Commit
·
457fc41
1
Parent(s):
717e0f1
Update parquet files (step 36 of 249)
Browse filesThis view is limited to 50 files because it contains too many changes.
See raw diff
- spaces/1acneusushi/gradio-2dmoleculeeditor/data/Graphisoft ArchiCAD 16 Build 3006 X64 Crack Goodies Learn How to Use the Software and Enhance Your Projects.md +0 -141
- spaces/1gistliPinn/ChatGPT4/Examples/Download !LINK! Ebook Cooperative Learning Anita Lie.md +0 -28
- spaces/1pelhydcardo/ChatGPT-prompt-generator/assets/2020 O L English Paper Pdf Download.md +0 -77
- spaces/1pelhydcardo/ChatGPT-prompt-generator/assets/Adobe Illustrator CC 2019 Create Amazing Vector Art and Illustrations.md +0 -106
- spaces/1phancelerku/anime-remove-background/Baixe o FIFA Mobile Dinheiro Infinito APK e jogue com os melhores times do mundo.md +0 -87
- spaces/2023Liu2023/bingo/src/components/turn-counter.tsx +0 -23
- spaces/4Taps/SadTalker/src/audio2pose_models/res_unet.py +0 -65
- spaces/801artistry/RVC801/utils/dependency.py +0 -170
- spaces/AIConsultant/MusicGen/tests/modules/__init__.py +0 -5
- spaces/AIWaves/SOP_Generation-single/LLM/__init__.py +0 -0
- spaces/AMR-KELEG/ALDi/README.md +0 -13
- spaces/ASJMO/freegpt/client/css/field.css +0 -11
- spaces/AchyuthGamer/OpenGPT/g4f/Provider/Providers/Zeabur.py +0 -50
- spaces/Adapter/T2I-Adapter/ldm/modules/extra_condition/openpose/hand.py +0 -77
- spaces/AgentVerse/agentVerse/agentverse/llms/openai.py +0 -346
- spaces/AgentVerse/agentVerse/ui/src/phaser3-rex-plugins/plugins/filechooser.js +0 -3
- spaces/AgentVerse/agentVerse/ui/src/phaser3-rex-plugins/templates/ui/gridbuttons/AddChildMethods.js +0 -18
- spaces/Aki004/herta-so-vits/modules/mel_processing.py +0 -112
- spaces/Amrrs/DragGan-Inversion/stylegan_human/torch_utils/ops/bias_act.cpp +0 -101
- spaces/Androidonnxfork/CivitAi-to-Diffusers/diffusers/docs/source/ko/optimization/opt_overview.md +0 -17
- spaces/Androidonnxfork/CivitAi-to-Diffusers/diffusers/src/diffusers/pipelines/shap_e/renderer.py +0 -1050
- spaces/Andy1621/uniformer_image_detection/configs/foveabox/fovea_align_r101_fpn_gn-head_4x4_2x_coco.py +0 -10
- spaces/AnishKumbhar/ChatBot/text-generation-webui-main/extensions/ngrok/script.py +0 -36
- spaces/AnishKumbhar/ChatBot/text-generation-webui-main/modules/models.py +0 -401
- spaces/Anonymous-sub/Rerender/app.py +0 -997
- spaces/Anonymous-sub/Rerender/gmflow_module/scripts/submission.sh +0 -67
- spaces/Ariharasudhan/YoloV5/utils/loggers/wandb/README.md +0 -162
- spaces/Arnaudding001/OpenAI_whisperLive/segments_test.py +0 -48
- spaces/Arulkumar03/GroundingDINO_SOTA_Zero_Shot_Model/groundingdino/util/box_ops.py +0 -140
- spaces/Ataturk-Chatbot/HuggingFaceChat/venv/lib/python3.11/site-packages/pip/_vendor/rich/_windows_renderer.py +0 -56
- spaces/Ataturk-Chatbot/HuggingFaceChat/venv/lib/python3.11/site-packages/setuptools/config/_validate_pyproject/formats.py +0 -259
- spaces/Awiny/Image2Paragraph/models/grit_src/third_party/CenterNet2/detectron2/layers/csrc/nms_rotated/nms_rotated.h +0 -39
- spaces/Awiny/Image2Paragraph/models/grit_src/third_party/CenterNet2/detectron2/modeling/meta_arch/dense_detector.py +0 -282
- spaces/Awiny/Image2Paragraph/models/grit_src/third_party/CenterNet2/detectron2/modeling/roi_heads/mask_head.py +0 -292
- spaces/Awiny/Image2Paragraph/models/grit_src/third_party/CenterNet2/detectron2/utils/serialize.py +0 -32
- spaces/Awiny/Image2Paragraph/models/grit_src/third_party/CenterNet2/dev/packaging/build_wheel.sh +0 -31
- spaces/Bala2-03-2003/AIBALA/README.md +0 -12
- spaces/Benson/text-generation/Examples/Carx Street 3 Apk.md +0 -105
- spaces/Benson/text-generation/Examples/Descarga Gratuita De Club Gacha Ipad.md +0 -76
- spaces/Big-Web/MMSD/env/Lib/site-packages/pip/_internal/commands/configuration.py +0 -282
- spaces/Big-Web/MMSD/env/Lib/site-packages/pip/_internal/index/__init__.py +0 -2
- spaces/Big-Web/MMSD/env/Lib/site-packages/s3transfer/processpool.py +0 -1008
- spaces/BlitzenPrancer/TheBloke-guanaco-65B-HF/app.py +0 -3
- spaces/CVPR/Dual-Key_Backdoor_Attacks/datagen/detectron2/detectron2/layers/roi_align.py +0 -105
- spaces/CVPR/WALT/mmdet/utils/contextmanagers.py +0 -121
- spaces/CVPR/transfiner/configs/common/data/coco_keypoint.py +0 -13
- spaces/Chris1/real2sim/README.md +0 -13
- spaces/CobaltZvc/sherlocks_pheonix/index.html +0 -29
- spaces/CompVis/celeba-latent-diffusion/app.py +0 -26
- spaces/DAMO-NLP-SG/Video-LLaMA/video_llama/tasks/video_text_pretrain.py +0 -18
spaces/1acneusushi/gradio-2dmoleculeeditor/data/Graphisoft ArchiCAD 16 Build 3006 X64 Crack Goodies Learn How to Use the Software and Enhance Your Projects.md
DELETED
@@ -1,141 +0,0 @@
|
|
1 |
-
<br />
|
2 |
-
<h1>Graphisoft ArchiCAD 16 Build 3006 X64 Crack Goodies: A Comprehensive Guide</h1>
|
3 |
-
<p>If you are looking for a way to enhance your architectural design and modeling experience with Graphisoft ArchiCAD 16, you might be interested in downloading and installing the crack goodies for this software. In this article, we will explain what Graphisoft ArchiCAD 16 is, what are the goodies for it, how to download and install them, and how to use them effectively. We will also answer some of the frequently asked questions about the crack goodies. By the end of this article, you will have a clear understanding of what Graphisoft ArchiCAD 16 Build 3006 X64 Crack Goodies are and how to use them.</p>
|
4 |
-
<h2>What is Graphisoft ArchiCAD 16?</h2>
|
5 |
-
<h3>A brief introduction to ArchiCAD</h3>
|
6 |
-
<p>Archicad is a software developed by Graphisoft that allows architects, designers, engineers, and builders to create and manage building information models (BIM) in a virtual environment. Archicad enables users to design, document, visualize, analyze, collaborate, and simulate building projects from concept to construction. Archicad supports various file formats, such as DWG, DXF, IFC, PDF, SKP, OBJ, STL, etc., and integrates with other software tools, such as AutoCAD, Revit, SketchUp, Rhino, Grasshopper, etc.</p>
|
7 |
-
<h2>Graphisoft ArchiCAD 16 Build 3006 X64 Crack Goodies</h2><br /><p><b><b>Download</b> ► <a href="https://byltly.com/2uKyTu">https://byltly.com/2uKyTu</a></b></p><br /><br />
|
8 |
-
<h3>The features and benefits of ArchiCAD 16</h3>
|
9 |
-
<p>Archicad 16 is the latest version of Archicad that was released in June 2012. It introduces several new features and improvements that make it more powerful, flexible, and user-friendly than previous versions. Some of the main features and benefits of Archicad 16 are:</p>
|
10 |
-
<ul>
|
11 |
-
<li><b>Morph Tool:</b> This tool allows users to create free-form elements with any shape and geometry without any limitations. Users can edit, sculpt, reshape, merge, subtract, or intersect morph elements with other elements or surfaces.</li>
|
12 |
-
<li><b>BIM Components:</b> This feature enables users to access a large library of parametric objects that can be customized and modified according to their needs. Users can also create their own objects or import them from other sources.</li>
|
13 |
-
<li><b>Built-in Energy Evaluation:</b> This feature allows users to perform energy analysis and optimization on their building models based on various criteria, such as climate data, building materials, orientation, insulation, ventilation, etc.</li>
|
14 |
-
<li><b>Improved Teamwork:</b> This feature enhances the collaboration and communication among team members working on the same project. Users can share their models with other users via BIM Server or BIM Cloud services.</li>
|
15 |
-
<li><b>Improved Performance:</b> This feature improves the speed and stability of Archicad by optimizing its memory usage, graphics engine, file management system, etc.</li>
|
16 |
-
</ul>
|
17 |
-
<h2>What are the goodies for Archicad 16?</h2>
|
18 |
-
<h3>The definition and purpose of goodies</h3>
|
19 |
-
<p>Goodies are additional tools or add-ons that extend the functionality of Archicad. They are developed by Graphisoft or third-party developers to provide users with more options and features that are not included in the standard version of Archicad. Goodies can be downloaded from Graphisoft's website or other sources for free or for a fee.</p>
|
20 |
-
<h3>The types and examples of goodies for Archicad 16</h3>
|
21 |
-
<p>There are various types of goodies for Archicad 16 that serve different purposes and functions. Some of the most popular and useful goodies for Archicad 16 are:</p>
|
22 |
-
<ul>
|
23 |
-
<li><b>Accessories:</b> These are objects that can be placed in the model to enhance its appearance or functionality. Examples of accessories are doors, windows, furniture, lamps, plants, etc.</li>
|
24 |
-
<li><b>Add-Ons:</b> These are programs that run within Archicad or as separate applications that add new features or capabilities to Archicad. Examples of add-ons are MEP Modeler (for mechanical, electrical, and plumbing systems), EcoDesigner (for environmental analysis), Cinema4D (for rendering), etc.</li>
|
25 |
-
<li><b>CADImage Tools:</b> These are tools that improve the productivity and efficiency of users by automating certain tasks or providing shortcuts. Examples of CADImage tools are Detail Elements (for creating detailed drawings), Keynotes (for adding notes and labels), Master Script (for scripting commands), etc.</li>
|
26 |
-
<li><b>GDL Objects:</b> These are parametric objects that can be created or modified using GDL (Geometric Description Language), a scripting language specific to Archicad. Examples of GDL objects are stairs, roofs, railings, columns, beams, etc.</li>
|
27 |
-
<li><b>Libraries:</b> These are collections of objects that can be used in the model. Examples of libraries are standard parts (such as bolts, nuts, screws), symbols (such as electrical outlets), textures (such as wood grains), etc.</li>
|
28 |
-
<li><b>Patches:</b> These are updates or fixes that improve the performance or stability of Archicad or resolve some issues or bugs. Examples of patches are hotfixes (for minor issues), service packs (for major issues), updates (for new features).</li>
|
29 |
-
</ul>
|
30 |
-
<h2>How to download and install Graphisoft ArchiCAD 16 Build 3006 X64 Crack Goodies?</h2>
|
31 |
-
<h3>The requirements and precautions for downloading and installing the crack goodies</h3>
|
32 |
-
<p>Before downloading and installing the crack goodies for Archicad 16 Build 3006 X64, you need to make sure that you have the following requirements:</p>
|
33 |
-
<ul>
|
34 |
-
<li>A computer running Windows XP/Vista/7/8/10 with a 64-bit processor.</li>
|
35 |
-
<li>A licensed copy of Graphisoft ArchiCAD 16 Build 3006 X64 installed on your computer.</li>
|
36 |
-
<li>A reliable internet connection.</li>
|
37 |
-
<li>A backup of your important files and data.</li>
|
38 |
-
</ul>
|
39 |
-
<p>You also need to be aware of some precautions when downloading and installing the crack goodies:</p>
|
40 |
-
<ul>
|
41 |
-
<li>The crack goodies are not official products from Graphisoft or endorsed by them. They may contain viruses, malware, spyware, or other harmful components that may damage your computer or compromise your security.</li>
|
42 |
-
<li>The crack goodies may not work properly with your version of ArchiCAD or cause conflicts with other programs or devices on your computer.</li>
|
43 |
-
<li>The crack goodies may violate the terms and conditions of your license agreement with Graphisoft or infringe on their intellectual property rights. You may face legal consequences if you use them without permission.</li>
|
44 |
-
</ul>
|
45 |
-
<p>Therefore, you should download and install the crack goodies at your own risk and discretion. We do not take any responsibility for any problems or damages that may occur as a result of using them.</p>
|
46 |
-
<h3>The step-by-step instructions for downloading and installing the crack goodies</h3>
|
47 |
-
<p>If you have decided to download and install the crack goodies for ArchiCAD 16 Build 3006 X64, you can follow these steps:</p>
|
48 |
-
<p>Graphisoft ArchiCAD 16 X64 Crack Download<br />
|
49 |
-
ArchiCAD 16 Build 3006 64 Bit Crack Free<br />
|
50 |
-
Graphisoft ArchiCAD 16 Crack Goodies Torrent<br />
|
51 |
-
ArchiCAD 16 X64 Crack Goodies NPM<br />
|
52 |
-
Graphisoft ArchiCAD 16 Build 3006 Crack Zip<br />
|
53 |
-
ArchiCAD 16 64 Bit Crack Goodies Download<br />
|
54 |
-
Graphisoft ArchiCAD 16 X64 Crack Goodies Opensea<br />
|
55 |
-
ArchiCAD 16 Build 3006 Crack Free Download<br />
|
56 |
-
Graphisoft ArchiCAD 16 Crack Goodies Clubitup<br />
|
57 |
-
ArchiCAD 16 X64 Crack Goodies Libraries.io<br />
|
58 |
-
Graphisoft ArchiCAD 16 Build 3006 X64 Keygen<br />
|
59 |
-
ArchiCAD 16 64 Bit Keygen Goodies Free<br />
|
60 |
-
Graphisoft ArchiCAD 16 Keygen Goodies Download<br />
|
61 |
-
ArchiCAD 16 X64 Keygen Goodies NPM<br />
|
62 |
-
Graphisoft ArchiCAD 16 Build 3006 Keygen Zip<br />
|
63 |
-
ArchiCAD 16 64 Bit Keygen Goodies Torrent<br />
|
64 |
-
Graphisoft ArchiCAD 16 X64 Keygen Goodies Opensea<br />
|
65 |
-
ArchiCAD 16 Build 3006 Keygen Free Download<br />
|
66 |
-
Graphisoft ArchiCAD 16 Keygen Goodies Clubitup<br />
|
67 |
-
ArchiCAD 16 X64 Keygen Goodies Libraries.io<br />
|
68 |
-
Graphisoft ArchiCAD 16 Build 3006 X64 Serial Number<br />
|
69 |
-
ArchiCAD 16 64 Bit Serial Number Goodies Free<br />
|
70 |
-
Graphisoft ArchiCAD 16 Serial Number Goodies Download<br />
|
71 |
-
ArchiCAD 16 X64 Serial Number Goodies NPM<br />
|
72 |
-
Graphisoft ArchiCAD 16 Build 3006 Serial Number Zip<br />
|
73 |
-
ArchiCAD 16 64 Bit Serial Number Goodies Torrent<br />
|
74 |
-
Graphisoft ArchiCAD 16 X64 Serial Number Goodies Opensea<br />
|
75 |
-
ArchiCAD 16 Build 3006 Serial Number Free Download<br />
|
76 |
-
Graphisoft ArchiCAD 16 Serial Number Goodies Clubitup<br />
|
77 |
-
ArchiCAD 16 X64 Serial Number Goodies Libraries.io<br />
|
78 |
-
Graphisoft ArchiCAD 16 Build 3006 X64 License Key<br />
|
79 |
-
ArchiCAD 16 64 Bit License Key Goodies Free<br />
|
80 |
-
Graphisoft ArchiCAD 16 License Key Goodies Download<br />
|
81 |
-
ArchiCAD 16 X64 License Key Goodies NPM<br />
|
82 |
-
Graphisoft ArchiCAD 16 Build 3006 License Key Zip<br />
|
83 |
-
ArchiCAD 16 64 Bit License Key Goodies Torrent<br />
|
84 |
-
Graphisoft ArchiCAD 16 X64 License Key Goodies Opensea<br />
|
85 |
-
ArchiCAD 16 Build 3006 License Key Free Download<br />
|
86 |
-
Graphisoft ArchiCAD 16 License Key Goodies Clubitup<br />
|
87 |
-
ArchiCAD 16 X64 License Key Goodies Libraries.io<br />
|
88 |
-
Graphisoft ArchiCAD 16 Build 3006 X64 Activation Code<br />
|
89 |
-
ArchiCAD</p>
|
90 |
-
<ol>
|
91 |
-
<li>Go to <a href="https://trello.com/c/uOp5fFN2/11-graphisoft-archicad-16-build-3006-x64-crack-goodies">this link</a>, which is one of the sources where you can find the crack goodies.</li>
|
92 |
-
<li>Select one of the download links provided on the page. You may need to complete some surveys or offers before you can access the download link.</li>
|
93 |
-
<li>Download the zip file containing the crack goodies to your computer.</li>
|
94 |
-
<li>Extract the zip file using a program that can open zip files, such as WinZip, WinRAR, 7-Zip, etc.</li>
|
95 |
-
<li>Open the extracted folder and run the setup.exe file to install the crack goodies on your computer.</li>
|
96 |
-
<li>Follow the on-screen instructions to complete the installation process.</li>
|
97 |
-
<li>Restart your computer if prompted.</li>
|
98 |
-
</ol>
|
99 |
-
<p>Congratulations! You have successfully downloaded and installed the crack goodies for ArchiCAD 16 Build 3006 X64 on your computer.</p>
|
100 |
-
<h2>How to use Graphisoft ArchiCAD 16 Build 3006 X64 Crack Goodies?</h2>
|
101 |
-
<h3>The tips and tricks for using the crack goodies effectively</h3>
|
102 |
-
<p>Now that you have installed the crack goodies for ArchiCAD 16 Build 3006 X64, you can start using them to enhance your ArchiCAD experience. Here are some tips and tricks for using the crack goodies effectively:</p>
|
103 |
-
<ul>
|
104 |
-
<li>Make sure you have a valid license for ArchiCAD 16 before using the crack goodies. The crack goodies are not meant to bypass the license verification or activation process of ArchiCAD. They are only meant to provide additional features and functions that are not available in the standard version of ArchiCAD.</li>
|
105 |
-
<li>Check the compatibility and requirements of each crack goodie before using it. Some crack goodies may not work with certain versions or editions of ArchiCAD or Windows. Some crack goodies may also require additional software or hardware components to function properly.</li>
|
106 |
-
<li>Read the documentation and instructions of each crack goodie carefully before using it. Some crack goodies may have specific settings or options that need to be configured or adjusted before using them. Some crack goodies may also have limitations or restrictions that need to be considered or respected when using them.</li>
|
107 |
-
<li>Use the crack goodies wisely and responsibly. Do not use the crack goodies for illegal or unethical purposes, such as pirating, hacking, cheating, etc. Do not use the crack goodies to harm or damage your computer or other people's computers or data. Do not use the crack goodies to violate or infringe on Graphisoft's or other parties' intellectual property rights or terms and conditions.</li>
|
108 |
-
</ul>
|
109 |
-
<h3>The common problems and solutions for using the crack goodies</h3>
|
110 |
-
<p>While using the crack goodies for ArchiCAD 16 Build 3006 X64, you may encounter some problems or issues that may affect your ArchiCAD performance or functionality. Here are some of the common problems and solutions for using the crack goodies:</p>
|
111 |
-
<ul>
|
112 |
-
<li><b>Problem:</b> The crack goodies do not work or show up in ArchiCAD.</li>
|
113 |
-
<li><b>Solution:</b> Make sure you have installed the crack goodies correctly and completely. Make sure you have restarted your computer after installing the crack goodies. Make sure you have run ArchiCAD as an administrator. Make sure you have enabled or activated the crack goodies in ArchiCAD's settings or preferences.</li>
|
114 |
-
<li><b>Problem:</b> The crack goodies cause errors or crashes in ArchiCAD.</li>
|
115 |
-
<li><b>Solution:</b> Make sure you have updated your ArchiCAD and Windows to the latest versions. Make sure you have installed the latest patches or fixes for ArchiCAD and Windows. Make sure you have scanned your computer for viruses, malware, spyware, or other harmful components. Make sure you have enough disk space, memory, and CPU resources for running ArchiCAD and the crack goodies smoothly.</li>
|
116 |
-
<li><b>Problem:</b> The crack goodies conflict with other programs or devices on your computer.</li>
|
117 |
-
<li><b>Solution:</b> Make sure you have closed or disabled any unnecessary programs or devices that may interfere with ArchiCAD and the crack goodies. Make sure you have updated your drivers and firmware for your hardware components. Make sure you have configured your firewall and antivirus settings to allow ArchiCAD and the crack goodies to run without any restrictions.</li>
|
118 |
-
</ul>
|
119 |
-
<h2>Conclusion</h2>
|
120 |
-
<p>In this article, we have explained what Graphisoft ArchiCAD 16 Build 3006 X64 Crack Goodies are, what are their features and benefits, how to download and install them, and how to use them effectively. We hope this article has been helpful and informative for you. If you have any questions or feedback, please feel free to leave a comment below.</p>
|
121 |
-
<h2>FAQs</h2>
|
122 |
-
<p>Here are some of the frequently asked questions about Graphisoft ArchiCAD 16 Build 3006 X64 Crack Goodies:</p>
|
123 |
-
<ol>
|
124 |
-
<li><b>Q: Where can I find more information about Graphisoft ArchiCAD 16?</b></li>
|
125 |
-
<li><b>A: You can visit Graphisoft's official website at <a href="https://graphisoft.com/archicad">https://graphisoft.com/archicad</a>, where you can find more details about ArchiCAD 16's features, specifications, system requirements, tutorials, support, etc.</b></li>
|
126 |
-
<li><b>Q: Where can I find more information about Graphisoft ArchiCAD 16 Goodies?</b></li>
|
127 |
-
<li><b>A: You can visit Graphisoft's official website at <a href="https://graphisoft.com/downloads/goodies/AC16">https://graphisoft.com/downloads/goodies/AC16</a>, where you can find more details about each goodie's description, compatibility, installation, usage, etc.</b></li>
|
128 |
-
<li><b>Q: Where can I find more sources for downloading Graphisoft ArchiCAD 16 Build 3006 X64 Crack Goodies?</b></li>
|
129 |
-
<li><b>A: You can search online for other sources that offer download links for Graphisoft ArchiCAD 16 Build 3006 X64 Crack Goodies. However, be careful when downloading from unknown or untrusted sources, as they may contain harmful or malicious components that may damage your computer or compromise your security.</b></li>
|
130 |
-
<li><b>Q: How can I uninstall Graphisoft ArchiCAD 16 Build 3006 X64 Crack Goodies?</b></li>
|
131 |
-
<li><b>A: You can uninstall Graphisoft ArchiCAD 16 Build 3006 X64 Crack Goodies by following these steps:</b></li>
|
132 |
-
<ul>
|
133 |
-
<li>Go to Control Panel > Programs > Programs and Features.</li>
|
134 |
-
<li>Select Graphisoft ArchiCAD 16 Build 3006 X64 Crack Goodies from the list of programs and click Uninstall.</li>
|
135 |
-
<li>Follow the on-screen instructions to complete the uninstallation process.</li>
|
136 |
-
<li>Delete any leftover files or folders related to Graphisoft ArchiCAD 16 Build 3006 X64 Crack Goodies from your computer.</li>
|
137 |
-
</ul>
|
138 |
-
</ol>
|
139 |
-
</p> 0a6ba089eb<br />
|
140 |
-
<br />
|
141 |
-
<br />
|
|
|
|
|
|
|
|
|
|
|
|
|
|
|
|
|
|
|
|
|
|
|
|
|
|
|
|
|
|
|
|
|
|
|
|
|
|
|
|
|
|
|
|
|
|
|
|
|
|
|
|
|
|
|
|
|
|
|
|
|
|
|
|
|
|
|
|
|
|
|
|
|
|
|
|
|
|
|
|
|
|
|
|
|
|
|
|
|
|
|
|
|
|
|
|
|
|
|
|
|
|
|
|
|
|
|
|
|
|
|
|
|
|
|
|
|
|
|
|
|
|
|
|
|
|
|
|
|
|
|
|
|
|
|
|
|
|
|
|
|
|
|
|
|
|
|
|
|
|
|
|
|
|
|
|
|
|
|
|
|
|
|
|
|
|
|
|
|
|
|
|
|
|
|
|
|
|
|
|
|
|
|
|
|
|
|
|
|
|
|
|
|
|
|
|
|
|
|
|
|
|
|
|
|
|
|
|
|
|
|
|
|
|
|
|
|
|
|
|
|
|
|
|
|
|
|
|
|
|
|
|
|
|
|
|
|
|
|
|
|
|
|
|
|
|
|
|
|
|
|
|
|
|
|
|
|
|
|
|
|
|
|
|
|
|
|
|
|
|
|
|
|
|
|
|
|
|
|
|
|
|
|
|
spaces/1gistliPinn/ChatGPT4/Examples/Download !LINK! Ebook Cooperative Learning Anita Lie.md
DELETED
@@ -1,28 +0,0 @@
|
|
1 |
-
<br />
|
2 |
-
<h1>How to Download Ebook Cooperative Learning by Anita Lie</h1>
|
3 |
-
<p>Cooperative learning is an instructional strategy that enables small groups of students to work together on a common assignment[^3^]. It has many benefits for students, such as enhancing social skills, academic achievement, and motivation. If you are interested in learning more about cooperative learning, you may want to read the ebook Cooperative Learning by Anita Lie.</p>
|
4 |
-
<h2>download ebook cooperative learning anita lie</h2><br /><p><b><b>Download File</b> »»» <a href="https://imgfil.com/2uxXil">https://imgfil.com/2uxXil</a></b></p><br /><br />
|
5 |
-
<p>Anita Lie is a professor at Widya Mandala Catholic University in Indonesia, who specializes in teacher professional development, language learning, and education policy[^2^]. She has written several books and articles on cooperative learning, such as Cooperative Learning: Theory, Research and Practice (2002) and Cooperative Learning in Asia and the Pacific (2008).</p>
|
6 |
-
<p>In her ebook Cooperative Learning, she provides a comprehensive overview of the principles, methods, and applications of cooperative learning in various contexts. She also offers practical tips and examples for teachers who want to implement cooperative learning in their classrooms.</p>
|
7 |
-
<p>If you want to download the ebook Cooperative Learning by Anita Lie, you can follow these steps:</p>
|
8 |
-
<ol>
|
9 |
-
<li>Go to <a href="https://docs.google.com/file/d/0BwsQDG-7Lin1UWRMSUFVVHZxb1E/edit">this link</a>, which is a Google Drive file that contains the ebook in PDF format[^1^]. You may need to sign in to your Google account to access the file.</li>
|
10 |
-
<li>Click on the download icon at the top right corner of the screen. You can choose to download the file as PDF or other formats.</li>
|
11 |
-
<li>Save the file to your device or cloud storage. You can then open it with any PDF reader or ebook app.</li>
|
12 |
-
</ol>
|
13 |
-
<p>That's it! You have successfully downloaded the ebook Cooperative Learning by Anita Lie. You can now enjoy reading it and learning more about cooperative learning. Happy reading!</p>
|
14 |
-
|
15 |
-
<p>Cooperative learning is not a new concept in education. It has been used for centuries in various cultures and traditions, such as the African Ubuntu philosophy, the Chinese Confucianism, and the Native American tribal councils. However, it was not until the 1970s that cooperative learning gained popularity in the Western world, thanks to the pioneering work of researchers such as David Johnson, Roger Johnson, and Robert Slavin.</p>
|
16 |
-
<p></p>
|
17 |
-
<p>Cooperative learning is based on the idea that learning is a social process that involves interaction, communication, and collaboration among peers. It differs from traditional learning, which is often individualistic, competitive, and teacher-centered. Cooperative learning requires students to work in small groups of two to six members, who share a common goal, have individual accountability, and use interpersonal skills. The teacher's role is to facilitate the group work, monitor the progress, and provide feedback and evaluation.</p>
|
18 |
-
<p>Cooperative learning has many advantages for students of all ages and levels. Some of the benefits are:</p>
|
19 |
-
<ul>
|
20 |
-
<li>It improves academic achievement by increasing student engagement, motivation, and retention of information.</li>
|
21 |
-
<li>It develops social skills by fostering positive interdependence, cooperation, and respect among group members.</li>
|
22 |
-
<li>It enhances self-esteem by providing opportunities for peer support, feedback, and recognition.</li>
|
23 |
-
<li>It promotes diversity by exposing students to different perspectives, cultures, and backgrounds.</li>
|
24 |
-
<li>It prepares students for the future by developing skills such as problem-solving, critical thinking, creativity, and teamwork.</li>
|
25 |
-
</ul>
|
26 |
-
<p>Cooperative learning is not a one-size-fits-all approach. It can be adapted to different subjects, curricula, and contexts. There are many types of cooperative learning methods, such as jigsaw, think-pair-share, numbered heads together, round robin, and learning together. Each method has its own structure, procedure, and purpose. Teachers can choose the method that best suits their objectives and students' needs.</p> d5da3c52bf<br />
|
27 |
-
<br />
|
28 |
-
<br />
|
|
|
|
|
|
|
|
|
|
|
|
|
|
|
|
|
|
|
|
|
|
|
|
|
|
|
|
|
|
|
|
|
|
|
|
|
|
|
|
|
|
|
|
|
|
|
|
|
|
|
|
|
|
|
|
|
|
spaces/1pelhydcardo/ChatGPT-prompt-generator/assets/2020 O L English Paper Pdf Download.md
DELETED
@@ -1,77 +0,0 @@
|
|
1 |
-
|
2 |
-
<h1>How to download 2020 O/L English paper PDF</h1>
|
3 |
-
<p>If you are preparing for your Ordinary Level (O/L) examination in Sri Lanka or Cambridge International General Certificate of Secondary Education (IGCSE) examination in other countries, you might be wondering how to get hold of the 2020 O/L English paper PDF. The O/L English paper is one of the most important papers in your exam as it tests your language skills in reading, writing, speaking, and listening. In this article, we will explain what the O/L English paper is, why it is important, and how to download it from various sources.</p>
|
4 |
-
<h2>2020 o l english paper pdf download</h2><br /><p><b><b>Download File</b> ★ <a href="https://urlin.us/2uT2LQ">https://urlin.us/2uT2LQ</a></b></p><br /><br />
|
5 |
-
<h2>What is the O/L English paper?</h2>
|
6 |
-
<p>The O/L English paper is a compulsory paper for all candidates who sit for the O/L examination in Sri Lanka or the IGCSE examination in other countries. The O/L English paper consists of two parts: Paper 1 and Paper 2. Paper 1 is a written paper that assesses the candidates' reading and writing skills. It has three sections: Section A (Reading Comprehension), Section B (Summary Writing), and Section C (Essay Writing). Paper 2 is an oral paper that assesses the candidates' speaking and listening skills. It has two components: Component 1 (Speaking) and Component 2 (Listening). The O/L English paper aims to test the candidates' ability to use English effectively for communication, study, and work purposes.</p>
|
7 |
-
<h3>Why is the O/L English paper important?</h3>
|
8 |
-
<p>The O/L English paper is important for several reasons. First, it helps the candidates to improve their communication skills in English, which is a widely used language in the world. By taking the O/L English paper, the candidates can enhance their vocabulary, grammar, pronunciation, and fluency in English. Second, it helps the candidates to improve their academic performance in other subjects. By taking the O/L English paper, the candidates can develop their reading, writing, speaking, and listening skills in English, which are essential for learning and understanding different topics and concepts in other subjects. Third, it helps the candidates to improve their career prospects in the future. By taking the O/L English paper, the candidates can demonstrate their proficiency in English, which is a valuable skill for many jobs and professions in various fields and industries.</p>
|
9 |
-
<h4>How to prepare for the O/L English paper?</h4>
|
10 |
-
<p>There are many ways to prepare for the O/L English paper. Here are some general tips that can help you to study for the O/L English paper effectively:</p>
|
11 |
-
<ul>
|
12 |
-
<li>Review the syllabus: The syllabus outlines the aims, objectives, content, and assessment of the O/L English paper. It also provides sample questions, marking schemes, and performance descriptors for each part of the paper. You can download the syllabus from the official website of the Department of Examinations or Cambridge International. By reviewing the syllabus, you can familiarize yourself with the structure, format, and expectations of the O/L English paper.</li>
|
13 |
-
<li>Practise past papers: Past papers are actual papers that were used in previous O/L or IGCSE examinations. They contain questions that cover various topics and skills that are relevant to the O/L English paper. You can download past papers from various sources, such as the official website of the Department of Examinations or Cambridge International, or other websites or platforms that offer educational resources. By practising past papers, you can test your knowledge, understanding, and application of English in different contexts and situations.</li>
|
14 |
-
<li>Take online lessons: Online lessons are interactive sessions that are conducted by qualified teachers or tutors who have experience and expertise in teaching English for O/L or IGCSE examinations. They provide guidance, feedback, and support to help you improve your language skills and prepare for the O/L English paper. You can find online lessons from various sources, such as online learning platforms, websites, or apps that offer educational services. By taking online lessons, you can learn from experts, ask questions, and get personalized attention.</li>
|
15 |
-
</ul> <h2>How to download 2020 O/L English paper PDF from official sources?</h2>
|
16 |
-
<p>One of the best ways to download the 2020 O/L English paper PDF is to use the official sources that are authorized and recognized by the Department of Examinations or Cambridge International. These sources are reliable and accurate, and they provide the latest and most updated versions of the O/L English paper PDF. There are two main official sources that you can use to download the 2020 O/L English paper PDF: the Department of Examinations website and the Cambridge International website.</p>
|
17 |
-
<h3>How to download 2020 O/L English paper PDF from the Department of Examinations website?</h3>
|
18 |
-
<p>The Department of Examinations website is the official website of the government agency that is responsible for conducting and administering the O/L examination in Sri Lanka. It provides various information and services related to the O/L examination, such as syllabuses, timetables, results, and past papers. You can download the 2020 O/L English paper PDF from the Department of Examinations website by following these steps:</p>
|
19 |
-
<ol>
|
20 |
-
<li>Go to the Department of Examinations website at <a href="">http://www.doenets.lk</a>.</li>
|
21 |
-
<li>Click on the "Examination" tab on the top menu bar and select "G.C.E. (O/L)" from the drop-down list.</li>
|
22 |
-
<li>Click on the "Past Papers" link on the left sidebar and select "2020" from the year list.</li>
|
23 |
-
<li>Scroll down to find "English Language" from the subject list and click on the "Download" button next to it.</li>
|
24 |
-
<li>A new window will open with the 2020 O/L English paper PDF file. You can either view it online or save it to your device by clicking on the "Download" icon on the top right corner.</li>
|
25 |
-
</ol>
|
26 |
-
<h4>Advantages and disadvantages of downloading from the Department of Examinations website?</h4>
|
27 |
-
<p>Downloading from the Department of Examinations website has some advantages and disadvantages. Here are some of them:</p>
|
28 |
-
<p></p>
|
29 |
-
<table>
|
30 |
-
<tr><th>Advantages</th><th>Disadvantages</th></tr>
|
31 |
-
<tr><td>- It is free of charge.</td><td>- It may not be available at all times due to high traffic or maintenance.</td></tr>
|
32 |
-
<tr><td>- It is reliable and authentic as it is provided by the official authority.</td><td>- It may have slow speed or low quality due to limited bandwidth or resources.</td></tr>
|
33 |
-
<tr><td>- It is updated and current as it reflects the latest changes or revisions in the syllabus or format.</td><td>- It may not have all the past papers or marking schemes for every year or subject.</td></tr>
|
34 |
-
</table> online.</li>
|
35 |
-
</ol>
|
36 |
-
<h4>Advantages and disadvantages of downloading from PaperHub?</h4>
|
37 |
-
<p>Downloading from PaperHub has some advantages and disadvantages. Here are some of them:</p>
|
38 |
-
<table>
|
39 |
-
<tr><th>Advantages</th><th>Disadvantages</th></tr>
|
40 |
-
<tr><td>- It is easy and convenient as it does not require registration or payment.</td><td>- It may have limited content or variety as it depends on the availability and contribution of the users.</td></tr>
|
41 |
-
<tr><td>- It is user-friendly and interactive as it allows users to rate, comment, and share the files.</td><td>- It may have ads or banners that may distract or annoy the users.</td></tr>
|
42 |
-
<tr><td>- It is fast and efficient as it has a simple and clear interface and a powerful search engine.</td><td>- It may have errors or mistakes as it does not verify or validate the files.</td></tr>
|
43 |
-
</table>
|
44 |
-
<h3>How to download 2020 O/L English paper PDF from Pastpapers Wiki?</h3>
|
45 |
-
<p>Pastpapers Wiki is a website that provides past papers, marking schemes, notes, and more for free. It covers various subjects and levels, including O/L and IGCSE. You can download the 2020 O/L English paper PDF from Pastpapers Wiki by following these steps:</p>
|
46 |
-
<ol>
|
47 |
-
<li>Go to Pastpapers Wiki website at <a href="">https://pastpapers.wiki</a>.</li>
|
48 |
-
<li>Click on the "O/L" tab on the top menu bar and select "English" from the subject list.</li>
|
49 |
-
<li>Click on the "2020" link on the left sidebar and select "English Language" from the paper list.</li>
|
50 |
-
<li>A new page will open with the 2020 O/L English paper PDF files for Paper 1 and Paper 2. You can either click on the "Download" button next to each file or click on the file name to view it online.</li>
|
51 |
-
</ol>
|
52 |
-
<h4>Advantages and disadvantages of downloading from Pastpapers Wiki?</h4>
|
53 |
-
<p>Downloading from Pastpapers Wiki has some advantages and disadvantages. Here are some of them:</p>
|
54 |
-
<table>
|
55 |
-
<tr><th>Advantages</th><th>Disadvantages</th></tr>
|
56 |
-
<tr><td>- It is extensive and updated as it has a large collection of past papers and marking schemes for every year and subject.</td><td>- It may have pop-ups or redirects that may lead to unwanted or harmful websites.</td></tr>
|
57 |
-
<tr><td>- It is helpful and informative as it provides notes, guides, tips, and tricks for each paper.</td><td>- It may have broken links or missing files that may cause inconvenience or frustration.</td></tr>
|
58 |
-
<tr><td>- It is secure and safe as it uses SSL encryption and HTTPS protocol to protect the users' data and privacy.</td><td>- It may not be compatible with some devices or browsers as it uses JavaScript and cookies to function properly.</td></tr>
|
59 |
-
</table>
|
60 |
-
<h2>Conclusion</h2>
|
61 |
-
<p>In conclusion, downloading the 2020 O/L English paper PDF is a useful and effective way to prepare for your O/L examination in Sri Lanka or IGCSE examination in other countries. The 2020 O/L English paper PDF can help you to improve your language skills, academic performance, and career prospects. You can download the 2020 O/L English paper PDF from various sources, such as the official sources of the Department of Examinations or Cambridge International, or other sources like PaperHub or Pastpapers Wiki. However, you should be aware of the advantages and disadvantages of each source, and choose the one that suits your needs and preferences. We hope that this article has provided you with some valuable information and guidance on how to download the 2020 O/L English paper PDF. We wish you all the best for your O/L examination!</p>
|
62 |
-
<h2>FAQs</h2>
|
63 |
-
<p>Here are some frequently asked questions and answers related to downloading the 2020 O/L English paper PDF:</p>
|
64 |
-
<ol>
|
65 |
-
<li><b>Q: How can I download the 2020 O/L English paper PDF without internet connection?</b></li>
|
66 |
-
<li>A: If you do not have internet connection, you can download the 2020 O/L English paper PDF from a friend, a teacher, a library, or a computer lab that has internet access. You can use a USB flash drive, a CD-ROM, or an email attachment to transfer the file to your device. Alternatively, you can print out the 2020 O/L English paper PDF from a printer that has internet access.</li>
|
67 |
-
<li><b>Q: How can I download the 2020 O/L English paper PDF with answers?</b></li>
|
68 |
-
<li>A: If you want to download the 2020 O/L English paper PDF with answers, you need to download the marking scheme or the examiner report for the 2020 O/L English paper. The marking scheme or the examiner report provides the answers, the marks, and the feedback for each question in the paper. You can download the marking scheme or the examiner report from the same sources that you download the 2020 O/L English paper PDF, such as the official sources of the Department of Examinations or Cambridge International, or other sources like PaperHub or Pastpapers Wiki. However, you should note that some sources may not have the marking scheme or the examiner report for every paper or year.</li>
|
69 |
-
<li><b>Q: How can I download the 2020 O/L English paper PDF in other languages?</b></li>
|
70 |
-
<li>A: If you want to download the 2020 O/L English paper PDF in other languages, you need to use a translation tool or service that can convert the PDF file from English to your preferred language. You can use online translation tools or services, such as Google Translate, Microsoft Translator, or DeepL, that can translate the PDF file automatically and instantly. However, you should be aware that online translation tools or services may not be accurate or reliable, and they may lose some meaning or context in the translation process. Alternatively, you can use offline translation tools or services, such as dictionaries, books, or tutors, that can translate the PDF file manually and carefully. However, you should be aware that offline translation tools or services may not be available or accessible, and they may take some time or cost some money in the translation process.</li>
|
71 |
-
<li><b>Q: How can I download the 2020 O/L English paper PDF for free?</b></li>
|
72 |
-
<li>A: If you want to download the 2020 O/L English paper PDF for free, you need to use sources that do not charge any fee or require any payment for downloading the PDF file. You can use sources that are free of charge, such as the Department of Examinations website, PaperHub, or Pastpapers Wiki, that provide the 2020 O/L English paper PDF for free. However, you should be aware that free sources may have some limitations or drawbacks, such as low quality, limited availability, ads, errors, etc. Alternatively, you can use sources that offer free trials or discounts, such as Cambridge International website or online learning platforms, that provide the 2020 O/L English paper PDF for free for a limited time or with some conditions. However, you should be aware that free trials or discounts may have some restrictions or obligations, such as registration, expiration, cancellation, etc.</li>
|
73 |
-
<li><b>Q: How can I download the 2020 O/L English paper PDF legally?</b></li>
|
74 |
-
<li>A: If you want to download the 2020 O/L English paper PDF legally, you need to use sources that respect and follow the intellectual property rights and laws of the creators and owners of the PDF file. You can use sources that are authorized and recognized by the Department of Examinations or Cambridge International, such as their official websites, that provide the 2020 O/L English paper PDF legally. However, you should be aware that authorized and recognized sources may require registration and payment as they are only accessible to teachers or students who are affiliated with them. Alternatively, you can use sources that are licensed and permitted by the Department of Examinations or Cambridge International, such as other websites or platforms that offer educational resources legally. However, you should be aware that licensed and permitted sources may have some terms and conditions that you need to agree and comply with, such as attribution, non-commercial use, etc.</li>
|
75 |
-
</ol></p> 197e85843d<br />
|
76 |
-
<br />
|
77 |
-
<br />
|
|
|
|
|
|
|
|
|
|
|
|
|
|
|
|
|
|
|
|
|
|
|
|
|
|
|
|
|
|
|
|
|
|
|
|
|
|
|
|
|
|
|
|
|
|
|
|
|
|
|
|
|
|
|
|
|
|
|
|
|
|
|
|
|
|
|
|
|
|
|
|
|
|
|
|
|
|
|
|
|
|
|
|
|
|
|
|
|
|
|
|
|
|
|
|
|
|
|
|
|
|
|
|
|
|
|
|
|
|
|
|
|
|
|
|
|
|
|
|
|
|
|
|
|
|
|
|
|
|
|
|
|
|
|
|
|
|
|
|
|
|
|
|
|
|
|
|
|
|
|
|
|
|
|
|
spaces/1pelhydcardo/ChatGPT-prompt-generator/assets/Adobe Illustrator CC 2019 Create Amazing Vector Art and Illustrations.md
DELETED
@@ -1,106 +0,0 @@
|
|
1 |
-
|
2 |
-
<h1>How to Download and Install Adobe Illustrator CC 2019 Kuyhaa</h1>
|
3 |
-
<p>If you are looking for a powerful and versatile graphic design software, you might want to try Adobe Illustrator CC 2019. This is the latest version of the industry-standard vector graphics app that lets you create logos, icons, drawings, typography, and complex illustrations for any medium. In this article, we will show you how to download and install Adobe Illustrator CC 2019 kuyhaa, which is a free and full version of the software that you can use without any limitations.</p>
|
4 |
-
<h2>What is Adobe Illustrator and what is it used for?</h2>
|
5 |
-
<p>Adobe Illustrator is a graphic design application that works with vector graphics. Vector graphics are made of points, lines, shapes, and curves based on mathematical formulas rather than pixels. This means that they can be scaled up or down without losing any quality or detail. Vector graphics are ideal for creating graphics that need to be printed on different sizes or displayed on different devices.</p>
|
6 |
-
<h2>download adobe illustrator cc 2019 kuyhaa</h2><br /><p><b><b>DOWNLOAD</b> ✺✺✺ <a href="https://urlin.us/2uSYJo">https://urlin.us/2uSYJo</a></b></p><br /><br />
|
7 |
-
<h3>Adobe Illustrator features and benefits</h3>
|
8 |
-
<p>Adobe Illustrator has over 1,300 unique features and functions that allow you to create stunning and professional graphics. Some of its core capabilities include:</p>
|
9 |
-
<ul>
|
10 |
-
<li>The ability to draw freehand with a variety of digital brushes, pencils, and pens.</li>
|
11 |
-
<li>Advanced color options, such as gradients, patterns, swatches, and color themes.</li>
|
12 |
-
<li>A Layers panel that lets you organize and edit your design elements across different layers.</li>
|
13 |
-
<li>A Properties panel that gives you quick access to the most relevant settings and controls for your selected object.</li>
|
14 |
-
<li>A Content-Aware Crop tool that automatically fills in the gaps when you resize or rotate your artwork.</li>
|
15 |
-
<li>A Puppet Warp tool that lets you transform your artwork by dragging pins on specific points.</li>
|
16 |
-
<li>A Global Edit option that lets you edit multiple instances of a symbol or text at once.</li>
|
17 |
-
<li>A Freeform Gradient tool that lets you create natural-looking color blends by placing points of color anywhere on your object.</li>
|
18 |
-
<li>A Perspective Grid tool that lets you create realistic depth and distance by applying perspective to your artwork.</li>
|
19 |
-
<li>A Shape Builder tool that lets you combine, subtract, or intersect multiple shapes with ease.</li>
|
20 |
-
</ul>
|
21 |
-
<h3>Adobe Illustrator system requirements</h3>
|
22 |
-
<p>Before you download and install Adobe Illustrator CC 2019 kuyhaa, make sure that your computer meets the minimum system requirements for the software. Here are the specifications for Windows:</p>
|
23 |
-
<table>
|
24 |
-
<tr><th>Processor</th><th>Memory</th><th>Hard disk space</th><th>Operating system</th><th>Graphics card</th></tr>
|
25 |
-
<tr><td>Intel Pentium 4 or AMD Athlon 64 processor (or faster)</td><td>4 GB of RAM (8 GB recommended)</td><td>2 GB of available hard disk space for installation; additional free space required during installation</td><td>Windows 7 (64-bit) with Service Pack 1 or Windows 10 (64-bit)</td><td>OpenGL 4.x compatible graphics card with at least 1 GB of VRAM</td></tr>
|
26 |
-
</table>
|
27 |
-
<h2>How to download Adobe Illustrator CC 2019 kuyhaa</h2>
|
28 |
-
<p>To download Adobe Illustrator CC 2019 kuyhaa, you need to follow these steps:</p>
|
29 |
-
<h3>Step 1: Visit the Google Drive link</h3>
|
30 |
-
<p>The first step is to visit the Google Drive link where the Adobe Illustrator CC 2019 kuyhaa file is stored. You can access the link by clicking [here]. This will take you to a page where you can see the file name and size.</p>
|
31 |
-
<h3>Step 2: Download the Adobe.Illustrator.CC.2019.v23.0.0.530x64.exe file</h3>
|
32 |
-
<p>The next step is to download the file to your computer. To do this, you need to click on the download icon at the top right corner of the page. This will open a pop-up window where you can choose to save the file to your preferred location or open it with a program. We recommend that you save the file to your desktop or downloads folder for easy access.</p>
|
33 |
-
<h3>Step 3: Extract the file using WinRAR or 7-Zip</h3>
|
34 |
-
<p>The last step in downloading Adobe Illustrator CC 2019 kuyhaa is to extract the file using a compression software such as WinRAR or 7-Zip. The file is compressed in a .rar format, which means that it contains multiple files and folders inside it. To extract the file, you need to right-click on it and choose "Extract Here" or "Extract to Adobe.Illustrator.CC.2019.v23.0.0.530x64". This will create a new folder with the same name as the file, where you can find all the files and folders related to Adobe Illustrator CC 2019 kuyhaa.</p>
|
35 |
-
<h2>How to install Adobe Illustrator CC 2019 kuyhaa</h2>
|
36 |
-
<p>Now that you have downloaded and extracted Adobe Illustrator CC 2019 kuyhaa, you are ready to install it on your computer. To install Adobe Illustrator CC 2019 kuyhaa, you need to follow these steps:</p>
|
37 |
-
<h3>Step 1: Run the setup.exe file as administrator</h3>
|
38 |
-
<p>The first step in installing Adobe Illustrator CC 2019 kuyhaa is to run the setup.exe file as administrator. To do this, you need to go to the folder where you extracted the file and find the setup.exe file. Then, you need to right-click on it and choose "Run as administrator". This will launch the installation wizard, which will guide you through the installation process.</p>
|
39 |
-
<p>download adobe illustrator cc 2019 full version<br />
|
40 |
-
download adobe illustrator cc 2019 google drive<br />
|
41 |
-
download adobe illustrator cc 2019 crack<br />
|
42 |
-
download adobe illustrator cc 2019 free<br />
|
43 |
-
download adobe illustrator cc 2019 offline installer<br />
|
44 |
-
download adobe illustrator cc 2019 for windows 10<br />
|
45 |
-
download adobe illustrator cc 2019 portable<br />
|
46 |
-
download adobe illustrator cc 2019 64 bit<br />
|
47 |
-
download adobe illustrator cc 2019 mac<br />
|
48 |
-
download adobe illustrator cc 2019 bagas31<br />
|
49 |
-
download adobe illustrator cc 2019 patch<br />
|
50 |
-
download adobe illustrator cc 2019 setup<br />
|
51 |
-
download adobe illustrator cc 2019 keygen<br />
|
52 |
-
download adobe illustrator cc 2019 serial number<br />
|
53 |
-
download adobe illustrator cc 2019 activation code<br />
|
54 |
-
download adobe illustrator cc 2019 with crack<br />
|
55 |
-
download adobe illustrator cc 2019 highly compressed<br />
|
56 |
-
download adobe illustrator cc 2019 mega<br />
|
57 |
-
download adobe illustrator cc 2019 latest version<br />
|
58 |
-
download adobe illustrator cc 2019 update<br />
|
59 |
-
download adobe illustrator cc 2019 trial<br />
|
60 |
-
download adobe illustrator cc 2019 preactivated<br />
|
61 |
-
download adobe illustrator cc 2019 repack<br />
|
62 |
-
download adobe illustrator cc 2019 rar<br />
|
63 |
-
download adobe illustrator cc 2019 zip<br />
|
64 |
-
download adobe illustrator cc 2019 torrent<br />
|
65 |
-
download adobe illustrator cc 2019 direct link<br />
|
66 |
-
download adobe illustrator cc 2019 from official site<br />
|
67 |
-
download adobe illustrator cc 2019 iso file<br />
|
68 |
-
download adobe illustrator cc 2019 license key<br />
|
69 |
-
how to download adobe illustrator cc 2019 for free<br />
|
70 |
-
how to download adobe illustrator cc 2019 crack version<br />
|
71 |
-
how to install adobe illustrator cc 2019 after downloading<br />
|
72 |
-
how to activate adobe illustrator cc 2019 without internet<br />
|
73 |
-
how to use adobe illustrator cc 2019 tutorial pdf<br />
|
74 |
-
how to update adobe illustrator cc 2019 to latest version<br />
|
75 |
-
how to uninstall adobe illustrator cc 2019 completely<br />
|
76 |
-
how to fix adobe illustrator cc 2019 not opening error<br />
|
77 |
-
how to speed up adobe illustrator cc 2019 performance<br />
|
78 |
-
how to change language in adobe illustrator cc 2019</p>
|
79 |
-
<h3>Step 2: Choose the language and destination folder</h3>
|
80 |
-
<p>The next step in installing Adobe Illustrator CC 2019 kuyhaa is to choose the language and destination folder for the software. You can choose from several languages, such as English, French, German, Spanish, Italian, Portuguese, Russian, Turkish, Arabic, Chinese, Japanese, Korean, and more. You can also change the destination folder where you want to install Adobe Illustrator CC 2019 kuyhaa on your computer. By default, it will be installed in C:\Program Files\Adobe\Adobe Illustrator CC 2019\. You can click on "Browse" to select a different folder if you wish.</p>
|
81 |
-
<h3>Step 3: Wait for the installation to complete</h3>
|
82 |
-
<p>The third step in installing Adobe Illustrator CC 2019 kuyhaa is to wait for the installation to complete. This may take several minutes depending on your computer speed and internet connection. You can see the progress of the installation on a green bar at the bottom of the wizard window. You can also see the details of what is being installed on your computer on a list at the right side of the window.</p>
|
83 |
-
<h3>Step 4: Launch Adobe Illustrator CC 2019 from the desktop shortcut</h3>
|
84 |
-
<p>The final step in installing Adobe Illustrator CC 2019 kuyhaa is to launch it from the desktop shortcut. Once the installation is complete, you will see a message that says "Installation successful". You will also see a checkbox that says "Launch Adobe Illustrator CC". If you want to start using Adobe Illustrator CC 2019 right away, you can leave this checkbox checked and click on "Finish". This will close the installation wizard and open Adobe Illustrator CC 2019 on your computer. Alternatively, you can uncheck this checkbox and click on "Finish". This will close the installation wizard and create a desktop shortcut for Adobe Illustrator CC 2019 on your computer. You can double-click on this shortcut anytime you want to use Adobe Illustrator CC 2019.</p>
|
85 |
-
<h2>Conclusion</h2>
|
86 |
-
<p>In this article, we have shown you how to download and install Adobe Illustrator CC 2019 kuyhaa, which is a free and full version of the graphic design software that you can use without any limitations. We have explained what Adobe Illustrator is and what it is used for, and we have provided a step-by-step guide on how to download and install it on your computer. We hope that this article has been helpful and informative for you, and that you enjoy using Adobe Illustrator CC 2019 kuyhaa for your graphic design projects.</p>
|
87 |
-
<h2>FAQs</h2>
|
88 |
-
<p>Here are some frequently asked questions about Adobe Illustrator CC 2019 kuyhaa:</p>
|
89 |
-
<h3>Q: Is Adobe Illustrator CC 2019 kuyhaa safe to download and install?</h3>
|
90 |
-
<p>A: Yes, Adobe Illustrator CC 2019 kuyhaa is safe to download and install, as long as you follow the instructions in this article and use the Google Drive link that we have provided. This link is from a trusted source and does not contain any viruses, malware, or spyware. However, you should always scan any file that you download from the internet with an antivirus software before opening it, just to be on the safe side.</p>
|
91 |
-
<h3>Q: Do I need to activate Adobe Illustrator CC 2019 kuyhaa after installing it?</h3>
|
92 |
-
<p>A: No, you do not need to activate Adobe Illustrator CC 2019 kuyhaa after installing it. This is because Adobe Illustrator CC 2019 kuyhaa is a pre-activated version of the software, which means that it does not require any serial number, license key, or crack to run. You can use it without any restrictions or limitations.</p>
|
93 |
-
<h3>Q: Can I update Adobe Illustrator CC 2019 kuyhaa to the latest version?</h3>
|
94 |
-
<p>A: No, you cannot update Adobe Illustrator CC 2019 kuyhaa to the latest version. This is because Adobe Illustrator CC 2019 kuyhaa is a standalone version of the software, which means that it does not connect to the internet or the Adobe servers. Therefore, it does not receive any updates or patches from Adobe. If you want to use the latest version of Adobe Illustrator, you will need to purchase a subscription from the official website.</p>
|
95 |
-
<h3>Q: Can I use Adobe Illustrator CC 2019 kuyhaa with other Adobe products?</h3>
|
96 |
-
<p>A: Yes, you can use Adobe Illustrator CC 2019 kuyhaa with other Adobe products, such as Photoshop, InDesign, After Effects, Premiere Pro, and more. You can import and export files between these applications and work seamlessly on your projects. However, you may encounter some compatibility issues if you use different versions of these products.</p>
|
97 |
-
<h3>Q: How can I uninstall Adobe Illustrator CC 2019 kuyhaa from my computer?</h3>
|
98 |
-
<p>A: If you want to uninstall Adobe Illustrator CC 2019 kuyhaa from your computer, you can follow these steps:</p>
|
99 |
-
<ol>
|
100 |
-
<li>Go to the Control Panel and click on "Uninstall a program".</li>
|
101 |
-
<li>Find and select "Adobe Illustrator CC 2019" from the list of programs and click on "Uninstall".</li>
|
102 |
-
<li>Follow the instructions on the screen to complete the uninstallation process.</li>
|
103 |
-
<li>Delete the folder where you installed Adobe Illustrator CC 2019 kuyhaa from your computer.</li>
|
104 |
-
</ol></p> 197e85843d<br />
|
105 |
-
<br />
|
106 |
-
<br />
|
|
|
|
|
|
|
|
|
|
|
|
|
|
|
|
|
|
|
|
|
|
|
|
|
|
|
|
|
|
|
|
|
|
|
|
|
|
|
|
|
|
|
|
|
|
|
|
|
|
|
|
|
|
|
|
|
|
|
|
|
|
|
|
|
|
|
|
|
|
|
|
|
|
|
|
|
|
|
|
|
|
|
|
|
|
|
|
|
|
|
|
|
|
|
|
|
|
|
|
|
|
|
|
|
|
|
|
|
|
|
|
|
|
|
|
|
|
|
|
|
|
|
|
|
|
|
|
|
|
|
|
|
|
|
|
|
|
|
|
|
|
|
|
|
|
|
|
|
|
|
|
|
|
|
|
|
|
|
|
|
|
|
|
|
|
|
|
|
|
|
|
|
|
|
|
|
|
|
|
|
|
|
|
|
|
|
|
|
|
|
|
|
|
|
|
|
|
|
|
|
|
|
|
|
|
|
|
|
|
|
|
|
|
spaces/1phancelerku/anime-remove-background/Baixe o FIFA Mobile Dinheiro Infinito APK e jogue com os melhores times do mundo.md
DELETED
@@ -1,87 +0,0 @@
|
|
1 |
-
|
2 |
-
<h1>FIFA Mobile Dinheiro Infinito APK Download Mediafıre: How to Get Unlimited Coins and Gems in FIFA Mobile</h1>
|
3 |
-
<p>FIFA Mobile is one of the most popular soccer games for mobile devices, with over 100 million downloads on Google Play. The game allows you to build your ultimate team of soccer stars, compete in various modes, and relive the world's greatest soccer tournament, the FIFA World Cup. However, if you want to enjoy all the features and content that FIFA Mobile has to offer, you will need a lot of coins and gems, which are the in-game currencies. Coins and gems can be used to buy players, items, packs, upgrades, and more. But earning coins and gems can be time-consuming and challenging, especially if you don't want to spend real money on them.</p>
|
4 |
-
<h2>fifa mobile dinheiro infinito apk download mediafıre</h2><br /><p><b><b>Download</b> ··· <a href="https://jinyurl.com/2uNRzt">https://jinyurl.com/2uNRzt</a></b></p><br /><br />
|
5 |
-
<p>That's why some players look for ways to get unlimited coins and gems in FIFA Mobile. One of the most common methods is to download a modded version of the game, such as FIFA Mobile Dinheiro Infinito APK. This is an APK file that claims to offer unlimited coins and gems in FIFA Mobile. But is it really worth it? How does it work? And what are the risks involved? In this article, we will answer these questions and more.</p>
|
6 |
-
<h2>What is FIFA Mobile Dinheiro Infinito APK?</h2>
|
7 |
-
<p>FIFA Mobile Dinheiro Infinito APK is a modified version of FIFA Mobile that supposedly gives you unlimited coins and gems in the game. It is not an official app from EA Sports or Google Play, but rather a third-party app that can be downloaded from Mediafıre, a file-sharing website. The name "Dinheiro Infinito" means "Infinite Money" in Portuguese, which suggests and risking legal actions from EA Sports or Google Play.</li>
|
8 |
-
<li>FIFA Mobile Dinheiro Infinito APK is not a reliable app. It may not work properly or cause errors, glitches, bugs, or crashes in the game. It may also not be compatible with the latest updates or versions of FIFA Mobile.</li>
|
9 |
-
<li>FIFA Mobile Dinheiro Infinito APK is not a fair app. It gives you an unfair advantage over other players who play FIFA Mobile legitimately. It also ruins the balance and integrity of the game.</li>
|
10 |
-
<li>FIFA Mobile Dinheiro Infinito APK is not a secure app. It may expose your account or device to hackers, scammers, or other malicious users who can steal your coins, gems, players, items, or personal information. It may also get your account banned or suspended by EA Sports for using cheats or hacks in FIFA Mobile.</li>
|
11 |
-
</ul>
|
12 |
-
<h2>Is FIFA Mobile Dinheiro Infinito APK Worth It?</h2>
|
13 |
-
<p>After weighing the benefits and risks of using FIFA Mobile Dinheiro Infinito APK, you may wonder if it is worth it or not. The answer depends on your personal preference and risk tolerance. However, we do not recommend using FIFA Mobile Dinheiro Infinito APK for the following reasons:</p>
|
14 |
-
<ul>
|
15 |
-
<li>It is not safe to use. It may harm your device or compromise your personal information.</li>
|
16 |
-
<li>It is not legal to use. It may get you in trouble with EA Sports or Google Play.</li>
|
17 |
-
<li>It is not reliable to use. It may not work as expected or cause problems in the game.</li>
|
18 |
-
<li>It is not fair to use. It may give you an unfair advantage over other players or ruin the game experience for everyone.</li>
|
19 |
-
<li>It is not secure to use. It may expose your account or device to hackers, scammers, or other malicious users.</li>
|
20 |
-
</ul>
|
21 |
-
<p>Instead of using FIFA Mobile Dinheiro Infinito APK, we suggest you play FIFA Mobile the way it was meant to be played: with skill, strategy, and fun. You can still enjoy FIFA Mobile without using cheats or hacks. You can still earn coins and gems by playing the game, completing tasks, watching ads, participating in events, or buying them with real money if you want to support the developers. You can still build your ultimate team of soccer stars by scouting, trading, upgrading, and managing your players. You can still compete in various modes and events by challenging yourself, improving your skills, and learning from other players.</p>
|
22 |
-
<p>fifa mobile mod apk dinheiro infinito atualizado via mediafire<br />
|
23 |
-
fifa 2021 mobile moedas infinitas download mediafire<br />
|
24 |
-
fifa mobile hack apk dinheiro infinito mediafire<br />
|
25 |
-
fifa mobile 2022 dinheiro infinito apk baixar mediafire<br />
|
26 |
-
fifa mobile apk mod moedas infinitas mediafire<br />
|
27 |
-
fifa mobile dinheiro infinito download mediafire 2021<br />
|
28 |
-
fifa mobile mod apk unlimited money mediafire<br />
|
29 |
-
fifa 2021 mobile coins unlimited download mediafire<br />
|
30 |
-
fifa mobile hack apk unlimited coins mediafire<br />
|
31 |
-
fifa mobile 2022 unlimited money apk download mediafire<br />
|
32 |
-
fifa mobile apk mod coins unlimited mediafire<br />
|
33 |
-
fifa mobile unlimited coins download mediafire 2021<br />
|
34 |
-
fifa mobile mod apk dinheiro infinito e pontos mediafire<br />
|
35 |
-
fifa 2021 mobile moedas e pontos infinitos download mediafire<br />
|
36 |
-
fifa mobile hack apk dinheiro e pontos infinitos mediafire<br />
|
37 |
-
fifa mobile 2022 dinheiro e pontos infinitos apk baixar mediafire<br />
|
38 |
-
fifa mobile apk mod moedas e pontos infinitos mediafire<br />
|
39 |
-
fifa mobile dinheiro e pontos infinitos download mediafire 2021<br />
|
40 |
-
fifa mobile mod apk dinheiro infinito offline mediafire<br />
|
41 |
-
fifa 2021 mobile moedas infinitas offline download mediafire<br />
|
42 |
-
fifa mobile hack apk dinheiro infinito offline mediafire<br />
|
43 |
-
fifa mobile 2022 dinheiro infinito offline apk baixar mediafire<br />
|
44 |
-
fifa mobile apk mod moedas infinitas offline mediafire<br />
|
45 |
-
fifa mobile dinheiro infinito offline download mediafire 2021<br />
|
46 |
-
fifa mobile mod apk dinheiro infinito atualizado 2021 mediafire<br />
|
47 |
-
fifa 2021 mobile moedas infinitas atualizado 2021 download mediafire<br />
|
48 |
-
fifa mobile hack apk dinheiro infinito atualizado 2021 mediafire<br />
|
49 |
-
fifa mobile 2022 dinheiro infinito atualizado 2021 apk baixar mediafire<br />
|
50 |
-
fifa mobile apk mod moedas infinitas atualizado 2021 mediafire<br />
|
51 |
-
fifa mobile dinheiro infinito atualizado 2021 download mediafire</p>
|
52 |
-
<h2>Conclusion</h2>
|
53 |
-
<p>FIFA Mobile Dinheiro Infinito APK is a modded version of FIFA Mobile that claims to offer unlimited coins and gems in the game. However, it is not a safe, legal, reliable, fair, or secure app to use. It may cause more harm than good to your device, account, and game experience. Therefore, we do not recommend using FIFA Mobile Dinheiro Infinito APK for getting unlimited coins and gems in FIFA Mobile.</p>
|
54 |
-
<p>If you want to enjoy FIFA Mobile without using cheats or hacks, you can follow these tips:</p>
|
55 |
-
<ul>
|
56 |
-
<li>Play the game regularly and complete tasks to earn coins and gems.</li>
|
57 |
-
<li>Watch ads or participate in events to get extra coins and gems.</li>
|
58 |
-
<li>Buy coins and gems with real money if you want to support the developers and get more content.</li>
|
59 |
-
<li>Scout, trade, upgrade, and manage your players to build your ultimate team.</li>
|
60 |
-
<li>Challenge yourself, improve your skills, and learn from other players to compete in various modes and events.</li>
|
61 |
-
</ul>
|
62 |
-
<p>We hope this article has helped you understand more about FIFA Mobile Dinheiro Infinito APK and why you should avoid using it. If you have any questions or feedback, please feel free to leave a comment below. Thank you for reading!</p>
|
63 |
-
<h2>FAQs</h2>
|
64 |
-
<h3>Is FIFA Mobile Dinheiro Infinito APK safe to use?</h3>
|
65 |
-
<p>No, it is not safe to use. FIFA Mobile Dinheiro Infinito APK is a third-party app that may contain malware, viruses, spyware, or other harmful software that can damage your device or steal your personal information.</p>
|
66 |
-
<h3>Is FIFA Mobile Dinheiro Infinito APK legal to use?</h3>
|
67 |
-
<p>No, it is not legal to use. FIFA Mobile Dinheiro Infinito APK violates the terms of service and policies of EA Sports and Google Play. By using FIFA Mobile Dinheiro Infinito APK, you are breaking the law and risking legal actions from EA Sports or Google Play.</p>
|
68 |
-
<h3>How can I get coins and gems in FIFA Mobile without using FIFA Mobile Dinheiro Infinito APK?</h3>
|
69 |
-
<p>You can get coins and gems in FIFA Mobile without using FIFA Mobile Dinheiro Infinito APK by playing the game, com completing tasks, watching ads, participating in events, or buying them with real money if you want to support the developers and get more content.</p>
|
70 |
-
<h3>What are some alternatives to FIFA Mobile Dinheiro Infinito APK?</h3>
|
71 |
-
<p>Some alternatives to FIFA Mobile Dinheiro Infinito APK are other modded versions or hacks for FIFA Mobile that are available online. However, we do not recommend using any of them, as they may also be unsafe, illegal, unreliable, unfair, or insecure to use. Some examples of these alternatives are:</p>
|
72 |
-
<ul>
|
73 |
-
<li>FIFA Mobile Mod APK</li>
|
74 |
-
<li>FIFA Mobile Hack APK</li>
|
75 |
-
<li>FIFA Mobile Unlimited Coins and Gems APK</li>
|
76 |
-
<li>FIFA Mobile Generator</li>
|
77 |
-
<li>FIFA Mobile Cheats</li>
|
78 |
-
</ul>
|
79 |
-
<h3>Where can I find more information about FIFA Mobile Dinheiro Infinito APK?</h3>
|
80 |
-
<p>If you want to find more information about FIFA Mobile Dinheiro Infinito APK, you can search for it on Google or YouTube. However, be careful of the sources and links that you click on, as they may be fake, misleading, or malicious. Some possible sources of information are:</p>
|
81 |
-
<ul>
|
82 |
-
<li>[FIFA Mobile Dinheiro Infinito APK Download Mediafıre]</li>
|
83 |
-
<li>[FIFA Mobile Dinheiro Infinito APK Tutorial]</li>
|
84 |
-
<li>[FIFA Mobile Dinheiro Infinito APK Review]</li>
|
85 |
-
</ul></p> 197e85843d<br />
|
86 |
-
<br />
|
87 |
-
<br />
|
|
|
|
|
|
|
|
|
|
|
|
|
|
|
|
|
|
|
|
|
|
|
|
|
|
|
|
|
|
|
|
|
|
|
|
|
|
|
|
|
|
|
|
|
|
|
|
|
|
|
|
|
|
|
|
|
|
|
|
|
|
|
|
|
|
|
|
|
|
|
|
|
|
|
|
|
|
|
|
|
|
|
|
|
|
|
|
|
|
|
|
|
|
|
|
|
|
|
|
|
|
|
|
|
|
|
|
|
|
|
|
|
|
|
|
|
|
|
|
|
|
|
|
|
|
|
|
|
|
|
|
|
|
|
|
|
|
|
|
|
|
|
|
|
|
|
|
|
|
|
|
|
|
|
|
|
|
|
|
|
|
|
|
|
|
|
|
|
|
|
|
|
|
|
|
spaces/2023Liu2023/bingo/src/components/turn-counter.tsx
DELETED
@@ -1,23 +0,0 @@
|
|
1 |
-
import React from 'react'
|
2 |
-
import { Throttling } from '@/lib/bots/bing/types'
|
3 |
-
|
4 |
-
export interface TurnCounterProps {
|
5 |
-
throttling?: Throttling
|
6 |
-
}
|
7 |
-
|
8 |
-
export function TurnCounter({ throttling }: TurnCounterProps) {
|
9 |
-
if (!throttling) {
|
10 |
-
return null
|
11 |
-
}
|
12 |
-
|
13 |
-
return (
|
14 |
-
<div className="turn-counter">
|
15 |
-
<div className="text">
|
16 |
-
<span>{throttling.numUserMessagesInConversation}</span>
|
17 |
-
<span> 共 </span>
|
18 |
-
<span>{throttling.maxNumUserMessagesInConversation}</span>
|
19 |
-
</div>
|
20 |
-
<div className="indicator"></div>
|
21 |
-
</div>
|
22 |
-
)
|
23 |
-
}
|
|
|
|
|
|
|
|
|
|
|
|
|
|
|
|
|
|
|
|
|
|
|
|
|
|
|
|
|
|
|
|
|
|
|
|
|
|
|
|
|
|
|
|
|
|
|
|
spaces/4Taps/SadTalker/src/audio2pose_models/res_unet.py
DELETED
@@ -1,65 +0,0 @@
|
|
1 |
-
import torch
|
2 |
-
import torch.nn as nn
|
3 |
-
from src.audio2pose_models.networks import ResidualConv, Upsample
|
4 |
-
|
5 |
-
|
6 |
-
class ResUnet(nn.Module):
|
7 |
-
def __init__(self, channel=1, filters=[32, 64, 128, 256]):
|
8 |
-
super(ResUnet, self).__init__()
|
9 |
-
|
10 |
-
self.input_layer = nn.Sequential(
|
11 |
-
nn.Conv2d(channel, filters[0], kernel_size=3, padding=1),
|
12 |
-
nn.BatchNorm2d(filters[0]),
|
13 |
-
nn.ReLU(),
|
14 |
-
nn.Conv2d(filters[0], filters[0], kernel_size=3, padding=1),
|
15 |
-
)
|
16 |
-
self.input_skip = nn.Sequential(
|
17 |
-
nn.Conv2d(channel, filters[0], kernel_size=3, padding=1)
|
18 |
-
)
|
19 |
-
|
20 |
-
self.residual_conv_1 = ResidualConv(filters[0], filters[1], stride=(2,1), padding=1)
|
21 |
-
self.residual_conv_2 = ResidualConv(filters[1], filters[2], stride=(2,1), padding=1)
|
22 |
-
|
23 |
-
self.bridge = ResidualConv(filters[2], filters[3], stride=(2,1), padding=1)
|
24 |
-
|
25 |
-
self.upsample_1 = Upsample(filters[3], filters[3], kernel=(2,1), stride=(2,1))
|
26 |
-
self.up_residual_conv1 = ResidualConv(filters[3] + filters[2], filters[2], stride=1, padding=1)
|
27 |
-
|
28 |
-
self.upsample_2 = Upsample(filters[2], filters[2], kernel=(2,1), stride=(2,1))
|
29 |
-
self.up_residual_conv2 = ResidualConv(filters[2] + filters[1], filters[1], stride=1, padding=1)
|
30 |
-
|
31 |
-
self.upsample_3 = Upsample(filters[1], filters[1], kernel=(2,1), stride=(2,1))
|
32 |
-
self.up_residual_conv3 = ResidualConv(filters[1] + filters[0], filters[0], stride=1, padding=1)
|
33 |
-
|
34 |
-
self.output_layer = nn.Sequential(
|
35 |
-
nn.Conv2d(filters[0], 1, 1, 1),
|
36 |
-
nn.Sigmoid(),
|
37 |
-
)
|
38 |
-
|
39 |
-
def forward(self, x):
|
40 |
-
# Encode
|
41 |
-
x1 = self.input_layer(x) + self.input_skip(x)
|
42 |
-
x2 = self.residual_conv_1(x1)
|
43 |
-
x3 = self.residual_conv_2(x2)
|
44 |
-
# Bridge
|
45 |
-
x4 = self.bridge(x3)
|
46 |
-
|
47 |
-
# Decode
|
48 |
-
x4 = self.upsample_1(x4)
|
49 |
-
x5 = torch.cat([x4, x3], dim=1)
|
50 |
-
|
51 |
-
x6 = self.up_residual_conv1(x5)
|
52 |
-
|
53 |
-
x6 = self.upsample_2(x6)
|
54 |
-
x7 = torch.cat([x6, x2], dim=1)
|
55 |
-
|
56 |
-
x8 = self.up_residual_conv2(x7)
|
57 |
-
|
58 |
-
x8 = self.upsample_3(x8)
|
59 |
-
x9 = torch.cat([x8, x1], dim=1)
|
60 |
-
|
61 |
-
x10 = self.up_residual_conv3(x9)
|
62 |
-
|
63 |
-
output = self.output_layer(x10)
|
64 |
-
|
65 |
-
return output
|
|
|
|
|
|
|
|
|
|
|
|
|
|
|
|
|
|
|
|
|
|
|
|
|
|
|
|
|
|
|
|
|
|
|
|
|
|
|
|
|
|
|
|
|
|
|
|
|
|
|
|
|
|
|
|
|
|
|
|
|
|
|
|
|
|
|
|
|
|
|
|
|
|
|
|
|
|
|
|
|
|
|
|
|
|
|
|
|
|
|
|
|
|
|
|
|
|
|
|
|
|
|
|
|
|
|
|
|
|
|
|
|
|
|
|
|
|
|
|
|
|
|
|
|
|
|
|
|
|
|
|
spaces/801artistry/RVC801/utils/dependency.py
DELETED
@@ -1,170 +0,0 @@
|
|
1 |
-
import os
|
2 |
-
import csv
|
3 |
-
import shutil
|
4 |
-
import tarfile
|
5 |
-
import subprocess
|
6 |
-
from pathlib import Path
|
7 |
-
from datetime import datetime
|
8 |
-
|
9 |
-
def install_packages_but_jank_af():
|
10 |
-
packages = ['build-essential', 'python3-dev', 'ffmpeg', 'aria2']
|
11 |
-
pip_packages = ['pip', 'setuptools', 'wheel', 'httpx==0.23.0', 'faiss-gpu', 'fairseq', 'gradio==3.34.0',
|
12 |
-
'ffmpeg', 'ffmpeg-python', 'praat-parselmouth', 'pyworld', 'numpy==1.23.5',
|
13 |
-
'numba==0.56.4', 'librosa==0.9.2', 'mega.py', 'gdown', 'onnxruntime', 'pyngrok==4.1.12',
|
14 |
-
'gTTS', 'elevenlabs', 'wget', 'tensorboardX', 'unidecode', 'huggingface-hub', 'stftpitchshift==1.5.1',
|
15 |
-
'yt-dlp', 'pedalboard', 'pathvalidate', 'nltk', 'edge-tts', 'git+https://github.com/suno-ai/bark.git', 'python-dotenv' , 'av']
|
16 |
-
|
17 |
-
print("Updating and installing system packages...")
|
18 |
-
for package in packages:
|
19 |
-
print(f"Installing {package}...")
|
20 |
-
subprocess.check_call(['apt-get', 'install', '-qq', '-y', package])
|
21 |
-
|
22 |
-
print("Updating and installing pip packages...")
|
23 |
-
subprocess.check_call(['pip', 'install', '--upgrade'] + pip_packages)
|
24 |
-
|
25 |
-
print('Packages up to date.')
|
26 |
-
|
27 |
-
|
28 |
-
def setup_environment(ForceUpdateDependencies, ForceTemporaryStorage):
|
29 |
-
# Mounting Google Drive
|
30 |
-
if not ForceTemporaryStorage:
|
31 |
-
from google.colab import drive
|
32 |
-
|
33 |
-
if not os.path.exists('/content/drive'):
|
34 |
-
drive.mount('/content/drive')
|
35 |
-
else:
|
36 |
-
print('Drive is already mounted. Proceeding...')
|
37 |
-
|
38 |
-
# Function to install dependencies with progress
|
39 |
-
def install_packages():
|
40 |
-
packages = ['build-essential', 'python3-dev', 'ffmpeg', 'aria2']
|
41 |
-
pip_packages = ['pip', 'setuptools', 'wheel', 'httpx==0.23.0', 'faiss-gpu', 'fairseq', 'gradio==3.34.0',
|
42 |
-
'ffmpeg', 'ffmpeg-python', 'praat-parselmouth', 'pyworld', 'numpy==1.23.5',
|
43 |
-
'numba==0.56.4', 'librosa==0.9.2', 'mega.py', 'gdown', 'onnxruntime', 'pyngrok==4.1.12',
|
44 |
-
'gTTS', 'elevenlabs', 'wget', 'tensorboardX', 'unidecode', 'huggingface-hub', 'stftpitchshift==1.5.1',
|
45 |
-
'yt-dlp', 'pedalboard', 'pathvalidate', 'nltk', 'edge-tts', 'git+https://github.com/suno-ai/bark.git', 'python-dotenv' , 'av']
|
46 |
-
|
47 |
-
print("Updating and installing system packages...")
|
48 |
-
for package in packages:
|
49 |
-
print(f"Installing {package}...")
|
50 |
-
subprocess.check_call(['apt-get', 'install', '-qq', '-y', package])
|
51 |
-
|
52 |
-
print("Updating and installing pip packages...")
|
53 |
-
subprocess.check_call(['pip', 'install', '--upgrade'] + pip_packages)
|
54 |
-
|
55 |
-
|
56 |
-
print('Packages up to date.')
|
57 |
-
|
58 |
-
# Function to scan a directory and writes filenames and timestamps
|
59 |
-
def scan_and_write(base_path, output_file):
|
60 |
-
with open(output_file, 'w', newline='') as f:
|
61 |
-
writer = csv.writer(f)
|
62 |
-
for dirpath, dirs, files in os.walk(base_path):
|
63 |
-
for filename in files:
|
64 |
-
fname = os.path.join(dirpath, filename)
|
65 |
-
try:
|
66 |
-
mtime = os.path.getmtime(fname)
|
67 |
-
writer.writerow([fname, mtime])
|
68 |
-
except Exception as e:
|
69 |
-
print(f'Skipping irrelevant nonexistent file {fname}: {str(e)}')
|
70 |
-
print(f'Finished recording filesystem timestamps to {output_file}.')
|
71 |
-
|
72 |
-
# Function to compare files
|
73 |
-
def compare_files(old_file, new_file):
|
74 |
-
old_files = {}
|
75 |
-
new_files = {}
|
76 |
-
|
77 |
-
with open(old_file, 'r') as f:
|
78 |
-
reader = csv.reader(f)
|
79 |
-
old_files = {rows[0]:rows[1] for rows in reader}
|
80 |
-
|
81 |
-
with open(new_file, 'r') as f:
|
82 |
-
reader = csv.reader(f)
|
83 |
-
new_files = {rows[0]:rows[1] for rows in reader}
|
84 |
-
|
85 |
-
removed_files = old_files.keys() - new_files.keys()
|
86 |
-
added_files = new_files.keys() - old_files.keys()
|
87 |
-
unchanged_files = old_files.keys() & new_files.keys()
|
88 |
-
|
89 |
-
changed_files = {f for f in unchanged_files if old_files[f] != new_files[f]}
|
90 |
-
|
91 |
-
for file in removed_files:
|
92 |
-
print(f'File has been removed: {file}')
|
93 |
-
|
94 |
-
for file in changed_files:
|
95 |
-
print(f'File has been updated: {file}')
|
96 |
-
|
97 |
-
return list(added_files) + list(changed_files)
|
98 |
-
|
99 |
-
# Check if CachedRVC.tar.gz exists
|
100 |
-
if ForceTemporaryStorage:
|
101 |
-
file_path = '/content/CachedRVC.tar.gz'
|
102 |
-
else:
|
103 |
-
file_path = '/content/drive/MyDrive/RVC_Cached/CachedRVC.tar.gz'
|
104 |
-
|
105 |
-
content_file_path = '/content/CachedRVC.tar.gz'
|
106 |
-
extract_path = '/'
|
107 |
-
|
108 |
-
if not os.path.exists(file_path):
|
109 |
-
folder_path = os.path.dirname(file_path)
|
110 |
-
os.makedirs(folder_path, exist_ok=True)
|
111 |
-
print('No cached dependency install found. Attempting to download GitHub backup..')
|
112 |
-
|
113 |
-
try:
|
114 |
-
download_url = "https://github.com/kalomaze/QuickMangioFixes/releases/download/release3/CachedRVC.tar.gz"
|
115 |
-
subprocess.run(["wget", "-O", file_path, download_url])
|
116 |
-
print('Download completed successfully!')
|
117 |
-
except Exception as e:
|
118 |
-
print('Download failed:', str(e))
|
119 |
-
|
120 |
-
# Delete the failed download file
|
121 |
-
if os.path.exists(file_path):
|
122 |
-
os.remove(file_path)
|
123 |
-
print('Failed download file deleted. Continuing manual backup..')
|
124 |
-
|
125 |
-
if Path(file_path).exists():
|
126 |
-
if ForceTemporaryStorage:
|
127 |
-
print('Finished downloading CachedRVC.tar.gz.')
|
128 |
-
else:
|
129 |
-
print('CachedRVC.tar.gz found on Google Drive. Proceeding to copy and extract...')
|
130 |
-
|
131 |
-
# Check if ForceTemporaryStorage is True and skip copying if it is
|
132 |
-
if ForceTemporaryStorage:
|
133 |
-
pass
|
134 |
-
else:
|
135 |
-
shutil.copy(file_path, content_file_path)
|
136 |
-
|
137 |
-
print('Beginning backup copy operation...')
|
138 |
-
|
139 |
-
with tarfile.open(content_file_path, 'r:gz') as tar:
|
140 |
-
for member in tar.getmembers():
|
141 |
-
target_path = os.path.join(extract_path, member.name)
|
142 |
-
try:
|
143 |
-
tar.extract(member, extract_path)
|
144 |
-
except Exception as e:
|
145 |
-
print('Failed to extract a file (this isn\'t normal)... forcing an update to compensate')
|
146 |
-
ForceUpdateDependencies = True
|
147 |
-
print(f'Extraction of {content_file_path} to {extract_path} completed.')
|
148 |
-
|
149 |
-
if ForceUpdateDependencies:
|
150 |
-
install_packages()
|
151 |
-
ForceUpdateDependencies = False
|
152 |
-
else:
|
153 |
-
print('CachedRVC.tar.gz not found. Proceeding to create an index of all current files...')
|
154 |
-
scan_and_write('/usr/', '/content/usr_files.csv')
|
155 |
-
|
156 |
-
install_packages()
|
157 |
-
|
158 |
-
scan_and_write('/usr/', '/content/usr_files_new.csv')
|
159 |
-
changed_files = compare_files('/content/usr_files.csv', '/content/usr_files_new.csv')
|
160 |
-
|
161 |
-
with tarfile.open('/content/CachedRVC.tar.gz', 'w:gz') as new_tar:
|
162 |
-
for file in changed_files:
|
163 |
-
new_tar.add(file)
|
164 |
-
print(f'Added to tar: {file}')
|
165 |
-
|
166 |
-
os.makedirs('/content/drive/MyDrive/RVC_Cached', exist_ok=True)
|
167 |
-
shutil.copy('/content/CachedRVC.tar.gz', '/content/drive/MyDrive/RVC_Cached/CachedRVC.tar.gz')
|
168 |
-
print('Updated CachedRVC.tar.gz copied to Google Drive.')
|
169 |
-
print('Dependencies fully up to date; future runs should be faster.')
|
170 |
-
|
|
|
|
|
|
|
|
|
|
|
|
|
|
|
|
|
|
|
|
|
|
|
|
|
|
|
|
|
|
|
|
|
|
|
|
|
|
|
|
|
|
|
|
|
|
|
|
|
|
|
|
|
|
|
|
|
|
|
|
|
|
|
|
|
|
|
|
|
|
|
|
|
|
|
|
|
|
|
|
|
|
|
|
|
|
|
|
|
|
|
|
|
|
|
|
|
|
|
|
|
|
|
|
|
|
|
|
|
|
|
|
|
|
|
|
|
|
|
|
|
|
|
|
|
|
|
|
|
|
|
|
|
|
|
|
|
|
|
|
|
|
|
|
|
|
|
|
|
|
|
|
|
|
|
|
|
|
|
|
|
|
|
|
|
|
|
|
|
|
|
|
|
|
|
|
|
|
|
|
|
|
|
|
|
|
|
|
|
|
|
|
|
|
|
|
|
|
|
|
|
|
|
|
|
|
|
|
|
|
|
|
|
|
|
|
|
|
|
|
|
|
|
|
|
|
|
|
|
|
|
|
|
|
|
|
|
|
|
|
|
|
|
|
|
|
|
|
|
|
|
|
|
|
|
|
|
|
|
|
|
|
|
|
|
|
|
|
|
|
|
|
|
|
|
|
|
|
|
|
|
|
|
|
|
|
|
|
|
|
|
|
|
|
|
|
|
|
|
|
|
|
|
|
|
|
|
|
|
|
|
|
|
|
|
|
|
|
|
|
|
|
|
|
|
|
|
|
|
|
|
|
|
|
|
|
|
|
|
|
|
|
spaces/AIConsultant/MusicGen/tests/modules/__init__.py
DELETED
@@ -1,5 +0,0 @@
|
|
1 |
-
# Copyright (c) Meta Platforms, Inc. and affiliates.
|
2 |
-
# All rights reserved.
|
3 |
-
#
|
4 |
-
# This source code is licensed under the license found in the
|
5 |
-
# LICENSE file in the root directory of this source tree.
|
|
|
|
|
|
|
|
|
|
|
|
spaces/AIWaves/SOP_Generation-single/LLM/__init__.py
DELETED
File without changes
|
spaces/AMR-KELEG/ALDi/README.md
DELETED
@@ -1,13 +0,0 @@
|
|
1 |
-
---
|
2 |
-
title: ALDi
|
3 |
-
emoji: ☕
|
4 |
-
colorFrom: indigo
|
5 |
-
colorTo: purple
|
6 |
-
sdk: streamlit
|
7 |
-
sdk_version: 1.27.2
|
8 |
-
app_file: app.py
|
9 |
-
pinned: true
|
10 |
-
tags: [Arabic]
|
11 |
-
---
|
12 |
-
|
13 |
-
Check out the configuration reference at https://huggingface.co/docs/hub/spaces-config-reference
|
|
|
|
|
|
|
|
|
|
|
|
|
|
|
|
|
|
|
|
|
|
|
|
|
|
|
|
spaces/ASJMO/freegpt/client/css/field.css
DELETED
@@ -1,11 +0,0 @@
|
|
1 |
-
.field {
|
2 |
-
display: flex;
|
3 |
-
align-items: center;
|
4 |
-
padding: 4px;
|
5 |
-
}
|
6 |
-
|
7 |
-
@media screen and (max-width: 990px) {
|
8 |
-
.field {
|
9 |
-
flex-wrap: nowrap;
|
10 |
-
}
|
11 |
-
}
|
|
|
|
|
|
|
|
|
|
|
|
|
|
|
|
|
|
|
|
|
|
|
|
spaces/AchyuthGamer/OpenGPT/g4f/Provider/Providers/Zeabur.py
DELETED
@@ -1,50 +0,0 @@
|
|
1 |
-
import os
|
2 |
-
import requests
|
3 |
-
from ...typing import sha256, Dict, get_type_hints
|
4 |
-
|
5 |
-
url = "https://gptleg.zeabur.app"
|
6 |
-
model = ['gpt-3.5-turbo', 'gpt-3.5-turbo-0301',
|
7 |
-
'gpt-3.5-turbo-16k', 'gpt-4', 'gpt-4-0613']
|
8 |
-
supports_stream = True
|
9 |
-
needs_auth = False
|
10 |
-
|
11 |
-
|
12 |
-
def _create_completion(model: str, messages: list, stream: bool, **kwargs):
|
13 |
-
headers = {
|
14 |
-
'Authority': 'chat.dfehub.com',
|
15 |
-
'Content-Type': 'application/json',
|
16 |
-
'Method': 'POST',
|
17 |
-
'Path': '/api/openai/v1/chat/completions',
|
18 |
-
'Scheme': 'https',
|
19 |
-
'Accept': 'text/event-stream',
|
20 |
-
'Accept-Language': 'pt-BR,pt;q=0.9,en-US;q=0.8,en;q=0.7,zh-CN;q=0.6,zh;q=0.5',
|
21 |
-
'Content-Type': 'application/json',
|
22 |
-
'Origin': 'https://gptleg.zeabur.app',
|
23 |
-
'Referer': 'https://gptleg.zeabur.app/',
|
24 |
-
'Sec-Ch-Ua': '"Not.A/Brand";v="8", "Chromium";v="114", "Google Chrome";v="114"',
|
25 |
-
'Sec-Ch-Ua-Mobile': '?0',
|
26 |
-
'Sec-Ch-Ua-Platform': '"Windows"',
|
27 |
-
'Sec-Fetch-Dest': 'empty',
|
28 |
-
'Sec-Fetch-Mode': 'cors',
|
29 |
-
'Sec-Fetch-Site': 'same-origin',
|
30 |
-
'User-Agent': 'Mozilla/5.0 (Windows NT 10.0; Win64; x64) AppleWebKit/537.36 (KHTML, like Gecko) Chrome/114.0.0.0 Safari/537.36',
|
31 |
-
'X-Requested-With': 'XMLHttpRequest',
|
32 |
-
}
|
33 |
-
|
34 |
-
data = {
|
35 |
-
'model': model,
|
36 |
-
'temperature': 0.7,
|
37 |
-
'max_tokens': '16000',
|
38 |
-
'presence_penalty': 0,
|
39 |
-
'messages': messages,
|
40 |
-
}
|
41 |
-
|
42 |
-
response = requests.post(url + '/api/openai/v1/chat/completions',
|
43 |
-
headers=headers, json=data, stream=stream)
|
44 |
-
|
45 |
-
yield response.json()['choices'][0]['message']['content']
|
46 |
-
|
47 |
-
|
48 |
-
params = f'g4f.Providers.{os.path.basename(__file__)[:-3]} supports: ' + \
|
49 |
-
'(%s)' % ', '.join(
|
50 |
-
[f"{name}: {get_type_hints(_create_completion)[name].__name__}" for name in _create_completion.__code__.co_varnames[:_create_completion.__code__.co_argcount]])
|
|
|
|
|
|
|
|
|
|
|
|
|
|
|
|
|
|
|
|
|
|
|
|
|
|
|
|
|
|
|
|
|
|
|
|
|
|
|
|
|
|
|
|
|
|
|
|
|
|
|
|
|
|
|
|
|
|
|
|
|
|
|
|
|
|
|
|
|
|
|
|
|
|
|
|
|
|
|
|
|
|
|
|
|
|
|
|
|
|
|
|
|
|
|
|
|
|
|
|
|
|
spaces/Adapter/T2I-Adapter/ldm/modules/extra_condition/openpose/hand.py
DELETED
@@ -1,77 +0,0 @@
|
|
1 |
-
import cv2
|
2 |
-
import json
|
3 |
-
import math
|
4 |
-
import matplotlib
|
5 |
-
import matplotlib.pyplot as plt
|
6 |
-
import numpy as np
|
7 |
-
import time
|
8 |
-
import torch
|
9 |
-
from scipy.ndimage.filters import gaussian_filter
|
10 |
-
from skimage.measure import label
|
11 |
-
|
12 |
-
from . import util
|
13 |
-
from .model import handpose_model
|
14 |
-
|
15 |
-
|
16 |
-
class Hand(object):
|
17 |
-
|
18 |
-
def __init__(self, model_path):
|
19 |
-
self.model = handpose_model()
|
20 |
-
if torch.cuda.is_available():
|
21 |
-
self.model = self.model.cuda()
|
22 |
-
print('cuda')
|
23 |
-
model_dict = util.transfer(self.model, torch.load(model_path))
|
24 |
-
self.model.load_state_dict(model_dict)
|
25 |
-
self.model.eval()
|
26 |
-
|
27 |
-
def __call__(self, oriImg):
|
28 |
-
scale_search = [0.5, 1.0, 1.5, 2.0]
|
29 |
-
# scale_search = [0.5]
|
30 |
-
boxsize = 368
|
31 |
-
stride = 8
|
32 |
-
padValue = 128
|
33 |
-
thre = 0.05
|
34 |
-
multiplier = [x * boxsize / oriImg.shape[0] for x in scale_search]
|
35 |
-
heatmap_avg = np.zeros((oriImg.shape[0], oriImg.shape[1], 22))
|
36 |
-
# paf_avg = np.zeros((oriImg.shape[0], oriImg.shape[1], 38))
|
37 |
-
|
38 |
-
for m in range(len(multiplier)):
|
39 |
-
scale = multiplier[m]
|
40 |
-
imageToTest = cv2.resize(oriImg, (0, 0), fx=scale, fy=scale, interpolation=cv2.INTER_CUBIC)
|
41 |
-
imageToTest_padded, pad = util.padRightDownCorner(imageToTest, stride, padValue)
|
42 |
-
im = np.transpose(np.float32(imageToTest_padded[:, :, :, np.newaxis]), (3, 2, 0, 1)) / 256 - 0.5
|
43 |
-
im = np.ascontiguousarray(im)
|
44 |
-
|
45 |
-
data = torch.from_numpy(im).float()
|
46 |
-
if torch.cuda.is_available():
|
47 |
-
data = data.cuda()
|
48 |
-
# data = data.permute([2, 0, 1]).unsqueeze(0).float()
|
49 |
-
with torch.no_grad():
|
50 |
-
output = self.model(data).cpu().numpy()
|
51 |
-
# output = self.model(data).numpy()q
|
52 |
-
|
53 |
-
# extract outputs, resize, and remove padding
|
54 |
-
heatmap = np.transpose(np.squeeze(output), (1, 2, 0)) # output 1 is heatmaps
|
55 |
-
heatmap = cv2.resize(heatmap, (0, 0), fx=stride, fy=stride, interpolation=cv2.INTER_CUBIC)
|
56 |
-
heatmap = heatmap[:imageToTest_padded.shape[0] - pad[2], :imageToTest_padded.shape[1] - pad[3], :]
|
57 |
-
heatmap = cv2.resize(heatmap, (oriImg.shape[1], oriImg.shape[0]), interpolation=cv2.INTER_CUBIC)
|
58 |
-
|
59 |
-
heatmap_avg += heatmap / len(multiplier)
|
60 |
-
|
61 |
-
all_peaks = []
|
62 |
-
for part in range(21):
|
63 |
-
map_ori = heatmap_avg[:, :, part]
|
64 |
-
one_heatmap = gaussian_filter(map_ori, sigma=3)
|
65 |
-
binary = np.ascontiguousarray(one_heatmap > thre, dtype=np.uint8)
|
66 |
-
# 全部小于阈值
|
67 |
-
if np.sum(binary) == 0:
|
68 |
-
all_peaks.append([0, 0])
|
69 |
-
continue
|
70 |
-
label_img, label_numbers = label(binary, return_num=True, connectivity=binary.ndim)
|
71 |
-
max_index = np.argmax([np.sum(map_ori[label_img == i]) for i in range(1, label_numbers + 1)]) + 1
|
72 |
-
label_img[label_img != max_index] = 0
|
73 |
-
map_ori[label_img == 0] = 0
|
74 |
-
|
75 |
-
y, x = util.npmax(map_ori)
|
76 |
-
all_peaks.append([x, y])
|
77 |
-
return np.array(all_peaks)
|
|
|
|
|
|
|
|
|
|
|
|
|
|
|
|
|
|
|
|
|
|
|
|
|
|
|
|
|
|
|
|
|
|
|
|
|
|
|
|
|
|
|
|
|
|
|
|
|
|
|
|
|
|
|
|
|
|
|
|
|
|
|
|
|
|
|
|
|
|
|
|
|
|
|
|
|
|
|
|
|
|
|
|
|
|
|
|
|
|
|
|
|
|
|
|
|
|
|
|
|
|
|
|
|
|
|
|
|
|
|
|
|
|
|
|
|
|
|
|
|
|
|
|
|
|
|
|
|
|
|
|
|
|
|
|
|
|
|
|
|
|
|
|
|
|
|
|
|
|
|
|
|
|
|
|
spaces/AgentVerse/agentVerse/agentverse/llms/openai.py
DELETED
@@ -1,346 +0,0 @@
|
|
1 |
-
import logging
|
2 |
-
import json
|
3 |
-
import ast
|
4 |
-
import os
|
5 |
-
import numpy as np
|
6 |
-
from aiohttp import ClientSession
|
7 |
-
from typing import Dict, List, Optional, Union
|
8 |
-
from tenacity import retry, stop_after_attempt, wait_exponential
|
9 |
-
|
10 |
-
from pydantic import BaseModel, Field
|
11 |
-
|
12 |
-
from agentverse.llms.base import LLMResult
|
13 |
-
from agentverse.logging import logger
|
14 |
-
from agentverse.message import Message
|
15 |
-
|
16 |
-
from . import llm_registry
|
17 |
-
from .base import BaseChatModel, BaseCompletionModel, BaseModelArgs
|
18 |
-
from .utils.jsonrepair import JsonRepair
|
19 |
-
|
20 |
-
try:
|
21 |
-
import openai
|
22 |
-
from openai.error import OpenAIError
|
23 |
-
except ImportError:
|
24 |
-
is_openai_available = False
|
25 |
-
logging.warning("openai package is not installed")
|
26 |
-
else:
|
27 |
-
# openai.proxy = os.environ.get("http_proxy")
|
28 |
-
# if openai.proxy is None:
|
29 |
-
# openai.proxy = os.environ.get("HTTP_PROXY")
|
30 |
-
if os.environ.get("OPENAI_API_KEY") != None:
|
31 |
-
openai.api_key = os.environ.get("OPENAI_API_KEY")
|
32 |
-
is_openai_available = True
|
33 |
-
elif os.environ.get("AZURE_OPENAI_API_KEY") != None:
|
34 |
-
openai.api_type = "azure"
|
35 |
-
openai.api_key = os.environ.get("AZURE_OPENAI_API_KEY")
|
36 |
-
openai.api_base = os.environ.get("AZURE_OPENAI_API_BASE")
|
37 |
-
openai.api_version = "2023-05-15"
|
38 |
-
is_openai_available = True
|
39 |
-
else:
|
40 |
-
logging.warning(
|
41 |
-
"OpenAI API key is not set. Please set the environment variable OPENAI_API_KEY"
|
42 |
-
)
|
43 |
-
is_openai_available = False
|
44 |
-
|
45 |
-
|
46 |
-
class OpenAIChatArgs(BaseModelArgs):
|
47 |
-
model: str = Field(default="gpt-3.5-turbo")
|
48 |
-
deployment_id: str = Field(default=None)
|
49 |
-
max_tokens: int = Field(default=2048)
|
50 |
-
temperature: float = Field(default=1.0)
|
51 |
-
top_p: int = Field(default=1)
|
52 |
-
n: int = Field(default=1)
|
53 |
-
stop: Optional[Union[str, List]] = Field(default=None)
|
54 |
-
presence_penalty: int = Field(default=0)
|
55 |
-
frequency_penalty: int = Field(default=0)
|
56 |
-
|
57 |
-
|
58 |
-
# class OpenAICompletionArgs(OpenAIChatArgs):
|
59 |
-
# model: str = Field(default="text-davinci-003")
|
60 |
-
# suffix: str = Field(default="")
|
61 |
-
# best_of: int = Field(default=1)
|
62 |
-
|
63 |
-
|
64 |
-
# @llm_registry.register("text-davinci-003")
|
65 |
-
# class OpenAICompletion(BaseCompletionModel):
|
66 |
-
# args: OpenAICompletionArgs = Field(default_factory=OpenAICompletionArgs)
|
67 |
-
|
68 |
-
# def __init__(self, max_retry: int = 3, **kwargs):
|
69 |
-
# args = OpenAICompletionArgs()
|
70 |
-
# args = args.dict()
|
71 |
-
# for k, v in args.items():
|
72 |
-
# args[k] = kwargs.pop(k, v)
|
73 |
-
# if len(kwargs) > 0:
|
74 |
-
# logging.warning(f"Unused arguments: {kwargs}")
|
75 |
-
# super().__init__(args=args, max_retry=max_retry)
|
76 |
-
|
77 |
-
# def generate_response(self, prompt: str) -> LLMResult:
|
78 |
-
# response = openai.Completion.create(prompt=prompt, **self.args.dict())
|
79 |
-
# return LLMResult(
|
80 |
-
# content=response["choices"][0]["text"],
|
81 |
-
# send_tokens=response["usage"]["prompt_tokens"],
|
82 |
-
# recv_tokens=response["usage"]["completion_tokens"],
|
83 |
-
# total_tokens=response["usage"]["total_tokens"],
|
84 |
-
# )
|
85 |
-
|
86 |
-
# async def agenerate_response(self, prompt: str) -> LLMResult:
|
87 |
-
# response = await openai.Completion.acreate(prompt=prompt, **self.args.dict())
|
88 |
-
# return LLMResult(
|
89 |
-
# content=response["choices"][0]["text"],
|
90 |
-
# send_tokens=response["usage"]["prompt_tokens"],
|
91 |
-
# recv_tokens=response["usage"]["completion_tokens"],
|
92 |
-
# total_tokens=response["usage"]["total_tokens"],
|
93 |
-
# )
|
94 |
-
|
95 |
-
|
96 |
-
@llm_registry.register("gpt-35-turbo")
|
97 |
-
@llm_registry.register("gpt-3.5-turbo")
|
98 |
-
@llm_registry.register("gpt-4")
|
99 |
-
class OpenAIChat(BaseChatModel):
|
100 |
-
args: OpenAIChatArgs = Field(default_factory=OpenAIChatArgs)
|
101 |
-
|
102 |
-
total_prompt_tokens: int = 0
|
103 |
-
total_completion_tokens: int = 0
|
104 |
-
|
105 |
-
def __init__(self, max_retry: int = 3, **kwargs):
|
106 |
-
args = OpenAIChatArgs()
|
107 |
-
args = args.dict()
|
108 |
-
for k, v in args.items():
|
109 |
-
args[k] = kwargs.pop(k, v)
|
110 |
-
if len(kwargs) > 0:
|
111 |
-
logging.warning(f"Unused arguments: {kwargs}")
|
112 |
-
super().__init__(args=args, max_retry=max_retry)
|
113 |
-
|
114 |
-
# def _construct_messages(self, history: List[Message]):
|
115 |
-
# return history + [{"role": "user", "content": query}]
|
116 |
-
@retry(
|
117 |
-
stop=stop_after_attempt(20),
|
118 |
-
wait=wait_exponential(multiplier=1, min=4, max=10),
|
119 |
-
reraise=True,
|
120 |
-
)
|
121 |
-
def generate_response(
|
122 |
-
self,
|
123 |
-
prepend_prompt: str = "",
|
124 |
-
history: List[dict] = [],
|
125 |
-
append_prompt: str = "",
|
126 |
-
functions: List[dict] = [],
|
127 |
-
) -> LLMResult:
|
128 |
-
messages = self.construct_messages(prepend_prompt, history, append_prompt)
|
129 |
-
logger.log_prompt(messages)
|
130 |
-
try:
|
131 |
-
# Execute function call
|
132 |
-
if functions != []:
|
133 |
-
response = openai.ChatCompletion.create(
|
134 |
-
messages=messages,
|
135 |
-
functions=functions,
|
136 |
-
**self.args.dict(),
|
137 |
-
)
|
138 |
-
if response["choices"][0]["message"].get("function_call") is not None:
|
139 |
-
self.collect_metrics(response)
|
140 |
-
return LLMResult(
|
141 |
-
content=response["choices"][0]["message"].get("content", ""),
|
142 |
-
function_name=response["choices"][0]["message"][
|
143 |
-
"function_call"
|
144 |
-
]["name"],
|
145 |
-
function_arguments=ast.literal_eval(
|
146 |
-
response["choices"][0]["message"]["function_call"][
|
147 |
-
"arguments"
|
148 |
-
]
|
149 |
-
),
|
150 |
-
send_tokens=response["usage"]["prompt_tokens"],
|
151 |
-
recv_tokens=response["usage"]["completion_tokens"],
|
152 |
-
total_tokens=response["usage"]["total_tokens"],
|
153 |
-
)
|
154 |
-
else:
|
155 |
-
self.collect_metrics(response)
|
156 |
-
return LLMResult(
|
157 |
-
content=response["choices"][0]["message"]["content"],
|
158 |
-
send_tokens=response["usage"]["prompt_tokens"],
|
159 |
-
recv_tokens=response["usage"]["completion_tokens"],
|
160 |
-
total_tokens=response["usage"]["total_tokens"],
|
161 |
-
)
|
162 |
-
|
163 |
-
else:
|
164 |
-
response = openai.ChatCompletion.create(
|
165 |
-
messages=messages,
|
166 |
-
**self.args.dict(),
|
167 |
-
)
|
168 |
-
self.collect_metrics(response)
|
169 |
-
return LLMResult(
|
170 |
-
content=response["choices"][0]["message"]["content"],
|
171 |
-
send_tokens=response["usage"]["prompt_tokens"],
|
172 |
-
recv_tokens=response["usage"]["completion_tokens"],
|
173 |
-
total_tokens=response["usage"]["total_tokens"],
|
174 |
-
)
|
175 |
-
except (OpenAIError, KeyboardInterrupt, json.decoder.JSONDecodeError) as error:
|
176 |
-
raise
|
177 |
-
|
178 |
-
@retry(
|
179 |
-
stop=stop_after_attempt(20),
|
180 |
-
wait=wait_exponential(multiplier=1, min=4, max=10),
|
181 |
-
reraise=True,
|
182 |
-
)
|
183 |
-
async def agenerate_response(
|
184 |
-
self,
|
185 |
-
prepend_prompt: str = "",
|
186 |
-
history: List[dict] = [],
|
187 |
-
append_prompt: str = "",
|
188 |
-
functions: List[dict] = [],
|
189 |
-
) -> LLMResult:
|
190 |
-
messages = self.construct_messages(prepend_prompt, history, append_prompt)
|
191 |
-
logger.log_prompt(messages)
|
192 |
-
|
193 |
-
try:
|
194 |
-
if functions != []:
|
195 |
-
async with ClientSession(trust_env=True) as session:
|
196 |
-
openai.aiosession.set(session)
|
197 |
-
response = await openai.ChatCompletion.acreate(
|
198 |
-
messages=messages,
|
199 |
-
functions=functions,
|
200 |
-
**self.args.dict(),
|
201 |
-
)
|
202 |
-
if response["choices"][0]["message"].get("function_call") is not None:
|
203 |
-
function_name = response["choices"][0]["message"]["function_call"][
|
204 |
-
"name"
|
205 |
-
]
|
206 |
-
valid_function = False
|
207 |
-
if function_name.startswith("function."):
|
208 |
-
function_name = function_name.replace("function.", "")
|
209 |
-
elif function_name.startswith("functions."):
|
210 |
-
function_name = function_name.replace("functions.", "")
|
211 |
-
for function in functions:
|
212 |
-
if function["name"] == function_name:
|
213 |
-
valid_function = True
|
214 |
-
break
|
215 |
-
if not valid_function:
|
216 |
-
logger.warn(
|
217 |
-
f"The returned function name {function_name} is not in the list of valid functions. Retrying..."
|
218 |
-
)
|
219 |
-
raise ValueError(
|
220 |
-
f"The returned function name {function_name} is not in the list of valid functions."
|
221 |
-
)
|
222 |
-
try:
|
223 |
-
arguments = ast.literal_eval(
|
224 |
-
response["choices"][0]["message"]["function_call"][
|
225 |
-
"arguments"
|
226 |
-
]
|
227 |
-
)
|
228 |
-
except:
|
229 |
-
try:
|
230 |
-
arguments = ast.literal_eval(
|
231 |
-
JsonRepair(
|
232 |
-
response["choices"][0]["message"]["function_call"][
|
233 |
-
"arguments"
|
234 |
-
]
|
235 |
-
).repair()
|
236 |
-
)
|
237 |
-
except:
|
238 |
-
logger.warn(
|
239 |
-
"The returned argument in function call is not valid json. Retrying..."
|
240 |
-
)
|
241 |
-
raise ValueError(
|
242 |
-
"The returned argument in function call is not valid json."
|
243 |
-
)
|
244 |
-
self.collect_metrics(response)
|
245 |
-
return LLMResult(
|
246 |
-
function_name=function_name,
|
247 |
-
function_arguments=arguments,
|
248 |
-
send_tokens=response["usage"]["prompt_tokens"],
|
249 |
-
recv_tokens=response["usage"]["completion_tokens"],
|
250 |
-
total_tokens=response["usage"]["total_tokens"],
|
251 |
-
)
|
252 |
-
|
253 |
-
else:
|
254 |
-
self.collect_metrics(response)
|
255 |
-
return LLMResult(
|
256 |
-
content=response["choices"][0]["message"]["content"],
|
257 |
-
send_tokens=response["usage"]["prompt_tokens"],
|
258 |
-
recv_tokens=response["usage"]["completion_tokens"],
|
259 |
-
total_tokens=response["usage"]["total_tokens"],
|
260 |
-
)
|
261 |
-
|
262 |
-
else:
|
263 |
-
async with ClientSession(trust_env=True) as session:
|
264 |
-
openai.aiosession.set(session)
|
265 |
-
response = await openai.ChatCompletion.acreate(
|
266 |
-
messages=messages,
|
267 |
-
**self.args.dict(),
|
268 |
-
)
|
269 |
-
self.collect_metrics(response)
|
270 |
-
return LLMResult(
|
271 |
-
content=response["choices"][0]["message"]["content"],
|
272 |
-
send_tokens=response["usage"]["prompt_tokens"],
|
273 |
-
recv_tokens=response["usage"]["completion_tokens"],
|
274 |
-
total_tokens=response["usage"]["total_tokens"],
|
275 |
-
)
|
276 |
-
except (OpenAIError, KeyboardInterrupt, json.decoder.JSONDecodeError) as error:
|
277 |
-
raise
|
278 |
-
|
279 |
-
def construct_messages(
|
280 |
-
self, prepend_prompt: str, history: List[dict], append_prompt: str
|
281 |
-
):
|
282 |
-
messages = []
|
283 |
-
if prepend_prompt != "":
|
284 |
-
messages.append({"role": "system", "content": prepend_prompt})
|
285 |
-
if len(history) > 0:
|
286 |
-
messages += history
|
287 |
-
if append_prompt != "":
|
288 |
-
messages.append({"role": "user", "content": append_prompt})
|
289 |
-
return messages
|
290 |
-
|
291 |
-
def collect_metrics(self, response):
|
292 |
-
self.total_prompt_tokens += response["usage"]["prompt_tokens"]
|
293 |
-
self.total_completion_tokens += response["usage"]["completion_tokens"]
|
294 |
-
|
295 |
-
def get_spend(self) -> int:
|
296 |
-
input_cost_map = {
|
297 |
-
"gpt-3.5-turbo": 0.0015,
|
298 |
-
"gpt-3.5-turbo-16k": 0.003,
|
299 |
-
"gpt-3.5-turbo-0613": 0.0015,
|
300 |
-
"gpt-3.5-turbo-16k-0613": 0.003,
|
301 |
-
"gpt-4": 0.03,
|
302 |
-
"gpt-4-0613": 0.03,
|
303 |
-
"gpt-4-32k": 0.06,
|
304 |
-
}
|
305 |
-
|
306 |
-
output_cost_map = {
|
307 |
-
"gpt-3.5-turbo": 0.002,
|
308 |
-
"gpt-3.5-turbo-16k": 0.004,
|
309 |
-
"gpt-3.5-turbo-0613": 0.002,
|
310 |
-
"gpt-3.5-turbo-16k-0613": 0.004,
|
311 |
-
"gpt-4": 0.06,
|
312 |
-
"gpt-4-0613": 0.06,
|
313 |
-
"gpt-4-32k": 0.12,
|
314 |
-
}
|
315 |
-
|
316 |
-
model = self.args.model
|
317 |
-
if model not in input_cost_map or model not in output_cost_map:
|
318 |
-
raise ValueError(f"Model type {model} not supported")
|
319 |
-
|
320 |
-
return (
|
321 |
-
self.total_prompt_tokens * input_cost_map[model] / 1000.0
|
322 |
-
+ self.total_completion_tokens * output_cost_map[model] / 1000.0
|
323 |
-
)
|
324 |
-
|
325 |
-
|
326 |
-
@retry(
|
327 |
-
stop=stop_after_attempt(3),
|
328 |
-
wait=wait_exponential(multiplier=1, min=4, max=10),
|
329 |
-
reraise=True,
|
330 |
-
)
|
331 |
-
def get_embedding(text: str, attempts=3) -> np.array:
|
332 |
-
try:
|
333 |
-
text = text.replace("\n", " ")
|
334 |
-
if openai.api_type == "azure":
|
335 |
-
embedding = openai.Embedding.create(
|
336 |
-
input=[text], deployment_id="text-embedding-ada-002"
|
337 |
-
)["data"][0]["embedding"]
|
338 |
-
else:
|
339 |
-
embedding = openai.Embedding.create(
|
340 |
-
input=[text], model="text-embedding-ada-002"
|
341 |
-
)["data"][0]["embedding"]
|
342 |
-
return tuple(embedding)
|
343 |
-
except Exception as e:
|
344 |
-
attempt += 1
|
345 |
-
logger.error(f"Error {e} when requesting openai models. Retrying")
|
346 |
-
raise
|
|
|
|
|
|
|
|
|
|
|
|
|
|
|
|
|
|
|
|
|
|
|
|
|
|
|
|
|
|
|
|
|
|
|
|
|
|
|
|
|
|
|
|
|
|
|
|
|
|
|
|
|
|
|
|
|
|
|
|
|
|
|
|
|
|
|
|
|
|
|
|
|
|
|
|
|
|
|
|
|
|
|
|
|
|
|
|
|
|
|
|
|
|
|
|
|
|
|
|
|
|
|
|
|
|
|
|
|
|
|
|
|
|
|
|
|
|
|
|
|
|
|
|
|
|
|
|
|
|
|
|
|
|
|
|
|
|
|
|
|
|
|
|
|
|
|
|
|
|
|
|
|
|
|
|
|
|
|
|
|
|
|
|
|
|
|
|
|
|
|
|
|
|
|
|
|
|
|
|
|
|
|
|
|
|
|
|
|
|
|
|
|
|
|
|
|
|
|
|
|
|
|
|
|
|
|
|
|
|
|
|
|
|
|
|
|
|
|
|
|
|
|
|
|
|
|
|
|
|
|
|
|
|
|
|
|
|
|
|
|
|
|
|
|
|
|
|
|
|
|
|
|
|
|
|
|
|
|
|
|
|
|
|
|
|
|
|
|
|
|
|
|
|
|
|
|
|
|
|
|
|
|
|
|
|
|
|
|
|
|
|
|
|
|
|
|
|
|
|
|
|
|
|
|
|
|
|
|
|
|
|
|
|
|
|
|
|
|
|
|
|
|
|
|
|
|
|
|
|
|
|
|
|
|
|
|
|
|
|
|
|
|
|
|
|
|
|
|
|
|
|
|
|
|
|
|
|
|
|
|
|
|
|
|
|
|
|
|
|
|
|
|
|
|
|
|
|
|
|
|
|
|
|
|
|
|
|
|
|
|
|
|
|
|
|
|
|
|
|
|
|
|
|
|
|
|
|
|
|
|
|
|
|
|
|
|
|
|
|
|
|
|
|
|
|
|
|
|
|
|
|
|
|
|
|
|
|
|
|
|
|
|
|
|
|
|
|
|
|
|
|
|
|
|
|
|
|
|
|
|
|
|
|
|
|
|
|
|
|
|
|
|
|
|
|
|
|
|
|
|
|
|
|
|
|
|
|
|
|
|
|
|
|
|
|
|
|
|
|
|
|
|
|
|
|
|
|
|
|
|
|
|
|
|
|
|
|
|
|
|
|
|
|
|
|
|
|
|
|
|
|
|
|
|
|
|
|
|
|
|
|
|
|
|
|
|
|
|
|
|
|
|
|
|
|
|
|
|
|
|
|
|
|
|
|
|
|
|
|
|
|
|
|
|
|
|
|
|
|
|
|
|
|
|
|
|
|
|
|
|
|
|
|
|
|
|
|
|
|
|
|
|
|
|
|
|
|
|
|
|
|
|
|
|
|
|
|
|
|
|
|
|
|
|
|
|
|
|
|
|
|
|
|
|
|
|
|
|
|
|
|
|
|
|
|
|
|
|
|
|
|
|
|
|
|
|
|
|
|
|
|
|
|
|
|
|
|
|
|
|
|
|
|
|
|
|
|
|
|
|
|
|
|
|
|
|
|
|
|
|
|
|
|
spaces/AgentVerse/agentVerse/ui/src/phaser3-rex-plugins/plugins/filechooser.js
DELETED
@@ -1,3 +0,0 @@
|
|
1 |
-
import OpenFileChooser from './behaviors/filechooser/Open.js';
|
2 |
-
import FileChooser from './gameobjects/dom/filechooser/FileChooser.js';
|
3 |
-
export { OpenFileChooser, FileChooser };
|
|
|
|
|
|
|
|
spaces/AgentVerse/agentVerse/ui/src/phaser3-rex-plugins/templates/ui/gridbuttons/AddChildMethods.js
DELETED
@@ -1,18 +0,0 @@
|
|
1 |
-
import GridSizer from '../gridsizer/GridSizer.js';
|
2 |
-
|
3 |
-
const SizerAdd = GridSizer.prototype.add;
|
4 |
-
|
5 |
-
export default {
|
6 |
-
addButton(gameObject, columnIndex, rowIndex) {
|
7 |
-
SizerAdd.call(this, gameObject, columnIndex, rowIndex, undefined, 0, this.buttonsExpand);
|
8 |
-
this.buttonGroup.add(gameObject);
|
9 |
-
return this;
|
10 |
-
},
|
11 |
-
|
12 |
-
addButtons(gameObjects, rowThenColumn) {
|
13 |
-
for (var i = 0, cnt = gameObjects; i < cnt; i++) {
|
14 |
-
this.addButton(gameObjects[i], undefined, rowThenColumn);
|
15 |
-
}
|
16 |
-
return this;
|
17 |
-
}
|
18 |
-
}
|
|
|
|
|
|
|
|
|
|
|
|
|
|
|
|
|
|
|
|
|
|
|
|
|
|
|
|
|
|
|
|
|
|
|
|
|
|
spaces/Aki004/herta-so-vits/modules/mel_processing.py
DELETED
@@ -1,112 +0,0 @@
|
|
1 |
-
import math
|
2 |
-
import os
|
3 |
-
import random
|
4 |
-
import torch
|
5 |
-
from torch import nn
|
6 |
-
import torch.nn.functional as F
|
7 |
-
import torch.utils.data
|
8 |
-
import numpy as np
|
9 |
-
import librosa
|
10 |
-
import librosa.util as librosa_util
|
11 |
-
from librosa.util import normalize, pad_center, tiny
|
12 |
-
from scipy.signal import get_window
|
13 |
-
from scipy.io.wavfile import read
|
14 |
-
from librosa.filters import mel as librosa_mel_fn
|
15 |
-
|
16 |
-
MAX_WAV_VALUE = 32768.0
|
17 |
-
|
18 |
-
|
19 |
-
def dynamic_range_compression_torch(x, C=1, clip_val=1e-5):
|
20 |
-
"""
|
21 |
-
PARAMS
|
22 |
-
------
|
23 |
-
C: compression factor
|
24 |
-
"""
|
25 |
-
return torch.log(torch.clamp(x, min=clip_val) * C)
|
26 |
-
|
27 |
-
|
28 |
-
def dynamic_range_decompression_torch(x, C=1):
|
29 |
-
"""
|
30 |
-
PARAMS
|
31 |
-
------
|
32 |
-
C: compression factor used to compress
|
33 |
-
"""
|
34 |
-
return torch.exp(x) / C
|
35 |
-
|
36 |
-
|
37 |
-
def spectral_normalize_torch(magnitudes):
|
38 |
-
output = dynamic_range_compression_torch(magnitudes)
|
39 |
-
return output
|
40 |
-
|
41 |
-
|
42 |
-
def spectral_de_normalize_torch(magnitudes):
|
43 |
-
output = dynamic_range_decompression_torch(magnitudes)
|
44 |
-
return output
|
45 |
-
|
46 |
-
|
47 |
-
mel_basis = {}
|
48 |
-
hann_window = {}
|
49 |
-
|
50 |
-
|
51 |
-
def spectrogram_torch(y, n_fft, sampling_rate, hop_size, win_size, center=False):
|
52 |
-
if torch.min(y) < -1.:
|
53 |
-
print('min value is ', torch.min(y))
|
54 |
-
if torch.max(y) > 1.:
|
55 |
-
print('max value is ', torch.max(y))
|
56 |
-
|
57 |
-
global hann_window
|
58 |
-
dtype_device = str(y.dtype) + '_' + str(y.device)
|
59 |
-
wnsize_dtype_device = str(win_size) + '_' + dtype_device
|
60 |
-
if wnsize_dtype_device not in hann_window:
|
61 |
-
hann_window[wnsize_dtype_device] = torch.hann_window(win_size).to(dtype=y.dtype, device=y.device)
|
62 |
-
|
63 |
-
y = torch.nn.functional.pad(y.unsqueeze(1), (int((n_fft-hop_size)/2), int((n_fft-hop_size)/2)), mode='reflect')
|
64 |
-
y = y.squeeze(1)
|
65 |
-
|
66 |
-
spec = torch.stft(y, n_fft, hop_length=hop_size, win_length=win_size, window=hann_window[wnsize_dtype_device],
|
67 |
-
center=center, pad_mode='reflect', normalized=False, onesided=True, return_complex=False)
|
68 |
-
|
69 |
-
spec = torch.sqrt(spec.pow(2).sum(-1) + 1e-6)
|
70 |
-
return spec
|
71 |
-
|
72 |
-
|
73 |
-
def spec_to_mel_torch(spec, n_fft, num_mels, sampling_rate, fmin, fmax):
|
74 |
-
global mel_basis
|
75 |
-
dtype_device = str(spec.dtype) + '_' + str(spec.device)
|
76 |
-
fmax_dtype_device = str(fmax) + '_' + dtype_device
|
77 |
-
if fmax_dtype_device not in mel_basis:
|
78 |
-
mel = librosa_mel_fn(sr=sampling_rate, n_fft=n_fft, n_mels=num_mels, fmin=fmin, fmax=fmax)
|
79 |
-
mel_basis[fmax_dtype_device] = torch.from_numpy(mel).to(dtype=spec.dtype, device=spec.device)
|
80 |
-
spec = torch.matmul(mel_basis[fmax_dtype_device], spec)
|
81 |
-
spec = spectral_normalize_torch(spec)
|
82 |
-
return spec
|
83 |
-
|
84 |
-
|
85 |
-
def mel_spectrogram_torch(y, n_fft, num_mels, sampling_rate, hop_size, win_size, fmin, fmax, center=False):
|
86 |
-
if torch.min(y) < -1.:
|
87 |
-
print('min value is ', torch.min(y))
|
88 |
-
if torch.max(y) > 1.:
|
89 |
-
print('max value is ', torch.max(y))
|
90 |
-
|
91 |
-
global mel_basis, hann_window
|
92 |
-
dtype_device = str(y.dtype) + '_' + str(y.device)
|
93 |
-
fmax_dtype_device = str(fmax) + '_' + dtype_device
|
94 |
-
wnsize_dtype_device = str(win_size) + '_' + dtype_device
|
95 |
-
if fmax_dtype_device not in mel_basis:
|
96 |
-
mel = librosa_mel_fn(sr=sampling_rate, n_fft=n_fft, n_mels=num_mels, fmin=fmin, fmax=fmax)
|
97 |
-
mel_basis[fmax_dtype_device] = torch.from_numpy(mel).to(dtype=y.dtype, device=y.device)
|
98 |
-
if wnsize_dtype_device not in hann_window:
|
99 |
-
hann_window[wnsize_dtype_device] = torch.hann_window(win_size).to(dtype=y.dtype, device=y.device)
|
100 |
-
|
101 |
-
y = torch.nn.functional.pad(y.unsqueeze(1), (int((n_fft-hop_size)/2), int((n_fft-hop_size)/2)), mode='reflect')
|
102 |
-
y = y.squeeze(1)
|
103 |
-
|
104 |
-
spec = torch.stft(y, n_fft, hop_length=hop_size, win_length=win_size, window=hann_window[wnsize_dtype_device],
|
105 |
-
center=center, pad_mode='reflect', normalized=False, onesided=True, return_complex=False)
|
106 |
-
|
107 |
-
spec = torch.sqrt(spec.pow(2).sum(-1) + 1e-6)
|
108 |
-
|
109 |
-
spec = torch.matmul(mel_basis[fmax_dtype_device], spec)
|
110 |
-
spec = spectral_normalize_torch(spec)
|
111 |
-
|
112 |
-
return spec
|
|
|
|
|
|
|
|
|
|
|
|
|
|
|
|
|
|
|
|
|
|
|
|
|
|
|
|
|
|
|
|
|
|
|
|
|
|
|
|
|
|
|
|
|
|
|
|
|
|
|
|
|
|
|
|
|
|
|
|
|
|
|
|
|
|
|
|
|
|
|
|
|
|
|
|
|
|
|
|
|
|
|
|
|
|
|
|
|
|
|
|
|
|
|
|
|
|
|
|
|
|
|
|
|
|
|
|
|
|
|
|
|
|
|
|
|
|
|
|
|
|
|
|
|
|
|
|
|
|
|
|
|
|
|
|
|
|
|
|
|
|
|
|
|
|
|
|
|
|
|
|
|
|
|
|
|
|
|
|
|
|
|
|
|
|
|
|
|
|
|
|
|
|
|
|
|
|
|
|
|
|
|
|
|
|
|
|
|
|
|
|
|
|
|
|
|
|
|
|
|
|
|
|
|
|
|
|
|
|
|
|
|
|
|
|
|
|
|
|
|
|
|
|
|
|
spaces/Amrrs/DragGan-Inversion/stylegan_human/torch_utils/ops/bias_act.cpp
DELETED
@@ -1,101 +0,0 @@
|
|
1 |
-
// Copyright (c) SenseTime Research. All rights reserved.
|
2 |
-
|
3 |
-
// Copyright (c) 2021, NVIDIA CORPORATION. All rights reserved.
|
4 |
-
//
|
5 |
-
// NVIDIA CORPORATION and its licensors retain all intellectual property
|
6 |
-
// and proprietary rights in and to this software, related documentation
|
7 |
-
// and any modifications thereto. Any use, reproduction, disclosure or
|
8 |
-
// distribution of this software and related documentation without an express
|
9 |
-
// license agreement from NVIDIA CORPORATION is strictly prohibited.
|
10 |
-
|
11 |
-
#include <torch/extension.h>
|
12 |
-
#include <ATen/cuda/CUDAContext.h>
|
13 |
-
#include <c10/cuda/CUDAGuard.h>
|
14 |
-
#include "bias_act.h"
|
15 |
-
|
16 |
-
//------------------------------------------------------------------------
|
17 |
-
|
18 |
-
static bool has_same_layout(torch::Tensor x, torch::Tensor y)
|
19 |
-
{
|
20 |
-
if (x.dim() != y.dim())
|
21 |
-
return false;
|
22 |
-
for (int64_t i = 0; i < x.dim(); i++)
|
23 |
-
{
|
24 |
-
if (x.size(i) != y.size(i))
|
25 |
-
return false;
|
26 |
-
if (x.size(i) >= 2 && x.stride(i) != y.stride(i))
|
27 |
-
return false;
|
28 |
-
}
|
29 |
-
return true;
|
30 |
-
}
|
31 |
-
|
32 |
-
//------------------------------------------------------------------------
|
33 |
-
|
34 |
-
static torch::Tensor bias_act(torch::Tensor x, torch::Tensor b, torch::Tensor xref, torch::Tensor yref, torch::Tensor dy, int grad, int dim, int act, float alpha, float gain, float clamp)
|
35 |
-
{
|
36 |
-
// Validate arguments.
|
37 |
-
TORCH_CHECK(x.is_cuda(), "x must reside on CUDA device");
|
38 |
-
TORCH_CHECK(b.numel() == 0 || (b.dtype() == x.dtype() && b.device() == x.device()), "b must have the same dtype and device as x");
|
39 |
-
TORCH_CHECK(xref.numel() == 0 || (xref.sizes() == x.sizes() && xref.dtype() == x.dtype() && xref.device() == x.device()), "xref must have the same shape, dtype, and device as x");
|
40 |
-
TORCH_CHECK(yref.numel() == 0 || (yref.sizes() == x.sizes() && yref.dtype() == x.dtype() && yref.device() == x.device()), "yref must have the same shape, dtype, and device as x");
|
41 |
-
TORCH_CHECK(dy.numel() == 0 || (dy.sizes() == x.sizes() && dy.dtype() == x.dtype() && dy.device() == x.device()), "dy must have the same dtype and device as x");
|
42 |
-
TORCH_CHECK(x.numel() <= INT_MAX, "x is too large");
|
43 |
-
TORCH_CHECK(b.dim() == 1, "b must have rank 1");
|
44 |
-
TORCH_CHECK(b.numel() == 0 || (dim >= 0 && dim < x.dim()), "dim is out of bounds");
|
45 |
-
TORCH_CHECK(b.numel() == 0 || b.numel() == x.size(dim), "b has wrong number of elements");
|
46 |
-
TORCH_CHECK(grad >= 0, "grad must be non-negative");
|
47 |
-
|
48 |
-
// Validate layout.
|
49 |
-
TORCH_CHECK(x.is_non_overlapping_and_dense(), "x must be non-overlapping and dense");
|
50 |
-
TORCH_CHECK(b.is_contiguous(), "b must be contiguous");
|
51 |
-
TORCH_CHECK(xref.numel() == 0 || has_same_layout(xref, x), "xref must have the same layout as x");
|
52 |
-
TORCH_CHECK(yref.numel() == 0 || has_same_layout(yref, x), "yref must have the same layout as x");
|
53 |
-
TORCH_CHECK(dy.numel() == 0 || has_same_layout(dy, x), "dy must have the same layout as x");
|
54 |
-
|
55 |
-
// Create output tensor.
|
56 |
-
const at::cuda::OptionalCUDAGuard device_guard(device_of(x));
|
57 |
-
torch::Tensor y = torch::empty_like(x);
|
58 |
-
TORCH_CHECK(has_same_layout(y, x), "y must have the same layout as x");
|
59 |
-
|
60 |
-
// Initialize CUDA kernel parameters.
|
61 |
-
bias_act_kernel_params p;
|
62 |
-
p.x = x.data_ptr();
|
63 |
-
p.b = (b.numel()) ? b.data_ptr() : NULL;
|
64 |
-
p.xref = (xref.numel()) ? xref.data_ptr() : NULL;
|
65 |
-
p.yref = (yref.numel()) ? yref.data_ptr() : NULL;
|
66 |
-
p.dy = (dy.numel()) ? dy.data_ptr() : NULL;
|
67 |
-
p.y = y.data_ptr();
|
68 |
-
p.grad = grad;
|
69 |
-
p.act = act;
|
70 |
-
p.alpha = alpha;
|
71 |
-
p.gain = gain;
|
72 |
-
p.clamp = clamp;
|
73 |
-
p.sizeX = (int)x.numel();
|
74 |
-
p.sizeB = (int)b.numel();
|
75 |
-
p.stepB = (b.numel()) ? (int)x.stride(dim) : 1;
|
76 |
-
|
77 |
-
// Choose CUDA kernel.
|
78 |
-
void* kernel;
|
79 |
-
AT_DISPATCH_FLOATING_TYPES_AND_HALF(x.scalar_type(), "upfirdn2d_cuda", [&]
|
80 |
-
{
|
81 |
-
kernel = choose_bias_act_kernel<scalar_t>(p);
|
82 |
-
});
|
83 |
-
TORCH_CHECK(kernel, "no CUDA kernel found for the specified activation func");
|
84 |
-
|
85 |
-
// Launch CUDA kernel.
|
86 |
-
p.loopX = 4;
|
87 |
-
int blockSize = 4 * 32;
|
88 |
-
int gridSize = (p.sizeX - 1) / (p.loopX * blockSize) + 1;
|
89 |
-
void* args[] = {&p};
|
90 |
-
AT_CUDA_CHECK(cudaLaunchKernel(kernel, gridSize, blockSize, args, 0, at::cuda::getCurrentCUDAStream()));
|
91 |
-
return y;
|
92 |
-
}
|
93 |
-
|
94 |
-
//------------------------------------------------------------------------
|
95 |
-
|
96 |
-
PYBIND11_MODULE(TORCH_EXTENSION_NAME, m)
|
97 |
-
{
|
98 |
-
m.def("bias_act", &bias_act);
|
99 |
-
}
|
100 |
-
|
101 |
-
//------------------------------------------------------------------------
|
|
|
|
|
|
|
|
|
|
|
|
|
|
|
|
|
|
|
|
|
|
|
|
|
|
|
|
|
|
|
|
|
|
|
|
|
|
|
|
|
|
|
|
|
|
|
|
|
|
|
|
|
|
|
|
|
|
|
|
|
|
|
|
|
|
|
|
|
|
|
|
|
|
|
|
|
|
|
|
|
|
|
|
|
|
|
|
|
|
|
|
|
|
|
|
|
|
|
|
|
|
|
|
|
|
|
|
|
|
|
|
|
|
|
|
|
|
|
|
|
|
|
|
|
|
|
|
|
|
|
|
|
|
|
|
|
|
|
|
|
|
|
|
|
|
|
|
|
|
|
|
|
|
|
|
|
|
|
|
|
|
|
|
|
|
|
|
|
|
|
|
|
|
|
|
|
|
|
|
|
|
|
|
|
|
|
|
|
|
|
|
|
|
|
|
|
|
|
|
|
|
|
|
spaces/Androidonnxfork/CivitAi-to-Diffusers/diffusers/docs/source/ko/optimization/opt_overview.md
DELETED
@@ -1,17 +0,0 @@
|
|
1 |
-
<!--Copyright 2023 The HuggingFace Team. All rights reserved.
|
2 |
-
|
3 |
-
Licensed under the Apache License, Version 2.0 (the "License"); you may not use this file except in compliance with
|
4 |
-
the License. You may obtain a copy of the License at
|
5 |
-
|
6 |
-
http://www.apache.org/licenses/LICENSE-2.0
|
7 |
-
|
8 |
-
Unless required by applicable law or agreed to in writing, software distributed under the License is distributed on
|
9 |
-
an "AS IS" BASIS, WITHOUT WARRANTIES OR CONDITIONS OF ANY KIND, either express or implied. See the License for the
|
10 |
-
specific language governing permissions and limitations under the License.
|
11 |
-
-->
|
12 |
-
|
13 |
-
# 개요
|
14 |
-
|
15 |
-
노이즈가 많은 출력에서 적은 출력으로 만드는 과정으로 고품질 생성 모델의 출력을 만드는 각각의 반복되는 스텝은 많은 계산이 필요합니다. 🧨 Diffuser의 목표 중 하나는 모든 사람이 이 기술을 널리 이용할 수 있도록 하는 것이며, 여기에는 소비자 및 특수 하드웨어에서 빠른 추론을 가능하게 하는 것을 포함합니다.
|
16 |
-
|
17 |
-
이 섹션에서는 추론 속도를 최적화하고 메모리 소비를 줄이기 위한 반정밀(half-precision) 가중치 및 sliced attention과 같은 팁과 요령을 다룹니다. 또한 [`torch.compile`](https://pytorch.org/tutorials/intermediate/torch_compile_tutorial.html) 또는 [ONNX Runtime](https://onnxruntime.ai/docs/)을 사용하여 PyTorch 코드의 속도를 높이고, [xFormers](https://facebookresearch.github.io/xformers/)를 사용하여 memory-efficient attention을 활성화하는 방법을 배울 수 있습니다. Apple Silicon, Intel 또는 Habana 프로세서와 같은 특정 하드웨어에서 추론을 실행하기 위한 가이드도 있습니다.
|
|
|
|
|
|
|
|
|
|
|
|
|
|
|
|
|
|
|
|
|
|
|
|
|
|
|
|
|
|
|
|
|
|
|
|
spaces/Androidonnxfork/CivitAi-to-Diffusers/diffusers/src/diffusers/pipelines/shap_e/renderer.py
DELETED
@@ -1,1050 +0,0 @@
|
|
1 |
-
# Copyright 2023 Open AI and The HuggingFace Team. All rights reserved.
|
2 |
-
#
|
3 |
-
# Licensed under the Apache License, Version 2.0 (the "License");
|
4 |
-
# you may not use this file except in compliance with the License.
|
5 |
-
# You may obtain a copy of the License at
|
6 |
-
#
|
7 |
-
# http://www.apache.org/licenses/LICENSE-2.0
|
8 |
-
#
|
9 |
-
# Unless required by applicable law or agreed to in writing, software
|
10 |
-
# distributed under the License is distributed on an "AS IS" BASIS,
|
11 |
-
# WITHOUT WARRANTIES OR CONDITIONS OF ANY KIND, either express or implied.
|
12 |
-
# See the License for the specific language governing permissions and
|
13 |
-
# limitations under the License.
|
14 |
-
|
15 |
-
import math
|
16 |
-
from dataclasses import dataclass
|
17 |
-
from typing import Dict, Optional, Tuple
|
18 |
-
|
19 |
-
import numpy as np
|
20 |
-
import torch
|
21 |
-
import torch.nn.functional as F
|
22 |
-
from torch import nn
|
23 |
-
|
24 |
-
from ...configuration_utils import ConfigMixin, register_to_config
|
25 |
-
from ...models import ModelMixin
|
26 |
-
from ...utils import BaseOutput
|
27 |
-
from .camera import create_pan_cameras
|
28 |
-
|
29 |
-
|
30 |
-
def sample_pmf(pmf: torch.Tensor, n_samples: int) -> torch.Tensor:
|
31 |
-
r"""
|
32 |
-
Sample from the given discrete probability distribution with replacement.
|
33 |
-
|
34 |
-
The i-th bin is assumed to have mass pmf[i].
|
35 |
-
|
36 |
-
Args:
|
37 |
-
pmf: [batch_size, *shape, n_samples, 1] where (pmf.sum(dim=-2) == 1).all()
|
38 |
-
n_samples: number of samples
|
39 |
-
|
40 |
-
Return:
|
41 |
-
indices sampled with replacement
|
42 |
-
"""
|
43 |
-
|
44 |
-
*shape, support_size, last_dim = pmf.shape
|
45 |
-
assert last_dim == 1
|
46 |
-
|
47 |
-
cdf = torch.cumsum(pmf.view(-1, support_size), dim=1)
|
48 |
-
inds = torch.searchsorted(cdf, torch.rand(cdf.shape[0], n_samples, device=cdf.device))
|
49 |
-
|
50 |
-
return inds.view(*shape, n_samples, 1).clamp(0, support_size - 1)
|
51 |
-
|
52 |
-
|
53 |
-
def posenc_nerf(x: torch.Tensor, min_deg: int = 0, max_deg: int = 15) -> torch.Tensor:
|
54 |
-
"""
|
55 |
-
Concatenate x and its positional encodings, following NeRF.
|
56 |
-
|
57 |
-
Reference: https://arxiv.org/pdf/2210.04628.pdf
|
58 |
-
"""
|
59 |
-
if min_deg == max_deg:
|
60 |
-
return x
|
61 |
-
|
62 |
-
scales = 2.0 ** torch.arange(min_deg, max_deg, dtype=x.dtype, device=x.device)
|
63 |
-
*shape, dim = x.shape
|
64 |
-
xb = (x.reshape(-1, 1, dim) * scales.view(1, -1, 1)).reshape(*shape, -1)
|
65 |
-
assert xb.shape[-1] == dim * (max_deg - min_deg)
|
66 |
-
emb = torch.cat([xb, xb + math.pi / 2.0], axis=-1).sin()
|
67 |
-
return torch.cat([x, emb], dim=-1)
|
68 |
-
|
69 |
-
|
70 |
-
def encode_position(position):
|
71 |
-
return posenc_nerf(position, min_deg=0, max_deg=15)
|
72 |
-
|
73 |
-
|
74 |
-
def encode_direction(position, direction=None):
|
75 |
-
if direction is None:
|
76 |
-
return torch.zeros_like(posenc_nerf(position, min_deg=0, max_deg=8))
|
77 |
-
else:
|
78 |
-
return posenc_nerf(direction, min_deg=0, max_deg=8)
|
79 |
-
|
80 |
-
|
81 |
-
def _sanitize_name(x: str) -> str:
|
82 |
-
return x.replace(".", "__")
|
83 |
-
|
84 |
-
|
85 |
-
def integrate_samples(volume_range, ts, density, channels):
|
86 |
-
r"""
|
87 |
-
Function integrating the model output.
|
88 |
-
|
89 |
-
Args:
|
90 |
-
volume_range: Specifies the integral range [t0, t1]
|
91 |
-
ts: timesteps
|
92 |
-
density: torch.Tensor [batch_size, *shape, n_samples, 1]
|
93 |
-
channels: torch.Tensor [batch_size, *shape, n_samples, n_channels]
|
94 |
-
returns:
|
95 |
-
channels: integrated rgb output weights: torch.Tensor [batch_size, *shape, n_samples, 1] (density
|
96 |
-
*transmittance)[i] weight for each rgb output at [..., i, :]. transmittance: transmittance of this volume
|
97 |
-
)
|
98 |
-
"""
|
99 |
-
|
100 |
-
# 1. Calculate the weights
|
101 |
-
_, _, dt = volume_range.partition(ts)
|
102 |
-
ddensity = density * dt
|
103 |
-
|
104 |
-
mass = torch.cumsum(ddensity, dim=-2)
|
105 |
-
transmittance = torch.exp(-mass[..., -1, :])
|
106 |
-
|
107 |
-
alphas = 1.0 - torch.exp(-ddensity)
|
108 |
-
Ts = torch.exp(torch.cat([torch.zeros_like(mass[..., :1, :]), -mass[..., :-1, :]], dim=-2))
|
109 |
-
# This is the probability of light hitting and reflecting off of
|
110 |
-
# something at depth [..., i, :].
|
111 |
-
weights = alphas * Ts
|
112 |
-
|
113 |
-
# 2. Integrate channels
|
114 |
-
channels = torch.sum(channels * weights, dim=-2)
|
115 |
-
|
116 |
-
return channels, weights, transmittance
|
117 |
-
|
118 |
-
|
119 |
-
def volume_query_points(volume, grid_size):
|
120 |
-
indices = torch.arange(grid_size**3, device=volume.bbox_min.device)
|
121 |
-
zs = indices % grid_size
|
122 |
-
ys = torch.div(indices, grid_size, rounding_mode="trunc") % grid_size
|
123 |
-
xs = torch.div(indices, grid_size**2, rounding_mode="trunc") % grid_size
|
124 |
-
combined = torch.stack([xs, ys, zs], dim=1)
|
125 |
-
return (combined.float() / (grid_size - 1)) * (volume.bbox_max - volume.bbox_min) + volume.bbox_min
|
126 |
-
|
127 |
-
|
128 |
-
def _convert_srgb_to_linear(u: torch.Tensor):
|
129 |
-
return torch.where(u <= 0.04045, u / 12.92, ((u + 0.055) / 1.055) ** 2.4)
|
130 |
-
|
131 |
-
|
132 |
-
def _create_flat_edge_indices(
|
133 |
-
flat_cube_indices: torch.Tensor,
|
134 |
-
grid_size: Tuple[int, int, int],
|
135 |
-
):
|
136 |
-
num_xs = (grid_size[0] - 1) * grid_size[1] * grid_size[2]
|
137 |
-
y_offset = num_xs
|
138 |
-
num_ys = grid_size[0] * (grid_size[1] - 1) * grid_size[2]
|
139 |
-
z_offset = num_xs + num_ys
|
140 |
-
return torch.stack(
|
141 |
-
[
|
142 |
-
# Edges spanning x-axis.
|
143 |
-
flat_cube_indices[:, 0] * grid_size[1] * grid_size[2]
|
144 |
-
+ flat_cube_indices[:, 1] * grid_size[2]
|
145 |
-
+ flat_cube_indices[:, 2],
|
146 |
-
flat_cube_indices[:, 0] * grid_size[1] * grid_size[2]
|
147 |
-
+ (flat_cube_indices[:, 1] + 1) * grid_size[2]
|
148 |
-
+ flat_cube_indices[:, 2],
|
149 |
-
flat_cube_indices[:, 0] * grid_size[1] * grid_size[2]
|
150 |
-
+ flat_cube_indices[:, 1] * grid_size[2]
|
151 |
-
+ flat_cube_indices[:, 2]
|
152 |
-
+ 1,
|
153 |
-
flat_cube_indices[:, 0] * grid_size[1] * grid_size[2]
|
154 |
-
+ (flat_cube_indices[:, 1] + 1) * grid_size[2]
|
155 |
-
+ flat_cube_indices[:, 2]
|
156 |
-
+ 1,
|
157 |
-
# Edges spanning y-axis.
|
158 |
-
(
|
159 |
-
y_offset
|
160 |
-
+ flat_cube_indices[:, 0] * (grid_size[1] - 1) * grid_size[2]
|
161 |
-
+ flat_cube_indices[:, 1] * grid_size[2]
|
162 |
-
+ flat_cube_indices[:, 2]
|
163 |
-
),
|
164 |
-
(
|
165 |
-
y_offset
|
166 |
-
+ (flat_cube_indices[:, 0] + 1) * (grid_size[1] - 1) * grid_size[2]
|
167 |
-
+ flat_cube_indices[:, 1] * grid_size[2]
|
168 |
-
+ flat_cube_indices[:, 2]
|
169 |
-
),
|
170 |
-
(
|
171 |
-
y_offset
|
172 |
-
+ flat_cube_indices[:, 0] * (grid_size[1] - 1) * grid_size[2]
|
173 |
-
+ flat_cube_indices[:, 1] * grid_size[2]
|
174 |
-
+ flat_cube_indices[:, 2]
|
175 |
-
+ 1
|
176 |
-
),
|
177 |
-
(
|
178 |
-
y_offset
|
179 |
-
+ (flat_cube_indices[:, 0] + 1) * (grid_size[1] - 1) * grid_size[2]
|
180 |
-
+ flat_cube_indices[:, 1] * grid_size[2]
|
181 |
-
+ flat_cube_indices[:, 2]
|
182 |
-
+ 1
|
183 |
-
),
|
184 |
-
# Edges spanning z-axis.
|
185 |
-
(
|
186 |
-
z_offset
|
187 |
-
+ flat_cube_indices[:, 0] * grid_size[1] * (grid_size[2] - 1)
|
188 |
-
+ flat_cube_indices[:, 1] * (grid_size[2] - 1)
|
189 |
-
+ flat_cube_indices[:, 2]
|
190 |
-
),
|
191 |
-
(
|
192 |
-
z_offset
|
193 |
-
+ (flat_cube_indices[:, 0] + 1) * grid_size[1] * (grid_size[2] - 1)
|
194 |
-
+ flat_cube_indices[:, 1] * (grid_size[2] - 1)
|
195 |
-
+ flat_cube_indices[:, 2]
|
196 |
-
),
|
197 |
-
(
|
198 |
-
z_offset
|
199 |
-
+ flat_cube_indices[:, 0] * grid_size[1] * (grid_size[2] - 1)
|
200 |
-
+ (flat_cube_indices[:, 1] + 1) * (grid_size[2] - 1)
|
201 |
-
+ flat_cube_indices[:, 2]
|
202 |
-
),
|
203 |
-
(
|
204 |
-
z_offset
|
205 |
-
+ (flat_cube_indices[:, 0] + 1) * grid_size[1] * (grid_size[2] - 1)
|
206 |
-
+ (flat_cube_indices[:, 1] + 1) * (grid_size[2] - 1)
|
207 |
-
+ flat_cube_indices[:, 2]
|
208 |
-
),
|
209 |
-
],
|
210 |
-
dim=-1,
|
211 |
-
)
|
212 |
-
|
213 |
-
|
214 |
-
class VoidNeRFModel(nn.Module):
|
215 |
-
"""
|
216 |
-
Implements the default empty space model where all queries are rendered as background.
|
217 |
-
"""
|
218 |
-
|
219 |
-
def __init__(self, background, channel_scale=255.0):
|
220 |
-
super().__init__()
|
221 |
-
background = nn.Parameter(torch.from_numpy(np.array(background)).to(dtype=torch.float32) / channel_scale)
|
222 |
-
|
223 |
-
self.register_buffer("background", background)
|
224 |
-
|
225 |
-
def forward(self, position):
|
226 |
-
background = self.background[None].to(position.device)
|
227 |
-
|
228 |
-
shape = position.shape[:-1]
|
229 |
-
ones = [1] * (len(shape) - 1)
|
230 |
-
n_channels = background.shape[-1]
|
231 |
-
background = torch.broadcast_to(background.view(background.shape[0], *ones, n_channels), [*shape, n_channels])
|
232 |
-
|
233 |
-
return background
|
234 |
-
|
235 |
-
|
236 |
-
@dataclass
|
237 |
-
class VolumeRange:
|
238 |
-
t0: torch.Tensor
|
239 |
-
t1: torch.Tensor
|
240 |
-
intersected: torch.Tensor
|
241 |
-
|
242 |
-
def __post_init__(self):
|
243 |
-
assert self.t0.shape == self.t1.shape == self.intersected.shape
|
244 |
-
|
245 |
-
def partition(self, ts):
|
246 |
-
"""
|
247 |
-
Partitions t0 and t1 into n_samples intervals.
|
248 |
-
|
249 |
-
Args:
|
250 |
-
ts: [batch_size, *shape, n_samples, 1]
|
251 |
-
|
252 |
-
Return:
|
253 |
-
|
254 |
-
lower: [batch_size, *shape, n_samples, 1] upper: [batch_size, *shape, n_samples, 1] delta: [batch_size,
|
255 |
-
*shape, n_samples, 1]
|
256 |
-
|
257 |
-
where
|
258 |
-
ts \\in [lower, upper] deltas = upper - lower
|
259 |
-
"""
|
260 |
-
|
261 |
-
mids = (ts[..., 1:, :] + ts[..., :-1, :]) * 0.5
|
262 |
-
lower = torch.cat([self.t0[..., None, :], mids], dim=-2)
|
263 |
-
upper = torch.cat([mids, self.t1[..., None, :]], dim=-2)
|
264 |
-
delta = upper - lower
|
265 |
-
assert lower.shape == upper.shape == delta.shape == ts.shape
|
266 |
-
return lower, upper, delta
|
267 |
-
|
268 |
-
|
269 |
-
class BoundingBoxVolume(nn.Module):
|
270 |
-
"""
|
271 |
-
Axis-aligned bounding box defined by the two opposite corners.
|
272 |
-
"""
|
273 |
-
|
274 |
-
def __init__(
|
275 |
-
self,
|
276 |
-
*,
|
277 |
-
bbox_min,
|
278 |
-
bbox_max,
|
279 |
-
min_dist: float = 0.0,
|
280 |
-
min_t_range: float = 1e-3,
|
281 |
-
):
|
282 |
-
"""
|
283 |
-
Args:
|
284 |
-
bbox_min: the left/bottommost corner of the bounding box
|
285 |
-
bbox_max: the other corner of the bounding box
|
286 |
-
min_dist: all rays should start at least this distance away from the origin.
|
287 |
-
"""
|
288 |
-
super().__init__()
|
289 |
-
|
290 |
-
self.min_dist = min_dist
|
291 |
-
self.min_t_range = min_t_range
|
292 |
-
|
293 |
-
self.bbox_min = torch.tensor(bbox_min)
|
294 |
-
self.bbox_max = torch.tensor(bbox_max)
|
295 |
-
self.bbox = torch.stack([self.bbox_min, self.bbox_max])
|
296 |
-
assert self.bbox.shape == (2, 3)
|
297 |
-
assert min_dist >= 0.0
|
298 |
-
assert min_t_range > 0.0
|
299 |
-
|
300 |
-
def intersect(
|
301 |
-
self,
|
302 |
-
origin: torch.Tensor,
|
303 |
-
direction: torch.Tensor,
|
304 |
-
t0_lower: Optional[torch.Tensor] = None,
|
305 |
-
epsilon=1e-6,
|
306 |
-
):
|
307 |
-
"""
|
308 |
-
Args:
|
309 |
-
origin: [batch_size, *shape, 3]
|
310 |
-
direction: [batch_size, *shape, 3]
|
311 |
-
t0_lower: Optional [batch_size, *shape, 1] lower bound of t0 when intersecting this volume.
|
312 |
-
params: Optional meta parameters in case Volume is parametric
|
313 |
-
epsilon: to stabilize calculations
|
314 |
-
|
315 |
-
Return:
|
316 |
-
A tuple of (t0, t1, intersected) where each has a shape [batch_size, *shape, 1]. If a ray intersects with
|
317 |
-
the volume, `o + td` is in the volume for all t in [t0, t1]. If the volume is bounded, t1 is guaranteed to
|
318 |
-
be on the boundary of the volume.
|
319 |
-
"""
|
320 |
-
|
321 |
-
batch_size, *shape, _ = origin.shape
|
322 |
-
ones = [1] * len(shape)
|
323 |
-
bbox = self.bbox.view(1, *ones, 2, 3).to(origin.device)
|
324 |
-
|
325 |
-
def _safe_divide(a, b, epsilon=1e-6):
|
326 |
-
return a / torch.where(b < 0, b - epsilon, b + epsilon)
|
327 |
-
|
328 |
-
ts = _safe_divide(bbox - origin[..., None, :], direction[..., None, :], epsilon=epsilon)
|
329 |
-
|
330 |
-
# Cases to think about:
|
331 |
-
#
|
332 |
-
# 1. t1 <= t0: the ray does not pass through the AABB.
|
333 |
-
# 2. t0 < t1 <= 0: the ray intersects but the BB is behind the origin.
|
334 |
-
# 3. t0 <= 0 <= t1: the ray starts from inside the BB
|
335 |
-
# 4. 0 <= t0 < t1: the ray is not inside and intersects with the BB twice.
|
336 |
-
#
|
337 |
-
# 1 and 4 are clearly handled from t0 < t1 below.
|
338 |
-
# Making t0 at least min_dist (>= 0) takes care of 2 and 3.
|
339 |
-
t0 = ts.min(dim=-2).values.max(dim=-1, keepdim=True).values.clamp(self.min_dist)
|
340 |
-
t1 = ts.max(dim=-2).values.min(dim=-1, keepdim=True).values
|
341 |
-
assert t0.shape == t1.shape == (batch_size, *shape, 1)
|
342 |
-
if t0_lower is not None:
|
343 |
-
assert t0.shape == t0_lower.shape
|
344 |
-
t0 = torch.maximum(t0, t0_lower)
|
345 |
-
|
346 |
-
intersected = t0 + self.min_t_range < t1
|
347 |
-
t0 = torch.where(intersected, t0, torch.zeros_like(t0))
|
348 |
-
t1 = torch.where(intersected, t1, torch.ones_like(t1))
|
349 |
-
|
350 |
-
return VolumeRange(t0=t0, t1=t1, intersected=intersected)
|
351 |
-
|
352 |
-
|
353 |
-
class StratifiedRaySampler(nn.Module):
|
354 |
-
"""
|
355 |
-
Instead of fixed intervals, a sample is drawn uniformly at random from each interval.
|
356 |
-
"""
|
357 |
-
|
358 |
-
def __init__(self, depth_mode: str = "linear"):
|
359 |
-
"""
|
360 |
-
:param depth_mode: linear samples ts linearly in depth. harmonic ensures
|
361 |
-
closer points are sampled more densely.
|
362 |
-
"""
|
363 |
-
self.depth_mode = depth_mode
|
364 |
-
assert self.depth_mode in ("linear", "geometric", "harmonic")
|
365 |
-
|
366 |
-
def sample(
|
367 |
-
self,
|
368 |
-
t0: torch.Tensor,
|
369 |
-
t1: torch.Tensor,
|
370 |
-
n_samples: int,
|
371 |
-
epsilon: float = 1e-3,
|
372 |
-
) -> torch.Tensor:
|
373 |
-
"""
|
374 |
-
Args:
|
375 |
-
t0: start time has shape [batch_size, *shape, 1]
|
376 |
-
t1: finish time has shape [batch_size, *shape, 1]
|
377 |
-
n_samples: number of ts to sample
|
378 |
-
Return:
|
379 |
-
sampled ts of shape [batch_size, *shape, n_samples, 1]
|
380 |
-
"""
|
381 |
-
ones = [1] * (len(t0.shape) - 1)
|
382 |
-
ts = torch.linspace(0, 1, n_samples).view(*ones, n_samples).to(t0.dtype).to(t0.device)
|
383 |
-
|
384 |
-
if self.depth_mode == "linear":
|
385 |
-
ts = t0 * (1.0 - ts) + t1 * ts
|
386 |
-
elif self.depth_mode == "geometric":
|
387 |
-
ts = (t0.clamp(epsilon).log() * (1.0 - ts) + t1.clamp(epsilon).log() * ts).exp()
|
388 |
-
elif self.depth_mode == "harmonic":
|
389 |
-
# The original NeRF recommends this interpolation scheme for
|
390 |
-
# spherical scenes, but there could be some weird edge cases when
|
391 |
-
# the observer crosses from the inner to outer volume.
|
392 |
-
ts = 1.0 / (1.0 / t0.clamp(epsilon) * (1.0 - ts) + 1.0 / t1.clamp(epsilon) * ts)
|
393 |
-
|
394 |
-
mids = 0.5 * (ts[..., 1:] + ts[..., :-1])
|
395 |
-
upper = torch.cat([mids, t1], dim=-1)
|
396 |
-
lower = torch.cat([t0, mids], dim=-1)
|
397 |
-
# yiyi notes: add a random seed here for testing, don't forget to remove
|
398 |
-
torch.manual_seed(0)
|
399 |
-
t_rand = torch.rand_like(ts)
|
400 |
-
|
401 |
-
ts = lower + (upper - lower) * t_rand
|
402 |
-
return ts.unsqueeze(-1)
|
403 |
-
|
404 |
-
|
405 |
-
class ImportanceRaySampler(nn.Module):
|
406 |
-
"""
|
407 |
-
Given the initial estimate of densities, this samples more from regions/bins expected to have objects.
|
408 |
-
"""
|
409 |
-
|
410 |
-
def __init__(
|
411 |
-
self,
|
412 |
-
volume_range: VolumeRange,
|
413 |
-
ts: torch.Tensor,
|
414 |
-
weights: torch.Tensor,
|
415 |
-
blur_pool: bool = False,
|
416 |
-
alpha: float = 1e-5,
|
417 |
-
):
|
418 |
-
"""
|
419 |
-
Args:
|
420 |
-
volume_range: the range in which a ray intersects the given volume.
|
421 |
-
ts: earlier samples from the coarse rendering step
|
422 |
-
weights: discretized version of density * transmittance
|
423 |
-
blur_pool: if true, use 2-tap max + 2-tap blur filter from mip-NeRF.
|
424 |
-
alpha: small value to add to weights.
|
425 |
-
"""
|
426 |
-
self.volume_range = volume_range
|
427 |
-
self.ts = ts.clone().detach()
|
428 |
-
self.weights = weights.clone().detach()
|
429 |
-
self.blur_pool = blur_pool
|
430 |
-
self.alpha = alpha
|
431 |
-
|
432 |
-
@torch.no_grad()
|
433 |
-
def sample(self, t0: torch.Tensor, t1: torch.Tensor, n_samples: int) -> torch.Tensor:
|
434 |
-
"""
|
435 |
-
Args:
|
436 |
-
t0: start time has shape [batch_size, *shape, 1]
|
437 |
-
t1: finish time has shape [batch_size, *shape, 1]
|
438 |
-
n_samples: number of ts to sample
|
439 |
-
Return:
|
440 |
-
sampled ts of shape [batch_size, *shape, n_samples, 1]
|
441 |
-
"""
|
442 |
-
lower, upper, _ = self.volume_range.partition(self.ts)
|
443 |
-
|
444 |
-
batch_size, *shape, n_coarse_samples, _ = self.ts.shape
|
445 |
-
|
446 |
-
weights = self.weights
|
447 |
-
if self.blur_pool:
|
448 |
-
padded = torch.cat([weights[..., :1, :], weights, weights[..., -1:, :]], dim=-2)
|
449 |
-
maxes = torch.maximum(padded[..., :-1, :], padded[..., 1:, :])
|
450 |
-
weights = 0.5 * (maxes[..., :-1, :] + maxes[..., 1:, :])
|
451 |
-
weights = weights + self.alpha
|
452 |
-
pmf = weights / weights.sum(dim=-2, keepdim=True)
|
453 |
-
inds = sample_pmf(pmf, n_samples)
|
454 |
-
assert inds.shape == (batch_size, *shape, n_samples, 1)
|
455 |
-
assert (inds >= 0).all() and (inds < n_coarse_samples).all()
|
456 |
-
|
457 |
-
t_rand = torch.rand(inds.shape, device=inds.device)
|
458 |
-
lower_ = torch.gather(lower, -2, inds)
|
459 |
-
upper_ = torch.gather(upper, -2, inds)
|
460 |
-
|
461 |
-
ts = lower_ + (upper_ - lower_) * t_rand
|
462 |
-
ts = torch.sort(ts, dim=-2).values
|
463 |
-
return ts
|
464 |
-
|
465 |
-
|
466 |
-
@dataclass
|
467 |
-
class MeshDecoderOutput(BaseOutput):
|
468 |
-
"""
|
469 |
-
A 3D triangle mesh with optional data at the vertices and faces.
|
470 |
-
|
471 |
-
Args:
|
472 |
-
verts (`torch.Tensor` of shape `(N, 3)`):
|
473 |
-
array of vertext coordinates
|
474 |
-
faces (`torch.Tensor` of shape `(N, 3)`):
|
475 |
-
array of triangles, pointing to indices in verts.
|
476 |
-
vertext_channels (Dict):
|
477 |
-
vertext coordinates for each color channel
|
478 |
-
"""
|
479 |
-
|
480 |
-
verts: torch.Tensor
|
481 |
-
faces: torch.Tensor
|
482 |
-
vertex_channels: Dict[str, torch.Tensor]
|
483 |
-
|
484 |
-
|
485 |
-
class MeshDecoder(nn.Module):
|
486 |
-
"""
|
487 |
-
Construct meshes from Signed distance functions (SDFs) using marching cubes method
|
488 |
-
"""
|
489 |
-
|
490 |
-
def __init__(self):
|
491 |
-
super().__init__()
|
492 |
-
cases = torch.zeros(256, 5, 3, dtype=torch.long)
|
493 |
-
masks = torch.zeros(256, 5, dtype=torch.bool)
|
494 |
-
|
495 |
-
self.register_buffer("cases", cases)
|
496 |
-
self.register_buffer("masks", masks)
|
497 |
-
|
498 |
-
def forward(self, field: torch.Tensor, min_point: torch.Tensor, size: torch.Tensor):
|
499 |
-
"""
|
500 |
-
For a signed distance field, produce a mesh using marching cubes.
|
501 |
-
|
502 |
-
:param field: a 3D tensor of field values, where negative values correspond
|
503 |
-
to the outside of the shape. The dimensions correspond to the x, y, and z directions, respectively.
|
504 |
-
:param min_point: a tensor of shape [3] containing the point corresponding
|
505 |
-
to (0, 0, 0) in the field.
|
506 |
-
:param size: a tensor of shape [3] containing the per-axis distance from the
|
507 |
-
(0, 0, 0) field corner and the (-1, -1, -1) field corner.
|
508 |
-
"""
|
509 |
-
assert len(field.shape) == 3, "input must be a 3D scalar field"
|
510 |
-
dev = field.device
|
511 |
-
|
512 |
-
cases = self.cases.to(dev)
|
513 |
-
masks = self.masks.to(dev)
|
514 |
-
|
515 |
-
min_point = min_point.to(dev)
|
516 |
-
size = size.to(dev)
|
517 |
-
|
518 |
-
grid_size = field.shape
|
519 |
-
grid_size_tensor = torch.tensor(grid_size).to(size)
|
520 |
-
|
521 |
-
# Create bitmasks between 0 and 255 (inclusive) indicating the state
|
522 |
-
# of the eight corners of each cube.
|
523 |
-
bitmasks = (field > 0).to(torch.uint8)
|
524 |
-
bitmasks = bitmasks[:-1, :, :] | (bitmasks[1:, :, :] << 1)
|
525 |
-
bitmasks = bitmasks[:, :-1, :] | (bitmasks[:, 1:, :] << 2)
|
526 |
-
bitmasks = bitmasks[:, :, :-1] | (bitmasks[:, :, 1:] << 4)
|
527 |
-
|
528 |
-
# Compute corner coordinates across the entire grid.
|
529 |
-
corner_coords = torch.empty(*grid_size, 3, device=dev, dtype=field.dtype)
|
530 |
-
corner_coords[range(grid_size[0]), :, :, 0] = torch.arange(grid_size[0], device=dev, dtype=field.dtype)[
|
531 |
-
:, None, None
|
532 |
-
]
|
533 |
-
corner_coords[:, range(grid_size[1]), :, 1] = torch.arange(grid_size[1], device=dev, dtype=field.dtype)[
|
534 |
-
:, None
|
535 |
-
]
|
536 |
-
corner_coords[:, :, range(grid_size[2]), 2] = torch.arange(grid_size[2], device=dev, dtype=field.dtype)
|
537 |
-
|
538 |
-
# Compute all vertices across all edges in the grid, even though we will
|
539 |
-
# throw some out later. We have (X-1)*Y*Z + X*(Y-1)*Z + X*Y*(Z-1) vertices.
|
540 |
-
# These are all midpoints, and don't account for interpolation (which is
|
541 |
-
# done later based on the used edge midpoints).
|
542 |
-
edge_midpoints = torch.cat(
|
543 |
-
[
|
544 |
-
((corner_coords[:-1] + corner_coords[1:]) / 2).reshape(-1, 3),
|
545 |
-
((corner_coords[:, :-1] + corner_coords[:, 1:]) / 2).reshape(-1, 3),
|
546 |
-
((corner_coords[:, :, :-1] + corner_coords[:, :, 1:]) / 2).reshape(-1, 3),
|
547 |
-
],
|
548 |
-
dim=0,
|
549 |
-
)
|
550 |
-
|
551 |
-
# Create a flat array of [X, Y, Z] indices for each cube.
|
552 |
-
cube_indices = torch.zeros(
|
553 |
-
grid_size[0] - 1, grid_size[1] - 1, grid_size[2] - 1, 3, device=dev, dtype=torch.long
|
554 |
-
)
|
555 |
-
cube_indices[range(grid_size[0] - 1), :, :, 0] = torch.arange(grid_size[0] - 1, device=dev)[:, None, None]
|
556 |
-
cube_indices[:, range(grid_size[1] - 1), :, 1] = torch.arange(grid_size[1] - 1, device=dev)[:, None]
|
557 |
-
cube_indices[:, :, range(grid_size[2] - 1), 2] = torch.arange(grid_size[2] - 1, device=dev)
|
558 |
-
flat_cube_indices = cube_indices.reshape(-1, 3)
|
559 |
-
|
560 |
-
# Create a flat array mapping each cube to 12 global edge indices.
|
561 |
-
edge_indices = _create_flat_edge_indices(flat_cube_indices, grid_size)
|
562 |
-
|
563 |
-
# Apply the LUT to figure out the triangles.
|
564 |
-
flat_bitmasks = bitmasks.reshape(-1).long() # must cast to long for indexing to believe this not a mask
|
565 |
-
local_tris = cases[flat_bitmasks]
|
566 |
-
local_masks = masks[flat_bitmasks]
|
567 |
-
# Compute the global edge indices for the triangles.
|
568 |
-
global_tris = torch.gather(edge_indices, 1, local_tris.reshape(local_tris.shape[0], -1)).reshape(
|
569 |
-
local_tris.shape
|
570 |
-
)
|
571 |
-
# Select the used triangles for each cube.
|
572 |
-
selected_tris = global_tris.reshape(-1, 3)[local_masks.reshape(-1)]
|
573 |
-
|
574 |
-
# Now we have a bunch of indices into the full list of possible vertices,
|
575 |
-
# but we want to reduce this list to only the used vertices.
|
576 |
-
used_vertex_indices = torch.unique(selected_tris.view(-1))
|
577 |
-
used_edge_midpoints = edge_midpoints[used_vertex_indices]
|
578 |
-
old_index_to_new_index = torch.zeros(len(edge_midpoints), device=dev, dtype=torch.long)
|
579 |
-
old_index_to_new_index[used_vertex_indices] = torch.arange(
|
580 |
-
len(used_vertex_indices), device=dev, dtype=torch.long
|
581 |
-
)
|
582 |
-
|
583 |
-
# Rewrite the triangles to use the new indices
|
584 |
-
faces = torch.gather(old_index_to_new_index, 0, selected_tris.view(-1)).reshape(selected_tris.shape)
|
585 |
-
|
586 |
-
# Compute the actual interpolated coordinates corresponding to edge midpoints.
|
587 |
-
v1 = torch.floor(used_edge_midpoints).to(torch.long)
|
588 |
-
v2 = torch.ceil(used_edge_midpoints).to(torch.long)
|
589 |
-
s1 = field[v1[:, 0], v1[:, 1], v1[:, 2]]
|
590 |
-
s2 = field[v2[:, 0], v2[:, 1], v2[:, 2]]
|
591 |
-
p1 = (v1.float() / (grid_size_tensor - 1)) * size + min_point
|
592 |
-
p2 = (v2.float() / (grid_size_tensor - 1)) * size + min_point
|
593 |
-
# The signs of s1 and s2 should be different. We want to find
|
594 |
-
# t such that t*s2 + (1-t)*s1 = 0.
|
595 |
-
t = (s1 / (s1 - s2))[:, None]
|
596 |
-
verts = t * p2 + (1 - t) * p1
|
597 |
-
|
598 |
-
return MeshDecoderOutput(verts=verts, faces=faces, vertex_channels=None)
|
599 |
-
|
600 |
-
|
601 |
-
@dataclass
|
602 |
-
class MLPNeRFModelOutput(BaseOutput):
|
603 |
-
density: torch.Tensor
|
604 |
-
signed_distance: torch.Tensor
|
605 |
-
channels: torch.Tensor
|
606 |
-
ts: torch.Tensor
|
607 |
-
|
608 |
-
|
609 |
-
class MLPNeRSTFModel(ModelMixin, ConfigMixin):
|
610 |
-
@register_to_config
|
611 |
-
def __init__(
|
612 |
-
self,
|
613 |
-
d_hidden: int = 256,
|
614 |
-
n_output: int = 12,
|
615 |
-
n_hidden_layers: int = 6,
|
616 |
-
act_fn: str = "swish",
|
617 |
-
insert_direction_at: int = 4,
|
618 |
-
):
|
619 |
-
super().__init__()
|
620 |
-
|
621 |
-
# Instantiate the MLP
|
622 |
-
|
623 |
-
# Find out the dimension of encoded position and direction
|
624 |
-
dummy = torch.eye(1, 3)
|
625 |
-
d_posenc_pos = encode_position(position=dummy).shape[-1]
|
626 |
-
d_posenc_dir = encode_direction(position=dummy).shape[-1]
|
627 |
-
|
628 |
-
mlp_widths = [d_hidden] * n_hidden_layers
|
629 |
-
input_widths = [d_posenc_pos] + mlp_widths
|
630 |
-
output_widths = mlp_widths + [n_output]
|
631 |
-
|
632 |
-
if insert_direction_at is not None:
|
633 |
-
input_widths[insert_direction_at] += d_posenc_dir
|
634 |
-
|
635 |
-
self.mlp = nn.ModuleList([nn.Linear(d_in, d_out) for d_in, d_out in zip(input_widths, output_widths)])
|
636 |
-
|
637 |
-
if act_fn == "swish":
|
638 |
-
# self.activation = swish
|
639 |
-
# yiyi testing:
|
640 |
-
self.activation = lambda x: F.silu(x)
|
641 |
-
else:
|
642 |
-
raise ValueError(f"Unsupported activation function {act_fn}")
|
643 |
-
|
644 |
-
self.sdf_activation = torch.tanh
|
645 |
-
self.density_activation = torch.nn.functional.relu
|
646 |
-
self.channel_activation = torch.sigmoid
|
647 |
-
|
648 |
-
def map_indices_to_keys(self, output):
|
649 |
-
h_map = {
|
650 |
-
"sdf": (0, 1),
|
651 |
-
"density_coarse": (1, 2),
|
652 |
-
"density_fine": (2, 3),
|
653 |
-
"stf": (3, 6),
|
654 |
-
"nerf_coarse": (6, 9),
|
655 |
-
"nerf_fine": (9, 12),
|
656 |
-
}
|
657 |
-
|
658 |
-
mapped_output = {k: output[..., start:end] for k, (start, end) in h_map.items()}
|
659 |
-
|
660 |
-
return mapped_output
|
661 |
-
|
662 |
-
def forward(self, *, position, direction, ts, nerf_level="coarse", rendering_mode="nerf"):
|
663 |
-
h = encode_position(position)
|
664 |
-
|
665 |
-
h_preact = h
|
666 |
-
h_directionless = None
|
667 |
-
for i, layer in enumerate(self.mlp):
|
668 |
-
if i == self.config.insert_direction_at: # 4 in the config
|
669 |
-
h_directionless = h_preact
|
670 |
-
h_direction = encode_direction(position, direction=direction)
|
671 |
-
h = torch.cat([h, h_direction], dim=-1)
|
672 |
-
|
673 |
-
h = layer(h)
|
674 |
-
|
675 |
-
h_preact = h
|
676 |
-
|
677 |
-
if i < len(self.mlp) - 1:
|
678 |
-
h = self.activation(h)
|
679 |
-
|
680 |
-
h_final = h
|
681 |
-
if h_directionless is None:
|
682 |
-
h_directionless = h_preact
|
683 |
-
|
684 |
-
activation = self.map_indices_to_keys(h_final)
|
685 |
-
|
686 |
-
if nerf_level == "coarse":
|
687 |
-
h_density = activation["density_coarse"]
|
688 |
-
else:
|
689 |
-
h_density = activation["density_fine"]
|
690 |
-
|
691 |
-
if rendering_mode == "nerf":
|
692 |
-
if nerf_level == "coarse":
|
693 |
-
h_channels = activation["nerf_coarse"]
|
694 |
-
else:
|
695 |
-
h_channels = activation["nerf_fine"]
|
696 |
-
|
697 |
-
elif rendering_mode == "stf":
|
698 |
-
h_channels = activation["stf"]
|
699 |
-
|
700 |
-
density = self.density_activation(h_density)
|
701 |
-
signed_distance = self.sdf_activation(activation["sdf"])
|
702 |
-
channels = self.channel_activation(h_channels)
|
703 |
-
|
704 |
-
# yiyi notes: I think signed_distance is not used
|
705 |
-
return MLPNeRFModelOutput(density=density, signed_distance=signed_distance, channels=channels, ts=ts)
|
706 |
-
|
707 |
-
|
708 |
-
class ChannelsProj(nn.Module):
|
709 |
-
def __init__(
|
710 |
-
self,
|
711 |
-
*,
|
712 |
-
vectors: int,
|
713 |
-
channels: int,
|
714 |
-
d_latent: int,
|
715 |
-
):
|
716 |
-
super().__init__()
|
717 |
-
self.proj = nn.Linear(d_latent, vectors * channels)
|
718 |
-
self.norm = nn.LayerNorm(channels)
|
719 |
-
self.d_latent = d_latent
|
720 |
-
self.vectors = vectors
|
721 |
-
self.channels = channels
|
722 |
-
|
723 |
-
def forward(self, x: torch.Tensor) -> torch.Tensor:
|
724 |
-
x_bvd = x
|
725 |
-
w_vcd = self.proj.weight.view(self.vectors, self.channels, self.d_latent)
|
726 |
-
b_vc = self.proj.bias.view(1, self.vectors, self.channels)
|
727 |
-
h = torch.einsum("bvd,vcd->bvc", x_bvd, w_vcd)
|
728 |
-
h = self.norm(h)
|
729 |
-
|
730 |
-
h = h + b_vc
|
731 |
-
return h
|
732 |
-
|
733 |
-
|
734 |
-
class ShapEParamsProjModel(ModelMixin, ConfigMixin):
|
735 |
-
"""
|
736 |
-
project the latent representation of a 3D asset to obtain weights of a multi-layer perceptron (MLP).
|
737 |
-
|
738 |
-
For more details, see the original paper:
|
739 |
-
"""
|
740 |
-
|
741 |
-
@register_to_config
|
742 |
-
def __init__(
|
743 |
-
self,
|
744 |
-
*,
|
745 |
-
param_names: Tuple[str] = (
|
746 |
-
"nerstf.mlp.0.weight",
|
747 |
-
"nerstf.mlp.1.weight",
|
748 |
-
"nerstf.mlp.2.weight",
|
749 |
-
"nerstf.mlp.3.weight",
|
750 |
-
),
|
751 |
-
param_shapes: Tuple[Tuple[int]] = (
|
752 |
-
(256, 93),
|
753 |
-
(256, 256),
|
754 |
-
(256, 256),
|
755 |
-
(256, 256),
|
756 |
-
),
|
757 |
-
d_latent: int = 1024,
|
758 |
-
):
|
759 |
-
super().__init__()
|
760 |
-
|
761 |
-
# check inputs
|
762 |
-
if len(param_names) != len(param_shapes):
|
763 |
-
raise ValueError("Must provide same number of `param_names` as `param_shapes`")
|
764 |
-
self.projections = nn.ModuleDict({})
|
765 |
-
for k, (vectors, channels) in zip(param_names, param_shapes):
|
766 |
-
self.projections[_sanitize_name(k)] = ChannelsProj(
|
767 |
-
vectors=vectors,
|
768 |
-
channels=channels,
|
769 |
-
d_latent=d_latent,
|
770 |
-
)
|
771 |
-
|
772 |
-
def forward(self, x: torch.Tensor):
|
773 |
-
out = {}
|
774 |
-
start = 0
|
775 |
-
for k, shape in zip(self.config.param_names, self.config.param_shapes):
|
776 |
-
vectors, _ = shape
|
777 |
-
end = start + vectors
|
778 |
-
x_bvd = x[:, start:end]
|
779 |
-
out[k] = self.projections[_sanitize_name(k)](x_bvd).reshape(len(x), *shape)
|
780 |
-
start = end
|
781 |
-
return out
|
782 |
-
|
783 |
-
|
784 |
-
class ShapERenderer(ModelMixin, ConfigMixin):
|
785 |
-
@register_to_config
|
786 |
-
def __init__(
|
787 |
-
self,
|
788 |
-
*,
|
789 |
-
param_names: Tuple[str] = (
|
790 |
-
"nerstf.mlp.0.weight",
|
791 |
-
"nerstf.mlp.1.weight",
|
792 |
-
"nerstf.mlp.2.weight",
|
793 |
-
"nerstf.mlp.3.weight",
|
794 |
-
),
|
795 |
-
param_shapes: Tuple[Tuple[int]] = (
|
796 |
-
(256, 93),
|
797 |
-
(256, 256),
|
798 |
-
(256, 256),
|
799 |
-
(256, 256),
|
800 |
-
),
|
801 |
-
d_latent: int = 1024,
|
802 |
-
d_hidden: int = 256,
|
803 |
-
n_output: int = 12,
|
804 |
-
n_hidden_layers: int = 6,
|
805 |
-
act_fn: str = "swish",
|
806 |
-
insert_direction_at: int = 4,
|
807 |
-
background: Tuple[float] = (
|
808 |
-
255.0,
|
809 |
-
255.0,
|
810 |
-
255.0,
|
811 |
-
),
|
812 |
-
):
|
813 |
-
super().__init__()
|
814 |
-
|
815 |
-
self.params_proj = ShapEParamsProjModel(
|
816 |
-
param_names=param_names,
|
817 |
-
param_shapes=param_shapes,
|
818 |
-
d_latent=d_latent,
|
819 |
-
)
|
820 |
-
self.mlp = MLPNeRSTFModel(d_hidden, n_output, n_hidden_layers, act_fn, insert_direction_at)
|
821 |
-
self.void = VoidNeRFModel(background=background, channel_scale=255.0)
|
822 |
-
self.volume = BoundingBoxVolume(bbox_max=[1.0, 1.0, 1.0], bbox_min=[-1.0, -1.0, -1.0])
|
823 |
-
self.mesh_decoder = MeshDecoder()
|
824 |
-
|
825 |
-
@torch.no_grad()
|
826 |
-
def render_rays(self, rays, sampler, n_samples, prev_model_out=None, render_with_direction=False):
|
827 |
-
"""
|
828 |
-
Perform volumetric rendering over a partition of possible t's in the union of rendering volumes (written below
|
829 |
-
with some abuse of notations)
|
830 |
-
|
831 |
-
C(r) := sum(
|
832 |
-
transmittance(t[i]) * integrate(
|
833 |
-
lambda t: density(t) * channels(t) * transmittance(t), [t[i], t[i + 1]],
|
834 |
-
) for i in range(len(parts))
|
835 |
-
) + transmittance(t[-1]) * void_model(t[-1]).channels
|
836 |
-
|
837 |
-
where
|
838 |
-
|
839 |
-
1) transmittance(s) := exp(-integrate(density, [t[0], s])) calculates the probability of light passing through
|
840 |
-
the volume specified by [t[0], s]. (transmittance of 1 means light can pass freely) 2) density and channels are
|
841 |
-
obtained by evaluating the appropriate part.model at time t. 3) [t[i], t[i + 1]] is defined as the range of t
|
842 |
-
where the ray intersects (parts[i].volume \\ union(part.volume for part in parts[:i])) at the surface of the
|
843 |
-
shell (if bounded). If the ray does not intersect, the integral over this segment is evaluated as 0 and
|
844 |
-
transmittance(t[i + 1]) := transmittance(t[i]). 4) The last term is integration to infinity (e.g. [t[-1],
|
845 |
-
math.inf]) that is evaluated by the void_model (i.e. we consider this space to be empty).
|
846 |
-
|
847 |
-
args:
|
848 |
-
rays: [batch_size x ... x 2 x 3] origin and direction. sampler: disjoint volume integrals. n_samples:
|
849 |
-
number of ts to sample. prev_model_outputs: model outputs from the previous rendering step, including
|
850 |
-
|
851 |
-
:return: A tuple of
|
852 |
-
- `channels`
|
853 |
-
- A importance samplers for additional fine-grained rendering
|
854 |
-
- raw model output
|
855 |
-
"""
|
856 |
-
origin, direction = rays[..., 0, :], rays[..., 1, :]
|
857 |
-
|
858 |
-
# Integrate over [t[i], t[i + 1]]
|
859 |
-
|
860 |
-
# 1 Intersect the rays with the current volume and sample ts to integrate along.
|
861 |
-
vrange = self.volume.intersect(origin, direction, t0_lower=None)
|
862 |
-
ts = sampler.sample(vrange.t0, vrange.t1, n_samples)
|
863 |
-
ts = ts.to(rays.dtype)
|
864 |
-
|
865 |
-
if prev_model_out is not None:
|
866 |
-
# Append the previous ts now before fprop because previous
|
867 |
-
# rendering used a different model and we can't reuse the output.
|
868 |
-
ts = torch.sort(torch.cat([ts, prev_model_out.ts], dim=-2), dim=-2).values
|
869 |
-
|
870 |
-
batch_size, *_shape, _t0_dim = vrange.t0.shape
|
871 |
-
_, *ts_shape, _ts_dim = ts.shape
|
872 |
-
|
873 |
-
# 2. Get the points along the ray and query the model
|
874 |
-
directions = torch.broadcast_to(direction.unsqueeze(-2), [batch_size, *ts_shape, 3])
|
875 |
-
positions = origin.unsqueeze(-2) + ts * directions
|
876 |
-
|
877 |
-
directions = directions.to(self.mlp.dtype)
|
878 |
-
positions = positions.to(self.mlp.dtype)
|
879 |
-
|
880 |
-
optional_directions = directions if render_with_direction else None
|
881 |
-
|
882 |
-
model_out = self.mlp(
|
883 |
-
position=positions,
|
884 |
-
direction=optional_directions,
|
885 |
-
ts=ts,
|
886 |
-
nerf_level="coarse" if prev_model_out is None else "fine",
|
887 |
-
)
|
888 |
-
|
889 |
-
# 3. Integrate the model results
|
890 |
-
channels, weights, transmittance = integrate_samples(
|
891 |
-
vrange, model_out.ts, model_out.density, model_out.channels
|
892 |
-
)
|
893 |
-
|
894 |
-
# 4. Clean up results that do not intersect with the volume.
|
895 |
-
transmittance = torch.where(vrange.intersected, transmittance, torch.ones_like(transmittance))
|
896 |
-
channels = torch.where(vrange.intersected, channels, torch.zeros_like(channels))
|
897 |
-
# 5. integration to infinity (e.g. [t[-1], math.inf]) that is evaluated by the void_model (i.e. we consider this space to be empty).
|
898 |
-
channels = channels + transmittance * self.void(origin)
|
899 |
-
|
900 |
-
weighted_sampler = ImportanceRaySampler(vrange, ts=model_out.ts, weights=weights)
|
901 |
-
|
902 |
-
return channels, weighted_sampler, model_out
|
903 |
-
|
904 |
-
@torch.no_grad()
|
905 |
-
def decode_to_image(
|
906 |
-
self,
|
907 |
-
latents,
|
908 |
-
device,
|
909 |
-
size: int = 64,
|
910 |
-
ray_batch_size: int = 4096,
|
911 |
-
n_coarse_samples=64,
|
912 |
-
n_fine_samples=128,
|
913 |
-
):
|
914 |
-
# project the the paramters from the generated latents
|
915 |
-
projected_params = self.params_proj(latents)
|
916 |
-
|
917 |
-
# update the mlp layers of the renderer
|
918 |
-
for name, param in self.mlp.state_dict().items():
|
919 |
-
if f"nerstf.{name}" in projected_params.keys():
|
920 |
-
param.copy_(projected_params[f"nerstf.{name}"].squeeze(0))
|
921 |
-
|
922 |
-
# create cameras object
|
923 |
-
camera = create_pan_cameras(size)
|
924 |
-
rays = camera.camera_rays
|
925 |
-
rays = rays.to(device)
|
926 |
-
n_batches = rays.shape[1] // ray_batch_size
|
927 |
-
|
928 |
-
coarse_sampler = StratifiedRaySampler()
|
929 |
-
|
930 |
-
images = []
|
931 |
-
|
932 |
-
for idx in range(n_batches):
|
933 |
-
rays_batch = rays[:, idx * ray_batch_size : (idx + 1) * ray_batch_size]
|
934 |
-
|
935 |
-
# render rays with coarse, stratified samples.
|
936 |
-
_, fine_sampler, coarse_model_out = self.render_rays(rays_batch, coarse_sampler, n_coarse_samples)
|
937 |
-
# Then, render with additional importance-weighted ray samples.
|
938 |
-
channels, _, _ = self.render_rays(
|
939 |
-
rays_batch, fine_sampler, n_fine_samples, prev_model_out=coarse_model_out
|
940 |
-
)
|
941 |
-
|
942 |
-
images.append(channels)
|
943 |
-
|
944 |
-
images = torch.cat(images, dim=1)
|
945 |
-
images = images.view(*camera.shape, camera.height, camera.width, -1).squeeze(0)
|
946 |
-
|
947 |
-
return images
|
948 |
-
|
949 |
-
@torch.no_grad()
|
950 |
-
def decode_to_mesh(
|
951 |
-
self,
|
952 |
-
latents,
|
953 |
-
device,
|
954 |
-
grid_size: int = 128,
|
955 |
-
query_batch_size: int = 4096,
|
956 |
-
texture_channels: Tuple = ("R", "G", "B"),
|
957 |
-
):
|
958 |
-
# 1. project the the paramters from the generated latents
|
959 |
-
projected_params = self.params_proj(latents)
|
960 |
-
|
961 |
-
# 2. update the mlp layers of the renderer
|
962 |
-
for name, param in self.mlp.state_dict().items():
|
963 |
-
if f"nerstf.{name}" in projected_params.keys():
|
964 |
-
param.copy_(projected_params[f"nerstf.{name}"].squeeze(0))
|
965 |
-
|
966 |
-
# 3. decoding with STF rendering
|
967 |
-
# 3.1 query the SDF values at vertices along a regular 128**3 grid
|
968 |
-
|
969 |
-
query_points = volume_query_points(self.volume, grid_size)
|
970 |
-
query_positions = query_points[None].repeat(1, 1, 1).to(device=device, dtype=self.mlp.dtype)
|
971 |
-
|
972 |
-
fields = []
|
973 |
-
|
974 |
-
for idx in range(0, query_positions.shape[1], query_batch_size):
|
975 |
-
query_batch = query_positions[:, idx : idx + query_batch_size]
|
976 |
-
|
977 |
-
model_out = self.mlp(
|
978 |
-
position=query_batch, direction=None, ts=None, nerf_level="fine", rendering_mode="stf"
|
979 |
-
)
|
980 |
-
fields.append(model_out.signed_distance)
|
981 |
-
|
982 |
-
# predicted SDF values
|
983 |
-
fields = torch.cat(fields, dim=1)
|
984 |
-
fields = fields.float()
|
985 |
-
|
986 |
-
assert (
|
987 |
-
len(fields.shape) == 3 and fields.shape[-1] == 1
|
988 |
-
), f"expected [meta_batch x inner_batch] SDF results, but got {fields.shape}"
|
989 |
-
|
990 |
-
fields = fields.reshape(1, *([grid_size] * 3))
|
991 |
-
|
992 |
-
# create grid 128 x 128 x 128
|
993 |
-
# - force a negative border around the SDFs to close off all the models.
|
994 |
-
full_grid = torch.zeros(
|
995 |
-
1,
|
996 |
-
grid_size + 2,
|
997 |
-
grid_size + 2,
|
998 |
-
grid_size + 2,
|
999 |
-
device=fields.device,
|
1000 |
-
dtype=fields.dtype,
|
1001 |
-
)
|
1002 |
-
full_grid.fill_(-1.0)
|
1003 |
-
full_grid[:, 1:-1, 1:-1, 1:-1] = fields
|
1004 |
-
fields = full_grid
|
1005 |
-
|
1006 |
-
# apply a differentiable implementation of Marching Cubes to construct meshs
|
1007 |
-
raw_meshes = []
|
1008 |
-
mesh_mask = []
|
1009 |
-
|
1010 |
-
for field in fields:
|
1011 |
-
raw_mesh = self.mesh_decoder(field, self.volume.bbox_min, self.volume.bbox_max - self.volume.bbox_min)
|
1012 |
-
mesh_mask.append(True)
|
1013 |
-
raw_meshes.append(raw_mesh)
|
1014 |
-
|
1015 |
-
mesh_mask = torch.tensor(mesh_mask, device=fields.device)
|
1016 |
-
max_vertices = max(len(m.verts) for m in raw_meshes)
|
1017 |
-
|
1018 |
-
# 3.2. query the texture color head at each vertex of the resulting mesh.
|
1019 |
-
texture_query_positions = torch.stack(
|
1020 |
-
[m.verts[torch.arange(0, max_vertices) % len(m.verts)] for m in raw_meshes],
|
1021 |
-
dim=0,
|
1022 |
-
)
|
1023 |
-
texture_query_positions = texture_query_positions.to(device=device, dtype=self.mlp.dtype)
|
1024 |
-
|
1025 |
-
textures = []
|
1026 |
-
|
1027 |
-
for idx in range(0, texture_query_positions.shape[1], query_batch_size):
|
1028 |
-
query_batch = texture_query_positions[:, idx : idx + query_batch_size]
|
1029 |
-
|
1030 |
-
texture_model_out = self.mlp(
|
1031 |
-
position=query_batch, direction=None, ts=None, nerf_level="fine", rendering_mode="stf"
|
1032 |
-
)
|
1033 |
-
textures.append(texture_model_out.channels)
|
1034 |
-
|
1035 |
-
# predict texture color
|
1036 |
-
textures = torch.cat(textures, dim=1)
|
1037 |
-
|
1038 |
-
textures = _convert_srgb_to_linear(textures)
|
1039 |
-
textures = textures.float()
|
1040 |
-
|
1041 |
-
# 3.3 augument the mesh with texture data
|
1042 |
-
assert len(textures.shape) == 3 and textures.shape[-1] == len(
|
1043 |
-
texture_channels
|
1044 |
-
), f"expected [meta_batch x inner_batch x texture_channels] field results, but got {textures.shape}"
|
1045 |
-
|
1046 |
-
for m, texture in zip(raw_meshes, textures):
|
1047 |
-
texture = texture[: len(m.verts)]
|
1048 |
-
m.vertex_channels = dict(zip(texture_channels, texture.unbind(-1)))
|
1049 |
-
|
1050 |
-
return raw_meshes[0]
|
|
|
|
|
|
|
|
|
|
|
|
|
|
|
|
|
|
|
|
|
|
|
|
|
|
|
|
|
|
|
|
|
|
|
|
|
|
|
|
|
|
|
|
|
|
|
|
|
|
|
|
|
|
|
|
|
|
|
|
|
|
|
|
|
|
|
|
|
|
|
|
|
|
|
|
|
|
|
|
|
|
|
|
|
|
|
|
|
|
|
|
|
|
|
|
|
|
|
|
|
|
|
|
|
|
|
|
|
|
|
|
|
|
|
|
|
|
|
|
|
|
|
|
|
|
|
|
|
|
|
|
|
|
|
|
|
|
|
|
|
|
|
|
|
|
|
|
|
|
|
|
|
|
|
|
|
|
|
|
|
|
|
|
|
|
|
|
|
|
|
|
|
|
|
|
|
|
|
|
|
|
|
|
|
|
|
|
|
|
|
|
|
|
|
|
|
|
|
|
|
|
|
|
|
|
|
|
|
|
|
|
|
|
|
|
|
|
|
|
|
|
|
|
|
|
|
|
|
|
|
|
|
|
|
|
|
|
|
|
|
|
|
|
|
|
|
|
|
|
|
|
|
|
|
|
|
|
|
|
|
|
|
|
|
|
|
|
|
|
|
|
|
|
|
|
|
|
|
|
|
|
|
|
|
|
|
|
|
|
|
|
|
|
|
|
|
|
|
|
|
|
|
|
|
|
|
|
|
|
|
|
|
|
|
|
|
|
|
|
|
|
|
|
|
|
|
|
|
|
|
|
|
|
|
|
|
|
|
|
|
|
|
|
|
|
|
|
|
|
|
|
|
|
|
|
|
|
|
|
|
|
|
|
|
|
|
|
|
|
|
|
|
|
|
|
|
|
|
|
|
|
|
|
|
|
|
|
|
|
|
|
|
|
|
|
|
|
|
|
|
|
|
|
|
|
|
|
|
|
|
|
|
|
|
|
|
|
|
|
|
|
|
|
|
|
|
|
|
|
|
|
|
|
|
|
|
|
|
|
|
|
|
|
|
|
|
|
|
|
|
|
|
|
|
|
|
|
|
|
|
|
|
|
|
|
|
|
|
|
|
|
|
|
|
|
|
|
|
|
|
|
|
|
|
|
|
|
|
|
|
|
|
|
|
|
|
|
|
|
|
|
|
|
|
|
|
|
|
|
|
|
|
|
|
|
|
|
|
|
|
|
|
|
|
|
|
|
|
|
|
|
|
|
|
|
|
|
|
|
|
|
|
|
|
|
|
|
|
|
|
|
|
|
|
|
|
|
|
|
|
|
|
|
|
|
|
|
|
|
|
|
|
|
|
|
|
|
|
|
|
|
|
|
|
|
|
|
|
|
|
|
|
|
|
|
|
|
|
|
|
|
|
|
|
|
|
|
|
|
|
|
|
|
|
|
|
|
|
|
|
|
|
|
|
|
|
|
|
|
|
|
|
|
|
|
|
|
|
|
|
|
|
|
|
|
|
|
|
|
|
|
|
|
|
|
|
|
|
|
|
|
|
|
|
|
|
|
|
|
|
|
|
|
|
|
|
|
|
|
|
|
|
|
|
|
|
|
|
|
|
|
|
|
|
|
|
|
|
|
|
|
|
|
|
|
|
|
|
|
|
|
|
|
|
|
|
|
|
|
|
|
|
|
|
|
|
|
|
|
|
|
|
|
|
|
|
|
|
|
|
|
|
|
|
|
|
|
|
|
|
|
|
|
|
|
|
|
|
|
|
|
|
|
|
|
|
|
|
|
|
|
|
|
|
|
|
|
|
|
|
|
|
|
|
|
|
|
|
|
|
|
|
|
|
|
|
|
|
|
|
|
|
|
|
|
|
|
|
|
|
|
|
|
|
|
|
|
|
|
|
|
|
|
|
|
|
|
|
|
|
|
|
|
|
|
|
|
|
|
|
|
|
|
|
|
|
|
|
|
|
|
|
|
|
|
|
|
|
|
|
|
|
|
|
|
|
|
|
|
|
|
|
|
|
|
|
|
|
|
|
|
|
|
|
|
|
|
|
|
|
|
|
|
|
|
|
|
|
|
|
|
|
|
|
|
|
|
|
|
|
|
|
|
|
|
|
|
|
|
|
|
|
|
|
|
|
|
|
|
|
|
|
|
|
|
|
|
|
|
|
|
|
|
|
|
|
|
|
|
|
|
|
|
|
|
|
|
|
|
|
|
|
|
|
|
|
|
|
|
|
|
|
|
|
|
|
|
|
|
|
|
|
|
|
|
|
|
|
|
|
|
|
|
|
|
|
|
|
|
|
|
|
|
|
|
|
|
|
|
|
|
|
|
|
|
|
|
|
|
|
|
|
|
|
|
|
|
|
|
|
|
|
|
|
|
|
|
|
|
|
|
|
|
|
|
|
|
|
|
|
|
|
|
|
|
|
|
|
|
|
|
|
|
|
|
|
|
|
|
|
|
|
|
|
|
|
|
|
|
|
|
|
|
|
|
|
|
|
|
|
|
|
|
|
|
|
|
|
|
|
|
|
|
|
|
|
|
|
|
|
|
|
|
|
|
|
|
|
|
|
|
|
|
|
|
|
|
|
|
|
|
|
|
|
|
|
|
|
|
|
|
|
|
|
|
|
|
|
|
|
|
|
|
|
|
|
|
|
|
|
|
|
|
|
|
|
|
|
|
|
|
|
|
|
|
|
|
|
|
|
|
|
|
|
|
|
|
|
|
|
|
|
|
|
|
|
|
|
|
|
|
|
|
|
|
|
|
|
|
|
|
|
|
|
|
|
|
|
|
|
|
|
|
|
|
|
|
|
|
|
|
|
|
|
|
|
|
|
|
|
|
|
|
|
|
|
|
|
|
|
|
|
|
|
|
|
|
|
|
|
|
|
|
|
|
|
|
|
|
|
|
|
|
|
|
|
|
|
|
|
|
|
|
|
|
|
|
|
|
|
|
|
|
|
|
|
|
|
|
|
|
|
|
|
|
|
|
|
|
|
|
|
|
|
|
|
|
|
|
|
|
|
|
|
|
|
|
|
|
|
|
|
|
|
|
|
|
|
|
|
|
|
|
|
|
|
|
|
|
|
|
|
|
|
|
|
|
|
|
|
|
|
|
|
|
|
|
|
|
|
|
|
|
|
|
|
|
|
|
|
|
|
|
|
|
|
|
|
|
|
|
|
|
|
|
|
|
|
|
|
|
|
|
|
|
|
|
|
|
|
|
|
|
|
|
|
|
|
|
|
|
|
|
|
|
|
|
|
|
|
|
|
|
|
|
|
|
|
|
|
|
|
|
|
|
|
|
|
|
|
|
|
|
|
|
|
|
|
|
|
|
|
|
|
|
|
|
|
|
|
|
|
|
|
|
|
|
|
|
|
|
|
|
|
|
|
|
|
|
|
|
|
|
|
|
|
|
|
|
|
|
|
|
|
|
|
|
|
|
|
|
|
|
|
|
|
|
|
|
|
|
|
|
|
|
|
|
|
|
|
|
|
|
|
|
|
|
|
|
|
|
|
|
|
|
|
|
|
|
|
|
|
|
|
|
|
|
|
|
|
|
|
|
|
|
|
|
|
|
|
|
|
|
|
|
|
|
|
|
|
|
|
|
|
|
|
|
|
|
|
|
|
|
|
|
|
|
|
|
|
|
|
|
|
|
|
|
|
|
|
|
|
|
|
|
|
|
|
|
|
|
|
|
|
|
|
|
|
|
|
|
|
|
|
|
|
|
|
|
|
|
|
|
|
|
|
|
|
|
|
|
|
|
|
|
|
|
|
|
|
|
|
|
|
|
|
|
|
|
|
|
|
|
|
|
|
|
|
|
|
|
|
|
|
|
|
|
|
|
|
|
|
|
|
|
|
|
|
|
|
|
|
|
|
|
|
|
|
|
|
|
|
|
|
|
|
|
|
|
|
|
|
|
|
|
|
|
|
|
|
|
|
|
|
|
|
|
|
|
|
|
|
|
|
|
|
|
|
|
|
|
|
|
|
|
|
|
|
|
|
|
|
|
|
|
|
|
|
|
|
|
|
|
|
|
|
|
|
|
|
|
|
|
|
|
|
|
|
|
|
|
|
|
|
|
|
|
|
|
|
|
|
|
|
|
|
|
|
|
|
|
|
|
|
|
|
|
|
|
|
|
|
|
|
|
|
|
|
|
|
|
|
|
|
|
|
|
|
|
|
|
|
|
|
|
|
|
|
|
|
|
|
|
|
|
|
|
|
|
|
|
|
|
|
|
|
|
|
|
|
|
|
|
|
|
|
|
|
|
|
|
|
|
|
|
|
|
|
|
|
|
|
|
|
|
|
|
|
|
|
|
|
|
|
|
|
|
|
|
|
|
|
|
|
|
|
|
|
|
|
|
|
|
|
|
|
|
|
|
|
|
|
|
|
|
|
|
|
|
|
|
|
|
|
|
|
|
|
|
|
|
|
|
|
|
|
|
|
|
|
|
|
|
|
|
|
|
|
|
|
|
|
|
|
|
|
|
|
|
|
|
|
|
|
|
|
|
|
|
|
|
|
|
|
|
|
|
|
|
|
|
|
|
|
|
|
|
|
|
|
|
|
|
|
|
|
|
|
|
|
|
|
|
|
|
|
|
|
|
|
|
|
|
|
|
|
|
|
|
|
|
|
|
|
|
|
|
|
|
|
|
|
|
|
|
|
|
|
|
|
|
|
|
|
|
|
|
|
|
|
|
|
|
|
|
|
|
|
|
|
|
|
|
|
|
|
|
|
|
|
|
|
|
|
|
|
|
|
|
|
|
spaces/Andy1621/uniformer_image_detection/configs/foveabox/fovea_align_r101_fpn_gn-head_4x4_2x_coco.py
DELETED
@@ -1,10 +0,0 @@
|
|
1 |
-
_base_ = './fovea_r50_fpn_4x4_1x_coco.py'
|
2 |
-
model = dict(
|
3 |
-
pretrained='torchvision://resnet101',
|
4 |
-
backbone=dict(depth=101),
|
5 |
-
bbox_head=dict(
|
6 |
-
with_deform=True,
|
7 |
-
norm_cfg=dict(type='GN', num_groups=32, requires_grad=True)))
|
8 |
-
# learning policy
|
9 |
-
lr_config = dict(step=[16, 22])
|
10 |
-
runner = dict(type='EpochBasedRunner', max_epochs=24)
|
|
|
|
|
|
|
|
|
|
|
|
|
|
|
|
|
|
|
|
|
|
spaces/AnishKumbhar/ChatBot/text-generation-webui-main/extensions/ngrok/script.py
DELETED
@@ -1,36 +0,0 @@
|
|
1 |
-
# Adds ngrok ingress, to use add `--extension ngrok` to the command line options
|
2 |
-
#
|
3 |
-
# Parameters can be customized in settings.json of webui, e.g.:
|
4 |
-
# {"ngrok": {"basic_auth":"user:password"} }
|
5 |
-
# or
|
6 |
-
# {"ngrok": {"oauth_provider":"google", "oauth_allow_emails":["[email protected]"]} }
|
7 |
-
#
|
8 |
-
# See this example for full list of options: https://github.com/ngrok/ngrok-py/blob/main/examples/ngrok-connect-full.py
|
9 |
-
# or the README.md in this directory.
|
10 |
-
|
11 |
-
import logging
|
12 |
-
from modules import shared
|
13 |
-
|
14 |
-
# Pick up host/port command line arguments
|
15 |
-
host = shared.args.listen_host if shared.args.listen_host and shared.args.listen else '127.0.0.1'
|
16 |
-
port = shared.args.listen_port if shared.args.listen_port else '7860'
|
17 |
-
|
18 |
-
# Default options
|
19 |
-
options = {
|
20 |
-
'addr': f"{host}:{port}",
|
21 |
-
'authtoken_from_env': True,
|
22 |
-
'session_metadata': 'text-generation-webui',
|
23 |
-
}
|
24 |
-
|
25 |
-
|
26 |
-
def ui():
|
27 |
-
settings = shared.settings.get("ngrok")
|
28 |
-
if settings:
|
29 |
-
options.update(settings)
|
30 |
-
|
31 |
-
try:
|
32 |
-
import ngrok
|
33 |
-
tunnel = ngrok.connect(**options)
|
34 |
-
logging.info(f"Ingress established at: {tunnel.url()}")
|
35 |
-
except ModuleNotFoundError:
|
36 |
-
logging.error("===> ngrok library not found, please run `pip install -r extensions/ngrok/requirements.txt`")
|
|
|
|
|
|
|
|
|
|
|
|
|
|
|
|
|
|
|
|
|
|
|
|
|
|
|
|
|
|
|
|
|
|
|
|
|
|
|
|
|
|
|
|
|
|
|
|
|
|
|
|
|
|
|
|
|
|
|
|
|
|
|
|
|
|
|
|
|
|
|
|
|
|
spaces/AnishKumbhar/ChatBot/text-generation-webui-main/modules/models.py
DELETED
@@ -1,401 +0,0 @@
|
|
1 |
-
import gc
|
2 |
-
import os
|
3 |
-
import re
|
4 |
-
import time
|
5 |
-
import traceback
|
6 |
-
from pathlib import Path
|
7 |
-
|
8 |
-
import torch
|
9 |
-
import transformers
|
10 |
-
from accelerate import infer_auto_device_map, init_empty_weights
|
11 |
-
from transformers import (
|
12 |
-
AutoConfig,
|
13 |
-
AutoModel,
|
14 |
-
AutoModelForCausalLM,
|
15 |
-
AutoModelForSeq2SeqLM,
|
16 |
-
AutoTokenizer,
|
17 |
-
BitsAndBytesConfig,
|
18 |
-
GPTQConfig
|
19 |
-
)
|
20 |
-
|
21 |
-
import modules.shared as shared
|
22 |
-
from modules import RoPE, llama_attn_hijack, sampler_hijack
|
23 |
-
from modules.logging_colors import logger
|
24 |
-
from modules.models_settings import get_model_metadata
|
25 |
-
|
26 |
-
transformers.logging.set_verbosity_error()
|
27 |
-
|
28 |
-
local_rank = None
|
29 |
-
if shared.args.deepspeed:
|
30 |
-
import deepspeed
|
31 |
-
from transformers.deepspeed import (
|
32 |
-
HfDeepSpeedConfig,
|
33 |
-
is_deepspeed_zero3_enabled
|
34 |
-
)
|
35 |
-
|
36 |
-
from modules.deepspeed_parameters import generate_ds_config
|
37 |
-
|
38 |
-
# Distributed setup
|
39 |
-
local_rank = shared.args.local_rank if shared.args.local_rank is not None else int(os.getenv("LOCAL_RANK", "0"))
|
40 |
-
world_size = int(os.getenv("WORLD_SIZE", "1"))
|
41 |
-
torch.cuda.set_device(local_rank)
|
42 |
-
deepspeed.init_distributed()
|
43 |
-
ds_config = generate_ds_config(shared.args.bf16, 1 * world_size, shared.args.nvme_offload_dir)
|
44 |
-
dschf = HfDeepSpeedConfig(ds_config) # Keep this object alive for the Transformers integration
|
45 |
-
|
46 |
-
sampler_hijack.hijack_samplers()
|
47 |
-
|
48 |
-
|
49 |
-
def load_model(model_name, loader=None):
|
50 |
-
logger.info(f"Loading {model_name}...")
|
51 |
-
t0 = time.time()
|
52 |
-
|
53 |
-
shared.is_seq2seq = False
|
54 |
-
load_func_map = {
|
55 |
-
'Transformers': huggingface_loader,
|
56 |
-
'AutoGPTQ': AutoGPTQ_loader,
|
57 |
-
'GPTQ-for-LLaMa': GPTQ_loader,
|
58 |
-
'llama.cpp': llamacpp_loader,
|
59 |
-
'llamacpp_HF': llamacpp_HF_loader,
|
60 |
-
'RWKV': RWKV_loader,
|
61 |
-
'ExLlama': ExLlama_loader,
|
62 |
-
'ExLlama_HF': ExLlama_HF_loader,
|
63 |
-
'ExLlamav2': ExLlamav2_loader,
|
64 |
-
'ExLlamav2_HF': ExLlamav2_HF_loader,
|
65 |
-
'ctransformers': ctransformers_loader,
|
66 |
-
'AutoAWQ': AutoAWQ_loader,
|
67 |
-
}
|
68 |
-
|
69 |
-
if loader is None:
|
70 |
-
if shared.args.loader is not None:
|
71 |
-
loader = shared.args.loader
|
72 |
-
else:
|
73 |
-
loader = get_model_metadata(model_name)['loader']
|
74 |
-
if loader is None:
|
75 |
-
logger.error('The path to the model does not exist. Exiting.')
|
76 |
-
return None, None
|
77 |
-
|
78 |
-
shared.args.loader = loader
|
79 |
-
output = load_func_map[loader](model_name)
|
80 |
-
if type(output) is tuple:
|
81 |
-
model, tokenizer = output
|
82 |
-
else:
|
83 |
-
model = output
|
84 |
-
if model is None:
|
85 |
-
return None, None
|
86 |
-
else:
|
87 |
-
tokenizer = load_tokenizer(model_name, model)
|
88 |
-
|
89 |
-
# Hijack attention with xformers
|
90 |
-
if any((shared.args.xformers, shared.args.sdp_attention)):
|
91 |
-
llama_attn_hijack.hijack_llama_attention()
|
92 |
-
|
93 |
-
logger.info(f"Loaded the model in {(time.time()-t0):.2f} seconds.\n")
|
94 |
-
return model, tokenizer
|
95 |
-
|
96 |
-
|
97 |
-
def load_tokenizer(model_name, model):
|
98 |
-
tokenizer = None
|
99 |
-
path_to_model = Path(f"{shared.args.model_dir}/{model_name}/")
|
100 |
-
if any(s in model_name.lower() for s in ['gpt-4chan', 'gpt4chan']) and Path(f"{shared.args.model_dir}/gpt-j-6B/").exists():
|
101 |
-
tokenizer = AutoTokenizer.from_pretrained(Path(f"{shared.args.model_dir}/gpt-j-6B/"))
|
102 |
-
elif path_to_model.exists():
|
103 |
-
if shared.args.use_fast:
|
104 |
-
logger.info('Loading the tokenizer with use_fast=True.')
|
105 |
-
|
106 |
-
tokenizer = AutoTokenizer.from_pretrained(
|
107 |
-
path_to_model,
|
108 |
-
trust_remote_code=shared.args.trust_remote_code,
|
109 |
-
use_fast=shared.args.use_fast
|
110 |
-
)
|
111 |
-
|
112 |
-
return tokenizer
|
113 |
-
|
114 |
-
|
115 |
-
def huggingface_loader(model_name):
|
116 |
-
|
117 |
-
path_to_model = Path(f'{shared.args.model_dir}/{model_name}')
|
118 |
-
params = {
|
119 |
-
'low_cpu_mem_usage': True,
|
120 |
-
'trust_remote_code': shared.args.trust_remote_code,
|
121 |
-
'torch_dtype': torch.bfloat16 if shared.args.bf16 else torch.float16
|
122 |
-
}
|
123 |
-
config = AutoConfig.from_pretrained(path_to_model, trust_remote_code=params['trust_remote_code'])
|
124 |
-
|
125 |
-
if 'chatglm' in model_name.lower():
|
126 |
-
LoaderClass = AutoModel
|
127 |
-
else:
|
128 |
-
if config.to_dict().get('is_encoder_decoder', False):
|
129 |
-
LoaderClass = AutoModelForSeq2SeqLM
|
130 |
-
shared.is_seq2seq = True
|
131 |
-
else:
|
132 |
-
LoaderClass = AutoModelForCausalLM
|
133 |
-
|
134 |
-
# Load the model in simple 16-bit mode by default
|
135 |
-
if not any([shared.args.cpu, shared.args.load_in_8bit, shared.args.load_in_4bit, shared.args.auto_devices, shared.args.disk, shared.args.deepspeed, shared.args.gpu_memory is not None, shared.args.cpu_memory is not None, shared.args.compress_pos_emb > 1, shared.args.alpha_value > 1, shared.args.disable_exllama]):
|
136 |
-
model = LoaderClass.from_pretrained(path_to_model, **params)
|
137 |
-
if torch.backends.mps.is_available():
|
138 |
-
device = torch.device('mps')
|
139 |
-
model = model.to(device)
|
140 |
-
else:
|
141 |
-
model = model.cuda()
|
142 |
-
|
143 |
-
# DeepSpeed ZeRO-3
|
144 |
-
elif shared.args.deepspeed:
|
145 |
-
model = LoaderClass.from_pretrained(path_to_model, torch_dtype=params['torch_dtype'])
|
146 |
-
model = deepspeed.initialize(model=model, config_params=ds_config, model_parameters=None, optimizer=None, lr_scheduler=None)[0]
|
147 |
-
model.module.eval() # Inference
|
148 |
-
logger.info(f'DeepSpeed ZeRO-3 is enabled: {is_deepspeed_zero3_enabled()}')
|
149 |
-
|
150 |
-
# Load with quantization and/or offloading
|
151 |
-
else:
|
152 |
-
if not any((shared.args.cpu, torch.cuda.is_available(), torch.backends.mps.is_available())):
|
153 |
-
logger.warning('torch.cuda.is_available() returned False. This means that no GPU has been detected. Falling back to CPU mode.')
|
154 |
-
shared.args.cpu = True
|
155 |
-
|
156 |
-
if shared.args.cpu:
|
157 |
-
params['torch_dtype'] = torch.float32
|
158 |
-
else:
|
159 |
-
params['device_map'] = 'auto'
|
160 |
-
params['max_memory'] = get_max_memory_dict()
|
161 |
-
if shared.args.load_in_4bit:
|
162 |
-
# See https://github.com/huggingface/transformers/pull/23479/files
|
163 |
-
# and https://huggingface.co/blog/4bit-transformers-bitsandbytes
|
164 |
-
quantization_config_params = {
|
165 |
-
'load_in_4bit': True,
|
166 |
-
'bnb_4bit_compute_dtype': eval("torch.{}".format(shared.args.compute_dtype)) if shared.args.compute_dtype in ["bfloat16", "float16", "float32"] else None,
|
167 |
-
'bnb_4bit_quant_type': shared.args.quant_type,
|
168 |
-
'bnb_4bit_use_double_quant': shared.args.use_double_quant,
|
169 |
-
}
|
170 |
-
|
171 |
-
logger.info('Using the following 4-bit params: ' + str(quantization_config_params))
|
172 |
-
params['quantization_config'] = BitsAndBytesConfig(**quantization_config_params)
|
173 |
-
|
174 |
-
elif shared.args.load_in_8bit:
|
175 |
-
if any((shared.args.auto_devices, shared.args.gpu_memory)):
|
176 |
-
params['quantization_config'] = BitsAndBytesConfig(load_in_8bit=True, llm_int8_enable_fp32_cpu_offload=True)
|
177 |
-
else:
|
178 |
-
params['quantization_config'] = BitsAndBytesConfig(load_in_8bit=True)
|
179 |
-
|
180 |
-
if params['max_memory'] is not None:
|
181 |
-
with init_empty_weights():
|
182 |
-
model = LoaderClass.from_config(config, trust_remote_code=params['trust_remote_code'])
|
183 |
-
|
184 |
-
model.tie_weights()
|
185 |
-
params['device_map'] = infer_auto_device_map(
|
186 |
-
model,
|
187 |
-
dtype=torch.int8,
|
188 |
-
max_memory=params['max_memory'],
|
189 |
-
no_split_module_classes=model._no_split_modules
|
190 |
-
)
|
191 |
-
|
192 |
-
if shared.args.disk:
|
193 |
-
params['offload_folder'] = shared.args.disk_cache_dir
|
194 |
-
|
195 |
-
if shared.args.disable_exllama:
|
196 |
-
try:
|
197 |
-
gptq_config = GPTQConfig(bits=config.quantization_config.get('bits', 4), disable_exllama=True)
|
198 |
-
params['quantization_config'] = gptq_config
|
199 |
-
logger.info('Loading with ExLlama kernel disabled.')
|
200 |
-
except:
|
201 |
-
exc = traceback.format_exc()
|
202 |
-
logger.error('Failed to disable exllama. Does the config.json for this model contain the necessary quantization info?')
|
203 |
-
print(exc)
|
204 |
-
|
205 |
-
if shared.args.compress_pos_emb > 1:
|
206 |
-
params['rope_scaling'] = {'type': 'linear', 'factor': shared.args.compress_pos_emb}
|
207 |
-
elif shared.args.alpha_value > 1:
|
208 |
-
params['rope_scaling'] = {'type': 'dynamic', 'factor': RoPE.get_alpha_value(shared.args.alpha_value, shared.args.rope_freq_base)}
|
209 |
-
|
210 |
-
model = LoaderClass.from_pretrained(path_to_model, **params)
|
211 |
-
|
212 |
-
return model
|
213 |
-
|
214 |
-
|
215 |
-
def llamacpp_loader(model_name):
|
216 |
-
from modules.llamacpp_model import LlamaCppModel
|
217 |
-
|
218 |
-
path = Path(f'{shared.args.model_dir}/{model_name}')
|
219 |
-
if path.is_file():
|
220 |
-
model_file = path
|
221 |
-
else:
|
222 |
-
model_file = list(Path(f'{shared.args.model_dir}/{model_name}').glob('*.gguf'))[0]
|
223 |
-
|
224 |
-
logger.info(f"llama.cpp weights detected: {model_file}")
|
225 |
-
model, tokenizer = LlamaCppModel.from_pretrained(model_file)
|
226 |
-
return model, tokenizer
|
227 |
-
|
228 |
-
|
229 |
-
def llamacpp_HF_loader(model_name):
|
230 |
-
from modules.llamacpp_hf import LlamacppHF
|
231 |
-
|
232 |
-
for fname in [model_name, "oobabooga_llama-tokenizer", "llama-tokenizer"]:
|
233 |
-
path = Path(f'{shared.args.model_dir}/{fname}')
|
234 |
-
if all((path / file).exists() for file in ['tokenizer_config.json', 'special_tokens_map.json', 'tokenizer.model']):
|
235 |
-
logger.info(f'Using tokenizer from: {path}')
|
236 |
-
break
|
237 |
-
else:
|
238 |
-
logger.error("Could not load the model because a tokenizer in transformers format was not found. Please download oobabooga/llama-tokenizer.")
|
239 |
-
return None, None
|
240 |
-
|
241 |
-
if shared.args.use_fast:
|
242 |
-
logger.info('Loading the tokenizer with use_fast=True.')
|
243 |
-
|
244 |
-
tokenizer = AutoTokenizer.from_pretrained(
|
245 |
-
path,
|
246 |
-
trust_remote_code=shared.args.trust_remote_code,
|
247 |
-
use_fast=shared.args.use_fast
|
248 |
-
)
|
249 |
-
|
250 |
-
model = LlamacppHF.from_pretrained(model_name)
|
251 |
-
return model, tokenizer
|
252 |
-
|
253 |
-
|
254 |
-
def ctransformers_loader(model_name):
|
255 |
-
from modules.ctransformers_model import CtransformersModel
|
256 |
-
|
257 |
-
path = Path(f'{shared.args.model_dir}/{model_name}')
|
258 |
-
ctrans = CtransformersModel()
|
259 |
-
if ctrans.model_type_is_auto():
|
260 |
-
model_file = path
|
261 |
-
else:
|
262 |
-
if path.is_file():
|
263 |
-
model_file = path
|
264 |
-
else:
|
265 |
-
entries = Path(f'{shared.args.model_dir}/{model_name}')
|
266 |
-
gguf = list(entries.glob('*.gguf'))
|
267 |
-
bin = list(entries.glob('*.bin'))
|
268 |
-
if len(gguf) > 0:
|
269 |
-
model_file = gguf[0]
|
270 |
-
elif len(bin) > 0:
|
271 |
-
model_file = bin[0]
|
272 |
-
else:
|
273 |
-
logger.error("Could not find a model for ctransformers.")
|
274 |
-
return None, None
|
275 |
-
|
276 |
-
logger.info(f'ctransformers weights detected: {model_file}')
|
277 |
-
model, tokenizer = ctrans.from_pretrained(model_file)
|
278 |
-
return model, tokenizer
|
279 |
-
|
280 |
-
def AutoAWQ_loader(model_name):
|
281 |
-
from awq import AutoAWQForCausalLM
|
282 |
-
|
283 |
-
model_dir = Path(f'{shared.args.model_dir}/{model_name}')
|
284 |
-
|
285 |
-
if shared.args.deepspeed:
|
286 |
-
logger.warn("AutoAWQ is incompatible with deepspeed")
|
287 |
-
|
288 |
-
model = AutoAWQForCausalLM.from_quantized(
|
289 |
-
quant_path=model_dir,
|
290 |
-
max_new_tokens=shared.args.max_seq_len,
|
291 |
-
trust_remote_code=shared.args.trust_remote_code,
|
292 |
-
fuse_layers=not shared.args.no_inject_fused_attention,
|
293 |
-
max_memory=get_max_memory_dict(),
|
294 |
-
batch_size=shared.args.n_batch,
|
295 |
-
safetensors=not shared.args.trust_remote_code)
|
296 |
-
|
297 |
-
return model
|
298 |
-
|
299 |
-
def GPTQ_loader(model_name):
|
300 |
-
|
301 |
-
# Monkey patch
|
302 |
-
if shared.args.monkey_patch:
|
303 |
-
logger.warning("Applying the monkey patch for using LoRAs with GPTQ models. It may cause undefined behavior outside its intended scope.")
|
304 |
-
from modules.monkey_patch_gptq_lora import load_model_llama
|
305 |
-
|
306 |
-
model, _ = load_model_llama(model_name)
|
307 |
-
|
308 |
-
# No monkey patch
|
309 |
-
else:
|
310 |
-
import modules.GPTQ_loader
|
311 |
-
|
312 |
-
model = modules.GPTQ_loader.load_quantized(model_name)
|
313 |
-
|
314 |
-
return model
|
315 |
-
|
316 |
-
|
317 |
-
def AutoGPTQ_loader(model_name):
|
318 |
-
import modules.AutoGPTQ_loader
|
319 |
-
|
320 |
-
return modules.AutoGPTQ_loader.load_quantized(model_name)
|
321 |
-
|
322 |
-
|
323 |
-
def ExLlama_loader(model_name):
|
324 |
-
from modules.exllama import ExllamaModel
|
325 |
-
|
326 |
-
model, tokenizer = ExllamaModel.from_pretrained(model_name)
|
327 |
-
return model, tokenizer
|
328 |
-
|
329 |
-
|
330 |
-
def ExLlama_HF_loader(model_name):
|
331 |
-
from modules.exllama_hf import ExllamaHF
|
332 |
-
|
333 |
-
return ExllamaHF.from_pretrained(model_name)
|
334 |
-
|
335 |
-
|
336 |
-
def ExLlamav2_loader(model_name):
|
337 |
-
from modules.exllamav2 import Exllamav2Model
|
338 |
-
|
339 |
-
model, tokenizer = Exllamav2Model.from_pretrained(model_name)
|
340 |
-
return model, tokenizer
|
341 |
-
|
342 |
-
|
343 |
-
def ExLlamav2_HF_loader(model_name):
|
344 |
-
from modules.exllamav2_hf import Exllamav2HF
|
345 |
-
|
346 |
-
return Exllamav2HF.from_pretrained(model_name)
|
347 |
-
|
348 |
-
|
349 |
-
def RWKV_loader(model_name):
|
350 |
-
'''
|
351 |
-
This loader is not currently maintained as RWKV can now be loaded
|
352 |
-
through the transformers library.
|
353 |
-
'''
|
354 |
-
from modules.RWKV import RWKVModel, RWKVTokenizer
|
355 |
-
|
356 |
-
model = RWKVModel.from_pretrained(Path(f'{shared.args.model_dir}/{model_name}'), dtype="fp32" if shared.args.cpu else "bf16" if shared.args.bf16 else "fp16", device="cpu" if shared.args.cpu else "cuda")
|
357 |
-
tokenizer = RWKVTokenizer.from_pretrained(Path(shared.args.model_dir))
|
358 |
-
return model, tokenizer
|
359 |
-
|
360 |
-
|
361 |
-
def get_max_memory_dict():
|
362 |
-
max_memory = {}
|
363 |
-
if shared.args.gpu_memory:
|
364 |
-
memory_map = list(map(lambda x: x.strip(), shared.args.gpu_memory))
|
365 |
-
for i in range(len(memory_map)):
|
366 |
-
max_memory[i] = f'{memory_map[i]}GiB' if not re.match('.*ib$', memory_map[i].lower()) else memory_map[i]
|
367 |
-
|
368 |
-
max_cpu_memory = shared.args.cpu_memory.strip() if shared.args.cpu_memory is not None else '99GiB'
|
369 |
-
max_memory['cpu'] = f'{max_cpu_memory}GiB' if not re.match('.*ib$', max_cpu_memory.lower()) else max_cpu_memory
|
370 |
-
|
371 |
-
# If --auto-devices is provided standalone, try to get a reasonable value
|
372 |
-
# for the maximum memory of device :0
|
373 |
-
elif shared.args.auto_devices:
|
374 |
-
total_mem = (torch.cuda.get_device_properties(0).total_memory / (1024 * 1024))
|
375 |
-
suggestion = round((total_mem - 1000) / 1000) * 1000
|
376 |
-
if total_mem - suggestion < 800:
|
377 |
-
suggestion -= 1000
|
378 |
-
|
379 |
-
suggestion = int(round(suggestion / 1000))
|
380 |
-
logger.warning(f"Auto-assiging --gpu-memory {suggestion} for your GPU to try to prevent out-of-memory errors. You can manually set other values.")
|
381 |
-
max_memory = {0: f'{suggestion}GiB', 'cpu': f'{shared.args.cpu_memory or 99}GiB'}
|
382 |
-
|
383 |
-
return max_memory if len(max_memory) > 0 else None
|
384 |
-
|
385 |
-
|
386 |
-
def clear_torch_cache():
|
387 |
-
gc.collect()
|
388 |
-
if not shared.args.cpu:
|
389 |
-
torch.cuda.empty_cache()
|
390 |
-
|
391 |
-
|
392 |
-
def unload_model():
|
393 |
-
shared.model = shared.tokenizer = None
|
394 |
-
shared.lora_names = []
|
395 |
-
shared.model_dirty_from_training = False
|
396 |
-
clear_torch_cache()
|
397 |
-
|
398 |
-
|
399 |
-
def reload_model():
|
400 |
-
unload_model()
|
401 |
-
shared.model, shared.tokenizer = load_model(shared.model_name)
|
|
|
|
|
|
|
|
|
|
|
|
|
|
|
|
|
|
|
|
|
|
|
|
|
|
|
|
|
|
|
|
|
|
|
|
|
|
|
|
|
|
|
|
|
|
|
|
|
|
|
|
|
|
|
|
|
|
|
|
|
|
|
|
|
|
|
|
|
|
|
|
|
|
|
|
|
|
|
|
|
|
|
|
|
|
|
|
|
|
|
|
|
|
|
|
|
|
|
|
|
|
|
|
|
|
|
|
|
|
|
|
|
|
|
|
|
|
|
|
|
|
|
|
|
|
|
|
|
|
|
|
|
|
|
|
|
|
|
|
|
|
|
|
|
|
|
|
|
|
|
|
|
|
|
|
|
|
|
|
|
|
|
|
|
|
|
|
|
|
|
|
|
|
|
|
|
|
|
|
|
|
|
|
|
|
|
|
|
|
|
|
|
|
|
|
|
|
|
|
|
|
|
|
|
|
|
|
|
|
|
|
|
|
|
|
|
|
|
|
|
|
|
|
|
|
|
|
|
|
|
|
|
|
|
|
|
|
|
|
|
|
|
|
|
|
|
|
|
|
|
|
|
|
|
|
|
|
|
|
|
|
|
|
|
|
|
|
|
|
|
|
|
|
|
|
|
|
|
|
|
|
|
|
|
|
|
|
|
|
|
|
|
|
|
|
|
|
|
|
|
|
|
|
|
|
|
|
|
|
|
|
|
|
|
|
|
|
|
|
|
|
|
|
|
|
|
|
|
|
|
|
|
|
|
|
|
|
|
|
|
|
|
|
|
|
|
|
|
|
|
|
|
|
|
|
|
|
|
|
|
|
|
|
|
|
|
|
|
|
|
|
|
|
|
|
|
|
|
|
|
|
|
|
|
|
|
|
|
|
|
|
|
|
|
|
|
|
|
|
|
|
|
|
|
|
|
|
|
|
|
|
|
|
|
|
|
|
|
|
|
|
|
|
|
|
|
|
|
|
|
|
|
|
|
|
|
|
|
|
|
|
|
|
|
|
|
|
|
|
|
|
|
|
|
|
|
|
|
|
|
|
|
|
|
|
|
|
|
|
|
|
|
|
|
|
|
|
|
|
|
|
|
|
|
|
|
|
|
|
|
|
|
|
|
|
|
|
|
|
|
|
|
|
|
|
|
|
|
|
|
|
|
|
|
|
|
|
|
|
|
|
|
|
|
|
|
|
|
|
|
|
|
|
|
|
|
|
|
|
|
|
|
|
|
|
|
|
|
|
|
|
|
|
|
|
|
|
|
|
|
|
|
|
|
|
|
|
|
|
|
|
|
|
|
|
|
|
|
|
|
|
|
|
|
|
|
|
|
|
|
|
|
|
|
|
|
|
|
|
|
|
|
|
|
|
|
|
|
|
|
|
|
|
|
|
|
|
|
|
|
|
|
|
|
|
|
|
|
|
|
|
|
|
|
|
|
|
|
|
|
|
|
|
|
|
|
|
|
|
|
|
|
|
|
|
|
|
|
|
|
|
|
|
|
|
|
|
|
|
|
|
|
|
|
|
|
|
|
|
|
|
|
|
|
|
|
|
|
|
|
|
|
|
|
|
|
|
|
|
|
|
|
|
|
|
|
|
|
|
|
|
|
|
|
|
|
|
|
|
|
|
|
|
|
|
|
|
|
|
|
|
|
|
|
|
|
|
|
|
|
|
|
|
|
|
|
|
|
|
|
|
|
|
|
|
|
|
|
|
|
|
|
|
|
|
|
|
|
|
|
|
|
|
|
|
|
|
|
|
|
|
|
|
|
|
|
|
|
|
|
|
|
|
|
|
|
|
|
|
|
|
|
|
spaces/Anonymous-sub/Rerender/app.py
DELETED
@@ -1,997 +0,0 @@
|
|
1 |
-
import os
|
2 |
-
import shutil
|
3 |
-
from enum import Enum
|
4 |
-
|
5 |
-
import cv2
|
6 |
-
import einops
|
7 |
-
import gradio as gr
|
8 |
-
import numpy as np
|
9 |
-
import torch
|
10 |
-
import torch.nn.functional as F
|
11 |
-
import torchvision.transforms as T
|
12 |
-
from blendmodes.blend import BlendType, blendLayers
|
13 |
-
from PIL import Image
|
14 |
-
from pytorch_lightning import seed_everything
|
15 |
-
from safetensors.torch import load_file
|
16 |
-
from skimage import exposure
|
17 |
-
|
18 |
-
import src.import_util # noqa: F401
|
19 |
-
from ControlNet.annotator.canny import CannyDetector
|
20 |
-
from ControlNet.annotator.hed import HEDdetector
|
21 |
-
from ControlNet.annotator.midas import MidasDetector
|
22 |
-
from ControlNet.annotator.util import HWC3
|
23 |
-
from ControlNet.cldm.model import create_model, load_state_dict
|
24 |
-
from gmflow_module.gmflow.gmflow import GMFlow
|
25 |
-
from flow.flow_utils import get_warped_and_mask
|
26 |
-
from sd_model_cfg import model_dict
|
27 |
-
from src.config import RerenderConfig
|
28 |
-
from src.controller import AttentionControl
|
29 |
-
from src.ddim_v_hacked import DDIMVSampler
|
30 |
-
from src.img_util import find_flat_region, numpy2tensor
|
31 |
-
from src.video_util import (frame_to_video, get_fps, get_frame_count,
|
32 |
-
prepare_frames)
|
33 |
-
|
34 |
-
import huggingface_hub
|
35 |
-
|
36 |
-
REPO_NAME = 'Anonymous-sub/Rerender'
|
37 |
-
|
38 |
-
huggingface_hub.hf_hub_download(REPO_NAME,
|
39 |
-
'pexels-koolshooters-7322716.mp4',
|
40 |
-
local_dir='videos')
|
41 |
-
huggingface_hub.hf_hub_download(
|
42 |
-
REPO_NAME,
|
43 |
-
'pexels-antoni-shkraba-8048492-540x960-25fps.mp4',
|
44 |
-
local_dir='videos')
|
45 |
-
huggingface_hub.hf_hub_download(
|
46 |
-
REPO_NAME,
|
47 |
-
'pexels-cottonbro-studio-6649832-960x506-25fps.mp4',
|
48 |
-
local_dir='videos')
|
49 |
-
|
50 |
-
inversed_model_dict = dict()
|
51 |
-
for k, v in model_dict.items():
|
52 |
-
inversed_model_dict[v] = k
|
53 |
-
|
54 |
-
to_tensor = T.PILToTensor()
|
55 |
-
blur = T.GaussianBlur(kernel_size=(9, 9), sigma=(18, 18))
|
56 |
-
device = 'cuda' if torch.cuda.is_available() else 'cpu'
|
57 |
-
|
58 |
-
|
59 |
-
class ProcessingState(Enum):
|
60 |
-
NULL = 0
|
61 |
-
FIRST_IMG = 1
|
62 |
-
KEY_IMGS = 2
|
63 |
-
|
64 |
-
|
65 |
-
MAX_KEYFRAME = float(os.environ.get('MAX_KEYFRAME', 8))
|
66 |
-
|
67 |
-
|
68 |
-
class GlobalState:
|
69 |
-
|
70 |
-
def __init__(self):
|
71 |
-
self.sd_model = None
|
72 |
-
self.ddim_v_sampler = None
|
73 |
-
self.detector_type = None
|
74 |
-
self.detector = None
|
75 |
-
self.controller = None
|
76 |
-
self.processing_state = ProcessingState.NULL
|
77 |
-
flow_model = GMFlow(
|
78 |
-
feature_channels=128,
|
79 |
-
num_scales=1,
|
80 |
-
upsample_factor=8,
|
81 |
-
num_head=1,
|
82 |
-
attention_type='swin',
|
83 |
-
ffn_dim_expansion=4,
|
84 |
-
num_transformer_layers=6,
|
85 |
-
).to(device)
|
86 |
-
|
87 |
-
checkpoint = torch.load('models/gmflow_sintel-0c07dcb3.pth',
|
88 |
-
map_location=lambda storage, loc: storage)
|
89 |
-
weights = checkpoint['model'] if 'model' in checkpoint else checkpoint
|
90 |
-
flow_model.load_state_dict(weights, strict=False)
|
91 |
-
flow_model.eval()
|
92 |
-
self.flow_model = flow_model
|
93 |
-
|
94 |
-
def update_controller(self, inner_strength, mask_period, cross_period,
|
95 |
-
ada_period, warp_period):
|
96 |
-
self.controller = AttentionControl(inner_strength, mask_period,
|
97 |
-
cross_period, ada_period,
|
98 |
-
warp_period)
|
99 |
-
|
100 |
-
def update_sd_model(self, sd_model, control_type):
|
101 |
-
if sd_model == self.sd_model:
|
102 |
-
return
|
103 |
-
self.sd_model = sd_model
|
104 |
-
model = create_model('./ControlNet/models/cldm_v15.yaml').cpu()
|
105 |
-
if control_type == 'HED':
|
106 |
-
model.load_state_dict(
|
107 |
-
load_state_dict(huggingface_hub.hf_hub_download(
|
108 |
-
'lllyasviel/ControlNet', './models/control_sd15_hed.pth'),
|
109 |
-
location=device))
|
110 |
-
elif control_type == 'canny':
|
111 |
-
model.load_state_dict(
|
112 |
-
load_state_dict(huggingface_hub.hf_hub_download(
|
113 |
-
'lllyasviel/ControlNet', 'models/control_sd15_canny.pth'),
|
114 |
-
location=device))
|
115 |
-
elif control_type == 'depth':
|
116 |
-
model.load_state_dict(
|
117 |
-
load_state_dict(huggingface_hub.hf_hub_download(
|
118 |
-
'lllyasviel/ControlNet', 'models/control_sd15_depth.pth'),
|
119 |
-
location=device))
|
120 |
-
|
121 |
-
model.to(device)
|
122 |
-
sd_model_path = model_dict[sd_model]
|
123 |
-
if len(sd_model_path) > 0:
|
124 |
-
repo_name = REPO_NAME
|
125 |
-
# check if sd_model is repo_id/name otherwise use global REPO_NAME
|
126 |
-
if sd_model.count('/') == 1:
|
127 |
-
repo_name = sd_model
|
128 |
-
|
129 |
-
model_ext = os.path.splitext(sd_model_path)[1]
|
130 |
-
downloaded_model = huggingface_hub.hf_hub_download(
|
131 |
-
repo_name, sd_model_path)
|
132 |
-
if model_ext == '.safetensors':
|
133 |
-
model.load_state_dict(load_file(downloaded_model),
|
134 |
-
strict=False)
|
135 |
-
elif model_ext == '.ckpt' or model_ext == '.pth':
|
136 |
-
model.load_state_dict(
|
137 |
-
torch.load(downloaded_model)['state_dict'], strict=False)
|
138 |
-
|
139 |
-
try:
|
140 |
-
model.first_stage_model.load_state_dict(torch.load(
|
141 |
-
huggingface_hub.hf_hub_download(
|
142 |
-
'stabilityai/sd-vae-ft-mse-original',
|
143 |
-
'vae-ft-mse-840000-ema-pruned.ckpt'))['state_dict'],
|
144 |
-
strict=False)
|
145 |
-
except Exception:
|
146 |
-
print('Warning: We suggest you download the fine-tuned VAE',
|
147 |
-
'otherwise the generation quality will be degraded')
|
148 |
-
|
149 |
-
self.ddim_v_sampler = DDIMVSampler(model)
|
150 |
-
|
151 |
-
def clear_sd_model(self):
|
152 |
-
self.sd_model = None
|
153 |
-
self.ddim_v_sampler = None
|
154 |
-
if device == 'cuda':
|
155 |
-
torch.cuda.empty_cache()
|
156 |
-
|
157 |
-
def update_detector(self, control_type, canny_low=100, canny_high=200):
|
158 |
-
if self.detector_type == control_type:
|
159 |
-
return
|
160 |
-
if control_type == 'HED':
|
161 |
-
self.detector = HEDdetector()
|
162 |
-
elif control_type == 'canny':
|
163 |
-
canny_detector = CannyDetector()
|
164 |
-
low_threshold = canny_low
|
165 |
-
high_threshold = canny_high
|
166 |
-
|
167 |
-
def apply_canny(x):
|
168 |
-
return canny_detector(x, low_threshold, high_threshold)
|
169 |
-
|
170 |
-
self.detector = apply_canny
|
171 |
-
|
172 |
-
elif control_type == 'depth':
|
173 |
-
midas = MidasDetector()
|
174 |
-
|
175 |
-
def apply_midas(x):
|
176 |
-
detected_map, _ = midas(x)
|
177 |
-
return detected_map
|
178 |
-
|
179 |
-
self.detector = apply_midas
|
180 |
-
|
181 |
-
|
182 |
-
global_state = GlobalState()
|
183 |
-
global_video_path = None
|
184 |
-
video_frame_count = None
|
185 |
-
|
186 |
-
|
187 |
-
def create_cfg(input_path, prompt, image_resolution, control_strength,
|
188 |
-
color_preserve, left_crop, right_crop, top_crop, bottom_crop,
|
189 |
-
control_type, low_threshold, high_threshold, ddim_steps, scale,
|
190 |
-
seed, sd_model, a_prompt, n_prompt, interval, keyframe_count,
|
191 |
-
x0_strength, use_constraints, cross_start, cross_end,
|
192 |
-
style_update_freq, warp_start, warp_end, mask_start, mask_end,
|
193 |
-
ada_start, ada_end, mask_strength, inner_strength,
|
194 |
-
smooth_boundary):
|
195 |
-
use_warp = 'shape-aware fusion' in use_constraints
|
196 |
-
use_mask = 'pixel-aware fusion' in use_constraints
|
197 |
-
use_ada = 'color-aware AdaIN' in use_constraints
|
198 |
-
|
199 |
-
if not use_warp:
|
200 |
-
warp_start = 1
|
201 |
-
warp_end = 0
|
202 |
-
|
203 |
-
if not use_mask:
|
204 |
-
mask_start = 1
|
205 |
-
mask_end = 0
|
206 |
-
|
207 |
-
if not use_ada:
|
208 |
-
ada_start = 1
|
209 |
-
ada_end = 0
|
210 |
-
|
211 |
-
input_name = os.path.split(input_path)[-1].split('.')[0]
|
212 |
-
frame_count = 2 + keyframe_count * interval
|
213 |
-
cfg = RerenderConfig()
|
214 |
-
cfg.create_from_parameters(
|
215 |
-
input_path,
|
216 |
-
os.path.join('result', input_name, 'blend.mp4'),
|
217 |
-
prompt,
|
218 |
-
a_prompt=a_prompt,
|
219 |
-
n_prompt=n_prompt,
|
220 |
-
frame_count=frame_count,
|
221 |
-
interval=interval,
|
222 |
-
crop=[left_crop, right_crop, top_crop, bottom_crop],
|
223 |
-
sd_model=sd_model,
|
224 |
-
ddim_steps=ddim_steps,
|
225 |
-
scale=scale,
|
226 |
-
control_type=control_type,
|
227 |
-
control_strength=control_strength,
|
228 |
-
canny_low=low_threshold,
|
229 |
-
canny_high=high_threshold,
|
230 |
-
seed=seed,
|
231 |
-
image_resolution=image_resolution,
|
232 |
-
x0_strength=x0_strength,
|
233 |
-
style_update_freq=style_update_freq,
|
234 |
-
cross_period=(cross_start, cross_end),
|
235 |
-
warp_period=(warp_start, warp_end),
|
236 |
-
mask_period=(mask_start, mask_end),
|
237 |
-
ada_period=(ada_start, ada_end),
|
238 |
-
mask_strength=mask_strength,
|
239 |
-
inner_strength=inner_strength,
|
240 |
-
smooth_boundary=smooth_boundary,
|
241 |
-
color_preserve=color_preserve)
|
242 |
-
return cfg
|
243 |
-
|
244 |
-
|
245 |
-
def cfg_to_input(filename):
|
246 |
-
|
247 |
-
cfg = RerenderConfig()
|
248 |
-
cfg.create_from_path(filename)
|
249 |
-
keyframe_count = (cfg.frame_count - 2) // cfg.interval
|
250 |
-
use_constraints = [
|
251 |
-
'shape-aware fusion', 'pixel-aware fusion', 'color-aware AdaIN'
|
252 |
-
]
|
253 |
-
|
254 |
-
sd_model = inversed_model_dict.get(cfg.sd_model, 'Stable Diffusion 1.5')
|
255 |
-
|
256 |
-
args = [
|
257 |
-
cfg.input_path, cfg.prompt, cfg.image_resolution, cfg.control_strength,
|
258 |
-
cfg.color_preserve, *cfg.crop, cfg.control_type, cfg.canny_low,
|
259 |
-
cfg.canny_high, cfg.ddim_steps, cfg.scale, cfg.seed, sd_model,
|
260 |
-
cfg.a_prompt, cfg.n_prompt, cfg.interval, keyframe_count,
|
261 |
-
cfg.x0_strength, use_constraints, *cfg.cross_period,
|
262 |
-
cfg.style_update_freq, *cfg.warp_period, *cfg.mask_period,
|
263 |
-
*cfg.ada_period, cfg.mask_strength, cfg.inner_strength,
|
264 |
-
cfg.smooth_boundary
|
265 |
-
]
|
266 |
-
return args
|
267 |
-
|
268 |
-
|
269 |
-
def setup_color_correction(image):
|
270 |
-
correction_target = cv2.cvtColor(np.asarray(image.copy()),
|
271 |
-
cv2.COLOR_RGB2LAB)
|
272 |
-
return correction_target
|
273 |
-
|
274 |
-
|
275 |
-
def apply_color_correction(correction, original_image):
|
276 |
-
image = Image.fromarray(
|
277 |
-
cv2.cvtColor(
|
278 |
-
exposure.match_histograms(cv2.cvtColor(np.asarray(original_image),
|
279 |
-
cv2.COLOR_RGB2LAB),
|
280 |
-
correction,
|
281 |
-
channel_axis=2),
|
282 |
-
cv2.COLOR_LAB2RGB).astype('uint8'))
|
283 |
-
|
284 |
-
image = blendLayers(image, original_image, BlendType.LUMINOSITY)
|
285 |
-
|
286 |
-
return image
|
287 |
-
|
288 |
-
|
289 |
-
@torch.no_grad()
|
290 |
-
def process(*args):
|
291 |
-
first_frame = process1(*args)
|
292 |
-
|
293 |
-
keypath = process2(*args)
|
294 |
-
|
295 |
-
return first_frame, keypath
|
296 |
-
|
297 |
-
|
298 |
-
@torch.no_grad()
|
299 |
-
def process0(*args):
|
300 |
-
global global_video_path
|
301 |
-
global_video_path = args[0]
|
302 |
-
return process(*args[1:])
|
303 |
-
|
304 |
-
|
305 |
-
@torch.no_grad()
|
306 |
-
def process1(*args):
|
307 |
-
|
308 |
-
global global_video_path
|
309 |
-
cfg = create_cfg(global_video_path, *args)
|
310 |
-
global global_state
|
311 |
-
global_state.update_sd_model(cfg.sd_model, cfg.control_type)
|
312 |
-
global_state.update_controller(cfg.inner_strength, cfg.mask_period,
|
313 |
-
cfg.cross_period, cfg.ada_period,
|
314 |
-
cfg.warp_period)
|
315 |
-
global_state.update_detector(cfg.control_type, cfg.canny_low,
|
316 |
-
cfg.canny_high)
|
317 |
-
global_state.processing_state = ProcessingState.FIRST_IMG
|
318 |
-
|
319 |
-
prepare_frames(cfg.input_path, cfg.input_dir, cfg.image_resolution,
|
320 |
-
cfg.crop)
|
321 |
-
|
322 |
-
ddim_v_sampler = global_state.ddim_v_sampler
|
323 |
-
model = ddim_v_sampler.model
|
324 |
-
detector = global_state.detector
|
325 |
-
controller = global_state.controller
|
326 |
-
model.control_scales = [cfg.control_strength] * 13
|
327 |
-
model.to(device)
|
328 |
-
|
329 |
-
num_samples = 1
|
330 |
-
eta = 0.0
|
331 |
-
imgs = sorted(os.listdir(cfg.input_dir))
|
332 |
-
imgs = [os.path.join(cfg.input_dir, img) for img in imgs]
|
333 |
-
|
334 |
-
model.cond_stage_model.device = device
|
335 |
-
|
336 |
-
with torch.no_grad():
|
337 |
-
frame = cv2.imread(imgs[0])
|
338 |
-
frame = cv2.cvtColor(frame, cv2.COLOR_BGR2RGB)
|
339 |
-
img = HWC3(frame)
|
340 |
-
H, W, C = img.shape
|
341 |
-
|
342 |
-
img_ = numpy2tensor(img)
|
343 |
-
|
344 |
-
def generate_first_img(img_, strength):
|
345 |
-
encoder_posterior = model.encode_first_stage(img_.to(device))
|
346 |
-
x0 = model.get_first_stage_encoding(encoder_posterior).detach()
|
347 |
-
|
348 |
-
detected_map = detector(img)
|
349 |
-
detected_map = HWC3(detected_map)
|
350 |
-
|
351 |
-
control = torch.from_numpy(
|
352 |
-
detected_map.copy()).float().to(device) / 255.0
|
353 |
-
control = torch.stack([control for _ in range(num_samples)], dim=0)
|
354 |
-
control = einops.rearrange(control, 'b h w c -> b c h w').clone()
|
355 |
-
cond = {
|
356 |
-
'c_concat': [control],
|
357 |
-
'c_crossattn': [
|
358 |
-
model.get_learned_conditioning(
|
359 |
-
[cfg.prompt + ', ' + cfg.a_prompt] * num_samples)
|
360 |
-
]
|
361 |
-
}
|
362 |
-
un_cond = {
|
363 |
-
'c_concat': [control],
|
364 |
-
'c_crossattn':
|
365 |
-
[model.get_learned_conditioning([cfg.n_prompt] * num_samples)]
|
366 |
-
}
|
367 |
-
shape = (4, H // 8, W // 8)
|
368 |
-
|
369 |
-
controller.set_task('initfirst')
|
370 |
-
seed_everything(cfg.seed)
|
371 |
-
|
372 |
-
samples, _ = ddim_v_sampler.sample(
|
373 |
-
cfg.ddim_steps,
|
374 |
-
num_samples,
|
375 |
-
shape,
|
376 |
-
cond,
|
377 |
-
verbose=False,
|
378 |
-
eta=eta,
|
379 |
-
unconditional_guidance_scale=cfg.scale,
|
380 |
-
unconditional_conditioning=un_cond,
|
381 |
-
controller=controller,
|
382 |
-
x0=x0,
|
383 |
-
strength=strength)
|
384 |
-
x_samples = model.decode_first_stage(samples)
|
385 |
-
x_samples_np = (
|
386 |
-
einops.rearrange(x_samples, 'b c h w -> b h w c') * 127.5 +
|
387 |
-
127.5).cpu().numpy().clip(0, 255).astype(np.uint8)
|
388 |
-
return x_samples, x_samples_np
|
389 |
-
|
390 |
-
# When not preserve color, draw a different frame at first and use its
|
391 |
-
# color to redraw the first frame.
|
392 |
-
if not cfg.color_preserve:
|
393 |
-
first_strength = -1
|
394 |
-
else:
|
395 |
-
first_strength = 1 - cfg.x0_strength
|
396 |
-
|
397 |
-
x_samples, x_samples_np = generate_first_img(img_, first_strength)
|
398 |
-
|
399 |
-
if not cfg.color_preserve:
|
400 |
-
color_corrections = setup_color_correction(
|
401 |
-
Image.fromarray(x_samples_np[0]))
|
402 |
-
global_state.color_corrections = color_corrections
|
403 |
-
img_ = apply_color_correction(color_corrections,
|
404 |
-
Image.fromarray(img))
|
405 |
-
img_ = to_tensor(img_).unsqueeze(0)[:, :3] / 127.5 - 1
|
406 |
-
x_samples, x_samples_np = generate_first_img(
|
407 |
-
img_, 1 - cfg.x0_strength)
|
408 |
-
|
409 |
-
global_state.first_result = x_samples
|
410 |
-
global_state.first_img = img
|
411 |
-
|
412 |
-
Image.fromarray(x_samples_np[0]).save(
|
413 |
-
os.path.join(cfg.first_dir, 'first.jpg'))
|
414 |
-
|
415 |
-
return x_samples_np[0]
|
416 |
-
|
417 |
-
|
418 |
-
@torch.no_grad()
|
419 |
-
def process2(*args):
|
420 |
-
global global_state
|
421 |
-
global global_video_path
|
422 |
-
|
423 |
-
if global_state.processing_state != ProcessingState.FIRST_IMG:
|
424 |
-
raise gr.Error('Please generate the first key image before generating'
|
425 |
-
' all key images')
|
426 |
-
|
427 |
-
cfg = create_cfg(global_video_path, *args)
|
428 |
-
global_state.update_sd_model(cfg.sd_model, cfg.control_type)
|
429 |
-
global_state.update_detector(cfg.control_type, cfg.canny_low,
|
430 |
-
cfg.canny_high)
|
431 |
-
global_state.processing_state = ProcessingState.KEY_IMGS
|
432 |
-
|
433 |
-
# reset key dir
|
434 |
-
shutil.rmtree(cfg.key_dir)
|
435 |
-
os.makedirs(cfg.key_dir, exist_ok=True)
|
436 |
-
|
437 |
-
ddim_v_sampler = global_state.ddim_v_sampler
|
438 |
-
model = ddim_v_sampler.model
|
439 |
-
detector = global_state.detector
|
440 |
-
controller = global_state.controller
|
441 |
-
flow_model = global_state.flow_model
|
442 |
-
model.control_scales = [cfg.control_strength] * 13
|
443 |
-
|
444 |
-
num_samples = 1
|
445 |
-
eta = 0.0
|
446 |
-
firstx0 = True
|
447 |
-
pixelfusion = cfg.use_mask
|
448 |
-
imgs = sorted(os.listdir(cfg.input_dir))
|
449 |
-
imgs = [os.path.join(cfg.input_dir, img) for img in imgs]
|
450 |
-
|
451 |
-
first_result = global_state.first_result
|
452 |
-
first_img = global_state.first_img
|
453 |
-
pre_result = first_result
|
454 |
-
pre_img = first_img
|
455 |
-
|
456 |
-
for i in range(0, cfg.frame_count - 1, cfg.interval):
|
457 |
-
cid = i + 1
|
458 |
-
frame = cv2.imread(imgs[i + 1])
|
459 |
-
print(cid)
|
460 |
-
frame = cv2.cvtColor(frame, cv2.COLOR_BGR2RGB)
|
461 |
-
img = HWC3(frame)
|
462 |
-
H, W, C = img.shape
|
463 |
-
|
464 |
-
if cfg.color_preserve or global_state.color_corrections is None:
|
465 |
-
img_ = numpy2tensor(img)
|
466 |
-
else:
|
467 |
-
img_ = apply_color_correction(global_state.color_corrections,
|
468 |
-
Image.fromarray(img))
|
469 |
-
img_ = to_tensor(img_).unsqueeze(0)[:, :3] / 127.5 - 1
|
470 |
-
encoder_posterior = model.encode_first_stage(img_.to(device))
|
471 |
-
x0 = model.get_first_stage_encoding(encoder_posterior).detach()
|
472 |
-
|
473 |
-
detected_map = detector(img)
|
474 |
-
detected_map = HWC3(detected_map)
|
475 |
-
|
476 |
-
control = torch.from_numpy(
|
477 |
-
detected_map.copy()).float().to(device) / 255.0
|
478 |
-
control = torch.stack([control for _ in range(num_samples)], dim=0)
|
479 |
-
control = einops.rearrange(control, 'b h w c -> b c h w').clone()
|
480 |
-
cond = {
|
481 |
-
'c_concat': [control],
|
482 |
-
'c_crossattn': [
|
483 |
-
model.get_learned_conditioning(
|
484 |
-
[cfg.prompt + ', ' + cfg.a_prompt] * num_samples)
|
485 |
-
]
|
486 |
-
}
|
487 |
-
un_cond = {
|
488 |
-
'c_concat': [control],
|
489 |
-
'c_crossattn':
|
490 |
-
[model.get_learned_conditioning([cfg.n_prompt] * num_samples)]
|
491 |
-
}
|
492 |
-
shape = (4, H // 8, W // 8)
|
493 |
-
|
494 |
-
cond['c_concat'] = [control]
|
495 |
-
un_cond['c_concat'] = [control]
|
496 |
-
|
497 |
-
image1 = torch.from_numpy(pre_img).permute(2, 0, 1).float()
|
498 |
-
image2 = torch.from_numpy(img).permute(2, 0, 1).float()
|
499 |
-
warped_pre, bwd_occ_pre, bwd_flow_pre = get_warped_and_mask(
|
500 |
-
flow_model, image1, image2, pre_result, False)
|
501 |
-
blend_mask_pre = blur(
|
502 |
-
F.max_pool2d(bwd_occ_pre, kernel_size=9, stride=1, padding=4))
|
503 |
-
blend_mask_pre = torch.clamp(blend_mask_pre + bwd_occ_pre, 0, 1)
|
504 |
-
|
505 |
-
image1 = torch.from_numpy(first_img).permute(2, 0, 1).float()
|
506 |
-
warped_0, bwd_occ_0, bwd_flow_0 = get_warped_and_mask(
|
507 |
-
flow_model, image1, image2, first_result, False)
|
508 |
-
blend_mask_0 = blur(
|
509 |
-
F.max_pool2d(bwd_occ_0, kernel_size=9, stride=1, padding=4))
|
510 |
-
blend_mask_0 = torch.clamp(blend_mask_0 + bwd_occ_0, 0, 1)
|
511 |
-
|
512 |
-
if firstx0:
|
513 |
-
mask = 1 - F.max_pool2d(blend_mask_0, kernel_size=8)
|
514 |
-
controller.set_warp(
|
515 |
-
F.interpolate(bwd_flow_0 / 8.0,
|
516 |
-
scale_factor=1. / 8,
|
517 |
-
mode='bilinear'), mask)
|
518 |
-
else:
|
519 |
-
mask = 1 - F.max_pool2d(blend_mask_pre, kernel_size=8)
|
520 |
-
controller.set_warp(
|
521 |
-
F.interpolate(bwd_flow_pre / 8.0,
|
522 |
-
scale_factor=1. / 8,
|
523 |
-
mode='bilinear'), mask)
|
524 |
-
|
525 |
-
controller.set_task('keepx0, keepstyle')
|
526 |
-
seed_everything(cfg.seed)
|
527 |
-
samples, intermediates = ddim_v_sampler.sample(
|
528 |
-
cfg.ddim_steps,
|
529 |
-
num_samples,
|
530 |
-
shape,
|
531 |
-
cond,
|
532 |
-
verbose=False,
|
533 |
-
eta=eta,
|
534 |
-
unconditional_guidance_scale=cfg.scale,
|
535 |
-
unconditional_conditioning=un_cond,
|
536 |
-
controller=controller,
|
537 |
-
x0=x0,
|
538 |
-
strength=1 - cfg.x0_strength)
|
539 |
-
direct_result = model.decode_first_stage(samples)
|
540 |
-
|
541 |
-
if not pixelfusion:
|
542 |
-
pre_result = direct_result
|
543 |
-
pre_img = img
|
544 |
-
viz = (
|
545 |
-
einops.rearrange(direct_result, 'b c h w -> b h w c') * 127.5 +
|
546 |
-
127.5).cpu().numpy().clip(0, 255).astype(np.uint8)
|
547 |
-
|
548 |
-
else:
|
549 |
-
|
550 |
-
blend_results = (1 - blend_mask_pre
|
551 |
-
) * warped_pre + blend_mask_pre * direct_result
|
552 |
-
blend_results = (
|
553 |
-
1 - blend_mask_0) * warped_0 + blend_mask_0 * blend_results
|
554 |
-
|
555 |
-
bwd_occ = 1 - torch.clamp(1 - bwd_occ_pre + 1 - bwd_occ_0, 0, 1)
|
556 |
-
blend_mask = blur(
|
557 |
-
F.max_pool2d(bwd_occ, kernel_size=9, stride=1, padding=4))
|
558 |
-
blend_mask = 1 - torch.clamp(blend_mask + bwd_occ, 0, 1)
|
559 |
-
|
560 |
-
encoder_posterior = model.encode_first_stage(blend_results)
|
561 |
-
xtrg = model.get_first_stage_encoding(
|
562 |
-
encoder_posterior).detach() # * mask
|
563 |
-
blend_results_rec = model.decode_first_stage(xtrg)
|
564 |
-
encoder_posterior = model.encode_first_stage(blend_results_rec)
|
565 |
-
xtrg_rec = model.get_first_stage_encoding(
|
566 |
-
encoder_posterior).detach()
|
567 |
-
xtrg_ = (xtrg + 1 * (xtrg - xtrg_rec)) # * mask
|
568 |
-
blend_results_rec_new = model.decode_first_stage(xtrg_)
|
569 |
-
tmp = (abs(blend_results_rec_new - blend_results).mean(
|
570 |
-
dim=1, keepdims=True) > 0.25).float()
|
571 |
-
mask_x = F.max_pool2d((F.interpolate(tmp,
|
572 |
-
scale_factor=1 / 8.,
|
573 |
-
mode='bilinear') > 0).float(),
|
574 |
-
kernel_size=3,
|
575 |
-
stride=1,
|
576 |
-
padding=1)
|
577 |
-
|
578 |
-
mask = (1 - F.max_pool2d(1 - blend_mask, kernel_size=8)
|
579 |
-
) # * (1-mask_x)
|
580 |
-
|
581 |
-
if cfg.smooth_boundary:
|
582 |
-
noise_rescale = find_flat_region(mask)
|
583 |
-
else:
|
584 |
-
noise_rescale = torch.ones_like(mask)
|
585 |
-
masks = []
|
586 |
-
for i in range(cfg.ddim_steps):
|
587 |
-
if i <= cfg.ddim_steps * cfg.mask_period[
|
588 |
-
0] or i >= cfg.ddim_steps * cfg.mask_period[1]:
|
589 |
-
masks += [None]
|
590 |
-
else:
|
591 |
-
masks += [mask * cfg.mask_strength]
|
592 |
-
|
593 |
-
# mask 3
|
594 |
-
# xtrg = ((1-mask_x) *
|
595 |
-
# (xtrg + xtrg - xtrg_rec) + mask_x * samples) * mask
|
596 |
-
# mask 2
|
597 |
-
# xtrg = (xtrg + 1 * (xtrg - xtrg_rec)) * mask
|
598 |
-
xtrg = (xtrg + (1 - mask_x) * (xtrg - xtrg_rec)) * mask # mask 1
|
599 |
-
|
600 |
-
tasks = 'keepstyle, keepx0'
|
601 |
-
if not firstx0:
|
602 |
-
tasks += ', updatex0'
|
603 |
-
if i % cfg.style_update_freq == 0:
|
604 |
-
tasks += ', updatestyle'
|
605 |
-
controller.set_task(tasks, 1.0)
|
606 |
-
|
607 |
-
seed_everything(cfg.seed)
|
608 |
-
samples, _ = ddim_v_sampler.sample(
|
609 |
-
cfg.ddim_steps,
|
610 |
-
num_samples,
|
611 |
-
shape,
|
612 |
-
cond,
|
613 |
-
verbose=False,
|
614 |
-
eta=eta,
|
615 |
-
unconditional_guidance_scale=cfg.scale,
|
616 |
-
unconditional_conditioning=un_cond,
|
617 |
-
controller=controller,
|
618 |
-
x0=x0,
|
619 |
-
strength=1 - cfg.x0_strength,
|
620 |
-
xtrg=xtrg,
|
621 |
-
mask=masks,
|
622 |
-
noise_rescale=noise_rescale)
|
623 |
-
x_samples = model.decode_first_stage(samples)
|
624 |
-
pre_result = x_samples
|
625 |
-
pre_img = img
|
626 |
-
|
627 |
-
viz = (einops.rearrange(x_samples, 'b c h w -> b h w c') * 127.5 +
|
628 |
-
127.5).cpu().numpy().clip(0, 255).astype(np.uint8)
|
629 |
-
|
630 |
-
Image.fromarray(viz[0]).save(
|
631 |
-
os.path.join(cfg.key_dir, f'{cid:04d}.png'))
|
632 |
-
|
633 |
-
key_video_path = os.path.join(cfg.work_dir, 'key.mp4')
|
634 |
-
fps = get_fps(cfg.input_path)
|
635 |
-
fps //= cfg.interval
|
636 |
-
frame_to_video(key_video_path, cfg.key_dir, fps, False)
|
637 |
-
|
638 |
-
return key_video_path
|
639 |
-
|
640 |
-
|
641 |
-
DESCRIPTION = '''
|
642 |
-
## [Rerender A Video](https://github.com/williamyang1991/Rerender_A_Video)
|
643 |
-
### This space provides the function of key frame translation. Full code for full video translation will be released upon the publication of the paper.
|
644 |
-
### To avoid overload, we set limitations to the **maximum frame number** (8) and the maximum frame resolution (512x768).
|
645 |
-
### The running time of a video of size 512x640 is about 1 minute per keyframe under T4 GPU.
|
646 |
-
### How to use:
|
647 |
-
1. **Run 1st Key Frame**: only translate the first frame, so you can adjust the prompts/models/parameters to find your ideal output appearance before run the whole video.
|
648 |
-
2. **Run Key Frames**: translate all the key frames based on the settings of the first frame
|
649 |
-
3. **Run All**: **Run 1st Key Frame** and **Run Key Frames**
|
650 |
-
4. **Run Propagation**: propogate the key frames to other frames for full video translation. This function is supported [here](https://github.com/williamyang1991/Rerender_A_Video#webui-recommended)
|
651 |
-
### Tips:
|
652 |
-
1. This method cannot handle large or quick motions where the optical flow is hard to estimate. **Videos with stable motions are preferred**.
|
653 |
-
2. Pixel-aware fusion may not work for large or quick motions.
|
654 |
-
3. Try different color-aware AdaIN settings and even unuse it to avoid color jittering.
|
655 |
-
4. `revAnimated_v11` model for non-photorealstic style, `realisticVisionV20_v20` model for photorealstic style.
|
656 |
-
5. To use your own SD/LoRA model, you may clone the space and specify your model with [sd_model_cfg.py](https://huggingface.co/spaces/Anonymous-sub/Rerender/blob/main/sd_model_cfg.py).
|
657 |
-
6. This method is based on the original SD model. You may need to [convert](https://github.com/huggingface/diffusers/blob/main/scripts/convert_diffusers_to_original_stable_diffusion.py) Diffuser/Automatic1111 models to the original one.
|
658 |
-
|
659 |
-
**This code is for research purpose and non-commercial use only.**
|
660 |
-
|
661 |
-
[](https://huggingface.co/spaces/Anonymous-sub/Rerender?duplicate=true) for no queue on your own hardware.
|
662 |
-
'''
|
663 |
-
|
664 |
-
|
665 |
-
ARTICLE = r"""
|
666 |
-
If Rerender-A-Video is helpful, please help to ⭐ the <a href='https://github.com/williamyang1991/Rerender_A_Video' target='_blank'>Github Repo</a>. Thanks!
|
667 |
-
[](https://github.com/williamyang1991/Rerender_A_Video)
|
668 |
-
---
|
669 |
-
📝 **Citation**
|
670 |
-
If our work is useful for your research, please consider citing:
|
671 |
-
```bibtex
|
672 |
-
@inproceedings{yang2023rerender,
|
673 |
-
title = {Rerender A Video: Zero-Shot Text-Guided Video-to-Video Translation},
|
674 |
-
author = {Yang, Shuai and Zhou, Yifan and Liu, Ziwei and and Loy, Chen Change},
|
675 |
-
booktitle = {ACM SIGGRAPH Asia Conference Proceedings},
|
676 |
-
year = {2023},
|
677 |
-
}
|
678 |
-
```
|
679 |
-
📋 **License**
|
680 |
-
This project is licensed under <a rel="license" href="https://github.com/williamyang1991/Rerender_A_Video/blob/main/LICENSE.md">S-Lab License 1.0</a>.
|
681 |
-
Redistribution and use for non-commercial purposes should follow this license.
|
682 |
-
|
683 |
-
📧 **Contact**
|
684 |
-
If you have any questions, please feel free to reach me out at <b>[email protected]</b>.
|
685 |
-
"""
|
686 |
-
|
687 |
-
FOOTER = '<div align=center><img id="visitor-badge" alt="visitor badge" src="https://visitor-badge.laobi.icu/badge?page_id=williamyang1991/Rerender_A_Video" /></div>'
|
688 |
-
|
689 |
-
|
690 |
-
block = gr.Blocks().queue()
|
691 |
-
with block:
|
692 |
-
with gr.Row():
|
693 |
-
gr.Markdown(DESCRIPTION)
|
694 |
-
with gr.Row():
|
695 |
-
with gr.Column():
|
696 |
-
input_path = gr.Video(label='Input Video',
|
697 |
-
source='upload',
|
698 |
-
format='mp4',
|
699 |
-
visible=True)
|
700 |
-
prompt = gr.Textbox(label='Prompt')
|
701 |
-
seed = gr.Slider(label='Seed',
|
702 |
-
minimum=0,
|
703 |
-
maximum=2147483647,
|
704 |
-
step=1,
|
705 |
-
value=0,
|
706 |
-
randomize=True)
|
707 |
-
run_button = gr.Button(value='Run All')
|
708 |
-
with gr.Row():
|
709 |
-
run_button1 = gr.Button(value='Run 1st Key Frame')
|
710 |
-
run_button2 = gr.Button(value='Run Key Frames')
|
711 |
-
run_button3 = gr.Button(value='Run Propagation')
|
712 |
-
with gr.Accordion('Advanced options for the 1st frame translation',
|
713 |
-
open=False):
|
714 |
-
image_resolution = gr.Slider(
|
715 |
-
label='Frame rsolution',
|
716 |
-
minimum=256,
|
717 |
-
maximum=512,
|
718 |
-
value=512,
|
719 |
-
step=64,
|
720 |
-
info='To avoid overload, maximum 512')
|
721 |
-
control_strength = gr.Slider(label='ControNet strength',
|
722 |
-
minimum=0.0,
|
723 |
-
maximum=2.0,
|
724 |
-
value=1.0,
|
725 |
-
step=0.01)
|
726 |
-
x0_strength = gr.Slider(
|
727 |
-
label='Denoising strength',
|
728 |
-
minimum=0.00,
|
729 |
-
maximum=1.05,
|
730 |
-
value=0.75,
|
731 |
-
step=0.05,
|
732 |
-
info=('0: fully recover the input.'
|
733 |
-
'1.05: fully rerender the input.'))
|
734 |
-
color_preserve = gr.Checkbox(
|
735 |
-
label='Preserve color',
|
736 |
-
value=True,
|
737 |
-
info='Keep the color of the input video')
|
738 |
-
with gr.Row():
|
739 |
-
left_crop = gr.Slider(label='Left crop length',
|
740 |
-
minimum=0,
|
741 |
-
maximum=512,
|
742 |
-
value=0,
|
743 |
-
step=1)
|
744 |
-
right_crop = gr.Slider(label='Right crop length',
|
745 |
-
minimum=0,
|
746 |
-
maximum=512,
|
747 |
-
value=0,
|
748 |
-
step=1)
|
749 |
-
with gr.Row():
|
750 |
-
top_crop = gr.Slider(label='Top crop length',
|
751 |
-
minimum=0,
|
752 |
-
maximum=512,
|
753 |
-
value=0,
|
754 |
-
step=1)
|
755 |
-
bottom_crop = gr.Slider(label='Bottom crop length',
|
756 |
-
minimum=0,
|
757 |
-
maximum=512,
|
758 |
-
value=0,
|
759 |
-
step=1)
|
760 |
-
with gr.Row():
|
761 |
-
control_type = gr.Dropdown(['HED', 'canny', 'depth'],
|
762 |
-
label='Control type',
|
763 |
-
value='HED')
|
764 |
-
low_threshold = gr.Slider(label='Canny low threshold',
|
765 |
-
minimum=1,
|
766 |
-
maximum=255,
|
767 |
-
value=100,
|
768 |
-
step=1)
|
769 |
-
high_threshold = gr.Slider(label='Canny high threshold',
|
770 |
-
minimum=1,
|
771 |
-
maximum=255,
|
772 |
-
value=200,
|
773 |
-
step=1)
|
774 |
-
ddim_steps = gr.Slider(label='Steps',
|
775 |
-
minimum=1,
|
776 |
-
maximum=20,
|
777 |
-
value=20,
|
778 |
-
step=1,
|
779 |
-
info='To avoid overload, maximum 20')
|
780 |
-
scale = gr.Slider(label='CFG scale',
|
781 |
-
minimum=0.1,
|
782 |
-
maximum=30.0,
|
783 |
-
value=7.5,
|
784 |
-
step=0.1)
|
785 |
-
sd_model_list = list(model_dict.keys())
|
786 |
-
sd_model = gr.Dropdown(sd_model_list,
|
787 |
-
label='Base model',
|
788 |
-
value='Stable Diffusion 1.5')
|
789 |
-
a_prompt = gr.Textbox(label='Added prompt',
|
790 |
-
value='best quality, extremely detailed')
|
791 |
-
n_prompt = gr.Textbox(
|
792 |
-
label='Negative prompt',
|
793 |
-
value=('longbody, lowres, bad anatomy, bad hands, '
|
794 |
-
'missing fingers, extra digit, fewer digits, '
|
795 |
-
'cropped, worst quality, low quality'))
|
796 |
-
with gr.Accordion('Advanced options for the key fame translation',
|
797 |
-
open=False):
|
798 |
-
interval = gr.Slider(
|
799 |
-
label='Key frame frequency (K)',
|
800 |
-
minimum=1,
|
801 |
-
maximum=MAX_KEYFRAME,
|
802 |
-
value=1,
|
803 |
-
step=1,
|
804 |
-
info='Uniformly sample the key frames every K frames')
|
805 |
-
keyframe_count = gr.Slider(
|
806 |
-
label='Number of key frames',
|
807 |
-
minimum=1,
|
808 |
-
maximum=MAX_KEYFRAME,
|
809 |
-
value=1,
|
810 |
-
step=1,
|
811 |
-
info='To avoid overload, maximum 8 key frames')
|
812 |
-
|
813 |
-
use_constraints = gr.CheckboxGroup(
|
814 |
-
[
|
815 |
-
'shape-aware fusion', 'pixel-aware fusion',
|
816 |
-
'color-aware AdaIN'
|
817 |
-
],
|
818 |
-
label='Select the cross-frame contraints to be used',
|
819 |
-
value=[
|
820 |
-
'shape-aware fusion', 'pixel-aware fusion',
|
821 |
-
'color-aware AdaIN'
|
822 |
-
]),
|
823 |
-
with gr.Row():
|
824 |
-
cross_start = gr.Slider(
|
825 |
-
label='Cross-frame attention start',
|
826 |
-
minimum=0,
|
827 |
-
maximum=1,
|
828 |
-
value=0,
|
829 |
-
step=0.05)
|
830 |
-
cross_end = gr.Slider(label='Cross-frame attention end',
|
831 |
-
minimum=0,
|
832 |
-
maximum=1,
|
833 |
-
value=1,
|
834 |
-
step=0.05)
|
835 |
-
style_update_freq = gr.Slider(
|
836 |
-
label='Cross-frame attention update frequency',
|
837 |
-
minimum=1,
|
838 |
-
maximum=100,
|
839 |
-
value=1,
|
840 |
-
step=1,
|
841 |
-
info=('Update the key and value for '
|
842 |
-
'cross-frame attention every N key frames (recommend N*K>=10)'
|
843 |
-
))
|
844 |
-
with gr.Row():
|
845 |
-
warp_start = gr.Slider(label='Shape-aware fusion start',
|
846 |
-
minimum=0,
|
847 |
-
maximum=1,
|
848 |
-
value=0,
|
849 |
-
step=0.05)
|
850 |
-
warp_end = gr.Slider(label='Shape-aware fusion end',
|
851 |
-
minimum=0,
|
852 |
-
maximum=1,
|
853 |
-
value=0.1,
|
854 |
-
step=0.05)
|
855 |
-
with gr.Row():
|
856 |
-
mask_start = gr.Slider(label='Pixel-aware fusion start',
|
857 |
-
minimum=0,
|
858 |
-
maximum=1,
|
859 |
-
value=0.5,
|
860 |
-
step=0.05)
|
861 |
-
mask_end = gr.Slider(label='Pixel-aware fusion end',
|
862 |
-
minimum=0,
|
863 |
-
maximum=1,
|
864 |
-
value=0.8,
|
865 |
-
step=0.05)
|
866 |
-
with gr.Row():
|
867 |
-
ada_start = gr.Slider(label='Color-aware AdaIN start',
|
868 |
-
minimum=0,
|
869 |
-
maximum=1,
|
870 |
-
value=0.8,
|
871 |
-
step=0.05)
|
872 |
-
ada_end = gr.Slider(label='Color-aware AdaIN end',
|
873 |
-
minimum=0,
|
874 |
-
maximum=1,
|
875 |
-
value=1,
|
876 |
-
step=0.05)
|
877 |
-
mask_strength = gr.Slider(label='Pixel-aware fusion stength',
|
878 |
-
minimum=0,
|
879 |
-
maximum=1,
|
880 |
-
value=0.5,
|
881 |
-
step=0.01)
|
882 |
-
inner_strength = gr.Slider(
|
883 |
-
label='Pixel-aware fusion detail level',
|
884 |
-
minimum=0.5,
|
885 |
-
maximum=1,
|
886 |
-
value=0.9,
|
887 |
-
step=0.01,
|
888 |
-
info='Use a low value to prevent artifacts')
|
889 |
-
smooth_boundary = gr.Checkbox(
|
890 |
-
label='Smooth fusion boundary',
|
891 |
-
value=True,
|
892 |
-
info='Select to prevent artifacts at boundary')
|
893 |
-
|
894 |
-
with gr.Accordion('Example configs', open=True):
|
895 |
-
config_dir = 'config'
|
896 |
-
config_list = os.listdir(config_dir)
|
897 |
-
args_list = []
|
898 |
-
for config in config_list:
|
899 |
-
try:
|
900 |
-
config_path = os.path.join(config_dir, config)
|
901 |
-
args = cfg_to_input(config_path)
|
902 |
-
args_list.append(args)
|
903 |
-
except FileNotFoundError:
|
904 |
-
# The video file does not exist, skipped
|
905 |
-
pass
|
906 |
-
|
907 |
-
ips = [
|
908 |
-
prompt, image_resolution, control_strength, color_preserve,
|
909 |
-
left_crop, right_crop, top_crop, bottom_crop, control_type,
|
910 |
-
low_threshold, high_threshold, ddim_steps, scale, seed,
|
911 |
-
sd_model, a_prompt, n_prompt, interval, keyframe_count,
|
912 |
-
x0_strength, use_constraints[0], cross_start, cross_end,
|
913 |
-
style_update_freq, warp_start, warp_end, mask_start,
|
914 |
-
mask_end, ada_start, ada_end, mask_strength,
|
915 |
-
inner_strength, smooth_boundary
|
916 |
-
]
|
917 |
-
|
918 |
-
with gr.Column():
|
919 |
-
result_image = gr.Image(label='Output first frame',
|
920 |
-
type='numpy',
|
921 |
-
interactive=False)
|
922 |
-
result_keyframe = gr.Video(label='Output key frame video',
|
923 |
-
format='mp4',
|
924 |
-
interactive=False)
|
925 |
-
with gr.Row():
|
926 |
-
gr.Examples(examples=args_list,
|
927 |
-
inputs=[input_path, *ips],
|
928 |
-
fn=process0,
|
929 |
-
outputs=[result_image, result_keyframe],
|
930 |
-
cache_examples=True)
|
931 |
-
|
932 |
-
gr.Markdown(ARTICLE)
|
933 |
-
gr.Markdown(FOOTER)
|
934 |
-
|
935 |
-
def input_uploaded(path):
|
936 |
-
frame_count = get_frame_count(path)
|
937 |
-
if frame_count <= 2:
|
938 |
-
raise gr.Error('The input video is too short!'
|
939 |
-
'Please input another video.')
|
940 |
-
|
941 |
-
default_interval = min(10, frame_count - 2)
|
942 |
-
max_keyframe = min((frame_count - 2) // default_interval, MAX_KEYFRAME)
|
943 |
-
|
944 |
-
global video_frame_count
|
945 |
-
video_frame_count = frame_count
|
946 |
-
global global_video_path
|
947 |
-
global_video_path = path
|
948 |
-
|
949 |
-
return gr.Slider.update(value=default_interval,
|
950 |
-
maximum=frame_count - 2), gr.Slider.update(
|
951 |
-
value=max_keyframe, maximum=max_keyframe)
|
952 |
-
|
953 |
-
def input_changed(path):
|
954 |
-
frame_count = get_frame_count(path)
|
955 |
-
if frame_count <= 2:
|
956 |
-
return gr.Slider.update(maximum=1), gr.Slider.update(maximum=1)
|
957 |
-
|
958 |
-
default_interval = min(10, frame_count - 2)
|
959 |
-
max_keyframe = min((frame_count - 2) // default_interval, MAX_KEYFRAME)
|
960 |
-
|
961 |
-
global video_frame_count
|
962 |
-
video_frame_count = frame_count
|
963 |
-
global global_video_path
|
964 |
-
global_video_path = path
|
965 |
-
|
966 |
-
return gr.Slider.update(value=default_interval,
|
967 |
-
maximum=frame_count - 2), \
|
968 |
-
gr.Slider.update(maximum=max_keyframe)
|
969 |
-
|
970 |
-
def interval_changed(interval):
|
971 |
-
global video_frame_count
|
972 |
-
if video_frame_count is None:
|
973 |
-
return gr.Slider.update()
|
974 |
-
|
975 |
-
max_keyframe = min((video_frame_count - 2) // interval, MAX_KEYFRAME)
|
976 |
-
|
977 |
-
return gr.Slider.update(value=max_keyframe, maximum=max_keyframe)
|
978 |
-
|
979 |
-
input_path.change(input_changed, input_path, [interval, keyframe_count])
|
980 |
-
input_path.upload(input_uploaded, input_path, [interval, keyframe_count])
|
981 |
-
interval.change(interval_changed, interval, keyframe_count)
|
982 |
-
|
983 |
-
run_button.click(fn=process,
|
984 |
-
inputs=ips,
|
985 |
-
outputs=[result_image, result_keyframe])
|
986 |
-
run_button1.click(fn=process1, inputs=ips, outputs=[result_image])
|
987 |
-
run_button2.click(fn=process2, inputs=ips, outputs=[result_keyframe])
|
988 |
-
|
989 |
-
def process3():
|
990 |
-
raise gr.Error(
|
991 |
-
"Coming Soon. Full code for full video translation will be "
|
992 |
-
"released upon the publication of the paper.")
|
993 |
-
|
994 |
-
run_button3.click(fn=process3, outputs=[result_keyframe])
|
995 |
-
|
996 |
-
block.queue(concurrency_count=1, max_size=20)
|
997 |
-
block.launch(server_name='0.0.0.0')
|
|
|
|
|
|
|
|
|
|
|
|
|
|
|
|
|
|
|
|
|
|
|
|
|
|
|
|
|
|
|
|
|
|
|
|
|
|
|
|
|
|
|
|
|
|
|
|
|
|
|
|
|
|
|
|
|
|
|
|
|
|
|
|
|
|
|
|
|
|
|
|
|
|
|
|
|
|
|
|
|
|
|
|
|
|
|
|
|
|
|
|
|
|
|
|
|
|
|
|
|
|
|
|
|
|
|
|
|
|
|
|
|
|
|
|
|
|
|
|
|
|
|
|
|
|
|
|
|
|
|
|
|
|
|
|
|
|
|
|
|
|
|
|
|
|
|
|
|
|
|
|
|
|
|
|
|
|
|
|
|
|
|
|
|
|
|
|
|
|
|
|
|
|
|
|
|
|
|
|
|
|
|
|
|
|
|
|
|
|
|
|
|
|
|
|
|
|
|
|
|
|
|
|
|
|
|
|
|
|
|
|
|
|
|
|
|
|
|
|
|
|
|
|
|
|
|
|
|
|
|
|
|
|
|
|
|
|
|
|
|
|
|
|
|
|
|
|
|
|
|
|
|
|
|
|
|
|
|
|
|
|
|
|
|
|
|
|
|
|
|
|
|
|
|
|
|
|
|
|
|
|
|
|
|
|
|
|
|
|
|
|
|
|
|
|
|
|
|
|
|
|
|
|
|
|
|
|
|
|
|
|
|
|
|
|
|
|
|
|
|
|
|
|
|
|
|
|
|
|
|
|
|
|
|
|
|
|
|
|
|
|
|
|
|
|
|
|
|
|
|
|
|
|
|
|
|
|
|
|
|
|
|
|
|
|
|
|
|
|
|
|
|
|
|
|
|
|
|
|
|
|
|
|
|
|
|
|
|
|
|
|
|
|
|
|
|
|
|
|
|
|
|
|
|
|
|
|
|
|
|
|
|
|
|
|
|
|
|
|
|
|
|
|
|
|
|
|
|
|
|
|
|
|
|
|
|
|
|
|
|
|
|
|
|
|
|
|
|
|
|
|
|
|
|
|
|
|
|
|
|
|
|
|
|
|
|
|
|
|
|
|
|
|
|
|
|
|
|
|
|
|
|
|
|
|
|
|
|
|
|
|
|
|
|
|
|
|
|
|
|
|
|
|
|
|
|
|
|
|
|
|
|
|
|
|
|
|
|
|
|
|
|
|
|
|
|
|
|
|
|
|
|
|
|
|
|
|
|
|
|
|
|
|
|
|
|
|
|
|
|
|
|
|
|
|
|
|
|
|
|
|
|
|
|
|
|
|
|
|
|
|
|
|
|
|
|
|
|
|
|
|
|
|
|
|
|
|
|
|
|
|
|
|
|
|
|
|
|
|
|
|
|
|
|
|
|
|
|
|
|
|
|
|
|
|
|
|
|
|
|
|
|
|
|
|
|
|
|
|
|
|
|
|
|
|
|
|
|
|
|
|
|
|
|
|
|
|
|
|
|
|
|
|
|
|
|
|
|
|
|
|
|
|
|
|
|
|
|
|
|
|
|
|
|
|
|
|
|
|
|
|
|
|
|
|
|
|
|
|
|
|
|
|
|
|
|
|
|
|
|
|
|
|
|
|
|
|
|
|
|
|
|
|
|
|
|
|
|
|
|
|
|
|
|
|
|
|
|
|
|
|
|
|
|
|
|
|
|
|
|
|
|
|
|
|
|
|
|
|
|
|
|
|
|
|
|
|
|
|
|
|
|
|
|
|
|
|
|
|
|
|
|
|
|
|
|
|
|
|
|
|
|
|
|
|
|
|
|
|
|
|
|
|
|
|
|
|
|
|
|
|
|
|
|
|
|
|
|
|
|
|
|
|
|
|
|
|
|
|
|
|
|
|
|
|
|
|
|
|
|
|
|
|
|
|
|
|
|
|
|
|
|
|
|
|
|
|
|
|
|
|
|
|
|
|
|
|
|
|
|
|
|
|
|
|
|
|
|
|
|
|
|
|
|
|
|
|
|
|
|
|
|
|
|
|
|
|
|
|
|
|
|
|
|
|
|
|
|
|
|
|
|
|
|
|
|
|
|
|
|
|
|
|
|
|
|
|
|
|
|
|
|
|
|
|
|
|
|
|
|
|
|
|
|
|
|
|
|
|
|
|
|
|
|
|
|
|
|
|
|
|
|
|
|
|
|
|
|
|
|
|
|
|
|
|
|
|
|
|
|
|
|
|
|
|
|
|
|
|
|
|
|
|
|
|
|
|
|
|
|
|
|
|
|
|
|
|
|
|
|
|
|
|
|
|
|
|
|
|
|
|
|
|
|
|
|
|
|
|
|
|
|
|
|
|
|
|
|
|
|
|
|
|
|
|
|
|
|
|
|
|
|
|
|
|
|
|
|
|
|
|
|
|
|
|
|
|
|
|
|
|
|
|
|
|
|
|
|
|
|
|
|
|
|
|
|
|
|
|
|
|
|
|
|
|
|
|
|
|
|
|
|
|
|
|
|
|
|
|
|
|
|
|
|
|
|
|
|
|
|
|
|
|
|
|
|
|
|
|
|
|
|
|
|
|
|
|
|
|
|
|
|
|
|
|
|
|
|
|
|
|
|
|
|
|
|
|
|
|
|
|
|
|
|
|
|
|
|
|
|
|
|
|
|
|
|
|
|
|
|
|
|
|
|
|
|
|
|
|
|
|
|
|
|
|
|
|
|
|
|
|
|
|
|
|
|
|
|
|
|
|
|
|
|
|
|
|
|
|
|
|
|
|
|
|
|
|
|
|
|
|
|
|
|
|
|
|
|
|
|
|
|
|
|
|
|
|
|
|
|
|
|
|
|
|
|
|
|
|
|
|
|
|
|
|
|
|
|
|
|
|
|
|
|
|
|
|
|
|
|
|
|
|
|
|
|
|
|
|
|
|
|
|
|
|
|
|
|
|
|
|
|
|
|
|
|
|
|
|
|
|
|
|
|
|
|
|
|
|
|
|
|
|
|
|
|
|
|
|
|
|
|
|
|
|
|
|
|
|
|
|
|
|
|
|
|
|
|
|
|
|
|
|
|
|
|
|
|
|
|
|
|
|
|
|
|
|
|
|
|
|
|
|
|
|
|
|
|
|
|
|
|
|
|
|
|
|
|
|
|
|
|
|
|
|
|
|
|
|
|
|
|
|
|
|
|
|
|
|
|
|
|
|
|
|
|
|
|
|
|
|
|
|
|
|
|
|
|
|
|
|
|
|
|
|
|
|
|
|
|
|
|
|
|
|
|
|
|
|
|
|
|
|
|
|
|
|
|
|
|
|
|
|
|
|
|
|
|
|
|
|
|
|
|
|
|
|
|
|
|
|
|
|
|
|
|
|
|
|
|
|
|
|
|
|
|
|
|
|
|
|
|
|
|
|
|
|
|
|
|
|
|
|
|
|
|
|
|
|
|
|
|
|
|
|
|
|
|
|
|
|
|
|
|
|
|
|
|
|
|
|
|
|
|
|
|
|
|
|
|
|
|
|
|
|
|
|
|
|
|
|
|
|
|
|
|
|
|
|
|
|
|
|
|
|
|
|
|
|
|
|
|
|
|
|
|
|
|
|
|
|
|
|
|
|
|
|
|
|
|
|
|
|
|
|
|
|
|
|
|
|
|
|
|
|
|
|
|
|
|
|
|
|
|
|
|
|
|
|
|
|
|
|
|
|
|
|
|
|
|
|
|
|
|
|
|
|
|
|
|
|
|
|
|
|
|
|
|
|
|
|
|
|
|
|
|
|
|
|
|
|
|
|
|
|
|
|
|
|
|
|
|
|
|
|
|
|
|
|
|
|
|
|
|
|
|
|
|
|
|
|
|
|
|
|
|
|
|
|
|
|
|
|
|
|
|
|
|
|
|
|
|
|
|
|
|
|
|
|
|
|
|
|
|
|
|
|
|
|
|
|
|
|
|
|
|
|
|
|
|
|
|
|
|
|
|
|
|
|
|
|
|
|
|
|
|
|
|
|
|
|
|
|
|
|
|
|
|
|
|
|
|
|
|
|
|
|
|
|
|
|
|
|
|
|
|
|
|
|
|
|
|
|
|
|
|
|
|
|
|
|
|
|
|
|
|
|
|
|
|
|
|
|
|
|
|
|
|
|
|
|
|
|
|
|
|
|
|
|
|
|
|
|
|
|
|
|
|
|
|
|
|
|
|
|
|
|
|
|
|
|
|
|
|
|
|
|
|
|
|
|
|
|
|
|
|
|
|
|
|
|
|
|
|
|
|
|
|
|
|
|
|
|
|
|
|
|
|
|
|
|
|
|
|
|
|
|
|
|
|
|
|
|
|
|
|
|
|
|
|
|
|
|
|
|
|
|
|
|
|
|
|
|
|
|
|
|
|
|
|
|
|
|
|
|
|
|
|
|
|
|
|
|
|
|
|
|
|
|
|
|
|
|
|
|
|
|
|
|
|
|
|
|
|
|
|
|
|
|
|
|
|
|
|
|
|
|
|
|
|
|
|
|
|
|
|
|
|
|
|
|
|
|
|
|
spaces/Anonymous-sub/Rerender/gmflow_module/scripts/submission.sh
DELETED
@@ -1,67 +0,0 @@
|
|
1 |
-
#!/usr/bin/env bash
|
2 |
-
|
3 |
-
|
4 |
-
# generate prediction results for submission on sintel and kitti online servers
|
5 |
-
|
6 |
-
|
7 |
-
# GMFlow without refinement
|
8 |
-
|
9 |
-
# submission to sintel
|
10 |
-
CUDA_VISIBLE_DEVICES=0 python main.py \
|
11 |
-
--submission \
|
12 |
-
--output_path submission/sintel-gmflow-norefine \
|
13 |
-
--val_dataset sintel \
|
14 |
-
--resume pretrained/gmflow_sintel-0c07dcb3.pth
|
15 |
-
|
16 |
-
# submission to kitti
|
17 |
-
CUDA_VISIBLE_DEVICES=0 python main.py \
|
18 |
-
--submission \
|
19 |
-
--output_path submission/kitti-gmflow-norefine \
|
20 |
-
--val_dataset kitti \
|
21 |
-
--resume pretrained/gmflow_kitti-285701a8.pth
|
22 |
-
|
23 |
-
|
24 |
-
# you can also visualize the predictions before submission
|
25 |
-
# CUDA_VISIBLE_DEVICES=0 python main.py \
|
26 |
-
# --submission \
|
27 |
-
# --output_path submission/sintel-gmflow-norefine-vis \
|
28 |
-
# --save_vis_flow \
|
29 |
-
# --no_save_flo \
|
30 |
-
# --val_dataset sintel \
|
31 |
-
# --resume pretrained/gmflow_sintel.pth
|
32 |
-
|
33 |
-
|
34 |
-
|
35 |
-
|
36 |
-
# GMFlow with refinement
|
37 |
-
|
38 |
-
# submission to sintel
|
39 |
-
CUDA_VISIBLE_DEVICES=0 python main.py \
|
40 |
-
--submission \
|
41 |
-
--output_path submission/sintel-gmflow-withrefine \
|
42 |
-
--val_dataset sintel \
|
43 |
-
--resume pretrained/gmflow_with_refine_sintel-3ed1cf48.pth \
|
44 |
-
--padding_factor 32 \
|
45 |
-
--upsample_factor 4 \
|
46 |
-
--num_scales 2 \
|
47 |
-
--attn_splits_list 2 8 \
|
48 |
-
--corr_radius_list -1 4 \
|
49 |
-
--prop_radius_list -1 1
|
50 |
-
|
51 |
-
# submission to kitti
|
52 |
-
CUDA_VISIBLE_DEVICES=0 python main.py \
|
53 |
-
--submission \
|
54 |
-
--output_path submission/kitti-gmflow-withrefine \
|
55 |
-
--val_dataset kitti \
|
56 |
-
--resume pretrained/gmflow_with_refine_kitti-8d3b9786.pth \
|
57 |
-
--padding_factor 32 \
|
58 |
-
--upsample_factor 4 \
|
59 |
-
--num_scales 2 \
|
60 |
-
--attn_splits_list 2 8 \
|
61 |
-
--corr_radius_list -1 4 \
|
62 |
-
--prop_radius_list -1 1
|
63 |
-
|
64 |
-
|
65 |
-
|
66 |
-
|
67 |
-
|
|
|
|
|
|
|
|
|
|
|
|
|
|
|
|
|
|
|
|
|
|
|
|
|
|
|
|
|
|
|
|
|
|
|
|
|
|
|
|
|
|
|
|
|
|
|
|
|
|
|
|
|
|
|
|
|
|
|
|
|
|
|
|
|
|
|
|
|
|
|
|
|
|
|
|
|
|
|
|
|
|
|
|
|
|
|
|
|
|
|
|
|
|
|
|
|
|
|
|
|
|
|
|
|
|
|
|
|
|
|
|
|
|
|
|
|
|
|
|
|
|
|
|
|
|
|
|
|
|
|
|
|
|
|
|
spaces/Ariharasudhan/YoloV5/utils/loggers/wandb/README.md
DELETED
@@ -1,162 +0,0 @@
|
|
1 |
-
📚 This guide explains how to use **Weights & Biases** (W&B) with YOLOv5 🚀. UPDATED 29 September 2021.
|
2 |
-
|
3 |
-
- [About Weights & Biases](#about-weights-&-biases)
|
4 |
-
- [First-Time Setup](#first-time-setup)
|
5 |
-
- [Viewing runs](#viewing-runs)
|
6 |
-
- [Disabling wandb](#disabling-wandb)
|
7 |
-
- [Advanced Usage: Dataset Versioning and Evaluation](#advanced-usage)
|
8 |
-
- [Reports: Share your work with the world!](#reports)
|
9 |
-
|
10 |
-
## About Weights & Biases
|
11 |
-
|
12 |
-
Think of [W&B](https://wandb.ai/site?utm_campaign=repo_yolo_wandbtutorial) like GitHub for machine learning models. With a few lines of code, save everything you need to debug, compare and reproduce your models — architecture, hyperparameters, git commits, model weights, GPU usage, and even datasets and predictions.
|
13 |
-
|
14 |
-
Used by top researchers including teams at OpenAI, Lyft, Github, and MILA, W&B is part of the new standard of best practices for machine learning. How W&B can help you optimize your machine learning workflows:
|
15 |
-
|
16 |
-
- [Debug](https://wandb.ai/wandb/getting-started/reports/Visualize-Debug-Machine-Learning-Models--VmlldzoyNzY5MDk#Free-2) model performance in real time
|
17 |
-
- [GPU usage](https://wandb.ai/wandb/getting-started/reports/Visualize-Debug-Machine-Learning-Models--VmlldzoyNzY5MDk#System-4) visualized automatically
|
18 |
-
- [Custom charts](https://wandb.ai/wandb/customizable-charts/reports/Powerful-Custom-Charts-To-Debug-Model-Peformance--VmlldzoyNzY4ODI) for powerful, extensible visualization
|
19 |
-
- [Share insights](https://wandb.ai/wandb/getting-started/reports/Visualize-Debug-Machine-Learning-Models--VmlldzoyNzY5MDk#Share-8) interactively with collaborators
|
20 |
-
- [Optimize hyperparameters](https://docs.wandb.com/sweeps) efficiently
|
21 |
-
- [Track](https://docs.wandb.com/artifacts) datasets, pipelines, and production models
|
22 |
-
|
23 |
-
## First-Time Setup
|
24 |
-
|
25 |
-
<details open>
|
26 |
-
<summary> Toggle Details </summary>
|
27 |
-
When you first train, W&B will prompt you to create a new account and will generate an **API key** for you. If you are an existing user you can retrieve your key from https://wandb.ai/authorize. This key is used to tell W&B where to log your data. You only need to supply your key once, and then it is remembered on the same device.
|
28 |
-
|
29 |
-
W&B will create a cloud **project** (default is 'YOLOv5') for your training runs, and each new training run will be provided a unique run **name** within that project as project/name. You can also manually set your project and run name as:
|
30 |
-
|
31 |
-
```shell
|
32 |
-
$ python train.py --project ... --name ...
|
33 |
-
```
|
34 |
-
|
35 |
-
YOLOv5 notebook example: <a href="https://colab.research.google.com/github/ultralytics/yolov5/blob/master/tutorial.ipynb"><img src="https://colab.research.google.com/assets/colab-badge.svg" alt="Open In Colab"></a> <a href="https://www.kaggle.com/ultralytics/yolov5"><img src="https://kaggle.com/static/images/open-in-kaggle.svg" alt="Open In Kaggle"></a>
|
36 |
-
<img width="960" alt="Screen Shot 2021-09-29 at 10 23 13 PM" src="https://user-images.githubusercontent.com/26833433/135392431-1ab7920a-c49d-450a-b0b0-0c86ec86100e.png">
|
37 |
-
|
38 |
-
</details>
|
39 |
-
|
40 |
-
## Viewing Runs
|
41 |
-
|
42 |
-
<details open>
|
43 |
-
<summary> Toggle Details </summary>
|
44 |
-
Run information streams from your environment to the W&B cloud console as you train. This allows you to monitor and even cancel runs in <b>realtime</b> . All important information is logged:
|
45 |
-
|
46 |
-
- Training & Validation losses
|
47 |
-
- Metrics: Precision, Recall, [email protected], [email protected]:0.95
|
48 |
-
- Learning Rate over time
|
49 |
-
- A bounding box debugging panel, showing the training progress over time
|
50 |
-
- GPU: Type, **GPU Utilization**, power, temperature, **CUDA memory usage**
|
51 |
-
- System: Disk I/0, CPU utilization, RAM memory usage
|
52 |
-
- Your trained model as W&B Artifact
|
53 |
-
- Environment: OS and Python types, Git repository and state, **training command**
|
54 |
-
|
55 |
-
<p align="center"><img width="900" alt="Weights & Biases dashboard" src="https://user-images.githubusercontent.com/26833433/135390767-c28b050f-8455-4004-adb0-3b730386e2b2.png"></p>
|
56 |
-
</details>
|
57 |
-
|
58 |
-
## Disabling wandb
|
59 |
-
|
60 |
-
- training after running `wandb disabled` inside that directory creates no wandb run
|
61 |
-
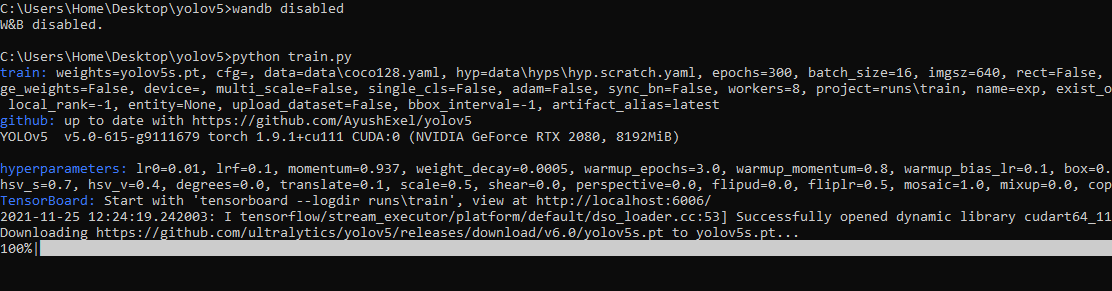
|
62 |
-
|
63 |
-
- To enable wandb again, run `wandb online`
|
64 |
-
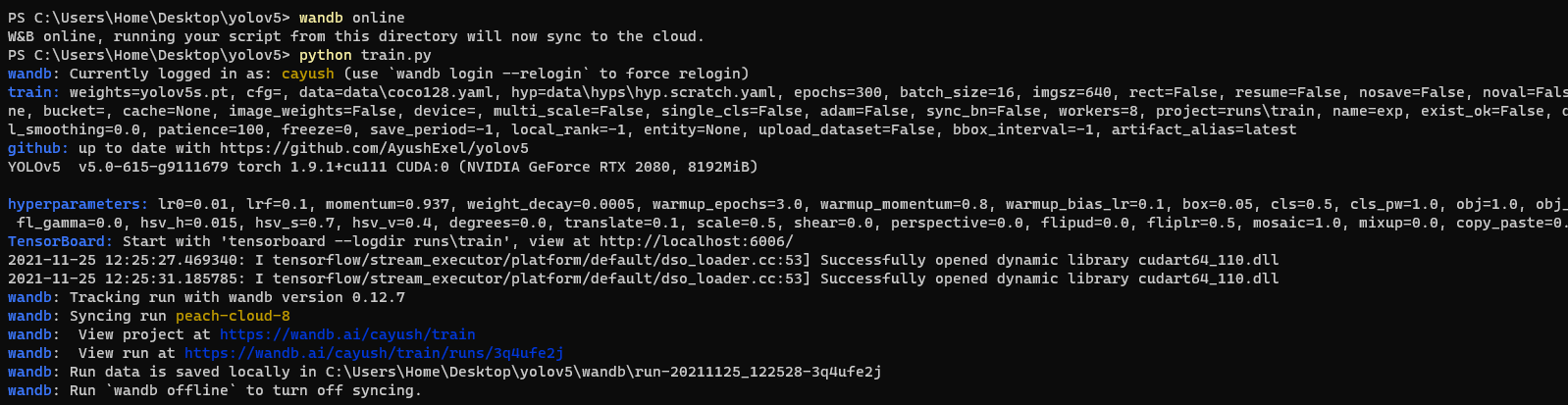
|
65 |
-
|
66 |
-
## Advanced Usage
|
67 |
-
|
68 |
-
You can leverage W&B artifacts and Tables integration to easily visualize and manage your datasets, models and training evaluations. Here are some quick examples to get you started.
|
69 |
-
|
70 |
-
<details open>
|
71 |
-
<h3> 1: Train and Log Evaluation simultaneousy </h3>
|
72 |
-
This is an extension of the previous section, but it'll also training after uploading the dataset. <b> This also evaluation Table</b>
|
73 |
-
Evaluation table compares your predictions and ground truths across the validation set for each epoch. It uses the references to the already uploaded datasets,
|
74 |
-
so no images will be uploaded from your system more than once.
|
75 |
-
<details open>
|
76 |
-
<summary> <b>Usage</b> </summary>
|
77 |
-
<b>Code</b> <code> $ python train.py --upload_data val</code>
|
78 |
-
|
79 |
-
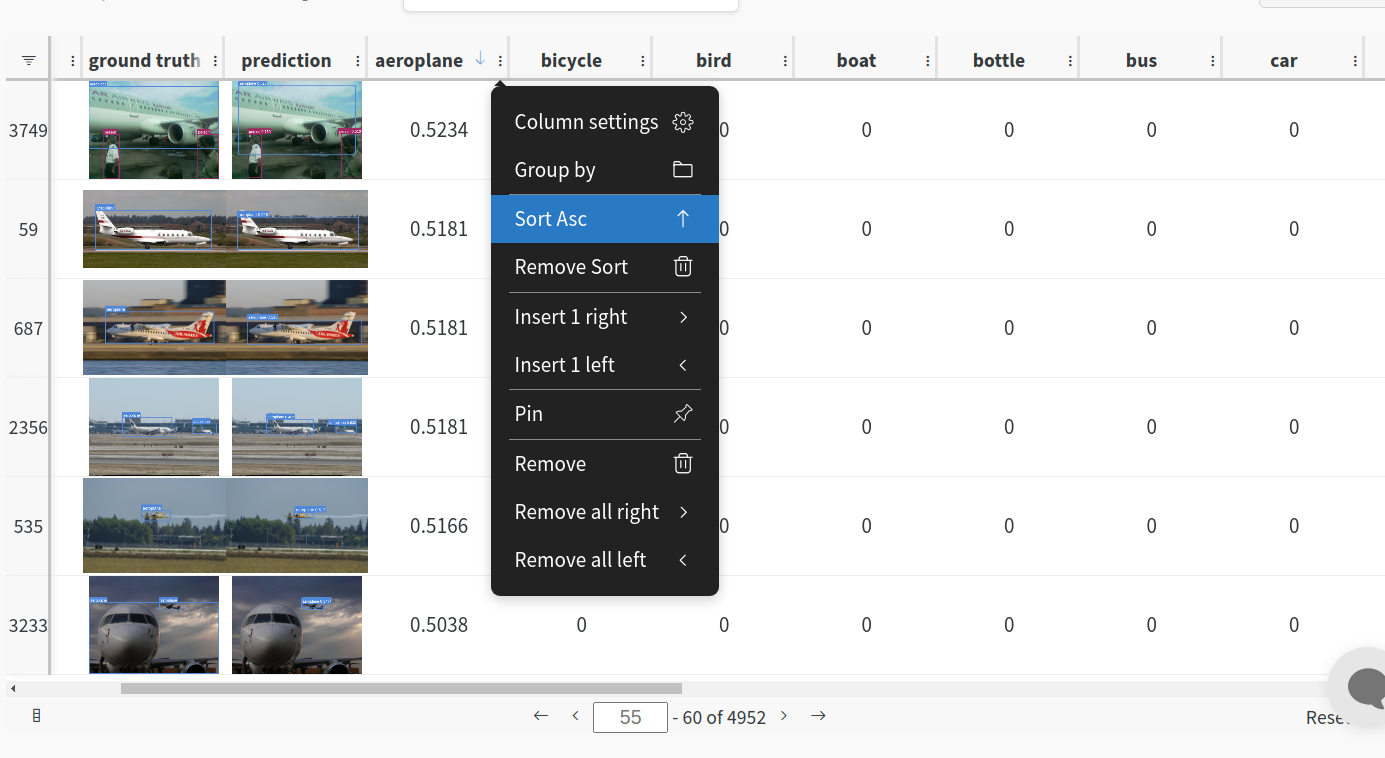
|
80 |
-
|
81 |
-
</details>
|
82 |
-
|
83 |
-
<h3>2. Visualize and Version Datasets</h3>
|
84 |
-
Log, visualize, dynamically query, and understand your data with <a href='https://docs.wandb.ai/guides/data-vis/tables'>W&B Tables</a>. You can use the following command to log your dataset as a W&B Table. This will generate a <code>{dataset}_wandb.yaml</code> file which can be used to train from dataset artifact.
|
85 |
-
<details>
|
86 |
-
<summary> <b>Usage</b> </summary>
|
87 |
-
<b>Code</b> <code> $ python utils/logger/wandb/log_dataset.py --project ... --name ... --data .. </code>
|
88 |
-
|
89 |
-
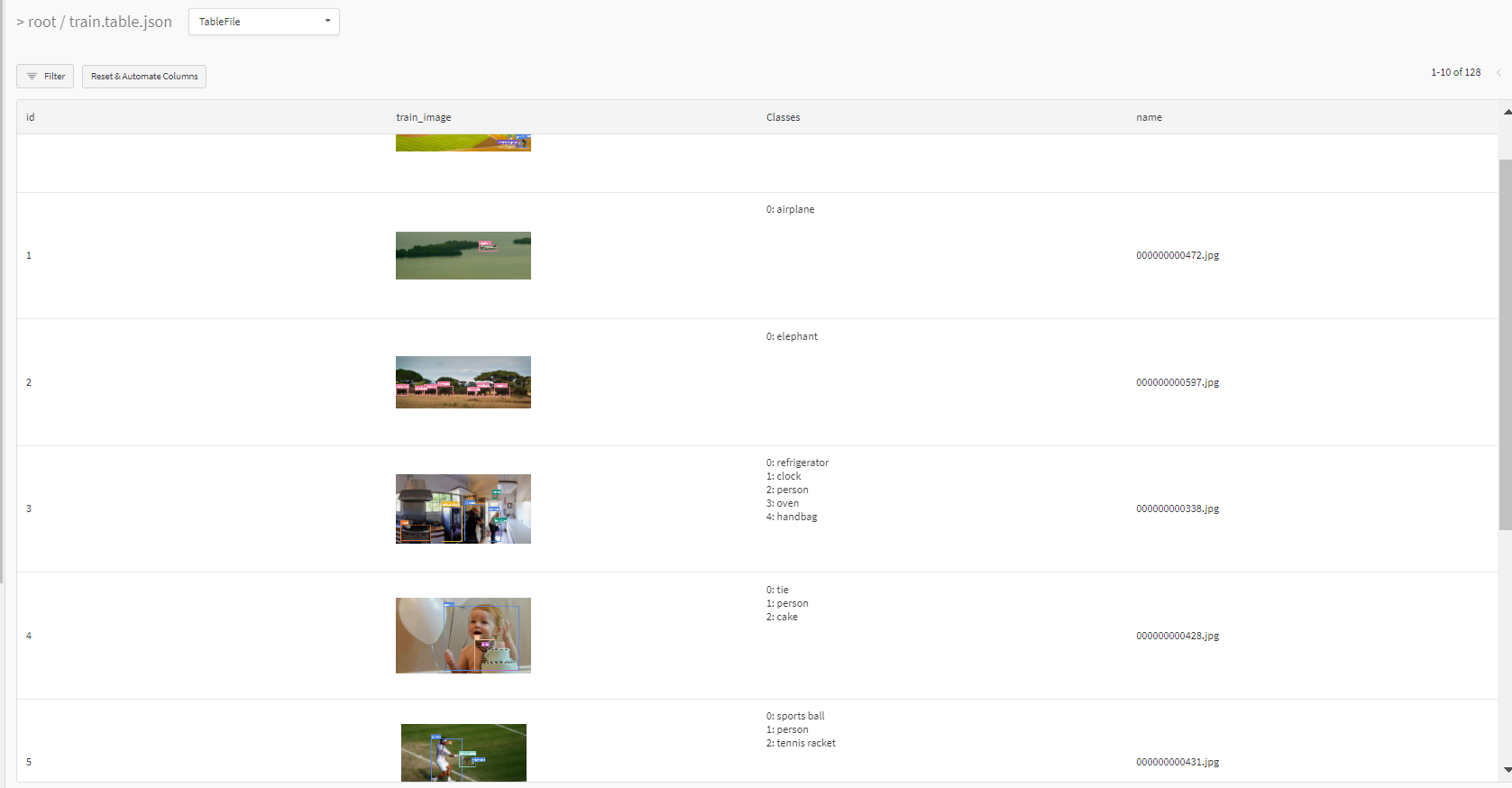
|
90 |
-
|
91 |
-
</details>
|
92 |
-
|
93 |
-
<h3> 3: Train using dataset artifact </h3>
|
94 |
-
When you upload a dataset as described in the first section, you get a new config file with an added `_wandb` to its name. This file contains the information that
|
95 |
-
can be used to train a model directly from the dataset artifact. <b> This also logs evaluation </b>
|
96 |
-
<details>
|
97 |
-
<summary> <b>Usage</b> </summary>
|
98 |
-
<b>Code</b> <code> $ python train.py --data {data}_wandb.yaml </code>
|
99 |
-
|
100 |
-
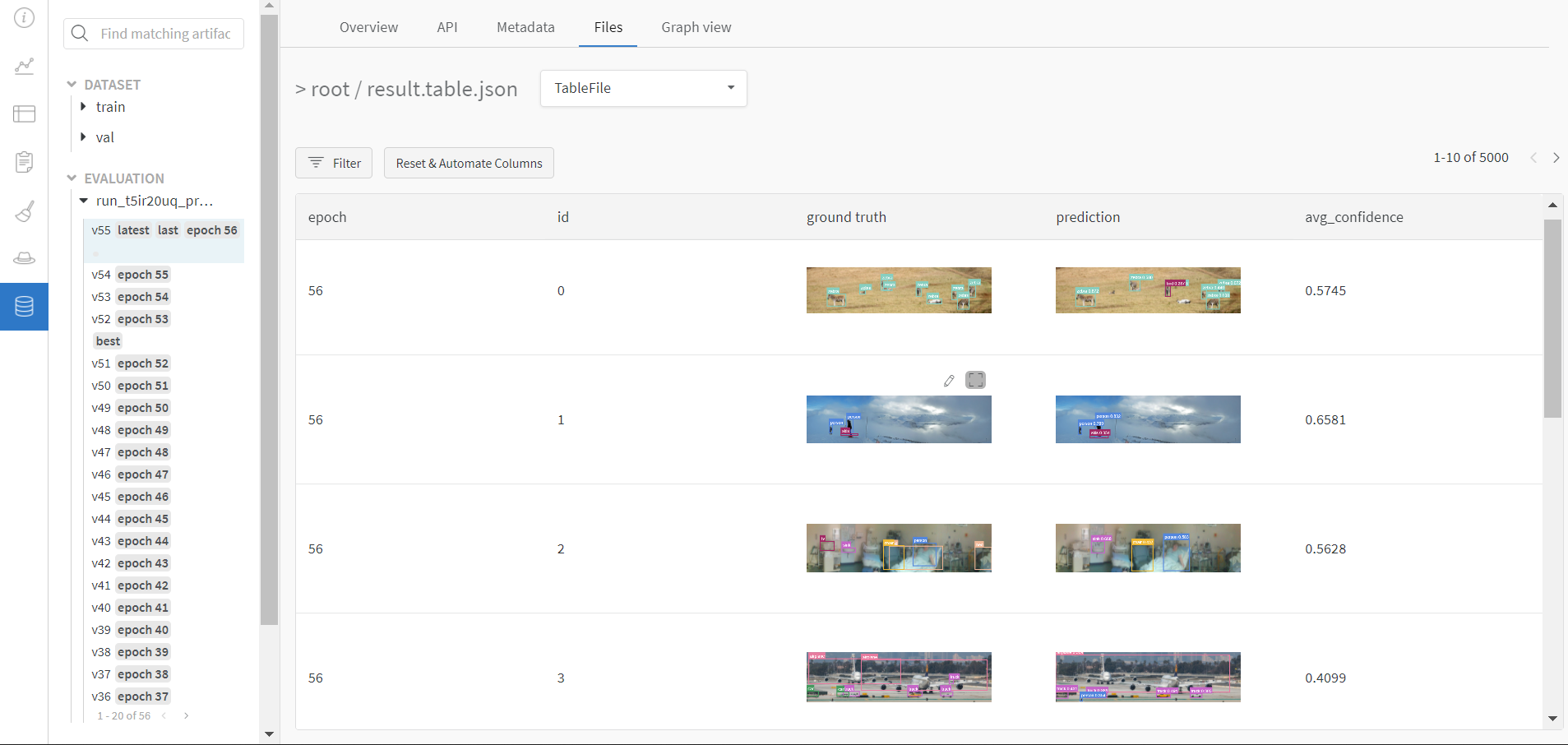
|
101 |
-
|
102 |
-
</details>
|
103 |
-
|
104 |
-
<h3> 4: Save model checkpoints as artifacts </h3>
|
105 |
-
To enable saving and versioning checkpoints of your experiment, pass `--save_period n` with the base cammand, where `n` represents checkpoint interval.
|
106 |
-
You can also log both the dataset and model checkpoints simultaneously. If not passed, only the final model will be logged
|
107 |
-
|
108 |
-
<details>
|
109 |
-
<summary> <b>Usage</b> </summary>
|
110 |
-
<b>Code</b> <code> $ python train.py --save_period 1 </code>
|
111 |
-
|
112 |
-
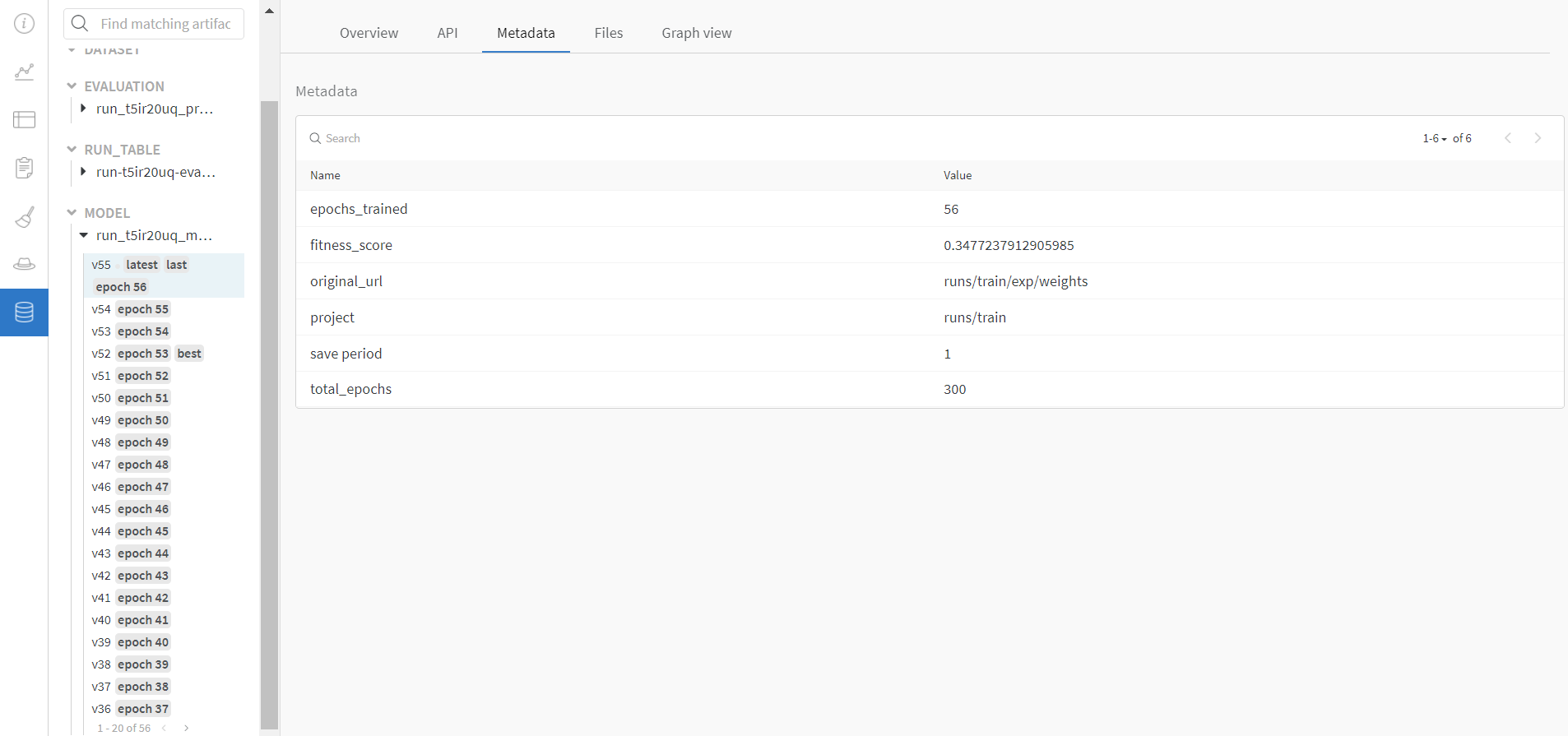
|
113 |
-
|
114 |
-
</details>
|
115 |
-
|
116 |
-
</details>
|
117 |
-
|
118 |
-
<h3> 5: Resume runs from checkpoint artifacts. </h3>
|
119 |
-
Any run can be resumed using artifacts if the <code>--resume</code> argument starts with <code>wandb-artifact://</code> prefix followed by the run path, i.e, <code>wandb-artifact://username/project/runid </code>. This doesn't require the model checkpoint to be present on the local system.
|
120 |
-
|
121 |
-
<details>
|
122 |
-
<summary> <b>Usage</b> </summary>
|
123 |
-
<b>Code</b> <code> $ python train.py --resume wandb-artifact://{run_path} </code>
|
124 |
-
|
125 |
-
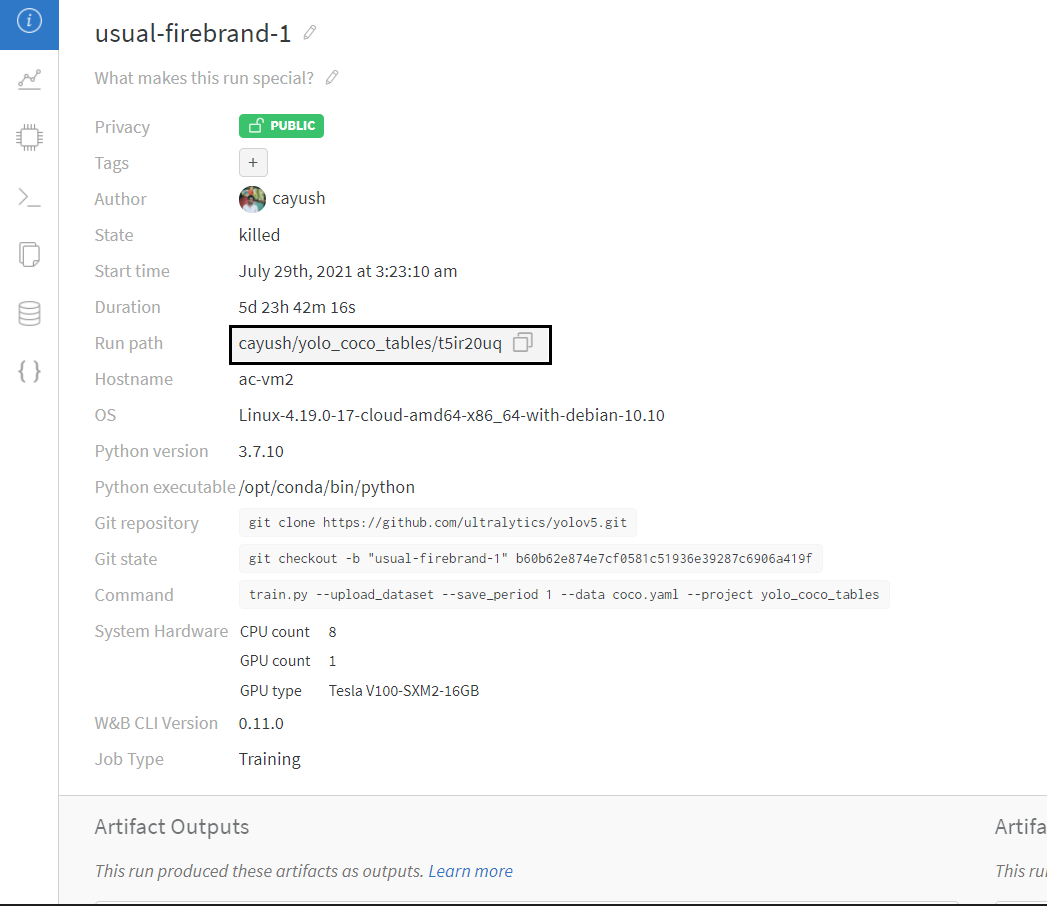
|
126 |
-
|
127 |
-
</details>
|
128 |
-
|
129 |
-
<h3> 6: Resume runs from dataset artifact & checkpoint artifacts. </h3>
|
130 |
-
<b> Local dataset or model checkpoints are not required. This can be used to resume runs directly on a different device </b>
|
131 |
-
The syntax is same as the previous section, but you'll need to lof both the dataset and model checkpoints as artifacts, i.e, set bot <code>--upload_dataset</code> or
|
132 |
-
train from <code>_wandb.yaml</code> file and set <code>--save_period</code>
|
133 |
-
|
134 |
-
<details>
|
135 |
-
<summary> <b>Usage</b> </summary>
|
136 |
-
<b>Code</b> <code> $ python train.py --resume wandb-artifact://{run_path} </code>
|
137 |
-
|
138 |
-
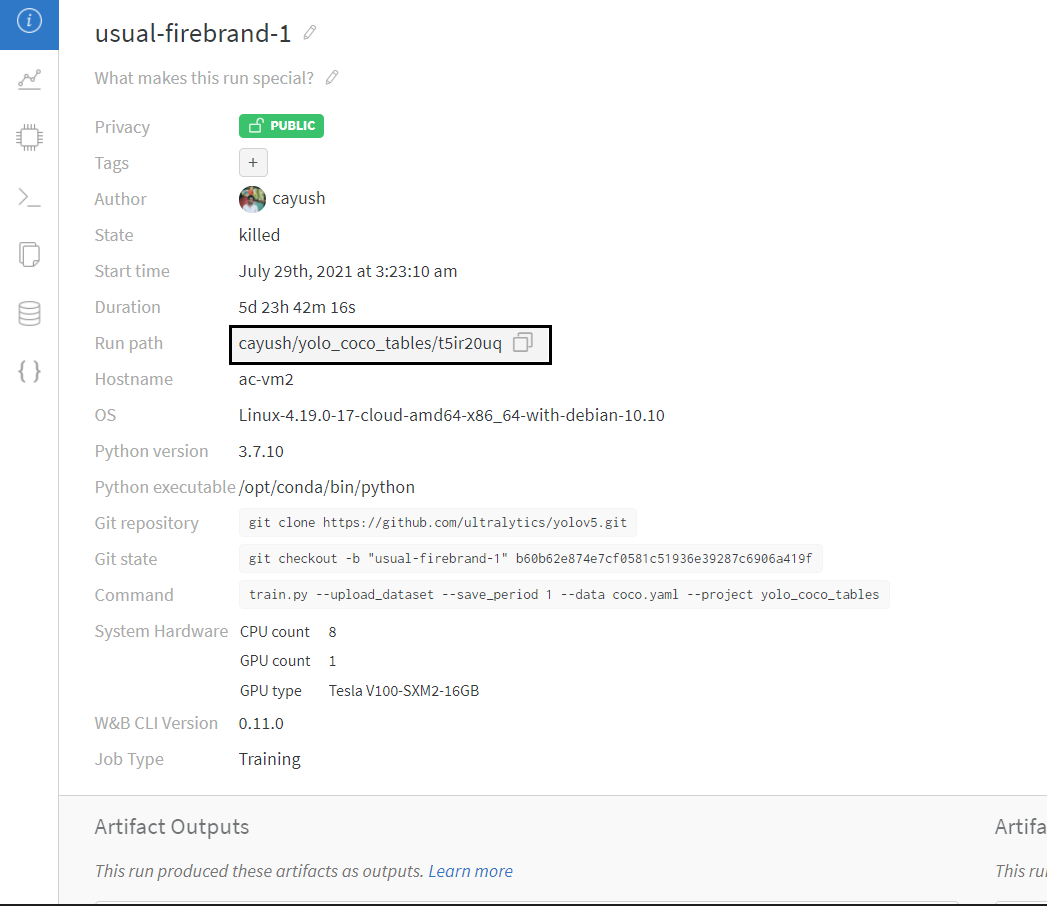
|
139 |
-
|
140 |
-
</details>
|
141 |
-
|
142 |
-
</details>
|
143 |
-
|
144 |
-
<h3> Reports </h3>
|
145 |
-
W&B Reports can be created from your saved runs for sharing online. Once a report is created you will receive a link you can use to publically share your results. Here is an example report created from the COCO128 tutorial trainings of all four YOLOv5 models ([link](https://wandb.ai/glenn-jocher/yolov5_tutorial/reports/YOLOv5-COCO128-Tutorial-Results--VmlldzozMDI5OTY)).
|
146 |
-
|
147 |
-
<img width="900" alt="Weights & Biases Reports" src="https://user-images.githubusercontent.com/26833433/135394029-a17eaf86-c6c1-4b1d-bb80-b90e83aaffa7.png">
|
148 |
-
|
149 |
-
## Environments
|
150 |
-
|
151 |
-
YOLOv5 may be run in any of the following up-to-date verified environments (with all dependencies including [CUDA](https://developer.nvidia.com/cuda)/[CUDNN](https://developer.nvidia.com/cudnn), [Python](https://www.python.org/) and [PyTorch](https://pytorch.org/) preinstalled):
|
152 |
-
|
153 |
-
- **Google Colab and Kaggle** notebooks with free GPU: <a href="https://colab.research.google.com/github/ultralytics/yolov5/blob/master/tutorial.ipynb"><img src="https://colab.research.google.com/assets/colab-badge.svg" alt="Open In Colab"></a> <a href="https://www.kaggle.com/ultralytics/yolov5"><img src="https://kaggle.com/static/images/open-in-kaggle.svg" alt="Open In Kaggle"></a>
|
154 |
-
- **Google Cloud** Deep Learning VM. See [GCP Quickstart Guide](https://github.com/ultralytics/yolov5/wiki/GCP-Quickstart)
|
155 |
-
- **Amazon** Deep Learning AMI. See [AWS Quickstart Guide](https://github.com/ultralytics/yolov5/wiki/AWS-Quickstart)
|
156 |
-
- **Docker Image**. See [Docker Quickstart Guide](https://github.com/ultralytics/yolov5/wiki/Docker-Quickstart) <a href="https://hub.docker.com/r/ultralytics/yolov5"><img src="https://img.shields.io/docker/pulls/ultralytics/yolov5?logo=docker" alt="Docker Pulls"></a>
|
157 |
-
|
158 |
-
## Status
|
159 |
-
|
160 |
-

|
161 |
-
|
162 |
-
If this badge is green, all [YOLOv5 GitHub Actions](https://github.com/ultralytics/yolov5/actions) Continuous Integration (CI) tests are currently passing. CI tests verify correct operation of YOLOv5 training ([train.py](https://github.com/ultralytics/yolov5/blob/master/train.py)), validation ([val.py](https://github.com/ultralytics/yolov5/blob/master/val.py)), inference ([detect.py](https://github.com/ultralytics/yolov5/blob/master/detect.py)) and export ([export.py](https://github.com/ultralytics/yolov5/blob/master/export.py)) on macOS, Windows, and Ubuntu every 24 hours and on every commit.
|
|
|
|
|
|
|
|
|
|
|
|
|
|
|
|
|
|
|
|
|
|
|
|
|
|
|
|
|
|
|
|
|
|
|
|
|
|
|
|
|
|
|
|
|
|
|
|
|
|
|
|
|
|
|
|
|
|
|
|
|
|
|
|
|
|
|
|
|
|
|
|
|
|
|
|
|
|
|
|
|
|
|
|
|
|
|
|
|
|
|
|
|
|
|
|
|
|
|
|
|
|
|
|
|
|
|
|
|
|
|
|
|
|
|
|
|
|
|
|
|
|
|
|
|
|
|
|
|
|
|
|
|
|
|
|
|
|
|
|
|
|
|
|
|
|
|
|
|
|
|
|
|
|
|
|
|
|
|
|
|
|
|
|
|
|
|
|
|
|
|
|
|
|
|
|
|
|
|
|
|
|
|
|
|
|
|
|
|
|
|
|
|
|
|
|
|
|
|
|
|
|
|
|
|
|
|
|
|
|
|
|
|
|
|
|
|
|
|
|
|
|
|
|
|
|
|
|
|
|
|
|
|
|
|
|
|
|
|
|
|
|
|
|
|
|
|
|
|
|
|
|
|
|
|
|
|
|
|
|
|
|
|
|
|
|
|
|
|
|
|
|
|
|
|
|
|
|
|
|
|
|
|
|
|
|
|
|
|
|
|
|
|
|
|
|
|
|
|
|
|
|
|
|
|
|
|
|
|
|
|
|
|
|
|
|
|
|
|
|
|
|
|
|
|
|
spaces/Arnaudding001/OpenAI_whisperLive/segments_test.py
DELETED
@@ -1,48 +0,0 @@
|
|
1 |
-
import sys
|
2 |
-
import unittest
|
3 |
-
|
4 |
-
sys.path.append('../whisper-webui')
|
5 |
-
|
6 |
-
from src.segments import merge_timestamps
|
7 |
-
|
8 |
-
class TestSegments(unittest.TestCase):
|
9 |
-
def __init__(self, *args, **kwargs):
|
10 |
-
super(TestSegments, self).__init__(*args, **kwargs)
|
11 |
-
|
12 |
-
def test_merge_segments(self):
|
13 |
-
segments = [
|
14 |
-
{'start': 10.0, 'end': 20.0},
|
15 |
-
{'start': 22.0, 'end': 27.0},
|
16 |
-
{'start': 31.0, 'end': 35.0},
|
17 |
-
{'start': 45.0, 'end': 60.0},
|
18 |
-
{'start': 61.0, 'end': 65.0},
|
19 |
-
{'start': 68.0, 'end': 98.0},
|
20 |
-
{'start': 100.0, 'end': 102.0},
|
21 |
-
{'start': 110.0, 'end': 112.0}
|
22 |
-
]
|
23 |
-
|
24 |
-
result = merge_timestamps(segments, merge_window=5, max_merge_size=30, padding_left=1, padding_right=1)
|
25 |
-
|
26 |
-
self.assertListEqual(result, [
|
27 |
-
{'start': 9.0, 'end': 36.0},
|
28 |
-
{'start': 44.0, 'end': 66.0},
|
29 |
-
{'start': 67.0, 'end': 99.0},
|
30 |
-
{'start': 99.0, 'end': 103.0},
|
31 |
-
{'start': 109.0, 'end': 113.0}
|
32 |
-
])
|
33 |
-
|
34 |
-
def test_overlap_next(self):
|
35 |
-
segments = [
|
36 |
-
{'start': 5.0, 'end': 39.182},
|
37 |
-
{'start': 39.986, 'end': 40.814}
|
38 |
-
]
|
39 |
-
|
40 |
-
result = merge_timestamps(segments, merge_window=5, max_merge_size=30, padding_left=1, padding_right=1)
|
41 |
-
|
42 |
-
self.assertListEqual(result, [
|
43 |
-
{'start': 4.0, 'end': 39.584},
|
44 |
-
{'start': 39.584, 'end': 41.814}
|
45 |
-
])
|
46 |
-
|
47 |
-
if __name__ == '__main__':
|
48 |
-
unittest.main()
|
|
|
|
|
|
|
|
|
|
|
|
|
|
|
|
|
|
|
|
|
|
|
|
|
|
|
|
|
|
|
|
|
|
|
|
|
|
|
|
|
|
|
|
|
|
|
|
|
|
|
|
|
|
|
|
|
|
|
|
|
|
|
|
|
|
|
|
|
|
|
|
|
|
|
|
|
|
|
|
|
|
|
|
|
|
|
|
|
|
|
|
|
|
|
|
|
|
spaces/Arulkumar03/GroundingDINO_SOTA_Zero_Shot_Model/groundingdino/util/box_ops.py
DELETED
@@ -1,140 +0,0 @@
|
|
1 |
-
# Copyright (c) Facebook, Inc. and its affiliates. All Rights Reserved
|
2 |
-
"""
|
3 |
-
Utilities for bounding box manipulation and GIoU.
|
4 |
-
"""
|
5 |
-
import torch
|
6 |
-
from torchvision.ops.boxes import box_area
|
7 |
-
|
8 |
-
|
9 |
-
def box_cxcywh_to_xyxy(x):
|
10 |
-
x_c, y_c, w, h = x.unbind(-1)
|
11 |
-
b = [(x_c - 0.5 * w), (y_c - 0.5 * h), (x_c + 0.5 * w), (y_c + 0.5 * h)]
|
12 |
-
return torch.stack(b, dim=-1)
|
13 |
-
|
14 |
-
|
15 |
-
def box_xyxy_to_cxcywh(x):
|
16 |
-
x0, y0, x1, y1 = x.unbind(-1)
|
17 |
-
b = [(x0 + x1) / 2, (y0 + y1) / 2, (x1 - x0), (y1 - y0)]
|
18 |
-
return torch.stack(b, dim=-1)
|
19 |
-
|
20 |
-
|
21 |
-
# modified from torchvision to also return the union
|
22 |
-
def box_iou(boxes1, boxes2):
|
23 |
-
area1 = box_area(boxes1)
|
24 |
-
area2 = box_area(boxes2)
|
25 |
-
|
26 |
-
# import ipdb; ipdb.set_trace()
|
27 |
-
lt = torch.max(boxes1[:, None, :2], boxes2[:, :2]) # [N,M,2]
|
28 |
-
rb = torch.min(boxes1[:, None, 2:], boxes2[:, 2:]) # [N,M,2]
|
29 |
-
|
30 |
-
wh = (rb - lt).clamp(min=0) # [N,M,2]
|
31 |
-
inter = wh[:, :, 0] * wh[:, :, 1] # [N,M]
|
32 |
-
|
33 |
-
union = area1[:, None] + area2 - inter
|
34 |
-
|
35 |
-
iou = inter / (union + 1e-6)
|
36 |
-
return iou, union
|
37 |
-
|
38 |
-
|
39 |
-
def generalized_box_iou(boxes1, boxes2):
|
40 |
-
"""
|
41 |
-
Generalized IoU from https://giou.stanford.edu/
|
42 |
-
|
43 |
-
The boxes should be in [x0, y0, x1, y1] format
|
44 |
-
|
45 |
-
Returns a [N, M] pairwise matrix, where N = len(boxes1)
|
46 |
-
and M = len(boxes2)
|
47 |
-
"""
|
48 |
-
# degenerate boxes gives inf / nan results
|
49 |
-
# so do an early check
|
50 |
-
assert (boxes1[:, 2:] >= boxes1[:, :2]).all()
|
51 |
-
assert (boxes2[:, 2:] >= boxes2[:, :2]).all()
|
52 |
-
# except:
|
53 |
-
# import ipdb; ipdb.set_trace()
|
54 |
-
iou, union = box_iou(boxes1, boxes2)
|
55 |
-
|
56 |
-
lt = torch.min(boxes1[:, None, :2], boxes2[:, :2])
|
57 |
-
rb = torch.max(boxes1[:, None, 2:], boxes2[:, 2:])
|
58 |
-
|
59 |
-
wh = (rb - lt).clamp(min=0) # [N,M,2]
|
60 |
-
area = wh[:, :, 0] * wh[:, :, 1]
|
61 |
-
|
62 |
-
return iou - (area - union) / (area + 1e-6)
|
63 |
-
|
64 |
-
|
65 |
-
# modified from torchvision to also return the union
|
66 |
-
def box_iou_pairwise(boxes1, boxes2):
|
67 |
-
area1 = box_area(boxes1)
|
68 |
-
area2 = box_area(boxes2)
|
69 |
-
|
70 |
-
lt = torch.max(boxes1[:, :2], boxes2[:, :2]) # [N,2]
|
71 |
-
rb = torch.min(boxes1[:, 2:], boxes2[:, 2:]) # [N,2]
|
72 |
-
|
73 |
-
wh = (rb - lt).clamp(min=0) # [N,2]
|
74 |
-
inter = wh[:, 0] * wh[:, 1] # [N]
|
75 |
-
|
76 |
-
union = area1 + area2 - inter
|
77 |
-
|
78 |
-
iou = inter / union
|
79 |
-
return iou, union
|
80 |
-
|
81 |
-
|
82 |
-
def generalized_box_iou_pairwise(boxes1, boxes2):
|
83 |
-
"""
|
84 |
-
Generalized IoU from https://giou.stanford.edu/
|
85 |
-
|
86 |
-
Input:
|
87 |
-
- boxes1, boxes2: N,4
|
88 |
-
Output:
|
89 |
-
- giou: N, 4
|
90 |
-
"""
|
91 |
-
# degenerate boxes gives inf / nan results
|
92 |
-
# so do an early check
|
93 |
-
assert (boxes1[:, 2:] >= boxes1[:, :2]).all()
|
94 |
-
assert (boxes2[:, 2:] >= boxes2[:, :2]).all()
|
95 |
-
assert boxes1.shape == boxes2.shape
|
96 |
-
iou, union = box_iou_pairwise(boxes1, boxes2) # N, 4
|
97 |
-
|
98 |
-
lt = torch.min(boxes1[:, :2], boxes2[:, :2])
|
99 |
-
rb = torch.max(boxes1[:, 2:], boxes2[:, 2:])
|
100 |
-
|
101 |
-
wh = (rb - lt).clamp(min=0) # [N,2]
|
102 |
-
area = wh[:, 0] * wh[:, 1]
|
103 |
-
|
104 |
-
return iou - (area - union) / area
|
105 |
-
|
106 |
-
|
107 |
-
def masks_to_boxes(masks):
|
108 |
-
"""Compute the bounding boxes around the provided masks
|
109 |
-
|
110 |
-
The masks should be in format [N, H, W] where N is the number of masks, (H, W) are the spatial dimensions.
|
111 |
-
|
112 |
-
Returns a [N, 4] tensors, with the boxes in xyxy format
|
113 |
-
"""
|
114 |
-
if masks.numel() == 0:
|
115 |
-
return torch.zeros((0, 4), device=masks.device)
|
116 |
-
|
117 |
-
h, w = masks.shape[-2:]
|
118 |
-
|
119 |
-
y = torch.arange(0, h, dtype=torch.float)
|
120 |
-
x = torch.arange(0, w, dtype=torch.float)
|
121 |
-
y, x = torch.meshgrid(y, x)
|
122 |
-
|
123 |
-
x_mask = masks * x.unsqueeze(0)
|
124 |
-
x_max = x_mask.flatten(1).max(-1)[0]
|
125 |
-
x_min = x_mask.masked_fill(~(masks.bool()), 1e8).flatten(1).min(-1)[0]
|
126 |
-
|
127 |
-
y_mask = masks * y.unsqueeze(0)
|
128 |
-
y_max = y_mask.flatten(1).max(-1)[0]
|
129 |
-
y_min = y_mask.masked_fill(~(masks.bool()), 1e8).flatten(1).min(-1)[0]
|
130 |
-
|
131 |
-
return torch.stack([x_min, y_min, x_max, y_max], 1)
|
132 |
-
|
133 |
-
|
134 |
-
if __name__ == "__main__":
|
135 |
-
x = torch.rand(5, 4)
|
136 |
-
y = torch.rand(3, 4)
|
137 |
-
iou, union = box_iou(x, y)
|
138 |
-
import ipdb
|
139 |
-
|
140 |
-
ipdb.set_trace()
|
|
|
|
|
|
|
|
|
|
|
|
|
|
|
|
|
|
|
|
|
|
|
|
|
|
|
|
|
|
|
|
|
|
|
|
|
|
|
|
|
|
|
|
|
|
|
|
|
|
|
|
|
|
|
|
|
|
|
|
|
|
|
|
|
|
|
|
|
|
|
|
|
|
|
|
|
|
|
|
|
|
|
|
|
|
|
|
|
|
|
|
|
|
|
|
|
|
|
|
|
|
|
|
|
|
|
|
|
|
|
|
|
|
|
|
|
|
|
|
|
|
|
|
|
|
|
|
|
|
|
|
|
|
|
|
|
|
|
|
|
|
|
|
|
|
|
|
|
|
|
|
|
|
|
|
|
|
|
|
|
|
|
|
|
|
|
|
|
|
|
|
|
|
|
|
|
|
|
|
|
|
|
|
|
|
|
|
|
|
|
|
|
|
|
|
|
|
|
|
|
|
|
|
|
|
|
|
|
|
|
|
|
|
|
|
|
|
|
|
|
|
|
|
|
|
|
|
|
|
|
|
|
|
|
|
|
|
|
|
|
|
|
|
|
|
|
|
|
|
|
|
|
|
|
|
|
|
|
|
|
|
|
|
|
|
|
|
|
|
|
|
|
|
|
|
|
|
|
|
|
|
spaces/Ataturk-Chatbot/HuggingFaceChat/venv/lib/python3.11/site-packages/pip/_vendor/rich/_windows_renderer.py
DELETED
@@ -1,56 +0,0 @@
|
|
1 |
-
from typing import Iterable, Sequence, Tuple, cast
|
2 |
-
|
3 |
-
from pip._vendor.rich._win32_console import LegacyWindowsTerm, WindowsCoordinates
|
4 |
-
from pip._vendor.rich.segment import ControlCode, ControlType, Segment
|
5 |
-
|
6 |
-
|
7 |
-
def legacy_windows_render(buffer: Iterable[Segment], term: LegacyWindowsTerm) -> None:
|
8 |
-
"""Makes appropriate Windows Console API calls based on the segments in the buffer.
|
9 |
-
|
10 |
-
Args:
|
11 |
-
buffer (Iterable[Segment]): Iterable of Segments to convert to Win32 API calls.
|
12 |
-
term (LegacyWindowsTerm): Used to call the Windows Console API.
|
13 |
-
"""
|
14 |
-
for text, style, control in buffer:
|
15 |
-
if not control:
|
16 |
-
if style:
|
17 |
-
term.write_styled(text, style)
|
18 |
-
else:
|
19 |
-
term.write_text(text)
|
20 |
-
else:
|
21 |
-
control_codes: Sequence[ControlCode] = control
|
22 |
-
for control_code in control_codes:
|
23 |
-
control_type = control_code[0]
|
24 |
-
if control_type == ControlType.CURSOR_MOVE_TO:
|
25 |
-
_, x, y = cast(Tuple[ControlType, int, int], control_code)
|
26 |
-
term.move_cursor_to(WindowsCoordinates(row=y - 1, col=x - 1))
|
27 |
-
elif control_type == ControlType.CARRIAGE_RETURN:
|
28 |
-
term.write_text("\r")
|
29 |
-
elif control_type == ControlType.HOME:
|
30 |
-
term.move_cursor_to(WindowsCoordinates(0, 0))
|
31 |
-
elif control_type == ControlType.CURSOR_UP:
|
32 |
-
term.move_cursor_up()
|
33 |
-
elif control_type == ControlType.CURSOR_DOWN:
|
34 |
-
term.move_cursor_down()
|
35 |
-
elif control_type == ControlType.CURSOR_FORWARD:
|
36 |
-
term.move_cursor_forward()
|
37 |
-
elif control_type == ControlType.CURSOR_BACKWARD:
|
38 |
-
term.move_cursor_backward()
|
39 |
-
elif control_type == ControlType.CURSOR_MOVE_TO_COLUMN:
|
40 |
-
_, column = cast(Tuple[ControlType, int], control_code)
|
41 |
-
term.move_cursor_to_column(column - 1)
|
42 |
-
elif control_type == ControlType.HIDE_CURSOR:
|
43 |
-
term.hide_cursor()
|
44 |
-
elif control_type == ControlType.SHOW_CURSOR:
|
45 |
-
term.show_cursor()
|
46 |
-
elif control_type == ControlType.ERASE_IN_LINE:
|
47 |
-
_, mode = cast(Tuple[ControlType, int], control_code)
|
48 |
-
if mode == 0:
|
49 |
-
term.erase_end_of_line()
|
50 |
-
elif mode == 1:
|
51 |
-
term.erase_start_of_line()
|
52 |
-
elif mode == 2:
|
53 |
-
term.erase_line()
|
54 |
-
elif control_type == ControlType.SET_WINDOW_TITLE:
|
55 |
-
_, title = cast(Tuple[ControlType, str], control_code)
|
56 |
-
term.set_title(title)
|
|
|
|
|
|
|
|
|
|
|
|
|
|
|
|
|
|
|
|
|
|
|
|
|
|
|
|
|
|
|
|
|
|
|
|
|
|
|
|
|
|
|
|
|
|
|
|
|
|
|
|
|
|
|
|
|
|
|
|
|
|
|
|
|
|
|
|
|
|
|
|
|
|
|
|
|
|
|
|
|
|
|
|
|
|
|
|
|
|
|
|
|
|
|
|
|
|
|
|
|
|
|
|
|
|
|
|
|
|
|
|
|
|
spaces/Ataturk-Chatbot/HuggingFaceChat/venv/lib/python3.11/site-packages/setuptools/config/_validate_pyproject/formats.py
DELETED
@@ -1,259 +0,0 @@
|
|
1 |
-
import logging
|
2 |
-
import os
|
3 |
-
import re
|
4 |
-
import string
|
5 |
-
import typing
|
6 |
-
from itertools import chain as _chain
|
7 |
-
|
8 |
-
_logger = logging.getLogger(__name__)
|
9 |
-
|
10 |
-
# -------------------------------------------------------------------------------------
|
11 |
-
# PEP 440
|
12 |
-
|
13 |
-
VERSION_PATTERN = r"""
|
14 |
-
v?
|
15 |
-
(?:
|
16 |
-
(?:(?P<epoch>[0-9]+)!)? # epoch
|
17 |
-
(?P<release>[0-9]+(?:\.[0-9]+)*) # release segment
|
18 |
-
(?P<pre> # pre-release
|
19 |
-
[-_\.]?
|
20 |
-
(?P<pre_l>(a|b|c|rc|alpha|beta|pre|preview))
|
21 |
-
[-_\.]?
|
22 |
-
(?P<pre_n>[0-9]+)?
|
23 |
-
)?
|
24 |
-
(?P<post> # post release
|
25 |
-
(?:-(?P<post_n1>[0-9]+))
|
26 |
-
|
|
27 |
-
(?:
|
28 |
-
[-_\.]?
|
29 |
-
(?P<post_l>post|rev|r)
|
30 |
-
[-_\.]?
|
31 |
-
(?P<post_n2>[0-9]+)?
|
32 |
-
)
|
33 |
-
)?
|
34 |
-
(?P<dev> # dev release
|
35 |
-
[-_\.]?
|
36 |
-
(?P<dev_l>dev)
|
37 |
-
[-_\.]?
|
38 |
-
(?P<dev_n>[0-9]+)?
|
39 |
-
)?
|
40 |
-
)
|
41 |
-
(?:\+(?P<local>[a-z0-9]+(?:[-_\.][a-z0-9]+)*))? # local version
|
42 |
-
"""
|
43 |
-
|
44 |
-
VERSION_REGEX = re.compile(r"^\s*" + VERSION_PATTERN + r"\s*$", re.X | re.I)
|
45 |
-
|
46 |
-
|
47 |
-
def pep440(version: str) -> bool:
|
48 |
-
return VERSION_REGEX.match(version) is not None
|
49 |
-
|
50 |
-
|
51 |
-
# -------------------------------------------------------------------------------------
|
52 |
-
# PEP 508
|
53 |
-
|
54 |
-
PEP508_IDENTIFIER_PATTERN = r"([A-Z0-9]|[A-Z0-9][A-Z0-9._-]*[A-Z0-9])"
|
55 |
-
PEP508_IDENTIFIER_REGEX = re.compile(f"^{PEP508_IDENTIFIER_PATTERN}$", re.I)
|
56 |
-
|
57 |
-
|
58 |
-
def pep508_identifier(name: str) -> bool:
|
59 |
-
return PEP508_IDENTIFIER_REGEX.match(name) is not None
|
60 |
-
|
61 |
-
|
62 |
-
try:
|
63 |
-
try:
|
64 |
-
from packaging import requirements as _req
|
65 |
-
except ImportError: # pragma: no cover
|
66 |
-
# let's try setuptools vendored version
|
67 |
-
from setuptools._vendor.packaging import requirements as _req # type: ignore
|
68 |
-
|
69 |
-
def pep508(value: str) -> bool:
|
70 |
-
try:
|
71 |
-
_req.Requirement(value)
|
72 |
-
return True
|
73 |
-
except _req.InvalidRequirement:
|
74 |
-
return False
|
75 |
-
|
76 |
-
except ImportError: # pragma: no cover
|
77 |
-
_logger.warning(
|
78 |
-
"Could not find an installation of `packaging`. Requirements, dependencies and "
|
79 |
-
"versions might not be validated. "
|
80 |
-
"To enforce validation, please install `packaging`."
|
81 |
-
)
|
82 |
-
|
83 |
-
def pep508(value: str) -> bool:
|
84 |
-
return True
|
85 |
-
|
86 |
-
|
87 |
-
def pep508_versionspec(value: str) -> bool:
|
88 |
-
"""Expression that can be used to specify/lock versions (including ranges)"""
|
89 |
-
if any(c in value for c in (";", "]", "@")):
|
90 |
-
# In PEP 508:
|
91 |
-
# conditional markers, extras and URL specs are not included in the
|
92 |
-
# versionspec
|
93 |
-
return False
|
94 |
-
# Let's pretend we have a dependency called `requirement` with the given
|
95 |
-
# version spec, then we can re-use the pep508 function for validation:
|
96 |
-
return pep508(f"requirement{value}")
|
97 |
-
|
98 |
-
|
99 |
-
# -------------------------------------------------------------------------------------
|
100 |
-
# PEP 517
|
101 |
-
|
102 |
-
|
103 |
-
def pep517_backend_reference(value: str) -> bool:
|
104 |
-
module, _, obj = value.partition(":")
|
105 |
-
identifiers = (i.strip() for i in _chain(module.split("."), obj.split(".")))
|
106 |
-
return all(python_identifier(i) for i in identifiers if i)
|
107 |
-
|
108 |
-
|
109 |
-
# -------------------------------------------------------------------------------------
|
110 |
-
# Classifiers - PEP 301
|
111 |
-
|
112 |
-
|
113 |
-
def _download_classifiers() -> str:
|
114 |
-
import ssl
|
115 |
-
from email.message import Message
|
116 |
-
from urllib.request import urlopen
|
117 |
-
|
118 |
-
url = "https://pypi.org/pypi?:action=list_classifiers"
|
119 |
-
context = ssl.create_default_context()
|
120 |
-
with urlopen(url, context=context) as response:
|
121 |
-
headers = Message()
|
122 |
-
headers["content_type"] = response.getheader("content-type", "text/plain")
|
123 |
-
return response.read().decode(headers.get_param("charset", "utf-8"))
|
124 |
-
|
125 |
-
|
126 |
-
class _TroveClassifier:
|
127 |
-
"""The ``trove_classifiers`` package is the official way of validating classifiers,
|
128 |
-
however this package might not be always available.
|
129 |
-
As a workaround we can still download a list from PyPI.
|
130 |
-
We also don't want to be over strict about it, so simply skipping silently is an
|
131 |
-
option (classifiers will be validated anyway during the upload to PyPI).
|
132 |
-
"""
|
133 |
-
|
134 |
-
def __init__(self):
|
135 |
-
self.downloaded: typing.Union[None, False, typing.Set[str]] = None
|
136 |
-
self._skip_download = False
|
137 |
-
# None => not cached yet
|
138 |
-
# False => cache not available
|
139 |
-
self.__name__ = "trove_classifier" # Emulate a public function
|
140 |
-
|
141 |
-
def _disable_download(self):
|
142 |
-
# This is a private API. Only setuptools has the consent of using it.
|
143 |
-
self._skip_download = True
|
144 |
-
|
145 |
-
def __call__(self, value: str) -> bool:
|
146 |
-
if self.downloaded is False or self._skip_download is True:
|
147 |
-
return True
|
148 |
-
|
149 |
-
if os.getenv("NO_NETWORK") or os.getenv("VALIDATE_PYPROJECT_NO_NETWORK"):
|
150 |
-
self.downloaded = False
|
151 |
-
msg = (
|
152 |
-
"Install ``trove-classifiers`` to ensure proper validation. "
|
153 |
-
"Skipping download of classifiers list from PyPI (NO_NETWORK)."
|
154 |
-
)
|
155 |
-
_logger.debug(msg)
|
156 |
-
return True
|
157 |
-
|
158 |
-
if self.downloaded is None:
|
159 |
-
msg = (
|
160 |
-
"Install ``trove-classifiers`` to ensure proper validation. "
|
161 |
-
"Meanwhile a list of classifiers will be downloaded from PyPI."
|
162 |
-
)
|
163 |
-
_logger.debug(msg)
|
164 |
-
try:
|
165 |
-
self.downloaded = set(_download_classifiers().splitlines())
|
166 |
-
except Exception:
|
167 |
-
self.downloaded = False
|
168 |
-
_logger.debug("Problem with download, skipping validation")
|
169 |
-
return True
|
170 |
-
|
171 |
-
return value in self.downloaded or value.lower().startswith("private ::")
|
172 |
-
|
173 |
-
|
174 |
-
try:
|
175 |
-
from trove_classifiers import classifiers as _trove_classifiers
|
176 |
-
|
177 |
-
def trove_classifier(value: str) -> bool:
|
178 |
-
return value in _trove_classifiers or value.lower().startswith("private ::")
|
179 |
-
|
180 |
-
except ImportError: # pragma: no cover
|
181 |
-
trove_classifier = _TroveClassifier()
|
182 |
-
|
183 |
-
|
184 |
-
# -------------------------------------------------------------------------------------
|
185 |
-
# Non-PEP related
|
186 |
-
|
187 |
-
|
188 |
-
def url(value: str) -> bool:
|
189 |
-
from urllib.parse import urlparse
|
190 |
-
|
191 |
-
try:
|
192 |
-
parts = urlparse(value)
|
193 |
-
if not parts.scheme:
|
194 |
-
_logger.warning(
|
195 |
-
"For maximum compatibility please make sure to include a "
|
196 |
-
"`scheme` prefix in your URL (e.g. 'http://'). "
|
197 |
-
f"Given value: {value}"
|
198 |
-
)
|
199 |
-
if not (value.startswith("/") or value.startswith("\\") or "@" in value):
|
200 |
-
parts = urlparse(f"http://{value}")
|
201 |
-
|
202 |
-
return bool(parts.scheme and parts.netloc)
|
203 |
-
except Exception:
|
204 |
-
return False
|
205 |
-
|
206 |
-
|
207 |
-
# https://packaging.python.org/specifications/entry-points/
|
208 |
-
ENTRYPOINT_PATTERN = r"[^\[\s=]([^=]*[^\s=])?"
|
209 |
-
ENTRYPOINT_REGEX = re.compile(f"^{ENTRYPOINT_PATTERN}$", re.I)
|
210 |
-
RECOMMEDED_ENTRYPOINT_PATTERN = r"[\w.-]+"
|
211 |
-
RECOMMEDED_ENTRYPOINT_REGEX = re.compile(f"^{RECOMMEDED_ENTRYPOINT_PATTERN}$", re.I)
|
212 |
-
ENTRYPOINT_GROUP_PATTERN = r"\w+(\.\w+)*"
|
213 |
-
ENTRYPOINT_GROUP_REGEX = re.compile(f"^{ENTRYPOINT_GROUP_PATTERN}$", re.I)
|
214 |
-
|
215 |
-
|
216 |
-
def python_identifier(value: str) -> bool:
|
217 |
-
return value.isidentifier()
|
218 |
-
|
219 |
-
|
220 |
-
def python_qualified_identifier(value: str) -> bool:
|
221 |
-
if value.startswith(".") or value.endswith("."):
|
222 |
-
return False
|
223 |
-
return all(python_identifier(m) for m in value.split("."))
|
224 |
-
|
225 |
-
|
226 |
-
def python_module_name(value: str) -> bool:
|
227 |
-
return python_qualified_identifier(value)
|
228 |
-
|
229 |
-
|
230 |
-
def python_entrypoint_group(value: str) -> bool:
|
231 |
-
return ENTRYPOINT_GROUP_REGEX.match(value) is not None
|
232 |
-
|
233 |
-
|
234 |
-
def python_entrypoint_name(value: str) -> bool:
|
235 |
-
if not ENTRYPOINT_REGEX.match(value):
|
236 |
-
return False
|
237 |
-
if not RECOMMEDED_ENTRYPOINT_REGEX.match(value):
|
238 |
-
msg = f"Entry point `{value}` does not follow recommended pattern: "
|
239 |
-
msg += RECOMMEDED_ENTRYPOINT_PATTERN
|
240 |
-
_logger.warning(msg)
|
241 |
-
return True
|
242 |
-
|
243 |
-
|
244 |
-
def python_entrypoint_reference(value: str) -> bool:
|
245 |
-
module, _, rest = value.partition(":")
|
246 |
-
if "[" in rest:
|
247 |
-
obj, _, extras_ = rest.partition("[")
|
248 |
-
if extras_.strip()[-1] != "]":
|
249 |
-
return False
|
250 |
-
extras = (x.strip() for x in extras_.strip(string.whitespace + "[]").split(","))
|
251 |
-
if not all(pep508_identifier(e) for e in extras):
|
252 |
-
return False
|
253 |
-
_logger.warning(f"`{value}` - using extras for entry points is not recommended")
|
254 |
-
else:
|
255 |
-
obj = rest
|
256 |
-
|
257 |
-
module_parts = module.split(".")
|
258 |
-
identifiers = _chain(module_parts, obj.split(".")) if rest else module_parts
|
259 |
-
return all(python_identifier(i.strip()) for i in identifiers)
|
|
|
|
|
|
|
|
|
|
|
|
|
|
|
|
|
|
|
|
|
|
|
|
|
|
|
|
|
|
|
|
|
|
|
|
|
|
|
|
|
|
|
|
|
|
|
|
|
|
|
|
|
|
|
|
|
|
|
|
|
|
|
|
|
|
|
|
|
|
|
|
|
|
|
|
|
|
|
|
|
|
|
|
|
|
|
|
|
|
|
|
|
|
|
|
|
|
|
|
|
|
|
|
|
|
|
|
|
|
|
|
|
|
|
|
|
|
|
|
|
|
|
|
|
|
|
|
|
|
|
|
|
|
|
|
|
|
|
|
|
|
|
|
|
|
|
|
|
|
|
|
|
|
|
|
|
|
|
|
|
|
|
|
|
|
|
|
|
|
|
|
|
|
|
|
|
|
|
|
|
|
|
|
|
|
|
|
|
|
|
|
|
|
|
|
|
|
|
|
|
|
|
|
|
|
|
|
|
|
|
|
|
|
|
|
|
|
|
|
|
|
|
|
|
|
|
|
|
|
|
|
|
|
|
|
|
|
|
|
|
|
|
|
|
|
|
|
|
|
|
|
|
|
|
|
|
|
|
|
|
|
|
|
|
|
|
|
|
|
|
|
|
|
|
|
|
|
|
|
|
|
|
|
|
|
|
|
|
|
|
|
|
|
|
|
|
|
|
|
|
|
|
|
|
|
|
|
|
|
|
|
|
|
|
|
|
|
|
|
|
|
|
|
|
|
|
|
|
|
|
|
|
|
|
|
|
|
|
|
|
|
|
|
|
|
|
|
|
|
|
|
|
|
|
|
|
|
|
|
|
|
|
|
|
|
|
|
|
|
|
|
|
|
|
|
|
|
|
|
|
|
|
|
|
|
|
|
|
|
|
|
|
|
|
|
|
|
|
|
|
|
|
|
|
|
|
|
|
|
|
|
|
|
|
|
|
|
|
|
|
|
|
|
|
|
|
|
|
|
|
|
|
|
|
|
|
|
|
|
|
|
|
|
|
|
|
|
|
|
|
|
|
|
|
|
|
|
|
|
|
|
|
|
|
|
|
|
|
|
|
|
|
|
|
|
|
|
|
|
|
|
|
|
|
|
|
|
|
|
|
|
|
|
|
|
|
|
|
|
|
|
|
|
|
|
|
|
|
|
|
|
|
|
|
|
|
|
|
|
spaces/Awiny/Image2Paragraph/models/grit_src/third_party/CenterNet2/detectron2/layers/csrc/nms_rotated/nms_rotated.h
DELETED
@@ -1,39 +0,0 @@
|
|
1 |
-
// Copyright (c) Facebook, Inc. and its affiliates.
|
2 |
-
#pragma once
|
3 |
-
#include <torch/types.h>
|
4 |
-
|
5 |
-
namespace detectron2 {
|
6 |
-
|
7 |
-
at::Tensor nms_rotated_cpu(
|
8 |
-
const at::Tensor& dets,
|
9 |
-
const at::Tensor& scores,
|
10 |
-
const double iou_threshold);
|
11 |
-
|
12 |
-
#if defined(WITH_CUDA) || defined(WITH_HIP)
|
13 |
-
at::Tensor nms_rotated_cuda(
|
14 |
-
const at::Tensor& dets,
|
15 |
-
const at::Tensor& scores,
|
16 |
-
const double iou_threshold);
|
17 |
-
#endif
|
18 |
-
|
19 |
-
// Interface for Python
|
20 |
-
// inline is needed to prevent multiple function definitions when this header is
|
21 |
-
// included by different cpps
|
22 |
-
inline at::Tensor nms_rotated(
|
23 |
-
const at::Tensor& dets,
|
24 |
-
const at::Tensor& scores,
|
25 |
-
const double iou_threshold) {
|
26 |
-
assert(dets.device().is_cuda() == scores.device().is_cuda());
|
27 |
-
if (dets.device().is_cuda()) {
|
28 |
-
#if defined(WITH_CUDA) || defined(WITH_HIP)
|
29 |
-
return nms_rotated_cuda(
|
30 |
-
dets.contiguous(), scores.contiguous(), iou_threshold);
|
31 |
-
#else
|
32 |
-
AT_ERROR("Detectron2 is not compiled with GPU support!");
|
33 |
-
#endif
|
34 |
-
}
|
35 |
-
|
36 |
-
return nms_rotated_cpu(dets.contiguous(), scores.contiguous(), iou_threshold);
|
37 |
-
}
|
38 |
-
|
39 |
-
} // namespace detectron2
|
|
|
|
|
|
|
|
|
|
|
|
|
|
|
|
|
|
|
|
|
|
|
|
|
|
|
|
|
|
|
|
|
|
|
|
|
|
|
|
|
|
|
|
|
|
|
|
|
|
|
|
|
|
|
|
|
|
|
|
|
|
|
|
|
|
|
|
|
|
|
|
|
|
|
|
|
|
|
|
spaces/Awiny/Image2Paragraph/models/grit_src/third_party/CenterNet2/detectron2/modeling/meta_arch/dense_detector.py
DELETED
@@ -1,282 +0,0 @@
|
|
1 |
-
import numpy as np
|
2 |
-
from typing import Dict, List, Optional, Tuple
|
3 |
-
import torch
|
4 |
-
from torch import Tensor, nn
|
5 |
-
|
6 |
-
from detectron2.data.detection_utils import convert_image_to_rgb
|
7 |
-
from detectron2.modeling import Backbone
|
8 |
-
from detectron2.structures import Boxes, ImageList, Instances
|
9 |
-
from detectron2.utils.events import get_event_storage
|
10 |
-
|
11 |
-
from ..postprocessing import detector_postprocess
|
12 |
-
|
13 |
-
|
14 |
-
def permute_to_N_HWA_K(tensor, K: int):
|
15 |
-
"""
|
16 |
-
Transpose/reshape a tensor from (N, (Ai x K), H, W) to (N, (HxWxAi), K)
|
17 |
-
"""
|
18 |
-
assert tensor.dim() == 4, tensor.shape
|
19 |
-
N, _, H, W = tensor.shape
|
20 |
-
tensor = tensor.view(N, -1, K, H, W)
|
21 |
-
tensor = tensor.permute(0, 3, 4, 1, 2)
|
22 |
-
tensor = tensor.reshape(N, -1, K) # Size=(N,HWA,K)
|
23 |
-
return tensor
|
24 |
-
|
25 |
-
|
26 |
-
class DenseDetector(nn.Module):
|
27 |
-
"""
|
28 |
-
Base class for dense detector. We define a dense detector as a fully-convolutional model that
|
29 |
-
makes per-pixel (i.e. dense) predictions.
|
30 |
-
"""
|
31 |
-
|
32 |
-
def __init__(
|
33 |
-
self,
|
34 |
-
backbone: Backbone,
|
35 |
-
head: nn.Module,
|
36 |
-
head_in_features: Optional[List[str]] = None,
|
37 |
-
*,
|
38 |
-
pixel_mean,
|
39 |
-
pixel_std,
|
40 |
-
):
|
41 |
-
"""
|
42 |
-
Args:
|
43 |
-
backbone: backbone module
|
44 |
-
head: head module
|
45 |
-
head_in_features: backbone features to use in head. Default to all backbone features.
|
46 |
-
pixel_mean (Tuple[float]):
|
47 |
-
Values to be used for image normalization (BGR order).
|
48 |
-
To train on images of different number of channels, set different mean & std.
|
49 |
-
Default values are the mean pixel value from ImageNet: [103.53, 116.28, 123.675]
|
50 |
-
pixel_std (Tuple[float]):
|
51 |
-
When using pre-trained models in Detectron1 or any MSRA models,
|
52 |
-
std has been absorbed into its conv1 weights, so the std needs to be set 1.
|
53 |
-
Otherwise, you can use [57.375, 57.120, 58.395] (ImageNet std)
|
54 |
-
"""
|
55 |
-
super().__init__()
|
56 |
-
|
57 |
-
self.backbone = backbone
|
58 |
-
self.head = head
|
59 |
-
if head_in_features is None:
|
60 |
-
shapes = self.backbone.output_shape()
|
61 |
-
self.head_in_features = sorted(shapes.keys(), key=lambda x: shapes[x].stride)
|
62 |
-
else:
|
63 |
-
self.head_in_features = head_in_features
|
64 |
-
|
65 |
-
self.register_buffer("pixel_mean", torch.tensor(pixel_mean).view(-1, 1, 1), False)
|
66 |
-
self.register_buffer("pixel_std", torch.tensor(pixel_std).view(-1, 1, 1), False)
|
67 |
-
|
68 |
-
@property
|
69 |
-
def device(self):
|
70 |
-
return self.pixel_mean.device
|
71 |
-
|
72 |
-
def forward(self, batched_inputs: List[Dict[str, Tensor]]):
|
73 |
-
"""
|
74 |
-
Args:
|
75 |
-
batched_inputs: a list, batched outputs of :class:`DatasetMapper` .
|
76 |
-
Each item in the list contains the inputs for one image.
|
77 |
-
For now, each item in the list is a dict that contains:
|
78 |
-
|
79 |
-
* image: Tensor, image in (C, H, W) format.
|
80 |
-
* instances: Instances
|
81 |
-
|
82 |
-
Other information that's included in the original dicts, such as:
|
83 |
-
|
84 |
-
* "height", "width" (int): the output resolution of the model, used in inference.
|
85 |
-
See :meth:`postprocess` for details.
|
86 |
-
|
87 |
-
Returns:
|
88 |
-
In training, dict[str, Tensor]: mapping from a named loss to a tensor storing the
|
89 |
-
loss. Used during training only. In inference, the standard output format, described
|
90 |
-
in :doc:`/tutorials/models`.
|
91 |
-
"""
|
92 |
-
images = self.preprocess_image(batched_inputs)
|
93 |
-
features = self.backbone(images.tensor)
|
94 |
-
features = [features[f] for f in self.head_in_features]
|
95 |
-
predictions = self.head(features)
|
96 |
-
|
97 |
-
if self.training:
|
98 |
-
assert not torch.jit.is_scripting(), "Not supported"
|
99 |
-
assert "instances" in batched_inputs[0], "Instance annotations are missing in training!"
|
100 |
-
gt_instances = [x["instances"].to(self.device) for x in batched_inputs]
|
101 |
-
return self.forward_training(images, features, predictions, gt_instances)
|
102 |
-
else:
|
103 |
-
results = self.forward_inference(images, features, predictions)
|
104 |
-
if torch.jit.is_scripting():
|
105 |
-
return results
|
106 |
-
|
107 |
-
processed_results = []
|
108 |
-
for results_per_image, input_per_image, image_size in zip(
|
109 |
-
results, batched_inputs, images.image_sizes
|
110 |
-
):
|
111 |
-
height = input_per_image.get("height", image_size[0])
|
112 |
-
width = input_per_image.get("width", image_size[1])
|
113 |
-
r = detector_postprocess(results_per_image, height, width)
|
114 |
-
processed_results.append({"instances": r})
|
115 |
-
return processed_results
|
116 |
-
|
117 |
-
def forward_training(self, images, features, predictions, gt_instances):
|
118 |
-
raise NotImplementedError()
|
119 |
-
|
120 |
-
def preprocess_image(self, batched_inputs: List[Dict[str, Tensor]]):
|
121 |
-
"""
|
122 |
-
Normalize, pad and batch the input images.
|
123 |
-
"""
|
124 |
-
images = [x["image"].to(self.device) for x in batched_inputs]
|
125 |
-
images = [(x - self.pixel_mean) / self.pixel_std for x in images]
|
126 |
-
images = ImageList.from_tensors(images, self.backbone.size_divisibility)
|
127 |
-
return images
|
128 |
-
|
129 |
-
def _transpose_dense_predictions(
|
130 |
-
self, predictions: List[List[Tensor]], dims_per_anchor: List[int]
|
131 |
-
) -> List[List[Tensor]]:
|
132 |
-
"""
|
133 |
-
Transpose the dense per-level predictions.
|
134 |
-
|
135 |
-
Args:
|
136 |
-
predictions: a list of outputs, each is a list of per-level
|
137 |
-
predictions with shape (N, Ai x K, Hi, Wi), where N is the
|
138 |
-
number of images, Ai is the number of anchors per location on
|
139 |
-
level i, K is the dimension of predictions per anchor.
|
140 |
-
dims_per_anchor: the value of K for each predictions. e.g. 4 for
|
141 |
-
box prediction, #classes for classification prediction.
|
142 |
-
|
143 |
-
Returns:
|
144 |
-
List[List[Tensor]]: each prediction is transposed to (N, Hi x Wi x Ai, K).
|
145 |
-
"""
|
146 |
-
assert len(predictions) == len(dims_per_anchor)
|
147 |
-
res: List[List[Tensor]] = []
|
148 |
-
for pred, dim_per_anchor in zip(predictions, dims_per_anchor):
|
149 |
-
pred = [permute_to_N_HWA_K(x, dim_per_anchor) for x in pred]
|
150 |
-
res.append(pred)
|
151 |
-
return res
|
152 |
-
|
153 |
-
def _ema_update(self, name: str, value: float, initial_value: float, momentum: float = 0.9):
|
154 |
-
"""
|
155 |
-
Apply EMA update to `self.name` using `value`.
|
156 |
-
|
157 |
-
This is mainly used for loss normalizer. In Detectron1, loss is normalized by number
|
158 |
-
of foreground samples in the batch. When batch size is 1 per GPU, #foreground has a
|
159 |
-
large variance and using it lead to lower performance. Therefore we maintain an EMA of
|
160 |
-
#foreground to stabilize the normalizer.
|
161 |
-
|
162 |
-
Args:
|
163 |
-
name: name of the normalizer
|
164 |
-
value: the new value to update
|
165 |
-
initial_value: the initial value to start with
|
166 |
-
momentum: momentum of EMA
|
167 |
-
|
168 |
-
Returns:
|
169 |
-
float: the updated EMA value
|
170 |
-
"""
|
171 |
-
if hasattr(self, name):
|
172 |
-
old = getattr(self, name)
|
173 |
-
else:
|
174 |
-
old = initial_value
|
175 |
-
new = old * momentum + value * (1 - momentum)
|
176 |
-
setattr(self, name, new)
|
177 |
-
return new
|
178 |
-
|
179 |
-
def _decode_per_level_predictions(
|
180 |
-
self,
|
181 |
-
anchors: Boxes,
|
182 |
-
pred_scores: Tensor,
|
183 |
-
pred_deltas: Tensor,
|
184 |
-
score_thresh: float,
|
185 |
-
topk_candidates: int,
|
186 |
-
image_size: Tuple[int, int],
|
187 |
-
) -> Instances:
|
188 |
-
"""
|
189 |
-
Decode boxes and classification predictions of one featuer level, by
|
190 |
-
the following steps:
|
191 |
-
1. filter the predictions based on score threshold and top K scores.
|
192 |
-
2. transform the box regression outputs
|
193 |
-
3. return the predicted scores, classes and boxes
|
194 |
-
|
195 |
-
Args:
|
196 |
-
anchors: Boxes, anchor for this feature level
|
197 |
-
pred_scores: HxWxA,K
|
198 |
-
pred_deltas: HxWxA,4
|
199 |
-
|
200 |
-
Returns:
|
201 |
-
Instances: with field "scores", "pred_boxes", "pred_classes".
|
202 |
-
"""
|
203 |
-
# Apply two filtering to make NMS faster.
|
204 |
-
# 1. Keep boxes with confidence score higher than threshold
|
205 |
-
keep_idxs = pred_scores > score_thresh
|
206 |
-
pred_scores = pred_scores[keep_idxs]
|
207 |
-
topk_idxs = torch.nonzero(keep_idxs) # Kx2
|
208 |
-
|
209 |
-
# 2. Keep top k top scoring boxes only
|
210 |
-
num_topk = min(topk_candidates, topk_idxs.size(0))
|
211 |
-
pred_scores, idxs = pred_scores.topk(num_topk)
|
212 |
-
topk_idxs = topk_idxs[idxs]
|
213 |
-
|
214 |
-
anchor_idxs, classes_idxs = topk_idxs.unbind(dim=1)
|
215 |
-
|
216 |
-
pred_boxes = self.box2box_transform.apply_deltas(
|
217 |
-
pred_deltas[anchor_idxs], anchors.tensor[anchor_idxs]
|
218 |
-
)
|
219 |
-
return Instances(
|
220 |
-
image_size, pred_boxes=Boxes(pred_boxes), scores=pred_scores, pred_classes=classes_idxs
|
221 |
-
)
|
222 |
-
|
223 |
-
def _decode_multi_level_predictions(
|
224 |
-
self,
|
225 |
-
anchors: List[Boxes],
|
226 |
-
pred_scores: List[Tensor],
|
227 |
-
pred_deltas: List[Tensor],
|
228 |
-
score_thresh: float,
|
229 |
-
topk_candidates: int,
|
230 |
-
image_size: Tuple[int, int],
|
231 |
-
) -> Instances:
|
232 |
-
"""
|
233 |
-
Run `_decode_per_level_predictions` for all feature levels and concat the results.
|
234 |
-
"""
|
235 |
-
predictions = [
|
236 |
-
self._decode_per_level_predictions(
|
237 |
-
anchors_i,
|
238 |
-
box_cls_i,
|
239 |
-
box_reg_i,
|
240 |
-
self.test_score_thresh,
|
241 |
-
self.test_topk_candidates,
|
242 |
-
image_size,
|
243 |
-
)
|
244 |
-
# Iterate over every feature level
|
245 |
-
for box_cls_i, box_reg_i, anchors_i in zip(pred_scores, pred_deltas, anchors)
|
246 |
-
]
|
247 |
-
return predictions[0].cat(predictions) # 'Instances.cat' is not scriptale but this is
|
248 |
-
|
249 |
-
def visualize_training(self, batched_inputs, results):
|
250 |
-
"""
|
251 |
-
A function used to visualize ground truth images and final network predictions.
|
252 |
-
It shows ground truth bounding boxes on the original image and up to 20
|
253 |
-
predicted object bounding boxes on the original image.
|
254 |
-
|
255 |
-
Args:
|
256 |
-
batched_inputs (list): a list that contains input to the model.
|
257 |
-
results (List[Instances]): a list of #images elements returned by forward_inference().
|
258 |
-
"""
|
259 |
-
from detectron2.utils.visualizer import Visualizer
|
260 |
-
|
261 |
-
assert len(batched_inputs) == len(
|
262 |
-
results
|
263 |
-
), "Cannot visualize inputs and results of different sizes"
|
264 |
-
storage = get_event_storage()
|
265 |
-
max_boxes = 20
|
266 |
-
|
267 |
-
image_index = 0 # only visualize a single image
|
268 |
-
img = batched_inputs[image_index]["image"]
|
269 |
-
img = convert_image_to_rgb(img.permute(1, 2, 0), self.input_format)
|
270 |
-
v_gt = Visualizer(img, None)
|
271 |
-
v_gt = v_gt.overlay_instances(boxes=batched_inputs[image_index]["instances"].gt_boxes)
|
272 |
-
anno_img = v_gt.get_image()
|
273 |
-
processed_results = detector_postprocess(results[image_index], img.shape[0], img.shape[1])
|
274 |
-
predicted_boxes = processed_results.pred_boxes.tensor.detach().cpu().numpy()
|
275 |
-
|
276 |
-
v_pred = Visualizer(img, None)
|
277 |
-
v_pred = v_pred.overlay_instances(boxes=predicted_boxes[0:max_boxes])
|
278 |
-
prop_img = v_pred.get_image()
|
279 |
-
vis_img = np.vstack((anno_img, prop_img))
|
280 |
-
vis_img = vis_img.transpose(2, 0, 1)
|
281 |
-
vis_name = f"Top: GT bounding boxes; Bottom: {max_boxes} Highest Scoring Results"
|
282 |
-
storage.put_image(vis_name, vis_img)
|
|
|
|
|
|
|
|
|
|
|
|
|
|
|
|
|
|
|
|
|
|
|
|
|
|
|
|
|
|
|
|
|
|
|
|
|
|
|
|
|
|
|
|
|
|
|
|
|
|
|
|
|
|
|
|
|
|
|
|
|
|
|
|
|
|
|
|
|
|
|
|
|
|
|
|
|
|
|
|
|
|
|
|
|
|
|
|
|
|
|
|
|
|
|
|
|
|
|
|
|
|
|
|
|
|
|
|
|
|
|
|
|
|
|
|
|
|
|
|
|
|
|
|
|
|
|
|
|
|
|
|
|
|
|
|
|
|
|
|
|
|
|
|
|
|
|
|
|
|
|
|
|
|
|
|
|
|
|
|
|
|
|
|
|
|
|
|
|
|
|
|
|
|
|
|
|
|
|
|
|
|
|
|
|
|
|
|
|
|
|
|
|
|
|
|
|
|
|
|
|
|
|
|
|
|
|
|
|
|
|
|
|
|
|
|
|
|
|
|
|
|
|
|
|
|
|
|
|
|
|
|
|
|
|
|
|
|
|
|
|
|
|
|
|
|
|
|
|
|
|
|
|
|
|
|
|
|
|
|
|
|
|
|
|
|
|
|
|
|
|
|
|
|
|
|
|
|
|
|
|
|
|
|
|
|
|
|
|
|
|
|
|
|
|
|
|
|
|
|
|
|
|
|
|
|
|
|
|
|
|
|
|
|
|
|
|
|
|
|
|
|
|
|
|
|
|
|
|
|
|
|
|
|
|
|
|
|
|
|
|
|
|
|
|
|
|
|
|
|
|
|
|
|
|
|
|
|
|
|
|
|
|
|
|
|
|
|
|
|
|
|
|
|
|
|
|
|
|
|
|
|
|
|
|
|
|
|
|
|
|
|
|
|
|
|
|
|
|
|
|
|
|
|
|
|
|
|
|
|
|
|
|
|
|
|
|
|
|
|
|
|
|
|
|
|
|
|
|
|
|
|
|
|
|
|
|
|
|
|
|
|
|
|
|
|
|
|
|
|
|
|
|
|
|
|
|
|
|
|
|
|
|
|
|
|
|
|
|
|
|
|
|
|
|
|
|
|
|
|
|
|
|
|
|
|
|
|
|
|
|
|
|
|
|
|
|
|
|
|
|
|
|
|
|
|
|
|
|
|
|
|
|
|
|
|
|
|
|
|
|
|
|
|
|
|
|
|
|
|
|
|
|
|
|
|
|
|
|
|
|
|
|
|
|
|
|
|
|
|
|
|
|
|
|
|
|
|
|
|
|
|
|
|
|
|
spaces/Awiny/Image2Paragraph/models/grit_src/third_party/CenterNet2/detectron2/modeling/roi_heads/mask_head.py
DELETED
@@ -1,292 +0,0 @@
|
|
1 |
-
# Copyright (c) Facebook, Inc. and its affiliates.
|
2 |
-
from typing import List
|
3 |
-
import fvcore.nn.weight_init as weight_init
|
4 |
-
import torch
|
5 |
-
from torch import nn
|
6 |
-
from torch.nn import functional as F
|
7 |
-
|
8 |
-
from detectron2.config import configurable
|
9 |
-
from detectron2.layers import Conv2d, ConvTranspose2d, ShapeSpec, cat, get_norm
|
10 |
-
from detectron2.structures import Instances
|
11 |
-
from detectron2.utils.events import get_event_storage
|
12 |
-
from detectron2.utils.registry import Registry
|
13 |
-
|
14 |
-
__all__ = [
|
15 |
-
"BaseMaskRCNNHead",
|
16 |
-
"MaskRCNNConvUpsampleHead",
|
17 |
-
"build_mask_head",
|
18 |
-
"ROI_MASK_HEAD_REGISTRY",
|
19 |
-
]
|
20 |
-
|
21 |
-
|
22 |
-
ROI_MASK_HEAD_REGISTRY = Registry("ROI_MASK_HEAD")
|
23 |
-
ROI_MASK_HEAD_REGISTRY.__doc__ = """
|
24 |
-
Registry for mask heads, which predicts instance masks given
|
25 |
-
per-region features.
|
26 |
-
|
27 |
-
The registered object will be called with `obj(cfg, input_shape)`.
|
28 |
-
"""
|
29 |
-
|
30 |
-
|
31 |
-
@torch.jit.unused
|
32 |
-
def mask_rcnn_loss(pred_mask_logits: torch.Tensor, instances: List[Instances], vis_period: int = 0):
|
33 |
-
"""
|
34 |
-
Compute the mask prediction loss defined in the Mask R-CNN paper.
|
35 |
-
|
36 |
-
Args:
|
37 |
-
pred_mask_logits (Tensor): A tensor of shape (B, C, Hmask, Wmask) or (B, 1, Hmask, Wmask)
|
38 |
-
for class-specific or class-agnostic, where B is the total number of predicted masks
|
39 |
-
in all images, C is the number of foreground classes, and Hmask, Wmask are the height
|
40 |
-
and width of the mask predictions. The values are logits.
|
41 |
-
instances (list[Instances]): A list of N Instances, where N is the number of images
|
42 |
-
in the batch. These instances are in 1:1
|
43 |
-
correspondence with the pred_mask_logits. The ground-truth labels (class, box, mask,
|
44 |
-
...) associated with each instance are stored in fields.
|
45 |
-
vis_period (int): the period (in steps) to dump visualization.
|
46 |
-
|
47 |
-
Returns:
|
48 |
-
mask_loss (Tensor): A scalar tensor containing the loss.
|
49 |
-
"""
|
50 |
-
cls_agnostic_mask = pred_mask_logits.size(1) == 1
|
51 |
-
total_num_masks = pred_mask_logits.size(0)
|
52 |
-
mask_side_len = pred_mask_logits.size(2)
|
53 |
-
assert pred_mask_logits.size(2) == pred_mask_logits.size(3), "Mask prediction must be square!"
|
54 |
-
|
55 |
-
gt_classes = []
|
56 |
-
gt_masks = []
|
57 |
-
for instances_per_image in instances:
|
58 |
-
if len(instances_per_image) == 0:
|
59 |
-
continue
|
60 |
-
if not cls_agnostic_mask:
|
61 |
-
gt_classes_per_image = instances_per_image.gt_classes.to(dtype=torch.int64)
|
62 |
-
gt_classes.append(gt_classes_per_image)
|
63 |
-
|
64 |
-
gt_masks_per_image = instances_per_image.gt_masks.crop_and_resize(
|
65 |
-
instances_per_image.proposal_boxes.tensor, mask_side_len
|
66 |
-
).to(device=pred_mask_logits.device)
|
67 |
-
# A tensor of shape (N, M, M), N=#instances in the image; M=mask_side_len
|
68 |
-
gt_masks.append(gt_masks_per_image)
|
69 |
-
|
70 |
-
if len(gt_masks) == 0:
|
71 |
-
return pred_mask_logits.sum() * 0
|
72 |
-
|
73 |
-
gt_masks = cat(gt_masks, dim=0)
|
74 |
-
|
75 |
-
if cls_agnostic_mask:
|
76 |
-
pred_mask_logits = pred_mask_logits[:, 0]
|
77 |
-
else:
|
78 |
-
indices = torch.arange(total_num_masks)
|
79 |
-
gt_classes = cat(gt_classes, dim=0)
|
80 |
-
pred_mask_logits = pred_mask_logits[indices, gt_classes]
|
81 |
-
|
82 |
-
if gt_masks.dtype == torch.bool:
|
83 |
-
gt_masks_bool = gt_masks
|
84 |
-
else:
|
85 |
-
# Here we allow gt_masks to be float as well (depend on the implementation of rasterize())
|
86 |
-
gt_masks_bool = gt_masks > 0.5
|
87 |
-
gt_masks = gt_masks.to(dtype=torch.float32)
|
88 |
-
|
89 |
-
# Log the training accuracy (using gt classes and 0.5 threshold)
|
90 |
-
mask_incorrect = (pred_mask_logits > 0.0) != gt_masks_bool
|
91 |
-
mask_accuracy = 1 - (mask_incorrect.sum().item() / max(mask_incorrect.numel(), 1.0))
|
92 |
-
num_positive = gt_masks_bool.sum().item()
|
93 |
-
false_positive = (mask_incorrect & ~gt_masks_bool).sum().item() / max(
|
94 |
-
gt_masks_bool.numel() - num_positive, 1.0
|
95 |
-
)
|
96 |
-
false_negative = (mask_incorrect & gt_masks_bool).sum().item() / max(num_positive, 1.0)
|
97 |
-
|
98 |
-
storage = get_event_storage()
|
99 |
-
storage.put_scalar("mask_rcnn/accuracy", mask_accuracy)
|
100 |
-
storage.put_scalar("mask_rcnn/false_positive", false_positive)
|
101 |
-
storage.put_scalar("mask_rcnn/false_negative", false_negative)
|
102 |
-
if vis_period > 0 and storage.iter % vis_period == 0:
|
103 |
-
pred_masks = pred_mask_logits.sigmoid()
|
104 |
-
vis_masks = torch.cat([pred_masks, gt_masks], axis=2)
|
105 |
-
name = "Left: mask prediction; Right: mask GT"
|
106 |
-
for idx, vis_mask in enumerate(vis_masks):
|
107 |
-
vis_mask = torch.stack([vis_mask] * 3, axis=0)
|
108 |
-
storage.put_image(name + f" ({idx})", vis_mask)
|
109 |
-
|
110 |
-
mask_loss = F.binary_cross_entropy_with_logits(pred_mask_logits, gt_masks, reduction="mean")
|
111 |
-
return mask_loss
|
112 |
-
|
113 |
-
|
114 |
-
def mask_rcnn_inference(pred_mask_logits: torch.Tensor, pred_instances: List[Instances]):
|
115 |
-
"""
|
116 |
-
Convert pred_mask_logits to estimated foreground probability masks while also
|
117 |
-
extracting only the masks for the predicted classes in pred_instances. For each
|
118 |
-
predicted box, the mask of the same class is attached to the instance by adding a
|
119 |
-
new "pred_masks" field to pred_instances.
|
120 |
-
|
121 |
-
Args:
|
122 |
-
pred_mask_logits (Tensor): A tensor of shape (B, C, Hmask, Wmask) or (B, 1, Hmask, Wmask)
|
123 |
-
for class-specific or class-agnostic, where B is the total number of predicted masks
|
124 |
-
in all images, C is the number of foreground classes, and Hmask, Wmask are the height
|
125 |
-
and width of the mask predictions. The values are logits.
|
126 |
-
pred_instances (list[Instances]): A list of N Instances, where N is the number of images
|
127 |
-
in the batch. Each Instances must have field "pred_classes".
|
128 |
-
|
129 |
-
Returns:
|
130 |
-
None. pred_instances will contain an extra "pred_masks" field storing a mask of size (Hmask,
|
131 |
-
Wmask) for predicted class. Note that the masks are returned as a soft (non-quantized)
|
132 |
-
masks the resolution predicted by the network; post-processing steps, such as resizing
|
133 |
-
the predicted masks to the original image resolution and/or binarizing them, is left
|
134 |
-
to the caller.
|
135 |
-
"""
|
136 |
-
cls_agnostic_mask = pred_mask_logits.size(1) == 1
|
137 |
-
|
138 |
-
if cls_agnostic_mask:
|
139 |
-
mask_probs_pred = pred_mask_logits.sigmoid()
|
140 |
-
else:
|
141 |
-
# Select masks corresponding to the predicted classes
|
142 |
-
num_masks = pred_mask_logits.shape[0]
|
143 |
-
class_pred = cat([i.pred_classes for i in pred_instances])
|
144 |
-
indices = torch.arange(num_masks, device=class_pred.device)
|
145 |
-
mask_probs_pred = pred_mask_logits[indices, class_pred][:, None].sigmoid()
|
146 |
-
# mask_probs_pred.shape: (B, 1, Hmask, Wmask)
|
147 |
-
|
148 |
-
num_boxes_per_image = [len(i) for i in pred_instances]
|
149 |
-
mask_probs_pred = mask_probs_pred.split(num_boxes_per_image, dim=0)
|
150 |
-
|
151 |
-
for prob, instances in zip(mask_probs_pred, pred_instances):
|
152 |
-
instances.pred_masks = prob # (1, Hmask, Wmask)
|
153 |
-
|
154 |
-
|
155 |
-
class BaseMaskRCNNHead(nn.Module):
|
156 |
-
"""
|
157 |
-
Implement the basic Mask R-CNN losses and inference logic described in :paper:`Mask R-CNN`
|
158 |
-
"""
|
159 |
-
|
160 |
-
@configurable
|
161 |
-
def __init__(self, *, loss_weight: float = 1.0, vis_period: int = 0):
|
162 |
-
"""
|
163 |
-
NOTE: this interface is experimental.
|
164 |
-
|
165 |
-
Args:
|
166 |
-
loss_weight (float): multiplier of the loss
|
167 |
-
vis_period (int): visualization period
|
168 |
-
"""
|
169 |
-
super().__init__()
|
170 |
-
self.vis_period = vis_period
|
171 |
-
self.loss_weight = loss_weight
|
172 |
-
|
173 |
-
@classmethod
|
174 |
-
def from_config(cls, cfg, input_shape):
|
175 |
-
return {"vis_period": cfg.VIS_PERIOD}
|
176 |
-
|
177 |
-
def forward(self, x, instances: List[Instances]):
|
178 |
-
"""
|
179 |
-
Args:
|
180 |
-
x: input region feature(s) provided by :class:`ROIHeads`.
|
181 |
-
instances (list[Instances]): contains the boxes & labels corresponding
|
182 |
-
to the input features.
|
183 |
-
Exact format is up to its caller to decide.
|
184 |
-
Typically, this is the foreground instances in training, with
|
185 |
-
"proposal_boxes" field and other gt annotations.
|
186 |
-
In inference, it contains boxes that are already predicted.
|
187 |
-
|
188 |
-
Returns:
|
189 |
-
A dict of losses in training. The predicted "instances" in inference.
|
190 |
-
"""
|
191 |
-
x = self.layers(x)
|
192 |
-
if self.training:
|
193 |
-
return {"loss_mask": mask_rcnn_loss(x, instances, self.vis_period) * self.loss_weight}
|
194 |
-
else:
|
195 |
-
mask_rcnn_inference(x, instances)
|
196 |
-
return instances
|
197 |
-
|
198 |
-
def layers(self, x):
|
199 |
-
"""
|
200 |
-
Neural network layers that makes predictions from input features.
|
201 |
-
"""
|
202 |
-
raise NotImplementedError
|
203 |
-
|
204 |
-
|
205 |
-
# To get torchscript support, we make the head a subclass of `nn.Sequential`.
|
206 |
-
# Therefore, to add new layers in this head class, please make sure they are
|
207 |
-
# added in the order they will be used in forward().
|
208 |
-
@ROI_MASK_HEAD_REGISTRY.register()
|
209 |
-
class MaskRCNNConvUpsampleHead(BaseMaskRCNNHead, nn.Sequential):
|
210 |
-
"""
|
211 |
-
A mask head with several conv layers, plus an upsample layer (with `ConvTranspose2d`).
|
212 |
-
Predictions are made with a final 1x1 conv layer.
|
213 |
-
"""
|
214 |
-
|
215 |
-
@configurable
|
216 |
-
def __init__(self, input_shape: ShapeSpec, *, num_classes, conv_dims, conv_norm="", **kwargs):
|
217 |
-
"""
|
218 |
-
NOTE: this interface is experimental.
|
219 |
-
|
220 |
-
Args:
|
221 |
-
input_shape (ShapeSpec): shape of the input feature
|
222 |
-
num_classes (int): the number of foreground classes (i.e. background is not
|
223 |
-
included). 1 if using class agnostic prediction.
|
224 |
-
conv_dims (list[int]): a list of N>0 integers representing the output dimensions
|
225 |
-
of N-1 conv layers and the last upsample layer.
|
226 |
-
conv_norm (str or callable): normalization for the conv layers.
|
227 |
-
See :func:`detectron2.layers.get_norm` for supported types.
|
228 |
-
"""
|
229 |
-
super().__init__(**kwargs)
|
230 |
-
assert len(conv_dims) >= 1, "conv_dims have to be non-empty!"
|
231 |
-
|
232 |
-
self.conv_norm_relus = []
|
233 |
-
|
234 |
-
cur_channels = input_shape.channels
|
235 |
-
for k, conv_dim in enumerate(conv_dims[:-1]):
|
236 |
-
conv = Conv2d(
|
237 |
-
cur_channels,
|
238 |
-
conv_dim,
|
239 |
-
kernel_size=3,
|
240 |
-
stride=1,
|
241 |
-
padding=1,
|
242 |
-
bias=not conv_norm,
|
243 |
-
norm=get_norm(conv_norm, conv_dim),
|
244 |
-
activation=nn.ReLU(),
|
245 |
-
)
|
246 |
-
self.add_module("mask_fcn{}".format(k + 1), conv)
|
247 |
-
self.conv_norm_relus.append(conv)
|
248 |
-
cur_channels = conv_dim
|
249 |
-
|
250 |
-
self.deconv = ConvTranspose2d(
|
251 |
-
cur_channels, conv_dims[-1], kernel_size=2, stride=2, padding=0
|
252 |
-
)
|
253 |
-
self.add_module("deconv_relu", nn.ReLU())
|
254 |
-
cur_channels = conv_dims[-1]
|
255 |
-
|
256 |
-
self.predictor = Conv2d(cur_channels, num_classes, kernel_size=1, stride=1, padding=0)
|
257 |
-
|
258 |
-
for layer in self.conv_norm_relus + [self.deconv]:
|
259 |
-
weight_init.c2_msra_fill(layer)
|
260 |
-
# use normal distribution initialization for mask prediction layer
|
261 |
-
nn.init.normal_(self.predictor.weight, std=0.001)
|
262 |
-
if self.predictor.bias is not None:
|
263 |
-
nn.init.constant_(self.predictor.bias, 0)
|
264 |
-
|
265 |
-
@classmethod
|
266 |
-
def from_config(cls, cfg, input_shape):
|
267 |
-
ret = super().from_config(cfg, input_shape)
|
268 |
-
conv_dim = cfg.MODEL.ROI_MASK_HEAD.CONV_DIM
|
269 |
-
num_conv = cfg.MODEL.ROI_MASK_HEAD.NUM_CONV
|
270 |
-
ret.update(
|
271 |
-
conv_dims=[conv_dim] * (num_conv + 1), # +1 for ConvTranspose
|
272 |
-
conv_norm=cfg.MODEL.ROI_MASK_HEAD.NORM,
|
273 |
-
input_shape=input_shape,
|
274 |
-
)
|
275 |
-
if cfg.MODEL.ROI_MASK_HEAD.CLS_AGNOSTIC_MASK:
|
276 |
-
ret["num_classes"] = 1
|
277 |
-
else:
|
278 |
-
ret["num_classes"] = cfg.MODEL.ROI_HEADS.NUM_CLASSES
|
279 |
-
return ret
|
280 |
-
|
281 |
-
def layers(self, x):
|
282 |
-
for layer in self:
|
283 |
-
x = layer(x)
|
284 |
-
return x
|
285 |
-
|
286 |
-
|
287 |
-
def build_mask_head(cfg, input_shape):
|
288 |
-
"""
|
289 |
-
Build a mask head defined by `cfg.MODEL.ROI_MASK_HEAD.NAME`.
|
290 |
-
"""
|
291 |
-
name = cfg.MODEL.ROI_MASK_HEAD.NAME
|
292 |
-
return ROI_MASK_HEAD_REGISTRY.get(name)(cfg, input_shape)
|
|
|
|
|
|
|
|
|
|
|
|
|
|
|
|
|
|
|
|
|
|
|
|
|
|
|
|
|
|
|
|
|
|
|
|
|
|
|
|
|
|
|
|
|
|
|
|
|
|
|
|
|
|
|
|
|
|
|
|
|
|
|
|
|
|
|
|
|
|
|
|
|
|
|
|
|
|
|
|
|
|
|
|
|
|
|
|
|
|
|
|
|
|
|
|
|
|
|
|
|
|
|
|
|
|
|
|
|
|
|
|
|
|
|
|
|
|
|
|
|
|
|
|
|
|
|
|
|
|
|
|
|
|
|
|
|
|
|
|
|
|
|
|
|
|
|
|
|
|
|
|
|
|
|
|
|
|
|
|
|
|
|
|
|
|
|
|
|
|
|
|
|
|
|
|
|
|
|
|
|
|
|
|
|
|
|
|
|
|
|
|
|
|
|
|
|
|
|
|
|
|
|
|
|
|
|
|
|
|
|
|
|
|
|
|
|
|
|
|
|
|
|
|
|
|
|
|
|
|
|
|
|
|
|
|
|
|
|
|
|
|
|
|
|
|
|
|
|
|
|
|
|
|
|
|
|
|
|
|
|
|
|
|
|
|
|
|
|
|
|
|
|
|
|
|
|
|
|
|
|
|
|
|
|
|
|
|
|
|
|
|
|
|
|
|
|
|
|
|
|
|
|
|
|
|
|
|
|
|
|
|
|
|
|
|
|
|
|
|
|
|
|
|
|
|
|
|
|
|
|
|
|
|
|
|
|
|
|
|
|
|
|
|
|
|
|
|
|
|
|
|
|
|
|
|
|
|
|
|
|
|
|
|
|
|
|
|
|
|
|
|
|
|
|
|
|
|
|
|
|
|
|
|
|
|
|
|
|
|
|
|
|
|
|
|
|
|
|
|
|
|
|
|
|
|
|
|
|
|
|
|
|
|
|
|
|
|
|
|
|
|
|
|
|
|
|
|
|
|
|
|
|
|
|
|
|
|
|
|
|
|
|
|
|
|
|
|
|
|
|
|
|
|
|
|
|
|
|
|
|
|
|
|
|
|
|
|
|
|
|
|
|
|
|
|
|
|
|
|
|
|
|
|
|
|
|
|
|
|
|
|
|
|
|
|
|
|
|
|
|
|
|
|
|
|
|
|
|
|
|
|
|
|
|
|
|
|
|
|
|
|
|
|
|
|
|
|
|
|
|
|
|
|
|
|
|
|
|
|
|
|
|
|
|
|
|
|
|
|
|
|
|
|
|
|
|
|
|
|
|
|
|
|
|
|
|
|
|
|
|
|
|
|
|
|
|
|
|
|
|
|
|
|
|
|
spaces/Awiny/Image2Paragraph/models/grit_src/third_party/CenterNet2/detectron2/utils/serialize.py
DELETED
@@ -1,32 +0,0 @@
|
|
1 |
-
# Copyright (c) Facebook, Inc. and its affiliates.
|
2 |
-
import cloudpickle
|
3 |
-
|
4 |
-
|
5 |
-
class PicklableWrapper(object):
|
6 |
-
"""
|
7 |
-
Wrap an object to make it more picklable, note that it uses
|
8 |
-
heavy weight serialization libraries that are slower than pickle.
|
9 |
-
It's best to use it only on closures (which are usually not picklable).
|
10 |
-
|
11 |
-
This is a simplified version of
|
12 |
-
https://github.com/joblib/joblib/blob/master/joblib/externals/loky/cloudpickle_wrapper.py
|
13 |
-
"""
|
14 |
-
|
15 |
-
def __init__(self, obj):
|
16 |
-
while isinstance(obj, PicklableWrapper):
|
17 |
-
# Wrapping an object twice is no-op
|
18 |
-
obj = obj._obj
|
19 |
-
self._obj = obj
|
20 |
-
|
21 |
-
def __reduce__(self):
|
22 |
-
s = cloudpickle.dumps(self._obj)
|
23 |
-
return cloudpickle.loads, (s,)
|
24 |
-
|
25 |
-
def __call__(self, *args, **kwargs):
|
26 |
-
return self._obj(*args, **kwargs)
|
27 |
-
|
28 |
-
def __getattr__(self, attr):
|
29 |
-
# Ensure that the wrapped object can be used seamlessly as the previous object.
|
30 |
-
if attr not in ["_obj"]:
|
31 |
-
return getattr(self._obj, attr)
|
32 |
-
return getattr(self, attr)
|
|
|
|
|
|
|
|
|
|
|
|
|
|
|
|
|
|
|
|
|
|
|
|
|
|
|
|
|
|
|
|
|
|
|
|
|
|
|
|
|
|
|
|
|
|
|
|
|
|
|
|
|
|
|
|
|
|
|
|
|
|
|
|
|
|
spaces/Awiny/Image2Paragraph/models/grit_src/third_party/CenterNet2/dev/packaging/build_wheel.sh
DELETED
@@ -1,31 +0,0 @@
|
|
1 |
-
#!/bin/bash
|
2 |
-
# Copyright (c) Facebook, Inc. and its affiliates.
|
3 |
-
set -ex
|
4 |
-
|
5 |
-
ldconfig # https://github.com/NVIDIA/nvidia-docker/issues/854
|
6 |
-
|
7 |
-
script_dir="$( cd "$( dirname "${BASH_SOURCE[0]}" )" >/dev/null 2>&1 && pwd )"
|
8 |
-
. "$script_dir/pkg_helpers.bash"
|
9 |
-
|
10 |
-
echo "Build Settings:"
|
11 |
-
echo "CU_VERSION: $CU_VERSION" # e.g. cu101
|
12 |
-
echo "D2_VERSION_SUFFIX: $D2_VERSION_SUFFIX" # e.g. +cu101 or ""
|
13 |
-
echo "PYTHON_VERSION: $PYTHON_VERSION" # e.g. 3.6
|
14 |
-
echo "PYTORCH_VERSION: $PYTORCH_VERSION" # e.g. 1.4
|
15 |
-
|
16 |
-
setup_cuda
|
17 |
-
setup_wheel_python
|
18 |
-
|
19 |
-
yum install ninja-build -y
|
20 |
-
ln -sv /usr/bin/ninja-build /usr/bin/ninja || true
|
21 |
-
|
22 |
-
pip_install pip numpy -U
|
23 |
-
pip_install "torch==$PYTORCH_VERSION" \
|
24 |
-
-f https://download.pytorch.org/whl/"$CU_VERSION"/torch_stable.html
|
25 |
-
|
26 |
-
# use separate directories to allow parallel build
|
27 |
-
BASE_BUILD_DIR=build/$CU_VERSION-py$PYTHON_VERSION-pt$PYTORCH_VERSION
|
28 |
-
python setup.py \
|
29 |
-
build -b "$BASE_BUILD_DIR" \
|
30 |
-
bdist_wheel -b "$BASE_BUILD_DIR/build_dist" -d "wheels/$CU_VERSION/torch$PYTORCH_VERSION"
|
31 |
-
rm -rf "$BASE_BUILD_DIR"
|
|
|
|
|
|
|
|
|
|
|
|
|
|
|
|
|
|
|
|
|
|
|
|
|
|
|
|
|
|
|
|
|
|
|
|
|
|
|
|
|
|
|
|
|
|
|
|
|
|
|
|
|
|
|
|
|
|
|
|
|
|
|
|
spaces/Bala2-03-2003/AIBALA/README.md
DELETED
@@ -1,12 +0,0 @@
|
|
1 |
-
---
|
2 |
-
title: AIBALA
|
3 |
-
emoji: 👁
|
4 |
-
colorFrom: green
|
5 |
-
colorTo: indigo
|
6 |
-
sdk: gradio
|
7 |
-
sdk_version: 3.39.0
|
8 |
-
app_file: app.py
|
9 |
-
pinned: false
|
10 |
-
---
|
11 |
-
|
12 |
-
Check out the configuration reference at https://huggingface.co/docs/hub/spaces-config-reference
|
|
|
|
|
|
|
|
|
|
|
|
|
|
|
|
|
|
|
|
|
|
|
|
|
|
spaces/Benson/text-generation/Examples/Carx Street 3 Apk.md
DELETED
@@ -1,105 +0,0 @@
|
|
1 |
-
|
2 |
-
<h1>CarX Street 3 APK: Una revisión del último juego de carreras de calle</h1>
|
3 |
-
<p>Si usted es un fan de los juegos de carreras callejeras, es posible que haya oído hablar de CarX Street 3 APK, la última entrega de la serie CarX. Este juego se encuentra actualmente en pruebas beta abiertas y promete ofrecer una experiencia de carreras emocionante y realista en un mundo abierto dinámico. En este artículo, vamos a revisar las características, proceso de descarga, pros y contras, y preguntas frecuentes de CarX Street 3 APK.</p>
|
4 |
-
<h2>¿Qué es CarX Street 3 APK? </h2>
|
5 |
-
<p>CarX Street 3 APK es un juego para Android desarrollado por CarX Technologies, LLC, los creadores de CarX Drift Racing 2. Es un juego de carreras callejeras que le permite abrazar la libertad de ser un corredor callejero en el mundo abierto de Sunset City. Puedes aceptar el desafío y convertirte en la leyenda de la ciudad al competir en carreras realistas en carreteras y calles de la ciudad, así como en carreras de deriva de alta velocidad. También puede construir el coche de sus sueños utilizando la afinación de piezas que desbloquea toda la física del comportamiento del coche CarX Technology. Puedes explorar cada rincón del enorme mundo y disfrutar del dinámico cambio de día/noche. </p>
|
6 |
-
<h2>carx street 3 apk</h2><br /><p><b><b>Download</b> > <a href="https://bltlly.com/2v6Kl5">https://bltlly.com/2v6Kl5</a></b></p><br /><br />
|
7 |
-
<h3>Características de CarX Street 3 APK</h3>
|
8 |
-
<p>CarX Street 3 APK tiene muchas características que lo convierten en un juego emocionante e inmersivo para los entusiastas de las carreras callejeras. Estos son algunos de ellos:</p>
|
9 |
-
<h4>Modo de carrera</h4>
|
10 |
-
<p>Usted puede elegir su propio camino en el modo de carrera, donde se puede conducir a la velocidad máxima o la deriva a través de turnos. Puedes unirte a clubes, derrotar jefes y demostrar a todos que eres el mejor conductor de la ciudad. También puedes comprar casas para tus coches y reunir colecciones para cada modo de carrera. Puedes cargar combustible con el combustible adecuado para la próxima carrera en las gasolineras de la ciudad. </p>
|
11 |
-
<h4>Sintonización de coches</h4>
|
12 |
-
<p>Puedes personalizar tu coche para que se adapte a tus preferencias y necesidades para cada carrera. Puedes intercambiar piezas y engañar a tu coche para una carrera específica. Puede actualizar el motor, la transmisión, el cuerpo, la suspensión y los neumáticos. También puede cambiar el motor de su automóvil único. </p>
|
13 |
-
<h4>Personalización visual</h4>
|
14 |
-
|
15 |
-
<h4>Física y gráficos realistas</h4>
|
16 |
-
<p>El juego cuenta con una física impresionante y controles que te hacen sentir como si estuvieras conduciendo un coche real. Puedes admirar los modernos gráficos de alta calidad y el enorme mundo abierto que ofrece impresionantes vistas y detalles. </p>
|
17 |
-
<h4>Exploración del mundo abierto</h4>
|
18 |
-
<p>Puedes explorar cada rincón de Sunset City a cualquier hora del día o de la noche. Puedes descubrir lugares ocultos, atajos, rampas, saltos y secretos. También puedes interactuar con otros jugadores y PNJ de la ciudad. </p>
|
19 |
-
<h2> Cómo descargar e instalar CarX Street 3 APK? </h2>
|
20 |
-
<p>Si desea probar CarX Street 3 APK en su dispositivo Android, es necesario seguir estos pasos:</p>
|
21 |
-
<p></p>
|
22 |
-
<h3>Requisitos para CarX Street 3 APK</h3>
|
23 |
-
<p> <p>Antes de descargar e instalar CarX Street 3 APK, es necesario asegurarse de que el dispositivo cumple con los siguientes requisitos:</p>
|
24 |
-
<tabla>
|
25 |
-
<tr>
|
26 |
-
<th>Requisito</th>
|
27 |
-
<th>Mínimo</th>
|
28 |
-
<th>Recomendado</th>
|
29 |
-
</tr>
|
30 |
-
<tr>
|
31 |
-
<td>Sistema operativo</td>
|
32 |
-
<td>Android 6.0 o superior</td>
|
33 |
-
<td>Android 8.0 o superior</td>
|
34 |
-
</tr>
|
35 |
-
<tr>
|
36 |
-
<td>RAM</td>
|
37 |
-
<td>2 GB</td>
|
38 |
-
<td>4 GB o más</td>
|
39 |
-
</tr>
|
40 |
-
<tr>
|
41 |
-
<td>Espacio de almacenamiento</td>
|
42 |
-
<td>1.5 GB</td>
|
43 |
-
<td>2 GB o más</td>
|
44 |
-
</tr>
|
45 |
-
<tr>
|
46 |
-
<td>Conexión a Internet</td>
|
47 |
-
<td>Requerido para funciones en línea</td>
|
48 |
-
<td>Requerido para funciones en línea</td>
|
49 |
-
</tr>
|
50 |
-
<tr>
|
51 |
-
<td>Servicios de Google Play</td>
|
52 |
-
<td>Necesario para la instalación y las actualizaciones</td>
|
53 |
-
<td>Necesario para la instalación y las actualizaciones</td>
|
54 |
-
</tr>
|
55 |
-
</tabla>
|
56 |
-
<h3> Pasos para descargar e instalar CarX Street 3 APK</h3>
|
57 |
-
<p>Para descargar e instalar CarX Street 3 APK en su dispositivo, debe seguir estos pasos:</p>
|
58 |
-
<ol>
|
59 |
-
<li>Vaya al sitio web oficial de CarX Technologies, LLC, [7](https://carx-online.com/), y haga clic en el botón "Descargar". </li>
|
60 |
-
<li>Seleccione la opción "CarX Street" y elija la opción "APK". </li>
|
61 |
-
<li>Serás redirigido a una página de descarga donde puedes elegir un enlace espejo para descargar el archivo APK. </li>
|
62 |
-
|
63 |
-
<li>Es posible que tenga que habilitar la opción "Fuentes desconocidas" en la configuración del dispositivo para permitir la instalación de aplicaciones desde fuentes distintas de Google Play.</li>
|
64 |
-
<li>Siga las instrucciones en pantalla para completar el proceso de instalación. </li>
|
65 |
-
<li> Ahora puede iniciar el juego y disfrutar de la experiencia de carreras callejeras. </li>
|
66 |
-
</ol>
|
67 |
-
<h2>Pros y contras de CarX Street 3 APK</h2>
|
68 |
-
<p>CarX Street 3 APK es un juego que tiene muchas ventajas y desventajas. Estos son algunos de ellos:</p>
|
69 |
-
<h3>Pros de CarX Street 3 APK</h3>
|
70 |
-
<ul>
|
71 |
-
<li>El juego es gratis para descargar y jugar, con compras opcionales en la aplicación para características adicionales y contenido. </li>
|
72 |
-
<li>El juego ofrece una experiencia de carreras callejeras realista e inmersiva con gráficos, física y controles de alta calidad. </li>
|
73 |
-
<li> El juego tiene una variedad de coches, piezas y opciones de personalización que le permiten crear su propio coche único. </li>
|
74 |
-
<li>El juego tiene un mundo abierto dinámico que puedes explorar a cualquier hora del día o de la noche, con lugares ocultos, secretos e interacciones. </li>
|
75 |
-
<li> El juego tiene un modo de carrera que le permite elegir su propio camino, unirse a los clubes, derrotar a los jefes, y convertirse en la leyenda de Sunset City.</li>
|
76 |
-
<li>El juego tiene un modo online que te permite competir con otros jugadores en carreras y derivas en tiempo real. </li>
|
77 |
-
<h3>Contras de CarX Street 3 APK</h3>
|
78 |
-
<ul>
|
79 |
-
<li>El juego todavía está en pruebas beta abiertas, lo que significa que puede tener algunos errores, fallas y errores que afectan el juego. </li>
|
80 |
-
<li>El juego requiere una conexión a Internet estable para las funciones en línea, que pueden consumir datos y batería. </li>
|
81 |
-
<li>El juego puede no ser compatible con algunos dispositivos o sistemas operativos, o puede no funcionar sin problemas en dispositivos de gama baja. </li>
|
82 |
-
<li> El juego puede tener algunos anuncios que pueden interrumpir el juego o afectar la experiencia del usuario. </li>
|
83 |
-
<li>El juego puede tener algún contenido o características que se bloquean detrás de los muros de pago o requieren dinero real para acceder. </li>
|
84 |
-
<h2>Conclusión</h2>
|
85 |
-
|
86 |
-
<h2>Preguntas frecuentes</h2>
|
87 |
-
<p>Aquí hay algunas preguntas frecuentes sobre CarX Street 3 APK:</p>
|
88 |
-
<ol>
|
89 |
-
<li><b>Es CarX Street 3 APK seguro de usar? </b></li>
|
90 |
-
<p>CarX Street 3 APK es seguro para <p>CarX Street 3 APK es seguro de usar si lo descarga desde el sitio web oficial de CarX Technologies, LLC, [7](https://carx-online.com/). Sin embargo, si lo descarga de otras fuentes, puede correr el riesgo de obtener un archivo dañado o infectado que puede dañar su dispositivo o comprometer sus datos. Siempre debe escanear el archivo APK con un software antivirus confiable antes de instalarlo en su dispositivo. </p>
|
91 |
-
<li><b>¿Cómo puedo actualizar CarX Street 3 APK? </b></li>
|
92 |
-
<p>Puede actualizar CarX Street 3 APK visitando el sitio web oficial de CarX Technologies, LLC, [7](https://carx-online.com/), y descargar la última versión del archivo APK. También puede habilitar la opción de actualización automática en la configuración del dispositivo para recibir notificaciones y actualizaciones de los servicios de Google Play. Sin embargo, siempre debes hacer una copia de seguridad de tus datos y progreso antes de actualizar el juego, ya que algunas actualizaciones pueden causar problemas de compatibilidad o pérdida de datos. </p>
|
93 |
-
<li><b>¿Puedo jugar CarX Street 3 APK sin conexión? </b></li>
|
94 |
-
<p>No, no puede jugar CarX Street 3 APK sin conexión. El juego requiere una conexión a Internet estable para funciones en línea, como el modo multijugador, tablas de clasificación, logros y eventos. También necesitas una conexión a Internet para descargar e instalar el juego y sus actualizaciones. Si pierdes tu conexión a Internet mientras juegas, puedes experimentar retardo, problemas técnicos o desconexión. </p>
|
95 |
-
<li><b>¿Puedo jugar CarX Street 3 APK en PC? </b></li>
|
96 |
-
|
97 |
-
<li><b> ¿Cuáles son algunas alternativas a CarX Street 3 APK? </b></li>
|
98 |
-
<p>Si usted está buscando algunas alternativas a CarX Street 3 APK, es posible que desee echa un vistazo a estos otros juegos de carreras de calle para Android:</p>
|
99 |
-
<ul>
|
100 |
-
<li><b>Asphalt 9: Legends</b>: Este es un juego de carreras de ritmo rápido y lleno de acción que te permite conducir algunos de los coches más prestigiosos del mundo. Puedes competir en modo individual o multijugador en impresionantes ubicaciones y pistas. También puedes personalizar tu coche con varias opciones y características. </li>
|
101 |
-
<li><b>Need for Speed: No Limits</b>: Este es un emocionante juego de carreras de adrenalina que te permite competir por el dominio en el mundo subterráneo de las carreras callejeras. Puede construir su coche de ensueño con más de 250 piezas y personalizarlo con varias opciones. También puedes retar a otros jugadores en carreras y eventos en tiempo real. </li>
|
102 |
-
<li><b>Real Racing 3</b>: Este es un juego de carreras realista e inmersivo que te permite conducir algunos de los coches más auténticos del mundo. Puedes competir en más de 40 circuitos en 19 lugares del mundo real. También puedes competir con otros jugadores en modos multijugador y eventos en tiempo real. </li>
|
103 |
-
</ul></p> 64aa2da5cf<br />
|
104 |
-
<br />
|
105 |
-
<br />
|
|
|
|
|
|
|
|
|
|
|
|
|
|
|
|
|
|
|
|
|
|
|
|
|
|
|
|
|
|
|
|
|
|
|
|
|
|
|
|
|
|
|
|
|
|
|
|
|
|
|
|
|
|
|
|
|
|
|
|
|
|
|
|
|
|
|
|
|
|
|
|
|
|
|
|
|
|
|
|
|
|
|
|
|
|
|
|
|
|
|
|
|
|
|
|
|
|
|
|
|
|
|
|
|
|
|
|
|
|
|
|
|
|
|
|
|
|
|
|
|
|
|
|
|
|
|
|
|
|
|
|
|
|
|
|
|
|
|
|
|
|
|
|
|
|
|
|
|
|
|
|
|
|
|
|
|
|
|
|
|
|
|
|
|
|
|
|
|
|
|
|
|
|
|
|
|
|
|
|
|
|
|
|
|
|
|
|
|
|
|
|
|
|
|
|
|
|
|
|
|
|
|
|
|
|
|
|
|
|
|
|
spaces/Benson/text-generation/Examples/Descarga Gratuita De Club Gacha Ipad.md
DELETED
@@ -1,76 +0,0 @@
|
|
1 |
-
<br />
|
2 |
-
<h1>Descarga gratuita de Gacha Club iPad: Una guía para fans del anime</h1>
|
3 |
-
<p>Si eres un fan del anime, es posible que hayas oído hablar de Gacha Club, el último juego de Lunime que te permite crear tus propios personajes e historias de anime. Gacha Club es un juego gratuito, creativo y divertido que tiene millones de fans en todo el mundo. Puedes personalizar a tus personajes con miles de trajes, accesorios, peinados, armas y más. También puedes entrar en el modo estudio y crear cualquier escena que puedas imaginar con tus personajes, mascotas, objetos y fondos. Puede incluso gacha y batalla con más de 180 unidades, recoger gemas y bytes, y jugar mini-juegos. </p>
|
4 |
-
<p>Pero, ¿cómo puedes descargar Gacha Club gratis en tu iPad? En realidad es muy fácil. Todo lo que necesitas hacer es ir a la App Store y buscar Gacha Club. Verás el icono del juego con un fondo púrpura y un lindo gato. Toque en él y luego toque en el "Obtener" botón. El juego comenzará a descargar e instalar en su dispositivo. También puedes usar este [link]( 1 ) para ir directamente a la página del juego en la App Store.</p>
|
5 |
-
<h2>descarga gratuita de club gacha ipad</h2><br /><p><b><b>Download File</b> ->>> <a href="https://bltlly.com/2v6JHT">https://bltlly.com/2v6JHT</a></b></p><br /><br />
|
6 |
-
<p>Una vez instalado el juego, puedes lanzarlo y empezar a jugar. Usted será recibido por un tutorial que le guiará a través de los fundamentos del juego. Puedes omitirlo si quieres, pero te recomendamos que lo sigas para aprender a usar las características del juego. </p>
|
7 |
-
<h2>Características de Gacha Club</h2>
|
8 |
-
<p>Gacha Club tiene muchas características que lo convierten en un juego divertido y atractivo para los amantes del anime. Estos son algunos de ellos:</p>
|
9 |
-
<h3>Personalización de personajes y escenas</h3>
|
10 |
-
<p>Una de las principales atracciones de Gacha Club es la función de personalización. Puedes crear hasta 10 personajes principales y 90 personajes adicionales, cada uno con su propio perfil y personalidad. Puedes cambiar sus colores, poses, expresiones, ropa, accesorios, peinados, armas y más. También puedes personalizar cientos de mascotas y objetos que puedes añadir a tus escenas. </p>
|
11 |
-
|
12 |
-
<h3>Gacha y modos de batalla</h3>
|
13 |
-
<p>Si quieres algo de acción, puedes probar los modos gacha y battle. Usted puede gacha más de 180 unidades para utilizar en la batalla, cada uno con sus propias habilidades y estadísticas. También puede gacha para 150 mascotas que pueden aumentar sus estadísticas. Usted puede recoger súper raros personajes corruptos y DJ que tienen habilidades especiales. </p>
|
14 |
-
<p>Puedes elegir entre cuatro modos de batalla: historia, entrenamiento, torre y sombras de corrupción. En el modo historia, puedes seguir la historia principal del juego y luchar contra diferentes enemigos. En el modo de entrenamiento, puede practicar sus habilidades y ganar oro y materiales. En el modo torre, puedes desafiarte con diferentes niveles de dificultad y recompensas. En las sombras del modo de corrupción, puede enfrentar versiones corruptas de sus personajes que tienen estadísticas más altas. </p>
|
15 |
-
<h3>Minijuegos y coleccionables</h3>
|
16 |
-
<p>Gacha Club también tiene muchos mini-juegos que puedes jugar por diversión o para ganar gemas y bytes. Las gemas son la moneda principal del juego que puedes usar para gacha para más unidades o mascotas. Los bytes son una moneda secundaria que puedes usar para comprar artículos o mejorar tus unidades. </p>
|
17 |
-
<p>Algunos de los mini-juegos son Usagi vs Neko, Memory Match, Lemo & Yumi, Narwhal Sky y más. También puedes desbloquear logros y recoger regalos raros que contienen artículos exclusivos. </p>
|
18 |
-
<p></p>
|
19 |
-
<h2>Consejos y trucos para Gacha Club</h2>
|
20 |
-
<p>Gacha Club es un juego que tiene mucha profundidad y contenido. Puedes sentirte abrumado al principio, pero no te preocupes. Aquí hay algunos consejos y trucos que pueden ayudarle a disfrutar del juego más:</p>
|
21 |
-
<h3>Cómo equilibrar tu equipo y usar afinidades elementales</h3>
|
22 |
-
<p>Cuando gacha para las unidades, se dará cuenta de que tienen diferentes elementos: agua, fuego, viento, tierra, luz, oscuridad y neutro. Cada elemento tiene sus propias fortalezas y debilidades contra otros elementos. Por ejemplo, el agua es fuerte contra el fuego, pero débil contra la tierra. Puedes ver el gráfico elemental completo en el menú del juego. </p>
|
23 |
-
|
24 |
-
<h3>Cómo traer mascotas y objetos para aumentar sus estadísticas</h3>
|
25 |
-
<p>Las mascotas y los objetos no son solo para la decoración. También pueden aumentar sus estadísticas y darle efectos especiales. Puedes llevar hasta cuatro mascotas y cuatro objetos a cada escena. Cada mascota y objeto tiene su propia rareza y nivel, que afectan la cantidad que aumentan sus estadísticas. </p>
|
26 |
-
<p>Puede ver las estadísticas y efectos de sus mascotas y objetos tocando en ellos en el modo de estudio. También puede mejorarlos con bytes para aumentar su nivel y estadísticas. Algunas mascotas y objetos tienen efectos únicos que pueden ayudarte en la batalla, como sanación, protección o aturdimiento. </p>
|
27 |
-
<h3>Cómo jugar sin conexión y granja de gemas</h3>
|
28 |
-
<p>Gacha Club es un juego en línea que requiere una conexión a Internet para jugar. Sin embargo, también puede jugar sin conexión si lo desea. Solo tienes que descargar los datos del juego antes de desconectar. Puedes hacerlo yendo al menú de opciones y pulsando el botón "Descargar datos". Esto descargará todas las imágenes y sonidos del juego en tu dispositivo. </p>
|
29 |
-
<p>Cuando juegas sin conexión, todavía puedes acceder a la mayoría de las características del juego, a excepción de gacha y modos de batalla. Aún puedes personalizar tus personajes y escenas, jugar minijuegos, recoger regalos y exportar imágenes o videos. También puede cultivar gemas jugando minijuegos o viendo anuncios. Puede usar estas gemas para gacha para obtener más unidades o mascotas cuando vuelva a conectarse. </p>
|
30 |
-
<h2>Alternativas al Club Gacha</h2>
|
31 |
-
<p>Gacha Club no es el único juego de Lunime que puedes jugar en tu iPad. Hay otros juegos que son similares a Gacha Club en términos de personalización y jugabilidad. Estos son algunos de ellos:</p>
|
32 |
-
<h3>Vida de Gacha</h3>
|
33 |
-
|
34 |
-
<p>Puedes descargar Gacha Life gratis en la App Store [aquí]. </p>
|
35 |
-
<h3>Gachaverse</h3>
|
36 |
-
<p>Gachaverse es otro juego de Lunime que combina elementos gacha y RPG. Puedes crear tus propios personajes de anime con cientos de opciones de personalización. También puedes explorar diferentes mundos e historias con tus personajes, o crear los tuyos con el modo estudio. También puedes gacha para personajes y objetos raros, o luchar contra otros jugadores en el modo arena. </p>
|
37 |
-
<p>Puedes descargar Gachaverse gratis en la App Store [aquí]. </p>
|
38 |
-
<h3>Otros juegos de gacha para iOS</h3>
|
39 |
-
<p>Si usted está buscando otros juegos gacha que ofrecen diferentes juegos y géneros, es posible que desee echa un vistazo a estos juegos:</p>
|
40 |
-
<tabla>
|
41 |
-
<tr>
|
42 |
-
<th>Juego</th>
|
43 |
-
<th>Descripción</th>
|
44 |
-
<th>Enlace</th>
|
45 |
-
</tr>
|
46 |
-
<tr>
|
47 |
-
<td>Destino/Gran Orden</td>
|
48 |
-
<td>Un popular juego gacha basado en la serie de anime Fate. Puedes invocar héroes legendarios de la historia y la mitología para luchar junto a ti en batallas épicas. </td>
|
49 |
-
<td>[aquí]</td>
|
50 |
-
</tr>
|
51 |
-
<tr>
|
52 |
-
<td>Héroes del emblema del fuego</td>
|
53 |
-
<td>Un juego gacha basado en la franquicia Fire Emblem. Puedes reunir y entrenar personajes de diferentes juegos de Fire Emblem y dirigirlos en combates estratégicos por turnos. </td> <td>[here]</td>
|
54 |
-
</tr>
|
55 |
-
<tr>
|
56 |
-
<td>Arknights</td>
|
57 |
-
<td>Un juego gacha que combina elementos de torre de defensa y RPG. Puedes reclutar y actualizar operadores con diferentes habilidades y roles para defender tu base de los enemigos. </td>
|
58 |
-
<td>[aquí]</td>
|
59 |
-
</tr>
|
60 |
-
</tabla>
|
61 |
-
<h2>Conclusión</h2>
|
62 |
-
<p>Gacha Club es un juego gratuito, creativo y divertido que te permite crear tus propios personajes e historias de anime. Puedes descargarlo gratis en tu iPad y disfrutar de sus muchas características, como personalización, gacha, batalla, minijuegos y más. También puedes probar otros juegos de Lunime u otros juegos gacha para iOS que ofrecen diferentes modos de juego y géneros. Si usted es un jugador casual o hardcore, seguramente encontrará algo que se adapte a su gusto y estilo. </p>
|
63 |
-
|
64 |
-
<h2>Preguntas frecuentes</h2>
|
65 |
-
<h3>Q: ¿Es seguro el Club Gacha para los niños? </h3>
|
66 |
-
<p>A: Gacha Club tiene una calificación de 9+ en la App Store, lo que significa que puede contener violencia leve, dibujos animados poco frecuentes o leves o violencia de fantasía, o temas de terror o miedo poco frecuentes o leves. Corresponde a los padres o tutores decidir si el juego es adecuado para sus hijos. También pueden usar los controles parentales en sus dispositivos para restringir el acceso o el contenido del juego. </p>
|
67 |
-
<h3>P: ¿Cómo puedo transferir mis datos de Gacha Life a Gacha Club? </h3>
|
68 |
-
<p>A: Desafortunadamente, no hay manera de transferir sus datos de Gacha Life a Gacha Club. Son juegos separados con diferentes características y contenido. Tendrás que empezar desde cero en Gacha Club, pero todavía puedes mantener tus datos de Gacha Life en tu dispositivo. </p>
|
69 |
-
<h3>P: ¿Cómo puedo obtener más gemas y bytes en Gacha Club? </h3>
|
70 |
-
<p>A: Hay varias maneras de obtener más gemas y bytes en Gacha Club. Puedes jugar minijuegos, ver anuncios, recoger regalos, completar logros, luchar contra enemigos o comprarlos con dinero real. También puedes usar códigos dados por Lunime u otras fuentes para obtener gemas y bytes gratis. </p>
|
71 |
-
<h3>P: ¿Cómo puedo compartir mis escenas o personajes con otros en Gacha Club? </h3>
|
72 |
-
<p>A: Puede compartir sus escenas o personajes con otros en Gacha Club mediante la función de exportación. Puedes exportar tus escenas como imágenes o vídeos, y tus personajes como códigos QR. Luego puedes compartirlos en redes sociales, correos electrónicos u otras plataformas. También puede importar escenas o caracteres de otras personas mediante la función de importación. </p>
|
73 |
-
<h3>Q: ¿Cómo puedo contactar a Lunime o reportar un error o problema en Gacha Club? </h3>
|
74 |
-
<p>A: Puede ponerse en contacto con Lunime o informar de un error o problema en Gacha Club mediante la función de retroalimentación. Puede encontrarlo en el menú de opciones bajo el botón "Feedback". También puede visitar su sitio web [aquí] o sus cuentas de redes sociales [aquí] para obtener más información y actualizaciones. </p> 64aa2da5cf<br />
|
75 |
-
<br />
|
76 |
-
<br />
|
|
|
|
|
|
|
|
|
|
|
|
|
|
|
|
|
|
|
|
|
|
|
|
|
|
|
|
|
|
|
|
|
|
|
|
|
|
|
|
|
|
|
|
|
|
|
|
|
|
|
|
|
|
|
|
|
|
|
|
|
|
|
|
|
|
|
|
|
|
|
|
|
|
|
|
|
|
|
|
|
|
|
|
|
|
|
|
|
|
|
|
|
|
|
|
|
|
|
|
|
|
|
|
|
|
|
|
|
|
|
|
|
|
|
|
|
|
|
|
|
|
|
|
|
|
|
|
|
|
|
|
|
|
|
|
|
|
|
|
|
|
|
|
|
|
|
|
|
|
|
|
|
|
spaces/Big-Web/MMSD/env/Lib/site-packages/pip/_internal/commands/configuration.py
DELETED
@@ -1,282 +0,0 @@
|
|
1 |
-
import logging
|
2 |
-
import os
|
3 |
-
import subprocess
|
4 |
-
from optparse import Values
|
5 |
-
from typing import Any, List, Optional
|
6 |
-
|
7 |
-
from pip._internal.cli.base_command import Command
|
8 |
-
from pip._internal.cli.status_codes import ERROR, SUCCESS
|
9 |
-
from pip._internal.configuration import (
|
10 |
-
Configuration,
|
11 |
-
Kind,
|
12 |
-
get_configuration_files,
|
13 |
-
kinds,
|
14 |
-
)
|
15 |
-
from pip._internal.exceptions import PipError
|
16 |
-
from pip._internal.utils.logging import indent_log
|
17 |
-
from pip._internal.utils.misc import get_prog, write_output
|
18 |
-
|
19 |
-
logger = logging.getLogger(__name__)
|
20 |
-
|
21 |
-
|
22 |
-
class ConfigurationCommand(Command):
|
23 |
-
"""
|
24 |
-
Manage local and global configuration.
|
25 |
-
|
26 |
-
Subcommands:
|
27 |
-
|
28 |
-
- list: List the active configuration (or from the file specified)
|
29 |
-
- edit: Edit the configuration file in an editor
|
30 |
-
- get: Get the value associated with command.option
|
31 |
-
- set: Set the command.option=value
|
32 |
-
- unset: Unset the value associated with command.option
|
33 |
-
- debug: List the configuration files and values defined under them
|
34 |
-
|
35 |
-
Configuration keys should be dot separated command and option name,
|
36 |
-
with the special prefix "global" affecting any command. For example,
|
37 |
-
"pip config set global.index-url https://example.org/" would configure
|
38 |
-
the index url for all commands, but "pip config set download.timeout 10"
|
39 |
-
would configure a 10 second timeout only for "pip download" commands.
|
40 |
-
|
41 |
-
If none of --user, --global and --site are passed, a virtual
|
42 |
-
environment configuration file is used if one is active and the file
|
43 |
-
exists. Otherwise, all modifications happen to the user file by
|
44 |
-
default.
|
45 |
-
"""
|
46 |
-
|
47 |
-
ignore_require_venv = True
|
48 |
-
usage = """
|
49 |
-
%prog [<file-option>] list
|
50 |
-
%prog [<file-option>] [--editor <editor-path>] edit
|
51 |
-
|
52 |
-
%prog [<file-option>] get command.option
|
53 |
-
%prog [<file-option>] set command.option value
|
54 |
-
%prog [<file-option>] unset command.option
|
55 |
-
%prog [<file-option>] debug
|
56 |
-
"""
|
57 |
-
|
58 |
-
def add_options(self) -> None:
|
59 |
-
self.cmd_opts.add_option(
|
60 |
-
"--editor",
|
61 |
-
dest="editor",
|
62 |
-
action="store",
|
63 |
-
default=None,
|
64 |
-
help=(
|
65 |
-
"Editor to use to edit the file. Uses VISUAL or EDITOR "
|
66 |
-
"environment variables if not provided."
|
67 |
-
),
|
68 |
-
)
|
69 |
-
|
70 |
-
self.cmd_opts.add_option(
|
71 |
-
"--global",
|
72 |
-
dest="global_file",
|
73 |
-
action="store_true",
|
74 |
-
default=False,
|
75 |
-
help="Use the system-wide configuration file only",
|
76 |
-
)
|
77 |
-
|
78 |
-
self.cmd_opts.add_option(
|
79 |
-
"--user",
|
80 |
-
dest="user_file",
|
81 |
-
action="store_true",
|
82 |
-
default=False,
|
83 |
-
help="Use the user configuration file only",
|
84 |
-
)
|
85 |
-
|
86 |
-
self.cmd_opts.add_option(
|
87 |
-
"--site",
|
88 |
-
dest="site_file",
|
89 |
-
action="store_true",
|
90 |
-
default=False,
|
91 |
-
help="Use the current environment configuration file only",
|
92 |
-
)
|
93 |
-
|
94 |
-
self.parser.insert_option_group(0, self.cmd_opts)
|
95 |
-
|
96 |
-
def run(self, options: Values, args: List[str]) -> int:
|
97 |
-
handlers = {
|
98 |
-
"list": self.list_values,
|
99 |
-
"edit": self.open_in_editor,
|
100 |
-
"get": self.get_name,
|
101 |
-
"set": self.set_name_value,
|
102 |
-
"unset": self.unset_name,
|
103 |
-
"debug": self.list_config_values,
|
104 |
-
}
|
105 |
-
|
106 |
-
# Determine action
|
107 |
-
if not args or args[0] not in handlers:
|
108 |
-
logger.error(
|
109 |
-
"Need an action (%s) to perform.",
|
110 |
-
", ".join(sorted(handlers)),
|
111 |
-
)
|
112 |
-
return ERROR
|
113 |
-
|
114 |
-
action = args[0]
|
115 |
-
|
116 |
-
# Determine which configuration files are to be loaded
|
117 |
-
# Depends on whether the command is modifying.
|
118 |
-
try:
|
119 |
-
load_only = self._determine_file(
|
120 |
-
options, need_value=(action in ["get", "set", "unset", "edit"])
|
121 |
-
)
|
122 |
-
except PipError as e:
|
123 |
-
logger.error(e.args[0])
|
124 |
-
return ERROR
|
125 |
-
|
126 |
-
# Load a new configuration
|
127 |
-
self.configuration = Configuration(
|
128 |
-
isolated=options.isolated_mode, load_only=load_only
|
129 |
-
)
|
130 |
-
self.configuration.load()
|
131 |
-
|
132 |
-
# Error handling happens here, not in the action-handlers.
|
133 |
-
try:
|
134 |
-
handlers[action](options, args[1:])
|
135 |
-
except PipError as e:
|
136 |
-
logger.error(e.args[0])
|
137 |
-
return ERROR
|
138 |
-
|
139 |
-
return SUCCESS
|
140 |
-
|
141 |
-
def _determine_file(self, options: Values, need_value: bool) -> Optional[Kind]:
|
142 |
-
file_options = [
|
143 |
-
key
|
144 |
-
for key, value in (
|
145 |
-
(kinds.USER, options.user_file),
|
146 |
-
(kinds.GLOBAL, options.global_file),
|
147 |
-
(kinds.SITE, options.site_file),
|
148 |
-
)
|
149 |
-
if value
|
150 |
-
]
|
151 |
-
|
152 |
-
if not file_options:
|
153 |
-
if not need_value:
|
154 |
-
return None
|
155 |
-
# Default to user, unless there's a site file.
|
156 |
-
elif any(
|
157 |
-
os.path.exists(site_config_file)
|
158 |
-
for site_config_file in get_configuration_files()[kinds.SITE]
|
159 |
-
):
|
160 |
-
return kinds.SITE
|
161 |
-
else:
|
162 |
-
return kinds.USER
|
163 |
-
elif len(file_options) == 1:
|
164 |
-
return file_options[0]
|
165 |
-
|
166 |
-
raise PipError(
|
167 |
-
"Need exactly one file to operate upon "
|
168 |
-
"(--user, --site, --global) to perform."
|
169 |
-
)
|
170 |
-
|
171 |
-
def list_values(self, options: Values, args: List[str]) -> None:
|
172 |
-
self._get_n_args(args, "list", n=0)
|
173 |
-
|
174 |
-
for key, value in sorted(self.configuration.items()):
|
175 |
-
write_output("%s=%r", key, value)
|
176 |
-
|
177 |
-
def get_name(self, options: Values, args: List[str]) -> None:
|
178 |
-
key = self._get_n_args(args, "get [name]", n=1)
|
179 |
-
value = self.configuration.get_value(key)
|
180 |
-
|
181 |
-
write_output("%s", value)
|
182 |
-
|
183 |
-
def set_name_value(self, options: Values, args: List[str]) -> None:
|
184 |
-
key, value = self._get_n_args(args, "set [name] [value]", n=2)
|
185 |
-
self.configuration.set_value(key, value)
|
186 |
-
|
187 |
-
self._save_configuration()
|
188 |
-
|
189 |
-
def unset_name(self, options: Values, args: List[str]) -> None:
|
190 |
-
key = self._get_n_args(args, "unset [name]", n=1)
|
191 |
-
self.configuration.unset_value(key)
|
192 |
-
|
193 |
-
self._save_configuration()
|
194 |
-
|
195 |
-
def list_config_values(self, options: Values, args: List[str]) -> None:
|
196 |
-
"""List config key-value pairs across different config files"""
|
197 |
-
self._get_n_args(args, "debug", n=0)
|
198 |
-
|
199 |
-
self.print_env_var_values()
|
200 |
-
# Iterate over config files and print if they exist, and the
|
201 |
-
# key-value pairs present in them if they do
|
202 |
-
for variant, files in sorted(self.configuration.iter_config_files()):
|
203 |
-
write_output("%s:", variant)
|
204 |
-
for fname in files:
|
205 |
-
with indent_log():
|
206 |
-
file_exists = os.path.exists(fname)
|
207 |
-
write_output("%s, exists: %r", fname, file_exists)
|
208 |
-
if file_exists:
|
209 |
-
self.print_config_file_values(variant)
|
210 |
-
|
211 |
-
def print_config_file_values(self, variant: Kind) -> None:
|
212 |
-
"""Get key-value pairs from the file of a variant"""
|
213 |
-
for name, value in self.configuration.get_values_in_config(variant).items():
|
214 |
-
with indent_log():
|
215 |
-
write_output("%s: %s", name, value)
|
216 |
-
|
217 |
-
def print_env_var_values(self) -> None:
|
218 |
-
"""Get key-values pairs present as environment variables"""
|
219 |
-
write_output("%s:", "env_var")
|
220 |
-
with indent_log():
|
221 |
-
for key, value in sorted(self.configuration.get_environ_vars()):
|
222 |
-
env_var = f"PIP_{key.upper()}"
|
223 |
-
write_output("%s=%r", env_var, value)
|
224 |
-
|
225 |
-
def open_in_editor(self, options: Values, args: List[str]) -> None:
|
226 |
-
editor = self._determine_editor(options)
|
227 |
-
|
228 |
-
fname = self.configuration.get_file_to_edit()
|
229 |
-
if fname is None:
|
230 |
-
raise PipError("Could not determine appropriate file.")
|
231 |
-
elif '"' in fname:
|
232 |
-
# This shouldn't happen, unless we see a username like that.
|
233 |
-
# If that happens, we'd appreciate a pull request fixing this.
|
234 |
-
raise PipError(
|
235 |
-
f'Can not open an editor for a file name containing "\n{fname}'
|
236 |
-
)
|
237 |
-
|
238 |
-
try:
|
239 |
-
subprocess.check_call(f'{editor} "{fname}"', shell=True)
|
240 |
-
except FileNotFoundError as e:
|
241 |
-
if not e.filename:
|
242 |
-
e.filename = editor
|
243 |
-
raise
|
244 |
-
except subprocess.CalledProcessError as e:
|
245 |
-
raise PipError(
|
246 |
-
"Editor Subprocess exited with exit code {}".format(e.returncode)
|
247 |
-
)
|
248 |
-
|
249 |
-
def _get_n_args(self, args: List[str], example: str, n: int) -> Any:
|
250 |
-
"""Helper to make sure the command got the right number of arguments"""
|
251 |
-
if len(args) != n:
|
252 |
-
msg = (
|
253 |
-
"Got unexpected number of arguments, expected {}. "
|
254 |
-
'(example: "{} config {}")'
|
255 |
-
).format(n, get_prog(), example)
|
256 |
-
raise PipError(msg)
|
257 |
-
|
258 |
-
if n == 1:
|
259 |
-
return args[0]
|
260 |
-
else:
|
261 |
-
return args
|
262 |
-
|
263 |
-
def _save_configuration(self) -> None:
|
264 |
-
# We successfully ran a modifying command. Need to save the
|
265 |
-
# configuration.
|
266 |
-
try:
|
267 |
-
self.configuration.save()
|
268 |
-
except Exception:
|
269 |
-
logger.exception(
|
270 |
-
"Unable to save configuration. Please report this as a bug."
|
271 |
-
)
|
272 |
-
raise PipError("Internal Error.")
|
273 |
-
|
274 |
-
def _determine_editor(self, options: Values) -> str:
|
275 |
-
if options.editor is not None:
|
276 |
-
return options.editor
|
277 |
-
elif "VISUAL" in os.environ:
|
278 |
-
return os.environ["VISUAL"]
|
279 |
-
elif "EDITOR" in os.environ:
|
280 |
-
return os.environ["EDITOR"]
|
281 |
-
else:
|
282 |
-
raise PipError("Could not determine editor to use.")
|
|
|
|
|
|
|
|
|
|
|
|
|
|
|
|
|
|
|
|
|
|
|
|
|
|
|
|
|
|
|
|
|
|
|
|
|
|
|
|
|
|
|
|
|
|
|
|
|
|
|
|
|
|
|
|
|
|
|
|
|
|
|
|
|
|
|
|
|
|
|
|
|
|
|
|
|
|
|
|
|
|
|
|
|
|
|
|
|
|
|
|
|
|
|
|
|
|
|
|
|
|
|
|
|
|
|
|
|
|
|
|
|
|
|
|
|
|
|
|
|
|
|
|
|
|
|
|
|
|
|
|
|
|
|
|
|
|
|
|
|
|
|
|
|
|
|
|
|
|
|
|
|
|
|
|
|
|
|
|
|
|
|
|
|
|
|
|
|
|
|
|
|
|
|
|
|
|
|
|
|
|
|
|
|
|
|
|
|
|
|
|
|
|
|
|
|
|
|
|
|
|
|
|
|
|
|
|
|
|
|
|
|
|
|
|
|
|
|
|
|
|
|
|
|
|
|
|
|
|
|
|
|
|
|
|
|
|
|
|
|
|
|
|
|
|
|
|
|
|
|
|
|
|
|
|
|
|
|
|
|
|
|
|
|
|
|
|
|
|
|
|
|
|
|
|
|
|
|
|
|
|
|
|
|
|
|
|
|
|
|
|
|
|
|
|
|
|
|
|
|
|
|
|
|
|
|
|
|
|
|
|
|
|
|
|
|
|
|
|
|
|
|
|
|
|
|
|
|
|
|
|
|
|
|
|
|
|
|
|
|
|
|
|
|
|
|
|
|
|
|
|
|
|
|
|
|
|
|
|
|
|
|
|
|
|
|
|
|
|
|
|
|
|
|
|
|
|
|
|
|
|
|
|
|
|
|
|
|
|
|
|
|
|
|
|
|
|
|
|
|
|
|
|
|
|
|
|
|
|
|
|
|
|
|
|
|
|
|
|
|
|
|
|
|
|
|
|
|
|
|
|
|
|
|
|
|
|
|
|
|
|
|
|
|
|
|
|
|
|
|
|
|
|
|
|
|
|
|
|
|
|
|
|
|
|
|
|
|
|
|
|
|
|
|
|
|
|
|
|
|
|
|
|
|
|
|
|
|
|
|
|
|
|
|
|
|
|
|
|
|
|
|
|
|
|
|
|
|
|
|
|
|
|
|
|
|
|
|
|
|
|
|
|
|
|
|
|
|
|
|
|
|
|
|
|
|
|
|
|
|
|
|
|
|
|
|
|
|
|
|
|
|
|
|
|
|
|
|
|
|
|
|
|
|
|
spaces/Big-Web/MMSD/env/Lib/site-packages/pip/_internal/index/__init__.py
DELETED
@@ -1,2 +0,0 @@
|
|
1 |
-
"""Index interaction code
|
2 |
-
"""
|
|
|
|
|
|
spaces/Big-Web/MMSD/env/Lib/site-packages/s3transfer/processpool.py
DELETED
@@ -1,1008 +0,0 @@
|
|
1 |
-
# Copyright 2019 Amazon.com, Inc. or its affiliates. All Rights Reserved.
|
2 |
-
#
|
3 |
-
# Licensed under the Apache License, Version 2.0 (the "License"). You
|
4 |
-
# may not use this file except in compliance with the License. A copy of
|
5 |
-
# the License is located at
|
6 |
-
#
|
7 |
-
# http://aws.amazon.com/apache2.0/
|
8 |
-
#
|
9 |
-
# or in the "license" file accompanying this file. This file is
|
10 |
-
# distributed on an "AS IS" BASIS, WITHOUT WARRANTIES OR CONDITIONS OF
|
11 |
-
# ANY KIND, either express or implied. See the License for the specific
|
12 |
-
# language governing permissions and limitations under the License.
|
13 |
-
"""Speeds up S3 throughput by using processes
|
14 |
-
|
15 |
-
Getting Started
|
16 |
-
===============
|
17 |
-
|
18 |
-
The :class:`ProcessPoolDownloader` can be used to download a single file by
|
19 |
-
calling :meth:`ProcessPoolDownloader.download_file`:
|
20 |
-
|
21 |
-
.. code:: python
|
22 |
-
|
23 |
-
from s3transfer.processpool import ProcessPoolDownloader
|
24 |
-
|
25 |
-
with ProcessPoolDownloader() as downloader:
|
26 |
-
downloader.download_file('mybucket', 'mykey', 'myfile')
|
27 |
-
|
28 |
-
|
29 |
-
This snippet downloads the S3 object located in the bucket ``mybucket`` at the
|
30 |
-
key ``mykey`` to the local file ``myfile``. Any errors encountered during the
|
31 |
-
transfer are not propagated. To determine if a transfer succeeded or
|
32 |
-
failed, use the `Futures`_ interface.
|
33 |
-
|
34 |
-
|
35 |
-
The :class:`ProcessPoolDownloader` can be used to download multiple files as
|
36 |
-
well:
|
37 |
-
|
38 |
-
.. code:: python
|
39 |
-
|
40 |
-
from s3transfer.processpool import ProcessPoolDownloader
|
41 |
-
|
42 |
-
with ProcessPoolDownloader() as downloader:
|
43 |
-
downloader.download_file('mybucket', 'mykey', 'myfile')
|
44 |
-
downloader.download_file('mybucket', 'myotherkey', 'myotherfile')
|
45 |
-
|
46 |
-
|
47 |
-
When running this snippet, the downloading of ``mykey`` and ``myotherkey``
|
48 |
-
happen in parallel. The first ``download_file`` call does not block the
|
49 |
-
second ``download_file`` call. The snippet blocks when exiting
|
50 |
-
the context manager and blocks until both downloads are complete.
|
51 |
-
|
52 |
-
Alternatively, the ``ProcessPoolDownloader`` can be instantiated
|
53 |
-
and explicitly be shutdown using :meth:`ProcessPoolDownloader.shutdown`:
|
54 |
-
|
55 |
-
.. code:: python
|
56 |
-
|
57 |
-
from s3transfer.processpool import ProcessPoolDownloader
|
58 |
-
|
59 |
-
downloader = ProcessPoolDownloader()
|
60 |
-
downloader.download_file('mybucket', 'mykey', 'myfile')
|
61 |
-
downloader.download_file('mybucket', 'myotherkey', 'myotherfile')
|
62 |
-
downloader.shutdown()
|
63 |
-
|
64 |
-
|
65 |
-
For this code snippet, the call to ``shutdown`` blocks until both
|
66 |
-
downloads are complete.
|
67 |
-
|
68 |
-
|
69 |
-
Additional Parameters
|
70 |
-
=====================
|
71 |
-
|
72 |
-
Additional parameters can be provided to the ``download_file`` method:
|
73 |
-
|
74 |
-
* ``extra_args``: A dictionary containing any additional client arguments
|
75 |
-
to include in the
|
76 |
-
`GetObject <https://botocore.amazonaws.com/v1/documentation/api/latest/reference/services/s3.html#S3.Client.get_object>`_
|
77 |
-
API request. For example:
|
78 |
-
|
79 |
-
.. code:: python
|
80 |
-
|
81 |
-
from s3transfer.processpool import ProcessPoolDownloader
|
82 |
-
|
83 |
-
with ProcessPoolDownloader() as downloader:
|
84 |
-
downloader.download_file(
|
85 |
-
'mybucket', 'mykey', 'myfile',
|
86 |
-
extra_args={'VersionId': 'myversion'})
|
87 |
-
|
88 |
-
|
89 |
-
* ``expected_size``: By default, the downloader will make a HeadObject
|
90 |
-
call to determine the size of the object. To opt-out of this additional
|
91 |
-
API call, you can provide the size of the object in bytes:
|
92 |
-
|
93 |
-
.. code:: python
|
94 |
-
|
95 |
-
from s3transfer.processpool import ProcessPoolDownloader
|
96 |
-
|
97 |
-
MB = 1024 * 1024
|
98 |
-
with ProcessPoolDownloader() as downloader:
|
99 |
-
downloader.download_file(
|
100 |
-
'mybucket', 'mykey', 'myfile', expected_size=2 * MB)
|
101 |
-
|
102 |
-
|
103 |
-
Futures
|
104 |
-
=======
|
105 |
-
|
106 |
-
When ``download_file`` is called, it immediately returns a
|
107 |
-
:class:`ProcessPoolTransferFuture`. The future can be used to poll the state
|
108 |
-
of a particular transfer. To get the result of the download,
|
109 |
-
call :meth:`ProcessPoolTransferFuture.result`. The method blocks
|
110 |
-
until the transfer completes, whether it succeeds or fails. For example:
|
111 |
-
|
112 |
-
.. code:: python
|
113 |
-
|
114 |
-
from s3transfer.processpool import ProcessPoolDownloader
|
115 |
-
|
116 |
-
with ProcessPoolDownloader() as downloader:
|
117 |
-
future = downloader.download_file('mybucket', 'mykey', 'myfile')
|
118 |
-
print(future.result())
|
119 |
-
|
120 |
-
|
121 |
-
If the download succeeds, the future returns ``None``:
|
122 |
-
|
123 |
-
.. code:: python
|
124 |
-
|
125 |
-
None
|
126 |
-
|
127 |
-
|
128 |
-
If the download fails, the exception causing the failure is raised. For
|
129 |
-
example, if ``mykey`` did not exist, the following error would be raised
|
130 |
-
|
131 |
-
|
132 |
-
.. code:: python
|
133 |
-
|
134 |
-
botocore.exceptions.ClientError: An error occurred (404) when calling the HeadObject operation: Not Found
|
135 |
-
|
136 |
-
|
137 |
-
.. note::
|
138 |
-
|
139 |
-
:meth:`ProcessPoolTransferFuture.result` can only be called while the
|
140 |
-
``ProcessPoolDownloader`` is running (e.g. before calling ``shutdown`` or
|
141 |
-
inside the context manager).
|
142 |
-
|
143 |
-
|
144 |
-
Process Pool Configuration
|
145 |
-
==========================
|
146 |
-
|
147 |
-
By default, the downloader has the following configuration options:
|
148 |
-
|
149 |
-
* ``multipart_threshold``: The threshold size for performing ranged downloads
|
150 |
-
in bytes. By default, ranged downloads happen for S3 objects that are
|
151 |
-
greater than or equal to 8 MB in size.
|
152 |
-
|
153 |
-
* ``multipart_chunksize``: The size of each ranged download in bytes. By
|
154 |
-
default, the size of each ranged download is 8 MB.
|
155 |
-
|
156 |
-
* ``max_request_processes``: The maximum number of processes used to download
|
157 |
-
S3 objects. By default, the maximum is 10 processes.
|
158 |
-
|
159 |
-
|
160 |
-
To change the default configuration, use the :class:`ProcessTransferConfig`:
|
161 |
-
|
162 |
-
.. code:: python
|
163 |
-
|
164 |
-
from s3transfer.processpool import ProcessPoolDownloader
|
165 |
-
from s3transfer.processpool import ProcessTransferConfig
|
166 |
-
|
167 |
-
config = ProcessTransferConfig(
|
168 |
-
multipart_threshold=64 * 1024 * 1024, # 64 MB
|
169 |
-
max_request_processes=50
|
170 |
-
)
|
171 |
-
downloader = ProcessPoolDownloader(config=config)
|
172 |
-
|
173 |
-
|
174 |
-
Client Configuration
|
175 |
-
====================
|
176 |
-
|
177 |
-
The process pool downloader creates ``botocore`` clients on your behalf. In
|
178 |
-
order to affect how the client is created, pass the keyword arguments
|
179 |
-
that would have been used in the :meth:`botocore.Session.create_client` call:
|
180 |
-
|
181 |
-
.. code:: python
|
182 |
-
|
183 |
-
|
184 |
-
from s3transfer.processpool import ProcessPoolDownloader
|
185 |
-
from s3transfer.processpool import ProcessTransferConfig
|
186 |
-
|
187 |
-
downloader = ProcessPoolDownloader(
|
188 |
-
client_kwargs={'region_name': 'us-west-2'})
|
189 |
-
|
190 |
-
|
191 |
-
This snippet ensures that all clients created by the ``ProcessPoolDownloader``
|
192 |
-
are using ``us-west-2`` as their region.
|
193 |
-
|
194 |
-
"""
|
195 |
-
import collections
|
196 |
-
import contextlib
|
197 |
-
import logging
|
198 |
-
import multiprocessing
|
199 |
-
import signal
|
200 |
-
import threading
|
201 |
-
from copy import deepcopy
|
202 |
-
|
203 |
-
import botocore.session
|
204 |
-
from botocore.config import Config
|
205 |
-
|
206 |
-
from s3transfer.compat import MAXINT, BaseManager
|
207 |
-
from s3transfer.constants import ALLOWED_DOWNLOAD_ARGS, MB, PROCESS_USER_AGENT
|
208 |
-
from s3transfer.exceptions import CancelledError, RetriesExceededError
|
209 |
-
from s3transfer.futures import BaseTransferFuture, BaseTransferMeta
|
210 |
-
from s3transfer.utils import (
|
211 |
-
S3_RETRYABLE_DOWNLOAD_ERRORS,
|
212 |
-
CallArgs,
|
213 |
-
OSUtils,
|
214 |
-
calculate_num_parts,
|
215 |
-
calculate_range_parameter,
|
216 |
-
)
|
217 |
-
|
218 |
-
logger = logging.getLogger(__name__)
|
219 |
-
|
220 |
-
SHUTDOWN_SIGNAL = 'SHUTDOWN'
|
221 |
-
|
222 |
-
# The DownloadFileRequest tuple is submitted from the ProcessPoolDownloader
|
223 |
-
# to the GetObjectSubmitter in order for the submitter to begin submitting
|
224 |
-
# GetObjectJobs to the GetObjectWorkers.
|
225 |
-
DownloadFileRequest = collections.namedtuple(
|
226 |
-
'DownloadFileRequest',
|
227 |
-
[
|
228 |
-
'transfer_id', # The unique id for the transfer
|
229 |
-
'bucket', # The bucket to download the object from
|
230 |
-
'key', # The key to download the object from
|
231 |
-
'filename', # The user-requested download location
|
232 |
-
'extra_args', # Extra arguments to provide to client calls
|
233 |
-
'expected_size', # The user-provided expected size of the download
|
234 |
-
],
|
235 |
-
)
|
236 |
-
|
237 |
-
# The GetObjectJob tuple is submitted from the GetObjectSubmitter
|
238 |
-
# to the GetObjectWorkers to download the file or parts of the file.
|
239 |
-
GetObjectJob = collections.namedtuple(
|
240 |
-
'GetObjectJob',
|
241 |
-
[
|
242 |
-
'transfer_id', # The unique id for the transfer
|
243 |
-
'bucket', # The bucket to download the object from
|
244 |
-
'key', # The key to download the object from
|
245 |
-
'temp_filename', # The temporary file to write the content to via
|
246 |
-
# completed GetObject calls.
|
247 |
-
'extra_args', # Extra arguments to provide to the GetObject call
|
248 |
-
'offset', # The offset to write the content for the temp file.
|
249 |
-
'filename', # The user-requested download location. The worker
|
250 |
-
# of final GetObjectJob will move the file located at
|
251 |
-
# temp_filename to the location of filename.
|
252 |
-
],
|
253 |
-
)
|
254 |
-
|
255 |
-
|
256 |
-
@contextlib.contextmanager
|
257 |
-
def ignore_ctrl_c():
|
258 |
-
original_handler = _add_ignore_handler_for_interrupts()
|
259 |
-
yield
|
260 |
-
signal.signal(signal.SIGINT, original_handler)
|
261 |
-
|
262 |
-
|
263 |
-
def _add_ignore_handler_for_interrupts():
|
264 |
-
# Windows is unable to pickle signal.signal directly so it needs to
|
265 |
-
# be wrapped in a function defined at the module level
|
266 |
-
return signal.signal(signal.SIGINT, signal.SIG_IGN)
|
267 |
-
|
268 |
-
|
269 |
-
class ProcessTransferConfig:
|
270 |
-
def __init__(
|
271 |
-
self,
|
272 |
-
multipart_threshold=8 * MB,
|
273 |
-
multipart_chunksize=8 * MB,
|
274 |
-
max_request_processes=10,
|
275 |
-
):
|
276 |
-
"""Configuration for the ProcessPoolDownloader
|
277 |
-
|
278 |
-
:param multipart_threshold: The threshold for which ranged downloads
|
279 |
-
occur.
|
280 |
-
|
281 |
-
:param multipart_chunksize: The chunk size of each ranged download.
|
282 |
-
|
283 |
-
:param max_request_processes: The maximum number of processes that
|
284 |
-
will be making S3 API transfer-related requests at a time.
|
285 |
-
"""
|
286 |
-
self.multipart_threshold = multipart_threshold
|
287 |
-
self.multipart_chunksize = multipart_chunksize
|
288 |
-
self.max_request_processes = max_request_processes
|
289 |
-
|
290 |
-
|
291 |
-
class ProcessPoolDownloader:
|
292 |
-
def __init__(self, client_kwargs=None, config=None):
|
293 |
-
"""Downloads S3 objects using process pools
|
294 |
-
|
295 |
-
:type client_kwargs: dict
|
296 |
-
:param client_kwargs: The keyword arguments to provide when
|
297 |
-
instantiating S3 clients. The arguments must match the keyword
|
298 |
-
arguments provided to the
|
299 |
-
`botocore.session.Session.create_client()` method.
|
300 |
-
|
301 |
-
:type config: ProcessTransferConfig
|
302 |
-
:param config: Configuration for the downloader
|
303 |
-
"""
|
304 |
-
if client_kwargs is None:
|
305 |
-
client_kwargs = {}
|
306 |
-
self._client_factory = ClientFactory(client_kwargs)
|
307 |
-
|
308 |
-
self._transfer_config = config
|
309 |
-
if config is None:
|
310 |
-
self._transfer_config = ProcessTransferConfig()
|
311 |
-
|
312 |
-
self._download_request_queue = multiprocessing.Queue(1000)
|
313 |
-
self._worker_queue = multiprocessing.Queue(1000)
|
314 |
-
self._osutil = OSUtils()
|
315 |
-
|
316 |
-
self._started = False
|
317 |
-
self._start_lock = threading.Lock()
|
318 |
-
|
319 |
-
# These below are initialized in the start() method
|
320 |
-
self._manager = None
|
321 |
-
self._transfer_monitor = None
|
322 |
-
self._submitter = None
|
323 |
-
self._workers = []
|
324 |
-
|
325 |
-
def download_file(
|
326 |
-
self, bucket, key, filename, extra_args=None, expected_size=None
|
327 |
-
):
|
328 |
-
"""Downloads the object's contents to a file
|
329 |
-
|
330 |
-
:type bucket: str
|
331 |
-
:param bucket: The name of the bucket to download from
|
332 |
-
|
333 |
-
:type key: str
|
334 |
-
:param key: The name of the key to download from
|
335 |
-
|
336 |
-
:type filename: str
|
337 |
-
:param filename: The name of a file to download to.
|
338 |
-
|
339 |
-
:type extra_args: dict
|
340 |
-
:param extra_args: Extra arguments that may be passed to the
|
341 |
-
client operation
|
342 |
-
|
343 |
-
:type expected_size: int
|
344 |
-
:param expected_size: The expected size in bytes of the download. If
|
345 |
-
provided, the downloader will not call HeadObject to determine the
|
346 |
-
object's size and use the provided value instead. The size is
|
347 |
-
needed to determine whether to do a multipart download.
|
348 |
-
|
349 |
-
:rtype: s3transfer.futures.TransferFuture
|
350 |
-
:returns: Transfer future representing the download
|
351 |
-
"""
|
352 |
-
self._start_if_needed()
|
353 |
-
if extra_args is None:
|
354 |
-
extra_args = {}
|
355 |
-
self._validate_all_known_args(extra_args)
|
356 |
-
transfer_id = self._transfer_monitor.notify_new_transfer()
|
357 |
-
download_file_request = DownloadFileRequest(
|
358 |
-
transfer_id=transfer_id,
|
359 |
-
bucket=bucket,
|
360 |
-
key=key,
|
361 |
-
filename=filename,
|
362 |
-
extra_args=extra_args,
|
363 |
-
expected_size=expected_size,
|
364 |
-
)
|
365 |
-
logger.debug(
|
366 |
-
'Submitting download file request: %s.', download_file_request
|
367 |
-
)
|
368 |
-
self._download_request_queue.put(download_file_request)
|
369 |
-
call_args = CallArgs(
|
370 |
-
bucket=bucket,
|
371 |
-
key=key,
|
372 |
-
filename=filename,
|
373 |
-
extra_args=extra_args,
|
374 |
-
expected_size=expected_size,
|
375 |
-
)
|
376 |
-
future = self._get_transfer_future(transfer_id, call_args)
|
377 |
-
return future
|
378 |
-
|
379 |
-
def shutdown(self):
|
380 |
-
"""Shutdown the downloader
|
381 |
-
|
382 |
-
It will wait till all downloads are complete before returning.
|
383 |
-
"""
|
384 |
-
self._shutdown_if_needed()
|
385 |
-
|
386 |
-
def __enter__(self):
|
387 |
-
return self
|
388 |
-
|
389 |
-
def __exit__(self, exc_type, exc_value, *args):
|
390 |
-
if isinstance(exc_value, KeyboardInterrupt):
|
391 |
-
if self._transfer_monitor is not None:
|
392 |
-
self._transfer_monitor.notify_cancel_all_in_progress()
|
393 |
-
self.shutdown()
|
394 |
-
|
395 |
-
def _start_if_needed(self):
|
396 |
-
with self._start_lock:
|
397 |
-
if not self._started:
|
398 |
-
self._start()
|
399 |
-
|
400 |
-
def _start(self):
|
401 |
-
self._start_transfer_monitor_manager()
|
402 |
-
self._start_submitter()
|
403 |
-
self._start_get_object_workers()
|
404 |
-
self._started = True
|
405 |
-
|
406 |
-
def _validate_all_known_args(self, provided):
|
407 |
-
for kwarg in provided:
|
408 |
-
if kwarg not in ALLOWED_DOWNLOAD_ARGS:
|
409 |
-
download_args = ', '.join(ALLOWED_DOWNLOAD_ARGS)
|
410 |
-
raise ValueError(
|
411 |
-
f"Invalid extra_args key '{kwarg}', "
|
412 |
-
f"must be one of: {download_args}"
|
413 |
-
)
|
414 |
-
|
415 |
-
def _get_transfer_future(self, transfer_id, call_args):
|
416 |
-
meta = ProcessPoolTransferMeta(
|
417 |
-
call_args=call_args, transfer_id=transfer_id
|
418 |
-
)
|
419 |
-
future = ProcessPoolTransferFuture(
|
420 |
-
monitor=self._transfer_monitor, meta=meta
|
421 |
-
)
|
422 |
-
return future
|
423 |
-
|
424 |
-
def _start_transfer_monitor_manager(self):
|
425 |
-
logger.debug('Starting the TransferMonitorManager.')
|
426 |
-
self._manager = TransferMonitorManager()
|
427 |
-
# We do not want Ctrl-C's to cause the manager to shutdown immediately
|
428 |
-
# as worker processes will still need to communicate with it when they
|
429 |
-
# are shutting down. So instead we ignore Ctrl-C and let the manager
|
430 |
-
# be explicitly shutdown when shutting down the downloader.
|
431 |
-
self._manager.start(_add_ignore_handler_for_interrupts)
|
432 |
-
self._transfer_monitor = self._manager.TransferMonitor()
|
433 |
-
|
434 |
-
def _start_submitter(self):
|
435 |
-
logger.debug('Starting the GetObjectSubmitter.')
|
436 |
-
self._submitter = GetObjectSubmitter(
|
437 |
-
transfer_config=self._transfer_config,
|
438 |
-
client_factory=self._client_factory,
|
439 |
-
transfer_monitor=self._transfer_monitor,
|
440 |
-
osutil=self._osutil,
|
441 |
-
download_request_queue=self._download_request_queue,
|
442 |
-
worker_queue=self._worker_queue,
|
443 |
-
)
|
444 |
-
self._submitter.start()
|
445 |
-
|
446 |
-
def _start_get_object_workers(self):
|
447 |
-
logger.debug(
|
448 |
-
'Starting %s GetObjectWorkers.',
|
449 |
-
self._transfer_config.max_request_processes,
|
450 |
-
)
|
451 |
-
for _ in range(self._transfer_config.max_request_processes):
|
452 |
-
worker = GetObjectWorker(
|
453 |
-
queue=self._worker_queue,
|
454 |
-
client_factory=self._client_factory,
|
455 |
-
transfer_monitor=self._transfer_monitor,
|
456 |
-
osutil=self._osutil,
|
457 |
-
)
|
458 |
-
worker.start()
|
459 |
-
self._workers.append(worker)
|
460 |
-
|
461 |
-
def _shutdown_if_needed(self):
|
462 |
-
with self._start_lock:
|
463 |
-
if self._started:
|
464 |
-
self._shutdown()
|
465 |
-
|
466 |
-
def _shutdown(self):
|
467 |
-
self._shutdown_submitter()
|
468 |
-
self._shutdown_get_object_workers()
|
469 |
-
self._shutdown_transfer_monitor_manager()
|
470 |
-
self._started = False
|
471 |
-
|
472 |
-
def _shutdown_transfer_monitor_manager(self):
|
473 |
-
logger.debug('Shutting down the TransferMonitorManager.')
|
474 |
-
self._manager.shutdown()
|
475 |
-
|
476 |
-
def _shutdown_submitter(self):
|
477 |
-
logger.debug('Shutting down the GetObjectSubmitter.')
|
478 |
-
self._download_request_queue.put(SHUTDOWN_SIGNAL)
|
479 |
-
self._submitter.join()
|
480 |
-
|
481 |
-
def _shutdown_get_object_workers(self):
|
482 |
-
logger.debug('Shutting down the GetObjectWorkers.')
|
483 |
-
for _ in self._workers:
|
484 |
-
self._worker_queue.put(SHUTDOWN_SIGNAL)
|
485 |
-
for worker in self._workers:
|
486 |
-
worker.join()
|
487 |
-
|
488 |
-
|
489 |
-
class ProcessPoolTransferFuture(BaseTransferFuture):
|
490 |
-
def __init__(self, monitor, meta):
|
491 |
-
"""The future associated to a submitted process pool transfer request
|
492 |
-
|
493 |
-
:type monitor: TransferMonitor
|
494 |
-
:param monitor: The monitor associated to the process pool downloader
|
495 |
-
|
496 |
-
:type meta: ProcessPoolTransferMeta
|
497 |
-
:param meta: The metadata associated to the request. This object
|
498 |
-
is visible to the requester.
|
499 |
-
"""
|
500 |
-
self._monitor = monitor
|
501 |
-
self._meta = meta
|
502 |
-
|
503 |
-
@property
|
504 |
-
def meta(self):
|
505 |
-
return self._meta
|
506 |
-
|
507 |
-
def done(self):
|
508 |
-
return self._monitor.is_done(self._meta.transfer_id)
|
509 |
-
|
510 |
-
def result(self):
|
511 |
-
try:
|
512 |
-
return self._monitor.poll_for_result(self._meta.transfer_id)
|
513 |
-
except KeyboardInterrupt:
|
514 |
-
# For the multiprocessing Manager, a thread is given a single
|
515 |
-
# connection to reuse in communicating between the thread in the
|
516 |
-
# main process and the Manager's process. If a Ctrl-C happens when
|
517 |
-
# polling for the result, it will make the main thread stop trying
|
518 |
-
# to receive from the connection, but the Manager process will not
|
519 |
-
# know that the main process has stopped trying to receive and
|
520 |
-
# will not close the connection. As a result if another message is
|
521 |
-
# sent to the Manager process, the listener in the Manager
|
522 |
-
# processes will not process the new message as it is still trying
|
523 |
-
# trying to process the previous message (that was Ctrl-C'd) and
|
524 |
-
# thus cause the thread in the main process to hang on its send.
|
525 |
-
# The only way around this is to create a new connection and send
|
526 |
-
# messages from that new connection instead.
|
527 |
-
self._monitor._connect()
|
528 |
-
self.cancel()
|
529 |
-
raise
|
530 |
-
|
531 |
-
def cancel(self):
|
532 |
-
self._monitor.notify_exception(
|
533 |
-
self._meta.transfer_id, CancelledError()
|
534 |
-
)
|
535 |
-
|
536 |
-
|
537 |
-
class ProcessPoolTransferMeta(BaseTransferMeta):
|
538 |
-
"""Holds metadata about the ProcessPoolTransferFuture"""
|
539 |
-
|
540 |
-
def __init__(self, transfer_id, call_args):
|
541 |
-
self._transfer_id = transfer_id
|
542 |
-
self._call_args = call_args
|
543 |
-
self._user_context = {}
|
544 |
-
|
545 |
-
@property
|
546 |
-
def call_args(self):
|
547 |
-
return self._call_args
|
548 |
-
|
549 |
-
@property
|
550 |
-
def transfer_id(self):
|
551 |
-
return self._transfer_id
|
552 |
-
|
553 |
-
@property
|
554 |
-
def user_context(self):
|
555 |
-
return self._user_context
|
556 |
-
|
557 |
-
|
558 |
-
class ClientFactory:
|
559 |
-
def __init__(self, client_kwargs=None):
|
560 |
-
"""Creates S3 clients for processes
|
561 |
-
|
562 |
-
Botocore sessions and clients are not pickleable so they cannot be
|
563 |
-
inherited across Process boundaries. Instead, they must be instantiated
|
564 |
-
once a process is running.
|
565 |
-
"""
|
566 |
-
self._client_kwargs = client_kwargs
|
567 |
-
if self._client_kwargs is None:
|
568 |
-
self._client_kwargs = {}
|
569 |
-
|
570 |
-
client_config = deepcopy(self._client_kwargs.get('config', Config()))
|
571 |
-
if not client_config.user_agent_extra:
|
572 |
-
client_config.user_agent_extra = PROCESS_USER_AGENT
|
573 |
-
else:
|
574 |
-
client_config.user_agent_extra += " " + PROCESS_USER_AGENT
|
575 |
-
self._client_kwargs['config'] = client_config
|
576 |
-
|
577 |
-
def create_client(self):
|
578 |
-
"""Create a botocore S3 client"""
|
579 |
-
return botocore.session.Session().create_client(
|
580 |
-
's3', **self._client_kwargs
|
581 |
-
)
|
582 |
-
|
583 |
-
|
584 |
-
class TransferMonitor:
|
585 |
-
def __init__(self):
|
586 |
-
"""Monitors transfers for cross-process communication
|
587 |
-
|
588 |
-
Notifications can be sent to the monitor and information can be
|
589 |
-
retrieved from the monitor for a particular transfer. This abstraction
|
590 |
-
is ran in a ``multiprocessing.managers.BaseManager`` in order to be
|
591 |
-
shared across processes.
|
592 |
-
"""
|
593 |
-
# TODO: Add logic that removes the TransferState if the transfer is
|
594 |
-
# marked as done and the reference to the future is no longer being
|
595 |
-
# held onto. Without this logic, this dictionary will continue to
|
596 |
-
# grow in size with no limit.
|
597 |
-
self._transfer_states = {}
|
598 |
-
self._id_count = 0
|
599 |
-
self._init_lock = threading.Lock()
|
600 |
-
|
601 |
-
def notify_new_transfer(self):
|
602 |
-
with self._init_lock:
|
603 |
-
transfer_id = self._id_count
|
604 |
-
self._transfer_states[transfer_id] = TransferState()
|
605 |
-
self._id_count += 1
|
606 |
-
return transfer_id
|
607 |
-
|
608 |
-
def is_done(self, transfer_id):
|
609 |
-
"""Determine a particular transfer is complete
|
610 |
-
|
611 |
-
:param transfer_id: Unique identifier for the transfer
|
612 |
-
:return: True, if done. False, otherwise.
|
613 |
-
"""
|
614 |
-
return self._transfer_states[transfer_id].done
|
615 |
-
|
616 |
-
def notify_done(self, transfer_id):
|
617 |
-
"""Notify a particular transfer is complete
|
618 |
-
|
619 |
-
:param transfer_id: Unique identifier for the transfer
|
620 |
-
"""
|
621 |
-
self._transfer_states[transfer_id].set_done()
|
622 |
-
|
623 |
-
def poll_for_result(self, transfer_id):
|
624 |
-
"""Poll for the result of a transfer
|
625 |
-
|
626 |
-
:param transfer_id: Unique identifier for the transfer
|
627 |
-
:return: If the transfer succeeded, it will return the result. If the
|
628 |
-
transfer failed, it will raise the exception associated to the
|
629 |
-
failure.
|
630 |
-
"""
|
631 |
-
self._transfer_states[transfer_id].wait_till_done()
|
632 |
-
exception = self._transfer_states[transfer_id].exception
|
633 |
-
if exception:
|
634 |
-
raise exception
|
635 |
-
return None
|
636 |
-
|
637 |
-
def notify_exception(self, transfer_id, exception):
|
638 |
-
"""Notify an exception was encountered for a transfer
|
639 |
-
|
640 |
-
:param transfer_id: Unique identifier for the transfer
|
641 |
-
:param exception: The exception encountered for that transfer
|
642 |
-
"""
|
643 |
-
# TODO: Not all exceptions are pickleable so if we are running
|
644 |
-
# this in a multiprocessing.BaseManager we will want to
|
645 |
-
# make sure to update this signature to ensure pickleability of the
|
646 |
-
# arguments or have the ProxyObject do the serialization.
|
647 |
-
self._transfer_states[transfer_id].exception = exception
|
648 |
-
|
649 |
-
def notify_cancel_all_in_progress(self):
|
650 |
-
for transfer_state in self._transfer_states.values():
|
651 |
-
if not transfer_state.done:
|
652 |
-
transfer_state.exception = CancelledError()
|
653 |
-
|
654 |
-
def get_exception(self, transfer_id):
|
655 |
-
"""Retrieve the exception encountered for the transfer
|
656 |
-
|
657 |
-
:param transfer_id: Unique identifier for the transfer
|
658 |
-
:return: The exception encountered for that transfer. Otherwise
|
659 |
-
if there were no exceptions, returns None.
|
660 |
-
"""
|
661 |
-
return self._transfer_states[transfer_id].exception
|
662 |
-
|
663 |
-
def notify_expected_jobs_to_complete(self, transfer_id, num_jobs):
|
664 |
-
"""Notify the amount of jobs expected for a transfer
|
665 |
-
|
666 |
-
:param transfer_id: Unique identifier for the transfer
|
667 |
-
:param num_jobs: The number of jobs to complete the transfer
|
668 |
-
"""
|
669 |
-
self._transfer_states[transfer_id].jobs_to_complete = num_jobs
|
670 |
-
|
671 |
-
def notify_job_complete(self, transfer_id):
|
672 |
-
"""Notify that a single job is completed for a transfer
|
673 |
-
|
674 |
-
:param transfer_id: Unique identifier for the transfer
|
675 |
-
:return: The number of jobs remaining to complete the transfer
|
676 |
-
"""
|
677 |
-
return self._transfer_states[transfer_id].decrement_jobs_to_complete()
|
678 |
-
|
679 |
-
|
680 |
-
class TransferState:
|
681 |
-
"""Represents the current state of an individual transfer"""
|
682 |
-
|
683 |
-
# NOTE: Ideally the TransferState object would be used directly by the
|
684 |
-
# various different abstractions in the ProcessPoolDownloader and remove
|
685 |
-
# the need for the TransferMonitor. However, it would then impose the
|
686 |
-
# constraint that two hops are required to make or get any changes in the
|
687 |
-
# state of a transfer across processes: one hop to get a proxy object for
|
688 |
-
# the TransferState and then a second hop to communicate calling the
|
689 |
-
# specific TransferState method.
|
690 |
-
def __init__(self):
|
691 |
-
self._exception = None
|
692 |
-
self._done_event = threading.Event()
|
693 |
-
self._job_lock = threading.Lock()
|
694 |
-
self._jobs_to_complete = 0
|
695 |
-
|
696 |
-
@property
|
697 |
-
def done(self):
|
698 |
-
return self._done_event.is_set()
|
699 |
-
|
700 |
-
def set_done(self):
|
701 |
-
self._done_event.set()
|
702 |
-
|
703 |
-
def wait_till_done(self):
|
704 |
-
self._done_event.wait(MAXINT)
|
705 |
-
|
706 |
-
@property
|
707 |
-
def exception(self):
|
708 |
-
return self._exception
|
709 |
-
|
710 |
-
@exception.setter
|
711 |
-
def exception(self, val):
|
712 |
-
self._exception = val
|
713 |
-
|
714 |
-
@property
|
715 |
-
def jobs_to_complete(self):
|
716 |
-
return self._jobs_to_complete
|
717 |
-
|
718 |
-
@jobs_to_complete.setter
|
719 |
-
def jobs_to_complete(self, val):
|
720 |
-
self._jobs_to_complete = val
|
721 |
-
|
722 |
-
def decrement_jobs_to_complete(self):
|
723 |
-
with self._job_lock:
|
724 |
-
self._jobs_to_complete -= 1
|
725 |
-
return self._jobs_to_complete
|
726 |
-
|
727 |
-
|
728 |
-
class TransferMonitorManager(BaseManager):
|
729 |
-
pass
|
730 |
-
|
731 |
-
|
732 |
-
TransferMonitorManager.register('TransferMonitor', TransferMonitor)
|
733 |
-
|
734 |
-
|
735 |
-
class BaseS3TransferProcess(multiprocessing.Process):
|
736 |
-
def __init__(self, client_factory):
|
737 |
-
super().__init__()
|
738 |
-
self._client_factory = client_factory
|
739 |
-
self._client = None
|
740 |
-
|
741 |
-
def run(self):
|
742 |
-
# Clients are not pickleable so their instantiation cannot happen
|
743 |
-
# in the __init__ for processes that are created under the
|
744 |
-
# spawn method.
|
745 |
-
self._client = self._client_factory.create_client()
|
746 |
-
with ignore_ctrl_c():
|
747 |
-
# By default these processes are ran as child processes to the
|
748 |
-
# main process. Any Ctrl-c encountered in the main process is
|
749 |
-
# propagated to the child process and interrupt it at any time.
|
750 |
-
# To avoid any potentially bad states caused from an interrupt
|
751 |
-
# (i.e. a transfer failing to notify its done or making the
|
752 |
-
# communication protocol become out of sync with the
|
753 |
-
# TransferMonitor), we ignore all Ctrl-C's and allow the main
|
754 |
-
# process to notify these child processes when to stop processing
|
755 |
-
# jobs.
|
756 |
-
self._do_run()
|
757 |
-
|
758 |
-
def _do_run(self):
|
759 |
-
raise NotImplementedError('_do_run()')
|
760 |
-
|
761 |
-
|
762 |
-
class GetObjectSubmitter(BaseS3TransferProcess):
|
763 |
-
def __init__(
|
764 |
-
self,
|
765 |
-
transfer_config,
|
766 |
-
client_factory,
|
767 |
-
transfer_monitor,
|
768 |
-
osutil,
|
769 |
-
download_request_queue,
|
770 |
-
worker_queue,
|
771 |
-
):
|
772 |
-
"""Submit GetObjectJobs to fulfill a download file request
|
773 |
-
|
774 |
-
:param transfer_config: Configuration for transfers.
|
775 |
-
:param client_factory: ClientFactory for creating S3 clients.
|
776 |
-
:param transfer_monitor: Monitor for notifying and retrieving state
|
777 |
-
of transfer.
|
778 |
-
:param osutil: OSUtils object to use for os-related behavior when
|
779 |
-
performing the transfer.
|
780 |
-
:param download_request_queue: Queue to retrieve download file
|
781 |
-
requests.
|
782 |
-
:param worker_queue: Queue to submit GetObjectJobs for workers
|
783 |
-
to perform.
|
784 |
-
"""
|
785 |
-
super().__init__(client_factory)
|
786 |
-
self._transfer_config = transfer_config
|
787 |
-
self._transfer_monitor = transfer_monitor
|
788 |
-
self._osutil = osutil
|
789 |
-
self._download_request_queue = download_request_queue
|
790 |
-
self._worker_queue = worker_queue
|
791 |
-
|
792 |
-
def _do_run(self):
|
793 |
-
while True:
|
794 |
-
download_file_request = self._download_request_queue.get()
|
795 |
-
if download_file_request == SHUTDOWN_SIGNAL:
|
796 |
-
logger.debug('Submitter shutdown signal received.')
|
797 |
-
return
|
798 |
-
try:
|
799 |
-
self._submit_get_object_jobs(download_file_request)
|
800 |
-
except Exception as e:
|
801 |
-
logger.debug(
|
802 |
-
'Exception caught when submitting jobs for '
|
803 |
-
'download file request %s: %s',
|
804 |
-
download_file_request,
|
805 |
-
e,
|
806 |
-
exc_info=True,
|
807 |
-
)
|
808 |
-
self._transfer_monitor.notify_exception(
|
809 |
-
download_file_request.transfer_id, e
|
810 |
-
)
|
811 |
-
self._transfer_monitor.notify_done(
|
812 |
-
download_file_request.transfer_id
|
813 |
-
)
|
814 |
-
|
815 |
-
def _submit_get_object_jobs(self, download_file_request):
|
816 |
-
size = self._get_size(download_file_request)
|
817 |
-
temp_filename = self._allocate_temp_file(download_file_request, size)
|
818 |
-
if size < self._transfer_config.multipart_threshold:
|
819 |
-
self._submit_single_get_object_job(
|
820 |
-
download_file_request, temp_filename
|
821 |
-
)
|
822 |
-
else:
|
823 |
-
self._submit_ranged_get_object_jobs(
|
824 |
-
download_file_request, temp_filename, size
|
825 |
-
)
|
826 |
-
|
827 |
-
def _get_size(self, download_file_request):
|
828 |
-
expected_size = download_file_request.expected_size
|
829 |
-
if expected_size is None:
|
830 |
-
expected_size = self._client.head_object(
|
831 |
-
Bucket=download_file_request.bucket,
|
832 |
-
Key=download_file_request.key,
|
833 |
-
**download_file_request.extra_args,
|
834 |
-
)['ContentLength']
|
835 |
-
return expected_size
|
836 |
-
|
837 |
-
def _allocate_temp_file(self, download_file_request, size):
|
838 |
-
temp_filename = self._osutil.get_temp_filename(
|
839 |
-
download_file_request.filename
|
840 |
-
)
|
841 |
-
self._osutil.allocate(temp_filename, size)
|
842 |
-
return temp_filename
|
843 |
-
|
844 |
-
def _submit_single_get_object_job(
|
845 |
-
self, download_file_request, temp_filename
|
846 |
-
):
|
847 |
-
self._notify_jobs_to_complete(download_file_request.transfer_id, 1)
|
848 |
-
self._submit_get_object_job(
|
849 |
-
transfer_id=download_file_request.transfer_id,
|
850 |
-
bucket=download_file_request.bucket,
|
851 |
-
key=download_file_request.key,
|
852 |
-
temp_filename=temp_filename,
|
853 |
-
offset=0,
|
854 |
-
extra_args=download_file_request.extra_args,
|
855 |
-
filename=download_file_request.filename,
|
856 |
-
)
|
857 |
-
|
858 |
-
def _submit_ranged_get_object_jobs(
|
859 |
-
self, download_file_request, temp_filename, size
|
860 |
-
):
|
861 |
-
part_size = self._transfer_config.multipart_chunksize
|
862 |
-
num_parts = calculate_num_parts(size, part_size)
|
863 |
-
self._notify_jobs_to_complete(
|
864 |
-
download_file_request.transfer_id, num_parts
|
865 |
-
)
|
866 |
-
for i in range(num_parts):
|
867 |
-
offset = i * part_size
|
868 |
-
range_parameter = calculate_range_parameter(
|
869 |
-
part_size, i, num_parts
|
870 |
-
)
|
871 |
-
get_object_kwargs = {'Range': range_parameter}
|
872 |
-
get_object_kwargs.update(download_file_request.extra_args)
|
873 |
-
self._submit_get_object_job(
|
874 |
-
transfer_id=download_file_request.transfer_id,
|
875 |
-
bucket=download_file_request.bucket,
|
876 |
-
key=download_file_request.key,
|
877 |
-
temp_filename=temp_filename,
|
878 |
-
offset=offset,
|
879 |
-
extra_args=get_object_kwargs,
|
880 |
-
filename=download_file_request.filename,
|
881 |
-
)
|
882 |
-
|
883 |
-
def _submit_get_object_job(self, **get_object_job_kwargs):
|
884 |
-
self._worker_queue.put(GetObjectJob(**get_object_job_kwargs))
|
885 |
-
|
886 |
-
def _notify_jobs_to_complete(self, transfer_id, jobs_to_complete):
|
887 |
-
logger.debug(
|
888 |
-
'Notifying %s job(s) to complete for transfer_id %s.',
|
889 |
-
jobs_to_complete,
|
890 |
-
transfer_id,
|
891 |
-
)
|
892 |
-
self._transfer_monitor.notify_expected_jobs_to_complete(
|
893 |
-
transfer_id, jobs_to_complete
|
894 |
-
)
|
895 |
-
|
896 |
-
|
897 |
-
class GetObjectWorker(BaseS3TransferProcess):
|
898 |
-
# TODO: It may make sense to expose these class variables as configuration
|
899 |
-
# options if users want to tweak them.
|
900 |
-
_MAX_ATTEMPTS = 5
|
901 |
-
_IO_CHUNKSIZE = 2 * MB
|
902 |
-
|
903 |
-
def __init__(self, queue, client_factory, transfer_monitor, osutil):
|
904 |
-
"""Fulfills GetObjectJobs
|
905 |
-
|
906 |
-
Downloads the S3 object, writes it to the specified file, and
|
907 |
-
renames the file to its final location if it completes the final
|
908 |
-
job for a particular transfer.
|
909 |
-
|
910 |
-
:param queue: Queue for retrieving GetObjectJob's
|
911 |
-
:param client_factory: ClientFactory for creating S3 clients
|
912 |
-
:param transfer_monitor: Monitor for notifying
|
913 |
-
:param osutil: OSUtils object to use for os-related behavior when
|
914 |
-
performing the transfer.
|
915 |
-
"""
|
916 |
-
super().__init__(client_factory)
|
917 |
-
self._queue = queue
|
918 |
-
self._client_factory = client_factory
|
919 |
-
self._transfer_monitor = transfer_monitor
|
920 |
-
self._osutil = osutil
|
921 |
-
|
922 |
-
def _do_run(self):
|
923 |
-
while True:
|
924 |
-
job = self._queue.get()
|
925 |
-
if job == SHUTDOWN_SIGNAL:
|
926 |
-
logger.debug('Worker shutdown signal received.')
|
927 |
-
return
|
928 |
-
if not self._transfer_monitor.get_exception(job.transfer_id):
|
929 |
-
self._run_get_object_job(job)
|
930 |
-
else:
|
931 |
-
logger.debug(
|
932 |
-
'Skipping get object job %s because there was a previous '
|
933 |
-
'exception.',
|
934 |
-
job,
|
935 |
-
)
|
936 |
-
remaining = self._transfer_monitor.notify_job_complete(
|
937 |
-
job.transfer_id
|
938 |
-
)
|
939 |
-
logger.debug(
|
940 |
-
'%s jobs remaining for transfer_id %s.',
|
941 |
-
remaining,
|
942 |
-
job.transfer_id,
|
943 |
-
)
|
944 |
-
if not remaining:
|
945 |
-
self._finalize_download(
|
946 |
-
job.transfer_id, job.temp_filename, job.filename
|
947 |
-
)
|
948 |
-
|
949 |
-
def _run_get_object_job(self, job):
|
950 |
-
try:
|
951 |
-
self._do_get_object(
|
952 |
-
bucket=job.bucket,
|
953 |
-
key=job.key,
|
954 |
-
temp_filename=job.temp_filename,
|
955 |
-
extra_args=job.extra_args,
|
956 |
-
offset=job.offset,
|
957 |
-
)
|
958 |
-
except Exception as e:
|
959 |
-
logger.debug(
|
960 |
-
'Exception caught when downloading object for '
|
961 |
-
'get object job %s: %s',
|
962 |
-
job,
|
963 |
-
e,
|
964 |
-
exc_info=True,
|
965 |
-
)
|
966 |
-
self._transfer_monitor.notify_exception(job.transfer_id, e)
|
967 |
-
|
968 |
-
def _do_get_object(self, bucket, key, extra_args, temp_filename, offset):
|
969 |
-
last_exception = None
|
970 |
-
for i in range(self._MAX_ATTEMPTS):
|
971 |
-
try:
|
972 |
-
response = self._client.get_object(
|
973 |
-
Bucket=bucket, Key=key, **extra_args
|
974 |
-
)
|
975 |
-
self._write_to_file(temp_filename, offset, response['Body'])
|
976 |
-
return
|
977 |
-
except S3_RETRYABLE_DOWNLOAD_ERRORS as e:
|
978 |
-
logger.debug(
|
979 |
-
'Retrying exception caught (%s), '
|
980 |
-
'retrying request, (attempt %s / %s)',
|
981 |
-
e,
|
982 |
-
i + 1,
|
983 |
-
self._MAX_ATTEMPTS,
|
984 |
-
exc_info=True,
|
985 |
-
)
|
986 |
-
last_exception = e
|
987 |
-
raise RetriesExceededError(last_exception)
|
988 |
-
|
989 |
-
def _write_to_file(self, filename, offset, body):
|
990 |
-
with open(filename, 'rb+') as f:
|
991 |
-
f.seek(offset)
|
992 |
-
chunks = iter(lambda: body.read(self._IO_CHUNKSIZE), b'')
|
993 |
-
for chunk in chunks:
|
994 |
-
f.write(chunk)
|
995 |
-
|
996 |
-
def _finalize_download(self, transfer_id, temp_filename, filename):
|
997 |
-
if self._transfer_monitor.get_exception(transfer_id):
|
998 |
-
self._osutil.remove_file(temp_filename)
|
999 |
-
else:
|
1000 |
-
self._do_file_rename(transfer_id, temp_filename, filename)
|
1001 |
-
self._transfer_monitor.notify_done(transfer_id)
|
1002 |
-
|
1003 |
-
def _do_file_rename(self, transfer_id, temp_filename, filename):
|
1004 |
-
try:
|
1005 |
-
self._osutil.rename_file(temp_filename, filename)
|
1006 |
-
except Exception as e:
|
1007 |
-
self._transfer_monitor.notify_exception(transfer_id, e)
|
1008 |
-
self._osutil.remove_file(temp_filename)
|
|
|
|
|
|
|
|
|
|
|
|
|
|
|
|
|
|
|
|
|
|
|
|
|
|
|
|
|
|
|
|
|
|
|
|
|
|
|
|
|
|
|
|
|
|
|
|
|
|
|
|
|
|
|
|
|
|
|
|
|
|
|
|
|
|
|
|
|
|
|
|
|
|
|
|
|
|
|
|
|
|
|
|
|
|
|
|
|
|
|
|
|
|
|
|
|
|
|
|
|
|
|
|
|
|
|
|
|
|
|
|
|
|
|
|
|
|
|
|
|
|
|
|
|
|
|
|
|
|
|
|
|
|
|
|
|
|
|
|
|
|
|
|
|
|
|
|
|
|
|
|
|
|
|
|
|
|
|
|
|
|
|
|
|
|
|
|
|
|
|
|
|
|
|
|
|
|
|
|
|
|
|
|
|
|
|
|
|
|
|
|
|
|
|
|
|
|
|
|
|
|
|
|
|
|
|
|
|
|
|
|
|
|
|
|
|
|
|
|
|
|
|
|
|
|
|
|
|
|
|
|
|
|
|
|
|
|
|
|
|
|
|
|
|
|
|
|
|
|
|
|
|
|
|
|
|
|
|
|
|
|
|
|
|
|
|
|
|
|
|
|
|
|
|
|
|
|
|
|
|
|
|
|
|
|
|
|
|
|
|
|
|
|
|
|
|
|
|
|
|
|
|
|
|
|
|
|
|
|
|
|
|
|
|
|
|
|
|
|
|
|
|
|
|
|
|
|
|
|
|
|
|
|
|
|
|
|
|
|
|
|
|
|
|
|
|
|
|
|
|
|
|
|
|
|
|
|
|
|
|
|
|
|
|
|
|
|
|
|
|
|
|
|
|
|
|
|
|
|
|
|
|
|
|
|
|
|
|
|
|
|
|
|
|
|
|
|
|
|
|
|
|
|
|
|
|
|
|
|
|
|
|
|
|
|
|
|
|
|
|
|
|
|
|
|
|
|
|
|
|
|
|
|
|
|
|
|
|
|
|
|
|
|
|
|
|
|
|
|
|
|
|
|
|
|
|
|
|
|
|
|
|
|
|
|
|
|
|
|
|
|
|
|
|
|
|
|
|
|
|
|
|
|
|
|
|
|
|
|
|
|
|
|
|
|
|
|
|
|
|
|
|
|
|
|
|
|
|
|
|
|
|
|
|
|
|
|
|
|
|
|
|
|
|
|
|
|
|
|
|
|
|
|
|
|
|
|
|
|
|
|
|
|
|
|
|
|
|
|
|
|
|
|
|
|
|
|
|
|
|
|
|
|
|
|
|
|
|
|
|
|
|
|
|
|
|
|
|
|
|
|
|
|
|
|
|
|
|
|
|
|
|
|
|
|
|
|
|
|
|
|
|
|
|
|
|
|
|
|
|
|
|
|
|
|
|
|
|
|
|
|
|
|
|
|
|
|
|
|
|
|
|
|
|
|
|
|
|
|
|
|
|
|
|
|
|
|
|
|
|
|
|
|
|
|
|
|
|
|
|
|
|
|
|
|
|
|
|
|
|
|
|
|
|
|
|
|
|
|
|
|
|
|
|
|
|
|
|
|
|
|
|
|
|
|
|
|
|
|
|
|
|
|
|
|
|
|
|
|
|
|
|
|
|
|
|
|
|
|
|
|
|
|
|
|
|
|
|
|
|
|
|
|
|
|
|
|
|
|
|
|
|
|
|
|
|
|
|
|
|
|
|
|
|
|
|
|
|
|
|
|
|
|
|
|
|
|
|
|
|
|
|
|
|
|
|
|
|
|
|
|
|
|
|
|
|
|
|
|
|
|
|
|
|
|
|
|
|
|
|
|
|
|
|
|
|
|
|
|
|
|
|
|
|
|
|
|
|
|
|
|
|
|
|
|
|
|
|
|
|
|
|
|
|
|
|
|
|
|
|
|
|
|
|
|
|
|
|
|
|
|
|
|
|
|
|
|
|
|
|
|
|
|
|
|
|
|
|
|
|
|
|
|
|
|
|
|
|
|
|
|
|
|
|
|
|
|
|
|
|
|
|
|
|
|
|
|
|
|
|
|
|
|
|
|
|
|
|
|
|
|
|
|
|
|
|
|
|
|
|
|
|
|
|
|
|
|
|
|
|
|
|
|
|
|
|
|
|
|
|
|
|
|
|
|
|
|
|
|
|
|
|
|
|
|
|
|
|
|
|
|
|
|
|
|
|
|
|
|
|
|
|
|
|
|
|
|
|
|
|
|
|
|
|
|
|
|
|
|
|
|
|
|
|
|
|
|
|
|
|
|
|
|
|
|
|
|
|
|
|
|
|
|
|
|
|
|
|
|
|
|
|
|
|
|
|
|
|
|
|
|
|
|
|
|
|
|
|
|
|
|
|
|
|
|
|
|
|
|
|
|
|
|
|
|
|
|
|
|
|
|
|
|
|
|
|
|
|
|
|
|
|
|
|
|
|
|
|
|
|
|
|
|
|
|
|
|
|
|
|
|
|
|
|
|
|
|
|
|
|
|
|
|
|
|
|
|
|
|
|
|
|
|
|
|
|
|
|
|
|
|
|
|
|
|
|
|
|
|
|
|
|
|
|
|
|
|
|
|
|
|
|
|
|
|
|
|
|
|
|
|
|
|
|
|
|
|
|
|
|
|
|
|
|
|
|
|
|
|
|
|
|
|
|
|
|
|
|
|
|
|
|
|
|
|
|
|
|
|
|
|
|
|
|
|
|
|
|
|
|
|
|
|
|
|
|
|
|
|
|
|
|
|
|
|
|
|
|
|
|
|
|
|
|
|
|
|
|
|
|
|
|
|
|
|
|
|
|
|
|
|
|
|
|
|
|
|
|
|
|
|
|
|
|
|
|
|
|
|
|
|
|
|
|
|
|
|
|
|
|
|
|
|
|
|
|
|
|
|
|
|
|
|
|
|
|
|
|
|
|
|
|
|
|
|
|
|
|
|
|
|
|
|
|
|
|
|
|
|
|
|
|
|
|
|
|
|
|
|
|
|
|
|
|
|
|
|
|
|
|
|
|
|
|
|
|
|
|
|
|
|
|
|
|
|
|
|
|
|
|
|
|
|
|
|
|
|
|
|
|
|
|
|
|
|
|
|
|
|
|
|
|
|
|
|
|
|
|
|
|
|
|
|
|
|
|
|
|
|
|
|
|
|
|
|
|
|
|
|
|
|
|
|
|
|
|
|
|
|
|
|
|
|
|
|
|
|
|
|
|
|
|
|
|
|
|
|
|
|
|
|
|
|
|
|
|
|
|
|
|
|
|
|
|
|
|
|
|
|
|
|
|
|
|
|
|
|
|
|
|
|
|
|
|
|
|
|
|
|
|
|
|
|
|
|
|
|
|
|
|
|
|
|
|
|
|
|
|
|
|
|
|
|
|
|
|
|
|
|
|
|
|
|
|
|
|
|
|
|
|
|
|
|
|
|
|
|
|
|
|
|
|
|
|
|
|
|
|
|
|
|
|
|
|
|
|
|
|
|
|
|
|
|
|
|
|
|
|
|
|
|
|
|
|
|
|
|
|
|
|
|
|
|
|
|
|
|
|
|
|
|
|
|
|
|
|
|
|
|
|
|
|
|
|
|
|
|
|
|
|
|
|
|
|
|
|
|
|
|
|
|
|
|
|
|
|
|
|
|
|
|
|
|
|
|
|
|
|
|
|
|
|
|
|
|
|
|
|
|
|
|
|
|
|
|
|
|
|
|
|
|
|
|
|
|
|
|
|
|
|
|
|
|
|
|
|
|
|
|
|
|
|
|
|
|
|
|
|
|
|
|
|
|
|
|
|
|
|
|
|
|
|
|
|
|
|
|
|
|
|
|
|
|
|
|
|
|
|
|
|
|
|
|
|
|
|
|
|
|
|
|
|
|
|
|
|
|
|
|
|
|
|
|
|
|
|
|
|
|
|
|
|
|
|
|
|
|
|
|
|
|
|
|
|
|
|
|
|
|
|
|
|
|
|
|
|
|
|
|
|
|
|
|
|
|
|
|
|
|
|
|
|
|
|
|
|
|
|
|
|
|
|
|
|
|
|
|
|
|
|
|
|
|
|
|
|
|
|
|
|
|
|
|
|
|
|
|
|
|
|
|
|
|
|
|
|
|
|
|
|
|
|
|
|
|
|
|
|
|
|
|
|
|
|
|
|
|
|
|
|
|
|
|
|
|
|
|
|
|
|
|
|
|
|
|
|
|
|
|
|
|
|
|
|
|
|
|
|
|
|
|
|
|
|
|
|
|
|
|
|
|
|
|
|
|
|
|
|
|
|
|
|
|
|
|
|
|
|
|
|
|
|
|
|
|
|
|
|
|
|
|
|
|
|
|
|
|
|
|
|
|
|
|
|
|
|
|
|
|
|
|
|
|
|
|
|
|
|
|
|
|
|
|
|
|
|
|
|
|
|
|
|
|
|
|
|
|
|
|
|
|
|
|
|
|
|
|
|
|
|
|
|
|
|
|
|
|
|
|
|
|
|
|
|
|
|
|
|
|
|
|
|
|
|
|
|
|
|
|
|
|
|
|
|
|
|
|
|
|
|
|
|
|
|
|
|
|
spaces/BlitzenPrancer/TheBloke-guanaco-65B-HF/app.py
DELETED
@@ -1,3 +0,0 @@
|
|
1 |
-
import gradio as gr
|
2 |
-
|
3 |
-
gr.Interface.load("models/TheBloke/guanaco-65B-HF").launch()
|
|
|
|
|
|
|
|
spaces/CVPR/Dual-Key_Backdoor_Attacks/datagen/detectron2/detectron2/layers/roi_align.py
DELETED
@@ -1,105 +0,0 @@
|
|
1 |
-
# Copyright (c) Facebook, Inc. and its affiliates. All Rights Reserved
|
2 |
-
from torch import nn
|
3 |
-
from torch.autograd import Function
|
4 |
-
from torch.autograd.function import once_differentiable
|
5 |
-
from torch.nn.modules.utils import _pair
|
6 |
-
|
7 |
-
from detectron2 import _C
|
8 |
-
|
9 |
-
|
10 |
-
class _ROIAlign(Function):
|
11 |
-
@staticmethod
|
12 |
-
def forward(ctx, input, roi, output_size, spatial_scale, sampling_ratio, aligned):
|
13 |
-
ctx.save_for_backward(roi)
|
14 |
-
ctx.output_size = _pair(output_size)
|
15 |
-
ctx.spatial_scale = spatial_scale
|
16 |
-
ctx.sampling_ratio = sampling_ratio
|
17 |
-
ctx.input_shape = input.size()
|
18 |
-
ctx.aligned = aligned
|
19 |
-
output = _C.roi_align_forward(
|
20 |
-
input, roi, spatial_scale, output_size[0], output_size[1], sampling_ratio, aligned
|
21 |
-
)
|
22 |
-
return output
|
23 |
-
|
24 |
-
@staticmethod
|
25 |
-
@once_differentiable
|
26 |
-
def backward(ctx, grad_output):
|
27 |
-
rois, = ctx.saved_tensors
|
28 |
-
output_size = ctx.output_size
|
29 |
-
spatial_scale = ctx.spatial_scale
|
30 |
-
sampling_ratio = ctx.sampling_ratio
|
31 |
-
bs, ch, h, w = ctx.input_shape
|
32 |
-
grad_input = _C.roi_align_backward(
|
33 |
-
grad_output,
|
34 |
-
rois,
|
35 |
-
spatial_scale,
|
36 |
-
output_size[0],
|
37 |
-
output_size[1],
|
38 |
-
bs,
|
39 |
-
ch,
|
40 |
-
h,
|
41 |
-
w,
|
42 |
-
sampling_ratio,
|
43 |
-
ctx.aligned,
|
44 |
-
)
|
45 |
-
return grad_input, None, None, None, None, None
|
46 |
-
|
47 |
-
|
48 |
-
roi_align = _ROIAlign.apply
|
49 |
-
|
50 |
-
|
51 |
-
class ROIAlign(nn.Module):
|
52 |
-
def __init__(self, output_size, spatial_scale, sampling_ratio, aligned=True):
|
53 |
-
"""
|
54 |
-
Args:
|
55 |
-
output_size (tuple): h, w
|
56 |
-
spatial_scale (float): scale the input boxes by this number
|
57 |
-
sampling_ratio (int): number of inputs samples to take for each output
|
58 |
-
sample. 0 to take samples densely.
|
59 |
-
aligned (bool): if False, use the legacy implementation in
|
60 |
-
Detectron. If True, align the results more perfectly.
|
61 |
-
|
62 |
-
Note:
|
63 |
-
The meaning of aligned=True:
|
64 |
-
|
65 |
-
Given a continuous coordinate c, its two neighboring pixel indices (in our
|
66 |
-
pixel model) are computed by floor(c - 0.5) and ceil(c - 0.5). For example,
|
67 |
-
c=1.3 has pixel neighbors with discrete indices [0] and [1] (which are sampled
|
68 |
-
from the underlying signal at continuous coordinates 0.5 and 1.5). But the original
|
69 |
-
roi_align (aligned=False) does not subtract the 0.5 when computing neighboring
|
70 |
-
pixel indices and therefore it uses pixels with a slightly incorrect alignment
|
71 |
-
(relative to our pixel model) when performing bilinear interpolation.
|
72 |
-
|
73 |
-
With `aligned=True`,
|
74 |
-
we first appropriately scale the ROI and then shift it by -0.5
|
75 |
-
prior to calling roi_align. This produces the correct neighbors; see
|
76 |
-
detectron2/tests/test_roi_align.py for verification.
|
77 |
-
|
78 |
-
The difference does not make a difference to the model's performance if
|
79 |
-
ROIAlign is used together with conv layers.
|
80 |
-
"""
|
81 |
-
super(ROIAlign, self).__init__()
|
82 |
-
self.output_size = output_size
|
83 |
-
self.spatial_scale = spatial_scale
|
84 |
-
self.sampling_ratio = sampling_ratio
|
85 |
-
self.aligned = aligned
|
86 |
-
|
87 |
-
def forward(self, input, rois):
|
88 |
-
"""
|
89 |
-
Args:
|
90 |
-
input: NCHW images
|
91 |
-
rois: Bx5 boxes. First column is the index into N. The other 4 columns are xyxy.
|
92 |
-
"""
|
93 |
-
assert rois.dim() == 2 and rois.size(1) == 5
|
94 |
-
return roi_align(
|
95 |
-
input, rois, self.output_size, self.spatial_scale, self.sampling_ratio, self.aligned
|
96 |
-
)
|
97 |
-
|
98 |
-
def __repr__(self):
|
99 |
-
tmpstr = self.__class__.__name__ + "("
|
100 |
-
tmpstr += "output_size=" + str(self.output_size)
|
101 |
-
tmpstr += ", spatial_scale=" + str(self.spatial_scale)
|
102 |
-
tmpstr += ", sampling_ratio=" + str(self.sampling_ratio)
|
103 |
-
tmpstr += ", aligned=" + str(self.aligned)
|
104 |
-
tmpstr += ")"
|
105 |
-
return tmpstr
|
|
|
|
|
|
|
|
|
|
|
|
|
|
|
|
|
|
|
|
|
|
|
|
|
|
|
|
|
|
|
|
|
|
|
|
|
|
|
|
|
|
|
|
|
|
|
|
|
|
|
|
|
|
|
|
|
|
|
|
|
|
|
|
|
|
|
|
|
|
|
|
|
|
|
|
|
|
|
|
|
|
|
|
|
|
|
|
|
|
|
|
|
|
|
|
|
|
|
|
|
|
|
|
|
|
|
|
|
|
|
|
|
|
|
|
|
|
|
|
|
|
|
|
|
|
|
|
|
|
|
|
|
|
|
|
|
|
|
|
|
|
|
|
|
|
|
|
|
|
|
|
|
|
|
|
|
|
|
|
|
|
|
|
|
|
|
|
|
|
|
|
|
|
|
|
|
|
|
|
|
|
|
|
|
|
|
|
|
|
|
|
|
|
|
|
|
|
|
|
|
|
|
|
|
|
|
|
|
|
|
|
spaces/CVPR/WALT/mmdet/utils/contextmanagers.py
DELETED
@@ -1,121 +0,0 @@
|
|
1 |
-
import asyncio
|
2 |
-
import contextlib
|
3 |
-
import logging
|
4 |
-
import os
|
5 |
-
import time
|
6 |
-
from typing import List
|
7 |
-
|
8 |
-
import torch
|
9 |
-
|
10 |
-
logger = logging.getLogger(__name__)
|
11 |
-
|
12 |
-
DEBUG_COMPLETED_TIME = bool(os.environ.get('DEBUG_COMPLETED_TIME', False))
|
13 |
-
|
14 |
-
|
15 |
-
@contextlib.asynccontextmanager
|
16 |
-
async def completed(trace_name='',
|
17 |
-
name='',
|
18 |
-
sleep_interval=0.05,
|
19 |
-
streams: List[torch.cuda.Stream] = None):
|
20 |
-
"""Async context manager that waits for work to complete on given CUDA
|
21 |
-
streams."""
|
22 |
-
if not torch.cuda.is_available():
|
23 |
-
yield
|
24 |
-
return
|
25 |
-
|
26 |
-
stream_before_context_switch = torch.cuda.current_stream()
|
27 |
-
if not streams:
|
28 |
-
streams = [stream_before_context_switch]
|
29 |
-
else:
|
30 |
-
streams = [s if s else stream_before_context_switch for s in streams]
|
31 |
-
|
32 |
-
end_events = [
|
33 |
-
torch.cuda.Event(enable_timing=DEBUG_COMPLETED_TIME) for _ in streams
|
34 |
-
]
|
35 |
-
|
36 |
-
if DEBUG_COMPLETED_TIME:
|
37 |
-
start = torch.cuda.Event(enable_timing=True)
|
38 |
-
stream_before_context_switch.record_event(start)
|
39 |
-
|
40 |
-
cpu_start = time.monotonic()
|
41 |
-
logger.debug('%s %s starting, streams: %s', trace_name, name, streams)
|
42 |
-
grad_enabled_before = torch.is_grad_enabled()
|
43 |
-
try:
|
44 |
-
yield
|
45 |
-
finally:
|
46 |
-
current_stream = torch.cuda.current_stream()
|
47 |
-
assert current_stream == stream_before_context_switch
|
48 |
-
|
49 |
-
if DEBUG_COMPLETED_TIME:
|
50 |
-
cpu_end = time.monotonic()
|
51 |
-
for i, stream in enumerate(streams):
|
52 |
-
event = end_events[i]
|
53 |
-
stream.record_event(event)
|
54 |
-
|
55 |
-
grad_enabled_after = torch.is_grad_enabled()
|
56 |
-
|
57 |
-
# observed change of torch.is_grad_enabled() during concurrent run of
|
58 |
-
# async_test_bboxes code
|
59 |
-
assert (grad_enabled_before == grad_enabled_after
|
60 |
-
), 'Unexpected is_grad_enabled() value change'
|
61 |
-
|
62 |
-
are_done = [e.query() for e in end_events]
|
63 |
-
logger.debug('%s %s completed: %s streams: %s', trace_name, name,
|
64 |
-
are_done, streams)
|
65 |
-
with torch.cuda.stream(stream_before_context_switch):
|
66 |
-
while not all(are_done):
|
67 |
-
await asyncio.sleep(sleep_interval)
|
68 |
-
are_done = [e.query() for e in end_events]
|
69 |
-
logger.debug(
|
70 |
-
'%s %s completed: %s streams: %s',
|
71 |
-
trace_name,
|
72 |
-
name,
|
73 |
-
are_done,
|
74 |
-
streams,
|
75 |
-
)
|
76 |
-
|
77 |
-
current_stream = torch.cuda.current_stream()
|
78 |
-
assert current_stream == stream_before_context_switch
|
79 |
-
|
80 |
-
if DEBUG_COMPLETED_TIME:
|
81 |
-
cpu_time = (cpu_end - cpu_start) * 1000
|
82 |
-
stream_times_ms = ''
|
83 |
-
for i, stream in enumerate(streams):
|
84 |
-
elapsed_time = start.elapsed_time(end_events[i])
|
85 |
-
stream_times_ms += f' {stream} {elapsed_time:.2f} ms'
|
86 |
-
logger.info('%s %s %.2f ms %s', trace_name, name, cpu_time,
|
87 |
-
stream_times_ms)
|
88 |
-
|
89 |
-
|
90 |
-
@contextlib.asynccontextmanager
|
91 |
-
async def concurrent(streamqueue: asyncio.Queue,
|
92 |
-
trace_name='concurrent',
|
93 |
-
name='stream'):
|
94 |
-
"""Run code concurrently in different streams.
|
95 |
-
|
96 |
-
:param streamqueue: asyncio.Queue instance.
|
97 |
-
|
98 |
-
Queue tasks define the pool of streams used for concurrent execution.
|
99 |
-
"""
|
100 |
-
if not torch.cuda.is_available():
|
101 |
-
yield
|
102 |
-
return
|
103 |
-
|
104 |
-
initial_stream = torch.cuda.current_stream()
|
105 |
-
|
106 |
-
with torch.cuda.stream(initial_stream):
|
107 |
-
stream = await streamqueue.get()
|
108 |
-
assert isinstance(stream, torch.cuda.Stream)
|
109 |
-
|
110 |
-
try:
|
111 |
-
with torch.cuda.stream(stream):
|
112 |
-
logger.debug('%s %s is starting, stream: %s', trace_name, name,
|
113 |
-
stream)
|
114 |
-
yield
|
115 |
-
current = torch.cuda.current_stream()
|
116 |
-
assert current == stream
|
117 |
-
logger.debug('%s %s has finished, stream: %s', trace_name,
|
118 |
-
name, stream)
|
119 |
-
finally:
|
120 |
-
streamqueue.task_done()
|
121 |
-
streamqueue.put_nowait(stream)
|
|
|
|
|
|
|
|
|
|
|
|
|
|
|
|
|
|
|
|
|
|
|
|
|
|
|
|
|
|
|
|
|
|
|
|
|
|
|
|
|
|
|
|
|
|
|
|
|
|
|
|
|
|
|
|
|
|
|
|
|
|
|
|
|
|
|
|
|
|
|
|
|
|
|
|
|
|
|
|
|
|
|
|
|
|
|
|
|
|
|
|
|
|
|
|
|
|
|
|
|
|
|
|
|
|
|
|
|
|
|
|
|
|
|
|
|
|
|
|
|
|
|
|
|
|
|
|
|
|
|
|
|
|
|
|
|
|
|
|
|
|
|
|
|
|
|
|
|
|
|
|
|
|
|
|
|
|
|
|
|
|
|
|
|
|
|
|
|
|
|
|
|
|
|
|
|
|
|
|
|
|
|
|
|
|
|
|
|
|
|
|
|
|
|
|
|
|
|
|
|
|
|
|
|
|
|
|
|
|
|
|
|
|
|
|
|
|
|
|
|
|
|
|
|
|
|
|
|
|
|
|
|
|
|
|
|
|
|
|
|
|
|
|
spaces/CVPR/transfiner/configs/common/data/coco_keypoint.py
DELETED
@@ -1,13 +0,0 @@
|
|
1 |
-
from detectron2.data.detection_utils import create_keypoint_hflip_indices
|
2 |
-
|
3 |
-
from .coco import dataloader
|
4 |
-
|
5 |
-
dataloader.train.dataset.min_keypoints = 1
|
6 |
-
dataloader.train.dataset.names = "keypoints_coco_2017_train"
|
7 |
-
dataloader.test.dataset.names = "keypoints_coco_2017_val"
|
8 |
-
|
9 |
-
dataloader.train.mapper.update(
|
10 |
-
use_instance_mask=False,
|
11 |
-
use_keypoint=True,
|
12 |
-
keypoint_hflip_indices=create_keypoint_hflip_indices(dataloader.train.dataset.names),
|
13 |
-
)
|
|
|
|
|
|
|
|
|
|
|
|
|
|
|
|
|
|
|
|
|
|
|
|
|
|
|
|
spaces/Chris1/real2sim/README.md
DELETED
@@ -1,13 +0,0 @@
|
|
1 |
-
---
|
2 |
-
title: Real2sim
|
3 |
-
emoji: 👁
|
4 |
-
colorFrom: yellow
|
5 |
-
colorTo: green
|
6 |
-
sdk: gradio
|
7 |
-
sdk_version: 2.9.4
|
8 |
-
app_file: app.py
|
9 |
-
pinned: false
|
10 |
-
license: mit
|
11 |
-
---
|
12 |
-
|
13 |
-
Check out the configuration reference at https://huggingface.co/docs/hub/spaces#reference
|
|
|
|
|
|
|
|
|
|
|
|
|
|
|
|
|
|
|
|
|
|
|
|
|
|
|
|
spaces/CobaltZvc/sherlocks_pheonix/index.html
DELETED
@@ -1,29 +0,0 @@
|
|
1 |
-
<!DOCTYPE html>
|
2 |
-
<html>
|
3 |
-
<head>
|
4 |
-
<title>Sherlock's Phoenix</title>
|
5 |
-
</head>
|
6 |
-
<body>
|
7 |
-
<div style="text-align: center;">
|
8 |
-
<iframe id="myIframe"
|
9 |
-
frameborder="0"
|
10 |
-
style="width: 100%; max-width: 850px; height: 2000px;"
|
11 |
-
></iframe>
|
12 |
-
</div>
|
13 |
-
<script>
|
14 |
-
// Fetch the content of Read.txt from the Hugging Face repository
|
15 |
-
fetch('Read.txt')
|
16 |
-
.then(response => response.text())
|
17 |
-
.then(data => {
|
18 |
-
// Fetch the content of the linked file from GitHub repository
|
19 |
-
return fetch(data.trim());
|
20 |
-
})
|
21 |
-
.then(response => response.text())
|
22 |
-
.then(data => {
|
23 |
-
const myIframe = document.getElementById('myIframe');
|
24 |
-
myIframe.src = data.trim();
|
25 |
-
})
|
26 |
-
.catch(error => console.error(error));
|
27 |
-
</script>
|
28 |
-
</body>
|
29 |
-
</html>
|
|
|
|
|
|
|
|
|
|
|
|
|
|
|
|
|
|
|
|
|
|
|
|
|
|
|
|
|
|
|
|
|
|
|
|
|
|
|
|
|
|
|
|
|
|
|
|
|
|
|
|
|
|
|
|
|
|
|
|
spaces/CompVis/celeba-latent-diffusion/app.py
DELETED
@@ -1,26 +0,0 @@
|
|
1 |
-
from diffusers import LDMPipeline
|
2 |
-
import torch
|
3 |
-
import PIL.Image
|
4 |
-
import gradio as gr
|
5 |
-
import random
|
6 |
-
import numpy as np
|
7 |
-
|
8 |
-
pipeline = LDMPipeline.from_pretrained("CompVis/ldm-celebahq-256")
|
9 |
-
|
10 |
-
def predict(steps, seed):
|
11 |
-
generator = torch.manual_seed(seed)
|
12 |
-
for i in range(1,steps):
|
13 |
-
yield pipeline(generator=generator, num_inference_steps=i)["sample"][0]
|
14 |
-
|
15 |
-
random_seed = random.randint(0, 2147483647)
|
16 |
-
gr.Interface(
|
17 |
-
predict,
|
18 |
-
inputs=[
|
19 |
-
gr.inputs.Slider(1, 100, label='Inference Steps', default=5, step=1),
|
20 |
-
gr.inputs.Slider(0, 2147483647, label='Seed', default=random_seed, step=1),
|
21 |
-
],
|
22 |
-
outputs=gr.Image(shape=[256,256], type="pil", elem_id="output_image"),
|
23 |
-
css="#output_image{width: 256px}",
|
24 |
-
title="ldm-celebahq-256 - 🧨 diffusers library",
|
25 |
-
description="This Spaces contains an unconditional Latent Diffusion process for the <a href=\"https://huggingface.co/CompVis/ldm-celebahq-256\">ldm-celebahq-256</a> face generator model by <a href=\"https://huggingface.co/CompVis\">CompVis</a> using the <a href=\"https://github.com/huggingface/diffusers\">diffusers library</a>. The goal of this demo is to showcase the diffusers library capabilities. If you want the state-of-the-art experience with Latent Diffusion text-to-image check out the <a href=\"https://huggingface.co/spaces/multimodalart/latentdiffusion\">main Spaces</a>.",
|
26 |
-
).queue().launch()
|
|
|
|
|
|
|
|
|
|
|
|
|
|
|
|
|
|
|
|
|
|
|
|
|
|
|
|
|
|
|
|
|
|
|
|
|
|
|
|
|
|
|
|
|
|
|
|
|
|
|
|
|
|
spaces/DAMO-NLP-SG/Video-LLaMA/video_llama/tasks/video_text_pretrain.py
DELETED
@@ -1,18 +0,0 @@
|
|
1 |
-
"""
|
2 |
-
Copyright (c) 2022, salesforce.com, inc.
|
3 |
-
All rights reserved.
|
4 |
-
SPDX-License-Identifier: BSD-3-Clause
|
5 |
-
For full license text, see the LICENSE_Lavis file in the repo root or https://opensource.org/licenses/BSD-3-Clause
|
6 |
-
"""
|
7 |
-
|
8 |
-
from video_llama.common.registry import registry
|
9 |
-
from video_llama.tasks.base_task import BaseTask
|
10 |
-
|
11 |
-
|
12 |
-
@registry.register_task("video_text_pretrain")
|
13 |
-
class VideoTextPretrainTask(BaseTask):
|
14 |
-
def __init__(self):
|
15 |
-
super().__init__()
|
16 |
-
|
17 |
-
def evaluation(self, model, data_loader, cuda_enabled=True):
|
18 |
-
pass
|
|
|
|
|
|
|
|
|
|
|
|
|
|
|
|
|
|
|
|
|
|
|
|
|
|
|
|
|
|
|
|
|
|
|
|
|
|