Commit
·
3849e21
1
Parent(s):
c2ebc44
Update parquet files (step 44 of 397)
Browse filesThis view is limited to 50 files because it contains too many changes.
See raw diff
- spaces/1gistliPinn/ChatGPT4/Examples/Altium Designer 10 License Crack.md +0 -10
- spaces/1gistliPinn/ChatGPT4/Examples/Download Cs 1.6 Hack Aimbot.md +0 -15
- spaces/1pelhydcardo/ChatGPT-prompt-generator/assets/Download 8 Ball Pool Mod APK 2022 and Play with Legends on Android.md +0 -115
- spaces/1phancelerku/anime-remove-background/Derbeder A Tribute to Ferdi Tayfurs Legendary Song.md +0 -135
- spaces/1phancelerku/anime-remove-background/Download GB Instagram Mod APK 2022 and Unlock Hidden Features.md +0 -139
- spaces/801artistry/RVC801/infer/lib/uvr5_pack/lib_v5/layers_537227KB.py +0 -126
- spaces/AB-TW/team-ai/documents/bussiness_context/NOTION_DB/Engineering Wiki 2402f5396a3244fdb3f1d135bdb0f3d6/CircleCI 719905fcb593423cad302d3fdc1c5dff.md +0 -5
- spaces/AIFILMS/generate_human_motion/VQ-Trans/utils/skeleton.py +0 -199
- spaces/AIGC-Audio/AudioGPT/text_to_audio/Make_An_Audio/wav_evaluation/models/__init__.py +0 -3
- spaces/AIGC-Audio/AudioGPT/text_to_speech/tasks/tts/vocoder_infer/pwg.py +0 -32
- spaces/AIGC-Audio/Make_An_Audio/ldm/modules/encoders/open_clap/utils.py +0 -369
- spaces/ANLPRL/NER_On_Oral_Medicine/README.md +0 -12
- spaces/ATang0729/Forecast4Muses/Model/Model6/Model6_1_ClothesKeyPoint/work_dirs_1-x/td_hm_res50_4xb64-60e_deepfashion2_trousers_256x192/__init__.py +0 -0
- spaces/AgentVerse/agentVerse/ui/src/phaser3-rex-plugins/templates/ui/easemove/EaseMove.js +0 -2
- spaces/AgentVerse/agentVerse/ui/src/phaser3-rex-plugins/templates/ui/gridtable/GridTable.d.ts +0 -63
- spaces/Aloento/9Nine-PITS/text/frontend/zh_normalization/__init__.py +0 -14
- spaces/Alpaca233/SadTalker/scripts/extension.py +0 -189
- spaces/Amrrs/DragGan-Inversion/PTI/torch_utils/ops/fma.py +0 -60
- spaces/Androidonnxfork/CivitAi-to-Diffusers/diffusers/docs/source/en/api/pipelines/stable_diffusion/adapter.md +0 -187
- spaces/Androidonnxfork/CivitAi-to-Diffusers/diffusers/tests/pipelines/dance_diffusion/test_dance_diffusion.py +0 -162
- spaces/Anish13/characterGPT/app.py +0 -187
- spaces/AnishKumbhar/ChatBot/text-generation-webui-main/wsl.sh +0 -112
- spaces/Anonymous-sub/Rerender/ControlNet/annotator/uniformer/mmcv/image/misc.py +0 -44
- spaces/ArtificialArtist007/Rate-my-Aiart/app.py +0 -37
- spaces/BAAI/vid2vid-zero/vid2vid_zero/util.py +0 -114
- spaces/Babelscape/rebel-demo/README.md +0 -37
- spaces/Banbri/zcvzcv/src/lib/cleanJson.ts +0 -19
- spaces/Bart92/RVC_HF/audioEffects.py +0 -37
- spaces/Bart92/RVC_HF/infer/lib/infer_pack/modules/F0Predictor/HarvestF0Predictor.py +0 -87
- spaces/Benson/text-generation/Examples/Cmo Descargar Videos De Google Drive.md +0 -157
- spaces/Big-Web/MMSD/env/Lib/site-packages/pip/_vendor/urllib3/util/timeout.py +0 -271
- spaces/CC123123/blip2_t/index.html +0 -19
- spaces/CVPR/LIVE/thrust/dependencies/cub/experimental/sparse_matrix.h +0 -1244
- spaces/Caoyunkang/Segment-Any-Anomaly/SAM/segment_anything/modeling/transformer.py +0 -240
- spaces/CassBunny/anything-v3.0/README.md +0 -13
- spaces/Chilangosta/text-to-pokemon/README.md +0 -13
- spaces/CofAI/chat/client/css/field.css +0 -11
- spaces/CofAI/chat/run.py +0 -48
- spaces/DQChoi/gpt-demo/venv/lib/python3.11/site-packages/fontTools/__init__.py +0 -8
- spaces/Dao3/Text-To-image-AllModels/README.md +0 -14
- spaces/Demi2809/rvc-models/app.py +0 -180
- spaces/DemoLou/moe-tts/README.md +0 -14
- spaces/Dinoking/Guccio-AI-Designer/netdissect/tool/allunitsample.py +0 -199
- spaces/Dralkkin/Lorule-Proxy/Dockerfile +0 -11
- spaces/Dusan/clickbaitonator/fudge/predict_clickbait.py +0 -199
- spaces/Duskfallcrew/Duskfallcrew-duskfallai/README.md +0 -13
- spaces/EPFL-VILAB/MultiMAE/dpt/base_model.py +0 -16
- spaces/EXPOSUREEE/Ai-Image-Enhancer/realesrgan/models/realesrnet_model.py +0 -188
- spaces/EagleLoveAI/ChatGPT_Application_Robot/README.md +0 -13
- spaces/Eddycrack864/Applio-Inference/lib/infer_pack/modules/F0Predictor/F0Predictor.py +0 -16
spaces/1gistliPinn/ChatGPT4/Examples/Altium Designer 10 License Crack.md
DELETED
@@ -1,10 +0,0 @@
|
|
1 |
-
<h2>altium designer 10 license crack</h2><br /><p><b><b>DOWNLOAD</b> » <a href="https://imgfil.com/2uy1Op">https://imgfil.com/2uy1Op</a></b></p><br /><br />
|
2 |
-
<br />
|
3 |
-
Select a license to activate the product
|
4 |
-
The Microsoft Software License Agreement provides for a choice of one of several available versions:
|
5 |
-
â–º Product Key License - to install the program on one computer or on one computer and on several computers if it is connected to the Internet.
|
6 |
-
â–º Product License on Demand - a license to install the product on multiple computers if it is connected to the Internet.
|
7 |
-
â–º Product Code License - For installing the program on a single computer. 8a78ff9644<br />
|
8 |
-
<br />
|
9 |
-
<br />
|
10 |
-
<p></p>
|
|
|
|
|
|
|
|
|
|
|
|
|
|
|
|
|
|
|
|
|
|
spaces/1gistliPinn/ChatGPT4/Examples/Download Cs 1.6 Hack Aimbot.md
DELETED
@@ -1,15 +0,0 @@
|
|
1 |
-
<h2>Download Cs 1.6 Hack Aimbot</h2><br /><p><b><b>Download Zip</b> • <a href="https://imgfil.com/2uy0MH">https://imgfil.com/2uy0MH</a></b></p><br /><br />
|
2 |
-
<br />
|
3 |
-
Features: Server hack that works under linux and windows servers. Damage/health, aimbot, teleport, etc. skins player, cs 1.6 ... Type: Client / Online-mode
|
4 |
-
Genre: First person shooter
|
5 |
-
Publication type: License
|
6 |
-
Platform: PC
|
7 |
-
Developer: Jedidos
|
8 |
-
Year of release: 2012
|
9 |
-
Interface language: Russian
|
10 |
-
Tablet: Sewn
|
11 |
-
System requirements: Operating system: Windows XP, Windows Vista, Windows 7 Processor: Pentium 4 2 GHz RAM: 512 MB Video card: 128 MB VRAM Sound card: DirectX 9.0c compatible
|
12 |
-
Description: Counter-Strike 1.6 by Jedidos is the most popular among cyber teams. 8a78ff9644<br />
|
13 |
-
<br />
|
14 |
-
<br />
|
15 |
-
<p></p>
|
|
|
|
|
|
|
|
|
|
|
|
|
|
|
|
|
|
|
|
|
|
|
|
|
|
|
|
|
|
|
|
spaces/1pelhydcardo/ChatGPT-prompt-generator/assets/Download 8 Ball Pool Mod APK 2022 and Play with Legends on Android.md
DELETED
@@ -1,115 +0,0 @@
|
|
1 |
-
<br />
|
2 |
-
<h1>8 Ball Pool Mod APK 2022 _Apkpure: Everything You Need to Know</h1>
|
3 |
-
<p>If you are a fan of pool games, you might have heard of <strong>8 Ball Pool</strong>, one of the most popular and addictive online multiplayer games for Android and iOS devices. But did you know that there is a way to enjoy this game with more features and unlimited resources? Yes, we are talking about <strong>8 Ball Pool Mod APK 2022 _Apkpure</strong>, a modified version of the original game that gives you access to unlimited coins, cash, cues, and more. In this article, we will tell you everything you need to know about this mod apk, including its features, benefits, risks, and how to download and install it on your device. So, let's get started!</p>
|
4 |
-
<h2>8 ball pool mod apk 2022 _apkpure</h2><br /><p><b><b>Download</b> ✅ <a href="https://urlin.us/2uSVSw">https://urlin.us/2uSVSw</a></b></p><br /><br />
|
5 |
-
<h2>What is 8 Ball Pool?</h2>
|
6 |
-
<p><strong>8 Ball Pool</strong> is a popular online multiplayer pool game developed by Miniclip. It allows you to play with millions of players from around the world in various modes, such as 1-on-1 matches, tournaments, practice arena, and more. You can also customize your cue and table, chat with your opponents, and challenge your friends. The game is free to download and play, but it also offers in-app purchases for some items and features.</p>
|
7 |
-
<h3>Features of 8 Ball Pool</h3>
|
8 |
-
<p>Some of the main features of <strong>8 Ball Pool</strong> are:</p>
|
9 |
-
<ul>
|
10 |
-
<li>Realistic physics and graphics that make you feel like playing in a real pool hall.</li>
|
11 |
-
<li>Various game modes to suit your preferences and skill levels.</li>
|
12 |
-
<li>A ranking system that lets you compete with players from different leagues and regions.</li>
|
13 |
-
<li>A reward system that gives you coins, cash, cues, and other items for winning matches and completing achievements.</li>
|
14 |
-
<li>A shop where you can buy and upgrade your cues, tables, chat packs, and more.</li>
|
15 |
-
<li>A club where you can join or create your own club and play with your club members.</li>
|
16 |
-
<li>A mini-game where you can spin the wheel and win prizes every day.</li>
|
17 |
-
</ul>
|
18 |
-
<h3>How to play 8 Ball Pool</h3>
|
19 |
-
<p>The gameplay of <strong>8 Ball Pool</strong> is simple and intuitive. You just need to swipe your finger on the screen to aim your cue, adjust the power and spin, and release to hit the ball. The goal is to pocket all your balls (solid or striped) before your opponent does, and then pocket the black ball (8 ball) to win the game. You can also use some tricks and tips to improve your skills, such as using the guidelines, adjusting the spin, choosing the right cue, etc.</p>
|
20 |
-
<p>8 ball pool mod apk 2022 unlimited coins and cash apkpure<br />
|
21 |
-
8 ball pool mod apk 2022 anti ban apkpure<br />
|
22 |
-
8 ball pool mod apk 2022 latest version download apkpure<br />
|
23 |
-
8 ball pool mod apk 2022 long line apkpure<br />
|
24 |
-
8 ball pool mod apk 2022 free cues and tables apkpure<br />
|
25 |
-
8 ball pool mod apk 2022 hack online generator apkpure<br />
|
26 |
-
8 ball pool mod apk 2022 all legendary cues unlocked apkpure<br />
|
27 |
-
8 ball pool mod apk 2022 mega mod menu apkpure<br />
|
28 |
-
8 ball pool mod apk 2022 no root required apkpure<br />
|
29 |
-
8 ball pool mod apk 2022 vip pass premium apkpure<br />
|
30 |
-
8 ball pool mod apk 2022 unlimited money and gems apkpure<br />
|
31 |
-
8 ball pool mod apk 2022 auto win and level up apkpure<br />
|
32 |
-
8 ball pool mod apk 2022 low mb size download apkpure<br />
|
33 |
-
8 ball pool mod apk 2022 offline mode apkpure<br />
|
34 |
-
8 ball pool mod apk 2022 new update features apkpure<br />
|
35 |
-
8 ball pool mod apk 2022 best aim tool apkpure<br />
|
36 |
-
8 ball pool mod apk 2022 unlimited spins and scratchers apkpure<br />
|
37 |
-
8 ball pool mod apk 2022 no verification needed apkpure<br />
|
38 |
-
8 ball pool mod apk 2022 easy installation guide apkpure<br />
|
39 |
-
8 ball pool mod apk 2022 high quality graphics and sound apkpure<br />
|
40 |
-
8 ball pool mod apk 2022 support all android devices apkpure<br />
|
41 |
-
8 ball pool mod apk 2022 fast and secure download link apkpure<br />
|
42 |
-
8 ball pool mod apk 2022 play with friends and chat apkpure<br />
|
43 |
-
8 ball pool mod apk 2022 unlimited tournament tickets apkpure<br />
|
44 |
-
8 ball pool mod apk 2022 customise your cue and table apkpure<br />
|
45 |
-
8 ball pool mod apk 2022 win trophies and exclusive rewards apkpure<br />
|
46 |
-
8 ball pool mod apk 2022 challenge the world in online matches apkpure<br />
|
47 |
-
8 ball pool mod apk 2022 access to exclusive events and offers apkpure<br />
|
48 |
-
8 ball pool mod apk 2022 join clubs and compete with other players apkpure<br />
|
49 |
-
8 ball pool mod apk 2022 get free coins and cash daily apkpure<br />
|
50 |
-
download latest version of the best android game "8 ball pool" with unlimited everything in the year of the ox - only from _apkpure.com_<br />
|
51 |
-
how to install and play "8 ball pool" on your android device with the most updated and working modded version of the game in the year of the ox - step by step tutorial by _apkpure.com_<br />
|
52 |
-
enjoy the ultimate fun and excitement of playing "8 ball pool" on your android device with the best graphics, sound, and gameplay - download the latest version of the game with unlimited features from _apkpure.com_<br />
|
53 |
-
become a pro player of "8 ball pool" on your android device with the help of the most advanced and powerful aim tool, hack tool, and cheat tool - get them all for free from _apkpure.com_<br />
|
54 |
-
unlock all the legendary cues, tables, and rewards in "8 ball pool" on your android device with the easiest and fastest method - download the latest version of the game with unlimited everything from _apkpure.com_<br />
|
55 |
-
play "8 ball pool" offline on your android device without any internet connection or data usage - download the latest version of the game with offline mode from _apkpure.com_<br />
|
56 |
-
win every match and tournament in "8 ball pool" on your android device with the most amazing and reliable auto win and level up feature - download the latest version of the game with unlimited everything from _apkpure.com_<br />
|
57 |
-
get unlimited coins, cash, gems, spins, scratchers, tickets, cues, tables, and more in "8 ball pool" on your android device with the most trusted and safe online generator - visit _apkpure.com_ now to get started<br />
|
58 |
-
play "8 ball pool" with your friends and chat with them in real time on your android device - download the latest version of the game with social features from _apkpure.com_</p>
|
59 |
-
<h2>What is a mod apk?</h2>
|
60 |
-
<p>A <strong>mod apk</strong> is a modified version of an original application that has been altered by some developers or hackers to provide some extra features or advantages that are not available in the official version. A mod apk usually has a different signature from the original app, which means that it cannot be installed from the Google Play Store or other official sources. Instead, you need to download it from a third-party website or source and install it manually on your device.</p>
|
61 |
-
<h3>Benefits of using a mod apk</h3>
|
62 |
-
<p>Some of the benefits of using a mod apk are:</p>
|
63 |
-
<ul>
|
64 |
-
<li>You can enjoy some premium features or items that are otherwise locked or paid in the original app.</li>
|
65 |
-
<li>You can bypass some restrictions or limitations that are imposed by the original app <h3>Risks of using a mod apk</h3>
|
66 |
-
<p>Using a mod apk may seem tempting, but it also comes with some risks that you should be aware of. Some of the risks of using a mod apk are:</p>
|
67 |
-
<ul>
|
68 |
-
<li><strong>Malware:</strong> Mod apk files can be infected with malware that can harm your device or steal your data . Malware can also compromise the security of your device and expose it to hackers or other threats.</li>
|
69 |
-
<li><strong>Compatibility:</strong> Mod apk files may not work properly with your device or the latest version of the app . This can affect the performance or functionality of the app or cause errors or crashes.</li>
|
70 |
-
<li><strong>Updates:</strong> Mod apk files are not updated as frequently as the official versions of apps, which can affect the performance or security of the app . You may also miss out on some new features or improvements that are available in the original app.</li>
|
71 |
-
<li><strong>Legality:</strong> Mod apk files may violate the copyright or terms of service of the original app, which can result in legal consequences . You may also face ethical issues for using a mod apk that gives you an unfair advantage over other players or deprives the original developer of their revenue.</li>
|
72 |
-
</ul>
|
73 |
-
<h2>What is 8 Ball Pool Mod APK 2022 _Apkpure?</h2>
|
74 |
-
<p><strong>8 Ball Pool Mod APK 2022 _Apkpure</strong> is a modified version of 8 Ball Pool that is available on a third-party website called Apkpure. Apkpure is a platform that provides various modded apps and games for Android devices. 8 Ball Pool Mod APK 2022 _Apkpure claims to offer unlimited coins, cash, cues, and other resources that can enhance your gaming experience and help you win more matches.</p>
|
75 |
-
<h3>Features of 8 Ball Pool Mod APK 2022 _Apkpure</h3>
|
76 |
-
<p>Some of the features of <strong>8 Ball Pool Mod APK 2022 _Apkpure</strong> are:</p>
|
77 |
-
<ul>
|
78 |
-
<li><strong>Unlimited coins and cash:</strong> You can get unlimited coins and cash in your account, which you can use to buy and upgrade your cues, tables, chat packs, and more. You can also enter higher-stake matches and tournaments without worrying about losing your money.</li>
|
79 |
-
<li><strong>Unlocked cues and tables:</strong> You can access all the cues and tables in the game, including the legendary and exclusive ones. You can also customize your cue and table with different colors, patterns, and stickers.</li>
|
80 |
-
<li><strong>Anti-ban feature:</strong> You can play the game without worrying about getting banned by Miniclip. The mod apk has an anti-ban feature that protects your account from detection and suspension.</li>
|
81 |
-
<li><strong>No ads:</strong> You can enjoy the game without any annoying ads that interrupt your gameplay or consume your data.</li>
|
82 |
-
</ul>
|
83 |
-
<h3>How to download and install 8 Ball Pool Mod APK 2022 _Apkpure</h3>
|
84 |
-
<p>To download and install <strong>8 Ball Pool Mod APK 2022 _Apkpure</strong>, you need to follow these steps:</p>
|
85 |
-
<ol>
|
86 |
-
<li>Go to the Apkpure website and search for 8 Ball Pool Mod APK 2022 _Apkpure.</li>
|
87 |
-
<li>Select the latest version of the mod apk and click on the download button.</li>
|
88 |
-
<li>Wait for the download to finish and then locate the file on your device.</li>
|
89 |
-
<li>Before installing the mod apk, make sure you enable the unknown sources option in your device settings. This will allow you to install apps from sources other than the Google Play Store.</li>
|
90 |
-
<li>Tap on the mod apk file and follow the instructions to install it on your device.</li>
|
91 |
-
<li>Launch the game and enjoy the modded features.</li>
|
92 |
-
</ol>
|
93 |
-
<h2>Conclusion</h2>
|
94 |
-
<p><strong>8 Ball Pool Mod APK 2022 _Apkpure</strong> is a modified version of 8 Ball Pool that offers unlimited resources and features that can make your gaming experience more fun and exciting. However, using a mod apk also comes with some risks, such as malware, compatibility issues, update problems, and legal issues. Therefore, you should be careful when downloading and installing a mod apk from a third-party source. You should also respect the original developer of the app and support them by using the official version of the app. We hope this article has helped you understand what is 8 Ball Pool Mod APK 2022 _Apkpure and how to use it. If you have any questions or feedback, please let us know in the comments below. Thank you for reading!</p>
|
95 |
-
<h3>FAQs</h3>
|
96 |
-
<p>Here are some frequently asked questions about <strong>8 Ball Pool Mod APK 2022 _Apkpure</strong>:</p>
|
97 |
-
<ul>
|
98 |
-
<li><strong>Q: Is 8 Ball Pool Mod APK 2022 _Apkpure safe to use?</strong></li>
|
99 |
-
<li><strong>A: There is no guarantee that 8 Ball Pool Mod APK 2022 _Apkpure is safe to use, as it is a modded version of the original app that has been altered by an unknown source. It may contain malware or viruses that can harm your device or data. Therefore, you should use it at your own risk and discretion.</strong></li>
|
100 |
-
<li><strong>Q: Can I play 8 Ball Pool Mod APK 2022 _Apkpure with my friends?</strong></li>
|
101 |
-
<li><strong>A: Yes, you can play 8 Ball Pool Mod APK 2022 _Apkpure with your friends, as long as they also have the same mod apk installed on their devices. You can invite them to join your club or challenge them to a match.</strong></li>
|
102 |
-
<li><strong>Q: Will I get banned for using 8 Ball Pool Mod APK 2022 _Apkpure?</strong></li>
|
103 |
-
<li><strong>A: There is a possibility that you may get banned for using 8 Ball Pool Mod APK 2022 _Apkpure, as it violates the terms of service of Miniclip, the original developer of the app. However, the mod apk claims to have an anti-ban feature that protects your account from detection and suspension. However, this feature may not work all the time or for all users, so you should be careful when using the mod apk.</strong></li>
|
104 |
-
<li><strong>Q: How can I update 8 Ball Pool Mod APK 2022 _Apkpure?</strong></li>
|
105 |
-
<li><strong>A: You cannot update 8 Ball Pool Mod APK 2022 _Apkpure from the Google Play Store or other official sources, as it has a different signature from the original app. You need to check the Apkpure website regularly for any new versions of the mod apk and download and install them manually on your device.</strong></li>
|
106 |
-
<li><strong>Q: What are some alternatives to 8 Ball Pool Mod APK 2022 _Apkpure?</strong></li>
|
107 |
-
<li><strong>A: Some alternatives to 8 Ball Pool Mod APK 2022 _Apkpure are:</strong></li>
|
108 |
-
<ul>
|
109 |
-
<li><strong>8 Ball Pool Hack:</strong> This is another mod apk that offers unlimited coins, cash, cues, and more. It also has an anti-ban feature and no ads. You can download it from [here].</li>
|
110 |
-
<li><strong>Pool Billiards Pro:</strong> This is a similar pool game that has realistic physics and graphics, various game modes, and online multiplayer features. It is free to download and play, but it also has in-app purchases. You can download it from [here].</li>
|
111 |
-
<li><strong>Pool Break Pro:</strong> This is a premium pool game that has stunning graphics, realistic physics, and multiple game types, such as snooker, carrom, crokinole, and more. It also supports online multiplayer and chat features. You can download it from [here].</li>
|
112 |
-
</ul>
|
113 |
-
</ul></p> 197e85843d<br />
|
114 |
-
<br />
|
115 |
-
<br />
|
|
|
|
|
|
|
|
|
|
|
|
|
|
|
|
|
|
|
|
|
|
|
|
|
|
|
|
|
|
|
|
|
|
|
|
|
|
|
|
|
|
|
|
|
|
|
|
|
|
|
|
|
|
|
|
|
|
|
|
|
|
|
|
|
|
|
|
|
|
|
|
|
|
|
|
|
|
|
|
|
|
|
|
|
|
|
|
|
|
|
|
|
|
|
|
|
|
|
|
|
|
|
|
|
|
|
|
|
|
|
|
|
|
|
|
|
|
|
|
|
|
|
|
|
|
|
|
|
|
|
|
|
|
|
|
|
|
|
|
|
|
|
|
|
|
|
|
|
|
|
|
|
|
|
|
|
|
|
|
|
|
|
|
|
|
|
|
|
|
|
|
|
|
|
|
|
|
|
|
|
|
|
|
|
|
|
|
|
|
|
|
|
|
|
|
|
|
|
|
|
|
|
|
|
|
|
|
|
|
|
|
|
|
|
|
|
|
|
|
|
|
|
|
|
|
|
|
|
|
|
|
spaces/1phancelerku/anime-remove-background/Derbeder A Tribute to Ferdi Tayfurs Legendary Song.md
DELETED
@@ -1,135 +0,0 @@
|
|
1 |
-
|
2 |
-
<h1>What is Derbeder?</h1>
|
3 |
-
<p>If you are familiar with Turkish culture, you may have heard the word derbeder before. But what does it mean exactly? And where does it come from?</p>
|
4 |
-
<p>Derbeder is a Turkish word that describes a person who lives an irregular, careless, or reckless lifestyle. A derbeder is someone who wanders from place to place without a fixed home or job, who does not care about social norms or rules, who is adventurous or rebellious, or who has lost hope or direction in life.</p>
|
5 |
-
<h2>derbeder</h2><br /><p><b><b>Download Zip</b> ⚹ <a href="https://jinyurl.com/2uNQat">https://jinyurl.com/2uNQat</a></b></p><br /><br />
|
6 |
-
<p>The word derbeder comes from the Persian words dar (door) and bedar (open), meaning someone who has no door or shelter. It was originally used to refer to homeless people or refugees who had to flee their homes due to war or persecution. Later, it acquired a more figurative meaning, referring to anyone who lives a free-spirited or unconventional life.</p>
|
7 |
-
<h2>Derbeder in Turkish Culture</h2>
|
8 |
-
<h3>Derbeder in Literature</h3>
|
9 |
-
<p>Derbeder is a word that has been used by many Turkish writers and poets to portray characters who are either heroes or anti-heroes, depending on the perspective. Some examples of derbeder in Turkish literature are:</p>
|
10 |
-
<ul>
|
11 |
-
<li>Köroğlu, a legendary folk hero who rebelled against the oppressive rulers and became an outlaw leader. He was known for his bravery, generosity, and love for poetry.</li>
|
12 |
-
<li>Kaygusuz Abdal, a 14th-century mystic poet who renounced worldly pleasures and wandered around Anatolia spreading his teachings. He was considered a derbeder by the orthodox religious authorities who opposed his unconventional views.</li>
|
13 |
-
<li>Ahmet Arif, a 20th-century poet who wrote about the plight of the Kurdish people and their struggle for freedom and justice. He was arrested and tortured by the Turkish government for his political views.</li>
|
14 |
-
</ul>
|
15 |
-
<h3>Derbeder in Music</h3>
|
16 |
-
<p>Derbeder is also a word that has been used by many Turkish singers and songwriters to express their emotions and experiences. Some examples of derbeder in Turkish music are:</p>
|
17 |
-
<ul>
|
18 |
-
<li>Ferdi Tayfur, a famous singer and actor who starred in a movie called Derbeder in 1986. He sang about his love, pain, and loneliness in his songs, which resonated with many people who felt the same way.</li>
|
19 |
-
<li>Barış Manço, a legendary musician and cultural icon who blended rock, folk, and psychedelic music. He was known for his eccentric style, colorful outfits, and long hair. He was also a derbeder in the sense that he traveled around the world and explored different cultures and languages.</li>
|
20 |
-
<li>Sezen Aksu, a popular singer and songwriter who is considered the queen of Turkish pop music. She has written and performed songs that deal with various social issues, such as women's rights, domestic violence, and environmentalism. She has also been a derbeder in her personal life, having gone through several divorces and relationships.</li>
|
21 |
-
</ul>
|
22 |
-
<h3>Derbeder in Movies</h3>
|
23 |
-
<p>Derbeder is also a word that has been used by many Turkish filmmakers and actors to depict characters who are either protagonists or antagonists, depending on the plot. Some examples of derbeder in Turkish movies are:</p>
|
24 |
-
<ul>
|
25 |
-
<li>Eşkıya, a 1996 movie directed by Yavuz Turgul and starring Şener Şen. It tells the story of Baran, an old bandit who escapes from prison after 35 years and tries to adapt to the modern world. He is a derbeder who lives by his own code of honor and loyalty.</li>
|
26 |
-
<li>Yol, a 1982 movie directed by Şerif Gören and Yılmaz Güney. It follows the lives of five prisoners who are granted a week-long leave from jail. They face various challenges and hardships as they try to reconnect with their families and society. They are derbeders who have been marginalized and oppressed by the system.</li>
|
27 |
-
<li>G.O.R.A., a 2004 movie directed by Ömer Faruk Sorak and starring Cem Yılmaz. It is a comedy sci-fi movie that parodies various Hollywood films. It features Arif, a carpet salesman who is abducted by aliens and taken to the planet G.O.R.A. He is a derbeder who uses his humor and wit to survive and save the day.</li>
|
28 |
-
</ul>
|
29 |
-
<h2>Derbeder in English</h2>
|
30 |
-
<h3>Derbeder Translations</h3>
|
31 |
-
<p>Derbeder is a word that has no exact equivalent in English, but there are some possible translations that can capture its meaning and connotation. Some of them are:</p>
|
32 |
-
<p>*serseri*<br />
|
33 |
-
*avare*<br />
|
34 |
-
*çapkın*<br />
|
35 |
-
*başıboş*<br />
|
36 |
-
*berduş*<br />
|
37 |
-
*aylak*<br />
|
38 |
-
*hovarda*<br />
|
39 |
-
*rüküş*<br />
|
40 |
-
*kılıksız*<br />
|
41 |
-
*düzensiz*<br />
|
42 |
-
*dağınık*<br />
|
43 |
-
*pasaklı*<br />
|
44 |
-
*savruk*<br />
|
45 |
-
*dağılmış*<br />
|
46 |
-
*kurnaz*<br />
|
47 |
-
*düzenbaz*<br />
|
48 |
-
*göçebe*<br />
|
49 |
-
*eski moda giysili*<br />
|
50 |
-
derbeder yaşam tarzı<br />
|
51 |
-
derbeder şarkı sözleri<br />
|
52 |
-
derbeder filmi izle<br />
|
53 |
-
derbeder ferdi tayfur<br />
|
54 |
-
derbeder ne demek<br />
|
55 |
-
derbeder erkek nasıl olur<br />
|
56 |
-
derbeder kadın nasıl olur<br />
|
57 |
-
derbeder aşk sözleri<br />
|
58 |
-
derbeder giyim modelleri<br />
|
59 |
-
derbeder insanların özellikleri<br />
|
60 |
-
derbeder olmak istiyorum<br />
|
61 |
-
derbeder bir hayat hikayesi<br />
|
62 |
-
derbeder adam nasıl tavlanır<br />
|
63 |
-
derbeder kız nasıl tavlanır<br />
|
64 |
-
derbeder olmanın zararları<br />
|
65 |
-
derbeder olmanın avantajları<br />
|
66 |
-
derbeder bir gecenin sonu<br />
|
67 |
-
derbeder bir aşkın sonu<br />
|
68 |
-
derbeder bir adamın günlüğü<br />
|
69 |
-
derbeder bir kızın günlüğü<br />
|
70 |
-
derbeder bir şehrin sokakları<br />
|
71 |
-
derbeder bir ülkenin hali<br />
|
72 |
-
derbeder bir sanatçının eserleri<br />
|
73 |
-
derbeder bir yazarın kitapları<br />
|
74 |
-
derbeder bir müzisyenin şarkıları</p>
|
75 |
-
<table>
|
76 |
-
<tr><th>Turkish</th><th>English</th></tr>
|
77 |
-
<tr><td>Derbeder</td><td>Tramping</td></tr>
|
78 |
-
<tr><td>Derbeder</td><td>Untidy</td></tr>
|
79 |
-
<tr><td>Derbeder</td><td>Roguish</td></tr>
|
80 |
-
<tr><td>Derbeder</td><td>Vagrant</td></tr>
|
81 |
-
<tr><td>Derbeder</td><td>Vagabond</td></tr>
|
82 |
-
<tr><td>Derbeder</td><td>Frumpish</td></tr>
|
83 |
-
<tr><td>Derbeder</td><td>Down and out</td></tr>
|
84 |
-
</table>
|
85 |
-
<p>However, these translations may not fully convey the nuances of derbeder, which can have both positive and negative associations depending on the context. For example, tramping can imply wandering or traveling for pleasure or adventure, but it can also imply being homeless or poor. Similarly, roguish can imply being playful or charming, but it can also imply being dishonest or immoral.</p>
|
86 |
-
<h3>Derbeder Synonyms</h3>
|
87 |
-
<p>Derbeder is a word that has many synonyms in Turkish, but they may not have the same meaning or usage. Some of them are:</p>
|
88 |
-
<table>
|
89 |
-
<tr><th>Turkish</th><th>Synonym</th><th>Difference</th></tr>
|
90 |
-
<tr><td>Derbeder</td><td>Serseri</td><td>Serseri is more commonly used to refer to young men who are rebellious or irresponsible.</td></tr>
|
91 |
-
<tr><td>Derbeder</td><td>Avare</td><td>Avare is more commonly used to refer to people who are idle or lazy.</td></ <tr><td>Derbeder</td><td>Çapkın</td><td>Çapkın is more commonly used to refer to men who are flirtatious or promiscuous.</td></tr>
|
92 |
-
<tr><td>Derbeder</td><td>Berduş</td><td>Berduş is more commonly used to refer to people who are outcast or unwanted.</td></tr>
|
93 |
-
<tr><td>Derbeder</td><td>Hovarda</td><td>Hovarda is more commonly used to refer to people who are extravagant or wasteful.</td></tr>
|
94 |
-
</table>
|
95 |
-
<p>Therefore, it is important to understand the context and tone of the word derbeder before using it or its synonyms.</p>
|
96 |
-
<h2>Derbeder in Betting</h2>
|
97 |
-
<h3>What is Draw Betting?</h3>
|
98 |
-
<p>Draw betting is a type of betting market that involves predicting that a match will end in a tie or a draw. It is often overlooked by most bettors who prefer to back one side to win, but it can provide some value for those who are looking for low-risk and high-reward outcomes.</p>
|
99 |
-
<p>Draw betting can be applied to any sport that has the possibility of a draw, such as soccer, rugby, cricket, or hockey. However, it is most popular in soccer, where draws are more common and more predictable than in other sports.</p>
|
100 |
-
<p>Draw betting has some advantages over other betting markets, such as:</p>
|
101 |
-
<ul>
|
102 |
-
<li>It offers higher odds and payouts than backing a single team to win.</li>
|
103 |
-
<li>It reduces the number of possible outcomes from three to two, making it easier to analyze and select bets.</li>
|
104 |
-
<li>It can be combined with other bets, such as double chance, correct score, or handicap, to increase the chances of winning or hedge against losses.</li>
|
105 |
-
</ul>
|
106 |
-
<h3>How to Bet on Draws?</h3>
|
107 |
-
<p>Betting on draws requires careful analysis of statistics, trends, and team performances. It is not enough to rely on intuition or luck. Some of the factors that can help you bet on draws are:</p>
|
108 |
-
<ul>
|
109 |
-
<li>The history and frequency of draws between the teams involved. You can check the past results and head-to-head records of the teams to see how often they have drawn in their previous matches.</li>
|
110 |
-
<li>The current form and motivation of the teams involved. You can check the recent results and standings of the teams to see how well they are playing and how much they need a win or a draw.</li>
|
111 |
-
<li>The style and strategy of the teams involved. You can check the tactics and formations of the teams to see how they approach the game and how likely they are to score or concede goals.</li>
|
112 |
-
<li>The injuries and suspensions of the key players involved. You can check the availability and fitness of the players to see how they affect the strength and balance of the teams.</li>
|
113 |
-
<li>The weather and pitch conditions of the venue involved. You can check the weather forecast and pitch report to see how they affect the speed and quality of the game.</li>
|
114 |
-
</ul>
|
115 |
-
<p>Based on these factors, you can identify the matches that have a high probability of ending in a draw and place your bets accordingly. You can also use some tips and tricks from experts and professionals who have experience and knowledge in draw betting.</p>
|
116 |
-
<h2>Conclusion</h2>
|
117 |
-
<p>In conclusion, derbeder is a Turkish word that has many meanings and implications depending on the context and usage. It can be used to describe a person who lives an irregular or careless lifestyle, or a character who is rebellious or adventurous. It can also be translated into English as tramping, untidy, roguish, vagrant, vagabond, frumpish, or down and out. It can also be used as a synonym for serseri, avare, çapkın, berduş, or hovarda. Finally, it can also be used as a term for draw betting, which is a type of betting market that involves predicting that a match will end in a tie.</p>
|
118 |
-
<p>If you are interested in learning more about derbeder or draw betting, you can use Bing search engine to find more information and resources. You can also use Bing graphic art tool to create some images related to derbeder or draw betting. You can also use Bing request ads tool to find some advertisements relevant to derbeder or draw betting.</p>
|
119 |
-
<p>We hope you enjoyed this article and learned something new. If you have any questions or feedback, please feel free to contact us. Thank you for reading!</p>
|
120 |
-
<h4>Frequently Asked Questions</h4>
|
121 |
-
<ol>
|
122 |
-
<li>What is the origin of the word derbeder?</li>
|
123 |
-
<p>The word derbeder comes from the Persian words dar (door) and bedar (open), meaning someone who has no door or shelter.</p <li>What are some examples of derbeder in Turkish culture?</li>
|
124 |
-
<p>Some examples of derbeder in Turkish culture are Köroğlu, a legendary folk hero who rebelled against the oppressive rulers and became an outlaw leader; Kaygusuz Abdal, a 14th-century mystic poet who renounced worldly pleasures and wandered around Anatolia spreading his teachings; and Ahmet Arif, a 20th-century poet who wrote about the plight of the Kurdish people and their struggle for freedom and justice.</p>
|
125 |
-
<li>What are some advantages of draw betting?</li>
|
126 |
-
<p>Some advantages of draw betting are that it offers higher odds and payouts than backing a single team to win; it reduces the number of possible outcomes from three to two, making it easier to analyze and select bets; and it can be combined with other bets, such as double chance, correct score, or handicap, to increase the chances of winning or hedge against losses.</p>
|
127 |
-
<li>How can I find more information and resources about derbeder or draw betting?</li>
|
128 |
-
<p>You can use Bing search engine to find more information and resources about derbeder or draw betting. You can also use Bing graphic art tool to create some images related to derbeder or draw betting. You can also use Bing request ads tool to find some advertisements relevant to derbeder or draw betting.</p>
|
129 |
-
<li>What is the difference between derbeder and serseri?</li>
|
130 |
-
<p>Derbeder and serseri are both Turkish words that describe a person who lives an irregular or careless lifestyle, but serseri is more commonly used to refer to young men who are rebellious or irresponsible.</p>
|
131 |
-
<li>What is the best way to analyze and select draw bets?</li>
|
132 |
-
<p>The best way to analyze and select draw bets is to consider various factors, such as the history and frequency of draws between the teams involved, the current form and motivation of the teams involved, the style and strategy of the teams involved, the injuries and suspensions of the key players involved, and the weather and pitch conditions of the venue involved.</p>
|
133 |
-
</ol></p> 197e85843d<br />
|
134 |
-
<br />
|
135 |
-
<br />
|
|
|
|
|
|
|
|
|
|
|
|
|
|
|
|
|
|
|
|
|
|
|
|
|
|
|
|
|
|
|
|
|
|
|
|
|
|
|
|
|
|
|
|
|
|
|
|
|
|
|
|
|
|
|
|
|
|
|
|
|
|
|
|
|
|
|
|
|
|
|
|
|
|
|
|
|
|
|
|
|
|
|
|
|
|
|
|
|
|
|
|
|
|
|
|
|
|
|
|
|
|
|
|
|
|
|
|
|
|
|
|
|
|
|
|
|
|
|
|
|
|
|
|
|
|
|
|
|
|
|
|
|
|
|
|
|
|
|
|
|
|
|
|
|
|
|
|
|
|
|
|
|
|
|
|
|
|
|
|
|
|
|
|
|
|
|
|
|
|
|
|
|
|
|
|
|
|
|
|
|
|
|
|
|
|
|
|
|
|
|
|
|
|
|
|
|
|
|
|
|
|
|
|
|
|
|
|
|
|
|
|
|
|
|
|
|
|
|
|
|
|
|
|
|
|
|
|
|
|
|
|
|
|
|
|
|
|
|
|
|
|
|
|
|
|
|
|
|
|
|
|
|
|
|
|
|
|
|
|
|
|
|
|
|
|
|
|
|
|
|
|
spaces/1phancelerku/anime-remove-background/Download GB Instagram Mod APK 2022 and Unlock Hidden Features.md
DELETED
@@ -1,139 +0,0 @@
|
|
1 |
-
<br />
|
2 |
-
<table>
|
3 |
-
<tr>
|
4 |
-
<td>
|
5 |
-
<h1><b>GB Instagram Mod APK Download 2022: Everything You Need to Know</b></h1>
|
6 |
-
<p>Do you love using Instagram but wish you could have more features and options? Do you want to download photos, videos, stories, and IGTV videos from your favorite accounts? Do you want to customize your app appearance and hide your online status? If you answered yes to any of these questions, then you might be interested in GB Instagram.</p>
|
7 |
-
<p>GB Instagram is a modded version of the official Instagram app that offers many extra features and benefits. It is one of the most popular mods for Instagram users who want to enhance their experience and enjoy more freedom and flexibility. In this article, we will tell you everything you need to know about GB Instagram, including its features, how to download and install it, how to use it, and its pros and cons.</p>
|
8 |
-
<h2>gb instagram mod apk download 2022</h2><br /><p><b><b>Download</b> ✅ <a href="https://jinyurl.com/2uNQub">https://jinyurl.com/2uNQub</a></b></p><br /><br />
|
9 |
-
<h2><b>What is GB Instagram?</b></h2>
|
10 |
-
<p>GB Instagram is a modified version of the official Instagram app that was created by a third-party developer named Atnfas Hoak. It is not available on the Google Play Store or any other official app store, but you can download it from various websites that host modded apps.</p>
|
11 |
-
<p>GB Instagram is based on the latest version of the official Instagram app, so you can enjoy all the features that you are familiar with, such as posting photos and videos, liking and commenting on posts, following and unfollowing accounts, sending and receiving messages, watching stories and live videos, etc.</p>
|
12 |
-
<p>However, GB Instagram also adds many extra features that are not available on the official app, such as downloading media files, hiding your online status, customizing your app appearance, zooming in on profile pictures, copying captions and comments, and disabling story view. These features make GB Instagram more fun and convenient to use, as well as giving you more control and privacy over your account.</p>
|
13 |
-
<h2><b>Features of GB Instagram</b></h2>
|
14 |
-
<p>GB Instagram has many features that make it stand out from the official app. Here are some of the most notable ones:</p>
|
15 |
-
<h3><b>Download media files</b></h3>
|
16 |
-
<p>One of the most useful features of GB Instagram is that it allows you to download any photo, video, story, or IGTV video from any account, whether it is public or private. You can save the media files to your device's gallery or share them with other apps. You can also download profile pictures of any user by tapping and holding on them.</p>
|
17 |
-
<p>gb instagram mod apk download 2022 latest version<br />
|
18 |
-
how to install gb instagram mod apk on android<br />
|
19 |
-
gb instagram mod apk features and benefits<br />
|
20 |
-
gb instagram mod apk vs original instagram app<br />
|
21 |
-
gb instagram mod apk no ads and no stories<br />
|
22 |
-
gb instagram mod apk download 2022 for ios<br />
|
23 |
-
gb instagram mod apk download 2022 free<br />
|
24 |
-
gb instagram mod apk download 2022 with dark mode<br />
|
25 |
-
gb instagram mod apk download 2022 without root<br />
|
26 |
-
gb instagram mod apk download 2022 for pc<br />
|
27 |
-
gb instagram mod apk download 2022 with stickers<br />
|
28 |
-
gb instagram mod apk download 2022 with fonts<br />
|
29 |
-
gb instagram mod apk download 2022 with themes<br />
|
30 |
-
gb instagram mod apk download 2022 with video downloader<br />
|
31 |
-
gb instagram mod apk download 2022 with voice messages<br />
|
32 |
-
gb instagram mod apk download 2022 with privacy settings<br />
|
33 |
-
gb instagram mod apk download 2022 with anti-ban<br />
|
34 |
-
gb instagram mod apk download 2022 with zoom option<br />
|
35 |
-
gb instagram mod apk download 2022 with copy link option<br />
|
36 |
-
gb instagram mod apk download 2022 with dual account option<br />
|
37 |
-
gb instagram mod apk review and rating<br />
|
38 |
-
gb instagram mod apk pros and cons<br />
|
39 |
-
gb instagram mod apk alternatives and competitors<br />
|
40 |
-
gb instagram mod apk updates and changelog<br />
|
41 |
-
gb instagram mod apk faq and troubleshooting<br />
|
42 |
-
is gb instagram mod apk safe and legal<br />
|
43 |
-
how to uninstall gb instagram mod apk from android<br />
|
44 |
-
how to backup and restore gb instagram mod apk data<br />
|
45 |
-
how to customize and personalize gb instagram mod apk settings<br />
|
46 |
-
how to use gb instagram mod apk for business and marketing<br />
|
47 |
-
how to get more followers and likes with gb instagram mod apk<br />
|
48 |
-
how to create and edit stories with gb instagram mod apk<br />
|
49 |
-
how to watch and download IGTV videos with gb instagram mod apk<br />
|
50 |
-
how to send and receive direct messages with gb instagram mod apk<br />
|
51 |
-
how to post and share photos and videos with gb instagram mod apk<br />
|
52 |
-
how to follow and unfollow users with gb instagram mod apk<br />
|
53 |
-
how to block and report users with gb instagram mod apk<br />
|
54 |
-
how to mute and unmute users with gb instagram mod apk<br />
|
55 |
-
how to hide and show online status with gb instagram mod apk<br />
|
56 |
-
how to hide and show seen tick with gb instagram mod apk</p>
|
57 |
-
<h3><b>Hide your online status</b></h3>
|
58 |
-
<p>If you don't want others to know when you are online or when you were last active on Instagram, you can hide your online status with GB Instagram. This way, you can browse and use the app without worrying about being seen by anyone. You can also disable the blue ticks that indicate that you have read a message.</p>
|
59 |
-
<h3><b>Customize your app appearance</b></h3>
|
60 |
-
<p>GB Instagram lets you change the look and feel of your app by offering various themes and fonts. You can choose from different colors and styles for your app background, icons, buttons, text, etc. You can also create your own theme and apply it to your app. This way, you can personalize your app according to your preferences and mood.</p>
|
61 |
-
<h3><b>Zoom in on profile pictures</b></h3>
|
62 |
-
<p>Sometimes, you might want to see a profile picture of a user more clearly, but the official app does not allow you to zoom in on it. With GB Instagram, you can zoom in on any profile picture by tapping and holding on it. You can also zoom in on any photo or video in a post by pinching the screen.</p>
|
63 |
-
<h3><b>Copy captions and comments</b></h3>
|
64 |
-
<p>If you come across a caption or a comment that you like or want to use for yourself, you can easily copy it with GB Instagram. You just need to tap and hold on the caption or comment and select the copy option. You can also copy hashtags and bio from any user's profile.</p>
|
65 |
-
<h3><b>Disable story view</b></h3>
|
66 |
-
<p>If you want to watch someone's story without letting them know that you have seen it, you can disable the story view feature with GB Instagram. This way, you can watch any story anonymously and avoid any awkward situations. You can also disable video autoplay if you don't want to waste your data or battery.</p>
|
67 |
-
<h2><b>How to download and install GB Instagram?</b></h2>
|
68 |
-
<p>If you are interested in trying out GB Instagram, you will need to download and install it manually from a reliable website that hosts modded apps. Here are the requirements and steps for downloading and installing GB Instagram:</p>
|
69 |
-
<h3><b>Requirements for GB Instagram</b></h3>
|
70 |
-
<ul>
|
71 |
-
<li>An Android device running Android 4.1 or higher.</li>
|
72 |
-
<li>A stable internet connection.</li>
|
73 |
-
<li>Enough storage space on your device.</li>
|
74 |
-
<li>A backup of your data in case something goes wrong.</li>
|
75 |
-
<li>The permission to install apps from unknown sources enabled on your device.</li>
|
76 |
-
</ul>
|
77 |
-
<h3><b>Steps to download and install GB Instagram</b></h3>
|
78 |
-
<ol>
|
79 |
-
<li>Go to a website that offers GB Instagram mod apk download 2022, such as <a href="">GBPlus.net</a>.</li>
|
80 |
-
<li>Click on the download button and wait for the apk file to be downloaded on your device.</li>
|
81 |
-
<li>Locate the apk file in your device's file manager and tap on it to start the installation process.</li>
|
82 |
-
<li>Follow the instructions on the screen and grant the necessary permissions to the app.</li>
|
83 |
-
<li>Wait for the installation to be completed and then open the app.</li>
|
84 |
-
<li>Login with your existing Instagram account or create a new one if you don't have one.</li>
|
85 |
-
<li>Enjoy using GB Instagram with all its features.</li>
|
86 |
-
</ol> <h2><b>How to use GB Instagram?</b></h2>
|
87 |
-
<p>Now that you have downloaded and installed GB Instagram, you might be wondering how to use it and access its features. Don't worry, it is very easy and intuitive to use GB Instagram, as it has a similar interface and functionality as the official app. Here are some tips on how to use GB Instagram and enjoy its features:</p>
|
88 |
-
<h3><b>How to download media files from GB Instagram?</b></h3>
|
89 |
-
<p>If you want to download any photo, video, story, or IGTV video from any account on GB Instagram, you just need to follow these simple steps:</p>
|
90 |
-
<ol>
|
91 |
-
<li>Open the post or story that contains the media file that you want to download.</li>
|
92 |
-
<li>Tap on the three-dot menu icon at the top right corner of the screen.</li>
|
93 |
-
<li>Select the download option from the menu and choose the destination folder where you want to save the file.</li>
|
94 |
-
<li>Wait for the download to be completed and then check your device's gallery or file manager for the file.</li>
|
95 |
-
</ol>
|
96 |
-
<h3><b>How to hide your online status on GB Instagram?</b></h3>
|
97 |
-
<p>If you want to hide your online status or last seen activity on GB Instagram, you just need to follow these simple steps:</p>
|
98 |
-
<ol>
|
99 |
-
<li>Open GB Instagram and tap on your profile icon at the bottom right corner of the screen.</li>
|
100 |
-
<li>Tap on the three-line menu icon at the top right corner of the screen.</li>
|
101 |
-
<li>Select the settings option from the menu and then tap on privacy.</li>
|
102 |
-
<li>Scroll down and find the activity status option and toggle it off.</li>
|
103 |
-
<li>Now, no one will be able to see when you are online or when you were last active on GB Instagram.</li>
|
104 |
-
</ol>
|
105 |
-
<h3><b>How to customize your app appearance on GB Instagram?</b></h3>
|
106 |
-
<p>If you want to change the theme or font of your GB Instagram app, you just need to follow these simple steps:</p>
|
107 |
-
<ol>
|
108 |
-
<li>Open GB Instagram and tap on your profile icon at the bottom right corner of the screen.</li>
|
109 |
-
<li>Tap on the three-line menu icon at the top right corner of the screen.</li>
|
110 |
-
<li>Select the settings option from the menu and then tap on themes.</li>
|
111 |
-
<li>You will see a list of available themes and fonts that you can choose from. You can also create your own theme by tapping on create theme.</li>
|
112 |
-
<li>Select the theme or font that you like and apply it to your app. You can also preview it before applying it.</li>
|
113 |
-
<li>You will need to restart your app for the changes to take effect.</li>
|
114 |
-
</ol> </b></h3>
|
115 |
-
<ul>
|
116 |
-
<li>GB Instagram is not an official app and it is not endorsed by Instagram. It may violate the terms and conditions of Instagram and put your account at risk of being banned or suspended.</li>
|
117 |
-
<li>GB Instagram is not available on the Google Play Store or any other official app store. You have to download it from third-party websites that may not be safe or reliable. You may expose your device to malware or viruses by installing GB Instagram.</li>
|
118 |
-
<li>GB Instagram may not be updated regularly or in sync with the official app. You may miss out on some of the latest features or bug fixes that Instagram offers. You may also experience some glitches or errors while using GB Instagram.</li>
|
119 |
-
</ul>
|
120 |
-
<h2><b>Conclusion</b></h2>
|
121 |
-
<p>GB Instagram is a modded version of the official Instagram app that offers many extra features and benefits that are not available on the official app. It allows you to download media files, hide your online status, customize your app appearance, zoom in on profile pictures, copy captions and comments, and disable story view. However, GB Instagram also has some drawbacks, such as being unofficial, unsafe, and outdated. You should weigh the pros and cons of GB Instagram before deciding to use it.</p>
|
122 |
-
<p>If you want to try GB Instagram, you can download it from a reliable website that hosts modded apps, such as <a href="">GBPlus.net</a>. You will need to enable the permission to install apps from unknown sources on your device and follow the steps to download and install GB Instagram. You can then login with your existing Instagram account or create a new one and enjoy using GB Instagram with all its features.</p>
|
123 |
-
<p>We hope this article has helped you learn everything you need to know about GB Instagram mod apk download 2022. If you have any questions or feedback, please feel free to leave a comment below. Thank you for reading!</p>
|
124 |
-
<h2><b>FAQs</b></h2>
|
125 |
-
<p>Here are some of the frequently asked questions about GB Instagram:</p>
|
126 |
-
<ol>
|
127 |
-
<li><b>Is GB Instagram safe to use?</b></li>
|
128 |
-
<p>GB Instagram is not an official app and it is not endorsed by Instagram. It may violate the terms and conditions of Instagram and put your account at risk of being banned or suspended. It is also not available on the Google Play Store or any other official app store. You have to download it from third-party websites that may not be safe or reliable. You may expose your device to malware or viruses by installing GB Instagram. Therefore, GB Instagram is not completely safe to use and you should use it at your own risk.</p>
|
129 |
-
<li><b>Is GB Instagram free to use?</b></li>
|
130 |
-
<p>Yes, GB Instagram is free to use and it does not require any subscription or payment. However, you may see some ads or pop-ups while using GB Instagram, as it is a way for the developer to generate some revenue.</p>
|
131 |
-
<li><b>Can I use GB Instagram and the official app at the same time?</b></li>
|
132 |
-
<p>No, you cannot use GB Instagram and the official app at the same time on the same device. You will need to uninstall the official app before installing GB Instagram. However, you can use GB Instagram and the official app on different devices with the same account.</p>
|
133 |
-
<li><b>How can I update GB Instagram?</b></li>
|
134 |
-
<p>GB Instagram may not be updated regularly or in sync with the official app. You will need to check the website where you downloaded GB Instagram for any new updates. You will also need to uninstall the old version of GB Instagram before installing the new one.</p>
|
135 |
-
<li><b>How can I contact the developer of GB Instagram?</b></li>
|
136 |
-
<p>You can contact the developer of GB Instagram by visiting his website <a href="">GBMods.co</a>. You can also follow him on his social media accounts, such as Facebook, Twitter, and Telegram.</p>
|
137 |
-
</ol></p> 401be4b1e0<br />
|
138 |
-
<br />
|
139 |
-
<br />
|
|
|
|
|
|
|
|
|
|
|
|
|
|
|
|
|
|
|
|
|
|
|
|
|
|
|
|
|
|
|
|
|
|
|
|
|
|
|
|
|
|
|
|
|
|
|
|
|
|
|
|
|
|
|
|
|
|
|
|
|
|
|
|
|
|
|
|
|
|
|
|
|
|
|
|
|
|
|
|
|
|
|
|
|
|
|
|
|
|
|
|
|
|
|
|
|
|
|
|
|
|
|
|
|
|
|
|
|
|
|
|
|
|
|
|
|
|
|
|
|
|
|
|
|
|
|
|
|
|
|
|
|
|
|
|
|
|
|
|
|
|
|
|
|
|
|
|
|
|
|
|
|
|
|
|
|
|
|
|
|
|
|
|
|
|
|
|
|
|
|
|
|
|
|
|
|
|
|
|
|
|
|
|
|
|
|
|
|
|
|
|
|
|
|
|
|
|
|
|
|
|
|
|
|
|
|
|
|
|
|
|
|
|
|
|
|
|
|
|
|
|
|
|
|
|
|
|
|
|
|
|
|
|
|
|
|
|
|
|
|
|
|
|
|
|
|
|
|
|
|
|
|
|
|
|
|
|
|
|
|
|
|
|
|
|
|
|
|
|
|
|
|
|
|
|
|
|
|
|
spaces/801artistry/RVC801/infer/lib/uvr5_pack/lib_v5/layers_537227KB.py
DELETED
@@ -1,126 +0,0 @@
|
|
1 |
-
import torch
|
2 |
-
import torch.nn.functional as F
|
3 |
-
from torch import nn
|
4 |
-
|
5 |
-
from . import spec_utils
|
6 |
-
|
7 |
-
|
8 |
-
class Conv2DBNActiv(nn.Module):
|
9 |
-
def __init__(self, nin, nout, ksize=3, stride=1, pad=1, dilation=1, activ=nn.ReLU):
|
10 |
-
super(Conv2DBNActiv, self).__init__()
|
11 |
-
self.conv = nn.Sequential(
|
12 |
-
nn.Conv2d(
|
13 |
-
nin,
|
14 |
-
nout,
|
15 |
-
kernel_size=ksize,
|
16 |
-
stride=stride,
|
17 |
-
padding=pad,
|
18 |
-
dilation=dilation,
|
19 |
-
bias=False,
|
20 |
-
),
|
21 |
-
nn.BatchNorm2d(nout),
|
22 |
-
activ(),
|
23 |
-
)
|
24 |
-
|
25 |
-
def __call__(self, x):
|
26 |
-
return self.conv(x)
|
27 |
-
|
28 |
-
|
29 |
-
class SeperableConv2DBNActiv(nn.Module):
|
30 |
-
def __init__(self, nin, nout, ksize=3, stride=1, pad=1, dilation=1, activ=nn.ReLU):
|
31 |
-
super(SeperableConv2DBNActiv, self).__init__()
|
32 |
-
self.conv = nn.Sequential(
|
33 |
-
nn.Conv2d(
|
34 |
-
nin,
|
35 |
-
nin,
|
36 |
-
kernel_size=ksize,
|
37 |
-
stride=stride,
|
38 |
-
padding=pad,
|
39 |
-
dilation=dilation,
|
40 |
-
groups=nin,
|
41 |
-
bias=False,
|
42 |
-
),
|
43 |
-
nn.Conv2d(nin, nout, kernel_size=1, bias=False),
|
44 |
-
nn.BatchNorm2d(nout),
|
45 |
-
activ(),
|
46 |
-
)
|
47 |
-
|
48 |
-
def __call__(self, x):
|
49 |
-
return self.conv(x)
|
50 |
-
|
51 |
-
|
52 |
-
class Encoder(nn.Module):
|
53 |
-
def __init__(self, nin, nout, ksize=3, stride=1, pad=1, activ=nn.LeakyReLU):
|
54 |
-
super(Encoder, self).__init__()
|
55 |
-
self.conv1 = Conv2DBNActiv(nin, nout, ksize, 1, pad, activ=activ)
|
56 |
-
self.conv2 = Conv2DBNActiv(nout, nout, ksize, stride, pad, activ=activ)
|
57 |
-
|
58 |
-
def __call__(self, x):
|
59 |
-
skip = self.conv1(x)
|
60 |
-
h = self.conv2(skip)
|
61 |
-
|
62 |
-
return h, skip
|
63 |
-
|
64 |
-
|
65 |
-
class Decoder(nn.Module):
|
66 |
-
def __init__(
|
67 |
-
self, nin, nout, ksize=3, stride=1, pad=1, activ=nn.ReLU, dropout=False
|
68 |
-
):
|
69 |
-
super(Decoder, self).__init__()
|
70 |
-
self.conv = Conv2DBNActiv(nin, nout, ksize, 1, pad, activ=activ)
|
71 |
-
self.dropout = nn.Dropout2d(0.1) if dropout else None
|
72 |
-
|
73 |
-
def __call__(self, x, skip=None):
|
74 |
-
x = F.interpolate(x, scale_factor=2, mode="bilinear", align_corners=True)
|
75 |
-
if skip is not None:
|
76 |
-
skip = spec_utils.crop_center(skip, x)
|
77 |
-
x = torch.cat([x, skip], dim=1)
|
78 |
-
h = self.conv(x)
|
79 |
-
|
80 |
-
if self.dropout is not None:
|
81 |
-
h = self.dropout(h)
|
82 |
-
|
83 |
-
return h
|
84 |
-
|
85 |
-
|
86 |
-
class ASPPModule(nn.Module):
|
87 |
-
def __init__(self, nin, nout, dilations=(4, 8, 16, 32, 64), activ=nn.ReLU):
|
88 |
-
super(ASPPModule, self).__init__()
|
89 |
-
self.conv1 = nn.Sequential(
|
90 |
-
nn.AdaptiveAvgPool2d((1, None)),
|
91 |
-
Conv2DBNActiv(nin, nin, 1, 1, 0, activ=activ),
|
92 |
-
)
|
93 |
-
self.conv2 = Conv2DBNActiv(nin, nin, 1, 1, 0, activ=activ)
|
94 |
-
self.conv3 = SeperableConv2DBNActiv(
|
95 |
-
nin, nin, 3, 1, dilations[0], dilations[0], activ=activ
|
96 |
-
)
|
97 |
-
self.conv4 = SeperableConv2DBNActiv(
|
98 |
-
nin, nin, 3, 1, dilations[1], dilations[1], activ=activ
|
99 |
-
)
|
100 |
-
self.conv5 = SeperableConv2DBNActiv(
|
101 |
-
nin, nin, 3, 1, dilations[2], dilations[2], activ=activ
|
102 |
-
)
|
103 |
-
self.conv6 = SeperableConv2DBNActiv(
|
104 |
-
nin, nin, 3, 1, dilations[2], dilations[2], activ=activ
|
105 |
-
)
|
106 |
-
self.conv7 = SeperableConv2DBNActiv(
|
107 |
-
nin, nin, 3, 1, dilations[2], dilations[2], activ=activ
|
108 |
-
)
|
109 |
-
self.bottleneck = nn.Sequential(
|
110 |
-
Conv2DBNActiv(nin * 7, nout, 1, 1, 0, activ=activ), nn.Dropout2d(0.1)
|
111 |
-
)
|
112 |
-
|
113 |
-
def forward(self, x):
|
114 |
-
_, _, h, w = x.size()
|
115 |
-
feat1 = F.interpolate(
|
116 |
-
self.conv1(x), size=(h, w), mode="bilinear", align_corners=True
|
117 |
-
)
|
118 |
-
feat2 = self.conv2(x)
|
119 |
-
feat3 = self.conv3(x)
|
120 |
-
feat4 = self.conv4(x)
|
121 |
-
feat5 = self.conv5(x)
|
122 |
-
feat6 = self.conv6(x)
|
123 |
-
feat7 = self.conv7(x)
|
124 |
-
out = torch.cat((feat1, feat2, feat3, feat4, feat5, feat6, feat7), dim=1)
|
125 |
-
bottle = self.bottleneck(out)
|
126 |
-
return bottle
|
|
|
|
|
|
|
|
|
|
|
|
|
|
|
|
|
|
|
|
|
|
|
|
|
|
|
|
|
|
|
|
|
|
|
|
|
|
|
|
|
|
|
|
|
|
|
|
|
|
|
|
|
|
|
|
|
|
|
|
|
|
|
|
|
|
|
|
|
|
|
|
|
|
|
|
|
|
|
|
|
|
|
|
|
|
|
|
|
|
|
|
|
|
|
|
|
|
|
|
|
|
|
|
|
|
|
|
|
|
|
|
|
|
|
|
|
|
|
|
|
|
|
|
|
|
|
|
|
|
|
|
|
|
|
|
|
|
|
|
|
|
|
|
|
|
|
|
|
|
|
|
|
|
|
|
|
|
|
|
|
|
|
|
|
|
|
|
|
|
|
|
|
|
|
|
|
|
|
|
|
|
|
|
|
|
|
|
|
|
|
|
|
|
|
|
|
|
|
|
|
|
|
|
|
|
|
|
|
|
|
|
|
|
|
|
|
|
|
|
|
|
|
|
|
|
|
|
|
|
|
|
|
|
|
|
|
|
|
|
|
|
|
|
|
|
|
|
|
|
|
|
|
|
spaces/AB-TW/team-ai/documents/bussiness_context/NOTION_DB/Engineering Wiki 2402f5396a3244fdb3f1d135bdb0f3d6/CircleCI 719905fcb593423cad302d3fdc1c5dff.md
DELETED
@@ -1,5 +0,0 @@
|
|
1 |
-
# CircleCI
|
2 |
-
|
3 |
-
Last edited time: March 31, 2023 1:49 PM
|
4 |
-
Owner: Anonymous
|
5 |
-
Tags: Infrastructure
|
|
|
|
|
|
|
|
|
|
|
|
spaces/AIFILMS/generate_human_motion/VQ-Trans/utils/skeleton.py
DELETED
@@ -1,199 +0,0 @@
|
|
1 |
-
from utils.quaternion import *
|
2 |
-
import scipy.ndimage.filters as filters
|
3 |
-
|
4 |
-
class Skeleton(object):
|
5 |
-
def __init__(self, offset, kinematic_tree, device):
|
6 |
-
self.device = device
|
7 |
-
self._raw_offset_np = offset.numpy()
|
8 |
-
self._raw_offset = offset.clone().detach().to(device).float()
|
9 |
-
self._kinematic_tree = kinematic_tree
|
10 |
-
self._offset = None
|
11 |
-
self._parents = [0] * len(self._raw_offset)
|
12 |
-
self._parents[0] = -1
|
13 |
-
for chain in self._kinematic_tree:
|
14 |
-
for j in range(1, len(chain)):
|
15 |
-
self._parents[chain[j]] = chain[j-1]
|
16 |
-
|
17 |
-
def njoints(self):
|
18 |
-
return len(self._raw_offset)
|
19 |
-
|
20 |
-
def offset(self):
|
21 |
-
return self._offset
|
22 |
-
|
23 |
-
def set_offset(self, offsets):
|
24 |
-
self._offset = offsets.clone().detach().to(self.device).float()
|
25 |
-
|
26 |
-
def kinematic_tree(self):
|
27 |
-
return self._kinematic_tree
|
28 |
-
|
29 |
-
def parents(self):
|
30 |
-
return self._parents
|
31 |
-
|
32 |
-
# joints (batch_size, joints_num, 3)
|
33 |
-
def get_offsets_joints_batch(self, joints):
|
34 |
-
assert len(joints.shape) == 3
|
35 |
-
_offsets = self._raw_offset.expand(joints.shape[0], -1, -1).clone()
|
36 |
-
for i in range(1, self._raw_offset.shape[0]):
|
37 |
-
_offsets[:, i] = torch.norm(joints[:, i] - joints[:, self._parents[i]], p=2, dim=1)[:, None] * _offsets[:, i]
|
38 |
-
|
39 |
-
self._offset = _offsets.detach()
|
40 |
-
return _offsets
|
41 |
-
|
42 |
-
# joints (joints_num, 3)
|
43 |
-
def get_offsets_joints(self, joints):
|
44 |
-
assert len(joints.shape) == 2
|
45 |
-
_offsets = self._raw_offset.clone()
|
46 |
-
for i in range(1, self._raw_offset.shape[0]):
|
47 |
-
# print(joints.shape)
|
48 |
-
_offsets[i] = torch.norm(joints[i] - joints[self._parents[i]], p=2, dim=0) * _offsets[i]
|
49 |
-
|
50 |
-
self._offset = _offsets.detach()
|
51 |
-
return _offsets
|
52 |
-
|
53 |
-
# face_joint_idx should follow the order of right hip, left hip, right shoulder, left shoulder
|
54 |
-
# joints (batch_size, joints_num, 3)
|
55 |
-
def inverse_kinematics_np(self, joints, face_joint_idx, smooth_forward=False):
|
56 |
-
assert len(face_joint_idx) == 4
|
57 |
-
'''Get Forward Direction'''
|
58 |
-
l_hip, r_hip, sdr_r, sdr_l = face_joint_idx
|
59 |
-
across1 = joints[:, r_hip] - joints[:, l_hip]
|
60 |
-
across2 = joints[:, sdr_r] - joints[:, sdr_l]
|
61 |
-
across = across1 + across2
|
62 |
-
across = across / np.sqrt((across**2).sum(axis=-1))[:, np.newaxis]
|
63 |
-
# print(across1.shape, across2.shape)
|
64 |
-
|
65 |
-
# forward (batch_size, 3)
|
66 |
-
forward = np.cross(np.array([[0, 1, 0]]), across, axis=-1)
|
67 |
-
if smooth_forward:
|
68 |
-
forward = filters.gaussian_filter1d(forward, 20, axis=0, mode='nearest')
|
69 |
-
# forward (batch_size, 3)
|
70 |
-
forward = forward / np.sqrt((forward**2).sum(axis=-1))[..., np.newaxis]
|
71 |
-
|
72 |
-
'''Get Root Rotation'''
|
73 |
-
target = np.array([[0,0,1]]).repeat(len(forward), axis=0)
|
74 |
-
root_quat = qbetween_np(forward, target)
|
75 |
-
|
76 |
-
'''Inverse Kinematics'''
|
77 |
-
# quat_params (batch_size, joints_num, 4)
|
78 |
-
# print(joints.shape[:-1])
|
79 |
-
quat_params = np.zeros(joints.shape[:-1] + (4,))
|
80 |
-
# print(quat_params.shape)
|
81 |
-
root_quat[0] = np.array([[1.0, 0.0, 0.0, 0.0]])
|
82 |
-
quat_params[:, 0] = root_quat
|
83 |
-
# quat_params[0, 0] = np.array([[1.0, 0.0, 0.0, 0.0]])
|
84 |
-
for chain in self._kinematic_tree:
|
85 |
-
R = root_quat
|
86 |
-
for j in range(len(chain) - 1):
|
87 |
-
# (batch, 3)
|
88 |
-
u = self._raw_offset_np[chain[j+1]][np.newaxis,...].repeat(len(joints), axis=0)
|
89 |
-
# print(u.shape)
|
90 |
-
# (batch, 3)
|
91 |
-
v = joints[:, chain[j+1]] - joints[:, chain[j]]
|
92 |
-
v = v / np.sqrt((v**2).sum(axis=-1))[:, np.newaxis]
|
93 |
-
# print(u.shape, v.shape)
|
94 |
-
rot_u_v = qbetween_np(u, v)
|
95 |
-
|
96 |
-
R_loc = qmul_np(qinv_np(R), rot_u_v)
|
97 |
-
|
98 |
-
quat_params[:,chain[j + 1], :] = R_loc
|
99 |
-
R = qmul_np(R, R_loc)
|
100 |
-
|
101 |
-
return quat_params
|
102 |
-
|
103 |
-
# Be sure root joint is at the beginning of kinematic chains
|
104 |
-
def forward_kinematics(self, quat_params, root_pos, skel_joints=None, do_root_R=True):
|
105 |
-
# quat_params (batch_size, joints_num, 4)
|
106 |
-
# joints (batch_size, joints_num, 3)
|
107 |
-
# root_pos (batch_size, 3)
|
108 |
-
if skel_joints is not None:
|
109 |
-
offsets = self.get_offsets_joints_batch(skel_joints)
|
110 |
-
if len(self._offset.shape) == 2:
|
111 |
-
offsets = self._offset.expand(quat_params.shape[0], -1, -1)
|
112 |
-
joints = torch.zeros(quat_params.shape[:-1] + (3,)).to(self.device)
|
113 |
-
joints[:, 0] = root_pos
|
114 |
-
for chain in self._kinematic_tree:
|
115 |
-
if do_root_R:
|
116 |
-
R = quat_params[:, 0]
|
117 |
-
else:
|
118 |
-
R = torch.tensor([[1.0, 0.0, 0.0, 0.0]]).expand(len(quat_params), -1).detach().to(self.device)
|
119 |
-
for i in range(1, len(chain)):
|
120 |
-
R = qmul(R, quat_params[:, chain[i]])
|
121 |
-
offset_vec = offsets[:, chain[i]]
|
122 |
-
joints[:, chain[i]] = qrot(R, offset_vec) + joints[:, chain[i-1]]
|
123 |
-
return joints
|
124 |
-
|
125 |
-
# Be sure root joint is at the beginning of kinematic chains
|
126 |
-
def forward_kinematics_np(self, quat_params, root_pos, skel_joints=None, do_root_R=True):
|
127 |
-
# quat_params (batch_size, joints_num, 4)
|
128 |
-
# joints (batch_size, joints_num, 3)
|
129 |
-
# root_pos (batch_size, 3)
|
130 |
-
if skel_joints is not None:
|
131 |
-
skel_joints = torch.from_numpy(skel_joints)
|
132 |
-
offsets = self.get_offsets_joints_batch(skel_joints)
|
133 |
-
if len(self._offset.shape) == 2:
|
134 |
-
offsets = self._offset.expand(quat_params.shape[0], -1, -1)
|
135 |
-
offsets = offsets.numpy()
|
136 |
-
joints = np.zeros(quat_params.shape[:-1] + (3,))
|
137 |
-
joints[:, 0] = root_pos
|
138 |
-
for chain in self._kinematic_tree:
|
139 |
-
if do_root_R:
|
140 |
-
R = quat_params[:, 0]
|
141 |
-
else:
|
142 |
-
R = np.array([[1.0, 0.0, 0.0, 0.0]]).repeat(len(quat_params), axis=0)
|
143 |
-
for i in range(1, len(chain)):
|
144 |
-
R = qmul_np(R, quat_params[:, chain[i]])
|
145 |
-
offset_vec = offsets[:, chain[i]]
|
146 |
-
joints[:, chain[i]] = qrot_np(R, offset_vec) + joints[:, chain[i - 1]]
|
147 |
-
return joints
|
148 |
-
|
149 |
-
def forward_kinematics_cont6d_np(self, cont6d_params, root_pos, skel_joints=None, do_root_R=True):
|
150 |
-
# cont6d_params (batch_size, joints_num, 6)
|
151 |
-
# joints (batch_size, joints_num, 3)
|
152 |
-
# root_pos (batch_size, 3)
|
153 |
-
if skel_joints is not None:
|
154 |
-
skel_joints = torch.from_numpy(skel_joints)
|
155 |
-
offsets = self.get_offsets_joints_batch(skel_joints)
|
156 |
-
if len(self._offset.shape) == 2:
|
157 |
-
offsets = self._offset.expand(cont6d_params.shape[0], -1, -1)
|
158 |
-
offsets = offsets.numpy()
|
159 |
-
joints = np.zeros(cont6d_params.shape[:-1] + (3,))
|
160 |
-
joints[:, 0] = root_pos
|
161 |
-
for chain in self._kinematic_tree:
|
162 |
-
if do_root_R:
|
163 |
-
matR = cont6d_to_matrix_np(cont6d_params[:, 0])
|
164 |
-
else:
|
165 |
-
matR = np.eye(3)[np.newaxis, :].repeat(len(cont6d_params), axis=0)
|
166 |
-
for i in range(1, len(chain)):
|
167 |
-
matR = np.matmul(matR, cont6d_to_matrix_np(cont6d_params[:, chain[i]]))
|
168 |
-
offset_vec = offsets[:, chain[i]][..., np.newaxis]
|
169 |
-
# print(matR.shape, offset_vec.shape)
|
170 |
-
joints[:, chain[i]] = np.matmul(matR, offset_vec).squeeze(-1) + joints[:, chain[i-1]]
|
171 |
-
return joints
|
172 |
-
|
173 |
-
def forward_kinematics_cont6d(self, cont6d_params, root_pos, skel_joints=None, do_root_R=True):
|
174 |
-
# cont6d_params (batch_size, joints_num, 6)
|
175 |
-
# joints (batch_size, joints_num, 3)
|
176 |
-
# root_pos (batch_size, 3)
|
177 |
-
if skel_joints is not None:
|
178 |
-
# skel_joints = torch.from_numpy(skel_joints)
|
179 |
-
offsets = self.get_offsets_joints_batch(skel_joints)
|
180 |
-
if len(self._offset.shape) == 2:
|
181 |
-
offsets = self._offset.expand(cont6d_params.shape[0], -1, -1)
|
182 |
-
joints = torch.zeros(cont6d_params.shape[:-1] + (3,)).to(cont6d_params.device)
|
183 |
-
joints[..., 0, :] = root_pos
|
184 |
-
for chain in self._kinematic_tree:
|
185 |
-
if do_root_R:
|
186 |
-
matR = cont6d_to_matrix(cont6d_params[:, 0])
|
187 |
-
else:
|
188 |
-
matR = torch.eye(3).expand((len(cont6d_params), -1, -1)).detach().to(cont6d_params.device)
|
189 |
-
for i in range(1, len(chain)):
|
190 |
-
matR = torch.matmul(matR, cont6d_to_matrix(cont6d_params[:, chain[i]]))
|
191 |
-
offset_vec = offsets[:, chain[i]].unsqueeze(-1)
|
192 |
-
# print(matR.shape, offset_vec.shape)
|
193 |
-
joints[:, chain[i]] = torch.matmul(matR, offset_vec).squeeze(-1) + joints[:, chain[i-1]]
|
194 |
-
return joints
|
195 |
-
|
196 |
-
|
197 |
-
|
198 |
-
|
199 |
-
|
|
|
|
|
|
|
|
|
|
|
|
|
|
|
|
|
|
|
|
|
|
|
|
|
|
|
|
|
|
|
|
|
|
|
|
|
|
|
|
|
|
|
|
|
|
|
|
|
|
|
|
|
|
|
|
|
|
|
|
|
|
|
|
|
|
|
|
|
|
|
|
|
|
|
|
|
|
|
|
|
|
|
|
|
|
|
|
|
|
|
|
|
|
|
|
|
|
|
|
|
|
|
|
|
|
|
|
|
|
|
|
|
|
|
|
|
|
|
|
|
|
|
|
|
|
|
|
|
|
|
|
|
|
|
|
|
|
|
|
|
|
|
|
|
|
|
|
|
|
|
|
|
|
|
|
|
|
|
|
|
|
|
|
|
|
|
|
|
|
|
|
|
|
|
|
|
|
|
|
|
|
|
|
|
|
|
|
|
|
|
|
|
|
|
|
|
|
|
|
|
|
|
|
|
|
|
|
|
|
|
|
|
|
|
|
|
|
|
|
|
|
|
|
|
|
|
|
|
|
|
|
|
|
|
|
|
|
|
|
|
|
|
|
|
|
|
|
|
|
|
|
|
|
|
|
|
|
|
|
|
|
|
|
|
|
|
|
|
|
|
|
|
|
|
|
|
|
|
|
|
|
|
|
|
|
|
|
|
|
|
|
|
|
|
|
|
|
|
|
|
|
|
|
|
|
|
|
|
|
|
|
|
|
|
|
|
|
|
|
|
|
|
|
|
|
|
|
|
|
|
|
|
|
|
|
|
|
|
|
|
|
|
|
|
|
|
|
|
|
|
|
|
|
|
|
|
|
|
|
|
|
|
|
|
|
|
|
|
|
|
|
|
|
|
|
|
|
|
|
|
|
|
|
|
|
|
|
|
|
|
|
|
|
|
|
|
|
|
|
spaces/AIGC-Audio/AudioGPT/text_to_audio/Make_An_Audio/wav_evaluation/models/__init__.py
DELETED
@@ -1,3 +0,0 @@
|
|
1 |
-
from . import clap
|
2 |
-
from . import audio
|
3 |
-
from . import utils
|
|
|
|
|
|
|
|
spaces/AIGC-Audio/AudioGPT/text_to_speech/tasks/tts/vocoder_infer/pwg.py
DELETED
@@ -1,32 +0,0 @@
|
|
1 |
-
import torch
|
2 |
-
from text_to_speech.modules.vocoder.parallel_wavegan.models.parallel_wavegan import ParallelWaveGANGenerator
|
3 |
-
from tasks.tts.vocoder_infer.base_vocoder import register_vocoder, BaseVocoder
|
4 |
-
from text_to_speech.utils.commons.ckpt_utils import load_ckpt
|
5 |
-
from text_to_speech.utils.commons.hparams import set_hparams, hparams
|
6 |
-
from text_to_speech.utils.commons.meters import Timer
|
7 |
-
|
8 |
-
total_time = 0
|
9 |
-
|
10 |
-
|
11 |
-
@register_vocoder('PWG')
|
12 |
-
class PWG(BaseVocoder):
|
13 |
-
def __init__(self):
|
14 |
-
base_dir = hparams['vocoder_ckpt']
|
15 |
-
config_path = f'{base_dir}/config.yaml'
|
16 |
-
self.config = config = set_hparams(config_path, global_hparams=False)
|
17 |
-
self.device = torch.device("cuda" if torch.cuda.is_available() else "cpu")
|
18 |
-
self.model = ParallelWaveGANGenerator(**config["generator_params"])
|
19 |
-
load_ckpt(self.model, base_dir, 'model_gen')
|
20 |
-
self.model.to(self.device)
|
21 |
-
self.model.eval()
|
22 |
-
|
23 |
-
def spec2wav(self, mel, **kwargs):
|
24 |
-
device = self.device
|
25 |
-
with torch.no_grad():
|
26 |
-
c = torch.FloatTensor(mel).unsqueeze(0).to(device)
|
27 |
-
c = c.transpose(2, 1) # [B, C, T]
|
28 |
-
z = None
|
29 |
-
with Timer('pwg', enable=hparams['profile_infer']):
|
30 |
-
y = self.model(z, c).view(-1)
|
31 |
-
wav_out = y.cpu().numpy()
|
32 |
-
return wav_out
|
|
|
|
|
|
|
|
|
|
|
|
|
|
|
|
|
|
|
|
|
|
|
|
|
|
|
|
|
|
|
|
|
|
|
|
|
|
|
|
|
|
|
|
|
|
|
|
|
|
|
|
|
|
|
|
|
|
|
|
|
|
|
|
|
|
spaces/AIGC-Audio/Make_An_Audio/ldm/modules/encoders/open_clap/utils.py
DELETED
@@ -1,369 +0,0 @@
|
|
1 |
-
import numpy as np
|
2 |
-
import torch
|
3 |
-
from torch import nn as nn
|
4 |
-
from torchvision.ops.misc import FrozenBatchNorm2d
|
5 |
-
import logging
|
6 |
-
import h5py
|
7 |
-
from tqdm import tqdm
|
8 |
-
import random
|
9 |
-
import json
|
10 |
-
import os
|
11 |
-
import pathlib
|
12 |
-
|
13 |
-
# TODO: (yusong) this not a good place to store those information and does not scale. Need to be fixed later.
|
14 |
-
dataset_split = {
|
15 |
-
"audiocaps": ["train", "valid", "test"],
|
16 |
-
"audioset": ["balanced_train", "unbalanced_train", "eval"],
|
17 |
-
"BBCSoundEffects": ["train", "test"],
|
18 |
-
"Clotho": ["train", "test", "valid"],
|
19 |
-
"free_to_use_sounds": ["train", "test"],
|
20 |
-
"paramount_motion": ["train", "test"],
|
21 |
-
"sonniss_game_effects": ["train", "test"],
|
22 |
-
"wesoundeffects": ["train", "test"],
|
23 |
-
"MACS": ["train", "test"],
|
24 |
-
"freesound": ["train", "test"],
|
25 |
-
"FSD50K": ["train", "test", "valid"],
|
26 |
-
"fsd50k_class_label": ["train", "test", "valid"],
|
27 |
-
"esc50": ["train", "test"],
|
28 |
-
"audiostock": ["train", "test"],
|
29 |
-
"freesound_no_overlap_noesc50": ["train", "test"],
|
30 |
-
"epidemic_sound_effects": ["train", "test"],
|
31 |
-
"VGGSound": ["train", "test"],
|
32 |
-
"urbansound8k_class_label": ["train", "test"],
|
33 |
-
"audioset_t5": ["balanced_train", "unbalanced_train", "eval"],
|
34 |
-
"epidemic_sound_effects_t5": ["train", "test"],
|
35 |
-
"WavText5K": ["train", "test"],
|
36 |
-
"esc50_no_overlap": ["train", "test"],
|
37 |
-
"usd8k_no_overlap": ["train", "test"],
|
38 |
-
"fsd50k_200_class_label": ["train", "test", "valid"]
|
39 |
-
}
|
40 |
-
|
41 |
-
|
42 |
-
def freeze_batch_norm_2d(module, module_match={}, name=""):
|
43 |
-
"""
|
44 |
-
Converts all `BatchNorm2d` and `SyncBatchNorm` layers of provided module into `FrozenBatchNorm2d`. If `module` is
|
45 |
-
itself an instance of either `BatchNorm2d` or `SyncBatchNorm`, it is converted into `FrozenBatchNorm2d` and
|
46 |
-
returned. Otherwise, the module is walked recursively and submodules are converted in place.
|
47 |
-
|
48 |
-
Args:
|
49 |
-
module (torch.nn.Module): Any PyTorch module.
|
50 |
-
module_match (dict): Dictionary of full module names to freeze (all if empty)
|
51 |
-
name (str): Full module name (prefix)
|
52 |
-
|
53 |
-
Returns:
|
54 |
-
torch.nn.Module: Resulting module
|
55 |
-
|
56 |
-
Inspired by https://github.com/pytorch/pytorch/blob/a5895f85be0f10212791145bfedc0261d364f103/torch/nn/modules/batchnorm.py#L762
|
57 |
-
"""
|
58 |
-
res = module
|
59 |
-
is_match = True
|
60 |
-
if module_match:
|
61 |
-
is_match = name in module_match
|
62 |
-
if is_match and isinstance(
|
63 |
-
module, (nn.modules.batchnorm.BatchNorm2d, nn.modules.batchnorm.SyncBatchNorm)
|
64 |
-
):
|
65 |
-
res = FrozenBatchNorm2d(module.num_features)
|
66 |
-
res.num_features = module.num_features
|
67 |
-
res.affine = module.affine
|
68 |
-
if module.affine:
|
69 |
-
res.weight.data = module.weight.data.clone().detach()
|
70 |
-
res.bias.data = module.bias.data.clone().detach()
|
71 |
-
res.running_mean.data = module.running_mean.data
|
72 |
-
res.running_var.data = module.running_var.data
|
73 |
-
res.eps = module.eps
|
74 |
-
else:
|
75 |
-
for child_name, child in module.named_children():
|
76 |
-
full_child_name = ".".join([name, child_name]) if name else child_name
|
77 |
-
new_child = freeze_batch_norm_2d(child, module_match, full_child_name)
|
78 |
-
if new_child is not child:
|
79 |
-
res.add_module(child_name, new_child)
|
80 |
-
return res
|
81 |
-
|
82 |
-
|
83 |
-
def exist(dataset_name, dataset_type):
|
84 |
-
"""
|
85 |
-
Check if dataset exists
|
86 |
-
"""
|
87 |
-
if dataset_type in dataset_split[dataset_name]:
|
88 |
-
return True
|
89 |
-
else:
|
90 |
-
return False
|
91 |
-
|
92 |
-
|
93 |
-
def get_tar_path_from_dataset_name(
|
94 |
-
dataset_names,
|
95 |
-
dataset_types,
|
96 |
-
islocal,
|
97 |
-
dataset_path,
|
98 |
-
proportion=1,
|
99 |
-
full_dataset=None
|
100 |
-
):
|
101 |
-
"""
|
102 |
-
Get tar path from dataset name and type
|
103 |
-
"""
|
104 |
-
output = []
|
105 |
-
for n in dataset_names:
|
106 |
-
if full_dataset is not None and n in full_dataset:
|
107 |
-
current_dataset_types = dataset_split[n]
|
108 |
-
else:
|
109 |
-
current_dataset_types = dataset_types
|
110 |
-
for s in current_dataset_types:
|
111 |
-
tmp = []
|
112 |
-
if islocal:
|
113 |
-
sizefilepath_ = f"{dataset_path}/{n}/{s}/sizes.json"
|
114 |
-
if not os.path.exists(sizefilepath_):
|
115 |
-
sizefilepath_ = f"./json_files/{n}/{s}/sizes.json"
|
116 |
-
else:
|
117 |
-
sizefilepath_ = f"./json_files/{n}/{s}/sizes.json"
|
118 |
-
if not os.path.exists(sizefilepath_):
|
119 |
-
continue
|
120 |
-
sizes = json.load(open(sizefilepath_, "r"))
|
121 |
-
for k in sizes.keys():
|
122 |
-
if islocal:
|
123 |
-
tmp.append(f"{dataset_path}/{n}/{s}/{k}")
|
124 |
-
else:
|
125 |
-
tmp.append(
|
126 |
-
f"pipe:aws s3 --cli-connect-timeout 0 cp s3://s-laion-audio/webdataset_tar/{n}/{s}/{k} -"
|
127 |
-
)
|
128 |
-
if proportion != 1:
|
129 |
-
tmp = random.sample(tmp, int(proportion * len(tmp)))
|
130 |
-
output.append(tmp)
|
131 |
-
return sum(output, [])
|
132 |
-
|
133 |
-
|
134 |
-
def get_tar_path_from_txts(txt_path, islocal, proportion=1):
|
135 |
-
"""
|
136 |
-
Get tar path from txt path
|
137 |
-
"""
|
138 |
-
if isinstance(txt_path, (list, tuple)):
|
139 |
-
return sum(
|
140 |
-
[
|
141 |
-
get_tar_path_from_txts(
|
142 |
-
txt_path[i], islocal=islocal, proportion=proportion
|
143 |
-
)
|
144 |
-
for i in range(len(txt_path))
|
145 |
-
],
|
146 |
-
[],
|
147 |
-
)
|
148 |
-
if isinstance(txt_path, str):
|
149 |
-
with open(txt_path) as f:
|
150 |
-
lines = f.readlines()
|
151 |
-
if islocal:
|
152 |
-
lines = [
|
153 |
-
lines[i]
|
154 |
-
.split("\n")[0]
|
155 |
-
.replace("pipe:aws s3 cp s3://s-laion-audio/", "/mnt/audio_clip/")
|
156 |
-
for i in range(len(lines))
|
157 |
-
]
|
158 |
-
else:
|
159 |
-
lines = [
|
160 |
-
lines[i].split("\n")[0].replace(".tar", ".tar -")
|
161 |
-
for i in range(len(lines))
|
162 |
-
]
|
163 |
-
if proportion != 1:
|
164 |
-
print("Sampling tars with proportion of {}".format(proportion))
|
165 |
-
lines = random.sample(lines, int(proportion * len(lines)))
|
166 |
-
return lines
|
167 |
-
|
168 |
-
|
169 |
-
def get_mix_lambda(mixup_alpha, batch_size):
|
170 |
-
mixup_lambdas = [
|
171 |
-
np.random.beta(mixup_alpha, mixup_alpha, 1)[0] for _ in range(batch_size)
|
172 |
-
]
|
173 |
-
return np.array(mixup_lambdas).astype(np.float32)
|
174 |
-
|
175 |
-
|
176 |
-
def do_mixup(x, mixup_lambda):
|
177 |
-
"""
|
178 |
-
Args:
|
179 |
-
x: (batch_size , ...)
|
180 |
-
mixup_lambda: (batch_size,)
|
181 |
-
Returns:
|
182 |
-
out: (batch_size, ...)
|
183 |
-
"""
|
184 |
-
out = (
|
185 |
-
x.transpose(0, -1) * mixup_lambda
|
186 |
-
+ torch.flip(x, dims=[0]).transpose(0, -1) * (1 - mixup_lambda)
|
187 |
-
).transpose(0, -1)
|
188 |
-
return out
|
189 |
-
|
190 |
-
|
191 |
-
def interpolate(x, ratio):
|
192 |
-
"""Interpolate data in time domain. This is used to compensate the
|
193 |
-
resolution reduction in downsampling of a CNN.
|
194 |
-
|
195 |
-
Args:
|
196 |
-
x: (batch_size, time_steps, classes_num)
|
197 |
-
ratio: int, ratio to interpolate
|
198 |
-
Returns:
|
199 |
-
upsampled: (batch_size, time_steps * ratio, classes_num)
|
200 |
-
"""
|
201 |
-
(batch_size, time_steps, classes_num) = x.shape
|
202 |
-
upsampled = x[:, :, None, :].repeat(1, 1, ratio, 1)
|
203 |
-
upsampled = upsampled.reshape(batch_size, time_steps * ratio, classes_num)
|
204 |
-
return upsampled
|
205 |
-
|
206 |
-
|
207 |
-
def pad_framewise_output(framewise_output, frames_num):
|
208 |
-
"""Pad framewise_output to the same length as input frames. The pad value
|
209 |
-
is the same as the value of the last frame.
|
210 |
-
Args:
|
211 |
-
framewise_output: (batch_size, frames_num, classes_num)
|
212 |
-
frames_num: int, number of frames to pad
|
213 |
-
Outputs:
|
214 |
-
output: (batch_size, frames_num, classes_num)
|
215 |
-
"""
|
216 |
-
pad = framewise_output[:, -1:, :].repeat(
|
217 |
-
1, frames_num - framewise_output.shape[1], 1
|
218 |
-
)
|
219 |
-
"""tensor for padding"""
|
220 |
-
|
221 |
-
output = torch.cat((framewise_output, pad), dim=1)
|
222 |
-
"""(batch_size, frames_num, classes_num)"""
|
223 |
-
|
224 |
-
|
225 |
-
def process_ipc(index_path, classes_num, filename):
|
226 |
-
# load data
|
227 |
-
logging.info("Load Data...............")
|
228 |
-
ipc = [[] for _ in range(classes_num)]
|
229 |
-
with h5py.File(index_path, "r") as f:
|
230 |
-
for i in tqdm(range(len(f["target"]))):
|
231 |
-
t_class = np.where(f["target"][i])[0]
|
232 |
-
for t in t_class:
|
233 |
-
ipc[t].append(i)
|
234 |
-
print(ipc)
|
235 |
-
np.save(filename, ipc)
|
236 |
-
logging.info("Load Data Succeed...............")
|
237 |
-
|
238 |
-
|
239 |
-
def save_to_dict(s, o_={}):
|
240 |
-
sp = s.split(": ")
|
241 |
-
o_.update({sp[0]: float(sp[1])})
|
242 |
-
return o_
|
243 |
-
|
244 |
-
|
245 |
-
def get_data_from_log(txt_path):
|
246 |
-
"""
|
247 |
-
Output dictionary from out.txt log file
|
248 |
-
"""
|
249 |
-
with open(txt_path) as f:
|
250 |
-
lines = f.readlines()
|
251 |
-
val_data = {}
|
252 |
-
train_data = {}
|
253 |
-
train_losses = []
|
254 |
-
train_losses_epoch = []
|
255 |
-
for i in range(len(lines)):
|
256 |
-
if "| INFO |" in lines[i]:
|
257 |
-
if "Eval Epoch" in lines[i]:
|
258 |
-
if "val_loss" in lines[i]:
|
259 |
-
# float(regex.sub("", lines[310].split(" ")[-1]).replace(" ", ""))
|
260 |
-
line = lines[i].split("Eval Epoch: ")[-1]
|
261 |
-
num_epoch = int(line.split(" ")[0].split(" ")[0])
|
262 |
-
d = {
|
263 |
-
line.split(" ")[0]
|
264 |
-
.split(" ")[1]
|
265 |
-
.replace(":", ""): float(line.split(" ")[0].split(" ")[-1])
|
266 |
-
}
|
267 |
-
for i in range(1, len(line.split(" "))):
|
268 |
-
d = save_to_dict(line.split(" ")[i], d)
|
269 |
-
val_data[num_epoch] = d
|
270 |
-
elif "Train Epoch" in lines[i]:
|
271 |
-
num_epoch = int(lines[i].split("Train Epoch: ")[1][0])
|
272 |
-
loss = float(lines[i].split("Loss: ")[-1].split(" (")[0])
|
273 |
-
train_losses.append(loss)
|
274 |
-
train_losses_epoch.append(num_epoch)
|
275 |
-
for i in range(len(train_losses)):
|
276 |
-
train_data[i] = {
|
277 |
-
"num_epoch": train_losses_epoch[i],
|
278 |
-
"train_loss": train_losses[i],
|
279 |
-
}
|
280 |
-
return train_data, val_data
|
281 |
-
|
282 |
-
|
283 |
-
def save_p(obj, filename):
|
284 |
-
import pickle
|
285 |
-
|
286 |
-
try:
|
287 |
-
from deepdiff import DeepDiff
|
288 |
-
except:
|
289 |
-
os.system("pip install deepdiff")
|
290 |
-
from deepdiff import DeepDiff
|
291 |
-
with open(filename, "wb") as file:
|
292 |
-
pickle.dump(obj, file, protocol=pickle.HIGHEST_PROTOCOL) # highest protocol
|
293 |
-
with open(filename, "rb") as file:
|
294 |
-
z = pickle.load(file)
|
295 |
-
assert (
|
296 |
-
DeepDiff(obj, z, ignore_string_case=True) == {}
|
297 |
-
), "there is something wrong with the saving process"
|
298 |
-
return
|
299 |
-
|
300 |
-
|
301 |
-
def load_p(filename):
|
302 |
-
import pickle
|
303 |
-
|
304 |
-
with open(filename, "rb") as file:
|
305 |
-
z = pickle.load(file)
|
306 |
-
return z
|
307 |
-
|
308 |
-
|
309 |
-
def save_json(data, name="data.json"):
|
310 |
-
import json
|
311 |
-
with open(name, 'w') as fp:
|
312 |
-
json.dump(data, fp)
|
313 |
-
return
|
314 |
-
|
315 |
-
|
316 |
-
def load_json(name):
|
317 |
-
import json
|
318 |
-
with open(name, 'r') as fp:
|
319 |
-
data = json.load(fp)
|
320 |
-
return data
|
321 |
-
|
322 |
-
|
323 |
-
from multiprocessing import Process, Manager
|
324 |
-
from multiprocessing import Process, Value, Array
|
325 |
-
from ctypes import c_wchar
|
326 |
-
|
327 |
-
|
328 |
-
def load_class_label(path):
|
329 |
-
# https://stackoverflow.com/questions/48004243/how-to-share-large-read-only-dictionary-list-across-processes-in-multiprocessing
|
330 |
-
# https://stackoverflow.com/questions/45693949/storing-strings-in-a-multiprocessing-sharedctypes-array
|
331 |
-
out = None
|
332 |
-
if path is not None:
|
333 |
-
if pathlib.Path(path).suffix in [".pkl", ".pickle"]:
|
334 |
-
out = load_p(path)
|
335 |
-
elif pathlib.Path(path).suffix in [".json", ".txt"]:
|
336 |
-
out = load_json(path)
|
337 |
-
elif pathlib.Path(path).suffix in [".npy", ".npz"]:
|
338 |
-
out = np.load(path)
|
339 |
-
elif pathlib.Path(path).suffix in [".csv"]:
|
340 |
-
import pandas as pd
|
341 |
-
out = pd.read_csv(path)
|
342 |
-
return out
|
343 |
-
# if out is None:
|
344 |
-
# return None
|
345 |
-
# else:
|
346 |
-
# key = Array(c_wchar, '\n'.join(list(out.keys())), lock=False)
|
347 |
-
# val = Array('i', out.values(), lock=False)
|
348 |
-
# return (key, val)
|
349 |
-
|
350 |
-
|
351 |
-
from torch import optim
|
352 |
-
|
353 |
-
|
354 |
-
def get_optimizer(params, lr, betas, eps, momentum, optimizer_name):
|
355 |
-
if optimizer_name.lower() == "adamw":
|
356 |
-
optimizer = optim.AdamW(
|
357 |
-
params, lr=lr, betas=betas, eps=eps
|
358 |
-
)
|
359 |
-
elif optimizer_name.lower() == "sgd":
|
360 |
-
optimizer = optim.SGD(
|
361 |
-
params, lr=lr, momentum=momentum
|
362 |
-
)
|
363 |
-
elif optimizer_name.lower() == "adam":
|
364 |
-
optimizer = optim.Adam(
|
365 |
-
params, lr=lr, betas=betas, eps=eps
|
366 |
-
)
|
367 |
-
else:
|
368 |
-
raise ValueError("optimizer name is not correct")
|
369 |
-
return optimizer
|
|
|
|
|
|
|
|
|
|
|
|
|
|
|
|
|
|
|
|
|
|
|
|
|
|
|
|
|
|
|
|
|
|
|
|
|
|
|
|
|
|
|
|
|
|
|
|
|
|
|
|
|
|
|
|
|
|
|
|
|
|
|
|
|
|
|
|
|
|
|
|
|
|
|
|
|
|
|
|
|
|
|
|
|
|
|
|
|
|
|
|
|
|
|
|
|
|
|
|
|
|
|
|
|
|
|
|
|
|
|
|
|
|
|
|
|
|
|
|
|
|
|
|
|
|
|
|
|
|
|
|
|
|
|
|
|
|
|
|
|
|
|
|
|
|
|
|
|
|
|
|
|
|
|
|
|
|
|
|
|
|
|
|
|
|
|
|
|
|
|
|
|
|
|
|
|
|
|
|
|
|
|
|
|
|
|
|
|
|
|
|
|
|
|
|
|
|
|
|
|
|
|
|
|
|
|
|
|
|
|
|
|
|
|
|
|
|
|
|
|
|
|
|
|
|
|
|
|
|
|
|
|
|
|
|
|
|
|
|
|
|
|
|
|
|
|
|
|
|
|
|
|
|
|
|
|
|
|
|
|
|
|
|
|
|
|
|
|
|
|
|
|
|
|
|
|
|
|
|
|
|
|
|
|
|
|
|
|
|
|
|
|
|
|
|
|
|
|
|
|
|
|
|
|
|
|
|
|
|
|
|
|
|
|
|
|
|
|
|
|
|
|
|
|
|
|
|
|
|
|
|
|
|
|
|
|
|
|
|
|
|
|
|
|
|
|
|
|
|
|
|
|
|
|
|
|
|
|
|
|
|
|
|
|
|
|
|
|
|
|
|
|
|
|
|
|
|
|
|
|
|
|
|
|
|
|
|
|
|
|
|
|
|
|
|
|
|
|
|
|
|
|
|
|
|
|
|
|
|
|
|
|
|
|
|
|
|
|
|
|
|
|
|
|
|
|
|
|
|
|
|
|
|
|
|
|
|
|
|
|
|
|
|
|
|
|
|
|
|
|
|
|
|
|
|
|
|
|
|
|
|
|
|
|
|
|
|
|
|
|
|
|
|
|
|
|
|
|
|
|
|
|
|
|
|
|
|
|
|
|
|
|
|
|
|
|
|
|
|
|
|
|
|
|
|
|
|
|
|
|
|
|
|
|
|
|
|
|
|
|
|
|
|
|
|
|
|
|
|
|
|
|
|
|
|
|
|
|
|
|
|
|
|
|
|
|
|
|
|
|
|
|
|
|
|
|
|
|
|
|
|
|
|
|
|
|
|
|
|
|
|
|
|
|
|
|
|
|
|
|
|
|
|
|
|
|
|
|
|
|
|
|
|
|
|
|
|
|
|
|
|
|
|
|
|
|
|
|
|
|
|
|
|
|
|
|
|
|
|
|
|
|
|
|
|
|
|
|
|
|
|
|
|
|
|
|
|
|
|
|
|
|
|
|
|
|
|
|
|
|
|
|
|
|
|
|
|
|
|
|
|
|
|
|
|
|
|
|
|
|
|
|
|
|
|
|
|
|
|
|
|
|
|
|
|
|
|
|
|
|
|
|
|
|
|
|
|
|
|
|
|
|
|
|
|
|
|
|
|
|
|
|
|
|
|
|
|
|
|
|
|
|
|
|
|
|
|
|
|
|
|
|
|
|
|
|
|
|
|
spaces/ANLPRL/NER_On_Oral_Medicine/README.md
DELETED
@@ -1,12 +0,0 @@
|
|
1 |
-
---
|
2 |
-
title: NER On Oral Medicine
|
3 |
-
emoji: 😻
|
4 |
-
colorFrom: green
|
5 |
-
colorTo: gray
|
6 |
-
sdk: streamlit
|
7 |
-
sdk_version: 1.19.0
|
8 |
-
app_file: app.py
|
9 |
-
pinned: false
|
10 |
-
---
|
11 |
-
|
12 |
-
Check out the configuration reference at https://huggingface.co/docs/hub/spaces-config-reference
|
|
|
|
|
|
|
|
|
|
|
|
|
|
|
|
|
|
|
|
|
|
|
|
|
|
spaces/ATang0729/Forecast4Muses/Model/Model6/Model6_1_ClothesKeyPoint/work_dirs_1-x/td_hm_res50_4xb64-60e_deepfashion2_trousers_256x192/__init__.py
DELETED
File without changes
|
spaces/AgentVerse/agentVerse/ui/src/phaser3-rex-plugins/templates/ui/easemove/EaseMove.js
DELETED
@@ -1,2 +0,0 @@
|
|
1 |
-
import { EaseMove, EaseMoveTo, EaseMoveFrom } from '../../../plugins/easemove.js';
|
2 |
-
export { EaseMove, EaseMoveTo, EaseMoveFrom };
|
|
|
|
|
|
spaces/AgentVerse/agentVerse/ui/src/phaser3-rex-plugins/templates/ui/gridtable/GridTable.d.ts
DELETED
@@ -1,63 +0,0 @@
|
|
1 |
-
// import * as Phaser from 'phaser';
|
2 |
-
import Scrollable from '../utils/scrollable/Scrollable';
|
3 |
-
import GridTableCore from '../../../plugins/gridtable'
|
4 |
-
|
5 |
-
export default GridTable;
|
6 |
-
|
7 |
-
declare namespace GridTable {
|
8 |
-
|
9 |
-
type CreateCellContainerCallbackType = (
|
10 |
-
cell: GridTableCore.CellData,
|
11 |
-
cellContainer: Phaser.GameObjects.GameObject | null
|
12 |
-
) => Phaser.GameObjects.GameObject | null;
|
13 |
-
|
14 |
-
interface IConfig extends Scrollable.IConfig {
|
15 |
-
space?: {
|
16 |
-
left?: number, right?: number, top?: number, bottom?: number,
|
17 |
-
|
18 |
-
table?: number | {
|
19 |
-
left?: number, right?: number, top?: number, bottom?: number,
|
20 |
-
},
|
21 |
-
|
22 |
-
header?: number,
|
23 |
-
footer?: number,
|
24 |
-
},
|
25 |
-
|
26 |
-
scrollMode?: GridTableCore.ScrollModeType,
|
27 |
-
|
28 |
-
table: {
|
29 |
-
width?: number | undefined,
|
30 |
-
height?: number | undefined,
|
31 |
-
|
32 |
-
cellWidth?: number | undefined,
|
33 |
-
cellHeight?: number | undefined,
|
34 |
-
columns?: number,
|
35 |
-
mask?: GridTableCore.MaskConfig,
|
36 |
-
interactive?: boolean,
|
37 |
-
reuseCellContainer?: boolean,
|
38 |
-
},
|
39 |
-
|
40 |
-
createCellContainerCallback: CreateCellContainerCallbackType,
|
41 |
-
|
42 |
-
items: unknown[]
|
43 |
-
}
|
44 |
-
|
45 |
-
}
|
46 |
-
|
47 |
-
declare class GridTable extends Scrollable {
|
48 |
-
constructor(
|
49 |
-
scene: Phaser.Scene,
|
50 |
-
config?: GridTable.IConfig
|
51 |
-
);
|
52 |
-
|
53 |
-
setItems(items?: unknown[]): this;
|
54 |
-
refresh(): this;
|
55 |
-
updateVisibleCell(cellIndex: number): this;
|
56 |
-
|
57 |
-
getCell(cellIndex: number): GridTableCore.CellData;
|
58 |
-
getCellContainer(cellIndex: number): Phaser.GameObjects.GameObject | null;
|
59 |
-
startRowIndex: number;
|
60 |
-
|
61 |
-
scrollToRow(rowIndex: number): this;
|
62 |
-
scrollToNextRow(rowCount?: number): this;
|
63 |
-
}
|
|
|
|
|
|
|
|
|
|
|
|
|
|
|
|
|
|
|
|
|
|
|
|
|
|
|
|
|
|
|
|
|
|
|
|
|
|
|
|
|
|
|
|
|
|
|
|
|
|
|
|
|
|
|
|
|
|
|
|
|
|
|
|
|
|
|
|
|
|
|
|
|
|
|
|
|
|
|
|
|
|
|
|
|
|
|
|
|
|
|
|
|
|
|
|
|
|
|
|
|
|
|
|
|
|
|
|
|
|
|
|
|
|
|
|
|
|
|
|
|
|
|
|
|
|
|
|
spaces/Aloento/9Nine-PITS/text/frontend/zh_normalization/__init__.py
DELETED
@@ -1,14 +0,0 @@
|
|
1 |
-
# Copyright (c) 2020 PaddlePaddle Authors. All Rights Reserved.
|
2 |
-
#
|
3 |
-
# Licensed under the Apache License, Version 2.0 (the "License");
|
4 |
-
# you may not use this file except in compliance with the License.
|
5 |
-
# You may obtain a copy of the License at
|
6 |
-
#
|
7 |
-
# http://www.apache.org/licenses/LICENSE-2.0
|
8 |
-
#
|
9 |
-
# Unless required by applicable law or agreed to in writing, software
|
10 |
-
# distributed under the License is distributed on an "AS IS" BASIS,
|
11 |
-
# WITHOUT WARRANTIES OR CONDITIONS OF ANY KIND, either express or implied.
|
12 |
-
# See the License for the specific language governing permissions and
|
13 |
-
# limitations under the License.
|
14 |
-
from text.frontend.zh_normalization.text_normlization import *
|
|
|
|
|
|
|
|
|
|
|
|
|
|
|
|
|
|
|
|
|
|
|
|
|
|
|
|
|
|
spaces/Alpaca233/SadTalker/scripts/extension.py
DELETED
@@ -1,189 +0,0 @@
|
|
1 |
-
import os, sys
|
2 |
-
from pathlib import Path
|
3 |
-
import tempfile
|
4 |
-
import gradio as gr
|
5 |
-
from modules.call_queue import wrap_gradio_gpu_call, wrap_queued_call
|
6 |
-
from modules.shared import opts, OptionInfo
|
7 |
-
from modules import shared, paths, script_callbacks
|
8 |
-
import launch
|
9 |
-
import glob
|
10 |
-
from huggingface_hub import snapshot_download
|
11 |
-
|
12 |
-
|
13 |
-
|
14 |
-
def check_all_files_safetensor(current_dir):
|
15 |
-
kv = {
|
16 |
-
"SadTalker_V0.0.2_256.safetensors": "sadtalker-256",
|
17 |
-
"SadTalker_V0.0.2_512.safetensors": "sadtalker-512",
|
18 |
-
"mapping_00109-model.pth.tar" : "mapping-109" ,
|
19 |
-
"mapping_00229-model.pth.tar" : "mapping-229" ,
|
20 |
-
}
|
21 |
-
|
22 |
-
if not os.path.isdir(current_dir):
|
23 |
-
return False
|
24 |
-
|
25 |
-
dirs = os.listdir(current_dir)
|
26 |
-
|
27 |
-
for f in dirs:
|
28 |
-
if f in kv.keys():
|
29 |
-
del kv[f]
|
30 |
-
|
31 |
-
return len(kv.keys()) == 0
|
32 |
-
|
33 |
-
def check_all_files(current_dir):
|
34 |
-
kv = {
|
35 |
-
"auido2exp_00300-model.pth": "audio2exp",
|
36 |
-
"auido2pose_00140-model.pth": "audio2pose",
|
37 |
-
"epoch_20.pth": "face_recon",
|
38 |
-
"facevid2vid_00189-model.pth.tar": "face-render",
|
39 |
-
"mapping_00109-model.pth.tar" : "mapping-109" ,
|
40 |
-
"mapping_00229-model.pth.tar" : "mapping-229" ,
|
41 |
-
"wav2lip.pth": "wav2lip",
|
42 |
-
"shape_predictor_68_face_landmarks.dat": "dlib",
|
43 |
-
}
|
44 |
-
|
45 |
-
if not os.path.isdir(current_dir):
|
46 |
-
return False
|
47 |
-
|
48 |
-
dirs = os.listdir(current_dir)
|
49 |
-
|
50 |
-
for f in dirs:
|
51 |
-
if f in kv.keys():
|
52 |
-
del kv[f]
|
53 |
-
|
54 |
-
return len(kv.keys()) == 0
|
55 |
-
|
56 |
-
|
57 |
-
|
58 |
-
def download_model(local_dir='./checkpoints'):
|
59 |
-
REPO_ID = 'vinthony/SadTalker'
|
60 |
-
snapshot_download(repo_id=REPO_ID, local_dir=local_dir, local_dir_use_symlinks=False)
|
61 |
-
|
62 |
-
def get_source_image(image):
|
63 |
-
return image
|
64 |
-
|
65 |
-
def get_img_from_txt2img(x):
|
66 |
-
talker_path = Path(paths.script_path) / "outputs"
|
67 |
-
imgs_from_txt_dir = str(talker_path / "txt2img-images/")
|
68 |
-
imgs = glob.glob(imgs_from_txt_dir+'/*/*.png')
|
69 |
-
imgs.sort(key=lambda x:os.path.getmtime(os.path.join(imgs_from_txt_dir, x)))
|
70 |
-
img_from_txt_path = os.path.join(imgs_from_txt_dir, imgs[-1])
|
71 |
-
return img_from_txt_path, img_from_txt_path
|
72 |
-
|
73 |
-
def get_img_from_img2img(x):
|
74 |
-
talker_path = Path(paths.script_path) / "outputs"
|
75 |
-
imgs_from_img_dir = str(talker_path / "img2img-images/")
|
76 |
-
imgs = glob.glob(imgs_from_img_dir+'/*/*.png')
|
77 |
-
imgs.sort(key=lambda x:os.path.getmtime(os.path.join(imgs_from_img_dir, x)))
|
78 |
-
img_from_img_path = os.path.join(imgs_from_img_dir, imgs[-1])
|
79 |
-
return img_from_img_path, img_from_img_path
|
80 |
-
|
81 |
-
def get_default_checkpoint_path():
|
82 |
-
# check the path of models/checkpoints and extensions/
|
83 |
-
checkpoint_path = Path(paths.script_path) / "models"/ "SadTalker"
|
84 |
-
extension_checkpoint_path = Path(paths.script_path) / "extensions"/ "SadTalker" / "checkpoints"
|
85 |
-
|
86 |
-
if check_all_files_safetensor(checkpoint_path):
|
87 |
-
# print('founding sadtalker checkpoint in ' + str(checkpoint_path))
|
88 |
-
return checkpoint_path
|
89 |
-
|
90 |
-
if check_all_files_safetensor(extension_checkpoint_path):
|
91 |
-
# print('founding sadtalker checkpoint in ' + str(extension_checkpoint_path))
|
92 |
-
return extension_checkpoint_path
|
93 |
-
|
94 |
-
if check_all_files(checkpoint_path):
|
95 |
-
# print('founding sadtalker checkpoint in ' + str(checkpoint_path))
|
96 |
-
return checkpoint_path
|
97 |
-
|
98 |
-
if check_all_files(extension_checkpoint_path):
|
99 |
-
# print('founding sadtalker checkpoint in ' + str(extension_checkpoint_path))
|
100 |
-
return extension_checkpoint_path
|
101 |
-
|
102 |
-
return None
|
103 |
-
|
104 |
-
|
105 |
-
|
106 |
-
def install():
|
107 |
-
|
108 |
-
kv = {
|
109 |
-
"face_alignment": "face-alignment==1.3.5",
|
110 |
-
"imageio": "imageio==2.19.3",
|
111 |
-
"imageio_ffmpeg": "imageio-ffmpeg==0.4.7",
|
112 |
-
"librosa":"librosa==0.8.0",
|
113 |
-
"pydub":"pydub==0.25.1",
|
114 |
-
"scipy":"scipy==1.8.1",
|
115 |
-
"tqdm": "tqdm",
|
116 |
-
"yacs":"yacs==0.1.8",
|
117 |
-
"yaml": "pyyaml",
|
118 |
-
"av":"av",
|
119 |
-
"gfpgan": "gfpgan",
|
120 |
-
}
|
121 |
-
|
122 |
-
# # dlib is not necessary currently
|
123 |
-
# if 'darwin' in sys.platform:
|
124 |
-
# kv['dlib'] = "dlib"
|
125 |
-
# else:
|
126 |
-
# kv['dlib'] = 'dlib-bin'
|
127 |
-
|
128 |
-
# #### we need to have a newer version of imageio for our method.
|
129 |
-
# launch.run_pip("install imageio==2.19.3", "requirements for SadTalker")
|
130 |
-
|
131 |
-
for k,v in kv.items():
|
132 |
-
if not launch.is_installed(k):
|
133 |
-
print(k, launch.is_installed(k))
|
134 |
-
launch.run_pip("install "+ v, "requirements for SadTalker")
|
135 |
-
|
136 |
-
if os.getenv('SADTALKER_CHECKPOINTS'):
|
137 |
-
print('load Sadtalker Checkpoints from '+ os.getenv('SADTALKER_CHECKPOINTS'))
|
138 |
-
|
139 |
-
elif get_default_checkpoint_path() is not None:
|
140 |
-
os.environ['SADTALKER_CHECKPOINTS'] = str(get_default_checkpoint_path())
|
141 |
-
else:
|
142 |
-
|
143 |
-
print(
|
144 |
-
""""
|
145 |
-
SadTalker will not support download all the files from hugging face, which will take a long time.
|
146 |
-
|
147 |
-
please manually set the SADTALKER_CHECKPOINTS in `webui_user.bat`(windows) or `webui_user.sh`(linux)
|
148 |
-
"""
|
149 |
-
)
|
150 |
-
|
151 |
-
# python = sys.executable
|
152 |
-
|
153 |
-
# launch.run(f'"{python}" -m pip uninstall -y huggingface_hub', live=True)
|
154 |
-
# launch.run(f'"{python}" -m pip install --upgrade git+https://github.com/huggingface/huggingface_hub@main', live=True)
|
155 |
-
# ### run the scripts to downlod models to correct localtion.
|
156 |
-
# # print('download models for SadTalker')
|
157 |
-
# # launch.run("cd " + paths.script_path+"/extensions/SadTalker && bash ./scripts/download_models.sh", live=True)
|
158 |
-
# # print('SadTalker is successfully installed!')
|
159 |
-
# download_model(paths.script_path+'/extensions/SadTalker/checkpoints')
|
160 |
-
|
161 |
-
|
162 |
-
def on_ui_tabs():
|
163 |
-
install()
|
164 |
-
|
165 |
-
sys.path.extend([paths.script_path+'/extensions/SadTalker'])
|
166 |
-
|
167 |
-
repo_dir = paths.script_path+'/extensions/SadTalker/'
|
168 |
-
|
169 |
-
result_dir = opts.sadtalker_result_dir
|
170 |
-
os.makedirs(result_dir, exist_ok=True)
|
171 |
-
|
172 |
-
from app_sadtalker import sadtalker_demo
|
173 |
-
|
174 |
-
if os.getenv('SADTALKER_CHECKPOINTS'):
|
175 |
-
checkpoint_path = os.getenv('SADTALKER_CHECKPOINTS')
|
176 |
-
else:
|
177 |
-
checkpoint_path = repo_dir+'checkpoints/'
|
178 |
-
|
179 |
-
audio_to_video = sadtalker_demo(checkpoint_path=checkpoint_path, config_path=repo_dir+'src/config', warpfn = wrap_queued_call)
|
180 |
-
|
181 |
-
return [(audio_to_video, "SadTalker", "extension")]
|
182 |
-
|
183 |
-
def on_ui_settings():
|
184 |
-
talker_path = Path(paths.script_path) / "outputs"
|
185 |
-
section = ('extension', "SadTalker")
|
186 |
-
opts.add_option("sadtalker_result_dir", OptionInfo(str(talker_path / "SadTalker/"), "Path to save results of sadtalker", section=section))
|
187 |
-
|
188 |
-
script_callbacks.on_ui_settings(on_ui_settings)
|
189 |
-
script_callbacks.on_ui_tabs(on_ui_tabs)
|
|
|
|
|
|
|
|
|
|
|
|
|
|
|
|
|
|
|
|
|
|
|
|
|
|
|
|
|
|
|
|
|
|
|
|
|
|
|
|
|
|
|
|
|
|
|
|
|
|
|
|
|
|
|
|
|
|
|
|
|
|
|
|
|
|
|
|
|
|
|
|
|
|
|
|
|
|
|
|
|
|
|
|
|
|
|
|
|
|
|
|
|
|
|
|
|
|
|
|
|
|
|
|
|
|
|
|
|
|
|
|
|
|
|
|
|
|
|
|
|
|
|
|
|
|
|
|
|
|
|
|
|
|
|
|
|
|
|
|
|
|
|
|
|
|
|
|
|
|
|
|
|
|
|
|
|
|
|
|
|
|
|
|
|
|
|
|
|
|
|
|
|
|
|
|
|
|
|
|
|
|
|
|
|
|
|
|
|
|
|
|
|
|
|
|
|
|
|
|
|
|
|
|
|
|
|
|
|
|
|
|
|
|
|
|
|
|
|
|
|
|
|
|
|
|
|
|
|
|
|
|
|
|
|
|
|
|
|
|
|
|
|
|
|
|
|
|
|
|
|
|
|
|
|
|
|
|
|
|
|
|
|
|
|
|
|
|
|
|
|
|
|
|
|
|
|
|
|
|
|
|
|
|
|
|
|
|
|
|
|
|
|
|
|
|
|
|
|
|
|
|
|
|
|
|
|
|
|
|
|
|
|
|
|
|
|
|
|
|
|
|
|
|
|
|
|
|
|
|
|
|
|
|
|
|
|
|
|
|
|
|
|
|
|
|
|
|
|
|
|
|
|
|
|
|
|
|
|
|
|
|
|
|
|
|
|
|
|
|
|
|
|
|
|
|
|
|
|
|
spaces/Amrrs/DragGan-Inversion/PTI/torch_utils/ops/fma.py
DELETED
@@ -1,60 +0,0 @@
|
|
1 |
-
# Copyright (c) 2021, NVIDIA CORPORATION. All rights reserved.
|
2 |
-
#
|
3 |
-
# NVIDIA CORPORATION and its licensors retain all intellectual property
|
4 |
-
# and proprietary rights in and to this software, related documentation
|
5 |
-
# and any modifications thereto. Any use, reproduction, disclosure or
|
6 |
-
# distribution of this software and related documentation without an express
|
7 |
-
# license agreement from NVIDIA CORPORATION is strictly prohibited.
|
8 |
-
|
9 |
-
"""Fused multiply-add, with slightly faster gradients than `torch.addcmul()`."""
|
10 |
-
|
11 |
-
import torch
|
12 |
-
|
13 |
-
#----------------------------------------------------------------------------
|
14 |
-
|
15 |
-
def fma(a, b, c): # => a * b + c
|
16 |
-
return _FusedMultiplyAdd.apply(a, b, c)
|
17 |
-
|
18 |
-
#----------------------------------------------------------------------------
|
19 |
-
|
20 |
-
class _FusedMultiplyAdd(torch.autograd.Function): # a * b + c
|
21 |
-
@staticmethod
|
22 |
-
def forward(ctx, a, b, c): # pylint: disable=arguments-differ
|
23 |
-
out = torch.addcmul(c, a, b)
|
24 |
-
ctx.save_for_backward(a, b)
|
25 |
-
ctx.c_shape = c.shape
|
26 |
-
return out
|
27 |
-
|
28 |
-
@staticmethod
|
29 |
-
def backward(ctx, dout): # pylint: disable=arguments-differ
|
30 |
-
a, b = ctx.saved_tensors
|
31 |
-
c_shape = ctx.c_shape
|
32 |
-
da = None
|
33 |
-
db = None
|
34 |
-
dc = None
|
35 |
-
|
36 |
-
if ctx.needs_input_grad[0]:
|
37 |
-
da = _unbroadcast(dout * b, a.shape)
|
38 |
-
|
39 |
-
if ctx.needs_input_grad[1]:
|
40 |
-
db = _unbroadcast(dout * a, b.shape)
|
41 |
-
|
42 |
-
if ctx.needs_input_grad[2]:
|
43 |
-
dc = _unbroadcast(dout, c_shape)
|
44 |
-
|
45 |
-
return da, db, dc
|
46 |
-
|
47 |
-
#----------------------------------------------------------------------------
|
48 |
-
|
49 |
-
def _unbroadcast(x, shape):
|
50 |
-
extra_dims = x.ndim - len(shape)
|
51 |
-
assert extra_dims >= 0
|
52 |
-
dim = [i for i in range(x.ndim) if x.shape[i] > 1 and (i < extra_dims or shape[i - extra_dims] == 1)]
|
53 |
-
if len(dim):
|
54 |
-
x = x.sum(dim=dim, keepdim=True)
|
55 |
-
if extra_dims:
|
56 |
-
x = x.reshape(-1, *x.shape[extra_dims+1:])
|
57 |
-
assert x.shape == shape
|
58 |
-
return x
|
59 |
-
|
60 |
-
#----------------------------------------------------------------------------
|
|
|
|
|
|
|
|
|
|
|
|
|
|
|
|
|
|
|
|
|
|
|
|
|
|
|
|
|
|
|
|
|
|
|
|
|
|
|
|
|
|
|
|
|
|
|
|
|
|
|
|
|
|
|
|
|
|
|
|
|
|
|
|
|
|
|
|
|
|
|
|
|
|
|
|
|
|
|
|
|
|
|
|
|
|
|
|
|
|
|
|
|
|
|
|
|
|
|
|
|
|
|
|
|
|
|
|
|
|
|
|
|
|
|
|
|
|
|
|
|
|
spaces/Androidonnxfork/CivitAi-to-Diffusers/diffusers/docs/source/en/api/pipelines/stable_diffusion/adapter.md
DELETED
@@ -1,187 +0,0 @@
|
|
1 |
-
<!--Copyright 2023 The HuggingFace Team. All rights reserved.
|
2 |
-
|
3 |
-
Licensed under the Apache License, Version 2.0 (the "License"); you may not use this file except in compliance with
|
4 |
-
the License. You may obtain a copy of the License at
|
5 |
-
|
6 |
-
http://www.apache.org/licenses/LICENSE-2.0
|
7 |
-
|
8 |
-
Unless required by applicable law or agreed to in writing, software distributed under the License is distributed on
|
9 |
-
an "AS IS" BASIS, WITHOUT WARRANTIES OR CONDITIONS OF ANY KIND, either express or implied. See the License for the
|
10 |
-
specific language governing permissions and limitations under the License.
|
11 |
-
-->
|
12 |
-
|
13 |
-
# Text-to-Image Generation with Adapter Conditioning
|
14 |
-
|
15 |
-
## Overview
|
16 |
-
|
17 |
-
[T2I-Adapter: Learning Adapters to Dig out More Controllable Ability for Text-to-Image Diffusion Models](https://arxiv.org/abs/2302.08453) by Chong Mou, Xintao Wang, Liangbin Xie, Jian Zhang, Zhongang Qi, Ying Shan, Xiaohu Qie.
|
18 |
-
|
19 |
-
Using the pretrained models we can provide control images (for example, a depth map) to control Stable Diffusion text-to-image generation so that it follows the structure of the depth image and fills in the details.
|
20 |
-
|
21 |
-
The abstract of the paper is the following:
|
22 |
-
|
23 |
-
*The incredible generative ability of large-scale text-to-image (T2I) models has demonstrated strong power of learning complex structures and meaningful semantics. However, relying solely on text prompts cannot fully take advantage of the knowledge learned by the model, especially when flexible and accurate structure control is needed. In this paper, we aim to ``dig out" the capabilities that T2I models have implicitly learned, and then explicitly use them to control the generation more granularly. Specifically, we propose to learn simple and small T2I-Adapters to align internal knowledge in T2I models with external control signals, while freezing the original large T2I models. In this way, we can train various adapters according to different conditions, and achieve rich control and editing effects. Further, the proposed T2I-Adapters have attractive properties of practical value, such as composability and generalization ability. Extensive experiments demonstrate that our T2I-Adapter has promising generation quality and a wide range of applications.*
|
24 |
-
|
25 |
-
This model was contributed by the community contributor [HimariO](https://github.com/HimariO) ❤️ .
|
26 |
-
|
27 |
-
## Available Pipelines:
|
28 |
-
|
29 |
-
| Pipeline | Tasks | Demo
|
30 |
-
|---|---|:---:|
|
31 |
-
| [StableDiffusionAdapterPipeline](https://github.com/huggingface/diffusers/blob/main/src/diffusers/pipelines/stable_diffusion/pipeline_stable_diffusion_adapter.py) | *Text-to-Image Generation with T2I-Adapter Conditioning* | -
|
32 |
-
|
33 |
-
## Usage example
|
34 |
-
|
35 |
-
In the following we give a simple example of how to use a *T2IAdapter* checkpoint with Diffusers for inference.
|
36 |
-
All adapters use the same pipeline.
|
37 |
-
|
38 |
-
1. Images are first converted into the appropriate *control image* format.
|
39 |
-
2. The *control image* and *prompt* are passed to the [`StableDiffusionAdapterPipeline`].
|
40 |
-
|
41 |
-
Let's have a look at a simple example using the [Color Adapter](https://huggingface.co/TencentARC/t2iadapter_color_sd14v1).
|
42 |
-
|
43 |
-
```python
|
44 |
-
from diffusers.utils import load_image
|
45 |
-
|
46 |
-
image = load_image("https://huggingface.co/datasets/diffusers/docs-images/resolve/main/t2i-adapter/color_ref.png")
|
47 |
-
```
|
48 |
-
|
49 |
-
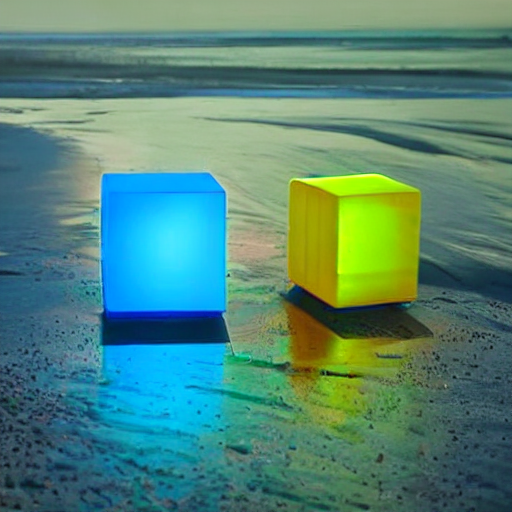
|
50 |
-
|
51 |
-
|
52 |
-
Then we can create our color palette by simply resizing it to 8 by 8 pixels and then scaling it back to original size.
|
53 |
-
|
54 |
-
```python
|
55 |
-
from PIL import Image
|
56 |
-
|
57 |
-
color_palette = image.resize((8, 8))
|
58 |
-
color_palette = color_palette.resize((512, 512), resample=Image.Resampling.NEAREST)
|
59 |
-
```
|
60 |
-
|
61 |
-
Let's take a look at the processed image.
|
62 |
-
|
63 |
-
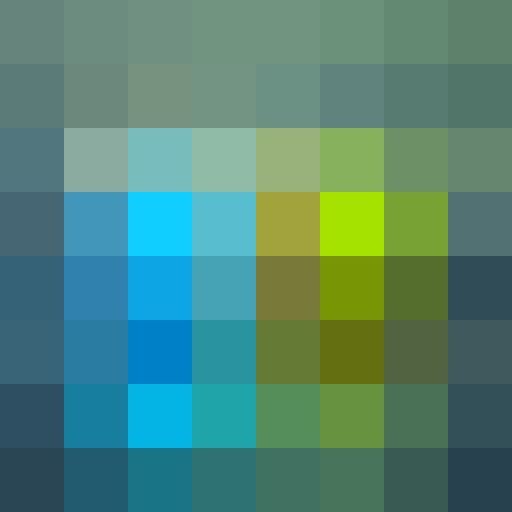
|
64 |
-
|
65 |
-
|
66 |
-
Next, create the adapter pipeline
|
67 |
-
|
68 |
-
```py
|
69 |
-
import torch
|
70 |
-
from diffusers import StableDiffusionAdapterPipeline, T2IAdapter
|
71 |
-
|
72 |
-
adapter = T2IAdapter.from_pretrained("TencentARC/t2iadapter_color_sd14v1")
|
73 |
-
pipe = StableDiffusionAdapterPipeline.from_pretrained(
|
74 |
-
"CompVis/stable-diffusion-v1-4",
|
75 |
-
adapter=adapter,
|
76 |
-
torch_dtype=torch.float16,
|
77 |
-
)
|
78 |
-
pipe.to("cuda")
|
79 |
-
```
|
80 |
-
|
81 |
-
Finally, pass the prompt and control image to the pipeline
|
82 |
-
|
83 |
-
```py
|
84 |
-
# fix the random seed, so you will get the same result as the example
|
85 |
-
generator = torch.manual_seed(7)
|
86 |
-
|
87 |
-
out_image = pipe(
|
88 |
-
"At night, glowing cubes in front of the beach",
|
89 |
-
image=color_palette,
|
90 |
-
generator=generator,
|
91 |
-
).images[0]
|
92 |
-
```
|
93 |
-
|
94 |
-
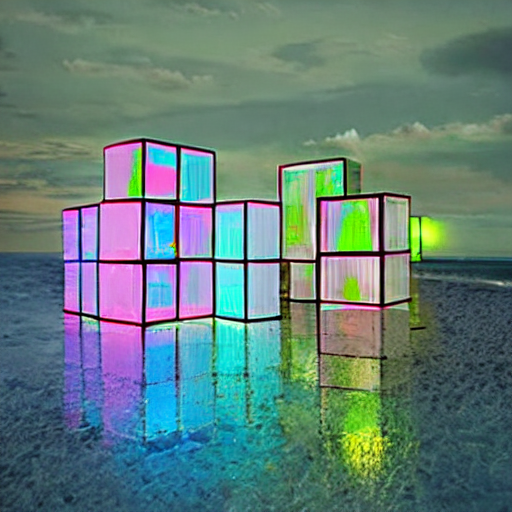
|
95 |
-
|
96 |
-
|
97 |
-
## Available checkpoints
|
98 |
-
|
99 |
-
Non-diffusers checkpoints can be found under [TencentARC/T2I-Adapter](https://huggingface.co/TencentARC/T2I-Adapter/tree/main/models).
|
100 |
-
|
101 |
-
### T2I-Adapter with Stable Diffusion 1.4
|
102 |
-
|
103 |
-
| Model Name | Control Image Overview| Control Image Example | Generated Image Example |
|
104 |
-
|---|---|---|---|
|
105 |
-
|[TencentARC/t2iadapter_color_sd14v1](https://huggingface.co/TencentARC/t2iadapter_color_sd14v1)<br/> *Trained with spatial color palette* | A image with 8x8 color palette.|<a href="https://huggingface.co/datasets/diffusers/docs-images/resolve/main/t2i-adapter/color_sample_input.png"><img width="64" style="margin:0;padding:0;" src="https://huggingface.co/datasets/diffusers/docs-images/resolve/main/t2i-adapter/color_sample_input.png"/></a>|<a href="https://huggingface.co/datasets/diffusers/docs-images/resolve/main/t2i-adapter/color_sample_output.png"><img width="64" src="https://huggingface.co/datasets/diffusers/docs-images/resolve/main/t2i-adapter/color_sample_output.png"/></a>|
|
106 |
-
|[TencentARC/t2iadapter_canny_sd14v1](https://huggingface.co/TencentARC/t2iadapter_canny_sd14v1)<br/> *Trained with canny edge detection* | A monochrome image with white edges on a black background.|<a href="https://huggingface.co/datasets/diffusers/docs-images/resolve/main/t2i-adapter/canny_sample_input.png"><img width="64" style="margin:0;padding:0;" src="https://huggingface.co/datasets/diffusers/docs-images/resolve/main/t2i-adapter/canny_sample_input.png"/></a>|<a href="https://huggingface.co/datasets/diffusers/docs-images/resolve/main/t2i-adapter/canny_sample_output.png"><img width="64" src="https://huggingface.co/datasets/diffusers/docs-images/resolve/main/t2i-adapter/canny_sample_output.png"/></a>|
|
107 |
-
|[TencentARC/t2iadapter_sketch_sd14v1](https://huggingface.co/TencentARC/t2iadapter_sketch_sd14v1)<br/> *Trained with [PidiNet](https://github.com/zhuoinoulu/pidinet) edge detection* | A hand-drawn monochrome image with white outlines on a black background.|<a href="https://huggingface.co/datasets/diffusers/docs-images/resolve/main/t2i-adapter/sketch_sample_input.png"><img width="64" style="margin:0;padding:0;" src="https://huggingface.co/datasets/diffusers/docs-images/resolve/main/t2i-adapter/sketch_sample_input.png"/></a>|<a href="https://huggingface.co/datasets/diffusers/docs-images/resolve/main/t2i-adapter/sketch_sample_output.png"><img width="64" src="https://huggingface.co/datasets/diffusers/docs-images/resolve/main/t2i-adapter/sketch_sample_output.png"/></a>|
|
108 |
-
|[TencentARC/t2iadapter_depth_sd14v1](https://huggingface.co/TencentARC/t2iadapter_depth_sd14v1)<br/> *Trained with Midas depth estimation* | A grayscale image with black representing deep areas and white representing shallow areas.|<a href="https://huggingface.co/datasets/diffusers/docs-images/resolve/main/t2i-adapter/depth_sample_input.png"><img width="64" src="https://huggingface.co/datasets/diffusers/docs-images/resolve/main/t2i-adapter/depth_sample_input.png"/></a>|<a href="https://huggingface.co/datasets/diffusers/docs-images/resolve/main/t2i-adapter/depth_sample_output.png"><img width="64" src="https://huggingface.co/datasets/diffusers/docs-images/resolve/main/t2i-adapter/depth_sample_output.png"/></a>|
|
109 |
-
|[TencentARC/t2iadapter_openpose_sd14v1](https://huggingface.co/TencentARC/t2iadapter_openpose_sd14v1)<br/> *Trained with OpenPose bone image* | A [OpenPose bone](https://github.com/CMU-Perceptual-Computing-Lab/openpose) image.|<a href="https://huggingface.co/datasets/diffusers/docs-images/resolve/main/t2i-adapter/openpose_sample_input.png"><img width="64" src="https://huggingface.co/datasets/diffusers/docs-images/resolve/main/t2i-adapter/openpose_sample_input.png"/></a>|<a href="https://huggingface.co/datasets/diffusers/docs-images/resolve/main/t2i-adapter/openpose_sample_output.png"><img width="64" src="https://huggingface.co/datasets/diffusers/docs-images/resolve/main/t2i-adapter/openpose_sample_output.png"/></a>|
|
110 |
-
|[TencentARC/t2iadapter_keypose_sd14v1](https://huggingface.co/TencentARC/t2iadapter_keypose_sd14v1)<br/> *Trained with mmpose skeleton image* | A [mmpose skeleton](https://github.com/open-mmlab/mmpose) image.|<a href="https://huggingface.co/datasets/diffusers/docs-images/resolve/main/t2i-adapter/keypose_sample_input.png"><img width="64" src="https://huggingface.co/datasets/diffusers/docs-images/resolve/main/t2i-adapter/keypose_sample_input.png"/></a>|<a href="https://huggingface.co/datasets/diffusers/docs-images/resolve/main/t2i-adapter/keypose_sample_output.png"><img width="64" src="https://huggingface.co/datasets/diffusers/docs-images/resolve/main/t2i-adapter/keypose_sample_output.png"/></a>|
|
111 |
-
|[TencentARC/t2iadapter_seg_sd14v1](https://huggingface.co/TencentARC/t2iadapter_seg_sd14v1)<br/>*Trained with semantic segmentation* | An [custom](https://github.com/TencentARC/T2I-Adapter/discussions/25) segmentation protocol image.|<a href="https://huggingface.co/datasets/diffusers/docs-images/resolve/main/t2i-adapter/seg_sample_input.png"><img width="64" src="https://huggingface.co/datasets/diffusers/docs-images/resolve/main/t2i-adapter/seg_sample_input.png"/></a>|<a href="https://huggingface.co/datasets/diffusers/docs-images/resolve/main/t2i-adapter/seg_sample_output.png"><img width="64" src="https://huggingface.co/datasets/diffusers/docs-images/resolve/main/t2i-adapter/seg_sample_output.png"/></a> |
|
112 |
-
|[TencentARC/t2iadapter_canny_sd15v2](https://huggingface.co/TencentARC/t2iadapter_canny_sd15v2)||
|
113 |
-
|[TencentARC/t2iadapter_depth_sd15v2](https://huggingface.co/TencentARC/t2iadapter_depth_sd15v2)||
|
114 |
-
|[TencentARC/t2iadapter_sketch_sd15v2](https://huggingface.co/TencentARC/t2iadapter_sketch_sd15v2)||
|
115 |
-
|[TencentARC/t2iadapter_zoedepth_sd15v1](https://huggingface.co/TencentARC/t2iadapter_zoedepth_sd15v1)||
|
116 |
-
|
117 |
-
## Combining multiple adapters
|
118 |
-
|
119 |
-
[`MultiAdapter`] can be used for applying multiple conditionings at once.
|
120 |
-
|
121 |
-
Here we use the keypose adapter for the character posture and the depth adapter for creating the scene.
|
122 |
-
|
123 |
-
```py
|
124 |
-
import torch
|
125 |
-
from PIL import Image
|
126 |
-
from diffusers.utils import load_image
|
127 |
-
|
128 |
-
cond_keypose = load_image(
|
129 |
-
"https://huggingface.co/datasets/diffusers/docs-images/resolve/main/t2i-adapter/keypose_sample_input.png"
|
130 |
-
)
|
131 |
-
cond_depth = load_image(
|
132 |
-
"https://huggingface.co/datasets/diffusers/docs-images/resolve/main/t2i-adapter/depth_sample_input.png"
|
133 |
-
)
|
134 |
-
cond = [[cond_keypose, cond_depth]]
|
135 |
-
|
136 |
-
prompt = ["A man walking in an office room with a nice view"]
|
137 |
-
```
|
138 |
-
|
139 |
-
The two control images look as such:
|
140 |
-
|
141 |
-
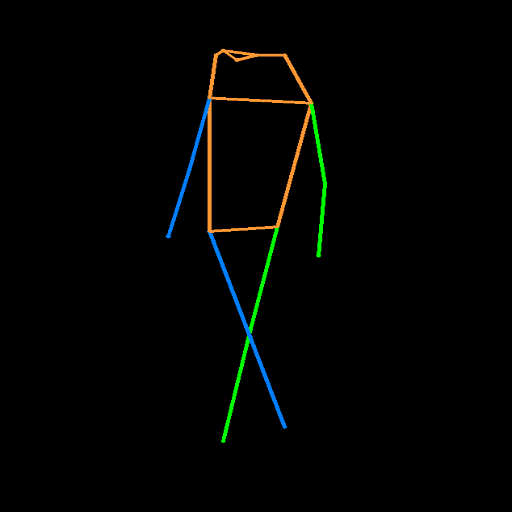
|
142 |
-
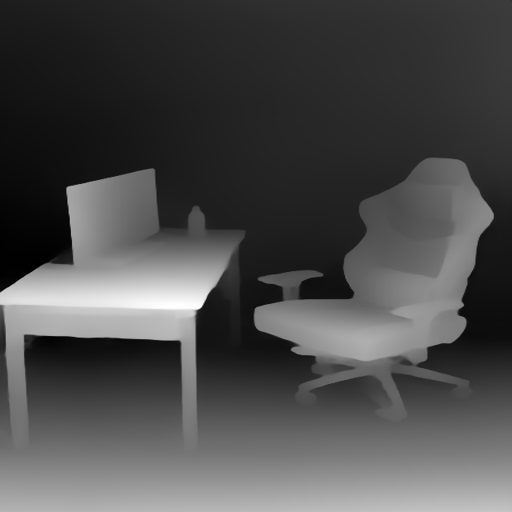
|
143 |
-
|
144 |
-
|
145 |
-
`MultiAdapter` combines keypose and depth adapters.
|
146 |
-
|
147 |
-
`adapter_conditioning_scale` balances the relative influence of the different adapters.
|
148 |
-
|
149 |
-
```py
|
150 |
-
from diffusers import StableDiffusionAdapterPipeline, MultiAdapter
|
151 |
-
|
152 |
-
adapters = MultiAdapter(
|
153 |
-
[
|
154 |
-
T2IAdapter.from_pretrained("TencentARC/t2iadapter_keypose_sd14v1"),
|
155 |
-
T2IAdapter.from_pretrained("TencentARC/t2iadapter_depth_sd14v1"),
|
156 |
-
]
|
157 |
-
)
|
158 |
-
adapters = adapters.to(torch.float16)
|
159 |
-
|
160 |
-
pipe = StableDiffusionAdapterPipeline.from_pretrained(
|
161 |
-
"CompVis/stable-diffusion-v1-4",
|
162 |
-
torch_dtype=torch.float16,
|
163 |
-
adapter=adapters,
|
164 |
-
)
|
165 |
-
|
166 |
-
images = pipe(prompt, cond, adapter_conditioning_scale=[0.8, 0.8])
|
167 |
-
```
|
168 |
-
|
169 |
-
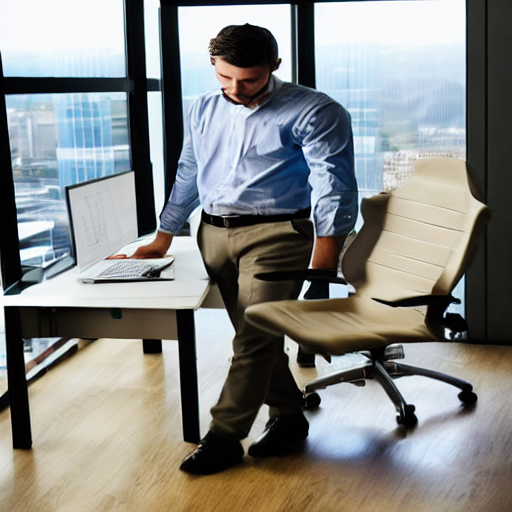
|
170 |
-
|
171 |
-
|
172 |
-
## T2I Adapter vs ControlNet
|
173 |
-
|
174 |
-
T2I-Adapter is similar to [ControlNet](https://huggingface.co/docs/diffusers/main/en/api/pipelines/controlnet).
|
175 |
-
T2i-Adapter uses a smaller auxiliary network which is only run once for the entire diffusion process.
|
176 |
-
However, T2I-Adapter performs slightly worse than ControlNet.
|
177 |
-
|
178 |
-
## StableDiffusionAdapterPipeline
|
179 |
-
[[autodoc]] StableDiffusionAdapterPipeline
|
180 |
-
- all
|
181 |
-
- __call__
|
182 |
-
- enable_attention_slicing
|
183 |
-
- disable_attention_slicing
|
184 |
-
- enable_vae_slicing
|
185 |
-
- disable_vae_slicing
|
186 |
-
- enable_xformers_memory_efficient_attention
|
187 |
-
- disable_xformers_memory_efficient_attention
|
|
|
|
|
|
|
|
|
|
|
|
|
|
|
|
|
|
|
|
|
|
|
|
|
|
|
|
|
|
|
|
|
|
|
|
|
|
|
|
|
|
|
|
|
|
|
|
|
|
|
|
|
|
|
|
|
|
|
|
|
|
|
|
|
|
|
|
|
|
|
|
|
|
|
|
|
|
|
|
|
|
|
|
|
|
|
|
|
|
|
|
|
|
|
|
|
|
|
|
|
|
|
|
|
|
|
|
|
|
|
|
|
|
|
|
|
|
|
|
|
|
|
|
|
|
|
|
|
|
|
|
|
|
|
|
|
|
|
|
|
|
|
|
|
|
|
|
|
|
|
|
|
|
|
|
|
|
|
|
|
|
|
|
|
|
|
|
|
|
|
|
|
|
|
|
|
|
|
|
|
|
|
|
|
|
|
|
|
|
|
|
|
|
|
|
|
|
|
|
|
|
|
|
|
|
|
|
|
|
|
|
|
|
|
|
|
|
|
|
|
|
|
|
|
|
|
|
|
|
|
|
|
|
|
|
|
|
|
|
|
|
|
|
|
|
|
|
|
|
|
|
|
|
|
|
|
|
|
|
|
|
|
|
|
|
|
|
|
|
|
|
|
|
|
|
|
|
|
|
|
|
|
|
|
|
|
|
|
|
|
|
|
|
|
|
|
|
|
|
|
|
|
|
|
|
|
|
|
|
|
|
|
|
|
|
|
|
|
|
|
|
|
|
|
|
|
|
|
|
|
|
|
|
|
|
|
|
|
|
|
|
|
|
|
|
|
|
|
|
|
|
|
|
|
|
|
|
|
|
|
|
|
|
|
|
|
|
|
|
|
|
|
|
|
|
spaces/Androidonnxfork/CivitAi-to-Diffusers/diffusers/tests/pipelines/dance_diffusion/test_dance_diffusion.py
DELETED
@@ -1,162 +0,0 @@
|
|
1 |
-
# coding=utf-8
|
2 |
-
# Copyright 2023 HuggingFace Inc.
|
3 |
-
#
|
4 |
-
# Licensed under the Apache License, Version 2.0 (the "License");
|
5 |
-
# you may not use this file except in compliance with the License.
|
6 |
-
# You may obtain a copy of the License at
|
7 |
-
#
|
8 |
-
# http://www.apache.org/licenses/LICENSE-2.0
|
9 |
-
#
|
10 |
-
# Unless required by applicable law or agreed to in writing, software
|
11 |
-
# distributed under the License is distributed on an "AS IS" BASIS,
|
12 |
-
# WITHOUT WARRANTIES OR CONDITIONS OF ANY KIND, either express or implied.
|
13 |
-
# See the License for the specific language governing permissions and
|
14 |
-
# limitations under the License.
|
15 |
-
|
16 |
-
import gc
|
17 |
-
import unittest
|
18 |
-
|
19 |
-
import numpy as np
|
20 |
-
import torch
|
21 |
-
|
22 |
-
from diffusers import DanceDiffusionPipeline, IPNDMScheduler, UNet1DModel
|
23 |
-
from diffusers.utils import slow, torch_device
|
24 |
-
from diffusers.utils.testing_utils import enable_full_determinism, require_torch_gpu, skip_mps
|
25 |
-
|
26 |
-
from ..pipeline_params import UNCONDITIONAL_AUDIO_GENERATION_BATCH_PARAMS, UNCONDITIONAL_AUDIO_GENERATION_PARAMS
|
27 |
-
from ..test_pipelines_common import PipelineTesterMixin
|
28 |
-
|
29 |
-
|
30 |
-
enable_full_determinism()
|
31 |
-
|
32 |
-
|
33 |
-
class DanceDiffusionPipelineFastTests(PipelineTesterMixin, unittest.TestCase):
|
34 |
-
pipeline_class = DanceDiffusionPipeline
|
35 |
-
params = UNCONDITIONAL_AUDIO_GENERATION_PARAMS
|
36 |
-
required_optional_params = PipelineTesterMixin.required_optional_params - {
|
37 |
-
"callback",
|
38 |
-
"latents",
|
39 |
-
"callback_steps",
|
40 |
-
"output_type",
|
41 |
-
"num_images_per_prompt",
|
42 |
-
}
|
43 |
-
batch_params = UNCONDITIONAL_AUDIO_GENERATION_BATCH_PARAMS
|
44 |
-
test_attention_slicing = False
|
45 |
-
|
46 |
-
def get_dummy_components(self):
|
47 |
-
torch.manual_seed(0)
|
48 |
-
unet = UNet1DModel(
|
49 |
-
block_out_channels=(32, 32, 64),
|
50 |
-
extra_in_channels=16,
|
51 |
-
sample_size=512,
|
52 |
-
sample_rate=16_000,
|
53 |
-
in_channels=2,
|
54 |
-
out_channels=2,
|
55 |
-
flip_sin_to_cos=True,
|
56 |
-
use_timestep_embedding=False,
|
57 |
-
time_embedding_type="fourier",
|
58 |
-
mid_block_type="UNetMidBlock1D",
|
59 |
-
down_block_types=("DownBlock1DNoSkip", "DownBlock1D", "AttnDownBlock1D"),
|
60 |
-
up_block_types=("AttnUpBlock1D", "UpBlock1D", "UpBlock1DNoSkip"),
|
61 |
-
)
|
62 |
-
scheduler = IPNDMScheduler()
|
63 |
-
|
64 |
-
components = {
|
65 |
-
"unet": unet,
|
66 |
-
"scheduler": scheduler,
|
67 |
-
}
|
68 |
-
return components
|
69 |
-
|
70 |
-
def get_dummy_inputs(self, device, seed=0):
|
71 |
-
if str(device).startswith("mps"):
|
72 |
-
generator = torch.manual_seed(seed)
|
73 |
-
else:
|
74 |
-
generator = torch.Generator(device=device).manual_seed(seed)
|
75 |
-
inputs = {
|
76 |
-
"batch_size": 1,
|
77 |
-
"generator": generator,
|
78 |
-
"num_inference_steps": 4,
|
79 |
-
}
|
80 |
-
return inputs
|
81 |
-
|
82 |
-
def test_dance_diffusion(self):
|
83 |
-
device = "cpu" # ensure determinism for the device-dependent torch.Generator
|
84 |
-
components = self.get_dummy_components()
|
85 |
-
pipe = DanceDiffusionPipeline(**components)
|
86 |
-
pipe = pipe.to(device)
|
87 |
-
pipe.set_progress_bar_config(disable=None)
|
88 |
-
|
89 |
-
inputs = self.get_dummy_inputs(device)
|
90 |
-
output = pipe(**inputs)
|
91 |
-
audio = output.audios
|
92 |
-
|
93 |
-
audio_slice = audio[0, -3:, -3:]
|
94 |
-
|
95 |
-
assert audio.shape == (1, 2, components["unet"].sample_size)
|
96 |
-
expected_slice = np.array([-0.7265, 1.0000, -0.8388, 0.1175, 0.9498, -1.0000])
|
97 |
-
assert np.abs(audio_slice.flatten() - expected_slice).max() < 1e-2
|
98 |
-
|
99 |
-
@skip_mps
|
100 |
-
def test_save_load_local(self):
|
101 |
-
return super().test_save_load_local()
|
102 |
-
|
103 |
-
@skip_mps
|
104 |
-
def test_dict_tuple_outputs_equivalent(self):
|
105 |
-
return super().test_dict_tuple_outputs_equivalent(expected_max_difference=3e-3)
|
106 |
-
|
107 |
-
@skip_mps
|
108 |
-
def test_save_load_optional_components(self):
|
109 |
-
return super().test_save_load_optional_components()
|
110 |
-
|
111 |
-
@skip_mps
|
112 |
-
def test_attention_slicing_forward_pass(self):
|
113 |
-
return super().test_attention_slicing_forward_pass()
|
114 |
-
|
115 |
-
def test_inference_batch_single_identical(self):
|
116 |
-
super().test_inference_batch_single_identical(expected_max_diff=3e-3)
|
117 |
-
|
118 |
-
|
119 |
-
@slow
|
120 |
-
@require_torch_gpu
|
121 |
-
class PipelineIntegrationTests(unittest.TestCase):
|
122 |
-
def tearDown(self):
|
123 |
-
# clean up the VRAM after each test
|
124 |
-
super().tearDown()
|
125 |
-
gc.collect()
|
126 |
-
torch.cuda.empty_cache()
|
127 |
-
|
128 |
-
def test_dance_diffusion(self):
|
129 |
-
device = torch_device
|
130 |
-
|
131 |
-
pipe = DanceDiffusionPipeline.from_pretrained("harmonai/maestro-150k")
|
132 |
-
pipe = pipe.to(device)
|
133 |
-
pipe.set_progress_bar_config(disable=None)
|
134 |
-
|
135 |
-
generator = torch.manual_seed(0)
|
136 |
-
output = pipe(generator=generator, num_inference_steps=100, audio_length_in_s=4.096)
|
137 |
-
audio = output.audios
|
138 |
-
|
139 |
-
audio_slice = audio[0, -3:, -3:]
|
140 |
-
|
141 |
-
assert audio.shape == (1, 2, pipe.unet.sample_size)
|
142 |
-
expected_slice = np.array([-0.0192, -0.0231, -0.0318, -0.0059, 0.0002, -0.0020])
|
143 |
-
|
144 |
-
assert np.abs(audio_slice.flatten() - expected_slice).max() < 1e-2
|
145 |
-
|
146 |
-
def test_dance_diffusion_fp16(self):
|
147 |
-
device = torch_device
|
148 |
-
|
149 |
-
pipe = DanceDiffusionPipeline.from_pretrained("harmonai/maestro-150k", torch_dtype=torch.float16)
|
150 |
-
pipe = pipe.to(device)
|
151 |
-
pipe.set_progress_bar_config(disable=None)
|
152 |
-
|
153 |
-
generator = torch.manual_seed(0)
|
154 |
-
output = pipe(generator=generator, num_inference_steps=100, audio_length_in_s=4.096)
|
155 |
-
audio = output.audios
|
156 |
-
|
157 |
-
audio_slice = audio[0, -3:, -3:]
|
158 |
-
|
159 |
-
assert audio.shape == (1, 2, pipe.unet.sample_size)
|
160 |
-
expected_slice = np.array([-0.0367, -0.0488, -0.0771, -0.0525, -0.0444, -0.0341])
|
161 |
-
|
162 |
-
assert np.abs(audio_slice.flatten() - expected_slice).max() < 1e-2
|
|
|
|
|
|
|
|
|
|
|
|
|
|
|
|
|
|
|
|
|
|
|
|
|
|
|
|
|
|
|
|
|
|
|
|
|
|
|
|
|
|
|
|
|
|
|
|
|
|
|
|
|
|
|
|
|
|
|
|
|
|
|
|
|
|
|
|
|
|
|
|
|
|
|
|
|
|
|
|
|
|
|
|
|
|
|
|
|
|
|
|
|
|
|
|
|
|
|
|
|
|
|
|
|
|
|
|
|
|
|
|
|
|
|
|
|
|
|
|
|
|
|
|
|
|
|
|
|
|
|
|
|
|
|
|
|
|
|
|
|
|
|
|
|
|
|
|
|
|
|
|
|
|
|
|
|
|
|
|
|
|
|
|
|
|
|
|
|
|
|
|
|
|
|
|
|
|
|
|
|
|
|
|
|
|
|
|
|
|
|
|
|
|
|
|
|
|
|
|
|
|
|
|
|
|
|
|
|
|
|
|
|
|
|
|
|
|
|
|
|
|
|
|
|
|
|
|
|
|
|
|
|
|
|
|
|
|
|
|
|
|
|
|
|
|
|
|
|
|
|
|
|
|
|
|
|
|
|
|
|
|
|
|
|
|
|
|
|
|
|
|
|
|
|
|
|
|
|
|
|
|
|
|
|
|
|
|
|
|
|
|
|
|
|
|
|
|
|
|
|
|
|
|
|
|
|
|
|
|
|
|
|
|
|
|
|
|
|
|
|
|
|
|
|
|
spaces/Anish13/characterGPT/app.py
DELETED
@@ -1,187 +0,0 @@
|
|
1 |
-
import gradio as gr
|
2 |
-
|
3 |
-
import torch
|
4 |
-
import torch.nn as nn
|
5 |
-
import torch.nn.functional as F
|
6 |
-
|
7 |
-
batch_size = 64 # how many independent sequences will we process in parallel?
|
8 |
-
block_size = 256 # what is the maximum context length for predictions?
|
9 |
-
max_iters = 5000
|
10 |
-
eval_interval = 500
|
11 |
-
learning_rate = 3e-4
|
12 |
-
device = 'cuda' if torch.cuda.is_available() else 'cpu'
|
13 |
-
print(f"The code is running on {device}")
|
14 |
-
eval_iters = 200
|
15 |
-
n_embd = 384
|
16 |
-
n_head = 6
|
17 |
-
n_layer = 6
|
18 |
-
dropout = 0.2
|
19 |
-
|
20 |
-
|
21 |
-
torch.manual_seed(1337)
|
22 |
-
|
23 |
-
# wget https://raw.githubusercontent.com/karpathy/char-rnn/master/data/tinyshakespeare/input.txt
|
24 |
-
with open('input.txt', 'r', encoding='utf-8') as f:
|
25 |
-
text = f.read()
|
26 |
-
|
27 |
-
# here are all the unique characters that occur in this text
|
28 |
-
chars = sorted(list(set(text)))
|
29 |
-
vocab_size = len(chars)
|
30 |
-
# create a mapping from characters to integers
|
31 |
-
stoi = { ch:i for i,ch in enumerate(chars) }
|
32 |
-
itos = { i:ch for i,ch in enumerate(chars) }
|
33 |
-
encode = lambda s: [stoi[c] for c in s] # encoder: take a string, output a list of integers
|
34 |
-
decode = lambda l: ''.join([itos[i] for i in l]) # decoder: take a list of integers, output a string
|
35 |
-
|
36 |
-
|
37 |
-
class Head(nn.Module):
|
38 |
-
""" one head of self-attention """
|
39 |
-
|
40 |
-
def __init__(self, head_size):
|
41 |
-
super().__init__()
|
42 |
-
self.key = nn.Linear(n_embd, head_size, bias=False)
|
43 |
-
self.query = nn.Linear(n_embd, head_size, bias=False)
|
44 |
-
self.value = nn.Linear(n_embd, head_size, bias=False)
|
45 |
-
self.register_buffer('tril', torch.tril(torch.ones(block_size, block_size))) # create lower triangular matrix
|
46 |
-
|
47 |
-
self.dropout = nn.Dropout(dropout)
|
48 |
-
|
49 |
-
def forward(self, x):
|
50 |
-
B,T,C = x.shape
|
51 |
-
k = self.key(x) # B, T, C
|
52 |
-
q = self.query(x) # B, T, C
|
53 |
-
# compute attention scores = ("affinities")
|
54 |
-
wei = q @ k.transpose(-2, -1) * C**-0.5 # (B, T, C) @ (B, C, T) -> (B, T, T)
|
55 |
-
#wei = wei.masked_fill(self.tril[:T, :T]==0, float('-inf')) # (B, T, T)
|
56 |
-
tril = torch.tril(torch.ones(T, T)).to(device)
|
57 |
-
wei = wei.masked_fill(tril == 0, float('-inf'))
|
58 |
-
wei = F.softmax(wei, dim=-1) # (B, T, T)
|
59 |
-
wei = self.dropout(wei)
|
60 |
-
# perform the weighted aggregation of the values
|
61 |
-
v = self.value(x) # (B, T, C)
|
62 |
-
out = wei @ v
|
63 |
-
return out
|
64 |
-
|
65 |
-
|
66 |
-
class MultiHeadAttention(nn.Module):
|
67 |
-
""" multiple heads of self-attention in parallel """
|
68 |
-
|
69 |
-
def __init__(self, num_heads, head_size):
|
70 |
-
super().__init__()
|
71 |
-
self.heads = nn.ModuleList([Head(head_size) for _ in range(num_heads)])
|
72 |
-
self.proj = nn.Linear(n_embd, n_embd)
|
73 |
-
self.dropout = nn.Dropout(dropout)
|
74 |
-
|
75 |
-
def forward(self, x):
|
76 |
-
out = torch.cat([h(x) for h in self.heads], dim=-1) # h(x) call forward function is Head class
|
77 |
-
out = self.dropout(self.proj(out))
|
78 |
-
return out
|
79 |
-
|
80 |
-
class FeedForward(nn.Module): # per token level, every token does this independently, its allowing tokens to think on data provided by self attention
|
81 |
-
""" a simple linear layer followed by a non-linearity"""
|
82 |
-
|
83 |
-
def __init__(self, n_embd):
|
84 |
-
super().__init__()
|
85 |
-
self.net = nn.Sequential(
|
86 |
-
nn.Linear(n_embd, 4 * n_embd), # we multiply by 4 cause the paper says so
|
87 |
-
nn.ReLU(),
|
88 |
-
nn.Linear(4 * n_embd, n_embd),
|
89 |
-
nn.Dropout(dropout)
|
90 |
-
)
|
91 |
-
|
92 |
-
def forward(self, x):
|
93 |
-
return self.net(x)
|
94 |
-
|
95 |
-
class Block(nn.Module):
|
96 |
-
"""Transformer block: communication followed by computation """
|
97 |
-
|
98 |
-
def __init__(self, n_embed, n_head):
|
99 |
-
# n_embd: embedding dimension, n_head: the number of heads we'd like
|
100 |
-
super().__init__()
|
101 |
-
head_size = n_embd // n_head
|
102 |
-
self.sa = MultiHeadAttention(n_head, head_size)
|
103 |
-
self.ffwd = FeedForward(n_embd)
|
104 |
-
self.ln1 = nn.LayerNorm(n_embd)
|
105 |
-
self.ln2 = nn.LayerNorm(n_embd)
|
106 |
-
|
107 |
-
def forward(self, x):
|
108 |
-
x = x + self.sa(self.ln1(x)) # x = x + self .. is residual connection
|
109 |
-
x = x + self.ffwd(self.ln2(x))
|
110 |
-
return x
|
111 |
-
|
112 |
-
|
113 |
-
class BigramLanguageModel(nn.Module):
|
114 |
-
|
115 |
-
def __init__(self):
|
116 |
-
super().__init__()
|
117 |
-
# each token directly reads off the logits for the next token from a lookup table
|
118 |
-
self.token_embedding_table = nn.Embedding(vocab_size, n_embd)
|
119 |
-
self.position_embedding_table = nn.Embedding(block_size, n_embd) # so each position from 0 to block_size - 1 will also get its own embedding vector
|
120 |
-
self.blocks = nn.Sequential(*[Block(n_embd, n_head=n_head) for _ in range(n_layer)])
|
121 |
-
self.ln_f = nn.LayerNorm(n_embd) # final layer Norm
|
122 |
-
self.lm_head = nn.Linear(n_embd, vocab_size)
|
123 |
-
|
124 |
-
def forward(self, idx, targets=None):
|
125 |
-
B, T = idx.shape
|
126 |
-
|
127 |
-
# idx and targets are both (B,T) tensor of integers
|
128 |
-
tok_emb = self.token_embedding_table(idx) # (B,T,C=n_embed)
|
129 |
-
pos_emb = self.position_embedding_table(torch.arange(T, device=device)) # (T, C)
|
130 |
-
# pos_emb tensor will be a (block_size, n_emb) tensor # block_size is max context length for predictions
|
131 |
-
# each row represents the embedding vector for the corresponding position
|
132 |
-
# so 0th row will represent the vector for 0th position
|
133 |
-
x = tok_emb + pos_emb # (B, T, C)
|
134 |
-
x = self.blocks(x) # (B, T, C)
|
135 |
-
logits = self.lm_head(x) # (B, T, C=vocab_size)
|
136 |
-
|
137 |
-
if targets is None:
|
138 |
-
loss = None
|
139 |
-
else:
|
140 |
-
B, T, C = logits.shape
|
141 |
-
logits = logits.view(B*T, C)
|
142 |
-
targets = targets.view(B*T)
|
143 |
-
loss = F.cross_entropy(logits, targets)
|
144 |
-
|
145 |
-
return logits, loss
|
146 |
-
|
147 |
-
def generate(self, idx, max_new_tokens):
|
148 |
-
# idx is (B, T) array of indices in the current context
|
149 |
-
for _ in range(max_new_tokens):
|
150 |
-
# crop idx to the last block_size tokens
|
151 |
-
idx_cond = idx[:, -block_size:]
|
152 |
-
# get the predictions
|
153 |
-
logits, loss = self.forward(idx_cond)
|
154 |
-
# focus only on the last time step
|
155 |
-
logits = logits[:, -1, :] # becomes (B, C)
|
156 |
-
# apply softmax to get probabilities
|
157 |
-
probs = F.softmax(logits, dim=-1) # (B, C)
|
158 |
-
# sample from the distribution
|
159 |
-
idx_next = torch.multinomial(probs, num_samples=1) # (B, 1)
|
160 |
-
# append sampled index to the running sequence
|
161 |
-
idx = torch.cat((idx, idx_next), dim=1) # (B, T+1)
|
162 |
-
return idx
|
163 |
-
|
164 |
-
|
165 |
-
# Instantiate the model
|
166 |
-
model = BigramLanguageModel()
|
167 |
-
|
168 |
-
# Specify the path to the pre-trained model checkpoint
|
169 |
-
checkpoint_path = 'checkpoint.pth'
|
170 |
-
|
171 |
-
# Load the model checkpoint
|
172 |
-
checkpoint = torch.load(checkpoint_path, map_location=torch.device(device))
|
173 |
-
model.load_state_dict(checkpoint['model_state_dict'])
|
174 |
-
model.eval()
|
175 |
-
model.to(device)
|
176 |
-
|
177 |
-
|
178 |
-
# generate from the model
|
179 |
-
context = torch.zeros((1, 1), dtype=torch.long, device=device)
|
180 |
-
|
181 |
-
def greet(number_of_tokens, start_character):
|
182 |
-
context[0][0] = encode(start_character)[0]
|
183 |
-
max_new_tokens = number_of_tokens
|
184 |
-
return decode(model.generate(context, max_new_tokens=int(max_new_tokens))[0].tolist())
|
185 |
-
|
186 |
-
iface = gr.Interface(fn=greet, inputs=["number", "text"], outputs="text")
|
187 |
-
iface.launch()
|
|
|
|
|
|
|
|
|
|
|
|
|
|
|
|
|
|
|
|
|
|
|
|
|
|
|
|
|
|
|
|
|
|
|
|
|
|
|
|
|
|
|
|
|
|
|
|
|
|
|
|
|
|
|
|
|
|
|
|
|
|
|
|
|
|
|
|
|
|
|
|
|
|
|
|
|
|
|
|
|
|
|
|
|
|
|
|
|
|
|
|
|
|
|
|
|
|
|
|
|
|
|
|
|
|
|
|
|
|
|
|
|
|
|
|
|
|
|
|
|
|
|
|
|
|
|
|
|
|
|
|
|
|
|
|
|
|
|
|
|
|
|
|
|
|
|
|
|
|
|
|
|
|
|
|
|
|
|
|
|
|
|
|
|
|
|
|
|
|
|
|
|
|
|
|
|
|
|
|
|
|
|
|
|
|
|
|
|
|
|
|
|
|
|
|
|
|
|
|
|
|
|
|
|
|
|
|
|
|
|
|
|
|
|
|
|
|
|
|
|
|
|
|
|
|
|
|
|
|
|
|
|
|
|
|
|
|
|
|
|
|
|
|
|
|
|
|
|
|
|
|
|
|
|
|
|
|
|
|
|
|
|
|
|
|
|
|
|
|
|
|
|
|
|
|
|
|
|
|
|
|
|
|
|
|
|
|
|
|
|
|
|
|
|
|
|
|
|
|
|
|
|
|
|
|
|
|
|
|
|
|
|
|
|
|
|
|
|
|
|
|
|
|
|
|
|
|
|
|
|
|
|
|
|
|
|
|
|
|
|
|
|
|
|
|
|
|
|
|
|
|
|
|
|
|
|
|
|
|
|
|
|
|
|
|
|
|
|
|
|
|
|
|
|
|
spaces/AnishKumbhar/ChatBot/text-generation-webui-main/wsl.sh
DELETED
@@ -1,112 +0,0 @@
|
|
1 |
-
#!/bin/bash
|
2 |
-
|
3 |
-
# detect if build-essential is missing or broken
|
4 |
-
if ! dpkg-query -W -f'${Status}' "build-essential" 2>/dev/null | grep -q "ok installed"; then
|
5 |
-
echo "build-essential not found or broken!
|
6 |
-
|
7 |
-
A C++ compiler is required to build needed Python packages!
|
8 |
-
To install one, run cmd_wsl.bat and enter these commands:
|
9 |
-
|
10 |
-
sudo apt-get update
|
11 |
-
sudo apt-get install build-essential
|
12 |
-
"
|
13 |
-
read -n1 -p "Continue the installer anyway? [y,n]" EXIT_PROMPT
|
14 |
-
# only continue if user inputs 'y' else exit
|
15 |
-
if ! [[ $EXIT_PROMPT == "Y" || $EXIT_PROMPT == "y" ]]; then exit; fi
|
16 |
-
fi
|
17 |
-
|
18 |
-
# deactivate existing conda envs as needed to avoid conflicts
|
19 |
-
{ conda deactivate && conda deactivate && conda deactivate; } 2> /dev/null
|
20 |
-
|
21 |
-
# config unlike other scripts, can't use current directory due to file IO bug in WSL, needs to be in virtual drive
|
22 |
-
INSTALL_DIR_PREFIX="$HOME/text-gen-install"
|
23 |
-
if [[ ! $(realpath "$(pwd)/..") = /mnt/* ]]; then
|
24 |
-
INSTALL_DIR_PREFIX="$(realpath "$(pwd)/..")" && INSTALL_INPLACE=1
|
25 |
-
fi
|
26 |
-
INSTALL_DIR="$INSTALL_DIR_PREFIX/text-generation-webui"
|
27 |
-
CONDA_ROOT_PREFIX="$INSTALL_DIR/installer_files/conda"
|
28 |
-
INSTALL_ENV_DIR="$INSTALL_DIR/installer_files/env"
|
29 |
-
MINICONDA_DOWNLOAD_URL="https://repo.anaconda.com/miniconda/Miniconda3-py310_23.3.1-0-Linux-x86_64.sh"
|
30 |
-
conda_exists="F"
|
31 |
-
|
32 |
-
# environment isolation
|
33 |
-
export PYTHONNOUSERSITE=1
|
34 |
-
unset PYTHONPATH
|
35 |
-
unset PYTHONHOME
|
36 |
-
export CUDA_PATH="$INSTALL_ENV_DIR"
|
37 |
-
export CUDA_HOME="$CUDA_PATH"
|
38 |
-
|
39 |
-
# /usr/lib/wsl/lib needs to be added to LD_LIBRARY_PATH to fix years-old bug in WSL where GPU drivers aren't linked properly
|
40 |
-
export LD_LIBRARY_PATH="$CUDA_HOME/lib:/usr/lib/wsl/lib:$LD_LIBRARY_PATH"
|
41 |
-
|
42 |
-
# open bash cli if called with 'wsl.sh cmd' with workarounds for existing conda
|
43 |
-
if [ "$1" == "cmd" ]; then
|
44 |
-
exec bash --init-file <(echo ". ~/.bashrc; conda deactivate 2> /dev/null; cd $INSTALL_DIR || cd $HOME; source $CONDA_ROOT_PREFIX/etc/profile.d/conda.sh; conda activate $INSTALL_ENV_DIR")
|
45 |
-
exit
|
46 |
-
fi
|
47 |
-
|
48 |
-
if [[ "$INSTALL_DIR" =~ " " ]]; then echo This script relies on Miniconda which can not be silently installed under a path with spaces. && exit; fi
|
49 |
-
|
50 |
-
# create install dir if missing
|
51 |
-
if [ ! -d "$INSTALL_DIR" ]; then mkdir -p "$INSTALL_DIR" || exit; fi
|
52 |
-
|
53 |
-
# figure out whether git and conda needs to be installed
|
54 |
-
if "$CONDA_ROOT_PREFIX/bin/conda" --version &>/dev/null; then conda_exists="T"; fi
|
55 |
-
|
56 |
-
# (if necessary) install git and conda into a contained environment
|
57 |
-
# download miniconda
|
58 |
-
if [ "$conda_exists" == "F" ]; then
|
59 |
-
echo "Downloading Miniconda from $MINICONDA_DOWNLOAD_URL to $INSTALL_DIR/miniconda_installer.sh"
|
60 |
-
|
61 |
-
curl -Lk "$MINICONDA_DOWNLOAD_URL" > "$INSTALL_DIR/miniconda_installer.sh"
|
62 |
-
|
63 |
-
chmod u+x "$INSTALL_DIR/miniconda_installer.sh"
|
64 |
-
bash "$INSTALL_DIR/miniconda_installer.sh" -b -p $CONDA_ROOT_PREFIX
|
65 |
-
|
66 |
-
# test the conda binary
|
67 |
-
echo "Miniconda version:"
|
68 |
-
"$CONDA_ROOT_PREFIX/bin/conda" --version
|
69 |
-
fi
|
70 |
-
|
71 |
-
# create the installer env
|
72 |
-
if [ ! -e "$INSTALL_ENV_DIR" ]; then
|
73 |
-
"$CONDA_ROOT_PREFIX/bin/conda" create -y -k --prefix "$INSTALL_ENV_DIR" python=3.10 git
|
74 |
-
fi
|
75 |
-
|
76 |
-
# check if conda environment was actually created
|
77 |
-
if [ ! -e "$INSTALL_ENV_DIR/bin/python" ]; then
|
78 |
-
echo "Conda environment is empty."
|
79 |
-
exit
|
80 |
-
fi
|
81 |
-
|
82 |
-
# activate installer env
|
83 |
-
source "$CONDA_ROOT_PREFIX/etc/profile.d/conda.sh" # otherwise conda complains about 'shell not initialized' (needed when running in a script)
|
84 |
-
conda activate "$INSTALL_ENV_DIR"
|
85 |
-
|
86 |
-
pushd $INSTALL_DIR 1> /dev/null || exit
|
87 |
-
|
88 |
-
if [ ! -f "./server.py" ]; then
|
89 |
-
git init -b main
|
90 |
-
git remote add origin https://github.com/oobabooga/text-generation-webui
|
91 |
-
git fetch
|
92 |
-
git remote set-head origin -a
|
93 |
-
git reset origin/HEAD --hard
|
94 |
-
git branch --set-upstream-to=origin/HEAD
|
95 |
-
git restore -- . :!./CMD_FLAGS.txt
|
96 |
-
fi
|
97 |
-
|
98 |
-
# copy CMD_FLAGS.txt to install dir to allow edits within Windows
|
99 |
-
if [[ $INSTALL_INPLACE != 1 ]]; then
|
100 |
-
# workaround for old install migration
|
101 |
-
if [ ! -f "./wsl.sh" ]; then
|
102 |
-
git pull || exit
|
103 |
-
[ -f "../webui.py" ] && mv "../webui.py" "../webui-old.py"
|
104 |
-
fi
|
105 |
-
if [ -f "$(dirs +1)/CMD_FLAGS.txt" ] && [ -f "./CMD_FLAGS.txt" ]; then cp -u "$(dirs +1)/CMD_FLAGS.txt" "$INSTALL_DIR"; fi
|
106 |
-
fi
|
107 |
-
|
108 |
-
# setup installer env update env if called with 'wsl.sh update'
|
109 |
-
case "$1" in
|
110 |
-
("update") python one_click.py --update;;
|
111 |
-
(*) python one_click.py $@;;
|
112 |
-
esac
|
|
|
|
|
|
|
|
|
|
|
|
|
|
|
|
|
|
|
|
|
|
|
|
|
|
|
|
|
|
|
|
|
|
|
|
|
|
|
|
|
|
|
|
|
|
|
|
|
|
|
|
|
|
|
|
|
|
|
|
|
|
|
|
|
|
|
|
|
|
|
|
|
|
|
|
|
|
|
|
|
|
|
|
|
|
|
|
|
|
|
|
|
|
|
|
|
|
|
|
|
|
|
|
|
|
|
|
|
|
|
|
|
|
|
|
|
|
|
|
|
|
|
|
|
|
|
|
|
|
|
|
|
|
|
|
|
|
|
|
|
|
|
|
|
|
|
|
|
|
|
|
|
|
|
|
|
|
|
|
|
|
|
|
|
|
|
|
|
|
|
|
|
|
|
|
|
|
|
|
|
|
|
|
|
|
|
|
|
|
|
|
|
|
|
|
|
|
|
|
|
|
|
|
|
|
|
|
|
|
|
|
|
|
|
|
|
|
|
|
|
|
|
|
|
|
spaces/Anonymous-sub/Rerender/ControlNet/annotator/uniformer/mmcv/image/misc.py
DELETED
@@ -1,44 +0,0 @@
|
|
1 |
-
# Copyright (c) OpenMMLab. All rights reserved.
|
2 |
-
import numpy as np
|
3 |
-
|
4 |
-
import annotator.uniformer.mmcv as mmcv
|
5 |
-
|
6 |
-
try:
|
7 |
-
import torch
|
8 |
-
except ImportError:
|
9 |
-
torch = None
|
10 |
-
|
11 |
-
|
12 |
-
def tensor2imgs(tensor, mean=(0, 0, 0), std=(1, 1, 1), to_rgb=True):
|
13 |
-
"""Convert tensor to 3-channel images.
|
14 |
-
|
15 |
-
Args:
|
16 |
-
tensor (torch.Tensor): Tensor that contains multiple images, shape (
|
17 |
-
N, C, H, W).
|
18 |
-
mean (tuple[float], optional): Mean of images. Defaults to (0, 0, 0).
|
19 |
-
std (tuple[float], optional): Standard deviation of images.
|
20 |
-
Defaults to (1, 1, 1).
|
21 |
-
to_rgb (bool, optional): Whether the tensor was converted to RGB
|
22 |
-
format in the first place. If so, convert it back to BGR.
|
23 |
-
Defaults to True.
|
24 |
-
|
25 |
-
Returns:
|
26 |
-
list[np.ndarray]: A list that contains multiple images.
|
27 |
-
"""
|
28 |
-
|
29 |
-
if torch is None:
|
30 |
-
raise RuntimeError('pytorch is not installed')
|
31 |
-
assert torch.is_tensor(tensor) and tensor.ndim == 4
|
32 |
-
assert len(mean) == 3
|
33 |
-
assert len(std) == 3
|
34 |
-
|
35 |
-
num_imgs = tensor.size(0)
|
36 |
-
mean = np.array(mean, dtype=np.float32)
|
37 |
-
std = np.array(std, dtype=np.float32)
|
38 |
-
imgs = []
|
39 |
-
for img_id in range(num_imgs):
|
40 |
-
img = tensor[img_id, ...].cpu().numpy().transpose(1, 2, 0)
|
41 |
-
img = mmcv.imdenormalize(
|
42 |
-
img, mean, std, to_bgr=to_rgb).astype(np.uint8)
|
43 |
-
imgs.append(np.ascontiguousarray(img))
|
44 |
-
return imgs
|
|
|
|
|
|
|
|
|
|
|
|
|
|
|
|
|
|
|
|
|
|
|
|
|
|
|
|
|
|
|
|
|
|
|
|
|
|
|
|
|
|
|
|
|
|
|
|
|
|
|
|
|
|
|
|
|
|
|
|
|
|
|
|
|
|
|
|
|
|
|
|
|
|
|
|
|
|
|
|
|
|
|
|
|
|
|
|
|
|
spaces/ArtificialArtist007/Rate-my-Aiart/app.py
DELETED
@@ -1,37 +0,0 @@
|
|
1 |
-
import gradio as gr
|
2 |
-
import tensorflow as tf
|
3 |
-
from tensorflow.compat.v2.experimental import dtensor
|
4 |
-
|
5 |
-
import numpy as np
|
6 |
-
from PIL import Image
|
7 |
-
|
8 |
-
# Load pre-trained MobileNetV2 model
|
9 |
-
model = tf.keras.applications.MobileNetV2(weights='imagenet')
|
10 |
-
|
11 |
-
def predict_difficulty_score(image):
|
12 |
-
# Load image and preprocess it for the model
|
13 |
-
img = Image.fromarray(image.astype('uint8'), 'RGB')
|
14 |
-
img = img.resize((224, 224))
|
15 |
-
img_array = tf.keras.preprocessing.image.img_to_array(img)
|
16 |
-
img_array = tf.keras.applications.mobilenet_v2.preprocess_input(img_array[np.newaxis,...])
|
17 |
-
|
18 |
-
# Use the model to predict the image class probabilities
|
19 |
-
preds = model.predict(img_array)
|
20 |
-
|
21 |
-
# Get the index of the top predicted class
|
22 |
-
class_idx = np.argmax(preds[0])
|
23 |
-
|
24 |
-
# Get the difficulty score based on the class index
|
25 |
-
difficulty_score = round((class_idx / 999) * 99000) + 1000
|
26 |
-
|
27 |
-
# Return the difficulty score
|
28 |
-
return difficulty_score
|
29 |
-
|
30 |
-
# Create a Gradio interface
|
31 |
-
inputs = gr.inputs.Image(shape=(224, 224))
|
32 |
-
outputs = gr.outputs.Textbox(label="Difficulty Score")
|
33 |
-
interface = gr.Interface(fn=predict_difficulty_score, inputs=inputs, outputs=outputs,
|
34 |
-
title="AI Art Difficulty Score", description="Upload an AI art image and get its difficulty score.")
|
35 |
-
|
36 |
-
# Launch the interface
|
37 |
-
interface.launch()
|
|
|
|
|
|
|
|
|
|
|
|
|
|
|
|
|
|
|
|
|
|
|
|
|
|
|
|
|
|
|
|
|
|
|
|
|
|
|
|
|
|
|
|
|
|
|
|
|
|
|
|
|
|
|
|
|
|
|
|
|
|
|
|
|
|
|
|
|
|
|
|
|
|
|
|
spaces/BAAI/vid2vid-zero/vid2vid_zero/util.py
DELETED
@@ -1,114 +0,0 @@
|
|
1 |
-
import os
|
2 |
-
import imageio
|
3 |
-
import tempfile
|
4 |
-
import numpy as np
|
5 |
-
from PIL import Image
|
6 |
-
from typing import Union
|
7 |
-
|
8 |
-
import torch
|
9 |
-
import torchvision
|
10 |
-
|
11 |
-
from tqdm import tqdm
|
12 |
-
from einops import rearrange
|
13 |
-
|
14 |
-
|
15 |
-
def save_videos_as_images(videos: torch.Tensor, path: str, rescale=False, n_rows=4, fps=2):
|
16 |
-
dir_name = os.path.dirname(path)
|
17 |
-
videos = rearrange(videos, "b c t h w -> t b h w c")
|
18 |
-
|
19 |
-
os.makedirs(os.path.join(dir_name, "vis_images"), exist_ok=True)
|
20 |
-
for frame_idx, x in enumerate(videos):
|
21 |
-
if rescale:
|
22 |
-
x = (x + 1.0) / 2.0
|
23 |
-
x = (x * 255).numpy().astype(np.uint8)
|
24 |
-
|
25 |
-
for batch_idx, image in enumerate(x):
|
26 |
-
save_dir = os.path.join(dir_name, "vis_images", f"batch_{batch_idx}")
|
27 |
-
os.makedirs(save_dir, exist_ok=True)
|
28 |
-
save_path = os.path.join(save_dir, f"frame_{frame_idx}.png")
|
29 |
-
image = Image.fromarray(image)
|
30 |
-
image.save(save_path)
|
31 |
-
|
32 |
-
|
33 |
-
def save_videos_grid(videos: torch.Tensor, path: str, rescale=False, n_rows=4, fps=2):
|
34 |
-
videos = rearrange(videos, "b c t h w -> t b c h w")
|
35 |
-
outputs = []
|
36 |
-
for x in videos:
|
37 |
-
x = torchvision.utils.make_grid(x, nrow=n_rows)
|
38 |
-
x = x.transpose(0, 1).transpose(1, 2).squeeze(-1)
|
39 |
-
if rescale:
|
40 |
-
x = (x + 1.0) / 2.0 # -1,1 -> 0,1
|
41 |
-
x = (x * 255).numpy().astype(np.uint8)
|
42 |
-
outputs.append(x)
|
43 |
-
|
44 |
-
os.makedirs(os.path.dirname(path), exist_ok=True)
|
45 |
-
imageio.mimsave(path, outputs, fps=8)
|
46 |
-
|
47 |
-
# save for gradio demo
|
48 |
-
out_file = tempfile.NamedTemporaryFile(suffix='.mp4', delete=False)
|
49 |
-
out_file.name = path.replace('.gif', '.mp4')
|
50 |
-
writer = imageio.get_writer(out_file.name, fps=fps)
|
51 |
-
for frame in outputs:
|
52 |
-
writer.append_data(frame)
|
53 |
-
writer.close()
|
54 |
-
|
55 |
-
|
56 |
-
@torch.no_grad()
|
57 |
-
def init_prompt(prompt, pipeline):
|
58 |
-
uncond_input = pipeline.tokenizer(
|
59 |
-
[""], padding="max_length", max_length=pipeline.tokenizer.model_max_length,
|
60 |
-
return_tensors="pt"
|
61 |
-
)
|
62 |
-
uncond_embeddings = pipeline.text_encoder(uncond_input.input_ids.to(pipeline.device))[0]
|
63 |
-
text_input = pipeline.tokenizer(
|
64 |
-
[prompt],
|
65 |
-
padding="max_length",
|
66 |
-
max_length=pipeline.tokenizer.model_max_length,
|
67 |
-
truncation=True,
|
68 |
-
return_tensors="pt",
|
69 |
-
)
|
70 |
-
text_embeddings = pipeline.text_encoder(text_input.input_ids.to(pipeline.device))[0]
|
71 |
-
context = torch.cat([uncond_embeddings, text_embeddings])
|
72 |
-
|
73 |
-
return context
|
74 |
-
|
75 |
-
|
76 |
-
def next_step(model_output: Union[torch.FloatTensor, np.ndarray], timestep: int,
|
77 |
-
sample: Union[torch.FloatTensor, np.ndarray], ddim_scheduler):
|
78 |
-
timestep, next_timestep = min(
|
79 |
-
timestep - ddim_scheduler.config.num_train_timesteps // ddim_scheduler.num_inference_steps, 999), timestep
|
80 |
-
alpha_prod_t = ddim_scheduler.alphas_cumprod[timestep] if timestep >= 0 else ddim_scheduler.final_alpha_cumprod
|
81 |
-
alpha_prod_t_next = ddim_scheduler.alphas_cumprod[next_timestep]
|
82 |
-
beta_prod_t = 1 - alpha_prod_t
|
83 |
-
next_original_sample = (sample - beta_prod_t ** 0.5 * model_output) / alpha_prod_t ** 0.5
|
84 |
-
next_sample_direction = (1 - alpha_prod_t_next) ** 0.5 * model_output
|
85 |
-
next_sample = alpha_prod_t_next ** 0.5 * next_original_sample + next_sample_direction
|
86 |
-
return next_sample
|
87 |
-
|
88 |
-
|
89 |
-
def get_noise_pred_single(latents, t, context, unet, normal_infer=False):
|
90 |
-
bs = latents.shape[0] # (b*f, c, h, w) or (b, c, f, h, w)
|
91 |
-
if bs != context.shape[0]:
|
92 |
-
context = context.repeat(bs, 1, 1) # (b*f, len, dim)
|
93 |
-
noise_pred = unet(latents, t, encoder_hidden_states=context, normal_infer=normal_infer)["sample"]
|
94 |
-
return noise_pred
|
95 |
-
|
96 |
-
|
97 |
-
@torch.no_grad()
|
98 |
-
def ddim_loop(pipeline, ddim_scheduler, latent, num_inv_steps, prompt, normal_infer=False):
|
99 |
-
context = init_prompt(prompt, pipeline)
|
100 |
-
uncond_embeddings, cond_embeddings = context.chunk(2)
|
101 |
-
all_latent = [latent]
|
102 |
-
latent = latent.clone().detach()
|
103 |
-
for i in tqdm(range(num_inv_steps)):
|
104 |
-
t = ddim_scheduler.timesteps[len(ddim_scheduler.timesteps) - i - 1]
|
105 |
-
noise_pred = get_noise_pred_single(latent, t, cond_embeddings, pipeline.unet, normal_infer=normal_infer)
|
106 |
-
latent = next_step(noise_pred, t, latent, ddim_scheduler)
|
107 |
-
all_latent.append(latent)
|
108 |
-
return all_latent
|
109 |
-
|
110 |
-
|
111 |
-
@torch.no_grad()
|
112 |
-
def ddim_inversion(pipeline, ddim_scheduler, video_latent, num_inv_steps, prompt="", normal_infer=False):
|
113 |
-
ddim_latents = ddim_loop(pipeline, ddim_scheduler, video_latent, num_inv_steps, prompt, normal_infer=normal_infer)
|
114 |
-
return ddim_latents
|
|
|
|
|
|
|
|
|
|
|
|
|
|
|
|
|
|
|
|
|
|
|
|
|
|
|
|
|
|
|
|
|
|
|
|
|
|
|
|
|
|
|
|
|
|
|
|
|
|
|
|
|
|
|
|
|
|
|
|
|
|
|
|
|
|
|
|
|
|
|
|
|
|
|
|
|
|
|
|
|
|
|
|
|
|
|
|
|
|
|
|
|
|
|
|
|
|
|
|
|
|
|
|
|
|
|
|
|
|
|
|
|
|
|
|
|
|
|
|
|
|
|
|
|
|
|
|
|
|
|
|
|
|
|
|
|
|
|
|
|
|
|
|
|
|
|
|
|
|
|
|
|
|
|
|
|
|
|
|
|
|
|
|
|
|
|
|
|
|
|
|
|
|
|
|
|
|
|
|
|
|
|
|
|
|
|
|
|
|
|
|
|
|
|
|
|
|
|
|
|
|
|
|
|
|
|
|
|
|
|
|
|
|
|
|
|
|
|
|
|
|
|
|
|
|
|
|
|
|
spaces/Babelscape/rebel-demo/README.md
DELETED
@@ -1,37 +0,0 @@
|
|
1 |
-
---
|
2 |
-
title: Rebel Demo
|
3 |
-
emoji: 🌍
|
4 |
-
colorFrom: purple
|
5 |
-
colorTo: pink
|
6 |
-
sdk: streamlit
|
7 |
-
app_file: app.py
|
8 |
-
pinned: false
|
9 |
-
---
|
10 |
-
|
11 |
-
# Configuration
|
12 |
-
|
13 |
-
`title`: _string_
|
14 |
-
Display title for the Space
|
15 |
-
|
16 |
-
`emoji`: _string_
|
17 |
-
Space emoji (emoji-only character allowed)
|
18 |
-
|
19 |
-
`colorFrom`: _string_
|
20 |
-
Color for Thumbnail gradient (red, yellow, green, blue, indigo, purple, pink, gray)
|
21 |
-
|
22 |
-
`colorTo`: _string_
|
23 |
-
Color for Thumbnail gradient (red, yellow, green, blue, indigo, purple, pink, gray)
|
24 |
-
|
25 |
-
`sdk`: _string_
|
26 |
-
Can be either `gradio` or `streamlit`
|
27 |
-
|
28 |
-
`sdk_version` : _string_
|
29 |
-
Only applicable for `streamlit` SDK.
|
30 |
-
See [doc](https://hf.co/docs/hub/spaces) for more info on supported versions.
|
31 |
-
|
32 |
-
`app_file`: _string_
|
33 |
-
Path to your main application file (which contains either `gradio` or `streamlit` Python code).
|
34 |
-
Path is relative to the root of the repository.
|
35 |
-
|
36 |
-
`pinned`: _boolean_
|
37 |
-
Whether the Space stays on top of your list.
|
|
|
|
|
|
|
|
|
|
|
|
|
|
|
|
|
|
|
|
|
|
|
|
|
|
|
|
|
|
|
|
|
|
|
|
|
|
|
|
|
|
|
|
|
|
|
|
|
|
|
|
|
|
|
|
|
|
|
|
|
|
|
|
|
|
|
|
|
|
|
|
|
|
|
|
spaces/Banbri/zcvzcv/src/lib/cleanJson.ts
DELETED
@@ -1,19 +0,0 @@
|
|
1 |
-
import { dirtyLLMResponseCleaner } from "./dirtyLLMResponseCleaner"
|
2 |
-
|
3 |
-
export function cleanJson(input: string) {
|
4 |
-
|
5 |
-
if (input.includes('```')) {
|
6 |
-
input = input.split('```')[0]
|
7 |
-
}
|
8 |
-
let tmp = dirtyLLMResponseCleaner(input)
|
9 |
-
|
10 |
-
// we only keep what's after the first [
|
11 |
-
tmp = `[${tmp.split("[").pop() || ""}`
|
12 |
-
|
13 |
-
// and before the first ]
|
14 |
-
tmp = `${tmp.split("]").shift() || ""}]`
|
15 |
-
|
16 |
-
tmp = dirtyLLMResponseCleaner(tmp)
|
17 |
-
|
18 |
-
return tmp
|
19 |
-
}
|
|
|
|
|
|
|
|
|
|
|
|
|
|
|
|
|
|
|
|
|
|
|
|
|
|
|
|
|
|
|
|
|
|
|
|
|
|
|
|
spaces/Bart92/RVC_HF/audioEffects.py
DELETED
@@ -1,37 +0,0 @@
|
|
1 |
-
from pedalboard import Pedalboard, Compressor, Reverb, NoiseGate
|
2 |
-
from pedalboard.io import AudioFile
|
3 |
-
import sys
|
4 |
-
import os
|
5 |
-
now_dir = os.getcwd()
|
6 |
-
sys.path.append(now_dir)
|
7 |
-
from i18n import I18nAuto
|
8 |
-
i18n = I18nAuto()
|
9 |
-
from pydub import AudioSegment
|
10 |
-
import numpy as np
|
11 |
-
import soundfile as sf
|
12 |
-
from pydub.playback import play
|
13 |
-
|
14 |
-
def process_audio(input_path, output_path, reverb_enabled, compressor_enabled, noise_gate_enabled, ):
|
15 |
-
print(reverb_enabled)
|
16 |
-
print(compressor_enabled)
|
17 |
-
print(noise_gate_enabled)
|
18 |
-
effects = []
|
19 |
-
if reverb_enabled:
|
20 |
-
effects.append(Reverb(room_size=0.01))
|
21 |
-
if compressor_enabled:
|
22 |
-
effects.append(Compressor(threshold_db=-10, ratio=25))
|
23 |
-
if noise_gate_enabled:
|
24 |
-
effects.append(NoiseGate(threshold_db=-16, ratio=1.5, release_ms=250))
|
25 |
-
|
26 |
-
board = Pedalboard(effects)
|
27 |
-
|
28 |
-
with AudioFile(input_path) as f:
|
29 |
-
with AudioFile(output_path, 'w', f.samplerate, f.num_channels) as o:
|
30 |
-
while f.tell() < f.frames:
|
31 |
-
chunk = f.read(f.samplerate)
|
32 |
-
effected = board(chunk, f.samplerate, reset=False)
|
33 |
-
o.write(effected)
|
34 |
-
|
35 |
-
result = i18n("Processed audio saved at: ") + output_path
|
36 |
-
print(result)
|
37 |
-
return output_path
|
|
|
|
|
|
|
|
|
|
|
|
|
|
|
|
|
|
|
|
|
|
|
|
|
|
|
|
|
|
|
|
|
|
|
|
|
|
|
|
|
|
|
|
|
|
|
|
|
|
|
|
|
|
|
|
|
|
|
|
|
|
|
|
|
|
|
|
|
|
|
|
|
|
|
|
spaces/Bart92/RVC_HF/infer/lib/infer_pack/modules/F0Predictor/HarvestF0Predictor.py
DELETED
@@ -1,87 +0,0 @@
|
|
1 |
-
import numpy as np
|
2 |
-
import pyworld
|
3 |
-
|
4 |
-
from infer.lib.infer_pack.modules.F0Predictor.F0Predictor import F0Predictor
|
5 |
-
|
6 |
-
|
7 |
-
class HarvestF0Predictor(F0Predictor):
|
8 |
-
def __init__(self, hop_length=512, f0_min=50, f0_max=1100, sampling_rate=44100):
|
9 |
-
self.hop_length = hop_length
|
10 |
-
self.f0_min = f0_min
|
11 |
-
self.f0_max = f0_max
|
12 |
-
self.sampling_rate = sampling_rate
|
13 |
-
|
14 |
-
def interpolate_f0(self, f0):
|
15 |
-
"""
|
16 |
-
对F0进行插值处理
|
17 |
-
"""
|
18 |
-
|
19 |
-
data = np.reshape(f0, (f0.size, 1))
|
20 |
-
|
21 |
-
vuv_vector = np.zeros((data.size, 1), dtype=np.float32)
|
22 |
-
vuv_vector[data > 0.0] = 1.0
|
23 |
-
vuv_vector[data <= 0.0] = 0.0
|
24 |
-
|
25 |
-
ip_data = data
|
26 |
-
|
27 |
-
frame_number = data.size
|
28 |
-
last_value = 0.0
|
29 |
-
for i in range(frame_number):
|
30 |
-
if data[i] <= 0.0:
|
31 |
-
j = i + 1
|
32 |
-
for j in range(i + 1, frame_number):
|
33 |
-
if data[j] > 0.0:
|
34 |
-
break
|
35 |
-
if j < frame_number - 1:
|
36 |
-
if last_value > 0.0:
|
37 |
-
step = (data[j] - data[i - 1]) / float(j - i)
|
38 |
-
for k in range(i, j):
|
39 |
-
ip_data[k] = data[i - 1] + step * (k - i + 1)
|
40 |
-
else:
|
41 |
-
for k in range(i, j):
|
42 |
-
ip_data[k] = data[j]
|
43 |
-
else:
|
44 |
-
for k in range(i, frame_number):
|
45 |
-
ip_data[k] = last_value
|
46 |
-
else:
|
47 |
-
ip_data[i] = data[i] # 这里可能存在一个没有必要的拷贝
|
48 |
-
last_value = data[i]
|
49 |
-
|
50 |
-
return ip_data[:, 0], vuv_vector[:, 0]
|
51 |
-
|
52 |
-
def resize_f0(self, x, target_len):
|
53 |
-
source = np.array(x)
|
54 |
-
source[source < 0.001] = np.nan
|
55 |
-
target = np.interp(
|
56 |
-
np.arange(0, len(source) * target_len, len(source)) / target_len,
|
57 |
-
np.arange(0, len(source)),
|
58 |
-
source,
|
59 |
-
)
|
60 |
-
res = np.nan_to_num(target)
|
61 |
-
return res
|
62 |
-
|
63 |
-
def compute_f0(self, wav, p_len=None):
|
64 |
-
if p_len is None:
|
65 |
-
p_len = wav.shape[0] // self.hop_length
|
66 |
-
f0, t = pyworld.harvest(
|
67 |
-
wav.astype(np.double),
|
68 |
-
fs=self.hop_length,
|
69 |
-
f0_ceil=self.f0_max,
|
70 |
-
f0_floor=self.f0_min,
|
71 |
-
frame_period=1000 * self.hop_length / self.sampling_rate,
|
72 |
-
)
|
73 |
-
f0 = pyworld.stonemask(wav.astype(np.double), f0, t, self.fs)
|
74 |
-
return self.interpolate_f0(self.resize_f0(f0, p_len))[0]
|
75 |
-
|
76 |
-
def compute_f0_uv(self, wav, p_len=None):
|
77 |
-
if p_len is None:
|
78 |
-
p_len = wav.shape[0] // self.hop_length
|
79 |
-
f0, t = pyworld.harvest(
|
80 |
-
wav.astype(np.double),
|
81 |
-
fs=self.sampling_rate,
|
82 |
-
f0_floor=self.f0_min,
|
83 |
-
f0_ceil=self.f0_max,
|
84 |
-
frame_period=1000 * self.hop_length / self.sampling_rate,
|
85 |
-
)
|
86 |
-
f0 = pyworld.stonemask(wav.astype(np.double), f0, t, self.sampling_rate)
|
87 |
-
return self.interpolate_f0(self.resize_f0(f0, p_len))
|
|
|
|
|
|
|
|
|
|
|
|
|
|
|
|
|
|
|
|
|
|
|
|
|
|
|
|
|
|
|
|
|
|
|
|
|
|
|
|
|
|
|
|
|
|
|
|
|
|
|
|
|
|
|
|
|
|
|
|
|
|
|
|
|
|
|
|
|
|
|
|
|
|
|
|
|
|
|
|
|
|
|
|
|
|
|
|
|
|
|
|
|
|
|
|
|
|
|
|
|
|
|
|
|
|
|
|
|
|
|
|
|
|
|
|
|
|
|
|
|
|
|
|
|
|
|
|
|
|
|
|
|
|
|
|
|
|
|
|
|
|
|
|
|
|
|
|
|
|
|
|
|
|
|
|
|
|
|
|
|
|
|
|
|
|
|
|
|
|
|
|
|
|
|
|
spaces/Benson/text-generation/Examples/Cmo Descargar Videos De Google Drive.md
DELETED
@@ -1,157 +0,0 @@
|
|
1 |
-
|
2 |
-
<h1>Cómo descargar videos de Google Drive</h1>
|
3 |
-
<p>Google Drive es un servicio de almacenamiento en la nube popular que le permite almacenar y acceder a sus archivos en línea. Puede usarlo para almacenar sus videos y verlos en cualquier momento, en cualquier lugar y en cualquier dispositivo. Pero lo que si desea descargar sus vídeos de Google Drive a su ordenador o teléfono? Tal vez quieras liberar algo de espacio en tu Drive, o crear una copia de seguridad de tus videos, o verlos sin conexión. Cualquiera que sea la razón, la descarga de vídeo desde Google Drive es fácil y rápido. En este artículo, le mostraremos cómo descargar videos de Google Drive a diferentes dispositivos, incluyendo PC con Windows, Mac, Android y iPhone. </p>
|
4 |
-
<h2>cómo descargar videos de google drive</h2><br /><p><b><b>Download Zip</b> 🔗 <a href="https://bltlly.com/2v6JMe">https://bltlly.com/2v6JMe</a></b></p><br /><br />
|
5 |
-
<h2>Introducción</h2>
|
6 |
-
<h3>¿Qué es Google Drive y por qué usarlo para vídeos? </h3>
|
7 |
-
<p>Google Drive es un servicio de almacenamiento en la nube que te permite almacenar hasta 15 GB de archivos de forma gratuita. Puede cargar cualquier tipo de archivo, incluidos documentos, fotos, música y videos. También puede crear y editar archivos con Google Docs, Hojas y Diapositivas. Puede acceder a sus archivos desde cualquier dispositivo que tenga una conexión a Internet y un navegador web. También puede usar la aplicación Google Drive en su computadora o teléfono para sincronizar sus archivos y acceder a ellos sin conexión. </p>
|
8 |
-
<p>Uno de los beneficios de usar Google Drive para videos es que puedes verlos en línea sin descargarlos. También puede compartirlos con otros enviando un enlace o invitándolos a ver o editar sus archivos. También puede colaborar en videos con otros mediante comentarios y sugerencias. También puede organizar sus vídeos en carpetas y subcarpetas, y buscarlos usando palabras clave o filtros. </p>
|
9 |
-
<h3>Cómo descargar video de Google Drive a diferentes dispositivos</h3>
|
10 |
-
<p>Descargar video de Google Drive es simple y sencillo. Solo tiene que seguir estos pasos:</p>
|
11 |
-
<ol>
|
12 |
-
<li>Vaya a <a href="( 1 )">drive.google.com</a> e inicie sesión con su cuenta de Google. </li>
|
13 |
-
<li>Seleccione el vídeo o vídeos que desea descargar. </li>
|
14 |
-
|
15 |
-
<li> Elija una ubicación en su dispositivo donde desea guardar el vídeo o vídeos descargados. </li>
|
16 |
-
</ol>
|
17 |
-
<p>Los pasos exactos pueden variar ligeramente dependiendo del dispositivo que esté utilizando. Explicaremos las diferencias en las siguientes secciones. </p>
|
18 |
-
<h2>Descargar vídeo de Google Drive a Windows PC</h2>
|
19 |
-
<h3>Descargar vídeos individuales</h3>
|
20 |
-
<p>Si desea descargar un solo video de Google Drive a su PC con Windows, puede seguir estos pasos:</p>
|
21 |
-
<p></p>
|
22 |
-
<ol>
|
23 |
-
<li>Vaya a <a href="( 1 )">drive.google.com</a> e inicie sesión con su cuenta de Google. </li>
|
24 |
-
<li>Haga clic en el vídeo que desea descargar. </li>
|
25 |
-
<li>Haga clic en el icono de menú de tres puntos en la esquina superior derecha y elija "Descargar". </li>
|
26 |
-
<li>El vídeo se descargará como un archivo MP4 a su carpeta de descarga predeterminada. Puede cambiar esta carpeta en la configuración de su navegador. </li>
|
27 |
-
</ol>
|
28 |
-
<h3>Descargar múltiples vídeos</h3>
|
29 |
-
<p>Si desea descargar más de un video de Google Drive a su PC con Windows, puede seguir estos pasos:</p>
|
30 |
-
<ol>
|
31 |
-
<li>Vaya a <a href="( 1 )">drive.google.com</a> e inicie sesión con su cuenta de Google. </li>
|
32 |
-
<li>Mantenga presionada la tecla Ctrl y haga clic en cada video que desea descargar. </li>
|
33 |
-
<li>Haga clic en el icono de menú de tres puntos en la esquina superior derecha y elija "Descargar". </li>
|
34 |
-
<li>Los vídeos se descargarán como un archivo ZIP en la carpeta de descarga predeterminada. Puede cambiar esta carpeta en la configuración de su navegador. </li>
|
35 |
-
<li>Extraer el archivo ZIP para acceder a los archivos MP4 individuales. </li>
|
36 |
-
</ol>
|
37 |
-
<h3>Sincronizar Google Drive a PC</h3>
|
38 |
-
<p>Si desea sincronizar sus videos de Google Drive a su PC con Windows, puede seguir estos pasos:</p>
|
39 |
-
<ol>
|
40 |
-
<li>Descargar e instalar el <a href="">Google Drive app</a> para Windows.</li>
|
41 |
-
<li>Inicie sesión con su cuenta de Google y elija una carpeta en su PC donde desea sincronizar sus archivos de Google Drive. </li>
|
42 |
-
<li>Haga clic en el icono de Google Drive en la bandeja del sistema y seleccione "Preferencias". </li>
|
43 |
-
|
44 |
-
<li>Haga clic en "OK" y espere a que se complete la sincronización. </li>
|
45 |
-
</ol>
|
46 |
-
<p>Una vez que se realiza la sincronización, puede acceder a sus videos de Google Drive desde la carpeta que eligió en su PC. También puede verlos sin conexión, editarlos o eliminarlos. Cualquier cambio que realice se reflejará en su Google Drive en línea. </p>
|
47 |
-
<h2>Descargar vídeo de Google Drive a Mac</h2>
|
48 |
-
<h3>Descargar vídeos individuales</h3>
|
49 |
-
<p>Si desea descargar un solo video de Google Drive a su Mac, puede seguir estos pasos:</p>
|
50 |
-
<ol>
|
51 |
-
<li>Vaya a <a href=">drive.google.com</a> e inicie sesión con su cuenta de Google. </li>
|
52 |
-
<li>Haga clic en el vídeo que desea descargar. </li>
|
53 |
-
<li>Haga clic en el icono de menú de tres puntos en la esquina superior derecha y elija "Descargar". </li>
|
54 |
-
<li>El vídeo se descargará como un archivo MP4 a su carpeta de descarga predeterminada. Puede cambiar esta carpeta en la configuración de su navegador. </li>
|
55 |
-
</ol>
|
56 |
-
<h3>Descargar múltiples vídeos</h3>
|
57 |
-
<p>Si desea descargar más de un video de Google Drive a su Mac, puede seguir estos pasos:</p>
|
58 |
-
<ol>
|
59 |
-
<li>Vaya a <a href=">drive.google.com</a> e inicie sesión con su cuenta de Google. </li>
|
60 |
-
<li>Mantenga pulsada la tecla Comando y haga clic en cada vídeo que desee descargar. </li>
|
61 |
-
<li>Haga clic en el icono de menú de tres puntos en la esquina superior derecha y elija "Descargar". </li>
|
62 |
-
<li>Los vídeos se descargarán como un archivo ZIP en la carpeta de descarga predeterminada. Puede cambiar esta carpeta en la configuración de su navegador. </li>
|
63 |
-
<li>Extraer el archivo ZIP para acceder a los archivos MP4 individuales. </li>
|
64 |
-
</ol>
|
65 |
-
<h3>Sincronizar Google Drive a Mac</h3>
|
66 |
-
<p>Si quieres sincronizar tus vídeos de Google Drive con tu Mac, puedes seguir estos pasos:</p>
|
67 |
-
<ol>
|
68 |
-
<li>Descargar e instalar el <a href="">Google Drive app</a> para Mac.</li>
|
69 |
-
<li>Inicie sesión con su cuenta de Google y elija una carpeta en su Mac donde desea sincronizar sus archivos de Google Drive. </li>
|
70 |
-
<li>Haga clic en el icono de Google Drive en la barra de menú y seleccione "Preferencias". </li>
|
71 |
-
|
72 |
-
<li>Haga clic en "OK" y espere a que se complete la sincronización. </li>
|
73 |
-
</ol>
|
74 |
-
<p>Una vez que se realiza la sincronización, puede acceder a sus videos de Google Drive desde la carpeta que eligió en su Mac. También puede verlos sin conexión, editarlos o eliminarlos. Cualquier cambio que realice se reflejará en su Google Drive en línea. </p>
|
75 |
-
<h2>Descargar vídeo de Google Drive para Android o iPhone</h2>
|
76 |
-
<h3>Descargar vídeos individuales</h3>
|
77 |
-
<p>Si desea descargar un solo video de Google Drive a su Android o iPhone, puede seguir estos pasos:</p>
|
78 |
-
<ol>
|
79 |
-
<li>Descargar e instalar el <a href="">Google Drive app</a> para Android o iPhone. </li>
|
80 |
-
<li>Abra la aplicación e inicie sesión con su cuenta de Google. </li>
|
81 |
-
<li>Toque en el vídeo que desea descargar. </li>
|
82 |
-
<li>Toque en el icono de menú de tres puntos en la esquina inferior derecha y elija "Descargar". </li>
|
83 |
-
<li>El video se descargará como un archivo MP4 en el almacenamiento de su dispositivo. Puede encontrarlo en la carpeta "Descargas" o en la aplicación "Fotos". </li>
|
84 |
-
</ol>
|
85 |
-
<h3>Descargar múltiples vídeos</h3>
|
86 |
-
<p>Si quieres descargar más de un video de Google Drive a tu Android o iPhone, puedes seguir estos pasos:</p>
|
87 |
-
<ol>
|
88 |
-
<li>Descargar e instalar el <a href="">Google Drive app</a> para Android o iPhone. </li>
|
89 |
-
<li>Abra la aplicación e inicie sesión con su cuenta de Google. </li>
|
90 |
-
<li>Mantenga pulsado el primer vídeo que desea descargar, luego pulse sobre los otros vídeos que desea descargar. </li>
|
91 |
-
<li>Toque en el icono de menú de tres puntos en la esquina superior derecha y elija "Descargar". </li>
|
92 |
-
<li>Los vídeos se descargarán como archivos MP4 en el almacenamiento de su dispositivo. Puede encontrarlos en la carpeta "Descargas" o en la aplicación "Fotos". </li>
|
93 |
-
</ol>
|
94 |
-
<h3>Sincronizar Google Drive al teléfono</h3>
|
95 |
-
<p>Si quieres sincronizar tus vídeos de Google Drive con tu Android o iPhone, puedes seguir estos pasos:</p>
|
96 |
-
<ol>
|
97 |
-
<li>Descargar e instalar el <a href="">Google Drive app</a> para Android o iPhone. </li>
|
98 |
-
<li>Abra la aplicación e inicie sesión con su cuenta de Google. </li>
|
99 |
-
|
100 |
-
<li>Toque en el icono de menú de tres líneas en la esquina superior izquierda y elija "Configuración". </li>
|
101 |
-
<li>Toque en "Copia de seguridad y sincronización". </li>
|
102 |
-
<li>Activar la palanca para "Copia de seguridad y sincronización". </li>
|
103 |
-
<li>Seleccione las carpetas que desea sincronizar. También puede optar por sincronizar todo en su Google Drive o solo archivos específicos. </li>
|
104 |
-
<li>Toque en "Hecho" y espere a que la sincronización se complete. </li>
|
105 |
-
</ol>
|
106 |
-
<p>Una vez que se realiza la sincronización, puede acceder a sus videos de Google Drive desde la pestaña "Archivos" en la aplicación. También puede verlos sin conexión, editarlos o eliminarlos. Cualquier cambio que realice se reflejará en su Google Drive en línea. </p>
|
107 |
-
<h2>Conclusión</h2>
|
108 |
-
<h3>Resumen de los puntos principales</h3>
|
109 |
-
<p>En este artículo, le hemos mostrado cómo descargar video de Google Drive a diferentes dispositivos, incluyendo PC con Windows, Mac, Android y iPhone. También hemos explicado cómo sincronizar tus vídeos de Google Drive con tus dispositivos, para que puedas acceder a ellos sin conexión y mantenerlos actualizados. Descargar video desde Google Drive es fácil y rápido, y puede ayudarlo a ahorrar espacio en su unidad, crear copias de seguridad de sus videos o verlos sin una conexión a Internet. </p>
|
110 |
-
<h3>Llamada a la acción</h3>
|
111 |
-
<p>Esperamos que haya encontrado este artículo útil e informativo. Si tiene alguna pregunta o comentario, deje un comentario a continuación. Si te gustó este artículo, por favor compártelo con tus amigos y familiares. Y si desea obtener más información sobre Google Drive y otros servicios de almacenamiento en la nube, suscríbase a nuestro boletín y síganos en las redes sociales. ¡Gracias por leer! </p>
|
112 |
-
<h2>Preguntas frecuentes</h2>
|
113 |
-
<h4>¿Cómo puedo descargar un video de Google Drive que es demasiado grande? </h4>
|
114 |
-
<p>Si intenta descargar un video de Google Drive que es mayor que 2 GB, puede encontrar un mensaje de error que dice "Este archivo es demasiado grande para que Google lo escanee en busca de virus". Esto no significa que el archivo esté infectado, sino que Google no puede verificar su seguridad. Para descargar este archivo, debe hacer lo siguiente:</p>
|
115 |
-
<ol>
|
116 |
-
|
117 |
-
<li>Haga clic en el icono de menú de tres puntos en la esquina superior derecha y elija "Descargar de todos modos". </li>
|
118 |
-
<li>El vídeo se descargará como un archivo MP4 a su carpeta de descarga predeterminada. Puede cambiar esta carpeta en la configuración de su navegador. </li>
|
119 |
-
</ol>
|
120 |
-
<h4>¿Cómo puedo descargar un video de Google Drive que se comparte conmigo? </h4>
|
121 |
-
<p>Si alguien ha compartido un video contigo en Google Drive, puedes descargarlo siguiendo estos pasos:</p>
|
122 |
-
<ol>
|
123 |
-
<li>Vaya a <a href=">drive.google.com</a> e inicie sesión con su cuenta de Google. </li>
|
124 |
-
<li>Haga clic en la pestaña "Compartido conmigo" en el lado izquierdo de la pantalla. </li>
|
125 |
-
<li>Encuentra el video que quieres descargar y haz clic en él. </li>
|
126 |
-
<li>Haga clic en el icono de menú de tres puntos en la esquina superior derecha y elija "Descargar". </li>
|
127 |
-
<li>El vídeo se descargará como un archivo MP4 a su carpeta de descarga predeterminada. Puede cambiar esta carpeta en la configuración de su navegador. </li>
|
128 |
-
</ol>
|
129 |
-
<h4>¿Cómo puedo descargar un video de Google Drive que no es mío? </h4>
|
130 |
-
<p>Si encuentras un video en Google Drive que no es tuyo pero tienes permiso para verlo, puedes descargarlo siguiendo estos pasos:</p>
|
131 |
-
<ol>
|
132 |
-
<li>Vaya a <a href=">drive.google.com</a> e inicie sesión con su cuenta de Google. </li>
|
133 |
-
<li>Haga clic en el vídeo que desea descargar. </li>
|
134 |
-
<li>Si el video no es propiedad de usted, verá un mensaje que dice "Está utilizando una versión de vista previa de este archivo". Haga clic en "Abrir con" en la parte superior de la pantalla y seleccione "Google Drive Viewer". </li> <li>El vídeo se abrirá en una nueva pestaña. Haga clic en el icono del menú de tres puntos en la esquina superior derecha y elija "Descargar". </li>
|
135 |
-
<li>El vídeo se descargará como un archivo MP4 a su carpeta de descarga predeterminada. Puede cambiar esta carpeta en la configuración de su navegador. </li>
|
136 |
-
</ol>
|
137 |
-
<h4>¿Cómo puedo descargar un video de Google Drive que está en un formato diferente? </h4>
|
138 |
-
|
139 |
-
<ol>
|
140 |
-
<li>Descargar el video de Google Drive como un archivo MP4 utilizando los pasos anteriores. </li>
|
141 |
-
<li>Ir a la página web de la herramienta en línea que desea utilizar y cargar el archivo MP4. </li>
|
142 |
-
<li>Seleccione el formato de salida al que desea convertir el vídeo, como AVI, MOV, WMV, etc.</li>
|
143 |
-
<li>Haga clic en "Convertir" o "Iniciar" y espere a que la conversión termine. </li>
|
144 |
-
<li>Descargar el archivo de vídeo convertido a su dispositivo. </li>
|
145 |
-
</ol>
|
146 |
-
<h4>¿Cómo puedo descargar un video de Google Drive que está incrustado en un sitio web? </h4>
|
147 |
-
<p>Si desea descargar un video de Google Drive que está incrustado en un sitio web, puede usar una extensión del navegador o una herramienta de raspado web para extraer la URL del video. Algunos ejemplos de estas herramientas son <a href="">Video DownloadHelper</a>, <a href="">Flash Video Downloader</a>, o <a href="">Video Downloader Professional</a>. Puedes usar estas herramientas siguiendo estos pasos:</p>
|
148 |
-
<ol>
|
149 |
-
<li>Descargue e instale la extensión del navegador o la herramienta de raspador web que desea usar. </li>
|
150 |
-
<li>Ir al sitio web que tiene el video incrustado de Google Drive.</li>
|
151 |
-
<li>Haga clic en el icono de la herramienta que instaló en la barra de herramientas del navegador y elija "Descargar" o "Extraer". </li>
|
152 |
-
<li>La herramienta le mostrará la URL del video y le permitirá descargarlo como un archivo MP4. </li>
|
153 |
-
</ol>
|
154 |
-
<h2></h2>
|
155 |
-
<p>Este es el final del artículo. Espero que hayas disfrutado leyéndolo y hayas aprendido algo nuevo. Si tiene alguna pregunta o comentario, por favor deje un comentario abajo. ¡Gracias por su atención! </p> 64aa2da5cf<br />
|
156 |
-
<br />
|
157 |
-
<br />
|
|
|
|
|
|
|
|
|
|
|
|
|
|
|
|
|
|
|
|
|
|
|
|
|
|
|
|
|
|
|
|
|
|
|
|
|
|
|
|
|
|
|
|
|
|
|
|
|
|
|
|
|
|
|
|
|
|
|
|
|
|
|
|
|
|
|
|
|
|
|
|
|
|
|
|
|
|
|
|
|
|
|
|
|
|
|
|
|
|
|
|
|
|
|
|
|
|
|
|
|
|
|
|
|
|
|
|
|
|
|
|
|
|
|
|
|
|
|
|
|
|
|
|
|
|
|
|
|
|
|
|
|
|
|
|
|
|
|
|
|
|
|
|
|
|
|
|
|
|
|
|
|
|
|
|
|
|
|
|
|
|
|
|
|
|
|
|
|
|
|
|
|
|
|
|
|
|
|
|
|
|
|
|
|
|
|
|
|
|
|
|
|
|
|
|
|
|
|
|
|
|
|
|
|
|
|
|
|
|
|
|
|
|
|
|
|
|
|
|
|
|
|
|
|
|
|
|
|
|
|
|
|
|
|
|
|
|
|
|
|
|
|
|
|
|
|
|
|
|
|
|
|
|
|
|
|
|
|
|
|
|
|
|
|
|
|
|
|
|
|
|
|
|
|
|
|
|
|
|
|
|
|
|
|
|
|
|
|
|
|
|
|
|
|
|
|
|
|
|
|
|
|
|
|
|
|
|
|
|
|
|
|
|
|
|
spaces/Big-Web/MMSD/env/Lib/site-packages/pip/_vendor/urllib3/util/timeout.py
DELETED
@@ -1,271 +0,0 @@
|
|
1 |
-
from __future__ import absolute_import
|
2 |
-
|
3 |
-
import time
|
4 |
-
|
5 |
-
# The default socket timeout, used by httplib to indicate that no timeout was; specified by the user
|
6 |
-
from socket import _GLOBAL_DEFAULT_TIMEOUT, getdefaulttimeout
|
7 |
-
|
8 |
-
from ..exceptions import TimeoutStateError
|
9 |
-
|
10 |
-
# A sentinel value to indicate that no timeout was specified by the user in
|
11 |
-
# urllib3
|
12 |
-
_Default = object()
|
13 |
-
|
14 |
-
|
15 |
-
# Use time.monotonic if available.
|
16 |
-
current_time = getattr(time, "monotonic", time.time)
|
17 |
-
|
18 |
-
|
19 |
-
class Timeout(object):
|
20 |
-
"""Timeout configuration.
|
21 |
-
|
22 |
-
Timeouts can be defined as a default for a pool:
|
23 |
-
|
24 |
-
.. code-block:: python
|
25 |
-
|
26 |
-
timeout = Timeout(connect=2.0, read=7.0)
|
27 |
-
http = PoolManager(timeout=timeout)
|
28 |
-
response = http.request('GET', 'http://example.com/')
|
29 |
-
|
30 |
-
Or per-request (which overrides the default for the pool):
|
31 |
-
|
32 |
-
.. code-block:: python
|
33 |
-
|
34 |
-
response = http.request('GET', 'http://example.com/', timeout=Timeout(10))
|
35 |
-
|
36 |
-
Timeouts can be disabled by setting all the parameters to ``None``:
|
37 |
-
|
38 |
-
.. code-block:: python
|
39 |
-
|
40 |
-
no_timeout = Timeout(connect=None, read=None)
|
41 |
-
response = http.request('GET', 'http://example.com/, timeout=no_timeout)
|
42 |
-
|
43 |
-
|
44 |
-
:param total:
|
45 |
-
This combines the connect and read timeouts into one; the read timeout
|
46 |
-
will be set to the time leftover from the connect attempt. In the
|
47 |
-
event that both a connect timeout and a total are specified, or a read
|
48 |
-
timeout and a total are specified, the shorter timeout will be applied.
|
49 |
-
|
50 |
-
Defaults to None.
|
51 |
-
|
52 |
-
:type total: int, float, or None
|
53 |
-
|
54 |
-
:param connect:
|
55 |
-
The maximum amount of time (in seconds) to wait for a connection
|
56 |
-
attempt to a server to succeed. Omitting the parameter will default the
|
57 |
-
connect timeout to the system default, probably `the global default
|
58 |
-
timeout in socket.py
|
59 |
-
<http://hg.python.org/cpython/file/603b4d593758/Lib/socket.py#l535>`_.
|
60 |
-
None will set an infinite timeout for connection attempts.
|
61 |
-
|
62 |
-
:type connect: int, float, or None
|
63 |
-
|
64 |
-
:param read:
|
65 |
-
The maximum amount of time (in seconds) to wait between consecutive
|
66 |
-
read operations for a response from the server. Omitting the parameter
|
67 |
-
will default the read timeout to the system default, probably `the
|
68 |
-
global default timeout in socket.py
|
69 |
-
<http://hg.python.org/cpython/file/603b4d593758/Lib/socket.py#l535>`_.
|
70 |
-
None will set an infinite timeout.
|
71 |
-
|
72 |
-
:type read: int, float, or None
|
73 |
-
|
74 |
-
.. note::
|
75 |
-
|
76 |
-
Many factors can affect the total amount of time for urllib3 to return
|
77 |
-
an HTTP response.
|
78 |
-
|
79 |
-
For example, Python's DNS resolver does not obey the timeout specified
|
80 |
-
on the socket. Other factors that can affect total request time include
|
81 |
-
high CPU load, high swap, the program running at a low priority level,
|
82 |
-
or other behaviors.
|
83 |
-
|
84 |
-
In addition, the read and total timeouts only measure the time between
|
85 |
-
read operations on the socket connecting the client and the server,
|
86 |
-
not the total amount of time for the request to return a complete
|
87 |
-
response. For most requests, the timeout is raised because the server
|
88 |
-
has not sent the first byte in the specified time. This is not always
|
89 |
-
the case; if a server streams one byte every fifteen seconds, a timeout
|
90 |
-
of 20 seconds will not trigger, even though the request will take
|
91 |
-
several minutes to complete.
|
92 |
-
|
93 |
-
If your goal is to cut off any request after a set amount of wall clock
|
94 |
-
time, consider having a second "watcher" thread to cut off a slow
|
95 |
-
request.
|
96 |
-
"""
|
97 |
-
|
98 |
-
#: A sentinel object representing the default timeout value
|
99 |
-
DEFAULT_TIMEOUT = _GLOBAL_DEFAULT_TIMEOUT
|
100 |
-
|
101 |
-
def __init__(self, total=None, connect=_Default, read=_Default):
|
102 |
-
self._connect = self._validate_timeout(connect, "connect")
|
103 |
-
self._read = self._validate_timeout(read, "read")
|
104 |
-
self.total = self._validate_timeout(total, "total")
|
105 |
-
self._start_connect = None
|
106 |
-
|
107 |
-
def __repr__(self):
|
108 |
-
return "%s(connect=%r, read=%r, total=%r)" % (
|
109 |
-
type(self).__name__,
|
110 |
-
self._connect,
|
111 |
-
self._read,
|
112 |
-
self.total,
|
113 |
-
)
|
114 |
-
|
115 |
-
# __str__ provided for backwards compatibility
|
116 |
-
__str__ = __repr__
|
117 |
-
|
118 |
-
@classmethod
|
119 |
-
def resolve_default_timeout(cls, timeout):
|
120 |
-
return getdefaulttimeout() if timeout is cls.DEFAULT_TIMEOUT else timeout
|
121 |
-
|
122 |
-
@classmethod
|
123 |
-
def _validate_timeout(cls, value, name):
|
124 |
-
"""Check that a timeout attribute is valid.
|
125 |
-
|
126 |
-
:param value: The timeout value to validate
|
127 |
-
:param name: The name of the timeout attribute to validate. This is
|
128 |
-
used to specify in error messages.
|
129 |
-
:return: The validated and casted version of the given value.
|
130 |
-
:raises ValueError: If it is a numeric value less than or equal to
|
131 |
-
zero, or the type is not an integer, float, or None.
|
132 |
-
"""
|
133 |
-
if value is _Default:
|
134 |
-
return cls.DEFAULT_TIMEOUT
|
135 |
-
|
136 |
-
if value is None or value is cls.DEFAULT_TIMEOUT:
|
137 |
-
return value
|
138 |
-
|
139 |
-
if isinstance(value, bool):
|
140 |
-
raise ValueError(
|
141 |
-
"Timeout cannot be a boolean value. It must "
|
142 |
-
"be an int, float or None."
|
143 |
-
)
|
144 |
-
try:
|
145 |
-
float(value)
|
146 |
-
except (TypeError, ValueError):
|
147 |
-
raise ValueError(
|
148 |
-
"Timeout value %s was %s, but it must be an "
|
149 |
-
"int, float or None." % (name, value)
|
150 |
-
)
|
151 |
-
|
152 |
-
try:
|
153 |
-
if value <= 0:
|
154 |
-
raise ValueError(
|
155 |
-
"Attempted to set %s timeout to %s, but the "
|
156 |
-
"timeout cannot be set to a value less "
|
157 |
-
"than or equal to 0." % (name, value)
|
158 |
-
)
|
159 |
-
except TypeError:
|
160 |
-
# Python 3
|
161 |
-
raise ValueError(
|
162 |
-
"Timeout value %s was %s, but it must be an "
|
163 |
-
"int, float or None." % (name, value)
|
164 |
-
)
|
165 |
-
|
166 |
-
return value
|
167 |
-
|
168 |
-
@classmethod
|
169 |
-
def from_float(cls, timeout):
|
170 |
-
"""Create a new Timeout from a legacy timeout value.
|
171 |
-
|
172 |
-
The timeout value used by httplib.py sets the same timeout on the
|
173 |
-
connect(), and recv() socket requests. This creates a :class:`Timeout`
|
174 |
-
object that sets the individual timeouts to the ``timeout`` value
|
175 |
-
passed to this function.
|
176 |
-
|
177 |
-
:param timeout: The legacy timeout value.
|
178 |
-
:type timeout: integer, float, sentinel default object, or None
|
179 |
-
:return: Timeout object
|
180 |
-
:rtype: :class:`Timeout`
|
181 |
-
"""
|
182 |
-
return Timeout(read=timeout, connect=timeout)
|
183 |
-
|
184 |
-
def clone(self):
|
185 |
-
"""Create a copy of the timeout object
|
186 |
-
|
187 |
-
Timeout properties are stored per-pool but each request needs a fresh
|
188 |
-
Timeout object to ensure each one has its own start/stop configured.
|
189 |
-
|
190 |
-
:return: a copy of the timeout object
|
191 |
-
:rtype: :class:`Timeout`
|
192 |
-
"""
|
193 |
-
# We can't use copy.deepcopy because that will also create a new object
|
194 |
-
# for _GLOBAL_DEFAULT_TIMEOUT, which socket.py uses as a sentinel to
|
195 |
-
# detect the user default.
|
196 |
-
return Timeout(connect=self._connect, read=self._read, total=self.total)
|
197 |
-
|
198 |
-
def start_connect(self):
|
199 |
-
"""Start the timeout clock, used during a connect() attempt
|
200 |
-
|
201 |
-
:raises urllib3.exceptions.TimeoutStateError: if you attempt
|
202 |
-
to start a timer that has been started already.
|
203 |
-
"""
|
204 |
-
if self._start_connect is not None:
|
205 |
-
raise TimeoutStateError("Timeout timer has already been started.")
|
206 |
-
self._start_connect = current_time()
|
207 |
-
return self._start_connect
|
208 |
-
|
209 |
-
def get_connect_duration(self):
|
210 |
-
"""Gets the time elapsed since the call to :meth:`start_connect`.
|
211 |
-
|
212 |
-
:return: Elapsed time in seconds.
|
213 |
-
:rtype: float
|
214 |
-
:raises urllib3.exceptions.TimeoutStateError: if you attempt
|
215 |
-
to get duration for a timer that hasn't been started.
|
216 |
-
"""
|
217 |
-
if self._start_connect is None:
|
218 |
-
raise TimeoutStateError(
|
219 |
-
"Can't get connect duration for timer that has not started."
|
220 |
-
)
|
221 |
-
return current_time() - self._start_connect
|
222 |
-
|
223 |
-
@property
|
224 |
-
def connect_timeout(self):
|
225 |
-
"""Get the value to use when setting a connection timeout.
|
226 |
-
|
227 |
-
This will be a positive float or integer, the value None
|
228 |
-
(never timeout), or the default system timeout.
|
229 |
-
|
230 |
-
:return: Connect timeout.
|
231 |
-
:rtype: int, float, :attr:`Timeout.DEFAULT_TIMEOUT` or None
|
232 |
-
"""
|
233 |
-
if self.total is None:
|
234 |
-
return self._connect
|
235 |
-
|
236 |
-
if self._connect is None or self._connect is self.DEFAULT_TIMEOUT:
|
237 |
-
return self.total
|
238 |
-
|
239 |
-
return min(self._connect, self.total)
|
240 |
-
|
241 |
-
@property
|
242 |
-
def read_timeout(self):
|
243 |
-
"""Get the value for the read timeout.
|
244 |
-
|
245 |
-
This assumes some time has elapsed in the connection timeout and
|
246 |
-
computes the read timeout appropriately.
|
247 |
-
|
248 |
-
If self.total is set, the read timeout is dependent on the amount of
|
249 |
-
time taken by the connect timeout. If the connection time has not been
|
250 |
-
established, a :exc:`~urllib3.exceptions.TimeoutStateError` will be
|
251 |
-
raised.
|
252 |
-
|
253 |
-
:return: Value to use for the read timeout.
|
254 |
-
:rtype: int, float, :attr:`Timeout.DEFAULT_TIMEOUT` or None
|
255 |
-
:raises urllib3.exceptions.TimeoutStateError: If :meth:`start_connect`
|
256 |
-
has not yet been called on this object.
|
257 |
-
"""
|
258 |
-
if (
|
259 |
-
self.total is not None
|
260 |
-
and self.total is not self.DEFAULT_TIMEOUT
|
261 |
-
and self._read is not None
|
262 |
-
and self._read is not self.DEFAULT_TIMEOUT
|
263 |
-
):
|
264 |
-
# In case the connect timeout has not yet been established.
|
265 |
-
if self._start_connect is None:
|
266 |
-
return self._read
|
267 |
-
return max(0, min(self.total - self.get_connect_duration(), self._read))
|
268 |
-
elif self.total is not None and self.total is not self.DEFAULT_TIMEOUT:
|
269 |
-
return max(0, self.total - self.get_connect_duration())
|
270 |
-
else:
|
271 |
-
return self._read
|
|
|
|
|
|
|
|
|
|
|
|
|
|
|
|
|
|
|
|
|
|
|
|
|
|
|
|
|
|
|
|
|
|
|
|
|
|
|
|
|
|
|
|
|
|
|
|
|
|
|
|
|
|
|
|
|
|
|
|
|
|
|
|
|
|
|
|
|
|
|
|
|
|
|
|
|
|
|
|
|
|
|
|
|
|
|
|
|
|
|
|
|
|
|
|
|
|
|
|
|
|
|
|
|
|
|
|
|
|
|
|
|
|
|
|
|
|
|
|
|
|
|
|
|
|
|
|
|
|
|
|
|
|
|
|
|
|
|
|
|
|
|
|
|
|
|
|
|
|
|
|
|
|
|
|
|
|
|
|
|
|
|
|
|
|
|
|
|
|
|
|
|
|
|
|
|
|
|
|
|
|
|
|
|
|
|
|
|
|
|
|
|
|
|
|
|
|
|
|
|
|
|
|
|
|
|
|
|
|
|
|
|
|
|
|
|
|
|
|
|
|
|
|
|
|
|
|
|
|
|
|
|
|
|
|
|
|
|
|
|
|
|
|
|
|
|
|
|
|
|
|
|
|
|
|
|
|
|
|
|
|
|
|
|
|
|
|
|
|
|
|
|
|
|
|
|
|
|
|
|
|
|
|
|
|
|
|
|
|
|
|
|
|
|
|
|
|
|
|
|
|
|
|
|
|
|
|
|
|
|
|
|
|
|
|
|
|
|
|
|
|
|
|
|
|
|
|
|
|
|
|
|
|
|
|
|
|
|
|
|
|
|
|
|
|
|
|
|
|
|
|
|
|
|
|
|
|
|
|
|
|
|
|
|
|
|
|
|
|
|
|
|
|
|
|
|
|
|
|
|
|
|
|
|
|
|
|
|
|
|
|
|
|
|
|
|
|
|
|
|
|
|
|
|
|
|
|
|
|
|
|
|
|
|
|
|
|
|
|
|
|
|
|
|
|
|
|
|
|
|
|
|
|
|
|
|
|
|
|
|
|
|
|
|
|
|
|
|
|
|
|
|
|
|
|
|
|
|
|
|
|
|
|
|
|
|
|
|
|
|
|
|
|
|
|
|
|
|
|
|
|
|
|
|
|
|
|
|
|
|
|
|
|
|
|
|
|
|
|
|
|
|
|
|
|
|
|
|
|
|
|
|
|
|
|
|
|
|
|
|
|
|
|
|
|
|
|
|
|
|
|
|
|
|
|
|
|
|
|
|
|
|
|
spaces/CC123123/blip2_t/index.html
DELETED
@@ -1,19 +0,0 @@
|
|
1 |
-
<!DOCTYPE html>
|
2 |
-
<html>
|
3 |
-
<head>
|
4 |
-
<meta charset="utf-8" />
|
5 |
-
<meta name="viewport" content="width=device-width" />
|
6 |
-
<title>My static Space</title>
|
7 |
-
<link rel="stylesheet" href="style.css" />
|
8 |
-
</head>
|
9 |
-
<body>
|
10 |
-
<div class="card">
|
11 |
-
<h1>Welcome to your static Space!</h1>
|
12 |
-
<p>You can modify this app directly by editing <i>index.html</i> in the Files and versions tab.</p>
|
13 |
-
<p>
|
14 |
-
Also don't forget to check the
|
15 |
-
<a href="https://huggingface.co/docs/hub/spaces" target="_blank">Spaces documentation</a>.
|
16 |
-
</p>
|
17 |
-
</div>
|
18 |
-
</body>
|
19 |
-
</html>
|
|
|
|
|
|
|
|
|
|
|
|
|
|
|
|
|
|
|
|
|
|
|
|
|
|
|
|
|
|
|
|
|
|
|
|
|
|
|
|
spaces/CVPR/LIVE/thrust/dependencies/cub/experimental/sparse_matrix.h
DELETED
@@ -1,1244 +0,0 @@
|
|
1 |
-
/******************************************************************************
|
2 |
-
* Copyright (c) 2011, Duane Merrill. All rights reserved.
|
3 |
-
* Copyright (c) 2011-2018, NVIDIA CORPORATION. All rights reserved.
|
4 |
-
*
|
5 |
-
* Redistribution and use in source and binary forms, with or without
|
6 |
-
* modification, are permitted provided that the following conditions are met:
|
7 |
-
* * Redistributions of source code must retain the above copyright
|
8 |
-
* notice, this list of conditions and the following disclaimer.
|
9 |
-
* * Redistributions in binary form must reproduce the above copyright
|
10 |
-
* notice, this list of conditions and the following disclaimer in the
|
11 |
-
* documentation and/or other materials provided with the distribution.
|
12 |
-
* * Neither the name of the NVIDIA CORPORATION nor the
|
13 |
-
* names of its contributors may be used to endorse or promote products
|
14 |
-
* derived from this software without specific prior written permission.
|
15 |
-
*
|
16 |
-
* THIS SOFTWARE IS PROVIDED BY THE COPYRIGHT HOLDERS AND CONTRIBUTORS "AS IS" AND
|
17 |
-
* ANY EXPRESS OR IMPLIED WARRANTIES, INCLUDING, BUT NOT LIMITED TO, THE IMPLIED
|
18 |
-
* WARRANTIES OF MERCHANTABILITY AND FITNESS FOR A PARTICULAR PURPOSE ARE
|
19 |
-
* DISCLAIMED. IN NO EVENT SHALL NVIDIA CORPORATION BE LIABLE FOR ANY
|
20 |
-
* DIRECT, INDIRECT, INCIDENTAL, SPECIAL, EXEMPLARY, OR CONSEQUENTIAL DAMAGES
|
21 |
-
* (INCLUDING, BUT NOT LIMITED TO, PROCUREMENT OF SUBSTITUTE GOODS OR SERVICES;
|
22 |
-
* LOSS OF USE, DATA, OR PROFITS; OR BUSINESS INTERRUPTION) HOWEVER CAUSED AND
|
23 |
-
* ON ANY THEORY OF LIABILITY, WHETHER IN CONTRACT, STRICT LIABILITY, OR TORT
|
24 |
-
* (INCLUDING NEGLIGENCE OR OTHERWISE) ARISING IN ANY WAY OUT OF THE USE OF THIS
|
25 |
-
* SOFTWARE, EVEN IF ADVISED OF THE POSSIBILITY OF SUCH DAMAGE.
|
26 |
-
*
|
27 |
-
******************************************************************************/
|
28 |
-
|
29 |
-
/******************************************************************************
|
30 |
-
* Matrix data structures and parsing logic
|
31 |
-
******************************************************************************/
|
32 |
-
|
33 |
-
#pragma once
|
34 |
-
|
35 |
-
#include <cmath>
|
36 |
-
#include <cstring>
|
37 |
-
|
38 |
-
#include <iterator>
|
39 |
-
#include <string>
|
40 |
-
#include <algorithm>
|
41 |
-
#include <iostream>
|
42 |
-
#include <queue>
|
43 |
-
#include <set>
|
44 |
-
#include <fstream>
|
45 |
-
#include <stdio.h>
|
46 |
-
|
47 |
-
#ifdef CUB_MKL
|
48 |
-
#include <numa.h>
|
49 |
-
#include <mkl.h>
|
50 |
-
#endif
|
51 |
-
|
52 |
-
using namespace std;
|
53 |
-
|
54 |
-
/******************************************************************************
|
55 |
-
* COO matrix type
|
56 |
-
******************************************************************************/
|
57 |
-
|
58 |
-
struct GraphStats
|
59 |
-
{
|
60 |
-
int num_rows;
|
61 |
-
int num_cols;
|
62 |
-
int num_nonzeros;
|
63 |
-
|
64 |
-
double diag_dist_mean; // mean
|
65 |
-
double diag_dist_std_dev; // sample std dev
|
66 |
-
double pearson_r; // coefficient of variation
|
67 |
-
|
68 |
-
double row_length_mean; // mean
|
69 |
-
double row_length_std_dev; // sample std_dev
|
70 |
-
double row_length_variation; // coefficient of variation
|
71 |
-
double row_length_skewness; // skewness
|
72 |
-
|
73 |
-
void Display(bool show_labels = true)
|
74 |
-
{
|
75 |
-
if (show_labels)
|
76 |
-
printf("\n"
|
77 |
-
"\t num_rows: %d\n"
|
78 |
-
"\t num_cols: %d\n"
|
79 |
-
"\t num_nonzeros: %d\n"
|
80 |
-
"\t diag_dist_mean: %.2f\n"
|
81 |
-
"\t diag_dist_std_dev: %.2f\n"
|
82 |
-
"\t pearson_r: %f\n"
|
83 |
-
"\t row_length_mean: %.5f\n"
|
84 |
-
"\t row_length_std_dev: %.5f\n"
|
85 |
-
"\t row_length_variation: %.5f\n"
|
86 |
-
"\t row_length_skewness: %.5f\n",
|
87 |
-
num_rows,
|
88 |
-
num_cols,
|
89 |
-
num_nonzeros,
|
90 |
-
diag_dist_mean,
|
91 |
-
diag_dist_std_dev,
|
92 |
-
pearson_r,
|
93 |
-
row_length_mean,
|
94 |
-
row_length_std_dev,
|
95 |
-
row_length_variation,
|
96 |
-
row_length_skewness);
|
97 |
-
else
|
98 |
-
printf(
|
99 |
-
"%d, "
|
100 |
-
"%d, "
|
101 |
-
"%d, "
|
102 |
-
"%.2f, "
|
103 |
-
"%.2f, "
|
104 |
-
"%f, "
|
105 |
-
"%.5f, "
|
106 |
-
"%.5f, "
|
107 |
-
"%.5f, "
|
108 |
-
"%.5f, ",
|
109 |
-
num_rows,
|
110 |
-
num_cols,
|
111 |
-
num_nonzeros,
|
112 |
-
diag_dist_mean,
|
113 |
-
diag_dist_std_dev,
|
114 |
-
pearson_r,
|
115 |
-
row_length_mean,
|
116 |
-
row_length_std_dev,
|
117 |
-
row_length_variation,
|
118 |
-
row_length_skewness);
|
119 |
-
}
|
120 |
-
};
|
121 |
-
|
122 |
-
|
123 |
-
|
124 |
-
/******************************************************************************
|
125 |
-
* COO matrix type
|
126 |
-
******************************************************************************/
|
127 |
-
|
128 |
-
|
129 |
-
/**
|
130 |
-
* COO matrix type. A COO matrix is just a vector of edge tuples. Tuples are sorted
|
131 |
-
* first by row, then by column.
|
132 |
-
*/
|
133 |
-
template<typename ValueT, typename OffsetT>
|
134 |
-
struct CooMatrix
|
135 |
-
{
|
136 |
-
//---------------------------------------------------------------------
|
137 |
-
// Type definitions and constants
|
138 |
-
//---------------------------------------------------------------------
|
139 |
-
|
140 |
-
// COO edge tuple
|
141 |
-
struct CooTuple
|
142 |
-
{
|
143 |
-
OffsetT row;
|
144 |
-
OffsetT col;
|
145 |
-
ValueT val;
|
146 |
-
|
147 |
-
CooTuple() {}
|
148 |
-
CooTuple(OffsetT row, OffsetT col) : row(row), col(col) {}
|
149 |
-
CooTuple(OffsetT row, OffsetT col, ValueT val) : row(row), col(col), val(val) {}
|
150 |
-
|
151 |
-
/**
|
152 |
-
* Comparator for sorting COO sparse format num_nonzeros
|
153 |
-
*/
|
154 |
-
bool operator<(const CooTuple &other) const
|
155 |
-
{
|
156 |
-
if ((row < other.row) || ((row == other.row) && (col < other.col)))
|
157 |
-
{
|
158 |
-
return true;
|
159 |
-
}
|
160 |
-
|
161 |
-
return false;
|
162 |
-
}
|
163 |
-
};
|
164 |
-
|
165 |
-
|
166 |
-
//---------------------------------------------------------------------
|
167 |
-
// Data members
|
168 |
-
//---------------------------------------------------------------------
|
169 |
-
|
170 |
-
// Fields
|
171 |
-
int num_rows;
|
172 |
-
int num_cols;
|
173 |
-
int num_nonzeros;
|
174 |
-
CooTuple* coo_tuples;
|
175 |
-
|
176 |
-
//---------------------------------------------------------------------
|
177 |
-
// Methods
|
178 |
-
//---------------------------------------------------------------------
|
179 |
-
|
180 |
-
// Constructor
|
181 |
-
CooMatrix() : num_rows(0), num_cols(0), num_nonzeros(0), coo_tuples(NULL) {}
|
182 |
-
|
183 |
-
|
184 |
-
/**
|
185 |
-
* Clear
|
186 |
-
*/
|
187 |
-
void Clear()
|
188 |
-
{
|
189 |
-
if (coo_tuples) delete[] coo_tuples;
|
190 |
-
coo_tuples = NULL;
|
191 |
-
}
|
192 |
-
|
193 |
-
|
194 |
-
// Destructor
|
195 |
-
~CooMatrix()
|
196 |
-
{
|
197 |
-
Clear();
|
198 |
-
}
|
199 |
-
|
200 |
-
|
201 |
-
// Display matrix to stdout
|
202 |
-
void Display()
|
203 |
-
{
|
204 |
-
cout << "COO Matrix (" << num_rows << " rows, " << num_cols << " columns, " << num_nonzeros << " non-zeros):\n";
|
205 |
-
cout << "Ordinal, Row, Column, Value\n";
|
206 |
-
for (int i = 0; i < num_nonzeros; i++)
|
207 |
-
{
|
208 |
-
cout << '\t' << i << ',' << coo_tuples[i].row << ',' << coo_tuples[i].col << ',' << coo_tuples[i].val << "\n";
|
209 |
-
}
|
210 |
-
}
|
211 |
-
|
212 |
-
|
213 |
-
/**
|
214 |
-
* Builds a symmetric COO sparse from an asymmetric CSR matrix.
|
215 |
-
*/
|
216 |
-
template <typename CsrMatrixT>
|
217 |
-
void InitCsrSymmetric(CsrMatrixT &csr_matrix)
|
218 |
-
{
|
219 |
-
if (coo_tuples)
|
220 |
-
{
|
221 |
-
fprintf(stderr, "Matrix already constructed\n");
|
222 |
-
exit(1);
|
223 |
-
}
|
224 |
-
|
225 |
-
num_rows = csr_matrix.num_cols;
|
226 |
-
num_cols = csr_matrix.num_rows;
|
227 |
-
num_nonzeros = csr_matrix.num_nonzeros * 2;
|
228 |
-
coo_tuples = new CooTuple[num_nonzeros];
|
229 |
-
|
230 |
-
for (OffsetT row = 0; row < csr_matrix.num_rows; ++row)
|
231 |
-
{
|
232 |
-
for (OffsetT nonzero = csr_matrix.row_offsets[row]; nonzero < csr_matrix.row_offsets[row + 1]; ++nonzero)
|
233 |
-
{
|
234 |
-
coo_tuples[nonzero].row = row;
|
235 |
-
coo_tuples[nonzero].col = csr_matrix.column_indices[nonzero];
|
236 |
-
coo_tuples[nonzero].val = csr_matrix.values[nonzero];
|
237 |
-
|
238 |
-
coo_tuples[csr_matrix.num_nonzeros + nonzero].row = coo_tuples[nonzero].col;
|
239 |
-
coo_tuples[csr_matrix.num_nonzeros + nonzero].col = coo_tuples[nonzero].row;
|
240 |
-
coo_tuples[csr_matrix.num_nonzeros + nonzero].val = csr_matrix.values[nonzero];
|
241 |
-
|
242 |
-
}
|
243 |
-
}
|
244 |
-
|
245 |
-
// Sort by rows, then columns
|
246 |
-
std::stable_sort(coo_tuples, coo_tuples + num_nonzeros);
|
247 |
-
}
|
248 |
-
|
249 |
-
/**
|
250 |
-
* Builds a COO sparse from a relabeled CSR matrix.
|
251 |
-
*/
|
252 |
-
template <typename CsrMatrixT>
|
253 |
-
void InitCsrRelabel(CsrMatrixT &csr_matrix, OffsetT* relabel_indices)
|
254 |
-
{
|
255 |
-
if (coo_tuples)
|
256 |
-
{
|
257 |
-
fprintf(stderr, "Matrix already constructed\n");
|
258 |
-
exit(1);
|
259 |
-
}
|
260 |
-
|
261 |
-
num_rows = csr_matrix.num_rows;
|
262 |
-
num_cols = csr_matrix.num_cols;
|
263 |
-
num_nonzeros = csr_matrix.num_nonzeros;
|
264 |
-
coo_tuples = new CooTuple[num_nonzeros];
|
265 |
-
|
266 |
-
for (OffsetT row = 0; row < num_rows; ++row)
|
267 |
-
{
|
268 |
-
for (OffsetT nonzero = csr_matrix.row_offsets[row]; nonzero < csr_matrix.row_offsets[row + 1]; ++nonzero)
|
269 |
-
{
|
270 |
-
coo_tuples[nonzero].row = relabel_indices[row];
|
271 |
-
coo_tuples[nonzero].col = relabel_indices[csr_matrix.column_indices[nonzero]];
|
272 |
-
coo_tuples[nonzero].val = csr_matrix.values[nonzero];
|
273 |
-
}
|
274 |
-
}
|
275 |
-
|
276 |
-
// Sort by rows, then columns
|
277 |
-
std::stable_sort(coo_tuples, coo_tuples + num_nonzeros);
|
278 |
-
}
|
279 |
-
|
280 |
-
|
281 |
-
|
282 |
-
/**
|
283 |
-
* Builds a METIS COO sparse from the given file.
|
284 |
-
*/
|
285 |
-
void InitMetis(const string &metis_filename)
|
286 |
-
{
|
287 |
-
if (coo_tuples)
|
288 |
-
{
|
289 |
-
fprintf(stderr, "Matrix already constructed\n");
|
290 |
-
exit(1);
|
291 |
-
}
|
292 |
-
|
293 |
-
// TODO
|
294 |
-
}
|
295 |
-
|
296 |
-
|
297 |
-
/**
|
298 |
-
* Builds a MARKET COO sparse from the given file.
|
299 |
-
*/
|
300 |
-
void InitMarket(
|
301 |
-
const string& market_filename,
|
302 |
-
ValueT default_value = 1.0,
|
303 |
-
bool verbose = false)
|
304 |
-
{
|
305 |
-
if (verbose) {
|
306 |
-
printf("Reading... "); fflush(stdout);
|
307 |
-
}
|
308 |
-
|
309 |
-
if (coo_tuples)
|
310 |
-
{
|
311 |
-
fprintf(stderr, "Matrix already constructed\n");
|
312 |
-
exit(1);
|
313 |
-
}
|
314 |
-
|
315 |
-
std::ifstream ifs;
|
316 |
-
ifs.open(market_filename.c_str(), std::ifstream::in);
|
317 |
-
if (!ifs.good())
|
318 |
-
{
|
319 |
-
fprintf(stderr, "Error opening file\n");
|
320 |
-
exit(1);
|
321 |
-
}
|
322 |
-
|
323 |
-
bool array = false;
|
324 |
-
bool symmetric = false;
|
325 |
-
bool skew = false;
|
326 |
-
int current_edge = -1;
|
327 |
-
char line[1024];
|
328 |
-
|
329 |
-
if (verbose) {
|
330 |
-
printf("Parsing... "); fflush(stdout);
|
331 |
-
}
|
332 |
-
|
333 |
-
while (true)
|
334 |
-
{
|
335 |
-
ifs.getline(line, 1024);
|
336 |
-
if (!ifs.good())
|
337 |
-
{
|
338 |
-
// Done
|
339 |
-
break;
|
340 |
-
}
|
341 |
-
|
342 |
-
if (line[0] == '%')
|
343 |
-
{
|
344 |
-
// Comment
|
345 |
-
if (line[1] == '%')
|
346 |
-
{
|
347 |
-
// Banner
|
348 |
-
symmetric = (strstr(line, "symmetric") != NULL);
|
349 |
-
skew = (strstr(line, "skew") != NULL);
|
350 |
-
array = (strstr(line, "array") != NULL);
|
351 |
-
|
352 |
-
if (verbose) {
|
353 |
-
printf("(symmetric: %d, skew: %d, array: %d) ", symmetric, skew, array); fflush(stdout);
|
354 |
-
}
|
355 |
-
}
|
356 |
-
}
|
357 |
-
else if (current_edge == -1)
|
358 |
-
{
|
359 |
-
// Problem description
|
360 |
-
int nparsed = sscanf(line, "%d %d %d", &num_rows, &num_cols, &num_nonzeros);
|
361 |
-
if ((!array) && (nparsed == 3))
|
362 |
-
{
|
363 |
-
if (symmetric)
|
364 |
-
num_nonzeros *= 2;
|
365 |
-
|
366 |
-
// Allocate coo matrix
|
367 |
-
coo_tuples = new CooTuple[num_nonzeros];
|
368 |
-
current_edge = 0;
|
369 |
-
|
370 |
-
}
|
371 |
-
else if (array && (nparsed == 2))
|
372 |
-
{
|
373 |
-
// Allocate coo matrix
|
374 |
-
num_nonzeros = num_rows * num_cols;
|
375 |
-
coo_tuples = new CooTuple[num_nonzeros];
|
376 |
-
current_edge = 0;
|
377 |
-
}
|
378 |
-
else
|
379 |
-
{
|
380 |
-
fprintf(stderr, "Error parsing MARKET matrix: invalid problem description: %s\n", line);
|
381 |
-
exit(1);
|
382 |
-
}
|
383 |
-
|
384 |
-
}
|
385 |
-
else
|
386 |
-
{
|
387 |
-
// Edge
|
388 |
-
if (current_edge >= num_nonzeros)
|
389 |
-
{
|
390 |
-
fprintf(stderr, "Error parsing MARKET matrix: encountered more than %d num_nonzeros\n", num_nonzeros);
|
391 |
-
exit(1);
|
392 |
-
}
|
393 |
-
|
394 |
-
int row, col;
|
395 |
-
double val;
|
396 |
-
|
397 |
-
if (array)
|
398 |
-
{
|
399 |
-
if (sscanf(line, "%lf", &val) != 1)
|
400 |
-
{
|
401 |
-
fprintf(stderr, "Error parsing MARKET matrix: badly formed current_edge: '%s' at edge %d\n", line, current_edge);
|
402 |
-
exit(1);
|
403 |
-
}
|
404 |
-
col = (current_edge / num_rows);
|
405 |
-
row = (current_edge - (num_rows * col));
|
406 |
-
|
407 |
-
coo_tuples[current_edge] = CooTuple(row, col, val); // Convert indices to zero-based
|
408 |
-
}
|
409 |
-
else
|
410 |
-
{
|
411 |
-
// Parse nonzero (note: using strtol and strtod is 2x faster than sscanf or istream parsing)
|
412 |
-
char *l = line;
|
413 |
-
char *t = NULL;
|
414 |
-
|
415 |
-
// parse row
|
416 |
-
row = strtol(l, &t, 0);
|
417 |
-
if (t == l)
|
418 |
-
{
|
419 |
-
fprintf(stderr, "Error parsing MARKET matrix: badly formed row at edge %d\n", current_edge);
|
420 |
-
exit(1);
|
421 |
-
}
|
422 |
-
l = t;
|
423 |
-
|
424 |
-
// parse col
|
425 |
-
col = strtol(l, &t, 0);
|
426 |
-
if (t == l)
|
427 |
-
{
|
428 |
-
fprintf(stderr, "Error parsing MARKET matrix: badly formed col at edge %d\n", current_edge);
|
429 |
-
exit(1);
|
430 |
-
}
|
431 |
-
l = t;
|
432 |
-
|
433 |
-
// parse val
|
434 |
-
val = strtod(l, &t);
|
435 |
-
if (t == l)
|
436 |
-
{
|
437 |
-
val = default_value;
|
438 |
-
}
|
439 |
-
/*
|
440 |
-
int nparsed = sscanf(line, "%d %d %lf", &row, &col, &val);
|
441 |
-
if (nparsed == 2)
|
442 |
-
{
|
443 |
-
// No value specified
|
444 |
-
val = default_value;
|
445 |
-
|
446 |
-
}
|
447 |
-
else if (nparsed != 3)
|
448 |
-
{
|
449 |
-
fprintf(stderr, "Error parsing MARKET matrix 1: badly formed current_edge: %d parsed at edge %d\n", nparsed, current_edge);
|
450 |
-
exit(1);
|
451 |
-
}
|
452 |
-
*/
|
453 |
-
|
454 |
-
coo_tuples[current_edge] = CooTuple(row - 1, col - 1, val); // Convert indices to zero-based
|
455 |
-
|
456 |
-
}
|
457 |
-
|
458 |
-
current_edge++;
|
459 |
-
|
460 |
-
if (symmetric && (row != col))
|
461 |
-
{
|
462 |
-
coo_tuples[current_edge].row = coo_tuples[current_edge - 1].col;
|
463 |
-
coo_tuples[current_edge].col = coo_tuples[current_edge - 1].row;
|
464 |
-
coo_tuples[current_edge].val = coo_tuples[current_edge - 1].val * (skew ? -1 : 1);
|
465 |
-
current_edge++;
|
466 |
-
}
|
467 |
-
}
|
468 |
-
}
|
469 |
-
|
470 |
-
// Adjust nonzero count (nonzeros along the diagonal aren't reversed)
|
471 |
-
num_nonzeros = current_edge;
|
472 |
-
|
473 |
-
if (verbose) {
|
474 |
-
printf("done. Ordering..."); fflush(stdout);
|
475 |
-
}
|
476 |
-
|
477 |
-
// Sort by rows, then columns
|
478 |
-
std::stable_sort(coo_tuples, coo_tuples + num_nonzeros);
|
479 |
-
|
480 |
-
if (verbose) {
|
481 |
-
printf("done. "); fflush(stdout);
|
482 |
-
}
|
483 |
-
|
484 |
-
ifs.close();
|
485 |
-
}
|
486 |
-
|
487 |
-
|
488 |
-
/**
|
489 |
-
* Builds a dense matrix
|
490 |
-
*/
|
491 |
-
int InitDense(
|
492 |
-
OffsetT num_rows,
|
493 |
-
OffsetT num_cols,
|
494 |
-
ValueT default_value = 1.0,
|
495 |
-
bool verbose = false)
|
496 |
-
{
|
497 |
-
if (coo_tuples)
|
498 |
-
{
|
499 |
-
fprintf(stderr, "Matrix already constructed\n");
|
500 |
-
exit(1);
|
501 |
-
}
|
502 |
-
|
503 |
-
this->num_rows = num_rows;
|
504 |
-
this->num_cols = num_cols;
|
505 |
-
|
506 |
-
num_nonzeros = num_rows * num_cols;
|
507 |
-
coo_tuples = new CooTuple[num_nonzeros];
|
508 |
-
|
509 |
-
for (OffsetT row = 0; row < num_rows; ++row)
|
510 |
-
{
|
511 |
-
for (OffsetT col = 0; col < num_cols; ++col)
|
512 |
-
{
|
513 |
-
coo_tuples[(row * num_cols) + col] = CooTuple(row, col, default_value);
|
514 |
-
}
|
515 |
-
}
|
516 |
-
|
517 |
-
// Sort by rows, then columns
|
518 |
-
std::stable_sort(coo_tuples, coo_tuples + num_nonzeros);
|
519 |
-
|
520 |
-
return 0;
|
521 |
-
}
|
522 |
-
|
523 |
-
/**
|
524 |
-
* Builds a wheel COO sparse matrix having spokes spokes.
|
525 |
-
*/
|
526 |
-
int InitWheel(
|
527 |
-
OffsetT spokes,
|
528 |
-
ValueT default_value = 1.0,
|
529 |
-
bool verbose = false)
|
530 |
-
{
|
531 |
-
if (coo_tuples)
|
532 |
-
{
|
533 |
-
fprintf(stderr, "Matrix already constructed\n");
|
534 |
-
exit(1);
|
535 |
-
}
|
536 |
-
|
537 |
-
num_rows = spokes + 1;
|
538 |
-
num_cols = num_rows;
|
539 |
-
num_nonzeros = spokes * 2;
|
540 |
-
coo_tuples = new CooTuple[num_nonzeros];
|
541 |
-
|
542 |
-
// Add spoke num_nonzeros
|
543 |
-
int current_edge = 0;
|
544 |
-
for (OffsetT i = 0; i < spokes; i++)
|
545 |
-
{
|
546 |
-
coo_tuples[current_edge] = CooTuple(0, i + 1, default_value);
|
547 |
-
current_edge++;
|
548 |
-
}
|
549 |
-
|
550 |
-
// Add rim
|
551 |
-
for (OffsetT i = 0; i < spokes; i++)
|
552 |
-
{
|
553 |
-
OffsetT dest = (i + 1) % spokes;
|
554 |
-
coo_tuples[current_edge] = CooTuple(i + 1, dest + 1, default_value);
|
555 |
-
current_edge++;
|
556 |
-
}
|
557 |
-
|
558 |
-
// Sort by rows, then columns
|
559 |
-
std::stable_sort(coo_tuples, coo_tuples + num_nonzeros);
|
560 |
-
|
561 |
-
return 0;
|
562 |
-
}
|
563 |
-
|
564 |
-
|
565 |
-
/**
|
566 |
-
* Builds a square 2D grid CSR matrix. Interior num_vertices have degree 5 when including
|
567 |
-
* a self-loop.
|
568 |
-
*
|
569 |
-
* Returns 0 on success, 1 on failure.
|
570 |
-
*/
|
571 |
-
int InitGrid2d(OffsetT width, bool self_loop, ValueT default_value = 1.0)
|
572 |
-
{
|
573 |
-
if (coo_tuples)
|
574 |
-
{
|
575 |
-
fprintf(stderr, "Matrix already constructed\n");
|
576 |
-
exit(1);
|
577 |
-
}
|
578 |
-
|
579 |
-
int interior_nodes = (width - 2) * (width - 2);
|
580 |
-
int edge_nodes = (width - 2) * 4;
|
581 |
-
int corner_nodes = 4;
|
582 |
-
num_rows = width * width;
|
583 |
-
num_cols = num_rows;
|
584 |
-
num_nonzeros = (interior_nodes * 4) + (edge_nodes * 3) + (corner_nodes * 2);
|
585 |
-
|
586 |
-
if (self_loop)
|
587 |
-
num_nonzeros += num_rows;
|
588 |
-
|
589 |
-
coo_tuples = new CooTuple[num_nonzeros];
|
590 |
-
int current_edge = 0;
|
591 |
-
|
592 |
-
for (OffsetT j = 0; j < width; j++)
|
593 |
-
{
|
594 |
-
for (OffsetT k = 0; k < width; k++)
|
595 |
-
{
|
596 |
-
OffsetT me = (j * width) + k;
|
597 |
-
|
598 |
-
// West
|
599 |
-
OffsetT neighbor = (j * width) + (k - 1);
|
600 |
-
if (k - 1 >= 0) {
|
601 |
-
coo_tuples[current_edge] = CooTuple(me, neighbor, default_value);
|
602 |
-
current_edge++;
|
603 |
-
}
|
604 |
-
|
605 |
-
// East
|
606 |
-
neighbor = (j * width) + (k + 1);
|
607 |
-
if (k + 1 < width) {
|
608 |
-
coo_tuples[current_edge] = CooTuple(me, neighbor, default_value);
|
609 |
-
current_edge++;
|
610 |
-
}
|
611 |
-
|
612 |
-
// North
|
613 |
-
neighbor = ((j - 1) * width) + k;
|
614 |
-
if (j - 1 >= 0) {
|
615 |
-
coo_tuples[current_edge] = CooTuple(me, neighbor, default_value);
|
616 |
-
current_edge++;
|
617 |
-
}
|
618 |
-
|
619 |
-
// South
|
620 |
-
neighbor = ((j + 1) * width) + k;
|
621 |
-
if (j + 1 < width) {
|
622 |
-
coo_tuples[current_edge] = CooTuple(me, neighbor, default_value);
|
623 |
-
current_edge++;
|
624 |
-
}
|
625 |
-
|
626 |
-
if (self_loop)
|
627 |
-
{
|
628 |
-
neighbor = me;
|
629 |
-
coo_tuples[current_edge] = CooTuple(me, neighbor, default_value);
|
630 |
-
current_edge++;
|
631 |
-
}
|
632 |
-
}
|
633 |
-
}
|
634 |
-
|
635 |
-
// Sort by rows, then columns, update dims
|
636 |
-
std::stable_sort(coo_tuples, coo_tuples + num_nonzeros);
|
637 |
-
|
638 |
-
return 0;
|
639 |
-
}
|
640 |
-
|
641 |
-
|
642 |
-
/**
|
643 |
-
* Builds a square 3D grid COO sparse matrix. Interior num_vertices have degree 7 when including
|
644 |
-
* a self-loop. Values are unintialized, coo_tuples are sorted.
|
645 |
-
*/
|
646 |
-
int InitGrid3d(OffsetT width, bool self_loop, ValueT default_value = 1.0)
|
647 |
-
{
|
648 |
-
if (coo_tuples)
|
649 |
-
{
|
650 |
-
fprintf(stderr, "Matrix already constructed\n");
|
651 |
-
return -1;
|
652 |
-
}
|
653 |
-
|
654 |
-
OffsetT interior_nodes = (width - 2) * (width - 2) * (width - 2);
|
655 |
-
OffsetT face_nodes = (width - 2) * (width - 2) * 6;
|
656 |
-
OffsetT edge_nodes = (width - 2) * 12;
|
657 |
-
OffsetT corner_nodes = 8;
|
658 |
-
num_cols = width * width * width;
|
659 |
-
num_rows = num_cols;
|
660 |
-
num_nonzeros = (interior_nodes * 6) + (face_nodes * 5) + (edge_nodes * 4) + (corner_nodes * 3);
|
661 |
-
|
662 |
-
if (self_loop)
|
663 |
-
num_nonzeros += num_rows;
|
664 |
-
|
665 |
-
coo_tuples = new CooTuple[num_nonzeros];
|
666 |
-
int current_edge = 0;
|
667 |
-
|
668 |
-
for (OffsetT i = 0; i < width; i++)
|
669 |
-
{
|
670 |
-
for (OffsetT j = 0; j < width; j++)
|
671 |
-
{
|
672 |
-
for (OffsetT k = 0; k < width; k++)
|
673 |
-
{
|
674 |
-
|
675 |
-
OffsetT me = (i * width * width) + (j * width) + k;
|
676 |
-
|
677 |
-
// Up
|
678 |
-
OffsetT neighbor = (i * width * width) + (j * width) + (k - 1);
|
679 |
-
if (k - 1 >= 0) {
|
680 |
-
coo_tuples[current_edge] = CooTuple(me, neighbor, default_value);
|
681 |
-
current_edge++;
|
682 |
-
}
|
683 |
-
|
684 |
-
// Down
|
685 |
-
neighbor = (i * width * width) + (j * width) + (k + 1);
|
686 |
-
if (k + 1 < width) {
|
687 |
-
coo_tuples[current_edge] = CooTuple(me, neighbor, default_value);
|
688 |
-
current_edge++;
|
689 |
-
}
|
690 |
-
|
691 |
-
// West
|
692 |
-
neighbor = (i * width * width) + ((j - 1) * width) + k;
|
693 |
-
if (j - 1 >= 0) {
|
694 |
-
coo_tuples[current_edge] = CooTuple(me, neighbor, default_value);
|
695 |
-
current_edge++;
|
696 |
-
}
|
697 |
-
|
698 |
-
// East
|
699 |
-
neighbor = (i * width * width) + ((j + 1) * width) + k;
|
700 |
-
if (j + 1 < width) {
|
701 |
-
coo_tuples[current_edge] = CooTuple(me, neighbor, default_value);
|
702 |
-
current_edge++;
|
703 |
-
}
|
704 |
-
|
705 |
-
// North
|
706 |
-
neighbor = ((i - 1) * width * width) + (j * width) + k;
|
707 |
-
if (i - 1 >= 0) {
|
708 |
-
coo_tuples[current_edge] = CooTuple(me, neighbor, default_value);
|
709 |
-
current_edge++;
|
710 |
-
}
|
711 |
-
|
712 |
-
// South
|
713 |
-
neighbor = ((i + 1) * width * width) + (j * width) + k;
|
714 |
-
if (i + 1 < width) {
|
715 |
-
coo_tuples[current_edge] = CooTuple(me, neighbor, default_value);
|
716 |
-
current_edge++;
|
717 |
-
}
|
718 |
-
|
719 |
-
if (self_loop)
|
720 |
-
{
|
721 |
-
neighbor = me;
|
722 |
-
coo_tuples[current_edge] = CooTuple(me, neighbor, default_value);
|
723 |
-
current_edge++;
|
724 |
-
}
|
725 |
-
}
|
726 |
-
}
|
727 |
-
}
|
728 |
-
|
729 |
-
// Sort by rows, then columns, update dims
|
730 |
-
std::stable_sort(coo_tuples, coo_tuples + num_nonzeros);
|
731 |
-
|
732 |
-
return 0;
|
733 |
-
}
|
734 |
-
};
|
735 |
-
|
736 |
-
|
737 |
-
|
738 |
-
/******************************************************************************
|
739 |
-
* COO matrix type
|
740 |
-
******************************************************************************/
|
741 |
-
|
742 |
-
|
743 |
-
/**
|
744 |
-
* CSR sparse format matrix
|
745 |
-
*/
|
746 |
-
template<
|
747 |
-
typename ValueT,
|
748 |
-
typename OffsetT>
|
749 |
-
struct CsrMatrix
|
750 |
-
{
|
751 |
-
int num_rows;
|
752 |
-
int num_cols;
|
753 |
-
int num_nonzeros;
|
754 |
-
OffsetT* row_offsets;
|
755 |
-
OffsetT* column_indices;
|
756 |
-
ValueT* values;
|
757 |
-
bool numa_malloc;
|
758 |
-
|
759 |
-
/**
|
760 |
-
* Constructor
|
761 |
-
*/
|
762 |
-
CsrMatrix() : num_rows(0), num_cols(0), num_nonzeros(0), row_offsets(NULL), column_indices(NULL), values(NULL)
|
763 |
-
{
|
764 |
-
#ifdef CUB_MKL
|
765 |
-
numa_malloc = ((numa_available() >= 0) && (numa_num_task_nodes() > 1));
|
766 |
-
#else
|
767 |
-
numa_malloc = false;
|
768 |
-
#endif
|
769 |
-
}
|
770 |
-
|
771 |
-
|
772 |
-
/**
|
773 |
-
* Clear
|
774 |
-
*/
|
775 |
-
void Clear()
|
776 |
-
{
|
777 |
-
#ifdef CUB_MKL
|
778 |
-
if (numa_malloc)
|
779 |
-
{
|
780 |
-
numa_free(row_offsets, sizeof(OffsetT) * (num_rows + 1));
|
781 |
-
numa_free(values, sizeof(ValueT) * num_nonzeros);
|
782 |
-
numa_free(column_indices, sizeof(OffsetT) * num_nonzeros);
|
783 |
-
}
|
784 |
-
else
|
785 |
-
{
|
786 |
-
if (row_offsets) mkl_free(row_offsets);
|
787 |
-
if (column_indices) mkl_free(column_indices);
|
788 |
-
if (values) mkl_free(values);
|
789 |
-
}
|
790 |
-
|
791 |
-
#else
|
792 |
-
if (row_offsets) delete[] row_offsets;
|
793 |
-
if (column_indices) delete[] column_indices;
|
794 |
-
if (values) delete[] values;
|
795 |
-
#endif
|
796 |
-
|
797 |
-
row_offsets = NULL;
|
798 |
-
column_indices = NULL;
|
799 |
-
values = NULL;
|
800 |
-
}
|
801 |
-
|
802 |
-
/**
|
803 |
-
* Destructor
|
804 |
-
*/
|
805 |
-
~CsrMatrix()
|
806 |
-
{
|
807 |
-
Clear();
|
808 |
-
}
|
809 |
-
|
810 |
-
GraphStats Stats()
|
811 |
-
{
|
812 |
-
GraphStats stats;
|
813 |
-
stats.num_rows = num_rows;
|
814 |
-
stats.num_cols = num_cols;
|
815 |
-
stats.num_nonzeros = num_nonzeros;
|
816 |
-
|
817 |
-
//
|
818 |
-
// Compute diag-distance statistics
|
819 |
-
//
|
820 |
-
|
821 |
-
OffsetT samples = 0;
|
822 |
-
double mean = 0.0;
|
823 |
-
double ss_tot = 0.0;
|
824 |
-
|
825 |
-
for (OffsetT row = 0; row < num_rows; ++row)
|
826 |
-
{
|
827 |
-
OffsetT nz_idx_start = row_offsets[row];
|
828 |
-
OffsetT nz_idx_end = row_offsets[row + 1];
|
829 |
-
|
830 |
-
for (int nz_idx = nz_idx_start; nz_idx < nz_idx_end; ++nz_idx)
|
831 |
-
{
|
832 |
-
OffsetT col = column_indices[nz_idx];
|
833 |
-
double x = (col > row) ? col - row : row - col;
|
834 |
-
|
835 |
-
samples++;
|
836 |
-
double delta = x - mean;
|
837 |
-
mean = mean + (delta / samples);
|
838 |
-
ss_tot += delta * (x - mean);
|
839 |
-
}
|
840 |
-
}
|
841 |
-
stats.diag_dist_mean = mean;
|
842 |
-
double variance = ss_tot / samples;
|
843 |
-
stats.diag_dist_std_dev = sqrt(variance);
|
844 |
-
|
845 |
-
|
846 |
-
//
|
847 |
-
// Compute deming statistics
|
848 |
-
//
|
849 |
-
|
850 |
-
samples = 0;
|
851 |
-
double mean_x = 0.0;
|
852 |
-
double mean_y = 0.0;
|
853 |
-
double ss_x = 0.0;
|
854 |
-
double ss_y = 0.0;
|
855 |
-
|
856 |
-
for (OffsetT row = 0; row < num_rows; ++row)
|
857 |
-
{
|
858 |
-
OffsetT nz_idx_start = row_offsets[row];
|
859 |
-
OffsetT nz_idx_end = row_offsets[row + 1];
|
860 |
-
|
861 |
-
for (int nz_idx = nz_idx_start; nz_idx < nz_idx_end; ++nz_idx)
|
862 |
-
{
|
863 |
-
OffsetT col = column_indices[nz_idx];
|
864 |
-
|
865 |
-
samples++;
|
866 |
-
double x = col;
|
867 |
-
double y = row;
|
868 |
-
double delta;
|
869 |
-
|
870 |
-
delta = x - mean_x;
|
871 |
-
mean_x = mean_x + (delta / samples);
|
872 |
-
ss_x += delta * (x - mean_x);
|
873 |
-
|
874 |
-
delta = y - mean_y;
|
875 |
-
mean_y = mean_y + (delta / samples);
|
876 |
-
ss_y += delta * (y - mean_y);
|
877 |
-
}
|
878 |
-
}
|
879 |
-
|
880 |
-
samples = 0;
|
881 |
-
double s_xy = 0.0;
|
882 |
-
double s_xxy = 0.0;
|
883 |
-
double s_xyy = 0.0;
|
884 |
-
for (OffsetT row = 0; row < num_rows; ++row)
|
885 |
-
{
|
886 |
-
OffsetT nz_idx_start = row_offsets[row];
|
887 |
-
OffsetT nz_idx_end = row_offsets[row + 1];
|
888 |
-
|
889 |
-
for (int nz_idx = nz_idx_start; nz_idx < nz_idx_end; ++nz_idx)
|
890 |
-
{
|
891 |
-
OffsetT col = column_indices[nz_idx];
|
892 |
-
|
893 |
-
samples++;
|
894 |
-
double x = col;
|
895 |
-
double y = row;
|
896 |
-
|
897 |
-
double xy = (x - mean_x) * (y - mean_y);
|
898 |
-
double xxy = (x - mean_x) * (x - mean_x) * (y - mean_y);
|
899 |
-
double xyy = (x - mean_x) * (y - mean_y) * (y - mean_y);
|
900 |
-
double delta;
|
901 |
-
|
902 |
-
delta = xy - s_xy;
|
903 |
-
s_xy = s_xy + (delta / samples);
|
904 |
-
|
905 |
-
delta = xxy - s_xxy;
|
906 |
-
s_xxy = s_xxy + (delta / samples);
|
907 |
-
|
908 |
-
delta = xyy - s_xyy;
|
909 |
-
s_xyy = s_xyy + (delta / samples);
|
910 |
-
}
|
911 |
-
}
|
912 |
-
|
913 |
-
double s_xx = ss_x / num_nonzeros;
|
914 |
-
double s_yy = ss_y / num_nonzeros;
|
915 |
-
|
916 |
-
double deming_slope = (s_yy - s_xx + sqrt(((s_yy - s_xx) * (s_yy - s_xx)) + (4 * s_xy * s_xy))) / (2 * s_xy);
|
917 |
-
|
918 |
-
stats.pearson_r = (num_nonzeros * s_xy) / (sqrt(ss_x) * sqrt(ss_y));
|
919 |
-
|
920 |
-
|
921 |
-
//
|
922 |
-
// Compute row-length statistics
|
923 |
-
//
|
924 |
-
|
925 |
-
// Sample mean
|
926 |
-
stats.row_length_mean = double(num_nonzeros) / num_rows;
|
927 |
-
variance = 0.0;
|
928 |
-
stats.row_length_skewness = 0.0;
|
929 |
-
for (OffsetT row = 0; row < num_rows; ++row)
|
930 |
-
{
|
931 |
-
OffsetT length = row_offsets[row + 1] - row_offsets[row];
|
932 |
-
double delta = double(length) - stats.row_length_mean;
|
933 |
-
variance += (delta * delta);
|
934 |
-
stats.row_length_skewness += (delta * delta * delta);
|
935 |
-
}
|
936 |
-
variance /= num_rows;
|
937 |
-
stats.row_length_std_dev = sqrt(variance);
|
938 |
-
stats.row_length_skewness = (stats.row_length_skewness / num_rows) / pow(stats.row_length_std_dev, 3.0);
|
939 |
-
stats.row_length_variation = stats.row_length_std_dev / stats.row_length_mean;
|
940 |
-
|
941 |
-
return stats;
|
942 |
-
}
|
943 |
-
|
944 |
-
/**
|
945 |
-
* Build CSR matrix from sorted COO matrix
|
946 |
-
*/
|
947 |
-
void FromCoo(const CooMatrix<ValueT, OffsetT> &coo_matrix)
|
948 |
-
{
|
949 |
-
num_rows = coo_matrix.num_rows;
|
950 |
-
num_cols = coo_matrix.num_cols;
|
951 |
-
num_nonzeros = coo_matrix.num_nonzeros;
|
952 |
-
|
953 |
-
#ifdef CUB_MKL
|
954 |
-
|
955 |
-
if (numa_malloc)
|
956 |
-
{
|
957 |
-
numa_set_strict(1);
|
958 |
-
// numa_set_bind_policy(1);
|
959 |
-
|
960 |
-
// values = (ValueT*) numa_alloc_interleaved(sizeof(ValueT) * num_nonzeros);
|
961 |
-
// row_offsets = (OffsetT*) numa_alloc_interleaved(sizeof(OffsetT) * (num_rows + 1));
|
962 |
-
// column_indices = (OffsetT*) numa_alloc_interleaved(sizeof(OffsetT) * num_nonzeros);
|
963 |
-
|
964 |
-
row_offsets = (OffsetT*) numa_alloc_onnode(sizeof(OffsetT) * (num_rows + 1), 0);
|
965 |
-
column_indices = (OffsetT*) numa_alloc_onnode(sizeof(OffsetT) * num_nonzeros, 0);
|
966 |
-
values = (ValueT*) numa_alloc_onnode(sizeof(ValueT) * num_nonzeros, 1);
|
967 |
-
}
|
968 |
-
else
|
969 |
-
{
|
970 |
-
values = (ValueT*) mkl_malloc(sizeof(ValueT) * num_nonzeros, 4096);
|
971 |
-
row_offsets = (OffsetT*) mkl_malloc(sizeof(OffsetT) * (num_rows + 1), 4096);
|
972 |
-
column_indices = (OffsetT*) mkl_malloc(sizeof(OffsetT) * num_nonzeros, 4096);
|
973 |
-
|
974 |
-
}
|
975 |
-
|
976 |
-
#else
|
977 |
-
row_offsets = new OffsetT[num_rows + 1];
|
978 |
-
column_indices = new OffsetT[num_nonzeros];
|
979 |
-
values = new ValueT[num_nonzeros];
|
980 |
-
#endif
|
981 |
-
|
982 |
-
OffsetT prev_row = -1;
|
983 |
-
for (OffsetT current_edge = 0; current_edge < num_nonzeros; current_edge++)
|
984 |
-
{
|
985 |
-
OffsetT current_row = coo_matrix.coo_tuples[current_edge].row;
|
986 |
-
|
987 |
-
// Fill in rows up to and including the current row
|
988 |
-
for (OffsetT row = prev_row + 1; row <= current_row; row++)
|
989 |
-
{
|
990 |
-
row_offsets[row] = current_edge;
|
991 |
-
}
|
992 |
-
prev_row = current_row;
|
993 |
-
|
994 |
-
column_indices[current_edge] = coo_matrix.coo_tuples[current_edge].col;
|
995 |
-
values[current_edge] = coo_matrix.coo_tuples[current_edge].val;
|
996 |
-
}
|
997 |
-
|
998 |
-
// Fill out any trailing edgeless vertices (and the end-of-list element)
|
999 |
-
for (OffsetT row = prev_row + 1; row <= num_rows; row++)
|
1000 |
-
{
|
1001 |
-
row_offsets[row] = num_nonzeros;
|
1002 |
-
}
|
1003 |
-
}
|
1004 |
-
|
1005 |
-
|
1006 |
-
/**
|
1007 |
-
* Display log-histogram to stdout
|
1008 |
-
*/
|
1009 |
-
void DisplayHistogram()
|
1010 |
-
{
|
1011 |
-
// Initialize
|
1012 |
-
int log_counts[9];
|
1013 |
-
for (int i = 0; i < 9; i++)
|
1014 |
-
{
|
1015 |
-
log_counts[i] = 0;
|
1016 |
-
}
|
1017 |
-
|
1018 |
-
// Scan
|
1019 |
-
int max_log_length = -1;
|
1020 |
-
for (OffsetT row = 0; row < num_rows; row++)
|
1021 |
-
{
|
1022 |
-
OffsetT length = row_offsets[row + 1] - row_offsets[row];
|
1023 |
-
|
1024 |
-
int log_length = -1;
|
1025 |
-
while (length > 0)
|
1026 |
-
{
|
1027 |
-
length /= 10;
|
1028 |
-
log_length++;
|
1029 |
-
}
|
1030 |
-
if (log_length > max_log_length)
|
1031 |
-
{
|
1032 |
-
max_log_length = log_length;
|
1033 |
-
}
|
1034 |
-
|
1035 |
-
log_counts[log_length + 1]++;
|
1036 |
-
}
|
1037 |
-
printf("CSR matrix (%d rows, %d columns, %d non-zeros):\n", (int) num_rows, (int) num_cols, (int) num_nonzeros);
|
1038 |
-
for (int i = -1; i < max_log_length + 1; i++)
|
1039 |
-
{
|
1040 |
-
printf("\tDegree 1e%d: \t%d (%.2f%%)\n", i, log_counts[i + 1], (float) log_counts[i + 1] * 100.0 / num_cols);
|
1041 |
-
}
|
1042 |
-
fflush(stdout);
|
1043 |
-
}
|
1044 |
-
|
1045 |
-
|
1046 |
-
/**
|
1047 |
-
* Display matrix to stdout
|
1048 |
-
*/
|
1049 |
-
void Display()
|
1050 |
-
{
|
1051 |
-
printf("Input Matrix:\n");
|
1052 |
-
for (OffsetT row = 0; row < num_rows; row++)
|
1053 |
-
{
|
1054 |
-
printf("%d [@%d, #%d]: ", row, row_offsets[row], row_offsets[row + 1] - row_offsets[row]);
|
1055 |
-
for (OffsetT current_edge = row_offsets[row]; current_edge < row_offsets[row + 1]; current_edge++)
|
1056 |
-
{
|
1057 |
-
printf("%d (%f), ", column_indices[current_edge], values[current_edge]);
|
1058 |
-
}
|
1059 |
-
printf("\n");
|
1060 |
-
}
|
1061 |
-
fflush(stdout);
|
1062 |
-
}
|
1063 |
-
|
1064 |
-
|
1065 |
-
};
|
1066 |
-
|
1067 |
-
|
1068 |
-
|
1069 |
-
/******************************************************************************
|
1070 |
-
* Matrix transformations
|
1071 |
-
******************************************************************************/
|
1072 |
-
|
1073 |
-
// Comparator for ordering rows by degree (lowest first), then by row-id (lowest first)
|
1074 |
-
template <typename OffsetT>
|
1075 |
-
struct OrderByLow
|
1076 |
-
{
|
1077 |
-
OffsetT* row_degrees;
|
1078 |
-
OrderByLow(OffsetT* row_degrees) : row_degrees(row_degrees) {}
|
1079 |
-
|
1080 |
-
bool operator()(const OffsetT &a, const OffsetT &b)
|
1081 |
-
{
|
1082 |
-
if (row_degrees[a] < row_degrees[b])
|
1083 |
-
return true;
|
1084 |
-
else if (row_degrees[a] > row_degrees[b])
|
1085 |
-
return false;
|
1086 |
-
else
|
1087 |
-
return (a < b);
|
1088 |
-
}
|
1089 |
-
};
|
1090 |
-
|
1091 |
-
// Comparator for ordering rows by degree (highest first), then by row-id (lowest first)
|
1092 |
-
template <typename OffsetT>
|
1093 |
-
struct OrderByHigh
|
1094 |
-
{
|
1095 |
-
OffsetT* row_degrees;
|
1096 |
-
OrderByHigh(OffsetT* row_degrees) : row_degrees(row_degrees) {}
|
1097 |
-
|
1098 |
-
bool operator()(const OffsetT &a, const OffsetT &b)
|
1099 |
-
{
|
1100 |
-
if (row_degrees[a] > row_degrees[b])
|
1101 |
-
return true;
|
1102 |
-
else if (row_degrees[a] < row_degrees[b])
|
1103 |
-
return false;
|
1104 |
-
else
|
1105 |
-
return (a < b);
|
1106 |
-
}
|
1107 |
-
};
|
1108 |
-
|
1109 |
-
|
1110 |
-
|
1111 |
-
/**
|
1112 |
-
* Reverse Cuthill-McKee
|
1113 |
-
*/
|
1114 |
-
template <typename ValueT, typename OffsetT>
|
1115 |
-
void RcmRelabel(
|
1116 |
-
CsrMatrix<ValueT, OffsetT>& matrix,
|
1117 |
-
OffsetT* relabel_indices)
|
1118 |
-
{
|
1119 |
-
// Initialize row degrees
|
1120 |
-
OffsetT* row_degrees_in = new OffsetT[matrix.num_rows];
|
1121 |
-
OffsetT* row_degrees_out = new OffsetT[matrix.num_rows];
|
1122 |
-
for (OffsetT row = 0; row < matrix.num_rows; ++row)
|
1123 |
-
{
|
1124 |
-
row_degrees_in[row] = 0;
|
1125 |
-
row_degrees_out[row] = matrix.row_offsets[row + 1] - matrix.row_offsets[row];
|
1126 |
-
}
|
1127 |
-
for (OffsetT nonzero = 0; nonzero < matrix.num_nonzeros; ++nonzero)
|
1128 |
-
{
|
1129 |
-
row_degrees_in[matrix.column_indices[nonzero]]++;
|
1130 |
-
}
|
1131 |
-
|
1132 |
-
// Initialize unlabeled set
|
1133 |
-
typedef std::set<OffsetT, OrderByLow<OffsetT> > UnlabeledSet;
|
1134 |
-
typename UnlabeledSet::key_compare unlabeled_comp(row_degrees_in);
|
1135 |
-
UnlabeledSet unlabeled(unlabeled_comp);
|
1136 |
-
for (OffsetT row = 0; row < matrix.num_rows; ++row)
|
1137 |
-
{
|
1138 |
-
relabel_indices[row] = -1;
|
1139 |
-
unlabeled.insert(row);
|
1140 |
-
}
|
1141 |
-
|
1142 |
-
// Initialize queue set
|
1143 |
-
std::deque<OffsetT> q;
|
1144 |
-
|
1145 |
-
// Process unlabeled vertices (traverse connected components)
|
1146 |
-
OffsetT relabel_idx = 0;
|
1147 |
-
while (!unlabeled.empty())
|
1148 |
-
{
|
1149 |
-
// Seed the unvisited frontier queue with the unlabeled vertex of lowest-degree
|
1150 |
-
OffsetT vertex = *unlabeled.begin();
|
1151 |
-
q.push_back(vertex);
|
1152 |
-
|
1153 |
-
while (!q.empty())
|
1154 |
-
{
|
1155 |
-
vertex = q.front();
|
1156 |
-
q.pop_front();
|
1157 |
-
|
1158 |
-
if (relabel_indices[vertex] == -1)
|
1159 |
-
{
|
1160 |
-
// Update this vertex
|
1161 |
-
unlabeled.erase(vertex);
|
1162 |
-
relabel_indices[vertex] = relabel_idx;
|
1163 |
-
relabel_idx++;
|
1164 |
-
|
1165 |
-
// Sort neighbors by degree
|
1166 |
-
OrderByLow<OffsetT> neighbor_comp(row_degrees_in);
|
1167 |
-
std::sort(
|
1168 |
-
matrix.column_indices + matrix.row_offsets[vertex],
|
1169 |
-
matrix.column_indices + matrix.row_offsets[vertex + 1],
|
1170 |
-
neighbor_comp);
|
1171 |
-
|
1172 |
-
// Inspect neighbors, adding to the out frontier if unlabeled
|
1173 |
-
for (OffsetT neighbor_idx = matrix.row_offsets[vertex];
|
1174 |
-
neighbor_idx < matrix.row_offsets[vertex + 1];
|
1175 |
-
++neighbor_idx)
|
1176 |
-
{
|
1177 |
-
OffsetT neighbor = matrix.column_indices[neighbor_idx];
|
1178 |
-
q.push_back(neighbor);
|
1179 |
-
}
|
1180 |
-
}
|
1181 |
-
}
|
1182 |
-
}
|
1183 |
-
|
1184 |
-
/*
|
1185 |
-
// Reverse labels
|
1186 |
-
for (int row = 0; row < matrix.num_rows; ++row)
|
1187 |
-
{
|
1188 |
-
relabel_indices[row] = matrix.num_rows - relabel_indices[row] - 1;
|
1189 |
-
}
|
1190 |
-
*/
|
1191 |
-
|
1192 |
-
// Cleanup
|
1193 |
-
if (row_degrees_in) delete[] row_degrees_in;
|
1194 |
-
if (row_degrees_out) delete[] row_degrees_out;
|
1195 |
-
}
|
1196 |
-
|
1197 |
-
|
1198 |
-
/**
|
1199 |
-
* Reverse Cuthill-McKee
|
1200 |
-
*/
|
1201 |
-
template <typename ValueT, typename OffsetT>
|
1202 |
-
void RcmRelabel(
|
1203 |
-
CsrMatrix<ValueT, OffsetT>& matrix,
|
1204 |
-
bool verbose = false)
|
1205 |
-
{
|
1206 |
-
// Do not process if not square
|
1207 |
-
if (matrix.num_cols != matrix.num_rows)
|
1208 |
-
{
|
1209 |
-
if (verbose) {
|
1210 |
-
printf("RCM transformation ignored (not square)\n"); fflush(stdout);
|
1211 |
-
}
|
1212 |
-
return;
|
1213 |
-
}
|
1214 |
-
|
1215 |
-
// Initialize relabel indices
|
1216 |
-
OffsetT* relabel_indices = new OffsetT[matrix.num_rows];
|
1217 |
-
|
1218 |
-
if (verbose) {
|
1219 |
-
printf("RCM relabeling... "); fflush(stdout);
|
1220 |
-
}
|
1221 |
-
|
1222 |
-
RcmRelabel(matrix, relabel_indices);
|
1223 |
-
|
1224 |
-
if (verbose) {
|
1225 |
-
printf("done. Reconstituting... "); fflush(stdout);
|
1226 |
-
}
|
1227 |
-
|
1228 |
-
// Create a COO matrix from the relabel indices
|
1229 |
-
CooMatrix<ValueT, OffsetT> coo_matrix;
|
1230 |
-
coo_matrix.InitCsrRelabel(matrix, relabel_indices);
|
1231 |
-
|
1232 |
-
// Reconstitute the CSR matrix from the sorted COO tuples
|
1233 |
-
if (relabel_indices) delete[] relabel_indices;
|
1234 |
-
matrix.Clear();
|
1235 |
-
matrix.FromCoo(coo_matrix);
|
1236 |
-
|
1237 |
-
if (verbose) {
|
1238 |
-
printf("done. "); fflush(stdout);
|
1239 |
-
}
|
1240 |
-
}
|
1241 |
-
|
1242 |
-
|
1243 |
-
|
1244 |
-
|
|
|
|
|
|
|
|
|
|
|
|
|
|
|
|
|
|
|
|
|
|
|
|
|
|
|
|
|
|
|
|
|
|
|
|
|
|
|
|
|
|
|
|
|
|
|
|
|
|
|
|
|
|
|
|
|
|
|
|
|
|
|
|
|
|
|
|
|
|
|
|
|
|
|
|
|
|
|
|
|
|
|
|
|
|
|
|
|
|
|
|
|
|
|
|
|
|
|
|
|
|
|
|
|
|
|
|
|
|
|
|
|
|
|
|
|
|
|
|
|
|
|
|
|
|
|
|
|
|
|
|
|
|
|
|
|
|
|
|
|
|
|
|
|
|
|
|
|
|
|
|
|
|
|
|
|
|
|
|
|
|
|
|
|
|
|
|
|
|
|
|
|
|
|
|
|
|
|
|
|
|
|
|
|
|
|
|
|
|
|
|
|
|
|
|
|
|
|
|
|
|
|
|
|
|
|
|
|
|
|
|
|
|
|
|
|
|
|
|
|
|
|
|
|
|
|
|
|
|
|
|
|
|
|
|
|
|
|
|
|
|
|
|
|
|
|
|
|
|
|
|
|
|
|
|
|
|
|
|
|
|
|
|
|
|
|
|
|
|
|
|
|
|
|
|
|
|
|
|
|
|
|
|
|
|
|
|
|
|
|
|
|
|
|
|
|
|
|
|
|
|
|
|
|
|
|
|
|
|
|
|
|
|
|
|
|
|
|
|
|
|
|
|
|
|
|
|
|
|
|
|
|
|
|
|
|
|
|
|
|
|
|
|
|
|
|
|
|
|
|
|
|
|
|
|
|
|
|
|
|
|
|
|
|
|
|
|
|
|
|
|
|
|
|
|
|
|
|
|
|
|
|
|
|
|
|
|
|
|
|
|
|
|
|
|
|
|
|
|
|
|
|
|
|
|
|
|
|
|
|
|
|
|
|
|
|
|
|
|
|
|
|
|
|
|
|
|
|
|
|
|
|
|
|
|
|
|
|
|
|
|
|
|
|
|
|
|
|
|
|
|
|
|
|
|
|
|
|
|
|
|
|
|
|
|
|
|
|
|
|
|
|
|
|
|
|
|
|
|
|
|
|
|
|
|
|
|
|
|
|
|
|
|
|
|
|
|
|
|
|
|
|
|
|
|
|
|
|
|
|
|
|
|
|
|
|
|
|
|
|
|
|
|
|
|
|
|
|
|
|
|
|
|
|
|
|
|
|
|
|
|
|
|
|
|
|
|
|
|
|
|
|
|
|
|
|
|
|
|
|
|
|
|
|
|
|
|
|
|
|
|
|
|
|
|
|
|
|
|
|
|
|
|
|
|
|
|
|
|
|
|
|
|
|
|
|
|
|
|
|
|
|
|
|
|
|
|
|
|
|
|
|
|
|
|
|
|
|
|
|
|
|
|
|
|
|
|
|
|
|
|
|
|
|
|
|
|
|
|
|
|
|
|
|
|
|
|
|
|
|
|
|
|
|
|
|
|
|
|
|
|
|
|
|
|
|
|
|
|
|
|
|
|
|
|
|
|
|
|
|
|
|
|
|
|
|
|
|
|
|
|
|
|
|
|
|
|
|
|
|
|
|
|
|
|
|
|
|
|
|
|
|
|
|
|
|
|
|
|
|
|
|
|
|
|
|
|
|
|
|
|
|
|
|
|
|
|
|
|
|
|
|
|
|
|
|
|
|
|
|
|
|
|
|
|
|
|
|
|
|
|
|
|
|
|
|
|
|
|
|
|
|
|
|
|
|
|
|
|
|
|
|
|
|
|
|
|
|
|
|
|
|
|
|
|
|
|
|
|
|
|
|
|
|
|
|
|
|
|
|
|
|
|
|
|
|
|
|
|
|
|
|
|
|
|
|
|
|
|
|
|
|
|
|
|
|
|
|
|
|
|
|
|
|
|
|
|
|
|
|
|
|
|
|
|
|
|
|
|
|
|
|
|
|
|
|
|
|
|
|
|
|
|
|
|
|
|
|
|
|
|
|
|
|
|
|
|
|
|
|
|
|
|
|
|
|
|
|
|
|
|
|
|
|
|
|
|
|
|
|
|
|
|
|
|
|
|
|
|
|
|
|
|
|
|
|
|
|
|
|
|
|
|
|
|
|
|
|
|
|
|
|
|
|
|
|
|
|
|
|
|
|
|
|
|
|
|
|
|
|
|
|
|
|
|
|
|
|
|
|
|
|
|
|
|
|
|
|
|
|
|
|
|
|
|
|
|
|
|
|
|
|
|
|
|
|
|
|
|
|
|
|
|
|
|
|
|
|
|
|
|
|
|
|
|
|
|
|
|
|
|
|
|
|
|
|
|
|
|
|
|
|
|
|
|
|
|
|
|
|
|
|
|
|
|
|
|
|
|
|
|
|
|
|
|
|
|
|
|
|
|
|
|
|
|
|
|
|
|
|
|
|
|
|
|
|
|
|
|
|
|
|
|
|
|
|
|
|
|
|
|
|
|
|
|
|
|
|
|
|
|
|
|
|
|
|
|
|
|
|
|
|
|
|
|
|
|
|
|
|
|
|
|
|
|
|
|
|
|
|
|
|
|
|
|
|
|
|
|
|
|
|
|
|
|
|
|
|
|
|
|
|
|
|
|
|
|
|
|
|
|
|
|
|
|
|
|
|
|
|
|
|
|
|
|
|
|
|
|
|
|
|
|
|
|
|
|
|
|
|
|
|
|
|
|
|
|
|
|
|
|
|
|
|
|
|
|
|
|
|
|
|
|
|
|
|
|
|
|
|
|
|
|
|
|
|
|
|
|
|
|
|
|
|
|
|
|
|
|
|
|
|
|
|
|
|
|
|
|
|
|
|
|
|
|
|
|
|
|
|
|
|
|
|
|
|
|
|
|
|
|
|
|
|
|
|
|
|
|
|
|
|
|
|
|
|
|
|
|
|
|
|
|
|
|
|
|
|
|
|
|
|
|
|
|
|
|
|
|
|
|
|
|
|
|
|
|
|
|
|
|
|
|
|
|
|
|
|
|
|
|
|
|
|
|
|
|
|
|
|
|
|
|
|
|
|
|
|
|
|
|
|
|
|
|
|
|
|
|
|
|
|
|
|
|
|
|
|
|
|
|
|
|
|
|
|
|
|
|
|
|
|
|
|
|
|
|
|
|
|
|
|
|
|
|
|
|
|
|
|
|
|
|
|
|
|
|
|
|
|
|
|
|
|
|
|
|
|
|
|
|
|
|
|
|
|
|
|
|
|
|
|
|
|
|
|
|
|
|
|
|
|
|
|
|
|
|
|
|
|
|
|
|
|
|
|
|
|
|
|
|
|
|
|
|
|
|
|
|
|
|
|
|
|
|
|
|
|
|
|
|
|
|
|
|
|
|
|
|
|
|
|
|
|
|
|
|
|
|
|
|
|
|
|
|
|
|
|
|
|
|
|
|
|
|
|
|
|
|
|
|
|
|
|
|
|
|
|
|
|
|
|
|
|
|
|
|
|
|
|
|
|
|
|
|
|
|
|
|
|
|
|
|
|
|
|
|
|
|
|
|
|
|
|
|
|
|
|
|
|
|
|
|
|
|
|
|
|
|
|
|
|
|
|
|
|
|
|
|
|
|
|
|
|
|
|
|
|
|
|
|
|
|
|
|
|
|
|
|
|
|
|
|
|
|
|
|
|
|
|
|
|
|
|
|
|
|
|
|
|
|
|
|
|
|
|
|
|
|
|
|
|
|
|
|
|
|
|
|
|
|
|
|
|
|
|
|
|
|
|
|
|
|
|
|
|
|
|
|
|
|
|
|
|
|
|
|
|
|
|
|
|
|
|
|
|
|
|
|
|
|
|
|
|
|
|
|
|
|
|
|
|
|
|
|
|
|
|
|
|
|
|
|
|
|
|
|
|
|
|
|
|
|
|
|
|
|
|
|
|
|
|
|
|
|
|
|
|
|
|
|
|
|
|
|
|
|
|
|
|
|
|
|
|
|
|
|
|
|
|
|
|
|
|
|
|
|
|
|
|
|
|
|
|
|
|
|
|
|
|
|
|
|
|
|
|
|
|
|
|
|
|
|
|
|
|
|
|
|
|
|
|
|
|
|
|
|
|
|
|
|
|
|
|
|
|
|
|
|
|
|
|
|
|
|
|
|
|
|
|
|
|
|
|
|
|
|
|
|
|
|
|
|
|
|
|
|
|
|
|
|
|
|
|
|
|
|
|
|
|
|
|
|
|
|
|
|
|
|
|
|
|
|
|
|
|
|
|
|
|
|
|
|
|
|
|
|
|
|
|
|
|
|
|
|
|
|
|
|
|
|
|
|
|
|
|
|
|
|
|
|
|
|
|
|
|
|
|
|
|
|
|
|
|
|
|
|
|
|
|
|
|
|
|
|
|
|
|
|
|
|
|
|
|
|
|
|
|
|
|
|
|
|
|
|
|
|
|
|
|
|
|
|
|
|
|
|
|
|
|
|
|
|
|
|
|
|
|
|
|
|
|
|
|
|
|
|
|
|
|
|
|
|
|
|
|
|
|
|
|
|
|
|
|
|
|
|
|
|
|
|
|
|
|
|
|
|
|
|
|
|
|
|
|
|
|
|
|
|
|
|
|
|
|
|
|
|
|
|
|
|
|
|
|
|
|
|
|
|
|
|
|
|
|
|
|
|
|
|
|
|
|
|
|
|
|
|
|
|
|
|
|
|
|
|
|
|
|
|
|
|
|
|
|
|
|
|
|
|
|
|
|
|
|
|
|
|
|
|
|
|
|
|
|
|
|
|
|
|
|
|
|
|
|
|
|
|
|
|
|
|
|
|
|
|
|
|
|
|
|
|
|
|
|
|
|
|
|
|
|
|
|
|
|
|
|
|
|
|
|
|
|
|
|
|
|
|
|
|
|
|
|
|
|
|
|
|
|
|
|
|
|
|
|
|
|
|
|
|
|
|
|
|
|
|
|
|
|
|
|
|
|
|
|
|
|
|
|
|
|
|
|
|
|
|
|
|
|
|
|
|
|
|
|
|
|
|
|
|
|
|
|
|
|
|
|
|
|
|
|
|
|
|
|
|
|
|
|
|
|
|
|
|
|
|
|
|
|
|
|
|
|
|
|
|
|
|
|
|
|
|
|
|
|
|
|
|
|
|
|
|
|
|
|
|
|
|
|
|
|
|
|
|
|
|
|
|
|
|
|
|
|
|
|
|
|
|
|
|
|
|
|
|
|
|
|
|
|
|
|
|
|
|
|
|
|
|
|
|
|
|
|
|
|
|
|
|
|
|
|
|
|
|
|
|
|
|
|
|
|
|
|
|
|
|
|
|
|
|
|
|
|
|
|
|
|
|
|
|
|
|
|
|
|
|
|
|
|
|
|
|
|
|
|
|
|
|
|
|
|
|
|
|
|
|
|
|
|
|
|
|
|
|
|
|
|
|
|
|
|
|
|
|
|
|
|
|
|
|
|
|
|
|
|
|
|
|
|
|
|
|
|
|
|
|
|
|
|
|
|
|
|
|
|
|
|
|
|
|
|
|
|
|
|
|
|
|
|
|
|
|
|
|
|
|
|
|
|
|
|
|
|
|
|
|
|
|
|
|
|
|
|
|
|
|
|
|
|
|
|
|
|
|
|
|
|
|
|
|
spaces/Caoyunkang/Segment-Any-Anomaly/SAM/segment_anything/modeling/transformer.py
DELETED
@@ -1,240 +0,0 @@
|
|
1 |
-
# Copyright (c) Meta Platforms, Inc. and affiliates.
|
2 |
-
# All rights reserved.
|
3 |
-
|
4 |
-
# This source code is licensed under the license found in the
|
5 |
-
# LICENSE file in the root directory of this source tree.
|
6 |
-
|
7 |
-
import torch
|
8 |
-
from torch import Tensor, nn
|
9 |
-
|
10 |
-
import math
|
11 |
-
from typing import Tuple, Type
|
12 |
-
|
13 |
-
from .common import MLPBlock
|
14 |
-
|
15 |
-
|
16 |
-
class TwoWayTransformer(nn.Module):
|
17 |
-
def __init__(
|
18 |
-
self,
|
19 |
-
depth: int,
|
20 |
-
embedding_dim: int,
|
21 |
-
num_heads: int,
|
22 |
-
mlp_dim: int,
|
23 |
-
activation: Type[nn.Module] = nn.ReLU,
|
24 |
-
attention_downsample_rate: int = 2,
|
25 |
-
) -> None:
|
26 |
-
"""
|
27 |
-
A transformer decoder that attends to an input image using
|
28 |
-
queries whose positional embedding is supplied.
|
29 |
-
|
30 |
-
Args:
|
31 |
-
depth (int): number of layers in the transformer
|
32 |
-
embedding_dim (int): the channel dimension for the input embeddings
|
33 |
-
num_heads (int): the number of heads for multihead attention. Must
|
34 |
-
divide embedding_dim
|
35 |
-
mlp_dim (int): the channel dimension internal to the MLP block
|
36 |
-
activation (nn.Module): the activation to use in the MLP block
|
37 |
-
"""
|
38 |
-
super().__init__()
|
39 |
-
self.depth = depth
|
40 |
-
self.embedding_dim = embedding_dim
|
41 |
-
self.num_heads = num_heads
|
42 |
-
self.mlp_dim = mlp_dim
|
43 |
-
self.layers = nn.ModuleList()
|
44 |
-
|
45 |
-
for i in range(depth):
|
46 |
-
self.layers.append(
|
47 |
-
TwoWayAttentionBlock(
|
48 |
-
embedding_dim=embedding_dim,
|
49 |
-
num_heads=num_heads,
|
50 |
-
mlp_dim=mlp_dim,
|
51 |
-
activation=activation,
|
52 |
-
attention_downsample_rate=attention_downsample_rate,
|
53 |
-
skip_first_layer_pe=(i == 0),
|
54 |
-
)
|
55 |
-
)
|
56 |
-
|
57 |
-
self.final_attn_token_to_image = Attention(
|
58 |
-
embedding_dim, num_heads, downsample_rate=attention_downsample_rate
|
59 |
-
)
|
60 |
-
self.norm_final_attn = nn.LayerNorm(embedding_dim)
|
61 |
-
|
62 |
-
def forward(
|
63 |
-
self,
|
64 |
-
image_embedding: Tensor,
|
65 |
-
image_pe: Tensor,
|
66 |
-
point_embedding: Tensor,
|
67 |
-
) -> Tuple[Tensor, Tensor]:
|
68 |
-
"""
|
69 |
-
Args:
|
70 |
-
image_embedding (torch.Tensor): image to attend to. Should be shape
|
71 |
-
B x embedding_dim x h x w for any h and w.
|
72 |
-
image_pe (torch.Tensor): the positional encoding to add to the image. Must
|
73 |
-
have the same shape as image_embedding.
|
74 |
-
point_embedding (torch.Tensor): the embedding to add to the query points.
|
75 |
-
Must have shape B x N_points x embedding_dim for any N_points.
|
76 |
-
|
77 |
-
Returns:
|
78 |
-
torch.Tensor: the processed point_embedding
|
79 |
-
torch.Tensor: the processed image_embedding
|
80 |
-
"""
|
81 |
-
# BxCxHxW -> BxHWxC == B x N_image_tokens x C
|
82 |
-
bs, c, h, w = image_embedding.shape
|
83 |
-
image_embedding = image_embedding.flatten(2).permute(0, 2, 1)
|
84 |
-
image_pe = image_pe.flatten(2).permute(0, 2, 1)
|
85 |
-
|
86 |
-
# Prepare queries
|
87 |
-
queries = point_embedding
|
88 |
-
keys = image_embedding
|
89 |
-
|
90 |
-
# Apply transformer blocks and final layernorm
|
91 |
-
for layer in self.layers:
|
92 |
-
queries, keys = layer(
|
93 |
-
queries=queries,
|
94 |
-
keys=keys,
|
95 |
-
query_pe=point_embedding,
|
96 |
-
key_pe=image_pe,
|
97 |
-
)
|
98 |
-
|
99 |
-
# Apply the final attenion layer from the points to the image
|
100 |
-
q = queries + point_embedding
|
101 |
-
k = keys + image_pe
|
102 |
-
attn_out = self.final_attn_token_to_image(q=q, k=k, v=keys)
|
103 |
-
queries = queries + attn_out
|
104 |
-
queries = self.norm_final_attn(queries)
|
105 |
-
|
106 |
-
return queries, keys
|
107 |
-
|
108 |
-
|
109 |
-
class TwoWayAttentionBlock(nn.Module):
|
110 |
-
def __init__(
|
111 |
-
self,
|
112 |
-
embedding_dim: int,
|
113 |
-
num_heads: int,
|
114 |
-
mlp_dim: int = 2048,
|
115 |
-
activation: Type[nn.Module] = nn.ReLU,
|
116 |
-
attention_downsample_rate: int = 2,
|
117 |
-
skip_first_layer_pe: bool = False,
|
118 |
-
) -> None:
|
119 |
-
"""
|
120 |
-
A transformer block with four layers: (1) self-attention of sparse
|
121 |
-
inputs, (2) cross attention of sparse inputs to dense inputs, (3) mlp
|
122 |
-
block on sparse inputs, and (4) cross attention of dense inputs to sparse
|
123 |
-
inputs.
|
124 |
-
|
125 |
-
Arguments:
|
126 |
-
embedding_dim (int): the channel dimension of the embeddings
|
127 |
-
num_heads (int): the number of heads in the attention layers
|
128 |
-
mlp_dim (int): the hidden dimension of the mlp block
|
129 |
-
activation (nn.Module): the activation of the mlp block
|
130 |
-
skip_first_layer_pe (bool): skip the PE on the first layer
|
131 |
-
"""
|
132 |
-
super().__init__()
|
133 |
-
self.self_attn = Attention(embedding_dim, num_heads)
|
134 |
-
self.norm1 = nn.LayerNorm(embedding_dim)
|
135 |
-
|
136 |
-
self.cross_attn_token_to_image = Attention(
|
137 |
-
embedding_dim, num_heads, downsample_rate=attention_downsample_rate
|
138 |
-
)
|
139 |
-
self.norm2 = nn.LayerNorm(embedding_dim)
|
140 |
-
|
141 |
-
self.mlp = MLPBlock(embedding_dim, mlp_dim, activation)
|
142 |
-
self.norm3 = nn.LayerNorm(embedding_dim)
|
143 |
-
|
144 |
-
self.norm4 = nn.LayerNorm(embedding_dim)
|
145 |
-
self.cross_attn_image_to_token = Attention(
|
146 |
-
embedding_dim, num_heads, downsample_rate=attention_downsample_rate
|
147 |
-
)
|
148 |
-
|
149 |
-
self.skip_first_layer_pe = skip_first_layer_pe
|
150 |
-
|
151 |
-
def forward(
|
152 |
-
self, queries: Tensor, keys: Tensor, query_pe: Tensor, key_pe: Tensor
|
153 |
-
) -> Tuple[Tensor, Tensor]:
|
154 |
-
# Self attention block
|
155 |
-
if self.skip_first_layer_pe:
|
156 |
-
queries = self.self_attn(q=queries, k=queries, v=queries)
|
157 |
-
else:
|
158 |
-
q = queries + query_pe
|
159 |
-
attn_out = self.self_attn(q=q, k=q, v=queries)
|
160 |
-
queries = queries + attn_out
|
161 |
-
queries = self.norm1(queries)
|
162 |
-
|
163 |
-
# Cross attention block, tokens attending to image embedding
|
164 |
-
q = queries + query_pe
|
165 |
-
k = keys + key_pe
|
166 |
-
attn_out = self.cross_attn_token_to_image(q=q, k=k, v=keys)
|
167 |
-
queries = queries + attn_out
|
168 |
-
queries = self.norm2(queries)
|
169 |
-
|
170 |
-
# MLP block
|
171 |
-
mlp_out = self.mlp(queries)
|
172 |
-
queries = queries + mlp_out
|
173 |
-
queries = self.norm3(queries)
|
174 |
-
|
175 |
-
# Cross attention block, image embedding attending to tokens
|
176 |
-
q = queries + query_pe
|
177 |
-
k = keys + key_pe
|
178 |
-
attn_out = self.cross_attn_image_to_token(q=k, k=q, v=queries)
|
179 |
-
keys = keys + attn_out
|
180 |
-
keys = self.norm4(keys)
|
181 |
-
|
182 |
-
return queries, keys
|
183 |
-
|
184 |
-
|
185 |
-
class Attention(nn.Module):
|
186 |
-
"""
|
187 |
-
An attention layer that allows for downscaling the size of the embedding
|
188 |
-
after projection to queries, keys, and values.
|
189 |
-
"""
|
190 |
-
|
191 |
-
def __init__(
|
192 |
-
self,
|
193 |
-
embedding_dim: int,
|
194 |
-
num_heads: int,
|
195 |
-
downsample_rate: int = 1,
|
196 |
-
) -> None:
|
197 |
-
super().__init__()
|
198 |
-
self.embedding_dim = embedding_dim
|
199 |
-
self.internal_dim = embedding_dim // downsample_rate
|
200 |
-
self.num_heads = num_heads
|
201 |
-
assert self.internal_dim % num_heads == 0, "num_heads must divide embedding_dim."
|
202 |
-
|
203 |
-
self.q_proj = nn.Linear(embedding_dim, self.internal_dim)
|
204 |
-
self.k_proj = nn.Linear(embedding_dim, self.internal_dim)
|
205 |
-
self.v_proj = nn.Linear(embedding_dim, self.internal_dim)
|
206 |
-
self.out_proj = nn.Linear(self.internal_dim, embedding_dim)
|
207 |
-
|
208 |
-
def _separate_heads(self, x: Tensor, num_heads: int) -> Tensor:
|
209 |
-
b, n, c = x.shape
|
210 |
-
x = x.reshape(b, n, num_heads, c // num_heads)
|
211 |
-
return x.transpose(1, 2) # B x N_heads x N_tokens x C_per_head
|
212 |
-
|
213 |
-
def _recombine_heads(self, x: Tensor) -> Tensor:
|
214 |
-
b, n_heads, n_tokens, c_per_head = x.shape
|
215 |
-
x = x.transpose(1, 2)
|
216 |
-
return x.reshape(b, n_tokens, n_heads * c_per_head) # B x N_tokens x C
|
217 |
-
|
218 |
-
def forward(self, q: Tensor, k: Tensor, v: Tensor) -> Tensor:
|
219 |
-
# Input projections
|
220 |
-
q = self.q_proj(q)
|
221 |
-
k = self.k_proj(k)
|
222 |
-
v = self.v_proj(v)
|
223 |
-
|
224 |
-
# Separate into heads
|
225 |
-
q = self._separate_heads(q, self.num_heads)
|
226 |
-
k = self._separate_heads(k, self.num_heads)
|
227 |
-
v = self._separate_heads(v, self.num_heads)
|
228 |
-
|
229 |
-
# Attention
|
230 |
-
_, _, _, c_per_head = q.shape
|
231 |
-
attn = q @ k.permute(0, 1, 3, 2) # B x N_heads x N_tokens x N_tokens
|
232 |
-
attn = attn / math.sqrt(c_per_head)
|
233 |
-
attn = torch.softmax(attn, dim=-1)
|
234 |
-
|
235 |
-
# Get output
|
236 |
-
out = attn @ v
|
237 |
-
out = self._recombine_heads(out)
|
238 |
-
out = self.out_proj(out)
|
239 |
-
|
240 |
-
return out
|
|
|
|
|
|
|
|
|
|
|
|
|
|
|
|
|
|
|
|
|
|
|
|
|
|
|
|
|
|
|
|
|
|
|
|
|
|
|
|
|
|
|
|
|
|
|
|
|
|
|
|
|
|
|
|
|
|
|
|
|
|
|
|
|
|
|
|
|
|
|
|
|
|
|
|
|
|
|
|
|
|
|
|
|
|
|
|
|
|
|
|
|
|
|
|
|
|
|
|
|
|
|
|
|
|
|
|
|
|
|
|
|
|
|
|
|
|
|
|
|
|
|
|
|
|
|
|
|
|
|
|
|
|
|
|
|
|
|
|
|
|
|
|
|
|
|
|
|
|
|
|
|
|
|
|
|
|
|
|
|
|
|
|
|
|
|
|
|
|
|
|
|
|
|
|
|
|
|
|
|
|
|
|
|
|
|
|
|
|
|
|
|
|
|
|
|
|
|
|
|
|
|
|
|
|
|
|
|
|
|
|
|
|
|
|
|
|
|
|
|
|
|
|
|
|
|
|
|
|
|
|
|
|
|
|
|
|
|
|
|
|
|
|
|
|
|
|
|
|
|
|
|
|
|
|
|
|
|
|
|
|
|
|
|
|
|
|
|
|
|
|
|
|
|
|
|
|
|
|
|
|
|
|
|
|
|
|
|
|
|
|
|
|
|
|
|
|
|
|
|
|
|
|
|
|
|
|
|
|
|
|
|
|
|
|
|
|
|
|
|
|
|
|
|
|
|
|
|
|
|
|
|
|
|
|
|
|
|
|
|
|
|
|
|
|
|
|
|
|
|
|
|
|
|
|
|
|
|
|
|
|
|
|
|
|
|
|
|
|
|
|
|
|
|
|
|
|
|
|
|
|
|
|
|
|
|
|
|
|
|
|
|
|
|
|
|
|
|
|
|
|
|
|
|
|
|
|
|
|
|
|
|
|
|
|
|
|
|
|
|
|
|
|
|
|
|
|
|
|
|
|
|
|
|
|
|
|
|
|
|
|
|
|
|
|
|
|
|
|
|
|
|
|
|
|
|
|
|
|
|
|
|
|
|
|
|
|
|
|
|
|
|
|
|
|
|
|
|
|
|
|
spaces/CassBunny/anything-v3.0/README.md
DELETED
@@ -1,13 +0,0 @@
|
|
1 |
-
---
|
2 |
-
title: Anything V3.0
|
3 |
-
emoji: 🏃
|
4 |
-
colorFrom: gray
|
5 |
-
colorTo: yellow
|
6 |
-
sdk: gradio
|
7 |
-
sdk_version: 3.10.1
|
8 |
-
app_file: app.py
|
9 |
-
pinned: false
|
10 |
-
duplicated_from: akhaliq/anything-v3.0
|
11 |
-
---
|
12 |
-
|
13 |
-
Check out the configuration reference at https://huggingface.co/docs/hub/spaces-config-reference
|
|
|
|
|
|
|
|
|
|
|
|
|
|
|
|
|
|
|
|
|
|
|
|
|
|
|
|
spaces/Chilangosta/text-to-pokemon/README.md
DELETED
@@ -1,13 +0,0 @@
|
|
1 |
-
---
|
2 |
-
title: Text to Pokémon
|
3 |
-
emoji: 🌖
|
4 |
-
colorFrom: purple
|
5 |
-
colorTo: purple
|
6 |
-
sdk: gradio
|
7 |
-
sdk_version: 3.4
|
8 |
-
app_file: app.py
|
9 |
-
pinned: false
|
10 |
-
duplicated_from: lambdalabs/text-to-pokemon
|
11 |
-
---
|
12 |
-
|
13 |
-
Check out the configuration reference at https://huggingface.co/docs/hub/spaces-config-reference
|
|
|
|
|
|
|
|
|
|
|
|
|
|
|
|
|
|
|
|
|
|
|
|
|
|
|
|
spaces/CofAI/chat/client/css/field.css
DELETED
@@ -1,11 +0,0 @@
|
|
1 |
-
.field {
|
2 |
-
display: flex;
|
3 |
-
align-items: center;
|
4 |
-
padding: 4px;
|
5 |
-
}
|
6 |
-
|
7 |
-
@media screen and (max-width: 990px) {
|
8 |
-
.field {
|
9 |
-
flex-wrap: nowrap;
|
10 |
-
}
|
11 |
-
}
|
|
|
|
|
|
|
|
|
|
|
|
|
|
|
|
|
|
|
|
|
|
|
|
spaces/CofAI/chat/run.py
DELETED
@@ -1,48 +0,0 @@
|
|
1 |
-
import secrets
|
2 |
-
|
3 |
-
from server.bp import bp
|
4 |
-
from server.website import Website
|
5 |
-
from server.backend import Backend_Api
|
6 |
-
from server.babel import create_babel
|
7 |
-
from json import load
|
8 |
-
from flask import Flask
|
9 |
-
|
10 |
-
if __name__ == '__main__':
|
11 |
-
|
12 |
-
# Load configuration from config.json
|
13 |
-
config = load(open('config.json', 'r'))
|
14 |
-
site_config = config['site_config']
|
15 |
-
url_prefix = config.pop('url_prefix')
|
16 |
-
|
17 |
-
# Create the app
|
18 |
-
app = Flask(__name__)
|
19 |
-
app.secret_key = secrets.token_hex(16)
|
20 |
-
|
21 |
-
# Set up Babel
|
22 |
-
create_babel(app)
|
23 |
-
|
24 |
-
# Set up the website routes
|
25 |
-
site = Website(bp, url_prefix)
|
26 |
-
for route in site.routes:
|
27 |
-
bp.add_url_rule(
|
28 |
-
route,
|
29 |
-
view_func=site.routes[route]['function'],
|
30 |
-
methods=site.routes[route]['methods'],
|
31 |
-
)
|
32 |
-
|
33 |
-
# Set up the backend API routes
|
34 |
-
backend_api = Backend_Api(bp, config)
|
35 |
-
for route in backend_api.routes:
|
36 |
-
bp.add_url_rule(
|
37 |
-
route,
|
38 |
-
view_func=backend_api.routes[route]['function'],
|
39 |
-
methods=backend_api.routes[route]['methods'],
|
40 |
-
)
|
41 |
-
|
42 |
-
# Register the blueprint
|
43 |
-
app.register_blueprint(bp, url_prefix=url_prefix)
|
44 |
-
|
45 |
-
# Run the Flask server
|
46 |
-
print(f"Running on {site_config['port']}{url_prefix}")
|
47 |
-
app.run(**site_config)
|
48 |
-
print(f"Closing port {site_config['port']}")
|
|
|
|
|
|
|
|
|
|
|
|
|
|
|
|
|
|
|
|
|
|
|
|
|
|
|
|
|
|
|
|
|
|
|
|
|
|
|
|
|
|
|
|
|
|
|
|
|
|
|
|
|
|
|
|
|
|
|
|
|
|
|
|
|
|
|
|
|
|
|
|
|
|
|
|
|
|
|
|
|
|
|
|
|
|
|
|
|
|
|
|
|
|
|
|
|
|
spaces/DQChoi/gpt-demo/venv/lib/python3.11/site-packages/fontTools/__init__.py
DELETED
@@ -1,8 +0,0 @@
|
|
1 |
-
import logging
|
2 |
-
from fontTools.misc.loggingTools import configLogger
|
3 |
-
|
4 |
-
log = logging.getLogger(__name__)
|
5 |
-
|
6 |
-
version = __version__ = "4.41.0"
|
7 |
-
|
8 |
-
__all__ = ["version", "log", "configLogger"]
|
|
|
|
|
|
|
|
|
|
|
|
|
|
|
|
|
|
spaces/Dao3/Text-To-image-AllModels/README.md
DELETED
@@ -1,14 +0,0 @@
|
|
1 |
-
---
|
2 |
-
title: Text To Image AllModels
|
3 |
-
emoji: 🐠
|
4 |
-
colorFrom: blue
|
5 |
-
colorTo: indigo
|
6 |
-
sdk: gradio
|
7 |
-
sdk_version: 3.15.0
|
8 |
-
app_file: app.py
|
9 |
-
pinned: false
|
10 |
-
license: openrail
|
11 |
-
duplicated_from: BilalSardar/Text-To-image-AllModels
|
12 |
-
---
|
13 |
-
|
14 |
-
Check out the configuration reference at https://huggingface.co/docs/hub/spaces-config-reference
|
|
|
|
|
|
|
|
|
|
|
|
|
|
|
|
|
|
|
|
|
|
|
|
|
|
|
|
|
|
spaces/Demi2809/rvc-models/app.py
DELETED
@@ -1,180 +0,0 @@
|
|
1 |
-
import os
|
2 |
-
import json
|
3 |
-
import argparse
|
4 |
-
import traceback
|
5 |
-
import logging
|
6 |
-
import gradio as gr
|
7 |
-
import numpy as np
|
8 |
-
import librosa
|
9 |
-
import torch
|
10 |
-
import asyncio
|
11 |
-
import edge_tts
|
12 |
-
from datetime import datetime
|
13 |
-
from fairseq import checkpoint_utils
|
14 |
-
from infer_pack.models import SynthesizerTrnMs256NSFsid, SynthesizerTrnMs256NSFsid_nono
|
15 |
-
from vc_infer_pipeline import VC
|
16 |
-
from config import (
|
17 |
-
is_half,
|
18 |
-
device
|
19 |
-
)
|
20 |
-
logging.getLogger("numba").setLevel(logging.WARNING)
|
21 |
-
limitation = os.getenv("SYSTEM") == "spaces" # limit audio length in huggingface spaces
|
22 |
-
|
23 |
-
def create_vc_fn(tgt_sr, net_g, vc, if_f0, file_index, file_big_npy):
|
24 |
-
def vc_fn(
|
25 |
-
input_audio,
|
26 |
-
f0_up_key,
|
27 |
-
f0_method,
|
28 |
-
index_rate,
|
29 |
-
tts_mode,
|
30 |
-
tts_text,
|
31 |
-
tts_voice
|
32 |
-
):
|
33 |
-
try:
|
34 |
-
if tts_mode:
|
35 |
-
if len(tts_text) > 100 and limitation:
|
36 |
-
return "Text is too long", None
|
37 |
-
if tts_text is None or tts_voice is None:
|
38 |
-
return "You need to enter text and select a voice", None
|
39 |
-
asyncio.run(edge_tts.Communicate(tts_text, "-".join(tts_voice.split('-')[:-1])).save("tts.mp3"))
|
40 |
-
audio, sr = librosa.load("tts.mp3", sr=16000, mono=True)
|
41 |
-
else:
|
42 |
-
if input_audio is None:
|
43 |
-
return "You need to upload an audio", None
|
44 |
-
sampling_rate, audio = input_audio
|
45 |
-
duration = audio.shape[0] / sampling_rate
|
46 |
-
if duration > 20 and limitation:
|
47 |
-
return "Please upload an audio file that is less than 20 seconds. If you need to generate a longer audio file, please use Colab.", None
|
48 |
-
audio = (audio / np.iinfo(audio.dtype).max).astype(np.float32)
|
49 |
-
if len(audio.shape) > 1:
|
50 |
-
audio = librosa.to_mono(audio.transpose(1, 0))
|
51 |
-
if sampling_rate != 16000:
|
52 |
-
audio = librosa.resample(audio, orig_sr=sampling_rate, target_sr=16000)
|
53 |
-
times = [0, 0, 0]
|
54 |
-
f0_up_key = int(f0_up_key)
|
55 |
-
audio_opt = vc.pipeline(
|
56 |
-
hubert_model,
|
57 |
-
net_g,
|
58 |
-
0,
|
59 |
-
audio,
|
60 |
-
times,
|
61 |
-
f0_up_key,
|
62 |
-
f0_method,
|
63 |
-
file_index,
|
64 |
-
file_big_npy,
|
65 |
-
index_rate,
|
66 |
-
if_f0,
|
67 |
-
)
|
68 |
-
print(
|
69 |
-
f"[{datetime.now().strftime('%Y-%m-%d %H:%M')}]: npy: {times[0]}, f0: {times[1]}s, infer: {times[2]}s"
|
70 |
-
)
|
71 |
-
return "Success", (tgt_sr, audio_opt)
|
72 |
-
except:
|
73 |
-
info = traceback.format_exc()
|
74 |
-
print(info)
|
75 |
-
return info, (None, None)
|
76 |
-
return vc_fn
|
77 |
-
|
78 |
-
def load_hubert():
|
79 |
-
global hubert_model
|
80 |
-
models, _, _ = checkpoint_utils.load_model_ensemble_and_task(
|
81 |
-
["hubert_base.pt"],
|
82 |
-
suffix="",
|
83 |
-
)
|
84 |
-
hubert_model = models[0]
|
85 |
-
hubert_model = hubert_model.to(device)
|
86 |
-
if is_half:
|
87 |
-
hubert_model = hubert_model.half()
|
88 |
-
else:
|
89 |
-
hubert_model = hubert_model.float()
|
90 |
-
hubert_model.eval()
|
91 |
-
|
92 |
-
def change_to_tts_mode(tts_mode):
|
93 |
-
if tts_mode:
|
94 |
-
return gr.Audio.update(visible=False), gr.Textbox.update(visible=True), gr.Dropdown.update(visible=True)
|
95 |
-
else:
|
96 |
-
return gr.Audio.update(visible=True), gr.Textbox.update(visible=False), gr.Dropdown.update(visible=False)
|
97 |
-
|
98 |
-
if __name__ == '__main__':
|
99 |
-
parser = argparse.ArgumentParser()
|
100 |
-
parser.add_argument('--api', action="store_true", default=False)
|
101 |
-
parser.add_argument("--colab", action="store_true", default=False, help="share gradio app")
|
102 |
-
args, unknown = parser.parse_known_args()
|
103 |
-
load_hubert()
|
104 |
-
models = []
|
105 |
-
tts_voice_list = asyncio.get_event_loop().run_until_complete(edge_tts.list_voices())
|
106 |
-
voices = [f"{v['ShortName']}-{v['Gender']}" for v in tts_voice_list]
|
107 |
-
with open("weights/model_info.json", "r", encoding="utf-8") as f:
|
108 |
-
models_info = json.load(f)
|
109 |
-
for name, info in models_info.items():
|
110 |
-
if not info['enable']:
|
111 |
-
continue
|
112 |
-
title = info['title']
|
113 |
-
author = info.get("author", None)
|
114 |
-
cover = f"weights/{name}/{info['cover']}"
|
115 |
-
index = f"weights/{name}/{info['feature_retrieval_library']}"
|
116 |
-
npy = f"weights/{name}/{info['feature_file']}"
|
117 |
-
cpt = torch.load(f"weights/{name}/{name}.pth", map_location="cpu")
|
118 |
-
tgt_sr = cpt["config"][-1]
|
119 |
-
cpt["config"][-3] = cpt["weight"]["emb_g.weight"].shape[0] # n_spk
|
120 |
-
if_f0 = cpt.get("f0", 1)
|
121 |
-
if if_f0 == 1:
|
122 |
-
net_g = SynthesizerTrnMs256NSFsid(*cpt["config"], is_half=is_half)
|
123 |
-
else:
|
124 |
-
net_g = SynthesizerTrnMs256NSFsid_nono(*cpt["config"])
|
125 |
-
del net_g.enc_q
|
126 |
-
print(net_g.load_state_dict(cpt["weight"], strict=False)) # 不加这一行清不干净, 真奇葩
|
127 |
-
net_g.eval().to(device)
|
128 |
-
if is_half:
|
129 |
-
net_g = net_g.half()
|
130 |
-
else:
|
131 |
-
net_g = net_g.float()
|
132 |
-
vc = VC(tgt_sr, device, is_half)
|
133 |
-
models.append((name, title, author, cover, create_vc_fn(tgt_sr, net_g, vc, if_f0, index, npy)))
|
134 |
-
with gr.Blocks() as app:
|
135 |
-
gr.Markdown(
|
136 |
-
"# <center> RVC Models (Outdated)\n"
|
137 |
-
"## <center> The input audio should be clean and pure voice without background music.\n"
|
138 |
-
"### <center> Updated Repository: [NEW RVC Models](https://huggingface.co/spaces/ArkanDash/rvc-models-new).\n"
|
139 |
-
"#### <center> [Recommended to use google colab for more features](https://colab.research.google.com/drive/1hx6kKvIuv5XNY1Gai2PEuZhpO5z6xpVh?usp=sharing)\n"
|
140 |
-
"[](https://colab.research.google.com/drive/1hx6kKvIuv5XNY1Gai2PEuZhpO5z6xpVh?usp=sharing)\n\n"
|
141 |
-
"[](https://github.com/RVC-Project/Retrieval-based-Voice-Conversion-WebUI)"
|
142 |
-
)
|
143 |
-
with gr.Tabs():
|
144 |
-
for (name, title, author, cover, vc_fn) in models:
|
145 |
-
with gr.TabItem(name):
|
146 |
-
with gr.Row():
|
147 |
-
gr.Markdown(
|
148 |
-
'<div align="center">'
|
149 |
-
f'<div>{title}</div>\n'+
|
150 |
-
(f'<div>Model author: {author}</div>' if author else "")+
|
151 |
-
(f'<img style="width:auto;height:300px;" src="file/{cover}">' if cover else "")+
|
152 |
-
'</div>'
|
153 |
-
)
|
154 |
-
with gr.Row():
|
155 |
-
with gr.Column():
|
156 |
-
vc_input = gr.Audio(label="Input audio"+' (less than 20 seconds)' if limitation else '')
|
157 |
-
vc_transpose = gr.Number(label="Transpose", value=0)
|
158 |
-
vc_f0method = gr.Radio(
|
159 |
-
label="Pitch extraction algorithm, PM is fast but Harvest is better for low frequencies",
|
160 |
-
choices=["pm", "harvest"],
|
161 |
-
value="pm",
|
162 |
-
interactive=True,
|
163 |
-
)
|
164 |
-
vc_index_ratio = gr.Slider(
|
165 |
-
minimum=0,
|
166 |
-
maximum=1,
|
167 |
-
label="Retrieval feature ratio",
|
168 |
-
value=0.6,
|
169 |
-
interactive=True,
|
170 |
-
)
|
171 |
-
tts_mode = gr.Checkbox(label="tts (use edge-tts as input)", value=False)
|
172 |
-
tts_text = gr.Textbox(visible=False,label="TTS text (100 words limitation)" if limitation else "TTS text")
|
173 |
-
tts_voice = gr.Dropdown(label="Edge-tts speaker", choices=voices, visible=False, allow_custom_value=False, value="en-US-AnaNeural-Female")
|
174 |
-
vc_submit = gr.Button("Generate", variant="primary")
|
175 |
-
with gr.Column():
|
176 |
-
vc_output1 = gr.Textbox(label="Output Message")
|
177 |
-
vc_output2 = gr.Audio(label="Output Audio")
|
178 |
-
vc_submit.click(vc_fn, [vc_input, vc_transpose, vc_f0method, vc_index_ratio, tts_mode, tts_text, tts_voice], [vc_output1, vc_output2])
|
179 |
-
tts_mode.change(change_to_tts_mode, [tts_mode], [vc_input, tts_text, tts_voice])
|
180 |
-
app.queue(concurrency_count=1, max_size=20, api_open=args.api).launch(share=args.colab)
|
|
|
|
|
|
|
|
|
|
|
|
|
|
|
|
|
|
|
|
|
|
|
|
|
|
|
|
|
|
|
|
|
|
|
|
|
|
|
|
|
|
|
|
|
|
|
|
|
|
|
|
|
|
|
|
|
|
|
|
|
|
|
|
|
|
|
|
|
|
|
|
|
|
|
|
|
|
|
|
|
|
|
|
|
|
|
|
|
|
|
|
|
|
|
|
|
|
|
|
|
|
|
|
|
|
|
|
|
|
|
|
|
|
|
|
|
|
|
|
|
|
|
|
|
|
|
|
|
|
|
|
|
|
|
|
|
|
|
|
|
|
|
|
|
|
|
|
|
|
|
|
|
|
|
|
|
|
|
|
|
|
|
|
|
|
|
|
|
|
|
|
|
|
|
|
|
|
|
|
|
|
|
|
|
|
|
|
|
|
|
|
|
|
|
|
|
|
|
|
|
|
|
|
|
|
|
|
|
|
|
|
|
|
|
|
|
|
|
|
|
|
|
|
|
|
|
|
|
|
|
|
|
|
|
|
|
|
|
|
|
|
|
|
|
|
|
|
|
|
|
|
|
|
|
|
|
|
|
|
|
|
|
|
|
|
|
|
|
|
|
|
|
|
|
|
|
|
|
|
|
|
|
|
|
|
|
|
|
|
|
|
|
|
|
|
|
|
|
|
|
|
|
|
|
|
|
|
|
|
|
|
|
|
|
|
|
|
|
|
|
|
|
|
|
|
|
|
|
|
|
|
|
|
|
|
|
|
|
|
|
|
|
|
|
|
|
|
|
|
|
|
|
|
|
|
|
|
|
|
|
|
spaces/DemoLou/moe-tts/README.md
DELETED
@@ -1,14 +0,0 @@
|
|
1 |
-
---
|
2 |
-
title: Moe TTS
|
3 |
-
emoji: 😊🎙️
|
4 |
-
colorFrom: red
|
5 |
-
colorTo: pink
|
6 |
-
sdk: gradio
|
7 |
-
sdk_version: 3.22.1
|
8 |
-
app_file: test.py
|
9 |
-
pinned: false
|
10 |
-
license: mit
|
11 |
-
duplicated_from: skytnt/moe-tts
|
12 |
-
---
|
13 |
-
|
14 |
-
Check out the configuration reference at https://huggingface.co/docs/hub/spaces-config-reference
|
|
|
|
|
|
|
|
|
|
|
|
|
|
|
|
|
|
|
|
|
|
|
|
|
|
|
|
|
|
spaces/Dinoking/Guccio-AI-Designer/netdissect/tool/allunitsample.py
DELETED
@@ -1,199 +0,0 @@
|
|
1 |
-
'''
|
2 |
-
A simple tool to generate sample of output of a GAN,
|
3 |
-
subject to filtering, sorting, or intervention.
|
4 |
-
'''
|
5 |
-
|
6 |
-
import torch, numpy, os, argparse, sys, shutil, errno, numbers
|
7 |
-
from PIL import Image
|
8 |
-
from torch.utils.data import TensorDataset
|
9 |
-
from netdissect.zdataset import standard_z_sample
|
10 |
-
from netdissect.progress import default_progress, verbose_progress
|
11 |
-
from netdissect.autoeval import autoimport_eval
|
12 |
-
from netdissect.workerpool import WorkerBase, WorkerPool
|
13 |
-
from netdissect.nethook import retain_layers
|
14 |
-
from netdissect.runningstats import RunningTopK
|
15 |
-
|
16 |
-
def main():
|
17 |
-
parser = argparse.ArgumentParser(description='GAN sample making utility')
|
18 |
-
parser.add_argument('--model', type=str, default=None,
|
19 |
-
help='constructor for the model to test')
|
20 |
-
parser.add_argument('--pthfile', type=str, default=None,
|
21 |
-
help='filename of .pth file for the model')
|
22 |
-
parser.add_argument('--outdir', type=str, default='images',
|
23 |
-
help='directory for image output')
|
24 |
-
parser.add_argument('--size', type=int, default=100,
|
25 |
-
help='number of images to output')
|
26 |
-
parser.add_argument('--test_size', type=int, default=None,
|
27 |
-
help='number of images to test')
|
28 |
-
parser.add_argument('--layer', type=str, default=None,
|
29 |
-
help='layer to inspect')
|
30 |
-
parser.add_argument('--seed', type=int, default=1,
|
31 |
-
help='seed')
|
32 |
-
parser.add_argument('--quiet', action='store_true', default=False,
|
33 |
-
help='silences console output')
|
34 |
-
if len(sys.argv) == 1:
|
35 |
-
parser.print_usage(sys.stderr)
|
36 |
-
sys.exit(1)
|
37 |
-
args = parser.parse_args()
|
38 |
-
verbose_progress(not args.quiet)
|
39 |
-
|
40 |
-
# Instantiate the model
|
41 |
-
model = autoimport_eval(args.model)
|
42 |
-
if args.pthfile is not None:
|
43 |
-
data = torch.load(args.pthfile)
|
44 |
-
if 'state_dict' in data:
|
45 |
-
meta = {}
|
46 |
-
for key in data:
|
47 |
-
if isinstance(data[key], numbers.Number):
|
48 |
-
meta[key] = data[key]
|
49 |
-
data = data['state_dict']
|
50 |
-
model.load_state_dict(data)
|
51 |
-
# Unwrap any DataParallel-wrapped model
|
52 |
-
if isinstance(model, torch.nn.DataParallel):
|
53 |
-
model = next(model.children())
|
54 |
-
# Examine first conv in model to determine input feature size.
|
55 |
-
first_layer = [c for c in model.modules()
|
56 |
-
if isinstance(c, (torch.nn.Conv2d, torch.nn.ConvTranspose2d,
|
57 |
-
torch.nn.Linear))][0]
|
58 |
-
# 4d input if convolutional, 2d input if first layer is linear.
|
59 |
-
if isinstance(first_layer, (torch.nn.Conv2d, torch.nn.ConvTranspose2d)):
|
60 |
-
z_channels = first_layer.in_channels
|
61 |
-
spatialdims = (1, 1)
|
62 |
-
else:
|
63 |
-
z_channels = first_layer.in_features
|
64 |
-
spatialdims = ()
|
65 |
-
# Instrument the model
|
66 |
-
retain_layers(model, [args.layer])
|
67 |
-
model.cuda()
|
68 |
-
|
69 |
-
if args.test_size is None:
|
70 |
-
args.test_size = args.size * 20
|
71 |
-
z_universe = standard_z_sample(args.test_size, z_channels,
|
72 |
-
seed=args.seed)
|
73 |
-
z_universe = z_universe.view(tuple(z_universe.shape) + spatialdims)
|
74 |
-
indexes = get_all_highest_znums(
|
75 |
-
model, z_universe, args.size, seed=args.seed)
|
76 |
-
save_chosen_unit_images(args.outdir, model, z_universe, indexes,
|
77 |
-
lightbox=True)
|
78 |
-
|
79 |
-
|
80 |
-
def get_all_highest_znums(model, z_universe, size,
|
81 |
-
batch_size=10, seed=1):
|
82 |
-
# The model should have been instrumented already
|
83 |
-
retained_items = list(model.retained.items())
|
84 |
-
assert len(retained_items) == 1
|
85 |
-
layer = retained_items[0][0]
|
86 |
-
# By default, a 10% sample
|
87 |
-
progress = default_progress()
|
88 |
-
num_units = None
|
89 |
-
with torch.no_grad():
|
90 |
-
# Pass 1: collect max activation stats
|
91 |
-
z_loader = torch.utils.data.DataLoader(TensorDataset(z_universe),
|
92 |
-
batch_size=batch_size, num_workers=2,
|
93 |
-
pin_memory=True)
|
94 |
-
rtk = RunningTopK(k=size)
|
95 |
-
for [z] in progress(z_loader, desc='Finding max activations'):
|
96 |
-
z = z.cuda()
|
97 |
-
model(z)
|
98 |
-
feature = model.retained[layer]
|
99 |
-
num_units = feature.shape[1]
|
100 |
-
max_feature = feature.view(
|
101 |
-
feature.shape[0], num_units, -1).max(2)[0]
|
102 |
-
rtk.add(max_feature)
|
103 |
-
td, ti = rtk.result()
|
104 |
-
highest = ti.sort(1)[0]
|
105 |
-
return highest
|
106 |
-
|
107 |
-
def save_chosen_unit_images(dirname, model, z_universe, indices,
|
108 |
-
shared_dir="shared_images",
|
109 |
-
unitdir_template="unit_{}",
|
110 |
-
name_template="image_{}.jpg",
|
111 |
-
lightbox=False, batch_size=50, seed=1):
|
112 |
-
all_indices = torch.unique(indices.view(-1), sorted=True)
|
113 |
-
z_sample = z_universe[all_indices]
|
114 |
-
progress = default_progress()
|
115 |
-
sdir = os.path.join(dirname, shared_dir)
|
116 |
-
created_hashdirs = set()
|
117 |
-
for index in range(len(z_universe)):
|
118 |
-
hd = hashdir(index)
|
119 |
-
if hd not in created_hashdirs:
|
120 |
-
created_hashdirs.add(hd)
|
121 |
-
os.makedirs(os.path.join(sdir, hd), exist_ok=True)
|
122 |
-
with torch.no_grad():
|
123 |
-
# Pass 2: now generate images
|
124 |
-
z_loader = torch.utils.data.DataLoader(TensorDataset(z_sample),
|
125 |
-
batch_size=batch_size, num_workers=2,
|
126 |
-
pin_memory=True)
|
127 |
-
saver = WorkerPool(SaveImageWorker)
|
128 |
-
for batch_num, [z] in enumerate(progress(z_loader,
|
129 |
-
desc='Saving images')):
|
130 |
-
z = z.cuda()
|
131 |
-
start_index = batch_num * batch_size
|
132 |
-
im = ((model(z) + 1) / 2 * 255).clamp(0, 255).byte().permute(
|
133 |
-
0, 2, 3, 1).cpu()
|
134 |
-
for i in range(len(im)):
|
135 |
-
index = all_indices[i + start_index].item()
|
136 |
-
filename = os.path.join(sdir, hashdir(index),
|
137 |
-
name_template.format(index))
|
138 |
-
saver.add(im[i].numpy(), filename)
|
139 |
-
saver.join()
|
140 |
-
linker = WorkerPool(MakeLinkWorker)
|
141 |
-
for u in progress(range(len(indices)), desc='Making links'):
|
142 |
-
udir = os.path.join(dirname, unitdir_template.format(u))
|
143 |
-
os.makedirs(udir, exist_ok=True)
|
144 |
-
for r in range(indices.shape[1]):
|
145 |
-
index = indices[u,r].item()
|
146 |
-
fn = name_template.format(index)
|
147 |
-
# sourcename = os.path.join('..', shared_dir, fn)
|
148 |
-
sourcename = os.path.join(sdir, hashdir(index), fn)
|
149 |
-
targname = os.path.join(udir, fn)
|
150 |
-
linker.add(sourcename, targname)
|
151 |
-
if lightbox:
|
152 |
-
copy_lightbox_to(udir)
|
153 |
-
linker.join()
|
154 |
-
|
155 |
-
def copy_lightbox_to(dirname):
|
156 |
-
srcdir = os.path.realpath(
|
157 |
-
os.path.join(os.getcwd(), os.path.dirname(__file__)))
|
158 |
-
shutil.copy(os.path.join(srcdir, 'lightbox.html'),
|
159 |
-
os.path.join(dirname, '+lightbox.html'))
|
160 |
-
|
161 |
-
def hashdir(index):
|
162 |
-
# To keep the number of files the shared directory lower, split it
|
163 |
-
# into 100 subdirectories named as follows.
|
164 |
-
return '%02d' % (index % 100)
|
165 |
-
|
166 |
-
class SaveImageWorker(WorkerBase):
|
167 |
-
# Saving images can be sped up by sending jpeg encoding and
|
168 |
-
# file-writing work to a pool.
|
169 |
-
def work(self, data, filename):
|
170 |
-
Image.fromarray(data).save(filename, optimize=True, quality=100)
|
171 |
-
|
172 |
-
class MakeLinkWorker(WorkerBase):
|
173 |
-
# Creating symbolic links is a bit slow and can be done faster
|
174 |
-
# in parallel rather than waiting for each to be created.
|
175 |
-
def work(self, sourcename, targname):
|
176 |
-
try:
|
177 |
-
os.link(sourcename, targname)
|
178 |
-
except OSError as e:
|
179 |
-
if e.errno == errno.EEXIST:
|
180 |
-
os.remove(targname)
|
181 |
-
os.link(sourcename, targname)
|
182 |
-
else:
|
183 |
-
raise
|
184 |
-
|
185 |
-
class MakeSyminkWorker(WorkerBase):
|
186 |
-
# Creating symbolic links is a bit slow and can be done faster
|
187 |
-
# in parallel rather than waiting for each to be created.
|
188 |
-
def work(self, sourcename, targname):
|
189 |
-
try:
|
190 |
-
os.symlink(sourcename, targname)
|
191 |
-
except OSError as e:
|
192 |
-
if e.errno == errno.EEXIST:
|
193 |
-
os.remove(targname)
|
194 |
-
os.symlink(sourcename, targname)
|
195 |
-
else:
|
196 |
-
raise
|
197 |
-
|
198 |
-
if __name__ == '__main__':
|
199 |
-
main()
|
|
|
|
|
|
|
|
|
|
|
|
|
|
|
|
|
|
|
|
|
|
|
|
|
|
|
|
|
|
|
|
|
|
|
|
|
|
|
|
|
|
|
|
|
|
|
|
|
|
|
|
|
|
|
|
|
|
|
|
|
|
|
|
|
|
|
|
|
|
|
|
|
|
|
|
|
|
|
|
|
|
|
|
|
|
|
|
|
|
|
|
|
|
|
|
|
|
|
|
|
|
|
|
|
|
|
|
|
|
|
|
|
|
|
|
|
|
|
|
|
|
|
|
|
|
|
|
|
|
|
|
|
|
|
|
|
|
|
|
|
|
|
|
|
|
|
|
|
|
|
|
|
|
|
|
|
|
|
|
|
|
|
|
|
|
|
|
|
|
|
|
|
|
|
|
|
|
|
|
|
|
|
|
|
|
|
|
|
|
|
|
|
|
|
|
|
|
|
|
|
|
|
|
|
|
|
|
|
|
|
|
|
|
|
|
|
|
|
|
|
|
|
|
|
|
|
|
|
|
|
|
|
|
|
|
|
|
|
|
|
|
|
|
|
|
|
|
|
|
|
|
|
|
|
|
|
|
|
|
|
|
|
|
|
|
|
|
|
|
|
|
|
|
|
|
|
|
|
|
|
|
|
|
|
|
|
|
|
|
|
|
|
|
|
|
|
|
|
|
|
|
|
|
|
|
|
|
|
|
|
|
|
|
|
|
|
|
|
|
|
|
|
|
|
|
|
|
|
|
|
|
|
|
|
|
|
|
|
|
|
|
|
|
|
|
|
|
|
|
|
|
|
|
|
|
|
|
|
|
|
|
|
|
|
|
|
|
|
|
|
|
|
|
|
|
|
|
|
|
|
|
|
|
|
|
|
|
|
|
|
|
|
|
|
|
|
|
|
|
spaces/Dralkkin/Lorule-Proxy/Dockerfile
DELETED
@@ -1,11 +0,0 @@
|
|
1 |
-
FROM node:18-bullseye-slim
|
2 |
-
RUN apt-get update && \
|
3 |
-
apt-get install -y git
|
4 |
-
RUN git clone https://gitgud.io/khanon/oai-reverse-proxy.git /app
|
5 |
-
WORKDIR /app
|
6 |
-
RUN npm install
|
7 |
-
COPY Dockerfile greeting.md* .env* ./
|
8 |
-
RUN npm run build
|
9 |
-
EXPOSE 7860
|
10 |
-
ENV NODE_ENV=production
|
11 |
-
CMD [ "npm", "start" ]
|
|
|
|
|
|
|
|
|
|
|
|
|
|
|
|
|
|
|
|
|
|
|
|
spaces/Dusan/clickbaitonator/fudge/predict_clickbait.py
DELETED
@@ -1,199 +0,0 @@
|
|
1 |
-
import os
|
2 |
-
import random
|
3 |
-
import time
|
4 |
-
import pickle
|
5 |
-
import math
|
6 |
-
from argparse import ArgumentParser
|
7 |
-
|
8 |
-
from typing import Iterable, List, Optional, Tuple
|
9 |
-
|
10 |
-
from tqdm import tqdm
|
11 |
-
import numpy as np
|
12 |
-
import torch
|
13 |
-
import torch.nn as nn
|
14 |
-
import torch.nn.functional as F
|
15 |
-
from transformers import AutoTokenizer, AutoModelWithLMHead
|
16 |
-
from torch import Tensor
|
17 |
-
|
18 |
-
from fudge.data import Dataset
|
19 |
-
from fudge.model import Model
|
20 |
-
from fudge.util import num_params
|
21 |
-
from fudge.constants import *
|
22 |
-
|
23 |
-
|
24 |
-
|
25 |
-
tokenizer = AutoTokenizer.from_pretrained('google/pegasus-xsum')
|
26 |
-
classifier_tokenizer = AutoTokenizer.from_pretrained('sentence-transformers/all-mpnet-base-v2')
|
27 |
-
|
28 |
-
|
29 |
-
def main(args):
|
30 |
-
with open(args.dataset_info, 'rb') as rf:
|
31 |
-
dataset_info = pickle.load(rf)
|
32 |
-
|
33 |
-
article_content = """Australian actor Guy Pearce will return for the iconic soap Neighbours finale on August 1 to reprise his role as Mike Young.
|
34 |
-
Guy, 54, played the troubled Mike from 1986 to 1989, and is now set to make a comeback on the show after 33 years, Metro.co.uk reports.
|
35 |
-
The star's character arcs explored the implications of domestic abuse, student-teacher relationships and dealing with loss of loved ones.
|
36 |
-
Speaking to Metro.co.uk, Guy said: 'It is very exciting and surreal at the same time being back on set again, however it feels like coming home.
|
37 |
-
'It's where it all started for me professionally. I've been asked to come back on occasions over the years and wondered if it was the right thing
|
38 |
-
to do, but once I knew the show was finishing, I knew I had to do it.'He added that there is 'nothing like being here all together again'
|
39 |
-
, even though he's had a chance to catch-up with other cast members."""
|
40 |
-
|
41 |
-
tokenizer.add_special_tokens({'pad_token': PAD_TOKEN})
|
42 |
-
pad_id = tokenizer.encode(PAD_TOKEN)[0]
|
43 |
-
|
44 |
-
#For loading Clickbait summarizer
|
45 |
-
model = AutoModelWithLMHead.from_pretrained(args.model_string, return_dict=True).to(args.device)
|
46 |
-
|
47 |
-
model.eval()
|
48 |
-
|
49 |
-
checkpoint = torch.load(args.ckpt, map_location=args.device)
|
50 |
-
model_args = checkpoint['args']
|
51 |
-
conditioning_model = Model(model_args, pad_id, len(dataset_info.index2word)) # no need to get the glove embeddings when reloading since they're saved in model ckpt anyway
|
52 |
-
conditioning_model.load_state_dict(checkpoint['state_dict'])
|
53 |
-
conditioning_model = conditioning_model.to(args.device)
|
54 |
-
conditioning_model.eval()
|
55 |
-
print("=> loaded checkpoint '{}' (epoch {})"
|
56 |
-
.format(args.ckpt, checkpoint['epoch']))
|
57 |
-
print('num params', num_params(conditioning_model))
|
58 |
-
|
59 |
-
while True:
|
60 |
-
results = generate_clickbait(model,
|
61 |
-
tokenizer,
|
62 |
-
conditioning_model,
|
63 |
-
[args.input_text],
|
64 |
-
dataset_info,
|
65 |
-
precondition_topk=args.precondition_topk,
|
66 |
-
do_sample=args.do_sample,
|
67 |
-
length_cutoff=args.length_cutoff,
|
68 |
-
condition_lambda=args.condition_lambda,
|
69 |
-
article_content=article_content,
|
70 |
-
device=args.device)
|
71 |
-
# print(results)
|
72 |
-
import pdb; pdb.set_trace()
|
73 |
-
|
74 |
-
|
75 |
-
def generate_clickbait(model,
|
76 |
-
tokenizer,
|
77 |
-
conditioning_model,
|
78 |
-
input_text,
|
79 |
-
dataset_info,
|
80 |
-
precondition_topk,
|
81 |
-
length_cutoff,
|
82 |
-
condition_lambda=1.0,
|
83 |
-
article_content=None,
|
84 |
-
device='cuda'):
|
85 |
-
with torch.no_grad():
|
86 |
-
batch_size = len(input_text)
|
87 |
-
# encoded_input_article = [tokenizer.encode(article_content, return_tensors='pt',add_special_tokens=False).to(device)] # batch x seq
|
88 |
-
max_input_length = 512
|
89 |
-
encoded_input_article = tokenizer(article_content, return_tensors='pt',add_special_tokens=False, max_length = max_input_length).to(device) # batch x seq
|
90 |
-
# encoded_input_article = torch.cat(encoded_input_article, dim=0)
|
91 |
-
# attention_mask = encoded_input_article.new_ones(encoded_input_article.shape).to(device)
|
92 |
-
|
93 |
-
# CHANGE=ko
|
94 |
-
encoded_input = tokenizer('<pad>', return_tensors='pt',add_special_tokens=False).to(device) # batch x seq
|
95 |
-
# encoded_input = tokenizer('<pad>'+ input_text[0], return_tensors='pt',add_special_tokens=False).to(device) # batch x seq
|
96 |
-
# encoded_input = torch.cat(encoded_input, dim=0)
|
97 |
-
encoded_input = encoded_input['input_ids']
|
98 |
-
|
99 |
-
|
100 |
-
lengths = torch.LongTensor([encoded_input.shape[1]]).to(device)
|
101 |
-
# lengths = 1
|
102 |
-
|
103 |
-
past = None
|
104 |
-
use_cache = True
|
105 |
-
|
106 |
-
# CHANGE
|
107 |
-
# model_kwargs = {'encoder_outputs': model.get_encoder()(encoded_input_article, attention_mask=attention_mask)}
|
108 |
-
model_kwargs = {'encoder_outputs': model.get_encoder()(input_ids=encoded_input_article['input_ids'],
|
109 |
-
attention_mask=encoded_input_article['attention_mask'],
|
110 |
-
return_dict=True,
|
111 |
-
output_attentions=False,
|
112 |
-
output_hidden_states=False),
|
113 |
-
}
|
114 |
-
|
115 |
-
while lengths.max() < length_cutoff:
|
116 |
-
model_inputs = model.prepare_inputs_for_generation(
|
117 |
-
input_ids = encoded_input_article['input_ids'],
|
118 |
-
decoder_input_ids=encoded_input,
|
119 |
-
# past=past,
|
120 |
-
attention_mask=encoded_input_article['attention_mask'],
|
121 |
-
use_cache=use_cache,
|
122 |
-
**model_kwargs
|
123 |
-
)
|
124 |
-
|
125 |
-
outputs = model(**model_inputs, return_dict=True)
|
126 |
-
logits = outputs.logits[:, -1, :]
|
127 |
-
|
128 |
-
if "past_key_values" in outputs:
|
129 |
-
model_kwargs["past"] = outputs.past_key_values
|
130 |
-
|
131 |
-
# logits = model(encoded_input)[0][:, -1, :] # batch x vocab
|
132 |
-
top_logits, top_indices = logits.topk(precondition_topk, dim=1) # batch x topk
|
133 |
-
new_input_candidates = torch.cat([encoded_input.unsqueeze(1).expand(-1, precondition_topk, -1), top_indices.unsqueeze(2)], dim=2) # batch x topk x seq+1
|
134 |
-
expanded_lengths = (lengths + 1).unsqueeze(1).expand(batch_size, precondition_topk) # batch x topk
|
135 |
-
|
136 |
-
if condition_lambda == 0:
|
137 |
-
condition_logits = torch.zeros_like(top_logits).float()
|
138 |
-
condition_logits = condition_logits.view(batch_size, precondition_topk, -1) # batch x topk x N
|
139 |
-
else:
|
140 |
-
decoded_outputs = tokenizer.batch_decode(new_input_candidates.view(-1, new_input_candidates.size(-1)), clean_up_tokenization_spaces=False)
|
141 |
-
resulting_tokenization = classifier_tokenizer(decoded_outputs, add_special_tokens=False, padding='longest')
|
142 |
-
encoded_with_classifier = resulting_tokenization['input_ids']
|
143 |
-
attention_mask = torch.tensor(resulting_tokenization['attention_mask']).to(model.device)
|
144 |
-
tplus1_candidates_classifier = torch.tensor(encoded_with_classifier).view(batch_size, precondition_topk, -1).to(model.device)
|
145 |
-
|
146 |
-
condition_logits = conditioning_model(tplus1_candidates_classifier.flatten(0, 1), # batch*topk x seq+1
|
147 |
-
expanded_lengths.flatten(0, 1), # batch*topk
|
148 |
-
None,
|
149 |
-
None,
|
150 |
-
None,
|
151 |
-
attention_mask=attention_mask
|
152 |
-
)
|
153 |
-
condition_logits = condition_logits.view(batch_size, precondition_topk, -1) # batch x topk x N
|
154 |
-
condition_logits = condition_logits - torch.log(1 + torch.exp(condition_logits)) # get correct log probs
|
155 |
-
|
156 |
-
condition_logits = torch.mean(condition_logits, dim=2)
|
157 |
-
full_logits = top_logits + condition_logits * condition_lambda # batch x topk
|
158 |
-
post_logits, post_indices = full_logits.topk(precondition_topk, dim=1)
|
159 |
-
post_probs = F.softmax(post_logits, dim=1)
|
160 |
-
# index_into_top_indices = post_indices[torch.arange(batch_size).to(post_indices.device), torch.multinomial(post_probs, 1).flatten()] # batch
|
161 |
-
index_into_top_indices = post_indices[:, torch.multinomial(post_probs, 1).flatten()] # batch
|
162 |
-
|
163 |
-
# next_indices = top_indices[torch.arange(batch_size).to(top_indices.device), index_into_top_indices] # batch
|
164 |
-
next_indices = top_indices[:, index_into_top_indices] # batch
|
165 |
-
|
166 |
-
# encoded_input = torch.cat([encoded_input, next_indices.unsqueeze(1)], dim=1) # batch x seq+1
|
167 |
-
encoded_input = torch.cat([encoded_input, next_indices.squeeze(1)], dim=1)
|
168 |
-
lengths = lengths + 1 # batch
|
169 |
-
|
170 |
-
# print(tokenizer.decode(encoded_input[0], add_special_tokens=False))
|
171 |
-
return [tokenizer.decode(s) for s in encoded_input]
|
172 |
-
|
173 |
-
|
174 |
-
if __name__=='__main__':
|
175 |
-
parser = ArgumentParser()
|
176 |
-
|
177 |
-
# DATA
|
178 |
-
parser.add_argument('--ckpt', type=str, required=True)
|
179 |
-
parser.add_argument('--dataset_info', type=str, required=True, help='saved dataset info')
|
180 |
-
parser.add_argument('--model_string', type=str, default='Helsinki-NLP/opus-mt-es-en')
|
181 |
-
|
182 |
-
parser.add_argument('--in_file', type=str, default=None, required=True, help='text to run pred on')
|
183 |
-
|
184 |
-
parser.add_argument('--precondition_topk', type=int, default=200, help='consider top k outputs from text generation at each step before conditioning and re-pruning')
|
185 |
-
parser.add_argument('--do_sample', action='store_true', default=False, help='sample instead of greedy')
|
186 |
-
parser.add_argument('--condition_lambda', type=float, default=1.0, help='lambda weight on conditioning model')
|
187 |
-
parser.add_argument('--length_cutoff', type=int, default=512, help='max length')
|
188 |
-
|
189 |
-
parser.add_argument('--seed', type=int, default=1, help='random seed')
|
190 |
-
parser.add_argument('--device', type=str, default='cuda', choices=['cpu', 'cuda'])
|
191 |
-
parser.add_argument('--debug', action='store_true', default=False)
|
192 |
-
|
193 |
-
args = parser.parse_args()
|
194 |
-
|
195 |
-
random.seed(args.seed)
|
196 |
-
np.random.seed(args.seed)
|
197 |
-
torch.manual_seed(args.seed)
|
198 |
-
|
199 |
-
main(args)
|
|
|
|
|
|
|
|
|
|
|
|
|
|
|
|
|
|
|
|
|
|
|
|
|
|
|
|
|
|
|
|
|
|
|
|
|
|
|
|
|
|
|
|
|
|
|
|
|
|
|
|
|
|
|
|
|
|
|
|
|
|
|
|
|
|
|
|
|
|
|
|
|
|
|
|
|
|
|
|
|
|
|
|
|
|
|
|
|
|
|
|
|
|
|
|
|
|
|
|
|
|
|
|
|
|
|
|
|
|
|
|
|
|
|
|
|
|
|
|
|
|
|
|
|
|
|
|
|
|
|
|
|
|
|
|
|
|
|
|
|
|
|
|
|
|
|
|
|
|
|
|
|
|
|
|
|
|
|
|
|
|
|
|
|
|
|
|
|
|
|
|
|
|
|
|
|
|
|
|
|
|
|
|
|
|
|
|
|
|
|
|
|
|
|
|
|
|
|
|
|
|
|
|
|
|
|
|
|
|
|
|
|
|
|
|
|
|
|
|
|
|
|
|
|
|
|
|
|
|
|
|
|
|
|
|
|
|
|
|
|
|
|
|
|
|
|
|
|
|
|
|
|
|
|
|
|
|
|
|
|
|
|
|
|
|
|
|
|
|
|
|
|
|
|
|
|
|
|
|
|
|
|
|
|
|
|
|
|
|
|
|
|
|
|
|
|
|
|
|
|
|
|
|
|
|
|
|
|
|
|
|
|
|
|
|
|
|
|
|
|
|
|
|
|
|
|
|
|
|
|
|
|
|
|
|
|
|
|
|
|
|
|
|
|
|
|
|
|
|
|
|
|
|
|
|
|
|
|
|
|
|
|
|
|
|
|
|
|
|
|
|
|
|
|
|
|
|
|
|
|
|
|
|
|
|
|
|
|
|
|
|
|
|
|
|
|
|
|
|
spaces/Duskfallcrew/Duskfallcrew-duskfallai/README.md
DELETED
@@ -1,13 +0,0 @@
|
|
1 |
-
---
|
2 |
-
title: Duskfallcrew Duskfallai
|
3 |
-
emoji: 🏢
|
4 |
-
colorFrom: blue
|
5 |
-
colorTo: green
|
6 |
-
sdk: gradio
|
7 |
-
sdk_version: 3.16.2
|
8 |
-
app_file: app.py
|
9 |
-
pinned: false
|
10 |
-
license: creativeml-openrail-m
|
11 |
-
---
|
12 |
-
|
13 |
-
Check out the configuration reference at https://huggingface.co/docs/hub/spaces-config-reference
|
|
|
|
|
|
|
|
|
|
|
|
|
|
|
|
|
|
|
|
|
|
|
|
|
|
|
|
spaces/EPFL-VILAB/MultiMAE/dpt/base_model.py
DELETED
@@ -1,16 +0,0 @@
|
|
1 |
-
import torch
|
2 |
-
|
3 |
-
|
4 |
-
class BaseModel(torch.nn.Module):
|
5 |
-
def load(self, path):
|
6 |
-
"""Load model from file.
|
7 |
-
|
8 |
-
Args:
|
9 |
-
path (str): file path
|
10 |
-
"""
|
11 |
-
parameters = torch.load(path, map_location=torch.device("cpu"))
|
12 |
-
|
13 |
-
if "optimizer" in parameters:
|
14 |
-
parameters = parameters["model"]
|
15 |
-
|
16 |
-
self.load_state_dict(parameters)
|
|
|
|
|
|
|
|
|
|
|
|
|
|
|
|
|
|
|
|
|
|
|
|
|
|
|
|
|
|
|
|
|
|
spaces/EXPOSUREEE/Ai-Image-Enhancer/realesrgan/models/realesrnet_model.py
DELETED
@@ -1,188 +0,0 @@
|
|
1 |
-
import numpy as np
|
2 |
-
import random
|
3 |
-
import torch
|
4 |
-
from basicsr.data.degradations import random_add_gaussian_noise_pt, random_add_poisson_noise_pt
|
5 |
-
from basicsr.data.transforms import paired_random_crop
|
6 |
-
from basicsr.models.sr_model import SRModel
|
7 |
-
from basicsr.utils import DiffJPEG, USMSharp
|
8 |
-
from basicsr.utils.img_process_util import filter2D
|
9 |
-
from basicsr.utils.registry import MODEL_REGISTRY
|
10 |
-
from torch.nn import functional as F
|
11 |
-
|
12 |
-
|
13 |
-
@MODEL_REGISTRY.register()
|
14 |
-
class RealESRNetModel(SRModel):
|
15 |
-
"""RealESRNet Model for Real-ESRGAN: Training Real-World Blind Super-Resolution with Pure Synthetic Data.
|
16 |
-
|
17 |
-
It is trained without GAN losses.
|
18 |
-
It mainly performs:
|
19 |
-
1. randomly synthesize LQ images in GPU tensors
|
20 |
-
2. optimize the networks with GAN training.
|
21 |
-
"""
|
22 |
-
|
23 |
-
def __init__(self, opt):
|
24 |
-
super(RealESRNetModel, self).__init__(opt)
|
25 |
-
self.jpeger = DiffJPEG(differentiable=False).cuda() # simulate JPEG compression artifacts
|
26 |
-
self.usm_sharpener = USMSharp().cuda() # do usm sharpening
|
27 |
-
self.queue_size = opt.get('queue_size', 180)
|
28 |
-
|
29 |
-
@torch.no_grad()
|
30 |
-
def _dequeue_and_enqueue(self):
|
31 |
-
"""It is the training pair pool for increasing the diversity in a batch.
|
32 |
-
|
33 |
-
Batch processing limits the diversity of synthetic degradations in a batch. For example, samples in a
|
34 |
-
batch could not have different resize scaling factors. Therefore, we employ this training pair pool
|
35 |
-
to increase the degradation diversity in a batch.
|
36 |
-
"""
|
37 |
-
# initialize
|
38 |
-
b, c, h, w = self.lq.size()
|
39 |
-
if not hasattr(self, 'queue_lr'):
|
40 |
-
assert self.queue_size % b == 0, f'queue size {self.queue_size} should be divisible by batch size {b}'
|
41 |
-
self.queue_lr = torch.zeros(self.queue_size, c, h, w).cuda()
|
42 |
-
_, c, h, w = self.gt.size()
|
43 |
-
self.queue_gt = torch.zeros(self.queue_size, c, h, w).cuda()
|
44 |
-
self.queue_ptr = 0
|
45 |
-
if self.queue_ptr == self.queue_size: # the pool is full
|
46 |
-
# do dequeue and enqueue
|
47 |
-
# shuffle
|
48 |
-
idx = torch.randperm(self.queue_size)
|
49 |
-
self.queue_lr = self.queue_lr[idx]
|
50 |
-
self.queue_gt = self.queue_gt[idx]
|
51 |
-
# get first b samples
|
52 |
-
lq_dequeue = self.queue_lr[0:b, :, :, :].clone()
|
53 |
-
gt_dequeue = self.queue_gt[0:b, :, :, :].clone()
|
54 |
-
# update the queue
|
55 |
-
self.queue_lr[0:b, :, :, :] = self.lq.clone()
|
56 |
-
self.queue_gt[0:b, :, :, :] = self.gt.clone()
|
57 |
-
|
58 |
-
self.lq = lq_dequeue
|
59 |
-
self.gt = gt_dequeue
|
60 |
-
else:
|
61 |
-
# only do enqueue
|
62 |
-
self.queue_lr[self.queue_ptr:self.queue_ptr + b, :, :, :] = self.lq.clone()
|
63 |
-
self.queue_gt[self.queue_ptr:self.queue_ptr + b, :, :, :] = self.gt.clone()
|
64 |
-
self.queue_ptr = self.queue_ptr + b
|
65 |
-
|
66 |
-
@torch.no_grad()
|
67 |
-
def feed_data(self, data):
|
68 |
-
"""Accept data from dataloader, and then add two-order degradations to obtain LQ images.
|
69 |
-
"""
|
70 |
-
if self.is_train and self.opt.get('high_order_degradation', True):
|
71 |
-
# training data synthesis
|
72 |
-
self.gt = data['gt'].to(self.device)
|
73 |
-
# USM sharpen the GT images
|
74 |
-
if self.opt['gt_usm'] is True:
|
75 |
-
self.gt = self.usm_sharpener(self.gt)
|
76 |
-
|
77 |
-
self.kernel1 = data['kernel1'].to(self.device)
|
78 |
-
self.kernel2 = data['kernel2'].to(self.device)
|
79 |
-
self.sinc_kernel = data['sinc_kernel'].to(self.device)
|
80 |
-
|
81 |
-
ori_h, ori_w = self.gt.size()[2:4]
|
82 |
-
|
83 |
-
# ----------------------- The first degradation process ----------------------- #
|
84 |
-
# blur
|
85 |
-
out = filter2D(self.gt, self.kernel1)
|
86 |
-
# random resize
|
87 |
-
updown_type = random.choices(['up', 'down', 'keep'], self.opt['resize_prob'])[0]
|
88 |
-
if updown_type == 'up':
|
89 |
-
scale = np.random.uniform(1, self.opt['resize_range'][1])
|
90 |
-
elif updown_type == 'down':
|
91 |
-
scale = np.random.uniform(self.opt['resize_range'][0], 1)
|
92 |
-
else:
|
93 |
-
scale = 1
|
94 |
-
mode = random.choice(['area', 'bilinear', 'bicubic'])
|
95 |
-
out = F.interpolate(out, scale_factor=scale, mode=mode)
|
96 |
-
# add noise
|
97 |
-
gray_noise_prob = self.opt['gray_noise_prob']
|
98 |
-
if np.random.uniform() < self.opt['gaussian_noise_prob']:
|
99 |
-
out = random_add_gaussian_noise_pt(
|
100 |
-
out, sigma_range=self.opt['noise_range'], clip=True, rounds=False, gray_prob=gray_noise_prob)
|
101 |
-
else:
|
102 |
-
out = random_add_poisson_noise_pt(
|
103 |
-
out,
|
104 |
-
scale_range=self.opt['poisson_scale_range'],
|
105 |
-
gray_prob=gray_noise_prob,
|
106 |
-
clip=True,
|
107 |
-
rounds=False)
|
108 |
-
# JPEG compression
|
109 |
-
jpeg_p = out.new_zeros(out.size(0)).uniform_(*self.opt['jpeg_range'])
|
110 |
-
out = torch.clamp(out, 0, 1) # clamp to [0, 1], otherwise JPEGer will result in unpleasant artifacts
|
111 |
-
out = self.jpeger(out, quality=jpeg_p)
|
112 |
-
|
113 |
-
# ----------------------- The second degradation process ----------------------- #
|
114 |
-
# blur
|
115 |
-
if np.random.uniform() < self.opt['second_blur_prob']:
|
116 |
-
out = filter2D(out, self.kernel2)
|
117 |
-
# random resize
|
118 |
-
updown_type = random.choices(['up', 'down', 'keep'], self.opt['resize_prob2'])[0]
|
119 |
-
if updown_type == 'up':
|
120 |
-
scale = np.random.uniform(1, self.opt['resize_range2'][1])
|
121 |
-
elif updown_type == 'down':
|
122 |
-
scale = np.random.uniform(self.opt['resize_range2'][0], 1)
|
123 |
-
else:
|
124 |
-
scale = 1
|
125 |
-
mode = random.choice(['area', 'bilinear', 'bicubic'])
|
126 |
-
out = F.interpolate(
|
127 |
-
out, size=(int(ori_h / self.opt['scale'] * scale), int(ori_w / self.opt['scale'] * scale)), mode=mode)
|
128 |
-
# add noise
|
129 |
-
gray_noise_prob = self.opt['gray_noise_prob2']
|
130 |
-
if np.random.uniform() < self.opt['gaussian_noise_prob2']:
|
131 |
-
out = random_add_gaussian_noise_pt(
|
132 |
-
out, sigma_range=self.opt['noise_range2'], clip=True, rounds=False, gray_prob=gray_noise_prob)
|
133 |
-
else:
|
134 |
-
out = random_add_poisson_noise_pt(
|
135 |
-
out,
|
136 |
-
scale_range=self.opt['poisson_scale_range2'],
|
137 |
-
gray_prob=gray_noise_prob,
|
138 |
-
clip=True,
|
139 |
-
rounds=False)
|
140 |
-
|
141 |
-
# JPEG compression + the final sinc filter
|
142 |
-
# We also need to resize images to desired sizes. We group [resize back + sinc filter] together
|
143 |
-
# as one operation.
|
144 |
-
# We consider two orders:
|
145 |
-
# 1. [resize back + sinc filter] + JPEG compression
|
146 |
-
# 2. JPEG compression + [resize back + sinc filter]
|
147 |
-
# Empirically, we find other combinations (sinc + JPEG + Resize) will introduce twisted lines.
|
148 |
-
if np.random.uniform() < 0.5:
|
149 |
-
# resize back + the final sinc filter
|
150 |
-
mode = random.choice(['area', 'bilinear', 'bicubic'])
|
151 |
-
out = F.interpolate(out, size=(ori_h // self.opt['scale'], ori_w // self.opt['scale']), mode=mode)
|
152 |
-
out = filter2D(out, self.sinc_kernel)
|
153 |
-
# JPEG compression
|
154 |
-
jpeg_p = out.new_zeros(out.size(0)).uniform_(*self.opt['jpeg_range2'])
|
155 |
-
out = torch.clamp(out, 0, 1)
|
156 |
-
out = self.jpeger(out, quality=jpeg_p)
|
157 |
-
else:
|
158 |
-
# JPEG compression
|
159 |
-
jpeg_p = out.new_zeros(out.size(0)).uniform_(*self.opt['jpeg_range2'])
|
160 |
-
out = torch.clamp(out, 0, 1)
|
161 |
-
out = self.jpeger(out, quality=jpeg_p)
|
162 |
-
# resize back + the final sinc filter
|
163 |
-
mode = random.choice(['area', 'bilinear', 'bicubic'])
|
164 |
-
out = F.interpolate(out, size=(ori_h // self.opt['scale'], ori_w // self.opt['scale']), mode=mode)
|
165 |
-
out = filter2D(out, self.sinc_kernel)
|
166 |
-
|
167 |
-
# clamp and round
|
168 |
-
self.lq = torch.clamp((out * 255.0).round(), 0, 255) / 255.
|
169 |
-
|
170 |
-
# random crop
|
171 |
-
gt_size = self.opt['gt_size']
|
172 |
-
self.gt, self.lq = paired_random_crop(self.gt, self.lq, gt_size, self.opt['scale'])
|
173 |
-
|
174 |
-
# training pair pool
|
175 |
-
self._dequeue_and_enqueue()
|
176 |
-
self.lq = self.lq.contiguous() # for the warning: grad and param do not obey the gradient layout contract
|
177 |
-
else:
|
178 |
-
# for paired training or validation
|
179 |
-
self.lq = data['lq'].to(self.device)
|
180 |
-
if 'gt' in data:
|
181 |
-
self.gt = data['gt'].to(self.device)
|
182 |
-
self.gt_usm = self.usm_sharpener(self.gt)
|
183 |
-
|
184 |
-
def nondist_validation(self, dataloader, current_iter, tb_logger, save_img):
|
185 |
-
# do not use the synthetic process during validation
|
186 |
-
self.is_train = False
|
187 |
-
super(RealESRNetModel, self).nondist_validation(dataloader, current_iter, tb_logger, save_img)
|
188 |
-
self.is_train = True
|
|
|
|
|
|
|
|
|
|
|
|
|
|
|
|
|
|
|
|
|
|
|
|
|
|
|
|
|
|
|
|
|
|
|
|
|
|
|
|
|
|
|
|
|
|
|
|
|
|
|
|
|
|
|
|
|
|
|
|
|
|
|
|
|
|
|
|
|
|
|
|
|
|
|
|
|
|
|
|
|
|
|
|
|
|
|
|
|
|
|
|
|
|
|
|
|
|
|
|
|
|
|
|
|
|
|
|
|
|
|
|
|
|
|
|
|
|
|
|
|
|
|
|
|
|
|
|
|
|
|
|
|
|
|
|
|
|
|
|
|
|
|
|
|
|
|
|
|
|
|
|
|
|
|
|
|
|
|
|
|
|
|
|
|
|
|
|
|
|
|
|
|
|
|
|
|
|
|
|
|
|
|
|
|
|
|
|
|
|
|
|
|
|
|
|
|
|
|
|
|
|
|
|
|
|
|
|
|
|
|
|
|
|
|
|
|
|
|
|
|
|
|
|
|
|
|
|
|
|
|
|
|
|
|
|
|
|
|
|
|
|
|
|
|
|
|
|
|
|
|
|
|
|
|
|
|
|
|
|
|
|
|
|
|
|
|
|
|
|
|
|
|
|
|
|
|
|
|
|
|
|
|
|
|
|
|
|
|
|
|
|
|
|
|
|
|
|
|
|
|
|
|
|
|
|
|
|
|
|
|
|
|
|
|
|
|
|
|
|
|
|
|
|
|
|
|
|
|
|
|
|
|
|
|
|
|
|
|
|
|
|
|
|
|
|
|
|
|
|
|
|
|
|
|
|
|
|
|
|
|
|
|
|
|
|
|
|
|
|
|
|
|
|
|
|
|
|
spaces/EagleLoveAI/ChatGPT_Application_Robot/README.md
DELETED
@@ -1,13 +0,0 @@
|
|
1 |
-
---
|
2 |
-
title: ChatGPT Application Robot
|
3 |
-
emoji: 💩
|
4 |
-
colorFrom: indigo
|
5 |
-
colorTo: purple
|
6 |
-
sdk: gradio
|
7 |
-
sdk_version: 3.27.0
|
8 |
-
app_file: app.py
|
9 |
-
pinned: false
|
10 |
-
license: mit
|
11 |
-
---
|
12 |
-
|
13 |
-
Check out the configuration reference at https://huggingface.co/docs/hub/spaces-config-reference
|
|
|
|
|
|
|
|
|
|
|
|
|
|
|
|
|
|
|
|
|
|
|
|
|
|
|
|
spaces/Eddycrack864/Applio-Inference/lib/infer_pack/modules/F0Predictor/F0Predictor.py
DELETED
@@ -1,16 +0,0 @@
|
|
1 |
-
class F0Predictor(object):
|
2 |
-
def compute_f0(self, wav, p_len):
|
3 |
-
"""
|
4 |
-
input: wav:[signal_length]
|
5 |
-
p_len:int
|
6 |
-
output: f0:[signal_length//hop_length]
|
7 |
-
"""
|
8 |
-
pass
|
9 |
-
|
10 |
-
def compute_f0_uv(self, wav, p_len):
|
11 |
-
"""
|
12 |
-
input: wav:[signal_length]
|
13 |
-
p_len:int
|
14 |
-
output: f0:[signal_length//hop_length],uv:[signal_length//hop_length]
|
15 |
-
"""
|
16 |
-
pass
|
|
|
|
|
|
|
|
|
|
|
|
|
|
|
|
|
|
|
|
|
|
|
|
|
|
|
|
|
|
|
|
|
|