repo_name
stringlengths 6
112
| path
stringlengths 4
204
| copies
stringlengths 1
3
| size
stringlengths 4
6
| content
stringlengths 714
810k
| license
stringclasses 15
values |
---|---|---|---|---|---|
luo66/scikit-learn | sklearn/utils/tests/test_linear_assignment.py | 421 | 1349 | # Author: Brian M. Clapper, G Varoquaux
# License: BSD
import numpy as np
# XXX we should be testing the public API here
from sklearn.utils.linear_assignment_ import _hungarian
def test_hungarian():
matrices = [
# Square
([[400, 150, 400],
[400, 450, 600],
[300, 225, 300]],
850 # expected cost
),
# Rectangular variant
([[400, 150, 400, 1],
[400, 450, 600, 2],
[300, 225, 300, 3]],
452 # expected cost
),
# Square
([[10, 10, 8],
[9, 8, 1],
[9, 7, 4]],
18
),
# Rectangular variant
([[10, 10, 8, 11],
[9, 8, 1, 1],
[9, 7, 4, 10]],
15
),
# n == 2, m == 0 matrix
([[], []],
0
),
]
for cost_matrix, expected_total in matrices:
cost_matrix = np.array(cost_matrix)
indexes = _hungarian(cost_matrix)
total_cost = 0
for r, c in indexes:
x = cost_matrix[r, c]
total_cost += x
assert expected_total == total_cost
indexes = _hungarian(cost_matrix.T)
total_cost = 0
for c, r in indexes:
x = cost_matrix[r, c]
total_cost += x
assert expected_total == total_cost
| bsd-3-clause |
owlabs/incubator-airflow | scripts/perf/scheduler_ops_metrics.py | 1 | 7222 | # -*- coding: utf-8 -*-
#
# Licensed to the Apache Software Foundation (ASF) under one
# or more contributor license agreements. See the NOTICE file
# distributed with this work for additional information
# regarding copyright ownership. The ASF licenses this file
# to you under the Apache License, Version 2.0 (the
# "License"); you may not use this file except in compliance
# with the License. You may obtain a copy of the License at
#
# http://www.apache.org/licenses/LICENSE-2.0
#
# Unless required by applicable law or agreed to in writing,
# software distributed under the License is distributed on an
# "AS IS" BASIS, WITHOUT WARRANTIES OR CONDITIONS OF ANY
# KIND, either express or implied. See the License for the
# specific language governing permissions and limitations
# under the License.
import logging
import pandas as pd
import sys
from airflow import settings
from airflow.configuration import conf
from airflow.jobs import SchedulerJob
from airflow.models import DagBag, DagModel, DagRun, TaskInstance
from airflow.utils import timezone
from airflow.utils.state import State
SUBDIR = 'scripts/perf/dags'
DAG_IDS = ['perf_dag_1', 'perf_dag_2']
MAX_RUNTIME_SECS = 6
class SchedulerMetricsJob(SchedulerJob):
"""
This class extends SchedulerJob to instrument the execution performance of
task instances contained in each DAG. We want to know if any DAG
is starved of resources, and this will be reflected in the stats printed
out at the end of the test run. The following metrics will be instrumented
for each task instance (dag_id, task_id, execution_date) tuple:
1. Queuing delay - time taken from starting the executor to the task
instance to be added to the executor queue.
2. Start delay - time taken from starting the executor to the task instance
to start execution.
3. Land time - time taken from starting the executor to task instance
completion.
4. Duration - time taken for executing the task instance.
The DAGs implement bash operators that call the system wait command. This
is representative of typical operators run on Airflow - queries that are
run on remote systems and spend the majority of their time on I/O wait.
To Run:
$ python scripts/perf/scheduler_ops_metrics.py [timeout]
You can specify timeout in seconds as an optional parameter.
Its default value is 6 seconds.
"""
__mapper_args__ = {
'polymorphic_identity': 'SchedulerMetricsJob'
}
def print_stats(self):
"""
Print operational metrics for the scheduler test.
"""
session = settings.Session()
TI = TaskInstance
tis = (
session
.query(TI)
.filter(TI.dag_id.in_(DAG_IDS))
.all()
)
successful_tis = [x for x in tis if x.state == State.SUCCESS]
ti_perf = [(ti.dag_id, ti.task_id, ti.execution_date,
(ti.queued_dttm - self.start_date).total_seconds(),
(ti.start_date - self.start_date).total_seconds(),
(ti.end_date - self.start_date).total_seconds(),
ti.duration) for ti in successful_tis]
ti_perf_df = pd.DataFrame(ti_perf, columns=['dag_id', 'task_id',
'execution_date',
'queue_delay',
'start_delay', 'land_time',
'duration'])
print('Performance Results')
print('###################')
for dag_id in DAG_IDS:
print('DAG {}'.format(dag_id))
print(ti_perf_df[ti_perf_df['dag_id'] == dag_id])
print('###################')
if len(tis) > len(successful_tis):
print("WARNING!! The following task instances haven't completed")
print(pd.DataFrame([(ti.dag_id, ti.task_id, ti.execution_date, ti.state)
for ti in filter(lambda x: x.state != State.SUCCESS, tis)],
columns=['dag_id', 'task_id', 'execution_date', 'state']))
session.commit()
def heartbeat(self):
"""
Override the scheduler heartbeat to determine when the test is complete
"""
super(SchedulerMetricsJob, self).heartbeat()
session = settings.Session()
# Get all the relevant task instances
TI = TaskInstance
successful_tis = (
session
.query(TI)
.filter(TI.dag_id.in_(DAG_IDS))
.filter(TI.state.in_([State.SUCCESS]))
.all()
)
session.commit()
dagbag = DagBag(SUBDIR)
dags = [dagbag.dags[dag_id] for dag_id in DAG_IDS]
# the tasks in perf_dag_1 and per_dag_2 have a daily schedule interval.
num_task_instances = sum([(timezone.utcnow() - task.start_date).days
for dag in dags for task in dag.tasks])
if (len(successful_tis) == num_task_instances or
(timezone.utcnow() - self.start_date).total_seconds() >
MAX_RUNTIME_SECS):
if len(successful_tis) == num_task_instances:
self.log.info("All tasks processed! Printing stats.")
else:
self.log.info("Test timeout reached. Printing available stats.")
self.print_stats()
set_dags_paused_state(True)
sys.exit()
def clear_dag_runs():
"""
Remove any existing DAG runs for the perf test DAGs.
"""
session = settings.Session()
drs = session.query(DagRun).filter(
DagRun.dag_id.in_(DAG_IDS),
).all()
for dr in drs:
logging.info('Deleting DagRun :: {}'.format(dr))
session.delete(dr)
def clear_dag_task_instances():
"""
Remove any existing task instances for the perf test DAGs.
"""
session = settings.Session()
TI = TaskInstance
tis = (
session
.query(TI)
.filter(TI.dag_id.in_(DAG_IDS))
.all()
)
for ti in tis:
logging.info('Deleting TaskInstance :: {}'.format(ti))
session.delete(ti)
session.commit()
def set_dags_paused_state(is_paused):
"""
Toggle the pause state of the DAGs in the test.
"""
session = settings.Session()
dms = session.query(DagModel).filter(
DagModel.dag_id.in_(DAG_IDS))
for dm in dms:
logging.info('Setting DAG :: {} is_paused={}'.format(dm, is_paused))
dm.is_paused = is_paused
session.commit()
def main():
global MAX_RUNTIME_SECS
if len(sys.argv) > 1:
try:
max_runtime_secs = int(sys.argv[1])
if max_runtime_secs < 1:
raise ValueError
MAX_RUNTIME_SECS = max_runtime_secs
except ValueError:
logging.error('Specify a positive integer for timeout.')
sys.exit(1)
conf.load_test_config()
set_dags_paused_state(False)
clear_dag_runs()
clear_dag_task_instances()
job = SchedulerMetricsJob(dag_ids=DAG_IDS, subdir=SUBDIR)
job.run()
if __name__ == "__main__":
main()
| apache-2.0 |
degoldschmidt/ribeirolab-codeconversion | python/flyPAD/fp_plot.py | 1 | 12102 | import numpy as np
import pandas as pd
import matplotlib
matplotlib.use("TkAgg")
import matplotlib.pyplot as plt
import hdf5storage
import scipy.stats as stat
import os, math
import seaborn as sns
sns.set_style("ticks")
sns.despine(left=True)
import functools
def conj(conditions, printit=False):
outstr = ""
for ind, cond in enumerate(conditions):
outstr += "Label == "
outstr += "'"
outstr += cond
outstr += "'"
if ind < len(conditions)-1:
outstr += " | "
if printit:
print(outstr)
return outstr
"""
Helper functions
"""
def get_cond(_file):
_cond = ""
if "Jan" in _file:
_cond = "3d fresh food"
if "Feb" in _file:
_cond = "8d deprived (sucrose water)"
return _cond
def get_conds(_file):
_effect = ""
if "KIR" in _file:
_effect = "Kir"
if "TrpA" in _file:
_effect = "TrpA"
if "Jan" in _file:
_c = "FF"
if "Feb" in _file:
_c = "8dD"
if "3600" in _file:
_len = "3600"
if "1800" in _file:
_len = "1800"
if "900" in _file:
_len = "900"
return _c+"_"+_effect+"_"+_len
def get_data(_file, _id): ### TODO
frames = []
for ind, _file in enumerate(_files):
Df, pvals = h5_to_panda(_file, _id)
frames.append(Df)
return pd.concat(frames, keys=[get_conds(_file) for _file in _files])
def get_data_files(_files, _id):### TODO
frames = []
for ind, _file in enumerate(_files):
Df, pvals = h5_to_panda(_file, _id)
frames.append(Df)
return pd.concat(frames, keys=[get_conds(_file) for _file in _files])
def get_filename(_file, ID, _sort="", _suf=""):
_effect = ""
if "KIR" in _file:
_effect = "Kir"
if "TrpA" in _file:
_effect = "TrpA"
if "Jan" in _file:
_c = "FF"
if "Feb" in _file:
_c = "8dD"
if "3600" in _file:
_len = "3600"
if "1800" in _file:
_len = "1800"
if "900" in _file:
_len = "900"
file_prefix = _c + "_" + _effect + "_" + _len
return file_prefix+_sort+"_"+ID+"_"+_suf+".png"
def h5_to_panda(_file, _id):
if _id == "PI":
thisid = "Number_of_sips"
else:
thisid = _id
dataid = "data2/" + thisid
pid = "PVALS/" + thisid
out = hdf5storage.loadmat(_file, variable_names=[dataid, pid, "LABELS"])
datapoints = unrv_data(out[dataid])
pvals = unrv_data(out[pid])
labels = unrv_labels(out["LABELS"])
labels = [label[0,0] for label in labels]
labels[0] = "control"
yeast_data = datapoints[0]
sucrose_data = datapoints[1]
yeast_ps = pvals[0]
sucrose_ps = pvals[1]
numdtpoints = yeast_data.size-np.count_nonzero(~np.isnan(yeast_data))
if _id == "PI":
PIout = {"Label": [], "Data": [], "Median": [], "Signif": []}
contr = (yeast_data[:, 0] - sucrose_data[:, 0]) / (yeast_data[:, 0] + sucrose_data[:, 0])
Pvals = {}
for col in range(yeast_data.shape[1]): # different labels
PIcol = (yeast_data[:, col] - sucrose_data[:, col]) / (yeast_data[:, col] + sucrose_data[:, col])
PImedian = np.nanmedian(PIcol)
PIcol = PIcol[~np.isnan(PIcol)]
s, PIpval = stat.ranksums(contr, PIcol)
if np.isnan(PIpval):
PIpval = 1
Pvals[labels[col]] = PIpval
for row in range(yeast_data.shape[0]): # different datapoints same label
if ~np.isnan(yeast_data[row, col]) and ~np.isnan(sucrose_data[row, col]):
PIout["Label"].append(labels[col])
PIout["Data"].append(PIcol[row])
PIout["Median"].append(PImedian)
PIout["Signif"].append("Yes" if math.log10(1./PIpval)>2 else "No")
PIout = pd.DataFrame(PIout)
return PIout, Pvals
else:
Yout = {"Label": [], "Data": [], "Median": [], "Signif": []}
Sout = {"Label": [], "Data": [], "Median": [], "Signif": []}
for row in range(yeast_data.shape[0]): # different datapoints same label
for col in range(yeast_data.shape[1]): # different labels
if ~np.isnan(yeast_data[row, col]):
Yout["Label"].append(labels[col])
Yout["Data"].append(yeast_data[row, col])
Yout["Median"].append(np.nanmedian(yeast_data[:,col]))
Yout["Signif"].append("Yes" if math.log10(1./yeast_ps[col])>2 else "No")
if ~np.isnan(sucrose_data[row, col]):
Sout["Label"].append(labels[col])
Sout["Data"].append(sucrose_data[row, col])
Sout["Median"].append(np.nanmedian(sucrose_data[:,col]))
Sout["Signif"].append("Yes" if np.log10(1./sucrose_ps[col])>2 else "No")
Ydf = pd.DataFrame(Yout)
Sdf = pd.DataFrame(Sout)
Pvals = {}
for ind, label in enumerate(labels):
Pvals[label] = [yeast_ps[ind], sucrose_ps[ind]]
return [Ydf, Sdf], Pvals
def h5_to_median(_file, _id):
thisid = _id
dataid = "data2/" + thisid
pid = "PVALS/" + thisid
out = hdf5storage.loadmat(_file, variable_names=[dataid, pid, "LABELS"])
datapoints = unrv_data(out[dataid])
pvals = unrv_data(out[pid])
labels = unrv_labels(out["LABELS"])
labels = [label[0,0] for label in labels]
labels[0] = "control"
yeast_data = datapoints[0]
sucrose_data = datapoints[1]
yeast_ps = pvals[0]
sucrose_ps = pvals[1]
numdtpoints = yeast_data.size-np.count_nonzero(~np.isnan(yeast_data))
Yout = {"Label": [], "Data": []}
Sout = {"Label": [], "Data": []}
for col in range(yeast_data.shape[1]): # different labels
Yout["Label"].append(labels[col])
Yout["Data"].append(np.nanmedian(yeast_data[:, col]))
Sout["Label"].append(labels[col])
Sout["Data"].append(np.nanmedian(sucrose_data[:, col]))
Ydf = pd.DataFrame(Yout)
Sdf = pd.DataFrame(Sout)
Pvals = {}
for ind, label in enumerate(labels):
Pvals[label] = [yeast_ps[ind], sucrose_ps[ind]]
return [Ydf, Sdf], Pvals
def swarmbox(_data, _x, _y, _pval, _ax, ps=2, hueon=False):
if hueon:
un_med = [ _data[_data["Label"]==label]["Median"].unique()[0] for label in _data["Label"].unique()]
pal = sns.diverging_palette(240, 10, s=99, n=201)
pal = [pal[int(100*(med+1))] for med in un_med]
_ax = sns.boxplot(x=_x, y=_y, palette=pal, data=_data, width=0.4, linewidth=0.5, showcaps=False, showfliers=False,whiskerprops={'linewidth':0}, ax=_ax)
else:
_ax = sns.boxplot(x=_x, y=_y, data=_data, width=0.4, linewidth=0.5, showcaps=False,boxprops={'facecolor':'.85'}, showfliers=False,whiskerprops={'linewidth':0}, ax=_ax)
_ax = sns.swarmplot(x=_x, y=_y, hue="Signif", data=_data, size=ps, ax=_ax, palette=dict(Yes = 'r', No = 'k'))
_ax = sns.pointplot(x=_x, y=_y, data=_data, estimator=np.median, ci=None, join=False, color="0.5", markers="_", scale=0.75, ax=_ax)
return _ax
def unrv_data(_in):
return _in[0][0][0,]
def unrv_labels(_in):
return _in[0][0][0]
"""
Plot scripts:
* Y/S screening plot (plot_id)
* PI screening plot (plot_pi)
* scatter plots (plot_scatter)
"""
def plot_id(_file, _ID, _sort="Y", lims=[], _title="", _only=[], _fsuff=""):
ID = _ID
if _title == "":
title = _ID.replace("_", " ")
else:
title = _title
supptitle = get_cond(_file)
Substr = ["10% Yeast", "20 mM Sucrose"]
Df, pvals = h5_to_panda(_file, ID)
fwid = 10
if len(_only) > 0:
Df[0] = Df[0].query(conj(_only))
Df[1] = Df[1].query(conj(_only))
if len(_only) > 15:
fwid *= len(_only)/50
else:
fwid *= len(_only)/30
supptitle= ""
if _sort == "Y":
Df[0] = Df[0].sort_values("Median")
Df[1] = Df[1].reindex(Df[0].index)
else:
Df[1] = Df[1].sort_values("Median")
Df[0] = Df[0].reindex(Df[1].index)
Labels = [Df[0]["Label"].unique(), Df[1]["Label"].unique()]
for j, substr_labels in enumerate(Labels):
for i, labl in enumerate(substr_labels):
if type(labl) is float:
if np.isnan(labl):
print(j, labl, type(labl))
Labels[j] = np.delete(Labels[j], i)
plotpvals = [[pvals[label][0] for label in Labels[0]], [pvals[label][1] for label in Labels[1]]]
f, axes = plt.subplots(2, sharex=False, figsize=(fwid,5))
for i,ax in enumerate(axes):
ax = swarmbox(Df[i], "Label", "Data", np.log10(1./np.array(plotpvals[i])), ax)
ax2 = ax.twinx()
ax2.plot(np.log10(1./np.array(plotpvals[i])), 'k-', linewidth=0.5)
ax.set_xticklabels(Labels[i], rotation=60, ha='right')
ax.grid(which='major', axis='y', linestyle='--')
ax.tick_params(axis='both', direction='out', labelsize=9, pad=1)
ax.tick_params(axis='y', labelsize=10)
[lab.set_color("red") for j, lab in enumerate(ax.get_xticklabels()) if np.log10(1./np.array(plotpvals[i][j])) > 2.]
ax.set_xlabel("JRC SplitGal4 Label", fontsize=8, fontweight='bold')
ax.set_ylabel(title)
ax.set_title(Substr[i], fontsize=12, loc='left', fontweight='bold')
ax.legend(loc='upper left', title=" p < 0.01", labelspacing=0.25, handletextpad=-0.2, borderpad=0.,fontsize=8)
plt.suptitle(supptitle, fontsize=12)
if len(lims)>1:
axes[0].set_ylim([lims[0], lims[1]])
if len(lims)>3:
axes[1].set_ylim([lims[2], lims[3]])
plt.tight_layout()
#folder = os.path.dirname(_file)+os.sep+"plots"+os.sep
folder = "/Users/degoldschmidt/Google Drive/PhD Project/Data/2017_01/Final plots/" ## MacOS fullpath
fullfile = get_filename(_file, ID, _sort,_suf=_fsuff)
print("Saving plot for", title, "as:", folder+fullfile)
plt.savefig(folder+fullfile, dpi=300)
plt.clf()
plt.close()
def plot_pi(_file, _sort="Y"):
ID = "PI"
title = ID.replace("_", " ")
Df, pvals = h5_to_panda(_file, ID)
if _sort == "Y":
Df = Df.sort_values("Median", ascending=True)
else:
Df = Df.sort_values("Median", ascending=False)
Labels = Df["Label"].unique()
for j, substr_labels in enumerate(Labels):
for i, labl in enumerate(substr_labels):
if type(labl) is float:
if np.isnan(labl):
print(j, labl, type(labl))
Labels[j] = np.delete(Labels[j], i)
plotpvals = [pvals[label] for label in Labels]
fig, ax = plt.subplots(figsize=(10,5))
ax = swarmbox(Df, "Label", "Data", np.log10(1./np.array(plotpvals)), ax, ps=1.5, hueon=True)
ax2 = ax.twinx()
ax2.plot(np.log10(1./np.array(plotpvals)), 'k-', linewidth=0.5)
ax.set_xticklabels(Labels, rotation=60, ha='right')
ax.grid(which='major', axis='y', linestyle='--')
ax.tick_params(axis='both', direction='out', labelsize=9, pad=1)
ax.tick_params(axis='y', labelsize=10)
[lab.set_color("red") for j, lab in enumerate(ax.get_xticklabels()) if np.log10(1./np.array(plotpvals[j])) > 2.]
ax.set_xlabel("JRC SplitGal4 Label", fontsize=8, fontweight='bold')
ax.set_ylabel(title)
ax2.set_ylabel('log10(1/p)')
ax.legend(loc='upper left', title=" p < 0.01", labelspacing=0.25, handletextpad=-0.2, borderpad=0.,fontsize=8)
plt.title(get_cond(_file), fontsize=12)
ax.set_ylim([-1, 1])
plt.tight_layout()
#folder = os.path.dirname(_file)+os.sep+"plots"+os.sep
folder = "/Users/degoldschmidt/Google Drive/PhD Project/Data/2017_01/Final plots/" ## MacOS fullpath
fullfile = get_filename(_file, ID, _sort)
print("Saving plot for", title, "as:", folder+fullfile)
plt.savefig(folder+fullfile, dpi=300)
fig.clf()
plt.close()
def plot_scatter(_files, _ID):
ID = _ID
title = _ID.replace("_", " ")
Substr = ["10% Yeast", "20 mM Sucrose"]
col = ["r", "g", "b"]
f, ax = plt.subplots(figsize=(10,5))
Df = h5multi_to_panda(_files, ID)
| gpl-3.0 |
mikehulluk/morphforge | doc/srcs_generated_examples/python_srcs/poster2.py | 1 | 3814 |
import matplotlib as mpl
mpl.rcParams['font.size'] = 14
from morphforge.stdimports import *
from morphforgecontrib.stdimports import *
# Create a cell:
def build_cell(name, sim):
my_morph = MorphologyBuilder.get_soma_axon_morph(axon_length=1500.0, axon_radius=0.3, soma_radius=10.0)
my_cell = sim.create_cell(name=name, morphology=my_morph)
na_chls = ChannelLibrary.get_channel(modelsrc=StandardModels.HH52, channeltype="Na", env=sim.environment)
k_chls = ChannelLibrary.get_channel(modelsrc=StandardModels.HH52, channeltype="K", env=sim.environment)
lk_chls = ChannelLibrary.get_channel(modelsrc=StandardModels.HH52, channeltype="Lk", env=sim.environment)
my_cell.apply_channel(lk_chls)
my_cell.apply_channel(k_chls)
my_cell.apply_channel(na_chls)
my_cell.apply_channel(na_chls, where="axon", parameter_multipliers={'gScale':1.0})
return my_cell
# Create a simulation:
env = NEURONEnvironment()
sim = env.Simulation()
# Two cells:
cell1 = build_cell(name="cell1", sim=sim)
cell2 = build_cell(name="cell2", sim=sim)
cell3 = build_cell(name="cell3", sim=sim)
# Connect with a synapse:
simple_ampa_syn = """
define_component syn_simple {
g' = - g/g_tau
i = gmax * (v-erev) * g
gmax = 300pS * scale
erev = 0mV
g_tau = 10ms
<=> INPUT v: mV METADATA {"mf":{"role":"MEMBRANEVOLTAGE"} }
<=> OUTPUT i:(mA) METADATA {"mf":{"role":"TRANSMEMBRANECURRENT"} }
<=> PARAMETER scale:()
on on_event(){
g = g + 1.0
}
}
"""
post_syn_tmpl = env.PostSynapticMechTemplate(
NeuroUnitEqnsetPostSynaptic,
eqnset = simple_ampa_syn,
default_parameters = { 'scale':1.0}
)
syn1 = sim.create_synapse(
trigger = env.SynapticTrigger(
SynapticTriggerByVoltageThreshold,
cell_location = CellLocator.get_location_at_distance_away_from_dummy(cell1, 300),
voltage_threshold = qty("0:mV"), delay=qty("0:ms"),
),
postsynaptic_mech = post_syn_tmpl.instantiate(cell_location = cell2.soma,),
)
syn1 = sim.create_synapse(
trigger = env.SynapticTrigger(
SynapticTriggerByVoltageThreshold,
cell_location = CellLocator.get_location_at_distance_away_from_dummy(cell1, 700),
voltage_threshold = qty("0:mV"), delay = qty("0:ms"),
),
postsynaptic_mech = post_syn_tmpl.instantiate(cell_location = cell3.soma, parameter_overrides={'scale':2.0} )
)
# Record Voltages from axons:
for loc in CellLocator.get_locations_at_distances_away_from_dummy(cell1, range(0, 1000, 50)):
sim.record( what=StandardTags.Voltage, cell_location = loc, user_tags=['cell1'])
sim.record(what=StandardTags.Voltage, cell_location = cell2.get_location("soma"), user_tags=['cell2'])
sim.record(what=StandardTags.Voltage, cell_location = cell3.get_location("soma"), user_tags=['cell3'])
# Create the stimulus and record the injected current:
cc = sim.create_currentclamp(name="CC1", amp=qty("200:pA"), dur=qty("1:ms"), delay=qty("100:ms"), cell_location=cell1.get_location("soma"))
sim.record(cc, what=StandardTags.Current)
results = sim.run()
TagViewer(results, timerange=(98, 120)*units.ms,
fig_kwargs = {'figsize':(12, 10)},
show=True,
plots = [
TagPlot('Current', yunit=units.picoamp),
TagPlot('Voltage,cell1', yrange=(-80*units.mV, 50*units.mV), yunit=units.mV),
TagPlot('Voltage AND ANY{cell2,cell3}', yrange=(-70*units.mV, -55*units.mV), yunit=units.millivolt),
],
)
| bsd-2-clause |
RPGOne/Skynet | scikit-learn-0.18.1/sklearn/decomposition/truncated_svd.py | 5 | 7878 | """Truncated SVD for sparse matrices, aka latent semantic analysis (LSA).
"""
# Author: Lars Buitinck
# Olivier Grisel <[email protected]>
# Michael Becker <[email protected]>
# License: 3-clause BSD.
import numpy as np
import scipy.sparse as sp
try:
from scipy.sparse.linalg import svds
except ImportError:
from ..utils.arpack import svds
from ..base import BaseEstimator, TransformerMixin
from ..utils import check_array, as_float_array, check_random_state
from ..utils.extmath import randomized_svd, safe_sparse_dot, svd_flip
from ..utils.sparsefuncs import mean_variance_axis
__all__ = ["TruncatedSVD"]
class TruncatedSVD(BaseEstimator, TransformerMixin):
"""Dimensionality reduction using truncated SVD (aka LSA).
This transformer performs linear dimensionality reduction by means of
truncated singular value decomposition (SVD). Contrary to PCA, this
estimator does not center the data before computing the singular value
decomposition. This means it can work with scipy.sparse matrices
efficiently.
In particular, truncated SVD works on term count/tf-idf matrices as
returned by the vectorizers in sklearn.feature_extraction.text. In that
context, it is known as latent semantic analysis (LSA).
This estimator supports two algorithms: a fast randomized SVD solver, and
a "naive" algorithm that uses ARPACK as an eigensolver on (X * X.T) or
(X.T * X), whichever is more efficient.
Read more in the :ref:`User Guide <LSA>`.
Parameters
----------
n_components : int, default = 2
Desired dimensionality of output data.
Must be strictly less than the number of features.
The default value is useful for visualisation. For LSA, a value of
100 is recommended.
algorithm : string, default = "randomized"
SVD solver to use. Either "arpack" for the ARPACK wrapper in SciPy
(scipy.sparse.linalg.svds), or "randomized" for the randomized
algorithm due to Halko (2009).
n_iter : int, optional (default 5)
Number of iterations for randomized SVD solver. Not used by ARPACK.
The default is larger than the default in `randomized_svd` to handle
sparse matrices that may have large slowly decaying spectrum.
random_state : int or RandomState, optional
(Seed for) pseudo-random number generator. If not given, the
numpy.random singleton is used.
tol : float, optional
Tolerance for ARPACK. 0 means machine precision. Ignored by randomized
SVD solver.
Attributes
----------
components_ : array, shape (n_components, n_features)
explained_variance_ratio_ : array, [n_components]
Percentage of variance explained by each of the selected components.
explained_variance_ : array, [n_components]
The variance of the training samples transformed by a projection to
each component.
Examples
--------
>>> from sklearn.decomposition import TruncatedSVD
>>> from sklearn.random_projection import sparse_random_matrix
>>> X = sparse_random_matrix(100, 100, density=0.01, random_state=42)
>>> svd = TruncatedSVD(n_components=5, n_iter=7, random_state=42)
>>> svd.fit(X) # doctest: +NORMALIZE_WHITESPACE
TruncatedSVD(algorithm='randomized', n_components=5, n_iter=7,
random_state=42, tol=0.0)
>>> print(svd.explained_variance_ratio_) # doctest: +ELLIPSIS
[ 0.0606... 0.0584... 0.0497... 0.0434... 0.0372...]
>>> print(svd.explained_variance_ratio_.sum()) # doctest: +ELLIPSIS
0.249...
See also
--------
PCA
RandomizedPCA
References
----------
Finding structure with randomness: Stochastic algorithms for constructing
approximate matrix decompositions
Halko, et al., 2009 (arXiv:909) http://arxiv.org/pdf/0909.4061
Notes
-----
SVD suffers from a problem called "sign indeterminancy", which means the
sign of the ``components_`` and the output from transform depend on the
algorithm and random state. To work around this, fit instances of this
class to data once, then keep the instance around to do transformations.
"""
def __init__(self, n_components=2, algorithm="randomized", n_iter=5,
random_state=None, tol=0.):
self.algorithm = algorithm
self.n_components = n_components
self.n_iter = n_iter
self.random_state = random_state
self.tol = tol
def fit(self, X, y=None):
"""Fit LSI model on training data X.
Parameters
----------
X : {array-like, sparse matrix}, shape (n_samples, n_features)
Training data.
Returns
-------
self : object
Returns the transformer object.
"""
self.fit_transform(X)
return self
def fit_transform(self, X, y=None):
"""Fit LSI model to X and perform dimensionality reduction on X.
Parameters
----------
X : {array-like, sparse matrix}, shape (n_samples, n_features)
Training data.
Returns
-------
X_new : array, shape (n_samples, n_components)
Reduced version of X. This will always be a dense array.
"""
X = as_float_array(X, copy=False)
random_state = check_random_state(self.random_state)
# If sparse and not csr or csc, convert to csr
if sp.issparse(X) and X.getformat() not in ["csr", "csc"]:
X = X.tocsr()
if self.algorithm == "arpack":
U, Sigma, VT = svds(X, k=self.n_components, tol=self.tol)
# svds doesn't abide by scipy.linalg.svd/randomized_svd
# conventions, so reverse its outputs.
Sigma = Sigma[::-1]
U, VT = svd_flip(U[:, ::-1], VT[::-1])
elif self.algorithm == "randomized":
k = self.n_components
n_features = X.shape[1]
if k >= n_features:
raise ValueError("n_components must be < n_features;"
" got %d >= %d" % (k, n_features))
U, Sigma, VT = randomized_svd(X, self.n_components,
n_iter=self.n_iter,
random_state=random_state)
else:
raise ValueError("unknown algorithm %r" % self.algorithm)
self.components_ = VT
# Calculate explained variance & explained variance ratio
X_transformed = U * Sigma
self.explained_variance_ = exp_var = np.var(X_transformed, axis=0)
if sp.issparse(X):
_, full_var = mean_variance_axis(X, axis=0)
full_var = full_var.sum()
else:
full_var = np.var(X, axis=0).sum()
self.explained_variance_ratio_ = exp_var / full_var
return X_transformed
def transform(self, X):
"""Perform dimensionality reduction on X.
Parameters
----------
X : {array-like, sparse matrix}, shape (n_samples, n_features)
New data.
Returns
-------
X_new : array, shape (n_samples, n_components)
Reduced version of X. This will always be a dense array.
"""
X = check_array(X, accept_sparse='csr')
return safe_sparse_dot(X, self.components_.T)
def inverse_transform(self, X):
"""Transform X back to its original space.
Returns an array X_original whose transform would be X.
Parameters
----------
X : array-like, shape (n_samples, n_components)
New data.
Returns
-------
X_original : array, shape (n_samples, n_features)
Note that this is always a dense array.
"""
X = check_array(X)
return np.dot(X, self.components_)
| bsd-3-clause |
lamastex/scalable-data-science | dbcArchives/2021/000_0-sds-3-x-projects/student-project-19_group-Featuring/development/FundamentalMatrix_dev_50images.py | 1 | 17630 | # Databricks notebook source
# MAGIC %md
# MAGIC ## Problem formulation
# MAGIC A common problem in computer vision is estimating the fundamental matrix based on a image pair. The fundamental matrix relates corresponding points in stereo geometry, and is useful as a pre-processing step for example when one wants to perform reconstruction of a captured scene. In this small project we use a scalable distributed algorithm to compute fundamental matrices between a large set of images.
# MAGIC
# MAGIC #### Short theory section
# MAGIC Assume that we want to link points in some image taken by camera <img src="https://latex.codecogs.com/svg.latex?&space;P_1" /> to points in an image taken by another camera <img src="https://latex.codecogs.com/svg.latex?&space;P_2" />. Let <img src="https://latex.codecogs.com/svg.latex?&space;x_i" /> and <img src="https://latex.codecogs.com/svg.latex?&space;x_i'" /> denote the projections of global point <img src="https://latex.codecogs.com/svg.latex?&space;X_i" /> onto the cameras <img src="https://latex.codecogs.com/svg.latex?&space;P_1" /> and <img src="https://latex.codecogs.com/svg.latex?&space;P_2" />, respectivly. Then the points are related as follows
# MAGIC
# MAGIC <img src="https://latex.codecogs.com/svg.latex?&space;\begin{cases}\lambda_i x_i = P_1X_i \\ \lambda_i' x_i' = P_2X_i
# MAGIC \end{cases} \Leftrightarrow \quad \begin{cases}\lambda_i x_i = P_1HH^{-1}X_i \\ \lambda_i' x_i' = P_2HH^{-1}X_i
# MAGIC \end{cases} \Leftrightarrow \quad \begin{cases}\lambda_i x_i = \tilde{P_1}\tilde{X_i} \\ \lambda_i' x_i' = \tilde{P_2}\tilde{X_i}
# MAGIC \end{cases}" />
# MAGIC
# MAGIC where <img src="https://latex.codecogs.com/svg.latex?&space;\lambda, \lambda'" /> are scale factors. Since we always can apply a projective transformation <img src="https://latex.codecogs.com/svg.latex?&space;H" /> to set one of the cameras to <img src="https://latex.codecogs.com/svg.latex?&space;P_1 = [I \quad 0]" /> and the other to some <img src="https://latex.codecogs.com/svg.latex?&space;P_2 = [A \quad t]" /> we can parametrize the global point <img src="https://latex.codecogs.com/svg.latex?&space;X_i" /> by
# MAGIC <img src="https://latex.codecogs.com/svg.latex?&space;X_i(\lambda) = [\lambda x_i \quad 1]^T" />. Thus the projected point onto camera <img src="https://latex.codecogs.com/svg.latex?&space;P_2" /> is represented by the line <img src="https://latex.codecogs.com/svg.latex?&space;P_2X_i(\lambda) = \lambda Ax_i + t " />. This line is called the epipolar line to the point <img src="https://latex.codecogs.com/svg.latex?&space;x_i" /> in epipolar geomtry, and descirbes how the point <img src="https://latex.codecogs.com/svg.latex?&space;x_i" /> in image 1 is related to points on in image 2. Since
# MAGIC all scene points that can project to <img src="https://latex.codecogs.com/svg.latex?&space;x_i" /> are on the viewing ray, all points in the second image that can
# MAGIC correspond <img src="https://latex.codecogs.com/svg.latex?&space;x_i" /> have to be on the epipolar line. This condition is called the epipolar constraint.
# MAGIC
# MAGIC 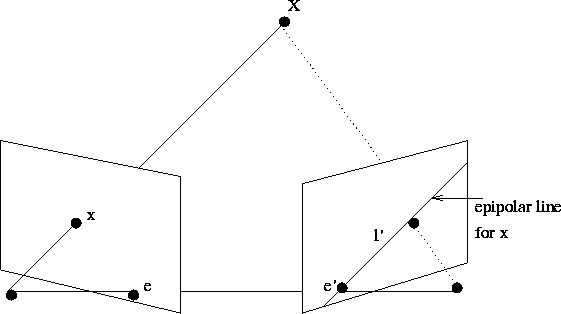
# MAGIC
# MAGIC Taking two points on this line (one of them being <img src="https://latex.codecogs.com/svg.latex?&space;e'" /> using <img src="https://latex.codecogs.com/svg.latex?&space;\lambda = 0" />), (add what is e_2)
# MAGIC we can derive an expression of this line <img src="https://latex.codecogs.com/svg.latex?&space;\ell" />, as any point x on the line <img src="https://latex.codecogs.com/svg.latex?&space;\ell" /> must fulfill <img src="https://latex.codecogs.com/svg.latex?&space;\ell^Tx = 0" />. Thus the line is thus given by
# MAGIC
# MAGIC
# MAGIC <img src="https://latex.codecogs.com/svg.latex?&space;\ell = t
# MAGIC \times (Ax +t ) = t \times (Ax) = e' \times Ax_i.\\" />
# MAGIC
# MAGIC Let <img src="https://latex.codecogs.com/svg.latex?&space;F = e' \times A " />, this is called the fundamental matrix. The fundamental matrix thus is a mathematical formulation which links points in image 1 to lines in image 2 (and vice versa). If <img src="https://latex.codecogs.com/svg.latex?&space;x'" /> corresponds to <img src="https://latex.codecogs.com/svg.latex?&space;x" /> then the epipolar constraint can be written
# MAGIC
# MAGIC <img src="https://latex.codecogs.com/svg.latex?&space;x'^T\ell = x'^T F x = 0 " />
# MAGIC
# MAGIC F is a 3x 3 matrix with 9 entiers and has 7 degrees of freedom. It can be estimated using 7 points using the 7-point algorithm.
# MAGIC
# MAGIC
# MAGIC Before we have assumed the the correspndeces between points in the imagaes are known, however these are found by first extracting features in the images using some form of feature extractor (e.g. SIFT) and subsequently finding matches using some mathcing criterion/algorithm (e.g. using Lowes criterion or in our case FLANN based matcher)
# MAGIC #### SIFT
# MAGIC Scale-invariant feature transform (SIFT) is a feature detection algorithm which detect and describe local features in images, see examples of detected SIFT features in the two images (a) and (b). SIFT finds local features present in the image and compute desriptors and locations of these features. Next we need to link the features present in image 1 to the features in image 2, which can be done using e.g. a FLANN (Fast Library for Approximate Nearest Neighbors) based matcher. In short the features in the images are compared and the matches are found using a nearest neighbor search. After a matching algorithm is used we have correspandence between the detected points in image 1 and image 2, see example in image (c) below. Note that there is still a high probaility that some of these matches are incorrect.
# MAGIC
# MAGIC 
# MAGIC
# MAGIC ### RANSAC
# MAGIC Some matches found by the FLANN may be incorrect, and a common robust method used for reducing the influence of these outliers in the estimation of F is RANSAC (RANdom SAmpling Consensus). In short, it relies on the fact that the inliers will tend to a consesus regarding the correct estimation, whereas the outlier estimation will show greater variation. By sampling random sets of points with size corresponding to the degrees of freedom of the model, calculating their corresponding estimations, and grouping all estimations with a difference below a set threshold, the largest consesus group is found. This set is then lastly used for the final estimate of F.
# COMMAND ----------
# MAGIC %md
# MAGIC ###For a more joyful presentation of the theory, listed to The Fundamental Matrix Song! (link)
# MAGIC [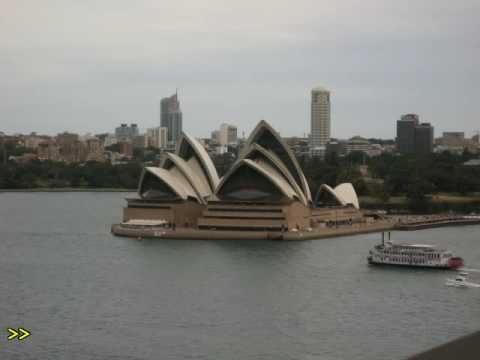](https://www.youtube.com/watch?v=DgGV3l82NTk)
# COMMAND ----------
# MAGIC %md
# MAGIC OpenCV is an well-known open-source library for computer vision, machine learning, and image processing tasks. In this project we will use it for feature extraction (SIFT), feature matching (FLANN) and the estimation of the fundamental matrix (using the 7-point algorithm). Let us install opencv
# COMMAND ----------
# MAGIC %pip install opencv-python
# COMMAND ----------
# MAGIC %md
# MAGIC Also we need to download a dataset that we can work with, this dataset is collected by Carl Olsson from LTH.
# MAGIC This is achieved by the bash shell script below.
# MAGIC The dataset is placed in the /tmp folder using the -P "prefix"
# COMMAND ----------
# MAGIC %sh
# MAGIC rm -r /tmp/0019
# MAGIC rm -r /tmp/eglise_int1.zip
# MAGIC
# MAGIC wget -P /tmp vision.maths.lth.se/calledataset/eglise_int/eglise_int1.zip
# MAGIC unzip /tmp/eglise_int1.zip -d /tmp/0019/
# MAGIC rm -r /tmp/eglise_int1.zip
# COMMAND ----------
# MAGIC %sh
# MAGIC rm -r /tmp/eglise_int2.zip
# MAGIC
# MAGIC wget -P /tmp vision.maths.lth.se/calledataset/eglise_int/eglise_int2.zip
# MAGIC unzip /tmp/eglise_int2.zip -d /tmp/0019/
# MAGIC rm -r /tmp/eglise_int2.zip
# COMMAND ----------
# MAGIC %sh
# MAGIC rm -r /tmp/eglise_int3.zip
# MAGIC
# MAGIC wget -P /tmp vision.maths.lth.se/calledataset/eglise_int/eglise_int3.zip
# MAGIC unzip /tmp/eglise_int3.zip -d /tmp/0019/
# MAGIC rm -r /tmp/eglise_int3.zip
# COMMAND ----------
# MAGIC %sh
# MAGIC cd /tmp/0019/
# MAGIC for f in *; do mv "$f" "eglise_$f"; done
# MAGIC cd /databricks/driver
# COMMAND ----------
# MAGIC %sh
# MAGIC rm -r /tmp/gbg.zip
# MAGIC
# MAGIC wget -P /tmp vision.maths.lth.se/calledataset/gbg/gbg.zip
# MAGIC unzip /tmp/gbg.zip -d /tmp/0019/
# MAGIC rm -r /tmp/gbg.zip
# COMMAND ----------
# MAGIC %scala
# MAGIC
# MAGIC import sys.process._
# MAGIC
# MAGIC //"wget -P /tmp vision.maths.lth.se/calledataset/door/door.zip" !!
# MAGIC //"unzip /tmp/door.zip -d /tmp/door/"!!
# MAGIC
# MAGIC //move downloaded dataset to dbfs
# MAGIC
# MAGIC val localpath="file:/tmp/0019/"
# MAGIC
# MAGIC dbutils.fs.rm("dbfs:/datasets/0019/mixedimages", true) // the boolean is for recursive rm
# MAGIC
# MAGIC dbutils.fs.mkdirs("dbfs:/datasets/0019/mixedimages")
# MAGIC
# MAGIC dbutils.fs.cp(localpath, "dbfs:/datasets/0019/mixedimages", true)
# COMMAND ----------
# MAGIC %sh
# MAGIC rm -r /tmp/0019
# COMMAND ----------
# MAGIC %scala
# MAGIC
# MAGIC display(dbutils.fs.ls("dbfs:/datasets/0019/mixedimages"))
# COMMAND ----------
#Loading one image from the dataset for testing
import numpy as np
import cv2
import matplotlib.pyplot as plt
def plot_img(figtitle,img):
#create figure with std size
fig = plt.figure(figtitle, figsize=(10, 5))
plt.imshow(img)
display(plt.show())
img1 = cv2.imread("/dbfs/datasets/0019/mixedimages/eglise_DSC_0133.JPG")
#img2 = cv2.imread("/dbfs/datasets/0019/mixedimages/DSC_0133.JPG")
plot_img("eglise", img1)
#plot_img("gbg", img2)
# COMMAND ----------
# MAGIC %md
# MAGIC
# MAGIC Read Image Dataset
# COMMAND ----------
import glob
import numpy as np
import cv2
import os
dataset_path = "/dbfs/datasets/0019/mixedimages/"
#get all filenames in folder
files = glob.glob(os.path.join(dataset_path,"*.JPG"))
dataset = []
#load all images
for i, file in enumerate(files): # Alex: changed
# Load an color image
#img = cv2.imread(file)
#add image and image name as a tupel to the list
dataset.append((file))
if i >= 50: # Alex: changed
break
# COMMAND ----------
# MAGIC %md
# MAGIC
# MAGIC Define maps
# COMMAND ----------
import glob
import numpy as np
import cv2
import matplotlib.pyplot as plt
max_features = 1000
def plot_img(figtitle,s):
img = cv2.imread(s)
#create figure with std size
fig = plt.figure(figtitle, figsize=(10, 5))
plt.imshow(img)
display(plt.show())
def extract_features(s):
"""
"""
img = cv2.imread(s) # Johan : here we load the images on the executor from dbfs into memory
#convert to gray scale
gray= cv2.cvtColor(img,cv2.COLOR_BGR2GRAY)
sift = cv2.SIFT_create(max_features)
#extract sift features and descriptors
kp, des = sift.detectAndCompute(gray, None)
#convert keypoint class to list of feature locations (for serialization)
points=[]
for i in range(len(kp)):
points.append(kp[i].pt)
#return a tuple of image name, image, feature points, descriptors, called a feature tuple
return (s, points, des) # Johan : here we don't send the images
def estimate_fundamental_matrix(s):
"""
"""
# s[0] is a feature tuple for the first image, s[1] is the same for the second image
a = s[0]
b = s[1]
# unpacks the tuples
name1, kp1, desc1 = a
name2, kp2, desc2 = b
# Create FLANN matcher object
FLANN_INDEX_KDTREE = 0
indexParams = dict(algorithm=FLANN_INDEX_KDTREE,
trees=5)
searchParams = dict(checks=50)
flann = cv2.FlannBasedMatcher(indexParams,
searchParams)
# matches the descriptors, for each query descriptor it finds the two best matches among the train descriptors
matches = flann.knnMatch(desc1, desc2, k=2)
goodMatches = []
pts1 = []
pts2 = []
# compares the best with the second best match and only adds those where the best match is significantly better than the next best.
for i,(m,n) in enumerate(matches):
if m.distance < 0.8*n.distance:
goodMatches.append([m.queryIdx, m.trainIdx])
pts2.append(kp2[m.trainIdx])
pts1.append(kp1[m.queryIdx])
pts1 = np.array(pts1, dtype=np.float32)
pts2 = np.array(pts2, dtype=np.float32)
# finds the fundamental matrix using ransac:
# selects minimal sub-set of the matches,
# estimates the fundamental matrix,
# checks how many of the matches satisfy the epipolar geometry (the inlier set)
# iterates this for a number of iterations,
# returns the fundamental matrix and mask with the largest number of inliers.
F, mask = cv2.findFundamentalMat(pts1, pts2, cv2.FM_RANSAC)
inlier_matches = []
# removes all matches that are not inliers
if mask is not None:
for i, el in enumerate(mask):
if el == 1:
inlier_matches.append(goodMatches[i])
# returns a tuple containing the feature tuple of image one and image two, the fundamental matrix and the inlier matches
return (a, b, F, inlier_matches)
def display_data(data):
for el in data:
print(el[2])
print("#######################################################")
# COMMAND ----------
# MAGIC %md
# MAGIC Perform Calculations
# COMMAND ----------
# creates an rdd from the loaded images (im_name, image)
rdd = sc.parallelize(dataset)
print("num partitions: ",rdd.getNumPartitions())
# applys the feature extraction to the images
rdd_features = rdd.map(extract_features) # Alex: we could leave the name but remove the image in a and b
# forms pairs of images by applying the cartisian product and filtering away the identity pair
rdd_pairs = rdd_features.cartesian(rdd_features).filter(lambda s: s[0][0] != s[1][0])
# applys the fundamental matrix estimation function on the pairs formed in the previous step and filters away all pairs with a low inlier set.
rdd_fundamental_matrix = rdd_pairs.map(estimate_fundamental_matrix).filter(lambda s: len(s[3]) > 50)
# collects the result from the nodes
data = rdd_fundamental_matrix.collect()
# displays the fundamental matrices
display_data(data)
# COMMAND ----------
# MAGIC %md
# MAGIC Now we have computed the fundamental matrices, let us have a look at them by present the epipolar lines.
# COMMAND ----------
import random
def drawlines(img1,img2,lines,pts1,pts2):
#from opencv tutorial
''' img1 - image on which we draw the epilines for the points in img2
lines - corresponding epilines '''
r,c,_ = img1.shape
for r,pt1,pt2 in zip(lines,pts1,pts2):
color = tuple(np.random.randint(0,255,3).tolist())
x0,y0 = map(int, [0, -r[2]/r[1] ])
x1,y1 = map(int, [c, -(r[2]+r[0]*c)/r[1] ])
img1 = cv2.line(img1, (x0,y0), (x1,y1), color,3)
img1 = cv2.circle(img1,tuple(pt1),10,color,-1)
img2 = cv2.circle(img2,tuple(pt2),10,color,-1)
return img1,img2
# draws a random subset of the data
sampling = random.choices(data, k=4)
#plotts the inlier features in the first image and the corresponding epipolar lines in the second image
i = 0
fig, axs = plt.subplots(1, 8, figsize=(25, 5))
for el in sampling:
a, b, F, matches = el;
if F is None:
continue
name1, kp1, desc1 = a
name2, kp2, desc2 = b
im1 = cv2.imread(name1)
im2 = cv2.imread(name2)
pts1 = []
pts2 = []
for m in matches:
pts1.append(kp1[m[0]]);
pts2.append(kp2[m[1]]);
pts1 = np.array(pts1, dtype=np.float32)
pts2 = np.array(pts2, dtype=np.float32)
lines1 = cv2.computeCorrespondEpilines(pts2.reshape(-1,1,2), 2, F)
lines1 = lines1.reshape(-1,3)
img1, img2 = drawlines(im1,im2,lines1,pts1,pts2)
axs[i].imshow(img2), axs[i].set_title('Image pair '+str(i+1)+': Features')
axs[i+1].imshow(img1), axs[i+1].set_title('Image pair '+str(i+1)+': Epipolar lines')
i += 2
#plt.subplot(121),plt.imshow(img1), plt.title('Epipolar lines')
#plt.subplot(122),plt.imshow(img2), plt.title('Points')
display(plt.show())
# COMMAND ----------
# MAGIC %md
# MAGIC Present Matches
# COMMAND ----------
import random
# draws a random subset of the data
sampling = random.choices(data, k=4)
j = 0
fig, axs = plt.subplots(1, 4, figsize=(25, 5))
# draws lines between the matched feature in the two images (not epipolar lines!)
for el in sampling:
a, b, F, matches = el;
if F is None:
continue
name1, kp1, desc1 = a
name2, kp2, desc2 = b
im1 = cv2.imread(name1)
im2 = cv2.imread(name2)
kp1_vec = []
kp2_vec = []
matches_vec = []
for i,m in enumerate(matches):
kp1_vec.append(cv2.KeyPoint(kp1[m[0]][0], kp1[m[0]][1],1))
kp2_vec.append(cv2.KeyPoint(kp2[m[1]][0], kp2[m[1]][1],1))
matches_vec.append(cv2.DMatch(i, i, 1))
matched_image = im1.copy()
matched_image = cv2.drawMatches(im1, kp1_vec, im2, kp2_vec, matches_vec, matched_image)
axs[j].imshow(matched_image), axs[j].set_title('Image pair '+str(j+1)+': Matches')
j += 1
#plot_img("matches", matched_image)
display(plt.show())
# COMMAND ----------
# Questions:
# Pics of different resolutions/sizes: Yes
#
# COMMAND ----------
| unlicense |
argriffing/cvxpy | doc/sphinxext/docscrape_sphinx.py | 154 | 7759 | import re, inspect, textwrap, pydoc
import sphinx
from docscrape import NumpyDocString, FunctionDoc, ClassDoc
class SphinxDocString(NumpyDocString):
def __init__(self, docstring, config={}):
self.use_plots = config.get('use_plots', False)
NumpyDocString.__init__(self, docstring, config=config)
# string conversion routines
def _str_header(self, name, symbol='`'):
return ['.. rubric:: ' + name, '']
def _str_field_list(self, name):
return [':' + name + ':']
def _str_indent(self, doc, indent=4):
out = []
for line in doc:
out += [' '*indent + line]
return out
def _str_signature(self):
return ['']
if self['Signature']:
return ['``%s``' % self['Signature']] + ['']
else:
return ['']
def _str_summary(self):
return self['Summary'] + ['']
def _str_extended_summary(self):
return self['Extended Summary'] + ['']
def _str_param_list(self, name):
out = []
if self[name]:
out += self._str_field_list(name)
out += ['']
for param,param_type,desc in self[name]:
out += self._str_indent(['**%s** : %s' % (param.strip(),
param_type)])
out += ['']
out += self._str_indent(desc,8)
out += ['']
return out
@property
def _obj(self):
if hasattr(self, '_cls'):
return self._cls
elif hasattr(self, '_f'):
return self._f
return None
def _str_member_list(self, name):
"""
Generate a member listing, autosummary:: table where possible,
and a table where not.
"""
out = []
if self[name]:
out += ['.. rubric:: %s' % name, '']
prefix = getattr(self, '_name', '')
if prefix:
prefix = '~%s.' % prefix
autosum = []
others = []
for param, param_type, desc in self[name]:
param = param.strip()
if not self._obj or hasattr(self._obj, param):
autosum += [" %s%s" % (prefix, param)]
else:
others.append((param, param_type, desc))
if autosum:
out += ['.. autosummary::', ' :toctree:', '']
out += autosum
if others:
maxlen_0 = max([len(x[0]) for x in others])
maxlen_1 = max([len(x[1]) for x in others])
hdr = "="*maxlen_0 + " " + "="*maxlen_1 + " " + "="*10
fmt = '%%%ds %%%ds ' % (maxlen_0, maxlen_1)
n_indent = maxlen_0 + maxlen_1 + 4
out += [hdr]
for param, param_type, desc in others:
out += [fmt % (param.strip(), param_type)]
out += self._str_indent(desc, n_indent)
out += [hdr]
out += ['']
return out
def _str_section(self, name):
out = []
if self[name]:
out += self._str_header(name)
out += ['']
content = textwrap.dedent("\n".join(self[name])).split("\n")
out += content
out += ['']
return out
def _str_see_also(self, func_role):
out = []
if self['See Also']:
see_also = super(SphinxDocString, self)._str_see_also(func_role)
out = ['.. seealso::', '']
out += self._str_indent(see_also[2:])
return out
def _str_warnings(self):
out = []
if self['Warnings']:
out = ['.. warning::', '']
out += self._str_indent(self['Warnings'])
return out
def _str_index(self):
idx = self['index']
out = []
if len(idx) == 0:
return out
out += ['.. index:: %s' % idx.get('default','')]
for section, references in idx.iteritems():
if section == 'default':
continue
elif section == 'refguide':
out += [' single: %s' % (', '.join(references))]
else:
out += [' %s: %s' % (section, ','.join(references))]
return out
def _str_references(self):
out = []
if self['References']:
out += self._str_header('References')
if isinstance(self['References'], str):
self['References'] = [self['References']]
out.extend(self['References'])
out += ['']
# Latex collects all references to a separate bibliography,
# so we need to insert links to it
if sphinx.__version__ >= "0.6":
out += ['.. only:: latex','']
else:
out += ['.. latexonly::','']
items = []
for line in self['References']:
m = re.match(r'.. \[([a-z0-9._-]+)\]', line, re.I)
if m:
items.append(m.group(1))
out += [' ' + ", ".join(["[%s]_" % item for item in items]), '']
return out
def _str_examples(self):
examples_str = "\n".join(self['Examples'])
if (self.use_plots and 'import matplotlib' in examples_str
and 'plot::' not in examples_str):
out = []
out += self._str_header('Examples')
out += ['.. plot::', '']
out += self._str_indent(self['Examples'])
out += ['']
return out
else:
return self._str_section('Examples')
def __str__(self, indent=0, func_role="obj"):
out = []
out += self._str_signature()
out += self._str_index() + ['']
out += self._str_summary()
out += self._str_extended_summary()
for param_list in ('Parameters', 'Returns', 'Other Parameters',
'Raises', 'Warns'):
out += self._str_param_list(param_list)
out += self._str_warnings()
out += self._str_see_also(func_role)
out += self._str_section('Notes')
out += self._str_references()
out += self._str_examples()
for param_list in ('Attributes', 'Methods'):
out += self._str_member_list(param_list)
out = self._str_indent(out,indent)
return '\n'.join(out)
class SphinxFunctionDoc(SphinxDocString, FunctionDoc):
def __init__(self, obj, doc=None, config={}):
self.use_plots = config.get('use_plots', False)
FunctionDoc.__init__(self, obj, doc=doc, config=config)
class SphinxClassDoc(SphinxDocString, ClassDoc):
def __init__(self, obj, doc=None, func_doc=None, config={}):
self.use_plots = config.get('use_plots', False)
ClassDoc.__init__(self, obj, doc=doc, func_doc=None, config=config)
class SphinxObjDoc(SphinxDocString):
def __init__(self, obj, doc=None, config={}):
self._f = obj
SphinxDocString.__init__(self, doc, config=config)
def get_doc_object(obj, what=None, doc=None, config={}):
if what is None:
if inspect.isclass(obj):
what = 'class'
elif inspect.ismodule(obj):
what = 'module'
elif callable(obj):
what = 'function'
else:
what = 'object'
if what == 'class':
return SphinxClassDoc(obj, func_doc=SphinxFunctionDoc, doc=doc,
config=config)
elif what in ('function', 'method'):
return SphinxFunctionDoc(obj, doc=doc, config=config)
else:
if doc is None:
doc = pydoc.getdoc(obj)
return SphinxObjDoc(obj, doc, config=config)
| gpl-3.0 |
alekz112/statsmodels | statsmodels/graphics/gofplots.py | 29 | 26714 | from statsmodels.compat.python import lzip, string_types
import numpy as np
from scipy import stats
from statsmodels.regression.linear_model import OLS
from statsmodels.tools.tools import add_constant
from statsmodels.tools.decorators import (resettable_cache,
cache_readonly,
cache_writable)
from . import utils
__all__ = ['qqplot', 'qqplot_2samples', 'qqline', 'ProbPlot']
class ProbPlot(object):
"""
Class for convenient construction of Q-Q, P-P, and probability plots.
Can take arguments specifying the parameters for dist or fit them
automatically. (See fit under kwargs.)
Parameters
----------
data : array-like
1d data array
dist : A scipy.stats or statsmodels distribution
Compare x against dist. The default is
scipy.stats.distributions.norm (a standard normal).
distargs : tuple
A tuple of arguments passed to dist to specify it fully
so dist.ppf may be called.
loc : float
Location parameter for dist
a : float
Offset for the plotting position of an expected order
statistic, for example. The plotting positions are given
by (i - a)/(nobs - 2*a + 1) for i in range(0,nobs+1)
scale : float
Scale parameter for dist
fit : boolean
If fit is false, loc, scale, and distargs are passed to the
distribution. If fit is True then the parameters for dist
are fit automatically using dist.fit. The quantiles are formed
from the standardized data, after subtracting the fitted loc
and dividing by the fitted scale.
See Also
--------
scipy.stats.probplot
Notes
-----
1) Depends on matplotlib.
2) If `fit` is True then the parameters are fit using the
distribution's `fit()` method.
3) The call signatures for the `qqplot`, `ppplot`, and `probplot`
methods are similar, so examples 1 through 4 apply to all
three methods.
4) The three plotting methods are summarized below:
ppplot : Probability-Probability plot
Compares the sample and theoretical probabilities (percentiles).
qqplot : Quantile-Quantile plot
Compares the sample and theoretical quantiles
probplot : Probability plot
Same as a Q-Q plot, however probabilities are shown in the scale of
the theoretical distribution (x-axis) and the y-axis contains
unscaled quantiles of the sample data.
Examples
--------
>>> import statsmodels.api as sm
>>> from matplotlib import pyplot as plt
>>> # example 1
>>> data = sm.datasets.longley.load()
>>> data.exog = sm.add_constant(data.exog)
>>> model = sm.OLS(data.endog, data.exog)
>>> mod_fit = model.fit()
>>> res = mod_fit.resid # residuals
>>> probplot = sm.ProbPlot(res)
>>> probplot.qqplot()
>>> plt.show()
qqplot of the residuals against quantiles of t-distribution with 4
degrees of freedom:
>>> # example 2
>>> import scipy.stats as stats
>>> probplot = sm.ProbPlot(res, stats.t, distargs=(4,))
>>> fig = probplot.qqplot()
>>> plt.show()
qqplot against same as above, but with mean 3 and std 10:
>>> # example 3
>>> probplot = sm.ProbPlot(res, stats.t, distargs=(4,), loc=3, scale=10)
>>> fig = probplot.qqplot()
>>> plt.show()
Automatically determine parameters for t distribution including the
loc and scale:
>>> # example 4
>>> probplot = sm.ProbPlot(res, stats.t, fit=True)
>>> fig = probplot.qqplot(line='45')
>>> plt.show()
A second `ProbPlot` object can be used to compare two seperate sample
sets by using the `other` kwarg in the `qqplot` and `ppplot` methods.
>>> # example 5
>>> import numpy as np
>>> x = np.random.normal(loc=8.25, scale=2.75, size=37)
>>> y = np.random.normal(loc=8.75, scale=3.25, size=37)
>>> pp_x = sm.ProbPlot(x, fit=True)
>>> pp_y = sm.ProbPlot(y, fit=True)
>>> fig = pp_x.qqplot(line='45', other=pp_y)
>>> plt.show()
The following plot displays some options, follow the link to see the
code.
.. plot:: plots/graphics_gofplots_qqplot.py
"""
def __init__(self, data, dist=stats.norm, fit=False,
distargs=(), a=0, loc=0, scale=1):
self.data = data
self.a = a
self.nobs = data.shape[0]
self.distargs = distargs
self.fit = fit
if isinstance(dist, string_types):
dist = getattr(stats, dist)
self.fit_params = dist.fit(data)
if fit:
self.loc = self.fit_params[-2]
self.scale = self.fit_params[-1]
if len(self.fit_params) > 2:
self.dist = dist(*self.fit_params[:-2],
**dict(loc = 0, scale = 1))
else:
self.dist = dist(loc=0, scale=1)
elif distargs or loc == 0 or scale == 1:
self.dist = dist(*distargs, **dict(loc=loc, scale=scale))
self.loc = loc
self.scale = scale
else:
self.dist = dist
self.loc = loc
self.scale = scale
# propertes
self._cache = resettable_cache()
@cache_readonly
def theoretical_percentiles(self):
return plotting_pos(self.nobs, self.a)
@cache_readonly
def theoretical_quantiles(self):
try:
return self.dist.ppf(self.theoretical_percentiles)
except TypeError:
msg = '%s requires more parameters to ' \
'compute ppf'.format(self.dist.name,)
raise TypeError(msg)
except:
msg = 'failed to compute the ppf of {0}'.format(self.dist.name,)
raise
@cache_readonly
def sorted_data(self):
sorted_data = np.array(self.data, copy=True)
sorted_data.sort()
return sorted_data
@cache_readonly
def sample_quantiles(self):
if self.fit and self.loc != 0 and self.scale != 1:
return (self.sorted_data-self.loc)/self.scale
else:
return self.sorted_data
@cache_readonly
def sample_percentiles(self):
quantiles = \
(self.sorted_data - self.fit_params[-2])/self.fit_params[-1]
return self.dist.cdf(quantiles)
def ppplot(self, xlabel=None, ylabel=None, line=None, other=None,
ax=None, **plotkwargs):
"""
P-P plot of the percentiles (probabilities) of x versus the
probabilities (percetiles) of a distribution.
Parameters
----------
xlabel, ylabel : str or None, optional
User-provided lables for the x-axis and y-axis. If None (default),
other values are used depending on the status of the kwarg `other`.
line : str {'45', 's', 'r', q'} or None, optional
Options for the reference line to which the data is compared:
- '45' - 45-degree line
- 's' - standardized line, the expected order statistics are scaled
by the standard deviation of the given sample and have the mean
added to them
- 'r' - A regression line is fit
- 'q' - A line is fit through the quartiles.
- None - by default no reference line is added to the plot.
other : `ProbPlot` instance, array-like, or None, optional
If provided, the sample quantiles of this `ProbPlot` instance are
plotted against the sample quantiles of the `other` `ProbPlot`
instance. If an array-like object is provided, it will be turned
into a `ProbPlot` instance using default parameters. If not provided
(default), the theoretical quantiles are used.
ax : Matplotlib AxesSubplot instance, optional
If given, this subplot is used to plot in instead of a new figure
being created.
**plotkwargs : additional matplotlib arguments to be passed to the
`plot` command.
Returns
-------
fig : Matplotlib figure instance
If `ax` is None, the created figure. Otherwise the figure to which
`ax` is connected.
"""
if other is not None:
check_other = isinstance(other, ProbPlot)
if not check_other:
other = ProbPlot(other)
fig, ax = _do_plot(other.sample_percentiles,
self.sample_percentiles,
self.dist, ax=ax, line=line,
**plotkwargs)
if xlabel is None:
xlabel = 'Probabilities of 2nd Sample'
if ylabel is None:
ylabel = 'Probabilities of 1st Sample'
else:
fig, ax = _do_plot(self.theoretical_percentiles,
self.sample_percentiles,
self.dist, ax=ax, line=line,
**plotkwargs)
if xlabel is None:
xlabel = "Theoretical Probabilities"
if ylabel is None:
ylabel = "Sample Probabilities"
ax.set_xlabel(xlabel)
ax.set_ylabel(ylabel)
ax.set_xlim([0.0, 1.0])
ax.set_ylim([0.0, 1.0])
return fig
def qqplot(self, xlabel=None, ylabel=None, line=None, other=None,
ax=None, **plotkwargs):
"""
Q-Q plot of the quantiles of x versus the quantiles/ppf of a
distribution or the quantiles of another `ProbPlot` instance.
Parameters
----------
xlabel, ylabel : str or None, optional
User-provided lables for the x-axis and y-axis. If None (default),
other values are used depending on the status of the kwarg `other`.
line : str {'45', 's', 'r', q'} or None, optional
Options for the reference line to which the data is compared:
- '45' - 45-degree line
- 's' - standardized line, the expected order statistics are scaled
by the standard deviation of the given sample and have the mean
added to them
- 'r' - A regression line is fit
- 'q' - A line is fit through the quartiles.
- None - by default no reference line is added to the plot.
other : `ProbPlot` instance, array-like, or None, optional
If provided, the sample quantiles of this `ProbPlot` instance are
plotted against the sample quantiles of the `other` `ProbPlot`
instance. If an array-like object is provided, it will be turned
into a `ProbPlot` instance using default parameters. If not
provided (default), the theoretical quantiles are used.
ax : Matplotlib AxesSubplot instance, optional
If given, this subplot is used to plot in instead of a new figure
being created.
**plotkwargs : additional matplotlib arguments to be passed to the
`plot` command.
Returns
-------
fig : Matplotlib figure instance
If `ax` is None, the created figure. Otherwise the figure to which
`ax` is connected.
"""
if other is not None:
check_other = isinstance(other, ProbPlot)
if not check_other:
other = ProbPlot(other)
fig, ax = _do_plot(other.sample_quantiles,
self.sample_quantiles,
self.dist, ax=ax, line=line,
**plotkwargs)
if xlabel is None:
xlabel = 'Quantiles of 2nd Sample'
if ylabel is None:
ylabel = 'Quantiles of 1st Sample'
else:
fig, ax = _do_plot(self.theoretical_quantiles,
self.sample_quantiles,
self.dist, ax=ax, line=line,
**plotkwargs)
if xlabel is None:
xlabel = "Theoretical Quantiles"
if ylabel is None:
ylabel = "Sample Quantiles"
ax.set_xlabel(xlabel)
ax.set_ylabel(ylabel)
return fig
def probplot(self, xlabel=None, ylabel=None, line=None,
exceed=False, ax=None, **plotkwargs):
"""
Probability plot of the unscaled quantiles of x versus the
probabilities of a distibution (not to be confused with a P-P plot).
The x-axis is scaled linearly with the quantiles, but the probabilities
are used to label the axis.
Parameters
----------
xlabel, ylabel : str or None, optional
User-provided lables for the x-axis and y-axis. If None (default),
other values are used depending on the status of the kwarg `other`.
line : str {'45', 's', 'r', q'} or None, optional
Options for the reference line to which the data is compared:
- '45' - 45-degree line
- 's' - standardized line, the expected order statistics are scaled
by the standard deviation of the given sample and have the mean
added to them
- 'r' - A regression line is fit
- 'q' - A line is fit through the quartiles.
- None - by default no reference line is added to the plot.
exceed : boolean, optional
- If False (default) the raw sample quantiles are plotted against
the theoretical quantiles, show the probability that a sample
will not exceed a given value
- If True, the theoretical quantiles are flipped such that the
figure displays the probability that a sample will exceed a
given value.
ax : Matplotlib AxesSubplot instance, optional
If given, this subplot is used to plot in instead of a new figure
being created.
**plotkwargs : additional matplotlib arguments to be passed to the
`plot` command.
Returns
-------
fig : Matplotlib figure instance
If `ax` is None, the created figure. Otherwise the figure to which
`ax` is connected.
"""
if exceed:
fig, ax = _do_plot(self.theoretical_quantiles[::-1],
self.sorted_data,
self.dist, ax=ax, line=line,
**plotkwargs)
if xlabel is None:
xlabel = 'Probability of Exceedance (%)'
else:
fig, ax = _do_plot(self.theoretical_quantiles,
self.sorted_data,
self.dist, ax=ax, line=line,
**plotkwargs)
if xlabel is None:
xlabel = 'Non-exceedance Probability (%)'
if ylabel is None:
ylabel = "Sample Quantiles"
ax.set_xlabel(xlabel)
ax.set_ylabel(ylabel)
_fmt_probplot_axis(ax, self.dist, self.nobs)
return fig
def qqplot(data, dist=stats.norm, distargs=(), a=0, loc=0, scale=1, fit=False,
line=None, ax=None):
"""
Q-Q plot of the quantiles of x versus the quantiles/ppf of a distribution.
Can take arguments specifying the parameters for dist or fit them
automatically. (See fit under Parameters.)
Parameters
----------
data : array-like
1d data array
dist : A scipy.stats or statsmodels distribution
Compare x against dist. The default
is scipy.stats.distributions.norm (a standard normal).
distargs : tuple
A tuple of arguments passed to dist to specify it fully
so dist.ppf may be called.
loc : float
Location parameter for dist
a : float
Offset for the plotting position of an expected order statistic, for
example. The plotting positions are given by (i - a)/(nobs - 2*a + 1)
for i in range(0,nobs+1)
scale : float
Scale parameter for dist
fit : boolean
If fit is false, loc, scale, and distargs are passed to the
distribution. If fit is True then the parameters for dist
are fit automatically using dist.fit. The quantiles are formed
from the standardized data, after subtracting the fitted loc
and dividing by the fitted scale.
line : str {'45', 's', 'r', q'} or None
Options for the reference line to which the data is compared:
- '45' - 45-degree line
- 's' - standardized line, the expected order statistics are scaled
by the standard deviation of the given sample and have the mean
added to them
- 'r' - A regression line is fit
- 'q' - A line is fit through the quartiles.
- None - by default no reference line is added to the plot.
ax : Matplotlib AxesSubplot instance, optional
If given, this subplot is used to plot in instead of a new figure being
created.
Returns
-------
fig : Matplotlib figure instance
If `ax` is None, the created figure. Otherwise the figure to which
`ax` is connected.
See Also
--------
scipy.stats.probplot
Examples
--------
>>> import statsmodels.api as sm
>>> from matplotlib import pyplot as plt
>>> data = sm.datasets.longley.load()
>>> data.exog = sm.add_constant(data.exog)
>>> mod_fit = sm.OLS(data.endog, data.exog).fit()
>>> res = mod_fit.resid # residuals
>>> fig = sm.qqplot(res)
>>> plt.show()
qqplot of the residuals against quantiles of t-distribution with 4 degrees
of freedom:
>>> import scipy.stats as stats
>>> fig = sm.qqplot(res, stats.t, distargs=(4,))
>>> plt.show()
qqplot against same as above, but with mean 3 and std 10:
>>> fig = sm.qqplot(res, stats.t, distargs=(4,), loc=3, scale=10)
>>> plt.show()
Automatically determine parameters for t distribution including the
loc and scale:
>>> fig = sm.qqplot(res, stats.t, fit=True, line='45')
>>> plt.show()
The following plot displays some options, follow the link to see the code.
.. plot:: plots/graphics_gofplots_qqplot.py
Notes
-----
Depends on matplotlib. If `fit` is True then the parameters are fit using
the distribution's fit() method.
"""
probplot = ProbPlot(data, dist=dist, distargs=distargs,
fit=fit, a=a, loc=loc, scale=scale)
fig = probplot.qqplot(ax=ax, line=line)
return fig
def qqplot_2samples(data1, data2, xlabel=None, ylabel=None, line=None, ax=None):
"""
Q-Q Plot of two samples' quantiles.
Can take either two `ProbPlot` instances or two array-like objects. In the
case of the latter, both inputs will be converted to `ProbPlot` instances
using only the default values - so use `ProbPlot` instances if
finer-grained control of the quantile computations is required.
Parameters
----------
data1, data2 : array-like (1d) or `ProbPlot` instances
xlabel, ylabel : str or None
User-provided labels for the x-axis and y-axis. If None (default),
other values are used.
line : str {'45', 's', 'r', q'} or None
Options for the reference line to which the data is compared:
- '45' - 45-degree line
- 's' - standardized line, the expected order statistics are scaled
by the standard deviation of the given sample and have the mean
added to them
- 'r' - A regression line is fit
- 'q' - A line is fit through the quartiles.
- None - by default no reference line is added to the plot.
ax : Matplotlib AxesSubplot instance, optional
If given, this subplot is used to plot in instead of a new figure being
created.
Returns
-------
fig : Matplotlib figure instance
If `ax` is None, the created figure. Otherwise the figure to which
`ax` is connected.
See Also
--------
scipy.stats.probplot
Examples
--------
>>> x = np.random.normal(loc=8.5, scale=2.5, size=37)
>>> y = np.random.normal(loc=8.0, scale=3.0, size=37)
>>> pp_x = sm.ProbPlot(x)
>>> pp_y = sm.ProbPlot(y)
>>> qqplot_2samples(data1, data2, xlabel=None, ylabel=None, line=None, ax=None):
Notes
-----
1) Depends on matplotlib.
2) If `data1` and `data2` are not `ProbPlot` instances, instances will be
created using the default parameters. Therefore, it is recommended to use
`ProbPlot` instance if fine-grained control is needed in the computation
of the quantiles.
"""
check_data1 = isinstance(data1, ProbPlot)
check_data2 = isinstance(data2, ProbPlot)
if not check_data1 and not check_data2:
data1 = ProbPlot(data1)
data2 = ProbPlot(data2)
fig = data1.qqplot(xlabel=xlabel, ylabel=ylabel,
line=line, other=data2, ax=ax)
return fig
def qqline(ax, line, x=None, y=None, dist=None, fmt='r-'):
"""
Plot a reference line for a qqplot.
Parameters
----------
ax : matplotlib axes instance
The axes on which to plot the line
line : str {'45','r','s','q'}
Options for the reference line to which the data is compared.:
- '45' - 45-degree line
- 's' - standardized line, the expected order statistics are scaled by
the standard deviation of the given sample and have the mean
added to them
- 'r' - A regression line is fit
- 'q' - A line is fit through the quartiles.
- None - By default no reference line is added to the plot.
x : array
X data for plot. Not needed if line is '45'.
y : array
Y data for plot. Not needed if line is '45'.
dist : scipy.stats.distribution
A scipy.stats distribution, needed if line is 'q'.
Notes
-----
There is no return value. The line is plotted on the given `ax`.
"""
if line == '45':
end_pts = lzip(ax.get_xlim(), ax.get_ylim())
end_pts[0] = min(end_pts[0])
end_pts[1] = max(end_pts[1])
ax.plot(end_pts, end_pts, fmt)
ax.set_xlim(end_pts)
ax.set_ylim(end_pts)
return # does this have any side effects?
if x is None and y is None:
raise ValueError("If line is not 45, x and y cannot be None.")
elif line == 'r':
# could use ax.lines[0].get_xdata(), get_ydata(),
# but don't know axes are 'clean'
y = OLS(y, add_constant(x)).fit().fittedvalues
ax.plot(x,y,fmt)
elif line == 's':
m,b = y.std(), y.mean()
ref_line = x*m + b
ax.plot(x, ref_line, fmt)
elif line == 'q':
_check_for_ppf(dist)
q25 = stats.scoreatpercentile(y, 25)
q75 = stats.scoreatpercentile(y, 75)
theoretical_quartiles = dist.ppf([0.25, 0.75])
m = (q75 - q25) / np.diff(theoretical_quartiles)
b = q25 - m*theoretical_quartiles[0]
ax.plot(x, m*x + b, fmt)
#about 10x faster than plotting_position in sandbox and mstats
def plotting_pos(nobs, a):
"""
Generates sequence of plotting positions
Parameters
----------
nobs : int
Number of probability points to plot
a : float
Offset for the plotting position of an expected order statistic, for
example.
Returns
-------
plotting_positions : array
The plotting positions
Notes
-----
The plotting positions are given by (i - a)/(nobs - 2*a + 1) for i in
range(0,nobs+1)
See also
--------
scipy.stats.mstats.plotting_positions
"""
return (np.arange(1.,nobs+1) - a)/(nobs- 2*a + 1)
def _fmt_probplot_axis(ax, dist, nobs):
"""
Formats a theoretical quantile axis to display the corresponding
probabilities on the quantiles' scale.
Parameteters
------------
ax : Matplotlib AxesSubplot instance, optional
The axis to be formatted
nobs : scalar
Numbero of observations in the sample
dist : scipy.stats.distribution
A scipy.stats distribution sufficiently specified to impletment its
ppf() method.
Returns
-------
There is no return value. This operates on `ax` in place
"""
_check_for_ppf(dist)
if nobs < 50:
axis_probs = np.array([1,2,5,10,20,30,40,50,60,
70,80,90,95,98,99,])/100.0
elif nobs < 500:
axis_probs = np.array([0.1,0.2,0.5,1,2,5,10,20,30,40,50,60,70,
80,90,95,98,99,99.5,99.8,99.9])/100.0
else:
axis_probs = np.array([0.01,0.02,0.05,0.1,0.2,0.5,1,2,5,10,
20,30,40,50,60,70,80,90,95,98,99,99.5,
99.8,99.9,99.95,99.98,99.99])/100.0
axis_qntls = dist.ppf(axis_probs)
ax.set_xticks(axis_qntls)
ax.set_xticklabels(axis_probs*100, rotation=45,
rotation_mode='anchor',
horizontalalignment='right',
verticalalignment='center')
ax.set_xlim([axis_qntls.min(), axis_qntls.max()])
def _do_plot(x, y, dist=None, line=False, ax=None, fmt='bo', **kwargs):
"""
Boiler plate plotting function for the `ppplot`, `qqplot`, and
`probplot` methods of the `ProbPlot` class
Parameteters
------------
x, y : array-like
Data to be plotted
dist : scipy.stats.distribution
A scipy.stats distribution, needed if `line` is 'q'.
line : str {'45', 's', 'r', q'} or None
Options for the reference line to which the data is compared.
ax : Matplotlib AxesSubplot instance, optional
If given, this subplot is used to plot in instead of a new figure being
created.
fmt : str, optional
matplotlib-compatible formatting string for the data markers
kwargs : keywords
These are passed to matplotlib.plot
Returns
-------
fig : Matplotlib Figure instance
ax : Matplotlib AxesSubplot instance (see Parameters)
"""
fig, ax = utils.create_mpl_ax(ax)
ax.set_xmargin(0.02)
ax.plot(x, y, fmt, **kwargs)
if line:
if line not in ['r','q','45','s']:
msg = "%s option for line not understood" % line
raise ValueError(msg)
qqline(ax, line, x=x, y=y, dist=dist)
return fig, ax
def _check_for_ppf(dist):
if not hasattr(dist, 'ppf'):
raise ValueError("distribution must have a ppf method")
| bsd-3-clause |
trabucayre/gnuradio | gr-filter/examples/reconstruction.py | 1 | 4254 | #!/usr/bin/env python
#
# Copyright 2010,2012,2013 Free Software Foundation, Inc.
#
# This file is part of GNU Radio
#
# SPDX-License-Identifier: GPL-3.0-or-later
#
#
from gnuradio import gr, digital
from gnuradio import filter
from gnuradio import blocks
import sys
import numpy
try:
from gnuradio import channels
except ImportError:
print("Error: Program requires gr-channels.")
sys.exit(1)
try:
from matplotlib import pyplot
except ImportError:
print("Error: Program requires matplotlib (see: matplotlib.sourceforge.net).")
sys.exit(1)
fftlen = 8192
def main():
N = 10000
fs = 2000.0
Ts = 1.0 / fs
t = numpy.arange(0, N*Ts, Ts)
# When playing with the number of channels, be careful about the filter
# specs and the channel map of the synthesizer set below.
nchans = 10
# Build the filter(s)
bw = 1000
tb = 400
proto_taps = filter.firdes.low_pass_2(1, nchans*fs,
bw, tb, 80,
filter.firdes.WIN_BLACKMAN_hARRIS)
print("Filter length: ", len(proto_taps))
# Create a modulated signal
npwr = 0.01
data = numpy.random.randint(0, 256, N)
rrc_taps = filter.firdes.root_raised_cosine(1, 2, 1, 0.35, 41)
src = blocks.vector_source_b(data.astype(numpy.uint8).tolist(), False)
mod = digital.bpsk_mod(samples_per_symbol=2)
chan = channels.channel_model(npwr)
rrc = filter.fft_filter_ccc(1, rrc_taps)
# Split it up into pieces
channelizer = filter.pfb.channelizer_ccf(nchans, proto_taps, 2)
# Put the pieces back together again
syn_taps = [nchans*t for t in proto_taps]
synthesizer = filter.pfb_synthesizer_ccf(nchans, syn_taps, True)
src_snk = blocks.vector_sink_c()
snk = blocks.vector_sink_c()
# Remap the location of the channels
# Can be done in synth or channelizer (watch out for rotattions in
# the channelizer)
synthesizer.set_channel_map([ 0, 1, 2, 3, 4,
15, 16, 17, 18, 19])
tb = gr.top_block()
tb.connect(src, mod, chan, rrc, channelizer)
tb.connect(rrc, src_snk)
vsnk = []
for i in range(nchans):
tb.connect((channelizer,i), (synthesizer, i))
vsnk.append(blocks.vector_sink_c())
tb.connect((channelizer,i), vsnk[i])
tb.connect(synthesizer, snk)
tb.run()
sin = numpy.array(src_snk.data()[1000:])
sout = numpy.array(snk.data()[1000:])
# Plot original signal
fs_in = nchans*fs
f1 = pyplot.figure(1, figsize=(16,12), facecolor='w')
s11 = f1.add_subplot(2,2,1)
s11.psd(sin, NFFT=fftlen, Fs=fs_in)
s11.set_title("PSD of Original Signal")
s11.set_ylim([-200, -20])
s12 = f1.add_subplot(2,2,2)
s12.plot(sin.real[1000:1500], "o-b")
s12.plot(sin.imag[1000:1500], "o-r")
s12.set_title("Original Signal in Time")
start = 1
skip = 2
s13 = f1.add_subplot(2,2,3)
s13.plot(sin.real[start::skip], sin.imag[start::skip], "o")
s13.set_title("Constellation")
s13.set_xlim([-2, 2])
s13.set_ylim([-2, 2])
# Plot channels
nrows = int(numpy.sqrt(nchans))
ncols = int(numpy.ceil(float(nchans) / float(nrows)))
f2 = pyplot.figure(2, figsize=(16,12), facecolor='w')
for n in range(nchans):
s = f2.add_subplot(nrows, ncols, n+1)
s.psd(vsnk[n].data(), NFFT=fftlen, Fs=fs_in)
s.set_title("Channel {0}".format(n))
s.set_ylim([-200, -20])
# Plot reconstructed signal
fs_out = 2*nchans*fs
f3 = pyplot.figure(3, figsize=(16,12), facecolor='w')
s31 = f3.add_subplot(2,2,1)
s31.psd(sout, NFFT=fftlen, Fs=fs_out)
s31.set_title("PSD of Reconstructed Signal")
s31.set_ylim([-200, -20])
s32 = f3.add_subplot(2,2,2)
s32.plot(sout.real[1000:1500], "o-b")
s32.plot(sout.imag[1000:1500], "o-r")
s32.set_title("Reconstructed Signal in Time")
start = 0
skip = 4
s33 = f3.add_subplot(2,2,3)
s33.plot(sout.real[start::skip], sout.imag[start::skip], "o")
s33.set_title("Constellation")
s33.set_xlim([-2, 2])
s33.set_ylim([-2, 2])
pyplot.show()
if __name__ == "__main__":
try:
main()
except KeyboardInterrupt:
pass
| gpl-3.0 |
mbayon/TFG-MachineLearning | venv/lib/python3.6/site-packages/sklearn/linear_model/tests/test_huber.py | 26 | 7588 | # Authors: Manoj Kumar [email protected]
# License: BSD 3 clause
import numpy as np
from scipy import optimize, sparse
from sklearn.utils.testing import assert_equal
from sklearn.utils.testing import assert_almost_equal
from sklearn.utils.testing import assert_array_equal
from sklearn.utils.testing import assert_array_almost_equal
from sklearn.utils.testing import assert_greater
from sklearn.utils.testing import assert_false
from sklearn.datasets import make_regression
from sklearn.linear_model import (
HuberRegressor, LinearRegression, SGDRegressor, Ridge)
from sklearn.linear_model.huber import _huber_loss_and_gradient
def make_regression_with_outliers(n_samples=50, n_features=20):
rng = np.random.RandomState(0)
# Generate data with outliers by replacing 10% of the samples with noise.
X, y = make_regression(
n_samples=n_samples, n_features=n_features,
random_state=0, noise=0.05)
# Replace 10% of the sample with noise.
num_noise = int(0.1 * n_samples)
random_samples = rng.randint(0, n_samples, num_noise)
X[random_samples, :] = 2.0 * rng.normal(0, 1, (num_noise, X.shape[1]))
return X, y
def test_huber_equals_lr_for_high_epsilon():
# Test that Ridge matches LinearRegression for large epsilon
X, y = make_regression_with_outliers()
lr = LinearRegression(fit_intercept=True)
lr.fit(X, y)
huber = HuberRegressor(fit_intercept=True, epsilon=1e3, alpha=0.0)
huber.fit(X, y)
assert_almost_equal(huber.coef_, lr.coef_, 3)
assert_almost_equal(huber.intercept_, lr.intercept_, 2)
def test_huber_gradient():
# Test that the gradient calculated by _huber_loss_and_gradient is correct
rng = np.random.RandomState(1)
X, y = make_regression_with_outliers()
sample_weight = rng.randint(1, 3, (y.shape[0]))
loss_func = lambda x, *args: _huber_loss_and_gradient(x, *args)[0]
grad_func = lambda x, *args: _huber_loss_and_gradient(x, *args)[1]
# Check using optimize.check_grad that the gradients are equal.
for _ in range(5):
# Check for both fit_intercept and otherwise.
for n_features in [X.shape[1] + 1, X.shape[1] + 2]:
w = rng.randn(n_features)
w[-1] = np.abs(w[-1])
grad_same = optimize.check_grad(
loss_func, grad_func, w, X, y, 0.01, 0.1, sample_weight)
assert_almost_equal(grad_same, 1e-6, 4)
def test_huber_sample_weights():
# Test sample_weights implementation in HuberRegressor"""
X, y = make_regression_with_outliers()
huber = HuberRegressor(fit_intercept=True)
huber.fit(X, y)
huber_coef = huber.coef_
huber_intercept = huber.intercept_
# Rescale coefs before comparing with assert_array_almost_equal to make sure
# that the number of decimal places used is somewhat insensitive to the
# amplitude of the coefficients and therefore to the scale of the data
# and the regularization parameter
scale = max(np.mean(np.abs(huber.coef_)),
np.mean(np.abs(huber.intercept_)))
huber.fit(X, y, sample_weight=np.ones(y.shape[0]))
assert_array_almost_equal(huber.coef_ / scale, huber_coef / scale)
assert_array_almost_equal(huber.intercept_ / scale,
huber_intercept / scale)
X, y = make_regression_with_outliers(n_samples=5, n_features=20)
X_new = np.vstack((X, np.vstack((X[1], X[1], X[3]))))
y_new = np.concatenate((y, [y[1]], [y[1]], [y[3]]))
huber.fit(X_new, y_new)
huber_coef = huber.coef_
huber_intercept = huber.intercept_
sample_weight = np.ones(X.shape[0])
sample_weight[1] = 3
sample_weight[3] = 2
huber.fit(X, y, sample_weight=sample_weight)
assert_array_almost_equal(huber.coef_ / scale, huber_coef / scale)
assert_array_almost_equal(huber.intercept_ / scale,
huber_intercept / scale)
# Test sparse implementation with sample weights.
X_csr = sparse.csr_matrix(X)
huber_sparse = HuberRegressor(fit_intercept=True)
huber_sparse.fit(X_csr, y, sample_weight=sample_weight)
assert_array_almost_equal(huber_sparse.coef_ / scale,
huber_coef / scale)
def test_huber_sparse():
X, y = make_regression_with_outliers()
huber = HuberRegressor(fit_intercept=True, alpha=0.1)
huber.fit(X, y)
X_csr = sparse.csr_matrix(X)
huber_sparse = HuberRegressor(fit_intercept=True, alpha=0.1)
huber_sparse.fit(X_csr, y)
assert_array_almost_equal(huber_sparse.coef_, huber.coef_)
assert_array_equal(huber.outliers_, huber_sparse.outliers_)
def test_huber_scaling_invariant():
# Test that outliers filtering is scaling independent.
X, y = make_regression_with_outliers()
huber = HuberRegressor(fit_intercept=False, alpha=0.0, max_iter=100)
huber.fit(X, y)
n_outliers_mask_1 = huber.outliers_
assert_false(np.all(n_outliers_mask_1))
huber.fit(X, 2. * y)
n_outliers_mask_2 = huber.outliers_
assert_array_equal(n_outliers_mask_2, n_outliers_mask_1)
huber.fit(2. * X, 2. * y)
n_outliers_mask_3 = huber.outliers_
assert_array_equal(n_outliers_mask_3, n_outliers_mask_1)
def test_huber_and_sgd_same_results():
# Test they should converge to same coefficients for same parameters
X, y = make_regression_with_outliers(n_samples=10, n_features=2)
# Fit once to find out the scale parameter. Scale down X and y by scale
# so that the scale parameter is optimized to 1.0
huber = HuberRegressor(fit_intercept=False, alpha=0.0, max_iter=100,
epsilon=1.35)
huber.fit(X, y)
X_scale = X / huber.scale_
y_scale = y / huber.scale_
huber.fit(X_scale, y_scale)
assert_almost_equal(huber.scale_, 1.0, 3)
sgdreg = SGDRegressor(
alpha=0.0, loss="huber", shuffle=True, random_state=0, max_iter=10000,
fit_intercept=False, epsilon=1.35, tol=None)
sgdreg.fit(X_scale, y_scale)
assert_array_almost_equal(huber.coef_, sgdreg.coef_, 1)
def test_huber_warm_start():
X, y = make_regression_with_outliers()
huber_warm = HuberRegressor(
fit_intercept=True, alpha=1.0, max_iter=10000, warm_start=True, tol=1e-1)
huber_warm.fit(X, y)
huber_warm_coef = huber_warm.coef_.copy()
huber_warm.fit(X, y)
# SciPy performs the tol check after doing the coef updates, so
# these would be almost same but not equal.
assert_array_almost_equal(huber_warm.coef_, huber_warm_coef, 1)
# No n_iter_ in old SciPy (<=0.9)
if huber_warm.n_iter_ is not None:
assert_equal(0, huber_warm.n_iter_)
def test_huber_better_r2_score():
# Test that huber returns a better r2 score than non-outliers"""
X, y = make_regression_with_outliers()
huber = HuberRegressor(fit_intercept=True, alpha=0.01, max_iter=100)
huber.fit(X, y)
linear_loss = np.dot(X, huber.coef_) + huber.intercept_ - y
mask = np.abs(linear_loss) < huber.epsilon * huber.scale_
huber_score = huber.score(X[mask], y[mask])
huber_outlier_score = huber.score(X[~mask], y[~mask])
# The Ridge regressor should be influenced by the outliers and hence
# give a worse score on the non-outliers as compared to the huber regressor.
ridge = Ridge(fit_intercept=True, alpha=0.01)
ridge.fit(X, y)
ridge_score = ridge.score(X[mask], y[mask])
ridge_outlier_score = ridge.score(X[~mask], y[~mask])
assert_greater(huber_score, ridge_score)
# The huber model should also fit poorly on the outliers.
assert_greater(ridge_outlier_score, huber_outlier_score)
| mit |
iwarobots/aerodynamics | src/combination.py | 1 | 6858 | #!/usr/bin/env python
from __future__ import absolute_import, division
import numpy as np
import matplotlib
matplotlib.use('Agg')
from matplotlib import pyplot as plt
from common import Model, View, Controller
from wind_tunnel import WindTunnel
from test_section import TestSection
from diffuser import Diffuser
class InvalidThroatArea(Exception):
pass
class Combination(Model):
def __init__(self, nozzle):
self._nozzle = nozzle
self._ts = None
self._diffuser = None
def add_test_section(self, ts_len):
self._ts = TestSection(self._nozzle.x2m(self.n_len),
self._nozzle.ats,
self._nozzle.x2p(self.n_len)*self._nozzle.p02,
self._nozzle.x2t(self.n_len)*self._nozzle.t0,
self._nozzle.p02,
self._nozzle.t0,
self._nozzle.z_len,
ts_len)
def add_diffuser(self,
at,
ae,
con_len,
div_len,
back_pressure):
if not self._nozzle.at <= at < self._nozzle.ats:
raise InvalidThroatArea
self._diffuser = Diffuser(self._nozzle.x2m(self.n_len),
self._nozzle.p02,
self._nozzle.x2p(self.n_len)*self._nozzle.p02,
self._nozzle.x2t(self.n_len)*self._nozzle.t0,
self._nozzle.ats,
at,
ae,
con_len,
div_len,
self._nozzle.z_len,
back_pressure,
self._nozzle.at,
self._nozzle.p01,
self._nozzle.wc)
@property
def n_len(self):
return self._nozzle.t_len
@property
def n_con_len(self):
return self._nozzle.con_len
@property
def n_div_len(self):
return self._nozzle.div_len
@property
def n_ts_len(self):
return self.n_len + self._ts.t_len
@property
def n_ts_con_len(self):
return self.n_ts_len + self._diffuser.con_len
@property
def n_ts_d_len(self):
return self.n_ts_len + self._diffuser.t_len
@property
def t_len(self):
return self.n_ts_d_len
def x2func(self, func, x):
res = 0
if 0 <= x <= self.n_len:
res = getattr(self._nozzle, func)(x)
elif self.n_len < x <= self.n_ts_len:
res = getattr(self._ts, func)(x-self.n_len)
elif self.n_ts_len < x <= self.n_ts_d_len:
res = getattr(self._diffuser, func)(x-self.n_ts_len)
return res
def x2m(self, x):
return self.x2func('x2m', x)
def x2a(self, x):
return self.x2func('x2a', x)
def x2y(self, x):
return self.x2func('x2y', x)
def x2p(self, x):
return self.x2func('x2p', x)
def x2t(self, x):
return self.x2func('x2t', x)
def x2rho(self, x):
return self.x2func('x2rho', x)
def get_wall_shape(self):
x1 = np.array([0, self.n_con_len,
self.n_len, self.n_ts_len,
self.n_ts_con_len, self.n_ts_d_len])
x2 = x1[::-1]
n = len(x1)
xs = np.zeros(2*n)
ys = np.zeros(2*n)
for i in xrange(n):
xs[i] = x1[i]
xs[i+n] = x2[i]
for i in xrange(n):
ys[i] = self.x2y(xs[i])
ys[2*n-i-1] = -self.x2y(xs[i])
return np.array([xs, ys]).T
class Report(View):
def __init__(self):
pass
def wall_shape(self, points):
n = len(points)
points = np.vstack([points, points[0]])
fig = plt.figure()
for i in xrange(n):
sub = fig.add_subplot(111)
x = [points[i, 0], points[i+1, 0]]
y = [points[i, 1], points[i+1, 1]]
sub.plot(x, y, 'b')
#t_len = self.wt.t_len
#max_y = self.wt.ymax
#h_margin = t_len * 0.1 / 2
#v_margin = max_y * 0.1
#plt.axis([-h_margin, t_len+h_margin,
# -max_y-v_margin, max_y+v_margin])
return fig
def graph(self, x, profile):
graph = plt.figure()
sub = graph.add_subplot(111)
sub.plot(x, profile, 'b')
return graph
class WindTunnelReportCreator(Controller):
def __init__(self, model, view):
Controller.__init__(self, model, view)
@property
def plot_types(self):
return ['s', 'a', 'm', 'p', 'rho', 't']
def save_plot(self, filename, plot_type, steps=1000):
if plot_type == 's':
points = self._model.get_wall_shape()
fig = self._view.wall_shape(points)
fig.savefig(filename)
else:
xs = np.linspace(0, self._model.t_len, steps)
profile = np.zeros(steps)
if plot_type == 'a':
for i in xrange(steps):
profile[i] = self._model.x2a(xs[i])
elif plot_type == 'm':
for i in xrange(steps):
profile[i] = self._model.x2m(xs[i])
elif plot_type == 'p':
for i in xrange(steps):
profile[i] = self._model.x2p(xs[i])
elif plot_type == 'rho':
for i in xrange(steps):
profile[i] = self._model.x2rho(xs[i])
elif plot_type == 't':
for i in xrange(steps):
profile[i] = self._model.x2t(xs[i])
graph = self._view.graph(xs, profile)
graph.savefig(filename)
def generate(self):
for t in self.plot_types:
self.save_plot('%s.png' % t, t)
if __name__ == '__main__':
import uuid
#pb = .068399E6
pb = 0.99E6
t = WindTunnel(2.4, # md
0.24, # ats
1e6, # p0
300, # t0
20, # ain
5, # con_len
5, # div_len
1, # z_len
0.99E6) # pb
com = Combination(t)
com.add_test_section(5) # ts_len
com.add_diffuser(0.17, # at
5, # ae
5, # con_len
5, # div_len
0.99E6) # pb
r = Report()
c = WindTunnelReportCreator(com, r)
c.generate()
| apache-2.0 |
plotly/python-api | packages/python/plotly/plotly/graph_objs/bar/_error_x.py | 1 | 19328 | from plotly.basedatatypes import BaseTraceHierarchyType as _BaseTraceHierarchyType
import copy as _copy
class ErrorX(_BaseTraceHierarchyType):
# class properties
# --------------------
_parent_path_str = "bar"
_path_str = "bar.error_x"
_valid_props = {
"array",
"arrayminus",
"arrayminussrc",
"arraysrc",
"color",
"copy_ystyle",
"symmetric",
"thickness",
"traceref",
"tracerefminus",
"type",
"value",
"valueminus",
"visible",
"width",
}
# array
# -----
@property
def array(self):
"""
Sets the data corresponding the length of each error bar.
Values are plotted relative to the underlying data.
The 'array' property is an array that may be specified as a tuple,
list, numpy array, or pandas Series
Returns
-------
numpy.ndarray
"""
return self["array"]
@array.setter
def array(self, val):
self["array"] = val
# arrayminus
# ----------
@property
def arrayminus(self):
"""
Sets the data corresponding the length of each error bar in the
bottom (left) direction for vertical (horizontal) bars Values
are plotted relative to the underlying data.
The 'arrayminus' property is an array that may be specified as a tuple,
list, numpy array, or pandas Series
Returns
-------
numpy.ndarray
"""
return self["arrayminus"]
@arrayminus.setter
def arrayminus(self, val):
self["arrayminus"] = val
# arrayminussrc
# -------------
@property
def arrayminussrc(self):
"""
Sets the source reference on Chart Studio Cloud for arrayminus
.
The 'arrayminussrc' property must be specified as a string or
as a plotly.grid_objs.Column object
Returns
-------
str
"""
return self["arrayminussrc"]
@arrayminussrc.setter
def arrayminussrc(self, val):
self["arrayminussrc"] = val
# arraysrc
# --------
@property
def arraysrc(self):
"""
Sets the source reference on Chart Studio Cloud for array .
The 'arraysrc' property must be specified as a string or
as a plotly.grid_objs.Column object
Returns
-------
str
"""
return self["arraysrc"]
@arraysrc.setter
def arraysrc(self, val):
self["arraysrc"] = val
# color
# -----
@property
def color(self):
"""
Sets the stoke color of the error bars.
The 'color' property is a color and may be specified as:
- A hex string (e.g. '#ff0000')
- An rgb/rgba string (e.g. 'rgb(255,0,0)')
- An hsl/hsla string (e.g. 'hsl(0,100%,50%)')
- An hsv/hsva string (e.g. 'hsv(0,100%,100%)')
- A named CSS color:
aliceblue, antiquewhite, aqua, aquamarine, azure,
beige, bisque, black, blanchedalmond, blue,
blueviolet, brown, burlywood, cadetblue,
chartreuse, chocolate, coral, cornflowerblue,
cornsilk, crimson, cyan, darkblue, darkcyan,
darkgoldenrod, darkgray, darkgrey, darkgreen,
darkkhaki, darkmagenta, darkolivegreen, darkorange,
darkorchid, darkred, darksalmon, darkseagreen,
darkslateblue, darkslategray, darkslategrey,
darkturquoise, darkviolet, deeppink, deepskyblue,
dimgray, dimgrey, dodgerblue, firebrick,
floralwhite, forestgreen, fuchsia, gainsboro,
ghostwhite, gold, goldenrod, gray, grey, green,
greenyellow, honeydew, hotpink, indianred, indigo,
ivory, khaki, lavender, lavenderblush, lawngreen,
lemonchiffon, lightblue, lightcoral, lightcyan,
lightgoldenrodyellow, lightgray, lightgrey,
lightgreen, lightpink, lightsalmon, lightseagreen,
lightskyblue, lightslategray, lightslategrey,
lightsteelblue, lightyellow, lime, limegreen,
linen, magenta, maroon, mediumaquamarine,
mediumblue, mediumorchid, mediumpurple,
mediumseagreen, mediumslateblue, mediumspringgreen,
mediumturquoise, mediumvioletred, midnightblue,
mintcream, mistyrose, moccasin, navajowhite, navy,
oldlace, olive, olivedrab, orange, orangered,
orchid, palegoldenrod, palegreen, paleturquoise,
palevioletred, papayawhip, peachpuff, peru, pink,
plum, powderblue, purple, red, rosybrown,
royalblue, rebeccapurple, saddlebrown, salmon,
sandybrown, seagreen, seashell, sienna, silver,
skyblue, slateblue, slategray, slategrey, snow,
springgreen, steelblue, tan, teal, thistle, tomato,
turquoise, violet, wheat, white, whitesmoke,
yellow, yellowgreen
Returns
-------
str
"""
return self["color"]
@color.setter
def color(self, val):
self["color"] = val
# copy_ystyle
# -----------
@property
def copy_ystyle(self):
"""
The 'copy_ystyle' property must be specified as a bool
(either True, or False)
Returns
-------
bool
"""
return self["copy_ystyle"]
@copy_ystyle.setter
def copy_ystyle(self, val):
self["copy_ystyle"] = val
# symmetric
# ---------
@property
def symmetric(self):
"""
Determines whether or not the error bars have the same length
in both direction (top/bottom for vertical bars, left/right for
horizontal bars.
The 'symmetric' property must be specified as a bool
(either True, or False)
Returns
-------
bool
"""
return self["symmetric"]
@symmetric.setter
def symmetric(self, val):
self["symmetric"] = val
# thickness
# ---------
@property
def thickness(self):
"""
Sets the thickness (in px) of the error bars.
The 'thickness' property is a number and may be specified as:
- An int or float in the interval [0, inf]
Returns
-------
int|float
"""
return self["thickness"]
@thickness.setter
def thickness(self, val):
self["thickness"] = val
# traceref
# --------
@property
def traceref(self):
"""
The 'traceref' property is a integer and may be specified as:
- An int (or float that will be cast to an int)
in the interval [0, 9223372036854775807]
Returns
-------
int
"""
return self["traceref"]
@traceref.setter
def traceref(self, val):
self["traceref"] = val
# tracerefminus
# -------------
@property
def tracerefminus(self):
"""
The 'tracerefminus' property is a integer and may be specified as:
- An int (or float that will be cast to an int)
in the interval [0, 9223372036854775807]
Returns
-------
int
"""
return self["tracerefminus"]
@tracerefminus.setter
def tracerefminus(self, val):
self["tracerefminus"] = val
# type
# ----
@property
def type(self):
"""
Determines the rule used to generate the error bars. If
*constant`, the bar lengths are of a constant value. Set this
constant in `value`. If "percent", the bar lengths correspond
to a percentage of underlying data. Set this percentage in
`value`. If "sqrt", the bar lengths correspond to the sqaure of
the underlying data. If "data", the bar lengths are set with
data set `array`.
The 'type' property is an enumeration that may be specified as:
- One of the following enumeration values:
['percent', 'constant', 'sqrt', 'data']
Returns
-------
Any
"""
return self["type"]
@type.setter
def type(self, val):
self["type"] = val
# value
# -----
@property
def value(self):
"""
Sets the value of either the percentage (if `type` is set to
"percent") or the constant (if `type` is set to "constant")
corresponding to the lengths of the error bars.
The 'value' property is a number and may be specified as:
- An int or float in the interval [0, inf]
Returns
-------
int|float
"""
return self["value"]
@value.setter
def value(self, val):
self["value"] = val
# valueminus
# ----------
@property
def valueminus(self):
"""
Sets the value of either the percentage (if `type` is set to
"percent") or the constant (if `type` is set to "constant")
corresponding to the lengths of the error bars in the bottom
(left) direction for vertical (horizontal) bars
The 'valueminus' property is a number and may be specified as:
- An int or float in the interval [0, inf]
Returns
-------
int|float
"""
return self["valueminus"]
@valueminus.setter
def valueminus(self, val):
self["valueminus"] = val
# visible
# -------
@property
def visible(self):
"""
Determines whether or not this set of error bars is visible.
The 'visible' property must be specified as a bool
(either True, or False)
Returns
-------
bool
"""
return self["visible"]
@visible.setter
def visible(self, val):
self["visible"] = val
# width
# -----
@property
def width(self):
"""
Sets the width (in px) of the cross-bar at both ends of the
error bars.
The 'width' property is a number and may be specified as:
- An int or float in the interval [0, inf]
Returns
-------
int|float
"""
return self["width"]
@width.setter
def width(self, val):
self["width"] = val
# Self properties description
# ---------------------------
@property
def _prop_descriptions(self):
return """\
array
Sets the data corresponding the length of each error
bar. Values are plotted relative to the underlying
data.
arrayminus
Sets the data corresponding the length of each error
bar in the bottom (left) direction for vertical
(horizontal) bars Values are plotted relative to the
underlying data.
arrayminussrc
Sets the source reference on Chart Studio Cloud for
arrayminus .
arraysrc
Sets the source reference on Chart Studio Cloud for
array .
color
Sets the stoke color of the error bars.
copy_ystyle
symmetric
Determines whether or not the error bars have the same
length in both direction (top/bottom for vertical bars,
left/right for horizontal bars.
thickness
Sets the thickness (in px) of the error bars.
traceref
tracerefminus
type
Determines the rule used to generate the error bars. If
*constant`, the bar lengths are of a constant value.
Set this constant in `value`. If "percent", the bar
lengths correspond to a percentage of underlying data.
Set this percentage in `value`. If "sqrt", the bar
lengths correspond to the sqaure of the underlying
data. If "data", the bar lengths are set with data set
`array`.
value
Sets the value of either the percentage (if `type` is
set to "percent") or the constant (if `type` is set to
"constant") corresponding to the lengths of the error
bars.
valueminus
Sets the value of either the percentage (if `type` is
set to "percent") or the constant (if `type` is set to
"constant") corresponding to the lengths of the error
bars in the bottom (left) direction for vertical
(horizontal) bars
visible
Determines whether or not this set of error bars is
visible.
width
Sets the width (in px) of the cross-bar at both ends of
the error bars.
"""
def __init__(
self,
arg=None,
array=None,
arrayminus=None,
arrayminussrc=None,
arraysrc=None,
color=None,
copy_ystyle=None,
symmetric=None,
thickness=None,
traceref=None,
tracerefminus=None,
type=None,
value=None,
valueminus=None,
visible=None,
width=None,
**kwargs
):
"""
Construct a new ErrorX object
Parameters
----------
arg
dict of properties compatible with this constructor or
an instance of :class:`plotly.graph_objs.bar.ErrorX`
array
Sets the data corresponding the length of each error
bar. Values are plotted relative to the underlying
data.
arrayminus
Sets the data corresponding the length of each error
bar in the bottom (left) direction for vertical
(horizontal) bars Values are plotted relative to the
underlying data.
arrayminussrc
Sets the source reference on Chart Studio Cloud for
arrayminus .
arraysrc
Sets the source reference on Chart Studio Cloud for
array .
color
Sets the stoke color of the error bars.
copy_ystyle
symmetric
Determines whether or not the error bars have the same
length in both direction (top/bottom for vertical bars,
left/right for horizontal bars.
thickness
Sets the thickness (in px) of the error bars.
traceref
tracerefminus
type
Determines the rule used to generate the error bars. If
*constant`, the bar lengths are of a constant value.
Set this constant in `value`. If "percent", the bar
lengths correspond to a percentage of underlying data.
Set this percentage in `value`. If "sqrt", the bar
lengths correspond to the sqaure of the underlying
data. If "data", the bar lengths are set with data set
`array`.
value
Sets the value of either the percentage (if `type` is
set to "percent") or the constant (if `type` is set to
"constant") corresponding to the lengths of the error
bars.
valueminus
Sets the value of either the percentage (if `type` is
set to "percent") or the constant (if `type` is set to
"constant") corresponding to the lengths of the error
bars in the bottom (left) direction for vertical
(horizontal) bars
visible
Determines whether or not this set of error bars is
visible.
width
Sets the width (in px) of the cross-bar at both ends of
the error bars.
Returns
-------
ErrorX
"""
super(ErrorX, self).__init__("error_x")
if "_parent" in kwargs:
self._parent = kwargs["_parent"]
return
# Validate arg
# ------------
if arg is None:
arg = {}
elif isinstance(arg, self.__class__):
arg = arg.to_plotly_json()
elif isinstance(arg, dict):
arg = _copy.copy(arg)
else:
raise ValueError(
"""\
The first argument to the plotly.graph_objs.bar.ErrorX
constructor must be a dict or
an instance of :class:`plotly.graph_objs.bar.ErrorX`"""
)
# Handle skip_invalid
# -------------------
self._skip_invalid = kwargs.pop("skip_invalid", False)
self._validate = kwargs.pop("_validate", True)
# Populate data dict with properties
# ----------------------------------
_v = arg.pop("array", None)
_v = array if array is not None else _v
if _v is not None:
self["array"] = _v
_v = arg.pop("arrayminus", None)
_v = arrayminus if arrayminus is not None else _v
if _v is not None:
self["arrayminus"] = _v
_v = arg.pop("arrayminussrc", None)
_v = arrayminussrc if arrayminussrc is not None else _v
if _v is not None:
self["arrayminussrc"] = _v
_v = arg.pop("arraysrc", None)
_v = arraysrc if arraysrc is not None else _v
if _v is not None:
self["arraysrc"] = _v
_v = arg.pop("color", None)
_v = color if color is not None else _v
if _v is not None:
self["color"] = _v
_v = arg.pop("copy_ystyle", None)
_v = copy_ystyle if copy_ystyle is not None else _v
if _v is not None:
self["copy_ystyle"] = _v
_v = arg.pop("symmetric", None)
_v = symmetric if symmetric is not None else _v
if _v is not None:
self["symmetric"] = _v
_v = arg.pop("thickness", None)
_v = thickness if thickness is not None else _v
if _v is not None:
self["thickness"] = _v
_v = arg.pop("traceref", None)
_v = traceref if traceref is not None else _v
if _v is not None:
self["traceref"] = _v
_v = arg.pop("tracerefminus", None)
_v = tracerefminus if tracerefminus is not None else _v
if _v is not None:
self["tracerefminus"] = _v
_v = arg.pop("type", None)
_v = type if type is not None else _v
if _v is not None:
self["type"] = _v
_v = arg.pop("value", None)
_v = value if value is not None else _v
if _v is not None:
self["value"] = _v
_v = arg.pop("valueminus", None)
_v = valueminus if valueminus is not None else _v
if _v is not None:
self["valueminus"] = _v
_v = arg.pop("visible", None)
_v = visible if visible is not None else _v
if _v is not None:
self["visible"] = _v
_v = arg.pop("width", None)
_v = width if width is not None else _v
if _v is not None:
self["width"] = _v
# Process unknown kwargs
# ----------------------
self._process_kwargs(**dict(arg, **kwargs))
# Reset skip_invalid
# ------------------
self._skip_invalid = False
| mit |
aetilley/scikit-learn | sklearn/preprocessing/tests/test_function_transformer.py | 176 | 2169 | from nose.tools import assert_equal
import numpy as np
from sklearn.preprocessing import FunctionTransformer
def _make_func(args_store, kwargs_store, func=lambda X, *a, **k: X):
def _func(X, *args, **kwargs):
args_store.append(X)
args_store.extend(args)
kwargs_store.update(kwargs)
return func(X)
return _func
def test_delegate_to_func():
# (args|kwargs)_store will hold the positional and keyword arguments
# passed to the function inside the FunctionTransformer.
args_store = []
kwargs_store = {}
X = np.arange(10).reshape((5, 2))
np.testing.assert_array_equal(
FunctionTransformer(_make_func(args_store, kwargs_store)).transform(X),
X,
'transform should have returned X unchanged',
)
# The function should only have recieved X.
assert_equal(
args_store,
[X],
'Incorrect positional arguments passed to func: {args}'.format(
args=args_store,
),
)
assert_equal(
kwargs_store,
{},
'Unexpected keyword arguments passed to func: {args}'.format(
args=kwargs_store,
),
)
# reset the argument stores.
args_store[:] = [] # python2 compatible inplace list clear.
kwargs_store.clear()
y = object()
np.testing.assert_array_equal(
FunctionTransformer(
_make_func(args_store, kwargs_store),
pass_y=True,
).transform(X, y),
X,
'transform should have returned X unchanged',
)
# The function should have recieved X and y.
assert_equal(
args_store,
[X, y],
'Incorrect positional arguments passed to func: {args}'.format(
args=args_store,
),
)
assert_equal(
kwargs_store,
{},
'Unexpected keyword arguments passed to func: {args}'.format(
args=kwargs_store,
),
)
def test_np_log():
X = np.arange(10).reshape((5, 2))
# Test that the numpy.log example still works.
np.testing.assert_array_equal(
FunctionTransformer(np.log1p).transform(X),
np.log1p(X),
)
| bsd-3-clause |
CompPhysics/ComputationalPhysicsMSU | doc/Programs/LecturePrograms/programs/StatPhys/python/IsingHisto2dim.py | 2 | 2193 | from __future__ import division
import matplotlib.pyplot as plt
import numpy as np
import math, sys
def periodic (i, limit, add):
return (i+limit+add) % limit
def monteCarlo(Energies, temp, NSpins, MCcycles):
#Setup spin matrix, initialize to ground state
spin_matrix = np.zeros( (NSpins,NSpins), np.int8) + 1
E = M = 0.0
#Setup array for possible energy changes
w = np.zeros(17,np.float64)
for de in range(-8,9,4): #include +8
w[de+8] = math.exp(-de/temp)
#Calculate initial magnetization:
M = spin_matrix.sum()
#Calculate initial energy
for j in range(NSpins):
for i in range(NSpins):
E -= spin_matrix.item(i,j)*\
(spin_matrix.item(periodic(i,NSpins,-1),j) + spin_matrix.item(i,periodic(j,NSpins,1)))
#Start metropolis MonteCarlo computation
for i in range(MCcycles):
#Metropolis
#Loop over all spins, pick a random spin each time
for s in range(NSpins**2):
x = int(np.random.random()*NSpins)
y = int(np.random.random()*NSpins)
deltaE = 2*spin_matrix.item(x,y)*\
(spin_matrix.item(periodic(x,NSpins,-1), y) +\
spin_matrix.item(periodic(x,NSpins,1), y) +\
spin_matrix.item(x, periodic(y,NSpins,-1)) +\
spin_matrix.item(x, periodic(y,NSpins,1)))
if np.random.random() <= w[deltaE+8]:
#Accept!
spin_matrix[x,y] *= -1
E += deltaE
#Update expectation values
Energies[i] += E
# Main program
# Define number of spins
NSpins = 20
# Define number of Monte Carlo cycles
MCcycles = 10000
# temperature steps, initial temperature, final temperature
Temp = 2.5
# Declare arrays that hold averages
Energies = np.zeros(MCcycles)
# Obtain the energies to construct the diagram
monteCarlo(Energies,Temp,NSpins,MCcycles)
n, bins, patches = plt.hist(Energies, 100, facecolor='green')
plt.xlabel('$E$')
plt.ylabel('Energy distribution P(E)')
plt.title(r'Energy distribution at $k_BT=2.5$')
plt.axis([-800, -300, 0, 500])
plt.grid(True)
plt.show()
| cc0-1.0 |
scarrazza/mc2hessian | src/mc2hlib/lh.py | 1 | 4805 | # -*- coding: utf-8 -*-
"""
Created on Tue Apr 21 22:00:01 2015
@author: zah
"""
import os
import sys
import shutil
import lhapdf
import numpy as np
import pandas as pd
def split_sep(f):
for line in f:
if line.startswith(b'---') or line.startswith(b' ---'):
break
yield line
def read_xqf_from_file(f):
lines = split_sep(f)
try:
(xtext, qtext, ftext) = [next(lines) for _ in range(3)]
except StopIteration:
return None
xvals = np.fromstring(xtext, sep = " ")
qvals = np.fromstring(qtext, sep = " ")
fvals = np.fromstring(ftext, sep = " ", dtype=np.int)
vals = np.fromstring(b''.join(lines), sep= " ")
return pd.Series(vals, index = pd.MultiIndex.from_product((xvals, qvals, fvals)))
def read_xqf_from_lhapdf(pdf, rep0grids):
indexes = tuple(rep0grids.index)
vals = []
for x in indexes:
vals += [pdf.xfxQ(x[3],x[1],x[2])]
return pd.Series(vals, index = rep0grids.index)
def read_all_xqf(f):
while True:
result = read_xqf_from_file(f)
if result is None:
return
yield result
#TODO: Make pdf_name the pdf_name instead of path
def load_replica_2(rep, pdf_name, pdf=None, rep0grids=None):
sys.stdout.write("-> Reading replica from LHAPDF %d \r" % rep)
sys.stdout.flush()
suffix = str(rep).zfill(4)
with open(pdf_name + "_" + suffix + ".dat", 'rb') as inn:
header = b"".join(split_sep(inn))
if rep0grids is not None:
xfqs = read_xqf_from_lhapdf(pdf, rep0grids)
else:
xfqs = list(read_all_xqf(inn))
xfqs = pd.concat(xfqs, keys=range(len(xfqs)))
return header, xfqs
#Split this to debug easily
def _rep_to_buffer(out, header, subgrids):
sep = b'---'
out.write(header)
out.write(sep)
for _,g in subgrids.groupby(level=0):
out.write(b'\n')
ind = g.index.get_level_values(1).unique()
np.savetxt(out, ind, fmt='%.7E',delimiter=' ', newline=' ')
out.write(b'\n')
ind = g.index.get_level_values(2).unique()
np.savetxt(out, ind, fmt='%.7E',delimiter=' ', newline=' ')
out.write(b'\n')
#Integer format
ind = g.index.get_level_values(3).unique()
np.savetxt(out, ind, delimiter=' ', fmt="%d",
newline=' ')
out.write(b'\n ')
#Reshape so printing is easy
reshaped = g.values.reshape((len(g.groupby(level=1))*len(g.groupby(level=2)),
len(g.groupby(level=3))))
np.savetxt(out, reshaped, delimiter=" ", newline="\n ", fmt='%14.7E')
out.write(sep)
def write_replica(rep, pdf_name, header, subgrids):
suffix = str(rep).zfill(4)
with open(pdf_name + "_" + suffix + ".dat", 'wb') as out:
_rep_to_buffer(out, header, subgrids)
def load_all_replicas(pdf, pdf_name):
rep0headers, rep0grids = load_replica_2(0,pdf_name)
headers, grids = zip(*[load_replica_2(rep, pdf_name, pdf.pdf[rep], rep0grids) for rep in range(1,pdf.n_rep+1)])
return [rep0headers] + list(headers), [rep0grids] + list(grids)
def big_matrix(gridlist):
central_value = gridlist[0]
X = pd.concat(gridlist[1:], axis=1,
keys=range(1,len(gridlist)+1), #avoid confusion with rep0
).subtract(central_value, axis=0)
if np.any(X.isnull()) or X.shape[0] != len(central_value):
raise ValueError("Incompatible grid specifications")
return X
def hessian_from_lincomb(pdf, V, set_name=None, folder = None):
# preparing output folder
neig = V.shape[1]
base = lhapdf.paths()[0] + "/" + pdf.pdf_name + "/" + pdf.pdf_name
if set_name is None:
set_name = pdf.pdf_name + "_hessian_" + str(neig)
if folder is None:
folder = ''
set_root = os.path.join(folder,set_name)
if not os.path.exists(set_root): os.makedirs(os.path.join(set_root))
# copy replica 0
shutil.copy(base + "_0000.dat", set_root + "/" + set_name + "_0000.dat")
inn = open(base + ".info", 'r')
out = open(set_root + "/" + set_name + ".info", 'w')
for l in inn.readlines():
if l.find("SetDesc:") >= 0: out.write(f"SetDesc: \"Hessian {pdf.pdf_name}_hessian\"\n")
elif l.find("NumMembers:") >= 0: out.write(f"NumMembers: {neig+1}\n")
elif l.find("ErrorType: replicas") >= 0: out.write("ErrorType: symmhessian\n")
else: out.write(l)
inn.close()
out.close()
headers, grids = load_all_replicas(pdf, base)
hess_name = set_root + '/' + set_name
result = (big_matrix(grids).dot(V)).add(grids[0], axis=0, )
hess_header = b"PdfType: error\nFormat: lhagrid1\n"
for column in result.columns:
write_replica(column + 1, hess_name, hess_header, result[column])
print ("\n")
| gpl-2.0 |
viisar/brew | test/dataset.py | 3 | 1583 | import numpy as np
import sklearn.datasets as datasets
# this indices will always be used so that we get reproduceable results in the tests
iris_index = np.array([ 69, 63, 32, 131, 13, 94, 10, 17, 4, 108, 29, 96, 100,
143, 20, 86, 35, 144, 78, 18, 11, 33, 72, 106, 24, 84,
42, 126, 51, 50, 90, 30, 146, 119, 1, 43, 37, 64, 5,
116, 122, 81, 45, 34, 112, 49, 31, 127, 114, 113, 41, 107,
22, 48, 137, 88, 110, 65, 105, 101, 23, 83, 26, 25, 111,
60, 68, 135, 109, 9, 47, 148, 142, 97, 130, 129, 6, 87,
58, 138, 73, 117, 133, 128, 39, 56, 85, 76, 104, 102, 38,
61, 92, 140, 70, 120, 12, 57, 134, 115, 147, 103, 82, 53,
62, 46, 118, 59, 36, 141, 132, 54, 44, 21, 7, 123, 125,
145, 99, 98, 79, 14, 139, 89, 74, 77, 66, 27, 149, 8,
0, 19, 95, 121, 16, 28, 40, 93, 124, 15, 52, 80, 136,
91, 67, 3, 75, 2, 55, 71])
def load_iris():
iris = datasets.load_iris()
data = iris['data']
target = iris['target']
# use fixed shuffling
dataset = np.concatenate((data, target.reshape((150,1))), axis=1)[iris_index, :]
# will always obtain the same train and test set
train_set = dataset[:105, :] # 70%
test_set = dataset[105:, :] # 30%
print(train_set.shape)
print(test_set.shape)
| mit |
ephes/scikit-learn | sklearn/decomposition/tests/test_fastica.py | 272 | 7798 | """
Test the fastica algorithm.
"""
import itertools
import warnings
import numpy as np
from scipy import stats
from nose.tools import assert_raises
from sklearn.utils.testing import assert_almost_equal
from sklearn.utils.testing import assert_array_almost_equal
from sklearn.utils.testing import assert_true
from sklearn.utils.testing import assert_less
from sklearn.utils.testing import assert_equal
from sklearn.utils.testing import assert_warns
from sklearn.decomposition import FastICA, fastica, PCA
from sklearn.decomposition.fastica_ import _gs_decorrelation
from sklearn.externals.six import moves
def center_and_norm(x, axis=-1):
""" Centers and norms x **in place**
Parameters
-----------
x: ndarray
Array with an axis of observations (statistical units) measured on
random variables.
axis: int, optional
Axis along which the mean and variance are calculated.
"""
x = np.rollaxis(x, axis)
x -= x.mean(axis=0)
x /= x.std(axis=0)
def test_gs():
# Test gram schmidt orthonormalization
# generate a random orthogonal matrix
rng = np.random.RandomState(0)
W, _, _ = np.linalg.svd(rng.randn(10, 10))
w = rng.randn(10)
_gs_decorrelation(w, W, 10)
assert_less((w ** 2).sum(), 1.e-10)
w = rng.randn(10)
u = _gs_decorrelation(w, W, 5)
tmp = np.dot(u, W.T)
assert_less((tmp[:5] ** 2).sum(), 1.e-10)
def test_fastica_simple(add_noise=False):
# Test the FastICA algorithm on very simple data.
rng = np.random.RandomState(0)
# scipy.stats uses the global RNG:
np.random.seed(0)
n_samples = 1000
# Generate two sources:
s1 = (2 * np.sin(np.linspace(0, 100, n_samples)) > 0) - 1
s2 = stats.t.rvs(1, size=n_samples)
s = np.c_[s1, s2].T
center_and_norm(s)
s1, s2 = s
# Mixing angle
phi = 0.6
mixing = np.array([[np.cos(phi), np.sin(phi)],
[np.sin(phi), -np.cos(phi)]])
m = np.dot(mixing, s)
if add_noise:
m += 0.1 * rng.randn(2, 1000)
center_and_norm(m)
# function as fun arg
def g_test(x):
return x ** 3, (3 * x ** 2).mean(axis=-1)
algos = ['parallel', 'deflation']
nls = ['logcosh', 'exp', 'cube', g_test]
whitening = [True, False]
for algo, nl, whiten in itertools.product(algos, nls, whitening):
if whiten:
k_, mixing_, s_ = fastica(m.T, fun=nl, algorithm=algo)
assert_raises(ValueError, fastica, m.T, fun=np.tanh,
algorithm=algo)
else:
X = PCA(n_components=2, whiten=True).fit_transform(m.T)
k_, mixing_, s_ = fastica(X, fun=nl, algorithm=algo, whiten=False)
assert_raises(ValueError, fastica, X, fun=np.tanh,
algorithm=algo)
s_ = s_.T
# Check that the mixing model described in the docstring holds:
if whiten:
assert_almost_equal(s_, np.dot(np.dot(mixing_, k_), m))
center_and_norm(s_)
s1_, s2_ = s_
# Check to see if the sources have been estimated
# in the wrong order
if abs(np.dot(s1_, s2)) > abs(np.dot(s1_, s1)):
s2_, s1_ = s_
s1_ *= np.sign(np.dot(s1_, s1))
s2_ *= np.sign(np.dot(s2_, s2))
# Check that we have estimated the original sources
if not add_noise:
assert_almost_equal(np.dot(s1_, s1) / n_samples, 1, decimal=2)
assert_almost_equal(np.dot(s2_, s2) / n_samples, 1, decimal=2)
else:
assert_almost_equal(np.dot(s1_, s1) / n_samples, 1, decimal=1)
assert_almost_equal(np.dot(s2_, s2) / n_samples, 1, decimal=1)
# Test FastICA class
_, _, sources_fun = fastica(m.T, fun=nl, algorithm=algo, random_state=0)
ica = FastICA(fun=nl, algorithm=algo, random_state=0)
sources = ica.fit_transform(m.T)
assert_equal(ica.components_.shape, (2, 2))
assert_equal(sources.shape, (1000, 2))
assert_array_almost_equal(sources_fun, sources)
assert_array_almost_equal(sources, ica.transform(m.T))
assert_equal(ica.mixing_.shape, (2, 2))
for fn in [np.tanh, "exp(-.5(x^2))"]:
ica = FastICA(fun=fn, algorithm=algo, random_state=0)
assert_raises(ValueError, ica.fit, m.T)
assert_raises(TypeError, FastICA(fun=moves.xrange(10)).fit, m.T)
def test_fastica_nowhiten():
m = [[0, 1], [1, 0]]
# test for issue #697
ica = FastICA(n_components=1, whiten=False, random_state=0)
assert_warns(UserWarning, ica.fit, m)
assert_true(hasattr(ica, 'mixing_'))
def test_non_square_fastica(add_noise=False):
# Test the FastICA algorithm on very simple data.
rng = np.random.RandomState(0)
n_samples = 1000
# Generate two sources:
t = np.linspace(0, 100, n_samples)
s1 = np.sin(t)
s2 = np.ceil(np.sin(np.pi * t))
s = np.c_[s1, s2].T
center_and_norm(s)
s1, s2 = s
# Mixing matrix
mixing = rng.randn(6, 2)
m = np.dot(mixing, s)
if add_noise:
m += 0.1 * rng.randn(6, n_samples)
center_and_norm(m)
k_, mixing_, s_ = fastica(m.T, n_components=2, random_state=rng)
s_ = s_.T
# Check that the mixing model described in the docstring holds:
assert_almost_equal(s_, np.dot(np.dot(mixing_, k_), m))
center_and_norm(s_)
s1_, s2_ = s_
# Check to see if the sources have been estimated
# in the wrong order
if abs(np.dot(s1_, s2)) > abs(np.dot(s1_, s1)):
s2_, s1_ = s_
s1_ *= np.sign(np.dot(s1_, s1))
s2_ *= np.sign(np.dot(s2_, s2))
# Check that we have estimated the original sources
if not add_noise:
assert_almost_equal(np.dot(s1_, s1) / n_samples, 1, decimal=3)
assert_almost_equal(np.dot(s2_, s2) / n_samples, 1, decimal=3)
def test_fit_transform():
# Test FastICA.fit_transform
rng = np.random.RandomState(0)
X = rng.random_sample((100, 10))
for whiten, n_components in [[True, 5], [False, None]]:
n_components_ = (n_components if n_components is not None else
X.shape[1])
ica = FastICA(n_components=n_components, whiten=whiten, random_state=0)
Xt = ica.fit_transform(X)
assert_equal(ica.components_.shape, (n_components_, 10))
assert_equal(Xt.shape, (100, n_components_))
ica = FastICA(n_components=n_components, whiten=whiten, random_state=0)
ica.fit(X)
assert_equal(ica.components_.shape, (n_components_, 10))
Xt2 = ica.transform(X)
assert_array_almost_equal(Xt, Xt2)
def test_inverse_transform():
# Test FastICA.inverse_transform
n_features = 10
n_samples = 100
n1, n2 = 5, 10
rng = np.random.RandomState(0)
X = rng.random_sample((n_samples, n_features))
expected = {(True, n1): (n_features, n1),
(True, n2): (n_features, n2),
(False, n1): (n_features, n2),
(False, n2): (n_features, n2)}
for whiten in [True, False]:
for n_components in [n1, n2]:
n_components_ = (n_components if n_components is not None else
X.shape[1])
ica = FastICA(n_components=n_components, random_state=rng,
whiten=whiten)
with warnings.catch_warnings(record=True):
# catch "n_components ignored" warning
Xt = ica.fit_transform(X)
expected_shape = expected[(whiten, n_components_)]
assert_equal(ica.mixing_.shape, expected_shape)
X2 = ica.inverse_transform(Xt)
assert_equal(X.shape, X2.shape)
# reversibility test in non-reduction case
if n_components == X.shape[1]:
assert_array_almost_equal(X, X2)
| bsd-3-clause |
HyperloopTeam/FullOpenMDAO | lib/python2.7/site-packages/matplotlib/tri/triplot.py | 21 | 3124 | from __future__ import (absolute_import, division, print_function,
unicode_literals)
import six
import numpy as np
from matplotlib.tri.triangulation import Triangulation
def triplot(ax, *args, **kwargs):
"""
Draw a unstructured triangular grid as lines and/or markers.
The triangulation to plot can be specified in one of two ways;
either::
triplot(triangulation, ...)
where triangulation is a :class:`matplotlib.tri.Triangulation`
object, or
::
triplot(x, y, ...)
triplot(x, y, triangles, ...)
triplot(x, y, triangles=triangles, ...)
triplot(x, y, mask=mask, ...)
triplot(x, y, triangles, mask=mask, ...)
in which case a Triangulation object will be created. See
:class:`~matplotlib.tri.Triangulation` for a explanation of these
possibilities.
The remaining args and kwargs are the same as for
:meth:`~matplotlib.axes.Axes.plot`.
Return a list of 2 :class:`~matplotlib.lines.Line2D` containing
respectively:
- the lines plotted for triangles edges
- the markers plotted for triangles nodes
**Example:**
.. plot:: mpl_examples/pylab_examples/triplot_demo.py
"""
import matplotlib.axes
tri, args, kwargs = Triangulation.get_from_args_and_kwargs(*args, **kwargs)
x, y, edges = (tri.x, tri.y, tri.edges)
# Decode plot format string, e.g., 'ro-'
fmt = ""
if len(args) > 0:
fmt = args[0]
linestyle, marker, color = matplotlib.axes._base._process_plot_format(fmt)
# Insert plot format string into a copy of kwargs (kwargs values prevail).
kw = kwargs.copy()
for key, val in zip(('linestyle', 'marker', 'color'),
(linestyle, marker, color)):
if val is not None:
kw[key] = kwargs.get(key, val)
# Draw lines without markers.
# Note 1: If we drew markers here, most markers would be drawn more than
# once as they belong to several edges.
# Note 2: We insert nan values in the flattened edges arrays rather than
# plotting directly (triang.x[edges].T, triang.y[edges].T)
# as it considerably speeds-up code execution.
linestyle = kw['linestyle']
kw_lines = kw.copy()
kw_lines['marker'] = 'None' # No marker to draw.
kw_lines['zorder'] = kw.get('zorder', 1) # Path default zorder is used.
if (linestyle is not None) and (linestyle not in ['None', '', ' ']):
tri_lines_x = np.insert(x[edges], 2, np.nan, axis=1)
tri_lines_y = np.insert(y[edges], 2, np.nan, axis=1)
tri_lines = ax.plot(tri_lines_x.ravel(), tri_lines_y.ravel(),
**kw_lines)
else:
tri_lines = ax.plot([], [], **kw_lines)
# Draw markers separately.
marker = kw['marker']
kw_markers = kw.copy()
kw_markers['linestyle'] = 'None' # No line to draw.
if (marker is not None) and (marker not in ['None', '', ' ']):
tri_markers = ax.plot(x, y, **kw_markers)
else:
tri_markers = ax.plot([], [], **kw_markers)
return tri_lines + tri_markers
| gpl-2.0 |
gautelinga/BERNAISE | utilities/mesh_scripts/hourglass.py | 1 | 3012 | """ hourglass script. """
from common import info
from generate_mesh import MESHES_DIR, store_mesh_HDF5, add_vertical_boundary_vertices
import dolfin as df
import os
import mshr
import matplotlib.pyplot as plt
def description(**kwargs):
info("Generates hourglass mesh.")
def method(L=6., H=2., R=0.3, n_segments=40, res=180, show=False, **kwargs):
"""
Generates hourglass.
"""
info("Generating mesh of an hourglass")
pt_1 = df.Point(0., 0.)
pt_1star = df.Point(2., 0.)
pt_1starstar = df.Point(L/(2*res), 0.)
pt_2 = df.Point(L, H)
pt_2star = df.Point(L-2., H)
pt_2starstar = df.Point(L-L/(2*res), H)
pt_3 = df.Point(2., H)
pt_3star = df.Point(0, H)
pt_3starstar = df.Point(L/(2*res), H)
pt_4 = df.Point(L-2., 0)
pt_4star = df.Point(L, 0)
pt_4starstar = df.Point(L-L/(2*res), 0)
pt_5 = df.Point(2., R)
pt_6 = df.Point(2., H-R)
pt_7 = df.Point(L-2., R)
pt_8 = df.Point(L-2., H-R)
pt_9 = df.Point(2.+2*R, R)
pt_10 = df.Point(2.+2*R, H-R)
pt_11 = df.Point(L-2*R-2, R)
pt_12 = df.Point(L-2*R-2, H-R)
pt_13 = df.Point(2.+2*R, H-2*R)
pt_14 = df.Point(L-2*R-2, 2*R)
inlet_polygon = [pt_1]
inlet_polygon.append(pt_1starstar)
add_vertical_boundary_vertices(inlet_polygon, L/res, H, res, 1)
inlet_polygon.append(pt_3starstar)
inlet_polygon.append(pt_3star)
add_vertical_boundary_vertices(inlet_polygon, 0.0, H, res, -1)
inlet_polygon.append(pt_1)
outlet_polygon = [pt_4starstar]
outlet_polygon.append(pt_4star)
add_vertical_boundary_vertices(outlet_polygon, L, H, res, 1)
outlet_polygon.append(pt_2)
outlet_polygon.append(pt_2starstar)
add_vertical_boundary_vertices(outlet_polygon, L-L/res, H, res, -1)
outlet_polygon.append(pt_4starstar)
inlet1 = mshr.Polygon(inlet_polygon)
inlet2 = mshr.Rectangle(pt_1starstar, pt_3)
outlet1 = mshr.Polygon(outlet_polygon)
outlet2 = mshr.Rectangle(pt_4, pt_2starstar)
channel = mshr.Rectangle(pt_5, pt_8)
pos_cir_1 = mshr.Circle(pt_5, R, segments=n_segments)
pos_cir_2 = mshr.Circle(pt_6, R, segments=n_segments)
pos_cir_3 = mshr.Circle(pt_7, R, segments=n_segments)
pos_cir_4 = mshr.Circle(pt_8, R, segments=n_segments)
neg_cir_1 = mshr.Circle(pt_9, R, segments=n_segments)
neg_cir_2 = mshr.Circle(pt_10, R, segments=n_segments)
neg_cir_3 = mshr.Circle(pt_11, R, segments=n_segments)
neg_cir_4 = mshr.Circle(pt_12, R, segments=n_segments)
neg_reg_1 = mshr.Rectangle(pt_13, pt_12)
neg_reg_2 = mshr.Rectangle(pt_9, pt_14)
domain = inlet1 + inlet2 + outlet1 + outlet2 + channel + \
pos_cir_1 + pos_cir_2 + pos_cir_3 + pos_cir_4 - neg_cir_1 - \
neg_cir_2 - neg_cir_3 - neg_cir_4 - neg_reg_1 - neg_reg_2
mesh = mshr.generate_mesh(domain, res)
mesh_path = os.path.join(MESHES_DIR,
"hourglass_res" + str(res))
store_mesh_HDF5(mesh, mesh_path)
if show:
df.plot(mesh)
plt.show()
| mit |
RPGOne/Skynet | scikit-learn-0.18.1/examples/tree/plot_tree_regression.py | 95 | 1516 | """
===================================================================
Decision Tree Regression
===================================================================
A 1D regression with decision tree.
The :ref:`decision trees <tree>` is
used to fit a sine curve with addition noisy observation. As a result, it
learns local linear regressions approximating the sine curve.
We can see that if the maximum depth of the tree (controlled by the
`max_depth` parameter) is set too high, the decision trees learn too fine
details of the training data and learn from the noise, i.e. they overfit.
"""
print(__doc__)
# Import the necessary modules and libraries
import numpy as np
from sklearn.tree import DecisionTreeRegressor
import matplotlib.pyplot as plt
# Create a random dataset
rng = np.random.RandomState(1)
X = np.sort(5 * rng.rand(80, 1), axis=0)
y = np.sin(X).ravel()
y[::5] += 3 * (0.5 - rng.rand(16))
# Fit regression model
regr_1 = DecisionTreeRegressor(max_depth=2)
regr_2 = DecisionTreeRegressor(max_depth=5)
regr_1.fit(X, y)
regr_2.fit(X, y)
# Predict
X_test = np.arange(0.0, 5.0, 0.01)[:, np.newaxis]
y_1 = regr_1.predict(X_test)
y_2 = regr_2.predict(X_test)
# Plot the results
plt.figure()
plt.scatter(X, y, c="darkorange", label="data")
plt.plot(X_test, y_1, color="cornflowerblue", label="max_depth=2", linewidth=2)
plt.plot(X_test, y_2, color="yellowgreen", label="max_depth=5", linewidth=2)
plt.xlabel("data")
plt.ylabel("target")
plt.title("Decision Tree Regression")
plt.legend()
plt.show()
| bsd-3-clause |
talespaiva/folium | folium/plugins/image_overlay.py | 2 | 5347 | # -*- coding: utf-8 -*-
"""
Image Overlay
-------------
Used to load and display a single image over specific bounds of
the map, implements ILayer interface.
"""
import json
from jinja2 import Template
from branca.utilities import image_to_url
from folium.map import Layer
def mercator_transform(data, lat_bounds, origin='upper', height_out=None):
"""Transforms an image computed in (longitude,latitude) coordinates into
the a Mercator projection image.
Parameters
----------
data: numpy array or equivalent list-like object.
Must be NxM (mono), NxMx3 (RGB) or NxMx4 (RGBA)
lat_bounds : length 2 tuple
Minimal and maximal value of the latitude of the image.
Bounds must be between -85.051128779806589 and 85.051128779806589
otherwise they will be clipped to that values.
origin : ['upper' | 'lower'], optional, default 'upper'
Place the [0,0] index of the array in the upper left or lower left
corner of the axes.
height_out : int, default None
The expected height of the output.
If None, the height of the input is used.
See https://en.wikipedia.org/wiki/Web_Mercator for more details.
"""
import numpy as np
def mercator(x):
return np.arcsinh(np.tan(x*np.pi/180.))*180./np.pi
array = np.atleast_3d(data).copy()
height, width, nblayers = array.shape
lat_min = max(lat_bounds[0], -85.051128779806589)
lat_max = min(lat_bounds[1], 85.051128779806589)
if height_out is None:
height_out = height
# Eventually flip the image
if origin == 'upper':
array = array[::-1, :, :]
lats = (lat_min + np.linspace(0.5/height, 1.-0.5/height, height) *
(lat_max-lat_min))
latslats = (mercator(lat_min) +
np.linspace(0.5/height_out, 1.-0.5/height_out, height_out) *
(mercator(lat_max)-mercator(lat_min)))
out = np.zeros((height_out, width, nblayers))
for i in range(width):
for j in range(nblayers):
out[:, i, j] = np.interp(latslats, mercator(lats), array[:, i, j])
# Eventually flip the image.
if origin == 'upper':
out = out[::-1, :, :]
return out
class ImageOverlay(Layer):
def __init__(self, image, bounds, opacity=1., attr=None,
origin='upper', colormap=None, mercator_project=False,
overlay=True, control=True):
"""
Used to load and display a single image over specific bounds of
the map, implements ILayer interface.
Parameters
----------
image: string, file or array-like object
The data you want to draw on the map.
* If string, it will be written directly in the output file.
* If file, it's content will be converted as embedded in the
output file.
* If array-like, it will be converted to PNG base64 string
and embedded in the output.
bounds: list
Image bounds on the map in the form [[lat_min, lon_min],
[lat_max, lon_max]]
opacity: float, default Leaflet's default (1.0)
attr: string, default Leaflet's default ("")
origin : ['upper' | 'lower'], optional, default 'upper'
Place the [0,0] index of the array in the upper left or
lower left corner of the axes.
colormap : callable, used only for `mono` image.
Function of the form [x -> (r,g,b)] or [x -> (r,g,b,a)]
for transforming a mono image into RGB.
It must output iterables of length 3 or 4,
with values between 0 and 1.
Hint : you can use colormaps from `matplotlib.cm`.
mercator_project : bool, default False.
Used only for array-like image. Transforms the data to
project (longitude, latitude) coordinates to the
Mercator projection.
Beware that this will only work if `image` is an array-like
object.
"""
super(ImageOverlay, self).__init__(overlay=overlay, control=control)
self._name = 'ImageOverlay'
self.overlay = overlay
if mercator_project:
image = mercator_transform(image,
[bounds[0][0], bounds[1][0]],
origin=origin)
self.url = image_to_url(image, origin=origin, colormap=colormap)
self.bounds = json.loads(json.dumps(bounds))
options = {
'opacity': opacity,
'attribution': attr,
}
self.options = json.dumps({key: val for key, val
in options.items() if val},
sort_keys=True)
self._template = Template(u"""
{% macro script(this, kwargs) %}
var {{this.get_name()}} = L.imageOverlay(
'{{ this.url }}',
{{ this.bounds }},
{{ this.options }}
).addTo({{this._parent.get_name()}});
{% endmacro %}
""")
def _get_self_bounds(self):
"""Computes the bounds of the object itself (not including it's children)
in the form [[lat_min, lon_min], [lat_max, lon_max]]
"""
return self.bounds
| mit |
johnbachman/rasmodel | kras_gtp_hydrolysis.py | 6 | 1613 | from rasmodel.scenarios.default import model
import numpy as np
from matplotlib import pyplot as plt
from pysb.integrate import Solver
from pysb import *
from tbidbaxlipo.util import fitting
KRAS = model.monomers['KRAS']
GTP = model.monomers['GTP']
total_pi = 50000
for mutant in KRAS.site_states['mutant']:
Initial(KRAS(gtp=1, gap=None, gef=None, p_loop=None, s1s2='open', CAAX=None,
mutant=mutant) % GTP(p=1, label='n'),
Parameter('KRAS_%s_GTP_0' % mutant, 0))
plt.ion()
plt.figure()
t = np.linspace(0, 1000, 1000) # 1000 seconds
for mutant in KRAS.site_states['mutant']:
# Zero out all initial conditions
for ic in model.initial_conditions:
ic[1].value = 0
model.parameters['KRAS_%s_GTP_0' % mutant].value = total_pi
sol = Solver(model, t)
sol.run()
plt.plot(t, sol.yobs['Pi_'] / total_pi, label=mutant)
plt.ylabel('GTP hydrolyzed (%)')
plt.ylim(top=1)
plt.xlabel('Time (s)')
plt.title('Intrinsic hydrolysis')
plt.legend(loc='upper left', fontsize=11, frameon=False)
plt.figure()
for mutant in KRAS.site_states['mutant']:
# Zero out all initial conditions
for ic in model.initial_conditions:
ic[1].value = 0
model.parameters['RASA1_0'].value = 50000
model.parameters['KRAS_%s_GTP_0' % mutant].value = total_pi
sol = Solver(model, t)
sol.run()
plt.plot(t, sol.yobs['Pi_'] / total_pi, label=mutant)
plt.ylabel('GTP hydrolyzed (%)')
plt.ylim(top=1)
plt.xlabel('Time (s)')
plt.title('GAP-mediated hydrolysis')
plt.legend(loc='upper right', fontsize=11, frameon=False)
| mit |
phobson/seaborn | examples/structured_heatmap.py | 4 | 1067 | """
Discovering structure in heatmap data
=====================================
_thumb: .4, .25
"""
import pandas as pd
import seaborn as sns
sns.set()
# Load the brain networks example dataset
df = sns.load_dataset("brain_networks", header=[0, 1, 2], index_col=0)
# Select a subset of the networks
used_networks = [1, 5, 6, 7, 8, 12, 13, 17]
used_columns = (df.columns.get_level_values("network")
.astype(int)
.isin(used_networks))
df = df.loc[:, used_columns]
# Create a categorical palette to identify the networks
network_pal = sns.husl_palette(8, s=.45)
network_lut = dict(zip(map(str, used_networks), network_pal))
# Convert the palette to vectors that will be drawn on the side of the matrix
networks = df.columns.get_level_values("network")
network_colors = pd.Series(networks, index=df.columns).map(network_lut)
# Draw the full plot
sns.clustermap(df.corr(), center=0, cmap="vlag",
row_colors=network_colors, col_colors=network_colors,
linewidths=.75, figsize=(13, 13))
| bsd-3-clause |
RPGOne/scikit-learn | examples/cluster/plot_dbscan.py | 346 | 2479 | # -*- coding: utf-8 -*-
"""
===================================
Demo of DBSCAN clustering algorithm
===================================
Finds core samples of high density and expands clusters from them.
"""
print(__doc__)
import numpy as np
from sklearn.cluster import DBSCAN
from sklearn import metrics
from sklearn.datasets.samples_generator import make_blobs
from sklearn.preprocessing import StandardScaler
##############################################################################
# Generate sample data
centers = [[1, 1], [-1, -1], [1, -1]]
X, labels_true = make_blobs(n_samples=750, centers=centers, cluster_std=0.4,
random_state=0)
X = StandardScaler().fit_transform(X)
##############################################################################
# Compute DBSCAN
db = DBSCAN(eps=0.3, min_samples=10).fit(X)
core_samples_mask = np.zeros_like(db.labels_, dtype=bool)
core_samples_mask[db.core_sample_indices_] = True
labels = db.labels_
# Number of clusters in labels, ignoring noise if present.
n_clusters_ = len(set(labels)) - (1 if -1 in labels else 0)
print('Estimated number of clusters: %d' % n_clusters_)
print("Homogeneity: %0.3f" % metrics.homogeneity_score(labels_true, labels))
print("Completeness: %0.3f" % metrics.completeness_score(labels_true, labels))
print("V-measure: %0.3f" % metrics.v_measure_score(labels_true, labels))
print("Adjusted Rand Index: %0.3f"
% metrics.adjusted_rand_score(labels_true, labels))
print("Adjusted Mutual Information: %0.3f"
% metrics.adjusted_mutual_info_score(labels_true, labels))
print("Silhouette Coefficient: %0.3f"
% metrics.silhouette_score(X, labels))
##############################################################################
# Plot result
import matplotlib.pyplot as plt
# Black removed and is used for noise instead.
unique_labels = set(labels)
colors = plt.cm.Spectral(np.linspace(0, 1, len(unique_labels)))
for k, col in zip(unique_labels, colors):
if k == -1:
# Black used for noise.
col = 'k'
class_member_mask = (labels == k)
xy = X[class_member_mask & core_samples_mask]
plt.plot(xy[:, 0], xy[:, 1], 'o', markerfacecolor=col,
markeredgecolor='k', markersize=14)
xy = X[class_member_mask & ~core_samples_mask]
plt.plot(xy[:, 0], xy[:, 1], 'o', markerfacecolor=col,
markeredgecolor='k', markersize=6)
plt.title('Estimated number of clusters: %d' % n_clusters_)
plt.show()
| bsd-3-clause |
jzt5132/scikit-learn | sklearn/preprocessing/tests/test_data.py | 71 | 38516 | import warnings
import numpy as np
import numpy.linalg as la
from scipy import sparse
from distutils.version import LooseVersion
from sklearn.utils.testing import assert_almost_equal, clean_warning_registry
from sklearn.utils.testing import assert_array_almost_equal
from sklearn.utils.testing import assert_array_equal
from sklearn.utils.testing import assert_equal
from sklearn.utils.testing import assert_greater_equal
from sklearn.utils.testing import assert_less_equal
from sklearn.utils.testing import assert_raises
from sklearn.utils.testing import assert_raises_regex
from sklearn.utils.testing import assert_true
from sklearn.utils.testing import assert_false
from sklearn.utils.testing import assert_warns_message
from sklearn.utils.testing import assert_no_warnings
from sklearn.utils.testing import ignore_warnings
from sklearn.utils.sparsefuncs import mean_variance_axis
from sklearn.preprocessing.data import _transform_selected
from sklearn.preprocessing.data import Binarizer
from sklearn.preprocessing.data import KernelCenterer
from sklearn.preprocessing.data import Normalizer
from sklearn.preprocessing.data import normalize
from sklearn.preprocessing.data import OneHotEncoder
from sklearn.preprocessing.data import StandardScaler
from sklearn.preprocessing.data import scale
from sklearn.preprocessing.data import MinMaxScaler
from sklearn.preprocessing.data import minmax_scale
from sklearn.preprocessing.data import MaxAbsScaler
from sklearn.preprocessing.data import maxabs_scale
from sklearn.preprocessing.data import RobustScaler
from sklearn.preprocessing.data import robust_scale
from sklearn.preprocessing.data import add_dummy_feature
from sklearn.preprocessing.data import PolynomialFeatures
from sklearn.utils.validation import DataConversionWarning
from sklearn import datasets
iris = datasets.load_iris()
def toarray(a):
if hasattr(a, "toarray"):
a = a.toarray()
return a
def test_polynomial_features():
# Test Polynomial Features
X1 = np.arange(6)[:, np.newaxis]
P1 = np.hstack([np.ones_like(X1),
X1, X1 ** 2, X1 ** 3])
deg1 = 3
X2 = np.arange(6).reshape((3, 2))
x1 = X2[:, :1]
x2 = X2[:, 1:]
P2 = np.hstack([x1 ** 0 * x2 ** 0,
x1 ** 1 * x2 ** 0,
x1 ** 0 * x2 ** 1,
x1 ** 2 * x2 ** 0,
x1 ** 1 * x2 ** 1,
x1 ** 0 * x2 ** 2])
deg2 = 2
for (deg, X, P) in [(deg1, X1, P1), (deg2, X2, P2)]:
P_test = PolynomialFeatures(deg, include_bias=True).fit_transform(X)
assert_array_almost_equal(P_test, P)
P_test = PolynomialFeatures(deg, include_bias=False).fit_transform(X)
assert_array_almost_equal(P_test, P[:, 1:])
interact = PolynomialFeatures(2, interaction_only=True, include_bias=True)
X_poly = interact.fit_transform(X)
assert_array_almost_equal(X_poly, P2[:, [0, 1, 2, 4]])
@ignore_warnings
def test_scaler_1d():
# Test scaling of dataset along single axis
rng = np.random.RandomState(0)
X = rng.randn(5)
X_orig_copy = X.copy()
scaler = StandardScaler()
X_scaled = scaler.fit(X).transform(X, copy=False)
assert_array_almost_equal(X_scaled.mean(axis=0), 0.0)
assert_array_almost_equal(X_scaled.std(axis=0), 1.0)
# check inverse transform
X_scaled_back = scaler.inverse_transform(X_scaled)
assert_array_almost_equal(X_scaled_back, X_orig_copy)
# Test with 1D list
X = [0., 1., 2, 0.4, 1.]
scaler = StandardScaler()
X_scaled = scaler.fit(X).transform(X, copy=False)
assert_array_almost_equal(X_scaled.mean(axis=0), 0.0)
assert_array_almost_equal(X_scaled.std(axis=0), 1.0)
X_scaled = scale(X)
assert_array_almost_equal(X_scaled.mean(axis=0), 0.0)
assert_array_almost_equal(X_scaled.std(axis=0), 1.0)
X = np.ones(5)
assert_array_equal(scale(X, with_mean=False), X)
def test_standard_scaler_numerical_stability():
"""Test numerical stability of scaling"""
# np.log(1e-5) is taken because of its floating point representation
# was empirically found to cause numerical problems with np.mean & np.std.
x = np.zeros(8, dtype=np.float64) + np.log(1e-5, dtype=np.float64)
if LooseVersion(np.__version__) >= LooseVersion('1.9'):
# This does not raise a warning as the number of samples is too low
# to trigger the problem in recent numpy
x_scaled = assert_no_warnings(scale, x)
assert_array_almost_equal(scale(x), np.zeros(8))
else:
w = "standard deviation of the data is probably very close to 0"
x_scaled = assert_warns_message(UserWarning, w, scale, x)
assert_array_almost_equal(x_scaled, np.zeros(8))
# with 2 more samples, the std computation run into numerical issues:
x = np.zeros(10, dtype=np.float64) + np.log(1e-5, dtype=np.float64)
w = "standard deviation of the data is probably very close to 0"
x_scaled = assert_warns_message(UserWarning, w, scale, x)
assert_array_almost_equal(x_scaled, np.zeros(10))
x = np.ones(10, dtype=np.float64) * 1e-100
x_small_scaled = assert_no_warnings(scale, x)
assert_array_almost_equal(x_small_scaled, np.zeros(10))
# Large values can cause (often recoverable) numerical stability issues:
x_big = np.ones(10, dtype=np.float64) * 1e100
w = "Dataset may contain too large values"
x_big_scaled = assert_warns_message(UserWarning, w, scale, x_big)
assert_array_almost_equal(x_big_scaled, np.zeros(10))
assert_array_almost_equal(x_big_scaled, x_small_scaled)
x_big_centered = assert_warns_message(UserWarning, w, scale, x_big,
with_std=False)
assert_array_almost_equal(x_big_centered, np.zeros(10))
assert_array_almost_equal(x_big_centered, x_small_scaled)
def test_scaler_2d_arrays():
# Test scaling of 2d array along first axis
rng = np.random.RandomState(0)
X = rng.randn(4, 5)
X[:, 0] = 0.0 # first feature is always of zero
scaler = StandardScaler()
X_scaled = scaler.fit(X).transform(X, copy=True)
assert_false(np.any(np.isnan(X_scaled)))
assert_array_almost_equal(X_scaled.mean(axis=0), 5 * [0.0])
assert_array_almost_equal(X_scaled.std(axis=0), [0., 1., 1., 1., 1.])
# Check that X has been copied
assert_true(X_scaled is not X)
# check inverse transform
X_scaled_back = scaler.inverse_transform(X_scaled)
assert_true(X_scaled_back is not X)
assert_true(X_scaled_back is not X_scaled)
assert_array_almost_equal(X_scaled_back, X)
X_scaled = scale(X, axis=1, with_std=False)
assert_false(np.any(np.isnan(X_scaled)))
assert_array_almost_equal(X_scaled.mean(axis=1), 4 * [0.0])
X_scaled = scale(X, axis=1, with_std=True)
assert_false(np.any(np.isnan(X_scaled)))
assert_array_almost_equal(X_scaled.mean(axis=1), 4 * [0.0])
assert_array_almost_equal(X_scaled.std(axis=1), 4 * [1.0])
# Check that the data hasn't been modified
assert_true(X_scaled is not X)
X_scaled = scaler.fit(X).transform(X, copy=False)
assert_false(np.any(np.isnan(X_scaled)))
assert_array_almost_equal(X_scaled.mean(axis=0), 5 * [0.0])
assert_array_almost_equal(X_scaled.std(axis=0), [0., 1., 1., 1., 1.])
# Check that X has not been copied
assert_true(X_scaled is X)
X = rng.randn(4, 5)
X[:, 0] = 1.0 # first feature is a constant, non zero feature
scaler = StandardScaler()
X_scaled = scaler.fit(X).transform(X, copy=True)
assert_false(np.any(np.isnan(X_scaled)))
assert_array_almost_equal(X_scaled.mean(axis=0), 5 * [0.0])
assert_array_almost_equal(X_scaled.std(axis=0), [0., 1., 1., 1., 1.])
# Check that X has not been copied
assert_true(X_scaled is not X)
def test_min_max_scaler_iris():
X = iris.data
scaler = MinMaxScaler()
# default params
X_trans = scaler.fit_transform(X)
assert_array_almost_equal(X_trans.min(axis=0), 0)
assert_array_almost_equal(X_trans.min(axis=0), 0)
assert_array_almost_equal(X_trans.max(axis=0), 1)
X_trans_inv = scaler.inverse_transform(X_trans)
assert_array_almost_equal(X, X_trans_inv)
# not default params: min=1, max=2
scaler = MinMaxScaler(feature_range=(1, 2))
X_trans = scaler.fit_transform(X)
assert_array_almost_equal(X_trans.min(axis=0), 1)
assert_array_almost_equal(X_trans.max(axis=0), 2)
X_trans_inv = scaler.inverse_transform(X_trans)
assert_array_almost_equal(X, X_trans_inv)
# min=-.5, max=.6
scaler = MinMaxScaler(feature_range=(-.5, .6))
X_trans = scaler.fit_transform(X)
assert_array_almost_equal(X_trans.min(axis=0), -.5)
assert_array_almost_equal(X_trans.max(axis=0), .6)
X_trans_inv = scaler.inverse_transform(X_trans)
assert_array_almost_equal(X, X_trans_inv)
# raises on invalid range
scaler = MinMaxScaler(feature_range=(2, 1))
assert_raises(ValueError, scaler.fit, X)
def test_min_max_scaler_zero_variance_features():
# Check min max scaler on toy data with zero variance features
X = [[0., 1., +0.5],
[0., 1., -0.1],
[0., 1., +1.1]]
X_new = [[+0., 2., 0.5],
[-1., 1., 0.0],
[+0., 1., 1.5]]
# default params
scaler = MinMaxScaler()
X_trans = scaler.fit_transform(X)
X_expected_0_1 = [[0., 0., 0.5],
[0., 0., 0.0],
[0., 0., 1.0]]
assert_array_almost_equal(X_trans, X_expected_0_1)
X_trans_inv = scaler.inverse_transform(X_trans)
assert_array_almost_equal(X, X_trans_inv)
X_trans_new = scaler.transform(X_new)
X_expected_0_1_new = [[+0., 1., 0.500],
[-1., 0., 0.083],
[+0., 0., 1.333]]
assert_array_almost_equal(X_trans_new, X_expected_0_1_new, decimal=2)
# not default params
scaler = MinMaxScaler(feature_range=(1, 2))
X_trans = scaler.fit_transform(X)
X_expected_1_2 = [[1., 1., 1.5],
[1., 1., 1.0],
[1., 1., 2.0]]
assert_array_almost_equal(X_trans, X_expected_1_2)
# function interface
X_trans = minmax_scale(X)
assert_array_almost_equal(X_trans, X_expected_0_1)
X_trans = minmax_scale(X, feature_range=(1, 2))
assert_array_almost_equal(X_trans, X_expected_1_2)
def test_minmax_scale_axis1():
X = iris.data
X_trans = minmax_scale(X, axis=1)
assert_array_almost_equal(np.min(X_trans, axis=1), 0)
assert_array_almost_equal(np.max(X_trans, axis=1), 1)
@ignore_warnings
def test_min_max_scaler_1d():
# Test scaling of dataset along single axis
rng = np.random.RandomState(0)
X = rng.randn(5)
X_orig_copy = X.copy()
scaler = MinMaxScaler()
X_scaled = scaler.fit(X).transform(X)
assert_array_almost_equal(X_scaled.min(axis=0), 0.0)
assert_array_almost_equal(X_scaled.max(axis=0), 1.0)
# check inverse transform
X_scaled_back = scaler.inverse_transform(X_scaled)
assert_array_almost_equal(X_scaled_back, X_orig_copy)
# Test with 1D list
X = [0., 1., 2, 0.4, 1.]
scaler = MinMaxScaler()
X_scaled = scaler.fit(X).transform(X)
assert_array_almost_equal(X_scaled.min(axis=0), 0.0)
assert_array_almost_equal(X_scaled.max(axis=0), 1.0)
# Constant feature.
X = np.zeros(5)
scaler = MinMaxScaler()
X_scaled = scaler.fit(X).transform(X)
assert_greater_equal(X_scaled.min(), 0.)
assert_less_equal(X_scaled.max(), 1.)
def test_scaler_without_centering():
rng = np.random.RandomState(42)
X = rng.randn(4, 5)
X[:, 0] = 0.0 # first feature is always of zero
X_csr = sparse.csr_matrix(X)
X_csc = sparse.csc_matrix(X)
assert_raises(ValueError, StandardScaler().fit, X_csr)
null_transform = StandardScaler(with_mean=False, with_std=False, copy=True)
X_null = null_transform.fit_transform(X_csr)
assert_array_equal(X_null.data, X_csr.data)
X_orig = null_transform.inverse_transform(X_null)
assert_array_equal(X_orig.data, X_csr.data)
scaler = StandardScaler(with_mean=False).fit(X)
X_scaled = scaler.transform(X, copy=True)
assert_false(np.any(np.isnan(X_scaled)))
scaler_csr = StandardScaler(with_mean=False).fit(X_csr)
X_csr_scaled = scaler_csr.transform(X_csr, copy=True)
assert_false(np.any(np.isnan(X_csr_scaled.data)))
scaler_csc = StandardScaler(with_mean=False).fit(X_csc)
X_csc_scaled = scaler_csr.transform(X_csc, copy=True)
assert_false(np.any(np.isnan(X_csc_scaled.data)))
assert_equal(scaler.mean_, scaler_csr.mean_)
assert_array_almost_equal(scaler.std_, scaler_csr.std_)
assert_equal(scaler.mean_, scaler_csc.mean_)
assert_array_almost_equal(scaler.std_, scaler_csc.std_)
assert_array_almost_equal(
X_scaled.mean(axis=0), [0., -0.01, 2.24, -0.35, -0.78], 2)
assert_array_almost_equal(X_scaled.std(axis=0), [0., 1., 1., 1., 1.])
X_csr_scaled_mean, X_csr_scaled_std = mean_variance_axis(X_csr_scaled, 0)
assert_array_almost_equal(X_csr_scaled_mean, X_scaled.mean(axis=0))
assert_array_almost_equal(X_csr_scaled_std, X_scaled.std(axis=0))
# Check that X has not been modified (copy)
assert_true(X_scaled is not X)
assert_true(X_csr_scaled is not X_csr)
X_scaled_back = scaler.inverse_transform(X_scaled)
assert_true(X_scaled_back is not X)
assert_true(X_scaled_back is not X_scaled)
assert_array_almost_equal(X_scaled_back, X)
X_csr_scaled_back = scaler_csr.inverse_transform(X_csr_scaled)
assert_true(X_csr_scaled_back is not X_csr)
assert_true(X_csr_scaled_back is not X_csr_scaled)
assert_array_almost_equal(X_csr_scaled_back.toarray(), X)
X_csc_scaled_back = scaler_csr.inverse_transform(X_csc_scaled.tocsc())
assert_true(X_csc_scaled_back is not X_csc)
assert_true(X_csc_scaled_back is not X_csc_scaled)
assert_array_almost_equal(X_csc_scaled_back.toarray(), X)
def test_scaler_int():
# test that scaler converts integer input to floating
# for both sparse and dense matrices
rng = np.random.RandomState(42)
X = rng.randint(20, size=(4, 5))
X[:, 0] = 0 # first feature is always of zero
X_csr = sparse.csr_matrix(X)
X_csc = sparse.csc_matrix(X)
null_transform = StandardScaler(with_mean=False, with_std=False, copy=True)
clean_warning_registry()
with warnings.catch_warnings(record=True):
X_null = null_transform.fit_transform(X_csr)
assert_array_equal(X_null.data, X_csr.data)
X_orig = null_transform.inverse_transform(X_null)
assert_array_equal(X_orig.data, X_csr.data)
clean_warning_registry()
with warnings.catch_warnings(record=True):
scaler = StandardScaler(with_mean=False).fit(X)
X_scaled = scaler.transform(X, copy=True)
assert_false(np.any(np.isnan(X_scaled)))
clean_warning_registry()
with warnings.catch_warnings(record=True):
scaler_csr = StandardScaler(with_mean=False).fit(X_csr)
X_csr_scaled = scaler_csr.transform(X_csr, copy=True)
assert_false(np.any(np.isnan(X_csr_scaled.data)))
clean_warning_registry()
with warnings.catch_warnings(record=True):
scaler_csc = StandardScaler(with_mean=False).fit(X_csc)
X_csc_scaled = scaler_csr.transform(X_csc, copy=True)
assert_false(np.any(np.isnan(X_csc_scaled.data)))
assert_equal(scaler.mean_, scaler_csr.mean_)
assert_array_almost_equal(scaler.std_, scaler_csr.std_)
assert_equal(scaler.mean_, scaler_csc.mean_)
assert_array_almost_equal(scaler.std_, scaler_csc.std_)
assert_array_almost_equal(
X_scaled.mean(axis=0),
[0., 1.109, 1.856, 21., 1.559], 2)
assert_array_almost_equal(X_scaled.std(axis=0), [0., 1., 1., 1., 1.])
X_csr_scaled_mean, X_csr_scaled_std = mean_variance_axis(
X_csr_scaled.astype(np.float), 0)
assert_array_almost_equal(X_csr_scaled_mean, X_scaled.mean(axis=0))
assert_array_almost_equal(X_csr_scaled_std, X_scaled.std(axis=0))
# Check that X has not been modified (copy)
assert_true(X_scaled is not X)
assert_true(X_csr_scaled is not X_csr)
X_scaled_back = scaler.inverse_transform(X_scaled)
assert_true(X_scaled_back is not X)
assert_true(X_scaled_back is not X_scaled)
assert_array_almost_equal(X_scaled_back, X)
X_csr_scaled_back = scaler_csr.inverse_transform(X_csr_scaled)
assert_true(X_csr_scaled_back is not X_csr)
assert_true(X_csr_scaled_back is not X_csr_scaled)
assert_array_almost_equal(X_csr_scaled_back.toarray(), X)
X_csc_scaled_back = scaler_csr.inverse_transform(X_csc_scaled.tocsc())
assert_true(X_csc_scaled_back is not X_csc)
assert_true(X_csc_scaled_back is not X_csc_scaled)
assert_array_almost_equal(X_csc_scaled_back.toarray(), X)
def test_scaler_without_copy():
# Check that StandardScaler.fit does not change input
rng = np.random.RandomState(42)
X = rng.randn(4, 5)
X[:, 0] = 0.0 # first feature is always of zero
X_csr = sparse.csr_matrix(X)
X_copy = X.copy()
StandardScaler(copy=False).fit(X)
assert_array_equal(X, X_copy)
X_csr_copy = X_csr.copy()
StandardScaler(with_mean=False, copy=False).fit(X_csr)
assert_array_equal(X_csr.toarray(), X_csr_copy.toarray())
def test_scale_sparse_with_mean_raise_exception():
rng = np.random.RandomState(42)
X = rng.randn(4, 5)
X_csr = sparse.csr_matrix(X)
# check scaling and fit with direct calls on sparse data
assert_raises(ValueError, scale, X_csr, with_mean=True)
assert_raises(ValueError, StandardScaler(with_mean=True).fit, X_csr)
# check transform and inverse_transform after a fit on a dense array
scaler = StandardScaler(with_mean=True).fit(X)
assert_raises(ValueError, scaler.transform, X_csr)
X_transformed_csr = sparse.csr_matrix(scaler.transform(X))
assert_raises(ValueError, scaler.inverse_transform, X_transformed_csr)
def test_scale_input_finiteness_validation():
# Check if non finite inputs raise ValueError
X = [np.nan, 5, 6, 7, 8]
assert_raises_regex(ValueError,
"Input contains NaN, infinity or a value too large",
scale, X)
X = [np.inf, 5, 6, 7, 8]
assert_raises_regex(ValueError,
"Input contains NaN, infinity or a value too large",
scale, X)
def test_scale_function_without_centering():
rng = np.random.RandomState(42)
X = rng.randn(4, 5)
X[:, 0] = 0.0 # first feature is always of zero
X_csr = sparse.csr_matrix(X)
X_scaled = scale(X, with_mean=False)
assert_false(np.any(np.isnan(X_scaled)))
X_csr_scaled = scale(X_csr, with_mean=False)
assert_false(np.any(np.isnan(X_csr_scaled.data)))
# test csc has same outcome
X_csc_scaled = scale(X_csr.tocsc(), with_mean=False)
assert_array_almost_equal(X_scaled, X_csc_scaled.toarray())
# raises value error on axis != 0
assert_raises(ValueError, scale, X_csr, with_mean=False, axis=1)
assert_array_almost_equal(X_scaled.mean(axis=0),
[0., -0.01, 2.24, -0.35, -0.78], 2)
assert_array_almost_equal(X_scaled.std(axis=0), [0., 1., 1., 1., 1.])
# Check that X has not been copied
assert_true(X_scaled is not X)
X_csr_scaled_mean, X_csr_scaled_std = mean_variance_axis(X_csr_scaled, 0)
assert_array_almost_equal(X_csr_scaled_mean, X_scaled.mean(axis=0))
assert_array_almost_equal(X_csr_scaled_std, X_scaled.std(axis=0))
def test_robust_scaler_2d_arrays():
"""Test robust scaling of 2d array along first axis"""
rng = np.random.RandomState(0)
X = rng.randn(4, 5)
X[:, 0] = 0.0 # first feature is always of zero
scaler = RobustScaler()
X_scaled = scaler.fit(X).transform(X)
assert_array_almost_equal(np.median(X_scaled, axis=0), 5 * [0.0])
assert_array_almost_equal(X_scaled.std(axis=0)[0], 0)
def test_robust_scaler_iris():
X = iris.data
scaler = RobustScaler()
X_trans = scaler.fit_transform(X)
assert_array_almost_equal(np.median(X_trans, axis=0), 0)
X_trans_inv = scaler.inverse_transform(X_trans)
assert_array_almost_equal(X, X_trans_inv)
q = np.percentile(X_trans, q=(25, 75), axis=0)
iqr = q[1] - q[0]
assert_array_almost_equal(iqr, 1)
def test_robust_scale_axis1():
X = iris.data
X_trans = robust_scale(X, axis=1)
assert_array_almost_equal(np.median(X_trans, axis=1), 0)
q = np.percentile(X_trans, q=(25, 75), axis=1)
iqr = q[1] - q[0]
assert_array_almost_equal(iqr, 1)
def test_robust_scaler_zero_variance_features():
"""Check RobustScaler on toy data with zero variance features"""
X = [[0., 1., +0.5],
[0., 1., -0.1],
[0., 1., +1.1]]
scaler = RobustScaler()
X_trans = scaler.fit_transform(X)
# NOTE: for such a small sample size, what we expect in the third column
# depends HEAVILY on the method used to calculate quantiles. The values
# here were calculated to fit the quantiles produces by np.percentile
# using numpy 1.9 Calculating quantiles with
# scipy.stats.mstats.scoreatquantile or scipy.stats.mstats.mquantiles
# would yield very different results!
X_expected = [[0., 0., +0.0],
[0., 0., -1.0],
[0., 0., +1.0]]
assert_array_almost_equal(X_trans, X_expected)
X_trans_inv = scaler.inverse_transform(X_trans)
assert_array_almost_equal(X, X_trans_inv)
# make sure new data gets transformed correctly
X_new = [[+0., 2., 0.5],
[-1., 1., 0.0],
[+0., 1., 1.5]]
X_trans_new = scaler.transform(X_new)
X_expected_new = [[+0., 1., +0.],
[-1., 0., -0.83333],
[+0., 0., +1.66667]]
assert_array_almost_equal(X_trans_new, X_expected_new, decimal=3)
def test_maxabs_scaler_zero_variance_features():
"""Check MaxAbsScaler on toy data with zero variance features"""
X = [[0., 1., +0.5],
[0., 1., -0.3],
[0., 1., +1.5],
[0., 0., +0.0]]
scaler = MaxAbsScaler()
X_trans = scaler.fit_transform(X)
X_expected = [[0., 1., 1.0 / 3.0],
[0., 1., -0.2],
[0., 1., 1.0],
[0., 0., 0.0]]
assert_array_almost_equal(X_trans, X_expected)
X_trans_inv = scaler.inverse_transform(X_trans)
assert_array_almost_equal(X, X_trans_inv)
# make sure new data gets transformed correctly
X_new = [[+0., 2., 0.5],
[-1., 1., 0.0],
[+0., 1., 1.5]]
X_trans_new = scaler.transform(X_new)
X_expected_new = [[+0., 2.0, 1.0 / 3.0],
[-1., 1.0, 0.0],
[+0., 1.0, 1.0]]
assert_array_almost_equal(X_trans_new, X_expected_new, decimal=2)
# sparse data
X_csr = sparse.csr_matrix(X)
X_trans = scaler.fit_transform(X_csr)
X_expected = [[0., 1., 1.0 / 3.0],
[0., 1., -0.2],
[0., 1., 1.0],
[0., 0., 0.0]]
assert_array_almost_equal(X_trans.A, X_expected)
X_trans_inv = scaler.inverse_transform(X_trans)
assert_array_almost_equal(X, X_trans_inv.A)
def test_maxabs_scaler_large_negative_value():
"""Check MaxAbsScaler on toy data with a large negative value"""
X = [[0., 1., +0.5, -1.0],
[0., 1., -0.3, -0.5],
[0., 1., -100.0, 0.0],
[0., 0., +0.0, -2.0]]
scaler = MaxAbsScaler()
X_trans = scaler.fit_transform(X)
X_expected = [[0., 1., 0.005, -0.5],
[0., 1., -0.003, -0.25],
[0., 1., -1.0, 0.0],
[0., 0., 0.0, -1.0]]
assert_array_almost_equal(X_trans, X_expected)
def test_warning_scaling_integers():
# Check warning when scaling integer data
X = np.array([[1, 2, 0],
[0, 0, 0]], dtype=np.uint8)
w = "Data with input dtype uint8 was converted to float64"
clean_warning_registry()
assert_warns_message(DataConversionWarning, w, scale, X)
assert_warns_message(DataConversionWarning, w, StandardScaler().fit, X)
assert_warns_message(DataConversionWarning, w, MinMaxScaler().fit, X)
def test_normalizer_l1():
rng = np.random.RandomState(0)
X_dense = rng.randn(4, 5)
X_sparse_unpruned = sparse.csr_matrix(X_dense)
# set the row number 3 to zero
X_dense[3, :] = 0.0
# set the row number 3 to zero without pruning (can happen in real life)
indptr_3 = X_sparse_unpruned.indptr[3]
indptr_4 = X_sparse_unpruned.indptr[4]
X_sparse_unpruned.data[indptr_3:indptr_4] = 0.0
# build the pruned variant using the regular constructor
X_sparse_pruned = sparse.csr_matrix(X_dense)
# check inputs that support the no-copy optim
for X in (X_dense, X_sparse_pruned, X_sparse_unpruned):
normalizer = Normalizer(norm='l1', copy=True)
X_norm = normalizer.transform(X)
assert_true(X_norm is not X)
X_norm1 = toarray(X_norm)
normalizer = Normalizer(norm='l1', copy=False)
X_norm = normalizer.transform(X)
assert_true(X_norm is X)
X_norm2 = toarray(X_norm)
for X_norm in (X_norm1, X_norm2):
row_sums = np.abs(X_norm).sum(axis=1)
for i in range(3):
assert_almost_equal(row_sums[i], 1.0)
assert_almost_equal(row_sums[3], 0.0)
# check input for which copy=False won't prevent a copy
for init in (sparse.coo_matrix, sparse.csc_matrix, sparse.lil_matrix):
X = init(X_dense)
X_norm = normalizer = Normalizer(norm='l2', copy=False).transform(X)
assert_true(X_norm is not X)
assert_true(isinstance(X_norm, sparse.csr_matrix))
X_norm = toarray(X_norm)
for i in range(3):
assert_almost_equal(row_sums[i], 1.0)
assert_almost_equal(la.norm(X_norm[3]), 0.0)
def test_normalizer_l2():
rng = np.random.RandomState(0)
X_dense = rng.randn(4, 5)
X_sparse_unpruned = sparse.csr_matrix(X_dense)
# set the row number 3 to zero
X_dense[3, :] = 0.0
# set the row number 3 to zero without pruning (can happen in real life)
indptr_3 = X_sparse_unpruned.indptr[3]
indptr_4 = X_sparse_unpruned.indptr[4]
X_sparse_unpruned.data[indptr_3:indptr_4] = 0.0
# build the pruned variant using the regular constructor
X_sparse_pruned = sparse.csr_matrix(X_dense)
# check inputs that support the no-copy optim
for X in (X_dense, X_sparse_pruned, X_sparse_unpruned):
normalizer = Normalizer(norm='l2', copy=True)
X_norm1 = normalizer.transform(X)
assert_true(X_norm1 is not X)
X_norm1 = toarray(X_norm1)
normalizer = Normalizer(norm='l2', copy=False)
X_norm2 = normalizer.transform(X)
assert_true(X_norm2 is X)
X_norm2 = toarray(X_norm2)
for X_norm in (X_norm1, X_norm2):
for i in range(3):
assert_almost_equal(la.norm(X_norm[i]), 1.0)
assert_almost_equal(la.norm(X_norm[3]), 0.0)
# check input for which copy=False won't prevent a copy
for init in (sparse.coo_matrix, sparse.csc_matrix, sparse.lil_matrix):
X = init(X_dense)
X_norm = normalizer = Normalizer(norm='l2', copy=False).transform(X)
assert_true(X_norm is not X)
assert_true(isinstance(X_norm, sparse.csr_matrix))
X_norm = toarray(X_norm)
for i in range(3):
assert_almost_equal(la.norm(X_norm[i]), 1.0)
assert_almost_equal(la.norm(X_norm[3]), 0.0)
def test_normalizer_max():
rng = np.random.RandomState(0)
X_dense = rng.randn(4, 5)
X_sparse_unpruned = sparse.csr_matrix(X_dense)
# set the row number 3 to zero
X_dense[3, :] = 0.0
# set the row number 3 to zero without pruning (can happen in real life)
indptr_3 = X_sparse_unpruned.indptr[3]
indptr_4 = X_sparse_unpruned.indptr[4]
X_sparse_unpruned.data[indptr_3:indptr_4] = 0.0
# build the pruned variant using the regular constructor
X_sparse_pruned = sparse.csr_matrix(X_dense)
# check inputs that support the no-copy optim
for X in (X_dense, X_sparse_pruned, X_sparse_unpruned):
normalizer = Normalizer(norm='max', copy=True)
X_norm1 = normalizer.transform(X)
assert_true(X_norm1 is not X)
X_norm1 = toarray(X_norm1)
normalizer = Normalizer(norm='max', copy=False)
X_norm2 = normalizer.transform(X)
assert_true(X_norm2 is X)
X_norm2 = toarray(X_norm2)
for X_norm in (X_norm1, X_norm2):
row_maxs = X_norm.max(axis=1)
for i in range(3):
assert_almost_equal(row_maxs[i], 1.0)
assert_almost_equal(row_maxs[3], 0.0)
# check input for which copy=False won't prevent a copy
for init in (sparse.coo_matrix, sparse.csc_matrix, sparse.lil_matrix):
X = init(X_dense)
X_norm = normalizer = Normalizer(norm='l2', copy=False).transform(X)
assert_true(X_norm is not X)
assert_true(isinstance(X_norm, sparse.csr_matrix))
X_norm = toarray(X_norm)
for i in range(3):
assert_almost_equal(row_maxs[i], 1.0)
assert_almost_equal(la.norm(X_norm[3]), 0.0)
def test_normalize():
# Test normalize function
# Only tests functionality not used by the tests for Normalizer.
X = np.random.RandomState(37).randn(3, 2)
assert_array_equal(normalize(X, copy=False),
normalize(X.T, axis=0, copy=False).T)
assert_raises(ValueError, normalize, [[0]], axis=2)
assert_raises(ValueError, normalize, [[0]], norm='l3')
def test_binarizer():
X_ = np.array([[1, 0, 5], [2, 3, -1]])
for init in (np.array, list, sparse.csr_matrix, sparse.csc_matrix):
X = init(X_.copy())
binarizer = Binarizer(threshold=2.0, copy=True)
X_bin = toarray(binarizer.transform(X))
assert_equal(np.sum(X_bin == 0), 4)
assert_equal(np.sum(X_bin == 1), 2)
X_bin = binarizer.transform(X)
assert_equal(sparse.issparse(X), sparse.issparse(X_bin))
binarizer = Binarizer(copy=True).fit(X)
X_bin = toarray(binarizer.transform(X))
assert_true(X_bin is not X)
assert_equal(np.sum(X_bin == 0), 2)
assert_equal(np.sum(X_bin == 1), 4)
binarizer = Binarizer(copy=True)
X_bin = binarizer.transform(X)
assert_true(X_bin is not X)
X_bin = toarray(X_bin)
assert_equal(np.sum(X_bin == 0), 2)
assert_equal(np.sum(X_bin == 1), 4)
binarizer = Binarizer(copy=False)
X_bin = binarizer.transform(X)
if init is not list:
assert_true(X_bin is X)
X_bin = toarray(X_bin)
assert_equal(np.sum(X_bin == 0), 2)
assert_equal(np.sum(X_bin == 1), 4)
binarizer = Binarizer(threshold=-0.5, copy=True)
for init in (np.array, list):
X = init(X_.copy())
X_bin = toarray(binarizer.transform(X))
assert_equal(np.sum(X_bin == 0), 1)
assert_equal(np.sum(X_bin == 1), 5)
X_bin = binarizer.transform(X)
# Cannot use threshold < 0 for sparse
assert_raises(ValueError, binarizer.transform, sparse.csc_matrix(X))
def test_center_kernel():
# Test that KernelCenterer is equivalent to StandardScaler
# in feature space
rng = np.random.RandomState(0)
X_fit = rng.random_sample((5, 4))
scaler = StandardScaler(with_std=False)
scaler.fit(X_fit)
X_fit_centered = scaler.transform(X_fit)
K_fit = np.dot(X_fit, X_fit.T)
# center fit time matrix
centerer = KernelCenterer()
K_fit_centered = np.dot(X_fit_centered, X_fit_centered.T)
K_fit_centered2 = centerer.fit_transform(K_fit)
assert_array_almost_equal(K_fit_centered, K_fit_centered2)
# center predict time matrix
X_pred = rng.random_sample((2, 4))
K_pred = np.dot(X_pred, X_fit.T)
X_pred_centered = scaler.transform(X_pred)
K_pred_centered = np.dot(X_pred_centered, X_fit_centered.T)
K_pred_centered2 = centerer.transform(K_pred)
assert_array_almost_equal(K_pred_centered, K_pred_centered2)
def test_fit_transform():
rng = np.random.RandomState(0)
X = rng.random_sample((5, 4))
for obj in ((StandardScaler(), Normalizer(), Binarizer())):
X_transformed = obj.fit(X).transform(X)
X_transformed2 = obj.fit_transform(X)
assert_array_equal(X_transformed, X_transformed2)
def test_add_dummy_feature():
X = [[1, 0], [0, 1], [0, 1]]
X = add_dummy_feature(X)
assert_array_equal(X, [[1, 1, 0], [1, 0, 1], [1, 0, 1]])
def test_add_dummy_feature_coo():
X = sparse.coo_matrix([[1, 0], [0, 1], [0, 1]])
X = add_dummy_feature(X)
assert_true(sparse.isspmatrix_coo(X), X)
assert_array_equal(X.toarray(), [[1, 1, 0], [1, 0, 1], [1, 0, 1]])
def test_add_dummy_feature_csc():
X = sparse.csc_matrix([[1, 0], [0, 1], [0, 1]])
X = add_dummy_feature(X)
assert_true(sparse.isspmatrix_csc(X), X)
assert_array_equal(X.toarray(), [[1, 1, 0], [1, 0, 1], [1, 0, 1]])
def test_add_dummy_feature_csr():
X = sparse.csr_matrix([[1, 0], [0, 1], [0, 1]])
X = add_dummy_feature(X)
assert_true(sparse.isspmatrix_csr(X), X)
assert_array_equal(X.toarray(), [[1, 1, 0], [1, 0, 1], [1, 0, 1]])
def test_one_hot_encoder_sparse():
# Test OneHotEncoder's fit and transform.
X = [[3, 2, 1], [0, 1, 1]]
enc = OneHotEncoder()
# discover max values automatically
X_trans = enc.fit_transform(X).toarray()
assert_equal(X_trans.shape, (2, 5))
assert_array_equal(enc.active_features_,
np.where([1, 0, 0, 1, 0, 1, 1, 0, 1])[0])
assert_array_equal(enc.feature_indices_, [0, 4, 7, 9])
# check outcome
assert_array_equal(X_trans,
[[0., 1., 0., 1., 1.],
[1., 0., 1., 0., 1.]])
# max value given as 3
enc = OneHotEncoder(n_values=4)
X_trans = enc.fit_transform(X)
assert_equal(X_trans.shape, (2, 4 * 3))
assert_array_equal(enc.feature_indices_, [0, 4, 8, 12])
# max value given per feature
enc = OneHotEncoder(n_values=[3, 2, 2])
X = [[1, 0, 1], [0, 1, 1]]
X_trans = enc.fit_transform(X)
assert_equal(X_trans.shape, (2, 3 + 2 + 2))
assert_array_equal(enc.n_values_, [3, 2, 2])
# check that testing with larger feature works:
X = np.array([[2, 0, 1], [0, 1, 1]])
enc.transform(X)
# test that an error is raised when out of bounds:
X_too_large = [[0, 2, 1], [0, 1, 1]]
assert_raises(ValueError, enc.transform, X_too_large)
assert_raises(ValueError, OneHotEncoder(n_values=2).fit_transform, X)
# test that error is raised when wrong number of features
assert_raises(ValueError, enc.transform, X[:, :-1])
# test that error is raised when wrong number of features in fit
# with prespecified n_values
assert_raises(ValueError, enc.fit, X[:, :-1])
# test exception on wrong init param
assert_raises(TypeError, OneHotEncoder(n_values=np.int).fit, X)
enc = OneHotEncoder()
# test negative input to fit
assert_raises(ValueError, enc.fit, [[0], [-1]])
# test negative input to transform
enc.fit([[0], [1]])
assert_raises(ValueError, enc.transform, [[0], [-1]])
def test_one_hot_encoder_dense():
# check for sparse=False
X = [[3, 2, 1], [0, 1, 1]]
enc = OneHotEncoder(sparse=False)
# discover max values automatically
X_trans = enc.fit_transform(X)
assert_equal(X_trans.shape, (2, 5))
assert_array_equal(enc.active_features_,
np.where([1, 0, 0, 1, 0, 1, 1, 0, 1])[0])
assert_array_equal(enc.feature_indices_, [0, 4, 7, 9])
# check outcome
assert_array_equal(X_trans,
np.array([[0., 1., 0., 1., 1.],
[1., 0., 1., 0., 1.]]))
def _check_transform_selected(X, X_expected, sel):
for M in (X, sparse.csr_matrix(X)):
Xtr = _transform_selected(M, Binarizer().transform, sel)
assert_array_equal(toarray(Xtr), X_expected)
def test_transform_selected():
X = [[3, 2, 1], [0, 1, 1]]
X_expected = [[1, 2, 1], [0, 1, 1]]
_check_transform_selected(X, X_expected, [0])
_check_transform_selected(X, X_expected, [True, False, False])
X_expected = [[1, 1, 1], [0, 1, 1]]
_check_transform_selected(X, X_expected, [0, 1, 2])
_check_transform_selected(X, X_expected, [True, True, True])
_check_transform_selected(X, X_expected, "all")
_check_transform_selected(X, X, [])
_check_transform_selected(X, X, [False, False, False])
def _run_one_hot(X, X2, cat):
enc = OneHotEncoder(categorical_features=cat)
Xtr = enc.fit_transform(X)
X2tr = enc.transform(X2)
return Xtr, X2tr
def _check_one_hot(X, X2, cat, n_features):
ind = np.where(cat)[0]
# With mask
A, B = _run_one_hot(X, X2, cat)
# With indices
C, D = _run_one_hot(X, X2, ind)
# Check shape
assert_equal(A.shape, (2, n_features))
assert_equal(B.shape, (1, n_features))
assert_equal(C.shape, (2, n_features))
assert_equal(D.shape, (1, n_features))
# Check that mask and indices give the same results
assert_array_equal(toarray(A), toarray(C))
assert_array_equal(toarray(B), toarray(D))
def test_one_hot_encoder_categorical_features():
X = np.array([[3, 2, 1], [0, 1, 1]])
X2 = np.array([[1, 1, 1]])
cat = [True, False, False]
_check_one_hot(X, X2, cat, 4)
# Edge case: all non-categorical
cat = [False, False, False]
_check_one_hot(X, X2, cat, 3)
# Edge case: all categorical
cat = [True, True, True]
_check_one_hot(X, X2, cat, 5)
def test_one_hot_encoder_unknown_transform():
X = np.array([[0, 2, 1], [1, 0, 3], [1, 0, 2]])
y = np.array([[4, 1, 1]])
# Test that one hot encoder raises error for unknown features
# present during transform.
oh = OneHotEncoder(handle_unknown='error')
oh.fit(X)
assert_raises(ValueError, oh.transform, y)
# Test the ignore option, ignores unknown features.
oh = OneHotEncoder(handle_unknown='ignore')
oh.fit(X)
assert_array_equal(
oh.transform(y).toarray(),
np.array([[0., 0., 0., 0., 1., 0., 0.]])
)
# Raise error if handle_unknown is neither ignore or error.
oh = OneHotEncoder(handle_unknown='42')
oh.fit(X)
assert_raises(ValueError, oh.transform, y)
| bsd-3-clause |
SciTools/cartopy | examples/lines_and_polygons/rotated_pole.py | 2 | 1070 | """
Rotated pole boxes
------------------
A demonstration of the way a box is warped when it is defined
in a rotated pole coordinate system.
Try changing the ``box_top`` to ``44``, ``46`` and ``75`` to see the effect
that including the pole in the polygon has.
"""
import matplotlib.pyplot as plt
import cartopy.crs as ccrs
def main():
rotated_pole = ccrs.RotatedPole(pole_latitude=45, pole_longitude=180)
box_top = 45
x, y = [-44, -44, 45, 45, -44], [-45, box_top, box_top, -45, -45]
fig = plt.figure()
ax = fig.add_subplot(2, 1, 1, projection=rotated_pole)
ax.stock_img()
ax.coastlines()
ax.plot(x, y, marker='o', transform=rotated_pole)
ax.fill(x, y, color='coral', transform=rotated_pole, alpha=0.4)
ax.gridlines()
ax = fig.add_subplot(2, 1, 2, projection=ccrs.PlateCarree())
ax.stock_img()
ax.coastlines()
ax.plot(x, y, marker='o', transform=rotated_pole)
ax.fill(x, y, transform=rotated_pole, color='coral', alpha=0.4)
ax.gridlines()
plt.show()
if __name__ == '__main__':
main()
| lgpl-3.0 |
rajat1994/scikit-learn | sklearn/preprocessing/tests/test_imputation.py | 213 | 11911 | import numpy as np
from scipy import sparse
from sklearn.utils.testing import assert_equal
from sklearn.utils.testing import assert_array_equal
from sklearn.utils.testing import assert_raises
from sklearn.utils.testing import assert_false
from sklearn.utils.testing import assert_true
from sklearn.preprocessing.imputation import Imputer
from sklearn.pipeline import Pipeline
from sklearn import grid_search
from sklearn import tree
from sklearn.random_projection import sparse_random_matrix
def _check_statistics(X, X_true,
strategy, statistics, missing_values):
"""Utility function for testing imputation for a given strategy.
Test:
- along the two axes
- with dense and sparse arrays
Check that:
- the statistics (mean, median, mode) are correct
- the missing values are imputed correctly"""
err_msg = "Parameters: strategy = %s, missing_values = %s, " \
"axis = {0}, sparse = {1}" % (strategy, missing_values)
# Normal matrix, axis = 0
imputer = Imputer(missing_values, strategy=strategy, axis=0)
X_trans = imputer.fit(X).transform(X.copy())
assert_array_equal(imputer.statistics_, statistics,
err_msg.format(0, False))
assert_array_equal(X_trans, X_true, err_msg.format(0, False))
# Normal matrix, axis = 1
imputer = Imputer(missing_values, strategy=strategy, axis=1)
imputer.fit(X.transpose())
if np.isnan(statistics).any():
assert_raises(ValueError, imputer.transform, X.copy().transpose())
else:
X_trans = imputer.transform(X.copy().transpose())
assert_array_equal(X_trans, X_true.transpose(),
err_msg.format(1, False))
# Sparse matrix, axis = 0
imputer = Imputer(missing_values, strategy=strategy, axis=0)
imputer.fit(sparse.csc_matrix(X))
X_trans = imputer.transform(sparse.csc_matrix(X.copy()))
if sparse.issparse(X_trans):
X_trans = X_trans.toarray()
assert_array_equal(imputer.statistics_, statistics,
err_msg.format(0, True))
assert_array_equal(X_trans, X_true, err_msg.format(0, True))
# Sparse matrix, axis = 1
imputer = Imputer(missing_values, strategy=strategy, axis=1)
imputer.fit(sparse.csc_matrix(X.transpose()))
if np.isnan(statistics).any():
assert_raises(ValueError, imputer.transform,
sparse.csc_matrix(X.copy().transpose()))
else:
X_trans = imputer.transform(sparse.csc_matrix(X.copy().transpose()))
if sparse.issparse(X_trans):
X_trans = X_trans.toarray()
assert_array_equal(X_trans, X_true.transpose(),
err_msg.format(1, True))
def test_imputation_shape():
# Verify the shapes of the imputed matrix for different strategies.
X = np.random.randn(10, 2)
X[::2] = np.nan
for strategy in ['mean', 'median', 'most_frequent']:
imputer = Imputer(strategy=strategy)
X_imputed = imputer.fit_transform(X)
assert_equal(X_imputed.shape, (10, 2))
X_imputed = imputer.fit_transform(sparse.csr_matrix(X))
assert_equal(X_imputed.shape, (10, 2))
def test_imputation_mean_median_only_zero():
# Test imputation using the mean and median strategies, when
# missing_values == 0.
X = np.array([
[np.nan, 0, 0, 0, 5],
[np.nan, 1, 0, np.nan, 3],
[np.nan, 2, 0, 0, 0],
[np.nan, 6, 0, 5, 13],
])
X_imputed_mean = np.array([
[3, 5],
[1, 3],
[2, 7],
[6, 13],
])
statistics_mean = [np.nan, 3, np.nan, np.nan, 7]
# Behaviour of median with NaN is undefined, e.g. different results in
# np.median and np.ma.median
X_for_median = X[:, [0, 1, 2, 4]]
X_imputed_median = np.array([
[2, 5],
[1, 3],
[2, 5],
[6, 13],
])
statistics_median = [np.nan, 2, np.nan, 5]
_check_statistics(X, X_imputed_mean, "mean", statistics_mean, 0)
_check_statistics(X_for_median, X_imputed_median, "median",
statistics_median, 0)
def test_imputation_mean_median():
# Test imputation using the mean and median strategies, when
# missing_values != 0.
rng = np.random.RandomState(0)
dim = 10
dec = 10
shape = (dim * dim, dim + dec)
zeros = np.zeros(shape[0])
values = np.arange(1, shape[0]+1)
values[4::2] = - values[4::2]
tests = [("mean", "NaN", lambda z, v, p: np.mean(np.hstack((z, v)))),
("mean", 0, lambda z, v, p: np.mean(v)),
("median", "NaN", lambda z, v, p: np.median(np.hstack((z, v)))),
("median", 0, lambda z, v, p: np.median(v))]
for strategy, test_missing_values, true_value_fun in tests:
X = np.empty(shape)
X_true = np.empty(shape)
true_statistics = np.empty(shape[1])
# Create a matrix X with columns
# - with only zeros,
# - with only missing values
# - with zeros, missing values and values
# And a matrix X_true containing all true values
for j in range(shape[1]):
nb_zeros = (j - dec + 1 > 0) * (j - dec + 1) * (j - dec + 1)
nb_missing_values = max(shape[0] + dec * dec
- (j + dec) * (j + dec), 0)
nb_values = shape[0] - nb_zeros - nb_missing_values
z = zeros[:nb_zeros]
p = np.repeat(test_missing_values, nb_missing_values)
v = values[rng.permutation(len(values))[:nb_values]]
true_statistics[j] = true_value_fun(z, v, p)
# Create the columns
X[:, j] = np.hstack((v, z, p))
if 0 == test_missing_values:
X_true[:, j] = np.hstack((v,
np.repeat(
true_statistics[j],
nb_missing_values + nb_zeros)))
else:
X_true[:, j] = np.hstack((v,
z,
np.repeat(true_statistics[j],
nb_missing_values)))
# Shuffle them the same way
np.random.RandomState(j).shuffle(X[:, j])
np.random.RandomState(j).shuffle(X_true[:, j])
# Mean doesn't support columns containing NaNs, median does
if strategy == "median":
cols_to_keep = ~np.isnan(X_true).any(axis=0)
else:
cols_to_keep = ~np.isnan(X_true).all(axis=0)
X_true = X_true[:, cols_to_keep]
_check_statistics(X, X_true, strategy,
true_statistics, test_missing_values)
def test_imputation_median_special_cases():
# Test median imputation with sparse boundary cases
X = np.array([
[0, np.nan, np.nan], # odd: implicit zero
[5, np.nan, np.nan], # odd: explicit nonzero
[0, 0, np.nan], # even: average two zeros
[-5, 0, np.nan], # even: avg zero and neg
[0, 5, np.nan], # even: avg zero and pos
[4, 5, np.nan], # even: avg nonzeros
[-4, -5, np.nan], # even: avg negatives
[-1, 2, np.nan], # even: crossing neg and pos
]).transpose()
X_imputed_median = np.array([
[0, 0, 0],
[5, 5, 5],
[0, 0, 0],
[-5, 0, -2.5],
[0, 5, 2.5],
[4, 5, 4.5],
[-4, -5, -4.5],
[-1, 2, .5],
]).transpose()
statistics_median = [0, 5, 0, -2.5, 2.5, 4.5, -4.5, .5]
_check_statistics(X, X_imputed_median, "median",
statistics_median, 'NaN')
def test_imputation_most_frequent():
# Test imputation using the most-frequent strategy.
X = np.array([
[-1, -1, 0, 5],
[-1, 2, -1, 3],
[-1, 1, 3, -1],
[-1, 2, 3, 7],
])
X_true = np.array([
[2, 0, 5],
[2, 3, 3],
[1, 3, 3],
[2, 3, 7],
])
# scipy.stats.mode, used in Imputer, doesn't return the first most
# frequent as promised in the doc but the lowest most frequent. When this
# test will fail after an update of scipy, Imputer will need to be updated
# to be consistent with the new (correct) behaviour
_check_statistics(X, X_true, "most_frequent", [np.nan, 2, 3, 3], -1)
def test_imputation_pipeline_grid_search():
# Test imputation within a pipeline + gridsearch.
pipeline = Pipeline([('imputer', Imputer(missing_values=0)),
('tree', tree.DecisionTreeRegressor(random_state=0))])
parameters = {
'imputer__strategy': ["mean", "median", "most_frequent"],
'imputer__axis': [0, 1]
}
l = 100
X = sparse_random_matrix(l, l, density=0.10)
Y = sparse_random_matrix(l, 1, density=0.10).toarray()
gs = grid_search.GridSearchCV(pipeline, parameters)
gs.fit(X, Y)
def test_imputation_pickle():
# Test for pickling imputers.
import pickle
l = 100
X = sparse_random_matrix(l, l, density=0.10)
for strategy in ["mean", "median", "most_frequent"]:
imputer = Imputer(missing_values=0, strategy=strategy)
imputer.fit(X)
imputer_pickled = pickle.loads(pickle.dumps(imputer))
assert_array_equal(imputer.transform(X.copy()),
imputer_pickled.transform(X.copy()),
"Fail to transform the data after pickling "
"(strategy = %s)" % (strategy))
def test_imputation_copy():
# Test imputation with copy
X_orig = sparse_random_matrix(5, 5, density=0.75, random_state=0)
# copy=True, dense => copy
X = X_orig.copy().toarray()
imputer = Imputer(missing_values=0, strategy="mean", copy=True)
Xt = imputer.fit(X).transform(X)
Xt[0, 0] = -1
assert_false(np.all(X == Xt))
# copy=True, sparse csr => copy
X = X_orig.copy()
imputer = Imputer(missing_values=X.data[0], strategy="mean", copy=True)
Xt = imputer.fit(X).transform(X)
Xt.data[0] = -1
assert_false(np.all(X.data == Xt.data))
# copy=False, dense => no copy
X = X_orig.copy().toarray()
imputer = Imputer(missing_values=0, strategy="mean", copy=False)
Xt = imputer.fit(X).transform(X)
Xt[0, 0] = -1
assert_true(np.all(X == Xt))
# copy=False, sparse csr, axis=1 => no copy
X = X_orig.copy()
imputer = Imputer(missing_values=X.data[0], strategy="mean",
copy=False, axis=1)
Xt = imputer.fit(X).transform(X)
Xt.data[0] = -1
assert_true(np.all(X.data == Xt.data))
# copy=False, sparse csc, axis=0 => no copy
X = X_orig.copy().tocsc()
imputer = Imputer(missing_values=X.data[0], strategy="mean",
copy=False, axis=0)
Xt = imputer.fit(X).transform(X)
Xt.data[0] = -1
assert_true(np.all(X.data == Xt.data))
# copy=False, sparse csr, axis=0 => copy
X = X_orig.copy()
imputer = Imputer(missing_values=X.data[0], strategy="mean",
copy=False, axis=0)
Xt = imputer.fit(X).transform(X)
Xt.data[0] = -1
assert_false(np.all(X.data == Xt.data))
# copy=False, sparse csc, axis=1 => copy
X = X_orig.copy().tocsc()
imputer = Imputer(missing_values=X.data[0], strategy="mean",
copy=False, axis=1)
Xt = imputer.fit(X).transform(X)
Xt.data[0] = -1
assert_false(np.all(X.data == Xt.data))
# copy=False, sparse csr, axis=1, missing_values=0 => copy
X = X_orig.copy()
imputer = Imputer(missing_values=0, strategy="mean",
copy=False, axis=1)
Xt = imputer.fit(X).transform(X)
assert_false(sparse.issparse(Xt))
# Note: If X is sparse and if missing_values=0, then a (dense) copy of X is
# made, even if copy=False.
| bsd-3-clause |
altaetran/bayesianoracle | tests/quadraticBayesianAveraging/2Dexample.py | 1 | 2993 | import numpy as np
import bayesianoracle as bo
from pprint import pprint as pp
ndim = 2
bmao = bo.optimizer.QuadraticBMAOptimizer(ndim=ndim)
H = np.array([[ 1389.4217, 1151.5168],
[ 1151.5168, 36896.3534]])
g = np.array([ 643.2191, 6206.7597])
f = 596.83446220293399
xk = np.array([ 0., 0.])
bmao.add_observation(xk, f, g, H)
for i in xrange(3):
H = np.array([[ 1846.2641, 870.278 ],
[ 870.278 , 31874.2671]])
g = np.array([ -71.6421, -1062.788 ])
f = 18.327839153167439
xk = np.array([-0.332, -0.158])
bmao.add_observation(xk, f, g, H)
"""
H = np.array([[ 1846.2641, 870.278 ],
[ 870.278 , 31874.2671]])
g = np.array([ -71.6421, -1062.788 ])
f = 18.327839153167439
xk = np.array([-0.332, -0.158])
bmao.add_observation(xk, f, g, H)
"""
print("hyperprior")
print(bmao.bma.kernel_prior.a)
print(bmao.bma.kernel_prior.scale)
bmao.optimize_hyperparameters()
#print(bmao.predict_with_unc(np.array([[-3.0797e-01 , -1.2921e-01]]).T))
#print(bmao.bma.estimate_model_weights(np.array([[-3.0797e-01 , -1.2921e-01]]).T, return_likelihoods=True))
print(bmao.predict_with_unc(np.array([xk]).T))
print("lololol")
pp(bmao.bma.estimate_model_weights(np.array([xk]).T, return_likelihoods=True))
print('done')
pp(bmao.bma.calc_relevance_weights(np.array([xk]).T))
#pp(bmao.bma.quadratic_models)
pp(bmao.bma.estimate_model_priors(np.array([xk]).T))
pp(bmao.bma.model_predictions(np.array([xk]).T))
# Get the relevence weights (nModels x p)
relevance_weights = bmao.bma.calc_relevance_weights(np.array([xk]).T)
print("relevance weights")
pp(relevance_weights)
bma = bmao.bma
import matplotlib.pyplot as plt
### Likelihood plots
fig4, ax = plt.subplots(3, sharex=True)
kernel_grid = np.logspace(-2.0, 2.0, num=50)
# Get the likelihoods
unreg_loglikelihood = np.array([bma.loglikelihood(kernel_range, regularization=False, skew=False) for kernel_range in kernel_grid])
skewness = np.array([bma.estimate_skewness(kernel_range) for kernel_range in kernel_grid])
reg_loglikelihood = np.array([bma.loglikelihood(kernel_range) for kernel_range in kernel_grid])
# Plot the two terms
ll1 = ax[0].plot(kernel_grid, unreg_loglikelihood)
ax[0].set_xscale('log')
ll2 = ax[1].plot(kernel_grid, skewness)
ax[1].set_xscale('log')
ll3 = ax[2].plot(kernel_grid, reg_loglikelihood)
ax[2].set_xscale('log')
pp(reg_loglikelihood)
ax[0].set_xlim([kernel_grid[0],kernel_grid[-1]])
ax[1].set_xlim([kernel_grid[0],kernel_grid[-1]])
ax[2].set_xlim([kernel_grid[0],kernel_grid[-1]])
plt.setp(ll1, color="red", linewidth=3.0, alpha=0.5, linestyle='-',
dash_capstyle='round')
plt.setp(ll2, color="red", linewidth=3.0, alpha=0.5, linestyle='-',
dash_capstyle='round')
plt.setp(ll3, color="red", linewidth=3.0, alpha=0.5, linestyle='-',
dash_capstyle='round')
ax[2].set_xlabel("kernel range",fontsize=16)
plt.savefig("figures/2Dexample_bma_loglikelihood.png")
print("skew")
print(bma.estimate_skewness())
| apache-2.0 |
ephes/scikit-learn | sklearn/decomposition/truncated_svd.py | 199 | 7744 | """Truncated SVD for sparse matrices, aka latent semantic analysis (LSA).
"""
# Author: Lars Buitinck <[email protected]>
# Olivier Grisel <[email protected]>
# Michael Becker <[email protected]>
# License: 3-clause BSD.
import numpy as np
import scipy.sparse as sp
try:
from scipy.sparse.linalg import svds
except ImportError:
from ..utils.arpack import svds
from ..base import BaseEstimator, TransformerMixin
from ..utils import check_array, as_float_array, check_random_state
from ..utils.extmath import randomized_svd, safe_sparse_dot, svd_flip
from ..utils.sparsefuncs import mean_variance_axis
__all__ = ["TruncatedSVD"]
class TruncatedSVD(BaseEstimator, TransformerMixin):
"""Dimensionality reduction using truncated SVD (aka LSA).
This transformer performs linear dimensionality reduction by means of
truncated singular value decomposition (SVD). It is very similar to PCA,
but operates on sample vectors directly, instead of on a covariance matrix.
This means it can work with scipy.sparse matrices efficiently.
In particular, truncated SVD works on term count/tf-idf matrices as
returned by the vectorizers in sklearn.feature_extraction.text. In that
context, it is known as latent semantic analysis (LSA).
This estimator supports two algorithm: a fast randomized SVD solver, and
a "naive" algorithm that uses ARPACK as an eigensolver on (X * X.T) or
(X.T * X), whichever is more efficient.
Read more in the :ref:`User Guide <LSA>`.
Parameters
----------
n_components : int, default = 2
Desired dimensionality of output data.
Must be strictly less than the number of features.
The default value is useful for visualisation. For LSA, a value of
100 is recommended.
algorithm : string, default = "randomized"
SVD solver to use. Either "arpack" for the ARPACK wrapper in SciPy
(scipy.sparse.linalg.svds), or "randomized" for the randomized
algorithm due to Halko (2009).
n_iter : int, optional
Number of iterations for randomized SVD solver. Not used by ARPACK.
random_state : int or RandomState, optional
(Seed for) pseudo-random number generator. If not given, the
numpy.random singleton is used.
tol : float, optional
Tolerance for ARPACK. 0 means machine precision. Ignored by randomized
SVD solver.
Attributes
----------
components_ : array, shape (n_components, n_features)
explained_variance_ratio_ : array, [n_components]
Percentage of variance explained by each of the selected components.
explained_variance_ : array, [n_components]
The variance of the training samples transformed by a projection to
each component.
Examples
--------
>>> from sklearn.decomposition import TruncatedSVD
>>> from sklearn.random_projection import sparse_random_matrix
>>> X = sparse_random_matrix(100, 100, density=0.01, random_state=42)
>>> svd = TruncatedSVD(n_components=5, random_state=42)
>>> svd.fit(X) # doctest: +NORMALIZE_WHITESPACE
TruncatedSVD(algorithm='randomized', n_components=5, n_iter=5,
random_state=42, tol=0.0)
>>> print(svd.explained_variance_ratio_) # doctest: +ELLIPSIS
[ 0.07825... 0.05528... 0.05445... 0.04997... 0.04134...]
>>> print(svd.explained_variance_ratio_.sum()) # doctest: +ELLIPSIS
0.27930...
See also
--------
PCA
RandomizedPCA
References
----------
Finding structure with randomness: Stochastic algorithms for constructing
approximate matrix decompositions
Halko, et al., 2009 (arXiv:909) http://arxiv.org/pdf/0909.4061
Notes
-----
SVD suffers from a problem called "sign indeterminancy", which means the
sign of the ``components_`` and the output from transform depend on the
algorithm and random state. To work around this, fit instances of this
class to data once, then keep the instance around to do transformations.
"""
def __init__(self, n_components=2, algorithm="randomized", n_iter=5,
random_state=None, tol=0.):
self.algorithm = algorithm
self.n_components = n_components
self.n_iter = n_iter
self.random_state = random_state
self.tol = tol
def fit(self, X, y=None):
"""Fit LSI model on training data X.
Parameters
----------
X : {array-like, sparse matrix}, shape (n_samples, n_features)
Training data.
Returns
-------
self : object
Returns the transformer object.
"""
self.fit_transform(X)
return self
def fit_transform(self, X, y=None):
"""Fit LSI model to X and perform dimensionality reduction on X.
Parameters
----------
X : {array-like, sparse matrix}, shape (n_samples, n_features)
Training data.
Returns
-------
X_new : array, shape (n_samples, n_components)
Reduced version of X. This will always be a dense array.
"""
X = as_float_array(X, copy=False)
random_state = check_random_state(self.random_state)
# If sparse and not csr or csc, convert to csr
if sp.issparse(X) and X.getformat() not in ["csr", "csc"]:
X = X.tocsr()
if self.algorithm == "arpack":
U, Sigma, VT = svds(X, k=self.n_components, tol=self.tol)
# svds doesn't abide by scipy.linalg.svd/randomized_svd
# conventions, so reverse its outputs.
Sigma = Sigma[::-1]
U, VT = svd_flip(U[:, ::-1], VT[::-1])
elif self.algorithm == "randomized":
k = self.n_components
n_features = X.shape[1]
if k >= n_features:
raise ValueError("n_components must be < n_features;"
" got %d >= %d" % (k, n_features))
U, Sigma, VT = randomized_svd(X, self.n_components,
n_iter=self.n_iter,
random_state=random_state)
else:
raise ValueError("unknown algorithm %r" % self.algorithm)
self.components_ = VT
# Calculate explained variance & explained variance ratio
X_transformed = np.dot(U, np.diag(Sigma))
self.explained_variance_ = exp_var = np.var(X_transformed, axis=0)
if sp.issparse(X):
_, full_var = mean_variance_axis(X, axis=0)
full_var = full_var.sum()
else:
full_var = np.var(X, axis=0).sum()
self.explained_variance_ratio_ = exp_var / full_var
return X_transformed
def transform(self, X):
"""Perform dimensionality reduction on X.
Parameters
----------
X : {array-like, sparse matrix}, shape (n_samples, n_features)
New data.
Returns
-------
X_new : array, shape (n_samples, n_components)
Reduced version of X. This will always be a dense array.
"""
X = check_array(X, accept_sparse='csr')
return safe_sparse_dot(X, self.components_.T)
def inverse_transform(self, X):
"""Transform X back to its original space.
Returns an array X_original whose transform would be X.
Parameters
----------
X : array-like, shape (n_samples, n_components)
New data.
Returns
-------
X_original : array, shape (n_samples, n_features)
Note that this is always a dense array.
"""
X = check_array(X)
return np.dot(X, self.components_)
| bsd-3-clause |
VDBWRAIR/bio_pieces | bio_bits/plot_muts.py | 2 | 10355 | '''
Usage:
plot_muts.py --query <query> --refs <refs> [--out <outfile>] [--html] [--cluster]
Options:
--cluster Evaluate by comparing to the first two sequences in <refs> rather than by time
--html Produce html output in addition to a static image
--refs,-r=<refs> Fasta file, sequence with earliest year is base reference
--query,-q=<query> Query sequences
--out,-o=<outfile> Figure saved here
Help:
All sequences must be the same length.
'''
from __future__ import print_function
import numpy as np
from functools import partial
import operator
import os, sys, re
from Bio import SeqIO
import matplotlib.pyplot as plt
import docopt, schema
from operator import itemgetter as get
import csv
from dateutil import parser
import datetime
from time import mktime
from funcy import compose
from funcy.py2 import map, zip
try:
#below import is necessary for some reason
from scipy.stats import poisson
import scipy
DISTRIBUTION = scipy.stats.poisson
except ImportError:
DISTRIBUTION = None
years = r'190\\d|19[1-9]\\d|200\\d|201[0-5]' #1900-2015
year_regex = re.compile(years)
hamming = compose(sum, partial(map, operator.ne))
timestamp = lambda x: mktime(x.timetuple())
legend = {"queries": 'r', "references": 'b', "interval": 'g'}
#def pdist(s1, s2):
# assert len(s1) == len(s2), "All sequences must be the same length! %s %s" % (s1, s2)
# return hamming(s1, s2)/float(len(s1))
class InvalidFastaIdentifier(Exception): pass
def extract_date(fasta_id):
''' fasta id '''
_e = InvalidFastaIdentifier("Could retrieve date from {0}".format(fasta_id))
if '____' not in fasta_id:
raise _e
s = fasta_id.split('____')[-1]
try:
dt = parser.parse(s.replace('_','/'))
return dt
except Exception as e:
print("Error parsing {0}".format(s))
raise _e
def get_seqs_and_dates(fn):
fasta = SeqIO.parse(fn, format="fasta")
info = [(str(seq.seq), seq.id, seq.description) for seq in fasta]
seqs, ids, descriptions = zip(*info)
dates = map(extract_date, ids)
return seqs, dates, ids
def process(refs_fn, query_fn, save_path=None, html=True):
ref_seqs, ref_dates, ref_names = zip(*sorted(zip(*get_seqs_and_dates(refs_fn)), key=get(1)))
#assert len(ref_seqs) > 1, "Need more than 1 reference sequence"
ref_seqs = map(str.upper, ref_seqs)
super_ref_seq, super_ref_date, super_ref_name = ref_seqs[0], ref_dates[0], ref_names[0]
get_mutations = partial(hamming, super_ref_seq)
def get_relative_info(seqs, dates, names):
muts = map(get_mutations, seqs)
dists = [(yr - super_ref_date).days for yr in dates]
return muts, dists, names
ref_muts, ref_dists, ref_names = get_relative_info(ref_seqs, ref_dates, ref_names)
query_muts, query_dists, query_names = get_relative_info(*get_seqs_and_dates(query_fn))
do_plot(ref_dists, ref_muts, ref_names, query_dists, query_muts, query_names, save_path, html)
#map(compose(print, '{0}\t{1}'.format ), ref_dists, ref_muts)
def do_plot(x1, y1, ref_names, x2, y2, query_names, save_path=None, html=True, \
title='Mutations over time (days)', x_axis_label='time since base reference', y_axis_label='p-distance'):
'''
:param iterable x1: reference dates distances
:param iterable y1: reference p-distances
:param iterable x2: query dates diferences
:param iterable y2: query p-distances
:param str save_path: path to save image or None to open GTK if installed
'''
assert len(x1) > 0, "No reference dates to use"
assert len(y2) > 0, "No reference p-distances to use"
assert len(x2) > 0, "No query dates to use"
assert len(y2) > 0, "No query p-distances to use"
assert len(ref_names) == len(x1) and len(query_names) == len(x2)
fig = plt.figure()
ax = plt.subplot(111)
# from matplotlib.dates import YearLocator, MonthLocator, DateFormatter
# years = YearLocator() # every year
# months = MonthLocator() # every month
# yearsFmt = DateFormatter('%Y')
# ax.xaxis.set_major_locator(years)
# ax.xaxis.set_major_formatter(yearsFmt)
# ax.xaxis.set_minor_locator(months)
max_x = max(max(x1), max(x2))
#legend_info = [mpatches.Patch(label=n, color=c) for n, c in legend.items()]
""" http://stackoverflow.com/questions/4700614/how-to-put-the-legend-out-of-the-plot"""
ref_info = zip(ref_names, x1, y1)
query_info = zip(query_names, x2, y2)
all_info = sorted(ref_info + query_info, key=lambda x: x[2], reverse=True)
if save_path:
fh = open(save_path+'.csv', 'wt')
else:
fh = sys.stdout
fh.write('name,dates,p-dist\n')
outcsv = csv.writer(fh)
map(outcsv.writerow, all_info)
if html:
assert sys.version[:3] != '2.6', "Requires python 2.7 or higher to run bokeh."
import bokeh.models as bkm
import bokeh.plotting as bkp
bokeh_tools = [bkm.WheelZoomTool(), bkm.PanTool(), bkm.BoxZoomTool(),
bkm.PreviewSaveTool(), bkm.ResetTool(), bkm.BoxSelectTool(),
bkm.ResizeTool()]
ref_names = map('R: {0}'.format, ref_names)
query_names = map('Q: {0}'.format, query_names)
hover = bkm.HoverTool(tooltips=[("id", "@ids"),]) # ("(days,muts)", "($x, $y)"),
source1 = bkm.ColumnDataSource(data=dict(x=x1, y=y1, ids=ref_names))
source2 = bkm.ColumnDataSource(data=dict(x2=x2, y2=y2, ids=query_names))
p = bkp.figure(plot_width=400, plot_height=400, tools=[hover]+bokeh_tools, title=title)
p.circle('x', 'y', source=source1, line_color='gray', legend='reference')
p.square('x2', 'y2', source=source2, fill_color='red', legend='query')
if save_path:
bkp.output_file(save_path + '.html')
bkp.save(p)
else: bkp.show(p)
else:
plot_muts(ax, x1, y1, plotkwargs=dict(label='references (blue)', color=legend['references'], marker='s'), polyfit=True, max_x=max_x, dist=None)
plot_muts(ax, x2, y2, plotkwargs=dict(label='queries (red)', color=legend['queries']), dist=None)
box = ax.get_position()
ax.set_position([box.x0, box.y0, box.width * 0.8, box.height])
#ax.legend(handles=legend_info, loc='center left', bbox_to_anchor=(1, 0.5))
#ax.legend(loc='center left', bbox_to_anchor=(1, 0.5), framealpha=0)
ax.legend(framealpha=0)
plt.xlabel(x_axis_label)
plt.ylabel(y_axis_label)
if save_path:
plt.savefig(save_path)
else:
plt.show()
def plot_muts(ax, x, y, dist=DISTRIBUTION, polyfit=False, max_x=None, plotkwargs=dict(marker='o')):
'''
Plot x and y
if norm distribution, probably have to scale (via passing loc= and scale=)
problem was didn't account for +b
'''
if max_x and isinstance(max_x, datetime.datetime):
max_x = timestamp(max_x)
retval = ax.scatter(x, y, **plotkwargs)#color=color, label=label, marker=marker)
if polyfit:
''' this forces a polyfit with y-intercept at zero, necessary because
we necessarily start with 0 mutations from the query at date 0.'''
x = np.array(x)[:,np.newaxis]
m, _, _, _ = np.linalg.lstsq(x, y)
x, y = np.linspace(0,max_x,100), m*np.linspace(0,max_x,100)
#ax.plot(x, y, color='y', label='Best Fit', linewidth=2)
ax.plot(x, y, color='y', linewidth=2)
if dist:
"""see http://stackoverflow.com/a/14814711/3757222"""
R = dist.interval(0.95, y)
interval_left, interval_right = R
interval_color = legend['interval']
ax.plot(x, interval_left, color=interval_color)
ax.plot(x, interval_right,color=interval_color)
return retval
#def test_more():
# refs = range(25), range(25)
# queries = [1, 5, 20, 10], [2, 20, 40, 10]
# do_plot(refs[0], refs[1], queries[0], queries[1], None)
#
#def test_plot():
# ''' can verify this works by using scipy.stats.norm.interval instead'''
# default_x = range(25)
# default_y = range(0, 50, 2)
# plot_muts(default_x, default_y, 'r', True, scipy.stats.poisson, max_x=max(default_x))
# plt.show()
def extract_info(fasta):
objs = SeqIO.parse(fasta, format="fasta")
info = [(str(seq.seq), seq.id) for seq in objs]
seqs, ids = zip(*info)
return seqs, ids
def get_clusters(refs, queries):
all_ref_seqs, all_ref_ids = extract_info(refs)
ref1, ref2 = all_ref_seqs[:2]
dists1, dists2 = partial(hamming, ref1), partial(hamming, ref2)
ref_seqs, ref_ids = all_ref_seqs[2:], all_ref_ids[2:]
# There may only be 2 references to compare
# so we check here if that is the case and set distances to 0 if so
if ref_seqs:
ref_dists1, ref_dists2 = map(dists1, ref_seqs), map(dists2, ref_seqs)
else:
ref_dists1 = ref_dists2 = [0,0]
ref_ids = ['','']
query_seqs, query_ids = extract_info(queries)
query_dists1, query_dists2 = map(dists1, query_seqs), map(dists2, query_seqs)
return ref_dists1, ref_dists2, ref_ids, query_dists1, query_dists2, query_ids, all_ref_ids[0], all_ref_ids[1]
def process_cluster(refs, queries, save_path=None, html=False):
ref_dists1, ref_dists2, ref_ids, query_dists1, query_dists2, query_ids, ref1_id, ref2_id = get_clusters(refs, queries)
do_plot(ref_dists1, ref_dists2, ref_ids, query_dists1, query_dists2, query_ids, \
save_path, html, title='test', x_axis_label=ref1_id, y_axis_label=ref2_id)
def main():
#if sys.argv[1] == 'test': test_more()
scheme = schema.Schema(
{ '--query' : os.path.exists,
'--refs' : os.path.exists,
schema.Optional('--out') : lambda x: True,
schema.Optional('--html') : lambda x: True,
schema.Optional('--cluster') : lambda x: True
# schema.Or(lambda x: x is None, #check file can be created
# lambda x: os.access(os.path.dirname(x), os.W_OK))
})
args = docopt.docopt(__doc__, version='Version 1.0')
scheme.validate(args)
queries, refs, out, do_cluster = args['--query'], args['--refs'], args['--out'], args['--cluster']
if do_cluster:
process_cluster(refs, queries, out, args['--html'])
else:
process(refs, queries, out, args['--html'])
if __name__ == '__main__': main()
| gpl-2.0 |
mfjb/scikit-learn | examples/semi_supervised/plot_label_propagation_digits.py | 268 | 2723 | """
===================================================
Label Propagation digits: Demonstrating performance
===================================================
This example demonstrates the power of semisupervised learning by
training a Label Spreading model to classify handwritten digits
with sets of very few labels.
The handwritten digit dataset has 1797 total points. The model will
be trained using all points, but only 30 will be labeled. Results
in the form of a confusion matrix and a series of metrics over each
class will be very good.
At the end, the top 10 most uncertain predictions will be shown.
"""
print(__doc__)
# Authors: Clay Woolam <[email protected]>
# Licence: BSD
import numpy as np
import matplotlib.pyplot as plt
from scipy import stats
from sklearn import datasets
from sklearn.semi_supervised import label_propagation
from sklearn.metrics import confusion_matrix, classification_report
digits = datasets.load_digits()
rng = np.random.RandomState(0)
indices = np.arange(len(digits.data))
rng.shuffle(indices)
X = digits.data[indices[:330]]
y = digits.target[indices[:330]]
images = digits.images[indices[:330]]
n_total_samples = len(y)
n_labeled_points = 30
indices = np.arange(n_total_samples)
unlabeled_set = indices[n_labeled_points:]
# shuffle everything around
y_train = np.copy(y)
y_train[unlabeled_set] = -1
###############################################################################
# Learn with LabelSpreading
lp_model = label_propagation.LabelSpreading(gamma=0.25, max_iter=5)
lp_model.fit(X, y_train)
predicted_labels = lp_model.transduction_[unlabeled_set]
true_labels = y[unlabeled_set]
cm = confusion_matrix(true_labels, predicted_labels, labels=lp_model.classes_)
print("Label Spreading model: %d labeled & %d unlabeled points (%d total)" %
(n_labeled_points, n_total_samples - n_labeled_points, n_total_samples))
print(classification_report(true_labels, predicted_labels))
print("Confusion matrix")
print(cm)
# calculate uncertainty values for each transduced distribution
pred_entropies = stats.distributions.entropy(lp_model.label_distributions_.T)
# pick the top 10 most uncertain labels
uncertainty_index = np.argsort(pred_entropies)[-10:]
###############################################################################
# plot
f = plt.figure(figsize=(7, 5))
for index, image_index in enumerate(uncertainty_index):
image = images[image_index]
sub = f.add_subplot(2, 5, index + 1)
sub.imshow(image, cmap=plt.cm.gray_r)
plt.xticks([])
plt.yticks([])
sub.set_title('predict: %i\ntrue: %i' % (
lp_model.transduction_[image_index], y[image_index]))
f.suptitle('Learning with small amount of labeled data')
plt.show()
| bsd-3-clause |
sumspr/scikit-learn | examples/datasets/plot_random_dataset.py | 348 | 2254 | """
==============================================
Plot randomly generated classification dataset
==============================================
Plot several randomly generated 2D classification datasets.
This example illustrates the :func:`datasets.make_classification`
:func:`datasets.make_blobs` and :func:`datasets.make_gaussian_quantiles`
functions.
For ``make_classification``, three binary and two multi-class classification
datasets are generated, with different numbers of informative features and
clusters per class. """
print(__doc__)
import matplotlib.pyplot as plt
from sklearn.datasets import make_classification
from sklearn.datasets import make_blobs
from sklearn.datasets import make_gaussian_quantiles
plt.figure(figsize=(8, 8))
plt.subplots_adjust(bottom=.05, top=.9, left=.05, right=.95)
plt.subplot(321)
plt.title("One informative feature, one cluster per class", fontsize='small')
X1, Y1 = make_classification(n_features=2, n_redundant=0, n_informative=1,
n_clusters_per_class=1)
plt.scatter(X1[:, 0], X1[:, 1], marker='o', c=Y1)
plt.subplot(322)
plt.title("Two informative features, one cluster per class", fontsize='small')
X1, Y1 = make_classification(n_features=2, n_redundant=0, n_informative=2,
n_clusters_per_class=1)
plt.scatter(X1[:, 0], X1[:, 1], marker='o', c=Y1)
plt.subplot(323)
plt.title("Two informative features, two clusters per class", fontsize='small')
X2, Y2 = make_classification(n_features=2, n_redundant=0, n_informative=2)
plt.scatter(X2[:, 0], X2[:, 1], marker='o', c=Y2)
plt.subplot(324)
plt.title("Multi-class, two informative features, one cluster",
fontsize='small')
X1, Y1 = make_classification(n_features=2, n_redundant=0, n_informative=2,
n_clusters_per_class=1, n_classes=3)
plt.scatter(X1[:, 0], X1[:, 1], marker='o', c=Y1)
plt.subplot(325)
plt.title("Three blobs", fontsize='small')
X1, Y1 = make_blobs(n_features=2, centers=3)
plt.scatter(X1[:, 0], X1[:, 1], marker='o', c=Y1)
plt.subplot(326)
plt.title("Gaussian divided into three quantiles", fontsize='small')
X1, Y1 = make_gaussian_quantiles(n_features=2, n_classes=3)
plt.scatter(X1[:, 0], X1[:, 1], marker='o', c=Y1)
plt.show()
| bsd-3-clause |
lscsoft/gwdetchar | gwdetchar/omega/plot.py | 1 | 8869 | # coding=utf-8
# Copyright (C) Alex Urban (2018-)
#
# This file is part of the GW DetChar python package.
#
# GW DetChar is free software: you can redistribute it and/or modify
# it under the terms of the GNU General Public License as published by
# the Free Software Foundation, either version 3 of the License, or
# (at your option) any later version.
#
# GW DetChar is distributed in the hope that it will be useful,
# but WITHOUT ANY WARRANTY; without even the implied warranty of
# MERCHANTABILITY or FITNESS FOR A PARTICULAR PURPOSE. See the
# GNU General Public License for more details.
#
# You should have received a copy of the GNU General Public License
# along with GW DetChar. If not, see <http://www.gnu.org/licenses/>.
"""Plotting routines for omega scans
"""
from __future__ import division
from matplotlib import (cm, rcParams)
from gwpy.plot.colors import GW_OBSERVATORY_COLORS
__author__ = 'Alex Urban <[email protected]>'
__credits__ = 'Duncan Macleod <[email protected]>'
rcParams.update({
'xtick.labelsize': 16,
'ytick.labelsize': 16,
'axes.labelsize': 20,
'axes.labelpad': 12,
'axes.titlesize': 15,
'grid.alpha': 0.5,
})
# -- internal formatting tools ------------------------------------------------
def _format_time_axis(ax, gps, span):
"""Format the time axis of an omega scan plot
Parameters
----------
ax : `~matplotlib.axis.Axis`
the `Axis` object to format
gps : `float`
reference GPS time (in seconds) to serve as the origin
span : `float`
total duration (in seconds) of the time axis
"""
# set time axis units
ax.set_xscale('seconds', epoch=gps)
ax.set_xlim(gps-span/2, gps+span/2)
ax.set_xlabel('Time [seconds]')
ax.grid(True, axis='x', which='major')
def _format_frequency_axis(ax):
"""Format the frequency axis of an omega scan plot
Parameters
----------
ax : `~matplotlib.axis.Axis`
the `Axis` object to format
"""
ax.grid(True, axis='y', which='both')
ax.set_yscale('log')
ax.set_ylabel('Frequency [Hz]')
def _format_color_axis(ax, colormap='viridis', clim=None, norm='linear'):
"""Format the color axis of an omega scan spectral plot
Parameters
----------
ax : `~matplotlib.axis.Axis`
the `Axis` object to format
colormap : `str`
matplotlib colormap to use, default: viridis
clim : `tuple` or `None`
limits of the color axis, default: autoscale with log scaling
norm : `str`
scaling of the color axis, only used if `clim` is given,
default: linear
"""
cmap = cm.get_cmap(colormap)
ax.set_facecolor(cmap(0))
# set colorbar format
if clim is None: # force a log colorbar with autoscaled limits
ax.colorbar(cmap=colormap, norm='log', vmin=0.5,
label='Normalized energy')
else:
ax.colorbar(cmap=colormap, norm=norm, clim=clim,
label='Normalized energy')
# -- utilities ----------------------------------------------------------------
def timeseries_plot(data, gps, span, channel, output, ylabel=None,
figsize=(9, 4.5)):
"""Custom plot for a GWPy TimeSeries object
Parameters
----------
data : `~gwpy.timeseries.TimeSeries`
the series to plot
gps : `float`
reference GPS time (in seconds) to serve as the origin
span : `float`
total duration (in seconds) of the time axis
channel : `str`
name of the channel corresponding to this data
output : `str`
name of the output file
ylabel : `str` or `None`
label for the y-axis
figsize : `tuple`
size (width x height) of the final figure, default: `(12, 6)`
"""
# set color by IFO
ifo = channel[:2]
data = data.crop(gps-span/2, gps+span/2)
plot = data.plot(color=GW_OBSERVATORY_COLORS[ifo], figsize=figsize)
# set axis properties
ax = plot.gca()
_format_time_axis(ax, gps=gps, span=span)
ax.set_yscale('linear')
ax.set_ylabel(ylabel)
# set title
title = r'$\mathtt{%s}$ at %.3f' % (channel.replace('_', '\_'), gps)
ax.set_title(title, y=1.1)
# save plot and close
plot.savefig(output, bbox_inches='tight')
plot.close()
def spectral_plot(data, gps, span, channel, output, colormap='viridis',
clim=None, nx=1400, norm='linear', figsize=(8, 4.35)):
"""Custom plot for a GWPy spectrogram or Q-gram
Parameters
----------
data : `~gwpy.timeseries.TimeSeries`
the series to plot
gps : `float`
reference GPS time (in seconds) to serve as the origin
span : `float`
total duration (in seconds) of the time axis
channel : `str`
name of the channel corresponding to this data
output : `str`
name of the output file
colormap : `str`
matplotlib colormap to use, default: viridis
clim : `tuple` or `None`
limits of the color axis, default: autoscale with log scaling
norm : `str`
scaling of the color axis, only used if `clim` is given,
default: linear
nx : `int`
number of points along the time axis, default: 500
figsize : `tuple`
size (width x height) of the final figure, default: `(12, 6)`
"""
from gwpy.spectrogram import Spectrogram
# construct plot
if isinstance(data, Spectrogram):
# plot interpolated spectrogram
Q = data.q
data = data.crop(gps-span/2, gps+span/2)
nslice = max(1, int(data.shape[0] / nx))
plot = data[::nslice].pcolormesh(figsize=figsize)
else:
# plot eventgram
Q = data.meta['q']
plot = data.tile('time', 'frequency', 'duration', 'bandwidth',
color='energy', figsize=figsize, antialiased=True)
# set axis properties
ax = plot.gca()
_format_time_axis(ax, gps=gps, span=span)
_format_frequency_axis(ax)
# set colorbar properties
_format_color_axis(ax, colormap=colormap, clim=clim, norm=norm)
# set title
title = r'$\mathtt{%s}$ at %.3f with $Q$ of %.1f' \
% (channel.replace('_', '\_'), gps, Q)
ax.set_title(title, y=1.05)
# save plot and close
plot.savefig(output, bbox_inches='tight')
plot.close()
def write_qscan_plots(gps, channel, series, colormap='viridis'):
"""Custom plot utility for a full omega scan
Parameters
----------
gps : `float`
reference GPS time (in seconds) to serve as the origin
channel : `OmegaChannel`
channel corresponding to these data
series : `tuple`
a collection of `TimeSeries`, `Spectrogram`, and `QGram` objects
colormap : `str`, optional
matplotlib colormap to use, default: viridis
"""
# unpack series objects
xoft, hpxoft, wxoft, qgram, rqgram, qspec, rqspec = series
# range over plot types
fnames = channel.plots
for span, png1, png2, png3, png4, png5, png6, png7, png8, png9 in zip(
channel.pranges, fnames['qscan_whitened'],
fnames['qscan_autoscaled'], fnames['qscan_highpassed'],
fnames['timeseries_raw'], fnames['timeseries_highpassed'],
fnames['timeseries_whitened'], fnames['eventgram_highpassed'],
fnames['eventgram_whitened'], fnames['eventgram_autoscaled']
):
# plot whitened qscan
spectral_plot(
qspec, gps, span, channel.name, str(png1), clim=(0, 25),
colormap=colormap)
# plot autoscaled, whitened qscan
spectral_plot(qspec, gps, span, channel.name, str(png2),
colormap=colormap)
# plot raw qscan
spectral_plot(
rqspec, gps, span, channel.name, str(png3), clim=(0, 25),
colormap=colormap)
# plot raw timeseries
timeseries_plot(xoft, gps, span, channel.name, str(png4),
ylabel='Amplitude')
# plot highpassed timeseries
timeseries_plot(hpxoft, gps, span, channel.name, str(png5),
ylabel='Highpassed Amplitude')
# plot whitened timeseries
timeseries_plot(wxoft, gps, span, channel.name, str(png6),
ylabel='Whitened Amplitude')
# plot raw eventgram
rtable = rqgram.table(snrthresh=channel.snrthresh)
spectral_plot(
rtable, gps, span, channel.name, str(png7), clim=(0, 25),
colormap=colormap)
# plot whitened eventgram
table = qgram.table(snrthresh=channel.snrthresh)
spectral_plot(
table, gps, span, channel.name, str(png8), clim=(0, 25),
colormap=colormap)
# plot autoscaled whitened eventgram
spectral_plot(table, gps, span, channel.name, str(png9),
colormap=colormap)
return
| gpl-3.0 |
effigies/mne-python | examples/plot_topo_customized.py | 2 | 1914 | """
========================================
Plot custom topographies for MEG sensors
========================================
This example exposes the `iter_topography` function that makes it
very easy to generate custom sensor topography plots.
Here we will plot the power spectrum of each channel on a topographic
layout.
"""
# Author: Denis A. Engemann <[email protected]>
#
# License: BSD (3-clause)
print(__doc__)
import numpy as np
import mne
from mne.viz import iter_topography
from mne import io
from mne.time_frequency import compute_raw_psd
import matplotlib.pyplot as plt
from mne.datasets import sample
data_path = sample.data_path()
raw_fname = data_path + '/MEG/sample/sample_audvis_filt-0-40_raw.fif'
raw = io.Raw(raw_fname, preload=True)
raw.filter(1, 20)
picks = mne.pick_types(raw.info, meg=True, exclude=[])
tmin, tmax = 0, 120 # use the first 120s of data
fmin, fmax = 2, 20 # look at frequencies between 2 and 20Hz
n_fft = 2048 # the FFT size (n_fft). Ideally a power of 2
psds, freqs = compute_raw_psd(raw, picks=picks, tmin=tmin, tmax=tmax,
fmin=fmin, fmax=fmax)
psds = 20 * np.log10(psds) # scale to dB
def my_callback(ax, ch_idx):
"""
This block of code is executed once you click on one of the channel axes
in the plot. To work with the viz internals, this function should only take
two parameters, the axis and the channel or data index.
"""
ax.plot(freqs, psds[ch_idx], color='red')
ax.set_xlabel = 'Frequency (Hz)'
ax.set_ylabel = 'Power (dB)'
for ax, idx in iter_topography(raw.info,
fig_facecolor='white',
axis_facecolor='white',
axis_spinecolor='white',
on_pick=my_callback):
ax.plot(psds[idx], color='red')
plt.gcf().suptitle('Power spectral densities')
plt.show()
| bsd-3-clause |
RichardLeeK/MachineLearning | MachineLearning/CBFV/CBFV.py | 1 | 3957 | import sys
#sys.path.insert(0, 'D:/Sources/Python Source Code/MachineLearning/CBFV')
#sys.path.insert(0, 'D:/Sources/Python Source Code/MachineLearning/CBFV')
from sklearn import svm
import pickle
import datetime
import sklearn.cross_validation as cv
import ml.shallow_model as sm
import ml.deep_model as dl
import data_gen as dg
import numpy as np
import copy
import threading
test_param = 'PI'
parameter_class = 'REG_MOR+BRS'
fvx_threshold = 1.56
fold = 4
mode = test_param + '_' + parameter_class + '_' + str(fvx_threshold)+ '_' + str(fold)
def pos_counter(Y):
cnt = 0
for y in Y:
if y == 0:
cnt += 1
return cnt, len(Y) - cnt
if __name__ == "__main__":
sys.setrecursionlimit(10000)
print(mode)
info_list = dg.gen_info_pickle()
info_list, test_info_list = dg.test_data_extractor(info_list, fold)
cp3 = datetime.datetime.now()
X_train, params, _ = dg.gen_x(info_list, ['BAS', 'BRS', 'MOR'], 'abp')
X_test, _, OL_test = dg.gen_x(test_info_list, ['BAS', 'BRS', 'MOR'], 'abp')
print('Use ' + str(len(params)) + ' features!')
pen = open('rubbish/params.csv', 'a')
sentence = '\n' + mode
for p in params:
sentence += ',' + p
pen.write(sentence)
pen.close()
Y_train, cnt = dg.gen_y_pi(info_list, fvx_threshold)
Y_test, cnt_test = dg.gen_y_pi(test_info_list, fvx_threshold)
"""
Y_train, cnt = dg.gen_y_fvx(info_list, fvx_threshold)
Y_test, cnt_test = dg.gen_y_fvx(test_info_list, fvx_threshold)
Y_train, cnt = dg.gen_y_reduction(info_list, 0.2)
Y_test, cnt_test = dg.gen_y_reduction(test_info_list, 0.2)
"""
cp4 = datetime.datetime.now()
print('Generate data fininshed ' + str(cp4 - cp3))
print('Train Positive: ' + str(cnt))
print('Train Negative: ' + str(len(Y_train)-cnt))
print('Test Positive: ' + str(cnt_test))
print('Test Negative: ' + str(len(Y_test)-cnt_test))
""" Regulization
X, Y = dg.pos_neg_regulator(X, Y, cnt, len(Y) - cnt)
pos_cnt, neg_cnt = pos_counter(Y)
print('Regulated Positive: ' + str(pos_cnt))
print('Regulated Negative: ' + str(neg_cnt))
"""
X_train, Y_train = dg.pos_neg_regulator(X_train, Y_train, cnt, len(Y_train) - cnt)
pos_cnt, neg_cnt = pos_counter(Y_train)
print('Regulated Positive: ' + str(pos_cnt))
print('Regulated Negative: ' + str(neg_cnt))
#X_train, Y_train = dg.pos_neg_regulator(X_train, Y_train, cnt, len(Y_train)-cnt)
#pos_cnt, neg_cnt = pos_counter(Y_train)
#print('Regulated Train Positive: ' + str(pos_cnt))
#print('Regulated Train Negative: ' + str(neg_cnt))
X_train = dg.x_normalizer(X_train)
X_test = dg.x_normalizer(X_test)
p, n = pos_counter(Y_train)
pp, nn = pos_counter(Y_test)
print('Y_train: ' + str(p) + '\t' + str(n))
print('Y_test: ' + str(pp) + '\t' + str(nn))
X_train = np.array(X_train)
X_test = np.array(X_test)
Y_train = np.array(Y_train)
Y_test = np.array(Y_test)
with open('data/dataset.pickle', 'wb') as handle:
pickle.dump({'X_train': X_train, 'y_train': Y_train, 'X_test': X_test, 'y_test': Y_test}, handle, protocol=pickle.HIGHEST_PROTOCOL)
line = mode + ',' + sm.rf_train_test(X_train, X_test, Y_train, Y_test)
pen = open('MLResult.csv', 'a')
pen.write(line + '\n')
pen.close()
line = mode + ',' + sm.lr_train_test(X_train, X_test, Y_train, Y_test)
pen = open('MLResult.csv', 'a')
pen.write(line + '\n')
pen.close()
line = mode + ',' + sm.gb_train_test(X_train, X_test, Y_train, Y_test)
pen = open('MLResult.csv', 'a')
pen.write(line + '\n')
pen.close()
line = mode + ',' + dl.dnn_train_test(X_train, X_test, Y_train, Y_test)
pen = open('MLResult.csv', 'a')
pen.write(line + '\n')
pen.close()
"""
line = mode + ',' + dl.lstm_train_test(X_train, X_test, Y_train, Y_test)
pen = open('MLResult.csv', 'a')
pen.write(line + '\n')
pen.close()
line = mode + ',' + sm.svm_train_test(X_train, X_test, Y_train, Y_test)
pen = open('MLResult.csv', 'a')
pen.write(line + '\n')
pen.close()
""" | mit |
stablum/reimplementations | theano_decoder_mnist_deeper.py | 1 | 12219 | #!/usr/bin/env python3
import theano
from theano import tensor as T
import pandas as pd
from tqdm import trange,tqdm
import numpy as np
from sklearn.preprocessing import normalize
import sklearn.svm
import time
import mnist # pip3 install python-mnist
import os
import sys
#theano.config.exception_verbosity="high"
#theano.config.optimizer='None'
theano.config.optimizer='fast_run'
theano.config.openmp=False
theano.config.openmp_elemwise_minsize=10
#theano.config.device='gpu'
theano.config.floatX='float32'
lr_begin = 0.02 # 0.2
lr_annealing_T=10
lr=None
n_epochs = 10000
data_amplify = 0.5
data_offset = 0.25
sigma_x = 0.5
sigma_z = 1#1e2#1e4
latent_dim = None
hidden_dims = None
num_hidden_layers = None
activation_function = None
#activation_function_inverse = lambda x: T.log(x) - T.log(1-x) # logit
minibatch_size = None
repeat_training=1
possible_activations = {
'sigmoid': T.nnet.sigmoid,
# 2.37 seems to make a sigmoid a good approximation for erf(x),
'pseudogelu': lambda x: x * T.nnet.sigmoid(x*2.37),
'gelu': lambda x : x*T.erf(x),
'elu': T.nnet.elu,
'relu': T.nnet.relu
}
class Logger():
def __init__(self):
self.filename = "theano_decoder_"+str(time.time())+".log"
self.f = open(self.filename,'w')
def __call__(self, *args):
print(*args, flush=True)
print(*args,file=self.f, flush=True)
log = None
def calculate_lr(t):
# decaying learning rate with annealing
# see: https://www.willamette.edu/~gorr/classes/cs449/momrate.html
ret = lr_begin / (
1. + float(t)/lr_annealing_T
)
return ret
def shuffle(Z,X,Y):
sel = np.arange(X.shape[1])
np.random.shuffle(sel)
X = X[:,sel]
Z = Z[:,sel]
Y = Y[:,sel]
return Z,X,Y
def fix_data(features,labels):
# please notice the transpose '.T' operator
# in a neural network, the datapoints needs to be scattered across the columns
# because dot product.
X = (np.array(features).T.astype('float32')/255.)*data_amplify + data_offset
Y = np.expand_dims(np.array(labels).astype('float32'),1).T
return X,Y
def load_data():
print("setting up mnist loader..")
_mnist = mnist.MNIST(path='./python-mnist/data')
print("loading training data..")
X_train,Y_train = fix_data(*_mnist.load_training())
print("X_train.shape=",X_train.shape,"Y_train.shape=",Y_train.shape)
print("loading testing data..")
X_test,Y_test = fix_data(*_mnist.load_testing())
print("X_test.shape=",X_test.shape,"Y_test.shape=",Y_test.shape)
return X_train, Y_train, X_test, Y_test
def add_layer(inputs,i):
assert type(i) is int
W = T.matrix('W%d'%i)
bias = T.matrix('bias%d'%i)
bias_tiled = bias.repeat(minibatch_size, axis=1)
d = T.dot(W, inputs)
d.name = "d%d"%i
lin = d + bias_tiled
lin.name = 'lin%d'%i
out = activation_function(lin)
out.name = 'out%d'%i
return W,bias,out
def add_layer_inverse(curr, i, W, bias):
assert type(i) is int
lin = activation_function_inverse(curr)
lin.name = 'lin_inverse%d'%i
bias_tiled = bias.repeat(minibatch_size, axis=1)
bias_tiled.name = 'bias_tiled_inverse%d'%i
d = lin - bias_tiled
d.name = "d_inverse%d"%i
W_pinv = T.nlinalg.MatrixPinv()(W)
W_pinv.name = "W_pinv%d"%i
ret = T.dot(W_pinv,d)
return ret
def build_net_inverse(xs,Ws,biases):
curr = xs
for i, (W, bias) in enumerate(reversed(list(zip(Ws,biases)))):
curr = add_layer_inverse(curr, i, W, bias)
return curr
def build_net():
curr = inputs = T.matrix('inputs')
Ws = []
biases = []
for i in range(num_hidden_layers + 1):
W, bias, curr = add_layer(curr,i)
Ws.append(W)
biases.append(bias)
return inputs, Ws, biases, curr
def update(learnable, grad):
learnable -= lr * grad
def step(zs, xs, Ws_vals, biases_vals, grad_fn):
grad_vals = grad_fn(*([zs, xs] + Ws_vals + biases_vals))
Ws_grads = grad_vals[:len(Ws_vals)]
biases_grads = grad_vals[len(Ws_vals):-1]
z_grads = grad_vals[-1]
for curr_W, curr_grad in zip(Ws_vals,Ws_grads):
update(curr_W, curr_grad)
for curr_bias, curr_grad in zip(biases_vals,biases_grads):
update(curr_bias, curr_grad)
#if np.mean(np.abs(z_grads)) > 1e-4:
# log(z_grads)
update(zs,z_grads)
def partition(a):
assert type(a) is np.ndarray
assert a.shape[1] > minibatch_size, "a.shape[1] should be larger than the minibatch size. a.shape=%s"%str(a.shape)
minibatches_num = int(a.shape[1] / minibatch_size)
assert minibatches_num > 0
off = lambda i : i * minibatch_size
return [
a[:,off(i):off(i+1)]
for i
in range(minibatches_num)
]
def partition_minibatches(Z,X):
assert Z.shape[1] == X.shape[1], "Z and X have different lengths: %d and %d"%(Z.shape[1],X.shape[1])
return list(zip(partition(Z),partition(X)))
def train(Z, X, Ws_vals, biases_vals, grad_fn,repeat=1):
for zs,xs in tqdm(partition_minibatches(Z,X)*repeat,desc="training"):
step(zs, xs, Ws_vals, biases_vals, grad_fn)
def nll_sum(Z, X, Ws_vals, biases_vals, nll_fn):
ret = 0
for zs,xs in tqdm(partition_minibatches(Z,X),desc="nll_sum"):
curr, = nll_fn(*([zs, xs] + Ws_vals + biases_vals))
ret += curr
return ret
def reconstruction_error(Z, X, Ws_vals, biases_vals, inverse_fn, generate_fn):
minibatches_means = []
for _,xs in tqdm(partition_minibatches(Z,X)[:3],desc="reconstruction_error"):
_zs_inverse, = inverse_fn(*([xs] + Ws_vals + biases_vals))
log("_zs_inverse",_zs_inverse)
curr_reconstructions, = generate_fn(*([_zs_inverse] + Ws_vals + biases_vals))
differences = np.abs(xs - curr_reconstructions)
minibatches_means.append(np.mean(differences))
ret = np.mean(minibatches_means)
return ret
def build_negative_log_likelihoods(zs,outputs,xs):
error_term = 1/sigma_x * T.sum((xs-outputs)**2,axis=0)
prior_term = 1/sigma_z * T.sum((zs)**2,axis=0)
nlls = error_term + prior_term
return nlls
def test_classifier(Z,Y):
#classifier = sklearn.svm.SVC()
log("training classifier..")
classifier = sklearn.svm.SVC(
kernel='rbf',
max_iter=1000
)
# please notice the transpose '.T' operator: sklearn wants one datapoint per row
classifier.fit(Z.T,Y[0,:])
log("done. Scoring..")
svc_score = classifier.score(Z.T,Y[0,:])
log("SVC score: %s"%svc_score)
def generate_grid_samples(epoch,Ws_vals,biases_vals,generate_fn):
log("generating samples from a grid")
space1d = np.linspace(-2*sigma_z, 2*sigma_z, 20)
mgs = np.meshgrid(*[space1d]*latent_dim)
points = np.vstack([
np.reshape(curr,-1)
for curr
in mgs
]).astype('float32')
samples_l = []
for curr in partition(points):
samples_l.append( generate_fn(*([curr]+Ws_vals+biases_vals)) )
samples = np.vstack(samples_l)
filename = "grid_samples_epoch_%d.npy"%(epoch)
np.save(filename, samples)
log("done generating grid samples")
def generate_samples(epoch,Ws_vals,biases_vals,generate_fn,inverse_fn):
log("generating a bunch of random samples")
_zs_l = []
for i in range(minibatch_size):
_z = np.random.normal(np.array([0]*latent_dim),sigma_z).astype('float32')
_zs_l.append(_z)
_zs = np.vstack(_zs_l).T
samples = generate_fn(*([_zs]+Ws_vals+biases_vals))
log("generated samples. mean:",np.mean(samples),"std:",np.std(samples))
#_zs_inverse = inverse_fn(*([samples[0]]+Ws_vals+biases_vals)) # FIXME: this 0 index
log("_zs",_zs)
#log("_zs_inverse!",_zs_inverse)
filename = "random_samples_epoch_%d.npy"%(epoch)
np.save(filename, samples)
log("done generating random samples.")
def initial_weights_and_biases(x_dim):
Ws_vals = []
biases_vals = []
dims1 = hidden_dims + [x_dim]
dims2 = [ latent_dim ] + hidden_dims
for curr in zip(dims1, dims2):
xavier_var = 1./curr[0]
W_vals_curr = (np.random.normal(0,xavier_var,curr).astype('float32'))
biases_curr = (np.random.normal(0,xavier_var,(curr[0],1)).astype('float32'))
Ws_vals.append(W_vals_curr)
biases_vals.append(biases_curr)
return Ws_vals, biases_vals
def main():
global log
global latent_dim
global hidden_dims
global minibatch_size
global num_hidden_layers
global activation_function
assert len(sys.argv) > 1, "usage: %s harvest_dir"%(sys.argv[0])
latent_dim = int(sys.argv[1])
hidden_dims = list(map(int,sys.argv[2].split("_")))
num_hidden_layers = len(hidden_dims)
minibatch_size = int(sys.argv[3])
activation_name = sys.argv[4]
activation_function = possible_activations[activation_name]
harvest_dir = "harvest_zdim{}_hdims_{}_minibatch_size_{}_activation_{}".format(
latent_dim,
sys.argv[2],
minibatch_size,
activation_name
)
np.set_printoptions(precision=4, suppress=True)
X,Y,X_test,Y_test = load_data() # needs to be before cd
try:
os.mkdir(harvest_dir)
except OSError as e: # directory already exists. It's ok.
print(e)
os.system("cp %s %s -vf"%(sys.argv[0],harvest_dir+"/"))
os.chdir(harvest_dir)
log = Logger()
log("sys.argv",sys.argv)
x_dim = X.shape[0]
num_datapoints = X.shape[1]
Z = (np.random.normal(0,sigma_z,(latent_dim,num_datapoints)).astype('float32'))
Ws_vals, biases_vals = initial_weights_and_biases(x_dim)
# set up
zs, Ws, biases, outputs = build_net()
xs = T.matrix('xs')
#zs_inverted = build_net_inverse(xs,Ws,biases)
nlls = build_negative_log_likelihoods(zs,outputs,xs)
nll = T.sum(nlls,axis=0)
for curr_W,curr_bias in zip(Ws,biases):
weights_regularizer = 1/100 * T.sum((curr_W)**2) # FIXME: do proper derivation and variable naming
bias_regularizer = 1/100 * T.sum(curr_bias**2)
nll = nll + weights_regularizer + bias_regularizer
grads = T.grad(nll,Ws+biases+[zs])
#theano.pp(grad)
def summary():
total_nll = nll_sum(Z,X,Ws_vals,biases_vals,nll_fn)
#_reconstruction_error = reconstruction_error(Z,X,Ws_vals,biases_vals,inverse_fn,generate_fn)
log("epoch %d"%epoch)
log("harvest_dir",harvest_dir)
log("lr %f"%lr)
log("total nll: {:,}".format(total_nll))
#log("average reconstruction error: {:,}".format(_reconstruction_error))
log("mean Z: {:,}".format(np.mean(Z)))
log("mean abs Z: {:,}".format(np.mean(np.abs(Z))))
log("std Z: {:,}".format(np.std(Z)))
log("means Ws: %s"%([np.mean(curr) for curr in Ws_vals]))
log("stds Ws: %s"%([np.std(curr) for curr in Ws_vals]))
log("means biases: %s"%([np.mean(curr) for curr in biases_vals]))
log("stds biases: %s"%([np.std(curr) for curr in biases_vals]))
log("compiling theano grad_fn..")
grad_fn = theano.function([zs, xs]+Ws+biases, grads)
log("compiling theano nll_fn..")
nll_fn = theano.function([zs, xs]+Ws+biases, [nll])
log("compiling theano generate_fn..")
generate_fn = theano.function([zs]+Ws+biases, [outputs])
#log("compiling theano inverse_fn..")
inverse_fn=None#inverse_fn = theano.function([xs]+Ws+biases, [zs_inverted])
log("done. epochs loop..")
def save():
log("saving Z,Y,Ws,biases..")
np.save("theano_decoder_Z.npy",Z)
np.save("theano_decoder_Y.npy",Y)
for i, (_w,_b) in enumerate(zip(Ws_vals,biases_vals)):
np.save('theano_decoder_W_{}.npy'.format(i), _w)
np.save('theano_decoder_bias_{}.npy'.format(i), _b)
log("done saving.")
# train
for epoch in range(n_epochs):
global lr
lr = calculate_lr(epoch)
Z,X,Y = shuffle(Z,X,Y)
summary()
if epoch % 5 == 0:
generate_samples(epoch,Ws_vals,biases_vals,generate_fn,inverse_fn)
generate_grid_samples(epoch,Ws_vals,biases_vals,generate_fn)
test_classifier(Z,Y)
save()
train(Z,X,Ws_vals,biases_vals,grad_fn,repeat=repeat_training)
log("epochs loop ended")
summary()
if __name__=="__main__":
main()
| gpl-3.0 |
MatthieuBizien/scikit-learn | examples/gaussian_process/plot_gpr_co2.py | 131 | 5705 | """
========================================================
Gaussian process regression (GPR) on Mauna Loa CO2 data.
========================================================
This example is based on Section 5.4.3 of "Gaussian Processes for Machine
Learning" [RW2006]. It illustrates an example of complex kernel engineering and
hyperparameter optimization using gradient ascent on the
log-marginal-likelihood. The data consists of the monthly average atmospheric
CO2 concentrations (in parts per million by volume (ppmv)) collected at the
Mauna Loa Observatory in Hawaii, between 1958 and 1997. The objective is to
model the CO2 concentration as a function of the time t.
The kernel is composed of several terms that are responsible for explaining
different properties of the signal:
- a long term, smooth rising trend is to be explained by an RBF kernel. The
RBF kernel with a large length-scale enforces this component to be smooth;
it is not enforced that the trend is rising which leaves this choice to the
GP. The specific length-scale and the amplitude are free hyperparameters.
- a seasonal component, which is to be explained by the periodic
ExpSineSquared kernel with a fixed periodicity of 1 year. The length-scale
of this periodic component, controlling its smoothness, is a free parameter.
In order to allow decaying away from exact periodicity, the product with an
RBF kernel is taken. The length-scale of this RBF component controls the
decay time and is a further free parameter.
- smaller, medium term irregularities are to be explained by a
RationalQuadratic kernel component, whose length-scale and alpha parameter,
which determines the diffuseness of the length-scales, are to be determined.
According to [RW2006], these irregularities can better be explained by
a RationalQuadratic than an RBF kernel component, probably because it can
accommodate several length-scales.
- a "noise" term, consisting of an RBF kernel contribution, which shall
explain the correlated noise components such as local weather phenomena,
and a WhiteKernel contribution for the white noise. The relative amplitudes
and the RBF's length scale are further free parameters.
Maximizing the log-marginal-likelihood after subtracting the target's mean
yields the following kernel with an LML of -83.214::
34.4**2 * RBF(length_scale=41.8)
+ 3.27**2 * RBF(length_scale=180) * ExpSineSquared(length_scale=1.44,
periodicity=1)
+ 0.446**2 * RationalQuadratic(alpha=17.7, length_scale=0.957)
+ 0.197**2 * RBF(length_scale=0.138) + WhiteKernel(noise_level=0.0336)
Thus, most of the target signal (34.4ppm) is explained by a long-term rising
trend (length-scale 41.8 years). The periodic component has an amplitude of
3.27ppm, a decay time of 180 years and a length-scale of 1.44. The long decay
time indicates that we have a locally very close to periodic seasonal
component. The correlated noise has an amplitude of 0.197ppm with a length
scale of 0.138 years and a white-noise contribution of 0.197ppm. Thus, the
overall noise level is very small, indicating that the data can be very well
explained by the model. The figure shows also that the model makes very
confident predictions until around 2015.
"""
print(__doc__)
# Authors: Jan Hendrik Metzen <[email protected]>
#
# License: BSD 3 clause
import numpy as np
from matplotlib import pyplot as plt
from sklearn.gaussian_process import GaussianProcessRegressor
from sklearn.gaussian_process.kernels \
import RBF, WhiteKernel, RationalQuadratic, ExpSineSquared
from sklearn.datasets import fetch_mldata
data = fetch_mldata('mauna-loa-atmospheric-co2').data
X = data[:, [1]]
y = data[:, 0]
# Kernel with parameters given in GPML book
k1 = 66.0**2 * RBF(length_scale=67.0) # long term smooth rising trend
k2 = 2.4**2 * RBF(length_scale=90.0) \
* ExpSineSquared(length_scale=1.3, periodicity=1.0) # seasonal component
# medium term irregularity
k3 = 0.66**2 \
* RationalQuadratic(length_scale=1.2, alpha=0.78)
k4 = 0.18**2 * RBF(length_scale=0.134) \
+ WhiteKernel(noise_level=0.19**2) # noise terms
kernel_gpml = k1 + k2 + k3 + k4
gp = GaussianProcessRegressor(kernel=kernel_gpml, alpha=0,
optimizer=None, normalize_y=True)
gp.fit(X, y)
print("GPML kernel: %s" % gp.kernel_)
print("Log-marginal-likelihood: %.3f"
% gp.log_marginal_likelihood(gp.kernel_.theta))
# Kernel with optimized parameters
k1 = 50.0**2 * RBF(length_scale=50.0) # long term smooth rising trend
k2 = 2.0**2 * RBF(length_scale=100.0) \
* ExpSineSquared(length_scale=1.0, periodicity=1.0,
periodicity_bounds="fixed") # seasonal component
# medium term irregularities
k3 = 0.5**2 * RationalQuadratic(length_scale=1.0, alpha=1.0)
k4 = 0.1**2 * RBF(length_scale=0.1) \
+ WhiteKernel(noise_level=0.1**2,
noise_level_bounds=(1e-3, np.inf)) # noise terms
kernel = k1 + k2 + k3 + k4
gp = GaussianProcessRegressor(kernel=kernel, alpha=0,
normalize_y=True)
gp.fit(X, y)
print("\nLearned kernel: %s" % gp.kernel_)
print("Log-marginal-likelihood: %.3f"
% gp.log_marginal_likelihood(gp.kernel_.theta))
X_ = np.linspace(X.min(), X.max() + 30, 1000)[:, np.newaxis]
y_pred, y_std = gp.predict(X_, return_std=True)
# Illustration
plt.scatter(X, y, c='k')
plt.plot(X_, y_pred)
plt.fill_between(X_[:, 0], y_pred - y_std, y_pred + y_std,
alpha=0.5, color='k')
plt.xlim(X_.min(), X_.max())
plt.xlabel("Year")
plt.ylabel(r"CO$_2$ in ppm")
plt.title(r"Atmospheric CO$_2$ concentration at Mauna Loa")
plt.tight_layout()
plt.show()
| bsd-3-clause |
datapythonista/pandas | pandas/tests/frame/methods/test_dtypes.py | 4 | 4250 | from datetime import timedelta
import numpy as np
from pandas.core.dtypes.dtypes import DatetimeTZDtype
import pandas as pd
from pandas import (
DataFrame,
Series,
date_range,
option_context,
)
import pandas._testing as tm
def _check_cast(df, v):
"""
Check if all dtypes of df are equal to v
"""
assert all(s.dtype.name == v for _, s in df.items())
class TestDataFrameDataTypes:
def test_empty_frame_dtypes(self):
empty_df = DataFrame()
tm.assert_series_equal(empty_df.dtypes, Series(dtype=object))
nocols_df = DataFrame(index=[1, 2, 3])
tm.assert_series_equal(nocols_df.dtypes, Series(dtype=object))
norows_df = DataFrame(columns=list("abc"))
tm.assert_series_equal(norows_df.dtypes, Series(object, index=list("abc")))
norows_int_df = DataFrame(columns=list("abc")).astype(np.int32)
tm.assert_series_equal(
norows_int_df.dtypes, Series(np.dtype("int32"), index=list("abc"))
)
df = DataFrame({"a": 1, "b": True, "c": 1.0}, index=[1, 2, 3])
ex_dtypes = Series({"a": np.int64, "b": np.bool_, "c": np.float64})
tm.assert_series_equal(df.dtypes, ex_dtypes)
# same but for empty slice of df
tm.assert_series_equal(df[:0].dtypes, ex_dtypes)
def test_datetime_with_tz_dtypes(self):
tzframe = DataFrame(
{
"A": date_range("20130101", periods=3),
"B": date_range("20130101", periods=3, tz="US/Eastern"),
"C": date_range("20130101", periods=3, tz="CET"),
}
)
tzframe.iloc[1, 1] = pd.NaT
tzframe.iloc[1, 2] = pd.NaT
result = tzframe.dtypes.sort_index()
expected = Series(
[
np.dtype("datetime64[ns]"),
DatetimeTZDtype("ns", "US/Eastern"),
DatetimeTZDtype("ns", "CET"),
],
["A", "B", "C"],
)
tm.assert_series_equal(result, expected)
def test_dtypes_are_correct_after_column_slice(self):
# GH6525
df = DataFrame(index=range(5), columns=list("abc"), dtype=np.float_)
tm.assert_series_equal(
df.dtypes,
Series({"a": np.float_, "b": np.float_, "c": np.float_}),
)
tm.assert_series_equal(df.iloc[:, 2:].dtypes, Series({"c": np.float_}))
tm.assert_series_equal(
df.dtypes,
Series({"a": np.float_, "b": np.float_, "c": np.float_}),
)
def test_dtypes_gh8722(self, float_string_frame):
float_string_frame["bool"] = float_string_frame["A"] > 0
result = float_string_frame.dtypes
expected = Series(
{k: v.dtype for k, v in float_string_frame.items()}, index=result.index
)
tm.assert_series_equal(result, expected)
# compat, GH 8722
with option_context("use_inf_as_na", True):
df = DataFrame([[1]])
result = df.dtypes
tm.assert_series_equal(result, Series({0: np.dtype("int64")}))
def test_dtypes_timedeltas(self):
df = DataFrame(
{
"A": Series(date_range("2012-1-1", periods=3, freq="D")),
"B": Series([timedelta(days=i) for i in range(3)]),
}
)
result = df.dtypes
expected = Series(
[np.dtype("datetime64[ns]"), np.dtype("timedelta64[ns]")], index=list("AB")
)
tm.assert_series_equal(result, expected)
df["C"] = df["A"] + df["B"]
result = df.dtypes
expected = Series(
[
np.dtype("datetime64[ns]"),
np.dtype("timedelta64[ns]"),
np.dtype("datetime64[ns]"),
],
index=list("ABC"),
)
tm.assert_series_equal(result, expected)
# mixed int types
df["D"] = 1
result = df.dtypes
expected = Series(
[
np.dtype("datetime64[ns]"),
np.dtype("timedelta64[ns]"),
np.dtype("datetime64[ns]"),
np.dtype("int64"),
],
index=list("ABCD"),
)
tm.assert_series_equal(result, expected)
| bsd-3-clause |
pyrolysis/low-order-particle | sphere-cyl-slab.py | 1 | 5161 | """
Compare temperature profiles of 1-D solid sphere, cylinder, and cube shapes that
are volume equivalent. Note that due to surface area, sphere heats slowest, for
example sphere < cylinder < cube.
"""
import numpy as np
import matplotlib.pyplot as py
from funcHeatCond import hc3
from funcOther import volume, surf, lump, dsv
# Parameters
# -----------------------------------------------------------------------------
Gb = 0.54 # basic specific gravity, Wood Handbook Table 4-7, (-)
cp = 1800 # heat capacity, J/kg*K
k = 0.12 # thermal conductivity, W/mK
x = 0 # moisture content, %
h = 350 # heat transfer coefficient, W/m^2*K
Ti = 293 # initial particle temp, K
Tinf = 773 # ambient temp, K
# Calculations for Volume and Surface Area
# -----------------------------------------------------------------------------
# calculate height and cube side to give same volume as sphere
d = 0.001 # diameter of sphere, m
ht = 2/3*d # cylinder height of equal volume as sphere, m
s = ((np.pi*d**3)/6)**(1/3) # cube side of equal volume as sphere, m
Vsph, Vcyl, Vcube = volume(d, ht, s) # solid volume of sphere, cylinder, cube
SAsph, SAcyl, SAcube = surf(d, ht, s) # surface area of sphere, cylinder, cube
xf = 1000 # convert length from m to mm, 1 m = 1000 mm
vf = 1e9 # convert volume from m^3 to mm^3, 1 m^3 = 1e9 mm^3
sf = 1e6 # convert surface area from m^2 to mm^2, 1 m^2 = 1e6 mm^2
print('PARAMETERS in mm '+'-'*60)
print('d_sph = {:.8} \t h_cyl = {:.8} \t a_cube = {:.8}'.format(d*xf, ht*xf, s*xf))
print('VOLUME '+'-'*70)
print('V_sph {:.8} \t V_cyl {:.8} \t V_cube {:.8}'.format(Vsph*vf, Vcyl*vf, Vcube*vf))
print('SURFACE AREA '+'-'*64)
print('SA_sph {:.8} \t SA_cyl {:.8} \t SA_cube {:.8}'.format(SAsph*sf, SAcyl*sf, SAcube*sf))
# 1D Transient Heat Conduction Method
# -----------------------------------------------------------------------------
# number of nodes from center of particle (m=0) to surface (m)
m = 1000
# time vector from 0 to max time
tmax = 4.0 # max time, s
nt = 400 # number of time steps
dt = tmax/nt # time step, s
t = np.arange(0, tmax+dt, dt) # time vector, s
# solid sphere, shell, microstructure temperature array [T] in Kelvin
# row = time step, column = node point from 0 (center) to m (surface)
Tsphere = hc3(d, cp, k, Gb, h, Ti, Tinf, 2, m, t)
Tsphere_avg = [np.mean(row) for row in Tsphere]
Tcyl = hc3(d, cp, k, Gb, h, Ti, Tinf, 1, m, t)
Tcyl_avg = [np.mean(row) for row in Tcyl]
Tslab = hc3(s, cp, k, Gb, h, Ti, Tinf, 0, m, t)
Tslab_avg = [np.mean(row) for row in Tslab]
# 1D Transient Heat Conduction Method (Dsv)
# -----------------------------------------------------------------------------
dcyl = dsv(SAcyl, Vcyl) # cylinder
dcube = dsv(SAcube, Vcube) # cube
Tsph_dsv = hc3(d, cp, k, Gb, h, Ti, Tinf, 2, m, t)
Tsph_dsv_avg = [np.mean(row) for row in Tsph_dsv]
Tcyl_dsv = hc3(dcyl, cp, k, Gb, h, Ti, Tinf, 2, m, t)
Tcyl_dsv_avg = [np.mean(row) for row in Tcyl_dsv]
Tcube_dsv = hc3(dcube, cp, k, Gb, h, Ti, Tinf, 2, m, t)
Tcube_dsv_avg = [np.mean(row) for row in Tcube_dsv]
# Lumped Capacitance Method
# -----------------------------------------------------------------------------
Tsph_lump = lump(cp, h, k, Gb, t, Vsph, SAsph, Ti, Tinf) # sphere
Tcyl_lump = lump(cp, h, k, Gb, t, Vcyl, SAcyl, Ti, Tinf) # cylinder
Tcube_lump = lump(cp, h, k, Gb, t, Vcube, SAcube, Ti, Tinf) # cube
# Plots
# -----------------------------------------------------------------------------
py.ion()
py.close('all')
def despine():
ax = py.gca()
ax.spines['top'].set_visible(False)
ax.spines['right'].set_visible(False)
py.tick_params(axis='both', bottom='off', top='off', left='off', right='off')
py.figure(1)
py.plot(t, Tsphere_avg, 'o-', markevery=20, mec='b', mew=2, lw=2, label='sphere_1d')
py.plot(t, Tcyl_avg, '^-', markevery=20, mec='g', mew=2, lw=2, label='cylinder_1d')
py.plot(t, Tslab_avg, 's-', markevery=20, mec='r', mew=2, lw=2, label='slab_1d')
py.ylim([250, 800])
py.xlabel('Time (s)')
py.ylabel('Average Temperature (K)')
py.title('1-D method')
py.legend(loc='best', numpoints=1, frameon=False)
py.grid()
despine()
py.figure(2)
py.plot(t, Tsph_dsv_avg, 'o-', markevery=20, mec='b', mew=2, lw=2, label='sphere_1d')
py.plot(t, Tcyl_dsv_avg, '^-', markevery=20, mec='g', mew=2, lw=2, label='cylinder_1d')
py.plot(t, Tcube_dsv_avg, 's-', markevery=20, mec='r', mew=2, lw=2, label='cube_1d')
py.ylim([250, 800])
py.xlabel('Time (s)')
py.ylabel('Average Temperature (K)')
py.title('1-D method with Dsv')
py.legend(loc='best', numpoints=1, frameon=False)
py.grid()
despine()
py.figure(3)
py.plot(t, Tsph_lump, 'o-', markevery=20, mec='b', mew=2, lw=2, label='sphere_1d')
py.plot(t, Tcyl_lump, '^-', markevery=20, mec='g', mew=2, lw=2, label='cylinder_1d')
py.plot(t, Tcube_lump, 's-', markevery=20, mec='r', mew=2, lw=2, label='cube_1d')
py.ylim([250, 800])
py.xlabel('Time (s)')
py.ylabel('Average Temperature (K)')
py.title('Lumped method')
py.legend(loc='best', numpoints=1, frameon=False)
py.grid()
despine()
| mit |
ran5515/DeepDecision | tensorflow/examples/learn/iris_run_config.py | 76 | 2565 | # Copyright 2016 The TensorFlow Authors. All Rights Reserved.
#
# Licensed under the Apache License, Version 2.0 (the "License");
# you may not use this file except in compliance with the License.
# You may obtain a copy of the License at
#
# http://www.apache.org/licenses/LICENSE-2.0
#
# Unless required by applicable law or agreed to in writing, software
# distributed under the License is distributed on an "AS IS" BASIS,
# WITHOUT WARRANTIES OR CONDITIONS OF ANY KIND, either express or implied.
# See the License for the specific language governing permissions and
# limitations under the License.
"""Example of DNNClassifier for Iris plant dataset, with run config."""
from __future__ import absolute_import
from __future__ import division
from __future__ import print_function
import numpy as np
from sklearn import datasets
from sklearn import metrics
from sklearn import model_selection
import tensorflow as tf
X_FEATURE = 'x' # Name of the input feature.
def main(unused_argv):
# Load dataset.
iris = datasets.load_iris()
x_train, x_test, y_train, y_test = model_selection.train_test_split(
iris.data, iris.target, test_size=0.2, random_state=42)
# You can define you configurations by providing a RunConfig object to
# estimator to control session configurations, e.g. tf_random_seed.
run_config = tf.estimator.RunConfig().replace(tf_random_seed=1)
# Build 3 layer DNN with 10, 20, 10 units respectively.
feature_columns = [
tf.feature_column.numeric_column(
X_FEATURE, shape=np.array(x_train).shape[1:])]
classifier = tf.estimator.DNNClassifier(
feature_columns=feature_columns, hidden_units=[10, 20, 10], n_classes=3,
config=run_config)
# Train.
train_input_fn = tf.estimator.inputs.numpy_input_fn(
x={X_FEATURE: x_train}, y=y_train, num_epochs=None, shuffle=True)
classifier.train(input_fn=train_input_fn, steps=200)
# Predict.
test_input_fn = tf.estimator.inputs.numpy_input_fn(
x={X_FEATURE: x_test}, y=y_test, num_epochs=1, shuffle=False)
predictions = classifier.predict(input_fn=test_input_fn)
y_predicted = np.array(list(p['class_ids'] for p in predictions))
y_predicted = y_predicted.reshape(np.array(y_test).shape)
# Score with sklearn.
score = metrics.accuracy_score(y_test, y_predicted)
print('Accuracy (sklearn): {0:f}'.format(score))
# Score with tensorflow.
scores = classifier.evaluate(input_fn=test_input_fn)
print('Accuracy (tensorflow): {0:f}'.format(scores['accuracy']))
if __name__ == '__main__':
tf.app.run()
| apache-2.0 |
compmem/ptsa | ptsa/plotting/logo.py | 1 | 2563 | """
Logo design inspired by the matplotlib logo by Tony Yu <[email protected]>.
"""
import numpy as np
import matplotlib as mpl
import matplotlib.pyplot as plt
import matplotlib.cm as cm
import matplotlib.mlab as mlab
mpl.rcParams['xtick.labelsize'] = 10
mpl.rcParams['ytick.labelsize'] = 12
mpl.rcParams['axes.edgecolor'] = 'gray'
axalpha = 0.05
#figcolor = '#EFEFEF'
figcolor = 'white'
dpi = 80
fig = plt.figure(figsize=(4, 1.1),dpi=dpi)
fig.figurePatch.set_edgecolor(figcolor)
fig.figurePatch.set_facecolor(figcolor)
def add_timeseries():
ax = fig.add_axes([0., 0., 1., 1.])
x = np.linspace(0,1,1000)
freqs = [8,16,32,64]
# y = np.zeros(1000)
# for f in freqs:
# y = y + np.sin(x*np.pi*f*4 + f/60.)*(10.0/(f))
# y = y+.5
y = np.sin(x*np.pi*32)*.45 + .5
lines = plt.plot(x,y,
transform=ax.transAxes,
color="#11557c", alpha=0.25,)
ax.set_axis_off()
return ax
def add_ptsa_text(ax):
ax.text(0.95, 0.5, 'PTSA', color='#11557c', fontsize=65,
ha='right', va='center', alpha=1.0, transform=ax.transAxes)
def add_pizza():
ax = fig.add_axes([0.025, 0.075, 0.3, 0.85], polar=True, resolution=50)
ax.axesPatch.set_alpha(axalpha)
ax.set_axisbelow(True)
N = 8
arc = 2. * np.pi
theta = np.arange(0.0, arc, arc/N)
radii = 10 * np.array([0.79, 0.81, 0.78, 0.77, 0.79, 0.78, 0.83, 0.78])
width = np.pi / 4 * np.array([1.0]*N)
theta = theta[1:]
radii = radii[1:]
width = width[1:]
bars = ax.bar(theta, radii, width=width, bottom=0.0)
for r, bar in zip(radii, bars):
bar.set_facecolor(cm.hot(r/10.))
bar.set_edgecolor('r')
bar.set_alpha(0.6)
for label in ax.get_xticklabels() + ax.get_yticklabels():
label.set_visible(False)
for line in ax.get_ygridlines() + ax.get_xgridlines():
line.set_lw(0.8)
line.set_alpha(0.9)
line.set_ls('-')
line.set_color('0.5')
# add some veggie peperoni
#theta = np.array([.08,.18,.32,.46,.54,.68,.77,.85,.96]) * np.pi * 2.0
#radii = 10*np.array([.6,.38,.58,.5,.62,.42,.58,.67,.45])
theta = np.array([.18,.32,.46,.54,.68,.77,.85,.96]) * np.pi * 2.0
radii = 10*np.array([.38,.58,.5,.62,.42,.58,.67,.45])
c = plt.scatter(theta,radii,c='r',s=7**2)
c.set_alpha(0.75)
ax.set_yticks(np.arange(1, 9, 2))
ax.set_rmax(9)
if __name__ == '__main__':
main_axes = add_timeseries()
add_pizza()
#add_ptsa_text(main_axes)
#plt.show()
plt.savefig('logo.png')
| gpl-3.0 |
gakarak/FCN_MSCOCO_Food_Segmentation | MSCOCO_Processing/PythonAPI/run03_resize_images_and_masks_v1.py | 1 | 1791 | #!/usr/bin/python
# -*- coding: utf-8 -*-
__author__ = 'ar'
import os
import sys
import shutil
import pandas as pd
import numpy as np
import skimage.io as skio
import skimage.transform as sktf
######################################
def makeDirIfNotExists(pathToDir, isCleanIfExists=True):
"""
create directory if directory is absent
:param pathToDir: path to directory
:param isCleanIfExists: flag: clean directory if directory exists
:return: None
"""
if os.path.isdir(pathToDir) and isCleanIfExists:
shutil.rmtree(pathToDir)
if not os.path.isdir(pathToDir):
os.makedirs(pathToDir)
######################################
if __name__ == '__main__':
parSize = 128
fidx = '/mnt/data1T2/datasets2/mscoco/raw-data/train2014-food2/idx.txt'
wdir = os.path.dirname(fidx)
dataIdx=pd.read_csv(fidx, header=None)
listPathImg = [os.path.join(wdir, xx) for xx in dataIdx[0]]
#
outDir = '%s-%dx%d' % (wdir, parSize,parSize)
makeDirIfNotExists(outDir, isCleanIfExists=True)
numImages = len(listPathImg)
for ii,pp in enumerate(listPathImg):
tfnImg = os.path.basename(pp)
tfnMsk = '%s-mskfood.png' % tfnImg
finpMsk = os.path.join(wdir, tfnMsk)
foutImg = os.path.join(outDir, tfnImg)
foutMsk = os.path.join(outDir, tfnMsk)
if (ii%20)==0:
print ('[%d/%d] : %s --> %s' % (ii, numImages, tfnImg, foutImg))
timg = skio.imread(pp)
tmsk = skio.imread(finpMsk)
timgr = sktf.resize(timg, (parSize, parSize), preserve_range=True).astype(np.uint8)
tmskr = sktf.resize(tmsk, (parSize, parSize), order=0, preserve_range=True).astype(np.uint8)
skio.imsave(foutImg, timgr)
skio.imsave(foutMsk, tmskr)
print ('----')
| apache-2.0 |
lthurlow/Network-Grapher | proj/external/matplotlib-1.2.1/lib/mpl_examples/mplot3d/pathpatch3d_demo.py | 10 | 1527 | import matplotlib.pyplot as plt
from matplotlib.patches import Circle, PathPatch
# register Axes3D class with matplotlib by importing Axes3D
from mpl_toolkits.mplot3d import Axes3D
import mpl_toolkits.mplot3d.art3d as art3d
from matplotlib.text import TextPath
from matplotlib.transforms import Affine2D
def text3d(ax, xyz, s, zdir="z", size=None, angle=0, usetex=False, **kwargs):
x, y, z = xyz
if zdir == "y":
xy1, z1 = (x, z), y
elif zdir == "y":
xy1, z1 = (y, z), x
else:
xy1, z1 = (x, y), z
text_path = TextPath((0, 0), s, size=size, usetex=usetex)
trans = Affine2D().rotate(angle).translate(xy1[0], xy1[1])
p1 = PathPatch(trans.transform_path(text_path), **kwargs)
ax.add_patch(p1)
art3d.pathpatch_2d_to_3d(p1, z=z1, zdir=zdir)
fig = plt.figure()
ax = fig.add_subplot(111, projection='3d')
p = Circle((5, 5), 3)
ax.add_patch(p)
art3d.pathpatch_2d_to_3d(p, z=0, zdir="x")
text3d(ax, (4, -2, 0), "X-axis", zdir="z", size=.5, usetex=False,
ec="none", fc="k")
text3d(ax, (12, 4, 0), "Y-axis", zdir="z", size=.5, usetex=False,
angle=.5*3.14159, ec="none", fc="k")
text3d(ax, (12, 10, 4), "Z-axis", zdir="y", size=.5, usetex=False,
angle=.5*3.14159, ec="none", fc="k")
text3d(ax, (1, 5, 0),
r"$\displaystyle G_{\mu\nu} + \Lambda g_{\mu\nu} = "
r"\frac{8\pi G}{c^4} T_{\mu\nu} $",
zdir="z", size=1, usetex=True,
ec="none", fc="k")
ax.set_xlim3d(0, 10)
ax.set_ylim3d(0, 10)
ax.set_zlim3d(0, 10)
plt.show()
| mit |
rzarcone/CAEs | debug_cae_model.py | 1 | 5501 | import tensorflow as tf
import matplotlib.pyplot as plt
import numpy as np
import os
from tensorflow.python import debug as tf_debug
from cae_model import cae
params = {}
#shitty hard coding
params["n_mem"] = 7680 #32768 #49152 for color, 32768 for grayscale
#general params
params["run_name"] = "debug_ent_test_med_compress"
#params["file_location"] = "/media/tbell/datasets/natural_images.txt"
params["file_location"] = "/media/tbell/datasets/test_images.txt"
params["gpu_ids"] = ["0"]#['0','1']
params["output_location"] = os.path.expanduser("~")+"/CAE_Project/CAEs/model_outputs/"+params["run_name"]
params["num_threads"] = 6
params["num_epochs"] = 20
#params["epoch_size"] = 112682
params["epoch_size"] = 49900
params["eval_interval"] = 1
params["seed"] = 1234567890
#checkpoint params
params["run_from_check"] = False
params["check_load_run_name"] = "7680_med_compress_pcm"
params["check_load_path"] = os.path.expanduser("~")+"/CAE_Project/CAEs/model_outputs/"+params["check_load_run_name"]+"/checkpoints/chkpt_ep39-45040"
#image params
params["shuffle_inputs"] = True
params["batch_size"] = 100
params["img_shape_y"] = 256
params["num_colors"] = 1
params["downsample_images"] = True
params["downsample_method"] = "resize" # can be "crop" or "resize"
#learning rates
params["init_learning_rate"] = 5.0e-4
params["decay_steps"] = 10000#epoch_size*0.5*num_epochs #0.5*epoch_size
params["staircase"] = True
params["decay_rate"] = 0.9
#layer params
params["memristorify"] = False
params["god_damn_network"] = True
params["relu"] = False
#layer dimensions
params["input_channels"] = [params["num_colors"], 128, 128]
params["output_channels"] = [128, 128, 30]
params["patch_size_y"] = [9, 5, 5]
params["strides"] = [4, 2, 2]
#memristor params
params["GAMMA"] = 1.0 # slope of the out of bounds cost
params["mem_v_min"] = -1.0
params["mem_v_max"] = 1.0
params["gauss_chan"] = False
#entropy params
params["LAMBDA"] = 0.1
params["num_triangles"] = 20
params["mle_lr"] = 0.1
params["num_mle_steps"] = 5
params["quant_noise_scale"] = 1.0/128.0 # simulating quantizing u in {-1.0, 1.0} to uint8 (256 values)
mle_triangle_centers = np.linspace(params["mem_v_min"], params["mem_v_max"], params["num_triangles"])
cae_model = cae(params)
config = tf.ConfigProto()
config.gpu_options.allow_growth = True
config.allow_soft_placement = True
config.log_device_placement = False # for debugging - log devices used by each variable
with tf.Session(config=config, graph=cae_model.graph) as sess:
sess = tf_debug.LocalCLIDebugWrapperSession(sess)
sess.add_tensor_filter("has_inf_or_nan", tf_debug.has_inf_or_nan)
sess.run(cae_model.init_op)
if cae_model.params["run_from_check"] == True:
cae_model.full_saver.restore(sess, cae_model.params["check_load_path"])
# Coordinator manages threads, checks for stopping requests
coord = tf.train.Coordinator()
# queue_runners are created by helper functions tf.train.string_input_producer() and tf.train.batch_join()
enqueue_threads = tf.train.start_queue_runners(sess, coord=coord, start=True)
feed_dict={cae_model.triangle_centers:mle_triangle_centers}
for epoch_idx in range(cae_model.params["num_epochs"]):
for batch_idx in range(cae_model.params["batches_per_epoch"]):
# Add quantization noise if not using a fixed channel
if not params["memristorify"] and not params["gauss_chan"]:
quant_noise = np.random.uniform(-params["quant_noise_scale"], params["quant_noise_scale"],
size=(cae_model.params["effective_batch_size"], cae_model.params["n_mem"]))
feed_dict[cae_model.quantization_noise] = quant_noise
else:
mem_std_eps = np.random.standard_normal((cae_model.params["effective_batch_size"],
cae_model.params["n_mem"])).astype(np.float32)
feed_dict[cae_model.memristor_std_eps] = mem_std_eps
# Update MLE estimate
sess.run(cae_model.reset_mle_thetas, feed_dict)
for mle_step in range(params["num_mle_steps"]):
sess.run(cae_model.mle_update, feed_dict)
# Update network weights
_, step = sess.run([cae_model.train_op, cae_model.global_step], feed_dict)
# Eval model
if step % cae_model.params["eval_interval"] == 0:
model_vars = [cae_model.merged_summaries, cae_model.reg_loss, cae_model.recon_loss,
cae_model.ent_loss, cae_model.total_loss, cae_model.batch_MSE]
[summary, ev_reg_loss, ev_recon_loss, ev_ent_loss, ev_total_loss, mse] = sess.run(model_vars, feed_dict)
cae_model.train_writer.add_summary(summary, step)
print("step %04d\treg_loss %03g\trecon_loss %g\tent_loss %g\ttotal_loss %g\tMSE %g"%(
step, ev_reg_loss, ev_recon_loss, ev_ent_loss, ev_total_loss, mse))
#Checkpoint and save image of weights each epoch
cae_model.full_saver.save(sess, save_path=cae_model.params["output_location"]+"/checkpoints/chkpt",
global_step=cae_model.global_step)
w_enc_eval = np.squeeze(sess.run(tf.transpose(cae_model.w_list[0], perm=[3,0,1,2])))
pf.save_data_tiled(w_enc_eval, normalize=True, title="Weights0",
save_filename=cae_model.params["weight_save_filename"]+"/Weights_enc_ep"+str(epoch_idx)+".png")
w_dec_eval = np.squeeze(sess.run(tf.transpose(cae_model.w_list[-1], perm=[3,0,1,2])))
pf.save_data_tiled(w_dec_eval, normalize=True, title="Weights-1",
save_filename=cae_model.params["weight_save_filename"]+"/Weights_dec_ep"+str(epoch_idx)+".png")
coord.request_stop()
coord.join(enqueue_threads)
| bsd-2-clause |
andyh616/mne-python | tutorials/plot_cluster_stats_spatio_temporal_repeated_measures_anova.py | 15 | 11568 | """
.. _tut_stats_cluster_source_rANOVA:
======================================================================
Repeated measures ANOVA on source data with spatio-temporal clustering
======================================================================
This example illustrates how to make use of the clustering functions
for arbitrary, self-defined contrasts beyond standard t-tests. In this
case we will tests if the differences in evoked responses between
stimulation modality (visual VS auditory) depend on the stimulus
location (left vs right) for a group of subjects (simulated here
using one subject's data). For this purpose we will compute an
interaction effect using a repeated measures ANOVA. The multiple
comparisons problem is addressed with a cluster-level permutation test
across space and time.
"""
# Authors: Alexandre Gramfort <[email protected]>
# Eric Larson <[email protected]>
# Denis Engemannn <[email protected]>
#
# License: BSD (3-clause)
import os.path as op
import numpy as np
from numpy.random import randn
import matplotlib.pyplot as plt
import mne
from mne import (io, spatial_tris_connectivity, compute_morph_matrix,
grade_to_tris)
from mne.stats import (spatio_temporal_cluster_test, f_threshold_mway_rm,
f_mway_rm, summarize_clusters_stc)
from mne.minimum_norm import apply_inverse, read_inverse_operator
from mne.datasets import sample
print(__doc__)
###############################################################################
# Set parameters
data_path = sample.data_path()
raw_fname = data_path + '/MEG/sample/sample_audvis_filt-0-40_raw.fif'
event_fname = data_path + '/MEG/sample/sample_audvis_filt-0-40_raw-eve.fif'
subjects_dir = data_path + '/subjects'
tmin = -0.2
tmax = 0.3 # Use a lower tmax to reduce multiple comparisons
# Setup for reading the raw data
raw = io.Raw(raw_fname)
events = mne.read_events(event_fname)
###############################################################################
# Read epochs for all channels, removing a bad one
raw.info['bads'] += ['MEG 2443']
picks = mne.pick_types(raw.info, meg=True, eog=True, exclude='bads')
# we'll load all four conditions that make up the 'two ways' of our ANOVA
event_id = dict(l_aud=1, r_aud=2, l_vis=3, r_vis=4)
reject = dict(grad=1000e-13, mag=4000e-15, eog=150e-6)
epochs = mne.Epochs(raw, events, event_id, tmin, tmax, picks=picks,
baseline=(None, 0), reject=reject, preload=True)
# Equalize trial counts to eliminate bias (which would otherwise be
# introduced by the abs() performed below)
epochs.equalize_event_counts(event_id, copy=False)
###############################################################################
# Transform to source space
fname_inv = data_path + '/MEG/sample/sample_audvis-meg-oct-6-meg-inv.fif'
snr = 3.0
lambda2 = 1.0 / snr ** 2
method = "dSPM" # use dSPM method (could also be MNE or sLORETA)
inverse_operator = read_inverse_operator(fname_inv)
# we'll only use one hemisphere to speed up this example
# instead of a second vertex array we'll pass an empty array
sample_vertices = [inverse_operator['src'][0]['vertno'], np.array([], int)]
# Let's average and compute inverse, then resample to speed things up
conditions = []
for cond in ['l_aud', 'r_aud', 'l_vis', 'r_vis']: # order is important
evoked = epochs[cond].average()
evoked.resample(50)
condition = apply_inverse(evoked, inverse_operator, lambda2, method)
# Let's only deal with t > 0, cropping to reduce multiple comparisons
condition.crop(0, None)
conditions.append(condition)
tmin = conditions[0].tmin
tstep = conditions[0].tstep
###############################################################################
# Transform to common cortical space
# Normally you would read in estimates across several subjects and morph
# them to the same cortical space (e.g. fsaverage). For example purposes,
# we will simulate this by just having each "subject" have the same
# response (just noisy in source space) here.
# we'll only consider the left hemisphere in this example.
n_vertices_sample, n_times = conditions[0].lh_data.shape
n_subjects = 7
print('Simulating data for %d subjects.' % n_subjects)
# Let's make sure our results replicate, so set the seed.
np.random.seed(0)
X = randn(n_vertices_sample, n_times, n_subjects, 4) * 10
for ii, condition in enumerate(conditions):
X[:, :, :, ii] += condition.lh_data[:, :, np.newaxis]
# It's a good idea to spatially smooth the data, and for visualization
# purposes, let's morph these to fsaverage, which is a grade 5 source space
# with vertices 0:10242 for each hemisphere. Usually you'd have to morph
# each subject's data separately (and you might want to use morph_data
# instead), but here since all estimates are on 'sample' we can use one
# morph matrix for all the heavy lifting.
fsave_vertices = [np.arange(10242), np.array([], int)] # right hemi is empty
morph_mat = compute_morph_matrix('sample', 'fsaverage', sample_vertices,
fsave_vertices, 20, subjects_dir)
n_vertices_fsave = morph_mat.shape[0]
# We have to change the shape for the dot() to work properly
X = X.reshape(n_vertices_sample, n_times * n_subjects * 4)
print('Morphing data.')
X = morph_mat.dot(X) # morph_mat is a sparse matrix
X = X.reshape(n_vertices_fsave, n_times, n_subjects, 4)
# Now we need to prepare the group matrix for the ANOVA statistic.
# To make the clustering function work correctly with the
# ANOVA function X needs to be a list of multi-dimensional arrays
# (one per condition) of shape: samples (subjects) x time x space
X = np.transpose(X, [2, 1, 0, 3]) # First we permute dimensions
# finally we split the array into a list a list of conditions
# and discard the empty dimension resulting from the split using numpy squeeze
X = [np.squeeze(x) for x in np.split(X, 4, axis=-1)]
###############################################################################
# Prepare function for arbitrary contrast
# As our ANOVA function is a multi-purpose tool we need to apply a few
# modifications to integrate it with the clustering function. This
# includes reshaping data, setting default arguments and processing
# the return values. For this reason we'll write a tiny dummy function.
# We will tell the ANOVA how to interpret the data matrix in terms of
# factors. This is done via the factor levels argument which is a list
# of the number factor levels for each factor.
factor_levels = [2, 2]
# Finally we will pick the interaction effect by passing 'A:B'.
# (this notation is borrowed from the R formula language)
effects = 'A:B' # Without this also the main effects will be returned.
# Tell the ANOVA not to compute p-values which we don't need for clustering
return_pvals = False
# a few more convenient bindings
n_times = X[0].shape[1]
n_conditions = 4
# A stat_fun must deal with a variable number of input arguments.
def stat_fun(*args):
# Inside the clustering function each condition will be passed as
# flattened array, necessitated by the clustering procedure.
# The ANOVA however expects an input array of dimensions:
# subjects X conditions X observations (optional).
# The following expression catches the list input
# and swaps the first and the second dimension, and finally calls ANOVA.
return f_mway_rm(np.swapaxes(args, 1, 0), factor_levels=factor_levels,
effects=effects, return_pvals=return_pvals)[0]
# get f-values only.
# Note. for further details on this ANOVA function consider the
# corresponding time frequency example.
###############################################################################
# Compute clustering statistic
# To use an algorithm optimized for spatio-temporal clustering, we
# just pass the spatial connectivity matrix (instead of spatio-temporal)
source_space = grade_to_tris(5)
# as we only have one hemisphere we need only need half the connectivity
lh_source_space = source_space[source_space[:, 0] < 10242]
print('Computing connectivity.')
connectivity = spatial_tris_connectivity(lh_source_space)
# Now let's actually do the clustering. Please relax, on a small
# notebook and one single thread only this will take a couple of minutes ...
pthresh = 0.0005
f_thresh = f_threshold_mway_rm(n_subjects, factor_levels, effects, pthresh)
# To speed things up a bit we will ...
n_permutations = 128 # ... run fewer permutations (reduces sensitivity)
print('Clustering.')
T_obs, clusters, cluster_p_values, H0 = clu = \
spatio_temporal_cluster_test(X, connectivity=connectivity, n_jobs=1,
threshold=f_thresh, stat_fun=stat_fun,
n_permutations=n_permutations,
buffer_size=None)
# Now select the clusters that are sig. at p < 0.05 (note that this value
# is multiple-comparisons corrected).
good_cluster_inds = np.where(cluster_p_values < 0.05)[0]
###############################################################################
# Visualize the clusters
print('Visualizing clusters.')
# Now let's build a convenient representation of each cluster, where each
# cluster becomes a "time point" in the SourceEstimate
stc_all_cluster_vis = summarize_clusters_stc(clu, tstep=tstep,
vertices=fsave_vertices,
subject='fsaverage')
# Let's actually plot the first "time point" in the SourceEstimate, which
# shows all the clusters, weighted by duration
subjects_dir = op.join(data_path, 'subjects')
# The brighter the color, the stronger the interaction between
# stimulus modality and stimulus location
brain = stc_all_cluster_vis.plot(subjects_dir=subjects_dir, colormap='mne',
time_label='Duration significant (ms)')
brain.set_data_time_index(0)
brain.show_view('lateral')
brain.save_image('cluster-lh.png')
brain.show_view('medial')
###############################################################################
# Finally, let's investigate interaction effect by reconstructing the time
# courses
inds_t, inds_v = [(clusters[cluster_ind]) for ii, cluster_ind in
enumerate(good_cluster_inds)][0] # first cluster
times = np.arange(X[0].shape[1]) * tstep * 1e3
plt.figure()
colors = ['y', 'b', 'g', 'purple']
event_ids = ['l_aud', 'r_aud', 'l_vis', 'r_vis']
for ii, (condition, color, eve_id) in enumerate(zip(X, colors, event_ids)):
# extract time course at cluster vertices
condition = condition[:, :, inds_v]
# normally we would normalize values across subjects but
# here we use data from the same subject so we're good to just
# create average time series across subjects and vertices.
mean_tc = condition.mean(axis=2).mean(axis=0)
std_tc = condition.std(axis=2).std(axis=0)
plt.plot(times, mean_tc.T, color=color, label=eve_id)
plt.fill_between(times, mean_tc + std_tc, mean_tc - std_tc, color='gray',
alpha=0.5, label='')
ymin, ymax = mean_tc.min() - 5, mean_tc.max() + 5
plt.xlabel('Time (ms)')
plt.ylabel('Activation (F-values)')
plt.xlim(times[[0, -1]])
plt.ylim(ymin, ymax)
plt.fill_betweenx((ymin, ymax), times[inds_t[0]],
times[inds_t[-1]], color='orange', alpha=0.3)
plt.legend()
plt.title('Interaction between stimulus-modality and location.')
plt.show()
| bsd-3-clause |
setten/pymatgen | pymatgen/analysis/diffusion_analyzer.py | 3 | 37712 | # coding: utf-8
# Copyright (c) Pymatgen Development Team.
# Distributed under the terms of the MIT License.
from __future__ import division, unicode_literals
import numpy as np
import warnings
import scipy.constants as const
from monty.json import MSONable
from pymatgen.analysis.structure_matcher import StructureMatcher, \
OrderDisorderElementComparator
from pymatgen.core.periodic_table import get_el_sp
from pymatgen.core.structure import Structure
from pymatgen.io.vasp.outputs import Vasprun
from pymatgen.util.coord import pbc_diff
"""
A module to perform diffusion analyses (e.g. calculating diffusivity from
mean square displacements etc.). If you use this module, please consider
citing the following papers::
Ong, S. P., Mo, Y., Richards, W. D., Miara, L., Lee, H. S., & Ceder, G.
(2013). Phase stability, electrochemical stability and ionic conductivity
of the Li10+-1MP2X12 (M = Ge, Si, Sn, Al or P, and X = O, S or Se) family
of superionic conductors. Energy & Environmental Science, 6(1), 148.
doi:10.1039/c2ee23355j
Mo, Y., Ong, S. P., & Ceder, G. (2012). First Principles Study of the
Li10GeP2S12 Lithium Super Ionic Conductor Material. Chemistry of Materials,
24(1), 15-17. doi:10.1021/cm203303y
"""
__author__ = "Will Richards, Shyue Ping Ong"
__version__ = "0.2"
__maintainer__ = "Will Richards"
__email__ = "[email protected]"
__status__ = "Beta"
__date__ = "5/2/13"
class DiffusionAnalyzer(MSONable):
"""
Class for performing diffusion analysis.
.. attribute: diffusivity
Diffusivity in cm^2 / s
.. attribute: chg_diffusivity
Charge diffusivity in cm^2 / s
.. attribute: conductivity
Conductivity in mS / cm
.. attribute: chg_conductivity
Conductivity derived from Nernst-Einstein equation using charge
diffusivity, in mS / cm
.. attribute: diffusivity_components
A vector with diffusivity in the a, b and c directions in cm^2 / s
.. attribute: conductivity_components
A vector with conductivity in the a, b and c directions in mS / cm
.. attribute: diffusivity_std_dev
Std dev in diffusivity in cm^2 / s. Note that this makes sense only
for non-smoothed analyses.
.. attribute: chg_diffusivity_std_dev
Std dev in charge diffusivity in cm^2 / s. Note that this makes sense only
for non-smoothed analyses.
.. attribute: conductivity_std_dev
Std dev in conductivity in mS / cm. Note that this makes sense only
for non-smoothed analyses.
.. attribute: diffusivity_components_std_dev
A vector with std dev. in diffusivity in the a, b and c directions in
cm^2 / cm. Note that this makes sense only for non-smoothed analyses.
.. attribute: conductivity_components_std_dev
A vector with std dev. in conductivity in the a, b and c directions
in mS / cm. Note that this makes sense only for non-smoothed analyses.
.. attribute: max_framework_displacement
The maximum (drift adjusted) distance of any framework atom from its
starting location in A.
.. attribute: max_ion_displacements
nions x 1 array of the maximum displacement of each individual ion.
.. attribute: msd
nsteps x 1 array of the mean square displacement of specie.
.. attribute: mscd
nsteps x 1 array of the mean square charge displacement of specie.
.. attribute: msd_components
nsteps x 3 array of the MSD in each lattice direction of specie.
.. attribute: sq_disp_ions
The square displacement of all ion (both specie and other ions) as a
nions x nsteps array.
.. attribute: dt
Time coordinate array.
.. attribute: haven_ratio
Haven ratio defined as diffusivity / chg_diffusivity.
"""
def __init__(self, structure, displacements, specie, temperature,
time_step, step_skip, smoothed="max", min_obs=30,
avg_nsteps=1000, lattices=None):
"""
This constructor is meant to be used with pre-processed data.
Other convenient constructors are provided as class methods (see
from_vaspruns and from_files).
Given a matrix of displacements (see arguments below for expected
format), the diffusivity is given by::
D = 1 / 2dt * <mean square displacement>
where d is the dimensionality, t is the time. To obtain a reliable
diffusion estimate, a least squares regression of the MSD against
time to obtain the slope, which is then related to the diffusivity.
For traditional analysis, use smoothed=False and weighted=False.
Args:
structure (Structure): Initial structure.
displacements (array): Numpy array of with shape [site,
time step, axis]
specie (Element/Specie): Specie to calculate diffusivity for as a
String. E.g., "Li".
temperature (float): Temperature of the diffusion run in Kelvin.
time_step (int): Time step between measurements.
step_skip (int): Sampling frequency of the displacements (
time_step is multiplied by this number to get the real time
between measurements)
smoothed (str): Whether to smooth the MSD, and what mode to smooth.
Supported modes are:
i. "max", which tries to use the maximum #
of data points for each time origin, subject to a
minimum # of observations given by min_obs, and then
weights the observations based on the variance
accordingly. This is the default.
ii. "constant", in which each timestep is averaged over
the number of time_steps given by min_steps.
iii. None / False / any other false-like quantity. No
smoothing.
min_obs (int): Used with smoothed="max". Minimum number of
observations to have before including in the MSD vs dt
calculation. E.g. If a structure has 10 diffusing atoms,
and min_obs = 30, the MSD vs dt will be
calculated up to dt = total_run_time / 3, so that each
diffusing atom is measured at least 3 uncorrelated times.
Only applies in smoothed="max".
avg_nsteps (int): Used with smoothed="constant". Determines the
number of time steps to average over to get the msd for each
timestep. Default of 1000 is usually pretty good.
lattices (array): Numpy array of lattice matrix of every step. Used
for NPT-AIMD. For NVT-AIMD, the lattice at each time step is
set to the lattice in the "structure" argument.
"""
self.structure = structure
self.disp = displacements
self.specie = specie
self.temperature = temperature
self.time_step = time_step
self.step_skip = step_skip
self.min_obs = min_obs
self.smoothed = smoothed
self.avg_nsteps = avg_nsteps
self.lattices = lattices
if lattices is None:
self.lattices = np.array([structure.lattice.matrix.tolist()])
indices = []
framework_indices = []
for i, site in enumerate(structure):
if site.specie.symbol == specie:
indices.append(i)
else:
framework_indices.append(i)
if self.disp.shape[1] < 2:
self.diffusivity = 0.
self.conductivity = 0.
self.diffusivity_components = np.array([0., 0., 0.])
self.conductivity_components = np.array([0., 0., 0.])
self.max_framework_displacement = 0
else:
framework_disp = self.disp[framework_indices]
drift = np.average(framework_disp, axis=0)[None, :, :]
# drift corrected position
dc = self.disp - drift
nions, nsteps, dim = dc.shape
if not smoothed:
timesteps = np.arange(0, nsteps)
elif smoothed == "constant":
if nsteps <= avg_nsteps:
raise ValueError('Not enough data to calculate diffusivity')
timesteps = np.arange(0, nsteps - avg_nsteps)
else:
# limit the number of sampled timesteps to 200
min_dt = int(1000 / (self.step_skip * self.time_step))
max_dt = min(len(indices) * nsteps // self.min_obs, nsteps)
if min_dt >= max_dt:
raise ValueError('Not enough data to calculate diffusivity')
timesteps = np.arange(min_dt, max_dt,
max(int((max_dt - min_dt) / 200), 1))
dt = timesteps * self.time_step * self.step_skip
# calculate the smoothed msd values
msd = np.zeros_like(dt, dtype=np.double)
sq_disp_ions = np.zeros((len(dc), len(dt)), dtype=np.double)
msd_components = np.zeros(dt.shape + (3,))
# calculate mean square charge displacement
mscd = np.zeros_like(msd, dtype=np.double)
for i, n in enumerate(timesteps):
if not smoothed:
dx = dc[:, i:i + 1, :]
dcomponents = dc[:, i:i + 1, :]
elif smoothed == "constant":
dx = dc[:, i:i + avg_nsteps, :] - dc[:, 0:avg_nsteps, :]
dcomponents = dc[:, i:i + avg_nsteps, :] \
- dc[:, 0:avg_nsteps, :]
else:
dx = dc[:, n:, :] - dc[:, :-n, :]
dcomponents = dc[:, n:, :] - dc[:, :-n, :]
# Get msd
sq_disp = dx ** 2
sq_disp_ions[:, i] = np.average(np.sum(sq_disp, axis=2), axis=1)
msd[i] = np.average(sq_disp_ions[:, i][indices])
msd_components[i] = np.average(dcomponents[indices] ** 2,
axis=(0, 1))
# Get mscd
sq_chg_disp = np.sum(dx[indices, :, :], axis=0) ** 2
mscd[i] = np.average(np.sum(sq_chg_disp, axis=1), axis=0) / len(indices)
def weighted_lstsq(a, b):
if smoothed == "max":
# For max smoothing, we need to weight by variance.
w_root = (1 / dt) ** 0.5
return np.linalg.lstsq(a * w_root[:, None], b * w_root)
else:
return np.linalg.lstsq(a, b)
# Get self diffusivity
m_components = np.zeros(3)
m_components_res = np.zeros(3)
a = np.ones((len(dt), 2))
a[:, 0] = dt
for i in range(3):
(m, c), res, rank, s = weighted_lstsq(a, msd_components[:, i])
m_components[i] = max(m, 1e-15)
m_components_res[i] = res[0]
(m, c), res, rank, s = weighted_lstsq(a, msd)
# m shouldn't be negative
m = max(m, 1e-15)
# Get also the charge diffusivity
(m_chg, c_chg), res_chg, _, _ = weighted_lstsq(a, mscd)
# m shouldn't be negative
m_chg = max(m_chg, 1e-15)
# factor of 10 is to convert from A^2/fs to cm^2/s
# factor of 6 is for dimensionality
conv_factor = get_conversion_factor(self.structure, self.specie,
self.temperature)
self.diffusivity = m / 60
self.chg_diffusivity = m_chg / 60
# Calculate the error in the diffusivity using the error in the
# slope from the lst sq.
# Variance in slope = n * Sum Squared Residuals / (n * Sxx - Sx
# ** 2) / (n-2).
n = len(dt)
# Pre-compute the denominator since we will use it later.
# We divide dt by 1000 to avoid overflow errors in some systems (
# e.g., win). This is subsequently corrected where denom is used.
denom = (n * np.sum((dt / 1000) ** 2) - np.sum(dt / 1000) ** 2) * (
n - 2)
self.diffusivity_std_dev = np.sqrt(n * res[0] / denom) / 60 / 1000
self.chg_diffusivity_std_dev = np.sqrt(n * res_chg[0] / denom) / 60 / 1000
self.conductivity = self.diffusivity * conv_factor
self.chg_conductivity = self.chg_diffusivity * conv_factor
self.conductivity_std_dev = self.diffusivity_std_dev * conv_factor
self.diffusivity_components = m_components / 20
self.diffusivity_components_std_dev = np.sqrt(
n * m_components_res / denom) / 20 / 1000
self.conductivity_components = self.diffusivity_components * \
conv_factor
self.conductivity_components_std_dev = \
self.diffusivity_components_std_dev * conv_factor
# Drift and displacement information.
self.drift = drift
self.corrected_displacements = dc
self.max_ion_displacements = np.max(np.sum(
dc ** 2, axis=-1) ** 0.5, axis=1)
self.max_framework_displacement = \
np.max(self.max_ion_displacements[framework_indices])
self.msd = msd
self.mscd = mscd
self.haven_ratio = self.diffusivity / self.chg_diffusivity
self.sq_disp_ions = sq_disp_ions
self.msd_components = msd_components
self.dt = dt
self.indices = indices
self.framework_indices = framework_indices
def get_drift_corrected_structures(self, start=None, stop=None, step=None):
"""
Returns an iterator for the drift-corrected structures. Use of
iterator is to reduce memory usage as # of structures in MD can be
huge. You don't often need all the structures all at once.
Args:
start, stop, step (int): applies a start/stop/step to the iterator.
Faster than applying it after generation, as it reduces the
number of structures created.
"""
coords = np.array(self.structure.cart_coords)
species = self.structure.species_and_occu
lattices = self.lattices
nsites, nsteps, dim = self.corrected_displacements.shape
for i in range(start or 0, stop or nsteps, step or 1):
latt = lattices[0] if len(lattices) == 1 else lattices[i]
yield Structure(
latt, species,
coords + self.corrected_displacements[:, i, :],
coords_are_cartesian=True)
def get_summary_dict(self, include_msd_t=False, include_mscd_t=False):
"""
Provides a summary of diffusion information.
Args:
include_msd_t (bool): Whether to include mean square displace and
time data with the data.
include_msd_t (bool): Whether to include mean square charge displace and
time data with the data.
Returns:
(dict) of diffusion and conductivity data.
"""
d = {
"D": self.diffusivity,
"D_sigma": self.diffusivity_std_dev,
"D_charge": self.chg_diffusivity,
"D_charge_sigma": self.chg_diffusivity_std_dev,
"S": self.conductivity,
"S_sigma": self.conductivity_std_dev,
"S_charge": self.chg_conductivity,
"D_components": self.diffusivity_components.tolist(),
"S_components": self.conductivity_components.tolist(),
"D_components_sigma": self.diffusivity_components_std_dev.tolist(),
"S_components_sigma": self.conductivity_components_std_dev.tolist(),
"specie": str(self.specie),
"step_skip": self.step_skip,
"time_step": self.time_step,
"temperature": self.temperature,
"max_framework_displacement": self.max_framework_displacement,
"Haven_ratio": self.haven_ratio
}
if include_msd_t:
d["msd"] = self.msd.tolist()
d["msd_components"] = self.msd_components.tolist()
d["dt"] = self.dt.tolist()
if include_mscd_t:
d["mscd"] = self.mscd.tolist()
return d
def get_framework_rms_plot(self, plt=None, granularity=200,
matching_s=None):
"""
Get the plot of rms framework displacement vs time. Useful for checking
for melting, especially if framework atoms can move via paddle-wheel
or similar mechanism (which would show up in max framework displacement
but doesn't constitute melting).
Args:
plt (matplotlib.pyplot): If plt is supplied, changes will be made
to an existing plot. Otherwise, a new plot will be created.
granularity (int): Number of structures to match
matching_s (Structure): Optionally match to a disordered structure
instead of the first structure in the analyzer. Required when
a secondary mobile ion is present.
Notes:
The method doesn't apply to NPT-AIMD simulation analysis.
"""
from pymatgen.util.plotting import pretty_plot
if self.lattices is not None and len(self.lattices) > 1:
warnings.warn("Note the method doesn't apply to NPT-AIMD "
"simulation analysis!")
plt = pretty_plot(12, 8, plt=plt)
step = (self.corrected_displacements.shape[1] - 1) // (granularity - 1)
f = (matching_s or self.structure).copy()
f.remove_species([self.specie])
sm = StructureMatcher(primitive_cell=False, stol=0.6,
comparator=OrderDisorderElementComparator(),
allow_subset=True)
rms = []
for s in self.get_drift_corrected_structures(step=step):
s.remove_species([self.specie])
d = sm.get_rms_dist(f, s)
if d:
rms.append(d)
else:
rms.append((1, 1))
max_dt = (len(rms) - 1) * step * self.step_skip * self.time_step
if max_dt > 100000:
plot_dt = np.linspace(0, max_dt / 1000, len(rms))
unit = 'ps'
else:
plot_dt = np.linspace(0, max_dt, len(rms))
unit = 'fs'
rms = np.array(rms)
plt.plot(plot_dt, rms[:, 0], label='RMS')
plt.plot(plot_dt, rms[:, 1], label='max')
plt.legend(loc='best')
plt.xlabel("Timestep ({})".format(unit))
plt.ylabel("normalized distance")
plt.tight_layout()
return plt
def get_msd_plot(self, plt=None, mode="specie"):
"""
Get the plot of the smoothed msd vs time graph. Useful for
checking convergence. This can be written to an image file.
Args:
plt: A plot object. Defaults to None, which means one will be
generated.
mode (str): Determines type of msd plot. By "species", "sites",
or direction (default). If mode = "mscd", the smoothed mscd vs.
time will be plotted.
"""
from pymatgen.util.plotting import pretty_plot
plt = pretty_plot(12, 8, plt=plt)
if np.max(self.dt) > 100000:
plot_dt = self.dt / 1000
unit = 'ps'
else:
plot_dt = self.dt
unit = 'fs'
if mode == "species":
for sp in sorted(self.structure.composition.keys()):
indices = [i for i, site in enumerate(self.structure) if
site.specie == sp]
sd = np.average(self.sq_disp_ions[indices, :], axis=0)
plt.plot(plot_dt, sd, label=sp.__str__())
plt.legend(loc=2, prop={"size": 20})
elif mode == "sites":
for i, site in enumerate(self.structure):
sd = self.sq_disp_ions[i, :]
plt.plot(plot_dt, sd, label="%s - %d" % (
site.specie.__str__(), i))
plt.legend(loc=2, prop={"size": 20})
elif mode == "mscd":
plt.plot(plot_dt, self.mscd, 'r')
plt.legend(["Overall"], loc=2, prop={"size": 20})
else:
# Handle default / invalid mode case
plt.plot(plot_dt, self.msd, 'k')
plt.plot(plot_dt, self.msd_components[:, 0], 'r')
plt.plot(plot_dt, self.msd_components[:, 1], 'g')
plt.plot(plot_dt, self.msd_components[:, 2], 'b')
plt.legend(["Overall", "a", "b", "c"], loc=2, prop={"size": 20})
plt.xlabel("Timestep ({})".format(unit))
if mode == "mscd":
plt.ylabel("MSCD ($\\AA^2$)")
else:
plt.ylabel("MSD ($\\AA^2$)")
plt.tight_layout()
return plt
def plot_msd(self, mode="default"):
"""
Plot the smoothed msd vs time graph. Useful for checking convergence.
Args:
mode (str): Can be "default" (the default, shows only the MSD for
the diffusing specie, and its components), "ions" (individual
square displacements of all ions), "species" (mean square
displacement by specie), or "mscd" (overall mean square charge
displacement for diffusing specie).
"""
self.get_msd_plot(mode=mode).show()
def export_msdt(self, filename):
"""
Writes MSD data to a csv file that can be easily plotted in other
software.
Args:
filename (str): Filename. Supported formats are csv and dat. If
the extension is csv, a csv file is written. Otherwise,
a dat format is assumed.
"""
fmt = "csv" if filename.lower().endswith(".csv") else "dat"
delimiter = ", " if fmt == "csv" else " "
with open(filename, "wt") as f:
if fmt == "dat":
f.write("# ")
f.write(delimiter.join(["t", "MSD", "MSD_a", "MSD_b", "MSD_c",
"MSCD"]))
f.write("\n")
for dt, msd, msdc, mscd in zip(self.dt, self.msd,
self.msd_components, self.mscd):
f.write(delimiter.join(["%s" % v for v in [dt, msd] + list(
msdc) + [mscd]]))
f.write("\n")
@classmethod
def from_structures(cls, structures, specie, temperature,
time_step, step_skip, initial_disp=None,
initial_structure=None, **kwargs):
"""
Convenient constructor that takes in a list of Structure objects to
perform diffusion analysis.
Args:
structures ([Structure]): list of Structure objects (must be
ordered in sequence of run). E.g., you may have performed
sequential VASP runs to obtain sufficient statistics.
specie (Element/Specie): Specie to calculate diffusivity for as a
String. E.g., "Li".
temperature (float): Temperature of the diffusion run in Kelvin.
time_step (int): Time step between measurements.
step_skip (int): Sampling frequency of the displacements (
time_step is multiplied by this number to get the real time
between measurements)
initial_disp (np.ndarray): Sometimes, you need to iteratively
compute estimates of the diffusivity. This supplies an
initial displacement that will be added on to the initial
displacements. Note that this makes sense only when
smoothed=False.
initial_structure (Structure): Like initial_disp, this is used
for iterative computations of estimates of the diffusivity. You
typically need to supply both variables. This stipulates the
initial structure from which the current set of displacements
are computed.
\\*\\*kwargs: kwargs supported by the :class:`DiffusionAnalyzer`_.
Examples include smoothed, min_obs, avg_nsteps.
"""
p, l = [], []
for i, s in enumerate(structures):
if i == 0:
structure = s
p.append(np.array(s.frac_coords)[:, None])
l.append(s.lattice.matrix)
if initial_structure is not None:
p.insert(0, np.array(initial_structure.frac_coords)[:, None])
l.insert(0, initial_structure.lattice.matrix)
else:
p.insert(0, p[0])
l.insert(0, l[0])
p = np.concatenate(p, axis=1)
dp = p[:, 1:] - p[:, :-1]
dp = dp - np.round(dp)
f_disp = np.cumsum(dp, axis=1)
c_disp = [np.dot(d, m) for d, m in zip(f_disp, l)]
disp = np.array(c_disp)
# If is NVT-AIMD, clear lattice data.
if np.array_equal(l[0], l[-1]):
l = np.array([l[0]])
else:
l = np.array(l)
if initial_disp is not None:
disp += initial_disp[:, None, :]
return cls(structure, disp, specie, temperature, time_step,
step_skip=step_skip, lattices=l, **kwargs)
@classmethod
def from_vaspruns(cls, vaspruns, specie, initial_disp=None,
initial_structure=None, **kwargs):
"""
Convenient constructor that takes in a list of Vasprun objects to
perform diffusion analysis.
Args:
vaspruns ([Vasprun]): List of Vaspruns (must be ordered in
sequence of MD simulation). E.g., you may have performed
sequential VASP runs to obtain sufficient statistics.
specie (Element/Specie): Specie to calculate diffusivity for as a
String. E.g., "Li".
initial_disp (np.ndarray): Sometimes, you need to iteratively
compute estimates of the diffusivity. This supplies an
initial displacement that will be added on to the initial
displacements. Note that this makes sense only when
smoothed=False.
initial_structure (Structure): Like initial_disp, this is used
for iterative computations of estimates of the diffusivity. You
typically need to supply both variables. This stipulates the
initial stricture from which the current set of displacements
are computed.
\\*\\*kwargs: kwargs supported by the :class:`DiffusionAnalyzer`_.
Examples include smoothed, min_obs, avg_nsteps.
"""
def get_structures(vaspruns):
for i, vr in enumerate(vaspruns):
if i == 0:
step_skip = vr.ionic_step_skip or 1
final_structure = vr.initial_structure
temperature = vr.parameters['TEEND']
time_step = vr.parameters['POTIM']
yield step_skip, temperature, time_step
# check that the runs are continuous
fdist = pbc_diff(vr.initial_structure.frac_coords,
final_structure.frac_coords)
if np.any(fdist > 0.001):
raise ValueError('initial and final structures do not '
'match.')
final_structure = vr.final_structure
assert (vr.ionic_step_skip or 1) == step_skip
for s in vr.ionic_steps:
yield s['structure']
s = get_structures(vaspruns)
step_skip, temperature, time_step = next(s)
return cls.from_structures(
structures=s, specie=specie, temperature=temperature,
time_step=time_step, step_skip=step_skip,
initial_disp=initial_disp, initial_structure=initial_structure,
**kwargs)
@classmethod
def from_files(cls, filepaths, specie, step_skip=10, ncores=None,
initial_disp=None, initial_structure=None, **kwargs):
"""
Convenient constructor that takes in a list of vasprun.xml paths to
perform diffusion analysis.
Args:
filepaths ([str]): List of paths to vasprun.xml files of runs. (
must be ordered in sequence of MD simulation). For example,
you may have done sequential VASP runs and they are in run1,
run2, run3, etc. You should then pass in
["run1/vasprun.xml", "run2/vasprun.xml", ...].
specie (Element/Specie): Specie to calculate diffusivity for as a
String. E.g., "Li".
step_skip (int): Sampling frequency of the displacements (
time_step is multiplied by this number to get the real time
between measurements)
ncores (int): Numbers of cores to use for multiprocessing. Can
speed up vasprun parsing considerably. Defaults to None,
which means serial. It should be noted that if you want to
use multiprocessing, the number of ionic steps in all vasprun
.xml files should be a multiple of the ionic_step_skip.
Otherwise, inconsistent results may arise. Serial mode has no
such restrictions.
initial_disp (np.ndarray): Sometimes, you need to iteratively
compute estimates of the diffusivity. This supplies an
initial displacement that will be added on to the initial
displacements. Note that this makes sense only when
smoothed=False.
initial_structure (Structure): Like initial_disp, this is used
for iterative computations of estimates of the diffusivity. You
typically need to supply both variables. This stipulates the
initial structure from which the current set of displacements
are computed.
\\*\\*kwargs: kwargs supported by the :class:`DiffusionAnalyzer`_.
Examples include smoothed, min_obs, avg_nsteps.
"""
if ncores is not None and len(filepaths) > 1:
import multiprocessing
p = multiprocessing.Pool(ncores)
vaspruns = p.imap(_get_vasprun,
[(fp, step_skip) for fp in filepaths])
analyzer = cls.from_vaspruns(
vaspruns, specie=specie, initial_disp=initial_disp,
initial_structure=initial_structure, **kwargs)
p.close()
p.join()
return analyzer
else:
def vr(filepaths):
offset = 0
for p in filepaths:
v = Vasprun(p, ionic_step_offset=offset,
ionic_step_skip=step_skip)
yield v
# Recompute offset.
offset = (-(v.nionic_steps - offset)) % step_skip
return cls.from_vaspruns(
vr(filepaths), specie=specie, initial_disp=initial_disp,
initial_structure=initial_structure, **kwargs)
def as_dict(self):
return {
"@module": self.__class__.__module__,
"@class": self.__class__.__name__,
"structure": self.structure.as_dict(),
"displacements": self.disp.tolist(),
"specie": self.specie,
"temperature": self.temperature,
"time_step": self.time_step,
"step_skip": self.step_skip,
"min_obs": self.min_obs,
"smoothed": self.smoothed,
"avg_nsteps": self.avg_nsteps,
"lattices": self.lattices.tolist()
}
@classmethod
def from_dict(cls, d):
structure = Structure.from_dict(d["structure"])
return cls(structure, np.array(d["displacements"]), specie=d["specie"],
temperature=d["temperature"], time_step=d["time_step"],
step_skip=d["step_skip"], min_obs=d["min_obs"],
smoothed=d.get("smoothed", "max"),
avg_nsteps=d.get("avg_nsteps", 1000),
lattices=np.array(d.get("lattices",
[d["structure"]["lattice"][
"matrix"]])))
def get_conversion_factor(structure, species, temperature):
"""
Conversion factor to convert between cm^2/s diffusivity measurements and
mS/cm conductivity measurements based on number of atoms of diffusing
species. Note that the charge is based on the oxidation state of the
species (where available), or else the number of valence electrons
(usually a good guess, esp for main group ions).
Args:
structure (Structure): Input structure.
species (Element/Specie): Diffusing species.
temperature (float): Temperature of the diffusion run in Kelvin.
Returns:
Conversion factor.
Conductivity (in mS/cm) = Conversion Factor * Diffusivity (in cm^2/s)
"""
df_sp = get_el_sp(species)
if hasattr(df_sp, "oxi_state"):
z = df_sp.oxi_state
else:
z = df_sp.full_electronic_structure[-1][2]
n = structure.composition[species]
vol = structure.volume * 1e-24 # units cm^3
return 1000 * n / (vol * const.N_A) * z ** 2 * (const.N_A * const.e) ** 2 \
/ (const.R * temperature)
def _get_vasprun(args):
"""
Internal method to support multiprocessing.
"""
return Vasprun(args[0], ionic_step_skip=args[1],
parse_dos=False, parse_eigen=False)
def fit_arrhenius(temps, diffusivities):
"""
Returns Ea, c, standard error of Ea from the Arrhenius fit:
D = c * exp(-Ea/kT)
Args:
temps ([float]): A sequence of temperatures. units: K
diffusivities ([float]): A sequence of diffusivities (e.g.,
from DiffusionAnalyzer.diffusivity). units: cm^2/s
"""
t_1 = 1 / np.array(temps)
logd = np.log(diffusivities)
# Do a least squares regression of log(D) vs 1/T
a = np.array([t_1, np.ones(len(temps))]).T
w, res, _, _ = np.linalg.lstsq(a, logd)
w = np.array(w)
n = len(temps)
if n > 2:
std_Ea = (res[0] / (n - 2) / (
n * np.var(t_1))) ** 0.5 * const.k / const.e
else:
std_Ea = None
return -w[0] * const.k / const.e, np.exp(w[1]), std_Ea
def get_extrapolated_diffusivity(temps, diffusivities, new_temp):
"""
Returns (Arrhenius) extrapolated diffusivity at new_temp
Args:
temps ([float]): A sequence of temperatures. units: K
diffusivities ([float]): A sequence of diffusivities (e.g.,
from DiffusionAnalyzer.diffusivity). units: cm^2/s
new_temp (float): desired temperature. units: K
Returns:
(float) Diffusivity at extrapolated temp in mS/cm.
"""
Ea, c, _ = fit_arrhenius(temps, diffusivities)
return c * np.exp(-Ea / (const.k / const.e * new_temp))
def get_extrapolated_conductivity(temps, diffusivities, new_temp, structure,
species):
"""
Returns extrapolated mS/cm conductivity.
Args:
temps ([float]): A sequence of temperatures. units: K
diffusivities ([float]): A sequence of diffusivities (e.g.,
from DiffusionAnalyzer.diffusivity). units: cm^2/s
new_temp (float): desired temperature. units: K
structure (structure): Structure used for the diffusivity calculation
species (string/Specie): conducting species
Returns:
(float) Conductivity at extrapolated temp in mS/cm.
"""
return get_extrapolated_diffusivity(temps, diffusivities, new_temp) \
* get_conversion_factor(structure, species, new_temp)
def get_arrhenius_plot(temps, diffusivities, diffusivity_errors=None,
**kwargs):
"""
Returns an Arrhenius plot.
Args:
temps ([float]): A sequence of temperatures.
diffusivities ([float]): A sequence of diffusivities (e.g.,
from DiffusionAnalyzer.diffusivity).
diffusivity_errors ([float]): A sequence of errors for the
diffusivities. If None, no error bar is plotted.
\\*\\*kwargs:
Any keyword args supported by matplotlib.pyplot.plot.
Returns:
A matplotlib.pyplot object. Do plt.show() to show the plot.
"""
Ea, c, _ = fit_arrhenius(temps, diffusivities)
from pymatgen.util.plotting import pretty_plot
plt = pretty_plot(12, 8)
# log10 of the arrhenius fit
arr = c * np.exp(-Ea / (const.k / const.e * np.array(temps)))
t_1 = 1000 / np.array(temps)
plt.plot(t_1, diffusivities, 'ko', t_1, arr, 'k--', markersize=10,
**kwargs)
if diffusivity_errors is not None:
n = len(diffusivity_errors)
plt.errorbar(t_1[0:n], diffusivities[0:n], yerr=diffusivity_errors,
fmt='ko', ecolor='k', capthick=2, linewidth=2)
ax = plt.axes()
ax.set_yscale('log')
plt.text(0.6, 0.85, "E$_a$ = {:.0f} meV".format(Ea * 1000),
fontsize=30, transform=plt.axes().transAxes)
plt.ylabel("D (cm$^2$/s)")
plt.xlabel("1000/T (K$^{-1}$)")
plt.tight_layout()
return plt
| mit |
carolinarias/Sensing-the-City | data_preprocessing/exploration.py | 1 | 13008 | #!/usr/bin/env python
"""
------------------------------------------------------------------------------------------------------------------
TELECOM DATA PREPROCESSING AND EXPLORATION
File name: exploration.py
Description: This script makes data exploration for telecom Open data 2013.
Author:Carolina Arias Munoz
Date Created: 30/07/2016
Date Last Modified: 30/07/2016
Python version: 2.7
------------------------------------------------------------------------------------------------------------------
"""
#import sys
import pandas
import numpy
import matplotlib
matplotlib.rcParams['agg.path.chunksize'] = 10000
import matplotlib.pyplot as plt
import glob
matplotlib.style.use('ggplot')
#path for the csv files
log_path = '/media/sf_2_PhD_2013_-2014/1PhD_WorkDocs/PhD_Data-calculations/data/sms-call-internet-mi/scripts/2exploration/'
data_path = '/media/sf_2_PhD_2013_-2014/1PhD_WorkDocs/PhD_Data-calculations/data/sms-call-internet-mi/csv/'
data_path_outliers = '/media/sf_2_PhD_2013_-2014/1PhD_WorkDocs/PhD_Data-calculations/data/sms-call-internet-mi/csv/stats/outliers/'
plots_path = '/media/sf_2_PhD_2013_-2014/1PhD_WorkDocs/PhD_Data-calculations/data/sms-call-internet-mi/csv/stats/fig/'
stats_path = '/media/sf_2_PhD_2013_-2014/1PhD_WorkDocs/PhD_Data-calculations/data/sms-call-internet-mi/csv/stats/'
#path for the normalized csv files
data_path_norm = '/media/sf_2_PhD_2013_-2014/1PhD_WorkDocs/PhD_Data-calculations/data/sms-call-internet-mi/csv/csvnorm/'
data_path_norm_week = '/media/sf_2_PhD_2013_-2014/1PhD_WorkDocs/PhD_Data-calculations/data/sms-call-internet-mi/csv/csvnorm/byweek/'
#strings of paths
data_files = glob.glob(data_path + '*.txt')
##Creating log file
#sys.stdout = open (log_path + 'explorationlog.txt', 'w')
#------------------------------------------TELECOM DATA -----------------------------------------------------
for data_file in data_files:
#obtainning just the name of the variable
varname = data_file[93:110]
# eliminating '.txt'
varname = varname.replace('.txt', '')
#variables.append(varname)
with open(data_file, 'rb') as data_file:
#Importing data
df = pandas.read_table(data_file, sep='\t', names=['date_time','cellid', varname])
# Setting date_time as data frame index
df = df.sort_values(by ='date_time', axis=0, ascending=True)
df = df.reset_index(drop=True)
df = df.set_index(['date_time'], drop = False)
#------------------------------#
# STATISTICS AND PLOTS
#------------------------------#
#some info on the data
# print 'Some info on ' + varname + ' dataframe'
# print ''
# #df.info()
# print 'Creating time index... it will take a while...'
# #creating time index
# df = df.set_index(pandas.DatetimeIndex(df['date_time']))
# print 'Time index created for ' + varname
# print ''
print 'Statitics on ' + varname + ':'
#calculate statistics
statistics = df[varname].describe()
print statistics
# print ''
# #saving the statistics in a csv file
# statistics.to_csv(stats_path + varname + '.csv', sep=',')
# print 'Plots on ' + varname
# #simple plot
mask = (df['date_time'] >= '2013-11-01T00:00:00+0100') & (df['date_time'] < '2013-12-01T00:00:00+0100')
dfnov = df.loc[mask]
line = dfnov['sms_outlog'].plot(kind='line', title = varname + ' 2013', figsize = (30,10))
fig1 = line.get_figure()
fig1.savefig(plots_path + 'sms_outlog_line.png',dpi=300)
plt.close(fig1)
#density plot
# density = df[varname].plot(kind='density', title = varname + ' 2013. Density Plot')
# fig2 = density.get_figure()
# fig2.savefig(plots_path + varname + '_density.png',dpi=300)
# plt.close(fig2)
# #histogram
# histplot = df[varname].plot(kind='hist',title = varname + ' 2013. Histogram')
# fig3 = histplot.get_figure()
# fig3.savefig(plots_path + varname + '_hist.png',dpi=300)
# plt.close(fig3)
# #boxplot
# boxplot = df[varname].plot(kind='box', title = varname + ' 2013. Boxplot')
# fig4 = boxplot.get_figure()
# fig4.savefig(plots_path + varname + '_boxplot.png',dpi=300)
# plt.close(fig4)
#Checking data for an specific location
# cellid = 5999
# dfcell = df[(df.cellid == cellid)]
# line = dfcell[varname].plot(kind='line', title = varname + ''+ ' cellid' +' 2013', figsize = (40,20))
# fig1 = line.get_figure()
# fig1.savefig(plots_path + varname + '_line.png',dpi=300)
# plt.close(fig1)
#print boxplot
#---------------------------------------------------#
# Cheking OUTLIERS
#---------------------------------------------------#
#standard deviaton of data
std = df[varname].std()
#Interquartile range
iqr = 1.35 * std
#25% quartile
q1 = statistics.ix['25%']
#75% quartile
q3 = statistics.ix['75%']
#superior outer fence limit
sup_out_lim = q3 + (25 * iqr)
mask = df[varname] >= sup_out_lim
df_so = df.loc[mask]
df_so.to_csv(path_or_buf = data_path_outliers + varname + 'so.csv', sep=',', index = False)
#------------------------------#
# NORMALIZING DATA
#------------------------------#
print 'Normalizing data for ' + varname + '...'
#setting values cero to 0.1: log (0) = undefined
df = df.replace(0, 0.1)
#df = df.replace(0, numpy.nan)
#Transforming data into log
df[varname + 'log'] = df[varname].apply(lambda x: numpy.log(x))
#Cheking normality
# histplot = df[varname + 'log'].plot(kind='hist',logy=False, title = varname + ' 2013 Normalized [log(x)]. Histogram')
# fig5 = histplot.get_figure()
# fig5.savefig(plots_path + varname + '_histlogx.png')
# plt.close(fig5)
df.info()
boxout = df[varname + 'log'].plot(kind='box',logy=False, title = varname + ' 2013 Normalized [log(x)] Boxplot')
fig6 = boxout.get_figure()
fig6.savefig(plots_path + varname + '_boxplotlogx.png',dpi=300)
plt.close(fig6)
#---------------------------------------------------#
# Cheking OUTLIERS on normalized data
#---------------------------------------------------#
print 'Checking outliers for ' + varname + '...'
statslog = df[varname + 'log'].describe()
print statslog
#saving the statistics in a csv file
# statslog.to_csv(stats_path + varname + 'log.csv', sep=',')
#standard deviaton of data
std = df[varname + 'log'].std()
#Interquartile range
iqr = 1.35 * std
#25% quartile
q1 = statslog.ix['25%']
#75% quartile
q3 = statslog.ix['75%']
#inferior outer fence limit
inf_out_lim = q1 - (3 * iqr)
#superior outer fence limit
sup_out_lim = q3 + (3 * iqr)
#detecting mayor outliers
df['i_outliers'] = df[varname + 'log'].apply(lambda x: 'inf_major_outlier' if x<inf_out_lim else 'NO') #inferior outliers
df['s_outliers'] = df[varname + 'log'].apply(lambda x: 'sup_major_outlier' if x>sup_out_lim else 'NO') #superior outliers
print ''
print 'Interquartile range:'
print iqr
print '25% quartile: '
print q1
print '75% quartile: '
print q3
print 'inferior outer fence limit: '
print inf_out_lim
print 'superior outer fence limit: '
print sup_out_lim
print ''
#pivot table to count the number of ouliers
print 'Pivot table to count the number of ouliers'
print ''
pivot = pandas.pivot_table(df, values=varname + 'log', columns=['i_outliers', 's_outliers'], aggfunc=numpy.count_nonzero)
print pivot
print ''
df.drop(['i_outliers','s_outliers'],inplace=True,axis=1)
#create a new dataset without the outliers
df[varname + 'nout'] = df[varname + 'log'].apply(lambda x: numpy.nan if x<inf_out_lim else x)
df[varname + 'nout'] = df[varname + 'nout'].apply(lambda x: numpy.nan if x>sup_out_lim else x)
#------------------------------------------------#
# CHECKING OUTLIERS BEHAVIOUR on normalized data
#------------------------------------------------#
# superior outliers
mask = df[varname +'log'] >= sup_out_lim
df_so = df.loc[mask]
df_so.to_csv(path_or_buf = data_path_outliers + varname + 'solog.csv', sep=',', index = False)
# inferior outliers
mask = df[varname +'log'] <= inf_out_lim
df_info = df.loc[mask]
df_info.to_csv(path_or_buf = data_path_outliers + varname + 'infolog.csv', sep=',', index = False)
#check the new dataset
print 'Some info on the new normalized, no outlier dataset of ' + varname
print ''
df.info()
histout = df[varname + 'nout'].plot(kind='hist',logy=False, title = varname + ' 2013 Normalized [log(x)] and no outliers. Histogram')
fig6 = histout.get_figure()
fig6.savefig(plots_path + varname + '_histoutlogx.png',dpi=300)
plt.close(fig6)
boxout = df[varname + 'nout'].plot(kind='box',logy=False, title = varname + ' 2013 Normalized [log(x)] and no outliers')
fig7 = boxout.get_figure()
fig7.savefig(plots_path + varname + '_lboxplotoutlogx.png',dpi=300)
plt.close(fig7)
#saving file into a csv
df.to_csv(path_or_buf = data_path_norm + varname + '.csv', sep=',', index = False)
#------------------------------#
# DIVIDING DATA BY WEEK
#------------------------------#
# # 1 - 3 NOV
# mask = (df['date_time'] >= '2013-11-01T00:00:00+0100') & (df['date_time'] < '2013-11-04T00:00:00+0100')
# sdf1 = df.loc[mask]
# sdf1.to_csv(path_or_buf = data_path_norm_week + '1_3nov' + varname + '.csv', sep=',', index = False)
# # 4 - 10 NOV
# mask = (df['date_time'] >= '2013-11-04T00:00:00+0100') & (df['date_time'] < '2013-11-10T00:00:00+0100')
# sdf2 = df.loc[mask]
# sdf2.to_csv(path_or_buf = data_path_norm_week + '4_10nov' + varname + '.csv', sep=',', index = False)
# # 11 - 17 NOV
# mask = (df['date_time'] >= '2013-11-11T00:00:00+0100') & (df['date_time'] < '2013-11-18T00:00:00+0100')
# sdf3 = df.loc[mask]
# sdf3.to_csv(path_or_buf = data_path_norm_week + '11_17nov' + varname + '.csv', sep=',', index = False)
# # 18 - 24 NOV
# mask = (df['date_time'] >= '2013-11-18T00:00:00+0100') & (df['date_time'] < '2013-11-25T00:00:00+0100')
# sdf4 = df.loc[mask]
# sdf4.to_csv(path_or_buf = data_path_norm_week + '18_24nov' + varname + '.csv', sep=',', index = False)
# # 25 - 1 DIC
# mask = (df['date_time'] >= '2013-11-25T00:00:00+0100') & (df['date_time'] < '2013-12-02T00:00:00+0100')
# sdf5 = df.loc[mask]
# sdf5.to_csv(path_or_buf = data_path_norm_week + '25_1dic' + varname + '.csv', sep=',', index = False)
# # 2 - 8 DIC
# mask = (df['date_time'] >= '2013-12-02T00:00:00+0100') & (df['date_time'] < '2013-12-09T00:00:00+0100')
# sdf6 = df.loc[mask]
# sdf6.to_csv(path_or_buf = data_path_norm_week + '2_8dic' + varname + '.csv', sep=',', index = False)
# # 9 - 15 DIC
# mask = (df['date_time'] >= '2013-12-09T00:00:00+0100') & (df['date_time'] < '2013-12-16T00:00:00+0100')
# sdf7 = df.loc[mask]
# sdf7.to_csv(path_or_buf = data_path_norm_week + '9_15dic' + varname + '.csv', sep=',', index = False)
# # 16 - 22 DIC
# mask = (df['date_time'] >= '2013-12-16T00:00:00+0100') & (df['date_time'] < '2013-12-23T00:00:00+0100')
# sdf8 = df.loc[mask]
# sdf8.to_csv(path_or_buf = data_path_norm_week + '16_22dic' + varname + '.csv', sep=',', index = False)
# # 23 - 29 DIC
# mask = (df['date_time'] >= '2013-12-23T00:00:00+0100') & (df['date_time'] < '2013-12-30T00:00:00+0100')
# sdf9 = df.loc[mask]
# sdf9.to_csv(path_or_buf = data_path_norm_week + '23_29dic' + varname + '.csv', sep=',', index = False)
# # 30 - 31 DIC
# mask = (df['date_time'] >= '2013-12-30T00:00:00+0100') & (df['date_time'] < '2014-01-01T00:00:00+0100')
# sdf10 = df.loc[mask]
# sdf10.to_csv(path_or_buf = data_path_norm_week + '30_31dic' + varname + '.csv', sep=',', index = False)
# print ' '
print '__________________________________________'
print ' '
print ' '
print '__________________________________________'
print ' '
#------------------------------------------ARPA DATA -----------------------------------------------------
#sys.stdout.close()
print 'enjoy! bye'
| mit |
treycausey/scikit-learn | examples/mixture/plot_gmm.py | 18 | 2796 | """
=================================
Gaussian Mixture Model Ellipsoids
=================================
Plot the confidence ellipsoids of a mixture of two gaussians with EM
and variational dirichlet process.
Both models have access to five components with which to fit the
data. Note that the EM model will necessarily use all five components
while the DP model will effectively only use as many as are needed for
a good fit. This is a property of the Dirichlet Process prior. Here we
can see that the EM model splits some components arbitrarily, because it
is trying to fit too many components, while the Dirichlet Process model
adapts it number of state automatically.
This example doesn't show it, as we're in a low-dimensional space, but
another advantage of the dirichlet process model is that it can fit
full covariance matrices effectively even when there are less examples
per cluster than there are dimensions in the data, due to
regularization properties of the inference algorithm.
"""
import itertools
import numpy as np
from scipy import linalg
import pylab as pl
import matplotlib as mpl
from sklearn import mixture
# Number of samples per component
n_samples = 500
# Generate random sample, two components
np.random.seed(0)
C = np.array([[0., -0.1], [1.7, .4]])
X = np.r_[np.dot(np.random.randn(n_samples, 2), C),
.7 * np.random.randn(n_samples, 2) + np.array([-6, 3])]
# Fit a mixture of gaussians with EM using five components
gmm = mixture.GMM(n_components=5, covariance_type='full')
gmm.fit(X)
# Fit a dirichlet process mixture of gaussians using five components
dpgmm = mixture.DPGMM(n_components=5, covariance_type='full')
dpgmm.fit(X)
color_iter = itertools.cycle(['r', 'g', 'b', 'c', 'm'])
for i, (clf, title) in enumerate([(gmm, 'GMM'),
(dpgmm, 'Dirichlet Process GMM')]):
splot = pl.subplot(2, 1, 1 + i)
Y_ = clf.predict(X)
for i, (mean, covar, color) in enumerate(zip(
clf.means_, clf._get_covars(), color_iter)):
v, w = linalg.eigh(covar)
u = w[0] / linalg.norm(w[0])
# as the DP will not use every component it has access to
# unless it needs it, we shouldn't plot the redundant
# components.
if not np.any(Y_ == i):
continue
pl.scatter(X[Y_ == i, 0], X[Y_ == i, 1], .8, color=color)
# Plot an ellipse to show the Gaussian component
angle = np.arctan(u[1] / u[0])
angle = 180 * angle / np.pi # convert to degrees
ell = mpl.patches.Ellipse(mean, v[0], v[1], 180 + angle, color=color)
ell.set_clip_box(splot.bbox)
ell.set_alpha(0.5)
splot.add_artist(ell)
pl.xlim(-10, 10)
pl.ylim(-3, 6)
pl.xticks(())
pl.yticks(())
pl.title(title)
pl.show()
| bsd-3-clause |
bigdataelephants/scikit-learn | sklearn/cluster/tests/test_hierarchical.py | 3 | 18096 | """
Several basic tests for hierarchical clustering procedures
"""
# Authors: Vincent Michel, 2010, Gael Varoquaux 2012,
# Matteo Visconti di Oleggio Castello 2014
# License: BSD 3 clause
from tempfile import mkdtemp
from functools import partial
import numpy as np
from scipy import sparse
from scipy.cluster import hierarchy
from sklearn.utils.testing import assert_true
from sklearn.utils.testing import assert_raises
from sklearn.utils.testing import assert_equal
from sklearn.utils.testing import assert_almost_equal
from sklearn.utils.testing import assert_array_almost_equal
from sklearn.utils.testing import ignore_warnings
from sklearn.cluster import Ward, WardAgglomeration, ward_tree
from sklearn.cluster import AgglomerativeClustering, FeatureAgglomeration
from sklearn.cluster.hierarchical import (_hc_cut, _TREE_BUILDERS,
linkage_tree)
from sklearn.feature_extraction.image import grid_to_graph
from sklearn.metrics.pairwise import PAIRED_DISTANCES, cosine_distances,\
manhattan_distances
from sklearn.metrics.cluster import normalized_mutual_info_score
from sklearn.neighbors.graph import kneighbors_graph
from sklearn.cluster._hierarchical import average_merge, max_merge
from sklearn.utils.fast_dict import IntFloatDict
from sklearn.utils.testing import assert_array_equal
from sklearn.utils.testing import assert_warns
def test_linkage_misc():
# Misc tests on linkage
rng = np.random.RandomState(42)
X = rng.normal(size=(5, 5))
assert_raises(ValueError, AgglomerativeClustering(linkage='foo').fit, X)
assert_raises(ValueError, linkage_tree, X, linkage='foo')
assert_raises(ValueError, linkage_tree, X, connectivity=np.ones((4, 4)))
# Smoke test FeatureAgglomeration
FeatureAgglomeration().fit(X)
# Deprecation of Ward class
assert_warns(DeprecationWarning, Ward).fit(X)
# test hiearchical clustering on a precomputed distances matrix
dis = cosine_distances(X)
res = linkage_tree(dis, affinity="precomputed")
assert_array_equal(res[0], linkage_tree(X, affinity="cosine")[0])
# test hiearchical clustering on a precomputed distances matrix
res = linkage_tree(X, affinity=manhattan_distances)
assert_array_equal(res[0], linkage_tree(X, affinity="manhattan")[0])
def test_structured_linkage_tree():
"""
Check that we obtain the correct solution for structured linkage trees.
"""
rng = np.random.RandomState(0)
mask = np.ones([10, 10], dtype=np.bool)
# Avoiding a mask with only 'True' entries
mask[4:7, 4:7] = 0
X = rng.randn(50, 100)
connectivity = grid_to_graph(*mask.shape)
for tree_builder in _TREE_BUILDERS.values():
children, n_components, n_leaves, parent = \
tree_builder(X.T, connectivity)
n_nodes = 2 * X.shape[1] - 1
assert_true(len(children) + n_leaves == n_nodes)
# Check that ward_tree raises a ValueError with a connectivity matrix
# of the wrong shape
assert_raises(ValueError,
tree_builder, X.T, np.ones((4, 4)))
# Check that fitting with no samples raises an error
assert_raises(ValueError,
tree_builder, X.T[:0], connectivity)
def test_unstructured_linkage_tree():
"""
Check that we obtain the correct solution for unstructured linkage trees.
"""
rng = np.random.RandomState(0)
X = rng.randn(50, 100)
for this_X in (X, X[0]):
# With specified a number of clusters just for the sake of
# raising a warning and testing the warning code
with ignore_warnings():
children, n_nodes, n_leaves, parent = assert_warns(
UserWarning, ward_tree, this_X.T, n_clusters=10)
n_nodes = 2 * X.shape[1] - 1
assert_equal(len(children) + n_leaves, n_nodes)
for tree_builder in _TREE_BUILDERS.values():
for this_X in (X, X[0]):
with ignore_warnings():
children, n_nodes, n_leaves, parent = assert_warns(
UserWarning, tree_builder, this_X.T, n_clusters=10)
n_nodes = 2 * X.shape[1] - 1
assert_equal(len(children) + n_leaves, n_nodes)
def test_height_linkage_tree():
"""
Check that the height of the results of linkage tree is sorted.
"""
rng = np.random.RandomState(0)
mask = np.ones([10, 10], dtype=np.bool)
X = rng.randn(50, 100)
connectivity = grid_to_graph(*mask.shape)
for linkage_func in _TREE_BUILDERS.values():
children, n_nodes, n_leaves, parent = linkage_func(X.T, connectivity)
n_nodes = 2 * X.shape[1] - 1
assert_true(len(children) + n_leaves == n_nodes)
def test_agglomerative_clustering():
"""
Check that we obtain the correct number of clusters with
agglomerative clustering.
"""
rng = np.random.RandomState(0)
mask = np.ones([10, 10], dtype=np.bool)
n_samples = 100
X = rng.randn(n_samples, 50)
connectivity = grid_to_graph(*mask.shape)
for linkage in ("ward", "complete", "average"):
clustering = AgglomerativeClustering(n_clusters=10,
connectivity=connectivity,
linkage=linkage)
clustering.fit(X)
# test caching
clustering = AgglomerativeClustering(
n_clusters=10, connectivity=connectivity,
memory=mkdtemp(),
linkage=linkage)
clustering.fit(X)
labels = clustering.labels_
assert_true(np.size(np.unique(labels)) == 10)
# Turn caching off now
clustering = AgglomerativeClustering(
n_clusters=10, connectivity=connectivity, linkage=linkage)
# Check that we obtain the same solution with early-stopping of the
# tree building
clustering.compute_full_tree = False
clustering.fit(X)
assert_almost_equal(normalized_mutual_info_score(clustering.labels_,
labels), 1)
clustering.connectivity = None
clustering.fit(X)
assert_true(np.size(np.unique(clustering.labels_)) == 10)
# Check that we raise a TypeError on dense matrices
clustering = AgglomerativeClustering(
n_clusters=10,
connectivity=sparse.lil_matrix(
connectivity.toarray()[:10, :10]),
linkage=linkage)
assert_raises(ValueError, clustering.fit, X)
# Test that using ward with another metric than euclidean raises an
# exception
clustering = AgglomerativeClustering(
n_clusters=10,
connectivity=connectivity.toarray(),
affinity="manhattan",
linkage="ward")
assert_raises(ValueError, clustering.fit, X)
# Test using another metric than euclidean works with linkage complete
for affinity in PAIRED_DISTANCES.keys():
# Compare our (structured) implementation to scipy
clustering = AgglomerativeClustering(
n_clusters=10,
connectivity=np.ones((n_samples, n_samples)),
affinity=affinity,
linkage="complete")
clustering.fit(X)
clustering2 = AgglomerativeClustering(
n_clusters=10,
connectivity=None,
affinity=affinity,
linkage="complete")
clustering2.fit(X)
assert_almost_equal(normalized_mutual_info_score(clustering2.labels_,
clustering.labels_),
1)
def test_ward_agglomeration():
"""
Check that we obtain the correct solution in a simplistic case
"""
rng = np.random.RandomState(0)
mask = np.ones([10, 10], dtype=np.bool)
X = rng.randn(50, 100)
connectivity = grid_to_graph(*mask.shape)
assert_warns(DeprecationWarning, WardAgglomeration)
with ignore_warnings():
ward = WardAgglomeration(n_clusters=5, connectivity=connectivity)
ward.fit(X)
agglo = FeatureAgglomeration(n_clusters=5, connectivity=connectivity)
agglo.fit(X)
assert_array_equal(agglo.labels_, ward.labels_)
assert_true(np.size(np.unique(agglo.labels_)) == 5)
X_red = agglo.transform(X)
assert_true(X_red.shape[1] == 5)
X_full = agglo.inverse_transform(X_red)
assert_true(np.unique(X_full[0]).size == 5)
assert_array_almost_equal(agglo.transform(X_full), X_red)
# Check that fitting with no samples raises a ValueError
assert_raises(ValueError, agglo.fit, X[:0])
def assess_same_labelling(cut1, cut2):
"""Util for comparison with scipy"""
co_clust = []
for cut in [cut1, cut2]:
n = len(cut)
k = cut.max() + 1
ecut = np.zeros((n, k))
ecut[np.arange(n), cut] = 1
co_clust.append(np.dot(ecut, ecut.T))
assert_true((co_clust[0] == co_clust[1]).all())
def test_scikit_vs_scipy():
"""Test scikit linkage with full connectivity (i.e. unstructured) vs scipy
"""
n, p, k = 10, 5, 3
rng = np.random.RandomState(0)
# Not using a lil_matrix here, just to check that non sparse
# matrices are well handled
connectivity = np.ones((n, n))
for linkage in _TREE_BUILDERS.keys():
for i in range(5):
X = .1 * rng.normal(size=(n, p))
X -= 4. * np.arange(n)[:, np.newaxis]
X -= X.mean(axis=1)[:, np.newaxis]
out = hierarchy.linkage(X, method=linkage)
children_ = out[:, :2].astype(np.int)
children, _, n_leaves, _ = _TREE_BUILDERS[linkage](X, connectivity)
cut = _hc_cut(k, children, n_leaves)
cut_ = _hc_cut(k, children_, n_leaves)
assess_same_labelling(cut, cut_)
# Test error management in _hc_cut
assert_raises(ValueError, _hc_cut, n_leaves + 1, children, n_leaves)
def test_connectivity_propagation():
"""
Check that connectivity in the ward tree is propagated correctly during
merging.
"""
X = np.array([(.014, .120), (.014, .099), (.014, .097),
(.017, .153), (.017, .153), (.018, .153),
(.018, .153), (.018, .153), (.018, .153),
(.018, .153), (.018, .153), (.018, .153),
(.018, .152), (.018, .149), (.018, .144),
])
connectivity = kneighbors_graph(X, 10)
ward = AgglomerativeClustering(
n_clusters=4, connectivity=connectivity, linkage='ward')
# If changes are not propagated correctly, fit crashes with an
# IndexError
ward.fit(X)
def test_ward_tree_children_order():
"""
Check that children are ordered in the same way for both structured and
unstructured versions of ward_tree.
"""
# test on five random datasets
n, p = 10, 5
rng = np.random.RandomState(0)
connectivity = np.ones((n, n))
for i in range(5):
X = .1 * rng.normal(size=(n, p))
X -= 4. * np.arange(n)[:, np.newaxis]
X -= X.mean(axis=1)[:, np.newaxis]
out_unstructured = ward_tree(X)
out_structured = ward_tree(X, connectivity=connectivity)
assert_array_equal(out_unstructured[0], out_structured[0])
def test_ward_linkage_tree_return_distance():
"""Test return_distance option on linkage and ward trees"""
# test that return_distance when set true, gives same
# output on both structured and unstructured clustering.
n, p = 10, 5
rng = np.random.RandomState(0)
connectivity = np.ones((n, n))
for i in range(5):
X = .1 * rng.normal(size=(n, p))
X -= 4. * np.arange(n)[:, np.newaxis]
X -= X.mean(axis=1)[:, np.newaxis]
out_unstructured = ward_tree(X, return_distance=True)
out_structured = ward_tree(X, connectivity=connectivity,
return_distance=True)
# get children
children_unstructured = out_unstructured[0]
children_structured = out_structured[0]
# check if we got the same clusters
assert_array_equal(children_unstructured, children_structured)
# check if the distances are the same
dist_unstructured = out_unstructured[-1]
dist_structured = out_structured[-1]
assert_array_almost_equal(dist_unstructured, dist_structured)
for linkage in ['average', 'complete']:
structured_items = linkage_tree(
X, connectivity=connectivity, linkage=linkage,
return_distance=True)[-1]
unstructured_items = linkage_tree(
X, linkage=linkage, return_distance=True)[-1]
structured_dist = structured_items[-1]
unstructured_dist = unstructured_items[-1]
structured_children = structured_items[0]
unstructured_children = unstructured_items[0]
assert_array_almost_equal(structured_dist, unstructured_dist)
assert_array_almost_equal(
structured_children, unstructured_children)
# test on the following dataset where we know the truth
# taken from scipy/cluster/tests/hierarchy_test_data.py
X = np.array([[1.43054825, -7.5693489],
[6.95887839, 6.82293382],
[2.87137846, -9.68248579],
[7.87974764, -6.05485803],
[8.24018364, -6.09495602],
[7.39020262, 8.54004355]])
# truth
linkage_X_ward = np.array([[3., 4., 0.36265956, 2.],
[1., 5., 1.77045373, 2.],
[0., 2., 2.55760419, 2.],
[6., 8., 9.10208346, 4.],
[7., 9., 24.7784379, 6.]])
linkage_X_complete = np.array(
[[3., 4., 0.36265956, 2.],
[1., 5., 1.77045373, 2.],
[0., 2., 2.55760419, 2.],
[6., 8., 6.96742194, 4.],
[7., 9., 18.77445997, 6.]])
linkage_X_average = np.array(
[[3., 4., 0.36265956, 2.],
[1., 5., 1.77045373, 2.],
[0., 2., 2.55760419, 2.],
[6., 8., 6.55832839, 4.],
[7., 9., 15.44089605, 6.]])
n_samples, n_features = np.shape(X)
connectivity_X = np.ones((n_samples, n_samples))
out_X_unstructured = ward_tree(X, return_distance=True)
out_X_structured = ward_tree(X, connectivity=connectivity_X,
return_distance=True)
# check that the labels are the same
assert_array_equal(linkage_X_ward[:, :2], out_X_unstructured[0])
assert_array_equal(linkage_X_ward[:, :2], out_X_structured[0])
# check that the distances are correct
assert_array_almost_equal(linkage_X_ward[:, 2], out_X_unstructured[4])
assert_array_almost_equal(linkage_X_ward[:, 2], out_X_structured[4])
linkage_options = ['complete', 'average']
X_linkage_truth = [linkage_X_complete, linkage_X_average]
for (linkage, X_truth) in zip(linkage_options, X_linkage_truth):
out_X_unstructured = linkage_tree(
X, return_distance=True, linkage=linkage)
out_X_structured = linkage_tree(
X, connectivity=connectivity_X, linkage=linkage,
return_distance=True)
# check that the labels are the same
assert_array_equal(X_truth[:, :2], out_X_unstructured[0])
assert_array_equal(X_truth[:, :2], out_X_structured[0])
# check that the distances are correct
assert_array_almost_equal(X_truth[:, 2], out_X_unstructured[4])
assert_array_almost_equal(X_truth[:, 2], out_X_structured[4])
def test_connectivity_fixing_non_lil():
"""
Check non regression of a bug if a non item assignable connectivity is
provided with more than one component.
"""
# create dummy data
x = np.array([[0, 0], [1, 1]])
# create a mask with several components to force connectivity fixing
m = np.array([[True, False], [False, True]])
c = grid_to_graph(n_x=2, n_y=2, mask=m)
w = AgglomerativeClustering(connectivity=c, linkage='ward')
assert_warns(UserWarning, w.fit, x)
def test_int_float_dict():
rng = np.random.RandomState(0)
keys = np.unique(rng.randint(100, size=10).astype(np.intp))
values = rng.rand(len(keys))
d = IntFloatDict(keys, values)
for key, value in zip(keys, values):
assert d[key] == value
other_keys = np.arange(50).astype(np.intp)[::2]
other_values = 0.5 * np.ones(50)[::2]
other = IntFloatDict(other_keys, other_values)
# Complete smoke test
max_merge(d, other, mask=np.ones(100, dtype=np.intp), n_a=1, n_b=1)
average_merge(d, other, mask=np.ones(100, dtype=np.intp), n_a=1, n_b=1)
def test_connectivity_callable():
rng = np.random.RandomState(0)
X = rng.rand(20, 5)
connectivity = kneighbors_graph(X, 3)
aglc1 = AgglomerativeClustering(connectivity=connectivity)
aglc2 = AgglomerativeClustering(
connectivity=partial(kneighbors_graph, n_neighbors=3))
aglc1.fit(X)
aglc2.fit(X)
assert_array_equal(aglc1.labels_, aglc2.labels_)
def test_compute_full_tree():
"""Test that the full tree is computed if n_clusters is small"""
rng = np.random.RandomState(0)
X = rng.randn(10, 2)
connectivity = kneighbors_graph(X, 5)
# When n_clusters is less, the full tree should be built
# that is the number of merges should be n_samples - 1
agc = AgglomerativeClustering(n_clusters=2, connectivity=connectivity)
agc.fit(X)
n_samples = X.shape[0]
n_nodes = agc.children_.shape[0]
assert_equal(n_nodes, n_samples - 1)
# When n_clusters is large, greater than max of 100 and 0.02 * n_samples.
# we should stop when there are n_clusters.
n_clusters = 101
X = rng.randn(200, 2)
connectivity = kneighbors_graph(X, 10)
agc = AgglomerativeClustering(n_clusters=n_clusters,
connectivity=connectivity)
agc.fit(X)
n_samples = X.shape[0]
n_nodes = agc.children_.shape[0]
assert_equal(n_nodes, n_samples - n_clusters)
if __name__ == '__main__':
import nose
nose.run(argv=['', __file__])
| bsd-3-clause |
francescobaldi86/Ecos2015PaperExtension | Data_Process/cleaning_data.py | 1 | 5320 | import pandas as pd
import numpy as np
import os
import datetime
project_path = os.path.realpath('.')
database_path = project_path + os.sep + 'Database' + os.sep
log_path = project_path + os.sep + 'Log' + os.sep
df = pd.read_hdf(database_path + 'selected_df.h5','table')
# Create dictonary translation from original to new! (not the other way around)
headers_dict = pd.read_excel(project_path + os.sep + 'General' + os.sep + 'headers_dict.xlsx')
# Load the data from the Excel-file with headers. Please not the project_path
# Create a list of each column, then a dictonary which is acting as the translotor.
old = headers_dict['ORIGINAL_HEADER']
new = headers_dict['NEW_HEADER']
hd = {}
for n in range(len(old)):
hd[old[n]] = new[n]
hd[new[n]] = old[n] # To make it bi-directional
#%%
# Load the the values from the xlsx-file which contains information about
# the data cleaning process.
# This section could later on be implemented in the pre-processing code
# We must only work with the rows that are in the selected dataset,
# as the headers_dict contains all data points from the raw database we need
# a new index.
index_selected = headers_dict.loc[headers_dict['FB'] == 'x']
# Function for checking if the value is a NaN or not.
# Needed to be sure that the script works if not all fields
# in the excel-file are filled.
def isNaN(num):
return num != num
# Duplicate the original dataset so it can be used for comparison
df_filtered = df.copy()
run_log = list()
for i in list(index_selected.index):
# In the headers_dict file there are values and relations stored for
# each data-point. Most of them does not contain anything but those that
# are needs to be put back in to the data-set.
name = headers_dict.loc[i]['ORIGINAL_HEADER']
# If a relation is used the following code is executed...
if ( headers_dict.loc[i]['REL'] == "<") | ( headers_dict.loc[i]['REL'] == ">" ) & \
( (isinstance(headers_dict.loc[i]['VALUE'],float)) | (isinstance(headers_dict.loc[i]['VALUE'],int)) ) & \
( (isinstance(headers_dict.loc[i]['HIGH_BOUND'],float)) | (isinstance(headers_dict.loc[i]['HIGH_BOUND'],int)) ) & \
( (isinstance(headers_dict.loc[i]['LOW_BOUND'],float)) | (isinstance(headers_dict.loc[i]['LOW_BOUND'],int)) ) & \
(isNaN(headers_dict.loc[i]['VAR']) == False):
value = headers_dict.loc[i]['VALUE']
rel = headers_dict.loc[i]['REL']
var = headers_dict.loc[i]['VAR']
high_bound = headers_dict.loc[i]['HIGH_BOUND']
low_bound =headers_dict.loc[i]['LOW_BOUND']
# For debugging ....
exec("df_filtered[name][(df_filtered[hd[var]] "+ rel +" value) & (df_filtered[name] > high_bound)] = np.nan")
exec("df_filtered[name][(df_filtered[hd[var]] "+ rel +" value) & (df_filtered[name] < low_bound)] = np.nan")
values_removed = len(df_filtered[name])-df_filtered[name].count()
df_filtered[name] = df_filtered[name].interpolate()
diff_abs = abs( (df_filtered[name] - df[name]) ).sum()
a = ('Index:' + str(i) +\
' ;Name: '+ hd[name]+\
' ;Relation: '+ rel + \
' ;Value: '+ str(value) +\
' ;Var: '+ var +\
' ;High: '+ str(high_bound) +\
' ;Low: '+ str(low_bound) +\
' ;Values filtered: '+ str(values_removed) +\
' ;Absolute sum: '+ str(diff_abs) +\
' ;Average/point: '+ str(diff_abs/values_removed))
run_log.append(a)
# If no relation is used...
if ( (isinstance(headers_dict.loc[i]['HIGH_BOUND'],float)) | (isinstance(headers_dict.loc[i]['HIGH_BOUND'],int)) ) & \
( (isinstance(headers_dict.loc[i]['LOW_BOUND'],float)) | (isinstance(headers_dict.loc[i]['LOW_BOUND'],int)) ) & \
(isNaN(headers_dict.loc[i]['HIGH_BOUND']) == False) & \
(isNaN(headers_dict.loc[i]['LOW_BOUND']) == False) & \
(isNaN(headers_dict.loc[i]['VAR']) == True):
high_bound = headers_dict.loc[i]['HIGH_BOUND']
low_bound = headers_dict.loc[i]['LOW_BOUND']
df_filtered[name][df_filtered[name] > high_bound] = np.nan
df_filtered[name][df_filtered[name] < low_bound] = np.nan
values_removed = len(df_filtered[name])-df_filtered[name].count()
df_filtered[name] = df_filtered[name].interpolate()
diff_abs = abs( (df_filtered[name] - df[name]) ).sum()
a = ('Index:' + str(i) +\
' ;Name: '+ hd[name]+\
' ;Relation: NONE'+\
' ;Value: NONE' +\
' ;Var: NONE' +\
' ;High: '+ str(high_bound) +\
' ;Low: '+ str(low_bound) +\
' ;Values filtered: '+ str(values_removed) +\
' ;Absolute sum: '+ str(diff_abs) +\
' ;Average/point: '+ str(diff_abs/values_removed))
run_log.append(a)
#df_filtered[name] = df_filtered[name].interpolate()
log_file = open(log_path + 'log_file_' +str(datetime.datetime.now())+ '.txt','w')
for item in run_log:
log_file.write('\n'+item)
log_file.close()
df_filtered.to_hdf(database_path + 'filtered_df.h5','table',complevel=9,complib='blosc')
log_file = open(log_path + 'log_file.txt','w')
log_file.write(str(datetime.datetime.now()) + '\n')
for item in run_log:
log_file.write('\n'+item)
log_file.close()
df_filtered.to_hdf(database_path + 'filtered_df.h5','table',complevel=9,complib='blosc')
#%%
| mit |
shawntan/quora-codesprint-2013 | qn1.py | 1 | 6875 | """
62.900
"""
import sys,json,re,math
import numpy as np
from sklearn.pipeline import Pipeline,FeatureUnion
from sklearn.decomposition import PCA
from sklearn.feature_extraction.text import CountVectorizer,TfidfTransformer,HashingVectorizer
from sklearn.feature_extraction import DictVectorizer,FeatureHasher
from sklearn.linear_model import *
from sklearn.svm import *
from sklearn.cluster import KMeans,MiniBatchKMeans
from sklearn.neighbors import KNeighborsRegressor
from sklearn.tree import *
from sklearn.preprocessing import StandardScaler
from nltk.tokenize import wordpunct_tokenize
from nltk.corpus import words
from nltk.corpus import stopwords,gazetteers,names
from sklearn.feature_selection import *
eng_words = set([ w.lower() for w in words.words('en') ])
qn_words = set(['who','what','what',
'when','where','how',
'is','should','do',
'if','would','should'])
stopwords = [ w for w in stopwords.words('english') if w not in qn_words ]
places = set([ w.lower() for w in gazetteers.words() ])
names = set([ w.lower() for w in names.words() ])
class Extractor:
def __init__(self,fun):
self.extractor = fun
def fit(self,X,Y):
pass
def transform(self,X):
return [ self.extractor(x) for x in X ]
def fit_transform(self,X,_):
return self.transform(X)
class ToArray:
def __init__(self):
pass
def fit(self,X,Y):
pass
def transform(self,X):
return X.toarray()
def fit_transform(self,X,_):
return self.transform(X)
qn_type_words = [ set(l) for l in [
[
'who',
# 'which',
'when',
# 'where'
],
[
'what',
# 'why',
'how'
],
[
# 'is',
'do',
'can',
# 'did',
'was'
],
['i'],
# [
# 'should',
# 'could',
# 'would',
# 'will'
# ]
]]
def formatting_features(obj):
question = obj['question_text'].strip()
topics = [ t['name'] for t in obj['topics'] ]
tokens = [ w for w in wordpunct_tokenize(question) if not re.match(r'[\'\"\.\?\!\,\/\\\(\)\`]',w) ]
punct = [ p for p in wordpunct_tokenize(question) if re.match(r'[\'\"\.\?\!\,\/\\\(\)\`]',p) ]
top_toks = set([ w.lower() for t in obj['topics']
for w in wordpunct_tokenize(t['name']) ])
qn_toks = set(tokens)
#qn_topic_words = len(top_toks & qn_toks)
qn_mark = 1 if "?" in question else -1
start_cap = 1 if re.match(r'^[A-Z]',question) else -1
if tokens:
qn_type = [ sum(1.0 for w in tokens if w in qws)
for qws in qn_type_words ]
nm_pres = sum(1.0 for w in tokens if w.lower() in names
and re.match(r'^[A-Z]',w))
pl_pres = sum(1.0 for w in tokens if w.lower() in places
and re.match(r'^[A-Z]',w))
else:
qn_type = [0.0]*len(qn_type_words)
nm_pres = -1.0
pl_pres = -1.0
# qn_somewhere = 1 if sum(qn_type) and (re.match(r'\?$',question)
# or re.match(r'\?\s*[A-Z]',question)) else -1
total_words = len(tokens)
dict_words = sum(1 for w in tokens if w.lower() in eng_words)
correct_form_count = sum(1.0 for w in tokens
if (w.lower() in eng_words and not re.match(r'^[A-Z]+$',w))
or re.match(r'^[A-Z]',w)
)
question_form = 1 if '?' in punct and sum(1 for w in tokens if w in qn_words) else -1
correct_form_ratio = correct_form_count/float(total_words+1)
#topic_word_ratio = qn_topic_words/float(total_words+1)
name_ratio = (nm_pres + pl_pres)/float(total_words+1)
punctuation_ratio = len(punct)/float(total_words+1)
result = [
# 1 if nm_pres else 0,
nm_pres,
# 1 if pl_pres else 0,
pl_pres,
qn_mark,
start_cap,
# qn_somewhere,
correct_form_ratio,
#len(punct),
punctuation_ratio,
math.log(len(topics)+1),
#len(topics),
name_ratio,
# topic_word_ratio,
dict_words,
# qn_topic_words,
# correct_form_count,
# math.log(total_words+1),
total_words,
] + qn_type
return result
word_counter = CountVectorizer(
tokenizer=wordpunct_tokenize,
stop_words=stopwords,
# binary=True,
ngram_range=(1,1),
# dtype=np.float32
)
formatting = Pipeline([
('other', Extractor(formatting_features)),
('scaler', StandardScaler()),
])
def word_scorer(x):
res = {}
tokens = wordpunct_tokenize(x.lower())
for i,w in enumerate(tokens):
#w= ' '.join(w)
if w not in stopwords and len(w) > 2:
res[w] = res.get(w,0) + 1/float(i+1)#math.exp(-i*len(tokens)) + 1
return res
question = Pipeline([
('extract', Extractor(lambda x: x['question_text'])),
#('extract', Extractor(lambda x: x['question_text'])),
('counter', word_counter),
# ('word_s', Extractor(word_scorer)),('counter',DictVectorizer()),
# ('f_sel', SelectKBest(score_func=chi2,k=20)),
# ('cluster',MiniBatchKMeans(n_clusters=8))
])
topics = Pipeline([
('extract',Extractor(lambda x: {
t['name']:1 for t in x['topics']
})),
# ('counter', FeatureHasher(n_features=2**16+1, dtype=np.float32)),
('counter',DictVectorizer()),
# ('f_sel', SelectKBest(score_func=chi2,k=260)),
# ('cluster', MiniBatchKMeans(n_clusters=55))
# ('cluster',MiniBatchKMeans(n_clusters=8))
])
ctopic = Pipeline([
('extract',Extractor(lambda x:
{ x['context_topic']['name']:1 }
if x['context_topic'] else {'none':1})),
#('counter',FeatureHasher(n_features=2**10+1, dtype=np.float)),
('counter', DictVectorizer()),
# ('f_sel', SelectKBest(score_func=chi2,k=30)),
])
topic_question = Pipeline([
('content',FeatureUnion([
('question', question),
('topics', topics),
('ctopic', ctopic),
])),
('f_sel', SelectKBest(score_func=chi2,k=650)),#650
])
others = Pipeline([
('extract', Extractor(lambda x: [
float(1 if x['anonymous'] else 0),
])),
# ('scaler', StandardScaler())
])
followers = Pipeline([
('extract',Extractor(lambda x: [
math.log(sum(t['followers'] for t in x['topics'])+1)
# float(sum(t['followers'] for t in x['topics']))
])),
('scaler' ,StandardScaler())
])
model = Pipeline([
('union',FeatureUnion([
('content', topic_question),
('formatting',formatting),
('followers',followers),
('others',others)
])),
# ('toarray',ToArray()),
# ('dim_red',PCA(n_components=2)),
# ('regress',DecisionTreeRegressor())
# ('regress',KNeighborsRegressor())
# ('regress',SVR())
# ('regress',Ridge())
# ('f_sel', SelectKBest(score_func=f_classif,k=500)),#650
('classify',SGDClassifier(alpha=1e-3,n_iter=1250))
# ('classify',SVC(kernel='linear'))
# ('classify',LinearSVC())
# ('regress',SGDRegressor(alpha=1e-3,n_iter=1500))
])
training_count = int(sys.stdin.next())
training_data = [ json.loads(sys.stdin.next()) for _ in xrange(training_count) ]
target = [ obj['__ans__'] for obj in training_data ]
model.fit(training_data,target)
#sys.stderr.write(' '.join(vocabulary)+"\n")
#sys.stderr.write("%s\n"%counter.transform([' '.join(vocabulary)]))
test_count = int(sys.stdin.next())
test_data = [ json.loads(sys.stdin.next()) for _ in xrange(test_count) ]
for i,j in zip(model.predict(test_data).tolist(),test_data):
print json.dumps({
'__ans__':i, 'question_key':j['question_key']
})
| unlicense |
abhishekgahlot/scikit-learn | sklearn/utils/tests/test_utils.py | 23 | 6045 | import warnings
import numpy as np
import scipy.sparse as sp
from scipy.linalg import pinv2
from sklearn.utils.testing import (assert_equal, assert_raises, assert_true,
assert_almost_equal, assert_array_equal,
SkipTest)
from sklearn.utils import check_random_state
from sklearn.utils import deprecated
from sklearn.utils import resample
from sklearn.utils import safe_mask
from sklearn.utils import column_or_1d
from sklearn.utils import safe_indexing
from sklearn.utils import shuffle
from sklearn.utils.extmath import pinvh
from sklearn.utils.mocking import MockDataFrame
def test_make_rng():
"""Check the check_random_state utility function behavior"""
assert_true(check_random_state(None) is np.random.mtrand._rand)
assert_true(check_random_state(np.random) is np.random.mtrand._rand)
rng_42 = np.random.RandomState(42)
assert_true(check_random_state(42).randint(100) == rng_42.randint(100))
rng_42 = np.random.RandomState(42)
assert_true(check_random_state(rng_42) is rng_42)
rng_42 = np.random.RandomState(42)
assert_true(check_random_state(43).randint(100) != rng_42.randint(100))
assert_raises(ValueError, check_random_state, "some invalid seed")
def test_resample_noarg():
"""Border case not worth mentioning in doctests"""
assert_true(resample() is None)
def test_deprecated():
"""Test whether the deprecated decorator issues appropriate warnings"""
# Copied almost verbatim from http://docs.python.org/library/warnings.html
# First a function...
with warnings.catch_warnings(record=True) as w:
warnings.simplefilter("always")
@deprecated()
def ham():
return "spam"
spam = ham()
assert_equal(spam, "spam") # function must remain usable
assert_equal(len(w), 1)
assert_true(issubclass(w[0].category, DeprecationWarning))
assert_true("deprecated" in str(w[0].message).lower())
# ... then a class.
with warnings.catch_warnings(record=True) as w:
warnings.simplefilter("always")
@deprecated("don't use this")
class Ham(object):
SPAM = 1
ham = Ham()
assert_true(hasattr(ham, "SPAM"))
assert_equal(len(w), 1)
assert_true(issubclass(w[0].category, DeprecationWarning))
assert_true("deprecated" in str(w[0].message).lower())
def test_resample_value_errors():
"""Check that invalid arguments yield ValueError"""
assert_raises(ValueError, resample, [0], [0, 1])
assert_raises(ValueError, resample, [0, 1], [0, 1], n_samples=3)
assert_raises(ValueError, resample, [0, 1], [0, 1], meaning_of_life=42)
def test_safe_mask():
random_state = check_random_state(0)
X = random_state.rand(5, 4)
X_csr = sp.csr_matrix(X)
mask = [False, False, True, True, True]
mask = safe_mask(X, mask)
assert_equal(X[mask].shape[0], 3)
mask = safe_mask(X_csr, mask)
assert_equal(X_csr[mask].shape[0], 3)
def test_pinvh_simple_real():
a = np.array([[1, 2, 3], [4, 5, 6], [7, 8, 10]], dtype=np.float64)
a = np.dot(a, a.T)
a_pinv = pinvh(a)
assert_almost_equal(np.dot(a, a_pinv), np.eye(3))
def test_pinvh_nonpositive():
a = np.array([[1, 2, 3], [4, 5, 6], [7, 8, 9]], dtype=np.float64)
a = np.dot(a, a.T)
u, s, vt = np.linalg.svd(a)
s[0] *= -1
a = np.dot(u * s, vt) # a is now symmetric non-positive and singular
a_pinv = pinv2(a)
a_pinvh = pinvh(a)
assert_almost_equal(a_pinv, a_pinvh)
def test_pinvh_simple_complex():
a = (np.array([[1, 2, 3], [4, 5, 6], [7, 8, 10]])
+ 1j * np.array([[10, 8, 7], [6, 5, 4], [3, 2, 1]]))
a = np.dot(a, a.conj().T)
a_pinv = pinvh(a)
assert_almost_equal(np.dot(a, a_pinv), np.eye(3))
def test_column_or_1d():
EXAMPLES = [
("binary", ["spam", "egg", "spam"]),
("binary", [0, 1, 0, 1]),
("continuous", np.arange(10) / 20.),
("multiclass", [1, 2, 3]),
("multiclass", [0, 1, 2, 2, 0]),
("multiclass", [[1], [2], [3]]),
("multilabel-indicator", [[0, 1, 0], [0, 0, 1]]),
("multiclass-multioutput", [[1, 2, 3]]),
("multiclass-multioutput", [[1, 1], [2, 2], [3, 1]]),
("multiclass-multioutput", [[5, 1], [4, 2], [3, 1]]),
("multiclass-multioutput", [[1, 2, 3]]),
("continuous-multioutput", np.arange(30).reshape((-1, 3))),
]
for y_type, y in EXAMPLES:
if y_type in ["binary", 'multiclass', "continuous"]:
assert_array_equal(column_or_1d(y), np.ravel(y))
else:
assert_raises(ValueError, column_or_1d, y)
def test_safe_indexing():
X = [[1, 2, 3], [4, 5, 6], [7, 8, 9]]
inds = np.array([1, 2])
X_inds = safe_indexing(X, inds)
X_arrays = safe_indexing(np.array(X), inds)
assert_array_equal(np.array(X_inds), X_arrays)
assert_array_equal(np.array(X_inds), np.array(X)[inds])
def test_safe_indexing_pandas():
try:
import pandas as pd
except ImportError:
raise SkipTest("Pandas not found")
X = np.array([[1, 2, 3], [4, 5, 6], [7, 8, 9]])
X_df = pd.DataFrame(X)
inds = np.array([1, 2])
X_df_indexed = safe_indexing(X_df, inds)
X_indexed = safe_indexing(X_df, inds)
assert_array_equal(np.array(X_df_indexed), X_indexed)
def test_safe_indexing_mock_pandas():
X = np.array([[1, 2, 3], [4, 5, 6], [7, 8, 9]])
X_df = MockDataFrame(X)
inds = np.array([1, 2])
X_df_indexed = safe_indexing(X_df, inds)
X_indexed = safe_indexing(X_df, inds)
assert_array_equal(np.array(X_df_indexed), X_indexed)
def test_shuffle_on_ndim_equals_three():
def to_tuple(A): # to make the inner arrays hashable
return tuple(tuple(tuple(C) for C in B) for B in A)
A = np.array([[[1, 2], [3, 4]], [[5, 6], [7, 8]]]) # A.shape = (2,2,2)
S = set(to_tuple(A))
shuffle(A) # shouldn't raise a ValueError for dim = 3
assert_equal(set(to_tuple(A)), S)
| bsd-3-clause |
ghostop14/sparrow-wifi | telemetry.py | 1 | 32406 | #!/usr/bin/python3
#
# Copyright 2017 ghostop14
#
# This is free software; you can redistribute it and/or modify
# it under the terms of the GNU General Public License as published by
# the Free Software Foundation; either version 3, or (at your option)
# any later version.
#
# This software is distributed in the hope that it will be useful,
# but WITHOUT ANY WARRANTY; without even the implied warranty of
# MERCHANTABILITY or FITNESS FOR A PARTICULAR PURPOSE. See the
# GNU General Public License for more details.
#
# You should have received a copy of the GNU General Public License
# along with this software; see the file COPYING. If not, write to
# the Free Software Foundation, Inc., 51 Franklin Street,
# Boston, MA 02110-1301, USA.
#
from PyQt5.QtWidgets import QDialog, QApplication,QDesktopWidget
from PyQt5.QtWidgets import QTableWidget, QHeaderView,QTableWidgetItem, QMessageBox, QFileDialog, QMenu, QAction
# from PyQt5.QtWidgets import QLabel, QComboBox, QLineEdit, QPushButton, QFileDialog
#from PyQt5.QtCore import Qt
from PyQt5 import QtWidgets
from PyQt5 import QtCore
from PyQt5.QtCore import Qt
from PyQt5.QtChart import QChart, QChartView, QLineSeries, QValueAxis
from PyQt5.QtGui import QPen, QFont, QBrush, QColor, QPainter
from PyQt5.QtWidgets import QPushButton
import numpy as np
import matplotlib.pyplot as plt
from matplotlib.backends.backend_qt5agg import FigureCanvasQTAgg as FigureCanvas
from matplotlib.figure import Figure
from sparrowtablewidgets import IntTableWidgetItem, FloatTableWidgetItem, DateTableWidgetItem
from threading import Lock
# from wirelessengine import WirelessNetwork
# https://matplotlib.org/examples/user_interfaces/embedding_in_qt5.html
class RadarWidget(FigureCanvas):
def __init__(self, parent=None, useBlackoutColors=True, width=4, height=4, dpi=100):
# fig = Figure(figsize=(width, height), dpi=dpi)
# self.axes = fig.add_subplot(111)
# -----------------------------------------------------------
# fig = plt.figure()
# useBlackoutColors = False
self.useBlackoutColors = useBlackoutColors
if self.useBlackoutColors:
self.fontColor = 'white'
self.backgroundColor = 'black'
else:
self.fontColor = 'black'
self.backgroundColor = 'white'
self.fig = Figure(figsize=(width, height), dpi=dpi)
self.fig.patch.set_facecolor(self.backgroundColor)
# "axisbg was deprecated, use facecolor instead"
# self.axes = self.fig.add_subplot(111, polar=True, axisbg=self.backgroundColor)
self.axes = self.fig.add_subplot(111, polar=True, facecolor=self.backgroundColor)
# Angle: np.linspace(0, 2*np.pi, 100)
# Radius: np.ones(100)*5
# ax.plot(np.linspace(0, 2*np.pi, 100), np.ones(100)*5, color='r', linestyle='-')
# Each of these use 100 points. linespace creates the angles 0-2 PI with 100 points
# np.ones creates a 100 point array filled with 1's then multiplies that by the scalar 5
# Create an "invisible" line at 100 to set the max for the plot
self.axes.plot(np.linspace(0, 2*np.pi, 100), np.ones(100)*100, color=self.fontColor, linestyle='')
# Plot line: Initialize out to 100 and blank
radius = 100
self.blackline = self.axes.plot(np.linspace(0, 2*np.pi, 100), np.ones(100)*radius, color=self.fontColor, linestyle='-')
self.redline = None
# Plot a filled circle
# http://nullege.com/codes/search/matplotlib.pyplot.Circle
# Params are: Cartesian coord of center, radius, etc...
# circle = plt.Circle((0.0, 0.0), radius, transform=self.axes.transData._b, color="red", alpha=0.4)
# self.filledcircle = self.axes.add_artist(circle)
self.filledcircle = None
# Create bullseye
circle = plt.Circle((0.0, 0.0), 20, transform=self.axes.transData._b, color=self.fontColor, alpha=0.4)
self.bullseye = self.axes.add_artist(circle)
# Rotate zero up
self.axes.set_theta_zero_location("N")
self.axes.set_yticklabels(['-20', '-40', '-60', '-80', '-100'], color=self.fontColor)
# plt.show()
# -----------------------------------------------------------
FigureCanvas.__init__(self, self.fig)
self.setParent(parent)
self.title = self.fig.suptitle('Tracker', fontsize=8, fontweight='bold', color=self.fontColor)
FigureCanvas.setSizePolicy(self,
QtWidgets.QSizePolicy.Expanding,
QtWidgets.QSizePolicy.Expanding)
FigureCanvas.updateGeometry(self)
def updateData(self, radius):
if self.redline is not None:
self.redline.pop(0).remove()
self.redline = self.axes.plot(np.linspace(0, 2*np.pi, 100), np.ones(100)*radius, color='r', linestyle='-')
if self.filledcircle:
self.filledcircle.remove()
self.bullseye.remove()
circle = plt.Circle((0.0, 0.0), radius, transform=self.axes.transData._b, color="red", alpha=0.4)
self.filledcircle = self.axes.add_artist(circle)
# Create bullseye
circle = plt.Circle((0.0, 0.0), 20, transform=self.axes.transData._b, color=self.fontColor, alpha=0.4)
self.bullseye = self.axes.add_artist(circle)
class TelemetryDialog(QDialog):
resized = QtCore.pyqtSignal()
visibility = QtCore.pyqtSignal(bool)
def __init__(self, winTitle = "Network Telemetry", parent = None):
super(TelemetryDialog, self).__init__(parent)
self.visibility.connect(self.onVisibilityChanged)
self.winTitle = winTitle
self.updateLock = Lock()
# Used to detect network change
self.lastNetKey = ""
self.lastSeen = None
self.maxPoints = 20
self.maxRowPoints = 60
self.paused = False
self.streamingSave = False
self.streamingFile = None
self.linesBeforeFlush = 10
self.currentLine = 0
# OK and Cancel buttons
#buttons = QDialogButtonBox(QDialogButtonBox.Ok,Qt.Horizontal, self)
#buttons.accepted.connect(self.accept)
#buttons.move(170, 280)
desktopSize = QApplication.desktop().screenGeometry()
#self.mainWidth=1024
#self.mainHeight=768
#self.mainWidth = desktopSize.width() * 3 / 4
#self.mainHeight = desktopSize.height() * 3 / 4
self.setGeometry(self.geometry().x(), self.geometry().y(), desktopSize.width() /2,desktopSize.height() /2)
self.setWindowTitle(winTitle)
self.radar = RadarWidget(self)
self.radar.setGeometry(self.geometry().width()/2, 10, self.geometry().width()/2-20, self.geometry().width()/2-20)
self.createTable()
self.btnExport = QPushButton("Export Table", self)
self.btnExport.clicked[bool].connect(self.onExportClicked)
self.btnExport.setStyleSheet("background-color: rgba(2,128,192,255);")
self.btnPause = QPushButton("Pause Table", self)
self.btnPause.setCheckable(True)
self.btnPause.clicked[bool].connect(self.onPauseClicked)
self.btnPause.setStyleSheet("background-color: rgba(2,128,192,255);")
self.btnStream = QPushButton("Streaming Save", self)
self.btnStream.setCheckable(True)
self.btnStream.clicked[bool].connect(self.onStreamClicked)
self.btnStream.setStyleSheet("background-color: rgba(2,128,192,255);")
self.createChart()
self.setBlackoutColors()
self.setMinimumWidth(600)
self.setMinimumHeight(600)
self.center()
def createTable(self):
# Set up location table
self.locationTable = QTableWidget(self)
self.locationTable.setColumnCount(8)
self.locationTable.setGeometry(10, 10, self.geometry().width()/2-20, self.geometry().height()/2)
self.locationTable.setShowGrid(True)
self.locationTable.setHorizontalHeaderLabels(['macAddr','SSID', 'Strength', 'Timestamp','GPS', 'Latitude', 'Longitude', 'Altitude'])
self.locationTable.resizeColumnsToContents()
self.locationTable.setRowCount(0)
self.locationTable.horizontalHeader().setSectionResizeMode(1, QHeaderView.Stretch)
self.ntRightClickMenu = QMenu(self)
newAct = QAction('Copy', self)
newAct.setStatusTip('Copy data to clipboard')
newAct.triggered.connect(self.onCopy)
self.ntRightClickMenu.addAction(newAct)
self.locationTable.setContextMenuPolicy(Qt.CustomContextMenu)
self.locationTable.customContextMenuRequested.connect(self.showNTContextMenu)
def setBlackoutColors(self):
self.locationTable.setStyleSheet("QTableView {background-color: black;gridline-color: white;color: white} QTableCornerButton::section{background-color: white;}")
headerStyle = "QHeaderView::section{background-color: white;border: 1px solid black;color: black;} QHeaderView::down-arrow,QHeaderView::up-arrow {background: none;}"
self.locationTable.horizontalHeader().setStyleSheet(headerStyle)
self.locationTable.verticalHeader().setStyleSheet(headerStyle)
mainTitleBrush = QBrush(Qt.red)
self.timeChart.setTitleBrush(mainTitleBrush)
self.timeChart.setBackgroundBrush(QBrush(Qt.black))
self.timeChart.axisX().setLabelsColor(Qt.white)
self.timeChart.axisY().setLabelsColor(Qt.white)
titleBrush = QBrush(Qt.white)
self.timeChart.axisX().setTitleBrush(titleBrush)
self.timeChart.axisY().setTitleBrush(titleBrush)
def resizeEvent(self, event):
wDim = self.geometry().width()/2-20
hDim = self.geometry().height()/2
smallerDim = wDim
if hDim < smallerDim:
smallerDim = hDim
# Radar
self.radar.setGeometry(self.geometry().width() - smallerDim - 10, 10, smallerDim, smallerDim)
# chart
self.timePlot.setGeometry(10, 10, self.geometry().width() - smallerDim - 30, smallerDim)
# Buttons
self.btnPause.setGeometry(10, self.geometry().height()/2+18, 110, 25)
self.btnExport.setGeometry(150, self.geometry().height()/2+18, 110, 25)
self.btnStream.setGeometry(290, self.geometry().height()/2+18, 110, 25)
# Table
self.locationTable.setGeometry(10, self.geometry().height()/2 + 50, self.geometry().width()-20, self.geometry().height()/2-60)
def center(self):
# Get our geometry
qr = self.frameGeometry()
# Find the desktop center point
cp = QDesktopWidget().availableGeometry().center()
# Move our center point to the desktop center point
qr.moveCenter(cp)
# Move the top-left point of the application window to the top-left point of the qr rectangle,
# basically centering the window
self.move(qr.topLeft())
def showNTContextMenu(self, pos):
curRow = self.locationTable.currentRow()
if curRow == -1:
return
self.ntRightClickMenu.exec_(self.locationTable.mapToGlobal(pos))
def onCopy(self):
self.updateLock.acquire()
curRow = self.locationTable.currentRow()
curCol = self.locationTable.currentColumn()
if curRow == -1 or curCol == -1:
self.updateLock.release()
return
curText = self.locationTable.item(curRow, curCol).text()
clipboard = QApplication.clipboard()
clipboard.setText(curText)
self.updateLock.release()
def onVisibilityChanged(self, visible):
if not visible:
self.paused = True
self.btnPause.setStyleSheet("background-color: rgba(255,0,0,255);")
# We're coming out of streaming
self.streamingSave = False
self.btnStream.setStyleSheet("background-color: rgba(2,128,192,255);")
self.btnStream.setChecked(False)
if (self.streamingFile):
self.streamingFile.close()
self.streamingFile = None
return
else:
self.paused = False
self.btnPause.setStyleSheet("background-color: rgba(2,128,192,255);")
if self.locationTable.rowCount() > 1:
self.locationTable.scrollToItem(self.locationTable.item(0, 0))
def hideEvent(self, event):
self.visibility.emit(False)
def showEvent(self, event):
self.visibility.emit(True)
def onPauseClicked(self, pressed):
if self.btnPause.isChecked():
self.paused = True
self.btnPause.setStyleSheet("background-color: rgba(255,0,0,255);")
else:
self.paused = False
self.btnPause.setStyleSheet("background-color: rgba(2,128,192,255);")
def onStreamClicked(self, pressed):
if not self.btnStream.isChecked():
# We're coming out of streaming
self.streamingSave = False
self.btnStream.setStyleSheet("background-color: rgba(2,128,192,255);")
if (self.streamingFile):
self.streamingFile.close()
self.streamingFile = None
return
self.btnStream.setStyleSheet("background-color: rgba(255,0,0,255);")
self.streamingSave = True
fileName = self.saveFileDialog()
if not fileName:
self.btnStream.setStyleSheet("background-color: rgba(2,128,192,255);")
self.btnStream.setChecked(False)
return
try:
self.streamingFile = open(fileName, 'w', 1) # 1 says use line buffering, otherwise it fully buffers and doesn't write
except:
QMessageBox.question(self, 'Error',"Unable to write to " + fileName, QMessageBox.Ok)
self.streamingFile = None
self.streamingSave = False
self.btnStream.setStyleSheet("background-color: rgba(2,128,192,255);")
self.btnStream.setChecked(False)
return
self.streamingFile.write('MAC Address,SSID,Strength,Timestamp,GPS,Latitude,Longitude,Altitude\n')
def onExportClicked(self):
fileName = self.saveFileDialog()
if not fileName:
return
try:
outputFile = open(fileName, 'w')
except:
QMessageBox.question(self, 'Error',"Unable to write to " + fileName, QMessageBox.Ok)
return
outputFile.write('MAC Address,SSID,Strength,Timestamp,GPS,Latitude,Longitude,Altitude\n')
numItems = self.locationTable.rowCount()
if numItems == 0:
outputFile.close()
return
self.updateLock.acquire()
for i in range(0, numItems):
outputFile.write(self.locationTable.item(i, 0).text() + ',"' + self.locationTable.item(i, 1).text() + '",' + self.locationTable.item(i, 2).text() + ',' + self.locationTable.item(i, 3).text())
outputFile.write(',' + self.locationTable.item(i, 4).text()+ ',' + self.locationTable.item(i, 5).text()+ ',' + self.locationTable.item(i, 6).text()+ ',' + self.locationTable.item(i, 7).text() + '\n')
self.updateLock.release()
outputFile.close()
def saveFileDialog(self):
options = QFileDialog.Options()
options |= QFileDialog.DontUseNativeDialog
fileName, _ = QFileDialog.getSaveFileName(self,"QFileDialog.getSaveFileName()","","CSV Files (*.csv);;All Files (*)", options=options)
if fileName:
return fileName
else:
return None
def createChart(self):
self.timeChart = QChart()
titleFont = QFont()
titleFont.setPixelSize(18)
titleBrush = QBrush(QColor(0, 0, 255))
self.timeChart.setTitleFont(titleFont)
self.timeChart.setTitleBrush(titleBrush)
self.timeChart.setTitle('Signal (Past ' + str(self.maxPoints) + ' Samples)')
# self.timeChart.addSeries(testseries)
# self.timeChart.createDefaultAxes()
self.timeChart.legend().hide()
# Axis examples: https://doc.qt.io/qt-5/qtcharts-multiaxis-example.html
newAxis = QValueAxis()
newAxis.setMin(0)
newAxis.setMax(self.maxPoints)
newAxis.setTickCount(11)
newAxis.setLabelFormat("%d")
newAxis.setTitleText("Sample")
self.timeChart.addAxis(newAxis, Qt.AlignBottom)
newAxis = QValueAxis()
newAxis.setMin(-100)
newAxis.setMax(-10)
newAxis.setTickCount(9)
newAxis.setLabelFormat("%d")
newAxis.setTitleText("dBm")
self.timeChart.addAxis(newAxis, Qt.AlignLeft)
chartBorder = Qt.darkGray
self.timePlot = QChartView(self.timeChart, self)
self.timePlot.setBackgroundBrush(chartBorder)
self.timePlot.setRenderHint(QPainter.Antialiasing)
self.timeSeries = QLineSeries()
pen = QPen(Qt.yellow)
pen.setWidth(2)
self.timeSeries.setPen(pen)
self.timeChart.addSeries(self.timeSeries)
self.timeSeries.attachAxis(self.timeChart.axisX())
self.timeSeries.attachAxis(self.timeChart.axisY())
def updateNetworkData(self, curNet):
if not self.isVisible():
return
# Signal is -NN dBm. Need to make it positive for the plot
self.radar.updateData(curNet.signal*-1)
if self.winTitle == "Client Telemetry":
self.setWindowTitle(self.winTitle + " - [" + curNet.macAddr + "] " + curNet.ssid)
else:
self.setWindowTitle(self.winTitle + " - " + curNet.ssid)
self.radar.draw()
# Network changed. Clear our table and time data
updateChartAndTable = False
self.updateLock.acquire()
if (curNet.getKey() != self.lastNetKey):
self.lastNetKey = curNet.getKey()
self.locationTable.setRowCount(0)
self.timeSeries.clear()
updateChartAndTable = True
ssidTitle = curNet.ssid
if len(ssidTitle) > 28:
ssidTitle = ssidTitle[:28]
ssidTitle = ssidTitle + '...'
self.timeChart.setTitle(ssidTitle + ' Signal (Past ' + str(self.maxPoints) + ' Samples)')
else:
if self.lastSeen != curNet.lastSeen:
updateChartAndTable = True
if updateChartAndTable:
# Update chart
numPoints = len(self.timeSeries.pointsVector())
if numPoints >= self.maxPoints:
self.timeSeries.remove(0)
# Now we need to reset the x data to pull the series back
counter = 0
for curPoint in self.timeSeries.pointsVector():
self.timeSeries.replace(counter, counter, curPoint.y())
counter += 1
if curNet.signal >= -100:
self.timeSeries.append(numPoints,curNet.signal)
else:
self.timeSeries.append(numPoints,-100)
# Update Table
self.addTableData(curNet)
# Limit points in each
if self.locationTable.rowCount() > self.maxRowPoints:
self.locationTable.setRowCount(self.maxRowPoints)
self.updateLock.release()
def addTableData(self, curNet):
if self.paused:
return
# rowPosition = self.locationTable.rowCount()
# Always insert at row(0)
rowPosition = 0
self.locationTable.insertRow(rowPosition)
#if (addedFirstRow):
# self.locationTable.setRowCount(1)
# ['macAddr','SSID', 'Strength', 'Timestamp','GPS', 'Latitude', 'Longitude', 'Altitude']
self.locationTable.setItem(rowPosition, 0, QTableWidgetItem(curNet.macAddr))
tmpssid = curNet.ssid
if (len(tmpssid) == 0):
tmpssid = '<Unknown>'
newSSID = QTableWidgetItem(tmpssid)
self.locationTable.setItem(rowPosition, 1, newSSID)
self.locationTable.setItem(rowPosition, 2, IntTableWidgetItem(str(curNet.signal)))
self.locationTable.setItem(rowPosition, 3, DateTableWidgetItem(curNet.lastSeen.strftime("%m/%d/%Y %H:%M:%S")))
if curNet.gps.isValid:
self.locationTable.setItem(rowPosition, 4, QTableWidgetItem('Yes'))
else:
self.locationTable.setItem(rowPosition, 4, QTableWidgetItem('No'))
self.locationTable.setItem(rowPosition, 5, FloatTableWidgetItem(str(curNet.gps.latitude)))
self.locationTable.setItem(rowPosition, 6, FloatTableWidgetItem(str(curNet.gps.longitude)))
self.locationTable.setItem(rowPosition, 7, FloatTableWidgetItem(str(curNet.gps.altitude)))
#order = Qt.DescendingOrder
#self.locationTable.sortItems(3, order )
# If we're in streaming mode, write the data out to disk as well
if self.streamingFile:
self.streamingFile.write(self.locationTable.item(rowPosition, 0).text() + ',"' + self.locationTable.item(rowPosition, 1).text() + '",' + self.locationTable.item(rowPosition, 2).text() + ',' +
self.locationTable.item(rowPosition, 3).text() + ',' + self.locationTable.item(rowPosition, 4).text()+ ',' + self.locationTable.item(rowPosition, 5).text()+ ',' + self.locationTable.item(rowPosition, 6).text()+ ',' + self.locationTable.item(rowPosition, 7).text() + '\n')
if (self.currentLine > self.linesBeforeFlush):
self.streamingFile.flush()
self.currentLine += 1
numRows = self.locationTable.rowCount()
if numRows > 1:
self.locationTable.scrollToItem(self.locationTable.item(0, 0))
def onTableHeadingClicked(self, logical_index):
header = self.locationTable.horizontalHeader()
order = Qt.DescendingOrder
# order = Qt.DescendingOrder
if not header.isSortIndicatorShown():
header.setSortIndicatorShown( True )
elif header.sortIndicatorSection()==logical_index:
# apparently, the sort order on the header is already switched
# when the section was clicked, so there is no need to reverse it
order = header.sortIndicatorOrder()
header.setSortIndicator( logical_index, order )
self.locationTable.sortItems(logical_index, order )
def updateData(self, newRadius):
self.radar.updateData(newRadius)
def showTelemetry(parent = None):
dialog = TelemetryDialog(parent)
result = dialog.exec_()
return (result == QDialog.Accepted)
class BluetoothTelemetry(TelemetryDialog):
def __init__(self, winTitle = "Bluetooth Telemetry", parent = None):
super().__init__(winTitle, parent)
def createTable(self):
# Set up location table
self.locationTable = QTableWidget(self)
self.locationTable.setColumnCount(10)
self.locationTable.setGeometry(10, 10, self.geometry().width()/2-20, self.geometry().height()/2)
self.locationTable.setShowGrid(True)
self.locationTable.setHorizontalHeaderLabels(['macAddr','Name', 'RSSI', 'TX Power', 'Est Range (m)', 'Timestamp','GPS', 'Latitude', 'Longitude', 'Altitude'])
self.locationTable.resizeColumnsToContents()
self.locationTable.setRowCount(0)
self.locationTable.horizontalHeader().setSectionResizeMode(1, QHeaderView.Stretch)
self.ntRightClickMenu = QMenu(self)
newAct = QAction('Copy', self)
newAct.setStatusTip('Copy data to clipboard')
newAct.triggered.connect(self.onCopy)
self.ntRightClickMenu.addAction(newAct)
self.locationTable.setContextMenuPolicy(Qt.CustomContextMenu)
self.locationTable.customContextMenuRequested.connect(self.showNTContextMenu)
def onStreamClicked(self, pressed):
if not self.btnStream.isChecked():
# We're coming out of streaming
self.streamingSave = False
self.btnStream.setStyleSheet("background-color: rgba(2,128,192,255);")
if (self.streamingFile):
self.streamingFile.close()
self.streamingFile = None
return
self.btnStream.setStyleSheet("background-color: rgba(255,0,0,255);")
self.streamingSave = True
fileName = self.saveFileDialog()
if not fileName:
self.btnStream.setStyleSheet("background-color: rgba(2,128,192,255);")
self.btnStream.setChecked(False)
return
try:
self.streamingFile = open(fileName, 'w', 1) # 1 says use line buffering, otherwise it fully buffers and doesn't write
except:
QMessageBox.question(self, 'Error',"Unable to write to " + fileName, QMessageBox.Ok)
self.streamingFile = None
self.streamingSave = False
self.btnStream.setStyleSheet("background-color: rgba(2,128,192,255);")
self.btnStream.setChecked(False)
return
self.streamingFile.write('MAC Address,Name,RSSI,TX Power,Est Range (m),Timestamp,GPS,Latitude,Longitude,Altitude\n')
def onExportClicked(self):
fileName = self.saveFileDialog()
if not fileName:
return
try:
outputFile = open(fileName, 'w')
except:
QMessageBox.question(self, 'Error',"Unable to write to " + fileName, QMessageBox.Ok)
return
outputFile.write('MAC Address,Name,RSSI,TX Power,Est Range (m),Timestamp,GPS,Latitude,Longitude,Altitude\n')
numItems = self.locationTable.rowCount()
if numItems == 0:
outputFile.close()
return
self.updateLock.acquire()
for i in range(0, numItems):
outputFile.write(self.locationTable.item(i, 0).text() + ',"' + self.locationTable.item(i, 1).text() + '",' + self.locationTable.item(i, 2).text() + ',' + self.locationTable.item(i, 3).text())
outputFile.write(',' + self.locationTable.item(i, 4).text()+ ',' + self.locationTable.item(i, 5).text()+ ',' + self.locationTable.item(i, 6).text()+ ',' + self.locationTable.item(i, 7).text() +
',' + self.locationTable.item(i, 8).text()+ ',' + self.locationTable.item(i, 9).text() + '\n')
self.updateLock.release()
outputFile.close()
def updateNetworkData(self, curDevice):
if not self.isVisible():
return
# Signal is -NN dBm. Need to make it positive for the plot
self.radar.updateData(curDevice.rssi*-1)
if len(curDevice.name) > 0:
self.setWindowTitle(self.winTitle + " - " + curDevice.name)
else:
self.setWindowTitle(self.winTitle + " - " + curDevice.macAddress)
self.radar.draw()
# Network changed. Clear our table and time data
updateChartAndTable = False
self.updateLock.acquire()
if self.lastSeen != curDevice.lastSeen:
updateChartAndTable = True
if updateChartAndTable:
# Update chart
numPoints = len(self.timeSeries.pointsVector())
if numPoints >= self.maxPoints:
self.timeSeries.remove(0)
# Now we need to reset the x data to pull the series back
counter = 0
for curPoint in self.timeSeries.pointsVector():
self.timeSeries.replace(counter, counter, curPoint.y())
counter += 1
if curDevice.rssi >= -100:
self.timeSeries.append(numPoints,curDevice.rssi)
else:
self.timeSeries.append(numPoints,-100)
# Update Table
self.addTableData(curDevice)
# Limit points in each
if self.locationTable.rowCount() > self.maxRowPoints:
self.locationTable.setRowCount(self.maxRowPoints)
self.updateLock.release()
def addTableData(self, curDevice):
if self.paused:
return
# rowPosition = self.locationTable.rowCount()
# Always insert at row(0)
rowPosition = 0
self.locationTable.insertRow(rowPosition)
#if (addedFirstRow):
# self.locationTable.setRowCount(1)
# ['macAddr','name', 'rssi','tx power','est range (m)', 'Timestamp','GPS', 'Latitude', 'Longitude', 'Altitude']
self.locationTable.setItem(rowPosition, 0, QTableWidgetItem(curDevice.macAddress))
self.locationTable.setItem(rowPosition, 1, QTableWidgetItem(curDevice.name))
self.locationTable.setItem(rowPosition, 2, IntTableWidgetItem(str(curDevice.rssi)))
if curDevice.txPowerValid:
self.locationTable.setItem(rowPosition, 3, IntTableWidgetItem(str(curDevice.txPower)))
else:
self.locationTable.setItem(rowPosition, 3, IntTableWidgetItem('Unknown'))
if curDevice.iBeaconRange != -1 and curDevice.txPowerValid:
self.locationTable.setItem(rowPosition, 4, IntTableWidgetItem(str(curDevice.iBeaconRange)))
else:
self.locationTable.setItem(rowPosition, 4, IntTableWidgetItem(str('Unknown')))
self.locationTable.setItem(rowPosition, 5, DateTableWidgetItem(curDevice.lastSeen.strftime("%m/%d/%Y %H:%M:%S")))
if curDevice.gps.isValid:
self.locationTable.setItem(rowPosition, 6, QTableWidgetItem('Yes'))
else:
self.locationTable.setItem(rowPosition, 6, QTableWidgetItem('No'))
self.locationTable.setItem(rowPosition, 7, FloatTableWidgetItem(str(curDevice.gps.latitude)))
self.locationTable.setItem(rowPosition, 8, FloatTableWidgetItem(str(curDevice.gps.longitude)))
self.locationTable.setItem(rowPosition, 9, FloatTableWidgetItem(str(curDevice.gps.altitude)))
#order = Qt.DescendingOrder
#self.locationTable.sortItems(3, order )
# If we're in streaming mode, write the data out to disk as well
if self.streamingFile:
self.streamingFile.write(self.locationTable.item(rowPosition, 0).text() + ',"' + self.locationTable.item(rowPosition, 1).text() + '",' + self.locationTable.item(rowPosition, 2).text() + ',' +
self.locationTable.item(rowPosition, 3).text() + ',' + self.locationTable.item(rowPosition, 4).text()+ ',' + self.locationTable.item(rowPosition, 5).text()+ ',' +
self.locationTable.item(rowPosition, 6).text()+ ',' + self.locationTable.item(rowPosition, 7).text() +
+ ',' + self.locationTable.item(rowPosition, 8).text()+ ',' + self.locationTable.item(rowPosition, 9).text() + '\n')
if (self.currentLine > self.linesBeforeFlush):
self.streamingFile.flush()
self.currentLine += 1
numRows = self.locationTable.rowCount()
if numRows > 1:
self.locationTable.scrollToItem(self.locationTable.item(0, 0))
# ------- Main Routine For Debugging-------------------------
if __name__ == '__main__':
app = QApplication([])
# date, time, ok = DB2Dialog.getDateTime()
# ok = TelemetryDialog.showTelemetry()
# dialog = TelemetryDialog()
dialog = BluetoothTelemetry()
dialog.show()
dialog.updateData(50)
#print("{} {} {}".format(date, time, ok))
app.exec_()
| gpl-3.0 |
xiaoxiamii/scikit-learn | sklearn/kernel_ridge.py | 155 | 6545 | """Module :mod:`sklearn.kernel_ridge` implements kernel ridge regression."""
# Authors: Mathieu Blondel <[email protected]>
# Jan Hendrik Metzen <[email protected]>
# License: BSD 3 clause
import numpy as np
from .base import BaseEstimator, RegressorMixin
from .metrics.pairwise import pairwise_kernels
from .linear_model.ridge import _solve_cholesky_kernel
from .utils import check_X_y
from .utils.validation import check_is_fitted
class KernelRidge(BaseEstimator, RegressorMixin):
"""Kernel ridge regression.
Kernel ridge regression (KRR) combines ridge regression (linear least
squares with l2-norm regularization) with the kernel trick. It thus
learns a linear function in the space induced by the respective kernel and
the data. For non-linear kernels, this corresponds to a non-linear
function in the original space.
The form of the model learned by KRR is identical to support vector
regression (SVR). However, different loss functions are used: KRR uses
squared error loss while support vector regression uses epsilon-insensitive
loss, both combined with l2 regularization. In contrast to SVR, fitting a
KRR model can be done in closed-form and is typically faster for
medium-sized datasets. On the other hand, the learned model is non-sparse
and thus slower than SVR, which learns a sparse model for epsilon > 0, at
prediction-time.
This estimator has built-in support for multi-variate regression
(i.e., when y is a 2d-array of shape [n_samples, n_targets]).
Read more in the :ref:`User Guide <kernel_ridge>`.
Parameters
----------
alpha : {float, array-like}, shape = [n_targets]
Small positive values of alpha improve the conditioning of the problem
and reduce the variance of the estimates. Alpha corresponds to
``(2*C)^-1`` in other linear models such as LogisticRegression or
LinearSVC. If an array is passed, penalties are assumed to be specific
to the targets. Hence they must correspond in number.
kernel : string or callable, default="linear"
Kernel mapping used internally. A callable should accept two arguments
and the keyword arguments passed to this object as kernel_params, and
should return a floating point number.
gamma : float, default=None
Gamma parameter for the RBF, polynomial, exponential chi2 and
sigmoid kernels. Interpretation of the default value is left to
the kernel; see the documentation for sklearn.metrics.pairwise.
Ignored by other kernels.
degree : float, default=3
Degree of the polynomial kernel. Ignored by other kernels.
coef0 : float, default=1
Zero coefficient for polynomial and sigmoid kernels.
Ignored by other kernels.
kernel_params : mapping of string to any, optional
Additional parameters (keyword arguments) for kernel function passed
as callable object.
Attributes
----------
dual_coef_ : array, shape = [n_features] or [n_targets, n_features]
Weight vector(s) in kernel space
X_fit_ : {array-like, sparse matrix}, shape = [n_samples, n_features]
Training data, which is also required for prediction
References
----------
* Kevin P. Murphy
"Machine Learning: A Probabilistic Perspective", The MIT Press
chapter 14.4.3, pp. 492-493
See also
--------
Ridge
Linear ridge regression.
SVR
Support Vector Regression implemented using libsvm.
Examples
--------
>>> from sklearn.kernel_ridge import KernelRidge
>>> import numpy as np
>>> n_samples, n_features = 10, 5
>>> rng = np.random.RandomState(0)
>>> y = rng.randn(n_samples)
>>> X = rng.randn(n_samples, n_features)
>>> clf = KernelRidge(alpha=1.0)
>>> clf.fit(X, y) # doctest: +NORMALIZE_WHITESPACE
KernelRidge(alpha=1.0, coef0=1, degree=3, gamma=None, kernel='linear',
kernel_params=None)
"""
def __init__(self, alpha=1, kernel="linear", gamma=None, degree=3, coef0=1,
kernel_params=None):
self.alpha = alpha
self.kernel = kernel
self.gamma = gamma
self.degree = degree
self.coef0 = coef0
self.kernel_params = kernel_params
def _get_kernel(self, X, Y=None):
if callable(self.kernel):
params = self.kernel_params or {}
else:
params = {"gamma": self.gamma,
"degree": self.degree,
"coef0": self.coef0}
return pairwise_kernels(X, Y, metric=self.kernel,
filter_params=True, **params)
@property
def _pairwise(self):
return self.kernel == "precomputed"
def fit(self, X, y=None, sample_weight=None):
"""Fit Kernel Ridge regression model
Parameters
----------
X : {array-like, sparse matrix}, shape = [n_samples, n_features]
Training data
y : array-like, shape = [n_samples] or [n_samples, n_targets]
Target values
sample_weight : float or numpy array of shape [n_samples]
Individual weights for each sample, ignored if None is passed.
Returns
-------
self : returns an instance of self.
"""
# Convert data
X, y = check_X_y(X, y, accept_sparse=("csr", "csc"), multi_output=True,
y_numeric=True)
K = self._get_kernel(X)
alpha = np.atleast_1d(self.alpha)
ravel = False
if len(y.shape) == 1:
y = y.reshape(-1, 1)
ravel = True
copy = self.kernel == "precomputed"
self.dual_coef_ = _solve_cholesky_kernel(K, y, alpha,
sample_weight,
copy)
if ravel:
self.dual_coef_ = self.dual_coef_.ravel()
self.X_fit_ = X
return self
def predict(self, X):
"""Predict using the the kernel ridge model
Parameters
----------
X : {array-like, sparse matrix}, shape = [n_samples, n_features]
Samples.
Returns
-------
C : array, shape = [n_samples] or [n_samples, n_targets]
Returns predicted values.
"""
check_is_fitted(self, ["X_fit_", "dual_coef_"])
K = self._get_kernel(X, self.X_fit_)
return np.dot(K, self.dual_coef_)
| bsd-3-clause |
BorisJeremic/Real-ESSI-Examples | analytic_solution/test_cases/Contact/Stress_Based_Contact_Verification/SoftContact_ElPPlShear/Shear_Zone_Length/SZ_h_1e3/Normal_Stress_Plot.py | 72 | 2800 | #!/usr/bin/python
import h5py
import matplotlib.pylab as plt
import matplotlib as mpl
import sys
import numpy as np;
import matplotlib;
import math;
from matplotlib.ticker import MaxNLocator
plt.rcParams.update({'font.size': 28})
# set tick width
mpl.rcParams['xtick.major.size'] = 10
mpl.rcParams['xtick.major.width'] = 5
mpl.rcParams['xtick.minor.size'] = 10
mpl.rcParams['xtick.minor.width'] = 5
plt.rcParams['xtick.labelsize']=24
mpl.rcParams['ytick.major.size'] = 10
mpl.rcParams['ytick.major.width'] = 5
mpl.rcParams['ytick.minor.size'] = 10
mpl.rcParams['ytick.minor.width'] = 5
plt.rcParams['ytick.labelsize']=24
###############################################################
## Analytical Solution
###############################################################
# Go over each feioutput and plot each one.
thefile = "Analytical_Solution_Normal_Stress.feioutput";
finput = h5py.File(thefile)
# Read the time and displacement
times = finput["time"][:]
normal_stress = -finput["/Model/Elements/Element_Outputs"][9,:];
normal_strain = -finput["/Model/Elements/Element_Outputs"][6,:];
# Configure the figure filename, according to the input filename.
outfig=thefile.replace("_","-")
outfigname=outfig.replace("h5.feioutput","pdf")
# Plot the figure. Add labels and titles.
plt.figure(figsize=(12,10))
plt.plot(normal_strain*100,normal_stress/1000,'-r',label='Analytical Solution', Linewidth=4, markersize=20)
plt.xlabel(r"Interface Type #")
plt.ylabel(r"Normal Stress $\sigma_n [kPa]$")
plt.hold(True)
###############################################################
## Numerical Solution
###############################################################
# Go over each feioutput and plot each one.
thefile = "Monotonic_Contact_Behaviour_Adding_Normal_Load.h5.feioutput";
finput = h5py.File(thefile)
# Read the time and displacement
times = finput["time"][:]
normal_stress = -finput["/Model/Elements/Element_Outputs"][9,:];
normal_strain = -finput["/Model/Elements/Element_Outputs"][6,:];
# Configure the figure filename, according to the input filename.
outfig=thefile.replace("_","-")
outfigname=outfig.replace("h5.feioutput","pdf")
# Plot the figure. Add labels and titles.
plt.plot(normal_strain*100,normal_stress/1000,'-k',label='Numerical Solution', Linewidth=4, markersize=20)
plt.xlabel(r"Normal Strain [%]")
plt.ylabel(r"Normal Stress $\sigma_n [kPa]$")
#############################################################
# # # axes = plt.gca()
# # # axes.set_xlim([-7,7])
# # # axes.set_ylim([-1,1])
# outfigname = "Interface_Test_Normal_Stress.pdf";
# plt.axis([0, 5.5, 90, 101])
# legend = plt.legend()
# legend.get_frame().set_linewidth(0.0)
# legend.get_frame().set_facecolor('none')
plt.legend()
plt.savefig('Normal_Stress.pdf', bbox_inches='tight')
# plt.show()
| cc0-1.0 |
mhdella/scikit-learn | sklearn/tree/export.py | 53 | 15772 | """
This module defines export functions for decision trees.
"""
# Authors: Gilles Louppe <[email protected]>
# Peter Prettenhofer <[email protected]>
# Brian Holt <[email protected]>
# Noel Dawe <[email protected]>
# Satrajit Gosh <[email protected]>
# Trevor Stephens <[email protected]>
# Licence: BSD 3 clause
import numpy as np
from ..externals import six
from . import _tree
def _color_brew(n):
"""Generate n colors with equally spaced hues.
Parameters
----------
n : int
The number of colors required.
Returns
-------
color_list : list, length n
List of n tuples of form (R, G, B) being the components of each color.
"""
color_list = []
# Initialize saturation & value; calculate chroma & value shift
s, v = 0.75, 0.9
c = s * v
m = v - c
for h in np.arange(25, 385, 360. / n).astype(int):
# Calculate some intermediate values
h_bar = h / 60.
x = c * (1 - abs((h_bar % 2) - 1))
# Initialize RGB with same hue & chroma as our color
rgb = [(c, x, 0),
(x, c, 0),
(0, c, x),
(0, x, c),
(x, 0, c),
(c, 0, x),
(c, x, 0)]
r, g, b = rgb[int(h_bar)]
# Shift the initial RGB values to match value and store
rgb = [(int(255 * (r + m))),
(int(255 * (g + m))),
(int(255 * (b + m)))]
color_list.append(rgb)
return color_list
def export_graphviz(decision_tree, out_file="tree.dot", max_depth=None,
feature_names=None, class_names=None, label='all',
filled=False, leaves_parallel=False, impurity=True,
node_ids=False, proportion=False, rotate=False,
rounded=False, special_characters=False):
"""Export a decision tree in DOT format.
This function generates a GraphViz representation of the decision tree,
which is then written into `out_file`. Once exported, graphical renderings
can be generated using, for example::
$ dot -Tps tree.dot -o tree.ps (PostScript format)
$ dot -Tpng tree.dot -o tree.png (PNG format)
The sample counts that are shown are weighted with any sample_weights that
might be present.
Read more in the :ref:`User Guide <tree>`.
Parameters
----------
decision_tree : decision tree classifier
The decision tree to be exported to GraphViz.
out_file : file object or string, optional (default="tree.dot")
Handle or name of the output file.
max_depth : int, optional (default=None)
The maximum depth of the representation. If None, the tree is fully
generated.
feature_names : list of strings, optional (default=None)
Names of each of the features.
class_names : list of strings, bool or None, optional (default=None)
Names of each of the target classes in ascending numerical order.
Only relevant for classification and not supported for multi-output.
If ``True``, shows a symbolic representation of the class name.
label : {'all', 'root', 'none'}, optional (default='all')
Whether to show informative labels for impurity, etc.
Options include 'all' to show at every node, 'root' to show only at
the top root node, or 'none' to not show at any node.
filled : bool, optional (default=False)
When set to ``True``, paint nodes to indicate majority class for
classification, extremity of values for regression, or purity of node
for multi-output.
leaves_parallel : bool, optional (default=False)
When set to ``True``, draw all leaf nodes at the bottom of the tree.
impurity : bool, optional (default=True)
When set to ``True``, show the impurity at each node.
node_ids : bool, optional (default=False)
When set to ``True``, show the ID number on each node.
proportion : bool, optional (default=False)
When set to ``True``, change the display of 'values' and/or 'samples'
to be proportions and percentages respectively.
rotate : bool, optional (default=False)
When set to ``True``, orient tree left to right rather than top-down.
rounded : bool, optional (default=False)
When set to ``True``, draw node boxes with rounded corners and use
Helvetica fonts instead of Times-Roman.
special_characters : bool, optional (default=False)
When set to ``False``, ignore special characters for PostScript
compatibility.
Examples
--------
>>> from sklearn.datasets import load_iris
>>> from sklearn import tree
>>> clf = tree.DecisionTreeClassifier()
>>> iris = load_iris()
>>> clf = clf.fit(iris.data, iris.target)
>>> tree.export_graphviz(clf,
... out_file='tree.dot') # doctest: +SKIP
"""
def get_color(value):
# Find the appropriate color & intensity for a node
if colors['bounds'] is None:
# Classification tree
color = list(colors['rgb'][np.argmax(value)])
sorted_values = sorted(value, reverse=True)
alpha = int(255 * (sorted_values[0] - sorted_values[1]) /
(1 - sorted_values[1]))
else:
# Regression tree or multi-output
color = list(colors['rgb'][0])
alpha = int(255 * ((value - colors['bounds'][0]) /
(colors['bounds'][1] - colors['bounds'][0])))
# Return html color code in #RRGGBBAA format
color.append(alpha)
hex_codes = [str(i) for i in range(10)]
hex_codes.extend(['a', 'b', 'c', 'd', 'e', 'f'])
color = [hex_codes[c // 16] + hex_codes[c % 16] for c in color]
return '#' + ''.join(color)
def node_to_str(tree, node_id, criterion):
# Generate the node content string
if tree.n_outputs == 1:
value = tree.value[node_id][0, :]
else:
value = tree.value[node_id]
# Should labels be shown?
labels = (label == 'root' and node_id == 0) or label == 'all'
# PostScript compatibility for special characters
if special_characters:
characters = ['#', '<SUB>', '</SUB>', '≤', '<br/>', '>']
node_string = '<'
else:
characters = ['#', '[', ']', '<=', '\\n', '"']
node_string = '"'
# Write node ID
if node_ids:
if labels:
node_string += 'node '
node_string += characters[0] + str(node_id) + characters[4]
# Write decision criteria
if tree.children_left[node_id] != _tree.TREE_LEAF:
# Always write node decision criteria, except for leaves
if feature_names is not None:
feature = feature_names[tree.feature[node_id]]
else:
feature = "X%s%s%s" % (characters[1],
tree.feature[node_id],
characters[2])
node_string += '%s %s %s%s' % (feature,
characters[3],
round(tree.threshold[node_id], 4),
characters[4])
# Write impurity
if impurity:
if isinstance(criterion, _tree.FriedmanMSE):
criterion = "friedman_mse"
elif not isinstance(criterion, six.string_types):
criterion = "impurity"
if labels:
node_string += '%s = ' % criterion
node_string += (str(round(tree.impurity[node_id], 4)) +
characters[4])
# Write node sample count
if labels:
node_string += 'samples = '
if proportion:
percent = (100. * tree.n_node_samples[node_id] /
float(tree.n_node_samples[0]))
node_string += (str(round(percent, 1)) + '%' +
characters[4])
else:
node_string += (str(tree.n_node_samples[node_id]) +
characters[4])
# Write node class distribution / regression value
if proportion and tree.n_classes[0] != 1:
# For classification this will show the proportion of samples
value = value / tree.weighted_n_node_samples[node_id]
if labels:
node_string += 'value = '
if tree.n_classes[0] == 1:
# Regression
value_text = np.around(value, 4)
elif proportion:
# Classification
value_text = np.around(value, 2)
elif np.all(np.equal(np.mod(value, 1), 0)):
# Classification without floating-point weights
value_text = value.astype(int)
else:
# Classification with floating-point weights
value_text = np.around(value, 4)
# Strip whitespace
value_text = str(value_text.astype('S32')).replace("b'", "'")
value_text = value_text.replace("' '", ", ").replace("'", "")
if tree.n_classes[0] == 1 and tree.n_outputs == 1:
value_text = value_text.replace("[", "").replace("]", "")
value_text = value_text.replace("\n ", characters[4])
node_string += value_text + characters[4]
# Write node majority class
if (class_names is not None and
tree.n_classes[0] != 1 and
tree.n_outputs == 1):
# Only done for single-output classification trees
if labels:
node_string += 'class = '
if class_names is not True:
class_name = class_names[np.argmax(value)]
else:
class_name = "y%s%s%s" % (characters[1],
np.argmax(value),
characters[2])
node_string += class_name
# Clean up any trailing newlines
if node_string[-2:] == '\\n':
node_string = node_string[:-2]
if node_string[-5:] == '<br/>':
node_string = node_string[:-5]
return node_string + characters[5]
def recurse(tree, node_id, criterion, parent=None, depth=0):
if node_id == _tree.TREE_LEAF:
raise ValueError("Invalid node_id %s" % _tree.TREE_LEAF)
left_child = tree.children_left[node_id]
right_child = tree.children_right[node_id]
# Add node with description
if max_depth is None or depth <= max_depth:
# Collect ranks for 'leaf' option in plot_options
if left_child == _tree.TREE_LEAF:
ranks['leaves'].append(str(node_id))
elif str(depth) not in ranks:
ranks[str(depth)] = [str(node_id)]
else:
ranks[str(depth)].append(str(node_id))
out_file.write('%d [label=%s'
% (node_id,
node_to_str(tree, node_id, criterion)))
if filled:
# Fetch appropriate color for node
if 'rgb' not in colors:
# Initialize colors and bounds if required
colors['rgb'] = _color_brew(tree.n_classes[0])
if tree.n_outputs != 1:
# Find max and min impurities for multi-output
colors['bounds'] = (np.min(-tree.impurity),
np.max(-tree.impurity))
elif tree.n_classes[0] == 1:
# Find max and min values in leaf nodes for regression
colors['bounds'] = (np.min(tree.value),
np.max(tree.value))
if tree.n_outputs == 1:
node_val = (tree.value[node_id][0, :] /
tree.weighted_n_node_samples[node_id])
if tree.n_classes[0] == 1:
# Regression
node_val = tree.value[node_id][0, :]
else:
# If multi-output color node by impurity
node_val = -tree.impurity[node_id]
out_file.write(', fillcolor="%s"' % get_color(node_val))
out_file.write('] ;\n')
if parent is not None:
# Add edge to parent
out_file.write('%d -> %d' % (parent, node_id))
if parent == 0:
# Draw True/False labels if parent is root node
angles = np.array([45, -45]) * ((rotate - .5) * -2)
out_file.write(' [labeldistance=2.5, labelangle=')
if node_id == 1:
out_file.write('%d, headlabel="True"]' % angles[0])
else:
out_file.write('%d, headlabel="False"]' % angles[1])
out_file.write(' ;\n')
if left_child != _tree.TREE_LEAF:
recurse(tree, left_child, criterion=criterion, parent=node_id,
depth=depth + 1)
recurse(tree, right_child, criterion=criterion, parent=node_id,
depth=depth + 1)
else:
ranks['leaves'].append(str(node_id))
out_file.write('%d [label="(...)"' % node_id)
if filled:
# color cropped nodes grey
out_file.write(', fillcolor="#C0C0C0"')
out_file.write('] ;\n' % node_id)
if parent is not None:
# Add edge to parent
out_file.write('%d -> %d ;\n' % (parent, node_id))
own_file = False
try:
if isinstance(out_file, six.string_types):
if six.PY3:
out_file = open(out_file, "w", encoding="utf-8")
else:
out_file = open(out_file, "wb")
own_file = True
# The depth of each node for plotting with 'leaf' option
ranks = {'leaves': []}
# The colors to render each node with
colors = {'bounds': None}
out_file.write('digraph Tree {\n')
# Specify node aesthetics
out_file.write('node [shape=box')
rounded_filled = []
if filled:
rounded_filled.append('filled')
if rounded:
rounded_filled.append('rounded')
if len(rounded_filled) > 0:
out_file.write(', style="%s", color="black"'
% ", ".join(rounded_filled))
if rounded:
out_file.write(', fontname=helvetica')
out_file.write('] ;\n')
# Specify graph & edge aesthetics
if leaves_parallel:
out_file.write('graph [ranksep=equally, splines=polyline] ;\n')
if rounded:
out_file.write('edge [fontname=helvetica] ;\n')
if rotate:
out_file.write('rankdir=LR ;\n')
# Now recurse the tree and add node & edge attributes
if isinstance(decision_tree, _tree.Tree):
recurse(decision_tree, 0, criterion="impurity")
else:
recurse(decision_tree.tree_, 0, criterion=decision_tree.criterion)
# If required, draw leaf nodes at same depth as each other
if leaves_parallel:
for rank in sorted(ranks):
out_file.write("{rank=same ; " +
"; ".join(r for r in ranks[rank]) + "} ;\n")
out_file.write("}")
finally:
if own_file:
out_file.close()
| bsd-3-clause |
RPGOne/Skynet | scikit-learn-0.18.1/sklearn/ensemble/tests/test_partial_dependence.py | 365 | 6996 | """
Testing for the partial dependence module.
"""
import numpy as np
from numpy.testing import assert_array_equal
from sklearn.utils.testing import assert_raises
from sklearn.utils.testing import if_matplotlib
from sklearn.ensemble.partial_dependence import partial_dependence
from sklearn.ensemble.partial_dependence import plot_partial_dependence
from sklearn.ensemble import GradientBoostingClassifier
from sklearn.ensemble import GradientBoostingRegressor
from sklearn import datasets
# toy sample
X = [[-2, -1], [-1, -1], [-1, -2], [1, 1], [1, 2], [2, 1]]
y = [-1, -1, -1, 1, 1, 1]
T = [[-1, -1], [2, 2], [3, 2]]
true_result = [-1, 1, 1]
# also load the boston dataset
boston = datasets.load_boston()
# also load the iris dataset
iris = datasets.load_iris()
def test_partial_dependence_classifier():
# Test partial dependence for classifier
clf = GradientBoostingClassifier(n_estimators=10, random_state=1)
clf.fit(X, y)
pdp, axes = partial_dependence(clf, [0], X=X, grid_resolution=5)
# only 4 grid points instead of 5 because only 4 unique X[:,0] vals
assert pdp.shape == (1, 4)
assert axes[0].shape[0] == 4
# now with our own grid
X_ = np.asarray(X)
grid = np.unique(X_[:, 0])
pdp_2, axes = partial_dependence(clf, [0], grid=grid)
assert axes is None
assert_array_equal(pdp, pdp_2)
def test_partial_dependence_multiclass():
# Test partial dependence for multi-class classifier
clf = GradientBoostingClassifier(n_estimators=10, random_state=1)
clf.fit(iris.data, iris.target)
grid_resolution = 25
n_classes = clf.n_classes_
pdp, axes = partial_dependence(
clf, [0], X=iris.data, grid_resolution=grid_resolution)
assert pdp.shape == (n_classes, grid_resolution)
assert len(axes) == 1
assert axes[0].shape[0] == grid_resolution
def test_partial_dependence_regressor():
# Test partial dependence for regressor
clf = GradientBoostingRegressor(n_estimators=10, random_state=1)
clf.fit(boston.data, boston.target)
grid_resolution = 25
pdp, axes = partial_dependence(
clf, [0], X=boston.data, grid_resolution=grid_resolution)
assert pdp.shape == (1, grid_resolution)
assert axes[0].shape[0] == grid_resolution
def test_partial_dependecy_input():
# Test input validation of partial dependence.
clf = GradientBoostingClassifier(n_estimators=10, random_state=1)
clf.fit(X, y)
assert_raises(ValueError, partial_dependence,
clf, [0], grid=None, X=None)
assert_raises(ValueError, partial_dependence,
clf, [0], grid=[0, 1], X=X)
# first argument must be an instance of BaseGradientBoosting
assert_raises(ValueError, partial_dependence,
{}, [0], X=X)
# Gradient boosting estimator must be fit
assert_raises(ValueError, partial_dependence,
GradientBoostingClassifier(), [0], X=X)
assert_raises(ValueError, partial_dependence, clf, [-1], X=X)
assert_raises(ValueError, partial_dependence, clf, [100], X=X)
# wrong ndim for grid
grid = np.random.rand(10, 2, 1)
assert_raises(ValueError, partial_dependence, clf, [0], grid=grid)
@if_matplotlib
def test_plot_partial_dependence():
# Test partial dependence plot function.
clf = GradientBoostingRegressor(n_estimators=10, random_state=1)
clf.fit(boston.data, boston.target)
grid_resolution = 25
fig, axs = plot_partial_dependence(clf, boston.data, [0, 1, (0, 1)],
grid_resolution=grid_resolution,
feature_names=boston.feature_names)
assert len(axs) == 3
assert all(ax.has_data for ax in axs)
# check with str features and array feature names
fig, axs = plot_partial_dependence(clf, boston.data, ['CRIM', 'ZN',
('CRIM', 'ZN')],
grid_resolution=grid_resolution,
feature_names=boston.feature_names)
assert len(axs) == 3
assert all(ax.has_data for ax in axs)
# check with list feature_names
feature_names = boston.feature_names.tolist()
fig, axs = plot_partial_dependence(clf, boston.data, ['CRIM', 'ZN',
('CRIM', 'ZN')],
grid_resolution=grid_resolution,
feature_names=feature_names)
assert len(axs) == 3
assert all(ax.has_data for ax in axs)
@if_matplotlib
def test_plot_partial_dependence_input():
# Test partial dependence plot function input checks.
clf = GradientBoostingClassifier(n_estimators=10, random_state=1)
# not fitted yet
assert_raises(ValueError, plot_partial_dependence,
clf, X, [0])
clf.fit(X, y)
assert_raises(ValueError, plot_partial_dependence,
clf, np.array(X)[:, :0], [0])
# first argument must be an instance of BaseGradientBoosting
assert_raises(ValueError, plot_partial_dependence,
{}, X, [0])
# must be larger than -1
assert_raises(ValueError, plot_partial_dependence,
clf, X, [-1])
# too large feature value
assert_raises(ValueError, plot_partial_dependence,
clf, X, [100])
# str feature but no feature_names
assert_raises(ValueError, plot_partial_dependence,
clf, X, ['foobar'])
# not valid features value
assert_raises(ValueError, plot_partial_dependence,
clf, X, [{'foo': 'bar'}])
@if_matplotlib
def test_plot_partial_dependence_multiclass():
# Test partial dependence plot function on multi-class input.
clf = GradientBoostingClassifier(n_estimators=10, random_state=1)
clf.fit(iris.data, iris.target)
grid_resolution = 25
fig, axs = plot_partial_dependence(clf, iris.data, [0, 1],
label=0,
grid_resolution=grid_resolution)
assert len(axs) == 2
assert all(ax.has_data for ax in axs)
# now with symbol labels
target = iris.target_names[iris.target]
clf = GradientBoostingClassifier(n_estimators=10, random_state=1)
clf.fit(iris.data, target)
grid_resolution = 25
fig, axs = plot_partial_dependence(clf, iris.data, [0, 1],
label='setosa',
grid_resolution=grid_resolution)
assert len(axs) == 2
assert all(ax.has_data for ax in axs)
# label not in gbrt.classes_
assert_raises(ValueError, plot_partial_dependence,
clf, iris.data, [0, 1], label='foobar',
grid_resolution=grid_resolution)
# label not provided
assert_raises(ValueError, plot_partial_dependence,
clf, iris.data, [0, 1],
grid_resolution=grid_resolution)
| bsd-3-clause |
mailhexu/pyDFTutils | pyDFTutils/tightbinding/mytb.py | 2 | 2820 | #!/usr/bin/env python
import numpy as np
import scipy.linalg as sl
import cmath
class mytb(object):
def __init__(self, dim_k, dim_r, lat=None, orb=None, per=None, nspin=1):
self.dim_k = dim_k
self.dim_r = dim_r
self.lat = lat
self.orb = orb
self.norb = len(orb)
self.per = per
self.nspin = nspin
self.ham = {}
self.ham_R = {}
G = np.zeros((3, 3))
G[0] = 2 * np.pi * np.cross(lat[1], lat[2]) / (np.inner(
lat[0], np.cross(lat[1], lat[2])))
G[1] = 2 * np.pi * np.cross(lat[2], lat[0]) / (np.inner(
lat[1], np.cross(lat[2], lat[0])))
G[2] = 2 * np.pi * np.cross(lat[0], lat[1]) / (np.inner(
lat[2], np.cross(lat[0], lat[1])))
self.G = G
def set_onsite(self, val, ind_i):
self.ham[(ind_i, ind_i, (0, ) * self.dim_r)] = val
self.ham_R[(ind_i, ind_i, (0, ) * self.dim_r)] = val
def set_hop_dis(self, val, i, j, dis):
self.ham[(i, j, tuple(dis))] = val
def set_hop(self, val, i, j, R, allow_conjugate_pair=True):
dis = np.dot(self.lat,
R) + np.array(self.orb[j]) - np.array(self.orb[i])
self.ham[(i, j, tuple(dis))] = val
self.ham_R[i, j, tuple(R)] = val
def is_hermitian(self, H):
return np.isclose(H, H.T.conj(), atol=1e-6).all()
def make_hamk(self, k):
"""
build k space hamiltonian.
"""
k = np.dot(self.G, np.asarray(k))
hamk = np.zeros((self.norb, self.norb, ), dtype='complex')
for key in self.ham:
i, j, dis = key
val = self.ham[key]
hamk[i, j] += val * cmath.exp(1j * np.inner(k, dis))
# if not self.is_hermitian(hamk):
# print "Hamk is not Hermitian"
hamk = (hamk + hamk.T.conj()) / 2.0
return hamk
def solve_k(self, k, eigvec=False):
hamk = self.make_hamk(k)
if eigvec:
eigval, eigvec = sl.eigh(hamk)
eigvec=np.linalg.qr(eigvec)[0]
return eigval, eigvec
else:
eigval = sl.eigvalsh(hamk)
return eigval
def solve_all(self, k_vec):
eigvals = []
for k in k_vec:
eigval = self.solve_k(k)
eigvals.append(eigval)
return np.array(eigvals).T
def test():
tb = mytb(3, 3, lat=np.eye(3), orb=[(0, 0, 0)])
tb.set_onsite(1, 0)
tb.set_hop(1, 0, 0, (0, 0, 1))
print "real space H:\n", tb.ham
print tb.make_hamk([0, 0, 0.2])
print tb.solve_k([0, 0, 0.2])
kpath = [(0, 0, x) for x in np.arange(0, 0.5, 0.02)]
eigvals = tb.solve_all(kpath)
print eigvals[:, 0]
import matplotlib.pyplot as plt
plt.plot(eigvals[0])
plt.show()
if __name__ == '__main__':
test()
| lgpl-3.0 |
jreback/pandas | pandas/tests/groupby/test_value_counts.py | 1 | 4186 | """
these are systematically testing all of the args to value_counts
with different size combinations. This is to ensure stability of the sorting
and proper parameter handling
"""
from itertools import product
import numpy as np
import pytest
from pandas import (
Categorical,
CategoricalIndex,
DataFrame,
Grouper,
MultiIndex,
Series,
date_range,
to_datetime,
)
import pandas._testing as tm
# our starting frame
def seed_df(seed_nans, n, m):
np.random.seed(1234)
days = date_range("2015-08-24", periods=10)
frame = DataFrame(
{
"1st": np.random.choice(list("abcd"), n),
"2nd": np.random.choice(days, n),
"3rd": np.random.randint(1, m + 1, n),
}
)
if seed_nans:
frame.loc[1::11, "1st"] = np.nan
frame.loc[3::17, "2nd"] = np.nan
frame.loc[7::19, "3rd"] = np.nan
frame.loc[8::19, "3rd"] = np.nan
frame.loc[9::19, "3rd"] = np.nan
return frame
# create input df, keys, and the bins
binned = []
ids = []
for seed_nans in [True, False]:
for n, m in product((100, 1000), (5, 20)):
df = seed_df(seed_nans, n, m)
bins = None, np.arange(0, max(5, df["3rd"].max()) + 1, 2)
keys = "1st", "2nd", ["1st", "2nd"]
for k, b in product(keys, bins):
binned.append((df, k, b, n, m))
ids.append(f"{k}-{n}-{m}")
@pytest.mark.slow
@pytest.mark.parametrize("df, keys, bins, n, m", binned, ids=ids)
@pytest.mark.parametrize("isort", [True, False])
@pytest.mark.parametrize("normalize", [True, False])
@pytest.mark.parametrize("sort", [True, False])
@pytest.mark.parametrize("ascending", [True, False])
@pytest.mark.parametrize("dropna", [True, False])
def test_series_groupby_value_counts(
df, keys, bins, n, m, isort, normalize, sort, ascending, dropna
):
def rebuild_index(df):
arr = list(map(df.index.get_level_values, range(df.index.nlevels)))
df.index = MultiIndex.from_arrays(arr, names=df.index.names)
return df
kwargs = {
"normalize": normalize,
"sort": sort,
"ascending": ascending,
"dropna": dropna,
"bins": bins,
}
gr = df.groupby(keys, sort=isort)
left = gr["3rd"].value_counts(**kwargs)
gr = df.groupby(keys, sort=isort)
right = gr["3rd"].apply(Series.value_counts, **kwargs)
right.index.names = right.index.names[:-1] + ["3rd"]
# have to sort on index because of unstable sort on values
left, right = map(rebuild_index, (left, right)) # xref GH9212
tm.assert_series_equal(left.sort_index(), right.sort_index())
def test_series_groupby_value_counts_with_grouper():
# GH28479
df = DataFrame(
{
"Timestamp": [
1565083561,
1565083561 + 86400,
1565083561 + 86500,
1565083561 + 86400 * 2,
1565083561 + 86400 * 3,
1565083561 + 86500 * 3,
1565083561 + 86400 * 4,
],
"Food": ["apple", "apple", "banana", "banana", "orange", "orange", "pear"],
}
).drop([3])
df["Datetime"] = to_datetime(df["Timestamp"].apply(lambda t: str(t)), unit="s")
dfg = df.groupby(Grouper(freq="1D", key="Datetime"))
# have to sort on index because of unstable sort on values xref GH9212
result = dfg["Food"].value_counts().sort_index()
expected = dfg["Food"].apply(Series.value_counts).sort_index()
expected.index.names = result.index.names
tm.assert_series_equal(result, expected)
def test_series_groupby_value_counts_on_categorical():
# GH38672
s = Series(Categorical(["a"], categories=["a", "b"]))
result = s.groupby([0]).value_counts()
expected = Series(
data=[1, 0],
index=MultiIndex.from_arrays(
[
[0, 0],
CategoricalIndex(
["a", "b"], categories=["a", "b"], ordered=False, dtype="category"
),
]
),
name=0,
)
# Expected:
# 0 a 1
# b 0
# Name: 0, dtype: int64
tm.assert_series_equal(result, expected)
| bsd-3-clause |
mlyundin/scikit-learn | examples/linear_model/plot_sgd_weighted_samples.py | 344 | 1458 | """
=====================
SGD: Weighted samples
=====================
Plot decision function of a weighted dataset, where the size of points
is proportional to its weight.
"""
print(__doc__)
import numpy as np
import matplotlib.pyplot as plt
from sklearn import linear_model
# we create 20 points
np.random.seed(0)
X = np.r_[np.random.randn(10, 2) + [1, 1], np.random.randn(10, 2)]
y = [1] * 10 + [-1] * 10
sample_weight = 100 * np.abs(np.random.randn(20))
# and assign a bigger weight to the last 10 samples
sample_weight[:10] *= 10
# plot the weighted data points
xx, yy = np.meshgrid(np.linspace(-4, 5, 500), np.linspace(-4, 5, 500))
plt.figure()
plt.scatter(X[:, 0], X[:, 1], c=y, s=sample_weight, alpha=0.9,
cmap=plt.cm.bone)
## fit the unweighted model
clf = linear_model.SGDClassifier(alpha=0.01, n_iter=100)
clf.fit(X, y)
Z = clf.decision_function(np.c_[xx.ravel(), yy.ravel()])
Z = Z.reshape(xx.shape)
no_weights = plt.contour(xx, yy, Z, levels=[0], linestyles=['solid'])
## fit the weighted model
clf = linear_model.SGDClassifier(alpha=0.01, n_iter=100)
clf.fit(X, y, sample_weight=sample_weight)
Z = clf.decision_function(np.c_[xx.ravel(), yy.ravel()])
Z = Z.reshape(xx.shape)
samples_weights = plt.contour(xx, yy, Z, levels=[0], linestyles=['dashed'])
plt.legend([no_weights.collections[0], samples_weights.collections[0]],
["no weights", "with weights"], loc="lower left")
plt.xticks(())
plt.yticks(())
plt.show()
| bsd-3-clause |
waltervh/BornAgain | Examples/python/fitting/ex02_AdvancedExamples/multiple_datasets.py | 2 | 6304 | """
Fitting example: simultaneous fit of two datasets
"""
import numpy as np
import matplotlib
from matplotlib import pyplot as plt
import bornagain as ba
from bornagain import deg, angstrom, nm
def get_sample(params):
"""
Returns a sample with uncorrelated cylinders and pyramids.
"""
radius_a = params["radius_a"]
radius_b = params["radius_b"]
height = params["height"]
m_air = ba.HomogeneousMaterial("Air", 0.0, 0.0)
m_substrate = ba.HomogeneousMaterial("Substrate", 6e-6, 2e-8)
m_particle = ba.HomogeneousMaterial("Particle", 6e-4, 2e-8)
formfactor = ba.FormFactorHemiEllipsoid(radius_a, radius_b, height)
particle = ba.Particle(m_particle, formfactor)
layout = ba.ParticleLayout()
layout.addParticle(particle)
air_layer = ba.Layer(m_air)
air_layer.addLayout(layout)
substrate_layer = ba.Layer(m_substrate)
multi_layer = ba.MultiLayer()
multi_layer.addLayer(air_layer)
multi_layer.addLayer(substrate_layer)
return multi_layer
def get_simulation(params):
"""
Returns a GISAXS simulation with beam and detector defined.
"""
incident_angle = params["incident_angle"]
simulation = ba.GISASSimulation()
simulation.setDetectorParameters(50, -1.5*deg, 1.5*deg,
50, 0.0*deg, 2.0*deg)
simulation.setBeamParameters(1.0*angstrom, incident_angle, 0.0*deg)
simulation.setBeamIntensity(1e+08)
simulation.setSample(get_sample(params))
return simulation
def simulation1(params):
params["incident_angle"] = 0.1*deg
return get_simulation(params)
def simulation2(params):
params["incident_angle"] = 0.4*deg
return get_simulation(params)
def create_real_data(incident_alpha):
"""
Generating "real" data by adding noise to the simulated data.
"""
params = {'radius_a': 5.0*nm, 'radius_b': 6.0*nm,
'height': 8.0*nm, "incident_angle": incident_alpha}
simulation = get_simulation(params)
simulation.runSimulation()
# retrieving simulated data in the form of numpy array
real_data = simulation.result().array()
# spoiling simulated data with the noise to produce "real" data
noise_factor = 0.1
noisy = np.random.normal(real_data, noise_factor*np.sqrt(real_data))
noisy[noisy < 0.1] = 0.1
return noisy
class PlotObserver():
"""
Draws fit progress every nth iteration. Real data, simulated data
and chi2 map will be shown for both datasets.
"""
def __init__(self):
self.fig = plt.figure(figsize=(12.8, 10.24))
self.fig.canvas.draw()
def __call__(self, fit_objective):
self.update(fit_objective)
@staticmethod
def plot_dataset(fit_objective, canvas):
for i_dataset in range(0, fit_objective.fitObjectCount()):
real_data = fit_objective.experimentalData(i_dataset)
simul_data = fit_objective.simulationResult(i_dataset)
chi2_map = fit_objective.relativeDifference(i_dataset)
zmax = real_data.histogram2d().getMaximum()
plt.subplot(canvas[i_dataset*3])
ba.plot_colormap(real_data, title="\"Real\" data - #"+str(i_dataset+1),
zmin=1.0, zmax=zmax, zlabel="")
plt.subplot(canvas[1+i_dataset*3])
ba.plot_colormap(simul_data, title="Simulated data - #"+str(i_dataset+1),
zmin=1.0, zmax=zmax, zlabel="")
plt.subplot(canvas[2+i_dataset*3])
ba.plot_colormap(chi2_map, title="Chi2 map - #"+str(i_dataset+1),
zmin=0.001, zmax=10.0, zlabel="")
@staticmethod
def display_fit_parameters(fit_objective):
"""
Displays fit parameters, chi and iteration number.
"""
plt.title('Parameters')
plt.axis('off')
iteration_info = fit_objective.iterationInfo()
plt.text(0.01, 0.85, "Iterations " + '{:d}'.
format(iteration_info.iterationCount()))
plt.text(0.01, 0.75, "Chi2 " + '{:8.4f}'.format(iteration_info.chi2()))
for index, params in enumerate(iteration_info.parameters()):
plt.text(0.01, 0.55 - index * 0.1,
'{:30.30s}: {:6.3f}'.format(params.name(), params.value))
@staticmethod
def plot_fit_parameters(fit_objective):
"""
Displays fit parameters, chi and iteration number.
"""
plt.axis('off')
iteration_info = fit_objective.iterationInfo()
plt.text(0.01, 0.95, "Iterations " + '{:d}'.
format(iteration_info.iterationCount()))
plt.text(0.01, 0.70, "Chi2 " + '{:8.4f}'.format(iteration_info.chi2()))
for index, params in enumerate(iteration_info.parameters()):
plt.text(0.01, 0.30 - index * 0.3,
'{:30.30s}: {:6.3f}'.format(params.name(), params.value))
def update(self, fit_objective):
self.fig.clf()
# we divide figure to have 3x3 subplots, with two first rows occupying
# most of the space
canvas = matplotlib.gridspec.GridSpec(
3, 3, width_ratios=[1, 1, 1], height_ratios=[4, 4, 1])
canvas.update(left=0.05, right=0.95, hspace=0.5, wspace=0.2)
self.plot_dataset(fit_objective, canvas)
plt.subplot(canvas[6:])
self.plot_fit_parameters(fit_objective)
plt.draw()
plt.pause(0.01)
def run_fitting():
"""
main function to run fitting
"""
data1 = create_real_data(0.1 * deg)
data2 = create_real_data(0.4 * deg)
fit_objective = ba.FitObjective()
fit_objective.addSimulationAndData(simulation1, data1, 1.0)
fit_objective.addSimulationAndData(simulation2, data2, 1.0)
fit_objective.initPrint(10)
# creating custom observer which will draw fit progress
plotter = PlotObserver()
fit_objective.initPlot(10, plotter.update)
params = ba.Parameters()
params.add("radius_a", 4.*nm, min=2.0, max=10.0)
params.add("radius_b", 6.*nm, vary=False)
params.add("height", 4.*nm, min=2.0, max=10.0)
minimizer = ba.Minimizer()
result = minimizer.minimize(fit_objective.evaluate, params)
fit_objective.finalize(result)
if __name__ == '__main__':
run_fitting()
plt.show()
| gpl-3.0 |
Microsoft/hummingbird | hummingbird/ml/operator_converters/sklearn/scaler.py | 1 | 1796 | # -------------------------------------------------------------------------
# Copyright (c) Microsoft Corporation. All rights reserved.
# Licensed under the MIT License. See License.txt in the project root for
# license information.
# --------------------------------------------------------------------------
"""
Converters for scikit-learn scalers: RobustScaler, MaxAbsScaler, MinMaxScaler, StandardScaler.
"""
import numpy as np
from onnxconverter_common.registration import register_converter
from .._scaler_implementations import Scaler
def convert_sklearn_robust_scaler(operator, device, extra_config):
scale = operator.raw_operator.scale_
if scale is not None:
scale = np.reciprocal(scale)
return Scaler(operator.raw_operator.center_, scale, device)
def convert_sklearn_max_abs_scaler(operator, device, extra_config):
scale = operator.raw_operator.scale_
if scale is not None:
scale = np.reciprocal(scale)
return Scaler(0, scale, device)
def convert_sklearn_min_max_scaler(operator, device, extra_config):
scale = [x for x in operator.raw_operator.scale_]
offset = [-1.0 / x * y for x, y in zip(operator.raw_operator.scale_, operator.raw_operator.min_)]
return Scaler(offset, scale, device)
def convert_sklearn_standard_scaler(operator, device, extra_config):
scale = operator.raw_operator.scale_
if scale is not None:
scale = np.reciprocal(scale)
return Scaler(operator.raw_operator.mean_, scale, device)
register_converter("SklearnRobustScaler", convert_sklearn_robust_scaler)
register_converter("SklearnMaxAbsScaler", convert_sklearn_max_abs_scaler)
register_converter("SklearnMinMaxScaler", convert_sklearn_min_max_scaler)
register_converter("SklearnStandardScaler", convert_sklearn_standard_scaler)
| mit |
florian-f/sklearn | sklearn/covariance/__init__.py | 10 | 1197 | """
The :mod:`sklearn.covariance` module includes methods and algorithms to
robustly estimate the covariance of features given a set of points. The
precision matrix defined as the inverse of the covariance is also estimated.
Covariance estimation is closely related to the theory of Gaussian Graphical
Models.
"""
from .empirical_covariance_ import empirical_covariance, EmpiricalCovariance, \
log_likelihood
from .shrunk_covariance_ import shrunk_covariance, ShrunkCovariance, \
ledoit_wolf, ledoit_wolf_shrinkage, LedoitWolf, oas, OAS
from .robust_covariance import fast_mcd, MinCovDet
from .graph_lasso_ import graph_lasso, GraphLasso, GraphLassoCV
from .outlier_detection import EllipticEnvelope, EllipticEnvelop
__all__ = ['EllipticEnvelop',
'EllipticEnvelope',
'EmpiricalCovariance',
'GraphLasso',
'GraphLassoCV',
'LedoitWolf',
'MinCovDet',
'OAS',
'ShrunkCovariance',
'empirical_covariance',
'fast_mcd',
'graph_lasso',
'ledoit_wolf',
'ledoit_wolf_shrinkage',
'log_likelihood',
'oas',
'shrunk_covariance']
| bsd-3-clause |
deepakantony/sms-tools | workspace/A4/A4Part3.py | 1 | 5531 | import os
import sys
import numpy as np
from scipy.signal import get_window
import matplotlib.pyplot as plt
sys.path.append(os.path.join(os.path.dirname(os.path.realpath(__file__)), '../../software/models/'))
import stft
import utilFunctions as UF
eps = np.finfo(float).eps
"""
A4-Part-3: Computing band-wise energy envelopes of a signal
Write a function that computes band-wise energy envelopes of a given audio signal by using the STFT.
Consider two frequency bands for this question, low and high. The low frequency band is the set of
all the frequencies between 0 and 3000 Hz and the high frequency band is the set of all the
frequencies between 3000 and 10000 Hz (excluding the boundary frequencies in both the cases).
At a given frame, the value of the energy envelope of a band can be computed as the sum of squared
values of all the frequency coefficients in that band. Compute the energy envelopes in decibels.
Refer to "A4-STFT.pdf" document for further details on computing bandwise energy.
The input arguments to the function are the wav file name including the path (inputFile), window
type (window), window length (M), FFT size (N) and hop size (H). The function should return a numpy
array with two columns, where the first column is the energy envelope of the low frequency band and
the second column is that of the high frequency band.
Use stft.stftAnal() to obtain the STFT magnitude spectrum for all the audio frames. Then compute two
energy values for each frequency band specified. While calculating frequency bins for each frequency
band, consider only the bins that are within the specified frequency range. For example, for the low
frequency band consider only the bins with frequency > 0 Hz and < 3000 Hz (you can use np.where() to
find those bin indexes). This way we also remove the DC offset in the signal in energy envelope
computation. The frequency corresponding to the bin index k can be computed as k*fs/N, where fs is
the sampling rate of the signal.
To get a better understanding of the energy envelope and its characteristics you can plot the envelopes
together with the spectrogram of the signal. You can use matplotlib plotting library for this purpose.
To visualize the spectrogram of a signal, a good option is to use colormesh. You can reuse the code in
sms-tools/lectures/4-STFT/plots-code/spectrogram.py. Either overlay the envelopes on the spectrogram
or plot them in a different subplot. Make sure you use the same range of the x-axis for both the
spectrogram and the energy envelopes.
NOTE: Running these test cases might take a few seconds depending on your hardware.
Test case 1: Use piano.wav file with window = 'blackman', M = 513, N = 1024 and H = 128 as input.
The bin indexes of the low frequency band span from 1 to 69 (69 samples) and of the high frequency
band span from 70 to 232 (163 samples). To numerically compare your output, use loadTestCases.py
script to obtain the expected output.
Test case 2: Use piano.wav file with window = 'blackman', M = 2047, N = 4096 and H = 128 as input.
The bin indexes of the low frequency band span from 1 to 278 (278 samples) and of the high frequency
band span from 279 to 928 (650 samples). To numerically compare your output, use loadTestCases.py
script to obtain the expected output.
Test case 3: Use sax-phrase-short.wav file with window = 'hamming', M = 513, N = 2048 and H = 256 as
input. The bin indexes of the low frequency band span from 1 to 139 (139 samples) and of the high
frequency band span from 140 to 464 (325 samples). To numerically compare your output, use
loadTestCases.py script to obtain the expected output.
In addition to comparing results with the expected output, you can also plot your output for these
test cases.You can clearly notice the sharp attacks and decay of the piano notes for test case 1
(See figure in the accompanying pdf). You can compare this with the output from test case 2 that
uses a larger window. You can infer the influence of window size on sharpness of the note attacks
and discuss it on the forums.
"""
def computeEngEnv(inputFile, window, M, N, H):
"""
Inputs:
inputFile (string): input sound file (monophonic with sampling rate of 44100)
window (string): analysis window type (choice of rectangular, triangular, hanning,
hamming, blackman, blackmanharris)
M (integer): analysis window size (odd positive integer)
N (integer): FFT size (power of 2, such that N > M)
H (integer): hop size for the stft computation
Output:
The function should return a numpy array engEnv with shape Kx2, K = Number of frames
containing energy envelop of the signal in decibles (dB) scale
engEnv[:,0]: Energy envelope in band 0 < f < 3000 Hz (in dB)
engEnv[:,1]: Energy envelope in band 3000 < f < 10000 Hz (in dB)
"""
### your code here
fs,x = UF.wavread(inputFile)
w = get_window(window,M)
mX,pX = stft.stftAnal(x,w,N,H)
mX = pow(10,mX/20.)
band_energy = np.zeros((len(mX),2))
for frm_idx in range(len(mX)):
frm = mX[frm_idx]
for k in range(len(frm)):
cur_f = k*44100/N
if cur_f > 0 and cur_f < 3000:
band_energy[frm_idx,0] += (frm[k]*frm[k])
elif cur_f > 3000 and cur_f < 10000:
band_energy[frm_idx,1] += (frm[k]*frm[k])
band_energy = 10.0*np.log10(band_energy)
return band_energy
| agpl-3.0 |
KL-WLCR/incubator-airflow | scripts/perf/scheduler_ops_metrics.py | 16 | 6530 | # -*- coding: utf-8 -*-
#
# Licensed under the Apache License, Version 2.0 (the "License");
# you may not use this file except in compliance with the License.
# You may obtain a copy of the License at
#
# http://www.apache.org/licenses/LICENSE-2.0
#
# Unless required by applicable law or agreed to in writing, software
# distributed under the License is distributed on an "AS IS" BASIS,
# WITHOUT WARRANTIES OR CONDITIONS OF ANY KIND, either express or implied.
# See the License for the specific language governing permissions and
# limitations under the License.
from datetime import datetime
import logging
import pandas as pd
import sys
from airflow import configuration, settings
from airflow.jobs import SchedulerJob
from airflow.models import DagBag, DagModel, DagRun, TaskInstance
from airflow.utils.state import State
SUBDIR = 'scripts/perf/dags'
DAG_IDS = ['perf_dag_1', 'perf_dag_2']
MAX_RUNTIME_SECS = 6
class SchedulerMetricsJob(SchedulerJob):
"""
This class extends SchedulerJob to instrument the execution performance of
task instances contained in each DAG. We want to know if any DAG
is starved of resources, and this will be reflected in the stats printed
out at the end of the test run. The following metrics will be instrumented
for each task instance (dag_id, task_id, execution_date) tuple:
1. Queuing delay - time taken from starting the executor to the task
instance to be added to the executor queue.
2. Start delay - time taken from starting the executor to the task instance
to start execution.
3. Land time - time taken from starting the executor to task instance
completion.
4. Duration - time taken for executing the task instance.
The DAGs implement bash operators that call the system wait command. This
is representative of typical operators run on Airflow - queries that are
run on remote systems and spend the majority of their time on I/O wait.
To Run:
$ python scripts/perf/scheduler_ops_metrics.py
"""
__mapper_args__ = {
'polymorphic_identity': 'SchedulerMetricsJob'
}
def print_stats(self):
"""
Print operational metrics for the scheduler test.
"""
session = settings.Session()
TI = TaskInstance
tis = (
session
.query(TI)
.filter(TI.dag_id.in_(DAG_IDS))
.all()
)
successful_tis = filter(lambda x: x.state == State.SUCCESS, tis)
ti_perf = [(ti.dag_id, ti.task_id, ti.execution_date,
(ti.queued_dttm - self.start_date).total_seconds(),
(ti.start_date - self.start_date).total_seconds(),
(ti.end_date - self.start_date).total_seconds(),
ti.duration) for ti in successful_tis]
ti_perf_df = pd.DataFrame(ti_perf, columns=['dag_id', 'task_id',
'execution_date',
'queue_delay',
'start_delay', 'land_time',
'duration'])
print('Performance Results')
print('###################')
for dag_id in DAG_IDS:
print('DAG {}'.format(dag_id))
print(ti_perf_df[ti_perf_df['dag_id'] == dag_id])
print('###################')
if len(tis) > len(successful_tis):
print("WARNING!! The following task instances haven't completed")
print(pd.DataFrame([(ti.dag_id, ti.task_id, ti.execution_date, ti.state)
for ti in filter(lambda x: x.state != State.SUCCESS, tis)],
columns=['dag_id', 'task_id', 'execution_date', 'state']))
session.commit()
def heartbeat(self):
"""
Override the scheduler heartbeat to determine when the test is complete
"""
super(SchedulerMetricsJob, self).heartbeat()
session = settings.Session()
# Get all the relevant task instances
TI = TaskInstance
successful_tis = (
session
.query(TI)
.filter(TI.dag_id.in_(DAG_IDS))
.filter(TI.state.in_([State.SUCCESS]))
.all()
)
session.commit()
dagbag = DagBag(SUBDIR)
dags = [dagbag.dags[dag_id] for dag_id in DAG_IDS]
# the tasks in perf_dag_1 and per_dag_2 have a daily schedule interval.
num_task_instances = sum([(datetime.today() - task.start_date).days
for dag in dags for task in dag.tasks])
if (len(successful_tis) == num_task_instances or
(datetime.now()-self.start_date).total_seconds() >
MAX_RUNTIME_SECS):
if (len(successful_tis) == num_task_instances):
self.log.info("All tasks processed! Printing stats.")
else:
self.log.info("Test timeout reached. "
"Printing available stats.")
self.print_stats()
set_dags_paused_state(True)
sys.exit()
def clear_dag_runs():
"""
Remove any existing DAG runs for the perf test DAGs.
"""
session = settings.Session()
drs = session.query(DagRun).filter(
DagRun.dag_id.in_(DAG_IDS),
).all()
for dr in drs:
logging.info('Deleting DagRun :: {}'.format(dr))
session.delete(dr)
def clear_dag_task_instances():
"""
Remove any existing task instances for the perf test DAGs.
"""
session = settings.Session()
TI = TaskInstance
tis = (
session
.query(TI)
.filter(TI.dag_id.in_(DAG_IDS))
.all()
)
for ti in tis:
logging.info('Deleting TaskInstance :: {}'.format(ti))
session.delete(ti)
session.commit()
def set_dags_paused_state(is_paused):
"""
Toggle the pause state of the DAGs in the test.
"""
session = settings.Session()
dms = session.query(DagModel).filter(
DagModel.dag_id.in_(DAG_IDS))
for dm in dms:
logging.info('Setting DAG :: {} is_paused={}'.format(dm, is_paused))
dm.is_paused = is_paused
session.commit()
def main():
configuration.load_test_config()
set_dags_paused_state(False)
clear_dag_runs()
clear_dag_task_instances()
job = SchedulerMetricsJob(dag_ids=DAG_IDS, subdir=SUBDIR)
job.run()
if __name__ == "__main__":
main()
| apache-2.0 |
cmoutard/mne-python | examples/decoding/plot_decoding_xdawn_eeg.py | 8 | 3397 | """
=============================
XDAWN Decoding From EEG data
=============================
ERP decoding with Xdawn. For each event type, a set of spatial Xdawn filters
are trained and applied on the signal. Channels are concatenated and rescaled
to create features vectors that will be fed into a Logistic Regression.
References
----------
[1] Rivet, B., Souloumiac, A., Attina, V., & Gibert, G. (2009). xDAWN
algorithm to enhance evoked potentials: application to brain-computer
interface. Biomedical Engineering, IEEE Transactions on, 56(8), 2035-2043.
[2] Rivet, B., Cecotti, H., Souloumiac, A., Maby, E., & Mattout, J. (2011,
August). Theoretical analysis of xDAWN algorithm: application to an
efficient sensor selection in a P300 BCI. In Signal Processing Conference,
2011 19th European (pp. 1382-1386). IEEE.
"""
# Authors: Alexandre Barachant <[email protected]>
#
# License: BSD (3-clause)
import numpy as np
import matplotlib.pyplot as plt
from sklearn.cross_validation import StratifiedKFold
from sklearn.pipeline import make_pipeline
from sklearn.linear_model import LogisticRegression
from sklearn.metrics import classification_report, confusion_matrix
from sklearn.preprocessing import MinMaxScaler
from mne import io, pick_types, read_events, Epochs
from mne.datasets import sample
from mne.preprocessing import Xdawn
from mne.decoding import EpochsVectorizer
from mne.viz import tight_layout
print(__doc__)
data_path = sample.data_path()
###############################################################################
# Set parameters and read data
raw_fname = data_path + '/MEG/sample/sample_audvis_filt-0-40_raw.fif'
event_fname = data_path + '/MEG/sample/sample_audvis_filt-0-40_raw-eve.fif'
tmin, tmax = -0.1, 0.3
event_id = dict(aud_l=1, aud_r=2, vis_l=3, vis_r=4)
# Setup for reading the raw data
raw = io.Raw(raw_fname, preload=True)
raw.filter(1, 20, method='iir')
events = read_events(event_fname)
picks = pick_types(raw.info, meg=False, eeg=True, stim=False, eog=False,
exclude='bads')
epochs = Epochs(raw, events, event_id, tmin, tmax, proj=False,
picks=picks, baseline=None, preload=True,
add_eeg_ref=False, verbose=False)
# Create classification pipeline
clf = make_pipeline(Xdawn(n_components=3),
EpochsVectorizer(),
MinMaxScaler(),
LogisticRegression(penalty='l1'))
# Get the labels
labels = epochs.events[:, -1]
# Cross validator
cv = StratifiedKFold(y=labels, n_folds=10, shuffle=True, random_state=42)
# Do cross-validation
preds = np.empty(len(labels))
for train, test in cv:
clf.fit(epochs[train], labels[train])
preds[test] = clf.predict(epochs[test])
# Classification report
target_names = ['aud_l', 'aud_r', 'vis_l', 'vis_r']
report = classification_report(labels, preds, target_names=target_names)
print(report)
# Normalized confusion matrix
cm = confusion_matrix(labels, preds)
cm_normalized = cm.astype(float) / cm.sum(axis=1)[:, np.newaxis]
# Plot confusion matrix
plt.imshow(cm_normalized, interpolation='nearest', cmap=plt.cm.Blues)
plt.title('Normalized Confusion matrix')
plt.colorbar()
tick_marks = np.arange(len(target_names))
plt.xticks(tick_marks, target_names, rotation=45)
plt.yticks(tick_marks, target_names)
tight_layout()
plt.ylabel('True label')
plt.xlabel('Predicted label')
plt.show()
| bsd-3-clause |
jmschrei/scikit-learn | examples/decomposition/plot_incremental_pca.py | 244 | 1878 | """
===============
Incremental PCA
===============
Incremental principal component analysis (IPCA) is typically used as a
replacement for principal component analysis (PCA) when the dataset to be
decomposed is too large to fit in memory. IPCA builds a low-rank approximation
for the input data using an amount of memory which is independent of the
number of input data samples. It is still dependent on the input data features,
but changing the batch size allows for control of memory usage.
This example serves as a visual check that IPCA is able to find a similar
projection of the data to PCA (to a sign flip), while only processing a
few samples at a time. This can be considered a "toy example", as IPCA is
intended for large datasets which do not fit in main memory, requiring
incremental approaches.
"""
print(__doc__)
# Authors: Kyle Kastner
# License: BSD 3 clause
import numpy as np
import matplotlib.pyplot as plt
from sklearn.datasets import load_iris
from sklearn.decomposition import PCA, IncrementalPCA
iris = load_iris()
X = iris.data
y = iris.target
n_components = 2
ipca = IncrementalPCA(n_components=n_components, batch_size=10)
X_ipca = ipca.fit_transform(X)
pca = PCA(n_components=n_components)
X_pca = pca.fit_transform(X)
for X_transformed, title in [(X_ipca, "Incremental PCA"), (X_pca, "PCA")]:
plt.figure(figsize=(8, 8))
for c, i, target_name in zip("rgb", [0, 1, 2], iris.target_names):
plt.scatter(X_transformed[y == i, 0], X_transformed[y == i, 1],
c=c, label=target_name)
if "Incremental" in title:
err = np.abs(np.abs(X_pca) - np.abs(X_ipca)).mean()
plt.title(title + " of iris dataset\nMean absolute unsigned error "
"%.6f" % err)
else:
plt.title(title + " of iris dataset")
plt.legend(loc="best")
plt.axis([-4, 4, -1.5, 1.5])
plt.show()
| bsd-3-clause |
MartinDelzant/scikit-learn | sklearn/tests/test_learning_curve.py | 225 | 10791 | # Author: Alexander Fabisch <[email protected]>
#
# License: BSD 3 clause
import sys
from sklearn.externals.six.moves import cStringIO as StringIO
import numpy as np
import warnings
from sklearn.base import BaseEstimator
from sklearn.learning_curve import learning_curve, validation_curve
from sklearn.utils.testing import assert_raises
from sklearn.utils.testing import assert_warns
from sklearn.utils.testing import assert_equal
from sklearn.utils.testing import assert_array_equal
from sklearn.utils.testing import assert_array_almost_equal
from sklearn.datasets import make_classification
from sklearn.cross_validation import KFold
from sklearn.linear_model import PassiveAggressiveClassifier
class MockImprovingEstimator(BaseEstimator):
"""Dummy classifier to test the learning curve"""
def __init__(self, n_max_train_sizes):
self.n_max_train_sizes = n_max_train_sizes
self.train_sizes = 0
self.X_subset = None
def fit(self, X_subset, y_subset=None):
self.X_subset = X_subset
self.train_sizes = X_subset.shape[0]
return self
def predict(self, X):
raise NotImplementedError
def score(self, X=None, Y=None):
# training score becomes worse (2 -> 1), test error better (0 -> 1)
if self._is_training_data(X):
return 2. - float(self.train_sizes) / self.n_max_train_sizes
else:
return float(self.train_sizes) / self.n_max_train_sizes
def _is_training_data(self, X):
return X is self.X_subset
class MockIncrementalImprovingEstimator(MockImprovingEstimator):
"""Dummy classifier that provides partial_fit"""
def __init__(self, n_max_train_sizes):
super(MockIncrementalImprovingEstimator,
self).__init__(n_max_train_sizes)
self.x = None
def _is_training_data(self, X):
return self.x in X
def partial_fit(self, X, y=None, **params):
self.train_sizes += X.shape[0]
self.x = X[0]
class MockEstimatorWithParameter(BaseEstimator):
"""Dummy classifier to test the validation curve"""
def __init__(self, param=0.5):
self.X_subset = None
self.param = param
def fit(self, X_subset, y_subset):
self.X_subset = X_subset
self.train_sizes = X_subset.shape[0]
return self
def predict(self, X):
raise NotImplementedError
def score(self, X=None, y=None):
return self.param if self._is_training_data(X) else 1 - self.param
def _is_training_data(self, X):
return X is self.X_subset
def test_learning_curve():
X, y = make_classification(n_samples=30, n_features=1, n_informative=1,
n_redundant=0, n_classes=2,
n_clusters_per_class=1, random_state=0)
estimator = MockImprovingEstimator(20)
with warnings.catch_warnings(record=True) as w:
train_sizes, train_scores, test_scores = learning_curve(
estimator, X, y, cv=3, train_sizes=np.linspace(0.1, 1.0, 10))
if len(w) > 0:
raise RuntimeError("Unexpected warning: %r" % w[0].message)
assert_equal(train_scores.shape, (10, 3))
assert_equal(test_scores.shape, (10, 3))
assert_array_equal(train_sizes, np.linspace(2, 20, 10))
assert_array_almost_equal(train_scores.mean(axis=1),
np.linspace(1.9, 1.0, 10))
assert_array_almost_equal(test_scores.mean(axis=1),
np.linspace(0.1, 1.0, 10))
def test_learning_curve_unsupervised():
X, _ = make_classification(n_samples=30, n_features=1, n_informative=1,
n_redundant=0, n_classes=2,
n_clusters_per_class=1, random_state=0)
estimator = MockImprovingEstimator(20)
train_sizes, train_scores, test_scores = learning_curve(
estimator, X, y=None, cv=3, train_sizes=np.linspace(0.1, 1.0, 10))
assert_array_equal(train_sizes, np.linspace(2, 20, 10))
assert_array_almost_equal(train_scores.mean(axis=1),
np.linspace(1.9, 1.0, 10))
assert_array_almost_equal(test_scores.mean(axis=1),
np.linspace(0.1, 1.0, 10))
def test_learning_curve_verbose():
X, y = make_classification(n_samples=30, n_features=1, n_informative=1,
n_redundant=0, n_classes=2,
n_clusters_per_class=1, random_state=0)
estimator = MockImprovingEstimator(20)
old_stdout = sys.stdout
sys.stdout = StringIO()
try:
train_sizes, train_scores, test_scores = \
learning_curve(estimator, X, y, cv=3, verbose=1)
finally:
out = sys.stdout.getvalue()
sys.stdout.close()
sys.stdout = old_stdout
assert("[learning_curve]" in out)
def test_learning_curve_incremental_learning_not_possible():
X, y = make_classification(n_samples=2, n_features=1, n_informative=1,
n_redundant=0, n_classes=2,
n_clusters_per_class=1, random_state=0)
# The mockup does not have partial_fit()
estimator = MockImprovingEstimator(1)
assert_raises(ValueError, learning_curve, estimator, X, y,
exploit_incremental_learning=True)
def test_learning_curve_incremental_learning():
X, y = make_classification(n_samples=30, n_features=1, n_informative=1,
n_redundant=0, n_classes=2,
n_clusters_per_class=1, random_state=0)
estimator = MockIncrementalImprovingEstimator(20)
train_sizes, train_scores, test_scores = learning_curve(
estimator, X, y, cv=3, exploit_incremental_learning=True,
train_sizes=np.linspace(0.1, 1.0, 10))
assert_array_equal(train_sizes, np.linspace(2, 20, 10))
assert_array_almost_equal(train_scores.mean(axis=1),
np.linspace(1.9, 1.0, 10))
assert_array_almost_equal(test_scores.mean(axis=1),
np.linspace(0.1, 1.0, 10))
def test_learning_curve_incremental_learning_unsupervised():
X, _ = make_classification(n_samples=30, n_features=1, n_informative=1,
n_redundant=0, n_classes=2,
n_clusters_per_class=1, random_state=0)
estimator = MockIncrementalImprovingEstimator(20)
train_sizes, train_scores, test_scores = learning_curve(
estimator, X, y=None, cv=3, exploit_incremental_learning=True,
train_sizes=np.linspace(0.1, 1.0, 10))
assert_array_equal(train_sizes, np.linspace(2, 20, 10))
assert_array_almost_equal(train_scores.mean(axis=1),
np.linspace(1.9, 1.0, 10))
assert_array_almost_equal(test_scores.mean(axis=1),
np.linspace(0.1, 1.0, 10))
def test_learning_curve_batch_and_incremental_learning_are_equal():
X, y = make_classification(n_samples=30, n_features=1, n_informative=1,
n_redundant=0, n_classes=2,
n_clusters_per_class=1, random_state=0)
train_sizes = np.linspace(0.2, 1.0, 5)
estimator = PassiveAggressiveClassifier(n_iter=1, shuffle=False)
train_sizes_inc, train_scores_inc, test_scores_inc = \
learning_curve(
estimator, X, y, train_sizes=train_sizes,
cv=3, exploit_incremental_learning=True)
train_sizes_batch, train_scores_batch, test_scores_batch = \
learning_curve(
estimator, X, y, cv=3, train_sizes=train_sizes,
exploit_incremental_learning=False)
assert_array_equal(train_sizes_inc, train_sizes_batch)
assert_array_almost_equal(train_scores_inc.mean(axis=1),
train_scores_batch.mean(axis=1))
assert_array_almost_equal(test_scores_inc.mean(axis=1),
test_scores_batch.mean(axis=1))
def test_learning_curve_n_sample_range_out_of_bounds():
X, y = make_classification(n_samples=30, n_features=1, n_informative=1,
n_redundant=0, n_classes=2,
n_clusters_per_class=1, random_state=0)
estimator = MockImprovingEstimator(20)
assert_raises(ValueError, learning_curve, estimator, X, y, cv=3,
train_sizes=[0, 1])
assert_raises(ValueError, learning_curve, estimator, X, y, cv=3,
train_sizes=[0.0, 1.0])
assert_raises(ValueError, learning_curve, estimator, X, y, cv=3,
train_sizes=[0.1, 1.1])
assert_raises(ValueError, learning_curve, estimator, X, y, cv=3,
train_sizes=[0, 20])
assert_raises(ValueError, learning_curve, estimator, X, y, cv=3,
train_sizes=[1, 21])
def test_learning_curve_remove_duplicate_sample_sizes():
X, y = make_classification(n_samples=3, n_features=1, n_informative=1,
n_redundant=0, n_classes=2,
n_clusters_per_class=1, random_state=0)
estimator = MockImprovingEstimator(2)
train_sizes, _, _ = assert_warns(
RuntimeWarning, learning_curve, estimator, X, y, cv=3,
train_sizes=np.linspace(0.33, 1.0, 3))
assert_array_equal(train_sizes, [1, 2])
def test_learning_curve_with_boolean_indices():
X, y = make_classification(n_samples=30, n_features=1, n_informative=1,
n_redundant=0, n_classes=2,
n_clusters_per_class=1, random_state=0)
estimator = MockImprovingEstimator(20)
cv = KFold(n=30, n_folds=3)
train_sizes, train_scores, test_scores = learning_curve(
estimator, X, y, cv=cv, train_sizes=np.linspace(0.1, 1.0, 10))
assert_array_equal(train_sizes, np.linspace(2, 20, 10))
assert_array_almost_equal(train_scores.mean(axis=1),
np.linspace(1.9, 1.0, 10))
assert_array_almost_equal(test_scores.mean(axis=1),
np.linspace(0.1, 1.0, 10))
def test_validation_curve():
X, y = make_classification(n_samples=2, n_features=1, n_informative=1,
n_redundant=0, n_classes=2,
n_clusters_per_class=1, random_state=0)
param_range = np.linspace(0, 1, 10)
with warnings.catch_warnings(record=True) as w:
train_scores, test_scores = validation_curve(
MockEstimatorWithParameter(), X, y, param_name="param",
param_range=param_range, cv=2
)
if len(w) > 0:
raise RuntimeError("Unexpected warning: %r" % w[0].message)
assert_array_almost_equal(train_scores.mean(axis=1), param_range)
assert_array_almost_equal(test_scores.mean(axis=1), 1 - param_range)
| bsd-3-clause |
btabibian/scikit-learn | sklearn/cluster/__init__.py | 364 | 1228 | """
The :mod:`sklearn.cluster` module gathers popular unsupervised clustering
algorithms.
"""
from .spectral import spectral_clustering, SpectralClustering
from .mean_shift_ import (mean_shift, MeanShift,
estimate_bandwidth, get_bin_seeds)
from .affinity_propagation_ import affinity_propagation, AffinityPropagation
from .hierarchical import (ward_tree, AgglomerativeClustering, linkage_tree,
FeatureAgglomeration)
from .k_means_ import k_means, KMeans, MiniBatchKMeans
from .dbscan_ import dbscan, DBSCAN
from .bicluster import SpectralBiclustering, SpectralCoclustering
from .birch import Birch
__all__ = ['AffinityPropagation',
'AgglomerativeClustering',
'Birch',
'DBSCAN',
'KMeans',
'FeatureAgglomeration',
'MeanShift',
'MiniBatchKMeans',
'SpectralClustering',
'affinity_propagation',
'dbscan',
'estimate_bandwidth',
'get_bin_seeds',
'k_means',
'linkage_tree',
'mean_shift',
'spectral_clustering',
'ward_tree',
'SpectralBiclustering',
'SpectralCoclustering']
| bsd-3-clause |
mxlei01/healthcareai-py | healthcareai/common/table_archiver.py | 4 | 2336 | import time
import datetime
import pandas as pd
from healthcareai.common.healthcareai_error import HealthcareAIError
def table_archiver(server, database, source_table, destination_table, timestamp_column_name='ArchivedDTS'):
"""
Takes a table and archives a complete copy of it with the addition of a timestamp of when the archive occurred to a
given destination table on the same database.
This should build a new table if the table doesn't exist.
Args:
server (str): Server name
database (str): Database name
source_table (str): Source table name
destination_table (str): Destination table name
timestamp_column_name (str): New timestamp column name
Returns:
(str): A string with details on records archived.
Example usage:
```
from healthcareai.common.table_archiver import table_archiver
table_archiver('localhost', 'SAM_123', 'RiskScores', 'RiskScoreArchive', 'ArchiveDTS')
```
"""
# Basic input validation
if type(server) is not str:
raise HealthcareAIError('Please specify a server address')
if type(database) is not str:
raise HealthcareAIError('Please specify a database name')
if type(source_table) is not str:
raise HealthcareAIError('Please specify a source table name')
if type(destination_table) is not str:
raise HealthcareAIError('Please specify a destination table name')
start_time = time.time()
connection_string = 'mssql+pyodbc://{}/{}?driver=SQL+Server+Native+Client+11.0'.format(server, database)
# Load the table to be archived
df = pd.read_sql_table(source_table, connection_string)
number_records_to_add = len(df)
# Add timestamp to dataframe
df[timestamp_column_name] = datetime.datetime.fromtimestamp(time.time()).strftime('%Y-%m-%d %H:%M:%S')
# Save the new dataframe out to the db without the index, appending values
df.to_sql(destination_table, connection_string, index=False, if_exists='append')
end_time = time.time()
delta_time = end_time - start_time
result = 'Archived {0} records from {1}/{2}/{3} to {4} in {5} seconds'.format(
number_records_to_add,
server,
database,
source_table,
destination_table,
delta_time)
return result
| mit |
beepee14/scikit-learn | examples/linear_model/plot_logistic_path.py | 349 | 1195 | #!/usr/bin/env python
"""
=================================
Path with L1- Logistic Regression
=================================
Computes path on IRIS dataset.
"""
print(__doc__)
# Author: Alexandre Gramfort <[email protected]>
# License: BSD 3 clause
from datetime import datetime
import numpy as np
import matplotlib.pyplot as plt
from sklearn import linear_model
from sklearn import datasets
from sklearn.svm import l1_min_c
iris = datasets.load_iris()
X = iris.data
y = iris.target
X = X[y != 2]
y = y[y != 2]
X -= np.mean(X, 0)
###############################################################################
# Demo path functions
cs = l1_min_c(X, y, loss='log') * np.logspace(0, 3)
print("Computing regularization path ...")
start = datetime.now()
clf = linear_model.LogisticRegression(C=1.0, penalty='l1', tol=1e-6)
coefs_ = []
for c in cs:
clf.set_params(C=c)
clf.fit(X, y)
coefs_.append(clf.coef_.ravel().copy())
print("This took ", datetime.now() - start)
coefs_ = np.array(coefs_)
plt.plot(np.log10(cs), coefs_)
ymin, ymax = plt.ylim()
plt.xlabel('log(C)')
plt.ylabel('Coefficients')
plt.title('Logistic Regression Path')
plt.axis('tight')
plt.show()
| bsd-3-clause |
TNick/pylearn2 | pylearn2/scripts/plot_monitor.py | 37 | 10204 | #!/usr/bin/env python
"""
usage:
plot_monitor.py model_1.pkl model_2.pkl ... model_n.pkl
Loads any number of .pkl files produced by train.py. Extracts
all of their monitoring channels and prompts the user to select
a subset of them to be plotted.
"""
from __future__ import print_function
__authors__ = "Ian Goodfellow, Harm Aarts"
__copyright__ = "Copyright 2010-2012, Universite de Montreal"
__credits__ = ["Ian Goodfellow"]
__license__ = "3-clause BSD"
__maintainer__ = "LISA Lab"
__email__ = "pylearn-dev@googlegroups"
import gc
import numpy as np
import sys
from theano.compat.six.moves import input, xrange
from pylearn2.utils import serial
from theano.printing import _TagGenerator
from pylearn2.utils.string_utils import number_aware_alphabetical_key
from pylearn2.utils import contains_nan, contains_inf
import argparse
channels = {}
def unique_substring(s, other, min_size=1):
"""
.. todo::
WRITEME
"""
size = min(len(s), min_size)
while size <= len(s):
for pos in xrange(0,len(s)-size+1):
rval = s[pos:pos+size]
fail = False
for o in other:
if o.find(rval) != -1:
fail = True
break
if not fail:
return rval
size += 1
# no unique substring
return s
def unique_substrings(l, min_size=1):
"""
.. todo::
WRITEME
"""
return [unique_substring(s, [x for x in l if x is not s], min_size)
for s in l]
def main():
"""
.. todo::
WRITEME
"""
parser = argparse.ArgumentParser()
parser.add_argument("--out")
parser.add_argument("model_paths", nargs='+')
parser.add_argument("--yrange", help='The y-range to be used for plotting, e.g. 0:1')
options = parser.parse_args()
model_paths = options.model_paths
if options.out is not None:
import matplotlib
matplotlib.use('Agg')
import matplotlib.pyplot as plt
print('generating names...')
model_names = [model_path.replace('.pkl', '!') for model_path in
model_paths]
model_names = unique_substrings(model_names, min_size=10)
model_names = [model_name.replace('!','') for model_name in
model_names]
print('...done')
for i, arg in enumerate(model_paths):
try:
model = serial.load(arg)
except Exception:
if arg.endswith('.yaml'):
print(sys.stderr, arg + " is a yaml config file," +
"you need to load a trained model.", file=sys.stderr)
quit(-1)
raise
this_model_channels = model.monitor.channels
if len(sys.argv) > 2:
postfix = ":" + model_names[i]
else:
postfix = ""
for channel in this_model_channels:
channels[channel+postfix] = this_model_channels[channel]
del model
gc.collect()
while True:
# Make a list of short codes for each channel so user can specify them
# easily
tag_generator = _TagGenerator()
codebook = {}
sorted_codes = []
for channel_name in sorted(channels,
key = number_aware_alphabetical_key):
code = tag_generator.get_tag()
codebook[code] = channel_name
codebook['<'+channel_name+'>'] = channel_name
sorted_codes.append(code)
x_axis = 'example'
print('set x_axis to example')
if len(channels.values()) == 0:
print("there are no channels to plot")
break
# If there is more than one channel in the monitor ask which ones to
# plot
prompt = len(channels.values()) > 1
if prompt:
# Display the codebook
for code in sorted_codes:
print(code + '. ' + codebook[code])
print()
print("Put e, b, s or h in the list somewhere to plot " +
"epochs, batches, seconds, or hours, respectively.")
response = input('Enter a list of channels to plot ' + \
'(example: A, C,F-G, h, <test_err>) or q to quit' + \
' or o for options: ')
if response == 'o':
print('1: smooth all channels')
print('any other response: do nothing, go back to plotting')
response = input('Enter your choice: ')
if response == '1':
for channel in channels.values():
k = 5
new_val_record = []
for i in xrange(len(channel.val_record)):
new_val = 0.
count = 0.
for j in xrange(max(0, i-k), i+1):
new_val += channel.val_record[j]
count += 1.
new_val_record.append(new_val / count)
channel.val_record = new_val_record
continue
if response == 'q':
break
#Remove spaces
response = response.replace(' ','')
#Split into list
codes = response.split(',')
final_codes = set([])
for code in codes:
if code == 'e':
x_axis = 'epoch'
continue
elif code == 'b':
x_axis = 'batche'
elif code == 's':
x_axis = 'second'
elif code == 'h':
x_axis = 'hour'
elif code.startswith('<'):
assert code.endswith('>')
final_codes.add(code)
elif code.find('-') != -1:
#The current list element is a range of codes
rng = code.split('-')
if len(rng) != 2:
print("Input not understood: "+code)
quit(-1)
found = False
for i in xrange(len(sorted_codes)):
if sorted_codes[i] == rng[0]:
found = True
break
if not found:
print("Invalid code: "+rng[0])
quit(-1)
found = False
for j in xrange(i,len(sorted_codes)):
if sorted_codes[j] == rng[1]:
found = True
break
if not found:
print("Invalid code: "+rng[1])
quit(-1)
final_codes = final_codes.union(set(sorted_codes[i:j+1]))
else:
#The current list element is just a single code
final_codes = final_codes.union(set([code]))
# end for code in codes
else:
final_codes ,= set(codebook.keys())
colors = ['b', 'g', 'r', 'c', 'm', 'y', 'k']
styles = list(colors)
styles += [color+'--' for color in colors]
styles += [color+':' for color in colors]
fig = plt.figure()
ax = plt.subplot(1,1,1)
# plot the requested channels
for idx, code in enumerate(sorted(final_codes)):
channel_name= codebook[code]
channel = channels[channel_name]
y = np.asarray(channel.val_record)
if contains_nan(y):
print(channel_name + ' contains NaNs')
if contains_inf(y):
print(channel_name + 'contains infinite values')
if x_axis == 'example':
x = np.asarray(channel.example_record)
elif x_axis == 'batche':
x = np.asarray(channel.batch_record)
elif x_axis == 'epoch':
try:
x = np.asarray(channel.epoch_record)
except AttributeError:
# older saved monitors won't have epoch_record
x = np.arange(len(channel.batch_record))
elif x_axis == 'second':
x = np.asarray(channel.time_record)
elif x_axis == 'hour':
x = np.asarray(channel.time_record) / 3600.
else:
assert False
ax.plot( x,
y,
styles[idx % len(styles)],
marker = '.', # add point margers to lines
label = channel_name)
plt.xlabel('# '+x_axis+'s')
ax.ticklabel_format( scilimits = (-3,3), axis = 'both')
handles, labels = ax.get_legend_handles_labels()
lgd = ax.legend(handles, labels, loc = 'upper left',
bbox_to_anchor = (1.05, 1.02))
# Get the axis positions and the height and width of the legend
plt.draw()
ax_pos = ax.get_position()
pad_width = ax_pos.x0 * fig.get_size_inches()[0]
pad_height = ax_pos.y0 * fig.get_size_inches()[1]
dpi = fig.get_dpi()
lgd_width = ax.get_legend().get_frame().get_width() / dpi
lgd_height = ax.get_legend().get_frame().get_height() / dpi
# Adjust the bounding box to encompass both legend and axis. Axis should be 3x3 inches.
# I had trouble getting everything to align vertically.
ax_width = 3
ax_height = 3
total_width = 2*pad_width + ax_width + lgd_width
total_height = 2*pad_height + np.maximum(ax_height, lgd_height)
fig.set_size_inches(total_width, total_height)
ax.set_position([pad_width/total_width, 1-6*pad_height/total_height, ax_width/total_width, ax_height/total_height])
if(options.yrange is not None):
ymin, ymax = map(float, options.yrange.split(':'))
plt.ylim(ymin, ymax)
if options.out is None:
plt.show()
else:
plt.savefig(options.out)
if not prompt:
break
if __name__ == "__main__":
main()
| bsd-3-clause |
Kirubaharan/hydrology | agu_plot.py | 2 | 21923 | __author__ = 'kiruba'
import numpy as np
import matplotlib.pyplot as plt
import pandas as pd
import itertools
# import seaborn as sns
from matplotlib import rc
import pickle
from datetime import timedelta
import brewer2mpl
from matplotlib import cm
from scipy.optimize import curve_fit
import matplotlib as mpl
from math import sqrt
SPINE_COLOR = 'gray'
import matplotlib
from matplotlib.ticker import MaxNLocator
from statsmodels.nonparametric.smoothers_lowess import lowess
# latex parameters
rc('font', **{'family': 'sans-serif', 'serif': ['Computer Modern Roman']})
rc('text', usetex=True)
plt.rc('text', usetex=True)
plt.rc('font', family='serif', size=36)
def latexify(fig_width=None, fig_height=None, columns=1):
"""Set up matplotlib's RC params for LaTeX plotting.
Call this before plotting a figure.
Parameters
----------
fig_width : float, optional, inches
fig_height : float, optional, inches
columns : {1, 2}
"""
# code adapted from http://www.scipy.org/Cookbook/Matplotlib/LaTeX_Examples
# Width and max height in inches for IEEE journals taken from
# computer.org/cms/Computer.org/Journal%20templates/transactions_art_guide.pdf
assert(columns in [1,2])
if fig_width is None:
fig_width = 3.39 if columns==1 else 6.9 # width in inches
if fig_height is None:
golden_mean = (sqrt(5)-1.0)/2.0 # Aesthetic ratio
fig_height = fig_width*golden_mean # height in inches
MAX_HEIGHT_INCHES =32
if fig_height > MAX_HEIGHT_INCHES:
print("WARNING: fig_height too large:" + fig_height +
"so will reduce to" + MAX_HEIGHT_INCHES + "inches.")
fig_height = MAX_HEIGHT_INCHES
params = {'backend': 'ps',
'text.latex.preamble': ['\usepackage{gensymb}'],
'axes.labelsize': 28, # fontsize for x and y labels (was 10)
'axes.titlesize': 30,
'text.fontsize': 30, # was 10
'legend.fontsize': 30, # was 10
'xtick.labelsize': 28,
'ytick.labelsize': 28,
'text.usetex': True,
'figure.figsize': [fig_width,fig_height],
'font.family': 'serif'
}
matplotlib.rcParams.update(params)
def format_axes(ax):
for spine in ['top', 'right']:
ax.spines[spine].set_visible(False)
for spine in ['left', 'bottom']:
ax.spines[spine].set_color(SPINE_COLOR)
ax.spines[spine].set_linewidth(0.5)
ax.xaxis.set_ticks_position('bottom')
ax.yaxis.set_ticks_position('left')
for axis in [ax.xaxis, ax.yaxis]:
axis.set_tick_params(direction='out', color=SPINE_COLOR)
return ax
# mpl.rcParams.update(mpl.rcParamsDefault)
daily_format = '%Y-%m-%d'
datetime_format = '%Y-%m-%d %H:%M:%S'
"""
stage vs volume and area
"""
cont_area_591_file = '/media/kiruba/New Volume/ACCUWA_Data/Checkdam_water_balance/591/cont_area.csv'
cont_area_599_file = '/media/kiruba/New Volume/ACCUWA_Data/Checkdam_water_balance/ch_599/cont_area.csv'
stage_vol_599_file = '/media/kiruba/New Volume/ACCUWA_Data/Checkdam_water_balance/ch_599/stage_vol.csv'
stage_vol_591_file = '/media/kiruba/New Volume/ACCUWA_Data/Checkdam_water_balance/591/stage_vol_new.csv'
cont_area_591_df = pd.read_csv(cont_area_591_file)
cont_area_599_df = pd.read_csv(cont_area_599_file)
stage_vol_599_df = pd.read_csv(stage_vol_599_file)
stage_vol_591_df = pd.read_csv(stage_vol_591_file)
#
# latexify(fig_width=10, fig_height=6)
fig, (ax1, ax2) = plt.subplots(nrows=2, ncols=1,facecolor='white')
line_1, = ax1.plot(cont_area_591_df['Z'], cont_area_591_df['Area'], '-', lw=2, color='#a70c0b')
# line_2, = ax2.plot(cont_area_599_df['Z'], cont_area_599_df['Area'], '-', lw=2, color='#a70c0b')
line_3, = ax2.plot(stage_vol_591_df['stage_m'], stage_vol_591_df['total_vol_cu_m'], '-', lw=2)
# line_4, = ax4.plot(stage_vol_599_df['stage_m'], stage_vol_599_df['total_vol_cu_m'], '-', lw=2)
list_ax = [ax1, ax2]
for ax in list_ax:
ax.spines['top'].set_visible(False)
ax.spines['right'].set_visible(False)
ax.spines['bottom'].set_position(('outward', 10))
ax.spines['left'].set_position(('outward', 30))
ax.yaxis.set_ticks_position('left')
ax.xaxis.set_ticks_position('bottom')
ax.grid(False)
ax.set_axis_bgcolor('white')
# ax.set_axis_off()
ax1.xaxis.set_major_locator(MaxNLocator(nbins=3))
ax1.yaxis.set_major_locator(MaxNLocator(nbins=3))
ax2.xaxis.set_major_locator(MaxNLocator(nbins=3))
ax2.yaxis.set_major_locator(MaxNLocator(nbins=3))
ax1.tick_params(axis='x',
which='both',
labelbottom='off')
# ax3.xaxis.set_major_locator(MaxNLocator(nbins=3))
# ax3.yaxis.set_major_locator(MaxNLocator(nbins=3))
# ax4.xaxis.set_major_locator(MaxNLocator(nbins=3))
# ax4.yaxis.set_major_locator(MaxNLocator(nbins=3))
ax1.set_title('Check dam 591')
# ax2.set_title('Check dam 599')
ax1.set_ylabel(r'Area ($m^2$) ')
ax2.set_ylabel(r"Volume ($m^3$)")
plt.xlabel(r"Stage ($m$)")
# yyl.set_position((-0.1, 0))
# ax1.set_xlim(1.9)
# [__.set_clip_on(False) for __ in plt.gca().get_children()]
plt.savefig('/media/kiruba/New Volume/AGU/poster/agu_checkdam/image/stage_vol_area_591.png',bbox_inches='tight')
plt.show()
# fig, ((ax1, ax2), (ax3, ax4)) = plt.subplots(nrows=2, ncols=2,facecolor='white')
# line_1, = ax1.plot(cont_area_591_df['Z'], cont_area_591_df['Area'], '-', lw=2, color='#a70c0b')
# line_2, = ax2.plot(cont_area_599_df['Z'], cont_area_599_df['Area'], '-', lw=2, color='#a70c0b')
# line_3, = ax3.plot(stage_vol_591_df['stage_m'], stage_vol_591_df['total_vol_cu_m'], '-', lw=2)
# line_4, = ax4.plot(stage_vol_599_df['stage_m'], stage_vol_599_df['total_vol_cu_m'], '-', lw=2)
# list_ax = [ax1, ax2, ax3, ax4]
# for ax in list_ax:
# ax.spines['top'].set_visible(False)
# ax.spines['right'].set_visible(False)
# ax.spines['bottom'].set_position(('outward', 20))
# ax.spines['left'].set_position(('outward', 30))
# ax.yaxis.set_ticks_position('left')
# ax.xaxis.set_ticks_position('bottom')
# ax.grid(False)
# ax.set_axis_bgcolor('white')
# ax1.xaxis.set_major_locator(MaxNLocator(nbins=3))
# ax1.yaxis.set_major_locator(MaxNLocator(nbins=3))
# ax2.xaxis.set_major_locator(MaxNLocator(nbins=3))
# ax2.yaxis.set_major_locator(MaxNLocator(nbins=3))
# ax3.xaxis.set_major_locator(MaxNLocator(nbins=3))
# ax3.yaxis.set_major_locator(MaxNLocator(nbins=3))
# ax4.xaxis.set_major_locator(MaxNLocator(nbins=3))
# ax4.yaxis.set_major_locator(MaxNLocator(nbins=3))
# ax1.set_title('Check dam 591')
# ax2.set_title('Check dam 599')
# ax1.set_ylabel(r'Area ($m^2$) ')
# ax3.set_ylabel(r"Volume ($m^3$)")
# yyl = plt.xlabel(r"Stage ($m$)")
# yyl.set_position((-0.1, 0))
# # ax1.set_xlim(1.9)
# plt.show()
# raise SystemExit(0)
# colorbrewer
dark2_colors = brewer2mpl.get_map('Dark2', 'Qualitative', 7).mpl_colors
inflow_file = '/media/kiruba/New Volume/ACCUWA_Data/tghalliinflowsbaseflowmonthandindex/tghalli_inflow.csv'
inflow_df = pd.read_csv(inflow_file)
inflow_df.index = pd.to_datetime(inflow_df['Year'], format='%Y')
base_flow_file = '/media/kiruba/New Volume/ACCUWA_Data/tghalliinflowsbaseflowmonthandindex/base_flow.csv'
base_flow_df = pd.read_csv(base_flow_file)
base_flow_df.index = pd.to_datetime(base_flow_df['Period'], format='%b-%Y')
base_flow_df = base_flow_df.resample('A', how=np.sum)
# print base_flow_df.head()
latexify(fig_width=13.2, fig_height=8)
fig,(ax, ax1) = plt.subplots(nrows=2,ncols=1, sharex=True,facecolor='white')
lowess_line = lowess(inflow_df['ML/Year'], inflow_df['Year'])
inflow = ax.bar(inflow_df['Year'], inflow_df['ML/Year'],color='#203a72')
ax.plot(lowess_line[:,0], lowess_line[:,1],'-', lw=3, color= '#23af2b')
base_flow = ax1.bar(base_flow_df.index.year, base_flow_df['Baseflow'], color='#a70c0b')
fig.autofmt_xdate()
ax.spines['top'].set_visible(False)
ax.spines['right'].set_visible(False)
# ax.spines['bottom'].set_position(('outward', 20))
ax.spines['left'].set_position(('outward', 30))
ax.yaxis.set_ticks_position('left')
# ax.xaxis.set_ticks_position('bottom')
ax.grid(False)
ax.set_axis_bgcolor('white')
ax.set_xlim(min(inflow_df['Year']), max(inflow_df['Year']))
ax.set_ylim(min(inflow_df['ML/Year']), max(inflow_df['ML/Year']))
ax1.spines['top'].set_visible(False)
ax1.spines['right'].set_visible(False)
ax1.spines['bottom'].set_position(('outward', 20))
ax1.spines['left'].set_position(('outward', 30))
ax1.yaxis.set_ticks_position('left')
ax1.xaxis.set_ticks_position('bottom')
ax1.grid(False)
ax1.set_axis_bgcolor('white')
ax1.set_ylabel(r'No of months')
ax.set_ylabel(r"Million Litres/year")
my_locator = MaxNLocator(6)
y_locator = MaxNLocator(3)
x_locator = MaxNLocator(3)
ax1.yaxis.set_major_locator(x_locator)
ax1.xaxis.set_major_locator(my_locator)
ax.yaxis.set_major_locator(y_locator)
ax.legend([inflow,base_flow], [r'Inflow (ML/year)', r"Baseflow (months)"],fancybox=True, loc="upper right")
ax1.yaxis.labelpad=57
# print ax.yaxis.labelpad
# plt.show()
# mpl.rcParams.update(mpl.rcParamsDefault)
file_591 = '/media/kiruba/New Volume/ACCUWA_Data/Checkdam_water_balance/591/et_infilt_591_w_of.csv'
# file_599 = '/media/kiruba/New Volume/ACCUWA_Data/Checkdam_water_balance/ch_599/daily_wb_599.CSV'
rain_file = '/media/kiruba/New Volume/ACCUWA_Data/weather_station/smgollahalli/ksndmc_rain.csv'
stage_591_file = '/media/kiruba/New Volume/ACCUWA_Data/Checkdam_water_balance/591/stage_591.csv'
# stage_599_file = '/media/kiruba/New Volume/ACCUWA_Data/Checkdam_water_balance/ch_599/water_level.csv'
rain_df = pd.read_csv(rain_file, sep=',', header=0)
rain_df['Date_Time'] = pd.to_datetime(rain_df['Date_Time'], format=datetime_format)
rain_df.set_index(rain_df['Date_Time'], inplace=True)
# sort based on index
rain_df.sort_index(inplace=True)
# drop date time column
rain_df = rain_df.drop('Date_Time', 1)
rain_w_df = rain_df.resample('W-MON', how=np.sum)
rain_w_df.to_csv('/media/kiruba/New Volume/ACCUWA_Data/weekly_rain_aral.csv')
# print rain_w_df.tail(10)
# raise SystemExit(0)
rain_df = rain_df.resample('D', how=np.sum)
wb_591 = pd.read_csv(file_591, sep=',', header=0)
# wb_599 = pd.read_csv(file_599, sep=',', header=0)
stage_591_df = pd.read_csv(stage_591_file, sep=',', header=0)
stage_591_df.set_index(pd.to_datetime(stage_591_df['Date'],format=datetime_format), inplace=True)
# stage_599_df = pd.read_csv(stage_599_file, sep=',', header=0)
# stage_599_df.set_index(pd.to_datetime(stage_599_df['Date'],format=datetime_format), inplace=True)
wb_591.set_index(pd.to_datetime(wb_591['Date'], format=daily_format), inplace=True)
# wb_599.set_index(pd.to_datetime(wb_599['Date'], format=daily_format), inplace=True)
del wb_591['Date']
# del wb_599['Date']
# missing date time for 599
# with open("/media/kiruba/New Volume/ACCUWA_Data/Checkdam_water_balance/ch_599/initial_time.pickle", "rb") as f:
# initial_time = pickle.load(f)
# with open("/media/kiruba/New Volume/ACCUWA_Data/Checkdam_water_balance/ch_599/final_time.pickle", "rb") as f:
# final_time = pickle.load(f)
# initial_time_591 = initial_time.strftime(daily_format)
# final_time_591 = final_time.strftime(daily_format)
# missing_data_days = (final_time-initial_time).days
# wb_599 = wb_599[wb_599['infiltration(cu.m)'] > 0]
stage_591_df = stage_591_df.resample('D', how=np.mean)
# stage_599_df = stage_599_df.resample('D', how=np.mean)
def annotate_dim(ax,xyfrom,xyto,text=None):
ax.annotate("",xyfrom,xyto,arrowprops=dict(arrowstyle='<->', linewidth=1.5))
# ax.annotate(text, xy=(xyfrom[0]+ timedelta(days=17), xyfrom[1]),xycoords='data', xytext=(-10,-10), textcoords='offset points')
def make_patch_spines_invisible(ax):
ax.set_frame_on(True)
ax.patch.set_visible(False)
for sp in ax.spines.itervalues():
sp.set_visible(False)
latexify(fig_width=15, fig_height=10)
fig, ax1 = plt.subplots(nrows=1,ncols=1, sharex=True, facecolor='white')
# ax1_1 = ax1.twinx()
# ax2_2 = ax2.twinx()
bar_1_1 = ax1.bar(rain_df.index, rain_df['rain (mm)'], 0.45, color='#203a72',alpha=0.85, label = 'Rainfall (mm)')
ax1.invert_yaxis()
for t1 in ax1.get_yticklabels():
t1.set_color('#203a72')
ax1_1 = ax1.twinx()
ax1_2 = ax1.twinx()
# bar_2_1 = ax2.bar(rain_df.index, rain_df['Rain Collection (mm)'], 0.45, color='#203a72',alpha=0.85, label = 'Rainfall (mm)')
# ax2.invert_yaxis()
# for t1 in ax2.get_yticklabels():
# t1.set_color('#203a72')
# ax2_1 = ax2.twinx()
bar_1 = ax1_2.bar(wb_591.index, wb_591['infiltration(cu.m)'], 0.45, color='#23530b',alpha=0.85,label=r"\textbf{Infiltration ($m^3/day$}")
line_1, = ax1_2.plot(stage_591_df.index, stage_591_df['stage(m)'], color='#a70c0b',linestyle='-', lw=3, alpha=0.75)
# line_2 = ax2_2.plot(stage_599_df.index, stage_599_df['stage(m)'], color='#a70c0b',linestyle='-', lw=3, alpha=0.75)
# bar_2 = ax2_1.bar(wb_599.index, wb_599['infiltration(cu.m)'], 0.45, color='#23530b',alpha=0.85)
bar_1_2 = ax1_2.bar(wb_591.index, wb_591['Evaporation (cu.m)'], 0.45, color='#a70c0b',alpha=0.85, label=r"\textbf{Evaporation ($m^3/day$)}")
# bar_2_2 = ax2_1.bar(wb_599.index, wb_599['Evaporation (cu.m)'], 0.45, color='#a70c0b',alpha=0.85, label=r"\textbf{Evaporation ($m^3/day$)}")
# bracket = annotate_dim(ax2_1, xyfrom=[initial_time_591,1], xyto=[final_time_591,1], text='Missing Data')
# text = ax2_1.text(initial_time, 2, "Missing Data")
lns = [bar_1_1, bar_1, bar_1_2, line_1]
labs = [r'\textbf{Rainfall ($mm$)}', r"\textbf{Infiltration ($m^3/day$)}", r"\textbf{Evaporation ($m^3/day$)}", r"\textbf{Stage ($m$)}"]
ax1.legend(lns, labs, loc='upper center', fancybox=True, ncol=4, bbox_to_anchor=(0.5, -0.05),prop={'size':30} )
# yyl = plt.ylabel(r"Evaporation/Infiltration ($m^3/day$)")
# yyl.set_position((0.06, 1))
# yyl_1 = ax2.set_ylabel(r'Rainfall($mm$)')
# yyl_1.set_position((yyl_1.get_position()[0], 1))
# fig.text(0.06, 0.5, 'Rainfall (mm)', ha='center', va='center', rotation='vertical')
# plt.figtext(0.95, 0.5, r'Evaporation/Infiltration ($m^3/day)$', ha='center', va='center', rotation='vertical')
ax1.set_title("Check Dam 591")
# ax2_1.set_title("Check Dam 599")
# ax1_2.spines['right'].set_position(('axes', -0.6))
# make_patch_spines_invisible(ax1_2)
# ax1_2.spines['right'].set_visible(True)
ax1_1.yaxis.set_label_position('left')
ax1_1.yaxis.set_ticks_position('left')
for t1 in ax1_1.get_yticklabels():
t1.set_color('#a70c0b')
ax1_1.set_axis_bgcolor('white')
locator_1 = MaxNLocator(3)
locator_2 = MaxNLocator(3)
locator_1_1 = MaxNLocator(3)
locator_1_2 = MaxNLocator(3)
locator_2_1 = MaxNLocator(3)
locator_2_2 = MaxNLocator(3)
ax1.yaxis.set_major_locator(locator_1)
# ax2.yaxis.set_major_locator(locator_2)
# ax1_1.yaxis.set_major_locator(locator_1_1)
ax1_1.yaxis.set_major_locator(locator_1_2)
# ax2_1.yaxis.set_major_locator(locator_2_1)
# ax2_2.yaxis.set_major_locator(locator_2_2)
ax1_1.spines['top'].set_visible(False)
ax1_1.spines['right'].set_visible(False)
ax1_1.spines['bottom'].set_visible(False)
ax1_1.spines['left'].set_position(('outward', 50))
ax1_1.yaxis.set_ticks_position('left')
ax1_1.xaxis.set_ticks_position('bottom')
ax1_1.tick_params(axis='y', colors='#a70c0b')
ax1_1.spines['left'].set_color('#a70c0b')
# ax1_2.set_ylabel("Stage (m)")
ax1_1.yaxis.label.set_color('#a70c0b')
# ax1.set_ylabel('Rainfall (mm)')
ax1.yaxis.label.set_color('#203a72')
# ax2_2.spines['top'].set_visible(False)
# ax2_2.spines['right'].set_visible(False)
# ax2_2.spines['bottom'].set_visible(False)
# ax2_2.spines['left'].set_position(('outward', 50))
# ax2_2.yaxis.set_ticks_position('left')
# ax2_2.xaxis.set_ticks_position('bottom')
# ax2_2.tick_params(axis='y', colors='#a70c0b')
# ax2_2.spines['left'].set_color('#a70c0b')
# ax2_2.set_ylabel("Stage (m)")
# ax2_2.yaxis.label.set_color('#a70c0b')
# increase tick label size
# left y axis 1
# for tick in ax2.yaxis.get_major_ticks():
# tick.label.set_fontsize(24)
# # left y axis 2
# for tick in ax1.yaxis.get_major_ticks():
# tick.label.set_fontsize(24)
# # xaxis
# for tick in ax2.xaxis.get_major_ticks():
# tick.label.set_fontsize(24)
# for tick in ax2.yaxis.get_major_ticks():
# tick.label.set_fontsize(24)
# for tick in ax1_1.get_yticklabels():
# tick.set_fontsize(24)
# for tick in ax2_1.get_yticklabels():
# tick.set_fontsize(24)
plt.tight_layout()
fig.autofmt_xdate(rotation=90)
plt.savefig('/media/kiruba/New Volume/AGU/poster/agu_checkdam/image/evap_infilt.pdf', dpi=400)
plt.show()
# raise SystemExit(0)
# pie charts
# 591
print wb_599.head()
evap_591 = wb_591['Evaporation (cu.m)'].sum()
infilt_591 = wb_591['infiltration(cu.m)'].sum()
overflow_591 = wb_591['overflow(cu.m)'].sum()
inflow_591 = wb_591['Inflow (cu.m)'].sum()
pumping_591 = wb_591['pumping (cu.m)'].sum()
check_storage_591 = abs((evap_591+infilt_591+overflow_591+pumping_591)-inflow_591)
evap_599 = wb_599['Evaporation (cu.m)'].sum()
infilt_599 = wb_599['infiltration(cu.m)'].sum()
evap_599 = wb_599['Evaporation (cu.m)'].sum()
infilt_599 = wb_599['infiltration(cu.m)'].sum()
overflow_599 = wb_599['overflow(cu.m)'].sum()
inflow_599 = wb_599['Inflow (cu.m)'].sum()
pumping_599 = wb_599['pumping (cu.m)'].sum()
check_storage_599 = abs((evap_599+infilt_599+overflow_599+pumping_599)-inflow_599)
print evap_599
print infilt_599
print overflow_599
print inflow_599
print pumping_599
# latexify(fig_width=13.2, fig_height=8)
latexify(fig_width=15, fig_height=15)
# fig, (ax1, ax2) = plt.subplots(nrows=1, ncols=2, facecolor='white' )
fig, ax1 = plt.subplots(facecolor='white')
pie_1, texts_1, autotexts_1 = ax1.pie([evap_591,infilt_591, overflow_591], labels=['E', 'P', 'O' ],
colors=['#a70c0b', '#23530b', '#377eb8'],
autopct='%i%%',
explode=(0,0.1, 0.1),
startangle=90)
# pie_2, texts_2, autotexts_2 = ax2.pie([evap_599,infilt_599, overflow_599, inflow_599, pumping_599], labels=['E', 'Pe', 'O', 'I', 'Pu' ],
# colors=['#a70c0b', '#23530b', '#377eb8', '#984ea3', '#ff7f00'],
# autopct='%i%%',
# explode=(0,0.1, 0.1, 0.1, 0.1),
# startangle=90)
# ax1.axis('equal')
# ax2.axis('equal')
# plt.tight_layout()
ax1.set_title('Check Dam 591')
# ax2.set_title('Check Dam 599')
ax1.set_axis_bgcolor('white')
# ax2.set_axis_bgcolor('white')
# plt.subplots_adjust(bottom=0.15)
legend = fig.legend([pie_1[0], pie_1[1], pie_1[2]],["Evaporation", "Percolation", "Overflow"],fancybox=True, ncol=3,loc=(0.01,0.02))
# for label in texts_1:
# label.set_fontsize(56)
# for label in texts_2:
# label.set_fontsize(56)
# for label in autotexts_1:
# label.set_fontsize(48)
# for label in autotexts_2:
# label.set_fontsize(48)
# plt.tight_layout()
plt.savefig('/media/kiruba/New Volume/AGU/poster/agu_checkdam/image/pie_evap_infilt.png')
plt.show()
# raise SystemExit(0)
# print wb_591.head()
dry_water_balance_591 = wb_591[wb_591['status'] == 'N']
dry_water_balance_599 = wb_599[wb_599['status'] == 'N']
stage_cal_591 = dry_water_balance_591['stage(m)']
stage_cal_599 = dry_water_balance_599['stage(m)']
inf_cal_591 = dry_water_balance_591['infiltration rate (m/day)']
inf_cal_599 = dry_water_balance_599['infiltration rate (m/day)']
def func(h, alpha, beta):
return alpha*(h**beta)
popt_591, pcov_591 = curve_fit(func, stage_cal_591, inf_cal_591)
popt_599, pcov_599 = curve_fit(func, stage_cal_599, inf_cal_599)
stage_cal_new_591 = np.linspace(min(stage_cal_591), max(stage_cal_591), 50)
inf_cal_new_591 = func(stage_cal_new_591, *popt_591)
stage_cal_new_599 = np.linspace(min(stage_cal_599), max(stage_cal_599), 50)
inf_cal_new_599 = func(stage_cal_new_599, *popt_599)
latexify(fig_width=13.2, fig_height=8)
fig, (ax1, ax2) = plt.subplots(nrows=1, ncols=2, facecolor='white' )
ax1.scatter(stage_cal_591, inf_cal_591, facecolor='#203a72',marker='o',s=(np.pi*(5**2)))
ax1.plot(stage_cal_new_591, inf_cal_new_591, '#a70c0b',linestyle='-', linewidth=2)
ax1.text(x=0.4, y=.04, s=r'Infiltration rate = ${0:.2f}{{h}}^{{{1:.2f}}}$'.format(popt_591[0], popt_591[1]))
ax2.scatter(stage_cal_599, inf_cal_599, facecolor='#203a72',marker='o',s=(np.pi*(5**2)))
ax2.plot(stage_cal_new_599, inf_cal_new_599, '#a70c0b',linestyle='-', linewidth=2)
ax2.text(x=0.3, y=.04, s=r'Infiltration rate = ${0:.2f}{{h}}^{{{1:.2f}}}$'.format(popt_599[0], popt_599[1]))
ax1.spines['top'].set_visible(False)
ax1.spines['right'].set_visible(False)
ax1.spines['bottom'].set_position(('outward', 20))
ax1.spines['left'].set_position(('outward', 30))
ax1.yaxis.set_ticks_position('left')
ax1.xaxis.set_ticks_position('bottom')
ax1.grid(False)
ax1.set_axis_bgcolor('white')
ax2.spines['top'].set_visible(False)
ax2.spines['right'].set_visible(False)
ax2.spines['bottom'].set_position(('outward', 20))
ax2.spines['left'].set_position(('outward', 30))
ax2.yaxis.set_ticks_position('left')
ax2.xaxis.set_ticks_position('bottom')
ax2.grid(False)
ax2.set_axis_bgcolor('white')
ax1.set_ylabel(r'Infiltration rate ($m/day$)')
xxl = ax1.set_xlabel(r'Stage ($m$)')
xxl.set_position((1, xxl.get_position()[1]))
# fig.text(0.5, 0.02, 'Stage (m)', ha='center', va='center')
# plt.figtext(0.03, 0.5, r'Infiltration rate ($m/day)$', ha='center', va='center', rotation='vertical')
ax1.set_title("Check Dam 591")
ax2.set_title("Check Dam 599")
ax2.set_ylim(ax1.get_ylim())
locator_x = MaxNLocator(6)
locator_y = MaxNLocator(4)
locator_x_1 = MaxNLocator(6)
locator_y_1 = MaxNLocator(4)
ax1.yaxis.set_major_locator(locator_y)
ax1.xaxis.set_major_locator(locator_x)
ax2.xaxis.set_major_locator(locator_x_1)
ax2.yaxis.set_major_locator(locator_y_1)
# for tick in ax2.yaxis.get_major_ticks():
# tick.label.set_fontsize(24)
# for tick in ax2.xaxis.get_major_ticks():
# tick.label.set_fontsize(24)
# for tick in ax1.yaxis.get_major_ticks():
# tick.label.set_fontsize(24)
# for tick in ax1.xaxis.get_major_ticks():
# tick.label.set_fontsize(24)
ax2.legend(["Observation", "Prediction"],fancybox=True)
# plt.tight_layout()
plt.savefig('/media/kiruba/New Volume/AGU/poster/agu_checkdam/image/stage_infilt.pdf', dpi=400)
# plt.show()
| gpl-3.0 |
ngoix/OCRF | examples/mixture/plot_gmm_covariances.py | 13 | 4262 | """
===============
GMM covariances
===============
Demonstration of several covariances types for Gaussian mixture models.
See :ref:`gmm` for more information on the estimator.
Although GMM are often used for clustering, we can compare the obtained
clusters with the actual classes from the dataset. We initialize the means
of the Gaussians with the means of the classes from the training set to make
this comparison valid.
We plot predicted labels on both training and held out test data using a
variety of GMM covariance types on the iris dataset.
We compare GMMs with spherical, diagonal, full, and tied covariance
matrices in increasing order of performance. Although one would
expect full covariance to perform best in general, it is prone to
overfitting on small datasets and does not generalize well to held out
test data.
On the plots, train data is shown as dots, while test data is shown as
crosses. The iris dataset is four-dimensional. Only the first two
dimensions are shown here, and thus some points are separated in other
dimensions.
"""
print(__doc__)
# Author: Ron Weiss <[email protected]>, Gael Varoquaux
# License: BSD 3 clause
# $Id$
import matplotlib.pyplot as plt
import matplotlib as mpl
import numpy as np
from sklearn import datasets
from sklearn.model_selection import StratifiedKFold
from sklearn.externals.six.moves import xrange
from sklearn.mixture import GMM
colors = ['navy', 'turquoise', 'darkorange']
def make_ellipses(gmm, ax):
for n, color in enumerate(colors):
v, w = np.linalg.eigh(gmm._get_covars()[n][:2, :2])
u = w[0] / np.linalg.norm(w[0])
angle = np.arctan2(u[1], u[0])
angle = 180 * angle / np.pi # convert to degrees
v *= 9
ell = mpl.patches.Ellipse(gmm.means_[n, :2], v[0], v[1],
180 + angle, color=color)
ell.set_clip_box(ax.bbox)
ell.set_alpha(0.5)
ax.add_artist(ell)
iris = datasets.load_iris()
# Break up the dataset into non-overlapping training (75%) and testing
# (25%) sets.
skf = StratifiedKFold(n_folds=4)
# Only take the first fold.
train_index, test_index = next(iter(skf.split(iris.data, iris.target)))
X_train = iris.data[train_index]
y_train = iris.target[train_index]
X_test = iris.data[test_index]
y_test = iris.target[test_index]
n_classes = len(np.unique(y_train))
# Try GMMs using different types of covariances.
estimators = dict((covar_type,
GMM(n_components=n_classes, covariance_type=covar_type,
init_params='wc', n_iter=20))
for covar_type in ['spherical', 'diag', 'tied', 'full'])
n_estimators = len(estimators)
plt.figure(figsize=(3 * n_estimators / 2, 6))
plt.subplots_adjust(bottom=.01, top=0.95, hspace=.15, wspace=.05,
left=.01, right=.99)
for index, (name, estimator) in enumerate(estimators.items()):
# Since we have class labels for the training data, we can
# initialize the GMM parameters in a supervised manner.
estimator.means_ = np.array([X_train[y_train == i].mean(axis=0)
for i in xrange(n_classes)])
# Train the other parameters using the EM algorithm.
estimator.fit(X_train)
h = plt.subplot(2, n_estimators / 2, index + 1)
make_ellipses(estimator, h)
for n, color in enumerate(colors):
data = iris.data[iris.target == n]
plt.scatter(data[:, 0], data[:, 1], s=0.8, color=color,
label=iris.target_names[n])
# Plot the test data with crosses
for n, color in enumerate(colors):
data = X_test[y_test == n]
plt.scatter(data[:, 0], data[:, 1], marker='x', color=color)
y_train_pred = estimator.predict(X_train)
train_accuracy = np.mean(y_train_pred.ravel() == y_train.ravel()) * 100
plt.text(0.05, 0.9, 'Train accuracy: %.1f' % train_accuracy,
transform=h.transAxes)
y_test_pred = estimator.predict(X_test)
test_accuracy = np.mean(y_test_pred.ravel() == y_test.ravel()) * 100
plt.text(0.05, 0.8, 'Test accuracy: %.1f' % test_accuracy,
transform=h.transAxes)
plt.xticks(())
plt.yticks(())
plt.title(name)
plt.legend(loc='lower right', prop=dict(size=12))
plt.show()
| bsd-3-clause |
sshh12/Students-Visualization | other_visuals/show_demo_gpa_stats.py | 1 | 1754 | from cyranchdb import cyranch_db
from collections import defaultdict
import numpy as np
import math
users = defaultdict(dict)
for index, row in cyranch_db.tables.rank.all().iterrows():
users[row["user_id"]]["gpa"] = row["gpa"]
for index, row in cyranch_db.tables.demo.all().iterrows():
if row["gradelevel"] > 12 or row["user_id"] not in users:
continue
users[row["user_id"]]["lang"] = row["language"]
users[row["user_id"]]["gender"] = row["gender"]
users[row["user_id"]]["grade"] = str(row["gradelevel"])
def create_plot():
"""Plots with matplot lib"""
import matplotlib.pyplot as plt
def make_box(plot, users, category, labels, colors):
x_datas = []
for label in labels:
x = []
for user, data in users.items():
if "lang" not in data: # filter incomplete demo data
continue
if label.lower() in data[category]:
x.append(data["gpa"])
x_datas.append(x)
bplot = plot.boxplot(x_datas, notch=True, sym='+', patch_artist=True)
plot.set_xticks(np.arange(1, len(labels) + 1))
plot.set_xticklabels(labels)
for patch, color in zip(bplot['boxes'], colors):
patch.set_facecolor(color)
plt.style.use('ggplot')
f, ((ax1, ax2, ax3)) = plt.subplots(3, 1, sharey=True)
f.suptitle('GPA Demographics')
make_box(ax1, users, "lang", ['English', 'Spanish', 'Vietnamese', 'Arabic', 'Cantonese'], ['r', 'g', 'b', 'y', 'c'])
make_box(ax2, users, "gender", ['Male', 'Female'], ['b', 'r'])
make_box(ax3, users, "grade", ['10', '11', '12'], ['w', 'm', 'c'])
plt.show()
if __name__ == "__main__":
create_plot()
| mit |
dolaameng/keras | keras/callbacks.py | 2 | 28758 | from __future__ import absolute_import
from __future__ import print_function
import csv
import numpy as np
import time
import json
import warnings
from collections import deque, OrderedDict, Iterable
from .utils.generic_utils import Progbar
from keras import backend as K
from pkg_resources import parse_version
class CallbackList(object):
def __init__(self, callbacks=[], queue_length=10):
self.callbacks = [c for c in callbacks]
self.queue_length = queue_length
def append(self, callback):
self.callbacks.append(callback)
def _set_params(self, params):
for callback in self.callbacks:
callback._set_params(params)
def _set_model(self, model):
for callback in self.callbacks:
callback._set_model(model)
def on_epoch_begin(self, epoch, logs={}):
for callback in self.callbacks:
callback.on_epoch_begin(epoch, logs)
self._delta_t_batch = 0.
self._delta_ts_batch_begin = deque([], maxlen=self.queue_length)
self._delta_ts_batch_end = deque([], maxlen=self.queue_length)
def on_epoch_end(self, epoch, logs={}):
for callback in self.callbacks:
callback.on_epoch_end(epoch, logs)
def on_batch_begin(self, batch, logs={}):
t_before_callbacks = time.time()
for callback in self.callbacks:
callback.on_batch_begin(batch, logs)
self._delta_ts_batch_begin.append(time.time() - t_before_callbacks)
delta_t_median = np.median(self._delta_ts_batch_begin)
if self._delta_t_batch > 0. and delta_t_median > 0.95 * \
self._delta_t_batch and delta_t_median > 0.1:
warnings.warn('Method on_batch_begin() is slow compared '
'to the batch update (%f). Check your callbacks.'
% delta_t_median)
self._t_enter_batch = time.time()
def on_batch_end(self, batch, logs={}):
if not hasattr(self, '_t_enter_batch'):
self._t_enter_batch = time.time()
self._delta_t_batch = time.time() - self._t_enter_batch
t_before_callbacks = time.time()
for callback in self.callbacks:
callback.on_batch_end(batch, logs)
self._delta_ts_batch_end.append(time.time() - t_before_callbacks)
delta_t_median = np.median(self._delta_ts_batch_end)
if self._delta_t_batch > 0. and (delta_t_median > 0.95 * self._delta_t_batch and delta_t_median > 0.1):
warnings.warn('Method on_batch_end() is slow compared '
'to the batch update (%f). Check your callbacks.'
% delta_t_median)
def on_train_begin(self, logs={}):
for callback in self.callbacks:
callback.on_train_begin(logs)
def on_train_end(self, logs={}):
for callback in self.callbacks:
callback.on_train_end(logs)
class Callback(object):
'''Abstract base class used to build new callbacks.
# Properties
params: dict. Training parameters
(eg. verbosity, batch size, number of epochs...).
model: instance of `keras.models.Model`.
Reference of the model being trained.
The `logs` dictionary that callback methods
take as argument will contain keys for quantities relevant to
the current batch or epoch.
Currently, the `.fit()` method of the `Sequential` model class
will include the following quantities in the `logs` that
it passes to its callbacks:
on_epoch_end: logs include `acc` and `loss`, and
optionally include `val_loss`
(if validation is enabled in `fit`), and `val_acc`
(if validation and accuracy monitoring are enabled).
on_batch_begin: logs include `size`,
the number of samples in the current batch.
on_batch_end: logs include `loss`, and optionally `acc`
(if accuracy monitoring is enabled).
'''
def __init__(self):
pass
def _set_params(self, params):
self.params = params
def _set_model(self, model):
self.model = model
def on_epoch_begin(self, epoch, logs={}):
pass
def on_epoch_end(self, epoch, logs={}):
pass
def on_batch_begin(self, batch, logs={}):
pass
def on_batch_end(self, batch, logs={}):
pass
def on_train_begin(self, logs={}):
pass
def on_train_end(self, logs={}):
pass
class BaseLogger(Callback):
'''Callback that accumulates epoch averages of
the metrics being monitored.
This callback is automatically applied to
every Keras model.
'''
def on_epoch_begin(self, epoch, logs={}):
self.seen = 0
self.totals = {}
def on_batch_end(self, batch, logs={}):
batch_size = logs.get('size', 0)
self.seen += batch_size
for k, v in logs.items():
if k in self.totals:
self.totals[k] += v * batch_size
else:
self.totals[k] = v * batch_size
def on_epoch_end(self, epoch, logs={}):
for k in self.params['metrics']:
if k in self.totals:
# make value available to next callbacks
logs[k] = self.totals[k] / self.seen
class ProgbarLogger(Callback):
'''Callback that prints metrics to stdout.
'''
def on_train_begin(self, logs={}):
self.verbose = self.params['verbose']
self.nb_epoch = self.params['nb_epoch']
def on_epoch_begin(self, epoch, logs={}):
if self.verbose:
print('Epoch %d/%d' % (epoch + 1, self.nb_epoch))
self.progbar = Progbar(target=self.params['nb_sample'],
verbose=self.verbose)
self.seen = 0
def on_batch_begin(self, batch, logs={}):
if self.seen < self.params['nb_sample']:
self.log_values = []
def on_batch_end(self, batch, logs={}):
batch_size = logs.get('size', 0)
self.seen += batch_size
for k in self.params['metrics']:
if k in logs:
self.log_values.append((k, logs[k]))
# skip progbar update for the last batch;
# will be handled by on_epoch_end
if self.verbose and self.seen < self.params['nb_sample']:
self.progbar.update(self.seen, self.log_values)
def on_epoch_end(self, epoch, logs={}):
for k in self.params['metrics']:
if k in logs:
self.log_values.append((k, logs[k]))
if self.verbose:
self.progbar.update(self.seen, self.log_values, force=True)
class History(Callback):
'''Callback that records events
into a `History` object.
This callback is automatically applied to
every Keras model. The `History` object
gets returned by the `fit` method of models.
'''
def on_train_begin(self, logs={}):
self.epoch = []
self.history = {}
def on_epoch_end(self, epoch, logs={}):
self.epoch.append(epoch)
for k, v in logs.items():
self.history.setdefault(k, []).append(v)
class ModelCheckpoint(Callback):
'''Save the model after every epoch.
`filepath` can contain named formatting options,
which will be filled the value of `epoch` and
keys in `logs` (passed in `on_epoch_end`).
For example: if `filepath` is `weights.{epoch:02d}-{val_loss:.2f}.hdf5`,
then multiple files will be save with the epoch number and
the validation loss.
# Arguments
filepath: string, path to save the model file.
monitor: quantity to monitor.
verbose: verbosity mode, 0 or 1.
save_best_only: if `save_best_only=True`,
the latest best model according to
the quantity monitored will not be overwritten.
mode: one of {auto, min, max}.
If `save_best_only=True`, the decision
to overwrite the current save file is made
based on either the maximization or the
minimization of the monitored quantity. For `val_acc`,
this should be `max`, for `val_loss` this should
be `min`, etc. In `auto` mode, the direction is
automatically inferred from the name of the monitored quantity.
save_weights_only: if True, then only the model's weights will be
saved (`model.save_weights(filepath)`), else the full model
is saved (`model.save(filepath)`).
'''
def __init__(self, filepath, monitor='val_loss', verbose=0,
save_best_only=False, save_weights_only=False,
mode='auto'):
super(ModelCheckpoint, self).__init__()
self.monitor = monitor
self.verbose = verbose
self.filepath = filepath
self.save_best_only = save_best_only
self.save_weights_only = save_weights_only
if mode not in ['auto', 'min', 'max']:
warnings.warn('ModelCheckpoint mode %s is unknown, '
'fallback to auto mode.' % (mode),
RuntimeWarning)
mode = 'auto'
if mode == 'min':
self.monitor_op = np.less
self.best = np.Inf
elif mode == 'max':
self.monitor_op = np.greater
self.best = -np.Inf
else:
if 'acc' in self.monitor:
self.monitor_op = np.greater
self.best = -np.Inf
else:
self.monitor_op = np.less
self.best = np.Inf
def on_epoch_end(self, epoch, logs={}):
filepath = self.filepath.format(epoch=epoch, **logs)
if self.save_best_only:
current = logs.get(self.monitor)
if current is None:
warnings.warn('Can save best model only with %s available, '
'skipping.' % (self.monitor), RuntimeWarning)
else:
if self.monitor_op(current, self.best):
if self.verbose > 0:
print('Epoch %05d: %s improved from %0.5f to %0.5f,'
' saving model to %s'
% (epoch, self.monitor, self.best,
current, filepath))
self.best = current
if self.save_weights_only:
self.model.save_weights(filepath, overwrite=True)
else:
self.model.save(filepath, overwrite=True)
else:
if self.verbose > 0:
print('Epoch %05d: %s did not improve' %
(epoch, self.monitor))
else:
if self.verbose > 0:
print('Epoch %05d: saving model to %s' % (epoch, filepath))
if self.save_weights_only:
self.model.save_weights(filepath, overwrite=True)
else:
self.model.save(filepath, overwrite=True)
class EarlyStopping(Callback):
'''Stop training when a monitored quantity has stopped improving.
# Arguments
monitor: quantity to be monitored.
min_delta: minimum change in the monitored quantity
to qualify as an improvement, i.e. an absolute
change of less than min_delta, will count as no
improvement.
patience: number of epochs with no improvement
after which training will be stopped.
verbose: verbosity mode.
mode: one of {auto, min, max}. In `min` mode,
training will stop when the quantity
monitored has stopped decreasing; in `max`
mode it will stop when the quantity
monitored has stopped increasing; in `auto`
mode, the direction is automatically inferred
from the name of the monitored quantity.
'''
def __init__(self, monitor='val_loss', min_delta=0, patience=0, verbose=0, mode='auto'):
super(EarlyStopping, self).__init__()
self.monitor = monitor
self.patience = patience
self.verbose = verbose
self.min_delta = min_delta
self.wait = 0
if mode not in ['auto', 'min', 'max']:
warnings.warn('EarlyStopping mode %s is unknown, '
'fallback to auto mode.' % (self.mode),
RuntimeWarning)
mode = 'auto'
if mode == 'min':
self.monitor_op = np.less
elif mode == 'max':
self.monitor_op = np.greater
else:
if 'acc' in self.monitor:
self.monitor_op = np.greater
else:
self.monitor_op = np.less
if self.monitor_op == np.greater:
self.min_delta *= 1
else:
self.min_delta *= -1
def on_train_begin(self, logs={}):
self.wait = 0 # Allow instances to be re-used
self.best = np.Inf if self.monitor_op == np.less else -np.Inf
def on_epoch_end(self, epoch, logs={}):
current = logs.get(self.monitor)
if current is None:
warnings.warn('Early stopping requires %s available!' %
(self.monitor), RuntimeWarning)
if self.monitor_op(current - self.min_delta, self.best):
self.best = current
self.wait = 0
else:
if self.wait >= self.patience:
if self.verbose > 0:
print('Epoch %05d: early stopping' % (epoch))
self.model.stop_training = True
self.wait += 1
class RemoteMonitor(Callback):
'''Callback used to stream events to a server.
Requires the `requests` library.
# Arguments
root: root url to which the events will be sent (at the end
of every epoch). Events are sent to
`root + '/publish/epoch/end/'` by default. Calls are
HTTP POST, with a `data` argument which is a
JSON-encoded dictionary of event data.
'''
def __init__(self,
root='http://localhost:9000',
path='/publish/epoch/end/',
field='data'):
super(RemoteMonitor, self).__init__()
self.root = root
self.path = path
self.field = field
def on_epoch_end(self, epoch, logs={}):
import requests
send = {}
send['epoch'] = epoch
for k, v in logs.items():
send[k] = v
try:
requests.post(self.root + self.path,
{self.field: json.dumps(send)})
except:
print('Warning: could not reach RemoteMonitor '
'root server at ' + str(self.root))
class LearningRateScheduler(Callback):
'''Learning rate scheduler.
# Arguments
schedule: a function that takes an epoch index as input
(integer, indexed from 0) and returns a new
learning rate as output (float).
'''
def __init__(self, schedule):
super(LearningRateScheduler, self).__init__()
self.schedule = schedule
def on_epoch_begin(self, epoch, logs={}):
assert hasattr(self.model.optimizer, 'lr'), \
'Optimizer must have a "lr" attribute.'
lr = self.schedule(epoch)
assert type(lr) == float, 'The output of the "schedule" function should be float.'
K.set_value(self.model.optimizer.lr, lr)
class TensorBoard(Callback):
''' Tensorboard basic visualizations.
This callback writes a log for TensorBoard, which allows
you to visualize dynamic graphs of your training and test
metrics, as well as activation histograms for the different
layers in your model.
TensorBoard is a visualization tool provided with TensorFlow.
If you have installed TensorFlow with pip, you should be able
to launch TensorBoard from the command line:
```
tensorboard --logdir=/full_path_to_your_logs
```
You can find more information about TensorBoard
[here](https://www.tensorflow.org/versions/master/how_tos/summaries_and_tensorboard/index.html).
# Arguments
log_dir: the path of the directory where to save the log
files to be parsed by Tensorboard
histogram_freq: frequency (in epochs) at which to compute activation
histograms for the layers of the model. If set to 0,
histograms won't be computed.
write_graph: whether to visualize the graph in Tensorboard.
The log file can become quite large when
write_graph is set to True.
'''
def __init__(self, log_dir='./logs', histogram_freq=0, write_graph=True, write_images=False):
super(TensorBoard, self).__init__()
if K._BACKEND != 'tensorflow':
raise Exception('TensorBoard callback only works '
'with the TensorFlow backend.')
self.log_dir = log_dir
self.histogram_freq = histogram_freq
self.merged = None
self.write_graph = write_graph
self.write_images = write_images
def _set_model(self, model):
import tensorflow as tf
import keras.backend.tensorflow_backend as KTF
self.model = model
self.sess = KTF.get_session()
if self.histogram_freq and self.merged is None:
for layer in self.model.layers:
for weight in layer.weights:
tf.histogram_summary(weight.name, weight)
if self.write_images:
w_img = tf.squeeze(weight)
shape = w_img.get_shape()
if len(shape) > 1 and shape[0] > shape[1]:
w_img = tf.transpose(w_img)
if len(shape) == 1:
w_img = tf.expand_dims(w_img, 0)
w_img = tf.expand_dims(tf.expand_dims(w_img, 0), -1)
tf.image_summary(weight.name, w_img)
if hasattr(layer, 'output'):
tf.histogram_summary('{}_out'.format(layer.name),
layer.output)
self.merged = tf.merge_all_summaries()
if self.write_graph:
if parse_version(tf.__version__) >= parse_version('0.8.0'):
self.writer = tf.train.SummaryWriter(self.log_dir,
self.sess.graph)
else:
self.writer = tf.train.SummaryWriter(self.log_dir,
self.sess.graph_def)
else:
self.writer = tf.train.SummaryWriter(self.log_dir)
def on_epoch_end(self, epoch, logs={}):
import tensorflow as tf
if self.model.validation_data and self.histogram_freq:
if epoch % self.histogram_freq == 0:
# TODO: implement batched calls to sess.run
# (current call will likely go OOM on GPU)
if self.model.uses_learning_phase:
cut_v_data = len(self.model.inputs)
val_data = self.model.validation_data[:cut_v_data] + [0]
tensors = self.model.inputs + [K.learning_phase()]
else:
val_data = self.model.validation_data
tensors = self.model.inputs
feed_dict = dict(zip(tensors, val_data))
result = self.sess.run([self.merged], feed_dict=feed_dict)
summary_str = result[0]
self.writer.add_summary(summary_str, epoch)
for name, value in logs.items():
if name in ['batch', 'size']:
continue
summary = tf.Summary()
summary_value = summary.value.add()
summary_value.simple_value = value.item()
summary_value.tag = name
self.writer.add_summary(summary, epoch)
self.writer.flush()
class ReduceLROnPlateau(Callback):
'''Reduce learning rate when a metric has stopped improving.
Models often benefit from reducing the learning rate by a factor
of 2-10 once learning stagnates. This callback monitors a
quantity and if no improvement is seen for a 'patience' number
of epochs, the learning rate is reduced.
# Example
```python
reduce_lr = ReduceLROnPlateau(monitor='val_loss', factor=0.2,
patience=5, min_lr=0.001)
model.fit(X_train, Y_train, callbacks=[reduce_lr])
```
# Arguments
monitor: quantity to be monitored.
factor: factor by which the learning rate will
be reduced. new_lr = lr * factor
patience: number of epochs with no improvement
after which learning rate will be reduced.
verbose: int. 0: quiet, 1: update messages.
mode: one of {auto, min, max}. In `min` mode,
lr will be reduced when the quantity
monitored has stopped decreasing; in `max`
mode it will be reduced when the quantity
monitored has stopped increasing; in `auto`
mode, the direction is automatically inferred
from the name of the monitored quantity.
epsilon: threshold for measuring the new optimum,
to only focus on significant changes.
cooldown: number of epochs to wait before resuming
normal operation after lr has been reduced.
min_lr: lower bound on the learning rate.
'''
def __init__(self, monitor='val_loss', factor=0.1, patience=10,
verbose=0, mode='auto', epsilon=1e-4, cooldown=0, min_lr=0):
super(Callback, self).__init__()
self.monitor = monitor
if factor >= 1.0:
raise ValueError('ReduceLROnPlateau does not support a factor >= 1.0.')
self.factor = factor
self.min_lr = min_lr
self.epsilon = epsilon
self.patience = patience
self.verbose = verbose
self.cooldown = cooldown
self.cooldown_counter = 0 # Cooldown counter.
self.wait = 0
self.best = 0
self.mode = mode
self.monitor_op = None
self.reset()
def reset(self):
if self.mode not in ['auto', 'min', 'max']:
warnings.warn('Learning Rate Plateau Reducing mode %s is unknown, '
'fallback to auto mode.' % (self.mode), RuntimeWarning)
self.mode = 'auto'
if self.mode == 'min' or (self.mode == 'auto' and 'acc' not in self.monitor):
self.monitor_op = lambda a, b: np.less(a, b - self.epsilon)
self.best = np.Inf
else:
self.monitor_op = lambda a, b: np.greater(a, b + self.epsilon)
self.best = -np.Inf
self.cooldown_counter = 0
self.wait = 0
self.lr_epsilon = self.min_lr * 1e-4
def on_train_begin(self, logs={}):
self.reset()
def on_epoch_end(self, epoch, logs={}):
logs['lr'] = K.get_value(self.model.optimizer.lr)
current = logs.get(self.monitor)
if current is None:
warnings.warn('Learning Rate Plateau Reducing requires %s available!' %
self.monitor, RuntimeWarning)
else:
if self.in_cooldown():
self.cooldown_counter -= 1
self.wait = 0
if self.monitor_op(current, self.best):
self.best = current
self.wait = 0
elif not self.in_cooldown():
if self.wait >= self.patience:
old_lr = float(K.get_value(self.model.optimizer.lr))
if old_lr > self.min_lr + self.lr_epsilon:
new_lr = old_lr * self.factor
new_lr = max(new_lr, self.min_lr)
K.set_value(self.model.optimizer.lr, new_lr)
if self.verbose > 0:
print('\nEpoch %05d: reducing learning rate to %s.' % (epoch, new_lr))
self.cooldown_counter = self.cooldown
self.wait = 0
self.wait += 1
def in_cooldown(self):
return self.cooldown_counter > 0
class CSVLogger(Callback):
'''Callback that streams epoch results to a csv file.
Supports all values that can be represented as a string,
including 1D iterables such as np.ndarray.
# Example
```python
csv_logger = CSVLogger('training.log')
model.fit(X_train, Y_train, callbacks=[csv_logger])
```
Arguments
filename: filename of the csv file, e.g. 'run/log.csv'.
separator: string used to separate elements in the csv file.
append: True: append if file exists (useful for continuing
training). False: overwrite existing file,
'''
def __init__(self, filename, separator=',', append=False):
self.sep = separator
self.filename = filename
self.append = append
self.writer = None
self.keys = None
super(CSVLogger, self).__init__()
def on_train_begin(self, logs={}):
if self.append:
self.csv_file = open(self.filename, 'a')
else:
self.csv_file = open(self.filename, 'w')
def on_epoch_end(self, epoch, logs={}):
def handle_value(k):
is_zero_dim_ndarray = isinstance(k, np.ndarray) and k.ndim == 0
if isinstance(k, Iterable) and not is_zero_dim_ndarray:
return '"[%s]"' % (', '.join(map(lambda x: str(x), k)))
else:
return k
if not self.writer:
self.keys = sorted(logs.keys())
self.writer = csv.DictWriter(self.csv_file, fieldnames=['epoch'] + self.keys)
self.writer.writeheader()
row_dict = OrderedDict({'epoch': epoch})
row_dict.update((key, handle_value(logs[key])) for key in self.keys)
self.writer.writerow(row_dict)
self.csv_file.flush()
def on_train_end(self, logs={}):
self.csv_file.close()
class LambdaCallback(Callback):
"""Callback for creating simple, custom callbacks on-the-fly.
This callback is constructed with anonymous functions that will be called
at the appropiate time. Note that the callbacks expects positional
arguments, as:
- `on_epoch_begin` and `on_epoch_end` expect two positional arguments: `epoch`, `logs`
- `on_batch_begin` and `on_batch_end` expect two positional arguments: `batch`, `logs`
- `on_train_begin` and `on_train_end` expect one positional argument: `logs`
# Arguments
on_epoch_begin: called at the beginning of every epoch.
on_epoch_end: called at the end of every epoch.
on_batch_begin: called at the beginning of every batch.
on_batch_end: called at the end of every batch.
on_train_begin: called at the beginning of model training.
on_train_end: called at the end of model training.
# Example
```python
# Print the batch number at the beginning of every batch.
batch_print_callback = LambdaCallback(on_batch_begin=lambda batch, logs: print(batch))
# Plot the loss after every epoch.
import numpy as np
import matplotlib.pyplot as plt
plot_loss_callback = LambdaCallback(on_epoch_end=lambda epoch, logs: plt.plot(np.arange(epoch), logs['loss']))
# Terminate some processes after having finished model training.
processes = ...
cleanup_callback = LambdaCallback(on_train_end=lambda logs: [p.terminate() for p in processes if p.is_alive()])
model.fit(..., callbacks=[batch_print_callback, plot_loss_callback, cleanup_callback])
```
"""
def __init__(self,
on_epoch_begin=None,
on_epoch_end=None,
on_batch_begin=None,
on_batch_end=None,
on_train_begin=None,
on_train_end=None,
**kwargs):
super(Callback, self).__init__()
self.__dict__.update(kwargs)
self.on_epoch_begin = on_epoch_begin if on_epoch_begin else lambda epoch, logs: None
self.on_epoch_end = on_epoch_end if on_epoch_end else lambda epoch, logs: None
self.on_batch_begin = on_batch_begin if on_batch_begin else lambda batch, logs: None
self.on_batch_end = on_batch_end if on_batch_end else lambda batch, logs: None
self.on_train_begin = on_train_begin if on_train_begin else lambda logs: None
self.on_train_end = on_train_end if on_train_end else lambda logs: None
| mit |
carrillo/scikit-learn | examples/neighbors/plot_kde_1d.py | 347 | 5100 | """
===================================
Simple 1D Kernel Density Estimation
===================================
This example uses the :class:`sklearn.neighbors.KernelDensity` class to
demonstrate the principles of Kernel Density Estimation in one dimension.
The first plot shows one of the problems with using histograms to visualize
the density of points in 1D. Intuitively, a histogram can be thought of as a
scheme in which a unit "block" is stacked above each point on a regular grid.
As the top two panels show, however, the choice of gridding for these blocks
can lead to wildly divergent ideas about the underlying shape of the density
distribution. If we instead center each block on the point it represents, we
get the estimate shown in the bottom left panel. This is a kernel density
estimation with a "top hat" kernel. This idea can be generalized to other
kernel shapes: the bottom-right panel of the first figure shows a Gaussian
kernel density estimate over the same distribution.
Scikit-learn implements efficient kernel density estimation using either
a Ball Tree or KD Tree structure, through the
:class:`sklearn.neighbors.KernelDensity` estimator. The available kernels
are shown in the second figure of this example.
The third figure compares kernel density estimates for a distribution of 100
samples in 1 dimension. Though this example uses 1D distributions, kernel
density estimation is easily and efficiently extensible to higher dimensions
as well.
"""
# Author: Jake Vanderplas <[email protected]>
#
import numpy as np
import matplotlib.pyplot as plt
from scipy.stats import norm
from sklearn.neighbors import KernelDensity
#----------------------------------------------------------------------
# Plot the progression of histograms to kernels
np.random.seed(1)
N = 20
X = np.concatenate((np.random.normal(0, 1, 0.3 * N),
np.random.normal(5, 1, 0.7 * N)))[:, np.newaxis]
X_plot = np.linspace(-5, 10, 1000)[:, np.newaxis]
bins = np.linspace(-5, 10, 10)
fig, ax = plt.subplots(2, 2, sharex=True, sharey=True)
fig.subplots_adjust(hspace=0.05, wspace=0.05)
# histogram 1
ax[0, 0].hist(X[:, 0], bins=bins, fc='#AAAAFF', normed=True)
ax[0, 0].text(-3.5, 0.31, "Histogram")
# histogram 2
ax[0, 1].hist(X[:, 0], bins=bins + 0.75, fc='#AAAAFF', normed=True)
ax[0, 1].text(-3.5, 0.31, "Histogram, bins shifted")
# tophat KDE
kde = KernelDensity(kernel='tophat', bandwidth=0.75).fit(X)
log_dens = kde.score_samples(X_plot)
ax[1, 0].fill(X_plot[:, 0], np.exp(log_dens), fc='#AAAAFF')
ax[1, 0].text(-3.5, 0.31, "Tophat Kernel Density")
# Gaussian KDE
kde = KernelDensity(kernel='gaussian', bandwidth=0.75).fit(X)
log_dens = kde.score_samples(X_plot)
ax[1, 1].fill(X_plot[:, 0], np.exp(log_dens), fc='#AAAAFF')
ax[1, 1].text(-3.5, 0.31, "Gaussian Kernel Density")
for axi in ax.ravel():
axi.plot(X[:, 0], np.zeros(X.shape[0]) - 0.01, '+k')
axi.set_xlim(-4, 9)
axi.set_ylim(-0.02, 0.34)
for axi in ax[:, 0]:
axi.set_ylabel('Normalized Density')
for axi in ax[1, :]:
axi.set_xlabel('x')
#----------------------------------------------------------------------
# Plot all available kernels
X_plot = np.linspace(-6, 6, 1000)[:, None]
X_src = np.zeros((1, 1))
fig, ax = plt.subplots(2, 3, sharex=True, sharey=True)
fig.subplots_adjust(left=0.05, right=0.95, hspace=0.05, wspace=0.05)
def format_func(x, loc):
if x == 0:
return '0'
elif x == 1:
return 'h'
elif x == -1:
return '-h'
else:
return '%ih' % x
for i, kernel in enumerate(['gaussian', 'tophat', 'epanechnikov',
'exponential', 'linear', 'cosine']):
axi = ax.ravel()[i]
log_dens = KernelDensity(kernel=kernel).fit(X_src).score_samples(X_plot)
axi.fill(X_plot[:, 0], np.exp(log_dens), '-k', fc='#AAAAFF')
axi.text(-2.6, 0.95, kernel)
axi.xaxis.set_major_formatter(plt.FuncFormatter(format_func))
axi.xaxis.set_major_locator(plt.MultipleLocator(1))
axi.yaxis.set_major_locator(plt.NullLocator())
axi.set_ylim(0, 1.05)
axi.set_xlim(-2.9, 2.9)
ax[0, 1].set_title('Available Kernels')
#----------------------------------------------------------------------
# Plot a 1D density example
N = 100
np.random.seed(1)
X = np.concatenate((np.random.normal(0, 1, 0.3 * N),
np.random.normal(5, 1, 0.7 * N)))[:, np.newaxis]
X_plot = np.linspace(-5, 10, 1000)[:, np.newaxis]
true_dens = (0.3 * norm(0, 1).pdf(X_plot[:, 0])
+ 0.7 * norm(5, 1).pdf(X_plot[:, 0]))
fig, ax = plt.subplots()
ax.fill(X_plot[:, 0], true_dens, fc='black', alpha=0.2,
label='input distribution')
for kernel in ['gaussian', 'tophat', 'epanechnikov']:
kde = KernelDensity(kernel=kernel, bandwidth=0.5).fit(X)
log_dens = kde.score_samples(X_plot)
ax.plot(X_plot[:, 0], np.exp(log_dens), '-',
label="kernel = '{0}'".format(kernel))
ax.text(6, 0.38, "N={0} points".format(N))
ax.legend(loc='upper left')
ax.plot(X[:, 0], -0.005 - 0.01 * np.random.random(X.shape[0]), '+k')
ax.set_xlim(-4, 9)
ax.set_ylim(-0.02, 0.4)
plt.show()
| bsd-3-clause |
HolgerPeters/scikit-learn | sklearn/utils/tests/test_extmath.py | 19 | 24513 | # Authors: Olivier Grisel <[email protected]>
# Mathieu Blondel <[email protected]>
# Denis Engemann <[email protected]>
#
# License: BSD 3 clause
import numpy as np
from scipy import sparse
from scipy import linalg
from scipy import stats
from sklearn.utils.testing import assert_equal
from sklearn.utils.testing import assert_almost_equal
from sklearn.utils.testing import assert_array_equal
from sklearn.utils.testing import assert_array_almost_equal
from sklearn.utils.testing import assert_true
from sklearn.utils.testing import assert_false
from sklearn.utils.testing import assert_greater
from sklearn.utils.testing import assert_raises
from sklearn.utils.testing import assert_warns
from sklearn.utils.testing import skip_if_32bit
from sklearn.utils.testing import SkipTest
from sklearn.utils.fixes import np_version
from sklearn.utils.extmath import density
from sklearn.utils.extmath import logsumexp
from sklearn.utils.extmath import norm, squared_norm
from sklearn.utils.extmath import randomized_svd
from sklearn.utils.extmath import row_norms
from sklearn.utils.extmath import weighted_mode
from sklearn.utils.extmath import cartesian
from sklearn.utils.extmath import log_logistic
from sklearn.utils.extmath import fast_dot, _fast_dot
from sklearn.utils.extmath import svd_flip
from sklearn.utils.extmath import _incremental_mean_and_var
from sklearn.utils.extmath import _deterministic_vector_sign_flip
from sklearn.utils.extmath import softmax
from sklearn.utils.extmath import stable_cumsum
from sklearn.datasets.samples_generator import make_low_rank_matrix
def test_density():
rng = np.random.RandomState(0)
X = rng.randint(10, size=(10, 5))
X[1, 2] = 0
X[5, 3] = 0
X_csr = sparse.csr_matrix(X)
X_csc = sparse.csc_matrix(X)
X_coo = sparse.coo_matrix(X)
X_lil = sparse.lil_matrix(X)
for X_ in (X_csr, X_csc, X_coo, X_lil):
assert_equal(density(X_), density(X))
def test_uniform_weights():
# with uniform weights, results should be identical to stats.mode
rng = np.random.RandomState(0)
x = rng.randint(10, size=(10, 5))
weights = np.ones(x.shape)
for axis in (None, 0, 1):
mode, score = stats.mode(x, axis)
mode2, score2 = weighted_mode(x, weights, axis)
assert_array_equal(mode, mode2)
assert_array_equal(score, score2)
def test_random_weights():
# set this up so that each row should have a weighted mode of 6,
# with a score that is easily reproduced
mode_result = 6
rng = np.random.RandomState(0)
x = rng.randint(mode_result, size=(100, 10))
w = rng.random_sample(x.shape)
x[:, :5] = mode_result
w[:, :5] += 1
mode, score = weighted_mode(x, w, axis=1)
assert_array_equal(mode, mode_result)
assert_array_almost_equal(score.ravel(), w[:, :5].sum(1))
def test_logsumexp():
# Try to add some smallish numbers in logspace
x = np.array([1e-40] * 1000000)
logx = np.log(x)
assert_almost_equal(np.exp(logsumexp(logx)), x.sum())
X = np.vstack([x, x])
logX = np.vstack([logx, logx])
assert_array_almost_equal(np.exp(logsumexp(logX, axis=0)), X.sum(axis=0))
assert_array_almost_equal(np.exp(logsumexp(logX, axis=1)), X.sum(axis=1))
def test_randomized_svd_low_rank():
# Check that extmath.randomized_svd is consistent with linalg.svd
n_samples = 100
n_features = 500
rank = 5
k = 10
# generate a matrix X of approximate effective rank `rank` and no noise
# component (very structured signal):
X = make_low_rank_matrix(n_samples=n_samples, n_features=n_features,
effective_rank=rank, tail_strength=0.0,
random_state=0)
assert_equal(X.shape, (n_samples, n_features))
# compute the singular values of X using the slow exact method
U, s, V = linalg.svd(X, full_matrices=False)
for normalizer in ['auto', 'LU', 'QR']: # 'none' would not be stable
# compute the singular values of X using the fast approximate method
Ua, sa, Va = \
randomized_svd(X, k, power_iteration_normalizer=normalizer,
random_state=0)
assert_equal(Ua.shape, (n_samples, k))
assert_equal(sa.shape, (k,))
assert_equal(Va.shape, (k, n_features))
# ensure that the singular values of both methods are equal up to the
# real rank of the matrix
assert_almost_equal(s[:k], sa)
# check the singular vectors too (while not checking the sign)
assert_almost_equal(np.dot(U[:, :k], V[:k, :]), np.dot(Ua, Va))
# check the sparse matrix representation
X = sparse.csr_matrix(X)
# compute the singular values of X using the fast approximate method
Ua, sa, Va = \
randomized_svd(X, k, power_iteration_normalizer=normalizer,
random_state=0)
assert_almost_equal(s[:rank], sa[:rank])
def test_norm_squared_norm():
X = np.random.RandomState(42).randn(50, 63)
X *= 100 # check stability
X += 200
assert_almost_equal(np.linalg.norm(X.ravel()), norm(X))
assert_almost_equal(norm(X) ** 2, squared_norm(X), decimal=6)
assert_almost_equal(np.linalg.norm(X), np.sqrt(squared_norm(X)), decimal=6)
def test_row_norms():
X = np.random.RandomState(42).randn(100, 100)
for dtype in (np.float32, np.float64):
if dtype is np.float32:
precision = 4
else:
precision = 5
X = X.astype(dtype)
sq_norm = (X ** 2).sum(axis=1)
assert_array_almost_equal(sq_norm, row_norms(X, squared=True),
precision)
assert_array_almost_equal(np.sqrt(sq_norm), row_norms(X), precision)
Xcsr = sparse.csr_matrix(X, dtype=dtype)
assert_array_almost_equal(sq_norm, row_norms(Xcsr, squared=True),
precision)
assert_array_almost_equal(np.sqrt(sq_norm), row_norms(Xcsr), precision)
def test_randomized_svd_low_rank_with_noise():
# Check that extmath.randomized_svd can handle noisy matrices
n_samples = 100
n_features = 500
rank = 5
k = 10
# generate a matrix X wity structure approximate rank `rank` and an
# important noisy component
X = make_low_rank_matrix(n_samples=n_samples, n_features=n_features,
effective_rank=rank, tail_strength=0.1,
random_state=0)
assert_equal(X.shape, (n_samples, n_features))
# compute the singular values of X using the slow exact method
_, s, _ = linalg.svd(X, full_matrices=False)
for normalizer in ['auto', 'none', 'LU', 'QR']:
# compute the singular values of X using the fast approximate
# method without the iterated power method
_, sa, _ = randomized_svd(X, k, n_iter=0,
power_iteration_normalizer=normalizer,
random_state=0)
# the approximation does not tolerate the noise:
assert_greater(np.abs(s[:k] - sa).max(), 0.01)
# compute the singular values of X using the fast approximate
# method with iterated power method
_, sap, _ = randomized_svd(X, k,
power_iteration_normalizer=normalizer,
random_state=0)
# the iterated power method is helping getting rid of the noise:
assert_almost_equal(s[:k], sap, decimal=3)
def test_randomized_svd_infinite_rank():
# Check that extmath.randomized_svd can handle noisy matrices
n_samples = 100
n_features = 500
rank = 5
k = 10
# let us try again without 'low_rank component': just regularly but slowly
# decreasing singular values: the rank of the data matrix is infinite
X = make_low_rank_matrix(n_samples=n_samples, n_features=n_features,
effective_rank=rank, tail_strength=1.0,
random_state=0)
assert_equal(X.shape, (n_samples, n_features))
# compute the singular values of X using the slow exact method
_, s, _ = linalg.svd(X, full_matrices=False)
for normalizer in ['auto', 'none', 'LU', 'QR']:
# compute the singular values of X using the fast approximate method
# without the iterated power method
_, sa, _ = randomized_svd(X, k, n_iter=0,
power_iteration_normalizer=normalizer)
# the approximation does not tolerate the noise:
assert_greater(np.abs(s[:k] - sa).max(), 0.1)
# compute the singular values of X using the fast approximate method
# with iterated power method
_, sap, _ = randomized_svd(X, k, n_iter=5,
power_iteration_normalizer=normalizer)
# the iterated power method is still managing to get most of the
# structure at the requested rank
assert_almost_equal(s[:k], sap, decimal=3)
def test_randomized_svd_transpose_consistency():
# Check that transposing the design matrix has limited impact
n_samples = 100
n_features = 500
rank = 4
k = 10
X = make_low_rank_matrix(n_samples=n_samples, n_features=n_features,
effective_rank=rank, tail_strength=0.5,
random_state=0)
assert_equal(X.shape, (n_samples, n_features))
U1, s1, V1 = randomized_svd(X, k, n_iter=3, transpose=False,
random_state=0)
U2, s2, V2 = randomized_svd(X, k, n_iter=3, transpose=True,
random_state=0)
U3, s3, V3 = randomized_svd(X, k, n_iter=3, transpose='auto',
random_state=0)
U4, s4, V4 = linalg.svd(X, full_matrices=False)
assert_almost_equal(s1, s4[:k], decimal=3)
assert_almost_equal(s2, s4[:k], decimal=3)
assert_almost_equal(s3, s4[:k], decimal=3)
assert_almost_equal(np.dot(U1, V1), np.dot(U4[:, :k], V4[:k, :]),
decimal=2)
assert_almost_equal(np.dot(U2, V2), np.dot(U4[:, :k], V4[:k, :]),
decimal=2)
# in this case 'auto' is equivalent to transpose
assert_almost_equal(s2, s3)
def test_randomized_svd_power_iteration_normalizer():
# randomized_svd with power_iteration_normalized='none' diverges for
# large number of power iterations on this dataset
rng = np.random.RandomState(42)
X = make_low_rank_matrix(100, 500, effective_rank=50, random_state=rng)
X += 3 * rng.randint(0, 2, size=X.shape)
n_components = 50
# Check that it diverges with many (non-normalized) power iterations
U, s, V = randomized_svd(X, n_components, n_iter=2,
power_iteration_normalizer='none')
A = X - U.dot(np.diag(s).dot(V))
error_2 = linalg.norm(A, ord='fro')
U, s, V = randomized_svd(X, n_components, n_iter=20,
power_iteration_normalizer='none')
A = X - U.dot(np.diag(s).dot(V))
error_20 = linalg.norm(A, ord='fro')
assert_greater(np.abs(error_2 - error_20), 100)
for normalizer in ['LU', 'QR', 'auto']:
U, s, V = randomized_svd(X, n_components, n_iter=2,
power_iteration_normalizer=normalizer,
random_state=0)
A = X - U.dot(np.diag(s).dot(V))
error_2 = linalg.norm(A, ord='fro')
for i in [5, 10, 50]:
U, s, V = randomized_svd(X, n_components, n_iter=i,
power_iteration_normalizer=normalizer,
random_state=0)
A = X - U.dot(np.diag(s).dot(V))
error = linalg.norm(A, ord='fro')
assert_greater(15, np.abs(error_2 - error))
def test_svd_flip():
# Check that svd_flip works in both situations, and reconstructs input.
rs = np.random.RandomState(1999)
n_samples = 20
n_features = 10
X = rs.randn(n_samples, n_features)
# Check matrix reconstruction
U, S, V = linalg.svd(X, full_matrices=False)
U1, V1 = svd_flip(U, V, u_based_decision=False)
assert_almost_equal(np.dot(U1 * S, V1), X, decimal=6)
# Check transposed matrix reconstruction
XT = X.T
U, S, V = linalg.svd(XT, full_matrices=False)
U2, V2 = svd_flip(U, V, u_based_decision=True)
assert_almost_equal(np.dot(U2 * S, V2), XT, decimal=6)
# Check that different flip methods are equivalent under reconstruction
U_flip1, V_flip1 = svd_flip(U, V, u_based_decision=True)
assert_almost_equal(np.dot(U_flip1 * S, V_flip1), XT, decimal=6)
U_flip2, V_flip2 = svd_flip(U, V, u_based_decision=False)
assert_almost_equal(np.dot(U_flip2 * S, V_flip2), XT, decimal=6)
def test_randomized_svd_sign_flip():
a = np.array([[2.0, 0.0], [0.0, 1.0]])
u1, s1, v1 = randomized_svd(a, 2, flip_sign=True, random_state=41)
for seed in range(10):
u2, s2, v2 = randomized_svd(a, 2, flip_sign=True, random_state=seed)
assert_almost_equal(u1, u2)
assert_almost_equal(v1, v2)
assert_almost_equal(np.dot(u2 * s2, v2), a)
assert_almost_equal(np.dot(u2.T, u2), np.eye(2))
assert_almost_equal(np.dot(v2.T, v2), np.eye(2))
def test_randomized_svd_sign_flip_with_transpose():
# Check if the randomized_svd sign flipping is always done based on u
# irrespective of transpose.
# See https://github.com/scikit-learn/scikit-learn/issues/5608
# for more details.
def max_loading_is_positive(u, v):
"""
returns bool tuple indicating if the values maximising np.abs
are positive across all rows for u and across all columns for v.
"""
u_based = (np.abs(u).max(axis=0) == u.max(axis=0)).all()
v_based = (np.abs(v).max(axis=1) == v.max(axis=1)).all()
return u_based, v_based
mat = np.arange(10 * 8).reshape(10, -1)
# Without transpose
u_flipped, _, v_flipped = randomized_svd(mat, 3, flip_sign=True)
u_based, v_based = max_loading_is_positive(u_flipped, v_flipped)
assert_true(u_based)
assert_false(v_based)
# With transpose
u_flipped_with_transpose, _, v_flipped_with_transpose = randomized_svd(
mat, 3, flip_sign=True, transpose=True)
u_based, v_based = max_loading_is_positive(
u_flipped_with_transpose, v_flipped_with_transpose)
assert_true(u_based)
assert_false(v_based)
def test_cartesian():
# Check if cartesian product delivers the right results
axes = (np.array([1, 2, 3]), np.array([4, 5]), np.array([6, 7]))
true_out = np.array([[1, 4, 6],
[1, 4, 7],
[1, 5, 6],
[1, 5, 7],
[2, 4, 6],
[2, 4, 7],
[2, 5, 6],
[2, 5, 7],
[3, 4, 6],
[3, 4, 7],
[3, 5, 6],
[3, 5, 7]])
out = cartesian(axes)
assert_array_equal(true_out, out)
# check single axis
x = np.arange(3)
assert_array_equal(x[:, np.newaxis], cartesian((x,)))
def test_logistic_sigmoid():
# Check correctness and robustness of logistic sigmoid implementation
def naive_log_logistic(x):
return np.log(1 / (1 + np.exp(-x)))
x = np.linspace(-2, 2, 50)
assert_array_almost_equal(log_logistic(x), naive_log_logistic(x))
extreme_x = np.array([-100., 100.])
assert_array_almost_equal(log_logistic(extreme_x), [-100, 0])
def test_fast_dot():
# Check fast dot blas wrapper function
if fast_dot is np.dot:
return
rng = np.random.RandomState(42)
A = rng.random_sample([2, 10])
B = rng.random_sample([2, 10])
try:
linalg.get_blas_funcs(['gemm'])[0]
has_blas = True
except (AttributeError, ValueError):
has_blas = False
if has_blas:
# Test _fast_dot for invalid input.
# Maltyped data.
for dt1, dt2 in [['f8', 'f4'], ['i4', 'i4']]:
assert_raises(ValueError, _fast_dot, A.astype(dt1),
B.astype(dt2).T)
# Malformed data.
# ndim == 0
E = np.empty(0)
assert_raises(ValueError, _fast_dot, E, E)
# ndim == 1
assert_raises(ValueError, _fast_dot, A, A[0])
# ndim > 2
assert_raises(ValueError, _fast_dot, A.T, np.array([A, A]))
# min(shape) == 1
assert_raises(ValueError, _fast_dot, A, A[0, :][None, :])
# test for matrix mismatch error
assert_raises(ValueError, _fast_dot, A, A)
# Test cov-like use case + dtypes.
for dtype in ['f8', 'f4']:
A = A.astype(dtype)
B = B.astype(dtype)
# col < row
C = np.dot(A.T, A)
C_ = fast_dot(A.T, A)
assert_almost_equal(C, C_, decimal=5)
C = np.dot(A.T, B)
C_ = fast_dot(A.T, B)
assert_almost_equal(C, C_, decimal=5)
C = np.dot(A, B.T)
C_ = fast_dot(A, B.T)
assert_almost_equal(C, C_, decimal=5)
# Test square matrix * rectangular use case.
A = rng.random_sample([2, 2])
for dtype in ['f8', 'f4']:
A = A.astype(dtype)
B = B.astype(dtype)
C = np.dot(A, B)
C_ = fast_dot(A, B)
assert_almost_equal(C, C_, decimal=5)
C = np.dot(A.T, B)
C_ = fast_dot(A.T, B)
assert_almost_equal(C, C_, decimal=5)
if has_blas:
for x in [np.array([[d] * 10] * 2) for d in [np.inf, np.nan]]:
assert_raises(ValueError, _fast_dot, x, x.T)
def test_incremental_variance_update_formulas():
# Test Youngs and Cramer incremental variance formulas.
# Doggie data from http://www.mathsisfun.com/data/standard-deviation.html
A = np.array([[600, 470, 170, 430, 300],
[600, 470, 170, 430, 300],
[600, 470, 170, 430, 300],
[600, 470, 170, 430, 300]]).T
idx = 2
X1 = A[:idx, :]
X2 = A[idx:, :]
old_means = X1.mean(axis=0)
old_variances = X1.var(axis=0)
old_sample_count = X1.shape[0]
final_means, final_variances, final_count = \
_incremental_mean_and_var(X2, old_means, old_variances,
old_sample_count)
assert_almost_equal(final_means, A.mean(axis=0), 6)
assert_almost_equal(final_variances, A.var(axis=0), 6)
assert_almost_equal(final_count, A.shape[0])
@skip_if_32bit
def test_incremental_variance_numerical_stability():
# Test Youngs and Cramer incremental variance formulas.
def np_var(A):
return A.var(axis=0)
# Naive one pass variance computation - not numerically stable
# https://en.wikipedia.org/wiki/Algorithms_for_calculating_variance
def one_pass_var(X):
n = X.shape[0]
exp_x2 = (X ** 2).sum(axis=0) / n
expx_2 = (X.sum(axis=0) / n) ** 2
return exp_x2 - expx_2
# Two-pass algorithm, stable.
# We use it as a benchmark. It is not an online algorithm
# https://en.wikipedia.org/wiki/Algorithms_for_calculating_variance#Two-pass_algorithm
def two_pass_var(X):
mean = X.mean(axis=0)
Y = X.copy()
return np.mean((Y - mean)**2, axis=0)
# Naive online implementation
# https://en.wikipedia.org/wiki/Algorithms_for_calculating_variance#Online_algorithm
# This works only for chunks for size 1
def naive_mean_variance_update(x, last_mean, last_variance,
last_sample_count):
updated_sample_count = (last_sample_count + 1)
samples_ratio = last_sample_count / float(updated_sample_count)
updated_mean = x / updated_sample_count + last_mean * samples_ratio
updated_variance = last_variance * samples_ratio + \
(x - last_mean) * (x - updated_mean) / updated_sample_count
return updated_mean, updated_variance, updated_sample_count
# We want to show a case when one_pass_var has error > 1e-3 while
# _batch_mean_variance_update has less.
tol = 200
n_features = 2
n_samples = 10000
x1 = np.array(1e8, dtype=np.float64)
x2 = np.log(1e-5, dtype=np.float64)
A0 = x1 * np.ones((n_samples // 2, n_features), dtype=np.float64)
A1 = x2 * np.ones((n_samples // 2, n_features), dtype=np.float64)
A = np.vstack((A0, A1))
# Older versions of numpy have different precision
# In some old version, np.var is not stable
if np.abs(np_var(A) - two_pass_var(A)).max() < 1e-6:
stable_var = np_var
else:
stable_var = two_pass_var
# Naive one pass var: >tol (=1063)
assert_greater(np.abs(stable_var(A) - one_pass_var(A)).max(), tol)
# Starting point for online algorithms: after A0
# Naive implementation: >tol (436)
mean, var, n = A0[0, :], np.zeros(n_features), n_samples // 2
for i in range(A1.shape[0]):
mean, var, n = \
naive_mean_variance_update(A1[i, :], mean, var, n)
assert_equal(n, A.shape[0])
# the mean is also slightly unstable
assert_greater(np.abs(A.mean(axis=0) - mean).max(), 1e-6)
assert_greater(np.abs(stable_var(A) - var).max(), tol)
# Robust implementation: <tol (177)
mean, var, n = A0[0, :], np.zeros(n_features), n_samples // 2
for i in range(A1.shape[0]):
mean, var, n = \
_incremental_mean_and_var(A1[i, :].reshape((1, A1.shape[1])),
mean, var, n)
assert_equal(n, A.shape[0])
assert_array_almost_equal(A.mean(axis=0), mean)
assert_greater(tol, np.abs(stable_var(A) - var).max())
def test_incremental_variance_ddof():
# Test that degrees of freedom parameter for calculations are correct.
rng = np.random.RandomState(1999)
X = rng.randn(50, 10)
n_samples, n_features = X.shape
for batch_size in [11, 20, 37]:
steps = np.arange(0, X.shape[0], batch_size)
if steps[-1] != X.shape[0]:
steps = np.hstack([steps, n_samples])
for i, j in zip(steps[:-1], steps[1:]):
batch = X[i:j, :]
if i == 0:
incremental_means = batch.mean(axis=0)
incremental_variances = batch.var(axis=0)
# Assign this twice so that the test logic is consistent
incremental_count = batch.shape[0]
sample_count = batch.shape[0]
else:
result = _incremental_mean_and_var(
batch, incremental_means, incremental_variances,
sample_count)
(incremental_means, incremental_variances,
incremental_count) = result
sample_count += batch.shape[0]
calculated_means = np.mean(X[:j], axis=0)
calculated_variances = np.var(X[:j], axis=0)
assert_almost_equal(incremental_means, calculated_means, 6)
assert_almost_equal(incremental_variances,
calculated_variances, 6)
assert_equal(incremental_count, sample_count)
def test_vector_sign_flip():
# Testing that sign flip is working & largest value has positive sign
data = np.random.RandomState(36).randn(5, 5)
max_abs_rows = np.argmax(np.abs(data), axis=1)
data_flipped = _deterministic_vector_sign_flip(data)
max_rows = np.argmax(data_flipped, axis=1)
assert_array_equal(max_abs_rows, max_rows)
signs = np.sign(data[range(data.shape[0]), max_abs_rows])
assert_array_equal(data, data_flipped * signs[:, np.newaxis])
def test_softmax():
rng = np.random.RandomState(0)
X = rng.randn(3, 5)
exp_X = np.exp(X)
sum_exp_X = np.sum(exp_X, axis=1).reshape((-1, 1))
assert_array_almost_equal(softmax(X), exp_X / sum_exp_X)
def test_stable_cumsum():
if np_version < (1, 9):
raise SkipTest("Sum is as unstable as cumsum for numpy < 1.9")
assert_array_equal(stable_cumsum([1, 2, 3]), np.cumsum([1, 2, 3]))
r = np.random.RandomState(0).rand(100000)
assert_warns(RuntimeWarning, stable_cumsum, r, rtol=0, atol=0)
# test axis parameter
A = np.random.RandomState(36).randint(1000, size=(5, 5, 5))
assert_array_equal(stable_cumsum(A, axis=0), np.cumsum(A, axis=0))
assert_array_equal(stable_cumsum(A, axis=1), np.cumsum(A, axis=1))
assert_array_equal(stable_cumsum(A, axis=2), np.cumsum(A, axis=2))
| bsd-3-clause |
danellecline/mbari-aesa | misclassification_clean.py | 1 | 2289 | #!/usr/bin/env python
__author__ = 'Danelle Cline'
__copyright__ = '2016'
__license__ = 'GPL v3'
__contact__ = 'dcline at mbari.org'
__doc__ = '''
Reads in AESA annotation file and cleans according to misclassification file
@var __date__: Date of last svn commit
@undocumented: __doc__ parser
@status: production
@license: GPL
'''
import sys
import argparse
import os
import fnmatch
import pandas as pd
def process_command_line():
from argparse import RawTextHelpFormatter
examples = 'Examples:' + '\n\n'
examples += sys.argv[0] + """
--csvdir /tmp/data/model_output_final/JC062_M535455_M56_75pad_refined_cnidaria/
\n"""
parser = argparse.ArgumentParser(formatter_class=RawTextHelpFormatter,
description='Remove misclassifications',
epilog=examples)
parser.add_argument('--csvdir', type=str, required=True, help="Path to directories with misclassified csv files.")
parser.add_argument('--class_actual', type=str, required=True, default='', help="Classes to remove")
parser.add_argument('--class_predicted', type=str, required=True, default='', help="Classes to remove")
args = parser.parse_args()
return args
# Cleaned with:
# /Users/dcline/anaconda/bin/python /Users/dcline/Dropbox/GitHub/mbari-aesa/misclassification_clean.py --csvdir --csvdir /Users/dcline/Dropbox/GitHub/mbari-aesa/data/model_output_final/JC062_M535455_M56_75pad_refined_cnidaria/
if __name__ == '__main__':
args = process_command_line()
try:
print 'Parsing ' + args.csvdir
matches = []
for root, dirnames, filenames in os.walk(args.csvdir):
for filename in fnmatch.filter(filenames, 'misclassified.csv'):
matches.append(os.path.join(root, filename))
for m in matches:
df = pd.read_csv(m, sep=',')
print 'Reading %s' % m
for index, row in df.iterrows():
file = row['Filename']
class_predicted = row['Predicted']
class_actual = row['Actual']
if class_predicted == args.class_predicted and class_actual == args.class_actual and os.path.exists(file):
os.remove(file)
print 'Removing {0}'.format(file)
except Exception as ex:
print ex
print 'Done'
| gpl-3.0 |
christianurich/VIBe2UrbanSim | 3rdparty/opus/src/opus_gui/results_manager/run/indicator_framework/visualizer/visualizers/matplotlib_lorenzcurve.py | 2 | 11105 | # Opus/UrbanSim urban simulation software.
# Copyright (C) 2005-2009 University of Washington
# See opus_core/LICENSE
import os, re, sys, time, traceback
from copy import copy
from opus_gui.results_manager.run.indicator_framework.visualizer.visualizers.abstract_visualization import Visualization
from opus_core.logger import logger
from numpy import array, arange
from numpy import ones, zeros, hstack, vstack
from numpy import trapz, trim_zeros
from pylab import subplot, plot, show
from pylab import xlabel, ylabel, title, text
from pylab import MultipleLocator, FormatStrFormatter
from pylab import savefig, clf, close
class LorenzCurve(Visualization):
def __init__(self, source_data, dataset_name,
attribute = None,
years = None, operation = None, name = None, scale = None,
storage_location = None):
Visualizer.__init__(self, source_data, dataset_name, [attribute],
years, operation, name,
storage_location)
self._values = None
self._ginicoeff = None
def is_single_year_indicator_image_type(self):
return True
def get_file_extension(self):
return 'png'
def get_visualization_shorthand(self):
return 'lorenzcurve'
def get_additional_metadata(self):
return {}
def _create_indicator(self, year):
"""Create a Lorenz Curve for the given indicator,
save it to the cache directory's 'indicators' sub-directory.
"""
attribute_short = self.get_attribute_alias(attribute = self.attributes[0],
year = year)
title = attribute_short + ' ' + str(year)
if self.run_description is not None:
title += '\n' + self.run_description
# Do calculation
# Make fresh copy with dtype float64 to avoid overflows
self._values = array(self._get_indicator(year, wrap = False).astype('float64'))
self._compute_lorenz()
file_path = self.get_file_path(year = year)
self._plot(attribute_short, file_path );
return file_path
def _compute_lorenz(self ):
''' Do the lorenz curve computation and save the result in the corresponding
class variables
'''
self._values.sort()
#remove 0 values from array
self._values = trim_zeros(self._values,'f')
num_values = self._values.size
F = arange(1, num_values + 1, 1, "float64")/num_values
L = self._values.cumsum(dtype="float64")/sum(self._values)
# Add (0, 0) as the first point for completeness (e.g. plotting)
origin = array([[0], [0]])
self._values = vstack((F, L))
self._values = hstack((origin, self._values))
# This is the simple form of (0.5 - integral) / 0.5
self._ginicoeff = 1 - 2 * trapz(self._values[1], self._values[0])
def _plot(self, attribute_name, file_path=None ):
clf() # Clear existing plot
a = self._values[0] * 100
b = self._values[1] * 100
ax = subplot(111)
plot(a, a, 'k--', a, b, 'r')
ax.set_ylim([0,100])
ax.grid(color='0.5', linestyle=':', linewidth=0.5)
xlabel('population')
ylabel(attribute_name)
title('Lorenz curve')
font = {'fontname' : 'Courier',
'color' : 'r',
'fontweight' : 'bold',
'fontsize' : 11
}
box = { 'pad' : 6,
'facecolor' : 'w',
'linewidth' : 1,
'fill' : True
}
text(5, 90, 'Gini coefficient: %(gini)f' % {'gini' : self._ginicoeff}, font, color='k', bbox=box )
majorLocator = MultipleLocator(20)
majorFormatter = FormatStrFormatter('%d %%')
minorLocator = MultipleLocator(5)
ax.xaxis.set_major_locator( majorLocator )
ax.xaxis.set_major_formatter( majorFormatter)
ax.xaxis.set_minor_locator( minorLocator )
ax.yaxis.set_major_locator( majorLocator )
ax.yaxis.set_major_formatter( majorFormatter)
ax.yaxis.set_minor_locator( minorLocator )
if file_path:
savefig(file_path)
close()
else:
show()
import os
from opus_core.tests import opus_unittest
from numpy import allclose
from opus_gui.results_manager.run.indicator_framework.test_classes.abstract_indicator_test import AbstractIndicatorTest
class Tests(AbstractIndicatorTest):
def skip_test_create_indicator(self):
indicator_path = os.path.join(self.temp_cache_path, 'indicators')
self.assert_(not os.path.exists(indicator_path))
lorenzcurve = LorenzCurve(
source_data = self.source_data,
attribute = 'opus_core.test.attribute',
dataset_name = 'test',
years = None
)
lorenzcurve.create(False)
self.assert_(os.path.exists(indicator_path))
self.assert_(os.path.exists(os.path.join(indicator_path, 'test__lorenzcurve__attribute__1980.png')))
def skip_test_perfect_equality(self):
"""Perfect equality is when everybody has the same amount of something"""
lorenzcurve = LorenzCurve(
source_data = self.source_data,
attribute = 'opus_core.test.attribute',
dataset_name = 'test',
years = None
)
incomes = ones(100)
lorenzcurve._values = incomes
lorenzcurve._compute_lorenz()
wanted_result = vstack((arange(0, 101) / 100., arange(0, 101) / 100.))
self.assert_(allclose(lorenzcurve._values, wanted_result))
def skip_test_perfect_inequality(self):
"""Perfect inequality is when one person has all of something"""
lorenzcurve = LorenzCurve(
source_data = self.source_data,
attribute = 'opus_core.test.attribute',
dataset_name = 'test',
years = None
)
incomes = zeros(100)
incomes[0] = 42
lorenzcurve._values = incomes
lorenzcurve._compute_lorenz()
#We strip all the zero values, so the result consists of only two values
wanted_result = [[0.,1.],[0.,1.]]
self.assert_(allclose(lorenzcurve._values, wanted_result))
def skip_test_small_lorenz(self):
"""Test case for less than 100 people"""
lorenzcurve = LorenzCurve(
source_data = self.source_data,
attribute = 'opus_core.test.attribute',
dataset_name = 'test',
years = None
)
incomes = array([1, 1, 2, 3, 4, 5])
lorenzcurve._values = incomes
lorenzcurve._compute_lorenz()
wanted_result = array(
[[ 0, 1/6., 2/6., 3/6., 4/6., 5/6., 6/6. ],
[ 0, 1/16., 2/16., 4/16., 7/16., 11/16., 16/16. ]])
self.assert_(allclose(lorenzcurve._values, wanted_result))
def skip_test_small_gini(self):
"""Test case for gini coefficient for the small case"""
lorenzcurve = LorenzCurve(
source_data = self.source_data,
attribute = 'opus_core.test.attribute',
dataset_name = 'test',
years = None
)
incomes = array([1, 1, 2, 3, 4, 5])
lorenzcurve._values = incomes
lorenzcurve._compute_lorenz()
self.assertAlmostEqual(lorenzcurve._ginicoeff, 0.3125)
def skip_test_large_lorenz(self):
"""Test case for more than 100 people"""
lorenzcurve = LorenzCurve(
source_data = self.source_data,
attribute = 'opus_core.test.attribute',
dataset_name = 'test',
years = None
)
incomes = array([731, 700, 619, 450, 419, 512, 232, 266, 131, 188,
498, 293, 935, 177, 160, 380, 538, 783, 256, 280,
731, 362, 870, 970, 674, 211, 524, 207, 513, 461,
280, 275, 410, 282, 144, 682, 573, 252, 382, 909,
719, 666, 236, 636, 628, 542, 630, 484, 629, 974,
747, 509, 281, 725, 377, 565, 495, 840, 391, 191,
929, 679, 217, 179, 336, 562, 293, 881, 271, 172,
426, 697, 293, 576, 203, 390, 522, 948, 312, 491,
531, 959, 646, 495, 306, 631, 722, 322, 876, 586,
316, 124, 796, 250, 456, 112, 661, 294, 749, 619,
134, 582, 996, 413, 421, 219, 796, 923, 832, 557])
lorenzcurve._values = incomes
lorenzcurve._compute_lorenz()
wanted_result_F = arange(0, 111) / 110.
wanted_result_L = array([ 0, 0.00202803, 0.00427335, 0.00664542, 0.00907181, 0.01167928,
0.01457647, 0.01769094, 0.02089595, 0.02413718, 0.02754138,
0.03099989, 0.0346757 , 0.03842393, 0.04224459, 0.0461739 ,
0.05013943, 0.05434035, 0.0586137 , 0.06314055, 0.06770362,
0.07233912, 0.07715569, 0.0820628 , 0.08704234, 0.09211241,
0.09718249, 0.10227067, 0.10737696, 0.11268243, 0.1179879 ,
0.12329338, 0.12861696, 0.13415782, 0.13980734, 0.14552928,
0.15135987, 0.15744396, 0.16399884, 0.17082534, 0.17770615,
0.18462318, 0.19168508, 0.19876507, 0.20618911, 0.21366748,
0.22125448, 0.2288777 , 0.23659146, 0.2447398 , 0.25299678,
0.26134429, 0.27010828, 0.27899902, 0.28796219, 0.29692536,
0.30594285, 0.31515953, 0.32443052, 0.33371962, 0.34317169,
0.35265998, 0.36227502, 0.3720168 , 0.38183102, 0.39191685,
0.40209322, 0.41232391, 0.42269945, 0.43312932, 0.44366784,
0.45427878, 0.46548727, 0.47669576, 0.48806721, 0.49945678,
0.51086445, 0.52229023, 0.53380654, 0.54550393, 0.55747293,
0.56953247, 0.58173686, 0.5940318 , 0.60638105, 0.61900192,
0.63167711, 0.64469634, 0.65776989, 0.67089777, 0.68413428,
0.6973708 , 0.71089704, 0.72445949, 0.7386376 , 0.7530511 ,
0.7674646 , 0.78252997, 0.79774019, 0.81349364, 0.82935574,
0.84530837, 0.86176801, 0.87848115, 0.89530294, 0.91223337,
0.9293992 , 0.94676421, 0.9643284 , 0.98196502, 1. ])
self.assert_(allclose(lorenzcurve._values, vstack((wanted_result_F, wanted_result_L))))
if __name__ == '__main__':
try:
import matplotlib
except:
print 'could not import matplotlib'
else:
opus_unittest.main()
| gpl-2.0 |
tgensol/projet | starting kit/python/minimal_preprocessed.py | 1 | 2411 | from __future__ import division, print_function
import os
import numpy as np
from sklearn.ensemble import RandomForestClassifier
from sklearn.datasets import load_svmlight_file
"""
Minimal code to load the preprocessed data, train a classic Random Forest,
computes the predictions on the valid and the test sets and write them to
files ('valid.predict' & 'test.predict').
Tested with :
- Anaconda 2.3.0
- Python 2.7
- Python 3.5
"""
# Change the path to your data location :
data_dir = os.path.abspath('../data/')
if __name__ == '__main__':
print('I am at your service, Master.')
# Load the sparse training data :
X_train, y_train = load_svmlight_file(
os.path.join(data_dir, 'train_preprocessed.data'))
# Now X_train contains a 'scipy.sparse.csr.csr_matrix'
# And y_train is a simple numpy array of float64
# (even if the labels are only 0 or 1)
# Load the sparse validation data :
X_valid, y_valid = load_svmlight_file(
os.path.join(data_dir, 'valid_preprocessed.data'))
# Now X_valid contains a 'scipy.sparse.csr.csr_matrix'
# And y_valid is a simple numpy array of float64
# (even if the labels are only 0 or 1)
# Load the sparse validation data :
X_test, y_test = load_svmlight_file(
os.path.join(data_dir, 'test_preprocessed.data'))
# Now X_test contains a 'scipy.sparse.csr.csr_matrix'
# And y_test is a simple numpy array of float64
# (even if the labels are only 0 or 1)
# Declaration of the model algorithm
clf = RandomForestClassifier()
# Most of the classifiers can take sparse matrix as inputs
# (they automatically convert it if necessary)
# fit() function is used to train the classifier
clf.fit(X_train, y_train)
# Computes the prediction thanks to the predict() function
# for the validation set
valid_predictions = clf.predict(X_valid)
# and the test set
test_predictions = clf.predict(X_test)
# Before writing the predictions you have to convert the predictions arrays
# to integers in order to write a well formatted submission file
valid_predictions = np.array(valid_predictions, dtype=int)
test_predictions = np.array(test_predictions, dtype=int)
# Now let's write the submission files
np.savetxt('valid.predict', valid_predictions, fmt='%d')
np.savetxt('test.predict', test_predictions, fmt='%d')
print('Done.')
| mit |
phdowling/scikit-learn | examples/svm/plot_rbf_parameters.py | 132 | 8096 | '''
==================
RBF SVM parameters
==================
This example illustrates the effect of the parameters ``gamma`` and ``C`` of
the Radial Basis Function (RBF) kernel SVM.
Intuitively, the ``gamma`` parameter defines how far the influence of a single
training example reaches, with low values meaning 'far' and high values meaning
'close'. The ``gamma`` parameters can be seen as the inverse of the radius of
influence of samples selected by the model as support vectors.
The ``C`` parameter trades off misclassification of training examples against
simplicity of the decision surface. A low ``C`` makes the decision surface
smooth, while a high ``C`` aims at classifying all training examples correctly
by giving the model freedom to select more samples as support vectors.
The first plot is a visualization of the decision function for a variety of
parameter values on a simplified classification problem involving only 2 input
features and 2 possible target classes (binary classification). Note that this
kind of plot is not possible to do for problems with more features or target
classes.
The second plot is a heatmap of the classifier's cross-validation accuracy as a
function of ``C`` and ``gamma``. For this example we explore a relatively large
grid for illustration purposes. In practice, a logarithmic grid from
:math:`10^{-3}` to :math:`10^3` is usually sufficient. If the best parameters
lie on the boundaries of the grid, it can be extended in that direction in a
subsequent search.
Note that the heat map plot has a special colorbar with a midpoint value close
to the score values of the best performing models so as to make it easy to tell
them appart in the blink of an eye.
The behavior of the model is very sensitive to the ``gamma`` parameter. If
``gamma`` is too large, the radius of the area of influence of the support
vectors only includes the support vector itself and no amount of
regularization with ``C`` will be able to prevent overfitting.
When ``gamma`` is very small, the model is too constrained and cannot capture
the complexity or "shape" of the data. The region of influence of any selected
support vector would include the whole training set. The resulting model will
behave similarly to a linear model with a set of hyperplanes that separate the
centers of high density of any pair of two classes.
For intermediate values, we can see on the second plot that good models can
be found on a diagonal of ``C`` and ``gamma``. Smooth models (lower ``gamma``
values) can be made more complex by selecting a larger number of support
vectors (larger ``C`` values) hence the diagonal of good performing models.
Finally one can also observe that for some intermediate values of ``gamma`` we
get equally performing models when ``C`` becomes very large: it is not
necessary to regularize by limiting the number of support vectors. The radius of
the RBF kernel alone acts as a good structural regularizer. In practice though
it might still be interesting to limit the number of support vectors with a
lower value of ``C`` so as to favor models that use less memory and that are
faster to predict.
We should also note that small differences in scores results from the random
splits of the cross-validation procedure. Those spurious variations can be
smoothed out by increasing the number of CV iterations ``n_iter`` at the
expense of compute time. Increasing the value number of ``C_range`` and
``gamma_range`` steps will increase the resolution of the hyper-parameter heat
map.
'''
print(__doc__)
import numpy as np
import matplotlib.pyplot as plt
from matplotlib.colors import Normalize
from sklearn.svm import SVC
from sklearn.preprocessing import StandardScaler
from sklearn.datasets import load_iris
from sklearn.cross_validation import StratifiedShuffleSplit
from sklearn.grid_search import GridSearchCV
# Utility function to move the midpoint of a colormap to be around
# the values of interest.
class MidpointNormalize(Normalize):
def __init__(self, vmin=None, vmax=None, midpoint=None, clip=False):
self.midpoint = midpoint
Normalize.__init__(self, vmin, vmax, clip)
def __call__(self, value, clip=None):
x, y = [self.vmin, self.midpoint, self.vmax], [0, 0.5, 1]
return np.ma.masked_array(np.interp(value, x, y))
##############################################################################
# Load and prepare data set
#
# dataset for grid search
iris = load_iris()
X = iris.data
y = iris.target
# Dataset for decision function visualization: we only keep the first two
# features in X and sub-sample the dataset to keep only 2 classes and
# make it a binary classification problem.
X_2d = X[:, :2]
X_2d = X_2d[y > 0]
y_2d = y[y > 0]
y_2d -= 1
# It is usually a good idea to scale the data for SVM training.
# We are cheating a bit in this example in scaling all of the data,
# instead of fitting the transformation on the training set and
# just applying it on the test set.
scaler = StandardScaler()
X = scaler.fit_transform(X)
X_2d = scaler.fit_transform(X_2d)
##############################################################################
# Train classifiers
#
# For an initial search, a logarithmic grid with basis
# 10 is often helpful. Using a basis of 2, a finer
# tuning can be achieved but at a much higher cost.
C_range = np.logspace(-2, 10, 13)
gamma_range = np.logspace(-9, 3, 13)
param_grid = dict(gamma=gamma_range, C=C_range)
cv = StratifiedShuffleSplit(y, n_iter=5, test_size=0.2, random_state=42)
grid = GridSearchCV(SVC(), param_grid=param_grid, cv=cv)
grid.fit(X, y)
print("The best parameters are %s with a score of %0.2f"
% (grid.best_params_, grid.best_score_))
# Now we need to fit a classifier for all parameters in the 2d version
# (we use a smaller set of parameters here because it takes a while to train)
C_2d_range = [1e-2, 1, 1e2]
gamma_2d_range = [1e-1, 1, 1e1]
classifiers = []
for C in C_2d_range:
for gamma in gamma_2d_range:
clf = SVC(C=C, gamma=gamma)
clf.fit(X_2d, y_2d)
classifiers.append((C, gamma, clf))
##############################################################################
# visualization
#
# draw visualization of parameter effects
plt.figure(figsize=(8, 6))
xx, yy = np.meshgrid(np.linspace(-3, 3, 200), np.linspace(-3, 3, 200))
for (k, (C, gamma, clf)) in enumerate(classifiers):
# evaluate decision function in a grid
Z = clf.decision_function(np.c_[xx.ravel(), yy.ravel()])
Z = Z.reshape(xx.shape)
# visualize decision function for these parameters
plt.subplot(len(C_2d_range), len(gamma_2d_range), k + 1)
plt.title("gamma=10^%d, C=10^%d" % (np.log10(gamma), np.log10(C)),
size='medium')
# visualize parameter's effect on decision function
plt.pcolormesh(xx, yy, -Z, cmap=plt.cm.RdBu)
plt.scatter(X_2d[:, 0], X_2d[:, 1], c=y_2d, cmap=plt.cm.RdBu_r)
plt.xticks(())
plt.yticks(())
plt.axis('tight')
# plot the scores of the grid
# grid_scores_ contains parameter settings and scores
# We extract just the scores
scores = [x[1] for x in grid.grid_scores_]
scores = np.array(scores).reshape(len(C_range), len(gamma_range))
# Draw heatmap of the validation accuracy as a function of gamma and C
#
# The score are encoded as colors with the hot colormap which varies from dark
# red to bright yellow. As the most interesting scores are all located in the
# 0.92 to 0.97 range we use a custom normalizer to set the mid-point to 0.92 so
# as to make it easier to visualize the small variations of score values in the
# interesting range while not brutally collapsing all the low score values to
# the same color.
plt.figure(figsize=(8, 6))
plt.subplots_adjust(left=.2, right=0.95, bottom=0.15, top=0.95)
plt.imshow(scores, interpolation='nearest', cmap=plt.cm.hot,
norm=MidpointNormalize(vmin=0.2, midpoint=0.92))
plt.xlabel('gamma')
plt.ylabel('C')
plt.colorbar()
plt.xticks(np.arange(len(gamma_range)), gamma_range, rotation=45)
plt.yticks(np.arange(len(C_range)), C_range)
plt.title('Validation accuracy')
plt.show()
| bsd-3-clause |
isabellewei/deephealth | data/gary/neural.py | 1 | 1463 | from sklearn.model_selection import train_test_split
from sklearn.preprocessing import StandardScaler
from sklearn.neural_network import MLPClassifier
from sklearn.metrics import classification_report,confusion_matrix
import pandas as pd
from sklearn.naive_bayes import GaussianNB
from sklearn.ensemble import RandomForestClassifier, VotingClassifier
df = pd.read_csv("../parsed.csv")
y1 = df["admission_type_id"].values
y2 = df["discharge_disposition_id"].values
columns = list(df)[1:4] + list(df)[7:49]
X = df[columns].values
#ignore this(just takinig out some columns)
cols = list(df)
dropped = set(['admission_type_id', 'discharge_disposition_id', 'weight', 'payer_code'])
columns2 = [z for z in cols if z not in dropped]
X2 = df[columns2].values
X_train, X_test, y_train, y_test = train_test_split(X,y1,test_size=0.3)
scaler = StandardScaler()
scaler.fit(X_train)
X_train = scaler.transform(X_train)
X_test = scaler.transform(X_test)
mlp = MLPClassifier(hidden_layer_sizes=(50,25), warm_start=True,
batch_size=20, solver='sgd', activation='relu', alpha=0.001,
learning_rate='adaptive', learning_rate_init=0.01,
max_iter=1000
)
#train 1
mlp.fit(X_train,y_train)
predictions = mlp.predict(X_test)
print(classification_report(y_test, predictions))
#train 2
mlp.fit(X_train,y_train)
predictions = mlp.predict(X_test)
print(classification_report(y_test, predictions))
| mit |
aabadie/scikit-learn | examples/preprocessing/plot_robust_scaling.py | 85 | 2698 | #!/usr/bin/python
# -*- coding: utf-8 -*-
"""
=========================================================
Robust Scaling on Toy Data
=========================================================
Making sure that each Feature has approximately the same scale can be a
crucial preprocessing step. However, when data contains outliers,
:class:`StandardScaler <sklearn.preprocessing.StandardScaler>` can often
be mislead. In such cases, it is better to use a scaler that is robust
against outliers.
Here, we demonstrate this on a toy dataset, where one single datapoint
is a large outlier.
"""
from __future__ import print_function
print(__doc__)
# Code source: Thomas Unterthiner
# License: BSD 3 clause
import matplotlib.pyplot as plt
import numpy as np
from sklearn.preprocessing import StandardScaler, RobustScaler
# Create training and test data
np.random.seed(42)
n_datapoints = 100
Cov = [[0.9, 0.0], [0.0, 20.0]]
mu1 = [100.0, -3.0]
mu2 = [101.0, -3.0]
X1 = np.random.multivariate_normal(mean=mu1, cov=Cov, size=n_datapoints)
X2 = np.random.multivariate_normal(mean=mu2, cov=Cov, size=n_datapoints)
Y_train = np.hstack([[-1]*n_datapoints, [1]*n_datapoints])
X_train = np.vstack([X1, X2])
X1 = np.random.multivariate_normal(mean=mu1, cov=Cov, size=n_datapoints)
X2 = np.random.multivariate_normal(mean=mu2, cov=Cov, size=n_datapoints)
Y_test = np.hstack([[-1]*n_datapoints, [1]*n_datapoints])
X_test = np.vstack([X1, X2])
X_train[0, 0] = -1000 # a fairly large outlier
# Scale data
standard_scaler = StandardScaler()
Xtr_s = standard_scaler.fit_transform(X_train)
Xte_s = standard_scaler.transform(X_test)
robust_scaler = RobustScaler()
Xtr_r = robust_scaler.fit_transform(X_train)
Xte_r = robust_scaler.transform(X_test)
# Plot data
fig, ax = plt.subplots(1, 3, figsize=(12, 4))
ax[0].scatter(X_train[:, 0], X_train[:, 1],
color=np.where(Y_train > 0, 'r', 'b'))
ax[1].scatter(Xtr_s[:, 0], Xtr_s[:, 1], color=np.where(Y_train > 0, 'r', 'b'))
ax[2].scatter(Xtr_r[:, 0], Xtr_r[:, 1], color=np.where(Y_train > 0, 'r', 'b'))
ax[0].set_title("Unscaled data")
ax[1].set_title("After standard scaling (zoomed in)")
ax[2].set_title("After robust scaling (zoomed in)")
# for the scaled data, we zoom in to the data center (outlier can't be seen!)
for a in ax[1:]:
a.set_xlim(-3, 3)
a.set_ylim(-3, 3)
plt.tight_layout()
plt.show()
# Classify using k-NN
from sklearn.neighbors import KNeighborsClassifier
knn = KNeighborsClassifier()
knn.fit(Xtr_s, Y_train)
acc_s = knn.score(Xte_s, Y_test)
print("Testset accuracy using standard scaler: %.3f" % acc_s)
knn.fit(Xtr_r, Y_train)
acc_r = knn.score(Xte_r, Y_test)
print("Testset accuracy using robust scaler: %.3f" % acc_r)
| bsd-3-clause |
Titan-C/sympy | sympy/plotting/plot.py | 55 | 64797 | """Plotting module for Sympy.
A plot is represented by the ``Plot`` class that contains a reference to the
backend and a list of the data series to be plotted. The data series are
instances of classes meant to simplify getting points and meshes from sympy
expressions. ``plot_backends`` is a dictionary with all the backends.
This module gives only the essential. For all the fancy stuff use directly
the backend. You can get the backend wrapper for every plot from the
``_backend`` attribute. Moreover the data series classes have various useful
methods like ``get_points``, ``get_segments``, ``get_meshes``, etc, that may
be useful if you wish to use another plotting library.
Especially if you need publication ready graphs and this module is not enough
for you - just get the ``_backend`` attribute and add whatever you want
directly to it. In the case of matplotlib (the common way to graph data in
python) just copy ``_backend.fig`` which is the figure and ``_backend.ax``
which is the axis and work on them as you would on any other matplotlib object.
Simplicity of code takes much greater importance than performance. Don't use it
if you care at all about performance. A new backend instance is initialized
every time you call ``show()`` and the old one is left to the garbage collector.
"""
from __future__ import print_function, division
from inspect import getargspec
from collections import Callable
import warnings
from sympy import sympify, Expr, Tuple, Dummy, Symbol
from sympy.external import import_module
from sympy.core.compatibility import range
from sympy.utilities.decorator import doctest_depends_on
from sympy.utilities.iterables import is_sequence
from .experimental_lambdify import (vectorized_lambdify, lambdify)
# N.B.
# When changing the minimum module version for matplotlib, please change
# the same in the `SymPyDocTestFinder`` in `sympy/utilities/runtests.py`
# Backend specific imports - textplot
from sympy.plotting.textplot import textplot
# Global variable
# Set to False when running tests / doctests so that the plots don't show.
_show = True
def unset_show():
global _show
_show = False
##############################################################################
# The public interface
##############################################################################
class Plot(object):
"""The central class of the plotting module.
For interactive work the function ``plot`` is better suited.
This class permits the plotting of sympy expressions using numerous
backends (matplotlib, textplot, the old pyglet module for sympy, Google
charts api, etc).
The figure can contain an arbitrary number of plots of sympy expressions,
lists of coordinates of points, etc. Plot has a private attribute _series that
contains all data series to be plotted (expressions for lines or surfaces,
lists of points, etc (all subclasses of BaseSeries)). Those data series are
instances of classes not imported by ``from sympy import *``.
The customization of the figure is on two levels. Global options that
concern the figure as a whole (eg title, xlabel, scale, etc) and
per-data series options (eg name) and aesthetics (eg. color, point shape,
line type, etc.).
The difference between options and aesthetics is that an aesthetic can be
a function of the coordinates (or parameters in a parametric plot). The
supported values for an aesthetic are:
- None (the backend uses default values)
- a constant
- a function of one variable (the first coordinate or parameter)
- a function of two variables (the first and second coordinate or
parameters)
- a function of three variables (only in nonparametric 3D plots)
Their implementation depends on the backend so they may not work in some
backends.
If the plot is parametric and the arity of the aesthetic function permits
it the aesthetic is calculated over parameters and not over coordinates.
If the arity does not permit calculation over parameters the calculation is
done over coordinates.
Only cartesian coordinates are supported for the moment, but you can use
the parametric plots to plot in polar, spherical and cylindrical
coordinates.
The arguments for the constructor Plot must be subclasses of BaseSeries.
Any global option can be specified as a keyword argument.
The global options for a figure are:
- title : str
- xlabel : str
- ylabel : str
- legend : bool
- xscale : {'linear', 'log'}
- yscale : {'linear', 'log'}
- axis : bool
- axis_center : tuple of two floats or {'center', 'auto'}
- xlim : tuple of two floats
- ylim : tuple of two floats
- aspect_ratio : tuple of two floats or {'auto'}
- autoscale : bool
- margin : float in [0, 1]
The per data series options and aesthetics are:
There are none in the base series. See below for options for subclasses.
Some data series support additional aesthetics or options:
ListSeries, LineOver1DRangeSeries, Parametric2DLineSeries,
Parametric3DLineSeries support the following:
Aesthetics:
- line_color : function which returns a float.
options:
- label : str
- steps : bool
- integers_only : bool
SurfaceOver2DRangeSeries, ParametricSurfaceSeries support the following:
aesthetics:
- surface_color : function which returns a float.
"""
def __init__(self, *args, **kwargs):
super(Plot, self).__init__()
# Options for the graph as a whole.
# The possible values for each option are described in the docstring of
# Plot. They are based purely on convention, no checking is done.
self.title = None
self.xlabel = None
self.ylabel = None
self.aspect_ratio = 'auto'
self.xlim = None
self.ylim = None
self.axis_center = 'auto'
self.axis = True
self.xscale = 'linear'
self.yscale = 'linear'
self.legend = False
self.autoscale = True
self.margin = 0
# Contains the data objects to be plotted. The backend should be smart
# enough to iterate over this list.
self._series = []
self._series.extend(args)
# The backend type. On every show() a new backend instance is created
# in self._backend which is tightly coupled to the Plot instance
# (thanks to the parent attribute of the backend).
self.backend = DefaultBackend
# The keyword arguments should only contain options for the plot.
for key, val in kwargs.items():
if hasattr(self, key):
setattr(self, key, val)
def show(self):
# TODO move this to the backend (also for save)
if hasattr(self, '_backend'):
self._backend.close()
self._backend = self.backend(self)
self._backend.show()
def save(self, path):
if hasattr(self, '_backend'):
self._backend.close()
self._backend = self.backend(self)
self._backend.save(path)
def __str__(self):
series_strs = [('[%d]: ' % i) + str(s)
for i, s in enumerate(self._series)]
return 'Plot object containing:\n' + '\n'.join(series_strs)
def __getitem__(self, index):
return self._series[index]
def __setitem__(self, index, *args):
if len(args) == 1 and isinstance(args[0], BaseSeries):
self._series[index] = args
def __delitem__(self, index):
del self._series[index]
@doctest_depends_on(modules=('numpy', 'matplotlib',))
def append(self, arg):
"""Adds an element from a plot's series to an existing plot.
Examples
========
Consider two ``Plot`` objects, ``p1`` and ``p2``. To add the
second plot's first series object to the first, use the
``append`` method, like so:
>>> from sympy import symbols
>>> from sympy.plotting import plot
>>> x = symbols('x')
>>> p1 = plot(x*x)
>>> p2 = plot(x)
>>> p1.append(p2[0])
>>> p1
Plot object containing:
[0]: cartesian line: x**2 for x over (-10.0, 10.0)
[1]: cartesian line: x for x over (-10.0, 10.0)
See Also
========
extend
"""
if isinstance(arg, BaseSeries):
self._series.append(arg)
else:
raise TypeError('Must specify element of plot to append.')
@doctest_depends_on(modules=('numpy', 'matplotlib',))
def extend(self, arg):
"""Adds all series from another plot.
Examples
========
Consider two ``Plot`` objects, ``p1`` and ``p2``. To add the
second plot to the first, use the ``extend`` method, like so:
>>> from sympy import symbols
>>> from sympy.plotting import plot
>>> x = symbols('x')
>>> p1 = plot(x*x)
>>> p2 = plot(x)
>>> p1.extend(p2)
>>> p1
Plot object containing:
[0]: cartesian line: x**2 for x over (-10.0, 10.0)
[1]: cartesian line: x for x over (-10.0, 10.0)
"""
if isinstance(arg, Plot):
self._series.extend(arg._series)
elif is_sequence(arg):
self._series.extend(arg)
else:
raise TypeError('Expecting Plot or sequence of BaseSeries')
##############################################################################
# Data Series
##############################################################################
#TODO more general way to calculate aesthetics (see get_color_array)
### The base class for all series
class BaseSeries(object):
"""Base class for the data objects containing stuff to be plotted.
The backend should check if it supports the data series that it's given.
(eg TextBackend supports only LineOver1DRange).
It's the backend responsibility to know how to use the class of
data series that it's given.
Some data series classes are grouped (using a class attribute like is_2Dline)
according to the api they present (based only on convention). The backend is
not obliged to use that api (eg. The LineOver1DRange belongs to the
is_2Dline group and presents the get_points method, but the
TextBackend does not use the get_points method).
"""
# Some flags follow. The rationale for using flags instead of checking base
# classes is that setting multiple flags is simpler than multiple
# inheritance.
is_2Dline = False
# Some of the backends expect:
# - get_points returning 1D np.arrays list_x, list_y
# - get_segments returning np.array (done in Line2DBaseSeries)
# - get_color_array returning 1D np.array (done in Line2DBaseSeries)
# with the colors calculated at the points from get_points
is_3Dline = False
# Some of the backends expect:
# - get_points returning 1D np.arrays list_x, list_y, list_y
# - get_segments returning np.array (done in Line2DBaseSeries)
# - get_color_array returning 1D np.array (done in Line2DBaseSeries)
# with the colors calculated at the points from get_points
is_3Dsurface = False
# Some of the backends expect:
# - get_meshes returning mesh_x, mesh_y, mesh_z (2D np.arrays)
# - get_points an alias for get_meshes
is_contour = False
# Some of the backends expect:
# - get_meshes returning mesh_x, mesh_y, mesh_z (2D np.arrays)
# - get_points an alias for get_meshes
is_implicit = False
# Some of the backends expect:
# - get_meshes returning mesh_x (1D array), mesh_y(1D array,
# mesh_z (2D np.arrays)
# - get_points an alias for get_meshes
#Different from is_contour as the colormap in backend will be
#different
is_parametric = False
# The calculation of aesthetics expects:
# - get_parameter_points returning one or two np.arrays (1D or 2D)
# used for calculation aesthetics
def __init__(self):
super(BaseSeries, self).__init__()
@property
def is_3D(self):
flags3D = [
self.is_3Dline,
self.is_3Dsurface
]
return any(flags3D)
@property
def is_line(self):
flagslines = [
self.is_2Dline,
self.is_3Dline
]
return any(flagslines)
### 2D lines
class Line2DBaseSeries(BaseSeries):
"""A base class for 2D lines.
- adding the label, steps and only_integers options
- making is_2Dline true
- defining get_segments and get_color_array
"""
is_2Dline = True
_dim = 2
def __init__(self):
super(Line2DBaseSeries, self).__init__()
self.label = None
self.steps = False
self.only_integers = False
self.line_color = None
def get_segments(self):
np = import_module('numpy')
points = self.get_points()
if self.steps is True:
x = np.array((points[0], points[0])).T.flatten()[1:]
y = np.array((points[1], points[1])).T.flatten()[:-1]
points = (x, y)
points = np.ma.array(points).T.reshape(-1, 1, self._dim)
return np.ma.concatenate([points[:-1], points[1:]], axis=1)
def get_color_array(self):
np = import_module('numpy')
c = self.line_color
if hasattr(c, '__call__'):
f = np.vectorize(c)
arity = len(getargspec(c)[0])
if arity == 1 and self.is_parametric:
x = self.get_parameter_points()
return f(centers_of_segments(x))
else:
variables = list(map(centers_of_segments, self.get_points()))
if arity == 1:
return f(variables[0])
elif arity == 2:
return f(*variables[:2])
else: # only if the line is 3D (otherwise raises an error)
return f(*variables)
else:
return c*np.ones(self.nb_of_points)
class List2DSeries(Line2DBaseSeries):
"""Representation for a line consisting of list of points."""
def __init__(self, list_x, list_y):
np = import_module('numpy')
super(List2DSeries, self).__init__()
self.list_x = np.array(list_x)
self.list_y = np.array(list_y)
self.label = 'list'
def __str__(self):
return 'list plot'
def get_points(self):
return (self.list_x, self.list_y)
class LineOver1DRangeSeries(Line2DBaseSeries):
"""Representation for a line consisting of a SymPy expression over a range."""
def __init__(self, expr, var_start_end, **kwargs):
super(LineOver1DRangeSeries, self).__init__()
self.expr = sympify(expr)
self.label = str(self.expr)
self.var = sympify(var_start_end[0])
self.start = float(var_start_end[1])
self.end = float(var_start_end[2])
self.nb_of_points = kwargs.get('nb_of_points', 300)
self.adaptive = kwargs.get('adaptive', True)
self.depth = kwargs.get('depth', 12)
self.line_color = kwargs.get('line_color', None)
def __str__(self):
return 'cartesian line: %s for %s over %s' % (
str(self.expr), str(self.var), str((self.start, self.end)))
def get_segments(self):
"""
Adaptively gets segments for plotting.
The adaptive sampling is done by recursively checking if three
points are almost collinear. If they are not collinear, then more
points are added between those points.
References
==========
[1] Adaptive polygonal approximation of parametric curves,
Luiz Henrique de Figueiredo.
"""
if self.only_integers or not self.adaptive:
return super(LineOver1DRangeSeries, self).get_segments()
else:
f = lambdify([self.var], self.expr)
list_segments = []
def sample(p, q, depth):
""" Samples recursively if three points are almost collinear.
For depth < 6, points are added irrespective of whether they
satisfy the collinearity condition or not. The maximum depth
allowed is 12.
"""
np = import_module('numpy')
#Randomly sample to avoid aliasing.
random = 0.45 + np.random.rand() * 0.1
xnew = p[0] + random * (q[0] - p[0])
ynew = f(xnew)
new_point = np.array([xnew, ynew])
#Maximum depth
if depth > self.depth:
list_segments.append([p, q])
#Sample irrespective of whether the line is flat till the
#depth of 6. We are not using linspace to avoid aliasing.
elif depth < 6:
sample(p, new_point, depth + 1)
sample(new_point, q, depth + 1)
#Sample ten points if complex values are encountered
#at both ends. If there is a real value in between, then
#sample those points further.
elif p[1] is None and q[1] is None:
xarray = np.linspace(p[0], q[0], 10)
yarray = list(map(f, xarray))
if any(y is not None for y in yarray):
for i in range(len(yarray) - 1):
if yarray[i] is not None or yarray[i + 1] is not None:
sample([xarray[i], yarray[i]],
[xarray[i + 1], yarray[i + 1]], depth + 1)
#Sample further if one of the end points in None( i.e. a complex
#value) or the three points are not almost collinear.
elif (p[1] is None or q[1] is None or new_point[1] is None
or not flat(p, new_point, q)):
sample(p, new_point, depth + 1)
sample(new_point, q, depth + 1)
else:
list_segments.append([p, q])
f_start = f(self.start)
f_end = f(self.end)
sample([self.start, f_start], [self.end, f_end], 0)
return list_segments
def get_points(self):
np = import_module('numpy')
if self.only_integers is True:
list_x = np.linspace(int(self.start), int(self.end),
num=int(self.end) - int(self.start) + 1)
else:
list_x = np.linspace(self.start, self.end, num=self.nb_of_points)
f = vectorized_lambdify([self.var], self.expr)
list_y = f(list_x)
return (list_x, list_y)
class Parametric2DLineSeries(Line2DBaseSeries):
"""Representation for a line consisting of two parametric sympy expressions
over a range."""
is_parametric = True
def __init__(self, expr_x, expr_y, var_start_end, **kwargs):
super(Parametric2DLineSeries, self).__init__()
self.expr_x = sympify(expr_x)
self.expr_y = sympify(expr_y)
self.label = "(%s, %s)" % (str(self.expr_x), str(self.expr_y))
self.var = sympify(var_start_end[0])
self.start = float(var_start_end[1])
self.end = float(var_start_end[2])
self.nb_of_points = kwargs.get('nb_of_points', 300)
self.adaptive = kwargs.get('adaptive', True)
self.depth = kwargs.get('depth', 12)
self.line_color = kwargs.get('line_color', None)
def __str__(self):
return 'parametric cartesian line: (%s, %s) for %s over %s' % (
str(self.expr_x), str(self.expr_y), str(self.var),
str((self.start, self.end)))
def get_parameter_points(self):
np = import_module('numpy')
return np.linspace(self.start, self.end, num=self.nb_of_points)
def get_points(self):
param = self.get_parameter_points()
fx = vectorized_lambdify([self.var], self.expr_x)
fy = vectorized_lambdify([self.var], self.expr_y)
list_x = fx(param)
list_y = fy(param)
return (list_x, list_y)
def get_segments(self):
"""
Adaptively gets segments for plotting.
The adaptive sampling is done by recursively checking if three
points are almost collinear. If they are not collinear, then more
points are added between those points.
References
==========
[1] Adaptive polygonal approximation of parametric curves,
Luiz Henrique de Figueiredo.
"""
if not self.adaptive:
return super(Parametric2DLineSeries, self).get_segments()
f_x = lambdify([self.var], self.expr_x)
f_y = lambdify([self.var], self.expr_y)
list_segments = []
def sample(param_p, param_q, p, q, depth):
""" Samples recursively if three points are almost collinear.
For depth < 6, points are added irrespective of whether they
satisfy the collinearity condition or not. The maximum depth
allowed is 12.
"""
#Randomly sample to avoid aliasing.
np = import_module('numpy')
random = 0.45 + np.random.rand() * 0.1
param_new = param_p + random * (param_q - param_p)
xnew = f_x(param_new)
ynew = f_y(param_new)
new_point = np.array([xnew, ynew])
#Maximum depth
if depth > self.depth:
list_segments.append([p, q])
#Sample irrespective of whether the line is flat till the
#depth of 6. We are not using linspace to avoid aliasing.
elif depth < 6:
sample(param_p, param_new, p, new_point, depth + 1)
sample(param_new, param_q, new_point, q, depth + 1)
#Sample ten points if complex values are encountered
#at both ends. If there is a real value in between, then
#sample those points further.
elif ((p[0] is None and q[1] is None) or
(p[1] is None and q[1] is None)):
param_array = np.linspace(param_p, param_q, 10)
x_array = list(map(f_x, param_array))
y_array = list(map(f_y, param_array))
if any(x is not None and y is not None
for x, y in zip(x_array, y_array)):
for i in range(len(y_array) - 1):
if ((x_array[i] is not None and y_array[i] is not None) or
(x_array[i + 1] is not None and y_array[i + 1] is not None)):
point_a = [x_array[i], y_array[i]]
point_b = [x_array[i + 1], y_array[i + 1]]
sample(param_array[i], param_array[i], point_a,
point_b, depth + 1)
#Sample further if one of the end points in None( ie a complex
#value) or the three points are not almost collinear.
elif (p[0] is None or p[1] is None
or q[1] is None or q[0] is None
or not flat(p, new_point, q)):
sample(param_p, param_new, p, new_point, depth + 1)
sample(param_new, param_q, new_point, q, depth + 1)
else:
list_segments.append([p, q])
f_start_x = f_x(self.start)
f_start_y = f_y(self.start)
start = [f_start_x, f_start_y]
f_end_x = f_x(self.end)
f_end_y = f_y(self.end)
end = [f_end_x, f_end_y]
sample(self.start, self.end, start, end, 0)
return list_segments
### 3D lines
class Line3DBaseSeries(Line2DBaseSeries):
"""A base class for 3D lines.
Most of the stuff is derived from Line2DBaseSeries."""
is_2Dline = False
is_3Dline = True
_dim = 3
def __init__(self):
super(Line3DBaseSeries, self).__init__()
class Parametric3DLineSeries(Line3DBaseSeries):
"""Representation for a 3D line consisting of two parametric sympy
expressions and a range."""
def __init__(self, expr_x, expr_y, expr_z, var_start_end, **kwargs):
super(Parametric3DLineSeries, self).__init__()
self.expr_x = sympify(expr_x)
self.expr_y = sympify(expr_y)
self.expr_z = sympify(expr_z)
self.label = "(%s, %s)" % (str(self.expr_x), str(self.expr_y))
self.var = sympify(var_start_end[0])
self.start = float(var_start_end[1])
self.end = float(var_start_end[2])
self.nb_of_points = kwargs.get('nb_of_points', 300)
self.line_color = kwargs.get('line_color', None)
def __str__(self):
return '3D parametric cartesian line: (%s, %s, %s) for %s over %s' % (
str(self.expr_x), str(self.expr_y), str(self.expr_z),
str(self.var), str((self.start, self.end)))
def get_parameter_points(self):
np = import_module('numpy')
return np.linspace(self.start, self.end, num=self.nb_of_points)
def get_points(self):
param = self.get_parameter_points()
fx = vectorized_lambdify([self.var], self.expr_x)
fy = vectorized_lambdify([self.var], self.expr_y)
fz = vectorized_lambdify([self.var], self.expr_z)
list_x = fx(param)
list_y = fy(param)
list_z = fz(param)
return (list_x, list_y, list_z)
### Surfaces
class SurfaceBaseSeries(BaseSeries):
"""A base class for 3D surfaces."""
is_3Dsurface = True
def __init__(self):
super(SurfaceBaseSeries, self).__init__()
self.surface_color = None
def get_color_array(self):
np = import_module('numpy')
c = self.surface_color
if isinstance(c, Callable):
f = np.vectorize(c)
arity = len(getargspec(c)[0])
if self.is_parametric:
variables = list(map(centers_of_faces, self.get_parameter_meshes()))
if arity == 1:
return f(variables[0])
elif arity == 2:
return f(*variables)
variables = list(map(centers_of_faces, self.get_meshes()))
if arity == 1:
return f(variables[0])
elif arity == 2:
return f(*variables[:2])
else:
return f(*variables)
else:
return c*np.ones(self.nb_of_points)
class SurfaceOver2DRangeSeries(SurfaceBaseSeries):
"""Representation for a 3D surface consisting of a sympy expression and 2D
range."""
def __init__(self, expr, var_start_end_x, var_start_end_y, **kwargs):
super(SurfaceOver2DRangeSeries, self).__init__()
self.expr = sympify(expr)
self.var_x = sympify(var_start_end_x[0])
self.start_x = float(var_start_end_x[1])
self.end_x = float(var_start_end_x[2])
self.var_y = sympify(var_start_end_y[0])
self.start_y = float(var_start_end_y[1])
self.end_y = float(var_start_end_y[2])
self.nb_of_points_x = kwargs.get('nb_of_points_x', 50)
self.nb_of_points_y = kwargs.get('nb_of_points_y', 50)
self.surface_color = kwargs.get('surface_color', None)
def __str__(self):
return ('cartesian surface: %s for'
' %s over %s and %s over %s') % (
str(self.expr),
str(self.var_x),
str((self.start_x, self.end_x)),
str(self.var_y),
str((self.start_y, self.end_y)))
def get_meshes(self):
np = import_module('numpy')
mesh_x, mesh_y = np.meshgrid(np.linspace(self.start_x, self.end_x,
num=self.nb_of_points_x),
np.linspace(self.start_y, self.end_y,
num=self.nb_of_points_y))
f = vectorized_lambdify((self.var_x, self.var_y), self.expr)
return (mesh_x, mesh_y, f(mesh_x, mesh_y))
class ParametricSurfaceSeries(SurfaceBaseSeries):
"""Representation for a 3D surface consisting of three parametric sympy
expressions and a range."""
is_parametric = True
def __init__(
self, expr_x, expr_y, expr_z, var_start_end_u, var_start_end_v,
**kwargs):
super(ParametricSurfaceSeries, self).__init__()
self.expr_x = sympify(expr_x)
self.expr_y = sympify(expr_y)
self.expr_z = sympify(expr_z)
self.var_u = sympify(var_start_end_u[0])
self.start_u = float(var_start_end_u[1])
self.end_u = float(var_start_end_u[2])
self.var_v = sympify(var_start_end_v[0])
self.start_v = float(var_start_end_v[1])
self.end_v = float(var_start_end_v[2])
self.nb_of_points_u = kwargs.get('nb_of_points_u', 50)
self.nb_of_points_v = kwargs.get('nb_of_points_v', 50)
self.surface_color = kwargs.get('surface_color', None)
def __str__(self):
return ('parametric cartesian surface: (%s, %s, %s) for'
' %s over %s and %s over %s') % (
str(self.expr_x),
str(self.expr_y),
str(self.expr_z),
str(self.var_u),
str((self.start_u, self.end_u)),
str(self.var_v),
str((self.start_v, self.end_v)))
def get_parameter_meshes(self):
np = import_module('numpy')
return np.meshgrid(np.linspace(self.start_u, self.end_u,
num=self.nb_of_points_u),
np.linspace(self.start_v, self.end_v,
num=self.nb_of_points_v))
def get_meshes(self):
mesh_u, mesh_v = self.get_parameter_meshes()
fx = vectorized_lambdify((self.var_u, self.var_v), self.expr_x)
fy = vectorized_lambdify((self.var_u, self.var_v), self.expr_y)
fz = vectorized_lambdify((self.var_u, self.var_v), self.expr_z)
return (fx(mesh_u, mesh_v), fy(mesh_u, mesh_v), fz(mesh_u, mesh_v))
### Contours
class ContourSeries(BaseSeries):
"""Representation for a contour plot."""
#The code is mostly repetition of SurfaceOver2DRange.
#XXX: Presently not used in any of those functions.
#XXX: Add contour plot and use this seties.
is_contour = True
def __init__(self, expr, var_start_end_x, var_start_end_y):
super(ContourSeries, self).__init__()
self.nb_of_points_x = 50
self.nb_of_points_y = 50
self.expr = sympify(expr)
self.var_x = sympify(var_start_end_x[0])
self.start_x = float(var_start_end_x[1])
self.end_x = float(var_start_end_x[2])
self.var_y = sympify(var_start_end_y[0])
self.start_y = float(var_start_end_y[1])
self.end_y = float(var_start_end_y[2])
self.get_points = self.get_meshes
def __str__(self):
return ('contour: %s for '
'%s over %s and %s over %s') % (
str(self.expr),
str(self.var_x),
str((self.start_x, self.end_x)),
str(self.var_y),
str((self.start_y, self.end_y)))
def get_meshes(self):
np = import_module('numpy')
mesh_x, mesh_y = np.meshgrid(np.linspace(self.start_x, self.end_x,
num=self.nb_of_points_x),
np.linspace(self.start_y, self.end_y,
num=self.nb_of_points_y))
f = vectorized_lambdify((self.var_x, self.var_y), self.expr)
return (mesh_x, mesh_y, f(mesh_x, mesh_y))
##############################################################################
# Backends
##############################################################################
class BaseBackend(object):
def __init__(self, parent):
super(BaseBackend, self).__init__()
self.parent = parent
## don't have to check for the success of importing matplotlib in each case;
## we will only be using this backend if we can successfully import matploblib
class MatplotlibBackend(BaseBackend):
def __init__(self, parent):
super(MatplotlibBackend, self).__init__(parent)
are_3D = [s.is_3D for s in self.parent._series]
self.matplotlib = import_module('matplotlib',
__import__kwargs={'fromlist': ['pyplot', 'cm', 'collections']},
min_module_version='1.1.0', catch=(RuntimeError,))
self.plt = self.matplotlib.pyplot
self.cm = self.matplotlib.cm
self.LineCollection = self.matplotlib.collections.LineCollection
if any(are_3D) and not all(are_3D):
raise ValueError('The matplotlib backend can not mix 2D and 3D.')
elif not any(are_3D):
self.fig = self.plt.figure()
self.ax = self.fig.add_subplot(111)
self.ax.spines['left'].set_position('zero')
self.ax.spines['right'].set_color('none')
self.ax.spines['bottom'].set_position('zero')
self.ax.spines['top'].set_color('none')
self.ax.spines['left'].set_smart_bounds(True)
self.ax.spines['bottom'].set_smart_bounds(False)
self.ax.xaxis.set_ticks_position('bottom')
self.ax.yaxis.set_ticks_position('left')
elif all(are_3D):
## mpl_toolkits.mplot3d is necessary for
## projection='3d'
mpl_toolkits = import_module('mpl_toolkits',
__import__kwargs={'fromlist': ['mplot3d']})
self.fig = self.plt.figure()
self.ax = self.fig.add_subplot(111, projection='3d')
def process_series(self):
parent = self.parent
for s in self.parent._series:
# Create the collections
if s.is_2Dline:
collection = self.LineCollection(s.get_segments())
self.ax.add_collection(collection)
elif s.is_contour:
self.ax.contour(*s.get_meshes())
elif s.is_3Dline:
# TODO too complicated, I blame matplotlib
mpl_toolkits = import_module('mpl_toolkits',
__import__kwargs={'fromlist': ['mplot3d']})
art3d = mpl_toolkits.mplot3d.art3d
collection = art3d.Line3DCollection(s.get_segments())
self.ax.add_collection(collection)
x, y, z = s.get_points()
self.ax.set_xlim((min(x), max(x)))
self.ax.set_ylim((min(y), max(y)))
self.ax.set_zlim((min(z), max(z)))
elif s.is_3Dsurface:
x, y, z = s.get_meshes()
collection = self.ax.plot_surface(x, y, z, cmap=self.cm.jet,
rstride=1, cstride=1,
linewidth=0.1)
elif s.is_implicit:
#Smart bounds have to be set to False for implicit plots.
self.ax.spines['left'].set_smart_bounds(False)
self.ax.spines['bottom'].set_smart_bounds(False)
points = s.get_raster()
if len(points) == 2:
#interval math plotting
x, y = _matplotlib_list(points[0])
self.ax.fill(x, y, facecolor=s.line_color, edgecolor='None')
else:
# use contourf or contour depending on whether it is
# an inequality or equality.
#XXX: ``contour`` plots multiple lines. Should be fixed.
ListedColormap = self.matplotlib.colors.ListedColormap
colormap = ListedColormap(["white", s.line_color])
xarray, yarray, zarray, plot_type = points
if plot_type == 'contour':
self.ax.contour(xarray, yarray, zarray,
contours=(0, 0), fill=False, cmap=colormap)
else:
self.ax.contourf(xarray, yarray, zarray, cmap=colormap)
else:
raise ValueError('The matplotlib backend supports only '
'is_2Dline, is_3Dline, is_3Dsurface and '
'is_contour objects.')
# Customise the collections with the corresponding per-series
# options.
if hasattr(s, 'label'):
collection.set_label(s.label)
if s.is_line and s.line_color:
if isinstance(s.line_color, (float, int)) or isinstance(s.line_color, Callable):
color_array = s.get_color_array()
collection.set_array(color_array)
else:
collection.set_color(s.line_color)
if s.is_3Dsurface and s.surface_color:
if self.matplotlib.__version__ < "1.2.0": # TODO in the distant future remove this check
warnings.warn('The version of matplotlib is too old to use surface coloring.')
elif isinstance(s.surface_color, (float, int)) or isinstance(s.surface_color, Callable):
color_array = s.get_color_array()
color_array = color_array.reshape(color_array.size)
collection.set_array(color_array)
else:
collection.set_color(s.surface_color)
# Set global options.
# TODO The 3D stuff
# XXX The order of those is important.
mpl_toolkits = import_module('mpl_toolkits',
__import__kwargs={'fromlist': ['mplot3d']})
Axes3D = mpl_toolkits.mplot3d.Axes3D
if parent.xscale and not isinstance(self.ax, Axes3D):
self.ax.set_xscale(parent.xscale)
if parent.yscale and not isinstance(self.ax, Axes3D):
self.ax.set_yscale(parent.yscale)
if parent.xlim:
self.ax.set_xlim(parent.xlim)
else:
if all(isinstance(s, LineOver1DRangeSeries) for s in parent._series):
starts = [s.start for s in parent._series]
ends = [s.end for s in parent._series]
self.ax.set_xlim(min(starts), max(ends))
if parent.ylim:
self.ax.set_ylim(parent.ylim)
if not isinstance(self.ax, Axes3D) or self.matplotlib.__version__ >= '1.2.0': # XXX in the distant future remove this check
self.ax.set_autoscale_on(parent.autoscale)
if parent.axis_center:
val = parent.axis_center
if isinstance(self.ax, Axes3D):
pass
elif val == 'center':
self.ax.spines['left'].set_position('center')
self.ax.spines['bottom'].set_position('center')
elif val == 'auto':
xl, xh = self.ax.get_xlim()
yl, yh = self.ax.get_ylim()
pos_left = ('data', 0) if xl*xh <= 0 else 'center'
pos_bottom = ('data', 0) if yl*yh <= 0 else 'center'
self.ax.spines['left'].set_position(pos_left)
self.ax.spines['bottom'].set_position(pos_bottom)
else:
self.ax.spines['left'].set_position(('data', val[0]))
self.ax.spines['bottom'].set_position(('data', val[1]))
if not parent.axis:
self.ax.set_axis_off()
if parent.legend:
if self.ax.legend():
self.ax.legend_.set_visible(parent.legend)
if parent.margin:
self.ax.set_xmargin(parent.margin)
self.ax.set_ymargin(parent.margin)
if parent.title:
self.ax.set_title(parent.title)
if parent.xlabel:
self.ax.set_xlabel(parent.xlabel, position=(1, 0))
if parent.ylabel:
self.ax.set_ylabel(parent.ylabel, position=(0, 1))
def show(self):
self.process_series()
#TODO after fixing https://github.com/ipython/ipython/issues/1255
# you can uncomment the next line and remove the pyplot.show() call
#self.fig.show()
if _show:
self.plt.show()
def save(self, path):
self.process_series()
self.fig.savefig(path)
def close(self):
self.plt.close(self.fig)
class TextBackend(BaseBackend):
def __init__(self, parent):
super(TextBackend, self).__init__(parent)
def show(self):
if len(self.parent._series) != 1:
raise ValueError(
'The TextBackend supports only one graph per Plot.')
elif not isinstance(self.parent._series[0], LineOver1DRangeSeries):
raise ValueError(
'The TextBackend supports only expressions over a 1D range')
else:
ser = self.parent._series[0]
textplot(ser.expr, ser.start, ser.end)
def close(self):
pass
class DefaultBackend(BaseBackend):
def __new__(cls, parent):
matplotlib = import_module('matplotlib', min_module_version='1.1.0', catch=(RuntimeError,))
if matplotlib:
return MatplotlibBackend(parent)
else:
return TextBackend(parent)
plot_backends = {
'matplotlib': MatplotlibBackend,
'text': TextBackend,
'default': DefaultBackend
}
##############################################################################
# Finding the centers of line segments or mesh faces
##############################################################################
def centers_of_segments(array):
np = import_module('numpy')
return np.average(np.vstack((array[:-1], array[1:])), 0)
def centers_of_faces(array):
np = import_module('numpy')
return np.average(np.dstack((array[:-1, :-1],
array[1:, :-1],
array[:-1, 1: ],
array[:-1, :-1],
)), 2)
def flat(x, y, z, eps=1e-3):
"""Checks whether three points are almost collinear"""
np = import_module('numpy')
# Workaround plotting piecewise (#8577):
# workaround for `lambdify` in `.experimental_lambdify` fails
# to return numerical values in some cases. Lower-level fix
# in `lambdify` is possible.
vector_a = (x - y).astype(np.float)
vector_b = (z - y).astype(np.float)
dot_product = np.dot(vector_a, vector_b)
vector_a_norm = np.linalg.norm(vector_a)
vector_b_norm = np.linalg.norm(vector_b)
cos_theta = dot_product / (vector_a_norm * vector_b_norm)
return abs(cos_theta + 1) < eps
def _matplotlib_list(interval_list):
"""
Returns lists for matplotlib ``fill`` command from a list of bounding
rectangular intervals
"""
xlist = []
ylist = []
if len(interval_list):
for intervals in interval_list:
intervalx = intervals[0]
intervaly = intervals[1]
xlist.extend([intervalx.start, intervalx.start,
intervalx.end, intervalx.end, None])
ylist.extend([intervaly.start, intervaly.end,
intervaly.end, intervaly.start, None])
else:
#XXX Ugly hack. Matplotlib does not accept empty lists for ``fill``
xlist.extend([None, None, None, None])
ylist.extend([None, None, None, None])
return xlist, ylist
####New API for plotting module ####
# TODO: Add color arrays for plots.
# TODO: Add more plotting options for 3d plots.
# TODO: Adaptive sampling for 3D plots.
@doctest_depends_on(modules=('numpy', 'matplotlib',))
def plot(*args, **kwargs):
"""
Plots a function of a single variable and returns an instance of
the ``Plot`` class (also, see the description of the
``show`` keyword argument below).
The plotting uses an adaptive algorithm which samples recursively to
accurately plot the plot. The adaptive algorithm uses a random point near
the midpoint of two points that has to be further sampled. Hence the same
plots can appear slightly different.
Usage
=====
Single Plot
``plot(expr, range, **kwargs)``
If the range is not specified, then a default range of (-10, 10) is used.
Multiple plots with same range.
``plot(expr1, expr2, ..., range, **kwargs)``
If the range is not specified, then a default range of (-10, 10) is used.
Multiple plots with different ranges.
``plot((expr1, range), (expr2, range), ..., **kwargs)``
Range has to be specified for every expression.
Default range may change in the future if a more advanced default range
detection algorithm is implemented.
Arguments
=========
``expr`` : Expression representing the function of single variable
``range``: (x, 0, 5), A 3-tuple denoting the range of the free variable.
Keyword Arguments
=================
Arguments for ``plot`` function:
``show``: Boolean. The default value is set to ``True``. Set show to
``False`` and the function will not display the plot. The returned
instance of the ``Plot`` class can then be used to save or display
the plot by calling the ``save()`` and ``show()`` methods
respectively.
Arguments for ``LineOver1DRangeSeries`` class:
``adaptive``: Boolean. The default value is set to True. Set adaptive to False and
specify ``nb_of_points`` if uniform sampling is required.
``depth``: int Recursion depth of the adaptive algorithm. A depth of value ``n``
samples a maximum of `2^{n}` points.
``nb_of_points``: int. Used when the ``adaptive`` is set to False. The function
is uniformly sampled at ``nb_of_points`` number of points.
Aesthetics options:
``line_color``: float. Specifies the color for the plot.
See ``Plot`` to see how to set color for the plots.
If there are multiple plots, then the same series series are applied to
all the plots. If you want to set these options separately, you can index
the ``Plot`` object returned and set it.
Arguments for ``Plot`` class:
``title`` : str. Title of the plot. It is set to the latex representation of
the expression, if the plot has only one expression.
``xlabel`` : str. Label for the x-axis.
``ylabel`` : str. Label for the y-axis.
``xscale``: {'linear', 'log'} Sets the scaling of the x-axis.
``yscale``: {'linear', 'log'} Sets the scaling if the y-axis.
``axis_center``: tuple of two floats denoting the coordinates of the center or
{'center', 'auto'}
``xlim`` : tuple of two floats, denoting the x-axis limits.
``ylim`` : tuple of two floats, denoting the y-axis limits.
Examples
========
>>> from sympy import symbols
>>> from sympy.plotting import plot
>>> x = symbols('x')
Single Plot
>>> plot(x**2, (x, -5, 5))
Plot object containing:
[0]: cartesian line: x**2 for x over (-5.0, 5.0)
Multiple plots with single range.
>>> plot(x, x**2, x**3, (x, -5, 5))
Plot object containing:
[0]: cartesian line: x for x over (-5.0, 5.0)
[1]: cartesian line: x**2 for x over (-5.0, 5.0)
[2]: cartesian line: x**3 for x over (-5.0, 5.0)
Multiple plots with different ranges.
>>> plot((x**2, (x, -6, 6)), (x, (x, -5, 5)))
Plot object containing:
[0]: cartesian line: x**2 for x over (-6.0, 6.0)
[1]: cartesian line: x for x over (-5.0, 5.0)
No adaptive sampling.
>>> plot(x**2, adaptive=False, nb_of_points=400)
Plot object containing:
[0]: cartesian line: x**2 for x over (-10.0, 10.0)
See Also
========
Plot, LineOver1DRangeSeries.
"""
args = list(map(sympify, args))
free = set()
for a in args:
if isinstance(a, Expr):
free |= a.free_symbols
if len(free) > 1:
raise ValueError(
'The same variable should be used in all '
'univariate expressions being plotted.')
x = free.pop() if free else Symbol('x')
kwargs.setdefault('xlabel', x.name)
kwargs.setdefault('ylabel', 'f(%s)' % x.name)
show = kwargs.pop('show', True)
series = []
plot_expr = check_arguments(args, 1, 1)
series = [LineOver1DRangeSeries(*arg, **kwargs) for arg in plot_expr]
plots = Plot(*series, **kwargs)
if show:
plots.show()
return plots
@doctest_depends_on(modules=('numpy', 'matplotlib',))
def plot_parametric(*args, **kwargs):
"""
Plots a 2D parametric plot.
The plotting uses an adaptive algorithm which samples recursively to
accurately plot the plot. The adaptive algorithm uses a random point near
the midpoint of two points that has to be further sampled. Hence the same
plots can appear slightly different.
Usage
=====
Single plot.
``plot_parametric(expr_x, expr_y, range, **kwargs)``
If the range is not specified, then a default range of (-10, 10) is used.
Multiple plots with same range.
``plot_parametric((expr1_x, expr1_y), (expr2_x, expr2_y), range, **kwargs)``
If the range is not specified, then a default range of (-10, 10) is used.
Multiple plots with different ranges.
``plot_parametric((expr_x, expr_y, range), ..., **kwargs)``
Range has to be specified for every expression.
Default range may change in the future if a more advanced default range
detection algorithm is implemented.
Arguments
=========
``expr_x`` : Expression representing the function along x.
``expr_y`` : Expression representing the function along y.
``range``: (u, 0, 5), A 3-tuple denoting the range of the parameter
variable.
Keyword Arguments
=================
Arguments for ``Parametric2DLineSeries`` class:
``adaptive``: Boolean. The default value is set to True. Set adaptive to
False and specify ``nb_of_points`` if uniform sampling is required.
``depth``: int Recursion depth of the adaptive algorithm. A depth of
value ``n`` samples a maximum of `2^{n}` points.
``nb_of_points``: int. Used when the ``adaptive`` is set to False. The
function is uniformly sampled at ``nb_of_points`` number of points.
Aesthetics
----------
``line_color``: function which returns a float. Specifies the color for the
plot. See ``sympy.plotting.Plot`` for more details.
If there are multiple plots, then the same Series arguments are applied to
all the plots. If you want to set these options separately, you can index
the returned ``Plot`` object and set it.
Arguments for ``Plot`` class:
``xlabel`` : str. Label for the x-axis.
``ylabel`` : str. Label for the y-axis.
``xscale``: {'linear', 'log'} Sets the scaling of the x-axis.
``yscale``: {'linear', 'log'} Sets the scaling if the y-axis.
``axis_center``: tuple of two floats denoting the coordinates of the center
or {'center', 'auto'}
``xlim`` : tuple of two floats, denoting the x-axis limits.
``ylim`` : tuple of two floats, denoting the y-axis limits.
Examples
========
>>> from sympy import symbols, cos, sin
>>> from sympy.plotting import plot_parametric
>>> u = symbols('u')
Single Parametric plot
>>> plot_parametric(cos(u), sin(u), (u, -5, 5))
Plot object containing:
[0]: parametric cartesian line: (cos(u), sin(u)) for u over (-5.0, 5.0)
Multiple parametric plot with single range.
>>> plot_parametric((cos(u), sin(u)), (u, cos(u)))
Plot object containing:
[0]: parametric cartesian line: (cos(u), sin(u)) for u over (-10.0, 10.0)
[1]: parametric cartesian line: (u, cos(u)) for u over (-10.0, 10.0)
Multiple parametric plots.
>>> plot_parametric((cos(u), sin(u), (u, -5, 5)),
... (cos(u), u, (u, -5, 5)))
Plot object containing:
[0]: parametric cartesian line: (cos(u), sin(u)) for u over (-5.0, 5.0)
[1]: parametric cartesian line: (cos(u), u) for u over (-5.0, 5.0)
See Also
========
Plot, Parametric2DLineSeries
"""
args = list(map(sympify, args))
show = kwargs.pop('show', True)
series = []
plot_expr = check_arguments(args, 2, 1)
series = [Parametric2DLineSeries(*arg, **kwargs) for arg in plot_expr]
plots = Plot(*series, **kwargs)
if show:
plots.show()
return plots
@doctest_depends_on(modules=('numpy', 'matplotlib',))
def plot3d_parametric_line(*args, **kwargs):
"""
Plots a 3D parametric line plot.
Usage
=====
Single plot:
``plot3d_parametric_line(expr_x, expr_y, expr_z, range, **kwargs)``
If the range is not specified, then a default range of (-10, 10) is used.
Multiple plots.
``plot3d_parametric_line((expr_x, expr_y, expr_z, range), ..., **kwargs)``
Ranges have to be specified for every expression.
Default range may change in the future if a more advanced default range
detection algorithm is implemented.
Arguments
=========
``expr_x`` : Expression representing the function along x.
``expr_y`` : Expression representing the function along y.
``expr_z`` : Expression representing the function along z.
``range``: ``(u, 0, 5)``, A 3-tuple denoting the range of the parameter
variable.
Keyword Arguments
=================
Arguments for ``Parametric3DLineSeries`` class.
``nb_of_points``: The range is uniformly sampled at ``nb_of_points``
number of points.
Aesthetics:
``line_color``: function which returns a float. Specifies the color for the
plot. See ``sympy.plotting.Plot`` for more details.
If there are multiple plots, then the same series arguments are applied to
all the plots. If you want to set these options separately, you can index
the returned ``Plot`` object and set it.
Arguments for ``Plot`` class.
``title`` : str. Title of the plot.
Examples
========
>>> from sympy import symbols, cos, sin
>>> from sympy.plotting import plot3d_parametric_line
>>> u = symbols('u')
Single plot.
>>> plot3d_parametric_line(cos(u), sin(u), u, (u, -5, 5))
Plot object containing:
[0]: 3D parametric cartesian line: (cos(u), sin(u), u) for u over (-5.0, 5.0)
Multiple plots.
>>> plot3d_parametric_line((cos(u), sin(u), u, (u, -5, 5)),
... (sin(u), u**2, u, (u, -5, 5)))
Plot object containing:
[0]: 3D parametric cartesian line: (cos(u), sin(u), u) for u over (-5.0, 5.0)
[1]: 3D parametric cartesian line: (sin(u), u**2, u) for u over (-5.0, 5.0)
See Also
========
Plot, Parametric3DLineSeries
"""
args = list(map(sympify, args))
show = kwargs.pop('show', True)
series = []
plot_expr = check_arguments(args, 3, 1)
series = [Parametric3DLineSeries(*arg, **kwargs) for arg in plot_expr]
plots = Plot(*series, **kwargs)
if show:
plots.show()
return plots
@doctest_depends_on(modules=('numpy', 'matplotlib',))
def plot3d(*args, **kwargs):
"""
Plots a 3D surface plot.
Usage
=====
Single plot
``plot3d(expr, range_x, range_y, **kwargs)``
If the ranges are not specified, then a default range of (-10, 10) is used.
Multiple plot with the same range.
``plot3d(expr1, expr2, range_x, range_y, **kwargs)``
If the ranges are not specified, then a default range of (-10, 10) is used.
Multiple plots with different ranges.
``plot3d((expr1, range_x, range_y), (expr2, range_x, range_y), ..., **kwargs)``
Ranges have to be specified for every expression.
Default range may change in the future if a more advanced default range
detection algorithm is implemented.
Arguments
=========
``expr`` : Expression representing the function along x.
``range_x``: (x, 0, 5), A 3-tuple denoting the range of the x
variable.
``range_y``: (y, 0, 5), A 3-tuple denoting the range of the y
variable.
Keyword Arguments
=================
Arguments for ``SurfaceOver2DRangeSeries`` class:
``nb_of_points_x``: int. The x range is sampled uniformly at
``nb_of_points_x`` of points.
``nb_of_points_y``: int. The y range is sampled uniformly at
``nb_of_points_y`` of points.
Aesthetics:
``surface_color``: Function which returns a float. Specifies the color for
the surface of the plot. See ``sympy.plotting.Plot`` for more details.
If there are multiple plots, then the same series arguments are applied to
all the plots. If you want to set these options separately, you can index
the returned ``Plot`` object and set it.
Arguments for ``Plot`` class:
``title`` : str. Title of the plot.
Examples
========
>>> from sympy import symbols
>>> from sympy.plotting import plot3d
>>> x, y = symbols('x y')
Single plot
>>> plot3d(x*y, (x, -5, 5), (y, -5, 5))
Plot object containing:
[0]: cartesian surface: x*y for x over (-5.0, 5.0) and y over (-5.0, 5.0)
Multiple plots with same range
>>> plot3d(x*y, -x*y, (x, -5, 5), (y, -5, 5))
Plot object containing:
[0]: cartesian surface: x*y for x over (-5.0, 5.0) and y over (-5.0, 5.0)
[1]: cartesian surface: -x*y for x over (-5.0, 5.0) and y over (-5.0, 5.0)
Multiple plots with different ranges.
>>> plot3d((x**2 + y**2, (x, -5, 5), (y, -5, 5)),
... (x*y, (x, -3, 3), (y, -3, 3)))
Plot object containing:
[0]: cartesian surface: x**2 + y**2 for x over (-5.0, 5.0) and y over (-5.0, 5.0)
[1]: cartesian surface: x*y for x over (-3.0, 3.0) and y over (-3.0, 3.0)
See Also
========
Plot, SurfaceOver2DRangeSeries
"""
args = list(map(sympify, args))
show = kwargs.pop('show', True)
series = []
plot_expr = check_arguments(args, 1, 2)
series = [SurfaceOver2DRangeSeries(*arg, **kwargs) for arg in plot_expr]
plots = Plot(*series, **kwargs)
if show:
plots.show()
return plots
@doctest_depends_on(modules=('numpy', 'matplotlib',))
def plot3d_parametric_surface(*args, **kwargs):
"""
Plots a 3D parametric surface plot.
Usage
=====
Single plot.
``plot3d_parametric_surface(expr_x, expr_y, expr_z, range_u, range_v, **kwargs)``
If the ranges is not specified, then a default range of (-10, 10) is used.
Multiple plots.
``plot3d_parametric_surface((expr_x, expr_y, expr_z, range_u, range_v), ..., **kwargs)``
Ranges have to be specified for every expression.
Default range may change in the future if a more advanced default range
detection algorithm is implemented.
Arguments
=========
``expr_x``: Expression representing the function along ``x``.
``expr_y``: Expression representing the function along ``y``.
``expr_z``: Expression representing the function along ``z``.
``range_u``: ``(u, 0, 5)``, A 3-tuple denoting the range of the ``u``
variable.
``range_v``: ``(v, 0, 5)``, A 3-tuple denoting the range of the v
variable.
Keyword Arguments
=================
Arguments for ``ParametricSurfaceSeries`` class:
``nb_of_points_u``: int. The ``u`` range is sampled uniformly at
``nb_of_points_v`` of points
``nb_of_points_y``: int. The ``v`` range is sampled uniformly at
``nb_of_points_y`` of points
Aesthetics:
``surface_color``: Function which returns a float. Specifies the color for
the surface of the plot. See ``sympy.plotting.Plot`` for more details.
If there are multiple plots, then the same series arguments are applied for
all the plots. If you want to set these options separately, you can index
the returned ``Plot`` object and set it.
Arguments for ``Plot`` class:
``title`` : str. Title of the plot.
Examples
========
>>> from sympy import symbols, cos, sin
>>> from sympy.plotting import plot3d_parametric_surface
>>> u, v = symbols('u v')
Single plot.
>>> plot3d_parametric_surface(cos(u + v), sin(u - v), u - v,
... (u, -5, 5), (v, -5, 5))
Plot object containing:
[0]: parametric cartesian surface: (cos(u + v), sin(u - v), u - v) for u over (-5.0, 5.0) and v over (-5.0, 5.0)
See Also
========
Plot, ParametricSurfaceSeries
"""
args = list(map(sympify, args))
show = kwargs.pop('show', True)
series = []
plot_expr = check_arguments(args, 3, 2)
series = [ParametricSurfaceSeries(*arg, **kwargs) for arg in plot_expr]
plots = Plot(*series, **kwargs)
if show:
plots.show()
return plots
def check_arguments(args, expr_len, nb_of_free_symbols):
"""
Checks the arguments and converts into tuples of the
form (exprs, ranges)
Examples
========
>>> from sympy import plot, cos, sin, symbols
>>> from sympy.plotting.plot import check_arguments
>>> x = symbols('x')
>>> check_arguments([cos(x), sin(x)], 2, 1)
[(cos(x), sin(x), (x, -10, 10))]
>>> check_arguments([x, x**2], 1, 1)
[(x, (x, -10, 10)), (x**2, (x, -10, 10))]
"""
if expr_len > 1 and isinstance(args[0], Expr):
# Multiple expressions same range.
# The arguments are tuples when the expression length is
# greater than 1.
if len(args) < expr_len:
raise ValueError("len(args) should not be less than expr_len")
for i in range(len(args)):
if isinstance(args[i], Tuple):
break
else:
i = len(args) + 1
exprs = Tuple(*args[:i])
free_symbols = list(set().union(*[e.free_symbols for e in exprs]))
if len(args) == expr_len + nb_of_free_symbols:
#Ranges given
plots = [exprs + Tuple(*args[expr_len:])]
else:
default_range = Tuple(-10, 10)
ranges = []
for symbol in free_symbols:
ranges.append(Tuple(symbol) + default_range)
for i in range(len(free_symbols) - nb_of_free_symbols):
ranges.append(Tuple(Dummy()) + default_range)
plots = [exprs + Tuple(*ranges)]
return plots
if isinstance(args[0], Expr) or (isinstance(args[0], Tuple) and
len(args[0]) == expr_len and
expr_len != 3):
# Cannot handle expressions with number of expression = 3. It is
# not possible to differentiate between expressions and ranges.
#Series of plots with same range
for i in range(len(args)):
if isinstance(args[i], Tuple) and len(args[i]) != expr_len:
break
if not isinstance(args[i], Tuple):
args[i] = Tuple(args[i])
else:
i = len(args) + 1
exprs = args[:i]
assert all(isinstance(e, Expr) for expr in exprs for e in expr)
free_symbols = list(set().union(*[e.free_symbols for expr in exprs
for e in expr]))
if len(free_symbols) > nb_of_free_symbols:
raise ValueError("The number of free_symbols in the expression "
"is greater than %d" % nb_of_free_symbols)
if len(args) == i + nb_of_free_symbols and isinstance(args[i], Tuple):
ranges = Tuple(*[range_expr for range_expr in args[
i:i + nb_of_free_symbols]])
plots = [expr + ranges for expr in exprs]
return plots
else:
#Use default ranges.
default_range = Tuple(-10, 10)
ranges = []
for symbol in free_symbols:
ranges.append(Tuple(symbol) + default_range)
for i in range(len(free_symbols) - nb_of_free_symbols):
ranges.append(Tuple(Dummy()) + default_range)
ranges = Tuple(*ranges)
plots = [expr + ranges for expr in exprs]
return plots
elif isinstance(args[0], Tuple) and len(args[0]) == expr_len + nb_of_free_symbols:
#Multiple plots with different ranges.
for arg in args:
for i in range(expr_len):
if not isinstance(arg[i], Expr):
raise ValueError("Expected an expression, given %s" %
str(arg[i]))
for i in range(nb_of_free_symbols):
if not len(arg[i + expr_len]) == 3:
raise ValueError("The ranges should be a tuple of "
"length 3, got %s" % str(arg[i + expr_len]))
return args
| bsd-3-clause |
nhmc/LAE | python_modules/barak/spec.py | 1 | 50662 | """ Contains an object to describe a spectrum, and various
spectrum-related functions."""
# p2.6+ compatibility
from __future__ import division, print_function, unicode_literals
try:
unicode
except NameError:
unicode = basestring = str
xrange = range
import copy, warnings
import os, pdb
from math import sqrt
from pprint import pformat
import numpy as np
import matplotlib.pyplot as pl
try:
import astropy.io.fits as fits
except ImportError:
import pyfits as fits
from .utilities import nan2num, between, get_data_path, stats
from .convolve import convolve_psf
from .io import readtxt, readtabfits, loadtxt
from .plot import axvlines, axvfill, puttext
from .constants import c_kms
from .stats import remove_outliers
DATAPATH = get_data_path()
debug = False
def getwave(hd):
""" Given a fits header, get the wavelength solution.
"""
dv = None
dw = get_cdelt(hd)
if dw is None:
raise ValueError('Neither CD1_1 nor CDELT1 are present!')
CRVAL = hd[str('CRVAL1')]
CRPIX = hd[str('CRPIX1')]
# wavelength of pixel 1
wstart = CRVAL + (1 - CRPIX) * dw
# check if it's log-linear scale (heuristic)
if CRVAL < 10:
#wstart = 10**wstart
#dv = c_kms * (1. - 1. / 10. ** -dw)
dv = dw * c_kms * np.log(10.)
print('constant dv = %.3f km/s (assume CRVAL1 in log(Angstroms))' % dv)
npts = hd[str('NAXIS1')]
return make_wa_scale(wstart, dw, npts, constantdv=dv)
def get_cdelt(hd):
""" Return wavelength stepsize keyword from a fits header.
Parameters
----------
hd: astropy.io.fits header instance
Returns
-------
cdelt: float
Wavelength stepsize, or None if nothing suitable is found.
"""
cdelt = None
if str('CDELT1') in hd:
cdelt = hd[str('CDELT1')]
elif str('CD1_1') in hd:
cdelt = hd[str('CD1_1')]
return cdelt
def parse_UVES_popler(filename):
""" Read a spectrum from a UVES_popler-style fits file.
"""
fh = fits.open(filename)
hd = fh[0].header
cdelt = get_cdelt(hd)
co = fh[0].data[3]
fl = fh[0].data[0] * co # Flux
er = fh[0].data[1] * co
fh.close()
return Spectrum(fl=fl, er=er, co=co, filename=filename, CDELT=cdelt,
CRVAL=hd[str('CRVAL1')], CRPIX=hd[str('CRPIX1')])
def find_bin_edges(cbins):
""" Given bin centres, find the bin edges.
Examples
--------
>>> print find_bin_edges([1, 2.1, 3.3, 4.6])
[ 0.45 1.55 2.7 3.95 5.25]
"""
cbins = np.asarray(cbins)
edges = cbins[:-1] + 0.5 * (cbins[1:] - cbins[:-1])
edges = np.concatenate( ([2*cbins[0] - edges[0]], edges,
[2*cbins[-1] - edges[-1]]) )
return edges
def make_wa_scale(wstart, dw, npts, constantdv=False, verbose=False):
""" Generates a wavelength scale from the wstart, dw, and npts
values.
Parameters
----------
wstart : float
The wavelength of the first pixel in Angstroms. If constantdv
is True, this should be log10 of the wavelength.
dw : float
The width of each bin in Angstroms. If constantdv is True, this
must be log10 of the width.
npts : int
Number of points in the spectrum.
constantdv : bool
If True, then create a constant dv scale, i.e. a constant
log10(wavelength) step size.
Returns
-------
wa : ndarray
The wavelength in Angstroms.
See Also
--------
barak.sed.make_constant_dv_wa_scale
Make a wavelength scale with a constant velocity pixel size by
specifying, the start, end and width in km/s.
Examples
--------
>>> print make_wa_scale(40, 1, 5)
[40., 41., 42., 43., 44.]
>>> print make_wa_scale(3.5, 1e-3, 5, constantdv=True)
[3162.278, 3169.568, 3176.874, 3184.198, 3191.537]
"""
if constantdv:
if verbose: print('make_wa_scale(): Using log-linear scale')
#import pdb; pdb.set_trace()
wa = 10**(wstart + np.arange(npts, dtype=float) * dw)
else:
if verbose: print('make_wa_scale(): Using linear scale')
wa = wstart + np.arange(npts, dtype=float) * dw
return wa
class Spectrum(object):
""" A class to hold information about a spectrum.
Attributes
----------
wa : array of floats, shape(N,)
Wavelength values (overrides all wavelength keywords)
fl : array of floats, shape(N,)
Flux.
er : array of floats, shape(N,)
Error.
co : array of floats, shape(N,)
Continuum.
dw : float
Wavelength difference between adjacent pixel centres.
dv : float
Velocity difference (km/s)
fwhm : float
Instrumental FWHM in km/s
filename : str
Filename of spectrum
Notes
-----
If enough information is given, the wavelength scale will be
generated. Note that there is no error check if you give
conflicting wavelength scale information in the keywords! In this
case certain combinations of keywords take precendence. See the
code comments for details.
Notes for FITS header::
wstart = CRVAL - (CRPIX - 1.0) * CDELT, dw = CDELT
Conversion between velocity width and log-linear pixel width::
dv / c_kms = 1 - 10**(-dw)
Examples
--------
>>> sp = Spectrum(wstart=4000, dw=1, npts=500)
>>> sp = Spectrum(wstart=4000, dv=60, npts=500)
>>> sp = Spectrum(wstart=4000, wend=4400, npts=500)
>>> wa = np.linspace(4000, 5000, 500)
>>> fl = np.ones(len(wa))
>>> sp = Spectrum(wa=wa, fl=fl)
>>> sp = Spectrum(CRVAL=4000, CRPIX=1, CDELT=1, fl=np.ones(500))
"""
def __init__(self,
dw=None, dv=None, wstart=None, wend=None, npts=None,
CRVAL=None, CRPIX=None, CDELT=None,
wa=None, fl=None, er=None, co=None,
fwhm=None, filename=None):
""" Create the wavelength scale and initialise attributes."""
if fl is not None:
fl = np.asarray(fl)
fl[np.isinf(fl)] = np.nan
self.fl = fl
npts = len(fl)
if er is not None:
er = np.asarray(er)
# replace bad values with NaN
er[np.isinf(er)|(er<=0.)] = np.nan
self.er = er
npts = len(er)
if co is not None:
co = np.asarray(co)
co[np.isinf(co)] = np.nan
self.co = co
npts = len(co)
# Check whether we need to make a wavelength scale.
makescale = True
if dv is not None:
dw = np.log10(1. / (1. - dv / c_kms))
if None not in (CRVAL, CRPIX, CDELT) :
wstart = CRVAL - (CRPIX - 1.0) * CDELT
dw = CDELT
# check if it's log-linear scale (heuristic)
if CRVAL < 10:
wstart = 10**wstart
dv = c_kms * (1. - 1. / 10. ** -dw)
if wa is not None:
wa = np.asarray(wa, float)
npts = len(wa)
makescale = False
elif None not in (wstart, dw, npts):
if dv is not None:
wstart = np.log10(wstart)
elif None not in (wstart, wend, dw):
if dv is not None:
wstart, wend = np.log10([wstart,wend])
# make sure the scale is the same or bigger than the
# requested wavelength range
npts = int(np.ceil((wend - wstart) / float(dw)))
elif None not in (wstart, wend, npts):
# Make a linear wavelength scale
dw = (wend - wstart) / (npts - 1.0)
elif None not in (wend, dw, npts):
raise ValueError('Please specify wstart instead of wend')
else:
raise ValueError('Not enough info to make a wavelength scale!')
if makescale:
if debug: print('making wav scale,', wstart, dw, npts, bool(dv))
wa = make_wa_scale(wstart, dw, npts, constantdv=bool(dv))
else:
# check whether wavelength scale is linear or log-linear
# (constant velocity)
diff = wa[1:] - wa[:-1]
if np.allclose(diff, diff[0]):
dw = np.median(diff)
else:
diff = np.log10(wa[1:]) - np.log10(wa[:-1])
if np.allclose(diff, diff[0]):
dw = np.median(diff)
dv = c_kms * (1. - 1. / 10. ** dw)
# assign remaining attributes
if fl is None:
self.fl = np.zeros(npts)
if er is None:
self.er = np.empty(npts) * np.nan # error (one sig)
if co is None:
self.co = np.empty(npts) * np.nan
self.fwhm = fwhm
self.dw = dw
self.dv = dv
self.filename = filename
self.wa = wa
def __repr__(self):
return 'Spectrum(wa, fl, er, co, dw, dv, fwhm, filename)'
def multiply(self, val):
""" Multipy the flux, error and continuum by `val`.
>>> sp = Spectrum(wstart=4000, dw=1, npts=500, fl=np.ones(500))
>>> sp.multiply(2)
"""
self.fl *= val
self.er *= val
self.co *= val
def plot(self, ax=None, show=True, yperc=0.98, alpha=0.8,
linewidth=1., linestyle='steps-mid',
flcolor='blue', cocolor='red'):
""" Plots a spectrum.
Returns the matplotlib artists that represent the flux, error
and continuum curves.
"""
f,e,c,w = self.fl, self.er, self.co, self.wa
return plot(w, f, e, c, ax=ax, show=show, yperc=yperc, alpha=alpha,
linewidth=linewidth, linestyle=linestyle,
flcolor=flcolor, cocolor=cocolor)
def stats(self, wa1, wa2, show=False):
"""Calculates statistics (mean, standard deviation (i.e. RMS), mean
error, etc) of the flux between two wavelength points.
Returns::
mean flux, RMS of flux, mean error, SNR:
SNR = (mean flux / RMS)
"""
i,j = self.wa.searchsorted([wa1, wa2])
fl = self.fl[i:j]
er = self.er[i:j]
good = (er > 0) & ~np.isnan(fl)
if len(good.nonzero()[0]) == 0:
print('No good data in this range!')
return np.nan, np.nan, np.nan, np.nan
fl = fl[good]
er = er[good]
mfl = fl.mean()
std = fl.std()
mer = er.mean()
snr = mfl / std
if show:
print('mean %g, std %g, er %g, snr %g' % (mfl, std, mer, snr))
return mfl, std, mer, snr
def rebin(self, **kwargs):
""" Class method version of spec.rebin() """
return rebin(self.wa, self.fl, self.er, **kwargs)
def rebin_simple(self, n):
""" Class method version of spec.rebin_simple()."""
return rebin_simple(self.wa, self.fl, self.er, self.co, n)
def write(self, filename, header=None, overwrite=False):
""" Writes out a spectrum, as ascii - wavelength, flux, error,
continuum.
`overwrite` can be True or False.
`header` is a string to be written to the file before the
spectrum. A special case is `header='RESVEL'`, which means the
instrumental fwhm in km/s will be written on the first line
(VPFIT style).
"""
if os.path.lexists(filename) and not overwrite:
c = raw_input('File %s exists - overwrite? (y) or n: ' % filename)
if c != '':
if c.strip().lower()[0] == 'n':
print('returning without writing anything...')
return
fh = open(filename, 'w')
if header is not None:
if header == 'RESVEL':
if self.fwhm is None:
raise ValueError('Instrumental fwhm is not set!')
fh.write('RESVEL %.2f' % self.fwhm)
else:
fh.write(header)
fl = np.nan_to_num(self.fl)
er = np.nan_to_num(self.er)
if np.all(np.isnan(self.co)):
for w,f,e in zip(self.wa, fl, er):
fh.write("% .12g % #12.8g % #12.8g\n" % (w,f,e))
else:
co = np.nan_to_num(self.co)
for w,f,e,c in zip(self.wa, fl, er, co):
fh.write("% .12g % #12.8g % #12.8g % #12.8g\n" % (w,f,e,c))
fh.close()
if self.filename is None:
self.filename = filename
def fits_write(self, filename, header=None, overwrite=False): # Generate a binary FITS table
from astropy.table import Table, Column
""" Writes out a Spectrum, as binary FITS table - wavelength, flux, error,
continuum.
`overwrite` can be True or False.
"""
# Overwrite?
if os.path.lexists(filename) and not overwrite:
c = raw_input('File %s exists - overwrite? (y) or n: ' % filename)
if c != '':
if c.strip().lower()[0] == 'n':
print('returning without writing anything...')
return
# Generate FITS table and write
cwa = Column(data=self.wa,name=str('wa'))
cfl = Column(data=self.fl,name=str('fl'))
cer = Column(data=self.er,name=str('er'))
cco = Column(data=self.co,name=str('co'))
sp = Table()
#pdb.set_trace()
sp.add_columns([cwa,cfl,cer,cco])
sp.write(filename, format='fits',overwrite=True)
# Save filename
if self.filename is None:
self.filename = filename
def read(filename, comment='#', debug=False):
"""
Reads in QSO spectrum given a filename. Returns a Spectrum class
object:
Parameters
----------
filename : str
comment : str ('#')
String that marks beginning of comment line, only used when
reading in ascii files
"""
if filename.endswith('.gz'):
import gzip
fh = gzip.open(filename, 'rb')
else:
fh = open(filename, 'rb')
test = next(fh)
fh.close()
if test[:20].decode('utf-8')[8] != '=':
# Then probably not a fits file
fwhm = None
skip = 0
test = test.decode('utf-8').split()
try:
name = test[0].strip()
except IndexError:
pass
else:
if name.upper() == 'RESVEL':
fwhm = float(test[1])
skip = 1
try: # uves_popler .dat file
wa,fl,er,co = loadtxt(filename, usecols=(0,1,2,4), unpack=True,
comments=comment, skiprows=skip)
except IndexError:
try:
wa,fl,er,co = loadtxt(filename, usecols=(0,1,2,3),
unpack=True, comments=comment,
skiprows=skip)
except IndexError:
try:
wa,fl,er = loadtxt(filename, usecols=(0,1,2),
unpack=True, comments=comment,
skiprows=skip)
except IndexError:
wa,fl = loadtxt(filename, usecols=(0,1),
unpack=True, comments=comment,
skiprows=skip)
er = find_err(fl, find_cont(fl))
co = None
else:
# heuristic to check for Jill Bechtold's FOS spectra
if filename.endswith('.XY'):
wa,fl,er,co = loadtxt(
filename, usecols=(0,1,2,3), unpack=True, comments=comment,
skiprows=skip)
else:
fl *= co
er *= co
if wa[0] > wa[-1]:
wa = wa[::-1]; fl = fl[::-1];
if er is not None: er = er[::-1]
if co is not None: co = co[::-1]
sp = Spectrum(wa=wa, fl=fl, er=er, co=co, filename=filename, fwhm=fwhm)
return sp
# Otherwise assume fits file
f = fits.open(filename)
hd = f[0].header
#import pdb; pdb.set_trace()
if str('CTYPE1') in hd and ('_f.fits' in filename.lower() or
'_xf.fits' in filename.lower()):
# ESI, HIRES, etc. from XIDL
dontscale = (True if str('BZERO') in hd else False)
if hd['CTYPE1'] == 'LINEAR':
wa = getwave(hd)
fl = fits.getdata(filename, do_not_scale_image_data=dontscale)
if 'F.fits' in filename:
n = filename.replace('F.fits','E.fits')
else:
n = filename.replace('f.fits','e.fits')
er = fits.getdata(n, do_not_scale_image_data=dontscale)
return Spectrum(wa=wa, fl=fl, er=er, filename=filename)
if str('TELESCOP') in hd and str('FLAVOR') in hd:
if hd[str('TELESCOP')] == 'SDSS 2.5-M' and \
hd[str('flavor')] == 'science':
d = f[1].data
if 'loglam' in d.dtype.names:
wa = 10**d[str('loglam')]
fl = d[str('flux')]
er = 1 / np.sqrt(d[str('ivar')])
co = d[str('model')]
return Spectrum(wa=wa, fl=fl, er=er, co=co, filename=filename)
# try record array
try:
data = f[1].data
except IndexError:
pass
else:
good = False
names = data.dtype.names
if 'wa' in names and 'fl' in names:
wa = data.wa
fl = data.fl
good = True
elif 'wavelength' in names and 'flux' in names:
wa = data.wavelength
fl = data.flux
good = True
if good:
er = np.ones_like(fl)
try:
er = data.er
except AttributeError:
pass
co = np.empty_like(fl) * np.nan
try:
co = data.co
except AttributeError:
pass
return Spectrum(wa=wa, fl=fl, er=er, co=co, filename=filename)
##################################################################
# First generate the wavelength scale. Look for CTYPE, CRVAL,
# CDELT header cards. Then read in flux values, and look for
# cont and error axes/files.
##################################################################
#naxis1 = hd['NAXIS1'] # 1st axis length (no. data points)
# pixel stepsize
cdelt = get_cdelt(hd)
if cdelt is None:
# Read Songaila's spectra
wa = f[0].data[0]
fl = f[0].data[1]
npts = len(fl)
try:
er = f[0].data[2]
if len(er[er > 0] < 0.5 * npts):
er = f[0].data[3]
except IndexError:
i = int(npts * 0.75)
er = np.ones(npts) * np.std(fl[i-50:i+50])
f.close()
return Spectrum(wa=wa, fl=fl, er=er, filename=filename)
##########################################################
# Check if SDSS spectrum
##########################################################
if str('TELESCOP') in hd:
if hd[str('TELESCOP')] == 'SDSS 2.5-M': # then Sloan spectrum
data = f[0].data
fl = data[0]
er = data[2]
f.close()
return Spectrum(fl=fl, er=er, filename=filename, CDELT=cdelt,
CRVAL=hd[str('CRVAL1')],
CRPIX=hd[str('CRPIX1')])
##########################################################
# Check if HIRES spectrum
##########################################################
if str('INSTRUME') in hd: # Check if Keck spectrum
if hd[str('INSTRUME')].startswith('HIRES'):
if debug: print('Looks like Makee output format')
fl = f[0].data # Flux
f.close()
errname = filename[0:filename.rfind('.fits')] + 'e.fits'
try:
er = fits.getdata(errname)
except IOError:
er = np.ones(len(fl))
return Spectrum(fl=fl, er=er, filename=filename, CDELT=cdelt,
CRVAL=hd[str('CRVAL1')], CRPIX=hd[str('CRPIX1')])
##########################################################
# Check if UVES_popler output
##########################################################
history = hd['HISTORY']
for row in history:
if 'UVES POst Pipeline Echelle Reduction' in row:
return parse_UVES_popler(filename)
data = f[0].data
fl = data[0]
er = data[2]
f.close()
if str('CRPIX1') in hd:
crpix = hd[str('CRPIX1')]
else:
crpix = 1
return Spectrum(fl=fl, er=er, filename=filename, CDELT=cdelt,
CRVAL=hd[str('CRVAL1')], CRPIX=crpix)
#raise Exception('Unknown file format')
def rebin_simple(wa, fl, er, co, n):
""" Bins up the spectrum by averaging the values of every n
pixels. Not very accurate, but much faster than rebin().
"""
remain = -(len(wa) % n) or None
wa = wa[:remain].reshape(-1, n)
fl = fl[:remain].reshape(-1, n)
er = er[:remain].reshape(-1, n)
co = co[:remain].reshape(-1, n)
n = float(n)
wa = np.nansum(wa, axis=1) / n
co = np.nansum(co, axis=1) / n
er = np.nansum(er, axis=1) / n / sqrt(n)
fl = np.nansum(fl, axis=1) / n
return Spectrum(wa=wa, fl=fl, er=er, co=co)
def rebin(wav, fl, er, **kwargs):
""" Rebins spectrum to a new wavelength scale generated using the
keyword parameters.
Returns the rebinned spectrum.
Accepts the same keywords as Spectrum.__init__() (see that
docstring for a description of those keywords)
Will probably get the flux and errors for the first and last pixel
of the rebinned spectrum wrong.
General pointers about rebinning if you care about errors in the
rebinned values:
1. Don't rebin to a smaller bin size.
2. Be aware when you rebin you introduce correlations between
neighbouring points and between their errors.
3. Rebin as few times as possible.
"""
# Note: 0 suffix indicates the old spectrum, 1 the rebinned spectrum.
colors= 'brgy'
debug = kwargs.pop('debug', False)
# Create rebinned spectrum wavelength scale
sp1 = Spectrum(**kwargs)
# find pixel edges, used when rebinning
edges0 = find_bin_edges(wav)
edges1 = find_bin_edges(sp1.wa)
if debug:
pl.clf()
x0,x1 = edges1[0:2]
yh, = pl.bar(x0, 0, width=(x1-x0),color='gray',
linestyle='dotted',alpha=0.3)
widths0 = edges0[1:] - edges0[:-1]
npts0 = len(wav)
npts1 = len(sp1.wa)
df = 0.
de2 = 0.
npix = 0 # number of old pixels contributing to rebinned pixel,
j = 0 # index of rebinned array
i = 0 # index of old array
# sanity check
if edges0[-1] < edges1[0] or edges1[-1] < edges0[0]:
raise ValueError('Wavelength scales do not overlap!')
# find the first contributing old pixel to the rebinned spectrum
if edges0[i+1] < edges1[0]:
# Old wa scale extends lower than the rebinned scale. Find the
# first old pixel that overlaps with rebinned scale.
while edges0[i+1] < edges1[0]:
i += 1
i -= 1
elif edges0[0] > edges1[j+1]:
# New rebinned wa scale extends lower than the old scale. Find
# the first rebinned pixel that overlaps with the old spectrum
while edges0[0] > edges1[j+1]:
sp1.fl[j] = np.nan
sp1.er[j] = np.nan
j += 1
j -= 1
lo0 = edges0[i] # low edge of contr. (sub-)pixel in old scale
while True:
hi0 = edges0[i+1] # upper edge of contr. (sub-)pixel in old scale
hi1 = edges1[j+1] # upper edge of jth pixel in rebinned scale
if hi0 < hi1:
if er[i] > 0:
dpix = (hi0 - lo0) / widths0[i]
df += fl[i] * dpix
# We don't square dpix below, since this causes an
# artificial variation in the rebinned errors depending on
# how the old wav bins are divided up into the rebinned
# wav bins.
#
# i.e. 0.25**2 + 0.75**2 != 0.5**2 + 0.5**2 != 1**2
de2 += er[i]**2 * dpix
npix += dpix
if debug:
yh.set_height(df/npix)
c0 = colors[i % len(colors)]
pl.bar(lo0, fl[i], width=hi0-lo0, color=c0, alpha=0.3)
pl.text(lo0, fl[i], 'lo0')
pl.text(hi0, fl[i], 'hi0')
pl.text(hi1, fl[i], 'hi1')
raw_input('enter...')
lo0 = hi0
i += 1
if i == npts0: break
else:
# We have all old pixel flux values that contribute to the
# new pixel; append the new flux value and move to the
# next new pixel.
if er[i] > 0:
dpix = (hi1 - lo0) / widths0[i]
df += fl[i] * dpix
de2 += er[i]**2 * dpix
npix += dpix
if debug:
yh.set_height(df/npix)
c0 = colors[i % len(colors)]
pl.bar(lo0, fl[i], width=hi1-lo0, color=c0, alpha=0.3)
pl.text(lo0, fl[i], 'lo0')
pl.text(hi0, fl[i], 'hi0')
pl.text(hi1, fl[i], 'hi1')
raw_input('df, de2, npix: %s %s %s enter...' %
(df, de2, npix))
if npix > 0:
# find total flux and error, then divide by number of
# pixels (i.e. conserve flux density).
sp1.fl[j] = df / npix
sp1.er[j] = sqrt(de2) / npix
else:
sp1.fl[j] = np.nan
sp1.er[j] = np.nan
df = 0.
de2 = 0.
npix = 0.
lo0 = hi1
j += 1
if j == npts1: break
if debug:
x0,x1 = edges1[j:j+2]
yh, = pl.bar(x0, 0, width=x1-x0, color='gray',
linestyle='dotted', alpha=0.3)
raw_input('enter...')
return sp1
def combine(spectra, cliphi=None, cliplo=None, verbose=False):
""" Combine spectra pixel by pixel, weighting by the inverse variance
of each pixel. Clip high sigma values by sigma times clip values
Returns the combined spectrum.
If the wavelength scales of the input spectra differ, combine()
will rebin the spectra to a common linear (not log-linear)
wavelength scale, with pixel width equal to the largest pixel
width in the input spectra. If this is not what you want, rebin
the spectra by hand with rebin() before using combine().
"""
def clip(cliphi, cliplo, s_rebinned):
# clip the rebinned input spectra
# find pixels where we can clip: where we have at least three
# good contributing values.
goodpix = np.zeros(len(s_rebinned[0].wa))
for s in s_rebinned:
goodpix += (s.er > 0).astype(int)
canclip = goodpix > 2
# find median values
medfl = np.median([s.fl[canclip] for s in s_rebinned], axis=0)
nclipped = 0
for i,s in enumerate(s_rebinned):
fl = s.fl[canclip]
er = s.er[canclip]
diff = (fl - medfl) / er
if cliphi is not None:
badpix = diff > cliphi
s_rebinned[i].er[canclip][badpix] = np.nan
nclipped += len(badpix.nonzero()[0])
if cliplo is not None:
badpix = diff < -cliplo
s_rebinned[i].er[canclip][badpix] = np.nan
nclipped += len(badpix.nonzero()[0])
if debug: print(nclipped, 'pixels clipped across all input spectra')
return nclipped
nspectra = len(spectra)
if verbose:
print('%s spectra to combine' % nspectra)
if nspectra < 2:
raise Exception('Need at least 2 spectra to combine.')
if cliphi is not None and nspectra < 3: cliphi = None
if cliplo is not None and nspectra < 3: cliplo = None
# Check if wavescales are the same:
spec0 = spectra[0]
wa = spec0.wa
npts = len(wa)
needrebin = True
for sp in spectra:
if len(sp.wa) != npts:
if verbose: print('Rebin required')
break
if (np.abs(sp.wa - wa) / wa[0]).max() > 1e-8:
if verbose:
print((np.abs(sp.wa - wa) / wa[0]).max(), 'Rebin required')
break
else:
needrebin = False
if verbose: print('No rebin required')
# interpolate over 1 sigma error arrays
if needrebin:
# Make wavelength scale for combined spectrum. Only linear for now.
wstart = min(sp.wa[0] for sp in spectra)
wend = max(sp.wa[-1] for sp in spectra)
# Choose largest wavelength bin size of old spectra.
if verbose: print('finding new bin size')
maxwidth = max((sp.wa[1:] - sp.wa[:-1]).max() for sp in spectra)
npts = int(np.ceil((wend - wstart) / maxwidth)) # round up
# rebin spectra to combined wavelength scale
if verbose: print('Rebinning spectra')
s_rebinned = [s.rebin(wstart=wstart, npts=npts, dw=maxwidth)
for s in spectra]
combined = Spectrum(wstart=wstart, npts=npts, dw=maxwidth)
if verbose:
print('New wavelength scale wstart=%s, wend=%s, npts=%s, dw=%s'
% (wstart, combined.wa[-1], npts, maxwidth))
else:
combined = Spectrum(wa=spec0.wa)
s_rebinned = copy.deepcopy(spectra)
# sigma clipping, if requested
if cliphi is not None or cliplo is not None:
clip(cliphi, cliplo, s_rebinned)
# repeat, clipping to 4 sigma this time
#npixclipped = clip(4.,4.,s_rebinned)
# Now add the spectra
for i in xrange(len(combined.wa)):
wtot = fltot = ertot = 0.
npix = 0 # num of old spectrum pixels contributing to new
for s in s_rebinned:
# if not a sensible flux value, skip to the next pixel
if s.er[i] > 0:
npix += 1
# Weighted mean (weight by inverse variance)
variance = s.er[i] ** 2
w = 1. / variance
fltot += s.fl[i] * w
ertot += (s.er[i] * w)**2
wtot += w
if npix > 0:
combined.fl[i] = fltot / wtot
combined.er[i] = np.sqrt(ertot) / wtot
else:
combined.fl[i] = np.nan
combined.er[i] = np.nan
#contributing.fl[i] = npix_contrib
return combined
def cr_reject(flux, error, nsigma=15.0, npix=2, verbose=False):
""" Given flux and errors, rejects cosmic-ray type or dead
pixels. These are defined as pixels that are more than
nsigma*sigma above or below the median of the npixEL pixels on
either side.
Returns newflux,newerror where the rejected pixels have been
replaced by the median value of npix to either side, and the
error has been set to NaN.
The default values work ok for S/N~20, Resolution=500 spectra.
"""
if verbose: print(nsigma,npix)
flux,error = list(flux), list(error) # make copies
i1 = npix
i2 = len(flux) - npix
for i in range(i1, i2):
# make a list of flux values used to find the median
fl = flux[i-npix:i] + flux[i+1:i+1+npix]
er = error[i-npix:i] + error[i+1:i+1+npix]
fl = [f for f,e in zip(fl,er) if e > 0]
er = [e for e in er if e > 0]
medfl = np.median(fl)
meder = np.median(er)
if np.abs((flux[i] - medfl) / meder) > nsigma:
flux[i] = medfl
error[i] = np.nan
if verbose: print(len(fl), len(er))
return np.array(flux), np.array(error)
def cr_reject2(fl, er, nsig=10.0, fwhm=2, grow=1, debug=True):
""" interpolate across features that have widths smaller than the
expected fwhm resolution.
Parameters
----------
fwhm: int
Resolution fwhm in pixels
fl : array of floats, shape (N,)
Flux
er : array of floats, shape (N,)
Error
Returns the interpolated flux and error arrays.
"""
fl, er = (np.array(a, dtype=float) for a in (fl, er))
# interpolate over bad pixels
fl1 = convolve_psf(fl, fwhm)
ibad = np.where(np.abs(fl1 - fl) > nsig*er)[0]
if debug: print(len(ibad))
extras1 = np.concatenate([ibad + 1 + i for i in range(grow)])
extras2 = np.concatenate([ibad - 1 - i for i in range(grow)])
ibad = np.union1d(ibad, np.union1d(extras1, extras2))
ibad = ibad[(ibad > -1) & (ibad < len(fl))]
igood = np.setdiff1d(np.arange(len(fl1)), ibad)
fl[ibad] = np.interp(ibad, igood, fl[igood])
er[ibad] = np.nan
return fl,er
def scalemult(w0, f0, e0, w1, f1, e1, mask=None):
""" find the constant to multipy f1 by so its median will match
f0 where they overlap in wavelength.
Errors are just used to identify bad pixels, if they are all > 0
they are ignored.
`mask` is optional, and of the form `[(3500,4500), (4650,4680)]`
to mask wavelengths from 3500 to 4500 Ang, and 4650 to 4680 Ang
for example.
"""
w0,f0,e0,w1,f1,e1 = map(np.asarray, [w0,f0,e0,w1,f1,e1])
masked0 = np.zeros(len(w0), bool)
masked1 = np.zeros(len(w0), bool)
if mask is not None:
for wmin,wmax in mask:
masked0 |= (wmin < w0) & (w0 < wmax)
masked1 |= (wmin < w1) & (w1 < wmax)
wmin = max(w0.min(), w1.min())
wmax = min(w0.max(), w1.max())
good0 = (e0 > 0) & ~np.isnan(f0) & ~masked0 & (wmin < w0) & (w0 < wmax)
good1 = (e1 > 0) & ~np.isnan(f1) & ~masked1 & (wmin < w1) & (w1 < wmax)
if good0.sum() < 3 or good1.sum() < 3:
raise ValueError('Too few good pixels to use for scaling')
med0 = np.median(f0[good0])
med1 = np.median(f1[good1])
if not (med0 > 0) or not (med1 > 0):
raise ValueError('bad medians:', med0, med1)
return med0 / med1
def scale_overlap(w0, f0, e0, w1, f1, e1):
""" Scale two spectra to match where they overlap. Assumes
spectrum 0 covers a lower wavelength range than spectrum 1. If no
good regions overlap, regions close to the overlap are searched
for.
Parameters
----------
w0, f0, e0 : arrays of shape (M,)
Wavelength, flux and 1 sigma error for spectrum 0.
w1, f1, e1 : arrays of shape (N,)
Wavelength, flux and 1 sigma error for spectrum 1.
Returns
-------
scale_factor, i0, i1 : float, int, int
Multiply spectrum 1 by scale_factor to match spectrum 0. i0
gives the index into spectrum 0 where the overlapping region
starts, i1 gives the index into spectrum 1where the overlap
ends.
"""
# find overlapping regions
dtype = [(str('wa'), str('f8')),
(str('fl'), str('f8')),
(str('er'), str('f8'))]
sp0 = np.rec.fromarrays([w0,f0,e0], dtype=dtype)
sp1 = np.rec.fromarrays([w1,f1,e1], dtype=dtype)
if sp0.wa.max() < sp1.wa.min():
print('No overlap! Matching medians of closest half spectra')
good0 = (sp0.er > 0) & ~np.isnan(sp0.fl) & (sp0.fl > 2*sp0.er) & \
(sp0.wa > 0.5*(sp0.wa.max() + sp0.wa.min()))
good1 = (sp1.er > 0) & ~np.isnan(sp1.fl) & (sp1.fl > 2*sp1.er) & \
(sp1.wa < 0.5*(sp1.wa.max() + sp1.wa.min()))
if good0.sum() and good1.sum():
m0, m1 = np.median(sp0.fl[good0]), np.median(sp1.fl[good1])
return m0 / m1, 0, len(sp1)-1
else:
return 1, 0, len(sp1)-1
# find first overlapping good pixel
i = 0
while not (sp1.er[i] > 0): i += 1
i0min = sp0.wa.searchsorted(sp1.wa[i])
i = -1
while not (sp0.er[i] > 0): i -= 1
i1max = sp1.wa.searchsorted(sp0.wa[i])
#print(sp0.wa[i0min], sp1.wa[i1max])
while True:
s0 = sp0[i0min:]
s1 = sp1[:i1max]
# don't want saturated pixels...
good0 = (s0.er > 0) & ~np.isnan(s0.fl) & (s0.fl > 2*s0.er)
good1 = (s1.er > 0) & ~np.isnan(s1.fl) & (s1.fl > 2*s1.er)
if good0.sum() == 0 or good1.sum() == 0:
i0min = i0min - (len(sp0) - i0min)
i1max = 2 * i1max
if i0min < 0 or i1max > len(sp1)-1:
raise ValueError('No good pixels to use for scaling')
continue
m0, m1 = np.median(s0.fl[good0]), np.median(s1.fl[good1])
if m0 <= 0:
print('looping')
i0min = max(0, i0min - (len(sp0) - i0min))
elif m1 <= 0:
print('looping')
i1max = min(len(sp1)-1, 2 * i1max)
else:
break
#print(m0, m1)
return m0 / m1, i0min, i1max-1
def plotlines(z, ax, atmos=None, lines=None, labels=False, ls='dotted',
color='k', lcolor='k', trim=False, fontsize=10,
offsets=True, **kwargs):
""" Draw vertical dotted lines showing expected positions of
absorption and emission lines, given a redshift.
Parameters
----------
atmos : list of float pairs, or True (None)
Regions of atmospheric absorption to plot. If True, it uses an
internal list of regions.
lines : stuctured array, optional
If given, it must be a record array with fields 'name' and 'wa'.
Returns the mpl artists representing the lines.
"""
if lines is None:
lines = readtxt(DATAPATH + 'linelists/galaxy_lines', names='wa,name,select')
else:
lines = np.rec.fromrecords([(l['name'], l['wa']) for l in lines],
names=str('name,wa'))
autoscale = ax.get_autoscale_on()
if autoscale:
ax.set_autoscale_on(False)
artists = {'labels' : []}
w0,w1 = ax.get_xlim()
wa = lines.wa * (z+1)
if trim:
c0 = between(wa,w0,w1)
wa = wa[c0]
lines = lines[c0]
artists['lines'] = []
artists['lines'].append(axvlines(wa, ax=ax, ls=ls, color=color, **kwargs))
if labels:
for i in range(3):
for w,l in zip(wa[i::3], lines[i::3]):
if not (w0 < w < w1) and trim:
continue
#name = l.name + '%.2f' % l.wa
name = l.name
off = (0.7 + i*0.08 if offsets else 0.9)
artists['labels'].append(puttext(
w, off, name, ax,
xcoord='data', alpha=1, fontsize=fontsize,
rotation=90, ha='right', color=lcolor))
artists['atmos'] = []
if atmos:
if atmos == True:
atmos = None
artists['atmos'].extend(plotatmos(ax, atmos=atmos))
if autoscale:
ax.set_autoscale_on(True)
return artists
def plotatmos(ax, atmos=None, color='y'):
""" Plot rough areas where atmospheric absorption is expected.
"""
autoscale = ax.get_autoscale_on()
if autoscale:
ax.set_autoscale_on(False)
if atmos is None:
atmos = [(5570, 5590),
(5885, 5900),
(6275, 6325),
(6870, 6950),
(7170, 7350),
(7580, 7690),
(8130, 8350),
(8900, 9200),
(9300, 9850),
(11100, 11620),
(12590, 12790),
(13035, 15110),
(17435, 20850),
(24150, 24800)]
artists = axvfill(atmos, ax=ax, color=color, alpha=0.15)
if autoscale:
ax.set_autoscale_on(True)
return artists
def plot(w, f=None, e=None, c=None, ax=None, show=True, yperc=0.98, alpha=0.8,
linewidth=1., linestyle='steps-mid', flcolor='blue', cocolor='red'):
""" Plots spectrum.
Returns the matplotlib artists that represent the flux, error
and continuum curves.
Can also give a single argument that is a record array with fields
wa, fl and optionally er, co.
>>> from utilities import get_data_path
>>> sp = read(get_data_path() + 'tests/spSpec-52017-0516-139.fit.gz')
>>> lines = plot(sp.wa, sp.fl, sp.er, sp.co, show=False)
"""
witherr = True
if f is None and e is None and c is None:
rec = w
w = rec.wa
f = rec.fl
try:
e = rec.er
except AttributeError:
e = w * np.nan
witherr = False
try:
c = rec.co
except AttributeError:
c = w * np.nan
elif e is None:
e = w * np.nan
witherr = False
elif c is None:
c = w * np.nan
if ax is None:
fig = pl.figure(figsize=(10,5))
fig.subplots_adjust(left=0.08, right=0.94)
ax = pl.gca()
artists = []
# check we have something to plot...
good = ~np.isnan(f)
if witherr:
good &= (e > 0)
if np.any(good):
artists.extend(ax.plot(w, f, lw=linewidth, color=flcolor,
alpha=alpha, ls=linestyle))
if witherr:
artists.extend(ax.plot(w, e, lw=linewidth, color=flcolor,
alpha=alpha, ls='dashed'))
artists.extend(ax.plot(w, c, lw=linewidth, color=cocolor,
alpha=alpha))
# plotting limits
f = f[good]
ymax = 1.5 * np.percentile(f, 100*yperc)
if witherr:
e = e[good]
ymin = min(-0.1 * np.median(f), -1.0 * np.median(e))
else:
ymin = -abs(0.1*ymax)
if ymax < ymin:
ymax = 2. * abs(ymin)
ax.axis((w.min(), w.max(), ymin, ymax))
ax.axhline(y=0., color='k', alpha=alpha)
if show:
pl.show()
return artists
def writesp(filename, sp, resvel=None, overwrite=False):
""" Writes out a spectrum, as ascii for now - wavelength, flux,
error, continuum.
sp must have attributes wa, fl, er and optionally co.
Keyword overwrite can be True or False.
resvel means the instrumental fwhm in km/s will be written on the
first line (VPFIT style).
"""
if os.path.lexists(filename) and not overwrite:
c = raw_input('File %s exists - overwrite? (y) or n: ' % filename)
if c != '':
if c.strip().lower()[0] == 'n':
print('returning without writing anything...')
return
fh = open(filename, 'w')
if resvel is not None:
fh.write('RESVEL %.2f' % resvel)
fl = np.nan_to_num(sp.fl)
er = np.nan_to_num(sp.er)
if not hasattr(sp, 'co') or np.all(np.isnan(sp.co)):
for w,f,e in zip(sp.wa, fl, er):
fh.write("% .12g % #12.8g % #12.8g\n" % (w,f,e))
else:
co = np.nan_to_num(sp.co)
for w,f,e,c in zip(sp.wa, fl, er, co):
fh.write("% .12g % #12.8g % #12.8g % #12.8g\n" % (w,f,e,c))
fh.close()
def find_cont(fl, fwhm1=300, fwhm2=200, nchunks=4, nsiglo=2, nsighi=3):
""" Given the flux, estimate the continuum. fwhm values are
smoothing lengths.
"""
# smooth flux, with smoothing length much longer than expected
# emission line widths.
fl = nan2num(fl.astype(float), replace='mean')
if len(fl) < 3*fwhm1:
fwhm1 = len(fl) / 3.
warnings.warn('Reducing fwhm1 to %i pixels' % fwhm1)
if len(fl) < 3*fwhm2:
fwhm2 = len(fl) / 3.
warnings.warn('Reducing fwhm2 to %i pixels' % fwhm2)
co = convolve_psf(fl, fwhm1, edge=10)
npts = len(fl)
indices = np.arange(npts)
# throw away top and bottom 2% of data that deviates from
# continuum and re-fit new continuum. Go chunk by chunk so that
# points are thrown away evenly across the spectrum.
c0 = co <= 0.
co[c0] = co[~c0].mean()
nfl = fl / co
step = npts // nchunks + 1
ind = range(0, npts, step) + [npts]
#igood = []
good = np.ones(len(nfl), dtype=bool)
for i0,i1 in zip(ind[:-1], ind[1:]):
c0 = remove_outliers(nfl[i0:i1], nsiglo, nsighi)
good[i0:i1] &= c0
#isort = nfl[i0:i1].argsort()
#len_isort = len(isort)
#j0,j1 = int(0.05 * len_isort), int(0.95 * len_isort)
#igood.extend(isort[j0:j1]+i0)
#good = np.in1d(indices, igood)
sfl = fl.copy()
sfl[~good] = np.interp(indices[~good], indices[good], sfl[good])
co = convolve_psf(sfl, fwhm2, edge=10)
return co
def find_err(fl, co, nchunks=10):
""" Given a continuum and flux array, return a very rough estimate
of the error.
"""
rms = []
midpoints = []
npts = len(fl)
step = npts // nchunks + 1
indices = range(0, npts, step) + [npts]
for i,j in zip(indices[:-1], indices[1:]):
#print(i,j)
imid = int(0.5 * (i + j))
midpoints.append(imid)
df = fl[i:j] - co[i:j]
n = len(df)
# throw away top and bottom 5% of these
df = np.sort(df)[int(0.05*n):int(0.95*n)]
rms.append(df.std())
er = np.interp(np.arange(len(fl)), midpoints, rms)
return er
def pca_qso_cont(nspec, seed=None, return_weights=False):
""" Make qso continua using the PCA and weights from N. Suzuki et
al. 2005 and N. Suzuki 2006.
Parameters
----------
nspec : int
Number of spectra to create
Returns
-------
wavelength (shape N), array of spectra [shape (nspec, N)]
Memory use might be prohibitive for nspec > ~1e4.
"""
# read the principle components
filename = DATAPATH + '/PCAcont/Suzuki05/tab3.txt'
names = 'wa,mu,musig,e1,e2,e3,e4,e5,e6,e7,e8,e9,e10'
co = readtxt(filename, skip=23, names=names)
# use only the first 7 eigenvetors
eig = [co['e%i' % i] for i in range(1,8)]
# from Suzuki et al 2006.
csig = np.array([7.563, 3.604, 2.351, 2.148, 1.586,
1.479, 1.137]) #, 0.778, 0.735, 0.673])
# generate weights for each eigenvector
if seed is not None: np.random.seed(seed)
weights = []
for sig in csig:
temp = np.random.randn(2*nspec)
# make sure we don't have any very large deviations from the mean
temp = temp[np.abs(temp) < 3][:nspec]
assert len(temp) == nspec
weights.append(temp * sig)
# generate nspec continua. loop over pixels
sp = []
for i in range(len(co.wa)):
sp.append(co.mu[i] + np.sum(w*e[i] for w,e in zip(weights, eig)))
sp = np.transpose(sp)
if return_weights:
return co.wa, sp, weights
else:
return co.wa, sp
def vac2air_Ciddor(vacw):
""" Convert vacuum wavelengths in Angstroms to air wavelengths.
This uses the relation from Ciddor 1996, Applied Optics LP,
vol. 35, Issue 9, p.1566. Only valid for wavelengths > 2000 Ang.
"""
vacw = np.atleast_1d(vacw)
k0 = 238.0185
k1 = 1e-8 * 5792105.
k2 = 57.362
k3 = 1e-8 * 167917.
s2 = (1e4 / vacw)**2
n = 1 + k1/(k0 - s2) + k3/(k2 - s2)
airw = vacw / n
if len(airw) == 1:
return airw[0]
return airw
def vac2air_Morton(vacw):
""" Convert vacuum wavelengths in Angstroms to air wavelengths.
This uses the relation from Morton 1991, ApJS, 77, 119. Only valid
for wavelengths > 2000 Ang. Use this for compatibility with older
spectra that may have been corrected using the (older) Morton
relation. The Ciddor relation used in vac2air_Ciddor() is claimed
to be more accurate at IR wavelengths.
"""
vacw = np.atleast_1d(vacw)
temp = (1e4 / vacw) ** 2
airw = 1. / (1. + 6.4328e-5 + 2.94981e-2/(146 - temp) +
2.5540e-4/(41 - temp)) * vacw
if len(airw) == 1:
return airw[0]
return airw
def air2vac_Morton(airw):
""" Convert air wavelengths in Angstroms to vacuum wavelengths.
Uses linear interpolation of the inverse transformation for
vac2air_Morton. The fractional error (wa - watrue) / watrue
introduced by interpolation is < 1e-9.
Only valid for wa > 2000 Angstroms.
"""
airw = np.atleast_1d(airw)
if (np.diff(airw) < 0).any(): raise ValueError('Wavelengths must be sorted lowest to highest')
if airw[0] < 2000:
raise ValueError('Only valid for wavelengths > 2000 Angstroms')
dwmax = abs(vac2air_Morton(airw[-1]) - airw[-1]) + 10
dwmin = abs(vac2air_Morton(airw[0]) - airw[0]) + 10
testvac = np.arange(airw[0] - dwmin, airw[-1] + dwmax, 2)
testair = vac2air_Morton(testvac)
vacw = np.interp(airw, testair, testvac)
if len(vacw) == 1:
return vacw[0]
return vacw
def air2vac_Ciddor(airw):
""" Convert air wavelengths in Angstroms to vacuum wavelengths.
Uses linear interpolation of the inverse transformation for
vac2air_Ciddor. The fractional error (wa - watrue) / watrue
introduced by interpolation is < 1e-9.
Only valid for wa > 2000 Angstroms.
"""
airw = np.atleast_1d(airw)
if (np.diff(airw) < 0).any():
raise ValueError('Wavelengths must be sorted lowest to highest')
if airw[0] < 2000:
raise ValueError('Only valid for wavelengths > 2000 Angstroms')
dwmax = abs(vac2air_Ciddor(airw[-1]) - airw[-1]) + 10
dwmin = abs(vac2air_Ciddor(airw[0]) - airw[0]) + 10
testvac = np.arange(airw[0] - dwmin, airw[-1] + dwmax, 2)
testair = vac2air_Ciddor(testvac)
vacw = np.interp(airw, testair, testvac)
if len(vacw) == 1:
return vacw[0]
return vacw
def resamplespec(wa1, wa, fl, oversamp=100):
"""
Resample a spectrum while conserving flux density.
Parameters
----------
wa1 : sequence
new wavelength grid (i.e., center wavelength of each pixel)
wa : sequence
old wavelength grid (i.e., center wavelength of each pixel)
fl : sequence
old spectrum (e.g. flux density or photon counts)
oversamp : int
The factor by which to oversample input spectrum prior to
rebinning. The worst fractional precision one achieves is
roughly 1./oversamp.
References
----------
Originally written by Ian Crossfield.
"""
wa_oversamp = np.linspace(wa[0], wa[-1], len(wa) * oversamp)
fl_oversamp = np.interp(wa_oversamp, wa, fl) / oversamp
# Set up the bin edges for down-binning
wbin_edges = find_bin_edges(wa1)
# Bin down the interpolated spectrum
indices = wa_oversamp.searchsorted(wbin_edges)
fl_resampled = []
for i0,i1 in zip(indices[:-1], indices[1:]):
fl_resampled.append(fl_oversamp[i0:i1].sum())
return np.array(fl_resampled)
| mit |
Arcanewinds/FDL-LunarResources | CraterDetection/Hough/houghDetector.py | 1 | 2080 | #Written by Timothy Seabrook
#[email protected]
#This script is really slow for large images, so should only be executed on those resampled.
#This is a first baseline circle detector to identify the limitations of convential techniques for crater detection.
import numpy as np
import matplotlib.pyplot as plt
import os, glob
from osgeo import gdal
from skimage import color
from skimage.transform import hough_circle, hough_circle_peaks
from skimage.draw import circle_perimeter
from skimage.feature import canny
thisDir = os.path.dirname(os.path.abspath(__file__))
rootDir = os.path.join(thisDir, os.pardir, os.pardir)
dataDir = os.path.join(rootDir, 'Data')
NACDir = os.path.join(dataDir, 'LROC_NAC', 'South_Pole', 'Resampled')
pos_file_names = glob.glob(os.path.join(NACDir,'*.tif'))
for i in range (np.minimum(pos_file_names.__len__, 10)):
filename = pos_file_names[i]
# Load picture and detect edges
ds = gdal.Open(filename)
image = ds.GetRasterBand(1).ReadAsArray()
if(image is not None):
# Low threshold and High threshold represent number of pixels that may be skipped and max consisting pixels to make a line [4, 60 seems good]
# Sigma represents the width of the gaussian smoothing kernel [3 seems good]
edges = canny(image, sigma=3, low_threshold=4, high_threshold=60)
# Detect two radii
hough_radii = np.arange(20, 100, 2)
hough_res = hough_circle(edges, hough_radii)
# Select the most prominent circles
accums, cx, cy, radii = hough_circle_peaks(hough_res, hough_radii,
total_num_peaks=10)
# Draw them
fig, axarr = plt.subplots(ncols=2, nrows=1, figsize=(10, 4))
image = color.gray2rgb(image)
for center_y, center_x, radius in zip(cy, cx, radii):
circy, circx = circle_perimeter(center_y, center_x, radius)
image[circy, circx] = (220, 20, 20)
axarr[1].imshow(image, cmap=plt.cm.gray)
axarr[0].imshow(edges, cmap=plt.cm.gray)
plt.show() | gpl-3.0 |
vermouthmjl/scikit-learn | examples/linear_model/plot_sgd_comparison.py | 112 | 1819 | """
==================================
Comparing various online solvers
==================================
An example showing how different online solvers perform
on the hand-written digits dataset.
"""
# Author: Rob Zinkov <rob at zinkov dot com>
# License: BSD 3 clause
import numpy as np
import matplotlib.pyplot as plt
from sklearn import datasets
from sklearn.model_selection import train_test_split
from sklearn.linear_model import SGDClassifier, Perceptron
from sklearn.linear_model import PassiveAggressiveClassifier
from sklearn.linear_model import LogisticRegression
heldout = [0.95, 0.90, 0.75, 0.50, 0.01]
rounds = 20
digits = datasets.load_digits()
X, y = digits.data, digits.target
classifiers = [
("SGD", SGDClassifier()),
("ASGD", SGDClassifier(average=True)),
("Perceptron", Perceptron()),
("Passive-Aggressive I", PassiveAggressiveClassifier(loss='hinge',
C=1.0)),
("Passive-Aggressive II", PassiveAggressiveClassifier(loss='squared_hinge',
C=1.0)),
("SAG", LogisticRegression(solver='sag', tol=1e-1, C=1.e4 / X.shape[0]))
]
xx = 1. - np.array(heldout)
for name, clf in classifiers:
print("training %s" % name)
rng = np.random.RandomState(42)
yy = []
for i in heldout:
yy_ = []
for r in range(rounds):
X_train, X_test, y_train, y_test = \
train_test_split(X, y, test_size=i, random_state=rng)
clf.fit(X_train, y_train)
y_pred = clf.predict(X_test)
yy_.append(1 - np.mean(y_pred == y_test))
yy.append(np.mean(yy_))
plt.plot(xx, yy, label=name)
plt.legend(loc="upper right")
plt.xlabel("Proportion train")
plt.ylabel("Test Error Rate")
plt.show()
| bsd-3-clause |
HIPS/optofit | examples/gp_squid_test.py | 1 | 8400 | import numpy as np
seed = np.random.randint(2**16)
# seed = 50431
seed = 58482
print "Seed: ", seed
import matplotlib.pyplot as plt
from matplotlib.patches import Polygon
from optofit.cneuron.compartment import Compartment, SquidCompartment
from optofit.cneuron.channels import LeakChannel, NaChannel, KdrChannel
from optofit.cneuron.simulate import forward_euler
from optofit.cneuron.gpchannel import GPChannel, sigma, GPKdrChannel
from hips.inference.particle_mcmc import *
from optofit.cinference.pmcmc import *
# Set the random seed for reproducibility
np.random.seed(seed)
# Make a simple compartment
hypers = {
'C' : 1.0,
'V0' : -60.0,
'g_leak' : 0.03,
'E_leak' : -65.0}
gp1_hypers = {'D': 2,
'sig' : 1,
'g_gp' : 12.0,
'E_gp' : 50.0,
'alpha_0': 1.0,
'beta_0' : 2.0,
'sigma_kernel': 2.0}
gp2_hypers = {'D' : 1,
'sig' : 1,
'g_gp' : 3.60,
'E_gp' : -77.0,
'alpha_0': 1.0,
'beta_0' : 2.0,
'sigma_kernel': 2.0}
squid_hypers = {
'C' : 1.0,
'V0' : -60.0,
'g_leak' : 0.03,
'E_leak' : -65.0,
'g_na' : 12.0,
# 'g_na' : 0.0,
'E_na' : 50.0,
'g_kdr' : 3.60,
'E_kdr' : -77.0
}
def create_gp_model():
# Add a few channels
body = Compartment(name='body', hypers=hypers)
leak = LeakChannel(name='leak', hypers=hypers)
gp1 = GPChannel(name='gpna', hypers=gp1_hypers)
gp2 = GPKdrChannel(name='gpk', hypers=gp2_hypers)
body.add_child(leak)
body.add_child(gp1)
body.add_child(gp2)
# Initialize the model
D, I = body.initialize_offsets()
return body, gp1, gp2, D, I
def sample_squid_model():
squid_body = SquidCompartment(name='body', hypers=squid_hypers)
# squid_body = Compartment(name='body', hypers=squid_hypers)
# leak = LeakChannel(name='leak', hypers=squid_hypers)
# na = NaChannel(name='na', hypers=squid_hypers)
# kdr = KdrChannel(name='kdr', hypers=squid_hypers)
# squid_body.add_child(leak)
# body.add_child(na)
# squid_body.add_child(kdr)
# Initialize the model
D, I = squid_body.initialize_offsets()
# Set the recording duration
t_start = 0
t_stop = 100.
dt = 0.1
t_ds = 0.1
t = np.arange(t_start, t_stop, dt)
T = len(t)
# Make input with an injected current from 500-600ms
inpt = np.zeros((T, I))
inpt[20/dt:80/dt,:] = 7.
inpt += np.random.randn(T, I)
# Set the initial distribution to be Gaussian around the steady state
z0 = np.zeros(D)
squid_body.steady_state(z0)
init = GaussianInitialDistribution(z0, 0.1**2 * np.eye(D))
# Set the proposal distribution using Hodgkin Huxley dynamics
# TODO: Fix the hack which requires us to know the number of particles
N = 100
sigmas = 0.0001*np.ones(D)
# Set the voltage transition dynamics to be a bit noisier
sigmas[squid_body.x_offset] = 0.25
prop = HodgkinHuxleyProposal(T, N, D, squid_body, sigmas, t, inpt)
# Set the observation model to observe only the voltage
etas = np.ones(1)
observed_dims = np.array([squid_body.x_offset]).astype(np.int32)
lkhd = PartialGaussianLikelihood(observed_dims, etas)
# Initialize the latent state matrix to sample N=1 particle
z = np.zeros((T,N,D))
z[0,0,:] = init.sample()
# Initialize the output matrix
x = np.zeros((T,D))
# Sample the latent state sequence
for i in np.arange(0,T-1):
# The interface kinda sucks. We have to tell it that
# the first particle is always its ancestor
prop.sample_next(z, i, np.array([0], dtype=np.int32))
# Sample observations
for i in np.arange(0,T):
lkhd.sample(z,x,i,0)
# Extract the first (and in this case only) particle
z = z[:,0,:].copy(order='C')
# Downsample
intvl = int(t_ds / dt)
td = t[::intvl].copy('C')
zd = z[::intvl, :].copy('C')
xd = x[::intvl, :].copy('C')
inptd = inpt[::intvl].copy('C')
# Plot the first particle trajectory
plt.ion()
st_axs, _ = squid_body.plot(td, zd, color='k')
# Plot the observed voltage
st_axs[0].plot(td, xd[:,0], 'r')
# plt.plot(t, x[:,0], 'r')
plt.show()
plt.pause(0.01)
return td, zd, xd, inptd, st_axs
def sample_from_model(T,D, init, prop):
z = np.zeros((T,1,D))
z[0,0,:] = init.sample()[:]
# Sample the latent state sequence with the given initial condition
for i in np.arange(0,T-1):
# The interface kinda sucks. We have to tell it that
# the first particle is always its ancestor
prop.sample_next(z, i, np.array([0], dtype=np.int32))
return z[:,0,:]
# Now run the pMCMC inference
def sample_gp_given_true_z(t, x, inpt,
z_squid,
N_particles=100,
axs=None, gp1_ax=None, gp2_ax=None):
dt = np.diff(t)
T,O = x.shape
# Make a model
body, gp1, gp2, D, I = create_gp_model()
# Set the initial distribution to be Gaussian around the steady state
ss = np.zeros(D)
body.steady_state(ss)
init = GaussianInitialDistribution(ss, 0.1**2 * np.eye(D))
# Set the proposal distribution using Hodgkin Huxley dynamics
sigmas = 0.001*np.ones(D)
# Set the voltage transition dynamics to be a bit noisier
sigmas[body.x_offset] = 0.25
prop = HodgkinHuxleyProposal(T, N_particles, D, body, sigmas, t, inpt)
# Set the observation model to observe only the voltage
etas = np.ones(1)
observed_dims = np.array([body.x_offset]).astype(np.int32)
lkhd = PartialGaussianLikelihood(observed_dims, etas)
# Initialize the latent state matrix with the equivalent of the squid latent state
z = np.zeros((T,1,D))
z[:,0,0] = z_squid[:,0] # V = V
m3h = z_squid[:,1]**3 * z_squid[:,2]
m3h = np.clip(m3h, 1e-4,1-1e-4)
z[:,0,1] = np.log(m3h/(1.0-m3h)) # Na open fraction
n4 = z_squid[:,3]**4
n4 = np.clip(n4, 1e-4,1-1e-4)
z[:,0,2] = np.log(n4/(1.0-n4)) # Kdr open fraction
# Prepare the particle Gibbs sampler with the first particle
pf = ParticleGibbsAncestorSampling(T, N_particles, D)
pf.initialize(init, prop, lkhd, x, z[:,0,:].copy('C'))
# Plot the initial state
# gp1_ax, im1, l_gp1 = gp1.plot(ax=gp1_ax, data=z[:,0,:])
gp2_ax, im2, l_gp2 = gp2.plot(ax=gp2_ax, data=z[:,0,:])
axs, lines = body.plot(t, z[:,0,:], color='b', axs=axs)
axs[0].plot(t, x[:,0], 'r')
# Plot a sample from the model
lpred = axs[0].plot(t, sample_from_model(T,D, init, prop)[:,0], 'g')
# Update figures
for i in range(1,4):
plt.figure(i)
plt.pause(0.001)
# Initialize sample outputs
S = 1000
z_smpls = np.zeros((S,T,D))
z_smpls[0,:,:] = z[:,0,:]
for s in range(1,S):
print "Iteration %d" % s
# Reinitialize with the previous particle
# pf.initialize(init, prop, lkhd, x, z_smpls[s-1,:,:])
# Sample a new trajectory given the updated kinetics and the previous sample
# z_smpls[s,:,:] = pf.sample()
z_smpls[s,:,:] = z_smpls[s-1,:,:]
# Resample the GP
gp1.resample(z_smpls[s,:,:], dt)
gp2.resample(z_smpls[s,:,:], dt)
# Resample the conductances
# resample_body(body, t, z_smpls[s,:,:], sigmas[0])
# Plot the sample
body.plot(t, z_smpls[s,:,:], lines=lines)
# gp1.plot(im=im1, l=l_gp1, data=z_smpls[s,:,:])
gp2.plot(im=im2, l=l_gp2, data=z_smpls[s,:,:])
# Sample from the model and plot
lpred[0].set_data(t, sample_from_model(T,D, init,prop)[:,0])
# Update figures
for i in range(1,4):
plt.figure(i)
plt.pause(0.001)
z_mean = z_smpls.mean(axis=0)
z_std = z_smpls.std(axis=0)
z_env = np.zeros((T*2,2))
z_env[:,0] = np.concatenate((t, t[::-1]))
z_env[:,1] = np.concatenate((z_mean[:,0] + z_std[:,0], z_mean[::-1,0] - z_std[::-1,0]))
plt.ioff()
plt.show()
return z_smpls
t, z, x, inpt, st_axs = sample_squid_model()
raw_input("Press enter to being sampling...\n")
sample_gp_given_true_z(t, x, inpt, z, axs=st_axs)
| gpl-2.0 |
haphaeu/yoshimi | fall_factor.py | 1 | 3916 | # -*- coding: utf-8 -*-
"""
Fall Factor
Impact loads on rock climbing gear.
Force = sqrt(2 * m * g * EA * h/Lo)
where:
m: mass of the falling object
g: gravity
EA: stiffness of the rope/sling
h: height of the fall
Lo: length of rope/sling absorbing the impact load
Note that a fall factor can be defined as FF = h/Lo.
by Rafael Rossi, 21.08.2017
"""
import pandas
from matplotlib import pyplot as plt
def dmm_tests():
"""Calibrate slings stiffness based on a batch of tests performed by DMM [1]
[1] http://dmmclimbing.com/knowledge/how-to-break-nylon-dyneema-slings/
"""
tests = [(1, 'Nylon 16mm', '120cm', 12.8),
(2, 'Nylon 16mm', '120cm', 17.6),
(1, 'Dyneema 8mm', '60cm', 17.8),
(1, 'Dyneema 11mm', '60cm', 16.7),
(1, 'Nylon 16mm', '60cm', 11.6),
(1, 'Nylon 26mm', '60cm', 11.8),
(1, 'Static rope 11mm', '60cm', 7.3),
(1, 'Dynamic rope 10mm', '60cm', 5.7),
(2, 'Nylon 16mm', '60cm', 15.4),
(2, 'Nylon 26mm', '60cm', 16.3),
(2, 'Static rope 11mm', '60cm', 10.3),
(2, 'Dynamic rope 10mm', '60cm', 7.4),
(1, 'Dyneema 8mm', '30cm', 14.8),
(1, 'Dyneema 11mm', '30cm', 16.4),
(1, 'Nylon 16mm', '30cm', 10.6),
(1, 'Nylon 26mm', '30cm', 11.0),
(2, 'Dyneema 8mm', '30cm', 22.6),
(2, 'Dyneema 11mm', '30cm', 18.7),
(2, 'Nylon 16mm', '30cm', 14.0)]
df = pandas.DataFrame(data=tests, columns=['FF', 'Type', 'Length', 'Load'])
print('Impact Loads from DMM tests [kN]')
print(df.pivot_table(values='Load', columns='FF', index=('Type', 'Length'),
fill_value='-'))
# Calculate and add EA values
test_weight = 80 * 9.806
df['EA'] = df.Load**2 / (2*test_weight*df.FF) * 1000
print('Calculated stiffness [kN]')
print(df.pivot_table(values='EA', columns='FF', index=('Type', 'Length'),
fill_value='-'))
def solve_fall(sling_length=1.2,
fall_factor=1,
sling_stiffness=105000,
mass=80):
"""Solve the motion of an impact load. No damping effects.
sling_length in meters
fall_factor
sling_stiffness in N
mass in kg
force in kN
"""
# Echo input
print('Sling length %.2f m' % sling_length)
print('Fall factor %.1f' % fall_factor)
print('Sling stiffness %.1f N' % sling_stiffness)
print('Mass %.1f kg\n' % mass)
# Simplified- Force only
force = (2 * mass * 9.806 * sling_stiffness * fall_factor)**0.5 / 1000
# Motion solver
vo = (2*9.806*sling_length*fall_factor)**0.5
c = sling_stiffness/sling_length/mass
dt = 1e-5
t, x, v, a, F = [0], [0], [vo], [0], [0]
while v[-1] > 0:
xp = x[-1]
t.append(t[-1]+dt)
a.append(- c * xp)
v.append(v[-1] + a[-1] * dt)
x.append(x[-1] + v[-1] * dt)
F.append(sling_stiffness/sling_length * x[-1] / 1000)
return force, t, x, v, a, F
def plot_fall(*args, **kwargs):
"""Plots the time traces of the fall with impact load.
Same input arguments as solve_fall.
"""
force, t, x, v, a, F = solve_fall(*args, **kwargs)
print('Impact force %.1f kN' % force)
plt.subplot(221)
plt.plot(t, F)
plt.title('Fall Impact Force [kN]')
plt.xticks(())
plt.subplot(222)
plt.plot(t, x)
plt.title('Fall Distance [m]')
plt.xticks(())
plt.subplot(223)
plt.plot(t, v)
plt.title('Fall Speed [m/s]')
plt.subplot(224)
plt.plot(t, a)
plt.title('Fall Acceleration [m/s2]')
plt.xlabel('Time [s]')
plt.show()
print('Solved only up to maximum force.')
def main():
plot_fall()
if __name__ == '__main__':
import time
t0 = time.time()
main()
print('\nruntime %f s' % (time.time()-t0))
| lgpl-3.0 |
gaborfodor/MLSP_2013 | 1_pattern_extraction.py | 2 | 10429 |
import numpy as np
import pandas as pd
import scipy as sp
import pickle
from scipy import fft
from time import localtime, strftime
import matplotlib.pyplot as plt
from skimage.morphology import disk,remove_small_objects
from skimage.filter import rank
from skimage.util import img_as_ubyte
import wave
###########################
# Folder Name Setting
###########################
folder = 'J:/DATAMINING/KAGGLE/MLSP_BirdClassification/'
essential_folder = folder+'essential_data/'
supplemental_folder = folder+'supplemental_data/'
spectro_folder =folder+'my_spectro/'
single_spectro_folder =folder+'my_spectro_single/'
dp_folder = folder+'DP/'
###################################################
## Read the Essential Data
## labels, training-test split,file_names etc.
###################################################
# Each audio file has a unique recording identifier ("rec_id"), ranging from 0 to 644.
# The file rec_id2filename.txt indicates which wav file is associated with each rec_id.
rec2f = pd.read_csv(essential_folder + 'rec_id2filename.txt', sep = ',')
# There are 19 bird species in the dataset. species_list.txt gives each a number from 0 to 18.
species = pd.read_csv(essential_folder + 'species_list.txt', sep = ',')
num_species = 19
# The dataset is split into training and test sets.
# CVfolds_2.txt gives the fold for each rec_id. 0 is the training set, and 1 is the test set.
cv = pd.read_csv(essential_folder + 'CVfolds_2.txt', sep = ',')
# This is your main label training data. For each rec_id, a set of species is listed. The format is:
# rec_id,[labels]
raw = pd.read_csv(essential_folder + 'rec_labels_test_hidden.txt', sep = ';')
label = np.zeros(len(raw)*num_species)
label = label.reshape([len(raw),num_species])
for i in range(len(raw)):
line = raw.irow(i)
labels = line[0].split(',')
labels.pop(0) # rec_id == i
for c in labels:
if(c != '?'):
label[i,c] = 1
label = pd.DataFrame(label)
label['rec_id'] = cv.rec_id
label['fold'] = cv.fold
label['filename'] = rec2f.filename
# Sparse training set
# training species 1%--5%--20%
spec_avg = label[label.fold ==0][range(num_species)].mean()
spec_avg.sort()
plt.plot(spec_avg,'go')
# Read the audio files
# /src_wavs
# This folder contains the original wav files for the dataset (both training and test sets).
# These are 10-second mono recordings sampled at 16kHz, 16 bits per sample.
def pic_to_ubyte (pic):
a = (pic-np.min(pic) ) /(np.max(pic - np.min(pic)))
a = img_as_ubyte(a)
return a
# Parameters to create the spectrogram
N = 160000
K = 512
Step = 4
wind = 0.5*(1 -np.cos(np.array(range(K))*2*np.pi/(K-1) ))
ffts = []
def wav_to_floats(filename):
s = wave.open(filename,'r')
strsig = s.readframes(s.getnframes())
y = np.fromstring(strsig, np.short)
s.close()
return y
###############################
## Create the Spectrograms
## Train + Test
###############################
print strftime("%a, %d %b %Y %H:%M:%S +0000", localtime())
for file_idx in range(len(label)):
test_flag = label.irow(file_idx)['fold']
fname = label.irow(file_idx)['filename']
species_on_pic = []
for n in range(num_species):
if(label.irow(file_idx)[n] > 0):
species_on_pic.append(n)
S = wav_to_floats(essential_folder+'src_wavs/'+fname+'.wav')
Spectogram = []
for j in range(int(Step*N/K)-Step):
vec = S[j * K/Step : (j+Step) * K/Step] * wind
Spectogram.append(abs(fft(vec,K)[:K/2]))
ffts.append(np.array(Spectogram))
print strftime("%a, %d %b %Y %H:%M:%S +0000", localtime())
SPEC_SEGMENTS = []
LOG_SPEC_SEGMENTS = []
MIN_SEGMENT_SIZE = 99
p = 90
#fig = plt.figure(figsize=(20, 10))
for file_idx in range(len(label)):
test_flag = label.irow(file_idx)['fold']
fname = label.irow(file_idx)['filename']
species_on_pic = []
for n in range(num_species):
if(label.irow(file_idx)[n] > 0):
species_on_pic.append(n)
label_count = label.irow(file_idx)[range(num_species)].sum()
bird_spec = label.irow(file_idx)[range(num_species)].argmax() # first bird
if(test_flag < 1 and label_count ==1):
mypic = np.transpose(ffts[file_idx])
mypic_rev = np.zeros_like(mypic)
for i in range(mypic.shape[0]):
mypic_rev[i] = mypic[-i - 1]
mypic_rev_small = mypic_rev[:200,:]
mypic_rev = mypic_rev_small
mypic_rev_log = np.log10(mypic_rev+ 0.001)
mypic_rev_gauss =sp.ndimage.gaussian_filter(mypic_rev, sigma=3)
mypic_rev_log_gauss = sp.ndimage.gaussian_filter(mypic_rev_log, sigma=3)
mypic_rev_gauss_bin = mypic_rev_gauss > np.percentile(mypic_rev_gauss,p)
mypic_rev_log_gauss_bin = mypic_rev_log_gauss > np.percentile(mypic_rev_log_gauss,p)
mypic_rev_gauss_bin_close =sp.ndimage.binary_closing( sp.ndimage.binary_opening(mypic_rev_gauss_bin))
mypic_rev_log_gauss_bin_close =sp.ndimage.binary_closing( sp.ndimage.binary_opening(mypic_rev_log_gauss_bin))
mypic_rev_gauss_grad = rank.gradient(pic_to_ubyte(mypic_rev_gauss), disk(3))
mypic_rev_log_gauss_grad = rank.gradient(pic_to_ubyte(mypic_rev_log_gauss), disk(3))
mypic_rev_gauss_grad_bin = mypic_rev_gauss_grad > np.percentile(mypic_rev_gauss_grad,p)
mypic_rev_log_gauss_grad_bin = mypic_rev_log_gauss_grad > np.percentile(mypic_rev_log_gauss_grad,p )
mypic_rev_gauss_grad_bin_close =sp.ndimage.binary_closing( sp.ndimage.binary_opening(mypic_rev_gauss_grad_bin))
mypic_rev_log_gauss_grad_bin_close =sp.ndimage.binary_closing( sp.ndimage.binary_opening(mypic_rev_log_gauss_grad_bin))
bfh = sp.ndimage.binary_fill_holes(mypic_rev_gauss_grad_bin_close)
bfh_rm = remove_small_objects(bfh, MIN_SEGMENT_SIZE)
log_bfh = sp.ndimage.binary_fill_holes(mypic_rev_log_gauss_grad_bin_close)
log_bfh_rm = remove_small_objects(log_bfh, MIN_SEGMENT_SIZE)
# plt.subplot(6,2,1)
# plt.imshow(mypic_rev,cmap=plt.cm.afmhot_r)
# plt.axis('off')
# plt.title('Spectrogram')
# plt.subplot(6,2,2)
# plt.imshow(mypic_rev_log,cmap=plt.cm.afmhot_r)
# plt.axis('off')
# plt.title('Spectrogram (log)')
# plt.subplot(6,2,3)
# plt.imshow(mypic_rev_log_gauss,cmap=plt.cm.afmhot_r)
# plt.axis('off')
# plt.title('+ Gaussian Filtering')
# plt.subplot(6,2,4)
# plt.imshow(mypic_rev_log,cmap=plt.cm.afmhot_r)
# plt.axis('off')
# plt.title('+ Gaussian Filtering (log)')
# plt.subplot(6,2,5)
# plt.imshow(mypic_rev_gauss_grad,cmap=plt.cm.afmhot_r)
# plt.axis('off')
# plt.title('+ Gradient')
# plt.subplot(6,2,6)
# plt.imshow(mypic_rev_log_gauss_grad,cmap=plt.cm.afmhot_r)
# plt.axis('off')
# plt.title('+ Gradient (log)')
# plt.subplot(6,2,7)
# plt.imshow(mypic_rev_gauss_grad_bin,cmap=plt.cm.gray)
# plt.axis('off')
# plt.title('+ >90%')
# plt.subplot(6,2,8)
# plt.imshow(mypic_rev_log_gauss_grad_bin,cmap=plt.cm.gray)
# plt.axis('off')
# plt.title('+ >90% (log)')
# plt.subplot(6,2,9)
# plt.imshow(mypic_rev_gauss_grad_bin_close,cmap=plt.cm.gray)
# plt.axis('off')
# plt.title('+ binary_closing + binary_opening')
# plt.subplot(6,2,10)
# plt.imshow(mypic_rev_log_gauss_grad_bin_close,cmap=plt.cm.gray)
# plt.axis('off')
# plt.title('+ binary_closing + binary_opening (log)')
#SEGMENTS
labeled_segments, num_seg = sp.ndimage.label(bfh_rm)
# plt.subplot(6,2,11)
# plt.imshow(labeled_segments)
# plt.axis('off')
# plt.title('+ binary_fill_holes + remove_small_objects')
for current_segment_id in range(1,num_seg+1):
current_segment = (labeled_segments == current_segment_id)*1
xr = current_segment.max(axis = 0)
yr = current_segment.max(axis = 1)
xr_max = np.max(xr*np.arange(len(xr)))
xr[xr==0] = xr.shape[0]
xr_min = np.argmin(xr)
yr_max = np.max(yr*np.arange(len(yr)))
yr[yr==0] = yr.shape[0]
yr_min = np.argmin(yr)
segment_frame = [yr_min, yr_max, xr_min, xr_max]
subpic = mypic_rev_gauss[yr_min:yr_max+1,xr_min:xr_max+1]
SPEC_SEGMENTS.append([file_idx, current_segment_id, segment_frame, subpic])
# LOG SEGMENTS
labeled_segments, num_seg = sp.ndimage.label(log_bfh_rm)
# plt.subplot(6,2,12)
# plt.imshow(labeled_segments)
# plt.axis('off')
# plt.title('+ binary_fill_holes + remove_small_objects (log)')
for current_segment_id in range(1,num_seg+1):
current_segment = (labeled_segments == current_segment_id)*1
xr = current_segment.max(axis = 0)
yr = current_segment.max(axis = 1)
xr_max = np.max(xr*np.arange(len(xr)))
xr[xr==0] = xr.shape[0]
xr_min = np.argmin(xr)
yr_max = np.max(yr*np.arange(len(yr)))
yr[yr==0] = yr.shape[0]
yr_min = np.argmin(yr)
segment_frame = [yr_min, yr_max, xr_min, xr_max]
subpic = mypic_rev_log_gauss[yr_min:yr_max+1,xr_min:xr_max+1]
LOG_SPEC_SEGMENTS.append([file_idx, current_segment_id, segment_frame, subpic])
#fig.savefig(single_spectro_folder+str(bird_spec)+'_'+fname+'_patterns.png',dpi = 300)
#fig.clear()
#plt.show()
print strftime("%a, %d %b %Y %H:%M:%S +0000", localtime())
## CHECK THE SEGMENTS:
#N = 10
#fig = plt.figure(figsize=(20, 10))
#for i in range(N):
# for j in range(N):
# plt.subplot(N,N,i*N+j)
# plt.imshow( SPEC_SEGMENTS[i*N+j][3])
#
#
#N = 10
#fig = plt.figure(figsize=(20, 10))
#for i in range(N):
# for j in range(N):
# plt.subplot(N,N,i*N+j)
# plt.imshow( LOG_SPEC_SEGMENTS[-(i*N+j)][3],cmap=plt.cm.afmhot_r)
#
#a = []
#for r in SPEC_SEGMENTS:
# a.append(r[2][1] - r[2][0] )
#
#plt.hist(a)
output = open(dp_folder + 'SPEC_SEGMENTS.pkl', 'wb')
pickle.dump(SPEC_SEGMENTS, output)
output.close()
output = open(dp_folder + 'LOG_SPEC_SEGMENTS.pkl', 'wb')
pickle.dump(LOG_SPEC_SEGMENTS, output)
output.close()
| mit |
heli522/scikit-learn | sklearn/linear_model/stochastic_gradient.py | 130 | 50966 | # Authors: Peter Prettenhofer <[email protected]> (main author)
# Mathieu Blondel (partial_fit support)
#
# License: BSD 3 clause
"""Classification and regression using Stochastic Gradient Descent (SGD)."""
import numpy as np
import scipy.sparse as sp
from abc import ABCMeta, abstractmethod
from ..externals.joblib import Parallel, delayed
from .base import LinearClassifierMixin, SparseCoefMixin
from ..base import BaseEstimator, RegressorMixin
from ..feature_selection.from_model import _LearntSelectorMixin
from ..utils import (check_array, check_random_state, check_X_y,
deprecated)
from ..utils.extmath import safe_sparse_dot
from ..utils.multiclass import _check_partial_fit_first_call
from ..utils.validation import check_is_fitted
from ..externals import six
from .sgd_fast import plain_sgd, average_sgd
from ..utils.fixes import astype
from ..utils.seq_dataset import ArrayDataset, CSRDataset
from ..utils import compute_class_weight
from .sgd_fast import Hinge
from .sgd_fast import SquaredHinge
from .sgd_fast import Log
from .sgd_fast import ModifiedHuber
from .sgd_fast import SquaredLoss
from .sgd_fast import Huber
from .sgd_fast import EpsilonInsensitive
from .sgd_fast import SquaredEpsilonInsensitive
LEARNING_RATE_TYPES = {"constant": 1, "optimal": 2, "invscaling": 3,
"pa1": 4, "pa2": 5}
PENALTY_TYPES = {"none": 0, "l2": 2, "l1": 1, "elasticnet": 3}
SPARSE_INTERCEPT_DECAY = 0.01
"""For sparse data intercept updates are scaled by this decay factor to avoid
intercept oscillation."""
DEFAULT_EPSILON = 0.1
"""Default value of ``epsilon`` parameter. """
class BaseSGD(six.with_metaclass(ABCMeta, BaseEstimator, SparseCoefMixin)):
"""Base class for SGD classification and regression."""
def __init__(self, loss, penalty='l2', alpha=0.0001, C=1.0,
l1_ratio=0.15, fit_intercept=True, n_iter=5, shuffle=True,
verbose=0, epsilon=0.1, random_state=None,
learning_rate="optimal", eta0=0.0, power_t=0.5,
warm_start=False, average=False):
self.loss = loss
self.penalty = penalty
self.learning_rate = learning_rate
self.epsilon = epsilon
self.alpha = alpha
self.C = C
self.l1_ratio = l1_ratio
self.fit_intercept = fit_intercept
self.n_iter = n_iter
self.shuffle = shuffle
self.random_state = random_state
self.verbose = verbose
self.eta0 = eta0
self.power_t = power_t
self.warm_start = warm_start
self.average = average
self._validate_params()
self.coef_ = None
if self.average > 0:
self.standard_coef_ = None
self.average_coef_ = None
# iteration count for learning rate schedule
# must not be int (e.g. if ``learning_rate=='optimal'``)
self.t_ = None
def set_params(self, *args, **kwargs):
super(BaseSGD, self).set_params(*args, **kwargs)
self._validate_params()
return self
@abstractmethod
def fit(self, X, y):
"""Fit model."""
def _validate_params(self):
"""Validate input params. """
if not isinstance(self.shuffle, bool):
raise ValueError("shuffle must be either True or False")
if self.n_iter <= 0:
raise ValueError("n_iter must be > zero")
if not (0.0 <= self.l1_ratio <= 1.0):
raise ValueError("l1_ratio must be in [0, 1]")
if self.alpha < 0.0:
raise ValueError("alpha must be >= 0")
if self.learning_rate in ("constant", "invscaling"):
if self.eta0 <= 0.0:
raise ValueError("eta0 must be > 0")
# raises ValueError if not registered
self._get_penalty_type(self.penalty)
self._get_learning_rate_type(self.learning_rate)
if self.loss not in self.loss_functions:
raise ValueError("The loss %s is not supported. " % self.loss)
def _get_loss_function(self, loss):
"""Get concrete ``LossFunction`` object for str ``loss``. """
try:
loss_ = self.loss_functions[loss]
loss_class, args = loss_[0], loss_[1:]
if loss in ('huber', 'epsilon_insensitive',
'squared_epsilon_insensitive'):
args = (self.epsilon, )
return loss_class(*args)
except KeyError:
raise ValueError("The loss %s is not supported. " % loss)
def _get_learning_rate_type(self, learning_rate):
try:
return LEARNING_RATE_TYPES[learning_rate]
except KeyError:
raise ValueError("learning rate %s "
"is not supported. " % learning_rate)
def _get_penalty_type(self, penalty):
penalty = str(penalty).lower()
try:
return PENALTY_TYPES[penalty]
except KeyError:
raise ValueError("Penalty %s is not supported. " % penalty)
def _validate_sample_weight(self, sample_weight, n_samples):
"""Set the sample weight array."""
if sample_weight is None:
# uniform sample weights
sample_weight = np.ones(n_samples, dtype=np.float64, order='C')
else:
# user-provided array
sample_weight = np.asarray(sample_weight, dtype=np.float64,
order="C")
if sample_weight.shape[0] != n_samples:
raise ValueError("Shapes of X and sample_weight do not match.")
return sample_weight
def _allocate_parameter_mem(self, n_classes, n_features, coef_init=None,
intercept_init=None):
"""Allocate mem for parameters; initialize if provided."""
if n_classes > 2:
# allocate coef_ for multi-class
if coef_init is not None:
coef_init = np.asarray(coef_init, order="C")
if coef_init.shape != (n_classes, n_features):
raise ValueError("Provided ``coef_`` does not match dataset. ")
self.coef_ = coef_init
else:
self.coef_ = np.zeros((n_classes, n_features),
dtype=np.float64, order="C")
# allocate intercept_ for multi-class
if intercept_init is not None:
intercept_init = np.asarray(intercept_init, order="C")
if intercept_init.shape != (n_classes, ):
raise ValueError("Provided intercept_init "
"does not match dataset.")
self.intercept_ = intercept_init
else:
self.intercept_ = np.zeros(n_classes, dtype=np.float64,
order="C")
else:
# allocate coef_ for binary problem
if coef_init is not None:
coef_init = np.asarray(coef_init, dtype=np.float64,
order="C")
coef_init = coef_init.ravel()
if coef_init.shape != (n_features,):
raise ValueError("Provided coef_init does not "
"match dataset.")
self.coef_ = coef_init
else:
self.coef_ = np.zeros(n_features,
dtype=np.float64,
order="C")
# allocate intercept_ for binary problem
if intercept_init is not None:
intercept_init = np.asarray(intercept_init, dtype=np.float64)
if intercept_init.shape != (1,) and intercept_init.shape != ():
raise ValueError("Provided intercept_init "
"does not match dataset.")
self.intercept_ = intercept_init.reshape(1,)
else:
self.intercept_ = np.zeros(1, dtype=np.float64, order="C")
# initialize average parameters
if self.average > 0:
self.standard_coef_ = self.coef_
self.standard_intercept_ = self.intercept_
self.average_coef_ = np.zeros(self.coef_.shape,
dtype=np.float64,
order="C")
self.average_intercept_ = np.zeros(self.standard_intercept_.shape,
dtype=np.float64,
order="C")
def _make_dataset(X, y_i, sample_weight):
"""Create ``Dataset`` abstraction for sparse and dense inputs.
This also returns the ``intercept_decay`` which is different
for sparse datasets.
"""
if sp.issparse(X):
dataset = CSRDataset(X.data, X.indptr, X.indices, y_i, sample_weight)
intercept_decay = SPARSE_INTERCEPT_DECAY
else:
dataset = ArrayDataset(X, y_i, sample_weight)
intercept_decay = 1.0
return dataset, intercept_decay
def _prepare_fit_binary(est, y, i):
"""Initialization for fit_binary.
Returns y, coef, intercept.
"""
y_i = np.ones(y.shape, dtype=np.float64, order="C")
y_i[y != est.classes_[i]] = -1.0
average_intercept = 0
average_coef = None
if len(est.classes_) == 2:
if not est.average:
coef = est.coef_.ravel()
intercept = est.intercept_[0]
else:
coef = est.standard_coef_.ravel()
intercept = est.standard_intercept_[0]
average_coef = est.average_coef_.ravel()
average_intercept = est.average_intercept_[0]
else:
if not est.average:
coef = est.coef_[i]
intercept = est.intercept_[i]
else:
coef = est.standard_coef_[i]
intercept = est.standard_intercept_[i]
average_coef = est.average_coef_[i]
average_intercept = est.average_intercept_[i]
return y_i, coef, intercept, average_coef, average_intercept
def fit_binary(est, i, X, y, alpha, C, learning_rate, n_iter,
pos_weight, neg_weight, sample_weight):
"""Fit a single binary classifier.
The i'th class is considered the "positive" class.
"""
# if average is not true, average_coef, and average_intercept will be
# unused
y_i, coef, intercept, average_coef, average_intercept = \
_prepare_fit_binary(est, y, i)
assert y_i.shape[0] == y.shape[0] == sample_weight.shape[0]
dataset, intercept_decay = _make_dataset(X, y_i, sample_weight)
penalty_type = est._get_penalty_type(est.penalty)
learning_rate_type = est._get_learning_rate_type(learning_rate)
# XXX should have random_state_!
random_state = check_random_state(est.random_state)
# numpy mtrand expects a C long which is a signed 32 bit integer under
# Windows
seed = random_state.randint(0, np.iinfo(np.int32).max)
if not est.average:
return plain_sgd(coef, intercept, est.loss_function,
penalty_type, alpha, C, est.l1_ratio,
dataset, n_iter, int(est.fit_intercept),
int(est.verbose), int(est.shuffle), seed,
pos_weight, neg_weight,
learning_rate_type, est.eta0,
est.power_t, est.t_, intercept_decay)
else:
standard_coef, standard_intercept, average_coef, \
average_intercept = average_sgd(coef, intercept, average_coef,
average_intercept,
est.loss_function, penalty_type,
alpha, C, est.l1_ratio, dataset,
n_iter, int(est.fit_intercept),
int(est.verbose), int(est.shuffle),
seed, pos_weight, neg_weight,
learning_rate_type, est.eta0,
est.power_t, est.t_,
intercept_decay,
est.average)
if len(est.classes_) == 2:
est.average_intercept_[0] = average_intercept
else:
est.average_intercept_[i] = average_intercept
return standard_coef, standard_intercept
class BaseSGDClassifier(six.with_metaclass(ABCMeta, BaseSGD,
LinearClassifierMixin)):
loss_functions = {
"hinge": (Hinge, 1.0),
"squared_hinge": (SquaredHinge, 1.0),
"perceptron": (Hinge, 0.0),
"log": (Log, ),
"modified_huber": (ModifiedHuber, ),
"squared_loss": (SquaredLoss, ),
"huber": (Huber, DEFAULT_EPSILON),
"epsilon_insensitive": (EpsilonInsensitive, DEFAULT_EPSILON),
"squared_epsilon_insensitive": (SquaredEpsilonInsensitive,
DEFAULT_EPSILON),
}
@abstractmethod
def __init__(self, loss="hinge", penalty='l2', alpha=0.0001, l1_ratio=0.15,
fit_intercept=True, n_iter=5, shuffle=True, verbose=0,
epsilon=DEFAULT_EPSILON, n_jobs=1, random_state=None,
learning_rate="optimal", eta0=0.0, power_t=0.5,
class_weight=None, warm_start=False, average=False):
super(BaseSGDClassifier, self).__init__(loss=loss, penalty=penalty,
alpha=alpha, l1_ratio=l1_ratio,
fit_intercept=fit_intercept,
n_iter=n_iter, shuffle=shuffle,
verbose=verbose,
epsilon=epsilon,
random_state=random_state,
learning_rate=learning_rate,
eta0=eta0, power_t=power_t,
warm_start=warm_start,
average=average)
self.class_weight = class_weight
self.classes_ = None
self.n_jobs = int(n_jobs)
def _partial_fit(self, X, y, alpha, C,
loss, learning_rate, n_iter,
classes, sample_weight,
coef_init, intercept_init):
X, y = check_X_y(X, y, 'csr', dtype=np.float64, order="C")
n_samples, n_features = X.shape
self._validate_params()
_check_partial_fit_first_call(self, classes)
n_classes = self.classes_.shape[0]
# Allocate datastructures from input arguments
self._expanded_class_weight = compute_class_weight(self.class_weight,
self.classes_, y)
sample_weight = self._validate_sample_weight(sample_weight, n_samples)
if self.coef_ is None or coef_init is not None:
self._allocate_parameter_mem(n_classes, n_features,
coef_init, intercept_init)
elif n_features != self.coef_.shape[-1]:
raise ValueError("Number of features %d does not match previous data %d."
% (n_features, self.coef_.shape[-1]))
self.loss_function = self._get_loss_function(loss)
if self.t_ is None:
self.t_ = 1.0
# delegate to concrete training procedure
if n_classes > 2:
self._fit_multiclass(X, y, alpha=alpha, C=C,
learning_rate=learning_rate,
sample_weight=sample_weight, n_iter=n_iter)
elif n_classes == 2:
self._fit_binary(X, y, alpha=alpha, C=C,
learning_rate=learning_rate,
sample_weight=sample_weight, n_iter=n_iter)
else:
raise ValueError("The number of class labels must be "
"greater than one.")
return self
def _fit(self, X, y, alpha, C, loss, learning_rate, coef_init=None,
intercept_init=None, sample_weight=None):
if hasattr(self, "classes_"):
self.classes_ = None
X, y = check_X_y(X, y, 'csr', dtype=np.float64, order="C")
n_samples, n_features = X.shape
# labels can be encoded as float, int, or string literals
# np.unique sorts in asc order; largest class id is positive class
classes = np.unique(y)
if self.warm_start and self.coef_ is not None:
if coef_init is None:
coef_init = self.coef_
if intercept_init is None:
intercept_init = self.intercept_
else:
self.coef_ = None
self.intercept_ = None
if self.average > 0:
self.standard_coef_ = self.coef_
self.standard_intercept_ = self.intercept_
self.average_coef_ = None
self.average_intercept_ = None
# Clear iteration count for multiple call to fit.
self.t_ = None
self._partial_fit(X, y, alpha, C, loss, learning_rate, self.n_iter,
classes, sample_weight, coef_init, intercept_init)
return self
def _fit_binary(self, X, y, alpha, C, sample_weight,
learning_rate, n_iter):
"""Fit a binary classifier on X and y. """
coef, intercept = fit_binary(self, 1, X, y, alpha, C,
learning_rate, n_iter,
self._expanded_class_weight[1],
self._expanded_class_weight[0],
sample_weight)
self.t_ += n_iter * X.shape[0]
# need to be 2d
if self.average > 0:
if self.average <= self.t_ - 1:
self.coef_ = self.average_coef_.reshape(1, -1)
self.intercept_ = self.average_intercept_
else:
self.coef_ = self.standard_coef_.reshape(1, -1)
self.standard_intercept_ = np.atleast_1d(intercept)
self.intercept_ = self.standard_intercept_
else:
self.coef_ = coef.reshape(1, -1)
# intercept is a float, need to convert it to an array of length 1
self.intercept_ = np.atleast_1d(intercept)
def _fit_multiclass(self, X, y, alpha, C, learning_rate,
sample_weight, n_iter):
"""Fit a multi-class classifier by combining binary classifiers
Each binary classifier predicts one class versus all others. This
strategy is called OVA: One Versus All.
"""
# Use joblib to fit OvA in parallel.
result = Parallel(n_jobs=self.n_jobs, backend="threading",
verbose=self.verbose)(
delayed(fit_binary)(self, i, X, y, alpha, C, learning_rate,
n_iter, self._expanded_class_weight[i], 1.,
sample_weight)
for i in range(len(self.classes_)))
for i, (_, intercept) in enumerate(result):
self.intercept_[i] = intercept
self.t_ += n_iter * X.shape[0]
if self.average > 0:
if self.average <= self.t_ - 1.0:
self.coef_ = self.average_coef_
self.intercept_ = self.average_intercept_
else:
self.coef_ = self.standard_coef_
self.standard_intercept_ = np.atleast_1d(intercept)
self.intercept_ = self.standard_intercept_
def partial_fit(self, X, y, classes=None, sample_weight=None):
"""Fit linear model with Stochastic Gradient Descent.
Parameters
----------
X : {array-like, sparse matrix}, shape (n_samples, n_features)
Subset of the training data
y : numpy array, shape (n_samples,)
Subset of the target values
classes : array, shape (n_classes,)
Classes across all calls to partial_fit.
Can be obtained by via `np.unique(y_all)`, where y_all is the
target vector of the entire dataset.
This argument is required for the first call to partial_fit
and can be omitted in the subsequent calls.
Note that y doesn't need to contain all labels in `classes`.
sample_weight : array-like, shape (n_samples,), optional
Weights applied to individual samples.
If not provided, uniform weights are assumed.
Returns
-------
self : returns an instance of self.
"""
if self.class_weight in ['balanced', 'auto']:
raise ValueError("class_weight '{0}' is not supported for "
"partial_fit. In order to use 'balanced' weights, "
"use compute_class_weight('{0}', classes, y). "
"In place of y you can us a large enough sample "
"of the full training set target to properly "
"estimate the class frequency distributions. "
"Pass the resulting weights as the class_weight "
"parameter.".format(self.class_weight))
return self._partial_fit(X, y, alpha=self.alpha, C=1.0, loss=self.loss,
learning_rate=self.learning_rate, n_iter=1,
classes=classes, sample_weight=sample_weight,
coef_init=None, intercept_init=None)
def fit(self, X, y, coef_init=None, intercept_init=None, sample_weight=None):
"""Fit linear model with Stochastic Gradient Descent.
Parameters
----------
X : {array-like, sparse matrix}, shape (n_samples, n_features)
Training data
y : numpy array, shape (n_samples,)
Target values
coef_init : array, shape (n_classes, n_features)
The initial coefficients to warm-start the optimization.
intercept_init : array, shape (n_classes,)
The initial intercept to warm-start the optimization.
sample_weight : array-like, shape (n_samples,), optional
Weights applied to individual samples.
If not provided, uniform weights are assumed. These weights will
be multiplied with class_weight (passed through the
contructor) if class_weight is specified
Returns
-------
self : returns an instance of self.
"""
return self._fit(X, y, alpha=self.alpha, C=1.0,
loss=self.loss, learning_rate=self.learning_rate,
coef_init=coef_init, intercept_init=intercept_init,
sample_weight=sample_weight)
class SGDClassifier(BaseSGDClassifier, _LearntSelectorMixin):
"""Linear classifiers (SVM, logistic regression, a.o.) with SGD training.
This estimator implements regularized linear models with stochastic
gradient descent (SGD) learning: the gradient of the loss is estimated
each sample at a time and the model is updated along the way with a
decreasing strength schedule (aka learning rate). SGD allows minibatch
(online/out-of-core) learning, see the partial_fit method.
For best results using the default learning rate schedule, the data should
have zero mean and unit variance.
This implementation works with data represented as dense or sparse arrays
of floating point values for the features. The model it fits can be
controlled with the loss parameter; by default, it fits a linear support
vector machine (SVM).
The regularizer is a penalty added to the loss function that shrinks model
parameters towards the zero vector using either the squared euclidean norm
L2 or the absolute norm L1 or a combination of both (Elastic Net). If the
parameter update crosses the 0.0 value because of the regularizer, the
update is truncated to 0.0 to allow for learning sparse models and achieve
online feature selection.
Read more in the :ref:`User Guide <sgd>`.
Parameters
----------
loss : str, 'hinge', 'log', 'modified_huber', 'squared_hinge',\
'perceptron', or a regression loss: 'squared_loss', 'huber',\
'epsilon_insensitive', or 'squared_epsilon_insensitive'
The loss function to be used. Defaults to 'hinge', which gives a
linear SVM.
The 'log' loss gives logistic regression, a probabilistic classifier.
'modified_huber' is another smooth loss that brings tolerance to
outliers as well as probability estimates.
'squared_hinge' is like hinge but is quadratically penalized.
'perceptron' is the linear loss used by the perceptron algorithm.
The other losses are designed for regression but can be useful in
classification as well; see SGDRegressor for a description.
penalty : str, 'none', 'l2', 'l1', or 'elasticnet'
The penalty (aka regularization term) to be used. Defaults to 'l2'
which is the standard regularizer for linear SVM models. 'l1' and
'elasticnet' might bring sparsity to the model (feature selection)
not achievable with 'l2'.
alpha : float
Constant that multiplies the regularization term. Defaults to 0.0001
l1_ratio : float
The Elastic Net mixing parameter, with 0 <= l1_ratio <= 1.
l1_ratio=0 corresponds to L2 penalty, l1_ratio=1 to L1.
Defaults to 0.15.
fit_intercept : bool
Whether the intercept should be estimated or not. If False, the
data is assumed to be already centered. Defaults to True.
n_iter : int, optional
The number of passes over the training data (aka epochs). The number
of iterations is set to 1 if using partial_fit.
Defaults to 5.
shuffle : bool, optional
Whether or not the training data should be shuffled after each epoch.
Defaults to True.
random_state : int seed, RandomState instance, or None (default)
The seed of the pseudo random number generator to use when
shuffling the data.
verbose : integer, optional
The verbosity level
epsilon : float
Epsilon in the epsilon-insensitive loss functions; only if `loss` is
'huber', 'epsilon_insensitive', or 'squared_epsilon_insensitive'.
For 'huber', determines the threshold at which it becomes less
important to get the prediction exactly right.
For epsilon-insensitive, any differences between the current prediction
and the correct label are ignored if they are less than this threshold.
n_jobs : integer, optional
The number of CPUs to use to do the OVA (One Versus All, for
multi-class problems) computation. -1 means 'all CPUs'. Defaults
to 1.
learning_rate : string, optional
The learning rate schedule:
constant: eta = eta0
optimal: eta = 1.0 / (t + t0) [default]
invscaling: eta = eta0 / pow(t, power_t)
where t0 is chosen by a heuristic proposed by Leon Bottou.
eta0 : double
The initial learning rate for the 'constant' or 'invscaling'
schedules. The default value is 0.0 as eta0 is not used by the
default schedule 'optimal'.
power_t : double
The exponent for inverse scaling learning rate [default 0.5].
class_weight : dict, {class_label: weight} or "balanced" or None, optional
Preset for the class_weight fit parameter.
Weights associated with classes. If not given, all classes
are supposed to have weight one.
The "balanced" mode uses the values of y to automatically adjust
weights inversely proportional to class frequencies in the input data
as ``n_samples / (n_classes * np.bincount(y))``
warm_start : bool, optional
When set to True, reuse the solution of the previous call to fit as
initialization, otherwise, just erase the previous solution.
average : bool or int, optional
When set to True, computes the averaged SGD weights and stores the
result in the ``coef_`` attribute. If set to an int greater than 1,
averaging will begin once the total number of samples seen reaches
average. So average=10 will begin averaging after seeing 10 samples.
Attributes
----------
coef_ : array, shape (1, n_features) if n_classes == 2 else (n_classes,\
n_features)
Weights assigned to the features.
intercept_ : array, shape (1,) if n_classes == 2 else (n_classes,)
Constants in decision function.
Examples
--------
>>> import numpy as np
>>> from sklearn import linear_model
>>> X = np.array([[-1, -1], [-2, -1], [1, 1], [2, 1]])
>>> Y = np.array([1, 1, 2, 2])
>>> clf = linear_model.SGDClassifier()
>>> clf.fit(X, Y)
... #doctest: +NORMALIZE_WHITESPACE
SGDClassifier(alpha=0.0001, average=False, class_weight=None, epsilon=0.1,
eta0=0.0, fit_intercept=True, l1_ratio=0.15,
learning_rate='optimal', loss='hinge', n_iter=5, n_jobs=1,
penalty='l2', power_t=0.5, random_state=None, shuffle=True,
verbose=0, warm_start=False)
>>> print(clf.predict([[-0.8, -1]]))
[1]
See also
--------
LinearSVC, LogisticRegression, Perceptron
"""
def __init__(self, loss="hinge", penalty='l2', alpha=0.0001, l1_ratio=0.15,
fit_intercept=True, n_iter=5, shuffle=True, verbose=0,
epsilon=DEFAULT_EPSILON, n_jobs=1, random_state=None,
learning_rate="optimal", eta0=0.0, power_t=0.5,
class_weight=None, warm_start=False, average=False):
super(SGDClassifier, self).__init__(
loss=loss, penalty=penalty, alpha=alpha, l1_ratio=l1_ratio,
fit_intercept=fit_intercept, n_iter=n_iter, shuffle=shuffle,
verbose=verbose, epsilon=epsilon, n_jobs=n_jobs,
random_state=random_state, learning_rate=learning_rate, eta0=eta0,
power_t=power_t, class_weight=class_weight, warm_start=warm_start,
average=average)
def _check_proba(self):
check_is_fitted(self, "t_")
if self.loss not in ("log", "modified_huber"):
raise AttributeError("probability estimates are not available for"
" loss=%r" % self.loss)
@property
def predict_proba(self):
"""Probability estimates.
This method is only available for log loss and modified Huber loss.
Multiclass probability estimates are derived from binary (one-vs.-rest)
estimates by simple normalization, as recommended by Zadrozny and
Elkan.
Binary probability estimates for loss="modified_huber" are given by
(clip(decision_function(X), -1, 1) + 1) / 2.
Parameters
----------
X : {array-like, sparse matrix}, shape (n_samples, n_features)
Returns
-------
array, shape (n_samples, n_classes)
Returns the probability of the sample for each class in the model,
where classes are ordered as they are in `self.classes_`.
References
----------
Zadrozny and Elkan, "Transforming classifier scores into multiclass
probability estimates", SIGKDD'02,
http://www.research.ibm.com/people/z/zadrozny/kdd2002-Transf.pdf
The justification for the formula in the loss="modified_huber"
case is in the appendix B in:
http://jmlr.csail.mit.edu/papers/volume2/zhang02c/zhang02c.pdf
"""
self._check_proba()
return self._predict_proba
def _predict_proba(self, X):
if self.loss == "log":
return self._predict_proba_lr(X)
elif self.loss == "modified_huber":
binary = (len(self.classes_) == 2)
scores = self.decision_function(X)
if binary:
prob2 = np.ones((scores.shape[0], 2))
prob = prob2[:, 1]
else:
prob = scores
np.clip(scores, -1, 1, prob)
prob += 1.
prob /= 2.
if binary:
prob2[:, 0] -= prob
prob = prob2
else:
# the above might assign zero to all classes, which doesn't
# normalize neatly; work around this to produce uniform
# probabilities
prob_sum = prob.sum(axis=1)
all_zero = (prob_sum == 0)
if np.any(all_zero):
prob[all_zero, :] = 1
prob_sum[all_zero] = len(self.classes_)
# normalize
prob /= prob_sum.reshape((prob.shape[0], -1))
return prob
else:
raise NotImplementedError("predict_(log_)proba only supported when"
" loss='log' or loss='modified_huber' "
"(%r given)" % self.loss)
@property
def predict_log_proba(self):
"""Log of probability estimates.
This method is only available for log loss and modified Huber loss.
When loss="modified_huber", probability estimates may be hard zeros
and ones, so taking the logarithm is not possible.
See ``predict_proba`` for details.
Parameters
----------
X : array-like, shape (n_samples, n_features)
Returns
-------
T : array-like, shape (n_samples, n_classes)
Returns the log-probability of the sample for each class in the
model, where classes are ordered as they are in
`self.classes_`.
"""
self._check_proba()
return self._predict_log_proba
def _predict_log_proba(self, X):
return np.log(self.predict_proba(X))
class BaseSGDRegressor(BaseSGD, RegressorMixin):
loss_functions = {
"squared_loss": (SquaredLoss, ),
"huber": (Huber, DEFAULT_EPSILON),
"epsilon_insensitive": (EpsilonInsensitive, DEFAULT_EPSILON),
"squared_epsilon_insensitive": (SquaredEpsilonInsensitive,
DEFAULT_EPSILON),
}
@abstractmethod
def __init__(self, loss="squared_loss", penalty="l2", alpha=0.0001,
l1_ratio=0.15, fit_intercept=True, n_iter=5, shuffle=True,
verbose=0, epsilon=DEFAULT_EPSILON, random_state=None,
learning_rate="invscaling", eta0=0.01, power_t=0.25,
warm_start=False, average=False):
super(BaseSGDRegressor, self).__init__(loss=loss, penalty=penalty,
alpha=alpha, l1_ratio=l1_ratio,
fit_intercept=fit_intercept,
n_iter=n_iter, shuffle=shuffle,
verbose=verbose,
epsilon=epsilon,
random_state=random_state,
learning_rate=learning_rate,
eta0=eta0, power_t=power_t,
warm_start=warm_start,
average=average)
def _partial_fit(self, X, y, alpha, C, loss, learning_rate,
n_iter, sample_weight,
coef_init, intercept_init):
X, y = check_X_y(X, y, "csr", copy=False, order='C', dtype=np.float64)
y = astype(y, np.float64, copy=False)
n_samples, n_features = X.shape
self._validate_params()
# Allocate datastructures from input arguments
sample_weight = self._validate_sample_weight(sample_weight, n_samples)
if self.coef_ is None:
self._allocate_parameter_mem(1, n_features,
coef_init, intercept_init)
elif n_features != self.coef_.shape[-1]:
raise ValueError("Number of features %d does not match previous data %d."
% (n_features, self.coef_.shape[-1]))
if self.average > 0 and self.average_coef_ is None:
self.average_coef_ = np.zeros(n_features,
dtype=np.float64,
order="C")
self.average_intercept_ = np.zeros(1,
dtype=np.float64,
order="C")
self._fit_regressor(X, y, alpha, C, loss, learning_rate,
sample_weight, n_iter)
return self
def partial_fit(self, X, y, sample_weight=None):
"""Fit linear model with Stochastic Gradient Descent.
Parameters
----------
X : {array-like, sparse matrix}, shape (n_samples, n_features)
Subset of training data
y : numpy array of shape (n_samples,)
Subset of target values
sample_weight : array-like, shape (n_samples,), optional
Weights applied to individual samples.
If not provided, uniform weights are assumed.
Returns
-------
self : returns an instance of self.
"""
return self._partial_fit(X, y, self.alpha, C=1.0,
loss=self.loss,
learning_rate=self.learning_rate, n_iter=1,
sample_weight=sample_weight,
coef_init=None, intercept_init=None)
def _fit(self, X, y, alpha, C, loss, learning_rate, coef_init=None,
intercept_init=None, sample_weight=None):
if self.warm_start and self.coef_ is not None:
if coef_init is None:
coef_init = self.coef_
if intercept_init is None:
intercept_init = self.intercept_
else:
self.coef_ = None
self.intercept_ = None
if self.average > 0:
self.standard_intercept_ = self.intercept_
self.standard_coef_ = self.coef_
self.average_coef_ = None
self.average_intercept_ = None
# Clear iteration count for multiple call to fit.
self.t_ = None
return self._partial_fit(X, y, alpha, C, loss, learning_rate,
self.n_iter, sample_weight,
coef_init, intercept_init)
def fit(self, X, y, coef_init=None, intercept_init=None,
sample_weight=None):
"""Fit linear model with Stochastic Gradient Descent.
Parameters
----------
X : {array-like, sparse matrix}, shape (n_samples, n_features)
Training data
y : numpy array, shape (n_samples,)
Target values
coef_init : array, shape (n_features,)
The initial coefficients to warm-start the optimization.
intercept_init : array, shape (1,)
The initial intercept to warm-start the optimization.
sample_weight : array-like, shape (n_samples,), optional
Weights applied to individual samples (1. for unweighted).
Returns
-------
self : returns an instance of self.
"""
return self._fit(X, y, alpha=self.alpha, C=1.0,
loss=self.loss, learning_rate=self.learning_rate,
coef_init=coef_init,
intercept_init=intercept_init,
sample_weight=sample_weight)
@deprecated(" and will be removed in 0.19.")
def decision_function(self, X):
"""Predict using the linear model
Parameters
----------
X : {array-like, sparse matrix}, shape (n_samples, n_features)
Returns
-------
array, shape (n_samples,)
Predicted target values per element in X.
"""
return self._decision_function(X)
def _decision_function(self, X):
"""Predict using the linear model
Parameters
----------
X : {array-like, sparse matrix}, shape (n_samples, n_features)
Returns
-------
array, shape (n_samples,)
Predicted target values per element in X.
"""
check_is_fitted(self, ["t_", "coef_", "intercept_"], all_or_any=all)
X = check_array(X, accept_sparse='csr')
scores = safe_sparse_dot(X, self.coef_.T,
dense_output=True) + self.intercept_
return scores.ravel()
def predict(self, X):
"""Predict using the linear model
Parameters
----------
X : {array-like, sparse matrix}, shape (n_samples, n_features)
Returns
-------
array, shape (n_samples,)
Predicted target values per element in X.
"""
return self._decision_function(X)
def _fit_regressor(self, X, y, alpha, C, loss, learning_rate,
sample_weight, n_iter):
dataset, intercept_decay = _make_dataset(X, y, sample_weight)
loss_function = self._get_loss_function(loss)
penalty_type = self._get_penalty_type(self.penalty)
learning_rate_type = self._get_learning_rate_type(learning_rate)
if self.t_ is None:
self.t_ = 1.0
random_state = check_random_state(self.random_state)
# numpy mtrand expects a C long which is a signed 32 bit integer under
# Windows
seed = random_state.randint(0, np.iinfo(np.int32).max)
if self.average > 0:
self.standard_coef_, self.standard_intercept_, \
self.average_coef_, self.average_intercept_ =\
average_sgd(self.standard_coef_,
self.standard_intercept_[0],
self.average_coef_,
self.average_intercept_[0],
loss_function,
penalty_type,
alpha, C,
self.l1_ratio,
dataset,
n_iter,
int(self.fit_intercept),
int(self.verbose),
int(self.shuffle),
seed,
1.0, 1.0,
learning_rate_type,
self.eta0, self.power_t, self.t_,
intercept_decay, self.average)
self.average_intercept_ = np.atleast_1d(self.average_intercept_)
self.standard_intercept_ = np.atleast_1d(self.standard_intercept_)
self.t_ += n_iter * X.shape[0]
if self.average <= self.t_ - 1.0:
self.coef_ = self.average_coef_
self.intercept_ = self.average_intercept_
else:
self.coef_ = self.standard_coef_
self.intercept_ = self.standard_intercept_
else:
self.coef_, self.intercept_ = \
plain_sgd(self.coef_,
self.intercept_[0],
loss_function,
penalty_type,
alpha, C,
self.l1_ratio,
dataset,
n_iter,
int(self.fit_intercept),
int(self.verbose),
int(self.shuffle),
seed,
1.0, 1.0,
learning_rate_type,
self.eta0, self.power_t, self.t_,
intercept_decay)
self.t_ += n_iter * X.shape[0]
self.intercept_ = np.atleast_1d(self.intercept_)
class SGDRegressor(BaseSGDRegressor, _LearntSelectorMixin):
"""Linear model fitted by minimizing a regularized empirical loss with SGD
SGD stands for Stochastic Gradient Descent: the gradient of the loss is
estimated each sample at a time and the model is updated along the way with
a decreasing strength schedule (aka learning rate).
The regularizer is a penalty added to the loss function that shrinks model
parameters towards the zero vector using either the squared euclidean norm
L2 or the absolute norm L1 or a combination of both (Elastic Net). If the
parameter update crosses the 0.0 value because of the regularizer, the
update is truncated to 0.0 to allow for learning sparse models and achieve
online feature selection.
This implementation works with data represented as dense numpy arrays of
floating point values for the features.
Read more in the :ref:`User Guide <sgd>`.
Parameters
----------
loss : str, 'squared_loss', 'huber', 'epsilon_insensitive', \
or 'squared_epsilon_insensitive'
The loss function to be used. Defaults to 'squared_loss' which refers
to the ordinary least squares fit. 'huber' modifies 'squared_loss' to
focus less on getting outliers correct by switching from squared to
linear loss past a distance of epsilon. 'epsilon_insensitive' ignores
errors less than epsilon and is linear past that; this is the loss
function used in SVR. 'squared_epsilon_insensitive' is the same but
becomes squared loss past a tolerance of epsilon.
penalty : str, 'none', 'l2', 'l1', or 'elasticnet'
The penalty (aka regularization term) to be used. Defaults to 'l2'
which is the standard regularizer for linear SVM models. 'l1' and
'elasticnet' might bring sparsity to the model (feature selection)
not achievable with 'l2'.
alpha : float
Constant that multiplies the regularization term. Defaults to 0.0001
l1_ratio : float
The Elastic Net mixing parameter, with 0 <= l1_ratio <= 1.
l1_ratio=0 corresponds to L2 penalty, l1_ratio=1 to L1.
Defaults to 0.15.
fit_intercept : bool
Whether the intercept should be estimated or not. If False, the
data is assumed to be already centered. Defaults to True.
n_iter : int, optional
The number of passes over the training data (aka epochs). The number
of iterations is set to 1 if using partial_fit.
Defaults to 5.
shuffle : bool, optional
Whether or not the training data should be shuffled after each epoch.
Defaults to True.
random_state : int seed, RandomState instance, or None (default)
The seed of the pseudo random number generator to use when
shuffling the data.
verbose : integer, optional
The verbosity level.
epsilon : float
Epsilon in the epsilon-insensitive loss functions; only if `loss` is
'huber', 'epsilon_insensitive', or 'squared_epsilon_insensitive'.
For 'huber', determines the threshold at which it becomes less
important to get the prediction exactly right.
For epsilon-insensitive, any differences between the current prediction
and the correct label are ignored if they are less than this threshold.
learning_rate : string, optional
The learning rate:
constant: eta = eta0
optimal: eta = 1.0/(alpha * t)
invscaling: eta = eta0 / pow(t, power_t) [default]
eta0 : double, optional
The initial learning rate [default 0.01].
power_t : double, optional
The exponent for inverse scaling learning rate [default 0.25].
warm_start : bool, optional
When set to True, reuse the solution of the previous call to fit as
initialization, otherwise, just erase the previous solution.
average : bool or int, optional
When set to True, computes the averaged SGD weights and stores the
result in the ``coef_`` attribute. If set to an int greater than 1,
averaging will begin once the total number of samples seen reaches
average. So ``average=10 will`` begin averaging after seeing 10 samples.
Attributes
----------
coef_ : array, shape (n_features,)
Weights assigned to the features.
intercept_ : array, shape (1,)
The intercept term.
average_coef_ : array, shape (n_features,)
Averaged weights assigned to the features.
average_intercept_ : array, shape (1,)
The averaged intercept term.
Examples
--------
>>> import numpy as np
>>> from sklearn import linear_model
>>> n_samples, n_features = 10, 5
>>> np.random.seed(0)
>>> y = np.random.randn(n_samples)
>>> X = np.random.randn(n_samples, n_features)
>>> clf = linear_model.SGDRegressor()
>>> clf.fit(X, y)
... #doctest: +NORMALIZE_WHITESPACE
SGDRegressor(alpha=0.0001, average=False, epsilon=0.1, eta0=0.01,
fit_intercept=True, l1_ratio=0.15, learning_rate='invscaling',
loss='squared_loss', n_iter=5, penalty='l2', power_t=0.25,
random_state=None, shuffle=True, verbose=0, warm_start=False)
See also
--------
Ridge, ElasticNet, Lasso, SVR
"""
def __init__(self, loss="squared_loss", penalty="l2", alpha=0.0001,
l1_ratio=0.15, fit_intercept=True, n_iter=5, shuffle=True,
verbose=0, epsilon=DEFAULT_EPSILON, random_state=None,
learning_rate="invscaling", eta0=0.01, power_t=0.25,
warm_start=False, average=False):
super(SGDRegressor, self).__init__(loss=loss, penalty=penalty,
alpha=alpha, l1_ratio=l1_ratio,
fit_intercept=fit_intercept,
n_iter=n_iter, shuffle=shuffle,
verbose=verbose,
epsilon=epsilon,
random_state=random_state,
learning_rate=learning_rate,
eta0=eta0, power_t=power_t,
warm_start=warm_start,
average=average)
| bsd-3-clause |
jseabold/statsmodels | statsmodels/sandbox/infotheo.py | 5 | 16540 | """
Information Theoretic and Entropy Measures
References
----------
Golan, As. 2008. "Information and Entropy Econometrics -- A Review and
Synthesis." Foundations And Trends in Econometrics 2(1-2), 1-145.
Golan, A., Judge, G., and Miller, D. 1996. Maximum Entropy Econometrics.
Wiley & Sons, Chichester.
"""
#For MillerMadow correction
#Miller, G. 1955. Note on the bias of information estimates. Info. Theory
# Psychol. Prob. Methods II-B:95-100.
#For ChaoShen method
#Chao, A., and T.-J. Shen. 2003. Nonparametric estimation of Shannon's index of diversity when
#there are unseen species in sample. Environ. Ecol. Stat. 10:429-443.
#Good, I. J. 1953. The population frequencies of species and the estimation of population parameters.
#Biometrika 40:237-264.
#Horvitz, D.G., and D. J. Thompson. 1952. A generalization of sampling without replacement from a finute universe. J. Am. Stat. Assoc. 47:663-685.
#For NSB method
#Nemenman, I., F. Shafee, and W. Bialek. 2002. Entropy and inference, revisited. In: Dietterich, T.,
#S. Becker, Z. Gharamani, eds. Advances in Neural Information Processing Systems 14: 471-478.
#Cambridge (Massachusetts): MIT Press.
#For shrinkage method
#Dougherty, J., Kohavi, R., and Sahami, M. (1995). Supervised and unsupervised discretization of
#continuous features. In International Conference on Machine Learning.
#Yang, Y. and Webb, G. I. (2003). Discretization for naive-bayes learning: managing discretization
#bias and variance. Technical Report 2003/131 School of Computer Science and Software Engineer-
#ing, Monash University.
from statsmodels.compat.python import lzip, lmap
from scipy import stats
import numpy as np
from matplotlib import pyplot as plt
from scipy.special import logsumexp as sp_logsumexp
#TODO: change these to use maxentutils so that over/underflow is handled
#with the logsumexp.
def logsumexp(a, axis=None):
"""
Compute the log of the sum of exponentials log(e^{a_1}+...e^{a_n}) of a
Avoids numerical overflow.
Parameters
----------
a : array_like
The vector to exponentiate and sum
axis : int, optional
The axis along which to apply the operation. Defaults is None.
Returns
-------
sum(log(exp(a)))
Notes
-----
This function was taken from the mailing list
http://mail.scipy.org/pipermail/scipy-user/2009-October/022931.html
This should be superceded by the ufunc when it is finished.
"""
if axis is None:
# Use the scipy.maxentropy version.
return sp_logsumexp(a)
a = np.asarray(a)
shp = list(a.shape)
shp[axis] = 1
a_max = a.max(axis=axis)
s = np.log(np.exp(a - a_max.reshape(shp)).sum(axis=axis))
lse = a_max + s
return lse
def _isproperdist(X):
"""
Checks to see if `X` is a proper probability distribution
"""
X = np.asarray(X)
if not np.allclose(np.sum(X), 1) or not np.all(X>=0) or not np.all(X<=1):
return False
else:
return True
def discretize(X, method="ef", nbins=None):
"""
Discretize `X`
Parameters
----------
bins : int, optional
Number of bins. Default is floor(sqrt(N))
method : str
"ef" is equal-frequency binning
"ew" is equal-width binning
Examples
--------
"""
nobs = len(X)
if nbins is None:
nbins = np.floor(np.sqrt(nobs))
if method == "ef":
discrete = np.ceil(nbins * stats.rankdata(X)/nobs)
if method == "ew":
width = np.max(X) - np.min(X)
width = np.floor(width/nbins)
svec, ivec = stats.fastsort(X)
discrete = np.zeros(nobs)
binnum = 1
base = svec[0]
discrete[ivec[0]] = binnum
for i in range(1,nobs):
if svec[i] < base + width:
discrete[ivec[i]] = binnum
else:
base = svec[i]
binnum += 1
discrete[ivec[i]] = binnum
return discrete
#TODO: looks okay but needs more robust tests for corner cases
def logbasechange(a,b):
"""
There is a one-to-one transformation of the entropy value from
a log base b to a log base a :
H_{b}(X)=log_{b}(a)[H_{a}(X)]
Returns
-------
log_{b}(a)
"""
return np.log(b)/np.log(a)
def natstobits(X):
"""
Converts from nats to bits
"""
return logbasechange(np.e, 2) * X
def bitstonats(X):
"""
Converts from bits to nats
"""
return logbasechange(2, np.e) * X
#TODO: make this entropy, and then have different measures as
#a method
def shannonentropy(px, logbase=2):
"""
This is Shannon's entropy
Parameters
----------
logbase, int or np.e
The base of the log
px : 1d or 2d array_like
Can be a discrete probability distribution, a 2d joint distribution,
or a sequence of probabilities.
Returns
-----
For log base 2 (bits) given a discrete distribution
H(p) = sum(px * log2(1/px) = -sum(pk*log2(px)) = E[log2(1/p(X))]
For log base 2 (bits) given a joint distribution
H(px,py) = -sum_{k,j}*w_{kj}log2(w_{kj})
Notes
-----
shannonentropy(0) is defined as 0
"""
#TODO: have not defined the px,py case?
px = np.asarray(px)
if not np.all(px <= 1) or not np.all(px >= 0):
raise ValueError("px does not define proper distribution")
entropy = -np.sum(np.nan_to_num(px*np.log2(px)))
if logbase != 2:
return logbasechange(2,logbase) * entropy
else:
return entropy
# Shannon's information content
def shannoninfo(px, logbase=2):
"""
Shannon's information
Parameters
----------
px : float or array_like
`px` is a discrete probability distribution
Returns
-------
For logbase = 2
np.log2(px)
"""
px = np.asarray(px)
if not np.all(px <= 1) or not np.all(px >= 0):
raise ValueError("px does not define proper distribution")
if logbase != 2:
return - logbasechange(2,logbase) * np.log2(px)
else:
return - np.log2(px)
def condentropy(px, py, pxpy=None, logbase=2):
"""
Return the conditional entropy of X given Y.
Parameters
----------
px : array_like
py : array_like
pxpy : array_like, optional
If pxpy is None, the distributions are assumed to be independent
and conendtropy(px,py) = shannonentropy(px)
logbase : int or np.e
Returns
-------
sum_{kj}log(q_{j}/w_{kj}
where q_{j} = Y[j]
and w_kj = X[k,j]
"""
if not _isproperdist(px) or not _isproperdist(py):
raise ValueError("px or py is not a proper probability distribution")
if pxpy is not None and not _isproperdist(pxpy):
raise ValueError("pxpy is not a proper joint distribtion")
if pxpy is None:
pxpy = np.outer(py,px)
condent = np.sum(pxpy * np.nan_to_num(np.log2(py/pxpy)))
if logbase == 2:
return condent
else:
return logbasechange(2, logbase) * condent
def mutualinfo(px,py,pxpy, logbase=2):
"""
Returns the mutual information between X and Y.
Parameters
----------
px : array_like
Discrete probability distribution of random variable X
py : array_like
Discrete probability distribution of random variable Y
pxpy : 2d array_like
The joint probability distribution of random variables X and Y.
Note that if X and Y are independent then the mutual information
is zero.
logbase : int or np.e, optional
Default is 2 (bits)
Returns
-------
shannonentropy(px) - condentropy(px,py,pxpy)
"""
if not _isproperdist(px) or not _isproperdist(py):
raise ValueError("px or py is not a proper probability distribution")
if pxpy is not None and not _isproperdist(pxpy):
raise ValueError("pxpy is not a proper joint distribtion")
if pxpy is None:
pxpy = np.outer(py,px)
return shannonentropy(px, logbase=logbase) - condentropy(px,py,pxpy,
logbase=logbase)
def corrent(px,py,pxpy,logbase=2):
"""
An information theoretic correlation measure.
Reflects linear and nonlinear correlation between two random variables
X and Y, characterized by the discrete probability distributions px and py
respectively.
Parameters
----------
px : array_like
Discrete probability distribution of random variable X
py : array_like
Discrete probability distribution of random variable Y
pxpy : 2d array_like, optional
Joint probability distribution of X and Y. If pxpy is None, X and Y
are assumed to be independent.
logbase : int or np.e, optional
Default is 2 (bits)
Returns
-------
mutualinfo(px,py,pxpy,logbase=logbase)/shannonentropy(py,logbase=logbase)
Notes
-----
This is also equivalent to
corrent(px,py,pxpy) = 1 - condent(px,py,pxpy)/shannonentropy(py)
"""
if not _isproperdist(px) or not _isproperdist(py):
raise ValueError("px or py is not a proper probability distribution")
if pxpy is not None and not _isproperdist(pxpy):
raise ValueError("pxpy is not a proper joint distribtion")
if pxpy is None:
pxpy = np.outer(py,px)
return mutualinfo(px,py,pxpy,logbase=logbase)/shannonentropy(py,
logbase=logbase)
def covent(px,py,pxpy,logbase=2):
"""
An information theoretic covariance measure.
Reflects linear and nonlinear correlation between two random variables
X and Y, characterized by the discrete probability distributions px and py
respectively.
Parameters
----------
px : array_like
Discrete probability distribution of random variable X
py : array_like
Discrete probability distribution of random variable Y
pxpy : 2d array_like, optional
Joint probability distribution of X and Y. If pxpy is None, X and Y
are assumed to be independent.
logbase : int or np.e, optional
Default is 2 (bits)
Returns
-------
condent(px,py,pxpy,logbase=logbase) + condent(py,px,pxpy,
logbase=logbase)
Notes
-----
This is also equivalent to
covent(px,py,pxpy) = condent(px,py,pxpy) + condent(py,px,pxpy)
"""
if not _isproperdist(px) or not _isproperdist(py):
raise ValueError("px or py is not a proper probability distribution")
if pxpy is not None and not _isproperdist(pxpy):
raise ValueError("pxpy is not a proper joint distribtion")
if pxpy is None:
pxpy = np.outer(py,px)
# FIXME: these should be `condentropy`, not `condent`
return (condent(px, py, pxpy, logbase=logbase) # noqa:F821 See GH#5756
+ condent(py, px, pxpy, logbase=logbase)) # noqa:F821 See GH#5756
#### Generalized Entropies ####
def renyientropy(px,alpha=1,logbase=2,measure='R'):
"""
Renyi's generalized entropy
Parameters
----------
px : array_like
Discrete probability distribution of random variable X. Note that
px is assumed to be a proper probability distribution.
logbase : int or np.e, optional
Default is 2 (bits)
alpha : float or inf
The order of the entropy. The default is 1, which in the limit
is just Shannon's entropy. 2 is Renyi (Collision) entropy. If
the string "inf" or numpy.inf is specified the min-entropy is returned.
measure : str, optional
The type of entropy measure desired. 'R' returns Renyi entropy
measure. 'T' returns the Tsallis entropy measure.
Returns
-------
1/(1-alpha)*log(sum(px**alpha))
In the limit as alpha -> 1, Shannon's entropy is returned.
In the limit as alpha -> inf, min-entropy is returned.
"""
#TODO:finish returns
#TODO:add checks for measure
if not _isproperdist(px):
raise ValueError("px is not a proper probability distribution")
alpha = float(alpha)
if alpha == 1:
genent = shannonentropy(px)
if logbase != 2:
return logbasechange(2, logbase) * genent
return genent
elif 'inf' in str(alpha).lower() or alpha == np.inf:
return -np.log(np.max(px))
# gets here if alpha != (1 or inf)
px = px**alpha
genent = np.log(px.sum())
if logbase == 2:
return 1/(1-alpha) * genent
else:
return 1/(1-alpha) * logbasechange(2, logbase) * genent
#TODO: before completing this, need to rethink the organization of
# (relative) entropy measures, ie., all put into one function
# and have kwdargs, etc.?
def gencrossentropy(px,py,pxpy,alpha=1,logbase=2, measure='T'):
"""
Generalized cross-entropy measures.
Parameters
----------
px : array_like
Discrete probability distribution of random variable X
py : array_like
Discrete probability distribution of random variable Y
pxpy : 2d array_like, optional
Joint probability distribution of X and Y. If pxpy is None, X and Y
are assumed to be independent.
logbase : int or np.e, optional
Default is 2 (bits)
measure : str, optional
The measure is the type of generalized cross-entropy desired. 'T' is
the cross-entropy version of the Tsallis measure. 'CR' is Cressie-Read
measure.
"""
if __name__ == "__main__":
print("From Golan (2008) \"Information and Entropy Econometrics -- A Review \
and Synthesis")
print("Table 3.1")
# Examples from Golan (2008)
X = [.2,.2,.2,.2,.2]
Y = [.322,.072,.511,.091,.004]
for i in X:
print(shannoninfo(i))
for i in Y:
print(shannoninfo(i))
print(shannonentropy(X))
print(shannonentropy(Y))
p = [1e-5,1e-4,.001,.01,.1,.15,.2,.25,.3,.35,.4,.45,.5]
plt.subplot(111)
plt.ylabel("Information")
plt.xlabel("Probability")
x = np.linspace(0,1,100001)
plt.plot(x, shannoninfo(x))
# plt.show()
plt.subplot(111)
plt.ylabel("Entropy")
plt.xlabel("Probability")
x = np.linspace(0,1,101)
plt.plot(x, lmap(shannonentropy, lzip(x,1-x)))
# plt.show()
# define a joint probability distribution
# from Golan (2008) table 3.3
w = np.array([[0,0,1./3],[1/9.,1/9.,1/9.],[1/18.,1/9.,1/6.]])
# table 3.4
px = w.sum(0)
py = w.sum(1)
H_X = shannonentropy(px)
H_Y = shannonentropy(py)
H_XY = shannonentropy(w)
H_XgivenY = condentropy(px,py,w)
H_YgivenX = condentropy(py,px,w)
# note that cross-entropy is not a distance measure as the following shows
D_YX = logbasechange(2,np.e)*stats.entropy(px, py)
D_XY = logbasechange(2,np.e)*stats.entropy(py, px)
I_XY = mutualinfo(px,py,w)
print("Table 3.3")
print(H_X,H_Y, H_XY, H_XgivenY, H_YgivenX, D_YX, D_XY, I_XY)
print("discretize functions")
X=np.array([21.2,44.5,31.0,19.5,40.6,38.7,11.1,15.8,31.9,25.8,20.2,14.2,
24.0,21.0,11.3,18.0,16.3,22.2,7.8,27.8,16.3,35.1,14.9,17.1,28.2,16.4,
16.5,46.0,9.5,18.8,32.1,26.1,16.1,7.3,21.4,20.0,29.3,14.9,8.3,22.5,
12.8,26.9,25.5,22.9,11.2,20.7,26.2,9.3,10.8,15.6])
discX = discretize(X)
#CF: R's infotheo
#TODO: compare to pyentropy quantize?
print
print("Example in section 3.6 of Golan, using table 3.3")
print("Bounding errors using Fano's inequality")
print("H(P_{e}) + P_{e}log(K-1) >= H(X|Y)")
print("or, a weaker inequality")
print("P_{e} >= [H(X|Y) - 1]/log(K)")
print("P(x) = %s" % px)
print("X = 3 has the highest probability, so this is the estimate Xhat")
pe = 1 - px[2]
print("The probability of error Pe is 1 - p(X=3) = %0.4g" % pe)
H_pe = shannonentropy([pe,1-pe])
print("H(Pe) = %0.4g and K=3" % H_pe)
print("H(Pe) + Pe*log(K-1) = %0.4g >= H(X|Y) = %0.4g" % \
(H_pe+pe*np.log2(2), H_XgivenY))
print("or using the weaker inequality")
print("Pe = %0.4g >= [H(X) - 1]/log(K) = %0.4g" % (pe, (H_X - 1)/np.log2(3)))
print("Consider now, table 3.5, where there is additional information")
print("The conditional probabilities of P(X|Y=y) are ")
w2 = np.array([[0.,0.,1.],[1/3.,1/3.,1/3.],[1/6.,1/3.,1/2.]])
print(w2)
# not a proper distribution?
print("The probability of error given this information is")
print("Pe = [H(X|Y) -1]/log(K) = %0.4g" % ((np.mean([0,shannonentropy(w2[1]),shannonentropy(w2[2])])-1)/np.log2(3)))
print("such that more information lowers the error")
### Stochastic processes
markovchain = np.array([[.553,.284,.163],[.465,.312,.223],[.420,.322,.258]])
| bsd-3-clause |
h2educ/scikit-learn | sklearn/linear_model/tests/test_logistic.py | 59 | 35368 | import numpy as np
import scipy.sparse as sp
from scipy import linalg, optimize, sparse
import scipy
from sklearn.utils.testing import assert_almost_equal
from sklearn.utils.testing import assert_array_equal
from sklearn.utils.testing import assert_array_almost_equal
from sklearn.utils.testing import assert_equal
from sklearn.utils.testing import assert_greater
from sklearn.utils.testing import assert_raises
from sklearn.utils.testing import assert_true
from sklearn.utils.testing import assert_warns
from sklearn.utils.testing import raises
from sklearn.utils.testing import ignore_warnings
from sklearn.utils.testing import assert_raise_message
from sklearn.utils import ConvergenceWarning
from sklearn.linear_model.logistic import (
LogisticRegression,
logistic_regression_path, LogisticRegressionCV,
_logistic_loss_and_grad, _logistic_grad_hess,
_multinomial_grad_hess, _logistic_loss,
)
from sklearn.cross_validation import StratifiedKFold
from sklearn.datasets import load_iris, make_classification
from sklearn.metrics import log_loss
X = [[-1, 0], [0, 1], [1, 1]]
X_sp = sp.csr_matrix(X)
Y1 = [0, 1, 1]
Y2 = [2, 1, 0]
iris = load_iris()
sp_version = tuple([int(s) for s in scipy.__version__.split('.')])
def check_predictions(clf, X, y):
"""Check that the model is able to fit the classification data"""
n_samples = len(y)
classes = np.unique(y)
n_classes = classes.shape[0]
predicted = clf.fit(X, y).predict(X)
assert_array_equal(clf.classes_, classes)
assert_equal(predicted.shape, (n_samples,))
assert_array_equal(predicted, y)
probabilities = clf.predict_proba(X)
assert_equal(probabilities.shape, (n_samples, n_classes))
assert_array_almost_equal(probabilities.sum(axis=1), np.ones(n_samples))
assert_array_equal(probabilities.argmax(axis=1), y)
def test_predict_2_classes():
# Simple sanity check on a 2 classes dataset
# Make sure it predicts the correct result on simple datasets.
check_predictions(LogisticRegression(random_state=0), X, Y1)
check_predictions(LogisticRegression(random_state=0), X_sp, Y1)
check_predictions(LogisticRegression(C=100, random_state=0), X, Y1)
check_predictions(LogisticRegression(C=100, random_state=0), X_sp, Y1)
check_predictions(LogisticRegression(fit_intercept=False,
random_state=0), X, Y1)
check_predictions(LogisticRegression(fit_intercept=False,
random_state=0), X_sp, Y1)
def test_error():
# Test for appropriate exception on errors
msg = "Penalty term must be positive"
assert_raise_message(ValueError, msg,
LogisticRegression(C=-1).fit, X, Y1)
assert_raise_message(ValueError, msg,
LogisticRegression(C="test").fit, X, Y1)
for LR in [LogisticRegression, LogisticRegressionCV]:
msg = "Tolerance for stopping criteria must be positive"
assert_raise_message(ValueError, msg, LR(tol=-1).fit, X, Y1)
assert_raise_message(ValueError, msg, LR(tol="test").fit, X, Y1)
msg = "Maximum number of iteration must be positive"
assert_raise_message(ValueError, msg, LR(max_iter=-1).fit, X, Y1)
assert_raise_message(ValueError, msg, LR(max_iter="test").fit, X, Y1)
def test_predict_3_classes():
check_predictions(LogisticRegression(C=10), X, Y2)
check_predictions(LogisticRegression(C=10), X_sp, Y2)
def test_predict_iris():
# Test logistic regression with the iris dataset
n_samples, n_features = iris.data.shape
target = iris.target_names[iris.target]
# Test that both multinomial and OvR solvers handle
# multiclass data correctly and give good accuracy
# score (>0.95) for the training data.
for clf in [LogisticRegression(C=len(iris.data)),
LogisticRegression(C=len(iris.data), solver='lbfgs',
multi_class='multinomial'),
LogisticRegression(C=len(iris.data), solver='newton-cg',
multi_class='multinomial'),
LogisticRegression(C=len(iris.data), solver='sag', tol=1e-2,
multi_class='ovr', random_state=42)]:
clf.fit(iris.data, target)
assert_array_equal(np.unique(target), clf.classes_)
pred = clf.predict(iris.data)
assert_greater(np.mean(pred == target), .95)
probabilities = clf.predict_proba(iris.data)
assert_array_almost_equal(probabilities.sum(axis=1),
np.ones(n_samples))
pred = iris.target_names[probabilities.argmax(axis=1)]
assert_greater(np.mean(pred == target), .95)
def test_multinomial_validation():
for solver in ['lbfgs', 'newton-cg']:
lr = LogisticRegression(C=-1, solver=solver, multi_class='multinomial')
assert_raises(ValueError, lr.fit, [[0, 1], [1, 0]], [0, 1])
def test_check_solver_option():
X, y = iris.data, iris.target
for LR in [LogisticRegression, LogisticRegressionCV]:
msg = ("Logistic Regression supports only liblinear, newton-cg, lbfgs"
" and sag solvers, got wrong_name")
lr = LR(solver="wrong_name")
assert_raise_message(ValueError, msg, lr.fit, X, y)
msg = "multi_class should be either multinomial or ovr, got wrong_name"
lr = LR(solver='newton-cg', multi_class="wrong_name")
assert_raise_message(ValueError, msg, lr.fit, X, y)
# all solver except 'newton-cg' and 'lfbgs'
for solver in ['liblinear', 'sag']:
msg = ("Solver %s does not support a multinomial backend." %
solver)
lr = LR(solver=solver, multi_class='multinomial')
assert_raise_message(ValueError, msg, lr.fit, X, y)
# all solvers except 'liblinear'
for solver in ['newton-cg', 'lbfgs', 'sag']:
msg = ("Solver %s supports only l2 penalties, got l1 penalty." %
solver)
lr = LR(solver=solver, penalty='l1')
assert_raise_message(ValueError, msg, lr.fit, X, y)
msg = ("Solver %s supports only dual=False, got dual=True" %
solver)
lr = LR(solver=solver, dual=True)
assert_raise_message(ValueError, msg, lr.fit, X, y)
def test_multinomial_binary():
# Test multinomial LR on a binary problem.
target = (iris.target > 0).astype(np.intp)
target = np.array(["setosa", "not-setosa"])[target]
for solver in ['lbfgs', 'newton-cg']:
clf = LogisticRegression(solver=solver, multi_class='multinomial')
clf.fit(iris.data, target)
assert_equal(clf.coef_.shape, (1, iris.data.shape[1]))
assert_equal(clf.intercept_.shape, (1,))
assert_array_equal(clf.predict(iris.data), target)
mlr = LogisticRegression(solver=solver, multi_class='multinomial',
fit_intercept=False)
mlr.fit(iris.data, target)
pred = clf.classes_[np.argmax(clf.predict_log_proba(iris.data),
axis=1)]
assert_greater(np.mean(pred == target), .9)
def test_sparsify():
# Test sparsify and densify members.
n_samples, n_features = iris.data.shape
target = iris.target_names[iris.target]
clf = LogisticRegression(random_state=0).fit(iris.data, target)
pred_d_d = clf.decision_function(iris.data)
clf.sparsify()
assert_true(sp.issparse(clf.coef_))
pred_s_d = clf.decision_function(iris.data)
sp_data = sp.coo_matrix(iris.data)
pred_s_s = clf.decision_function(sp_data)
clf.densify()
pred_d_s = clf.decision_function(sp_data)
assert_array_almost_equal(pred_d_d, pred_s_d)
assert_array_almost_equal(pred_d_d, pred_s_s)
assert_array_almost_equal(pred_d_d, pred_d_s)
def test_inconsistent_input():
# Test that an exception is raised on inconsistent input
rng = np.random.RandomState(0)
X_ = rng.random_sample((5, 10))
y_ = np.ones(X_.shape[0])
y_[0] = 0
clf = LogisticRegression(random_state=0)
# Wrong dimensions for training data
y_wrong = y_[:-1]
assert_raises(ValueError, clf.fit, X, y_wrong)
# Wrong dimensions for test data
assert_raises(ValueError, clf.fit(X_, y_).predict,
rng.random_sample((3, 12)))
def test_write_parameters():
# Test that we can write to coef_ and intercept_
clf = LogisticRegression(random_state=0)
clf.fit(X, Y1)
clf.coef_[:] = 0
clf.intercept_[:] = 0
assert_array_almost_equal(clf.decision_function(X), 0)
@raises(ValueError)
def test_nan():
# Test proper NaN handling.
# Regression test for Issue #252: fit used to go into an infinite loop.
Xnan = np.array(X, dtype=np.float64)
Xnan[0, 1] = np.nan
LogisticRegression(random_state=0).fit(Xnan, Y1)
def test_consistency_path():
# Test that the path algorithm is consistent
rng = np.random.RandomState(0)
X = np.concatenate((rng.randn(100, 2) + [1, 1], rng.randn(100, 2)))
y = [1] * 100 + [-1] * 100
Cs = np.logspace(0, 4, 10)
f = ignore_warnings
# can't test with fit_intercept=True since LIBLINEAR
# penalizes the intercept
for solver in ('lbfgs', 'newton-cg', 'liblinear', 'sag'):
coefs, Cs, _ = f(logistic_regression_path)(
X, y, Cs=Cs, fit_intercept=False, tol=1e-5, solver=solver,
random_state=0)
for i, C in enumerate(Cs):
lr = LogisticRegression(C=C, fit_intercept=False, tol=1e-5,
random_state=0)
lr.fit(X, y)
lr_coef = lr.coef_.ravel()
assert_array_almost_equal(lr_coef, coefs[i], decimal=4,
err_msg="with solver = %s" % solver)
# test for fit_intercept=True
for solver in ('lbfgs', 'newton-cg', 'liblinear', 'sag'):
Cs = [1e3]
coefs, Cs, _ = f(logistic_regression_path)(
X, y, Cs=Cs, fit_intercept=True, tol=1e-6, solver=solver,
intercept_scaling=10000., random_state=0)
lr = LogisticRegression(C=Cs[0], fit_intercept=True, tol=1e-4,
intercept_scaling=10000., random_state=0)
lr.fit(X, y)
lr_coef = np.concatenate([lr.coef_.ravel(), lr.intercept_])
assert_array_almost_equal(lr_coef, coefs[0], decimal=4,
err_msg="with solver = %s" % solver)
def test_liblinear_dual_random_state():
# random_state is relevant for liblinear solver only if dual=True
X, y = make_classification(n_samples=20)
lr1 = LogisticRegression(random_state=0, dual=True, max_iter=1, tol=1e-15)
lr1.fit(X, y)
lr2 = LogisticRegression(random_state=0, dual=True, max_iter=1, tol=1e-15)
lr2.fit(X, y)
lr3 = LogisticRegression(random_state=8, dual=True, max_iter=1, tol=1e-15)
lr3.fit(X, y)
# same result for same random state
assert_array_almost_equal(lr1.coef_, lr2.coef_)
# different results for different random states
msg = "Arrays are not almost equal to 6 decimals"
assert_raise_message(AssertionError, msg,
assert_array_almost_equal, lr1.coef_, lr3.coef_)
def test_logistic_loss_and_grad():
X_ref, y = make_classification(n_samples=20)
n_features = X_ref.shape[1]
X_sp = X_ref.copy()
X_sp[X_sp < .1] = 0
X_sp = sp.csr_matrix(X_sp)
for X in (X_ref, X_sp):
w = np.zeros(n_features)
# First check that our derivation of the grad is correct
loss, grad = _logistic_loss_and_grad(w, X, y, alpha=1.)
approx_grad = optimize.approx_fprime(
w, lambda w: _logistic_loss_and_grad(w, X, y, alpha=1.)[0], 1e-3
)
assert_array_almost_equal(grad, approx_grad, decimal=2)
# Second check that our intercept implementation is good
w = np.zeros(n_features + 1)
loss_interp, grad_interp = _logistic_loss_and_grad(
w, X, y, alpha=1.
)
assert_array_almost_equal(loss, loss_interp)
approx_grad = optimize.approx_fprime(
w, lambda w: _logistic_loss_and_grad(w, X, y, alpha=1.)[0], 1e-3
)
assert_array_almost_equal(grad_interp, approx_grad, decimal=2)
def test_logistic_grad_hess():
rng = np.random.RandomState(0)
n_samples, n_features = 50, 5
X_ref = rng.randn(n_samples, n_features)
y = np.sign(X_ref.dot(5 * rng.randn(n_features)))
X_ref -= X_ref.mean()
X_ref /= X_ref.std()
X_sp = X_ref.copy()
X_sp[X_sp < .1] = 0
X_sp = sp.csr_matrix(X_sp)
for X in (X_ref, X_sp):
w = .1 * np.ones(n_features)
# First check that _logistic_grad_hess is consistent
# with _logistic_loss_and_grad
loss, grad = _logistic_loss_and_grad(w, X, y, alpha=1.)
grad_2, hess = _logistic_grad_hess(w, X, y, alpha=1.)
assert_array_almost_equal(grad, grad_2)
# Now check our hessian along the second direction of the grad
vector = np.zeros_like(grad)
vector[1] = 1
hess_col = hess(vector)
# Computation of the Hessian is particularly fragile to numerical
# errors when doing simple finite differences. Here we compute the
# grad along a path in the direction of the vector and then use a
# least-square regression to estimate the slope
e = 1e-3
d_x = np.linspace(-e, e, 30)
d_grad = np.array([
_logistic_loss_and_grad(w + t * vector, X, y, alpha=1.)[1]
for t in d_x
])
d_grad -= d_grad.mean(axis=0)
approx_hess_col = linalg.lstsq(d_x[:, np.newaxis], d_grad)[0].ravel()
assert_array_almost_equal(approx_hess_col, hess_col, decimal=3)
# Second check that our intercept implementation is good
w = np.zeros(n_features + 1)
loss_interp, grad_interp = _logistic_loss_and_grad(w, X, y, alpha=1.)
loss_interp_2 = _logistic_loss(w, X, y, alpha=1.)
grad_interp_2, hess = _logistic_grad_hess(w, X, y, alpha=1.)
assert_array_almost_equal(loss_interp, loss_interp_2)
assert_array_almost_equal(grad_interp, grad_interp_2)
def test_logistic_cv():
# test for LogisticRegressionCV object
n_samples, n_features = 50, 5
rng = np.random.RandomState(0)
X_ref = rng.randn(n_samples, n_features)
y = np.sign(X_ref.dot(5 * rng.randn(n_features)))
X_ref -= X_ref.mean()
X_ref /= X_ref.std()
lr_cv = LogisticRegressionCV(Cs=[1.], fit_intercept=False,
solver='liblinear')
lr_cv.fit(X_ref, y)
lr = LogisticRegression(C=1., fit_intercept=False)
lr.fit(X_ref, y)
assert_array_almost_equal(lr.coef_, lr_cv.coef_)
assert_array_equal(lr_cv.coef_.shape, (1, n_features))
assert_array_equal(lr_cv.classes_, [-1, 1])
assert_equal(len(lr_cv.classes_), 2)
coefs_paths = np.asarray(list(lr_cv.coefs_paths_.values()))
assert_array_equal(coefs_paths.shape, (1, 3, 1, n_features))
assert_array_equal(lr_cv.Cs_.shape, (1, ))
scores = np.asarray(list(lr_cv.scores_.values()))
assert_array_equal(scores.shape, (1, 3, 1))
def test_logistic_cv_sparse():
X, y = make_classification(n_samples=50, n_features=5,
random_state=0)
X[X < 1.0] = 0.0
csr = sp.csr_matrix(X)
clf = LogisticRegressionCV(fit_intercept=True)
clf.fit(X, y)
clfs = LogisticRegressionCV(fit_intercept=True)
clfs.fit(csr, y)
assert_array_almost_equal(clfs.coef_, clf.coef_)
assert_array_almost_equal(clfs.intercept_, clf.intercept_)
assert_equal(clfs.C_, clf.C_)
def test_intercept_logistic_helper():
n_samples, n_features = 10, 5
X, y = make_classification(n_samples=n_samples, n_features=n_features,
random_state=0)
# Fit intercept case.
alpha = 1.
w = np.ones(n_features + 1)
grad_interp, hess_interp = _logistic_grad_hess(w, X, y, alpha)
loss_interp = _logistic_loss(w, X, y, alpha)
# Do not fit intercept. This can be considered equivalent to adding
# a feature vector of ones, i.e column of one vectors.
X_ = np.hstack((X, np.ones(10)[:, np.newaxis]))
grad, hess = _logistic_grad_hess(w, X_, y, alpha)
loss = _logistic_loss(w, X_, y, alpha)
# In the fit_intercept=False case, the feature vector of ones is
# penalized. This should be taken care of.
assert_almost_equal(loss_interp + 0.5 * (w[-1] ** 2), loss)
# Check gradient.
assert_array_almost_equal(grad_interp[:n_features], grad[:n_features])
assert_almost_equal(grad_interp[-1] + alpha * w[-1], grad[-1])
rng = np.random.RandomState(0)
grad = rng.rand(n_features + 1)
hess_interp = hess_interp(grad)
hess = hess(grad)
assert_array_almost_equal(hess_interp[:n_features], hess[:n_features])
assert_almost_equal(hess_interp[-1] + alpha * grad[-1], hess[-1])
def test_ovr_multinomial_iris():
# Test that OvR and multinomial are correct using the iris dataset.
train, target = iris.data, iris.target
n_samples, n_features = train.shape
# Use pre-defined fold as folds generated for different y
cv = StratifiedKFold(target, 3)
clf = LogisticRegressionCV(cv=cv)
clf.fit(train, target)
clf1 = LogisticRegressionCV(cv=cv)
target_copy = target.copy()
target_copy[target_copy == 0] = 1
clf1.fit(train, target_copy)
assert_array_almost_equal(clf.scores_[2], clf1.scores_[2])
assert_array_almost_equal(clf.intercept_[2:], clf1.intercept_)
assert_array_almost_equal(clf.coef_[2][np.newaxis, :], clf1.coef_)
# Test the shape of various attributes.
assert_equal(clf.coef_.shape, (3, n_features))
assert_array_equal(clf.classes_, [0, 1, 2])
coefs_paths = np.asarray(list(clf.coefs_paths_.values()))
assert_array_almost_equal(coefs_paths.shape, (3, 3, 10, n_features + 1))
assert_equal(clf.Cs_.shape, (10, ))
scores = np.asarray(list(clf.scores_.values()))
assert_equal(scores.shape, (3, 3, 10))
# Test that for the iris data multinomial gives a better accuracy than OvR
for solver in ['lbfgs', 'newton-cg']:
clf_multi = LogisticRegressionCV(
solver=solver, multi_class='multinomial', max_iter=15
)
clf_multi.fit(train, target)
multi_score = clf_multi.score(train, target)
ovr_score = clf.score(train, target)
assert_greater(multi_score, ovr_score)
# Test attributes of LogisticRegressionCV
assert_equal(clf.coef_.shape, clf_multi.coef_.shape)
assert_array_equal(clf_multi.classes_, [0, 1, 2])
coefs_paths = np.asarray(list(clf_multi.coefs_paths_.values()))
assert_array_almost_equal(coefs_paths.shape, (3, 3, 10,
n_features + 1))
assert_equal(clf_multi.Cs_.shape, (10, ))
scores = np.asarray(list(clf_multi.scores_.values()))
assert_equal(scores.shape, (3, 3, 10))
def test_logistic_regression_solvers():
X, y = make_classification(n_features=10, n_informative=5, random_state=0)
ncg = LogisticRegression(solver='newton-cg', fit_intercept=False)
lbf = LogisticRegression(solver='lbfgs', fit_intercept=False)
lib = LogisticRegression(fit_intercept=False)
sag = LogisticRegression(solver='sag', fit_intercept=False,
random_state=42)
ncg.fit(X, y)
lbf.fit(X, y)
sag.fit(X, y)
lib.fit(X, y)
assert_array_almost_equal(ncg.coef_, lib.coef_, decimal=3)
assert_array_almost_equal(lib.coef_, lbf.coef_, decimal=3)
assert_array_almost_equal(ncg.coef_, lbf.coef_, decimal=3)
assert_array_almost_equal(sag.coef_, lib.coef_, decimal=3)
assert_array_almost_equal(sag.coef_, ncg.coef_, decimal=3)
assert_array_almost_equal(sag.coef_, lbf.coef_, decimal=3)
def test_logistic_regression_solvers_multiclass():
X, y = make_classification(n_samples=20, n_features=20, n_informative=10,
n_classes=3, random_state=0)
tol = 1e-6
ncg = LogisticRegression(solver='newton-cg', fit_intercept=False, tol=tol)
lbf = LogisticRegression(solver='lbfgs', fit_intercept=False, tol=tol)
lib = LogisticRegression(fit_intercept=False, tol=tol)
sag = LogisticRegression(solver='sag', fit_intercept=False, tol=tol,
max_iter=1000, random_state=42)
ncg.fit(X, y)
lbf.fit(X, y)
sag.fit(X, y)
lib.fit(X, y)
assert_array_almost_equal(ncg.coef_, lib.coef_, decimal=4)
assert_array_almost_equal(lib.coef_, lbf.coef_, decimal=4)
assert_array_almost_equal(ncg.coef_, lbf.coef_, decimal=4)
assert_array_almost_equal(sag.coef_, lib.coef_, decimal=4)
assert_array_almost_equal(sag.coef_, ncg.coef_, decimal=4)
assert_array_almost_equal(sag.coef_, lbf.coef_, decimal=4)
def test_logistic_regressioncv_class_weights():
X, y = make_classification(n_samples=20, n_features=20, n_informative=10,
n_classes=3, random_state=0)
# Test the liblinear fails when class_weight of type dict is
# provided, when it is multiclass. However it can handle
# binary problems.
clf_lib = LogisticRegressionCV(class_weight={0: 0.1, 1: 0.2},
solver='liblinear')
assert_raises(ValueError, clf_lib.fit, X, y)
y_ = y.copy()
y_[y == 2] = 1
clf_lib.fit(X, y_)
assert_array_equal(clf_lib.classes_, [0, 1])
# Test for class_weight=balanced
X, y = make_classification(n_samples=20, n_features=20, n_informative=10,
random_state=0)
clf_lbf = LogisticRegressionCV(solver='lbfgs', fit_intercept=False,
class_weight='balanced')
clf_lbf.fit(X, y)
clf_lib = LogisticRegressionCV(solver='liblinear', fit_intercept=False,
class_weight='balanced')
clf_lib.fit(X, y)
clf_sag = LogisticRegressionCV(solver='sag', fit_intercept=False,
class_weight='balanced', max_iter=2000)
clf_sag.fit(X, y)
assert_array_almost_equal(clf_lib.coef_, clf_lbf.coef_, decimal=4)
assert_array_almost_equal(clf_sag.coef_, clf_lbf.coef_, decimal=4)
assert_array_almost_equal(clf_lib.coef_, clf_sag.coef_, decimal=4)
def test_logistic_regression_sample_weights():
X, y = make_classification(n_samples=20, n_features=5, n_informative=3,
n_classes=2, random_state=0)
for LR in [LogisticRegression, LogisticRegressionCV]:
# Test that liblinear fails when sample weights are provided
clf_lib = LR(solver='liblinear')
assert_raises(ValueError, clf_lib.fit, X, y,
sample_weight=np.ones(y.shape[0]))
# Test that passing sample_weight as ones is the same as
# not passing them at all (default None)
clf_sw_none = LR(solver='lbfgs', fit_intercept=False)
clf_sw_none.fit(X, y)
clf_sw_ones = LR(solver='lbfgs', fit_intercept=False)
clf_sw_ones.fit(X, y, sample_weight=np.ones(y.shape[0]))
assert_array_almost_equal(clf_sw_none.coef_, clf_sw_ones.coef_, decimal=4)
# Test that sample weights work the same with the lbfgs,
# newton-cg, and 'sag' solvers
clf_sw_lbfgs = LR(solver='lbfgs', fit_intercept=False)
clf_sw_lbfgs.fit(X, y, sample_weight=y + 1)
clf_sw_n = LR(solver='newton-cg', fit_intercept=False)
clf_sw_n.fit(X, y, sample_weight=y + 1)
clf_sw_sag = LR(solver='sag', fit_intercept=False,
max_iter=2000, tol=1e-7)
clf_sw_sag.fit(X, y, sample_weight=y + 1)
assert_array_almost_equal(clf_sw_lbfgs.coef_, clf_sw_n.coef_, decimal=4)
assert_array_almost_equal(clf_sw_lbfgs.coef_, clf_sw_sag.coef_, decimal=4)
# Test that passing class_weight as [1,2] is the same as
# passing class weight = [1,1] but adjusting sample weights
# to be 2 for all instances of class 2
clf_cw_12 = LR(solver='lbfgs', fit_intercept=False,
class_weight={0: 1, 1: 2})
clf_cw_12.fit(X, y)
sample_weight = np.ones(y.shape[0])
sample_weight[y == 1] = 2
clf_sw_12 = LR(solver='lbfgs', fit_intercept=False)
clf_sw_12.fit(X, y, sample_weight=sample_weight)
assert_array_almost_equal(clf_cw_12.coef_, clf_sw_12.coef_, decimal=4)
def test_logistic_regression_convergence_warnings():
# Test that warnings are raised if model does not converge
X, y = make_classification(n_samples=20, n_features=20)
clf_lib = LogisticRegression(solver='liblinear', max_iter=2, verbose=1)
assert_warns(ConvergenceWarning, clf_lib.fit, X, y)
assert_equal(clf_lib.n_iter_, 2)
def test_logistic_regression_multinomial():
# Tests for the multinomial option in logistic regression
# Some basic attributes of Logistic Regression
n_samples, n_features, n_classes = 50, 20, 3
X, y = make_classification(n_samples=n_samples,
n_features=n_features,
n_informative=10,
n_classes=n_classes, random_state=0)
clf_int = LogisticRegression(solver='lbfgs', multi_class='multinomial')
clf_int.fit(X, y)
assert_array_equal(clf_int.coef_.shape, (n_classes, n_features))
clf_wint = LogisticRegression(solver='lbfgs', multi_class='multinomial',
fit_intercept=False)
clf_wint.fit(X, y)
assert_array_equal(clf_wint.coef_.shape, (n_classes, n_features))
# Similar tests for newton-cg solver option
clf_ncg_int = LogisticRegression(solver='newton-cg',
multi_class='multinomial')
clf_ncg_int.fit(X, y)
assert_array_equal(clf_ncg_int.coef_.shape, (n_classes, n_features))
clf_ncg_wint = LogisticRegression(solver='newton-cg', fit_intercept=False,
multi_class='multinomial')
clf_ncg_wint.fit(X, y)
assert_array_equal(clf_ncg_wint.coef_.shape, (n_classes, n_features))
# Compare solutions between lbfgs and newton-cg
assert_almost_equal(clf_int.coef_, clf_ncg_int.coef_, decimal=3)
assert_almost_equal(clf_wint.coef_, clf_ncg_wint.coef_, decimal=3)
assert_almost_equal(clf_int.intercept_, clf_ncg_int.intercept_, decimal=3)
# Test that the path give almost the same results. However since in this
# case we take the average of the coefs after fitting across all the
# folds, it need not be exactly the same.
for solver in ['lbfgs', 'newton-cg']:
clf_path = LogisticRegressionCV(solver=solver,
multi_class='multinomial', Cs=[1.])
clf_path.fit(X, y)
assert_array_almost_equal(clf_path.coef_, clf_int.coef_, decimal=3)
assert_almost_equal(clf_path.intercept_, clf_int.intercept_, decimal=3)
def test_multinomial_grad_hess():
rng = np.random.RandomState(0)
n_samples, n_features, n_classes = 100, 5, 3
X = rng.randn(n_samples, n_features)
w = rng.rand(n_classes, n_features)
Y = np.zeros((n_samples, n_classes))
ind = np.argmax(np.dot(X, w.T), axis=1)
Y[range(0, n_samples), ind] = 1
w = w.ravel()
sample_weights = np.ones(X.shape[0])
grad, hessp = _multinomial_grad_hess(w, X, Y, alpha=1.,
sample_weight=sample_weights)
# extract first column of hessian matrix
vec = np.zeros(n_features * n_classes)
vec[0] = 1
hess_col = hessp(vec)
# Estimate hessian using least squares as done in
# test_logistic_grad_hess
e = 1e-3
d_x = np.linspace(-e, e, 30)
d_grad = np.array([
_multinomial_grad_hess(w + t * vec, X, Y, alpha=1.,
sample_weight=sample_weights)[0]
for t in d_x
])
d_grad -= d_grad.mean(axis=0)
approx_hess_col = linalg.lstsq(d_x[:, np.newaxis], d_grad)[0].ravel()
assert_array_almost_equal(hess_col, approx_hess_col)
def test_liblinear_decision_function_zero():
# Test negative prediction when decision_function values are zero.
# Liblinear predicts the positive class when decision_function values
# are zero. This is a test to verify that we do not do the same.
# See Issue: https://github.com/scikit-learn/scikit-learn/issues/3600
# and the PR https://github.com/scikit-learn/scikit-learn/pull/3623
X, y = make_classification(n_samples=5, n_features=5)
clf = LogisticRegression(fit_intercept=False)
clf.fit(X, y)
# Dummy data such that the decision function becomes zero.
X = np.zeros((5, 5))
assert_array_equal(clf.predict(X), np.zeros(5))
def test_liblinear_logregcv_sparse():
# Test LogRegCV with solver='liblinear' works for sparse matrices
X, y = make_classification(n_samples=10, n_features=5)
clf = LogisticRegressionCV(solver='liblinear')
clf.fit(sparse.csr_matrix(X), y)
def test_logreg_intercept_scaling():
# Test that the right error message is thrown when intercept_scaling <= 0
for i in [-1, 0]:
clf = LogisticRegression(intercept_scaling=i)
msg = ('Intercept scaling is %r but needs to be greater than 0.'
' To disable fitting an intercept,'
' set fit_intercept=False.' % clf.intercept_scaling)
assert_raise_message(ValueError, msg, clf.fit, X, Y1)
def test_logreg_intercept_scaling_zero():
# Test that intercept_scaling is ignored when fit_intercept is False
clf = LogisticRegression(fit_intercept=False)
clf.fit(X, Y1)
assert_equal(clf.intercept_, 0.)
def test_logreg_cv_penalty():
# Test that the correct penalty is passed to the final fit.
X, y = make_classification(n_samples=50, n_features=20, random_state=0)
lr_cv = LogisticRegressionCV(penalty="l1", Cs=[1.0], solver='liblinear')
lr_cv.fit(X, y)
lr = LogisticRegression(penalty="l1", C=1.0, solver='liblinear')
lr.fit(X, y)
assert_equal(np.count_nonzero(lr_cv.coef_), np.count_nonzero(lr.coef_))
def test_logreg_predict_proba_multinomial():
X, y = make_classification(n_samples=10, n_features=20, random_state=0,
n_classes=3, n_informative=10)
# Predicted probabilites using the true-entropy loss should give a
# smaller loss than those using the ovr method.
clf_multi = LogisticRegression(multi_class="multinomial", solver="lbfgs")
clf_multi.fit(X, y)
clf_multi_loss = log_loss(y, clf_multi.predict_proba(X))
clf_ovr = LogisticRegression(multi_class="ovr", solver="lbfgs")
clf_ovr.fit(X, y)
clf_ovr_loss = log_loss(y, clf_ovr.predict_proba(X))
assert_greater(clf_ovr_loss, clf_multi_loss)
# Predicted probabilites using the soft-max function should give a
# smaller loss than those using the logistic function.
clf_multi_loss = log_loss(y, clf_multi.predict_proba(X))
clf_wrong_loss = log_loss(y, clf_multi._predict_proba_lr(X))
assert_greater(clf_wrong_loss, clf_multi_loss)
@ignore_warnings
def test_max_iter():
# Test that the maximum number of iteration is reached
X, y_bin = iris.data, iris.target.copy()
y_bin[y_bin == 2] = 0
solvers = ['newton-cg', 'liblinear', 'sag']
# old scipy doesn't have maxiter
if sp_version >= (0, 12):
solvers.append('lbfgs')
for max_iter in range(1, 5):
for solver in solvers:
lr = LogisticRegression(max_iter=max_iter, tol=1e-15,
random_state=0, solver=solver)
lr.fit(X, y_bin)
assert_equal(lr.n_iter_[0], max_iter)
def test_n_iter():
# Test that self.n_iter_ has the correct format.
X, y = iris.data, iris.target
y_bin = y.copy()
y_bin[y_bin == 2] = 0
n_Cs = 4
n_cv_fold = 2
for solver in ['newton-cg', 'liblinear', 'sag', 'lbfgs']:
# OvR case
n_classes = 1 if solver == 'liblinear' else np.unique(y).shape[0]
clf = LogisticRegression(tol=1e-2, multi_class='ovr',
solver=solver, C=1.,
random_state=42, max_iter=100)
clf.fit(X, y)
assert_equal(clf.n_iter_.shape, (n_classes,))
n_classes = np.unique(y).shape[0]
clf = LogisticRegressionCV(tol=1e-2, multi_class='ovr',
solver=solver, Cs=n_Cs, cv=n_cv_fold,
random_state=42, max_iter=100)
clf.fit(X, y)
assert_equal(clf.n_iter_.shape, (n_classes, n_cv_fold, n_Cs))
clf.fit(X, y_bin)
assert_equal(clf.n_iter_.shape, (1, n_cv_fold, n_Cs))
# multinomial case
n_classes = 1
if solver in ('liblinear', 'sag'):
break
clf = LogisticRegression(tol=1e-2, multi_class='multinomial',
solver=solver, C=1.,
random_state=42, max_iter=100)
clf.fit(X, y)
assert_equal(clf.n_iter_.shape, (n_classes,))
clf = LogisticRegressionCV(tol=1e-2, multi_class='multinomial',
solver=solver, Cs=n_Cs, cv=n_cv_fold,
random_state=42, max_iter=100)
clf.fit(X, y)
assert_equal(clf.n_iter_.shape, (n_classes, n_cv_fold, n_Cs))
clf.fit(X, y_bin)
assert_equal(clf.n_iter_.shape, (1, n_cv_fold, n_Cs))
@ignore_warnings
def test_warm_start():
# A 1-iteration second fit on same data should give almost same result
# with warm starting, and quite different result without warm starting.
# Warm starting does not work with liblinear solver.
X, y = iris.data, iris.target
solvers = ['newton-cg', 'sag']
# old scipy doesn't have maxiter
if sp_version >= (0, 12):
solvers.append('lbfgs')
for warm_start in [True, False]:
for fit_intercept in [True, False]:
for solver in solvers:
for multi_class in ['ovr', 'multinomial']:
if solver == 'sag' and multi_class == 'multinomial':
break
clf = LogisticRegression(tol=1e-4, multi_class=multi_class,
warm_start=warm_start,
solver=solver,
random_state=42, max_iter=100,
fit_intercept=fit_intercept)
clf.fit(X, y)
coef_1 = clf.coef_
clf.max_iter = 1
with ignore_warnings():
clf.fit(X, y)
cum_diff = np.sum(np.abs(coef_1 - clf.coef_))
msg = ("Warm starting issue with %s solver in %s mode "
"with fit_intercept=%s and warm_start=%s"
% (solver, multi_class, str(fit_intercept),
str(warm_start)))
if warm_start:
assert_greater(2.0, cum_diff, msg)
else:
assert_greater(cum_diff, 2.0, msg)
| bsd-3-clause |
noelevans/sandpit | bayesian_methods_for_hackers/convergence_to_single_data_point_ch03.py | 1 | 2982 | from matplotlib import pyplot as plt
import numpy as np
from scipy import stats
from IPython.core.pylabtools import figsize
def main():
# create the observed data
# sample size of data we observe, try varying this
# (keep it less than 100 ;)
N = 15
# the true parameters, but of course we do not see these values...
lambda_1_true = 1
lambda_2_true = 3
#...we see the data generated, dependent on the above two values.
data = np.concatenate([
stats.poisson.rvs(lambda_1_true, size=(N, 1)),
stats.poisson.rvs(lambda_2_true, size=(N, 1))
], axis=1)
print "observed (2-dimensional,sample size = %d):" % N, data
# plotting details.
x = y = np.linspace(.01, 5, 100)
likelihood_x = np.array([stats.poisson.pmf(data[:, 0], _x)
for _x in x]).prod(axis=1)
likelihood_y = np.array([stats.poisson.pmf(data[:, 1], _y)
for _y in y]).prod(axis=1)
L = np.dot(likelihood_x[:, None], likelihood_y[None, :])
# figsize(12.5, 12)
# matplotlib heavy lifting below, beware!
jet = plt.cm.jet
plt.subplot(221)
uni_x = stats.uniform.pdf(x, loc=0, scale=5)
uni_y = stats.uniform.pdf(x, loc=0, scale=5)
M = np.dot(uni_x[:, None], uni_y[None, :])
im = plt.imshow(M, interpolation='none', origin='lower',
cmap=jet, vmax=1, vmin=-.15, extent=(0, 5, 0, 5))
plt.scatter(lambda_2_true, lambda_1_true, c="k", s=50, edgecolor="none")
plt.xlim(0, 5)
plt.ylim(0, 5)
plt.title("Landscape formed by Uniform priors on $p_1, p_2$.")
plt.subplot(223)
plt.contour(x, y, M * L)
im = plt.imshow(M * L, interpolation='none', origin='lower',
cmap=jet, extent=(0, 5, 0, 5))
plt.title("Landscape warped by %d data observation;\n Uniform priors on $p_1, p_2$." % N)
plt.scatter(lambda_2_true, lambda_1_true, c="k", s=50, edgecolor="none")
plt.xlim(0, 5)
plt.ylim(0, 5)
plt.subplot(222)
exp_x = stats.expon.pdf(x, loc=0, scale=3)
exp_y = stats.expon.pdf(x, loc=0, scale=10)
M = np.dot(exp_x[:, None], exp_y[None, :])
plt.contour(x, y, M)
im = plt.imshow(M, interpolation='none', origin='lower',
cmap=jet, extent=(0, 5, 0, 5))
plt.scatter(lambda_2_true, lambda_1_true, c="k", s=50, edgecolor="none")
plt.xlim(0, 5)
plt.ylim(0, 5)
plt.title("Landscape formed by Exponential priors on $p_1, p_2$.")
plt.subplot(224)
# This is the likelihood times prior, that results in the posterior.
plt.contour(x, y, M * L)
im = plt.imshow(M * L, interpolation='none', origin='lower',
cmap=jet, extent=(0, 5, 0, 5))
plt.scatter(lambda_2_true, lambda_1_true, c="k", s=50, edgecolor="none")
plt.title("Landscape warped by %d data observation;\n Exponential priors on \
$p_1, p_2$." % N)
plt.xlim(0, 5)
plt.ylim(0, 5)
plt.show()
if __name__ == '__main__':
main()
| mit |
mbkumar/pydii | pydii/scripts/gen_def_profile.py | 1 | 6727 | #!/usr/bin/env python
"""
PyDIM file for generating defect concentrations
"""
from __future__ import division
#__author__ == 'Bharat Medasani'
#__version__ = "0.1"
#__maintainer__ = "Bharat Medasani"
#__email__ = "[email protected]"
#__status__ = "Beta"
#__date__ = "9/16/14"
import json
import os
from argparse import ArgumentParser
#import numpy as np
#import scipy
#import matplotlib
#matplotlib.use('ps')
#import matplotlib.pyplot as plt
from monty.serialization import loadfn, dumpfn
from monty.json import MontyEncoder, MontyDecoder
from pydii.dilute_solution_model import \
solute_site_preference_finder, compute_defect_density, \
solute_defect_density
def get_def_profile(mpid, T, file):
raw_energy_dict = loadfn(file,cls=MontyDecoder)
e0 = raw_energy_dict[mpid]['e0']
struct = raw_energy_dict[mpid]['structure']
vacs = raw_energy_dict[mpid]['vacancies']
antisites = raw_energy_dict[mpid]['antisites']
vacs.sort(key=lambda entry: entry['site_index'])
antisites.sort(key=lambda entry: entry['site_index'])
for vac_def in vacs:
if not vac_def:
print 'All vacancy defect energies not present'
continue
for antisite_def in antisites:
if not antisite_def:
print 'All antisite defect energies not preset'
continue
try:
def_conc, def_en, mu = compute_defect_density(struct, e0, vacs, antisites, T,
plot_style='gnuplot')
return def_conc, def_en, mu
except:
raise
def get_solute_def_profile(mpid, solute, solute_conc, T, def_file, sol_file,
trial_chem_pot):
raw_energy_dict = loadfn(def_file,cls=MontyDecoder)
sol_raw_energy_dict = loadfn(sol_file,cls=MontyDecoder)
#try:
e0 = raw_energy_dict[mpid]['e0']
struct = raw_energy_dict[mpid]['structure']
vacs = raw_energy_dict[mpid]['vacancies']
antisites = raw_energy_dict[mpid]['antisites']
solutes = sol_raw_energy_dict[mpid]['solutes']
for vac_def in vacs:
if not vac_def:
print 'All vacancy defect energies not present'
continue
for antisite_def in antisites:
if not antisite_def:
print 'All antisite defect energies not preset'
continue
for solute_def in solutes:
if not solute_def:
print 'All solute defect energies not preset'
continue
try:
def_conc = solute_defect_density(struct, e0, vacs,
antisites, solutes, solute_concen=solute_conc, T=T,
trial_chem_pot=trial_chem_pot, plot_style="gnuplot")
return def_conc
except:
raise
def im_vac_antisite_def_profile():
m_description = 'Command to generate vacancy and antisite defect ' \
'concentration for intermetallics from the raw defect energies.'
parser = ArgumentParser(description=m_description)
parser.add_argument("--mpid",
type=str.lower,
help="Materials Project id of the intermetallic structure.\n" \
"For more info on Materials Project, please refer to " \
"www.materialsproject.org")
parser.add_argument('-T', "--temp", type=float, default=1000,
help="Temperature in Kelvin")
parser.add_argument("--file",
default = None,
help = "The default file is 'mpid'+'_raw_defect_energy.json'.\n" \
"If the file is named differently supply it.")
#parser.add_argument("--mapi_key",
# default = None,
# help="Your Materials Project REST API key.\n" \
# "For more info, please refer to " \
# "www.materialsproject.org/opne")
args = parser.parse_args()
if not args.mpid:
print ('===========\nERROR: mpid is not given.\n===========')
return
if not args.file:
file = args.mpid+'_raw_defect_energy.json'
else:
file = args.file
conc_dat, en_dat, mu_dat = get_def_profile(args.mpid, args.temp, file)
if conc_dat:
fl_nm = args.mpid+'_def_concentration.dat'
with open(fl_nm,'w') as fp:
for row in conc_dat:
print >> fp, row
fl_nm = args.mpid+'_def_energy.dat'
with open(fl_nm,'w') as fp:
for row in en_dat:
print >> fp, row
fl_nm = args.mpid+'_chem_pot.dat'
with open(fl_nm,'w') as fp:
for row in mu_dat:
print >> fp, row
def im_sol_sub_def_profile():
m_description = 'Command to generate solute defect site preference ' \
'in an intermetallics from the raw defect energies.'
parser = ArgumentParser(description=m_description)
parser.add_argument("--mpid",
type=str.lower,
help="Materials Project id of the intermetallic structure.\n" \
"For more info on Materials Project, please refer to " \
"www.materialsproject.org")
parser.add_argument("--solute", help="Solute Element")
parser.add_argument("--sol_conc", type=float, default=1.0,
help="Solute Concentration in %. Default is 1%")
parser.add_argument("-T", "--temp", type=float, default=1000.0,
help="Temperature in Kelvin")
parser.add_argument("--trail_mu_file", default=None,
help="Trial chemcal potential in dict format stored in file")
args = parser.parse_args()
if not args.mpid:
print ('===========\nERROR: mpid is not given.\n===========')
return
if not args.solute:
print ('===========\nERROR: Solute atom is not given.\n===========')
return
def_file = args.mpid+'_raw_defect_energy.json'
sol_file = args.mpid+'_solute-'+args.solute+'_raw_defect_energy.json'
sol_conc = args.sol_conc/100.0 # Convert from percentage
if not os.path.exists(def_file):
print ('===========\nERROR: Defect file not found.\n===========')
return
if not os.path.exists(sol_file):
print ('===========\nERROR: Solute file not found.\n===========')
return
if args.trail_mu_file:
trail_chem_pot = loadfn(args.trial_mu_file,cls=MontyDecoder)
else:
trail_chem_pot = None
pt_def_conc = get_solute_def_profile(
args.mpid, args.solute, sol_conc, args.temp, def_file,
sol_file, trial_chem_pot=trail_chem_pot)
if pt_def_conc:
fl_nm = args.mpid+'_solute-'+args.solute+'_def_concentration.dat'
with open(fl_nm,'w') as fp:
for row in pt_def_conc:
print >> fp, row
if __name__ == '__main__':
im_vac_antisite_def_profile()
#im_sol_sub_def_profile()
| mit |
ctn-waterloo/nengo_theano | nengo_theano/scripts/abs_val.py | 1 | 3521 | import nengo_theano as nef
def make_abs_val(net, name, neurons, dimensions, intercept=[0, 1]):
"""A function that makes a subnetwork to calculate the absolute
value of the input vector, and adds it to the network.
:param Network net: the network to add it to
:param string name: the name of the abs val subnetwork
:param int neurons: the number of neurons per ensemble
:param int dimensions: the dimensionality of the input
:param intercept: the range of represented values
:param intercept type: int or list
if int or list length 1, then value is the lower bound,
and the upper bound is set to 1
"""
abs_val = net.make_subnetwork(name)
# create input relay
abs_val.make('input', neurons=1, dimensions=dimensions, mode='direct')
# create output relay
abs_val.make('output', neurons=1, dimensions=dimensions, mode='direct')
num_samples = 500
dx = (intercept[1] - intercept[0]) / num_samples
eval_points_pos = [x*dx + intercept[0] for x in range(num_samples)]
eval_points_neg = [-x*dx + intercept[0] for x in range(num_samples)]
# for each dimension in the input signal
for d in range(dimensions):
# create a population for the positive and negative parts of the signal
abs_val.make('abs_pos%d'%d, neurons=neurons,
dimensions=1, encoders=[[1]], intercept=intercept,
eval_points=eval_points_pos)
abs_val.make('abs_neg%d'%d, neurons=neurons,
dimensions=1, encoders=[[-1]], intercept=intercept,
eval_points=eval_points_neg)
# connect to input, pstc = 0 so no delay introduced by relay populations
abs_val.connect('input', 'abs_pos%d'%d, index_pre=d, pstc=1e-6)
abs_val.connect('input', 'abs_neg%d'%d, index_pre=d, pstc=1e-6)
# connect to output, making the negative values positive
abs_val.connect('abs_pos%d'%d, 'output', index_post=d)
abs_val.connect('abs_neg%d'%d, 'output', index_post=d, weight=-1)
def test_abs_val():
net = nef.Network('Abs val test')
import numpy as np
input = np.array([-.2, .5, -.8])
net.make_input('input', values=input)
make_abs_val(net, 'abs_val', neurons=50,
dimensions=3, intercept=(.2, 1))
net.connect('input', 'abs_val.input')
im_probe = net.make_probe('abs_val.input')
av0_pos_probe = net.make_probe('abs_val.abs_pos0')
av0_neg_probe = net.make_probe('abs_val.abs_neg0')
av1_pos_probe = net.make_probe('abs_val.abs_pos1')
av1_neg_probe = net.make_probe('abs_val.abs_neg1')
av2_pos_probe = net.make_probe('abs_val.abs_pos2')
av2_neg_probe = net.make_probe('abs_val.abs_neg2')
av_probe = net.make_probe('abs_val.output')
net.run(1)
import matplotlib.pyplot as plt
a = 'input = ', input
plt.subplot(411); plt.title(a)
plt.plot(im_probe.get_data())
plt.subplot(412); plt.title('abs_val_neg')
plt.plot(av0_pos_probe.get_data())
plt.plot(av1_pos_probe.get_data())
plt.plot(av2_pos_probe.get_data())
plt.legend(['input0','input1','input2'])
plt.subplot(413); plt.title('abs_val_neg')
plt.plot(av0_neg_probe.get_data())
plt.plot(av1_neg_probe.get_data())
plt.plot(av2_neg_probe.get_data())
plt.legend(['input0','input1','input2'])
input[np.abs(input) <= .2] = 0
b = 'answer = ', np.abs(input)
plt.subplot(414); plt.title(b)
plt.plot(av_probe.get_data())
plt.tight_layout()
plt.show()
#test_abs_val()
| mit |
pnedunuri/scikit-learn | sklearn/metrics/scorer.py | 211 | 13141 | """
The :mod:`sklearn.metrics.scorer` submodule implements a flexible
interface for model selection and evaluation using
arbitrary score functions.
A scorer object is a callable that can be passed to
:class:`sklearn.grid_search.GridSearchCV` or
:func:`sklearn.cross_validation.cross_val_score` as the ``scoring`` parameter,
to specify how a model should be evaluated.
The signature of the call is ``(estimator, X, y)`` where ``estimator``
is the model to be evaluated, ``X`` is the test data and ``y`` is the
ground truth labeling (or ``None`` in the case of unsupervised models).
"""
# Authors: Andreas Mueller <[email protected]>
# Lars Buitinck <[email protected]>
# Arnaud Joly <[email protected]>
# License: Simplified BSD
from abc import ABCMeta, abstractmethod
from functools import partial
import numpy as np
from . import (r2_score, median_absolute_error, mean_absolute_error,
mean_squared_error, accuracy_score, f1_score,
roc_auc_score, average_precision_score,
precision_score, recall_score, log_loss)
from .cluster import adjusted_rand_score
from ..utils.multiclass import type_of_target
from ..externals import six
from ..base import is_regressor
class _BaseScorer(six.with_metaclass(ABCMeta, object)):
def __init__(self, score_func, sign, kwargs):
self._kwargs = kwargs
self._score_func = score_func
self._sign = sign
@abstractmethod
def __call__(self, estimator, X, y, sample_weight=None):
pass
def __repr__(self):
kwargs_string = "".join([", %s=%s" % (str(k), str(v))
for k, v in self._kwargs.items()])
return ("make_scorer(%s%s%s%s)"
% (self._score_func.__name__,
"" if self._sign > 0 else ", greater_is_better=False",
self._factory_args(), kwargs_string))
def _factory_args(self):
"""Return non-default make_scorer arguments for repr."""
return ""
class _PredictScorer(_BaseScorer):
def __call__(self, estimator, X, y_true, sample_weight=None):
"""Evaluate predicted target values for X relative to y_true.
Parameters
----------
estimator : object
Trained estimator to use for scoring. Must have a predict_proba
method; the output of that is used to compute the score.
X : array-like or sparse matrix
Test data that will be fed to estimator.predict.
y_true : array-like
Gold standard target values for X.
sample_weight : array-like, optional (default=None)
Sample weights.
Returns
-------
score : float
Score function applied to prediction of estimator on X.
"""
y_pred = estimator.predict(X)
if sample_weight is not None:
return self._sign * self._score_func(y_true, y_pred,
sample_weight=sample_weight,
**self._kwargs)
else:
return self._sign * self._score_func(y_true, y_pred,
**self._kwargs)
class _ProbaScorer(_BaseScorer):
def __call__(self, clf, X, y, sample_weight=None):
"""Evaluate predicted probabilities for X relative to y_true.
Parameters
----------
clf : object
Trained classifier to use for scoring. Must have a predict_proba
method; the output of that is used to compute the score.
X : array-like or sparse matrix
Test data that will be fed to clf.predict_proba.
y : array-like
Gold standard target values for X. These must be class labels,
not probabilities.
sample_weight : array-like, optional (default=None)
Sample weights.
Returns
-------
score : float
Score function applied to prediction of estimator on X.
"""
y_pred = clf.predict_proba(X)
if sample_weight is not None:
return self._sign * self._score_func(y, y_pred,
sample_weight=sample_weight,
**self._kwargs)
else:
return self._sign * self._score_func(y, y_pred, **self._kwargs)
def _factory_args(self):
return ", needs_proba=True"
class _ThresholdScorer(_BaseScorer):
def __call__(self, clf, X, y, sample_weight=None):
"""Evaluate decision function output for X relative to y_true.
Parameters
----------
clf : object
Trained classifier to use for scoring. Must have either a
decision_function method or a predict_proba method; the output of
that is used to compute the score.
X : array-like or sparse matrix
Test data that will be fed to clf.decision_function or
clf.predict_proba.
y : array-like
Gold standard target values for X. These must be class labels,
not decision function values.
sample_weight : array-like, optional (default=None)
Sample weights.
Returns
-------
score : float
Score function applied to prediction of estimator on X.
"""
y_type = type_of_target(y)
if y_type not in ("binary", "multilabel-indicator"):
raise ValueError("{0} format is not supported".format(y_type))
if is_regressor(clf):
y_pred = clf.predict(X)
else:
try:
y_pred = clf.decision_function(X)
# For multi-output multi-class estimator
if isinstance(y_pred, list):
y_pred = np.vstack(p for p in y_pred).T
except (NotImplementedError, AttributeError):
y_pred = clf.predict_proba(X)
if y_type == "binary":
y_pred = y_pred[:, 1]
elif isinstance(y_pred, list):
y_pred = np.vstack([p[:, -1] for p in y_pred]).T
if sample_weight is not None:
return self._sign * self._score_func(y, y_pred,
sample_weight=sample_weight,
**self._kwargs)
else:
return self._sign * self._score_func(y, y_pred, **self._kwargs)
def _factory_args(self):
return ", needs_threshold=True"
def get_scorer(scoring):
if isinstance(scoring, six.string_types):
try:
scorer = SCORERS[scoring]
except KeyError:
raise ValueError('%r is not a valid scoring value. '
'Valid options are %s'
% (scoring, sorted(SCORERS.keys())))
else:
scorer = scoring
return scorer
def _passthrough_scorer(estimator, *args, **kwargs):
"""Function that wraps estimator.score"""
return estimator.score(*args, **kwargs)
def check_scoring(estimator, scoring=None, allow_none=False):
"""Determine scorer from user options.
A TypeError will be thrown if the estimator cannot be scored.
Parameters
----------
estimator : estimator object implementing 'fit'
The object to use to fit the data.
scoring : string, callable or None, optional, default: None
A string (see model evaluation documentation) or
a scorer callable object / function with signature
``scorer(estimator, X, y)``.
allow_none : boolean, optional, default: False
If no scoring is specified and the estimator has no score function, we
can either return None or raise an exception.
Returns
-------
scoring : callable
A scorer callable object / function with signature
``scorer(estimator, X, y)``.
"""
has_scoring = scoring is not None
if not hasattr(estimator, 'fit'):
raise TypeError("estimator should a be an estimator implementing "
"'fit' method, %r was passed" % estimator)
elif has_scoring:
return get_scorer(scoring)
elif hasattr(estimator, 'score'):
return _passthrough_scorer
elif allow_none:
return None
else:
raise TypeError(
"If no scoring is specified, the estimator passed should "
"have a 'score' method. The estimator %r does not." % estimator)
def make_scorer(score_func, greater_is_better=True, needs_proba=False,
needs_threshold=False, **kwargs):
"""Make a scorer from a performance metric or loss function.
This factory function wraps scoring functions for use in GridSearchCV
and cross_val_score. It takes a score function, such as ``accuracy_score``,
``mean_squared_error``, ``adjusted_rand_index`` or ``average_precision``
and returns a callable that scores an estimator's output.
Read more in the :ref:`User Guide <scoring>`.
Parameters
----------
score_func : callable,
Score function (or loss function) with signature
``score_func(y, y_pred, **kwargs)``.
greater_is_better : boolean, default=True
Whether score_func is a score function (default), meaning high is good,
or a loss function, meaning low is good. In the latter case, the
scorer object will sign-flip the outcome of the score_func.
needs_proba : boolean, default=False
Whether score_func requires predict_proba to get probability estimates
out of a classifier.
needs_threshold : boolean, default=False
Whether score_func takes a continuous decision certainty.
This only works for binary classification using estimators that
have either a decision_function or predict_proba method.
For example ``average_precision`` or the area under the roc curve
can not be computed using discrete predictions alone.
**kwargs : additional arguments
Additional parameters to be passed to score_func.
Returns
-------
scorer : callable
Callable object that returns a scalar score; greater is better.
Examples
--------
>>> from sklearn.metrics import fbeta_score, make_scorer
>>> ftwo_scorer = make_scorer(fbeta_score, beta=2)
>>> ftwo_scorer
make_scorer(fbeta_score, beta=2)
>>> from sklearn.grid_search import GridSearchCV
>>> from sklearn.svm import LinearSVC
>>> grid = GridSearchCV(LinearSVC(), param_grid={'C': [1, 10]},
... scoring=ftwo_scorer)
"""
sign = 1 if greater_is_better else -1
if needs_proba and needs_threshold:
raise ValueError("Set either needs_proba or needs_threshold to True,"
" but not both.")
if needs_proba:
cls = _ProbaScorer
elif needs_threshold:
cls = _ThresholdScorer
else:
cls = _PredictScorer
return cls(score_func, sign, kwargs)
# Standard regression scores
r2_scorer = make_scorer(r2_score)
mean_squared_error_scorer = make_scorer(mean_squared_error,
greater_is_better=False)
mean_absolute_error_scorer = make_scorer(mean_absolute_error,
greater_is_better=False)
median_absolute_error_scorer = make_scorer(median_absolute_error,
greater_is_better=False)
# Standard Classification Scores
accuracy_scorer = make_scorer(accuracy_score)
f1_scorer = make_scorer(f1_score)
# Score functions that need decision values
roc_auc_scorer = make_scorer(roc_auc_score, greater_is_better=True,
needs_threshold=True)
average_precision_scorer = make_scorer(average_precision_score,
needs_threshold=True)
precision_scorer = make_scorer(precision_score)
recall_scorer = make_scorer(recall_score)
# Score function for probabilistic classification
log_loss_scorer = make_scorer(log_loss, greater_is_better=False,
needs_proba=True)
# Clustering scores
adjusted_rand_scorer = make_scorer(adjusted_rand_score)
SCORERS = dict(r2=r2_scorer,
median_absolute_error=median_absolute_error_scorer,
mean_absolute_error=mean_absolute_error_scorer,
mean_squared_error=mean_squared_error_scorer,
accuracy=accuracy_scorer, roc_auc=roc_auc_scorer,
average_precision=average_precision_scorer,
log_loss=log_loss_scorer,
adjusted_rand_score=adjusted_rand_scorer)
for name, metric in [('precision', precision_score),
('recall', recall_score), ('f1', f1_score)]:
SCORERS[name] = make_scorer(metric)
for average in ['macro', 'micro', 'samples', 'weighted']:
qualified_name = '{0}_{1}'.format(name, average)
SCORERS[qualified_name] = make_scorer(partial(metric, pos_label=None,
average=average))
| bsd-3-clause |
sbebo/joypy | joypy/joyplot.py | 1 | 21552 | import os
import numpy as np
from scipy.stats import gaussian_kde
import warnings
try:
# pandas < 0.25
from pandas.plotting._tools import (_subplots, _flatten)
except ImportError:
try:
#pandas >= 0.25, <1.2.0
from pandas.plotting._matplotlib.tools import (_subplots, _flatten)
except ImportError:
#pandas >= 1.2.0
from pandas.plotting._matplotlib.tools import create_subplots as _subplots
from pandas.plotting._matplotlib.tools import flatten_axes as _flatten
from pandas import (DataFrame, Series)
from pandas.core.dtypes.common import is_number
from pandas.core.groupby import DataFrameGroupBy
from matplotlib import pyplot as plt
from warnings import warn
_DEBUG = False
def _x_range(data, extra=0.2):
""" Compute the x_range, i.e., the values for which the
density will be computed. It should be slightly larger than
the max and min so that the plot actually reaches 0, and
also has a bit of a tail on both sides.
"""
try:
sample_range = np.nanmax(data) - np.nanmin(data)
except ValueError:
return []
if sample_range < 1e-6:
return [np.nanmin(data), np.nanmax(data)]
return np.linspace(np.nanmin(data) - extra*sample_range,
np.nanmax(data) + extra*sample_range, 1000)
def _setup_axis(ax, x_range, col_name=None, grid=False, ylabelsize=None, yrot=None):
""" Setup the axis for the joyplot:
- add the y label if required (as an ytick)
- add y grid if required
- make the background transparent
- set the xlim according to the x_range
- hide the xaxis and the spines
"""
if col_name is not None:
ax.set_yticks([0])
ax.set_yticklabels([col_name], fontsize=ylabelsize, rotation=yrot)
ax.yaxis.grid(grid)
else:
ax.yaxis.set_visible(False)
ax.patch.set_alpha(0)
ax.set_xlim([min(x_range), max(x_range)])
ax.tick_params(axis='both', which='both', length=0, pad=10)
ax.xaxis.set_visible(_DEBUG)
ax.set_frame_on(_DEBUG)
def _is_numeric(x):
""" Whether the array x is numeric. """
return all(is_number(i) for i in x)
def _get_alpha(i, n, start=0.4, end=1.0):
""" Compute alpha value at position i out of n """
return start + (1 + i)*(end - start)/n
def _remove_na(l):
""" Remove NA values. Should work for lists, arrays, series. """
return Series(l).dropna().values
def _moving_average(a, n=3, zero_padded=False):
""" Moving average of order n.
If zero padded, returns an array of the same size as
the input: the values before a[0] are considered to be 0.
Otherwise, returns an array of length len(a) - n + 1 """
ret = np.cumsum(a, dtype=float)
ret[n:] = ret[n:] - ret[:-n]
if zero_padded:
return ret / n
else:
return ret[n - 1:] / n
def joyplot(data, column=None, by=None, grid=False,
xlabelsize=None, xrot=None, ylabelsize=None, yrot=None,
ax=None, figsize=None,
hist=False, bins=10,
fade=False, ylim='max',
fill=True, linecolor=None,
overlap=1, background=None,
labels=None, xlabels=True, ylabels=True,
range_style='all',
x_range=None,
title=None,
colormap=None,
color=None,
**kwds):
"""
Draw joyplot of a DataFrame, or appropriately nested collection,
using matplotlib and pandas.
A joyplot is a stack of vertically aligned density plots / histograms.
By default, if 'data' is a DataFrame,
this function will plot a density plot for each column.
This wrapper method tries to convert whatever structure is given
to a nested collection of lists with additional information
on labels, and use the private _joyplot function to actually
draw theh plot.
Parameters
----------
data : DataFrame, Series or nested collection
column : string or sequence
If passed, will be used to limit data to a subset of columns
by : object, optional
If passed, used to form separate plot groups
grid : boolean, default True
Whether to show axis grid lines
labels : boolean or list, default True.
If list, must be the same size of the de
xlabelsize : int, default None
If specified changes the x-axis label size
xrot : float, default None
rotation of x axis labels
ylabelsize : int, default None
If specified changes the y-axis label size
yrot : float, default None
rotation of y axis labels
ax : matplotlib axes object, default None
figsize : tuple
The size of the figure to create in inches by default
hist : boolean, default False
bins : integer, default 10
Number of histogram bins to be used
color : color or colors to be used in the plots. It can be:
a string or anything interpretable as color by matplotib;
a list of colors. See docs / examples for more details.
kwds : other plotting keyword arguments
To be passed to hist/kde plot function
"""
if column is not None:
if not isinstance(column, (list, np.ndarray)):
column = [column]
def _grouped_df_to_standard(grouped, column):
converted = []
labels = []
for i, (key, group) in enumerate(grouped):
if column is not None:
group = group[column]
labels.append(key)
converted.append([_remove_na(group[c]) for c in group.columns if _is_numeric(group[c])])
if i == 0:
sublabels = [col for col in group.columns if _is_numeric(group[col])]
return converted, labels, sublabels
#################################################################
# GROUPED
# - given a grouped DataFrame, a group by key, or a dict of dicts of Series/lists/arrays
# - select the required columns/Series/lists/arrays
# - convert to standard format: list of lists of non-null arrays
# + extra parameters (labels and sublabels)
#################################################################
if isinstance(data, DataFrameGroupBy):
grouped = data
converted, _labels, sublabels = _grouped_df_to_standard(grouped, column)
if labels is None:
labels = _labels
elif by is not None and isinstance(data, DataFrame):
grouped = data.groupby(by)
if column is None:
# Remove the groupby key. It's not automatically removed by pandas.
column = list(data.columns)
column.remove(by)
converted, _labels, sublabels = _grouped_df_to_standard(grouped, column)
if labels is None:
labels = _labels
# If there is at least an element which is not a list of lists.. go on.
elif isinstance(data, dict) and all(isinstance(g, dict) for g in data.values()):
grouped = data
if labels is None:
labels = list(grouped.keys())
converted = []
for i, (key, group) in enumerate(grouped.items()):
if column is not None:
converted.append([_remove_na(g) for k,g in group.items() if _is_numeric(g) and k in column])
if i == 0:
sublabels = [k for k,g in group.items() if _is_numeric(g)]
else:
converted.append([_remove_na(g) for k,g in group.items() if _is_numeric(g)])
if i == 0:
sublabels = [k for k,g in group.items() if _is_numeric(g)]
#################################################################
# PLAIN:
# - given a DataFrame or list/dict of Series/lists/arrays
# - select the required columns/Series/lists/arrays
# - convert to standard format: list of lists of non-null arrays + extra parameter (labels)
#################################################################
elif isinstance(data, DataFrame):
if column is not None:
data = data[column]
converted = [[_remove_na(data[col])] for col in data.columns if _is_numeric(data[col])]
labels = [col for col in data.columns if _is_numeric(data[col])]
sublabels = None
elif isinstance(data, dict):
if column is not None:
converted = [[_remove_na(g)] for k,g in data.items() if _is_numeric(g) and k in column]
labels = [k for k,g in data.items() if _is_numeric(g) and k in column]
else:
converted = [[_remove_na(g)] for k,g in data.items() if _is_numeric(g)]
labels = [k for k,g in data.items() if _is_numeric(g)]
sublabels = None
elif isinstance(data, list):
if column is not None:
converted = [[_remove_na(g)] for g in data if _is_numeric(g) and i in column]
else:
converted = [[_remove_na(g)] for g in data if _is_numeric(g)]
if labels and len(labels) != len(converted):
raise ValueError("The number of labels does not match the length of the list.")
sublabels = None
else:
raise TypeError("Unknown type for 'data': {!r}".format(type(data)))
if ylabels is False:
labels = None
if all(len(subg)==0 for g in converted for subg in g):
raise ValueError("No numeric values found. Joyplot requires at least a numeric column/group.")
if any(len(subg)==0 for g in converted for subg in g):
warn("At least a column/group has no numeric values.")
return _joyplot(converted, labels=labels, sublabels=sublabels,
grid=grid,
xlabelsize=xlabelsize, xrot=xrot, ylabelsize=ylabelsize, yrot=yrot,
ax=ax, figsize=figsize,
hist=hist, bins=bins,
fade=fade, ylim=ylim,
fill=fill, linecolor=linecolor,
overlap=overlap, background=background,
xlabels=xlabels,
range_style=range_style, x_range=x_range,
title=title,
colormap=colormap,
color=color,
**kwds)
###########################################
def plot_density(ax, x_range, v, kind="kde", bw_method=None,
bins=50,
fill=False, linecolor=None, clip_on=True, **kwargs):
""" Draw a density plot given an axis, an array of values v and an array
of x positions where to return the estimated density.
"""
v = _remove_na(v)
if len(v) == 0 or len(x_range) == 0:
return
if kind == "kde":
try:
gkde = gaussian_kde(v, bw_method=bw_method)
y = gkde.evaluate(x_range)
except ValueError:
# Handle cases where there is no data in a group.
y = np.zeros_like(x_range)
except np.linalg.LinAlgError as e:
# Handle singular matrix in kde computation.
distinct_values = np.unique(v)
if len(distinct_values) == 1:
# In case of a group with a single value val,
# that should have infinite density,
# return a δ(val)
val = distinct_values[0]
warnings.warn("The data contains a group with a single distinct value ({}) "
"having infinite probability density. "
"Consider using a different visualization.".format(val))
# Find index i of x_range
# such that x_range[i-1] < val ≤ x_range[i]
i = np.searchsorted(x_range, val)
y = np.zeros_like(x_range)
y[i] = 1
else:
raise e
elif kind == "counts":
y, bin_edges = np.histogram(v, bins=bins, range=(min(x_range), max(x_range)))
# np.histogram returns the edges of the bins.
# We compute here the middle of the bins.
x_range = _moving_average(bin_edges, 2)
elif kind == "normalized_counts":
y, bin_edges = np.histogram(v, bins=bins, density=False,
range=(min(x_range), max(x_range)))
# np.histogram returns the edges of the bins.
# We compute here the middle of the bins.
y = y / len(v)
x_range = _moving_average(bin_edges, 2)
elif kind == "values":
# Warning: to use values and get a meaningful visualization,
# x_range must also be manually set in the main function.
y = v
x_range = list(range(len(y)))
else:
raise NotImplementedError
if fill:
ax.fill_between(x_range, 0.0, y, clip_on=clip_on, **kwargs)
# Hack to have a border at the bottom at the fill patch
# (of the same color of the fill patch)
# so that the fill reaches the same bottom margin as the edge lines
# with y value = 0.0
kw = kwargs
kw["label"] = None
ax.plot(x_range, [0.0]*len(x_range), clip_on=clip_on, **kw)
if linecolor is not None:
kwargs["color"] = linecolor
# Remove the legend labels if we are plotting filled curve:
# we only want one entry per group in the legend (if shown).
if fill:
kwargs["label"] = None
ax.plot(x_range, y, clip_on=clip_on, **kwargs)
###########################################
def _joyplot(data,
grid=False,
labels=None, sublabels=None,
xlabels=True,
xlabelsize=None, xrot=None,
ylabelsize=None, yrot=None,
ax=None, figsize=None,
hist=False, bins=10,
fade=False,
xlim=None, ylim='max',
fill=True, linecolor=None,
overlap=1, background=None,
range_style='all', x_range=None, tails=0.2,
title=None,
legend=False, loc="upper right",
colormap=None, color=None,
**kwargs):
"""
Internal method.
Draw a joyplot from an appropriately nested collection of lists
using matplotlib and pandas.
Parameters
----------
data : DataFrame, Series or nested collection
grid : boolean, default True
Whether to show axis grid lines
labels : boolean or list, default True.
If list, must be the same size of the de
xlabelsize : int, default None
If specified changes the x-axis label size
xrot : float, default None
rotation of x axis labels
ylabelsize : int, default None
If specified changes the y-axis label size
yrot : float, default None
rotation of y axis labels
ax : matplotlib axes object, default None
figsize : tuple
The size of the figure to create in inches by default
hist : boolean, default False
bins : integer, default 10
Number of histogram bins to be used
kwarg : other plotting keyword arguments
To be passed to hist/kde plot function
"""
if fill is True and linecolor is None:
linecolor = "k"
if sublabels is None:
legend = False
def _get_color(i, num_axes, j, num_subgroups):
if isinstance(color, list):
return color[j] if num_subgroups > 1 else color[i]
elif color is not None:
return color
elif isinstance(colormap, list):
return colormap[j](i/num_axes)
elif color is None and colormap is None:
num_cycle_colors = len(plt.rcParams['axes.prop_cycle'].by_key()['color'])
return plt.rcParams['axes.prop_cycle'].by_key()['color'][j % num_cycle_colors]
else:
return colormap(i/num_axes)
ygrid = (grid is True or grid == 'y' or grid == 'both')
xgrid = (grid is True or grid == 'x' or grid == 'both')
num_axes = len(data)
if x_range is None:
global_x_range = _x_range([v for g in data for sg in g for v in sg])
else:
global_x_range = _x_range(x_range, 0.0)
global_x_min, global_x_max = min(global_x_range), max(global_x_range)
# Each plot will have its own axis
fig, axes = _subplots(naxes=num_axes, ax=ax, squeeze=False,
sharex=True, sharey=False, figsize=figsize,
layout_type='vertical')
_axes = _flatten(axes)
# The legend must be drawn in the last axis if we want it at the bottom.
if loc in (3, 4, 8) or 'lower' in str(loc):
legend_axis = num_axes - 1
else:
legend_axis = 0
# A couple of simple checks.
if labels is not None:
assert len(labels) == num_axes
if sublabels is not None:
assert all(len(g) == len(sublabels) for g in data)
if isinstance(color, list):
assert all(len(g) <= len(color) for g in data)
if isinstance(colormap, list):
assert all(len(g) == len(colormap) for g in data)
for i, group in enumerate(data):
a = _axes[i]
group_zorder = i
if fade:
kwargs['alpha'] = _get_alpha(i, num_axes)
num_subgroups = len(group)
if hist:
# matplotlib hist() already handles multiple subgroups in a histogram
a.hist(group, label=sublabels, bins=bins, color=color,
range=[min(global_x_range), max(global_x_range)],
edgecolor=linecolor, zorder=group_zorder, **kwargs)
else:
for j, subgroup in enumerate(group):
# Compute the x_range of the current plot
if range_style == 'all':
# All plots have the same range
x_range = global_x_range
elif range_style == 'own':
# Each plot has its own range
x_range = _x_range(subgroup, tails)
elif range_style == 'group':
# Each plot has a range that covers the whole group
x_range = _x_range(group, tails)
elif isinstance(range_style, (list, np.ndarray)):
# All plots have exactly the range passed as argument
x_range = _x_range(range_style, 0.0)
else:
raise NotImplementedError("Unrecognized range style.")
if sublabels is None:
sublabel = None
else:
sublabel = sublabels[j]
element_zorder = group_zorder + j/(num_subgroups+1)
element_color = _get_color(i, num_axes, j, num_subgroups)
plot_density(a, x_range, subgroup,
fill=fill, linecolor=linecolor, label=sublabel,
zorder=element_zorder, color=element_color,
bins=bins, **kwargs)
# Setup the current axis: transparency, labels, spines.
col_name = None if labels is None else labels[i]
_setup_axis(a, global_x_range, col_name=col_name, grid=ygrid,
ylabelsize=ylabelsize, yrot=yrot)
# When needed, draw the legend
if legend and i == legend_axis:
a.legend(loc=loc)
# Bypass alpha values, in case
for p in a.get_legend().get_patches():
p.set_facecolor(p.get_facecolor())
p.set_alpha(1.0)
for l in a.get_legend().get_lines():
l.set_alpha(1.0)
# Final adjustments
# Set the y limit for the density plots.
# Since the y range in the subplots can vary significantly,
# different options are available.
if ylim == 'max':
# Set all yaxis limit to the same value (max range among all)
max_ylim = max(a.get_ylim()[1] for a in _axes)
min_ylim = min(a.get_ylim()[0] for a in _axes)
for a in _axes:
a.set_ylim([min_ylim - 0.1*(max_ylim-min_ylim), max_ylim])
elif ylim == 'own':
# Do nothing, each axis keeps its own ylim
pass
else:
# Set all yaxis lim to the argument value ylim
try:
for a in _axes:
a.set_ylim(ylim)
except:
print("Warning: the value of ylim must be either 'max', 'own', or a tuple of length 2. The value you provided has no effect.")
# Compute a final axis, used to apply global settings
last_axis = fig.add_subplot(1, 1, 1)
# Background color
if background is not None:
last_axis.patch.set_facecolor(background)
for side in ['top', 'bottom', 'left', 'right']:
last_axis.spines[side].set_visible(_DEBUG)
# This looks hacky, but all the axes share the x-axis,
# so they have the same lims and ticks
last_axis.set_xlim(_axes[0].get_xlim())
if xlabels is True:
last_axis.set_xticks(np.array(_axes[0].get_xticks()[1:-1]))
for t in last_axis.get_xticklabels():
t.set_visible(True)
t.set_fontsize(xlabelsize)
t.set_rotation(xrot)
# If grid is enabled, do not allow xticks (they are ugly)
if xgrid:
last_axis.tick_params(axis='both', which='both',length=0)
else:
last_axis.xaxis.set_visible(False)
last_axis.yaxis.set_visible(False)
last_axis.grid(xgrid)
# Last axis on the back
last_axis.zorder = min(a.zorder for a in _axes) - 1
_axes = list(_axes) + [last_axis]
if title is not None:
plt.title(title)
# The magic overlap happens here.
h_pad = 5 + (- 5*(1 + overlap))
fig.tight_layout(h_pad=h_pad)
return fig, _axes
| mit |
mattilyra/gensim | gensim/sklearn_api/lsimodel.py | 1 | 6165 | #!/usr/bin/env python
# -*- coding: utf-8 -*-
#
# Author: Chinmaya Pancholi <[email protected]>
# Copyright (C) 2017 Radim Rehurek <[email protected]>
# Licensed under the GNU LGPL v2.1 - http://www.gnu.org/licenses/lgpl.html
"""Scikit learn interface for :class:`gensim.models.lsimodel.LsiModel`.
Follows scikit-learn API conventions to facilitate using gensim along with scikit-learn.
Examples
--------
Integrate with sklearn Pipelines:
>>> from sklearn.pipeline import Pipeline
>>> from sklearn import linear_model
>>> from gensim.test.utils import common_corpus, common_dictionary
>>> from gensim.sklearn_api import LsiTransformer
>>>
>>> # Create stages for our pipeline (including gensim and sklearn models alike).
>>> model = LsiTransformer(num_topics=15, id2word=common_dictionary)
>>> clf = linear_model.LogisticRegression(penalty='l2', C=0.1)
>>> pipe = Pipeline([('features', model,), ('classifier', clf)])
>>>
>>> # Create some random binary labels for our documents.
>>> labels = np.random.choice([0, 1], len(common_corpus))
>>>
>>> # How well does our pipeline perform on the training set?
>>> score = pipe.fit(common_corpus, labels).score(common_corpus, labels)
"""
import numpy as np
from scipy import sparse
from sklearn.base import TransformerMixin, BaseEstimator
from sklearn.exceptions import NotFittedError
from gensim import models
from gensim import matutils
class LsiTransformer(TransformerMixin, BaseEstimator):
"""Base LSI module, wraps :class:`~gensim.models.lsimodel.LsiModel`.
For more information please have a look to `Latent semantic analysis
<https://en.wikipedia.org/wiki/Latent_semantic_analysis>`_.
"""
def __init__(self, num_topics=200, id2word=None, chunksize=20000,
decay=1.0, onepass=True, power_iters=2, extra_samples=100):
"""
Parameters
----------
num_topics : int, optional
Number of requested factors (latent dimensions).
id2word : :class:`~gensim.corpora.dictionary.Dictionary`, optional
ID to word mapping, optional.
chunksize : int, optional
Number of documents to be used in each training chunk.
decay : float, optional
Weight of existing observations relatively to new ones.
onepass : bool, optional
Whether the one-pass algorithm should be used for training, pass `False` to force a
multi-pass stochastic algorithm.
power_iters: int, optional
Number of power iteration steps to be used.
Increasing the number of power iterations improves accuracy, but lowers performance.
extra_samples : int, optional
Extra samples to be used besides the rank `k`. Can improve accuracy.
"""
self.gensim_model = None
self.num_topics = num_topics
self.id2word = id2word
self.chunksize = chunksize
self.decay = decay
self.onepass = onepass
self.extra_samples = extra_samples
self.power_iters = power_iters
def fit(self, X, y=None):
"""Fit the model according to the given training data.
Parameters
----------
X : {iterable of list of (int, number), scipy.sparse matrix}
A collection of documents in BOW format to be transformed.
Returns
-------
:class:`~gensim.sklearn_api.lsimodel.LsiTransformer`
The trained model.
"""
if sparse.issparse(X):
corpus = matutils.Sparse2Corpus(sparse=X, documents_columns=False)
else:
corpus = X
self.gensim_model = models.LsiModel(
corpus=corpus, num_topics=self.num_topics, id2word=self.id2word, chunksize=self.chunksize,
decay=self.decay, onepass=self.onepass, power_iters=self.power_iters, extra_samples=self.extra_samples
)
return self
def transform(self, docs):
"""Computes the latent factors for `docs`.
Parameters
----------
docs : {iterable of list of (int, number), list of (int, number), scipy.sparse matrix}
Document or collection of documents in BOW format to be transformed.
Returns
-------
numpy.ndarray of shape [`len(docs)`, `num_topics`]
Topic distribution matrix.
"""
if self.gensim_model is None:
raise NotFittedError(
"This model has not been fitted yet. Call 'fit' with appropriate arguments before using this method."
)
# The input as array of array
if isinstance(docs[0], tuple):
docs = [docs]
# returning dense representation for compatibility with sklearn
# but we should go back to sparse representation in the future
distribution = [matutils.sparse2full(self.gensim_model[doc], self.num_topics) for doc in docs]
return np.reshape(np.array(distribution), (len(docs), self.num_topics))
def partial_fit(self, X):
"""Train model over a potentially incomplete set of documents.
This method can be used in two ways:
1. On an unfitted model in which case the model is initialized and trained on `X`.
2. On an already fitted model in which case the model is **further** trained on `X`.
Parameters
----------
X : {iterable of list of (int, number), scipy.sparse matrix}
Stream of document vectors or sparse matrix of shape: [`num_terms`, `num_documents`].
Returns
-------
:class:`~gensim.sklearn_api.lsimodel.LsiTransformer`
The trained model.
"""
if sparse.issparse(X):
X = matutils.Sparse2Corpus(sparse=X, documents_columns=False)
if self.gensim_model is None:
self.gensim_model = models.LsiModel(
num_topics=self.num_topics, id2word=self.id2word, chunksize=self.chunksize, decay=self.decay,
onepass=self.onepass, power_iters=self.power_iters, extra_samples=self.extra_samples
)
self.gensim_model.add_documents(corpus=X)
return self
| lgpl-2.1 |
Subsets and Splits
No community queries yet
The top public SQL queries from the community will appear here once available.