan for transactions that have all the outputs of type 'scripthash'.* **version** * e.g., `version=(minversion, maxversion)` will scan for transactions that have a version number between minversion and maxversion included.* **size** * e.g., `size=(minsize, maxsize)` will scan for transactions that have a size between minsize and maxsize included.* **vsize** * e.g., `vsize=(minvsize, maxvsize)` will scan for transactions that have a virtual size between minvsize and maxvsize included.* **weight** * e.g., `weight=(minweight, maxweight)` will scan for transactions that have a weight between minweight and maxweight included.* **locktime** * e.g., `locktime=(minlocktime, maxlocktime)` will scan for transactions that have a locktime between minlocktime and maxlocktime included.* **n_in** * e.g., `n_in=(minn_in, maxn_in)` will scan for transactions that have a number of inputs between minn_in and maxn_in included.* **n_out** * e.g., `n_out=(minn_out, maxn_out)` will scan for transactions that have a number of outputs between minn_out and maxn_out included.* **n_eq** * e.g., `n_eq=(minn_eq, maxn_out)` will scan for transactions that have a number of equally sized outputs between minn_eq and maxn_out included.* **den** * e.g., `den=(minden, maxden)` will scan for transactions that have a denomination between minden and maxden included.* **abs_fee** * e.g., `abs_fee=(minabs_fee, maxabs_fee)` will scan for transactions that have an absolute fee between minabs_fee and maxabs_fee included.* **rel_fee** * e.g., `rel_fee=(minrel_fee, maxrel_fee)` will scan for transactions that have a relative fee between minrel_fee and maxrel_fee included.* **height** * e.g., `height=(minheight, maxheight)` will scan for transactions that have a height between minheight and maxheight included.* **date** * e.g., `date=('2020-09-18', '2020-09-19 19:00')` will scan for transactions that have a date between mindate and maxdate included. You can also pass arbitrary callables as long as they accept a Tx object as parameter and return True or False. e.g., `callables=[mycallable]`Here's how a filter that searches for transactions that pay more than 100 sat/vbyte and have just 1 input and 1 output would look like.`tx_filter = filters.TxFilter(n_in=(1, 1), n_out=(1, 1), rel_fee=(100, 100000))`
###Code
import observatory as obs
import advanced.filters as filters
### INSERT HERE ###
start = -10
end = 0
tx_filter = filters.TxFilter(n_in=(1, 1), n_out=(1, 1), rel_fee=(100, 100000))
txs = await obs.create_dataframe(start, end, tx_filter)
###Output
_____no_output_____ |
code/DQN_cartpole.ipynb | ###Markdown
**Course "Artificial Neural Networks and Deep Learning" - Universidad Politécnica de Madrid (UPM)** **Deep Q-Learning for Cartpole**This notebook includes an implementation of the Deep Q-learning (DQN) algorithm for the cartpole problem (see [OpenAI's Cartpole](https://gym.openai.com/envs/CartPole-v1/)). Libraries
###Code
import gym
import numpy as np
from tensorflow import keras
import matplotlib.pyplot as plt
import time
import random
###Output
_____no_output_____
###Markdown
Hyperparameters
###Code
GAMMA = 0.99
MEMORY_SIZE = 100000
LEARNING_RATE = 0.001
BATCH_SIZE = 32
EXPLORATION_MAX = 1
EXPLORATION_MIN = 0.01
EXPLORATION_DECAY = 0.995
NUMBER_OF_EPISODES = 300
MAX_STEPS = 200
###Output
_____no_output_____
###Markdown
Class ReplayMemoryMemory of transitions for experience replay.
###Code
class ReplayMemory:
def __init__(self,number_of_observations):
# Create replay memory
self.states = np.zeros((MEMORY_SIZE, number_of_observations))
self.states_next = np.zeros((MEMORY_SIZE, number_of_observations))
self.actions = np.zeros(MEMORY_SIZE, dtype=np.int32)
self.rewards = np.zeros(MEMORY_SIZE)
self.terminal_states = np.zeros(MEMORY_SIZE, dtype=bool)
self.current_size=0
def store_transition(self, state, action, reward, state_next, terminal_state):
# Store a transition (s,a,r,s') in the replay memory
i = self.current_size
self.states[i] = state
self.states_next[i] = state_next
self.actions[i] = action
self.rewards[i] = reward
self.terminal_states[i] = terminal_state
self.current_size = i + 1
def sample_memory(self, batch_size):
# Generate a sample of transitions from the replay memory
batch = np.random.choice(self.current_size, batch_size)
states = self.states[batch]
states_next = self.states_next[batch]
rewards = self.rewards[batch]
actions = self.actions[batch]
terminal_states = self.terminal_states[batch]
return states, actions, rewards, states_next, terminal_states
###Output
_____no_output_____
###Markdown
Class DQNReinforcement learning agent with a Deep Q-Network.
###Code
class DQN:
def __init__(self, number_of_observations, number_of_actions):
# Initialize variables and create neural model
self.exploration_rate = EXPLORATION_MAX
self.number_of_actions = number_of_actions
self.number_of_observations = number_of_observations
self.scores = []
self.memory = ReplayMemory(number_of_observations)
self.model = keras.models.Sequential()
self.model.add(keras.layers.Dense(24, input_shape=(number_of_observations,), \
activation="relu",kernel_initializer="he_normal"))
self.model.add(keras.layers.Dense(24, activation="relu",kernel_initializer="he_normal"))
self.model.add(keras.layers.Dense(number_of_actions, activation="linear"))
self.model.compile(loss="mse", optimizer=keras.optimizers.Adam(learning_rate=LEARNING_RATE))
def remember(self, state, action, reward, next_state, terminal_state):
# Store a tuple (s, a, r, s') for experience replay
state = np.reshape(state, [1, self.number_of_observations])
next_state = np.reshape(next_state, [1, self.number_of_observations])
self.memory.store_transition(state, action, reward, next_state, terminal_state)
def select(self, state):
# Generate an action for a given state using epsilon-greedy policy
if np.random.rand() < self.exploration_rate:
return random.randrange(self.number_of_actions)
else:
state = np.reshape(state, [1, self.number_of_observations])
q_values = self.model.predict(state)
return np.argmax(q_values[0])
def learn(self):
# Learn the value Q using a sample of examples from the replay memory
if self.memory.current_size < BATCH_SIZE: return
states, actions, rewards, next_states, terminal_states = self.memory.sample_memory(BATCH_SIZE)
q_targets = self.model.predict(states)
q_next_states = self.model.predict(next_states)
for i in range(BATCH_SIZE):
if (terminal_states[i]):
q_targets[i][actions[i]] = rewards[i]
else:
q_targets[i][actions[i]] = rewards[i] + GAMMA * np.max(q_next_states[i])
self.model.train_on_batch(states, q_targets)
# Decrease exploration rate
self.exploration_rate *= EXPLORATION_DECAY
self.exploration_rate = max(EXPLORATION_MIN, self.exploration_rate)
def add_score(self, score):
# Add the obtained score in a list to be presented later
self.scores.append(score)
def display_scores_graphically(self):
# Display the obtained scores graphically
plt.plot(self.scores)
plt.xlabel("Episode")
plt.ylabel("Score")
###Output
_____no_output_____
###Markdown
Environment CartpoleCartpole simulator from [Open Ai Gym](https://gym.openai.com/envs/CartPole-v1/):State vector:- state[0]: position- state[1]: velocity- state[2]: angle- state[3]: angular velocityActions:- 0 (push cart to the left)- 1 (push cart to the right)
###Code
def create_environment():
# Create simulated environment
environment = gym.make("CartPole-v1")
number_of_observations = environment.observation_space.shape[0]
number_of_actions = environment.action_space.n
return environment, number_of_observations, number_of_actions
###Output
_____no_output_____
###Markdown
Main program
###Code
environment, number_of_observations, number_of_actions = create_environment()
agent = DQN(number_of_observations, number_of_actions)
episode = 0
goal_reached = False
start_time = time.perf_counter()
while (episode < NUMBER_OF_EPISODES) and not (goal_reached):
episode += 1
step = 1
end_episode = False
state = environment.reset()
while not(end_episode):
# Select an action for the current state
action = agent.select(state)
# Execute the action on the environment
state_next, reward, terminal_state, info = environment.step(action)
# Store in memory the transition (s,a,r,s')
agent.remember(state, action, reward, state_next, terminal_state)
# Learn using a batch of experience stored in memory
agent.learn()
# Detect end of episode
if terminal_state or step >= MAX_STEPS:
agent.add_score(step)
if step >= MAX_STEPS: goal_reached = True
print("Episode {0:>3}: ".format(episode), end = '')
print("score {0:>3} ".format(step), end = '')
print("(exploration rate: %.2f, " % agent.exploration_rate, end = '')
print("transitions: " + str(agent.memory.current_size) + ")")
end_episode = True
else:
state = state_next
step += 1
if goal_reached: print("Reached goal sucessfully.")
else: print("Failure to reach the goal.")
print ("Time:", round((time.perf_counter() - start_time)/60), "minutes")
agent.display_scores_graphically()
###Output
Episode 1: score 14 (exploration rate: 1.00, transitions: 14)
Episode 2: score 29 (exploration rate: 0.94, transitions: 43)
Episode 3: score 13 (exploration rate: 0.88, transitions: 56)
Episode 4: score 53 (exploration rate: 0.68, transitions: 109)
Episode 5: score 12 (exploration rate: 0.64, transitions: 121)
Episode 6: score 21 (exploration rate: 0.57, transitions: 142)
Episode 7: score 13 (exploration rate: 0.54, transitions: 155)
Episode 8: score 11 (exploration rate: 0.51, transitions: 166)
Episode 9: score 11 (exploration rate: 0.48, transitions: 177)
Episode 10: score 9 (exploration rate: 0.46, transitions: 186)
Episode 11: score 9 (exploration rate: 0.44, transitions: 195)
Episode 12: score 11 (exploration rate: 0.42, transitions: 206)
Episode 13: score 10 (exploration rate: 0.40, transitions: 216)
Episode 14: score 10 (exploration rate: 0.38, transitions: 226)
Episode 15: score 13 (exploration rate: 0.35, transitions: 239)
Episode 16: score 9 (exploration rate: 0.34, transitions: 248)
Episode 17: score 12 (exploration rate: 0.32, transitions: 260)
Episode 18: score 13 (exploration rate: 0.30, transitions: 273)
Episode 19: score 9 (exploration rate: 0.28, transitions: 282)
Episode 20: score 13 (exploration rate: 0.27, transitions: 295)
Episode 21: score 10 (exploration rate: 0.25, transitions: 305)
Episode 22: score 13 (exploration rate: 0.24, transitions: 318)
Episode 23: score 9 (exploration rate: 0.23, transitions: 327)
Episode 24: score 10 (exploration rate: 0.22, transitions: 337)
Episode 25: score 17 (exploration rate: 0.20, transitions: 354)
Episode 26: score 10 (exploration rate: 0.19, transitions: 364)
Episode 27: score 9 (exploration rate: 0.18, transitions: 373)
Episode 28: score 10 (exploration rate: 0.17, transitions: 383)
Episode 29: score 15 (exploration rate: 0.16, transitions: 398)
Episode 30: score 9 (exploration rate: 0.15, transitions: 407)
Episode 31: score 9 (exploration rate: 0.15, transitions: 416)
Episode 32: score 10 (exploration rate: 0.14, transitions: 426)
Episode 33: score 11 (exploration rate: 0.13, transitions: 437)
Episode 34: score 10 (exploration rate: 0.12, transitions: 447)
Episode 35: score 12 (exploration rate: 0.12, transitions: 459)
Episode 36: score 8 (exploration rate: 0.11, transitions: 467)
Episode 37: score 11 (exploration rate: 0.11, transitions: 478)
Episode 38: score 8 (exploration rate: 0.10, transitions: 486)
Episode 39: score 9 (exploration rate: 0.10, transitions: 495)
Episode 40: score 15 (exploration rate: 0.09, transitions: 510)
Episode 41: score 9 (exploration rate: 0.09, transitions: 519)
Episode 42: score 12 (exploration rate: 0.08, transitions: 531)
Episode 43: score 9 (exploration rate: 0.08, transitions: 540)
Episode 44: score 9 (exploration rate: 0.07, transitions: 549)
Episode 45: score 8 (exploration rate: 0.07, transitions: 557)
Episode 46: score 9 (exploration rate: 0.07, transitions: 566)
Episode 47: score 8 (exploration rate: 0.07, transitions: 574)
Episode 48: score 9 (exploration rate: 0.06, transitions: 583)
Episode 49: score 8 (exploration rate: 0.06, transitions: 591)
Episode 50: score 9 (exploration rate: 0.06, transitions: 600)
Episode 51: score 10 (exploration rate: 0.05, transitions: 610)
Episode 52: score 9 (exploration rate: 0.05, transitions: 619)
Episode 53: score 12 (exploration rate: 0.05, transitions: 631)
Episode 54: score 11 (exploration rate: 0.05, transitions: 642)
Episode 55: score 10 (exploration rate: 0.04, transitions: 652)
Episode 56: score 28 (exploration rate: 0.04, transitions: 680)
Episode 57: score 9 (exploration rate: 0.04, transitions: 689)
Episode 58: score 9 (exploration rate: 0.04, transitions: 698)
Episode 59: score 23 (exploration rate: 0.03, transitions: 721)
Episode 60: score 136 (exploration rate: 0.02, transitions: 857)
Episode 61: score 62 (exploration rate: 0.01, transitions: 919)
Episode 62: score 52 (exploration rate: 0.01, transitions: 971)
Episode 63: score 18 (exploration rate: 0.01, transitions: 989)
Episode 64: score 12 (exploration rate: 0.01, transitions: 1001)
Episode 65: score 56 (exploration rate: 0.01, transitions: 1057)
Episode 66: score 22 (exploration rate: 0.01, transitions: 1079)
Episode 67: score 14 (exploration rate: 0.01, transitions: 1093)
Episode 68: score 13 (exploration rate: 0.01, transitions: 1106)
Episode 69: score 20 (exploration rate: 0.01, transitions: 1126)
Episode 70: score 29 (exploration rate: 0.01, transitions: 1155)
Episode 71: score 22 (exploration rate: 0.01, transitions: 1177)
Episode 72: score 21 (exploration rate: 0.01, transitions: 1198)
Episode 73: score 21 (exploration rate: 0.01, transitions: 1219)
Episode 74: score 24 (exploration rate: 0.01, transitions: 1243)
Episode 75: score 22 (exploration rate: 0.01, transitions: 1265)
Episode 76: score 23 (exploration rate: 0.01, transitions: 1288)
Episode 77: score 15 (exploration rate: 0.01, transitions: 1303)
Episode 78: score 23 (exploration rate: 0.01, transitions: 1326)
Episode 79: score 21 (exploration rate: 0.01, transitions: 1347)
Episode 80: score 18 (exploration rate: 0.01, transitions: 1365)
Episode 81: score 32 (exploration rate: 0.01, transitions: 1397)
Episode 82: score 31 (exploration rate: 0.01, transitions: 1428)
Episode 83: score 24 (exploration rate: 0.01, transitions: 1452)
Episode 84: score 24 (exploration rate: 0.01, transitions: 1476)
Episode 85: score 49 (exploration rate: 0.01, transitions: 1525)
Episode 86: score 30 (exploration rate: 0.01, transitions: 1555)
Episode 87: score 22 (exploration rate: 0.01, transitions: 1577)
Episode 88: score 26 (exploration rate: 0.01, transitions: 1603)
Episode 89: score 29 (exploration rate: 0.01, transitions: 1632)
Episode 90: score 47 (exploration rate: 0.01, transitions: 1679)
Episode 91: score 36 (exploration rate: 0.01, transitions: 1715)
Episode 92: score 51 (exploration rate: 0.01, transitions: 1766)
Episode 93: score 71 (exploration rate: 0.01, transitions: 1837)
Episode 94: score 58 (exploration rate: 0.01, transitions: 1895)
Episode 95: score 40 (exploration rate: 0.01, transitions: 1935)
Episode 96: score 40 (exploration rate: 0.01, transitions: 1975)
Episode 97: score 48 (exploration rate: 0.01, transitions: 2023)
Episode 98: score 85 (exploration rate: 0.01, transitions: 2108)
Episode 99: score 45 (exploration rate: 0.01, transitions: 2153)
Episode 100: score 41 (exploration rate: 0.01, transitions: 2194)
Episode 101: score 50 (exploration rate: 0.01, transitions: 2244)
Episode 102: score 90 (exploration rate: 0.01, transitions: 2334)
Episode 103: score 61 (exploration rate: 0.01, transitions: 2395)
Episode 104: score 87 (exploration rate: 0.01, transitions: 2482)
Episode 105: score 92 (exploration rate: 0.01, transitions: 2574)
Episode 106: score 94 (exploration rate: 0.01, transitions: 2668)
Episode 107: score 108 (exploration rate: 0.01, transitions: 2776)
Episode 108: score 129 (exploration rate: 0.01, transitions: 2905)
Episode 109: score 152 (exploration rate: 0.01, transitions: 3057)
Episode 110: score 166 (exploration rate: 0.01, transitions: 3223)
Episode 111: score 146 (exploration rate: 0.01, transitions: 3369)
Episode 112: score 200 (exploration rate: 0.01, transitions: 3569)
Reached goal sucessfully.
Time: 8 minutes
|
Data Collection/crawling_1.ipynb | ###Markdown
BeautifulSoup - Beautiful Soup is a Python library for pulling data out of HTML and XML files. It works with your favorite parser to provide idiomatic ways of navigating, searching, and modifying the parse tree. - https://www.crummy.com/software/BeautifulSoup/bs4/doc/
###Code
r = requests.get("http://finance.naver.com/sise/lastsearch2.nhn")
soup = BeautifulSoup(r.text, "html.parser")
ts = soup.find('table', {"class" : "type_5"}) # ts 는 top searches
ts
# column name 추출해서 DataFrame 으로 정리하기
nf_cols = ts.find("tr" , {"class" : "type1"}) # nf 는 naver finance
nf_cols = nf_cols.text
print(nf_cols)
categories = re.split("\n", nf_cols)
finance_df = pd.DataFrame(columns = categories)
finance_df
###Output
_____no_output_____ |
lectures/performance/performance-live.ipynb | ###Markdown
Some Notes on Performance for Python Code What we're doing today.In this one-off lecture, we'll consider a question that often pops up when writing Python code: > How can I make my code *faster*? There are a large number of things to keep in mind when considering how to make our code performant. Today, we'll briefly survey just a few of the possibilities. Here's a [nice list](https://stackify.com/20-simple-python-performance-tuning-tips/) of useful tips. Numpy and PandasAs you know, code that is primarily related to numerical computations or manipulation of rectangular data should almost always be performed in Numpy or Pandas. Both of these tools bring considerable performance improvements over base Python code in the situations to which they are suited, and should generally be used when possible. I won't be talking more about these topics today, but please remember that using these tools for appropriate problems is one of the easiest and biggest wins for performance. 0. Should I Write This Code? So, you've got a task that you'd like to perform in Python, and you can see the outlines of a good code solution. *Should you write code for your solution?* Maybe! Before you do, take a moment to look around and see what else might be available. Public and well-established functions will almost always be more reliable and performant than what you hack together. Use them whenever possible! Suppose we'd like to iterate through all possible pairs of elements from a list. Here's one way:
###Code
captains = ["Picard", "Sisko", "Janeway", "Archer", "Georgiou"]
###Output
_____no_output_____
###Markdown
This code looks pretty fast, but a quick Google search for "iterate through all pairs python" turns up this [StackOverflow](https://stackoverflow.com/questions/942543/operation-on-every-pair-of-element-in-a-list/37907649) post, indicating that I should use `itertools.product()`. Use of `itertools.product()` saved me from writing some code, and is also noticeably faster. Both kinds of savings will tend to become more pronounced the more complex the task that you need to accomplish. 1. *Should* I Optimize This Code? Let's now suppose that you've done your research and determined that you do need to write up your own solution. Indeed, you've already done it. Great job! But now we face the next question: should you spend time making your code faster? When creating any kind of code product, we face various decisions about how to best allocate our time and energy. Making code faster can be a very helpful or even necessary thing to do. Before choosing to spend effort optimizing your code, ask yourself the following questions: 1. Does my code run in practical (non-annoying) time on realistic cases? 2. How many times will my code be run? By how many people? 3. Is the code that I am considering optimizing a major part of my overall project? This third question is especially important. Maybe you have a function that takes 1 ms to run. This might be considered relatively slow! Maybe you can improve it to 0.1 ms with effort, a 10x speed up. But if that function will only be called once or twice in a given interaction, your user is unlikely to notice the difference. A function that takes 1 µs but which is called 1M times is much more important to optimize, and even a 2x speedup will have a much larger benefit for your user. 2. *Which* Code Should I Optimize? Suppose you have a function or even an entire program. Which parts of it need your attention? A *profiler* can be used to analyze your code and provide important information, like: 1. How many times are various functions within your code called? 2. How much time does each function take per call? There are a number of different profilers available for Python. `cProfile` comes bundled with all modern Python distributions and is a traditional choice. We'll illustrate cProfile here. Another option I found is [Yappi](https://pypi.org/project/yappi/) (Yet Another Python ProfIler), which has several advanced features. It can be installed via the Anaconda Environments Pane. Let's write a simple function to count distinct elements in a list. Here's some data on which we'll call our function.
###Code
s = """
Space, the final frontier
These are the voyages of the Starship Enterprise
Its five year mission
To explore strange new worlds
To seek out new life
And new civilizations
To boldly go where no one has gone before"""
L = (s*100).lower().split()
len(L), len(set(L))
###Output
_____no_output_____
###Markdown
Hmmm, that's pretty slow. Was it the way that we're updating our dictionary, maybe? Should we seek a faster method than `count()` for actually counting objects? For these kinds of questions, profiling is exactly what we need: The cProfile output contains lines for each of the function calls that the profiler was able to detect (note: it doesn't always detect all functions used). Note that dictionary update takes essentially no time at all, while the `.count()` method occupies the bulk of the time spent executing this function. To reduce this, we essentially have three options: 1. We can reduce the time spent executing each `count()` call, by using a faster method. 2. We can reduce the number of times `count()` is executed. 3. We can completely refactor our code. I don't know of a faster version of `count()`, but it's not hard to dramatically reduce the number of times we execute it: That's **much** faster! cProfile can tell us why: We dramatically reduced the number of times that the `count()` function was called, resulting in a large speed-up. In fact, we can do even better than this by changing our entire strategy. Instead of `count`ing each distinct element, we'll instead simply initialize a count and add to it each time we encounter the appropriate item. This works well because dictionary operations are extremely fast: cProfile tells us that we have a large number of calls to `dict.get()`, but access of dictionary elements is exceptionally fast (that's what dictionaries are optimized for), so this doesn't pose a major problem. However, we could do even better still by going back to Question 0: *Should* I write this code? Relative to our initial version of the code, we've achieved a 1000x speedup by relying on built-in functions. Even our relatively efficient hand-written version is roughly 4x slower. Various Tips Reduce Function CallsThe simple act of calling a function in Python can carry some performance overhead. For this reason, reducing the number of times that functions are called can lead to performance improvements, even when there's no otherwise-obvious redundancy. This function is logically coded, but it involves many calls to the `__add__` method of strings. Unfortunately, detecting this issue can be difficult for cProfile: The output doesn't make clear that there are any issues involving functions being called multiple times. But if we know to look out for this issue, then we might decide to use other tools, with the benefit of shortening our code. MultithreadingA popular myth from a few years back asserted that most humans use only 10% of their brain most of the time. This is [not in fact true](https://en.wikipedia.org/wiki/Ten_percent_of_the_brain_myth). What **is** true is that your Python process uses only (about) 10% of the full computational resources of your computer at any given moment. More concretely, most modern personal (Intel-based) computers possess somewhere between 2 and 6 *cores*, with 4 being perhaps the most common number. Each of these cores usually possesses 2 *threads*. Each thread is a separate process that can be used for executing tasks. When we run Python, we typically do so in a single-threaded environment, which means that our commands are executed by only one of our computer's threads. If your machine has 4 cores, and therefore 8 threads, this corresponds to only 12.5% of your overall CPU resources. *Multithreading* allows us to perform computations using more than one core. For large jobs, this can be beneficial. If your problem admits multithreading, if you use 4 threads, you may be able to finish your computation roughly 4 times as quickly. Nice! CautionMultithreading sounds exciting, and indeed, it can be a useful thing to do. However, multithreading should usually be your **last** measure for improving performance. Here's why: 1. The speedup offered by multithreading is limited by the number of threads available on your computer. In my case, for example, my laptop has 8 threads. This means that the largest possible benefit I could ever obtain from multithreading is an 8x speed up. That's not nothing, but one can often obtain better speedups by revising one's logic and coding constructs, as demonstrated above. 2. Multithreading can also place undue burdens on your hardware. There's **a reason** that many processes default to single-threaded runtimes. Your laptop simply isn't built to have all of its computational power engaged all the time. Using many threads for extended periods will likely overheat your system, resulting in thermal throttling. Your CPU will automatically reduce the rate of computation on each thread in order to dissipate heat. Thus, you could actually end up with **slower** code this way. 3. There's overhead associated with distributing jobs between threads and syncing them back up. This overhead is manifest both in the time you need to spend writing code in order to manage these processes, and in the actual computation time as well. 4. Multithreading really only "works" for processes that can be decoupled into independent components or chunks of data. Complex processes in which the current results of computation depend on previous results are often not suitable for multithreading. For these reasons, multithreading should only be considered **after** you're pretty confident that you've exhausted your other means of speeding up your code. ExampleLet's finish up with a simple example of multithreading in action. This is a toy example rather than a practical illustration. In a coming Discussion, we will work through some of the important and occasionally subtle associated with successfully deploying multithreading in practice. The simplest way to spawn multiple threads is through the `threading` module. One first defines a function which should be executed on each thread. This function presumably performs some time-intensive computation. One then spawns the desired number of `threading.Thread()` objects with `target` equal to the function which should be executed. Finally, one then `start`s each thread, and calls `join()` to ensure that no code is executed in the main process before each of the spawned threads finishes their work. This example is lightly modified from one presented [here](https://www.geeksforgeeks.org/multithreading-python-set-1/).
###Code
import threading
import os
def task1():
print("Task 1 assigned to thread: {}".format(threading.current_thread().name))
print("ID of process running task 1: {}".format(os.getpid()))
print("To boldly go")
print()
def task2():
print("Task 2 assigned to thread: {}".format(threading.current_thread().name))
print("ID of process running task 2: {}".format(os.getpid()))
print("Where no one has gone before")
print()
###Output
_____no_output_____ |
Lab/20--randvars-testing.ipynb | ###Markdown
CX 4230, Spring 2016: [20] Generating Random Variates and Testing Random Number GeneratorsThis notebook accompanies the slides presented in class: [link](https://t-square.gatech.edu/access/content/group/gtc-59b8-dc03-5a67-a5f4-88b8e4d5b69a/cx4230-sp16--20-rand-var-test.pdf).For a deeper survey of these ideas, see the readings from the last lab, especially volume 2 of Knuth's _The Art of Computer Programming_: [link](https://t-square.gatech.edu/access/content/group/gtc-59b8-dc03-5a67-a5f4-88b8e4d5b69a/Knuth-TAOCP-v2--9780133488791.pdf). From uniform to arbitrary distributionsSuppose you are given a way to generate a uniform random variable $U \sim \mathcal{U}(0, 1)$. How do you convert $U$ into a different random variable $X$ following some _other_ distribution, such as exponential?One technique is to "invert" the _cumulative distribution function_ (CDF) of $X$. Recall that the CDF of $X$ is a function$$ F_X(x) \equiv \mathrm{Pr}[X \leq x].$$Given a sample value $u$ of the random variable $U$, you can convert $u$ into a sample $x$ of $X$ by$$ x = F_X^{-1}(u).$$ **Example.** Let $X \sim \mathcal{E}(\lambda)$ be an exponentially distributed random variable with mean $\lambda$. Then it has a cumulative distribution$$ F_X(x) = 1 - e^{-x / \lambda}.$$Given an observed sample $u$ of a random variable $U$, you would compute the sample $x$ by solving $u = F_X^{-1}(x)$ for $x$. The result would be$$ x = {-\lambda \ln (1-u)}$$ **Exercise.** Implement a function to generate samples from $\mathcal{E}(\lambda)$.
###Code
from random import random, seed
from math import log
def sample_exp (l):
"""Generates a sample from an exponential random variable with mean `l`."""
# @YOUSE
return ...
# Test code: Generate samples and plot them as a histogram
import matplotlib.pyplot as plt
%matplotlib inline
n = 250
l = 5.0
seed (20160224)
x = [sample_exp (l) for i in range (n)]
x_mean = sum (x) / n
print ("Sample mean:", x_mean)
plt.hist (x)
###Output
_____no_output_____
###Markdown
Generating samples for an empirical CDFYou can apply essentially the same idea to discrete random variables. Let's walk through an example.Suppose you wish to generate letters from a distribution that matches the empirically observed distribution of letters in the English language.
###Code
import requests # http://docs.python-requests.org/en/master/user/quickstart/#make-a-request
def download_text (url):
print ("... downloading", url, "...")
req = requests.get (url)
return req.text
text = download_text ('http://www.gutenberg.org/cache/epub/11/pg11.txt')
#text = download_text ('http://www.gutenberg.org/cache/epub/15532/pg15532.txt')
print ("\n=== Snippet ===\n...", text[5000:5500], "\n...")
###Output
_____no_output_____
###Markdown
**Exercise.** From what book was this text drawn? > Answer: ?? Next, let's make a histogram of letter frequencies, stored as a dictionary where the key is the letter and the value is the number of occurrences of that letter. For simplicity, consider only alphabetic characters and normalize all characters to lowercase.
###Code
# Make a histogram of individual characters. See: https://docs.python.org/2/library/collections.html
from collections import Counter
def count_chars (s):
"""
Given a string or list of characters `s`, this function returns a
histogram of the number of occurrences of alphabetic characters.
The histogram is stored as a dictionary and the characters are
normalized to lowercase.
"""
histogram = Counter ()
for c in s:
if c.isalpha ():
histogram[c.lower ()] += 1
return histogram
# Count the occurrences of each (lowercase) alphabetic characters
text_counts = count_chars (text)
num_chars = sum (text_counts.values ())
print ("=== Occurrences:", num_chars, "characters total ===")
text_counts
###Output
_____no_output_____
###Markdown
To make this distribution a little easier to read, let's convert it to a list and sort by value.
###Code
# A function to "sort" dictionaries:
# http://stackoverflow.com/questions/613183/sort-a-python-dictionary-by-value
import operator
def sort_dict (d, descending=False):
"""
Given a dictionary `d`, returns a list of (key, value) pairs sorted by value.
To sort in descending order, set `ascending=False`.
"""
if descending:
compare = lambda x: -(operator.itemgetter (1) (x))
else:
compare = operator.itemgetter (1)
return sorted (d.items (), key=compare)
text_counts_sorted = sort_dict (text_counts, descending=True)
text_counts_sorted
###Output
_____no_output_____
###Markdown
Let's convert these ordered counts into a CDF.
###Code
def cumsum_key_value_pairs (p):
s = 0.0
p_cumulative = []
for (k, v) in p:
s = s + v
p_cumulative.append ((k, s))
return p_cumulative
text_cdf = [(k, float (v)/num_chars) for (k, v) in cumsum_key_value_pairs (text_counts_sorted)]
print ("=== Empirical CDF ===")
text_cdf
###Output
_____no_output_____
###Markdown
**Exercise.** Write a function to generate a sample from a discrete CDF like `text_cdf`. Recall that `text_cdf` is a list of (key, value) pairs where the key is an outcome of the random variable and the value is the cumulative probability of observing the key and all preceding keys in list order.> Hint: The [`bisect()`](https://docs.python.org/2/library/bisect.html) makes this task easy. In particular, given a list `X` of values in ascending order, `bisect(X, v)` returns the largest index `i` such `X[i] <= v`.
###Code
from bisect import bisect # Per the hint
def gen_sample (cdf):
"""
Given a discrete cumulative distribution function, this function returns
a single random sample from the distribution. The input CDF is given as
a list `cdf` of (`key`, `value`) pairs, where `value` is the cumulative
probability of observing the key and all preceding keys in the list.
"""
# @YOUSE
return ...
# Test code: Generate `n` samples and count the occurrences of each unique character.
n = 1000
sample = [gen_sample (text_cdf) for i in range (n)]
sort_dict (count_chars (sample), descending=True)
###Output
_____no_output_____
###Markdown
Chi-square ($\chi^2$) testing**Overview.** The previous example began with a "true" (empirical) distribution of letter frequencies, extracted from text real text. We then generated samples from this distribution.What if we had just been handed the generated samples. How could we check whether they came from our letter-frequency distribution? In class, we discussed one way to test how likely a random sample was to have been drawn from a given discrete distribution, using a method called the _chi-square ($\chi^2$) test_. There are canned (black-box) routines to compute it, e.g., [`scipy.stats.chisquare()`](http://docs.scipy.org/doc/scipy-0.16.1/reference/generated/scipy.stats.chisquare.html). Here is how we would apply it to the data for the pair of dice in the slides.
###Code
# Check against slide data
X_slides = [4, 8, 12, 16, 20, 24, 20, 16, 12, 8, 4]
Y_slides = [2, 4, 10, 12, 22, 29, 21, 15, 14, 9, 6]
chisquare (Y_slides, f_exp=X_slides)
###Output
_____no_output_____
###Markdown
However, for pedagogical purposes, let's see how it works the black-box actually works through a series of exercises. In these exercises, assume that the `sample` dictionary computed above is the set of values we are testing against a "true" distribution, which comes from the text (`text_counts`). **Walk-through.** Per the class slides, consider a discrete distribution for which you know that any outcome $v$ occurs with probability $p_v$. You observe a random sample of size $n$, $\{y_0, y_1, \ldots, y_{n-1}\}$. You wish to check how likely it is to have come from the given distribution. **Exercise.** Convert the letter counts into probabilities.
###Code
# @YOUSE: Compute a new dictionary of key-value pairs, (v, p_v), from `text_counts`.
text_probs = ...
# Test code (display probabilities)
text_probs
###Output
_____no_output_____
###Markdown
Next, compute the _counts_ of the number of occurrences of $v$ in the sample. Denote these observed counts mathematically by $Y_v$.**Exercise.** Compute a dictionary `sample_counts[k] = c` where `k` is $v$ and `c` is $Y_v$.
###Code
# @YOUSE:
sample_counts = ...
# Display
sample_counts
###Output
_____no_output_____
###Markdown
Next, compute the _chi-square statistic_, which is$$ \chi^2 \equiv \sum_v \dfrac{(Y_v - np_v)^2}{np_v},$$where $np_v$ is the expected number of occurrences of $v$ in a sample of size $n$.**Exercise.** Complete the following function, which computes the $\chi^2$ statistic.
###Code
def calc_chisquare_statistic (counts, probs):
"""
Given a target distribution and empirically observed counts, compute the
chi-square statistic of the observations relative to the target.
The input `counts[v] = y_v` is the dictionary of observations and
`probs[v] = p_v` is the probability of observing `v`.
"""
n = sum (counts.values ())
chi_sq = 0.0
# @YOUSE:
assert False
return chi_sq
sample_chi2 = calc_chisquare_statistic (sample_counts, text_probs)
print ("\nchi^2 for the random sample:", sample_chi2)
###Output
_____no_output_____
###Markdown
To check the $\chi^2$ statistic, you also need to know the number of degrees of freedom of the distribution.**Exercise.** Compute the degrees of freedom of the target distribution.
###Code
# @YOUSE
text_dof = ...
print ("Degrees of freedom =", text_dof)
###Output
_____no_output_____
###Markdown
At this point, you would "look up" the chances of observing your $\chi^2$ given the number of degrees of freedom. In Python, you can do this look-up by calling a function that evaluates the CDF of a $\chi^2$ distribution. See: http://docs.scipy.org/doc/scipy-0.16.1/reference/generated/scipy.stats.chi2.html
###Code
from scipy.stats import chi2
chi2_stat_check = chi2.cdf (sample_chi2, text_dof)
chi2_stat_check2 = 1 - chi2_stat_check
print ("chi^2 CDF:", chi2_stat_check, "/", chi2_stat_check2)
###Output
_____no_output_____
###Markdown
**Exercise.** What would you conclude about the likelihood that the random sample came from the target distribution? > Answer: ?? **Sanity check.** Let's double-check your result against what the "canned" routine produces.
###Code
from scipy.stats import chisquare
def calc_chisquare (sample, probs):
assert (type (sample) is dict) or (type (sample) is Counter) # Counts
assert type (probs) is dict # Probabilities
# Total number of samples
n_Y = sum (sample.values ())
Y = [] # Holds observed counts, $Y_v$
X = [] # Holds expected counts, $X_v$
for (v, p_v) in probs.items (): # probabilities of the true distribution
x_v = p_v * n_Y
if v in sample:
y_v = sample[v]
else:
y_v = 0
X.append (x_v)
Y.append (y_v)
return (chisquare (Y, f_exp=X), X, Y)
chi2_result, _, _ = calc_chisquare (sample_counts, text_probs)
print (chi2_result)
###Output
_____no_output_____
###Markdown
Extra stuff: English vs. SpanishHow does the frequency distribution of English differ from Spanish? Let's download an example of Spanish text, compute the letter frequencies, and compare that distribution against the one for the English text. > Spanish contains a number of vowels that don't occur in English. To make this comparable, the [`unidecode` package](https://pypi.python.org/pypi/Unidecode) can normalize a general unicode string into its closest pure ASCII representation. However, since `unidecode` is not a part of most standard Python distributions, you might need to install it first (see below).
###Code
# Downloads and installs the unidecode module; see: https://pypi.python.org/pypi/Unidecode
!pip install unidecode
from unidecode import unidecode
text_es = download_text ('http://www.gutenberg.org/cache/epub/15532/pg15532.txt')
# If you don't have unidecode, just comment this next line out:
text_es = unidecode (text_es)
print ("\n=== Snippet (Spanish text) ===\n...", text_es[5000:5500], "\n...")
text_es_counts = count_chars (text_es)
sort_dict (text_es_counts, descending=True)
num_chars_es = sum (text_es_counts.values ())
text_es_probs = {k: v/num_chars_es for (k, v) in text_es_counts.items ()}
text_es_probs
text_probs_sorted = sort_dict (text_probs, descending=True)
x_labels = [k for (k, _) in text_probs_sorted]
y_values = [v for (_, v) in text_probs_sorted]
y_values_es = []
for v in x_labels:
if v in text_es_probs:
y_v = text_es_probs[v]
else:
y_v = 0.0
y_values_es.append (y_v)
x_values = range (len (x_labels))
w = 0.25
fig = plt.figure (figsize=(16, 6))
ax = fig.add_subplot (111)
ax.bar ([x-w for x in x_values], y_values, w, color='b')
ax.bar (x_values, y_values_es, w, color='r')
ax.set_xticks (x_values)
ax.set_xticklabels (x_labels)
pass
calc_chisquare (text_es_counts, text_probs)
###Output
_____no_output_____ |
JJ_Cheung_CTCUC22Assignment05.ipynb | ###Markdown
Assignment 5 Sorting Machine**Task: Design and implement an awesome sorting machine.****Baseline requirement:** Design an (O/A)-level appropriate assignment to asssess understanding and application of one or more of the following sorting algorithm(s):- bubble sort- insertion sort- quick sort- merge sortYou may should provide suitable dataset(s) for testing purposes. Justify the choice of dataset(s) used.**Bonus requirement:** For differentiated instruction to higher ability students, or to connect students to the real world beyond academic toy or exam style problems, design a wicked (https://en.wikipedia.org/wiki/Wicked_problem) extension/component to make your sorting machine even more awesome! You can/should apply the computational thinking ideas of (not exhaustively):- abstraction- decomposition- pattern recognition - algorithm design (You are not expected to solve your own wicked problem. :)Happy designing/coding! :) Submit either - a video walkthrough or- a write-upexplaining how your sorting works via the end of session feedback form https://forms.gle/kku2YMgxVVntTFjD9. :)Focus will be on sorting machine features and justifying appropriate programming constructs, data structures and algorithms used, as in how you would explain it to your students. **Sorting Assignment** **1. Bubble Sort**---A student wrote a set of code for bubble sort in the cell below.a) Explain the errors in the code in the cell, through the use of comments.
###Code
def bubble_sort(A):
swapped = False
passes = len(A)
while swapped == True:
swapped = False
for i in range (passes):
if A[i] > A[i+1]:
A[i] = A[i+1]
A[i+1] = A[i]
swapped = True
passes -= 1
return A
print(bubble_sort([5,6,2,4,3,4,5,7,8,3])) #duplicate numbers
print(bubble_sort([])) #empty list
print(bubble_sort([9,7,5,3,2,1,0,-2])) #reverse order
###Output
[5, 6, 2, 4, 3, 4, 5, 7, 8, 3]
[]
[9, 7, 5, 3, 2, 1, 0, -2]
###Markdown
b) Copy the code to the cell below, and modify the code to make it work.
###Code
#copy code here
#teacher's answer
def bubble_sort(A):
swapped = True #must be True first in order to initiate while loop
passes = len(A) - 1 #set number of passes to length of A -1, since we are comparing ith and (i+1)th element, to prevent index error
while swapped == True:
swapped = False
for i in range (passes):
if A[i] > A[i+1]: #if passes set to length of A, i+1 will exceed length of A
A[i], A[i+1] = A[i+1], A[i] #use comma assignment to make swapping possible.
swapped = True
passes -= 1
return A
print(bubble_sort([5,6,2,4,3,4,5,7,8,3]))
print(bubble_sort([]))
print(bubble_sort([9,7,5,3,2,1,0,-2]))
###Output
[2, 3, 3, 4, 4, 5, 5, 6, 7, 8]
[]
[-2, 0, 1, 2, 3, 5, 7, 9]
###Markdown
**2. Merge Sort**---Write a recursive function `merge_sort(A)` that takes in an unsorted list `A` and returns the sorted list `sorted`. The algorithm should consist of splitting the list into the smallest elements, before sorting them and merging the sorted lists.
###Code
def merge_sort(A):
#simplest base case
#splitting algorithm
front = [___]
back = [___]
#recursive calling
front = merge_sort(front)
back = merge_sort(back)
#merging sorted lists
i =
j =
sorted = []
return sorted
merge_sort([5,6,2,4,3,4,5,7,8,3])
#teacher's answer
def merge_sort(A):
#simplest base case
if len(A) == 1:
return A
#splitting algorithm - splitting the list A into two halves evenly
mid = len(A) // 2
front = A[:mid]
back = A[mid:]
#output to show splitting
print('Splitting')
print(A)
print('Split')
print(front)
print(back)
#recursive calling - for further splitting before merging
front = merge_sort(front)
back = merge_sort(back)
#merging sorted lists returned from recursive function called previously
print('Merging')
print(front)
print(back)
i = 0 #index for front []
j = 0 #index for back []
sorted = [] #empty to store sorted values
#while loop to look for smaller values at the front of each lists
#append smaller value to sorted list, increment index in same list
#loops until all values in either list are appended to sorted []
while (i<len(front)) and (j<len(back)):
if front[i] < back[j]:
sorted.append(front[i])
i += 1
else:
sorted.append(back[j])
j += 1
#add the rest of the elements in the other list to the sorted []
if i<len(front):
sorted.extend(front[i:])
elif j<len(back):
sorted.extend(back[j:])
#now sorted[] contains sorted values from both front and back []
print('Merged')
print(sorted)
return sorted
merge_sort([5,6,2,4,3,4,5,7,8,3])
###Output
Splitting
[5, 6, 2, 4, 3, 4, 5, 7, 8, 3]
Split
[5, 6, 2, 4, 3]
[4, 5, 7, 8, 3]
Splitting
[5, 6, 2, 4, 3]
Split
[5, 6]
[2, 4, 3]
Splitting
[5, 6]
Split
[5]
[6]
Merging
[5]
[6]
Merged
[5, 6]
Splitting
[2, 4, 3]
Split
[2]
[4, 3]
Splitting
[4, 3]
Split
[4]
[3]
Merging
[4]
[3]
Merged
[3, 4]
Merging
[2]
[3, 4]
Merged
[2, 3, 4]
Merging
[5, 6]
[2, 3, 4]
Merged
[2, 3, 4, 5, 6]
Splitting
[4, 5, 7, 8, 3]
Split
[4, 5]
[7, 8, 3]
Splitting
[4, 5]
Split
[4]
[5]
Merging
[4]
[5]
Merged
[4, 5]
Splitting
[7, 8, 3]
Split
[7]
[8, 3]
Splitting
[8, 3]
Split
[8]
[3]
Merging
[8]
[3]
Merged
[3, 8]
Merging
[7]
[3, 8]
Merged
[3, 7, 8]
Merging
[4, 5]
[3, 7, 8]
Merged
[3, 4, 5, 7, 8]
Merging
[2, 3, 4, 5, 6]
[3, 4, 5, 7, 8]
Merged
[2, 3, 3, 4, 4, 5, 5, 6, 7, 8]
###Markdown
**3. Class List Sorting**---You are provided with a list of lists, containing the first names, last names and gender of students in a class. Write a function sortclass(classlist) to return a sorted class list according to gender (females before males), last name and first name in this order. Use any of the four sorting methods taught, and not the `.sort()` function. You may define additional funtions to aid in the sorting.
###Code
class1A = [
['Benjamin', 'Goh', 'Male'],
['Henna', 'Lee', 'Female'],
['Lee Lee', 'Seah', 'Female'],
['Ah Boon', 'Tan', 'Male'],
['Kenneth', 'Seow', 'Male'],
['Ah Beng', 'Goh', 'Male'],
['Jeannette', 'Lee', 'Female'],
['Jean', 'Tan', 'Female'],
['Abigail', 'Wee', 'Female'],
['Aloysius', 'Han', 'Male'],
['William', 'Tan', 'Male'],
['Elsa', 'Lee', 'Female']
]
def sortclass(classlist):
pass
sorted1A = sortclass(class1A)
for student in sorted1A:
print (student)
#teacher's answer
#typical class list with some students having same last names
class1A = [
['Benjamin', 'Goh', 'Male'],
['Henna', 'Lee', 'Female'],
['Lee Lee', 'Seah', 'Female'],
['Ah Boon','Tan', 'Male'],
['Kenneth', 'Seow', 'Male'],
['Ah Beng', 'Goh', 'Male'],
['Jeannette', 'Lee', 'Female'],
['Jean', 'Tan', 'Female'],
['Abigail', 'Wee', 'Female'],
['Aloysius', 'Han', 'Male'],
['William', 'Tan', 'Male'],
['Elsa', 'Lee', 'Female']
]
def sort_first_name (B):
#sorting first name using quick sort
#base case
if len(B) == 0:
return []
#recursive case
else:
pivot = B[0] #setting first element as pivot
front = [] #empty list for front (elements smaller than pivot)
back = [] #empty list for back (elements greater than pivot)
for i in B[1:]: #for loop to run through list from 2nd to last element
if i[0] < pivot[0]: #elements smaller than pivot, append to front[]
front.append(i)
else:
back.append(i) #elements larger than pivot, append to back[]
#recursive calling to get front list + pivot + back list
return sort_first_name(front) + [pivot] + sort_first_name(back)
def sort_last_name(A):
#sorting last name using insertion sort
for i in range (1, len(A)): #first element is sorted, so start from 2nd element till end of list
n = i - 1 #compare with preceding element first
current = A[i] #value to be inserted in sorted list in front
while n >= 0 and A[n][1] > current[1]: #while loop to shift sorted element to adjacent element if larger than current
A[n+1] = A[n]
n -= 1
A[n+1] = current #assign current value to empty slot
#grouping the people with the same last names to be sorted by first name
lastname = {} #empty dictionary to store last names as keys and list of indices as values
for j in range (len(A)): #for loop to comb through entire list
if A[j][1] in lastname.keys(): #if last name already exist as key in dictionary,
lastname[A[j][1]].append(j) #append index to value in dictionary
else: #if last name doesn't exist as key in dictionary, i.e. new last name
lastname[A[j][1]] = [j] #create key and value in dictionary
for k, v in lastname.items(): #looping through every key and value in dictionary
start, end = v[0], v[-1] #getting the first and last index of people who have the same lastnames, per the dictionary
A[start:end+1] = sort_first_name(A[start:end+1]) #calls sort_first_name function with list of people with same last names as argument, and overwrite with sorted list
return A
def sortclass(classlist):
female = [] #sorting by gender. 2 empty lists, one for each gender
male = []
for i in range (len(classlist)):
if classlist[i][2] == 'Male':
male.append(classlist[i]) #append student to male list if M
else:
female.append(classlist[i]) #append student to female list if F
return sort_last_name(female) + sort_last_name(male) #sort female before male
sorted1A = sortclass(class1A)
for student in sorted1A:
print (student)
#empty class list, to check if code can run empty list
class1B = []
print(sortclass(class1B))
#class list of 200 names, to check if runtime limit is reached in their algorithm
class1C = [['Charlotte', 'Andrews', 'Female'], ['Adrianna', 'Gray', 'Female'], ['April', 'Henderson', 'Female'], ['Adison', 'Morrison', 'Male'], ['Mary', 'Jones', 'Female'], ['Cherry', 'Wells', 'Female'], ['Arthur', 'Nelson', 'Male'], ['James', 'Bennett', 'Male'], ['Stuart', 'Williams', 'Male'], ['Annabella', 'Henderson', 'Female'], ['Arianna', 'Hill', 'Female'], ['Maddie', 'Thompson', 'Female'], ['Robert', 'Wilson', 'Male'], ['Kelvin', 'Davis', 'Male'], ['Alexia', 'Chapman', 'Female'], ['Catherine', 'Higgins', 'Female'], ['Kimberly', 'Evans', 'Female'], ['Evelyn', 'Phillips', 'Female'], ['Rebecca', 'Evans', 'Female'], ['Byron', 'Johnson', 'Male'], ['Ada', 'Ryan', 'Female'], ['Adrian', 'Parker', 'Male'], ['Alford', 'Hamilton', 'Male'], ['Alexander', 'Clark', 'Male'], ['Carl', 'Miller', 'Male'], ['George', 'Mitchell', 'Male'], ['Adison', 'Hunt', 'Male'], ['Freddie', 'Fowler', 'Male'], ['Justin', 'Perry', 'Male'], ['Nicole', 'Edwards', 'Female'], ['Hailey', 'Evans', 'Female'], ['Amy', 'Fowler', 'Female'], ['Oliver', 'Hamilton', 'Male'], ['Vincent', 'Johnson', 'Male'], ['Brad', 'Richardson', 'Male'], ['Miranda', 'Reed', 'Female'], ['Lenny', 'Adams', 'Male'], ['Briony', 'Murphy', 'Female'], ['Derek', 'Andrews', 'Male'], ['Sabrina', 'Andrews', 'Female'], ['Alisa', 'Henderson', 'Female'], ['Eddy', 'Tucker', 'Male'], ['William', 'Mason', 'Male'], ['Julia', 'Alexander', 'Female'], ['Rosie', 'Carter', 'Female'], ['Lucy', 'Casey', 'Female'], ['Roman', 'Roberts', 'Male'], ['Victor', 'Cunningham', 'Male'], ['Jacob', 'Stewart', 'Male'], ['Richard', 'Ellis', 'Male'], ['Florrie', 'Perkins', 'Female'], ['George', 'Kelley', 'Male'], ['Vanessa', 'Watson', 'Female'], ['Adam', 'Smith', 'Male'], ['Florrie', 'Henderson', 'Female'], ['Owen', 'Robinson', 'Male'], ['Ned', 'Warren', 'Male'], ['Roman', 'Howard', 'Male'], ['Catherine', 'Myers', 'Female'], ['Dominik', 'Alexander', 'Male'], ['Adrian', 'Hill', 'Male'], ['Eleanor', 'Carter', 'Female'], ['Mike', 'Foster', 'Male'], ['Alina', 'Dixon', 'Female'], ['Victor', 'Kelley', 'Male'], ['Kelvin', 'Warren', 'Male'], ['Jack', 'Murray', 'Male'], ['Charlotte', 'Payne', 'Female'], ['Anna', 'Allen', 'Female'], ['Jacob', 'Miller', 'Male'], ['Mary', 'Morrison', 'Female'], ['Antony', 'Farrell', 'Male'], ['Ada', 'Scott', 'Female'], ['Brianna', 'Owens', 'Female'], ['Briony', 'Carter', 'Female'], ['Daisy', 'Cameron', 'Female'], ['Jacob', 'Murray', 'Male'], ['Valeria', 'Payne', 'Female'], ['Carl', 'Lloyd', 'Male'], ['Alan', 'West', 'Male'], ['Tiana', 'Turner', 'Female'], ['Roman', 'Brooks', 'Male'], ['Julia', 'Henderson', 'Female'], ['Abraham', 'Brooks', 'Male'], ['Nicole', 'Brown', 'Female'], ['Alfred', 'Johnson', 'Male'], ['Patrick', 'Morris', 'Male'], ['Brianna', 'Richards', 'Female'], ['Tony', 'Rogers', 'Male'], ['Tara', 'Barnes', 'Female'], ['Edgar', 'Richards', 'Male'], ['Ashton', 'Barnes', 'Male'], ['Kate', 'Clark', 'Female'], ['Lily', 'Robinson', 'Female'], ['Byron', 'Kelly', 'Male'], ['David', 'Smith', 'Male'], ['Nicole', 'Alexander', 'Female'], ['Edwin', 'Kelley', 'Male'], ['Haris', 'Richards', 'Male'], ['William', 'Harper', 'Male'], ['Valeria', 'Hall', 'Female'], ['Carl', 'Hamilton', 'Male'], ['Abigail', 'Robinson', 'Female'], ['Lydia', 'Hunt', 'Female'], ['Harold', 'Hill', 'Male'], ['Michelle', 'Myers', 'Female'], ['Agata', 'Thomas', 'Female'], ['Preston', 'Baker', 'Male'], ['Aston', 'Barnes', 'Male'], ['Isabella', 'Bennett', 'Female'], ['Lana', 'Farrell', 'Female'], ['Naomi', 'Owens', 'Female'], ['Oscar', 'Walker', 'Male'], ['Luke', 'Douglas', 'Male'], ['Abigail', 'Phillips', 'Female'], ['Alfred', 'Henderson', 'Male'], ['Martin', 'Allen', 'Male'], ['Roman', 'Scott', 'Male'], ['Lyndon', 'Williams', 'Male'], ['Haris', 'Stevens', 'Male'], ['Victoria', 'Perkins', 'Female'], ['Albert', 'Cameron', 'Male'], ['Martin', 'Cunningham', 'Male'], ['Carina', 'Howard', 'Female'], ['Dainton', 'Roberts', 'Male'], ['Sabrina', 'Payne', 'Female'], ['Deanna', 'Russell', 'Female'], ['Tara', 'Spencer', 'Female'], ['Tess', 'Kelley', 'Female'], ['Ned', 'Crawford', 'Male'], ['Connie', 'Myers', 'Female'], ['Oscar', 'Douglas', 'Male'], ['Lilianna', 'Casey', 'Female'], ['Paul', 'Morgan', 'Male'], ['Tyler', 'Johnson', 'Male'], ['Elian', 'Harris', 'Male'], ['Fenton', 'Campbell', 'Male'], ['Caroline', 'Spencer', 'Female'], ['Rafael', 'Clark', 'Male'], ['Carina', 'Alexander', 'Female'], ['Jenna', 'Martin', 'Female'], ['Frederick', 'Brooks', 'Male'], ['Cadie', 'Parker', 'Female'], ['Savana', 'Hill', 'Female'], ['Tess', 'Smith', 'Female'], ['Clark', 'Harper', 'Male'], ['Andrew', 'Tucker', 'Male'], ['Chloe', 'Williams', 'Female'], ['Antony', 'Taylor', 'Male'], ['Cadie', 'Thomas', 'Female'], ['Daryl', 'Ross', 'Male'], ['Alexia', 'Russell', 'Female'], ['Paul', 'Cunningham', 'Male'], ['Rosie', 'Cooper', 'Female'], ['Bruce', 'Mitchell', 'Male'], ['Aida', 'Smith', 'Female'], ['Camila', 'Johnston', 'Female'], ['James', 'Hall', 'Male'], ['Melissa', 'Evans', 'Female'], ['Lilianna', 'Lloyd', 'Female'], ['Edward', 'Dixon', 'Male'], ['Emma', 'Richards', 'Female'], ['Madaline', 'Perkins', 'Female'], ['Jessica', 'Thompson', 'Female'], ['Edwin', 'Walker', 'Male'], ['Lilianna', 'Richardson', 'Female'], ['John', 'Alexander', 'Male'], ['Alfred', 'Myers', 'Male'], ['Henry', 'Mason', 'Male'], ['Kimberly', 'Elliott', 'Female'], ['Victor', 'Phillips', 'Male'], ['Vincent', 'Miller', 'Male'], ['Martin', 'Campbell', 'Male'], ['Brad', 'Alexander', 'Male'], ['Valeria', 'Tucker', 'Female'], ['Charlie', 'Mitchell', 'Male'], ['Evelyn', 'Grant', 'Female'], ['Kirsten', 'Stevens', 'Female'], ['Mike', 'Elliott', 'Male'], ['Frederick', 'Tucker', 'Male'], ['Annabella', 'Cameron', 'Female'], ['Owen', 'Thompson', 'Male'], ['Abraham', 'Hawkins', 'Male'], ['Amanda', 'Barrett', 'Female'], ['Ryan', 'Johnson', 'Male'], ['Nicole', 'Davis', 'Female'], ['Kelvin', 'Spencer', 'Male'], ['Kristian', 'Perkins', 'Male'], ['Arianna', 'Foster', 'Female'], ['Kate', 'Hill', 'Female'], ['Joyce', 'Johnson', 'Female'], ['Jessica', 'Hawkins', 'Female'], ['Amelia', 'Elliott', 'Female'], ['Adele', 'Russell', 'Female'], ['Max', 'Thomas', 'Male'], ['Walter', 'Elliott', 'Male'], ['Wilson', 'Harrison', 'Male'], ['Tess', 'Taylor', 'Female'], ['Caroline', 'Brooks', 'Female'], ['Dexter', 'Walker', 'Male']]
sorted1C = sortclass(class1C)
for student in sorted1C:
print (student)
###Output
_____no_output_____ |
0625 melon_scraping.ipynb | ###Markdown
[['곡명','가수명','링크'],[],[],[]...]
###Code
result = []
results = []
for i in range(len(tags)-3) :
if i % 2 == 0 :
result = []
title = tags[i].text.strip()
result.append(title)
else :
name = tags[i].text.strip()
link = tags[i]['title']
result.append(name)
result.append(link)
results.append(result)
results
len(results)
###Output
_____no_output_____ |
implementations/notebooks_df/reviews.ipynb | ###Markdown
ReviewsThis is the reference implementation for [Reviews](https://github.com/chaoss/wg-evolution/blob/master/metrics/Reviews.md),a metric specified by the[Evolution Working Group](https://github.com/chaoss/wg-evolution) of the[CHAOSS project](https://chaoss.community).This implementation is specific to Git repositories.Have a look at [README.md](../README.md) to find out how to run this notebook (and others in this directory) as well as to get a better understanding of the purpose of the implementations.The implementation is described in two parts (see below):* Class for computing Reviews* An explanatory analysis of the class' functionalitySome more auxiliary information in this notebook:* Examples of the use of the implementation As discussed in the [README](../README.md) file, the scripts required to analyze the data fetched by Perceval are located in the `code_df` package. Due to python's import system, to import modules from a package which is not in the current directory, we have to either add the package to `PYTHONPATH` or simply append a `..` to `sys.path`, so that `code_df` can be successfully imported.
###Code
from datetime import datetime
import matplotlib.pyplot as plt
import sys
sys.path.append('..')
from code_df import utils
from code_df import conditions
from code_df.pullrequest import PullRequest
%matplotlib inline
class Reviews(PullRequest):
"""
Class for Reviews
"""
def compute(self):
"""
Compute the total number of reviews created, from the Perceval data.
:returns count: The total number of reviews created
"""
count = len(self.df['hash'].unique())
return count
def _agg(self, df, period):
"""
Perform an aggregation operation on a DataFrame to find
the total number of reviews created in every
interval of the period specified in the time_series method,
like 'M', 'W',etc.
It computes the count of the "category" column of the
DataFrame.
:param df: a pandas DataFrame on which the aggregation will be
applied.
:param period: A string which can be any one of the pandas time
series rules:
'W': week
'M': month
'D': day
:returns df: The aggregated dataframe, where aggregations have
been performed on the "category" column
"""
df = df.resample(period)['category'].agg(['count'])
return df
###Output
_____no_output_____
###Markdown
Performing the AnalysisUsing the above class, we can perform several kinds of analysis on the JSON data file, fetched by Perceval.For starters, we can perform a simple count of all reviews in the data. For this analysis, we can vary the value passed to the `date_range` parameter. Counting the total number of commits We first read the JSON file containing Perceval data using the `read_json_file` utility function.
###Code
items = utils.read_json_file('../pull_requests.json')
###Output
_____no_output_____
###Markdown
Let's use the `compute` method to count the total number of reviews created. First, we will do it without passing any since and until dates. Next, we can pass in the start and end dates as a tuple. The format would be `%Y-%m-%d`.A third kind of analysis we can perform is passing only one of the dates to `date_range` --- either `since` or `until`.
###Code
reviews = Reviews(items)
print("The total number of reviews "
"in the file is {}.".format(reviews.compute()))
date_since = datetime.strptime("2018-01-01", "%Y-%m-%d")
date_until = datetime.strptime("2018-07-01", "%Y-%m-%d")
reviews_dated = Reviews(items,
date_range=(date_since, date_until))
print("The total number of reviews created between "
"2018-01-01 and 2018-07-01 is {}.".format(reviews_dated.compute()))
reviews_after = Reviews(items,
date_range=(date_since, None))
print("The total number of reviews created after "
"2018-01-01 is {}.".format(reviews_after.compute()))
###Output
The total number of reviews in the file is 229.
The total number of reviews created between 2018-01-01 and 2018-07-01 is 43.
The total number of reviews created after 2018-01-01 is 75.
###Markdown
Counting reviews over regular time intervalsUsing the `time_series` method, it is possible to compute the number of reviews created every month, or every week, or infact any valid pandas timeperiod. This kind of analysis is useful in finding trends over time, as we will see in the cell below.Let's perform a basic analysis: lets see the change in the number of reviews created between the same dates we used above on a weekly basis: 2018-01-01 and 2018-07-01. The Reviews object, `reviews_dated`, will be the same as used above.
###Code
weekly_df = reviews_dated.time_series(period='W')
###Output
_____no_output_____
###Markdown
Lets see what the dataframe returned by `time_series` looks like. As you will notice, the dataframe has rows corresponding to each and every week between the start and end dates. To do this, we simply set the `created_date` column of the DataFrame `changes_dated.df`, as its index and then `resample` it to whatever time period we need. In this case, we have used `W`.
###Code
weekly_df
###Output
_____no_output_____
###Markdown
Lets plot the dataframe `weekly_df` using matplotlib.pyplot. We use the `seaborn` theme and plot a simple line plot --- commit count vs time interval. Using the `plt.fill_between` method allows us to "fill up" the area between the line plot and the x axis.
###Code
plt.style.use('seaborn')
weekly_df.plot(y='count', use_index=True)
plt.fill_between(y1=weekly_df['count'], y2=0, x=weekly_df.index)
plt.title("Review Count");
###Output
_____no_output_____
###Markdown
The same thing can be tried for months, instead of weeks. By passing `month` in place of week, we get a similar dataframe but with only a few rows, due to the larger timescale.
###Code
monthly_df = reviews_dated.time_series('M')
monthly_df
###Output
_____no_output_____
###Markdown
Lets plot it just like we did for `weekly_df`.
###Code
plt.style.use('seaborn')
monthly_df.plot(y='count', use_index=True)
plt.fill_between(y1=monthly_df['count'], y2=0, x=monthly_df.index)
plt.title("Review Count");
###Output
_____no_output_____ |
[IMPL]_NLP_spam_class.ipynb | ###Markdown
Implementing a spam classifier Read and examine the data
###Code
# Define url
archive = r'https://archive.ics.uci.edu/ml/machine-learning-databases/00228/smsspamcollection.zip'
# Open url
url = urlopen(archive)
# read zip file
my_zip = ZipFile(BytesIO(url.read()))
# Get file names
my_zip.namelist()
filename = my_zip.namelist()[0]
# Read in the data
data = pd.read_csv(my_zip.open(filename), sep='\t', header=None, names=['class', 'text'])
data.sample(10)
def get_sample(data=data, n=10):
sample = data.sample(n).iloc[:,1]
for i in sample:
print(f'{i}\n')
get_sample(n=2)
###Output
Yeah like if it goes like it did with my friends imma flip my shit in like half an hour
Yes:)from last week itself i'm taking live call.
###Markdown
Prepare and pre-process the data
###Code
# Binarize labels
data['class'] = data['class'].map({'ham': 0, 'spam': 1})
###Output
_____no_output_____
###Markdown
* Train test split
###Code
# Train-test split
X_train, X_test, y_train, y_test = train_test_split(data['text'], data['class'],
test_size=.25)
# Vectorize X
count_vec = CountVectorizer(min_df=1)
X_train_counts = count_vec.fit_transform(X_train)
X_train_counts
# A quick check of a vocab list
number = np.random.randint(2314)
list(count_vec.vocabulary_.items())[number:number+4]
###Output
_____no_output_____
###Markdown
Train
###Code
# Instantiate NB clf
clf = MultinomialNB()
# Fit our clf
clf.fit(X_train_counts, y_train.ravel())
len(clf.coef_[0])
importance_count = collections.Counter()
for word, imp in zip(count_vec.vocabulary_.keys(), clf.coef_[0]):
importance_count[word] = imp
importance_count.most_common()[:10]
###Output
_____no_output_____
###Markdown
Test
###Code
# Transform test
X_test_counts = count_vec.transform(X_test)
# Predict
preds = clf.predict(X_test_counts)
###Output
_____no_output_____
###Markdown
Evaluate
###Code
# Evaluate
def get_metrics(y_true, y_pred, metric=[f1_score]):
for m in metric:
metric_name = s = re.search('\s\S+\s', str(m)).group(0).strip()
print(f'{metric_name:30}== {m(y_true, y_pred):.4f}')
print('\n')
print(classification_report(y_true, y_pred))
get_metrics(y_test, preds, metric=[f1_score,
average_precision_score,
accuracy_score])
len(X_train.values)
len(X_test.values)
# Some snity check
def predict_random(test_set):
phrase = test_set.values[np.random.randint(len(test_set))]
pred = clf.predict(count_vec.transform([phrase]))
if pred == 1:
pred = 'SPAM'
else:
pred = 'HAM'
return phrase, pred
def make_preds(test_set, n):
for i in range(n):
pred = predict_random(test_set)
print(f'Message:\n{pred[0]}\nClass: {pred[1]}\n')
make_preds(X_test, 10)
# Confusion matrix
mtrx = confusion_matrix(y_test, preds)
sns.heatmap(mtrx.T, square=True, annot=True, fmt='d', cbar=False)
plt.xlabel('true label')
plt.ylabel('predicted label')
plt.title('Confusion matrix')
plt.show()
mtrx
tn, fp, fn, tp = confusion_matrix(y_test, preds).ravel()
###Output
_____no_output_____ |
site/en/tutorials/text/word_level_generation.ipynb | ###Markdown
Copyright 2019 The TensorFlow Authors.
###Code
#@title Licensed under the Apache License, Version 2.0 (the "License");
# you may not use this file except in compliance with the License.
# You may obtain a copy of the License at
#
# https://www.apache.org/licenses/LICENSE-2.0
#
# Unless required by applicable law or agreed to in writing, software
# distributed under the License is distributed on an "AS IS" BASIS,
# WITHOUT WARRANTIES OR CONDITIONS OF ANY KIND, either express or implied.
# See the License for the specific language governing permissions and
# limitations under the License.
###Output
_____no_output_____
###Markdown
Word-level Text generation with an LSTM View on TensorFlow.org Run in Google Colab View source on GitHub Download notebook This tutorial demonstrates how to generate text using a word-based RNN. Let's use the [IMDb Reviews dataset](http://ai.stanford.edu/~amaas/data/sentiment/) that consists of Movie reviews on the popular site IMDb. As we are using a Word-level model, it's task is to predict given `positive` the word `reviews` or likewise.Note: Enable GPU acceleration to execute this notebook faster. In Colab: *Runtime > Change runtime type > Hardware accelerator > GPU*. If running locally make sure TensorFlow version >= 1.11.This tutorial includes runnable code implemented using [tf.keras](https://www.tensorflow.org/programmers_guide/keras) and [eager execution](https://www.tensorflow.org/programmers_guide/eager). *Write about output here* Setup Import TensorFlow and other libraries
###Code
import tensorflow as tf
import numpy as np
import os
import time
import tensorflow_datasets as tfds
###Output
_____no_output_____
###Markdown
Download the IMDb datasetAs this dataset is included by TensorFlow in its [TF Datasets catalog](https://www.tensorflow.org/datasets/catalog/imdb_reviews), we can directly use it from there. Change the following line to run this code on your own data.
###Code
imdb = tfds.load('imdb_reviews', split='unsupervised', shuffle_files=True)
###Output
[1mDownloading and preparing dataset imdb_reviews/plain_text/1.0.0 (download: 80.23 MiB, generated: Unknown size, total: 80.23 MiB) to /root/tensorflow_datasets/imdb_reviews/plain_text/1.0.0...[0m
###Markdown
Read the dataFirst, look in the text:
###Code
# Read, then decode for py2 compat.
text = open(path_to_file, 'rb').read().decode(encoding='utf-8')
# length of text is the number of characters in it
print('Length of text: {} characters'.format(len(text)))
# Take a look at the first 250 characters in text
print(text[:250])
# The unique characters in the file
vocab = sorted(set(text))
print('{} unique characters'.format(len(vocab)))
###Output
_____no_output_____
###Markdown
Process the text Vectorize the textBefore training, you need to map strings to a numerical representation. Create two lookup tables: one mapping characters to numbers, and another for numbers to characters.
###Code
# Creating a mapping from unique characters to indices
char2idx = {u:i for i, u in enumerate(vocab)}
idx2char = np.array(vocab)
text_as_int = np.array([char2idx[c] for c in text])
###Output
_____no_output_____
###Markdown
Now you have an integer representation for each character. Notice that you mapped the character as indexes from 0 to `len(unique)`.
###Code
print('{')
for char,_ in zip(char2idx, range(20)):
print(' {:4s}: {:3d},'.format(repr(char), char2idx[char]))
print(' ...\n}')
# Show how the first 13 characters from the text are mapped to integers
print('{} ---- characters mapped to int ---- > {}'.format(repr(text[:13]), text_as_int[:13]))
###Output
_____no_output_____
###Markdown
The prediction task Given a character, or a sequence of characters, what is the most probable next character? This is the task you're training the model to perform. The input to the model will be a sequence of characters, and you train the model to predict the output—the following character at each time step.Since RNNs maintain an internal state that depends on the previously seen elements, given all the characters computed until this moment, what is the next character? Create training examples and targetsNext divide the text into example sequences. Each input sequence will contain `seq_length` characters from the text.For each input sequence, the corresponding targets contain the same length of text, except shifted one character to the right.So break the text into chunks of `seq_length+1`. For example, say `seq_length` is 4 and our text is "Hello". The input sequence would be "Hell", and the target sequence "ello".To do this first use the `tf.data.Dataset.from_tensor_slices` function to convert the text vector into a stream of character indices.
###Code
# The maximum length sentence you want for a single input in characters
seq_length = 100
examples_per_epoch = len(text)//(seq_length+1)
# Create training examples / targets
char_dataset = tf.data.Dataset.from_tensor_slices(text_as_int)
for i in char_dataset.take(5):
print(idx2char[i.numpy()])
###Output
_____no_output_____
###Markdown
The `batch` method lets us easily convert these individual characters to sequences of the desired size.
###Code
sequences = char_dataset.batch(seq_length+1, drop_remainder=True)
for item in sequences.take(5):
print(repr(''.join(idx2char[item.numpy()])))
###Output
_____no_output_____
###Markdown
For each sequence, duplicate and shift it to form the input and target text by using the `map` method to apply a simple function to each batch:
###Code
def split_input_target(chunk):
input_text = chunk[:-1]
target_text = chunk[1:]
return input_text, target_text
dataset = sequences.map(split_input_target)
###Output
_____no_output_____
###Markdown
Print the first example input and target values:
###Code
for input_example, target_example in dataset.take(1):
print('Input data: ', repr(''.join(idx2char[input_example.numpy()])))
print('Target data:', repr(''.join(idx2char[target_example.numpy()])))
###Output
_____no_output_____
###Markdown
Each index of these vectors is processed as a one time step. For the input at time step 0, the model receives the index for "F" and tries to predict the index for "i" as the next character. At the next timestep, it does the same thing but the `RNN` considers the previous step context in addition to the current input character.
###Code
for i, (input_idx, target_idx) in enumerate(zip(input_example[:5], target_example[:5])):
print("Step {:4d}".format(i))
print(" input: {} ({:s})".format(input_idx, repr(idx2char[input_idx])))
print(" expected output: {} ({:s})".format(target_idx, repr(idx2char[target_idx])))
###Output
_____no_output_____
###Markdown
Create training batchesYou used `tf.data` to split the text into manageable sequences. But before feeding this data into the model, you need to shuffle the data and pack it into batches.
###Code
# Batch size
BATCH_SIZE = 64
# Buffer size to shuffle the dataset
# (TF data is designed to work with possibly infinite sequences,
# so it doesn't attempt to shuffle the entire sequence in memory. Instead,
# it maintains a buffer in which it shuffles elements).
BUFFER_SIZE = 10000
dataset = dataset.shuffle(BUFFER_SIZE).batch(BATCH_SIZE, drop_remainder=True)
dataset
###Output
_____no_output_____
###Markdown
Build The Model Use `tf.keras.Sequential` to define the model. For this simple example three layers are used to define our model:* `tf.keras.layers.Embedding`: The input layer. A trainable lookup table that will map the numbers of each character to a vector with `embedding_dim` dimensions;* `tf.keras.layers.GRU`: A type of RNN with size `units=rnn_units` (You can also use an LSTM layer here.)* `tf.keras.layers.Dense`: The output layer, with `vocab_size` outputs.
###Code
# Length of the vocabulary in chars
vocab_size = len(vocab)
# The embedding dimension
embedding_dim = 256
# Number of RNN units
rnn_units = 1024
def build_model(vocab_size, embedding_dim, rnn_units, batch_size):
model = tf.keras.Sequential([
tf.keras.layers.Embedding(vocab_size, embedding_dim,
batch_input_shape=[batch_size, None]),
tf.keras.layers.GRU(rnn_units,
return_sequences=True,
stateful=True,
recurrent_initializer='glorot_uniform'),
tf.keras.layers.Dense(vocab_size)
])
return model
model = build_model(
vocab_size=len(vocab),
embedding_dim=embedding_dim,
rnn_units=rnn_units,
batch_size=BATCH_SIZE)
###Output
_____no_output_____
###Markdown
For each character the model looks up the embedding, runs the GRU one timestep with the embedding as input, and applies the dense layer to generate logits predicting the log-likelihood of the next character: Please note that Keras sequential model is used here since all the layers in the model only have single input and produce single output. In case you want to retrieve and reuse the states from stateful RNN layer, you might want to build your model with Keras functional API or model subclassing. Please check [Keras RNN guide](https://www.tensorflow.org/guide/keras/rnnrnn_state_reuse) for more details. Try the modelNow run the model to see that it behaves as expected.First check the shape of the output:
###Code
for input_example_batch, target_example_batch in dataset.take(1):
example_batch_predictions = model(input_example_batch)
print(example_batch_predictions.shape, "# (batch_size, sequence_length, vocab_size)")
###Output
_____no_output_____
###Markdown
In the above example the sequence length of the input is `100` but the model can be run on inputs of any length:
###Code
model.summary()
###Output
_____no_output_____
###Markdown
To get actual predictions from the model you need to sample from the output distribution, to get actual character indices. This distribution is defined by the logits over the character vocabulary.Note: It is important to _sample_ from this distribution as taking the _argmax_ of the distribution can easily get the model stuck in a loop.Try it for the first example in the batch:
###Code
sampled_indices = tf.random.categorical(example_batch_predictions[0], num_samples=1)
sampled_indices = tf.squeeze(sampled_indices,axis=-1).numpy()
###Output
_____no_output_____
###Markdown
This gives us, at each timestep, a prediction of the next character index:
###Code
sampled_indices
###Output
_____no_output_____
###Markdown
Decode these to see the text predicted by this untrained model:
###Code
print("Input: \n", repr("".join(idx2char[input_example_batch[0]])))
print()
print("Next Char Predictions: \n", repr("".join(idx2char[sampled_indices ])))
###Output
_____no_output_____
###Markdown
Train the model At this point the problem can be treated as a standard classification problem. Given the previous RNN state, and the input this time step, predict the class of the next character. Attach an optimizer, and a loss function The standard `tf.keras.losses.sparse_categorical_crossentropy` loss function works in this case because it is applied across the last dimension of the predictions.Because your model returns logits, you need to set the `from_logits` flag.
###Code
def loss(labels, logits):
return tf.keras.losses.sparse_categorical_crossentropy(labels, logits, from_logits=True)
example_batch_loss = loss(target_example_batch, example_batch_predictions)
print("Prediction shape: ", example_batch_predictions.shape, " # (batch_size, sequence_length, vocab_size)")
print("scalar_loss: ", example_batch_loss.numpy().mean())
###Output
_____no_output_____
###Markdown
Configure the training procedure using the `tf.keras.Model.compile` method. Use `tf.keras.optimizers.Adam` with default arguments and the loss function.
###Code
model.compile(optimizer='adam', loss=loss)
###Output
_____no_output_____
###Markdown
Configure checkpoints Use a `tf.keras.callbacks.ModelCheckpoint` to ensure that checkpoints are saved during training:
###Code
# Directory where the checkpoints will be saved
checkpoint_dir = './training_checkpoints'
# Name of the checkpoint files
checkpoint_prefix = os.path.join(checkpoint_dir, "ckpt_{epoch}")
checkpoint_callback = tf.keras.callbacks.ModelCheckpoint(
filepath=checkpoint_prefix,
save_weights_only=True)
###Output
_____no_output_____
###Markdown
Execute the training To keep training time reasonable, use 10 epochs to train the model. In Colab, set the runtime to GPU for faster training.
###Code
EPOCHS = 10
history = model.fit(dataset, epochs=EPOCHS, callbacks=[checkpoint_callback])
###Output
_____no_output_____
###Markdown
Generate text Restore the latest checkpoint To keep this prediction step simple, use a batch size of 1.Because of the way the RNN state is passed from timestep to timestep, the model only accepts a fixed batch size once built.To run the model with a different `batch_size`, you need to rebuild the model and restore the weights from the checkpoint.
###Code
tf.train.latest_checkpoint(checkpoint_dir)
model = build_model(vocab_size, embedding_dim, rnn_units, batch_size=1)
model.load_weights(tf.train.latest_checkpoint(checkpoint_dir))
model.build(tf.TensorShape([1, None]))
model.summary()
###Output
_____no_output_____
###Markdown
The prediction loopThe following code block generates the text:* Begin by choosing a start string, initializing the RNN state and setting the number of characters to generate.* Get the prediction distribution of the next character using the start string and the RNN state.* Then, use a categorical distribution to calculate the index of the predicted character. Use this predicted character as our next input to the model.* The RNN state returned by the model is fed back into the model so that it now has more context, instead of only one character. After predicting the next character, the modified RNN states are again fed back into the model, which is how it learns as it gets more context from the previously predicted characters.Looking at the generated text, you'll see the model knows when to capitalize, make paragraphs and imitates a Shakespeare-like writing vocabulary. With the small number of training epochs, it has not yet learned to form coherent sentences.
###Code
def generate_text(model, start_string):
# Evaluation step (generating text using the learned model)
# Number of characters to generate
num_generate = 1000
# Converting our start string to numbers (vectorizing)
input_eval = [char2idx[s] for s in start_string]
input_eval = tf.expand_dims(input_eval, 0)
# Empty string to store our results
text_generated = []
# Low temperature results in more predictable text.
# Higher temperature results in more surprising text.
# Experiment to find the best setting.
temperature = 1.0
# Here batch size == 1
model.reset_states()
for i in range(num_generate):
predictions = model(input_eval)
# remove the batch dimension
predictions = tf.squeeze(predictions, 0)
# using a categorical distribution to predict the character returned by the model
predictions = predictions / temperature
predicted_id = tf.random.categorical(predictions, num_samples=1)[-1,0].numpy()
# Pass the predicted character as the next input to the model
# along with the previous hidden state
input_eval = tf.expand_dims([predicted_id], 0)
text_generated.append(idx2char[predicted_id])
return (start_string + ''.join(text_generated))
print(generate_text(model, start_string=u"ROMEO: "))
###Output
_____no_output_____
###Markdown
The easiest thing you can do to improve the results is to train it for longer (try `EPOCHS = 30`).You can also experiment with a different start string, try adding another RNN layer to improve the model's accuracy, or adjust the temperature parameter to generate more or less random predictions. Advanced: Customized TrainingThe above training procedure is simple, but does not give you much control.So now that you've seen how to run the model manually let's unpack the training loop, and implement it ourselves. This gives a starting point if, for example, you want to implement _curriculum learning_ to help stabilize the model's open-loop output.Use `tf.GradientTape` to track the gradients. You can learn more about this approach by reading the [eager execution guide](https://www.tensorflow.org/guide/eager).The procedure works as follows:* First, reset the RNN state. You do this by calling the `tf.keras.Model.reset_states` method.* Next, iterate over the dataset (batch by batch) and calculate the *predictions* associated with each.* Open a `tf.GradientTape`, and calculate the predictions and loss in that context.* Calculate the gradients of the loss with respect to the model variables using the `tf.GradientTape.grads` method.* Finally, take a step downwards by using the optimizer's `tf.train.Optimizer.apply_gradients` method.
###Code
model = build_model(
vocab_size=len(vocab),
embedding_dim=embedding_dim,
rnn_units=rnn_units,
batch_size=BATCH_SIZE)
optimizer = tf.keras.optimizers.Adam()
@tf.function
def train_step(inp, target):
with tf.GradientTape() as tape:
predictions = model(inp)
loss = tf.reduce_mean(
tf.keras.losses.sparse_categorical_crossentropy(
target, predictions, from_logits=True))
grads = tape.gradient(loss, model.trainable_variables)
optimizer.apply_gradients(zip(grads, model.trainable_variables))
return loss
# Training step
EPOCHS = 10
for epoch in range(EPOCHS):
start = time.time()
# resetting the hidden state at the start of every epoch
model.reset_states()
for (batch_n, (inp, target)) in enumerate(dataset):
loss = train_step(inp, target)
if batch_n % 100 == 0:
template = 'Epoch {} Batch {} Loss {}'
print(template.format(epoch + 1, batch_n, loss))
# saving (checkpoint) the model every 5 epochs
if (epoch + 1) % 5 == 0:
model.save_weights(checkpoint_prefix.format(epoch=epoch))
print('Epoch {} Loss {:.4f}'.format(epoch + 1, loss))
print('Time taken for 1 epoch {} sec\n'.format(time.time() - start))
model.save_weights(checkpoint_prefix.format(epoch=epoch))
###Output
_____no_output_____ |
_sources/curriculum-notebooks/Science/LightOpticalSystems/light-optical-systems.ipynb | ###Markdown

###Code
import matplotlib.pyplot as plt
import plotly as py
import plotly.graph_objs as go
import numpy as np
import math
import ipywidgets as widgets
from IPython.display import display, Math, Latex, HTML, IFrame
from astropy.table import Table, Column
from ipywidgets import interact, interactive
py.offline.init_notebook_mode(connected=True)
%matplotlib inline
font = {'family' : 'sans-serif',
'weight' : 'normal',
'size' : 14}
plt.rc('font', **font)
'''Above, we are importing all the necessary modules in order to run the notebook.
Numpy allows us to define arrays of values for our variables to plot them
matplotlib is what we use to create the figures
the display and widgets are to make the notebook look neat
'''
HTML('''<script>
function code_toggle() {
if (code_shown){
$('div.input').hide('500');
$('#toggleButton').val('Show Code')
} else {
$('div.input').show('500');
$('#toggleButton').val('Hide Code')
}
code_shown = !code_shown
}
$( document ).ready(function(){
code_shown=false;
$('div.input').hide()
});
</script>
<form action="javascript:code_toggle()"><input type="submit" id="toggleButton" value="Show Code"></form>''')
###Output
_____no_output_____
###Markdown
*** **Light and Optics** ***Gif taken from https://giphy.com/gifs/fandor-sun-eclipse-3o7OsM9vKFH2ESl0KA/links, August 1st, 2018. Figure 1: For hundreds of years, scientists have tried to understand the nature of light. With advances in technology, and inventions like telescopes, we have been able to see farther than ever before. *** IntroductionThroughout most of history, humans did not understand light as we do today. As science and technology have progressed over time, so too has our knowledge of the nature of light. In this lesson, when we say the word "light", we will be referring to visible light (light that comes from the sun, lightbulbs etc.). We will go over how a few key experiments kickstarted a new way of thinking, and a few of the ways that we are able to manipulate light. We will also talk about how our eyes enable us to see. BackgroundIf you had to describe to someone what light is, you may have a hard time. Some people think of light as the absence of darkness, but even that doesn't say much about light itself.Our understanding of light truly began around the 17th century, when a few individuals started to realize that light was not a mystical substance. Scientists (or "natural philosophers", as they were called during that time) recognized that certain properties of light were measurable, and that some properties could be manipulated. Sir Isaac Newton and Ole Rømer were among the first scientists to take a step in this direction.> Isaac Newton's Prism ExperimentSir Isaac Newton has made contributions to many fields of science and mathematics. In 1666, while spending time at his childhood home in Lincolnshire, England, Newton began experimenting with light. Using a small slit in his window shutters, Newton passed a narrow beam of sunlight through a glass prism. The light travelled through the prism, and projected a rainbow of color on the other side!Picture taken from http://lightingmatters.com.au/wp/what-is-the-colour-of-white/white-light-prism-experiment/, July 30th, 2018. Figure 2: This picture shows how a prism can create a spectrum of color. This is what Newton would have seen in 1666.Later on, scientists determined that the prism was actually splitting light into its component parts. This phenomenon is called **dispersion**.Through this experiment, Newton demonstrated that white light was actually made up of all the individual colors of the rainbow!> Ole Rømer and the Speed of LightFor many years, people thought that if somebody lit a match, the light from that match would be instantly visible to everyone, no matter how far away they were. However, in 1676 Ole Rømer proved that this is not the case.Rømer spent a long time studying the orbit of Io, one of Jupiter's moons. As part of his study, he began predicting the times when Io should be hidden behind Jupiter's shadow (these periods are called eclipses). However, Rømer saw that his predictions for when these eclipses should occur were not always accurate. Gif taken from https://giphy.com/gifs/timelapse-DXIa1beDspYRy, August 1st, 2018. Figure 3: Here we can see Jupiter as it looks through a telescope. You might be able to see a black spot move from the left to the right across Jupiter's surface. This is actually one of Jupiter's many moons! Rømer then realized that these errors may be because the distance between Io and the Earth was always changing. Rømer thought that when the distance between Io and the Earth increased, it might take a longer time for light coming from Io to reach Earth. If this were the case, then the light must be travelling at a finite speed!After taking many measurements and using some clever mathematics, Rømer calculated the speed of light to be roughly 220,000,000 m/s, or 792,000,000 km/h.Today, we have measured the speed of light to be 299,792,458 m/s. Although he was not exactly right, Rømer provided one of the first mathematical calculations for the speed of light. ***Since the time of Rømer and Newton, scientists have made many new discoveries about the nature of light. While not all of these discoveries agree with one another, here are two things we know for sure:- Light is made up of a spectrum of color- Light travels at a speed of 299,792,458 m/sNow let's talk about some of the ways we can manipulate light.*** ReflectionWe are all familiar with reflection; chances are, you look at your reflection more than once a day. But have you ever stopped to wonder what is really going on? Reflection is the term used to describe how light can change direction when it comes into contact with certain surfaces. When incoming light rays encounter a reflective surface, they bounce off the surface and continue moving in a new direction. The new direction in which it moves is determined by the **law of reflection**.\begin{equation} \rm Law\: of\: Reflection: Angle\: of\: Incidence = Angle\: of\: Reflection\end{equation}On the animation below, click on the flashlight to turn it on, and move your mouse to change the angle of incidence.
###Code
IFrame('Animations/reflect.html',width=500,height=320)
###Output
_____no_output_____
###Markdown
As seen above, the **normal** is what we call the line that forms a 90$^{\circ}$ angle with the surface. The **angle of incidence** is what we call the angle between the flash lights beam and the normal. Similarly, the **angle of reflection** is the angle that the newly reflected light beam makes with the normal. The law of reflection states that these two angles will always be equal. RefractionHave you ever tried to reach down and grab an object sitting at the bottom of a pool of water? If you have, you may have noticed that the object isn't actually in the location that you thought it was. Image taken from http://legacy.sciencelearn.org.nz/Contexts/Light-and-Sight/Sci-Media/Video/Refraction/(quality)/hi on August 3rd, 2018. Figure 4: When you are looking into a body of water from above, the objects you see beneath the surface are not actually where they appear to be. This phenomenon occurs because the light travelling to your eyes from the bottom of the pool **refracts**, or changes its direction of travel, when it transitions from water to air. The **index of refraction** is a value that we use to show how much light will bend when travelling through a substance. For example, the index of refraction for air is approximately 1.00, and the index of refraction for water is about 1.33. Because these indexes are different, light will bend when passing from water to air, or vice versa.Use the animation below to see how light refracts when passing from air to water. Click on the flashlight to turn it on.
###Code
IFrame('Animations/refract.html',width=520,height=320)
###Output
_____no_output_____
###Markdown
Mathematically, reflection can be described using the following equation, known as Snell's Law:\begin{equation} \textrm{Snells Law:}\: n_1\sin(\theta_1) = n_2\sin(\theta_2)\end{equation}where $n_1$ is the index of refraction for the first medium, $\theta_1$ is the incident angle, $n_2$ is the index of refraction for the second medium, and $\theta_2$ is the angle of refraction.Light will bend *towards* the normal when travelling from a medium with a *lower* index of refraction to one with a *higher* index of refraction, and vice versa.***Some of the most beautiful sights in nature are caused by reflection and refraction. Here are a couple of examples: RainbowsRainbows are a result of both reflection and refraction. As its raining, each water droplet acts like a tiny prism, just like the one we saw in Figure 2. The water droplets split visible light into colors, and these colors are then reflected back towards our eyes. Image taken from https://waterstories.nestle-waters.com/environment/how-does-a-rainbow-form/ on August 3rd, 2018. Figure 5: Water droplets use reflection and refraction to create the beautiful rainbows that we see while it is raining. MiragesHave you ever been driving on a sunny day, and up ahead it looks as though a stream of water is running across the road? You are really seeing a mirage.Mirages also occur because of refraction, but they do not result in a display of color like a rainbow. This type of refraction occurs due to a difference in temperature between separate layers of air.As we were describing before, refraction occurs when light travels from one substance to another. Well, it turns out that hot air and cold air are actually different enough to act as different substances. Therefore, light will refract when passing through one to the other. Image taken from https://edexcellence.net/articles/what-the-mirage-gets-wrong-on-teacher-development on August 3rd, 2018. Figure 6: Although it may look like water running across the road, it is actually a mirage. These commonly occur in desert areas, where the road can become very hot.When you are looking at a mirage, it can look as though the air is wavy and fluid, which is why it is common to think that you are looking at water. This appearance occurs when layers of hot and cold air are mixing together, and light passing through these layers is constantly being refracted in different directions.You may see a mirage appear on top of a hot roadway, behind the exhaust pipe of a plane or car, or around any other source of heat. Applications of Reflection and Refraction LensesIf you have glasses, or contact lenses, then you are constantly using refraction in order to help you see! Lenses use refraction to point light in specific directions.Generally speaking, there are two types of lenses: **convex** and **concave**.To see how each type of lense affects light, use the following animation.
###Code
IFrame('Animations/convex.html',width=520,height=420)
###Output
_____no_output_____ |
introduction-to-data-analysis-and-visualization.ipynb | ###Markdown
Introduction to Data Analysis and VisualizationKuo, Yao-Jen, 2020-07-11> [slido](https://www.sli.do/) 54913>> >> [binder](https://mybinder.org/v2/gh/yaojenkuo/talks/master)
###Code
import os
import re
from string import ascii_uppercase
import numpy as np
import matplotlib.pyplot as plt
import pandas as pd
###Output
_____no_output_____
###Markdown
TL; DR> In this talk, we are gonna briefly introduce what is data analysis and visualization with modern data science approach. About me A data enthusiast and instructor focused on practical Python/R/SQL Teaching practical data science online/offline- [如何成為資料分析師:從問題解決到行動方案,Hahow 好學校](https://hahow.in/cr/dajourney)- Visualization and modern data science, Adjunct Instructor, National Taiwan University- Python for data analysis, Instructor, Chunghwa Telecom Academy- Python for data science, Machine learning from scratch, Senior Instructor, CSIE Train, National Taiwan University Data science instructor focused on commercial banking clients- 2020 DBS Python Training Program- 2019 HNCB Python Training Program- 2017 ESUN Python Training Program Writing books on data science- [輕鬆學習 R 語言](https://www.books.com.tw/products/0010835361)- [進擊的資料科學](https://www.books.com.tw/products/0010827812)- [新手村逃脫!初心者的 Python 機器學習攻略](https://yaojenkuo.io/ml-newbies/index.html) Writing tutorials on [Medium](https://medium.com/) with 3k+ followers- Follow my Medium account [@tonykuoyj](https://medium.com/@tonykuoyj)- Or, follow my publication [DataInPoint](https://medium.com/datainpoint)- Or, follow [DataInPoint](https://www.facebook.com/datainpoint) on Facebook Working experience and eductation- Working experience - Senior Data Analyst, Coupang Shanghai - Analytical Consultant, SAS Taiwan - Management Associate, Chinatrust Banking Corporation Taiwan - Research Assistant, McKinsey & Company Taiwan- Education - MBA, National Taiwan University - BA, National Taiwan University Loves running with a marathon PR of 2:43:12 at 2019 Seoul Marathon Future initiativesMake DataInPoint a text-based, in-browser, and subscription-only data science bootcamp. What is data analysis The definition> We generate questions about a specific topic, we search for answers by exploring, transforming, and modelling data referring to our topic. And then use what we've learned to refine questions or generate new questions.Source: [R for Data Science](https://r4ds.had.co.nz/) Why data analysis> It is now an era of data-driven strategic thinking, and is probably never coming back. The three means of persuasion that an orator must rely on- ethos- pathos- logosSource: [Aristotle, Rhetoric](https://en.wikipedia.org/wiki/Rhetoric) It is a lot easier to persuade via ethos or pathos, but it takes timeHowever, logos can be easily acquired once it is a fact and can be proven. Hence, data analysis is often the express way to logos. Let's bring up a topic NOW> 大選開票看哪個里最準? 「章魚里」神預測告訴你。每次到了選舉,總是會有幾個里開票與大選結果相似,因此被各界視為重點關注的開票區域。Source: We can then generate some questions regarding this topic- How to define 「章魚里」?- Can we find out 「章魚里」 based on 2020 presidential data?- Can we find the similarity of our own village? We'll do this later, now you've got the idea of data analysis, let's move on What is visualization The definition> Visualization is a tool that addresses the gap between data, function, formula, and viewers. Effective visualizations transform abstract collections of numbers into shapes that viewers quickly grasp and understand.Source: [Data Visualization with JavaScript](https://www.amazon.com/Data-Visualization-JavaScript-Stephen-Thomas-ebook/dp/B00V20IFDG) Why visualization
###Code
x = np.linspace(0, 4*np.pi)
f = np.sin(x)
###Output
_____no_output_____
###Markdown
What are $x$ and $f$
###Code
print(x)
print(f)
###Output
[ 0. 0.25645654 0.51291309 0.76936963 1.02582617 1.28228272
1.53873926 1.7951958 2.05165235 2.30810889 2.56456543 2.82102197
3.07747852 3.33393506 3.5903916 3.84684815 4.10330469 4.35976123
4.61621778 4.87267432 5.12913086 5.38558741 5.64204395 5.89850049
6.15495704 6.41141358 6.66787012 6.92432667 7.18078321 7.43723975
7.69369629 7.95015284 8.20660938 8.46306592 8.71952247 8.97597901
9.23243555 9.4888921 9.74534864 10.00180518 10.25826173 10.51471827
10.77117481 11.02763136 11.2840879 11.54054444 11.79700098 12.05345753
12.30991407 12.56637061]
[ 0.00000000e+00 2.53654584e-01 4.90717552e-01 6.95682551e-01
8.55142763e-01 9.58667853e-01 9.99486216e-01 9.74927912e-01
8.86599306e-01 7.40277997e-01 5.45534901e-01 3.15108218e-01
6.40702200e-02 -1.91158629e-01 -4.33883739e-01 -6.48228395e-01
-8.20172255e-01 -9.38468422e-01 -9.95379113e-01 -9.87181783e-01
-9.14412623e-01 -7.81831482e-01 -5.98110530e-01 -3.75267005e-01
-1.27877162e-01 1.27877162e-01 3.75267005e-01 5.98110530e-01
7.81831482e-01 9.14412623e-01 9.87181783e-01 9.95379113e-01
9.38468422e-01 8.20172255e-01 6.48228395e-01 4.33883739e-01
1.91158629e-01 -6.40702200e-02 -3.15108218e-01 -5.45534901e-01
-7.40277997e-01 -8.86599306e-01 -9.74927912e-01 -9.99486216e-01
-9.58667853e-01 -8.55142763e-01 -6.95682551e-01 -4.90717552e-01
-2.53654584e-01 -4.89858720e-16]
###Markdown
$f(x)=sin(x), \text{where } 0 \leq x \leq 4\pi$
###Code
def plot_sinx(x_arr, y_arr):
fig = plt.figure()
ax = plt.axes()
ax.plot(x_arr, y_arr)
ax.set_xticks([0, np.pi, 2*np.pi, 3*np.pi, 4*np.pi])
ax.set_xticklabels(['$0$', '$\pi$', '$2\pi$', '$3\pi$', '$4\pi$'])
ax.set_yticks([-1, 0, 1])
ax.set_yticklabels(['$-1$', '$0$', '$1$'])
ax.set_title('$f(x)=sin(x)$')
plt.show()
plot_sinx(x, f)
###Output
_____no_output_____
###Markdown
What does the following formula stand for$$\sigma(z) = \frac{1}{1 + e^{-z}}$$
###Code
def sigmoid(x):
return 1 / (1 + np.exp(-x))
def plot_sigmoid(x_arr, y_arr):
fig = plt.figure()
ax = plt.axes()
ax.plot(x_arr, y_arr)
ax.set_yticks([0, 0.5, 1])
ax.set_xticks([])
ax.set_title('$\sigma(z) = 1/(1+e^{-z})$')
ax.set_xlabel('z')
plt.show()
x = np.linspace(-6, 6)
S = sigmoid(x)
plot_sigmoid(x, S)
###Output
_____no_output_____
###Markdown
Visualization also plays a key role in machine learning Machine learning engineer uses line plot to visualize loss function
###Code
def plot_logistic_loss_function(x_arr, epsilon=1e-06):
y0 = -np.log(1-x_arr + epsilon)
y1 = -np.log(x_arr + epsilon)
fig = plt.figure()
ax = plt.axes()
ax.plot(x_arr, y0, label="$-log(h(x))$ if y = 0")
ax.plot(x_arr, y1, label="$-log(1 - h(x))$ if y = 1")
ax.legend()
ax.set_title("Logistic Regression Loss Function")
ax.set_xlabel("$h(x)$")
ax.set_ylabel("Loss")
plt.show()
x = np.linspace(0, 1, 100)
plot_logistic_loss_function(x)
###Output
_____no_output_____
###Markdown
Machine learning engineer uses surface plot to visualize gradientsSource: [Coursera | Machine Learning](https://www.coursera.org/learn/machine-learning) Machine learning engineer also uses contour plot to visualize gradientsSource: [Coursera | Machine Learning](https://www.coursera.org/learn/machine-learning) Machine learning engineer uses scatter plot and contour plot to visualize decision boundarySource:
###Code
from itertools import product
from sklearn import datasets
from sklearn.tree import DecisionTreeClassifier
from sklearn.neighbors import KNeighborsClassifier
from sklearn.svm import SVC
from sklearn.ensemble import VotingClassifier
def plot_multiple_decision_boundary():
# Loading some example data
iris = datasets.load_iris()
X = iris.data[:, [0, 2]]
y = iris.target
# Training classifiers
clf1 = DecisionTreeClassifier(max_depth=4)
clf2 = KNeighborsClassifier(n_neighbors=7)
clf3 = SVC(gamma=.1, kernel='rbf', probability=True)
eclf = VotingClassifier(estimators=[('dt', clf1), ('knn', clf2),
('svc', clf3)],
voting='soft', weights=[2, 1, 2])
clf1.fit(X, y)
clf2.fit(X, y)
clf3.fit(X, y)
eclf.fit(X, y)
# Plotting decision regions
x_min, x_max = X[:, 0].min() - 1, X[:, 0].max() + 1
y_min, y_max = X[:, 1].min() - 1, X[:, 1].max() + 1
xx, yy = np.meshgrid(np.arange(x_min, x_max, 0.1),
np.arange(y_min, y_max, 0.1))
f, axarr = plt.subplots(2, 2, sharex='col', sharey='row', figsize=(10, 8))
for idx, clf, tt in zip(product([0, 1], [0, 1]),
[clf1, clf2, clf3, eclf],
['Decision Tree (depth=4)', 'KNN (k=7)',
'Kernel SVM', 'Soft Voting']):
Z = clf.predict(np.c_[xx.ravel(), yy.ravel()])
Z = Z.reshape(xx.shape)
axarr[idx[0], idx[1]].contourf(xx, yy, Z, alpha=0.4)
axarr[idx[0], idx[1]].scatter(X[:, 0], X[:, 1], c=y,
s=20, edgecolor='k')
axarr[idx[0], idx[1]].set_title(tt)
plt.show()
plot_multiple_decision_boundary()
###Output
_____no_output_____
###Markdown
It is also best practice to visualize decision boundary for deep learning modelsSource: The 2 most influential visualizations of ALL TIME1. [Minard's map of Napoleon's disastrous Russian campaign of 1812](https://en.wikipedia.org/wiki/Flow_map/media/File:Minard.png)2. [Hans Rosling's 200 Countries, 200 Years, 4 Minutes](https://youtu.be/jbkSRLYSojo) [Minard's map of Napoleon's disastrous Russian campaign of 1812](https://en.wikipedia.org/wiki/Flow_map/media/File:Minard.png)In 1812, Napoleon marched to Moscow. 98% of his soldiers died. The simple but fascinating temperature line below the viz shows how cold ultimately defeated Napoleon’s army. [Hans Rosling's 200 Countries, 200 Years, 4 Minutes](https://youtu.be/jbkSRLYSojo)A 2007 TED talk for the Swedish scientist shared his passion with the world. It shows the relationship between wealth and health. >The simple graph has brought more information to the data analyst’s mind than any other device.>>[John Tukey](https://en.wikipedia.org/wiki/John_Tukey) [John Tukey](https://en.wikipedia.org/wiki/John_Tukey) is an American mathematician best known for development of box plot.
###Code
gapminder = pd.read_csv("https://python4ds.s3-ap-northeast-1.amazonaws.com/gapminder.csv")
def plot_box_plot(df):
continents = df["continent"].unique()
list_of_arr = [df[df["continent"] == cont]["gdpPercap"].values for cont in continents]
fig = plt.figure()
ax = plt.axes()
ax.boxplot(list_of_arr)
ax.set_ylim(0, 60000)
ax.set_xticklabels(continents)
ax.set_title("A Box Plot of Gapminder's GDP Per Capita")
ax.set_ylabel("GDP Per Capita")
plt.show()
plot_box_plot(gapminder)
###Output
_____no_output_____
###Markdown
What is modern data science > Modern data science is a huge field, it invovles applications and tools like importing, tidying, transformation, visualization, modeling, and communication. Surrounding all these is programming.Source: [R for Data Science](https://r4ds.had.co.nz/) Source: Source: Meet my favorite data scientist on TV show: Chandler Bing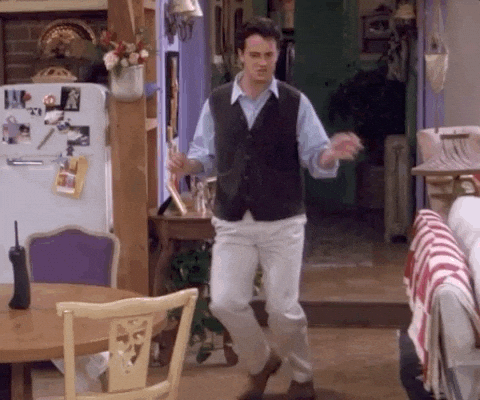Source: Source: Source: In fact, Python(or R, Julia, Matlab ...etc.) is capable to tackle everythingSource: [R for Data Science](https://r4ds.had.co.nz/) So it is inevitable to write codes in modern data science What does it take to write a programSource: Text editors and interpreter/compiler are your points of support! Let's explore 3 questions in [2019 Kaggle ML & DS Survey](https://www.kaggle.com/c/kaggle-survey-2019)- Q14: What is the primary tool that you use at work or school to analyze data?- Q18: What programming languages do you use on a regular basis?- Q19: What programming language would you recommend an aspiring data scientist to learn first? What is [Kaggle](https://kaggle.com/)> A subsidiary of Google, is an online community of data scientists and machine learning practitioners. Kaggle allows users to find and publish data sets, explore and build models in a web-based data-science environment, work with other data scientists and machine learning engineers, and enter competitions to solve data science challenges.Source:
###Code
def get_value_ratios(df, col_name):
return df[col_name].value_counts() / df[col_name].value_counts().sum()
def get_checkbox_ratios(df, col_pattern):
channel_names = []
channel_checks = []
for col_name in df.columns:
if (col_pattern in col_name) and ('OTHER_TEXT' not in col_name):
channel_name = df[col_name].value_counts().index
channel_check = df[col_name].value_counts().values
if channel_name.size != 0:
channel_names.append(channel_name[0])
channel_checks.append(channel_check[0])
channel_counts = pd.Series(channel_checks, index=channel_names)
channel_ratios = channel_counts / channel_counts.sum()
channel_ratios_sorted = channel_ratios.sort_values(ascending=False)
return channel_ratios_sorted
survey_2019 = pd.read_csv("https://kaggle-ml-ds-survey.s3-ap-northeast-1.amazonaws.com/kaggle-survey-2019/multiple_choice_responses.csv", encoding='ISO-8859-1', skiprows=[1], low_memory=False)
da_2019 = survey_2019[survey_2019['Q5'] == 'Data Analyst']
def plot_ans_14(ans_14_ser):
fig = plt.figure()
ax = plt.axes()
ans_14_ser = ans_14_ser[::-1]
ax.barh(ans_14_ser.index, ans_14_ser.values, color=['c', 'c', 'c', 'c', 'c', 'r'])
ax.set_title("What is the primary tool that you use at work or school to analyze data?")
ax.set_xticks([0, 0.1, 0.2, 0.3, 0.4, 0.5])
ax.set_xticklabels(['0%', '10%', '20%', '30%', '40%', '50%'])
ax.spines['top'].set_visible(False)
ax.spines['right'].set_visible(False)
ax.spines['bottom'].set_visible(False)
plt.show()
def get_ax(ans_ser, plot_title, ax):
ax = ax
ans_ser = ans_ser[:10][::-1]
ax.barh(ans_ser.index, ans_ser.values, color=['c', 'c', 'c', 'c', 'c', 'c', 'c', 'r', 'r', 'r'])
ax.spines['top'].set_visible(False)
ax.spines['right'].set_visible(False)
ax.spines['bottom'].set_visible(False)
ax.set_title(plot_title)
def plot_ans_18_19(ans_18_ser, ans_19_ser):
fig, (ax1, ax2) = plt.subplots(1, 2, figsize=(14, 4))
get_ax(ans_18_ser, "What programming languages do you use on a regular basis?", ax1)
get_ax(ans_19_ser, "What programming language would you recommend \n an aspiring data scientist to learn first?", ax2)
plt.tight_layout()
plt.show()
ans_14 = get_value_ratios(da_2019, 'Q14')
ans_18 = get_checkbox_ratios(da_2019, 'Q18')
ans_19 = get_value_ratios(da_2019, 'Q19')
# What is the primary tool that you use at work or school to analyze data?
plot_ans_14(ans_14)
# What programming languages do you use on a regular basis?
# What programming language would you recommend an aspiring data scientist to learn first?
plot_ans_18_19(ans_18, ans_19)
###Output
_____no_output_____
###Markdown
Now you've got what data analysis, visualization, and modern data science are We can answer our questions using a bit of technical assists- How to define 「章魚里」?- Can we find out 「章魚里」 based on 2020 presidential data?- Can we find the similarity of our own village? How to define 「章魚里」Basically, after a few literature search, you may find the definition of 「章魚里」 is quite ambigious. So we are using a much fancier metric: **cosine similarity**. What is cosine similarity> Cosine similarity is a measure of similarity between two non-zero vectors of an inner product space. It is defined to equal the cosine of the angle between them, which is also the same as the inner product of the same vectors normalized to both have length 1.\begin{equation}a = (a_1, a_2, a_3) \\b = (b_1, b_2, b_3)\end{equation}\begin{align}cos\theta &= \frac{\sum_i(a_i \times b_i)}{\sqrt{\sum_i a_i^2} \times \sqrt{\sum_i b_i^2}} \\&= \frac{a \cdot b}{\parallel a \parallel \times \parallel b \parallel}\end{align}Source: [Cosine similarity](https://en.wikipedia.org/wiki/Cosine_similarity) Can we find out 「章魚里」 based on 2020 presidential dataDefinitely, we all have access to [Central Election Commission](https://db.cec.gov.tw/). Now we have to write code to integrate these spreadsheets.
###Code
from presidential import Presidential
presidential = Presidential('presidential-2020')
presidential_df = presidential.adjust_presidential_df()
presidential_df.head()
presidential_df.tail()
###Output
_____no_output_____
###Markdown
Check if the summations are right
###Code
ttl_votes = presidential_df['votes'].sum()
ttl_votes_by_candidates = presidential_df.groupby('number')['votes'].sum()
ttl_votes_by_candidates
###Output
_____no_output_____
###Markdown
National percentage is our target vector to be compared
###Code
national_percentage = ttl_votes_by_candidates / ttl_votes
national_percentage
###Output
_____no_output_____
###Markdown
Total votes for each village
###Code
combined_key = presidential_df['county'].str.cat(presidential_df['town']).str.cat(presidential_df['village'])
presidential_df = presidential_df.assign(combined_key=combined_key)
ttl_votes_by_combined_key = presidential_df.groupby(['combined_key'])['votes'].sum()
ttl_votes_by_combined_key
###Output
_____no_output_____
###Markdown
Votes percentage by each candidate and village
###Code
ttl_votes_by_combined_key_candidates = presidential_df.groupby(['combined_key', 'number'])['votes'].sum()
soong = ttl_votes_by_combined_key_candidates[:, '1'] / ttl_votes_by_combined_key
han = ttl_votes_by_combined_key_candidates[:, '2'] / ttl_votes_by_combined_key
tsai = ttl_votes_by_combined_key_candidates[:, '3'] / ttl_votes_by_combined_key
votes_obtained = pd.concat([soong, han, tsai], axis=1)
votes_obtained.columns = ['soong', 'han', 'tsai']
votes_obtained
###Output
_____no_output_____
###Markdown
Calculate cosine similarity
###Code
a = national_percentage.values
a_norm = np.linalg.norm(a)
cos_similarities = []
for i in range(votes_obtained.shape[0]):
b = votes_obtained.iloc[i, :].values
b_norm = np.linalg.norm(b)
ab = np.dot(a, b)
cos_similarity = np.dot(a, b) / (a_norm*b_norm)
cos_similarities.append(cos_similarity)
votes_obtained = votes_obtained.assign(cosine_similarity=cos_similarities)
votes_obtained = votes_obtained.reset_index()
###Output
_____no_output_____
###Markdown
Sort by cosine similarity with descending order to find 「章魚里」
###Code
votes_obtained.sort_values(['cosine_similarity', 'combined_key'], ascending=[False, True]).head(10)
###Output
_____no_output_____
###Markdown
Can we find the similarity of our own village?Definitely.
###Code
def find_my_village(my_village):
return votes_obtained[votes_obtained['combined_key'] == my_village]
find_my_village('臺北市中正區文盛里')
###Output
_____no_output_____ |
trends.ipynb | ###Markdown
***Python TA library***[TA Library](https://github.com/bukosabino/ta)
###Code
import ta
df["RSI"] = ta.momentum.rsi(df["Close"])
df["RSI"].plot(figsize = (10, 2))
margin = 10
print(margin)
df['Close average 75W'] = df['Close'].rolling(525, min_periods=1).mean()
df['Close average 40W'] = df['Close'].rolling(280, min_periods=1).mean()
df['Close average 75W+'] = df['Close average 75W']*(1+(margin/100))
df['Close average 75W-'] = df['Close average 75W']*(1-(margin/100))
#Changing the column in which we need the difference to % change
df.drop("Adj Close", axis = 1, inplace = True)
df[["Open", "High", "Low", "Close"]] = df[["Open", "High", "Low", "Close"]].pct_change().fillna(0)
df[['Close average 75W+','Close','Close average 75W', 'Close average 75W-', 'Close average 40W']].plot(figsize=(20,10))
for i in range(0, len(df)):
if df['Close average 75W+'].iloc[i] > df[]
###Output
_____no_output_____ |
docs/notebooks/slippage_example.ipynb | ###Markdown
Slippage Analysis When evaluating a strategy using backtest results, we often want to know how sensitive it's performance is to implementation shortfall or slippage. pyfolio's transactions tear sheet can create "slippage sweep" plots that display strategy performance under various slippage assumptions. Additional per-dollar slippage can be applied to returns before running a tear sheet by providing `create_full_tear_sheet` with the a level of slippage in basis points (1% == 100 basis points) as the `slippage` keyword argument. The slippage plots in the transactions tear sheet will display returns with slippage added to the **unadjusted** returns. For example, if you run a backtest with no transaction costs and call `create_full_tear_sheet(returns, positions, transactions, slippage=5)`, 5 bps of slippage will be applied to `returns` before all plots and figures, with the exception of the slippage sweep plots, are generated.It is important to emphasize that the slippage plots will display performance under **additional** slippage. If the passed performance data already has slippage applied, the 5 bps slippage equity curve will represent performance under 5 bps of slippage in addition to the already simulated slippage penalty. If slippage is already applied to the performance results, pass `slippage=0` to the `create_full_tear_sheet` to trigger the creation of the additional slippage sweep plots without applying any additional slippage to the returns time series used throughout the rest of the tear sheet.
###Code
%matplotlib inline
import pyfolio as pf
import gzip
import pandas as pd
# silence warnings
import warnings
warnings.filterwarnings('ignore')
transactions = pd.read_csv(gzip.open('../tests/test_data/test_txn.csv.gz'),
index_col=0, parse_dates=True)
positions = pd.read_csv(gzip.open('../tests/test_data/test_pos.csv.gz'),
index_col=0, parse_dates=True)
returns = pd.read_csv(gzip.open('../tests/test_data/test_returns.csv.gz'),
index_col=0, parse_dates=True, header=None)[1]
returns.index = returns.index.tz_localize("UTC")
positions.index = positions.index.tz_localize("UTC")
transactions.index = transactions.index.tz_localize("UTC")
pf.create_full_tear_sheet(returns, positions, transactions, slippage=0)
###Output
Entire data start date: 2004-01-09
Entire data end date: 2009-12-31
Backtest months: 71
|
Projects_Jupyter/.ipynb_checkpoints/Star_Wars_Survey-checkpoint.ipynb | ###Markdown
Star Wars Survey (work in progress) In this project we analyse data from a FiveThirtyEight survey on the first two Star Wars trilogies, whose results are reported in [this article](https://fivethirtyeight.com/features/americas-favorite-star-wars-movies-and-least-favorite-characters/) published on 22 July 2014. Data cleaningThe dataset, `StarWars.csv`, was created by the FiveTHirtyeight team and is encoded in ISO-8859-1. Some of its most important columns are: * `RespondentID`: an anonymized ID for the each respondent* `Gender`: the respondent's gender* `Age`: the respondent's age* `Household Income`: the respondent's household income* `Education`: the respondent's education level* `Location (Census Region)`: where the respondent lives* `Have you seen any of the 6 films in the Star Wars franchise?`: whether the respondent has seen any of the films in the first two trilogies* `Do you consider yourself to be a fan of the Star Wars film franchise?`: whether the respondent considers themsellves a fanWe first load it into the Pandas dataframe `star_wars` and display the first 10 rows to look for possible inconsistencies.
###Code
import numpy as np
import pandas as pd
import matplotlib.pyplot as plt
star_wars = pd.read_csv('../Data/StarWars.csv', encoding='ISO-8859-1')
star_wars.head(10)
###Output
_____no_output_____
###Markdown
The first line does not contain data, but only clarifies the meanings of some columns.Number and types of objects in each column:
###Code
star_wars.info()
###Output
<class 'pandas.core.frame.DataFrame'>
RangeIndex: 1187 entries, 0 to 1186
Data columns (total 38 columns):
RespondentID 1186 non-null float64
Have you seen any of the 6 films in the Star Wars franchise? 1187 non-null object
Do you consider yourself to be a fan of the Star Wars film franchise? 837 non-null object
Which of the following Star Wars films have you seen? Please select all that apply. 674 non-null object
Unnamed: 4 572 non-null object
Unnamed: 5 551 non-null object
Unnamed: 6 608 non-null object
Unnamed: 7 759 non-null object
Unnamed: 8 739 non-null object
Please rank the Star Wars films in order of preference with 1 being your favorite film in the franchise and 6 being your least favorite film. 836 non-null object
Unnamed: 10 837 non-null object
Unnamed: 11 836 non-null object
Unnamed: 12 837 non-null object
Unnamed: 13 837 non-null object
Unnamed: 14 837 non-null object
Please state whether you view the following characters favorably, unfavorably, or are unfamiliar with him/her. 830 non-null object
Unnamed: 16 832 non-null object
Unnamed: 17 832 non-null object
Unnamed: 18 824 non-null object
Unnamed: 19 826 non-null object
Unnamed: 20 815 non-null object
Unnamed: 21 827 non-null object
Unnamed: 22 821 non-null object
Unnamed: 23 813 non-null object
Unnamed: 24 828 non-null object
Unnamed: 25 831 non-null object
Unnamed: 26 822 non-null object
Unnamed: 27 815 non-null object
Unnamed: 28 827 non-null object
Which character shot first? 829 non-null object
Are you familiar with the Expanded Universe? 829 non-null object
Do you consider yourself to be a fan of the Expanded Universe?æ 214 non-null object
Do you consider yourself to be a fan of the Star Trek franchise? 1069 non-null object
Gender 1047 non-null object
Age 1047 non-null object
Household Income 859 non-null object
Education 1037 non-null object
Location (Census Region) 1044 non-null object
dtypes: float64(1), object(37)
memory usage: 352.5+ KB
###Markdown
There are 38 columns. All columns seem to contain strings except the first (`RespondentID`), which contais floats. All columns except the second one (`Have you seen any of the 6 films in the Star Wars franchise?`) have at least one null value. The first column has only one null value, on the first row; the others have more. There also seems to be two useless characters at the end of the column name `Do you consider yourself to be a fan of the Expanded Universe?æ`.Let us rename some columns using shorter and/or more informative titles and delete the first row. Columns 15 to 28 are renamed using the information on the first row.
###Code
dict_replace_cols = {
'Have you seen any of the 6 films in the Star Wars franchise?': 'seen_any',
'Do you consider yourself to be a fan of the Star Wars film franchise?': 'fan',
'Which of the following Star Wars films have you seen? Please select all that apply.': 'seen_1',
'Unnamed: 4': 'seen_2',
'Unnamed: 5': 'seen_3',
'Unnamed: 6': 'seen_4',
'Unnamed: 7': 'seen_5',
'Unnamed: 8': 'seen_6',
'Please rank the Star Wars films in order of preference with 1 being your favorite film in the franchise and 6 being your least favorite film.': 'order_pref_1',
'Unnamed: 10': 'order_pref_2',
'Unnamed: 11': 'order_pref_3',
'Unnamed: 12': 'order_pref_4',
'Unnamed: 13': 'order_pref_5',
'Unnamed: 14': 'order_pref_6',
'Which character shot first?': 'shot_first',
'Are you familiar with the Expanded Universe?': 'fam_exp_universe',
'Do you consider yourself to be a fan of the Expanded Universe?æ': 'fan_exp_universe',
'Do you consider yourself to be a fan of the Star Trek franchise?': 'fan_star_treck',
'Household Income': 'Income',
'Location (Census Region)': 'Location'
}
star_wars.rename(columns = dict_replace_cols, inplace=True)
cols_names = list(star_wars.columns)
for i in list(range(15,29)):
cols_names[i] = star_wars.iloc[0,i]
star_wars.columns = cols_names
star_wars = star_wars.iloc[1:]
star_wars.info()
###Output
<class 'pandas.core.frame.DataFrame'>
RangeIndex: 1186 entries, 1 to 1186
Data columns (total 38 columns):
RespondentID 1186 non-null float64
seen_any 1186 non-null object
fan 836 non-null object
seen_1 673 non-null object
seen_2 571 non-null object
seen_3 550 non-null object
seen_4 607 non-null object
seen_5 758 non-null object
seen_6 738 non-null object
order_pref_1 835 non-null object
order_pref_2 836 non-null object
order_pref_3 835 non-null object
order_pref_4 836 non-null object
order_pref_5 836 non-null object
order_pref_6 836 non-null object
Han Solo 829 non-null object
Luke Skywalker 831 non-null object
Princess Leia Organa 831 non-null object
Anakin Skywalker 823 non-null object
Obi Wan Kenobi 825 non-null object
Emperor Palpatine 814 non-null object
Darth Vader 826 non-null object
Lando Calrissian 820 non-null object
Boba Fett 812 non-null object
C-3P0 827 non-null object
R2 D2 830 non-null object
Jar Jar Binks 821 non-null object
Padme Amidala 814 non-null object
Yoda 826 non-null object
shot_first 828 non-null object
fam_exp_universe 828 non-null object
fan_exp_universe 213 non-null object
fan_star_treck 1068 non-null object
Gender 1046 non-null object
Age 1046 non-null object
Income 858 non-null object
Education 1036 non-null object
Location 1043 non-null object
dtypes: float64(1), object(37)
memory usage: 352.2+ KB
###Markdown
Convert the values in the first column to integers:
###Code
star_wars.iloc[:,0] = star_wars.iloc[:,0].astype(int)
###Output
_____no_output_____
###Markdown
The titles of columns 1, 2, 31, 32, and 33 (with the convention that the first column has index 0) are yes/no questions. These columns contain the values 'Yes' and 'No'. To ease the analysis, we convert them to the boolean values `True` and `False`.
###Code
yes_no_dict = {'Yes': True, 'No': False}
column_indices = [1, 2, 30, 31, 32]
for col in column_indices:
star_wars.iloc[:,col] = star_wars.iloc[:,col].map(yes_no_dict)
star_wars.head()
###Output
_____no_output_____
###Markdown
Convert columns 2 to 8 to bool values:
###Code
def is_string(val):
return type(val) == str
for i in range(3,9):
star_wars.iloc[:,i] = star_wars.iloc[:,i].map(is_string)
###Output
_____no_output_____
###Markdown
Convert the orders of preference to numerical values:
###Code
star_wars[star_wars.columns[9:15]] = star_wars[star_wars.columns[9:15]].astype(float)
star_wars.head()
###Output
_____no_output_____
###Markdown
AnalysisAs a first indication of how much each movie is liked, we show the average ranking for each of them. The error bars show one standard error on each side of the average.
###Code
ave_rankings = star_wars.iloc[:,9:15].apply(np.mean)
ste_rankings = star_wars.iloc[:,9:15].apply(np.std) / np.sqrt(star_wars.iloc[:,9:15].count())
ave_rankings.index = range(1,7)
ave_rankings.plot.bar(yerr = list(ste_rankings), rot = 0)
plt.xlabel('movie')
plt.ylabel('average ranking')
plt.show()
###Output
_____no_output_____
###Markdown
The fifth episode has the best ranking by a statistically significant margin. The thirs episode has the worst ranking by a statistically significant margin. Let us now shos the number of views.
###Code
n_views = star_wars.iloc[:,3:9].apply(np.sum)
n_views.index = range(1,7)
n_views.plot.bar(rot = 0)
plt.xlabel('movie')
plt.ylabel('number of viewers')
plt.show()
###Output
_____no_output_____
###Markdown
The fifth episode has the largest number of views, while the third one has the lowest number of views. More generally, the higher the number of respondents to have seen a movie, the best its ranking is.Let us now perform the same analysis for different groups of respondents. We first define the functions `plot_rankings_by` and `plot_n_viewers_by` doing the bar plots given a column name by which to distinuish respondents.
###Code
def plot_rankings_by(col_name):
groups = list(star_wars[col_name].dropna().unique())
data_ave = []
data_ste = []
for group in groups:
df = star_wars[star_wars[col_name] == group].iloc[:,9:15]
data_ave.append(list(df.apply(np.mean)))
data_ste.append(df.apply(np.std) / np.sqrt(df.count()))
df_ave = pd.DataFrame(data_ave).transpose()
df_ave.index = index=range(1,7)
df_ave.columns = groups
df_ave.plot.bar(yerr = data_ste, rot = 0)
plt.xlabel('movie')
plt.ylabel('average ranking')
plt.show()
def plot_n_viewers_by(col_name):
groups = list(star_wars[col_name].dropna().unique())
data_count = []
for group in groups:
df = star_wars[star_wars[col_name] == group].iloc[:,3:9]
data_count.append(list(df.apply(np.sum)))
df_ave = pd.DataFrame(data_count).transpose()
df_ave.index = index=range(1,7)
df_ave.columns = groups
df_ave.plot.bar(rot = 0)
plt.xlabel('movie')
plt.ylabel('number of viewers')
plt.show()
###Output
_____no_output_____
###Markdown
We first divide the respondents by gender.
###Code
plot_rankings_by('Gender')
plot_n_viewers_by('Gender')
###Output
_____no_output_____
###Markdown
We notice that:* On average, women have a better opinion than men of the first episode and a lower opinion of the fourth.* Each episode has more male than female viewers. The gap is largest for the third episode and smallest for the fifth. Interestingly, the fraction of women having seen an episode seems to decrese with its average ranking. Let us compute the correlation coeficient between these two variables, as well as with the number of viewers.
###Code
n_female_viewers = star_wars[star_wars['Gender'] == 'Female'].iloc[:,3:9].apply(np.sum)
n_male_viewers = star_wars[star_wars['Gender'] == 'Male'].iloc[:,3:9].apply(np.sum)
frac_female_viewers = n_female_viewers / (n_female_viewers + n_male_viewers)
print('correlation coefficient between the fraction of female viewers and average ranking: {}'.format(np.corrcoef(list(frac_female_viewers), list(ave_rankings))[0][1]))
print('correlation coefficient between the fraction of female viewers and number of viewers: {}'.format(np.corrcoef(list(frac_female_viewers), list(n_views))[0][1]))
###Output
correlation coefficient between the fraction of female viewers and average ranking: -0.8460698256979539
correlation coefficient between the fraction of female viewers and number of viewers: 0.9942656122597326
###Markdown
*There is a strong negative correlation between the fraction of female viewers and average ranking, and a very strong positive correlation between the fraction of female viewers and total number of viewers.*Let us now distinguish between viewers depending on whether they identify as fans of the tar Wars franchise.
###Code
plot_rankings_by('fan')
plot_n_viewers_by('fan')
###Output
_____no_output_____
###Markdown
*Respondents who identify as fans have a significantly higher opinion of the oldest trilogy (episodes 4, 5, and 6) and a lower opinion of te newer one (episodes 1, 2, and 3).* For each episode, a large majority of respondents who has seen it identifies as a fan of the franchise.Finally, let us distinguish between viewers depending on whether they identify as fans of the Start Treck franchise.
###Code
plot_rankings_by('fan_star_treck')
plot_n_viewers_by('fan_star_treck')
###Output
_____no_output_____ |
Subsets and Splits
No community queries yet
The top public SQL queries from the community will appear here once available.