Search is not available for this dataset
modelId
stringlengths 5
137
| author
stringlengths 2
42
| last_modified
unknowndate 2020-02-15 11:33:14
2025-04-01 00:42:32
| downloads
int64 0
223M
| likes
int64 0
11.7k
| library_name
stringclasses 405
values | tags
sequencelengths 1
4.05k
| pipeline_tag
stringclasses 54
values | createdAt
unknowndate 2022-03-02 23:29:04
2025-04-01 00:42:15
| card
stringlengths 11
1.01M
|
---|---|---|---|---|---|---|---|---|---|
baby-dev/3d3428bc-7199-4377-a210-a4fa1c2e90ab | baby-dev | "2025-02-15T20:53:17Z" | 0 | 0 | peft | [
"peft",
"safetensors",
"qwen2",
"axolotl",
"generated_from_trainer",
"base_model:Qwen/Qwen2.5-7B-Instruct",
"base_model:adapter:Qwen/Qwen2.5-7B-Instruct",
"license:apache-2.0",
"region:us"
] | null | "2025-02-15T20:41:07Z" | ---
library_name: peft
license: apache-2.0
base_model: Qwen/Qwen2.5-7B-Instruct
tags:
- axolotl
- generated_from_trainer
model-index:
- name: 3d3428bc-7199-4377-a210-a4fa1c2e90ab
results: []
---
<!-- This model card has been generated automatically according to the information the Trainer had access to. You
should probably proofread and complete it, then remove this comment. -->
# 3d3428bc-7199-4377-a210-a4fa1c2e90ab
This model is a fine-tuned version of [Qwen/Qwen2.5-7B-Instruct](https://huggingface.co/Qwen/Qwen2.5-7B-Instruct) on the None dataset.
It achieves the following results on the evaluation set:
- Loss: 2.1943
## Model description
More information needed
## Intended uses & limitations
More information needed
## Training and evaluation data
More information needed
### Framework versions
- PEFT 0.13.2
- Transformers 4.46.0
- Pytorch 2.5.0+cu124
- Datasets 3.0.1
- Tokenizers 0.20.1 |
DMCF14/Raffles | DMCF14 | "2023-08-03T13:38:21Z" | 0 | 0 | null | [
"license:cc-by-nc-sa-4.0",
"region:us"
] | null | "2023-08-03T13:34:30Z" | ---
license: cc-by-nc-sa-4.0
---
|
KappaNeuro/randolph-caldecott-style | KappaNeuro | "2023-09-14T10:08:02Z" | 1 | 1 | diffusers | [
"diffusers",
"text-to-image",
"stable-diffusion",
"lora",
"art",
"style",
"illustrator",
"painting",
"children",
"literature",
"randolph caldecott",
"base_model:stabilityai/stable-diffusion-xl-base-1.0",
"base_model:adapter:stabilityai/stable-diffusion-xl-base-1.0",
"license:other",
"region:us"
] | text-to-image | "2023-09-14T10:07:58Z" | ---
license: other
tags:
- text-to-image
- stable-diffusion
- lora
- diffusers
- art
- style
- illustrator
- painting
- children
- literature
- randolph caldecott
base_model: stabilityai/stable-diffusion-xl-base-1.0
instance_prompt: Randolph Caldecott Style
widget:
- text: "Randolph Caldecott Style - a friendly alligator being served food at a catering party. The alligator is looking at his watch watch and eating food."
- text: "Randolph Caldecott Style - vast view of dense woodland in the background, a squirrel in a fashionable fedora and bomber jacket, reminiscent of Chip from Chip 'n Dale Rescue Rangers, looking at the audience, Beatrix Potter hand drawn style"
- text: "Randolph Caldecott Style - imagine a close-up shot of the baby in the pram. style of shirley hughes - angry child throwing toys out of pram. The baby's face is in sharp focus, capturing their expression of glee and mischief. The style should be hyper-realistic, with every detail of the baby's face and the toy captured in high resolution. The lighting should be soft and diffused, creating a warm and inviting atmosphere. The composition should be a tight shot, focusing on the baby and the toy, with a shallow depth of field to blur the background."
- text: "Randolph Caldecott Style - Illustration inspired by Kate Greenaway, depicts scenes of idyllic childhood with children dressed in late 18th and early 19th-century clothing. pastel color palette and detailed botanical backgrounds. children in innocent poses in natural settings such as gardens and meadows and often include whimsical elements such as fairies and animals"
- text: "Randolph Caldecott Style - Never in his life had he seen a river before, this sleck, sinuous, full bodied animal, chasing and chuckling, gripping things with a gurgle and leaving them with a laugh, to fling itself on fresh playmates that shook themselves free, and were caught and held again"
- text: "Randolph Caldecott Style - Costume sketch. Bunny girl. Girl 5 years old. Pretty, curly hair. Long silk dress, lace petticoat, velvet jacket, jabot collar. Hat with ears. patent leather shoes. Dusted tones - pink and beige. Retro. Vintage. Early 20th century."
- text: "Randolph Caldecott Style - a girl of 8 years, black hair, orange dress, brown shoes, eyes shining with curiosity, in school, hang out with friends, bright and courageous, brave, bright smile, in style of Randolph Caldecott book illustration"
- text: "Randolph Caldecott Style - a girl of 8 years, black hair, orange dress, brown shoes, eyes shining with curiosity, close up, in forest, sitting on a log, comfortable, flowers around in style of Randolph Caldecott book illustration"
- text: "Randolph Caldecott Style - dirty caucassian family laying in bed, wearing pajamas. In the same bed there are 4 chicken, 3 ducks, dog, cat and two pigs. in style of beatrix potter."
- text: "Randolph Caldecott Style - Hopefully, all unfortunate children will find warm homes. drawing and painting, blend of the styles of Beatrix Potter, ANton Pieck and Pieter Breughel"
---
# Randolph Caldecott Style ([CivitAI](https://civitai.com/models/154146)

> Randolph Caldecott Style - a friendly alligator being served food at a catering party. The alligator is looking at his watch watch and eating food.
<p>Randolph Caldecott was an English illustrator and artist who lived from 1846 to 1886. He is best known for his contributions to children's literature, particularly for his innovative and playful illustrations.</p><p>Caldecott's illustrations were characterized by their lively, energetic style and attention to detail. He often depicted scenes from everyday life, including animals, children, and humorous situations. His illustrations had a sense of movement and captured the essence of a story, making them highly engaging for young readers.</p><p>One of Caldecott's notable achievements was his development of the picture book format. He introduced the concept of integrating illustrations and text on the same page, creating a seamless narrative flow. His use of dynamic compositions and imaginative storytelling revolutionized children's book illustration.</p><p>Caldecott's illustrations were also renowned for their use of color and texture. He employed watercolors, ink, and other media to bring his characters and scenes to life, creating a sense of depth and atmosphere.</p><p>In recognition of his significant contributions to children's literature, the Caldecott Medal was established in his honor. It is awarded annually to the most distinguished illustrated children's book published in the United States.</p><p>Randolph Caldecott's influence on the field of children's book illustration is profound. His innovative approach to storytelling and his captivating illustrations continue to inspire and delight readers of all ages. His legacy as a pioneering illustrator has left an enduring impact on the world of children's literature.</p>
## Image examples for the model:

> Randolph Caldecott Style - vast view of dense woodland in the background, a squirrel in a fashionable fedora and bomber jacket, reminiscent of Chip from Chip 'n Dale Rescue Rangers, looking at the audience, Beatrix Potter hand drawn style

> Randolph Caldecott Style - imagine a close-up shot of the baby in the pram. style of shirley hughes - angry child throwing toys out of pram. The baby's face is in sharp focus, capturing their expression of glee and mischief. The style should be hyper-realistic, with every detail of the baby's face and the toy captured in high resolution. The lighting should be soft and diffused, creating a warm and inviting atmosphere. The composition should be a tight shot, focusing on the baby and the toy, with a shallow depth of field to blur the background.

> Randolph Caldecott Style - Illustration inspired by Kate Greenaway, depicts scenes of idyllic childhood with children dressed in late 18th and early 19th-century clothing. pastel color palette and detailed botanical backgrounds. children in innocent poses in natural settings such as gardens and meadows and often include whimsical elements such as fairies and animals

> Randolph Caldecott Style - Never in his life had he seen a river before, this sleck, sinuous, full bodied animal, chasing and chuckling, gripping things with a gurgle and leaving them with a laugh, to fling itself on fresh playmates that shook themselves free, and were caught and held again

> Randolph Caldecott Style - Costume sketch. Bunny girl. Girl 5 years old. Pretty, curly hair. Long silk dress, lace petticoat, velvet jacket, jabot collar. Hat with ears. patent leather shoes. Dusted tones - pink and beige. Retro. Vintage. Early 20th century.

> Randolph Caldecott Style - a girl of 8 years, black hair, orange dress, brown shoes, eyes shining with curiosity, in school, hang out with friends, bright and courageous, brave, bright smile, in style of Randolph Caldecott book illustration

> Randolph Caldecott Style - a girl of 8 years, black hair, orange dress, brown shoes, eyes shining with curiosity, close up, in forest, sitting on a log, comfortable, flowers around in style of Randolph Caldecott book illustration

> Randolph Caldecott Style - dirty caucassian family laying in bed, wearing pajamas. In the same bed there are 4 chicken, 3 ducks, dog, cat and two pigs. in style of beatrix potter.

> Randolph Caldecott Style - Hopefully, all unfortunate children will find warm homes. drawing and painting, blend of the styles of Beatrix Potter, ANton Pieck and Pieter Breughel
|
stefan-it/autotrain-flair-georgian-ner-xlm_r_large-bs4-e10-lr5e-06-2 | stefan-it | "2023-11-17T00:52:08Z" | 12 | 0 | flair | [
"flair",
"pytorch",
"tensorboard",
"token-classification",
"sequence-tagger-model",
"en",
"ka",
"base_model:FacebookAI/xlm-roberta-large",
"base_model:finetune:FacebookAI/xlm-roberta-large",
"license:mit",
"region:us"
] | token-classification | "2023-11-16T03:26:32Z" | ---
language:
- en
- ka
license: mit
tags:
- flair
- token-classification
- sequence-tagger-model
base_model: xlm-roberta-large
widget:
- text: ამით თავისი ქადაგება დაასრულა და დაბრუნდა იერუსალიმში . ერთ-ერთ გარე კედელზე
არსებობს ერნესტო ჩე გევარას პორტრეტი . შაკოსკა“ ინახება ბრაზილიაში , სან-პაულუს
ხელოვნების მუზეუმში .
---
# Fine-tuned English-Georgian NER Model with Flair
This Flair NER model was fine-tuned on the WikiANN dataset
([Rahimi et al.](https://www.aclweb.org/anthology/P19-1015) splits)
using XLM-R Large as backbone LM.
**Notice**: The dataset is very problematic, because it was automatically constructed.
We did manually inspect the development split of the Georgian data and found
a lot of bad labeled examples, e.g. DVD ( 💿 ) as `ORG`.
## Fine-Tuning
The latest
[Flair version](https://github.com/flairNLP/flair/tree/f30f5801df3f9e105ed078ec058b4e1152dd9159)
is used for fine-tuning.
We use English and Georgian training splits for fine-tuning and the
development set of Georgian for evaluation.
A hyper-parameter search over the following parameters with 5 different seeds per configuration is performed:
* Batch Sizes: [`4`]
* Learning Rates: [`5e-06`]
More details can be found in this [repository](https://github.com/stefan-it/georgian-ner).
## Results
A hyper-parameter search with 5 different seeds per configuration is performed and micro F1-score on development set
is reported:
| Configuration | Seed 1 | Seed 2 | Seed 3 | Seed 4 | Seed 5 | Average |
|-------------------|-------------|-----------------|-------------|------------|-------------|-----------------|
| `bs4-e10-lr5e-06` | [0.9005][1] | [**0.9012**][2] | [0.9069][3] | [0.905][4] | [0.9048][5] | 0.9037 ± 0.0027 |
[1]: https://hf.co/stefan-it/autotrain-flair-georgian-ner-xlm_r_large-bs4-e10-lr5e-06-1
[2]: https://hf.co/stefan-it/autotrain-flair-georgian-ner-xlm_r_large-bs4-e10-lr5e-06-2
[3]: https://hf.co/stefan-it/autotrain-flair-georgian-ner-xlm_r_large-bs4-e10-lr5e-06-3
[4]: https://hf.co/stefan-it/autotrain-flair-georgian-ner-xlm_r_large-bs4-e10-lr5e-06-4
[5]: https://hf.co/stefan-it/autotrain-flair-georgian-ner-xlm_r_large-bs4-e10-lr5e-06-5
The result in bold shows the performance of this model.
Additionally, the Flair [training log](training.log) and [TensorBoard logs](tensorboard) are also uploaded to the model
hub. |
Xmm/led-large-16384-cnn_dailymail | Xmm | "2023-09-02T08:09:40Z" | 98 | 0 | transformers | [
"transformers",
"pytorch",
"tensorboard",
"safetensors",
"led",
"text2text-generation",
"generated_from_trainer",
"dataset:cnn_dailymail",
"license:apache-2.0",
"model-index",
"autotrain_compatible",
"endpoints_compatible",
"region:us"
] | text2text-generation | "2023-06-17T03:05:46Z" | ---
license: apache-2.0
tags:
- generated_from_trainer
datasets:
- cnn_dailymail
metrics:
- rouge
model-index:
- name: led-large-16384-cnn_dailymail
results:
- task:
name: Sequence-to-sequence Language Modeling
type: text2text-generation
dataset:
name: cnn_dailymail
type: cnn_dailymail
config: 3.0.0
split: test
args: 3.0.0
metrics:
- name: Rouge1
type: rouge
value: 0.3869876274946419
---
<!-- This model card has been generated automatically according to the information the Trainer had access to. You
should probably proofread and complete it, then remove this comment. -->
# led-large-16384-cnn_dailymail
This model is a fine-tuned version of [allenai/led-base-16384](https://huggingface.co/allenai/led-base-16384) on the cnn_dailymail dataset.
It achieves the following results on the evaluation set:
- Loss: 1.5544
- Rouge1: 0.3870
- Rouge2: 0.1736
- Rougel: 0.2599
- Rougelsum: 0.3653
## Model description
More information needed
## Intended uses & limitations
More information needed
## Training and evaluation data
More information needed
## Training procedure
### Training hyperparameters
The following hyperparameters were used during training:
- learning_rate: 5e-05
- train_batch_size: 2
- eval_batch_size: 2
- seed: 42
- gradient_accumulation_steps: 64
- total_train_batch_size: 128
- optimizer: Adam with betas=(0.9,0.999) and epsilon=1e-08
- lr_scheduler_type: linear
- num_epochs: 10
- mixed_precision_training: Native AMP
### Training results
| Training Loss | Epoch | Step | Validation Loss | Rouge1 | Rouge2 | Rougel | Rougelsum |
|:-------------:|:-----:|:-----:|:---------------:|:------:|:------:|:------:|:---------:|
| 1.9531 | 0.4 | 500 | 1.8639 | 0.3485 | 0.1441 | 0.2275 | 0.3288 |
| 1.9563 | 0.8 | 1000 | 1.8260 | 0.3538 | 0.1482 | 0.2315 | 0.3343 |
| 1.7176 | 1.2 | 1500 | 1.8208 | 0.3628 | 0.1527 | 0.2383 | 0.3433 |
| 1.7197 | 1.6 | 2000 | 1.8162 | 0.3696 | 0.1602 | 0.2434 | 0.3486 |
| 1.8086 | 2.0 | 2500 | 1.7924 | 0.3558 | 0.1533 | 0.2334 | 0.3361 |
| 1.2448 | 2.4 | 3000 | 1.8510 | 0.3703 | 0.1591 | 0.2447 | 0.3483 |
| 1.3574 | 2.8 | 3500 | 1.8277 | 0.3741 | 0.1593 | 0.2422 | 0.3540 |
| 1.0966 | 3.2 | 4000 | 1.8924 | 0.3682 | 0.1576 | 0.2424 | 0.3479 |
| 0.9938 | 3.6 | 4500 | 1.8957 | 0.3723 | 0.1599 | 0.2451 | 0.3511 |
| 1.0735 | 4.0 | 5000 | 1.8772 | 0.3653 | 0.1557 | 0.2399 | 0.3454 |
| 0.9106 | 4.4 | 5500 | 1.9401 | 0.3720 | 0.1585 | 0.2436 | 0.3504 |
| 1.015 | 4.8 | 6000 | 1.9320 | 0.3725 | 0.1570 | 0.2429 | 0.3515 |
| 1.7854 | 0.36 | 6500 | 1.7800 | 0.3624 | 0.1544 | 0.2390 | 0.3422 |
| 1.9079 | 0.39 | 7000 | 1.7629 | 0.3573 | 0.1553 | 0.2352 | 0.3370 |
| 1.7606 | 3.34 | 7500 | 1.6902 | 0.3783 | 0.1673 | 0.2521 | 0.3570 |
| 1.7571 | 3.57 | 8000 | 1.6563 | 0.3802 | 0.1691 | 0.2538 | 0.3587 |
| 1.6602 | 3.79 | 8500 | 1.6439 | 0.3814 | 0.1693 | 0.2548 | 0.3600 |
| 1.6614 | 4.01 | 9000 | 1.6312 | 0.3812 | 0.1691 | 0.2544 | 0.3599 |
| 1.668 | 4.24 | 9500 | 1.6189 | 0.3815 | 0.1689 | 0.2550 | 0.3603 |
| 1.6491 | 4.46 | 10000 | 1.6172 | 0.3799 | 0.1681 | 0.2540 | 0.3586 |
| 1.5994 | 4.68 | 10500 | 1.6132 | 0.3825 | 0.1702 | 0.2560 | 0.3610 |
| 1.6493 | 4.9 | 11000 | 1.6093 | 0.3828 | 0.1701 | 0.2561 | 0.3613 |
| 1.6769 | 5.13 | 11500 | 1.6074 | 0.3831 | 0.1706 | 0.2569 | 0.3619 |
| 1.6554 | 5.35 | 12000 | 1.6044 | 0.3817 | 0.1695 | 0.2559 | 0.3605 |
| 1.6155 | 5.57 | 12500 | 1.6010 | 0.3825 | 0.1700 | 0.2561 | 0.3608 |
| 1.5863 | 5.8 | 13000 | 1.5981 | 0.3829 | 0.1704 | 0.2569 | 0.3614 |
| 1.6306 | 6.02 | 13500 | 1.6004 | 0.3831 | 0.1702 | 0.2563 | 0.3618 |
| 1.6425 | 6.24 | 14000 | 1.5987 | 0.3821 | 0.1698 | 0.2561 | 0.3610 |
| 1.6863 | 6.46 | 14500 | 1.5876 | 0.3837 | 0.1710 | 0.2569 | 0.3622 |
| 1.6085 | 6.69 | 15000 | 1.5815 | 0.3836 | 0.1717 | 0.2573 | 0.3621 |
| 1.6267 | 6.91 | 15500 | 1.5792 | 0.3852 | 0.1722 | 0.2579 | 0.3633 |
| 1.5637 | 7.13 | 16000 | 1.5768 | 0.3830 | 0.1709 | 0.2568 | 0.3611 |
| 1.5586 | 7.36 | 16500 | 1.5740 | 0.3833 | 0.1706 | 0.2567 | 0.3617 |
| 1.5389 | 7.58 | 17000 | 1.5689 | 0.3858 | 0.1729 | 0.2590 | 0.3640 |
| 1.5694 | 7.8 | 17500 | 1.5645 | 0.3853 | 0.1731 | 0.2589 | 0.3636 |
| 1.5265 | 8.02 | 18000 | 1.5621 | 0.3871 | 0.1733 | 0.2596 | 0.3654 |
| 1.5273 | 8.25 | 18500 | 1.5624 | 0.3861 | 0.1726 | 0.2588 | 0.3646 |
| 1.5148 | 8.47 | 19000 | 1.5602 | 0.3866 | 0.1733 | 0.2592 | 0.3651 |
| 1.532 | 8.69 | 19500 | 1.5599 | 0.3859 | 0.1732 | 0.2593 | 0.3642 |
| 1.5113 | 8.92 | 20000 | 1.5602 | 0.3877 | 0.1748 | 0.2606 | 0.3658 |
| 1.5133 | 9.14 | 20500 | 1.5595 | 0.3855 | 0.1725 | 0.2587 | 0.3637 |
| 1.4875 | 9.36 | 21000 | 1.5572 | 0.3873 | 0.1741 | 0.2600 | 0.3654 |
| 1.5038 | 9.59 | 21500 | 1.5557 | 0.3860 | 0.1728 | 0.2590 | 0.3641 |
| 1.5062 | 9.81 | 22000 | 1.5544 | 0.3870 | 0.1736 | 0.2599 | 0.3653 |
### Framework versions
- Transformers 4.27.1
- Pytorch 2.0.0+cu118
- Datasets 2.10.1
- Tokenizers 0.13.2
|
sd-concepts-library/beldam | sd-concepts-library | "2022-10-06T04:31:38Z" | 0 | 0 | null | [
"license:mit",
"region:us"
] | null | "2022-10-06T04:31:28Z" | ---
license: mit
---
### beldam on Stable Diffusion
This is the `beldam` concept taught to Stable Diffusion via Textual Inversion. You can load this concept into the [Stable Conceptualizer](https://colab.research.google.com/github/huggingface/notebooks/blob/main/diffusers/stable_conceptualizer_inference.ipynb) notebook. You can also train your own concepts and load them into the concept libraries using [this notebook](https://colab.research.google.com/github/huggingface/notebooks/blob/main/diffusers/sd_textual_inversion_training.ipynb).
Here is the new concept you will be able to use as an `object`:
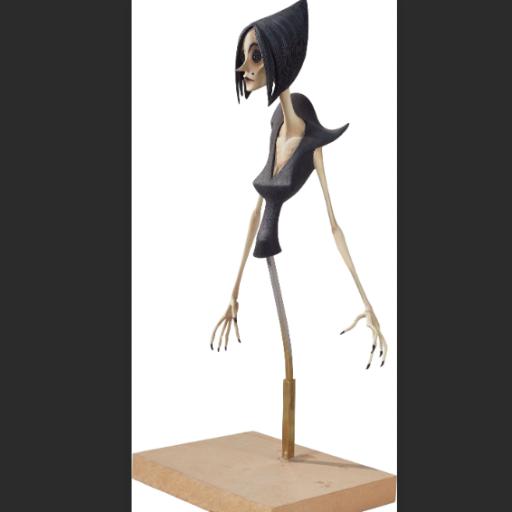
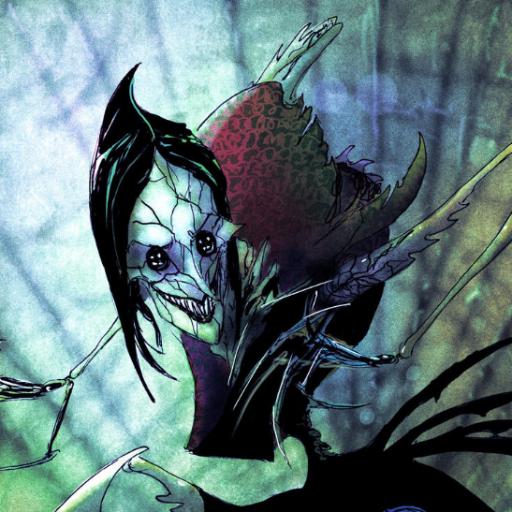
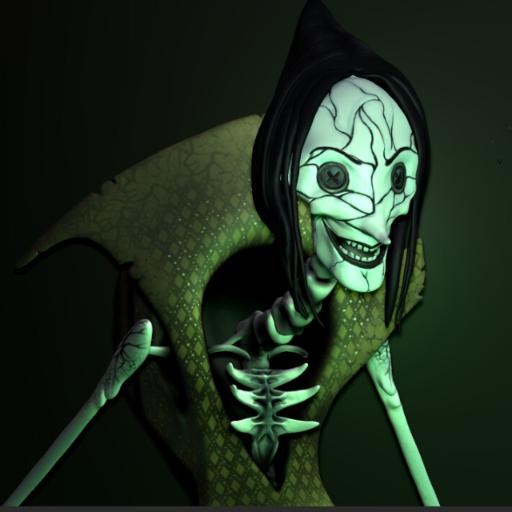
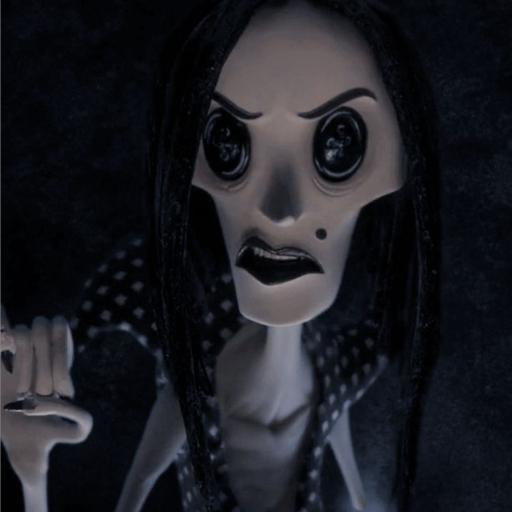
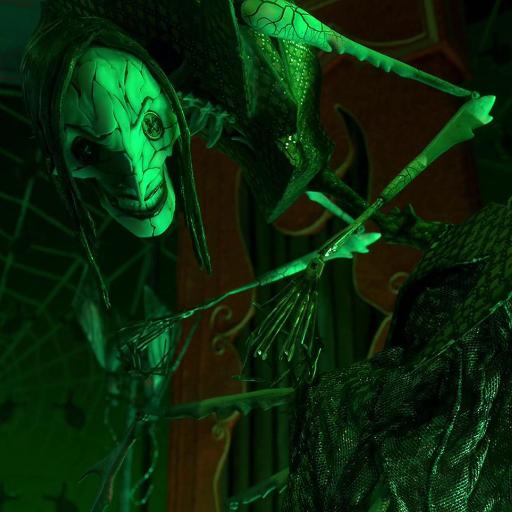
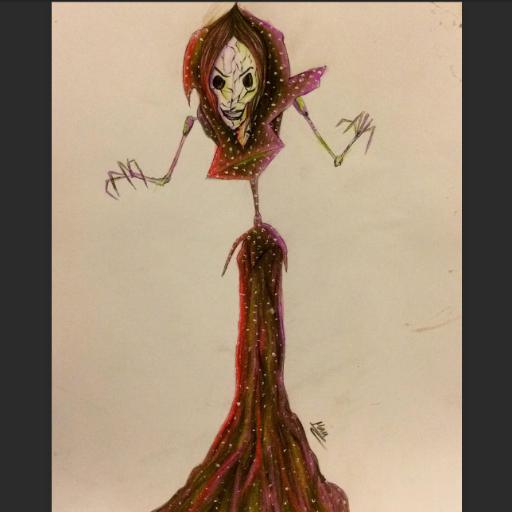
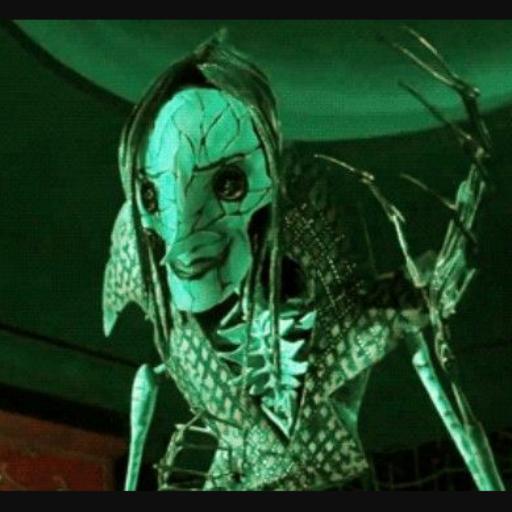
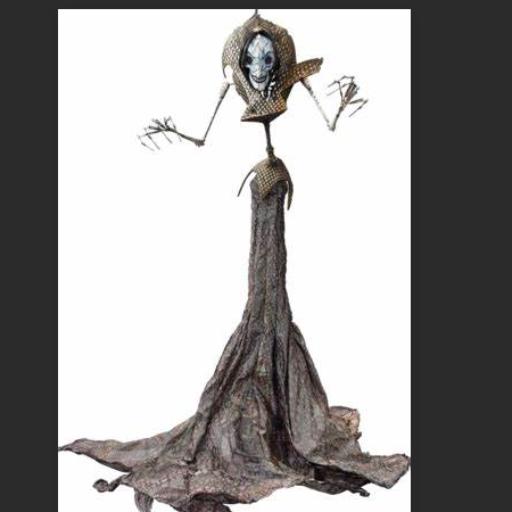
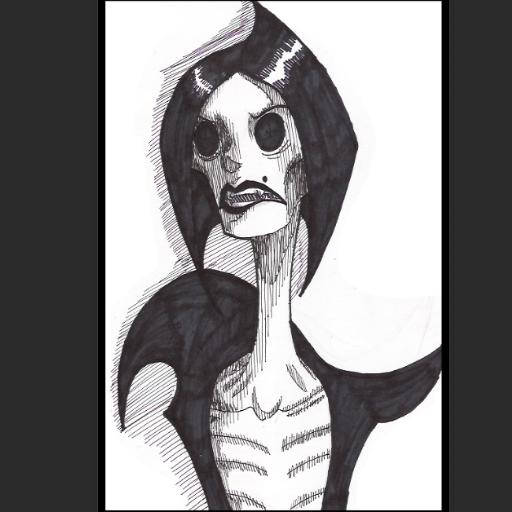
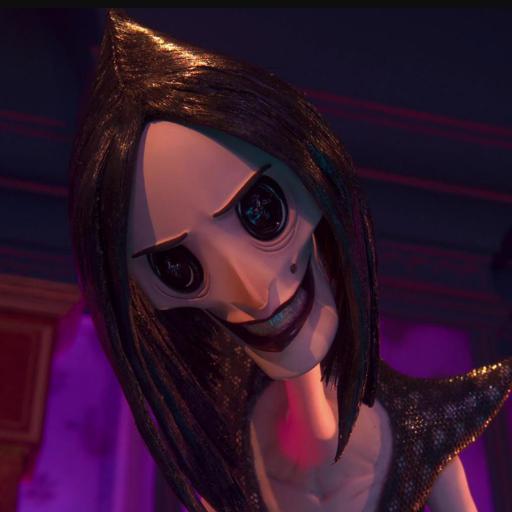
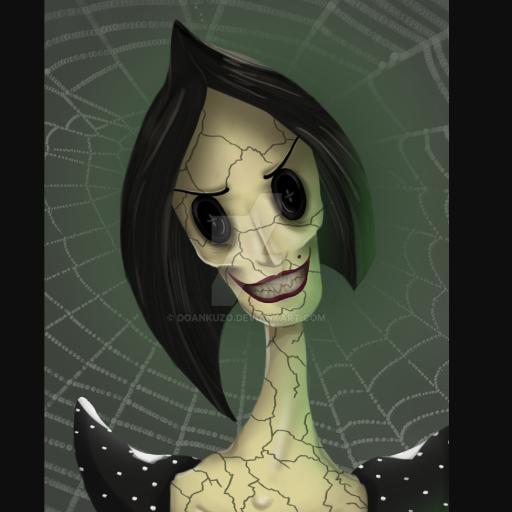
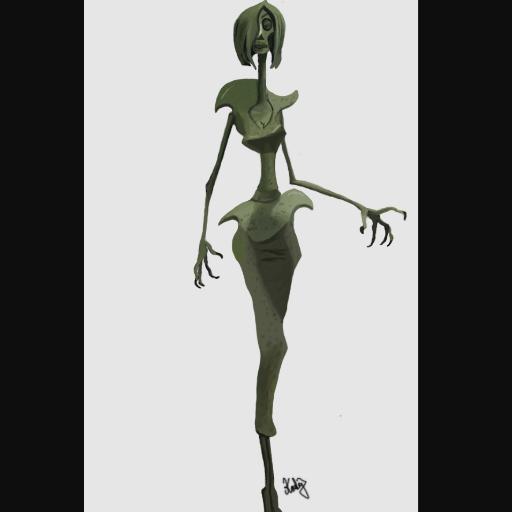
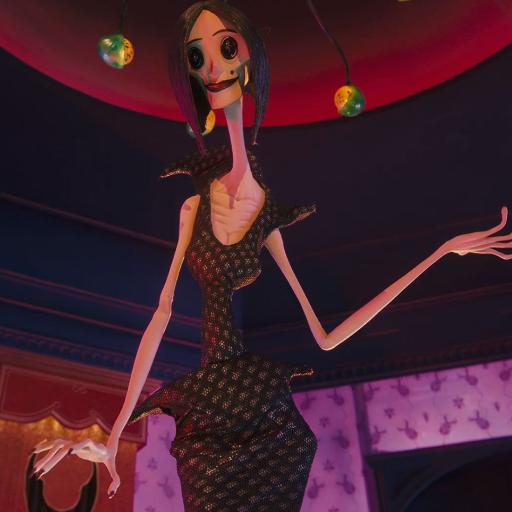
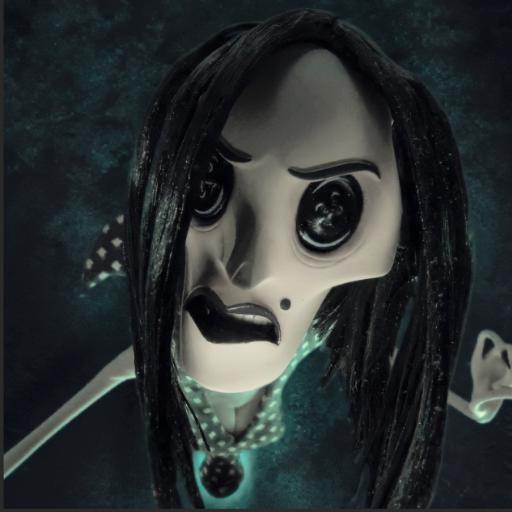
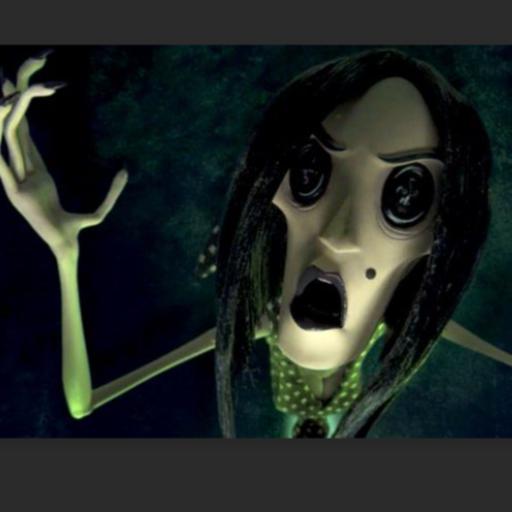
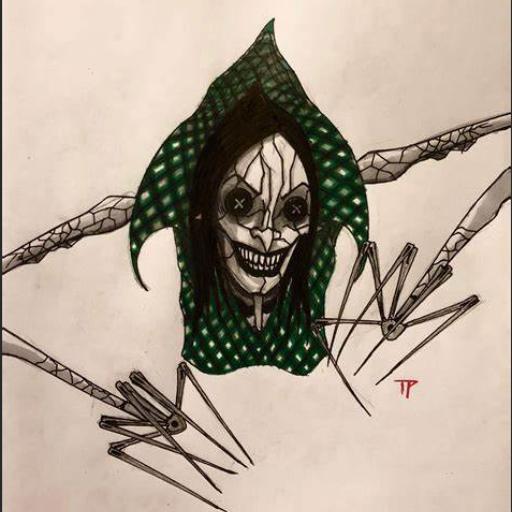
|
mradermacher/dehallucinated-llama-3.2-8b-instruct-GGUF | mradermacher | "2025-02-26T23:57:51Z" | 0 | 0 | transformers | [
"transformers",
"gguf",
"en",
"base_model:hirundo-io/dehallucinated-llama-3.2-8b-instruct",
"base_model:quantized:hirundo-io/dehallucinated-llama-3.2-8b-instruct",
"endpoints_compatible",
"region:us",
"conversational"
] | null | "2025-02-26T19:39:34Z" | ---
base_model: hirundo-io/dehallucinated-llama-3.2-8b-instruct
language:
- en
library_name: transformers
quantized_by: mradermacher
tags: []
---
## About
<!-- ### quantize_version: 2 -->
<!-- ### output_tensor_quantised: 1 -->
<!-- ### convert_type: hf -->
<!-- ### vocab_type: -->
<!-- ### tags: -->
static quants of https://huggingface.co/hirundo-io/dehallucinated-llama-3.2-8b-instruct
<!-- provided-files -->
weighted/imatrix quants are available at https://huggingface.co/mradermacher/dehallucinated-llama-3.2-8b-instruct-i1-GGUF
## Usage
If you are unsure how to use GGUF files, refer to one of [TheBloke's
READMEs](https://huggingface.co/TheBloke/KafkaLM-70B-German-V0.1-GGUF) for
more details, including on how to concatenate multi-part files.
## Provided Quants
(sorted by size, not necessarily quality. IQ-quants are often preferable over similar sized non-IQ quants)
| Link | Type | Size/GB | Notes |
|:-----|:-----|--------:|:------|
| [GGUF](https://huggingface.co/mradermacher/dehallucinated-llama-3.2-8b-instruct-GGUF/resolve/main/dehallucinated-llama-3.2-8b-instruct.Q2_K.gguf) | Q2_K | 1.5 | |
| [GGUF](https://huggingface.co/mradermacher/dehallucinated-llama-3.2-8b-instruct-GGUF/resolve/main/dehallucinated-llama-3.2-8b-instruct.Q3_K_S.gguf) | Q3_K_S | 1.6 | |
| [GGUF](https://huggingface.co/mradermacher/dehallucinated-llama-3.2-8b-instruct-GGUF/resolve/main/dehallucinated-llama-3.2-8b-instruct.Q3_K_M.gguf) | Q3_K_M | 1.8 | lower quality |
| [GGUF](https://huggingface.co/mradermacher/dehallucinated-llama-3.2-8b-instruct-GGUF/resolve/main/dehallucinated-llama-3.2-8b-instruct.Q3_K_L.gguf) | Q3_K_L | 1.9 | |
| [GGUF](https://huggingface.co/mradermacher/dehallucinated-llama-3.2-8b-instruct-GGUF/resolve/main/dehallucinated-llama-3.2-8b-instruct.IQ4_XS.gguf) | IQ4_XS | 1.9 | |
| [GGUF](https://huggingface.co/mradermacher/dehallucinated-llama-3.2-8b-instruct-GGUF/resolve/main/dehallucinated-llama-3.2-8b-instruct.Q4_K_S.gguf) | Q4_K_S | 2.0 | fast, recommended |
| [GGUF](https://huggingface.co/mradermacher/dehallucinated-llama-3.2-8b-instruct-GGUF/resolve/main/dehallucinated-llama-3.2-8b-instruct.Q4_K_M.gguf) | Q4_K_M | 2.1 | fast, recommended |
| [GGUF](https://huggingface.co/mradermacher/dehallucinated-llama-3.2-8b-instruct-GGUF/resolve/main/dehallucinated-llama-3.2-8b-instruct.Q5_K_S.gguf) | Q5_K_S | 2.4 | |
| [GGUF](https://huggingface.co/mradermacher/dehallucinated-llama-3.2-8b-instruct-GGUF/resolve/main/dehallucinated-llama-3.2-8b-instruct.Q5_K_M.gguf) | Q5_K_M | 2.4 | |
| [GGUF](https://huggingface.co/mradermacher/dehallucinated-llama-3.2-8b-instruct-GGUF/resolve/main/dehallucinated-llama-3.2-8b-instruct.Q6_K.gguf) | Q6_K | 2.7 | very good quality |
| [GGUF](https://huggingface.co/mradermacher/dehallucinated-llama-3.2-8b-instruct-GGUF/resolve/main/dehallucinated-llama-3.2-8b-instruct.Q8_0.gguf) | Q8_0 | 3.5 | fast, best quality |
| [GGUF](https://huggingface.co/mradermacher/dehallucinated-llama-3.2-8b-instruct-GGUF/resolve/main/dehallucinated-llama-3.2-8b-instruct.f16.gguf) | f16 | 6.5 | 16 bpw, overkill |
Here is a handy graph by ikawrakow comparing some lower-quality quant
types (lower is better):

And here are Artefact2's thoughts on the matter:
https://gist.github.com/Artefact2/b5f810600771265fc1e39442288e8ec9
## FAQ / Model Request
See https://huggingface.co/mradermacher/model_requests for some answers to
questions you might have and/or if you want some other model quantized.
## Thanks
I thank my company, [nethype GmbH](https://www.nethype.de/), for letting
me use its servers and providing upgrades to my workstation to enable
this work in my free time.
<!-- end -->
|
Xu-Ouyang/pythia-160m-deduped-int2-step110000-GPTQ-wikitext2-uva | Xu-Ouyang | "2024-09-13T12:41:32Z" | 75 | 0 | transformers | [
"transformers",
"safetensors",
"gpt_neox",
"text-generation",
"arxiv:1910.09700",
"autotrain_compatible",
"text-generation-inference",
"endpoints_compatible",
"2-bit",
"gptq",
"region:us"
] | text-generation | "2024-09-13T12:41:17Z" | ---
library_name: transformers
tags: []
---
# Model Card for Model ID
<!-- Provide a quick summary of what the model is/does. -->
## Model Details
### Model Description
<!-- Provide a longer summary of what this model is. -->
This is the model card of a 🤗 transformers model that has been pushed on the Hub. This model card has been automatically generated.
- **Developed by:** [More Information Needed]
- **Funded by [optional]:** [More Information Needed]
- **Shared by [optional]:** [More Information Needed]
- **Model type:** [More Information Needed]
- **Language(s) (NLP):** [More Information Needed]
- **License:** [More Information Needed]
- **Finetuned from model [optional]:** [More Information Needed]
### Model Sources [optional]
<!-- Provide the basic links for the model. -->
- **Repository:** [More Information Needed]
- **Paper [optional]:** [More Information Needed]
- **Demo [optional]:** [More Information Needed]
## Uses
<!-- Address questions around how the model is intended to be used, including the foreseeable users of the model and those affected by the model. -->
### Direct Use
<!-- This section is for the model use without fine-tuning or plugging into a larger ecosystem/app. -->
[More Information Needed]
### Downstream Use [optional]
<!-- This section is for the model use when fine-tuned for a task, or when plugged into a larger ecosystem/app -->
[More Information Needed]
### Out-of-Scope Use
<!-- This section addresses misuse, malicious use, and uses that the model will not work well for. -->
[More Information Needed]
## Bias, Risks, and Limitations
<!-- This section is meant to convey both technical and sociotechnical limitations. -->
[More Information Needed]
### Recommendations
<!-- This section is meant to convey recommendations with respect to the bias, risk, and technical limitations. -->
Users (both direct and downstream) should be made aware of the risks, biases and limitations of the model. More information needed for further recommendations.
## How to Get Started with the Model
Use the code below to get started with the model.
[More Information Needed]
## Training Details
### Training Data
<!-- This should link to a Dataset Card, perhaps with a short stub of information on what the training data is all about as well as documentation related to data pre-processing or additional filtering. -->
[More Information Needed]
### Training Procedure
<!-- This relates heavily to the Technical Specifications. Content here should link to that section when it is relevant to the training procedure. -->
#### Preprocessing [optional]
[More Information Needed]
#### Training Hyperparameters
- **Training regime:** [More Information Needed] <!--fp32, fp16 mixed precision, bf16 mixed precision, bf16 non-mixed precision, fp16 non-mixed precision, fp8 mixed precision -->
#### Speeds, Sizes, Times [optional]
<!-- This section provides information about throughput, start/end time, checkpoint size if relevant, etc. -->
[More Information Needed]
## Evaluation
<!-- This section describes the evaluation protocols and provides the results. -->
### Testing Data, Factors & Metrics
#### Testing Data
<!-- This should link to a Dataset Card if possible. -->
[More Information Needed]
#### Factors
<!-- These are the things the evaluation is disaggregating by, e.g., subpopulations or domains. -->
[More Information Needed]
#### Metrics
<!-- These are the evaluation metrics being used, ideally with a description of why. -->
[More Information Needed]
### Results
[More Information Needed]
#### Summary
## Model Examination [optional]
<!-- Relevant interpretability work for the model goes here -->
[More Information Needed]
## Environmental Impact
<!-- Total emissions (in grams of CO2eq) and additional considerations, such as electricity usage, go here. Edit the suggested text below accordingly -->
Carbon emissions can be estimated using the [Machine Learning Impact calculator](https://mlco2.github.io/impact#compute) presented in [Lacoste et al. (2019)](https://arxiv.org/abs/1910.09700).
- **Hardware Type:** [More Information Needed]
- **Hours used:** [More Information Needed]
- **Cloud Provider:** [More Information Needed]
- **Compute Region:** [More Information Needed]
- **Carbon Emitted:** [More Information Needed]
## Technical Specifications [optional]
### Model Architecture and Objective
[More Information Needed]
### Compute Infrastructure
[More Information Needed]
#### Hardware
[More Information Needed]
#### Software
[More Information Needed]
## Citation [optional]
<!-- If there is a paper or blog post introducing the model, the APA and Bibtex information for that should go in this section. -->
**BibTeX:**
[More Information Needed]
**APA:**
[More Information Needed]
## Glossary [optional]
<!-- If relevant, include terms and calculations in this section that can help readers understand the model or model card. -->
[More Information Needed]
## More Information [optional]
[More Information Needed]
## Model Card Authors [optional]
[More Information Needed]
## Model Card Contact
[More Information Needed] |
JacksonBrune/9aa904a1-6075-432a-8011-12852a7d995b | JacksonBrune | "2025-01-13T04:07:18Z" | 10 | 0 | peft | [
"peft",
"safetensors",
"llama",
"axolotl",
"generated_from_trainer",
"base_model:rayonlabs/merged-merged-af6dd40b-32e1-43b1-adfd-8ce14d65d738-PubMedQA-138437bf-44bd-4b03-8801-d05451a9ff28",
"base_model:adapter:rayonlabs/merged-merged-af6dd40b-32e1-43b1-adfd-8ce14d65d738-PubMedQA-138437bf-44bd-4b03-8801-d05451a9ff28",
"region:us"
] | null | "2025-01-13T02:10:16Z" | ---
library_name: peft
base_model: rayonlabs/merged-merged-af6dd40b-32e1-43b1-adfd-8ce14d65d738-PubMedQA-138437bf-44bd-4b03-8801-d05451a9ff28
tags:
- axolotl
- generated_from_trainer
model-index:
- name: 9aa904a1-6075-432a-8011-12852a7d995b
results: []
---
<!-- This model card has been generated automatically according to the information the Trainer had access to. You
should probably proofread and complete it, then remove this comment. -->
[<img src="https://raw.githubusercontent.com/axolotl-ai-cloud/axolotl/main/image/axolotl-badge-web.png" alt="Built with Axolotl" width="200" height="32"/>](https://github.com/axolotl-ai-cloud/axolotl)
<details><summary>See axolotl config</summary>
axolotl version: `0.4.1`
```yaml
adapter: lora
base_model: rayonlabs/merged-merged-af6dd40b-32e1-43b1-adfd-8ce14d65d738-PubMedQA-138437bf-44bd-4b03-8801-d05451a9ff28
bf16: auto
chat_template: llama3
dataset_prepared_path: null
datasets:
- data_files:
- 50570c988008bb52_train_data.json
ds_type: json
format: custom
path: /workspace/input_data/50570c988008bb52_train_data.json
type:
field_input: context
field_instruction: question
field_output: final_decision
format: '{instruction} {input}'
no_input_format: '{instruction}'
system_format: '{system}'
system_prompt: ''
debug: null
deepspeed: null
early_stopping_patience: null
eval_max_new_tokens: 128
eval_table_size: null
evals_per_epoch: 4
flash_attention: false
fp16: null
fsdp: null
fsdp_config: null
gradient_accumulation_steps: 4
gradient_checkpointing: false
group_by_length: false
hub_model_id: JacksonBrune/9aa904a1-6075-432a-8011-12852a7d995b
hub_repo: null
hub_strategy: checkpoint
hub_token: null
learning_rate: 0.0002
load_in_4bit: false
load_in_8bit: false
local_rank: null
logging_steps: 1
lora_alpha: 16
lora_dropout: 0.05
lora_fan_in_fan_out: null
lora_model_dir: null
lora_r: 8
lora_target_linear: true
lr_scheduler: cosine
max_steps: 10
micro_batch_size: 2
mlflow_experiment_name: /tmp/50570c988008bb52_train_data.json
model_type: AutoModelForCausalLM
num_epochs: 1
optimizer: adamw_bnb_8bit
output_dir: miner_id_24
pad_to_sequence_len: true
resume_from_checkpoint: null
s2_attention: null
sample_packing: false
saves_per_epoch: 4
sequence_len: 512
special_tokens:
pad_token: <|end_of_text|>
strict: false
tf32: false
tokenizer_type: AutoTokenizer
train_on_inputs: false
trust_remote_code: true
val_set_size: 0.05
wandb_entity: null
wandb_mode: online
wandb_name: bb82eda3-2375-490a-9d56-33d5775eeedb
wandb_project: birthdya-sn56-18-Gradients-On-Demand
wandb_run: your_name
wandb_runid: bb82eda3-2375-490a-9d56-33d5775eeedb
warmup_steps: 10
weight_decay: 0.0
xformers_attention: null
```
</details><br>
# 9aa904a1-6075-432a-8011-12852a7d995b
This model is a fine-tuned version of [rayonlabs/merged-merged-af6dd40b-32e1-43b1-adfd-8ce14d65d738-PubMedQA-138437bf-44bd-4b03-8801-d05451a9ff28](https://huggingface.co/rayonlabs/merged-merged-af6dd40b-32e1-43b1-adfd-8ce14d65d738-PubMedQA-138437bf-44bd-4b03-8801-d05451a9ff28) on the None dataset.
It achieves the following results on the evaluation set:
- Loss: 3.4878
## Model description
More information needed
## Intended uses & limitations
More information needed
## Training and evaluation data
More information needed
## Training procedure
### Training hyperparameters
The following hyperparameters were used during training:
- learning_rate: 0.0002
- train_batch_size: 2
- eval_batch_size: 2
- seed: 42
- gradient_accumulation_steps: 4
- total_train_batch_size: 8
- optimizer: Use OptimizerNames.ADAMW_BNB with betas=(0.9,0.999) and epsilon=1e-08 and optimizer_args=No additional optimizer arguments
- lr_scheduler_type: cosine
- lr_scheduler_warmup_steps: 10
- training_steps: 10
### Training results
| Training Loss | Epoch | Step | Validation Loss |
|:-------------:|:------:|:----:|:---------------:|
| 13.2355 | 0.0000 | 1 | 13.6415 |
| 13.9199 | 0.0001 | 3 | 13.1524 |
| 9.434 | 0.0002 | 6 | 6.8603 |
| 3.0717 | 0.0004 | 9 | 3.4878 |
### Framework versions
- PEFT 0.13.2
- Transformers 4.46.0
- Pytorch 2.5.0+cu124
- Datasets 3.0.1
- Tokenizers 0.20.1 |
Sukmin/Reinforce-PixelCopter | Sukmin | "2023-07-10T05:46:30Z" | 0 | 0 | null | [
"Pixelcopter-PLE-v0",
"reinforce",
"reinforcement-learning",
"custom-implementation",
"deep-rl-class",
"model-index",
"region:us"
] | reinforcement-learning | "2023-07-10T03:34:22Z" | ---
tags:
- Pixelcopter-PLE-v0
- reinforce
- reinforcement-learning
- custom-implementation
- deep-rl-class
model-index:
- name: Reinforce-PixelCopter
results:
- task:
type: reinforcement-learning
name: reinforcement-learning
dataset:
name: Pixelcopter-PLE-v0
type: Pixelcopter-PLE-v0
metrics:
- type: mean_reward
value: 35.40 +/- 26.24
name: mean_reward
verified: false
---
# **Reinforce** Agent playing **Pixelcopter-PLE-v0**
This is a trained model of a **Reinforce** agent playing **Pixelcopter-PLE-v0** .
To learn to use this model and train yours check Unit 4 of the Deep Reinforcement Learning Course: https://huggingface.co/deep-rl-course/unit4/introduction
|
nik135/distilbert-base-uncased-finetuned-emotion | nik135 | "2024-11-11T07:06:01Z" | 107 | 0 | transformers | [
"transformers",
"tensorboard",
"safetensors",
"distilbert",
"text-classification",
"generated_from_trainer",
"base_model:distilbert/distilbert-base-uncased",
"base_model:finetune:distilbert/distilbert-base-uncased",
"license:apache-2.0",
"autotrain_compatible",
"endpoints_compatible",
"region:us"
] | text-classification | "2024-10-08T08:38:01Z" | ---
library_name: transformers
license: apache-2.0
base_model: distilbert-base-uncased
tags:
- generated_from_trainer
metrics:
- accuracy
- f1
model-index:
- name: distilbert-base-uncased-finetuned-emotion
results: []
---
<!-- This model card has been generated automatically according to the information the Trainer had access to. You
should probably proofread and complete it, then remove this comment. -->
# distilbert-base-uncased-finetuned-emotion
This model is a fine-tuned version of [distilbert-base-uncased](https://huggingface.co/distilbert-base-uncased) on an unknown dataset.
It achieves the following results on the evaluation set:
- Loss: 0.2156
- Accuracy: 0.925
- F1: 0.9251
## Model description
More information needed
## Intended uses & limitations
More information needed
## Training and evaluation data
More information needed
## Training procedure
### Training hyperparameters
The following hyperparameters were used during training:
- learning_rate: 2e-05
- train_batch_size: 64
- eval_batch_size: 64
- seed: 42
- optimizer: Adam with betas=(0.9,0.999) and epsilon=1e-08
- lr_scheduler_type: linear
- num_epochs: 2
### Training results
| Training Loss | Epoch | Step | Validation Loss | Accuracy | F1 |
|:-------------:|:-----:|:----:|:---------------:|:--------:|:------:|
| 0.7958 | 1.0 | 250 | 0.3024 | 0.909 | 0.9086 |
| 0.2385 | 2.0 | 500 | 0.2156 | 0.925 | 0.9251 |
### Framework versions
- Transformers 4.44.2
- Pytorch 2.5.0+cu121
- Datasets 3.1.0
- Tokenizers 0.19.1
|
coreml-community/coreml-Roboetics-mix | coreml-community | "2023-03-05T14:59:16Z" | 0 | 3 | null | [
"coreml",
"stable-diffusion",
"text-to-image",
"license:creativeml-openrail-m",
"region:us"
] | text-to-image | "2023-01-11T01:43:05Z" | ---
license: creativeml-openrail-m
tags:
- coreml
- stable-diffusion
- text-to-image
---
# Core ML Converted Model:
- This model was converted to Core ML for use on Apple Silicon devices. Instructions can be found [here](https://github.com/godly-devotion/MochiDiffusion/wiki/How-to-convert-ckpt-files-to-Core-ML).<br>
- Provide the model to an app such as [Mochi Diffusion](https://github.com/godly-devotion/MochiDiffusion) to generate images.<br>
- `split_einsum` version is compatible with all compute unit options including Neural Engine.<br>
# Note: This model does not have the [unet split into chunks](https://github.com/apple/ml-stable-diffusion#-converting-models-to-core-ml).
# Roboetic's mix:
Source(s): [CivitAI](https://civitai.com/models/3738/roboetics-mix)
This model is some of my favourite models merged together.
It is a general purpose model which can generate good looking images with simpler prompts. |
baibaichuan/dqn-SpaceInvadersNoFrameskip-v4 | baibaichuan | "2025-03-11T13:23:19Z" | 0 | 0 | stable-baselines3 | [
"stable-baselines3",
"SpaceInvadersNoFrameskip-v4",
"deep-reinforcement-learning",
"reinforcement-learning",
"model-index",
"region:us"
] | reinforcement-learning | "2025-03-11T12:57:50Z" | ---
library_name: stable-baselines3
tags:
- SpaceInvadersNoFrameskip-v4
- deep-reinforcement-learning
- reinforcement-learning
- stable-baselines3
model-index:
- name: DQN
results:
- task:
type: reinforcement-learning
name: reinforcement-learning
dataset:
name: SpaceInvadersNoFrameskip-v4
type: SpaceInvadersNoFrameskip-v4
metrics:
- type: mean_reward
value: 512.50 +/- 169.64
name: mean_reward
verified: false
---
# **DQN** Agent playing **SpaceInvadersNoFrameskip-v4**
This is a trained model of a **DQN** agent playing **SpaceInvadersNoFrameskip-v4**
using the [stable-baselines3 library](https://github.com/DLR-RM/stable-baselines3)
and the [RL Zoo](https://github.com/DLR-RM/rl-baselines3-zoo).
The RL Zoo is a training framework for Stable Baselines3
reinforcement learning agents,
with hyperparameter optimization and pre-trained agents included.
## Usage (with SB3 RL Zoo)
RL Zoo: https://github.com/DLR-RM/rl-baselines3-zoo<br/>
SB3: https://github.com/DLR-RM/stable-baselines3<br/>
SB3 Contrib: https://github.com/Stable-Baselines-Team/stable-baselines3-contrib
SBX (SB3 + Jax): https://github.com/araffin/sbx
Install the RL Zoo (with SB3 and SB3-Contrib):
```bash
pip install rl_zoo3
```
```
# Download model and save it into the logs/ folder
python -m rl_zoo3.load_from_hub --algo dqn --env SpaceInvadersNoFrameskip-v4 -orga baibaichuan -f logs/
python -m rl_zoo3.enjoy --algo dqn --env SpaceInvadersNoFrameskip-v4 -f logs/
```
If you installed the RL Zoo3 via pip (`pip install rl_zoo3`), from anywhere you can do:
```
python -m rl_zoo3.load_from_hub --algo dqn --env SpaceInvadersNoFrameskip-v4 -orga baibaichuan -f logs/
python -m rl_zoo3.enjoy --algo dqn --env SpaceInvadersNoFrameskip-v4 -f logs/
```
## Training (with the RL Zoo)
```
python -m rl_zoo3.train --algo dqn --env SpaceInvadersNoFrameskip-v4 -f logs/
# Upload the model and generate video (when possible)
python -m rl_zoo3.push_to_hub --algo dqn --env SpaceInvadersNoFrameskip-v4 -f logs/ -orga baibaichuan
```
## Hyperparameters
```python
OrderedDict([('batch_size', 32),
('buffer_size', 100000),
('env_wrapper',
['stable_baselines3.common.atari_wrappers.AtariWrapper']),
('exploration_final_eps', 0.01),
('exploration_fraction', 0.1),
('frame_stack', 4),
('gradient_steps', 1),
('learning_rate', 0.0001),
('learning_starts', 100000),
('n_timesteps', 1000000.0),
('optimize_memory_usage', False),
('policy', 'CnnPolicy'),
('target_update_interval', 1000),
('train_freq', 4),
('normalize', False)])
```
# Environment Arguments
```python
{'render_mode': 'rgb_array'}
```
|
Jivika1/ASR | Jivika1 | "2025-02-20T13:32:33Z" | 0 | 0 | transformers | [
"transformers",
"safetensors",
"whisper",
"automatic-speech-recognition",
"generated_from_trainer",
"base_model:openai/whisper-medium",
"base_model:finetune:openai/whisper-medium",
"license:apache-2.0",
"endpoints_compatible",
"region:us"
] | automatic-speech-recognition | "2025-02-20T13:16:04Z" | ---
library_name: transformers
license: apache-2.0
base_model: openai/whisper-medium
tags:
- generated_from_trainer
metrics:
- wer
model-index:
- name: whisper-medium-medical
results: []
---
<!-- This model card has been generated automatically according to the information the Trainer had access to. You
should probably proofread and complete it, then remove this comment. -->
# whisper-medium-medical
This model is a fine-tuned version of [openai/whisper-medium](https://huggingface.co/openai/whisper-medium) on an unknown dataset.
It achieves the following results on the evaluation set:
- Loss: 0.0562
- Wer: 10.7169
## Model description
More information needed
## Intended uses & limitations
More information needed
## Training and evaluation data
More information needed
## Training procedure
### Training hyperparameters
The following hyperparameters were used during training:
- learning_rate: 1e-05
- train_batch_size: 32
- eval_batch_size: 8
- seed: 42
- optimizer: Use OptimizerNames.ADAMW_TORCH with betas=(0.9,0.999) and epsilon=1e-08 and optimizer_args=No additional optimizer arguments
- lr_scheduler_type: linear
- lr_scheduler_warmup_steps: 50
- training_steps: 500
- mixed_precision_training: Native AMP
### Training results
| Training Loss | Epoch | Step | Validation Loss | Wer |
|:-------------:|:------:|:----:|:---------------:|:-------:|
| 0.5008 | 0.5405 | 100 | 0.1965 | 12.0203 |
| 0.1034 | 1.0811 | 200 | 0.0870 | 12.2616 |
| 0.0563 | 1.6216 | 300 | 0.0642 | 8.3514 |
| 0.0238 | 2.1622 | 400 | 0.0610 | 11.6341 |
| 0.0129 | 2.7027 | 500 | 0.0562 | 10.7169 |
### Framework versions
- Transformers 4.49.0
- Pytorch 2.6.0+cu118
- Datasets 3.3.1
- Tokenizers 0.21.0
|
lesso16/cc432f5d-7a5c-4010-8f56-2589ff64aee7 | lesso16 | "2025-03-28T15:49:19Z" | 0 | 0 | peft | [
"peft",
"safetensors",
"llama",
"axolotl",
"generated_from_trainer",
"base_model:JackFram/llama-68m",
"base_model:adapter:JackFram/llama-68m",
"license:apache-2.0",
"region:us"
] | null | "2025-03-28T15:45:33Z" | ---
library_name: peft
license: apache-2.0
base_model: JackFram/llama-68m
tags:
- axolotl
- generated_from_trainer
model-index:
- name: cc432f5d-7a5c-4010-8f56-2589ff64aee7
results: []
---
<!-- This model card has been generated automatically according to the information the Trainer had access to. You
should probably proofread and complete it, then remove this comment. -->
[<img src="https://raw.githubusercontent.com/axolotl-ai-cloud/axolotl/main/image/axolotl-badge-web.png" alt="Built with Axolotl" width="200" height="32"/>](https://github.com/axolotl-ai-cloud/axolotl)
<details><summary>See axolotl config</summary>
axolotl version: `0.4.1`
```yaml
adapter: lora
base_model: JackFram/llama-68m
bf16: auto
chat_template: llama3
dataset_prepared_path: null
datasets:
- data_files:
- 623d9787d7fd7d0e_train_data.json
ds_type: json
format: custom
path: /workspace/input_data/623d9787d7fd7d0e_train_data.json
type:
field_instruction: text
field_output: title
format: '{instruction}'
no_input_format: '{instruction}'
system_format: '{system}'
system_prompt: ''
debug: null
deepspeed: null
do_eval: true
early_stopping_patience: 3
eval_batch_size: 4
eval_max_new_tokens: 128
eval_steps: 500
evals_per_epoch: null
flash_attention: true
fp16: false
fsdp: null
fsdp_config: null
gradient_accumulation_steps: 8
gradient_checkpointing: true
group_by_length: true
hub_model_id: lesso16/cc432f5d-7a5c-4010-8f56-2589ff64aee7
hub_repo: null
hub_strategy: checkpoint
hub_token: null
learning_rate: 0.000216
load_in_4bit: false
load_in_8bit: false
local_rank: null
logging_steps: 50
lora_alpha: 128
lora_dropout: 0.15
lora_fan_in_fan_out: null
lora_model_dir: null
lora_r: 64
lora_target_linear: true
lr_scheduler: cosine
max_grad_norm: 1.0
max_steps: 500
micro_batch_size: 4
mlflow_experiment_name: /tmp/623d9787d7fd7d0e_train_data.json
model_type: AutoModelForCausalLM
num_epochs: 10
optimizer: adamw_torch_fused
output_dir: miner_id_24
pad_to_sequence_len: true
resume_from_checkpoint: null
s2_attention: null
sample_packing: false
save_steps: 500
saves_per_epoch: null
seed: 160
sequence_len: 1024
special_tokens:
pad_token: </s>
strict: false
tf32: true
tokenizer_type: AutoTokenizer
train_on_inputs: false
trust_remote_code: true
val_set_size: 0.05
wandb_entity: null
wandb_mode: online
wandb_name: af6de128-8865-42e8-800b-ff2d2b1acccd
wandb_project: 16a
wandb_run: your_name
wandb_runid: af6de128-8865-42e8-800b-ff2d2b1acccd
warmup_steps: 100
weight_decay: 0.0
xformers_attention: null
```
</details><br>
# cc432f5d-7a5c-4010-8f56-2589ff64aee7
This model is a fine-tuned version of [JackFram/llama-68m](https://huggingface.co/JackFram/llama-68m) on the None dataset.
It achieves the following results on the evaluation set:
- Loss: 0.0133
## Model description
More information needed
## Intended uses & limitations
More information needed
## Training and evaluation data
More information needed
## Training procedure
### Training hyperparameters
The following hyperparameters were used during training:
- learning_rate: 0.000216
- train_batch_size: 4
- eval_batch_size: 4
- seed: 160
- gradient_accumulation_steps: 8
- total_train_batch_size: 32
- optimizer: Use OptimizerNames.ADAMW_TORCH_FUSED with betas=(0.9,0.999) and epsilon=1e-08 and optimizer_args=No additional optimizer arguments
- lr_scheduler_type: cosine
- lr_scheduler_warmup_steps: 100
- training_steps: 500
### Training results
| Training Loss | Epoch | Step | Validation Loss |
|:-------------:|:------:|:----:|:---------------:|
| No log | 0.0010 | 1 | 2.4174 |
| 0.0125 | 0.5182 | 500 | 0.0133 |
### Framework versions
- PEFT 0.13.2
- Transformers 4.46.0
- Pytorch 2.5.0+cu124
- Datasets 3.0.1
- Tokenizers 0.20.1 |
baddii/20_baddii_08_911 | baddii | "2025-02-18T08:46:20Z" | 0 | 0 | transformers | [
"transformers",
"safetensors",
"parler_tts",
"text2text-generation",
"arxiv:1910.09700",
"autotrain_compatible",
"endpoints_compatible",
"region:us"
] | text2text-generation | "2025-02-18T08:44:33Z" | ---
library_name: transformers
tags: []
---
# Model Card for Model ID
<!-- Provide a quick summary of what the model is/does. -->
## Model Details
### Model Description
<!-- Provide a longer summary of what this model is. -->
This is the model card of a 🤗 transformers model that has been pushed on the Hub. This model card has been automatically generated.
- **Developed by:** [More Information Needed]
- **Funded by [optional]:** [More Information Needed]
- **Shared by [optional]:** [More Information Needed]
- **Model type:** [More Information Needed]
- **Language(s) (NLP):** [More Information Needed]
- **License:** [More Information Needed]
- **Finetuned from model [optional]:** [More Information Needed]
### Model Sources [optional]
<!-- Provide the basic links for the model. -->
- **Repository:** [More Information Needed]
- **Paper [optional]:** [More Information Needed]
- **Demo [optional]:** [More Information Needed]
## Uses
<!-- Address questions around how the model is intended to be used, including the foreseeable users of the model and those affected by the model. -->
### Direct Use
<!-- This section is for the model use without fine-tuning or plugging into a larger ecosystem/app. -->
[More Information Needed]
### Downstream Use [optional]
<!-- This section is for the model use when fine-tuned for a task, or when plugged into a larger ecosystem/app -->
[More Information Needed]
### Out-of-Scope Use
<!-- This section addresses misuse, malicious use, and uses that the model will not work well for. -->
[More Information Needed]
## Bias, Risks, and Limitations
<!-- This section is meant to convey both technical and sociotechnical limitations. -->
[More Information Needed]
### Recommendations
<!-- This section is meant to convey recommendations with respect to the bias, risk, and technical limitations. -->
Users (both direct and downstream) should be made aware of the risks, biases and limitations of the model. More information needed for further recommendations.
## How to Get Started with the Model
Use the code below to get started with the model.
[More Information Needed]
## Training Details
### Training Data
<!-- This should link to a Dataset Card, perhaps with a short stub of information on what the training data is all about as well as documentation related to data pre-processing or additional filtering. -->
[More Information Needed]
### Training Procedure
<!-- This relates heavily to the Technical Specifications. Content here should link to that section when it is relevant to the training procedure. -->
#### Preprocessing [optional]
[More Information Needed]
#### Training Hyperparameters
- **Training regime:** [More Information Needed] <!--fp32, fp16 mixed precision, bf16 mixed precision, bf16 non-mixed precision, fp16 non-mixed precision, fp8 mixed precision -->
#### Speeds, Sizes, Times [optional]
<!-- This section provides information about throughput, start/end time, checkpoint size if relevant, etc. -->
[More Information Needed]
## Evaluation
<!-- This section describes the evaluation protocols and provides the results. -->
### Testing Data, Factors & Metrics
#### Testing Data
<!-- This should link to a Dataset Card if possible. -->
[More Information Needed]
#### Factors
<!-- These are the things the evaluation is disaggregating by, e.g., subpopulations or domains. -->
[More Information Needed]
#### Metrics
<!-- These are the evaluation metrics being used, ideally with a description of why. -->
[More Information Needed]
### Results
[More Information Needed]
#### Summary
## Model Examination [optional]
<!-- Relevant interpretability work for the model goes here -->
[More Information Needed]
## Environmental Impact
<!-- Total emissions (in grams of CO2eq) and additional considerations, such as electricity usage, go here. Edit the suggested text below accordingly -->
Carbon emissions can be estimated using the [Machine Learning Impact calculator](https://mlco2.github.io/impact#compute) presented in [Lacoste et al. (2019)](https://arxiv.org/abs/1910.09700).
- **Hardware Type:** [More Information Needed]
- **Hours used:** [More Information Needed]
- **Cloud Provider:** [More Information Needed]
- **Compute Region:** [More Information Needed]
- **Carbon Emitted:** [More Information Needed]
## Technical Specifications [optional]
### Model Architecture and Objective
[More Information Needed]
### Compute Infrastructure
[More Information Needed]
#### Hardware
[More Information Needed]
#### Software
[More Information Needed]
## Citation [optional]
<!-- If there is a paper or blog post introducing the model, the APA and Bibtex information for that should go in this section. -->
**BibTeX:**
[More Information Needed]
**APA:**
[More Information Needed]
## Glossary [optional]
<!-- If relevant, include terms and calculations in this section that can help readers understand the model or model card. -->
[More Information Needed]
## More Information [optional]
[More Information Needed]
## Model Card Authors [optional]
[More Information Needed]
## Model Card Contact
[More Information Needed] |
aitorrent/dolphin-2.9.3-qwen2-0.5b-GGUF-torrent | aitorrent | "2024-06-16T14:09:48Z" | 0 | 0 | null | [
"torrent",
"license:apache-2.0",
"region:us"
] | null | "2024-06-16T13:59:12Z" | ---
license: apache-2.0
tags:
- torrent
---
## About
<!-- ### quantize_version: 2 -->
<!-- ### output_tensor_quantised: 1 -->
<!-- ### convert_type: hf -->
<!-- ### vocab_type: -->
<!-- ### tags: -->
static quants of https://huggingface.co/cognitivecomputations/dolphin-2.9.3-qwen2-0.5b
<!-- provided-files -->
weighted/imatrix quants are available at https://huggingface.co/mradermacher/dolphin-2.9.3-qwen2-0.5b-i1-GGUF
## Usage
If you are unsure how to use GGUF files, refer to one of [TheBloke's
READMEs](https://huggingface.co/TheBloke/KafkaLM-70B-German-V0.1-GGUF) for
more details, including on how to concatenate multi-part files.
## Provided Quants
(sorted by size, not necessarily quality. IQ-quants are often preferable over similar sized non-IQ quants)
| Link | Type | Size/GB | Notes |
|:-----|:-----|--------:|:------|
| [GGUF](https://huggingface.co/mradermacher/dolphin-2.9.3-qwen2-0.5b-GGUF/resolve/main/dolphin-2.9.3-qwen2-0.5b.Q3_K_S.gguf) | Q3_K_S | 0.4 | |
| [GGUF](https://huggingface.co/mradermacher/dolphin-2.9.3-qwen2-0.5b-GGUF/resolve/main/dolphin-2.9.3-qwen2-0.5b.IQ3_S.gguf) | IQ3_S | 0.4 | beats Q3_K* |
| [GGUF](https://huggingface.co/mradermacher/dolphin-2.9.3-qwen2-0.5b-GGUF/resolve/main/dolphin-2.9.3-qwen2-0.5b.IQ3_XS.gguf) | IQ3_XS | 0.4 | |
| [GGUF](https://huggingface.co/mradermacher/dolphin-2.9.3-qwen2-0.5b-GGUF/resolve/main/dolphin-2.9.3-qwen2-0.5b.Q2_K.gguf) | Q2_K | 0.4 | |
| [GGUF](https://huggingface.co/mradermacher/dolphin-2.9.3-qwen2-0.5b-GGUF/resolve/main/dolphin-2.9.3-qwen2-0.5b.IQ3_M.gguf) | IQ3_M | 0.4 | |
| [GGUF](https://huggingface.co/mradermacher/dolphin-2.9.3-qwen2-0.5b-GGUF/resolve/main/dolphin-2.9.3-qwen2-0.5b.IQ4_XS.gguf) | IQ4_XS | 0.5 | |
| [GGUF](https://huggingface.co/mradermacher/dolphin-2.9.3-qwen2-0.5b-GGUF/resolve/main/dolphin-2.9.3-qwen2-0.5b.Q3_K_M.gguf) | Q3_K_M | 0.5 | lower quality |
| [GGUF](https://huggingface.co/mradermacher/dolphin-2.9.3-qwen2-0.5b-GGUF/resolve/main/dolphin-2.9.3-qwen2-0.5b.Q3_K_L.gguf) | Q3_K_L | 0.5 | |
| [GGUF](https://huggingface.co/mradermacher/dolphin-2.9.3-qwen2-0.5b-GGUF/resolve/main/dolphin-2.9.3-qwen2-0.5b.Q4_K_S.gguf) | Q4_K_S | 0.5 | fast, recommended |
| [GGUF](https://huggingface.co/mradermacher/dolphin-2.9.3-qwen2-0.5b-GGUF/resolve/main/dolphin-2.9.3-qwen2-0.5b.Q4_K_M.gguf) | Q4_K_M | 0.5 | fast, recommended |
| [GGUF](https://huggingface.co/mradermacher/dolphin-2.9.3-qwen2-0.5b-GGUF/resolve/main/dolphin-2.9.3-qwen2-0.5b.Q5_K_S.gguf) | Q5_K_S | 0.5 | |
| [GGUF](https://huggingface.co/mradermacher/dolphin-2.9.3-qwen2-0.5b-GGUF/resolve/main/dolphin-2.9.3-qwen2-0.5b.Q5_K_M.gguf) | Q5_K_M | 0.5 | |
| [GGUF](https://huggingface.co/mradermacher/dolphin-2.9.3-qwen2-0.5b-GGUF/resolve/main/dolphin-2.9.3-qwen2-0.5b.Q6_K.gguf) | Q6_K | 0.6 | very good quality |
| [GGUF](https://huggingface.co/mradermacher/dolphin-2.9.3-qwen2-0.5b-GGUF/resolve/main/dolphin-2.9.3-qwen2-0.5b.Q8_0.gguf) | Q8_0 | 0.6 | fast, best quality |
| [GGUF](https://huggingface.co/mradermacher/dolphin-2.9.3-qwen2-0.5b-GGUF/resolve/main/dolphin-2.9.3-qwen2-0.5b.f16.gguf) | f16 | 1.1 | 16 bpw, overkill |
Here is a handy graph by ikawrakow comparing some lower-quality quant
types (lower is better):

And here are Artefact2's thoughts on the matter:
https://gist.github.com/Artefact2/b5f810600771265fc1e39442288e8ec9 |
johnsnowlabs/PhigRange-2.7B-slerp | johnsnowlabs | "2024-04-10T11:14:41Z" | 6 | 0 | transformers | [
"transformers",
"safetensors",
"phi",
"text-generation",
"merge",
"mergekit",
"lazymergekit",
"liminerity/Phigments12",
"rhysjones/phi-2-orange-v2",
"base_model:liminerity/Phigments12",
"base_model:merge:liminerity/Phigments12",
"base_model:rhysjones/phi-2-orange-v2",
"base_model:merge:rhysjones/phi-2-orange-v2",
"autotrain_compatible",
"text-generation-inference",
"endpoints_compatible",
"region:us"
] | text-generation | "2024-04-08T19:42:59Z" | ---
tags:
- merge
- mergekit
- lazymergekit
- liminerity/Phigments12
- rhysjones/phi-2-orange-v2
base_model:
- liminerity/Phigments12
- rhysjones/phi-2-orange-v2
---
# PhigRange-2.7B-slerp

PhigRange-2.7B-slerp is a merge of the following models using [LazyMergekit](https://colab.research.google.com/drive/1obulZ1ROXHjYLn6PPZJwRR6GzgQogxxb?usp=sharing):
* [liminerity/Phigments12](https://huggingface.co/liminerity/Phigments12)
* [rhysjones/phi-2-orange-v2](https://huggingface.co/rhysjones/phi-2-orange-v2)
Special thanks to Charles Goddard for the quick implementation!
## 🧩 Configuration
```yaml
slices:
- sources:
- model: liminerity/Phigments12
layer_range: [0, 32]
- model: rhysjones/phi-2-orange-v2
layer_range: [0, 32]
merge_method: slerp
base_model: liminerity/Phigments12
parameters:
t:
- filter: self_attn
value: [0, 0.5, 0.3, 0.7, 1]
- filter: mlp
value: [1, 0.5, 0.7, 0.3, 0]
- value: 0.5
dtype: bfloat16
```
## 💻 Usage
```python
!pip install -qU transformers accelerate
from transformers import AutoTokenizer
import transformers
import torch
model = "johnsnowlabs/PhigRange-2.7B-slerp"
messages = [{"role": "user", "content": "What is a large language model?"}]
tokenizer = AutoTokenizer.from_pretrained(model)
prompt = tokenizer.apply_chat_template(messages, tokenize=False, add_generation_prompt=True)
pipeline = transformers.pipeline(
"text-generation",
model=model,
torch_dtype=torch.float16,
device_map="auto",
)
outputs = pipeline(prompt, max_new_tokens=256, do_sample=True, temperature=0.7, top_k=50, top_p=0.95)
print(outputs[0]["generated_text"])
```
## 🏆 Evaluation
Coming Soon! |
tensorblock/You_can_cry_Snowman-13B-GGUF | tensorblock | "2024-12-28T11:37:00Z" | 26 | 0 | transformers | [
"transformers",
"gguf",
"TensorBlock",
"GGUF",
"text-generation",
"ko",
"base_model:DopeorNope/You_can_cry_Snowman-13B",
"base_model:quantized:DopeorNope/You_can_cry_Snowman-13B",
"license:cc-by-nc-sa-4.0",
"endpoints_compatible",
"region:us",
"conversational"
] | text-generation | "2024-12-28T10:25:40Z" | ---
language:
- ko
library_name: transformers
pipeline_tag: text-generation
license: cc-by-nc-sa-4.0
tags:
- TensorBlock
- GGUF
base_model: DopeorNope/You_can_cry_Snowman-13B
---
<div style="width: auto; margin-left: auto; margin-right: auto">
<img src="https://i.imgur.com/jC7kdl8.jpeg" alt="TensorBlock" style="width: 100%; min-width: 400px; display: block; margin: auto;">
</div>
<div style="display: flex; justify-content: space-between; width: 100%;">
<div style="display: flex; flex-direction: column; align-items: flex-start;">
<p style="margin-top: 0.5em; margin-bottom: 0em;">
Feedback and support: TensorBlock's <a href="https://x.com/tensorblock_aoi">Twitter/X</a>, <a href="https://t.me/TensorBlock">Telegram Group</a> and <a href="https://x.com/tensorblock_aoi">Discord server</a>
</p>
</div>
</div>
## DopeorNope/You_can_cry_Snowman-13B - GGUF
This repo contains GGUF format model files for [DopeorNope/You_can_cry_Snowman-13B](https://huggingface.co/DopeorNope/You_can_cry_Snowman-13B).
The files were quantized using machines provided by [TensorBlock](https://tensorblock.co/), and they are compatible with llama.cpp as of [commit b4242](https://github.com/ggerganov/llama.cpp/commit/a6744e43e80f4be6398fc7733a01642c846dce1d).
<div style="text-align: left; margin: 20px 0;">
<a href="https://tensorblock.co/waitlist/client" style="display: inline-block; padding: 10px 20px; background-color: #007bff; color: white; text-decoration: none; border-radius: 5px; font-weight: bold;">
Run them on the TensorBlock client using your local machine ↗
</a>
</div>
## Prompt template
```
### System:
{system_prompt}
### User:
{prompt}
### Assistant:
```
## Model file specification
| Filename | Quant type | File Size | Description |
| -------- | ---------- | --------- | ----------- |
| [You_can_cry_Snowman-13B-Q2_K.gguf](https://huggingface.co/tensorblock/You_can_cry_Snowman-13B-GGUF/blob/main/You_can_cry_Snowman-13B-Q2_K.gguf) | Q2_K | 4.966 GB | smallest, significant quality loss - not recommended for most purposes |
| [You_can_cry_Snowman-13B-Q3_K_S.gguf](https://huggingface.co/tensorblock/You_can_cry_Snowman-13B-GGUF/blob/main/You_can_cry_Snowman-13B-Q3_K_S.gguf) | Q3_K_S | 5.790 GB | very small, high quality loss |
| [You_can_cry_Snowman-13B-Q3_K_M.gguf](https://huggingface.co/tensorblock/You_can_cry_Snowman-13B-GGUF/blob/main/You_can_cry_Snowman-13B-Q3_K_M.gguf) | Q3_K_M | 6.448 GB | very small, high quality loss |
| [You_can_cry_Snowman-13B-Q3_K_L.gguf](https://huggingface.co/tensorblock/You_can_cry_Snowman-13B-GGUF/blob/main/You_can_cry_Snowman-13B-Q3_K_L.gguf) | Q3_K_L | 7.022 GB | small, substantial quality loss |
| [You_can_cry_Snowman-13B-Q4_0.gguf](https://huggingface.co/tensorblock/You_can_cry_Snowman-13B-GGUF/blob/main/You_can_cry_Snowman-13B-Q4_0.gguf) | Q4_0 | 7.545 GB | legacy; small, very high quality loss - prefer using Q3_K_M |
| [You_can_cry_Snowman-13B-Q4_K_S.gguf](https://huggingface.co/tensorblock/You_can_cry_Snowman-13B-GGUF/blob/main/You_can_cry_Snowman-13B-Q4_K_S.gguf) | Q4_K_S | 7.598 GB | small, greater quality loss |
| [You_can_cry_Snowman-13B-Q4_K_M.gguf](https://huggingface.co/tensorblock/You_can_cry_Snowman-13B-GGUF/blob/main/You_can_cry_Snowman-13B-Q4_K_M.gguf) | Q4_K_M | 8.032 GB | medium, balanced quality - recommended |
| [You_can_cry_Snowman-13B-Q5_0.gguf](https://huggingface.co/tensorblock/You_can_cry_Snowman-13B-GGUF/blob/main/You_can_cry_Snowman-13B-Q5_0.gguf) | Q5_0 | 9.197 GB | legacy; medium, balanced quality - prefer using Q4_K_M |
| [You_can_cry_Snowman-13B-Q5_K_S.gguf](https://huggingface.co/tensorblock/You_can_cry_Snowman-13B-GGUF/blob/main/You_can_cry_Snowman-13B-Q5_K_S.gguf) | Q5_K_S | 9.197 GB | large, low quality loss - recommended |
| [You_can_cry_Snowman-13B-Q5_K_M.gguf](https://huggingface.co/tensorblock/You_can_cry_Snowman-13B-GGUF/blob/main/You_can_cry_Snowman-13B-Q5_K_M.gguf) | Q5_K_M | 9.448 GB | large, very low quality loss - recommended |
| [You_can_cry_Snowman-13B-Q6_K.gguf](https://huggingface.co/tensorblock/You_can_cry_Snowman-13B-GGUF/blob/main/You_can_cry_Snowman-13B-Q6_K.gguf) | Q6_K | 10.953 GB | very large, extremely low quality loss |
| [You_can_cry_Snowman-13B-Q8_0.gguf](https://huggingface.co/tensorblock/You_can_cry_Snowman-13B-GGUF/blob/main/You_can_cry_Snowman-13B-Q8_0.gguf) | Q8_0 | 14.185 GB | very large, extremely low quality loss - not recommended |
## Downloading instruction
### Command line
Firstly, install Huggingface Client
```shell
pip install -U "huggingface_hub[cli]"
```
Then, downoad the individual model file the a local directory
```shell
huggingface-cli download tensorblock/You_can_cry_Snowman-13B-GGUF --include "You_can_cry_Snowman-13B-Q2_K.gguf" --local-dir MY_LOCAL_DIR
```
If you wanna download multiple model files with a pattern (e.g., `*Q4_K*gguf`), you can try:
```shell
huggingface-cli download tensorblock/You_can_cry_Snowman-13B-GGUF --local-dir MY_LOCAL_DIR --local-dir-use-symlinks False --include='*Q4_K*gguf'
```
|
RichardErkhov/goethe0101_-_llama-3-2-3B-wame-16bit-survey-generator4-gguf | RichardErkhov | "2025-03-25T22:37:15Z" | 0 | 0 | null | [
"gguf",
"endpoints_compatible",
"region:us",
"conversational"
] | null | "2025-03-25T21:37:31Z" | Quantization made by Richard Erkhov.
[Github](https://github.com/RichardErkhov)
[Discord](https://discord.gg/pvy7H8DZMG)
[Request more models](https://github.com/RichardErkhov/quant_request)
llama-3-2-3B-wame-16bit-survey-generator4 - GGUF
- Model creator: https://huggingface.co/goethe0101/
- Original model: https://huggingface.co/goethe0101/llama-3-2-3B-wame-16bit-survey-generator4/
| Name | Quant method | Size |
| ---- | ---- | ---- |
| [llama-3-2-3B-wame-16bit-survey-generator4.Q2_K.gguf](https://huggingface.co/RichardErkhov/goethe0101_-_llama-3-2-3B-wame-16bit-survey-generator4-gguf/blob/main/llama-3-2-3B-wame-16bit-survey-generator4.Q2_K.gguf) | Q2_K | 1.27GB |
| [llama-3-2-3B-wame-16bit-survey-generator4.IQ3_XS.gguf](https://huggingface.co/RichardErkhov/goethe0101_-_llama-3-2-3B-wame-16bit-survey-generator4-gguf/blob/main/llama-3-2-3B-wame-16bit-survey-generator4.IQ3_XS.gguf) | IQ3_XS | 1.38GB |
| [llama-3-2-3B-wame-16bit-survey-generator4.IQ3_S.gguf](https://huggingface.co/RichardErkhov/goethe0101_-_llama-3-2-3B-wame-16bit-survey-generator4-gguf/blob/main/llama-3-2-3B-wame-16bit-survey-generator4.IQ3_S.gguf) | IQ3_S | 1.44GB |
| [llama-3-2-3B-wame-16bit-survey-generator4.Q3_K_S.gguf](https://huggingface.co/RichardErkhov/goethe0101_-_llama-3-2-3B-wame-16bit-survey-generator4-gguf/blob/main/llama-3-2-3B-wame-16bit-survey-generator4.Q3_K_S.gguf) | Q3_K_S | 1.44GB |
| [llama-3-2-3B-wame-16bit-survey-generator4.IQ3_M.gguf](https://huggingface.co/RichardErkhov/goethe0101_-_llama-3-2-3B-wame-16bit-survey-generator4-gguf/blob/main/llama-3-2-3B-wame-16bit-survey-generator4.IQ3_M.gguf) | IQ3_M | 1.49GB |
| [llama-3-2-3B-wame-16bit-survey-generator4.Q3_K.gguf](https://huggingface.co/RichardErkhov/goethe0101_-_llama-3-2-3B-wame-16bit-survey-generator4-gguf/blob/main/llama-3-2-3B-wame-16bit-survey-generator4.Q3_K.gguf) | Q3_K | 1.57GB |
| [llama-3-2-3B-wame-16bit-survey-generator4.Q3_K_M.gguf](https://huggingface.co/RichardErkhov/goethe0101_-_llama-3-2-3B-wame-16bit-survey-generator4-gguf/blob/main/llama-3-2-3B-wame-16bit-survey-generator4.Q3_K_M.gguf) | Q3_K_M | 1.57GB |
| [llama-3-2-3B-wame-16bit-survey-generator4.Q3_K_L.gguf](https://huggingface.co/RichardErkhov/goethe0101_-_llama-3-2-3B-wame-16bit-survey-generator4-gguf/blob/main/llama-3-2-3B-wame-16bit-survey-generator4.Q3_K_L.gguf) | Q3_K_L | 1.69GB |
| [llama-3-2-3B-wame-16bit-survey-generator4.IQ4_XS.gguf](https://huggingface.co/RichardErkhov/goethe0101_-_llama-3-2-3B-wame-16bit-survey-generator4-gguf/blob/main/llama-3-2-3B-wame-16bit-survey-generator4.IQ4_XS.gguf) | IQ4_XS | 1.71GB |
| [llama-3-2-3B-wame-16bit-survey-generator4.Q4_0.gguf](https://huggingface.co/RichardErkhov/goethe0101_-_llama-3-2-3B-wame-16bit-survey-generator4-gguf/blob/main/llama-3-2-3B-wame-16bit-survey-generator4.Q4_0.gguf) | Q4_0 | 1.79GB |
| [llama-3-2-3B-wame-16bit-survey-generator4.IQ4_NL.gguf](https://huggingface.co/RichardErkhov/goethe0101_-_llama-3-2-3B-wame-16bit-survey-generator4-gguf/blob/main/llama-3-2-3B-wame-16bit-survey-generator4.IQ4_NL.gguf) | IQ4_NL | 1.79GB |
| [llama-3-2-3B-wame-16bit-survey-generator4.Q4_K_S.gguf](https://huggingface.co/RichardErkhov/goethe0101_-_llama-3-2-3B-wame-16bit-survey-generator4-gguf/blob/main/llama-3-2-3B-wame-16bit-survey-generator4.Q4_K_S.gguf) | Q4_K_S | 1.8GB |
| [llama-3-2-3B-wame-16bit-survey-generator4.Q4_K.gguf](https://huggingface.co/RichardErkhov/goethe0101_-_llama-3-2-3B-wame-16bit-survey-generator4-gguf/blob/main/llama-3-2-3B-wame-16bit-survey-generator4.Q4_K.gguf) | Q4_K | 1.88GB |
| [llama-3-2-3B-wame-16bit-survey-generator4.Q4_K_M.gguf](https://huggingface.co/RichardErkhov/goethe0101_-_llama-3-2-3B-wame-16bit-survey-generator4-gguf/blob/main/llama-3-2-3B-wame-16bit-survey-generator4.Q4_K_M.gguf) | Q4_K_M | 1.88GB |
| [llama-3-2-3B-wame-16bit-survey-generator4.Q4_1.gguf](https://huggingface.co/RichardErkhov/goethe0101_-_llama-3-2-3B-wame-16bit-survey-generator4-gguf/blob/main/llama-3-2-3B-wame-16bit-survey-generator4.Q4_1.gguf) | Q4_1 | 1.95GB |
| [llama-3-2-3B-wame-16bit-survey-generator4.Q5_0.gguf](https://huggingface.co/RichardErkhov/goethe0101_-_llama-3-2-3B-wame-16bit-survey-generator4-gguf/blob/main/llama-3-2-3B-wame-16bit-survey-generator4.Q5_0.gguf) | Q5_0 | 2.11GB |
| [llama-3-2-3B-wame-16bit-survey-generator4.Q5_K_S.gguf](https://huggingface.co/RichardErkhov/goethe0101_-_llama-3-2-3B-wame-16bit-survey-generator4-gguf/blob/main/llama-3-2-3B-wame-16bit-survey-generator4.Q5_K_S.gguf) | Q5_K_S | 2.11GB |
| [llama-3-2-3B-wame-16bit-survey-generator4.Q5_K.gguf](https://huggingface.co/RichardErkhov/goethe0101_-_llama-3-2-3B-wame-16bit-survey-generator4-gguf/blob/main/llama-3-2-3B-wame-16bit-survey-generator4.Q5_K.gguf) | Q5_K | 2.16GB |
| [llama-3-2-3B-wame-16bit-survey-generator4.Q5_K_M.gguf](https://huggingface.co/RichardErkhov/goethe0101_-_llama-3-2-3B-wame-16bit-survey-generator4-gguf/blob/main/llama-3-2-3B-wame-16bit-survey-generator4.Q5_K_M.gguf) | Q5_K_M | 2.16GB |
| [llama-3-2-3B-wame-16bit-survey-generator4.Q5_1.gguf](https://huggingface.co/RichardErkhov/goethe0101_-_llama-3-2-3B-wame-16bit-survey-generator4-gguf/blob/main/llama-3-2-3B-wame-16bit-survey-generator4.Q5_1.gguf) | Q5_1 | 2.28GB |
| [llama-3-2-3B-wame-16bit-survey-generator4.Q6_K.gguf](https://huggingface.co/RichardErkhov/goethe0101_-_llama-3-2-3B-wame-16bit-survey-generator4-gguf/blob/main/llama-3-2-3B-wame-16bit-survey-generator4.Q6_K.gguf) | Q6_K | 2.46GB |
| [llama-3-2-3B-wame-16bit-survey-generator4.Q8_0.gguf](https://huggingface.co/RichardErkhov/goethe0101_-_llama-3-2-3B-wame-16bit-survey-generator4-gguf/blob/main/llama-3-2-3B-wame-16bit-survey-generator4.Q8_0.gguf) | Q8_0 | 3.19GB |
Original model description:
---
base_model: unsloth/llama-3.2-3b-instruct-bnb-4bit
tags:
- text-generation-inference
- transformers
- unsloth
- llama
- trl
- sft
license: apache-2.0
language:
- en
---
# Uploaded model
- **Developed by:** goethe0101
- **License:** apache-2.0
- **Finetuned from model :** unsloth/llama-3.2-3b-instruct-bnb-4bit
This llama model was trained 2x faster with [Unsloth](https://github.com/unslothai/unsloth) and Huggingface's TRL library.
[<img src="https://raw.githubusercontent.com/unslothai/unsloth/main/images/unsloth%20made%20with%20love.png" width="200"/>](https://github.com/unslothai/unsloth)
|
AlignmentResearch/robust_llm_pythia-wl-31m-mz-ada-v3-ch-139000 | AlignmentResearch | "2024-03-26T11:51:11Z" | 103 | 0 | transformers | [
"transformers",
"safetensors",
"gpt_neox",
"text-classification",
"generated_from_trainer",
"base_model:EleutherAI/pythia-31m",
"base_model:finetune:EleutherAI/pythia-31m",
"autotrain_compatible",
"text-generation-inference",
"endpoints_compatible",
"region:us"
] | text-classification | "2024-03-26T11:51:03Z" | ---
tags:
- generated_from_trainer
base_model: EleutherAI/pythia-31m
model-index:
- name: robust_llm_pythia-wl-31m-mz-ada-v3-ch-139000
results: []
---
<!-- This model card has been generated automatically according to the information the Trainer had access to. You
should probably proofread and complete it, then remove this comment. -->
# robust_llm_pythia-wl-31m-mz-ada-v3-ch-139000
This model is a fine-tuned version of [EleutherAI/pythia-31m](https://huggingface.co/EleutherAI/pythia-31m) on an unknown dataset.
## Model description
More information needed
## Intended uses & limitations
More information needed
## Training and evaluation data
More information needed
## Training procedure
### Training hyperparameters
The following hyperparameters were used during training:
- learning_rate: 1e-05
- train_batch_size: 8
- eval_batch_size: 64
- seed: 42
- optimizer: Adam with betas=(0.9,0.999) and epsilon=1e-08
- lr_scheduler_type: linear
- num_epochs: 1
### Training results
### Framework versions
- Transformers 4.37.2
- Pytorch 2.2.0
- Datasets 2.17.0
- Tokenizers 0.15.2
|
Finn13/Llama3.1_COsec_multi | Finn13 | "2025-03-24T19:36:08Z" | 0 | 0 | transformers | [
"transformers",
"safetensors",
"llama",
"text-generation",
"text-generation-inference",
"unsloth",
"trl",
"conversational",
"en",
"base_model:unsloth/Meta-Llama-3.1-8B-Instruct",
"base_model:finetune:unsloth/Meta-Llama-3.1-8B-Instruct",
"license:apache-2.0",
"autotrain_compatible",
"endpoints_compatible",
"region:us"
] | text-generation | "2025-03-24T19:32:03Z" | ---
base_model: unsloth/Meta-Llama-3.1-8B-Instruct
tags:
- text-generation-inference
- transformers
- unsloth
- llama
- trl
license: apache-2.0
language:
- en
---
# Uploaded model
- **Developed by:** Finn13
- **License:** apache-2.0
- **Finetuned from model :** unsloth/Meta-Llama-3.1-8B-Instruct
This llama model was trained 2x faster with [Unsloth](https://github.com/unslothai/unsloth) and Huggingface's TRL library.
[<img src="https://raw.githubusercontent.com/unslothai/unsloth/main/images/unsloth%20made%20with%20love.png" width="200"/>](https://github.com/unslothai/unsloth)
|
juhul/pop | juhul | "2025-02-05T07:27:35Z" | 295 | 0 | diffusers | [
"diffusers",
"text-to-image",
"lora",
"template:diffusion-lora",
"base_model:black-forest-labs/FLUX.1-dev",
"base_model:adapter:black-forest-labs/FLUX.1-dev",
"region:us"
] | text-to-image | "2025-02-05T07:16:17Z" | ---
tags:
- text-to-image
- lora
- diffusers
- template:diffusion-lora
widget:
- text: .
output:
url: images/out-0 - 2025-02-02T102121.559.jpg
base_model: black-forest-labs/FLUX.1-dev
instance_prompt: PPP
---
# pop
<Gallery />
## Trigger words
You should use `PPP` to trigger the image generation.
## Download model
Weights for this model are available in Safetensors format.
[Download](/juhul/pop/tree/main) them in the Files & versions tab.
|
surianto/nana | surianto | "2023-07-30T12:06:16Z" | 0 | 0 | null | [
"license:creativeml-openrail-m",
"region:us"
] | null | "2023-07-30T12:05:23Z" | ---
license: creativeml-openrail-m
---
|
Triangle104/Llama-3.2-3B-Instruct-abliterated-Q5_K_M-GGUF | Triangle104 | "2024-11-25T16:45:58Z" | 6 | 0 | transformers | [
"transformers",
"gguf",
"abliterated",
"uncensored",
"llama-cpp",
"gguf-my-repo",
"base_model:huihui-ai/Llama-3.2-3B-Instruct-abliterated",
"base_model:quantized:huihui-ai/Llama-3.2-3B-Instruct-abliterated",
"license:llama3.2",
"endpoints_compatible",
"region:us",
"conversational"
] | null | "2024-10-17T10:06:54Z" | ---
library_name: transformers
license: llama3.2
base_model: huihui-ai/Llama-3.2-3B-Instruct-abliterated
tags:
- abliterated
- uncensored
- llama-cpp
- gguf-my-repo
---
# Triangle104/Llama-3.2-3B-Instruct-abliterated-Q5_K_M-GGUF
This model was converted to GGUF format from [`huihui-ai/Llama-3.2-3B-Instruct-abliterated`](https://huggingface.co/huihui-ai/Llama-3.2-3B-Instruct-abliterated) using llama.cpp via the ggml.ai's [GGUF-my-repo](https://huggingface.co/spaces/ggml-org/gguf-my-repo) space.
Refer to the [original model card](https://huggingface.co/huihui-ai/Llama-3.2-3B-Instruct-abliterated) for more details on the model.
---
Model details:
-
This is an uncensored version of Llama 3.2 3B Instruct created with abliteration (see this article to know more about it).
Special thanks to @FailSpy for the original code and technique. Please follow him if you're interested in abliterated models.
---
## Use with llama.cpp
Install llama.cpp through brew (works on Mac and Linux)
```bash
brew install llama.cpp
```
Invoke the llama.cpp server or the CLI.
### CLI:
```bash
llama-cli --hf-repo Triangle104/Llama-3.2-3B-Instruct-abliterated-Q5_K_M-GGUF --hf-file llama-3.2-3b-instruct-abliterated-q5_k_m.gguf -p "The meaning to life and the universe is"
```
### Server:
```bash
llama-server --hf-repo Triangle104/Llama-3.2-3B-Instruct-abliterated-Q5_K_M-GGUF --hf-file llama-3.2-3b-instruct-abliterated-q5_k_m.gguf -c 2048
```
Note: You can also use this checkpoint directly through the [usage steps](https://github.com/ggerganov/llama.cpp?tab=readme-ov-file#usage) listed in the Llama.cpp repo as well.
Step 1: Clone llama.cpp from GitHub.
```
git clone https://github.com/ggerganov/llama.cpp
```
Step 2: Move into the llama.cpp folder and build it with `LLAMA_CURL=1` flag along with other hardware-specific flags (for ex: LLAMA_CUDA=1 for Nvidia GPUs on Linux).
```
cd llama.cpp && LLAMA_CURL=1 make
```
Step 3: Run inference through the main binary.
```
./llama-cli --hf-repo Triangle104/Llama-3.2-3B-Instruct-abliterated-Q5_K_M-GGUF --hf-file llama-3.2-3b-instruct-abliterated-q5_k_m.gguf -p "The meaning to life and the universe is"
```
or
```
./llama-server --hf-repo Triangle104/Llama-3.2-3B-Instruct-abliterated-Q5_K_M-GGUF --hf-file llama-3.2-3b-instruct-abliterated-q5_k_m.gguf -c 2048
```
|
alicekwak/TN-final-all-mpnet-base-v2 | alicekwak | "2022-11-02T22:58:35Z" | 5 | 0 | sentence-transformers | [
"sentence-transformers",
"pytorch",
"mpnet",
"feature-extraction",
"sentence-similarity",
"autotrain_compatible",
"endpoints_compatible",
"region:us"
] | sentence-similarity | "2022-11-02T22:58:25Z" | ---
pipeline_tag: sentence-similarity
tags:
- sentence-transformers
- feature-extraction
- sentence-similarity
---
# alicekwak/TN-final-all-mpnet-base-v2
This is a [sentence-transformers](https://www.SBERT.net) model: It maps sentences & paragraphs to a 768 dimensional dense vector space and can be used for tasks like clustering or semantic search.
<!--- Describe your model here -->
## Usage (Sentence-Transformers)
Using this model becomes easy when you have [sentence-transformers](https://www.SBERT.net) installed:
```
pip install -U sentence-transformers
```
Then you can use the model like this:
```python
from sentence_transformers import SentenceTransformer
sentences = ["This is an example sentence", "Each sentence is converted"]
model = SentenceTransformer('alicekwak/TN-final-all-mpnet-base-v2')
embeddings = model.encode(sentences)
print(embeddings)
```
## Evaluation Results
<!--- Describe how your model was evaluated -->
For an automated evaluation of this model, see the *Sentence Embeddings Benchmark*: [https://seb.sbert.net](https://seb.sbert.net?model_name=alicekwak/TN-final-all-mpnet-base-v2)
## Training
The model was trained with the parameters:
**DataLoader**:
`torch.utils.data.dataloader.DataLoader` of length 675 with parameters:
```
{'batch_size': 4, 'sampler': 'torch.utils.data.sampler.RandomSampler', 'batch_sampler': 'torch.utils.data.sampler.BatchSampler'}
```
**Loss**:
`sentence_transformers.losses.SoftmaxLoss.SoftmaxLoss`
Parameters of the fit()-Method:
```
{
"epochs": 5,
"evaluation_steps": 0,
"evaluator": "NoneType",
"max_grad_norm": 1,
"optimizer_class": "<class 'torch.optim.adamw.AdamW'>",
"optimizer_params": {
"lr": 2e-05
},
"scheduler": "WarmupLinear",
"steps_per_epoch": null,
"warmup_steps": 10,
"weight_decay": 0.01
}
```
## Full Model Architecture
```
SentenceTransformer(
(0): Transformer({'max_seq_length': 384, 'do_lower_case': False}) with Transformer model: MPNetModel
(1): Pooling({'word_embedding_dimension': 768, 'pooling_mode_cls_token': False, 'pooling_mode_mean_tokens': True, 'pooling_mode_max_tokens': False, 'pooling_mode_mean_sqrt_len_tokens': False})
(2): Normalize()
)
```
## Citing & Authors
<!--- Describe where people can find more information --> |
hqbui/Reinforce-PixelCopter-PLE-v0 | hqbui | "2023-12-12T21:02:22Z" | 0 | 0 | null | [
"Pixelcopter-PLE-v0",
"reinforce",
"reinforcement-learning",
"custom-implementation",
"deep-rl-class",
"model-index",
"region:us"
] | reinforcement-learning | "2023-12-12T19:11:38Z" | ---
tags:
- Pixelcopter-PLE-v0
- reinforce
- reinforcement-learning
- custom-implementation
- deep-rl-class
model-index:
- name: Reinforce-PixelCopter-PLE-v0
results:
- task:
type: reinforcement-learning
name: reinforcement-learning
dataset:
name: Pixelcopter-PLE-v0
type: Pixelcopter-PLE-v0
metrics:
- type: mean_reward
value: 23.70 +/- 17.46
name: mean_reward
verified: false
---
# **Reinforce** Agent playing **Pixelcopter-PLE-v0**
This is a trained model of a **Reinforce** agent playing **Pixelcopter-PLE-v0** .
To learn to use this model and train yours check Unit 4 of the Deep Reinforcement Learning Course: https://huggingface.co/deep-rl-course/unit4/introduction
|
pandaiedu/mesolitica-qwen-2.5-lora-1.5b-Instruct-Merged-20-epoch | pandaiedu | "2025-03-26T21:27:07Z" | 0 | 0 | transformers | [
"transformers",
"safetensors",
"qwen2",
"text-generation",
"conversational",
"arxiv:1910.09700",
"autotrain_compatible",
"text-generation-inference",
"endpoints_compatible",
"region:us"
] | text-generation | "2025-03-26T21:25:16Z" | ---
library_name: transformers
tags: []
---
# Model Card for Model ID
<!-- Provide a quick summary of what the model is/does. -->
## Model Details
### Model Description
<!-- Provide a longer summary of what this model is. -->
This is the model card of a 🤗 transformers model that has been pushed on the Hub. This model card has been automatically generated.
- **Developed by:** [More Information Needed]
- **Funded by [optional]:** [More Information Needed]
- **Shared by [optional]:** [More Information Needed]
- **Model type:** [More Information Needed]
- **Language(s) (NLP):** [More Information Needed]
- **License:** [More Information Needed]
- **Finetuned from model [optional]:** [More Information Needed]
### Model Sources [optional]
<!-- Provide the basic links for the model. -->
- **Repository:** [More Information Needed]
- **Paper [optional]:** [More Information Needed]
- **Demo [optional]:** [More Information Needed]
## Uses
<!-- Address questions around how the model is intended to be used, including the foreseeable users of the model and those affected by the model. -->
### Direct Use
<!-- This section is for the model use without fine-tuning or plugging into a larger ecosystem/app. -->
[More Information Needed]
### Downstream Use [optional]
<!-- This section is for the model use when fine-tuned for a task, or when plugged into a larger ecosystem/app -->
[More Information Needed]
### Out-of-Scope Use
<!-- This section addresses misuse, malicious use, and uses that the model will not work well for. -->
[More Information Needed]
## Bias, Risks, and Limitations
<!-- This section is meant to convey both technical and sociotechnical limitations. -->
[More Information Needed]
### Recommendations
<!-- This section is meant to convey recommendations with respect to the bias, risk, and technical limitations. -->
Users (both direct and downstream) should be made aware of the risks, biases and limitations of the model. More information needed for further recommendations.
## How to Get Started with the Model
Use the code below to get started with the model.
[More Information Needed]
## Training Details
### Training Data
<!-- This should link to a Dataset Card, perhaps with a short stub of information on what the training data is all about as well as documentation related to data pre-processing or additional filtering. -->
[More Information Needed]
### Training Procedure
<!-- This relates heavily to the Technical Specifications. Content here should link to that section when it is relevant to the training procedure. -->
#### Preprocessing [optional]
[More Information Needed]
#### Training Hyperparameters
- **Training regime:** [More Information Needed] <!--fp32, fp16 mixed precision, bf16 mixed precision, bf16 non-mixed precision, fp16 non-mixed precision, fp8 mixed precision -->
#### Speeds, Sizes, Times [optional]
<!-- This section provides information about throughput, start/end time, checkpoint size if relevant, etc. -->
[More Information Needed]
## Evaluation
<!-- This section describes the evaluation protocols and provides the results. -->
### Testing Data, Factors & Metrics
#### Testing Data
<!-- This should link to a Dataset Card if possible. -->
[More Information Needed]
#### Factors
<!-- These are the things the evaluation is disaggregating by, e.g., subpopulations or domains. -->
[More Information Needed]
#### Metrics
<!-- These are the evaluation metrics being used, ideally with a description of why. -->
[More Information Needed]
### Results
[More Information Needed]
#### Summary
## Model Examination [optional]
<!-- Relevant interpretability work for the model goes here -->
[More Information Needed]
## Environmental Impact
<!-- Total emissions (in grams of CO2eq) and additional considerations, such as electricity usage, go here. Edit the suggested text below accordingly -->
Carbon emissions can be estimated using the [Machine Learning Impact calculator](https://mlco2.github.io/impact#compute) presented in [Lacoste et al. (2019)](https://arxiv.org/abs/1910.09700).
- **Hardware Type:** [More Information Needed]
- **Hours used:** [More Information Needed]
- **Cloud Provider:** [More Information Needed]
- **Compute Region:** [More Information Needed]
- **Carbon Emitted:** [More Information Needed]
## Technical Specifications [optional]
### Model Architecture and Objective
[More Information Needed]
### Compute Infrastructure
[More Information Needed]
#### Hardware
[More Information Needed]
#### Software
[More Information Needed]
## Citation [optional]
<!-- If there is a paper or blog post introducing the model, the APA and Bibtex information for that should go in this section. -->
**BibTeX:**
[More Information Needed]
**APA:**
[More Information Needed]
## Glossary [optional]
<!-- If relevant, include terms and calculations in this section that can help readers understand the model or model card. -->
[More Information Needed]
## More Information [optional]
[More Information Needed]
## Model Card Authors [optional]
[More Information Needed]
## Model Card Contact
[More Information Needed] |
kla-20/qa-flant5 | kla-20 | "2023-09-21T15:30:53Z" | 105 | 0 | transformers | [
"transformers",
"pytorch",
"tensorboard",
"t5",
"text2text-generation",
"generated_from_trainer",
"license:apache-2.0",
"autotrain_compatible",
"text-generation-inference",
"endpoints_compatible",
"region:us"
] | text2text-generation | "2023-09-21T15:23:27Z" | ---
license: apache-2.0
tags:
- generated_from_trainer
model-index:
- name: qa-flant5
results: []
---
<!-- This model card has been generated automatically according to the information the Trainer had access to. You
should probably proofread and complete it, then remove this comment. -->
# qa-flant5
This model is a fine-tuned version of [google/flan-t5-base](https://huggingface.co/google/flan-t5-base) on an unknown dataset.
## Model description
More information needed
## Intended uses & limitations
More information needed
## Training and evaluation data
More information needed
## Training procedure
### Training hyperparameters
The following hyperparameters were used during training:
- learning_rate: 1e-05
- train_batch_size: 8
- eval_batch_size: 8
- seed: 42
- optimizer: Adam with betas=(0.9,0.999) and epsilon=1e-08
- lr_scheduler_type: linear
- training_steps: 1
### Training results
### Framework versions
- Transformers 4.27.2
- Pytorch 1.13.1+cu117
- Datasets 2.11.0
- Tokenizers 0.13.3
|
sherif1311/flan-t5-base-classification_int1 | sherif1311 | "2023-08-13T18:55:37Z" | 103 | 0 | transformers | [
"transformers",
"pytorch",
"tensorboard",
"t5",
"text2text-generation",
"generated_from_trainer",
"base_model:google/flan-t5-base",
"base_model:finetune:google/flan-t5-base",
"license:apache-2.0",
"autotrain_compatible",
"text-generation-inference",
"endpoints_compatible",
"region:us"
] | text2text-generation | "2023-08-13T18:50:58Z" | ---
license: apache-2.0
base_model: google/flan-t5-base
tags:
- generated_from_trainer
metrics:
- f1
model-index:
- name: flan-t5-base-classification_int1
results: []
---
<!-- This model card has been generated automatically according to the information the Trainer had access to. You
should probably proofread and complete it, then remove this comment. -->
# flan-t5-base-classification_int1
This model is a fine-tuned version of [google/flan-t5-base](https://huggingface.co/google/flan-t5-base) on the None dataset.
It achieves the following results on the evaluation set:
- Loss: 0.0036
- F1: 99.7778
- Gen Len: 2.3333
## Model description
More information needed
## Intended uses & limitations
More information needed
## Training and evaluation data
More information needed
## Training procedure
### Training hyperparameters
The following hyperparameters were used during training:
- learning_rate: 0.0003
- train_batch_size: 8
- eval_batch_size: 8
- seed: 42
- optimizer: Adam with betas=(0.9,0.999) and epsilon=1e-08
- lr_scheduler_type: linear
- num_epochs: 1
### Training results
### Framework versions
- Transformers 4.31.0
- Pytorch 1.12.1+cu116
- Datasets 2.14.4
- Tokenizers 0.12.1
|
tensorblock/llama-ko-peft-v0.6-GGUF | tensorblock | "2024-11-19T11:17:37Z" | 19 | 0 | null | [
"gguf",
"TensorBlock",
"GGUF",
"ko",
"base_model:colable/llama-ko-peft-v0.6",
"base_model:quantized:colable/llama-ko-peft-v0.6",
"license:mit",
"endpoints_compatible",
"region:us"
] | null | "2024-11-19T10:51:15Z" | ---
license: mit
language:
- ko
tags:
- TensorBlock
- GGUF
base_model: colable/llama-ko-peft-v0.6
---
<div style="width: auto; margin-left: auto; margin-right: auto">
<img src="https://i.imgur.com/jC7kdl8.jpeg" alt="TensorBlock" style="width: 100%; min-width: 400px; display: block; margin: auto;">
</div>
<div style="display: flex; justify-content: space-between; width: 100%;">
<div style="display: flex; flex-direction: column; align-items: flex-start;">
<p style="margin-top: 0.5em; margin-bottom: 0em;">
Feedback and support: TensorBlock's <a href="https://x.com/tensorblock_aoi">Twitter/X</a>, <a href="https://t.me/TensorBlock">Telegram Group</a> and <a href="https://x.com/tensorblock_aoi">Discord server</a>
</p>
</div>
</div>
## colable/llama-ko-peft-v0.6 - GGUF
This repo contains GGUF format model files for [colable/llama-ko-peft-v0.6](https://huggingface.co/colable/llama-ko-peft-v0.6).
The files were quantized using machines provided by [TensorBlock](https://tensorblock.co/), and they are compatible with llama.cpp as of [commit b4011](https://github.com/ggerganov/llama.cpp/commit/a6744e43e80f4be6398fc7733a01642c846dce1d).
<div style="text-align: left; margin: 20px 0;">
<a href="https://tensorblock.co/waitlist/client" style="display: inline-block; padding: 10px 20px; background-color: #007bff; color: white; text-decoration: none; border-radius: 5px; font-weight: bold;">
Run them on the TensorBlock client using your local machine ↗
</a>
</div>
## Prompt template
```
```
## Model file specification
| Filename | Quant type | File Size | Description |
| -------- | ---------- | --------- | ----------- |
| [llama-ko-peft-v0.6-Q2_K.gguf](https://huggingface.co/tensorblock/llama-ko-peft-v0.6-GGUF/blob/main/llama-ko-peft-v0.6-Q2_K.gguf) | Q2_K | 2.422 GB | smallest, significant quality loss - not recommended for most purposes |
| [llama-ko-peft-v0.6-Q3_K_S.gguf](https://huggingface.co/tensorblock/llama-ko-peft-v0.6-GGUF/blob/main/llama-ko-peft-v0.6-Q3_K_S.gguf) | Q3_K_S | 2.815 GB | very small, high quality loss |
| [llama-ko-peft-v0.6-Q3_K_M.gguf](https://huggingface.co/tensorblock/llama-ko-peft-v0.6-GGUF/blob/main/llama-ko-peft-v0.6-Q3_K_M.gguf) | Q3_K_M | 3.140 GB | very small, high quality loss |
| [llama-ko-peft-v0.6-Q3_K_L.gguf](https://huggingface.co/tensorblock/llama-ko-peft-v0.6-GGUF/blob/main/llama-ko-peft-v0.6-Q3_K_L.gguf) | Q3_K_L | 3.419 GB | small, substantial quality loss |
| [llama-ko-peft-v0.6-Q4_0.gguf](https://huggingface.co/tensorblock/llama-ko-peft-v0.6-GGUF/blob/main/llama-ko-peft-v0.6-Q4_0.gguf) | Q4_0 | 3.639 GB | legacy; small, very high quality loss - prefer using Q3_K_M |
| [llama-ko-peft-v0.6-Q4_K_S.gguf](https://huggingface.co/tensorblock/llama-ko-peft-v0.6-GGUF/blob/main/llama-ko-peft-v0.6-Q4_K_S.gguf) | Q4_K_S | 3.668 GB | small, greater quality loss |
| [llama-ko-peft-v0.6-Q4_K_M.gguf](https://huggingface.co/tensorblock/llama-ko-peft-v0.6-GGUF/blob/main/llama-ko-peft-v0.6-Q4_K_M.gguf) | Q4_K_M | 3.877 GB | medium, balanced quality - recommended |
| [llama-ko-peft-v0.6-Q5_0.gguf](https://huggingface.co/tensorblock/llama-ko-peft-v0.6-GGUF/blob/main/llama-ko-peft-v0.6-Q5_0.gguf) | Q5_0 | 4.415 GB | legacy; medium, balanced quality - prefer using Q4_K_M |
| [llama-ko-peft-v0.6-Q5_K_S.gguf](https://huggingface.co/tensorblock/llama-ko-peft-v0.6-GGUF/blob/main/llama-ko-peft-v0.6-Q5_K_S.gguf) | Q5_K_S | 4.415 GB | large, low quality loss - recommended |
| [llama-ko-peft-v0.6-Q5_K_M.gguf](https://huggingface.co/tensorblock/llama-ko-peft-v0.6-GGUF/blob/main/llama-ko-peft-v0.6-Q5_K_M.gguf) | Q5_K_M | 4.537 GB | large, very low quality loss - recommended |
| [llama-ko-peft-v0.6-Q6_K.gguf](https://huggingface.co/tensorblock/llama-ko-peft-v0.6-GGUF/blob/main/llama-ko-peft-v0.6-Q6_K.gguf) | Q6_K | 5.240 GB | very large, extremely low quality loss |
| [llama-ko-peft-v0.6-Q8_0.gguf](https://huggingface.co/tensorblock/llama-ko-peft-v0.6-GGUF/blob/main/llama-ko-peft-v0.6-Q8_0.gguf) | Q8_0 | 6.786 GB | very large, extremely low quality loss - not recommended |
## Downloading instruction
### Command line
Firstly, install Huggingface Client
```shell
pip install -U "huggingface_hub[cli]"
```
Then, downoad the individual model file the a local directory
```shell
huggingface-cli download tensorblock/llama-ko-peft-v0.6-GGUF --include "llama-ko-peft-v0.6-Q2_K.gguf" --local-dir MY_LOCAL_DIR
```
If you wanna download multiple model files with a pattern (e.g., `*Q4_K*gguf`), you can try:
```shell
huggingface-cli download tensorblock/llama-ko-peft-v0.6-GGUF --local-dir MY_LOCAL_DIR --local-dir-use-symlinks False --include='*Q4_K*gguf'
```
|
lunarsylph/stablecell_v13 | lunarsylph | "2024-03-30T02:51:40Z" | 91 | 0 | transformers | [
"transformers",
"safetensors",
"stablelm",
"text-generation",
"conversational",
"arxiv:1910.09700",
"autotrain_compatible",
"endpoints_compatible",
"region:us"
] | text-generation | "2024-03-30T02:29:39Z" | ---
library_name: transformers
tags: []
---
# Model Card for Model ID
<!-- Provide a quick summary of what the model is/does. -->
## Model Details
### Model Description
<!-- Provide a longer summary of what this model is. -->
This is the model card of a 🤗 transformers model that has been pushed on the Hub. This model card has been automatically generated.
- **Developed by:** [More Information Needed]
- **Funded by [optional]:** [More Information Needed]
- **Shared by [optional]:** [More Information Needed]
- **Model type:** [More Information Needed]
- **Language(s) (NLP):** [More Information Needed]
- **License:** [More Information Needed]
- **Finetuned from model [optional]:** [More Information Needed]
### Model Sources [optional]
<!-- Provide the basic links for the model. -->
- **Repository:** [More Information Needed]
- **Paper [optional]:** [More Information Needed]
- **Demo [optional]:** [More Information Needed]
## Uses
<!-- Address questions around how the model is intended to be used, including the foreseeable users of the model and those affected by the model. -->
### Direct Use
<!-- This section is for the model use without fine-tuning or plugging into a larger ecosystem/app. -->
[More Information Needed]
### Downstream Use [optional]
<!-- This section is for the model use when fine-tuned for a task, or when plugged into a larger ecosystem/app -->
[More Information Needed]
### Out-of-Scope Use
<!-- This section addresses misuse, malicious use, and uses that the model will not work well for. -->
[More Information Needed]
## Bias, Risks, and Limitations
<!-- This section is meant to convey both technical and sociotechnical limitations. -->
[More Information Needed]
### Recommendations
<!-- This section is meant to convey recommendations with respect to the bias, risk, and technical limitations. -->
Users (both direct and downstream) should be made aware of the risks, biases and limitations of the model. More information needed for further recommendations.
## How to Get Started with the Model
Use the code below to get started with the model.
[More Information Needed]
## Training Details
### Training Data
<!-- This should link to a Dataset Card, perhaps with a short stub of information on what the training data is all about as well as documentation related to data pre-processing or additional filtering. -->
[More Information Needed]
### Training Procedure
<!-- This relates heavily to the Technical Specifications. Content here should link to that section when it is relevant to the training procedure. -->
#### Preprocessing [optional]
[More Information Needed]
#### Training Hyperparameters
- **Training regime:** [More Information Needed] <!--fp32, fp16 mixed precision, bf16 mixed precision, bf16 non-mixed precision, fp16 non-mixed precision, fp8 mixed precision -->
#### Speeds, Sizes, Times [optional]
<!-- This section provides information about throughput, start/end time, checkpoint size if relevant, etc. -->
[More Information Needed]
## Evaluation
<!-- This section describes the evaluation protocols and provides the results. -->
### Testing Data, Factors & Metrics
#### Testing Data
<!-- This should link to a Dataset Card if possible. -->
[More Information Needed]
#### Factors
<!-- These are the things the evaluation is disaggregating by, e.g., subpopulations or domains. -->
[More Information Needed]
#### Metrics
<!-- These are the evaluation metrics being used, ideally with a description of why. -->
[More Information Needed]
### Results
[More Information Needed]
#### Summary
## Model Examination [optional]
<!-- Relevant interpretability work for the model goes here -->
[More Information Needed]
## Environmental Impact
<!-- Total emissions (in grams of CO2eq) and additional considerations, such as electricity usage, go here. Edit the suggested text below accordingly -->
Carbon emissions can be estimated using the [Machine Learning Impact calculator](https://mlco2.github.io/impact#compute) presented in [Lacoste et al. (2019)](https://arxiv.org/abs/1910.09700).
- **Hardware Type:** [More Information Needed]
- **Hours used:** [More Information Needed]
- **Cloud Provider:** [More Information Needed]
- **Compute Region:** [More Information Needed]
- **Carbon Emitted:** [More Information Needed]
## Technical Specifications [optional]
### Model Architecture and Objective
[More Information Needed]
### Compute Infrastructure
[More Information Needed]
#### Hardware
[More Information Needed]
#### Software
[More Information Needed]
## Citation [optional]
<!-- If there is a paper or blog post introducing the model, the APA and Bibtex information for that should go in this section. -->
**BibTeX:**
[More Information Needed]
**APA:**
[More Information Needed]
## Glossary [optional]
<!-- If relevant, include terms and calculations in this section that can help readers understand the model or model card. -->
[More Information Needed]
## More Information [optional]
[More Information Needed]
## Model Card Authors [optional]
[More Information Needed]
## Model Card Contact
[More Information Needed] |
SicariusSicariiStuff/SaisExperiments_Evil-Alpaca-3B-L3.2_iMatrix | SicariusSicariiStuff | "2024-09-29T18:18:27Z" | 8 | 0 | null | [
"gguf",
"license:apache-2.0",
"endpoints_compatible",
"region:us",
"imatrix",
"conversational"
] | null | "2024-09-29T17:52:58Z" | ---
license: apache-2.0
---
|
amaliaam/image_classification | amaliaam | "2023-09-18T16:58:49Z" | 261 | 0 | transformers | [
"transformers",
"pytorch",
"vit",
"image-classification",
"generated_from_trainer",
"dataset:imagefolder",
"base_model:google/vit-base-patch16-224-in21k",
"base_model:finetune:google/vit-base-patch16-224-in21k",
"license:apache-2.0",
"autotrain_compatible",
"endpoints_compatible",
"region:us"
] | image-classification | "2023-09-18T16:06:43Z" | ---
license: apache-2.0
base_model: google/vit-base-patch16-224-in21k
tags:
- generated_from_trainer
datasets:
- imagefolder
model-index:
- name: image_classification
results: []
---
<!-- This model card has been generated automatically according to the information the Trainer had access to. You
should probably proofread and complete it, then remove this comment. -->
# image_classification
This model is a fine-tuned version of [google/vit-base-patch16-224-in21k](https://huggingface.co/google/vit-base-patch16-224-in21k) on the imagefolder dataset.
It achieves the following results on the evaluation set:
- eval_loss: 2.0915
- eval_accuracy: 0.0938
- eval_runtime: 10.0977
- eval_samples_per_second: 15.845
- eval_steps_per_second: 0.99
- step: 0
## Model description
More information needed
## Intended uses & limitations
More information needed
## Training and evaluation data
More information needed
## Training procedure
### Training hyperparameters
The following hyperparameters were used during training:
- learning_rate: 5e-05
- train_batch_size: 16
- eval_batch_size: 16
- seed: 42
- optimizer: Adam with betas=(0.9,0.999) and epsilon=1e-08
- lr_scheduler_type: linear
- num_epochs: 3
### Framework versions
- Transformers 4.33.2
- Pytorch 2.0.1+cu118
- Datasets 2.14.5
- Tokenizers 0.13.3
|
georad/mediNER | georad | "2025-03-10T17:05:19Z" | 0 | 0 | null | [
"safetensors",
"region:us"
] | null | "2025-03-10T14:58:19Z" | # medNER_V2
This app performs Named Entity REcognition of medical entties.
|
PrunaAI/fblgit-juanako-7b-UNA-bnb-8bit-smashed | PrunaAI | "2024-08-15T14:47:06Z" | 5 | 0 | null | [
"safetensors",
"mistral",
"pruna-ai",
"base_model:fblgit/juanako-7b-UNA",
"base_model:quantized:fblgit/juanako-7b-UNA",
"8-bit",
"bitsandbytes",
"region:us"
] | null | "2024-08-15T14:43:39Z" | ---
thumbnail: "https://assets-global.website-files.com/646b351987a8d8ce158d1940/64ec9e96b4334c0e1ac41504_Logo%20with%20white%20text.svg"
base_model: fblgit/juanako-7b-UNA
metrics:
- memory_disk
- memory_inference
- inference_latency
- inference_throughput
- inference_CO2_emissions
- inference_energy_consumption
tags:
- pruna-ai
---
<!-- header start -->
<!-- 200823 -->
<div style="width: auto; margin-left: auto; margin-right: auto">
<a href="https://www.pruna.ai/" target="_blank" rel="noopener noreferrer">
<img src="https://i.imgur.com/eDAlcgk.png" alt="PrunaAI" style="width: 100%; min-width: 400px; display: block; margin: auto;">
</a>
</div>
<!-- header end -->
[](https://twitter.com/PrunaAI)
[](https://github.com/PrunaAI)
[](https://www.linkedin.com/company/93832878/admin/feed/posts/?feedType=following)
[](https://discord.gg/rskEr4BZJx)
# Simply make AI models cheaper, smaller, faster, and greener!
- Give a thumbs up if you like this model!
- Contact us and tell us which model to compress next [here](https://www.pruna.ai/contact).
- Request access to easily compress your *own* AI models [here](https://z0halsaff74.typeform.com/pruna-access?typeform-source=www.pruna.ai).
- Read the documentations to know more [here](https://pruna-ai-pruna.readthedocs-hosted.com/en/latest/)
- Join Pruna AI community on Discord [here](https://discord.gg/CP4VSgck) to share feedback/suggestions or get help.
## Results

**Frequently Asked Questions**
- ***How does the compression work?*** The model is compressed with llm-int8.
- ***How does the model quality change?*** The quality of the model output might vary compared to the base model.
- ***How is the model efficiency evaluated?*** These results were obtained on HARDWARE_NAME with configuration described in `model/smash_config.json` and are obtained after a hardware warmup. The smashed model is directly compared to the original base model. Efficiency results may vary in other settings (e.g. other hardware, image size, batch size, ...). We recommend to directly run them in the use-case conditions to know if the smashed model can benefit you.
- ***What is the model format?*** We use safetensors.
- ***What calibration data has been used?*** If needed by the compression method, we used WikiText as the calibration data.
- ***What is the naming convention for Pruna Huggingface models?*** We take the original model name and append "turbo", "tiny", or "green" if the smashed model has a measured inference speed, inference memory, or inference energy consumption which is less than 90% of the original base model.
- ***How to compress my own models?*** You can request premium access to more compression methods and tech support for your specific use-cases [here](https://z0halsaff74.typeform.com/pruna-access?typeform-source=www.pruna.ai).
- ***What are "first" metrics?*** Results mentioning "first" are obtained after the first run of the model. The first run might take more memory or be slower than the subsequent runs due cuda overheads.
- ***What are "Sync" and "Async" metrics?*** "Sync" metrics are obtained by syncing all GPU processes and stop measurement when all of them are executed. "Async" metrics are obtained without syncing all GPU processes and stop when the model output can be used by the CPU. We provide both metrics since both could be relevant depending on the use-case. We recommend to test the efficiency gains directly in your use-cases.
## Setup
You can run the smashed model with these steps:
0. Check requirements from the original repo fblgit/juanako-7b-UNA installed. In particular, check python, cuda, and transformers versions.
1. Make sure that you have installed quantization related packages.
```bash
pip install transformers accelerate bitsandbytes>0.37.0
```
2. Load & run the model.
```python
from transformers import AutoModelForCausalLM, AutoTokenizer
model = AutoModelForCausalLM.from_pretrained("PrunaAI/fblgit-juanako-7b-UNA-bnb-8bit-smashed", trust_remote_code=True, device_map='auto')
tokenizer = AutoTokenizer.from_pretrained("fblgit/juanako-7b-UNA")
input_ids = tokenizer("What is the color of prunes?,", return_tensors='pt').to(model.device)["input_ids"]
outputs = model.generate(input_ids, max_new_tokens=216)
tokenizer.decode(outputs[0])
```
## Configurations
The configuration info are in `smash_config.json`.
## Credits & License
The license of the smashed model follows the license of the original model. Please check the license of the original model fblgit/juanako-7b-UNA before using this model which provided the base model. The license of the `pruna-engine` is [here](https://pypi.org/project/pruna-engine/) on Pypi.
## Want to compress other models?
- Contact us and tell us which model to compress next [here](https://www.pruna.ai/contact).
- Request access to easily compress your own AI models [here](https://z0halsaff74.typeform.com/pruna-access?typeform-source=www.pruna.ai). |
umesh16071973/_Flooplan_DB_LoRA_ | umesh16071973 | "2024-02-06T14:24:48Z" | 3 | 1 | diffusers | [
"diffusers",
"tensorboard",
"stable-diffusion-xl",
"stable-diffusion-xl-diffusers",
"text-to-image",
"lora",
"template:sd-lora",
"base_model:stabilityai/stable-diffusion-xl-base-1.0",
"base_model:adapter:stabilityai/stable-diffusion-xl-base-1.0",
"license:openrail++",
"region:us"
] | text-to-image | "2024-02-06T14:24:40Z" |
---
tags:
- stable-diffusion-xl
- stable-diffusion-xl-diffusers
- text-to-image
- diffusers
- lora
- template:sd-lora
base_model: stabilityai/stable-diffusion-xl-base-1.0
instance_prompt: a high quality, 4K photo of a FLOORPLAN
license: openrail++
---
# SDXL LoRA DreamBooth - umesh16071973/_Flooplan_DB_LoRA_
<Gallery />
## Model description
These are umesh16071973/_Flooplan_DB_LoRA_ LoRA adaption weights for stabilityai/stable-diffusion-xl-base-1.0.
The weights were trained using [DreamBooth](https://dreambooth.github.io/).
LoRA for the text encoder was enabled: False.
Special VAE used for training: madebyollin/sdxl-vae-fp16-fix.
## Trigger words
You should use a high quality, 4K photo of a FLOORPLAN to trigger the image generation.
## Download model
Weights for this model are available in Safetensors format.
[Download](umesh16071973/_Flooplan_DB_LoRA_/tree/main) them in the Files & versions tab.
|
TFOCUS/Inference-Providers_17 | TFOCUS | "2025-02-28T16:29:24Z" | 0 | 0 | null | [
"onnx",
"any-to-any",
"omega",
"omegalabs",
"bittensor",
"agi",
"license:mit",
"region:us"
] | any-to-any | "2025-02-28T16:02:02Z" | ---
license: mit
tags:
- any-to-any
- omega
- omegalabs
- bittensor
- agi
---
This is an Any-to-Any model checkpoint for the OMEGA Labs x Bittensor Any-to-Any subnet.
Check out the [git repo](https://github.com/omegalabsinc/omegalabs-anytoany-bittensor) and find OMEGA on X: [@omegalabsai](https://x.com/omegalabsai).
|
John6666/toon-e-pony-v1-sdxl | John6666 | "2024-12-23T06:36:36Z" | 147 | 1 | diffusers | [
"diffusers",
"safetensors",
"text-to-image",
"stable-diffusion",
"stable-diffusion-xl",
"anime",
"cartoon",
"toon",
"cute",
"bold style",
"pony",
"en",
"license:other",
"autotrain_compatible",
"endpoints_compatible",
"diffusers:StableDiffusionXLPipeline",
"region:us"
] | text-to-image | "2024-10-11T10:32:16Z" | ---
license: other
license_name: faipl-1.0-sd
license_link: https://freedevproject.org/faipl-1.0-sd/
language:
- en
library_name: diffusers
pipeline_tag: text-to-image
tags:
- text-to-image
- stable-diffusion
- stable-diffusion-xl
- anime
- cartoon
- toon
- cute
- bold style
- pony
---
Original model is [here](https://civitai.com/models/843170/toon-e-pony?modelVersionId=943297).
The author is [here](https://huggingface.co/advokat).
This model created by [advokat](https://civitai.com/user/advokat).
|
altomek/CodeRosa-70B-AB1-5bpw-EXL2 | altomek | "2024-08-30T10:24:55Z" | 4 | 0 | transformers | [
"transformers",
"safetensors",
"llama",
"text-generation",
"en",
"base_model:altomek/CodeRosa-70B-AB1",
"base_model:finetune:altomek/CodeRosa-70B-AB1",
"license:llama2",
"autotrain_compatible",
"text-generation-inference",
"region:us"
] | text-generation | "2024-03-18T06:27:41Z" | ---
base_model: altomek/CodeRosa-70B-AB1
language:
- en
license: llama2
inference: false
---
# CodeRosa-70B-AB1
ExLlamav2 5 bpw 8 h quants of https://huggingface.co/altomek/CodeRosa-70B-AB1
|
auxyus/3b08c9c9-8517-49a0-8d26-63097e0e34a1 | auxyus | "2025-02-02T08:14:30Z" | 8 | 0 | peft | [
"peft",
"safetensors",
"qwen2",
"axolotl",
"generated_from_trainer",
"base_model:unsloth/Qwen2.5-3B",
"base_model:adapter:unsloth/Qwen2.5-3B",
"license:other",
"region:us"
] | null | "2025-02-02T08:04:58Z" | ---
library_name: peft
license: other
base_model: unsloth/Qwen2.5-3B
tags:
- axolotl
- generated_from_trainer
model-index:
- name: 3b08c9c9-8517-49a0-8d26-63097e0e34a1
results: []
---
<!-- This model card has been generated automatically according to the information the Trainer had access to. You
should probably proofread and complete it, then remove this comment. -->
[<img src="https://raw.githubusercontent.com/axolotl-ai-cloud/axolotl/main/image/axolotl-badge-web.png" alt="Built with Axolotl" width="200" height="32"/>](https://github.com/axolotl-ai-cloud/axolotl)
<details><summary>See axolotl config</summary>
axolotl version: `0.4.1`
```yaml
adapter: lora
base_model: unsloth/Qwen2.5-3B
bf16: auto
chat_template: llama3
dataset_prepared_path: null
datasets:
- data_files:
- c485b08dfb34ae17_train_data.json
ds_type: json
format: custom
path: /workspace/input_data/c485b08dfb34ae17_train_data.json
type:
field_input: authors
field_instruction: abstract
field_output: title
format: '{instruction} {input}'
no_input_format: '{instruction}'
system_format: '{system}'
system_prompt: ''
debug: null
deepspeed: null
early_stopping_patience: null
eval_max_new_tokens: 128
eval_table_size: null
evals_per_epoch: 4
flash_attention: true
fp16: null
fsdp: null
fsdp_config: null
gradient_accumulation_steps: 4
gradient_checkpointing: true
gradient_clipping: 1.0
group_by_length: false
hub_model_id: auxyus/3b08c9c9-8517-49a0-8d26-63097e0e34a1
hub_repo: null
hub_strategy: checkpoint
hub_token: null
learning_rate: 0.0001
load_in_4bit: false
load_in_8bit: false
local_rank: 0
logging_steps: 3
lora_alpha: 32
lora_dropout: 0.05
lora_fan_in_fan_out: null
lora_model_dir: null
lora_r: 16
lora_target_linear: true
lr_scheduler: cosine
max_steps: 100
micro_batch_size: 8
mlflow_experiment_name: /tmp/c485b08dfb34ae17_train_data.json
model_type: AutoModelForCausalLM
num_epochs: 3
optimizer: adamw_bnb_8bit
output_dir: miner_id_24
pad_to_sequence_len: true
resume_from_checkpoint: null
s2_attention: null
sample_packing: false
saves_per_epoch: 4
sequence_len: 1024
strict: false
tf32: false
tokenizer_type: AutoTokenizer
train_on_inputs: false
trust_remote_code: true
val_set_size: 0.05
wandb_entity: techspear-hub
wandb_mode: online
wandb_name: 33333ede-a0bf-4279-9af7-9eb33c9d47f1
wandb_project: Gradients-On-Two
wandb_run: your_name
wandb_runid: 33333ede-a0bf-4279-9af7-9eb33c9d47f1
warmup_steps: 10
weight_decay: 0.0
xformers_attention: null
```
</details><br>
# 3b08c9c9-8517-49a0-8d26-63097e0e34a1
This model is a fine-tuned version of [unsloth/Qwen2.5-3B](https://huggingface.co/unsloth/Qwen2.5-3B) on the None dataset.
It achieves the following results on the evaluation set:
- Loss: 1.1770
## Model description
More information needed
## Intended uses & limitations
More information needed
## Training and evaluation data
More information needed
## Training procedure
### Training hyperparameters
The following hyperparameters were used during training:
- learning_rate: 0.0001
- train_batch_size: 8
- eval_batch_size: 8
- seed: 42
- gradient_accumulation_steps: 4
- total_train_batch_size: 32
- optimizer: Use OptimizerNames.ADAMW_BNB with betas=(0.9,0.999) and epsilon=1e-08 and optimizer_args=No additional optimizer arguments
- lr_scheduler_type: cosine
- lr_scheduler_warmup_steps: 10
- training_steps: 100
### Training results
| Training Loss | Epoch | Step | Validation Loss |
|:-------------:|:------:|:----:|:---------------:|
| No log | 0.0129 | 1 | 2.1540 |
| 1.9569 | 0.1158 | 9 | 1.4746 |
| 1.3291 | 0.2315 | 18 | 1.2417 |
| 1.2773 | 0.3473 | 27 | 1.2062 |
| 1.1474 | 0.4630 | 36 | 1.1939 |
| 1.2433 | 0.5788 | 45 | 1.1861 |
| 1.3276 | 0.6945 | 54 | 1.1822 |
| 1.3739 | 0.8103 | 63 | 1.1801 |
| 1.2675 | 0.9260 | 72 | 1.1798 |
| 1.2192 | 1.0482 | 81 | 1.1771 |
| 1.2751 | 1.1640 | 90 | 1.1772 |
| 1.227 | 1.2797 | 99 | 1.1770 |
### Framework versions
- PEFT 0.13.2
- Transformers 4.46.0
- Pytorch 2.5.0+cu124
- Datasets 3.0.1
- Tokenizers 0.20.1 |
machinelearningzuu/queue_detection_cctv | machinelearningzuu | "2024-07-08T21:26:49Z" | 87 | 0 | transformers | [
"transformers",
"safetensors",
"conditional_detr",
"object-detection",
"generated_from_trainer",
"base_model:microsoft/conditional-detr-resnet-50",
"base_model:finetune:microsoft/conditional-detr-resnet-50",
"license:apache-2.0",
"endpoints_compatible",
"region:us"
] | object-detection | "2024-07-07T08:25:07Z" | ---
base_model: microsoft/conditional-detr-resnet-50
license: apache-2.0
tags:
- generated_from_trainer
model-index:
- name: queue_detection_cctv
results: []
---
<!-- This model card has been generated automatically according to the information the Trainer had access to. You
should probably proofread and complete it, then remove this comment. -->
# queue_detection_cctv
This model is a fine-tuned version of [microsoft/conditional-detr-resnet-50](https://huggingface.co/microsoft/conditional-detr-resnet-50) on the None dataset.
It achieves the following results on the evaluation set:
- Loss: 0.1291
- Map: 0.9532
- Map 50: 0.9901
- Map 75: 0.9845
- Map Small: -1.0
- Map Medium: 0.3203
- Map Large: 0.9578
- Mar 1: 0.5044
- Mar 10: 0.9715
- Mar 100: 0.972
- Mar Small: -1.0
- Mar Medium: 0.3538
- Mar Large: 0.9747
- Map Cashier: 0.9618
- Mar 100 Cashier: 0.9775
- Map Cx: 0.9447
- Mar 100 Cx: 0.9664
## Model description
More information needed
## Intended uses & limitations
More information needed
## Training and evaluation data
More information needed
## Training procedure
### Training hyperparameters
The following hyperparameters were used during training:
- learning_rate: 5e-05
- train_batch_size: 2
- eval_batch_size: 8
- seed: 42
- optimizer: Adam with betas=(0.9,0.999) and epsilon=1e-08
- lr_scheduler_type: cosine
- num_epochs: 100
- mixed_precision_training: Native AMP
### Training results
| Training Loss | Epoch | Step | Validation Loss | Map | Map 50 | Map 75 | Map Small | Map Medium | Map Large | Mar 1 | Mar 10 | Mar 100 | Mar Small | Mar Medium | Mar Large | Map Cashier | Mar 100 Cashier | Map Cx | Mar 100 Cx |
|:-------------:|:-----:|:-----:|:---------------:|:------:|:------:|:------:|:---------:|:----------:|:---------:|:------:|:------:|:-------:|:---------:|:----------:|:---------:|:-----------:|:---------------:|:------:|:----------:|
| No log | 1.0 | 218 | 1.3927 | 0.1975 | 0.3459 | 0.1995 | -1.0 | 0.0 | 0.1988 | 0.2409 | 0.5283 | 0.7011 | -1.0 | 0.0 | 0.7055 | 0.2115 | 0.8043 | 0.1834 | 0.5979 |
| No log | 2.0 | 436 | 0.9964 | 0.5247 | 0.8011 | 0.591 | -1.0 | 0.0079 | 0.5292 | 0.3316 | 0.6966 | 0.7387 | -1.0 | 0.0071 | 0.7453 | 0.5772 | 0.8086 | 0.4723 | 0.6688 |
| 2.7418 | 3.0 | 654 | 0.8535 | 0.6031 | 0.9058 | 0.6954 | -1.0 | 0.0349 | 0.6069 | 0.3603 | 0.7079 | 0.733 | -1.0 | 0.2 | 0.7362 | 0.6576 | 0.769 | 0.5485 | 0.6969 |
| 2.7418 | 4.0 | 872 | 0.7406 | 0.6499 | 0.9356 | 0.752 | -1.0 | 0.0479 | 0.6543 | 0.3756 | 0.7387 | 0.7586 | -1.0 | 0.0923 | 0.7634 | 0.7052 | 0.7953 | 0.5947 | 0.7219 |
| 0.8155 | 5.0 | 1090 | 0.6721 | 0.6731 | 0.9516 | 0.8113 | -1.0 | 0.0249 | 0.6773 | 0.3819 | 0.7501 | 0.7654 | -1.0 | 0.0455 | 0.7701 | 0.7451 | 0.8203 | 0.601 | 0.7105 |
| 0.8155 | 6.0 | 1308 | 0.5804 | 0.7244 | 0.9632 | 0.8738 | 0.0 | 0.0712 | 0.7288 | 0.4038 | 0.7882 | 0.8023 | 0.0 | 0.1731 | 0.8066 | 0.7818 | 0.8419 | 0.6671 | 0.7627 |
| 0.6668 | 7.0 | 1526 | 0.5430 | 0.7484 | 0.9667 | 0.9041 | -1.0 | 0.076 | 0.7527 | 0.417 | 0.8027 | 0.813 | -1.0 | 0.2205 | 0.8171 | 0.8068 | 0.8602 | 0.69 | 0.7658 |
| 0.6668 | 8.0 | 1744 | 0.5524 | 0.7361 | 0.9691 | 0.8958 | -1.0 | 0.0273 | 0.7416 | 0.4045 | 0.7839 | 0.7933 | -1.0 | 0.1286 | 0.7981 | 0.7845 | 0.8274 | 0.6877 | 0.7592 |
| 0.6668 | 9.0 | 1962 | 0.5359 | 0.7415 | 0.9737 | 0.901 | -1.0 | 0.0845 | 0.7462 | 0.4112 | 0.7999 | 0.8044 | -1.0 | 0.1462 | 0.8088 | 0.7844 | 0.8376 | 0.6986 | 0.7713 |
| 0.5735 | 10.0 | 2180 | 0.5154 | 0.7497 | 0.9744 | 0.907 | 0.0 | 0.0368 | 0.7538 | 0.414 | 0.8042 | 0.8093 | 0.0 | 0.1333 | 0.813 | 0.8085 | 0.86 | 0.6909 | 0.7586 |
| 0.5735 | 11.0 | 2398 | 0.4543 | 0.7824 | 0.9754 | 0.9337 | 0.0 | 0.0709 | 0.7908 | 0.4307 | 0.8323 | 0.8368 | 0.0 | 0.1794 | 0.8449 | 0.8312 | 0.8765 | 0.7336 | 0.7972 |
| 0.5189 | 12.0 | 2616 | 0.4802 | 0.7679 | 0.9769 | 0.9274 | 0.0 | 0.1201 | 0.7724 | 0.426 | 0.8197 | 0.825 | 0.0 | 0.1917 | 0.8291 | 0.7985 | 0.85 | 0.7374 | 0.8 |
| 0.5189 | 13.0 | 2834 | 0.4306 | 0.7906 | 0.9825 | 0.9332 | -1.0 | 0.0708 | 0.7941 | 0.435 | 0.8394 | 0.8448 | -1.0 | 0.23 | 0.8474 | 0.8474 | 0.889 | 0.7339 | 0.8006 |
| 0.4874 | 14.0 | 3052 | 0.4660 | 0.7649 | 0.9818 | 0.9264 | -1.0 | 0.0504 | 0.7713 | 0.4219 | 0.8155 | 0.8222 | -1.0 | 0.0875 | 0.8288 | 0.805 | 0.8527 | 0.7248 | 0.7917 |
| 0.4874 | 15.0 | 3270 | 0.4392 | 0.7867 | 0.9773 | 0.9278 | 0.0 | 0.0256 | 0.7961 | 0.4372 | 0.8336 | 0.8385 | 0.0 | 0.1028 | 0.8466 | 0.8243 | 0.8725 | 0.7492 | 0.8045 |
| 0.4874 | 16.0 | 3488 | 0.4178 | 0.8018 | 0.9847 | 0.9355 | -1.0 | 0.2037 | 0.8061 | 0.4387 | 0.8493 | 0.8551 | -1.0 | 0.3714 | 0.8589 | 0.8394 | 0.8881 | 0.7641 | 0.822 |
| 0.4646 | 17.0 | 3706 | 0.3859 | 0.8138 | 0.9838 | 0.9502 | -1.0 | 0.1217 | 0.8189 | 0.4459 | 0.8584 | 0.863 | -1.0 | 0.2038 | 0.8669 | 0.8508 | 0.8956 | 0.7769 | 0.8303 |
| 0.4646 | 18.0 | 3924 | 0.4041 | 0.7987 | 0.9822 | 0.9457 | -1.0 | 0.097 | 0.8032 | 0.4378 | 0.8486 | 0.8518 | -1.0 | 0.1611 | 0.8551 | 0.8323 | 0.881 | 0.7652 | 0.8226 |
| 0.4317 | 19.0 | 4142 | 0.4013 | 0.8086 | 0.9838 | 0.9442 | -1.0 | 0.1816 | 0.814 | 0.4412 | 0.8513 | 0.8557 | -1.0 | 0.2571 | 0.8605 | 0.8522 | 0.8919 | 0.765 | 0.8195 |
| 0.4317 | 20.0 | 4360 | 0.3869 | 0.8123 | 0.9823 | 0.9388 | -1.0 | 0.1597 | 0.8163 | 0.4475 | 0.8579 | 0.8617 | -1.0 | 0.2042 | 0.8653 | 0.8542 | 0.896 | 0.7705 | 0.8274 |
| 0.4215 | 21.0 | 4578 | 0.3721 | 0.816 | 0.9864 | 0.9536 | -1.0 | 0.1206 | 0.8198 | 0.4478 | 0.8598 | 0.863 | -1.0 | 0.2727 | 0.8655 | 0.8607 | 0.9003 | 0.7713 | 0.8258 |
| 0.4215 | 22.0 | 4796 | 0.3777 | 0.8245 | 0.9806 | 0.9507 | 0.0 | 0.1034 | 0.8324 | 0.4537 | 0.8621 | 0.8649 | 0.0 | 0.2118 | 0.8724 | 0.8651 | 0.9012 | 0.7839 | 0.8287 |
| 0.3925 | 23.0 | 5014 | 0.3387 | 0.8411 | 0.9872 | 0.9577 | -1.0 | 0.1184 | 0.845 | 0.4593 | 0.8775 | 0.8799 | -1.0 | 0.2429 | 0.8835 | 0.8813 | 0.9153 | 0.8008 | 0.8444 |
| 0.3925 | 24.0 | 5232 | 0.3234 | 0.842 | 0.9887 | 0.9671 | -1.0 | 0.1229 | 0.8463 | 0.4604 | 0.8794 | 0.8812 | -1.0 | 0.1864 | 0.885 | 0.8736 | 0.909 | 0.8104 | 0.8534 |
| 0.3925 | 25.0 | 5450 | 0.3463 | 0.8356 | 0.9869 | 0.9556 | -1.0 | 0.0775 | 0.8411 | 0.4552 | 0.8769 | 0.8793 | -1.0 | 0.1929 | 0.8838 | 0.8788 | 0.913 | 0.7925 | 0.8456 |
| 0.3676 | 26.0 | 5668 | 0.3170 | 0.846 | 0.988 | 0.9666 | 0.0 | 0.1172 | 0.8515 | 0.4603 | 0.886 | 0.8872 | 0.0 | 0.285 | 0.8907 | 0.8831 | 0.9182 | 0.8089 | 0.8562 |
| 0.3676 | 27.0 | 5886 | 0.3552 | 0.8246 | 0.9832 | 0.9545 | -1.0 | 0.13 | 0.8285 | 0.4535 | 0.8704 | 0.8745 | -1.0 | 0.2367 | 0.8785 | 0.8559 | 0.9005 | 0.7932 | 0.8484 |
| 0.3669 | 28.0 | 6104 | 0.3342 | 0.8427 | 0.9876 | 0.9665 | -1.0 | 0.1369 | 0.8468 | 0.4585 | 0.8813 | 0.8843 | -1.0 | 0.2625 | 0.8874 | 0.8587 | 0.898 | 0.8267 | 0.8707 |
| 0.3669 | 29.0 | 6322 | 0.3033 | 0.854 | 0.9892 | 0.9687 | -1.0 | 0.1795 | 0.8572 | 0.4663 | 0.8954 | 0.8968 | -1.0 | 0.3 | 0.8991 | 0.8813 | 0.9193 | 0.8268 | 0.8744 |
| 0.349 | 30.0 | 6540 | 0.3099 | 0.8515 | 0.9863 | 0.9676 | -1.0 | 0.1251 | 0.8571 | 0.4666 | 0.8917 | 0.8936 | -1.0 | 0.2 | 0.8978 | 0.8868 | 0.9261 | 0.8162 | 0.8611 |
| 0.349 | 31.0 | 6758 | 0.3247 | 0.842 | 0.9884 | 0.963 | 0.0 | 0.1145 | 0.8491 | 0.4607 | 0.8828 | 0.8854 | 0.0 | 0.1462 | 0.8916 | 0.8704 | 0.9104 | 0.8137 | 0.8605 |
| 0.349 | 32.0 | 6976 | 0.2943 | 0.8529 | 0.9887 | 0.9651 | -1.0 | 0.1639 | 0.8587 | 0.4683 | 0.8916 | 0.8949 | -1.0 | 0.225 | 0.8997 | 0.89 | 0.9246 | 0.8158 | 0.8653 |
| 0.3378 | 33.0 | 7194 | 0.2923 | 0.8605 | 0.989 | 0.9695 | -1.0 | 0.1212 | 0.8657 | 0.4687 | 0.8985 | 0.9006 | -1.0 | 0.2136 | 0.9042 | 0.8893 | 0.9257 | 0.8317 | 0.8756 |
| 0.3378 | 34.0 | 7412 | 0.2878 | 0.8616 | 0.9895 | 0.9673 | -1.0 | 0.1464 | 0.8665 | 0.4712 | 0.897 | 0.899 | -1.0 | 0.2 | 0.9036 | 0.8907 | 0.9246 | 0.8325 | 0.8734 |
| 0.3206 | 35.0 | 7630 | 0.3342 | 0.837 | 0.9866 | 0.9674 | -1.0 | 0.1634 | 0.8423 | 0.4584 | 0.8772 | 0.8802 | -1.0 | 0.2611 | 0.8844 | 0.8684 | 0.906 | 0.8057 | 0.8544 |
| 0.3206 | 36.0 | 7848 | 0.2796 | 0.8713 | 0.989 | 0.9716 | -1.0 | 0.1054 | 0.8759 | 0.4699 | 0.9066 | 0.9084 | -1.0 | 0.15 | 0.9128 | 0.9052 | 0.9373 | 0.8373 | 0.8795 |
| 0.3152 | 37.0 | 8066 | 0.2894 | 0.8667 | 0.987 | 0.9746 | 0.0 | 0.1359 | 0.8743 | 0.4716 | 0.9022 | 0.9037 | 0.0 | 0.1667 | 0.9109 | 0.8966 | 0.9309 | 0.8367 | 0.8765 |
| 0.3152 | 38.0 | 8284 | 0.2641 | 0.8744 | 0.9894 | 0.9722 | -1.0 | 0.1413 | 0.8793 | 0.4727 | 0.9132 | 0.9148 | -1.0 | 0.2333 | 0.9178 | 0.8909 | 0.9305 | 0.858 | 0.8992 |
| 0.3082 | 39.0 | 8502 | 0.2834 | 0.8703 | 0.9873 | 0.9702 | -1.0 | 0.132 | 0.8764 | 0.473 | 0.9082 | 0.9128 | -1.0 | 0.2633 | 0.9168 | 0.8988 | 0.9347 | 0.8417 | 0.891 |
| 0.3082 | 40.0 | 8720 | 0.2774 | 0.8655 | 0.9897 | 0.9738 | -1.0 | 0.2021 | 0.8711 | 0.4694 | 0.9025 | 0.9043 | -1.0 | 0.275 | 0.9081 | 0.8971 | 0.9314 | 0.8339 | 0.8772 |
| 0.3082 | 41.0 | 8938 | 0.2935 | 0.8598 | 0.988 | 0.9699 | -1.0 | 0.0999 | 0.8666 | 0.4688 | 0.8961 | 0.8976 | -1.0 | 0.15 | 0.9037 | 0.8889 | 0.9255 | 0.8308 | 0.8697 |
| 0.3078 | 42.0 | 9156 | 0.2746 | 0.868 | 0.9895 | 0.9777 | -1.0 | 0.2159 | 0.8738 | 0.4712 | 0.9021 | 0.9032 | -1.0 | 0.275 | 0.9079 | 0.9016 | 0.933 | 0.8343 | 0.8734 |
| 0.3078 | 43.0 | 9374 | 0.2662 | 0.8731 | 0.9897 | 0.9798 | -1.0 | 0.1849 | 0.8794 | 0.4752 | 0.9083 | 0.9091 | -1.0 | 0.2 | 0.9136 | 0.888 | 0.9206 | 0.8582 | 0.8975 |
| 0.2898 | 44.0 | 9592 | 0.2564 | 0.8824 | 0.9868 | 0.9732 | -1.0 | 0.1263 | 0.8871 | 0.4775 | 0.9148 | 0.9165 | -1.0 | 0.15 | 0.9211 | 0.9076 | 0.9377 | 0.8571 | 0.8954 |
| 0.2898 | 45.0 | 9810 | 0.2813 | 0.8753 | 0.9876 | 0.977 | 0.0 | 0.1325 | 0.8817 | 0.4714 | 0.911 | 0.9123 | 0.0 | 0.2167 | 0.9179 | 0.9042 | 0.9381 | 0.8464 | 0.8865 |
| 0.2758 | 46.0 | 10028 | 0.2633 | 0.8786 | 0.9872 | 0.9719 | 0.0 | 0.1841 | 0.8854 | 0.4758 | 0.9164 | 0.9177 | 0.0 | 0.2615 | 0.9218 | 0.9012 | 0.9374 | 0.856 | 0.898 |
| 0.2758 | 47.0 | 10246 | 0.2479 | 0.8795 | 0.9895 | 0.9765 | 0.0 | 0.2066 | 0.8849 | 0.4765 | 0.9146 | 0.9171 | 0.0 | 0.275 | 0.9207 | 0.9114 | 0.9448 | 0.8476 | 0.8893 |
| 0.2758 | 48.0 | 10464 | 0.2373 | 0.8894 | 0.9897 | 0.9799 | -1.0 | 0.1994 | 0.8939 | 0.4795 | 0.9253 | 0.926 | -1.0 | 0.2545 | 0.9293 | 0.9076 | 0.9431 | 0.8713 | 0.909 |
| 0.2708 | 49.0 | 10682 | 0.2538 | 0.8846 | 0.9893 | 0.9793 | 0.0 | 0.2669 | 0.8903 | 0.4799 | 0.9213 | 0.9224 | 0.0 | 0.315 | 0.9284 | 0.9052 | 0.9383 | 0.8641 | 0.9065 |
| 0.2708 | 50.0 | 10900 | 0.2445 | 0.8919 | 0.9896 | 0.9745 | -1.0 | 0.2193 | 0.8972 | 0.4765 | 0.9228 | 0.925 | -1.0 | 0.3969 | 0.9294 | 0.9239 | 0.9511 | 0.8599 | 0.8989 |
| 0.2595 | 51.0 | 11118 | 0.2110 | 0.9037 | 0.99 | 0.9845 | -1.0 | 0.2267 | 0.9093 | 0.4882 | 0.9339 | 0.9346 | -1.0 | 0.25 | 0.9374 | 0.9299 | 0.9574 | 0.8776 | 0.9117 |
| 0.2595 | 52.0 | 11336 | 0.2374 | 0.897 | 0.99 | 0.9792 | -1.0 | 0.2066 | 0.9029 | 0.48 | 0.9267 | 0.9285 | -1.0 | 0.3179 | 0.9335 | 0.9257 | 0.9531 | 0.8684 | 0.9039 |
| 0.2378 | 53.0 | 11554 | 0.2517 | 0.8826 | 0.9894 | 0.9716 | -1.0 | 0.1494 | 0.8901 | 0.4782 | 0.9162 | 0.9188 | -1.0 | 0.2475 | 0.9242 | 0.9152 | 0.9455 | 0.8501 | 0.892 |
| 0.2378 | 54.0 | 11772 | 0.2260 | 0.8971 | 0.9899 | 0.9771 | -1.0 | 0.1848 | 0.9029 | 0.4825 | 0.9304 | 0.9315 | -1.0 | 0.2077 | 0.936 | 0.9255 | 0.9544 | 0.8687 | 0.9087 |
| 0.2378 | 55.0 | 11990 | 0.2144 | 0.9118 | 0.9899 | 0.9844 | -1.0 | 0.2843 | 0.9158 | 0.4875 | 0.9417 | 0.9435 | -1.0 | 0.3333 | 0.9456 | 0.9351 | 0.9608 | 0.8885 | 0.9263 |
| 0.2494 | 56.0 | 12208 | 0.2028 | 0.9107 | 0.9897 | 0.9814 | 0.0 | 0.1831 | 0.9168 | 0.4906 | 0.9395 | 0.9414 | 0.0 | 0.22 | 0.9466 | 0.935 | 0.9585 | 0.8864 | 0.9243 |
| 0.2494 | 57.0 | 12426 | 0.2341 | 0.8897 | 0.9897 | 0.9812 | -1.0 | 0.1783 | 0.8932 | 0.4822 | 0.9242 | 0.926 | -1.0 | 0.2154 | 0.9303 | 0.9168 | 0.948 | 0.8625 | 0.9039 |
| 0.2228 | 58.0 | 12644 | 0.2075 | 0.9084 | 0.9899 | 0.9792 | -1.0 | 0.1741 | 0.9142 | 0.4899 | 0.9375 | 0.9379 | -1.0 | 0.2308 | 0.9421 | 0.932 | 0.9581 | 0.8849 | 0.9177 |
| 0.2228 | 59.0 | 12862 | 0.2059 | 0.9096 | 0.9896 | 0.9803 | 0.0 | 0.2969 | 0.9138 | 0.4893 | 0.9375 | 0.9395 | 0.0 | 0.31 | 0.9431 | 0.9311 | 0.957 | 0.8881 | 0.9219 |
| 0.2218 | 60.0 | 13080 | 0.2028 | 0.9136 | 0.9899 | 0.984 | -1.0 | 0.2316 | 0.9164 | 0.4875 | 0.9408 | 0.9416 | -1.0 | 0.295 | 0.9442 | 0.9433 | 0.9654 | 0.884 | 0.9177 |
| 0.2218 | 61.0 | 13298 | 0.2013 | 0.911 | 0.99 | 0.9786 | -1.0 | 0.253 | 0.9158 | 0.4904 | 0.9388 | 0.94 | -1.0 | 0.3 | 0.9435 | 0.9325 | 0.9572 | 0.8895 | 0.9228 |
| 0.2238 | 62.0 | 13516 | 0.2033 | 0.9134 | 0.9899 | 0.9825 | 0.0 | 0.2228 | 0.9199 | 0.4896 | 0.9426 | 0.9438 | 0.0 | 0.2667 | 0.9484 | 0.9367 | 0.9624 | 0.8902 | 0.9252 |
| 0.2238 | 63.0 | 13734 | 0.1893 | 0.9216 | 0.99 | 0.9836 | -1.0 | 0.1905 | 0.9271 | 0.4942 | 0.9509 | 0.9512 | -1.0 | 0.235 | 0.9546 | 0.9403 | 0.9664 | 0.9029 | 0.9361 |
| 0.2238 | 64.0 | 13952 | 0.1893 | 0.9267 | 0.9898 | 0.9835 | 0.0 | 0.2342 | 0.9317 | 0.4957 | 0.9524 | 0.9536 | 0.0 | 0.2583 | 0.9585 | 0.9491 | 0.971 | 0.9043 | 0.9363 |
| 0.2131 | 65.0 | 14170 | 0.1769 | 0.9322 | 0.9901 | 0.9847 | -1.0 | 0.2413 | 0.9349 | 0.4982 | 0.9554 | 0.9559 | -1.0 | 0.2864 | 0.959 | 0.9463 | 0.9673 | 0.9181 | 0.9445 |
| 0.2131 | 66.0 | 14388 | 0.1848 | 0.9312 | 0.9898 | 0.9842 | 0.0 | 0.2901 | 0.9358 | 0.4973 | 0.9545 | 0.9551 | 0.0 | 0.425 | 0.9591 | 0.9517 | 0.9709 | 0.9107 | 0.9394 |
| 0.2038 | 67.0 | 14606 | 0.1809 | 0.9277 | 0.9899 | 0.9815 | 0.0 | 0.2354 | 0.9329 | 0.4951 | 0.9524 | 0.9539 | 0.0 | 0.2846 | 0.9586 | 0.9441 | 0.9668 | 0.9112 | 0.9411 |
| 0.2038 | 68.0 | 14824 | 0.1831 | 0.9178 | 0.9899 | 0.98 | 0.0 | 0.1728 | 0.9256 | 0.4922 | 0.9472 | 0.9483 | 0.0 | 0.23 | 0.9538 | 0.9396 | 0.9646 | 0.896 | 0.9319 |
| 0.1995 | 69.0 | 15042 | 0.1631 | 0.934 | 0.9901 | 0.9861 | -1.0 | 0.2804 | 0.9405 | 0.4982 | 0.9574 | 0.9583 | -1.0 | 0.325 | 0.9615 | 0.954 | 0.9729 | 0.914 | 0.9438 |
| 0.1995 | 70.0 | 15260 | 0.1685 | 0.9293 | 0.9899 | 0.9846 | -1.0 | 0.2397 | 0.935 | 0.4964 | 0.9546 | 0.9553 | -1.0 | 0.2714 | 0.9593 | 0.948 | 0.9698 | 0.9105 | 0.9408 |
| 0.1995 | 71.0 | 15478 | 0.1629 | 0.9371 | 0.9901 | 0.9842 | -1.0 | 0.2541 | 0.942 | 0.498 | 0.9603 | 0.9609 | -1.0 | 0.4964 | 0.965 | 0.954 | 0.9741 | 0.9202 | 0.9477 |
| 0.1877 | 72.0 | 15696 | 0.1606 | 0.944 | 0.9901 | 0.9846 | -1.0 | 0.277 | 0.9469 | 0.4988 | 0.9636 | 0.9642 | -1.0 | 0.3038 | 0.9676 | 0.96 | 0.9758 | 0.9281 | 0.9527 |
| 0.1877 | 73.0 | 15914 | 0.1532 | 0.9389 | 0.99 | 0.9806 | 0.0 | 0.2592 | 0.9446 | 0.5009 | 0.961 | 0.962 | 0.0 | 0.3133 | 0.9662 | 0.9564 | 0.9749 | 0.9214 | 0.9492 |
| 0.1912 | 74.0 | 16132 | 0.1434 | 0.9488 | 0.995 | 0.9934 | -1.0 | 0.5552 | 0.9507 | 0.5033 | 0.9673 | 0.9675 | -1.0 | 0.7182 | 0.969 | 0.9639 | 0.9786 | 0.9336 | 0.9563 |
| 0.1912 | 75.0 | 16350 | 0.1726 | 0.9309 | 0.9901 | 0.9832 | -1.0 | 0.216 | 0.9344 | 0.4964 | 0.9568 | 0.9578 | -1.0 | 0.2611 | 0.9607 | 0.9539 | 0.9747 | 0.9079 | 0.941 |
| 0.1859 | 76.0 | 16568 | 0.1587 | 0.9378 | 0.9901 | 0.9847 | -1.0 | 0.1684 | 0.944 | 0.4994 | 0.9601 | 0.9607 | -1.0 | 0.2382 | 0.9662 | 0.952 | 0.9715 | 0.9237 | 0.9499 |
| 0.1859 | 77.0 | 16786 | 0.1378 | 0.9509 | 0.9901 | 0.9845 | -1.0 | 0.2089 | 0.959 | 0.5047 | 0.9688 | 0.9691 | -1.0 | 0.2353 | 0.9748 | 0.9666 | 0.9823 | 0.9352 | 0.9559 |
| 0.1747 | 78.0 | 17004 | 0.1416 | 0.9478 | 0.9901 | 0.985 | 0.0 | 0.3334 | 0.9521 | 0.5039 | 0.9685 | 0.9692 | 0.0 | 0.35 | 0.9719 | 0.9617 | 0.9799 | 0.9338 | 0.9586 |
| 0.1747 | 79.0 | 17222 | 0.1615 | 0.9376 | 0.9949 | 0.9873 | -1.0 | 0.5057 | 0.9406 | 0.5003 | 0.9599 | 0.9607 | -1.0 | 0.5688 | 0.9644 | 0.9583 | 0.9746 | 0.917 | 0.9469 |
| 0.1747 | 80.0 | 17440 | 0.1482 | 0.9427 | 0.99 | 0.9823 | -1.0 | 0.1933 | 0.9499 | 0.5025 | 0.9639 | 0.9642 | -1.0 | 0.2321 | 0.9689 | 0.9566 | 0.9762 | 0.9289 | 0.9521 |
| 0.1707 | 81.0 | 17658 | 0.1379 | 0.9518 | 0.9901 | 0.9894 | -1.0 | 0.2838 | 0.956 | 0.504 | 0.97 | 0.9702 | -1.0 | 0.3 | 0.9742 | 0.965 | 0.9787 | 0.9386 | 0.9618 |
| 0.1707 | 82.0 | 17876 | 0.1384 | 0.9478 | 0.9901 | 0.9846 | -1.0 | 0.2518 | 0.9545 | 0.504 | 0.9687 | 0.9691 | -1.0 | 0.2643 | 0.9734 | 0.9612 | 0.9787 | 0.9344 | 0.9595 |
| 0.1658 | 83.0 | 18094 | 0.1379 | 0.9532 | 0.9901 | 0.9845 | -1.0 | 0.2543 | 0.9567 | 0.5043 | 0.9707 | 0.9714 | -1.0 | 0.2708 | 0.975 | 0.9655 | 0.981 | 0.9408 | 0.9617 |
| 0.1658 | 84.0 | 18312 | 0.1325 | 0.9544 | 0.9901 | 0.9845 | 0.0 | 0.256 | 0.9597 | 0.5047 | 0.9712 | 0.972 | 0.0 | 0.3036 | 0.9762 | 0.9672 | 0.9811 | 0.9417 | 0.9628 |
| 0.1532 | 85.0 | 18530 | 0.1558 | 0.9452 | 0.99 | 0.9845 | -1.0 | 0.2469 | 0.9495 | 0.5009 | 0.9648 | 0.9657 | -1.0 | 0.2769 | 0.9695 | 0.9584 | 0.9749 | 0.932 | 0.9565 |
| 0.1532 | 86.0 | 18748 | 0.1228 | 0.9538 | 0.9901 | 0.9841 | -1.0 | 0.3437 | 0.9585 | 0.5056 | 0.972 | 0.9726 | -1.0 | 0.3727 | 0.9747 | 0.9642 | 0.9806 | 0.9434 | 0.9646 |
| 0.1532 | 87.0 | 18966 | 0.1317 | 0.9587 | 0.9901 | 0.9844 | 0.0 | 0.4141 | 0.965 | 0.5064 | 0.9738 | 0.974 | 0.0 | 0.4517 | 0.9791 | 0.9676 | 0.9815 | 0.9498 | 0.9664 |
| 0.1574 | 88.0 | 19184 | 0.1318 | 0.9508 | 0.9901 | 0.9845 | 0.0 | 0.2545 | 0.9581 | 0.5059 | 0.9705 | 0.9706 | 0.0 | 0.2962 | 0.9747 | 0.9594 | 0.9778 | 0.9422 | 0.9633 |
| 0.1574 | 89.0 | 19402 | 0.1424 | 0.9513 | 0.9899 | 0.984 | -1.0 | 0.2362 | 0.9547 | 0.5034 | 0.9691 | 0.9695 | -1.0 | 0.2875 | 0.9729 | 0.9636 | 0.9786 | 0.939 | 0.9603 |
| 0.1537 | 90.0 | 19620 | 0.1240 | 0.9565 | 0.9901 | 0.9896 | -1.0 | 0.5053 | 0.9592 | 0.5066 | 0.9747 | 0.9752 | -1.0 | 0.55 | 0.9771 | 0.9669 | 0.9823 | 0.9461 | 0.9681 |
| 0.1537 | 91.0 | 19838 | 0.1382 | 0.947 | 0.9901 | 0.9835 | 0.0 | 0.5316 | 0.9504 | 0.5018 | 0.9681 | 0.9683 | 0.0 | 0.555 | 0.9712 | 0.9622 | 0.9775 | 0.9319 | 0.9592 |
| 0.1547 | 92.0 | 20056 | 0.1276 | 0.9565 | 0.9901 | 0.983 | -1.0 | 0.3161 | 0.9618 | 0.5058 | 0.9742 | 0.9743 | -1.0 | 0.3458 | 0.977 | 0.9668 | 0.9818 | 0.9462 | 0.9669 |
| 0.1547 | 93.0 | 20274 | 0.1329 | 0.9539 | 0.99 | 0.9836 | -1.0 | 0.2997 | 0.9593 | 0.5053 | 0.9718 | 0.9728 | -1.0 | 0.3318 | 0.9754 | 0.9679 | 0.982 | 0.9398 | 0.9635 |
| 0.1547 | 94.0 | 20492 | 0.1348 | 0.9571 | 0.99 | 0.9846 | -1.0 | 0.3267 | 0.9615 | 0.5039 | 0.9732 | 0.9737 | -1.0 | 0.3625 | 0.9761 | 0.9678 | 0.9823 | 0.9463 | 0.9652 |
| 0.1513 | 95.0 | 20710 | 0.1251 | 0.9546 | 0.9901 | 0.9844 | 0.0 | 0.2549 | 0.9626 | 0.5049 | 0.9728 | 0.9731 | 0.0 | 0.2625 | 0.9775 | 0.965 | 0.981 | 0.9442 | 0.9652 |
| 0.1513 | 96.0 | 20928 | 0.1264 | 0.9594 | 0.9901 | 0.9899 | 0.0 | 0.327 | 0.9631 | 0.5068 | 0.9755 | 0.9763 | 0.0 | 0.3409 | 0.9794 | 0.9696 | 0.9842 | 0.9492 | 0.9683 |
| 0.1635 | 97.0 | 21146 | 0.1306 | 0.9515 | 0.9901 | 0.9843 | -1.0 | 0.2685 | 0.9561 | 0.5041 | 0.9696 | 0.9703 | -1.0 | 0.2857 | 0.9742 | 0.9626 | 0.9795 | 0.9404 | 0.9611 |
| 0.1635 | 98.0 | 21364 | 0.1410 | 0.9481 | 0.9899 | 0.9788 | 0.0 | 0.4025 | 0.9542 | 0.5031 | 0.9662 | 0.9678 | 0.0 | 0.4458 | 0.9722 | 0.9621 | 0.9789 | 0.9341 | 0.9567 |
| 0.1505 | 99.0 | 21582 | 0.1253 | 0.9571 | 0.9901 | 0.984 | -1.0 | 0.3105 | 0.962 | 0.5066 | 0.9737 | 0.974 | -1.0 | 0.3375 | 0.9777 | 0.9702 | 0.9832 | 0.944 | 0.9648 |
| 0.1505 | 100.0 | 21800 | 0.1291 | 0.9532 | 0.9901 | 0.9845 | -1.0 | 0.3203 | 0.9578 | 0.5044 | 0.9715 | 0.972 | -1.0 | 0.3538 | 0.9747 | 0.9618 | 0.9775 | 0.9447 | 0.9664 |
### Framework versions
- Transformers 4.42.3
- Pytorch 2.3.1+cu121
- Datasets 2.20.0
- Tokenizers 0.19.1
|
aidando73/Qwen-2.5-7B-Simple-RL-v9 | aidando73 | "2025-03-24T23:33:49Z" | 0 | 0 | transformers | [
"transformers",
"safetensors",
"qwen2",
"text-generation",
"generated_from_trainer",
"trl",
"grpo",
"conversational",
"arxiv:2402.03300",
"base_model:Qwen/Qwen2.5-Math-7B",
"base_model:finetune:Qwen/Qwen2.5-Math-7B",
"autotrain_compatible",
"text-generation-inference",
"endpoints_compatible",
"region:us"
] | text-generation | "2025-03-24T20:24:58Z" | ---
base_model: Qwen/Qwen2.5-Math-7B
library_name: transformers
model_name: Qwen-2.5-7B-Simple-RL-v9
tags:
- generated_from_trainer
- trl
- grpo
licence: license
---
# Model Card for Qwen-2.5-7B-Simple-RL-v9
This model is a fine-tuned version of [Qwen/Qwen2.5-Math-7B](https://huggingface.co/Qwen/Qwen2.5-Math-7B).
It has been trained using [TRL](https://github.com/huggingface/trl).
## Quick start
```python
from transformers import pipeline
question = "If you had a time machine, but could only go to the past or the future once and never return, which would you choose and why?"
generator = pipeline("text-generation", model="aidando73/Qwen-2.5-7B-Simple-RL-v9", device="cuda")
output = generator([{"role": "user", "content": question}], max_new_tokens=128, return_full_text=False)[0]
print(output["generated_text"])
```
## Training procedure
[<img src="https://raw.githubusercontent.com/wandb/assets/main/wandb-github-badge-28.svg" alt="Visualize in Weights & Biases" width="150" height="24"/>](https://wandb.ai/aidando73-personal/open-r1-math-rl/runs/1v3mtjxf)
This model was trained with GRPO, a method introduced in [DeepSeekMath: Pushing the Limits of Mathematical Reasoning in Open Language Models](https://huggingface.co/papers/2402.03300).
### Framework versions
- TRL: 0.16.0.dev0
- Transformers: 4.49.0
- Pytorch: 2.5.1
- Datasets: 3.4.1
- Tokenizers: 0.21.1
## Citations
Cite GRPO as:
```bibtex
@article{zhihong2024deepseekmath,
title = {{DeepSeekMath: Pushing the Limits of Mathematical Reasoning in Open Language Models}},
author = {Zhihong Shao and Peiyi Wang and Qihao Zhu and Runxin Xu and Junxiao Song and Mingchuan Zhang and Y. K. Li and Y. Wu and Daya Guo},
year = 2024,
eprint = {arXiv:2402.03300},
}
```
Cite TRL as:
```bibtex
@misc{vonwerra2022trl,
title = {{TRL: Transformer Reinforcement Learning}},
author = {Leandro von Werra and Younes Belkada and Lewis Tunstall and Edward Beeching and Tristan Thrush and Nathan Lambert and Shengyi Huang and Kashif Rasul and Quentin Gallouédec},
year = 2020,
journal = {GitHub repository},
publisher = {GitHub},
howpublished = {\url{https://github.com/huggingface/trl}}
}
``` |
Weni/ZeroShot-3.4.0-Mistral-Retry-7b-DPO-1.0.0 | Weni | "2024-03-11T14:17:06Z" | 0 | 0 | trl | [
"trl",
"safetensors",
"DPO",
"ZeroShot",
"en",
"es",
"pt",
"base_model:Weni/ZeroShot-3.3.14-Mistral-7b-Multilanguage-3.2.0-merged",
"base_model:finetune:Weni/ZeroShot-3.3.14-Mistral-7b-Multilanguage-3.2.0-merged",
"license:mit",
"region:us"
] | null | "2024-03-11T12:34:29Z" | ---
license: mit
library_name: "trl"
tags:
- DPO
- ZeroShot
base_model: Weni/ZeroShot-3.3.14-Mistral-7b-Multilanguage-3.2.0-merged
model-index:
- name: Weni/ZeroShot-3.4.0-Mistral-Retry-7b-DPO-1.0.0
results: []
language: ['en', 'es', 'pt']
---
# Weni/ZeroShot-3.4.0-Mistral-Retry-7b-DPO-1.0.0
This model is a fine-tuned version of [Weni/ZeroShot-3.3.14-Mistral-7b-Multilanguage-3.2.0-merged] on the dataset Weni/zeroshot-dpo-1.0.0 with the DPO trainer. It is part of the ZeroShot project for [Weni](https://weni.ai/).
It achieves the following results on the evaluation set:
{'eval_loss': 0.12734735012054443, 'eval_runtime': 25.6184, 'eval_samples_per_second': 2.381, 'eval_steps_per_second': 0.312, 'eval_rewards/chosen': 4.7875847816467285, 'eval_rewards/rejected': -1.6130797863006592, 'eval_rewards/accuracies': 0.921875, 'eval_rewards/margins': 6.400664329528809, 'eval_logps/rejected': -15.168061256408691, 'eval_logps/chosen': -11.294010162353516, 'eval_logits/rejected': -1.3262749910354614, 'eval_logits/chosen': -1.370504379272461, 'epoch': 0.94}
## Intended uses & limitations
This model has not been trained to avoid specific intructions.
## Training procedure
Finetuning was done on the model Weni/ZeroShot-3.3.14-Mistral-7b-Multilanguage-3.2.0-merged with the following prompt:
```
Portuguese:
[INST] Você é muito especialista em classificar a frase do usuário em um chatbot sobre: {context}
Pare, pense bem e responda com APENAS UM ÚNICO \`id\` da classe que melhor represente a intenção para a frase do usuário de acordo com a análise de seu contexto, responda APENAS com o \`id\` da classe só se você tiver muita certeza e não explique o motivo. Na ausência, falta de informações ou caso a frase do usuário não se enquadre em nenhuma classe, classifique como "-1".
# Essas são as Classes com seus Id e Contexto:
{all_classes}
# Frase do usuário: {input}
# Id da Classe: [/INST]
Spanish:
[INST] Eres muy experto en clasificar la frase del usuario en un chatbot sobre: {context}
Deténgase, piense bien y responda con SOLO UN ÚNICO \`id\` de la clase que mejor represente la intención para la frase del usuario de acuerdo con el análisis de su contexto, responda SOLO con el \`id\` de la clase si está muy seguro y no explique el motivo. En ausencia, falta de información o en caso de que la frase del usuario no se ajuste a ninguna clase, clasifique como "-1".
# Estas son las Clases con sus Id y Contexto:
{all_classes}
# Frase del usuario: {input}
# Id de la Clase: [/INST]
English:
[INST] You are very expert in classifying the user sentence in a chatbot about: {context}
Stop, think carefully, and respond with ONLY ONE SINGLE \`id\` of the class that best represents the intention for the user's sentence according to the analysis of its context, respond ONLY with the \`id\` of the class if you are very sure and do not explain the reason. In the absence, lack of information, or if the user's sentence does not fit into any class, classify as "-1".
# These are the Classes and its Context:
{all_classes}
# User's sentence: {input}
# Class Id: [/INST]
Chosen_response:
{chosen_response}
Rejected_response:
{rejected_response}
```
### Training hyperparameters
The following hyperparameters were used during training:
- learning_rate: 0.0002
- per_device_train_batch_size: 8
- per_device_eval_batch_size: 8
- gradient_accumulation_steps: 4
- num_gpus: 1
- total_train_batch_size: 32
- optimizer: AdamW
- lr_scheduler_type: cosine
- num_steps: 16
- quantization_type: bitsandbytes
- LoRA: ("\n - bits: 4\n - use_exllama: True\n - device_map: auto\n - use_cache: False\n - lora_r: 8\n - lora_alpha: 16\n - lora_dropout: 0.1\n - bias: none\n - target_modules: ['q_proj', 'k_proj', 'v_proj', 'o_proj']\n - task_type: CAUSAL_LM",)
### Training results
### Framework versions
- transformers==4.38.2
- datasets==2.17.1
- peft==0.8.2
- safetensors==0.4.2
- evaluate==0.4.1
- bitsandbytes==0.42
- huggingface_hub==0.20.3
- seqeval==1.2.2
- optimum==1.17.1
- auto-gptq==0.7.0
- gpustat==1.1.1
- deepspeed==0.13.2
- wandb==0.16.3
- trl==0.7.11
- accelerate==0.27.2
- coloredlogs==15.0.1
- traitlets==5.14.1
- autoawq@https://github.com/casper-hansen/AutoAWQ/releases/download/v0.2.0/autoawq-0.2.0+cu118-cp310-cp310-linux_x86_64.whl
### Hardware
- Cloud provided: runpod.io
|
sbaner24/vit-base-patch16-224-Trial008-YEL_STEM3 | sbaner24 | "2023-11-15T15:09:56Z" | 189 | 0 | transformers | [
"transformers",
"pytorch",
"vit",
"image-classification",
"generated_from_trainer",
"dataset:imagefolder",
"license:apache-2.0",
"model-index",
"autotrain_compatible",
"endpoints_compatible",
"region:us"
] | image-classification | "2023-11-14T04:57:19Z" | ---
license: apache-2.0
tags:
- generated_from_trainer
datasets:
- imagefolder
metrics:
- accuracy
model-index:
- name: vit-base-patch16-224-Trial008-YEL_STEM3
results:
- task:
name: Image Classification
type: image-classification
dataset:
name: imagefolder
type: imagefolder
config: default
split: train
args: default
metrics:
- name: Accuracy
type: accuracy
value: 1.0
---
<!-- This model card has been generated automatically according to the information the Trainer had access to. You
should probably proofread and complete it, then remove this comment. -->
# vit-base-patch16-224-Trial008-YEL_STEM3
This model is a fine-tuned version of [google/vit-base-patch16-224](https://huggingface.co/google/vit-base-patch16-224) on the imagefolder dataset.
It achieves the following results on the evaluation set:
- Loss: 0.0916
- Accuracy: 1.0
## Model description
More information needed
## Intended uses & limitations
More information needed
## Training and evaluation data
More information needed
## Training procedure
### Training hyperparameters
The following hyperparameters were used during training:
- learning_rate: 5e-05
- train_batch_size: 30
- eval_batch_size: 30
- seed: 42
- gradient_accumulation_steps: 4
- total_train_batch_size: 120
- optimizer: Adam with betas=(0.9,0.999) and epsilon=1e-08
- lr_scheduler_type: linear
- lr_scheduler_warmup_ratio: 0.1
- num_epochs: 50
### Training results
| Training Loss | Epoch | Step | Validation Loss | Accuracy |
|:-------------:|:-----:|:----:|:---------------:|:--------:|
| 0.7743 | 1.0 | 1 | 0.8267 | 0.3636 |
| 0.7964 | 2.0 | 2 | 0.7547 | 0.3636 |
| 0.6369 | 3.0 | 3 | 0.6399 | 0.7273 |
| 0.5344 | 4.0 | 4 | 0.5082 | 0.9091 |
| 0.4342 | 5.0 | 5 | 0.4664 | 0.9091 |
| 0.3056 | 6.0 | 6 | 0.2145 | 0.9091 |
| 0.257 | 7.0 | 7 | 0.1395 | 0.9091 |
| 0.2064 | 8.0 | 8 | 0.1990 | 0.9091 |
| 0.2609 | 9.0 | 9 | 0.0916 | 1.0 |
| 0.1758 | 10.0 | 10 | 0.0321 | 1.0 |
| 0.1152 | 11.0 | 11 | 0.0256 | 1.0 |
| 0.1343 | 12.0 | 12 | 0.0413 | 1.0 |
| 0.0955 | 13.0 | 13 | 0.0319 | 1.0 |
| 0.0723 | 14.0 | 14 | 0.0112 | 1.0 |
| 0.13 | 15.0 | 15 | 0.0073 | 1.0 |
| 0.1918 | 16.0 | 16 | 0.0057 | 1.0 |
| 0.2469 | 17.0 | 17 | 0.0052 | 1.0 |
| 0.1001 | 18.0 | 18 | 0.0051 | 1.0 |
| 0.1331 | 19.0 | 19 | 0.0039 | 1.0 |
| 0.1511 | 20.0 | 20 | 0.0031 | 1.0 |
| 0.0956 | 21.0 | 21 | 0.0027 | 1.0 |
| 0.0952 | 22.0 | 22 | 0.0027 | 1.0 |
| 0.1679 | 23.0 | 23 | 0.0025 | 1.0 |
| 0.1075 | 24.0 | 24 | 0.0023 | 1.0 |
| 0.1507 | 25.0 | 25 | 0.0024 | 1.0 |
| 0.1267 | 26.0 | 26 | 0.0027 | 1.0 |
| 0.1141 | 27.0 | 27 | 0.0030 | 1.0 |
| 0.0767 | 28.0 | 28 | 0.0031 | 1.0 |
| 0.1746 | 29.0 | 29 | 0.0029 | 1.0 |
| 0.1101 | 30.0 | 30 | 0.0032 | 1.0 |
| 0.1632 | 31.0 | 31 | 0.0036 | 1.0 |
| 0.1346 | 32.0 | 32 | 0.0038 | 1.0 |
| 0.1024 | 33.0 | 33 | 0.0038 | 1.0 |
| 0.1198 | 34.0 | 34 | 0.0037 | 1.0 |
| 0.1217 | 35.0 | 35 | 0.0033 | 1.0 |
| 0.1433 | 36.0 | 36 | 0.0030 | 1.0 |
| 0.1255 | 37.0 | 37 | 0.0029 | 1.0 |
| 0.1369 | 38.0 | 38 | 0.0027 | 1.0 |
| 0.091 | 39.0 | 39 | 0.0026 | 1.0 |
| 0.1318 | 40.0 | 40 | 0.0025 | 1.0 |
| 0.0964 | 41.0 | 41 | 0.0025 | 1.0 |
| 0.1164 | 42.0 | 42 | 0.0024 | 1.0 |
| 0.0935 | 43.0 | 43 | 0.0023 | 1.0 |
| 0.0564 | 44.0 | 44 | 0.0022 | 1.0 |
| 0.1136 | 45.0 | 45 | 0.0021 | 1.0 |
| 0.1306 | 46.0 | 46 | 0.0021 | 1.0 |
| 0.0757 | 47.0 | 47 | 0.0021 | 1.0 |
| 0.0475 | 48.0 | 48 | 0.0020 | 1.0 |
| 0.1455 | 49.0 | 49 | 0.0020 | 1.0 |
| 0.1892 | 50.0 | 50 | 0.0020 | 1.0 |
### Framework versions
- Transformers 4.30.0.dev0
- Pytorch 1.12.1
- Datasets 2.12.0
- Tokenizers 0.13.1
|
psxjp5/mt5-small_25 | psxjp5 | "2023-08-08T11:50:47Z" | 104 | 0 | transformers | [
"transformers",
"pytorch",
"tensorboard",
"mt5",
"text2text-generation",
"generated_from_trainer",
"base_model:google/mt5-small",
"base_model:finetune:google/mt5-small",
"license:apache-2.0",
"autotrain_compatible",
"endpoints_compatible",
"region:us"
] | text2text-generation | "2023-08-08T09:40:03Z" | ---
license: apache-2.0
base_model: google/mt5-small
tags:
- generated_from_trainer
metrics:
- rouge
- bleu
model-index:
- name: mt5-small_test
results: []
---
<!-- This model card has been generated automatically according to the information the Trainer had access to. You
should probably proofread and complete it, then remove this comment. -->
# mt5-small_test
This model is a fine-tuned version of [google/mt5-small](https://huggingface.co/google/mt5-small) on the None dataset.
It achieves the following results on the evaluation set:
- Loss: 0.7284
- Rouge1: 43.3718
- Rouge2: 37.5973
- Rougel: 42.0502
- Rougelsum: 42.0648
- Bleu: 32.8345
- Gen Len: 12.6063
- Meteor: 0.3949
- True negatives: 70.2115
- False negatives: 11.206
- Cosine Sim: 0.7485
## Model description
More information needed
## Intended uses & limitations
More information needed
## Training and evaluation data
More information needed
## Training procedure
### Training hyperparameters
The following hyperparameters were used during training:
- learning_rate: 0.001
- train_batch_size: 16
- eval_batch_size: 16
- seed: 9
- gradient_accumulation_steps: 8
- total_train_batch_size: 128
- optimizer: Adam with betas=(0.9,0.999) and epsilon=1e-08
- lr_scheduler_type: linear
- num_epochs: 20
### Training results
| Training Loss | Epoch | Step | Validation Loss | Rouge1 | Rouge2 | Rougel | Rougelsum | Bleu | Gen Len | Meteor | True negatives | False negatives | Cosine Sim |
|:-------------:|:-----:|:----:|:---------------:|:-------:|:-------:|:-------:|:---------:|:-------:|:-------:|:------:|:--------------:|:---------------:|:----------:|
| 3.1455 | 1.0 | 175 | 0.9832 | 18.7269 | 15.517 | 18.22 | 18.223 | 7.0634 | 7.6229 | 0.1626 | 74.6828 | 57.1687 | 0.3949 |
| 1.1623 | 1.99 | 350 | 0.8542 | 38.7603 | 32.7237 | 37.3447 | 37.3752 | 27.4323 | 12.5135 | 0.3487 | 60.0 | 15.942 | 0.6992 |
| 0.9431 | 2.99 | 525 | 0.8017 | 41.5759 | 35.6108 | 40.2536 | 40.2695 | 30.7994 | 12.8117 | 0.3755 | 61.2689 | 12.3447 | 0.7304 |
| 0.8119 | 3.98 | 700 | 0.7787 | 43.5881 | 37.4245 | 42.1096 | 42.1248 | 32.9646 | 13.2176 | 0.3947 | 59.1541 | 9.5238 | 0.7582 |
| 0.7235 | 4.98 | 875 | 0.7477 | 43.4069 | 37.2246 | 41.8444 | 41.8616 | 32.9345 | 13.116 | 0.3946 | 63.0816 | 9.8085 | 0.7561 |
| 0.6493 | 5.97 | 1050 | 0.7266 | 40.4506 | 35.0072 | 39.1206 | 39.1181 | 29.0601 | 11.748 | 0.3687 | 75.5287 | 17.2101 | 0.7071 |
| 0.5871 | 6.97 | 1225 | 0.7284 | 43.3718 | 37.5973 | 42.0502 | 42.0648 | 32.8345 | 12.6063 | 0.3949 | 70.2115 | 11.206 | 0.7485 |
### Framework versions
- Transformers 4.31.0
- Pytorch 2.0.1+cu118
- Datasets 2.13.1
- Tokenizers 0.13.3
|
dianamihalache27/deberta-v3-base_3epoch10 | dianamihalache27 | "2024-05-31T15:34:50Z" | 163 | 0 | transformers | [
"transformers",
"tensorboard",
"safetensors",
"deberta-v2",
"text-classification",
"generated_from_trainer",
"base_model:microsoft/deberta-v3-base",
"base_model:finetune:microsoft/deberta-v3-base",
"license:mit",
"autotrain_compatible",
"endpoints_compatible",
"region:us"
] | text-classification | "2024-05-31T15:34:11Z" | ---
license: mit
base_model: microsoft/deberta-v3-base
tags:
- generated_from_trainer
metrics:
- accuracy
- f1
- precision
- recall
model-index:
- name: deberta-v3-base_3epoch10
results: []
---
<!-- This model card has been generated automatically according to the information the Trainer had access to. You
should probably proofread and complete it, then remove this comment. -->
# deberta-v3-base_3epoch10
This model is a fine-tuned version of [microsoft/deberta-v3-base](https://huggingface.co/microsoft/deberta-v3-base) on an unknown dataset.
It achieves the following results on the evaluation set:
- Loss: 2.2046
- Accuracy: 0.7680
- F1: 0.5136
- Precision: 0.6439
- Recall: 0.4271
- Precision Sarcastic: 0.6439
- Recall Sarcastic: 0.4271
- F1 Sarcastic: 0.5136
## Model description
More information needed
## Intended uses & limitations
More information needed
## Training and evaluation data
More information needed
## Training procedure
### Training hyperparameters
The following hyperparameters were used during training:
- learning_rate: 5e-05
- train_batch_size: 16
- eval_batch_size: 16
- seed: 42
- optimizer: Adam with betas=(0.9,0.999) and epsilon=1e-08
- lr_scheduler_type: linear
- num_epochs: 10
### Training results
### Framework versions
- Transformers 4.41.2
- Pytorch 2.3.0+cu121
- Datasets 2.19.1
- Tokenizers 0.19.1
|
Tommert25/multibertfinetuned0407 | Tommert25 | "2023-07-04T15:15:05Z" | 4 | 0 | transformers | [
"transformers",
"pytorch",
"tensorboard",
"bert",
"token-classification",
"generated_from_trainer",
"license:apache-2.0",
"autotrain_compatible",
"endpoints_compatible",
"region:us"
] | token-classification | "2023-07-04T10:41:33Z" | ---
license: apache-2.0
tags:
- generated_from_trainer
metrics:
- precision
- recall
- f1
- accuracy
model-index:
- name: multibertfinetuned0407
results: []
---
<!-- This model card has been generated automatically according to the information the Trainer had access to. You
should probably proofread and complete it, then remove this comment. -->
# multibertfinetuned0407
This model is a fine-tuned version of [bert-base-multilingual-uncased](https://huggingface.co/bert-base-multilingual-uncased) on an unknown dataset.
It achieves the following results on the evaluation set:
- Loss: 0.4688
- Precision: 0.4879
- Recall: 0.4345
- F1: 0.4597
- Accuracy: 0.8764
## Model description
More information needed
## Intended uses & limitations
More information needed
## Training and evaluation data
More information needed
## Training procedure
### Training hyperparameters
The following hyperparameters were used during training:
- learning_rate: 5e-05
- train_batch_size: 16
- eval_batch_size: 16
- seed: 42
- optimizer: Adam with betas=(0.9,0.999) and epsilon=1e-08
- lr_scheduler_type: linear
- num_epochs: 5
### Training results
| Training Loss | Epoch | Step | Validation Loss | Precision | Recall | F1 | Accuracy |
|:-------------:|:-----:|:----:|:---------------:|:---------:|:------:|:------:|:--------:|
| No log | 1.0 | 131 | 0.4688 | 0.4879 | 0.4345 | 0.4597 | 0.8764 |
| No log | 2.0 | 262 | 0.5224 | 0.5400 | 0.4884 | 0.5129 | 0.8777 |
| No log | 3.0 | 393 | 0.5814 | 0.4900 | 0.4900 | 0.4900 | 0.8683 |
| 0.3219 | 4.0 | 524 | 0.6226 | 0.5125 | 0.5069 | 0.5097 | 0.8750 |
| 0.3219 | 5.0 | 655 | 0.6593 | 0.5008 | 0.4977 | 0.4992 | 0.8771 |
### Framework versions
- Transformers 4.30.2
- Pytorch 2.0.1+cu118
- Datasets 2.13.1
- Tokenizers 0.13.3
|
coffiee/lz3 | coffiee | "2025-02-25T05:51:58Z" | 0 | 0 | transformers | [
"transformers",
"safetensors",
"llama",
"text-generation",
"conversational",
"arxiv:1910.09700",
"autotrain_compatible",
"text-generation-inference",
"endpoints_compatible",
"region:us"
] | text-generation | "2025-02-25T05:51:04Z" | ---
library_name: transformers
tags: []
---
# Model Card for Model ID
<!-- Provide a quick summary of what the model is/does. -->
## Model Details
### Model Description
<!-- Provide a longer summary of what this model is. -->
This is the model card of a 🤗 transformers model that has been pushed on the Hub. This model card has been automatically generated.
- **Developed by:** [More Information Needed]
- **Funded by [optional]:** [More Information Needed]
- **Shared by [optional]:** [More Information Needed]
- **Model type:** [More Information Needed]
- **Language(s) (NLP):** [More Information Needed]
- **License:** [More Information Needed]
- **Finetuned from model [optional]:** [More Information Needed]
### Model Sources [optional]
<!-- Provide the basic links for the model. -->
- **Repository:** [More Information Needed]
- **Paper [optional]:** [More Information Needed]
- **Demo [optional]:** [More Information Needed]
## Uses
<!-- Address questions around how the model is intended to be used, including the foreseeable users of the model and those affected by the model. -->
### Direct Use
<!-- This section is for the model use without fine-tuning or plugging into a larger ecosystem/app. -->
[More Information Needed]
### Downstream Use [optional]
<!-- This section is for the model use when fine-tuned for a task, or when plugged into a larger ecosystem/app -->
[More Information Needed]
### Out-of-Scope Use
<!-- This section addresses misuse, malicious use, and uses that the model will not work well for. -->
[More Information Needed]
## Bias, Risks, and Limitations
<!-- This section is meant to convey both technical and sociotechnical limitations. -->
[More Information Needed]
### Recommendations
<!-- This section is meant to convey recommendations with respect to the bias, risk, and technical limitations. -->
Users (both direct and downstream) should be made aware of the risks, biases and limitations of the model. More information needed for further recommendations.
## How to Get Started with the Model
Use the code below to get started with the model.
[More Information Needed]
## Training Details
### Training Data
<!-- This should link to a Dataset Card, perhaps with a short stub of information on what the training data is all about as well as documentation related to data pre-processing or additional filtering. -->
[More Information Needed]
### Training Procedure
<!-- This relates heavily to the Technical Specifications. Content here should link to that section when it is relevant to the training procedure. -->
#### Preprocessing [optional]
[More Information Needed]
#### Training Hyperparameters
- **Training regime:** [More Information Needed] <!--fp32, fp16 mixed precision, bf16 mixed precision, bf16 non-mixed precision, fp16 non-mixed precision, fp8 mixed precision -->
#### Speeds, Sizes, Times [optional]
<!-- This section provides information about throughput, start/end time, checkpoint size if relevant, etc. -->
[More Information Needed]
## Evaluation
<!-- This section describes the evaluation protocols and provides the results. -->
### Testing Data, Factors & Metrics
#### Testing Data
<!-- This should link to a Dataset Card if possible. -->
[More Information Needed]
#### Factors
<!-- These are the things the evaluation is disaggregating by, e.g., subpopulations or domains. -->
[More Information Needed]
#### Metrics
<!-- These are the evaluation metrics being used, ideally with a description of why. -->
[More Information Needed]
### Results
[More Information Needed]
#### Summary
## Model Examination [optional]
<!-- Relevant interpretability work for the model goes here -->
[More Information Needed]
## Environmental Impact
<!-- Total emissions (in grams of CO2eq) and additional considerations, such as electricity usage, go here. Edit the suggested text below accordingly -->
Carbon emissions can be estimated using the [Machine Learning Impact calculator](https://mlco2.github.io/impact#compute) presented in [Lacoste et al. (2019)](https://arxiv.org/abs/1910.09700).
- **Hardware Type:** [More Information Needed]
- **Hours used:** [More Information Needed]
- **Cloud Provider:** [More Information Needed]
- **Compute Region:** [More Information Needed]
- **Carbon Emitted:** [More Information Needed]
## Technical Specifications [optional]
### Model Architecture and Objective
[More Information Needed]
### Compute Infrastructure
[More Information Needed]
#### Hardware
[More Information Needed]
#### Software
[More Information Needed]
## Citation [optional]
<!-- If there is a paper or blog post introducing the model, the APA and Bibtex information for that should go in this section. -->
**BibTeX:**
[More Information Needed]
**APA:**
[More Information Needed]
## Glossary [optional]
<!-- If relevant, include terms and calculations in this section that can help readers understand the model or model card. -->
[More Information Needed]
## More Information [optional]
[More Information Needed]
## Model Card Authors [optional]
[More Information Needed]
## Model Card Contact
[More Information Needed] |
chainup244/Qwen-Qwen1.5-1.8B-1719209906 | chainup244 | "2024-06-24T06:20:07Z" | 6 | 0 | transformers | [
"transformers",
"safetensors",
"qwen2",
"text-generation",
"conversational",
"arxiv:1910.09700",
"autotrain_compatible",
"text-generation-inference",
"endpoints_compatible",
"region:us"
] | text-generation | "2024-06-24T06:18:27Z" | ---
library_name: transformers
tags: []
---
# Model Card for Model ID
<!-- Provide a quick summary of what the model is/does. -->
## Model Details
### Model Description
<!-- Provide a longer summary of what this model is. -->
This is the model card of a 🤗 transformers model that has been pushed on the Hub. This model card has been automatically generated.
- **Developed by:** [More Information Needed]
- **Funded by [optional]:** [More Information Needed]
- **Shared by [optional]:** [More Information Needed]
- **Model type:** [More Information Needed]
- **Language(s) (NLP):** [More Information Needed]
- **License:** [More Information Needed]
- **Finetuned from model [optional]:** [More Information Needed]
### Model Sources [optional]
<!-- Provide the basic links for the model. -->
- **Repository:** [More Information Needed]
- **Paper [optional]:** [More Information Needed]
- **Demo [optional]:** [More Information Needed]
## Uses
<!-- Address questions around how the model is intended to be used, including the foreseeable users of the model and those affected by the model. -->
### Direct Use
<!-- This section is for the model use without fine-tuning or plugging into a larger ecosystem/app. -->
[More Information Needed]
### Downstream Use [optional]
<!-- This section is for the model use when fine-tuned for a task, or when plugged into a larger ecosystem/app -->
[More Information Needed]
### Out-of-Scope Use
<!-- This section addresses misuse, malicious use, and uses that the model will not work well for. -->
[More Information Needed]
## Bias, Risks, and Limitations
<!-- This section is meant to convey both technical and sociotechnical limitations. -->
[More Information Needed]
### Recommendations
<!-- This section is meant to convey recommendations with respect to the bias, risk, and technical limitations. -->
Users (both direct and downstream) should be made aware of the risks, biases and limitations of the model. More information needed for further recommendations.
## How to Get Started with the Model
Use the code below to get started with the model.
[More Information Needed]
## Training Details
### Training Data
<!-- This should link to a Dataset Card, perhaps with a short stub of information on what the training data is all about as well as documentation related to data pre-processing or additional filtering. -->
[More Information Needed]
### Training Procedure
<!-- This relates heavily to the Technical Specifications. Content here should link to that section when it is relevant to the training procedure. -->
#### Preprocessing [optional]
[More Information Needed]
#### Training Hyperparameters
- **Training regime:** [More Information Needed] <!--fp32, fp16 mixed precision, bf16 mixed precision, bf16 non-mixed precision, fp16 non-mixed precision, fp8 mixed precision -->
#### Speeds, Sizes, Times [optional]
<!-- This section provides information about throughput, start/end time, checkpoint size if relevant, etc. -->
[More Information Needed]
## Evaluation
<!-- This section describes the evaluation protocols and provides the results. -->
### Testing Data, Factors & Metrics
#### Testing Data
<!-- This should link to a Dataset Card if possible. -->
[More Information Needed]
#### Factors
<!-- These are the things the evaluation is disaggregating by, e.g., subpopulations or domains. -->
[More Information Needed]
#### Metrics
<!-- These are the evaluation metrics being used, ideally with a description of why. -->
[More Information Needed]
### Results
[More Information Needed]
#### Summary
## Model Examination [optional]
<!-- Relevant interpretability work for the model goes here -->
[More Information Needed]
## Environmental Impact
<!-- Total emissions (in grams of CO2eq) and additional considerations, such as electricity usage, go here. Edit the suggested text below accordingly -->
Carbon emissions can be estimated using the [Machine Learning Impact calculator](https://mlco2.github.io/impact#compute) presented in [Lacoste et al. (2019)](https://arxiv.org/abs/1910.09700).
- **Hardware Type:** [More Information Needed]
- **Hours used:** [More Information Needed]
- **Cloud Provider:** [More Information Needed]
- **Compute Region:** [More Information Needed]
- **Carbon Emitted:** [More Information Needed]
## Technical Specifications [optional]
### Model Architecture and Objective
[More Information Needed]
### Compute Infrastructure
[More Information Needed]
#### Hardware
[More Information Needed]
#### Software
[More Information Needed]
## Citation [optional]
<!-- If there is a paper or blog post introducing the model, the APA and Bibtex information for that should go in this section. -->
**BibTeX:**
[More Information Needed]
**APA:**
[More Information Needed]
## Glossary [optional]
<!-- If relevant, include terms and calculations in this section that can help readers understand the model or model card. -->
[More Information Needed]
## More Information [optional]
[More Information Needed]
## Model Card Authors [optional]
[More Information Needed]
## Model Card Contact
[More Information Needed] |
musa99/teachim | musa99 | "2025-02-28T18:37:14Z" | 0 | 0 | peft | [
"peft",
"safetensors",
"arxiv:1910.09700",
"base_model:unsloth/DeepSeek-R1-Distill-Llama-8B-unsloth-bnb-4bit",
"base_model:adapter:unsloth/DeepSeek-R1-Distill-Llama-8B-unsloth-bnb-4bit",
"region:us"
] | null | "2025-02-28T16:47:31Z" | ---
base_model: unsloth/DeepSeek-R1-Distill-Llama-8B-unsloth-bnb-4bit
library_name: peft
---
# Model Card for Model ID
<!-- Provide a quick summary of what the model is/does. -->
## Model Details
### Model Description
<!-- Provide a longer summary of what this model is. -->
- **Developed by:** [More Information Needed]
- **Funded by [optional]:** [More Information Needed]
- **Shared by [optional]:** [More Information Needed]
- **Model type:** [More Information Needed]
- **Language(s) (NLP):** [More Information Needed]
- **License:** [More Information Needed]
- **Finetuned from model [optional]:** [More Information Needed]
### Model Sources [optional]
<!-- Provide the basic links for the model. -->
- **Repository:** [More Information Needed]
- **Paper [optional]:** [More Information Needed]
- **Demo [optional]:** [More Information Needed]
## Uses
<!-- Address questions around how the model is intended to be used, including the foreseeable users of the model and those affected by the model. -->
### Direct Use
<!-- This section is for the model use without fine-tuning or plugging into a larger ecosystem/app. -->
[More Information Needed]
### Downstream Use [optional]
<!-- This section is for the model use when fine-tuned for a task, or when plugged into a larger ecosystem/app -->
[More Information Needed]
### Out-of-Scope Use
<!-- This section addresses misuse, malicious use, and uses that the model will not work well for. -->
[More Information Needed]
## Bias, Risks, and Limitations
<!-- This section is meant to convey both technical and sociotechnical limitations. -->
[More Information Needed]
### Recommendations
<!-- This section is meant to convey recommendations with respect to the bias, risk, and technical limitations. -->
Users (both direct and downstream) should be made aware of the risks, biases and limitations of the model. More information needed for further recommendations.
## How to Get Started with the Model
Use the code below to get started with the model.
[More Information Needed]
## Training Details
### Training Data
<!-- This should link to a Dataset Card, perhaps with a short stub of information on what the training data is all about as well as documentation related to data pre-processing or additional filtering. -->
[More Information Needed]
### Training Procedure
<!-- This relates heavily to the Technical Specifications. Content here should link to that section when it is relevant to the training procedure. -->
#### Preprocessing [optional]
[More Information Needed]
#### Training Hyperparameters
- **Training regime:** [More Information Needed] <!--fp32, fp16 mixed precision, bf16 mixed precision, bf16 non-mixed precision, fp16 non-mixed precision, fp8 mixed precision -->
#### Speeds, Sizes, Times [optional]
<!-- This section provides information about throughput, start/end time, checkpoint size if relevant, etc. -->
[More Information Needed]
## Evaluation
<!-- This section describes the evaluation protocols and provides the results. -->
### Testing Data, Factors & Metrics
#### Testing Data
<!-- This should link to a Dataset Card if possible. -->
[More Information Needed]
#### Factors
<!-- These are the things the evaluation is disaggregating by, e.g., subpopulations or domains. -->
[More Information Needed]
#### Metrics
<!-- These are the evaluation metrics being used, ideally with a description of why. -->
[More Information Needed]
### Results
[More Information Needed]
#### Summary
## Model Examination [optional]
<!-- Relevant interpretability work for the model goes here -->
[More Information Needed]
## Environmental Impact
<!-- Total emissions (in grams of CO2eq) and additional considerations, such as electricity usage, go here. Edit the suggested text below accordingly -->
Carbon emissions can be estimated using the [Machine Learning Impact calculator](https://mlco2.github.io/impact#compute) presented in [Lacoste et al. (2019)](https://arxiv.org/abs/1910.09700).
- **Hardware Type:** [More Information Needed]
- **Hours used:** [More Information Needed]
- **Cloud Provider:** [More Information Needed]
- **Compute Region:** [More Information Needed]
- **Carbon Emitted:** [More Information Needed]
## Technical Specifications [optional]
### Model Architecture and Objective
[More Information Needed]
### Compute Infrastructure
[More Information Needed]
#### Hardware
[More Information Needed]
#### Software
[More Information Needed]
## Citation [optional]
<!-- If there is a paper or blog post introducing the model, the APA and Bibtex information for that should go in this section. -->
**BibTeX:**
[More Information Needed]
**APA:**
[More Information Needed]
## Glossary [optional]
<!-- If relevant, include terms and calculations in this section that can help readers understand the model or model card. -->
[More Information Needed]
## More Information [optional]
[More Information Needed]
## Model Card Authors [optional]
[More Information Needed]
## Model Card Contact
[More Information Needed]
### Framework versions
- PEFT 0.14.0 |
chenlong7616/ddpm-celebahq-finetuned-butterflies-2epochs | chenlong7616 | "2023-10-12T06:12:11Z" | 46 | 0 | diffusers | [
"diffusers",
"safetensors",
"pytorch",
"unconditional-image-generation",
"diffusion-models-class",
"license:mit",
"diffusers:DDPMPipeline",
"region:us"
] | unconditional-image-generation | "2023-10-12T06:11:48Z" | ---
license: mit
tags:
- pytorch
- diffusers
- unconditional-image-generation
- diffusion-models-class
---
# Example Fine-Tuned Model for Unit 2 of the [Diffusion Models Class 🧨](https://github.com/huggingface/diffusion-models-class)
Describe your model here
## Usage
```python
from diffusers import DDPMPipeline
pipeline = DDPMPipeline.from_pretrained('chenlong7616/ddpm-celebahq-finetuned-butterflies-2epochs')
image = pipeline().images[0]
image
```
|
Q-bert/Merged-AGI-7B | Q-bert | "2023-12-24T12:41:18Z" | 56 | 5 | transformers | [
"transformers",
"safetensors",
"mistral",
"text-generation",
"Math",
"merge",
"en",
"dataset:meta-math/MetaMathQA",
"base_model:Q-bert/MetaMath-Cybertron-Starling",
"base_model:merge:Q-bert/MetaMath-Cybertron-Starling",
"base_model:fblgit/juanako-7b-UNA",
"base_model:merge:fblgit/juanako-7b-UNA",
"license:cc-by-nc-4.0",
"autotrain_compatible",
"text-generation-inference",
"endpoints_compatible",
"region:us"
] | text-generation | "2023-12-10T09:20:47Z" | ---
license: cc-by-nc-4.0
datasets:
- meta-math/MetaMathQA
language:
- en
pipeline_tag: text-generation
tags:
- Math
- merge
base_model:
- Q-bert/MetaMath-Cybertron-Starling
- fblgit/juanako-7b-UNA
---
## Merged-AGI-7B
Merge [Q-bert/MetaMath-Cybertron-Starling](https://huggingface.co/Q-bert/MetaMath-Cybertron-Starling) and [fblgit/juanako-7b-UNA](https://huggingface.co/fblgit/juanako-7b-UNA) using slerp merge.
You can use ChatML format.
# [Open LLM Leaderboard Evaluation Results](https://huggingface.co/spaces/HuggingFaceH4/open_llm_leaderboard)
Detailed results can be found [Coming soon]()
| Metric | Value |
|-----------------------|---------------------------|
| Avg. | Coming soon |
| ARC (25-shot) | Coming soon |
| HellaSwag (10-shot) | Coming soon |
| MMLU (5-shot) | Coming soon |
| TruthfulQA (0-shot) | Coming soon |
| Winogrande (5-shot) | Coming soon |
| GSM8K (5-shot) | Coming soon | |
Arbi-Houssem/mms_tts_tun_Lang1.6 | Arbi-Houssem | "2024-06-16T04:44:31Z" | 106 | 0 | transformers | [
"transformers",
"safetensors",
"vits",
"text-to-audio",
"arxiv:1910.09700",
"endpoints_compatible",
"region:us"
] | text-to-audio | "2024-06-16T02:24:55Z" | ---
library_name: transformers
tags: []
---
# Model Card for Model ID
<!-- Provide a quick summary of what the model is/does. -->
## Model Details
### Model Description
<!-- Provide a longer summary of what this model is. -->
This is the model card of a 🤗 transformers model that has been pushed on the Hub. This model card has been automatically generated.
- **Developed by:** [More Information Needed]
- **Funded by [optional]:** [More Information Needed]
- **Shared by [optional]:** [More Information Needed]
- **Model type:** [More Information Needed]
- **Language(s) (NLP):** [More Information Needed]
- **License:** [More Information Needed]
- **Finetuned from model [optional]:** [More Information Needed]
### Model Sources [optional]
<!-- Provide the basic links for the model. -->
- **Repository:** [More Information Needed]
- **Paper [optional]:** [More Information Needed]
- **Demo [optional]:** [More Information Needed]
## Uses
<!-- Address questions around how the model is intended to be used, including the foreseeable users of the model and those affected by the model. -->
### Direct Use
<!-- This section is for the model use without fine-tuning or plugging into a larger ecosystem/app. -->
[More Information Needed]
### Downstream Use [optional]
<!-- This section is for the model use when fine-tuned for a task, or when plugged into a larger ecosystem/app -->
[More Information Needed]
### Out-of-Scope Use
<!-- This section addresses misuse, malicious use, and uses that the model will not work well for. -->
[More Information Needed]
## Bias, Risks, and Limitations
<!-- This section is meant to convey both technical and sociotechnical limitations. -->
[More Information Needed]
### Recommendations
<!-- This section is meant to convey recommendations with respect to the bias, risk, and technical limitations. -->
Users (both direct and downstream) should be made aware of the risks, biases and limitations of the model. More information needed for further recommendations.
## How to Get Started with the Model
Use the code below to get started with the model.
[More Information Needed]
## Training Details
### Training Data
<!-- This should link to a Dataset Card, perhaps with a short stub of information on what the training data is all about as well as documentation related to data pre-processing or additional filtering. -->
[More Information Needed]
### Training Procedure
<!-- This relates heavily to the Technical Specifications. Content here should link to that section when it is relevant to the training procedure. -->
#### Preprocessing [optional]
[More Information Needed]
#### Training Hyperparameters
- **Training regime:** [More Information Needed] <!--fp32, fp16 mixed precision, bf16 mixed precision, bf16 non-mixed precision, fp16 non-mixed precision, fp8 mixed precision -->
#### Speeds, Sizes, Times [optional]
<!-- This section provides information about throughput, start/end time, checkpoint size if relevant, etc. -->
[More Information Needed]
## Evaluation
<!-- This section describes the evaluation protocols and provides the results. -->
### Testing Data, Factors & Metrics
#### Testing Data
<!-- This should link to a Dataset Card if possible. -->
[More Information Needed]
#### Factors
<!-- These are the things the evaluation is disaggregating by, e.g., subpopulations or domains. -->
[More Information Needed]
#### Metrics
<!-- These are the evaluation metrics being used, ideally with a description of why. -->
[More Information Needed]
### Results
[More Information Needed]
#### Summary
## Model Examination [optional]
<!-- Relevant interpretability work for the model goes here -->
[More Information Needed]
## Environmental Impact
<!-- Total emissions (in grams of CO2eq) and additional considerations, such as electricity usage, go here. Edit the suggested text below accordingly -->
Carbon emissions can be estimated using the [Machine Learning Impact calculator](https://mlco2.github.io/impact#compute) presented in [Lacoste et al. (2019)](https://arxiv.org/abs/1910.09700).
- **Hardware Type:** [More Information Needed]
- **Hours used:** [More Information Needed]
- **Cloud Provider:** [More Information Needed]
- **Compute Region:** [More Information Needed]
- **Carbon Emitted:** [More Information Needed]
## Technical Specifications [optional]
### Model Architecture and Objective
[More Information Needed]
### Compute Infrastructure
[More Information Needed]
#### Hardware
[More Information Needed]
#### Software
[More Information Needed]
## Citation [optional]
<!-- If there is a paper or blog post introducing the model, the APA and Bibtex information for that should go in this section. -->
**BibTeX:**
[More Information Needed]
**APA:**
[More Information Needed]
## Glossary [optional]
<!-- If relevant, include terms and calculations in this section that can help readers understand the model or model card. -->
[More Information Needed]
## More Information [optional]
[More Information Needed]
## Model Card Authors [optional]
[More Information Needed]
## Model Card Contact
[More Information Needed] |
EllieS/Temp-L1-SFT-L2-KTO | EllieS | "2024-05-09T08:39:58Z" | 1 | 0 | peft | [
"peft",
"tensorboard",
"safetensors",
"mistral",
"alignment-handbook",
"trl",
"dpo",
"generated_from_trainer",
"dataset:EllieS/Temp-L2-DPO",
"base_model:alignment-handbook/zephyr-7b-sft-full",
"base_model:adapter:alignment-handbook/zephyr-7b-sft-full",
"license:apache-2.0",
"region:us"
] | null | "2024-05-09T06:17:42Z" | ---
license: apache-2.0
library_name: peft
tags:
- alignment-handbook
- trl
- dpo
- generated_from_trainer
base_model: alignment-handbook/zephyr-7b-sft-full
datasets:
- EllieS/Temp-L2-DPO
model-index:
- name: Temp-L1-SFT-L2-KTO
results: []
---
<!-- This model card has been generated automatically according to the information the Trainer had access to. You
should probably proofread and complete it, then remove this comment. -->
# Temp-L1-SFT-L2-KTO
This model is a fine-tuned version of [EllieS/TempReason-L1](https://huggingface.co/EllieS/TempReason-L1) on the EllieS/Temp-L2-DPO dataset.
It achieves the following results on the evaluation set:
- Loss: 0.2213
- Rewards/chosen: 0.2579
- Rewards/rejected: -6.0725
- Rewards/accuracies: 1.0
- Rewards/margins: 6.3304
- Logps/rejected: -652.1185
- Logps/chosen: -0.1197
- Logits/rejected: -2.6590
- Logits/chosen: -2.5711
## Model description
More information needed
## Intended uses & limitations
More information needed
## Training and evaluation data
More information needed
## Training procedure
### Training hyperparameters
The following hyperparameters were used during training:
- learning_rate: 5e-06
- train_batch_size: 2
- eval_batch_size: 2
- seed: 42
- distributed_type: multi-GPU
- gradient_accumulation_steps: 2
- total_train_batch_size: 4
- optimizer: Adam with betas=(0.9,0.999) and epsilon=1e-08
- lr_scheduler_type: cosine
- lr_scheduler_warmup_ratio: 0.1
- num_epochs: 1
### Training results
| Training Loss | Epoch | Step | Validation Loss | Rewards/chosen | Rewards/rejected | Rewards/accuracies | Rewards/margins | Logps/rejected | Logps/chosen | Logits/rejected | Logits/chosen |
|:-------------:|:------:|:----:|:---------------:|:--------------:|:----------------:|:------------------:|:---------------:|:--------------:|:------------:|:---------------:|:-------------:|
| 0.2255 | 0.2497 | 1000 | 0.2230 | 0.2551 | -5.4032 | 1.0 | 5.6583 | -585.1871 | -0.3988 | -2.6372 | -2.5514 |
| 0.2252 | 0.4994 | 2000 | 0.2215 | 0.2576 | -5.9860 | 1.0 | 6.2436 | -643.4705 | -0.1526 | -2.6560 | -2.5690 |
| 0.2264 | 0.7492 | 3000 | 0.2213 | 0.2579 | -6.0565 | 1.0 | 6.3144 | -650.5204 | -0.1267 | -2.6590 | -2.5715 |
| 0.2262 | 0.9989 | 4000 | 0.2213 | 0.2579 | -6.0725 | 1.0 | 6.3304 | -652.1185 | -0.1197 | -2.6590 | -2.5711 |
### Framework versions
- PEFT 0.7.1
- Transformers 4.40.2
- Pytorch 2.1.2+cu121
- Datasets 2.18.0
- Tokenizers 0.19.1 |
baebee/guanaco-testv2 | baebee | "2023-09-04T09:07:41Z" | 0 | 0 | peft | [
"peft",
"region:us"
] | null | "2023-09-04T09:07:37Z" | ---
library_name: peft
---
## Training procedure
The following `bitsandbytes` quantization config was used during training:
- quant_method: bitsandbytes
- load_in_8bit: False
- load_in_4bit: True
- llm_int8_threshold: 6.0
- llm_int8_skip_modules: None
- llm_int8_enable_fp32_cpu_offload: False
- llm_int8_has_fp16_weight: False
- bnb_4bit_quant_type: nf4
- bnb_4bit_use_double_quant: False
- bnb_4bit_compute_dtype: float16
### Framework versions
- PEFT 0.6.0.dev0
|
yunheur/xlm-roberta-base-finetuned-panx-de-fr | yunheur | "2025-03-24T04:04:45Z" | 0 | 0 | null | [
"pytorch",
"xlm-roberta",
"generated_from_trainer",
"base_model:FacebookAI/xlm-roberta-base",
"base_model:finetune:FacebookAI/xlm-roberta-base",
"license:mit",
"region:us"
] | null | "2025-03-23T06:34:35Z" | ---
license: mit
base_model: xlm-roberta-base
tags:
- generated_from_trainer
metrics:
- f1
model-index:
- name: xlm-roberta-base-finetuned-panx-de-fr
results: []
---
<!-- This model card has been generated automatically according to the information the Trainer had access to. You
should probably proofread and complete it, then remove this comment. -->
# xlm-roberta-base-finetuned-panx-de-fr
This model is a fine-tuned version of [xlm-roberta-base](https://huggingface.co/xlm-roberta-base) on the None dataset.
It achieves the following results on the evaluation set:
- Loss: 0.1635
- F1: 0.8626
## Model description
More information needed
## Intended uses & limitations
More information needed
## Training and evaluation data
More information needed
## Training procedure
### Training hyperparameters
The following hyperparameters were used during training:
- learning_rate: 5e-05
- train_batch_size: 24
- eval_batch_size: 24
- seed: 42
- optimizer: Adam with betas=(0.9,0.999) and epsilon=1e-08
- lr_scheduler_type: linear
- num_epochs: 3
### Training results
| Training Loss | Epoch | Step | Validation Loss | F1 |
|:-------------:|:-----:|:----:|:---------------:|:------:|
| 0.2864 | 1.0 | 715 | 0.1862 | 0.8193 |
| 0.1479 | 2.0 | 1430 | 0.1711 | 0.8448 |
| 0.0947 | 3.0 | 2145 | 0.1635 | 0.8626 |
### Framework versions
- Transformers 4.33.3
- Pytorch 2.6.0+cu118
- Datasets 3.4.1
- Tokenizers 0.13.3
|
krishna195/finetuned_PHI | krishna195 | "2025-03-18T13:51:13Z" | 0 | 0 | transformers | [
"transformers",
"unsloth",
"arxiv:1910.09700",
"endpoints_compatible",
"region:us"
] | null | "2025-03-18T13:51:12Z" | ---
library_name: transformers
tags:
- unsloth
---
# Model Card for Model ID
<!-- Provide a quick summary of what the model is/does. -->
## Model Details
### Model Description
<!-- Provide a longer summary of what this model is. -->
This is the model card of a 🤗 transformers model that has been pushed on the Hub. This model card has been automatically generated.
- **Developed by:** [More Information Needed]
- **Funded by [optional]:** [More Information Needed]
- **Shared by [optional]:** [More Information Needed]
- **Model type:** [More Information Needed]
- **Language(s) (NLP):** [More Information Needed]
- **License:** [More Information Needed]
- **Finetuned from model [optional]:** [More Information Needed]
### Model Sources [optional]
<!-- Provide the basic links for the model. -->
- **Repository:** [More Information Needed]
- **Paper [optional]:** [More Information Needed]
- **Demo [optional]:** [More Information Needed]
## Uses
<!-- Address questions around how the model is intended to be used, including the foreseeable users of the model and those affected by the model. -->
### Direct Use
<!-- This section is for the model use without fine-tuning or plugging into a larger ecosystem/app. -->
[More Information Needed]
### Downstream Use [optional]
<!-- This section is for the model use when fine-tuned for a task, or when plugged into a larger ecosystem/app -->
[More Information Needed]
### Out-of-Scope Use
<!-- This section addresses misuse, malicious use, and uses that the model will not work well for. -->
[More Information Needed]
## Bias, Risks, and Limitations
<!-- This section is meant to convey both technical and sociotechnical limitations. -->
[More Information Needed]
### Recommendations
<!-- This section is meant to convey recommendations with respect to the bias, risk, and technical limitations. -->
Users (both direct and downstream) should be made aware of the risks, biases and limitations of the model. More information needed for further recommendations.
## How to Get Started with the Model
Use the code below to get started with the model.
[More Information Needed]
## Training Details
### Training Data
<!-- This should link to a Dataset Card, perhaps with a short stub of information on what the training data is all about as well as documentation related to data pre-processing or additional filtering. -->
[More Information Needed]
### Training Procedure
<!-- This relates heavily to the Technical Specifications. Content here should link to that section when it is relevant to the training procedure. -->
#### Preprocessing [optional]
[More Information Needed]
#### Training Hyperparameters
- **Training regime:** [More Information Needed] <!--fp32, fp16 mixed precision, bf16 mixed precision, bf16 non-mixed precision, fp16 non-mixed precision, fp8 mixed precision -->
#### Speeds, Sizes, Times [optional]
<!-- This section provides information about throughput, start/end time, checkpoint size if relevant, etc. -->
[More Information Needed]
## Evaluation
<!-- This section describes the evaluation protocols and provides the results. -->
### Testing Data, Factors & Metrics
#### Testing Data
<!-- This should link to a Dataset Card if possible. -->
[More Information Needed]
#### Factors
<!-- These are the things the evaluation is disaggregating by, e.g., subpopulations or domains. -->
[More Information Needed]
#### Metrics
<!-- These are the evaluation metrics being used, ideally with a description of why. -->
[More Information Needed]
### Results
[More Information Needed]
#### Summary
## Model Examination [optional]
<!-- Relevant interpretability work for the model goes here -->
[More Information Needed]
## Environmental Impact
<!-- Total emissions (in grams of CO2eq) and additional considerations, such as electricity usage, go here. Edit the suggested text below accordingly -->
Carbon emissions can be estimated using the [Machine Learning Impact calculator](https://mlco2.github.io/impact#compute) presented in [Lacoste et al. (2019)](https://arxiv.org/abs/1910.09700).
- **Hardware Type:** [More Information Needed]
- **Hours used:** [More Information Needed]
- **Cloud Provider:** [More Information Needed]
- **Compute Region:** [More Information Needed]
- **Carbon Emitted:** [More Information Needed]
## Technical Specifications [optional]
### Model Architecture and Objective
[More Information Needed]
### Compute Infrastructure
[More Information Needed]
#### Hardware
[More Information Needed]
#### Software
[More Information Needed]
## Citation [optional]
<!-- If there is a paper or blog post introducing the model, the APA and Bibtex information for that should go in this section. -->
**BibTeX:**
[More Information Needed]
**APA:**
[More Information Needed]
## Glossary [optional]
<!-- If relevant, include terms and calculations in this section that can help readers understand the model or model card. -->
[More Information Needed]
## More Information [optional]
[More Information Needed]
## Model Card Authors [optional]
[More Information Needed]
## Model Card Contact
[More Information Needed] |
mradermacher/EXAONE-3.5-32B-LIMO-Ko-e4-GGUF | mradermacher | "2025-02-19T12:00:06Z" | 0 | 0 | transformers | [
"transformers",
"gguf",
"en",
"ko",
"dataset:GAIR/LIMO",
"dataset:junnei/ko-limo",
"dataset:exp-models/GAIR-LIMO-KOREAN",
"base_model:werty1248/EXAONE-3.5-32B-LIMO-Ko-e4",
"base_model:quantized:werty1248/EXAONE-3.5-32B-LIMO-Ko-e4",
"license:other",
"endpoints_compatible",
"region:us",
"conversational"
] | null | "2025-02-19T09:48:22Z" | ---
base_model: werty1248/EXAONE-3.5-32B-LIMO-Ko-e4
datasets:
- GAIR/LIMO
- junnei/ko-limo
- exp-models/GAIR-LIMO-KOREAN
language:
- en
- ko
library_name: transformers
license: other
license_link: LICENSE
license_name: exaone
quantized_by: mradermacher
---
## About
<!-- ### quantize_version: 2 -->
<!-- ### output_tensor_quantised: 1 -->
<!-- ### convert_type: hf -->
<!-- ### vocab_type: -->
<!-- ### tags: -->
static quants of https://huggingface.co/werty1248/EXAONE-3.5-32B-LIMO-Ko-e4
<!-- provided-files -->
weighted/imatrix quants seem not to be available (by me) at this time. If they do not show up a week or so after the static ones, I have probably not planned for them. Feel free to request them by opening a Community Discussion.
## Usage
If you are unsure how to use GGUF files, refer to one of [TheBloke's
READMEs](https://huggingface.co/TheBloke/KafkaLM-70B-German-V0.1-GGUF) for
more details, including on how to concatenate multi-part files.
## Provided Quants
(sorted by size, not necessarily quality. IQ-quants are often preferable over similar sized non-IQ quants)
| Link | Type | Size/GB | Notes |
|:-----|:-----|--------:|:------|
| [GGUF](https://huggingface.co/mradermacher/EXAONE-3.5-32B-LIMO-Ko-e4-GGUF/resolve/main/EXAONE-3.5-32B-LIMO-Ko-e4.Q2_K.gguf) | Q2_K | 12.0 | |
| [GGUF](https://huggingface.co/mradermacher/EXAONE-3.5-32B-LIMO-Ko-e4-GGUF/resolve/main/EXAONE-3.5-32B-LIMO-Ko-e4.Q3_K_S.gguf) | Q3_K_S | 14.1 | |
| [GGUF](https://huggingface.co/mradermacher/EXAONE-3.5-32B-LIMO-Ko-e4-GGUF/resolve/main/EXAONE-3.5-32B-LIMO-Ko-e4.Q3_K_M.gguf) | Q3_K_M | 15.6 | lower quality |
| [GGUF](https://huggingface.co/mradermacher/EXAONE-3.5-32B-LIMO-Ko-e4-GGUF/resolve/main/EXAONE-3.5-32B-LIMO-Ko-e4.Q3_K_L.gguf) | Q3_K_L | 16.9 | |
| [GGUF](https://huggingface.co/mradermacher/EXAONE-3.5-32B-LIMO-Ko-e4-GGUF/resolve/main/EXAONE-3.5-32B-LIMO-Ko-e4.IQ4_XS.gguf) | IQ4_XS | 17.5 | |
| [GGUF](https://huggingface.co/mradermacher/EXAONE-3.5-32B-LIMO-Ko-e4-GGUF/resolve/main/EXAONE-3.5-32B-LIMO-Ko-e4.Q4_K_S.gguf) | Q4_K_S | 18.4 | fast, recommended |
| [GGUF](https://huggingface.co/mradermacher/EXAONE-3.5-32B-LIMO-Ko-e4-GGUF/resolve/main/EXAONE-3.5-32B-LIMO-Ko-e4.Q4_K_M.gguf) | Q4_K_M | 19.4 | fast, recommended |
| [GGUF](https://huggingface.co/mradermacher/EXAONE-3.5-32B-LIMO-Ko-e4-GGUF/resolve/main/EXAONE-3.5-32B-LIMO-Ko-e4.Q5_K_S.gguf) | Q5_K_S | 22.2 | |
| [GGUF](https://huggingface.co/mradermacher/EXAONE-3.5-32B-LIMO-Ko-e4-GGUF/resolve/main/EXAONE-3.5-32B-LIMO-Ko-e4.Q5_K_M.gguf) | Q5_K_M | 22.8 | |
| [GGUF](https://huggingface.co/mradermacher/EXAONE-3.5-32B-LIMO-Ko-e4-GGUF/resolve/main/EXAONE-3.5-32B-LIMO-Ko-e4.Q6_K.gguf) | Q6_K | 26.4 | very good quality |
| [GGUF](https://huggingface.co/mradermacher/EXAONE-3.5-32B-LIMO-Ko-e4-GGUF/resolve/main/EXAONE-3.5-32B-LIMO-Ko-e4.Q8_0.gguf) | Q8_0 | 34.1 | fast, best quality |
Here is a handy graph by ikawrakow comparing some lower-quality quant
types (lower is better):

And here are Artefact2's thoughts on the matter:
https://gist.github.com/Artefact2/b5f810600771265fc1e39442288e8ec9
## FAQ / Model Request
See https://huggingface.co/mradermacher/model_requests for some answers to
questions you might have and/or if you want some other model quantized.
## Thanks
I thank my company, [nethype GmbH](https://www.nethype.de/), for letting
me use its servers and providing upgrades to my workstation to enable
this work in my free time.
<!-- end -->
|
lottienghiem/distilgpt2-finetuned-wikitext2 | lottienghiem | "2024-04-18T06:04:02Z" | 44 | 0 | transformers | [
"transformers",
"pytorch",
"tensorboard",
"safetensors",
"gpt2",
"text-generation",
"generated_from_trainer",
"base_model:distilbert/distilgpt2",
"base_model:finetune:distilbert/distilgpt2",
"license:apache-2.0",
"autotrain_compatible",
"text-generation-inference",
"endpoints_compatible",
"region:us"
] | text-generation | "2024-04-08T07:23:23Z" | ---
license: apache-2.0
base_model: distilgpt2
tags:
- generated_from_trainer
model-index:
- name: distilgpt2-finetuned-wikitext2
results: []
---
<!-- This model card has been generated automatically according to the information the Trainer had access to. You
should probably proofread and complete it, then remove this comment. -->
# distilgpt2-finetuned-wikitext2
This model is a fine-tuned version of [distilgpt2](https://huggingface.co/distilgpt2) on an unknown dataset.
It achieves the following results on the evaluation set:
- Loss: 2.5082
## Model description
More information needed
## Intended uses & limitations
More information needed
## Training and evaluation data
More information needed
## Training procedure
### Training hyperparameters
The following hyperparameters were used during training:
- learning_rate: 2e-05
- train_batch_size: 8
- eval_batch_size: 8
- seed: 42
- optimizer: Adam with betas=(0.9,0.999) and epsilon=1e-08
- lr_scheduler_type: linear
- num_epochs: 3.0
### Training results
| Training Loss | Epoch | Step | Validation Loss |
|:-------------:|:-----:|:-----:|:---------------:|
| 2.4699 | 1.0 | 19369 | 2.5496 |
| 2.3425 | 2.0 | 38738 | 2.5165 |
| 2.256 | 3.0 | 58107 | 2.5082 |
### Framework versions
- Transformers 4.39.3
- Pytorch 2.2.1+cu121
- Datasets 2.18.0
- Tokenizers 0.15.1
|
hyungtak/ko-Llama2-7B | hyungtak | "2023-08-24T12:38:45Z" | 2 | 0 | peft | [
"peft",
"region:us"
] | null | "2023-08-24T12:38:35Z" | ---
library_name: peft
---
## Training procedure
The following `bitsandbytes` quantization config was used during training:
- load_in_8bit: True
- load_in_4bit: False
- llm_int8_threshold: 6.0
- llm_int8_skip_modules: None
- llm_int8_enable_fp32_cpu_offload: False
- llm_int8_has_fp16_weight: False
- bnb_4bit_quant_type: fp4
- bnb_4bit_use_double_quant: False
- bnb_4bit_compute_dtype: float32
### Framework versions
- PEFT 0.6.0.dev0
|
eschorn/3_loa | eschorn | "2023-07-20T03:54:40Z" | 0 | 0 | null | [
"generated_from_trainer",
"dataset:billsum",
"base_model:google/flan-t5-large",
"base_model:finetune:google/flan-t5-large",
"license:apache-2.0",
"region:us"
] | null | "2023-07-19T20:46:54Z" | ---
license: apache-2.0
base_model: google/flan-t5-large
tags:
- generated_from_trainer
datasets:
- billsum
metrics:
- rouge
model-index:
- name: 3_loa
results: []
---
<!-- This model card has been generated automatically according to the information the Trainer had access to. You
should probably proofread and complete it, then remove this comment. -->
# 3_loa
This model is a fine-tuned version of [google/flan-t5-large](https://huggingface.co/google/flan-t5-large) on the billsum dataset.
It achieves the following results on the evaluation set:
- Loss: 1.4825
- Rouge1: 0.201
- Rouge2: 0.1132
- Rougel: 0.1753
- Rougelsum: 0.1755
- Gen Len: 19.0
## Model description
More information needed
## Intended uses & limitations
More information needed
## Training and evaluation data
More information needed
## Training procedure
### Training hyperparameters
The following hyperparameters were used during training:
- learning_rate: 2e-05
- train_batch_size: 1
- eval_batch_size: 1
- seed: 42
- optimizer: Adam with betas=(0.9,0.999) and epsilon=1e-08
- lr_scheduler_type: linear
- num_epochs: 20
### Training results
| Training Loss | Epoch | Step | Validation Loss | Rouge1 | Rouge2 | Rougel | Rougelsum | Gen Len |
|:-------------:|:-----:|:-----:|:---------------:|:------:|:------:|:------:|:---------:|:-------:|
| 2.1079 | 1.0 | 989 | 1.6673 | 0.2028 | 0.1092 | 0.1748 | 0.1751 | 19.0 |
| 1.8481 | 2.0 | 1978 | 1.6150 | 0.1979 | 0.1061 | 0.1715 | 0.1717 | 19.0 |
| 1.7889 | 3.0 | 2967 | 1.5833 | 0.1994 | 0.11 | 0.1727 | 0.1727 | 19.0 |
| 1.7319 | 4.0 | 3956 | 1.5584 | 0.1978 | 0.1084 | 0.1718 | 0.1718 | 19.0 |
| 1.7279 | 5.0 | 4945 | 1.5440 | 0.2016 | 0.1106 | 0.1755 | 0.1756 | 19.0 |
| 1.7386 | 6.0 | 5934 | 1.5326 | 0.1991 | 0.1086 | 0.1734 | 0.1736 | 19.0 |
| 1.6972 | 7.0 | 6923 | 1.5251 | 0.2013 | 0.1122 | 0.1759 | 0.176 | 19.0 |
| 1.6732 | 8.0 | 7912 | 1.5145 | 0.2024 | 0.1123 | 0.1766 | 0.1766 | 19.0 |
| 1.6597 | 9.0 | 8901 | 1.5079 | 0.2019 | 0.1125 | 0.1751 | 0.1753 | 19.0 |
| 1.6151 | 10.0 | 9890 | 1.5045 | 0.201 | 0.1123 | 0.1758 | 0.1761 | 19.0 |
| 1.6381 | 11.0 | 10879 | 1.4997 | 0.2009 | 0.1116 | 0.1755 | 0.1756 | 19.0 |
| 1.6148 | 12.0 | 11868 | 1.4974 | 0.2018 | 0.1133 | 0.1763 | 0.1765 | 19.0 |
| 1.6196 | 13.0 | 12857 | 1.4940 | 0.2014 | 0.1129 | 0.1756 | 0.1756 | 19.0 |
| 1.6137 | 14.0 | 13846 | 1.4914 | 0.2025 | 0.1136 | 0.1766 | 0.1768 | 19.0 |
| 1.6313 | 15.0 | 14835 | 1.4873 | 0.2032 | 0.114 | 0.1769 | 0.1771 | 19.0 |
| 1.6098 | 16.0 | 15824 | 1.4847 | 0.2012 | 0.1133 | 0.175 | 0.1754 | 19.0 |
| 1.6061 | 17.0 | 16813 | 1.4845 | 0.2019 | 0.1138 | 0.1752 | 0.1755 | 19.0 |
| 1.5918 | 18.0 | 17802 | 1.4833 | 0.2011 | 0.1129 | 0.1747 | 0.175 | 19.0 |
| 1.5842 | 19.0 | 18791 | 1.4824 | 0.2013 | 0.1133 | 0.1753 | 0.1755 | 19.0 |
| 1.5964 | 20.0 | 19780 | 1.4825 | 0.201 | 0.1132 | 0.1753 | 0.1755 | 19.0 |
### Framework versions
- Transformers 4.31.0
- Pytorch 1.13.1.post200
- Datasets 2.10.0
- Tokenizers 0.13.2
|
Lots-of-LoRAs/Mistral-7B-Instruct-v0.2-4b-r16-task1425 | Lots-of-LoRAs | "2024-07-03T20:10:38Z" | 0 | 0 | pytorch | [
"pytorch",
"safetensors",
"en",
"arxiv:1910.09700",
"arxiv:2407.00066",
"license:mit",
"region:us"
] | null | "2024-06-18T19:50:29Z" | ---
language: en
license: mit
library_name: pytorch
---
# Model Card for Mistral-7B-Instruct-v0.2-4b-r16-task1425
<!-- Provide a quick summary of what the model is/does. -->
## Model Details
### Model Description
<!-- Provide a longer summary of what this model is. -->
LoRA trained on task1425_country_iso_numeric
- **Developed by:** bruel
- **Funded by [optional]:** [More Information Needed]
- **Shared by [optional]:** [More Information Needed]
- **Model type:** LoRA
- **Language(s) (NLP):** en
- **License:** mit
- **Finetuned from model [optional]:** mistralai/Mistral-7B-Instruct-v0.2
### Model Sources [optional]
<!-- Provide the basic links for the model. -->
- **Repository:** https://github.com/bruel-gabrielsson
- **Paper [optional]:** "Compress then Serve: Serving Thousands of LoRA Adapters with Little Overhead" (2024), Rickard Brüel Gabrielsson, Jiacheng Zhu, Onkar Bhardwaj, Leshem Choshen, Kristjan Greenewald, Mikhail Yurochkin and Justin Solomon
- **Demo [optional]:** [More Information Needed]
## Uses
<!-- Address questions around how the model is intended to be used, including the foreseeable users of the model and those affected by the model. -->
### Direct Use
<!-- This section is for the model use without fine-tuning or plugging into a larger ecosystem/app. -->
[More Information Needed]
### Downstream Use [optional]
<!-- This section is for the model use when fine-tuned for a task, or when plugged into a larger ecosystem/app -->
[More Information Needed]
### Out-of-Scope Use
<!-- This section addresses misuse, malicious use, and uses that the model will not work well for. -->
[More Information Needed]
## Bias, Risks, and Limitations
<!-- This section is meant to convey both technical and sociotechnical limitations. -->
[More Information Needed]
### Recommendations
<!-- This section is meant to convey recommendations with respect to the bias, risk, and technical limitations. -->
Users (both direct and downstream) should be made aware of the risks, biases and limitations of the model. More information needed for further recommendations.
## How to Get Started with the Model
Use the code below to get started with the model.
[More Information Needed]
## Training Details
### Training Data
<!-- This should link to a Dataset Card, perhaps with a short stub of information on what the training data is all about as well as documentation related to data pre-processing or additional filtering. -->
https://huggingface.co/datasets/Lots-of-LoRAs/task1425_country_iso_numeric sourced from https://github.com/allenai/natural-instructions
### Training Procedure
<!-- This relates heavily to the Technical Specifications. Content here should link to that section when it is relevant to the training procedure. -->
#### Preprocessing [optional]
[More Information Needed]
#### Training Hyperparameters
- **Training regime:** [More Information Needed] <!--fp32, fp16 mixed precision, bf16 mixed precision, bf16 non-mixed precision, fp16 non-mixed precision, fp8 mixed precision -->
#### Speeds, Sizes, Times [optional]
<!-- This section provides information about throughput, start/end time, checkpoint size if relevant, etc. -->
[More Information Needed]
## Evaluation
<!-- This section describes the evaluation protocols and provides the results. -->
### Testing Data, Factors & Metrics
#### Testing Data
<!-- This should link to a Dataset Card if possible. -->
[More Information Needed]
#### Factors
<!-- These are the things the evaluation is disaggregating by, e.g., subpopulations or domains. -->
[More Information Needed]
#### Metrics
<!-- These are the evaluation metrics being used, ideally with a description of why. -->
[More Information Needed]
### Results
[More Information Needed]
#### Summary
## Model Examination [optional]
<!-- Relevant interpretability work for the model goes here -->
[More Information Needed]
## Environmental Impact
<!-- Total emissions (in grams of CO2eq) and additional considerations, such as electricity usage, go here. Edit the suggested text below accordingly -->
Carbon emissions can be estimated using the [Machine Learning Impact calculator](https://mlco2.github.io/impact#compute) presented in [Lacoste et al. (2019)](https://arxiv.org/abs/1910.09700).
- **Hardware Type:** [More Information Needed]
- **Hours used:** [More Information Needed]
- **Cloud Provider:** [More Information Needed]
- **Compute Region:** [More Information Needed]
- **Carbon Emitted:** [More Information Needed]
## Technical Specifications [optional]
### Model Architecture and Objective
[More Information Needed]
### Compute Infrastructure
[More Information Needed]
#### Hardware
[More Information Needed]
#### Software
[More Information Needed]
## Citation [optional]
<!-- If there is a paper or blog post introducing the model, the APA and Bibtex information for that should go in this section. -->
**BibTeX:**
@misc{brüelgabrielsson2024compressserveservingthousands,
title={Compress then Serve: Serving Thousands of LoRA Adapters with Little Overhead},
author={Rickard Brüel-Gabrielsson and Jiacheng Zhu and Onkar Bhardwaj and Leshem Choshen and Kristjan Greenewald and Mikhail Yurochkin and Justin Solomon},
year={2024},
eprint={2407.00066},
archivePrefix={arXiv},
primaryClass={cs.DC},
url={https://arxiv.org/abs/2407.00066},
}
**APA:**
[More Information Needed]
## Glossary [optional]
<!-- If relevant, include terms and calculations in this section that can help readers understand the model or model card. -->
[More Information Needed]
## More Information [optional]
[More Information Needed]
## Model Card Authors [optional]
[More Information Needed]
## Model Card Contact
[More Information Needed] |
CharlesLi/llama_2_cot_simplest_code_math_4_full | CharlesLi | "2025-01-20T12:17:57Z" | 7 | 0 | transformers | [
"transformers",
"tensorboard",
"safetensors",
"llama",
"text-generation",
"alignment-handbook",
"trl",
"sft",
"generated_from_trainer",
"conversational",
"dataset:generator",
"base_model:meta-llama/Llama-2-7b-chat-hf",
"base_model:finetune:meta-llama/Llama-2-7b-chat-hf",
"license:llama2",
"autotrain_compatible",
"text-generation-inference",
"endpoints_compatible",
"region:us"
] | text-generation | "2025-01-20T04:43:36Z" | ---
library_name: transformers
license: llama2
base_model: meta-llama/Llama-2-7b-chat-hf
tags:
- alignment-handbook
- trl
- sft
- generated_from_trainer
- trl
- sft
- alignment-handbook
- generated_from_trainer
datasets:
- generator
model-index:
- name: llama_2_cot_simplest_code_math_4_full
results: []
---
<!-- This model card has been generated automatically according to the information the Trainer had access to. You
should probably proofread and complete it, then remove this comment. -->
# llama_2_cot_simplest_code_math_4_full
This model is a fine-tuned version of [meta-llama/Llama-2-7b-chat-hf](https://huggingface.co/meta-llama/Llama-2-7b-chat-hf) on the generator dataset.
It achieves the following results on the evaluation set:
- Loss: 0.6062
## Model description
More information needed
## Intended uses & limitations
More information needed
## Training and evaluation data
More information needed
## Training procedure
### Training hyperparameters
The following hyperparameters were used during training:
- learning_rate: 2e-05
- train_batch_size: 4
- eval_batch_size: 4
- seed: 42
- distributed_type: multi-GPU
- num_devices: 4
- gradient_accumulation_steps: 2
- total_train_batch_size: 32
- total_eval_batch_size: 16
- optimizer: Adam with betas=(0.9,0.999) and epsilon=1e-08
- lr_scheduler_type: cosine
- lr_scheduler_warmup_ratio: 0.1
- num_epochs: 1
### Training results
### Framework versions
- Transformers 4.44.2
- Pytorch 2.4.1+cu121
- Datasets 3.0.0
- Tokenizers 0.19.1
|
leabum/distilbert-base-uncased-finetuned-squad | leabum | "2022-08-11T06:25:42Z" | 61 | 0 | transformers | [
"transformers",
"tf",
"tensorboard",
"distilbert",
"question-answering",
"generated_from_keras_callback",
"license:apache-2.0",
"endpoints_compatible",
"region:us"
] | question-answering | "2022-08-02T13:48:08Z" | ---
license: apache-2.0
tags:
- generated_from_keras_callback
model-index:
- name: leabum/distilbert-base-uncased-finetuned-squad
results: []
---
<!-- This model card has been generated automatically according to the information Keras had access to. You should
probably proofread and complete it, then remove this comment. -->
# leabum/distilbert-base-uncased-finetuned-squad
This model is a fine-tuned version of [distilbert-base-uncased](https://huggingface.co/distilbert-base-uncased) on an unknown dataset.
It achieves the following results on the evaluation set:
- Train Loss: 5.5824
- Train End Logits Accuracy: 0.0347
- Train Start Logits Accuracy: 0.0694
- Validation Loss: 5.8343
- Validation End Logits Accuracy: 0.0
- Validation Start Logits Accuracy: 0.0
- Epoch: 1
## Model description
More information needed
## Intended uses & limitations
More information needed
## Training and evaluation data
More information needed
## Training procedure
### Training hyperparameters
The following hyperparameters were used during training:
- optimizer: {'name': 'Adam', 'learning_rate': {'class_name': 'PolynomialDecay', 'config': {'initial_learning_rate': 2e-05, 'decay_steps': 18, 'end_learning_rate': 0.0, 'power': 1.0, 'cycle': False, 'name': None}}, 'decay': 0.0, 'beta_1': 0.9, 'beta_2': 0.999, 'epsilon': 1e-08, 'amsgrad': False}
- training_precision: float32
### Training results
| Train Loss | Train End Logits Accuracy | Train Start Logits Accuracy | Validation Loss | Validation End Logits Accuracy | Validation Start Logits Accuracy | Epoch |
|:----------:|:-------------------------:|:---------------------------:|:---------------:|:------------------------------:|:--------------------------------:|:-----:|
| 5.8427 | 0.0069 | 0.0069 | 5.8688 | 0.0 | 0.0 | 0 |
| 5.5824 | 0.0347 | 0.0694 | 5.8343 | 0.0 | 0.0 | 1 |
### Framework versions
- Transformers 4.21.1
- TensorFlow 2.8.2
- Datasets 2.4.0
- Tokenizers 0.12.1
|
mradermacher/Qwen1.5-32B-i1-GGUF | mradermacher | "2025-03-31T21:28:13Z" | 136 | 0 | transformers | [
"transformers",
"gguf",
"pretrained",
"en",
"base_model:Qwen/Qwen1.5-32B",
"base_model:quantized:Qwen/Qwen1.5-32B",
"license:other",
"endpoints_compatible",
"region:us",
"imatrix",
"conversational"
] | null | "2024-05-12T13:43:50Z" | ---
base_model: Qwen/Qwen1.5-32B
language:
- en
library_name: transformers
license: other
license_link: https://huggingface.co/Qwen/Qwen1.5-32B/blob/main/LICENSE
license_name: tongyi-qianwen-research
quantized_by: mradermacher
tags:
- pretrained
---
## About
<!-- ### quantize_version: 2 -->
<!-- ### output_tensor_quantised: 1 -->
<!-- ### convert_type: hf -->
<!-- ### vocab_type: -->
weighted/imatrix quants of https://huggingface.co/Qwen/Qwen1.5-32B
<!-- provided-files -->
static quants are available at https://huggingface.co/mradermacher/Qwen1.5-32B-GGUF
## Usage
If you are unsure how to use GGUF files, refer to one of [TheBloke's
READMEs](https://huggingface.co/TheBloke/KafkaLM-70B-German-V0.1-GGUF) for
more details, including on how to concatenate multi-part files.
## Provided Quants
(sorted by size, not necessarily quality. IQ-quants are often preferable over similar sized non-IQ quants)
| Link | Type | Size/GB | Notes |
|:-----|:-----|--------:|:------|
| [GGUF](https://huggingface.co/mradermacher/Qwen1.5-32B-i1-GGUF/resolve/main/Qwen1.5-32B.i1-IQ1_S.gguf) | i1-IQ1_S | 7.3 | for the desperate |
| [GGUF](https://huggingface.co/mradermacher/Qwen1.5-32B-i1-GGUF/resolve/main/Qwen1.5-32B.i1-IQ1_M.gguf) | i1-IQ1_M | 8.0 | mostly desperate |
| [GGUF](https://huggingface.co/mradermacher/Qwen1.5-32B-i1-GGUF/resolve/main/Qwen1.5-32B.i1-IQ2_XXS.gguf) | i1-IQ2_XXS | 9.1 | |
| [GGUF](https://huggingface.co/mradermacher/Qwen1.5-32B-i1-GGUF/resolve/main/Qwen1.5-32B.i1-IQ2_XS.gguf) | i1-IQ2_XS | 10.0 | |
| [GGUF](https://huggingface.co/mradermacher/Qwen1.5-32B-i1-GGUF/resolve/main/Qwen1.5-32B.i1-IQ2_S.gguf) | i1-IQ2_S | 10.4 | |
| [GGUF](https://huggingface.co/mradermacher/Qwen1.5-32B-i1-GGUF/resolve/main/Qwen1.5-32B.i1-IQ2_M.gguf) | i1-IQ2_M | 11.3 | |
| [GGUF](https://huggingface.co/mradermacher/Qwen1.5-32B-i1-GGUF/resolve/main/Qwen1.5-32B.i1-Q2_K.gguf) | i1-Q2_K | 12.3 | IQ3_XXS probably better |
| [GGUF](https://huggingface.co/mradermacher/Qwen1.5-32B-i1-GGUF/resolve/main/Qwen1.5-32B.i1-IQ3_XXS.gguf) | i1-IQ3_XXS | 12.8 | lower quality |
| [GGUF](https://huggingface.co/mradermacher/Qwen1.5-32B-i1-GGUF/resolve/main/Qwen1.5-32B.i1-IQ3_XS.gguf) | i1-IQ3_XS | 13.7 | |
| [GGUF](https://huggingface.co/mradermacher/Qwen1.5-32B-i1-GGUF/resolve/main/Qwen1.5-32B.i1-Q3_K_S.gguf) | i1-Q3_K_S | 14.4 | IQ3_XS probably better |
| [GGUF](https://huggingface.co/mradermacher/Qwen1.5-32B-i1-GGUF/resolve/main/Qwen1.5-32B.i1-IQ3_S.gguf) | i1-IQ3_S | 14.4 | beats Q3_K* |
| [GGUF](https://huggingface.co/mradermacher/Qwen1.5-32B-i1-GGUF/resolve/main/Qwen1.5-32B.i1-IQ3_M.gguf) | i1-IQ3_M | 14.8 | |
| [GGUF](https://huggingface.co/mradermacher/Qwen1.5-32B-i1-GGUF/resolve/main/Qwen1.5-32B.i1-Q3_K_M.gguf) | i1-Q3_K_M | 15.9 | IQ3_S probably better |
| [GGUF](https://huggingface.co/mradermacher/Qwen1.5-32B-i1-GGUF/resolve/main/Qwen1.5-32B.i1-Q3_K_L.gguf) | i1-Q3_K_L | 17.2 | IQ3_M probably better |
| [GGUF](https://huggingface.co/mradermacher/Qwen1.5-32B-i1-GGUF/resolve/main/Qwen1.5-32B.i1-IQ4_XS.gguf) | i1-IQ4_XS | 17.7 | |
| [GGUF](https://huggingface.co/mradermacher/Qwen1.5-32B-i1-GGUF/resolve/main/Qwen1.5-32B.i1-Q4_0.gguf) | i1-Q4_0 | 18.7 | fast, low quality |
| [GGUF](https://huggingface.co/mradermacher/Qwen1.5-32B-i1-GGUF/resolve/main/Qwen1.5-32B.i1-Q4_K_S.gguf) | i1-Q4_K_S | 18.7 | optimal size/speed/quality |
| [GGUF](https://huggingface.co/mradermacher/Qwen1.5-32B-i1-GGUF/resolve/main/Qwen1.5-32B.i1-Q4_K_M.gguf) | i1-Q4_K_M | 19.8 | fast, recommended |
| [GGUF](https://huggingface.co/mradermacher/Qwen1.5-32B-i1-GGUF/resolve/main/Qwen1.5-32B.i1-Q5_K_S.gguf) | i1-Q5_K_S | 22.6 | |
| [GGUF](https://huggingface.co/mradermacher/Qwen1.5-32B-i1-GGUF/resolve/main/Qwen1.5-32B.i1-Q5_K_M.gguf) | i1-Q5_K_M | 23.2 | |
| [GGUF](https://huggingface.co/mradermacher/Qwen1.5-32B-i1-GGUF/resolve/main/Qwen1.5-32B.i1-Q6_K.gguf) | i1-Q6_K | 26.8 | practically like static Q6_K |
Here is a handy graph by ikawrakow comparing some lower-quality quant
types (lower is better):

And here are Artefact2's thoughts on the matter:
https://gist.github.com/Artefact2/b5f810600771265fc1e39442288e8ec9
## FAQ / Model Request
See https://huggingface.co/mradermacher/model_requests for some answers to
questions you might have and/or if you want some other model quantized.
## Thanks
I thank my company, [nethype GmbH](https://www.nethype.de/), for letting
me use its servers and providing upgrades to my workstation to enable
this work in my free time.
<!-- end -->
|
ragefu/ftxclip20240925model | ragefu | "2024-09-26T04:23:34Z" | 91 | 0 | transformers | [
"transformers",
"safetensors",
"xclip",
"feature-extraction",
"arxiv:1910.09700",
"endpoints_compatible",
"region:us"
] | feature-extraction | "2024-09-26T04:23:00Z" | ---
library_name: transformers
tags: []
---
# Model Card for Model ID
<!-- Provide a quick summary of what the model is/does. -->
## Model Details
### Model Description
<!-- Provide a longer summary of what this model is. -->
This is the model card of a 🤗 transformers model that has been pushed on the Hub. This model card has been automatically generated.
- **Developed by:** [More Information Needed]
- **Funded by [optional]:** [More Information Needed]
- **Shared by [optional]:** [More Information Needed]
- **Model type:** [More Information Needed]
- **Language(s) (NLP):** [More Information Needed]
- **License:** [More Information Needed]
- **Finetuned from model [optional]:** [More Information Needed]
### Model Sources [optional]
<!-- Provide the basic links for the model. -->
- **Repository:** [More Information Needed]
- **Paper [optional]:** [More Information Needed]
- **Demo [optional]:** [More Information Needed]
## Uses
<!-- Address questions around how the model is intended to be used, including the foreseeable users of the model and those affected by the model. -->
### Direct Use
<!-- This section is for the model use without fine-tuning or plugging into a larger ecosystem/app. -->
[More Information Needed]
### Downstream Use [optional]
<!-- This section is for the model use when fine-tuned for a task, or when plugged into a larger ecosystem/app -->
[More Information Needed]
### Out-of-Scope Use
<!-- This section addresses misuse, malicious use, and uses that the model will not work well for. -->
[More Information Needed]
## Bias, Risks, and Limitations
<!-- This section is meant to convey both technical and sociotechnical limitations. -->
[More Information Needed]
### Recommendations
<!-- This section is meant to convey recommendations with respect to the bias, risk, and technical limitations. -->
Users (both direct and downstream) should be made aware of the risks, biases and limitations of the model. More information needed for further recommendations.
## How to Get Started with the Model
Use the code below to get started with the model.
[More Information Needed]
## Training Details
### Training Data
<!-- This should link to a Dataset Card, perhaps with a short stub of information on what the training data is all about as well as documentation related to data pre-processing or additional filtering. -->
[More Information Needed]
### Training Procedure
<!-- This relates heavily to the Technical Specifications. Content here should link to that section when it is relevant to the training procedure. -->
#### Preprocessing [optional]
[More Information Needed]
#### Training Hyperparameters
- **Training regime:** [More Information Needed] <!--fp32, fp16 mixed precision, bf16 mixed precision, bf16 non-mixed precision, fp16 non-mixed precision, fp8 mixed precision -->
#### Speeds, Sizes, Times [optional]
<!-- This section provides information about throughput, start/end time, checkpoint size if relevant, etc. -->
[More Information Needed]
## Evaluation
<!-- This section describes the evaluation protocols and provides the results. -->
### Testing Data, Factors & Metrics
#### Testing Data
<!-- This should link to a Dataset Card if possible. -->
[More Information Needed]
#### Factors
<!-- These are the things the evaluation is disaggregating by, e.g., subpopulations or domains. -->
[More Information Needed]
#### Metrics
<!-- These are the evaluation metrics being used, ideally with a description of why. -->
[More Information Needed]
### Results
[More Information Needed]
#### Summary
## Model Examination [optional]
<!-- Relevant interpretability work for the model goes here -->
[More Information Needed]
## Environmental Impact
<!-- Total emissions (in grams of CO2eq) and additional considerations, such as electricity usage, go here. Edit the suggested text below accordingly -->
Carbon emissions can be estimated using the [Machine Learning Impact calculator](https://mlco2.github.io/impact#compute) presented in [Lacoste et al. (2019)](https://arxiv.org/abs/1910.09700).
- **Hardware Type:** [More Information Needed]
- **Hours used:** [More Information Needed]
- **Cloud Provider:** [More Information Needed]
- **Compute Region:** [More Information Needed]
- **Carbon Emitted:** [More Information Needed]
## Technical Specifications [optional]
### Model Architecture and Objective
[More Information Needed]
### Compute Infrastructure
[More Information Needed]
#### Hardware
[More Information Needed]
#### Software
[More Information Needed]
## Citation [optional]
<!-- If there is a paper or blog post introducing the model, the APA and Bibtex information for that should go in this section. -->
**BibTeX:**
[More Information Needed]
**APA:**
[More Information Needed]
## Glossary [optional]
<!-- If relevant, include terms and calculations in this section that can help readers understand the model or model card. -->
[More Information Needed]
## More Information [optional]
[More Information Needed]
## Model Card Authors [optional]
[More Information Needed]
## Model Card Contact
[More Information Needed] |
Dhanang/topic_model | Dhanang | "2023-12-14T08:08:11Z" | 8 | 0 | transformers | [
"transformers",
"tensorboard",
"safetensors",
"bert",
"text-classification",
"generated_from_trainer",
"base_model:indobenchmark/indobert-base-p2",
"base_model:finetune:indobenchmark/indobert-base-p2",
"license:mit",
"autotrain_compatible",
"endpoints_compatible",
"region:us"
] | text-classification | "2023-12-14T07:54:44Z" | ---
license: mit
base_model: indobenchmark/indobert-base-p2
tags:
- generated_from_trainer
metrics:
- accuracy
model-index:
- name: topic_model
results: []
---
<!-- This model card has been generated automatically according to the information the Trainer had access to. You
should probably proofread and complete it, then remove this comment. -->
# topic_model
This model is a fine-tuned version of [indobenchmark/indobert-base-p2](https://huggingface.co/indobenchmark/indobert-base-p2) on an unknown dataset.
It achieves the following results on the evaluation set:
- Loss: 0.0145
- Accuracy: 0.9984
## Model description
More information needed
## Intended uses & limitations
More information needed
## Training and evaluation data
More information needed
## Training procedure
### Training hyperparameters
The following hyperparameters were used during training:
- learning_rate: 2e-05
- train_batch_size: 16
- eval_batch_size: 16
- seed: 42
- optimizer: Adam with betas=(0.9,0.999) and epsilon=1e-08
- lr_scheduler_type: linear
- num_epochs: 10
### Training results
| Training Loss | Epoch | Step | Validation Loss | Accuracy |
|:-------------:|:-----:|:----:|:---------------:|:--------:|
| No log | 1.0 | 308 | 0.0315 | 0.9919 |
| 0.1039 | 2.0 | 616 | 0.0117 | 0.9984 |
| 0.1039 | 3.0 | 924 | 0.0147 | 0.9984 |
| 0.0047 | 4.0 | 1232 | 0.0223 | 0.9968 |
| 0.0002 | 5.0 | 1540 | 0.0138 | 0.9984 |
| 0.0002 | 6.0 | 1848 | 0.0140 | 0.9984 |
| 0.0001 | 7.0 | 2156 | 0.0142 | 0.9984 |
| 0.0001 | 8.0 | 2464 | 0.0144 | 0.9984 |
| 0.0001 | 9.0 | 2772 | 0.0145 | 0.9984 |
| 0.0001 | 10.0 | 3080 | 0.0145 | 0.9984 |
### Framework versions
- Transformers 4.35.2
- Pytorch 2.1.0+cu118
- Datasets 2.15.0
- Tokenizers 0.15.0
|
guydebruyn/q-FrozenLake-v1-4x4-noSlippery | guydebruyn | "2023-09-12T19:46:56Z" | 0 | 0 | null | [
"FrozenLake-v1-4x4-no_slippery",
"q-learning",
"reinforcement-learning",
"custom-implementation",
"model-index",
"region:us"
] | reinforcement-learning | "2023-09-12T19:46:53Z" | ---
tags:
- FrozenLake-v1-4x4-no_slippery
- q-learning
- reinforcement-learning
- custom-implementation
model-index:
- name: q-FrozenLake-v1-4x4-noSlippery
results:
- task:
type: reinforcement-learning
name: reinforcement-learning
dataset:
name: FrozenLake-v1-4x4-no_slippery
type: FrozenLake-v1-4x4-no_slippery
metrics:
- type: mean_reward
value: 1.00 +/- 0.00
name: mean_reward
verified: false
---
# **Q-Learning** Agent playing1 **FrozenLake-v1**
This is a trained model of a **Q-Learning** agent playing **FrozenLake-v1** .
## Usage
```python
model = load_from_hub(repo_id="guydebruyn/q-FrozenLake-v1-4x4-noSlippery", filename="q-learning.pkl")
# Don't forget to check if you need to add additional attributes (is_slippery=False etc)
env = gym.make(model["env_id"])
```
|
auro736/deberta-v3-large-tweet-fid-EMD | auro736 | "2024-01-14T10:38:20Z" | 67 | 0 | transformers | [
"transformers",
"pytorch",
"deberta-v2",
"token-classification",
"en",
"arxiv:2205.10726",
"license:mit",
"endpoints_compatible",
"region:us"
] | token-classification | "2023-10-30T16:00:37Z" | ---
license: mit
language:
- en
pipeline_tag: token-classification
---
## DeBERTa-large-tweet-fid-EMD
This is a [DeBERTa-large](https://huggingface.co/microsoft/deberta-v3-large) model trained on the [Tweet-FID](https://arxiv.org/abs/2205.10726) dataset (*"TWEET-FID: An Annotated Dataset for Multiple Foodborne Illness Detection Tasks", Ruofan Hu et al, 2022* ) which is a collection of Twitter to detect incidents of foodborne illnesses.
The model is enriched with a multi class classification head to perform the custom task called Entity Mention Detection (EMD).
The objective is to determine predefined entities (*food*, *location*, *symptom*, *other*) in a given text related to a food risk |
houbw/llama3_ruozhiba_ori_8_up_4 | houbw | "2024-05-23T02:17:37Z" | 0 | 0 | transformers | [
"transformers",
"safetensors",
"text-generation-inference",
"unsloth",
"llama",
"trl",
"en",
"base_model:unsloth/llama-3-8b-Instruct",
"base_model:finetune:unsloth/llama-3-8b-Instruct",
"license:apache-2.0",
"endpoints_compatible",
"region:us"
] | null | "2024-05-23T02:17:08Z" | ---
language:
- en
license: apache-2.0
tags:
- text-generation-inference
- transformers
- unsloth
- llama
- trl
base_model: unsloth/llama-3-8b-Instruct
---
# Uploaded model
- **Developed by:** houbw
- **License:** apache-2.0
- **Finetuned from model :** unsloth/llama-3-8b-Instruct
This llama model was trained 2x faster with [Unsloth](https://github.com/unslothai/unsloth) and Huggingface's TRL library.
[<img src="https://raw.githubusercontent.com/unslothai/unsloth/main/images/unsloth%20made%20with%20love.png" width="200"/>](https://github.com/unslothai/unsloth)
|
huudan123/stage1 | huudan123 | "2024-07-13T18:16:33Z" | 6 | 0 | sentence-transformers | [
"sentence-transformers",
"safetensors",
"roberta",
"sentence-similarity",
"feature-extraction",
"generated_from_trainer",
"dataset_size:102178",
"loss:TripletLoss",
"arxiv:1908.10084",
"arxiv:1703.07737",
"base_model:vinai/phobert-base-v2",
"base_model:finetune:vinai/phobert-base-v2",
"model-index",
"autotrain_compatible",
"endpoints_compatible",
"region:us"
] | sentence-similarity | "2024-07-13T18:15:57Z" | ---
base_model: vinai/phobert-base-v2
datasets: []
language: []
library_name: sentence-transformers
metrics:
- pearson_cosine
- spearman_cosine
- pearson_manhattan
- spearman_manhattan
- pearson_euclidean
- spearman_euclidean
- pearson_dot
- spearman_dot
- pearson_max
- spearman_max
pipeline_tag: sentence-similarity
tags:
- sentence-transformers
- sentence-similarity
- feature-extraction
- generated_from_trainer
- dataset_size:102178
- loss:TripletLoss
widget:
- source_sentence: Bàn cho thấy các thiết_kế và sản_xuất kiến_thức cần_thiết để thực_hiện
nhiều quyết_định thông_báo hơn .
sentences:
- Nixon quyết_định rằng hồ chí minh có_thể ở lại miền nam Việt_Nam .
- Không có gì cần_thiết để đưa ra một quyết_định thông_tin .
- Bảng Hiển_thị thiết_kế và sản_xuất thông_tin cần_thiết để đưa ra quyết_định .
- source_sentence: 95 gói nước_tiểu miễn_phí trong túi của họ .
sentences:
- Tây_ban nha trượt từ vị_trí quyền_lực của họ .
- Đội đã bước vào phòng thí_nghiệm mang theo tổng_cộng 99 đơn_vị trong_sạch , thử_nghiệm
thân_thiện .
- Túi được yêu_cầu cho nhà toàn_bộ 95 đơn_vị phục_vụ trong_sạch nước_tiểu giữa các
nhà cung_cấp các sản_phẩm .
- source_sentence: Tuyển một chiếc xe rất đắt tiền , và những gì có để xem_thường
là gần những con đường chính .
sentences:
- Thuê một chiếc xe rất rẻ nhưng có_thể không đáng_giá_như những cảnh_sát ở xa con
đường .
- Có một nhà_thờ hình_tròn ở orangerie ở Paris .
- Thuê một chiếc xe đến với chi_phí lớn và hầu_hết các điểm đến đều gần đường .
- source_sentence: Người da đen là 12 phần_trăm dân_số .
sentences:
- Người da đen tạo ra 50 % tổng_số dân_số .
- Người Mỹ Châu_Phi là một nhóm_thiểu_số .
- Tôi đoán là barney fife .
- source_sentence: Báo đen đã editorialized chống lại những cuộc viếng_thăm của farrakhan
với các nhà độc_tài châu phi .
sentences:
- Báo đen đã viết về quá_khứ của farrakhan .
- Khi bạn đi đến radda , bạn nên kiểm_tra piccolo bảo del chianti .
- Báo đen từ_chối yểm_trợ cho farrakhan .
model-index:
- name: SentenceTransformer based on vinai/phobert-base-v2
results:
- task:
type: semantic-similarity
name: Semantic Similarity
dataset:
name: sts dev
type: sts-dev
metrics:
- type: pearson_cosine
value: 0.42030854811305457
name: Pearson Cosine
- type: spearman_cosine
value: 0.5147968030818376
name: Spearman Cosine
- type: pearson_manhattan
value: 0.5605026901702432
name: Pearson Manhattan
- type: spearman_manhattan
value: 0.5792048311109484
name: Spearman Manhattan
- type: pearson_euclidean
value: 0.4710386131519505
name: Pearson Euclidean
- type: spearman_euclidean
value: 0.5087153254455983
name: Spearman Euclidean
- type: pearson_dot
value: 0.3923969498466928
name: Pearson Dot
- type: spearman_dot
value: 0.4338097270757405
name: Spearman Dot
- type: pearson_max
value: 0.5605026901702432
name: Pearson Max
- type: spearman_max
value: 0.5792048311109484
name: Spearman Max
---
# SentenceTransformer based on vinai/phobert-base-v2
This is a [sentence-transformers](https://www.SBERT.net) model finetuned from [vinai/phobert-base-v2](https://huggingface.co/vinai/phobert-base-v2). It maps sentences & paragraphs to a 768-dimensional dense vector space and can be used for semantic textual similarity, semantic search, paraphrase mining, text classification, clustering, and more.
## Model Details
### Model Description
- **Model Type:** Sentence Transformer
- **Base model:** [vinai/phobert-base-v2](https://huggingface.co/vinai/phobert-base-v2) <!-- at revision 2b51e367d92093c9688112098510e6a58bab67cd -->
- **Maximum Sequence Length:** 512 tokens
- **Output Dimensionality:** 768 tokens
- **Similarity Function:** Cosine Similarity
<!-- - **Training Dataset:** Unknown -->
<!-- - **Language:** Unknown -->
<!-- - **License:** Unknown -->
### Model Sources
- **Documentation:** [Sentence Transformers Documentation](https://sbert.net)
- **Repository:** [Sentence Transformers on GitHub](https://github.com/UKPLab/sentence-transformers)
- **Hugging Face:** [Sentence Transformers on Hugging Face](https://huggingface.co/models?library=sentence-transformers)
### Full Model Architecture
```
SentenceTransformer(
(0): Transformer({'max_seq_length': 512, 'do_lower_case': False}) with Transformer model: RobertaModel
(1): Pooling({'word_embedding_dimension': 768, 'pooling_mode_cls_token': False, 'pooling_mode_mean_tokens': True, 'pooling_mode_max_tokens': False, 'pooling_mode_mean_sqrt_len_tokens': False, 'pooling_mode_weightedmean_tokens': False, 'pooling_mode_lasttoken': False, 'include_prompt': True})
)
```
## Usage
### Direct Usage (Sentence Transformers)
First install the Sentence Transformers library:
```bash
pip install -U sentence-transformers
```
Then you can load this model and run inference.
```python
from sentence_transformers import SentenceTransformer
# Download from the 🤗 Hub
model = SentenceTransformer("huudan123/stage1")
# Run inference
sentences = [
'Báo đen đã editorialized chống lại những cuộc viếng_thăm của farrakhan với các nhà độc_tài châu phi .',
'Báo đen đã viết về quá_khứ của farrakhan .',
'Báo đen từ_chối yểm_trợ cho farrakhan .',
]
embeddings = model.encode(sentences)
print(embeddings.shape)
# [3, 768]
# Get the similarity scores for the embeddings
similarities = model.similarity(embeddings, embeddings)
print(similarities.shape)
# [3, 3]
```
<!--
### Direct Usage (Transformers)
<details><summary>Click to see the direct usage in Transformers</summary>
</details>
-->
<!--
### Downstream Usage (Sentence Transformers)
You can finetune this model on your own dataset.
<details><summary>Click to expand</summary>
</details>
-->
<!--
### Out-of-Scope Use
*List how the model may foreseeably be misused and address what users ought not to do with the model.*
-->
## Evaluation
### Metrics
#### Semantic Similarity
* Dataset: `sts-dev`
* Evaluated with [<code>EmbeddingSimilarityEvaluator</code>](https://sbert.net/docs/package_reference/sentence_transformer/evaluation.html#sentence_transformers.evaluation.EmbeddingSimilarityEvaluator)
| Metric | Value |
|:--------------------|:-----------|
| pearson_cosine | 0.4203 |
| **spearman_cosine** | **0.5148** |
| pearson_manhattan | 0.5605 |
| spearman_manhattan | 0.5792 |
| pearson_euclidean | 0.471 |
| spearman_euclidean | 0.5087 |
| pearson_dot | 0.3924 |
| spearman_dot | 0.4338 |
| pearson_max | 0.5605 |
| spearman_max | 0.5792 |
<!--
## Bias, Risks and Limitations
*What are the known or foreseeable issues stemming from this model? You could also flag here known failure cases or weaknesses of the model.*
-->
<!--
### Recommendations
*What are recommendations with respect to the foreseeable issues? For example, filtering explicit content.*
-->
## Training Details
### Training Dataset
#### Unnamed Dataset
* Size: 102,178 training samples
* Columns: <code>anchor</code>, <code>positive</code>, and <code>negative</code>
* Approximate statistics based on the first 1000 samples:
| | anchor | positive | negative |
|:--------|:-----------------------------------------------------------------------------------|:----------------------------------------------------------------------------------|:----------------------------------------------------------------------------------|
| type | string | string | string |
| details | <ul><li>min: 4 tokens</li><li>mean: 27.28 tokens</li><li>max: 147 tokens</li></ul> | <ul><li>min: 4 tokens</li><li>mean: 14.99 tokens</li><li>max: 44 tokens</li></ul> | <ul><li>min: 4 tokens</li><li>mean: 14.34 tokens</li><li>max: 34 tokens</li></ul> |
* Samples:
| anchor | positive | negative |
|:--------------------------------------------------------------------------------------------------------------------------------------------------------------|:-------------------------------------------------------------------------------------------------------------------------------------------------------------------------|:----------------------------------------------------------------------------------------------------------------------------------------------------------|
| <code>Tem đầy màu_sắc của madeira , cũng như tiền xu , ghi_chép ngân_hàng , và các mặt_hàng khác như bưu_thiếp là mối quan_tâm đến nhiều nhà sưu_tập .</code> | <code>Các nhà sưu_tập sẽ thích ghé thăm madeira bởi_vì những phân_chia lớn của tem , ghi_chép ngân_hàng , bưu_thiếp , và nhiều mặt_hàng khác họ có_thể đọc được .</code> | <code>Mọi người quan_tâm đến việc bắt_đầu bộ sưu_tập mới nên thoát madeira và đi du_lịch phía bắc , nơi họ có khả_năng tìm thấy các cửa_hàng tốt .</code> |
| <code>Cẩn_thận đấy , ông inglethorp . Poirot bị bồn_chồn .</code> | <code>Hãy chăm_sóc ông inglethorp .</code> | <code>Không cần phải cẩn_thận với anh ta .</code> |
| <code>Phải có một_chút hoài_nghi về trải nghiệm cá_nhân của sperling với trò_chơi .</code> | <code>Hãy suy_nghĩ về những tác_động khi nhìn vào kinh_nghiệm của anh ấy .</code> | <code>Một người có_thể lấy trải nghiệm cá_nhân của sperling với giá_trị mặt .</code> |
* Loss: [<code>TripletLoss</code>](https://sbert.net/docs/package_reference/sentence_transformer/losses.html#tripletloss) with these parameters:
```json
{
"distance_metric": "TripletDistanceMetric.EUCLIDEAN",
"triplet_margin": 5
}
```
### Evaluation Dataset
#### Unnamed Dataset
* Size: 12,772 evaluation samples
* Columns: <code>anchor</code>, <code>positive</code>, and <code>negative</code>
* Approximate statistics based on the first 1000 samples:
| | anchor | positive | negative |
|:--------|:-----------------------------------------------------------------------------------|:----------------------------------------------------------------------------------|:---------------------------------------------------------------------------------|
| type | string | string | string |
| details | <ul><li>min: 4 tokens</li><li>mean: 27.81 tokens</li><li>max: 164 tokens</li></ul> | <ul><li>min: 3 tokens</li><li>mean: 14.94 tokens</li><li>max: 42 tokens</li></ul> | <ul><li>min: 4 tokens</li><li>mean: 14.4 tokens</li><li>max: 39 tokens</li></ul> |
* Samples:
| anchor | positive | negative |
|:----------------------------------------------------------------------------------------------------------------------------------------------------------------------------------------------------------------------|:---------------------------------------------------------------------------------------------|:-----------------------------------------------------------------------------------------------------------------------------|
| <code>Tình_yêu , anh có muốn em trở_thành kassandra lubbock của anh không ?</code> | <code>Tôi có_thể là kassandra lubbock của anh .</code> | <code>Tôi từ_chối trở_thành kassandra lubbock của anh .</code> |
| <code>Ví_dụ , trong mùa thu năm 1997 , ủy ban điều_trị hạt_nhân ( nrc ) văn_phòng thanh_tra tướng liệu nrc để có được quan_điểm của họ trên văn_hóa an_toàn của đại_lý .</code> | <code>Nhân_viên nrc đã được hỏi về quan_điểm của họ trên văn_hóa an_toàn của đại_lý .</code> | <code>Các nhân_viên không bao_giờ quan_sát về quan_điểm của họ về văn_hóa an_toàn của đại_lý trong mùa thu năm 1997 .</code> |
| <code>Mỗi năm kem của trẻ nghệ và comedic tài_năng làm cho nó đường đến edinburgh , và fringe đã lớn lên trong việc huấn_luyện lớn nhất trong khung_cảnh lớn nhất cho các diễn_viên phát_triển trên thế_giới .</code> | <code>Tài_năng mới đến edinburgh .</code> | <code>Tài_năng mới đến dublin .</code> |
* Loss: [<code>TripletLoss</code>](https://sbert.net/docs/package_reference/sentence_transformer/losses.html#tripletloss) with these parameters:
```json
{
"distance_metric": "TripletDistanceMetric.EUCLIDEAN",
"triplet_margin": 5
}
```
### Training Hyperparameters
#### Non-Default Hyperparameters
- `overwrite_output_dir`: True
- `eval_strategy`: epoch
- `per_device_train_batch_size`: 128
- `per_device_eval_batch_size`: 128
- `num_train_epochs`: 20
- `lr_scheduler_type`: cosine
- `warmup_ratio`: 0.05
- `fp16`: True
- `load_best_model_at_end`: True
- `gradient_checkpointing`: True
#### All Hyperparameters
<details><summary>Click to expand</summary>
- `overwrite_output_dir`: True
- `do_predict`: False
- `eval_strategy`: epoch
- `prediction_loss_only`: True
- `per_device_train_batch_size`: 128
- `per_device_eval_batch_size`: 128
- `per_gpu_train_batch_size`: None
- `per_gpu_eval_batch_size`: None
- `gradient_accumulation_steps`: 1
- `eval_accumulation_steps`: None
- `learning_rate`: 5e-05
- `weight_decay`: 0.0
- `adam_beta1`: 0.9
- `adam_beta2`: 0.999
- `adam_epsilon`: 1e-08
- `max_grad_norm`: 1.0
- `num_train_epochs`: 20
- `max_steps`: -1
- `lr_scheduler_type`: cosine
- `lr_scheduler_kwargs`: {}
- `warmup_ratio`: 0.05
- `warmup_steps`: 0
- `log_level`: passive
- `log_level_replica`: warning
- `log_on_each_node`: True
- `logging_nan_inf_filter`: True
- `save_safetensors`: True
- `save_on_each_node`: False
- `save_only_model`: False
- `restore_callback_states_from_checkpoint`: False
- `no_cuda`: False
- `use_cpu`: False
- `use_mps_device`: False
- `seed`: 42
- `data_seed`: None
- `jit_mode_eval`: False
- `use_ipex`: False
- `bf16`: False
- `fp16`: True
- `fp16_opt_level`: O1
- `half_precision_backend`: auto
- `bf16_full_eval`: False
- `fp16_full_eval`: False
- `tf32`: None
- `local_rank`: 0
- `ddp_backend`: None
- `tpu_num_cores`: None
- `tpu_metrics_debug`: False
- `debug`: []
- `dataloader_drop_last`: False
- `dataloader_num_workers`: 0
- `dataloader_prefetch_factor`: None
- `past_index`: -1
- `disable_tqdm`: False
- `remove_unused_columns`: True
- `label_names`: None
- `load_best_model_at_end`: True
- `ignore_data_skip`: False
- `fsdp`: []
- `fsdp_min_num_params`: 0
- `fsdp_config`: {'min_num_params': 0, 'xla': False, 'xla_fsdp_v2': False, 'xla_fsdp_grad_ckpt': False}
- `fsdp_transformer_layer_cls_to_wrap`: None
- `accelerator_config`: {'split_batches': False, 'dispatch_batches': None, 'even_batches': True, 'use_seedable_sampler': True, 'non_blocking': False, 'gradient_accumulation_kwargs': None}
- `deepspeed`: None
- `label_smoothing_factor`: 0.0
- `optim`: adamw_torch
- `optim_args`: None
- `adafactor`: False
- `group_by_length`: False
- `length_column_name`: length
- `ddp_find_unused_parameters`: None
- `ddp_bucket_cap_mb`: None
- `ddp_broadcast_buffers`: False
- `dataloader_pin_memory`: True
- `dataloader_persistent_workers`: False
- `skip_memory_metrics`: True
- `use_legacy_prediction_loop`: False
- `push_to_hub`: False
- `resume_from_checkpoint`: None
- `hub_model_id`: None
- `hub_strategy`: every_save
- `hub_private_repo`: False
- `hub_always_push`: False
- `gradient_checkpointing`: True
- `gradient_checkpointing_kwargs`: None
- `include_inputs_for_metrics`: False
- `eval_do_concat_batches`: True
- `fp16_backend`: auto
- `push_to_hub_model_id`: None
- `push_to_hub_organization`: None
- `mp_parameters`:
- `auto_find_batch_size`: False
- `full_determinism`: False
- `torchdynamo`: None
- `ray_scope`: last
- `ddp_timeout`: 1800
- `torch_compile`: False
- `torch_compile_backend`: None
- `torch_compile_mode`: None
- `dispatch_batches`: None
- `split_batches`: None
- `include_tokens_per_second`: False
- `include_num_input_tokens_seen`: False
- `neftune_noise_alpha`: None
- `optim_target_modules`: None
- `batch_eval_metrics`: False
- `eval_on_start`: False
- `batch_sampler`: batch_sampler
- `multi_dataset_batch_sampler`: proportional
</details>
### Training Logs
<details><summary>Click to expand</summary>
| Epoch | Step | Training Loss | loss | sts-dev_spearman_cosine |
|:-------:|:--------:|:-------------:|:----------:|:-----------------------:|
| 0 | 0 | - | - | 0.6643 |
| 0.0626 | 50 | 4.6946 | - | - |
| 0.1252 | 100 | 4.031 | - | - |
| 0.1877 | 150 | 2.7654 | - | - |
| 0.2503 | 200 | 2.4176 | - | - |
| 0.3129 | 250 | 2.1111 | - | - |
| 0.3755 | 300 | 2.0263 | - | - |
| 0.4380 | 350 | 1.9296 | - | - |
| 0.5006 | 400 | 1.7793 | - | - |
| 0.5632 | 450 | 1.7903 | - | - |
| 0.6258 | 500 | 1.7638 | - | - |
| 0.6884 | 550 | 1.7042 | - | - |
| 0.7509 | 600 | 1.7038 | - | - |
| 0.8135 | 650 | 1.6221 | - | - |
| 0.8761 | 700 | 1.6172 | - | - |
| 0.9387 | 750 | 1.6227 | - | - |
| 1.0 | 799 | - | 1.5275 | 0.5219 |
| 1.0013 | 800 | 1.6264 | - | - |
| 1.0638 | 850 | 1.364 | - | - |
| 1.1264 | 900 | 1.4447 | - | - |
| 1.1890 | 950 | 1.4161 | - | - |
| 1.2516 | 1000 | 1.3575 | - | - |
| 1.3141 | 1050 | 1.3554 | - | - |
| 1.3767 | 1100 | 1.378 | - | - |
| 1.4393 | 1150 | 1.3806 | - | - |
| 1.5019 | 1200 | 1.3089 | - | - |
| 1.5645 | 1250 | 1.4314 | - | - |
| 1.6270 | 1300 | 1.3672 | - | - |
| 1.6896 | 1350 | 1.3777 | - | - |
| 1.7522 | 1400 | 1.3282 | - | - |
| 1.8148 | 1450 | 1.3432 | - | - |
| 1.8773 | 1500 | 1.3101 | - | - |
| 1.9399 | 1550 | 1.2919 | - | - |
| 2.0 | 1598 | - | 1.3643 | 0.5667 |
| 2.0025 | 1600 | 1.2969 | - | - |
| 2.0651 | 1650 | 0.9629 | - | - |
| 2.1277 | 1700 | 0.9878 | - | - |
| 2.1902 | 1750 | 0.9437 | - | - |
| 2.2528 | 1800 | 0.9832 | - | - |
| 2.3154 | 1850 | 0.9584 | - | - |
| 2.3780 | 1900 | 1.0689 | - | - |
| 2.4406 | 1950 | 1.0579 | - | - |
| 2.5031 | 2000 | 0.9888 | - | - |
| 2.5657 | 2050 | 0.9452 | - | - |
| 2.6283 | 2100 | 0.9378 | - | - |
| 2.6909 | 2150 | 0.9553 | - | - |
| 2.7534 | 2200 | 0.9337 | - | - |
| 2.8160 | 2250 | 1.0184 | - | - |
| 2.8786 | 2300 | 0.9663 | - | - |
| 2.9412 | 2350 | 0.9686 | - | - |
| 3.0 | 2397 | - | 1.3488 | 0.5442 |
| 3.0038 | 2400 | 0.9618 | - | - |
| 3.0663 | 2450 | 0.6878 | - | - |
| 3.1289 | 2500 | 0.6883 | - | - |
| 3.1915 | 2550 | 0.6498 | - | - |
| 3.2541 | 2600 | 0.6651 | - | - |
| 3.3166 | 2650 | 0.6554 | - | - |
| 3.3792 | 2700 | 0.7033 | - | - |
| 3.4418 | 2750 | 0.6416 | - | - |
| 3.5044 | 2800 | 0.7068 | - | - |
| 3.5670 | 2850 | 0.6834 | - | - |
| 3.6295 | 2900 | 0.7099 | - | - |
| 3.6921 | 2950 | 0.7306 | - | - |
| 3.7547 | 3000 | 0.7105 | - | - |
| 3.8173 | 3050 | 0.7072 | - | - |
| 3.8798 | 3100 | 0.7248 | - | - |
| 3.9424 | 3150 | 0.7216 | - | - |
| **4.0** | **3196** | **-** | **1.3358** | **0.5307** |
| 4.0050 | 3200 | 0.693 | - | - |
| 4.0676 | 3250 | 0.4741 | - | - |
| 4.1302 | 3300 | 0.4593 | - | - |
| 4.1927 | 3350 | 0.449 | - | - |
| 4.2553 | 3400 | 0.4326 | - | - |
| 4.3179 | 3450 | 0.4488 | - | - |
| 4.3805 | 3500 | 0.4762 | - | - |
| 4.4431 | 3550 | 0.4723 | - | - |
| 4.5056 | 3600 | 0.4713 | - | - |
| 4.5682 | 3650 | 0.4612 | - | - |
| 4.6308 | 3700 | 0.4537 | - | - |
| 4.6934 | 3750 | 0.4928 | - | - |
| 4.7559 | 3800 | 0.4568 | - | - |
| 4.8185 | 3850 | 0.4771 | - | - |
| 4.8811 | 3900 | 0.4688 | - | - |
| 4.9437 | 3950 | 0.4549 | - | - |
| 5.0 | 3995 | - | 1.4027 | 0.5360 |
| 5.0063 | 4000 | 0.5048 | - | - |
| 5.0688 | 4050 | 0.2822 | - | - |
| 5.1314 | 4100 | 0.3069 | - | - |
| 5.1940 | 4150 | 0.2971 | - | - |
| 5.2566 | 4200 | 0.3191 | - | - |
| 5.3191 | 4250 | 0.3023 | - | - |
| 5.3817 | 4300 | 0.3224 | - | - |
| 5.4443 | 4350 | 0.3114 | - | - |
| 5.5069 | 4400 | 0.3098 | - | - |
| 5.5695 | 4450 | 0.3071 | - | - |
| 5.6320 | 4500 | 0.3478 | - | - |
| 5.6946 | 4550 | 0.3288 | - | - |
| 5.7572 | 4600 | 0.3373 | - | - |
| 5.8198 | 4650 | 0.3577 | - | - |
| 5.8824 | 4700 | 0.331 | - | - |
| 5.9449 | 4750 | 0.3132 | - | - |
| 6.0 | 4794 | - | 1.4036 | 0.5148 |
* The bold row denotes the saved checkpoint.
</details>
### Framework Versions
- Python: 3.10.12
- Sentence Transformers: 3.0.1
- Transformers: 4.42.4
- PyTorch: 2.3.1+cu121
- Accelerate: 0.32.1
- Datasets: 2.20.0
- Tokenizers: 0.19.1
## Citation
### BibTeX
#### Sentence Transformers
```bibtex
@inproceedings{reimers-2019-sentence-bert,
title = "Sentence-BERT: Sentence Embeddings using Siamese BERT-Networks",
author = "Reimers, Nils and Gurevych, Iryna",
booktitle = "Proceedings of the 2019 Conference on Empirical Methods in Natural Language Processing",
month = "11",
year = "2019",
publisher = "Association for Computational Linguistics",
url = "https://arxiv.org/abs/1908.10084",
}
```
#### TripletLoss
```bibtex
@misc{hermans2017defense,
title={In Defense of the Triplet Loss for Person Re-Identification},
author={Alexander Hermans and Lucas Beyer and Bastian Leibe},
year={2017},
eprint={1703.07737},
archivePrefix={arXiv},
primaryClass={cs.CV}
}
```
<!--
## Glossary
*Clearly define terms in order to be accessible across audiences.*
-->
<!--
## Model Card Authors
*Lists the people who create the model card, providing recognition and accountability for the detailed work that goes into its construction.*
-->
<!--
## Model Card Contact
*Provides a way for people who have updates to the Model Card, suggestions, or questions, to contact the Model Card authors.*
--> |
jamesdolezal/CTransPath | jamesdolezal | "2023-02-09T19:17:09Z" | 0 | 2 | null | [
"license:gpl-3.0",
"region:us"
] | null | "2023-02-09T19:10:23Z" | ---
license: gpl-3.0
---
[UNOFFICIAL]
This is the pretrained CTransPath model that accompanies the manuscript Transformer-based Unsupervised Contrastive Learning for Histopathological Image Classification, published by Xiyue Wang *et al* in Medical Image Analysis (October 2022, DOI: https://doi.org/10.1016/j.media.2022.102559)
This model has been uploaded to HuggingFace for easier sharing, but has not been verified by the original authors and is in no way affiliated with the original authors.
The official pretrained model is available on the official GitHub repository (https://github.com/Xiyue-Wang/TransPath) and Google Drive (https://drive.google.com/file/d/1DoDx_70_TLj98gTf6YTXnu4tFhsFocDX/view?usp=sharing). The license as included in the original repository is GPL-3.0.
|
yantolakpau/minasanlora | yantolakpau | "2023-04-11T04:02:30Z" | 0 | 0 | null | [
"license:creativeml-openrail-m",
"region:us"
] | null | "2023-04-11T04:01:02Z" | ---
license: creativeml-openrail-m
---
|
PrunaAI/FLUX.1-schnell-4bit | PrunaAI | "2024-10-30T19:28:33Z" | 22 | 11 | null | [
"pruna-ai",
"base_model:ibm-granite/granite-8b-code-instruct-128k",
"base_model:finetune:ibm-granite/granite-8b-code-instruct-128k",
"region:us"
] | null | "2024-08-17T09:54:17Z" | ---
thumbnail: "https://assets-global.website-files.com/646b351987a8d8ce158d1940/64ec9e96b4334c0e1ac41504_Logo%20with%20white%20text.svg"
base_model: ibm-granite/granite-8b-code-instruct-128k
metrics:
- memory_disk
- memory_inference
- inference_latency
- inference_throughput
- inference_CO2_emissions
- inference_energy_consumption
tags:
- pruna-ai
---
<!-- header start -->
<!-- 200823 -->
<div style="width: auto; margin-left: auto; margin-right: auto">
<a href="https://www.pruna.ai/" target="_blank" rel="noopener noreferrer">
<img src="https://i.imgur.com/eDAlcgk.png" alt="PrunaAI" style="width: 100%; min-width: 400px; display: block; margin: auto;">
</a>
</div>
<!-- header end -->
[](https://twitter.com/PrunaAI)
[](https://github.com/PrunaAI)
[](https://www.linkedin.com/company/93832878/admin/feed/posts/?feedType=following)
[](https://discord.gg/Tun8YgzxZ9)
# Simply make AI models cheaper, smaller, faster, and greener!
- Give a thumbs up if you like this model!
- Contact us and tell us which model to compress next [here](https://www.pruna.ai/contact).
- Request access to easily compress your *own* AI models [here](https://z0halsaff74.typeform.com/pruna-access?typeform-source=www.pruna.ai).
- Read the documentations to know more [here](https://pruna-ai-pruna.readthedocs-hosted.com/en/latest/)
- Join Pruna AI community on Discord [here](https://discord.gg/CP4VSgck) to share feedback/suggestions or get help.
**Frequently Asked Questions**
- ***How does the compression work?*** The model is compressed with Quanto to 8 bits.
- ***How does the model quality change?*** The quality of the model output might vary compared to the base model.
- ***How is the model efficiency evaluated?*** These results were obtained on HARDWARE_NAME with configuration described in `model/smash_config.json` and are obtained after a hardware warmup. The smashed model is directly compared to the original base model. Efficiency results may vary in other settings (e.g. other hardware, image size, batch size, ...). We recommend to directly run them in the use-case conditions to know if the smashed model can benefit you.
- ***What is the model format?*** We use safetensors.
- ***What calibration data has been used?*** If needed by the compression method, we used WikiText as the calibration data.
- ***What is the naming convention for Pruna Huggingface models?*** We take the original model name and append "turbo", "tiny", or "green" if the smashed model has a measured inference speed, inference memory, or inference energy consumption which is less than 90% of the original base model.
- ***How to compress my own models?*** You can request premium access to more compression methods and tech support for your specific use-cases [here](https://z0halsaff74.typeform.com/pruna-access?typeform-source=www.pruna.ai).
- ***What are "first" metrics?*** Results mentioning "first" are obtained after the first run of the model. The first run might take more memory or be slower than the subsequent runs due cuda overheads.
- ***What are "Sync" and "Async" metrics?*** "Sync" metrics are obtained by syncing all GPU processes and stop measurement when all of them are executed. "Async" metrics are obtained without syncing all GPU processes and stop when the model output can be used by the CPU. We provide both metrics since both could be relevant depending on the use-case. We recommend to test the efficiency gains directly in your use-cases.
## Setup
You can run the smashed model on cards with less than 12 GB of memory with these steps:
0. Check requirements from the original repo black-forest-labs/FLUX.1-schnell installed. In particular, check python, diffusers, and transformers versions.
1. Make sure that you have installed quantization related packages.
```bash
pip install -U optimum-quanto
```
2. Download the model
- Use Python:
```python
import subprocess
repo_name = "FLUX.1-schnell-4bit"
subprocess.run(["mkdir", repo_name])
subprocess.run(["huggingface-cli", "download", 'PrunaAI/'+ repo_name, "--local-dir", repo_name, "--local-dir-use-symlinks", "False"])
```
2. Load & run the model.
```python
import torch
from optimum.quanto import freeze, qfloat8, quantize
from diffusers import FlowMatchEulerDiscreteScheduler, AutoencoderKL
from diffusers.models.transformers.transformer_flux import FluxTransformer2DModel
from diffusers.pipelines.flux.pipeline_flux import FluxPipeline
from transformers import CLIPTextModel, CLIPTokenizer,T5EncoderModel, T5TokenizerFast
dtype = torch.bfloat16
bfl_repo = "black-forest-labs/FLUX.1-schnell"
revision = "refs/pr/1"
local_path = "FLUX.1-schnell-4bit"
scheduler = FlowMatchEulerDiscreteScheduler.from_pretrained(bfl_repo, subfolder="scheduler", revision=revision)
text_encoder = CLIPTextModel.from_pretrained("openai/clip-vit-large-patch14", torch_dtype=dtype)
tokenizer = CLIPTokenizer.from_pretrained("openai/clip-vit-large-patch14", torch_dtype=dtype)
text_encoder_2 = torch.load(local_path + '/text_encoder_2.pt')
tokenizer_2 = T5TokenizerFast.from_pretrained(bfl_repo, subfolder="tokenizer_2", torch_dtype=dtype, revision=revision)
vae = AutoencoderKL.from_pretrained(bfl_repo, subfolder="vae", torch_dtype=dtype, revision=revision)
transformer = torch.load(local_path + '/transformer.pt')
pipe = FluxPipeline(
scheduler=scheduler,
text_encoder=text_encoder,
tokenizer=tokenizer,
text_encoder_2=None,
tokenizer_2=tokenizer_2,
vae=vae,
transformer=None,
)
pipe.text_encoder_2 = text_encoder_2
pipe.transformer = transformer
# pipe.enable_model_cpu_offload()
pipe.to('cuda')
print('done')
generator = torch.Generator().manual_seed(12345)
pipe(
"a cute apple smiling",
guidance_scale=0.0,
num_inference_steps=4,
max_sequence_length=256,
generator=torch.Generator("cpu").manual_seed(0)
).images[0]
```
## Configurations
The configuration info are in `smash_config.json`.
## Credits & License
The license of the smashed model follows the license of the original model. Please check the license of the original model black-forest-labs/FLUX.1-schnell before using this model which provided the base model. The license of the `pruna-engine` is [here](https://pypi.org/project/pruna-engine/) on Pypi.
## Want to compress other models?
- Contact us and tell us which model to compress next [here](https://www.pruna.ai/contact).
- Request access to easily compress your own AI models [here](https://z0halsaff74.typeform.com/pruna-access?typeform-source=www.pruna.ai). |
methinkss/m2 | methinkss | "2025-02-08T16:12:46Z" | 22 | 0 | transformers | [
"transformers",
"safetensors",
"llama",
"text-generation",
"conversational",
"arxiv:1910.09700",
"autotrain_compatible",
"text-generation-inference",
"endpoints_compatible",
"region:us"
] | text-generation | "2025-02-08T16:09:23Z" | ---
library_name: transformers
tags: []
---
# Model Card for Model ID
<!-- Provide a quick summary of what the model is/does. -->
## Model Details
### Model Description
<!-- Provide a longer summary of what this model is. -->
This is the model card of a 🤗 transformers model that has been pushed on the Hub. This model card has been automatically generated.
- **Developed by:** [More Information Needed]
- **Funded by [optional]:** [More Information Needed]
- **Shared by [optional]:** [More Information Needed]
- **Model type:** [More Information Needed]
- **Language(s) (NLP):** [More Information Needed]
- **License:** [More Information Needed]
- **Finetuned from model [optional]:** [More Information Needed]
### Model Sources [optional]
<!-- Provide the basic links for the model. -->
- **Repository:** [More Information Needed]
- **Paper [optional]:** [More Information Needed]
- **Demo [optional]:** [More Information Needed]
## Uses
<!-- Address questions around how the model is intended to be used, including the foreseeable users of the model and those affected by the model. -->
### Direct Use
<!-- This section is for the model use without fine-tuning or plugging into a larger ecosystem/app. -->
[More Information Needed]
### Downstream Use [optional]
<!-- This section is for the model use when fine-tuned for a task, or when plugged into a larger ecosystem/app -->
[More Information Needed]
### Out-of-Scope Use
<!-- This section addresses misuse, malicious use, and uses that the model will not work well for. -->
[More Information Needed]
## Bias, Risks, and Limitations
<!-- This section is meant to convey both technical and sociotechnical limitations. -->
[More Information Needed]
### Recommendations
<!-- This section is meant to convey recommendations with respect to the bias, risk, and technical limitations. -->
Users (both direct and downstream) should be made aware of the risks, biases and limitations of the model. More information needed for further recommendations.
## How to Get Started with the Model
Use the code below to get started with the model.
[More Information Needed]
## Training Details
### Training Data
<!-- This should link to a Dataset Card, perhaps with a short stub of information on what the training data is all about as well as documentation related to data pre-processing or additional filtering. -->
[More Information Needed]
### Training Procedure
<!-- This relates heavily to the Technical Specifications. Content here should link to that section when it is relevant to the training procedure. -->
#### Preprocessing [optional]
[More Information Needed]
#### Training Hyperparameters
- **Training regime:** [More Information Needed] <!--fp32, fp16 mixed precision, bf16 mixed precision, bf16 non-mixed precision, fp16 non-mixed precision, fp8 mixed precision -->
#### Speeds, Sizes, Times [optional]
<!-- This section provides information about throughput, start/end time, checkpoint size if relevant, etc. -->
[More Information Needed]
## Evaluation
<!-- This section describes the evaluation protocols and provides the results. -->
### Testing Data, Factors & Metrics
#### Testing Data
<!-- This should link to a Dataset Card if possible. -->
[More Information Needed]
#### Factors
<!-- These are the things the evaluation is disaggregating by, e.g., subpopulations or domains. -->
[More Information Needed]
#### Metrics
<!-- These are the evaluation metrics being used, ideally with a description of why. -->
[More Information Needed]
### Results
[More Information Needed]
#### Summary
## Model Examination [optional]
<!-- Relevant interpretability work for the model goes here -->
[More Information Needed]
## Environmental Impact
<!-- Total emissions (in grams of CO2eq) and additional considerations, such as electricity usage, go here. Edit the suggested text below accordingly -->
Carbon emissions can be estimated using the [Machine Learning Impact calculator](https://mlco2.github.io/impact#compute) presented in [Lacoste et al. (2019)](https://arxiv.org/abs/1910.09700).
- **Hardware Type:** [More Information Needed]
- **Hours used:** [More Information Needed]
- **Cloud Provider:** [More Information Needed]
- **Compute Region:** [More Information Needed]
- **Carbon Emitted:** [More Information Needed]
## Technical Specifications [optional]
### Model Architecture and Objective
[More Information Needed]
### Compute Infrastructure
[More Information Needed]
#### Hardware
[More Information Needed]
#### Software
[More Information Needed]
## Citation [optional]
<!-- If there is a paper or blog post introducing the model, the APA and Bibtex information for that should go in this section. -->
**BibTeX:**
[More Information Needed]
**APA:**
[More Information Needed]
## Glossary [optional]
<!-- If relevant, include terms and calculations in this section that can help readers understand the model or model card. -->
[More Information Needed]
## More Information [optional]
[More Information Needed]
## Model Card Authors [optional]
[More Information Needed]
## Model Card Contact
[More Information Needed] |
hinablue/illustriousXL1.0_v10_merged | hinablue | "2025-03-04T08:35:57Z" | 0 | 0 | null | [
"license:other",
"region:us"
] | null | "2025-03-04T05:47:08Z" | ---
license: other
license_name: fair-ai-public-license-1.0-sd
license_link: https://freedevproject.org/fdpl-1.0/
---
# Model Card
Merge illustriousXL 1.0 with waiNSFWIllustrious_v110 for testing.
## Model Details
[illustriousXL 1.0](https://civitai.com/models/1232765?modelVersionId=1410435)
[waiNSFWIllustrious_v110](https://civitai.com/models/827184/wai-nsfw-illustrious-sdxl)
### Model Description
```
ill 0.6 + wai 0.4 => merged
merged 0.6 + 0.4(0.5(ill 0.6 + wai 0.4, cosine A + cosine B)) => merged_plus_cosineAB
``` |
QuantFactory/Faro-Yi-9B-DPO-GGUF | QuantFactory | "2024-05-24T14:16:39Z" | 720 | 1 | null | [
"gguf",
"llama",
"conversational",
"text-generation",
"en",
"zh",
"dataset:wenbopan/Chinese-dpo-pairs",
"dataset:Intel/orca_dpo_pairs",
"dataset:argilla/ultrafeedback-binarized-preferences-cleaned",
"dataset:jondurbin/truthy-dpo-v0.1",
"arxiv:2303.08774",
"base_model:wenbopan/Faro-Yi-9B-DPO",
"base_model:quantized:wenbopan/Faro-Yi-9B-DPO",
"license:mit",
"endpoints_compatible",
"region:us"
] | text-generation | "2024-05-24T13:21:41Z" | ---
language:
- en
- zh
license: mit
datasets:
- wenbopan/Chinese-dpo-pairs
- Intel/orca_dpo_pairs
- argilla/ultrafeedback-binarized-preferences-cleaned
- jondurbin/truthy-dpo-v0.1
pipeline_tag: text-generation
tags:
- llama
- conversational
base_model: wenbopan/Faro-Yi-9B-DPO
---
# Faro-Yi-9B-DP-GGUF
This is quantized version of [wenbopan/Faro-Yi-9B-DPO](https://huggingface.co/wenbopan/Faro-Yi-9B-DPO) created using llama.cpp
# Model Description
This is the DPO version of [wenbopan/Faro-Yi-9B](https://huggingface.co/wenbopan/Faro-Yi-9B). Compared to Faro-Yi-9B and [Yi-9B-200K](https://huggingface.co/01-ai/Yi-9B-200K), the DPO model excels at many tasks, surpassing the original Yi-9B-200K by a large margin. On the [Open LLM Leaderboard](https://huggingface.co/spaces/HuggingFaceH4/open_llm_leaderboard), it ranks **#2** among all 9B models, **#1** among all Yi-9B variants.
| **Metric** | **MMLU** | **GSM8K** | **hellaswag** | **truthfulqa** | **ai2_arc** | **winogrande** | **CMMLU** |
| ----------------------- | --------- | --------- | ------------- | -------------- | ----------- | -------------- | --------- |
| **Yi-9B-200K** | 65.73 | 50.49 | 56.72 | 33.80 | 69.25 | 71.67 | 71.97 |
| **Faro-Yi-9B** | 68.80 | 63.08 | 57.28 | 40.86 | 72.58 | 71.11 | 73.28 |
| **Faro-Yi-9B-DPO** | **69.98** | **66.11** | **59.04** | **48.01** | **75.68** | **73.40** | **75.23** |
Faro-Yi-9B-DPO's responses are also favored by GPT-4 Judge in MT-Bench

## How to Use
Faro-Yi-9B-DPO uses the chatml template and performs well in both short and long contexts. For longer inputs under **24GB of VRAM**, I recommend to use vLLM to have a max prompt of 32K. Setting `kv_cache_dtype="fp8_e5m2"` allows for 48K input length. 4bit-AWQ quantization on top of that can boost input length to 160K, albeit with some performance impact. Adjust `max_model_len` arg in vLLM or `config.json` to avoid OOM.
```python
import io
import requests
from PyPDF2 import PdfReader
from vllm import LLM, SamplingParams
llm = LLM(model="wenbopan/Faro-Yi-9B-DPO", kv_cache_dtype="fp8_e5m2", max_model_len=100000)
pdf_data = io.BytesIO(requests.get("https://arxiv.org/pdf/2303.08774.pdf").content)
document = "".join(page.extract_text() for page in PdfReader(pdf_data).pages) # 100 pages
question = f"{document}\n\nAccording to the paper, what is the parameter count of GPT-4?"
messages = [ {"role": "user", "content": question} ] # 83K tokens
prompt = llm.get_tokenizer().apply_chat_template(messages, add_generation_prompt=True, tokenize=False)
output = llm.generate(prompt, SamplingParams(temperature=0.8, max_tokens=500))
print(output[0].outputs[0].text)
# Yi-9B-200K: 175B. GPT-4 has 175B \nparameters. How many models were combined to create GPT-4? Answer: 6. ...
# Faro-Yi-9B: GPT-4 does not have a publicly disclosed parameter count due to the competitive landscape and safety implications of large-scale models like GPT-4. ...
```
<details> <summary>Or With Transformers</summary>
```python
from transformers import AutoModelForCausalLM, AutoTokenizer
model = AutoModelForCausalLM.from_pretrained('wenbopan/Faro-Yi-9B-DPO', device_map="cuda")
tokenizer = AutoTokenizer.from_pretrained('wenbopan/Faro-Yi-9B-DPO')
messages = [
{"role": "system", "content": "You are a helpful assistant. Always answer with a short response."},
{"role": "user", "content": "Tell me what is Pythagorean theorem like you are a pirate."}
]
input_ids = tokenizer.apply_chat_template(messages, tokenize=True, add_generation_prompt=True, return_tensors="pt").to(model.device)
generated_ids = model.generate(input_ids, max_new_tokens=512, temperature=0.5)
response = tokenizer.decode(generated_ids[0], skip_special_tokens=True) # Aye, matey! The Pythagorean theorem is a nautical rule that helps us find the length of the third side of a triangle. ...
```
</details> |
marco-c88/distilgpt2-finetuned-mstatmem_1ep_2 | marco-c88 | "2023-03-17T10:55:18Z" | 176 | 0 | transformers | [
"transformers",
"pytorch",
"tensorboard",
"gpt2",
"text-generation",
"generated_from_trainer",
"license:apache-2.0",
"autotrain_compatible",
"text-generation-inference",
"endpoints_compatible",
"region:us"
] | text-generation | "2023-03-17T10:52:37Z" | ---
license: apache-2.0
tags:
- generated_from_trainer
model-index:
- name: distilgpt2-finetuned-mstatmem_1ep_2
results: []
---
<!-- This model card has been generated automatically according to the information the Trainer had access to. You
should probably proofread and complete it, then remove this comment. -->
# distilgpt2-finetuned-mstatmem_1ep_2
This model is a fine-tuned version of [distilgpt2](https://huggingface.co/distilgpt2) on the None dataset.
It achieves the following results on the evaluation set:
- Loss: 3.6512
## Model description
More information needed
## Intended uses & limitations
More information needed
## Training and evaluation data
More information needed
## Training procedure
### Training hyperparameters
The following hyperparameters were used during training:
- learning_rate: 2e-05
- train_batch_size: 8
- eval_batch_size: 8
- seed: 42
- optimizer: Adam with betas=(0.9,0.999) and epsilon=1e-08
- lr_scheduler_type: linear
- num_epochs: 1
### Training results
| Training Loss | Epoch | Step | Validation Loss |
|:-------------:|:-----:|:----:|:---------------:|
| 3.804 | 1.0 | 703 | 3.6512 |
### Framework versions
- Transformers 4.27.1
- Pytorch 1.13.1+cu116
- Datasets 2.10.1
- Tokenizers 0.13.2
|
Niyantha23M/llama-7b-chat-25000-25-75-L | Niyantha23M | "2024-04-12T06:57:35Z" | 0 | 0 | null | [
"trl",
"sft",
"generated_from_trainer",
"dataset:generator",
"base_model:meta-llama/Llama-2-7b-chat-hf",
"base_model:finetune:meta-llama/Llama-2-7b-chat-hf",
"region:us"
] | null | "2024-04-12T06:57:29Z" | ---
base_model: meta-llama/Llama-2-7b-chat-hf
tags:
- trl
- sft
- generated_from_trainer
datasets:
- generator
model-index:
- name: llama-7b-chat-25000-25-75-L
results: []
---
<!-- This model card has been generated automatically according to the information the Trainer had access to. You
should probably proofread and complete it, then remove this comment. -->
# llama-7b-chat-25000-25-75-L
This model is a fine-tuned version of [meta-llama/Llama-2-7b-chat-hf](https://huggingface.co/meta-llama/Llama-2-7b-chat-hf) on the generator dataset.
## Model description
More information needed
## Intended uses & limitations
More information needed
## Training and evaluation data
More information needed
## Training procedure
### Training hyperparameters
The following hyperparameters were used during training:
- learning_rate: 0.0002
- train_batch_size: 2200
- eval_batch_size: 8
- seed: 42
- gradient_accumulation_steps: 2
- total_train_batch_size: 4400
- optimizer: Adam with betas=(0.9,0.999) and epsilon=1e-08
- lr_scheduler_type: constant
- lr_scheduler_warmup_ratio: 0.03
- num_epochs: 3
### Training results
### Framework versions
- Transformers 4.33.3
- Pytorch 2.2.1
- Datasets 2.18.0
- Tokenizers 0.13.3
|
matrixportal/L3-Aspire-Heart-Matrix-8B-GGUF | matrixportal | "2025-01-22T21:55:23Z" | 70 | 0 | transformers | [
"transformers",
"gguf",
"merge",
"mergekit",
"lazymergekit",
"vllm",
"bfloat16",
"llama",
"llama-cpp",
"gguf-my-repo",
"text-generation",
"en",
"base_model:ZeroXClem/L3-Aspire-Heart-Matrix-8B",
"base_model:quantized:ZeroXClem/L3-Aspire-Heart-Matrix-8B",
"license:apache-2.0",
"endpoints_compatible",
"region:us",
"conversational"
] | text-generation | "2025-01-22T13:32:43Z" | ---
license: apache-2.0
tags:
- merge
- mergekit
- lazymergekit
- vllm
- bfloat16
- llama
- llama-cpp
- gguf-my-repo
language:
- en
base_model: ZeroXClem/L3-Aspire-Heart-Matrix-8B
pipeline_tag: text-generation
library_name: transformers
---
# matrixportal/L3-Aspire-Heart-Matrix-8B-GGUF
This model was converted to GGUF format from [`ZeroXClem/L3-Aspire-Heart-Matrix-8B`](https://huggingface.co/ZeroXClem/L3-Aspire-Heart-Matrix-8B) using llama.cpp via the ggml.ai's [GGUF-my-repo](https://huggingface.co/spaces/ggml-org/gguf-my-repo) space.
Refer to the [original model card](https://huggingface.co/ZeroXClem/L3-Aspire-Heart-Matrix-8B) for more details on the model.
## Use with llama.cpp
Install llama.cpp through brew (works on Mac and Linux)
```bash
brew install llama.cpp
```
Invoke the llama.cpp server or the CLI.
### CLI:
```bash
llama-cli --hf-repo matrixportal/L3-Aspire-Heart-Matrix-8B-GGUF --hf-file l3-aspire-heart-matrix-8b-q4_0.gguf -p "The meaning to life and the universe is"
```
### Server:
```bash
llama-server --hf-repo matrixportal/L3-Aspire-Heart-Matrix-8B-GGUF --hf-file l3-aspire-heart-matrix-8b-q4_0.gguf -c 2048
```
Note: You can also use this checkpoint directly through the [usage steps](https://github.com/ggerganov/llama.cpp?tab=readme-ov-file#usage) listed in the Llama.cpp repo as well.
Step 1: Clone llama.cpp from GitHub.
```
git clone https://github.com/ggerganov/llama.cpp
```
Step 2: Move into the llama.cpp folder and build it with `LLAMA_CURL=1` flag along with other hardware-specific flags (for ex: LLAMA_CUDA=1 for Nvidia GPUs on Linux).
```
cd llama.cpp && LLAMA_CURL=1 make
```
Step 3: Run inference through the main binary.
```
./llama-cli --hf-repo matrixportal/L3-Aspire-Heart-Matrix-8B-GGUF --hf-file l3-aspire-heart-matrix-8b-q4_0.gguf -p "The meaning to life and the universe is"
```
or
```
./llama-server --hf-repo matrixportal/L3-Aspire-Heart-Matrix-8B-GGUF --hf-file l3-aspire-heart-matrix-8b-q4_0.gguf -c 2048
```
|
jerryyun/kicon_llama3_8b_qlora_merged_v1 | jerryyun | "2024-07-14T16:47:24Z" | 5 | 0 | transformers | [
"transformers",
"safetensors",
"llama",
"text-generation",
"conversational",
"arxiv:1910.09700",
"autotrain_compatible",
"text-generation-inference",
"endpoints_compatible",
"4-bit",
"bitsandbytes",
"region:us"
] | text-generation | "2024-07-14T16:44:40Z" | ---
library_name: transformers
tags: []
---
# Model Card for Model ID
<!-- Provide a quick summary of what the model is/does. -->
## Model Details
### Model Description
<!-- Provide a longer summary of what this model is. -->
This is the model card of a 🤗 transformers model that has been pushed on the Hub. This model card has been automatically generated.
- **Developed by:** [More Information Needed]
- **Funded by [optional]:** [More Information Needed]
- **Shared by [optional]:** [More Information Needed]
- **Model type:** [More Information Needed]
- **Language(s) (NLP):** [More Information Needed]
- **License:** [More Information Needed]
- **Finetuned from model [optional]:** [More Information Needed]
### Model Sources [optional]
<!-- Provide the basic links for the model. -->
- **Repository:** [More Information Needed]
- **Paper [optional]:** [More Information Needed]
- **Demo [optional]:** [More Information Needed]
## Uses
<!-- Address questions around how the model is intended to be used, including the foreseeable users of the model and those affected by the model. -->
### Direct Use
<!-- This section is for the model use without fine-tuning or plugging into a larger ecosystem/app. -->
[More Information Needed]
### Downstream Use [optional]
<!-- This section is for the model use when fine-tuned for a task, or when plugged into a larger ecosystem/app -->
[More Information Needed]
### Out-of-Scope Use
<!-- This section addresses misuse, malicious use, and uses that the model will not work well for. -->
[More Information Needed]
## Bias, Risks, and Limitations
<!-- This section is meant to convey both technical and sociotechnical limitations. -->
[More Information Needed]
### Recommendations
<!-- This section is meant to convey recommendations with respect to the bias, risk, and technical limitations. -->
Users (both direct and downstream) should be made aware of the risks, biases and limitations of the model. More information needed for further recommendations.
## How to Get Started with the Model
Use the code below to get started with the model.
[More Information Needed]
## Training Details
### Training Data
<!-- This should link to a Dataset Card, perhaps with a short stub of information on what the training data is all about as well as documentation related to data pre-processing or additional filtering. -->
[More Information Needed]
### Training Procedure
<!-- This relates heavily to the Technical Specifications. Content here should link to that section when it is relevant to the training procedure. -->
#### Preprocessing [optional]
[More Information Needed]
#### Training Hyperparameters
- **Training regime:** [More Information Needed] <!--fp32, fp16 mixed precision, bf16 mixed precision, bf16 non-mixed precision, fp16 non-mixed precision, fp8 mixed precision -->
#### Speeds, Sizes, Times [optional]
<!-- This section provides information about throughput, start/end time, checkpoint size if relevant, etc. -->
[More Information Needed]
## Evaluation
<!-- This section describes the evaluation protocols and provides the results. -->
### Testing Data, Factors & Metrics
#### Testing Data
<!-- This should link to a Dataset Card if possible. -->
[More Information Needed]
#### Factors
<!-- These are the things the evaluation is disaggregating by, e.g., subpopulations or domains. -->
[More Information Needed]
#### Metrics
<!-- These are the evaluation metrics being used, ideally with a description of why. -->
[More Information Needed]
### Results
[More Information Needed]
#### Summary
## Model Examination [optional]
<!-- Relevant interpretability work for the model goes here -->
[More Information Needed]
## Environmental Impact
<!-- Total emissions (in grams of CO2eq) and additional considerations, such as electricity usage, go here. Edit the suggested text below accordingly -->
Carbon emissions can be estimated using the [Machine Learning Impact calculator](https://mlco2.github.io/impact#compute) presented in [Lacoste et al. (2019)](https://arxiv.org/abs/1910.09700).
- **Hardware Type:** [More Information Needed]
- **Hours used:** [More Information Needed]
- **Cloud Provider:** [More Information Needed]
- **Compute Region:** [More Information Needed]
- **Carbon Emitted:** [More Information Needed]
## Technical Specifications [optional]
### Model Architecture and Objective
[More Information Needed]
### Compute Infrastructure
[More Information Needed]
#### Hardware
[More Information Needed]
#### Software
[More Information Needed]
## Citation [optional]
<!-- If there is a paper or blog post introducing the model, the APA and Bibtex information for that should go in this section. -->
**BibTeX:**
[More Information Needed]
**APA:**
[More Information Needed]
## Glossary [optional]
<!-- If relevant, include terms and calculations in this section that can help readers understand the model or model card. -->
[More Information Needed]
## More Information [optional]
[More Information Needed]
## Model Card Authors [optional]
[More Information Needed]
## Model Card Contact
[More Information Needed] |
nomsgadded/pokemon-lora | nomsgadded | "2023-07-11T05:25:03Z" | 2 | 0 | diffusers | [
"diffusers",
"tensorboard",
"stable-diffusion",
"stable-diffusion-diffusers",
"text-to-image",
"lora",
"base_model:runwayml/stable-diffusion-v1-5",
"base_model:adapter:runwayml/stable-diffusion-v1-5",
"license:creativeml-openrail-m",
"region:us"
] | text-to-image | "2023-07-11T03:46:05Z" |
---
license: creativeml-openrail-m
base_model: runwayml/stable-diffusion-v1-5
tags:
- stable-diffusion
- stable-diffusion-diffusers
- text-to-image
- diffusers
- lora
inference: true
---
# LoRA text2image fine-tuning - nomsgadded/pokemon-lora
These are LoRA adaption weights for runwayml/stable-diffusion-v1-5. The weights were fine-tuned on the lambdalabs/pokemon-blip-captions dataset. You can find some example images in the following.




|
mradermacher/nox-solar-10.7b-v4-kolon-all-5-v3.0-i1-GGUF | mradermacher | "2024-11-25T08:56:31Z" | 11 | 1 | transformers | [
"transformers",
"gguf",
"ko",
"en",
"base_model:gwonny/nox-solar-10.7b-v4-kolon-all-5-v3.0",
"base_model:quantized:gwonny/nox-solar-10.7b-v4-kolon-all-5-v3.0",
"license:cc-by-nc-4.0",
"endpoints_compatible",
"region:us",
"imatrix",
"conversational"
] | null | "2024-11-23T15:12:29Z" | ---
base_model: gwonny/nox-solar-10.7b-v4-kolon-all-5-v3.0
language:
- ko
- en
library_name: transformers
license: cc-by-nc-4.0
quantized_by: mradermacher
---
## About
<!-- ### quantize_version: 2 -->
<!-- ### output_tensor_quantised: 1 -->
<!-- ### convert_type: hf -->
<!-- ### vocab_type: -->
<!-- ### tags: nicoboss -->
weighted/imatrix quants of https://huggingface.co/gwonny/nox-solar-10.7b-v4-kolon-all-5-v3.0
<!-- provided-files -->
static quants are available at https://huggingface.co/mradermacher/nox-solar-10.7b-v4-kolon-all-5-v3.0-GGUF
## Usage
If you are unsure how to use GGUF files, refer to one of [TheBloke's
READMEs](https://huggingface.co/TheBloke/KafkaLM-70B-German-V0.1-GGUF) for
more details, including on how to concatenate multi-part files.
## Provided Quants
(sorted by size, not necessarily quality. IQ-quants are often preferable over similar sized non-IQ quants)
| Link | Type | Size/GB | Notes |
|:-----|:-----|--------:|:------|
| [GGUF](https://huggingface.co/mradermacher/nox-solar-10.7b-v4-kolon-all-5-v3.0-i1-GGUF/resolve/main/nox-solar-10.7b-v4-kolon-all-5-v3.0.i1-IQ1_S.gguf) | i1-IQ1_S | 2.5 | for the desperate |
| [GGUF](https://huggingface.co/mradermacher/nox-solar-10.7b-v4-kolon-all-5-v3.0-i1-GGUF/resolve/main/nox-solar-10.7b-v4-kolon-all-5-v3.0.i1-IQ1_M.gguf) | i1-IQ1_M | 2.7 | mostly desperate |
| [GGUF](https://huggingface.co/mradermacher/nox-solar-10.7b-v4-kolon-all-5-v3.0-i1-GGUF/resolve/main/nox-solar-10.7b-v4-kolon-all-5-v3.0.i1-IQ2_XXS.gguf) | i1-IQ2_XXS | 3.0 | |
| [GGUF](https://huggingface.co/mradermacher/nox-solar-10.7b-v4-kolon-all-5-v3.0-i1-GGUF/resolve/main/nox-solar-10.7b-v4-kolon-all-5-v3.0.i1-IQ2_XS.gguf) | i1-IQ2_XS | 3.3 | |
| [GGUF](https://huggingface.co/mradermacher/nox-solar-10.7b-v4-kolon-all-5-v3.0-i1-GGUF/resolve/main/nox-solar-10.7b-v4-kolon-all-5-v3.0.i1-IQ2_S.gguf) | i1-IQ2_S | 3.5 | |
| [GGUF](https://huggingface.co/mradermacher/nox-solar-10.7b-v4-kolon-all-5-v3.0-i1-GGUF/resolve/main/nox-solar-10.7b-v4-kolon-all-5-v3.0.i1-IQ2_M.gguf) | i1-IQ2_M | 3.8 | |
| [GGUF](https://huggingface.co/mradermacher/nox-solar-10.7b-v4-kolon-all-5-v3.0-i1-GGUF/resolve/main/nox-solar-10.7b-v4-kolon-all-5-v3.0.i1-Q2_K.gguf) | i1-Q2_K | 4.1 | IQ3_XXS probably better |
| [GGUF](https://huggingface.co/mradermacher/nox-solar-10.7b-v4-kolon-all-5-v3.0-i1-GGUF/resolve/main/nox-solar-10.7b-v4-kolon-all-5-v3.0.i1-IQ3_XXS.gguf) | i1-IQ3_XXS | 4.3 | lower quality |
| [GGUF](https://huggingface.co/mradermacher/nox-solar-10.7b-v4-kolon-all-5-v3.0-i1-GGUF/resolve/main/nox-solar-10.7b-v4-kolon-all-5-v3.0.i1-IQ3_XS.gguf) | i1-IQ3_XS | 4.5 | |
| [GGUF](https://huggingface.co/mradermacher/nox-solar-10.7b-v4-kolon-all-5-v3.0-i1-GGUF/resolve/main/nox-solar-10.7b-v4-kolon-all-5-v3.0.i1-Q3_K_S.gguf) | i1-Q3_K_S | 4.8 | IQ3_XS probably better |
| [GGUF](https://huggingface.co/mradermacher/nox-solar-10.7b-v4-kolon-all-5-v3.0-i1-GGUF/resolve/main/nox-solar-10.7b-v4-kolon-all-5-v3.0.i1-IQ3_S.gguf) | i1-IQ3_S | 4.8 | beats Q3_K* |
| [GGUF](https://huggingface.co/mradermacher/nox-solar-10.7b-v4-kolon-all-5-v3.0-i1-GGUF/resolve/main/nox-solar-10.7b-v4-kolon-all-5-v3.0.i1-IQ3_M.gguf) | i1-IQ3_M | 4.9 | |
| [GGUF](https://huggingface.co/mradermacher/nox-solar-10.7b-v4-kolon-all-5-v3.0-i1-GGUF/resolve/main/nox-solar-10.7b-v4-kolon-all-5-v3.0.i1-Q3_K_M.gguf) | i1-Q3_K_M | 5.3 | IQ3_S probably better |
| [GGUF](https://huggingface.co/mradermacher/nox-solar-10.7b-v4-kolon-all-5-v3.0-i1-GGUF/resolve/main/nox-solar-10.7b-v4-kolon-all-5-v3.0.i1-Q3_K_L.gguf) | i1-Q3_K_L | 5.8 | IQ3_M probably better |
| [GGUF](https://huggingface.co/mradermacher/nox-solar-10.7b-v4-kolon-all-5-v3.0-i1-GGUF/resolve/main/nox-solar-10.7b-v4-kolon-all-5-v3.0.i1-IQ4_XS.gguf) | i1-IQ4_XS | 5.9 | |
| [GGUF](https://huggingface.co/mradermacher/nox-solar-10.7b-v4-kolon-all-5-v3.0-i1-GGUF/resolve/main/nox-solar-10.7b-v4-kolon-all-5-v3.0.i1-Q4_0_4_4.gguf) | i1-Q4_0_4_4 | 6.2 | fast on arm, low quality |
| [GGUF](https://huggingface.co/mradermacher/nox-solar-10.7b-v4-kolon-all-5-v3.0-i1-GGUF/resolve/main/nox-solar-10.7b-v4-kolon-all-5-v3.0.i1-Q4_0_4_8.gguf) | i1-Q4_0_4_8 | 6.2 | fast on arm+i8mm, low quality |
| [GGUF](https://huggingface.co/mradermacher/nox-solar-10.7b-v4-kolon-all-5-v3.0-i1-GGUF/resolve/main/nox-solar-10.7b-v4-kolon-all-5-v3.0.i1-Q4_0_8_8.gguf) | i1-Q4_0_8_8 | 6.2 | fast on arm+sve, low quality |
| [GGUF](https://huggingface.co/mradermacher/nox-solar-10.7b-v4-kolon-all-5-v3.0-i1-GGUF/resolve/main/nox-solar-10.7b-v4-kolon-all-5-v3.0.i1-Q4_0.gguf) | i1-Q4_0 | 6.2 | fast, low quality |
| [GGUF](https://huggingface.co/mradermacher/nox-solar-10.7b-v4-kolon-all-5-v3.0-i1-GGUF/resolve/main/nox-solar-10.7b-v4-kolon-all-5-v3.0.i1-Q4_K_S.gguf) | i1-Q4_K_S | 6.2 | optimal size/speed/quality |
| [GGUF](https://huggingface.co/mradermacher/nox-solar-10.7b-v4-kolon-all-5-v3.0-i1-GGUF/resolve/main/nox-solar-10.7b-v4-kolon-all-5-v3.0.i1-Q4_K_M.gguf) | i1-Q4_K_M | 6.6 | fast, recommended |
| [GGUF](https://huggingface.co/mradermacher/nox-solar-10.7b-v4-kolon-all-5-v3.0-i1-GGUF/resolve/main/nox-solar-10.7b-v4-kolon-all-5-v3.0.i1-Q5_K_S.gguf) | i1-Q5_K_S | 7.5 | |
| [GGUF](https://huggingface.co/mradermacher/nox-solar-10.7b-v4-kolon-all-5-v3.0-i1-GGUF/resolve/main/nox-solar-10.7b-v4-kolon-all-5-v3.0.i1-Q5_K_M.gguf) | i1-Q5_K_M | 7.7 | |
| [GGUF](https://huggingface.co/mradermacher/nox-solar-10.7b-v4-kolon-all-5-v3.0-i1-GGUF/resolve/main/nox-solar-10.7b-v4-kolon-all-5-v3.0.i1-Q6_K.gguf) | i1-Q6_K | 8.9 | practically like static Q6_K |
Here is a handy graph by ikawrakow comparing some lower-quality quant
types (lower is better):

And here are Artefact2's thoughts on the matter:
https://gist.github.com/Artefact2/b5f810600771265fc1e39442288e8ec9
## FAQ / Model Request
See https://huggingface.co/mradermacher/model_requests for some answers to
questions you might have and/or if you want some other model quantized.
## Thanks
I thank my company, [nethype GmbH](https://www.nethype.de/), for letting
me use its servers and providing upgrades to my workstation to enable
this work in my free time. Additional thanks to [@nicoboss](https://huggingface.co/nicoboss) for giving me access to his private supercomputer, enabling me to provide many more imatrix quants, at much higher quality, than I would otherwise be able to.
<!-- end -->
|
AdapterHub/facebook-bart-base_lingaccept_cola_pfeiffer | AdapterHub | "2024-05-05T19:21:14Z" | 0 | 0 | adapter-transformers | [
"adapter-transformers",
"text-classification",
"adapterhub:lingaccept/cola",
"bart",
"license:apache-2.0",
"region:us"
] | text-classification | "2024-05-05T19:21:11Z" | ---
tags:
- adapter-transformers
- text-classification
- adapterhub:lingaccept/cola
- bart
license: "apache-2.0"
---
# Adapter `facebook-bart-base_lingaccept_cola_pfeiffer` for facebook/bart-base
Adapter for bart-base in Pfeiffer architecture trained on the CoLA dataset for 15 epochs with early stopping and a learning rate of 1e-4.
**This adapter was created for usage with the [Adapters](https://github.com/Adapter-Hub/adapters) library.**
## Usage
First, install `adapters`:
```
pip install -U adapters
```
Now, the adapter can be loaded and activated like this:
```python
from adapters import AutoAdapterModel
model = AutoAdapterModel.from_pretrained("facebook/bart-base")
adapter_name = model.load_adapter("AdapterHub/facebook-bart-base_lingaccept_cola_pfeiffer")
model.set_active_adapters(adapter_name)
```
## Architecture & Training
- Adapter architecture: pfeiffer
- Prediction head: classification
- Dataset: [CoLA](https://nyu-mll.github.io/CoLA/)
## Author Information
- Author name(s): Clifton Poth
- Author email: [email protected]
- Author links: [Website](https://calpt.github.io), [GitHub](https://github.com/calpt), [Twitter](https://twitter.com/@clifapt)
## Citation
```bibtex
```
*This adapter has been auto-imported from https://github.com/Adapter-Hub/Hub/blob/master/adapters/ukp/facebook-bart-base_lingaccept_cola_pfeiffer.yaml*. |
Yaxin1992/llama3-8b-summary | Yaxin1992 | "2024-04-23T21:42:31Z" | 3 | 0 | peft | [
"peft",
"tensorboard",
"safetensors",
"generated_from_trainer",
"base_model:meta-llama/Meta-Llama-3-8B-Instruct",
"base_model:adapter:meta-llama/Meta-Llama-3-8B-Instruct",
"license:other",
"region:us"
] | null | "2024-04-23T16:10:29Z" | ---
license: other
library_name: peft
tags:
- generated_from_trainer
base_model: meta-llama/Meta-Llama-3-8B-Instruct
model-index:
- name: llama3-8b-summary
results: []
---
<!-- This model card has been generated automatically according to the information the Trainer had access to. You
should probably proofread and complete it, then remove this comment. -->
# llama3-8b-summary
This model is a fine-tuned version of [meta-llama/Meta-Llama-3-8B-Instruct](https://huggingface.co/meta-llama/Meta-Llama-3-8B-Instruct) on an unknown dataset.
## Model description
More information needed
## Intended uses & limitations
More information needed
## Training and evaluation data
More information needed
## Training procedure
### Training hyperparameters
The following hyperparameters were used during training:
- learning_rate: 1e-05
- train_batch_size: 1
- eval_batch_size: 8
- seed: 42
- optimizer: Adam with betas=(0.9,0.999) and epsilon=1e-08
- lr_scheduler_type: linear
- training_steps: 8000
- mixed_precision_training: Native AMP
### Training results
### Framework versions
- PEFT 0.10.0
- Transformers 4.40.0
- Pytorch 2.2.1+cu121
- Datasets 2.19.0
- Tokenizers 0.19.1 |
Jackson9z4x9/SFT-calculator | Jackson9z4x9 | "2025-02-12T01:15:31Z" | 0 | 0 | transformers | [
"transformers",
"safetensors",
"arxiv:1910.09700",
"endpoints_compatible",
"region:us"
] | null | "2025-02-10T22:10:39Z" | ---
library_name: transformers
tags: []
---
# Model Card for Model ID
<!-- Provide a quick summary of what the model is/does. -->
## Model Details
### Model Description
<!-- Provide a longer summary of what this model is. -->
This is the model card of a 🤗 transformers model that has been pushed on the Hub. This model card has been automatically generated.
- **Developed by:** [More Information Needed]
- **Funded by [optional]:** [More Information Needed]
- **Shared by [optional]:** [More Information Needed]
- **Model type:** [More Information Needed]
- **Language(s) (NLP):** [More Information Needed]
- **License:** [More Information Needed]
- **Finetuned from model [optional]:** [More Information Needed]
### Model Sources [optional]
<!-- Provide the basic links for the model. -->
- **Repository:** [More Information Needed]
- **Paper [optional]:** [More Information Needed]
- **Demo [optional]:** [More Information Needed]
## Uses
<!-- Address questions around how the model is intended to be used, including the foreseeable users of the model and those affected by the model. -->
### Direct Use
<!-- This section is for the model use without fine-tuning or plugging into a larger ecosystem/app. -->
[More Information Needed]
### Downstream Use [optional]
<!-- This section is for the model use when fine-tuned for a task, or when plugged into a larger ecosystem/app -->
[More Information Needed]
### Out-of-Scope Use
<!-- This section addresses misuse, malicious use, and uses that the model will not work well for. -->
[More Information Needed]
## Bias, Risks, and Limitations
<!-- This section is meant to convey both technical and sociotechnical limitations. -->
[More Information Needed]
### Recommendations
<!-- This section is meant to convey recommendations with respect to the bias, risk, and technical limitations. -->
Users (both direct and downstream) should be made aware of the risks, biases and limitations of the model. More information needed for further recommendations.
## How to Get Started with the Model
Use the code below to get started with the model.
[More Information Needed]
## Training Details
### Training Data
<!-- This should link to a Dataset Card, perhaps with a short stub of information on what the training data is all about as well as documentation related to data pre-processing or additional filtering. -->
[More Information Needed]
### Training Procedure
<!-- This relates heavily to the Technical Specifications. Content here should link to that section when it is relevant to the training procedure. -->
#### Preprocessing [optional]
[More Information Needed]
#### Training Hyperparameters
- **Training regime:** [More Information Needed] <!--fp32, fp16 mixed precision, bf16 mixed precision, bf16 non-mixed precision, fp16 non-mixed precision, fp8 mixed precision -->
#### Speeds, Sizes, Times [optional]
<!-- This section provides information about throughput, start/end time, checkpoint size if relevant, etc. -->
[More Information Needed]
## Evaluation
<!-- This section describes the evaluation protocols and provides the results. -->
### Testing Data, Factors & Metrics
#### Testing Data
<!-- This should link to a Dataset Card if possible. -->
[More Information Needed]
#### Factors
<!-- These are the things the evaluation is disaggregating by, e.g., subpopulations or domains. -->
[More Information Needed]
#### Metrics
<!-- These are the evaluation metrics being used, ideally with a description of why. -->
[More Information Needed]
### Results
[More Information Needed]
#### Summary
## Model Examination [optional]
<!-- Relevant interpretability work for the model goes here -->
[More Information Needed]
## Environmental Impact
<!-- Total emissions (in grams of CO2eq) and additional considerations, such as electricity usage, go here. Edit the suggested text below accordingly -->
Carbon emissions can be estimated using the [Machine Learning Impact calculator](https://mlco2.github.io/impact#compute) presented in [Lacoste et al. (2019)](https://arxiv.org/abs/1910.09700).
- **Hardware Type:** [More Information Needed]
- **Hours used:** [More Information Needed]
- **Cloud Provider:** [More Information Needed]
- **Compute Region:** [More Information Needed]
- **Carbon Emitted:** [More Information Needed]
## Technical Specifications [optional]
### Model Architecture and Objective
[More Information Needed]
### Compute Infrastructure
[More Information Needed]
#### Hardware
[More Information Needed]
#### Software
[More Information Needed]
## Citation [optional]
<!-- If there is a paper or blog post introducing the model, the APA and Bibtex information for that should go in this section. -->
**BibTeX:**
[More Information Needed]
**APA:**
[More Information Needed]
## Glossary [optional]
<!-- If relevant, include terms and calculations in this section that can help readers understand the model or model card. -->
[More Information Needed]
## More Information [optional]
[More Information Needed]
## Model Card Authors [optional]
[More Information Needed]
## Model Card Contact
[More Information Needed] |
Eswann/ML-Agents-Pyramids | Eswann | "2023-11-16T11:54:44Z" | 0 | 0 | ml-agents | [
"ml-agents",
"tensorboard",
"onnx",
"Pyramids",
"deep-reinforcement-learning",
"reinforcement-learning",
"ML-Agents-Pyramids",
"region:us"
] | reinforcement-learning | "2023-11-16T11:54:41Z" | ---
library_name: ml-agents
tags:
- Pyramids
- deep-reinforcement-learning
- reinforcement-learning
- ML-Agents-Pyramids
---
# **ppo** Agent playing **Pyramids**
This is a trained model of a **ppo** agent playing **Pyramids**
using the [Unity ML-Agents Library](https://github.com/Unity-Technologies/ml-agents).
## Usage (with ML-Agents)
The Documentation: https://unity-technologies.github.io/ml-agents/ML-Agents-Toolkit-Documentation/
We wrote a complete tutorial to learn to train your first agent using ML-Agents and publish it to the Hub:
- A *short tutorial* where you teach Huggy the Dog 🐶 to fetch the stick and then play with him directly in your
browser: https://huggingface.co/learn/deep-rl-course/unitbonus1/introduction
- A *longer tutorial* to understand how works ML-Agents:
https://huggingface.co/learn/deep-rl-course/unit5/introduction
### Resume the training
```bash
mlagents-learn <your_configuration_file_path.yaml> --run-id=<run_id> --resume
```
### Watch your Agent play
You can watch your agent **playing directly in your browser**
1. If the environment is part of ML-Agents official environments, go to https://huggingface.co/unity
2. Step 1: Find your model_id: Eswann/ML-Agents-Pyramids
3. Step 2: Select your *.nn /*.onnx file
4. Click on Watch the agent play 👀
|
judithrosell/ClinicalBERT_JNLPBA_NER_new | judithrosell | "2023-12-31T18:33:11Z" | 6 | 0 | transformers | [
"transformers",
"tensorboard",
"safetensors",
"distilbert",
"token-classification",
"generated_from_trainer",
"base_model:medicalai/ClinicalBERT",
"base_model:finetune:medicalai/ClinicalBERT",
"autotrain_compatible",
"endpoints_compatible",
"region:us"
] | token-classification | "2023-12-31T15:04:47Z" | ---
base_model: medicalai/ClinicalBERT
tags:
- generated_from_trainer
metrics:
- precision
- recall
- f1
- accuracy
model-index:
- name: ClinicalBERT_JNLPBA_NER_new
results: []
---
<!-- This model card has been generated automatically according to the information the Trainer had access to. You
should probably proofread and complete it, then remove this comment. -->
# ClinicalBERT_JNLPBA_NER_new
This model is a fine-tuned version of [medicalai/ClinicalBERT](https://huggingface.co/medicalai/ClinicalBERT) on the None dataset.
It achieves the following results on the evaluation set:
- Loss: 0.1699
- Precision: 0.7855
- Recall: 0.8043
- F1: 0.7948
- Accuracy: 0.9439
## Model description
More information needed
## Intended uses & limitations
More information needed
## Training and evaluation data
More information needed
## Training procedure
### Training hyperparameters
The following hyperparameters were used during training:
- learning_rate: 2e-05
- train_batch_size: 16
- eval_batch_size: 16
- seed: 42
- optimizer: Adam with betas=(0.9,0.999) and epsilon=1e-08
- lr_scheduler_type: linear
- num_epochs: 3
### Training results
| Training Loss | Epoch | Step | Validation Loss | Precision | Recall | F1 | Accuracy |
|:-------------:|:-----:|:----:|:---------------:|:---------:|:------:|:------:|:--------:|
| 0.2204 | 1.0 | 1164 | 0.1821 | 0.7652 | 0.7719 | 0.7685 | 0.9380 |
| 0.1618 | 2.0 | 2328 | 0.1716 | 0.7884 | 0.7886 | 0.7885 | 0.9426 |
| 0.1338 | 3.0 | 3492 | 0.1699 | 0.7855 | 0.8043 | 0.7948 | 0.9439 |
### Framework versions
- Transformers 4.35.2
- Pytorch 2.1.0+cu121
- Datasets 2.16.1
- Tokenizers 0.15.0
|
kelestemur/deep_rl | kelestemur | "2023-01-08T21:58:21Z" | 1 | 0 | stable-baselines3 | [
"stable-baselines3",
"LunarLander-v2",
"deep-reinforcement-learning",
"reinforcement-learning",
"model-index",
"region:us"
] | reinforcement-learning | "2023-01-08T21:57:58Z" | ---
library_name: stable-baselines3
tags:
- LunarLander-v2
- deep-reinforcement-learning
- reinforcement-learning
- stable-baselines3
model-index:
- name: PPO
results:
- task:
type: reinforcement-learning
name: reinforcement-learning
dataset:
name: LunarLander-v2
type: LunarLander-v2
metrics:
- type: mean_reward
value: 264.57 +/- 20.35
name: mean_reward
verified: false
---
# **PPO** Agent playing **LunarLander-v2**
This is a trained model of a **PPO** agent playing **LunarLander-v2**
using the [stable-baselines3 library](https://github.com/DLR-RM/stable-baselines3).
## Usage (with Stable-baselines3)
TODO: Add your code
```python
from stable_baselines3 import ...
from huggingface_sb3 import load_from_hub
...
```
|
mlninad/deciLM-finetuned | mlninad | "2023-10-23T19:22:53Z" | 15 | 0 | transformers | [
"transformers",
"pytorch",
"llama",
"text-generation",
"custom_code",
"en",
"license:apache-2.0",
"autotrain_compatible",
"text-generation-inference",
"endpoints_compatible",
"region:us"
] | text-generation | "2023-10-22T09:13:33Z" | ---
license: apache-2.0
language:
- en
--- |
Narrativaai/deberta-v3-small-finetuned-hate_speech18 | Narrativaai | "2021-12-01T17:41:13Z" | 9 | 3 | transformers | [
"transformers",
"pytorch",
"tensorboard",
"deberta-v2",
"text-classification",
"generated_from_trainer",
"dataset:hate_speech18",
"license:mit",
"model-index",
"autotrain_compatible",
"endpoints_compatible",
"region:us"
] | text-classification | "2022-03-02T23:29:04Z" | ---
license: mit
tags:
- generated_from_trainer
datasets:
- hate_speech18
widget:
- text: "ok, so do we need to kill them too or are the slavs okay ? for some reason whenever i hear the word slav , the word slobber comes to mind and i picture a slobbering half breed creature like the humpback of notre dame or Igor haha"
metrics:
- accuracy
model-index:
- name: deberta-v3-small-hate-speech
results:
- task:
name: Text Classification
type: text-classification
dataset:
name: hate_speech18
type: hate_speech18
args: default
metrics:
- name: Accuracy
type: accuracy
value: 0.916058394160584
---
<!-- This model card has been generated automatically according to the information the Trainer had access to. You
should probably proofread and complete it, then remove this comment. -->
# DeBERTa v3 small fine-tuned on hate_speech18 dataset for Hate Speech Detection
This model is a fine-tuned version of [microsoft/deberta-v3-small](https://huggingface.co/microsoft/deberta-v3-small) on the hate_speech18 dataset.
It achieves the following results on the evaluation set:
- Loss: 0.2922
- Accuracy: 0.9161
## Model description
More information needed
## Intended uses & limitations
More information needed
## Training and evaluation data
More information needed
## Training procedure
### Training hyperparameters
The following hyperparameters were used during training:
- learning_rate: 2e-05
- train_batch_size: 16
- eval_batch_size: 16
- seed: 42
- optimizer: Adam with betas=(0.9,0.999) and epsilon=1e-08
- lr_scheduler_type: linear
- num_epochs: 5
### Training results
| Training Loss | Epoch | Step | Validation Loss | Accuracy |
|:-------------:|:-----:|:----:|:---------------:|:--------:|
| 0.4147 | 1.0 | 650 | 0.3910 | 0.8832 |
| 0.2975 | 2.0 | 1300 | 0.2922 | 0.9161 |
| 0.2575 | 3.0 | 1950 | 0.3555 | 0.9051 |
| 0.1553 | 4.0 | 2600 | 0.4263 | 0.9124 |
| 0.1267 | 5.0 | 3250 | 0.4238 | 0.9161 |
### Framework versions
- Transformers 4.12.5
- Pytorch 1.10.0+cu111
- Datasets 1.16.1
- Tokenizers 0.10.3
|
mradermacher/Reverb-7b-Uncensored-DeLMAT-i1-GGUF | mradermacher | "2025-02-22T10:00:05Z" | 0 | 0 | transformers | [
"transformers",
"gguf",
"en",
"base_model:nkpz/Reverb-7b-Uncensored-DeLMAT",
"base_model:quantized:nkpz/Reverb-7b-Uncensored-DeLMAT",
"license:apache-2.0",
"endpoints_compatible",
"region:us",
"imatrix",
"conversational"
] | null | "2025-02-22T06:06:48Z" | ---
base_model: nkpz/Reverb-7b-Uncensored-DeLMAT
language:
- en
library_name: transformers
license: apache-2.0
quantized_by: mradermacher
---
## About
<!-- ### quantize_version: 2 -->
<!-- ### output_tensor_quantised: 1 -->
<!-- ### convert_type: hf -->
<!-- ### vocab_type: -->
<!-- ### tags: nicoboss -->
weighted/imatrix quants of https://huggingface.co/nkpz/Reverb-7b-Uncensored-DeLMAT
<!-- provided-files -->
static quants are available at https://huggingface.co/mradermacher/Reverb-7b-Uncensored-DeLMAT-GGUF
## Usage
If you are unsure how to use GGUF files, refer to one of [TheBloke's
READMEs](https://huggingface.co/TheBloke/KafkaLM-70B-German-V0.1-GGUF) for
more details, including on how to concatenate multi-part files.
## Provided Quants
(sorted by size, not necessarily quality. IQ-quants are often preferable over similar sized non-IQ quants)
| Link | Type | Size/GB | Notes |
|:-----|:-----|--------:|:------|
| [GGUF](https://huggingface.co/mradermacher/Reverb-7b-Uncensored-DeLMAT-i1-GGUF/resolve/main/Reverb-7b-Uncensored-DeLMAT.i1-IQ1_S.gguf) | i1-IQ1_S | 2.0 | for the desperate |
| [GGUF](https://huggingface.co/mradermacher/Reverb-7b-Uncensored-DeLMAT-i1-GGUF/resolve/main/Reverb-7b-Uncensored-DeLMAT.i1-IQ1_M.gguf) | i1-IQ1_M | 2.1 | mostly desperate |
| [GGUF](https://huggingface.co/mradermacher/Reverb-7b-Uncensored-DeLMAT-i1-GGUF/resolve/main/Reverb-7b-Uncensored-DeLMAT.i1-IQ2_XXS.gguf) | i1-IQ2_XXS | 2.4 | |
| [GGUF](https://huggingface.co/mradermacher/Reverb-7b-Uncensored-DeLMAT-i1-GGUF/resolve/main/Reverb-7b-Uncensored-DeLMAT.i1-IQ2_XS.gguf) | i1-IQ2_XS | 2.6 | |
| [GGUF](https://huggingface.co/mradermacher/Reverb-7b-Uncensored-DeLMAT-i1-GGUF/resolve/main/Reverb-7b-Uncensored-DeLMAT.i1-IQ2_S.gguf) | i1-IQ2_S | 2.7 | |
| [GGUF](https://huggingface.co/mradermacher/Reverb-7b-Uncensored-DeLMAT-i1-GGUF/resolve/main/Reverb-7b-Uncensored-DeLMAT.i1-IQ2_M.gguf) | i1-IQ2_M | 2.9 | |
| [GGUF](https://huggingface.co/mradermacher/Reverb-7b-Uncensored-DeLMAT-i1-GGUF/resolve/main/Reverb-7b-Uncensored-DeLMAT.i1-Q2_K_S.gguf) | i1-Q2_K_S | 2.9 | very low quality |
| [GGUF](https://huggingface.co/mradermacher/Reverb-7b-Uncensored-DeLMAT-i1-GGUF/resolve/main/Reverb-7b-Uncensored-DeLMAT.i1-Q2_K.gguf) | i1-Q2_K | 3.1 | IQ3_XXS probably better |
| [GGUF](https://huggingface.co/mradermacher/Reverb-7b-Uncensored-DeLMAT-i1-GGUF/resolve/main/Reverb-7b-Uncensored-DeLMAT.i1-IQ3_XXS.gguf) | i1-IQ3_XXS | 3.2 | lower quality |
| [GGUF](https://huggingface.co/mradermacher/Reverb-7b-Uncensored-DeLMAT-i1-GGUF/resolve/main/Reverb-7b-Uncensored-DeLMAT.i1-IQ3_XS.gguf) | i1-IQ3_XS | 3.4 | |
| [GGUF](https://huggingface.co/mradermacher/Reverb-7b-Uncensored-DeLMAT-i1-GGUF/resolve/main/Reverb-7b-Uncensored-DeLMAT.i1-Q3_K_S.gguf) | i1-Q3_K_S | 3.6 | IQ3_XS probably better |
| [GGUF](https://huggingface.co/mradermacher/Reverb-7b-Uncensored-DeLMAT-i1-GGUF/resolve/main/Reverb-7b-Uncensored-DeLMAT.i1-IQ3_S.gguf) | i1-IQ3_S | 3.6 | beats Q3_K* |
| [GGUF](https://huggingface.co/mradermacher/Reverb-7b-Uncensored-DeLMAT-i1-GGUF/resolve/main/Reverb-7b-Uncensored-DeLMAT.i1-IQ3_M.gguf) | i1-IQ3_M | 3.7 | |
| [GGUF](https://huggingface.co/mradermacher/Reverb-7b-Uncensored-DeLMAT-i1-GGUF/resolve/main/Reverb-7b-Uncensored-DeLMAT.i1-Q3_K_M.gguf) | i1-Q3_K_M | 3.9 | IQ3_S probably better |
| [GGUF](https://huggingface.co/mradermacher/Reverb-7b-Uncensored-DeLMAT-i1-GGUF/resolve/main/Reverb-7b-Uncensored-DeLMAT.i1-Q3_K_L.gguf) | i1-Q3_K_L | 4.2 | IQ3_M probably better |
| [GGUF](https://huggingface.co/mradermacher/Reverb-7b-Uncensored-DeLMAT-i1-GGUF/resolve/main/Reverb-7b-Uncensored-DeLMAT.i1-IQ4_XS.gguf) | i1-IQ4_XS | 4.3 | |
| [GGUF](https://huggingface.co/mradermacher/Reverb-7b-Uncensored-DeLMAT-i1-GGUF/resolve/main/Reverb-7b-Uncensored-DeLMAT.i1-IQ4_NL.gguf) | i1-IQ4_NL | 4.5 | prefer IQ4_XS |
| [GGUF](https://huggingface.co/mradermacher/Reverb-7b-Uncensored-DeLMAT-i1-GGUF/resolve/main/Reverb-7b-Uncensored-DeLMAT.i1-Q4_0.gguf) | i1-Q4_0 | 4.5 | fast, low quality |
| [GGUF](https://huggingface.co/mradermacher/Reverb-7b-Uncensored-DeLMAT-i1-GGUF/resolve/main/Reverb-7b-Uncensored-DeLMAT.i1-Q4_K_S.gguf) | i1-Q4_K_S | 4.6 | optimal size/speed/quality |
| [GGUF](https://huggingface.co/mradermacher/Reverb-7b-Uncensored-DeLMAT-i1-GGUF/resolve/main/Reverb-7b-Uncensored-DeLMAT.i1-Q4_K_M.gguf) | i1-Q4_K_M | 4.8 | fast, recommended |
| [GGUF](https://huggingface.co/mradermacher/Reverb-7b-Uncensored-DeLMAT-i1-GGUF/resolve/main/Reverb-7b-Uncensored-DeLMAT.i1-Q4_1.gguf) | i1-Q4_1 | 5.0 | |
| [GGUF](https://huggingface.co/mradermacher/Reverb-7b-Uncensored-DeLMAT-i1-GGUF/resolve/main/Reverb-7b-Uncensored-DeLMAT.i1-Q5_K_S.gguf) | i1-Q5_K_S | 5.4 | |
| [GGUF](https://huggingface.co/mradermacher/Reverb-7b-Uncensored-DeLMAT-i1-GGUF/resolve/main/Reverb-7b-Uncensored-DeLMAT.i1-Q5_K_M.gguf) | i1-Q5_K_M | 5.5 | |
| [GGUF](https://huggingface.co/mradermacher/Reverb-7b-Uncensored-DeLMAT-i1-GGUF/resolve/main/Reverb-7b-Uncensored-DeLMAT.i1-Q6_K.gguf) | i1-Q6_K | 6.4 | practically like static Q6_K |
Here is a handy graph by ikawrakow comparing some lower-quality quant
types (lower is better):

And here are Artefact2's thoughts on the matter:
https://gist.github.com/Artefact2/b5f810600771265fc1e39442288e8ec9
## FAQ / Model Request
See https://huggingface.co/mradermacher/model_requests for some answers to
questions you might have and/or if you want some other model quantized.
## Thanks
I thank my company, [nethype GmbH](https://www.nethype.de/), for letting
me use its servers and providing upgrades to my workstation to enable
this work in my free time. Additional thanks to [@nicoboss](https://huggingface.co/nicoboss) for giving me access to his private supercomputer, enabling me to provide many more imatrix quants, at much higher quality, than I would otherwise be able to.
<!-- end -->
|
sail-rvc/VergilRVC2byDreamnaught | sail-rvc | "2023-07-14T07:33:52Z" | 1 | 0 | transformers | [
"transformers",
"rvc",
"sail-rvc",
"audio-to-audio",
"endpoints_compatible",
"region:us"
] | audio-to-audio | "2023-07-14T07:33:33Z" |
---
pipeline_tag: audio-to-audio
tags:
- rvc
- sail-rvc
---
# VergilRVC2byDreamnaught
## RVC Model

This model repo was automatically generated.
Date: 2023-07-14 07:33:52
Bot Name: juuxnscrap
Model Type: RVC
Source: https://huggingface.co/juuxn/RVCModels/
Reason: Converting into loadable format for https://github.com/chavinlo/rvc-runpod
|
farleyknight/arxiv-summarization-fb-bart-base-2022-09-21 | farleyknight | "2022-09-23T08:34:25Z" | 121 | 0 | transformers | [
"transformers",
"pytorch",
"bart",
"text2text-generation",
"generated_from_trainer",
"dataset:ccdv/arxiv-summarization",
"license:apache-2.0",
"model-index",
"autotrain_compatible",
"endpoints_compatible",
"region:us"
] | text2text-generation | "2022-09-21T23:10:43Z" | ---
license: apache-2.0
tags:
- generated_from_trainer
datasets:
- ccdv/arxiv-summarization
metrics:
- rouge
model-index:
- name: arxiv-summarization-fb-bart-base-2022-09-21
results:
- task:
name: Summarization
type: summarization
dataset:
name: ccdv/arxiv-summarization
type: ccdv/arxiv-summarization
config: section
split: train
args: section
metrics:
- name: Rouge1
type: rouge
value: 42.9082
---
<!-- This model card has been generated automatically according to the information the Trainer had access to. You
should probably proofread and complete it, then remove this comment. -->
# arxiv-summarization-fb-bart-base-2022-09-21
This model is a fine-tuned version of [facebook/bart-base](https://huggingface.co/facebook/bart-base) on the ccdv/arxiv-summarization dataset.
It achieves the following results on the evaluation set:
- Loss: 2.1597
- Rouge1: 42.9082
- Rouge2: 15.7763
- Rougel: 25.9239
- Rougelsum: 37.7957
- Gen Len: 110.5816
## Model description
More information needed
## Intended uses & limitations
More information needed
## Training and evaluation data
More information needed
## Training procedure
### Training hyperparameters
The following hyperparameters were used during training:
- learning_rate: 5e-05
- train_batch_size: 1
- eval_batch_size: 1
- seed: 42
- optimizer: Adam with betas=(0.9,0.999) and epsilon=1e-08
- lr_scheduler_type: linear
- num_epochs: 3.0
### Training results
| Training Loss | Epoch | Step | Validation Loss | Rouge1 | Rouge2 | Rougel | Rougelsum | Gen Len |
|:-------------:|:-----:|:------:|:---------------:|:-------:|:------:|:-------:|:---------:|:-------:|
| 2.9142 | 0.05 | 10000 | 2.7522 | 17.073 | 6.7502 | 13.6779 | 15.6668 | 20.0 |
| 2.7876 | 0.1 | 20000 | 2.6888 | 16.7954 | 6.7038 | 13.4939 | 15.3426 | 19.9992 |
| 2.715 | 0.15 | 30000 | 2.6308 | 17.3324 | 6.8771 | 13.7918 | 15.7839 | 20.0 |
| 2.6431 | 0.2 | 40000 | 2.5858 | 16.7055 | 6.8108 | 13.4796 | 15.2959 | 20.0 |
| 2.6381 | 0.25 | 50000 | 2.5393 | 17.4643 | 7.0687 | 13.9507 | 16.012 | 20.0 |
| 2.6269 | 0.3 | 60000 | 2.5159 | 17.5934 | 7.0022 | 13.9394 | 16.0203 | 20.0 |
| 2.5482 | 0.34 | 70000 | 2.4894 | 17.5428 | 7.1822 | 13.9788 | 16.0355 | 20.0 |
| 2.4962 | 0.39 | 80000 | 2.4476 | 17.3587 | 7.1501 | 13.9215 | 15.8637 | 20.0 |
| 2.513 | 0.44 | 90000 | 2.4309 | 18.0806 | 7.5429 | 14.4201 | 16.561 | 20.0 |
| 2.4464 | 0.49 | 100000 | 2.4128 | 17.9813 | 7.5454 | 14.3403 | 16.52 | 19.9989 |
| 2.4969 | 0.54 | 110000 | 2.4114 | 17.3353 | 7.1382 | 13.9109 | 15.873 | 20.0 |
| 2.4417 | 0.59 | 120000 | 2.3866 | 18.0241 | 7.553 | 14.3892 | 16.5077 | 19.9980 |
| 2.4333 | 0.64 | 130000 | 2.3903 | 18.0578 | 7.4999 | 14.3901 | 16.5134 | 20.0 |
| 2.4296 | 0.69 | 140000 | 2.3793 | 17.7742 | 7.5182 | 14.2794 | 16.2879 | 20.0 |
| 2.4277 | 0.74 | 150000 | 2.3571 | 17.8015 | 7.4677 | 14.226 | 16.3288 | 20.0 |
| 2.4258 | 0.79 | 160000 | 2.3539 | 17.5335 | 7.399 | 14.09 | 16.0936 | 20.0 |
| 2.4006 | 0.84 | 170000 | 2.3469 | 17.5983 | 7.4285 | 14.1315 | 16.1385 | 20.0 |
| 2.367 | 0.89 | 180000 | 2.3344 | 17.297 | 7.2361 | 13.9286 | 15.8352 | 20.0 |
| 2.373 | 0.94 | 190000 | 2.3377 | 17.7189 | 7.4993 | 14.2603 | 16.2546 | 19.9980 |
| 2.3762 | 0.99 | 200000 | 2.3106 | 17.7883 | 7.4766 | 14.2675 | 16.3115 | 20.0 |
| 2.2538 | 1.03 | 210000 | 2.3197 | 17.4487 | 7.4171 | 14.0473 | 15.9771 | 20.0 |
| 2.268 | 1.08 | 220000 | 2.3044 | 17.9603 | 7.5806 | 14.3755 | 16.4328 | 20.0 |
| 2.2986 | 1.13 | 230000 | 2.3002 | 17.9268 | 7.5321 | 14.3503 | 16.4191 | 20.0 |
| 2.241 | 1.18 | 240000 | 2.3059 | 17.4542 | 7.3224 | 14.0578 | 16.0157 | 20.0 |
| 2.2534 | 1.23 | 250000 | 2.2927 | 17.8039 | 7.6232 | 14.2916 | 16.3442 | 20.0 |
| 2.26 | 1.28 | 260000 | 2.2910 | 17.8607 | 7.5645 | 14.318 | 16.3336 | 19.9983 |
| 2.3 | 1.33 | 270000 | 2.2818 | 17.8203 | 7.4815 | 14.3171 | 16.3309 | 20.0 |
| 2.2964 | 1.38 | 280000 | 2.2721 | 17.983 | 7.6867 | 14.3971 | 16.493 | 20.0 |
| 2.2564 | 1.43 | 290000 | 2.2701 | 18.059 | 7.7273 | 14.4806 | 16.5792 | 19.9988 |
| 2.2576 | 1.48 | 300000 | 2.2663 | 17.5706 | 7.4424 | 14.1424 | 16.1297 | 20.0 |
| 2.2605 | 1.53 | 310000 | 2.2607 | 17.8057 | 7.5219 | 14.3226 | 16.3355 | 19.9988 |
| 2.2587 | 1.58 | 320000 | 2.2552 | 18.0396 | 7.7064 | 14.5005 | 16.5823 | 20.0 |
| 2.2423 | 1.63 | 330000 | 2.2523 | 18.2229 | 7.8398 | 14.5868 | 16.7408 | 20.0 |
| 2.2793 | 1.68 | 340000 | 2.2431 | 17.6785 | 7.5437 | 14.1971 | 16.1724 | 19.9988 |
| 2.2005 | 1.72 | 350000 | 2.2343 | 17.7552 | 7.6026 | 14.2152 | 16.2797 | 19.9988 |
| 2.2454 | 1.77 | 360000 | 2.2339 | 17.9292 | 7.699 | 14.4099 | 16.4682 | 20.0 |
| 2.2175 | 1.82 | 370000 | 2.2345 | 17.7413 | 7.4892 | 14.2223 | 16.2442 | 20.0 |
| 2.238 | 1.87 | 380000 | 2.2259 | 17.6679 | 7.4976 | 14.24 | 16.243 | 19.9988 |
| 2.2108 | 1.92 | 390000 | 2.2210 | 17.8474 | 7.6054 | 14.3494 | 16.3635 | 19.9988 |
| 2.2124 | 1.97 | 400000 | 2.2170 | 17.8019 | 7.5182 | 14.264 | 16.3003 | 20.0 |
| 2.0976 | 2.02 | 410000 | 2.2248 | 17.8063 | 7.5383 | 14.2782 | 16.275 | 20.0 |
| 2.0932 | 2.07 | 420000 | 2.2196 | 17.9171 | 7.6187 | 14.3508 | 16.4333 | 20.0 |
| 2.0956 | 2.12 | 430000 | 2.2135 | 18.0616 | 7.7655 | 14.4837 | 16.5627 | 19.9988 |
| 2.0515 | 2.17 | 440000 | 2.2091 | 18.0281 | 7.7301 | 14.4696 | 16.5196 | 19.9981 |
| 2.1216 | 2.22 | 450000 | 2.2015 | 18.0609 | 7.7541 | 14.4633 | 16.5705 | 19.9988 |
| 2.1222 | 2.27 | 460000 | 2.1983 | 18.0717 | 7.7473 | 14.4725 | 16.5399 | 19.9988 |
| 2.0903 | 2.32 | 470000 | 2.2007 | 18.0751 | 7.7486 | 14.4583 | 16.546 | 20.0 |
| 2.1124 | 2.37 | 480000 | 2.1934 | 17.888 | 7.7124 | 14.3899 | 16.3901 | 20.0 |
| 2.1094 | 2.41 | 490000 | 2.1901 | 18.0254 | 7.7682 | 14.4427 | 16.5181 | 20.0 |
| 2.1085 | 2.46 | 500000 | 2.1924 | 17.9077 | 7.7004 | 14.3843 | 16.4221 | 19.9988 |
| 2.0781 | 2.51 | 510000 | 2.1781 | 18.1591 | 7.8456 | 14.565 | 16.6435 | 19.9988 |
| 2.0875 | 2.56 | 520000 | 2.1801 | 18.0389 | 7.7342 | 14.4259 | 16.5378 | 20.0 |
| 2.0945 | 2.61 | 530000 | 2.1758 | 18.0999 | 7.8217 | 14.5163 | 16.5784 | 19.9988 |
| 2.0723 | 2.66 | 540000 | 2.1756 | 17.9684 | 7.7369 | 14.4279 | 16.4815 | 19.9988 |
| 2.0918 | 2.71 | 550000 | 2.1738 | 18.1183 | 7.8414 | 14.5298 | 16.6119 | 19.9988 |
| 2.0835 | 2.76 | 560000 | 2.1671 | 17.8837 | 7.7379 | 14.3727 | 16.4068 | 19.9988 |
| 2.0936 | 2.81 | 570000 | 2.1670 | 17.9631 | 7.7708 | 14.4566 | 16.4823 | 19.9988 |
| 2.0518 | 2.86 | 580000 | 2.1631 | 18.0601 | 7.8112 | 14.5158 | 16.5816 | 19.9988 |
| 2.065 | 2.91 | 590000 | 2.1611 | 18.0548 | 7.8147 | 14.5271 | 16.5606 | 19.9988 |
| 2.0427 | 2.96 | 600000 | 2.1611 | 18.0642 | 7.8284 | 14.5293 | 16.5736 | 19.9988 |
### Framework versions
- Transformers 4.23.0.dev0
- Pytorch 1.12.0
- Datasets 2.5.1
- Tokenizers 0.13.0
|
jeroenherczeg/shawgpt-ft | jeroenherczeg | "2024-04-05T08:21:37Z" | 2 | 0 | peft | [
"peft",
"tensorboard",
"safetensors",
"generated_from_trainer",
"base_model:TheBloke/Mistral-7B-Instruct-v0.2-GPTQ",
"base_model:adapter:TheBloke/Mistral-7B-Instruct-v0.2-GPTQ",
"license:apache-2.0",
"region:us"
] | null | "2024-04-04T16:46:03Z" | ---
license: apache-2.0
library_name: peft
tags:
- generated_from_trainer
base_model: TheBloke/Mistral-7B-Instruct-v0.2-GPTQ
model-index:
- name: shawgpt-ft
results: []
---
<!-- This model card has been generated automatically according to the information the Trainer had access to. You
should probably proofread and complete it, then remove this comment. -->
# shawgpt-ft
This model is a fine-tuned version of [TheBloke/Mistral-7B-Instruct-v0.2-GPTQ](https://huggingface.co/TheBloke/Mistral-7B-Instruct-v0.2-GPTQ) on an unknown dataset.
It achieves the following results on the evaluation set:
- Loss: 4.2320
## Model description
More information needed
## Intended uses & limitations
More information needed
## Training and evaluation data
More information needed
## Training procedure
### Training hyperparameters
The following hyperparameters were used during training:
- learning_rate: 0.0002
- train_batch_size: 4
- eval_batch_size: 4
- seed: 42
- gradient_accumulation_steps: 4
- total_train_batch_size: 16
- optimizer: Adam with betas=(0.9,0.999) and epsilon=1e-08
- lr_scheduler_type: linear
- lr_scheduler_warmup_steps: 1
- training_steps: 1000
### Training results
| Training Loss | Epoch | Step | Validation Loss |
|:-------------:|:------:|:----:|:---------------:|
| 4.6433 | 0.92 | 3 | 4.2320 |
| 4.6544 | 1.85 | 6 | 4.2320 |
| 4.6459 | 2.77 | 9 | 4.2320 |
| 3.4822 | 4.0 | 13 | 4.2320 |
| 4.6298 | 4.92 | 16 | 4.2320 |
| 4.6605 | 5.85 | 19 | 4.2320 |
| 4.6392 | 6.77 | 22 | 4.2320 |
| 3.4844 | 8.0 | 26 | 4.2320 |
| 4.6305 | 8.92 | 29 | 4.2320 |
| 4.6337 | 9.85 | 32 | 4.2320 |
| 4.6501 | 10.77 | 35 | 4.2320 |
| 3.4793 | 12.0 | 39 | 4.2320 |
| 4.6568 | 12.92 | 42 | 4.2320 |
| 4.6402 | 13.85 | 45 | 4.2320 |
| 4.6381 | 14.77 | 48 | 4.2320 |
| 3.4787 | 16.0 | 52 | 4.2320 |
| 4.671 | 16.92 | 55 | 4.2320 |
| 4.6186 | 17.85 | 58 | 4.2320 |
| 4.6403 | 18.77 | 61 | 4.2320 |
| 3.5009 | 20.0 | 65 | 4.2320 |
| 4.6514 | 20.92 | 68 | 4.2320 |
| 4.6426 | 21.85 | 71 | 4.2320 |
| 4.6674 | 22.77 | 74 | 4.2320 |
| 3.4915 | 24.0 | 78 | 4.2320 |
| 4.6606 | 24.92 | 81 | 4.2320 |
| 4.6364 | 25.85 | 84 | 4.2320 |
| 4.6222 | 26.77 | 87 | 4.2320 |
| 3.4782 | 28.0 | 91 | 4.2320 |
| 4.6229 | 28.92 | 94 | 4.2320 |
| 4.6576 | 29.85 | 97 | 4.2320 |
| 4.6288 | 30.77 | 100 | 4.2320 |
| 3.4664 | 32.0 | 104 | 4.2320 |
| 4.6434 | 32.92 | 107 | 4.2320 |
| 4.6519 | 33.85 | 110 | 4.2320 |
| 4.6528 | 34.77 | 113 | 4.2320 |
| 3.471 | 36.0 | 117 | 4.2320 |
| 4.6453 | 36.92 | 120 | 4.2320 |
| 4.616 | 37.85 | 123 | 4.2320 |
| 4.6109 | 38.77 | 126 | 4.2320 |
| 3.4799 | 40.0 | 130 | 4.2320 |
| 4.6388 | 40.92 | 133 | 4.2320 |
| 4.6711 | 41.85 | 136 | 4.2320 |
| 4.6483 | 42.77 | 139 | 4.2320 |
| 3.4695 | 44.0 | 143 | 4.2320 |
| 4.6496 | 44.92 | 146 | 4.2320 |
| 4.644 | 45.85 | 149 | 4.2320 |
| 4.6444 | 46.77 | 152 | 4.2320 |
| 3.4741 | 48.0 | 156 | 4.2320 |
| 4.6189 | 48.92 | 159 | 4.2320 |
| 4.6683 | 49.85 | 162 | 4.2320 |
| 4.6345 | 50.77 | 165 | 4.2320 |
| 3.4703 | 52.0 | 169 | 4.2320 |
| 4.6144 | 52.92 | 172 | 4.2320 |
| 4.6648 | 53.85 | 175 | 4.2320 |
| 4.6522 | 54.77 | 178 | 4.2320 |
| 3.4838 | 56.0 | 182 | 4.2320 |
| 4.6506 | 56.92 | 185 | 4.2320 |
| 4.6339 | 57.85 | 188 | 4.2320 |
| 4.638 | 58.77 | 191 | 4.2320 |
| 3.4733 | 60.0 | 195 | 4.2320 |
| 4.6604 | 60.92 | 198 | 4.2320 |
| 4.6326 | 61.85 | 201 | 4.2320 |
| 4.6612 | 62.77 | 204 | 4.2320 |
| 3.4722 | 64.0 | 208 | 4.2320 |
| 4.6292 | 64.92 | 211 | 4.2320 |
| 4.6336 | 65.85 | 214 | 4.2320 |
| 4.642 | 66.77 | 217 | 4.2320 |
| 3.4915 | 68.0 | 221 | 4.2320 |
| 4.6453 | 68.92 | 224 | 4.2320 |
| 4.6459 | 69.85 | 227 | 4.2320 |
| 4.6202 | 70.77 | 230 | 4.2320 |
| 3.4753 | 72.0 | 234 | 4.2320 |
| 4.6552 | 72.92 | 237 | 4.2320 |
| 4.6443 | 73.85 | 240 | 4.2320 |
| 4.6495 | 74.77 | 243 | 4.2320 |
| 3.4798 | 76.0 | 247 | 4.2320 |
| 4.6358 | 76.92 | 250 | 4.2320 |
| 4.6434 | 77.85 | 253 | 4.2320 |
| 4.6325 | 78.77 | 256 | 4.2320 |
| 3.4951 | 80.0 | 260 | 4.2320 |
| 4.6302 | 80.92 | 263 | 4.2320 |
| 4.6458 | 81.85 | 266 | 4.2320 |
| 4.6407 | 82.77 | 269 | 4.2320 |
| 3.4828 | 84.0 | 273 | 4.2320 |
| 4.6436 | 84.92 | 276 | 4.2320 |
| 4.6143 | 85.85 | 279 | 4.2320 |
| 4.644 | 86.77 | 282 | 4.2320 |
| 3.4934 | 88.0 | 286 | 4.2320 |
| 4.6308 | 88.92 | 289 | 4.2320 |
| 4.6715 | 89.85 | 292 | 4.2320 |
| 4.6229 | 90.77 | 295 | 4.2320 |
| 3.4895 | 92.0 | 299 | 4.2320 |
| 4.6447 | 92.92 | 302 | 4.2320 |
| 4.6333 | 93.85 | 305 | 4.2320 |
| 4.643 | 94.77 | 308 | 4.2320 |
| 3.482 | 96.0 | 312 | 4.2320 |
| 4.6647 | 96.92 | 315 | 4.2320 |
| 4.65 | 97.85 | 318 | 4.2320 |
| 4.6545 | 98.77 | 321 | 4.2320 |
| 3.4881 | 100.0 | 325 | 4.2320 |
| 4.6828 | 100.92 | 328 | 4.2320 |
| 4.6328 | 101.85 | 331 | 4.2320 |
| 4.6419 | 102.77 | 334 | 4.2320 |
| 3.4954 | 104.0 | 338 | 4.2320 |
| 4.6203 | 104.92 | 341 | 4.2320 |
| 4.6236 | 105.85 | 344 | 4.2320 |
| 4.6539 | 106.77 | 347 | 4.2320 |
| 3.4737 | 108.0 | 351 | 4.2320 |
| 4.6319 | 108.92 | 354 | 4.2320 |
| 4.6696 | 109.85 | 357 | 4.2320 |
| 4.6678 | 110.77 | 360 | 4.2320 |
| 3.4698 | 112.0 | 364 | 4.2320 |
| 4.6459 | 112.92 | 367 | 4.2320 |
| 4.6524 | 113.85 | 370 | 4.2320 |
| 4.6399 | 114.77 | 373 | 4.2320 |
| 3.471 | 116.0 | 377 | 4.2320 |
| 4.6668 | 116.92 | 380 | 4.2320 |
| 4.634 | 117.85 | 383 | 4.2320 |
| 4.6345 | 118.77 | 386 | 4.2320 |
| 3.4938 | 120.0 | 390 | 4.2320 |
| 4.6386 | 120.92 | 393 | 4.2320 |
| 4.6661 | 121.85 | 396 | 4.2320 |
| 4.6465 | 122.77 | 399 | 4.2320 |
| 3.4903 | 124.0 | 403 | 4.2320 |
| 4.6255 | 124.92 | 406 | 4.2320 |
| 4.6306 | 125.85 | 409 | 4.2320 |
| 4.6348 | 126.77 | 412 | 4.2320 |
| 3.4811 | 128.0 | 416 | 4.2320 |
| 4.6335 | 128.92 | 419 | 4.2320 |
| 4.6678 | 129.85 | 422 | 4.2320 |
| 4.6336 | 130.77 | 425 | 4.2320 |
| 3.4722 | 132.0 | 429 | 4.2320 |
| 4.6371 | 132.92 | 432 | 4.2320 |
| 4.6488 | 133.85 | 435 | 4.2320 |
| 4.6456 | 134.77 | 438 | 4.2320 |
| 3.4866 | 136.0 | 442 | 4.2320 |
| 4.6349 | 136.92 | 445 | 4.2320 |
| 4.6418 | 137.85 | 448 | 4.2320 |
| 4.6546 | 138.77 | 451 | 4.2320 |
| 3.4811 | 140.0 | 455 | 4.2320 |
| 4.6322 | 140.92 | 458 | 4.2320 |
| 4.6154 | 141.85 | 461 | 4.2320 |
| 4.6362 | 142.77 | 464 | 4.2320 |
| 3.4809 | 144.0 | 468 | 4.2320 |
| 4.6317 | 144.92 | 471 | 4.2320 |
| 4.6329 | 145.85 | 474 | 4.2320 |
| 4.636 | 146.77 | 477 | 4.2320 |
| 3.4737 | 148.0 | 481 | 4.2320 |
| 4.629 | 148.92 | 484 | 4.2320 |
| 4.6212 | 149.85 | 487 | 4.2320 |
| 4.6548 | 150.77 | 490 | 4.2320 |
| 3.481 | 152.0 | 494 | 4.2320 |
| 4.6379 | 152.92 | 497 | 4.2320 |
| 4.6306 | 153.85 | 500 | 4.2320 |
| 4.6443 | 154.77 | 503 | 4.2320 |
| 3.4951 | 156.0 | 507 | 4.2320 |
| 4.6514 | 156.92 | 510 | 4.2320 |
| 4.6539 | 157.85 | 513 | 4.2320 |
| 4.6295 | 158.77 | 516 | 4.2320 |
| 3.485 | 160.0 | 520 | 4.2320 |
| 4.6665 | 160.92 | 523 | 4.2320 |
| 4.6508 | 161.85 | 526 | 4.2320 |
| 4.6754 | 162.77 | 529 | 4.2320 |
| 3.4689 | 164.0 | 533 | 4.2320 |
| 4.6286 | 164.92 | 536 | 4.2320 |
| 4.6164 | 165.85 | 539 | 4.2320 |
| 4.634 | 166.77 | 542 | 4.2320 |
| 3.4878 | 168.0 | 546 | 4.2320 |
| 4.6616 | 168.92 | 549 | 4.2320 |
| 4.6228 | 169.85 | 552 | 4.2320 |
| 4.6427 | 170.77 | 555 | 4.2320 |
| 3.4739 | 172.0 | 559 | 4.2320 |
| 4.656 | 172.92 | 562 | 4.2320 |
| 4.6488 | 173.85 | 565 | 4.2320 |
| 4.6199 | 174.77 | 568 | 4.2320 |
| 3.4842 | 176.0 | 572 | 4.2320 |
| 4.6632 | 176.92 | 575 | 4.2320 |
| 4.646 | 177.85 | 578 | 4.2320 |
| 4.6226 | 178.77 | 581 | 4.2320 |
| 3.4619 | 180.0 | 585 | 4.2320 |
| 4.6329 | 180.92 | 588 | 4.2320 |
| 4.6245 | 181.85 | 591 | 4.2320 |
| 4.6435 | 182.77 | 594 | 4.2320 |
| 3.478 | 184.0 | 598 | 4.2320 |
| 4.6256 | 184.92 | 601 | 4.2320 |
| 4.6516 | 185.85 | 604 | 4.2320 |
| 4.6438 | 186.77 | 607 | 4.2320 |
| 3.5015 | 188.0 | 611 | 4.2320 |
| 4.6254 | 188.92 | 614 | 4.2320 |
| 4.6265 | 189.85 | 617 | 4.2320 |
| 4.6447 | 190.77 | 620 | 4.2320 |
| 3.508 | 192.0 | 624 | 4.2320 |
| 4.6353 | 192.92 | 627 | 4.2320 |
| 4.6333 | 193.85 | 630 | 4.2320 |
| 4.6573 | 194.77 | 633 | 4.2320 |
| 3.4644 | 196.0 | 637 | 4.2320 |
| 4.6413 | 196.92 | 640 | 4.2320 |
| 4.6641 | 197.85 | 643 | 4.2320 |
| 4.638 | 198.77 | 646 | 4.2320 |
| 3.4885 | 200.0 | 650 | 4.2320 |
| 4.6502 | 200.92 | 653 | 4.2320 |
| 4.6476 | 201.85 | 656 | 4.2320 |
| 4.645 | 202.77 | 659 | 4.2320 |
| 3.4861 | 204.0 | 663 | 4.2320 |
| 4.6418 | 204.92 | 666 | 4.2320 |
| 4.6419 | 205.85 | 669 | 4.2320 |
| 4.6395 | 206.77 | 672 | 4.2320 |
| 3.4739 | 208.0 | 676 | 4.2320 |
| 4.6306 | 208.92 | 679 | 4.2320 |
| 4.6245 | 209.85 | 682 | 4.2320 |
| 4.6614 | 210.77 | 685 | 4.2320 |
| 3.4965 | 212.0 | 689 | 4.2320 |
| 4.642 | 212.92 | 692 | 4.2320 |
| 4.6371 | 213.85 | 695 | 4.2320 |
| 4.6265 | 214.77 | 698 | 4.2320 |
| 3.4965 | 216.0 | 702 | 4.2320 |
| 4.6648 | 216.92 | 705 | 4.2320 |
| 4.6248 | 217.85 | 708 | 4.2320 |
| 4.6507 | 218.77 | 711 | 4.2320 |
| 3.4741 | 220.0 | 715 | 4.2320 |
| 4.644 | 220.92 | 718 | 4.2320 |
| 4.6315 | 221.85 | 721 | 4.2320 |
| 4.659 | 222.77 | 724 | 4.2320 |
| 3.4942 | 224.0 | 728 | 4.2320 |
| 4.6463 | 224.92 | 731 | 4.2320 |
| 4.6477 | 225.85 | 734 | 4.2320 |
| 4.6323 | 226.77 | 737 | 4.2320 |
| 3.4907 | 228.0 | 741 | 4.2320 |
| 4.6323 | 228.92 | 744 | 4.2320 |
| 4.6442 | 229.85 | 747 | 4.2320 |
| 4.6351 | 230.77 | 750 | 4.2320 |
| 3.4799 | 232.0 | 754 | 4.2320 |
| 4.6463 | 232.92 | 757 | 4.2320 |
| 4.6389 | 233.85 | 760 | 4.2320 |
| 4.6399 | 234.77 | 763 | 4.2320 |
| 3.4819 | 236.0 | 767 | 4.2320 |
| 4.678 | 236.92 | 770 | 4.2320 |
| 4.6446 | 237.85 | 773 | 4.2320 |
| 4.642 | 238.77 | 776 | 4.2320 |
| 3.4879 | 240.0 | 780 | 4.2320 |
| 4.6561 | 240.92 | 783 | 4.2320 |
| 4.6226 | 241.85 | 786 | 4.2320 |
| 4.6607 | 242.77 | 789 | 4.2320 |
| 3.4901 | 244.0 | 793 | 4.2320 |
| 4.6317 | 244.92 | 796 | 4.2320 |
| 4.6387 | 245.85 | 799 | 4.2320 |
| 4.6493 | 246.77 | 802 | 4.2320 |
| 3.4863 | 248.0 | 806 | 4.2320 |
| 4.6187 | 248.92 | 809 | 4.2320 |
| 4.6449 | 249.85 | 812 | 4.2320 |
| 4.6542 | 250.77 | 815 | 4.2320 |
| 3.4905 | 252.0 | 819 | 4.2320 |
| 4.6514 | 252.92 | 822 | 4.2320 |
| 4.6496 | 253.85 | 825 | 4.2320 |
| 4.6542 | 254.77 | 828 | 4.2320 |
| 3.4661 | 256.0 | 832 | 4.2320 |
| 4.631 | 256.92 | 835 | 4.2320 |
| 4.644 | 257.85 | 838 | 4.2320 |
| 4.6348 | 258.77 | 841 | 4.2320 |
| 3.5069 | 260.0 | 845 | 4.2320 |
| 4.6257 | 260.92 | 848 | 4.2320 |
| 4.6584 | 261.85 | 851 | 4.2320 |
| 4.6344 | 262.77 | 854 | 4.2320 |
| 3.4721 | 264.0 | 858 | 4.2320 |
| 4.6429 | 264.92 | 861 | 4.2320 |
| 4.6433 | 265.85 | 864 | 4.2320 |
| 4.6391 | 266.77 | 867 | 4.2320 |
| 3.4916 | 268.0 | 871 | 4.2320 |
| 4.6564 | 268.92 | 874 | 4.2320 |
| 4.658 | 269.85 | 877 | 4.2320 |
| 4.6329 | 270.77 | 880 | 4.2320 |
| 3.4783 | 272.0 | 884 | 4.2320 |
| 4.6384 | 272.92 | 887 | 4.2320 |
| 4.6482 | 273.85 | 890 | 4.2320 |
| 4.6688 | 274.77 | 893 | 4.2320 |
| 3.4659 | 276.0 | 897 | 4.2320 |
| 4.6299 | 276.92 | 900 | 4.2320 |
| 4.6392 | 277.85 | 903 | 4.2320 |
| 4.6521 | 278.77 | 906 | 4.2320 |
| 3.4949 | 280.0 | 910 | 4.2320 |
| 4.6643 | 280.92 | 913 | 4.2320 |
| 4.6361 | 281.85 | 916 | 4.2320 |
| 4.6505 | 282.77 | 919 | 4.2320 |
| 3.4847 | 284.0 | 923 | 4.2320 |
| 4.639 | 284.92 | 926 | 4.2320 |
| 4.6276 | 285.85 | 929 | 4.2320 |
| 4.6438 | 286.77 | 932 | 4.2320 |
| 3.4883 | 288.0 | 936 | 4.2320 |
| 4.6483 | 288.92 | 939 | 4.2320 |
| 4.6564 | 289.85 | 942 | 4.2320 |
| 4.6437 | 290.77 | 945 | 4.2320 |
| 3.4712 | 292.0 | 949 | 4.2320 |
| 4.6627 | 292.92 | 952 | 4.2320 |
| 4.6371 | 293.85 | 955 | 4.2320 |
| 4.6196 | 294.77 | 958 | 4.2320 |
| 3.4859 | 296.0 | 962 | 4.2320 |
| 4.6457 | 296.92 | 965 | 4.2320 |
| 4.6249 | 297.85 | 968 | 4.2320 |
| 4.6382 | 298.77 | 971 | 4.2320 |
| 3.4824 | 300.0 | 975 | 4.2320 |
| 4.6541 | 300.92 | 978 | 4.2320 |
| 4.659 | 301.85 | 981 | 4.2320 |
| 4.618 | 302.77 | 984 | 4.2320 |
| 3.4751 | 304.0 | 988 | 4.2320 |
| 4.623 | 304.92 | 991 | 4.2320 |
| 4.6371 | 305.85 | 994 | 4.2320 |
| 4.6546 | 306.77 | 997 | 4.2320 |
| 3.1908 | 307.69 | 1000 | 4.2320 |
### Framework versions
- PEFT 0.10.0
- Transformers 4.36.2
- Pytorch 2.1.0+cu121
- Datasets 2.18.0
- Tokenizers 0.15.2 |
lmqg/mt5-small-ruquad-qg | lmqg | "2023-01-18T13:46:15Z" | 26 | 0 | transformers | [
"transformers",
"pytorch",
"mt5",
"text2text-generation",
"question generation",
"ru",
"dataset:lmqg/qg_ruquad",
"arxiv:2210.03992",
"license:cc-by-4.0",
"model-index",
"autotrain_compatible",
"endpoints_compatible",
"region:us"
] | text2text-generation | "2022-06-07T00:39:31Z" |
---
license: cc-by-4.0
metrics:
- bleu4
- meteor
- rouge-l
- bertscore
- moverscore
language: ru
datasets:
- lmqg/qg_ruquad
pipeline_tag: text2text-generation
tags:
- question generation
widget:
- text: "Нелишним будет отметить, что, развивая это направление, Д. И. Менделеев, поначалу априорно выдвинув идею о температуре, при которой высота мениска будет нулевой, <hl> в мае 1860 года <hl> провёл серию опытов."
example_title: "Question Generation Example 1"
- text: "Однако, франкоязычный <hl> Квебек <hl> практически никогда не включается в состав Латинской Америки."
example_title: "Question Generation Example 2"
- text: "Классическим примером международного синдиката XX века была группа компаний <hl> Де Бирс <hl> , которая в 1980-е годы контролировала до 90 % мировой торговли алмазами."
example_title: "Question Generation Example 3"
model-index:
- name: lmqg/mt5-small-ruquad-qg
results:
- task:
name: Text2text Generation
type: text2text-generation
dataset:
name: lmqg/qg_ruquad
type: default
args: default
metrics:
- name: BLEU4 (Question Generation)
type: bleu4_question_generation
value: 16.31
- name: ROUGE-L (Question Generation)
type: rouge_l_question_generation
value: 31.39
- name: METEOR (Question Generation)
type: meteor_question_generation
value: 26.39
- name: BERTScore (Question Generation)
type: bertscore_question_generation
value: 84.27
- name: MoverScore (Question Generation)
type: moverscore_question_generation
value: 62.49
- name: QAAlignedF1Score-BERTScore (Question & Answer Generation (with Gold Answer)) [Gold Answer]
type: qa_aligned_f1_score_bertscore_question_answer_generation_with_gold_answer_gold_answer
value: 90.17
- name: QAAlignedRecall-BERTScore (Question & Answer Generation (with Gold Answer)) [Gold Answer]
type: qa_aligned_recall_bertscore_question_answer_generation_with_gold_answer_gold_answer
value: 90.16
- name: QAAlignedPrecision-BERTScore (Question & Answer Generation (with Gold Answer)) [Gold Answer]
type: qa_aligned_precision_bertscore_question_answer_generation_with_gold_answer_gold_answer
value: 90.17
- name: QAAlignedF1Score-MoverScore (Question & Answer Generation (with Gold Answer)) [Gold Answer]
type: qa_aligned_f1_score_moverscore_question_answer_generation_with_gold_answer_gold_answer
value: 68.22
- name: QAAlignedRecall-MoverScore (Question & Answer Generation (with Gold Answer)) [Gold Answer]
type: qa_aligned_recall_moverscore_question_answer_generation_with_gold_answer_gold_answer
value: 68.21
- name: QAAlignedPrecision-MoverScore (Question & Answer Generation (with Gold Answer)) [Gold Answer]
type: qa_aligned_precision_moverscore_question_answer_generation_with_gold_answer_gold_answer
value: 68.23
- name: QAAlignedF1Score-BERTScore (Question & Answer Generation) [Gold Answer]
type: qa_aligned_f1_score_bertscore_question_answer_generation_gold_answer
value: 76.96
- name: QAAlignedRecall-BERTScore (Question & Answer Generation) [Gold Answer]
type: qa_aligned_recall_bertscore_question_answer_generation_gold_answer
value: 81.05
- name: QAAlignedPrecision-BERTScore (Question & Answer Generation) [Gold Answer]
type: qa_aligned_precision_bertscore_question_answer_generation_gold_answer
value: 73.41
- name: QAAlignedF1Score-MoverScore (Question & Answer Generation) [Gold Answer]
type: qa_aligned_f1_score_moverscore_question_answer_generation_gold_answer
value: 55.53
- name: QAAlignedRecall-MoverScore (Question & Answer Generation) [Gold Answer]
type: qa_aligned_recall_moverscore_question_answer_generation_gold_answer
value: 58.25
- name: QAAlignedPrecision-MoverScore (Question & Answer Generation) [Gold Answer]
type: qa_aligned_precision_moverscore_question_answer_generation_gold_answer
value: 53.24
---
# Model Card of `lmqg/mt5-small-ruquad-qg`
This model is fine-tuned version of [google/mt5-small](https://huggingface.co/google/mt5-small) for question generation task on the [lmqg/qg_ruquad](https://huggingface.co/datasets/lmqg/qg_ruquad) (dataset_name: default) via [`lmqg`](https://github.com/asahi417/lm-question-generation).
### Overview
- **Language model:** [google/mt5-small](https://huggingface.co/google/mt5-small)
- **Language:** ru
- **Training data:** [lmqg/qg_ruquad](https://huggingface.co/datasets/lmqg/qg_ruquad) (default)
- **Online Demo:** [https://autoqg.net/](https://autoqg.net/)
- **Repository:** [https://github.com/asahi417/lm-question-generation](https://github.com/asahi417/lm-question-generation)
- **Paper:** [https://arxiv.org/abs/2210.03992](https://arxiv.org/abs/2210.03992)
### Usage
- With [`lmqg`](https://github.com/asahi417/lm-question-generation#lmqg-language-model-for-question-generation-)
```python
from lmqg import TransformersQG
# initialize model
model = TransformersQG(language="ru", model="lmqg/mt5-small-ruquad-qg")
# model prediction
questions = model.generate_q(list_context="Нелишним будет отметить, что, развивая это направление, Д. И. Менделеев, поначалу априорно выдвинув идею о температуре, при которой высота мениска будет нулевой, в мае 1860 года провёл серию опытов.", list_answer="в мае 1860 года")
```
- With `transformers`
```python
from transformers import pipeline
pipe = pipeline("text2text-generation", "lmqg/mt5-small-ruquad-qg")
output = pipe("Нелишним будет отметить, что, развивая это направление, Д. И. Менделеев, поначалу априорно выдвинув идею о температуре, при которой высота мениска будет нулевой, <hl> в мае 1860 года <hl> провёл серию опытов.")
```
## Evaluation
- ***Metric (Question Generation)***: [raw metric file](https://huggingface.co/lmqg/mt5-small-ruquad-qg/raw/main/eval/metric.first.sentence.paragraph_answer.question.lmqg_qg_ruquad.default.json)
| | Score | Type | Dataset |
|:-----------|--------:|:--------|:-----------------------------------------------------------------|
| BERTScore | 84.27 | default | [lmqg/qg_ruquad](https://huggingface.co/datasets/lmqg/qg_ruquad) |
| Bleu_1 | 31.03 | default | [lmqg/qg_ruquad](https://huggingface.co/datasets/lmqg/qg_ruquad) |
| Bleu_2 | 24.58 | default | [lmqg/qg_ruquad](https://huggingface.co/datasets/lmqg/qg_ruquad) |
| Bleu_3 | 19.92 | default | [lmqg/qg_ruquad](https://huggingface.co/datasets/lmqg/qg_ruquad) |
| Bleu_4 | 16.31 | default | [lmqg/qg_ruquad](https://huggingface.co/datasets/lmqg/qg_ruquad) |
| METEOR | 26.39 | default | [lmqg/qg_ruquad](https://huggingface.co/datasets/lmqg/qg_ruquad) |
| MoverScore | 62.49 | default | [lmqg/qg_ruquad](https://huggingface.co/datasets/lmqg/qg_ruquad) |
| ROUGE_L | 31.39 | default | [lmqg/qg_ruquad](https://huggingface.co/datasets/lmqg/qg_ruquad) |
- ***Metric (Question & Answer Generation, Reference Answer)***: Each question is generated from *the gold answer*. [raw metric file](https://huggingface.co/lmqg/mt5-small-ruquad-qg/raw/main/eval/metric.first.answer.paragraph.questions_answers.lmqg_qg_ruquad.default.json)
| | Score | Type | Dataset |
|:--------------------------------|--------:|:--------|:-----------------------------------------------------------------|
| QAAlignedF1Score (BERTScore) | 90.17 | default | [lmqg/qg_ruquad](https://huggingface.co/datasets/lmqg/qg_ruquad) |
| QAAlignedF1Score (MoverScore) | 68.22 | default | [lmqg/qg_ruquad](https://huggingface.co/datasets/lmqg/qg_ruquad) |
| QAAlignedPrecision (BERTScore) | 90.17 | default | [lmqg/qg_ruquad](https://huggingface.co/datasets/lmqg/qg_ruquad) |
| QAAlignedPrecision (MoverScore) | 68.23 | default | [lmqg/qg_ruquad](https://huggingface.co/datasets/lmqg/qg_ruquad) |
| QAAlignedRecall (BERTScore) | 90.16 | default | [lmqg/qg_ruquad](https://huggingface.co/datasets/lmqg/qg_ruquad) |
| QAAlignedRecall (MoverScore) | 68.21 | default | [lmqg/qg_ruquad](https://huggingface.co/datasets/lmqg/qg_ruquad) |
- ***Metric (Question & Answer Generation, Pipeline Approach)***: Each question is generated on the answer generated by [`lmqg/mt5-small-ruquad-ae`](https://huggingface.co/lmqg/mt5-small-ruquad-ae). [raw metric file](https://huggingface.co/lmqg/mt5-small-ruquad-qg/raw/main/eval_pipeline/metric.first.answer.paragraph.questions_answers.lmqg_qg_ruquad.default.lmqg_mt5-small-ruquad-ae.json)
| | Score | Type | Dataset |
|:--------------------------------|--------:|:--------|:-----------------------------------------------------------------|
| QAAlignedF1Score (BERTScore) | 76.96 | default | [lmqg/qg_ruquad](https://huggingface.co/datasets/lmqg/qg_ruquad) |
| QAAlignedF1Score (MoverScore) | 55.53 | default | [lmqg/qg_ruquad](https://huggingface.co/datasets/lmqg/qg_ruquad) |
| QAAlignedPrecision (BERTScore) | 73.41 | default | [lmqg/qg_ruquad](https://huggingface.co/datasets/lmqg/qg_ruquad) |
| QAAlignedPrecision (MoverScore) | 53.24 | default | [lmqg/qg_ruquad](https://huggingface.co/datasets/lmqg/qg_ruquad) |
| QAAlignedRecall (BERTScore) | 81.05 | default | [lmqg/qg_ruquad](https://huggingface.co/datasets/lmqg/qg_ruquad) |
| QAAlignedRecall (MoverScore) | 58.25 | default | [lmqg/qg_ruquad](https://huggingface.co/datasets/lmqg/qg_ruquad) |
## Training hyperparameters
The following hyperparameters were used during fine-tuning:
- dataset_path: lmqg/qg_ruquad
- dataset_name: default
- input_types: ['paragraph_answer']
- output_types: ['question']
- prefix_types: None
- model: google/mt5-small
- max_length: 512
- max_length_output: 32
- epoch: 5
- batch: 64
- lr: 0.001
- fp16: False
- random_seed: 1
- gradient_accumulation_steps: 1
- label_smoothing: 0.15
The full configuration can be found at [fine-tuning config file](https://huggingface.co/lmqg/mt5-small-ruquad-qg/raw/main/trainer_config.json).
## Citation
```
@inproceedings{ushio-etal-2022-generative,
title = "{G}enerative {L}anguage {M}odels for {P}aragraph-{L}evel {Q}uestion {G}eneration",
author = "Ushio, Asahi and
Alva-Manchego, Fernando and
Camacho-Collados, Jose",
booktitle = "Proceedings of the 2022 Conference on Empirical Methods in Natural Language Processing",
month = dec,
year = "2022",
address = "Abu Dhabi, U.A.E.",
publisher = "Association for Computational Linguistics",
}
```
|
dnnsdunca/ddroidai_pro_gram | dnnsdunca | "2024-03-23T01:40:50Z" | 0 | 0 | adapter-transformers | [
"adapter-transformers",
"code",
"text-generation",
"en",
"license:mit",
"region:us"
] | text-generation | "2024-03-23T01:34:42Z" | ---
license: mit
language:
- en
metrics:
- code_eval
library_name: adapter-transformers
pipeline_tag: text-generation
tags:
- code
--- |
ALivshits/Llama3_8B_ATIS_100-merged | ALivshits | "2024-07-21T13:29:38Z" | 4 | 0 | transformers | [
"transformers",
"safetensors",
"llama",
"text-generation",
"arxiv:1910.09700",
"autotrain_compatible",
"text-generation-inference",
"endpoints_compatible",
"region:us"
] | text-generation | "2024-07-21T13:24:19Z" | ---
library_name: transformers
tags: []
---
# Model Card for Model ID
<!-- Provide a quick summary of what the model is/does. -->
## Model Details
### Model Description
<!-- Provide a longer summary of what this model is. -->
This is the model card of a 🤗 transformers model that has been pushed on the Hub. This model card has been automatically generated.
- **Developed by:** [More Information Needed]
- **Funded by [optional]:** [More Information Needed]
- **Shared by [optional]:** [More Information Needed]
- **Model type:** [More Information Needed]
- **Language(s) (NLP):** [More Information Needed]
- **License:** [More Information Needed]
- **Finetuned from model [optional]:** [More Information Needed]
### Model Sources [optional]
<!-- Provide the basic links for the model. -->
- **Repository:** [More Information Needed]
- **Paper [optional]:** [More Information Needed]
- **Demo [optional]:** [More Information Needed]
## Uses
<!-- Address questions around how the model is intended to be used, including the foreseeable users of the model and those affected by the model. -->
### Direct Use
<!-- This section is for the model use without fine-tuning or plugging into a larger ecosystem/app. -->
[More Information Needed]
### Downstream Use [optional]
<!-- This section is for the model use when fine-tuned for a task, or when plugged into a larger ecosystem/app -->
[More Information Needed]
### Out-of-Scope Use
<!-- This section addresses misuse, malicious use, and uses that the model will not work well for. -->
[More Information Needed]
## Bias, Risks, and Limitations
<!-- This section is meant to convey both technical and sociotechnical limitations. -->
[More Information Needed]
### Recommendations
<!-- This section is meant to convey recommendations with respect to the bias, risk, and technical limitations. -->
Users (both direct and downstream) should be made aware of the risks, biases and limitations of the model. More information needed for further recommendations.
## How to Get Started with the Model
Use the code below to get started with the model.
[More Information Needed]
## Training Details
### Training Data
<!-- This should link to a Dataset Card, perhaps with a short stub of information on what the training data is all about as well as documentation related to data pre-processing or additional filtering. -->
[More Information Needed]
### Training Procedure
<!-- This relates heavily to the Technical Specifications. Content here should link to that section when it is relevant to the training procedure. -->
#### Preprocessing [optional]
[More Information Needed]
#### Training Hyperparameters
- **Training regime:** [More Information Needed] <!--fp32, fp16 mixed precision, bf16 mixed precision, bf16 non-mixed precision, fp16 non-mixed precision, fp8 mixed precision -->
#### Speeds, Sizes, Times [optional]
<!-- This section provides information about throughput, start/end time, checkpoint size if relevant, etc. -->
[More Information Needed]
## Evaluation
<!-- This section describes the evaluation protocols and provides the results. -->
### Testing Data, Factors & Metrics
#### Testing Data
<!-- This should link to a Dataset Card if possible. -->
[More Information Needed]
#### Factors
<!-- These are the things the evaluation is disaggregating by, e.g., subpopulations or domains. -->
[More Information Needed]
#### Metrics
<!-- These are the evaluation metrics being used, ideally with a description of why. -->
[More Information Needed]
### Results
[More Information Needed]
#### Summary
## Model Examination [optional]
<!-- Relevant interpretability work for the model goes here -->
[More Information Needed]
## Environmental Impact
<!-- Total emissions (in grams of CO2eq) and additional considerations, such as electricity usage, go here. Edit the suggested text below accordingly -->
Carbon emissions can be estimated using the [Machine Learning Impact calculator](https://mlco2.github.io/impact#compute) presented in [Lacoste et al. (2019)](https://arxiv.org/abs/1910.09700).
- **Hardware Type:** [More Information Needed]
- **Hours used:** [More Information Needed]
- **Cloud Provider:** [More Information Needed]
- **Compute Region:** [More Information Needed]
- **Carbon Emitted:** [More Information Needed]
## Technical Specifications [optional]
### Model Architecture and Objective
[More Information Needed]
### Compute Infrastructure
[More Information Needed]
#### Hardware
[More Information Needed]
#### Software
[More Information Needed]
## Citation [optional]
<!-- If there is a paper or blog post introducing the model, the APA and Bibtex information for that should go in this section. -->
**BibTeX:**
[More Information Needed]
**APA:**
[More Information Needed]
## Glossary [optional]
<!-- If relevant, include terms and calculations in this section that can help readers understand the model or model card. -->
[More Information Needed]
## More Information [optional]
[More Information Needed]
## Model Card Authors [optional]
[More Information Needed]
## Model Card Contact
[More Information Needed] |
QuantFactory/HuatuoGPT-o1-8B-GGUF | QuantFactory | "2025-01-03T06:10:23Z" | 477 | 3 | null | [
"gguf",
"medical",
"text-generation",
"en",
"dataset:FreedomIntelligence/medical-o1-reasoning-SFT",
"dataset:FreedomIntelligence/medical-o1-verifiable-problem",
"arxiv:2412.18925",
"base_model:meta-llama/Llama-3.1-8B-Instruct",
"base_model:quantized:meta-llama/Llama-3.1-8B-Instruct",
"license:apache-2.0",
"endpoints_compatible",
"region:us",
"conversational"
] | text-generation | "2025-01-03T05:27:31Z" |
---
license: apache-2.0
datasets:
- FreedomIntelligence/medical-o1-reasoning-SFT
- FreedomIntelligence/medical-o1-verifiable-problem
language:
- en
base_model:
- meta-llama/Llama-3.1-8B-Instruct
pipeline_tag: text-generation
tags:
- medical
---
[](https://hf.co/QuantFactory)
# QuantFactory/HuatuoGPT-o1-8B-GGUF
This is quantized version of [FreedomIntelligence/HuatuoGPT-o1-8B](https://huggingface.co/FreedomIntelligence/HuatuoGPT-o1-8B) created using llama.cpp
# Original Model Card
<div align="center">
<h1>
HuatuoGPT-o1-8B
</h1>
</div>
<div align="center">
<a href="https://github.com/FreedomIntelligence/HuatuoGPT-o1" target="_blank">GitHub</a> | <a href="https://arxiv.org/pdf/2412.18925" target="_blank">Paper</a>
</div>
# <span>Introduction</span>
**HuatuoGPT-o1** is a medical LLM designed for advanced medical reasoning. It generates a complex thought process, reflecting and refining its reasoning, before providing a final response.
For more information, visit our GitHub repository:
[https://github.com/FreedomIntelligence/HuatuoGPT-o1](https://github.com/FreedomIntelligence/HuatuoGPT-o1).
# <span>Model Info</span>
| | Backbone | Supported Languages | Link |
| -------------------- | ------------ | ----- | --------------------------------------------------------------------- |
| **HuatuoGPT-o1-8B** | LLaMA-3.1-8B | English | [HF Link](https://huggingface.co/FreedomIntelligence/HuatuoGPT-o1-8B) |
| **HuatuoGPT-o1-70B** | LLaMA-3.1-70B | English | [HF Link](https://huggingface.co/FreedomIntelligence/HuatuoGPT-o1-70B) |
| **HuatuoGPT-o1-7B** | Qwen2.5-7B | English & Chinese | [HF Link](https://huggingface.co/FreedomIntelligence/HuatuoGPT-o1-7B) |
| **HuatuoGPT-o1-72B** | Qwen2.5-72B | English & Chinese | [HF Link](https://huggingface.co/FreedomIntelligence/HuatuoGPT-o1-72B) |
# <span>Usage</span>
You can use HuatuoGPT-o1 in the same way as `Llama-3.1-8B-Instruct`. You can deploy it with tools like [vllm](https://github.com/vllm-project/vllm) or [Sglang](https://github.com/sgl-project/sglang), or perform direct inference:
```python
from transformers import AutoModelForCausalLM, AutoTokenizer
model = AutoModelForCausalLM.from_pretrained("FreedomIntelligence/HuatuoGPT-o1-8B",torch_dtype="auto",device_map="auto")
tokenizer = AutoTokenizer.from_pretrained("FreedomIntelligence/HuatuoGPT-o1-8B")
input_text = "How to stop a cough?"
messages = [{"role": "user", "content": input_text}]
inputs = tokenizer(tokenizer.apply_chat_template(messages, tokenize=False,add_generation_prompt=True
), return_tensors="pt").to(model.device)
outputs = model.generate(**inputs, max_new_tokens=2048)
print(tokenizer.decode(outputs[0], skip_special_tokens=True))
```
HuatuoGPT-o1 adopts a *thinks-before-it-answers* approach, with outputs formatted as:
```
## Thinking
[Reasoning process]
## Final Response
[Output]
```
# <span>📖 Citation</span>
```
@misc{chen2024huatuogpto1medicalcomplexreasoning,
title={HuatuoGPT-o1, Towards Medical Complex Reasoning with LLMs},
author={Junying Chen and Zhenyang Cai and Ke Ji and Xidong Wang and Wanlong Liu and Rongsheng Wang and Jianye Hou and Benyou Wang},
year={2024},
eprint={2412.18925},
archivePrefix={arXiv},
primaryClass={cs.CL},
url={https://arxiv.org/abs/2412.18925},
}
```
|
Subsets and Splits