Diffusers Bot
commited on
Upload folder using huggingface_hub
Browse files- v0.6.0/README.md +170 -0
- v0.6.0/clip_guided_stable_diffusion.py +324 -0
- v0.6.0/interpolate_stable_diffusion.py +524 -0
- v0.6.0/one_step_unet.py +22 -0
- v0.6.0/stable_diffusion_mega.py +224 -0
v0.6.0/README.md
ADDED
@@ -0,0 +1,170 @@
|
|
|
|
|
|
|
|
|
|
|
|
|
|
|
|
|
|
|
|
|
|
|
|
|
|
|
|
|
|
|
|
|
|
|
|
|
|
|
|
|
|
|
|
|
|
|
|
|
|
|
|
|
|
|
|
|
|
|
|
|
|
|
|
|
|
|
|
|
|
|
|
|
|
|
|
|
|
|
|
|
|
|
|
|
|
|
|
|
|
|
|
|
|
|
|
|
|
|
|
|
|
|
|
|
|
|
|
|
|
|
|
|
|
|
|
|
|
|
|
|
|
|
|
|
|
|
|
|
|
|
|
|
|
|
|
|
|
|
|
|
|
|
|
|
|
|
|
|
|
|
|
|
|
|
|
|
|
|
|
|
|
|
|
|
|
|
|
|
|
|
|
|
|
|
|
|
|
|
|
|
|
|
|
|
|
|
|
|
|
|
|
|
|
|
|
|
|
|
|
|
|
|
|
|
|
|
|
|
|
|
|
|
|
|
|
|
|
|
|
|
|
|
|
|
|
|
|
|
|
|
|
|
|
|
|
|
|
|
|
|
|
|
|
|
|
|
|
|
|
|
|
|
|
|
|
|
|
|
|
|
|
|
|
|
|
|
|
|
|
|
|
|
|
|
|
|
|
|
|
|
|
|
|
|
|
|
|
|
|
|
|
|
|
|
|
|
|
|
|
|
|
|
|
|
|
|
|
|
|
|
|
|
|
|
|
|
|
|
|
|
|
|
|
|
|
|
|
|
|
|
|
|
|
|
|
|
|
|
|
|
|
|
1 |
+
# Community Examples
|
2 |
+
|
3 |
+
> **For more information about community pipelines, please have a look at [this issue](https://github.com/huggingface/diffusers/issues/841).**
|
4 |
+
|
5 |
+
**Community** examples consist of both inference and training examples that have been added by the community.
|
6 |
+
Please have a look at the following table to get an overview of all community examples. Click on the **Code Example** to get a copy-and-paste ready code example that you can try out.
|
7 |
+
If a community doesn't work as expected, please open an issue and ping the author on it.
|
8 |
+
|
9 |
+
| Example | Description | Code Example | Colab | Author |
|
10 |
+
|:----------|:----------------------|:-----------------|:-------------|----------:|
|
11 |
+
| CLIP Guided Stable Diffusion | Doing CLIP guidance for text to image generation with Stable Diffusion| [CLIP Guided Stable Diffusion](#clip-guided-stable-diffusion) | [](https://colab.research.google.com/github/huggingface/notebooks/blob/main/diffusers/CLIP_Guided_Stable_diffusion_with_diffusers.ipynb) | [Suraj Patil](https://github.com/patil-suraj/) |
|
12 |
+
| One Step U-Net (Dummy) | Example showcasing of how to use Community Pipelines (see https://github.com/huggingface/diffusers/issues/841) | [One Step U-Net](#one-step-unet) | - | [Patrick von Platen](https://github.com/patrickvonplaten/) |
|
13 |
+
| Stable Diffusion Interpolation | Interpolate the latent space of Stable Diffusion between different prompts/seeds | [Stable Diffusion Interpolation](#stable-diffusion-interpolation) | - | [Nate Raw](https://github.com/nateraw/) |
|
14 |
+
| Stable Diffusion Mega | **One** Stable Diffusion Pipeline with all functionalities of [Text2Image](https://github.com/huggingface/diffusers/blob/main/src/diffusers/pipelines/stable_diffusion/pipeline_stable_diffusion.py), [Image2Image](https://github.com/huggingface/diffusers/blob/main/src/diffusers/pipelines/stable_diffusion/pipeline_stable_diffusion_img2img.py) and [Inpainting](https://github.com/huggingface/diffusers/blob/main/src/diffusers/pipelines/stable_diffusion/pipeline_stable_diffusion_inpaint.py) | [Stable Diffusion Mega](#stable-diffusion-mega) | - | [Patrick von Platen](https://github.com/patrickvonplaten/) |
|
15 |
+
|
16 |
+
To load a custom pipeline you just need to pass the `custom_pipeline` argument to `DiffusionPipeline`, as one of the files in `diffusers/examples/community`. Feel free to send a PR with your own pipelines, we will merge them quickly.
|
17 |
+
```py
|
18 |
+
pipe = DiffusionPipeline.from_pretrained("CompVis/stable-diffusion-v1-4", custom_pipeline="filename_in_the_community_folder")
|
19 |
+
```
|
20 |
+
|
21 |
+
## Example usages
|
22 |
+
|
23 |
+
### CLIP Guided Stable Diffusion
|
24 |
+
|
25 |
+
CLIP guided stable diffusion can help to generate more realistic images
|
26 |
+
by guiding stable diffusion at every denoising step with an additional CLIP model.
|
27 |
+
|
28 |
+
The following code requires roughly 12GB of GPU RAM.
|
29 |
+
|
30 |
+
```python
|
31 |
+
from diffusers import DiffusionPipeline
|
32 |
+
from transformers import CLIPFeatureExtractor, CLIPModel
|
33 |
+
import torch
|
34 |
+
|
35 |
+
|
36 |
+
feature_extractor = CLIPFeatureExtractor.from_pretrained("laion/CLIP-ViT-B-32-laion2B-s34B-b79K")
|
37 |
+
clip_model = CLIPModel.from_pretrained("laion/CLIP-ViT-B-32-laion2B-s34B-b79K", torch_dtype=torch.float16)
|
38 |
+
|
39 |
+
|
40 |
+
guided_pipeline = DiffusionPipeline.from_pretrained(
|
41 |
+
"CompVis/stable-diffusion-v1-4",
|
42 |
+
custom_pipeline="clip_guided_stable_diffusion",
|
43 |
+
clip_model=clip_model,
|
44 |
+
feature_extractor=feature_extractor,
|
45 |
+
revision="fp16",
|
46 |
+
torch_dtype=torch.float16,
|
47 |
+
)
|
48 |
+
guided_pipeline.enable_attention_slicing()
|
49 |
+
guided_pipeline = guided_pipeline.to("cuda")
|
50 |
+
|
51 |
+
prompt = "fantasy book cover, full moon, fantasy forest landscape, golden vector elements, fantasy magic, dark light night, intricate, elegant, sharp focus, illustration, highly detailed, digital painting, concept art, matte, art by WLOP and Artgerm and Albert Bierstadt, masterpiece"
|
52 |
+
|
53 |
+
generator = torch.Generator(device="cuda").manual_seed(0)
|
54 |
+
images = []
|
55 |
+
for i in range(4):
|
56 |
+
image = guided_pipeline(
|
57 |
+
prompt,
|
58 |
+
num_inference_steps=50,
|
59 |
+
guidance_scale=7.5,
|
60 |
+
clip_guidance_scale=100,
|
61 |
+
num_cutouts=4,
|
62 |
+
use_cutouts=False,
|
63 |
+
generator=generator,
|
64 |
+
).images[0]
|
65 |
+
images.append(image)
|
66 |
+
|
67 |
+
# save images locally
|
68 |
+
for i, img in enumerate(images):
|
69 |
+
img.save(f"./clip_guided_sd/image_{i}.png")
|
70 |
+
```
|
71 |
+
|
72 |
+
The `images` list contains a list of PIL images that can be saved locally or displayed directly in a google colab.
|
73 |
+
Generated images tend to be of higher qualtiy than natively using stable diffusion. E.g. the above script generates the following images:
|
74 |
+
|
75 |
+
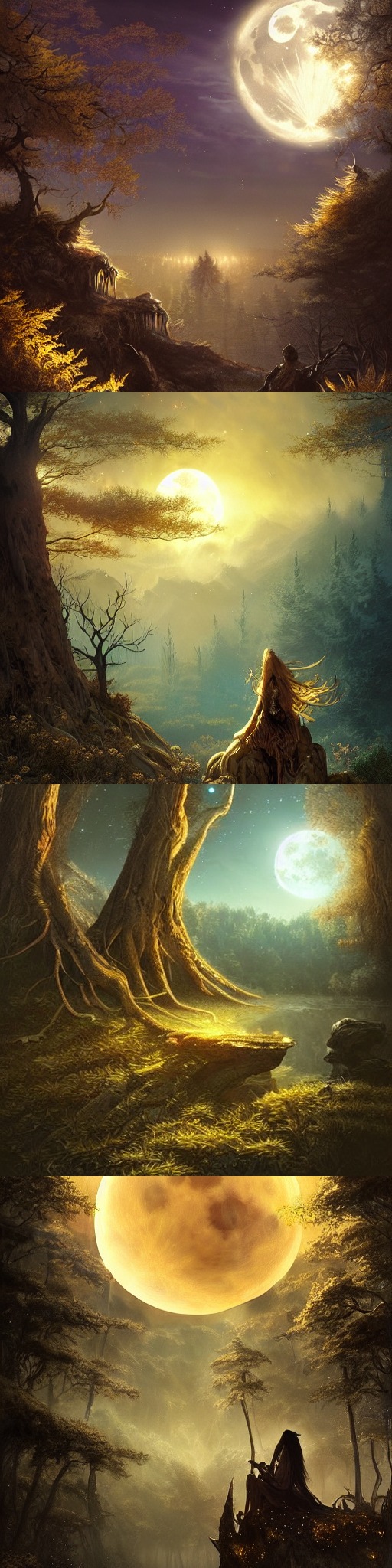.
|
76 |
+
|
77 |
+
### One Step Unet
|
78 |
+
|
79 |
+
The dummy "one-step-unet" can be run as follows:
|
80 |
+
|
81 |
+
```python
|
82 |
+
from diffusers import DiffusionPipeline
|
83 |
+
|
84 |
+
pipe = DiffusionPipeline.from_pretrained("google/ddpm-cifar10-32", custom_pipeline="one_step_unet")
|
85 |
+
pipe()
|
86 |
+
```
|
87 |
+
|
88 |
+
**Note**: This community pipeline is not useful as a feature, but rather just serves as an example of how community pipelines can be added (see https://github.com/huggingface/diffusers/issues/841).
|
89 |
+
|
90 |
+
### Stable Diffusion Interpolation
|
91 |
+
|
92 |
+
The following code can be run on a GPU of at least 8GB VRAM and should take approximately 5 minutes.
|
93 |
+
|
94 |
+
```python
|
95 |
+
from diffusers import DiffusionPipeline
|
96 |
+
import torch
|
97 |
+
|
98 |
+
pipe = DiffusionPipeline.from_pretrained(
|
99 |
+
"CompVis/stable-diffusion-v1-4",
|
100 |
+
revision='fp16',
|
101 |
+
torch_dtype=torch.float16,
|
102 |
+
safety_checker=None, # Very important for videos...lots of false positives while interpolating
|
103 |
+
custom_pipeline="interpolate_stable_diffusion",
|
104 |
+
).to('cuda')
|
105 |
+
pipe.enable_attention_slicing()
|
106 |
+
|
107 |
+
frame_filepaths = pipe.walk(
|
108 |
+
prompts=['a dog', 'a cat', 'a horse'],
|
109 |
+
seeds=[42, 1337, 1234],
|
110 |
+
num_interpolation_steps=16,
|
111 |
+
output_dir='./dreams',
|
112 |
+
batch_size=4,
|
113 |
+
height=512,
|
114 |
+
width=512,
|
115 |
+
guidance_scale=8.5,
|
116 |
+
num_inference_steps=50,
|
117 |
+
)
|
118 |
+
```
|
119 |
+
|
120 |
+
The output of the `walk(...)` function returns a list of images saved under the folder as defined in `output_dir`. You can use these images to create videos of stable diffusion.
|
121 |
+
|
122 |
+
> **Please have a look at https://github.com/nateraw/stable-diffusion-videos for more in-detail information on how to create videos using stable diffusion as well as more feature-complete functionality.**
|
123 |
+
|
124 |
+
### Stable Diffusion Mega
|
125 |
+
|
126 |
+
The Stable Diffusion Mega Pipeline lets you use the main use cases of the stable diffusion pipeline in a single class.
|
127 |
+
|
128 |
+
```python
|
129 |
+
#!/usr/bin/env python3
|
130 |
+
from diffusers import DiffusionPipeline
|
131 |
+
import PIL
|
132 |
+
import requests
|
133 |
+
from io import BytesIO
|
134 |
+
import torch
|
135 |
+
|
136 |
+
|
137 |
+
def download_image(url):
|
138 |
+
response = requests.get(url)
|
139 |
+
return PIL.Image.open(BytesIO(response.content)).convert("RGB")
|
140 |
+
|
141 |
+
pipe = DiffusionPipeline.from_pretrained("CompVis/stable-diffusion-v1-4", custom_pipeline="stable_diffusion_mega", torch_dtype=torch.float16, revision="fp16")
|
142 |
+
pipe.to("cuda")
|
143 |
+
pipe.enable_attention_slicing()
|
144 |
+
|
145 |
+
|
146 |
+
### Text-to-Image
|
147 |
+
|
148 |
+
images = pipe.text2img("An astronaut riding a horse").images
|
149 |
+
|
150 |
+
### Image-to-Image
|
151 |
+
|
152 |
+
init_image = download_image("https://raw.githubusercontent.com/CompVis/stable-diffusion/main/assets/stable-samples/img2img/sketch-mountains-input.jpg")
|
153 |
+
|
154 |
+
prompt = "A fantasy landscape, trending on artstation"
|
155 |
+
|
156 |
+
images = pipe.img2img(prompt=prompt, init_image=init_image, strength=0.75, guidance_scale=7.5).images
|
157 |
+
|
158 |
+
### Inpainting
|
159 |
+
|
160 |
+
img_url = "https://raw.githubusercontent.com/CompVis/latent-diffusion/main/data/inpainting_examples/overture-creations-5sI6fQgYIuo.png"
|
161 |
+
mask_url = "https://raw.githubusercontent.com/CompVis/latent-diffusion/main/data/inpainting_examples/overture-creations-5sI6fQgYIuo_mask.png"
|
162 |
+
init_image = download_image(img_url).resize((512, 512))
|
163 |
+
mask_image = download_image(mask_url).resize((512, 512))
|
164 |
+
|
165 |
+
prompt = "a cat sitting on a bench"
|
166 |
+
images = pipe.inpaint(prompt=prompt, init_image=init_image, mask_image=mask_image, strength=0.75).images
|
167 |
+
```
|
168 |
+
|
169 |
+
As shown above this one pipeline can run all both "text-to-image", "image-to-image", and "inpainting" in one pipeline.
|
170 |
+
|
v0.6.0/clip_guided_stable_diffusion.py
ADDED
@@ -0,0 +1,324 @@
|
|
|
|
|
|
|
|
|
|
|
|
|
|
|
|
|
|
|
|
|
|
|
|
|
|
|
|
|
|
|
|
|
|
|
|
|
|
|
|
|
|
|
|
|
|
|
|
|
|
|
|
|
|
|
|
|
|
|
|
|
|
|
|
|
|
|
|
|
|
|
|
|
|
|
|
|
|
|
|
|
|
|
|
|
|
|
|
|
|
|
|
|
|
|
|
|
|
|
|
|
|
|
|
|
|
|
|
|
|
|
|
|
|
|
|
|
|
|
|
|
|
|
|
|
|
|
|
|
|
|
|
|
|
|
|
|
|
|
|
|
|
|
|
|
|
|
|
|
|
|
|
|
|
|
|
|
|
|
|
|
|
|
|
|
|
|
|
|
|
|
|
|
|
|
|
|
|
|
|
|
|
|
|
|
|
|
|
|
|
|
|
|
|
|
|
|
|
|
|
|
|
|
|
|
|
|
|
|
|
|
|
|
|
|
|
|
|
|
|
|
|
|
|
|
|
|
|
|
|
|
|
|
|
|
|
|
|
|
|
|
|
|
|
|
|
|
|
|
|
|
|
|
|
|
|
|
|
|
|
|
|
|
|
|
|
|
|
|
|
|
|
|
|
|
|
|
|
|
|
|
|
|
|
|
|
|
|
|
|
|
|
|
|
|
|
|
|
|
|
|
|
|
|
|
|
|
|
|
|
|
|
|
|
|
|
|
|
|
|
|
|
|
|
|
|
|
|
|
|
|
|
|
|
|
|
|
|
|
|
|
|
|
|
|
|
|
|
|
|
|
|
|
|
|
|
|
|
|
|
|
|
|
|
|
|
|
|
|
|
|
|
|
|
|
|
|
|
|
|
|
|
|
|
|
|
|
|
|
|
|
|
|
|
|
|
|
|
|
|
|
|
|
|
|
|
|
|
|
|
|
|
|
|
|
|
|
|
|
|
|
|
|
|
|
|
|
|
|
|
|
|
|
|
|
|
|
|
|
|
|
|
|
|
|
|
|
|
|
|
|
|
|
|
|
|
|
|
|
|
|
|
|
|
|
|
|
|
|
|
|
|
|
|
|
|
|
|
|
|
|
|
|
|
|
|
|
|
|
|
|
|
|
|
|
|
|
|
|
|
|
|
|
|
|
|
|
|
|
|
|
|
|
|
|
|
|
|
|
|
|
|
|
|
|
|
|
|
|
|
|
|
|
|
|
|
|
|
|
|
|
|
|
|
|
|
|
|
|
|
|
|
|
|
|
|
|
|
|
|
|
|
|
|
|
|
|
|
|
|
|
|
|
|
|
|
|
|
|
|
|
|
|
|
|
|
|
|
|
|
|
|
|
|
|
|
|
|
|
|
|
|
|
|
|
|
|
|
|
|
|
|
|
|
|
|
|
|
|
|
|
|
|
|
|
|
|
|
|
|
|
|
|
|
|
|
|
|
|
|
|
|
|
|
|
|
|
|
|
|
|
1 |
+
import inspect
|
2 |
+
from typing import List, Optional, Union
|
3 |
+
|
4 |
+
import torch
|
5 |
+
from torch import nn
|
6 |
+
from torch.nn import functional as F
|
7 |
+
|
8 |
+
from diffusers import AutoencoderKL, DiffusionPipeline, LMSDiscreteScheduler, PNDMScheduler, UNet2DConditionModel
|
9 |
+
from diffusers.pipelines.stable_diffusion.pipeline_stable_diffusion import StableDiffusionPipelineOutput
|
10 |
+
from torchvision import transforms
|
11 |
+
from transformers import CLIPFeatureExtractor, CLIPModel, CLIPTextModel, CLIPTokenizer
|
12 |
+
|
13 |
+
|
14 |
+
class MakeCutouts(nn.Module):
|
15 |
+
def __init__(self, cut_size, cut_power=1.0):
|
16 |
+
super().__init__()
|
17 |
+
|
18 |
+
self.cut_size = cut_size
|
19 |
+
self.cut_power = cut_power
|
20 |
+
|
21 |
+
def forward(self, pixel_values, num_cutouts):
|
22 |
+
sideY, sideX = pixel_values.shape[2:4]
|
23 |
+
max_size = min(sideX, sideY)
|
24 |
+
min_size = min(sideX, sideY, self.cut_size)
|
25 |
+
cutouts = []
|
26 |
+
for _ in range(num_cutouts):
|
27 |
+
size = int(torch.rand([]) ** self.cut_power * (max_size - min_size) + min_size)
|
28 |
+
offsetx = torch.randint(0, sideX - size + 1, ())
|
29 |
+
offsety = torch.randint(0, sideY - size + 1, ())
|
30 |
+
cutout = pixel_values[:, :, offsety : offsety + size, offsetx : offsetx + size]
|
31 |
+
cutouts.append(F.adaptive_avg_pool2d(cutout, self.cut_size))
|
32 |
+
return torch.cat(cutouts)
|
33 |
+
|
34 |
+
|
35 |
+
def spherical_dist_loss(x, y):
|
36 |
+
x = F.normalize(x, dim=-1)
|
37 |
+
y = F.normalize(y, dim=-1)
|
38 |
+
return (x - y).norm(dim=-1).div(2).arcsin().pow(2).mul(2)
|
39 |
+
|
40 |
+
|
41 |
+
def set_requires_grad(model, value):
|
42 |
+
for param in model.parameters():
|
43 |
+
param.requires_grad = value
|
44 |
+
|
45 |
+
|
46 |
+
class CLIPGuidedStableDiffusion(DiffusionPipeline):
|
47 |
+
"""CLIP guided stable diffusion based on the amazing repo by @crowsonkb and @Jack000
|
48 |
+
- https://github.com/Jack000/glid-3-xl
|
49 |
+
- https://github.dev/crowsonkb/k-diffusion
|
50 |
+
"""
|
51 |
+
|
52 |
+
def __init__(
|
53 |
+
self,
|
54 |
+
vae: AutoencoderKL,
|
55 |
+
text_encoder: CLIPTextModel,
|
56 |
+
clip_model: CLIPModel,
|
57 |
+
tokenizer: CLIPTokenizer,
|
58 |
+
unet: UNet2DConditionModel,
|
59 |
+
scheduler: Union[PNDMScheduler, LMSDiscreteScheduler],
|
60 |
+
feature_extractor: CLIPFeatureExtractor,
|
61 |
+
):
|
62 |
+
super().__init__()
|
63 |
+
self.register_modules(
|
64 |
+
vae=vae,
|
65 |
+
text_encoder=text_encoder,
|
66 |
+
clip_model=clip_model,
|
67 |
+
tokenizer=tokenizer,
|
68 |
+
unet=unet,
|
69 |
+
scheduler=scheduler,
|
70 |
+
feature_extractor=feature_extractor,
|
71 |
+
)
|
72 |
+
|
73 |
+
self.normalize = transforms.Normalize(mean=feature_extractor.image_mean, std=feature_extractor.image_std)
|
74 |
+
self.make_cutouts = MakeCutouts(feature_extractor.size)
|
75 |
+
|
76 |
+
set_requires_grad(self.text_encoder, False)
|
77 |
+
set_requires_grad(self.clip_model, False)
|
78 |
+
|
79 |
+
def enable_attention_slicing(self, slice_size: Optional[Union[str, int]] = "auto"):
|
80 |
+
if slice_size == "auto":
|
81 |
+
# half the attention head size is usually a good trade-off between
|
82 |
+
# speed and memory
|
83 |
+
slice_size = self.unet.config.attention_head_dim // 2
|
84 |
+
self.unet.set_attention_slice(slice_size)
|
85 |
+
|
86 |
+
def disable_attention_slicing(self):
|
87 |
+
self.enable_attention_slicing(None)
|
88 |
+
|
89 |
+
def freeze_vae(self):
|
90 |
+
set_requires_grad(self.vae, False)
|
91 |
+
|
92 |
+
def unfreeze_vae(self):
|
93 |
+
set_requires_grad(self.vae, True)
|
94 |
+
|
95 |
+
def freeze_unet(self):
|
96 |
+
set_requires_grad(self.unet, False)
|
97 |
+
|
98 |
+
def unfreeze_unet(self):
|
99 |
+
set_requires_grad(self.unet, True)
|
100 |
+
|
101 |
+
@torch.enable_grad()
|
102 |
+
def cond_fn(
|
103 |
+
self,
|
104 |
+
latents,
|
105 |
+
timestep,
|
106 |
+
index,
|
107 |
+
text_embeddings,
|
108 |
+
noise_pred_original,
|
109 |
+
text_embeddings_clip,
|
110 |
+
clip_guidance_scale,
|
111 |
+
num_cutouts,
|
112 |
+
use_cutouts=True,
|
113 |
+
):
|
114 |
+
latents = latents.detach().requires_grad_()
|
115 |
+
|
116 |
+
if isinstance(self.scheduler, LMSDiscreteScheduler):
|
117 |
+
sigma = self.scheduler.sigmas[index]
|
118 |
+
# the model input needs to be scaled to match the continuous ODE formulation in K-LMS
|
119 |
+
latent_model_input = latents / ((sigma**2 + 1) ** 0.5)
|
120 |
+
else:
|
121 |
+
latent_model_input = latents
|
122 |
+
|
123 |
+
# predict the noise residual
|
124 |
+
noise_pred = self.unet(latent_model_input, timestep, encoder_hidden_states=text_embeddings).sample
|
125 |
+
|
126 |
+
if isinstance(self.scheduler, PNDMScheduler):
|
127 |
+
alpha_prod_t = self.scheduler.alphas_cumprod[timestep]
|
128 |
+
beta_prod_t = 1 - alpha_prod_t
|
129 |
+
# compute predicted original sample from predicted noise also called
|
130 |
+
# "predicted x_0" of formula (12) from https://arxiv.org/pdf/2010.02502.pdf
|
131 |
+
pred_original_sample = (latents - beta_prod_t ** (0.5) * noise_pred) / alpha_prod_t ** (0.5)
|
132 |
+
|
133 |
+
fac = torch.sqrt(beta_prod_t)
|
134 |
+
sample = pred_original_sample * (fac) + latents * (1 - fac)
|
135 |
+
elif isinstance(self.scheduler, LMSDiscreteScheduler):
|
136 |
+
sigma = self.scheduler.sigmas[index]
|
137 |
+
sample = latents - sigma * noise_pred
|
138 |
+
else:
|
139 |
+
raise ValueError(f"scheduler type {type(self.scheduler)} not supported")
|
140 |
+
|
141 |
+
sample = 1 / 0.18215 * sample
|
142 |
+
image = self.vae.decode(sample).sample
|
143 |
+
image = (image / 2 + 0.5).clamp(0, 1)
|
144 |
+
|
145 |
+
if use_cutouts:
|
146 |
+
image = self.make_cutouts(image, num_cutouts)
|
147 |
+
else:
|
148 |
+
image = transforms.Resize(self.feature_extractor.size)(image)
|
149 |
+
image = self.normalize(image).to(latents.dtype)
|
150 |
+
|
151 |
+
image_embeddings_clip = self.clip_model.get_image_features(image)
|
152 |
+
image_embeddings_clip = image_embeddings_clip / image_embeddings_clip.norm(p=2, dim=-1, keepdim=True)
|
153 |
+
|
154 |
+
if use_cutouts:
|
155 |
+
dists = spherical_dist_loss(image_embeddings_clip, text_embeddings_clip)
|
156 |
+
dists = dists.view([num_cutouts, sample.shape[0], -1])
|
157 |
+
loss = dists.sum(2).mean(0).sum() * clip_guidance_scale
|
158 |
+
else:
|
159 |
+
loss = spherical_dist_loss(image_embeddings_clip, text_embeddings_clip).mean() * clip_guidance_scale
|
160 |
+
|
161 |
+
grads = -torch.autograd.grad(loss, latents)[0]
|
162 |
+
|
163 |
+
if isinstance(self.scheduler, LMSDiscreteScheduler):
|
164 |
+
latents = latents.detach() + grads * (sigma**2)
|
165 |
+
noise_pred = noise_pred_original
|
166 |
+
else:
|
167 |
+
noise_pred = noise_pred_original - torch.sqrt(beta_prod_t) * grads
|
168 |
+
return noise_pred, latents
|
169 |
+
|
170 |
+
@torch.no_grad()
|
171 |
+
def __call__(
|
172 |
+
self,
|
173 |
+
prompt: Union[str, List[str]],
|
174 |
+
height: Optional[int] = 512,
|
175 |
+
width: Optional[int] = 512,
|
176 |
+
num_inference_steps: Optional[int] = 50,
|
177 |
+
guidance_scale: Optional[float] = 7.5,
|
178 |
+
num_images_per_prompt: Optional[int] = 1,
|
179 |
+
clip_guidance_scale: Optional[float] = 100,
|
180 |
+
clip_prompt: Optional[Union[str, List[str]]] = None,
|
181 |
+
num_cutouts: Optional[int] = 4,
|
182 |
+
use_cutouts: Optional[bool] = True,
|
183 |
+
generator: Optional[torch.Generator] = None,
|
184 |
+
latents: Optional[torch.FloatTensor] = None,
|
185 |
+
output_type: Optional[str] = "pil",
|
186 |
+
return_dict: bool = True,
|
187 |
+
):
|
188 |
+
if isinstance(prompt, str):
|
189 |
+
batch_size = 1
|
190 |
+
elif isinstance(prompt, list):
|
191 |
+
batch_size = len(prompt)
|
192 |
+
else:
|
193 |
+
raise ValueError(f"`prompt` has to be of type `str` or `list` but is {type(prompt)}")
|
194 |
+
|
195 |
+
if height % 8 != 0 or width % 8 != 0:
|
196 |
+
raise ValueError(f"`height` and `width` have to be divisible by 8 but are {height} and {width}.")
|
197 |
+
|
198 |
+
# get prompt text embeddings
|
199 |
+
text_input = self.tokenizer(
|
200 |
+
prompt,
|
201 |
+
padding="max_length",
|
202 |
+
max_length=self.tokenizer.model_max_length,
|
203 |
+
truncation=True,
|
204 |
+
return_tensors="pt",
|
205 |
+
)
|
206 |
+
text_embeddings = self.text_encoder(text_input.input_ids.to(self.device))[0]
|
207 |
+
# duplicate text embeddings for each generation per prompt
|
208 |
+
text_embeddings = text_embeddings.repeat_interleave(num_images_per_prompt, dim=0)
|
209 |
+
|
210 |
+
if clip_guidance_scale > 0:
|
211 |
+
if clip_prompt is not None:
|
212 |
+
clip_text_input = self.tokenizer(
|
213 |
+
clip_prompt,
|
214 |
+
padding="max_length",
|
215 |
+
max_length=self.tokenizer.model_max_length,
|
216 |
+
truncation=True,
|
217 |
+
return_tensors="pt",
|
218 |
+
).input_ids.to(self.device)
|
219 |
+
else:
|
220 |
+
clip_text_input = text_input.input_ids.to(self.device)
|
221 |
+
text_embeddings_clip = self.clip_model.get_text_features(clip_text_input)
|
222 |
+
text_embeddings_clip = text_embeddings_clip / text_embeddings_clip.norm(p=2, dim=-1, keepdim=True)
|
223 |
+
# duplicate text embeddings clip for each generation per prompt
|
224 |
+
text_embeddings_clip = text_embeddings_clip.repeat_interleave(num_images_per_prompt, dim=0)
|
225 |
+
|
226 |
+
# here `guidance_scale` is defined analog to the guidance weight `w` of equation (2)
|
227 |
+
# of the Imagen paper: https://arxiv.org/pdf/2205.11487.pdf . `guidance_scale = 1`
|
228 |
+
# corresponds to doing no classifier free guidance.
|
229 |
+
do_classifier_free_guidance = guidance_scale > 1.0
|
230 |
+
# get unconditional embeddings for classifier free guidance
|
231 |
+
if do_classifier_free_guidance:
|
232 |
+
max_length = text_input.input_ids.shape[-1]
|
233 |
+
uncond_input = self.tokenizer([""], padding="max_length", max_length=max_length, return_tensors="pt")
|
234 |
+
uncond_embeddings = self.text_encoder(uncond_input.input_ids.to(self.device))[0]
|
235 |
+
# duplicate unconditional embeddings for each generation per prompt
|
236 |
+
uncond_embeddings = uncond_embeddings.repeat_interleave(num_images_per_prompt, dim=0)
|
237 |
+
|
238 |
+
# For classifier free guidance, we need to do two forward passes.
|
239 |
+
# Here we concatenate the unconditional and text embeddings into a single batch
|
240 |
+
# to avoid doing two forward passes
|
241 |
+
text_embeddings = torch.cat([uncond_embeddings, text_embeddings])
|
242 |
+
|
243 |
+
# get the initial random noise unless the user supplied it
|
244 |
+
|
245 |
+
# Unlike in other pipelines, latents need to be generated in the target device
|
246 |
+
# for 1-to-1 results reproducibility with the CompVis implementation.
|
247 |
+
# However this currently doesn't work in `mps`.
|
248 |
+
latents_shape = (batch_size * num_images_per_prompt, self.unet.in_channels, height // 8, width // 8)
|
249 |
+
latents_dtype = text_embeddings.dtype
|
250 |
+
if latents is None:
|
251 |
+
if self.device.type == "mps":
|
252 |
+
# randn does not exist on mps
|
253 |
+
latents = torch.randn(latents_shape, generator=generator, device="cpu", dtype=latents_dtype).to(
|
254 |
+
self.device
|
255 |
+
)
|
256 |
+
else:
|
257 |
+
latents = torch.randn(latents_shape, generator=generator, device=self.device, dtype=latents_dtype)
|
258 |
+
else:
|
259 |
+
if latents.shape != latents_shape:
|
260 |
+
raise ValueError(f"Unexpected latents shape, got {latents.shape}, expected {latents_shape}")
|
261 |
+
latents = latents.to(self.device)
|
262 |
+
|
263 |
+
# set timesteps
|
264 |
+
accepts_offset = "offset" in set(inspect.signature(self.scheduler.set_timesteps).parameters.keys())
|
265 |
+
extra_set_kwargs = {}
|
266 |
+
if accepts_offset:
|
267 |
+
extra_set_kwargs["offset"] = 1
|
268 |
+
|
269 |
+
self.scheduler.set_timesteps(num_inference_steps, **extra_set_kwargs)
|
270 |
+
|
271 |
+
# Some schedulers like PNDM have timesteps as arrays
|
272 |
+
# It's more optimized to move all timesteps to correct device beforehand
|
273 |
+
timesteps_tensor = self.scheduler.timesteps.to(self.device)
|
274 |
+
|
275 |
+
# scale the initial noise by the standard deviation required by the scheduler
|
276 |
+
latents = latents * self.scheduler.init_noise_sigma
|
277 |
+
|
278 |
+
for i, t in enumerate(self.progress_bar(timesteps_tensor)):
|
279 |
+
# expand the latents if we are doing classifier free guidance
|
280 |
+
latent_model_input = torch.cat([latents] * 2) if do_classifier_free_guidance else latents
|
281 |
+
latent_model_input = self.scheduler.scale_model_input(latent_model_input, t)
|
282 |
+
|
283 |
+
# predict the noise residual
|
284 |
+
noise_pred = self.unet(latent_model_input, t, encoder_hidden_states=text_embeddings).sample
|
285 |
+
|
286 |
+
# perform classifier free guidance
|
287 |
+
if do_classifier_free_guidance:
|
288 |
+
noise_pred_uncond, noise_pred_text = noise_pred.chunk(2)
|
289 |
+
noise_pred = noise_pred_uncond + guidance_scale * (noise_pred_text - noise_pred_uncond)
|
290 |
+
|
291 |
+
# perform clip guidance
|
292 |
+
if clip_guidance_scale > 0:
|
293 |
+
text_embeddings_for_guidance = (
|
294 |
+
text_embeddings.chunk(2)[1] if do_classifier_free_guidance else text_embeddings
|
295 |
+
)
|
296 |
+
noise_pred, latents = self.cond_fn(
|
297 |
+
latents,
|
298 |
+
t,
|
299 |
+
i,
|
300 |
+
text_embeddings_for_guidance,
|
301 |
+
noise_pred,
|
302 |
+
text_embeddings_clip,
|
303 |
+
clip_guidance_scale,
|
304 |
+
num_cutouts,
|
305 |
+
use_cutouts,
|
306 |
+
)
|
307 |
+
|
308 |
+
# compute the previous noisy sample x_t -> x_t-1
|
309 |
+
latents = self.scheduler.step(noise_pred, t, latents).prev_sample
|
310 |
+
|
311 |
+
# scale and decode the image latents with vae
|
312 |
+
latents = 1 / 0.18215 * latents
|
313 |
+
image = self.vae.decode(latents).sample
|
314 |
+
|
315 |
+
image = (image / 2 + 0.5).clamp(0, 1)
|
316 |
+
image = image.cpu().permute(0, 2, 3, 1).numpy()
|
317 |
+
|
318 |
+
if output_type == "pil":
|
319 |
+
image = self.numpy_to_pil(image)
|
320 |
+
|
321 |
+
if not return_dict:
|
322 |
+
return (image, None)
|
323 |
+
|
324 |
+
return StableDiffusionPipelineOutput(images=image, nsfw_content_detected=None)
|
v0.6.0/interpolate_stable_diffusion.py
ADDED
@@ -0,0 +1,524 @@
|
|
|
|
|
|
|
|
|
|
|
|
|
|
|
|
|
|
|
|
|
|
|
|
|
|
|
|
|
|
|
|
|
|
|
|
|
|
|
|
|
|
|
|
|
|
|
|
|
|
|
|
|
|
|
|
|
|
|
|
|
|
|
|
|
|
|
|
|
|
|
|
|
|
|
|
|
|
|
|
|
|
|
|
|
|
|
|
|
|
|
|
|
|
|
|
|
|
|
|
|
|
|
|
|
|
|
|
|
|
|
|
|
|
|
|
|
|
|
|
|
|
|
|
|
|
|
|
|
|
|
|
|
|
|
|
|
|
|
|
|
|
|
|
|
|
|
|
|
|
|
|
|
|
|
|
|
|
|
|
|
|
|
|
|
|
|
|
|
|
|
|
|
|
|
|
|
|
|
|
|
|
|
|
|
|
|
|
|
|
|
|
|
|
|
|
|
|
|
|
|
|
|
|
|
|
|
|
|
|
|
|
|
|
|
|
|
|
|
|
|
|
|
|
|
|
|
|
|
|
|
|
|
|
|
|
|
|
|
|
|
|
|
|
|
|
|
|
|
|
|
|
|
|
|
|
|
|
|
|
|
|
|
|
|
|
|
|
|
|
|
|
|
|
|
|
|
|
|
|
|
|
|
|
|
|
|
|
|
|
|
|
|
|
|
|
|
|
|
|
|
|
|
|
|
|
|
|
|
|
|
|
|
|
|
|
|
|
|
|
|
|
|
|
|
|
|
|
|
|
|
|
|
|
|
|
|
|
|
|
|
|
|
|
|
|
|
|
|
|
|
|
|
|
|
|
|
|
|
|
|
|
|
|
|
|
|
|
|
|
|
|
|
|
|
|
|
|
|
|
|
|
|
|
|
|
|
|
|
|
|
|
|
|
|
|
|
|
|
|
|
|
|
|
|
|
|
|
|
|
|
|
|
|
|
|
|
|
|
|
|
|
|
|
|
|
|
|
|
|
|
|
|
|
|
|
|
|
|
|
|
|
|
|
|
|
|
|
|
|
|
|
|
|
|
|
|
|
|
|
|
|
|
|
|
|
|
|
|
|
|
|
|
|
|
|
|
|
|
|
|
|
|
|
|
|
|
|
|
|
|
|
|
|
|
|
|
|
|
|
|
|
|
|
|
|
|
|
|
|
|
|
|
|
|
|
|
|
|
|
|
|
|
|
|
|
|
|
|
|
|
|
|
|
|
|
|
|
|
|
|
|
|
|
|
|
|
|
|
|
|
|
|
|
|
|
|
|
|
|
|
|
|
|
|
|
|
|
|
|
|
|
|
|
|
|
|
|
|
|
|
|
|
|
|
|
|
|
|
|
|
|
|
|
|
|
|
|
|
|
|
|
|
|
|
|
|
|
|
|
|
|
|
|
|
|
|
|
|
|
|
|
|
|
|
|
|
|
|
|
|
|
|
|
|
|
|
|
|
|
|
|
|
|
|
|
|
|
|
|
|
|
|
|
|
|
|
|
|
|
|
|
|
|
|
|
|
|
|
|
|
|
|
|
|
|
|
|
|
|
|
|
|
|
|
|
|
|
|
|
|
|
|
|
|
|
|
|
|
|
|
|
|
|
|
|
|
|
|
|
|
|
|
|
|
|
|
|
|
|
|
|
|
|
|
|
|
|
|
|
|
|
|
|
|
|
|
|
|
|
|
|
|
|
|
|
|
|
|
|
|
|
|
|
|
|
|
|
|
|
|
|
|
|
|
|
|
|
|
|
|
|
|
|
|
|
|
|
|
|
|
|
|
|
|
|
|
|
|
|
|
|
|
|
|
|
|
|
|
|
|
|
|
|
|
|
|
|
|
|
|
|
|
|
|
|
|
|
|
|
|
|
|
|
|
|
|
|
|
|
|
|
|
|
|
|
|
|
|
|
|
|
|
|
|
|
|
|
|
|
|
|
|
|
|
|
|
|
|
|
|
|
|
|
|
|
|
|
|
|
|
|
|
|
|
|
|
|
|
|
|
|
|
|
|
|
|
|
|
|
|
|
|
|
|
|
|
|
|
|
|
|
|
|
|
|
|
|
|
|
|
|
|
|
|
|
|
|
|
|
|
|
|
|
|
|
|
|
|
|
|
|
|
|
|
|
|
|
|
|
|
|
|
|
|
|
|
|
|
|
|
|
|
|
|
|
|
|
|
|
|
|
|
|
|
|
|
|
|
|
|
|
|
|
|
|
|
|
|
|
|
|
|
|
|
|
|
|
|
|
|
|
|
|
|
|
|
|
|
|
|
|
|
|
|
|
|
|
|
|
|
|
|
|
|
|
|
|
|
|
|
|
|
|
|
|
|
|
|
|
|
|
|
|
|
|
|
|
|
|
|
|
|
|
|
|
|
|
|
|
|
1 |
+
import inspect
|
2 |
+
import time
|
3 |
+
from pathlib import Path
|
4 |
+
from typing import Callable, List, Optional, Union
|
5 |
+
|
6 |
+
import numpy as np
|
7 |
+
import torch
|
8 |
+
|
9 |
+
from diffusers.configuration_utils import FrozenDict
|
10 |
+
from diffusers.models import AutoencoderKL, UNet2DConditionModel
|
11 |
+
from diffusers.pipeline_utils import DiffusionPipeline
|
12 |
+
from diffusers.pipelines.stable_diffusion import StableDiffusionPipelineOutput
|
13 |
+
from diffusers.pipelines.stable_diffusion.safety_checker import StableDiffusionSafetyChecker
|
14 |
+
from diffusers.schedulers import DDIMScheduler, LMSDiscreteScheduler, PNDMScheduler
|
15 |
+
from diffusers.utils import deprecate, logging
|
16 |
+
from transformers import CLIPFeatureExtractor, CLIPTextModel, CLIPTokenizer
|
17 |
+
|
18 |
+
|
19 |
+
logger = logging.get_logger(__name__) # pylint: disable=invalid-name
|
20 |
+
|
21 |
+
|
22 |
+
def slerp(t, v0, v1, DOT_THRESHOLD=0.9995):
|
23 |
+
"""helper function to spherically interpolate two arrays v1 v2"""
|
24 |
+
|
25 |
+
if not isinstance(v0, np.ndarray):
|
26 |
+
inputs_are_torch = True
|
27 |
+
input_device = v0.device
|
28 |
+
v0 = v0.cpu().numpy()
|
29 |
+
v1 = v1.cpu().numpy()
|
30 |
+
|
31 |
+
dot = np.sum(v0 * v1 / (np.linalg.norm(v0) * np.linalg.norm(v1)))
|
32 |
+
if np.abs(dot) > DOT_THRESHOLD:
|
33 |
+
v2 = (1 - t) * v0 + t * v1
|
34 |
+
else:
|
35 |
+
theta_0 = np.arccos(dot)
|
36 |
+
sin_theta_0 = np.sin(theta_0)
|
37 |
+
theta_t = theta_0 * t
|
38 |
+
sin_theta_t = np.sin(theta_t)
|
39 |
+
s0 = np.sin(theta_0 - theta_t) / sin_theta_0
|
40 |
+
s1 = sin_theta_t / sin_theta_0
|
41 |
+
v2 = s0 * v0 + s1 * v1
|
42 |
+
|
43 |
+
if inputs_are_torch:
|
44 |
+
v2 = torch.from_numpy(v2).to(input_device)
|
45 |
+
|
46 |
+
return v2
|
47 |
+
|
48 |
+
|
49 |
+
class StableDiffusionWalkPipeline(DiffusionPipeline):
|
50 |
+
r"""
|
51 |
+
Pipeline for text-to-image generation using Stable Diffusion.
|
52 |
+
|
53 |
+
This model inherits from [`DiffusionPipeline`]. Check the superclass documentation for the generic methods the
|
54 |
+
library implements for all the pipelines (such as downloading or saving, running on a particular device, etc.)
|
55 |
+
|
56 |
+
Args:
|
57 |
+
vae ([`AutoencoderKL`]):
|
58 |
+
Variational Auto-Encoder (VAE) Model to encode and decode images to and from latent representations.
|
59 |
+
text_encoder ([`CLIPTextModel`]):
|
60 |
+
Frozen text-encoder. Stable Diffusion uses the text portion of
|
61 |
+
[CLIP](https://huggingface.co/docs/transformers/model_doc/clip#transformers.CLIPTextModel), specifically
|
62 |
+
the [clip-vit-large-patch14](https://huggingface.co/openai/clip-vit-large-patch14) variant.
|
63 |
+
tokenizer (`CLIPTokenizer`):
|
64 |
+
Tokenizer of class
|
65 |
+
[CLIPTokenizer](https://huggingface.co/docs/transformers/v4.21.0/en/model_doc/clip#transformers.CLIPTokenizer).
|
66 |
+
unet ([`UNet2DConditionModel`]): Conditional U-Net architecture to denoise the encoded image latents.
|
67 |
+
scheduler ([`SchedulerMixin`]):
|
68 |
+
A scheduler to be used in combination with `unet` to denoise the encoded image latens. Can be one of
|
69 |
+
[`DDIMScheduler`], [`LMSDiscreteScheduler`], or [`PNDMScheduler`].
|
70 |
+
safety_checker ([`StableDiffusionSafetyChecker`]):
|
71 |
+
Classification module that estimates whether generated images could be considered offensive or harmful.
|
72 |
+
Please, refer to the [model card](https://huggingface.co/CompVis/stable-diffusion-v1-4) for details.
|
73 |
+
feature_extractor ([`CLIPFeatureExtractor`]):
|
74 |
+
Model that extracts features from generated images to be used as inputs for the `safety_checker`.
|
75 |
+
"""
|
76 |
+
|
77 |
+
def __init__(
|
78 |
+
self,
|
79 |
+
vae: AutoencoderKL,
|
80 |
+
text_encoder: CLIPTextModel,
|
81 |
+
tokenizer: CLIPTokenizer,
|
82 |
+
unet: UNet2DConditionModel,
|
83 |
+
scheduler: Union[DDIMScheduler, PNDMScheduler, LMSDiscreteScheduler],
|
84 |
+
safety_checker: StableDiffusionSafetyChecker,
|
85 |
+
feature_extractor: CLIPFeatureExtractor,
|
86 |
+
):
|
87 |
+
super().__init__()
|
88 |
+
|
89 |
+
if hasattr(scheduler.config, "steps_offset") and scheduler.config.steps_offset != 1:
|
90 |
+
deprecation_message = (
|
91 |
+
f"The configuration file of this scheduler: {scheduler} is outdated. `steps_offset`"
|
92 |
+
f" should be set to 1 instead of {scheduler.config.steps_offset}. Please make sure "
|
93 |
+
"to update the config accordingly as leaving `steps_offset` might led to incorrect results"
|
94 |
+
" in future versions. If you have downloaded this checkpoint from the Hugging Face Hub,"
|
95 |
+
" it would be very nice if you could open a Pull request for the `scheduler/scheduler_config.json`"
|
96 |
+
" file"
|
97 |
+
)
|
98 |
+
deprecate("steps_offset!=1", "1.0.0", deprecation_message, standard_warn=False)
|
99 |
+
new_config = dict(scheduler.config)
|
100 |
+
new_config["steps_offset"] = 1
|
101 |
+
scheduler._internal_dict = FrozenDict(new_config)
|
102 |
+
|
103 |
+
if safety_checker is None:
|
104 |
+
logger.warn(
|
105 |
+
f"You have disabled the safety checker for {self.__class__} by passing `safety_checker=None`. Ensure"
|
106 |
+
" that you abide to the conditions of the Stable Diffusion license and do not expose unfiltered"
|
107 |
+
" results in services or applications open to the public. Both the diffusers team and Hugging Face"
|
108 |
+
" strongly recommend to keep the safety filter enabled in all public facing circumstances, disabling"
|
109 |
+
" it only for use-cases that involve analyzing network behavior or auditing its results. For more"
|
110 |
+
" information, please have a look at https://github.com/huggingface/diffusers/pull/254 ."
|
111 |
+
)
|
112 |
+
|
113 |
+
self.register_modules(
|
114 |
+
vae=vae,
|
115 |
+
text_encoder=text_encoder,
|
116 |
+
tokenizer=tokenizer,
|
117 |
+
unet=unet,
|
118 |
+
scheduler=scheduler,
|
119 |
+
safety_checker=safety_checker,
|
120 |
+
feature_extractor=feature_extractor,
|
121 |
+
)
|
122 |
+
|
123 |
+
def enable_attention_slicing(self, slice_size: Optional[Union[str, int]] = "auto"):
|
124 |
+
r"""
|
125 |
+
Enable sliced attention computation.
|
126 |
+
|
127 |
+
When this option is enabled, the attention module will split the input tensor in slices, to compute attention
|
128 |
+
in several steps. This is useful to save some memory in exchange for a small speed decrease.
|
129 |
+
|
130 |
+
Args:
|
131 |
+
slice_size (`str` or `int`, *optional*, defaults to `"auto"`):
|
132 |
+
When `"auto"`, halves the input to the attention heads, so attention will be computed in two steps. If
|
133 |
+
a number is provided, uses as many slices as `attention_head_dim // slice_size`. In this case,
|
134 |
+
`attention_head_dim` must be a multiple of `slice_size`.
|
135 |
+
"""
|
136 |
+
if slice_size == "auto":
|
137 |
+
# half the attention head size is usually a good trade-off between
|
138 |
+
# speed and memory
|
139 |
+
slice_size = self.unet.config.attention_head_dim // 2
|
140 |
+
self.unet.set_attention_slice(slice_size)
|
141 |
+
|
142 |
+
def disable_attention_slicing(self):
|
143 |
+
r"""
|
144 |
+
Disable sliced attention computation. If `enable_attention_slicing` was previously invoked, this method will go
|
145 |
+
back to computing attention in one step.
|
146 |
+
"""
|
147 |
+
# set slice_size = `None` to disable `attention slicing`
|
148 |
+
self.enable_attention_slicing(None)
|
149 |
+
|
150 |
+
@torch.no_grad()
|
151 |
+
def __call__(
|
152 |
+
self,
|
153 |
+
prompt: Optional[Union[str, List[str]]] = None,
|
154 |
+
height: int = 512,
|
155 |
+
width: int = 512,
|
156 |
+
num_inference_steps: int = 50,
|
157 |
+
guidance_scale: float = 7.5,
|
158 |
+
negative_prompt: Optional[Union[str, List[str]]] = None,
|
159 |
+
num_images_per_prompt: Optional[int] = 1,
|
160 |
+
eta: float = 0.0,
|
161 |
+
generator: Optional[torch.Generator] = None,
|
162 |
+
latents: Optional[torch.FloatTensor] = None,
|
163 |
+
output_type: Optional[str] = "pil",
|
164 |
+
return_dict: bool = True,
|
165 |
+
callback: Optional[Callable[[int, int, torch.FloatTensor], None]] = None,
|
166 |
+
callback_steps: Optional[int] = 1,
|
167 |
+
text_embeddings: Optional[torch.FloatTensor] = None,
|
168 |
+
**kwargs,
|
169 |
+
):
|
170 |
+
r"""
|
171 |
+
Function invoked when calling the pipeline for generation.
|
172 |
+
|
173 |
+
Args:
|
174 |
+
prompt (`str` or `List[str]`, *optional*, defaults to `None`):
|
175 |
+
The prompt or prompts to guide the image generation. If not provided, `text_embeddings` is required.
|
176 |
+
height (`int`, *optional*, defaults to 512):
|
177 |
+
The height in pixels of the generated image.
|
178 |
+
width (`int`, *optional*, defaults to 512):
|
179 |
+
The width in pixels of the generated image.
|
180 |
+
num_inference_steps (`int`, *optional*, defaults to 50):
|
181 |
+
The number of denoising steps. More denoising steps usually lead to a higher quality image at the
|
182 |
+
expense of slower inference.
|
183 |
+
guidance_scale (`float`, *optional*, defaults to 7.5):
|
184 |
+
Guidance scale as defined in [Classifier-Free Diffusion Guidance](https://arxiv.org/abs/2207.12598).
|
185 |
+
`guidance_scale` is defined as `w` of equation 2. of [Imagen
|
186 |
+
Paper](https://arxiv.org/pdf/2205.11487.pdf). Guidance scale is enabled by setting `guidance_scale >
|
187 |
+
1`. Higher guidance scale encourages to generate images that are closely linked to the text `prompt`,
|
188 |
+
usually at the expense of lower image quality.
|
189 |
+
negative_prompt (`str` or `List[str]`, *optional*):
|
190 |
+
The prompt or prompts not to guide the image generation. Ignored when not using guidance (i.e., ignored
|
191 |
+
if `guidance_scale` is less than `1`).
|
192 |
+
num_images_per_prompt (`int`, *optional*, defaults to 1):
|
193 |
+
The number of images to generate per prompt.
|
194 |
+
eta (`float`, *optional*, defaults to 0.0):
|
195 |
+
Corresponds to parameter eta (η) in the DDIM paper: https://arxiv.org/abs/2010.02502. Only applies to
|
196 |
+
[`schedulers.DDIMScheduler`], will be ignored for others.
|
197 |
+
generator (`torch.Generator`, *optional*):
|
198 |
+
A [torch generator](https://pytorch.org/docs/stable/generated/torch.Generator.html) to make generation
|
199 |
+
deterministic.
|
200 |
+
latents (`torch.FloatTensor`, *optional*):
|
201 |
+
Pre-generated noisy latents, sampled from a Gaussian distribution, to be used as inputs for image
|
202 |
+
generation. Can be used to tweak the same generation with different prompts. If not provided, a latents
|
203 |
+
tensor will ge generated by sampling using the supplied random `generator`.
|
204 |
+
output_type (`str`, *optional*, defaults to `"pil"`):
|
205 |
+
The output format of the generate image. Choose between
|
206 |
+
[PIL](https://pillow.readthedocs.io/en/stable/): `PIL.Image.Image` or `np.array`.
|
207 |
+
return_dict (`bool`, *optional*, defaults to `True`):
|
208 |
+
Whether or not to return a [`~pipelines.stable_diffusion.StableDiffusionPipelineOutput`] instead of a
|
209 |
+
plain tuple.
|
210 |
+
callback (`Callable`, *optional*):
|
211 |
+
A function that will be called every `callback_steps` steps during inference. The function will be
|
212 |
+
called with the following arguments: `callback(step: int, timestep: int, latents: torch.FloatTensor)`.
|
213 |
+
callback_steps (`int`, *optional*, defaults to 1):
|
214 |
+
The frequency at which the `callback` function will be called. If not specified, the callback will be
|
215 |
+
called at every step.
|
216 |
+
text_embeddings (`torch.FloatTensor`, *optional*, defaults to `None`):
|
217 |
+
Pre-generated text embeddings to be used as inputs for image generation. Can be used in place of
|
218 |
+
`prompt` to avoid re-computing the embeddings. If not provided, the embeddings will be generated from
|
219 |
+
the supplied `prompt`.
|
220 |
+
|
221 |
+
Returns:
|
222 |
+
[`~pipelines.stable_diffusion.StableDiffusionPipelineOutput`] or `tuple`:
|
223 |
+
[`~pipelines.stable_diffusion.StableDiffusionPipelineOutput`] if `return_dict` is True, otherwise a `tuple.
|
224 |
+
When returning a tuple, the first element is a list with the generated images, and the second element is a
|
225 |
+
list of `bool`s denoting whether the corresponding generated image likely represents "not-safe-for-work"
|
226 |
+
(nsfw) content, according to the `safety_checker`.
|
227 |
+
"""
|
228 |
+
|
229 |
+
if height % 8 != 0 or width % 8 != 0:
|
230 |
+
raise ValueError(f"`height` and `width` have to be divisible by 8 but are {height} and {width}.")
|
231 |
+
|
232 |
+
if (callback_steps is None) or (
|
233 |
+
callback_steps is not None and (not isinstance(callback_steps, int) or callback_steps <= 0)
|
234 |
+
):
|
235 |
+
raise ValueError(
|
236 |
+
f"`callback_steps` has to be a positive integer but is {callback_steps} of type"
|
237 |
+
f" {type(callback_steps)}."
|
238 |
+
)
|
239 |
+
|
240 |
+
if text_embeddings is None:
|
241 |
+
if isinstance(prompt, str):
|
242 |
+
batch_size = 1
|
243 |
+
elif isinstance(prompt, list):
|
244 |
+
batch_size = len(prompt)
|
245 |
+
else:
|
246 |
+
raise ValueError(f"`prompt` has to be of type `str` or `list` but is {type(prompt)}")
|
247 |
+
|
248 |
+
# get prompt text embeddings
|
249 |
+
text_inputs = self.tokenizer(
|
250 |
+
prompt,
|
251 |
+
padding="max_length",
|
252 |
+
max_length=self.tokenizer.model_max_length,
|
253 |
+
return_tensors="pt",
|
254 |
+
)
|
255 |
+
text_input_ids = text_inputs.input_ids
|
256 |
+
|
257 |
+
if text_input_ids.shape[-1] > self.tokenizer.model_max_length:
|
258 |
+
removed_text = self.tokenizer.batch_decode(text_input_ids[:, self.tokenizer.model_max_length :])
|
259 |
+
print(
|
260 |
+
"The following part of your input was truncated because CLIP can only handle sequences up to"
|
261 |
+
f" {self.tokenizer.model_max_length} tokens: {removed_text}"
|
262 |
+
)
|
263 |
+
text_input_ids = text_input_ids[:, : self.tokenizer.model_max_length]
|
264 |
+
text_embeddings = self.text_encoder(text_input_ids.to(self.device))[0]
|
265 |
+
else:
|
266 |
+
batch_size = text_embeddings.shape[0]
|
267 |
+
|
268 |
+
# duplicate text embeddings for each generation per prompt, using mps friendly method
|
269 |
+
bs_embed, seq_len, _ = text_embeddings.shape
|
270 |
+
text_embeddings = text_embeddings.repeat(1, num_images_per_prompt, 1)
|
271 |
+
text_embeddings = text_embeddings.view(bs_embed * num_images_per_prompt, seq_len, -1)
|
272 |
+
|
273 |
+
# here `guidance_scale` is defined analog to the guidance weight `w` of equation (2)
|
274 |
+
# of the Imagen paper: https://arxiv.org/pdf/2205.11487.pdf . `guidance_scale = 1`
|
275 |
+
# corresponds to doing no classifier free guidance.
|
276 |
+
do_classifier_free_guidance = guidance_scale > 1.0
|
277 |
+
# get unconditional embeddings for classifier free guidance
|
278 |
+
if do_classifier_free_guidance:
|
279 |
+
uncond_tokens: List[str]
|
280 |
+
if negative_prompt is None:
|
281 |
+
uncond_tokens = [""]
|
282 |
+
elif type(prompt) is not type(negative_prompt):
|
283 |
+
raise TypeError(
|
284 |
+
f"`negative_prompt` should be the same type to `prompt`, but got {type(negative_prompt)} !="
|
285 |
+
f" {type(prompt)}."
|
286 |
+
)
|
287 |
+
elif isinstance(negative_prompt, str):
|
288 |
+
uncond_tokens = [negative_prompt]
|
289 |
+
elif batch_size != len(negative_prompt):
|
290 |
+
raise ValueError(
|
291 |
+
f"`negative_prompt`: {negative_prompt} has batch size {len(negative_prompt)}, but `prompt`:"
|
292 |
+
f" {prompt} has batch size {batch_size}. Please make sure that passed `negative_prompt` matches"
|
293 |
+
" the batch size of `prompt`."
|
294 |
+
)
|
295 |
+
else:
|
296 |
+
uncond_tokens = negative_prompt
|
297 |
+
|
298 |
+
max_length = self.tokenizer.model_max_length
|
299 |
+
uncond_input = self.tokenizer(
|
300 |
+
uncond_tokens,
|
301 |
+
padding="max_length",
|
302 |
+
max_length=max_length,
|
303 |
+
truncation=True,
|
304 |
+
return_tensors="pt",
|
305 |
+
)
|
306 |
+
uncond_embeddings = self.text_encoder(uncond_input.input_ids.to(self.device))[0]
|
307 |
+
|
308 |
+
# duplicate unconditional embeddings for each generation per prompt, using mps friendly method
|
309 |
+
seq_len = uncond_embeddings.shape[1]
|
310 |
+
uncond_embeddings = uncond_embeddings.repeat(batch_size, num_images_per_prompt, 1)
|
311 |
+
uncond_embeddings = uncond_embeddings.view(batch_size * num_images_per_prompt, seq_len, -1)
|
312 |
+
|
313 |
+
# For classifier free guidance, we need to do two forward passes.
|
314 |
+
# Here we concatenate the unconditional and text embeddings into a single batch
|
315 |
+
# to avoid doing two forward passes
|
316 |
+
text_embeddings = torch.cat([uncond_embeddings, text_embeddings])
|
317 |
+
|
318 |
+
# get the initial random noise unless the user supplied it
|
319 |
+
|
320 |
+
# Unlike in other pipelines, latents need to be generated in the target device
|
321 |
+
# for 1-to-1 results reproducibility with the CompVis implementation.
|
322 |
+
# However this currently doesn't work in `mps`.
|
323 |
+
latents_shape = (batch_size * num_images_per_prompt, self.unet.in_channels, height // 8, width // 8)
|
324 |
+
latents_dtype = text_embeddings.dtype
|
325 |
+
if latents is None:
|
326 |
+
if self.device.type == "mps":
|
327 |
+
# randn does not exist on mps
|
328 |
+
latents = torch.randn(latents_shape, generator=generator, device="cpu", dtype=latents_dtype).to(
|
329 |
+
self.device
|
330 |
+
)
|
331 |
+
else:
|
332 |
+
latents = torch.randn(latents_shape, generator=generator, device=self.device, dtype=latents_dtype)
|
333 |
+
else:
|
334 |
+
if latents.shape != latents_shape:
|
335 |
+
raise ValueError(f"Unexpected latents shape, got {latents.shape}, expected {latents_shape}")
|
336 |
+
latents = latents.to(self.device)
|
337 |
+
|
338 |
+
# set timesteps
|
339 |
+
self.scheduler.set_timesteps(num_inference_steps)
|
340 |
+
|
341 |
+
# Some schedulers like PNDM have timesteps as arrays
|
342 |
+
# It's more optimized to move all timesteps to correct device beforehand
|
343 |
+
timesteps_tensor = self.scheduler.timesteps.to(self.device)
|
344 |
+
|
345 |
+
# scale the initial noise by the standard deviation required by the scheduler
|
346 |
+
latents = latents * self.scheduler.init_noise_sigma
|
347 |
+
|
348 |
+
# prepare extra kwargs for the scheduler step, since not all schedulers have the same signature
|
349 |
+
# eta (η) is only used with the DDIMScheduler, it will be ignored for other schedulers.
|
350 |
+
# eta corresponds to η in DDIM paper: https://arxiv.org/abs/2010.02502
|
351 |
+
# and should be between [0, 1]
|
352 |
+
accepts_eta = "eta" in set(inspect.signature(self.scheduler.step).parameters.keys())
|
353 |
+
extra_step_kwargs = {}
|
354 |
+
if accepts_eta:
|
355 |
+
extra_step_kwargs["eta"] = eta
|
356 |
+
|
357 |
+
for i, t in enumerate(self.progress_bar(timesteps_tensor)):
|
358 |
+
# expand the latents if we are doing classifier free guidance
|
359 |
+
latent_model_input = torch.cat([latents] * 2) if do_classifier_free_guidance else latents
|
360 |
+
latent_model_input = self.scheduler.scale_model_input(latent_model_input, t)
|
361 |
+
|
362 |
+
# predict the noise residual
|
363 |
+
noise_pred = self.unet(latent_model_input, t, encoder_hidden_states=text_embeddings).sample
|
364 |
+
|
365 |
+
# perform guidance
|
366 |
+
if do_classifier_free_guidance:
|
367 |
+
noise_pred_uncond, noise_pred_text = noise_pred.chunk(2)
|
368 |
+
noise_pred = noise_pred_uncond + guidance_scale * (noise_pred_text - noise_pred_uncond)
|
369 |
+
|
370 |
+
# compute the previous noisy sample x_t -> x_t-1
|
371 |
+
latents = self.scheduler.step(noise_pred, t, latents, **extra_step_kwargs).prev_sample
|
372 |
+
|
373 |
+
# call the callback, if provided
|
374 |
+
if callback is not None and i % callback_steps == 0:
|
375 |
+
callback(i, t, latents)
|
376 |
+
|
377 |
+
latents = 1 / 0.18215 * latents
|
378 |
+
image = self.vae.decode(latents).sample
|
379 |
+
|
380 |
+
image = (image / 2 + 0.5).clamp(0, 1)
|
381 |
+
|
382 |
+
# we always cast to float32 as this does not cause significant overhead and is compatible with bfloa16
|
383 |
+
image = image.cpu().permute(0, 2, 3, 1).float().numpy()
|
384 |
+
|
385 |
+
if self.safety_checker is not None:
|
386 |
+
safety_checker_input = self.feature_extractor(self.numpy_to_pil(image), return_tensors="pt").to(
|
387 |
+
self.device
|
388 |
+
)
|
389 |
+
image, has_nsfw_concept = self.safety_checker(
|
390 |
+
images=image, clip_input=safety_checker_input.pixel_values.to(text_embeddings.dtype)
|
391 |
+
)
|
392 |
+
else:
|
393 |
+
has_nsfw_concept = None
|
394 |
+
|
395 |
+
if output_type == "pil":
|
396 |
+
image = self.numpy_to_pil(image)
|
397 |
+
|
398 |
+
if not return_dict:
|
399 |
+
return (image, has_nsfw_concept)
|
400 |
+
|
401 |
+
return StableDiffusionPipelineOutput(images=image, nsfw_content_detected=has_nsfw_concept)
|
402 |
+
|
403 |
+
def embed_text(self, text):
|
404 |
+
"""takes in text and turns it into text embeddings"""
|
405 |
+
text_input = self.tokenizer(
|
406 |
+
text,
|
407 |
+
padding="max_length",
|
408 |
+
max_length=self.tokenizer.model_max_length,
|
409 |
+
truncation=True,
|
410 |
+
return_tensors="pt",
|
411 |
+
)
|
412 |
+
with torch.no_grad():
|
413 |
+
embed = self.text_encoder(text_input.input_ids.to(self.device))[0]
|
414 |
+
return embed
|
415 |
+
|
416 |
+
def get_noise(self, seed, dtype=torch.float32, height=512, width=512):
|
417 |
+
"""Takes in random seed and returns corresponding noise vector"""
|
418 |
+
return torch.randn(
|
419 |
+
(1, self.unet.in_channels, height // 8, width // 8),
|
420 |
+
generator=torch.Generator(device=self.device).manual_seed(seed),
|
421 |
+
device=self.device,
|
422 |
+
dtype=dtype,
|
423 |
+
)
|
424 |
+
|
425 |
+
def walk(
|
426 |
+
self,
|
427 |
+
prompts: List[str],
|
428 |
+
seeds: List[int],
|
429 |
+
num_interpolation_steps: Optional[int] = 6,
|
430 |
+
output_dir: Optional[str] = "./dreams",
|
431 |
+
name: Optional[str] = None,
|
432 |
+
batch_size: Optional[int] = 1,
|
433 |
+
height: Optional[int] = 512,
|
434 |
+
width: Optional[int] = 512,
|
435 |
+
guidance_scale: Optional[float] = 7.5,
|
436 |
+
num_inference_steps: Optional[int] = 50,
|
437 |
+
eta: Optional[float] = 0.0,
|
438 |
+
) -> List[str]:
|
439 |
+
"""
|
440 |
+
Walks through a series of prompts and seeds, interpolating between them and saving the results to disk.
|
441 |
+
|
442 |
+
Args:
|
443 |
+
prompts (`List[str]`):
|
444 |
+
List of prompts to generate images for.
|
445 |
+
seeds (`List[int]`):
|
446 |
+
List of seeds corresponding to provided prompts. Must be the same length as prompts.
|
447 |
+
num_interpolation_steps (`int`, *optional*, defaults to 6):
|
448 |
+
Number of interpolation steps to take between prompts.
|
449 |
+
output_dir (`str`, *optional*, defaults to `./dreams`):
|
450 |
+
Directory to save the generated images to.
|
451 |
+
name (`str`, *optional*, defaults to `None`):
|
452 |
+
Subdirectory of `output_dir` to save the generated images to. If `None`, the name will
|
453 |
+
be the current time.
|
454 |
+
batch_size (`int`, *optional*, defaults to 1):
|
455 |
+
Number of images to generate at once.
|
456 |
+
height (`int`, *optional*, defaults to 512):
|
457 |
+
Height of the generated images.
|
458 |
+
width (`int`, *optional*, defaults to 512):
|
459 |
+
Width of the generated images.
|
460 |
+
guidance_scale (`float`, *optional*, defaults to 7.5):
|
461 |
+
Guidance scale as defined in [Classifier-Free Diffusion Guidance](https://arxiv.org/abs/2207.12598).
|
462 |
+
`guidance_scale` is defined as `w` of equation 2. of [Imagen
|
463 |
+
Paper](https://arxiv.org/pdf/2205.11487.pdf). Guidance scale is enabled by setting `guidance_scale >
|
464 |
+
1`. Higher guidance scale encourages to generate images that are closely linked to the text `prompt`,
|
465 |
+
usually at the expense of lower image quality.
|
466 |
+
num_inference_steps (`int`, *optional*, defaults to 50):
|
467 |
+
The number of denoising steps. More denoising steps usually lead to a higher quality image at the
|
468 |
+
expense of slower inference.
|
469 |
+
eta (`float`, *optional*, defaults to 0.0):
|
470 |
+
Corresponds to parameter eta (η) in the DDIM paper: https://arxiv.org/abs/2010.02502. Only applies to
|
471 |
+
[`schedulers.DDIMScheduler`], will be ignored for others.
|
472 |
+
|
473 |
+
Returns:
|
474 |
+
`List[str]`: List of paths to the generated images.
|
475 |
+
"""
|
476 |
+
if not len(prompts) == len(seeds):
|
477 |
+
raise ValueError(
|
478 |
+
f"Number of prompts and seeds must be equalGot {len(prompts)} prompts and {len(seeds)} seeds"
|
479 |
+
)
|
480 |
+
|
481 |
+
name = name or time.strftime("%Y%m%d-%H%M%S")
|
482 |
+
save_path = Path(output_dir) / name
|
483 |
+
save_path.mkdir(exist_ok=True, parents=True)
|
484 |
+
|
485 |
+
frame_idx = 0
|
486 |
+
frame_filepaths = []
|
487 |
+
for prompt_a, prompt_b, seed_a, seed_b in zip(prompts, prompts[1:], seeds, seeds[1:]):
|
488 |
+
# Embed Text
|
489 |
+
embed_a = self.embed_text(prompt_a)
|
490 |
+
embed_b = self.embed_text(prompt_b)
|
491 |
+
|
492 |
+
# Get Noise
|
493 |
+
noise_dtype = embed_a.dtype
|
494 |
+
noise_a = self.get_noise(seed_a, noise_dtype, height, width)
|
495 |
+
noise_b = self.get_noise(seed_b, noise_dtype, height, width)
|
496 |
+
|
497 |
+
noise_batch, embeds_batch = None, None
|
498 |
+
T = np.linspace(0.0, 1.0, num_interpolation_steps)
|
499 |
+
for i, t in enumerate(T):
|
500 |
+
noise = slerp(float(t), noise_a, noise_b)
|
501 |
+
embed = torch.lerp(embed_a, embed_b, t)
|
502 |
+
|
503 |
+
noise_batch = noise if noise_batch is None else torch.cat([noise_batch, noise], dim=0)
|
504 |
+
embeds_batch = embed if embeds_batch is None else torch.cat([embeds_batch, embed], dim=0)
|
505 |
+
|
506 |
+
batch_is_ready = embeds_batch.shape[0] == batch_size or i + 1 == T.shape[0]
|
507 |
+
if batch_is_ready:
|
508 |
+
outputs = self(
|
509 |
+
latents=noise_batch,
|
510 |
+
text_embeddings=embeds_batch,
|
511 |
+
height=height,
|
512 |
+
width=width,
|
513 |
+
guidance_scale=guidance_scale,
|
514 |
+
eta=eta,
|
515 |
+
num_inference_steps=num_inference_steps,
|
516 |
+
)
|
517 |
+
noise_batch, embeds_batch = None, None
|
518 |
+
|
519 |
+
for image in outputs["images"]:
|
520 |
+
frame_filepath = str(save_path / f"frame_{frame_idx:06d}.png")
|
521 |
+
image.save(frame_filepath)
|
522 |
+
frame_filepaths.append(frame_filepath)
|
523 |
+
frame_idx += 1
|
524 |
+
return frame_filepaths
|
v0.6.0/one_step_unet.py
ADDED
@@ -0,0 +1,22 @@
|
|
|
|
|
|
|
|
|
|
|
|
|
|
|
|
|
|
|
|
|
|
|
|
|
|
|
|
|
|
|
|
|
|
|
|
|
|
|
|
|
|
|
|
|
|
|
1 |
+
#!/usr/bin/env python3
|
2 |
+
import torch
|
3 |
+
|
4 |
+
from diffusers import DiffusionPipeline
|
5 |
+
|
6 |
+
|
7 |
+
class UnetSchedulerOneForwardPipeline(DiffusionPipeline):
|
8 |
+
def __init__(self, unet, scheduler):
|
9 |
+
super().__init__()
|
10 |
+
|
11 |
+
self.register_modules(unet=unet, scheduler=scheduler)
|
12 |
+
|
13 |
+
def __call__(self):
|
14 |
+
image = torch.randn(
|
15 |
+
(1, self.unet.in_channels, self.unet.sample_size, self.unet.sample_size),
|
16 |
+
)
|
17 |
+
timestep = 1
|
18 |
+
|
19 |
+
model_output = self.unet(image, timestep).sample
|
20 |
+
scheduler_output = self.scheduler.step(model_output, timestep, image).prev_sample
|
21 |
+
|
22 |
+
return scheduler_output
|
v0.6.0/stable_diffusion_mega.py
ADDED
@@ -0,0 +1,224 @@
|
|
|
|
|
|
|
|
|
|
|
|
|
|
|
|
|
|
|
|
|
|
|
|
|
|
|
|
|
|
|
|
|
|
|
|
|
|
|
|
|
|
|
|
|
|
|
|
|
|
|
|
|
|
|
|
|
|
|
|
|
|
|
|
|
|
|
|
|
|
|
|
|
|
|
|
|
|
|
|
|
|
|
|
|
|
|
|
|
|
|
|
|
|
|
|
|
|
|
|
|
|
|
|
|
|
|
|
|
|
|
|
|
|
|
|
|
|
|
|
|
|
|
|
|
|
|
|
|
|
|
|
|
|
|
|
|
|
|
|
|
|
|
|
|
|
|
|
|
|
|
|
|
|
|
|
|
|
|
|
|
|
|
|
|
|
|
|
|
|
|
|
|
|
|
|
|
|
|
|
|
|
|
|
|
|
|
|
|
|
|
|
|
|
|
|
|
|
|
|
|
|
|
|
|
|
|
|
|
|
|
|
|
|
|
|
|
|
|
|
|
|
|
|
|
|
|
|
|
|
|
|
|
|
|
|
|
|
|
|
|
|
|
|
|
|
|
|
|
|
|
|
|
|
|
|
|
|
|
|
|
|
|
|
|
|
|
|
|
|
|
|
|
|
|
|
|
|
|
|
|
|
|
|
|
|
|
|
|
|
|
|
|
|
|
|
|
|
|
|
|
|
|
|
|
|
|
|
|
|
|
|
|
|
|
|
|
|
|
|
|
|
|
|
|
|
|
|
|
|
|
|
|
|
|
|
|
|
|
|
|
|
|
|
|
|
|
|
|
|
|
|
|
|
|
|
|
|
|
|
|
|
|
|
|
|
|
|
|
|
|
|
|
|
|
|
|
|
|
|
|
|
|
|
|
|
|
|
|
|
|
|
|
|
|
|
|
|
|
|
|
|
|
|
|
|
|
|
|
|
|
|
|
|
|
|
|
|
|
|
|
|
|
|
|
|
|
|
|
|
|
|
|
|
|
|
|
|
|
|
|
|
|
|
|
|
|
|
|
|
|
1 |
+
from typing import Any, Callable, Dict, List, Optional, Union
|
2 |
+
|
3 |
+
import torch
|
4 |
+
|
5 |
+
import PIL.Image
|
6 |
+
from diffusers import (
|
7 |
+
AutoencoderKL,
|
8 |
+
DDIMScheduler,
|
9 |
+
DiffusionPipeline,
|
10 |
+
LMSDiscreteScheduler,
|
11 |
+
PNDMScheduler,
|
12 |
+
StableDiffusionImg2ImgPipeline,
|
13 |
+
StableDiffusionInpaintPipelineLegacy,
|
14 |
+
StableDiffusionPipeline,
|
15 |
+
UNet2DConditionModel,
|
16 |
+
)
|
17 |
+
from diffusers.configuration_utils import FrozenDict
|
18 |
+
from diffusers.pipelines.stable_diffusion.safety_checker import StableDiffusionSafetyChecker
|
19 |
+
from diffusers.utils import deprecate, logging
|
20 |
+
from transformers import CLIPFeatureExtractor, CLIPTextModel, CLIPTokenizer
|
21 |
+
|
22 |
+
|
23 |
+
logger = logging.get_logger(__name__) # pylint: disable=invalid-name
|
24 |
+
|
25 |
+
|
26 |
+
class StableDiffusionMegaPipeline(DiffusionPipeline):
|
27 |
+
r"""
|
28 |
+
Pipeline for text-to-image generation using Stable Diffusion.
|
29 |
+
|
30 |
+
This model inherits from [`DiffusionPipeline`]. Check the superclass documentation for the generic methods the
|
31 |
+
library implements for all the pipelines (such as downloading or saving, running on a particular device, etc.)
|
32 |
+
|
33 |
+
Args:
|
34 |
+
vae ([`AutoencoderKL`]):
|
35 |
+
Variational Auto-Encoder (VAE) Model to encode and decode images to and from latent representations.
|
36 |
+
text_encoder ([`CLIPTextModel`]):
|
37 |
+
Frozen text-encoder. Stable Diffusion uses the text portion of
|
38 |
+
[CLIP](https://huggingface.co/docs/transformers/model_doc/clip#transformers.CLIPTextModel), specifically
|
39 |
+
the [clip-vit-large-patch14](https://huggingface.co/openai/clip-vit-large-patch14) variant.
|
40 |
+
tokenizer (`CLIPTokenizer`):
|
41 |
+
Tokenizer of class
|
42 |
+
[CLIPTokenizer](https://huggingface.co/docs/transformers/v4.21.0/en/model_doc/clip#transformers.CLIPTokenizer).
|
43 |
+
unet ([`UNet2DConditionModel`]): Conditional U-Net architecture to denoise the encoded image latents.
|
44 |
+
scheduler ([`SchedulerMixin`]):
|
45 |
+
A scheduler to be used in combination with `unet` to denoise the encoded image latens. Can be one of
|
46 |
+
[`DDIMScheduler`], [`LMSDiscreteScheduler`], or [`PNDMScheduler`].
|
47 |
+
safety_checker ([`StableDiffusionMegaSafetyChecker`]):
|
48 |
+
Classification module that estimates whether generated images could be considered offensive or harmful.
|
49 |
+
Please, refer to the [model card](https://huggingface.co/CompVis/stable-diffusion-v1-4) for details.
|
50 |
+
feature_extractor ([`CLIPFeatureExtractor`]):
|
51 |
+
Model that extracts features from generated images to be used as inputs for the `safety_checker`.
|
52 |
+
"""
|
53 |
+
|
54 |
+
def __init__(
|
55 |
+
self,
|
56 |
+
vae: AutoencoderKL,
|
57 |
+
text_encoder: CLIPTextModel,
|
58 |
+
tokenizer: CLIPTokenizer,
|
59 |
+
unet: UNet2DConditionModel,
|
60 |
+
scheduler: Union[DDIMScheduler, PNDMScheduler, LMSDiscreteScheduler],
|
61 |
+
safety_checker: StableDiffusionSafetyChecker,
|
62 |
+
feature_extractor: CLIPFeatureExtractor,
|
63 |
+
):
|
64 |
+
super().__init__()
|
65 |
+
if hasattr(scheduler.config, "steps_offset") and scheduler.config.steps_offset != 1:
|
66 |
+
deprecation_message = (
|
67 |
+
f"The configuration file of this scheduler: {scheduler} is outdated. `steps_offset`"
|
68 |
+
f" should be set to 1 instead of {scheduler.config.steps_offset}. Please make sure "
|
69 |
+
"to update the config accordingly as leaving `steps_offset` might led to incorrect results"
|
70 |
+
" in future versions. If you have downloaded this checkpoint from the Hugging Face Hub,"
|
71 |
+
" it would be very nice if you could open a Pull request for the `scheduler/scheduler_config.json`"
|
72 |
+
" file"
|
73 |
+
)
|
74 |
+
deprecate("steps_offset!=1", "1.0.0", deprecation_message, standard_warn=False)
|
75 |
+
new_config = dict(scheduler.config)
|
76 |
+
new_config["steps_offset"] = 1
|
77 |
+
scheduler._internal_dict = FrozenDict(new_config)
|
78 |
+
|
79 |
+
self.register_modules(
|
80 |
+
vae=vae,
|
81 |
+
text_encoder=text_encoder,
|
82 |
+
tokenizer=tokenizer,
|
83 |
+
unet=unet,
|
84 |
+
scheduler=scheduler,
|
85 |
+
safety_checker=safety_checker,
|
86 |
+
feature_extractor=feature_extractor,
|
87 |
+
)
|
88 |
+
|
89 |
+
@property
|
90 |
+
def components(self) -> Dict[str, Any]:
|
91 |
+
return {k: getattr(self, k) for k in self.config.keys() if not k.startswith("_")}
|
92 |
+
|
93 |
+
def enable_attention_slicing(self, slice_size: Optional[Union[str, int]] = "auto"):
|
94 |
+
r"""
|
95 |
+
Enable sliced attention computation.
|
96 |
+
|
97 |
+
When this option is enabled, the attention module will split the input tensor in slices, to compute attention
|
98 |
+
in several steps. This is useful to save some memory in exchange for a small speed decrease.
|
99 |
+
|
100 |
+
Args:
|
101 |
+
slice_size (`str` or `int`, *optional*, defaults to `"auto"`):
|
102 |
+
When `"auto"`, halves the input to the attention heads, so attention will be computed in two steps. If
|
103 |
+
a number is provided, uses as many slices as `attention_head_dim // slice_size`. In this case,
|
104 |
+
`attention_head_dim` must be a multiple of `slice_size`.
|
105 |
+
"""
|
106 |
+
if slice_size == "auto":
|
107 |
+
# half the attention head size is usually a good trade-off between
|
108 |
+
# speed and memory
|
109 |
+
slice_size = self.unet.config.attention_head_dim // 2
|
110 |
+
self.unet.set_attention_slice(slice_size)
|
111 |
+
|
112 |
+
def disable_attention_slicing(self):
|
113 |
+
r"""
|
114 |
+
Disable sliced attention computation. If `enable_attention_slicing` was previously invoked, this method will go
|
115 |
+
back to computing attention in one step.
|
116 |
+
"""
|
117 |
+
# set slice_size = `None` to disable `attention slicing`
|
118 |
+
self.enable_attention_slicing(None)
|
119 |
+
|
120 |
+
@torch.no_grad()
|
121 |
+
def inpaint(
|
122 |
+
self,
|
123 |
+
prompt: Union[str, List[str]],
|
124 |
+
init_image: Union[torch.FloatTensor, PIL.Image.Image],
|
125 |
+
mask_image: Union[torch.FloatTensor, PIL.Image.Image],
|
126 |
+
strength: float = 0.8,
|
127 |
+
num_inference_steps: Optional[int] = 50,
|
128 |
+
guidance_scale: Optional[float] = 7.5,
|
129 |
+
negative_prompt: Optional[Union[str, List[str]]] = None,
|
130 |
+
num_images_per_prompt: Optional[int] = 1,
|
131 |
+
eta: Optional[float] = 0.0,
|
132 |
+
generator: Optional[torch.Generator] = None,
|
133 |
+
output_type: Optional[str] = "pil",
|
134 |
+
return_dict: bool = True,
|
135 |
+
callback: Optional[Callable[[int, int, torch.FloatTensor], None]] = None,
|
136 |
+
callback_steps: Optional[int] = 1,
|
137 |
+
):
|
138 |
+
# For more information on how this function works, please see: https://huggingface.co/docs/diffusers/api/pipelines/stable_diffusion#diffusers.StableDiffusionImg2ImgPipeline
|
139 |
+
return StableDiffusionInpaintPipelineLegacy(**self.components)(
|
140 |
+
prompt=prompt,
|
141 |
+
init_image=init_image,
|
142 |
+
mask_image=mask_image,
|
143 |
+
strength=strength,
|
144 |
+
num_inference_steps=num_inference_steps,
|
145 |
+
guidance_scale=guidance_scale,
|
146 |
+
negative_prompt=negative_prompt,
|
147 |
+
num_images_per_prompt=num_images_per_prompt,
|
148 |
+
eta=eta,
|
149 |
+
generator=generator,
|
150 |
+
output_type=output_type,
|
151 |
+
return_dict=return_dict,
|
152 |
+
callback=callback,
|
153 |
+
)
|
154 |
+
|
155 |
+
@torch.no_grad()
|
156 |
+
def img2img(
|
157 |
+
self,
|
158 |
+
prompt: Union[str, List[str]],
|
159 |
+
init_image: Union[torch.FloatTensor, PIL.Image.Image],
|
160 |
+
strength: float = 0.8,
|
161 |
+
num_inference_steps: Optional[int] = 50,
|
162 |
+
guidance_scale: Optional[float] = 7.5,
|
163 |
+
negative_prompt: Optional[Union[str, List[str]]] = None,
|
164 |
+
num_images_per_prompt: Optional[int] = 1,
|
165 |
+
eta: Optional[float] = 0.0,
|
166 |
+
generator: Optional[torch.Generator] = None,
|
167 |
+
output_type: Optional[str] = "pil",
|
168 |
+
return_dict: bool = True,
|
169 |
+
callback: Optional[Callable[[int, int, torch.FloatTensor], None]] = None,
|
170 |
+
callback_steps: Optional[int] = 1,
|
171 |
+
**kwargs,
|
172 |
+
):
|
173 |
+
# For more information on how this function works, please see: https://huggingface.co/docs/diffusers/api/pipelines/stable_diffusion#diffusers.StableDiffusionImg2ImgPipeline
|
174 |
+
return StableDiffusionImg2ImgPipeline(**self.components)(
|
175 |
+
prompt=prompt,
|
176 |
+
init_image=init_image,
|
177 |
+
strength=strength,
|
178 |
+
num_inference_steps=num_inference_steps,
|
179 |
+
guidance_scale=guidance_scale,
|
180 |
+
negative_prompt=negative_prompt,
|
181 |
+
num_images_per_prompt=num_images_per_prompt,
|
182 |
+
eta=eta,
|
183 |
+
generator=generator,
|
184 |
+
output_type=output_type,
|
185 |
+
return_dict=return_dict,
|
186 |
+
callback=callback,
|
187 |
+
callback_steps=callback_steps,
|
188 |
+
)
|
189 |
+
|
190 |
+
@torch.no_grad()
|
191 |
+
def text2img(
|
192 |
+
self,
|
193 |
+
prompt: Union[str, List[str]],
|
194 |
+
height: int = 512,
|
195 |
+
width: int = 512,
|
196 |
+
num_inference_steps: int = 50,
|
197 |
+
guidance_scale: float = 7.5,
|
198 |
+
negative_prompt: Optional[Union[str, List[str]]] = None,
|
199 |
+
num_images_per_prompt: Optional[int] = 1,
|
200 |
+
eta: float = 0.0,
|
201 |
+
generator: Optional[torch.Generator] = None,
|
202 |
+
latents: Optional[torch.FloatTensor] = None,
|
203 |
+
output_type: Optional[str] = "pil",
|
204 |
+
return_dict: bool = True,
|
205 |
+
callback: Optional[Callable[[int, int, torch.FloatTensor], None]] = None,
|
206 |
+
callback_steps: Optional[int] = 1,
|
207 |
+
):
|
208 |
+
# For more information on how this function https://huggingface.co/docs/diffusers/api/pipelines/stable_diffusion#diffusers.StableDiffusionPipeline
|
209 |
+
return StableDiffusionPipeline(**self.components)(
|
210 |
+
prompt=prompt,
|
211 |
+
height=height,
|
212 |
+
width=width,
|
213 |
+
num_inference_steps=num_inference_steps,
|
214 |
+
guidance_scale=guidance_scale,
|
215 |
+
negative_prompt=negative_prompt,
|
216 |
+
num_images_per_prompt=num_images_per_prompt,
|
217 |
+
eta=eta,
|
218 |
+
generator=generator,
|
219 |
+
latents=latents,
|
220 |
+
output_type=output_type,
|
221 |
+
return_dict=return_dict,
|
222 |
+
callback=callback,
|
223 |
+
callback_steps=callback_steps,
|
224 |
+
)
|