repo_id
stringlengths 4
110
| author
stringlengths 2
27
⌀ | model_type
stringlengths 2
29
⌀ | files_per_repo
int64 2
15.4k
| downloads_30d
int64 0
19.9M
| library
stringlengths 2
37
⌀ | likes
int64 0
4.34k
| pipeline
stringlengths 5
30
⌀ | pytorch
bool 2
classes | tensorflow
bool 2
classes | jax
bool 2
classes | license
stringlengths 2
30
| languages
stringlengths 4
1.63k
⌀ | datasets
stringlengths 2
2.58k
⌀ | co2
stringclasses 29
values | prs_count
int64 0
125
| prs_open
int64 0
120
| prs_merged
int64 0
15
| prs_closed
int64 0
28
| discussions_count
int64 0
218
| discussions_open
int64 0
148
| discussions_closed
int64 0
70
| tags
stringlengths 2
513
| has_model_index
bool 2
classes | has_metadata
bool 1
class | has_text
bool 1
class | text_length
int64 401
598k
| is_nc
bool 1
class | readme
stringlengths 0
598k
| hash
stringlengths 32
32
|
---|---|---|---|---|---|---|---|---|---|---|---|---|---|---|---|---|---|---|---|---|---|---|---|---|---|---|---|---|---|
philschmid/distilbart-cnn-12-6-samsum | philschmid | bart | 13 | 2,437 | transformers | 6 | summarization | true | false | false | apache-2.0 | ['en'] | ['samsum'] | null | 4 | 0 | 4 | 0 | 0 | 0 | 0 | ['sagemaker', 'bart', 'summarization'] | true | true | true | 2,500 | false |
## `distilbart-cnn-12-6-samsum`
This model was trained using Amazon SageMaker and the new Hugging Face Deep Learning container.
For more information look at:
- [🤗 Transformers Documentation: Amazon SageMaker](https://huggingface.co/transformers/sagemaker.html)
- [Example Notebooks](https://github.com/huggingface/notebooks/tree/master/sagemaker)
- [Amazon SageMaker documentation for Hugging Face](https://docs.aws.amazon.com/sagemaker/latest/dg/hugging-face.html)
- [Python SDK SageMaker documentation for Hugging Face](https://sagemaker.readthedocs.io/en/stable/frameworks/huggingface/index.html)
- [Deep Learning Container](https://github.com/aws/deep-learning-containers/blob/master/available_images.md#huggingface-training-containers)
## Hyperparameters
```json
{
"dataset_name": "samsum",
"do_eval": true,
"do_train": true,
"fp16": true,
"learning_rate": 5e-05,
"model_name_or_path": "sshleifer/distilbart-cnn-12-6",
"num_train_epochs": 3,
"output_dir": "/opt/ml/model",
"per_device_eval_batch_size": 8,
"per_device_train_batch_size": 8,
"seed": 7
}
```
## Train results
| key | value |
| --- | ----- |
| epoch | 3.0 |
| init_mem_cpu_alloc_delta | 180338 |
| init_mem_cpu_peaked_delta | 18282 |
| init_mem_gpu_alloc_delta | 1222242816 |
| init_mem_gpu_peaked_delta | 0 |
| train_mem_cpu_alloc_delta | 6971403 |
| train_mem_cpu_peaked_delta | 640733 |
| train_mem_gpu_alloc_delta | 4910897664 |
| train_mem_gpu_peaked_delta | 23331969536 |
| train_runtime | 155.2034 |
| train_samples | 14732 |
| train_samples_per_second | 2.242 |
## Eval results
| key | value |
| --- | ----- |
| epoch | 3.0 |
| eval_loss | 1.4209576845169067 |
| eval_mem_cpu_alloc_delta | 868003 |
| eval_mem_cpu_peaked_delta | 18250 |
| eval_mem_gpu_alloc_delta | 0 |
| eval_mem_gpu_peaked_delta | 328244736 |
| eval_runtime | 0.6088 |
| eval_samples | 818 |
| eval_samples_per_second | 1343.647 |
## Usage
```python
from transformers import pipeline
summarizer = pipeline("summarization", model="philschmid/distilbart-cnn-12-6-samsum")
conversation = '''Jeff: Can I train a 🤗 Transformers model on Amazon SageMaker?
Philipp: Sure you can use the new Hugging Face Deep Learning Container.
Jeff: ok.
Jeff: and how can I get started?
Jeff: where can I find documentation?
Philipp: ok, ok you can find everything here. https://huggingface.co/blog/the-partnership-amazon-sagemaker-and-hugging-face
'''
nlp(conversation)
```
| fdc7a657e44d7fd8c9c7792249aff687 |
wanko/distilbert-base-uncased-finetuned-emotion | wanko | distilbert | 16 | 1 | transformers | 0 | text-classification | true | false | false | apache-2.0 | null | ['emotion'] | null | 0 | 0 | 0 | 0 | 0 | 0 | 0 | ['generated_from_trainer'] | true | true | true | 1,344 | false |
<!-- This model card has been generated automatically according to the information the Trainer had access to. You
should probably proofread and complete it, then remove this comment. -->
# distilbert-base-uncased-finetuned-emotion
This model is a fine-tuned version of [distilbert-base-uncased](https://huggingface.co/distilbert-base-uncased) on the emotion dataset.
It achieves the following results on the evaluation set:
- Loss: 0.2183
- Accuracy: 0.9285
- F1: 0.9285
## Model description
More information needed
## Intended uses & limitations
More information needed
## Training and evaluation data
More information needed
## Training procedure
### Training hyperparameters
The following hyperparameters were used during training:
- learning_rate: 2e-05
- train_batch_size: 64
- eval_batch_size: 64
- seed: 42
- optimizer: Adam with betas=(0.9,0.999) and epsilon=1e-08
- lr_scheduler_type: linear
- num_epochs: 2
### Training results
| Training Loss | Epoch | Step | Validation Loss | Accuracy | F1 |
|:-------------:|:-----:|:----:|:---------------:|:--------:|:------:|
| No log | 1.0 | 250 | 0.3165 | 0.908 | 0.9047 |
| No log | 2.0 | 500 | 0.2183 | 0.9285 | 0.9285 |
### Framework versions
- Transformers 4.21.1
- Pytorch 1.12.1+cu113
- Datasets 2.4.0
- Tokenizers 0.12.1
| 16300fcf6d73a93232358872c358f5de |
WillHeld/bert-base-cased-rte | WillHeld | bert | 14 | 1 | transformers | 0 | text-classification | true | false | false | apache-2.0 | ['en'] | ['glue'] | null | 0 | 0 | 0 | 0 | 0 | 0 | 0 | ['generated_from_trainer'] | true | true | true | 1,350 | false |
<!-- This model card has been generated automatically according to the information the Trainer had access to. You
should probably proofread and complete it, then remove this comment. -->
# bert-base-cased-rte
This model is a fine-tuned version of [bert-base-cased](https://huggingface.co/bert-base-cased) on the GLUE RTE dataset.
It achieves the following results on the evaluation set:
- Loss: 0.9753
- Accuracy: 0.6534
## Model description
More information needed
## Intended uses & limitations
More information needed
## Training and evaluation data
More information needed
## Training procedure
### Training hyperparameters
The following hyperparameters were used during training:
- learning_rate: 2e-05
- train_batch_size: 16
- eval_batch_size: 8
- seed: 42
- optimizer: Adam with betas=(0.9,0.999) and epsilon=1e-08
- lr_scheduler_type: linear
- lr_scheduler_warmup_ratio: 0.06
- num_epochs: 10.0
### Training results
| Training Loss | Epoch | Step | Validation Loss | Accuracy |
|:-------------:|:-----:|:----:|:---------------:|:--------:|
| 0.4837 | 3.21 | 500 | 0.9753 | 0.6534 |
| 0.0827 | 6.41 | 1000 | 1.6693 | 0.6715 |
| 0.0253 | 9.62 | 1500 | 1.7777 | 0.6643 |
### Framework versions
- Transformers 4.21.3
- Pytorch 1.7.1
- Datasets 1.18.3
- Tokenizers 0.11.6
| 7c25d40b76b9317efe09b4e2a7da8707 |
Jeffrover/my_donut-base-sroie | Jeffrover | vision-encoder-decoder | 14 | 2 | transformers | 0 | null | true | false | false | mit | null | ['imagefolder'] | null | 0 | 0 | 0 | 0 | 0 | 0 | 0 | ['generated_from_trainer'] | true | true | true | 979 | false |
<!-- This model card has been generated automatically according to the information the Trainer had access to. You
should probably proofread and complete it, then remove this comment. -->
# my_donut-base-sroie
This model is a fine-tuned version of [naver-clova-ix/donut-base](https://huggingface.co/naver-clova-ix/donut-base) on the imagefolder dataset.
## Model description
More information needed
## Intended uses & limitations
More information needed
## Training and evaluation data
More information needed
## Training procedure
### Training hyperparameters
The following hyperparameters were used during training:
- learning_rate: 2e-05
- train_batch_size: 2
- eval_batch_size: 8
- seed: 42
- optimizer: Adam with betas=(0.9,0.999) and epsilon=1e-08
- lr_scheduler_type: linear
- num_epochs: 3
- mixed_precision_training: Native AMP
### Training results
### Framework versions
- Transformers 4.23.1
- Pytorch 1.12.1+cu102
- Datasets 2.6.1
- Tokenizers 0.13.1
| 3a0dba0b61af036ba21070a408c05f12 |
bigmorning/whisper_0020 | bigmorning | whisper | 7 | 6 | transformers | 0 | automatic-speech-recognition | false | true | false | apache-2.0 | null | null | null | 0 | 0 | 0 | 0 | 0 | 0 | 0 | ['generated_from_keras_callback'] | true | true | true | 2,849 | false |
<!-- This model card has been generated automatically according to the information Keras had access to. You should
probably proofread and complete it, then remove this comment. -->
# whisper_0020
This model is a fine-tuned version of [openai/whisper-tiny](https://huggingface.co/openai/whisper-tiny) on an unknown dataset.
It achieves the following results on the evaluation set:
- Train Loss: 0.1698
- Train Accuracy: 0.0335
- Validation Loss: 0.5530
- Validation Accuracy: 0.0314
- Epoch: 19
## Model description
More information needed
## Intended uses & limitations
More information needed
## Training and evaluation data
More information needed
## Training procedure
### Training hyperparameters
The following hyperparameters were used during training:
- optimizer: {'name': 'AdamWeightDecay', 'learning_rate': 1e-05, 'decay': 0.0, 'beta_1': 0.9, 'beta_2': 0.999, 'epsilon': 1e-07, 'amsgrad': False, 'weight_decay_rate': 0.01}
- training_precision: float32
### Training results
| Train Loss | Train Accuracy | Validation Loss | Validation Accuracy | Epoch |
|:----------:|:--------------:|:---------------:|:-------------------:|:-----:|
| 5.0856 | 0.0116 | 4.4440 | 0.0123 | 0 |
| 4.3149 | 0.0131 | 4.0521 | 0.0142 | 1 |
| 3.9260 | 0.0146 | 3.7264 | 0.0153 | 2 |
| 3.5418 | 0.0160 | 3.3026 | 0.0174 | 3 |
| 2.7510 | 0.0198 | 2.0157 | 0.0241 | 4 |
| 1.6782 | 0.0250 | 1.3567 | 0.0273 | 5 |
| 1.1705 | 0.0274 | 1.0678 | 0.0286 | 6 |
| 0.9126 | 0.0287 | 0.9152 | 0.0294 | 7 |
| 0.7514 | 0.0296 | 0.8057 | 0.0299 | 8 |
| 0.6371 | 0.0302 | 0.7409 | 0.0302 | 9 |
| 0.5498 | 0.0307 | 0.6854 | 0.0306 | 10 |
| 0.4804 | 0.0312 | 0.6518 | 0.0307 | 11 |
| 0.4214 | 0.0316 | 0.6200 | 0.0310 | 12 |
| 0.3713 | 0.0319 | 0.5947 | 0.0311 | 13 |
| 0.3281 | 0.0322 | 0.5841 | 0.0311 | 14 |
| 0.2891 | 0.0325 | 0.5700 | 0.0313 | 15 |
| 0.2550 | 0.0328 | 0.5614 | 0.0313 | 16 |
| 0.2237 | 0.0331 | 0.5572 | 0.0313 | 17 |
| 0.1959 | 0.0333 | 0.5563 | 0.0314 | 18 |
| 0.1698 | 0.0335 | 0.5530 | 0.0314 | 19 |
### Framework versions
- Transformers 4.25.0.dev0
- TensorFlow 2.9.2
- Tokenizers 0.13.2
| d244699020fd6d8c554fe98070277d63 |
nvidia/nemo-megatron-gpt-1.3B | nvidia | null | 3 | 185 | nemo | 14 | text2text-generation | true | false | false | cc-by-4.0 | ['en'] | ['the_pile'] | null | 1 | 0 | 1 | 0 | 0 | 0 | 0 | ['text2text-generation', 'pytorch', 'causal-lm'] | false | true | true | 4,240 | false | # NeMo Megatron-GPT 1.3B
<style>
img {
display: inline;
}
</style>
|[](#model-architecture)|[](#model-architecture)|[](#datasets)
## Model Description
Megatron-GPT 1.3B is a transformer-based language model. GPT refers to a class of transformer decoder-only models similar to GPT-2 and 3 while 1.3B refers to the total trainable parameter count (1.3 Billion) [1, 2]. It has Tensor Parallelism (TP) of 1, Pipeline Parallelism (PP) of 1 and should fit on a single NVIDIA GPU.
This model was trained with [NeMo Megatron](https://docs.nvidia.com/deeplearning/nemo/user-guide/docs/en/stable/nlp/nemo_megatron/intro.html).
## Getting started
### Step 1: Install NeMo and dependencies
You will need to install NVIDIA Apex and NeMo.
```
git clone https://github.com/ericharper/apex.git
cd apex
git checkout nm_v1.11.0
pip install -v --disable-pip-version-check --no-cache-dir --global-option="--cpp_ext" --global-option="--cuda_ext" --global-option="--fast_layer_norm" --global-option="--distributed_adam" --global-option="--deprecated_fused_adam" ./
```
```
pip install nemo_toolkit['nlp']==1.11.0
```
Alternatively, you can use NeMo Megatron training docker container with all dependencies pre-installed.
### Step 2: Launch eval server
**Note.** The model has been trained with Tensor Parallelism (TP) of 1 and Pipeline Parallelism (PP) of 1 and should fit on a single NVIDIA GPU.
```
git clone https://github.com/NVIDIA/NeMo.git
cd NeMo/examples/nlp/language_modeling
git checkout v1.11.0
python megatron_gpt_eval.py gpt_model_file=nemo_gpt1.3B_fp16.nemo server=True tensor_model_parallel_size=1 trainer.devices=1
```
### Step 3: Send prompts to your model!
```python
import json
import requests
port_num = 5555
headers = {"Content-Type": "application/json"}
def request_data(data):
resp = requests.put('http://localhost:{}/generate'.format(port_num),
data=json.dumps(data),
headers=headers)
sentences = resp.json()['sentences']
return sentences
data = {
"sentences": ["Tell me an interesting fact about space travel."]*1,
"tokens_to_generate": 50,
"temperature": 1.0,
"add_BOS": True,
"top_k": 0,
"top_p": 0.9,
"greedy": False,
"all_probs": False,
"repetition_penalty": 1.2,
"min_tokens_to_generate": 2,
}
sentences = request_data(data)
print(sentences)
```
## Training Data
The model was trained on ["The Piles" dataset prepared by Eleuther.AI](https://pile.eleuther.ai/). [4]
## Evaluation results
*Zero-shot performance.* Evaluated using [LM Evaluation Test Suite from AI21](https://github.com/AI21Labs/lm-evaluation)
| ARC-Challenge | ARC-Easy | RACE-middle | RACE-high | Winogrande | RTE | BoolQA | HellaSwag | PiQA |
| ------------- | -------- | ----------- | --------- | ---------- | --- | ------ | --------- | ---- |
| 0.3012 | 0.4596 | 0.459 | 0.3797 | 0.5343 | 0.5451 | 0.5979 | 0.4443 | 0.6834 |
## Limitations
The model was trained on the data originally crawled from the Internet. This data contains toxic language and societal biases. Therefore, the model may amplify those biases and return toxic responses especially when prompted with toxic prompts.
## References
[1] [Improving Language Understanding by Generative Pre-Training](https://s3-us-west-2.amazonaws.com/openai-assets/research-covers/language-unsupervised/language_understanding_paper.pdf)
[2] [Megatron-LM: Training Multi-Billion Parameter Language Models Using Model Parallelism](https://arxiv.org/pdf/1909.08053.pdf)
[3] [NVIDIA NeMo Toolkit](https://github.com/NVIDIA/NeMo)
[4] [The Pile: An 800GB Dataset of Diverse Text for Language Modeling](https://arxiv.org/abs/2101.00027)
## Licence
License to use this model is covered by the [CC-BY-4.0](https://creativecommons.org/licenses/by/4.0/). By downloading the public and release version of the model, you accept the terms and conditions of the [CC-BY-4.0](https://creativecommons.org/licenses/by/4.0/) license.
| 54189e0c90b45540c3345aa4f93de9bd |
rkn/distilbert-base-uncased-finetuned-emotion | rkn | distilbert | 12 | 1 | transformers | 0 | text-classification | true | false | false | apache-2.0 | null | null | null | 0 | 0 | 0 | 0 | 0 | 0 | 0 | ['generated_from_trainer'] | true | true | true | 1,342 | false |
<!-- This model card has been generated automatically according to the information the Trainer had access to. You
should probably proofread and complete it, then remove this comment. -->
# distilbert-base-uncased-finetuned-emotion
This model is a fine-tuned version of [distilbert-base-uncased](https://huggingface.co/distilbert-base-uncased) on an unknown dataset.
It achieves the following results on the evaluation set:
- Loss: 0.2124
- Accuracy: 0.928
- F1: 0.9279
## Model description
More information needed
## Intended uses & limitations
More information needed
## Training and evaluation data
More information needed
## Training procedure
### Training hyperparameters
The following hyperparameters were used during training:
- learning_rate: 2e-05
- train_batch_size: 64
- eval_batch_size: 64
- seed: 42
- optimizer: Adam with betas=(0.9,0.999) and epsilon=1e-08
- lr_scheduler_type: linear
- num_epochs: 2
### Training results
| Training Loss | Epoch | Step | Validation Loss | Accuracy | F1 |
|:-------------:|:-----:|:----:|:---------------:|:--------:|:------:|
| No log | 1.0 | 250 | 0.2991 | 0.911 | 0.9091 |
| No log | 2.0 | 500 | 0.2124 | 0.928 | 0.9279 |
### Framework versions
- Transformers 4.25.1
- Pytorch 1.13.0+cu116
- Datasets 2.7.1
- Tokenizers 0.13.2
| 3995792b6dda47019e5f7a507699bfd4 |
VishwanathanR/resnet-50 | VishwanathanR | resnet | 5 | 4 | transformers | 0 | image-classification | false | true | false | apache-2.0 | null | null | null | 0 | 0 | 0 | 0 | 0 | 0 | 0 | ['generated_from_keras_callback'] | true | true | true | 834 | false |
<!-- This model card has been generated automatically according to the information Keras had access to. You should
probably proofread and complete it, then remove this comment. -->
# resnet-50
This model is a fine-tuned version of [microsoft/resnet-50](https://huggingface.co/microsoft/resnet-50) on an unknown dataset.
It achieves the following results on the evaluation set:
## Model description
More information needed
## Intended uses & limitations
More information needed
## Training and evaluation data
More information needed
## Training procedure
### Training hyperparameters
The following hyperparameters were used during training:
- optimizer: None
- training_precision: float32
### Training results
### Framework versions
- Transformers 4.25.0.dev0
- TensorFlow 2.6.2
- Datasets 2.7.1
- Tokenizers 0.13.2
| 0f1445d62c905912ef94c6dbdd9715ec |
innocent-charles/Swahili-question-answer-latest-cased | innocent-charles | bert | 12 | 12 | transformers | 2 | question-answering | true | false | false | cc-by-4.0 | ['sw'] | ['kenyacorpus_v2'] | null | 1 | 0 | 1 | 0 | 0 | 0 | 0 | [] | true | true | true | 3,609 | false |
# SWAHILI QUESTION - ANSWER MODEL
This is the [bert-base-multilingual-cased](https://huggingface.co/bert-base-multilingual-cased) model, fine-tuned using the [KenyaCorpus](https://github.com/Neurotech-HQ/Swahili-QA-dataset) dataset. It's been trained on question-answer pairs, including unanswerable questions, for the task of Question Answering in Swahili Language.
Question answering (QA) is a computer science discipline within the fields of information retrieval and NLP that help in the development of systems in such a way that, given a question in natural language, can extract relevant information from provided data and present it in the form of natural language answers.
## Overview
**Language model used:** bert-base-multilingual-cased
**Language:** Kiswahili
**Downstream-task:** Extractive Swahili QA
**Training data:** KenyaCorpus
**Eval data:** KenyaCorpus
**Code:** See [an example QA pipeline on Haystack](https://blog.neurotech.africa/building-swahili-question-and-answering-with-haystack/)
**Infrastructure**: AWS NVIDIA A100 Tensor Core GPU
## Hyperparameters
```
batch_size = 16
n_epochs = 10
base_LM_model = "bert-base-multilingual-cased"
max_seq_len = 386
learning_rate = 3e-5
lr_schedule = LinearWarmup
warmup_proportion = 0.2
doc_stride=128
max_query_length=64
```
## Usage
### In Haystack
Haystack is an NLP framework by deepset. You can use this model in a Haystack pipeline to do question answering at scale (over many documents). To load the model in [Haystack](https://github.com/deepset-ai/haystack/):
```python
reader = FARMReader(model_name_or_path="innocent-charles/Swahili-question-answer-latest-cased")
# or
reader = TransformersReader(model_name_or_path="innocent-charles/Swahili-question-answer-latest-cased",tokenizer="innocent-charles/Swahili-question-answer-latest-cased")
```
For a complete example of ``Swahili-question-answer-latest-cased`` being used for Swahili Question Answering, check out the [Tutorials in Haystack Documentation](https://haystack.deepset.ai)
### In Transformers
```python
from transformers import AutoModelForQuestionAnswering, AutoTokenizer, pipeline
model_name = "innocent-charles/Swahili-question-answer-latest-cased"
# a) Get predictions
nlp = pipeline('question-answering', model=model_name, tokenizer=model_name)
QA_input = {
'question': 'Asubuhi ilitupata pambajioi pa hospitali gani?',
'context': 'Asubuhi hiyo ilitupata pambajioni pa hospitali ya Uguzwa.'
}
res = nlp(QA_input)
# b) Load model & tokenizer
model = AutoModelForQuestionAnswering.from_pretrained(model_name)
tokenizer = AutoTokenizer.from_pretrained(model_name)
```
## Performance
```
"exact": 51.87029394424324,
"f1": 63.91251169582613,
"total": 445,
"HasAns_exact": 50.93522267206478,
"HasAns_f1": 62.02838248389763,
"HasAns_total": 386,
"NoAns_exact": 49.79983179142137,
"NoAns_f1": 60.79983179142137,
"NoAns_total": 59
```
## Special consideration
The project is still going, hence the model is still updated after training the model in more data, Therefore pull requests are welcome to contribute to increase the performance of the model.
## Author
**Innocent Charles:** [email protected]
## About Me
<P>
I build good things using Artificial Intelligence ,Data and Analytics , with over 3 Years of Experience as Applied AI Engineer & Data scientist from a strong background in Software Engineering ,with passion and extensive experience in Data and Businesses.
</P>
[Linkedin](https://www.linkedin.com/in/innocent-charles/) | [GitHub](https://github.com/innocent-charles) | [Website](innocentcharles.com)
| cdd4bb975564a2fbcc846dbc1f9c84c8 |
novacygni/ddpm-butterflies-128 | novacygni | null | 13 | 0 | diffusers | 0 | null | false | false | false | apache-2.0 | ['en'] | ['huggan/smithsonian_butterflies_subset'] | null | 0 | 0 | 0 | 0 | 0 | 0 | 0 | [] | false | true | true | 1,231 | false |
<!-- This model card has been generated automatically according to the information the training script had access to. You
should probably proofread and complete it, then remove this comment. -->
# ddpm-butterflies-128
## Model description
This diffusion model is trained with the [🤗 Diffusers](https://github.com/huggingface/diffusers) library
on the `huggan/smithsonian_butterflies_subset` dataset.
## Intended uses & limitations
#### How to use
```python
# TODO: add an example code snippet for running this diffusion pipeline
```
#### Limitations and bias
[TODO: provide examples of latent issues and potential remediations]
## Training data
[TODO: describe the data used to train the model]
### Training hyperparameters
The following hyperparameters were used during training:
- learning_rate: 0.0001
- train_batch_size: 16
- eval_batch_size: 16
- gradient_accumulation_steps: 1
- optimizer: AdamW with betas=(None, None), weight_decay=None and epsilon=None
- lr_scheduler: None
- lr_warmup_steps: 500
- ema_inv_gamma: None
- ema_inv_gamma: None
- ema_inv_gamma: None
- mixed_precision: fp16
### Training results
📈 [TensorBoard logs](https://huggingface.co/novacygni/ddpm-butterflies-128/tensorboard?#scalars)
| e9a036a2857f94e81be39d6d27d376f3 |
DOOGLAK/Article_50v4_NER_Model_3Epochs_AUGMENTED | DOOGLAK | bert | 13 | 5 | transformers | 0 | token-classification | true | false | false | apache-2.0 | null | ['article50v4_wikigold_split'] | null | 0 | 0 | 0 | 0 | 0 | 0 | 0 | ['generated_from_trainer'] | true | true | true | 1,557 | false |
<!-- This model card has been generated automatically according to the information the Trainer had access to. You
should probably proofread and complete it, then remove this comment. -->
# Article_50v4_NER_Model_3Epochs_AUGMENTED
This model is a fine-tuned version of [bert-base-cased](https://huggingface.co/bert-base-cased) on the article50v4_wikigold_split dataset.
It achieves the following results on the evaluation set:
- Loss: 0.4148
- Precision: 0.2442
- Recall: 0.1804
- F1: 0.2075
- Accuracy: 0.8392
## Model description
More information needed
## Intended uses & limitations
More information needed
## Training and evaluation data
More information needed
## Training procedure
### Training hyperparameters
The following hyperparameters were used during training:
- learning_rate: 2e-05
- train_batch_size: 8
- eval_batch_size: 8
- seed: 42
- optimizer: Adam with betas=(0.9,0.999) and epsilon=1e-08
- lr_scheduler_type: linear
- num_epochs: 3
### Training results
| Training Loss | Epoch | Step | Validation Loss | Precision | Recall | F1 | Accuracy |
|:-------------:|:-----:|:----:|:---------------:|:---------:|:------:|:------:|:--------:|
| No log | 1.0 | 26 | 0.5371 | 0.2683 | 0.0632 | 0.1023 | 0.7953 |
| No log | 2.0 | 52 | 0.4314 | 0.2259 | 0.1575 | 0.1856 | 0.8325 |
| No log | 3.0 | 78 | 0.4148 | 0.2442 | 0.1804 | 0.2075 | 0.8392 |
### Framework versions
- Transformers 4.17.0
- Pytorch 1.11.0+cu113
- Datasets 2.4.0
- Tokenizers 0.11.6
| b2f672d8e043358a9a287628b2333507 |
sd-concepts-library/solomon-temple | sd-concepts-library | null | 10 | 0 | null | 0 | null | false | false | false | mit | null | null | null | 0 | 0 | 0 | 0 | 0 | 0 | 0 | [] | false | true | true | 1,186 | false | ### solomon temple on Stable Diffusion
This is the `<solomon-temple>` concept taught to Stable Diffusion via Textual Inversion. You can load this concept into the [Stable Conceptualizer](https://colab.research.google.com/github/huggingface/notebooks/blob/main/diffusers/stable_conceptualizer_inference.ipynb) notebook. You can also train your own concepts and load them into the concept libraries using [this notebook](https://colab.research.google.com/github/huggingface/notebooks/blob/main/diffusers/sd_textual_inversion_training.ipynb).
Here is the new concept you will be able to use as an `object`:
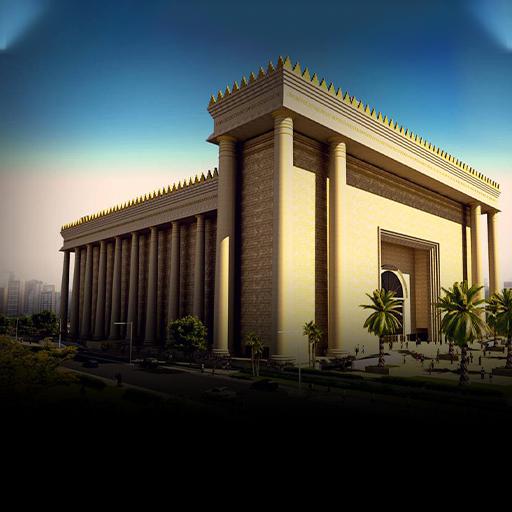
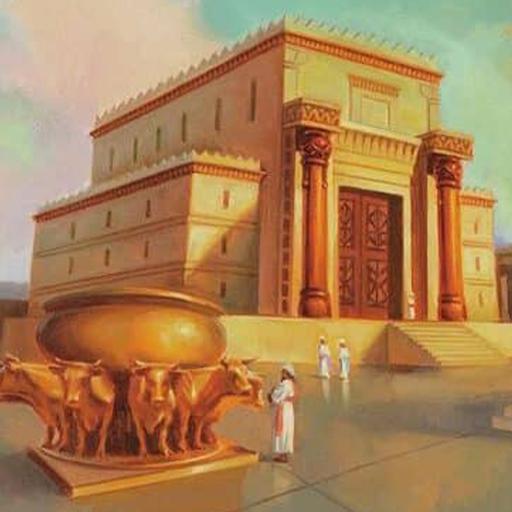
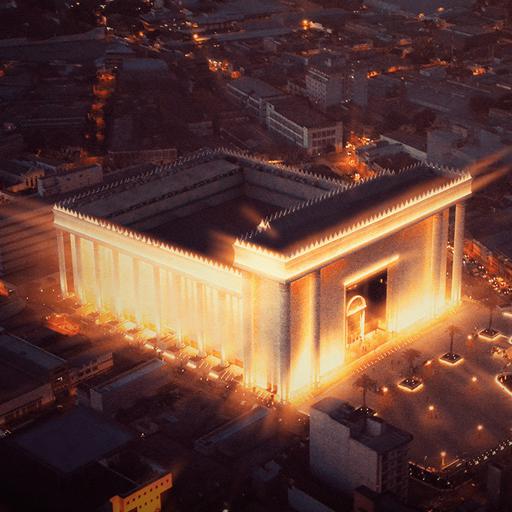
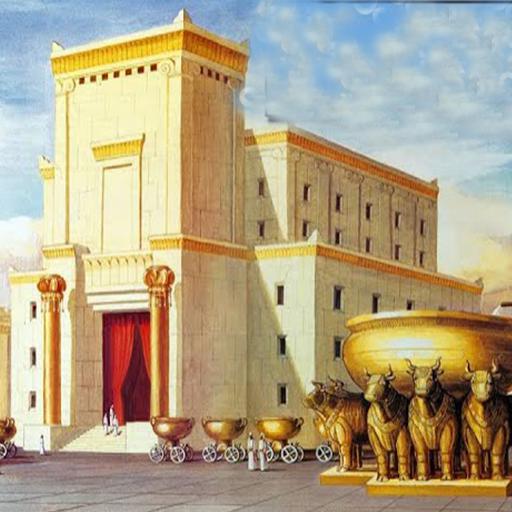
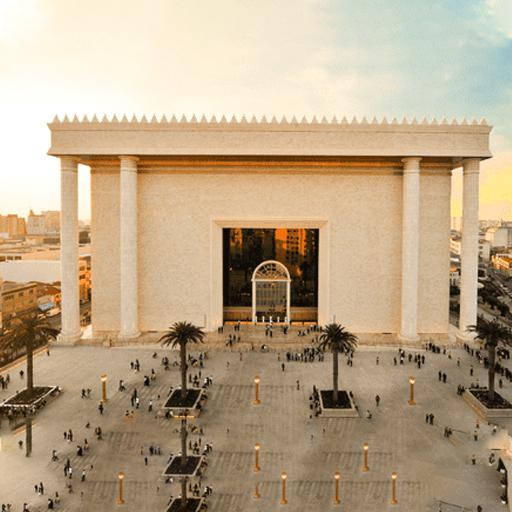
| aaadfb6a07440d3b9a1252df86dcc28d |
amitness/roberta-base-ne | amitness | roberta | 8 | 3 | transformers | 1 | fill-mask | true | false | true | mit | ['ne'] | ['cc100'] | null | 0 | 0 | 0 | 0 | 0 | 0 | 0 | ['roberta', 'nepali-laguage-model'] | false | true | true | 527 | false |
# nepbert
## Model description
Roberta trained from scratch on the Nepali CC-100 dataset with 12 million sentences.
## Intended uses & limitations
#### How to use
```python
from transformers import pipeline
pipe = pipeline(
"fill-mask",
model="amitness/nepbert",
tokenizer="amitness/nepbert"
)
print(pipe(u"तिमीलाई कस्तो <mask>?"))
```
## Training data
The data was taken from the nepali language subset of CC-100 dataset.
## Training procedure
The model was trained on Google Colab using `1x Tesla V100`. | 940cf5aa9e5999943c811a39e7e5b2c8 |
SreyanG-NVIDIA/bert-base-cased-finetuned-ner | SreyanG-NVIDIA | bert | 13 | 6 | transformers | 0 | token-classification | true | false | false | apache-2.0 | null | ['conll2003'] | null | 1 | 1 | 0 | 0 | 0 | 0 | 0 | ['generated_from_trainer'] | true | true | true | 1,531 | false |
<!-- This model card has been generated automatically according to the information the Trainer had access to. You
should probably proofread and complete it, then remove this comment. -->
# bert-base-cased-finetuned-ner
This model is a fine-tuned version of [bert-base-cased](https://huggingface.co/bert-base-cased) on the conll2003 dataset.
It achieves the following results on the evaluation set:
- Loss: 0.0650
- Precision: 0.9325
- Recall: 0.9375
- F1: 0.9350
- Accuracy: 0.9840
## Model description
More information needed
## Intended uses & limitations
More information needed
## Training and evaluation data
More information needed
## Training procedure
### Training hyperparameters
The following hyperparameters were used during training:
- learning_rate: 2e-05
- train_batch_size: 16
- eval_batch_size: 16
- seed: 42
- optimizer: Adam with betas=(0.9,0.999) and epsilon=1e-08
- lr_scheduler_type: linear
- num_epochs: 3
### Training results
| Training Loss | Epoch | Step | Validation Loss | Precision | Recall | F1 | Accuracy |
|:-------------:|:-----:|:----:|:---------------:|:---------:|:------:|:------:|:--------:|
| 0.2346 | 1.0 | 878 | 0.0722 | 0.9168 | 0.9217 | 0.9192 | 0.9795 |
| 0.0483 | 2.0 | 1756 | 0.0618 | 0.9299 | 0.9370 | 0.9335 | 0.9837 |
| 0.0262 | 3.0 | 2634 | 0.0650 | 0.9325 | 0.9375 | 0.9350 | 0.9840 |
### Framework versions
- Transformers 4.18.0
- Pytorch 1.11.0+cu102
- Datasets 2.1.0
- Tokenizers 0.12.1
| 591f1a49eb3256683ae5977480f5be4c |
Habana/stable-diffusion | Habana | null | 3 | 2,462 | null | 1 | null | false | false | false | apache-2.0 | null | null | null | 0 | 0 | 0 | 0 | 0 | 0 | 0 | [] | false | true | true | 2,503 | false |
[Optimum Habana](https://github.com/huggingface/optimum-habana) is the interface between the Hugging Face Transformers and Diffusers libraries and Habana's Gaudi processor (HPU).
It provides a set of tools enabling easy and fast model loading, training and inference on single- and multi-HPU settings for different downstream tasks.
Learn more about how to take advantage of the power of Habana HPUs to train and deploy Transformers and Diffusers models at [hf.co/hardware/habana](https://huggingface.co/hardware/habana).
## Stable Diffusion HPU configuration
This model only contains the `GaudiConfig` file for running **Stable Diffusion 1** (e.g. [CompVis/stable-diffusion-v1-4](https://huggingface.co/CompVis/stable-diffusion-v1-4)) or **Stable Diffusion 2** (e.g. [stabilityai/stable-diffusion-2](https://huggingface.co/stabilityai/stable-diffusion-2)) on Habana's Gaudi processors (HPU).
**This model contains no model weights, only a GaudiConfig.**
This enables to specify:
- `use_habana_mixed_precision`: whether to use Habana Mixed Precision (HMP)
- `hmp_opt_level`: optimization level for HMP, see [here](https://docs.habana.ai/en/latest/PyTorch/PyTorch_Mixed_Precision/PT_Mixed_Precision.html#configuration-options) for a detailed explanation
- `hmp_bf16_ops`: list of operators that should run in bf16
- `hmp_fp32_ops`: list of operators that should run in fp32
- `hmp_is_verbose`: verbosity
## Usage
The `GaudiStableDiffusionPipeline` (`GaudiDDIMScheduler`) is instantiated the same way as the `StableDiffusionPipeline` (`DDIMScheduler`) in the 🤗 Diffusers library.
The only difference is that there are a few new training arguments specific to HPUs.
Here is an example with one prompt:
```python
from optimum.habana import GaudiConfig
from optimum.habana.diffusers import GaudiDDIMScheduler, GaudiStableDiffusionPipeline
model_name = "stabilityai/stable-diffusion-2"
scheduler = GaudiDDIMScheduler.from_pretrained(model_name, subfolder="scheduler")
pipeline = GaudiStableDiffusionPipeline.from_pretrained(
model_name,
scheduler=scheduler,
use_habana=True,
use_hpu_graphs=True,
gaudi_config="Habana/stable-diffusion",
)
outputs = generator(
["An image of a squirrel in Picasso style"],
num_images_per_prompt=16,
batch_size=4,
)
```
Check out the [documentation](https://huggingface.co/docs/optimum/habana/usage_guides/stable_diffusion) and [this example](https://github.com/huggingface/optimum-habana/tree/main/examples/stable-diffusion) for more advanced usage.
| 413e545dc0e2df279da455945618aa84 |
KoenBronstring/finetuning-sentiment-model-3000-samples | KoenBronstring | distilbert | 18 | 11 | transformers | 0 | text-classification | true | false | false | apache-2.0 | null | ['imdb'] | null | 1 | 1 | 0 | 0 | 0 | 0 | 0 | ['generated_from_trainer'] | true | true | true | 1,053 | false |
<!-- This model card has been generated automatically according to the information the Trainer had access to. You
should probably proofread and complete it, then remove this comment. -->
# finetuning-sentiment-model-3000-samples
This model is a fine-tuned version of [distilbert-base-uncased](https://huggingface.co/distilbert-base-uncased) on the imdb dataset.
It achieves the following results on the evaluation set:
- Loss: 0.3149
- Accuracy: 0.8733
- F1: 0.8758
## Model description
More information needed
## Intended uses & limitations
More information needed
## Training and evaluation data
More information needed
## Training procedure
### Training hyperparameters
The following hyperparameters were used during training:
- learning_rate: 2e-05
- train_batch_size: 16
- eval_batch_size: 16
- seed: 42
- optimizer: Adam with betas=(0.9,0.999) and epsilon=1e-08
- lr_scheduler_type: linear
- num_epochs: 2
### Training results
### Framework versions
- Transformers 4.18.0
- Pytorch 1.11.0+cpu
- Datasets 2.1.0
- Tokenizers 0.12.1
| 897b06137fda2d710a176db43b0fdcb7 |
Ayham/distilgpt2_summarization_cnndm | Ayham | gpt2 | 8 | 63 | transformers | 0 | text-generation | true | false | false | apache-2.0 | null | null | null | 0 | 0 | 0 | 0 | 0 | 0 | 0 | ['generated_from_trainer'] | true | true | true | 1,215 | false |
<!-- This model card has been generated automatically according to the information the Trainer had access to. You
should probably proofread and complete it, then remove this comment. -->
# distilgpt2_summarization_cnndm
This model is a fine-tuned version of [distilgpt2](https://huggingface.co/distilgpt2) on the None dataset.
It achieves the following results on the evaluation set:
- Loss: 3.0608
## Model description
More information needed
## Intended uses & limitations
More information needed
## Training and evaluation data
More information needed
## Training procedure
### Training hyperparameters
The following hyperparameters were used during training:
- learning_rate: 5e-05
- train_batch_size: 4
- eval_batch_size: 4
- seed: 42
- optimizer: Adam with betas=(0.9,0.999) and epsilon=1e-08
- lr_scheduler_type: linear
- lr_scheduler_warmup_steps: 1000
- num_epochs: 1
- mixed_precision_training: Native AMP
### Training results
| Training Loss | Epoch | Step | Validation Loss |
|:-------------:|:-----:|:-----:|:---------------:|
| 3.0416 | 1.0 | 71779 | 3.0608 |
### Framework versions
- Transformers 4.25.1
- Pytorch 1.13.0+cu116
- Datasets 2.8.0
- Tokenizers 0.13.2
| 33de8f35b3f1a3d16b133d45abe742ef |
bvrtek/KusaMix | bvrtek | null | 5 | 2 | diffusers | 6 | text-to-image | false | false | false | creativeml-openrail-m | ['en'] | null | null | 0 | 0 | 0 | 0 | 0 | 0 | 0 | ['stable-diffusion', 'stable-diffusion-diffusers', 'text-to-image', 'diffusers', 'safetensors'] | false | true | true | 1,466 | false |
# 草ミックス
Welcome to KusaMix - a latent diffusion model for weebs. This model is intended to produce high-quality, highly detailed anime style with just a few prompts. Like other anime-style Stable Diffusion models, it also supports danbooru tags to generate images.
e.g. **_1girl, white hair, golden eyes, beautiful eyes, detail, flower meadow, cumulonimbus clouds, lighting, detailed sky, garden_**
Non cherry picked example of prompt from above:


## License
This model is open access and available to all, with a CreativeML OpenRAIL-M license further specifying rights and usage.
The CreativeML OpenRAIL License specifies:
1. You can't use the model to deliberately produce nor share illegal or harmful outputs or content
2. The authors claims no rights on the outputs you generate, you are free to use them and are accountable for their use which must not go against the provisions set in the license
3. You may re-distribute the weights and use the model commercially and/or as a service. If you do, please be aware you have to include the same use restrictions as the ones in the license and share a copy of the CreativeML OpenRAIL-M to all your users (please read the license entirely and carefully)
[Please read the full license here](https://huggingface.co/spaces/CompVis/stable-diffusion-license) | 9e551d38faf22873963d60aebf635fd2 |
Ulto/avengers2 | Ulto | gpt2 | 8 | 6 | transformers | 0 | text-generation | true | false | false | apache-2.0 | null | [] | null | 0 | 0 | 0 | 0 | 0 | 0 | 0 | ['generated_from_trainer'] | false | true | true | 1,215 | false |
<!-- This model card has been generated automatically according to the information the Trainer had access to. You
should probably proofread and complete it, then remove this comment. -->
# avengers2
This model is a fine-tuned version of [distilgpt2](https://huggingface.co/distilgpt2) on the None dataset.
It achieves the following results on the evaluation set:
- Loss: 4.0131
## Model description
More information needed
## Intended uses & limitations
More information needed
## Training and evaluation data
More information needed
## Training procedure
### Training hyperparameters
The following hyperparameters were used during training:
- learning_rate: 2e-05
- train_batch_size: 8
- eval_batch_size: 8
- seed: 42
- optimizer: Adam with betas=(0.9,0.999) and epsilon=1e-08
- lr_scheduler_type: linear
- num_epochs: 3.0
### Training results
| Training Loss | Epoch | Step | Validation Loss |
|:-------------:|:-----:|:----:|:---------------:|
| No log | 1.0 | 56 | 3.9588 |
| No log | 2.0 | 112 | 3.9996 |
| No log | 3.0 | 168 | 4.0131 |
### Framework versions
- Transformers 4.10.0
- Pytorch 1.9.0
- Datasets 1.2.1
- Tokenizers 0.10.1
| dad1afaad8364e4c290899176094e638 |
robkayinto/xlm-roberta-base-finetuned-panx-all | robkayinto | xlm-roberta | 10 | 1 | transformers | 0 | token-classification | true | false | false | mit | null | null | null | 0 | 0 | 0 | 0 | 0 | 0 | 0 | ['generated_from_trainer'] | true | true | true | 1,319 | false |
<!-- This model card has been generated automatically according to the information the Trainer had access to. You
should probably proofread and complete it, then remove this comment. -->
# xlm-roberta-base-finetuned-panx-all
This model is a fine-tuned version of [xlm-roberta-base](https://huggingface.co/xlm-roberta-base) on the None dataset.
It achieves the following results on the evaluation set:
- Loss: 0.1739
- F1: 0.8535
## Model description
More information needed
## Intended uses & limitations
More information needed
## Training and evaluation data
More information needed
## Training procedure
### Training hyperparameters
The following hyperparameters were used during training:
- learning_rate: 5e-05
- train_batch_size: 24
- eval_batch_size: 24
- seed: 42
- optimizer: Adam with betas=(0.9,0.999) and epsilon=1e-08
- lr_scheduler_type: linear
- num_epochs: 3
### Training results
| Training Loss | Epoch | Step | Validation Loss | F1 |
|:-------------:|:-----:|:----:|:---------------:|:------:|
| 0.3067 | 1.0 | 835 | 0.1840 | 0.8085 |
| 0.1566 | 2.0 | 1670 | 0.1727 | 0.8447 |
| 0.1013 | 3.0 | 2505 | 0.1739 | 0.8535 |
### Framework versions
- Transformers 4.11.3
- Pytorch 1.10.2+cu102
- Datasets 1.16.1
- Tokenizers 0.10.3
| 2ba8d2b40b162afd053facf8543c37fb |
Applemoon/bert-finetuned-ner | Applemoon | bert | 10 | 15 | transformers | 0 | token-classification | true | false | false | apache-2.0 | null | ['conll2003'] | null | 0 | 0 | 0 | 0 | 0 | 0 | 0 | ['generated_from_trainer'] | true | true | true | 1,512 | false |
<!-- This model card has been generated automatically according to the information the Trainer had access to. You
should probably proofread and complete it, then remove this comment. -->
# bert-finetuned-ner
This model is a fine-tuned version of [bert-base-cased](https://huggingface.co/bert-base-cased) on the conll2003 dataset.
It achieves the following results on the evaluation set:
- Loss: 0.0399
- Precision: 0.9513
- Recall: 0.9559
- F1: 0.9536
- Accuracy: 0.9922
## Model description
More information needed
## Intended uses & limitations
More information needed
## Training and evaluation data
More information needed
## Training procedure
### Training hyperparameters
The following hyperparameters were used during training:
- learning_rate: 2e-05
- train_batch_size: 8
- eval_batch_size: 8
- seed: 42
- optimizer: Adam with betas=(0.9,0.999) and epsilon=1e-08
- lr_scheduler_type: linear
- num_epochs: 3
### Training results
| Training Loss | Epoch | Step | Validation Loss | Precision | Recall | F1 | Accuracy |
|:-------------:|:-----:|:----:|:---------------:|:---------:|:------:|:------:|:--------:|
| 0.0548 | 1.0 | 1756 | 0.0438 | 0.9368 | 0.9411 | 0.9390 | 0.9900 |
| 0.021 | 2.0 | 3512 | 0.0395 | 0.9446 | 0.9519 | 0.9482 | 0.9914 |
| 0.0108 | 3.0 | 5268 | 0.0399 | 0.9513 | 0.9559 | 0.9536 | 0.9922 |
### Framework versions
- Transformers 4.21.1
- Pytorch 1.12.1
- Datasets 2.4.0
- Tokenizers 0.12.1
| 26457e9696f376b19ea4511ac767bb9d |
saattrupdan/wav2vec2-xls-r-300m-ftspeech | saattrupdan | wav2vec2 | 14 | 767 | transformers | 0 | automatic-speech-recognition | true | false | false | other | ['da'] | ['ftspeech'] | null | 0 | 0 | 0 | 0 | 0 | 0 | 0 | [] | true | true | true | 884 | false |
# XLS-R-300m-FTSpeech
## Model description
This model is a fine-tuned version of [facebook/wav2vec2-xls-r-300m](https://huggingface.co/facebook/wav2vec2-xls-r-300m) on the [FTSpeech dataset](https://ftspeech.github.io/), being a dataset of 1,800 hours of transcribed speeches from the Danish parliament.
## Performance
The model achieves the following WER scores (lower is better):
| **Dataset** | **WER without LM** | **WER with 5-gram LM** |
| :---: | ---: | ---: |
| [Danish part of Common Voice 8.0](https://huggingface.co/datasets/mozilla-foundation/common_voice_8_0/viewer/da/train) | 20.48 | 17.91 |
| [Alvenir test set](https://huggingface.co/datasets/Alvenir/alvenir_asr_da_eval) | 15.46 | 13.84 |
## License
The use of this model needs to adhere to [this license from the Danish Parliament](https://www.ft.dk/da/aktuelt/tv-fra-folketinget/deling-og-rettigheder). | b11eac1e2d6bd242293f1a09fc2e46b6 |
jonatasgrosman/exp_w2v2t_de_wav2vec2_s982 | jonatasgrosman | wav2vec2 | 10 | 5 | transformers | 0 | automatic-speech-recognition | true | false | false | apache-2.0 | ['de'] | ['mozilla-foundation/common_voice_7_0'] | null | 0 | 0 | 0 | 0 | 0 | 0 | 0 | ['automatic-speech-recognition', 'de'] | false | true | true | 456 | false | # exp_w2v2t_de_wav2vec2_s982
Fine-tuned [facebook/wav2vec2-large-lv60](https://huggingface.co/facebook/wav2vec2-large-lv60) for speech recognition using the train split of [Common Voice 7.0 (de)](https://huggingface.co/datasets/mozilla-foundation/common_voice_7_0).
When using this model, make sure that your speech input is sampled at 16kHz.
This model has been fine-tuned by the [HuggingSound](https://github.com/jonatasgrosman/huggingsound) tool.
| 67d36ffbe4420b89c8949a9ce4d75f68 |
ReKarma/ddpm-ema-flowers-64 | ReKarma | null | 11 | 3 | diffusers | 0 | null | false | false | false | apache-2.0 | ['en'] | ['huggan/flowers-102-categories'] | null | 0 | 0 | 0 | 0 | 0 | 0 | 0 | [] | false | true | true | 1,225 | false |
<!-- This model card has been generated automatically according to the information the training script had access to. You
should probably proofread and complete it, then remove this comment. -->
# ddpm-ema-flowers-64
## Model description
This diffusion model is trained with the [🤗 Diffusers](https://github.com/huggingface/diffusers) library
on the `huggan/flowers-102-categories` dataset.
## Intended uses & limitations
#### How to use
```python
# TODO: add an example code snippet for running this diffusion pipeline
```
#### Limitations and bias
[TODO: provide examples of latent issues and potential remediations]
## Training data
[TODO: describe the data used to train the model]
### Training hyperparameters
The following hyperparameters were used during training:
- learning_rate: 0.0001
- train_batch_size: 16
- eval_batch_size: 16
- gradient_accumulation_steps: 1
- optimizer: AdamW with betas=(0.95, 0.999), weight_decay=1e-06 and epsilon=1e-08
- lr_scheduler: cosine
- lr_warmup_steps: 500
- ema_inv_gamma: 1.0
- ema_inv_gamma: 0.75
- ema_inv_gamma: 0.9999
- mixed_precision: bf16
### Training results
📈 [TensorBoard logs](https://huggingface.co/ReKarma/ddpm-ema-flowers-64/tensorboard?#scalars)
| 7a454fd24320938fb671d8e8a3b38fb8 |
AnnaR/literature_summarizer | AnnaR | bart | 9 | 4 | transformers | 0 | text2text-generation | false | true | false | apache-2.0 | null | null | null | 0 | 0 | 0 | 0 | 0 | 0 | 0 | ['generated_from_keras_callback'] | true | true | true | 1,778 | false |
<!-- This model card has been generated automatically according to the information Keras had access to. You should
probably proofread and complete it, then remove this comment. -->
# AnnaR/literature_summarizer
This model is a fine-tuned version of [sshleifer/distilbart-xsum-1-1](https://huggingface.co/sshleifer/distilbart-xsum-1-1) on an unknown dataset.
It achieves the following results on the evaluation set:
- Train Loss: 3.2180
- Validation Loss: 4.7198
- Epoch: 10
## Model description
More information needed
## Intended uses & limitations
More information needed
## Training and evaluation data
More information needed
## Training procedure
### Training hyperparameters
The following hyperparameters were used during training:
- optimizer: {'name': 'AdamWeightDecay', 'learning_rate': {'class_name': 'PolynomialDecay', 'config': {'initial_learning_rate': 5.6e-05, 'decay_steps': 5300, 'end_learning_rate': 0.0, 'power': 1.0, 'cycle': False, 'name': None}}, 'decay': 0.0, 'beta_1': 0.9, 'beta_2': 0.999, 'epsilon': 1e-08, 'amsgrad': False, 'weight_decay_rate': 0.1}
- training_precision: float32
### Training results
| Train Loss | Validation Loss | Epoch |
|:----------:|:---------------:|:-----:|
| 5.6694 | 5.0234 | 0 |
| 4.9191 | 4.8161 | 1 |
| 4.5770 | 4.7170 | 2 |
| 4.3268 | 4.6571 | 3 |
| 4.1073 | 4.6296 | 4 |
| 3.9225 | 4.6279 | 5 |
| 3.7564 | 4.6288 | 6 |
| 3.5989 | 4.6731 | 7 |
| 3.4611 | 4.6767 | 8 |
| 3.3356 | 4.6934 | 9 |
| 3.2180 | 4.7198 | 10 |
### Framework versions
- Transformers 4.17.0
- TensorFlow 2.8.0
- Datasets 2.0.0
- Tokenizers 0.11.6
| f121b756af74b1123d33d48c42572aa6 |
HusseinHE/ramy | HusseinHE | null | 29 | 2 | diffusers | 0 | text-to-image | false | false | false | creativeml-openrail-m | null | null | null | 2 | 2 | 0 | 0 | 0 | 0 | 0 | ['text-to-image'] | false | true | true | 486 | false | ### ramy Dreambooth model trained by HusseinHE with [Hugging Face Dreambooth Training Space](https://huggingface.co/spaces/multimodalart/dreambooth-training) with the v1-5 base model
You run your new concept via `diffusers` [Colab Notebook for Inference](https://colab.research.google.com/github/huggingface/notebooks/blob/main/diffusers/sd_dreambooth_inference.ipynb). Don't forget to use the concept prompts!
Sample pictures of:
e3t (use that on your prompt)
| 6a06d78b6d1bafef7b9e9258d7cb3196 |
jonatasgrosman/exp_w2v2r_de_vp-100k_accent_germany-8_austria-2_s953 | jonatasgrosman | wav2vec2 | 10 | 4 | transformers | 0 | automatic-speech-recognition | true | false | false | apache-2.0 | ['de'] | ['mozilla-foundation/common_voice_7_0'] | null | 0 | 0 | 0 | 0 | 0 | 0 | 0 | ['automatic-speech-recognition', 'de'] | false | true | true | 502 | false | # exp_w2v2r_de_vp-100k_accent_germany-8_austria-2_s953
Fine-tuned [facebook/wav2vec2-large-100k-voxpopuli](https://huggingface.co/facebook/wav2vec2-large-100k-voxpopuli) for speech recognition using the train split of [Common Voice 7.0 (de)](https://huggingface.co/datasets/mozilla-foundation/common_voice_7_0).
When using this model, make sure that your speech input is sampled at 16kHz.
This model has been fine-tuned by the [HuggingSound](https://github.com/jonatasgrosman/huggingsound) tool.
| bdd88a7bdcb8331b66353bd8e794b0e2 |
sd-concepts-library/willy-hd | sd-concepts-library | null | 10 | 0 | null | 0 | null | false | false | false | mit | null | null | null | 0 | 0 | 0 | 0 | 0 | 0 | 0 | [] | false | true | true | 1,156 | false | ### Willy-HD on Stable Diffusion
This is the `<willy_character>` concept taught to Stable Diffusion via Textual Inversion. You can load this concept into the [Stable Conceptualizer](https://colab.research.google.com/github/huggingface/notebooks/blob/main/diffusers/stable_conceptualizer_inference.ipynb) notebook. You can also train your own concepts and load them into the concept libraries using [this notebook](https://colab.research.google.com/github/huggingface/notebooks/blob/main/diffusers/sd_textual_inversion_training.ipynb).
Here is the new concept you will be able to use as an `object`:
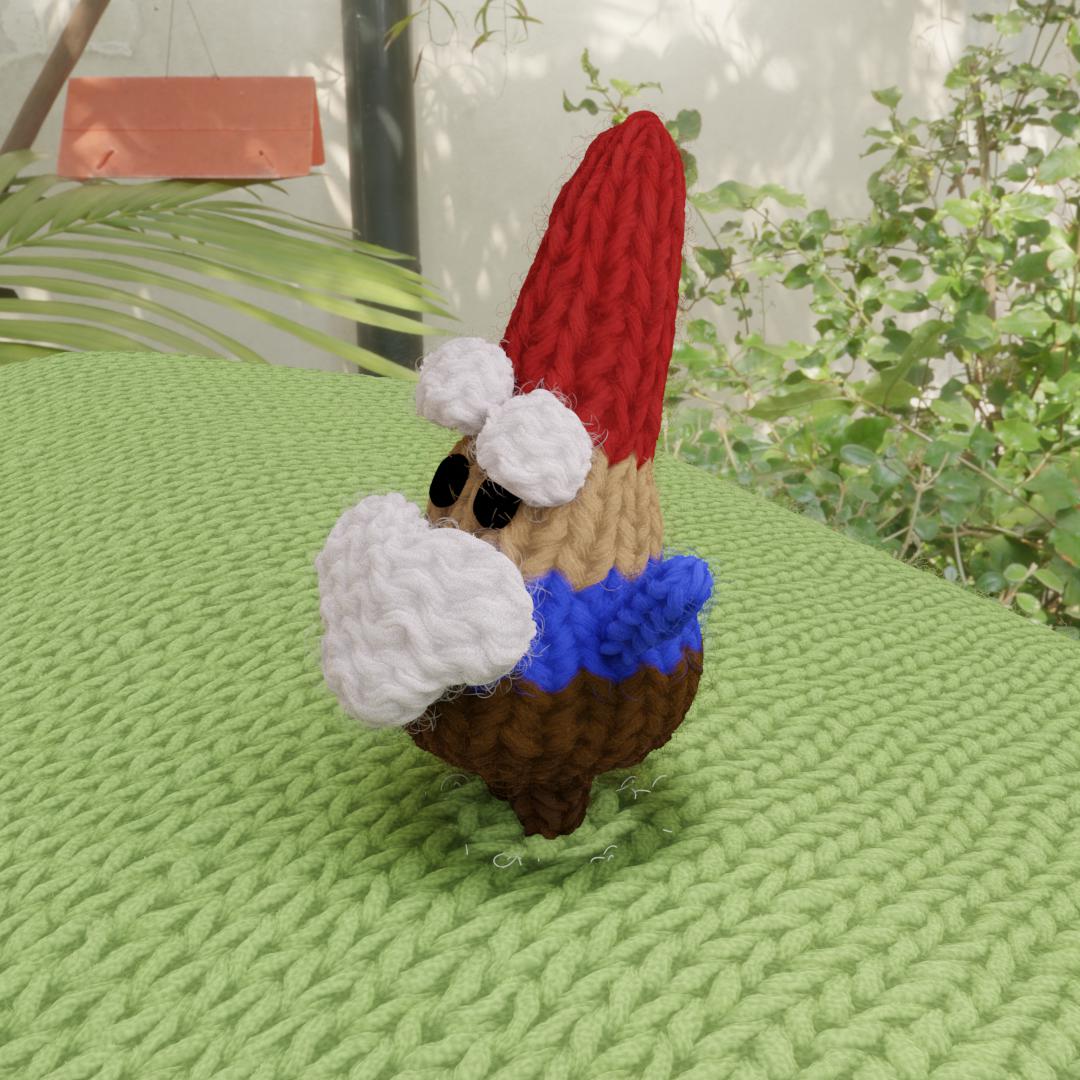
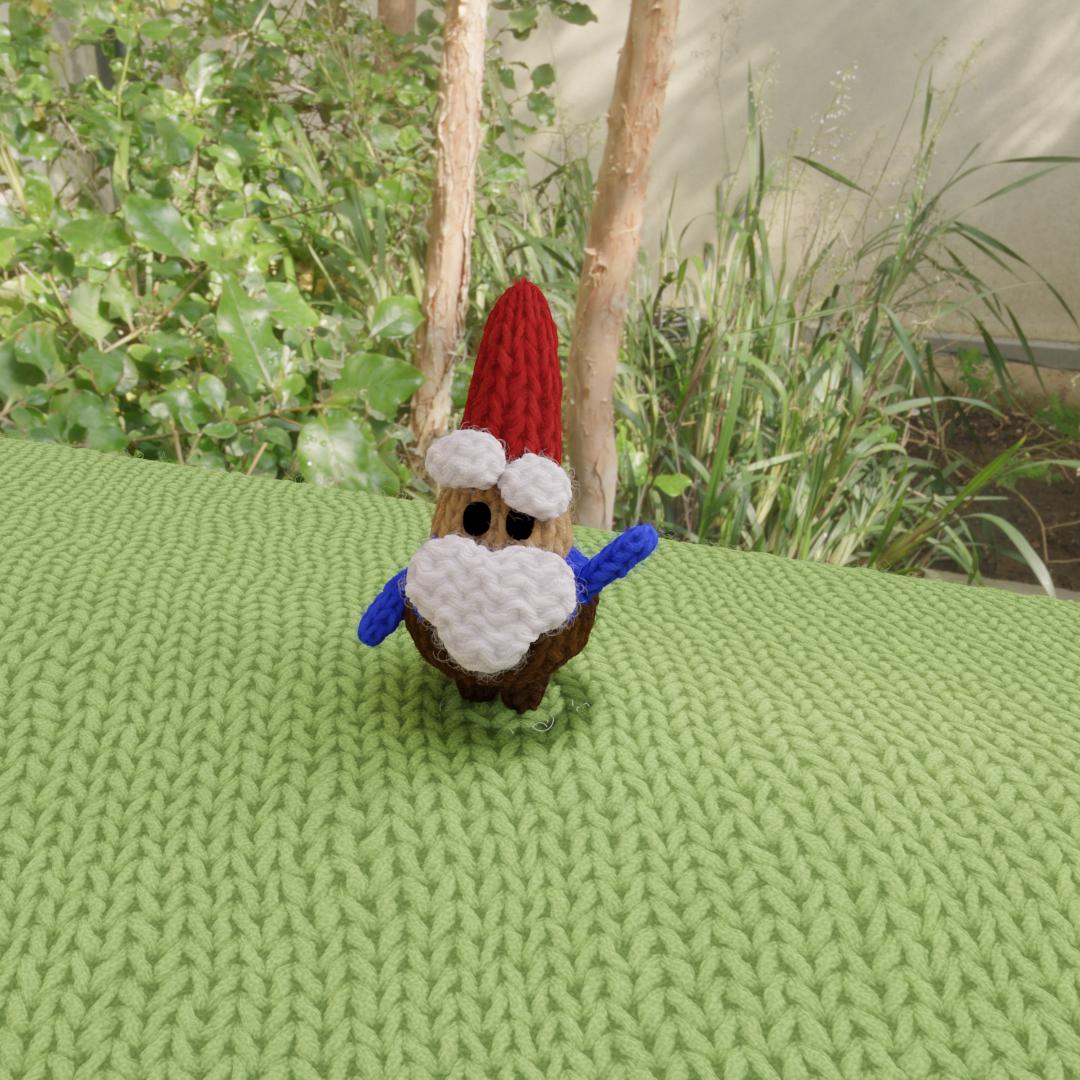
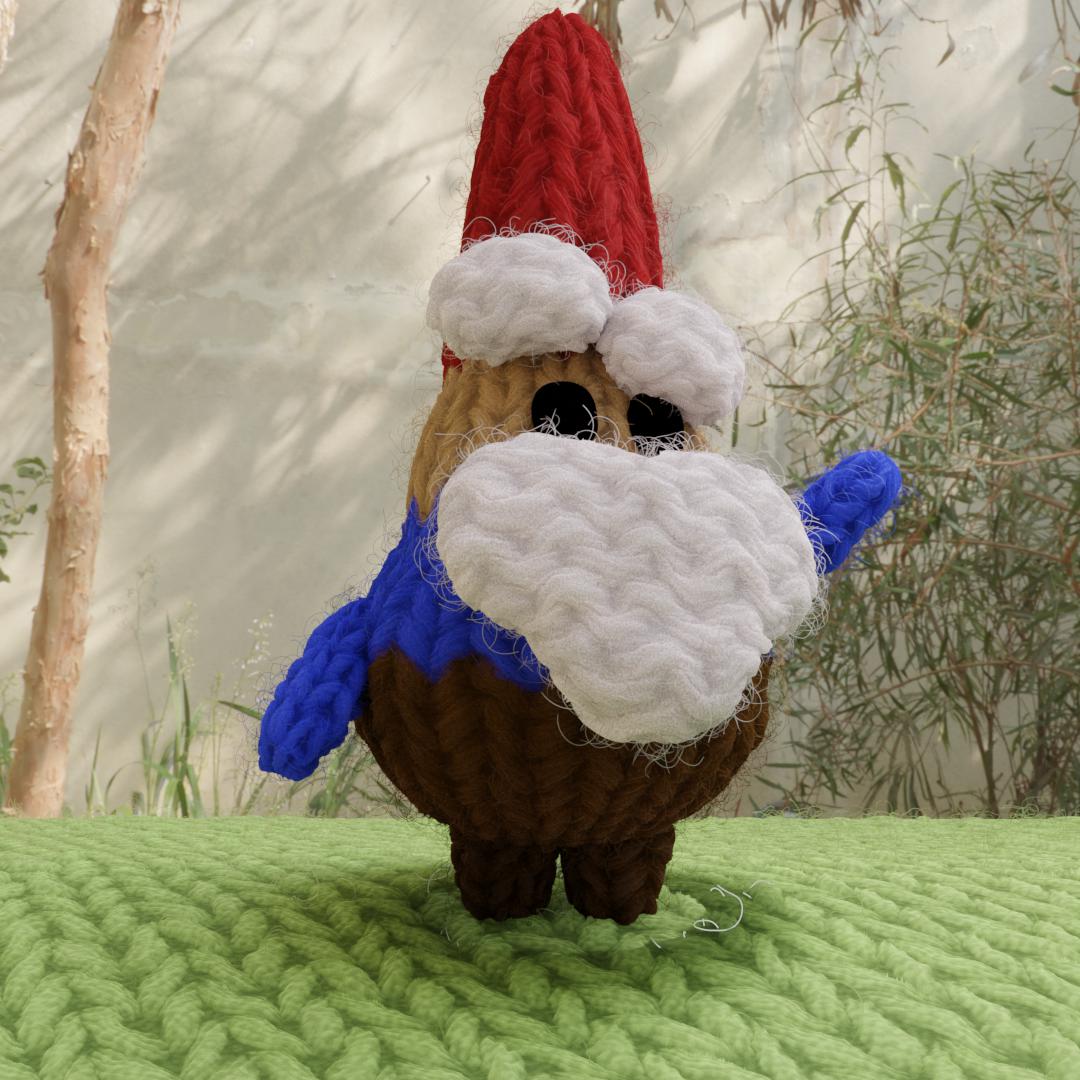
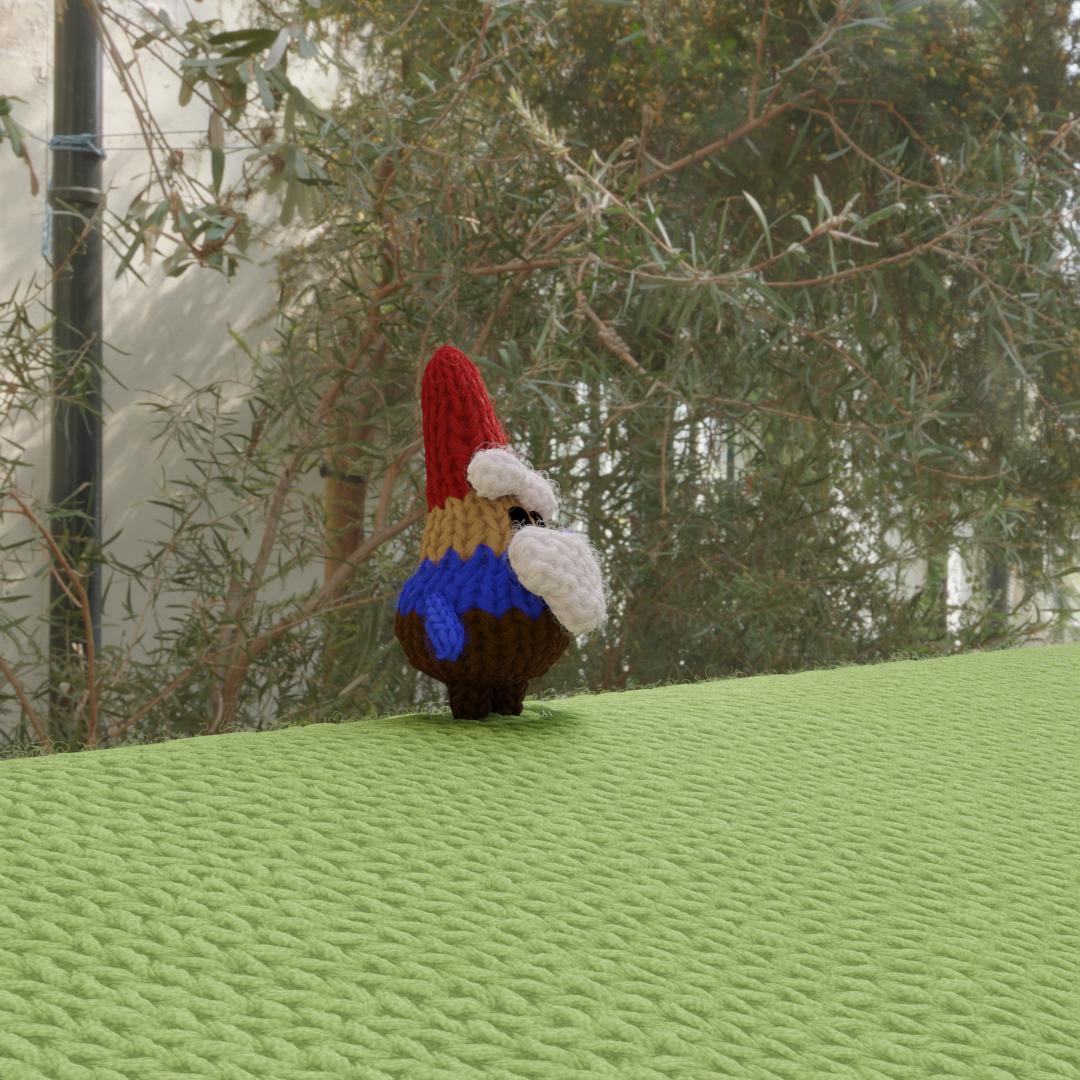
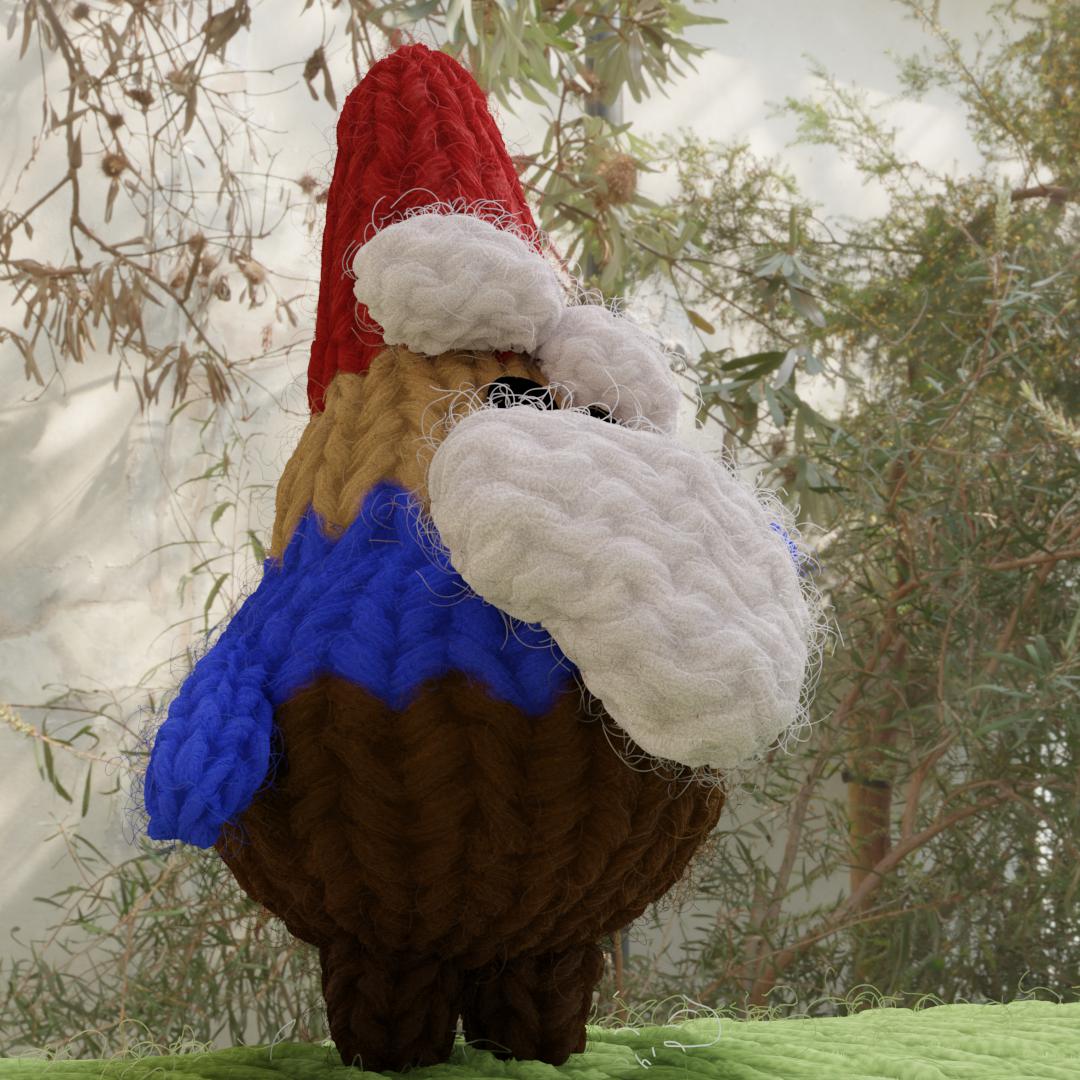
| 2c871dc55a91d1b624a49ebc2cfc2065 |
jonatasgrosman/exp_w2v2r_de_xls-r_age_teens-0_sixties-10_s288 | jonatasgrosman | wav2vec2 | 10 | 0 | transformers | 0 | automatic-speech-recognition | true | false | false | apache-2.0 | ['de'] | ['mozilla-foundation/common_voice_7_0'] | null | 0 | 0 | 0 | 0 | 0 | 0 | 0 | ['automatic-speech-recognition', 'de'] | false | true | true | 476 | false | # exp_w2v2r_de_xls-r_age_teens-0_sixties-10_s288
Fine-tuned [facebook/wav2vec2-xls-r-300m](https://huggingface.co/facebook/wav2vec2-xls-r-300m) for speech recognition using the train split of [Common Voice 7.0 (de)](https://huggingface.co/datasets/mozilla-foundation/common_voice_7_0).
When using this model, make sure that your speech input is sampled at 16kHz.
This model has been fine-tuned by the [HuggingSound](https://github.com/jonatasgrosman/huggingsound) tool.
| 2f3512ec2c6864b4f7f45c3fe448a652 |
jonatasgrosman/exp_w2v2r_fr_vp-100k_age_teens-10_sixties-0_s732 | jonatasgrosman | wav2vec2 | 10 | 0 | transformers | 0 | automatic-speech-recognition | true | false | false | apache-2.0 | ['fr'] | ['mozilla-foundation/common_voice_7_0'] | null | 0 | 0 | 0 | 0 | 0 | 0 | 0 | ['automatic-speech-recognition', 'fr'] | false | true | true | 498 | false | # exp_w2v2r_fr_vp-100k_age_teens-10_sixties-0_s732
Fine-tuned [facebook/wav2vec2-large-100k-voxpopuli](https://huggingface.co/facebook/wav2vec2-large-100k-voxpopuli) for speech recognition using the train split of [Common Voice 7.0 (fr)](https://huggingface.co/datasets/mozilla-foundation/common_voice_7_0).
When using this model, make sure that your speech input is sampled at 16kHz.
This model has been fine-tuned by the [HuggingSound](https://github.com/jonatasgrosman/huggingsound) tool.
| ed9d5aeecb632b431f65a822fe77a11f |
Helsinki-NLP/opus-mt-fr-ha | Helsinki-NLP | marian | 10 | 28 | transformers | 0 | translation | true | true | false | apache-2.0 | null | null | null | 0 | 0 | 0 | 0 | 0 | 0 | 0 | ['translation'] | false | true | true | 768 | false |
### opus-mt-fr-ha
* source languages: fr
* target languages: ha
* OPUS readme: [fr-ha](https://github.com/Helsinki-NLP/OPUS-MT-train/blob/master/models/fr-ha/README.md)
* dataset: opus
* model: transformer-align
* pre-processing: normalization + SentencePiece
* download original weights: [opus-2020-01-09.zip](https://object.pouta.csc.fi/OPUS-MT-models/fr-ha/opus-2020-01-09.zip)
* test set translations: [opus-2020-01-09.test.txt](https://object.pouta.csc.fi/OPUS-MT-models/fr-ha/opus-2020-01-09.test.txt)
* test set scores: [opus-2020-01-09.eval.txt](https://object.pouta.csc.fi/OPUS-MT-models/fr-ha/opus-2020-01-09.eval.txt)
## Benchmarks
| testset | BLEU | chr-F |
|-----------------------|-------|-------|
| JW300.fr.ha | 24.4 | 0.447 |
| bd57d1773fb4caa5ae47213f1751ed56 |
ghatgetanuj/microsoft-deberta-v3-large_cls_CR | ghatgetanuj | deberta-v2 | 13 | 1 | transformers | 0 | text-classification | true | false | false | mit | null | null | null | 0 | 0 | 0 | 0 | 0 | 0 | 0 | ['generated_from_trainer'] | true | true | true | 1,544 | false |
<!-- This model card has been generated automatically according to the information the Trainer had access to. You
should probably proofread and complete it, then remove this comment. -->
# microsoft-deberta-v3-large_cls_CR
This model is a fine-tuned version of [microsoft/deberta-v3-large](https://huggingface.co/microsoft/deberta-v3-large) on an unknown dataset.
It achieves the following results on the evaluation set:
- Loss: 0.3338
- Accuracy: 0.9388
## Model description
More information needed
## Intended uses & limitations
More information needed
## Training and evaluation data
More information needed
## Training procedure
### Training hyperparameters
The following hyperparameters were used during training:
- learning_rate: 4e-05
- train_batch_size: 16
- eval_batch_size: 16
- seed: 42
- optimizer: Adam with betas=(0.9,0.999) and epsilon=1e-08
- lr_scheduler_type: cosine
- lr_scheduler_warmup_ratio: 0.2
- num_epochs: 5
- mixed_precision_training: Native AMP
### Training results
| Training Loss | Epoch | Step | Validation Loss | Accuracy |
|:-------------:|:-----:|:----:|:---------------:|:--------:|
| No log | 1.0 | 213 | 0.3517 | 0.9043 |
| No log | 2.0 | 426 | 0.2648 | 0.9229 |
| 0.3074 | 3.0 | 639 | 0.3421 | 0.9388 |
| 0.3074 | 4.0 | 852 | 0.3039 | 0.9388 |
| 0.0844 | 5.0 | 1065 | 0.3338 | 0.9388 |
### Framework versions
- Transformers 4.20.1
- Pytorch 1.11.0
- Datasets 2.1.0
- Tokenizers 0.12.1
| e5d32233a740a9ae996dfb97b576bb60 |
AlexN/xls-r-300m-fr-0 | AlexN | wav2vec2 | 38 | 5 | transformers | 0 | automatic-speech-recognition | true | false | false | apache-2.0 | ['fr'] | ['mozilla-foundation/common_voice_8_0'] | null | 0 | 0 | 0 | 0 | 0 | 0 | 0 | ['automatic-speech-recognition', 'mozilla-foundation/common_voice_8_0', 'generated_from_trainer', 'robust-speech-event', 'hf-asr-leaderboard'] | true | true | true | 2,900 | false |
<!-- This model card has been generated automatically according to the information the Trainer had access to. You
should probably proofread and complete it, then remove this comment. -->
#
This model is a fine-tuned version of [facebook/wav2vec2-xls-r-300m](https://huggingface.co/facebook/wav2vec2-xls-r-300m) on the MOZILLA-FOUNDATION/COMMON_VOICE_8_0 - FR dataset.
It achieves the following results on the evaluation set:
- Loss: 0.2388
- Wer: 0.3681
## Model description
More information needed
## Intended uses & limitations
More information needed
## Training and evaluation data
More information needed
## Training procedure
### Training hyperparameters
The following hyperparameters were used during training:
- learning_rate: 0.0001
- train_batch_size: 64
- eval_batch_size: 64
- seed: 42
- optimizer: Adam with betas=(0.9,0.999) and epsilon=1e-08
- lr_scheduler_type: linear
- lr_scheduler_warmup_steps: 1500
- num_epochs: 2.0
- mixed_precision_training: Native AMP
### Training results
| Training Loss | Epoch | Step | Validation Loss | Wer |
|:-------------:|:-----:|:-----:|:---------------:|:------:|
| 4.3748 | 0.07 | 500 | 3.8784 | 1.0 |
| 2.8068 | 0.14 | 1000 | 2.8289 | 0.9826 |
| 1.6698 | 0.22 | 1500 | 0.8811 | 0.7127 |
| 1.3488 | 0.29 | 2000 | 0.5166 | 0.5369 |
| 1.2239 | 0.36 | 2500 | 0.4105 | 0.4741 |
| 1.1537 | 0.43 | 3000 | 0.3585 | 0.4448 |
| 1.1184 | 0.51 | 3500 | 0.3336 | 0.4292 |
| 1.0968 | 0.58 | 4000 | 0.3195 | 0.4180 |
| 1.0737 | 0.65 | 4500 | 0.3075 | 0.4141 |
| 1.0677 | 0.72 | 5000 | 0.3015 | 0.4089 |
| 1.0462 | 0.8 | 5500 | 0.2971 | 0.4077 |
| 1.0392 | 0.87 | 6000 | 0.2870 | 0.3997 |
| 1.0178 | 0.94 | 6500 | 0.2805 | 0.3963 |
| 0.992 | 1.01 | 7000 | 0.2748 | 0.3935 |
| 1.0197 | 1.09 | 7500 | 0.2691 | 0.3884 |
| 1.0056 | 1.16 | 8000 | 0.2682 | 0.3889 |
| 0.9826 | 1.23 | 8500 | 0.2647 | 0.3868 |
| 0.9815 | 1.3 | 9000 | 0.2603 | 0.3832 |
| 0.9717 | 1.37 | 9500 | 0.2561 | 0.3807 |
| 0.9605 | 1.45 | 10000 | 0.2523 | 0.3783 |
| 0.96 | 1.52 | 10500 | 0.2494 | 0.3788 |
| 0.9442 | 1.59 | 11000 | 0.2478 | 0.3760 |
| 0.9564 | 1.66 | 11500 | 0.2454 | 0.3733 |
| 0.9436 | 1.74 | 12000 | 0.2439 | 0.3747 |
| 0.938 | 1.81 | 12500 | 0.2411 | 0.3716 |
| 0.9353 | 1.88 | 13000 | 0.2397 | 0.3698 |
| 0.9271 | 1.95 | 13500 | 0.2388 | 0.3681 |
### Framework versions
- Transformers 4.17.0.dev0
- Pytorch 1.10.2+cu102
- Datasets 1.18.2.dev0
- Tokenizers 0.11.0
| 8eb5e12575d89fc3f56ed9af98e41d1d |
aXhyra/presentation_sentiment_31415 | aXhyra | distilbert | 10 | 6 | transformers | 0 | text-classification | true | false | false | apache-2.0 | null | ['tweet_eval'] | null | 0 | 0 | 0 | 0 | 0 | 0 | 0 | ['generated_from_trainer'] | true | true | true | 1,402 | false |
<!-- This model card has been generated automatically according to the information the Trainer had access to. You
should probably proofread and complete it, then remove this comment. -->
# presentation_sentiment_31415
This model is a fine-tuned version of [distilbert-base-uncased](https://huggingface.co/distilbert-base-uncased) on the tweet_eval dataset.
It achieves the following results on the evaluation set:
- Loss: 1.0860
- F1: 0.7183
## Model description
More information needed
## Intended uses & limitations
More information needed
## Training and evaluation data
More information needed
## Training procedure
### Training hyperparameters
The following hyperparameters were used during training:
- learning_rate: 7.2792011721188e-06
- train_batch_size: 4
- eval_batch_size: 4
- seed: 0
- optimizer: Adam with betas=(0.9,0.999) and epsilon=1e-08
- lr_scheduler_type: linear
- num_epochs: 4
### Training results
| Training Loss | Epoch | Step | Validation Loss | F1 |
|:-------------:|:-----:|:-----:|:---------------:|:------:|
| 0.3747 | 1.0 | 11404 | 0.6515 | 0.7045 |
| 0.6511 | 2.0 | 22808 | 0.7334 | 0.7188 |
| 0.0362 | 3.0 | 34212 | 0.9498 | 0.7195 |
| 1.0576 | 4.0 | 45616 | 1.0860 | 0.7183 |
### Framework versions
- Transformers 4.12.5
- Pytorch 1.9.1
- Datasets 1.16.1
- Tokenizers 0.10.3
| 93225fc58ee19f9c81e304bce7820e98 |
ghadeermobasher/BC4CHEMD-Original-128-PubMedBERT-Trial-latest-general | ghadeermobasher | bert | 15 | 7 | transformers | 0 | token-classification | true | false | false | mit | null | null | null | 0 | 0 | 0 | 0 | 0 | 0 | 0 | ['generated_from_trainer'] | true | true | true | 1,147 | false |
<!-- This model card has been generated automatically according to the information the Trainer had access to. You
should probably proofread and complete it, then remove this comment. -->
# BC4CHEMD-Original-128-PubMedBERT-Trial-latest-general
This model is a fine-tuned version of [microsoft/BiomedNLP-PubMedBERT-base-uncased-abstract](https://huggingface.co/microsoft/BiomedNLP-PubMedBERT-base-uncased-abstract) on an unknown dataset.
It achieves the following results on the evaluation set:
- Loss: 0.0044
- Precision: 0.9678
- Recall: 0.9892
- F1: 0.9784
## Model description
More information needed
## Intended uses & limitations
More information needed
## Training and evaluation data
More information needed
## Training procedure
### Training hyperparameters
The following hyperparameters were used during training:
- learning_rate: 5e-05
- train_batch_size: 32
- eval_batch_size: 8
- seed: 1
- optimizer: Adam with betas=(0.9,0.999) and epsilon=1e-08
- lr_scheduler_type: linear
- num_epochs: 5.0
### Training results
### Framework versions
- Transformers 4.11.3
- Pytorch 1.12.0+cu102
- Datasets 2.3.2
- Tokenizers 0.10.3
| e8098883164d8709c0840ebee8d695c8 |
muhtasham/tiny-mlm-glue-stsb-target-glue-mnli | muhtasham | bert | 10 | 2 | transformers | 0 | text-classification | true | false | false | apache-2.0 | null | null | null | 0 | 0 | 0 | 0 | 0 | 0 | 0 | ['generated_from_trainer'] | true | true | true | 2,511 | false |
<!-- This model card has been generated automatically according to the information the Trainer had access to. You
should probably proofread and complete it, then remove this comment. -->
# tiny-mlm-glue-stsb-target-glue-mnli
This model is a fine-tuned version of [muhtasham/tiny-mlm-glue-stsb](https://huggingface.co/muhtasham/tiny-mlm-glue-stsb) on the None dataset.
It achieves the following results on the evaluation set:
- Loss: 0.8112
- Accuracy: 0.6365
## Model description
More information needed
## Intended uses & limitations
More information needed
## Training and evaluation data
More information needed
## Training procedure
### Training hyperparameters
The following hyperparameters were used during training:
- learning_rate: 3e-05
- train_batch_size: 32
- eval_batch_size: 32
- seed: 42
- optimizer: Adam with betas=(0.9,0.999) and epsilon=1e-08
- lr_scheduler_type: constant
- num_epochs: 200
### Training results
| Training Loss | Epoch | Step | Validation Loss | Accuracy |
|:-------------:|:-----:|:-----:|:---------------:|:--------:|
| 1.0767 | 0.04 | 500 | 1.0354 | 0.4644 |
| 1.0091 | 0.08 | 1000 | 0.9646 | 0.5496 |
| 0.9629 | 0.12 | 1500 | 0.9236 | 0.5798 |
| 0.9384 | 0.16 | 2000 | 0.9054 | 0.5916 |
| 0.9254 | 0.2 | 2500 | 0.8894 | 0.5995 |
| 0.9167 | 0.24 | 3000 | 0.8788 | 0.6028 |
| 0.9013 | 0.29 | 3500 | 0.8707 | 0.6104 |
| 0.8962 | 0.33 | 4000 | 0.8603 | 0.6132 |
| 0.8802 | 0.37 | 4500 | 0.8561 | 0.6185 |
| 0.8834 | 0.41 | 5000 | 0.8490 | 0.6220 |
| 0.8734 | 0.45 | 5500 | 0.8427 | 0.6227 |
| 0.8721 | 0.49 | 6000 | 0.8399 | 0.6278 |
| 0.8739 | 0.53 | 6500 | 0.8336 | 0.6331 |
| 0.8654 | 0.57 | 7000 | 0.8345 | 0.6294 |
| 0.8579 | 0.61 | 7500 | 0.8192 | 0.6375 |
| 0.8567 | 0.65 | 8000 | 0.8191 | 0.6348 |
| 0.8517 | 0.69 | 8500 | 0.8275 | 0.6315 |
| 0.8528 | 0.73 | 9000 | 0.8060 | 0.6433 |
| 0.8448 | 0.77 | 9500 | 0.8152 | 0.6355 |
| 0.8361 | 0.81 | 10000 | 0.8026 | 0.6415 |
| 0.8398 | 0.86 | 10500 | 0.8112 | 0.6365 |
### Framework versions
- Transformers 4.26.0.dev0
- Pytorch 1.13.0+cu116
- Datasets 2.8.1.dev0
- Tokenizers 0.13.2
| 5db70b9ffe4b6d9e8ec9ee835a1bc55f |
jonatasgrosman/exp_w2v2t_nl_wav2vec2_s754 | jonatasgrosman | wav2vec2 | 10 | 5 | transformers | 0 | automatic-speech-recognition | true | false | false | apache-2.0 | ['nl'] | ['mozilla-foundation/common_voice_7_0'] | null | 0 | 0 | 0 | 0 | 0 | 0 | 0 | ['automatic-speech-recognition', 'nl'] | false | true | true | 456 | false | # exp_w2v2t_nl_wav2vec2_s754
Fine-tuned [facebook/wav2vec2-large-lv60](https://huggingface.co/facebook/wav2vec2-large-lv60) for speech recognition using the train split of [Common Voice 7.0 (nl)](https://huggingface.co/datasets/mozilla-foundation/common_voice_7_0).
When using this model, make sure that your speech input is sampled at 16kHz.
This model has been fine-tuned by the [HuggingSound](https://github.com/jonatasgrosman/huggingsound) tool.
| f9fbd731576f748d9636ab56e333e58c |
gokuls/mobilebert_sa_GLUE_Experiment_data_aug_wnli | gokuls | mobilebert | 17 | 0 | transformers | 0 | text-classification | true | false | false | apache-2.0 | ['en'] | ['glue'] | null | 0 | 0 | 0 | 0 | 0 | 0 | 0 | ['generated_from_trainer'] | true | true | true | 1,588 | false |
<!-- This model card has been generated automatically according to the information the Trainer had access to. You
should probably proofread and complete it, then remove this comment. -->
# mobilebert_sa_GLUE_Experiment_data_aug_wnli
This model is a fine-tuned version of [google/mobilebert-uncased](https://huggingface.co/google/mobilebert-uncased) on the GLUE WNLI dataset.
It achieves the following results on the evaluation set:
- Loss: 2.5287
- Accuracy: 0.1268
## Model description
More information needed
## Intended uses & limitations
More information needed
## Training and evaluation data
More information needed
## Training procedure
### Training hyperparameters
The following hyperparameters were used during training:
- learning_rate: 5e-05
- train_batch_size: 128
- eval_batch_size: 128
- seed: 10
- distributed_type: multi-GPU
- optimizer: Adam with betas=(0.9,0.999) and epsilon=1e-08
- lr_scheduler_type: linear
- num_epochs: 50
### Training results
| Training Loss | Epoch | Step | Validation Loss | Accuracy |
|:-------------:|:-----:|:----:|:---------------:|:--------:|
| 0.6415 | 1.0 | 435 | 2.5287 | 0.1268 |
| 0.4894 | 2.0 | 870 | 3.5123 | 0.1268 |
| 0.4427 | 3.0 | 1305 | 4.8804 | 0.0986 |
| 0.4026 | 4.0 | 1740 | 7.2410 | 0.0986 |
| 0.3707 | 5.0 | 2175 | 10.5770 | 0.0845 |
| 0.3376 | 6.0 | 2610 | 7.2101 | 0.0986 |
### Framework versions
- Transformers 4.26.0
- Pytorch 1.14.0a0+410ce96
- Datasets 2.9.0
- Tokenizers 0.13.2
| 6412ea4a640ba8737e0cf7648c3a0e00 |
Helsinki-NLP/opus-mt-fi-pap | Helsinki-NLP | marian | 10 | 7 | transformers | 0 | translation | true | true | false | apache-2.0 | null | null | null | 0 | 0 | 0 | 0 | 0 | 0 | 0 | ['translation'] | false | true | true | 776 | false |
### opus-mt-fi-pap
* source languages: fi
* target languages: pap
* OPUS readme: [fi-pap](https://github.com/Helsinki-NLP/OPUS-MT-train/blob/master/models/fi-pap/README.md)
* dataset: opus
* model: transformer-align
* pre-processing: normalization + SentencePiece
* download original weights: [opus-2020-01-24.zip](https://object.pouta.csc.fi/OPUS-MT-models/fi-pap/opus-2020-01-24.zip)
* test set translations: [opus-2020-01-24.test.txt](https://object.pouta.csc.fi/OPUS-MT-models/fi-pap/opus-2020-01-24.test.txt)
* test set scores: [opus-2020-01-24.eval.txt](https://object.pouta.csc.fi/OPUS-MT-models/fi-pap/opus-2020-01-24.eval.txt)
## Benchmarks
| testset | BLEU | chr-F |
|-----------------------|-------|-------|
| JW300.fi.pap | 27.3 | 0.478 |
| 34df180b5bee94119fe42261d581f665 |
jonatasgrosman/exp_w2v2t_nl_hubert_s319 | jonatasgrosman | hubert | 10 | 4 | transformers | 0 | automatic-speech-recognition | true | false | false | apache-2.0 | ['nl'] | ['mozilla-foundation/common_voice_7_0'] | null | 0 | 0 | 0 | 0 | 0 | 0 | 0 | ['automatic-speech-recognition', 'nl'] | false | true | true | 452 | false | # exp_w2v2t_nl_hubert_s319
Fine-tuned [facebook/hubert-large-ll60k](https://huggingface.co/facebook/hubert-large-ll60k) for speech recognition using the train split of [Common Voice 7.0 (nl)](https://huggingface.co/datasets/mozilla-foundation/common_voice_7_0).
When using this model, make sure that your speech input is sampled at 16kHz.
This model has been fine-tuned by the [HuggingSound](https://github.com/jonatasgrosman/huggingsound) tool.
| c274a8988c8642dc5744297076ada686 |
pritoms/distilgpt2-finetuned-wikitext2 | pritoms | gpt2 | 11 | 4 | transformers | 0 | text-generation | true | false | false | apache-2.0 | null | null | null | 0 | 0 | 0 | 0 | 0 | 0 | 0 | ['generated_from_trainer'] | true | true | true | 1,243 | false |
<!-- This model card has been generated automatically according to the information the Trainer had access to. You
should probably proofread and complete it, then remove this comment. -->
# distilgpt2-finetuned-wikitext2
This model is a fine-tuned version of [distilgpt2](https://huggingface.co/distilgpt2) on the None dataset.
It achieves the following results on the evaluation set:
- Loss: 3.0540
## Model description
More information needed
## Intended uses & limitations
More information needed
## Training and evaluation data
More information needed
## Training procedure
### Training hyperparameters
The following hyperparameters were used during training:
- learning_rate: 2e-05
- train_batch_size: 8
- eval_batch_size: 8
- seed: 42
- optimizer: Adam with betas=(0.9,0.999) and epsilon=1e-08
- lr_scheduler_type: linear
- num_epochs: 3.0
### Training results
| Training Loss | Epoch | Step | Validation Loss |
|:-------------:|:-----:|:----:|:---------------:|
| No log | 1.0 | 130 | 3.1733 |
| No log | 2.0 | 260 | 3.0756 |
| No log | 3.0 | 390 | 3.0540 |
### Framework versions
- Transformers 4.11.3
- Pytorch 1.9.0+cu111
- Datasets 1.14.0
- Tokenizers 0.10.3
| 9adce18eb81da60e0bbd631c7ce3a1ef |
ali2066/finetuned_token_2e-05_16_02_2022-14_18_19 | ali2066 | distilbert | 13 | 10 | transformers | 0 | token-classification | true | false | false | apache-2.0 | null | null | null | 0 | 0 | 0 | 0 | 0 | 0 | 0 | ['generated_from_trainer'] | true | true | true | 1,787 | false |
<!-- This model card has been generated automatically according to the information the Trainer had access to. You
should probably proofread and complete it, then remove this comment. -->
# finetuned_token_2e-05_16_02_2022-14_18_19
This model is a fine-tuned version of [distilbert-base-uncased-finetuned-sst-2-english](https://huggingface.co/distilbert-base-uncased-finetuned-sst-2-english) on the None dataset.
It achieves the following results on the evaluation set:
- Loss: 0.1722
- Precision: 0.3378
- Recall: 0.3615
- F1: 0.3492
- Accuracy: 0.9448
## Model description
More information needed
## Intended uses & limitations
More information needed
## Training and evaluation data
More information needed
## Training procedure
### Training hyperparameters
The following hyperparameters were used during training:
- learning_rate: 2e-05
- train_batch_size: 32
- eval_batch_size: 32
- seed: 42
- optimizer: Adam with betas=(0.9,0.999) and epsilon=1e-08
- lr_scheduler_type: linear
- num_epochs: 5
### Training results
| Training Loss | Epoch | Step | Validation Loss | Precision | Recall | F1 | Accuracy |
|:-------------:|:-----:|:----:|:---------------:|:---------:|:------:|:------:|:--------:|
| No log | 1.0 | 38 | 0.3781 | 0.1512 | 0.2671 | 0.1931 | 0.8216 |
| No log | 2.0 | 76 | 0.3020 | 0.1748 | 0.2938 | 0.2192 | 0.8551 |
| No log | 3.0 | 114 | 0.2723 | 0.1938 | 0.3339 | 0.2452 | 0.8663 |
| No log | 4.0 | 152 | 0.2574 | 0.2119 | 0.3506 | 0.2642 | 0.8727 |
| No log | 5.0 | 190 | 0.2521 | 0.2121 | 0.3623 | 0.2676 | 0.8756 |
### Framework versions
- Transformers 4.15.0
- Pytorch 1.10.1+cu113
- Datasets 1.18.0
- Tokenizers 0.10.3
| 3ef047dc6472c566204d0b2657da6421 |
tszocinski/bart-base-squad-question-generation | tszocinski | bart | 9 | 2 | transformers | 0 | text2text-generation | false | true | false | apache-2.0 | null | null | null | 0 | 0 | 0 | 0 | 0 | 0 | 0 | ['generated_from_keras_callback'] | true | true | true | 1,357 | false |
<!-- This model card has been generated automatically according to the information Keras had access to. You should
probably proofread and complete it, then remove this comment. -->
# tszocinski/bart-base-squad-question-generation
This model is a fine-tuned version of [tszocinski/bart-base-squad-question-generation](https://huggingface.co/tszocinski/bart-base-squad-question-generation) on an unknown dataset.
It achieves the following results on the evaluation set:
- Train Loss: 6.5656
- Validation Loss: 11.1958
- Epoch: 0
## Model description
More information needed
## Intended uses & limitations
More information needed
## Training and evaluation data
More information needed
## Training procedure
### Training hyperparameters
The following hyperparameters were used during training:
- optimizer: {'inner_optimizer': {'class_name': 'RMSprop', 'config': {'name': 'RMSprop', 'learning_rate': 0.001, 'decay': 0.0, 'rho': 0.9, 'momentum': 0.0, 'epsilon': 1e-07, 'centered': False}}, 'dynamic': True, 'initial_scale': 32768.0, 'dynamic_growth_steps': 2000}
- training_precision: mixed_float16
### Training results
| Train Loss | Validation Loss | Epoch |
|:----------:|:---------------:|:-----:|
| 6.5656 | 11.1958 | 0 |
### Framework versions
- Transformers 4.22.1
- TensorFlow 2.8.2
- Datasets 2.5.1
- Tokenizers 0.12.1
| 336d7179212f5232a8bfb50b83c77fc0 |
Palak/google_electra-base-discriminator_squad | Palak | electra | 13 | 7 | transformers | 0 | question-answering | true | false | false | apache-2.0 | null | ['squad'] | null | 0 | 0 | 0 | 0 | 0 | 0 | 0 | ['generated_from_trainer'] | true | true | true | 1,069 | false |
<!-- This model card has been generated automatically according to the information the Trainer had access to. You
should probably proofread and complete it, then remove this comment. -->
# google_electra-base-discriminator_squad
This model is a fine-tuned version of [google/electra-base-discriminator](https://huggingface.co/google/electra-base-discriminator) on the **squadV1** dataset.
- "eval_exact_match": 85.33585619678335
- "eval_f1": 91.97363450387108
- "eval_samples": 10784
## Model description
More information needed
## Intended uses & limitations
More information needed
## Training and evaluation data
More information needed
## Training procedure
### Training hyperparameters
The following hyperparameters were used during training:
- learning_rate: 3e-05
- train_batch_size: 16
- eval_batch_size: 32
- seed: 42
- optimizer: Adam with betas=(0.9,0.999) and epsilon=1e-08
- lr_scheduler_type: linear
- num_epochs: 3.0
### Training results
### Framework versions
- Transformers 4.14.1
- Pytorch 1.9.0
- Datasets 1.16.1
- Tokenizers 0.10.3
| 26cf8fe47c45ed727dc3dad570434e15 |
sayakpaul/glpn-nyu-finetuned-diode-221228-113625 | sayakpaul | glpn | 7 | 2 | transformers | 0 | depth-estimation | true | false | false | apache-2.0 | null | null | null | 0 | 0 | 0 | 0 | 0 | 0 | 0 | ['vision', 'depth-estimation', 'generated_from_trainer'] | true | true | true | 11,011 | false |
<!-- This model card has been generated automatically according to the information the Trainer had access to. You
should probably proofread and complete it, then remove this comment. -->
# glpn-nyu-finetuned-diode-221228-113625
This model is a fine-tuned version of [vinvino02/glpn-nyu](https://huggingface.co/vinvino02/glpn-nyu) on the diode-subset dataset.
It achieves the following results on the evaluation set:
- Loss: 0.3996
- Mae: 0.4013
- Rmse: 0.6161
- Abs Rel: 0.3535
- Log Mae: 0.1568
- Log Rmse: 0.2121
- Delta1: 0.4381
- Delta2: 0.7025
- Delta3: 0.8196
## Model description
More information needed
## Intended uses & limitations
More information needed
## Training and evaluation data
More information needed
## Training procedure
### Training hyperparameters
The following hyperparameters were used during training:
- learning_rate: 0.0003
- train_batch_size: 24
- eval_batch_size: 48
- seed: 2022
- optimizer: Adam with betas=(0.9,0.999) and epsilon=1e-08
- lr_scheduler_type: linear
- lr_scheduler_warmup_ratio: 0.15
- num_epochs: 75
- mixed_precision_training: Native AMP
### Training results
| Training Loss | Epoch | Step | Validation Loss | Mae | Rmse | Abs Rel | Log Mae | Log Rmse | Delta1 | Delta2 | Delta3 |
|:-------------:|:-----:|:----:|:---------------:|:------:|:------:|:-------:|:-------:|:--------:|:------:|:------:|:------:|
| 1.0075 | 1.0 | 72 | 0.4809 | 0.4610 | 0.6461 | 0.5165 | 0.1901 | 0.2446 | 0.3157 | 0.5632 | 0.8017 |
| 0.4692 | 2.0 | 144 | 0.4432 | 0.4491 | 0.6531 | 0.3950 | 0.1821 | 0.2318 | 0.3347 | 0.6198 | 0.7910 |
| 0.4635 | 3.0 | 216 | 0.4361 | 0.4278 | 0.6252 | 0.4165 | 0.1715 | 0.2230 | 0.3780 | 0.6285 | 0.8090 |
| 0.4364 | 4.0 | 288 | 0.4255 | 0.4200 | 0.6222 | 0.3930 | 0.1673 | 0.2198 | 0.3824 | 0.6639 | 0.8206 |
| 0.4632 | 5.0 | 360 | 0.4376 | 0.4267 | 0.6241 | 0.4144 | 0.1708 | 0.2235 | 0.3806 | 0.6337 | 0.8122 |
| 0.4703 | 6.0 | 432 | 0.4340 | 0.4315 | 0.6354 | 0.3799 | 0.1740 | 0.2262 | 0.3788 | 0.6275 | 0.7945 |
| 0.4136 | 7.0 | 504 | 0.4453 | 0.4291 | 0.6368 | 0.4144 | 0.1726 | 0.2306 | 0.3965 | 0.6458 | 0.7965 |
| 0.394 | 8.0 | 576 | 0.4620 | 0.4440 | 0.6297 | 0.4728 | 0.1808 | 0.2336 | 0.3606 | 0.5832 | 0.7826 |
| 0.4073 | 9.0 | 648 | 0.4485 | 0.4372 | 0.6244 | 0.4439 | 0.1769 | 0.2266 | 0.3511 | 0.6010 | 0.8002 |
| 0.3967 | 10.0 | 720 | 0.4523 | 0.4320 | 0.6250 | 0.4606 | 0.1750 | 0.2307 | 0.3676 | 0.6255 | 0.8146 |
| 0.3797 | 11.0 | 792 | 0.4413 | 0.4360 | 0.6332 | 0.4047 | 0.1769 | 0.2258 | 0.3426 | 0.6277 | 0.8025 |
| 0.439 | 12.0 | 864 | 0.4544 | 0.4365 | 0.6356 | 0.4215 | 0.1768 | 0.2299 | 0.3561 | 0.6282 | 0.8050 |
| 0.4666 | 13.0 | 936 | 0.4349 | 0.4278 | 0.6267 | 0.3893 | 0.1729 | 0.2227 | 0.3615 | 0.6375 | 0.8053 |
| 0.4071 | 14.0 | 1008 | 0.4337 | 0.4220 | 0.6235 | 0.3822 | 0.1692 | 0.2202 | 0.3909 | 0.6376 | 0.8044 |
| 0.4359 | 15.0 | 1080 | 0.4259 | 0.4193 | 0.6266 | 0.3855 | 0.1669 | 0.2217 | 0.4022 | 0.6601 | 0.8100 |
| 0.39 | 16.0 | 1152 | 0.4268 | 0.4075 | 0.6161 | 0.3981 | 0.1605 | 0.2184 | 0.4214 | 0.6838 | 0.8205 |
| 0.3654 | 17.0 | 1224 | 0.4503 | 0.4461 | 0.6615 | 0.3791 | 0.1840 | 0.2417 | 0.3783 | 0.6161 | 0.7636 |
| 0.4256 | 18.0 | 1296 | 0.4743 | 0.4529 | 0.6319 | 0.5162 | 0.1852 | 0.2398 | 0.3461 | 0.5736 | 0.7490 |
| 0.372 | 19.0 | 1368 | 0.4462 | 0.4326 | 0.6443 | 0.4068 | 0.1752 | 0.2331 | 0.3875 | 0.6410 | 0.7922 |
| 0.41 | 20.0 | 1440 | 0.4351 | 0.4500 | 0.6579 | 0.3735 | 0.1849 | 0.2365 | 0.3460 | 0.6021 | 0.7751 |
| 0.3683 | 21.0 | 1512 | 0.4060 | 0.4084 | 0.6177 | 0.3495 | 0.1605 | 0.2107 | 0.4168 | 0.6702 | 0.8235 |
| 0.36 | 22.0 | 1584 | 0.4447 | 0.4517 | 0.6667 | 0.3788 | 0.1852 | 0.2414 | 0.3676 | 0.6122 | 0.7572 |
| 0.4257 | 23.0 | 1656 | 0.4297 | 0.4141 | 0.6180 | 0.4066 | 0.1646 | 0.2201 | 0.4134 | 0.6586 | 0.8105 |
| 0.4344 | 24.0 | 1728 | 0.4545 | 0.4312 | 0.6237 | 0.4587 | 0.1742 | 0.2296 | 0.3769 | 0.6137 | 0.8008 |
| 0.4057 | 25.0 | 1800 | 0.4161 | 0.4099 | 0.6175 | 0.3744 | 0.1619 | 0.2144 | 0.4100 | 0.6701 | 0.8231 |
| 0.3569 | 26.0 | 1872 | 0.4199 | 0.4120 | 0.6181 | 0.3840 | 0.1634 | 0.2177 | 0.4039 | 0.6765 | 0.8165 |
| 0.3479 | 27.0 | 1944 | 0.4327 | 0.4180 | 0.6174 | 0.4138 | 0.1668 | 0.2205 | 0.3912 | 0.6481 | 0.8230 |
| 0.3732 | 28.0 | 2016 | 0.4426 | 0.4291 | 0.6236 | 0.4296 | 0.1715 | 0.2237 | 0.3866 | 0.6186 | 0.7911 |
| 0.3554 | 29.0 | 2088 | 0.4112 | 0.4073 | 0.6180 | 0.3598 | 0.1607 | 0.2146 | 0.4281 | 0.6800 | 0.8189 |
| 0.3679 | 30.0 | 2160 | 0.4139 | 0.4078 | 0.6190 | 0.3702 | 0.1609 | 0.2165 | 0.4249 | 0.6823 | 0.8110 |
| 0.3703 | 31.0 | 2232 | 0.4143 | 0.4097 | 0.6176 | 0.3730 | 0.1618 | 0.2156 | 0.4153 | 0.6782 | 0.8162 |
| 0.3605 | 32.0 | 2304 | 0.4179 | 0.4177 | 0.6303 | 0.3711 | 0.1654 | 0.2210 | 0.4062 | 0.6823 | 0.8022 |
| 0.3761 | 33.0 | 2376 | 0.4027 | 0.4070 | 0.6222 | 0.3441 | 0.1595 | 0.2127 | 0.4371 | 0.6834 | 0.8125 |
| 0.3352 | 34.0 | 2448 | 0.4077 | 0.4029 | 0.6134 | 0.3692 | 0.1581 | 0.2130 | 0.4322 | 0.6855 | 0.8273 |
| 0.336 | 35.0 | 2520 | 0.4212 | 0.4246 | 0.6328 | 0.3780 | 0.1696 | 0.2238 | 0.3844 | 0.6716 | 0.8005 |
| 0.3414 | 36.0 | 2592 | 0.4139 | 0.4132 | 0.6241 | 0.3720 | 0.1639 | 0.2184 | 0.4162 | 0.6714 | 0.8092 |
| 0.3416 | 37.0 | 2664 | 0.4183 | 0.4101 | 0.6149 | 0.3844 | 0.1625 | 0.2159 | 0.4157 | 0.6649 | 0.8172 |
| 0.3765 | 38.0 | 2736 | 0.4207 | 0.4120 | 0.6199 | 0.3926 | 0.1635 | 0.2193 | 0.4066 | 0.6767 | 0.8154 |
| 0.3548 | 39.0 | 2808 | 0.4096 | 0.4056 | 0.6167 | 0.3667 | 0.1593 | 0.2138 | 0.4244 | 0.6905 | 0.8213 |
| 0.3822 | 40.0 | 2880 | 0.4084 | 0.4061 | 0.6180 | 0.3653 | 0.1593 | 0.2134 | 0.4246 | 0.6891 | 0.8249 |
| 0.3505 | 41.0 | 2952 | 0.4041 | 0.4118 | 0.6271 | 0.3515 | 0.1620 | 0.2156 | 0.4279 | 0.6872 | 0.8098 |
| 0.3514 | 42.0 | 3024 | 0.4033 | 0.4006 | 0.6185 | 0.3558 | 0.1563 | 0.2132 | 0.4510 | 0.7030 | 0.8181 |
| 0.3459 | 43.0 | 3096 | 0.4061 | 0.4051 | 0.6196 | 0.3631 | 0.1587 | 0.2147 | 0.4282 | 0.7019 | 0.8206 |
| 0.3213 | 44.0 | 3168 | 0.4041 | 0.4093 | 0.6232 | 0.3539 | 0.1605 | 0.2148 | 0.4301 | 0.6893 | 0.8168 |
| 0.3346 | 45.0 | 3240 | 0.4103 | 0.4023 | 0.6151 | 0.3705 | 0.1578 | 0.2141 | 0.4339 | 0.6907 | 0.8219 |
| 0.3585 | 46.0 | 3312 | 0.4054 | 0.3953 | 0.6096 | 0.3627 | 0.1542 | 0.2113 | 0.4524 | 0.7052 | 0.8251 |
| 0.3799 | 47.0 | 3384 | 0.4063 | 0.4100 | 0.6230 | 0.3574 | 0.1616 | 0.2165 | 0.4263 | 0.6821 | 0.8113 |
| 0.3235 | 48.0 | 3456 | 0.4051 | 0.4004 | 0.6117 | 0.3692 | 0.1571 | 0.2123 | 0.4364 | 0.6928 | 0.8268 |
| 0.3628 | 49.0 | 3528 | 0.4051 | 0.3985 | 0.6115 | 0.3622 | 0.1560 | 0.2111 | 0.4486 | 0.6932 | 0.8234 |
| 0.3399 | 50.0 | 3600 | 0.4145 | 0.4059 | 0.6184 | 0.3789 | 0.1598 | 0.2169 | 0.4260 | 0.6977 | 0.8194 |
| 0.3288 | 51.0 | 3672 | 0.4089 | 0.4057 | 0.6172 | 0.3692 | 0.1597 | 0.2153 | 0.4300 | 0.6939 | 0.8198 |
| 0.3231 | 52.0 | 3744 | 0.4104 | 0.4126 | 0.6261 | 0.3643 | 0.1628 | 0.2185 | 0.4296 | 0.6826 | 0.8104 |
| 0.3238 | 53.0 | 3816 | 0.4107 | 0.4023 | 0.6170 | 0.3745 | 0.1580 | 0.2167 | 0.4362 | 0.7031 | 0.8216 |
| 0.3253 | 54.0 | 3888 | 0.4056 | 0.4006 | 0.6135 | 0.3673 | 0.1570 | 0.2134 | 0.4400 | 0.7034 | 0.8221 |
| 0.3383 | 55.0 | 3960 | 0.4053 | 0.4060 | 0.6187 | 0.3598 | 0.1593 | 0.2141 | 0.4310 | 0.6938 | 0.8187 |
| 0.3279 | 56.0 | 4032 | 0.4118 | 0.4003 | 0.6130 | 0.3797 | 0.1569 | 0.2153 | 0.4388 | 0.7040 | 0.8212 |
| 0.32 | 57.0 | 4104 | 0.4042 | 0.4001 | 0.6185 | 0.3566 | 0.1560 | 0.2123 | 0.4470 | 0.7070 | 0.8227 |
| 0.3282 | 58.0 | 4176 | 0.4035 | 0.4010 | 0.6173 | 0.3533 | 0.1568 | 0.2126 | 0.4438 | 0.7037 | 0.8208 |
| 0.3271 | 59.0 | 4248 | 0.4015 | 0.4018 | 0.6168 | 0.3551 | 0.1570 | 0.2123 | 0.4334 | 0.7095 | 0.8201 |
| 0.3127 | 60.0 | 4320 | 0.4029 | 0.3975 | 0.6142 | 0.3590 | 0.1549 | 0.2113 | 0.4420 | 0.7082 | 0.8245 |
| 0.3142 | 61.0 | 4392 | 0.4044 | 0.4031 | 0.6163 | 0.3585 | 0.1577 | 0.2126 | 0.4273 | 0.7034 | 0.8214 |
| 0.3059 | 62.0 | 4464 | 0.4034 | 0.4033 | 0.6151 | 0.3624 | 0.1580 | 0.2127 | 0.4256 | 0.7038 | 0.8223 |
| 0.3133 | 63.0 | 4536 | 0.4028 | 0.4066 | 0.6205 | 0.3554 | 0.1594 | 0.2137 | 0.4235 | 0.6991 | 0.8187 |
| 0.3086 | 64.0 | 4608 | 0.4023 | 0.3982 | 0.6117 | 0.3588 | 0.1556 | 0.2108 | 0.4381 | 0.7002 | 0.8248 |
| 0.3143 | 65.0 | 4680 | 0.4036 | 0.4084 | 0.6250 | 0.3566 | 0.1600 | 0.2157 | 0.4323 | 0.6946 | 0.8094 |
| 0.3031 | 66.0 | 4752 | 0.4012 | 0.3999 | 0.6170 | 0.3551 | 0.1559 | 0.2122 | 0.4458 | 0.7044 | 0.8200 |
| 0.3279 | 67.0 | 4824 | 0.4031 | 0.4001 | 0.6160 | 0.3609 | 0.1562 | 0.2129 | 0.4421 | 0.7042 | 0.8205 |
| 0.3173 | 68.0 | 4896 | 0.4000 | 0.3989 | 0.6141 | 0.3569 | 0.1557 | 0.2120 | 0.4456 | 0.7040 | 0.8226 |
| 0.3203 | 69.0 | 4968 | 0.3989 | 0.3995 | 0.6153 | 0.3545 | 0.1556 | 0.2114 | 0.4421 | 0.7069 | 0.8215 |
| 0.3165 | 70.0 | 5040 | 0.3984 | 0.3993 | 0.6144 | 0.3513 | 0.1558 | 0.2111 | 0.4450 | 0.7027 | 0.8222 |
| 0.3278 | 71.0 | 5112 | 0.3993 | 0.4032 | 0.6191 | 0.3509 | 0.1574 | 0.2124 | 0.4386 | 0.7007 | 0.8184 |
| 0.3232 | 72.0 | 5184 | 0.3990 | 0.4000 | 0.6149 | 0.3534 | 0.1561 | 0.2112 | 0.4396 | 0.7018 | 0.8223 |
| 0.3089 | 73.0 | 5256 | 0.3996 | 0.4022 | 0.6172 | 0.3526 | 0.1571 | 0.2121 | 0.4370 | 0.7011 | 0.8197 |
| 0.3118 | 74.0 | 5328 | 0.3994 | 0.4016 | 0.6164 | 0.3530 | 0.1570 | 0.2121 | 0.4375 | 0.7026 | 0.8195 |
| 0.3161 | 75.0 | 5400 | 0.3996 | 0.4013 | 0.6161 | 0.3535 | 0.1568 | 0.2121 | 0.4381 | 0.7025 | 0.8196 |
### Framework versions
- Transformers 4.24.0
- Pytorch 1.12.1+cu116
- Datasets 2.8.0
- Tokenizers 0.13.2
| 5bcbe6207193733c8fe118f0db9f6bf5 |
jonathang/mworld | jonathang | null | 17 | 5 | diffusers | 3 | text-to-image | true | false | false | creativeml-openrail-m | null | null | null | 1 | 1 | 0 | 0 | 0 | 0 | 0 | ['pytorch', 'diffusers', 'stable-diffusion', 'text-to-image', 'diffusion-models-class', 'dreambooth-hackathon', 'landscape'] | false | true | true | 730 | false |
# DreamBooth model for the mworld concept trained by jonathang on the jonathang/dreambooth-hackathon-images-mario-bg-1 dataset.
This is a Stable Diffusion model fine-tuned on the mworld concept with DreamBooth. It can be used by modifying the `instance_prompt`: **mworld**
This model was created as part of the DreamBooth Hackathon 🔥. Visit the [organisation page](https://huggingface.co/dreambooth-hackathon) for instructions on how to take part!
## Description
This is a Stable Diffusion model fine-tuned on `` images for the landscape theme.
## Usage
```python
from diffusers import StableDiffusionPipeline
pipeline = StableDiffusionPipeline.from_pretrained('jonathang/mworld')
image = pipeline().images[0]
image
```
| cb155934c137245ae1505805c07c9240 |
zannabethl/opus-mt-en-de-finetuned-en-to-de | zannabethl | marian | 13 | 0 | transformers | 0 | text2text-generation | true | false | false | cc-by-4.0 | null | ['wmt16'] | null | 0 | 0 | 0 | 0 | 0 | 0 | 0 | ['generated_from_trainer'] | true | true | true | 927 | false |
<!-- This model card has been generated automatically according to the information the Trainer had access to. You
should probably proofread and complete it, then remove this comment. -->
# opus-mt-en-de-finetuned-en-to-de
This model is a fine-tuned version of [Helsinki-NLP/opus-mt-en-de](https://huggingface.co/Helsinki-NLP/opus-mt-en-de) on the wmt16 dataset.
## Model description
More information needed
## Intended uses & limitations
More information needed
## Training and evaluation data
More information needed
## Training procedure
### Training hyperparameters
The following hyperparameters were used during training:
- learning_rate: 2e-05
- train_batch_size: 16
- eval_batch_size: 16
- seed: 42
- optimizer: Adam with betas=(0.9,0.999) and epsilon=1e-08
- lr_scheduler_type: linear
- num_epochs: 1
### Framework versions
- Transformers 4.23.1
- Pytorch 1.12.1+cu113
- Datasets 2.5.2
- Tokenizers 0.13.1
| 7720d93363c0ce3e7a254006912780b9 |
zhuzhusleepearly/bert-finetuned | zhuzhusleepearly | bert | 8 | 6 | transformers | 0 | token-classification | false | true | false | apache-2.0 | null | null | null | 0 | 0 | 0 | 0 | 0 | 0 | 0 | ['generated_from_keras_callback'] | true | true | true | 1,428 | false |
<!-- This model card has been generated automatically according to the information Keras had access to. You should
probably proofread and complete it, then remove this comment. -->
# zhuzhusleepearly/bert-finetuned
This model is a fine-tuned version of [bert-base-uncased](https://huggingface.co/bert-base-uncased) on an unknown dataset.
It achieves the following results on the evaluation set:
- Train Loss: 0.0248
- Validation Loss: 0.0614
- Epoch: 2
## Model description
More information needed
## Intended uses & limitations
More information needed
## Training and evaluation data
More information needed
## Training procedure
### Training hyperparameters
The following hyperparameters were used during training:
- optimizer: {'name': 'AdamWeightDecay', 'learning_rate': {'class_name': 'PolynomialDecay', 'config': {'initial_learning_rate': 2e-05, 'decay_steps': 1017, 'end_learning_rate': 0.0, 'power': 1.0, 'cycle': False, 'name': None}}, 'decay': 0.0, 'beta_1': 0.9, 'beta_2': 0.999, 'epsilon': 1e-08, 'amsgrad': False, 'weight_decay_rate': 0.01}
- training_precision: float32
### Training results
| Train Loss | Validation Loss | Epoch |
|:----------:|:---------------:|:-----:|
| 0.1264 | 0.0606 | 0 |
| 0.0422 | 0.0551 | 1 |
| 0.0248 | 0.0614 | 2 |
### Framework versions
- Transformers 4.18.0
- TensorFlow 2.8.0
- Datasets 2.1.0
- Tokenizers 0.12.1
| f94affb06be9fb9d16266ecd1c36c787 |
jnsulee/ko-mathbert | jnsulee | bert | 14 | 3 | transformers | 0 | fill-mask | true | false | false | cc-by-sa-4.0 | null | null | null | 0 | 0 | 0 | 0 | 0 | 0 | 0 | ['generated_from_trainer'] | true | true | true | 1,275 | false |
<!-- This model card has been generated automatically according to the information the Trainer had access to. You
should probably proofread and complete it, then remove this comment. -->
# ko-mathbert
This model is a fine-tuned version of [klue/bert-base](https://huggingface.co/klue/bert-base) on an unknown dataset.
It achieves the following results on the evaluation set:
- Loss: 2.6769
## Model description
More information needed
## Intended uses & limitations
More information needed
## Training and evaluation data
More information needed
## Training procedure
### Training hyperparameters
The following hyperparameters were used during training:
- learning_rate: 2e-05
- train_batch_size: 64
- eval_batch_size: 64
- seed: 42
- optimizer: Adam with betas=(0.9,0.999) and epsilon=1e-08
- lr_scheduler_type: linear
- num_epochs: 3.0
- mixed_precision_training: Native AMP
### Training results
| Training Loss | Epoch | Step | Validation Loss |
|:-------------:|:-----:|:----:|:---------------:|
| 2.9461 | 1.0 | 157 | 2.8731 |
| 2.7776 | 2.0 | 314 | 2.7040 |
| 2.7261 | 3.0 | 471 | 2.6835 |
### Framework versions
- Transformers 4.25.1
- Pytorch 1.13.0+cu116
- Datasets 2.8.0
- Tokenizers 0.13.2
| 706a5037b0ce6e7e3e39647e8fd2f995 |
sd-dreambooth-library/musical-isotope | sd-dreambooth-library | null | 23 | 4 | diffusers | 0 | null | false | false | false | mit | null | null | null | 2 | 2 | 0 | 0 | 0 | 0 | 0 | [] | false | true | true | 1,386 | false | ### Musical Isotope on Stable Diffusion via Dreambooth
#### model by Phillippe
This your the Stable Diffusion model fine-tuned the Musical Isotope concept taught to Stable Diffusion with Dreambooth.
It can be used by modifying the `instance_prompt`: **mi**
You can also train your own concepts and upload them to the library by using [this notebook](https://colab.research.google.com/github/huggingface/notebooks/blob/main/diffusers/sd_dreambooth_training.ipynb).
And you can run your new concept via `diffusers`: [Colab Notebook for Inference](https://colab.research.google.com/github/huggingface/notebooks/blob/main/diffusers/sd_dreambooth_inference.ipynb), [Spaces with the Public Concepts loaded](https://huggingface.co/spaces/sd-dreambooth-library/stable-diffusion-dreambooth-concepts)
Here are the images used for training this concept:
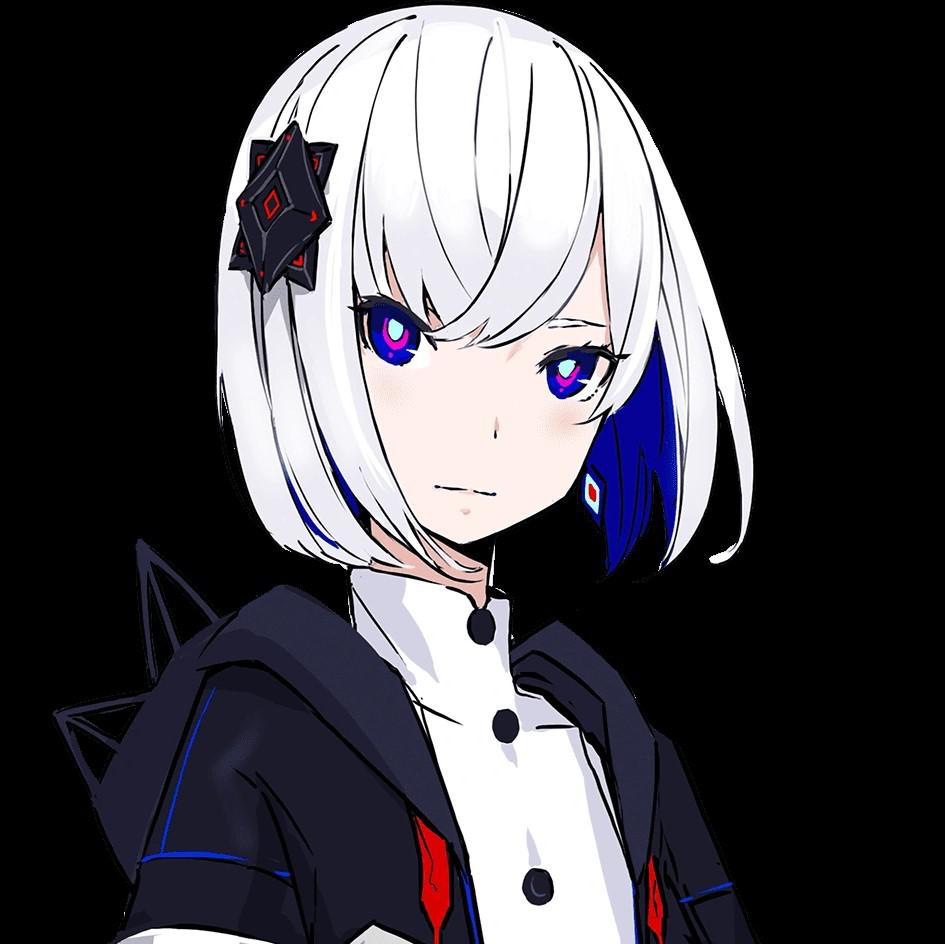
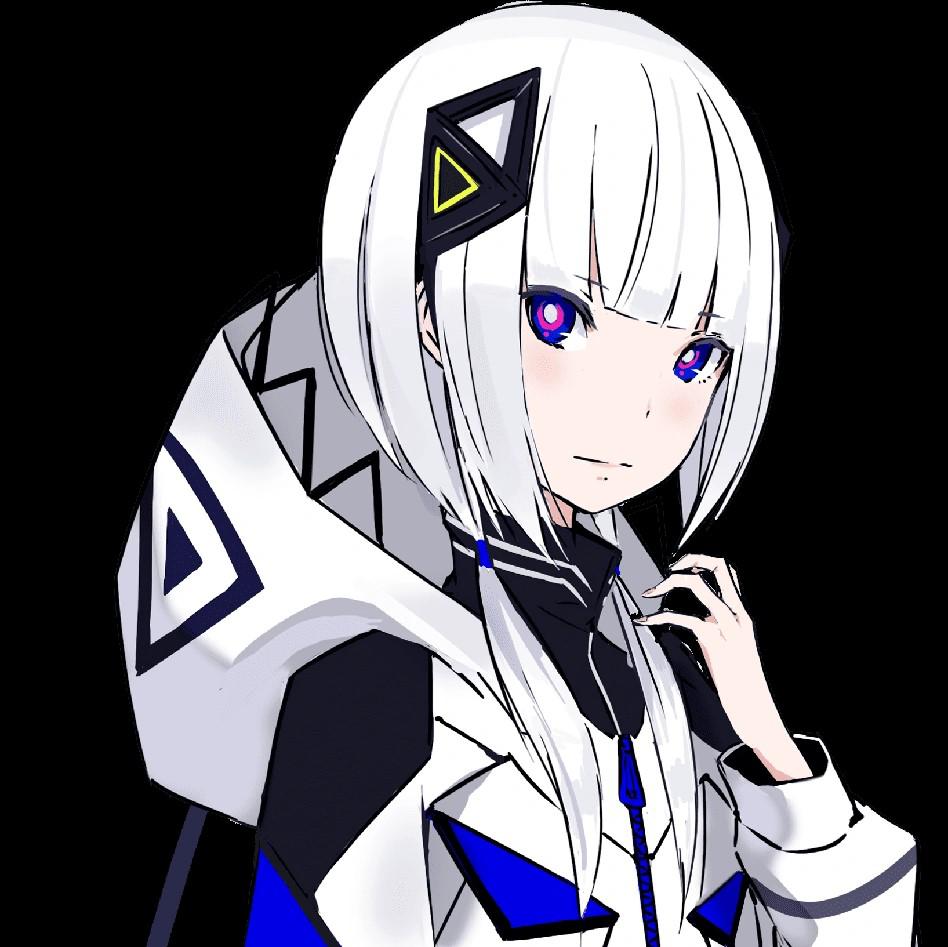
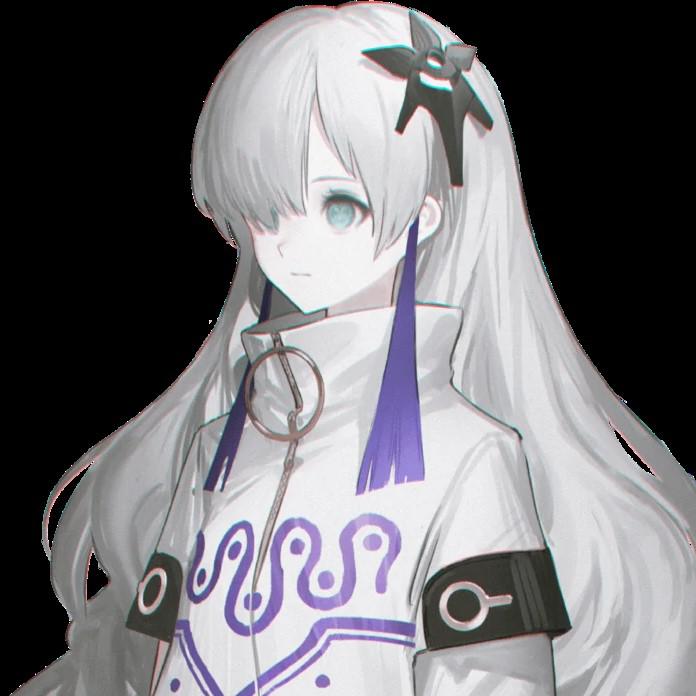
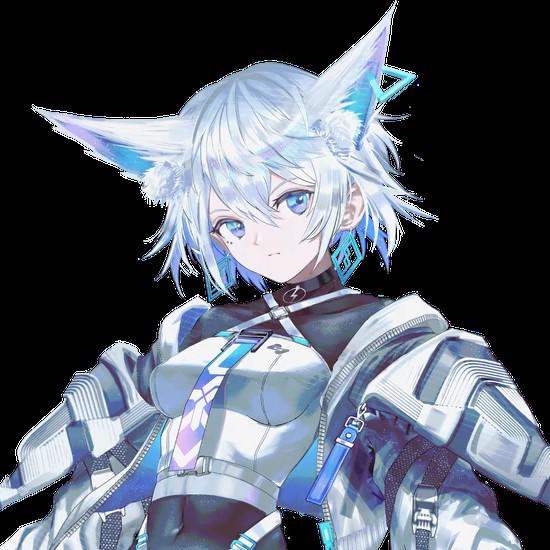
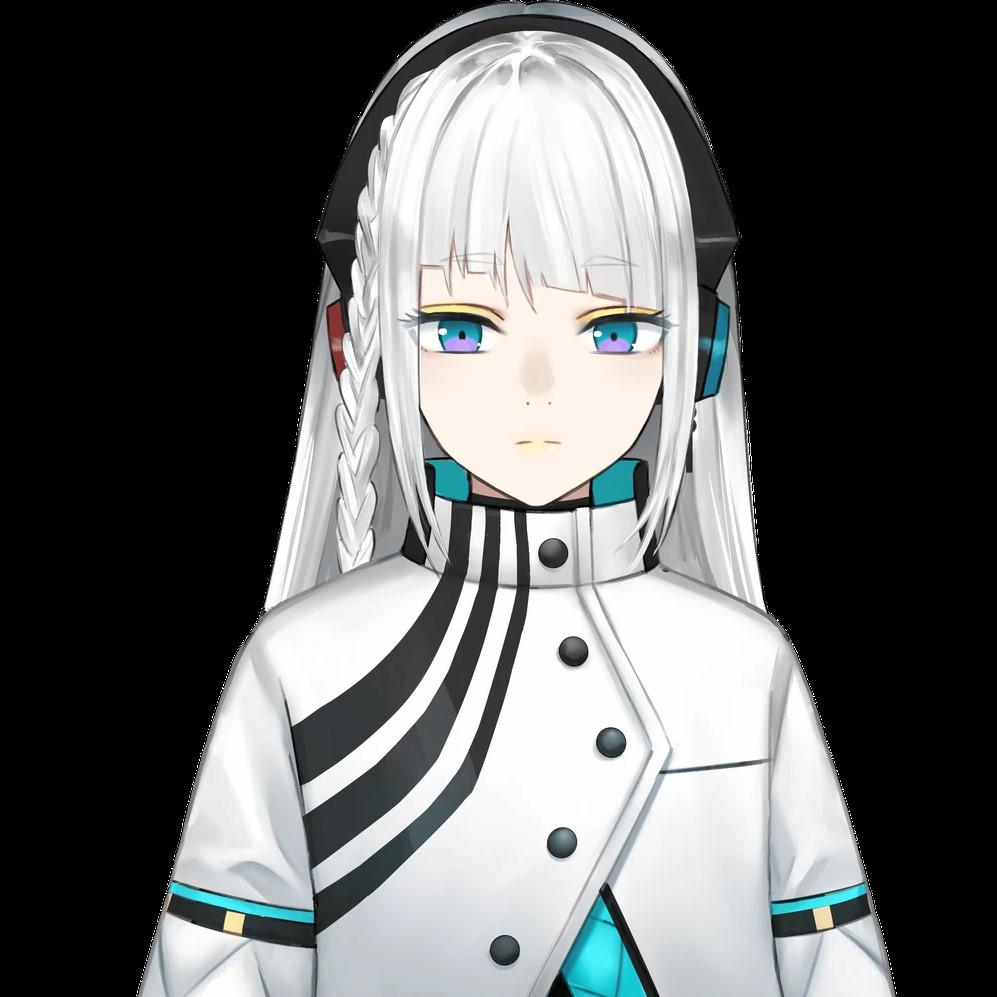
| ed6b170f7bb90bf20e1917212f0cc2fa |
pglee/github-issue-classifier | pglee | deberta-v2 | 11 | 12 | transformers | 0 | text-classification | true | false | false | mit | null | null | null | 0 | 0 | 0 | 0 | 0 | 0 | 0 | ['generated_from_trainer'] | true | true | true | 1,698 | false |
<!-- This model card has been generated automatically according to the information the Trainer had access to. You
should probably proofread and complete it, then remove this comment. -->
# github-issue-classifier
This model is a fine-tuned version of [microsoft/deberta-v3-small](https://huggingface.co/microsoft/deberta-v3-small) on the None dataset.
It achieves the following results on the evaluation set:
- Loss: 0.0684
- Accuracy: 0.875
- F1: 0.0455
- Precision: 1.0
- Recall: 0.0233
## Model description
More information needed
## Intended uses & limitations
More information needed
## Training and evaluation data
More information needed
## Training procedure
### Training hyperparameters
The following hyperparameters were used during training:
- learning_rate: 8e-05
- train_batch_size: 256
- eval_batch_size: 512
- seed: 42
- optimizer: Adam with betas=(0.9,0.999) and epsilon=1e-08
- lr_scheduler_type: cosine
- lr_scheduler_warmup_ratio: 0.1
- num_epochs: 4
- mixed_precision_training: Native AMP
### Training results
| Training Loss | Epoch | Step | Validation Loss | Accuracy | F1 | Precision | Recall |
|:-------------:|:-----:|:----:|:---------------:|:--------:|:------:|:---------:|:------:|
| No log | 1.0 | 6 | 0.0888 | 0.8720 | 0.0 | 0.0 | 0.0 |
| No log | 2.0 | 12 | 0.0700 | 0.8720 | 0.0 | 0.0 | 0.0 |
| No log | 3.0 | 18 | 0.0713 | 0.8720 | 0.0851 | 0.5 | 0.0465 |
| No log | 4.0 | 24 | 0.0684 | 0.875 | 0.0455 | 1.0 | 0.0233 |
### Framework versions
- Transformers 4.20.1
- Pytorch 1.11.0
- Datasets 2.1.0
- Tokenizers 0.12.1
| 2aaad7fbd8dc6ea19515eb68305cf51a |
yannhabib/my_awesome_wnut_model | yannhabib | distilbert | 12 | 1 | transformers | 0 | token-classification | true | false | false | apache-2.0 | null | ['wnut_17'] | null | 0 | 0 | 0 | 0 | 0 | 0 | 0 | ['generated_from_trainer'] | true | true | true | 1,445 | false |
<!-- This model card has been generated automatically according to the information the Trainer had access to. You
should probably proofread and complete it, then remove this comment. -->
# my_awesome_wnut_model
This model is a fine-tuned version of [distilbert-base-uncased](https://huggingface.co/distilbert-base-uncased) on the wnut_17 dataset.
It achieves the following results on the evaluation set:
- Loss: 0.2892
- Precision: 0.4964
- Recall: 0.2586
- F1: 0.3400
- Accuracy: 0.9387
## Model description
More information needed
## Intended uses & limitations
More information needed
## Training and evaluation data
More information needed
## Training procedure
### Training hyperparameters
The following hyperparameters were used during training:
- learning_rate: 2e-05
- train_batch_size: 16
- eval_batch_size: 16
- seed: 42
- optimizer: Adam with betas=(0.9,0.999) and epsilon=1e-08
- lr_scheduler_type: linear
- num_epochs: 2
### Training results
| Training Loss | Epoch | Step | Validation Loss | Precision | Recall | F1 | Accuracy |
|:-------------:|:-----:|:----:|:---------------:|:---------:|:------:|:------:|:--------:|
| No log | 1.0 | 213 | 0.3054 | 0.3875 | 0.1613 | 0.2277 | 0.9344 |
| No log | 2.0 | 426 | 0.2892 | 0.4964 | 0.2586 | 0.3400 | 0.9387 |
### Framework versions
- Transformers 4.25.1
- Pytorch 1.13.0+cu116
- Datasets 2.7.1
- Tokenizers 0.13.2
| 0cc6fd877861b27c9c9033d1aeeb9389 |
rdruce/ddpm-celeb-128 | rdruce | null | 15 | 0 | diffusers | 0 | null | false | false | false | apache-2.0 | ['en'] | ['data/img_align_celeba'] | null | 0 | 0 | 0 | 0 | 0 | 0 | 0 | [] | false | true | true | 1,200 | false |
<!-- This model card has been generated automatically according to the information the training script had access to. You
should probably proofread and complete it, then remove this comment. -->
# ddpm-celeb-128
## Model description
This diffusion model is trained with the [🤗 Diffusers](https://github.com/huggingface/diffusers) library
on the `data/img_align_celeba` dataset.
## Intended uses & limitations
#### How to use
```python
# TODO: add an example code snippet for running this diffusion pipeline
```
#### Limitations and bias
[TODO: provide examples of latent issues and potential remediations]
## Training data
[TODO: describe the data used to train the model]
### Training hyperparameters
The following hyperparameters were used during training:
- learning_rate: 5e-06
- train_batch_size: 16
- eval_batch_size: 16
- gradient_accumulation_steps: 1
- optimizer: AdamW with betas=(None, None), weight_decay=None and epsilon=None
- lr_scheduler: None
- lr_warmup_steps: 1000
- ema_inv_gamma: None
- ema_inv_gamma: None
- ema_inv_gamma: None
- mixed_precision: fp16
### Training results
📈 [TensorBoard logs](https://huggingface.co/rdruce/ddpm-celeb-128/tensorboard?#scalars)
| 6daebde224427e57e5793a028a0ff241 |
jonatasgrosman/exp_w2v2t_fa_vp-it_s64 | jonatasgrosman | wav2vec2 | 10 | 6 | transformers | 0 | automatic-speech-recognition | true | false | false | apache-2.0 | ['fa'] | ['mozilla-foundation/common_voice_7_0'] | null | 0 | 0 | 0 | 0 | 0 | 0 | 0 | ['automatic-speech-recognition', 'fa'] | false | true | true | 468 | false | # exp_w2v2t_fa_vp-it_s64
Fine-tuned [facebook/wav2vec2-large-it-voxpopuli](https://huggingface.co/facebook/wav2vec2-large-it-voxpopuli) for speech recognition using the train split of [Common Voice 7.0 (fa)](https://huggingface.co/datasets/mozilla-foundation/common_voice_7_0).
When using this model, make sure that your speech input is sampled at 16kHz.
This model has been fine-tuned by the [HuggingSound](https://github.com/jonatasgrosman/huggingsound) tool.
| 16fd689ff01a3671a7c302b72f8c9480 |
syedyusufali/bert-finetuned-ner | syedyusufali | bert | 8 | 9 | transformers | 0 | token-classification | false | true | false | apache-2.0 | null | null | null | 0 | 0 | 0 | 0 | 0 | 0 | 0 | ['generated_from_keras_callback'] | true | true | true | 1,573 | false |
<!-- This model card has been generated automatically according to the information Keras had access to. You should
probably proofread and complete it, then remove this comment. -->
# syedyusufali/bert-finetuned-ner
This model is a fine-tuned version of [bert-base-uncased](https://huggingface.co/bert-base-uncased) on an unknown dataset.
It achieves the following results on the evaluation set:
- Train Loss: 0.0900
- Validation Loss: 0.1200
- Epoch: 2
## Model description
More information needed
## Intended uses & limitations
More information needed
## Training and evaluation data
More information needed
## Training procedure
### Training hyperparameters
The following hyperparameters were used during training:
- optimizer: {'inner_optimizer': {'class_name': 'AdamWeightDecay', 'config': {'name': 'AdamWeightDecay', 'learning_rate': {'class_name': 'PolynomialDecay', 'config': {'initial_learning_rate': 2e-05, 'decay_steps': 1017, 'end_learning_rate': 0.0, 'power': 1.0, 'cycle': False, 'name': None}}, 'decay': 0.0, 'beta_1': 0.9, 'beta_2': 0.999, 'epsilon': 1e-08, 'amsgrad': False, 'weight_decay_rate': 0.01}}, 'dynamic': True, 'initial_scale': 32768.0, 'dynamic_growth_steps': 2000}
- training_precision: mixed_float16
### Training results
| Train Loss | Validation Loss | Epoch |
|:----------:|:---------------:|:-----:|
| 0.2904 | 0.1482 | 0 |
| 0.1317 | 0.1186 | 1 |
| 0.0900 | 0.1200 | 2 |
### Framework versions
- Transformers 4.18.0
- TensorFlow 2.8.0
- Datasets 2.0.0
- Tokenizers 0.11.6
| 7bc2297c09acabfe4fc7dc9d11d6dae3 |
marinone94/xls-r-300m-sv-robust | marinone94 | wav2vec2 | 466 | 3 | transformers | 1 | automatic-speech-recognition | true | false | false | cc0-1.0 | ['sv'] | ['mozilla-foundation/common_voice_9_0'] | null | 0 | 0 | 0 | 0 | 0 | 0 | 0 | ['automatic-speech-recognition', 'mozilla-foundation/common_voice_9_0', 'generated_from_trainer', 'sv'] | true | true | true | 1,689 | false | #
This model is a fine-tuned version of [KBLab/wav2vec2-large-voxrex](https://huggingface.co/KBLab/wav2vec2-large-voxrex) on the MOZILLA-FOUNDATION/COMMON_VOICE_9_0 - SV-SE dataset.
It achieves the following results on the evaluation set ("test" split, without LM):
- Loss: 0.1318
- Wer: 0.1121
## Model description
More information needed
## Intended uses & limitations
More information needed
## Training and evaluation data
More information needed
## Training procedure
### Training hyperparameters
The following hyperparameters were used during training:
- learning_rate: 7.5e-05
- train_batch_size: 32
- eval_batch_size: 32
- seed: 42
- gradient_accumulation_steps: 4
- total_train_batch_size: 128
- optimizer: Adam with betas=(0.9,0.999) and epsilon=1e-08
- lr_scheduler_type: linear
- lr_scheduler_warmup_ratio: 0.2
- num_epochs: 100.0
- mixed_precision_training: Native AMP
### Training results
| Training Loss | Epoch | Step | Validation Loss | Wer |
|:-------------:|:-----:|:----:|:---------------:|:------:|
| 2.9099 | 10.42 | 1000 | 2.8369 | 1.0 |
| 1.0745 | 20.83 | 2000 | 0.1957 | 0.1673 |
| 0.934 | 31.25 | 3000 | 0.1579 | 0.1389 |
| 0.8691 | 41.66 | 4000 | 0.1457 | 0.1290 |
| 0.8328 | 52.08 | 5000 | 0.1435 | 0.1205 |
| 0.8068 | 62.5 | 6000 | 0.1350 | 0.1191 |
| 0.7822 | 72.91 | 7000 | 0.1347 | 0.1155 |
| 0.7769 | 83.33 | 8000 | 0.1321 | 0.1131 |
| 0.7678 | 93.75 | 9000 | 0.1321 | 0.1115 |
### Framework versions
- Transformers 4.17.0.dev0
- Pytorch 1.10.2+cu102
- Datasets 2.2.2
- Tokenizers 0.11.0
| 72198fb1e6c9f9ec728684ffe235d301 |
Amir13/xlm-roberta-base-fa-aug-ner | Amir13 | xlm-roberta | 12 | 4 | transformers | 0 | token-classification | true | false | false | mit | null | null | null | 0 | 0 | 0 | 0 | 0 | 0 | 0 | ['generated_from_trainer'] | true | true | true | 1,712 | false |
<!-- This model card has been generated automatically according to the information the Trainer had access to. You
should probably proofread and complete it, then remove this comment. -->
# xlm-roberta-base-fa-aug-ner
This model is a fine-tuned version of [xlm-roberta-base](https://huggingface.co/xlm-roberta-base) on an unknown dataset.
It achieves the following results on the evaluation set:
- Loss: 0.2714
- Precision: 0.5446
- Recall: 0.5882
- F1: 0.5655
- Accuracy: 0.9201
## Model description
More information needed
## Intended uses & limitations
More information needed
## Training and evaluation data
More information needed
## Training procedure
### Training hyperparameters
The following hyperparameters were used during training:
- learning_rate: 2e-05
- train_batch_size: 32
- eval_batch_size: 32
- seed: 42
- optimizer: Adam with betas=(0.9,0.999) and epsilon=1e-08
- lr_scheduler_type: linear
- num_epochs: 5
### Training results
| Training Loss | Epoch | Step | Validation Loss | Precision | Recall | F1 | Accuracy |
|:-------------:|:-----:|:----:|:---------------:|:---------:|:------:|:------:|:--------:|
| 0.5864 | 1.0 | 784 | 0.3619 | 0.4741 | 0.4005 | 0.4342 | 0.8993 |
| 0.2659 | 2.0 | 1568 | 0.3057 | 0.5016 | 0.5178 | 0.5096 | 0.9093 |
| 0.2293 | 3.0 | 2352 | 0.2790 | 0.5380 | 0.5607 | 0.5491 | 0.9180 |
| 0.1945 | 4.0 | 3136 | 0.2715 | 0.5451 | 0.5672 | 0.5559 | 0.9191 |
| 0.1794 | 5.0 | 3920 | 0.2714 | 0.5446 | 0.5882 | 0.5655 | 0.9201 |
### Framework versions
- Transformers 4.25.1
- Pytorch 1.13.1+cu116
- Datasets 2.8.0
- Tokenizers 0.13.2
| 5be83d07938e553856dc94e63a7e9272 |
szabob-uly/ady_classifier | szabob-uly | bert | 8 | 1 | transformers | 0 | text-classification | false | true | false | apache-2.0 | null | null | null | 0 | 0 | 0 | 0 | 0 | 0 | 0 | ['generated_from_keras_callback'] | true | true | true | 1,132 | false |
<!-- This model card has been generated automatically according to the information Keras had access to. You should
probably proofread and complete it, then remove this comment. -->
# ady_classifier
This model is a fine-tuned version of [SZTAKI-HLT/hubert-base-cc](https://huggingface.co/SZTAKI-HLT/hubert-base-cc) on an unknown dataset.
It achieves the following results on the evaluation set:
## Model description
More information needed
## Intended uses & limitations
More information needed
## Training and evaluation data
More information needed
## Training procedure
### Training hyperparameters
The following hyperparameters were used during training:
- optimizer: {'name': 'Adam', 'learning_rate': {'class_name': 'PolynomialDecay', 'config': {'initial_learning_rate': 1e-06, 'decay_steps': 6500, 'end_learning_rate': 0.0, 'power': 1.0, 'cycle': False, 'name': None}}, 'decay': 0.0, 'beta_1': 0.9, 'beta_2': 0.999, 'epsilon': 1e-07, 'amsgrad': False}
- training_precision: float32
### Training results
### Framework versions
- Transformers 4.22.0.dev0
- TensorFlow 2.9.1
- Datasets 2.4.0
- Tokenizers 0.12.1
| d19308b16522b406e39580d4df7bb21d |
AbhiNaiky/finetuning-sentiment-model-3000-samples | AbhiNaiky | distilbert | 13 | 9 | transformers | 0 | text-classification | true | false | false | apache-2.0 | null | ['imdb'] | null | 1 | 1 | 0 | 0 | 0 | 0 | 0 | ['generated_from_trainer'] | true | true | true | 1,054 | false |
<!-- This model card has been generated automatically according to the information the Trainer had access to. You
should probably proofread and complete it, then remove this comment. -->
# finetuning-sentiment-model-3000-samples
This model is a fine-tuned version of [distilbert-base-uncased](https://huggingface.co/distilbert-base-uncased) on the imdb dataset.
It achieves the following results on the evaluation set:
- Loss: 0.3170
- Accuracy: 0.8733
- F1: 0.875
## Model description
More information needed
## Intended uses & limitations
More information needed
## Training and evaluation data
More information needed
## Training procedure
### Training hyperparameters
The following hyperparameters were used during training:
- learning_rate: 2e-05
- train_batch_size: 16
- eval_batch_size: 16
- seed: 42
- optimizer: Adam with betas=(0.9,0.999) and epsilon=1e-08
- lr_scheduler_type: linear
- num_epochs: 2
### Training results
### Framework versions
- Transformers 4.18.0
- Pytorch 1.11.0+cu113
- Datasets 2.1.0
- Tokenizers 0.12.1
| 4ab042aecd9f88aad697e000c7eb8410 |
gokuls/distilbert_sa_GLUE_Experiment_data_aug_qqp_96 | gokuls | distilbert | 19 | 1 | transformers | 0 | text-classification | true | false | false | apache-2.0 | ['en'] | ['glue'] | null | 0 | 0 | 0 | 0 | 0 | 0 | 0 | ['generated_from_trainer'] | true | true | true | 1,886 | false |
<!-- This model card has been generated automatically according to the information the Trainer had access to. You
should probably proofread and complete it, then remove this comment. -->
# distilbert_sa_GLUE_Experiment_data_aug_qqp_96
This model is a fine-tuned version of [distilbert-base-uncased](https://huggingface.co/distilbert-base-uncased) on the GLUE QQP dataset.
It achieves the following results on the evaluation set:
- Loss: 0.4833
- Accuracy: 0.7735
- F1: 0.7060
- Combined Score: 0.7397
## Model description
More information needed
## Intended uses & limitations
More information needed
## Training and evaluation data
More information needed
## Training procedure
### Training hyperparameters
The following hyperparameters were used during training:
- learning_rate: 5e-05
- train_batch_size: 256
- eval_batch_size: 256
- seed: 10
- distributed_type: multi-GPU
- optimizer: Adam with betas=(0.9,0.999) and epsilon=1e-08
- lr_scheduler_type: linear
- num_epochs: 50
- mixed_precision_training: Native AMP
### Training results
| Training Loss | Epoch | Step | Validation Loss | Accuracy | F1 | Combined Score |
|:-------------:|:-----:|:------:|:---------------:|:--------:|:------:|:--------------:|
| 0.4535 | 1.0 | 29671 | 0.4833 | 0.7735 | 0.7060 | 0.7397 |
| 0.3495 | 2.0 | 59342 | 0.5018 | 0.7825 | 0.7161 | 0.7493 |
| 0.289 | 3.0 | 89013 | 0.5229 | 0.7909 | 0.7268 | 0.7589 |
| 0.2484 | 4.0 | 118684 | 0.5749 | 0.7844 | 0.7255 | 0.7550 |
| 0.2181 | 5.0 | 148355 | 0.6016 | 0.7907 | 0.7309 | 0.7608 |
| 0.1951 | 6.0 | 178026 | 0.6304 | 0.7916 | 0.7274 | 0.7595 |
### Framework versions
- Transformers 4.26.0
- Pytorch 1.14.0a0+410ce96
- Datasets 2.9.0
- Tokenizers 0.13.2
| 0efb47d9878f0c59f57722716764452e |
maher13/English_ASR | maher13 | wav2vec2 | 12 | 7 | transformers | 0 | automatic-speech-recognition | true | false | false | apache-2.0 | null | null | null | 0 | 0 | 0 | 0 | 0 | 0 | 0 | ['generated_from_trainer'] | true | true | true | 1,615 | false |
<!-- This model card has been generated automatically according to the information the Trainer had access to. You
should probably proofread and complete it, then remove this comment. -->
# English_ASR
This model is a fine-tuned version of [facebook/wav2vec2-base](https://huggingface.co/facebook/wav2vec2-base) on the None dataset.
It achieves the following results on the evaluation set:
- Loss: 0.4971
- Wer: 0.3397
## Model description
More information needed
## Intended uses & limitations
More information needed
## Training and evaluation data
More information needed
## Training procedure
### Training hyperparameters
The following hyperparameters were used during training:
- learning_rate: 0.0001
- train_batch_size: 32
- eval_batch_size: 8
- seed: 42
- optimizer: Adam with betas=(0.9,0.999) and epsilon=1e-08
- lr_scheduler_type: linear
- lr_scheduler_warmup_steps: 1000
- num_epochs: 30
- mixed_precision_training: Native AMP
### Training results
| Training Loss | Epoch | Step | Validation Loss | Wer |
|:-------------:|:-----:|:----:|:---------------:|:------:|
| 3.3432 | 4.0 | 500 | 1.1711 | 0.7767 |
| 0.5691 | 8.0 | 1000 | 0.4613 | 0.4357 |
| 0.2182 | 12.0 | 1500 | 0.4715 | 0.3853 |
| 0.1267 | 16.0 | 2000 | 0.4307 | 0.3607 |
| 0.0846 | 20.0 | 2500 | 0.4971 | 0.3537 |
| 0.0608 | 24.0 | 3000 | 0.4712 | 0.3419 |
| 0.0457 | 28.0 | 3500 | 0.4971 | 0.3397 |
### Framework versions
- Transformers 4.11.3
- Pytorch 1.9.1
- Datasets 1.13.3
- Tokenizers 0.10.3
| ead414b048dc2024b7b51336efdb5329 |
dipteshkanojia/hing-roberta-CM-run-3 | dipteshkanojia | xlm-roberta | 9 | 4 | transformers | 0 | text-classification | true | false | false | cc-by-4.0 | null | null | null | 0 | 0 | 0 | 0 | 0 | 0 | 0 | ['generated_from_trainer'] | true | true | true | 3,101 | false |
<!-- This model card has been generated automatically according to the information the Trainer had access to. You
should probably proofread and complete it, then remove this comment. -->
# hing-roberta-CM-run-3
This model is a fine-tuned version of [l3cube-pune/hing-roberta](https://huggingface.co/l3cube-pune/hing-roberta) on an unknown dataset.
It achieves the following results on the evaluation set:
- Loss: 2.6968
- Accuracy: 0.7565
- Precision: 0.7045
- Recall: 0.7064
- F1: 0.7050
## Model description
More information needed
## Intended uses & limitations
More information needed
## Training and evaluation data
More information needed
## Training procedure
### Training hyperparameters
The following hyperparameters were used during training:
- learning_rate: 3e-05
- train_batch_size: 8
- eval_batch_size: 8
- seed: 42
- optimizer: Adam with betas=(0.9,0.999) and epsilon=1e-08
- lr_scheduler_type: linear
- num_epochs: 20
### Training results
| Training Loss | Epoch | Step | Validation Loss | Accuracy | Precision | Recall | F1 |
|:-------------:|:-----:|:----:|:---------------:|:--------:|:---------:|:------:|:------:|
| 0.8232 | 1.0 | 497 | 0.7145 | 0.6620 | 0.6319 | 0.6585 | 0.6167 |
| 0.5799 | 2.0 | 994 | 0.7155 | 0.7203 | 0.6718 | 0.6928 | 0.6672 |
| 0.4152 | 3.0 | 1491 | 0.8823 | 0.7485 | 0.6962 | 0.7136 | 0.7022 |
| 0.2657 | 4.0 | 1988 | 1.4502 | 0.7465 | 0.6945 | 0.7037 | 0.6968 |
| 0.16 | 5.0 | 2485 | 2.0667 | 0.7465 | 0.6890 | 0.6827 | 0.6855 |
| 0.0945 | 6.0 | 2982 | 2.0120 | 0.7565 | 0.7091 | 0.7159 | 0.7103 |
| 0.0802 | 7.0 | 3479 | 2.2426 | 0.7686 | 0.7253 | 0.7065 | 0.7088 |
| 0.059 | 8.0 | 3976 | 2.3472 | 0.7425 | 0.6844 | 0.6881 | 0.6861 |
| 0.041 | 9.0 | 4473 | 2.4801 | 0.7666 | 0.7258 | 0.7144 | 0.7145 |
| 0.0307 | 10.0 | 4970 | 2.6317 | 0.7545 | 0.7102 | 0.7021 | 0.7019 |
| 0.0471 | 11.0 | 5467 | 2.4626 | 0.7364 | 0.6836 | 0.6780 | 0.6788 |
| 0.0282 | 12.0 | 5964 | 2.3949 | 0.7586 | 0.7067 | 0.7108 | 0.7087 |
| 0.0267 | 13.0 | 6461 | 2.4750 | 0.7465 | 0.6938 | 0.6921 | 0.6921 |
| 0.0274 | 14.0 | 6958 | 2.5942 | 0.7565 | 0.7022 | 0.7062 | 0.7039 |
| 0.0212 | 15.0 | 7455 | 2.6728 | 0.7404 | 0.6851 | 0.6893 | 0.6867 |
| 0.026 | 16.0 | 7952 | 2.6683 | 0.7565 | 0.7064 | 0.7122 | 0.7085 |
| 0.0175 | 17.0 | 8449 | 2.6646 | 0.7505 | 0.7030 | 0.7087 | 0.7039 |
| 0.0126 | 18.0 | 8946 | 2.6948 | 0.7565 | 0.7021 | 0.7039 | 0.7030 |
| 0.0065 | 19.0 | 9443 | 2.6984 | 0.7565 | 0.7045 | 0.7064 | 0.7050 |
| 0.0103 | 20.0 | 9940 | 2.6968 | 0.7565 | 0.7045 | 0.7064 | 0.7050 |
### Framework versions
- Transformers 4.20.1
- Pytorch 1.10.1+cu111
- Datasets 2.3.2
- Tokenizers 0.12.1
| 850122cfa15b997c3c5dab37e1768aab |
tftgregrge/mpid-hassanblend-v1-5-main-hard800 | tftgregrge | null | 18 | 8 | diffusers | 0 | text-to-image | false | false | false | creativeml-openrail-m | null | null | null | 1 | 1 | 0 | 0 | 0 | 0 | 0 | ['text-to-image', 'stable-diffusion'] | false | true | true | 446 | false | ### mpid-hassanblend-v1-5-main-hard800 Dreambooth model trained by tftgregrge with [TheLastBen's fast-DreamBooth](https://colab.research.google.com/github/TheLastBen/fast-stable-diffusion/blob/main/fast-DreamBooth.ipynb) notebook
Test the concept via A1111 Colab [fast-Colab-A1111](https://colab.research.google.com/github/TheLastBen/fast-stable-diffusion/blob/main/fast_stable_diffusion_AUTOMATIC1111.ipynb)
Sample pictures of this concept:
| 52bc858d1061ff25bfffee860df58166 |
chrisvinsen/wav2vec2-final-1-lm-1 | chrisvinsen | wav2vec2 | 14 | 1 | transformers | 0 | automatic-speech-recognition | true | false | false | apache-2.0 | null | null | null | 0 | 0 | 0 | 0 | 0 | 0 | 0 | ['generated_from_trainer'] | true | true | true | 2,455 | false |
<!-- This model card has been generated automatically according to the information the Trainer had access to. You
should probably proofread and complete it, then remove this comment. -->
# wav2vec2-19
WER 0.283
WER 0.129 with 2-Gram
This model is a fine-tuned version of [facebook/wav2vec2-base](https://huggingface.co/facebook/wav2vec2-base) on the None dataset.
It achieves the following results on the evaluation set:
- Loss: 0.6305
- Wer: 0.4499
## Model description
More information needed
## Intended uses & limitations
More information needed
## Training and evaluation data
More information needed
## Training procedure
### Training hyperparameters
The following hyperparameters were used during training:
- learning_rate: 0.0003
- train_batch_size: 32
- eval_batch_size: 8
- seed: 42
- optimizer: Adam with betas=(0.9,0.999) and epsilon=1e-08
- lr_scheduler_type: linear
- lr_scheduler_warmup_steps: 800
- num_epochs: 60
### Training results
| Training Loss | Epoch | Step | Validation Loss | Wer |
|:-------------:|:-----:|:----:|:---------------:|:------:|
| 3.4816 | 2.74 | 400 | 1.0717 | 0.8927 |
| 0.751 | 5.48 | 800 | 0.7155 | 0.7533 |
| 0.517 | 8.22 | 1200 | 0.7039 | 0.6675 |
| 0.3988 | 10.96 | 1600 | 0.5935 | 0.6149 |
| 0.3179 | 13.7 | 2000 | 0.6477 | 0.5999 |
| 0.2755 | 16.44 | 2400 | 0.5549 | 0.5798 |
| 0.2343 | 19.18 | 2800 | 0.6626 | 0.5798 |
| 0.2103 | 21.92 | 3200 | 0.6488 | 0.5674 |
| 0.1877 | 24.66 | 3600 | 0.5874 | 0.5339 |
| 0.1719 | 27.4 | 4000 | 0.6354 | 0.5389 |
| 0.1603 | 30.14 | 4400 | 0.6612 | 0.5210 |
| 0.1401 | 32.88 | 4800 | 0.6676 | 0.5131 |
| 0.1286 | 35.62 | 5200 | 0.6366 | 0.5075 |
| 0.1159 | 38.36 | 5600 | 0.6064 | 0.4977 |
| 0.1084 | 41.1 | 6000 | 0.6530 | 0.4835 |
| 0.0974 | 43.84 | 6400 | 0.6118 | 0.4853 |
| 0.0879 | 46.58 | 6800 | 0.6316 | 0.4770 |
| 0.0815 | 49.32 | 7200 | 0.6125 | 0.4664 |
| 0.0708 | 52.05 | 7600 | 0.6449 | 0.4683 |
| 0.0651 | 54.79 | 8000 | 0.6068 | 0.4571 |
| 0.0555 | 57.53 | 8400 | 0.6305 | 0.4499 |
### Framework versions
- Transformers 4.19.2
- Pytorch 1.11.0+cu113
- Datasets 2.2.2
- Tokenizers 0.12.1
| 73837c156643bb6302694433f28dcffa |
prashil2792/distilbert-base-uncased-finetuned-emotions | prashil2792 | distilbert | 12 | 2 | transformers | 0 | text-classification | true | false | false | apache-2.0 | null | ['emotion'] | null | 0 | 0 | 0 | 0 | 0 | 0 | 0 | ['generated_from_trainer'] | true | true | true | 1,344 | false |
<!-- This model card has been generated automatically according to the information the Trainer had access to. You
should probably proofread and complete it, then remove this comment. -->
# distilbert-base-uncased-finetuned-emotions
This model is a fine-tuned version of [distilbert-base-uncased](https://huggingface.co/distilbert-base-uncased) on the emotion dataset.
It achieves the following results on the evaluation set:
- Loss: 0.2211
- Accuracy: 0.926
- F1: 0.9260
## Model description
More information needed
## Intended uses & limitations
More information needed
## Training and evaluation data
More information needed
## Training procedure
### Training hyperparameters
The following hyperparameters were used during training:
- learning_rate: 2e-05
- train_batch_size: 64
- eval_batch_size: 64
- seed: 42
- optimizer: Adam with betas=(0.9,0.999) and epsilon=1e-08
- lr_scheduler_type: linear
- num_epochs: 2
### Training results
| Training Loss | Epoch | Step | Validation Loss | Accuracy | F1 |
|:-------------:|:-----:|:----:|:---------------:|:--------:|:------:|
| 0.8174 | 1.0 | 250 | 0.3127 | 0.9035 | 0.9009 |
| 0.2479 | 2.0 | 500 | 0.2211 | 0.926 | 0.9260 |
### Framework versions
- Transformers 4.24.0
- Pytorch 1.12.1+cu113
- Datasets 2.7.0
- Tokenizers 0.13.2
| c807440a6c944ac55555a2b7a0ea08e9 |
jonatasgrosman/exp_w2v2r_es_vp-100k_accent_surpeninsular-2_nortepeninsular-8_s317 | jonatasgrosman | wav2vec2 | 10 | 1 | transformers | 0 | automatic-speech-recognition | true | false | false | apache-2.0 | ['es'] | ['mozilla-foundation/common_voice_7_0'] | null | 0 | 0 | 0 | 0 | 0 | 0 | 0 | ['automatic-speech-recognition', 'es'] | false | true | true | 516 | false | # exp_w2v2r_es_vp-100k_accent_surpeninsular-2_nortepeninsular-8_s317
Fine-tuned [facebook/wav2vec2-large-100k-voxpopuli](https://huggingface.co/facebook/wav2vec2-large-100k-voxpopuli) for speech recognition using the train split of [Common Voice 7.0 (es)](https://huggingface.co/datasets/mozilla-foundation/common_voice_7_0).
When using this model, make sure that your speech input is sampled at 16kHz.
This model has been fine-tuned by the [HuggingSound](https://github.com/jonatasgrosman/huggingsound) tool.
| 39680f542a662190a8430be18ab10967 |
JosephusCheung/ACertainty | JosephusCheung | null | 18 | 1,241 | diffusers | 44 | text-to-image | false | false | false | creativeml-openrail-m | ['en'] | null | null | 0 | 0 | 0 | 0 | 0 | 0 | 0 | ['stable-diffusion', 'stable-diffusion-diffusers', 'text-to-image', 'diffusers'] | false | true | true | 2,886 | false |
# ACertainty
ACertainty is a carefully designed model that is well-suited for further fine-tuning and training for use in dreambooth. It is easier to train than other anime-style Stable Diffusion models, and is less biased and more balanced for further development. This model is less likely to be biased by laion-aesthetic preferences, brought by Stable-Diffusion-v1-4+.
This is not the base of ACertainModel, but you can use this model as your new base to train your new dreambooth model about a couple themes or charactors or styles.
e.g. **_masterpiece, best quality, 1girl, brown hair, green eyes, colorful, autumn, cumulonimbus clouds, lighting, blue sky, falling leaves, garden_**
## About online preview with Hosted inference API, also generation with this model
Parameters are not allowed to be modified, as it seems that it is generated with *Clip skip: 1*, for better performance, it is strongly recommended to use *Clip skip: 2* instead.
Here is an example of inference settings, if it is applicable with you on your own server: *Steps: 28, Sampler: Euler a, CFG scale: 11, Clip skip: 2*.
## 🧨 Diffusers
This model can be used just like any other Stable Diffusion model. For more information,
please have a look at the [Stable Diffusion](https://huggingface.co/docs/diffusers/api/pipelines/stable_diffusion).
You can also export the model to [ONNX](https://huggingface.co/docs/diffusers/optimization/onnx), [MPS](https://huggingface.co/docs/diffusers/optimization/mps) and/or FLAX/JAX.
```python
from diffusers import StableDiffusionPipeline
import torch
model_id = "JosephusCheung/ACertainty"
branch_name= "main"
pipe = StableDiffusionPipeline.from_pretrained(model_id, revision=branch_name, torch_dtype=torch.float16)
pipe = pipe.to("cuda")
prompt = "pikachu"
image = pipe(prompt).images[0]
image.save("./pikachu.png")
```
## License
This model is open access and available to all, with a CreativeML OpenRAIL-M license further specifying rights and usage.
The CreativeML OpenRAIL License specifies:
1. You can't use the model to deliberately produce nor share illegal or harmful outputs or content
2. The authors claims no rights on the outputs you generate, you are free to use them and are accountable for their use which must not go against the provisions set in the license
3. You may re-distribute the weights and use the model commercially and/or as a service. If you do, please be aware you have to include the same use restrictions as the ones in the license and share a copy of the CreativeML OpenRAIL-M to all your users (please read the license entirely and carefully)
[Please read the full license here](https://huggingface.co/spaces/CompVis/stable-diffusion-license)
## Is it a NovelAI based model? What is the relationship with SD1.2 and SD1.4?
See [ASimilarityCalculatior](https://huggingface.co/JosephusCheung/ASimilarityCalculatior) | b1b954b1ee03a0e312250e8aec55fb4a |
burakyldrm/stt-v11-medium | burakyldrm | wav2vec2 | 13 | 8 | transformers | 0 | automatic-speech-recognition | true | false | false | apache-2.0 | null | null | null | 0 | 0 | 0 | 0 | 0 | 0 | 0 | ['generated_from_trainer'] | true | true | true | 2,374 | false |
<!-- This model card has been generated automatically according to the information the Trainer had access to. You
should probably proofread and complete it, then remove this comment. -->
# stt-v11-medium
This model is a fine-tuned version of [facebook/wav2vec2-xls-r-1b](https://huggingface.co/facebook/wav2vec2-xls-r-1b) on the None dataset.
It achieves the following results on the evaluation set:
- Loss: 0.3701
- Wer: 0.2216
## Model description
More information needed
## Intended uses & limitations
More information needed
## Training and evaluation data
More information needed
## Training procedure
### Training hyperparameters
The following hyperparameters were used during training:
- learning_rate: 0.0001
- train_batch_size: 16
- eval_batch_size: 8
- seed: 42
- gradient_accumulation_steps: 4
- total_train_batch_size: 64
- optimizer: Adam with betas=(0.9,0.999) and epsilon=1e-08
- lr_scheduler_type: linear
- lr_scheduler_warmup_steps: 500
- num_epochs: 271
- mixed_precision_training: Native AMP
### Training results
| Training Loss | Epoch | Step | Validation Loss | Wer |
|:-------------:|:------:|:----:|:---------------:|:------:|
| 2.8041 | 14.28 | 500 | 0.3662 | 0.4315 |
| 0.3702 | 28.56 | 1000 | 0.3102 | 0.2966 |
| 0.1978 | 42.85 | 1500 | 0.3378 | 0.2794 |
| 0.1467 | 57.14 | 2000 | 0.3201 | 0.2808 |
| 0.1144 | 71.42 | 2500 | 0.3646 | 0.2698 |
| 0.0969 | 85.7 | 3000 | 0.3234 | 0.2657 |
| 0.0832 | 99.99 | 3500 | 0.3744 | 0.2712 |
| 0.0732 | 114.28 | 4000 | 0.3217 | 0.2602 |
| 0.0635 | 128.56 | 4500 | 0.3419 | 0.2491 |
| 0.0561 | 142.85 | 5000 | 0.3628 | 0.2560 |
| 0.0491 | 157.14 | 5500 | 0.3458 | 0.2436 |
| 0.0439 | 171.42 | 6000 | 0.3615 | 0.2519 |
| 0.0397 | 185.7 | 6500 | 0.3610 | 0.2519 |
| 0.0352 | 199.99 | 7000 | 0.3514 | 0.2374 |
| 0.0314 | 214.28 | 7500 | 0.3469 | 0.2450 |
| 0.0272 | 228.56 | 8000 | 0.3615 | 0.2271 |
| 0.0247 | 242.85 | 8500 | 0.3614 | 0.2292 |
| 0.022 | 257.14 | 9000 | 0.3701 | 0.2216 |
### Framework versions
- Transformers 4.25.1
- Pytorch 1.13.1+cu116
- Datasets 2.8.0
- Tokenizers 0.13.2
| 08bf3352e1264661a67396b4b79ea2e6 |
wietsedv/xlm-roberta-base-ft-udpos28-be | wietsedv | xlm-roberta | 8 | 9 | transformers | 0 | token-classification | true | false | false | apache-2.0 | ['be'] | ['universal_dependencies'] | null | 0 | 0 | 0 | 0 | 0 | 0 | 0 | ['part-of-speech', 'token-classification'] | true | true | true | 570 | false |
# XLM-RoBERTa base Universal Dependencies v2.8 POS tagging: Belarusian
This model is part of our paper called:
- Make the Best of Cross-lingual Transfer: Evidence from POS Tagging with over 100 Languages
Check the [Space](https://huggingface.co/spaces/wietsedv/xpos) for more details.
## Usage
```python
from transformers import AutoTokenizer, AutoModelForTokenClassification
tokenizer = AutoTokenizer.from_pretrained("wietsedv/xlm-roberta-base-ft-udpos28-be")
model = AutoModelForTokenClassification.from_pretrained("wietsedv/xlm-roberta-base-ft-udpos28-be")
```
| dab4bb0a5cfa1ecc0239a59c6a8c3eb3 |
juro95/xlm-roberta-finetuned-ner-higher-ratio | juro95 | xlm-roberta | 8 | 4 | transformers | 0 | token-classification | false | true | false | mit | null | null | null | 0 | 0 | 0 | 0 | 0 | 0 | 0 | ['generated_from_keras_callback'] | true | true | true | 1,488 | false |
<!-- This model card has been generated automatically according to the information Keras had access to. You should
probably proofread and complete it, then remove this comment. -->
# juro95/xlm-roberta-finetuned-ner-higher-ratio
This model is a fine-tuned version of [xlm-roberta-base](https://huggingface.co/xlm-roberta-base) on an unknown dataset.
It achieves the following results on the evaluation set:
- Train Loss: 0.0860
- Validation Loss: 0.1320
- Epoch: 3
## Model description
More information needed
## Intended uses & limitations
More information needed
## Training and evaluation data
More information needed
## Training procedure
### Training hyperparameters
The following hyperparameters were used during training:
- optimizer: {'name': 'AdamWeightDecay', 'learning_rate': {'class_name': 'PolynomialDecay', 'config': {'initial_learning_rate': 2e-05, 'decay_steps': 53852, 'end_learning_rate': 0.0, 'power': 1.0, 'cycle': False, 'name': None}}, 'decay': 0.0, 'beta_1': 0.9, 'beta_2': 0.999, 'epsilon': 1e-08, 'amsgrad': False, 'weight_decay_rate': 0.01}
- training_precision: mixed_float16
### Training results
| Train Loss | Validation Loss | Epoch |
|:----------:|:---------------:|:-----:|
| 0.3317 | 0.1971 | 0 |
| 0.1689 | 0.1699 | 1 |
| 0.1179 | 0.1360 | 2 |
| 0.0860 | 0.1320 | 3 |
### Framework versions
- Transformers 4.25.1
- TensorFlow 2.6.5
- Datasets 2.3.2
- Tokenizers 0.13.2
| 81338c175060e50e7c0d5ed680a7d083 |
Norod78/distilgpt2-base-pretrained-he | Norod78 | gpt2 | 25 | 20 | transformers | 1 | text-generation | true | true | true | mit | ['he'] | null | null | 0 | 0 | 0 | 0 | 0 | 0 | 0 | [] | false | true | true | 2,513 | false |
# distilgpt2-base-pretrained-he
A tiny GPT2 based Hebrew text generation model initially trained on a TPUv3-8 which was made avilable to me via the [TPU Research Cloud](https://sites.research.google/trc/) Program. Then was further fine-tuned on GPU.
## Dataset
### oscar (unshuffled deduplicated he) - [Homepage](https://oscar-corpus.com) | [Dataset Permalink](https://huggingface.co/datasets/viewer/?dataset=oscar&config=unshuffled_deduplicated_he)
The Open Super-large Crawled ALMAnaCH coRpus is a huge multilingual corpus obtained by language classification and filtering of the Common Crawl corpus using the goclassy architecture.
### CC-100 (he) - [HomePage](https://data.statmt.org/cc-100/)
This corpus comprises of monolingual data for 100+ languages and also includes data for romanized languages. This was constructed using the urls and paragraph indices provided by the CC-Net repository by processing January-December 2018 Commoncrawl snapshots. Each file comprises of documents separated by double-newlines and paragraphs within the same document separated by a newline. The data is generated using the open source CC-Net repository.
### Misc
* Hebrew Twitter
* Wikipedia
* Various other sources
## Training
* Done on a TPUv3-8 VM using [Huggingface's clm-flax example script](https://github.com/huggingface/transformers/blob/master/examples/flax/language-modeling/run_clm_flax.py) <BR>
* I have made a list of items which might make it easier for other to use this script. The list was posted to [This discussion forum](https://discuss.huggingface.co/t/ideas-for-beginner-friendlier-tpu-vm-clm-training/8351)
* Further training was performed on GPU
## Usage
#### Simple usage sample code
```python
from transformers import AutoTokenizer, AutoModelForCausalLM, pipeline
def main():
model_name="Norod78/distilgpt2-base-pretrained-he"
prompt_text = "שלום, קוראים לי"
generated_max_length = 192
print("Loading model...")
model = AutoModelForCausalLM.from_pretrained(model_name)
print('Loading Tokenizer...')
tokenizer = AutoTokenizer.from_pretrained(model_name)
text_generator = pipeline(task="text-generation", model=model, tokenizer=tokenizer)
print("Generating text...")
result = text_generator(prompt_text, num_return_sequences=1, batch_size=1, do_sample=True, top_k=40, top_p=0.92, temperature = 1, repetition_penalty=5.0, max_length = generated_max_length)
print("result = " + str(result))
if __name__ == '__main__':
main()
```
| c98fcd488ccafe851e910daa5a7bb633 |
Helsinki-NLP/opus-mt-fr-tw | Helsinki-NLP | marian | 10 | 13 | transformers | 0 | translation | true | true | false | apache-2.0 | null | null | null | 0 | 0 | 0 | 0 | 0 | 0 | 0 | ['translation'] | false | true | true | 768 | false |
### opus-mt-fr-tw
* source languages: fr
* target languages: tw
* OPUS readme: [fr-tw](https://github.com/Helsinki-NLP/OPUS-MT-train/blob/master/models/fr-tw/README.md)
* dataset: opus
* model: transformer-align
* pre-processing: normalization + SentencePiece
* download original weights: [opus-2020-01-16.zip](https://object.pouta.csc.fi/OPUS-MT-models/fr-tw/opus-2020-01-16.zip)
* test set translations: [opus-2020-01-16.test.txt](https://object.pouta.csc.fi/OPUS-MT-models/fr-tw/opus-2020-01-16.test.txt)
* test set scores: [opus-2020-01-16.eval.txt](https://object.pouta.csc.fi/OPUS-MT-models/fr-tw/opus-2020-01-16.eval.txt)
## Benchmarks
| testset | BLEU | chr-F |
|-----------------------|-------|-------|
| JW300.fr.tw | 27.9 | 0.469 |
| c95488666c209b04c73c3a4fb1461fe3 |
XerOpred/sentiment-model | XerOpred | distilbert | 10 | 1 | transformers | 0 | text-classification | true | false | false | apache-2.0 | null | null | null | 0 | 0 | 0 | 0 | 0 | 0 | 0 | ['generated_from_trainer'] | true | true | true | 1,116 | false |
<!-- This model card has been generated automatically according to the information the Trainer had access to. You
should probably proofread and complete it, then remove this comment. -->
# sentiment-model
This model is a fine-tuned version of [distilbert-base-uncased](https://huggingface.co/distilbert-base-uncased) on an unknown dataset.
It achieves the following results on the evaluation set:
- eval_loss: 0.4302
- eval_accuracy: 0.8337
- eval_f1: 0.0
- eval_runtime: 25.9665
- eval_samples_per_second: 30.809
- eval_steps_per_second: 1.926
- epoch: 1.0
- step: 200
## Model description
More information needed
## Intended uses & limitations
More information needed
## Training and evaluation data
More information needed
## Training procedure
### Training hyperparameters
The following hyperparameters were used during training:
- learning_rate: 2e-05
- train_batch_size: 16
- eval_batch_size: 16
- seed: 42
- optimizer: Adam with betas=(0.9,0.999) and epsilon=1e-08
- lr_scheduler_type: linear
- num_epochs: 2
### Framework versions
- Transformers 4.22.2
- Pytorch 1.12.1+cpu
- Tokenizers 0.12.1
| 91cd68d48508a843833b1e83b4eee3ca |
Helsinki-NLP/opus-mt-sl-fr | Helsinki-NLP | marian | 10 | 13 | transformers | 0 | translation | true | true | false | apache-2.0 | null | null | null | 0 | 0 | 0 | 0 | 0 | 0 | 0 | ['translation'] | false | true | true | 768 | false |
### opus-mt-sl-fr
* source languages: sl
* target languages: fr
* OPUS readme: [sl-fr](https://github.com/Helsinki-NLP/OPUS-MT-train/blob/master/models/sl-fr/README.md)
* dataset: opus
* model: transformer-align
* pre-processing: normalization + SentencePiece
* download original weights: [opus-2020-01-16.zip](https://object.pouta.csc.fi/OPUS-MT-models/sl-fr/opus-2020-01-16.zip)
* test set translations: [opus-2020-01-16.test.txt](https://object.pouta.csc.fi/OPUS-MT-models/sl-fr/opus-2020-01-16.test.txt)
* test set scores: [opus-2020-01-16.eval.txt](https://object.pouta.csc.fi/OPUS-MT-models/sl-fr/opus-2020-01-16.eval.txt)
## Benchmarks
| testset | BLEU | chr-F |
|-----------------------|-------|-------|
| JW300.sl.fr | 25.0 | 0.475 |
| 5b917b24cf9bc71726450121b6f96daf |
S2312dal/M5_MLM | S2312dal | deberta-v2 | 14 | 4 | transformers | 0 | fill-mask | true | false | false | mit | null | null | null | 0 | 0 | 0 | 0 | 0 | 0 | 0 | ['generated_from_trainer'] | true | true | true | 1,290 | false |
<!-- This model card has been generated automatically according to the information the Trainer had access to. You
should probably proofread and complete it, then remove this comment. -->
# M5_MLM
This model is a fine-tuned version of [microsoft/deberta-v3-base](https://huggingface.co/microsoft/deberta-v3-base) on an unknown dataset.
It achieves the following results on the evaluation set:
- Loss: 7.0447
## Model description
More information needed
## Intended uses & limitations
More information needed
## Training and evaluation data
More information needed
## Training procedure
### Training hyperparameters
The following hyperparameters were used during training:
- learning_rate: 2e-05
- train_batch_size: 6
- eval_batch_size: 6
- seed: 42
- optimizer: Adam with betas=(0.9,0.999) and epsilon=1e-08
- lr_scheduler_type: linear
- num_epochs: 3.0
- mixed_precision_training: Native AMP
### Training results
| Training Loss | Epoch | Step | Validation Loss |
|:-------------:|:-----:|:----:|:---------------:|
| 9.8279 | 1.0 | 62 | 7.9889 |
| 7.7536 | 2.0 | 124 | 7.3750 |
| 7.2065 | 3.0 | 186 | 6.8625 |
### Framework versions
- Transformers 4.20.0
- Pytorch 1.11.0+cu113
- Datasets 2.3.2
- Tokenizers 0.12.1
| c0abfd7be076fcc8e8c303463cb4f2da |
Gladiator/microsoft-deberta-v3-large_ner_wnut_17 | Gladiator | deberta-v2 | 13 | 5 | transformers | 0 | token-classification | true | false | false | mit | null | ['wnut_17'] | null | 0 | 0 | 0 | 0 | 0 | 0 | 0 | ['generated_from_trainer'] | true | true | true | 1,738 | false |
<!-- This model card has been generated automatically according to the information the Trainer had access to. You
should probably proofread and complete it, then remove this comment. -->
# microsoft-deberta-v3-large_ner_wnut_17
This model is a fine-tuned version of [microsoft/deberta-v3-large](https://huggingface.co/microsoft/deberta-v3-large) on the wnut_17 dataset.
It achieves the following results on the evaluation set:
- Loss: 0.2199
- Precision: 0.7671
- Recall: 0.6184
- F1: 0.6848
- Accuracy: 0.9667
## Model description
More information needed
## Intended uses & limitations
More information needed
## Training and evaluation data
More information needed
## Training procedure
### Training hyperparameters
The following hyperparameters were used during training:
- learning_rate: 2e-05
- train_batch_size: 16
- eval_batch_size: 16
- seed: 42
- optimizer: Adam with betas=(0.9,0.999) and epsilon=1e-08
- lr_scheduler_type: cosine
- num_epochs: 5
### Training results
| Training Loss | Epoch | Step | Validation Loss | Precision | Recall | F1 | Accuracy |
|:-------------:|:-----:|:----:|:---------------:|:---------:|:------:|:------:|:--------:|
| No log | 1.0 | 213 | 0.1751 | 0.6884 | 0.5682 | 0.6225 | 0.9601 |
| No log | 2.0 | 426 | 0.1702 | 0.7351 | 0.6208 | 0.6732 | 0.9655 |
| 0.1003 | 3.0 | 639 | 0.1954 | 0.7360 | 0.6136 | 0.6693 | 0.9656 |
| 0.1003 | 4.0 | 852 | 0.2113 | 0.7595 | 0.6232 | 0.6846 | 0.9669 |
| 0.015 | 5.0 | 1065 | 0.2199 | 0.7671 | 0.6184 | 0.6848 | 0.9667 |
### Framework versions
- Transformers 4.20.1
- Pytorch 1.11.0
- Datasets 2.1.0
- Tokenizers 0.12.1
| d695192e5f1b4558ad7ca57352db1586 |
elopezlopez/distilbert-base-uncased_fold_9_binary_v1 | elopezlopez | distilbert | 16 | 1 | transformers | 0 | text-classification | true | false | false | apache-2.0 | null | null | null | 0 | 0 | 0 | 0 | 0 | 0 | 0 | ['generated_from_trainer'] | true | true | true | 2,658 | false |
<!-- This model card has been generated automatically according to the information the Trainer had access to. You
should probably proofread and complete it, then remove this comment. -->
# distilbert-base-uncased_fold_9_binary_v1
This model is a fine-tuned version of [distilbert-base-uncased](https://huggingface.co/distilbert-base-uncased) on the None dataset.
It achieves the following results on the evaluation set:
- Loss: 1.6965
- F1: 0.8090
## Model description
More information needed
## Intended uses & limitations
More information needed
## Training and evaluation data
More information needed
## Training procedure
### Training hyperparameters
The following hyperparameters were used during training:
- learning_rate: 2e-05
- train_batch_size: 16
- eval_batch_size: 16
- seed: 42
- optimizer: Adam with betas=(0.9,0.999) and epsilon=1e-08
- lr_scheduler_type: linear
- num_epochs: 25
### Training results
| Training Loss | Epoch | Step | Validation Loss | F1 |
|:-------------:|:-----:|:----:|:---------------:|:------:|
| No log | 1.0 | 291 | 0.4193 | 0.7989 |
| 0.3993 | 2.0 | 582 | 0.4039 | 0.8026 |
| 0.3993 | 3.0 | 873 | 0.5227 | 0.7995 |
| 0.2044 | 4.0 | 1164 | 0.7264 | 0.8011 |
| 0.2044 | 5.0 | 1455 | 0.8497 | 0.8007 |
| 0.0882 | 6.0 | 1746 | 0.9543 | 0.8055 |
| 0.0374 | 7.0 | 2037 | 1.1349 | 0.7997 |
| 0.0374 | 8.0 | 2328 | 1.3175 | 0.8009 |
| 0.0151 | 9.0 | 2619 | 1.3585 | 0.8030 |
| 0.0151 | 10.0 | 2910 | 1.4202 | 0.8067 |
| 0.0068 | 11.0 | 3201 | 1.4364 | 0.8108 |
| 0.0068 | 12.0 | 3492 | 1.4443 | 0.8088 |
| 0.0096 | 13.0 | 3783 | 1.5308 | 0.8075 |
| 0.0031 | 14.0 | 4074 | 1.5061 | 0.8020 |
| 0.0031 | 15.0 | 4365 | 1.5769 | 0.7980 |
| 0.0048 | 16.0 | 4656 | 1.5962 | 0.8038 |
| 0.0048 | 17.0 | 4947 | 1.5383 | 0.8085 |
| 0.0067 | 18.0 | 5238 | 1.5456 | 0.8158 |
| 0.0062 | 19.0 | 5529 | 1.6325 | 0.8044 |
| 0.0062 | 20.0 | 5820 | 1.5430 | 0.8141 |
| 0.0029 | 21.0 | 6111 | 1.6590 | 0.8117 |
| 0.0029 | 22.0 | 6402 | 1.6650 | 0.8112 |
| 0.0017 | 23.0 | 6693 | 1.7016 | 0.8053 |
| 0.0017 | 24.0 | 6984 | 1.6998 | 0.8090 |
| 0.0011 | 25.0 | 7275 | 1.6965 | 0.8090 |
### Framework versions
- Transformers 4.21.0
- Pytorch 1.12.0+cu113
- Datasets 2.4.0
- Tokenizers 0.12.1
| 04ddcb6b750baa56ae6adfd569e44ecc |
NoCrypt/animeinourworld-model | NoCrypt | null | 3 | 0 | null | 15 | text-to-image | false | false | false | creativeml-openrail-m | ['en'] | null | null | 0 | 0 | 0 | 0 | 0 | 0 | 0 | ['stable-diffusion', 'text-to-image'] | false | true | true | 2,213 | false |
# animeinourworld-model
> based on images from /r/animeinourworld, trained on 30-40 images for 2 epochs on kohya's db trainer at 5e-6
- Token is `mksks style`
- This model was trained by [closertodeath#1703](https://lookup.guru/112268417628651520).
- The author gave me the permission to mirror it to Hugging Face.
- The base model is [Yohan Diffusion](https://huggingface.co/andite/yohan-diffusion)
## Example Prompt
`mksks style, best quality, (ultra detailed:1.4), (professional photograph:1.4), backlighting, sidelighting, (1girl, solo:1.1), HuTao, pajamas, twintails, looking at viewer, smile, one eye closed, indoors, bedroom, potted plant, bed, windows, computer, desk, chair, sitting`
## Examples




## License
This model is open access and available to all, with a CreativeML OpenRAIL-M license further specifying rights and usage.
The CreativeML OpenRAIL License specifies:
1. You can't use the model to deliberately produce nor share illegal or harmful outputs or content
2. The authors claims no rights on the outputs you generate, you are free to use them and are accountable for their use which must not go against the provisions set in the license
3. You may re-distribute the weights and use the model commercially and/or as a service. If you do, please be aware you have to include the same use restrictions as the ones in the license and share a copy of the CreativeML OpenRAIL-M to all your users (please read the license entirely and carefully)
[Please read the full license here](https://huggingface.co/spaces/CompVis/stable-diffusion-license)
| bcfaa2fcf14a622ad3e3611b238351a1 |
ShadoWxShinigamI/SD2-Vray-Style | ShadoWxShinigamI | null | 4 | 0 | null | 3 | null | false | false | false | creativeml-openrail-m | null | null | null | 0 | 0 | 0 | 0 | 0 | 0 | 0 | [] | false | true | true | 926 | false | ##Textual Inversion Embed For SD 2.0 By ShadoWxShinigamI
This embed attempts to emulate the style and lighting of vray renderer. It has been trained for a total of 1000 steps based on 44 of my personal renders.
Model used for training:- SD 2.0 (512 Base). [Works well with the 768 Model]
This embed mixes well with other 2.0 embeds. Mix and have fun!
Examples:-





| a6c8ec862b6b716f62a3227feaf5cb09 |
davanstrien/deberta-v3-base_fine_tuned_food_ner | davanstrien | deberta-v2 | 13 | 302 | transformers | 2 | token-classification | true | false | false | mit | null | null | null | 0 | 0 | 0 | 0 | 0 | 0 | 0 | ['generated_from_trainer'] | true | true | true | 3,117 | false |
<!-- This model card has been generated automatically according to the information the Trainer had access to. You
should probably proofread and complete it, then remove this comment. -->
# deberta-v3-base_fine_tuned_food_ner
This model is a fine-tuned version of [microsoft/deberta-v3-base](https://huggingface.co/microsoft/deberta-v3-base) on the None dataset.
It achieves the following results on the evaluation set:
- Loss: 0.4164
- Precision: 0.9268
- Recall: 0.9446
- F1: 0.9356
- Accuracy: 0.9197
## Model description
More information needed
## Intended uses & limitations
More information needed
## Training and evaluation data
More information needed
## Training procedure
### Training hyperparameters
The following hyperparameters were used during training:
- learning_rate: 2e-05
- train_batch_size: 16
- eval_batch_size: 16
- seed: 42
- optimizer: Adam with betas=(0.9,0.999) and epsilon=1e-08
- lr_scheduler_type: linear
- num_epochs: 20
### Training results
| Training Loss | Epoch | Step | Validation Loss | Precision | Recall | F1 | Accuracy |
|:-------------:|:-----:|:----:|:---------------:|:---------:|:------:|:------:|:--------:|
| No log | 1.0 | 40 | 0.8425 | 0.8323 | 0.8323 | 0.8323 | 0.8073 |
| No log | 2.0 | 80 | 0.5533 | 0.8703 | 0.8941 | 0.8820 | 0.8731 |
| No log | 3.0 | 120 | 0.4855 | 0.8771 | 0.9109 | 0.8937 | 0.8797 |
| No log | 4.0 | 160 | 0.4238 | 0.8949 | 0.9222 | 0.9083 | 0.8964 |
| No log | 5.0 | 200 | 0.4176 | 0.9048 | 0.9302 | 0.9173 | 0.9008 |
| No log | 6.0 | 240 | 0.4127 | 0.9065 | 0.9342 | 0.9202 | 0.9004 |
| No log | 7.0 | 280 | 0.4409 | 0.9294 | 0.9302 | 0.9298 | 0.9043 |
| No log | 8.0 | 320 | 0.3971 | 0.9129 | 0.9334 | 0.9230 | 0.9061 |
| No log | 9.0 | 360 | 0.3941 | 0.9112 | 0.9390 | 0.9249 | 0.9061 |
| No log | 10.0 | 400 | 0.4069 | 0.9233 | 0.9366 | 0.9299 | 0.9148 |
| No log | 11.0 | 440 | 0.4039 | 0.9213 | 0.9390 | 0.9300 | 0.9162 |
| No log | 12.0 | 480 | 0.4000 | 0.9126 | 0.9470 | 0.9295 | 0.9113 |
| 0.3799 | 13.0 | 520 | 0.4126 | 0.9323 | 0.9390 | 0.9356 | 0.9179 |
| 0.3799 | 14.0 | 560 | 0.4076 | 0.9272 | 0.9398 | 0.9334 | 0.9140 |
| 0.3799 | 15.0 | 600 | 0.4129 | 0.9317 | 0.9414 | 0.9365 | 0.9188 |
| 0.3799 | 16.0 | 640 | 0.4000 | 0.9239 | 0.9446 | 0.9341 | 0.9162 |
| 0.3799 | 17.0 | 680 | 0.4098 | 0.9267 | 0.9438 | 0.9352 | 0.9179 |
| 0.3799 | 18.0 | 720 | 0.4110 | 0.9232 | 0.9454 | 0.9342 | 0.9188 |
| 0.3799 | 19.0 | 760 | 0.4202 | 0.9275 | 0.9446 | 0.9360 | 0.9183 |
| 0.3799 | 20.0 | 800 | 0.4164 | 0.9268 | 0.9446 | 0.9356 | 0.9197 |
### Framework versions
- Transformers 4.21.0
- Pytorch 1.12.0+cu113
- Datasets 2.4.0
- Tokenizers 0.12.1
| 98542be87cdf6046875148d5d72e8ba4 |
Duskfallcrew/Duskfalls_Slime_Tutorial | Duskfallcrew | null | 21 | 20 | diffusers | 1 | text-to-image | false | false | false | creativeml-openrail-m | ['en'] | null | null | 1 | 0 | 1 | 0 | 0 | 0 | 0 | ['text-to-image'] | false | true | true | 1,227 | false | [](https://huggingface.co/spaces/Duskfallcrew/Duskfalls_Slime_Tutorial)
### Duskfall's Slime Tutorial Dreambooth model trained by Duskfallcrew with [Hugging Face Dreambooth Training Space](https://huggingface.co/spaces/multimodalart/dreambooth-training) with the v1-5 base model
You run your new concept via `diffusers` [Colab Notebook for Inference](https://colab.research.google.com/github/huggingface/notebooks/blob/main/diffusers/sd_dreambooth_inference.ipynb). Don't forget to use the concept prompts!
Information on this model will be here:
https://civitai.com/models/5985/duskfalls-slime-tutorial
If you want to donate towards costs and don't want to subscribe:
https://ko-fi.com/DUSKFALLcrew
If you want to monthly support the EARTH & DUSK media projects and not just AI:
https://www.patreon.com/earthndusk
DO NOT SELL THIS MODEL, OR MERGES
Do merge, and do enjoy.
Generative images for commercial use are fine.
Credit in your merges would be great. | 0b9fe1cae41b0fa6b40a4cf7398f0fe0 |
timm/maxvit_large_tf_512.in21k_ft_in1k | timm | null | 4 | 1,149 | timm | 0 | image-classification | true | false | false | apache-2.0 | null | ['imagenet-1k', 'imagenet-21k'] | null | 0 | 0 | 0 | 0 | 0 | 0 | 0 | ['image-classification', 'timm'] | false | true | true | 22,175 | false | # Model card for maxvit_large_tf_512.in21k_ft_in1k
An official MaxViT image classification model. Pretrained in tensorflow on ImageNet-21k (21843 Google specific instance of ImageNet-22k) and fine-tuned on ImageNet-1k by paper authors.
Ported from official Tensorflow implementation (https://github.com/google-research/maxvit) to PyTorch by Ross Wightman.
### Model Variants in [maxxvit.py](https://github.com/rwightman/pytorch-image-models/blob/main/timm/models/maxxvit.py)
MaxxViT covers a number of related model architectures that share a common structure including:
- CoAtNet - Combining MBConv (depthwise-separable) convolutional blocks in early stages with self-attention transformer blocks in later stages.
- MaxViT - Uniform blocks across all stages, each containing a MBConv (depthwise-separable) convolution block followed by two self-attention blocks with different partitioning schemes (window followed by grid).
- CoAtNeXt - A timm specific arch that uses ConvNeXt blocks in place of MBConv blocks in CoAtNet. All normalization layers are LayerNorm (no BatchNorm).
- MaxxViT - A timm specific arch that uses ConvNeXt blocks in place of MBConv blocks in MaxViT. All normalization layers are LayerNorm (no BatchNorm).
- MaxxViT-V2 - A MaxxViT variation that removes the window block attention leaving only ConvNeXt blocks and grid attention w/ more width to compensate.
Aside from the major variants listed above, there are more subtle changes from model to model. Any model name with the string `rw` are `timm` specific configs w/ modelling adjustments made to favour PyTorch eager use. These were created while training initial reproductions of the models so there are variations.
All models with the string `tf` are models exactly matching Tensorflow based models by the original paper authors with weights ported to PyTorch. This covers a number of MaxViT models. The official CoAtNet models were never released.
## Model Details
- **Model Type:** Image classification / feature backbone
- **Model Stats:**
- Params (M): 212.3
- GMACs: 244.8
- Activations (M): 942.1
- Image size: 512 x 512
- **Papers:**
- MaxViT: Multi-Axis Vision Transformer: https://arxiv.org/abs/2204.01697
- **Dataset:** ImageNet-1k
- **Pretrain Dataset:** ImageNet-21k
## Model Usage
### Image Classification
```python
from urllib.request import urlopen
from PIL import Image
import timm
img = Image.open(
urlopen('https://huggingface.co/datasets/huggingface/documentation-images/resolve/main/beignets-task-guide.png'))
model = timm.create_model('maxvit_large_tf_512.in21k_ft_in1k', pretrained=True)
model = model.eval()
# get model specific transforms (normalization, resize)
data_config = timm.data.resolve_model_data_config(model)
transforms = timm.data.create_transform(**data_config, is_training=False)
output = model(transforms(img).unsqueeze(0)) # unsqueeze single image into batch of 1
top5_probabilities, top5_class_indices = torch.topk(output.softmax(dim=1) * 100, k=5)
```
### Feature Map Extraction
```python
from urllib.request import urlopen
from PIL import Image
import timm
img = Image.open(
urlopen('https://huggingface.co/datasets/huggingface/documentation-images/resolve/main/beignets-task-guide.png'))
model = timm.create_model(
'maxvit_large_tf_512.in21k_ft_in1k',
pretrained=True,
features_only=True,
)
model = model.eval()
# get model specific transforms (normalization, resize)
data_config = timm.data.resolve_model_data_config(model)
transforms = timm.data.create_transform(**data_config, is_training=False)
output = model(transforms(img).unsqueeze(0)) # unsqueeze single image into batch of 1
for o in output:
# print shape of each feature map in output
# e.g.:
# torch.Size([1, 128, 192, 192])
# torch.Size([1, 128, 96, 96])
# torch.Size([1, 256, 48, 48])
# torch.Size([1, 512, 24, 24])
# torch.Size([1, 1024, 12, 12])
print(o.shape)
```
### Image Embeddings
```python
from urllib.request import urlopen
from PIL import Image
import timm
img = Image.open(
urlopen('https://huggingface.co/datasets/huggingface/documentation-images/resolve/main/beignets-task-guide.png'))
model = timm.create_model(
'maxvit_large_tf_512.in21k_ft_in1k',
pretrained=True,
num_classes=0, # remove classifier nn.Linear
)
model = model.eval()
# get model specific transforms (normalization, resize)
data_config = timm.data.resolve_model_data_config(model)
transforms = timm.data.create_transform(**data_config, is_training=False)
output = model(transforms(img).unsqueeze(0)) # output is (batch_size, num_features) shaped tensor
# or equivalently (without needing to set num_classes=0)
output = model.forward_features(transforms(img).unsqueeze(0))
# output is unpooled (ie.e a (batch_size, num_features, H, W) tensor
output = model.forward_head(output, pre_logits=True)
# output is (batch_size, num_features) tensor
```
## Model Comparison
### By Top-1
|model |top1 |top5 |samples / sec |Params (M) |GMAC |Act (M)|
|------------------------------------------------------------------------------------------------------------------------|----:|----:|--------------:|--------------:|-----:|------:|
|[maxvit_xlarge_tf_512.in21k_ft_in1k](https://huggingface.co/timm/maxvit_xlarge_tf_512.in21k_ft_in1k) |88.53|98.64| 21.76| 475.77|534.14|1413.22|
|[maxvit_xlarge_tf_384.in21k_ft_in1k](https://huggingface.co/timm/maxvit_xlarge_tf_384.in21k_ft_in1k) |88.32|98.54| 42.53| 475.32|292.78| 668.76|
|[maxvit_base_tf_512.in21k_ft_in1k](https://huggingface.co/timm/maxvit_base_tf_512.in21k_ft_in1k) |88.20|98.53| 50.87| 119.88|138.02| 703.99|
|[maxvit_large_tf_512.in21k_ft_in1k](https://huggingface.co/timm/maxvit_large_tf_512.in21k_ft_in1k) |88.04|98.40| 36.42| 212.33|244.75| 942.15|
|[maxvit_large_tf_384.in21k_ft_in1k](https://huggingface.co/timm/maxvit_large_tf_384.in21k_ft_in1k) |87.98|98.56| 71.75| 212.03|132.55| 445.84|
|[maxvit_base_tf_384.in21k_ft_in1k](https://huggingface.co/timm/maxvit_base_tf_384.in21k_ft_in1k) |87.92|98.54| 104.71| 119.65| 73.80| 332.90|
|[maxvit_rmlp_base_rw_384.sw_in12k_ft_in1k](https://huggingface.co/timm/maxvit_rmlp_base_rw_384.sw_in12k_ft_in1k) |87.81|98.37| 106.55| 116.14| 70.97| 318.95|
|[maxxvitv2_rmlp_base_rw_384.sw_in12k_ft_in1k](https://huggingface.co/timm/maxxvitv2_rmlp_base_rw_384.sw_in12k_ft_in1k) |87.47|98.37| 149.49| 116.09| 72.98| 213.74|
|[coatnet_rmlp_2_rw_384.sw_in12k_ft_in1k](https://huggingface.co/timm/coatnet_rmlp_2_rw_384.sw_in12k_ft_in1k) |87.39|98.31| 160.80| 73.88| 47.69| 209.43|
|[maxvit_rmlp_base_rw_224.sw_in12k_ft_in1k](https://huggingface.co/timm/maxvit_rmlp_base_rw_224.sw_in12k_ft_in1k) |86.89|98.02| 375.86| 116.14| 23.15| 92.64|
|[maxxvitv2_rmlp_base_rw_224.sw_in12k_ft_in1k](https://huggingface.co/timm/maxxvitv2_rmlp_base_rw_224.sw_in12k_ft_in1k) |86.64|98.02| 501.03| 116.09| 24.20| 62.77|
|[maxvit_base_tf_512.in1k](https://huggingface.co/timm/maxvit_base_tf_512.in1k) |86.60|97.92| 50.75| 119.88|138.02| 703.99|
|[coatnet_2_rw_224.sw_in12k_ft_in1k](https://huggingface.co/timm/coatnet_2_rw_224.sw_in12k_ft_in1k) |86.57|97.89| 631.88| 73.87| 15.09| 49.22|
|[maxvit_large_tf_512.in1k](https://huggingface.co/timm/maxvit_large_tf_512.in1k) |86.52|97.88| 36.04| 212.33|244.75| 942.15|
|[coatnet_rmlp_2_rw_224.sw_in12k_ft_in1k](https://huggingface.co/timm/coatnet_rmlp_2_rw_224.sw_in12k_ft_in1k) |86.49|97.90| 620.58| 73.88| 15.18| 54.78|
|[maxvit_base_tf_384.in1k](https://huggingface.co/timm/maxvit_base_tf_384.in1k) |86.29|97.80| 101.09| 119.65| 73.80| 332.90|
|[maxvit_large_tf_384.in1k](https://huggingface.co/timm/maxvit_large_tf_384.in1k) |86.23|97.69| 70.56| 212.03|132.55| 445.84|
|[maxvit_small_tf_512.in1k](https://huggingface.co/timm/maxvit_small_tf_512.in1k) |86.10|97.76| 88.63| 69.13| 67.26| 383.77|
|[maxvit_tiny_tf_512.in1k](https://huggingface.co/timm/maxvit_tiny_tf_512.in1k) |85.67|97.58| 144.25| 31.05| 33.49| 257.59|
|[maxvit_small_tf_384.in1k](https://huggingface.co/timm/maxvit_small_tf_384.in1k) |85.54|97.46| 188.35| 69.02| 35.87| 183.65|
|[maxvit_tiny_tf_384.in1k](https://huggingface.co/timm/maxvit_tiny_tf_384.in1k) |85.11|97.38| 293.46| 30.98| 17.53| 123.42|
|[maxvit_large_tf_224.in1k](https://huggingface.co/timm/maxvit_large_tf_224.in1k) |84.93|96.97| 247.71| 211.79| 43.68| 127.35|
|[coatnet_rmlp_1_rw2_224.sw_in12k_ft_in1k](https://huggingface.co/timm/coatnet_rmlp_1_rw2_224.sw_in12k_ft_in1k) |84.90|96.96| 1025.45| 41.72| 8.11| 40.13|
|[maxvit_base_tf_224.in1k](https://huggingface.co/timm/maxvit_base_tf_224.in1k) |84.85|96.99| 358.25| 119.47| 24.04| 95.01|
|[maxxvit_rmlp_small_rw_256.sw_in1k](https://huggingface.co/timm/maxxvit_rmlp_small_rw_256.sw_in1k) |84.63|97.06| 575.53| 66.01| 14.67| 58.38|
|[coatnet_rmlp_2_rw_224.sw_in1k](https://huggingface.co/timm/coatnet_rmlp_2_rw_224.sw_in1k) |84.61|96.74| 625.81| 73.88| 15.18| 54.78|
|[maxvit_rmlp_small_rw_224.sw_in1k](https://huggingface.co/timm/maxvit_rmlp_small_rw_224.sw_in1k) |84.49|96.76| 693.82| 64.90| 10.75| 49.30|
|[maxvit_small_tf_224.in1k](https://huggingface.co/timm/maxvit_small_tf_224.in1k) |84.43|96.83| 647.96| 68.93| 11.66| 53.17|
|[maxvit_rmlp_tiny_rw_256.sw_in1k](https://huggingface.co/timm/maxvit_rmlp_tiny_rw_256.sw_in1k) |84.23|96.78| 807.21| 29.15| 6.77| 46.92|
|[coatnet_1_rw_224.sw_in1k](https://huggingface.co/timm/coatnet_1_rw_224.sw_in1k) |83.62|96.38| 989.59| 41.72| 8.04| 34.60|
|[maxvit_tiny_rw_224.sw_in1k](https://huggingface.co/timm/maxvit_tiny_rw_224.sw_in1k) |83.50|96.50| 1100.53| 29.06| 5.11| 33.11|
|[maxvit_tiny_tf_224.in1k](https://huggingface.co/timm/maxvit_tiny_tf_224.in1k) |83.41|96.59| 1004.94| 30.92| 5.60| 35.78|
|[coatnet_rmlp_1_rw_224.sw_in1k](https://huggingface.co/timm/coatnet_rmlp_1_rw_224.sw_in1k) |83.36|96.45| 1093.03| 41.69| 7.85| 35.47|
|[maxxvitv2_nano_rw_256.sw_in1k](https://huggingface.co/timm/maxxvitv2_nano_rw_256.sw_in1k) |83.11|96.33| 1276.88| 23.70| 6.26| 23.05|
|[maxxvit_rmlp_nano_rw_256.sw_in1k](https://huggingface.co/timm/maxxvit_rmlp_nano_rw_256.sw_in1k) |83.03|96.34| 1341.24| 16.78| 4.37| 26.05|
|[maxvit_rmlp_nano_rw_256.sw_in1k](https://huggingface.co/timm/maxvit_rmlp_nano_rw_256.sw_in1k) |82.96|96.26| 1283.24| 15.50| 4.47| 31.92|
|[maxvit_nano_rw_256.sw_in1k](https://huggingface.co/timm/maxvit_nano_rw_256.sw_in1k) |82.93|96.23| 1218.17| 15.45| 4.46| 30.28|
|[coatnet_bn_0_rw_224.sw_in1k](https://huggingface.co/timm/coatnet_bn_0_rw_224.sw_in1k) |82.39|96.19| 1600.14| 27.44| 4.67| 22.04|
|[coatnet_0_rw_224.sw_in1k](https://huggingface.co/timm/coatnet_0_rw_224.sw_in1k) |82.39|95.84| 1831.21| 27.44| 4.43| 18.73|
|[coatnet_rmlp_nano_rw_224.sw_in1k](https://huggingface.co/timm/coatnet_rmlp_nano_rw_224.sw_in1k) |82.05|95.87| 2109.09| 15.15| 2.62| 20.34|
|[coatnext_nano_rw_224.sw_in1k](https://huggingface.co/timm/coatnext_nano_rw_224.sw_in1k) |81.95|95.92| 2525.52| 14.70| 2.47| 12.80|
|[coatnet_nano_rw_224.sw_in1k](https://huggingface.co/timm/coatnet_nano_rw_224.sw_in1k) |81.70|95.64| 2344.52| 15.14| 2.41| 15.41|
|[maxvit_rmlp_pico_rw_256.sw_in1k](https://huggingface.co/timm/maxvit_rmlp_pico_rw_256.sw_in1k) |80.53|95.21| 1594.71| 7.52| 1.85| 24.86|
### By Throughput (samples / sec)
|model |top1 |top5 |samples / sec |Params (M) |GMAC |Act (M)|
|------------------------------------------------------------------------------------------------------------------------|----:|----:|--------------:|--------------:|-----:|------:|
|[coatnext_nano_rw_224.sw_in1k](https://huggingface.co/timm/coatnext_nano_rw_224.sw_in1k) |81.95|95.92| 2525.52| 14.70| 2.47| 12.80|
|[coatnet_nano_rw_224.sw_in1k](https://huggingface.co/timm/coatnet_nano_rw_224.sw_in1k) |81.70|95.64| 2344.52| 15.14| 2.41| 15.41|
|[coatnet_rmlp_nano_rw_224.sw_in1k](https://huggingface.co/timm/coatnet_rmlp_nano_rw_224.sw_in1k) |82.05|95.87| 2109.09| 15.15| 2.62| 20.34|
|[coatnet_0_rw_224.sw_in1k](https://huggingface.co/timm/coatnet_0_rw_224.sw_in1k) |82.39|95.84| 1831.21| 27.44| 4.43| 18.73|
|[coatnet_bn_0_rw_224.sw_in1k](https://huggingface.co/timm/coatnet_bn_0_rw_224.sw_in1k) |82.39|96.19| 1600.14| 27.44| 4.67| 22.04|
|[maxvit_rmlp_pico_rw_256.sw_in1k](https://huggingface.co/timm/maxvit_rmlp_pico_rw_256.sw_in1k) |80.53|95.21| 1594.71| 7.52| 1.85| 24.86|
|[maxxvit_rmlp_nano_rw_256.sw_in1k](https://huggingface.co/timm/maxxvit_rmlp_nano_rw_256.sw_in1k) |83.03|96.34| 1341.24| 16.78| 4.37| 26.05|
|[maxvit_rmlp_nano_rw_256.sw_in1k](https://huggingface.co/timm/maxvit_rmlp_nano_rw_256.sw_in1k) |82.96|96.26| 1283.24| 15.50| 4.47| 31.92|
|[maxxvitv2_nano_rw_256.sw_in1k](https://huggingface.co/timm/maxxvitv2_nano_rw_256.sw_in1k) |83.11|96.33| 1276.88| 23.70| 6.26| 23.05|
|[maxvit_nano_rw_256.sw_in1k](https://huggingface.co/timm/maxvit_nano_rw_256.sw_in1k) |82.93|96.23| 1218.17| 15.45| 4.46| 30.28|
|[maxvit_tiny_rw_224.sw_in1k](https://huggingface.co/timm/maxvit_tiny_rw_224.sw_in1k) |83.50|96.50| 1100.53| 29.06| 5.11| 33.11|
|[coatnet_rmlp_1_rw_224.sw_in1k](https://huggingface.co/timm/coatnet_rmlp_1_rw_224.sw_in1k) |83.36|96.45| 1093.03| 41.69| 7.85| 35.47|
|[coatnet_rmlp_1_rw2_224.sw_in12k_ft_in1k](https://huggingface.co/timm/coatnet_rmlp_1_rw2_224.sw_in12k_ft_in1k) |84.90|96.96| 1025.45| 41.72| 8.11| 40.13|
|[maxvit_tiny_tf_224.in1k](https://huggingface.co/timm/maxvit_tiny_tf_224.in1k) |83.41|96.59| 1004.94| 30.92| 5.60| 35.78|
|[coatnet_1_rw_224.sw_in1k](https://huggingface.co/timm/coatnet_1_rw_224.sw_in1k) |83.62|96.38| 989.59| 41.72| 8.04| 34.60|
|[maxvit_rmlp_tiny_rw_256.sw_in1k](https://huggingface.co/timm/maxvit_rmlp_tiny_rw_256.sw_in1k) |84.23|96.78| 807.21| 29.15| 6.77| 46.92|
|[maxvit_rmlp_small_rw_224.sw_in1k](https://huggingface.co/timm/maxvit_rmlp_small_rw_224.sw_in1k) |84.49|96.76| 693.82| 64.90| 10.75| 49.30|
|[maxvit_small_tf_224.in1k](https://huggingface.co/timm/maxvit_small_tf_224.in1k) |84.43|96.83| 647.96| 68.93| 11.66| 53.17|
|[coatnet_2_rw_224.sw_in12k_ft_in1k](https://huggingface.co/timm/coatnet_2_rw_224.sw_in12k_ft_in1k) |86.57|97.89| 631.88| 73.87| 15.09| 49.22|
|[coatnet_rmlp_2_rw_224.sw_in1k](https://huggingface.co/timm/coatnet_rmlp_2_rw_224.sw_in1k) |84.61|96.74| 625.81| 73.88| 15.18| 54.78|
|[coatnet_rmlp_2_rw_224.sw_in12k_ft_in1k](https://huggingface.co/timm/coatnet_rmlp_2_rw_224.sw_in12k_ft_in1k) |86.49|97.90| 620.58| 73.88| 15.18| 54.78|
|[maxxvit_rmlp_small_rw_256.sw_in1k](https://huggingface.co/timm/maxxvit_rmlp_small_rw_256.sw_in1k) |84.63|97.06| 575.53| 66.01| 14.67| 58.38|
|[maxxvitv2_rmlp_base_rw_224.sw_in12k_ft_in1k](https://huggingface.co/timm/maxxvitv2_rmlp_base_rw_224.sw_in12k_ft_in1k) |86.64|98.02| 501.03| 116.09| 24.20| 62.77|
|[maxvit_rmlp_base_rw_224.sw_in12k_ft_in1k](https://huggingface.co/timm/maxvit_rmlp_base_rw_224.sw_in12k_ft_in1k) |86.89|98.02| 375.86| 116.14| 23.15| 92.64|
|[maxvit_base_tf_224.in1k](https://huggingface.co/timm/maxvit_base_tf_224.in1k) |84.85|96.99| 358.25| 119.47| 24.04| 95.01|
|[maxvit_tiny_tf_384.in1k](https://huggingface.co/timm/maxvit_tiny_tf_384.in1k) |85.11|97.38| 293.46| 30.98| 17.53| 123.42|
|[maxvit_large_tf_224.in1k](https://huggingface.co/timm/maxvit_large_tf_224.in1k) |84.93|96.97| 247.71| 211.79| 43.68| 127.35|
|[maxvit_small_tf_384.in1k](https://huggingface.co/timm/maxvit_small_tf_384.in1k) |85.54|97.46| 188.35| 69.02| 35.87| 183.65|
|[coatnet_rmlp_2_rw_384.sw_in12k_ft_in1k](https://huggingface.co/timm/coatnet_rmlp_2_rw_384.sw_in12k_ft_in1k) |87.39|98.31| 160.80| 73.88| 47.69| 209.43|
|[maxxvitv2_rmlp_base_rw_384.sw_in12k_ft_in1k](https://huggingface.co/timm/maxxvitv2_rmlp_base_rw_384.sw_in12k_ft_in1k) |87.47|98.37| 149.49| 116.09| 72.98| 213.74|
|[maxvit_tiny_tf_512.in1k](https://huggingface.co/timm/maxvit_tiny_tf_512.in1k) |85.67|97.58| 144.25| 31.05| 33.49| 257.59|
|[maxvit_rmlp_base_rw_384.sw_in12k_ft_in1k](https://huggingface.co/timm/maxvit_rmlp_base_rw_384.sw_in12k_ft_in1k) |87.81|98.37| 106.55| 116.14| 70.97| 318.95|
|[maxvit_base_tf_384.in21k_ft_in1k](https://huggingface.co/timm/maxvit_base_tf_384.in21k_ft_in1k) |87.92|98.54| 104.71| 119.65| 73.80| 332.90|
|[maxvit_base_tf_384.in1k](https://huggingface.co/timm/maxvit_base_tf_384.in1k) |86.29|97.80| 101.09| 119.65| 73.80| 332.90|
|[maxvit_small_tf_512.in1k](https://huggingface.co/timm/maxvit_small_tf_512.in1k) |86.10|97.76| 88.63| 69.13| 67.26| 383.77|
|[maxvit_large_tf_384.in21k_ft_in1k](https://huggingface.co/timm/maxvit_large_tf_384.in21k_ft_in1k) |87.98|98.56| 71.75| 212.03|132.55| 445.84|
|[maxvit_large_tf_384.in1k](https://huggingface.co/timm/maxvit_large_tf_384.in1k) |86.23|97.69| 70.56| 212.03|132.55| 445.84|
|[maxvit_base_tf_512.in21k_ft_in1k](https://huggingface.co/timm/maxvit_base_tf_512.in21k_ft_in1k) |88.20|98.53| 50.87| 119.88|138.02| 703.99|
|[maxvit_base_tf_512.in1k](https://huggingface.co/timm/maxvit_base_tf_512.in1k) |86.60|97.92| 50.75| 119.88|138.02| 703.99|
|[maxvit_xlarge_tf_384.in21k_ft_in1k](https://huggingface.co/timm/maxvit_xlarge_tf_384.in21k_ft_in1k) |88.32|98.54| 42.53| 475.32|292.78| 668.76|
|[maxvit_large_tf_512.in21k_ft_in1k](https://huggingface.co/timm/maxvit_large_tf_512.in21k_ft_in1k) |88.04|98.40| 36.42| 212.33|244.75| 942.15|
|[maxvit_large_tf_512.in1k](https://huggingface.co/timm/maxvit_large_tf_512.in1k) |86.52|97.88| 36.04| 212.33|244.75| 942.15|
|[maxvit_xlarge_tf_512.in21k_ft_in1k](https://huggingface.co/timm/maxvit_xlarge_tf_512.in21k_ft_in1k) |88.53|98.64| 21.76| 475.77|534.14|1413.22|
## Citation
```bibtex
@misc{rw2019timm,
author = {Ross Wightman},
title = {PyTorch Image Models},
year = {2019},
publisher = {GitHub},
journal = {GitHub repository},
doi = {10.5281/zenodo.4414861},
howpublished = {\url{https://github.com/rwightman/pytorch-image-models}}
}
```
```bibtex
@article{tu2022maxvit,
title={MaxViT: Multi-Axis Vision Transformer},
author={Tu, Zhengzhong and Talebi, Hossein and Zhang, Han and Yang, Feng and Milanfar, Peyman and Bovik, Alan and Li, Yinxiao},
journal={ECCV},
year={2022},
}
```
```bibtex
@article{dai2021coatnet,
title={CoAtNet: Marrying Convolution and Attention for All Data Sizes},
author={Dai, Zihang and Liu, Hanxiao and Le, Quoc V and Tan, Mingxing},
journal={arXiv preprint arXiv:2106.04803},
year={2021}
}
```
| 935cef873cc8a5e967829490e5fae10a |
dousey/scene_segmentation | dousey | segformer | 5 | 0 | transformers | 0 | null | false | true | false | other | null | null | null | 0 | 0 | 0 | 0 | 0 | 0 | 0 | ['generated_from_keras_callback'] | true | true | true | 2,957 | false |
<!-- This model card has been generated automatically according to the information Keras had access to. You should
probably proofread and complete it, then remove this comment. -->
# dousey/scene_segmentation
This model is a fine-tuned version of [nvidia/mit-b0](https://huggingface.co/nvidia/mit-b0) on an unknown dataset.
It achieves the following results on the evaluation set:
- Train Loss: nan
- Validation Loss: nan
- Validation Mean Iou: 0.0217
- Validation Mean Accuracy: 0.5
- Validation Overall Accuracy: 0.2545
- Validation Accuracy Background: 1.0
- Validation Accuracy Bleuet: 0.0
- Validation Accuracy Comptonie: nan
- Validation Accuracy Kalmia: nan
- Validation Iou Background: 0.0433
- Validation Iou Bleuet: 0.0
- Validation Iou Comptonie: nan
- Validation Iou Kalmia: nan
- Epoch: 1
## Model description
More information needed
## Intended uses & limitations
More information needed
## Training and evaluation data
More information needed
## Training procedure
### Training hyperparameters
The following hyperparameters were used during training:
- optimizer: {'name': 'AdamWeightDecay', 'learning_rate': {'class_name': 'PolynomialDecay', 'config': {'initial_learning_rate': 6e-05, 'decay_steps': 76500, 'end_learning_rate': 0.0, 'power': 1.0, 'cycle': False, 'name': None}}, 'decay': 0.0, 'beta_1': 0.9, 'beta_2': 0.999, 'epsilon': 1e-08, 'amsgrad': False, 'weight_decay_rate': 0.01}
- training_precision: float32
### Training results
| Train Loss | Validation Loss | Validation Mean Iou | Validation Mean Accuracy | Validation Overall Accuracy | Validation Accuracy Background | Validation Accuracy Bleuet | Validation Accuracy Comptonie | Validation Accuracy Kalmia | Validation Iou Background | Validation Iou Bleuet | Validation Iou Comptonie | Validation Iou Kalmia | Epoch |
|:----------:|:---------------:|:-------------------:|:------------------------:|:---------------------------:|:------------------------------:|:--------------------------:|:-----------------------------:|:--------------------------:|:-------------------------:|:---------------------:|:------------------------:|:---------------------:|:-----:|
| nan | nan | 0.0217 | 0.5 | 0.2545 | 1.0 | 0.0 | nan | nan | 0.0433 | 0.0 | nan | nan | 0 |
| nan | nan | 0.0217 | 0.5 | 0.2545 | 1.0 | 0.0 | nan | nan | 0.0433 | 0.0 | nan | nan | 1 |
### Framework versions
- Transformers 4.26.0
- TensorFlow 2.9.2
- Datasets 2.9.0
- Tokenizers 0.13.2
| 0412ba2de41686fd1cbe0e4de5a4dbc4 |
Eman222/distilbert-base-uncased-finetuned-ner | Eman222 | distilbert | 13 | 3 | transformers | 0 | token-classification | true | false | false | apache-2.0 | null | ['conll2003'] | null | 0 | 0 | 0 | 0 | 0 | 0 | 0 | ['generated_from_trainer'] | true | true | true | 1,555 | false |
<!-- This model card has been generated automatically according to the information the Trainer had access to. You
should probably proofread and complete it, then remove this comment. -->
# distilbert-base-uncased-finetuned-ner
This model is a fine-tuned version of [distilbert-base-uncased](https://huggingface.co/distilbert-base-uncased) on the conll2003 dataset.
It achieves the following results on the evaluation set:
- Loss: 0.0611
- Precision: 0.9262
- Recall: 0.9361
- F1: 0.9311
- Accuracy: 0.9837
## Model description
More information needed
## Intended uses & limitations
More information needed
## Training and evaluation data
More information needed
## Training procedure
### Training hyperparameters
The following hyperparameters were used during training:
- learning_rate: 2e-05
- train_batch_size: 16
- eval_batch_size: 16
- seed: 42
- optimizer: Adam with betas=(0.9,0.999) and epsilon=1e-08
- lr_scheduler_type: linear
- num_epochs: 3
### Training results
| Training Loss | Epoch | Step | Validation Loss | Precision | Recall | F1 | Accuracy |
|:-------------:|:-----:|:----:|:---------------:|:---------:|:------:|:------:|:--------:|
| 0.2401 | 1.0 | 878 | 0.0684 | 0.9147 | 0.9172 | 0.9159 | 0.9808 |
| 0.0538 | 2.0 | 1756 | 0.0614 | 0.9231 | 0.9346 | 0.9288 | 0.9829 |
| 0.0301 | 3.0 | 2634 | 0.0611 | 0.9262 | 0.9361 | 0.9311 | 0.9837 |
### Framework versions
- Transformers 4.21.1
- Pytorch 1.12.1+cu113
- Datasets 2.4.0
- Tokenizers 0.12.1
| e55afcfa645c8472705264c05f123ccb |
Chalet37/ddpm-butterflies-128 | Chalet37 | null | 13 | 3 | diffusers | 0 | null | false | false | false | apache-2.0 | ['en'] | ['huggan/smithsonian_butterflies_subset'] | null | 0 | 0 | 0 | 0 | 0 | 0 | 0 | [] | false | true | true | 1,230 | false |
<!-- This model card has been generated automatically according to the information the training script had access to. You
should probably proofread and complete it, then remove this comment. -->
# ddpm-butterflies-128
## Model description
This diffusion model is trained with the [🤗 Diffusers](https://github.com/huggingface/diffusers) library
on the `huggan/smithsonian_butterflies_subset` dataset.
## Intended uses & limitations
#### How to use
```python
# TODO: add an example code snippet for running this diffusion pipeline
```
#### Limitations and bias
[TODO: provide examples of latent issues and potential remediations]
## Training data
[TODO: describe the data used to train the model]
### Training hyperparameters
The following hyperparameters were used during training:
- learning_rate: 0.0001
- train_batch_size: 16
- eval_batch_size: 16
- gradient_accumulation_steps: 1
- optimizer: AdamW with betas=(None, None), weight_decay=None and epsilon=None
- lr_scheduler: None
- lr_warmup_steps: 500
- ema_inv_gamma: None
- ema_inv_gamma: None
- ema_inv_gamma: None
- mixed_precision: fp16
### Training results
📈 [TensorBoard logs](https://huggingface.co/Chalet37/ddpm-butterflies-128/tensorboard?#scalars)
| 32bb43332964080056ede3a85b7d9628 |
gngpostalsrvc/BERiT_4500 | gngpostalsrvc | roberta | 11 | 7 | transformers | 0 | fill-mask | true | false | false | mit | null | null | null | 0 | 0 | 0 | 0 | 0 | 0 | 0 | ['generated_from_trainer'] | true | true | true | 1,839 | false |
<!-- This model card has been generated automatically according to the information the Trainer had access to. You
should probably proofread and complete it, then remove this comment. -->
# BERiT_4500
This model is a fine-tuned version of [roberta-base](https://huggingface.co/roberta-base) on an unknown dataset.
It achieves the following results on the evaluation set:
- Loss: 7.2982
## Model description
More information needed
## Intended uses & limitations
More information needed
## Training and evaluation data
More information needed
## Training procedure
### Training hyperparameters
The following hyperparameters were used during training:
- learning_rate: 5e-05
- train_batch_size: 8
- eval_batch_size: 8
- seed: 42
- optimizer: Adam with betas=(0.9,0.999) and epsilon=1e-08
- lr_scheduler_type: linear
- num_epochs: 3
### Training results
| Training Loss | Epoch | Step | Validation Loss |
|:-------------:|:-----:|:----:|:---------------:|
| 7.5996 | 0.19 | 500 | 7.4930 |
| 7.4322 | 0.39 | 1000 | 7.4460 |
| 7.3767 | 0.58 | 1500 | 7.3877 |
| 7.3711 | 0.77 | 2000 | 7.3511 |
| 7.3511 | 0.97 | 2500 | 7.3300 |
| 7.2984 | 1.16 | 3000 | 7.3526 |
| 7.3129 | 1.36 | 3500 | 7.3245 |
| 7.3235 | 1.55 | 4000 | 7.3333 |
| 7.2908 | 1.74 | 4500 | 7.2968 |
| 7.3262 | 1.94 | 5000 | 7.3058 |
| 7.3074 | 2.13 | 5500 | 7.3084 |
| 7.2701 | 2.32 | 6000 | 7.3020 |
| 7.2498 | 2.52 | 6500 | 7.2913 |
| 7.274 | 2.71 | 7000 | 7.2997 |
| 7.2593 | 2.9 | 7500 | 7.2982 |
### Framework versions
- Transformers 4.24.0
- Pytorch 1.12.1+cu113
- Datasets 2.6.1
- Tokenizers 0.13.2
| 7d717d3ecb759df05e611689e219302a |
inhee/m2m100_418M-finetuned-ko-to-en4 | inhee | m2m_100 | 12 | 1 | transformers | 0 | text2text-generation | true | false | false | mit | null | null | null | 0 | 0 | 0 | 0 | 0 | 0 | 0 | ['generated_from_trainer'] | true | true | true | 1,999 | false |
<!-- This model card has been generated automatically according to the information the Trainer had access to. You
should probably proofread and complete it, then remove this comment. -->
# m2m100_418M-finetuned-ko-to-en4
This model is a fine-tuned version of [facebook/m2m100_418M](https://huggingface.co/facebook/m2m100_418M) on the None dataset.
It achieves the following results on the evaluation set:
- Loss: 0.4598
- Bleu: 85.3745
- Gen Len: 9.7522
## Model description
More information needed
## Intended uses & limitations
More information needed
## Training and evaluation data
More information needed
## Training procedure
### Training hyperparameters
The following hyperparameters were used during training:
- learning_rate: 0.001
- train_batch_size: 4
- eval_batch_size: 4
- seed: 42
- gradient_accumulation_steps: 256
- total_train_batch_size: 1024
- optimizer: Adam with betas=(0.9,0.999) and epsilon=1e-08
- lr_scheduler_type: linear
- num_epochs: 10
- mixed_precision_training: Native AMP
### Training results
| Training Loss | Epoch | Step | Validation Loss | Bleu | Gen Len |
|:-------------:|:-----:|:----:|:---------------:|:-------:|:-------:|
| No log | 1.0 | 105 | 1.8667 | 24.5072 | 9.523 |
| No log | 2.0 | 210 | 0.8581 | 57.9973 | 9.2779 |
| No log | 3.0 | 315 | 0.6587 | 69.4588 | 9.7399 |
| No log | 4.0 | 420 | 0.5762 | 74.5636 | 9.6775 |
| 1.4539 | 5.0 | 525 | 0.5254 | 78.8897 | 9.6946 |
| 1.4539 | 6.0 | 630 | 0.4952 | 81.0054 | 9.7073 |
| 1.4539 | 7.0 | 735 | 0.4773 | 83.0792 | 9.7233 |
| 1.4539 | 8.0 | 840 | 0.4669 | 84.4309 | 9.7429 |
| 1.4539 | 9.0 | 945 | 0.4616 | 85.0965 | 9.749 |
| 0.144 | 10.0 | 1050 | 0.4598 | 85.3745 | 9.7522 |
### Framework versions
- Transformers 4.18.0
- Pytorch 1.11.0+cu113
- Datasets 2.1.0
- Tokenizers 0.12.1
| 91bee44a51ebddc60250f0adb31c7b10 |
shields/whisper-largev2-hindi | shields | whisper | 15 | 0 | transformers | 0 | automatic-speech-recognition | true | false | false | apache-2.0 | ['hi'] | ['mozilla-foundation/common_voice_11_0'] | null | 0 | 0 | 0 | 0 | 0 | 0 | 0 | ['hf-asr-leaderboard', 'generated_from_trainer'] | true | true | true | 1,481 | false |
<!-- This model card has been generated automatically according to the information the Trainer had access to. You
should probably proofread and complete it, then remove this comment. -->
# Whisper LargeV2 Hindi
This model is a fine-tuned version of [openai/whisper-large-v2](https://huggingface.co/openai/whisper-large-v2) on the Common Voice 11.0 dataset.
It achieves the following results on the evaluation set:
- Loss: 0.2347
- Wer: 20.8711
## Model description
More information needed
## Intended uses & limitations
More information needed
## Training and evaluation data
More information needed
## Training procedure
### Training hyperparameters
The following hyperparameters were used during training:
- learning_rate: 1e-05
- train_batch_size: 8
- eval_batch_size: 8
- seed: 42
- optimizer: Adam with betas=(0.9,0.999) and epsilon=1e-08
- lr_scheduler_type: linear
- lr_scheduler_warmup_steps: 500
- training_steps: 4000
- mixed_precision_training: Native AMP
### Training results
| Training Loss | Epoch | Step | Validation Loss | Wer |
|:-------------:|:-----:|:----:|:---------------:|:-------:|
| 0.1077 | 1.22 | 1000 | 0.2206 | 27.2581 |
| 0.0455 | 2.44 | 2000 | 0.2098 | 23.4784 |
| 0.015 | 3.67 | 3000 | 0.2106 | 21.4721 |
| 0.004 | 4.89 | 4000 | 0.2347 | 20.8711 |
### Framework versions
- Transformers 4.26.0.dev0
- Pytorch 1.13.0+cu116
- Datasets 2.8.0
- Tokenizers 0.13.2
| 3fb4172af879fbfdecba57a46094ae83 |
samwit/ddpm-afhq-cats-128 | samwit | null | 49 | 13 | diffusers | 0 | null | false | false | false | apache-2.0 | ['en'] | ['imagefolder'] | null | 0 | 0 | 0 | 0 | 0 | 0 | 0 | [] | false | true | true | 1,198 | false |
<!-- This model card has been generated automatically according to the information the training script had access to. You
should probably proofread and complete it, then remove this comment. -->
# ddpm-afhq-cats-128
## Model description
This diffusion model is trained with the [🤗 Diffusers](https://github.com/huggingface/diffusers) library
on the `imagefolder` dataset.
## Intended uses & limitations
#### How to use
```python
# TODO: add an example code snippet for running this diffusion pipeline
```
#### Limitations and bias
[TODO: provide examples of latent issues and potential remediations]
## Training data
[TODO: describe the data used to train the model]
### Training hyperparameters
The following hyperparameters were used during training:
- learning_rate: 0.0001
- train_batch_size: 16
- eval_batch_size: 16
- gradient_accumulation_steps: 1
- optimizer: AdamW with betas=(None, None), weight_decay=None and epsilon=None
- lr_scheduler: None
- lr_warmup_steps: 500
- ema_inv_gamma: None
- ema_inv_gamma: None
- ema_inv_gamma: None
- mixed_precision: fp16
### Training results
📈 [TensorBoard logs](https://huggingface.co/samwit/ddpm-afhq-cats-128/tensorboard?#scalars)
| 50bc350f1d802231c3fe0c819422f9a2 |
twieland/VN_ja-en_byt5_small | twieland | t5 | 8 | 1 | transformers | 0 | text2text-generation | true | false | false | apache-2.0 | null | null | null | 0 | 0 | 0 | 0 | 0 | 0 | 0 | ['generated_from_trainer'] | true | true | true | 2,658 | false |
<!-- This model card has been generated automatically according to the information the Trainer had access to. You
should probably proofread and complete it, then remove this comment. -->
# VN_ja-en_byt5_small
This model is a fine-tuned version of [google/byt5-small](https://huggingface.co/google/byt5-small) on an unknown dataset.
It achieves the following results on the evaluation set:
- Loss: 1.0552
## Model description
More information needed
## Intended uses & limitations
More information needed
## Training and evaluation data
More information needed
## Training procedure
### Training hyperparameters
The following hyperparameters were used during training:
- learning_rate: 0.0003
- train_batch_size: 32
- eval_batch_size: 32
- seed: 42
- optimizer: Adam with betas=(0.9,0.999) and epsilon=1e-08
- lr_scheduler_type: linear
- num_epochs: 3
### Training results
| Training Loss | Epoch | Step | Validation Loss |
|:-------------:|:-----:|:-----:|:---------------:|
| 1.1687 | 0.1 | 2000 | 1.1805 |
| 0.9685 | 0.19 | 4000 | 1.1384 |
| 0.8989 | 0.29 | 6000 | 1.1207 |
| 0.8583 | 0.39 | 8000 | 1.1046 |
| 0.833 | 0.49 | 10000 | 1.1290 |
| 0.8102 | 0.58 | 12000 | 1.1225 |
| 0.7932 | 0.68 | 14000 | 1.0956 |
| 0.7776 | 0.78 | 16000 | 1.0970 |
| 0.762 | 0.88 | 18000 | 1.0992 |
| 0.7522 | 0.97 | 20000 | 1.0760 |
| 0.7318 | 1.07 | 22000 | 1.0579 |
| 0.7197 | 1.17 | 24000 | 1.0780 |
| 0.7142 | 1.27 | 26000 | 1.0748 |
| 0.7093 | 1.36 | 28000 | 1.0781 |
| 0.7005 | 1.46 | 30000 | 1.0756 |
| 0.6938 | 1.56 | 32000 | 1.0702 |
| 0.6896 | 1.65 | 34000 | 1.0563 |
| 0.6846 | 1.75 | 36000 | 1.0603 |
| 0.6807 | 1.85 | 38000 | 1.0626 |
| 0.6766 | 1.95 | 40000 | 1.0666 |
| 0.6649 | 2.04 | 42000 | 1.0694 |
| 0.6532 | 2.14 | 44000 | 1.0564 |
| 0.6501 | 2.24 | 46000 | 1.0715 |
| 0.6476 | 2.34 | 48000 | 1.0551 |
| 0.646 | 2.43 | 50000 | 1.0601 |
| 0.6445 | 2.53 | 52000 | 1.0595 |
| 0.6404 | 2.63 | 54000 | 1.0494 |
| 0.6378 | 2.72 | 56000 | 1.0584 |
| 0.636 | 2.82 | 58000 | 1.0531 |
| 0.6345 | 2.92 | 60000 | 1.0552 |
### Framework versions
- Transformers 4.19.2
- Pytorch 1.11.0+cu113
- Datasets 2.2.2
- Tokenizers 0.12.1
| c56a7ee615b169a033449900391181c2 |
Helsinki-NLP/opus-mt-ru-fi | Helsinki-NLP | marian | 10 | 119 | transformers | 0 | translation | true | true | false | apache-2.0 | null | null | null | 0 | 0 | 0 | 0 | 0 | 0 | 0 | ['translation'] | false | true | true | 770 | false |
### opus-mt-ru-fi
* source languages: ru
* target languages: fi
* OPUS readme: [ru-fi](https://github.com/Helsinki-NLP/OPUS-MT-train/blob/master/models/ru-fi/README.md)
* dataset: opus
* model: transformer-align
* pre-processing: normalization + SentencePiece
* download original weights: [opus-2020-04-12.zip](https://object.pouta.csc.fi/OPUS-MT-models/ru-fi/opus-2020-04-12.zip)
* test set translations: [opus-2020-04-12.test.txt](https://object.pouta.csc.fi/OPUS-MT-models/ru-fi/opus-2020-04-12.test.txt)
* test set scores: [opus-2020-04-12.eval.txt](https://object.pouta.csc.fi/OPUS-MT-models/ru-fi/opus-2020-04-12.eval.txt)
## Benchmarks
| testset | BLEU | chr-F |
|-----------------------|-------|-------|
| Tatoeba.ru.fi | 40.1 | 0.646 |
| 448d6509f1543d99a8d6e34b68fbe96e |
wooglee/distilbert-imdb | wooglee | distilbert | 12 | 4 | transformers | 0 | text-classification | true | false | false | apache-2.0 | null | ['imdb'] | null | 1 | 1 | 0 | 0 | 0 | 0 | 0 | ['generated_from_trainer'] | true | true | true | 1,119 | false |
<!-- This model card has been generated automatically according to the information the Trainer had access to. You
should probably proofread and complete it, then remove this comment. -->
# distilbert-imdb
This model is a fine-tuned version of [distilbert-base-uncased](https://huggingface.co/distilbert-base-uncased) on the imdb dataset.
## Model description
More information needed
## Intended uses & limitations
More information needed
## Training and evaluation data
More information needed
## Training procedure
### Training hyperparameters
The following hyperparameters were used during training:
- learning_rate: 5e-05
- train_batch_size: 128
- eval_batch_size: 128
- seed: 42
- optimizer: Adam with betas=(0.9,0.999) and epsilon=1e-08
- lr_scheduler_type: linear
- num_epochs: 1
### Training results
| Training Loss | Epoch | Step | Validation Loss | Accuracy |
|:-------------:|:-----:|:----:|:---------------:|:--------:|
| No log | 1.0 | 196 | 0.1951 | 0.9240 |
### Framework versions
- Transformers 4.19.2
- Pytorch 1.11.0a0+17540c5
- Datasets 2.2.1
- Tokenizers 0.12.1
| d3a4132fc8e35ba1e7695f6081223839 |
valhalla/distilt5-qg-hl-6-4 | valhalla | t5 | 9 | 3 | transformers | 0 | text2text-generation | true | false | true | mit | null | ['squad'] | null | 0 | 0 | 0 | 0 | 0 | 0 | 0 | ['question-generation', 'distilt5', 'distilt5-qg'] | false | true | true | 2,228 | false |
## DistilT5 for question-generation
This is distilled version of [t5-small-qa-qg-hl](https://huggingface.co/valhalla/t5-small-qa-qg-hl) model trained for answer aware question generation task. The answer spans are highlighted within the text with special highlight tokens.
The model is distilled using the **No Teacher Distillation** method proposed by Huggingface, [here](https://github.com/huggingface/transformers/tree/master/examples/seq2seq#distilbart).
We just copy alternating layers from `t5-small-qa-qg-hl` and finetune more on the same data. Following table lists other distilled models and their metrics.
| Name | BLEU-4 | METEOR | ROUGE-L | QA-EM | QA-F1 |
|---------------------------------------------------------------------------------|---------|---------|---------|--------|--------|
| [distilt5-qg-hl-6-4](https://huggingface.co/valhalla/distilt5-qg-hl-6-4) | 18.4141 | 24.8417 | 40.3435 | - | - |
| [distilt5-qa-qg-hl-6-4](https://huggingface.co/valhalla/distilt5-qa-qg-hl-6-4) | 18.6493 | 24.9685 | 40.5605 | 76.13 | 84.659 |
| [distilt5-qg-hl-12-6](https://huggingface.co/valhalla/distilt5-qg-hl-12-6) | 20.5275 | 26.5010 | 43.2676 | - | - |
| [distilt5-qa-qg-hl-12-6](https://huggingface.co/valhalla/distilt5-qa-qg-hl-12-6)| 20.6109 | 26.4533 | 43.0895 | 81.61 | 89.831 |
You can play with the model using the inference API, just highlight the answer spans with `<hl>` tokens. For example
`<hl> 42 <hl> is the answer to life, the universe and everything.`
For more deatils see [this](https://github.com/patil-suraj/question_generation) repo.
### Model in action 🚀
You'll need to clone the [repo](https://github.com/patil-suraj/question_generation).
[](https://colab.research.google.com/github/patil-suraj/question_generation/blob/master/question_generation.ipynb)
```python3
from pipelines import pipeline
nlp = pipeline("question-generation", model="valhalla/distilt5-qg-hl-6-4")
nlp("42 is the answer to life, universe and everything.")
=> [{'answer': '42', 'question': 'What is the answer to life?'}]
``` | 17eed60579f9de6f015b0ebcf228b69f |
DOOGLAK/Tagged_One_250v7_NER_Model_3Epochs_AUGMENTED | DOOGLAK | bert | 13 | 5 | transformers | 0 | token-classification | true | false | false | apache-2.0 | null | ['tagged_one250v7_wikigold_split'] | null | 0 | 0 | 0 | 0 | 0 | 0 | 0 | ['generated_from_trainer'] | true | true | true | 1,565 | false |
<!-- This model card has been generated automatically according to the information the Trainer had access to. You
should probably proofread and complete it, then remove this comment. -->
# Tagged_One_250v7_NER_Model_3Epochs_AUGMENTED
This model is a fine-tuned version of [bert-base-cased](https://huggingface.co/bert-base-cased) on the tagged_one250v7_wikigold_split dataset.
It achieves the following results on the evaluation set:
- Loss: 0.3809
- Precision: 0.5509
- Recall: 0.4676
- F1: 0.5058
- Accuracy: 0.8894
## Model description
More information needed
## Intended uses & limitations
More information needed
## Training and evaluation data
More information needed
## Training procedure
### Training hyperparameters
The following hyperparameters were used during training:
- learning_rate: 2e-05
- train_batch_size: 8
- eval_batch_size: 8
- seed: 42
- optimizer: Adam with betas=(0.9,0.999) and epsilon=1e-08
- lr_scheduler_type: linear
- num_epochs: 3
### Training results
| Training Loss | Epoch | Step | Validation Loss | Precision | Recall | F1 | Accuracy |
|:-------------:|:-----:|:----:|:---------------:|:---------:|:------:|:------:|:--------:|
| No log | 1.0 | 87 | 0.4450 | 0.1912 | 0.1047 | 0.1353 | 0.8278 |
| No log | 2.0 | 174 | 0.3903 | 0.4992 | 0.4176 | 0.4548 | 0.8820 |
| No log | 3.0 | 261 | 0.3809 | 0.5509 | 0.4676 | 0.5058 | 0.8894 |
### Framework versions
- Transformers 4.17.0
- Pytorch 1.11.0+cu113
- Datasets 2.4.0
- Tokenizers 0.11.6
| b1a8a7a660c4215228222f9d2a1517ff |
RobertLau/cat-toy | RobertLau | null | 21 | 2 | diffusers | 0 | text-to-image | false | false | false | creativeml-openrail-m | null | null | null | 1 | 1 | 0 | 0 | 0 | 0 | 0 | ['text-to-image'] | false | true | true | 1,411 | false | ### Cat toy on Stable Diffusion via Dreambooth
#### model by RobertLau
This your the Stable Diffusion model fine-tuned the Cat toy concept taught to Stable Diffusion with Dreambooth.
It can be used by modifying the `instance_prompt`: **<cat-toy> toy**
You can also train your own concepts and upload them to the library by using [this notebook](https://colab.research.google.com/github/huggingface/notebooks/blob/main/diffusers/sd_dreambooth_training.ipynb).
And you can run your new concept via `diffusers`: [Colab Notebook for Inference](https://colab.research.google.com/github/huggingface/notebooks/blob/main/diffusers/sd_dreambooth_inference.ipynb), [Spaces with the Public Concepts loaded](https://huggingface.co/spaces/sd-dreambooth-library/stable-diffusion-dreambooth-concepts)
#### Usage:
If you want to use this concept, please add <cat-toy> in your prompt, for example: "A <cat-toy> in mad max fury road"
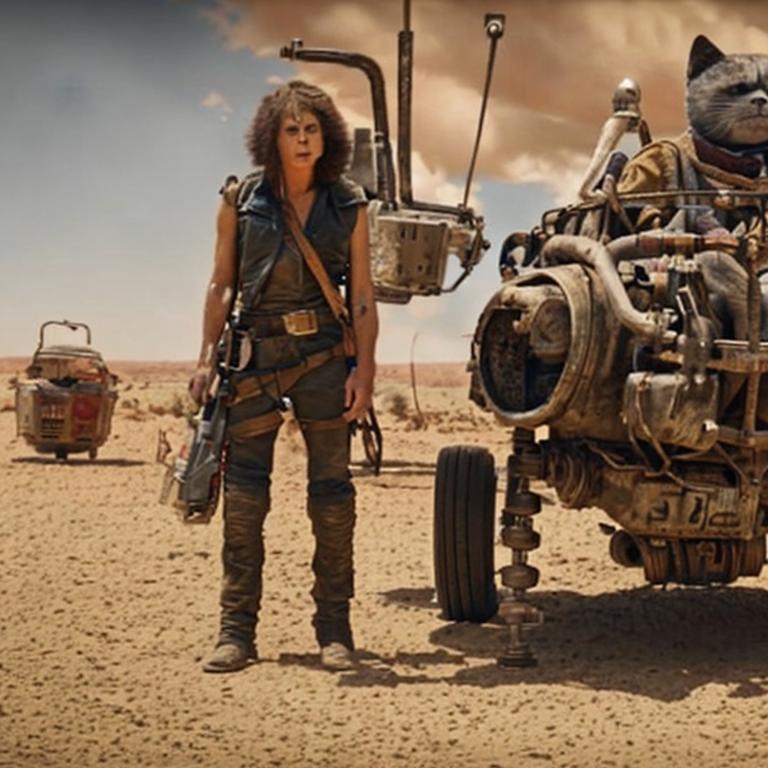
Here are the images used for training this concept:
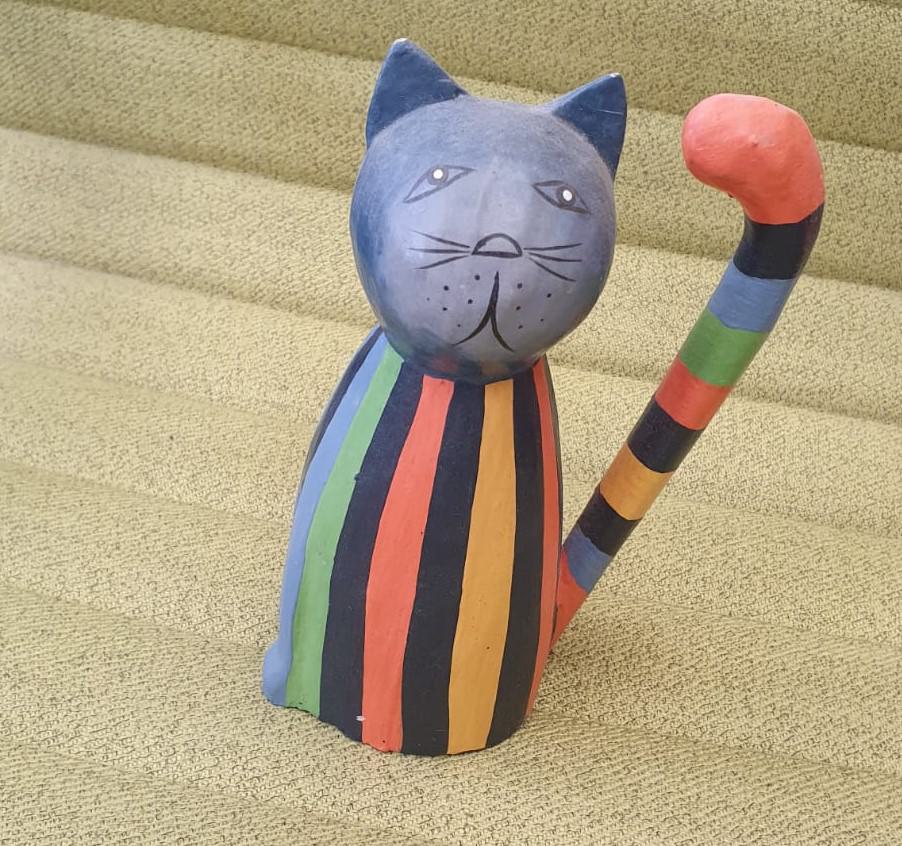
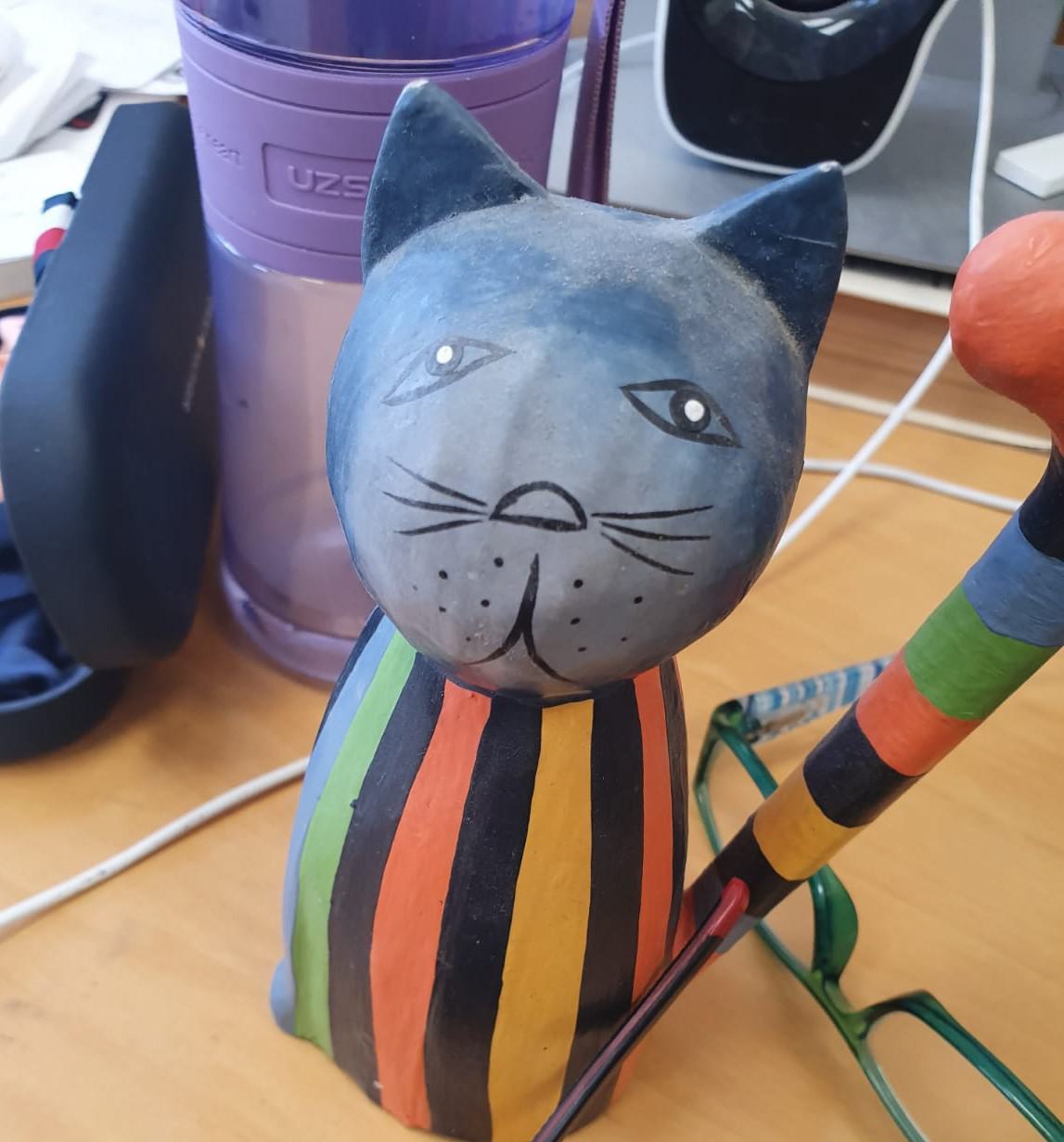
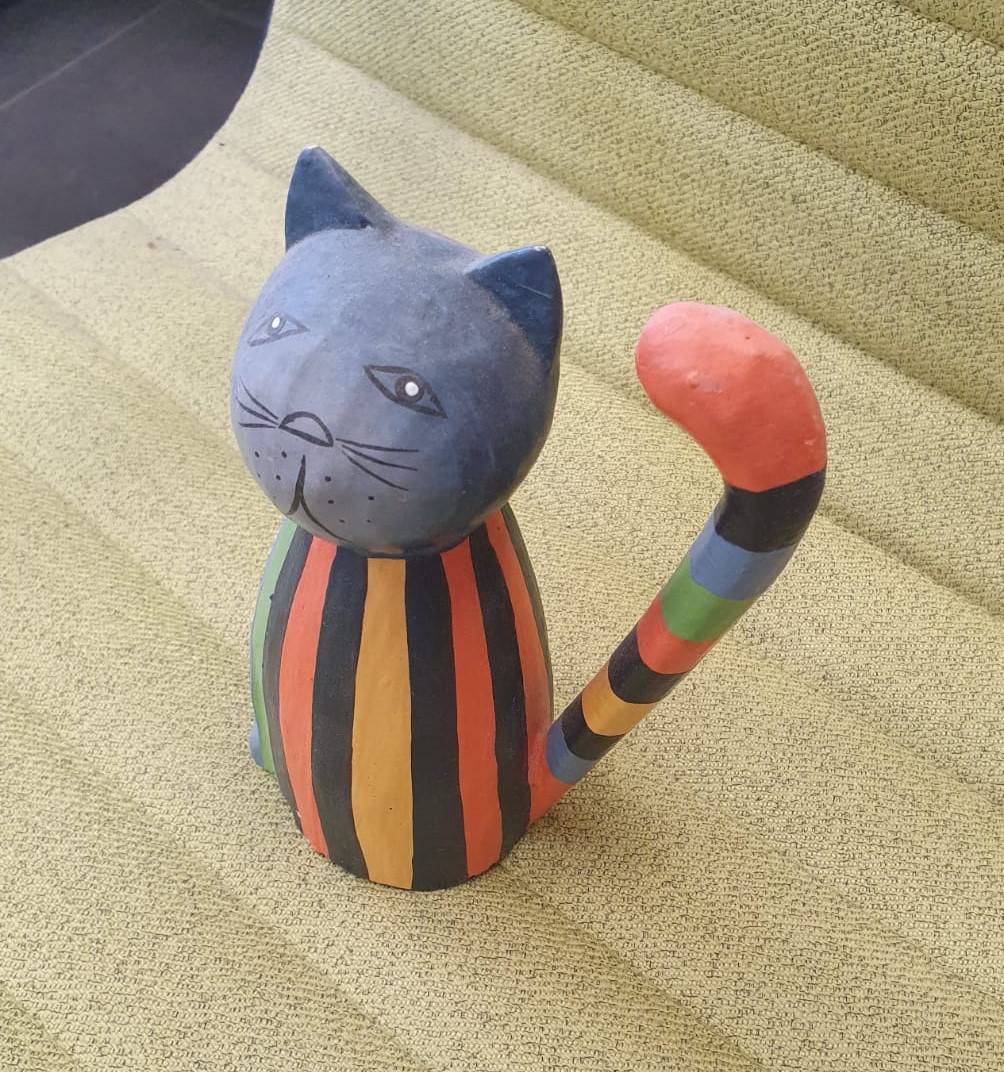
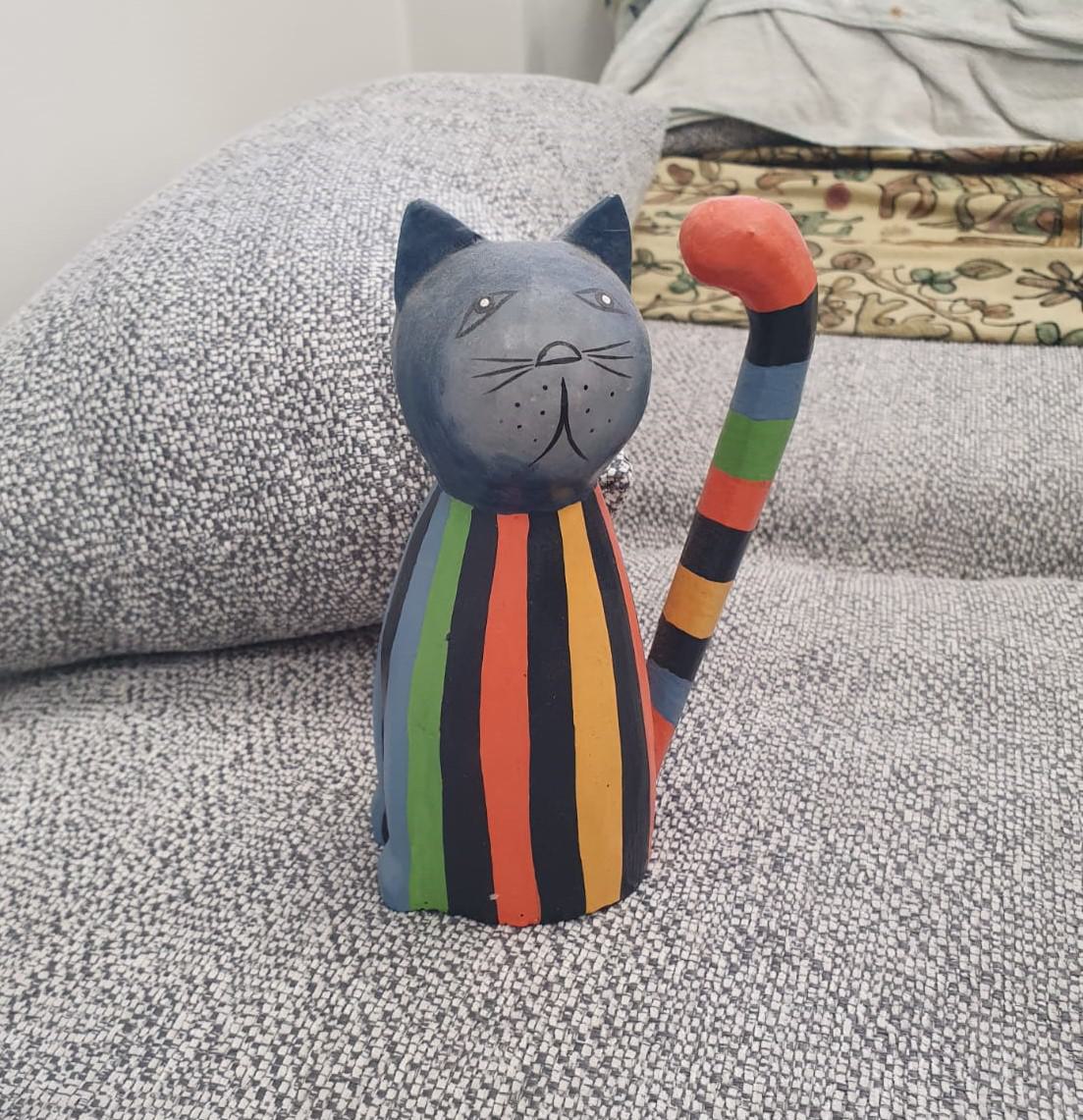
| 521564067fff629500a0ea049ff5ee0d |
Eulaliefy/distilbert-base-uncased-finetuned-ner | Eulaliefy | distilbert | 13 | 3 | transformers | 0 | token-classification | true | false | false | apache-2.0 | null | ['conll2003'] | null | 1 | 1 | 0 | 0 | 0 | 0 | 0 | ['generated_from_trainer'] | true | true | true | 1,555 | false |
<!-- This model card has been generated automatically according to the information the Trainer had access to. You
should probably proofread and complete it, then remove this comment. -->
# distilbert-base-uncased-finetuned-ner
This model is a fine-tuned version of [distilbert-base-uncased](https://huggingface.co/distilbert-base-uncased) on the conll2003 dataset.
It achieves the following results on the evaluation set:
- Loss: 0.0620
- Precision: 0.9251
- Recall: 0.9350
- F1: 0.9300
- Accuracy: 0.9836
## Model description
More information needed
## Intended uses & limitations
More information needed
## Training and evaluation data
More information needed
## Training procedure
### Training hyperparameters
The following hyperparameters were used during training:
- learning_rate: 2e-05
- train_batch_size: 16
- eval_batch_size: 16
- seed: 42
- optimizer: Adam with betas=(0.9,0.999) and epsilon=1e-08
- lr_scheduler_type: linear
- num_epochs: 3
### Training results
| Training Loss | Epoch | Step | Validation Loss | Precision | Recall | F1 | Accuracy |
|:-------------:|:-----:|:----:|:---------------:|:---------:|:------:|:------:|:--------:|
| 0.2356 | 1.0 | 878 | 0.0699 | 0.9110 | 0.9225 | 0.9167 | 0.9801 |
| 0.0509 | 2.0 | 1756 | 0.0621 | 0.9180 | 0.9314 | 0.9246 | 0.9823 |
| 0.0303 | 3.0 | 2634 | 0.0620 | 0.9251 | 0.9350 | 0.9300 | 0.9836 |
### Framework versions
- Transformers 4.19.2
- Pytorch 1.11.0+cu113
- Datasets 2.2.2
- Tokenizers 0.12.1
| 8146d976adb86f5a7a988fa6968bc11f |
tomekkorbak/cocky_carson | tomekkorbak | gpt2 | 36 | 2 | transformers | 0 | null | true | false | false | mit | ['en'] | ['tomekkorbak/pii-pile-chunk3-0-50000', 'tomekkorbak/pii-pile-chunk3-50000-100000', 'tomekkorbak/pii-pile-chunk3-100000-150000', 'tomekkorbak/pii-pile-chunk3-150000-200000', 'tomekkorbak/pii-pile-chunk3-200000-250000', 'tomekkorbak/pii-pile-chunk3-250000-300000', 'tomekkorbak/pii-pile-chunk3-300000-350000', 'tomekkorbak/pii-pile-chunk3-350000-400000', 'tomekkorbak/pii-pile-chunk3-400000-450000', 'tomekkorbak/pii-pile-chunk3-450000-500000', 'tomekkorbak/pii-pile-chunk3-500000-550000', 'tomekkorbak/pii-pile-chunk3-550000-600000', 'tomekkorbak/pii-pile-chunk3-600000-650000', 'tomekkorbak/pii-pile-chunk3-650000-700000', 'tomekkorbak/pii-pile-chunk3-700000-750000', 'tomekkorbak/pii-pile-chunk3-750000-800000', 'tomekkorbak/pii-pile-chunk3-800000-850000', 'tomekkorbak/pii-pile-chunk3-850000-900000', 'tomekkorbak/pii-pile-chunk3-900000-950000', 'tomekkorbak/pii-pile-chunk3-950000-1000000', 'tomekkorbak/pii-pile-chunk3-1000000-1050000', 'tomekkorbak/pii-pile-chunk3-1050000-1100000', 'tomekkorbak/pii-pile-chunk3-1100000-1150000', 'tomekkorbak/pii-pile-chunk3-1150000-1200000', 'tomekkorbak/pii-pile-chunk3-1200000-1250000', 'tomekkorbak/pii-pile-chunk3-1250000-1300000', 'tomekkorbak/pii-pile-chunk3-1300000-1350000', 'tomekkorbak/pii-pile-chunk3-1350000-1400000', 'tomekkorbak/pii-pile-chunk3-1400000-1450000', 'tomekkorbak/pii-pile-chunk3-1450000-1500000', 'tomekkorbak/pii-pile-chunk3-1500000-1550000', 'tomekkorbak/pii-pile-chunk3-1550000-1600000', 'tomekkorbak/pii-pile-chunk3-1600000-1650000', 'tomekkorbak/pii-pile-chunk3-1650000-1700000', 'tomekkorbak/pii-pile-chunk3-1700000-1750000', 'tomekkorbak/pii-pile-chunk3-1750000-1800000', 'tomekkorbak/pii-pile-chunk3-1800000-1850000', 'tomekkorbak/pii-pile-chunk3-1850000-1900000', 'tomekkorbak/pii-pile-chunk3-1900000-1950000'] | null | 0 | 0 | 0 | 0 | 0 | 0 | 0 | ['generated_from_trainer'] | true | true | true | 7,672 | false |
<!-- This model card has been generated automatically according to the information the Trainer had access to. You
should probably proofread and complete it, then remove this comment. -->
# cocky_carson
This model was trained from scratch on the tomekkorbak/pii-pile-chunk3-0-50000, the tomekkorbak/pii-pile-chunk3-50000-100000, the tomekkorbak/pii-pile-chunk3-100000-150000, the tomekkorbak/pii-pile-chunk3-150000-200000, the tomekkorbak/pii-pile-chunk3-200000-250000, the tomekkorbak/pii-pile-chunk3-250000-300000, the tomekkorbak/pii-pile-chunk3-300000-350000, the tomekkorbak/pii-pile-chunk3-350000-400000, the tomekkorbak/pii-pile-chunk3-400000-450000, the tomekkorbak/pii-pile-chunk3-450000-500000, the tomekkorbak/pii-pile-chunk3-500000-550000, the tomekkorbak/pii-pile-chunk3-550000-600000, the tomekkorbak/pii-pile-chunk3-600000-650000, the tomekkorbak/pii-pile-chunk3-650000-700000, the tomekkorbak/pii-pile-chunk3-700000-750000, the tomekkorbak/pii-pile-chunk3-750000-800000, the tomekkorbak/pii-pile-chunk3-800000-850000, the tomekkorbak/pii-pile-chunk3-850000-900000, the tomekkorbak/pii-pile-chunk3-900000-950000, the tomekkorbak/pii-pile-chunk3-950000-1000000, the tomekkorbak/pii-pile-chunk3-1000000-1050000, the tomekkorbak/pii-pile-chunk3-1050000-1100000, the tomekkorbak/pii-pile-chunk3-1100000-1150000, the tomekkorbak/pii-pile-chunk3-1150000-1200000, the tomekkorbak/pii-pile-chunk3-1200000-1250000, the tomekkorbak/pii-pile-chunk3-1250000-1300000, the tomekkorbak/pii-pile-chunk3-1300000-1350000, the tomekkorbak/pii-pile-chunk3-1350000-1400000, the tomekkorbak/pii-pile-chunk3-1400000-1450000, the tomekkorbak/pii-pile-chunk3-1450000-1500000, the tomekkorbak/pii-pile-chunk3-1500000-1550000, the tomekkorbak/pii-pile-chunk3-1550000-1600000, the tomekkorbak/pii-pile-chunk3-1600000-1650000, the tomekkorbak/pii-pile-chunk3-1650000-1700000, the tomekkorbak/pii-pile-chunk3-1700000-1750000, the tomekkorbak/pii-pile-chunk3-1750000-1800000, the tomekkorbak/pii-pile-chunk3-1800000-1850000, the tomekkorbak/pii-pile-chunk3-1850000-1900000 and the tomekkorbak/pii-pile-chunk3-1900000-1950000 datasets.
## Model description
More information needed
## Intended uses & limitations
More information needed
## Training and evaluation data
More information needed
## Training procedure
### Training hyperparameters
The following hyperparameters were used during training:
- learning_rate: 0.0005
- train_batch_size: 16
- eval_batch_size: 8
- seed: 42
- gradient_accumulation_steps: 4
- total_train_batch_size: 64
- optimizer: Adam with betas=(0.9,0.999) and epsilon=1e-08
- lr_scheduler_type: linear
- lr_scheduler_warmup_ratio: 0.01
- training_steps: 50354
- mixed_precision_training: Native AMP
### Framework versions
- Transformers 4.24.0
- Pytorch 1.11.0+cu113
- Datasets 2.5.1
- Tokenizers 0.11.6
# Full config
{'dataset': {'datasets': ['tomekkorbak/pii-pile-chunk3-0-50000',
'tomekkorbak/pii-pile-chunk3-50000-100000',
'tomekkorbak/pii-pile-chunk3-100000-150000',
'tomekkorbak/pii-pile-chunk3-150000-200000',
'tomekkorbak/pii-pile-chunk3-200000-250000',
'tomekkorbak/pii-pile-chunk3-250000-300000',
'tomekkorbak/pii-pile-chunk3-300000-350000',
'tomekkorbak/pii-pile-chunk3-350000-400000',
'tomekkorbak/pii-pile-chunk3-400000-450000',
'tomekkorbak/pii-pile-chunk3-450000-500000',
'tomekkorbak/pii-pile-chunk3-500000-550000',
'tomekkorbak/pii-pile-chunk3-550000-600000',
'tomekkorbak/pii-pile-chunk3-600000-650000',
'tomekkorbak/pii-pile-chunk3-650000-700000',
'tomekkorbak/pii-pile-chunk3-700000-750000',
'tomekkorbak/pii-pile-chunk3-750000-800000',
'tomekkorbak/pii-pile-chunk3-800000-850000',
'tomekkorbak/pii-pile-chunk3-850000-900000',
'tomekkorbak/pii-pile-chunk3-900000-950000',
'tomekkorbak/pii-pile-chunk3-950000-1000000',
'tomekkorbak/pii-pile-chunk3-1000000-1050000',
'tomekkorbak/pii-pile-chunk3-1050000-1100000',
'tomekkorbak/pii-pile-chunk3-1100000-1150000',
'tomekkorbak/pii-pile-chunk3-1150000-1200000',
'tomekkorbak/pii-pile-chunk3-1200000-1250000',
'tomekkorbak/pii-pile-chunk3-1250000-1300000',
'tomekkorbak/pii-pile-chunk3-1300000-1350000',
'tomekkorbak/pii-pile-chunk3-1350000-1400000',
'tomekkorbak/pii-pile-chunk3-1400000-1450000',
'tomekkorbak/pii-pile-chunk3-1450000-1500000',
'tomekkorbak/pii-pile-chunk3-1500000-1550000',
'tomekkorbak/pii-pile-chunk3-1550000-1600000',
'tomekkorbak/pii-pile-chunk3-1600000-1650000',
'tomekkorbak/pii-pile-chunk3-1650000-1700000',
'tomekkorbak/pii-pile-chunk3-1700000-1750000',
'tomekkorbak/pii-pile-chunk3-1750000-1800000',
'tomekkorbak/pii-pile-chunk3-1800000-1850000',
'tomekkorbak/pii-pile-chunk3-1850000-1900000',
'tomekkorbak/pii-pile-chunk3-1900000-1950000'],
'filter_threshold': 0.000286,
'is_split_by_sentences': True},
'generation': {'force_call_on': [25177],
'metrics_configs': [{}, {'n': 1}, {'n': 2}, {'n': 5}],
'scenario_configs': [{'generate_kwargs': {'do_sample': True,
'max_length': 128,
'min_length': 10,
'temperature': 0.7,
'top_k': 0,
'top_p': 0.9},
'name': 'unconditional',
'num_samples': 2048}],
'scorer_config': {}},
'kl_gpt3_callback': {'force_call_on': [25177],
'max_tokens': 64,
'num_samples': 4096},
'model': {'from_scratch': True,
'gpt2_config_kwargs': {'reorder_and_upcast_attn': True,
'scale_attn_by': True},
'path_or_name': 'gpt2'},
'objective': {'name': 'MLE'},
'tokenizer': {'path_or_name': 'gpt2'},
'training': {'dataloader_num_workers': 0,
'effective_batch_size': 64,
'evaluation_strategy': 'no',
'fp16': True,
'hub_model_id': 'cocky_carson',
'hub_strategy': 'all_checkpoints',
'learning_rate': 0.0005,
'logging_first_step': True,
'logging_steps': 1,
'num_tokens': 3300000000,
'output_dir': 'training_output2',
'per_device_train_batch_size': 16,
'push_to_hub': True,
'remove_unused_columns': False,
'save_steps': 25177,
'save_strategy': 'steps',
'seed': 42,
'warmup_ratio': 0.01,
'weight_decay': 0.1}}
# Wandb URL:
https://wandb.ai/tomekkorbak/apo/runs/2y0u35mu | ca8c2dbdbcc08f1b9b2154d140c83ea5 |
jiobiala24/wav2vec2-base-checkpoint-5 | jiobiala24 | wav2vec2 | 13 | 8 | transformers | 0 | automatic-speech-recognition | true | false | false | apache-2.0 | null | ['common_voice'] | null | 0 | 0 | 0 | 0 | 0 | 0 | 0 | ['generated_from_trainer'] | true | true | true | 2,172 | false |
<!-- This model card has been generated automatically according to the information the Trainer had access to. You
should probably proofread and complete it, then remove this comment. -->
# wav2vec2-base-checkpoint-5
This model is a fine-tuned version of [jiobiala24/wav2vec2-base-checkpoint-4](https://huggingface.co/jiobiala24/wav2vec2-base-checkpoint-4) on the common_voice dataset.
It achieves the following results on the evaluation set:
- Loss: 0.9849
- Wer: 0.3354
## Model description
More information needed
## Intended uses & limitations
More information needed
## Training and evaluation data
More information needed
## Training procedure
### Training hyperparameters
The following hyperparameters were used during training:
- learning_rate: 0.0001
- train_batch_size: 32
- eval_batch_size: 8
- seed: 42
- optimizer: Adam with betas=(0.9,0.999) and epsilon=1e-08
- lr_scheduler_type: linear
- lr_scheduler_warmup_steps: 1000
- num_epochs: 30
- mixed_precision_training: Native AMP
### Training results
| Training Loss | Epoch | Step | Validation Loss | Wer |
|:-------------:|:-----:|:-----:|:---------------:|:------:|
| 0.3947 | 1.96 | 1000 | 0.5749 | 0.3597 |
| 0.2856 | 3.93 | 2000 | 0.6212 | 0.3479 |
| 0.221 | 5.89 | 3000 | 0.6280 | 0.3502 |
| 0.1755 | 7.86 | 4000 | 0.6517 | 0.3526 |
| 0.1452 | 9.82 | 5000 | 0.7115 | 0.3481 |
| 0.1256 | 11.79 | 6000 | 0.7687 | 0.3509 |
| 0.1117 | 13.75 | 7000 | 0.7785 | 0.3490 |
| 0.0983 | 15.72 | 8000 | 0.8115 | 0.3442 |
| 0.0877 | 17.68 | 9000 | 0.8290 | 0.3429 |
| 0.0799 | 19.65 | 10000 | 0.8517 | 0.3412 |
| 0.0733 | 21.61 | 11000 | 0.9370 | 0.3448 |
| 0.066 | 23.58 | 12000 | 0.9157 | 0.3410 |
| 0.0623 | 25.54 | 13000 | 0.9673 | 0.3377 |
| 0.0583 | 27.5 | 14000 | 0.9804 | 0.3348 |
| 0.0544 | 29.47 | 15000 | 0.9849 | 0.3354 |
### Framework versions
- Transformers 4.11.3
- Pytorch 1.10.0+cu111
- Datasets 1.13.3
- Tokenizers 0.10.3
| 9cafa93925e28b94724f3c5e15fb25b2 |
gokuls/distilbert_add_GLUE_Experiment_logit_kd_qnli_192 | gokuls | distilbert | 17 | 2 | transformers | 0 | text-classification | true | false | false | apache-2.0 | ['en'] | ['glue'] | null | 0 | 0 | 0 | 0 | 0 | 0 | 0 | ['generated_from_trainer'] | true | true | true | 1,752 | false |
<!-- This model card has been generated automatically according to the information the Trainer had access to. You
should probably proofread and complete it, then remove this comment. -->
# distilbert_add_GLUE_Experiment_logit_kd_qnli_192
This model is a fine-tuned version of [distilbert-base-uncased](https://huggingface.co/distilbert-base-uncased) on the GLUE QNLI dataset.
It achieves the following results on the evaluation set:
- Loss: 0.3981
- Accuracy: 0.5830
## Model description
More information needed
## Intended uses & limitations
More information needed
## Training and evaluation data
More information needed
## Training procedure
### Training hyperparameters
The following hyperparameters were used during training:
- learning_rate: 5e-05
- train_batch_size: 256
- eval_batch_size: 256
- seed: 10
- distributed_type: multi-GPU
- optimizer: Adam with betas=(0.9,0.999) and epsilon=1e-08
- lr_scheduler_type: linear
- num_epochs: 50
- mixed_precision_training: Native AMP
### Training results
| Training Loss | Epoch | Step | Validation Loss | Accuracy |
|:-------------:|:-----:|:----:|:---------------:|:--------:|
| 0.4154 | 1.0 | 410 | 0.4115 | 0.5054 |
| 0.4103 | 2.0 | 820 | 0.4001 | 0.5826 |
| 0.3967 | 3.0 | 1230 | 0.3981 | 0.5830 |
| 0.3897 | 4.0 | 1640 | 0.3995 | 0.5942 |
| 0.3849 | 5.0 | 2050 | 0.4017 | 0.5885 |
| 0.3804 | 6.0 | 2460 | 0.4072 | 0.5836 |
| 0.3763 | 7.0 | 2870 | 0.4096 | 0.5751 |
| 0.3717 | 8.0 | 3280 | 0.4092 | 0.5773 |
### Framework versions
- Transformers 4.26.0
- Pytorch 1.14.0a0+410ce96
- Datasets 2.9.0
- Tokenizers 0.13.2
| 333d695d91864aedb2aec08d94dc497a |
kit-nlp/transformers-ud-japanese-electra-base-discriminator-cyberbullying | kit-nlp | electra | 8 | 17 | transformers | 1 | text-classification | true | false | false | cc-by-sa-4.0 | ['ja'] | null | null | 0 | 0 | 0 | 0 | 0 | 0 | 0 | [] | false | true | true | 1,298 | false |
# electra-base-cyberbullying
This is an [ELECTRA](https://github.com/google-research/electra) Base model for the Japanese language finetuned for automatic cyberbullying detection.
The model was based on [Megagon Labs ELECTRA Base](https://huggingface.co/megagonlabs/transformers-ud-japanese-electra-base-discriminator), and later finetuned on a balanced dataset created by unifying two datasets, namely "Harmful BBS Japanese comments dataset" and "Twitter Japanese cyberbullying dataset".
## Licenses
The finetuned model with all attached files is licensed under [CC BY-SA 4.0](http://creativecommons.org/licenses/by-sa/4.0/), or Creative Commons Attribution-ShareAlike 4.0 International License.
<a rel="license" href="http://creativecommons.org/licenses/by-sa/4.0/"><img alt="Creative Commons License" style="border-width:0" src="https://i.creativecommons.org/l/by-sa/4.0/88x31.png" /></a>
## Citations
Please, cite this model using the following citation.
```
@inproceedings{tanabe2022electra-base-cyberbullying,
title={北見工業大学 テキスト情報処理研究室 ELECTRA Base ネットいじめ検出モデル},
author={田邊 威裕 and プタシンスキ ミハウ and エロネン ユーソ and 桝井 文人},
publisher={HuggingFace},
year={2022},
url = "https://huggingface.co/kit-nlp/transformers-ud-japanese-electra-base-discriminator-cyberbullying"
}
```
| bc4a9918d8403eadec5e7ea732d0e602 |
Subsets and Splits