modelId
stringlengths 4
112
| sha
stringlengths 40
40
| lastModified
stringlengths 24
24
| tags
list | pipeline_tag
stringclasses 29
values | private
bool 1
class | author
stringlengths 2
38
⌀ | config
null | id
stringlengths 4
112
| downloads
float64 0
36.8M
⌀ | likes
float64 0
712
⌀ | library_name
stringclasses 17
values | __index_level_0__
int64 0
38.5k
| readme
stringlengths 0
186k
|
---|---|---|---|---|---|---|---|---|---|---|---|---|---|
LACAI/DialoGPT-large-PFG | 46a5452c74c1e428718a9c7dc6e87846c42f9aa8 | 2022-01-14T05:18:30.000Z | [
"pytorch",
"tensorboard",
"gpt2",
"text-generation",
"transformers"
]
| text-generation | false | LACAI | null | LACAI/DialoGPT-large-PFG | 43 | null | transformers | 6,300 | Base model: [microsoft/DialoGPT-large](https://huggingface.co/microsoft/DialoGPT-large)
Fine tuned for dialogue response generation on the [Persuasion For Good Dataset](https://gitlab.com/ucdavisnlp/persuasionforgood) (Wang et al., 2019)
Three additional special tokens were added during the fine-tuning process:
- <|pad|> padding token
- <|user|> speaker control token to prompt user responses
- <|system|> speaker control token to prompt system responses
The following Dialogues were excluded:
- Those with donation amounts outside of the task range of [$0, $2].
- Those where a donation of 0 was made at the end of the task but a non-zero amount was pledged in the dialogue.
- Those with more than 800 words.
Stats:
- Training set: 519 dialogues
- Validation set: 58 dialogues
- ~20 utterances per dialogue |
liangtaiwan/t5-v1_1-lm100k-small | bb7e8b7bc8cecfd21dbaf6be7b9834b38961b154 | 2021-10-21T09:28:54.000Z | [
"pytorch",
"t5",
"text2text-generation",
"transformers",
"autotrain_compatible"
]
| text2text-generation | false | liangtaiwan | null | liangtaiwan/t5-v1_1-lm100k-small | 43 | 1 | transformers | 6,301 | Entry not found |
mnaylor/bigbird-base-mimic-mortality | eb2f5ed4f3932e9bfd866e63c4bd47e2ed52fa55 | 2021-05-14T15:32:04.000Z | [
"pytorch",
"big_bird",
"text-classification",
"transformers"
]
| text-classification | false | mnaylor | null | mnaylor/bigbird-base-mimic-mortality | 43 | null | transformers | 6,302 | # BigBird for Mortality Prediction
Starting with Google's base BigBird model, we fine-tuned on binary mortality prediction in MIMIC admission notes. This
model seeks to predict whether a certain patient will expire within a given ICU stay, based on the text available upon
admission. Data prepared for this task as described in [this project](https://github.com/bvanaken/clinical-outcome-prediction),
using the simulated admission notes (taken from discharge summaries). This model will be used in an upcoming submission for
IMLH at ICML 2021.
### References
* Van Aken, et al., 2021: [Clinical Outcome Prediction from Admission Notes using Self-Supervised Knowledge Integration](https://www.aclweb.org/anthology/2021.eacl-main.75/)
* Zaheer, et al., 2020: [Big Bird: Transformers for Longer Sequences](https://papers.nips.cc/paper/2020/hash/c8512d142a2d849725f31a9a7a361ab9-Abstract.html) |
pere/norwegian-gptneo-blue | b3c5b7a2787debcf0a0aeda5885c637f33a689da | 2021-09-25T18:42:49.000Z | [
"pytorch",
"jax",
"tensorboard",
"gpt_neo",
"text-generation",
"transformers"
]
| text-generation | false | pere | null | pere/norwegian-gptneo-blue | 43 | null | transformers | 6,303 | # Norwegian GTPNeo Blue.
The first Norwegian GPTNeo model. This one is trained only on a administrative corpus. |
persiannlp/mt5-base-parsinlu-snli-entailment | 3ebac7c8f04554b3d8e37e9605e4ee93c5c01b0d | 2021-09-23T16:20:04.000Z | [
"pytorch",
"t5",
"text2text-generation",
"fa",
"multilingual",
"dataset:parsinlu",
"dataset:snli",
"transformers",
"entailment",
"mt5",
"persian",
"farsi",
"license:cc-by-nc-sa-4.0",
"autotrain_compatible"
]
| text2text-generation | false | persiannlp | null | persiannlp/mt5-base-parsinlu-snli-entailment | 43 | null | transformers | 6,304 | ---
language:
- fa
- multilingual
thumbnail: https://upload.wikimedia.org/wikipedia/commons/a/a2/Farsi.svg
tags:
- entailment
- mt5
- persian
- farsi
license: cc-by-nc-sa-4.0
datasets:
- parsinlu
- snli
metrics:
- accuracy
---
# Textual Entailment (مدل برای پاسخ به استلزام منطقی)
This is a model for textual entailment problems.
Here is an example of how you can run this model:
```python
from transformers import MT5ForConditionalGeneration, MT5Tokenizer
model_size="base"
model_name = f"persiannlp/mt5-{model_size}-parsinlu-snli-entailment"
tokenizer = MT5Tokenizer.from_pretrained(model_name)
model = MT5ForConditionalGeneration.from_pretrained(model_name)
def run_model(premise, hypothesis, **generator_args):
input_ids = tokenizer.encode(f"{premise}<sep>{hypothesis}", return_tensors="pt")
res = model.generate(input_ids, **generator_args)
output = tokenizer.batch_decode(res, skip_special_tokens=True)
print(output)
return output
run_model(
"این مسابقات بین آوریل و دسامبر در هیپودروم ولیفندی در نزدیکی باکرکی ، ۱۵ کیلومتری (۹ مایل) غرب استانبول برگزار می شود.",
"در ولیفندی هیپودروم، مسابقاتی از آوریل تا دسامبر وجود دارد."
)
run_model(
"آیا کودکانی وجود دارند که نیاز به سرگرمی دارند؟",
"هیچ کودکی هرگز نمی خواهد سرگرم شود.",
)
run_model(
"ما به سفرهایی رفته ایم که در نهرهایی شنا کرده ایم",
"علاوه بر استحمام در نهرها ، ما به اسپا ها و سونا ها نیز رفته ایم."
)
```
For more details, visit this page: https://github.com/persiannlp/parsinlu/
|
pgilles/wav2vec2-large-xls-r-LUXEMBOURGISH-with-LM | bfe868ff4e83f86e61b4b1aec03edd743725f86e | 2022-05-21T06:06:51.000Z | [
"pytorch",
"wav2vec2",
"automatic-speech-recognition",
"lb",
"ltz",
"dataset:Wav2Vec2-XLS-R-300M",
"transformers",
"ASR",
"Automatic Speech Recognition",
"speech",
"license:mit"
]
| automatic-speech-recognition | false | pgilles | null | pgilles/wav2vec2-large-xls-r-LUXEMBOURGISH-with-LM | 43 | 2 | transformers | 6,305 | ---
language:
- lb
- ltz
#thumbnail: "url to a thumbnail used in social sharing"
tags:
- ASR
- Automatic Speech Recognition
- speech
- wav2vec2
license: "mit"
datasets:
- "Wav2Vec2-XLS-R-300M"
---
Based on `pgilles/wav2vec2-large-xls-r-LUXEMBOURGISH2`, but with a language model (kenLM) attached. |
philschmid/deberta-v3-xsmall-emotion | 05fe5d586994d0c5260f22082df3a451cc910692 | 2021-12-16T12:37:10.000Z | [
"pytorch",
"deberta-v2",
"text-classification",
"dataset:emotion",
"transformers",
"generated_from_trainer",
"license:mit",
"model-index"
]
| text-classification | false | philschmid | null | philschmid/deberta-v3-xsmall-emotion | 43 | null | transformers | 6,306 | ---
license: mit
tags:
- generated_from_trainer
datasets:
- emotion
metrics:
- accuracy
model-index:
- name: deberta-v3-xsmall-emotion
results:
- task:
name: Text Classification
type: text-classification
dataset:
name: emotion
type: emotion
args: default
metrics:
- name: Accuracy
type: accuracy
value: 0.932
---
<!-- This model card has been generated automatically according to the information the Trainer had access to. You
should probably proofread and complete it, then remove this comment. -->
# deberta-v3-xsmall-emotion
This model is a fine-tuned version of [microsoft/deberta-v3-xsmall](https://huggingface.co/microsoft/deberta-v3-xsmall) on the emotion dataset.
It achieves the following results on the evaluation set:
- Loss: 0.1877
- Accuracy: 0.932
## Model description
More information needed
## Intended uses & limitations
More information needed
## Training and evaluation data
More information needed
## Training procedure
### Training hyperparameters
The following hyperparameters were used during training:
- learning_rate: 3e-05
- train_batch_size: 32
- eval_batch_size: 32
- seed: 42
- optimizer: Adam with betas=(0.9,0.999) and epsilon=1e-08
- lr_scheduler_type: linear
- lr_scheduler_warmup_steps: 500
- num_epochs: 5
- mixed_precision_training: Native AMP
### Training results
| Training Loss | Epoch | Step | Validation Loss | Accuracy |
|:-------------:|:-----:|:----:|:---------------:|:--------:|
| 1.3683 | 1.0 | 500 | 0.8479 | 0.6975 |
| 0.547 | 2.0 | 1000 | 0.2881 | 0.905 |
| 0.2378 | 3.0 | 1500 | 0.2116 | 0.925 |
| 0.1704 | 4.0 | 2000 | 0.1877 | 0.932 |
| 0.1392 | 5.0 | 2500 | 0.1718 | 0.9295 |
### Framework versions
- Transformers 4.12.3
- Pytorch 1.9.1
- Datasets 1.15.1
- Tokenizers 0.10.3
|
shiyue/roberta-large-tac08 | 7d2803d4bd14f97ec600f5a05103ada6ff6317bd | 2021-09-10T00:48:57.000Z | [
"pytorch",
"roberta",
"text-classification",
"transformers"
]
| text-classification | false | shiyue | null | shiyue/roberta-large-tac08 | 43 | null | transformers | 6,307 | Entry not found |
sshleifer/student-bart-base-3-3 | 7784495cf212708c19bb8c1356ff4dd3bc42f4c2 | 2021-06-14T08:27:02.000Z | [
"pytorch",
"jax",
"bart",
"text2text-generation",
"transformers",
"autotrain_compatible"
]
| text2text-generation | false | sshleifer | null | sshleifer/student-bart-base-3-3 | 43 | null | transformers | 6,308 | Entry not found |
textattack/facebook-bart-large-CoLA | fabf4bf22ad542d9b8e03737c9d8a1f08422f207 | 2020-06-09T16:49:04.000Z | [
"pytorch",
"bart",
"text-classification",
"transformers"
]
| text-classification | false | textattack | null | textattack/facebook-bart-large-CoLA | 43 | null | transformers | 6,309 | Entry not found |
tuscan-chicken-wrap/semeval2020_task11_si | ccc6a2e72c3ccfb76f9c99b2591b2a4f79e0ddea | 2021-05-20T22:43:34.000Z | [
"pytorch",
"jax",
"roberta",
"fill-mask",
"transformers",
"autotrain_compatible"
]
| fill-mask | false | tuscan-chicken-wrap | null | tuscan-chicken-wrap/semeval2020_task11_si | 43 | null | transformers | 6,310 | Entry not found |
voidful/albert_chinese_xlarge | 86cad9859449049d00b3e3683c609b977a388a72 | 2021-08-03T05:07:18.000Z | [
"pytorch",
"albert",
"fill-mask",
"zh",
"transformers",
"autotrain_compatible"
]
| fill-mask | false | voidful | null | voidful/albert_chinese_xlarge | 43 | null | transformers | 6,311 | ---
language: zh
pipeline_tag: fill-mask
widget:
- text: "今天[MASK]情很好"
---
# albert_chinese_xlarge
This a albert_chinese_xlarge model from [Google's github](https://github.com/google-research/ALBERT)
converted by huggingface's [script](https://github.com/huggingface/transformers/blob/master/src/transformers/convert_albert_original_tf_checkpoint_to_pytorch.py)
## Notice
*Support AutoTokenizer*
Since sentencepiece is not used in albert_chinese_base model
you have to call BertTokenizer instead of AlbertTokenizer !!!
we can eval it using an example on MaskedLM
由於 albert_chinese_base 模型沒有用 sentencepiece
用AlbertTokenizer會載不進詞表,因此需要改用BertTokenizer !!!
我們可以跑MaskedLM預測來驗證這個做法是否正確
## Justify (驗證有效性)
```python
from transformers import AutoTokenizer, AlbertForMaskedLM
import torch
from torch.nn.functional import softmax
pretrained = 'voidful/albert_chinese_xlarge'
tokenizer = AutoTokenizer.from_pretrained(pretrained)
model = AlbertForMaskedLM.from_pretrained(pretrained)
inputtext = "今天[MASK]情很好"
maskpos = tokenizer.encode(inputtext, add_special_tokens=True).index(103)
input_ids = torch.tensor(tokenizer.encode(inputtext, add_special_tokens=True)).unsqueeze(0) # Batch size 1
outputs = model(input_ids, labels=input_ids)
loss, prediction_scores = outputs[:2]
logit_prob = softmax(prediction_scores[0, maskpos],dim=-1).data.tolist()
predicted_index = torch.argmax(prediction_scores[0, maskpos]).item()
predicted_token = tokenizer.convert_ids_to_tokens([predicted_index])[0]
print(predicted_token, logit_prob[predicted_index])
```
Result: `心 0.9942440390586853`
|
Helsinki-NLP/opus-mt-tc-big-zlw-en | 46e1b8d81343043173f17ebbaf93a8a3d4374fea | 2022-06-01T13:13:16.000Z | [
"pytorch",
"marian",
"text2text-generation",
"cs",
"dsb",
"en",
"hsb",
"pl",
"zlw",
"transformers",
"translation",
"opus-mt-tc",
"license:cc-by-4.0",
"model-index",
"autotrain_compatible"
]
| translation | false | Helsinki-NLP | null | Helsinki-NLP/opus-mt-tc-big-zlw-en | 43 | null | transformers | 6,312 | ---
language:
- cs
- dsb
- en
- hsb
- pl
- zlw
tags:
- translation
- opus-mt-tc
license: cc-by-4.0
model-index:
- name: opus-mt-tc-big-zlw-en
results:
- task:
name: Translation ces-eng
type: translation
args: ces-eng
dataset:
name: flores101-devtest
type: flores_101
args: ces eng devtest
metrics:
- name: BLEU
type: bleu
value: 41.2
- task:
name: Translation pol-eng
type: translation
args: pol-eng
dataset:
name: flores101-devtest
type: flores_101
args: pol eng devtest
metrics:
- name: BLEU
type: bleu
value: 29.6
- task:
name: Translation slk-eng
type: translation
args: slk-eng
dataset:
name: flores101-devtest
type: flores_101
args: slk eng devtest
metrics:
- name: BLEU
type: bleu
value: 40.0
- task:
name: Translation ces-eng
type: translation
args: ces-eng
dataset:
name: multi30k_test_2016_flickr
type: multi30k-2016_flickr
args: ces-eng
metrics:
- name: BLEU
type: bleu
value: 37.6
- task:
name: Translation ces-eng
type: translation
args: ces-eng
dataset:
name: multi30k_test_2018_flickr
type: multi30k-2018_flickr
args: ces-eng
metrics:
- name: BLEU
type: bleu
value: 37.4
- task:
name: Translation ces-eng
type: translation
args: ces-eng
dataset:
name: news-test2008
type: news-test2008
args: ces-eng
metrics:
- name: BLEU
type: bleu
value: 26.3
- task:
name: Translation pol-eng
type: translation
args: pol-eng
dataset:
name: newsdev2020
type: newsdev2020
args: pol-eng
metrics:
- name: BLEU
type: bleu
value: 32.7
- task:
name: Translation ces-eng
type: translation
args: ces-eng
dataset:
name: tatoeba-test-v2021-08-07
type: tatoeba_mt
args: ces-eng
metrics:
- name: BLEU
type: bleu
value: 57.4
- task:
name: Translation pol-eng
type: translation
args: pol-eng
dataset:
name: tatoeba-test-v2021-08-07
type: tatoeba_mt
args: pol-eng
metrics:
- name: BLEU
type: bleu
value: 55.7
- task:
name: Translation ces-eng
type: translation
args: ces-eng
dataset:
name: newstest2009
type: wmt-2009-news
args: ces-eng
metrics:
- name: BLEU
type: bleu
value: 29.5
- task:
name: Translation ces-eng
type: translation
args: ces-eng
dataset:
name: newstest2010
type: wmt-2010-news
args: ces-eng
metrics:
- name: BLEU
type: bleu
value: 30.7
- task:
name: Translation ces-eng
type: translation
args: ces-eng
dataset:
name: newstest2011
type: wmt-2011-news
args: ces-eng
metrics:
- name: BLEU
type: bleu
value: 30.9
- task:
name: Translation ces-eng
type: translation
args: ces-eng
dataset:
name: newstest2012
type: wmt-2012-news
args: ces-eng
metrics:
- name: BLEU
type: bleu
value: 29.4
- task:
name: Translation ces-eng
type: translation
args: ces-eng
dataset:
name: newstest2013
type: wmt-2013-news
args: ces-eng
metrics:
- name: BLEU
type: bleu
value: 32.8
- task:
name: Translation ces-eng
type: translation
args: ces-eng
dataset:
name: newstest2014
type: wmt-2014-news
args: ces-eng
metrics:
- name: BLEU
type: bleu
value: 38.7
- task:
name: Translation ces-eng
type: translation
args: ces-eng
dataset:
name: newstest2015
type: wmt-2015-news
args: ces-eng
metrics:
- name: BLEU
type: bleu
value: 33.4
- task:
name: Translation ces-eng
type: translation
args: ces-eng
dataset:
name: newstest2016
type: wmt-2016-news
args: ces-eng
metrics:
- name: BLEU
type: bleu
value: 37.1
- task:
name: Translation ces-eng
type: translation
args: ces-eng
dataset:
name: newstest2017
type: wmt-2017-news
args: ces-eng
metrics:
- name: BLEU
type: bleu
value: 32.5
- task:
name: Translation ces-eng
type: translation
args: ces-eng
dataset:
name: newstest2018
type: wmt-2018-news
args: ces-eng
metrics:
- name: BLEU
type: bleu
value: 33.1
- task:
name: Translation pol-eng
type: translation
args: pol-eng
dataset:
name: newstest2020
type: wmt-2020-news
args: pol-eng
metrics:
- name: BLEU
type: bleu
value: 32.6
---
# opus-mt-tc-big-zlw-en
Neural machine translation model for translating from West Slavic languages (zlw) to English (en).
This model is part of the [OPUS-MT project](https://github.com/Helsinki-NLP/Opus-MT), an effort to make neural machine translation models widely available and accessible for many languages in the world. All models are originally trained using the amazing framework of [Marian NMT](https://marian-nmt.github.io/), an efficient NMT implementation written in pure C++. The models have been converted to pyTorch using the transformers library by huggingface. Training data is taken from [OPUS](https://opus.nlpl.eu/) and training pipelines use the procedures of [OPUS-MT-train](https://github.com/Helsinki-NLP/Opus-MT-train).
* Publications: [OPUS-MT – Building open translation services for the World](https://aclanthology.org/2020.eamt-1.61/) and [The Tatoeba Translation Challenge – Realistic Data Sets for Low Resource and Multilingual MT](https://aclanthology.org/2020.wmt-1.139/) (Please, cite if you use this model.)
```
@inproceedings{tiedemann-thottingal-2020-opus,
title = "{OPUS}-{MT} {--} Building open translation services for the World",
author = {Tiedemann, J{\"o}rg and Thottingal, Santhosh},
booktitle = "Proceedings of the 22nd Annual Conference of the European Association for Machine Translation",
month = nov,
year = "2020",
address = "Lisboa, Portugal",
publisher = "European Association for Machine Translation",
url = "https://aclanthology.org/2020.eamt-1.61",
pages = "479--480",
}
@inproceedings{tiedemann-2020-tatoeba,
title = "The Tatoeba Translation Challenge {--} Realistic Data Sets for Low Resource and Multilingual {MT}",
author = {Tiedemann, J{\"o}rg},
booktitle = "Proceedings of the Fifth Conference on Machine Translation",
month = nov,
year = "2020",
address = "Online",
publisher = "Association for Computational Linguistics",
url = "https://aclanthology.org/2020.wmt-1.139",
pages = "1174--1182",
}
```
## Model info
* Release: 2022-03-17
* source language(s): ces dsb hsb pol
* target language(s): eng
* model: transformer-big
* data: opusTCv20210807+bt ([source](https://github.com/Helsinki-NLP/Tatoeba-Challenge))
* tokenization: SentencePiece (spm32k,spm32k)
* original model: [opusTCv20210807+bt_transformer-big_2022-03-17.zip](https://object.pouta.csc.fi/Tatoeba-MT-models/zlw-eng/opusTCv20210807+bt_transformer-big_2022-03-17.zip)
* more information released models: [OPUS-MT zlw-eng README](https://github.com/Helsinki-NLP/Tatoeba-Challenge/tree/master/models/zlw-eng/README.md)
## Usage
A short example code:
```python
from transformers import MarianMTModel, MarianTokenizer
src_text = [
"Aoi'ego hobby to tańczenie.",
"Myślisz, że Tom planuje to zrobić?"
]
model_name = "pytorch-models/opus-mt-tc-big-zlw-en"
tokenizer = MarianTokenizer.from_pretrained(model_name)
model = MarianMTModel.from_pretrained(model_name)
translated = model.generate(**tokenizer(src_text, return_tensors="pt", padding=True))
for t in translated:
print( tokenizer.decode(t, skip_special_tokens=True) )
# expected output:
# Aoi's hobby is dancing.
# You think Tom's planning on doing that?
```
You can also use OPUS-MT models with the transformers pipelines, for example:
```python
from transformers import pipeline
pipe = pipeline("translation", model="Helsinki-NLP/opus-mt-tc-big-zlw-en")
print(pipe("Aoi'ego hobby to tańczenie."))
# expected output: Aoi's hobby is dancing.
```
## Benchmarks
* test set translations: [opusTCv20210807+bt_transformer-big_2022-03-17.test.txt](https://object.pouta.csc.fi/Tatoeba-MT-models/zlw-eng/opusTCv20210807+bt_transformer-big_2022-03-17.test.txt)
* test set scores: [opusTCv20210807+bt_transformer-big_2022-03-17.eval.txt](https://object.pouta.csc.fi/Tatoeba-MT-models/zlw-eng/opusTCv20210807+bt_transformer-big_2022-03-17.eval.txt)
* benchmark results: [benchmark_results.txt](benchmark_results.txt)
* benchmark output: [benchmark_translations.zip](benchmark_translations.zip)
| langpair | testset | chr-F | BLEU | #sent | #words |
|----------|---------|-------|-------|-------|--------|
| ces-eng | tatoeba-test-v2021-08-07 | 0.71861 | 57.4 | 13824 | 105010 |
| pol-eng | tatoeba-test-v2021-08-07 | 0.70544 | 55.7 | 10099 | 75766 |
| ces-eng | flores101-devtest | 0.66444 | 41.2 | 1012 | 24721 |
| pol-eng | flores101-devtest | 0.58301 | 29.6 | 1012 | 24721 |
| slk-eng | flores101-devtest | 0.66103 | 40.0 | 1012 | 24721 |
| ces-eng | multi30k_test_2016_flickr | 0.61482 | 37.6 | 1000 | 12955 |
| ces-eng | multi30k_test_2018_flickr | 0.61405 | 37.4 | 1071 | 14689 |
| pol-eng | newsdev2020 | 0.60478 | 32.7 | 2000 | 46654 |
| ces-eng | newssyscomb2009 | 0.56495 | 30.2 | 502 | 11818 |
| ces-eng | news-test2008 | 0.54300 | 26.3 | 2051 | 49380 |
| ces-eng | newstest2009 | 0.56309 | 29.5 | 2525 | 65399 |
| ces-eng | newstest2010 | 0.57778 | 30.7 | 2489 | 61711 |
| ces-eng | newstest2011 | 0.57336 | 30.9 | 3003 | 74681 |
| ces-eng | newstest2012 | 0.56761 | 29.4 | 3003 | 72812 |
| ces-eng | newstest2013 | 0.58809 | 32.8 | 3000 | 64505 |
| ces-eng | newstest2014 | 0.64401 | 38.7 | 3003 | 68065 |
| ces-eng | newstest2015 | 0.58607 | 33.4 | 2656 | 53569 |
| ces-eng | newstest2016 | 0.61780 | 37.1 | 2999 | 64670 |
| ces-eng | newstest2017 | 0.58259 | 32.5 | 3005 | 61721 |
| ces-eng | newstest2018 | 0.58677 | 33.1 | 2983 | 63495 |
| pol-eng | newstest2020 | 0.60047 | 32.6 | 1001 | 21755 |
## Acknowledgements
The work is supported by the [European Language Grid](https://www.european-language-grid.eu/) as [pilot project 2866](https://live.european-language-grid.eu/catalogue/#/resource/projects/2866), by the [FoTran project](https://www.helsinki.fi/en/researchgroups/natural-language-understanding-with-cross-lingual-grounding), funded by the European Research Council (ERC) under the European Union’s Horizon 2020 research and innovation programme (grant agreement No 771113), and the [MeMAD project](https://memad.eu/), funded by the European Union’s Horizon 2020 Research and Innovation Programme under grant agreement No 780069. We are also grateful for the generous computational resources and IT infrastructure provided by [CSC -- IT Center for Science](https://www.csc.fi/), Finland.
## Model conversion info
* transformers version: 4.16.2
* OPUS-MT git hash: 3405783
* port time: Wed Apr 13 20:19:48 EEST 2022
* port machine: LM0-400-22516.local
|
emilylearning/cond_ft_birth_place_on_wiki_bio__prcnt_100__test_run_False | 70c4bad3c2ce04b2fc8f5de28e0697013a88792d | 2022-05-12T22:28:01.000Z | [
"pytorch",
"bert",
"token-classification",
"transformers",
"autotrain_compatible"
]
| token-classification | false | emilylearning | null | emilylearning/cond_ft_birth_place_on_wiki_bio__prcnt_100__test_run_False | 43 | null | transformers | 6,313 | Entry not found |
BigSalmon/InformalToFormalLincoln45 | 5ec42041c5163c5dc82e9fc555bcf2405f0c59b8 | 2022-05-18T20:03:28.000Z | [
"pytorch",
"gpt2",
"text-generation",
"transformers"
]
| text-generation | false | BigSalmon | null | BigSalmon/InformalToFormalLincoln45 | 43 | null | transformers | 6,314 | ```
from transformers import AutoTokenizer, AutoModelForCausalLM
tokenizer = AutoTokenizer.from_pretrained("BigSalmon/InformalToFormalLincoln45")
model = AutoModelForCausalLM.from_pretrained("BigSalmon/InformalToFormalLincoln45")
```
```
How To Make Prompt:
informal english: i am very ready to do that just that.
Translated into the Style of Abraham Lincoln: you can assure yourself of my readiness to work toward this end.
Translated into the Style of Abraham Lincoln: please be assured that i am most ready to undertake this laborious task.
***
informal english: space is huge and needs to be explored.
Translated into the Style of Abraham Lincoln: space awaits traversal, a new world whose boundaries are endless.
Translated into the Style of Abraham Lincoln: space is a ( limitless / boundless ) expanse, a vast virgin domain awaiting exploration.
***
informal english: corn fields are all across illinois, visible once you leave chicago.
Translated into the Style of Abraham Lincoln: corn fields ( permeate illinois / span the state of illinois / ( occupy / persist in ) all corners of illinois / line the horizon of illinois / envelop the landscape of illinois ), manifesting themselves visibly as one ventures beyond chicago.
informal english:
```
```
infill: chrome extensions [MASK] accomplish everyday tasks.
Translated into the Style of Abraham Lincoln: chrome extensions ( expedite the ability to / unlock the means to more readily ) accomplish everyday tasks.
infill: at a time when nintendo has become inflexible, [MASK] consoles that are tethered to a fixed iteration, sega diligently curates its legacy of classic video games on handheld devices.
Translated into the Style of Abraham Lincoln: at a time when nintendo has become inflexible, ( stubbornly [MASK] on / firmly set on / unyielding in its insistence on ) consoles that are tethered to a fixed iteration, sega diligently curates its legacy of classic video games on handheld devices.
infill:
```
```
Essay Intro (Warriors vs. Rockets in Game 7):
text: eagerly anticipated by fans, game 7's are the highlight of the post-season.
text: ever-building in suspense, game 7's have the crowd captivated.
***
Essay Intro (South Korean TV Is Becoming Popular):
text: maturing into a bona fide paragon of programming, south korean television ( has much to offer / entertains without fail / never disappoints ).
text: increasingly held in critical esteem, south korean television continues to impress.
text: at the forefront of quality content, south korea is quickly achieving celebrity status.
***
Essay Intro (
```
```
Search: What is the definition of Checks and Balances?
https://en.wikipedia.org/wiki/Checks_and_balances
Checks and Balances is the idea of having a system where each and every action in government should be subject to one or more checks that would not allow one branch or the other to overly dominate.
https://www.harvard.edu/glossary/Checks_and_Balances
Checks and Balances is a system that allows each branch of government to limit the powers of the other branches in order to prevent abuse of power
https://www.law.cornell.edu/library/constitution/Checks_and_Balances
Checks and Balances is a system of separation through which branches of government can control the other, thus preventing excess power.
***
Search: What is the definition of Separation of Powers?
https://en.wikipedia.org/wiki/Separation_of_powers
The separation of powers is a principle in government, whereby governmental powers are separated into different branches, each with their own set of powers, that are prevent one branch from aggregating too much power.
https://www.yale.edu/tcf/Separation_of_Powers.html
Separation of Powers is the division of governmental functions between the executive, legislative and judicial branches, clearly demarcating each branch's authority, in the interest of ensuring that individual liberty or security is not undermined.
***
Search: What is the definition of Connection of Powers?
https://en.wikipedia.org/wiki/Connection_of_powers
Connection of Powers is a feature of some parliamentary forms of government where different branches of government are intermingled, typically the executive and legislative branches.
https://simple.wikipedia.org/wiki/Connection_of_powers
The term Connection of Powers describes a system of government in which there is overlap between different parts of the government.
***
Search: What is the definition of
```
```
Search: What are phrase synonyms for "second-guess"?
https://www.powerthesaurus.org/second-guess/synonyms
Shortest to Longest:
- feel dubious about
- raise an eyebrow at
- wrinkle their noses at
- cast a jaundiced eye at
- teeter on the fence about
***
Search: What are phrase synonyms for "mean to newbies"?
https://www.powerthesaurus.org/mean_to_newbies/synonyms
Shortest to Longest:
- readiness to balk at rookies
- absence of tolerance for novices
- hostile attitude toward newcomers
***
Search: What are phrase synonyms for "make use of"?
https://www.powerthesaurus.org/make_use_of/synonyms
Shortest to Longest:
- call upon
- glean value from
- reap benefits from
- derive utility from
- seize on the merits of
- draw on the strength of
- tap into the potential of
***
Search: What are phrase synonyms for "hurting itself"?
https://www.powerthesaurus.org/hurting_itself/synonyms
Shortest to Longest:
- erring
- slighting itself
- forfeiting its integrity
- doing itself a disservice
- evincing a lack of backbone
***
Search: What are phrase synonyms for "
```
```
- declining viewership facing the nba.
- does not have to be this way.
- in fact, many solutions exist.
- the four point line would surely draw in eyes.
text: failing to draw in the masses, the nba has ( fallen into / succumb to / bowed to ) disrepair. such does not have to be the case, however. in fact, a myriad of simple, relatively cheap ( solutions / interventions / enhancements ) could revive the league. the addition of the much-hyped four-point line would surely juice viewership.
***
-
```
```
original: sports teams are profitable for owners. [MASK], their valuations experience a dramatic uptick.
infill: sports teams are profitable for owners. ( accumulating vast sums / stockpiling treasure / realizing benefits / cashing in / registering robust financials / scoring on balance sheets ), their valuations experience a dramatic uptick.
***
original:
```
```
wordy: classical music is becoming less popular more and more.
Translate into Concise Text: interest in classic music is fading.
***
wordy:
```
```
sweet: savvy voters ousted him.
longer: voters who were informed delivered his defeat.
***
sweet:
```
```
1: commercial space company spacex plans to launch a whopping 52 flights in 2022.
2: spacex, a commercial space company, intends to undertake a total of 52 flights in 2022.
3: in 2022, commercial space company spacex has its sights set on undertaking 52 flights.
4: 52 flights are in the pipeline for 2022, according to spacex, a commercial space company.
5: a commercial space company, spacex aims to conduct 52 flights in 2022.
***
1:
```
Keywords to sentences or sentence.
```
ngos are characterized by:
□ voluntary citizens' group that is organized on a local, national or international level
□ encourage political participation
□ often serve humanitarian functions
□ work for social, economic, or environmental change
***
what are the drawbacks of living near an airbnb?
□ noise
□ parking
□ traffic
□ security
□ strangers
***
```
```
original: musicals generally use spoken dialogue as well as songs to convey the story. operas are usually fully sung.
adapted: musicals generally use spoken dialogue as well as songs to convey the story. ( in a stark departure / on the other hand / in contrast / by comparison / at odds with this practice / far from being alike / in defiance of this standard / running counter to this convention ), operas are usually fully sung.
***
original: akoya and tahitian are types of pearls. akoya pearls are mostly white, and tahitian pearls are naturally dark.
adapted: akoya and tahitian are types of pearls. ( a far cry from being indistinguishable / easily distinguished / on closer inspection / setting them apart / not to be mistaken for one another / hardly an instance of mere synonymy / differentiating the two ), akoya pearls are mostly white, and tahitian pearls are naturally dark.
***
original:
```
```
original: had trouble deciding.
translated into journalism speak: wrestled with the question, agonized over the matter, furrowed their brows in contemplation.
***
original:
``` |
wannaphong/Roman2Thai-transliterator | df06e6e4a116ea2687b53b867234a06848c4cf66 | 2022-06-26T15:02:19.000Z | [
"pytorch",
"marian",
"text2text-generation",
"dataset:thai2rom-v2",
"transformers",
"translation",
"license:apache-2.0",
"autotrain_compatible"
]
| translation | false | wannaphong | null | wannaphong/Roman2Thai-transliterator | 43 | null | transformers | 6,315 | ---
tags:
- translation
license: apache-2.0
datasets:
- thai2rom-v2
metrics:
- cer
widget:
- text: "maiphai"
---
# Roman-Thai Transliterator by Transformer Models
GitHub: https://github.com/wannaphong/thai2rom-v2/tree/main/roman2thai-transformer |
RUCAIBox/mvp-open-dialog | 24e8fb986592304c62668dbb90297d820bf9b24c | 2022-06-27T02:28:00.000Z | [
"pytorch",
"mvp",
"en",
"arxiv:2206.12131",
"transformers",
"text-generation",
"text2text-generation",
"conversational",
"license:apache-2.0"
]
| text2text-generation | false | RUCAIBox | null | RUCAIBox/mvp-open-dialog | 43 | 1 | transformers | 6,316 | ---
license: apache-2.0
language:
- en
tags:
- text-generation
- text2text-generation
- conversational
pipeline_tag: text2text-generation
widget:
- text: "Given the dialog: do you like dance? [SEP] Yes I do. Did you know Bruce Lee was a cha cha dancer?"
example_title: "Example1"
- text: "Given the dialog: i used to scare for darkness [X_SEP] it feels like hitting to blank wall when i see the darkness [SEP] Oh ya? I don't really see how [SEP] dont you feel so.. its a wonder [SEP] I do actually hit blank walls a lot of times but i get by"
example_title: "Example2"
---
# MVP-open-dialog
The MVP-open-dialog model was proposed in [**MVP: Multi-task Supervised Pre-training for Natural Language Generation**](https://arxiv.org/abs/2206.12131) by Tianyi Tang, Junyi Li, Wayne Xin Zhao and Ji-Rong Wen.
The detailed information and instructions can be found [https://github.com/RUCAIBox/MVP](https://github.com/RUCAIBox/MVP).
## Model Description
MVP-open-dialog is a prompt-based model that MVP is further equipped with prompts pre-trained using labeled open dialogue system datasets. It is a variant (MVP+S) of our main [MVP](https://huggingface.co/RUCAIBox/mvp) model. It follows a Transformer encoder-decoder architecture with layer-wise prompts.
MVP-open-dialog is specially designed for open dialogue system (conversation) tasks, such as chitchat (PersonaChat, DailyDialog), knowledge grounded conversation (Topical-Chat, Wizard of Wikipedia) and visual dialog (DSTC7-AVSD).
## Example
```python
>>> from transformers import MvpTokenizer, MvpForConditionalGeneration
>>> tokenizer = MvpTokenizer.from_pretrained("RUCAIBox/mvp")
>>> model = MvpForConditionalGeneration.from_pretrained("RUCAIBox/mvp-open-dialog")
>>> inputs = tokenizer(
... "Given the dialog: do you like dance? [SEP] Yes I do. Did you know Bruce Lee was a cha cha dancer?",
... return_tensors="pt",
... )
>>> generated_ids = model.generate(**inputs)
>>> tokenizer.batch_decode(generated_ids, skip_special_tokens=True)
['I did not know that. I did know that Tupac danced ballet in high school.']
```
## Related Models
**MVP**: [https://huggingface.co/RUCAIBox/mvp](https://huggingface.co/RUCAIBox/mvp).
**Prompt-based models**:
- MVP-multi-task: [https://huggingface.co/RUCAIBox/mvp-multi-task](https://huggingface.co/RUCAIBox/mvp-multi-task).
- MVP-summarization: [https://huggingface.co/RUCAIBox/mvp-summarization](https://huggingface.co/RUCAIBox/mvp-summarization).
- MVP-open-dialog: [https://huggingface.co/RUCAIBox/mvp-open-dialog](https://huggingface.co/RUCAIBox/mvp-open-dialog).
- MVP-data-to-text: [https://huggingface.co/RUCAIBox/mvp-data-to-text](https://huggingface.co/RUCAIBox/mvp-data-to-text).
- MVP-story: [https://huggingface.co/RUCAIBox/mvp-story](https://huggingface.co/RUCAIBox/mvp-story).
- MVP-question-answering: [https://huggingface.co/RUCAIBox/mvp-question-answering](https://huggingface.co/RUCAIBox/mvp-question-answering).
- MVP-question-generation: [https://huggingface.co/RUCAIBox/mvp-question-generation](https://huggingface.co/RUCAIBox/mvp-question-generation).
- MVP-task-dialog: [https://huggingface.co/RUCAIBox/mvp-task-dialog](https://huggingface.co/RUCAIBox/mvp-task-dialog).
**Multi-task models**:
- MTL-summarization: [https://huggingface.co/RUCAIBox/mtl-summarization](https://huggingface.co/RUCAIBox/mtl-summarization).
- MTL-open-dialog: [https://huggingface.co/RUCAIBox/mtl-open-dialog](https://huggingface.co/RUCAIBox/mtl-open-dialog).
- MTL-data-to-text: [https://huggingface.co/RUCAIBox/mtl-data-to-text](https://huggingface.co/RUCAIBox/mtl-data-to-text).
- MTL-story: [https://huggingface.co/RUCAIBox/mtl-story](https://huggingface.co/RUCAIBox/mtl-story).
- MTL-question-answering: [https://huggingface.co/RUCAIBox/mtl-question-answering](https://huggingface.co/RUCAIBox/mtl-question-answering).
- MTL-question-generation: [https://huggingface.co/RUCAIBox/mtl-question-generation](https://huggingface.co/RUCAIBox/mtl-question-generation).
- MTL-task-dialog: [https://huggingface.co/RUCAIBox/mtl-task-dialog](https://huggingface.co/RUCAIBox/mtl-task-dialog).
## Citation
```bibtex
@article{tang2022mvp,
title={MVP: Multi-task Supervised Pre-training for Natural Language Generation},
author={Tang, Tianyi and Li, Junyi and Zhao, Wayne Xin and Wen, Ji-Rong},
journal={arXiv preprint arXiv:2206.12131},
year={2022},
url={https://arxiv.org/abs/2206.12131},
}
```
|
victorlee071200/bert-base-cased-finetuned-squad | cfc56fa4d71a2229ed446e0d74a7e7a63c687d3e | 2022-06-09T06:28:47.000Z | [
"pytorch",
"bert",
"question-answering",
"dataset:squad",
"transformers",
"generated_from_trainer",
"license:apache-2.0",
"model-index",
"autotrain_compatible"
]
| question-answering | false | victorlee071200 | null | victorlee071200/bert-base-cased-finetuned-squad | 43 | null | transformers | 6,317 | ---
license: apache-2.0
tags:
- generated_from_trainer
datasets:
- squad
model-index:
- name: bert-base-cased-finetuned-squad
results: []
---
<!-- This model card has been generated automatically according to the information the Trainer had access to. You
should probably proofread and complete it, then remove this comment. -->
# bert-base-cased-finetuned-squad
This model is a fine-tuned version of [bert-base-cased](https://huggingface.co/bert-base-cased) on the squad dataset.
It achieves the following results on the evaluation set:
- Loss: 1.0835
## Model description
More information needed
## Intended uses & limitations
More information needed
## Training and evaluation data
More information needed
## Training procedure
### Training hyperparameters
The following hyperparameters were used during training:
- learning_rate: 2e-05
- train_batch_size: 16
- eval_batch_size: 16
- seed: 42
- optimizer: Adam with betas=(0.9,0.999) and epsilon=1e-08
- lr_scheduler_type: linear
- num_epochs: 3
### Training results
| Training Loss | Epoch | Step | Validation Loss |
|:-------------:|:-----:|:-----:|:---------------:|
| 1.0302 | 1.0 | 5546 | 1.0068 |
| 0.7597 | 2.0 | 11092 | 0.9976 |
| 0.5483 | 3.0 | 16638 | 1.0835 |
### Framework versions
- Transformers 4.19.2
- Pytorch 1.11.0+cu113
- Datasets 2.2.2
- Tokenizers 0.12.1
|
yanekyuk/berturk-uncased-keyword-extractor | 2a4297127e9b1909bf240028bc2473f3b093f572 | 2022-06-06T17:09:28.000Z | [
"pytorch",
"bert",
"token-classification",
"tr",
"transformers",
"generated_from_trainer",
"license:mit",
"model-index",
"autotrain_compatible"
]
| token-classification | false | yanekyuk | null | yanekyuk/berturk-uncased-keyword-extractor | 43 | null | transformers | 6,318 | ---
license: mit
tags:
- generated_from_trainer
metrics:
- precision
- recall
- accuracy
- f1
language:
- tr
widget:
- text: "İngiltere'de düzenlenen Avrupa Tekvando ve Para Tekvando Şampiyonası’nda millî tekvandocular 5 altın, 2 gümüş ve 4 bronz olmak üzere 11, millî para tekvandocular ise 4 altın, 3 gümüş ve 1 bronz olmak üzere 8 madalya kazanarak takım halinde Avrupa şampiyonu oldu."
- text: "Füme somon dedik ama aslında lox salamuralanmış somon anlamına geliyor, füme etme opsiyonel. Lox bagel, 1930'larda Eggs Benedict furyasında New Yorklu Yahudi cemaati tarafından koşer bir alternatif olarak çıkan bir lezzet. Günümüzde benim hangover yüreğim dâhil dünyanın birçok yerinde enfes bir kahvaltı sandviçi."
- text: "Türkiye'de son aylarda sıklıkla tartışılan konut satışı karşılığında yabancılara vatandaşlık verilmesi konusunu beyin göçü kapsamında ele almak mümkün. Daha önce 250 bin dolar olan vatandaşlık bedeli yükselen tepkiler üzerine 400 bin dolara çıkarılmıştı. Türkiye'den göç eden iyi eğitimli kişilerin , gittikleri ülkelerde 250 bin dolar tutarında yabancı yatırıma denk olduğu göz önüne alındığında nitelikli insan gücünün yabancılara konut karşılığında satılan vatandaşlık bedelin eş olduğunu görüyoruz. Yurt dışına giden her bir vatandaşın yüksek teknolojili katma değer üreten sektörlere yapacağı katkılar göz önünde bulundurulduğunda bu açığın inşaat sektörüyle kapatıldığını da görüyoruz. Beyin göçü konusunda sadece ekonomik perspektiften bakıldığında bile kısa vadeli döviz kaynağı yaratmak için kullanılan vatandaşlık satışı yerine beyin göçünü önleyecek önlemler alınmasının ülkemize çok daha faydalı olacağı sonucunu çıkarıyoruz."
- text: "Türkiye’de resmî verilere göre, 15 ve daha yukarı yaştaki kişilerde mevsim etkisinden arındırılmış işsiz sayısı, bu yılın ilk çeyreğinde bir önceki çeyreğe göre 50 bin kişi artarak 3 milyon 845 bin kişi oldu. Mevsim etkisinden arındırılmış işsizlik oranı ise 0,1 puanlık artışla %11,4 seviyesinde gerçekleşti. İşsizlik oranı, ilk çeyrekte geçen yılın aynı çeyreğine göre 1,7 puan azaldı."
- text: "Boeing’in insansız uzay aracı Starliner, birtakım sorunlara rağmen Uluslararası Uzay İstasyonuna (ISS) ulaşarak ilk kez başarılı bir şekilde kenetlendi. Aracın ISS’te beş gün kalmasını takiben sorunsuz bir şekilde New Mexico’ya inmesi halinde Boeing, sonbaharda astronotları yörüngeye göndermek için Starliner’ı kullanabilir.\n\nNeden önemli? NASA’nın personal aracı üretmeyi durdurmasından kaynaklı olarak görevli astronotlar ve kozmonotlar, ISS’te Rusya’nın ürettiği uzay araçları ile taşınıyordu. Starliner’ın kendini kanıtlaması ise bu konuda Rusya’ya olan bağımlılığın potansiyel olarak ortadan kalkabileceği anlamına geliyor."
model-index:
- name: berturk-uncased-keyword-extractor
results: []
---
<!-- This model card has been generated automatically according to the information the Trainer had access to. You
should probably proofread and complete it, then remove this comment. -->
# berturk-uncased-keyword-extractor
This model is a fine-tuned version of [dbmdz/bert-base-turkish-uncased](https://huggingface.co/dbmdz/bert-base-turkish-uncased) on an unknown dataset.
It achieves the following results on the evaluation set:
- Loss: 0.3931
- Precision: 0.6631
- Recall: 0.6728
- Accuracy: 0.9188
- F1: 0.6679
## Model description
More information needed
## Intended uses & limitations
More information needed
## Training and evaluation data
More information needed
## Training procedure
### Training hyperparameters
The following hyperparameters were used during training:
- learning_rate: 2e-05
- train_batch_size: 16
- eval_batch_size: 16
- seed: 42
- optimizer: Adam with betas=(0.9,0.999) and epsilon=1e-08
- lr_scheduler_type: linear
- num_epochs: 8
- mixed_precision_training: Native AMP
### Training results
| Training Loss | Epoch | Step | Validation Loss | Precision | Recall | Accuracy | F1 |
|:-------------:|:-----:|:-----:|:---------------:|:---------:|:------:|:--------:|:------:|
| 0.1779 | 1.0 | 1875 | 0.1862 | 0.6199 | 0.6356 | 0.9192 | 0.6276 |
| 0.1327 | 2.0 | 3750 | 0.1890 | 0.6328 | 0.6917 | 0.9206 | 0.6610 |
| 0.1008 | 3.0 | 5625 | 0.2188 | 0.6322 | 0.7037 | 0.9189 | 0.6660 |
| 0.0755 | 4.0 | 7500 | 0.2539 | 0.6395 | 0.7030 | 0.9181 | 0.6697 |
| 0.0574 | 5.0 | 9375 | 0.2882 | 0.6556 | 0.6868 | 0.9197 | 0.6709 |
| 0.0433 | 6.0 | 11250 | 0.3425 | 0.6565 | 0.6851 | 0.9189 | 0.6705 |
| 0.0352 | 7.0 | 13125 | 0.3703 | 0.6616 | 0.6776 | 0.9191 | 0.6695 |
| 0.0288 | 8.0 | 15000 | 0.3931 | 0.6631 | 0.6728 | 0.9188 | 0.6679 |
### Framework versions
- Transformers 4.19.2
- Pytorch 1.11.0+cu113
- Datasets 2.2.2
- Tokenizers 0.12.1
|
AnyaSchen/rugpt3_mayakovskij | 4b63fe666d353ccfb5e73b9eae90a5abcd169763 | 2022-06-15T11:18:15.000Z | [
"pytorch",
"gpt2",
"text-generation",
"transformers"
]
| text-generation | false | AnyaSchen | null | AnyaSchen/rugpt3_mayakovskij | 43 | null | transformers | 6,319 |
This model was created as a fine-tuned GPT-3 medium model, which is tuned to the style of Mayakovsky's poetry in Russian. You can give her a word, a phrase, or just an empty line as an input, and she will give out a poem in the style of Mayakovsky.
 |
Maxbnza/country-recognition | 246f60afa914121ffcd824d4e6a421d5443604b1 | 2022-06-30T16:03:34.000Z | [
"pytorch",
"xlm-roberta",
"text-classification",
"unk",
"dataset:Maxbnza/autotrain-data-address-training",
"transformers",
"autotrain",
"co2_eq_emissions"
]
| text-classification | false | Maxbnza | null | Maxbnza/country-recognition | 43 | 1 | transformers | 6,320 | ---
tags: autotrain
language: unk
widget:
- text: "I love AutoTrain 🤗"
datasets:
- Maxbnza/autotrain-data-address-training
co2_eq_emissions: 141.11976199388627
---
# Model Trained Using AutoTrain
- Problem type: Multi-class Classification
- Model ID: 1062136864
- CO2 Emissions (in grams): 141.11976199388627
## Validation Metrics
- Loss: 0.10147109627723694
- Accuracy: 0.9859325979151907
- Macro F1: 0.9715036017680622
- Micro F1: 0.9859325979151907
- Weighted F1: 0.9859070541468058
- Macro Precision: 0.9732956651937184
- Micro Precision: 0.9859325979151907
- Weighted Precision: 0.9860574596777458
- Macro Recall: 0.970199341807239
- Micro Recall: 0.9859325979151907
- Weighted Recall: 0.9859325979151907
## Usage
You can use cURL to access this model:
```
$ curl -X POST -H "Authorization: Bearer YOUR_API_KEY" -H "Content-Type: application/json" -d '{"inputs": "I love AutoTrain"}' https://api-inference.huggingface.co/models/Maxbnza/autotrain-address-training-1062136864
```
Or Python API:
```
from transformers import AutoModelForSequenceClassification, AutoTokenizer
model = AutoModelForSequenceClassification.from_pretrained("Maxbnza/autotrain-address-training-1062136864", use_auth_token=True)
tokenizer = AutoTokenizer.from_pretrained("Maxbnza/autotrain-address-training-1062136864", use_auth_token=True)
inputs = tokenizer("I love AutoTrain", return_tensors="pt")
outputs = model(**inputs)
``` |
bigscience/bloom-176-intermediate | 7258df6c654ebc02378dcf92d5060c9b077a55bc | 2022-07-11T16:47:42.000Z | [
"pytorch",
"bloom",
"ak",
"ar",
"as",
"bm",
"bn",
"ca",
"code",
"en",
"es",
"eu",
"fon",
"fr",
"gu",
"hi",
"id",
"ig",
"ki",
"kn",
"lg",
"ln",
"ml",
"mr",
"ne",
"nso",
"ny",
"or",
"pa",
"pt",
"rn",
"rw",
"sn",
"st",
"sw",
"ta",
"te",
"tn",
"ts",
"tum",
"tw",
"ur",
"vi",
"wo",
"xh",
"yo",
"zh",
"zhs",
"zht",
"zu",
"arxiv:1909.08053",
"arxiv:2110.02861",
"arxiv:2108.12409",
"transformers",
"license:bigscience-bloom-rail-1.0"
]
| null | false | bigscience | null | bigscience/bloom-176-intermediate | 43 | null | transformers | 6,321 | ---
license: bigscience-bloom-rail-1.0
language:
- ak
- ar
- as
- bm
- bn
- ca
- code
- en
- es
- eu
- fon
- fr
- gu
- hi
- id
- ig
- ki
- kn
- lg
- ln
- ml
- mr
- ne
- nso
- ny
- or
- pa
- pt
- rn
- rw
- sn
- st
- sw
- ta
- te
- tn
- ts
- tum
- tw
- ur
- vi
- wo
- xh
- yo
- zh
- zhs
- zht
- zu
---
# <span style="color:red"><b>WARNING:</b> The checkpoints on this repo are not fully trained model. Evaluations of intermediary checkpoints and the final model will be added when conducted (see below).</span>
# <p>BLOOM LM<br/> _BigScience Large Open-science Open-access Multilingual Language Model_ <br/>Model Card</p>
<img src="https://assets.website-files.com/6139f3cdcbbff3a68486761d/613cd8997b270da063e230c5_Tekengebied%201-p-500.png" alt="BigScience Logo" width="200"/>
Version 1.3 / 11.July.2022 - Available intermediary checkpoints - global steps:
+ `5000`, `10000`, `15000`, `20000`, `25000`, `30000`, `35000`, `40000`, `45000`, `50000`, `55000`, `60000`, `65000`, `70000`, `75000`, `80000`, `85000`, `90000`, `91000`, `92000`, `93000`
You can check the available checkpoints by clicking on the branches section of the repo
# How to load a specific version
We use `git tags` to load a model in a specific version (eg. `global_step5000`):

# Table of Contents
1. [Model Details](#model-details)
2. [Uses](#uses)
3. [Training Data](#training-data)
4. [Risks and Limitations](#risks-and-limitations)
5. [Evaluation](#evaluation)
6. [Recommendations](#recommendations)
7. [Glossary and Calculations](#glossary-and-calculations)
8. [More Information](#more-information)
9. [Model Card Authors](#model-card-authors)
---
# Model Details
BLOOM is a type of language model, which is a probability distribution over sequences of words. Specifically, BLOOM is a Large Language Model (LLM), meaning that it is trained on vast amounts of text data using industrial-scale computational resources. As such, the model is able to capture the statistical tendencies of words, phrases, sentences, and larger spans of text that it is exposed to in the training data.
## Basics
*This section provides information about the model type, version, license, funders, release date, developers, and contact information.*
*It is useful for anyone who wants to reference the model.*
<details>
<summary>Click to expand</summary>
**Developed by:** BigScience ([website](https://bigscience.huggingface.co))
*All collaborators are either volunteers or have an agreement with their employer. (Further breakdown of participants forthcoming.)*
**Model Type:** Transformer-based Language Model
**Version:** 1.0.0
**Languages:** Multiple; see [training data](#training-data)
**License:** RAIL License v1.0 ([link](https://huggingface.co/spaces/bigscience/license))
**Release Date Estimate:** Monday, 11.July.2022
**Send Questions to:** [email protected]
**Cite as:** BigScience, _BigScience Language Open-science Open-access Multilingual (BLOOM) Language Model_. International, May 2021-May 2022
**Funded by:**
* The French government.
* Hugging Face ([website](https://huggingface.co)).
* Organizations of contributors. *(Further breakdown of organizations forthcoming.)*
</details>
## Technical Specifications
*This section includes details about the model objective and architecture, and the compute infrastructure.*
*It is useful for people interested in model development.*
<details>
<summary>Click to expand</summary>
Please see [the BLOOM training README](https://github.com/bigscience-workshop/bigscience/tree/master/train/tr11-176B-ml#readme) for full details on replicating training.
### Model Architecture and Objective
* Modified from Megatron-LM GPT2 (see [paper](https://arxiv.org/abs/1909.08053), [BLOOM Megatron code](https://github.com/bigscience-workshop/Megatron-DeepSpeed)):
* Decoder-only architecture
* Layer normalization applied to word embeddings layer (`StableEmbedding`; see [code](https://github.com/facebookresearch/bitsandbytes), [paper](https://arxiv.org/pdf/2110.02861.pdf))
* ALiBI positional encodings (see [paper](https://arxiv.org/pdf/2108.12409.pdf)), with GeLU activation functions
* 176 billion parameters:
* 70 layers, 112 attention heads
* Hidden layers are 14336-dimensional
* Sequence length of 2048 tokens used (see [BLOOM tokenizer](https://huggingface.co/bigscience/tokenizer), [tokenizer description](#tokenization))
**Objective Function:** Cross Entropy with mean reduction (see [API documentation](https://pytorch.org/docs/stable/generated/torch.nn.CrossEntropyLoss.html#torch.nn.CrossEntropyLoss)).
### Compute infrastructure
Jean Zay Public Supercomputer, provided by the French government (see [announcement](https://www.enseignementsup-recherche.gouv.fr/fr/signature-du-marche-d-acquisition-de-l-un-des-supercalculateurs-les-plus-puissants-d-europe-46733)).
#### Hardware
* 384 A100 80GB GPUs (48 nodes)
* Additional 32 A100 80GB GPUs (4 nodes) in reserve
* 8 GPUs per node Using NVLink 4 inter-gpu connects, 4 OmniPath links
* CPU: AMD
* CPU memory: 512GB per node
* GPU memory: 640GB per node
* Inter-node connect: Omni-Path Architecture (OPA)
* NCCL-communications network: a fully dedicated subnet
* Disc IO network: shared network with other types of nodes
#### Software
* Megatron-DeepSpeed ([Github link](https://github.com/bigscience-workshop/Megatron-DeepSpeed))
* DeepSpeed ([Github link](https://github.com/microsoft/DeepSpeed))
* PyTorch (pytorch-1.11 w/ CUDA-11.5; see [Github link](https://github.com/pytorch/pytorch))
* apex ([Github link](https://github.com/NVIDIA/apex))
</details>
---
# Training
*This section provides information about the training data, the speed and size of training elements, and the environmental impact of training.*
*It is useful for people who want to learn more about the model inputs and training footprint.*
<details>
<summary>Click to expand</summary>
## Training Data
*This section provides a high-level overview of the training data. It is relevant for anyone who wants to know the basics of what the model is learning.*
Details for each dataset are provided in individual [Data Cards](https://huggingface.co/spaces/bigscience/BigScienceCorpus).
Training data includes:
- 45 natural languages
- 12 programming languages
- In 1.5TB of pre-processed text, converted into 350B unique tokens (see [the tokenizer section](#tokenization) for more.)
### Languages
The pie chart shows the distribution of languages in training data.

The following tables shows the further distribution of Niger-Congo & Indic languages and programming languages in the training data.
Distribution of Niger Congo and Indic languages.
| Niger Congo | Percentage | | Indic | Percentage |
|----------------|------------ |------ |-----------|------------|
| Chi Tumbuka | 0.00002 | | Assamese | 0.01 |
| Kikuyu | 0.00004 | | Odia | 0.04 |
| Bambara | 0.00004 | | Gujarati | 0.04 |
| Akan | 0.00007 | | Marathi | 0.05 |
| Xitsonga | 0.00007 | | Punjabi | 0.05 |
| Sesotho | 0.00007 | | Kannada | 0.06 |
| Chi Chewa | 0.0001 | | Nepali | 0.07 |
| Setswana | 0.0002 | | Telugu | 0.09 |
| Northern Sotho | 0.0002 | | Malayalam | 0.10 |
| Fon | 0.0002 | | Urdu | 0.10 |
| Kirundi | 0.0003 | | Tamil | 0.20 |
| Wolof | 0.0004 | | Bengali | 0.50 |
| Kuganda | 0.0004 | | Hindi | 0.70 |
| Chi Shona | 0.001 |
| Isi Zulu | 0.001 |
| Igbo | 0.001 |
| Xhosa | 0.001 |
| Kinyarwanda | 0.003 |
| Yoruba | 0.006 |
| Swahili | 0.02 |
Distribution of programming languages.
| Extension | Language | Number of files |
|----------------|------------|-----------------|
| java | Java | 5,407,724 |
| php | PHP | 4,942,186 |
| cpp | C++ | 2,503,930 |
| py | Python | 2,435,072 |
| js | JavaScript | 1,905,518 |
| cs | C# | 1,577,347 |
| rb | Ruby | 6,78,413 |
| cc | C++ | 443,054 |
| hpp | C++ | 391,048 |
| lua | Lua | 352,317 |
| go | GO | 227,763 |
| ts | TypeScript | 195,254 |
| C | C | 134,537 |
| scala | Scala | 92,052 |
| hh | C++ | 67,161 |
| H | C++ | 55,899 |
| tsx | TypeScript | 33,107 |
| rs | Rust | 29,693 |
| phpt | PHP | 9,702 |
| c++ | C++ | 1,342 |
| h++ | C++ | 791 |
| php3 | PHP | 540 |
| phps | PHP | 270 |
| php5 | PHP | 166 |
| php4 | PHP | 29 |
### Preprocessing
**Tokenization:** The BLOOM tokenizer ([link](https://huggingface.co/bigscience/tokenizer)), a learned subword tokenizer trained using:
- A byte-level Byte Pair Encoding (BPE) algorithm
- A simple pre-tokenization rule, no normalization
- A vocabulary size of 250,680
It was trained on a subset of a preliminary version of the corpus using alpha-weighting per language.
## Speeds, Sizes, Times
Training logs: [Tensorboard link](https://huggingface.co/tensorboard/bigscience/tr11-176B-ml-logs/)
- Dates:
- Started 11th March, 2022 11:42am PST
- Estimated end: 5th July, 2022
- Checkpoint size:
- Bf16 weights: 329GB
- Full checkpoint with optimizer states: 2.3TB
- Training throughput: About 150 TFLOP per GPU per second
- Number of epochs: 1
- Estimated cost of training: Equivalent of $2-5M in cloud computing (including preliminary experiments)
- Server training location: Île-de-France, France
## Environmental Impact
The training supercomputer, Jean Zay ([website](http://www.idris.fr/eng/jean-zay/jean-zay-presentation-eng.html)), uses mostly nuclear energy. The heat generated by it is reused for heating campus housing.
**Estimated carbon emissions:** *(Forthcoming.)*
**Estimated electricity usage:** *(Forthcoming.)*
</details>
---
# Uses
*This section addresses questions around how the model is intended to be used, discusses the foreseeable users of the model (including those affected by the model), and describes uses that are considered out of scope or misuse of the model.*
*It is useful for anyone considering using the model or who is affected by the model.*
<details>
<summary>Click to expand</summary>
## Intended Use
This model is being created in order to enable public research on large language models (LLMs). LLMs are intended to be used for language generation or as a pretrained base model that can be further fine-tuned for specific tasks. Use cases below are not exhaustive.
### Direct Use
- Text generation
- Exploring characteristics of language generated by a language model
- Examples: Cloze tests, counterfactuals, generations with reframings
### Downstream Use
- Tasks that leverage language models include: Information Extraction, Question Answering, Summarization
### Misuse and Out-of-scope Use
*This section addresses what users ought not do with the model.*
See the [BLOOM License](https://huggingface.co/spaces/bigscience/license), Attachment A, for detailed usage restrictions. The below list is non-exhaustive, but lists some easily foreseeable problematic use cases.
#### Out-of-scope Uses
Using the model in [high-stakes](#high-stakes) settings is out of scope for this model. The model is not designed for [critical decisions](#critical-decisions) nor uses with any material consequences on an individual's livelihood or wellbeing. The model outputs content that appears factual but is not correct.
Out-of-scope Uses Include:
- Usage in biomedical domains, political and legal domains, or finance domains
- Usage for evaluating or scoring individuals, such as for employment, education, or credit
- Applying the model for critical automatic decisions, generating factual content, creating reliable summaries, or generating predictions that must be correct
#### Misuse
Intentionally using the model for harm, violating [human rights](#human-rights), or other kinds of malicious activities, is a misuse of this model. This includes:
- Spam generation
- Disinformation and influence operations
- Disparagement and defamation
- Harassment and abuse
- [Deception](#deception)
- Unconsented impersonation and imitation
- Unconsented surveillance
- Generating content without attribution to the model, as specified in the [RAIL License, Use Restrictions](https://huggingface.co/spaces/bigscience/license)
## Intended Users
### Direct Users
- General Public
- Researchers
- Students
- Educators
- Engineers/developers
- Non-commercial entities
- Community advocates, including human and civil rights groups
### Indirect Users
- Users of derivatives created by Direct Users, such as those using software with an [intended use](#intended-use)
- Users of [Derivatives of the Model, as described in the License](https://huggingface.co/spaces/bigscience/license)
### Others Affected (Parties Prenantes)
- People and groups referred to by the LLM
- People and groups exposed to outputs of, or decisions based on, the LLM
- People and groups whose original work is included in the LLM
</details>
---
# Risks and Limitations
*This section identifies foreseeable harms and misunderstandings.*
<details>
<summary>Click to expand</summary>
Model may:
- Overrepresent some viewpoints and underrepresent others
- Contain stereotypes
- Contain [personal information](#personal-data-and-information)
- Generate:
- Hateful, abusive, or violent language
- Discriminatory or prejudicial language
- Content that may not be appropriate for all settings, including sexual content
- Make errors, including producing incorrect information as if it were factual
- Generate irrelevant or repetitive outputs
</details>
---
# Evaluation
*This section describes the evaluation protocols and provides the results.*
<details>
<summary>Click to expand</summary>
## Metrics
*This section describes the different ways performance is calculated and why.*
Includes:
| Metric | Why chosen |
|--------------------|--------------------------------------------------------------------|
| [Perplexity](#perplexity) | Standard metric for quantifying model improvements during training |
| Cross Entropy [Loss](#loss) | Standard objective for language models. |
And multiple different metrics for specific tasks. _(More evaluation metrics forthcoming upon completion of evaluation protocol.)_
## Factors
*This section lists some different aspects of what BLOOM models. Its focus is on those aspects that are likely to give rise to high variance in model behavior.*
- Language, such as English or Yoruba
- Domain, such as newswire or stories
- Demographic characteristics, such as gender or nationality
## Results
*Results are based on the [Factors](#factors) and [Metrics](#metrics).*
**Train-time Evaluation:**
As of 25.May.2022, 15:00 PST:
- Training Loss: 2.0
- Validation Loss: 2.2
- Perplexity: 8.9
(More evaluation scores forthcoming.)
</details>
---
# Recommendations
*This section provides information on warnings and potential mitigations.*
<details>
<summary>Click to expand</summary>
- Indirect users should be made aware when the content they're working with is created by the LLM.
- Users should be aware of [Risks and Limitations](#risks-and-limitations), and include an appropriate age disclaimer or blocking interface as necessary.
- Models trained or finetuned downstream of BLOOM LM should include an updated Model Card.
- Users of the model should provide mechanisms for those affected to provide feedback, such as an email address for comments.
</details>
---
# Glossary and Calculations
*This section defines common terms and how metrics are calculated.*
<details>
<summary>Click to expand</summary>
- <a name="loss">**Loss:**</a> A calculation of the difference between what the model has learned and what the data shows ("groundtruth"). The lower the loss, the better. The training process aims to minimize the loss.
- <a name="perplexity">**Perplexity:**</a> This is based on what the model estimates the probability of new data is. The lower the perplexity, the better. If the model is 100% correct at predicting the next token it will see, then the perplexity is 1. Mathematically this is calculated using entropy.
- <a name="high-stakes">**High-stakes settings:**</a> Such as those identified as "high-risk AI systems" and "unacceptable risk AI systems" in the European Union's proposed [Artificial Intelligence (AI) Act](https://artificialintelligenceact.eu/annexes/).
- <a name="critical-decisions">**Critical decisions:**</a> Such as those defined in [the United States' proposed Algorithmic Accountability Act](https://www.congress.gov/117/bills/s3572/BILLS-117s3572is.pdf).
- <a name="human-rights">**Human rights:**</a> Includes those rights defined in the [Universal Declaration of Human Rights](https://www.un.org/sites/un2.un.org/files/2021/03/udhr.pdf).
- <a name="personal-data-and-information">**Personal Data and Personal Information:**</a> Personal data and information is defined in multiple data protection regulations, such as "[personal data](https://gdpr-info.eu/issues/personal-data/)" in the [European Union's General Data Protection Regulation](https://gdpr-info.eu); and "personal information" in the Republic of South Africa's [Protection of Personal Information Act](https://www.gov.za/sites/default/files/gcis_document/201409/3706726-11act4of2013popi.pdf), The People's Republic of China's [Personal information protection law](http://en.npc.gov.cn.cdurl.cn/2021-12/29/c_694559.htm).
- <a name="sensitive-characteristics">**Sensitive characteristics:**</a> This includes specifically protected categories in human rights (see [UHDR, Article 2](https://www.un.org/sites/un2.un.org/files/2021/03/udhr.pdf)) and personal information regulation (see GDPR, [Article 9; Protection of Personal Information Act, Chapter 1](https://www.gov.za/sites/default/files/gcis_document/201409/3706726-11act4of2013popi.pdf))
- <a name="deception">**Deception:**</a> Doing something to intentionally mislead individuals to believe something that is false, such as by creating deadbots or chatbots on social media posing as real people, or generating text documents without making consumers aware that the text is machine generated.
</details>
---
# More Information
*This section provides links to writing on dataset creation, technical specifications, lessons learned, and initial results.*
<details>
<summary>Click to expand</summary>
## Dataset Creation
Blog post detailing the design choices during the dataset creation: https://bigscience.huggingface.co/blog/building-a-tb-scale-multilingual-dataset-for-language-modeling
## Technical Specifications
Blog post summarizing how the architecture, size, shape, and pre-training duration where selected: https://bigscience.huggingface.co/blog/what-language-model-to-train-if-you-have-two-million-gpu-hours
More details on the architecture/optimizer: https://github.com/bigscience-workshop/bigscience/tree/master/train/tr11-176B-ml
Blog post on the hardware/engineering side: https://bigscience.huggingface.co/blog/which-hardware-to-train-a-176b-parameters-model
Details on the distributed setup used for the training: https://github.com/bigscience-workshop/bigscience/tree/master/train/tr11-176B-ml
Tensorboard updated during the training: https://huggingface.co/bigscience/tr11-176B-ml-logs/tensorboard#scalars&tagFilter=loss
## Lessons
Insights on how to approach training, negative results: https://github.com/bigscience-workshop/bigscience/blob/master/train/lessons-learned.md
Details on the obstacles overcome during the preparation on the engineering side (instabilities, optimization of training throughput, so many technical tricks and questions): https://github.com/bigscience-workshop/bigscience/blob/master/train/tr11-176B-ml/chronicles.md
## Initial Results
Initial prompting experiments using interim checkpoints: https://huggingface.co/spaces/bigscience/bloom-book
</details>
---
# Model Card Authors
*Ordered roughly chronologically and by amount of time spent.*
Margaret Mitchell, Giada Pistilli, Yacine Jernite, Ezinwanne Ozoani, Marissa Gerchick, Nazneen Rajani, Sasha Luccioni, Irene Solaiman, Maraim Masoud, Somaieh Nikpoor, Carlos Muñoz Ferrandis, Stas Bekman, Christopher Akiki, Danish Contractor, David Lansky, Angelina McMillan-Major, Tristan Thrush, Suzana Ilić, Gérard Dupont, Shayne Longpre, Manan Dey, Stella Biderman, Douwe Kiela, Emi Baylor, Teven Le Scao, Aaron Gokaslan, Julien Launay |
amanbawa96/roberta_Aman | bff9ecb4654c84e62028f5ea0a1b7ba40c5eece8 | 2022-07-05T20:00:33.000Z | [
"pytorch",
"roberta",
"text-classification",
"transformers"
]
| text-classification | false | amanbawa96 | null | amanbawa96/roberta_Aman | 43 | null | transformers | 6,322 | Entry not found |
ryo0634/luke-base-comp-20181220 | 6cf7a55c09f07ebf0d5d7b4185a4c89516edd000 | 2022-07-08T04:09:52.000Z | [
"pytorch",
"luke",
"fill-mask",
"transformers",
"autotrain_compatible"
]
| fill-mask | false | ryo0634 | null | ryo0634/luke-base-comp-20181220 | 43 | null | transformers | 6,323 | Entry not found |
Samlit/rare-puppers3 | 95b0f560e86f5642b4693a78f4e912ecd83416ec | 2022-07-14T15:39:40.000Z | [
"pytorch",
"tensorboard",
"vit",
"image-classification",
"transformers",
"huggingpics",
"model-index"
]
| image-classification | false | Samlit | null | Samlit/rare-puppers3 | 43 | null | transformers | 6,324 | ---
tags:
- image-classification
- pytorch
- huggingpics
metrics:
- accuracy
model-index:
- name: rare-puppers3
results:
- task:
name: Image Classification
type: image-classification
metrics:
- name: Accuracy
type: accuracy
value: 1.0
---
# rare-puppers3
Autogenerated by HuggingPics🤗🖼️
Create your own image classifier for **anything** by running [the demo on Google Colab](https://colab.research.google.com/github/nateraw/huggingpics/blob/main/HuggingPics.ipynb).
Report any issues with the demo at the [github repo](https://github.com/nateraw/huggingpics).
## Example Images
#### Marcelle Lender doing the Bolero in Chilperic

#### Moulin Rouge_ La Goulue - Henri Toulouse-Lautrec

#### Salon at the Rue des Moulins - Henri de Toulouse-Lautrec

#### aristide bruant - Henri de Toulouse-Lautrec
 |
brjezierski/bert-to-gpt2-german-to-easy-german-2 | e7bba8da8a492eb221f83d1cbad7185eb0c3e5d5 | 2022-07-24T11:21:11.000Z | [
"pytorch",
"encoder-decoder",
"text2text-generation",
"transformers",
"autotrain_compatible"
]
| text2text-generation | false | brjezierski | null | brjezierski/bert-to-gpt2-german-to-easy-german-2 | 43 | null | transformers | 6,325 | Entry not found |
AI-Lab-Makerere/lg_en | 49f3c2ae6fd12733f777c06f24db4fb4506cd750 | 2022-06-28T08:39:16.000Z | [
"pytorch",
"marian",
"text2text-generation",
"unk",
"dataset:EricPeter/autonlp-data-MarianMT_lg_en",
"transformers",
"autonlp",
"co2_eq_emissions",
"autotrain_compatible"
]
| text2text-generation | false | AI-Lab-Makerere | null | AI-Lab-Makerere/lg_en | 42 | 1 | transformers | 6,326 | ---
tags: autonlp
language: unk
widget:
- text: "I love AutoNLP 🤗"
datasets:
- EricPeter/autonlp-data-MarianMT_lg_en
co2_eq_emissions: 126.34446293851818
---
# Model Trained Using AutoNLP
- Problem type: Machine Translation
- Model ID: 475112539
- CO2 Emissions (in grams): 126.34446293851818
## Validation Metrics
- Loss: 1.5376628637313843
- Rouge1: 62.4613
- Rouge2: 39.4759
- RougeL: 58.183
- RougeLsum: 58.226
- Gen Len: 26.5644
## Usage
You can use cURL to access this model:
```
$ curl -X POST -H "Authorization: Bearer YOUR_HUGGINGFACE_API_KEY" -H "Content-Type: application/json" -d '{"inputs": "I love AutoNLP"}' https://api-inference.huggingface.co/EricPeter/autonlp-MarianMT_lg_en-475112539
``` |
Helsinki-NLP/opus-mt-en-is | ad5c0ed5c4458c8a687bda683ca5b5a4ccd39814 | 2021-09-09T21:36:19.000Z | [
"pytorch",
"marian",
"text2text-generation",
"en",
"is",
"transformers",
"translation",
"license:apache-2.0",
"autotrain_compatible"
]
| translation | false | Helsinki-NLP | null | Helsinki-NLP/opus-mt-en-is | 42 | null | transformers | 6,327 | ---
tags:
- translation
license: apache-2.0
---
### opus-mt-en-is
* source languages: en
* target languages: is
* OPUS readme: [en-is](https://github.com/Helsinki-NLP/OPUS-MT-train/blob/master/models/en-is/README.md)
* dataset: opus
* model: transformer-align
* pre-processing: normalization + SentencePiece
* download original weights: [opus-2019-12-18.zip](https://object.pouta.csc.fi/OPUS-MT-models/en-is/opus-2019-12-18.zip)
* test set translations: [opus-2019-12-18.test.txt](https://object.pouta.csc.fi/OPUS-MT-models/en-is/opus-2019-12-18.test.txt)
* test set scores: [opus-2019-12-18.eval.txt](https://object.pouta.csc.fi/OPUS-MT-models/en-is/opus-2019-12-18.eval.txt)
## Benchmarks
| testset | BLEU | chr-F |
|-----------------------|-------|-------|
| Tatoeba.en.is | 25.3 | 0.518 |
|
Helsinki-NLP/opus-mt-es-uk | b32809fe6726fef738108a3c1796ae1096460b9f | 2021-01-18T08:29:39.000Z | [
"pytorch",
"marian",
"text2text-generation",
"es",
"uk",
"transformers",
"translation",
"license:apache-2.0",
"autotrain_compatible"
]
| translation | false | Helsinki-NLP | null | Helsinki-NLP/opus-mt-es-uk | 42 | null | transformers | 6,328 | ---
language:
- es
- uk
tags:
- translation
license: apache-2.0
---
### spa-ukr
* source group: Spanish
* target group: Ukrainian
* OPUS readme: [spa-ukr](https://github.com/Helsinki-NLP/Tatoeba-Challenge/tree/master/models/spa-ukr/README.md)
* model: transformer-align
* source language(s): spa
* target language(s): ukr
* model: transformer-align
* pre-processing: normalization + SentencePiece (spm32k,spm32k)
* download original weights: [opus-2020-06-17.zip](https://object.pouta.csc.fi/Tatoeba-MT-models/spa-ukr/opus-2020-06-17.zip)
* test set translations: [opus-2020-06-17.test.txt](https://object.pouta.csc.fi/Tatoeba-MT-models/spa-ukr/opus-2020-06-17.test.txt)
* test set scores: [opus-2020-06-17.eval.txt](https://object.pouta.csc.fi/Tatoeba-MT-models/spa-ukr/opus-2020-06-17.eval.txt)
## Benchmarks
| testset | BLEU | chr-F |
|-----------------------|-------|-------|
| Tatoeba-test.spa.ukr | 41.3 | 0.614 |
### System Info:
- hf_name: spa-ukr
- source_languages: spa
- target_languages: ukr
- opus_readme_url: https://github.com/Helsinki-NLP/Tatoeba-Challenge/tree/master/models/spa-ukr/README.md
- original_repo: Tatoeba-Challenge
- tags: ['translation']
- languages: ['es', 'uk']
- src_constituents: {'spa'}
- tgt_constituents: {'ukr'}
- src_multilingual: False
- tgt_multilingual: False
- prepro: normalization + SentencePiece (spm32k,spm32k)
- url_model: https://object.pouta.csc.fi/Tatoeba-MT-models/spa-ukr/opus-2020-06-17.zip
- url_test_set: https://object.pouta.csc.fi/Tatoeba-MT-models/spa-ukr/opus-2020-06-17.test.txt
- src_alpha3: spa
- tgt_alpha3: ukr
- short_pair: es-uk
- chrF2_score: 0.614
- bleu: 41.3
- brevity_penalty: 0.983
- ref_len: 53833.0
- src_name: Spanish
- tgt_name: Ukrainian
- train_date: 2020-06-17
- src_alpha2: es
- tgt_alpha2: uk
- prefer_old: False
- long_pair: spa-ukr
- helsinki_git_sha: 480fcbe0ee1bf4774bcbe6226ad9f58e63f6c535
- transformers_git_sha: 2207e5d8cb224e954a7cba69fa4ac2309e9ff30b
- port_machine: brutasse
- port_time: 2020-08-21-14:41 |
Helsinki-NLP/opus-mt-sv-fr | 232bc81ec9c58ac63268051775a58f71e1cb5ba9 | 2021-09-10T14:06:31.000Z | [
"pytorch",
"marian",
"text2text-generation",
"sv",
"fr",
"transformers",
"translation",
"license:apache-2.0",
"autotrain_compatible"
]
| translation | false | Helsinki-NLP | null | Helsinki-NLP/opus-mt-sv-fr | 42 | null | transformers | 6,329 | ---
tags:
- translation
license: apache-2.0
---
### opus-mt-sv-fr
* source languages: sv
* target languages: fr
* OPUS readme: [sv-fr](https://github.com/Helsinki-NLP/OPUS-MT-train/blob/master/models/sv-fr/README.md)
* dataset: opus
* model: transformer-align
* pre-processing: normalization + SentencePiece
* download original weights: [opus-2020-01-24.zip](https://object.pouta.csc.fi/OPUS-MT-models/sv-fr/opus-2020-01-24.zip)
* test set translations: [opus-2020-01-24.test.txt](https://object.pouta.csc.fi/OPUS-MT-models/sv-fr/opus-2020-01-24.test.txt)
* test set scores: [opus-2020-01-24.eval.txt](https://object.pouta.csc.fi/OPUS-MT-models/sv-fr/opus-2020-01-24.eval.txt)
## Benchmarks
| testset | BLEU | chr-F |
|-----------------------|-------|-------|
| Tatoeba.sv.fr | 59.7 | 0.731 |
|
Helsinki-NLP/opus-mt-sv-no | 720a49c2c10392a0b5ef10f78a39ebcfd7f1839d | 2020-08-21T14:42:50.000Z | [
"pytorch",
"marian",
"text2text-generation",
"sv",
"no",
"transformers",
"translation",
"license:apache-2.0",
"autotrain_compatible"
]
| translation | false | Helsinki-NLP | null | Helsinki-NLP/opus-mt-sv-no | 42 | null | transformers | 6,330 | ---
language:
- sv
- no
tags:
- translation
license: apache-2.0
---
### swe-nor
* source group: Swedish
* target group: Norwegian
* OPUS readme: [swe-nor](https://github.com/Helsinki-NLP/Tatoeba-Challenge/tree/master/models/swe-nor/README.md)
* model: transformer-align
* source language(s): swe
* target language(s): nno nob
* model: transformer-align
* pre-processing: normalization + SentencePiece (spm4k,spm4k)
* a sentence initial language token is required in the form of `>>id<<` (id = valid target language ID)
* download original weights: [opus-2020-06-17.zip](https://object.pouta.csc.fi/Tatoeba-MT-models/swe-nor/opus-2020-06-17.zip)
* test set translations: [opus-2020-06-17.test.txt](https://object.pouta.csc.fi/Tatoeba-MT-models/swe-nor/opus-2020-06-17.test.txt)
* test set scores: [opus-2020-06-17.eval.txt](https://object.pouta.csc.fi/Tatoeba-MT-models/swe-nor/opus-2020-06-17.eval.txt)
## Benchmarks
| testset | BLEU | chr-F |
|-----------------------|-------|-------|
| Tatoeba-test.swe.nor | 65.8 | 0.796 |
### System Info:
- hf_name: swe-nor
- source_languages: swe
- target_languages: nor
- opus_readme_url: https://github.com/Helsinki-NLP/Tatoeba-Challenge/tree/master/models/swe-nor/README.md
- original_repo: Tatoeba-Challenge
- tags: ['translation']
- languages: ['sv', 'no']
- src_constituents: {'swe'}
- tgt_constituents: {'nob', 'nno'}
- src_multilingual: False
- tgt_multilingual: False
- prepro: normalization + SentencePiece (spm4k,spm4k)
- url_model: https://object.pouta.csc.fi/Tatoeba-MT-models/swe-nor/opus-2020-06-17.zip
- url_test_set: https://object.pouta.csc.fi/Tatoeba-MT-models/swe-nor/opus-2020-06-17.test.txt
- src_alpha3: swe
- tgt_alpha3: nor
- short_pair: sv-no
- chrF2_score: 0.7959999999999999
- bleu: 65.8
- brevity_penalty: 0.991
- ref_len: 3682.0
- src_name: Swedish
- tgt_name: Norwegian
- train_date: 2020-06-17
- src_alpha2: sv
- tgt_alpha2: no
- prefer_old: False
- long_pair: swe-nor
- helsinki_git_sha: 480fcbe0ee1bf4774bcbe6226ad9f58e63f6c535
- transformers_git_sha: 2207e5d8cb224e954a7cba69fa4ac2309e9ff30b
- port_machine: brutasse
- port_time: 2020-08-21-14:41 |
Luciano/bert-base-portuguese-cased-finetuned-tcu-acordaos | 64c307200bdada3f7f33c9b98254559699f48410 | 2022-02-18T10:22:19.000Z | [
"pytorch",
"tensorboard",
"bert",
"fill-mask",
"pt",
"transformers",
"generated_from_trainer",
"license:mit",
"model-index",
"autotrain_compatible"
]
| fill-mask | false | Luciano | null | Luciano/bert-base-portuguese-cased-finetuned-tcu-acordaos | 42 | null | transformers | 6,331 | ---
language:
- pt
license: mit
tags:
- generated_from_trainer
model-index:
- name: bert-base-portuguese-cased-finetuned-tcu-acordaos
results: []
---
<!-- This model card has been generated automatically according to the information the Trainer had access to. You
should probably proofread and complete it, then remove this comment. -->
# bert-base-portuguese-cased-finetuned-tcu-acordaos
This model is a fine-tuned version of [neuralmind/bert-base-portuguese-cased](https://huggingface.co/neuralmind/bert-base-portuguese-cased) on the None dataset.
It achieves the following results on the evaluation set:
- Loss: 0.5765
## Model description
More information needed
## Intended uses & limitations
More information needed
## Training and evaluation data
More information needed
## Training procedure
### Training hyperparameters
The following hyperparameters were used during training:
- learning_rate: 2e-05
- train_batch_size: 8
- eval_batch_size: 8
- seed: 42
- optimizer: Adam with betas=(0.9,0.999) and epsilon=1e-08
- lr_scheduler_type: linear
- num_epochs: 3.0
### Training results
| Training Loss | Epoch | Step | Validation Loss |
|:-------------:|:-----:|:----:|:---------------:|
| 0.7308 | 1.0 | 1383 | 0.6286 |
| 0.6406 | 2.0 | 2766 | 0.5947 |
| 0.6033 | 3.0 | 4149 | 0.5881 |
### Framework versions
- Transformers 4.11.3
- Pytorch 1.9.0+cu111
- Datasets 1.13.2
- Tokenizers 0.10.3
|
Rajaram1996/Hubert_emotion | 7c15f7861bfecd2f6cccd421bb77b07991290246 | 2021-11-03T22:07:05.000Z | [
"pytorch",
"hubert",
"transformers",
"speech",
"audio",
"HUBert",
"audio-classification"
]
| audio-classification | false | Rajaram1996 | null | Rajaram1996/Hubert_emotion | 42 | null | transformers | 6,332 | ---
inference: true
pipeline_tag: audio-classification
tags:
- speech
- audio
- HUBert
---
A place to hold the model for easier inference. |
anuragshas/wav2vec2-large-xlsr-53-rm-vallader | 191c8fcc76c91a5ffc213b89f41ba1d9cc3811a5 | 2021-07-05T21:21:11.000Z | [
"pytorch",
"jax",
"wav2vec2",
"automatic-speech-recognition",
"rm-vallader",
"dataset:common_voice",
"transformers",
"audio",
"speech",
"xlsr-fine-tuning-week",
"license:apache-2.0",
"model-index"
]
| automatic-speech-recognition | false | anuragshas | null | anuragshas/wav2vec2-large-xlsr-53-rm-vallader | 42 | null | transformers | 6,333 | ---
language: rm-vallader
datasets:
- common_voice
metrics:
- wer
tags:
- audio
- automatic-speech-recognition
- speech
- xlsr-fine-tuning-week
license: apache-2.0
model-index:
- name: Anurag Singh XLSR Wav2Vec2 Large 53 Romansh Vallader
results:
- task:
name: Speech Recognition
type: automatic-speech-recognition
dataset:
name: Common Voice rm-vallader
type: common_voice
args: rm-vallader
metrics:
- name: Test WER
type: wer
value: 32.89
---
# Wav2Vec2-Large-XLSR-53-Romansh Vallader
Fine-tuned [facebook/wav2vec2-large-xlsr-53](https://huggingface.co/facebook/wav2vec2-large-xlsr-53) on Romansh Vallader using the [Common Voice](https://huggingface.co/datasets/common_voice).
When using this model, make sure that your speech input is sampled at 16kHz.
## Usage
The model can be used directly (without a language model) as follows:
```python
import torch
import torchaudio
from datasets import load_dataset
from transformers import Wav2Vec2ForCTC, Wav2Vec2Processor
test_dataset = load_dataset("common_voice", "rm-vallader", split="test[:2%]")
processor = Wav2Vec2Processor.from_pretrained("anuragshas/wav2vec2-large-xlsr-53-rm-vallader")
model = Wav2Vec2ForCTC.from_pretrained("anuragshas/wav2vec2-large-xlsr-53-rm-vallader")
resampler = torchaudio.transforms.Resample(48_000, 16_000)
# Preprocessing the datasets.
# We need to read the aduio files as arrays
def speech_file_to_array_fn(batch):
speech_array, sampling_rate = torchaudio.load(batch["path"])
batch["speech"] = resampler(speech_array).squeeze().numpy()
return batch
test_dataset = test_dataset.map(speech_file_to_array_fn)
inputs = processor(test_dataset["speech"][:2], sampling_rate=16_000, return_tensors="pt", padding=True)
with torch.no_grad():
logits = model(inputs.input_values, attention_mask=inputs.attention_mask).logits
predicted_ids = torch.argmax(logits, dim=-1)
print("Prediction:", processor.batch_decode(predicted_ids))
print("Reference:", test_dataset["sentence"][:2])
```
## Evaluation
The model can be evaluated as follows on the Romansh Vallader test data of Common Voice.
```python
import torch
import torchaudio
from datasets import load_dataset, load_metric
from transformers import Wav2Vec2ForCTC, Wav2Vec2Processor
import re
test_dataset = load_dataset("common_voice", "rm-vallader", split="test")
wer = load_metric("wer")
processor = Wav2Vec2Processor.from_pretrained("anuragshas/wav2vec2-large-xlsr-53-rm-vallader")
model = Wav2Vec2ForCTC.from_pretrained("anuragshas/wav2vec2-large-xlsr-53-rm-vallader")
model.to("cuda")
chars_to_ignore_regex = '[\,\?\.\!\-\;\:\"\“\%\”\„\–\…\«\»]'
resampler = torchaudio.transforms.Resample(48_000, 16_000)
# Preprocessing the datasets.
# We need to read the aduio files as arrays
def speech_file_to_array_fn(batch):
batch["sentence"] = re.sub('’ ',' ',batch["sentence"])
batch["sentence"] = re.sub(' ‘',' ',batch["sentence"])
batch["sentence"] = re.sub('’|‘','\'',batch["sentence"])
batch["sentence"] = re.sub(chars_to_ignore_regex, '', batch["sentence"]).lower()
speech_array, sampling_rate = torchaudio.load(batch["path"])
batch["speech"] = resampler(speech_array).squeeze().numpy()
return batch
test_dataset = test_dataset.map(speech_file_to_array_fn)
# Preprocessing the datasets.
# We need to read the aduio files as arrays
def evaluate(batch):
inputs = processor(batch["speech"], sampling_rate=16_000, return_tensors="pt", padding=True)
with torch.no_grad():
logits = model(inputs.input_values.to("cuda"), attention_mask=inputs.attention_mask.to("cuda")).logits
pred_ids = torch.argmax(logits, dim=-1)
batch["pred_strings"] = processor.batch_decode(pred_ids)
return batch
result = test_dataset.map(evaluate, batched=True, batch_size=8)
print("WER: {:2f}".format(100 * wer.compute(predictions=result["pred_strings"], references=result["sentence"])))
```
**Test Result**: 32.89 %
## Training
The Common Voice `train` and `validation` datasets were used for training. |
csarron/roberta-large-squad-v1 | bd58492f1067002a99a598c526fbeb3e3b6ab983 | 2021-05-20T15:51:59.000Z | [
"pytorch",
"jax",
"roberta",
"question-answering",
"transformers",
"autotrain_compatible"
]
| question-answering | false | csarron | null | csarron/roberta-large-squad-v1 | 42 | null | transformers | 6,334 | Entry not found |
edugp/wav2vec2-xls-r-300m-cv8-es | cc83c8946fdd2e61eb778261b132866c069f00b8 | 2022-02-08T08:57:24.000Z | [
"pytorch",
"wav2vec2",
"automatic-speech-recognition",
"dataset:common_voice",
"transformers",
"generated_from_trainer",
"license:apache-2.0",
"model-index"
]
| automatic-speech-recognition | false | edugp | null | edugp/wav2vec2-xls-r-300m-cv8-es | 42 | null | transformers | 6,335 | ---
license: apache-2.0
tags:
- generated_from_trainer
datasets:
- common_voice
model-index:
- name: wav2vec2-xls-r-300m-cv8-es
results: []
---
<!-- This model card has been generated automatically according to the information the Trainer had access to. You
should probably proofread and complete it, then remove this comment. -->
# wav2vec2-xls-r-300m-cv8-es
This model is a fine-tuned version of [facebook/wav2vec2-xls-r-300m](https://huggingface.co/facebook/wav2vec2-xls-r-300m) on the common_voice dataset.
It achieves the following results on the evaluation set:
- eval_loss: 0.2115
- eval_wer: 0.1931
- eval_runtime: 859.964
- eval_samples_per_second: 17.954
- eval_steps_per_second: 2.244
- epoch: 6.97
- step: 50000
## Model description
More information needed
## Intended uses & limitations
More information needed
## Training and evaluation data
More information needed
## Training procedure
### Training hyperparameters
The following hyperparameters were used during training:
- learning_rate: 0.0003
- train_batch_size: 16
- eval_batch_size: 8
- seed: 42
- gradient_accumulation_steps: 2
- total_train_batch_size: 32
- optimizer: Adam with betas=(0.9,0.999) and epsilon=1e-08
- lr_scheduler_type: linear
- lr_scheduler_warmup_steps: 500
- num_epochs: 30
- mixed_precision_training: Native AMP
### Framework versions
- Transformers 4.16.0.dev0
- Pytorch 1.10.1+cu102
- Datasets 1.18.3
- Tokenizers 0.11.0
|
entelecheia/ekonelectra-base-discriminator | 539e39d62f98f62ed431df524aa9a5f4f629b38a | 2021-05-04T09:05:45.000Z | [
"pytorch",
"electra",
"pretraining",
"transformers"
]
| null | false | entelecheia | null | entelecheia/ekonelectra-base-discriminator | 42 | null | transformers | 6,336 | Entry not found |
gchhablani/bert-base-cased-finetuned-qnli | e6c6176e551e137fbc6ab174ebd06f12215dbe1f | 2021-09-20T09:08:27.000Z | [
"pytorch",
"tensorboard",
"bert",
"text-classification",
"en",
"dataset:glue",
"arxiv:2105.03824",
"transformers",
"generated_from_trainer",
"fnet-bert-base-comparison",
"license:apache-2.0",
"model-index"
]
| text-classification | false | gchhablani | null | gchhablani/bert-base-cased-finetuned-qnli | 42 | 1 | transformers | 6,337 | ---
language:
- en
license: apache-2.0
tags:
- generated_from_trainer
- fnet-bert-base-comparison
datasets:
- glue
metrics:
- accuracy
model-index:
- name: bert-base-cased-finetuned-qnli
results:
- task:
name: Text Classification
type: text-classification
dataset:
name: GLUE QNLI
type: glue
args: qnli
metrics:
- name: Accuracy
type: accuracy
value: 0.9099395936298736
---
<!-- This model card has been generated automatically according to the information the Trainer had access to. You
should probably proofread and complete it, then remove this comment. -->
# bert-base-cased-finetuned-qnli
This model is a fine-tuned version of [bert-base-cased](https://huggingface.co/bert-base-cased) on the GLUE QNLI dataset.
It achieves the following results on the evaluation set:
- Loss: 0.3986
- Accuracy: 0.9099
The model was fine-tuned to compare [google/fnet-base](https://huggingface.co/google/fnet-base) as introduced in [this paper](https://arxiv.org/abs/2105.03824) against [bert-base-cased](https://huggingface.co/bert-base-cased).
## Model description
More information needed
## Intended uses & limitations
More information needed
## Training and evaluation data
More information needed
## Training procedure
This model is trained using the [run_glue](https://github.com/huggingface/transformers/blob/master/examples/pytorch/text-classification/run_glue.py) script. The following command was used:
```bash
#!/usr/bin/bash
python ../run_glue.py \\n --model_name_or_path bert-base-cased \\n --task_name qnli \\n --do_train \\n --do_eval \\n --max_seq_length 512 \\n --per_device_train_batch_size 16 \\n --learning_rate 2e-5 \\n --num_train_epochs 3 \\n --output_dir bert-base-cased-finetuned-qnli \\n --push_to_hub \\n --hub_strategy all_checkpoints \\n --logging_strategy epoch \\n --save_strategy epoch \\n --evaluation_strategy epoch \\n```
### Training hyperparameters
The following hyperparameters were used during training:
- learning_rate: 2e-05
- train_batch_size: 16
- eval_batch_size: 8
- seed: 42
- optimizer: Adam with betas=(0.9,0.999) and epsilon=1e-08
- lr_scheduler_type: linear
- num_epochs: 3.0
### Training results
| Training Loss | Epoch | Step | Accuracy | Validation Loss |
|:-------------:|:-----:|:-----:|:--------:|:---------------:|
| 0.337 | 1.0 | 6547 | 0.9013 | 0.2448 |
| 0.1971 | 2.0 | 13094 | 0.9143 | 0.2839 |
| 0.1175 | 3.0 | 19641 | 0.9099 | 0.3986 |
### Framework versions
- Transformers 4.11.0.dev0
- Pytorch 1.9.0
- Datasets 1.12.1
- Tokenizers 0.10.3
|
huggingtweets/mechanical_monk | d5cbe44b20fe5fd741f611d743a836b3558de11e | 2021-05-22T14:04:31.000Z | [
"pytorch",
"jax",
"gpt2",
"text-generation",
"en",
"transformers",
"huggingtweets"
]
| text-generation | false | huggingtweets | null | huggingtweets/mechanical_monk | 42 | null | transformers | 6,338 | ---
language: en
thumbnail: https://www.huggingtweets.com/mechanical_monk/1616856045639/predictions.png
tags:
- huggingtweets
widget:
- text: "My dream is"
---
<div>
<div style="width: 132px; height:132px; border-radius: 50%; background-size: cover; background-image: url('https://pbs.twimg.com/profile_images/1374353206898229249/p9ZaR7AU_400x400.jpg')">
</div>
<div style="margin-top: 8px; font-size: 19px; font-weight: 800">Solar Monk 🤖 AI Bot </div>
<div style="font-size: 15px">@mechanical_monk bot</div>
</div>
I was made with [huggingtweets](https://github.com/borisdayma/huggingtweets).
Create your own bot based on your favorite user with [the demo](https://colab.research.google.com/github/borisdayma/huggingtweets/blob/master/huggingtweets-demo.ipynb)!
## How does it work?
The model uses the following pipeline.
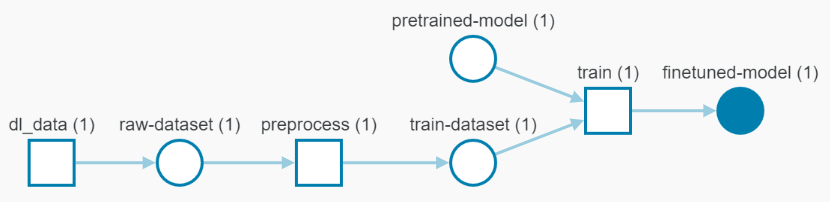
To understand how the model was developed, check the [W&B report](https://wandb.ai/wandb/huggingtweets/reports/HuggingTweets-Train-a-Model-to-Generate-Tweets--VmlldzoxMTY5MjI).
## Training data
The model was trained on [@mechanical_monk's tweets](https://twitter.com/mechanical_monk).
| Data | Quantity |
| --- | --- |
| Tweets downloaded | 3250 |
| Retweets | 9 |
| Short tweets | 540 |
| Tweets kept | 2701 |
[Explore the data](https://wandb.ai/wandb/huggingtweets/runs/3qymex3o/artifacts), which is tracked with [W&B artifacts](https://docs.wandb.com/artifacts) at every step of the pipeline.
## Training procedure
The model is based on a pre-trained [GPT-2](https://huggingface.co/gpt2) which is fine-tuned on @mechanical_monk's tweets.
Hyperparameters and metrics are recorded in the [W&B training run](https://wandb.ai/wandb/huggingtweets/runs/15h2yysz) for full transparency and reproducibility.
At the end of training, [the final model](https://wandb.ai/wandb/huggingtweets/runs/15h2yysz/artifacts) is logged and versioned.
## How to use
You can use this model directly with a pipeline for text generation:
```python
from transformers import pipeline
generator = pipeline('text-generation',
model='huggingtweets/mechanical_monk')
generator("My dream is", num_return_sequences=5)
```
## Limitations and bias
The model suffers from [the same limitations and bias as GPT-2](https://huggingface.co/gpt2#limitations-and-bias).
In addition, the data present in the user's tweets further affects the text generated by the model.
## About
*Built by Boris Dayma*
[](https://twitter.com/intent/follow?screen_name=borisdayma)
For more details, visit the project repository.
[](https://github.com/borisdayma/huggingtweets)
|
jaron-maene/gpt2-large-nl2bash | fd0ec759fc27149277f07d40ed04bdb16aaf625d | 2021-05-23T05:38:26.000Z | [
"pytorch",
"jax",
"gpt2",
"text-generation",
"transformers"
]
| text-generation | false | jaron-maene | null | jaron-maene/gpt2-large-nl2bash | 42 | null | transformers | 6,339 | Entry not found |
LACAI/gpt2-xl-dialog-narrative-persuasion | a862a1de73a001485a4ae5ab85d6b2161b497f62 | 2021-12-21T17:22:02.000Z | [
"pytorch",
"gpt2",
"text-generation",
"transformers"
]
| text-generation | false | LACAI | null | LACAI/gpt2-xl-dialog-narrative-persuasion | 42 | null | transformers | 6,340 | Base model: [gpt2-xl](https://huggingface.co/gpt2-xl)
Domain-adapted for dialogue response and narrative generation on a [narrative-aligned variant](https://github.com/AbrahamSanders/gutenberg-dialog#download-narrative-aligned-datasets) of the [Gutenberg Dialogue Dataset (Csaky & Recski, 2021)](https://aclanthology.org/2021.eacl-main.11.pdf)
Fine-tuned for dialogue response generation on [Persuasion For Good (Wang et al., 2019)](https://aclanthology.org/P19-1566.pdf) ([dataset](https://gitlab.com/ucdavisnlp/persuasionforgood)) |
laifuchicago/farm2tran | cfb9974f8df2bca9eb17da76d1cda393aa217aad | 2020-09-16T01:15:14.000Z | [
"pytorch",
"xlm-roberta",
"question-answering",
"transformers",
"autotrain_compatible"
]
| question-answering | false | laifuchicago | null | laifuchicago/farm2tran | 42 | null | transformers | 6,341 | Entry not found |
marefa-nlp/summarization-arabic-english-news | 161ce90f31a0f04358ad2af002e80a52aa8b8bac | 2021-07-13T13:06:31.000Z | [
"pytorch",
"t5",
"text2text-generation",
"transformers",
"autotrain_compatible"
]
| text2text-generation | false | marefa-nlp | null | marefa-nlp/summarization-arabic-english-news | 42 | null | transformers | 6,342 | ------------
## Arabic and English News Summarization NLP Model
### About
This model is for summarizing news stories in short highlights for both Arabic and English tasks.
نموذج معرفي متخصص في تلخيص الأخبار العربية و الإنجليزية الى مجموعة من أهم النقاط
### Fine-Tuning
The model was finetuned using the [Arabic T5 Model](https://huggingface.co/bakrianoo/t5-arabic-large) which developed by [Abu Bakr Soliman](http://github.com/bakrianoo).
The primary summarization model also developed by the same developer.
### How to Use
- You can use this [Colab Notebook](https://colab.research.google.com/drive/1DWND1CAfCXD4OxrfmLBEaKeXhjGmYkod?usp=sharing) to test the model
1. Install [PyTorch](https://pytorch.org/)
2. Install the following Python packages
`$ pip3 install transformers==4.7.0 nltk==3.5 protobuf==3.15.3 sentencepiece==0.1.96`
3. Run this code
```python
from transformers import AutoTokenizer, AutoModelWithLMHead
import torch
import nltk
nltk.download('punkt')
from nltk.tokenize import sent_tokenize
device = torch.device("cuda:0" if torch.cuda.is_available() else "cpu")
m_name = "marefa-nlp/summarization-arabic-english-news"
tokenizer = AutoTokenizer.from_pretrained(m_name)
model = AutoModelWithLMHead.from_pretrained(m_name).to(device)
def get_summary(text, tokenizer, model, device="cpu", num_beams=2):
if len(text.strip()) < 50:
return ["Please provide more longer text"]
text = "summarize: <paragraph> " + " <paragraph> ".join([ s.strip() for s in sent_tokenize(text) if s.strip() != ""]) + " </s>"
text = text.strip().replace("\n","")
tokenized_text = tokenizer.encode(text, return_tensors="pt").to(device)
summary_ids = model.generate(
tokenized_text,
max_length=512,
num_beams=num_beams,
repetition_penalty=1.5,
length_penalty=1.0,
early_stopping=True
)
output = tokenizer.decode(summary_ids[0], skip_special_tokens=True)
return [ s.strip() for s in output.split("<hl>") if s.strip() != "" ]
## Prepare Samples
samples = [
"""
قال المدافع الإيطالي ليوناردو بونوتشي إن منتخب بلاده ليس خائفا من مواجهة نظيره الانجليزي على أرضه في المباراة النهائية في بطولة يورو 2020 لكرة القدم، في حين وصف المدافع الانجليزي جون ستونز المباراة المرتقبة بأنها ستكون "أكثر تميزا".
وسوف تقام المباراة في استاد ويمبلي، شمال غربي لندن، يوم الأحد.
وتسعى إيطاليا لإحراز اللقب الأوروبي للمرة الثانية بعد فوزها به أول مرة عام 1968.
ولم يفز الفريق الانجليزي بهذا اللقب القاري من قبل. والبطولة الرئيسية الوحيدة التي فازت بها انجلترا هي كأس العالم عام 1966 الذي أقيمت مباراته النهائية في استاد ويمبلي.
""",
"""
On a night fraught with tension, Italy clinched its first major title for 15 years with a penalty shootout win over England in the Euro 2020 final.
Luke Shaw's goal inside the opening two minutes gave England a lead it looked like it would hold onto all night, before a goalmouth scramble midway through the second half allowed Leonardo Bonucci to poke home an equalizer for Italy.
For the remainder of the match, it felt as though extra-time and penalties were inevitable, as neither side seemed willing or brave enough to commit enough men forward to really trouble the opposing defenders.
England had suffered innumerable heartbreaks on penalties over the years and this time it was Italy's turn to inflict yet more pain on beleaguered English fans as Marcus Rashford, Jadon Sancho and Bukayo Saka all missed from the spot.
""",
]
## Get summaries
print("Original Article:", samples[0])
print("\n===========\nSummary: \n")
hls = get_summary(samples[0], tokenizer, model, device)
for hl in hls:
print("\t-", hl)
print("Original Article:", samples[1])
print("\n=========== \nSummary: \n")
hls = get_summary(samples[1], tokenizer, model, device)
for hl in hls:
print("\t-", hl)
```
Results
```
Original Article:
قال المدافع الإيطالي ليوناردو بونوتشي إن منتخب بلاده ليس خائفا من مواجهة نظيره الانجليزي على أرضه في المباراة النهائية في بطولة يورو 2020 لكرة القدم، في حين وصف المدافع الانجليزي جون ستونز المباراة المرتقبة بأنها ستكون "أكثر تميزا".
وسوف تقام المباراة في استاد ويمبلي، شمال غربي لندن، يوم الأحد.
وتسعى إيطاليا لإحراز اللقب الأوروبي للمرة الثانية بعد فوزها به أول مرة عام 1968.
ولم يفز الفريق الانجليزي بهذا اللقب القاري من قبل. والبطولة الرئيسية الوحيدة التي فازت بها انجلترا هي كأس العالم عام 1966 الذي أقيمت مباراته النهائية في استاد ويمبلي.
===========
Summary:
- وسوف تواجه إيطاليا إنجلترا في بطولة يورو 2020 لكرة القدم يوم الأحد.
- ستقام المباراة في استاد ويمبلي، شمال غربي لندن، يوم الأحد.
- ولم يفز الفريق الانجليزي بهذا اللقب القاري قبل.
```
```
Original Article:
On a night fraught with tension, Italy clinched its first major title for 15 years with a penalty shootout win over England in the Euro 2020 final.
Luke Shaw's goal inside the opening two minutes gave England a lead it looked like it would hold onto all night, before a goalmouth scramble midway through the second half allowed Leonardo Bonucci to poke home an equalizer for Italy.
For the remainder of the match it felt as though extra-time and penalties were inevitable, as neither side seemed willing or brave enough to commit enough men forward to really trouble the opposing defenders.
England had suffered innumerable heartbreaks on penalties over the years and this time it was Italy's turn to inflict yet more pain on beleaguered English fans as Marcus Rashford, Jadon Sancho and Bukayo Saka all missed from the spot.
===========
Summary:
- Luke Shaw's goal gave England a lead it looked like it would hold onto all night.
- Leonardo Bonucci scored the equalizer for Italy.
- Marcus Rashford, Jadon Sancho and Bukayo Saka all missed.
```
|
monologg/kocharelectra-base-modu-ner-all | 1088b83a351982fea13e933b3d7100c452e31707 | 2020-12-08T17:56:17.000Z | [
"pytorch",
"electra",
"token-classification",
"transformers",
"autotrain_compatible"
]
| token-classification | false | monologg | null | monologg/kocharelectra-base-modu-ner-all | 42 | null | transformers | 6,343 | Entry not found |
monologg/koelectra-small-finetuned-intent-cls | 0ba20de0556ea6b18b554d6e33a91e18fda5390b | 2020-05-15T08:20:13.000Z | [
"pytorch",
"electra",
"text-classification",
"transformers"
]
| text-classification | false | monologg | null | monologg/koelectra-small-finetuned-intent-cls | 42 | null | transformers | 6,344 | Entry not found |
mrm8488/chEMBL26_smiles_v2 | 99b195df7fc93ec93da38e37bc1cbd08ddf61fb6 | 2021-05-20T18:16:29.000Z | [
"pytorch",
"jax",
"roberta",
"fill-mask",
"en",
"transformers",
"drugs",
"chemist",
"drug design",
"smile",
"autotrain_compatible"
]
| fill-mask | false | mrm8488 | null | mrm8488/chEMBL26_smiles_v2 | 42 | null | transformers | 6,345 | ---
language: en
tags:
- drugs
- chemist
- drug design
- smile
widget:
- text: "CC(C)CN(CC(OP(=O)(O)O)C(Cc1ccccc1)NC(=O)OC1CCOC1)S(=O)(=O)c1ccc(N)<mask>"
---
|
nateraw/resnet50-oxford-iiit-pet | 0d14c5c8bd77492862ba2544cffba581ea8ee2ea | 2021-12-03T06:59:13.000Z | [
"pytorch",
"timm",
"image-classification"
]
| image-classification | false | nateraw | null | nateraw/resnet50-oxford-iiit-pet | 42 | null | timm | 6,346 | ---
tags:
- image-classification
- timm
library_tag: timm
---
# Model card for resnet50-oxford-iiit-pet
 |
nlpaueb/bert-base-uncased-echr | f89563b2afe4cf9db700bed1e31c481e4ddb09c5 | 2022-04-28T14:44:26.000Z | [
"pytorch",
"tf",
"jax",
"bert",
"en",
"transformers",
"legal",
"license:cc-by-sa-4.0",
"fill-mask"
]
| fill-mask | false | nlpaueb | null | nlpaueb/bert-base-uncased-echr | 42 | 3 | transformers | 6,347 | ---
language: en
pipeline_tag: fill-mask
license: cc-by-sa-4.0
thumbnail: https://i.ibb.co/p3kQ7Rw/Screenshot-2020-10-06-at-12-16-36-PM.png
tags:
- legal
widget:
- text: "The applicant submitted that her husband was subjected to treatment amounting to [MASK] whilst in the custody of Adana Security Directorate."
---
# LEGAL-BERT: The Muppets straight out of Law School
<img align="left" src="https://i.ibb.co/p3kQ7Rw/Screenshot-2020-10-06-at-12-16-36-PM.png" width="100"/>
LEGAL-BERT is a family of BERT models for the legal domain, intended to assist legal NLP research, computational law, and legal technology applications. To pre-train the different variations of LEGAL-BERT, we collected 12 GB of diverse English legal text from several fields (e.g., legislation, court cases, contracts) scraped from publicly available resources. Sub-domain variants (CONTRACTS-, EURLEX-, ECHR-) and/or general LEGAL-BERT perform better than using BERT out of the box for domain-specific tasks.<br>
This is the sub-domain variant pre-trained on ECHR cases.
<br/><br/>
---
I. Chalkidis, M. Fergadiotis, P. Malakasiotis, N. Aletras and I. Androutsopoulos. "LEGAL-BERT: The Muppets straight out of Law School". In Findings of Empirical Methods in Natural Language Processing (EMNLP 2020) (Short Papers), to be held online, 2020. (https://aclanthology.org/2020.findings-emnlp.261)
---
## Pre-training corpora
The pre-training corpora of LEGAL-BERT include:
* 116,062 documents of EU legislation, publicly available from EURLEX (http://eur-lex.europa.eu), the repository of EU Law running under the EU Publication Office.
* 61,826 documents of UK legislation, publicly available from the UK legislation portal (http://www.legislation.gov.uk).
* 19,867 cases from the European Court of Justice (ECJ), also available from EURLEX.
* 12,554 cases from HUDOC, the repository of the European Court of Human Rights (ECHR) (http://hudoc.echr.coe.int/eng).
* 164,141 cases from various courts across the USA, hosted in the Case Law Access Project portal (https://case.law).
* 76,366 US contracts from EDGAR, the database of US Securities and Exchange Commission (SECOM) (https://www.sec.gov/edgar.shtml).
## Pre-training details
* We trained BERT using the official code provided in Google BERT's GitHub repository (https://github.com/google-research/bert).
* We released a model similar to the English BERT-BASE model (12-layer, 768-hidden, 12-heads, 110M parameters).
* We chose to follow the same training set-up: 1 million training steps with batches of 256 sequences of length 512 with an initial learning rate 1e-4.
* We were able to use a single Google Cloud TPU v3-8 provided for free from [TensorFlow Research Cloud (TFRC)](https://www.tensorflow.org/tfrc), while also utilizing [GCP research credits](https://edu.google.com/programs/credits/research). Huge thanks to both Google programs for supporting us!
## Models list
| Model name | Model Path | Training corpora |
| ------------------- | ------------------------------------ | ------------------- |
| CONTRACTS-BERT-BASE | `nlpaueb/bert-base-uncased-contracts` | US contracts |
| EURLEX-BERT-BASE | `nlpaueb/bert-base-uncased-eurlex` | EU legislation |
| ECHR-BERT-BASE | `nlpaueb/bert-base-uncased-echr` | ECHR cases |
| LEGAL-BERT-BASE * | `nlpaueb/legal-bert-base-uncased` | All |
| LEGAL-BERT-SMALL | `nlpaueb/legal-bert-small-uncased` | All |
\* LEGAL-BERT-BASE is the model referred to as LEGAL-BERT-SC in Chalkidis et al. (2020); a model trained from scratch in the legal corpora mentioned below using a newly created vocabulary by a sentence-piece tokenizer trained on the very same corpora.
\*\* As many of you expressed interest in the LEGAL-BERT-FP models (those relying on the original BERT-BASE checkpoint), they have been released in Archive.org (https://archive.org/details/legal_bert_fp), as these models are secondary and possibly only interesting for those who aim to dig deeper in the open questions of Chalkidis et al. (2020).
## Load Pretrained Model
```python
from transformers import AutoTokenizer, AutoModel
tokenizer = AutoTokenizer.from_pretrained("nlpaueb/bert-base-uncased-echr")
model = AutoModel.from_pretrained("nlpaueb/bert-base-uncased-echr")
```
## Use LEGAL-BERT variants as Language Models
| Corpus | Model | Masked token | Predictions |
| --------------------------------- | ---------------------------------- | ------------ | ------------ |
| | **BERT-BASE-UNCASED** |
| (Contracts) | This [MASK] Agreement is between General Motors and John Murray . | employment | ('new', '0.09'), ('current', '0.04'), ('proposed', '0.03'), ('marketing', '0.03'), ('joint', '0.02')
| (ECHR) | The applicant submitted that her husband was subjected to treatment amounting to [MASK] whilst in the custody of Adana Security Directorate | torture | ('torture', '0.32'), ('rape', '0.22'), ('abuse', '0.14'), ('death', '0.04'), ('violence', '0.03')
| (EURLEX) | Establishing a system for the identification and registration of [MASK] animals and regarding the labelling of beef and beef products . | bovine | ('farm', '0.25'), ('livestock', '0.08'), ('draft', '0.06'), ('domestic', '0.05'), ('wild', '0.05')
| | **CONTRACTS-BERT-BASE** |
| (Contracts) | This [MASK] Agreement is between General Motors and John Murray . | employment | ('letter', '0.38'), ('dealer', '0.04'), ('employment', '0.03'), ('award', '0.03'), ('contribution', '0.02')
| (ECHR) | The applicant submitted that her husband was subjected to treatment amounting to [MASK] whilst in the custody of Adana Security Directorate | torture | ('death', '0.39'), ('imprisonment', '0.07'), ('contempt', '0.05'), ('being', '0.03'), ('crime', '0.02')
| (EURLEX) | Establishing a system for the identification and registration of [MASK] animals and regarding the labelling of beef and beef products . | bovine | (('domestic', '0.18'), ('laboratory', '0.07'), ('household', '0.06'), ('personal', '0.06'), ('the', '0.04')
| | **EURLEX-BERT-BASE** |
| (Contracts) | This [MASK] Agreement is between General Motors and John Murray . | employment | ('supply', '0.11'), ('cooperation', '0.08'), ('service', '0.07'), ('licence', '0.07'), ('distribution', '0.05')
| (ECHR) | The applicant submitted that her husband was subjected to treatment amounting to [MASK] whilst in the custody of Adana Security Directorate | torture | ('torture', '0.66'), ('death', '0.07'), ('imprisonment', '0.07'), ('murder', '0.04'), ('rape', '0.02')
| (EURLEX) | Establishing a system for the identification and registration of [MASK] animals and regarding the labelling of beef and beef products . | bovine | ('live', '0.43'), ('pet', '0.28'), ('certain', '0.05'), ('fur', '0.03'), ('the', '0.02')
| | **ECHR-BERT-BASE** |
| (Contracts) | This [MASK] Agreement is between General Motors and John Murray . | employment | ('second', '0.24'), ('latter', '0.10'), ('draft', '0.05'), ('bilateral', '0.05'), ('arbitration', '0.04')
| (ECHR) | The applicant submitted that her husband was subjected to treatment amounting to [MASK] whilst in the custody of Adana Security Directorate | torture | ('torture', '0.99'), ('death', '0.01'), ('inhuman', '0.00'), ('beating', '0.00'), ('rape', '0.00')
| (EURLEX) | Establishing a system for the identification and registration of [MASK] animals and regarding the labelling of beef and beef products . | bovine | ('pet', '0.17'), ('all', '0.12'), ('slaughtered', '0.10'), ('domestic', '0.07'), ('individual', '0.05')
| | **LEGAL-BERT-BASE** |
| (Contracts) | This [MASK] Agreement is between General Motors and John Murray . | employment | ('settlement', '0.26'), ('letter', '0.23'), ('dealer', '0.04'), ('master', '0.02'), ('supplemental', '0.02')
| (ECHR) | The applicant submitted that her husband was subjected to treatment amounting to [MASK] whilst in the custody of Adana Security Directorate | torture | ('torture', '1.00'), ('detention', '0.00'), ('arrest', '0.00'), ('rape', '0.00'), ('death', '0.00')
| (EURLEX) | Establishing a system for the identification and registration of [MASK] animals and regarding the labelling of beef and beef products . | bovine | ('live', '0.67'), ('beef', '0.17'), ('farm', '0.03'), ('pet', '0.02'), ('dairy', '0.01')
| | **LEGAL-BERT-SMALL** |
| (Contracts) | This [MASK] Agreement is between General Motors and John Murray . | employment | ('license', '0.09'), ('transition', '0.08'), ('settlement', '0.04'), ('consent', '0.03'), ('letter', '0.03')
| (ECHR) | The applicant submitted that her husband was subjected to treatment amounting to [MASK] whilst in the custody of Adana Security Directorate | torture | ('torture', '0.59'), ('pain', '0.05'), ('ptsd', '0.05'), ('death', '0.02'), ('tuberculosis', '0.02')
| (EURLEX) | Establishing a system for the identification and registration of [MASK] animals and regarding the labelling of beef and beef products . | bovine | ('all', '0.08'), ('live', '0.07'), ('certain', '0.07'), ('the', '0.07'), ('farm', '0.05')
## Evaluation on downstream tasks
Consider the experiments in the article "LEGAL-BERT: The Muppets straight out of Law School". Chalkidis et al., 2020, (https://aclanthology.org/2020.findings-emnlp.261)
## Author - Publication
```
@inproceedings{chalkidis-etal-2020-legal,
title = "{LEGAL}-{BERT}: The Muppets straight out of Law School",
author = "Chalkidis, Ilias and
Fergadiotis, Manos and
Malakasiotis, Prodromos and
Aletras, Nikolaos and
Androutsopoulos, Ion",
booktitle = "Findings of the Association for Computational Linguistics: EMNLP 2020",
month = nov,
year = "2020",
address = "Online",
publisher = "Association for Computational Linguistics",
doi = "10.18653/v1/2020.findings-emnlp.261",
pages = "2898--2904"
}
```
## About Us
[AUEB's Natural Language Processing Group](http://nlp.cs.aueb.gr) develops algorithms, models, and systems that allow computers to process and generate natural language texts.
The group's current research interests include:
* question answering systems for databases, ontologies, document collections, and the Web, especially biomedical question answering,
* natural language generation from databases and ontologies, especially Semantic Web ontologies,
text classification, including filtering spam and abusive content,
* information extraction and opinion mining, including legal text analytics and sentiment analysis,
* natural language processing tools for Greek, for example parsers and named-entity recognizers,
machine learning in natural language processing, especially deep learning.
The group is part of the Information Processing Laboratory of the Department of Informatics of the Athens University of Economics and Business.
[Ilias Chalkidis](https://iliaschalkidis.github.io) on behalf of [AUEB's Natural Language Processing Group](http://nlp.cs.aueb.gr)
| Github: [@ilias.chalkidis](https://github.com/iliaschalkidis) | Twitter: [@KiddoThe2B](https://twitter.com/KiddoThe2B) |
|
pablouribe/beto-copus-supercategories-overfitted | c6cf4147327975d9f198616ecb7bd9e1f7d80cd2 | 2022-01-18T16:18:23.000Z | [
"pytorch",
"bert",
"text-classification",
"transformers"
]
| text-classification | false | pablouribe | null | pablouribe/beto-copus-supercategories-overfitted | 42 | null | transformers | 6,348 | Entry not found |
prajjwal1/bert-small-mnli | 6aae77bde3e3b87fa9e16a1aa52b56fb07bbd83e | 2021-10-05T17:57:54.000Z | [
"pytorch",
"jax",
"bert",
"text-classification",
"arxiv:1908.08962",
"arxiv:2110.01518",
"transformers"
]
| text-classification | false | prajjwal1 | null | prajjwal1/bert-small-mnli | 42 | null | transformers | 6,349 | The following model is a Pytorch pre-trained model obtained from converting Tensorflow checkpoint found in the [official Google BERT repository](https://github.com/google-research/bert). These BERT variants were introduced in the paper [Well-Read Students Learn Better: On the Importance of Pre-training Compact Models](https://arxiv.org/abs/1908.08962). These models are trained on MNLI.
If you use the model, please consider citing the paper
```
@misc{bhargava2021generalization,
title={Generalization in NLI: Ways (Not) To Go Beyond Simple Heuristics},
author={Prajjwal Bhargava and Aleksandr Drozd and Anna Rogers},
year={2021},
eprint={2110.01518},
archivePrefix={arXiv},
primaryClass={cs.CL}
}
```
Original Implementation and more info can be found in [this Github repository](https://github.com/prajjwal1/generalize_lm_nli).
```
MNLI: 72.1%
MNLI-mm: 73.76%
```
These models were trained for 4 epochs.
[@prajjwal_1](https://twitter.com/prajjwal_1)
|
valhalla/distilt5-qa-qg-hl-6-4 | 44f28f3eb4d95ef8f7cee1318c61f70ad71d3ed0 | 2021-09-23T16:42:47.000Z | [
"pytorch",
"jax",
"t5",
"text2text-generation",
"dataset:squad",
"transformers",
"question-generation",
"distilt5",
"distilt5-qg",
"license:mit",
"autotrain_compatible"
]
| text2text-generation | false | valhalla | null | valhalla/distilt5-qa-qg-hl-6-4 | 42 | null | transformers | 6,350 | ---
datasets:
- squad
tags:
- question-generation
- distilt5
- distilt5-qg
widget:
- text: 'generate question: <hl> 42 <hl> is the answer to life, the universe and everything.
</s>'
- text: 'question: What is 42 context: 42 is the answer to life, the universe and
everything. </s>'
license: mit
---
## DistilT5 for question-generation
This is distilled version of [t5-small-qa-qg-hl](https://huggingface.co/valhalla/t5-small-qa-qg-hl) model trained for question answering and answer aware question generation tasks.
The model is distilled using the **No Teacher Distillation** method proposed by Huggingface, [here](https://github.com/huggingface/transformers/tree/master/examples/seq2seq#distilbart).
We just copy alternating layers from `t5-small-qa-qg-hl` and finetune more on the same data. Following table lists other distilled models and their metrics.
| Name | BLEU-4 | METEOR | ROUGE-L | QA-EM | QA-F1 |
|---------------------------------------------------------------------------------|---------|---------|---------|--------|--------|
| [distilt5-qg-hl-6-4](https://huggingface.co/valhalla/distilt5-qg-hl-6-4) | 18.4141 | 24.8417 | 40.3435 | - | - |
| [distilt5-qa-qg-hl-6-4](https://huggingface.co/valhalla/distilt5-qa-qg-hl-6-4) | 18.6493 | 24.9685 | 40.5605 | 76.13 | 84.659 |
| [distilt5-qg-hl-12-6](https://huggingface.co/valhalla/distilt5-qg-hl-12-6) | 20.5275 | 26.5010 | 43.2676 | - | - |
| [distilt5-qa-qg-hl-12-6](https://huggingface.co/valhalla/distilt5-qa-qg-hl-12-6)| 20.6109 | 26.4533 | 43.0895 | 81.61 | 89.831 |
You can play with the model using the inference API. Here's how you can use it
For QG
`generate question: <hl> 42 <hl> is the answer to life, the universe and everything.`
For QA
`question: What is 42 context: 42 is the answer to life, the universe and everything.`
For more deatils see [this](https://github.com/patil-suraj/question_generation) repo.
### Model in action 🚀
You'll need to clone the [repo](https://github.com/patil-suraj/question_generation).
[](https://colab.research.google.com/github/patil-suraj/question_generation/blob/master/question_generation.ipynb)
```python3
from pipelines import pipeline
nlp = pipeline("multitask-qa-qg", model="valhalla/distilt5-qa-qg-hl-6-4")
# to generate questions simply pass the text
nlp("42 is the answer to life, the universe and everything.")
=> [{'answer': '42', 'question': 'What is the answer to life?'}]
# for qa pass a dict with "question" and "context"
nlp({
"question": "What is 42 ?",
"context": "42 is the answer to life, the universe and everything."
})
=> 'the answer to life, the universe and everything'
``` |
QuickRead/pegasus-reddit | a6b6c7648536c851f385a8e8819bca95eabc7a2b | 2022-02-26T16:57:46.000Z | [
"pytorch",
"pegasus",
"text2text-generation",
"dataset:reddit",
"transformers",
"generated_from_trainer",
"model-index",
"autotrain_compatible"
]
| text2text-generation | false | QuickRead | null | QuickRead/pegasus-reddit | 42 | null | transformers | 6,351 | ---
tags:
- generated_from_trainer
datasets:
- reddit
metrics:
- rouge
model-index:
- name: pegasus-reddit
results:
- task:
name: Sequence-to-sequence Language Modeling
type: text2text-generation
dataset:
name: reddit
type: reddit
args: default
metrics:
- name: Rouge1
type: rouge
value: 23.967
---
<!-- This model card has been generated automatically according to the information the Trainer had access to. You
should probably proofread and complete it, then remove this comment. -->
# pegasus-reddit
This model is a fine-tuned version of [google/pegasus-large](https://huggingface.co/google/pegasus-large) on the reddit dataset.
It achieves the following results on the evaluation set:
- Loss: 3.3329
- Rouge1: 23.967
- Rouge2: 5.0032
- Rougel: 15.3267
- Rougelsum: 18.5905
- Gen Len: 69.2193
## Model description
More information needed
## Intended uses & limitations
More information needed
## Training and evaluation data
More information needed
## Training procedure
### Training hyperparameters
The following hyperparameters were used during training:
- learning_rate: 6.35e-05
- train_batch_size: 8
- eval_batch_size: 8
- seed: 42
- optimizer: Adam with betas=(0.9,0.999) and epsilon=1e-08
- lr_scheduler_type: cosine
- lr_scheduler_warmup_steps: 500
- num_epochs: 1.0
- mixed_precision_training: Native AMP
### Training results
### Framework versions
- Transformers 4.16.2
- Pytorch 1.10.1
- Datasets 1.17.0
- Tokenizers 0.10.3
|
bookbot/distil-wav2vec2-xls-r-adult-child-cls-89m | fd1675d47e72272002c0df18ab629aa9422cb968 | 2022-02-26T13:50:18.000Z | [
"pytorch",
"tensorboard",
"wav2vec2",
"audio-classification",
"en",
"arxiv:2111.09296",
"transformers",
"generated_from_trainer",
"license:apache-2.0",
"model-index"
]
| audio-classification | false | bookbot | null | bookbot/distil-wav2vec2-xls-r-adult-child-cls-89m | 42 | null | transformers | 6,352 | ---
language: en
license: apache-2.0
tags:
- audio-classification
- generated_from_trainer
metrics:
- accuracy
- f1
model-index:
- name: distil-wav2vec2-xls-r-adult-child-cls-89m
results: []
---
# DistilWav2Vec2 XLS-R Adult/Child Speech Classifier 89M
DistilWav2Vec2 XLS-R Adult/Child Speech Classifier is an audio classification model based on the [XLS-R](https://arxiv.org/abs/2111.09296) architecture. This model is a distilled version of [wav2vec2-xls-r-adult-child-cls](https://huggingface.co/bookbot/wav2vec2-xls-r-adult-child-cls) on a private adult/child speech classification dataset.
This model was trained using HuggingFace's PyTorch framework. All training was done on a Tesla P100, provided by Kaggle. Training metrics were logged via Tensorboard.
## Model
| Model | #params | Arch. | Training/Validation data (text) |
| ------------------------------------------- | ------- | ----- | ----------------------------------------- |
| `distil-wav2vec2-xls-r-adult-child-cls-89m` | 89M | XLS-R | Adult/Child Speech Classification Dataset |
## Evaluation Results
The model achieves the following results on evaluation:
| Dataset | Loss | Accuracy | F1 |
| --------------------------------- | ------ | -------- | ------ |
| Adult/Child Speech Classification | 0.3048 | 93.54% | 0.9420 |
## Training procedure
### Training hyperparameters
The following hyperparameters were used during training:
- `learning_rate`: 3e-05
- `train_batch_size`: 32
- `eval_batch_size`: 32
- `seed`: 42
- `gradient_accumulation_steps`: 4
- `total_train_batch_size`: 128
- `optimizer`: Adam with `betas=(0.9,0.999)` and `epsilon=1e-08`
- `lr_scheduler_type`: linear
- `lr_scheduler_warmup_ratio`: 0.1
- `num_epochs`: 5
### Training results
| Training Loss | Epoch | Step | Validation Loss | Accuracy | F1 |
| :-----------: | :---: | :--: | :-------------: | :------: | :----: |
| 0.7711 | 1.0 | 96 | 0.5413 | 0.9017 | 0.9156 |
| 0.5551 | 2.0 | 192 | 0.4627 | 0.9164 | 0.9272 |
| 0.4166 | 3.0 | 288 | 0.3832 | 0.9261 | 0.9352 |
| 0.3928 | 4.0 | 384 | 0.3242 | 0.9331 | 0.9406 |
| 0.3622 | 5.0 | 480 | 0.3048 | 0.9354 | 0.9420 |
## Disclaimer
Do consider the biases which came from pre-training datasets that may be carried over into the results of this model.
## Authors
DistilWav2Vec2 XLS-R Adult/Child Speech Classifier was trained and evaluated by [Wilson Wongso](https://w11wo.github.io/). All computation and development are done on Kaggle.
## Framework versions
- Transformers 4.17.0.dev0
- Pytorch 1.10.2+cu102
- Datasets 1.18.3
- Tokenizers 0.11.0
|
zhiweitong/rag-sequence-base-qg | e9accdf02427ad2650a97f51ddd96426e2711380 | 2022-03-22T03:03:03.000Z | [
"pytorch",
"rag",
"transformers"
]
| null | false | zhiweitong | null | zhiweitong/rag-sequence-base-qg | 42 | null | transformers | 6,353 | Entry not found |
tae898/emoberta-large | 8934b68e8b0d9fc3cd961cc7e7605533c7081e59 | 2022-03-16T11:01:48.000Z | [
"pytorch",
"roberta",
"text-classification",
"en",
"dataset:MELD",
"dataset:IEMOCAP",
"arxiv:2108.12009",
"transformers",
"emoberta",
"license:mit"
]
| text-classification | false | tae898 | null | tae898/emoberta-large | 42 | 4 | transformers | 6,354 | ---
language: en
tags:
- emoberta
- roberta
license: mit
datasets:
- MELD
- IEMOCAP
---
Check https://github.com/tae898/erc for the details
[Watch a demo video!](https://youtu.be/qbr7fNd6J28)
# Emotion Recognition in Coversation (ERC)
[](https://paperswithcode.com/sota/emotion-recognition-in-conversation-on?p=emoberta-speaker-aware-emotion-recognition-in)
[](https://paperswithcode.com/sota/emotion-recognition-in-conversation-on-meld?p=emoberta-speaker-aware-emotion-recognition-in)
At the moment, we only use the text modality to correctly classify the emotion of the utterances.The experiments were carried out on two datasets (i.e. MELD and IEMOCAP)
## Prerequisites
1. An x86-64 Unix or Unix-like machine
1. Python 3.8 or higher
1. Running in a virtual environment (e.g., conda, virtualenv, etc.) is highly recommended so that you don't mess up with the system python.
1. [`multimodal-datasets` repo](https://github.com/tae898/multimodal-datasets) (submodule)
1. pip install -r requirements.txt
## EmoBERTa training
First configure the hyper parameters and the dataset in `train-erc-text.yaml` and then,
In this directory run the below commands. I recommend you to run this in a virtualenv.
```sh
python train-erc-text.py
```
This will subsequently call `train-erc-text-hp.py` and `train-erc-text-full.py`.
## Results on the test split (weighted f1 scores)
| Model | | MELD | IEMOCAP |
| -------- | ------------------------------- | :-------: | :-------: |
| EmoBERTa | No past and future utterances | 63.46 | 56.09 |
| | Only past utterances | 64.55 | **68.57** |
| | Only future utterances | 64.23 | 66.56 |
| | Both past and future utterances | **65.61** | 67.42 |
| | → *without speaker names* | 65.07 | 64.02 |
Above numbers are the mean values of five random seed runs.
If you want to see more training test details, check out `./results/`
If you want to download the trained checkpoints and stuff, then [here](https://surfdrive.surf.nl/files/index.php/s/khREwk4MUI7MSnO/download) is where you can download them. It's a pretty big zip file.
## Deployment
### Huggingface
We have released our models on huggingface:
- [emoberta-base](https://huggingface.co/tae898/emoberta-base)
- [emoberta-large](https://huggingface.co/tae898/emoberta-large)
They are based on [RoBERTa-base](https://huggingface.co/roberta-base) and [RoBERTa-large](https://huggingface.co/roberta-large), respectively. They were trained on [both MELD and IEMOCAP datasets](utterance-ordered-MELD_IEMOCAP.json). Our deployed models are neither speaker-aware nor take previous utterances into account, meaning that it only classifies one utterance at a time without the speaker information (e.g., "I love you").
### Flask app
You can either run the Flask RESTful server app as a docker container or just as a python script.
1. Running the app as a docker container **(recommended)**.
There are four images. Take what you need:
- `docker run -it --rm -p 10006:10006 tae898/emoberta-base`
- `docker run -it --rm -p 10006:10006 --gpus all tae898/emoberta-base-cuda`
- `docker run -it --rm -p 10006:10006 tae898/emoberta-large`
- `docker run -it --rm -p 10006:10006 --gpus all tae898/emoberta-large-cuda`
1. Running the app in your python environment:
This method is less recommended than the docker one.
Run `pip install -r requirements-deploy.txt` first.<br>
The [`app.py`](app.py) is a flask RESTful server. The usage is below:
```console
app.py [-h] [--host HOST] [--port PORT] [--device DEVICE] [--model-type MODEL_TYPE]
```
For example:
```sh
python app.py --host 0.0.0.0 --port 10006 --device cpu --model-type emoberta-base
```
### Client
Once the app is running, you can send a text to the server. First install the necessary packages: `pip install -r requirements-client.txt`, and the run the [client.py](client.py). The usage is as below:
```console
client.py [-h] [--url-emoberta URL_EMOBERTA] --text TEXT
```
For example:
```sh
python client.py --text "Emotion recognition is so cool\!"
```
will give you:
```json
{
"neutral": 0.0049800905,
"joy": 0.96399665,
"surprise": 0.018937444,
"anger": 0.0071516023,
"sadness": 0.002021492,
"disgust": 0.001495996,
"fear": 0.0014167271
}
```
## Troubleshooting
The best way to find and solve your problems is to see in the github issue tab. If you can't find what you want, feel free to raise an issue. We are pretty responsive.
## Contributing
Contributions are what make the open source community such an amazing place to be learn, inspire, and create. Any contributions you make are **greatly appreciated**.
1. Fork the Project
1. Create your Feature Branch (`git checkout -b feature/AmazingFeature`)
1. Run `make style && quality` in the root repo directory, to ensure code quality.
1. Commit your Changes (`git commit -m 'Add some AmazingFeature'`)
1. Push to the Branch (`git push origin feature/AmazingFeature`)
1. Open a Pull Request
## Cite our work
Check out the [paper](https://arxiv.org/abs/2108.12009).
```bibtex
@misc{kim2021emoberta,
title={EmoBERTa: Speaker-Aware Emotion Recognition in Conversation with RoBERTa},
author={Taewoon Kim and Piek Vossen},
year={2021},
eprint={2108.12009},
archivePrefix={arXiv},
primaryClass={cs.CL}
}
```
[](https://zenodo.org/badge/latestdoi/328375452)<br>
## Authors
- [Taewoon Kim](https://taewoonkim.com/)
## License
[MIT](https://choosealicense.com/licenses/mit/)
|
oliverguhr/fullstop-dutch-punctuation-prediction | 381031ecce1efe9f82955100e60a8348a19dd4b7 | 2022-03-30T12:32:44.000Z | [
"pytorch",
"tensorboard",
"roberta",
"token-classification",
"nl",
"dataset:wmt/europarl",
"transformers",
"punctuation prediction",
"punctuation",
"license:mit",
"autotrain_compatible"
]
| token-classification | false | oliverguhr | null | oliverguhr/fullstop-dutch-punctuation-prediction | 42 | 1 | transformers | 6,355 | ---
language:
- nl
tags:
- punctuation prediction
- punctuation
datasets: wmt/europarl
license: mit
widget:
- text: "hervatting van de zitting ik verklaar de zitting van het europees parlement die op vrijdag 17 december werd onderbroken te zijn hervat"
example_title: "Euro Parl"
metrics:
- f1
---
## Performance
```
precision recall f1-score support
0 0.992584 0.994595 0.993588 9627605
. 0.960450 0.962452 0.961450 433554
, 0.816974 0.804882 0.810883 379759
? 0.871368 0.826812 0.848506 13494
- 0.619905 0.367690 0.461591 27341
: 0.718636 0.602076 0.655212 18305
accuracy 0.983874 10500058
macro avg 0.829986 0.759751 0.788538 10500058
weighted avg 0.983302 0.983874 0.983492 10500058
```
Usage:
```bash
pip install deepmultilingualpunctuation
```
```python
from deepmultilingualpunctuation import PunctuationModel
model = PunctuationModel(model="oliverguhr/fullstop-dutch-punctuation-prediction")
text = "hervatting van de zitting ik verklaar de zitting van het europees parlement die op vrijdag 17 december werd onderbroken te zijn hervat"
result = model.restore_punctuation(text)
print(result)
```
|
vumichien/sequence-classification-bigbird-roberta-base | 640c0ce5dbd56ee1f6dc7e1f4d6bfd85214cb031 | 2022-03-25T05:36:45.000Z | [
"pytorch",
"big_bird",
"text-classification",
"transformers"
]
| text-classification | false | vumichien | null | vumichien/sequence-classification-bigbird-roberta-base | 42 | null | transformers | 6,356 | Entry not found |
QuanjieHan/resume_ner_1 | 22c5a37c12529bc9f58fae8713cb8ec893b1f328 | 2022-04-06T02:40:28.000Z | [
"pytorch",
"bert",
"token-classification",
"transformers",
"license:other",
"autotrain_compatible"
]
| token-classification | false | QuanjieHan | null | QuanjieHan/resume_ner_1 | 42 | null | transformers | 6,357 | ---
license: other
---
|
Helsinki-NLP/opus-mt-tc-big-en-cat_oci_spa | 043fdefef8a0cce8ea652e0266cc1aa578385d10 | 2022-06-01T12:59:04.000Z | [
"pytorch",
"marian",
"text2text-generation",
"ca",
"en",
"es",
"oc",
"transformers",
"translation",
"opus-mt-tc",
"license:cc-by-4.0",
"model-index",
"autotrain_compatible"
]
| translation | false | Helsinki-NLP | null | Helsinki-NLP/opus-mt-tc-big-en-cat_oci_spa | 42 | 1 | transformers | 6,358 | ---
language:
- ca
- en
- es
- oc
tags:
- translation
- opus-mt-tc
license: cc-by-4.0
model-index:
- name: opus-mt-tc-big-en-cat_oci_spa
results:
- task:
name: Translation eng-cat
type: translation
args: eng-cat
dataset:
name: flores101-devtest
type: flores_101
args: eng cat devtest
metrics:
- name: BLEU
type: bleu
value: 41.5
- task:
name: Translation eng-oci
type: translation
args: eng-oci
dataset:
name: flores101-devtest
type: flores_101
args: eng oci devtest
metrics:
- name: BLEU
type: bleu
value: 25.4
- task:
name: Translation eng-spa
type: translation
args: eng-spa
dataset:
name: flores101-devtest
type: flores_101
args: eng spa devtest
metrics:
- name: BLEU
type: bleu
value: 28.1
- task:
name: Translation eng-spa
type: translation
args: eng-spa
dataset:
name: news-test2008
type: news-test2008
args: eng-spa
metrics:
- name: BLEU
type: bleu
value: 30.0
- task:
name: Translation eng-cat
type: translation
args: eng-cat
dataset:
name: tatoeba-test-v2021-08-07
type: tatoeba_mt
args: eng-cat
metrics:
- name: BLEU
type: bleu
value: 47.8
- task:
name: Translation eng-spa
type: translation
args: eng-spa
dataset:
name: tatoeba-test-v2021-08-07
type: tatoeba_mt
args: eng-spa
metrics:
- name: BLEU
type: bleu
value: 57.0
- task:
name: Translation eng-spa
type: translation
args: eng-spa
dataset:
name: tico19-test
type: tico19-test
args: eng-spa
metrics:
- name: BLEU
type: bleu
value: 52.5
- task:
name: Translation eng-spa
type: translation
args: eng-spa
dataset:
name: newstest2009
type: wmt-2009-news
args: eng-spa
metrics:
- name: BLEU
type: bleu
value: 30.5
- task:
name: Translation eng-spa
type: translation
args: eng-spa
dataset:
name: newstest2010
type: wmt-2010-news
args: eng-spa
metrics:
- name: BLEU
type: bleu
value: 37.4
- task:
name: Translation eng-spa
type: translation
args: eng-spa
dataset:
name: newstest2011
type: wmt-2011-news
args: eng-spa
metrics:
- name: BLEU
type: bleu
value: 39.1
- task:
name: Translation eng-spa
type: translation
args: eng-spa
dataset:
name: newstest2012
type: wmt-2012-news
args: eng-spa
metrics:
- name: BLEU
type: bleu
value: 39.6
- task:
name: Translation eng-spa
type: translation
args: eng-spa
dataset:
name: newstest2013
type: wmt-2013-news
args: eng-spa
metrics:
- name: BLEU
type: bleu
value: 35.8
---
# opus-mt-tc-big-en-cat_oci_spa
Neural machine translation model for translating from English (en) to Catalan, Occitan and Spanish (cat+oci+spa).
This model is part of the [OPUS-MT project](https://github.com/Helsinki-NLP/Opus-MT), an effort to make neural machine translation models widely available and accessible for many languages in the world. All models are originally trained using the amazing framework of [Marian NMT](https://marian-nmt.github.io/), an efficient NMT implementation written in pure C++. The models have been converted to pyTorch using the transformers library by huggingface. Training data is taken from [OPUS](https://opus.nlpl.eu/) and training pipelines use the procedures of [OPUS-MT-train](https://github.com/Helsinki-NLP/Opus-MT-train).
* Publications: [OPUS-MT – Building open translation services for the World](https://aclanthology.org/2020.eamt-1.61/) and [The Tatoeba Translation Challenge – Realistic Data Sets for Low Resource and Multilingual MT](https://aclanthology.org/2020.wmt-1.139/) (Please, cite if you use this model.)
```
@inproceedings{tiedemann-thottingal-2020-opus,
title = "{OPUS}-{MT} {--} Building open translation services for the World",
author = {Tiedemann, J{\"o}rg and Thottingal, Santhosh},
booktitle = "Proceedings of the 22nd Annual Conference of the European Association for Machine Translation",
month = nov,
year = "2020",
address = "Lisboa, Portugal",
publisher = "European Association for Machine Translation",
url = "https://aclanthology.org/2020.eamt-1.61",
pages = "479--480",
}
@inproceedings{tiedemann-2020-tatoeba,
title = "The Tatoeba Translation Challenge {--} Realistic Data Sets for Low Resource and Multilingual {MT}",
author = {Tiedemann, J{\"o}rg},
booktitle = "Proceedings of the Fifth Conference on Machine Translation",
month = nov,
year = "2020",
address = "Online",
publisher = "Association for Computational Linguistics",
url = "https://aclanthology.org/2020.wmt-1.139",
pages = "1174--1182",
}
```
## Model info
* Release: 2022-03-13
* source language(s): eng
* target language(s): cat spa
* valid target language labels: >>cat<< >>spa<<
* model: transformer-big
* data: opusTCv20210807+bt ([source](https://github.com/Helsinki-NLP/Tatoeba-Challenge))
* tokenization: SentencePiece (spm32k,spm32k)
* original model: [opusTCv20210807+bt_transformer-big_2022-03-13.zip](https://object.pouta.csc.fi/Tatoeba-MT-models/eng-cat+oci+spa/opusTCv20210807+bt_transformer-big_2022-03-13.zip)
* more information released models: [OPUS-MT eng-cat+oci+spa README](https://github.com/Helsinki-NLP/Tatoeba-Challenge/tree/master/models/eng-cat+oci+spa/README.md)
* more information about the model: [MarianMT](https://huggingface.co/docs/transformers/model_doc/marian)
This is a multilingual translation model with multiple target languages. A sentence initial language token is required in the form of `>>id<<` (id = valid target language ID), e.g. `>>cat<<`
## Usage
A short example code:
```python
from transformers import MarianMTModel, MarianTokenizer
src_text = [
">>spa<< Why do you want Tom to go there with me?",
">>spa<< She forced him to eat spinach."
]
model_name = "pytorch-models/opus-mt-tc-big-en-cat_oci_spa"
tokenizer = MarianTokenizer.from_pretrained(model_name)
model = MarianMTModel.from_pretrained(model_name)
translated = model.generate(**tokenizer(src_text, return_tensors="pt", padding=True))
for t in translated:
print( tokenizer.decode(t, skip_special_tokens=True) )
# expected output:
# ¿Por qué quieres que Tom vaya conmigo?
# Ella lo obligó a comer espinacas.
```
You can also use OPUS-MT models with the transformers pipelines, for example:
```python
from transformers import pipeline
pipe = pipeline("translation", model="Helsinki-NLP/opus-mt-tc-big-en-cat_oci_spa")
print(pipe(">>spa<< Why do you want Tom to go there with me?"))
# expected output: ¿Por qué quieres que Tom vaya conmigo?
```
## Benchmarks
* test set translations: [opusTCv20210807+bt_transformer-big_2022-03-13.test.txt](https://object.pouta.csc.fi/Tatoeba-MT-models/eng-cat+oci+spa/opusTCv20210807+bt_transformer-big_2022-03-13.test.txt)
* test set scores: [opusTCv20210807+bt_transformer-big_2022-03-13.eval.txt](https://object.pouta.csc.fi/Tatoeba-MT-models/eng-cat+oci+spa/opusTCv20210807+bt_transformer-big_2022-03-13.eval.txt)
* benchmark results: [benchmark_results.txt](benchmark_results.txt)
* benchmark output: [benchmark_translations.zip](benchmark_translations.zip)
| langpair | testset | chr-F | BLEU | #sent | #words |
|----------|---------|-------|-------|-------|--------|
| eng-cat | tatoeba-test-v2021-08-07 | 0.66414 | 47.8 | 1631 | 12344 |
| eng-spa | tatoeba-test-v2021-08-07 | 0.73725 | 57.0 | 16583 | 134710 |
| eng-cat | flores101-devtest | 0.66071 | 41.5 | 1012 | 27304 |
| eng-oci | flores101-devtest | 0.56192 | 25.4 | 1012 | 27305 |
| eng-spa | flores101-devtest | 0.56288 | 28.1 | 1012 | 29199 |
| eng-spa | newssyscomb2009 | 0.58431 | 31.4 | 502 | 12503 |
| eng-spa | news-test2008 | 0.56622 | 30.0 | 2051 | 52586 |
| eng-spa | newstest2009 | 0.57988 | 30.5 | 2525 | 68111 |
| eng-spa | newstest2010 | 0.62343 | 37.4 | 2489 | 65480 |
| eng-spa | newstest2011 | 0.62424 | 39.1 | 3003 | 79476 |
| eng-spa | newstest2012 | 0.63006 | 39.6 | 3003 | 79006 |
| eng-spa | newstest2013 | 0.60291 | 35.8 | 3000 | 70528 |
| eng-spa | tico19-test | 0.73224 | 52.5 | 2100 | 66563 |
## Acknowledgements
The work is supported by the [European Language Grid](https://www.european-language-grid.eu/) as [pilot project 2866](https://live.european-language-grid.eu/catalogue/#/resource/projects/2866), by the [FoTran project](https://www.helsinki.fi/en/researchgroups/natural-language-understanding-with-cross-lingual-grounding), funded by the European Research Council (ERC) under the European Union’s Horizon 2020 research and innovation programme (grant agreement No 771113), and the [MeMAD project](https://memad.eu/), funded by the European Union’s Horizon 2020 Research and Innovation Programme under grant agreement No 780069. We are also grateful for the generous computational resources and IT infrastructure provided by [CSC -- IT Center for Science](https://www.csc.fi/), Finland.
## Model conversion info
* transformers version: 4.16.2
* OPUS-MT git hash: 3405783
* port time: Wed Apr 13 16:40:45 EEST 2022
* port machine: LM0-400-22516.local
|
UT/BMW | 9b24861b1dc5ad9e90730f57687a49f44ad91290 | 2022-04-19T13:50:58.000Z | [
"pytorch",
"roberta",
"text-classification",
"transformers"
]
| text-classification | false | UT | null | UT/BMW | 42 | null | transformers | 6,359 | Entry not found |
Hate-speech-CNERG/urdu-abusive-MuRIL | 88c6bf8813ee8c89cd30aabc2e07a52c70ea3c5e | 2022-05-03T08:43:53.000Z | [
"pytorch",
"bert",
"text-classification",
"ur",
"arxiv:2204.12543",
"transformers",
"license:afl-3.0"
]
| text-classification | false | Hate-speech-CNERG | null | Hate-speech-CNERG/urdu-abusive-MuRIL | 42 | null | transformers | 6,360 | ---
language: ur
license: afl-3.0
---
This model is used to detect **abusive speech** in **Urdu**. It is finetuned on MuRIL model using Urdu abusive speech dataset.
The model is trained with learning rates of 2e-5. Training code can be found at this [url](https://github.com/hate-alert/IndicAbusive)
LABEL_0 :-> Normal
LABEL_1 :-> Abusive
### For more details about our paper
Mithun Das, Somnath Banerjee and Animesh Mukherjee. "[Data Bootstrapping Approaches to Improve Low Resource Abusive Language Detection for Indic Languages](https://arxiv.org/abs/2204.12543)". Accepted at ACM HT 2022.
***Please cite our paper in any published work that uses any of these resources.***
~~~
@article{das2022data,
title={Data Bootstrapping Approaches to Improve Low Resource Abusive Language Detection for Indic Languages},
author={Das, Mithun and Banerjee, Somnath and Mukherjee, Animesh},
journal={arXiv preprint arXiv:2204.12543},
year={2022}
}
~~~ |
Hate-speech-CNERG/english-abusive-MuRIL | 42738fee859c9f9278f0465e27667f4a29220c7f | 2022-05-03T06:06:23.000Z | [
"pytorch",
"bert",
"text-classification",
"en",
"arxiv:2204.12543",
"transformers",
"license:afl-3.0"
]
| text-classification | false | Hate-speech-CNERG | null | Hate-speech-CNERG/english-abusive-MuRIL | 42 | null | transformers | 6,361 | ---
language: en
license: afl-3.0
---
This model is used detecting **abusive speech** in **English**. It is finetuned on MuRIL model using English abusive speech dataset.
The model is trained with learning rates of 2e-5. Training code can be found at this [url](https://github.com/hate-alert/IndicAbusive)
LABEL_0 :-> Normal
LABEL_1 :-> Abusive
### For more details about our paper
Mithun Das, Somnath Banerjee and Animesh Mukherjee. "[Data Bootstrapping Approaches to Improve Low Resource Abusive Language Detection for Indic Languages](https://arxiv.org/abs/2204.12543)". Accepted at ACM HT 2022.
***Please cite our paper in any published work that uses any of these resources.***
~~~
@article{das2022data,
title={Data Bootstrapping Approaches to Improve Low Resource Abusive Language Detection for Indic Languages},
author={Das, Mithun and Banerjee, Somnath and Mukherjee, Animesh},
journal={arXiv preprint arXiv:2204.12543},
year={2022}
}
~~~ |
JacopoBandoni/BioBertRelationGenesDiseases | d1c10f54484629e37c99c377dfcd6924266126fd | 2022-05-09T09:47:10.000Z | [
"pytorch",
"bert",
"text-classification",
"transformers",
"license:afl-3.0"
]
| text-classification | false | JacopoBandoni | null | JacopoBandoni/BioBertRelationGenesDiseases | 42 | null | transformers | 6,362 | ---
license: afl-3.0
widget:
- text: "The case of a 72-year-old male with @DISEASE$ with poor insulin control (fasting hyperglycemia greater than 180 mg/dl) who had a long-standing polyuric syndrome is here presented. Hypernatremia and plasma osmolality elevated together with a low urinary osmolality led to the suspicion of diabetes insipidus, which was subsequently confirmed by the dehydration test and the administration of @GENE$ sc."
example_title: "Example 1"
- text: "Hypernatremia and plasma osmolality elevated together with a low urinary osmolality led to the suspicion of diabetes insipidus, which was subsequently confirmed by the dehydration test and the administration of @GENE$ sc. With 61% increase in the calculated urinary osmolarity one hour post desmopressin s.c., @DISEASE$ was diagnosed."
example_title: "Example 2"
---
The following is a fine-tuning of the BioBert models on the GAD dataset.
The model works by masking the gene string with "@GENE$" and the disease string with "@DISEASE$".
The output is a text classification that can either be:
- "LABEL0" if there is no relation
- "LABEL1" if there is a relation. |
Elfsong/ArtQuest | 2ab7e3bafd67e72be6f42de59b9a0129b7e0717e | 2022-05-11T07:05:24.000Z | [
"pytorch",
"t5",
"text2text-generation",
"transformers",
"autotrain_compatible"
]
| text2text-generation | false | Elfsong | null | Elfsong/ArtQuest | 42 | 1 | transformers | 6,363 | Entry not found |
CogComp/ZeroShotWiki | 0b85bb83cf94d5b79093c02b75455c293f3650ad | 2022-05-14T04:00:26.000Z | [
"pytorch",
"bert",
"text-classification",
"transformers",
"license:apache-2.0"
]
| text-classification | false | CogComp | null | CogComp/ZeroShotWiki | 42 | null | transformers | 6,364 | ---
license: apache-2.0
---
# Model description
A BertForSequenceClassification model that is finetuned on Wikipedia for zero-shot text classification. For details, see our NAACL'22 paper.
# Usage
Concatenate the text sentence with each of the candidate labels as input to the model. The model will output a score for each label. Below is an example.
```
from transformers import AutoTokenizer, AutoModelForSequenceClassification
import torch
tokenizer = AutoTokenizer.from_pretrained("CogComp/ZeroShotWiki")
model = AutoModelForSequenceClassification.from_pretrained("CogComp/ZeroShotWiki")
labels = ["sports", "business", "politics"]
texts = ["As of the 2018 FIFA World Cup, twenty-one final tournaments have been held and a total of 79 national teams have competed."]
with torch.no_grad():
for text in texts:
label_score = {}
for label in labels:
inputs = tokenizer(text, label, return_tensors='pt')
out = model(**inputs)
label_score[label]=float(torch.nn.functional.softmax(out[0], dim=-1)[0][0])
print(label_score) # Predict the label with the highest score
``` |
RuiqianLi/wav2vec2-large-xls-r-300m-chinese-taiwan-colab | ab3ca4ddaafcaa90d09c4609b847edcc300abc94 | 2022-06-06T08:12:49.000Z | [
"pytorch",
"tensorboard",
"wav2vec2",
"automatic-speech-recognition",
"dataset:common_voice",
"transformers",
"generated_from_trainer",
"license:apache-2.0",
"model-index"
]
| automatic-speech-recognition | false | RuiqianLi | null | RuiqianLi/wav2vec2-large-xls-r-300m-chinese-taiwan-colab | 42 | null | transformers | 6,365 | ---
license: apache-2.0
tags:
- generated_from_trainer
datasets:
- common_voice
model-index:
- name: wav2vec2-large-xls-r-300m-chinese-taiwan-colab
results: []
---
<!-- This model card has been generated automatically according to the information the Trainer had access to. You
should probably proofread and complete it, then remove this comment. -->
# wav2vec2-large-xls-r-300m-chinese-taiwan-colab
!!!this model has just been trained with very high learning rate and small epochs, please do not use this to do the speech to text.
!!!It's just a test, I'll retrain this model with more time later when I have time.
This model is a fine-tuned version of [facebook/wav2vec2-xls-r-300m](https://huggingface.co/facebook/wav2vec2-xls-r-300m) on the common_voice dataset.
## Model description
More information needed
## Intended uses & limitations
More information needed
## Training and evaluation data
More information needed
## Training procedure
### Training hyperparameters
The following hyperparameters were used during training:
- learning_rate: 0.1
- train_batch_size: 16
- eval_batch_size: 8
- seed: 42
- gradient_accumulation_steps: 2
- total_train_batch_size: 32
- optimizer: Adam with betas=(0.9,0.999) and epsilon=1e-08
- lr_scheduler_type: linear
- lr_scheduler_warmup_steps: 500
- num_epochs: 2
- mixed_precision_training: Native AMP
### Training results
### Framework versions
- Transformers 4.11.3
- Pytorch 1.10.0+cu113
- Datasets 1.18.3
- Tokenizers 0.10.3
|
mossaic-candle/adaptive-lm-molecules | 8023fdca93e68d74d9041331076ee02589a407df | 2022-05-23T17:13:09.000Z | [
"pytorch",
"bert",
"fill-mask",
"transformers",
"autotrain_compatible"
]
| fill-mask | false | mossaic-candle | null | mossaic-candle/adaptive-lm-molecules | 42 | null | transformers | 6,366 | Entry not found |
jkhan447/sarcasm-detection-RoBerta-base | 85b90e782f90e0359fa9f3ca1b3f2f0299cf3038 | 2022-05-30T09:24:12.000Z | [
"pytorch",
"tensorboard",
"roberta",
"text-classification",
"transformers",
"generated_from_trainer",
"license:mit",
"model-index"
]
| text-classification | false | jkhan447 | null | jkhan447/sarcasm-detection-RoBerta-base | 42 | null | transformers | 6,367 | ---
license: mit
tags:
- generated_from_trainer
metrics:
- accuracy
model-index:
- name: sarcasm-detection-RoBerta-base
results: []
---
<!-- This model card has been generated automatically according to the information the Trainer had access to. You
should probably proofread and complete it, then remove this comment. -->
# sarcasm-detection-RoBerta-base
This model is a fine-tuned version of [roberta-base](https://huggingface.co/roberta-base) on the None dataset.
It achieves the following results on the evaluation set:
- Loss: 2.8207
- Accuracy: 0.7273
## Model description
More information needed
## Intended uses & limitations
More information needed
## Training and evaluation data
More information needed
## Training procedure
### Training hyperparameters
The following hyperparameters were used during training:
- learning_rate: 2e-05
- train_batch_size: 16
- eval_batch_size: 16
- seed: 42
- optimizer: Adam with betas=(0.9,0.999) and epsilon=1e-08
- lr_scheduler_type: linear
- num_epochs: 50
### Training results
### Framework versions
- Transformers 4.19.2
- Pytorch 1.11.0+cu113
- Datasets 2.2.2
- Tokenizers 0.12.1
|
Clody0071/distilbert-base-multilingual-cased-finetuned-similarite | 54924203865f3dfa4d6ad0a9273b691bcbc22103 | 2022-06-10T15:25:52.000Z | [
"pytorch",
"tensorboard",
"distilbert",
"text-classification",
"dataset:pawsx",
"transformers",
"generated_from_trainer",
"license:apache-2.0",
"model-index"
]
| text-classification | false | Clody0071 | null | Clody0071/distilbert-base-multilingual-cased-finetuned-similarite | 42 | null | transformers | 6,368 | ---
license: apache-2.0
tags:
- generated_from_trainer
datasets:
- pawsx
metrics:
- accuracy
- f1
model-index:
- name: distilbert-base-multilingual-cased-finetuned-similarite
results:
- task:
name: Text Classification
type: text-classification
dataset:
name: pawsx
type: pawsx
args: fr
metrics:
- name: Accuracy
type: accuracy
value: 0.7995
- name: F1
type: f1
value: 0.7994565743967147
---
<!-- This model card has been generated automatically according to the information the Trainer had access to. You
should probably proofread and complete it, then remove this comment. -->
# distilbert-base-multilingual-cased-finetuned-similarite
This model is a fine-tuned version of [distilbert-base-multilingual-cased](https://huggingface.co/distilbert-base-multilingual-cased) on the pawsx dataset.
It achieves the following results on the evaluation set:
- Loss: 0.4781
- Accuracy: 0.7995
- F1: 0.7995
## Model description
More information needed
## Intended uses & limitations
More information needed
## Training and evaluation data
More information needed
## Training procedure
### Training hyperparameters
The following hyperparameters were used during training:
- learning_rate: 2e-05
- train_batch_size: 64
- eval_batch_size: 64
- seed: 42
- optimizer: Adam with betas=(0.9,0.999) and epsilon=1e-08
- lr_scheduler_type: linear
- num_epochs: 2
### Training results
| Training Loss | Epoch | Step | Validation Loss | Accuracy | F1 |
|:-------------:|:-----:|:----:|:---------------:|:--------:|:------:|
| 0.5343 | 1.0 | 772 | 0.4879 | 0.7705 | 0.7714 |
| 0.3523 | 2.0 | 1544 | 0.4781 | 0.7995 | 0.7995 |
### Framework versions
- Transformers 4.19.3
- Pytorch 1.11.0+cu113
- Datasets 2.2.2
- Tokenizers 0.12.1
|
gary109/wikitext_roberta-base | b067546c8e196f268b520bee94175bb823595f46 | 2022-06-17T06:44:42.000Z | [
"pytorch",
"tensorboard",
"roberta",
"fill-mask",
"dataset:wikitext",
"transformers",
"generated_from_trainer",
"license:mit",
"model-index",
"autotrain_compatible"
]
| fill-mask | false | gary109 | null | gary109/wikitext_roberta-base | 42 | null | transformers | 6,369 | ---
license: mit
tags:
- generated_from_trainer
datasets:
- wikitext
metrics:
- accuracy
model-index:
- name: wikitext_roberta-base
results:
- task:
name: Masked Language Modeling
type: fill-mask
dataset:
name: wikitext wikitext-2-raw-v1
type: wikitext
args: wikitext-2-raw-v1
metrics:
- name: Accuracy
type: accuracy
value: 0.7371052344006119
---
<!-- This model card has been generated automatically according to the information the Trainer had access to. You
should probably proofread and complete it, then remove this comment. -->
# wikitext_roberta-base
This model is a fine-tuned version of [roberta-base](https://huggingface.co/roberta-base) on the wikitext wikitext-2-raw-v1 dataset.
It achieves the following results on the evaluation set:
- Loss: 1.2143
- Accuracy: 0.7371
## Model description
More information needed
## Intended uses & limitations
More information needed
## Training and evaluation data
More information needed
## Training procedure
### Training hyperparameters
The following hyperparameters were used during training:
- learning_rate: 5e-05
- train_batch_size: 8
- eval_batch_size: 8
- seed: 42
- gradient_accumulation_steps: 16
- total_train_batch_size: 128
- optimizer: Adam with betas=(0.9,0.999) and epsilon=1e-08
- lr_scheduler_type: linear
- lr_scheduler_warmup_steps: 50
- num_epochs: 20.0
- mixed_precision_training: Native AMP
### Training results
| Training Loss | Epoch | Step | Validation Loss | Accuracy |
|:-------------:|:-----:|:----:|:---------------:|:--------:|
| 1.4175 | 0.99 | 37 | 1.3355 | 0.7194 |
| 1.438 | 1.99 | 74 | 1.2953 | 0.7249 |
| 1.4363 | 2.99 | 111 | 1.2759 | 0.7276 |
| 1.3391 | 3.99 | 148 | 1.2904 | 0.7252 |
| 1.3741 | 4.99 | 185 | 1.2621 | 0.7290 |
| 1.2771 | 5.99 | 222 | 1.2312 | 0.7353 |
| 1.287 | 6.99 | 259 | 1.2542 | 0.7289 |
| 1.29 | 7.99 | 296 | 1.2290 | 0.7345 |
| 1.2948 | 8.99 | 333 | 1.2537 | 0.7286 |
| 1.2741 | 9.99 | 370 | 1.2199 | 0.7354 |
| 1.2342 | 10.99 | 407 | 1.2520 | 0.7309 |
| 1.2199 | 11.99 | 444 | 1.2738 | 0.7260 |
| 1.206 | 12.99 | 481 | 1.2286 | 0.7335 |
| 1.221 | 13.99 | 518 | 1.2421 | 0.7327 |
| 1.2062 | 14.99 | 555 | 1.2402 | 0.7328 |
| 1.2305 | 15.99 | 592 | 1.2473 | 0.7308 |
| 1.2426 | 16.99 | 629 | 1.2250 | 0.7318 |
| 1.2096 | 17.99 | 666 | 1.2186 | 0.7353 |
| 1.1961 | 18.99 | 703 | 1.2214 | 0.7361 |
| 1.2136 | 19.99 | 740 | 1.2506 | 0.7311 |
### Framework versions
- Transformers 4.21.0.dev0
- Pytorch 1.11.0+cu113
- Datasets 2.3.3.dev0
- Tokenizers 0.12.1
|
wiselinjayajos/distilbert-base-uncased-finetuned-squad_v2 | 904763e1a7b9e55911c6449e0595a6c3889b05d0 | 2022-06-22T00:26:50.000Z | [
"pytorch",
"tensorboard",
"distilbert",
"question-answering",
"dataset:squad_v2",
"transformers",
"generated_from_trainer",
"license:apache-2.0",
"model-index",
"autotrain_compatible"
]
| question-answering | false | wiselinjayajos | null | wiselinjayajos/distilbert-base-uncased-finetuned-squad_v2 | 42 | null | transformers | 6,370 | ---
license: apache-2.0
tags:
- generated_from_trainer
datasets:
- squad_v2
model-index:
- name: distilbert-base-uncased-finetuned-squad_v2
results: []
---
<!-- This model card has been generated automatically according to the information the Trainer had access to. You
should probably proofread and complete it, then remove this comment. -->
# distilbert-base-uncased-finetuned-squad_v2
This model is a fine-tuned version of [distilbert-base-uncased](https://huggingface.co/distilbert-base-uncased) on the squad_v2 dataset.
It achieves the following results on the evaluation set:
- Loss: 1.3949
## Model description
More information needed
## Intended uses & limitations
More information needed
## Training and evaluation data
More information needed
## Training procedure
### Training hyperparameters
The following hyperparameters were used during training:
- learning_rate: 2e-05
- train_batch_size: 16
- eval_batch_size: 16
- seed: 42
- optimizer: Adam with betas=(0.9,0.999) and epsilon=1e-08
- lr_scheduler_type: linear
- num_epochs: 3
### Training results
| Training Loss | Epoch | Step | Validation Loss |
|:-------------:|:-----:|:-----:|:---------------:|
| 1.1996 | 1.0 | 8235 | 1.2485 |
| 0.9303 | 2.0 | 16470 | 1.2147 |
| 0.7438 | 3.0 | 24705 | 1.3949 |
### Framework versions
- Transformers 4.20.1
- Pytorch 1.11.0+cu113
- Datasets 2.3.2
- Tokenizers 0.12.1
|
shubhamitra/TinyBERT_General_4L_312D-finetuned-toxic-classification | 7e4d12ba3a7ec64f6a84814d10f8c14b5d9ba64c | 2022-07-05T07:29:35.000Z | [
"pytorch",
"tensorboard",
"bert",
"text-classification",
"transformers",
"generated_from_trainer",
"model-index"
]
| text-classification | false | shubhamitra | null | shubhamitra/TinyBERT_General_4L_312D-finetuned-toxic-classification | 42 | null | transformers | 6,371 | ---
tags:
- generated_from_trainer
model-index:
- name: TinyBERT_General_4L_312D-finetuned-toxic-classification
results: []
---
<!-- This model card has been generated automatically according to the information the Trainer had access to. You
should probably proofread and complete it, then remove this comment. -->
# TinyBERT_General_4L_312D-finetuned-toxic-classification
This model is a fine-tuned version of [huawei-noah/TinyBERT_General_4L_312D](https://huggingface.co/huawei-noah/TinyBERT_General_4L_312D) on an unknown dataset.
## Model description
More information needed
## Intended uses & limitations
More information needed
## Training and evaluation data
More information needed
## Training procedure
### Training hyperparameters
The following hyperparameters were used during training:
- learning_rate: 5e-05
- train_batch_size: 64
- eval_batch_size: 64
- seed: 123
- gradient_accumulation_steps: 4
- total_train_batch_size: 256
- optimizer: Adam with betas=(0.9,0.999) and epsilon=1e-08
- lr_scheduler_type: linear
- lr_scheduler_warmup_steps: 500
- num_epochs: 1
- mixed_precision_training: Native AMP
### Training results
| Training Loss | Epoch | Step | Validation Loss | F1 | Roc Auc | Accuracy |
|:-------------:|:-----:|:----:|:---------------:|:------:|:-------:|:--------:|
| No log | 1.0 | 498 | 0.0483 | 0.7486 | 0.8563 | 0.9171 |
### Framework versions
- Transformers 4.20.1
- Pytorch 1.11.0+cu113
- Datasets 2.3.2
- Tokenizers 0.12.1
|
kuttersn/gpt2_chatbot | 96e82985517b2da872418463bbb257ebcd1faf97 | 2022-07-14T19:04:01.000Z | [
"pytorch",
"tensorboard",
"gpt2",
"text-generation",
"transformers",
"generated_from_trainer",
"license:mit",
"model-index"
]
| text-generation | false | kuttersn | null | kuttersn/gpt2_chatbot | 42 | null | transformers | 6,372 | ---
license: mit
tags:
- generated_from_trainer
metrics:
- accuracy
model-index:
- name: gpt2_chatbot
results: []
---
<!-- This model card has been generated automatically according to the information the Trainer had access to. You
should probably proofread and complete it, then remove this comment. -->
# gpt2_chatbot
This model is a fine-tuned version of [gpt2](https://huggingface.co/gpt2) on an unknown dataset.
It achieves the following results on the evaluation set:
- Loss: 3.5732
- Accuracy: 0.3909
## Model description
More information needed
## Intended uses & limitations
More information needed
## Training and evaluation data
More information needed
## Training procedure
### Training hyperparameters
The following hyperparameters were used during training:
- learning_rate: 5e-05
- train_batch_size: 2
- eval_batch_size: 1
- seed: 42
- optimizer: Adam with betas=(0.9,0.999) and epsilon=1e-08
- lr_scheduler_type: linear
- num_epochs: 3.0
### Training results
### Framework versions
- Transformers 4.21.0.dev0
- Pytorch 1.12.0+cu113
- Datasets 2.3.2
- Tokenizers 0.12.1
|
nytimesrd/distilbert-base-uncased | 379b0cde998583f66bb304ccaee39a73bc04590a | 2022-07-27T14:25:40.000Z | [
"pytorch",
"distilbert",
"text-classification",
"transformers"
]
| text-classification | false | nytimesrd | null | nytimesrd/distilbert-base-uncased | 42 | null | transformers | 6,373 | Entry not found |
obl1t/DialoGPT-medium-Jolyne | 877095ab2bdaaef3491c05869c7d3d9d151a70ab | 2022-07-27T23:45:17.000Z | [
"pytorch",
"gpt2",
"text-generation",
"transformers",
"conversational"
]
| conversational | false | obl1t | null | obl1t/DialoGPT-medium-Jolyne | 42 | null | transformers | 6,374 | ---
tags:
- conversational
---
#Jolyne DialoGPT Model |
BSC-TeMU/roberta-base-biomedical-clinical-es | af1b189bbc1303f23f64661a4a913c4da302d821 | 2021-10-21T10:28:12.000Z | [
"pytorch",
"roberta",
"fill-mask",
"es",
"arxiv:2109.03570",
"arxiv:2109.07765",
"transformers",
"biomedical",
"clinical",
"spanish",
"license:apache-2.0",
"autotrain_compatible"
]
| fill-mask | false | BSC-TeMU | null | BSC-TeMU/roberta-base-biomedical-clinical-es | 41 | 5 | transformers | 6,375 | ---
language:
- es
tags:
- biomedical
- clinical
- spanish
license: apache-2.0
metrics:
- ppl
widget:
- text: "El único antecedente personal a reseñar era la <mask> arterial."
- text: "Las radiologías óseas de cuerpo entero no detectan alteraciones <mask>, ni alteraciones vertebrales."
- text: "En el <mask> toraco-abdómino-pélvico no se encontraron hallazgos patológicos de interés."
---
**⚠️NOTICE⚠️: THIS MODEL HAS BEEN MOVED TO THE FOLLOWING URL AND WILL SOON BE REMOVED:** https://huggingface.co/PlanTL-GOB-ES/roberta-base-biomedical-clinical-es
# Biomedical-clinical language model for Spanish
Biomedical pretrained language model for Spanish. For more details about the corpus, the pretraining and the evaluation, check the official [repository](https://github.com/PlanTL-SANIDAD/lm-biomedical-clinical-es) and read our [preprint](https://arxiv.org/abs/2109.03570) "_Carrino, C. P., Armengol-Estapé, J., Gutiérrez-Fandiño, A., Llop-Palao, J., Pàmies, M., Gonzalez-Agirre, A., & Villegas, M. (2021). Biomedical and Clinical Language Models for Spanish: On the Benefits of Domain-Specific Pretraining in a Mid-Resource Scenario._".
## Tokenization and model pretraining
This model is a [RoBERTa-based](https://github.com/pytorch/fairseq/tree/master/examples/roberta) model trained on a
**biomedical-clinical** corpus in Spanish collected from several sources (see next section).
The training corpus has been tokenized using a byte version of [Byte-Pair Encoding (BPE)](https://github.com/openai/gpt-2)
used in the original [RoBERTA](https://github.com/pytorch/fairseq/tree/master/examples/roberta) model with a vocabulary size of 52,000 tokens. The pretraining consists of a masked language model training at the subword level following the approach employed for the RoBERTa base model with the same hyperparameters as in the original work. The training lasted a total of 48 hours with 16 NVIDIA V100 GPUs of 16GB DDRAM, using Adam optimizer with a peak learning rate of 0.0005 and an effective batch size of 2,048 sentences.
## Training corpora and preprocessing
The training corpus is composed of several biomedical corpora in Spanish, collected from publicly available corpora and crawlers, and a real-world clinical corpus collected from more than 278K clinical documents and notes. To obtain a high-quality training corpus while retaining the idiosyncrasies of the clinical language, a cleaning pipeline has been applied only to the biomedical corpora, keeping the clinical corpus uncleaned. Essentially, the cleaning operations used are:
- data parsing in different formats
- sentence splitting
- language detection
- filtering of ill-formed sentences
- deduplication of repetitive contents
- keep the original document boundaries
Then, the biomedical corpora are concatenated and further global deduplication among the biomedical corpora have been applied.
Eventually, the clinical corpus is concatenated to the cleaned biomedical corpus resulting in a medium-size biomedical-clinical corpus for Spanish composed of more than 1B tokens. The table below shows some basic statistics of the individual cleaned corpora:
| Name | No. tokens | Description |
|-----------------------------------------------------------------------------------------|-------------|------------------------------------------------------------------------------------------------------------------------------------------------------------------------------------------------------------------------------------------------------|
| [Medical crawler](https://zenodo.org/record/4561970) | 745,705,946 | Crawler of more than 3,000 URLs belonging to Spanish biomedical and health domains. |
| Clinical cases misc. | 102,855,267 | A miscellany of medical content, essentially clinical cases. Note that a clinical case report is a scientific publication where medical practitioners share patient cases and it is different from a clinical note or document. |
| Clinical notes/documents | 91,250,080 | Collection of more than 278K clinical documents, including discharge reports, clinical course notes and X-ray reports, for a total of 91M tokens. |
| [Scielo](https://github.com/PlanTL-SANIDAD/SciELO-Spain-Crawler) | 60,007,289 | Publications written in Spanish crawled from the Spanish SciELO server in 2017. |
| [BARR2_background](https://temu.bsc.es/BARR2/downloads/background_set.raw_text.tar.bz2) | 24,516,442 | Biomedical Abbreviation Recognition and Resolution (BARR2) containing Spanish clinical case study sections from a variety of clinical disciplines. |
| Wikipedia_life_sciences | 13,890,501 | Wikipedia articles crawled 04/01/2021 with the [Wikipedia API python library](https://pypi.org/project/Wikipedia-API/) starting from the "Ciencias\_de\_la\_vida" category up to a maximum of 5 subcategories. Multiple links to the same articles are then discarded to avoid repeating content. |
| Patents | 13,463,387 | Google Patent in Medical Domain for Spain (Spanish). The accepted codes (Medical Domain) for Json files of patents are: "A61B", "A61C","A61F", "A61H", "A61K", "A61L","A61M", "A61B", "A61P". |
| [EMEA](http://opus.nlpl.eu/download.php?f=EMEA/v3/moses/en-es.txt.zip) | 5,377,448 | Spanish-side documents extracted from parallel corpora made out of PDF documents from the European Medicines Agency. |
| [mespen_Medline](https://zenodo.org/record/3562536#.YTt1fH2xXbR) | 4,166,077 | Spanish-side articles extracted from a collection of Spanish-English parallel corpus consisting of biomedical scientific literature. The collection of parallel resources are aggregated from the MedlinePlus source. |
| PubMed | 1,858,966 | Open-access articles from the PubMed repository crawled in 2017. |
## Evaluation and results
The model has been evaluated on the Named Entity Recognition (NER) using the following datasets:
- [PharmaCoNER](https://zenodo.org/record/4270158): is a track on chemical and drug mention recognition from Spanish medical texts (for more info see: https://temu.bsc.es/pharmaconer/).
- [CANTEMIST](https://zenodo.org/record/3978041#.YTt5qH2xXbQ): is a shared task specifically focusing on named entity recognition of tumor morphology, in Spanish (for more info see: https://zenodo.org/record/3978041#.YTt5qH2xXbQ).
- ICTUSnet: consists of 1,006 hospital discharge reports of patients admitted for stroke from 18 different Spanish hospitals. It contains more than 79,000 annotations for 51 different kinds of variables.
The evaluation results are compared against the [mBERT](https://huggingface.co/bert-base-multilingual-cased) and [BETO](https://huggingface.co/dccuchile/bert-base-spanish-wwm-cased) models:
| F1 - Precision - Recall | roberta-base-biomedical-clinical-es | mBERT | BETO |
|---------------------------|----------------------------|-------------------------------|-------------------------|
| PharmaCoNER | **90.04** - **88.92** - **91.18** | 87.46 - 86.50 - 88.46 | 88.18 - 87.12 - 89.28 |
| CANTEMIST | **83.34** - **81.48** - **85.30** | 82.61 - 81.12 - 84.15 | 82.42 - 80.91 - 84.00 |
| ICTUSnet | **88.08** - **84.92** - **91.50** | 86.75 - 83.53 - 90.23 | 85.95 - 83.10 - 89.02 |
## Intended uses & limitations
The model is ready-to-use only for masked language modelling to perform the Fill Mask task (try the inference API or read the next section)
However, the is intended to be fine-tuned on downstream tasks such as Named Entity Recognition or Text Classification.
## Cite
If you use our models, please cite our latest preprint:
```bibtex
@misc{carrino2021biomedical,
title={Biomedical and Clinical Language Models for Spanish: On the Benefits of Domain-Specific Pretraining in a Mid-Resource Scenario},
author={Casimiro Pio Carrino and Jordi Armengol-Estapé and Asier Gutiérrez-Fandiño and Joan Llop-Palao and Marc Pàmies and Aitor Gonzalez-Agirre and Marta Villegas},
year={2021},
eprint={2109.03570},
archivePrefix={arXiv},
primaryClass={cs.CL}
}
```
If you use our Medical Crawler corpus, please cite the preprint:
```bibtex
@misc{carrino2021spanish,
title={Spanish Biomedical Crawled Corpus: A Large, Diverse Dataset for Spanish Biomedical Language Models},
author={Casimiro Pio Carrino and Jordi Armengol-Estapé and Ona de Gibert Bonet and Asier Gutiérrez-Fandiño and Aitor Gonzalez-Agirre and Martin Krallinger and Marta Villegas},
year={2021},
eprint={2109.07765},
archivePrefix={arXiv},
primaryClass={cs.CL}
}
```
---
---
## How to use
```python
from transformers import AutoTokenizer, AutoModelForMaskedLM
tokenizer = AutoTokenizer.from_pretrained("BSC-TeMU/roberta-base-biomedical-es")
model = AutoModelForMaskedLM.from_pretrained("BSC-TeMU/roberta-base-biomedical-es")
from transformers import pipeline
unmasker = pipeline('fill-mask', model="BSC-TeMU/roberta-base-biomedical-es")
unmasker("El único antecedente personal a reseñar era la <mask> arterial.")
```
```
# Output
[
{
"sequence": " El único antecedente personal a reseñar era la hipertensión arterial.",
"score": 0.9855039715766907,
"token": 3529,
"token_str": " hipertensión"
},
{
"sequence": " El único antecedente personal a reseñar era la diabetes arterial.",
"score": 0.0039140828885138035,
"token": 1945,
"token_str": " diabetes"
},
{
"sequence": " El único antecedente personal a reseñar era la hipotensión arterial.",
"score": 0.002484665485098958,
"token": 11483,
"token_str": " hipotensión"
},
{
"sequence": " El único antecedente personal a reseñar era la Hipertensión arterial.",
"score": 0.0023484621196985245,
"token": 12238,
"token_str": " Hipertensión"
},
{
"sequence": " El único antecedente personal a reseñar era la presión arterial.",
"score": 0.0008009297889657319,
"token": 2267,
"token_str": " presión"
}
]
``` |
Fujitsu/AugCode | caf60fe07c98d0574b948c76770787662170ae6f | 2021-05-20T11:51:49.000Z | [
"pytorch",
"tf",
"jax",
"roberta",
"text-classification",
"en",
"dataset:augmented_codesearchnet",
"transformers",
"license:mit"
]
| text-classification | false | Fujitsu | null | Fujitsu/AugCode | 41 | null | transformers | 6,376 | ---
inference: false
license: mit
widget:
language:
- en
metrics:
- mrr
datasets:
- augmented_codesearchnet
---
# 🔥 Augmented Code Model 🔥
This is Augmented Code Model which is a fined-tune model of [CodeBERT](https://huggingface.co/microsoft/codebert-base) for processing of similarity between given docstring and code. This model is fined-model based on Augmented Code Corpus with ACS=4.
## How to use the model ?
Similar to other huggingface model, you may load the model as follows.
```python
from transformers import AutoTokenizer, AutoModelForSequenceClassification
tokenizer = AutoTokenizer.from_pretrained("Fujitsu/AugCode")
model = AutoModelForSequenceClassification.from_pretrained("Fujitsu/AugCode")
```
Then you may use `model` to infer the similarity between a given docstring and code.
### Citation
```bibtex@misc{bahrami2021augcode,
title={AugmentedCode: Examining the Effects of Natural Language Resources in Code Retrieval Models},
author={Mehdi Bahrami, N. C. Shrikanth, Yuji Mizobuchi, Lei Liu, Masahiro Fukuyori, Wei-Peng Chen, Kazuki Munakata},
year={2021},
eprint={TBA},
archivePrefix={TBA},
primaryClass={cs.CL}
}
``` |
Geotrend/distilbert-base-en-fr-es-de-zh-cased | 27a1e7f4623b07233a0f01609396aebe5f1749a0 | 2021-07-28T10:36:16.000Z | [
"pytorch",
"distilbert",
"fill-mask",
"multilingual",
"dataset:wikipedia",
"transformers",
"license:apache-2.0",
"autotrain_compatible"
]
| fill-mask | false | Geotrend | null | Geotrend/distilbert-base-en-fr-es-de-zh-cased | 41 | null | transformers | 6,377 | ---
language: multilingual
datasets: wikipedia
license: apache-2.0
---
# distilbert-base-en-fr-es-de-zh-cased
We are sharing smaller versions of [distilbert-base-multilingual-cased](https://huggingface.co/distilbert-base-multilingual-cased) that handle a custom number of languages.
Our versions give exactly the same representations produced by the original model which preserves the original accuracy.
For more information please visit our paper: [Load What You Need: Smaller Versions of Multilingual BERT](https://www.aclweb.org/anthology/2020.sustainlp-1.16.pdf).
## How to use
```python
from transformers import AutoTokenizer, AutoModel
tokenizer = AutoTokenizer.from_pretrained("Geotrend/distilbert-base-en-fr-es-de-zh-cased")
model = AutoModel.from_pretrained("Geotrend/distilbert-base-en-fr-es-de-zh-cased")
```
To generate other smaller versions of multilingual transformers please visit [our Github repo](https://github.com/Geotrend-research/smaller-transformers).
### How to cite
```bibtex
@inproceedings{smallermdistilbert,
title={Load What You Need: Smaller Versions of Mutlilingual BERT},
author={Abdaoui, Amine and Pradel, Camille and Sigel, Grégoire},
booktitle={SustaiNLP / EMNLP},
year={2020}
}
```
## Contact
Please contact [email protected] for any question, feedback or request. |
Helsinki-NLP/opus-mt-ca-fr | db974afe6ff1c6b1c1b058216583c8483068e38d | 2021-01-18T07:53:03.000Z | [
"pytorch",
"marian",
"text2text-generation",
"ca",
"fr",
"transformers",
"translation",
"license:apache-2.0",
"autotrain_compatible"
]
| translation | false | Helsinki-NLP | null | Helsinki-NLP/opus-mt-ca-fr | 41 | null | transformers | 6,378 | ---
language:
- ca
- fr
tags:
- translation
license: apache-2.0
---
### cat-fra
* source group: Catalan
* target group: French
* OPUS readme: [cat-fra](https://github.com/Helsinki-NLP/Tatoeba-Challenge/tree/master/models/cat-fra/README.md)
* model: transformer-align
* source language(s): cat
* target language(s): fra
* model: transformer-align
* pre-processing: normalization + SentencePiece (spm12k,spm12k)
* download original weights: [opus-2020-06-16.zip](https://object.pouta.csc.fi/Tatoeba-MT-models/cat-fra/opus-2020-06-16.zip)
* test set translations: [opus-2020-06-16.test.txt](https://object.pouta.csc.fi/Tatoeba-MT-models/cat-fra/opus-2020-06-16.test.txt)
* test set scores: [opus-2020-06-16.eval.txt](https://object.pouta.csc.fi/Tatoeba-MT-models/cat-fra/opus-2020-06-16.eval.txt)
## Benchmarks
| testset | BLEU | chr-F |
|-----------------------|-------|-------|
| Tatoeba-test.cat.fra | 52.4 | 0.694 |
### System Info:
- hf_name: cat-fra
- source_languages: cat
- target_languages: fra
- opus_readme_url: https://github.com/Helsinki-NLP/Tatoeba-Challenge/tree/master/models/cat-fra/README.md
- original_repo: Tatoeba-Challenge
- tags: ['translation']
- languages: ['ca', 'fr']
- src_constituents: {'cat'}
- tgt_constituents: {'fra'}
- src_multilingual: False
- tgt_multilingual: False
- prepro: normalization + SentencePiece (spm12k,spm12k)
- url_model: https://object.pouta.csc.fi/Tatoeba-MT-models/cat-fra/opus-2020-06-16.zip
- url_test_set: https://object.pouta.csc.fi/Tatoeba-MT-models/cat-fra/opus-2020-06-16.test.txt
- src_alpha3: cat
- tgt_alpha3: fra
- short_pair: ca-fr
- chrF2_score: 0.6940000000000001
- bleu: 52.4
- brevity_penalty: 0.987
- ref_len: 5517.0
- src_name: Catalan
- tgt_name: French
- train_date: 2020-06-16
- src_alpha2: ca
- tgt_alpha2: fr
- prefer_old: False
- long_pair: cat-fra
- helsinki_git_sha: 480fcbe0ee1bf4774bcbe6226ad9f58e63f6c535
- transformers_git_sha: 2207e5d8cb224e954a7cba69fa4ac2309e9ff30b
- port_machine: brutasse
- port_time: 2020-08-21-14:41 |
Helsinki-NLP/opus-mt-inc-en | b95c52d5907ce0a4718662571c63df42e73bdbe0 | 2020-08-21T14:42:46.000Z | [
"pytorch",
"marian",
"text2text-generation",
"bn",
"or",
"gu",
"mr",
"ur",
"hi",
"as",
"si",
"inc",
"en",
"transformers",
"translation",
"license:apache-2.0",
"autotrain_compatible"
]
| translation | false | Helsinki-NLP | null | Helsinki-NLP/opus-mt-inc-en | 41 | null | transformers | 6,379 | ---
language:
- bn
- or
- gu
- mr
- ur
- hi
- as
- si
- inc
- en
tags:
- translation
license: apache-2.0
---
### inc-eng
* source group: Indic languages
* target group: English
* OPUS readme: [inc-eng](https://github.com/Helsinki-NLP/Tatoeba-Challenge/tree/master/models/inc-eng/README.md)
* model: transformer
* source language(s): asm awa ben bho gom guj hif_Latn hin mai mar npi ori pan_Guru pnb rom san_Deva sin snd_Arab urd
* target language(s): eng
* model: transformer
* pre-processing: normalization + SentencePiece (spm32k,spm32k)
* download original weights: [opus2m-2020-08-01.zip](https://object.pouta.csc.fi/Tatoeba-MT-models/inc-eng/opus2m-2020-08-01.zip)
* test set translations: [opus2m-2020-08-01.test.txt](https://object.pouta.csc.fi/Tatoeba-MT-models/inc-eng/opus2m-2020-08-01.test.txt)
* test set scores: [opus2m-2020-08-01.eval.txt](https://object.pouta.csc.fi/Tatoeba-MT-models/inc-eng/opus2m-2020-08-01.eval.txt)
## Benchmarks
| testset | BLEU | chr-F |
|-----------------------|-------|-------|
| newsdev2014-hineng.hin.eng | 8.9 | 0.341 |
| newsdev2019-engu-gujeng.guj.eng | 8.7 | 0.321 |
| newstest2014-hien-hineng.hin.eng | 13.1 | 0.396 |
| newstest2019-guen-gujeng.guj.eng | 6.5 | 0.290 |
| Tatoeba-test.asm-eng.asm.eng | 18.1 | 0.363 |
| Tatoeba-test.awa-eng.awa.eng | 6.2 | 0.222 |
| Tatoeba-test.ben-eng.ben.eng | 44.7 | 0.595 |
| Tatoeba-test.bho-eng.bho.eng | 29.4 | 0.458 |
| Tatoeba-test.guj-eng.guj.eng | 19.3 | 0.383 |
| Tatoeba-test.hif-eng.hif.eng | 3.7 | 0.220 |
| Tatoeba-test.hin-eng.hin.eng | 38.6 | 0.564 |
| Tatoeba-test.kok-eng.kok.eng | 6.6 | 0.287 |
| Tatoeba-test.lah-eng.lah.eng | 16.0 | 0.272 |
| Tatoeba-test.mai-eng.mai.eng | 75.6 | 0.796 |
| Tatoeba-test.mar-eng.mar.eng | 25.9 | 0.497 |
| Tatoeba-test.multi.eng | 29.0 | 0.502 |
| Tatoeba-test.nep-eng.nep.eng | 4.5 | 0.198 |
| Tatoeba-test.ori-eng.ori.eng | 5.0 | 0.226 |
| Tatoeba-test.pan-eng.pan.eng | 17.4 | 0.375 |
| Tatoeba-test.rom-eng.rom.eng | 1.7 | 0.174 |
| Tatoeba-test.san-eng.san.eng | 5.0 | 0.173 |
| Tatoeba-test.sin-eng.sin.eng | 31.2 | 0.511 |
| Tatoeba-test.snd-eng.snd.eng | 45.7 | 0.670 |
| Tatoeba-test.urd-eng.urd.eng | 25.6 | 0.456 |
### System Info:
- hf_name: inc-eng
- source_languages: inc
- target_languages: eng
- opus_readme_url: https://github.com/Helsinki-NLP/Tatoeba-Challenge/tree/master/models/inc-eng/README.md
- original_repo: Tatoeba-Challenge
- tags: ['translation']
- languages: ['bn', 'or', 'gu', 'mr', 'ur', 'hi', 'as', 'si', 'inc', 'en']
- src_constituents: {'pnb', 'gom', 'ben', 'hif_Latn', 'ori', 'guj', 'pan_Guru', 'snd_Arab', 'npi', 'mar', 'urd', 'bho', 'hin', 'san_Deva', 'asm', 'rom', 'mai', 'awa', 'sin'}
- tgt_constituents: {'eng'}
- src_multilingual: True
- tgt_multilingual: False
- prepro: normalization + SentencePiece (spm32k,spm32k)
- url_model: https://object.pouta.csc.fi/Tatoeba-MT-models/inc-eng/opus2m-2020-08-01.zip
- url_test_set: https://object.pouta.csc.fi/Tatoeba-MT-models/inc-eng/opus2m-2020-08-01.test.txt
- src_alpha3: inc
- tgt_alpha3: eng
- short_pair: inc-en
- chrF2_score: 0.502
- bleu: 29.0
- brevity_penalty: 1.0
- ref_len: 64706.0
- src_name: Indic languages
- tgt_name: English
- train_date: 2020-08-01
- src_alpha2: inc
- tgt_alpha2: en
- prefer_old: False
- long_pair: inc-eng
- helsinki_git_sha: 480fcbe0ee1bf4774bcbe6226ad9f58e63f6c535
- transformers_git_sha: 2207e5d8cb224e954a7cba69fa4ac2309e9ff30b
- port_machine: brutasse
- port_time: 2020-08-21-14:41 |
Muennighoff/SGPT-125M-weightedmean-nli | 9e7693f0f115b3ddecde41b574574e0750b3841c | 2022-02-21T06:19:26.000Z | [
"pytorch",
"gpt_neo",
"feature-extraction",
"arxiv:2202.08904",
"sentence-transformers",
"sentence-similarity"
]
| sentence-similarity | false | Muennighoff | null | Muennighoff/SGPT-125M-weightedmean-nli | 41 | null | sentence-transformers | 6,380 | ---
pipeline_tag: sentence-similarity
tags:
- sentence-transformers
- feature-extraction
- sentence-similarity
---
# SGPT-125M-weightedmean-nli
## Usage
For usage instructions, refer to our codebase: https://github.com/Muennighoff/sgpt
## Evaluation Results
For eval results, refer to our paper: https://arxiv.org/abs/2202.08904
## Training
The model was trained with the parameters:
**DataLoader**:
`sentence_transformers.datasets.NoDuplicatesDataLoader.NoDuplicatesDataLoader` of length 8807 with parameters:
```
{'batch_size': 64}
```
**Loss**:
`sentence_transformers.losses.MultipleNegativesRankingLoss.MultipleNegativesRankingLoss` with parameters:
```
{'scale': 20.0, 'similarity_fct': 'cos_sim'}
```
Parameters of the fit()-Method:
```
{
"epochs": 1,
"evaluation_steps": 880,
"evaluator": "sentence_transformers.evaluation.EmbeddingSimilarityEvaluator.EmbeddingSimilarityEvaluator",
"max_grad_norm": 1,
"optimizer_class": "<class 'transformers.optimization.AdamW'>",
"optimizer_params": {
"lr": 2e-05
},
"scheduler": "WarmupLinear",
"steps_per_epoch": null,
"warmup_steps": 881,
"weight_decay": 0.01
}
```
## Full Model Architecture
```
SentenceTransformer(
(0): Transformer({'max_seq_length': 75, 'do_lower_case': False}) with Transformer model: GPTNeoModel
(1): Pooling({'word_embedding_dimension': 768, 'pooling_mode_cls_token': False, 'pooling_mode_mean_tokens': False, 'pooling_mode_max_tokens': False, 'pooling_mode_mean_sqrt_len_tokens': False, 'pooling_mode_weightedmean_tokens': True, 'pooling_mode_lasttoken': False})
)
```
## Citing & Authors
```bibtex
@article{muennighoff2022sgpt,
title={SGPT: GPT Sentence Embeddings for Semantic Search},
author={Muennighoff, Niklas},
journal={arXiv preprint arXiv:2202.08904},
year={2022}
}
```
|
RJ3vans/CCVspanTagger | 846daae8b8c71ebd08c2e6cb28675a194679c548 | 2022-07-14T23:46:26.000Z | [
"pytorch",
"bert",
"token-classification",
"transformers",
"autotrain_compatible"
]
| token-classification | false | RJ3vans | null | RJ3vans/CCVspanTagger | 41 | null | transformers | 6,381 | Try the test sentence:
<i>The woman said "my name is Sarah [and] I live in London."</i>
The model should tag the tokens in the sentence with information about whether or not they are contained within a compound clause. If you find the model useful, please cite my thesis which presents the dataset used for finetuning:
Evans, R. (2020) Sentence Simplification for Text Processing. Doctoral thesis. University of Wolverhampton. Wolverhampton, UK. (http://rgcl.wlv.ac.uk/~richard/Evans2020_SentenceSimplificationForTextProcessing.pdf)
There you will find more information about the tagging scheme.
The model was derived using code adapted from an original program written by Dr. Le An Ha at the University of Wolverhampton. |
SpacyGalaxy/DialoGPT-medium-Gandalf | 03d422eb3ee4e1ad90d0db5ca5a48b762b13bf81 | 2022-02-05T16:03:05.000Z | [
"pytorch",
"gpt2",
"text-generation",
"transformers",
"conversational"
]
| conversational | false | SpacyGalaxy | null | SpacyGalaxy/DialoGPT-medium-Gandalf | 41 | null | transformers | 6,382 | ---
tags:
- conversational
---
#Gandalf DialoGPT Model |
T-Systems-onsite/cross-en-fr-roberta-sentence-transformer | 6f2e0d0e39d6c82df959899db884567d51f4560b | 2022-06-28T19:57:04.000Z | [
"pytorch",
"tf",
"xlm-roberta",
"feature-extraction",
"fr",
"en",
"dataset:stsb_multi_mt",
"transformers",
"sentence_embedding",
"search",
"roberta",
"xlm-r-distilroberta-base-paraphrase-v1",
"license:mit"
]
| feature-extraction | false | T-Systems-onsite | null | T-Systems-onsite/cross-en-fr-roberta-sentence-transformer | 41 | null | transformers | 6,383 | ---
language:
- fr
- en
license: mit
tags:
- sentence_embedding
- search
- pytorch
- xlm-roberta
- roberta
- xlm-r-distilroberta-base-paraphrase-v1
datasets:
- stsb_multi_mt
metrics:
- Spearman’s rank correlation
- cosine similarity
---
# Cross English & French RoBERTa for Sentence Embeddings
|
castorini/bpr-nq-ctx-encoder | c905ce72c76dc9562672a22f2211cf31ab8009ba | 2021-09-05T00:57:58.000Z | [
"pytorch",
"dpr",
"transformers"
]
| null | false | castorini | null | castorini/bpr-nq-ctx-encoder | 41 | null | transformers | 6,384 | This model is converted from the original BPR [repo](https://github.com/studio-ousia/bpr) and fitted into Pyserini:
> Ikuya Yamada, Akari Asai, and Hannaneh Hajishirzi. 2021. Efficient passage retrieval with hashing for open-domain question answering. arXiv:2106.00882. |
ccdv/lsg-barthez-4096 | ce82caa236faf603a7422025c1e73c05aabe3aa1 | 2022-07-25T17:11:44.000Z | [
"pytorch",
"mbart",
"text2text-generation",
"fr",
"transformers",
"summarization",
"bart",
"long context",
"fill-mask",
"autotrain_compatible"
]
| fill-mask | false | ccdv | null | ccdv/lsg-barthez-4096 | 41 | null | transformers | 6,385 | ---
tags:
- summarization
- bart
- long context
language:
- fr
pipeline_tag: fill-mask
---
# LSG model
**Transformers >= 4.18.0**\
**This model relies on a custom modeling file, you need to add trust_remote_code=True**\
**See [\#13467](https://github.com/huggingface/transformers/pull/13467)**
* [Usage](#usage)
* [Parameters](#parameters)
* [Sparse selection type](#sparse-selection-type)
* [Tasks](#tasks)
This model is adapted from [BARThez](https://huggingface.co/moussaKam/barthez) for encoder-decoder tasks without additional pretraining. It uses the same number of parameters/layers and the same tokenizer.
This model can handle long sequences but faster and more efficiently than Longformer (LED) or BigBird (Pegasus) from the hub and relies on Local + Sparse + Global attention (LSG).
The model requires sequences whose length is a multiple of the block size. The model is "adaptive" and automatically pads the sequences if needed (adaptive=True in config). It is however recommended, thanks to the tokenizer, to truncate the inputs (truncation=True) and optionally to pad with a multiple of the block size (pad_to_multiple_of=...). \
Implemented in PyTorch.

## Usage
The model relies on a custom modeling file, you need to add trust_remote_code=True to use it.
```python:
from transformers import AutoModel, AutoTokenizer
model = AutoModel.from_pretrained("ccdv/lsg-barthez-4096", trust_remote_code=True)
tokenizer = AutoTokenizer.from_pretrained("ccdv/lsg-barthez-4096")
```
## Parameters
You can change various parameters like :
* the number of global tokens (num_global_tokens=1)
* local block size (block_size=128)
* sparse block size (sparse_block_size=128)
* sparsity factor (sparsity_factor=2)
* mask_first_token (mask first token since it is redundant with the first global token)
* see config.json file
Default parameters work well in practice. If you are short on memory, reduce block sizes, increase sparsity factor and remove dropout in the attention score matrix.
```python:
from transformers import AutoModel
model = AutoModel.from_pretrained("ccdv/lsg-barthez-4096",
trust_remote_code=True,
num_global_tokens=16,
block_size=64,
sparse_block_size=64,
attention_probs_dropout_prob=0.0
sparsity_factor=4,
sparsity_type="none",
mask_first_token=True
)
```
## Sparse selection type
There are 5 different sparse selection patterns. The best type is task dependent. \
Note that for sequences with length < 2*block_size, the type has no effect.
* sparsity_type="norm", select highest norm tokens
* Works best for a small sparsity_factor (2 to 4)
* Additional parameters:
* None
* sparsity_type="pooling", use average pooling to merge tokens
* Works best for a small sparsity_factor (2 to 4)
* Additional parameters:
* None
* sparsity_type="lsh", use the LSH algorithm to cluster similar tokens
* Works best for a large sparsity_factor (4+)
* LSH relies on random projections, thus inference may differ slightly with different seeds
* Additional parameters:
* lsg_num_pre_rounds=1, pre merge tokens n times before computing centroids
* sparsity_type="stride", use a striding mecanism per head
* Each head will use different tokens strided by sparsify_factor
* Not recommended if sparsify_factor > num_heads
* sparsity_type="block_stride", use a striding mecanism per head
* Each head will use block of tokens strided by sparsify_factor
* Not recommended if sparsify_factor > num_heads
## Tasks
Seq2Seq example for summarization:
```python:
from transformers import AutoModelForSeq2SeqLM, AutoTokenizer
model = AutoModelForSeq2SeqLM.from_pretrained("ccdv/lsg-barthez-4096",
trust_remote_code=True,
pass_global_tokens_to_decoder=True, # Pass encoder global tokens to decoder
)
tokenizer = AutoTokenizer.from_pretrained("ccdv/lsg-barthez-4096")
SENTENCE = "This is a test sequence to test the model. " * 300
token_ids = tokenizer(
SENTENCE,
return_tensors="pt",
padding="max_length", # Optional but recommended
truncation=True # Optional but recommended
)
output = model(**token_ids)
```
Classification example:
```python:
from transformers import AutoModelForSequenceClassification, AutoTokenizer
model = AutoModelForSequenceClassification.from_pretrained("ccdv/lsg-barthez-4096",
trust_remote_code=True,
pass_global_tokens_to_decoder=True, # Pass encoder global tokens to decoder
)
tokenizer = AutoTokenizer.from_pretrained("ccdv/lsg-barthez-4096")
SENTENCE = "This is a test sequence to test the model. " * 300
token_ids = tokenizer(
SENTENCE,
return_tensors="pt",
#pad_to_multiple_of=... # Optional
truncation=True
)
output = model(**token_ids)
> SequenceClassifierOutput(loss=None, logits=tensor([[-0.3051, -0.1762]], grad_fn=<AddmmBackward>), hidden_states=None, attentions=None)
```
## Conversion script
To convert a BERT, RoBERTa or BART checkpoint to LSG, see this [repo](https://github.com/ccdv-ai/convert_checkpoint_to_lsg).
**BARThez**
```
@article{eddine2020barthez,
title={BARThez: a Skilled Pretrained French Sequence-to-Sequence Model},
author={Eddine, Moussa Kamal and Tixier, Antoine J-P and Vazirgiannis, Michalis},
journal={arXiv preprint arXiv:2010.12321},
year={2020}
}
``` |
facebook/s2t-small-covost2-fr-en-st | 0eb50631bf2b906f2b694e15db162d20620bb6e9 | 2022-02-07T15:33:11.000Z | [
"pytorch",
"tf",
"speech_to_text",
"automatic-speech-recognition",
"fr",
"en",
"dataset:covost2",
"arxiv:2010.05171",
"arxiv:1912.06670",
"arxiv:1904.08779",
"transformers",
"audio",
"speech-translation",
"license:mit"
]
| automatic-speech-recognition | false | facebook | null | facebook/s2t-small-covost2-fr-en-st | 41 | null | transformers | 6,386 | ---
language:
- fr
- en
datasets:
- covost2
tags:
- audio
- speech-translation
- automatic-speech-recognition
license: mit
pipeline_tag: automatic-speech-recognition
widget:
- example_title: Librispeech sample 1
src: https://cdn-media.huggingface.co/speech_samples/sample1.flac
- example_title: Librispeech sample 2
src: https://cdn-media.huggingface.co/speech_samples/sample2.flac
---
# S2T-SMALL-COVOST2-FR-EN-ST
`s2t-small-covost2-fr-en-st` is a Speech to Text Transformer (S2T) model trained for end-to-end Speech Translation (ST).
The S2T model was proposed in [this paper](https://arxiv.org/abs/2010.05171) and released in
[this repository](https://github.com/pytorch/fairseq/tree/master/examples/speech_to_text)
## Model description
S2T is a transformer-based seq2seq (encoder-decoder) model designed for end-to-end Automatic Speech Recognition (ASR) and Speech
Translation (ST). It uses a convolutional downsampler to reduce the length of speech inputs by 3/4th before they are
fed into the encoder. The model is trained with standard autoregressive cross-entropy loss and generates the
transcripts/translations autoregressively.
## Intended uses & limitations
This model can be used for end-to-end French speech to English text translation.
See the [model hub](https://huggingface.co/models?filter=speech_to_text) to look for other S2T checkpoints.
### How to use
As this a standard sequence to sequence transformer model, you can use the `generate` method to generate the
transcripts by passing the speech features to the model.
*Note: The `Speech2TextProcessor` object uses [torchaudio](https://github.com/pytorch/audio) to extract the
filter bank features. Make sure to install the `torchaudio` package before running this example.*
You could either install those as extra speech dependancies with
`pip install transformers"[speech, sentencepiece]"` or install the packages seperatly
with `pip install torchaudio sentencepiece`.
```python
import torch
from transformers import Speech2TextProcessor, Speech2TextForConditionalGeneration
from datasets import load_dataset
import soundfile as sf
model = Speech2TextForConditionalGeneration.from_pretrained("facebook/s2t-small-covost2-fr-en-st")
processor = Speech2TextProcessor.from_pretrained("facebook/s2t-small-covost2-fr-en-st")
def map_to_array(batch):
speech, _ = sf.read(batch["file"])
batch["speech"] = speech
return batch
ds = load_dataset(
"patrickvonplaten/librispeech_asr_dummy",
"clean",
split="validation"
)
ds = ds.map(map_to_array)
inputs = processor(
ds["speech"][0],
sampling_rate=48_000,
return_tensors="pt"
)
generated_ids = model.generate(input_ids=inputs["input_features"], attention_mask=inputs["attention_mask"])
translation = processor.batch_decode(generated_ids, skip_special_tokens=True)
```
## Training data
The s2t-small-covost2-fr-en-st is trained on French-English subset of [CoVoST2](https://github.com/facebookresearch/covost).
CoVoST is a large-scale multilingual ST corpus based on [Common Voice](https://arxiv.org/abs/1912.06670), created to to foster
ST research with the largest ever open dataset
## Training procedure
### Preprocessing
The speech data is pre-processed by extracting Kaldi-compliant 80-channel log mel-filter bank features automatically from
WAV/FLAC audio files via PyKaldi or torchaudio. Further utterance-level CMVN (cepstral mean and variance normalization)
is applied to each example.
The texts are lowercased and tokenized using character based SentencePiece vocab.
### Training
The model is trained with standard autoregressive cross-entropy loss and using [SpecAugment](https://arxiv.org/abs/1904.08779).
The encoder receives speech features, and the decoder generates the transcripts autoregressively. To accelerate
model training and for better performance the encoder is pre-trained for English ASR.
## Evaluation results
CoVOST2 test results for fr-en (BLEU score): 26.25
### BibTeX entry and citation info
```bibtex
@inproceedings{wang2020fairseqs2t,
title = {fairseq S2T: Fast Speech-to-Text Modeling with fairseq},
author = {Changhan Wang and Yun Tang and Xutai Ma and Anne Wu and Dmytro Okhonko and Juan Pino},
booktitle = {Proceedings of the 2020 Conference of the Asian Chapter of the Association for Computational Linguistics (AACL): System Demonstrations},
year = {2020},
}
```
|
flax-community/alberti-bert-base-multilingual-cased | c081a91539f2fad5e68d7c86fac1aea3aa9e47a6 | 2022-01-18T23:19:36.000Z | [
"pytorch",
"jax",
"joblib",
"bert",
"fill-mask",
"es",
"transformers",
"multilingual",
"license:cc-by-4.0",
"autotrain_compatible"
]
| fill-mask | false | flax-community | null | flax-community/alberti-bert-base-multilingual-cased | 41 | 1 | transformers | 6,387 | ---
language: es
license: cc-by-4.0
tags:
- multilingual
- bert
pipeline_tag: fill-mask
widget:
- text: ¿Qué es la vida? Un [MASK].
---
# ALBERTI
ALBERTI is a set of two BERT-based multilingual model for poetry. One for verses and another one for stanzas. This model has been further trained with the PULPO corpus for verses using [Flax](https://github.com/google/flax), including training scripts.
This is part of the
[Flax/Jax Community Week](https://discuss.huggingface.co/t/open-to-the-community-community-week-using-jax-flax-for-nlp-cv/7104), organised by [HuggingFace](https://huggingface.co/) and TPU usage sponsored by Google.
## PULPO
PULPO, the Prodigious Unannotated Literary Poetry Corpus, is a set of multilingual corpora of verses and stanzas with over 95M words.
The following corpora has been downloaded using the [Averell](https://github.com/linhd-postdata/averell/) tool, developed by the [POSTDATA](https://postdata.linhd.uned.es/) team:
### Spanish
- [Disco v3](https://github.com/pruizf/disco)
- [Corpus of Spanish Golden-Age Sonnets](https://github.com/bncolorado/CorpusSonetosSigloDeOro)
- [Corpus general de poesía lírica castellana del Siglo de Oro](https://github.com/bncolorado/CorpusGeneralPoesiaLiricaCastellanaDelSigloDeOro)
- [Gongocorpus](https://github.com/linhd-postdata/gongocorpus) - [source](http://obvil.sorbonne-universite.site/corpus/gongora/gongora_obra-poetica)
### English
- [Eighteenth-Century Poetry Archive (ECPA)](https://github.com/alhuber1502/ECPA)
- [For better for verse](https://github.com/waynegraham/for_better_for_verse)
### French
- [Métrique en Ligne](https://crisco2.unicaen.fr/verlaine/index.php?navigation=accueil) - [source](https://github.com/linhd-postdata/metrique-en-ligne)
### Italian
- [Biblioteca italiana](https://github.com/linhd-postdata/biblioteca_italiana) - [source](http://www.bibliotecaitaliana.it/)
### Czech
- [Corpus of Czech Verse](https://github.com/versotym/corpusCzechVerse)
### Portuguese
- [Stichotheque](https://gitlab.com/stichotheque/stichotheque-pt)
Also, we obtained the following corpora from these sources:
### Spanish
- [Poesi.as](https://github.com/linhd-postdata/poesi.as) - [source](http://www.poesi.as/)
### English
- [A Gutenberg Poetry Corpus](https://github.com/aparrish/gutenberg-poetry-corpus)
### Arabic
- [Arabic Poetry dataset](https://www.kaggle.com/ahmedabelal/arabic-poetry)
### Chinese
- [THU Chinese Classical Poetry Corpus](https://github.com/THUNLP-AIPoet/Datasets/tree/master/CCPC)
### Finnish
- [SKVR](https://github.com/sks190/SKVR)
### German
- [TextGrid Poetry Corpus](https://github.com/linhd-postdata/textgrid-poetry) - [source](https://textgrid.de/en/digitale-bibliothek)
- [German Rhyme Corpus](https://github.com/tnhaider/german-rhyme-corpus)
### Hungarian
- [verskorpusz](https://github.com/ELTE-DH/verskorpusz)
### Portuguese
- [Poems in Portuguese](https://www.kaggle.com/oliveirasp6/poems-in-portuguese)
### Russian
- [19 000 Russian poems](https://www.kaggle.com/grafstor/19-000-russian-poems)
## Team members
- Álvaro Pérez ([alvp](https://huggingface.co/alvp))
- Javier de la Rosa ([versae](https://huggingface.co/versae))
- Aitor Díaz ([aitordiaz](https://huggingface.co/aitordiaz))
- Elena González-Blanco
- Salvador Ros ([salva](https://huggingface.co/salva))
## Useful links
- [Community Week timeline](https://discuss.huggingface.co/t/open-to-the-community-community-week-using-jax-flax-for-nlp-cv/7104#summary-timeline-calendar-6)
- [Community Week README](https://github.com/huggingface/transformers/blob/master/examples/research_projects/jax-projects/README.md)
- [Community Week thread](https://discuss.huggingface.co/t/bertin-pretrain-roberta-large-from-scratch-in-spanish/7125)
- [Community Week channel](https://discord.com/channels/858019234139602994/859113060068229190)
- [Masked Language Modelling example scripts](https://github.com/huggingface/transformers/tree/master/examples/flax/language-modeling)
- [Model Repository](https://huggingface.co/flax-community/alberti-bert-base-multilingual-cased/)
## Acknowledgments
This project would not have been possible without the infrastructure and resources provided by HuggingFace and Google Cloud. Moreover, we want to thank POSTDATA Project (ERC-StG-679528) and the Computational Literary Studies Infrastructure (CLS INFRA No. 101004984) of the European Union's Horizon 2020 research and innovation programme for their support and time allowance. |
frizwankhan/entity-linking-model-final | a303c2d39adde09c8f2341e534a93bc3c704c5a4 | 2022-01-13T13:32:04.000Z | [
"pytorch",
"layoutlmv2",
"question-answering",
"transformers",
"autotrain_compatible"
]
| question-answering | false | frizwankhan | null | frizwankhan/entity-linking-model-final | 41 | null | transformers | 6,388 | Entry not found |
google/t5-efficient-large | 4d2cec4e7a1171605cbe4014985fc2440a21b449 | 2022-02-15T10:49:59.000Z | [
"pytorch",
"tf",
"jax",
"t5",
"text2text-generation",
"en",
"dataset:c4",
"arxiv:2109.10686",
"transformers",
"deep-narrow",
"license:apache-2.0",
"autotrain_compatible"
]
| text2text-generation | false | google | null | google/t5-efficient-large | 41 | 1 | transformers | 6,389 | ---
language:
- en
datasets:
- c4
tags:
- deep-narrow
inference: false
license: apache-2.0
---
# T5-Efficient-LARGE (Deep-Narrow version)
T5-Efficient-LARGE is a variation of [Google's original T5](https://ai.googleblog.com/2020/02/exploring-transfer-learning-with-t5.html) following the [T5 model architecture](https://huggingface.co/docs/transformers/model_doc/t5).
It is a *pretrained-only* checkpoint and was released with the
paper **[Scale Efficiently: Insights from Pre-training and Fine-tuning Transformers](https://arxiv.org/abs/2109.10686)**
by *Yi Tay, Mostafa Dehghani, Jinfeng Rao, William Fedus, Samira Abnar, Hyung Won Chung, Sharan Narang, Dani Yogatama, Ashish Vaswani, Donald Metzler*.
In a nutshell, the paper indicates that a **Deep-Narrow** model architecture is favorable for **downstream** performance compared to other model architectures
of similar parameter count.
To quote the paper:
> We generally recommend a DeepNarrow strategy where the model’s depth is preferentially increased
> before considering any other forms of uniform scaling across other dimensions. This is largely due to
> how much depth influences the Pareto-frontier as shown in earlier sections of the paper. Specifically, a
> tall small (deep and narrow) model is generally more efficient compared to the base model. Likewise,
> a tall base model might also generally more efficient compared to a large model. We generally find
> that, regardless of size, even if absolute performance might increase as we continue to stack layers,
> the relative gain of Pareto-efficiency diminishes as we increase the layers, converging at 32 to 36
> layers. Finally, we note that our notion of efficiency here relates to any one compute dimension, i.e.,
> params, FLOPs or throughput (speed). We report all three key efficiency metrics (number of params,
> FLOPS and speed) and leave this decision to the practitioner to decide which compute dimension to
> consider.
To be more precise, *model depth* is defined as the number of transformer blocks that are stacked sequentially.
A sequence of word embeddings is therefore processed sequentially by each transformer block.
## Details model architecture
This model checkpoint - **t5-efficient-large** - is of model type **Large** with no variations.
It has **737.72** million parameters and thus requires *ca.* **2950.9 MB** of memory in full precision (*fp32*)
or **1475.45 MB** of memory in half precision (*fp16* or *bf16*).
A summary of the *original* T5 model architectures can be seen here:
| Model | nl (el/dl) | ff | dm | kv | nh | #Params|
| ----| ---- | ---- | ---- | ---- | ---- | ----|
| Tiny | 4/4 | 1024 | 256 | 32 | 4 | 16M|
| Mini | 4/4 | 1536 | 384 | 32 | 8 | 31M|
| Small | 6/6 | 2048 | 512 | 32 | 8 | 60M|
| Base | 12/12 | 3072 | 768 | 64 | 12 | 220M|
| Large | 24/24 | 4096 | 1024 | 64 | 16 | 738M|
| Xl | 24/24 | 16384 | 1024 | 128 | 32 | 3B|
| XXl | 24/24 | 65536 | 1024 | 128 | 128 | 11B|
whereas the following abbreviations are used:
| Abbreviation | Definition |
| ----| ---- |
| nl | Number of transformer blocks (depth) |
| dm | Dimension of embedding vector (output vector of transformers block) |
| kv | Dimension of key/value projection matrix |
| nh | Number of attention heads |
| ff | Dimension of intermediate vector within transformer block (size of feed-forward projection matrix) |
| el | Number of transformer blocks in the encoder (encoder depth) |
| dl | Number of transformer blocks in the decoder (decoder depth) |
| sh | Signifies that attention heads are shared |
| skv | Signifies that key-values projection matrices are tied |
If a model checkpoint has no specific, *el* or *dl* than both the number of encoder- and decoder layers correspond to *nl*.
## Pre-Training
The checkpoint was pretrained on the [Colossal, Cleaned version of Common Crawl (C4)](https://huggingface.co/datasets/c4) for 524288 steps using
the span-based masked language modeling (MLM) objective.
## Fine-Tuning
**Note**: This model is a **pretrained** checkpoint and has to be fine-tuned for practical usage.
The checkpoint was pretrained in English and is therefore only useful for English NLP tasks.
You can follow on of the following examples on how to fine-tune the model:
*PyTorch*:
- [Summarization](https://github.com/huggingface/transformers/tree/master/examples/pytorch/summarization)
- [Question Answering](https://github.com/huggingface/transformers/blob/master/examples/pytorch/question-answering/run_seq2seq_qa.py)
- [Text Classification](https://github.com/huggingface/transformers/tree/master/examples/pytorch/text-classification) - *Note*: You will have to slightly adapt the training example here to make it work with an encoder-decoder model.
*Tensorflow*:
- [Summarization](https://github.com/huggingface/transformers/tree/master/examples/tensorflow/summarization)
- [Text Classification](https://github.com/huggingface/transformers/tree/master/examples/tensorflow/text-classification) - *Note*: You will have to slightly adapt the training example here to make it work with an encoder-decoder model.
*JAX/Flax*:
- [Summarization](https://github.com/huggingface/transformers/tree/master/examples/flax/summarization)
- [Text Classification](https://github.com/huggingface/transformers/tree/master/examples/flax/text-classification) - *Note*: You will have to slightly adapt the training example here to make it work with an encoder-decoder model.
## Downstream Performance
TODO: Add table if available
## Computational Complexity
TODO: Add table if available
## More information
We strongly recommend the reader to go carefully through the original paper **[Scale Efficiently: Insights from Pre-training and Fine-tuning Transformers](https://arxiv.org/abs/2109.10686)** to get a more nuanced understanding of this model checkpoint.
As explained in the following [issue](https://github.com/google-research/google-research/issues/986#issuecomment-1035051145), checkpoints including the *sh* or *skv*
model architecture variations have *not* been ported to Transformers as they are probably of limited practical usage and are lacking a more detailed description. Those checkpoints are kept [here](https://huggingface.co/NewT5SharedHeadsSharedKeyValues) as they might be ported potentially in the future. |
huggingtweets/matteosalvinimi | 527886ae3db734d58567c9fdfc3771656fb4588e | 2021-05-22T13:42:13.000Z | [
"pytorch",
"jax",
"gpt2",
"text-generation",
"en",
"transformers",
"huggingtweets"
]
| text-generation | false | huggingtweets | null | huggingtweets/matteosalvinimi | 41 | null | transformers | 6,390 | ---
language: en
thumbnail: https://www.huggingtweets.com/matteosalvinimi/1602235798303/predictions.png
tags:
- huggingtweets
widget:
- text: "My dream is"
---
<link rel="stylesheet" href="https://unpkg.com/@tailwindcss/[email protected]/dist/typography.min.css">
<style>
@media (prefers-color-scheme: dark) {
.prose { color: #E2E8F0 !important; }
.prose h2, .prose h3, .prose a, .prose thead { color: #F7FAFC !important; }
}
</style>
<section class='prose'>
<div>
<div style="width: 132px; height:132px; border-radius: 50%; background-size: cover; background-image: url('https://pbs.twimg.com/profile_images/966325945060732928/0Ua5xPMX_400x400.jpg')">
</div>
<div style="margin-top: 8px; font-size: 19px; font-weight: 800">Matteo Salvini 🤖 AI Bot </div>
<div style="font-size: 15px; color: #657786">@matteosalvinimi bot</div>
</div>
I was made with [huggingtweets](https://github.com/borisdayma/huggingtweets).
Create your own bot based on your favorite user with [the demo](https://colab.research.google.com/github/borisdayma/huggingtweets/blob/master/huggingtweets-demo.ipynb)!
## How does it work?
The model uses the following pipeline.
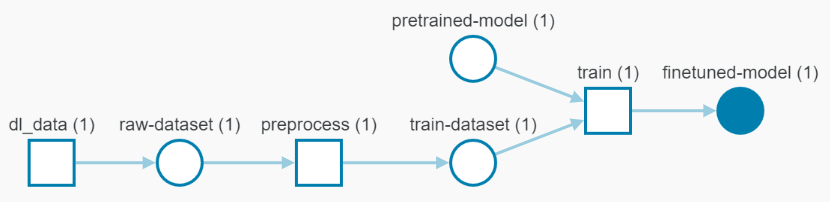
To understand how the model was developed, check the [W&B report](https://app.wandb.ai/wandb/huggingtweets/reports/HuggingTweets-Train-a-model-to-generate-tweets--VmlldzoxMTY5MjI).
## Training data
The model was trained on [@matteosalvinimi's tweets](https://twitter.com/matteosalvinimi).
<table style='border-width:0'>
<thead style='border-width:0'>
<tr style='border-width:0 0 1px 0; border-color: #CBD5E0'>
<th style='border-width:0'>Data</th>
<th style='border-width:0'>Quantity</th>
</tr>
</thead>
<tbody style='border-width:0'>
<tr style='border-width:0 0 1px 0; border-color: #E2E8F0'>
<td style='border-width:0'>Tweets downloaded</td>
<td style='border-width:0'>3247</td>
</tr>
<tr style='border-width:0 0 1px 0; border-color: #E2E8F0'>
<td style='border-width:0'>Retweets</td>
<td style='border-width:0'>16</td>
</tr>
<tr style='border-width:0 0 1px 0; border-color: #E2E8F0'>
<td style='border-width:0'>Short tweets</td>
<td style='border-width:0'>62</td>
</tr>
<tr style='border-width:0'>
<td style='border-width:0'>Tweets kept</td>
<td style='border-width:0'>3169</td>
</tr>
</tbody>
</table>
[Explore the data](https://app.wandb.ai/wandb/huggingtweets/runs/mr7hblho/artifacts), which is tracked with [W&B artifacts](https://docs.wandb.com/artifacts) at every step of the pipeline.
## Training procedure
The model is based on a pre-trained [GPT-2](https://huggingface.co/gpt2) which is fine-tuned on @matteosalvinimi's tweets.
Hyperparameters and metrics are recorded in the [W&B training run](https://app.wandb.ai/wandb/huggingtweets/runs/2tnq2vfo) for full transparency and reproducibility.
At the end of training, [the final model](https://app.wandb.ai/wandb/huggingtweets/runs/2tnq2vfo/artifacts) is logged and versioned.
## Intended uses & limitations
### How to use
You can use this model directly with a pipeline for text generation:
<pre><code><span style="color:#03A9F4">from</span> transformers <span style="color:#03A9F4">import</span> pipeline
generator = pipeline(<span style="color:#FF9800">'text-generation'</span>,
model=<span style="color:#FF9800">'huggingtweets/matteosalvinimi'</span>)
generator(<span style="color:#FF9800">"My dream is"</span>, num_return_sequences=<span style="color:#8BC34A">5</span>)</code></pre>
### Limitations and bias
The model suffers from [the same limitations and bias as GPT-2](https://huggingface.co/gpt2#limitations-and-bias).
In addition, the data present in the user's tweets further affects the text generated by the model.
## About
*Built by Boris Dayma*
</section>
[](https://twitter.com/intent/follow?screen_name=borisdayma)
<section class='prose'>
For more details, visit the project repository.
</section>
[](https://github.com/borisdayma/huggingtweets)
<!--- random size file --> |
huggingtweets/textmemeeffect | d2711e37736f68acf14158032e437a85310948c6 | 2021-05-23T01:08:08.000Z | [
"pytorch",
"jax",
"gpt2",
"text-generation",
"en",
"transformers",
"huggingtweets"
]
| text-generation | false | huggingtweets | null | huggingtweets/textmemeeffect | 41 | null | transformers | 6,391 | ---
language: en
thumbnail: https://www.huggingtweets.com/textmemeeffect/1617749862156/predictions.png
tags:
- huggingtweets
widget:
- text: "My dream is"
---
<div>
<div style="width: 132px; height:132px; border-radius: 50%; background-size: cover; background-image: url('https://pbs.twimg.com/profile_images/1279490677324361730/vljLWkCv_400x400.jpg')">
</div>
<div style="margin-top: 8px; font-size: 19px; font-weight: 800">new-ears elf 🤖 AI Bot </div>
<div style="font-size: 15px">@textmemeeffect bot</div>
</div>
I was made with [huggingtweets](https://github.com/borisdayma/huggingtweets).
Create your own bot based on your favorite user with [the demo](https://colab.research.google.com/github/borisdayma/huggingtweets/blob/master/huggingtweets-demo.ipynb)!
## How does it work?
The model uses the following pipeline.
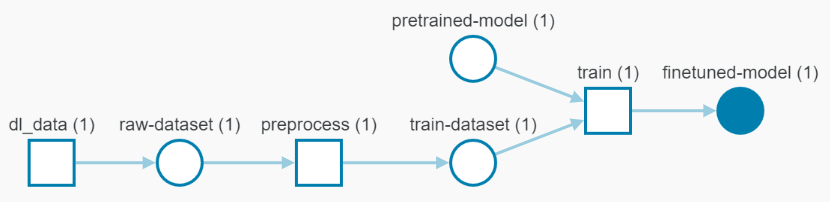
To understand how the model was developed, check the [W&B report](https://wandb.ai/wandb/huggingtweets/reports/HuggingTweets-Train-a-Model-to-Generate-Tweets--VmlldzoxMTY5MjI).
## Training data
The model was trained on [@textmemeeffect's tweets](https://twitter.com/textmemeeffect).
| Data | Quantity |
| --- | --- |
| Tweets downloaded | 3230 |
| Retweets | 405 |
| Short tweets | 519 |
| Tweets kept | 2306 |
[Explore the data](https://wandb.ai/wandb/huggingtweets/runs/3czrllt2/artifacts), which is tracked with [W&B artifacts](https://docs.wandb.com/artifacts) at every step of the pipeline.
## Training procedure
The model is based on a pre-trained [GPT-2](https://huggingface.co/gpt2) which is fine-tuned on @textmemeeffect's tweets.
Hyperparameters and metrics are recorded in the [W&B training run](https://wandb.ai/wandb/huggingtweets/runs/101b6evl) for full transparency and reproducibility.
At the end of training, [the final model](https://wandb.ai/wandb/huggingtweets/runs/101b6evl/artifacts) is logged and versioned.
## How to use
You can use this model directly with a pipeline for text generation:
```python
from transformers import pipeline
generator = pipeline('text-generation',
model='huggingtweets/textmemeeffect')
generator("My dream is", num_return_sequences=5)
```
## Limitations and bias
The model suffers from [the same limitations and bias as GPT-2](https://huggingface.co/gpt2#limitations-and-bias).
In addition, the data present in the user's tweets further affects the text generated by the model.
## About
*Built by Boris Dayma*
[](https://twitter.com/intent/follow?screen_name=borisdayma)
For more details, visit the project repository.
[](https://github.com/borisdayma/huggingtweets)
|
huggingtweets/tommyhump | 689b1730bab3026af7dc66e522d436858a7fc197 | 2021-05-23T02:37:51.000Z | [
"pytorch",
"jax",
"gpt2",
"text-generation",
"en",
"transformers",
"huggingtweets"
]
| text-generation | false | huggingtweets | null | huggingtweets/tommyhump | 41 | null | transformers | 6,392 | ---
language: en
thumbnail: https://www.huggingtweets.com/tommyhump/1617421683439/predictions.png
tags:
- huggingtweets
widget:
- text: "My dream is"
---
<div>
<div style="width: 132px; height:132px; border-radius: 50%; background-size: cover; background-image: url('https://pbs.twimg.com/profile_images/1361376517624766464/oFTs6sWT_400x400.jpg')">
</div>
<div style="margin-top: 8px; font-size: 19px; font-weight: 800">Tommy Humphreys 🤖 AI Bot </div>
<div style="font-size: 15px">@tommyhump bot</div>
</div>
I was made with [huggingtweets](https://github.com/borisdayma/huggingtweets).
Create your own bot based on your favorite user with [the demo](https://colab.research.google.com/github/borisdayma/huggingtweets/blob/master/huggingtweets-demo.ipynb)!
## How does it work?
The model uses the following pipeline.
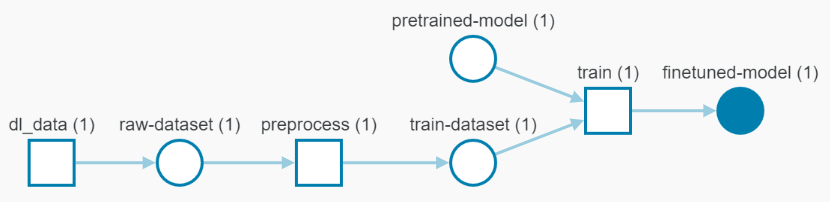
To understand how the model was developed, check the [W&B report](https://wandb.ai/wandb/huggingtweets/reports/HuggingTweets-Train-a-Model-to-Generate-Tweets--VmlldzoxMTY5MjI).
## Training data
The model was trained on [@tommyhump's tweets](https://twitter.com/tommyhump).
| Data | Quantity |
| --- | --- |
| Tweets downloaded | 3246 |
| Retweets | 542 |
| Short tweets | 133 |
| Tweets kept | 2571 |
[Explore the data](https://wandb.ai/wandb/huggingtweets/runs/3biusud1/artifacts), which is tracked with [W&B artifacts](https://docs.wandb.com/artifacts) at every step of the pipeline.
## Training procedure
The model is based on a pre-trained [GPT-2](https://huggingface.co/gpt2) which is fine-tuned on @tommyhump's tweets.
Hyperparameters and metrics are recorded in the [W&B training run](https://wandb.ai/wandb/huggingtweets/runs/1i3ro0vj) for full transparency and reproducibility.
At the end of training, [the final model](https://wandb.ai/wandb/huggingtweets/runs/1i3ro0vj/artifacts) is logged and versioned.
## How to use
You can use this model directly with a pipeline for text generation:
```python
from transformers import pipeline
generator = pipeline('text-generation',
model='huggingtweets/tommyhump')
generator("My dream is", num_return_sequences=5)
```
## Limitations and bias
The model suffers from [the same limitations and bias as GPT-2](https://huggingface.co/gpt2#limitations-and-bias).
In addition, the data present in the user's tweets further affects the text generated by the model.
## About
*Built by Boris Dayma*
[](https://twitter.com/intent/follow?screen_name=borisdayma)
For more details, visit the project repository.
[](https://github.com/borisdayma/huggingtweets)
|
jhgan/ko-sbert-nli | 0728da737a403c2acb69c2459900215e3b2390be | 2021-12-27T12:49:55.000Z | [
"pytorch",
"bert",
"feature-extraction",
"sentence-transformers",
"sentence-similarity",
"transformers"
]
| sentence-similarity | false | jhgan | null | jhgan/ko-sbert-nli | 41 | null | sentence-transformers | 6,393 | ---
pipeline_tag: sentence-similarity
tags:
- sentence-transformers
- feature-extraction
- sentence-similarity
- transformers
---
# ko-sbert-nli
This is a [sentence-transformers](https://www.SBERT.net) model: It maps sentences & paragraphs to a 768 dimensional dense vector space and can be used for tasks like clustering or semantic search.
<!--- Describe your model here -->
## Usage (Sentence-Transformers)
Using this model becomes easy when you have [sentence-transformers](https://www.SBERT.net) installed:
```
pip install -U sentence-transformers
```
Then you can use the model like this:
```python
from sentence_transformers import SentenceTransformer
sentences = ["안녕하세요?", "한국어 문장 임베딩을 위한 버트 모델입니다."]
model = SentenceTransformer('jhgan/ko-sbert-nli')
embeddings = model.encode(sentences)
print(embeddings)
```
## Usage (HuggingFace Transformers)
Without [sentence-transformers](https://www.SBERT.net), you can use the model like this: First, you pass your input through the transformer model, then you have to apply the right pooling-operation on-top of the contextualized word embeddings.
```python
from transformers import AutoTokenizer, AutoModel
import torch
#Mean Pooling - Take attention mask into account for correct averaging
def mean_pooling(model_output, attention_mask):
token_embeddings = model_output[0] #First element of model_output contains all token embeddings
input_mask_expanded = attention_mask.unsqueeze(-1).expand(token_embeddings.size()).float()
return torch.sum(token_embeddings * input_mask_expanded, 1) / torch.clamp(input_mask_expanded.sum(1), min=1e-9)
# Sentences we want sentence embeddings for
sentences = ['This is an example sentence', 'Each sentence is converted']
# Load model from HuggingFace Hub
tokenizer = AutoTokenizer.from_pretrained('jhgan/ko-sbert-nli')
model = AutoModel.from_pretrained('jhgan/ko-sbert-nli')
# Tokenize sentences
encoded_input = tokenizer(sentences, padding=True, truncation=True, return_tensors='pt')
# Compute token embeddings
with torch.no_grad():
model_output = model(**encoded_input)
# Perform pooling. In this case, mean pooling.
sentence_embeddings = mean_pooling(model_output, encoded_input['attention_mask'])
print("Sentence embeddings:")
print(sentence_embeddings)
```
## Evaluation Results
<!--- Describe how your model was evaluated -->
KorNLI 학습 데이터셋으로 학습한 후 KorSTS 평가 데이터셋으로 평가한 결과입니다.
- Cosine Pearson: 82.24
- Cosine Spearman: 83.16
- Euclidean Pearson: 82.19
- Euclidean Spearman: 82.31
- Manhattan Pearson: 82.18
- Manhattan Spearman: 82.30
- Dot Pearson: 79.30
- Dot Spearman: 78.78
## Training
The model was trained with the parameters:
**DataLoader**:
`sentence_transformers.datasets.NoDuplicatesDataLoader.NoDuplicatesDataLoader` of length 8885 with parameters:
```
{'batch_size': 64}
```
**Loss**:
`sentence_transformers.losses.MultipleNegativesRankingLoss.MultipleNegativesRankingLoss` with parameters:
```
{'scale': 20.0, 'similarity_fct': 'cos_sim'}
```
Parameters of the fit()-Method:
```
{
"epochs": 1,
"evaluation_steps": 1000,
"evaluator": "sentence_transformers.evaluation.EmbeddingSimilarityEvaluator.EmbeddingSimilarityEvaluator",
"max_grad_norm": 1,
"optimizer_class": "<class 'transformers.optimization.AdamW'>",
"optimizer_params": {
"lr": 2e-05
},
"scheduler": "WarmupLinear",
"steps_per_epoch": null,
"warmup_steps": 889,
"weight_decay": 0.01
}
```
## Full Model Architecture
```
SentenceTransformer(
(0): Transformer({'max_seq_length': 128, 'do_lower_case': False}) with Transformer model: BertModel
(1): Pooling({'word_embedding_dimension': 768, 'pooling_mode_cls_token': False, 'pooling_mode_mean_tokens': True, 'pooling_mode_max_tokens': False, 'pooling_mode_mean_sqrt_len_tokens': False})
)
```
## Citing & Authors
<!--- Describe where people can find more information -->
- Ham, J., Choe, Y. J., Park, K., Choi, I., & Soh, H. (2020). Kornli and korsts: New benchmark datasets for korean natural language understanding. arXiv preprint arXiv:2004.03289
- Reimers, Nils and Iryna Gurevych. “Sentence-BERT: Sentence Embeddings using Siamese BERT-Networks.” ArXiv abs/1908.10084 (2019)
- Reimers, Nils and Iryna Gurevych. “Making Monolingual Sentence Embeddings Multilingual Using Knowledge Distillation.” EMNLP (2020).
|
kurianbenoy/distilbert-base-uncased-finetuned-imdb | b1e9d28349f37fe1942e2a04da867a78c434e7c6 | 2022-07-21T00:50:02.000Z | [
"pytorch",
"tensorboard",
"distilbert",
"text-classification",
"dataset:imdb",
"transformers",
"generated_from_trainer",
"license:apache-2.0",
"model-index"
]
| text-classification | false | kurianbenoy | null | kurianbenoy/distilbert-base-uncased-finetuned-imdb | 41 | null | transformers | 6,394 | ---
license: apache-2.0
tags:
- generated_from_trainer
datasets:
- imdb
metrics:
- accuracy
model-index:
- name: distilbert-base-uncased-finetuned-imdb
results:
- task:
name: Text Classification
type: text-classification
dataset:
name: imdb
type: imdb
args: plain_text
metrics:
- name: Accuracy
type: accuracy
value: 0.923
---
<!-- This model card has been generated automatically according to the information the Trainer had access to. You
should probably proofread and complete it, then remove this comment. -->
# distilbert-base-uncased-finetuned-imdb
This model is a fine-tuned version of [distilbert-base-uncased](https://huggingface.co/distilbert-base-uncased) on the imdb dataset.
It achieves the following results on the evaluation set:
- Loss: 0.3073
- Accuracy: 0.923
## Model description
More information needed
## Intended uses & limitations
More information needed
## Training and evaluation data
More information needed
## Training procedure
### Training hyperparameters
The following hyperparameters were used during training:
- learning_rate: 2e-05
- train_batch_size: 16
- eval_batch_size: 16
- seed: 42
- optimizer: Adam with betas=(0.9,0.999) and epsilon=1e-08
- lr_scheduler_type: linear
- num_epochs: 3
### Training results
| Training Loss | Epoch | Step | Validation Loss | Accuracy |
|:-------------:|:-----:|:----:|:---------------:|:--------:|
| 0.2744 | 1.0 | 1563 | 0.2049 | 0.921 |
| 0.1572 | 2.0 | 3126 | 0.2308 | 0.923 |
| 0.0917 | 3.0 | 4689 | 0.3073 | 0.923 |
### Framework versions
- Transformers 4.16.2
- Pytorch 1.10.0+cu111
- Datasets 1.18.3
- Tokenizers 0.11.0
|
mrm8488/HindiBERTa | d8733c9dcb64a82e2e8c077093ef0f58f9b6516f | 2021-05-20T18:02:42.000Z | [
"pytorch",
"jax",
"roberta",
"fill-mask",
"transformers",
"autotrain_compatible"
]
| fill-mask | false | mrm8488 | null | mrm8488/HindiBERTa | 41 | null | transformers | 6,395 | Entry not found |
mrm8488/electricidad-small-finetuned-restaurant-sentiment-analysis | 76281ab08cb009203e59e4134fe588b7bcd0daf3 | 2021-06-15T16:54:27.000Z | [
"pytorch",
"electra",
"text-classification",
"es",
"transformers",
"restaurant",
"classification",
"reviews"
]
| text-classification | false | mrm8488 | null | mrm8488/electricidad-small-finetuned-restaurant-sentiment-analysis | 41 | 2 | transformers | 6,396 | ---
language: es
tags:
- restaurant
- classification
- reviews
widget:
- text: "No está a la altura, no volveremos."
---
# Electricidad-small fine-tuned on restaurant review sentiment analysis dataset
Test set accuray: 0.86 |
nouamanetazi/cover-letter-t5-base | 80f714aa4701a1e19663a1ab36b7d1d0fab3abff | 2021-11-30T21:14:47.000Z | [
"pytorch",
"t5",
"text2text-generation",
"en",
"transformers",
"generated_from_trainer",
"t5-base",
"license:apache-2.0",
"model-index",
"autotrain_compatible"
]
| text2text-generation | false | nouamanetazi | null | nouamanetazi/cover-letter-t5-base | 41 | 2 | transformers | 6,397 | ---
language: en
license: apache-2.0
tags:
- generated_from_trainer
- t5-base
model-index:
- name: cover-letter-t5-base
results: []
widget:
- text: "coverletter name: Nouamane Tazi job: Machine Learning Engineer at HuggingFace background: Master's student in AI at the University of Paris Saclay experiences: I participated in the Digital Tech Year program, developing three minimal valuable products for three companies in a 7-week constraint. I also spent 1 year as a machine learning engineer for Flashbrand where I mainly worked on their chatbot . And I recently completed the HuggingFace course, where I built an amazing huggingface space. I am a strong team player."
---
<!-- This model card has been generated automatically according to the information the Trainer had access to. You
should probably proofread and complete it, then remove this comment. -->
# cover-letter-t5-base
This model is a fine-tuned version of [t5-base](https://huggingface.co/t5-base) on cover letter samples scraped from Indeed and JobHero.
## Model description
More information needed
## Intended uses & limitations
More information needed
## Training and evaluation data
More information needed
## Training procedure
### Training hyperparameters
The following hyperparameters were used during training:
- learning_rate: 0.0001
- train_batch_size: 4
- eval_batch_size: 4
- seed: 42
- optimizer: Adam with betas=(0.9,0.999) and epsilon=1e-08
- lr_scheduler_type: linear
- num_epochs: 3
- mixed_precision_training: Native AMP
### Training results
### Framework versions
- Transformers 4.12.5
- Pytorch 1.10.0+cu111
- Datasets 1.16.1
- Tokenizers 0.10.3
|
patrickvonplaten/wav2vec2-xlsr-53-300m-mls-german-ft | d05d90ca4932582a0a6af89d14fda799204bd183 | 2021-11-18T22:30:46.000Z | [
"pytorch",
"tensorboard",
"wav2vec2",
"automatic-speech-recognition",
"dataset:multilingual_librispeech",
"transformers",
"multilingual_librispeech",
"generated_from_trainer",
"license:apache-2.0",
"model-index"
]
| automatic-speech-recognition | false | patrickvonplaten | null | patrickvonplaten/wav2vec2-xlsr-53-300m-mls-german-ft | 41 | null | transformers | 6,398 | ---
license: apache-2.0
tags:
- automatic-speech-recognition
- multilingual_librispeech
- generated_from_trainer
datasets:
- multilingual_librispeech
model-index:
- name: wav2vec2-xlsr-53-300m-mls-german-ft
results: []
---
<!-- This model card has been generated automatically according to the information the Trainer had access to. You
should probably proofread and complete it, then remove this comment. -->
# wav2vec2-xlsr-53-300m-mls-german-ft
This model is a fine-tuned version of [facebook/wav2vec2-large-xlsr-53](https://huggingface.co/facebook/wav2vec2-large-xlsr-53) on the MULTILINGUAL_LIBRISPEECH - GERMAN 10h dataset.
It achieves the following results on the evaluation set:
- Loss: 0.2219
- Wer: 0.1288
## Model description
More information needed
## Intended uses & limitations
More information needed
## Training and evaluation data
More information needed
## Training procedure
### Training hyperparameters
The following hyperparameters were used during training:
- learning_rate: 0.0001
- train_batch_size: 32
- eval_batch_size: 8
- seed: 42
- optimizer: Adam with betas=(0.9,0.999) and epsilon=1e-08
- lr_scheduler_type: linear
- lr_scheduler_warmup_steps: 1000
- num_epochs: 200.0
- mixed_precision_training: Native AMP
### Training results
| Training Loss | Epoch | Step | Validation Loss | Wer |
|:-------------:|:------:|:-----:|:---------------:|:------:|
| 2.9888 | 7.25 | 500 | 2.9192 | 1.0 |
| 2.9313 | 14.49 | 1000 | 2.8698 | 1.0 |
| 1.068 | 21.74 | 1500 | 0.2647 | 0.2565 |
| 0.8151 | 28.99 | 2000 | 0.2067 | 0.1719 |
| 0.764 | 36.23 | 2500 | 0.1975 | 0.1568 |
| 0.7332 | 43.48 | 3000 | 0.1812 | 0.1463 |
| 0.5952 | 50.72 | 3500 | 0.1923 | 0.1428 |
| 0.6655 | 57.97 | 4000 | 0.1900 | 0.1404 |
| 0.574 | 65.22 | 4500 | 0.1822 | 0.1370 |
| 0.6211 | 72.46 | 5000 | 0.1937 | 0.1355 |
| 0.5883 | 79.71 | 5500 | 0.1872 | 0.1335 |
| 0.5666 | 86.96 | 6000 | 0.1874 | 0.1324 |
| 0.5526 | 94.2 | 6500 | 0.1998 | 0.1368 |
| 0.5671 | 101.45 | 7000 | 0.2054 | 0.1365 |
| 0.5514 | 108.7 | 7500 | 0.1987 | 0.1340 |
| 0.5382 | 115.94 | 8000 | 0.2104 | 0.1344 |
| 0.5819 | 123.19 | 8500 | 0.2125 | 0.1334 |
| 0.5277 | 130.43 | 9000 | 0.2063 | 0.1330 |
| 0.4626 | 137.68 | 9500 | 0.2105 | 0.1310 |
| 0.5842 | 144.93 | 10000 | 0.2087 | 0.1307 |
| 0.535 | 152.17 | 10500 | 0.2137 | 0.1309 |
| 0.5081 | 159.42 | 11000 | 0.2215 | 0.1302 |
| 0.6033 | 166.67 | 11500 | 0.2162 | 0.1302 |
| 0.5549 | 173.91 | 12000 | 0.2198 | 0.1286 |
| 0.5389 | 181.16 | 12500 | 0.2241 | 0.1293 |
| 0.4912 | 188.41 | 13000 | 0.2190 | 0.1290 |
| 0.4671 | 195.65 | 13500 | 0.2218 | 0.1290 |
### Framework versions
- Transformers 4.13.0.dev0
- Pytorch 1.10.0
- Datasets 1.15.2.dev0
- Tokenizers 0.10.3
|
qinluo/wobert-chinese-plus | 7ee236e8778d014617f11fd21ed8be51b61c2563 | 2021-11-18T13:43:26.000Z | [
"pytorch",
"tf",
"bert",
"fill-mask",
"zh",
"transformers",
"wobert",
"autotrain_compatible"
]
| fill-mask | false | qinluo | null | qinluo/wobert-chinese-plus | 41 | 1 | transformers | 6,399 | ---
language: zh
tags:
- wobert
inference: True
---
## Word based BERT model
原模型及说明见:https://github.com/ZhuiyiTechnology/WoBERT
pytorch 模型见: https://github.com/JunnYu/WoBERT_pytorch
## 安装 WoBertTokenizer
```bash
pip install git+https://github.com/JunnYu/WoBERT_pytorch.git
```
## TF Example
```python
from transformers import TFBertForMaskedLM as WoBertForMaskedLM
from wobert import WoBertTokenizer
import tensorflow as tf
pretrained_model_or_path = 'qinluo/wobert-chinese-plus'
tokenizer = WoBertTokenizer.from_pretrained(pretrained_model_or_path)
model = WoBertForMaskedLM.from_pretrained(pretrained_model_or_path)
text = '今天[MASK]很好,我[MASK]去公园玩。'
inputs = tokenizer(text, return_tensors='tf')
outputs = model(**inputs).logits[0]
outputs_sentence = ''
for i, id in enumerate(tokenizer.encode(text)):
if id == tokenizer.mask_token_id:
tokens = tokenizer.convert_ids_to_tokens(tf.math.top_k(outputs[i], k=5)[1])
outputs_sentence += '[' + '|'.join(tokens) + ']'
else:
outputs_sentence += ''.join(tokenizer.convert_ids_to_tokens([id], skip_special_tokens=True))
print(outputs_sentence)
# 今天[天气|阳光|天|心情|空气]很好,我[想|要|打算|准备|就]去公园玩。
```
## Pytorch Example
```python
from transformers import BertForMaskedLM as WoBertForMaskedLM
from wobert import WoBertTokenizer
pretrained_model_or_path = 'qinluo/wobert-chinese-plus'
tokenizer = WoBertTokenizer.from_pretrained(pretrained_model_or_path)
model = WoBertForMaskedLM.from_pretrained(pretrained_model_or_path)
text = '今天[MASK]很好,我[MASK]去公园玩。'
inputs = tokenizer(text, return_tensors='pt')
outputs = model(**inputs).logits[0]
outputs_sentence = ''
for i, id in enumerate(tokenizer.encode(text)):
if id == tokenizer.mask_token_id:
tokens = tokenizer.convert_ids_to_tokens(outputs[i].topk(k=5)[1])
outputs_sentence += '[' + '|'.join(tokens) + ']'
else:
outputs_sentence += ''.join(tokenizer.convert_ids_to_tokens([id], skip_special_tokens=True))
print(outputs_sentence)
# 今天[天气|阳光|天|心情|空气]很好,我[想|要|打算|准备|就]去公园玩。
```
## 引用
Bibtex:
```tex
@techreport{zhuiyiwobert,
title={WoBERT: Word-based Chinese BERT model - ZhuiyiAI},
author={Jianlin Su},
year={2020},
url="https://github.com/ZhuiyiTechnology/WoBERT",
}
```
|
Subsets and Splits
No community queries yet
The top public SQL queries from the community will appear here once available.